hexsha
stringlengths 40
40
| size
int64 6
14.9M
| ext
stringclasses 1
value | lang
stringclasses 1
value | max_stars_repo_path
stringlengths 6
260
| max_stars_repo_name
stringlengths 6
119
| max_stars_repo_head_hexsha
stringlengths 40
41
| max_stars_repo_licenses
sequence | max_stars_count
int64 1
191k
⌀ | max_stars_repo_stars_event_min_datetime
stringlengths 24
24
⌀ | max_stars_repo_stars_event_max_datetime
stringlengths 24
24
⌀ | max_issues_repo_path
stringlengths 6
260
| max_issues_repo_name
stringlengths 6
119
| max_issues_repo_head_hexsha
stringlengths 40
41
| max_issues_repo_licenses
sequence | max_issues_count
int64 1
67k
⌀ | max_issues_repo_issues_event_min_datetime
stringlengths 24
24
⌀ | max_issues_repo_issues_event_max_datetime
stringlengths 24
24
⌀ | max_forks_repo_path
stringlengths 6
260
| max_forks_repo_name
stringlengths 6
119
| max_forks_repo_head_hexsha
stringlengths 40
41
| max_forks_repo_licenses
sequence | max_forks_count
int64 1
105k
⌀ | max_forks_repo_forks_event_min_datetime
stringlengths 24
24
⌀ | max_forks_repo_forks_event_max_datetime
stringlengths 24
24
⌀ | avg_line_length
float64 2
1.04M
| max_line_length
int64 2
11.2M
| alphanum_fraction
float64 0
1
| cells
sequence | cell_types
sequence | cell_type_groups
sequence |
---|---|---|---|---|---|---|---|---|---|---|---|---|---|---|---|---|---|---|---|---|---|---|---|---|---|---|---|---|---|---|
e774b569852818264b1cba384cda023678c0607d | 141,533 | ipynb | Jupyter Notebook | notebooks/machine_learning_deploy.ipynb | MieleSantos/bootcamp_ds | e470368877d1a2316fbe9ee2b0cc54c33fac7f7f | [
"MIT"
] | null | null | null | notebooks/machine_learning_deploy.ipynb | MieleSantos/bootcamp_ds | e470368877d1a2316fbe9ee2b0cc54c33fac7f7f | [
"MIT"
] | null | null | null | notebooks/machine_learning_deploy.ipynb | MieleSantos/bootcamp_ds | e470368877d1a2316fbe9ee2b0cc54c33fac7f7f | [
"MIT"
] | null | null | null | 49.26314 | 23,956 | 0.509026 | [
[
[
"## Stack - Bootcamp de Data Science",
"_____no_output_____"
],
[
"### Machine Learning.",
"_____no_output_____"
]
],
[
[
"import pandas as pd\nimport datetime\nimport glob\nfrom minio import Minio\nimport numpy as np\nimport matplotlib.pyplot as plt",
"_____no_output_____"
],
[
"client = Minio(\n \"localhost:9000\",\n access_key=\"minioadmin\",\n secret_key=\"minioadmin\",\n secure=False\n )",
"_____no_output_____"
]
],
[
[
"### Baixando o Dataset do Data Lake.",
"_____no_output_____"
]
],
[
[
"client.fget_object(\n \"processing\",\n \"employees_dataset.parquet\",\n \"temp_.parquet\",\n)\ndf = pd.read_parquet(\"temp_.parquet\")",
"_____no_output_____"
],
[
"df.head()",
"_____no_output_____"
]
],
[
[
"#### Organizando o dataset.",
"_____no_output_____"
]
],
[
[
"df = df[['department', 'salary', 'mean_work_last_3_months',\n 'number_projects', 'satisfaction_level', 'last_evaluation',\n 'time_in_company', 'work_accident','left']]",
"_____no_output_____"
],
[
"df.head()",
"_____no_output_____"
]
],
[
[
"#### Verificando os registros missing.",
"_____no_output_____"
]
],
[
[
"df.isnull().sum()",
"_____no_output_____"
],
[
"df[df.notnull()]",
"_____no_output_____"
],
[
"df = df[:14998]",
"_____no_output_____"
]
],
[
[
"#### Alterando os tipos de dados.",
"_____no_output_____"
]
],
[
[
"df[\"number_projects\"] = df[\"number_projects\"].astype(int)\ndf[\"mean_work_last_3_months\"] = df[\"mean_work_last_3_months\"].astype(int)\ndf[\"time_in_company\"] = df[\"time_in_company\"].astype(int)\ndf[\"work_accident\"] = df[\"work_accident\"].astype(int)\ndf[\"left\"] = df[\"left\"].astype(int)",
"_____no_output_____"
],
[
"df.info()",
"<class 'pandas.core.frame.DataFrame'>\nRangeIndex: 14998 entries, 0 to 14997\nData columns (total 9 columns):\n # Column Non-Null Count Dtype \n--- ------ -------------- ----- \n 0 department 14998 non-null object \n 1 salary 14998 non-null object \n 2 mean_work_last_3_months 14998 non-null int64 \n 3 number_projects 14998 non-null int64 \n 4 satisfaction_level 14998 non-null float64\n 5 last_evaluation 14998 non-null float64\n 6 time_in_company 14998 non-null int64 \n 7 work_accident 14998 non-null int64 \n 8 left 14998 non-null int64 \ndtypes: float64(2), int64(5), object(2)\nmemory usage: 1.0+ MB\n"
],
[
"df.head()",
"_____no_output_____"
],
[
"df = df[:14998]",
"_____no_output_____"
]
],
[
[
"#### Renomeando atributos",
"_____no_output_____"
]
],
[
[
"df = df.rename(columns={'satisfaction_level': 'satisfaction', \n 'last_evaluation': 'evaluation',\n 'number_projects': 'projectCount',\n 'mean_work_last_3_months': 'averageMonthlyHours',\n 'time_in_company': 'yearsAtCompany',\n 'work_accident': 'workAccident',\n 'left' : 'turnover'\n })",
"_____no_output_____"
],
[
"df.head()",
"_____no_output_____"
]
],
[
[
"### Importancia de Features",
"_____no_output_____"
],
[
"#### Converte os atributos em categoricos.",
"_____no_output_____"
]
],
[
[
"df[\"department\"] = df[\"department\"].astype('category').cat.codes\ndf[\"salary\"] = df[\"salary\"].astype('category').cat.codes",
"_____no_output_____"
],
[
"df.head()",
"_____no_output_____"
]
],
[
[
"#### Separando os conjuntos de dados em features e em classe\nNosso problema é um de `classificação`",
"_____no_output_____"
]
],
[
[
"# nosso atributo classe é o turnover\ntarget_name = 'turnover'\n# o x vai representa nossa features sem o turnover\nX = df.drop('turnover', axis=1)\ny = df[target_name]",
"_____no_output_____"
]
],
[
[
"#### Transformando os dados.",
"_____no_output_____"
]
],
[
[
"from sklearn.preprocessing import MinMaxScaler",
"_____no_output_____"
]
],
[
[
"#### MinMaxScaler\nPara cada valor em um recurso, `MinMaxScaler` subtrai o valor mínimo no recurso e, em seguida, divide pelo intervalo. \n - O intervalo é a diferença entre o máximo original e o mínimo original.\n - MinMaxScaler preserva a forma da distribuição original. Isso não altera significativamente as informações incorporadas nos dados originais.\n - Observe que MinMaxScaler não reduz a importância de outliers.\n - O intervalo padrão para o recurso retornado por MinMaxScaler é de 0 a 1.\n\n",
"_____no_output_____"
]
],
[
[
"scaler = MinMaxScaler()",
"_____no_output_____"
],
[
"# Fazendo a transformação\nX = scaler.fit_transform(X)",
"_____no_output_____"
],
[
"X",
"_____no_output_____"
]
],
[
[
"#### Separando os conjuntos de treino e testes",
"_____no_output_____"
]
],
[
[
"from sklearn.model_selection import train_test_split",
"_____no_output_____"
],
[
"X_train, X_test, y_train, y_test = train_test_split(\n X # conjunto das features\n ,y # é a classe\n ,test_size = 0.2 # 20% do conjunto, vai ser usado para testes\n ,random_state = 123 # semente aleatoria\n ,stratify = y # separação dos dados mantendo um numeros de classes(fazendo um balanceamento de classes) \n)",
"_____no_output_____"
]
],
[
[
"#### Treinando o algoritmo de arvore de decisão.",
"_____no_output_____"
]
],
[
[
"from sklearn.tree import DecisionTreeClassifier",
"_____no_output_____"
],
[
"dtree = DecisionTreeClassifier()\ndtree = dtree.fit(X_train,y_train)",
"_____no_output_____"
],
[
"importances = dtree.feature_importances_\nfeat_names = df.drop(['turnover'],axis=1).columns",
"_____no_output_____"
],
[
"indices = np.argsort(importances)[::-1]\nplt.figure(figsize=(12,4))\nplt.title(\"Feature importances by DecisionTreeClassifier\")\nplt.bar(range(len(indices)), importances[indices], color='lightblue', align=\"center\")\nplt.xticks(range(len(indices)), feat_names[indices], rotation='vertical',fontsize=14)\nplt.xlim([-1, len(indices)])\nplt.show()",
"_____no_output_____"
]
],
[
[
"#### Filtrando apenas os atributos relevantes.",
"_____no_output_____"
]
],
[
[
"X = df[[\"satisfaction\",\"evaluation\",\"averageMonthlyHours\",\"yearsAtCompany\"]]",
"_____no_output_____"
]
],
[
[
"#### Separando os conjuntos de dados.",
"_____no_output_____"
]
],
[
[
"scaler = MinMaxScaler()",
"_____no_output_____"
],
[
"X = scaler.fit_transform(X)",
"_____no_output_____"
],
[
"X_train, X_test, y_train, y_test = train_test_split(\n X\n ,y\n ,test_size = 0.2\n ,random_state = 123\n ,stratify = y\n)",
"_____no_output_____"
],
[
"X_train",
"_____no_output_____"
]
],
[
[
"#### Função do modelo de base.",
"_____no_output_____"
]
],
[
[
"def base_rate_model(X) :\n y = np.zeros(X.shape[0])\n return y",
"_____no_output_____"
]
],
[
[
"#### Importando métodos de métrica de avaliação.",
"_____no_output_____"
]
],
[
[
"from sklearn.metrics import roc_auc_score\nfrom sklearn.metrics import accuracy_score\nfrom sklearn.metrics import classification_report",
"_____no_output_____"
],
[
"def accuracy_result(y_test,y_predict):\n acc = accuracy_score(y_test, y_predict)\n print (\"Accuracy = %2.2f\" % acc)",
"_____no_output_____"
],
[
"def roc_classification_report_results(model,y_test,y_predict):\n roc_ = roc_auc_score(y_test, y_predict)\n classfication_report = classification_report(y_test, y_predict)\n \n print (\"\\n{} AUC = {}\\n\".format(model, roc_))\n print(classfication_report)",
"_____no_output_____"
]
],
[
[
"#### Análise do modelo de baseline",
"_____no_output_____"
]
],
[
[
"y_predict = base_rate_model(X_test)",
"_____no_output_____"
],
[
"accuracy_result(y_test, y_predict)",
"Accuracy = 0.76\n"
],
[
"roc_classification_report_results(\"Base Model\", y_test, y_predict)",
"\nBase Model AUC = 0.5\n\n precision recall f1-score support\n\n 0 0.76 1.00 0.86 2286\n 1 0.00 0.00 0.00 714\n\n accuracy 0.76 3000\n macro avg 0.38 0.50 0.43 3000\nweighted avg 0.58 0.76 0.66 3000\n\n"
]
],
[
[
"### Modelo de Regressão Logística.",
"_____no_output_____"
],
[
"#### Instânciando o algoritmo.",
"_____no_output_____"
]
],
[
[
"from sklearn.linear_model import LogisticRegression\nlogis = LogisticRegression()",
"_____no_output_____"
]
],
[
[
"#### Realizando o treinamento.",
"_____no_output_____"
]
],
[
[
"logis.fit(X_train, y_train)",
"_____no_output_____"
]
],
[
[
"#### Calculando as predições.",
"_____no_output_____"
]
],
[
[
"y_predict = logis.predict(X_test)",
"_____no_output_____"
]
],
[
[
"#### Avaliando o resultado.",
"_____no_output_____"
]
],
[
[
"accuracy_result(y_test, y_predict)",
"Accuracy = 0.76\n"
],
[
"roc_classification_report_results(\"Logistic Regression\", y_test, y_predict)",
"\nLogistic Regression AUC = 0.5897884088018409\n\n precision recall f1-score support\n\n 0 0.80 0.92 0.85 2286\n 1 0.50 0.26 0.34 714\n\n accuracy 0.76 3000\n macro avg 0.65 0.59 0.60 3000\nweighted avg 0.73 0.76 0.73 3000\n\n"
]
],
[
[
"### Modelo de Arvore de decisão.",
"_____no_output_____"
],
[
"#### Instânciando o algoritmo.",
"_____no_output_____"
]
],
[
[
"from sklearn.tree import DecisionTreeClassifier\ndtree = DecisionTreeClassifier()",
"_____no_output_____"
]
],
[
[
"#### Realizando o treinamento.",
"_____no_output_____"
]
],
[
[
"dtree = dtree.fit(X_train,y_train)",
"_____no_output_____"
]
],
[
[
"#### Calculando as predições.",
"_____no_output_____"
]
],
[
[
"y_predict = dtree.predict(X_test)",
"_____no_output_____"
]
],
[
[
"#### Avaliando o resultado.",
"_____no_output_____"
]
],
[
[
"accuracy_result(y_test, y_predict)",
"Accuracy = 0.96\n"
],
[
"roc_classification_report_results(\"Decision Tree\", y_test, y_predict)",
"\nDecision Tree AUC = 0.9462622319268915\n\n precision recall f1-score support\n\n 0 0.98 0.97 0.97 2286\n 1 0.90 0.93 0.91 714\n\n accuracy 0.96 3000\n macro avg 0.94 0.95 0.94 3000\nweighted avg 0.96 0.96 0.96 3000\n\n"
]
],
[
[
"### Modelo de Arvore Aleatória (Random Forest)",
"_____no_output_____"
],
[
"#### Instânciando o algoritmo.",
"_____no_output_____"
]
],
[
[
"from sklearn.ensemble import RandomForestClassifier",
"_____no_output_____"
],
[
"rf = RandomForestClassifier()",
"_____no_output_____"
]
],
[
[
"#### Realizando o treinamento.",
"_____no_output_____"
]
],
[
[
"rf = rf.fit(X_train,y_train)",
"_____no_output_____"
]
],
[
[
"#### Calculando as predições.",
"_____no_output_____"
]
],
[
[
"y_predict = rf.predict(X_test)",
"_____no_output_____"
]
],
[
[
"#### Avaliando o resultado.",
"_____no_output_____"
]
],
[
[
"accuracy_result(y_test, y_predict)",
"Accuracy = 0.97\n"
],
[
"roc_classification_report_results(\"Random Forest\", y_test, y_predict)",
"\nRandom Forest AUC = 0.9535664659564612\n\n precision recall f1-score support\n\n 0 0.98 0.99 0.98 2286\n 1 0.95 0.92 0.94 714\n\n accuracy 0.97 3000\n macro avg 0.96 0.95 0.96 3000\nweighted avg 0.97 0.97 0.97 3000\n\n"
]
],
[
[
"### Pycaret",
"_____no_output_____"
]
],
[
[
"#pip install pycaret",
"_____no_output_____"
]
],
[
[
"#### Importando os métodos.",
"_____no_output_____"
]
],
[
[
"from pycaret.classification import *",
"_____no_output_____"
]
],
[
[
"#### Definindo o Setup.",
"_____no_output_____"
]
],
[
[
"s = setup( df[[\"satisfaction\",\"evaluation\",\"averageMonthlyHours\",\"yearsAtCompany\",\"turnover\"]]\n ,target = \"turnover\"\n ,numeric_features = [\"yearsAtCompany\"]\n ,normalize = True\n ,normalize_method = \"minmax\"\n ,data_split_stratify = True\n ,fix_imbalance = True,\n )",
"_____no_output_____"
]
],
[
[
"#### Comparando diferentes modelos.",
"_____no_output_____"
]
],
[
[
"best = compare_models(fold = 5,sort = 'AUC',)",
"_____no_output_____"
]
],
[
[
"#### Criando o modelo.",
"_____no_output_____"
]
],
[
[
"gbc = create_model('gbc', fold = 5)",
"_____no_output_____"
]
],
[
[
"#### Realizando o tunning do modelo.",
"_____no_output_____"
]
],
[
[
"tuned_gbc = tune_model(gbc\n ,fold = 5\n ,custom_grid = {\"learning_rate\":[0.1,0.2,0.5]\n ,\"n_estimators\":[100,500,1000]\n ,\"min_samples_split\":[1,2,5,10]\n ,\"max_depth\":[1,3,9]\n }\n ,optimize = 'AUC')",
"_____no_output_____"
]
],
[
[
"#### Finalizando o modelo.",
"_____no_output_____"
]
],
[
[
"final_model = finalize_model(tuned_gbc)",
"_____no_output_____"
],
[
"save_model(final_model,'model')",
"Transformation Pipeline and Model Successfully Saved\n"
]
],
[
[
"#### Transferindo os arquivos para o Data Lake.",
"_____no_output_____"
],
[
"#### Modelo de Classificação.",
"_____no_output_____"
]
],
[
[
"client.fput_object(\n \"curated\",\n \"model.pkl\",\n \"model.pkl\"\n)",
"_____no_output_____"
]
],
[
[
"#### Exportando o conjunto de dados para o disco.",
"_____no_output_____"
]
],
[
[
"df.to_csv(\"dataset.csv\",index=False)",
"_____no_output_____"
],
[
"client.fput_object(\n \"curated\",\n \"dataset.csv\",\n \"dataset.csv\"\n)",
"_____no_output_____"
]
]
] | [
"markdown",
"code",
"markdown",
"code",
"markdown",
"code",
"markdown",
"code",
"markdown",
"code",
"markdown",
"code",
"markdown",
"code",
"markdown",
"code",
"markdown",
"code",
"markdown",
"code",
"markdown",
"code",
"markdown",
"code",
"markdown",
"code",
"markdown",
"code",
"markdown",
"code",
"markdown",
"code",
"markdown",
"code",
"markdown",
"code",
"markdown",
"code",
"markdown",
"code",
"markdown",
"code",
"markdown",
"code",
"markdown",
"code",
"markdown",
"code",
"markdown",
"code",
"markdown",
"code",
"markdown",
"code",
"markdown",
"code",
"markdown",
"code",
"markdown",
"code",
"markdown",
"code",
"markdown",
"code",
"markdown",
"code",
"markdown",
"code",
"markdown",
"code",
"markdown",
"code",
"markdown",
"code",
"markdown",
"code"
] | [
[
"markdown",
"markdown"
],
[
"code",
"code"
],
[
"markdown"
],
[
"code",
"code"
],
[
"markdown"
],
[
"code",
"code"
],
[
"markdown"
],
[
"code",
"code",
"code"
],
[
"markdown"
],
[
"code",
"code",
"code",
"code"
],
[
"markdown"
],
[
"code",
"code"
],
[
"markdown",
"markdown"
],
[
"code",
"code"
],
[
"markdown"
],
[
"code"
],
[
"markdown"
],
[
"code"
],
[
"markdown"
],
[
"code",
"code",
"code"
],
[
"markdown"
],
[
"code",
"code"
],
[
"markdown"
],
[
"code",
"code",
"code",
"code"
],
[
"markdown"
],
[
"code"
],
[
"markdown"
],
[
"code",
"code",
"code",
"code"
],
[
"markdown"
],
[
"code"
],
[
"markdown"
],
[
"code",
"code",
"code"
],
[
"markdown"
],
[
"code",
"code",
"code"
],
[
"markdown",
"markdown"
],
[
"code"
],
[
"markdown"
],
[
"code"
],
[
"markdown"
],
[
"code"
],
[
"markdown"
],
[
"code",
"code"
],
[
"markdown",
"markdown"
],
[
"code"
],
[
"markdown"
],
[
"code"
],
[
"markdown"
],
[
"code"
],
[
"markdown"
],
[
"code",
"code"
],
[
"markdown",
"markdown"
],
[
"code",
"code"
],
[
"markdown"
],
[
"code"
],
[
"markdown"
],
[
"code"
],
[
"markdown"
],
[
"code",
"code"
],
[
"markdown"
],
[
"code"
],
[
"markdown"
],
[
"code"
],
[
"markdown"
],
[
"code"
],
[
"markdown"
],
[
"code"
],
[
"markdown"
],
[
"code"
],
[
"markdown"
],
[
"code"
],
[
"markdown"
],
[
"code",
"code"
],
[
"markdown",
"markdown"
],
[
"code"
],
[
"markdown"
],
[
"code",
"code"
]
] |
e774b74b6a337b1b7ae9a662a12a6a36d3b8fb9f | 9,101 | ipynb | Jupyter Notebook | examples/ctrl/basic/lead_lag.ipynb | pydae/pydae | 8076bcfeb2cdc865a5fc58561ff8d246d0ed7d9d | [
"MIT"
] | 1 | 2020-12-20T03:45:26.000Z | 2020-12-20T03:45:26.000Z | examples/ctrl/basic/lead_lag.ipynb | pydae/pydae | 8076bcfeb2cdc865a5fc58561ff8d246d0ed7d9d | [
"MIT"
] | null | null | null | examples/ctrl/basic/lead_lag.ipynb | pydae/pydae | 8076bcfeb2cdc865a5fc58561ff8d246d0ed7d9d | [
"MIT"
] | null | null | null | 26.002857 | 120 | 0.492583 | [
[
[
"import numpy as np\nimport matplotlib.pyplot as plt\nfrom IPython.core.display import HTML\nimport pydae.svg_tools as svgt\n%config InlineBackend.figure_format = 'svg'",
"_____no_output_____"
],
[
"%matplotlib widget",
"_____no_output_____"
],
[
"from pydae import ssa\nfrom lead_lag import lead_lag_class",
"_____no_output_____"
],
[
"syst = lead_lag_class()",
"_____no_output_____"
],
[
"\nsyst.initialize([{ # parameters setting\n # initial desired angle = 0º\n }],-1) # here -1 means that -1 is considered as initial gess for\n # dynamic and algebraic states",
"_____no_output_____"
],
[
"syst.report_y()",
"z_l = 0.00\n"
],
[
"syst.simulate([{'t_end':1.0, 'u_l':0.0, 'T_1':0.1, 'T_2':0.1},\n {'t_end':5.0, 'u_l':0.5}],0);",
"_____no_output_____"
],
[
"fig, axes = plt.subplots(nrows=1,ncols=1, figsize=(6, 3), dpi=100)\n\naxes.plot(syst.T, syst.get_values('z_l'), label=f'z_l')\naxes.set_ylabel('$\\\\theta (º)$')\naxes.set_xlabel('Time (s)')\nfig.tight_layout()",
"_____no_output_____"
],
[
"Δt = 0.001\nsyst = lead_lag_class()\ntimes = np.arange(0,5,Δt)\nsyst.initialize([{'u_l':0.0, 'T_1':1.0, 'T_2':0.1}],0)\n\nfor t in times:\n syst.run([{'t_end':t,'u_l':np.sin(1*np.pi*2*t)}])\n \nsyst.post();\n",
"_____no_output_____"
],
[
"fig, axes = plt.subplots(nrows=1,ncols=1, figsize=(6, 3), dpi=100)\n\naxes.plot(syst.T, syst.get_values('u_l'), label=f'u_l')\naxes.plot(syst.T, syst.get_values('z_l'), label=f'z_l')\naxes.legend()\naxes.set_xlabel('Time (s)')\nfig.tight_layout()",
"_____no_output_____"
],
[
"import ipywidgets\n%matplotlib widget",
"_____no_output_____"
],
[
"plt.ioff()\nplt.clf()\nsyst = lead_lag_class()\nsyst.initialize([{'u_l':0.0, 'T_1':1.0, 'T_2':0.1}],0)\nfig, axes = plt.subplots(nrows=1,ncols=1, figsize=(6, 3), dpi=100)\n\nline_u_l = axes.plot(syst.T, syst.get_values('u_l'), label=f'u_l')\nline_z_l = axes.plot(syst.T, syst.get_values('z_l'), label=f'z_l')\naxes.legend()\naxes.set_xlabel('Time (s)')\nfig.tight_layout()\n\n\n\naxes.set_ylim((-2,2))\naxes.set_xlim((0,50))\n\naxes.grid(True)\naxes.legend(loc='best')\nfig.tight_layout()\n\n\nsld_T_1 = ipywidgets.FloatSlider(orientation='horizontal',description = \"$\\sf T_1$\", \n value=0.1, min=-0.5,max= 2.0, \n step=0.01,continuous_update=False)\n\n\nsld_T_2 = ipywidgets.FloatSlider(orientation='horizontal',description = \"$\\sf T_2$\", \n value=0.1, min=0.05,max= 10.0, \n step=0.01,continuous_update=False)\n\nsld_freq = ipywidgets.FloatSlider(orientation='horizontal',description = \"$\\sf freq$\", \n value=1.0, min=0.1,max= 2.0, \n step=0.1)\n\nckbox_gain = ipywidgets.Checkbox(\n value=False,\n description='Gain compensation',\n disabled=False\n)\n\ntxt_phase = ipywidgets.Text(\n value='1∠0º',\n placeholder='Type something',\n description='gain∠phase:',\n disabled=False)\n\ndrop_signal = ipywidgets.Dropdown(\n options=['sin(wt)', 'step'],\n value='sin(wt)',\n description='Input:',\n disabled=False,\n)\n\n\n\nΔt = 0.01\ntimes = np.arange(0,5,Δt)\n\ndef update(change):\n \n T_1 = sld_T_1.value\n T_2 = sld_T_2.value\n freq = sld_freq.value\n \n cplx_ = (1j*2*np.pi*freq*T_1 + 1)/(1j*2*np.pi*freq*T_2 + 1)\n phase_wo = np.angle(cplx_,deg=True)\n \n gain_wo = 1.0\n if ckbox_gain.value:\n gain_wo = np.abs(cplx_)\n \n \n txt_phase.value = f'{np.abs(cplx_):4.1f}∠{phase_wo:4.1f}º'\n \n syst.initialize([{'u_l':0.0, 'T_1':T_1, 'T_2':T_2}],0)\n \n if drop_signal.value == 'sin(wt)':\n for t in times:\n syst.run([{'t_end':t,'u_l':np.sin(2*np.pi*freq*t)}])\n if drop_signal.value == 'step':\n syst.run([{'t_end':50,'u_l':1.0, 'Dt':0.01}])\n \n\n syst.post();\n\n line_u_l[0].set_data(syst.T, syst.get_values('u_l'))\n line_z_l[0].set_data(syst.T, syst.get_values('z_l')/gain_wo)\n\n fig.canvas.draw_idle()\n \nsld_T_1.observe(update, names='value')\nsld_T_2.observe(update, names='value')\nsld_freq.observe(update, names='value')\nckbox_gain.observe(update, names='value')\ndrop_signal.observe(update, names='value')\n\nlayout_row1 = ipywidgets.HBox([fig.canvas])\nlayout_row2 = ipywidgets.HBox([sld_T_1,sld_T_2, txt_phase])\nlayout_row3 = ipywidgets.HBox([sld_freq,ckbox_gain,drop_signal])\nlayout = ipywidgets.VBox([layout_row1,layout_row2,layout_row3])\nlayout",
"_____no_output_____"
]
]
] | [
"code"
] | [
[
"code",
"code",
"code",
"code",
"code",
"code",
"code",
"code",
"code",
"code",
"code",
"code"
]
] |
e774bbb48b1188a57665609ed4b8736009bcf627 | 2,998 | ipynb | Jupyter Notebook | PlanetMaps_Earth.ipynb | tobyrsmith/BasemapPlots | 1bcd5c57313d9d3716dfaa300772cf7329bf3592 | [
"MIT"
] | null | null | null | PlanetMaps_Earth.ipynb | tobyrsmith/BasemapPlots | 1bcd5c57313d9d3716dfaa300772cf7329bf3592 | [
"MIT"
] | null | null | null | PlanetMaps_Earth.ipynb | tobyrsmith/BasemapPlots | 1bcd5c57313d9d3716dfaa300772cf7329bf3592 | [
"MIT"
] | null | null | null | 20.256757 | 101 | 0.508005 | [
[
[
"import numpy as np\nimport matplotlib.pyplot as plt\n\nplt.rc('axes', grid=False) # turn off the background grid for images",
"_____no_output_____"
],
[
"Topo = plt.imread('Earth_50.png')\nprint Topo.shape, Topo.dtype",
"_____no_output_____"
]
],
[
[
"### Impact Craters",
"_____no_output_____"
]
],
[
[
"TestLat = np.array([0,45,60,-45,-60])\nTestLon = np.array([0,-45,-90,45,90])\n\nLatD,LatM,LonD,LonM = np.loadtxt('World.csv', delimiter=',', usecols=(2,3,4,5), unpack=True)\n\nImY,ImX = Topo.shape[0:-1]\n\nMapY = -ImY/180.0 * LatD + ImY/2.0\nMapX = (ImX/360.0 * LonD) + ImX/2.0\n\n#mask1 = np.where(MapX > ImX)\n#MapX[mask1] -= ImX",
"_____no_output_____"
],
[
"#fig = plt.figure()\n#ax = fig.add_axes([0.0, 0.0, 1.0, 1.0])\n\nfig, ax = plt.subplots(1,1)\nfig.set_size_inches(10,5)\nfig.tight_layout()\n\nax.autoscale_view('tight')\nax.set_axis_off()\n\nax.scatter(MapX,MapY,marker='o',s=30,color='y', edgecolor='k')\nax.imshow(Topo)\n",
"_____no_output_____"
],
[
"fig.savefig('EarthCraters_RAW.png', dpi=300, bbox_inches='tight',pad_inches=0)",
"_____no_output_____"
]
]
] | [
"code",
"markdown",
"code"
] | [
[
"code",
"code"
],
[
"markdown"
],
[
"code",
"code",
"code"
]
] |
e774d3ce0c94cd5d478edfa5d3a7460cc3f30602 | 103,979 | ipynb | Jupyter Notebook | site/en/r2/tutorials/estimators/boosted_trees.ipynb | NexusXi/docs | 7c8aea728a4eca4ee719fe6cca36ee95eb7ad40d | [
"Apache-2.0"
] | 4 | 2019-08-20T11:59:23.000Z | 2020-01-12T13:42:50.000Z | site/en/r2/tutorials/estimators/boosted_trees.ipynb | NexusXi/docs | 7c8aea728a4eca4ee719fe6cca36ee95eb7ad40d | [
"Apache-2.0"
] | null | null | null | site/en/r2/tutorials/estimators/boosted_trees.ipynb | NexusXi/docs | 7c8aea728a4eca4ee719fe6cca36ee95eb7ad40d | [
"Apache-2.0"
] | null | null | null | 95.04479 | 17,392 | 0.818771 | [
[
[
"##### Copyright 2019 The TensorFlow Authors.",
"_____no_output_____"
]
],
[
[
"#@title Licensed under the Apache License, Version 2.0 (the \"License\");\n# you may not use this file except in compliance with the License.\n# You may obtain a copy of the License at\n#\n# https://www.apache.org/licenses/LICENSE-2.0\n#\n# Unless required by applicable law or agreed to in writing, software\n# distributed under the License is distributed on an \"AS IS\" BASIS,\n# WITHOUT WARRANTIES OR CONDITIONS OF ANY KIND, either express or implied.\n# See the License for the specific language governing permissions and\n# limitations under the License.",
"_____no_output_____"
]
],
[
[
"# How to train Boosted Trees models in TensorFlow",
"_____no_output_____"
],
[
"<table class=\"tfo-notebook-buttons\" align=\"left\">\n <td>\n <a target=\"_blank\" href=\"https://www.tensorflow.org/beta/tutorials/estimators/boosted_trees\"><img src=\"https://www.tensorflow.org/images/tf_logo_32px.png\" />View on TensorFlow.org</a>\n </td>\n <td>\n <a target=\"_blank\" href=\"https://colab.research.google.com/github/tensorflow/docs/blob/master/site/en/r2/tutorials/estimators/boosted_trees.ipynb\"><img src=\"https://www.tensorflow.org/images/colab_logo_32px.png\" />Run in Google Colab</a>\n </td>\n <td>\n <a target=\"_blank\" href=\"https://github.com/tensorflow/docs/blob/master/site/en/r2/tutorials/estimators/boosted_trees.ipynb\"><img src=\"https://www.tensorflow.org/images/GitHub-Mark-32px.png\">View source on GitHub</a>\n </td>\n</table>",
"_____no_output_____"
],
[
"This tutorial is an end-to-end walkthrough of training a Gradient Boosting model using decision trees with the `tf.estimator` API. Boosted Trees models are among the most popular and effective machine learning approaches for both regression and classification. It is an ensemble technique that combines the predictions from several (think 10s, 100s or even 1000s) tree models.\n\nBoosted Trees models are popular with many machine learning practitioners as they can achieve impressive performance with minimal hyperparameter tuning.",
"_____no_output_____"
],
[
"## Load the titanic dataset\nYou will be using the titanic dataset, where the (rather morbid) goal is to predict passenger survival, given characteristics such as gender, age, class, etc.",
"_____no_output_____"
]
],
[
[
"from __future__ import absolute_import, division, print_function, unicode_literals\n\nimport numpy as np\nimport pandas as pd\nfrom IPython.display import clear_output\nfrom matplotlib import pyplot as plt\n\n# Load dataset.\ndftrain = pd.read_csv('https://storage.googleapis.com/tf-datasets/titanic/train.csv')\ndfeval = pd.read_csv('https://storage.googleapis.com/tf-datasets/titanic/eval.csv')\ny_train = dftrain.pop('survived')\ny_eval = dfeval.pop('survived')",
"_____no_output_____"
],
[
"try:\n !pip install tf-nightly-2.0-preview\nexcept Exception:\n pass\nimport tensorflow as tf\ntf.random.set_seed(123)",
"_____no_output_____"
]
],
[
[
"The dataset consists of a training set and an evaluation set:\n\n* `dftrain` and `y_train` are the *training set*—the data the model uses to learn.\n* The model is tested against the *eval set*, `dfeval`, and `y_eval`.\n\nFor training you will use the following features:\n\n\n<table>\n <tr>\n <th>Feature Name</th>\n <th>Description</th>\n </tr>\n <tr>\n <td>sex</td>\n <td>Gender of passenger</td>\n </tr>\n <tr>\n <td>age</td>\n <td>Age of passenger</td>\n </tr>\n <tr>\n <td>n_siblings_spouses</td>\n <td># siblings and partners aboard</td>\n </tr>\n <tr>\n <td>parch</td>\n <td># of parents and children aboard</td>\n </tr>\n <tr>\n <td>fare</td>\n <td>Fare passenger paid.</td>\n </tr>\n <tr>\n <td>class</td>\n <td>Passenger's class on ship</td>\n </tr>\n <tr>\n <td>deck</td>\n <td>Which deck passenger was on</td>\n </tr>\n <tr>\n <td>embark_town</td>\n <td>Which town passenger embarked from</td>\n </tr>\n <tr>\n <td>alone</td>\n <td>If passenger was alone</td>\n </tr>\n</table>",
"_____no_output_____"
],
[
"## Explore the data",
"_____no_output_____"
],
[
"Let's first preview some of the data and create summary statistics on the training set.",
"_____no_output_____"
]
],
[
[
"dftrain.head()",
"_____no_output_____"
],
[
"dftrain.describe()",
"_____no_output_____"
]
],
[
[
"There are 627 and 264 examples in the training and evaluation sets, respectively.",
"_____no_output_____"
]
],
[
[
"dftrain.shape[0], dfeval.shape[0]",
"_____no_output_____"
]
],
[
[
"The majority of passengers are in their 20's and 30's.",
"_____no_output_____"
]
],
[
[
"dftrain.age.hist(bins=20)\nplt.show()",
"_____no_output_____"
]
],
[
[
"There are approximately twice as male passengers as female passengers aboard.",
"_____no_output_____"
]
],
[
[
"dftrain.sex.value_counts().plot(kind='barh')\nplt.show()",
"_____no_output_____"
]
],
[
[
"The majority of passengers were in the \"third\" class.",
"_____no_output_____"
]
],
[
[
"dftrain['class'].value_counts().plot(kind='barh')\nplt.show()",
"_____no_output_____"
]
],
[
[
"Most passengers embarked from Southampton.",
"_____no_output_____"
]
],
[
[
"dftrain['embark_town'].value_counts().plot(kind='barh')\nplt.show()",
"_____no_output_____"
]
],
[
[
"Females have a much higher chance of surviving vs. males. This will clearly be a predictive feature for the model.",
"_____no_output_____"
]
],
[
[
"pd.concat([dftrain, y_train], axis=1).groupby('sex').survived.mean().plot(kind='barh').set_xlabel('% survive')\nplt.show()",
"_____no_output_____"
]
],
[
[
"## Create feature columns and input functions\nThe Gradient Boosting estimator can utilize both numeric and categorical features. Feature columns work with all TensorFlow estimators and their purpose is to define the features used for modeling. Additionally they provide some feature engineering capabilities like one-hot-encoding, normalization, and bucketization. In this tutorial, the fields in `CATEGORICAL_COLUMNS` are transformed from categorical columns to one-hot-encoded columns ([indicator column](https://www.tensorflow.org/api_docs/python/tf/feature_column/indicator_column)):",
"_____no_output_____"
]
],
[
[
"fc = tf.feature_column\nCATEGORICAL_COLUMNS = ['sex', 'n_siblings_spouses', 'parch', 'class', 'deck',\n 'embark_town', 'alone']\nNUMERIC_COLUMNS = ['age', 'fare']\n\ndef one_hot_cat_column(feature_name, vocab):\n return tf.feature_column.indicator_column(\n tf.feature_column.categorical_column_with_vocabulary_list(feature_name,\n vocab))\nfeature_columns = []\nfor feature_name in CATEGORICAL_COLUMNS:\n # Need to one-hot encode categorical features.\n vocabulary = dftrain[feature_name].unique()\n feature_columns.append(one_hot_cat_column(feature_name, vocabulary))\n\nfor feature_name in NUMERIC_COLUMNS:\n feature_columns.append(tf.feature_column.numeric_column(feature_name,\n dtype=tf.float32))",
"_____no_output_____"
]
],
[
[
"You can view the transformation that a feature column produces. For example, here is the output when using the `indicator_column` on a single example:",
"_____no_output_____"
]
],
[
[
"example = dict(dftrain.head(1))\nclass_fc = tf.feature_column.indicator_column(tf.feature_column.categorical_column_with_vocabulary_list('class', ('First', 'Second', 'Third')))\nprint('Feature value: \"{}\"'.format(example['class'].iloc[0]))\nprint('One-hot encoded: ', tf.keras.layers.DenseFeatures([class_fc])(example).numpy())",
"Feature value: \"Third\"\nOne-hot encoded: [[ 0. 0. 1.]]\n"
]
],
[
[
"Additionally, you can view all of the feature column transformations together:",
"_____no_output_____"
]
],
[
[
"tf.keras.layers.DenseFeatures(feature_columns)(example).numpy()",
"_____no_output_____"
]
],
[
[
"Next you need to create the input functions. These will specify how data will be read into our model for both training and inference. You will use the `from_tensor_slices` method in the [`tf.data`](https://www.tensorflow.org/api_docs/python/tf/data) API to read in data directly from Pandas. This is suitable for smaller, in-memory datasets. For larger datasets, the tf.data API supports a variety of file formats (including [csv](https://www.tensorflow.org/api_docs/python/tf/data/experimental/make_csv_dataset)) so that you can process datasets that do not fit in memory.",
"_____no_output_____"
]
],
[
[
"# Use entire batch since this is such a small dataset.\nNUM_EXAMPLES = len(y_train)\n\ndef make_input_fn(X, y, n_epochs=None, shuffle=True):\n def input_fn():\n dataset = tf.data.Dataset.from_tensor_slices((dict(X), y))\n if shuffle:\n dataset = dataset.shuffle(NUM_EXAMPLES)\n # For training, cycle thru dataset as many times as need (n_epochs=None).\n dataset = dataset.repeat(n_epochs)\n # In memory training doesn't use batching.\n dataset = dataset.batch(NUM_EXAMPLES)\n return dataset\n return input_fn\n\n# Training and evaluation input functions.\ntrain_input_fn = make_input_fn(dftrain, y_train)\neval_input_fn = make_input_fn(dfeval, y_eval, shuffle=False, n_epochs=1)",
"_____no_output_____"
]
],
[
[
"## Train and evaluate the model\n\nBelow you will do the following steps:\n\n1. Initialize the model, specifying the features and hyperparameters.\n2. Feed the training data to the model using the `train_input_fn` and train the model using the `train` function.\n3. You will assess model performance using the evaluation set—in this example, the `dfeval` DataFrame. You will verify that the predictions match the labels from the `y_eval` array.\n\nBefore training a Boosted Trees model, let's first train a linear classifier (logistic regression model). It is best practice to start with simpler model to establish a benchmark.",
"_____no_output_____"
]
],
[
[
"linear_est = tf.estimator.LinearClassifier(feature_columns)\n\n# Train model.\nlinear_est.train(train_input_fn, max_steps=100)\n\n# Evaluation.\nresult = linear_est.evaluate(eval_input_fn)\nclear_output()\nprint(pd.Series(result))",
"accuracy 0.765152\naccuracy_baseline 0.625000\nauc 0.832844\nauc_precision_recall 0.789631\naverage_loss 0.478908\nglobal_step 100.000000\nlabel/mean 0.375000\nloss 0.478908\nprecision 0.703297\nprediction/mean 0.350790\nrecall 0.646465\ndtype: float64\n"
]
],
[
[
"Next let's train a Boosted Trees model. For boosted trees, regression (`BoostedTreesRegressor`) and classification (`BoostedTreesClassifier`) are supported. Since the goal is to predict a class - survive or not survive, you will use the `BoostedTreesClassifier`.\n\n\n",
"_____no_output_____"
]
],
[
[
"# Since data fits into memory, use entire dataset per layer. It will be faster.\n# Above one batch is defined as the entire dataset.\nn_batches = 1\nest = tf.estimator.BoostedTreesClassifier(feature_columns,\n n_batches_per_layer=n_batches)\n\n# The model will stop training once the specified number of trees is built, not\n# based on the number of steps.\nest.train(train_input_fn, max_steps=100)\n\n# Eval.\nresult = est.evaluate(eval_input_fn)\nclear_output()\nprint(pd.Series(result))",
"accuracy 0.829545\naccuracy_baseline 0.625000\nauc 0.872788\nauc_precision_recall 0.857807\naverage_loss 0.411839\nglobal_step 100.000000\nlabel/mean 0.375000\nloss 0.411839\nprecision 0.793478\nprediction/mean 0.381942\nrecall 0.737374\ndtype: float64\n"
]
],
[
[
"Now you can use the train model to make predictions on a passenger from the evaluation set. TensorFlow models are optimized to make predictions on a batch, or collection, of examples at once. Earlier, the `eval_input_fn` is defined using the entire evaluation set.",
"_____no_output_____"
]
],
[
[
"pred_dicts = list(est.predict(eval_input_fn))\nprobs = pd.Series([pred['probabilities'][1] for pred in pred_dicts])\n\nprobs.plot(kind='hist', bins=20, title='predicted probabilities')\nplt.show()",
"_____no_output_____"
]
],
[
[
"Finally you can also look at the receiver operating characteristic (ROC) of the results, which will give us a better idea of the tradeoff between the true positive rate and false positive rate.",
"_____no_output_____"
]
],
[
[
"from sklearn.metrics import roc_curve\n\nfpr, tpr, _ = roc_curve(y_eval, probs)\nplt.plot(fpr, tpr)\nplt.title('ROC curve')\nplt.xlabel('false positive rate')\nplt.ylabel('true positive rate')\nplt.xlim(0,)\nplt.ylim(0,)\nplt.show()",
"_____no_output_____"
]
]
] | [
"markdown",
"code",
"markdown",
"code",
"markdown",
"code",
"markdown",
"code",
"markdown",
"code",
"markdown",
"code",
"markdown",
"code",
"markdown",
"code",
"markdown",
"code",
"markdown",
"code",
"markdown",
"code",
"markdown",
"code",
"markdown",
"code",
"markdown",
"code",
"markdown",
"code",
"markdown",
"code",
"markdown",
"code"
] | [
[
"markdown"
],
[
"code"
],
[
"markdown",
"markdown",
"markdown",
"markdown"
],
[
"code",
"code"
],
[
"markdown",
"markdown",
"markdown"
],
[
"code",
"code"
],
[
"markdown"
],
[
"code"
],
[
"markdown"
],
[
"code"
],
[
"markdown"
],
[
"code"
],
[
"markdown"
],
[
"code"
],
[
"markdown"
],
[
"code"
],
[
"markdown"
],
[
"code"
],
[
"markdown"
],
[
"code"
],
[
"markdown"
],
[
"code"
],
[
"markdown"
],
[
"code"
],
[
"markdown"
],
[
"code"
],
[
"markdown"
],
[
"code"
],
[
"markdown"
],
[
"code"
],
[
"markdown"
],
[
"code"
],
[
"markdown"
],
[
"code"
]
] |
e774eecf643ad95364f737395d5b1129eda02b10 | 14,762 | ipynb | Jupyter Notebook | notebooks/demo_Hangul.ipynb | Badissane/TensorFlow-101 | 582cc1d946f0ecbce078e493b8ccb1d7b40684df | [
"MIT"
] | 2,879 | 2016-05-09T16:39:39.000Z | 2022-03-30T11:44:52.000Z | notebooks/demo_Hangul.ipynb | mihirkelkar/Tensorflow-101 | 582cc1d946f0ecbce078e493b8ccb1d7b40684df | [
"MIT"
] | 11 | 2016-05-16T02:28:28.000Z | 2021-08-11T03:30:23.000Z | notebooks/demo_Hangul.ipynb | mihirkelkar/Tensorflow-101 | 582cc1d946f0ecbce078e493b8ccb1d7b40684df | [
"MIT"
] | 933 | 2016-05-09T16:39:42.000Z | 2022-03-30T16:44:56.000Z | 26.987203 | 1,076 | 0.445942 | [
[
[
"# \"data/nine_dreams/ninedreams.txt\" IS REQUIRED",
"_____no_output_____"
],
[
"# SPECIFY FILE ENCODING TYOE IN PYTHON",
"_____no_output_____"
]
],
[
[
"# -*- coding: utf-8 -*-\nprint (\"UTF-8 ENCODING\")",
"UTF-8 ENCODING\n"
]
],
[
[
"# LOAD PACKAGES",
"_____no_output_____"
]
],
[
[
"import chardet # https://github.com/chardet/chardet\nimport glob\nimport codecs\nimport sys\nimport os\nfrom TextLoader import *\nfrom Hangulpy3 import *\nprint (\"PACKAGES LOADED\") ",
"PACKAGES LOADED\n"
]
],
[
[
"# CONVERT UTF8-ENCODED TXT FILE",
"_____no_output_____"
]
],
[
[
"def conv_file(fromfile, tofile):\n with open(fromfile, \"rb\") as f:\n sample_text=f.read(10240)\n pred = chardet.detect(sample_text)\n if not pred['encoding'] in ('EUC-KR', 'UTF-8', 'CP949', 'UTF-16LE'):\n print (\"WARNING! Unknown encoding! : %s = %s\") % (fromfile, pred['encoding'])\n pred['encoding'] = \"CP949\" # 못찾으면 기본이 CP949\n formfile = fromfile + \".unknown\"\n elif pred['confidence'] < 0.9:\n print (\"WARNING! Unsured encofing! : %s = %s / %s\")\n % (fromfile, pred['confidence'], pred['encoding'])\n formfile = fromfile + \".notsure\"\n with codecs.open(fromfile, \"r\", encoding=pred['encoding'], errors=\"ignore\") as f:\n with codecs.open(tofile, \"w+\", encoding=\"utf8\") as t:\n all_text = f.read()\n t.write(all_text)",
"_____no_output_____"
]
],
[
[
"# \"data/nine_dreams/ninedreams_utf8.txt\" IS GENERATED",
"_____no_output_____"
]
],
[
[
"# SOURCE TXT FILE\nfromfile = \"data/nine_dreams/ninedreams.txt\"\n# TARGET TXT FILE\ntofile = \"data/nine_dreams/ninedreams_utf8.txt\"\nconv_file(fromfile, tofile)\nprint (\"UTF8-CONVERTING DONE\")\nprint (\" [%s] IS GENERATED\" % (tofile))",
"UTF8-CONVERTING DONE\n [data/nine_dreams/ninedreams_utf8.txt] IS GENERATED\n"
]
],
[
[
"# DECOMPOSE HANGUL (THIS PART IS IMPORTANT!)",
"_____no_output_____"
]
],
[
[
"def dump_file(filename):\n result=u\"\" # <= UNICODE STRING \n with codecs.open(filename,\"r\", encoding=\"UTF8\") as f:\n for line in f.readlines():\n line = tuple(line)\n result = result + decompose_text(line)\n return result\nprint (\"FUNCTION READY\")",
"FUNCTION READY\n"
]
],
[
[
"# PYTHON 2 AND 3 COMPATIBILITY",
"_____no_output_____"
]
],
[
[
"if sys.version_info.major == 2:\n parsed_txt = dump_file(tofile).encode(\"utf8\") \nelse:\n parsed_txt = dump_file(tofile) \n\nprint (\"Parsing %s done\" % (tofile))\n# PRINT FIRST 100 CHARACTERS\nprint (parsed_txt[:100])",
"Parsing data/nine_dreams/ninedreams_utf8.txt done\n\n\n ㅎㅏㄴᴥㄱㅜㄱᴥ ㄱㅜㄱᴥㅁㅜㄴᴥㅎㅏㄱᴥㅅㅏᴥㅅㅏㅇᴥ ㅇㅕㅇᴥ�\n"
]
],
[
[
"# \"data/nine_dreams/input.txt\" IS GENERATED",
"_____no_output_____"
]
],
[
[
"with open(\"data/nine_dreams/input.txt\", \"w\") as text_file:\n text_file.write(parsed_txt)\nprint (\"Saved to a txt file\")\nprint (text_file)",
"Saved to a txt file\n<closed file 'data/nine_dreams/input.txt', mode 'w' at 0x7f62ae9a58a0>\n"
]
],
[
[
"# COMPOSE HANGUL CHARACTER FROM PHONEME ",
"_____no_output_____"
]
],
[
[
"data=[u'\\u3147', u'\\u3157', u'\\u1d25', u'\\u3134', u'\\u3161', u'\\u3139', u'\\u1d25'\n , u' ', u'\\u314f', u'\\u3147', u'\\u3145', u'\\u314f', u'\\u1d25', u'\\u1d25'\n , u'\\u3163', u'\\u1d25', u' ', u'\\u3147', u'\\u1d25', u'\\u3155', u'\\u1d25'\n , u'\\u3134', u'\\u314f', u'\\u1d25', u'\\u3155', u'\\u3147', u'\\u1d25'\n , u'\\u315b', u'\\u3131', u'\\u1d25', u'\\u3147', u'\\u3139', u'\\u3146'\n , u'\\u1d25', u'\\u3137', u'\\u314f', u'\\u314e', u'\\u3139', u'\\u1d25'\n , u'\\u3134', u'\\u1d25', u'\\u3145', u'\\u3163', u'\\u1d25', u'\\u1d25'\n , u'\\u314f', u'\\u1d25', u'\\u314e', u'\\u314f', u'\\u3147', u'\\u3131'\n , u'\\u3157', u'\\u3134', u'\\u1d25', u'\\u1d25', u'\\u315b', u'\\u1d25'\n , u'\\u3148', u'\\u3153', u'\\u3136', u'\\u1d25', u' ', u'\\u3145', u'\\u3150'\n , u'\\u3141', u'\\u3136', u'\\u3161', u'\\u3134', u'\\u3163', u'\\u1d25', u'.'\n , u'\\u3148', u'\\u3153', u'\\u3134', u'\\u314e', u'\\u3153', u'\\u1d25', u'\\u1d25'\n , u'\\u3147', u'\\u314f', u'\\u3134', u'\\u3148', u'\\u314f', u'\\u3139', u'\\u315d'\n , u'\\u314c', u'\\u1d25', u'\\u3161', u'\\u3134', u'\\u3148', u'\\u3163', u'\\u313a'\n , u'\\u1d25', u' ', u'\\u3147', u'\\u3161', u'\\u3146', u'\\u1d25', u'?', u'\\u3134'\n , u'\\u1d25', u'\\u314e', u'\\u3163', u'\\u1d25', u'\\u3147', u'\\u3148', u'\\u314f'\n ]\nprint automata(\"\".join(data))\n",
"오늘 ㅏㅇ사ㅣ ㅇㅕ나ㅕㅇㅛㄱㅇㄹㅆ닿ㄹㄴ시ㅏ항곤ㅛ젆 샘ㄶㅡ니.젆ㅓ앉ㅏ뤝ㅡㄴ짉 읐?ㄴ히ㅇ\n"
]
],
[
[
"# GENERATE \"vocab.pkl\" and \"data.npy\" in \"data/nine_dreams/\" FROM \"data/nine_dreams/input.txt\" ",
"_____no_output_____"
]
],
[
[
"data_dir = \"data/nine_dreams\"\nbatch_size = 50\nseq_length = 50\ndata_loader = TextLoader(data_dir, batch_size, seq_length)",
"loading preprocessed files\n"
]
],
[
[
"# DATA_LOADER IS:",
"_____no_output_____"
]
],
[
[
"print ( \"type of 'data_loader' is %s, length is %d\" \n % (type(data_loader.vocab), len(data_loader.vocab)) )",
"type of 'data_loader' is <type 'dict'>, length is 76\n"
]
],
[
[
"# DATA_LOADER.VOCAB IS:",
"_____no_output_____"
]
],
[
[
"print (\"data_loader.vocab looks like \\n%s \" % (data_loader.vocab,))",
"data_loader.vocab looks like \n{u'_': 69, u'6': 59, u':': 57, u'\\n': 19, u'4': 67, u'5': 63, u'>': 75, u'!': 52, u' ': 1, u'\"': 28, u'\\u1d25': 0, u\"'\": 49, u')': 46, u'(': 45, u'-': 65, u',': 27, u'.': 24, u'\\u3131': 7, u'0': 73, u'\\u3133': 60, u'\\u3132': 29, u'\\u3135': 50, u'\\u3134': 4, u'\\u3137': 13, u'\\u3136': 44, u'\\u3139': 5, u'\\u3138': 32, u'\\u313b': 55, u'\\u313a': 48, u'\\u313c': 54, u'?': 41, u'3': 66, u'\\u3141': 12, u'\\u3140': 51, u'\\u3143': 47, u'\\u3142': 17, u'\\u3145': 10, u'\\u3144': 43, u'\\u3147': 2, u'\\u3146': 22, u'\\u3149': 40, u'\\u3148': 15, u'\\u314b': 42, u'\\u314a': 23, u'\\u314d': 31, u'\\u314c': 30, u'\\u314f': 3, u'\\u314e': 14, u'\\u3151': 34, u'\\u3150': 21, u'\\u3153': 11, u'\\u3152': 74, u'\\u3155': 18, u'\\u3154': 20, u'\\u3157': 9, u'\\u3156': 39, u'\\u3159': 53, u'\\u3158': 26, u'\\u315b': 38, u'\\u315a': 33, u'\\u315d': 36, u'\\u315c': 16, u'\\u315f': 35, u'\\u315e': 61, u'\\u3161': 8, u'\\u3160': 37, u'\\u3163': 6, u'\\u3162': 25, u'\\x1a': 72, u'9': 64, u'7': 71, u'2': 62, u'1': 58, u'\\u313f': 56, u'\\u313e': 70, u'8': 68} \n"
]
],
[
[
"# DATA_LOADER.CHARS IS:",
"_____no_output_____"
]
],
[
[
"print ( \"type of 'data_loader.chars' is %s, length is %d\" \n % (type(data_loader.chars), len(data_loader.chars)) )",
"type of 'data_loader.chars' is <type 'tuple'>, length is 76\n"
]
],
[
[
"# CHARS CONVERTS INDEX -> CHAR",
"_____no_output_____"
]
],
[
[
"print (\"data_loader.chars looks like \\n%s \" % (data_loader.chars,))",
"data_loader.chars looks like \n(u'\\u1d25', u' ', u'\\u3147', u'\\u314f', u'\\u3134', u'\\u3139', u'\\u3163', u'\\u3131', u'\\u3161', u'\\u3157', u'\\u3145', u'\\u3153', u'\\u3141', u'\\u3137', u'\\u314e', u'\\u3148', u'\\u315c', u'\\u3142', u'\\u3155', u'\\n', u'\\u3154', u'\\u3150', u'\\u3146', u'\\u314a', u'.', u'\\u3162', u'\\u3158', u',', u'\"', u'\\u3132', u'\\u314c', u'\\u314d', u'\\u3138', u'\\u315a', u'\\u3151', u'\\u315f', u'\\u315d', u'\\u3160', u'\\u315b', u'\\u3156', u'\\u3149', u'?', u'\\u314b', u'\\u3144', u'\\u3136', u'(', u')', u'\\u3143', u'\\u313a', u\"'\", u'\\u3135', u'\\u3140', u'!', u'\\u3159', u'\\u313c', u'\\u313b', u'\\u313f', u':', u'1', u'6', u'\\u3133', u'\\u315e', u'2', u'5', u'9', u'-', u'3', u'4', u'8', u'_', u'\\u313e', u'7', u'\\x1a', u'0', u'\\u3152', u'>') \n"
],
[
"for i, char in enumerate(data_loader.chars):\n # GET INDEX OF THE CHARACTER\n idx = data_loader.vocab[char]\n print (\"[%02d] %03s (%02d)\" \n % (i, automata(\"\".join(char)), idx))",
"[00] (00)\n[01] (01)\n[02] (02)\n[03] ㅏ (03)\n[04] (04)\n[05] (05)\n[06] ㅣ (06)\n[07] (07)\n[08] ㅡ (08)\n[09] ㅗ (09)\n[10] (10)\n[11] ㅓ (11)\n[12] (12)\n[13] (13)\n[14] (14)\n[15] (15)\n[16] ㅜ (16)\n[17] (17)\n[18] ㅕ (18)\n[19] \n (19)\n[20] ㅔ (20)\n[21] ㅐ (21)\n[22] (22)\n[23] (23)\n[24] . (24)\n[25] ㅢ (25)\n[26] ㅘ (26)\n[27] , (27)\n[28] \" (28)\n[29] (29)\n[30] (30)\n[31] (31)\n[32] (32)\n[33] ㅚ (33)\n[34] ㅑ (34)\n[35] ㅟ (35)\n[36] ㅝ (36)\n[37] ㅠ (37)\n[38] ㅛ (38)\n[39] ㅖ (39)\n[40] (40)\n[41] ? (41)\n[42] (42)\n[43] ㅄ (43)\n[44] ㄶ (44)\n[45] ( (45)\n[46] ) (46)\n[47] (47)\n[48] ㄺ (48)\n[49] ' (49)\n[50] ㄵ (50)\n[51] ㅀ (51)\n[52] ! (52)\n[53] ㅙ (53)\n[54] ㄼ (54)\n[55] ㄻ (55)\n[56] ㄿ (56)\n[57] : (57)\n[58] 1 (58)\n[59] 6 (59)\n[60] ㄳ (60)\n[61] ㅞ (61)\n[62] 2 (62)\n[63] 5 (63)\n[64] 9 (64)\n[65] - (65)\n[66] 3 (66)\n[67] 4 (67)\n[68] 8 (68)\n[69] _ (69)\n[70] ㄾ (70)\n[71] 7 (71)\n[72] \u001a (72)\n[73] 0 (73)\n[74] ㅒ (74)\n[75] > (75)\n"
]
]
] | [
"markdown",
"code",
"markdown",
"code",
"markdown",
"code",
"markdown",
"code",
"markdown",
"code",
"markdown",
"code",
"markdown",
"code",
"markdown",
"code",
"markdown",
"code",
"markdown",
"code",
"markdown",
"code",
"markdown",
"code",
"markdown",
"code"
] | [
[
"markdown",
"markdown"
],
[
"code"
],
[
"markdown"
],
[
"code"
],
[
"markdown"
],
[
"code"
],
[
"markdown"
],
[
"code"
],
[
"markdown"
],
[
"code"
],
[
"markdown"
],
[
"code"
],
[
"markdown"
],
[
"code"
],
[
"markdown"
],
[
"code"
],
[
"markdown"
],
[
"code"
],
[
"markdown"
],
[
"code"
],
[
"markdown"
],
[
"code"
],
[
"markdown"
],
[
"code"
],
[
"markdown"
],
[
"code",
"code"
]
] |
e774f3514c349b721117068d041c19dfb47abbdb | 25,759 | ipynb | Jupyter Notebook | nbs/06_cli.ipynb | maarten990/nbdev | 53f9c4cce1ed943d905d15bdf6617745dd8e4123 | [
"Apache-2.0"
] | null | null | null | nbs/06_cli.ipynb | maarten990/nbdev | 53f9c4cce1ed943d905d15bdf6617745dd8e4123 | [
"Apache-2.0"
] | null | null | null | nbs/06_cli.ipynb | maarten990/nbdev | 53f9c4cce1ed943d905d15bdf6617745dd8e4123 | [
"Apache-2.0"
] | null | null | null | 34.208499 | 490 | 0.564657 | [
[
[
"# Command line functions\n\n> Console commands added by the nbdev library",
"_____no_output_____"
]
],
[
[
"# default_exp cli",
"_____no_output_____"
],
[
"# export\nfrom nbdev.imports import *\nfrom nbdev.export import *\nfrom nbdev.sync import *\nfrom nbdev.merge import *\nfrom nbdev.export2html import *\nfrom nbdev.test import *\nfrom fastscript import call_parse,Param,bool_arg",
"_____no_output_____"
]
],
[
[
"`nbdev` comes with the following commands. To use any of them, you muse be in one of the subfolder of your project: they will search for the `settings.ini` recursively in the parent directory but need to accessit to be able to work. Their names all begin by nbdev so you can easily get a list with tab completion.\n- `nbdev_build_lib` builds the library from the notebooks\n- `nbdev_update_lib` propagates any change in the library back to the notebooks\n- `nbdev_diff_nbs` gives you the diff between the notebooks and the exported library\n- `nbdev_build_docs` builds the documentation from the notebooks\n- `nbdev_nb2md` to convert a notebook to a markdown file\n- `nbdev_clean_nbs` removes all superfluous metadata form the notebooks, to avoid merge conflicts\n- `nbdev_read_nbs` read all notebooks to make sure none are broken\n- `nbdev_trust_nbs` trust all notebooks (so that the HTML content is shown)\n- `nbdev_fix_merge` will fix merge conflicts in a notebook file\n- `nbdev_install_git_hooks` install the git hooks that use the last two command automatically on each commit/merge.",
"_____no_output_____"
],
[
"## Navigating from notebooks to script and back",
"_____no_output_____"
]
],
[
[
"#export\n@call_parse\ndef nbdev_build_lib(fname:Param(\"A notebook name or glob to convert\", str)=None):\n \"Export notebooks matching `fname` to python modules\"\n write_tmpls()\n notebook2script(fname=fname)",
"_____no_output_____"
]
],
[
[
"By default (`fname` left to `None`), the whole library is built from the notebooks in the `lib_folder` set in your `settings.ini`.",
"_____no_output_____"
]
],
[
[
"#export\n@call_parse\ndef nbdev_update_lib(fname:Param(\"A notebook name or glob to convert\", str)=None):\n \"Propagates any change in the modules matching `fname` to the notebooks that created them\"\n script2notebook(fname=fname)",
"_____no_output_____"
]
],
[
[
"By default (`fname` left to `None`), the whole library is treated. Note that this tool is only designed for small changes such as typo or small bug fixes. You can't add new cells in notebook from the library.",
"_____no_output_____"
]
],
[
[
"#export\n@call_parse\ndef nbdev_diff_nbs(): \n \"Prints the diff between an export of the library in notebooks and the actual modules\"\n diff_nb_script()",
"_____no_output_____"
]
],
[
[
"## Extracting tests",
"_____no_output_____"
]
],
[
[
"# export\ndef _test_one(fname, flags=None, verbose=True):\n print(f\"testing: {fname}\")\n start = time.time()\n try: \n test_nb(fname, flags=flags)\n return True,time.time()-start\n except Exception as e: \n if \"Kernel died before replying to kernel_info\" in str(e):\n time.sleep(random.random())\n _test_one(fname, flags=flags)\n if verbose: print(f'Error in {fname}:\\n{e}')\n return False,time.time()-start",
"_____no_output_____"
],
[
"# export\n@call_parse\ndef nbdev_test_nbs(fname:Param(\"A notebook name or glob to convert\", str)=None,\n flags:Param(\"Space separated list of flags\", str)=None,\n n_workers:Param(\"Number of workers to use\", int)=None,\n verbose:Param(\"Print errors along the way\", bool)=True,\n timing:Param(\"Timing each notebook to see the ones are slow\", bool)=False):\n \"Test in parallel the notebooks matching `fname`, passing along `flags`\"\n if flags is not None: flags = flags.split(' ')\n if fname is None: \n files = [f for f in Config().nbs_path.glob('*.ipynb') if not f.name.startswith('_')]\n else: files = glob.glob(fname)\n files = [Path(f).absolute() for f in sorted(files)]\n if len(files)==1 and n_workers is None: n_workers=0\n # make sure we are inside the notebook folder of the project\n os.chdir(Config().nbs_path)\n results = parallel(_test_one, files, flags=flags, verbose=verbose, n_workers=n_workers)\n passed,times = [r[0] for r in results],[r[1] for r in results]\n if all(passed): print(\"All tests are passing!\")\n else:\n msg = \"The following notebooks failed:\\n\"\n raise Exception(msg + '\\n'.join([f.name for p,f in zip(passed,files) if not p]))\n if timing:\n for i,t in sorted(enumerate(times), key=lambda o:o[1], reverse=True): \n print(f\"Notebook {files[i].name} took {int(t)} seconds\")",
"_____no_output_____"
]
],
[
[
"By default (`fname` left to `None`), the whole library is tested from the notebooks in the `lib_folder` set in your `settings.ini`.",
"_____no_output_____"
],
[
"## Building documentation",
"_____no_output_____"
],
[
"The following functions complete the ones in `export2html` to fully build the documentation of your library.",
"_____no_output_____"
]
],
[
[
"#export\nimport time,random,warnings",
"_____no_output_____"
],
[
"#export\ndef _leaf(k,v):\n url = 'external_url' if \"http\" in v else 'url'\n #if url=='url': v=v+'.html'\n return {'title':k, url:v, 'output':'web,pdf'}",
"_____no_output_____"
],
[
"#export\n_k_names = ['folders', 'folderitems', 'subfolders', 'subfolderitems']\ndef _side_dict(title, data, level=0):\n k_name = _k_names[level]\n level += 1\n res = [(_side_dict(k, v, level) if isinstance(v,dict) else _leaf(k,v))\n for k,v in data.items()]\n return ({k_name:res} if not title\n else res if title.startswith('empty')\n else {'title': title, 'output':'web', k_name: res})",
"_____no_output_____"
],
[
"#export\n_re_catch_title = re.compile('^title\\s*:\\s*(\\S+.*)$', re.MULTILINE)",
"_____no_output_____"
],
[
"#export\ndef _get_title(fname):\n \"Grabs the title of html file `fname`\"\n with open(fname, 'r') as f: code = f.read()\n src = _re_catch_title.search(code)\n return fname.stem if src is None else src.groups()[0]",
"_____no_output_____"
],
[
"#hide\ntest_eq(_get_title(Config().doc_path/'export.html'), \"Export to modules\")",
"_____no_output_____"
],
[
"#export\nfrom nbdev.export2html import _nb2htmlfname",
"_____no_output_____"
],
[
"#export\ndef create_default_sidebar():\n \"Create the default sidebar for the docs website\"\n dic = {\"Overview\": \"/\"}\n files = [f for f in Config().nbs_path.glob('*.ipynb') if not f.name.startswith('_')]\n fnames = [_nb2htmlfname(f) for f in sorted(files)]\n titles = [_get_title(f) for f in fnames if 'index' not in f.stem!='index']\n if len(titles) > len(set(titles)): print(f\"Warning: Some of your Notebooks use the same title ({titles}).\")\n dic.update({_get_title(f):f'/{f.stem}' for f in fnames if f.stem!='index'})\n dic = {Config().lib_name: dic}\n json.dump(dic, open(Config().doc_path/'sidebar.json', 'w'), indent=2)",
"_____no_output_____"
]
],
[
[
"The default sidebar lists all html pages with their respective title, except the index that is named \"Overview\". To build a custom sidebar, set the flag `custom_sidebar` in your `settings.ini` to `True` then change the `sidebar.json` file in the `doc_folder` to your liking. Otherwise, the sidebar is updated at each doc build.",
"_____no_output_____"
]
],
[
[
"#hide\n#create_default_sidebar()",
"_____no_output_____"
],
[
"#export\ndef make_sidebar():\n \"Making sidebar for the doc website form the content of `doc_folder/sidebar.json`\"\n if not (Config().doc_path/'sidebar.json').exists() or Config().custom_sidebar == 'False': create_default_sidebar()\n sidebar_d = json.load(open(Config().doc_path/'sidebar.json', 'r'))\n res = _side_dict('Sidebar', sidebar_d)\n res = {'entries': [res]}\n res_s = yaml.dump(res, default_flow_style=False)\n res_s = res_s.replace('- subfolders:', ' subfolders:').replace(' - - ', ' - ')\n res_s = f\"\"\"\n#################################################\n### THIS FILE WAS AUTOGENERATED! DO NOT EDIT! ###\n#################################################\n# Instead edit {'../../sidebar.json'}\n\"\"\"+res_s\n open(Config().doc_path/'_data/sidebars/home_sidebar.yml', 'w').write(res_s)",
"_____no_output_____"
],
[
"# export\n_re_index = re.compile(r'^(?:\\d*_|)index\\.ipynb$')",
"_____no_output_____"
],
[
"# export\ndef make_readme():\n \"Convert the index notebook to README.md\"\n index_fn = None\n for f in Config().nbs_path.glob('*.ipynb'):\n if _re_index.match(f.name): index_fn = f\n assert index_fn is not None, \"Could not locate index notebook\"\n print(f\"converting {index_fn} to README.md\")\n convert_md(index_fn, Config().config_file.parent, jekyll=False)\n n = Config().config_file.parent/index_fn.with_suffix('.md').name\n shutil.move(n, Config().config_file.parent/'README.md')",
"_____no_output_____"
],
[
"# export\n@call_parse\ndef nbdev_build_docs(fname:Param(\"A notebook name or glob to convert\", str)=None,\n force_all:Param(\"Rebuild even notebooks that haven't changed\", bool)=False,\n mk_readme:Param(\"Also convert the index notebook to README\", bool)=True,\n n_workers:Param(\"Number of workers to use\", int)=None):\n \"Build the documentation by converting notebooks mathing `fname` to html\"\n notebook2html(fname=fname, force_all=force_all, n_workers=n_workers)\n if fname is None: make_sidebar()\n if mk_readme: make_readme()",
"_____no_output_____"
]
],
[
[
"By default (`fname` left to `None`), the whole documentation is build from the notebooks in the `lib_folder` set in your `settings.ini`, only converting the ones that have been modified since the their corresponding html was last touched unless you pass `force_all=True`. The index is also converted to make the README file, unless you pass along `mk_readme=False`.",
"_____no_output_____"
]
],
[
[
"# export\n@call_parse\ndef nbdev_nb2md(fname:Param(\"A notebook file name to convert\", str),\n dest:Param(\"The destination folder\", str)='.',\n img_path:Param(\"Folder to export images to\")=\"\",\n jekyll:Param(\"To use jekyll metadata for your markdown file or not\", bool_arg)=False,):\n \"Convert the notebook in `fname` to a markdown file\"\n nb_detach_cells(fname, dest=img_path)\n convert_md(fname, dest, jekyll=jekyll, img_path=img_path)",
"_____no_output_____"
],
[
"# export\n@call_parse\ndef nbdev_detach(path_nb:Param(\"Path to notebook\"),\n dest:Param(\"Destination folder\", str)=\"\"):\n \"Export cell attachments to `dest` and update references\"\n nb_detach_cells(path_nb, dest=dest)",
"_____no_output_____"
]
],
[
[
"## Other utils",
"_____no_output_____"
]
],
[
[
"# export\n@call_parse\ndef nbdev_read_nbs(fname:Param(\"A notebook name or glob to convert\", str)=None):\n \"Check all notebooks matching `fname` can be opened\"\n files = Config().nbs_path.glob('**/*.ipynb') if fname is None else glob.glob(fname)\n for nb in files:\n try: _ = read_nb(nb)\n except Exception as e:\n print(f\"{nb} is corrupted and can't be opened.\")\n raise e",
"_____no_output_____"
]
],
[
[
"By default (`fname` left to `None`), the all the notebooks in `lib_folder` are checked.",
"_____no_output_____"
]
],
[
[
"# export\n@call_parse\ndef nbdev_trust_nbs(fname:Param(\"A notebook name or glob to convert\", str)=None,\n force_all:Param(\"Trust even notebooks that haven't changed\", bool)=False):\n \"Trust noteboks matching `fname`\"\n check_fname = Config().nbs_path/\".last_checked\"\n last_checked = os.path.getmtime(check_fname) if check_fname.exists() else None\n files = Config().nbs_path.glob('**/*.ipynb') if fname is None else glob.glob(fname)\n for fn in files:\n if last_checked and not force_all:\n last_changed = os.path.getmtime(fn)\n if last_changed < last_checked: continue\n nb = read_nb(fn)\n if not NotebookNotary().check_signature(nb): NotebookNotary().sign(nb)\n check_fname.touch(exist_ok=True)",
"_____no_output_____"
]
],
[
[
"By default (`fname` left to `None`), the all the notebooks in `lib_folder` are trusted. To speed things up, only the ones touched since the last time this command was run are trusted unless you pass along `force_all=True`.",
"_____no_output_____"
]
],
[
[
"# export\n@call_parse\ndef nbdev_fix_merge(fname:Param(\"A notebook filename to fix\", str),\n fast:Param(\"Fast fix: automatically fix the merge conflicts in outputs or metadata\", bool)=True,\n trust_us:Param(\"Use local outputs/metadata when fast mergning\", bool)=True):\n \"Fix merge conflicts in notebook `fname`\"\n fix_conflicts(fname, fast=fast, trust_us=trust_us)",
"_____no_output_____"
]
],
[
[
"When you have merge conflicts after a `git pull`, the notebook file will be broken and won't open in jupyter notebook anymore. This command fixes this by changing the notebook to a proper json file again and add markdown cells to signal the conflict, you just have to open that notebook again and look for `>>>>>>>` to see those conflicts and manually fix them. The old broken file is copied with a `.ipynb.bak` extension, so is still accessible in case the merge wasn't sucessful.\n\nMoreover, if `fast=True`, conflicts in outputs and metadata will automatically be fixed by using the local version if `trust_us=True`, the remote one if `trust_us=False`. With this option, it's very likely you won't have anything to do, unless there is a real conflict.",
"_____no_output_____"
]
],
[
[
"#export\ndef bump_version(version, part=2):\n version = version.split('.')\n version[part] = str(int(version[part]) + 1)\n for i in range(part+1, 3): version[i] = '0'\n return '.'.join(version)",
"_____no_output_____"
],
[
"test_eq(bump_version('0.1.1' ), '0.1.2')\ntest_eq(bump_version('0.1.1', 1), '0.2.0')",
"_____no_output_____"
],
[
"# export\n@call_parse\ndef nbdev_bump_version(part:Param(\"Part of version to bump\", int)=2):\n \"Increment version in `settings.py` by one\"\n cfg = Config()\n print(f'Old version: {cfg.version}')\n cfg.d['version'] = bump_version(Config().version, part)\n cfg.save()\n update_version()\n print(f'New version: {cfg.version}')",
"_____no_output_____"
]
],
[
[
"## Git hooks",
"_____no_output_____"
]
],
[
[
"# export\nimport subprocess",
"_____no_output_____"
],
[
"# export\n@call_parse\ndef nbdev_install_git_hooks():\n \"Install git hooks to clean/trust notebooks automatically\"\n path = Config().config_file.parent\n fn = path/'.git'/'hooks'/'post-merge'\n #Trust notebooks after merge\n with open(fn, 'w') as f:\n f.write(\"\"\"#!/bin/bash\necho \"Trusting notebooks\"\nnbdev_trust_nbs\n\"\"\"\n )\n os.chmod(fn, os.stat(fn).st_mode | stat.S_IEXEC)\n #Clean notebooks on commit/diff\n with open(path/'.gitconfig', 'w') as f:\n f.write(\"\"\"# Generated by nbdev_install_git_hooks\n#\n# If you need to disable this instrumentation do:\n#\n# git config --local --unset include.path\n#\n# To restore the filter\n#\n# git config --local include.path .gitconfig\n#\n# If you see notebooks not stripped, checked the filters are applied in .gitattributes\n#\n[filter \"clean-nbs\"]\n clean = nbdev_clean_nbs --read_input_stream True\n smudge = cat\n required = true\n[diff \"ipynb\"]\n textconv = nbdev_clean_nbs --disp True --fname\n\"\"\")\n cmd = \"git config --local include.path ../.gitconfig\"\n print(f\"Executing: {cmd}\")\n result = subprocess.run(cmd.split(), shell=False, check=False, stderr=subprocess.PIPE)\n if result.returncode == 0:\n print(\"Success: hooks are installed and repo's .gitconfig is now trusted\")\n else:\n print(\"Failed to trust repo's .gitconfig\")\n if result.stderr: print(f\"Error: {result.stderr.decode('utf-8')}\")\n with open(Config().nbs_path/'.gitattributes', 'w') as f:\n f.write(\"\"\"**/*.ipynb filter=clean-nbs\n**/*.ipynb diff=ipynb\n\"\"\"\n )",
"_____no_output_____"
]
],
[
[
"This command installs git hooks to make sure notebooks are cleaned before you commit them to GitHub and automatically trusted at each merge. To be more specific, this creates:\n- an executable '.git/hooks/post-merge' file that contains the command `nbdev_trust_nbs`\n- a `.gitconfig` file that uses `nbev_clean_nbs` has a filter/diff on all notebook files inside `nbs_folder` and a `.gitattributes` file generated in this folder (copy this file in other folders where you might have notebooks you want cleaned as well)",
"_____no_output_____"
],
[
"## Starting a new project",
"_____no_output_____"
]
],
[
[
"#export\n_template_git_repo = \"https://github.com/fastai/nbdev_template.git\"",
"_____no_output_____"
],
[
"#export\n@call_parse\ndef nbdev_new(name: Param(\"A directory to create the project in\", str)):\n \"Create a new nbdev project with a given name.\"\n \n path = Path(f\"./{name}\").absolute()\n \n if path.is_dir():\n print(f\"Directory {path} already exists. Aborting.\")\n return\n \n print(f\"Creating a new nbdev project {name}.\")\n \n try:\n subprocess.run(f\"git clone {_template_git_repo} {path}\".split(), check=True, timeout=5000)\n shutil.rmtree(path/\".git\")\n subprocess.run(\"git init\".split(), cwd=path, check=True)\n subprocess.run(\"git add .\".split(), cwd=path, check=True)\n subprocess.run(\"git commit -am \\\"Initial\\\"\".split(), cwd=path, check=True)\n \n print(f\"Created a new repo for project {name}. Please edit settings.ini and run nbdev_build_lib to get started.\")\n except Exception as e:\n print(\"An error occured while copying nbdev project template:\")\n print(e)\n if os.path.isdir(path): shutil.rmtree(path)",
"_____no_output_____"
]
],
[
[
"`nbdev_new` is a command line tool that creates a new nbdev project based on the [nbdev_template repo](https://github.com/fastai/nbdev_template). It'll initialize a new git repository and commit the new project.\n\nAfter you run `nbdev_new`, please edit `settings.ini` and run `nbdev_build_lib`.",
"_____no_output_____"
],
[
"## Export",
"_____no_output_____"
]
],
[
[
"#hide\nfrom nbdev.export import *\nnotebook2script()",
"Converted 00_export.ipynb.\nConverted 01_sync.ipynb.\nConverted 02_showdoc.ipynb.\nConverted 03_export2html.ipynb.\nConverted 04_test.ipynb.\nConverted 05_merge.ipynb.\nConverted 06_cli.ipynb.\nConverted 07_clean.ipynb.\nConverted 99_search.ipynb.\nConverted index.ipynb.\nConverted tutorial.ipynb.\n"
]
]
] | [
"markdown",
"code",
"markdown",
"code",
"markdown",
"code",
"markdown",
"code",
"markdown",
"code",
"markdown",
"code",
"markdown",
"code",
"markdown",
"code",
"markdown",
"code",
"markdown",
"code",
"markdown",
"code",
"markdown",
"code",
"markdown",
"code",
"markdown",
"code",
"markdown",
"code"
] | [
[
"markdown"
],
[
"code",
"code"
],
[
"markdown",
"markdown"
],
[
"code"
],
[
"markdown"
],
[
"code"
],
[
"markdown"
],
[
"code"
],
[
"markdown"
],
[
"code",
"code"
],
[
"markdown",
"markdown",
"markdown"
],
[
"code",
"code",
"code",
"code",
"code",
"code",
"code",
"code"
],
[
"markdown"
],
[
"code",
"code",
"code",
"code",
"code"
],
[
"markdown"
],
[
"code",
"code"
],
[
"markdown"
],
[
"code"
],
[
"markdown"
],
[
"code"
],
[
"markdown"
],
[
"code"
],
[
"markdown"
],
[
"code",
"code",
"code"
],
[
"markdown"
],
[
"code",
"code"
],
[
"markdown",
"markdown"
],
[
"code",
"code"
],
[
"markdown",
"markdown"
],
[
"code"
]
] |
e7750dd960996739429c0eef81fb7a54e76f90a4 | 27,322 | ipynb | Jupyter Notebook | lecture02.ingestion/lecture02.ingestion.ipynb | philmui/algorithmic-bias-2019 | eada3d32f80a400ee4712237ff06c1c75abaed54 | [
"MIT"
] | 1 | 2019-06-22T18:33:50.000Z | 2019-06-22T18:33:50.000Z | lecture02.ingestion/lecture02.ingestion.ipynb | philmui/algorithmic-bias-2019 | eada3d32f80a400ee4712237ff06c1c75abaed54 | [
"MIT"
] | 2 | 2021-03-31T19:17:51.000Z | 2021-12-13T20:01:23.000Z | lecture02.ingestion/lecture02.ingestion.ipynb | philmui/algorithmic-bias-2019 | eada3d32f80a400ee4712237ff06c1c75abaed54 | [
"MIT"
] | null | null | null | 23.594128 | 476 | 0.566503 | [
[
[
"# Lecture 01 : intro, inputs, numpy, pandas",
"_____no_output_____"
],
[
"## 1. Inputs: CSV / Text",
"_____no_output_____"
],
[
"We will start by ingesting plain text.",
"_____no_output_____"
]
],
[
[
"from __future__ import print_function\nimport csv",
"_____no_output_____"
],
[
"my_reader = csv.DictReader(open('data/eu_revolving_loans.csv', 'r'))",
"_____no_output_____"
]
],
[
[
"DicReader returns a \"generator\" -- which means that we only have 1 chance to read the returning row dictionaries.\n\nLet's just print out line by line to see what we are reading in:",
"_____no_output_____"
]
],
[
[
"for line in my_reader:\n print(line)",
"_____no_output_____"
]
],
[
[
"Since the data is tabular format, pandas is ideally suited for such data. There are convenient pandas import functions for reading in tabular data.\n\nPandas provides direct csv ingestion into \"data frames\":",
"_____no_output_____"
]
],
[
[
"import pandas as pd\ndf = pd.read_csv('data/eu_revolving_loans.csv')\ndf.head()",
"_____no_output_____"
]
],
[
[
"As we briefly discussed last week, simply reading in without any configuration generates a fairly message data frame. We should try to specify some helping hints to pandas as to where the header rows are and which is the index colum:",
"_____no_output_____"
]
],
[
[
"df = pd.read_csv('data/eu_revolving_loans.csv', header=[1,2,4], index_col=0)\ndf.head()",
"_____no_output_____"
]
],
[
[
"## 2. Inputs: Excel",
"_____no_output_____"
],
[
"Many organizations still use Excel as the common medium for communicating data and analysis. We will look quickly at how to ingest Excel data. There are many packages available to read Excel files. We will use one popular one here.",
"_____no_output_____"
]
],
[
[
"from __future__ import print_function\nfrom openpyxl import load_workbook",
"_____no_output_____"
]
],
[
[
"Let's take a look at the excel file that want to read into Jupyter",
"_____no_output_____"
]
],
[
[
"!open 'data/climate_change_download_0.xlsx'",
"_____no_output_____"
]
],
[
[
"Here is how we can read the Excel file into the Jupyter environment.",
"_____no_output_____"
]
],
[
[
"wb = load_workbook(filename='data/climate_change_download_0.xlsx')",
"_____no_output_____"
]
],
[
[
"What are the \"sheets\" in this workbook?",
"_____no_output_____"
]
],
[
[
"wb.get_sheet_names()`",
"_____no_output_____"
]
],
[
[
"We will focus on the sheet 'Data':",
"_____no_output_____"
]
],
[
[
"ws = wb.get_sheet_by_name('Data')",
"_____no_output_____"
]
],
[
[
"For the sheet \"Data\", let's print out the content cell-by-cell to view the content.",
"_____no_output_____"
]
],
[
[
"for row in ws.rows:\n for cell in row:\n print(cell.value)",
"_____no_output_____"
]
],
[
[
"Pandas also provides direct Excel data ingest:",
"_____no_output_____"
]
],
[
[
"import pandas as pd\ndf = pd.read_excel('data/climate_change_download_0.xlsx')\ndf.head()",
"_____no_output_____"
]
],
[
[
"Here is another example with multiple sheets:",
"_____no_output_____"
]
],
[
[
"df = pd.read_excel('data/GHE_DALY_Global_2000_2012.xls', sheetname='Global2012', header=[4,5])",
"_____no_output_____"
]
],
[
[
"This dataframe has a \"multi-level\" index:",
"_____no_output_____"
]
],
[
[
"df.columns",
"_____no_output_____"
]
],
[
[
"How do we export a dataframe back to Excel?",
"_____no_output_____"
]
],
[
[
"df.to_excel('data/my_excel.xlsx')",
"_____no_output_____"
],
[
"!open 'data/my_excel.xlsx'",
"_____no_output_____"
]
],
[
[
"## 3. Inputs: PDF",
"_____no_output_____"
],
[
"PDF is also a common communication medium about data and analysis. Let's look at how one can read data from PDF into Python.",
"_____no_output_____"
]
],
[
[
"import pdftables\n\nmy_pdf = open('data/WEF_GlobalCompetitivenessReport_2014-15.pdf', 'rb')\nchart_page = pdftables.get_pdf_page(my_pdf, 29)",
"_____no_output_____"
]
],
[
[
"PDF is a proprietary file format with specific tagging that has been reverse engineered. Let's take a look at some structures in this file.",
"_____no_output_____"
]
],
[
[
"table = pdftables.page_to_tables(chart_page)\ntitles = zip(table[0][0], table[0][1])[:5]\ntitles = [''.join([title[0], title[1]]) for title in titles]\nprint(titles)",
"_____no_output_____"
]
],
[
[
"There is a table with structured data that we can peel out:",
"_____no_output_____"
]
],
[
[
"all_rows = []\nfor row_data in table[0][2:]:\n all_rows.extend([row_data[:5], row_data[5:]])\n\nprint(all_rows)",
"_____no_output_____"
]
],
[
[
"## 4. Configurations",
"_____no_output_____"
]
],
[
[
"from ConfigParser import ConfigParser\nconfig = ConfigParser()\nconfig.read('../cfg/sample.cfg')",
"_____no_output_____"
],
[
"config.sections()",
"_____no_output_____"
]
],
[
[
"## 5. APIs",
"_____no_output_____"
],
[
"### Getting Twitter data from API\n\nRelevant links to the exercise here:\n\n- Twitter Streaming: https://dev/twitter.com/streaming/overview\n- API client: https://github.com/tweepy/tweepy\n- Twitter app: https://apps.twitter.com\n",
"_____no_output_____"
],
[
"#### Create an authentication handler",
"_____no_output_____"
]
],
[
[
"import tweepy\nauth = tweepy.OAuthHandler(config.get('twitter', 'consumer_key'), config.get('twitter', 'consumer_secret'))\nauth.set_access_token(config.get('twitter','access_token'), config.get('twitter','access_token_secret'))\nauth",
"_____no_output_____"
]
],
[
[
"#### Create an API endpoint",
"_____no_output_____"
]
],
[
[
"api = tweepy.API(auth)",
"_____no_output_____"
]
],
[
[
"#### Try REST-ful API call to Twitter",
"_____no_output_____"
]
],
[
[
"python_tweets = api.search('turkey')",
"_____no_output_____"
],
[
"for tweet in python_tweets:\n print(tweet.text)",
"_____no_output_____"
]
],
[
[
"#### For streaming API call, we should run a standalone python program: tweetering.py",
"_____no_output_____"
],
[
"### Input & Output to OpenWeatherMap API\n\nRelevant links to the exercise here:\n\n- http://openweathermap.org/\n- http://openweathermap.org/current\n\nAPI call:\n\n```\napi.openweathermap.org/data/2.5/weather?q={city name}\n\napi.openweathermap.org/data/2.5/weather?q={city name},{country code}\n```\n\nParameters:\n\n> q city name and country code divided by comma, use ISO 3166 country codes\n\nExamples of API calls:\n```\napi.openweathermap.org/data/2.5/weather?q=London\n\napi.openweathermap.org/data/2.5/weather?q=London,uk\n```",
"_____no_output_____"
]
],
[
[
"from pprint import pprint\nimport requests\nweather_key = config.get('openweathermap', 'api_key')\nres = requests.get(\"http://api.openweathermap.org/data/2.5/weather\",\n params={\"q\": \"San Francisco\", \"appid\": weather_key, \"units\": \"metric\"})",
"_____no_output_____"
],
[
"pprint(res.json())",
"_____no_output_____"
]
],
[
[
"## 6. Python requests",
"_____no_output_____"
],
[
"\"requests\" is a wonderful HTTP library for Python, with the right level of abstraction to avoid lots of tedious plumbing (manually add query strings to your URLs, or to form-encode your POST data). Keep-alive and HTTP connection pooling are 100% automatic, powered by urllib3, which is embedded within Requests)\n\n```\n>>> r = requests.get('https://api.github.com/user', auth=('user', 'pass'))\n>>> r.status_code\n200\n>>> r.headers['content-type']\n'application/json; charset=utf8'\n>>> r.encoding\n'utf-8'\n>>> r.text\nu'{\"type\":\"User\"...'\n>>> r.json()\n{u'private_gists': 419, u'total_private_repos': 77, ...}\n```\n\nThere is a lot of great documentation at the python-requests [site](http://docs.python-requests.org/en/master/) -- we are extracting selected highlights from there for your convenience here.\n\n### Making a request\n\nMaking a request with Requests is very simple.\n\nBegin by importing the Requests module:",
"_____no_output_____"
]
],
[
[
"import requests",
"_____no_output_____"
]
],
[
[
"Now, let's try to get a webpage. For this example, let's get GitHub's public timeline",
"_____no_output_____"
]
],
[
[
"r = requests.get('https://api.github.com/events')",
"_____no_output_____"
]
],
[
[
"Now, we have a Response object called r. We can get all the information we need from this object.\n\nRequests' simple API means that all forms of HTTP request are as obvious. For example, this is how you make an HTTP POST request:\n",
"_____no_output_____"
]
],
[
[
"r = requests.post('http://httpbin.org/post', data = {'key':'value'})",
"_____no_output_____"
]
],
[
[
"What about the other HTTP request types: PUT, DELETE, HEAD and OPTIONS? These are all just as simple:",
"_____no_output_____"
]
],
[
[
"r = requests.put('http://httpbin.org/put', data = {'key':'value'})\nr = requests.delete('http://httpbin.org/delete')\nr = requests.head('http://httpbin.org/get')\nr = requests.options('http://httpbin.org/get')",
"_____no_output_____"
]
],
[
[
"### Passing Parameters In URLs\n\nYou often want to send some sort of data in the URL's query string. If you were constructing the URL by hand, this data would be given as key/value pairs in the URL after a question mark, e.g. httpbin.org/get?key=val. Requests allows you to provide these arguments as a dictionary, using the params keyword argument. As an example, if you wanted to pass key1=value1 and key2=value2 to httpbin.org/get, you would use the following code:",
"_____no_output_____"
]
],
[
[
"payload = {'key1': 'value1', 'key2': 'value2'}\nr = requests.get('http://httpbin.org/get', params=payload)",
"_____no_output_____"
]
],
[
[
"You can see that the URL has been correctly encoded by printing the URL:",
"_____no_output_____"
]
],
[
[
"print(r.url)",
"_____no_output_____"
]
],
[
[
"Note that any dictionary key whose value is None will not be added to the URL's query string.\n\nYou can also pass a list of items as a value:",
"_____no_output_____"
]
],
[
[
"payload = {'key1': 'value1', 'key2': ['value2', 'value3']}\n\nr = requests.get('http://httpbin.org/get', params=payload)\nprint(r.url)",
"_____no_output_____"
]
],
[
[
"### Response Content\n\nWe can read the content of the server's response. Consider the GitHub timeline again:",
"_____no_output_____"
]
],
[
[
"import requests\n\nr = requests.get('https://api.github.com/events')\nr.text",
"_____no_output_____"
]
],
[
[
"Requests will automatically decode content from the server. Most unicode charsets are seamlessly decoded.\n\nWhen you make a request, Requests makes educated guesses about the encoding of the response based on the HTTP headers. The text encoding guessed by Requests is used when you access r.text. You can find out what encoding Requests is using, and change it, using the r.encoding property:",
"_____no_output_____"
]
],
[
[
"r.encoding",
"_____no_output_____"
],
[
"r.encoding = 'ISO-8859-1'",
"_____no_output_____"
]
],
[
[
"If you change the encoding, Requests will use the new value of r.encoding whenever you call r.text. You might want to do this in any situation where you can apply special logic to work out what the encoding of the content will be. For example, HTTP and XML have the ability to specify their encoding in their body. In situations like this, you should use r.content to find the encoding, and then set r.encoding. This will let you use r.text with the correct encoding.\n\nRequests will also use custom encodings in the event that you need them. If you have created your own encoding and registered it with the codecs module, you can simply use the codec name as the value of r.encoding and Requests will handle the decoding for you.",
"_____no_output_____"
],
[
"### JSON Response Content\n\nThere's also a builtin JSON decoder, in case you're dealing with JSON data:",
"_____no_output_____"
]
],
[
[
"import requests\n\nr = requests.get('https://api.github.com/events')\nr.json()",
"_____no_output_____"
]
],
[
[
"In case the JSON decoding fails, r.json raises an exception. For example, if the response gets a 204 (No Content), or if the response contains invalid JSON, attempting r.json raises ValueError: No JSON object could be decoded.\n\nIt should be noted that the success of the call to r.json does not indicate the success of the response. Some servers may return a JSON object in a failed response (e.g. error details with HTTP 500). Such JSON will be decoded and returned. To check that a request is successful, use r.raise_for_status() or check r.status_code is what you expect.",
"_____no_output_____"
]
],
[
[
"r.status_code",
"_____no_output_____"
]
],
[
[
"### Custom Headers\nIf you'd like to add HTTP headers to a request, simply pass in a dict to the headers parameter.\n\nFor example, we didn't specify our user-agent in the previous example:",
"_____no_output_____"
]
],
[
[
"url = 'https://api.github.com/some/endpoint'\nheaders = {'user-agent': 'my-app/0.0.1'}\n\nr = requests.get(url, headers=headers)",
"_____no_output_____"
]
],
[
[
"Note: Custom headers are given less precedence than more specific sources of information. For instance:\n\n- Authorization headers set with headers= will be overridden if credentials are specified in .netrc, which in turn will be overridden by the auth= parameter.\n- Authorization headers will be removed if you get redirected off-host.\n- Proxy-Authorization headers will be overridden by proxy credentials provided in the URL.\n- Content-Length headers will be overridden when we can determine the length of the content.",
"_____no_output_____"
],
[
"### Response Headers\nWe can view the server's response headers using a Python dictionary:",
"_____no_output_____"
]
],
[
[
"r.headers",
"_____no_output_____"
]
],
[
[
"The dictionary is special, though: it's made just for HTTP headers. According to RFC 7230, HTTP Header names are case-insensitive.\n\nSo, we can access the headers using any capitalization we want:",
"_____no_output_____"
]
],
[
[
"r.headers['Content-Type']",
"_____no_output_____"
],
[
"r.headers.get('content-type')",
"_____no_output_____"
]
],
[
[
"### Cookies\nIf a response contains some Cookies, you can quickly access them:\n",
"_____no_output_____"
]
],
[
[
"url = 'http://www.cnn.com'\nr = requests.get(url)\nprint(r.cookies.items())",
"_____no_output_____"
]
],
[
[
"To send your own cookies to the server, you can use the cookies parameter:",
"_____no_output_____"
]
],
[
[
"url = 'http://httpbin.org/cookies'\ncookies = dict(cookies_are='working')\nr = requests.get(url, cookies=cookies)\nr.text\n",
"_____no_output_____"
]
],
[
[
"### Redirection and History\nBy default Requests will perform location redirection for all verbs except HEAD.\n\nWe can use the history property of the Response object to track redirection.\n\nThe Response.history list contains the Response objects that were created in order to complete the request. The list is sorted from the oldest to the most recent response.\n\nFor example, GitHub redirects all HTTP requests to HTTPS:\n",
"_____no_output_____"
]
],
[
[
"r = requests.get('http://github.com')\nr.url",
"_____no_output_____"
],
[
"r.status_code",
"_____no_output_____"
],
[
"r.history",
"_____no_output_____"
]
],
[
[
"If you're using GET, OPTIONS, POST, PUT, PATCH or DELETE, you can disable redirection handling with the allow_redirects parameter:",
"_____no_output_____"
]
],
[
[
"r = requests.get('http://github.com', allow_redirects=False)\n\nr.status_code",
"_____no_output_____"
],
[
"r.history",
"_____no_output_____"
]
],
[
[
"If you're using HEAD, you can enable redirection as well:",
"_____no_output_____"
]
],
[
[
"r = requests.head('http://github.com', allow_redirects=True)\nr.url",
"_____no_output_____"
],
[
"r.history",
"_____no_output_____"
]
],
[
[
"### Timeouts\nYou can tell Requests to stop waiting for a response after a given number of seconds with the timeout parameter:",
"_____no_output_____"
]
],
[
[
"requests.get('http://github.com', timeout=1)",
"_____no_output_____"
]
],
[
[
"> Note\n> ----\n> timeout is not a time limit on the entire response download; rather, an exception is raised if the server has not issued a response for timeout seconds (more precisely, if no bytes have been received on the underlying socket for timeout seconds).",
"_____no_output_____"
],
[
"### Errors and Exceptions\nIn the event of a network problem (e.g. DNS failure, refused connection, etc), Requests will raise a ConnectionError exception.\n\nResponse.raise_for_status() will raise an HTTPError if the HTTP request returned an unsuccessful status code.\n\nIf a request times out, a Timeout exception is raised.\n\nIf a request exceeds the configured number of maximum redirections, a TooManyRedirects exception is raised.\n\nAll exceptions that Requests explicitly raises inherit from requests.exceptions.RequestException.",
"_____no_output_____"
]
]
] | [
"markdown",
"code",
"markdown",
"code",
"markdown",
"code",
"markdown",
"code",
"markdown",
"code",
"markdown",
"code",
"markdown",
"code",
"markdown",
"code",
"markdown",
"code",
"markdown",
"code",
"markdown",
"code",
"markdown",
"code",
"markdown",
"code",
"markdown",
"code",
"markdown",
"code",
"markdown",
"code",
"markdown",
"code",
"markdown",
"code",
"markdown",
"code",
"markdown",
"code",
"markdown",
"code",
"markdown",
"code",
"markdown",
"code",
"markdown",
"code",
"markdown",
"code",
"markdown",
"code",
"markdown",
"code",
"markdown",
"code",
"markdown",
"code",
"markdown",
"code",
"markdown",
"code",
"markdown",
"code",
"markdown",
"code",
"markdown",
"code",
"markdown",
"code",
"markdown",
"code",
"markdown",
"code",
"markdown",
"code",
"markdown",
"code",
"markdown",
"code",
"markdown",
"code",
"markdown",
"code",
"markdown"
] | [
[
"markdown",
"markdown",
"markdown"
],
[
"code",
"code"
],
[
"markdown"
],
[
"code"
],
[
"markdown"
],
[
"code"
],
[
"markdown"
],
[
"code"
],
[
"markdown",
"markdown"
],
[
"code"
],
[
"markdown"
],
[
"code"
],
[
"markdown"
],
[
"code"
],
[
"markdown"
],
[
"code"
],
[
"markdown"
],
[
"code"
],
[
"markdown"
],
[
"code"
],
[
"markdown"
],
[
"code"
],
[
"markdown"
],
[
"code"
],
[
"markdown"
],
[
"code"
],
[
"markdown"
],
[
"code",
"code"
],
[
"markdown",
"markdown"
],
[
"code"
],
[
"markdown"
],
[
"code"
],
[
"markdown"
],
[
"code"
],
[
"markdown"
],
[
"code",
"code"
],
[
"markdown",
"markdown",
"markdown"
],
[
"code"
],
[
"markdown"
],
[
"code"
],
[
"markdown"
],
[
"code",
"code"
],
[
"markdown",
"markdown"
],
[
"code",
"code"
],
[
"markdown",
"markdown"
],
[
"code"
],
[
"markdown"
],
[
"code"
],
[
"markdown"
],
[
"code"
],
[
"markdown"
],
[
"code"
],
[
"markdown"
],
[
"code"
],
[
"markdown"
],
[
"code"
],
[
"markdown"
],
[
"code"
],
[
"markdown"
],
[
"code"
],
[
"markdown"
],
[
"code",
"code"
],
[
"markdown",
"markdown"
],
[
"code"
],
[
"markdown"
],
[
"code"
],
[
"markdown"
],
[
"code"
],
[
"markdown",
"markdown"
],
[
"code"
],
[
"markdown"
],
[
"code",
"code"
],
[
"markdown"
],
[
"code"
],
[
"markdown"
],
[
"code"
],
[
"markdown"
],
[
"code",
"code",
"code"
],
[
"markdown"
],
[
"code",
"code"
],
[
"markdown"
],
[
"code",
"code"
],
[
"markdown"
],
[
"code"
],
[
"markdown",
"markdown"
]
] |
e7751562c30a6498b8da4c827840b456740905b9 | 293,256 | ipynb | Jupyter Notebook | EDA_IPL_sport.ipynb | mohit421/EDA_IPL-The_Sparks_foundation | e877514bc38fb022e93d9159203c6cede896b6f4 | [
"MIT"
] | null | null | null | EDA_IPL_sport.ipynb | mohit421/EDA_IPL-The_Sparks_foundation | e877514bc38fb022e93d9159203c6cede896b6f4 | [
"MIT"
] | null | null | null | EDA_IPL_sport.ipynb | mohit421/EDA_IPL-The_Sparks_foundation | e877514bc38fb022e93d9159203c6cede896b6f4 | [
"MIT"
] | null | null | null | 126.567113 | 43,780 | 0.844709 | [
[
[
"# IPL_Sport_Analysis\n\nTask - 3\n",
"_____no_output_____"
],
[
"# Introduction",
"_____no_output_____"
],
[
"The Indian Premier League (IPL),is a professional Twenty20 \ncricket league in India contested during April and May of \nevery year by teams representing Indian cities. The league \nwas founded by the Board of Control for Cricket in India (BCCI) \nin 2007. The IPL is the most-attended cricket league in the world \nand ranks sixth among all sports league.",
"_____no_output_____"
],
[
"The data consists of two datasets : matches and deliveries.",
"_____no_output_____"
],
[
"matches dataset contains data of all IPL matches.\n\ndeliveries dataset contains ball by ball data of each IPL match.",
"_____no_output_____"
],
[
"# Objective",
"_____no_output_____"
],
[
"Aim is to provide some interesting insights by analyzing the IPL data.",
"_____no_output_____"
]
],
[
[
"## Importing Required Libraries\nimport pandas as pd\nimport numpy as np\nimport matplotlib.pyplot as plt\nimport seaborn as sns\nimport warnings\nwarnings.filterwarnings('ignore')\n%matplotlib inline",
"C:\\Users\\hp\\Anaconda3\\lib\\site-packages\\statsmodels\\tools\\_testing.py:19: FutureWarning: pandas.util.testing is deprecated. Use the functions in the public API at pandas.testing instead.\n import pandas.util.testing as tm\n"
],
[
"## function to add data to plot\ndef annot_plot(ax,w,h):\n ax.spines['top'].set_visible(False)\n ax.spines['right'].set_visible(False)\n for p in ax.patches:\n ax.annotate('{}'.format(p.get_height()), (p.get_x()+w, p.get_height()+h))",
"_____no_output_____"
]
],
[
[
"# Reading Data",
"_____no_output_____"
]
],
[
[
"match_data=pd.read_csv('matches.csv')\ndeliveries_data=pd.read_csv('deliveries.csv')",
"_____no_output_____"
],
[
"season_data=match_data[['id','season','winner']]\n\ncomplete_data=deliveries_data.merge(season_data,how='inner',left_on='match_id',right_on='id')",
"_____no_output_____"
],
[
"match_data.head()",
"_____no_output_____"
],
[
"match_data['win_by']=np.where(match_data['win_by_runs']>0,'Bat first','Bowl first')",
"_____no_output_____"
],
[
"match_data.shape",
"_____no_output_____"
],
[
"deliveries_data.head(5)",
"_____no_output_____"
],
[
"deliveries_data['runs']=deliveries_data['total_runs'].cumsum()",
"_____no_output_____"
],
[
"deliveries_data.shape",
"_____no_output_____"
]
],
[
[
"# Number of Matches played in each IPL season",
"_____no_output_____"
]
],
[
[
"ax=sns.countplot('season',data=match_data,palette=\"Set2\")\nplt.ylabel('Matches')\nannot_plot(ax,0.08,1)",
"_____no_output_____"
]
],
[
[
"# Matches Won By the Teams",
"_____no_output_____"
],
[
"Mumbai Indians won maximum number of matches followed by Chennai Super Kings.",
"_____no_output_____"
]
],
[
[
"match_data.groupby('winner')['winner'].agg(\n['count']).sort_values('count').reset_index().plot(x='winner',y='count',kind='barh')",
"_____no_output_____"
],
[
"ax=sns.countplot(x='winner',data=match_data)\nplt.ylabel('Match')\nplt.xticks(rotation=80)\nannot_plot(ax,0.05,1)",
"_____no_output_____"
]
],
[
[
"# Win Percentage",
"_____no_output_____"
]
],
[
[
"match=match_data.win_by.value_counts()\nlabels=np.array(match.index)\nsizes = match.values\ncolors = ['gold', 'lightskyblue']\n \n# Plot\nplt.pie(sizes, labels=labels, colors=colors,\n autopct='%1.1f%%', shadow=True,startangle=90)\n\nplt.title('Match Result')\nplt.axis('equal')\nplt.show()",
"_____no_output_____"
],
[
"sns.countplot('season',hue='win_by',data=match_data,palette=\"Set1\")",
"_____no_output_____"
]
],
[
[
"# Toss Decisions so far",
"_____no_output_____"
]
],
[
[
"toss=match_data.toss_decision.value_counts()\nlabels=np.array(toss.index)\nsizes = toss.values\ncolors = ['red', 'gold']\n#explode = (0.1, 0, 0, 0) # explode 1st slice\n \n# Plot\nplt.pie(sizes, labels=labels, colors=colors,\n autopct='%1.1f%%', shadow=True,startangle=90)\n\nplt.title('Toss Result')\nplt.axis('equal')\nplt.show()",
"_____no_output_____"
],
[
"sns.countplot('season',hue='toss_decision',data=match_data,palette=\"Set2\")",
"_____no_output_____"
]
],
[
[
"# IPL Winners",
"_____no_output_____"
]
],
[
[
"final_matches=match_data.drop_duplicates(subset=['season'], keep='last')\n\nfinal_matches[['season','winner']].reset_index(drop=True).sort_values('season')",
"_____no_output_____"
]
],
[
[
"# IPL Finals",
"_____no_output_____"
],
[
"IPL Finals venues and winners along with the number of wins.",
"_____no_output_____"
]
],
[
[
"final_matches.groupby(['city','winner']).size()",
"_____no_output_____"
]
],
[
[
"# Number of IPL seasons won by teams",
"_____no_output_____"
]
],
[
[
"final_matches['winner'].value_counts()",
"_____no_output_____"
]
],
[
[
"# Win Percentage in Finals",
"_____no_output_____"
]
],
[
[
"match=final_matches.win_by.value_counts()\nlabels=np.array(match.index)\nsizes = match.values\ncolors = ['gold', 'lightskyblue']\n \n# Plot\nplt.pie(sizes, labels=labels, colors=colors,\n autopct='%1.1f%%', shadow=True,startangle=90)\n\nplt.title('Match Result')\nplt.axis('equal')\nplt.show()",
"_____no_output_____"
]
],
[
[
"# Toss Decision in Finals",
"_____no_output_____"
]
],
[
[
"toss=final_matches.toss_decision.value_counts()\nlabels=np.array(toss.index)\nsizes = toss.values\ncolors = ['gold', 'lightskyblue']\n#explode = (0.1, 0, 0, 0) # explode 1st slice\n \n# Plot\nplt.pie(sizes, labels=labels, colors=colors,\n autopct='%1.1f%%', shadow=True,startangle=90)\n\nplt.title('Toss Result')\nplt.axis('equal')\nplt.show()",
"_____no_output_____"
],
[
"final_matches[['toss_winner','toss_decision','winner']].reset_index(drop=True)",
"_____no_output_____"
]
],
[
[
"# Man of the Match in final match",
"_____no_output_____"
]
],
[
[
"final_matches[['winner','player_of_match']].reset_index(drop=True)",
"_____no_output_____"
],
[
"len(final_matches[final_matches['toss_winner']==final_matches['winner']]['winner'])",
"_____no_output_____"
]
],
[
[
"# IPL leading Run Scorer¶\nSuresh Raina is at the top with 4548 Runs. There are 3 foreign players in this list. Among them Chris Gayle is the leading run scorer.",
"_____no_output_____"
]
],
[
[
"batsman_score=deliveries_data.groupby('batsman')['batsman_runs'].\nagg(['sum']).reset_index().sort_values('sum',ascending=False).reset_index(drop=True)\nbatsman_score=batsman_score.rename(columns={'sum':'batsman_runs'})\nprint(\"*** Top 10 Leading Run Scorer in IPL ***\")\nbatsman_score.iloc[:10,:]",
"*** Top 10 Leading Run Scorer in IPL ***\n"
],
[
"No_Matches_player_dismissed = deliveries_data[[\"match_id\",\"player_dismissed\"]]\nNo_Matches_player_dismissed =No_Matches_player_dismissed .groupby(\"player_dismissed\")[\"match_id\"].count().reset_index().sort_values(by=\"match_id\",ascending=False).reset_index(drop=True)\nNo_Matches_player_dismissed.columns=[\"batsman\",\"No_of Matches\"]\nNo_Matches_player_dismissed .head(5)",
"_____no_output_____"
]
],
[
[
"# Batting Average",
"_____no_output_____"
]
],
[
[
"Batsman_Average=pd.merge(batsman_score,No_Matches_player_dismissed ,on=\"batsman\")\n#merging the score and match played by batsman\nBatsman_Average=Batsman_Average[Batsman_Average[\"batsman_runs\"]>=500]\n# taking Average for those player for having more than 500 runs under thier belt\nBatsman_Average[\"Average\"]=Batsman_Average[\"batsman_runs\"]/Batsman_Average[\"No_of Matches\"]\nBatsman_Average['Average']=Batsman_Average['Average'].apply(lambda x: round(x,2))\nBatsman_Average=Batsman_Average.sort_values(by=\"Average\",ascending=False).reset_index(drop=True)\n\ntop_bat_avg=Batsman_Average.iloc[:10,:]\nax=top_bat_avg.plot('batsman','Average',color='green',kind='bar')\nplt.ylabel('Average')\nplt.xticks(rotation=80)\nannot_plot(ax,0,1)",
"_____no_output_____"
]
],
[
[
"Amla is at the top of this list with the batting average of 44.38.",
"_____no_output_____"
],
[
"# Dismissals in IPL",
"_____no_output_____"
]
],
[
[
"plt.figure(figsize=(12,6))\nax=sns.countplot(deliveries_data.dismissal_kind)\nplt.xticks(rotation=90)\nannot_plot(ax,0.2,100)",
"_____no_output_____"
]
],
[
[
"# Dismissal by Teams and their distribution",
"_____no_output_____"
]
],
[
[
"out=deliveries_data.groupby(['batting_team','dismissal_kind'])['dismissal_kind'].agg(['count'])\n\nout.groupby(level=0).apply(lambda x: round(100 * x / float(x.sum()),2)).reset_index().sort_values(['batting_team','count'],ascending=[1,0]).set_index(['batting_team','dismissal_kind'])",
"_____no_output_____"
],
[
"wicket_data=deliveries_data.dropna(subset=['dismissal_kind'])",
"_____no_output_____"
],
[
"wicket_data=wicket_data[~wicket_data['dismissal_kind'].isin(['run out','retired hurt','obstructing the field'])]",
"_____no_output_____"
]
],
[
[
"# IPL Most Wicket-Taking Bowlers",
"_____no_output_____"
]
],
[
[
"wicket_data.groupby('bowler')['dismissal_kind'].agg(['count']).reset_index().sort_values('count',ascending=False).reset_index(drop=True).iloc[:10,:]",
"_____no_output_____"
]
],
[
[
"Malinga is at the top of this list with 170 wickets",
"_____no_output_____"
],
[
"# Powerplays",
"_____no_output_____"
],
[
"In IPL the Powerplay consists of first 6 overs.\n\nDuring the first six overs, a maximum of two fielders can be outside the 30-yard circle.",
"_____no_output_____"
]
],
[
[
"powerplay_data=complete_data[complete_data['over']<=6]",
"_____no_output_____"
]
],
[
[
"# Runs in Powerplays",
"_____no_output_____"
]
],
[
[
"powerplay_data[ powerplay_data['inning']==1].groupby('match_id')['total_runs'].agg(['sum']).reset_index().plot(x='match_id',y='sum',title='Batting First')\npowerplay_data[ powerplay_data['inning']==2].groupby('match_id')['total_runs'].agg(['sum']).reset_index().plot(x='match_id',y='sum',title='Batting Second')",
"_____no_output_____"
]
],
[
[
"# Higgest Runs in PowerPlays",
"_____no_output_____"
]
],
[
[
"powerplay_data.groupby(['season','match_id','inning'])['total_runs'].agg(['sum']).reset_index().groupby('season')['sum'].max()",
"_____no_output_____"
]
],
[
[
"# Highest Runs in Powerplay :Batting First",
"_____no_output_____"
]
],
[
[
"pi1=powerplay_data[ powerplay_data['inning']==1].groupby(['season','match_id'])['total_runs'].agg(['sum'])\npi1.reset_index().groupby('season')['sum'].max()\n",
"_____no_output_____"
]
],
[
[
"# Highest Runs in Powerplay :Batting Second",
"_____no_output_____"
]
],
[
[
"pi2=powerplay_data[ powerplay_data['inning']==2].groupby(['season','match_id'])['total_runs'].agg(['sum'])\n\npi2.reset_index().groupby('season')['sum'].max()",
"_____no_output_____"
]
],
[
[
"# Maximum Wickets Fall in PowerPlay",
"_____no_output_____"
]
],
[
[
"powerplay_data.dropna(subset=['dismissal_kind']).groupby(['season','match_id','inning'])['dismissal_kind'].agg(['count']).reset_index().groupby('season')['count'].max()",
"_____no_output_____"
]
],
[
[
"# First Innings",
"_____no_output_____"
]
],
[
[
"powerplay_data[ powerplay_data['inning']==1].dropna(\n subset=['dismissal_kind']).groupby(\n ['season','match_id','inning'])['dismissal_kind'].agg(['count']).reset_index().groupby('season')['count'].max()\n",
"_____no_output_____"
]
],
[
[
"# Second Innings",
"_____no_output_____"
]
],
[
[
"powerplay_data[ powerplay_data['inning']==2].dropna(\n subset=['dismissal_kind']).groupby(['season','match_id','inning'])['dismissal_kind'].agg(\n ['count']).reset_index().groupby('season')['count'].max()",
"_____no_output_____"
]
],
[
[
"# Thank you",
"_____no_output_____"
]
]
] | [
"markdown",
"code",
"markdown",
"code",
"markdown",
"code",
"markdown",
"code",
"markdown",
"code",
"markdown",
"code",
"markdown",
"code",
"markdown",
"code",
"markdown",
"code",
"markdown",
"code",
"markdown",
"code",
"markdown",
"code",
"markdown",
"code",
"markdown",
"code",
"markdown",
"code",
"markdown",
"code",
"markdown",
"code",
"markdown",
"code",
"markdown",
"code",
"markdown",
"code",
"markdown",
"code",
"markdown",
"code",
"markdown",
"code",
"markdown",
"code",
"markdown",
"code",
"markdown"
] | [
[
"markdown",
"markdown",
"markdown",
"markdown",
"markdown",
"markdown",
"markdown"
],
[
"code",
"code"
],
[
"markdown"
],
[
"code",
"code",
"code",
"code",
"code",
"code",
"code",
"code"
],
[
"markdown"
],
[
"code"
],
[
"markdown",
"markdown"
],
[
"code",
"code"
],
[
"markdown"
],
[
"code",
"code"
],
[
"markdown"
],
[
"code",
"code"
],
[
"markdown"
],
[
"code"
],
[
"markdown",
"markdown"
],
[
"code"
],
[
"markdown"
],
[
"code"
],
[
"markdown"
],
[
"code"
],
[
"markdown"
],
[
"code",
"code"
],
[
"markdown"
],
[
"code",
"code"
],
[
"markdown"
],
[
"code",
"code"
],
[
"markdown"
],
[
"code"
],
[
"markdown",
"markdown"
],
[
"code"
],
[
"markdown"
],
[
"code",
"code",
"code"
],
[
"markdown"
],
[
"code"
],
[
"markdown",
"markdown",
"markdown"
],
[
"code"
],
[
"markdown"
],
[
"code"
],
[
"markdown"
],
[
"code"
],
[
"markdown"
],
[
"code"
],
[
"markdown"
],
[
"code"
],
[
"markdown"
],
[
"code"
],
[
"markdown"
],
[
"code"
],
[
"markdown"
],
[
"code"
],
[
"markdown"
]
] |
e77518186ce580a93708e2364d7442afcc9435e6 | 4,123 | ipynb | Jupyter Notebook | Hierarchical.ipynb | VladimirsHisamutdinovs/data-mining | 42d8e20f14f740c783d8ad4d1edbbd51e292fa61 | [
"Apache-2.0"
] | null | null | null | Hierarchical.ipynb | VladimirsHisamutdinovs/data-mining | 42d8e20f14f740c783d8ad4d1edbbd51e292fa61 | [
"Apache-2.0"
] | null | null | null | Hierarchical.ipynb | VladimirsHisamutdinovs/data-mining | 42d8e20f14f740c783d8ad4d1edbbd51e292fa61 | [
"Apache-2.0"
] | null | null | null | 31 | 424 | 0.587194 | [
[
[
"# HIERARCHICAL CLUSTERING\n**File:** hierarchical.ipynb\n\n**Course:** Data Science Foundations: Data Mining in Python",
"_____no_output_____"
],
[
"# IMPORT LIBRARIES",
"_____no_output_____"
]
],
[
[
"import pandas as pd # For dataframes\nimport matplotlib.pyplot as plt # For plotting data\nimport seaborn as sns # For plotting data\nfrom sklearn.cluster import AgglomerativeClustering # For clustering\nfrom scipy.cluster.hierarchy import dendrogram, linkage # For clustering and visualization",
"_____no_output_____"
]
],
[
[
"# LOAD AND PREPARE DATA\nRead the `penguins.csv` file from the `data` directory into variable `df`. Select a random sample of 75 cases of the dataset for easy visualization. Keep all features in variable `df` and store the class variable in `y`.",
"_____no_output_____"
]
],
[
[
"# Reads the .csv file into variable df\ndf = pd.read_csv('data/penguins.csv')\n\n# Selects a random sample of 75 cases\ndf = df.sample(n=75, random_state=1)\n\n# Separates the class variable in y\ny = df.y\n\n# Removes the y column from df\ndf = df.drop('y', axis=1)\n\n# Displays the first 5 rows of df\ndf.head()",
"_____no_output_____"
]
],
[
[
"# HIERARCHICAL CLUSTERING \nIn this demonstration, we'll use `SciPy` to perform hierarchical clustering. (Another common choice is `scikit-learn`.)\n\nThe `scipy.cluster.hierarchy` package contains two functions, i.e., `linkage()` and `dendogram()` for hierarchical clustering. The `linkage()` function performs agglomerative clustering and the `dendogram()` function displays the clusters. Various `linkage` methods are possible. Here we'll use the `ward` linkage method that merges clusters so that variance of the clusters is minimized. Other linkage options are:\n\n- `average`\n- `single` \n- `complete` \n\nThe `linkage()` function returns a linkage matrix with information about clusters. This matrix can be viewed using the `dendogram()` function. The code below performs clustering using the `euclidean` metric and displays the clusters.",
"_____no_output_____"
]
],
[
[
"# Performs agglomerative clustering using `ward` linkage and `euclidean` metric\nhc = linkage(df, method='ward', metric='euclidean')\n\n# Sets the figure size\nfig = plt.figure(figsize=(15, 15))\n\n# Displays the dendogram\n# The lambda function sets the labels of each leaf\ndn = dendrogram(\n hc,\n leaf_label_func=lambda id: y.values[id],\n leaf_font_size=10)",
"_____no_output_____"
]
],
[
[
"# CLEAN UP\n\n- If desired, clear the results with Cell > All Output > Clear. \n- Save your work by selecting File > Save and Checkpoint.\n- Shut down the Python kernel and close the file by selecting File > Close and Halt.",
"_____no_output_____"
]
]
] | [
"markdown",
"code",
"markdown",
"code",
"markdown",
"code",
"markdown"
] | [
[
"markdown",
"markdown"
],
[
"code"
],
[
"markdown"
],
[
"code"
],
[
"markdown"
],
[
"code"
],
[
"markdown"
]
] |
e7752d5475a97ee039f1e174a3412d61a56118d1 | 10,315 | ipynb | Jupyter Notebook | notebook/eda/Suraiya-Project/old-scrap-nltk/Indonesia-Translation.ipynb | langsari/quran-dataset | b41e2930216ef68b16771a96c62665565fc37c85 | [
"MIT"
] | 5 | 2020-10-08T05:16:39.000Z | 2020-12-09T03:47:17.000Z | notebook/eda/Suraiya-Project/old-scrap-nltk/Indonesia-Translation.ipynb | langsari/quran-dataset | b41e2930216ef68b16771a96c62665565fc37c85 | [
"MIT"
] | 4 | 2020-09-16T09:59:43.000Z | 2020-12-01T14:18:34.000Z | notebook/eda/Suraiya-Project/old-scrap-nltk/Indonesia-Translation.ipynb | langsari/quran-dataset | b41e2930216ef68b16771a96c62665565fc37c85 | [
"MIT"
] | 6 | 2020-09-09T09:29:58.000Z | 2022-03-17T08:59:34.000Z | 28.893557 | 119 | 0.385652 | [
[
[
"import pandas as pd\nimport numpy as np\nimport seaborn as sns\nimport matplotlib.pyplot as plt\nimport nltk",
"_____no_output_____"
],
[
"data = pd.read_json('https://raw.githubusercontent.com/risan/quran-json/master/json/translations/id.pretty.json')",
"_____no_output_____"
],
[
"data",
"_____no_output_____"
],
[
"data.drop(['surah_number'],axis=1,inplace=True)",
"_____no_output_____"
],
[
"data",
"_____no_output_____"
],
[
"data.rename(columns={'content':'translation'},inplace=True)",
"_____no_output_____"
],
[
"data",
"_____no_output_____"
],
[
"data.info()",
"<class 'pandas.core.frame.DataFrame'>\nRangeIndex: 6236 entries, 0 to 6235\nData columns (total 2 columns):\nverse_number 6236 non-null int64\ntranslation 6236 non-null object\ndtypes: int64(1), object(1)\nmemory usage: 97.6+ KB\n"
],
[
"data.to_json('DATAMAINING2020/Indonesia.json')",
"_____no_output_____"
],
[
"data.to_csv('DATAMAINING2020/Indonesia.csv',index=False)",
"_____no_output_____"
]
]
] | [
"code"
] | [
[
"code",
"code",
"code",
"code",
"code",
"code",
"code",
"code",
"code",
"code"
]
] |
e7753d5554b3cf85aae4b643c9ead1aecae95aec | 49,532 | ipynb | Jupyter Notebook | SurfsUp_Challenge.ipynb | jenv5507/surfs_up | 5e6bca83650b74020eec34a195ede1b48e22fa81 | [
"MIT"
] | null | null | null | SurfsUp_Challenge.ipynb | jenv5507/surfs_up | 5e6bca83650b74020eec34a195ede1b48e22fa81 | [
"MIT"
] | null | null | null | SurfsUp_Challenge.ipynb | jenv5507/surfs_up | 5e6bca83650b74020eec34a195ede1b48e22fa81 | [
"MIT"
] | null | null | null | 31.81246 | 118 | 0.36223 | [
[
[
"# Dependencies\nimport numpy as np\nimport pandas as pd\n\n# Python SQL toolkit and Object Relational Mapper\nimport sqlalchemy\nfrom sqlalchemy.ext.automap import automap_base\nfrom sqlalchemy.orm import Session\nfrom sqlalchemy import create_engine, func",
"_____no_output_____"
],
[
"engine = create_engine(\"sqlite:///hawaii.sqlite\")\n\n# reflect an existing database into a new model\nBase = automap_base()\n# reflect the tables\nBase.prepare(engine, reflect=True)\n\n# Save references to each table\nMeasurement = Base.classes.measurement\nStation = Base.classes.station",
"_____no_output_____"
],
[
"# Create our session (link) from Python to the DB\nsession = Session(engine)",
"_____no_output_____"
]
],
[
[
"## D1: Determine the Summary Statistics for June",
"_____no_output_____"
]
],
[
[
"# 1. Import the sqlalchemy extract function.\nfrom sqlalchemy import extract\n\n# 2. Write a query that filters the Measurement table to retrieve the temperatures for the month of June. \njune_temps = session.query(Measurement.date, Measurement.tobs).\\\nfilter(func.strftime(\"%m\", Measurement.date) == \"06\")\n\n ",
"_____no_output_____"
],
[
"# 3. Convert the June temperatures to a list.\njune_temps = session.query(Measurement.date, Measurement.tobs).\\\nfilter(func.strftime(\"%m\", Measurement.date) == \"06\").all()\njune_temps",
"_____no_output_____"
],
[
"# 4. Create a DataFrame from the list of temperatures for the month of June. \ndf = pd.DataFrame(june_temps, columns=['date', 'June temperature'])",
"_____no_output_____"
],
[
"# 5. Calculate and print out the summary statistics for the June temperature DataFrame.\ndf.describe()",
"_____no_output_____"
],
[
"#Calculate precipitation for June and put into list\njune_prcp = session.query(Measurement.date, Measurement.prcp).\\\nfilter(func.strftime(\"%m\", Measurement.date) == \"06\").all()",
"_____no_output_____"
],
[
"df = pd.DataFrame(june_prcp, columns=['date', 'June precipitation'])",
"_____no_output_____"
],
[
"df.describe()",
"_____no_output_____"
]
],
[
[
"## D2: Determine the Summary Statistics for December",
"_____no_output_____"
]
],
[
[
"# 6. Write a query that filters the Measurement table to retrieve the temperatures for the month of December.\ndec_temps = session.query(Measurement.date, Measurement.tobs).\\\nfilter(func.strftime(\"%m\", Measurement.date) == \"12\")",
"_____no_output_____"
],
[
"# 7. Convert the December temperatures to a list.\ndec_temps = session.query(Measurement.date, Measurement.tobs).\\\nfilter(func.strftime(\"%m\", Measurement.date) == \"12\").all()",
"_____no_output_____"
],
[
"# 8. Create a DataFrame from the list of temperatures for the month of December. \ndf = pd.DataFrame(dec_temps, columns=['date', 'December temperature'])",
"_____no_output_____"
],
[
"# 9. Calculate and print out the summary statistics for the Decemeber temperature DataFrame.\ndf.describe()",
"_____no_output_____"
],
[
"#Calculate precipitation for December and put into list\ndec_prcp = session.query(Measurement.date, Measurement.prcp).\\\nfilter(func.strftime(\"%m\", Measurement.date) == \"12\").all()",
"_____no_output_____"
],
[
"#Create a DataFrame for December precipitation\ndf = pd.DataFrame(dec_prcp, columns=['date', 'December precipitation'])",
"_____no_output_____"
],
[
"df.describe()",
"_____no_output_____"
]
]
] | [
"code",
"markdown",
"code",
"markdown",
"code"
] | [
[
"code",
"code",
"code"
],
[
"markdown"
],
[
"code",
"code",
"code",
"code",
"code",
"code",
"code"
],
[
"markdown"
],
[
"code",
"code",
"code",
"code",
"code",
"code",
"code"
]
] |
e7755316e7f3c28b7480f0bcc7581ab66f2d945f | 306,138 | ipynb | Jupyter Notebook | diffusion_simple.ipynb | BenGravell/gridtoy | 6e2ea208803d81fee739473ab5dcfbab46c64d0a | [
"MIT"
] | null | null | null | diffusion_simple.ipynb | BenGravell/gridtoy | 6e2ea208803d81fee739473ab5dcfbab46c64d0a | [
"MIT"
] | null | null | null | diffusion_simple.ipynb | BenGravell/gridtoy | 6e2ea208803d81fee739473ab5dcfbab46c64d0a | [
"MIT"
] | null | null | null | 85.68094 | 145 | 0.83205 | [
[
[
"# Simulation of diffusion with noise",
"_____no_output_____"
],
[
"## Package imports",
"_____no_output_____"
]
],
[
[
"import numpy as np\nimport numpy.random as npr\nfrom scipy.signal import convolve2d\nimport matplotlib.pyplot as plt\nfrom matplotlib.animation import FuncAnimation\nfrom IPython.display import HTML",
"_____no_output_____"
]
],
[
[
"## Settings",
"_____no_output_____"
]
],
[
[
"# Number of timesteps to simulate\nduration = 500\n\n# Dimension of simulation domain\nn = 100\n\n# Sampling time\ndt = 0.1\n\n# Initialize the state and noise\nstate = npr.rand(n, n)\nnoise = np.zeros([n, n])\n\n# Define the diffusion rate - ensure rate*dt < 0.25 for numerical stability using Euler integration\nrate = 2.0\n\n# Define the force and noise amount\nforce_amount = 0.005\nnoise_amount = 0.040\n\n# Define the force frequency\nforce_freq = 0.001\n\n# Define the noise inertia (between 0 and 1, 0 is fully white noise, 1 is a constant signal)\nnoise_inertia = 0.9",
"_____no_output_____"
]
],
[
[
"## Simulation",
"_____no_output_____"
]
],
[
[
"# Compute the convolution kernel for diffusion dynamics\ndiffusion_kernel = np.array([[ 0, rate, 0],\n [rate, -4*rate, rate],\n [ 0, rate, 0]])\n# Compute the force kernel\ns = np.linspace(-1, 1, n)\nx, y = np.meshgrid(s, s)\nforce_kernel = x**2 + y**2 < 0.2\n\n\ndef physics_update(state, noise, t):\n # Linear diffusion dynamics using Euler integration\n state = state + dt*convolve2d(state, diffusion_kernel, mode='same', boundary='wrap')\n\n # Periodic forcing\n amplitude = np.sin(force_freq*2*np.pi*t)**21\n force = amplitude*force_kernel\n state += force_amount*force\n\n # Random time-varying Gaussian colored noise\n noise = (1-noise_inertia)*npr.randn(*noise.shape) + noise_inertia*noise\n state += noise_amount*noise\n\n return state, noise",
"_____no_output_____"
]
],
[
[
"## Plotting",
"_____no_output_____"
]
],
[
[
"# Initialize the plot\nplt.ioff()\nfig, ax = plt.subplots()\nim = plt.imshow(state, vmin=0, vmax=1)\nax.axis('off')\nfig.tight_layout()\n\n\ndef update(t):\n global state, noise\n state, noise = physics_update(state, noise, t)\n im.set_data(state)\n return [im]\n\n\n# Create the animation\nanimation = FuncAnimation(fig, update, frames=duration, interval=20, blit=True)\nHTML(animation.to_html5_video()) # simple video\n# HTML(animation.to_jshtml()) # interactive video player",
"_____no_output_____"
]
]
] | [
"markdown",
"code",
"markdown",
"code",
"markdown",
"code",
"markdown",
"code"
] | [
[
"markdown",
"markdown"
],
[
"code"
],
[
"markdown"
],
[
"code"
],
[
"markdown"
],
[
"code"
],
[
"markdown"
],
[
"code"
]
] |
e775552b7529669431d724de10d85d23ab1da893 | 5,369 | ipynb | Jupyter Notebook | image_segmention/lowres/balancing_make_duplicates_lowres_1100_normal.ipynb | UVA-DSI-2019-Capstones/CHRC | 3b89fb6039e435f383754f933537201402391a07 | [
"MIT"
] | null | null | null | image_segmention/lowres/balancing_make_duplicates_lowres_1100_normal.ipynb | UVA-DSI-2019-Capstones/CHRC | 3b89fb6039e435f383754f933537201402391a07 | [
"MIT"
] | null | null | null | image_segmention/lowres/balancing_make_duplicates_lowres_1100_normal.ipynb | UVA-DSI-2019-Capstones/CHRC | 3b89fb6039e435f383754f933537201402391a07 | [
"MIT"
] | 1 | 2019-09-07T14:01:14.000Z | 2019-09-07T14:01:14.000Z | 40.674242 | 209 | 0.569566 | [
[
[
"import csv\nimport os\nimport glob\nimport re\nfrom pandas import DataFrame, Series\nfrom PIL import Image\nimport timeit\nimport time",
"_____no_output_____"
],
[
"## CHANGE THESE TO POINT TO VALID AND TRAIN FOR ALL CATEGORIES\n## PRESERVE THE ORDER\ntrain_paths = [\"/scratch/kk4ze/data_lowres_1100x1100_augmented/valid/Celiac\", \"/scratch/kk4ze/data_lowres_1100x1100_augmented/valid/EE\", \n \"/scratch/kk4ze/data_lowres_1100x1100_augmented/valid/Normal\", \"/scratch/kk4ze/data_lowres_1100x1100_augmented/train/Celiac\", \n \"/scratch/kk4ze/data_lowres_1100x1100_augmented/train/EE\", \"/scratch/kk4ze/data_lowres_1100x1100_augmented/train/Normal\"]\n\nimages = {}\nimages_by_folder = {}\nfor train_path in train_paths:\n images_by_folder[str(train_path)] = []\n files = glob.glob(os.path.join(train_path, '*.jpg'))\n for fl in files:\n flbase = os.path.basename(fl)\n flbase_noext = os.path.splitext(flbase)[0]\n images[flbase_noext]=fl\n images_by_folder[str(train_path)].append(flbase_noext)",
"_____no_output_____"
],
[
"from shutil import copyfile\n\n# CHANGE THESE PATHS TO POINT TO TRAIN AND VALID FOLDER FOR Normal RESPECTIVELY \ntrain_paths = [\"/scratch/kk4ze/data_lowres_1100x1100_augmented/train/Normal\"]\nvalid_paths = [\"/scratch/kk4ze/data_lowres_1100x1100_augmented/valid/Normal\"]\n\n## CHANGE THESE TO POINT TO TRAIN AND VALID FOLDER WITH THE MAXIMUM NUMBER OF FILES IN BOTH\n## EXAMPLE CHANGE max_train PATH TO POINT TO train/EE or train/Celiac or train/Normal. WHICHEVER HAS MAXIMUM NUMBER OF FILES\n## SIMILARLY FOR max_valid. BOTH COULD POINT TO DIFFERENT CATEGORIES!\n### MAKE SURE THESE ARE THE SAME IN ALL THREE SCRIPTS\nmax_train = len(images_by_folder[\"/scratch/kk4ze/data_lowres_1100x1100_augmented/train/Celiac\"])\nmax_valid = len(images_by_folder[\"/scratch/kk4ze/data_lowres_1100x1100_augmented/valid/Celiac\"])\n\nfor path in train_paths:\n mult = int(round(max_train / len(images_by_folder[str(path)])))\n print (str(path) + \" Multiply index: \" + str(mult) + \" Original SIze: \" + str(len(images_by_folder[str(path)])))\n counter = 0\n for img in images_by_folder[str(path)]:\n counter = counter + 1\n if (counter % 10000 == 0):\n print(counter)\n src = str(path) + \"/\" + str(img) + '.jpg'\n# dst = '../data_segments/' + 'data_balanced_duplicate_sample/' + str(path.split('/')[-2].strip()) + '/' + str(path.split('/')[-1].strip()) + '/' + str(img)\n for i in range(mult):\n dst = '/scratch/kk4ze/' + 'data_lowres_1100x1100_augmented_duplicated/' + str(path.split('/')[-2].strip()) + '/' + str(path.split('/')[-1].strip()) + '/' + str(img) + '_' + str(i) + '.jpg'\n copyfile(src, dst)\n \nprint ('Doing Valid now!')\nfor path in valid_paths:\n mult = int(round(max_valid / len(images_by_folder[str(path)])))\n print (str(path) + \" Multiply index: \" + str(mult) + \" Original Size: \" + str(len(images_by_folder[str(path)])))\n counter = 0\n for img in images_by_folder[str(path)]:\n counter = counter + 1\n if (counter % 10000 == 0):\n print(counter)\n src = str(path) + \"/\" + str(img) + '.jpg'\n# dst = '../data_segments/' + 'data_balanced_duplicate_sample/' + str(path.split('/')[-2].strip()) + '/' + str(path.split('/')[-1].strip()) + '/' + str(img) \n for i in range(mult):\n dst = '/scratch/kk4ze/' + 'data_lowres_1100x1100_augmented_duplicated/' + str(path.split('/')[-2].strip()) + '/' + str(path.split('/')[-1].strip()) + '/' + str(img) + '_' + str(i) + '.jpg'\n copyfile(src, dst)",
"/scratch/kk4ze/data_lowres_1100x1100_augmented/train/Normal Multiply index: 1 Original SIze: 283\nDoing Valid now!\n/scratch/kk4ze/data_lowres_1100x1100_augmented/valid/Normal Multiply index: 1 Original Size: 76\n"
]
]
] | [
"code"
] | [
[
"code",
"code",
"code"
]
] |
e77556e7cbc29fa12fc4557fb67c89f8090f8ed8 | 12,668 | ipynb | Jupyter Notebook | resources/nlp/ChineseNlpCorpus/datasets/waimai_10k/intro.ipynb | aicanhelp/ai-datasets | 2fbd175748a92a87ffe89ab0964d90ab154e39e1 | [
"MIT"
] | 9 | 2020-07-17T03:38:07.000Z | 2021-07-15T06:33:08.000Z | resources/nlp/ChineseNlpCorpus/datasets/waimai_10k/intro.ipynb | aicanhelp/ai-datasets | 2fbd175748a92a87ffe89ab0964d90ab154e39e1 | [
"MIT"
] | null | null | null | resources/nlp/ChineseNlpCorpus/datasets/waimai_10k/intro.ipynb | aicanhelp/ai-datasets | 2fbd175748a92a87ffe89ab0964d90ab154e39e1 | [
"MIT"
] | 8 | 2020-08-24T13:20:29.000Z | 2022-01-25T18:34:59.000Z | 29.667447 | 124 | 0.368093 | [
[
[
"# waimai_10k 说明\n0. **下载地址:** [Github](https://github.com/SophonPlus/ChineseNlpCorpus/raw/master/datasets/waimai_10k/waimai_10k.csv)\n1. **数据概览:** 某外卖平台收集的用户评价,正向 4000 条,负向 约 8000 条\n2. **推荐实验:** 情感/观点/评论 倾向性分析\n2. **数据来源:** 某外卖平台\n3. **原数据集:** [中文短文本情感分析语料 外卖评价](https://download.csdn.net/download/cstkl/10236683),网上搜集,具体作者、来源不详\n4. **加工处理:**\n 1. 将原来 2 个文件整合到 1 个文件中\n 2. 去重",
"_____no_output_____"
]
],
[
[
"import pandas as pd",
"_____no_output_____"
],
[
"path = 'waimai_10k_文件夹_所在_路径'",
"_____no_output_____"
]
],
[
[
"# 1. waimai_10k.csv",
"_____no_output_____"
],
[
"## 加载数据",
"_____no_output_____"
]
],
[
[
"pd_all = pd.read_csv(path + 'waimai_10k.csv')\n\nprint('评论数目(总体):%d' % pd_all.shape[0])\nprint('评论数目(正向):%d' % pd_all[pd_all.label==1].shape[0])\nprint('评论数目(负向):%d' % pd_all[pd_all.label==0].shape[0])",
"评论数目(总体):11987\n评论数目(正向):4000\n评论数目(负向):7987\n"
]
],
[
[
"## 字段说明\n\n| 字段 | 说明 |\n| ---- | ---- |\n| label | 1 表示正向评论,0 表示负向评论 |\n| review | 评论内容 |",
"_____no_output_____"
]
],
[
[
"pd_all.sample(20)",
"_____no_output_____"
]
],
[
[
"# 2. 构造平衡语料",
"_____no_output_____"
]
],
[
[
"pd_positive = pd_all[pd_all.label==1]\npd_negative = pd_all[pd_all.label==0]\n\ndef get_balance_corpus(corpus_size, corpus_pos, corpus_neg):\n sample_size = corpus_size // 2\n pd_corpus_balance = pd.concat([corpus_pos.sample(sample_size, replace=corpus_pos.shape[0]<sample_size), \\\n corpus_neg.sample(sample_size, replace=corpus_neg.shape[0]<sample_size)])\n \n print('评论数目(总体):%d' % pd_corpus_balance.shape[0])\n print('评论数目(正向):%d' % pd_corpus_balance[pd_corpus_balance.label==1].shape[0])\n print('评论数目(负向):%d' % pd_corpus_balance[pd_corpus_balance.label==0].shape[0]) \n \n return pd_corpus_balance",
"_____no_output_____"
],
[
"waimai_10k_ba_4000 = get_balance_corpus(4000, pd_positive, pd_negative)\n\nwaimai_10k_ba_4000.sample(10)",
"评论数目(总体):4000\n评论数目(正向):2000\n评论数目(负向):2000\n"
]
]
] | [
"markdown",
"code",
"markdown",
"code",
"markdown",
"code",
"markdown",
"code"
] | [
[
"markdown"
],
[
"code",
"code"
],
[
"markdown",
"markdown"
],
[
"code"
],
[
"markdown"
],
[
"code"
],
[
"markdown"
],
[
"code",
"code"
]
] |
e7756bd3176bc6a6b3f5ef1b8aaa74971a8e0549 | 2,540 | ipynb | Jupyter Notebook | data_augmentation.ipynb | arjunjanamatti/deep_learning | 44bf4d43641559c6cff0ea828815f311c7f423c7 | [
"MIT"
] | null | null | null | data_augmentation.ipynb | arjunjanamatti/deep_learning | 44bf4d43641559c6cff0ea828815f311c7f423c7 | [
"MIT"
] | null | null | null | data_augmentation.ipynb | arjunjanamatti/deep_learning | 44bf4d43641559c6cff0ea828815f311c7f423c7 | [
"MIT"
] | null | null | null | 25.918367 | 124 | 0.555118 | [
[
[
"# # Data augmentation using python and keras\n\nfrom keras.preprocessing.image import ImageDataGenerator, array_to_img, img_to_array\nfrom keras.preprocessing.image import load_img\n\ndatagen = ImageDataGenerator(\n rotation_range = 40,\n width_shift_range=0.2,\n height_shift_range=0.2,\n shear_range=0.2,\n zoom_range=0.2,\n horizontal_flip=True,\n fill_mode='nearest')\nimg = load_img('tales_4.png')\nimage_to_array_conversion = img_to_array(img)\nimage_to_array_conversion = image_to_array_conversion.reshape((1,)+image_to_array_conversion.shape)\n# the .flow() command generates batches of randomly transformed images and saves the results to 'preview' directory\ni = 0\nfor batch in datagen.flow(image_to_array_conversion, batch_size=1,\n save_to_dir='tales', save_prefix='tale', save_format='jpeg'):\n i = i + 1\n if i >100:\n break\n",
"_____no_output_____"
]
]
] | [
"code"
] | [
[
"code"
]
] |
e7756ff5c2c2be93f98377264c385ead33ba170f | 529 | ipynb | Jupyter Notebook | index.ipynb | tchambon/tcdeeptools | 351caf2aed14207e8ae7f6ed858fd75d1386c8de | [
"Apache-2.0"
] | null | null | null | index.ipynb | tchambon/tcdeeptools | 351caf2aed14207e8ae7f6ed858fd75d1386c8de | [
"Apache-2.0"
] | 2 | 2021-09-28T01:24:12.000Z | 2022-02-26T06:58:04.000Z | index.ipynb | tchambon/tcdeeptools | 351caf2aed14207e8ae7f6ed858fd75d1386c8de | [
"Apache-2.0"
] | null | null | null | 16.030303 | 75 | 0.521739 | [
[
[
"#hide\nfrom tcdeeptools.core import *",
"_____no_output_____"
]
],
[
[
"# Tcdeeptools\n\n> Simple tools for deep learning development in Jupyter environments.",
"_____no_output_____"
]
]
] | [
"code",
"markdown"
] | [
[
"code"
],
[
"markdown"
]
] |
e7758860ce576e9059d05dad62fcfa2b5f58f0bf | 189,083 | ipynb | Jupyter Notebook | Exo6PopulationDepartement.ipynb | ms2020bgd/ErwanFloch | 13739f192c0170e7da4249688945281c0310e395 | [
"MIT"
] | null | null | null | Exo6PopulationDepartement.ipynb | ms2020bgd/ErwanFloch | 13739f192c0170e7da4249688945281c0310e395 | [
"MIT"
] | null | null | null | Exo6PopulationDepartement.ipynb | ms2020bgd/ErwanFloch | 13739f192c0170e7da4249688945281c0310e395 | [
"MIT"
] | null | null | null | 34.687764 | 218 | 0.284298 | [
[
[
"**Exercice sur les populations municipales et totales par département**",
"_____no_output_____"
],
[
"À partir des datasets population_communes.csv et surface_departements.csv, créer un nouveau dataset qui contient une ligne par département, avec ces colonnes:\\\n- la somme des \"Population municipale\" du département\\\n- la somme des \"Population totale\" du département (pour l'explication de la distinction entre \"Population municipale\" et \"Population totale\", voir: https://www.insee.fr/fr/metadonnees/definition/c1270)\\\n- la part (en pourcentage) de la population municipale par rapport à la population totale\\\n- la part (en pourcentage) de la population (municipale) du département au sein de sa région\\\n- la densité de la population (municipale) en nb d'habitants / km2\\\n(Le dataset final devrait ressembler à result-exo-cc.csv)",
"_____no_output_____"
],
[
"**import and some data on the data sets**",
"_____no_output_____"
]
],
[
[
"import pandas as pd",
"_____no_output_____"
],
[
"communes = pd.read_csv('population_communes.csv')\nsurfaces = pd.read_csv('surface_departements.csv')",
"_____no_output_____"
],
[
"communes.head(5).reset_index()",
"_____no_output_____"
],
[
"communes.info()",
"<class 'pandas.core.frame.DataFrame'>\nRangeIndex: 70764 entries, 0 to 70763\nData columns (total 9 columns):\nCode département 70764 non-null object\nCode canton 70724 non-null float64\nCode arrondissement 70764 non-null int64\nCode région 70764 non-null int64\nNom de la commune 70764 non-null object\nCode commune 70764 non-null int64\nNom de la région 70764 non-null object\nvariable 70764 non-null object\nvalue 70764 non-null int64\ndtypes: float64(1), int64(4), object(4)\nmemory usage: 4.9+ MB\n"
]
],
[
[
"**Somme des variables \"Population municipale\" du département**",
"_____no_output_____"
]
],
[
[
"municipale = communes[communes['variable'] == 'Population municipale']",
"_____no_output_____"
],
[
"municipale = municipale.groupby(['Code région', 'Code département', 'variable']).sum().reset_index()\nmunicipale = municipale.rename(columns = {'value':'Population municipale', 'Code département': 'Département'})\nmunicipale[['Code région', 'Département', 'Population municipale']]",
"_____no_output_____"
]
],
[
[
" ",
"_____no_output_____"
],
[
"**Somme des variables \"Population totale\" du département**",
"_____no_output_____"
]
],
[
[
"totale = communes[communes.variable == 'Population totale']\ntotale.head(3)",
"_____no_output_____"
],
[
"totale = totale.groupby(['Code région', 'Code département', 'variable']).sum().reset_index()\ntotale = totale.rename(columns = {'value':'Population totale', 'Code département': 'Département'})\ntotale[['Code région', 'Département', 'Population totale']]",
"_____no_output_____"
]
],
[
[
" ",
"_____no_output_____"
],
[
"**Part (en pourcentage) de la population municipale par rapport à la population totale**",
"_____no_output_____"
]
],
[
[
"municipale['pourcentage municipale / totale']=100 * municipale['Population municipale'] / totale['Population totale']\nmunicipale[['Code région', 'Département', 'pourcentage municipale / totale']]",
"_____no_output_____"
]
],
[
[
" ",
"_____no_output_____"
],
[
"**Part (en pourcentage) de la population (municipale) du département au sein de sa région**",
"_____no_output_____"
]
],
[
[
"population_région = municipale.groupby('Code région').transform('sum')['Population municipale']",
"_____no_output_____"
],
[
"municipale['% pop. municipale / région'] = 100*municipale['Population municipale'] / population_région\nmunicipale[['Code région', 'Département', '% pop. municipale / région']]",
"_____no_output_____"
]
],
[
[
" ",
"_____no_output_____"
],
[
"**Densité de la population (municipale) en nb d'habitants / km2**",
"_____no_output_____"
]
],
[
[
"surfaces.info()",
"<class 'pandas.core.frame.DataFrame'>\nRangeIndex: 101 entries, 0 to 100\nData columns (total 3 columns):\ncode_insee 101 non-null object\nnom 101 non-null object\nsurf_km2 101 non-null float64\ndtypes: float64(1), object(2)\nmemory usage: 2.4+ KB\n"
],
[
"surfaces",
"_____no_output_____"
],
[
"surfaces = surfaces.rename(columns = {'code_insee' : 'Département'})\nsurfaces.head(5)",
"_____no_output_____"
],
[
"df = municipale.merge(surfaces, left_on='Département', right_on = 'Département')\ndf",
"_____no_output_____"
],
[
"df['densité'] = df['Population municipale'] / df['surf_km2']\ndf[['Code région', 'Département', 'densité']]",
"_____no_output_____"
],
[
"dfres = pd.read_csv('result-exo-cc.csv')",
"_____no_output_____"
],
[
"dfres",
"_____no_output_____"
]
]
] | [
"markdown",
"code",
"markdown",
"code",
"markdown",
"code",
"markdown",
"code",
"markdown",
"code",
"markdown",
"code"
] | [
[
"markdown",
"markdown",
"markdown"
],
[
"code",
"code",
"code",
"code"
],
[
"markdown"
],
[
"code",
"code"
],
[
"markdown",
"markdown"
],
[
"code",
"code"
],
[
"markdown",
"markdown"
],
[
"code"
],
[
"markdown",
"markdown"
],
[
"code",
"code"
],
[
"markdown",
"markdown"
],
[
"code",
"code",
"code",
"code",
"code",
"code",
"code"
]
] |
e7758eb9fe3bee0e56a0b1a2be30a81a29878ddd | 26,379 | ipynb | Jupyter Notebook | 2_Text Analysis.ipynb | laserkelvin/PythonExercises | b941c9aa1c8b3bbb0bded6c769e6ce1a5a44f2f7 | [
"MIT"
] | null | null | null | 2_Text Analysis.ipynb | laserkelvin/PythonExercises | b941c9aa1c8b3bbb0bded6c769e6ce1a5a44f2f7 | [
"MIT"
] | null | null | null | 2_Text Analysis.ipynb | laserkelvin/PythonExercises | b941c9aa1c8b3bbb0bded6c769e6ce1a5a44f2f7 | [
"MIT"
] | null | null | null | 104.678571 | 19,002 | 0.849236 | [
[
[
"# Text Analysis\n\n## Introduction\n\nEvery now and then you may want to analyze text that you have mined or even written yourself. For example, you may want to see (out of curiousity) what word occurs the most in a body of text.\n\nIn this notebook, we are going to analyze a well-cited astrochemistry article, titled \"Rotational Excitation of CO by Collisions with He, H, and H$_2$ Under Conditions in Interstellar Clouds\" by Green and Thaddeus, published in _The Astrophysical Journal_ in 1976.\n\nNormally you would have to mine the text out of a PDF - I've already done this step for you (albeit poorly). The data is located in the `data` directory.\n\nTo make sure the comparison is consistent throughout the analysis, we have to make sure we remove as much of the special characters and lower/upper casing as possible.\n\n## Aim\n\nThe objective in this notebook is to open the text file in Python, parse out every word, and generate a histogram of word occurances. The scope will be to pick up all of the words __longer than 5 characters__, and count the number of times they appear.\n\n__Note that your partner will have to perform the same analysis on a different text! Make sure your code is clean and well documented!__\n\nThese are the steps you need to take:\n\n1. Open the text file for reading\n2. Remove special characters from the text and remove case-sensitivity\n - I recommend replacing special characters with spaces!\n3. Loop through the words, and incrementing each time you find the same word again.\n4. Histogram count the words\n - This can be done with the `Counter` function from `collections`, or with `pandas DataFrame` built-in methods.\n5. Plot up the histogram with `matplotlib`\n\nThis is the preamble you probably need:",
"_____no_output_____"
]
],
[
[
"%matplotlib inline\n# This function will count the occurances in a list\nfrom collections import Counter\n\n# For your histogram needs\nimport numpy as np\n\n# Optional, if you're courageous!\nimport pandas as pd\n\n# For the plotting\nfrom matplotlib import pyplot as plt",
"_____no_output_____"
]
],
[
[
"## Method\n\n1. Open a text file and read in its contents in a \"Pythonic\" way.",
"_____no_output_____"
]
],
[
[
"with open(\"data/GreenThaddeus-1976-ApJ.txt\") as read_file:\n lines = read_file.read()",
"_____no_output_____"
]
],
[
[
"2. Clean up the text so that it's more easily processed, i.e. removing newline characters and other special characters",
"_____no_output_____"
]
],
[
[
"for character in [\"\\n\", \",\", \".\", \"\"\"(\"\"\", \"\"\")\"\"\", \"\"\":\"\"\", \"\"\"*\"\"\"]:\n lines = lines.replace(character, \" \")",
"_____no_output_____"
]
],
[
[
"3. I chose to store the data in a Python dictionary, where the key corresponds to the word, and the value is the count.",
"_____no_output_____"
]
],
[
[
"word_dict = dict()",
"_____no_output_____"
],
[
"for word in lines.split(\" \"):\n # If there are more than 5 characters, and is not a number then we count\n if len(word) > 5 and word.isdigit() is False:\n # If the word is not already in the dictionary, add it in\n if word.lower() not in word_dict:\n word_dict[word.lower()] = 1\n # Otherwise just increment the counter\n else:\n word_dict[word.lower()]+=1",
"_____no_output_____"
]
],
[
[
"4. The way I chose to analyze the data was to use `pandas`. You can easily convert the dictionary into a `pandas` `DataFrame`, which handles in a SQL-like fashion. I've oriented the `DataFrame` such that the words are in the index, and column 0 is the occurance.",
"_____no_output_____"
]
],
[
[
"df = pd.DataFrame.from_dict(word_dict, orient=\"index\")",
"_____no_output_____"
]
],
[
[
"5. The values are sorted in descending order, and in place (so nothing is returned from the function call)",
"_____no_output_____"
]
],
[
[
"df.sort_values([0], ascending=False, inplace=True)",
"_____no_output_____"
]
],
[
[
"6. Since I didn't want to swamp the figure, I am plotting only the top 10 occurances of a word. The `iloc` method will let you slice/select indices of a dataframe. I code below simply chooses the first 10 values of a dataframe.",
"_____no_output_____"
]
],
[
[
"cut_df = df.iloc[:10]",
"_____no_output_____"
],
[
"plt.style.use(\"seaborn\")\n\nfig, ax = plt.subplots(figsize=(10,6))\n\nax.bar(cut_df.index, cut_df[0])\n\nax.set_title(\"Top 10 words in Green & Thaddeus, 1976\")",
"_____no_output_____"
],
[
"fig.savefig(\"figures/Green1976-top10.png\", dpi=300)",
"_____no_output_____"
]
],
[
[
"## Partner objective\n\nSend your partner your notebook and the other text file (data/Schlegel-arxiv.txt). Tell them to run your notebook, and then ask them to perform the same analysis with the __top 5 words with 4 or more characters!__",
"_____no_output_____"
]
]
] | [
"markdown",
"code",
"markdown",
"code",
"markdown",
"code",
"markdown",
"code",
"markdown",
"code",
"markdown",
"code",
"markdown",
"code",
"markdown"
] | [
[
"markdown"
],
[
"code"
],
[
"markdown"
],
[
"code"
],
[
"markdown"
],
[
"code"
],
[
"markdown"
],
[
"code",
"code"
],
[
"markdown"
],
[
"code"
],
[
"markdown"
],
[
"code"
],
[
"markdown"
],
[
"code",
"code",
"code"
],
[
"markdown"
]
] |
e775906e0268f3b2fe757c3753daadd9e1422112 | 6,502 | ipynb | Jupyter Notebook | research_experiments/gqa/experiments.ipynb | aravind-arunachalam/masters-ljmu | f4b11d18b8aa760c85fe8ce1a3a8d560427a2e1e | [
"MIT"
] | null | null | null | research_experiments/gqa/experiments.ipynb | aravind-arunachalam/masters-ljmu | f4b11d18b8aa760c85fe8ce1a3a8d560427a2e1e | [
"MIT"
] | 1 | 2020-02-20T13:41:25.000Z | 2020-02-20T13:41:25.000Z | research_experiments/gqa/experiments.ipynb | aravind-arunachalam/masters-ljmu | f4b11d18b8aa760c85fe8ce1a3a8d560427a2e1e | [
"MIT"
] | 1 | 2021-09-29T16:12:44.000Z | 2021-09-29T16:12:44.000Z | 31.872549 | 73 | 0.319748 | [
[
[
"import h5py",
"_____no_output_____"
],
[
"f = h5py.File('./spatial/gqa_spatial_15.h5', 'r')",
"_____no_output_____"
],
[
"list(f.keys())",
"_____no_output_____"
],
[
"dset = f['features']",
"_____no_output_____"
],
[
"dset.shape",
"_____no_output_____"
],
[
"dset[581]",
"_____no_output_____"
]
]
] | [
"code"
] | [
[
"code",
"code",
"code",
"code",
"code",
"code"
]
] |
e77593e6d46dfff9a9c5ea6fb4d70bd838ba714e | 176,310 | ipynb | Jupyter Notebook | albert_finetune_optimization.ipynb | luanps/albert | 944dc3db8313e823219c34c9ebddb1915843df42 | [
"Apache-2.0"
] | null | null | null | albert_finetune_optimization.ipynb | luanps/albert | 944dc3db8313e823219c34c9ebddb1915843df42 | [
"Apache-2.0"
] | null | null | null | albert_finetune_optimization.ipynb | luanps/albert | 944dc3db8313e823219c34c9ebddb1915843df42 | [
"Apache-2.0"
] | null | null | null | 96.449672 | 30,137 | 0.491736 | [
[
[
"\n<a href=\"https://colab.research.google.com/github/luanps/albert/blob/master/albert_finetune_optimization.ipynb\" target=\"_parent\"><img src=\"https://colab.research.google.com/assets/colab-badge.svg\" alt=\"Open In Colab\"/></a>",
"_____no_output_____"
]
],
[
[
"# @title Copyright 2020 The ALBERT Authors. All Rights Reserved.\n#\n# Licensed under the Apache License, Version 2.0 (the \"License\");\n# you may not use this file except in compliance with the License.\n# You may obtain a copy of the License at\n#\n# http://www.apache.org/licenses/LICENSE-2.0\n#\n# Unless required by applicable law or agreed to in writing, software\n# distributed under the License is distributed on an \"AS IS\" BASIS,\n# WITHOUT WARRANTIES OR CONDITIONS OF ANY KIND, either express or implied.\n# See the License for the specific language governing permissions and\n# limitations under the License.\n# ==============================================================================",
"_____no_output_____"
]
],
[
[
"# ALBERT End to End (Fine-tuning + Predicting) with Cloud TPU",
"_____no_output_____"
],
[
"## Overview\n\nALBERT is \"A Lite\" version of BERT, a popular unsupervised language representation learning algorithm. ALBERT uses parameter-reduction techniques that allow for large-scale configurations, overcome previous memory limitations, and achieve better behavior with respect to model degradation.\n\nFor a technical description of the algorithm, see our paper:\n\nhttps://arxiv.org/abs/1909.11942\n\nZhenzhong Lan, Mingda Chen, Sebastian Goodman, Kevin Gimpel, Piyush Sharma, Radu Soricut\n\nThis Colab demonstates using a free Colab Cloud TPU to fine-tune GLUE tasks built on top of pretrained ALBERT models and \nrun predictions on tuned model. The colab demonsrates loading pretrained ALBERT models from both [TF Hub](https://www.tensorflow.org/hub) and checkpoints.\n\n**Note:** You will need a GCP (Google Compute Engine) account and a GCS (Google Cloud \nStorage) bucket for this Colab to run.\n\nPlease follow the [Google Cloud TPU quickstart](https://cloud.google.com/tpu/docs/quickstart) for how to create GCP account and GCS bucket. You have [$300 free credit](https://cloud.google.com/free/) to get started with any GCP product. You can learn more about Cloud TPU at https://cloud.google.com/tpu/docs.\n\nThis notebook is hosted on GitHub. To view it in its original repository, after opening the notebook, select **File > View on GitHub**.",
"_____no_output_____"
],
[
"### Instructions",
"_____no_output_____"
],
[
"<h3><a href=\"https://cloud.google.com/tpu/\"><img valign=\"middle\" src=\"https://raw.githubusercontent.com/GoogleCloudPlatform/tensorflow-without-a-phd/master/tensorflow-rl-pong/images/tpu-hexagon.png\" width=\"50\"></a> Train on TPU</h3>\n\n 1. Create a Cloud Storage bucket for your TensorBoard logs at http://console.cloud.google.com/storage and fill in the BUCKET parameter in the \"Parameters\" section below.\n \n 1. On the main menu, click Runtime and select **Change runtime type**. Set \"TPU\" as the hardware accelerator.\n 1. Click Runtime again and select **Runtime > Run All** (Watch out: the \"Colab-only auth for this notebook and the TPU\" cell requires user input). You can also run the cells manually with Shift-ENTER.",
"_____no_output_____"
],
[
"### Set up your TPU environment\n\nIn this section, you perform the following tasks:\n\n* Set up a Colab TPU running environment\n* Verify that you are connected to a TPU device\n* Upload your credentials to TPU to access your GCS bucket.",
"_____no_output_____"
]
],
[
[
"# TODO(lanzhzh): Add support for 2.x.\n%tensorflow_version 1.x\nimport os\nimport pprint\nimport json\nimport tensorflow as tf\n\nassert \"COLAB_TPU_ADDR\" in os.environ, \"ERROR: Not connected to a TPU runtime; please see the first cell in this notebook for instructions!\"\nTPU_ADDRESS = \"grpc://\" + os.environ[\"COLAB_TPU_ADDR\"] \nTPU_TOPOLOGY = \"2x2\"\nprint(\"TPU address is\", TPU_ADDRESS)\n\nfrom google.colab import auth\nauth.authenticate_user()\nwith tf.Session(TPU_ADDRESS) as session:\n print('TPU devices:')\n pprint.pprint(session.list_devices())\n\n # Upload credentials to TPU.\n with open('/content/adc.json', 'r') as f:\n auth_info = json.load(f)\n tf.contrib.cloud.configure_gcs(session, credentials=auth_info)\n # Now credentials are set for all future sessions on this TPU.",
"TensorFlow 1.x selected.\nTPU address is grpc://10.109.125.66:8470\nWARNING:tensorflow:\nThe TensorFlow contrib module will not be included in TensorFlow 2.0.\nFor more information, please see:\n * https://github.com/tensorflow/community/blob/master/rfcs/20180907-contrib-sunset.md\n * https://github.com/tensorflow/addons\n * https://github.com/tensorflow/io (for I/O related ops)\nIf you depend on functionality not listed there, please file an issue.\n\nTPU devices:\n[_DeviceAttributes(/job:tpu_worker/replica:0/task:0/device:CPU:0, CPU, -1, 2409099261969407911),\n _DeviceAttributes(/job:tpu_worker/replica:0/task:0/device:XLA_CPU:0, XLA_CPU, 17179869184, 1549954337002144741),\n _DeviceAttributes(/job:tpu_worker/replica:0/task:0/device:TPU:0, TPU, 17179869184, 5510839357321454835),\n _DeviceAttributes(/job:tpu_worker/replica:0/task:0/device:TPU:1, TPU, 17179869184, 873393571816079649),\n _DeviceAttributes(/job:tpu_worker/replica:0/task:0/device:TPU:2, TPU, 17179869184, 9117514880373904260),\n _DeviceAttributes(/job:tpu_worker/replica:0/task:0/device:TPU:3, TPU, 17179869184, 12704941682957268373),\n _DeviceAttributes(/job:tpu_worker/replica:0/task:0/device:TPU:4, TPU, 17179869184, 10623130967391006998),\n _DeviceAttributes(/job:tpu_worker/replica:0/task:0/device:TPU:5, TPU, 17179869184, 17893873024629234993),\n _DeviceAttributes(/job:tpu_worker/replica:0/task:0/device:TPU:6, TPU, 17179869184, 9214549767924212172),\n _DeviceAttributes(/job:tpu_worker/replica:0/task:0/device:TPU:7, TPU, 17179869184, 6427061617775819593),\n _DeviceAttributes(/job:tpu_worker/replica:0/task:0/device:TPU_SYSTEM:0, TPU_SYSTEM, 8589934592, 2138631231408532535)]\n"
]
],
[
[
"### Prepare and import ALBERT modules\n\nWith your environment configured, you can now prepare and import the ALBERT modules. The following step clones the source code from GitHub.",
"_____no_output_____"
]
],
[
[
"import sys\n\n!test -d albert || git clone https://github.com/google-research/albert albert\nif not 'albert' in sys.path:\n sys.path += ['albert']\n \n!pip install sentencepiece\n",
"Cloning into 'albert'...\nremote: Enumerating objects: 367, done.\u001b[K\nremote: Counting objects: 100% (14/14), done.\u001b[K\nremote: Compressing objects: 100% (11/11), done.\u001b[K\nremote: Total 367 (delta 5), reused 6 (delta 3), pack-reused 353\u001b[K\nReceiving objects: 100% (367/367), 262.46 KiB | 3.50 MiB/s, done.\nResolving deltas: 100% (237/237), done.\nCollecting sentencepiece\n Downloading sentencepiece-0.1.96-cp37-cp37m-manylinux_2_17_x86_64.manylinux2014_x86_64.whl (1.2 MB)\n\u001b[K |████████████████████████████████| 1.2 MB 5.2 MB/s \n\u001b[?25hInstalling collected packages: sentencepiece\nSuccessfully installed sentencepiece-0.1.96\n"
]
],
[
[
"## Prepare for training\n\nThis next section of code performs the following tasks:\n\n* Specify GS bucket, create output directory for model checkpoints and eval results.\n* Specify task and download training data.\n* Specify ALBERT pretrained model\n\n\n\n",
"_____no_output_____"
]
],
[
[
"#Download GLUE data\n!git clone https://github.com/nyu-mll/GLUE-baselines download_glue\n\nGLUE_DIR='glue_data'\n!python download_glue/download_glue_data.py --data_dir $GLUE_DIR --tasks all",
"_____no_output_____"
],
[
"# Please find the full list of tasks and their fintuning hyperparameters\n# here https://github.com/google-research/albert/blob/master/run_glue.sh\n\nBUCKET = \"luanps\" #@param { type: \"string\" }\nTASK = 'RTE' #@param {type:\"string\"}\n# Available pretrained model checkpoints:\n# base, large, xlarge, xxlarge\nALBERT_MODEL = 'base' #@param {type:\"string\"}\n\nTASK_DATA_DIR = 'glue_data'\n\nBASE_DIR = \"gs://\" + BUCKET\nif not BASE_DIR or BASE_DIR == \"gs://\":\n raise ValueError(\"You must enter a BUCKET.\")\nDATA_DIR = os.path.join(BASE_DIR, \"data\")\nMODELS_DIR = os.path.join(BASE_DIR, \"models\")\nOUTPUT_DIR = 'gs://{}/albert-tfhub/models/{}'.format(BUCKET, TASK)\ntf.gfile.MakeDirs(OUTPUT_DIR)\nprint('***** Model output directory: {} *****'.format(OUTPUT_DIR))\n\n# Download glue data.\n#! test -d download_glue_repo || git clone https://gist.github.com/60c2bdb54d156a41194446737ce03e2e.git download_glue_repo\n#!python download_glue_repo/download_glue_data.py --data_dir=$TASK_DATA_DIR --tasks=$TASK\n#print('***** Task data directory: {} *****'.format(TASK_DATA_DIR))\n\nALBERT_MODEL_HUB = 'https://tfhub.dev/google/albert_' + ALBERT_MODEL + '/3'",
"***** Model output directory: gs://luanps/albert-tfhub/models/RTE *****\n"
]
],
[
[
"Now let's run the fine-tuning scripts. If you use the default MRPC task, this should be finished in around 10 mintues and you will get an accuracy of around 86.5.",
"_____no_output_____"
],
[
"## Choose hyperparameters using [Optuna](https://optuna.readthedocs.io/en/stable/index.html)",
"_____no_output_____"
]
],
[
[
"#Install Optuna optimzation lib\n!pip install optuna",
"_____no_output_____"
],
[
"import optuna\nimport uuid",
"_____no_output_____"
],
[
"def get_last_acc_from_file(result_file):\n f = open(result_file,'r')\n results = f.readlines()\n result_dict = dict()\n for r in results:\n if 'eval_accuracy' in r:\n k,v = r.split(' = ')\n return float(v)",
"_____no_output_____"
],
[
"def objective(trial):\n\n #hyperparameter setting: RTE task\n warmup_steps = trial.suggest_int('warmup_steps', 100, 500,100)\n train_steps = trial.suggest_int('train_steps', 400, 2000,100)\n learning_rate = trial.suggest_loguniform(\"learning_rate\", 1e-5, 5e-5)\n batch_size = trial.suggest_int('batch_size', 16, 128,16)\n\n #Tmp config\n id = str(uuid.uuid4()).split('-')[0]\n OUTPUT_TMP = f'{OUTPUT_DIR}/{id}'\n os.environ['TFHUB_CACHE_DIR'] = OUTPUT_TMP\n\n !python -m albert.run_classifier \\\n --data_dir=\"glue_data/\" \\\n --output_dir=$OUTPUT_TMP \\\n --albert_hub_module_handle=$ALBERT_MODEL_HUB \\\n --spm_model_file=\"from_tf_hub\" \\\n --do_train=True \\\n --do_eval=True \\\n --do_predict=False \\\n --max_seq_length=512 \\\n --optimizer=adamw \\\n --task_name=$TASK \\\n --warmup_step=$warmup_steps \\\n --learning_rate=$learning_rate \\\n --train_step=$train_steps \\\n --save_checkpoints_steps=100 \\\n --train_batch_size=$batch_size\\\n --tpu_name=$TPU_ADDRESS \\\n --use_tpu=True\n\n #Download results and load model accuracy\n !mkdir $id\n !gsutil cp $OUTPUT_TMP/eval_results.txt $id\n model_acc = get_last_acc_from_file(f'{id}/eval_results.txt')\n return model_acc",
"_____no_output_____"
],
[
"#Run Optuna optimization\nstudy = optuna.create_study(direction='maximize',study_name=TASK)\nstudy.optimize(objective, n_trials=20)",
"_____no_output_____"
],
[
"#Pack Optuna results and save to Bucket\nimport joblib\n\nstudy_file = f'{TASK}_study.pkl'\n!rm $study_file\njoblib.dump(study, study_file)\n!gsutil cp $study_file $OUTPUT_DIR",
"Copying file://RTE_study.pkl [Content-Type=application/octet-stream]...\n/ [1 files][ 13.6 KiB/ 13.6 KiB] \nOperation completed over 1 objects/13.6 KiB. \n"
],
[
"study.trials_dataframe()",
"_____no_output_____"
]
],
[
[
"## Analyzing Optimization Results",
"_____no_output_____"
]
],
[
[
"#Download pkl file from GCP\nimport joblib\n\nstudy_file = f'{TASK}_study.pkl'\n!gsutil cp $OUTPUT_DIR/$study_file .\n\nstudy = joblib.load(study_file)\n",
"Copying gs://luanps/albert-tfhub/models/RTE/RTE_study.pkl...\n/ [1 files][ 7.8 KiB/ 7.8 KiB] \nOperation completed over 1 objects/7.8 KiB. \n"
],
[
"study.trials_dataframe()",
"_____no_output_____"
],
[
"import optuna\nfrom optuna.visualization import plot_contour\nfrom optuna.visualization import plot_edf\nfrom optuna.visualization import plot_intermediate_values\nfrom optuna.visualization import plot_optimization_history\nfrom optuna.visualization import plot_parallel_coordinate\nfrom optuna.visualization import plot_param_importances\nfrom optuna.visualization import plot_slice\n",
"_____no_output_____"
],
[
"plot_optimization_history(study)",
"_____no_output_____"
],
[
"plot_parallel_coordinate(study)",
"_____no_output_____"
],
[
"plot_contour(study)",
"_____no_output_____"
],
[
"plot_slice(study)",
"_____no_output_____"
],
[
"plot_param_importances(study)",
"_____no_output_____"
],
[
"plot_edf(study)",
"_____no_output_____"
]
]
] | [
"markdown",
"code",
"markdown",
"code",
"markdown",
"code",
"markdown",
"code",
"markdown",
"code",
"markdown",
"code"
] | [
[
"markdown"
],
[
"code"
],
[
"markdown",
"markdown",
"markdown",
"markdown",
"markdown"
],
[
"code"
],
[
"markdown"
],
[
"code"
],
[
"markdown"
],
[
"code",
"code"
],
[
"markdown",
"markdown"
],
[
"code",
"code",
"code",
"code",
"code",
"code",
"code"
],
[
"markdown"
],
[
"code",
"code",
"code",
"code",
"code",
"code",
"code",
"code",
"code"
]
] |
e775a16d83e794b851756a66508ac536ae7c408b | 43,141 | ipynb | Jupyter Notebook | Informatics/Reinforcement Learning/Practical RL - HSE/week6_exploration/bandits.ipynb | MarcosSalib/Cocktail_MOOC | 46279c2ec642554537c639702ed8e540ea49afdf | [
"MIT"
] | null | null | null | Informatics/Reinforcement Learning/Practical RL - HSE/week6_exploration/bandits.ipynb | MarcosSalib/Cocktail_MOOC | 46279c2ec642554537c639702ed8e540ea49afdf | [
"MIT"
] | null | null | null | Informatics/Reinforcement Learning/Practical RL - HSE/week6_exploration/bandits.ipynb | MarcosSalib/Cocktail_MOOC | 46279c2ec642554537c639702ed8e540ea49afdf | [
"MIT"
] | null | null | null | 98.047727 | 29,136 | 0.82286 | [
[
[
"import sys, os\nif 'google.colab' in sys.modules and not os.path.exists('.setup_complete'):\n !wget -q https://raw.githubusercontent.com/yandexdataschool/Practical_RL/spring20/setup_colab.sh -O- | bash\n\n !wget -q https://raw.githubusercontent.com/yandexdataschool/Practical_RL/coursera/grading.py -O ../grading.py\n !wget -q https://raw.githubusercontent.com/yandexdataschool/Practical_RL/coursera/week6_outro/submit.py\n\n !touch .setup_complete\n\n# This code creates a virtual display to draw game images on.\n# It will have no effect if your machine has a monitor.\nif type(os.environ.get(\"DISPLAY\")) is not str or len(os.environ.get(\"DISPLAY\")) == 0:\n !bash ../xvfb start\n os.environ['DISPLAY'] = ':1'",
"_____no_output_____"
],
[
"from abc import ABCMeta, abstractmethod, abstractproperty\nimport enum\n\nimport numpy as np\nnp.set_printoptions(precision=3)\nnp.set_printoptions(suppress=True)\n\nimport pandas\n\nfrom matplotlib import pyplot as plt\n%matplotlib inline",
"_____no_output_____"
]
],
[
[
"## Bernoulli Bandit\n\nWe are going to implement several exploration strategies for simplest problem - bernoulli bandit.\n\nThe bandit has $K$ actions. Action produce 1.0 reward $r$ with probability $0 \\le \\theta_k \\le 1$ which is unknown to agent, but fixed over time. Agent's objective is to minimize regret over fixed number $T$ of action selections:\n\n$$\\rho = T\\theta^* - \\sum_{t=1}^T r_t$$\n\nWhere $\\theta^* = \\max_k\\{\\theta_k\\}$\n\n**Real-world analogy:**\n\nClinical trials - we have $K$ pills and $T$ ill patient. After taking pill, patient is cured with probability $\\theta_k$. Task is to find most efficient pill.\n\nA research on clinical trials - https://arxiv.org/pdf/1507.08025.pdf",
"_____no_output_____"
]
],
[
[
"class BernoulliBandit:\n def __init__(self, n_actions=5):\n self._probs = np.random.random(n_actions)\n\n @property\n def action_count(self):\n return len(self._probs)\n\n def pull(self, action):\n if np.any(np.random.random() > self._probs[action]):\n return 0.0\n return 1.0\n\n def optimal_reward(self):\n \"\"\" Used for regret calculation\n \"\"\"\n return np.max(self._probs)\n\n def step(self):\n \"\"\" Used in nonstationary version\n \"\"\"\n pass\n\n def reset(self):\n \"\"\" Used in nonstationary version\n \"\"\"",
"_____no_output_____"
],
[
"class AbstractAgent(metaclass=ABCMeta):\n def init_actions(self, n_actions):\n self._successes = np.zeros(n_actions)\n self._failures = np.zeros(n_actions)\n self._total_pulls = 0\n\n @abstractmethod\n def get_action(self):\n \"\"\"\n Get current best action\n :rtype: int\n \"\"\"\n pass\n\n def update(self, action, reward):\n \"\"\"\n Observe reward from action and update agent's internal parameters\n :type action: int\n :type reward: int\n \"\"\"\n self._total_pulls += 1\n if reward == 1:\n self._successes[action] += 1\n else:\n self._failures[action] += 1\n\n @property\n def name(self):\n return self.__class__.__name__\n\n\nclass RandomAgent(AbstractAgent):\n def get_action(self):\n return np.random.randint(0, len(self._successes))",
"_____no_output_____"
]
],
[
[
"### Epsilon-greedy agent\n\n**for** $t = 1,2,...$ **do**\n\n **for** $k = 1,...,K$ **do**\n\n $\\hat\\theta_k \\leftarrow \\alpha_k / (\\alpha_k + \\beta_k)$\n\n **end for** \n\n $x_t \\leftarrow argmax_{k}\\hat\\theta$ with probability $1 - \\epsilon$ or random action with probability $\\epsilon$\n\n Apply $x_t$ and observe $r_t$\n\n $(\\alpha_{x_t}, \\beta_{x_t}) \\leftarrow (\\alpha_{x_t}, \\beta_{x_t}) + (r_t, 1-r_t)$\n\n**end for**\n\nImplement the algorithm above in the cell below:",
"_____no_output_____"
]
],
[
[
"class EpsilonGreedyAgent(AbstractAgent):\n def __init__(self, epsilon=0.01):\n self._epsilon = epsilon\n\n def get_action(self):\n # <YOUR CODE>\n alpha = self._successes\n beta = self._failures\n \n theta_ = alpha / (alpha + beta)\n if np.random.random() < self._epsilon:\n return np.random.randint(0, len(alpha))\n else:\n return np.argmax(theta_)\n\n @property\n def name(self):\n return self.__class__.__name__ + \"(epsilon={})\".format(self._epsilon)",
"_____no_output_____"
]
],
[
[
"### UCB Agent\nEpsilon-greedy strategy heve no preference for actions. It would be better to select among actions that are uncertain or have potential to be optimal. One can come up with idea of index for each action that represents otimality and uncertainty at the same time. One efficient way to do it is to use UCB1 algorithm:\n\n**for** $t = 1,2,...$ **do**\n\n **for** $k = 1,...,K$ **do**\n\n $w_k \\leftarrow \\alpha_k / (\\alpha_k + \\beta_k) + \\sqrt{2log\\ t \\ / \\ (\\alpha_k + \\beta_k)}$\n\n **end for** \n\n **end for** \n $x_t \\leftarrow argmax_{k}w$\n\n Apply $x_t$ and observe $r_t$\n\n $(\\alpha_{x_t}, \\beta_{x_t}) \\leftarrow (\\alpha_{x_t}, \\beta_{x_t}) + (r_t, 1-r_t)$\n\n**end for**\n\n__Note:__ in practice, one can multiply $\\sqrt{2log\\ t \\ / \\ (\\alpha_k + \\beta_k)}$ by some tunable parameter to regulate agent's optimism and wilingness to abandon non-promising actions.\n\nMore versions and optimality analysis - https://homes.di.unimi.it/~cesabian/Pubblicazioni/ml-02.pdf",
"_____no_output_____"
]
],
[
[
"class UCBAgent(AbstractAgent):\n def __init__(self, gamma=0.01):\n self._gamma = gamma\n \n def get_action(self):\n # <YOUR CODE>\n alpha = self._successes\n beta = self._failures\n t = self._total_pulls\n \n omega_ = alpha / (alpha + beta) + self._gamma * np.sqrt(2*np.log(t) / (alpha+beta))\n return np.argmax(omega_)\n \n @property\n def name(self):\n return self.__class__.__name__ + \"(gamma={})\".format(self._gamma)",
"_____no_output_____"
]
],
[
[
"### Thompson sampling\n\nUCB1 algorithm does not take into account actual distribution of rewards. If we know the distribution - we can do much better by using Thompson sampling:\n\n**for** $t = 1,2,...$ **do**\n\n **for** $k = 1,...,K$ **do**\n\n Sample $\\hat\\theta_k \\sim beta(\\alpha_k, \\beta_k)$\n\n **end for** \n\n $x_t \\leftarrow argmax_{k}\\hat\\theta$\n\n Apply $x_t$ and observe $r_t$\n\n $(\\alpha_{x_t}, \\beta_{x_t}) \\leftarrow (\\alpha_{x_t}, \\beta_{x_t}) + (r_t, 1-r_t)$\n\n**end for**\n \n\nMore on Thompson Sampling:\nhttps://web.stanford.edu/~bvr/pubs/TS_Tutorial.pdf",
"_____no_output_____"
]
],
[
[
"class ThompsonSamplingAgent(AbstractAgent):\n def get_action(self):\n # <YOUR CODE>\n alpha = self._successes\n beta = self._failures\n \n theta_ = np.random.beta(alpha+1, beta+1)\n return np.argmax(theta_)\n",
"_____no_output_____"
],
[
"from collections import OrderedDict\n\ndef get_regret(env, agents, n_steps=5000, n_trials=50):\n scores = OrderedDict({\n agent.name: [0.0 for step in range(n_steps)] for agent in agents\n })\n\n for trial in range(n_trials):\n env.reset()\n\n for a in agents:\n a.init_actions(env.action_count)\n\n for i in range(n_steps):\n optimal_reward = env.optimal_reward()\n\n for agent in agents:\n action = agent.get_action()\n reward = env.pull(action)\n agent.update(action, reward)\n scores[agent.name][i] += optimal_reward - reward\n\n env.step() # change bandit's state if it is unstationary\n\n for agent in agents:\n scores[agent.name] = np.cumsum(scores[agent.name]) / n_trials\n\n return scores\n\ndef plot_regret(agents, scores):\n for agent in agents:\n plt.plot(scores[agent.name])\n\n plt.legend([agent.name for agent in agents])\n\n plt.ylabel(\"regret\")\n plt.xlabel(\"steps\")\n\n plt.show()",
"_____no_output_____"
],
[
"# Uncomment agents\nagents = [\n EpsilonGreedyAgent(),\n UCBAgent(0.1),\n UCBAgent(0.2),\n ThompsonSamplingAgent()\n]\n\nregret = get_regret(BernoulliBandit(), agents, n_steps=10000, n_trials=10)\nplot_regret(agents, regret)",
"/home/x/anaconda3/envs/rl/lib/python3.7/site-packages/ipykernel_launcher.py:10: RuntimeWarning: invalid value encountered in true_divide\n # Remove the CWD from sys.path while we load stuff.\n/home/x/anaconda3/envs/rl/lib/python3.7/site-packages/ipykernel_launcher.py:11: RuntimeWarning: invalid value encountered in true_divide\n # This is added back by InteractiveShellApp.init_path()\n/home/x/anaconda3/envs/rl/lib/python3.7/site-packages/ipykernel_launcher.py:11: RuntimeWarning: divide by zero encountered in log\n # This is added back by InteractiveShellApp.init_path()\n/home/x/anaconda3/envs/rl/lib/python3.7/site-packages/ipykernel_launcher.py:11: RuntimeWarning: invalid value encountered in sqrt\n # This is added back by InteractiveShellApp.init_path()\n/home/x/anaconda3/envs/rl/lib/python3.7/site-packages/ipykernel_launcher.py:11: RuntimeWarning: divide by zero encountered in true_divide\n # This is added back by InteractiveShellApp.init_path()\n"
]
],
[
[
"### Submit to coursera",
"_____no_output_____"
]
],
[
[
"from submit import submit_bandits\n\nsubmit_bandits(agents, regret, '', '')",
"Submitted to Coursera platform. See results on assignment page!\n"
]
]
] | [
"code",
"markdown",
"code",
"markdown",
"code",
"markdown",
"code",
"markdown",
"code",
"markdown",
"code"
] | [
[
"code",
"code"
],
[
"markdown"
],
[
"code",
"code"
],
[
"markdown"
],
[
"code"
],
[
"markdown"
],
[
"code"
],
[
"markdown"
],
[
"code",
"code",
"code"
],
[
"markdown"
],
[
"code"
]
] |
e775c590e3adefd5ab93590a52580ddfea0be4f4 | 5,280 | ipynb | Jupyter Notebook | Tarea_7_Recursividad.ipynb | Sahp59/daa_2021_1 | d5da553329137a934c4e94921ebbbd7099a757b9 | [
"MIT"
] | 1 | 2020-09-28T14:37:18.000Z | 2020-09-28T14:37:18.000Z | Tarea_7_Recursividad.ipynb | Sahp59/daa_2021_1 | d5da553329137a934c4e94921ebbbd7099a757b9 | [
"MIT"
] | null | null | null | Tarea_7_Recursividad.ipynb | Sahp59/daa_2021_1 | d5da553329137a934c4e94921ebbbd7099a757b9 | [
"MIT"
] | null | null | null | 30.520231 | 154 | 0.391288 | [
[
[
"Realizar:\n1. Crear una lista de enteros en Python y realizar la suma con recursividad, el caso base es cuando la lista este vacía.\n2. Hacer un contador regresivo con recursión.\n3. Sacar de un ADT pila el valor en la posición media.",
"_____no_output_____"
]
],
[
[
"class Pila:\n def __init__(self):\n self.items = []\n \n def imprimirCompleto(self):\n for x in range(0,len(self.items),1):\n print(self.items[x],end=\",\")\n \n def estaVacia(self):\n return self.items == []\n\n def incluir(self, item):\n self.items.append(item)\n \n def extraerPrimero(self):\n return self.items.pop(0)\n \n def extraerUltimo(self):\n return self.items.pop()\n\n def inspeccionarUltimo(self):\n return self.items[len(self.items)-1]\n\n def tamano(self):\n return len(self.items)\n \ndef sumaRec(lista,longitud,suma = 0):\n if longitud != 0:\n suma += lista[longitud-1]\n print(suma)\n sumaRec(lista,longitud-1,suma)\n \ndef contador(maximo):\n if maximo != 0:\n print(maximo)\n contador(maximo-1)\n \ndef posicionRec(pila):\n lon = pila.tamano()\n if lon == 1:\n print(\"\\nEl elemento de la posicicon media es: \",pila.inspeccionarUltimo())\n else:\n pila.extraerPrimero()\n pila.extraerUltimo()\n posicionRec(pila)\n \ndef main():\n print(\"1. Crear una lista de enteros en Python y realizar \\nla suma con recursividad, el caso base es cuando \\nla lista este vacía.\")\n listNum = [1,2,3,4,5,6,7,8,9]\n print(listNum)\n sumaRec(listNum,len(listNum))\n \n print(\"2. Hacer un contador regresivo con recursión.\")\n print(\"INICIA CONTEO REGRESIVO\")\n contador(30)\n print(\"FINALIZA CONTEO REGRESIVO\")\n \n print(\"3. Sacar de un ADT pila el valor en la posición media\")\n p = Pila()\n p.incluir(1)\n p.incluir(2)\n p.incluir(3)\n p.incluir(4)\n p.incluir(5)\n p.incluir(6)\n p.incluir(7)\n p.incluir(8)\n p.incluir(9)\n p.imprimirCompleto()\n try:\n posicionRec(p)\n except:\n print(\"La lista no tiene posicion Media\")\nmain()\n\n",
"1. Crear una lista de enteros en Python y realizar \nla suma con recursividad, el caso base es cuando \nla lista este vacía.\n[1, 2, 3, 4, 5, 6, 7, 8, 9]\n9\n17\n24\n30\n35\n39\n42\n44\n45\n2. Hacer un contador regresivo con recursión.\nINICIA CONTEO REGRESIVO\n30\n29\n28\n27\n26\n25\n24\n23\n22\n21\n20\n19\n18\n17\n16\n15\n14\n13\n12\n11\n10\n9\n8\n7\n6\n5\n4\n3\n2\n1\nFINALIZA CONTEO REGRESIVO\n3. Sacar de un ADT pila el valor en la posición media\n1,2,3,4,5,6,7,8,9,\nEl elemento de la posicicon media es: 5\n"
]
]
] | [
"markdown",
"code"
] | [
[
"markdown"
],
[
"code"
]
] |
e775c7538b53b35b0d604f218efbf94888b2fcf6 | 30,897 | ipynb | Jupyter Notebook | how-to-use-azureml/machine-learning-pipelines/intro-to-pipelines/aml-pipelines-getting-started.ipynb | alla15747/MachineLearningNotebooks | cf7bbd693ac956a9c3c6692addf84db3e58df23a | [
"MIT"
] | 3 | 2020-09-10T15:02:56.000Z | 2020-09-13T17:37:47.000Z | how-to-use-azureml/machine-learning-pipelines/intro-to-pipelines/aml-pipelines-getting-started.ipynb | alla15747/MachineLearningNotebooks | cf7bbd693ac956a9c3c6692addf84db3e58df23a | [
"MIT"
] | null | null | null | how-to-use-azureml/machine-learning-pipelines/intro-to-pipelines/aml-pipelines-getting-started.ipynb | alla15747/MachineLearningNotebooks | cf7bbd693ac956a9c3c6692addf84db3e58df23a | [
"MIT"
] | 5 | 2019-05-03T20:20:53.000Z | 2019-05-04T13:01:49.000Z | 47.533846 | 960 | 0.609347 | [
[
[
"Copyright (c) Microsoft Corporation. All rights reserved. \nLicensed under the MIT License.",
"_____no_output_____"
],
[
"",
"_____no_output_____"
],
[
"# Azure Machine Learning Pipelines: Getting Started\n\n## Overview\n\n\nA common scenario when using machine learning components is to have a data workflow that includes the following steps:\n\n- Preparing/preprocessing a given dataset for training, followed by\n- Training a machine learning model on this data, and then\n- Deploying this trained model in a separate environment, and finally\n- Running a batch scoring task on another data set, using the trained model.\n\nAzure's Machine Learning pipelines give you a way to combine multiple steps like these into one configurable workflow, so that multiple agents/users can share and/or reuse this workflow. Machine learning pipelines thus provide a consistent, reproducible mechanism for building, evaluating, deploying, and running ML systems.\n\nTo get more information about Azure machine learning pipelines, please read our [Azure Machine Learning Pipelines](https://aka.ms/pl-concept) overview, or the [readme article](https://aka.ms/pl-readme).\n\nIn this notebook, we provide a gentle introduction to Azure machine learning pipelines. We build a pipeline that runs jobs unattended on different compute clusters; in this notebook, you'll see how to use the basic Azure ML SDK APIs for constructing this pipeline.\n ",
"_____no_output_____"
],
[
"## Prerequisites and Azure Machine Learning Basics\nIf you are using an Azure Machine Learning Notebook VM, you are all set. Otherwise, make sure you go through the [configuration notebook](https://aka.ms/pl-config) first if you haven't. This sets you up with a working config file that has information on your workspace, subscription id, etc. \n",
"_____no_output_____"
],
[
"### Azure Machine Learning Imports\n\nIn this first code cell, we import key Azure Machine Learning modules that we will use below. ",
"_____no_output_____"
]
],
[
[
"import os\nimport azureml.core\nfrom azureml.core import Workspace, Experiment, Datastore\nfrom azureml.widgets import RunDetails\n\n# Check core SDK version number\nprint(\"SDK version:\", azureml.core.VERSION)",
"_____no_output_____"
]
],
[
[
"### Pipeline-specific SDK imports\n\nHere, we import key pipeline modules, whose use will be illustrated in the examples below.",
"_____no_output_____"
]
],
[
[
"from azureml.pipeline.core import Pipeline\nfrom azureml.pipeline.steps import PythonScriptStep\n\nprint(\"Pipeline SDK-specific imports completed\")",
"_____no_output_____"
]
],
[
[
"### Initialize Workspace\n\nInitialize a [workspace](https://docs.microsoft.com/en-us/python/api/azureml-core/azureml.core.workspace(class%29) object from persisted configuration.",
"_____no_output_____"
]
],
[
[
"ws = Workspace.from_config()\nprint(ws.name, ws.resource_group, ws.location, ws.subscription_id, sep = '\\n')\n\n# Default datastore\ndef_blob_store = ws.get_default_datastore() \n# The following call GETS the Azure Blob Store associated with your workspace.\n# Note that workspaceblobstore is **the name of this store and CANNOT BE CHANGED and must be used as is** \ndef_blob_store = Datastore(ws, \"workspaceblobstore\")\nprint(\"Blobstore's name: {}\".format(def_blob_store.name))",
"_____no_output_____"
]
],
[
[
"### Required data and script files for the the tutorial\nSample files required to finish this tutorial are already copied to the corresponding source_directory locations. Even though the .py provided in the samples does not have much \"ML work\" as a data scientist, you will work on this extensively as part of your work. To complete this tutorial, the contents of these files are not very important. The one-line files are for demostration purpose only.",
"_____no_output_____"
],
[
"### Datastore concepts\nA [Datastore](https://docs.microsoft.com/en-us/python/api/azureml-core/azureml.core.datastore.datastore?view=azure-ml-py) is a place where data can be stored that is then made accessible to a compute either by means of mounting or copying the data to the compute target. \n\nA Datastore can either be backed by an Azure File Storage (default) or by an Azure Blob Storage.\n\nIn this next step, we will upload the training and test set into the workspace's default storage (File storage), and another piece of data to Azure Blob Storage. When to use [Azure Blobs](https://docs.microsoft.com/en-us/azure/storage/blobs/storage-blobs-introduction), [Azure Files](https://docs.microsoft.com/en-us/azure/storage/files/storage-files-introduction), or [Azure Disks](https://docs.microsoft.com/en-us/azure/virtual-machines/linux/managed-disks-overview) is [detailed here](https://docs.microsoft.com/en-us/azure/storage/common/storage-decide-blobs-files-disks).\n\n**Please take good note of the concept of the datastore.**",
"_____no_output_____"
],
[
"#### Upload data to default datastore\nDefault datastore on workspace is the Azure File storage. The workspace has a Blob storage associated with it as well. Let's upload a file to each of these storages.",
"_____no_output_____"
]
],
[
[
"# get_default_datastore() gets the default Azure Blob Store associated with your workspace.\n# Here we are reusing the def_blob_store object we obtained earlier\ndef_blob_store.upload_files([\"./20news.pkl\"], target_path=\"20newsgroups\", overwrite=True)\nprint(\"Upload call completed\")",
"_____no_output_____"
]
],
[
[
"#### (Optional) See your files using Azure Portal\nOnce you successfully uploaded the files, you can browse to them (or upload more files) using [Azure Portal](https://portal.azure.com). At the portal, make sure you have selected your subscription (click *Resource Groups* and then select the subscription). Then look for your **Machine Learning Workspace** name. It has a link to your storage. Click on the storage link. It will take you to a page where you can see [Blobs](https://docs.microsoft.com/en-us/azure/storage/blobs/storage-blobs-introduction), [Files](https://docs.microsoft.com/en-us/azure/storage/files/storage-files-introduction), [Tables](https://docs.microsoft.com/en-us/azure/storage/tables/table-storage-overview), and [Queues](https://docs.microsoft.com/en-us/azure/storage/queues/storage-queues-introduction). We have uploaded a file each to the Blob storage and to the File storage in the above step. You should be able to see both of these files in their respective locations. ",
"_____no_output_____"
],
[
"### Compute Targets\nA compute target specifies where to execute your program such as a remote Docker on a VM, or a cluster. A compute target needs to be addressable and accessible by you.\n\n**You need at least one compute target to send your payload to. We are planning to use Azure Machine Learning Compute exclusively for this tutorial for all steps. However in some cases you may require multiple compute targets as some steps may run in one compute target like Azure Machine Learning Compute, and some other steps in the same pipeline could run in a different compute target.**\n\n*The example belows show creating/retrieving/attaching to an Azure Machine Learning Compute instance.*",
"_____no_output_____"
],
[
"#### List of Compute Targets on the workspace",
"_____no_output_____"
]
],
[
[
"cts = ws.compute_targets\nfor ct in cts:\n print(ct)",
"_____no_output_____"
]
],
[
[
"#### Retrieve or create a Azure Machine Learning compute\nAzure Machine Learning Compute is a service for provisioning and managing clusters of Azure virtual machines for running machine learning workloads. Let's create a new Azure Machine Learning Compute in the current workspace, if it doesn't already exist. We will then run the training script on this compute target.\n\nIf we could not find the compute with the given name in the previous cell, then we will create a new compute here. We will create an Azure Machine Learning Compute containing **STANDARD_D2_V2 CPU VMs**. This process is broken down into the following steps:\n\n1. Create the configuration\n2. Create the Azure Machine Learning compute\n\n**This process will take about 3 minutes and is providing only sparse output in the process. Please make sure to wait until the call returns before moving to the next cell.**",
"_____no_output_____"
]
],
[
[
"from azureml.core.compute import ComputeTarget, AmlCompute\nfrom azureml.core.compute_target import ComputeTargetException\n\naml_compute_target = \"cpu-cluster\"\ntry:\n aml_compute = AmlCompute(ws, aml_compute_target)\n print(\"found existing compute target.\")\nexcept ComputeTargetException:\n print(\"creating new compute target\")\n \n provisioning_config = AmlCompute.provisioning_configuration(vm_size = \"STANDARD_D2_V2\",\n min_nodes = 1, \n max_nodes = 4) \n aml_compute = ComputeTarget.create(ws, aml_compute_target, provisioning_config)\n aml_compute.wait_for_completion(show_output=True, min_node_count=None, timeout_in_minutes=20)\n \nprint(\"Azure Machine Learning Compute attached\")\n",
"_____no_output_____"
],
[
"# For a more detailed view of current Azure Machine Learning Compute status, use get_status()\n# example: un-comment the following line.\n# print(aml_compute.get_status().serialize())",
"_____no_output_____"
]
],
[
[
"**Wait for this call to finish before proceeding (you will see the asterisk turning to a number).**\n\nNow that you have created the compute target, let's see what the workspace's compute_targets() function returns. You should now see one entry named 'amlcompute' of type AmlCompute.",
"_____no_output_____"
],
[
"**Now that we have completed learning the basics of Azure Machine Learning (AML), let's go ahead and start understanding the Pipeline concepts.**",
"_____no_output_____"
],
[
"## Creating a Step in a Pipeline\nA Step is a unit of execution. Step typically needs a target of execution (compute target), a script to execute, and may require script arguments and inputs, and can produce outputs. The step also could take a number of other parameters. Azure Machine Learning Pipelines provides the following built-in Steps:\n\n- [**PythonScriptStep**](https://docs.microsoft.com/en-us/python/api/azureml-pipeline-steps/azureml.pipeline.steps.python_script_step.pythonscriptstep?view=azure-ml-py): Adds a step to run a Python script in a Pipeline.\n- [**AdlaStep**](https://docs.microsoft.com/en-us/python/api/azureml-pipeline-steps/azureml.pipeline.steps.adla_step.adlastep?view=azure-ml-py): Adds a step to run U-SQL script using Azure Data Lake Analytics.\n- [**DataTransferStep**](https://docs.microsoft.com/en-us/python/api/azureml-pipeline-steps/azureml.pipeline.steps.data_transfer_step.datatransferstep?view=azure-ml-py): Transfers data between Azure Blob and Data Lake accounts.\n- [**DatabricksStep**](https://docs.microsoft.com/en-us/python/api/azureml-pipeline-steps/azureml.pipeline.steps.databricks_step.databricksstep?view=azure-ml-py): Adds a DataBricks notebook as a step in a Pipeline.\n- [**HyperDriveStep**](https://docs.microsoft.com/en-us/python/api/azureml-pipeline-steps/azureml.pipeline.steps.hyper_drive_step.hyperdrivestep?view=azure-ml-py): Creates a Hyper Drive step for Hyper Parameter Tuning in a Pipeline.\n- [**AzureBatchStep**](https://docs.microsoft.com/en-us/python/api/azureml-pipeline-steps/azureml.pipeline.steps.azurebatch_step.azurebatchstep?view=azure-ml-py): Creates a step for submitting jobs to Azure Batch\n- [**EstimatorStep**](https://docs.microsoft.com/en-us/python/api/azureml-pipeline-steps/azureml.pipeline.steps.estimator_step.estimatorstep?view=azure-ml-py): Adds a step to run Estimator in a Pipeline.\n- [**MpiStep**](https://docs.microsoft.com/en-us/python/api/azureml-pipeline-steps/azureml.pipeline.steps.mpi_step.mpistep?view=azure-ml-py): Adds a step to run a MPI job in a Pipeline.\n- [**AutoMLStep**](https://docs.microsoft.com/en-us/python/api/azureml-train-automl/azureml.train.automl.automlstep?view=azure-ml-py): Creates a AutoML step in a Pipeline.\n\nThe following code will create a PythonScriptStep to be executed in the Azure Machine Learning Compute we created above using train.py, one of the files already made available in the `source_directory`.\n\nA **PythonScriptStep** is a basic, built-in step to run a Python Script on a compute target. It takes a script name and optionally other parameters like arguments for the script, compute target, inputs and outputs. If no compute target is specified, default compute target for the workspace is used. You can also use a [**RunConfiguration**](https://docs.microsoft.com/en-us/python/api/azureml-core/azureml.core.runconfiguration?view=azure-ml-py) to specify requirements for the PythonScriptStep, such as conda dependencies and docker image.\n> The best practice is to use separate folders for scripts and its dependent files for each step and specify that folder as the `source_directory` for the step. This helps reduce the size of the snapshot created for the step (only the specific folder is snapshotted). Since changes in any files in the `source_directory` would trigger a re-upload of the snapshot, this helps keep the reuse of the step when there are no changes in the `source_directory` of the step.",
"_____no_output_____"
]
],
[
[
"# Uses default values for PythonScriptStep construct.\n\nsource_directory = './train'\nprint('Source directory for the step is {}.'.format(os.path.realpath(source_directory)))\n\n# Syntax\n# PythonScriptStep(\n# script_name, \n# name=None, \n# arguments=None, \n# compute_target=None, \n# runconfig=None, \n# inputs=None, \n# outputs=None, \n# params=None, \n# source_directory=None, \n# allow_reuse=True, \n# version=None, \n# hash_paths=None)\n# This returns a Step\nstep1 = PythonScriptStep(name=\"train_step\",\n script_name=\"train.py\", \n compute_target=aml_compute, \n source_directory=source_directory,\n allow_reuse=True)\nprint(\"Step1 created\")",
"_____no_output_____"
]
],
[
[
"**Note:** In the above call to PythonScriptStep(), the flag *allow_reuse* determines whether the step should reuse previous results when run with the same settings/inputs. This flag's default value is *True*; the default is set to *True* because, when inputs and parameters have not changed, we typically do not want to re-run a given pipeline step. \n\nIf *allow_reuse* is set to *False*, a new run will always be generated for this step during pipeline execution. The *allow_reuse* flag can come in handy in situations where you do *not* want to re-run a pipeline step.",
"_____no_output_____"
],
[
"## Running a few steps in parallel\nHere we are looking at a simple scenario where we are running a few steps (all involving PythonScriptStep) in parallel. Running nodes in **parallel** is the default behavior for steps in a pipeline.\n\nWe already have one step defined earlier. Let's define few more steps.",
"_____no_output_____"
]
],
[
[
"# For this step, we use a different source_directory\nsource_directory = './compare'\nprint('Source directory for the step is {}.'.format(os.path.realpath(source_directory)))\n\n# All steps use the same Azure Machine Learning compute target as well\nstep2 = PythonScriptStep(name=\"compare_step\",\n script_name=\"compare.py\", \n compute_target=aml_compute, \n source_directory=source_directory)\n\n# Use a RunConfiguration to specify some additional requirements for this step.\nfrom azureml.core.runconfig import RunConfiguration\nfrom azureml.core.conda_dependencies import CondaDependencies\nfrom azureml.core.runconfig import DEFAULT_CPU_IMAGE\n\n# create a new runconfig object\nrun_config = RunConfiguration()\n\n# enable Docker \nrun_config.environment.docker.enabled = True\n\n# set Docker base image to the default CPU-based image\nrun_config.environment.docker.base_image = DEFAULT_CPU_IMAGE\n\n# use conda_dependencies.yml to create a conda environment in the Docker image for execution\nrun_config.environment.python.user_managed_dependencies = False\n\n# specify CondaDependencies obj\nrun_config.environment.python.conda_dependencies = CondaDependencies.create(conda_packages=['scikit-learn'])\n\n# For this step, we use yet another source_directory\nsource_directory = './extract'\nprint('Source directory for the step is {}.'.format(os.path.realpath(source_directory)))\n\nstep3 = PythonScriptStep(name=\"extract_step\",\n script_name=\"extract.py\", \n compute_target=aml_compute, \n source_directory=source_directory,\n runconfig=run_config)\n\n# list of steps to run\nsteps = [step1, step2, step3]\nprint(\"Step lists created\")",
"_____no_output_____"
]
],
[
[
"### Build the pipeline\nOnce we have the steps (or steps collection), we can build the [pipeline](https://docs.microsoft.com/en-us/python/api/azureml-pipeline-core/azureml.pipeline.core.pipeline.pipeline?view=azure-ml-py). By deafult, all these steps will run in **parallel** once we submit the pipeline for run.\n\nA pipeline is created with a list of steps and a workspace. Submit a pipeline using [submit](https://docs.microsoft.com/en-us/python/api/azureml-core/azureml.core.experiment(class)?view=azure-ml-py#submit-config--tags-none----kwargs-). When submit is called, a [PipelineRun](https://docs.microsoft.com/en-us/python/api/azureml-pipeline-core/azureml.pipeline.core.pipelinerun?view=azure-ml-py) is created which in turn creates [StepRun](https://docs.microsoft.com/en-us/python/api/azureml-pipeline-core/azureml.pipeline.core.steprun?view=azure-ml-py) objects for each step in the workflow.",
"_____no_output_____"
]
],
[
[
"# Syntax\n# Pipeline(workspace, \n# steps, \n# description=None, \n# default_datastore_name=None, \n# default_source_directory=None, \n# resolve_closure=True, \n# _workflow_provider=None, \n# _service_endpoint=None)\n\npipeline1 = Pipeline(workspace=ws, steps=steps)\nprint (\"Pipeline is built\")",
"_____no_output_____"
]
],
[
[
"### Validate the pipeline\nYou have the option to [validate](https://docs.microsoft.com/en-us/python/api/azureml-pipeline-core/azureml.pipeline.core.pipeline.pipeline?view=azure-ml-py#validate--) the pipeline prior to submitting for run. The platform runs validation steps such as checking for circular dependencies and parameter checks etc. even if you do not explicitly call validate method.",
"_____no_output_____"
]
],
[
[
"pipeline1.validate()\nprint(\"Pipeline validation complete\")",
"_____no_output_____"
]
],
[
[
"### Submit the pipeline\n[Submitting](https://docs.microsoft.com/en-us/python/api/azureml-pipeline-core/azureml.pipeline.core.pipeline.pipeline?view=azure-ml-py#submit) the pipeline involves creating an [Experiment](https://docs.microsoft.com/en-us/python/api/azureml-core/azureml.core.experiment?view=azure-ml-py) object and providing the built pipeline for submission. ",
"_____no_output_____"
]
],
[
[
"# Submit syntax\n# submit(experiment_name, \n# pipeline_parameters=None, \n# continue_on_step_failure=False, \n# regenerate_outputs=False)\n\npipeline_run1 = Experiment(ws, 'Hello_World1').submit(pipeline1, regenerate_outputs=False)\nprint(\"Pipeline is submitted for execution\")",
"_____no_output_____"
]
],
[
[
"**Note:** If regenerate_outputs is set to True, a new submit will always force generation of all step outputs, and disallow data reuse for any step of this run. Once this run is complete, however, subsequent runs may reuse the results of this run.\n",
"_____no_output_____"
],
[
"### Examine the pipeline run\n\n#### Use RunDetails Widget\nWe are going to use the RunDetails widget to examine the run of the pipeline. You can click each row below to get more details on the step runs.",
"_____no_output_____"
]
],
[
[
"RunDetails(pipeline_run1).show()",
"_____no_output_____"
]
],
[
[
"#### Use Pipeline SDK objects\nYou can cycle through the node_run objects and examine job logs, stdout, and stderr of each of the steps.",
"_____no_output_____"
]
],
[
[
"step_runs = pipeline_run1.get_children()\nfor step_run in step_runs:\n status = step_run.get_status()\n print('Script:', step_run.name, 'status:', status)\n \n # Change this if you want to see details even if the Step has succeeded.\n if status == \"Failed\":\n joblog = step_run.get_job_log()\n print('job log:', joblog)",
"_____no_output_____"
]
],
[
[
"#### Get additonal run details\nIf you wait until the pipeline_run is finished, you may be able to get additional details on the run. **Since this is a blocking call, the following code is commented out.**",
"_____no_output_____"
]
],
[
[
"#pipeline_run1.wait_for_completion()\n#for step_run in pipeline_run1.get_children():\n# print(\"{}: {}\".format(step_run.name, step_run.get_metrics()))",
"_____no_output_____"
]
],
[
[
"## Running a few steps in sequence\nNow let's see how we run a few steps in sequence. We already have three steps defined earlier. Let's *reuse* those steps for this part.\n\nWe will reuse step1, step2, step3, but build the pipeline in such a way that we chain step3 after step2 and step2 after step1. Note that there is no explicit data dependency between these steps, but still steps can be made dependent by using the [run_after](https://docs.microsoft.com/en-us/python/api/azureml-pipeline-core/azureml.pipeline.core.builder.pipelinestep?view=azure-ml-py#run-after-step-) construct.",
"_____no_output_____"
]
],
[
[
"step2.run_after(step1)\nstep3.run_after(step2)\n\n# Try a loop\n#step2.run_after(step3)\n\n# Now, construct the pipeline using the steps.\n\n# We can specify the \"final step\" in the chain, \n# Pipeline will take care of \"transitive closure\" and \n# figure out the implicit or explicit dependencies\n# https://www.geeksforgeeks.org/transitive-closure-of-a-graph/\npipeline2 = Pipeline(workspace=ws, steps=[step3])\nprint (\"Pipeline is built\")\n\npipeline2.validate()\nprint(\"Simple validation complete\")",
"_____no_output_____"
],
[
"pipeline_run2 = Experiment(ws, 'Hello_World2').submit(pipeline2)\nprint(\"Pipeline is submitted for execution\")",
"_____no_output_____"
],
[
"RunDetails(pipeline_run2).show()",
"_____no_output_____"
]
],
[
[
"# Next: Pipelines with data dependency\nThe next [notebook](https://aka.ms/pl-data-dep) demostrates how to construct a pipeline with data dependency.",
"_____no_output_____"
]
]
] | [
"markdown",
"code",
"markdown",
"code",
"markdown",
"code",
"markdown",
"code",
"markdown",
"code",
"markdown",
"code",
"markdown",
"code",
"markdown",
"code",
"markdown",
"code",
"markdown",
"code",
"markdown",
"code",
"markdown",
"code",
"markdown",
"code",
"markdown",
"code",
"markdown",
"code",
"markdown"
] | [
[
"markdown",
"markdown",
"markdown",
"markdown",
"markdown"
],
[
"code"
],
[
"markdown"
],
[
"code"
],
[
"markdown"
],
[
"code"
],
[
"markdown",
"markdown",
"markdown"
],
[
"code"
],
[
"markdown",
"markdown",
"markdown"
],
[
"code"
],
[
"markdown"
],
[
"code",
"code"
],
[
"markdown",
"markdown",
"markdown"
],
[
"code"
],
[
"markdown",
"markdown"
],
[
"code"
],
[
"markdown"
],
[
"code"
],
[
"markdown"
],
[
"code"
],
[
"markdown"
],
[
"code"
],
[
"markdown",
"markdown"
],
[
"code"
],
[
"markdown"
],
[
"code"
],
[
"markdown"
],
[
"code"
],
[
"markdown"
],
[
"code",
"code",
"code"
],
[
"markdown"
]
] |
e775cbde7d78f0476e972a64e08fff53cfd1c3d7 | 33,154 | ipynb | Jupyter Notebook | PyTorch/Visual-Audio/Torchscript/dcgan_faces_tutorial.ipynb | MitchellTesla/Quantm | 57045e0ea9ee7b965ecd26e4a8d0c1902df65245 | [
"MIT"
] | 7 | 2021-02-15T06:43:23.000Z | 2022-01-13T10:43:32.000Z | PyTorch/Visual-Audio/Torchscript/dcgan_faces_tutorial.ipynb | MitchellTesla/Quantm | 57045e0ea9ee7b965ecd26e4a8d0c1902df65245 | [
"MIT"
] | 1 | 2021-04-19T12:32:49.000Z | 2021-04-19T12:32:49.000Z | PyTorch/Visual-Audio/Torchscript/dcgan_faces_tutorial.ipynb | MitchellTesla/Quantm | 57045e0ea9ee7b965ecd26e4a8d0c1902df65245 | [
"MIT"
] | 1 | 2021-02-14T23:10:58.000Z | 2021-02-14T23:10:58.000Z | 113.931271 | 5,478 | 0.660373 | [
[
[
"%matplotlib inline",
"_____no_output_____"
]
],
[
[
"\nDCGAN Tutorial\n==============\n\n**Author**: `Nathan Inkawhich <https://github.com/inkawhich>`__\n\n\n",
"_____no_output_____"
],
[
"Introduction\n------------\n\nThis tutorial will give an introduction to DCGANs through an example. We\nwill train a generative adversarial network (GAN) to generate new\ncelebrities after showing it pictures of many real celebrities. Most of\nthe code here is from the dcgan implementation in\n`pytorch/examples <https://github.com/pytorch/examples>`__, and this\ndocument will give a thorough explanation of the implementation and shed\nlight on how and why this model works. But don’t worry, no prior\nknowledge of GANs is required, but it may require a first-timer to spend\nsome time reasoning about what is actually happening under the hood.\nAlso, for the sake of time it will help to have a GPU, or two. Lets\nstart from the beginning.\n\nGenerative Adversarial Networks\n-------------------------------\n\nWhat is a GAN?\n~~~~~~~~~~~~~~\n\nGANs are a framework for teaching a DL model to capture the training\ndata’s distribution so we can generate new data from that same\ndistribution. GANs were invented by Ian Goodfellow in 2014 and first\ndescribed in the paper `Generative Adversarial\nNets <https://papers.nips.cc/paper/5423-generative-adversarial-nets.pdf>`__.\nThey are made of two distinct models, a *generator* and a\n*discriminator*. The job of the generator is to spawn ‘fake’ images that\nlook like the training images. The job of the discriminator is to look\nat an image and output whether or not it is a real training image or a\nfake image from the generator. During training, the generator is\nconstantly trying to outsmart the discriminator by generating better and\nbetter fakes, while the discriminator is working to become a better\ndetective and correctly classify the real and fake images. The\nequilibrium of this game is when the generator is generating perfect\nfakes that look as if they came directly from the training data, and the\ndiscriminator is left to always guess at 50% confidence that the\ngenerator output is real or fake.\n\nNow, lets define some notation to be used throughout tutorial starting\nwith the discriminator. Let $x$ be data representing an image.\n$D(x)$ is the discriminator network which outputs the (scalar)\nprobability that $x$ came from training data rather than the\ngenerator. Here, since we are dealing with images the input to\n$D(x)$ is an image of CHW size 3x64x64. Intuitively, $D(x)$\nshould be HIGH when $x$ comes from training data and LOW when\n$x$ comes from the generator. $D(x)$ can also be thought of\nas a traditional binary classifier.\n\nFor the generator’s notation, let $z$ be a latent space vector\nsampled from a standard normal distribution. $G(z)$ represents the\ngenerator function which maps the latent vector $z$ to data-space.\nThe goal of $G$ is to estimate the distribution that the training\ndata comes from ($p_{data}$) so it can generate fake samples from\nthat estimated distribution ($p_g$).\n\nSo, $D(G(z))$ is the probability (scalar) that the output of the\ngenerator $G$ is a real image. As described in `Goodfellow’s\npaper <https://papers.nips.cc/paper/5423-generative-adversarial-nets.pdf>`__,\n$D$ and $G$ play a minimax game in which $D$ tries to\nmaximize the probability it correctly classifies reals and fakes\n($logD(x)$), and $G$ tries to minimize the probability that\n$D$ will predict its outputs are fake ($log(1-D(G(x)))$).\nFrom the paper, the GAN loss function is\n\n\\begin{align}\\underset{G}{\\text{min}} \\underset{D}{\\text{max}}V(D,G) = \\mathbb{E}_{x\\sim p_{data}(x)}\\big[logD(x)\\big] + \\mathbb{E}_{z\\sim p_{z}(z)}\\big[log(1-D(G(z)))\\big]\\end{align}\n\nIn theory, the solution to this minimax game is where\n$p_g = p_{data}$, and the discriminator guesses randomly if the\ninputs are real or fake. However, the convergence theory of GANs is\nstill being actively researched and in reality models do not always\ntrain to this point.\n\nWhat is a DCGAN?\n~~~~~~~~~~~~~~~~\n\nA DCGAN is a direct extension of the GAN described above, except that it\nexplicitly uses convolutional and convolutional-transpose layers in the\ndiscriminator and generator, respectively. It was first described by\nRadford et. al. in the paper `Unsupervised Representation Learning With\nDeep Convolutional Generative Adversarial\nNetworks <https://arxiv.org/pdf/1511.06434.pdf>`__. The discriminator\nis made up of strided\n`convolution <https://pytorch.org/docs/stable/nn.html#torch.nn.Conv2d>`__\nlayers, `batch\nnorm <https://pytorch.org/docs/stable/nn.html#torch.nn.BatchNorm2d>`__\nlayers, and\n`LeakyReLU <https://pytorch.org/docs/stable/nn.html#torch.nn.LeakyReLU>`__\nactivations. The input is a 3x64x64 input image and the output is a\nscalar probability that the input is from the real data distribution.\nThe generator is comprised of\n`convolutional-transpose <https://pytorch.org/docs/stable/nn.html#torch.nn.ConvTranspose2d>`__\nlayers, batch norm layers, and\n`ReLU <https://pytorch.org/docs/stable/nn.html#relu>`__ activations. The\ninput is a latent vector, $z$, that is drawn from a standard\nnormal distribution and the output is a 3x64x64 RGB image. The strided\nconv-transpose layers allow the latent vector to be transformed into a\nvolume with the same shape as an image. In the paper, the authors also\ngive some tips about how to setup the optimizers, how to calculate the\nloss functions, and how to initialize the model weights, all of which\nwill be explained in the coming sections.\n\n\n",
"_____no_output_____"
]
],
[
[
"from __future__ import print_function\n#%matplotlib inline\nimport argparse\nimport os\nimport random\nimport torch\nimport torch.nn as nn\nimport torch.nn.parallel\nimport torch.backends.cudnn as cudnn\nimport torch.optim as optim\nimport torch.utils.data\nimport torchvision.datasets as dset\nimport torchvision.transforms as transforms\nimport torchvision.utils as vutils\nimport numpy as np\nimport matplotlib.pyplot as plt\nimport matplotlib.animation as animation\nfrom IPython.display import HTML\n\n# Set random seed for reproducibility\nmanualSeed = 999\n#manualSeed = random.randint(1, 10000) # use if you want new results\nprint(\"Random Seed: \", manualSeed)\nrandom.seed(manualSeed)\ntorch.manual_seed(manualSeed)",
"_____no_output_____"
]
],
[
[
"Inputs\n------\n\nLet’s define some inputs for the run:\n\n- **dataroot** - the path to the root of the dataset folder. We will\n talk more about the dataset in the next section\n- **workers** - the number of worker threads for loading the data with\n the DataLoader\n- **batch_size** - the batch size used in training. The DCGAN paper\n uses a batch size of 128\n- **image_size** - the spatial size of the images used for training.\n This implementation defaults to 64x64. If another size is desired,\n the structures of D and G must be changed. See\n `here <https://github.com/pytorch/examples/issues/70>`__ for more\n details\n- **nc** - number of color channels in the input images. For color\n images this is 3\n- **nz** - length of latent vector\n- **ngf** - relates to the depth of feature maps carried through the\n generator\n- **ndf** - sets the depth of feature maps propagated through the\n discriminator\n- **num_epochs** - number of training epochs to run. Training for\n longer will probably lead to better results but will also take much\n longer\n- **lr** - learning rate for training. As described in the DCGAN paper,\n this number should be 0.0002\n- **beta1** - beta1 hyperparameter for Adam optimizers. As described in\n paper, this number should be 0.5\n- **ngpu** - number of GPUs available. If this is 0, code will run in\n CPU mode. If this number is greater than 0 it will run on that number\n of GPUs\n\n\n",
"_____no_output_____"
]
],
[
[
"# Root directory for dataset\ndataroot = \"data/celeba\"\n\n# Number of workers for dataloader\nworkers = 2\n\n# Batch size during training\nbatch_size = 128\n\n# Spatial size of training images. All images will be resized to this\n# size using a transformer.\nimage_size = 64\n\n# Number of channels in the training images. For color images this is 3\nnc = 3\n\n# Size of z latent vector (i.e. size of generator input)\nnz = 100\n\n# Size of feature maps in generator\nngf = 64\n\n# Size of feature maps in discriminator\nndf = 64\n\n# Number of training epochs\nnum_epochs = 5\n\n# Learning rate for optimizers\nlr = 0.0002\n\n# Beta1 hyperparam for Adam optimizers\nbeta1 = 0.5\n\n# Number of GPUs available. Use 0 for CPU mode.\nngpu = 1",
"_____no_output_____"
]
],
[
[
"Data\n----\n\nIn this tutorial we will use the `Celeb-A Faces\ndataset <http://mmlab.ie.cuhk.edu.hk/projects/CelebA.html>`__ which can\nbe downloaded at the linked site, or in `Google\nDrive <https://drive.google.com/drive/folders/0B7EVK8r0v71pTUZsaXdaSnZBZzg>`__.\nThe dataset will download as a file named *img_align_celeba.zip*. Once\ndownloaded, create a directory named *celeba* and extract the zip file\ninto that directory. Then, set the *dataroot* input for this notebook to\nthe *celeba* directory you just created. The resulting directory\nstructure should be:\n\n::\n\n /path/to/celeba\n -> img_align_celeba \n -> 188242.jpg\n -> 173822.jpg\n -> 284702.jpg\n -> 537394.jpg\n ...\n\nThis is an important step because we will be using the ImageFolder\ndataset class, which requires there to be subdirectories in the\ndataset’s root folder. Now, we can create the dataset, create the\ndataloader, set the device to run on, and finally visualize some of the\ntraining data.\n\n\n",
"_____no_output_____"
]
],
[
[
"# We can use an image folder dataset the way we have it setup.\n# Create the dataset\ndataset = dset.ImageFolder(root=dataroot,\n transform=transforms.Compose([\n transforms.Resize(image_size),\n transforms.CenterCrop(image_size),\n transforms.ToTensor(),\n transforms.Normalize((0.5, 0.5, 0.5), (0.5, 0.5, 0.5)),\n ]))\n# Create the dataloader\ndataloader = torch.utils.data.DataLoader(dataset, batch_size=batch_size,\n shuffle=True, num_workers=workers)\n\n# Decide which device we want to run on\ndevice = torch.device(\"cuda:0\" if (torch.cuda.is_available() and ngpu > 0) else \"cpu\")\n\n# Plot some training images\nreal_batch = next(iter(dataloader))\nplt.figure(figsize=(8,8))\nplt.axis(\"off\")\nplt.title(\"Training Images\")\nplt.imshow(np.transpose(vutils.make_grid(real_batch[0].to(device)[:64], padding=2, normalize=True).cpu(),(1,2,0)))",
"_____no_output_____"
]
],
[
[
"Implementation\n--------------\n\nWith our input parameters set and the dataset prepared, we can now get\ninto the implementation. We will start with the weigth initialization\nstrategy, then talk about the generator, discriminator, loss functions,\nand training loop in detail.\n\nWeight Initialization\n~~~~~~~~~~~~~~~~~~~~~\n\nFrom the DCGAN paper, the authors specify that all model weights shall\nbe randomly initialized from a Normal distribution with mean=0,\nstdev=0.02. The ``weights_init`` function takes an initialized model as\ninput and reinitializes all convolutional, convolutional-transpose, and\nbatch normalization layers to meet this criteria. This function is\napplied to the models immediately after initialization.\n\n\n",
"_____no_output_____"
]
],
[
[
"# custom weights initialization called on netG and netD\ndef weights_init(m):\n classname = m.__class__.__name__\n if classname.find('Conv') != -1:\n nn.init.normal_(m.weight.data, 0.0, 0.02)\n elif classname.find('BatchNorm') != -1:\n nn.init.normal_(m.weight.data, 1.0, 0.02)\n nn.init.constant_(m.bias.data, 0)",
"_____no_output_____"
]
],
[
[
"Generator\n~~~~~~~~~\n\nThe generator, $G$, is designed to map the latent space vector\n($z$) to data-space. Since our data are images, converting\n$z$ to data-space means ultimately creating a RGB image with the\nsame size as the training images (i.e. 3x64x64). In practice, this is\naccomplished through a series of strided two dimensional convolutional\ntranspose layers, each paired with a 2d batch norm layer and a relu\nactivation. The output of the generator is fed through a tanh function\nto return it to the input data range of $[-1,1]$. It is worth\nnoting the existence of the batch norm functions after the\nconv-transpose layers, as this is a critical contribution of the DCGAN\npaper. These layers help with the flow of gradients during training. An\nimage of the generator from the DCGAN paper is shown below.\n\n.. figure:: /_static/img/dcgan_generator.png\n :alt: dcgan_generator\n\nNotice, the how the inputs we set in the input section (*nz*, *ngf*, and\n*nc*) influence the generator architecture in code. *nz* is the length\nof the z input vector, *ngf* relates to the size of the feature maps\nthat are propagated through the generator, and *nc* is the number of\nchannels in the output image (set to 3 for RGB images). Below is the\ncode for the generator.\n\n\n",
"_____no_output_____"
]
],
[
[
"# Generator Code\n\nclass Generator(nn.Module):\n def __init__(self, ngpu):\n super(Generator, self).__init__()\n self.ngpu = ngpu\n self.main = nn.Sequential(\n # input is Z, going into a convolution\n nn.ConvTranspose2d( nz, ngf * 8, 4, 1, 0, bias=False),\n nn.BatchNorm2d(ngf * 8),\n nn.ReLU(True),\n # state size. (ngf*8) x 4 x 4\n nn.ConvTranspose2d(ngf * 8, ngf * 4, 4, 2, 1, bias=False),\n nn.BatchNorm2d(ngf * 4),\n nn.ReLU(True),\n # state size. (ngf*4) x 8 x 8\n nn.ConvTranspose2d( ngf * 4, ngf * 2, 4, 2, 1, bias=False),\n nn.BatchNorm2d(ngf * 2),\n nn.ReLU(True),\n # state size. (ngf*2) x 16 x 16\n nn.ConvTranspose2d( ngf * 2, ngf, 4, 2, 1, bias=False),\n nn.BatchNorm2d(ngf),\n nn.ReLU(True),\n # state size. (ngf) x 32 x 32\n nn.ConvTranspose2d( ngf, nc, 4, 2, 1, bias=False),\n nn.Tanh()\n # state size. (nc) x 64 x 64\n )\n\n def forward(self, input):\n return self.main(input)",
"_____no_output_____"
]
],
[
[
"Now, we can instantiate the generator and apply the ``weights_init``\nfunction. Check out the printed model to see how the generator object is\nstructured.\n\n\n",
"_____no_output_____"
]
],
[
[
"# Create the generator\nnetG = Generator(ngpu).to(device)\n\n# Handle multi-gpu if desired\nif (device.type == 'cuda') and (ngpu > 1):\n netG = nn.DataParallel(netG, list(range(ngpu)))\n\n# Apply the weights_init function to randomly initialize all weights\n# to mean=0, stdev=0.2.\nnetG.apply(weights_init)\n\n# Print the model\nprint(netG)",
"_____no_output_____"
]
],
[
[
"Discriminator\n~~~~~~~~~~~~~\n\nAs mentioned, the discriminator, $D$, is a binary classification\nnetwork that takes an image as input and outputs a scalar probability\nthat the input image is real (as opposed to fake). Here, $D$ takes\na 3x64x64 input image, processes it through a series of Conv2d,\nBatchNorm2d, and LeakyReLU layers, and outputs the final probability\nthrough a Sigmoid activation function. This architecture can be extended\nwith more layers if necessary for the problem, but there is significance\nto the use of the strided convolution, BatchNorm, and LeakyReLUs. The\nDCGAN paper mentions it is a good practice to use strided convolution\nrather than pooling to downsample because it lets the network learn its\nown pooling function. Also batch norm and leaky relu functions promote\nhealthy gradient flow which is critical for the learning process of both\n$G$ and $D$.\n\n\n",
"_____no_output_____"
],
[
"Discriminator Code\n\n",
"_____no_output_____"
]
],
[
[
"class Discriminator(nn.Module):\n def __init__(self, ngpu):\n super(Discriminator, self).__init__()\n self.ngpu = ngpu\n self.main = nn.Sequential(\n # input is (nc) x 64 x 64\n nn.Conv2d(nc, ndf, 4, 2, 1, bias=False),\n nn.LeakyReLU(0.2, inplace=True),\n # state size. (ndf) x 32 x 32\n nn.Conv2d(ndf, ndf * 2, 4, 2, 1, bias=False),\n nn.BatchNorm2d(ndf * 2),\n nn.LeakyReLU(0.2, inplace=True),\n # state size. (ndf*2) x 16 x 16\n nn.Conv2d(ndf * 2, ndf * 4, 4, 2, 1, bias=False),\n nn.BatchNorm2d(ndf * 4),\n nn.LeakyReLU(0.2, inplace=True),\n # state size. (ndf*4) x 8 x 8\n nn.Conv2d(ndf * 4, ndf * 8, 4, 2, 1, bias=False),\n nn.BatchNorm2d(ndf * 8),\n nn.LeakyReLU(0.2, inplace=True),\n # state size. (ndf*8) x 4 x 4\n nn.Conv2d(ndf * 8, 1, 4, 1, 0, bias=False),\n nn.Sigmoid()\n )\n\n def forward(self, input):\n return self.main(input)",
"_____no_output_____"
]
],
[
[
"Now, as with the generator, we can create the discriminator, apply the\n``weights_init`` function, and print the model’s structure.\n\n\n",
"_____no_output_____"
]
],
[
[
"# Create the Discriminator\nnetD = Discriminator(ngpu).to(device)\n\n# Handle multi-gpu if desired\nif (device.type == 'cuda') and (ngpu > 1):\n netD = nn.DataParallel(netD, list(range(ngpu)))\n \n# Apply the weights_init function to randomly initialize all weights\n# to mean=0, stdev=0.2.\nnetD.apply(weights_init)\n\n# Print the model\nprint(netD)",
"_____no_output_____"
]
],
[
[
"Loss Functions and Optimizers\n~~~~~~~~~~~~~~~~~~~~~~~~~~~~~\n\nWith $D$ and $G$ setup, we can specify how they learn\nthrough the loss functions and optimizers. We will use the Binary Cross\nEntropy loss\n(`BCELoss <https://pytorch.org/docs/stable/nn.html#torch.nn.BCELoss>`__)\nfunction which is defined in PyTorch as:\n\n\\begin{align}\\ell(x, y) = L = \\{l_1,\\dots,l_N\\}^\\top, \\quad l_n = - \\left[ y_n \\cdot \\log x_n + (1 - y_n) \\cdot \\log (1 - x_n) \\right]\\end{align}\n\nNotice how this function provides the calculation of both log components\nin the objective function (i.e. $log(D(x))$ and\n$log(1-D(G(z)))$). We can specify what part of the BCE equation to\nuse with the $y$ input. This is accomplished in the training loop\nwhich is coming up soon, but it is important to understand how we can\nchoose which component we wish to calculate just by changing $y$\n(i.e. GT labels).\n\nNext, we define our real label as 1 and the fake label as 0. These\nlabels will be used when calculating the losses of $D$ and\n$G$, and this is also the convention used in the original GAN\npaper. Finally, we set up two separate optimizers, one for $D$ and\none for $G$. As specified in the DCGAN paper, both are Adam\noptimizers with learning rate 0.0002 and Beta1 = 0.5. For keeping track\nof the generator’s learning progression, we will generate a fixed batch\nof latent vectors that are drawn from a Gaussian distribution\n(i.e. fixed_noise) . In the training loop, we will periodically input\nthis fixed_noise into $G$, and over the iterations we will see\nimages form out of the noise.\n\n\n",
"_____no_output_____"
]
],
[
[
"# Initialize BCELoss function\ncriterion = nn.BCELoss()\n\n# Create batch of latent vectors that we will use to visualize\n# the progression of the generator\nfixed_noise = torch.randn(64, nz, 1, 1, device=device)\n\n# Establish convention for real and fake labels during training\nreal_label = 1.\nfake_label = 0.\n\n# Setup Adam optimizers for both G and D\noptimizerD = optim.Adam(netD.parameters(), lr=lr, betas=(beta1, 0.999))\noptimizerG = optim.Adam(netG.parameters(), lr=lr, betas=(beta1, 0.999))",
"_____no_output_____"
]
],
[
[
"Training\n~~~~~~~~\n\nFinally, now that we have all of the parts of the GAN framework defined,\nwe can train it. Be mindful that training GANs is somewhat of an art\nform, as incorrect hyperparameter settings lead to mode collapse with\nlittle explanation of what went wrong. Here, we will closely follow\nAlgorithm 1 from Goodfellow’s paper, while abiding by some of the best\npractices shown in `ganhacks <https://github.com/soumith/ganhacks>`__.\nNamely, we will “construct different mini-batches for real and fake”\nimages, and also adjust G’s objective function to maximize\n$logD(G(z))$. Training is split up into two main parts. Part 1\nupdates the Discriminator and Part 2 updates the Generator.\n\n**Part 1 - Train the Discriminator**\n\nRecall, the goal of training the discriminator is to maximize the\nprobability of correctly classifying a given input as real or fake. In\nterms of Goodfellow, we wish to “update the discriminator by ascending\nits stochastic gradient”. Practically, we want to maximize\n$log(D(x)) + log(1-D(G(z)))$. Due to the separate mini-batch\nsuggestion from ganhacks, we will calculate this in two steps. First, we\nwill construct a batch of real samples from the training set, forward\npass through $D$, calculate the loss ($log(D(x))$), then\ncalculate the gradients in a backward pass. Secondly, we will construct\na batch of fake samples with the current generator, forward pass this\nbatch through $D$, calculate the loss ($log(1-D(G(z)))$),\nand *accumulate* the gradients with a backward pass. Now, with the\ngradients accumulated from both the all-real and all-fake batches, we\ncall a step of the Discriminator’s optimizer.\n\n**Part 2 - Train the Generator**\n\nAs stated in the original paper, we want to train the Generator by\nminimizing $log(1-D(G(z)))$ in an effort to generate better fakes.\nAs mentioned, this was shown by Goodfellow to not provide sufficient\ngradients, especially early in the learning process. As a fix, we\ninstead wish to maximize $log(D(G(z)))$. In the code we accomplish\nthis by: classifying the Generator output from Part 1 with the\nDiscriminator, computing G’s loss *using real labels as GT*, computing\nG’s gradients in a backward pass, and finally updating G’s parameters\nwith an optimizer step. It may seem counter-intuitive to use the real\nlabels as GT labels for the loss function, but this allows us to use the\n$log(x)$ part of the BCELoss (rather than the $log(1-x)$\npart) which is exactly what we want.\n\nFinally, we will do some statistic reporting and at the end of each\nepoch we will push our fixed_noise batch through the generator to\nvisually track the progress of G’s training. The training statistics\nreported are:\n\n- **Loss_D** - discriminator loss calculated as the sum of losses for\n the all real and all fake batches ($log(D(x)) + log(D(G(z)))$).\n- **Loss_G** - generator loss calculated as $log(D(G(z)))$\n- **D(x)** - the average output (across the batch) of the discriminator\n for the all real batch. This should start close to 1 then\n theoretically converge to 0.5 when G gets better. Think about why\n this is.\n- **D(G(z))** - average discriminator outputs for the all fake batch.\n The first number is before D is updated and the second number is\n after D is updated. These numbers should start near 0 and converge to\n 0.5 as G gets better. Think about why this is.\n\n**Note:** This step might take a while, depending on how many epochs you\nrun and if you removed some data from the dataset.\n\n\n",
"_____no_output_____"
]
],
[
[
"# Training Loop\n\n# Lists to keep track of progress\nimg_list = []\nG_losses = []\nD_losses = []\niters = 0\n\nprint(\"Starting Training Loop...\")\n# For each epoch\nfor epoch in range(num_epochs):\n # For each batch in the dataloader\n for i, data in enumerate(dataloader, 0):\n \n ############################\n # (1) Update D network: maximize log(D(x)) + log(1 - D(G(z)))\n ###########################\n ## Train with all-real batch\n netD.zero_grad()\n # Format batch\n real_cpu = data[0].to(device)\n b_size = real_cpu.size(0)\n label = torch.full((b_size,), real_label, dtype=torch.float, device=device)\n # Forward pass real batch through D\n output = netD(real_cpu).view(-1)\n # Calculate loss on all-real batch\n errD_real = criterion(output, label)\n # Calculate gradients for D in backward pass\n errD_real.backward()\n D_x = output.mean().item()\n\n ## Train with all-fake batch\n # Generate batch of latent vectors\n noise = torch.randn(b_size, nz, 1, 1, device=device)\n # Generate fake image batch with G\n fake = netG(noise)\n label.fill_(fake_label)\n # Classify all fake batch with D\n output = netD(fake.detach()).view(-1)\n # Calculate D's loss on the all-fake batch\n errD_fake = criterion(output, label)\n # Calculate the gradients for this batch\n errD_fake.backward()\n D_G_z1 = output.mean().item()\n # Add the gradients from the all-real and all-fake batches\n errD = errD_real + errD_fake\n # Update D\n optimizerD.step()\n\n ############################\n # (2) Update G network: maximize log(D(G(z)))\n ###########################\n netG.zero_grad()\n label.fill_(real_label) # fake labels are real for generator cost\n # Since we just updated D, perform another forward pass of all-fake batch through D\n output = netD(fake).view(-1)\n # Calculate G's loss based on this output\n errG = criterion(output, label)\n # Calculate gradients for G\n errG.backward()\n D_G_z2 = output.mean().item()\n # Update G\n optimizerG.step()\n \n # Output training stats\n if i % 50 == 0:\n print('[%d/%d][%d/%d]\\tLoss_D: %.4f\\tLoss_G: %.4f\\tD(x): %.4f\\tD(G(z)): %.4f / %.4f'\n % (epoch, num_epochs, i, len(dataloader),\n errD.item(), errG.item(), D_x, D_G_z1, D_G_z2))\n \n # Save Losses for plotting later\n G_losses.append(errG.item())\n D_losses.append(errD.item())\n \n # Check how the generator is doing by saving G's output on fixed_noise\n if (iters % 500 == 0) or ((epoch == num_epochs-1) and (i == len(dataloader)-1)):\n with torch.no_grad():\n fake = netG(fixed_noise).detach().cpu()\n img_list.append(vutils.make_grid(fake, padding=2, normalize=True))\n \n iters += 1",
"_____no_output_____"
]
],
[
[
"Results\n-------\n\nFinally, lets check out how we did. Here, we will look at three\ndifferent results. First, we will see how D and G’s losses changed\nduring training. Second, we will visualize G’s output on the fixed_noise\nbatch for every epoch. And third, we will look at a batch of real data\nnext to a batch of fake data from G.\n\n**Loss versus training iteration**\n\nBelow is a plot of D & G’s losses versus training iterations.\n\n\n",
"_____no_output_____"
]
],
[
[
"plt.figure(figsize=(10,5))\nplt.title(\"Generator and Discriminator Loss During Training\")\nplt.plot(G_losses,label=\"G\")\nplt.plot(D_losses,label=\"D\")\nplt.xlabel(\"iterations\")\nplt.ylabel(\"Loss\")\nplt.legend()\nplt.show()",
"_____no_output_____"
]
],
[
[
"**Visualization of G’s progression**\n\nRemember how we saved the generator’s output on the fixed_noise batch\nafter every epoch of training. Now, we can visualize the training\nprogression of G with an animation. Press the play button to start the\nanimation.\n\n\n",
"_____no_output_____"
]
],
[
[
"#%%capture\nfig = plt.figure(figsize=(8,8))\nplt.axis(\"off\")\nims = [[plt.imshow(np.transpose(i,(1,2,0)), animated=True)] for i in img_list]\nani = animation.ArtistAnimation(fig, ims, interval=1000, repeat_delay=1000, blit=True)\n\nHTML(ani.to_jshtml())",
"_____no_output_____"
]
],
[
[
"**Real Images vs. Fake Images**\n\nFinally, lets take a look at some real images and fake images side by\nside.\n\n\n",
"_____no_output_____"
]
],
[
[
"# Grab a batch of real images from the dataloader\nreal_batch = next(iter(dataloader))\n\n# Plot the real images\nplt.figure(figsize=(15,15))\nplt.subplot(1,2,1)\nplt.axis(\"off\")\nplt.title(\"Real Images\")\nplt.imshow(np.transpose(vutils.make_grid(real_batch[0].to(device)[:64], padding=5, normalize=True).cpu(),(1,2,0)))\n\n# Plot the fake images from the last epoch\nplt.subplot(1,2,2)\nplt.axis(\"off\")\nplt.title(\"Fake Images\")\nplt.imshow(np.transpose(img_list[-1],(1,2,0)))\nplt.show()",
"_____no_output_____"
]
],
[
[
"Where to Go Next\n----------------\n\nWe have reached the end of our journey, but there are several places you\ncould go from here. You could:\n\n- Train for longer to see how good the results get\n- Modify this model to take a different dataset and possibly change the\n size of the images and the model architecture\n- Check out some other cool GAN projects\n `here <https://github.com/nashory/gans-awesome-applications>`__\n- Create GANs that generate\n `music <https://deepmind.com/blog/wavenet-generative-model-raw-audio/>`__\n\n\n",
"_____no_output_____"
]
]
] | [
"code",
"markdown",
"code",
"markdown",
"code",
"markdown",
"code",
"markdown",
"code",
"markdown",
"code",
"markdown",
"code",
"markdown",
"code",
"markdown",
"code",
"markdown",
"code",
"markdown",
"code",
"markdown",
"code",
"markdown",
"code",
"markdown",
"code",
"markdown"
] | [
[
"code"
],
[
"markdown",
"markdown"
],
[
"code"
],
[
"markdown"
],
[
"code"
],
[
"markdown"
],
[
"code"
],
[
"markdown"
],
[
"code"
],
[
"markdown"
],
[
"code"
],
[
"markdown"
],
[
"code"
],
[
"markdown",
"markdown"
],
[
"code"
],
[
"markdown"
],
[
"code"
],
[
"markdown"
],
[
"code"
],
[
"markdown"
],
[
"code"
],
[
"markdown"
],
[
"code"
],
[
"markdown"
],
[
"code"
],
[
"markdown"
],
[
"code"
],
[
"markdown"
]
] |
e775dc194d2ffc5fb389f1ff8ae9efd68cfb5986 | 41,347 | ipynb | Jupyter Notebook | YOLOv3RBC.ipynb | worldstar/keras-yolo3 | 4ae4908e84b988e732d6fea75f3df1c231922392 | [
"MIT"
] | null | null | null | YOLOv3RBC.ipynb | worldstar/keras-yolo3 | 4ae4908e84b988e732d6fea75f3df1c231922392 | [
"MIT"
] | null | null | null | YOLOv3RBC.ipynb | worldstar/keras-yolo3 | 4ae4908e84b988e732d6fea75f3df1c231922392 | [
"MIT"
] | 4 | 2021-02-25T08:21:15.000Z | 2021-02-25T08:56:39.000Z | 75.727106 | 2,476 | 0.563281 | [
[
[
"!pip install tensorflow-gpu==1.15.3",
"Collecting tensorflow-gpu==1.15.3\n\u001b[?25l Downloading https://files.pythonhosted.org/packages/32/d9/f977cb032856d7f65cc42541da1ff12dafc4ff35fddb819836cf5c4cab60/tensorflow_gpu-1.15.3-cp37-cp37m-manylinux2010_x86_64.whl (411.0MB)\n\u001b[K |████████████████████████████████| 411.0MB 43kB/s \n\u001b[?25hRequirement already satisfied: astor>=0.6.0 in /usr/local/lib/python3.7/dist-packages (from tensorflow-gpu==1.15.3) (0.8.1)\nCollecting tensorboard<1.16.0,>=1.15.0\n\u001b[?25l Downloading https://files.pythonhosted.org/packages/1e/e9/d3d747a97f7188f48aa5eda486907f3b345cd409f0a0850468ba867db246/tensorboard-1.15.0-py3-none-any.whl (3.8MB)\n\u001b[K |████████████████████████████████| 3.8MB 44.6MB/s \n\u001b[?25hRequirement already satisfied: google-pasta>=0.1.6 in /usr/local/lib/python3.7/dist-packages (from tensorflow-gpu==1.15.3) (0.2.0)\nRequirement already satisfied: absl-py>=0.7.0 in /usr/local/lib/python3.7/dist-packages (from tensorflow-gpu==1.15.3) (0.10.0)\nRequirement already satisfied: keras-preprocessing>=1.0.5 in /usr/local/lib/python3.7/dist-packages (from tensorflow-gpu==1.15.3) (1.1.2)\nCollecting tensorflow-estimator==1.15.1\n\u001b[?25l Downloading https://files.pythonhosted.org/packages/de/62/2ee9cd74c9fa2fa450877847ba560b260f5d0fb70ee0595203082dafcc9d/tensorflow_estimator-1.15.1-py2.py3-none-any.whl (503kB)\n\u001b[K |████████████████████████████████| 512kB 52.7MB/s \n\u001b[?25hRequirement already satisfied: six>=1.10.0 in /usr/local/lib/python3.7/dist-packages (from tensorflow-gpu==1.15.3) (1.15.0)\nRequirement already satisfied: protobuf>=3.6.1 in /usr/local/lib/python3.7/dist-packages (from tensorflow-gpu==1.15.3) (3.12.4)\nRequirement already satisfied: numpy<2.0,>=1.16.0 in /usr/local/lib/python3.7/dist-packages (from tensorflow-gpu==1.15.3) (1.19.5)\nCollecting keras-applications>=1.0.8\n\u001b[?25l Downloading https://files.pythonhosted.org/packages/71/e3/19762fdfc62877ae9102edf6342d71b28fbfd9dea3d2f96a882ce099b03f/Keras_Applications-1.0.8-py3-none-any.whl (50kB)\n\u001b[K |████████████████████████████████| 51kB 9.5MB/s \n\u001b[?25hRequirement already satisfied: grpcio>=1.8.6 in /usr/local/lib/python3.7/dist-packages (from tensorflow-gpu==1.15.3) (1.32.0)\nRequirement already satisfied: wrapt>=1.11.1 in /usr/local/lib/python3.7/dist-packages (from tensorflow-gpu==1.15.3) (1.12.1)\nRequirement already satisfied: opt-einsum>=2.3.2 in /usr/local/lib/python3.7/dist-packages (from tensorflow-gpu==1.15.3) (3.3.0)\nRequirement already satisfied: wheel>=0.26; python_version >= \"3\" in /usr/local/lib/python3.7/dist-packages (from tensorflow-gpu==1.15.3) (0.36.2)\nCollecting gast==0.2.2\n Downloading https://files.pythonhosted.org/packages/4e/35/11749bf99b2d4e3cceb4d55ca22590b0d7c2c62b9de38ac4a4a7f4687421/gast-0.2.2.tar.gz\nRequirement already satisfied: termcolor>=1.1.0 in /usr/local/lib/python3.7/dist-packages (from tensorflow-gpu==1.15.3) (1.1.0)\nRequirement already satisfied: werkzeug>=0.11.15 in /usr/local/lib/python3.7/dist-packages (from tensorboard<1.16.0,>=1.15.0->tensorflow-gpu==1.15.3) (1.0.1)\nRequirement already satisfied: markdown>=2.6.8 in /usr/local/lib/python3.7/dist-packages (from tensorboard<1.16.0,>=1.15.0->tensorflow-gpu==1.15.3) (3.3.3)\nRequirement already satisfied: setuptools>=41.0.0 in /usr/local/lib/python3.7/dist-packages (from tensorboard<1.16.0,>=1.15.0->tensorflow-gpu==1.15.3) (53.0.0)\nRequirement already satisfied: h5py in /usr/local/lib/python3.7/dist-packages (from keras-applications>=1.0.8->tensorflow-gpu==1.15.3) (2.10.0)\nRequirement already satisfied: importlib-metadata; python_version < \"3.8\" in /usr/local/lib/python3.7/dist-packages (from markdown>=2.6.8->tensorboard<1.16.0,>=1.15.0->tensorflow-gpu==1.15.3) (3.4.0)\nRequirement already satisfied: zipp>=0.5 in /usr/local/lib/python3.7/dist-packages (from importlib-metadata; python_version < \"3.8\"->markdown>=2.6.8->tensorboard<1.16.0,>=1.15.0->tensorflow-gpu==1.15.3) (3.4.0)\nRequirement already satisfied: typing-extensions>=3.6.4; python_version < \"3.8\" in /usr/local/lib/python3.7/dist-packages (from importlib-metadata; python_version < \"3.8\"->markdown>=2.6.8->tensorboard<1.16.0,>=1.15.0->tensorflow-gpu==1.15.3) (3.7.4.3)\nBuilding wheels for collected packages: gast\n Building wheel for gast (setup.py) ... \u001b[?25l\u001b[?25hdone\n Created wheel for gast: filename=gast-0.2.2-cp37-none-any.whl size=7540 sha256=4f77183c26d45864469072876cb7997af7c3d564abebfcb08b153eb5eecf2359\n Stored in directory: /root/.cache/pip/wheels/5c/2e/7e/a1d4d4fcebe6c381f378ce7743a3ced3699feb89bcfbdadadd\nSuccessfully built gast\n\u001b[31mERROR: tensorflow 2.4.1 has requirement gast==0.3.3, but you'll have gast 0.2.2 which is incompatible.\u001b[0m\n\u001b[31mERROR: tensorflow 2.4.1 has requirement tensorboard~=2.4, but you'll have tensorboard 1.15.0 which is incompatible.\u001b[0m\n\u001b[31mERROR: tensorflow 2.4.1 has requirement tensorflow-estimator<2.5.0,>=2.4.0, but you'll have tensorflow-estimator 1.15.1 which is incompatible.\u001b[0m\n\u001b[31mERROR: tensorflow-probability 0.12.1 has requirement gast>=0.3.2, but you'll have gast 0.2.2 which is incompatible.\u001b[0m\nInstalling collected packages: tensorboard, tensorflow-estimator, keras-applications, gast, tensorflow-gpu\n Found existing installation: tensorboard 2.4.1\n Uninstalling tensorboard-2.4.1:\n Successfully uninstalled tensorboard-2.4.1\n Found existing installation: tensorflow-estimator 2.4.0\n Uninstalling tensorflow-estimator-2.4.0:\n Successfully uninstalled tensorflow-estimator-2.4.0\n Found existing installation: gast 0.3.3\n Uninstalling gast-0.3.3:\n Successfully uninstalled gast-0.3.3\nSuccessfully installed gast-0.2.2 keras-applications-1.0.8 tensorboard-1.15.0 tensorflow-estimator-1.15.1 tensorflow-gpu-1.15.3\n"
],
[
"!pip install Keras==2.1.5",
"Collecting Keras==2.1.5\n\u001b[?25l Downloading https://files.pythonhosted.org/packages/ba/65/e4aff762b8696ec0626a6654b1e73b396fcc8b7cc6b98d78a1bc53b85b48/Keras-2.1.5-py2.py3-none-any.whl (334kB)\n\r\u001b[K |█ | 10kB 20.7MB/s eta 0:00:01\r\u001b[K |██ | 20kB 14.1MB/s eta 0:00:01\r\u001b[K |███ | 30kB 12.9MB/s eta 0:00:01\r\u001b[K |████ | 40kB 12.0MB/s eta 0:00:01\r\u001b[K |█████ | 51kB 7.5MB/s eta 0:00:01\r\u001b[K |█████▉ | 61kB 7.9MB/s eta 0:00:01\r\u001b[K |██████▉ | 71kB 8.9MB/s eta 0:00:01\r\u001b[K |███████▉ | 81kB 9.2MB/s eta 0:00:01\r\u001b[K |████████▉ | 92kB 9.1MB/s eta 0:00:01\r\u001b[K |█████████▉ | 102kB 8.4MB/s eta 0:00:01\r\u001b[K |██████████▊ | 112kB 8.4MB/s eta 0:00:01\r\u001b[K |███████████▊ | 122kB 8.4MB/s eta 0:00:01\r\u001b[K |████████████▊ | 133kB 8.4MB/s eta 0:00:01\r\u001b[K |█████████████▊ | 143kB 8.4MB/s eta 0:00:01\r\u001b[K |██████████████▊ | 153kB 8.4MB/s eta 0:00:01\r\u001b[K |███████████████▋ | 163kB 8.4MB/s eta 0:00:01\r\u001b[K |████████████████▋ | 174kB 8.4MB/s eta 0:00:01\r\u001b[K |█████████████████▋ | 184kB 8.4MB/s eta 0:00:01\r\u001b[K |██████████████████▋ | 194kB 8.4MB/s eta 0:00:01\r\u001b[K |███████████████████▋ | 204kB 8.4MB/s eta 0:00:01\r\u001b[K |████████████████████▌ | 215kB 8.4MB/s eta 0:00:01\r\u001b[K |█████████████████████▌ | 225kB 8.4MB/s eta 0:00:01\r\u001b[K |██████████████████████▌ | 235kB 8.4MB/s eta 0:00:01\r\u001b[K |███████████████████████▌ | 245kB 8.4MB/s eta 0:00:01\r\u001b[K |████████████████████████▌ | 256kB 8.4MB/s eta 0:00:01\r\u001b[K |█████████████████████████▍ | 266kB 8.4MB/s eta 0:00:01\r\u001b[K |██████████████████████████▍ | 276kB 8.4MB/s eta 0:00:01\r\u001b[K |███████████████████████████▍ | 286kB 8.4MB/s eta 0:00:01\r\u001b[K |████████████████████████████▍ | 296kB 8.4MB/s eta 0:00:01\r\u001b[K |█████████████████████████████▍ | 307kB 8.4MB/s eta 0:00:01\r\u001b[K |██████████████████████████████▎ | 317kB 8.4MB/s eta 0:00:01\r\u001b[K |███████████████████████████████▎| 327kB 8.4MB/s eta 0:00:01\r\u001b[K |████████████████████████████████| 337kB 8.4MB/s \n\u001b[?25hRequirement already satisfied: six>=1.9.0 in /usr/local/lib/python3.7/dist-packages (from Keras==2.1.5) (1.15.0)\nRequirement already satisfied: pyyaml in /usr/local/lib/python3.7/dist-packages (from Keras==2.1.5) (3.13)\nRequirement already satisfied: numpy>=1.9.1 in /usr/local/lib/python3.7/dist-packages (from Keras==2.1.5) (1.19.5)\nRequirement already satisfied: scipy>=0.14 in /usr/local/lib/python3.7/dist-packages (from Keras==2.1.5) (1.4.1)\nInstalling collected packages: Keras\n Found existing installation: Keras 2.4.3\n Uninstalling Keras-2.4.3:\n Successfully uninstalled Keras-2.4.3\nSuccessfully installed Keras-2.1.5\n"
],
[
"!git clone https://github.com/worldstar/keras-yolo3.git",
"Cloning into 'keras-yolo3'...\nremote: Enumerating objects: 144, done.\u001b[K\nremote: Total 144 (delta 0), reused 0 (delta 0), pack-reused 144\u001b[K\nReceiving objects: 100% (144/144), 151.08 KiB | 604.00 KiB/s, done.\nResolving deltas: 100% (65/65), done.\n"
],
[
"%cd keras-yolo3",
"/content/keras-yolo3\n"
],
[
"!wget https://pjreddie.com/media/files/yolov3.weights yolov3.weights\n!python convert.py yolov3.cfg yolov3.weights model_data/yolo_weights.h5",
"_____no_output_____"
],
[
"!git clone https://github.com/worldstar/dataset.git",
"Cloning into 'dataset'...\nremote: Enumerating objects: 713, done.\u001b[K\nremote: Total 713 (delta 0), reused 0 (delta 0), pack-reused 713\u001b[K\nReceiving objects: 100% (713/713), 7.26 MiB | 12.63 MiB/s, done.\nResolving deltas: 100% (341/341), done.\n"
],
[
"fw = open('model_data/voc_classes.txt', \"w\")\nfw.write('RBC')\nfw.close()",
"_____no_output_____"
],
[
"import xml.etree.ElementTree as ET\nimport os\nfrom os import getcwd\nimport sys\n\ndef generateAnnotations(path, imagePath, writePath, voc_classes):\n # path = sys.argv[1] #\"./Data/Annotations/\"\n # imagePath = sys.argv[2]#\"./Data/JPEGImages/\"\n # writePath = sys.argv[3]#\"./model_data/train.txt\"\n deputyFileName = \"jpg\"\n # classes = [\"bicycle\",\"car\",\"cat\",\"dog\",\"person\"]\n fr = open(voc_classes, 'r')#\"model_data/voc_classes.txt\"\n classes = fr.read().split(\"\\n\")\n fr.close()\n\n fw = open(writePath, \"w\")\n for fileName in os.listdir(path):\n if fileName in \".gitignore\":\n continue\n print(\"readFile:\",(path+fileName))\n convertResult = convert_annotation((path+fileName),classes,imagePath,deputyFileName)\n fw.write(convertResult)\n fw.close()\n\ndef dataUs(infos):\n return infos.split(\".\")[0]\n\ndef convert_annotation(path,classes,imagePath,deputyFileName): \n try: \n xmlFile = open(path) \n except:\n xmlFile = open(path,encoding=\"utf-8\") \n xmlTree = ET.parse(xmlFile)\n xmlRoot = xmlTree.getroot()\n width,height,depth = -1,-1,-1\n hasClass = False\n result = \"\"\n for xmlObj in xmlRoot.iter('size'):\n width = xmlObj.find('width').text.replace(\" \", \"\").replace(\"\\t\", \"\").replace(\"\\n\", \"\")\n height = xmlObj.find('height').text.replace(\" \", \"\").replace(\"\\t\", \"\").replace(\"\\n\", \"\").replace(\" \", \"\").replace(\"\\t\", \"\").replace(\"\\n\", \"\")\n depth = xmlObj.find('depth').text.replace(\" \", \"\").replace(\"\\t\", \"\").replace(\"\\n\", \"\")\n # print(width,height,depth)\n for xmlObj in xmlRoot.iter('object'):\n name = xmlObj.find('name').text.replace(\" \", \"\").replace(\"\\t\", \"\").replace(\"\\n\", \"\")\n isClass = False\n classNum = -1\n for i in range(0,len(classes),1):\n if(name == classes[i]):\n isClass = True\n hasClass = True\n classNum = i\n if(isClass):\n xmin , ymin , xmax , ymax = -1 , -1 , -1 , -1\n for xmlObj2 in xmlObj.iter('bndbox'):\n xmin = int(dataUs(xmlObj2.find('xmin').text.replace(\" \", \"\").replace(\"\\t\", \"\").replace(\"\\n\", \"\").replace(\" \", \"\").replace(\"\\t\", \"\").replace(\"\\n\", \"\")))\n ymin = int(dataUs(xmlObj2.find('ymin').text.replace(\" \", \"\").replace(\"\\t\", \"\").replace(\"\\n\", \"\")))\n xmax = int(dataUs(xmlObj2.find('xmax').text.replace(\" \", \"\").replace(\"\\t\", \"\").replace(\"\\n\", \"\")))\n ymax = int(dataUs(xmlObj2.find('ymax').text.replace(\" \", \"\").replace(\"\\t\", \"\").replace(\"\\n\", \"\")))\n result += \" %s,%s,%s,%s,%d\"%(xmin,ymin,xmax,ymax,classNum)\n if(hasClass):\n FileName = os.path.basename(path)\n FileName = os.path.splitext(FileName)[0]\n FileName = FileName+\".\"+deputyFileName\n result = \"%s%s\\n\"%((imagePath + FileName),result)\n return result",
"_____no_output_____"
],
[
"generateAnnotations('dataset/Annotations/', 'dataset/JPEGImages/', 'train.txt', 'model_data/voc_classes.txt')",
"_____no_output_____"
],
[
"!python train.py #WPlease note there are two stages, the first stage runs 20 epochs and the 2nd stage executes 100 epochs.",
"Using TensorFlow backend.\nWARNING:tensorflow:From /usr/local/lib/python3.7/dist-packages/keras/backend/tensorflow_backend.py:88: The name tf.reset_default_graph is deprecated. Please use tf.compat.v1.reset_default_graph instead.\n\nWARNING:tensorflow:From /usr/local/lib/python3.7/dist-packages/keras/backend/tensorflow_backend.py:91: The name tf.placeholder_with_default is deprecated. Please use tf.compat.v1.placeholder_with_default instead.\n\nWARNING:tensorflow:From /usr/local/lib/python3.7/dist-packages/keras/backend/tensorflow_backend.py:95: The name tf.get_default_graph is deprecated. Please use tf.compat.v1.get_default_graph instead.\n\nWARNING:tensorflow:From /usr/local/lib/python3.7/dist-packages/keras/backend/tensorflow_backend.py:507: The name tf.placeholder is deprecated. Please use tf.compat.v1.placeholder instead.\n\nWARNING:tensorflow:From /usr/local/lib/python3.7/dist-packages/keras/backend/tensorflow_backend.py:3831: The name tf.random_uniform is deprecated. Please use tf.random.uniform instead.\n\nWARNING:tensorflow:From /usr/local/lib/python3.7/dist-packages/keras/backend/tensorflow_backend.py:167: The name tf.get_default_session is deprecated. Please use tf.compat.v1.get_default_session instead.\n\nWARNING:tensorflow:From /usr/local/lib/python3.7/dist-packages/keras/backend/tensorflow_backend.py:174: The name tf.ConfigProto is deprecated. Please use tf.compat.v1.ConfigProto instead.\n\nWARNING:tensorflow:From /usr/local/lib/python3.7/dist-packages/keras/backend/tensorflow_backend.py:179: The name tf.Session is deprecated. Please use tf.compat.v1.Session instead.\n\n2021-02-25 03:44:13.524316: I tensorflow/core/platform/cpu_feature_guard.cc:142] Your CPU supports instructions that this TensorFlow binary was not compiled to use: AVX2 FMA\n2021-02-25 03:44:13.529010: I tensorflow/core/platform/profile_utils/cpu_utils.cc:94] CPU Frequency: 2199995000 Hz\n2021-02-25 03:44:13.529214: I tensorflow/compiler/xla/service/service.cc:168] XLA service 0x562fbe8fea00 initialized for platform Host (this does not guarantee that XLA will be used). Devices:\n2021-02-25 03:44:13.529243: I tensorflow/compiler/xla/service/service.cc:176] StreamExecutor device (0): Host, Default Version\n2021-02-25 03:44:13.530934: I tensorflow/stream_executor/platform/default/dso_loader.cc:44] Successfully opened dynamic library libcuda.so.1\n2021-02-25 03:44:13.666199: I tensorflow/stream_executor/cuda/cuda_gpu_executor.cc:983] successful NUMA node read from SysFS had negative value (-1), but there must be at least one NUMA node, so returning NUMA node zero\n2021-02-25 03:44:13.666920: I tensorflow/compiler/xla/service/service.cc:168] XLA service 0x562fbe8ffb80 initialized for platform CUDA (this does not guarantee that XLA will be used). Devices:\n2021-02-25 03:44:13.666956: I tensorflow/compiler/xla/service/service.cc:176] StreamExecutor device (0): Tesla T4, Compute Capability 7.5\n2021-02-25 03:44:13.667113: I tensorflow/stream_executor/cuda/cuda_gpu_executor.cc:983] successful NUMA node read from SysFS had negative value (-1), but there must be at least one NUMA node, so returning NUMA node zero\n2021-02-25 03:44:13.667645: I tensorflow/core/common_runtime/gpu/gpu_device.cc:1639] Found device 0 with properties: \nname: Tesla T4 major: 7 minor: 5 memoryClockRate(GHz): 1.59\npciBusID: 0000:00:04.0\n2021-02-25 03:44:13.667952: I tensorflow/stream_executor/platform/default/dso_loader.cc:44] Successfully opened dynamic library libcudart.so.10.0\n2021-02-25 03:44:13.669073: I tensorflow/stream_executor/platform/default/dso_loader.cc:44] Successfully opened dynamic library libcublas.so.10.0\n2021-02-25 03:44:13.670101: I tensorflow/stream_executor/platform/default/dso_loader.cc:44] Successfully opened dynamic library libcufft.so.10.0\n2021-02-25 03:44:13.670565: I tensorflow/stream_executor/platform/default/dso_loader.cc:44] Successfully opened dynamic library libcurand.so.10.0\n2021-02-25 03:44:13.671984: I tensorflow/stream_executor/platform/default/dso_loader.cc:44] Successfully opened dynamic library libcusolver.so.10.0\n2021-02-25 03:44:13.672965: I tensorflow/stream_executor/platform/default/dso_loader.cc:44] Successfully opened dynamic library libcusparse.so.10.0\n2021-02-25 03:44:13.675952: I tensorflow/stream_executor/platform/default/dso_loader.cc:44] Successfully opened dynamic library libcudnn.so.7\n2021-02-25 03:44:13.676052: I tensorflow/stream_executor/cuda/cuda_gpu_executor.cc:983] successful NUMA node read from SysFS had negative value (-1), but there must be at least one NUMA node, so returning NUMA node zero\n2021-02-25 03:44:13.676586: I tensorflow/stream_executor/cuda/cuda_gpu_executor.cc:983] successful NUMA node read from SysFS had negative value (-1), but there must be at least one NUMA node, so returning NUMA node zero\n2021-02-25 03:44:13.677097: I tensorflow/core/common_runtime/gpu/gpu_device.cc:1767] Adding visible gpu devices: 0\n2021-02-25 03:44:13.677152: I tensorflow/stream_executor/platform/default/dso_loader.cc:44] Successfully opened dynamic library libcudart.so.10.0\n2021-02-25 03:44:13.678101: I tensorflow/core/common_runtime/gpu/gpu_device.cc:1180] Device interconnect StreamExecutor with strength 1 edge matrix:\n2021-02-25 03:44:13.678127: I tensorflow/core/common_runtime/gpu/gpu_device.cc:1186] 0 \n2021-02-25 03:44:13.678139: I tensorflow/core/common_runtime/gpu/gpu_device.cc:1199] 0: N \n2021-02-25 03:44:13.678241: I tensorflow/stream_executor/cuda/cuda_gpu_executor.cc:983] successful NUMA node read from SysFS had negative value (-1), but there must be at least one NUMA node, so returning NUMA node zero\n2021-02-25 03:44:13.678792: I tensorflow/stream_executor/cuda/cuda_gpu_executor.cc:983] successful NUMA node read from SysFS had negative value (-1), but there must be at least one NUMA node, so returning NUMA node zero\n2021-02-25 03:44:13.679385: W tensorflow/core/common_runtime/gpu/gpu_bfc_allocator.cc:39] Overriding allow_growth setting because the TF_FORCE_GPU_ALLOW_GROWTH environment variable is set. Original config value was 0.\n2021-02-25 03:44:13.679440: I tensorflow/core/common_runtime/gpu/gpu_device.cc:1325] Created TensorFlow device (/job:localhost/replica:0/task:0/device:GPU:0 with 14257 MB memory) -> physical GPU (device: 0, name: Tesla T4, pci bus id: 0000:00:04.0, compute capability: 7.5)\nWARNING:tensorflow:From /usr/local/lib/python3.7/dist-packages/keras/backend/tensorflow_backend.py:183: The name tf.global_variables is deprecated. Please use tf.compat.v1.global_variables instead.\n\nWARNING:tensorflow:From /usr/local/lib/python3.7/dist-packages/keras/backend/tensorflow_backend.py:192: The name tf.is_variable_initialized is deprecated. Please use tf.compat.v1.is_variable_initialized instead.\n\nWARNING:tensorflow:From /usr/local/lib/python3.7/dist-packages/keras/backend/tensorflow_backend.py:199: The name tf.variables_initializer is deprecated. Please use tf.compat.v1.variables_initializer instead.\n\nWARNING:tensorflow:From /usr/local/lib/python3.7/dist-packages/keras/backend/tensorflow_backend.py:1797: The name tf.nn.fused_batch_norm is deprecated. Please use tf.compat.v1.nn.fused_batch_norm instead.\n\nWARNING:tensorflow:From /usr/local/lib/python3.7/dist-packages/keras/backend/tensorflow_backend.py:1940: The name tf.image.resize_nearest_neighbor is deprecated. Please use tf.compat.v1.image.resize_nearest_neighbor instead.\n\nCreate YOLOv3 model with 9 anchors and 1 classes.\n/usr/local/lib/python3.7/dist-packages/keras/engine/topology.py:3462: UserWarning: Skipping loading of weights for layer conv2d_59 due to mismatch in shape ((1, 1, 1024, 18) vs (255, 1024, 1, 1)).\n weight_values[i].shape))\n/usr/local/lib/python3.7/dist-packages/keras/engine/topology.py:3462: UserWarning: Skipping loading of weights for layer conv2d_59 due to mismatch in shape ((18,) vs (255,)).\n weight_values[i].shape))\n/usr/local/lib/python3.7/dist-packages/keras/engine/topology.py:3462: UserWarning: Skipping loading of weights for layer conv2d_67 due to mismatch in shape ((1, 1, 512, 18) vs (255, 512, 1, 1)).\n weight_values[i].shape))\n/usr/local/lib/python3.7/dist-packages/keras/engine/topology.py:3462: UserWarning: Skipping loading of weights for layer conv2d_67 due to mismatch in shape ((18,) vs (255,)).\n weight_values[i].shape))\n/usr/local/lib/python3.7/dist-packages/keras/engine/topology.py:3462: UserWarning: Skipping loading of weights for layer conv2d_75 due to mismatch in shape ((1, 1, 256, 18) vs (255, 256, 1, 1)).\n weight_values[i].shape))\n/usr/local/lib/python3.7/dist-packages/keras/engine/topology.py:3462: UserWarning: Skipping loading of weights for layer conv2d_75 due to mismatch in shape ((18,) vs (255,)).\n weight_values[i].shape))\nLoad weights model_data/yolo_weights.h5.\nFreeze the first 249 layers of total 252 layers.\nWARNING:tensorflow:From /usr/local/lib/python3.7/dist-packages/keras/backend/tensorflow_backend.py:1486: The name tf.log is deprecated. Please use tf.math.log instead.\n\nWARNING:tensorflow:From /usr/local/lib/python3.7/dist-packages/keras/backend/tensorflow_backend.py:2836: where (from tensorflow.python.ops.array_ops) is deprecated and will be removed in a future version.\nInstructions for updating:\nUse tf.where in 2.0, which has the same broadcast rule as np.where\nWARNING:tensorflow:From /usr/local/lib/python3.7/dist-packages/keras/optimizers.py:757: The name tf.train.Optimizer is deprecated. Please use tf.compat.v1.train.Optimizer instead.\n\nTrain on 309 samples, val on 34 samples, with batch size 16.\nWARNING:tensorflow:From /usr/local/lib/python3.7/dist-packages/keras/backend/tensorflow_backend.py:976: The name tf.assign_add is deprecated. Please use tf.compat.v1.assign_add instead.\n\nWARNING:tensorflow:From /usr/local/lib/python3.7/dist-packages/keras/backend/tensorflow_backend.py:963: The name tf.assign is deprecated. Please use tf.compat.v1.assign instead.\n\nWARNING:tensorflow:From /usr/local/lib/python3.7/dist-packages/keras/callbacks.py:774: The name tf.summary.merge_all is deprecated. Please use tf.compat.v1.summary.merge_all instead.\n\nWARNING:tensorflow:From /usr/local/lib/python3.7/dist-packages/keras/callbacks.py:777: The name tf.summary.FileWriter is deprecated. Please use tf.compat.v1.summary.FileWriter instead.\n\nEpoch 1/20\n2021-02-25 03:44:28.128617: E tensorflow/core/grappler/optimizers/meta_optimizer.cc:533] shape_optimizer failed: Invalid argument: Subshape must have computed start >= end since stride is negative, but is 0 and 2 (computed from start 0 and end 9223372036854775807 over shape with rank 2 and stride-1)\n2021-02-25 03:44:28.420652: E tensorflow/core/grappler/optimizers/meta_optimizer.cc:533] layout failed: Invalid argument: Subshape must have computed start >= end since stride is negative, but is 0 and 2 (computed from start 0 and end 9223372036854775807 over shape with rank 2 and stride-1)\n2021-02-25 03:44:28.702620: E tensorflow/core/grappler/optimizers/meta_optimizer.cc:533] shape_optimizer failed: Invalid argument: Subshape must have computed start >= end since stride is negative, but is 0 and 2 (computed from start 0 and end 9223372036854775807 over shape with rank 2 and stride-1)\n2021-02-25 03:44:29.701408: I tensorflow/stream_executor/platform/default/dso_loader.cc:44] Successfully opened dynamic library libcudnn.so.7\n2021-02-25 03:44:31.382347: I tensorflow/stream_executor/platform/default/dso_loader.cc:44] Successfully opened dynamic library libcublas.so.10.0\n18/19 [===========================>..] - ETA: 1s - loss: 3457.29042021-02-25 03:44:52.449103: E tensorflow/core/grappler/optimizers/meta_optimizer.cc:533] layout failed: Invalid argument: Subshape must have computed start >= end since stride is negative, but is 0 and 2 (computed from start 0 and end 9223372036854775807 over shape with rank 2 and stride-1)\n19/19 [==============================] - 29s 2s/step - loss: 3334.5453 - val_loss: 993.4081\nWARNING:tensorflow:From /usr/local/lib/python3.7/dist-packages/keras/callbacks.py:860: The name tf.Summary is deprecated. Please use tf.compat.v1.Summary instead.\n\nEpoch 2/20\n19/19 [==============================] - 23s 1s/step - loss: 625.5499 - val_loss: 372.0052\nEpoch 3/20\n19/19 [==============================] - 23s 1s/step - loss: 324.7218 - val_loss: 260.7542\nEpoch 4/20\n19/19 [==============================] - 16s 866ms/step - loss: 244.0774 - val_loss: 219.9932\nEpoch 5/20\n19/19 [==============================] - 23s 1s/step - loss: 207.5831 - val_loss: 180.8676\nEpoch 6/20\n19/19 [==============================] - 23s 1s/step - loss: 182.1875 - val_loss: 161.9958\nEpoch 7/20\n19/19 [==============================] - 23s 1s/step - loss: 167.5140 - val_loss: 148.1277\nEpoch 8/20\n19/19 [==============================] - 23s 1s/step - loss: 151.2248 - val_loss: 143.1213\nEpoch 9/20\n19/19 [==============================] - 23s 1s/step - loss: 142.6097 - val_loss: 136.3236\nEpoch 10/20\n19/19 [==============================] - 23s 1s/step - loss: 135.1423 - val_loss: 124.0489\nEpoch 11/20\n19/19 [==============================] - 23s 1s/step - loss: 128.4324 - val_loss: 119.1641\nEpoch 12/20\n19/19 [==============================] - 23s 1s/step - loss: 125.2811 - val_loss: 117.8455\nEpoch 13/20\n19/19 [==============================] - 23s 1s/step - loss: 121.7488 - val_loss: 110.1949\nEpoch 14/20\n19/19 [==============================] - 23s 1s/step - loss: 114.2375 - val_loss: 109.3775\nEpoch 15/20\n19/19 [==============================] - 23s 1s/step - loss: 112.9564 - val_loss: 106.6571\nEpoch 16/20\n19/19 [==============================] - 23s 1s/step - loss: 111.4869 - val_loss: 105.0343\nEpoch 17/20\n19/19 [==============================] - 23s 1s/step - loss: 108.5488 - val_loss: 98.9898\nEpoch 18/20\n19/19 [==============================] - 23s 1s/step - loss: 105.6998 - val_loss: 99.1945\nEpoch 19/20\n19/19 [==============================] - 23s 1s/step - loss: 104.0442 - val_loss: 94.9579\nEpoch 20/20\n19/19 [==============================] - 23s 1s/step - loss: 101.2193 - val_loss: 100.2676\nUnfreeze all of the layers.\nTrain on 309 samples, val on 34 samples, with batch size 16.\nEpoch 21/100\n2021-02-25 03:52:57.336490: E tensorflow/core/grappler/optimizers/meta_optimizer.cc:533] shape_optimizer failed: Invalid argument: Subshape must have computed start >= end since stride is negative, but is 0 and 2 (computed from start 0 and end 9223372036854775807 over shape with rank 2 and stride-1)\n2021-02-25 03:52:59.403652: E tensorflow/core/grappler/optimizers/meta_optimizer.cc:533] layout failed: Invalid argument: Subshape must have computed start >= end since stride is negative, but is 0 and 2 (computed from start 0 and end 9223372036854775807 over shape with rank 2 and stride-1)\n2021-02-25 03:53:00.909915: E tensorflow/core/grappler/optimizers/meta_optimizer.cc:533] shape_optimizer failed: Invalid argument: Subshape must have computed start >= end since stride is negative, but is 0 and 2 (computed from start 0 and end 9223372036854775807 over shape with rank 2 and stride-1)\n18/19 [===========================>..] - ETA: 1s - loss: 73.33162021-02-25 03:53:31.074251: E tensorflow/core/grappler/optimizers/meta_optimizer.cc:533] layout failed: Invalid argument: Subshape must have computed start >= end since stride is negative, but is 0 and 2 (computed from start 0 and end 9223372036854775807 over shape with rank 2 and stride-1)\n19/19 [==============================] - 40s 2s/step - loss: 72.7146 - val_loss: 67.2190\nEpoch 22/100\n19/19 [==============================] - 26s 1s/step - loss: 63.0159 - val_loss: 64.6116\nEpoch 23/100\n19/19 [==============================] - 26s 1s/step - loss: 58.7156 - val_loss: 57.9178\nEpoch 24/100\n19/19 [==============================] - 28s 1s/step - loss: 58.1431 - val_loss: 55.3014\nEpoch 25/100\n19/19 [==============================] - 28s 1s/step - loss: 56.7163 - val_loss: 58.5629\nEpoch 26/100\n19/19 [==============================] - 28s 1s/step - loss: 54.6510 - val_loss: 54.9712\nEpoch 27/100\n19/19 [==============================] - 28s 1s/step - loss: 54.4398 - val_loss: 51.6599\nEpoch 28/100\n19/19 [==============================] - 28s 1s/step - loss: 53.8172 - val_loss: 54.9441\nEpoch 29/100\n19/19 [==============================] - 28s 1s/step - loss: 52.9516 - val_loss: 55.0698\nEpoch 30/100\n19/19 [==============================] - 28s 1s/step - loss: 53.5554 - val_loss: 53.2384\nEpoch 31/100\n19/19 [==============================] - 28s 1s/step - loss: 52.9168 - val_loss: 54.8878\n\nEpoch 00031: ReduceLROnPlateau reducing learning rate to 9.999999747378752e-06.\nEpoch 32/100\n19/19 [==============================] - 27s 1s/step - loss: 51.4068 - val_loss: 54.6203\nEpoch 33/100\n19/19 [==============================] - 28s 1s/step - loss: 50.7900 - val_loss: 53.3654\nEpoch 34/100\n19/19 [==============================] - 28s 1s/step - loss: 51.1515 - val_loss: 53.7723\n\nEpoch 00034: ReduceLROnPlateau reducing learning rate to 9.999999747378752e-07.\nEpoch 35/100\n19/19 [==============================] - 28s 1s/step - loss: 50.9989 - val_loss: 49.4049\nEpoch 36/100\n19/19 [==============================] - 28s 1s/step - loss: 51.5877 - val_loss: 51.6969\nEpoch 37/100\n19/19 [==============================] - 28s 1s/step - loss: 50.6508 - val_loss: 52.0544\nEpoch 38/100\n19/19 [==============================] - 28s 1s/step - loss: 51.2635 - val_loss: 48.1271\nEpoch 39/100\n19/19 [==============================] - 28s 1s/step - loss: 51.0648 - val_loss: 52.7201\nEpoch 40/100\n19/19 [==============================] - 28s 1s/step - loss: 51.0443 - val_loss: 53.4587\nEpoch 41/100\n19/19 [==============================] - 28s 1s/step - loss: 50.3224 - val_loss: 50.5345\nEpoch 42/100\n19/19 [==============================] - 28s 1s/step - loss: 51.3830 - val_loss: 49.8061\n\nEpoch 00042: ReduceLROnPlateau reducing learning rate to 9.999999974752428e-08.\nEpoch 43/100\n19/19 [==============================] - 28s 1s/step - loss: 51.0624 - val_loss: 51.8863\nEpoch 44/100\n19/19 [==============================] - 28s 1s/step - loss: 51.0018 - val_loss: 51.3152\nEpoch 45/100\n19/19 [==============================] - 28s 1s/step - loss: 50.3419 - val_loss: 53.4304\n\nEpoch 00045: ReduceLROnPlateau reducing learning rate to 1.0000000116860975e-08.\nEpoch 46/100\n19/19 [==============================] - 28s 1s/step - loss: 50.4961 - val_loss: 50.0752\nEpoch 47/100\n19/19 [==============================] - 28s 1s/step - loss: 50.7682 - val_loss: 51.7997\nEpoch 48/100\n19/19 [==============================] - 28s 1s/step - loss: 50.8429 - val_loss: 53.9532\n\nEpoch 00048: ReduceLROnPlateau reducing learning rate to 9.999999939225292e-10.\nEpoch 00048: early stopping\n"
]
]
] | [
"code"
] | [
[
"code",
"code",
"code",
"code",
"code",
"code",
"code",
"code",
"code",
"code"
]
] |
e775e2f280d8bd1dc0b97bfa47c4b2d693dfb9b6 | 251,531 | ipynb | Jupyter Notebook | DMLCT/8x8/DMLCT.ipynb | Hiroya-W/Python_DCT | 5acb7553792335e178d8b99ca1ee42431cc26f92 | [
"MIT"
] | null | null | null | DMLCT/8x8/DMLCT.ipynb | Hiroya-W/Python_DCT | 5acb7553792335e178d8b99ca1ee42431cc26f92 | [
"MIT"
] | 2 | 2020-01-06T14:12:55.000Z | 2020-02-06T07:00:31.000Z | DMLCT/8x8/DMLCT.ipynb | Hiroya-W/TPHLCT_and_DMLCT | 5acb7553792335e178d8b99ca1ee42431cc26f92 | [
"MIT"
] | null | null | null | 191.715701 | 91,688 | 0.884523 | [
[
[
"import numpy as np\nimport scipy.misc\nfrom scipy.fftpack import dct, idct\nimport sys\nfrom PIL import Image\nimport matplotlib\nimport matplotlib.pyplot as plt\nimport random\nfrom tqdm._tqdm_notebook import tqdm_notebook\nfrom scipy.fftpack import dct, idct\nimport seaborn as sns\nfrom skimage.metrics import structural_similarity as ssim\nimport pandas as pd\nimport sympy\n\n%matplotlib inline\n\n\nclass ImageLoader:\n def __init__(self, FILE_PATH):\n self.img = np.array(Image.open(FILE_PATH))\n # 行数\n self.row_blocks_count = self.img.shape[0] // 8\n # 列数\n self.col_blocks_count = self.img.shape[1] // 8\n\n def get_points(self, POINT):\n Row = random.randint(0, len(self.img) - POINT - 1)\n Col = random.randint(0, len(self.img) - 1)\n return self.img[Row : Row + POINT, Col]\n\n def get_block(self, col, row):\n return self.img[col * 8 : (col + 1) * 8, row * 8 : (row + 1) * 8]\n\n\n# plt.rcParams['font.family'] ='sans-serif'#使用するフォント\n# plt.rcParams[\"font.sans-serif\"] = \"Source Han Sans\"\nplt.rcParams[\"font.family\"] = \"Source Han Sans JP\" # 使用するフォント\nplt.rcParams[\"xtick.direction\"] = \"in\" # x軸の目盛線が内向き('in')か外向き('out')か双方向か('inout')\nplt.rcParams[\"ytick.direction\"] = \"in\" # y軸の目盛線が内向き('in')か外向き('out')か双方向か('inout')\nplt.rcParams[\"xtick.major.width\"] = 1.0 # x軸主目盛り線の線幅\nplt.rcParams[\"ytick.major.width\"] = 1.0 # y軸主目盛り線の線幅\nplt.rcParams[\"font.size\"] = 12 # フォントの大きさ\nplt.rcParams[\"axes.linewidth\"] = 1.0 # 軸の線幅edge linewidth。囲みの太さ\nmatplotlib.font_manager._rebuild()\n\nMONO_DIR_PATH = \"../../Mono/\"\nAIRPLANE = ImageLoader(MONO_DIR_PATH + \"airplane512.bmp\")\nBARBARA = ImageLoader(MONO_DIR_PATH + \"barbara512.bmp\")\nBOAT = ImageLoader(MONO_DIR_PATH + \"boat512.bmp\")\nGOLDHILL = ImageLoader(MONO_DIR_PATH + \"goldhill512.bmp\")\nLENNA = ImageLoader(MONO_DIR_PATH + \"lenna512.bmp\")\nMANDRILL = ImageLoader(MONO_DIR_PATH + \"mandrill512.bmp\")\nMILKDROP = ImageLoader(MONO_DIR_PATH + \"milkdrop512.bmp\")\nSAILBOAT = ImageLoader(MONO_DIR_PATH + \"sailboat512.bmp\")",
"_____no_output_____"
],
[
"n_bar = 4\nN = 8",
"_____no_output_____"
]
],
[
[
"# MSDS",
"_____no_output_____"
]
],
[
[
"def msds(N,arr):\n w_e = 0\n e_e = 0\n n_e = 0\n s_e = 0\n nw_e = 0\n ne_e = 0\n sw_e = 0\n se_e = 0\n for row in range(arr.shape[0] // N):\n for col in range(arr.shape[1] // N):\n f_block = arr[row * N : (row + 1) * N, col * N : (col + 1) * N]\n # w\n if col == 0:\n w_block = np.fliplr(f_block)\n else:\n w_block = arr[row * N : (row + 1) * N, (col - 1) * N : col * N]\n # e\n if col == arr.shape[1] // N - 1:\n e_block = np.fliplr(f_block)\n else:\n e_block = arr[row * N : (row + 1) * N, (col + 1) * N : (col + 2) * N]\n # n\n if row == 0:\n n_block = np.flipud(f_block)\n else:\n n_block = arr[(row - 1) * N : row * N, col * N : (col + 1) * N]\n # s\n if row == arr.shape[0] // N - 1:\n s_block = np.flipud(f_block)\n else:\n s_block = arr[(row + 1) * N : (row + 2) * N, col * N : (col + 1) * N]\n\n w_d1 = f_block[:, 0] - w_block[:, N-1]\n e_d1 = f_block[:, N-1] - e_block[:, 0]\n n_d1 = f_block[0, :] - n_block[N-1, :]\n s_d1 = f_block[N-1, :] - s_block[0, :]\n\n w_d2 = (w_block[:, N-1] - w_block[:, N-2] + f_block[:, 1] - f_block[:, 0]) / 2\n e_d2 = (e_block[:, 1] - e_block[:, 0] + f_block[:, N-1] - f_block[:, N-2]) / 2\n n_d2 = (n_block[N-1, :] - n_block[N-2, :] + f_block[1, :] - f_block[0, :]) / 2\n s_d2 = (s_block[1, :] - s_block[0, :] + f_block[N-1, :] - f_block[N-2, :]) / 2\n\n w_e += np.sum((w_d1 - w_d2) ** 2 )\n e_e += np.sum((e_d1 - e_d2) ** 2 )\n n_e += np.sum((n_d1 - n_d2) ** 2)\n s_e += np.sum((s_d1 - s_d2) ** 2)\n\n # nw\n if row == 0 or col == 0:\n nw_block = np.flipud(np.fliplr(f_block))\n else:\n nw_block = arr[(row - 1) * N : row * N, (col - 1) * N : col * N]\n # ne\n if row == 0 or col == arr.shape[1] // N - 1:\n ne_block = np.flipud(np.fliplr(f_block))\n else:\n ne_block = arr[(row-1) * N : row * N, (col + 1) * N : (col + 2) * N]\n # sw\n if row == arr.shape[0] // N -1 or col == 0:\n sw_block = np.flipud(np.fliplr(f_block))\n else:\n sw_block = arr[row * N : (row+1) * N, (col-1) * N : col * N]\n # se\n if row == arr.shape[0]//N-1 or col == arr.shape[0] // N -1:\n se_block = np.flipud(np.fliplr(f_block))\n else:\n se_block = arr[(row + 1) * N : (row + 2) * N, (col+1) * N : (col + 2) * N]\n\n nw_g1 = f_block[0, 0] - nw_block[N-1, N-1]\n ne_g1 = f_block[0, N-1] - ne_block[N-1, 0]\n sw_g1 = f_block[N-1, 0] - sw_block[0, N-1]\n se_g1 = f_block[N-1, N-1] - se_block[0, 0]\n\n nw_g2 = (nw_block[N-1,N-1] - nw_block[N-2,N-2] + f_block[1,1] - f_block[0,0])/2\n ne_g2 = (ne_block[N-1,0] - ne_block[N-2,1] + f_block[1,N-2] - f_block[0,N-1])/2\n sw_g2 = (sw_block[0,N-1] - nw_block[1,N-2] + f_block[N-2,1] - f_block[N-1,0])/2\n se_g2 = (nw_block[0,0] - nw_block[1,1] + f_block[N-2,N-2] - f_block[N-1,N-1])/2\n\n nw_e += (nw_g1 - nw_g2) ** 2 \n ne_e += (ne_g1 - ne_g2) ** 2 \n sw_e += (sw_g1 - sw_g2) ** 2 \n se_e += (se_g1 - se_g2) ** 2 \n\n MSDSt = (w_e + e_e + n_e + s_e + nw_e + ne_e + sw_e + se_e)/ ((arr.shape[0]/N)**2)\n MSDS1 = (w_e + e_e + n_e + s_e)/ ((arr.shape[0]/N)**2)\n MSDS2 = (nw_e + ne_e + sw_e + se_e)/ ((arr.shape[0]/N)**2)\n return MSDSt, MSDS1, MSDS2",
"_____no_output_____"
],
[
"class DMLCT:\n def __init__(self, n_bar, N):\n self.n_bar = n_bar\n self.N = N\n\n self.x_l = (2 * np.arange(N) + 1) / (2 * N)\n self.s_l = np.arange(n_bar) / (n_bar - 1)\n self.xi = (np.arange(n_bar + 1) - 0.5) / (n_bar - 1)\n\n self.lambda_kh = self.get_lambda_kh(self.n_bar)\n\n self.w_k_j = self.get_w_k_j(self.n_bar, self.N)\n self.W_L_k_kh = self.get_W_L_k_kh(self.n_bar, self.N)\n self.W_k_kh = self.get_W_k_kh(self.n_bar, self.N)\n self.W_R_k_kh = self.get_W_R_k_kh(self.n_bar, self.N)\n\n def Lagrange_j(self, j):\n x = sympy.Symbol(\"x\")\n L_x = 1.0\n for l in range(self.n_bar):\n if l != j:\n L_x *= (x - self.s_l[l]) / (self.s_l[j] - self.s_l[l])\n return sympy.integrate(L_x)\n\n def get_lambda_kh(self, n_bar):\n lambda_kh = np.ones(n_bar)\n lambda_kh[0] = np.sqrt(1 / 2)\n return lambda_kh\n\n def get_w_k_j(self, n_bar, N):\n L_j = np.zeros((n_bar, N))\n x = sympy.Symbol(\"x\")\n for j in range(n_bar):\n temp = []\n Lj = self.Lagrange_j(j)\n for k in range(N):\n temp.append(Lj.subs(x, self.x_l[k]))\n L_j[j] = np.array(temp)\n\n w_k_j = np.zeros((n_bar, N))\n for j in range(n_bar):\n w_k_j[j] = scipy.fftpack.dct(L_j[j], norm=\"ortho\")\n return w_k_j\n\n def get_W_L_k_kh(self, n_bar, N):\n W_L_k_kh = np.zeros((n_bar - 1, N))\n lambda_kh = self.get_lambda_kh(n_bar)\n\n for kh in range(n_bar - 1):\n W_L_k_kh[kh] = (\n (1 - n_bar)\n * np.sqrt(2 / N)\n * lambda_kh[kh]\n * np.cos(np.pi * kh * (self.xi[0] + 1))\n * self.w_k_j[0]\n )\n return W_L_k_kh\n\n def get_W_k_kh(self, n_bar, N):\n W_k_kh = np.zeros((n_bar - 1, N))\n for kh in range(n_bar - 1):\n sum_sin = np.zeros(N)\n for j in range(1, n_bar - 2 + 1):\n sum_sin += np.sin(np.pi * kh * self.s_l[j]) * self.w_k_j[j]\n\n W_k_kh[kh] = (\n (n_bar - 1)\n * np.sqrt(2 / N)\n * self.lambda_kh[kh]\n * (\n np.cos(np.pi * kh * self.xi[1])\n * (self.w_k_j[0] - (-1) ** (kh) * self.w_k_j[n_bar - 1])\n - 2 * np.sin((np.pi * kh) / (2 * (n_bar - 1))) * sum_sin\n )\n )\n return W_k_kh\n\n def get_W_R_k_kh(self, n_bar, N):\n W_R_k_kh = np.zeros((n_bar - 1, N))\n for kh in range(n_bar - 1):\n W_R_k_kh[kh] = (\n (n_bar - 1)\n * np.sqrt(2 / N)\n * self.lambda_kh[kh]\n * np.cos(np.pi * kh * (self.xi[n_bar] - 1))\n * self.w_k_j[n_bar - 1]\n )\n return W_R_k_kh",
"_____no_output_____"
],
[
"def get_F_L_k_horizontal(arr, N, row, col):\n # w\n if col == 0:\n# w_block = np.zeros(N)\n w_block = arr[row, col * N : (col + 1) * N]\n else:\n w_block = arr[row, (col - 1) * N : col * N]\n\n return w_block",
"_____no_output_____"
],
[
"def get_F_R_k_horizontal(arr, N, row, col):\n # e\n if col == arr.shape[1] // N - 1:\n# e_block = np.zeros(N)\n e_block = arr[row, col * N : (col + 1) * N]\n else:\n e_block = arr[row, (col + 1) * N : (col + 2) * N]\n\n return e_block",
"_____no_output_____"
],
[
"def get_F_L_k_vertical(arr, N, row, col):\n # n\n if row == 0:\n# n_block = np.zeros(N)\n n_block = arr[row * N : (row + 1) * N, col]\n else:\n n_block = arr[(row - 1) * N : row * N, col]\n\n return n_block",
"_____no_output_____"
],
[
"def get_F_R_k_vertical(arr, N, row, col):\n # s\n if row == arr.shape[0] // N - 1:\n# s_block = np.zeros(N)\n s_block = arr[row * N : (row + 1) * N, col]\n else:\n s_block = arr[(row + 1) * N : (row + 2) * N, col]\n\n return s_block",
"_____no_output_____"
],
[
"# dmlct = DMLCT(n_bar, N)",
"_____no_output_____"
],
[
"IMG = AIRPLANE\n# IMG = ImageLoader(MONO_DIR_PATH + \"LENNA.bmp\")",
"_____no_output_____"
],
[
"Fk = np.zeros(IMG.img.shape)",
"_____no_output_____"
]
],
[
[
"# 順変換",
"_____no_output_____"
],
[
"## 縦方向",
"_____no_output_____"
],
[
"### DCT",
"_____no_output_____"
]
],
[
[
"for row in range(IMG.img.shape[0] // N):\n for col in range(IMG.img.shape[1]):\n eight_points = IMG.img[N * row : N * (row + 1), col]\n c = scipy.fftpack.dct(eight_points, norm=\"ortho\")\n Fk[N * row : N * (row + 1), col] = c",
"_____no_output_____"
]
],
[
[
"### 残差",
"_____no_output_____"
]
],
[
[
"dmlct = DMLCT(n_bar, N)\nfor row in range(IMG.img.shape[0] // N):\n for col in range(IMG.img.shape[1]):\n # ビューなら直接いじっちゃう\n F = Fk[N * row : N * (row + 1), col]\n F_L = get_F_L_k_vertical(Fk, N, row, col)\n F_R = get_F_R_k_vertical(Fk, N, row, col)\n\n U_k_n_bar = np.zeros(N)\n for kh in range(n_bar - 2 + 1):\n U_k_n_bar += (\n F_L[kh] * dmlct.W_L_k_kh[kh]\n + F[kh] * dmlct.W_k_kh[kh]\n + F_R[kh] * dmlct.W_R_k_kh[kh]\n )\n\n # n_bar = 4 なら 0,1,2は残す 3,4,5,6,7を書き換える\n F[n_bar - 2 + 1 :] -= U_k_n_bar[n_bar - 2 + 1 :]",
"_____no_output_____"
],
[
"# 0を残す\nfor k in reversed(range(1, n_bar - 2 + 1)):\n dmlct = DMLCT(k+1, N)\n for row in range(IMG.img.shape[0] // N):\n for col in range(IMG.img.shape[1]):\n # ビューなら直接いじっちゃう\n F = Fk[N * row : N * (row + 1), col]\n F_L = get_F_L_k_vertical(Fk, N, row, col)\n F_R = get_F_R_k_vertical(Fk, N, row, col)\n\n U_k_n_bar = np.zeros(N)\n\n for kh in range((k + 1) - 2 + 1):\n U_k_n_bar += (\n F_L[kh] * dmlct.W_L_k_kh[kh]\n + F[kh] * dmlct.W_k_kh[kh]\n + F_R[kh] * dmlct.W_R_k_kh[kh]\n )\n\n F[k] -= U_k_n_bar[k]",
"_____no_output_____"
]
],
[
[
"## 横方向",
"_____no_output_____"
],
[
"### DCT",
"_____no_output_____"
]
],
[
[
"for row in range(Fk.shape[0]):\n for col in range(Fk.shape[1] // N):\n eight_points = Fk[row, N * col : N * (col + 1)]\n c = scipy.fftpack.dct(eight_points, norm=\"ortho\")\n Fk[row, N * col : N * (col + 1)] = c",
"_____no_output_____"
]
],
[
[
"### 残差",
"_____no_output_____"
]
],
[
[
"dmlct = DMLCT(n_bar, N)\nfor row in range(IMG.img.shape[0]):\n for col in range(IMG.img.shape[1] // N):\n F = Fk[row, N * col : N * (col + 1)]\n F_L = get_F_L_k_horizontal(Fk, N, row, col)\n F_R = get_F_R_k_horizontal(Fk, N, row, col)\n\n U_k_n_bar = np.zeros(N)\n for kh in range(n_bar - 2 + 1):\n U_k_n_bar += (\n F_L[kh] * dmlct.W_L_k_kh[kh]\n + F[kh] * dmlct.W_k_kh[kh]\n + F_R[kh] * dmlct.W_R_k_kh[kh]\n )\n\n # n_bar = 4 なら 0,1,2は残す 3,4,5,6,7を書き換える\n F[n_bar - 2 + 1 :] -= U_k_n_bar[n_bar - 2 + 1 :]",
"_____no_output_____"
],
[
"# 0を残す\nfor k in reversed(range(1, n_bar - 2 + 1)):\n dmlct = DMLCT(k+1, N)\n for row in range(IMG.img.shape[0]):\n for col in range(IMG.img.shape[1] // N):\n F = Fk[row, N * col : N * (col + 1)]\n F_L = get_F_L_k_horizontal(Fk, N, row, col)\n F_R = get_F_R_k_horizontal(Fk, N, row, col)\n\n U_k_n_bar = np.zeros(N)\n\n for kh in range((k + 1) - 2 + 1):\n U_k_n_bar += (\n F_L[kh] * dmlct.W_L_k_kh[kh]\n + F[kh] * dmlct.W_k_kh[kh]\n + F_R[kh] * dmlct.W_R_k_kh[kh]\n )\n\n F[k] -= U_k_n_bar[k]",
"_____no_output_____"
],
[
"plt.imshow(Fk)\npd.DataFrame(Fk).to_csv(\"DMLCT_lenna_hiroya_coef.csv\",header=False,index=False)",
"_____no_output_____"
]
],
[
[
"# 係数の確保",
"_____no_output_____"
]
],
[
[
"Fk_Ori = np.copy(Fk)",
"_____no_output_____"
]
],
[
[
"# 逆変換",
"_____no_output_____"
]
],
[
[
"recover = np.zeros(IMG.img.shape)",
"_____no_output_____"
]
],
[
[
"## 横方向",
"_____no_output_____"
],
[
"### 残差",
"_____no_output_____"
]
],
[
[
"for k in range(1, n_bar - 2 + 1):\n dmlct = DMLCT(k+1, N)\n for row in range(IMG.img.shape[0]):\n for col in range(IMG.img.shape[1] // N):\n F = Fk[row, N * col : N * (col + 1)]\n F_L = get_F_L_k_horizontal(Fk, N, row, col)\n F_R = get_F_R_k_horizontal(Fk, N, row, col)\n\n U_k_n_bar = np.zeros(N)\n for kh in range((k + 1) - 2 + 1):\n U_k_n_bar += (\n F_L[kh] * dmlct.W_L_k_kh[kh]\n + F[kh] * dmlct.W_k_kh[kh]\n + F_R[kh] * dmlct.W_R_k_kh[kh]\n )\n\n F[k] += U_k_n_bar[k]",
"_____no_output_____"
],
[
"dmlct = DMLCT(n_bar, N)\nfor row in range(IMG.img.shape[0]):\n for col in range(IMG.img.shape[1] // N):\n F = Fk[row, N * col : N * (col + 1)]\n F_L = get_F_L_k_horizontal(Fk, N, row, col)\n F_R = get_F_R_k_horizontal(Fk, N, row, col)\n\n U_k_n_bar = np.zeros(N)\n for kh in range(n_bar - 2 + 1):\n U_k_n_bar += (\n F_L[kh] * dmlct.W_L_k_kh[kh]\n + F[kh] * dmlct.W_k_kh[kh]\n + F_R[kh] * dmlct.W_R_k_kh[kh]\n )\n\n # n_bar = 4 なら 0,1,2は残す 3,4,5,6,7を書き換える\n F[n_bar - 2 + 1 :] += U_k_n_bar[n_bar - 2 + 1 :]",
"_____no_output_____"
]
],
[
[
"### IDCT",
"_____no_output_____"
]
],
[
[
"for row in range(Fk.shape[0]):\n for col in range(Fk.shape[1] // N):\n F = Fk[row, N * col : N * col + N]\n data = scipy.fftpack.idct(F, norm=\"ortho\")\n # Fkに代入した後、縦方向に対して処理\n Fk[row, N * col : N * col + N] = data",
"_____no_output_____"
]
],
[
[
"## 縦方向",
"_____no_output_____"
],
[
"### 残差",
"_____no_output_____"
]
],
[
[
"for k in range(1, n_bar - 2 + 1):\n dmlct = DMLCT(k+1, N)\n for row in range(IMG.img.shape[0] // N):\n for col in range(IMG.img.shape[1]):\n # ビューなら直接いじっちゃう\n F = Fk[N * row : N * (row + 1), col]\n F_L = get_F_L_k_vertical(Fk, N, row, col)\n F_R = get_F_R_k_vertical(Fk, N, row, col)\n\n U_k_n_bar = np.zeros(N)\n\n for kh in range((k + 1) - 2 + 1):\n U_k_n_bar += (\n F_L[kh] * dmlct.W_L_k_kh[kh]\n + F[kh] * dmlct.W_k_kh[kh]\n + F_R[kh] * dmlct.W_R_k_kh[kh]\n )\n\n F[k] += U_k_n_bar[k]",
"_____no_output_____"
],
[
"dmlct = DMLCT(n_bar, N)\nfor row in range(IMG.img.shape[0] // N):\n for col in range(IMG.img.shape[1]):\n # ビューなら直接いじっちゃう\n F = Fk[N * row : N * (row + 1), col]\n F_L = get_F_L_k_vertical(Fk, N, row, col)\n F_R = get_F_R_k_vertical(Fk, N, row, col)\n\n U_k_n_bar = np.zeros(N)\n for kh in range(n_bar - 2 + 1):\n U_k_n_bar += (\n F_L[kh] * dmlct.W_L_k_kh[kh]\n + F[kh] * dmlct.W_k_kh[kh]\n + F_R[kh] * dmlct.W_R_k_kh[kh]\n )\n\n # n_bar = 4 なら 0,1,2は残す 3,4,5,6,7を書き換える\n F[n_bar - 2 + 1 :] += U_k_n_bar[n_bar - 2 + 1 :]",
"_____no_output_____"
]
],
[
[
"### IDCT",
"_____no_output_____"
]
],
[
[
"for row in range(Fk.shape[0] // N):\n for col in range(Fk.shape[1]):\n F = Fk[N * row : N * (row + 1), col]\n data = scipy.fftpack.idct(F, norm=\"ortho\")\n # 復元画像\n recover[N * row : N * (row + 1), col] = data",
"_____no_output_____"
],
[
"plt.imshow(recover.astype(\"u8\"), cmap=\"gray\")",
"_____no_output_____"
]
],
[
[
"もどった...!",
"_____no_output_____"
],
[
"# 量子化テーブル",
"_____no_output_____"
]
],
[
[
"Q50_Luminance = np.array(\n [\n [16, 11, 10, 16, 24, 40, 51, 61],\n [12, 12, 14, 19, 26, 58, 60, 55],\n [14, 13, 16, 24, 40, 57, 69, 56],\n [14, 17, 22, 29, 51, 87, 80, 62],\n [18, 22, 37, 56, 68, 109, 103, 77],\n [24, 35, 55, 64, 81, 104, 113, 92],\n [49, 64, 78, 87, 103, 121, 120, 101],\n [72, 92, 95, 98, 112, 100, 103, 99],\n ]\n)",
"_____no_output_____"
],
[
"for i in tqdm_notebook(np.arange(1,10000,1)):\n Q = i / 100\n Q_Luminance = np.copy(Q50_Luminance)\n if Q < 50:\n Q_Luminance = Q_Luminance * 50 / Q\n Q_Luminance = np.where(Q_Luminance > 255, 255, Q_Luminance)\n else:\n Q_Luminance = (100 - Q) * Q50_Luminance / 50\n Q_Luminance = np.where(Q_Luminance < 1, 1, Q_Luminance)",
"_____no_output_____"
]
],
[
[
"# 量子化",
"_____no_output_____"
]
],
[
[
"Fk = np.copy(Fk_Ori)\nQ_Fk = np.zeros(Fk.shape)\nfor row in range(IMG.img.shape[0] // N):\n for col in range(IMG.img.shape[1] // N):\n block = Fk[row * N : (row + 1) * N, col * N : (col + 1) * N]\n # 量子化\n block = np.round(block / Q_Luminance)\n # 逆量子化\n block = block * Q_Luminance\n Q_Fk[row * N : (row+1)*N, col * N : (col+1)*N] = block",
"_____no_output_____"
],
[
"Fk = np.copy(Q_Fk)\nQ_recover = np.zeros(Q_Fk.shape)",
"_____no_output_____"
]
],
[
[
"## 横方向",
"_____no_output_____"
],
[
"### 残差",
"_____no_output_____"
]
],
[
[
"for k in range(1, n_bar - 2 + 1):\n dmlct = DMLCT(k+1, N)\n for row in range(IMG.img.shape[0]):\n for col in range(IMG.img.shape[1] // N):\n F = Fk[row, N * col : N * (col + 1)]\n F_L = get_F_L_k_horizontal(Fk, N, row, col)\n F_R = get_F_R_k_horizontal(Fk, N, row, col)\n\n U_k_n_bar = np.zeros(N)\n for kh in range((k + 1) - 2 + 1):\n U_k_n_bar += (\n F_L[kh] * dmlct.W_L_k_kh[kh]\n + F[kh] * dmlct.W_k_kh[kh]\n + F_R[kh] * dmlct.W_R_k_kh[kh]\n )\n\n F[k] += U_k_n_bar[k]",
"_____no_output_____"
],
[
"dmlct = DMLCT(n_bar, N)\nfor row in range(IMG.img.shape[0]):\n for col in range(IMG.img.shape[1] // N):\n F = Fk[row, N * col : N * (col + 1)]\n F_L = get_F_L_k_horizontal(Fk, N, row, col)\n F_R = get_F_R_k_horizontal(Fk, N, row, col)\n\n U_k_n_bar = np.zeros(N)\n for kh in range(n_bar - 2 + 1):\n U_k_n_bar += (\n F_L[kh] * dmlct.W_L_k_kh[kh]\n + F[kh] * dmlct.W_k_kh[kh]\n + F_R[kh] * dmlct.W_R_k_kh[kh]\n )\n\n # n_bar = 4 なら 0,1,2は残す 3,4,5,6,7を書き換える\n F[n_bar - 2 + 1 :] += U_k_n_bar[n_bar - 2 + 1 :]",
"_____no_output_____"
]
],
[
[
"### IDCT",
"_____no_output_____"
]
],
[
[
"for row in range(Fk.shape[0]):\n for col in range(Fk.shape[1] // N):\n F = Fk[row, N * col : N * col + N]\n data = scipy.fftpack.idct(F, norm=\"ortho\")\n # Fkに代入した後、縦方向に対して処理\n Fk[row, N * col : N * col + N] = data",
"_____no_output_____"
]
],
[
[
"## 縦方向",
"_____no_output_____"
],
[
"### 残差",
"_____no_output_____"
]
],
[
[
"for k in range(1, n_bar - 2 + 1):\n dmlct = DMLCT(k+1, N)\n for row in range(IMG.img.shape[0] // N):\n for col in range(IMG.img.shape[1]):\n # ビューなら直接いじっちゃう\n F = Fk[N * row : N * (row + 1), col]\n F_L = get_F_L_k_vertical(Fk, N, row, col)\n F_R = get_F_R_k_vertical(Fk, N, row, col)\n\n U_k_n_bar = np.zeros(N)\n\n for kh in range((k + 1) - 2 + 1):\n U_k_n_bar += (\n F_L[kh] * dmlct.W_L_k_kh[kh]\n + F[kh] * dmlct.W_k_kh[kh]\n + F_R[kh] * dmlct.W_R_k_kh[kh]\n )\n\n F[k] += U_k_n_bar[k]",
"_____no_output_____"
],
[
"dmlct = DMLCT(n_bar, N)\nfor row in range(IMG.img.shape[0] // N):\n for col in range(IMG.img.shape[1]):\n # ビューなら直接いじっちゃう\n F = Fk[N * row : N * (row + 1), col]\n F_L = get_F_L_k_vertical(Fk, N, row, col)\n F_R = get_F_R_k_vertical(Fk, N, row, col)\n\n U_k_n_bar = np.zeros(N)\n for kh in range(n_bar - 2 + 1):\n U_k_n_bar += (\n F_L[kh] * dmlct.W_L_k_kh[kh]\n + F[kh] * dmlct.W_k_kh[kh]\n + F_R[kh] * dmlct.W_R_k_kh[kh]\n )\n\n # n_bar = 4 なら 0,1,2は残す 3,4,5,6,7を書き換える\n F[n_bar - 2 + 1 :] += U_k_n_bar[n_bar - 2 + 1 :]",
"_____no_output_____"
]
],
[
[
"### IDCT",
"_____no_output_____"
]
],
[
[
"for row in range(Fk.shape[0] // N):\n for col in range(Fk.shape[1]):\n F = Fk[N * row : N * (row + 1), col]\n data = scipy.fftpack.idct(F, norm=\"ortho\")\n # 復元画像\n Q_recover[N * row : N * (row + 1), col] = data",
"_____no_output_____"
],
[
"Q_recover = np.round(Q_recover)",
"_____no_output_____"
],
[
"plt.imshow(Q_recover, cmap=\"gray\")\nplt.imsave(\"DMLCT_8x8_n\"+str(n_bar)+ \"_LENNA.png\",Q_recover,cmap=\"gray\")",
"_____no_output_____"
]
],
[
[
"# 情報量",
"_____no_output_____"
]
],
[
[
"qfk = pd.Series(Q_Fk.flatten())\npro = qfk.value_counts() / qfk.value_counts().sum()\npro.head()",
"_____no_output_____"
],
[
"S = 0\nfor pi in pro:\n S -= pi * np.log2(pi)\nS",
"_____no_output_____"
]
],
[
[
"# PSNR",
"_____no_output_____"
]
],
[
[
"MSE = np.sum(np.sum(np.power((IMG.img - Q_recover),2)))/(Q_recover.shape[0] * Q_recover.shape[1])\nPSNR = 10 * np.log10(255 * 255 / MSE)\nPSNR",
"_____no_output_____"
]
],
[
[
"# MSSIM",
"_____no_output_____"
]
],
[
[
"MSSIM = ssim(IMG.img,Q_recover.astype(IMG.img.dtype),gaussian_weights=True,sigma=1.5,K1=0.01,K2=0.03)\nMSSIM",
"_____no_output_____"
],
[
"dmlct = DMLCT(n_bar, N)",
"_____no_output_____"
]
],
[
[
"# MSDS",
"_____no_output_____"
]
],
[
[
"MSDSt, MSDS1, MSDS2 = msds(N,Q_recover)",
"_____no_output_____"
],
[
"MSDS1",
"_____no_output_____"
],
[
"MSDS2",
"_____no_output_____"
]
]
] | [
"code",
"markdown",
"code",
"markdown",
"code",
"markdown",
"code",
"markdown",
"code",
"markdown",
"code",
"markdown",
"code",
"markdown",
"code",
"markdown",
"code",
"markdown",
"code",
"markdown",
"code",
"markdown",
"code",
"markdown",
"code",
"markdown",
"code",
"markdown",
"code",
"markdown",
"code",
"markdown",
"code",
"markdown",
"code",
"markdown",
"code",
"markdown",
"code",
"markdown",
"code",
"markdown",
"code"
] | [
[
"code",
"code"
],
[
"markdown"
],
[
"code",
"code",
"code",
"code",
"code",
"code",
"code",
"code",
"code"
],
[
"markdown",
"markdown",
"markdown"
],
[
"code"
],
[
"markdown"
],
[
"code",
"code"
],
[
"markdown",
"markdown"
],
[
"code"
],
[
"markdown"
],
[
"code",
"code",
"code"
],
[
"markdown"
],
[
"code"
],
[
"markdown"
],
[
"code"
],
[
"markdown",
"markdown"
],
[
"code",
"code"
],
[
"markdown"
],
[
"code"
],
[
"markdown",
"markdown"
],
[
"code",
"code"
],
[
"markdown"
],
[
"code",
"code"
],
[
"markdown",
"markdown"
],
[
"code",
"code"
],
[
"markdown"
],
[
"code",
"code"
],
[
"markdown",
"markdown"
],
[
"code",
"code"
],
[
"markdown"
],
[
"code"
],
[
"markdown",
"markdown"
],
[
"code",
"code"
],
[
"markdown"
],
[
"code",
"code",
"code"
],
[
"markdown"
],
[
"code",
"code"
],
[
"markdown"
],
[
"code"
],
[
"markdown"
],
[
"code",
"code"
],
[
"markdown"
],
[
"code",
"code",
"code"
]
] |
e7763920c1c05943f5a03939b26937ae47899acb | 468,385 | ipynb | Jupyter Notebook | 02 Line charts.ipynb | Bluelord/DataCamp_courses | 647d2fc4f5f691f7c7516ec4da79063efdea2d59 | [
"MIT"
] | null | null | null | 02 Line charts.ipynb | Bluelord/DataCamp_courses | 647d2fc4f5f691f7c7516ec4da79063efdea2d59 | [
"MIT"
] | null | null | null | 02 Line charts.ipynb | Bluelord/DataCamp_courses | 647d2fc4f5f691f7c7516ec4da79063efdea2d59 | [
"MIT"
] | null | null | null | 625.34713 | 137,674 | 0.935747 | [
[
[
"<a href=\"https://colab.research.google.com/github/Bluelord/DataCamp_Courses/blob/main/02%20Line%20charts.ipynb\" target=\"_parent\"><img src=\"https://colab.research.google.com/assets/colab-badge.svg\" alt=\"Open In Colab\"/></a>",
"_____no_output_____"
],
[
"# Line Charts\n\n---\n\n\n\n---\n\n",
"_____no_output_____"
],
[
"## Tutorial\n\n---",
"_____no_output_____"
]
],
[
[
"# mounting drive\nfrom google.colab import drive\ndrive.mount(\"/content/drive\")",
"Mounted at /content/drive\n"
],
[
"# Importing libraries\nimport pandas as pd\npd.plotting.register_matplotlib_converters()\nimport matplotlib.pyplot as plt\n%matplotlib inline\nimport seaborn as sns",
"_____no_output_____"
],
[
"# Loading Data\nspotify_data = pd.read_csv(\"/content/drive/MyDrive/Colab Notebooks/Kaggle_Courses/03 Data Visualization/spotify.csv\",\n index_col=\"Date\", parse_dates=True)\nspotify_data",
"_____no_output_____"
]
],
[
[
"### Plot the data",
"_____no_output_____"
]
],
[
[
"# Sixe of plot\nplt.figure(figsize=(15,8))\n\n# Line chart showing daily global streams of each song \nsns.lineplot(data=spotify_data)",
"_____no_output_____"
]
],
[
[
"As you can see above, the line of code is relatively short and has two main components:\n- `sns.lineplot` tells the notebook that we want to create a line chart. \n - _Every command that you learn about in this course will start with `sns`, which indicates that the command comes from the [seaborn](https://seaborn.pydata.org/) package. For instance, we use `sns.lineplot` to make line charts. Soon, you'll learn that we use `sns.barplot` and `sns.heatmap` to make bar charts and heatmaps, respectively._\n- `data=spotify_data` selects the data that will be used to create the chart.\n\nNote that you will always use this same format when you create a line chart, and **_the only thing that changes with a new dataset is the name of the dataset_**. So, if you were working with a different dataset named `financial_data`, for instance, the line of code would appear as follows:\n```\nsns.lineplot(data=financial_data)\n```\n\nSometimes there are additional details we'd like to modify, like the size of the figure and the title of the chart. Each of these options can easily be set with a single line of code.",
"_____no_output_____"
]
],
[
[
"# Set the width and height of the figure\nplt.figure(figsize=(14,6))\n# Add title\nplt.title(\"Daily Global Streams of Popular Songs in 2017-2018\")\n# Line chart showing daily global streams of each song \nsns.lineplot(data=spotify_data)",
"_____no_output_____"
]
],
[
[
"The first line of code sets the size of the figure to `14` inches (in width) by `6` inches (in height). To set the size of _any figure_, you need only copy the same line of code as it appears. Then, if you'd like to use a custom size, change the provided values of `14` and `6` to the desired width and height.\nThe second line of code sets the title of the figure. Note that the title must *always* be enclosed in quotation marks (`\"...\"`)!\n\n",
"_____no_output_____"
],
[
"### Plot a subset of the data\n\nSo far, you've learned how to plot a line for _every_ column in the dataset. In this section, you'll learn how to plot a _subset_ of the columns.\n\nWe'll begin by printing the names of all columns. This is done with one line of code and can be adapted for any dataset by just swapping out the name of the dataset (in this case, `spotify_data`).",
"_____no_output_____"
]
],
[
[
"list(spotify_data.columns)",
"_____no_output_____"
],
[
"# Set the width and height of the figure\nplt.figure(figsize=(14,6))\n# Add title\nplt.title(\"Daily Global Streams of Popular Songs in 2017-2018\")\n\n# Line chart showing daily global streams of 'Shape of You'\nsns.lineplot(data=spotify_data['Shape of You'], label=\"Shape of You\")\n# Line chart showing daily global streams of 'Despacito'\nsns.lineplot(data=spotify_data['Despacito'], label=\"Despacito\")\n# Add label for horizontal axis\nplt.xlabel(\"Date\")",
"_____no_output_____"
]
],
[
[
"## Exercise \n\n---",
"_____no_output_____"
]
],
[
[
"data = pd.read_csv(\"/content/drive/MyDrive/Colab Notebooks/Kaggle_Courses/03 Data Visualization/museum_visitors.csv\",\n index_col=\"Date\", parse_dates=True)\n\n# Print the last five rows of the data \ndata.tail(5)",
"_____no_output_____"
],
[
"# How many visitors did the Chinese American Museum \n# receive in July 2018? \nca_museum_jul18 = 2620\n# In October 2018, how many more visitors did Avila \n# Adobe receive than the Firehouse Museum?\navila_oct18 = 19280-4622",
"_____no_output_____"
],
[
"# Line chart showing the number of visitors to each museum over time\nplt.figure(figsize = (12,5))\n# Title\nplt.title('Line chart showing the number of visitors')\nsns.lineplot(data=data)",
"_____no_output_____"
],
[
"# Line plot showing the number of visitors to Avila Adobe over time\nplt.figure(figsize = (12,5))\nsns.lineplot(data = data['Avila Adobe'])\nplt.title('number of visitors to Avila Adobe')\nplt.xlabel('Date')\nplt.ylabel('No. of Visitors')\nplt.show()",
"_____no_output_____"
]
],
[
[
"Does Avila Adobe get more visitors:\n\n* in September-February (in LA, the fall and winter months), OR\n* in March-August (in LA, the spring and summer)?\n\nThe line chart generally dips to relatively low values around the early part of each year in December and January, and reaches its highest values in the middle of the year, especially around May and June. Thus, Avila Adobe usually gets more visitors in March-August, Avila Adobe could definitely benefit from hiring more seasonal employees to help with the extra work in March-August.",
"_____no_output_____"
]
]
] | [
"markdown",
"code",
"markdown",
"code",
"markdown",
"code",
"markdown",
"code",
"markdown",
"code",
"markdown"
] | [
[
"markdown",
"markdown",
"markdown"
],
[
"code",
"code",
"code"
],
[
"markdown"
],
[
"code"
],
[
"markdown"
],
[
"code"
],
[
"markdown",
"markdown"
],
[
"code",
"code"
],
[
"markdown"
],
[
"code",
"code",
"code",
"code"
],
[
"markdown"
]
] |
e776411d9ca6863931d4778a5d8133210f413eb8 | 4,783 | ipynb | Jupyter Notebook | nbplot/templates/nbplot-numpy.ipynb | nburrus/nbplot | 017a3448b70ea22104086db4eeff7c6c307ea511 | [
"MIT"
] | 3 | 2021-04-01T21:14:54.000Z | 2021-04-03T00:10:35.000Z | nbplot/templates/nbplot-numpy.ipynb | nburrus/nbplot | 017a3448b70ea22104086db4eeff7c6c307ea511 | [
"MIT"
] | null | null | null | nbplot/templates/nbplot-numpy.ipynb | nburrus/nbplot | 017a3448b70ea22104086db4eeff7c6c307ea511 | [
"MIT"
] | null | null | null | 34.410072 | 135 | 0.560527 | [
[
[
"# Numpy template\n\n* Cells before the `# [[nbplot]] template` are ignored.\n* Cells starting with `# [[nbplot]] ignore` are also ignored.\n\n* Some variables are substituted in every cell:\n * `${root_path}`: the working directory when `nbplot` was called. Input files will be relative to this.\n\n* Some variables are subtituted in the `[[nbplot]] for i,input in enumerate(inputs)` blocks:\n * `${i}`: index of the input in the list\n * `${input.pretty_name}`: truncated path of the file, or 'stdin'\n * `${input.rel_path}`: path of the file relative to the `root_path`, or `stdin`\n * `${input.abs_path_or_io}`: full filepath or StringIO when the data comes from stdin\n * `${input.guessed_sep}`: separator guessed by nbplot for this file. Usually space or comma.",
"_____no_output_____"
]
],
[
[
"# [[nbplot]] template\n# Note: don't change that first line, it tells nbplot that the notebook below is a template\n# This cell will be executed and the metadata dictionary loaded, but not included in the output.\n\ntemplate_metadata = {\n 'name': 'numpy',\n 'format_version': '0.1'\n}",
"_____no_output_____"
],
[
"import io, math, os, sys\nfrom base64 import b64decode # for stdin\nfrom pathlib import Path\n\nimport matplotlib.pyplot as plt\nimport numpy as np\nimport mplcursors # for interactive plot cursors\n\n# Transform x,y into a smooth x,y with splines (similar to gnuplot csplines)\n# Sample usage: ax.plot(*csplines(x,y)) # Don't forget the * to expand the output (x,y) tuple!\ndef csplines(x,y):\n from scipy.interpolate import make_interp_spline\n spl = make_interp_spline(x, y, 3)\n x_smooth = np.linspace(x[0], x[len(x)-1], max(300, len(x)*10)) # at least 10x the number of points\n return x_smooth, spl(x_smooth)\n\n# None will skip multiple spaces, ' ' will not.\ndef np_delim(delim): return None if delim == ' ' else delim",
"_____no_output_____"
]
],
[
[
"## <center> Cheatsheet gnuplot <> matplotlib </center>\n\n|Gnuplot | Matplotlib|\n| :-- | :-- |\n| `with lines` | `default` or `ax.plot(..., '-')` |\n| `with linespoints` | `ax.plot(..., '.-')` |\n| `with points` | `ax.plot(..., '.')` |\n| `smooth csplines` | `ax.plot(*csplines(x,y))` |\n| `using 1:2` | `ax.plot(data[:,0], data[:,1])` |\n| `using 0:1` | `ax.plot(data[:,0])` |",
"_____no_output_____"
]
],
[
[
"# interactive mode by default\n%matplotlib notebook\n#%matplotlib inline\nplt.ioff() # show the figure only at the end to avoid postponing potential loading errors\n\nfig,ax = plt.subplots(figsize=(8,6), num='MyWindow')\n#fig.suptitle('MyPlot')\n#ax.set_title('My Title')\n#ax.set_xlabel('x')\n#ax.set_ylabel('y')\n\nroot_path = Path(\"$root_path\")\n\n# [[nbplot]] for i,input in enumerate(inputs)\nname${i} = \"${input.pretty_name}\"; file_or_io${i} = ${input.abs_path_or_io}\ndata${i} = np.genfromtxt(file_or_io${i}, dtype=float, comments='#', delimiter=np_delim('${input.guessed_sep}'), skip_header=0)\ndisplay(data0[:4])\nx, y = (data${i}[:,0], data${i}[:,1]) if data${i}.ndim > 1 else (np.arange(0,data${i}.shape[0]), data${i})\nax.plot(x, y, label=name${i})\n# [[nbplot]] endfor\n\nax.legend()\nmplcursors.cursor() # enable the cursors, left click to annotate a point, right click to hide it.\nplt.show() # show the plot\nplt.ion(); # restore interactive mode",
"_____no_output_____"
]
]
] | [
"markdown",
"code",
"markdown",
"code"
] | [
[
"markdown"
],
[
"code",
"code"
],
[
"markdown"
],
[
"code"
]
] |
e7764ee707c7faf856ba3855c1715fc66e838fc1 | 21,340 | ipynb | Jupyter Notebook | 04.20-Missing-Category-Imputation-Feature-Engine.ipynb | sri-spirited/feature-engineering-for-ml | 607c376cf92efd0ca9cc0f4f4959f639f793dedc | [
"BSD-3-Clause"
] | null | null | null | 04.20-Missing-Category-Imputation-Feature-Engine.ipynb | sri-spirited/feature-engineering-for-ml | 607c376cf92efd0ca9cc0f4f4959f639f793dedc | [
"BSD-3-Clause"
] | null | null | null | 04.20-Missing-Category-Imputation-Feature-Engine.ipynb | sri-spirited/feature-engineering-for-ml | 607c376cf92efd0ca9cc0f4f4959f639f793dedc | [
"BSD-3-Clause"
] | null | null | null | 27.012658 | 145 | 0.409606 | [
[
[
"## Missing Category Imputation ==> Feature-Engine\n\n\n### What is Feature-Engine\n\nFeature-Engine is an open source python package that I created at the back of this course. \n\n- Feature-Engine includes all the feature engineering techniques described in the course\n- Feature-Engine works like to Scikit-learn, so it is easy to learn\n- Feature-Engine allows you to implement specific engineering steps to specific feature subsets\n- Feature-Engine can be integrated with the Scikit-learn pipeline allowing for smooth model building\n- \n**Feature-Engine allows you to design and store a feature engineering pipeline with bespoke procedures for different variable groups.**\n\n-------------------------------------------------------------------\nFeature-Engine can be installed via pip ==> pip install feature-engine\n\n- Make sure you have installed feature-engine before running this notebook\n\nFor more information visit:\nmy website\n\n## In this demo\n\nWe will use Feature-Engine to perform mean or median imputation using the Ames House Price Dataset.\n\n- To download the dataset visit the lecture **Datasets** in **Section 1** of the course.",
"_____no_output_____"
]
],
[
[
"import pandas as pd\nimport numpy as np\n\nimport matplotlib.pyplot as plt\n\n# to split the datasets\nfrom sklearn.model_selection import train_test_split\nfrom sklearn.pipeline import Pipeline\n\n# from feature-engine\nfrom feature_engine.imputation import CategoricalImputer",
"_____no_output_____"
],
[
"# let's load the dataset with a selected group of variables\n\ncols_to_use = [\n 'BsmtQual', 'FireplaceQu', 'LotFrontage', 'MasVnrArea', 'GarageYrBlt',\n 'SalePrice'\n]\n\ndata = pd.read_csv('../houseprice.csv', usecols=cols_to_use)\ndata.head()",
"_____no_output_____"
],
[
"data.isnull().mean()",
"_____no_output_____"
],
[
"# let's separate into training and testing set\n\n# first drop the target from the feature list\ncols_to_use.remove('SalePrice')\n\nX_train, X_test, y_train, y_test = train_test_split(data[cols_to_use],\n data['SalePrice'],\n test_size=0.3,\n random_state=0)\nX_train.shape, X_test.shape",
"_____no_output_____"
]
],
[
[
"### Feature-Engine captures the categorical variables automatically",
"_____no_output_____"
]
],
[
[
"# we call the imputer from featur- engine\n# we don't need to specify anything \n\nimputer = CategoricalImputer()",
"_____no_output_____"
],
[
"# we fit the imputer\n\nimputer.fit(X_train)",
"_____no_output_____"
],
[
"# we see that the imputer found the categorical variables to\n# impute with the frequent category\n\nimputer.variables",
"_____no_output_____"
]
],
[
[
"**This imputer will replace missing data in categorical variables by 'Missing'**",
"_____no_output_____"
]
],
[
[
"# feature-engine returns a dataframe\n\ntmp = imputer.transform(X_train)\ntmp.head()",
"_____no_output_____"
],
[
"# let's check that the numerical variables don't\n# contain NA any more\n\ntmp[imputer.variables].isnull().mean()",
"_____no_output_____"
]
],
[
[
"## Feature-engine allows you to specify variable groups easily",
"_____no_output_____"
]
],
[
[
"# let's do it imputation but this time\n# and let's do it over 1 of the 2 categorical variables\n\nimputer = CategoricalImputer(variables=['BsmtQual'])\n\nimputer.fit(X_train)",
"_____no_output_____"
],
[
"# now the imputer uses only the variables we indicated\n\nimputer.variables",
"_____no_output_____"
],
[
"# transform data set\n\ntmp = imputer.transform(X_train)\ntmp.head()",
"_____no_output_____"
],
[
"tmp[imputer.variables].isnull().mean()",
"_____no_output_____"
]
],
[
[
"## Feature-engine can be used with the Scikit-learn pipeline",
"_____no_output_____"
]
],
[
[
"# let's check the percentage of NA in each categorical variable\n\nX_train.isnull().mean()",
"_____no_output_____"
]
],
[
[
"- BsmtQual: 0.023 ==> frequent category imputation\n- FirePlaceQu: 0.46 ==> missing category imputation",
"_____no_output_____"
]
],
[
[
"pipe = Pipeline([\n ('imputer_mode', CategoricalImputer(imputation_method='frequent', variables=['BsmtQual'])),\n ('imputer_missing', CategoricalImputer(variables=['FireplaceQu'])),\n])",
"_____no_output_____"
],
[
"pipe.fit(X_train)",
"_____no_output_____"
],
[
"pipe.named_steps['imputer_mode'].variables",
"_____no_output_____"
],
[
"pipe.named_steps['imputer_missing'].variables",
"_____no_output_____"
],
[
"# let's transform the data with the pipeline\ntmp = pipe.transform(X_train)\n\n# let's check null values are gone\ntmp.isnull().mean()",
"_____no_output_____"
]
]
] | [
"markdown",
"code",
"markdown",
"code",
"markdown",
"code",
"markdown",
"code",
"markdown",
"code",
"markdown",
"code"
] | [
[
"markdown"
],
[
"code",
"code",
"code",
"code"
],
[
"markdown"
],
[
"code",
"code",
"code"
],
[
"markdown"
],
[
"code",
"code"
],
[
"markdown"
],
[
"code",
"code",
"code",
"code"
],
[
"markdown"
],
[
"code"
],
[
"markdown"
],
[
"code",
"code",
"code",
"code",
"code"
]
] |
e7765a2d74a4b028fa3f2534c511fa743113ea2f | 4,773 | ipynb | Jupyter Notebook | content/lessons/06/Now-You-Code/NYC1-Number.ipynb | cspelz-su/Final-Project | 01c4703ea69eb1f215800e4b21939e4836bc4c8c | [
"MIT"
] | null | null | null | content/lessons/06/Now-You-Code/NYC1-Number.ipynb | cspelz-su/Final-Project | 01c4703ea69eb1f215800e4b21939e4836bc4c8c | [
"MIT"
] | null | null | null | content/lessons/06/Now-You-Code/NYC1-Number.ipynb | cspelz-su/Final-Project | 01c4703ea69eb1f215800e4b21939e4836bc4c8c | [
"MIT"
] | null | null | null | 29.645963 | 406 | 0.591452 | [
[
[
"# Now You Code 1: Number\n\nIn this now you code we will learn to re-factor a program into a function. This is the most common way to write a function when you are a beginner. *Re-factoring* is the act of re-writing code without changing its functionality. We commonly do re-factoring to improve performance or readability of our code.\n\nThe way you do this is rather simple. First you write a program to solve the problem, then you re-write that program as a function and finally test the function to make sure it works as expected. \n\nThis helps train you to think abstractly about problems, but leverages what you understand currently about programming.\n\n## First we write the program \n\nWrite a program to take an input string and convert to a float. If the string cannot be converted to a float it returns the string \"NaN\" which means \"Not a Number\" We did this first part for you.\n\n## Step 1: Problem Analysis\n\nInputs: Any value\n\nOutputs: whether that value is a number\n\nAlgorithm:\n\n1. input a value\n2. try to convert the value to a number\n3. if you can convert it, print the number\n4. if you cannot print 'NaN' for Not a number.",
"_____no_output_____"
]
],
[
[
"## STEP 2 : Write the program\ntext = input(\"Enter a number: \")\ntry:\n number = float(text)\nexcept ValueError:\n number = \"NaN\"\n \nprint(number)",
"Enter a number: 5\n5.0\n"
]
],
[
[
"## Next we refactor it into a function\n\nComplete this function. It should be similar to the program above, but it should not have any `input()` or `print()` functions as those are reserved for the main program. Functions should take variables as input and return a variable as output. When the function executes, the variables are replaced with actual values. Our function in question takes `text` as input and returns `number` as output.\n",
"_____no_output_____"
]
],
[
[
"# Step 3: write the function\n## Function: Number\n## Argument (input): text value\n## Returns (output): float of text value or \"NaN\"\n\ndef number(text):\n # TODO Write code here\n ",
"_____no_output_____"
]
],
[
[
"## Rewrite the program to use the function\n\nFinally re-write the original program to use the new function. The program now works the same as STEP1 but it now uses a function!",
"_____no_output_____"
]
],
[
[
"## Step 4: write the program from step 2 again, but this time use the function\n\n",
"_____no_output_____"
]
],
[
[
"## Step 3: Questions\n\n1. Can you define a function with the same name more than once?\n2. Can you call a function with the name name more than once?\n3. What is the input to the number function? What is the output?\n",
"_____no_output_____"
],
[
"## Reminder of Evaluation Criteria\n\n1. What the problem attempted (analysis, code, and answered questions) ?\n2. What the problem analysis thought out? (does the program match the plan?)\n3. Does the code execute without syntax error?\n4. Does the code solve the intended problem?\n5. Is the code well written? (easy to understand, modular, and self-documenting, handles errors)",
"_____no_output_____"
]
]
] | [
"markdown",
"code",
"markdown",
"code",
"markdown",
"code",
"markdown"
] | [
[
"markdown"
],
[
"code"
],
[
"markdown"
],
[
"code"
],
[
"markdown"
],
[
"code"
],
[
"markdown",
"markdown"
]
] |
e776716ff7eca9d5dede13d0d89c9a5fe395b648 | 8,447 | ipynb | Jupyter Notebook | notebooks/SelfBuildingModel.ipynb | hammer-mt/VEX-MMM | 414ee95f0876e3a258edece5e455dd0889a0bd66 | [
"MIT"
] | 15 | 2021-09-30T08:42:01.000Z | 2022-03-21T16:00:47.000Z | notebooks/SelfBuildingModel.ipynb | hammer-mt/VEX-MMM | 414ee95f0876e3a258edece5e455dd0889a0bd66 | [
"MIT"
] | null | null | null | notebooks/SelfBuildingModel.ipynb | hammer-mt/VEX-MMM | 414ee95f0876e3a258edece5e455dd0889a0bd66 | [
"MIT"
] | 5 | 2021-10-10T23:45:32.000Z | 2022-03-08T19:20:21.000Z | 33.653386 | 155 | 0.485024 | [
[
[
"# SelfBuildingModel\n[www.vexpower.com](www.vexpower.com)",
"_____no_output_____"
]
],
[
[
"# Set the right folder\n\nimport sys\nimport os\n\nif not os.path.isdir(\"mmm\"):\n module_path = os.path.abspath(os.path.join('..'))\n if module_path not in sys.path:\n sys.path.append(module_path)\n\nimport mmm",
"_____no_output_____"
],
[
"import pandas as pd\npd.set_option('display.float_format', lambda x: '%.3f' % x) # suppress scientific notation\n\n# Load dataset\n\nfile_name = \"GoolyBib-ABT - Sheet1.csv\"\ndata = pd.read_csv('../data/'+file_name)\ndata.head()",
"_____no_output_____"
],
[
"from IPython.display import display\n\nfrom mmm.clean import make_column_index\nfrom mmm.engineer import add_constant\nfrom mmm.select import get_all_X_labels, guess_date_column, guess_y_column, guess_media_columns, backwards_feature_elimination, find_best_feature \nfrom mmm.build import run_regression, create_results_df, create_pred_df\nfrom mmm.validate import calculate_r2\nfrom mmm.display import display_accuracy_chart, save_model, display_contrib_chart, display_decomp_chart\n\n\nclass SelfBuildingModel():\n def __init__(self, file_name):\n # load data\n self.df = pd.read_csv('../data/'+file_name)\n \n # Guess labels\n self.date_label = guess_date_column(self.df)\n make_column_index(self.df, self.date_label)\n add_constant(self.df)\n self.y_label = guess_y_column(self.df)\n self.X_labels = get_all_X_labels(self.df, self.y_label)\n self.media_labels = guess_media_columns(self.df)\n self.base_labels = [l for l in self.X_labels if l not in self.media_labels]\n \n # Set placeholders\n self.coefficients = None\n self.p_values = None\n self.error_label = \"R2\"\n self.error_func = calculate_r2\n self.error_value = None\n self.y_actual = None\n self.y_pred = None\n self.pred_df = None \n \n # Self-build model\n self._ffs()\n \n def _ffs(self):\n self.find()\n y_label, error_value, X_labels, coefficients = self.fit()\n save_model(y_label, error_value, X_labels, coefficients)\n self.show()\n \n def find(self):\n # bfe on base variables\n base_keep = backwards_feature_elimination(self.df, self.y_label, self.base_labels)\n \n # find best adstock and diminishing return rate of each media variable\n best_media_labels = []\n for m in self.media_variables:\n adstock_columns = add_adstocks(self.df, m)\n best_adstock_column = find_best_feature(self.df, self.y_label, adstock_columns, base_keep)\n \n diminishing_columns = add_diminishing_returns(self.df, best_adstock_column)\n \n best_diminishing_column = find_best_feature(self.df, self.y_label, diminishing_columns, base_keep)\n best_media_labels.append(best_diminishing_column)\n \n self.best_X_labels = base_keep + best_media_labels\n \n def fit(self):\n y_actual, y_pred, coefficients, p_values = run_regression(self.df, self.y_label, self.best_X_labels)\n self.y_actual, self.y_pred, self.coefficients, self.p_values = y_actual, y_pred, coefficients, p_values\n \n self.error_value = self.error_func(self.y_actual, self.y_pred)\n \n self.results_df = create_results_df(self.X_labels, self.coefficients, self.p_values)\n \n self.pred_df = create_pred_df(self.df, self.results_df)\n \n return self.y_label, self.error_value, self.X_labels, self.coefficients\n \n def show(self):\n display(self.results_df)\n\n display_accuracy_chart(self.y_actual, self.y_pred, self.y_label, \n accuracy=(self.error_label, self.error_value))\n \n display_contrib_chart(self.pred_df)\n display_decomp_chart(self.pred_df)",
"_____no_output_____"
]
]
] | [
"markdown",
"code"
] | [
[
"markdown"
],
[
"code",
"code",
"code"
]
] |
e776794fdc8909d40e47e9119c697a603937cea0 | 24,442 | ipynb | Jupyter Notebook | 1.yolo2_pytorch_onnx_save_model_v2.ipynb | chenyu36/singleshot6Dpose | 0f5bad42d8a01bf4330b414359d169653d09516a | [
"MIT"
] | null | null | null | 1.yolo2_pytorch_onnx_save_model_v2.ipynb | chenyu36/singleshot6Dpose | 0f5bad42d8a01bf4330b414359d169653d09516a | [
"MIT"
] | null | null | null | 1.yolo2_pytorch_onnx_save_model_v2.ipynb | chenyu36/singleshot6Dpose | 0f5bad42d8a01bf4330b414359d169653d09516a | [
"MIT"
] | null | null | null | 68.083565 | 7,976 | 0.79793 | [
[
[
"# pytorch-yolo2",
"_____no_output_____"
],
[
"ref: https://github.com/longcw/yolo2-pytorch",
"_____no_output_____"
],
[
"### get model",
"_____no_output_____"
]
],
[
[
"from darknet import Darknet",
"_____no_output_____"
],
[
"cfgfile = './cfg/yolo-pose.cfg' \nweightfile = './backup/cargo/model_backup.weights'\nweightfile2 = './backup/cargo/model.weights'",
"_____no_output_____"
],
[
"m = Darknet(cfgfile)\nm2 = Darknet(cfgfile)\nm.load_weights(weightfile)\nm2.load_weights(weightfile2)\nprint('Loading weights from %s... Done!' % (weightfile))\nprint('Loading weights from %s... Done!' % (weightfile2))",
"_____no_output_____"
]
],
[
[
"### save detection information",
"_____no_output_____"
]
],
[
[
"import pickle\nop_dict = {\n 'num_classes':m.num_classes,\n 'anchors':m.anchors,\n 'num_anchors':m.num_anchors\n}\npickle.dump(op_dict, open('detection_information.pkl','wb'))",
"_____no_output_____"
]
],
[
[
"# use Onnx to convert model",
"_____no_output_____"
],
[
"ref: https://github.com/onnx/tutorials/blob/master/tutorials/PytorchOnnxExport.ipynb",
"_____no_output_____"
]
],
[
[
"import torch.onnx\nfrom torch.autograd import Variable\n\n# Standard ImageNet input - 3 channels, 224x224,\n# values don't matter as we care about network structure.\n# But they can also be real inputs.\ndummy_input = Variable(torch.randn(1, 3, 416, 416))\n# Obtain your model, it can be also constructed in your script explicitly\nmodel = m\nmodel2 = m2\n# Invoke export\ntorch.onnx.export(model, dummy_input, \"cargo_yolo2.onnx\")\ntorch.onnx.export(model2, dummy_input, \"cargo_yolo2_v2.onnx\")",
"_____no_output_____"
]
],
[
[
"# Build TensorRT engine and serialize it",
"_____no_output_____"
]
],
[
[
"import cv2\nimport numpy as np\nfrom numpy import array\n\nimport pycuda.driver as cuda\nimport pycuda.autoinit\n\nimport tensorrt as trt\n\nimport sys, os\nsys.path.insert(1, os.path.join(sys.path[0], \"..\"))\nimport common\n\n# You can set the logger severity higher to suppress messages (or lower to display more messages).\nTRT_LOGGER = trt.Logger(trt.Logger.WARNING)",
"_____no_output_____"
],
[
"import matplotlib\nimport matplotlib.pyplot as plt\n%matplotlib inline",
"_____no_output_____"
],
[
"def get_engine(onnx_file_path, engine_file_path=\"\"):\n \"\"\"Attempts to load a serialized engine if available, otherwise builds a new TensorRT engine and saves it.\"\"\"\n def build_engine():\n \"\"\"Takes an ONNX file and creates a TensorRT engine to run inference with\"\"\"\n with trt.Builder(TRT_LOGGER) as builder, builder.create_network() as network, trt.OnnxParser(network, TRT_LOGGER) as parser:\n builder.max_workspace_size = 1 << 30 # 1GB\n builder.max_batch_size = 1\n # Parse model file\n if not os.path.exists(onnx_file_path):\n print('ONNX file {} not found, please run yolov3_to_onnx.py first to generate it.'.format(onnx_file_path))\n exit(0)\n print('Loading ONNX file from path {}...'.format(onnx_file_path))\n with open(onnx_file_path, 'rb') as model:\n print('Beginning ONNX file parsing')\n parser.parse(model.read())\n print('Completed parsing of ONNX file')\n print('Building an engine from file {}; this may take a while...'.format(onnx_file_path))\n engine = builder.build_cuda_engine(network)\n print(\"Completed creating Engine\")\n with open(engine_file_path, \"wb\") as f:\n f.write(engine.serialize())\n return engine\n\n if os.path.exists(engine_file_path):\n # If a serialized engine exists, use it instead of building an engine.\n print(\"Reading engine from file {}\".format(engine_file_path))\n with open(engine_file_path, \"rb\") as f, trt.Runtime(TRT_LOGGER) as runtime:\n return runtime.deserialize_cuda_engine(f.read())\n else:\n return build_engine()",
"_____no_output_____"
],
[
"# Try to load a previously generated yolo network graph in ONNX format:\nonnx_file_path = './cargo_yolo2.onnx'\nonnx_file_v2_path = './cargo_yolo2_v2.onnx'\nengine_file_path = './cargo_yolo2.trt'\nengine_file_v2_path = './cargo_yolo2_v2.trt'\ninput_image_path = './cargo_sample.jpg'",
"_____no_output_____"
],
[
"def preprosess_img(img_path):\n frame = cv2.imread(img_path,0)\n img = cv2.cvtColor(frame, cv2.COLOR_BGR2RGB)\n yolo_img =cv2.resize(img, (416, 416), interpolation=cv2.INTER_AREA)\n plt.imshow(img)\n return yolo_img",
"_____no_output_____"
],
[
"# Do inference with TensorRT\ntrt_outputs = []\nwith get_engine(onnx_file_v2_path, engine_file_v2_path) as engine, engine.create_execution_context() as context:\n inputs, outputs, bindings, stream = common.allocate_buffers(engine)\n print(type(engine))\n img = preprosess_img(input_image_path)\n # Do inference\n print('Running inference on image {}...'.format(input_image_path))\n # Set host input to the image. The common.do_inference function will copy the input to the GPU before executing.\n inputs[0].host = img\n trt_outputs = common.do_inference(context, bindings=bindings, inputs=inputs, outputs=outputs, stream=stream)\n print(trt_outputs)\n print(type(trt_outputs))",
"Reading engine from file ./cargo_yolo2_v2.trt\n<class 'tensorrt.tensorrt.ICudaEngine'>\nRunning inference on image ./cargo_sample.jpg...\n[array([ -inf, -inf, -inf, ..., -250.57047 ,\n -136.78687 , -2.5162568], dtype=float32)]\n<class 'list'>\n"
],
[
"plt.imshow(img)",
"_____no_output_____"
],
[
"output_shapes = [(1,20,13,13)]\n#trt_outputs = [output.reshape(shape) for output, shape in zip(trt_outputs, output_shapes)]\ntrt_outputs = array(trt_outputs).reshape(1,20,13,13)\n# print('trt_outputs type', type(trt_outputs))",
"_____no_output_____"
],
[
"print('trt outputs shape ', trt_outputs.shape)",
"trt outputs shape (1, 20, 13, 13)\n"
]
]
] | [
"markdown",
"code",
"markdown",
"code",
"markdown",
"code",
"markdown",
"code"
] | [
[
"markdown",
"markdown",
"markdown"
],
[
"code",
"code",
"code"
],
[
"markdown"
],
[
"code"
],
[
"markdown",
"markdown"
],
[
"code"
],
[
"markdown"
],
[
"code",
"code",
"code",
"code",
"code",
"code",
"code",
"code",
"code"
]
] |
e7767e72fde08c382e63b118437aeb0b38accf1f | 11,104 | ipynb | Jupyter Notebook | notebooks/20 Feature Selection.ipynb | jlgorman/scipy-2016-sklearn | 39568d726e0676fde2a6a53e99d71368868934e7 | [
"CC0-1.0"
] | 23 | 2016-04-09T17:30:53.000Z | 2022-03-25T04:31:25.000Z | notebooks/20 Feature Selection.ipynb | jlgorman/scipy-2016-sklearn | 39568d726e0676fde2a6a53e99d71368868934e7 | [
"CC0-1.0"
] | null | null | null | notebooks/20 Feature Selection.ipynb | jlgorman/scipy-2016-sklearn | 39568d726e0676fde2a6a53e99d71368868934e7 | [
"CC0-1.0"
] | 27 | 2016-04-12T22:02:47.000Z | 2020-08-15T14:05:53.000Z | 31.278873 | 483 | 0.634096 | [
[
[
"import matplotlib.pyplot as plt\nimport numpy as np\n%matplotlib inline",
"_____no_output_____"
]
],
[
[
"## Automatic Feature Selection\nOften we collected many features that might be related to a supervised prediction task, but we don't know which of them are actually predictive. To improve interpretability, and sometimes also generalization performance, we can use automatic feature selection to select a subset of the original features. There are several types of feature selection methods available, which we'll explain in order of increasing complexity.\n\nFor a given supervised model, the best feature selection strategy would be to try out each possible subset of the features, and evaluate generalization performance using this subset. However, there are exponentially many subsets of features, so this exhaustive search is generally infeasible. The strategies discussed below can be thought of as proxies for this infeasible computation.\n\n### Univariate statistics\nThe simplest method to select features is using univariate statistics, that is by looking at each feature individually and running a statistical test to see whether it is related to the target. This kind of test is also known as analysis of variance (ANOVA).\n\nWe create a synthetic dataset that consists of the breast cancer data, with an addition of 50 completely random features.",
"_____no_output_____"
]
],
[
[
"from sklearn.datasets import load_breast_cancer, load_digits\nfrom sklearn.model_selection import train_test_split\n\ncancer = load_breast_cancer()\n\n# get deterministic random numbers\nrng = np.random.RandomState(42)\nnoise = rng.normal(size=(len(cancer.data), 50))\n# add noise features to the data\n# the first 30 features are from the dataset, the next 50 are noise\nX_w_noise = np.hstack([cancer.data, noise])\n\nX_train, X_test, y_train, y_test = train_test_split(\n X_w_noise, cancer.target, random_state=0, test_size=.5)\n",
"_____no_output_____"
]
],
[
[
"We have to define a threshold on the p-value of the statistical test to decide how many features to keep. There are several strategies implemented in scikit-learn, a straight-forward one being ``SelectPercentile``, which selects a percentile of the original features (we select 50% below):",
"_____no_output_____"
]
],
[
[
"from sklearn.feature_selection import SelectPercentile\n\n# use f_classif (the default) and SelectPercentile to select 50% of features:\nselect = SelectPercentile(percentile=50)\nselect.fit(X_train, y_train)\n# transform training set:\nX_train_selected = select.transform(X_train)\n\nprint(X_train.shape)\nprint(X_train_selected.shape)",
"_____no_output_____"
]
],
[
[
"We can also use the test statistic directly to see how relevant each feature is. As the breast cancer dataset is a classification task, we use f_classif, the F-test for classification. Below we plot the p-values associated with each of the 80 features (30 original features + 50 noise features). Low p-values indicate informative features.",
"_____no_output_____"
]
],
[
[
"from sklearn.feature_selection import f_classif, f_regression, chi2",
"_____no_output_____"
],
[
"F, p = f_classif(X_train, y_train)",
"_____no_output_____"
],
[
"plt.figure()\nplt.plot(p, 'o')",
"_____no_output_____"
]
],
[
[
"Clearly most of the first 30 features have very small p-values.\n\nGoing back to the SelectPercentile transformer, we can obtain the features that are selected using the ``get_support`` method:",
"_____no_output_____"
]
],
[
[
"mask = select.get_support()\nprint(mask)\n# visualize the mask. black is True, white is False\nplt.matshow(mask.reshape(1, -1), cmap='gray_r')",
"_____no_output_____"
]
],
[
[
"Nearly all of the original 30 features were recovered.\nWe can also analize the utility of the feature selection by training a supervised model on the data.\nIt's important to learn the feature selection only on the training set!",
"_____no_output_____"
]
],
[
[
"from sklearn.linear_model import LogisticRegression\n\n# transform test data:\nX_test_selected = select.transform(X_test)\n\nlr = LogisticRegression()\nlr.fit(X_train, y_train)\nprint(\"Score with all features: %f\" % lr.score(X_test, y_test))\nlr.fit(X_train_selected, y_train)\nprint(\"Score with only selected features: %f\" % lr.score(X_test_selected, y_test))",
"_____no_output_____"
]
],
[
[
"### Model-based Feature Selection\nA somewhat more sophisticated method for feature selection is using a supervised machine learning model, and selecting features based on how important they were deemed by the model. This requires the model to provide some way to rank the features by importance. This can be done for all tree-based models (which implement ``get_feature_importances``) and all linear models, for which the coefficients can be used to determine how much influence a feature has on the outcome.\n\nAny of these models can be made into a transformer that does feature selection by wrapping it with the ``SelectFromModel`` class:",
"_____no_output_____"
]
],
[
[
"from sklearn.feature_selection import SelectFromModel\nfrom sklearn.ensemble import RandomForestClassifier\nselect = SelectFromModel(RandomForestClassifier(n_estimators=100, random_state=42), threshold=\"median\")",
"_____no_output_____"
],
[
"select.fit(X_train, y_train)\nX_train_rf = select.transform(X_train)\nprint(X_train.shape)\nprint(X_train_rf.shape)",
"_____no_output_____"
],
[
"mask = select.get_support()\n# visualize the mask. black is True, white is False\nplt.matshow(mask.reshape(1, -1), cmap='gray_r')",
"_____no_output_____"
],
[
"X_test_rf = select.transform(X_test)\nLogisticRegression().fit(X_train_rf, y_train).score(X_test_rf, y_test)",
"_____no_output_____"
]
],
[
[
"This method builds a single model (in this case a random forest) and uses the feature importances from this model.\nWe can do a somewhat more elaborate search by training multiple models on subsets of the data. One particular strategy is recursive feature elimination:",
"_____no_output_____"
],
[
"### Recursive Feature Elimination\nRecursive feature elimination builds a model on the full set of features, and similar to the method above selects a subset of features that are deemed most important by the model. However, usually only a single feature is dropped from the dataset, and a new model is build on the remaining features. The process of dropping features and model building is repeated until there are only a pre-specified number of features left:",
"_____no_output_____"
]
],
[
[
"from sklearn.feature_selection import RFE\nselect = RFE(RandomForestClassifier(n_estimators=100, random_state=42), n_features_to_select=40)\n\nselect.fit(X_train, y_train)\n# visualize the selected features:\nmask = select.get_support()\nplt.matshow(mask.reshape(1, -1), cmap='gray_r')",
"_____no_output_____"
],
[
"X_train_rfe = select.transform(X_train)\nX_test_rfe = select.transform(X_test)\n\nLogisticRegression().fit(X_train_rfe, y_train).score(X_test_rfe, y_test)",
"_____no_output_____"
],
[
"select.score(X_test, y_test)",
"_____no_output_____"
]
],
[
[
"# Exercises",
"_____no_output_____"
],
[
"Plot the \"XOR\" dataset which is created like this:",
"_____no_output_____"
]
],
[
[
"xx, yy = np.meshgrid(np.linspace(-3, 3, 50),\n np.linspace(-3, 3, 50))\nrng = np.random.RandomState(0)\nX = rng.randn(200, 2)\nY = np.logical_xor(X[:, 0] > 0, X[:, 1] > 0)",
"_____no_output_____"
]
],
[
[
"Add random features to it and compare how univariate selection compares to model based selection using a Random Forest in recovering the original features.",
"_____no_output_____"
]
]
] | [
"code",
"markdown",
"code",
"markdown",
"code",
"markdown",
"code",
"markdown",
"code",
"markdown",
"code",
"markdown",
"code",
"markdown",
"code",
"markdown",
"code",
"markdown"
] | [
[
"code"
],
[
"markdown"
],
[
"code"
],
[
"markdown"
],
[
"code"
],
[
"markdown"
],
[
"code",
"code",
"code"
],
[
"markdown"
],
[
"code"
],
[
"markdown"
],
[
"code"
],
[
"markdown"
],
[
"code",
"code",
"code",
"code"
],
[
"markdown",
"markdown"
],
[
"code",
"code",
"code"
],
[
"markdown",
"markdown"
],
[
"code"
],
[
"markdown"
]
] |
e776865fb9744ba5ea3612a9640aebbfa0bef666 | 102,905 | ipynb | Jupyter Notebook | Notebooks/custom_NER_training_notebook.ipynb | streamlit-badge-bot/Resume-analyzer | 51f50be0a712e4c064689f50407dbb6678654ca6 | [
"MIT"
] | 3 | 2020-11-12T08:33:08.000Z | 2021-06-24T04:39:03.000Z | Notebooks/custom_NER_training_notebook.ipynb | streamlit-badge-bot/Resume-analyzer | 51f50be0a712e4c064689f50407dbb6678654ca6 | [
"MIT"
] | null | null | null | Notebooks/custom_NER_training_notebook.ipynb | streamlit-badge-bot/Resume-analyzer | 51f50be0a712e4c064689f50407dbb6678654ca6 | [
"MIT"
] | 4 | 2020-11-28T19:01:27.000Z | 2022-03-19T07:28:18.000Z | 92.374327 | 4,101 | 0.66402 | [
[
[
"import spacy\nimport pickle\nimport random",
"_____no_output_____"
],
[
"train_data = pickle.load(open('train_data.pkl', 'rb'))\ntrain_data[0]",
"_____no_output_____"
],
[
"nlp = spacy.blank('en')\n\ndef train_model(train_data):\n if 'ner' not in nlp.pipe_names:\n ner = nlp.create_pipe('ner')\n nlp.add_pipe(ner, last = True)\n \n for _, annotation in train_data:\n for ent in annotation['entities']:\n ner.add_label(ent[2])\n \n \n other_pipes = [pipe for pipe in nlp.pipe_names if pipe != 'ner']\n with nlp.disable_pipes(*other_pipes): # only train NER\n optimizer = nlp.begin_training()\n for itn in range(100):\n print(\"Statring iteration \" + str(itn))\n random.shuffle(train_data)\n losses = {}\n index = 0\n for text, annotations in train_data:\n try:\n nlp.update(\n [text], # batch of texts\n [annotations], # batch of annotations\n drop=0.2, # dropout - make it harder to memorise data\n sgd=optimizer, # callable to update weights\n losses=losses)\n except Exception as e:\n pass\n \n print(losses)\n \n ",
"_____no_output_____"
],
[
"train_model(train_data)",
"D:\\Users\\USER\\Anaconda3\\lib\\site-packages\\spacy\\language.py:639: UserWarning: [W033] Training a new parser or NER using a model with no lexeme normalization table. This may degrade the performance of the model to some degree. If this is intentional or the language you're using doesn't have a normalization table, please ignore this warning. If this is surprising, make sure you have the spacy-lookups-data package installed. The languages with lexeme normalization tables are currently: da, de, el, en, id, lb, pt, ru, sr, ta, th.\n **kwargs\n"
],
[
"import fitz\ndoc = fitz.open(r'D:/Documents/GSoC/resumes/dhmkrwtksdgy.pdf')\ntext = \"\"\n\nfor page in doc:\n text = text + str(page.getText())\n\ntxt = \" \".join(text.split(\"\\n\"))\ntype(txt)",
"_____no_output_____"
],
[
"nlp.to_disk('resume_sum1')",
"_____no_output_____"
],
[
"!pwd",
"/d/Documents/My_projects\n"
],
[
"model = spacy.load('resume_sum1')",
"_____no_output_____"
],
[
"doc = model(txt)\nfor ent in doc.ents:\n print(ent.label_.upper(), ent.text)",
"NAME Anubhav Singh\nCOLLEGE NAME Subhash Engineering College Kolkata\nCOMPANIES WORKED AT Information Technology\nLOCATION Machine\nSKILLS Languages: Python, PHP, C++, JavaScript, SQL, Bash, JAVA • Frameworks: Scikit, NLTK, SpaCy, TensorFlow, Keras, Django, Flask, NodeJS, LAMP • Tools: Kubernetes, Docker, GIT, PostgreSQL, MySQL, SQLite • Platforms: Linux, Web, Windows, Arduino, Raspberry, AWS, GCP, Alibaba Cloud, IBM Cloud • Soft Skills: Leadership, Event Management, Writing, Public Speaking, Time Management Experience • Google Summer of Code - Submitty Remote Student Developer (Full-time) May 2019 - Sep 2019 ◦ Discussion Forum Upgrades: Refactor forum for performance to handle large databases. ◦ REST\nGRADUATION YEAR 2018\nLOCATION Kolkata\n"
],
[
"doc.ents",
"_____no_output_____"
],
[
"doc.ents[1]",
"_____no_output_____"
],
[
"print(\"ent.label_.upper()\" + \" ent.text\")",
"ent.label_.upper() ent.text\n"
],
[
"type(doc.ents)",
"_____no_output_____"
],
[
"cv1 = \"Alice Clark AI / Machine Learning Delhi, India Email me on Indeed • 20+ years of experience in data handling, design, and development • Data Warehouse: Data analysis, star/snow flake scema data modelling and design specific to data warehousing and business intelligence • Database: Experience in database designing, scalability, back-up and recovery, writing and optimizing SQL code and Stored Procedures, creating functions, views, triggers and indexes. Cloud platform: Worked on Microsoft Azure cloud services like Document DB, SQL Azure, Stream Analytics, Event hub, Power BI, Web Job, Web App, Power BI, Azure data lake analytics(U-SQL) Willing to relocate anywhere WORK EXPERIENCE Software Engineer Microsoft – Bangalore, Karnataka January 2000 to Present 1. Microsoft Rewards Live dashboards: Description: - Microsoft rewards is loyalty program that rewards Users for browsing and shopping online. Microsoft Rewards members can earn points when searching with Bing, browsing with Microsoft Edge and making purchases at the Xbox Store, the Windows Store and the Microsoft Store. Plus, user can pick up bonus points for taking daily quizzes and tours on the Microsoft rewards website. Rewards live dashboards gives a live picture of usage world-wide and by markets like US, Canada, Australia, new user registration count, top/bottom performing rewards offers, orders stats and weekly trends of user activities, orders and new user registrations. the PBI tiles gets refreshed in different frequencies starting from 5 seconds to 30 minutes. Technology/Tools used EDUCATION Indian Institute of Technology – Mumbai 2001 SKILLS Machine Learning, Natural Language Processing, and Big Data Handling ADDITIONAL INFORMATION Professional Skills • Excellent analytical, problem solving, communication, knowledge transfer and interpersonal skills with ability to interact with individuals at all the levels • Quick learner and maintains cordial relationship with project manager and team members and good performer both in team and independent job environments • Positive attitude towards superiors & peers • Supervised junior developers throughout project lifecycle and provided technical assistance \"",
"_____no_output_____"
],
[
"doc = model(cv1)\nfor ent in doc.ents:\n print(f'{ent.label_.upper():{30}}- {ent.text}')",
"NAME - Alice Clark \nCOMPANIES WORKED AT - Microsoft\nDESIGNATION - Software Engineer\nCOMPANIES WORKED AT - Microsoft\nCOMPANIES WORKED AT - Microsoft\nCOMPANIES WORKED AT - Microsoft\nCOMPANIES WORKED AT - Microsoft\nDEGREE - Indian Institute of Technology – Mumbai\nSKILLS - Machine Learning, Natural Language Processing, and Big Data Handling ADDITIONAL INFORMATION\n"
],
[
"a",
"_____no_output_____"
],
[
"import re\nimport spacy\nimport os\nfrom flask import Flask, request, redirect, url_for, render_template, send_from_directory\nimport fitz\nfrom werkzeug.utils import secure_filename\nimport pickle\nimport nltk \nimport numpy as np #for large and multi-dimensional arrays\nimport pandas as pd\nfrom nltk.corpus import stopwords\nfrom sklearn.ensemble import RandomForestClassifier\nfrom nltk.stem.porter import PorterStemmer\nfrom sklearn.feature_extraction.text import TfidfVectorizer \nimport pdb\n\n#r'D:/Documents/GSoC/resumes/dhmkrwtksdgy.pdf'\npath = r'D:/Documents/GSoC/resumes'\nfilename = 'dhmkrwtksdgy.pdf'\n\n\n\ndef red_pdf(path,filename):\n doc = fitz.open((os.path.join(path,filename)))\n text = \"\"\n \n for pages in doc:\n text = text + str(pages.getText())\n txt = \" \".join(text.split(\"\\n\"))\n return txt\n \n\n\ndef gen_test_data_for_pred(path,filename):\n test = red_pdf(path,filename)\n snow = nltk.stem.SnowballStemmer('english')\n corpus_test = []\n # for i in range(0, len(df)):\n review = re.sub('[^a-zA-Z]', ' ', test)\n review = review.lower()\n review = review.split()\n \n review = [snow.stem(word) for word in review if not word in stopwords.words('english')]\n review = ' '.join(review)\n corpus_test.append(review)\n\n final_tf_test = corpus_test\n # tf_idf = TfidfVectorizer(ngram_range=(1,2),max_features=5000)\n tf_idf = pickle.load(open('tfidf_vectorizer.pkl','rb'))\n test_data = tf_idf.transform(final_tf_test)\n # tf_data_test.get_shape()\n return test_data\n\n \ndef prediction(path,filename):\n clf_model = pickle.load(open('rf_score_model.pkl','rb'))\n result = clf_model.predict(gen_test_data_for_pred(path,filename))\n return result\n \n \ndef custom_NER(path,filename):\n model = spacy.load('resume_sum')\n txt=red_pdf(path,filename)\n \n# snow = nltk.stem.SnowballStemmer('english')\n# corpus_test_ner = []\n# review = re.sub('[^a-zA-Z]', ' ', txt)\n# review = review.lower()\n# review = review.split()\n \n# review = [snow.stem(word) for word in review if not word in stopwords.words('english')]\n# review = ' '.join(review)\n# corpus_test_ner.append(review)\n# corpus_test_ner = str(corpus_test_ner)\n doc = model(txt)\n abc = doc.ents\n return abc\n \n \n \n \n \n \n \n \nif __name__=='__main__':\n txt = red_pdf(path,filename)\n print(type(txt))\n print(txt)\n# s = custom_NER(path,filename)\n# lists = []\n# s=list(s)\n# for i in range(len(s)):\n# lists.append(str(s[i]))\n# print(tuple(lists))\n# # s[i] = str(s[i])\n \n\n# # print(type(s[i]))\n \n ",
"<class 'str'>\nAnubhav Singh Email: [email protected] Portfolio: xprilion.com Mobile: +91-700-3944-046 Github: github.com/xprilion Education • Netaji Subhash Engineering College Kolkata, India Bachelor of Technology - Information Technology; GPA: 7.27 July 2016 - June 2020 Courses: Operating Systems, Data Structures, Analysis Of Algorithms, Artificial Intelligence, Machine Learning, Networking, Databases Skills Summary • Languages: Python, PHP, C++, JavaScript, SQL, Bash, JAVA • Frameworks: Scikit, NLTK, SpaCy, TensorFlow, Keras, Django, Flask, NodeJS, LAMP • Tools: Kubernetes, Docker, GIT, PostgreSQL, MySQL, SQLite • Platforms: Linux, Web, Windows, Arduino, Raspberry, AWS, GCP, Alibaba Cloud, IBM Cloud • Soft Skills: Leadership, Event Management, Writing, Public Speaking, Time Management Experience • Google Summer of Code - Submitty Remote Student Developer (Full-time) May 2019 - Sep 2019 ◦ Discussion Forum Upgrades: Refactor forum for performance to handle large databases. ◦ REST API for Discussion Forum: Symphony & Twig based Forum parts converted to API-first interface. ◦ Ratchet PHP WebSocket: Implemented a WebSocket for low-latency real time exchange of posts and thread updates. • DataCamp Inc. Remote Instructor (Part-time, Contractual) Dec 2018 - Present ◦ Project Course - Find Movie Similarity from Plot Summaries: Created project based course using Unsupervised learning and natural language processing. ◦ Tutorial - Introduction to Reinforcement Learning: Created tutorial for Q-learning RL algorithm and concepts. ◦ Impact: Course has been taken by 250+ students so far with 4.65 average rating. Projects • Vison - multimedia search engine (NLP, Search Engine, Web Crawlers, Multimedia Processing): (Work in progress) Research oriented, open source, search engine for bringing reverse multimedia search to small & mid scale enterprises. Tech: Python, NodeJS, Intel OpenVino Toolkit, Selenium, TensorFlow (October ’18) • Reinforcement Learning based Traffic Control System (Reinforcement Learning, Computer Vision): AI model to resolve city traffic around 50% faster. Tech: Python, Alibaba Cloud, Raspberry Pi, Arduino, SUMO & OpenCV. (August ’18) • Panorama from Satellite Imagery using Distributed Computing (Distributed Computing, Image Processing): Images clicked using drones, provided by ISRO were stitched together using distributed public compute nodes, effectively bringing down processing time exponentially. Tech: PHP, C++, Java, Python (March ’18) • Drag-n-drop machine learning learning environment (Web Development, Machine Learning): Scratch like tool for implementing machine learning pipelines along with built in tutorial for each concept. Tech: Python, JavaScript (September ’18) • Search Engine and Social Network(Web Development, Web Crawler, Search): Created from scratch a social network and a search engine based on the idea of integrating Facebook and Google. The launched website was among top 1000 websites in India during 2012-2013. Tech: PHP, MySQL, HTML, CSS, WebSockets, JavaScript, RSS, XML ( May ’12) Publications • Book: Deep Learning on Web (Web Development, Deep Learning): Work in Progress book to be published by Packt Publishing in late 2019. Tech: Django, Python, AWS, GCP, Azure (November ’18) • Book: Deep Learning on Mobile Devices (Flutter App Development, Deep Learning): Work in Progress book to be published by Packt Publishing in late 2019. Tech: Flutter, Android, Firebase, TensorFlow, Python, Dart (December ’18) Honors and Awards • Awarded title of Intel Software Innovator - May, 2019 • Second Runner’s Up at TCS EngiNx Engineering Project Innovation Content - September, 2018 • Runner’s Up at Facebook Developers Circle Hackathon - August, 2017 Volunteer Experience • Community Lead at Developer Student Clubs NSEC Kolkata, India Conducted online and offline technical & soft-skills training impacting over 3000 students. Jan 2019 - Present • Event Organizer at Google Developers Group Kolkata Kolkata, India Organized events, conducted workshops and delivered workshops reaching over 7000 developers. Jan 2018 - Present \n"
],
[
"os.path.join(path, filename)",
"_____no_output_____"
],
[
"absja=('Anubhav Singh', 'Netaji Subhash Engineering', 'Kolkata', 'Community Lead')\n",
"_____no_output_____"
],
[
"absja='ada'\nprint(absja)",
"ada\n"
],
[
"len(Anubhav Singh, Netaji Subhash Engineering, Kolkata, Community Lead)",
"_____no_output_____"
]
]
] | [
"code"
] | [
[
"code",
"code",
"code",
"code",
"code",
"code",
"code",
"code",
"code",
"code",
"code",
"code",
"code",
"code",
"code",
"code",
"code",
"code",
"code",
"code",
"code"
]
] |
e7768ed727d53019d675da3abab943a663c19181 | 26,390 | ipynb | Jupyter Notebook | 001 - Feature Engineering/.ipynb_checkpoints/Titanic_Categorical_Enconding-checkpoint.ipynb | howardroatti/Machine_Learning | 702f03f8e71ac2e0af16dee12cbdbcc9688c5fe5 | [
"MIT"
] | null | null | null | 001 - Feature Engineering/.ipynb_checkpoints/Titanic_Categorical_Enconding-checkpoint.ipynb | howardroatti/Machine_Learning | 702f03f8e71ac2e0af16dee12cbdbcc9688c5fe5 | [
"MIT"
] | null | null | null | 001 - Feature Engineering/.ipynb_checkpoints/Titanic_Categorical_Enconding-checkpoint.ipynb | howardroatti/Machine_Learning | 702f03f8e71ac2e0af16dee12cbdbcc9688c5fe5 | [
"MIT"
] | null | null | null | 35.47043 | 1,596 | 0.451156 | [
[
[
"import pandas as pd\nimport numpy as np\nimport matplotlib.pyplot as plt\n%matplotlib inline",
"_____no_output_____"
],
[
"df_train = pd.read_csv(\"train.csv\")\ndf_test = pd.read_csv(\"test.csv\")",
"_____no_output_____"
],
[
"df_train.info()",
"<class 'pandas.core.frame.DataFrame'>\nRangeIndex: 891 entries, 0 to 890\nData columns (total 12 columns):\nPassengerId 891 non-null int64\nSurvived 891 non-null int64\nPclass 891 non-null int64\nName 891 non-null object\nSex 891 non-null object\nAge 714 non-null float64\nSibSp 891 non-null int64\nParch 891 non-null int64\nTicket 891 non-null object\nFare 891 non-null float64\nCabin 204 non-null object\nEmbarked 889 non-null object\ndtypes: float64(2), int64(5), object(5)\nmemory usage: 83.6+ KB\n"
],
[
"df_train.drop(labels=['Ticket', 'Cabin', 'Name'], inplace=True, axis=1)\ndf_test.drop(labels=['Ticket', 'Cabin', 'Name'], inplace=True, axis=1)\n\ndf_train.dropna(subset=['Embarked'], inplace=True)#Exists value nan\ndf_test.dropna(subset=['Embarked'], inplace=True)",
"_____no_output_____"
],
[
"lista = (df_train.dtypes == 'object')\nobject_cols = list(lista[lista].index)\n\nprint(\"Variáveis Categóricas:\")\nprint(object_cols)",
"Variáveis Categóricas:\n['Sex', 'Embarked']\n"
],
[
"df_train.fillna(value={'Age': df_train['Age'].median()}, inplace=True)\ndf_test.fillna(value={'Age': df_test['Age'].median()}, inplace=True)\n\ndf_test.fillna(value={'Fare': df_test['Fare'].median()}, inplace=True)",
"_____no_output_____"
],
[
"df_train.info()",
"<class 'pandas.core.frame.DataFrame'>\nInt64Index: 889 entries, 0 to 890\nData columns (total 9 columns):\nPassengerId 889 non-null int64\nSurvived 889 non-null int64\nPclass 889 non-null int64\nSex 889 non-null object\nAge 889 non-null float64\nSibSp 889 non-null int64\nParch 889 non-null int64\nFare 889 non-null float64\nEmbarked 889 non-null object\ndtypes: float64(2), int64(5), object(2)\nmemory usage: 69.5+ KB\n"
],
[
"from sklearn.ensemble import RandomForestClassifier\nfrom sklearn.model_selection import train_test_split\nfrom sklearn import metrics\n\nX = df_train[['Sex', 'Pclass', 'SibSp', 'Embarked', 'PassengerId', 'Age', 'Parch', 'Fare']]\ny = df_train['Survived']\n\nX_train, X_test, y_train, y_test = train_test_split(X, y, test_size=0.33, random_state=42)\n\nclassifier = RandomForestClassifier(n_jobs=4, random_state=12)",
"C:\\Anaconda3\\lib\\site-packages\\sklearn\\ensemble\\weight_boosting.py:29: DeprecationWarning: numpy.core.umath_tests is an internal NumPy module and should not be imported. It will be removed in a future NumPy release.\n from numpy.core.umath_tests import inner1d\n"
],
[
"def score_dataset(X_train, X_valid, y_train, y_valid):\n classifier.fit(X_train, y_train)\n predicoes = classifier.predict(X_valid) \n print(metrics.classification_report(y_test, predicoes, target_names=['No', 'Yes']))",
"_____no_output_____"
],
[
"drop_X_train = X_train.select_dtypes(exclude=['object'])\ndrop_X_valid = X_test.select_dtypes(exclude=['object'])\n\nprint(\"Approach 1 (Drop categorical variables):\")\nprint(score_dataset(drop_X_train, drop_X_valid, y_train, y_test))",
"Approach 1 (Drop categorical variables):\n precision recall f1-score support\n\n No 0.71 0.79 0.75 184\n Yes 0.56 0.45 0.50 110\n\navg / total 0.65 0.66 0.65 294\n\nNone\n"
],
[
"from sklearn.preprocessing import LabelEncoder\n\n# Make copy to avoid changing original data \nlabel_X_train = X_train.copy()\nlabel_X_valid = X_test.copy()\n\n# Apply label encoder to each column with categorical data\nlabel_encoder = LabelEncoder()\nfor col in object_cols:\n label_X_train[col] = label_encoder.fit_transform(X_train[col])\n label_X_valid[col] = label_encoder.transform(X_test[col])\n\nprint(\"Approach 2 (Label Encoding):\") \nprint(score_dataset(label_X_train, label_X_valid, y_train, y_test))",
"Approach 2 (Label Encoding):\n precision recall f1-score support\n\n No 0.81 0.87 0.84 184\n Yes 0.75 0.65 0.70 110\n\navg / total 0.79 0.79 0.79 294\n\nNone\n"
],
[
"X_train[object_cols]",
"_____no_output_____"
],
[
"from sklearn.preprocessing import OneHotEncoder\n\n# Apply one-hot encoder to each column with categorical data\nOH_encoder = OneHotEncoder(handle_unknown='ignore', sparse=False, categories='auto')\n#OH_cols_train = pd.DataFrame(OH_encoder.fit_transform(X_train[object_cols]))\n#OH_cols_valid = pd.DataFrame(OH_encoder.transform(X_test[object_cols]))\n\nfor col in object_cols:\n X_train[col] = pd.Categorical(X_train[col])\n OH_cols_train[col] = OH_encoder.fit_transform(X_train[col].reshape(-1, 1))\n OH_cols_valid[col] = OH_encoder.transform(X_test[col])\n\n# One-hot encoding removed index; put it back\nOH_cols_train.index = X_train.index\nOH_cols_valid.index = X_test.index\n\n# Remove categorical columns (will replace with one-hot encoding)\nnum_X_train = X_train.drop(object_cols, axis=1)\nnum_X_valid = X_test.drop(object_cols, axis=1)\n\n# Add one-hot encoded columns to numerical features\nOH_X_train = pd.concat([num_X_train, OH_cols_train], axis=1)\nOH_X_valid = pd.concat([num_X_valid, OH_cols_valid], axis=1)\n\nprint(\"MAE from Approach 3 (One-Hot Encoding):\") \nprint(score_dataset(OH_X_train, OH_X_valid, y_train, y_test))",
"C:\\Anaconda3\\lib\\site-packages\\ipykernel_launcher.py:9: SettingWithCopyWarning: \nA value is trying to be set on a copy of a slice from a DataFrame.\nTry using .loc[row_indexer,col_indexer] = value instead\n\nSee the caveats in the documentation: http://pandas.pydata.org/pandas-docs/stable/indexing.html#indexing-view-versus-copy\n if __name__ == '__main__':\n"
]
]
] | [
"code"
] | [
[
"code",
"code",
"code",
"code",
"code",
"code",
"code",
"code",
"code",
"code",
"code",
"code",
"code"
]
] |
e77694c980b169544fec83f78f1c7d05d0aacf61 | 221,823 | ipynb | Jupyter Notebook | notebooks/09_wrapped_single_run_process.ipynb | TUDelft-CITG/Hydraulic-Infrastructure-Realisation | c5888ef8f8bd1676536e268701dbb974e6f87c40 | [
"MIT"
] | 6 | 2019-11-14T08:12:08.000Z | 2021-04-08T11:13:35.000Z | notebooks/09_wrapped_single_run_process.ipynb | TUDelft-CITG/Hydraulic-Infrastructure-Realisation | c5888ef8f8bd1676536e268701dbb974e6f87c40 | [
"MIT"
] | 16 | 2019-06-25T16:44:13.000Z | 2022-02-15T18:05:28.000Z | notebooks/09_wrapped_single_run_process.ipynb | TUDelft-CITG/Hydraulic-Infrastructure-Realisation | c5888ef8f8bd1676536e268701dbb974e6f87c40 | [
"MIT"
] | 8 | 2019-07-03T08:28:26.000Z | 2021-07-12T08:11:53.000Z | 55.070258 | 60,305 | 0.578853 | [
[
[
"## Demo: Wrapped single_run_process\nThe basic steps to set up an OpenCLSim simulation are:\n* Import libraries\n* Initialise simpy environment\n* Define object classes\n* Create objects\n * Create sites\n * Create vessels\n * Create activities\n* Register processes and run simpy\n\n----\n\nThis notebook provides an example of a single_run_process. In the single_run_process a typical \"loading-sailing full-unloading-sailing empty\" is pre-packed in a while activity. ",
"_____no_output_____"
],
[
"#### 0. Import libraries",
"_____no_output_____"
]
],
[
[
"import datetime, time\nimport simpy\n\nimport shapely.geometry\nimport pandas as pd\n\nimport openclsim.core as core\nimport openclsim.model as model\nimport openclsim.plot as plot",
"_____no_output_____"
]
],
[
[
"#### 1. Initialise simpy environment",
"_____no_output_____"
]
],
[
[
"# setup environment\nsimulation_start = 0\nmy_env = simpy.Environment(initial_time=simulation_start)",
"_____no_output_____"
]
],
[
[
"#### 2. Define object classes",
"_____no_output_____"
]
],
[
[
"# create a Site object based on desired mixin classes\nSite = type(\n \"Site\",\n (\n core.Identifiable,\n core.Log,\n core.Locatable,\n core.HasContainer,\n core.HasResource,\n ),\n {},\n)\n\n# create a TransportProcessingResource object based on desired mixin classes\nTransportProcessingResource = type(\n \"TransportProcessingResource\",\n (\n core.Identifiable,\n core.Log,\n core.ContainerDependentMovable,\n core.Processor,\n core.HasResource,\n core.LoadingFunction,\n core.UnloadingFunction,\n ),\n {},\n)",
"_____no_output_____"
]
],
[
[
"#### 3. Create objects\n##### 3.1. Create site object(s)",
"_____no_output_____"
]
],
[
[
"# prepare input data for from_site\nlocation_from_site = shapely.geometry.Point(4.18055556, 52.18664444)\ndata_from_site = {\"env\": my_env,\n \"name\": \"from_site\",\n \"geometry\": location_from_site,\n \"capacity\": 10_000,\n \"level\": 10_000\n }\n# instantiate from_site \nfrom_site = Site(**data_from_site)\n\n# prepare input data for to_site\nlocation_to_site = shapely.geometry.Point(4.25222222, 52.11428333)\ndata_to_site = {\"env\": my_env,\n \"name\": \"to_site\",\n \"geometry\": location_to_site,\n \"capacity\": 10_000,\n \"level\": 0\n }\n# instantiate to_site \nto_site = Site(**data_to_site)",
"_____no_output_____"
]
],
[
[
"##### 3.2. Create vessel object(s)",
"_____no_output_____"
]
],
[
[
"# prepare input data for vessel_01\ndata_vessel01 = {\"env\": my_env,\n \"name\": \"vessel01\",\n \"geometry\": location_from_site, \n \"loading_rate\": 1,\n \"unloading_rate\": 5,\n \"capacity\": 1_000,\n \"compute_v\": lambda x: 10 + 2 * x\n }\n# instantiate vessel_01 \nvessel01 = TransportProcessingResource(**data_vessel01)",
"_____no_output_____"
]
],
[
[
"##### 3.3 Create activity/activities",
"_____no_output_____"
]
],
[
[
"# initialise registry\nregistry = {}",
"_____no_output_____"
],
[
"# create a 'while activity' that contains a pre-packed set of 'sub_processes'\nsingle_run, while_activity = model.single_run_process(\n name=\"single_run\",\n registry={},\n env=my_env,\n origin=from_site,\n destination=to_site,\n mover=vessel01,\n loader=vessel01,\n unloader=vessel01\n)",
"_____no_output_____"
]
],
[
[
"#### 4. Register processes and run simpy",
"_____no_output_____"
]
],
[
[
"# initate the simpy processes defined in the 'while activity' and run simpy\nmodel.register_processes([while_activity])\nmy_env.run()",
"_____no_output_____"
]
],
[
[
"#### 5. Inspect results\n##### 5.1 Inspect logs",
"_____no_output_____"
]
],
[
[
"display(plot.get_log_dataframe(vessel01, [*single_run, while_activity]))",
"_____no_output_____"
],
[
"display(plot.get_log_dataframe(from_site, [*single_run]))",
"_____no_output_____"
],
[
"display(plot.get_log_dataframe(to_site, [*single_run]))",
"_____no_output_____"
]
],
[
[
"##### 5.2 Visualise gantt charts",
"_____no_output_____"
]
],
[
[
"plot.get_gantt_chart([while_activity, vessel01, *single_run])",
"_____no_output_____"
]
],
[
[
"##### 5.3 Visualise step charts",
"_____no_output_____"
]
],
[
[
"fig = plot.get_step_chart([from_site, to_site, vessel01])",
"_____no_output_____"
]
],
[
[
"You can observe in the step chart that the loading rate of vessel01 is 1 and the unloading rate is 5. This is clearly visible in the longer time it takes to transfer the cargo from from_site to vessel01, than it takes to transfer the cargo from vessel_1 to to_site. ",
"_____no_output_____"
]
]
] | [
"markdown",
"code",
"markdown",
"code",
"markdown",
"code",
"markdown",
"code",
"markdown",
"code",
"markdown",
"code",
"markdown",
"code",
"markdown",
"code",
"markdown",
"code",
"markdown",
"code",
"markdown"
] | [
[
"markdown",
"markdown"
],
[
"code"
],
[
"markdown"
],
[
"code"
],
[
"markdown"
],
[
"code"
],
[
"markdown"
],
[
"code"
],
[
"markdown"
],
[
"code"
],
[
"markdown"
],
[
"code",
"code"
],
[
"markdown"
],
[
"code"
],
[
"markdown"
],
[
"code",
"code",
"code"
],
[
"markdown"
],
[
"code"
],
[
"markdown"
],
[
"code"
],
[
"markdown"
]
] |
e776a9c6f438549dce416af05f3749b360b2076b | 183,013 | ipynb | Jupyter Notebook | Project 2/PROJECT 2.ipynb | ParadoxPD/Intro-to-machine-learning | 3dc4e662e2f7a3ca2099114eb3eb2d80cd5c3f4b | [
"MIT"
] | null | null | null | Project 2/PROJECT 2.ipynb | ParadoxPD/Intro-to-machine-learning | 3dc4e662e2f7a3ca2099114eb3eb2d80cd5c3f4b | [
"MIT"
] | null | null | null | Project 2/PROJECT 2.ipynb | ParadoxPD/Intro-to-machine-learning | 3dc4e662e2f7a3ca2099114eb3eb2d80cd5c3f4b | [
"MIT"
] | null | null | null | 44.067662 | 20,248 | 0.44481 | [
[
[
"# a) By using Logistic Regression Algorithm",
"_____no_output_____"
],
[
"# Part A: Data Preprocessing",
"_____no_output_____"
],
[
"# Step1 : importing the libraries",
"_____no_output_____"
]
],
[
[
"import numpy as np\nimport matplotlib.pyplot as plt\nimport pandas as pd",
"_____no_output_____"
]
],
[
[
"# step2: import data set",
"_____no_output_____"
]
],
[
[
"dataset=pd.read_csv('Logistic Data.csv')",
"_____no_output_____"
],
[
"dataset",
"_____no_output_____"
]
],
[
[
"# step3: to create feature matrix and dependent variable vector",
"_____no_output_____"
]
],
[
[
"a=dataset.iloc[:,:-1].values\nb=dataset.iloc[:,-1].values",
"_____no_output_____"
],
[
"a",
"_____no_output_____"
],
[
"b",
"_____no_output_____"
]
],
[
[
"# step4: replace the missing data",
"_____no_output_____"
]
],
[
[
"from sklearn.impute import SimpleImputer\nimputer=SimpleImputer(missing_values=np.nan,strategy='mean')\nimputer.fit(a[:,:])\na[:,:]=imputer.transform(a[:,:])",
"_____no_output_____"
],
[
"a",
"_____no_output_____"
],
[
"b",
"_____no_output_____"
]
],
[
[
"# Step5: Encoding(not required)",
"_____no_output_____"
],
[
"# step6 : spiliting of data set into training and testing set",
"_____no_output_____"
]
],
[
[
"from sklearn.model_selection import train_test_split\natrain,atest,btrain,btest=train_test_split(a,b,test_size=0.2,random_state=1)",
"_____no_output_____"
],
[
"atrain",
"_____no_output_____"
]
],
[
[
"# step7 : Feature scaling",
"_____no_output_____"
]
],
[
[
"from sklearn.preprocessing import StandardScaler\nsc=StandardScaler()\natrain=sc.fit_transform(atrain)\natest=sc.fit_transform(atest)",
"_____no_output_____"
],
[
"atrain",
"_____no_output_____"
]
],
[
[
"# Part B: build my first linear model",
"_____no_output_____"
],
[
"# step 1: training the classification model",
"_____no_output_____"
]
],
[
[
"from sklearn.linear_model import LogisticRegression\nLoR=LogisticRegression(random_state=0)\nLoR.fit(atrain,btrain)",
"_____no_output_____"
]
],
[
[
"# step 2: testing the linear model",
"_____no_output_____"
]
],
[
[
"bestimated=LoR.predict(atest)\nprint(np.concatenate((bestimated.reshape(len(bestimated),1),btest.reshape(len(btest),1)),1))",
"[[0 0]\n [0 0]\n [0 1]\n [1 1]\n [0 0]\n [0 0]\n [0 0]\n [1 1]\n [0 0]\n [1 0]\n [0 0]\n [0 0]\n [0 0]\n [1 1]\n [1 1]\n [1 1]\n [1 1]\n [0 0]\n [0 0]\n [1 1]\n [0 0]\n [1 1]\n [1 1]\n [0 0]\n [0 1]\n [0 0]\n [1 1]\n [1 0]\n [1 1]\n [1 0]\n [0 0]\n [0 0]\n [0 0]\n [1 1]\n [0 0]\n [0 0]\n [0 0]\n [0 0]\n [0 1]\n [0 0]\n [1 1]\n [1 1]\n [0 0]\n [0 0]\n [1 1]\n [0 1]\n [0 1]\n [1 1]\n [0 0]\n [1 1]\n [0 0]\n [0 0]\n [0 1]\n [0 1]\n [0 1]\n [0 0]\n [1 1]\n [0 0]\n [1 1]\n [1 1]\n [0 0]\n [0 0]\n [0 0]\n [0 0]\n [0 1]\n [1 1]\n [0 0]\n [0 0]\n [1 0]\n [0 0]\n [1 0]\n [0 0]\n [0 1]\n [0 0]\n [0 0]\n [1 1]\n [0 0]\n [0 0]\n [0 0]\n [0 0]]\n"
]
],
[
[
"# step C: performance matrix",
"_____no_output_____"
]
],
[
[
"from sklearn.metrics import confusion_matrix,accuracy_score,precision_score\ncm=confusion_matrix(btest,bestimated)\nprint(cm)\nprint(accuracy_score(btest,bestimated))\nprint(precision_score(btest,bestimated))",
"[[43 5]\n [10 22]]\n0.8125\n0.8148148148148148\n"
],
[
"np.mean((True,True,False))",
"_____no_output_____"
],
[
"error_rate=[]\nfor i in range(1,30):\n KC=KNeighborsClassifier(n_neighbors=i)\n KC.fit(atrain,btrain)\n bpred_i=KC.predict(atest)\n error_rate.append(np.mean(bpred_i!=btest))",
"_____no_output_____"
],
[
"plt.plot(range(1,30),error_rate,marker='o',markerfacecolor='red',markersize=5)\nplt.xlabel('K value')\nplt.ylabel('Error rate')",
"_____no_output_____"
]
],
[
[
"# b) By using KNN Algorithm",
"_____no_output_____"
],
[
"# Part A: Data Preprocessing",
"_____no_output_____"
],
[
"# Step1 : importing the libraries",
"_____no_output_____"
]
],
[
[
"import numpy as np\nimport matplotlib.pyplot as plt\nimport pandas as pd",
"_____no_output_____"
]
],
[
[
"# step2: import data set",
"_____no_output_____"
]
],
[
[
"dataset=pd.read_csv('Logistic Data.csv')",
"_____no_output_____"
],
[
"dataset",
"_____no_output_____"
]
],
[
[
"# step3: to create feature matrix and dependent variable vector",
"_____no_output_____"
]
],
[
[
"a=dataset.iloc[:,:-1].values\nb=dataset.iloc[:,-1].values",
"_____no_output_____"
],
[
"a",
"_____no_output_____"
],
[
"b",
"_____no_output_____"
]
],
[
[
"# step4: replace the missing data",
"_____no_output_____"
]
],
[
[
"from sklearn.impute import SimpleImputer\nimputer=SimpleImputer(missing_values=np.nan,strategy='mean')\nimputer.fit(a[:,:])\na[:,:]=imputer.transform(a[:,:])",
"_____no_output_____"
],
[
"a",
"_____no_output_____"
]
],
[
[
"# Step5: Encoding(not required)",
"_____no_output_____"
],
[
"# step6 : spiliting of data set into training and testing set",
"_____no_output_____"
]
],
[
[
"from sklearn.model_selection import train_test_split\natrain,atest,btrain,btest=train_test_split(a,b,test_size=0.2,random_state=1)",
"_____no_output_____"
],
[
"atrain",
"_____no_output_____"
]
],
[
[
"# step7 : Feature scaling",
"_____no_output_____"
]
],
[
[
"from sklearn.preprocessing import StandardScaler\nsc=StandardScaler()\natrain=sc.fit_transform(atrain)\natest=sc.fit_transform(atest)",
"_____no_output_____"
],
[
"atrain",
"_____no_output_____"
]
],
[
[
"# Part B: build my KNN classification model",
"_____no_output_____"
],
[
"# step 1: training the classification model",
"_____no_output_____"
]
],
[
[
"from sklearn.neighbors import KNeighborsClassifier\nKC=KNeighborsClassifier(n_neighbors=7,weights='uniform',p=2)\nKC.fit(atrain,btrain)",
"_____no_output_____"
]
],
[
[
"# step 2: testing the linear model",
"_____no_output_____"
]
],
[
[
"bestimated=KC.predict(atest)",
"_____no_output_____"
]
],
[
[
"# step C: performance matrix",
"_____no_output_____"
]
],
[
[
"from sklearn.metrics import confusion_matrix,accuracy_score,precision_score\ncm=confusion_matrix(btest,bestimated)\nprint(cm)\nprint(accuracy_score(btest,bestimated))\nprint(precision_score(btest,bestimated))",
"[[42 6]\n [ 2 30]]\n0.9\n0.8333333333333334\n"
],
[
"np.mean((True,True,False))",
"_____no_output_____"
],
[
"error_rate=[]\nfor i in range(1,30):\n KC=KNeighborsClassifier(n_neighbors=i)\n KC.fit(atrain,btrain)\n bpred_i=KC.predict(atest)\n error_rate.append(np.mean(bpred_i!=btest))",
"_____no_output_____"
],
[
"plt.plot(range(1,30),error_rate,marker='o',markerfacecolor='red',markersize=5)\nplt.xlabel('K value')\nplt.ylabel('Error rate')",
"_____no_output_____"
]
]
] | [
"markdown",
"code",
"markdown",
"code",
"markdown",
"code",
"markdown",
"code",
"markdown",
"code",
"markdown",
"code",
"markdown",
"code",
"markdown",
"code",
"markdown",
"code",
"markdown",
"code",
"markdown",
"code",
"markdown",
"code",
"markdown",
"code",
"markdown",
"code",
"markdown",
"code",
"markdown",
"code",
"markdown",
"code",
"markdown",
"code"
] | [
[
"markdown",
"markdown",
"markdown"
],
[
"code"
],
[
"markdown"
],
[
"code",
"code"
],
[
"markdown"
],
[
"code",
"code",
"code"
],
[
"markdown"
],
[
"code",
"code",
"code"
],
[
"markdown",
"markdown"
],
[
"code",
"code"
],
[
"markdown"
],
[
"code",
"code"
],
[
"markdown",
"markdown"
],
[
"code"
],
[
"markdown"
],
[
"code"
],
[
"markdown"
],
[
"code",
"code",
"code",
"code"
],
[
"markdown",
"markdown",
"markdown"
],
[
"code"
],
[
"markdown"
],
[
"code",
"code"
],
[
"markdown"
],
[
"code",
"code",
"code"
],
[
"markdown"
],
[
"code",
"code"
],
[
"markdown",
"markdown"
],
[
"code",
"code"
],
[
"markdown"
],
[
"code",
"code"
],
[
"markdown",
"markdown"
],
[
"code"
],
[
"markdown"
],
[
"code"
],
[
"markdown"
],
[
"code",
"code",
"code",
"code"
]
] |
e776af174eece8840260e1eab3fb86995accf0a4 | 5,800 | ipynb | Jupyter Notebook | vignere_cipher.ipynb | indradhar/fileCryption | 1a74fff0b0f07d8bc83f739fc89f6e6db8bf619e | [
"MIT"
] | null | null | null | vignere_cipher.ipynb | indradhar/fileCryption | 1a74fff0b0f07d8bc83f739fc89f6e6db8bf619e | [
"MIT"
] | null | null | null | vignere_cipher.ipynb | indradhar/fileCryption | 1a74fff0b0f07d8bc83f739fc89f6e6db8bf619e | [
"MIT"
] | null | null | null | 40.277778 | 807 | 0.553103 | [
[
[
"def number_value(text):\n tempo=[]\n alpha=\"abcdefghijklmnopqrstuvwxyz\"\n alphacap=\"ABCDEFGHIJKLMNOPQRSTUVWXYZ\"\n for i in range(len(text)):\n value=alpha.find(text[i])\n if value == -1:\n value=alphacap.find(text[i])\n tempo.append(value)\n return tempo\ndef alpha_value(number):\n alpha=\"abcdefghijklmnopqrstuvwxyz\"\n return alpha[number]",
"_____no_output_____"
],
[
"def cipher_encryption(text,key):\n tempo1=[]\n text_numbered=number_value(text)\n key_numbered=number_value(key)\n for i in range(len(text_numbered)):\n if text_numbered[i]==-1:\n tempo1.append(' ')\n else:\n calc=((text_numbered[i]+key_numbered[i])%26)\n alpha=alpha_value(calc)\n tempo1.append(alpha)\n return tempo1",
"_____no_output_____"
],
[
"def cipher_decryption(text,key):\n tempo2=[]\n text_numbered=number_value(text)\n key_numbered=number_value(key)\n for i in range(len(text_numbered)):\n if text_numbered[i]==-1:\n tempo2.append(' ')\n else:\n calc=((text_numbered[i]-key_numbered[i])%26)\n alpha=alpha_value(calc)\n tempo2.append(alpha)\n return tempo2",
"_____no_output_____"
],
[
"print(\"This is Vignere Cipher Encrtption and Decryption \\nEnter \\n1. For Encryption \\n2. For Decryption\")\nchoice=int(input())\nif choice == 1:\n plain_text=input(\"plain text and Enter Key\\n\")\n key=input()\n if len(key)<len(plain_text):\n size=len(plain_text)-len(key)\n for i in range(size):\n key += (key[i])\n cipher_text=cipher_encryption(plain_text,key)\n out=''\n for i in cipher_text:\n out+=str(i)\n print(out) \n\nelif choice == 2:\n cipher_text=input(\"cipher text and Enter Key\\n\")\n key=input()\n if len(key)<len(cipher_text):\n size=len(cipher_text)-len(key)\n for i in range(size):\n key += (key[i])\n plain_text=cipher_decryption(cipher_text,key)\n out1=''\n for i in plain_text:\n out1 +=str(i)\n print(out1) \n \nelse: print(\"invalid\")",
"This is Vignere Cipher Encrtption and Decryption \nEnter \n1. For Encryption \n2. For Decryption\n1\nplain text and Enter Key\nEducation can be thought of as the transmission of the values and accumulated knowledge of a society. In this sense, it is equivalent to what social scientists term socialization or enculturation. Children—whether conceived among New Guinea tribespeople, the Renaissance Florentines, or the middle classes of Manhattan—are born without culture. Education is designed to guide them in learning a culture, molding their behaviour in the ways of adulthood, and directing them toward their eventual role in society. In the most primitive cultures, there is often little formal learning—little of what one would ordinarily call school or classes or teachers. Instead, the entire environment and all activities are frequently viewed as school and classes, and many or all adults act as teachers. \nbin\nflhdigjwa kno of giwhhpg ws if buf gsiatuvtavpv pn upr dnmcrt nol bkpvuhmigfl lvbxtreor ws i twpjmgz jv upvt ffvff jb ja fyhjdnmmau gp jiig abdqnm fdqrobvtbf brsu twpjiyjhnuqbo bs rokhmbhsigjwa piqyezro jimgime kbokrjdre nnwah afe hcvomn bejjrtxrpxyf upr zroivtanokr nypzrobvomf bs gim nqqetr kybaffa pn niaiiguia ief opza evupbvb dcyucef flhdigjwa qf lrtqtomq bb ohjlr bufu jv mmnsvvoo b pvtgvzr zptqjvt bufqe jriiijwhs vo gim xilt bg necyupbpl iae qjzrdbvoo uprn gpensl uprjz fdrobhbt swyf vo fpkvfbl vo gim nwfu csqzjbvwm dcyucefa bufzr qf wsuma tvubyf spzzbt mmnsvvoo mqgutr ws eubb pvr ebvtq weeqabzvmg diym fdpbpt pz dtntart bs gfipimet jvfumne upr mauqef rodvswanmau nol bty ipuqijbvfa bzr nefyhfvgmg wqrxmq if apiwbm nol dtntart bvq unog pz bty iqvtgt ndb ba umndprsa \n"
]
]
] | [
"code"
] | [
[
"code",
"code",
"code",
"code"
]
] |
e776af276b858368efa460735092f381b362d795 | 146,677 | ipynb | Jupyter Notebook | _notebooks/2020-10-07-01-CNN-Basic.ipynb | AntonovMikhail/chans_jupyter | c2cd1675408238ad5be81ba98994611d8c4e48ae | [
"Apache-2.0"
] | 8 | 2020-06-26T23:48:52.000Z | 2021-02-27T22:26:31.000Z | _notebooks/2020-10-07-01-CNN-Basic.ipynb | AntonovMikhail/chans_jupyter | c2cd1675408238ad5be81ba98994611d8c4e48ae | [
"Apache-2.0"
] | 46 | 2020-06-30T00:45:37.000Z | 2021-03-07T14:47:10.000Z | _notebooks/2020-10-07-01-CNN-Basic.ipynb | AntonovMikhail/chans_jupyter | c2cd1675408238ad5be81ba98994611d8c4e48ae | [
"Apache-2.0"
] | 26 | 2020-07-24T17:30:15.000Z | 2021-02-19T10:19:25.000Z | 202.592541 | 32,232 | 0.899623 | [
[
[
"# CNN Basic\n> In this post, We will dig into the basic operation of Convolutional Neural Network, and explain about what each layer look like. And we will simply implement the basic CNN archiecture with tensorflow.\n\n- toc: true \n- badges: true\n- comments: true\n- author: Chanseok Kang\n- categories: [Python, Deep_Learning, Tensorflow-Keras]\n- image: images/cnn_stacked.png",
"_____no_output_____"
]
],
[
[
"import tensorflow as tf\nimport numpy as np\nimport matplotlib.pyplot as plt\nimport pandas as pd\n\nplt.rcParams['figure.figsize'] = (16, 10)\nplt.rc('font', size=15)",
"_____no_output_____"
]
],
[
[
"## Convolutional Neural Network\n [Convolutional Neural Network](https://en.wikipedia.org/wiki/Convolutional_neural_network) (CNN for short) is the most widely used for image classification. Previously, we handled image classification problem (Fashion-MNIST) with Multi Layer Perceptron, and we found that works. At that time, the information containing each image is very simple, and definitely classified with several parameters. But if the dataset contains high resolution image and several channels, it may require huge amount of parameters with mlp in image classification. How can we effectively classify the image without huge amount of parameters? And that's why the CNN exists.\n \n\n*Fig 1. Example of Convolutional Neural Network*\n \n Generally, it consists of **Convolution layer**, **Pooling layer**, and **Fully-connected layer**. Usually, Convolution layer and Pooling layer is used for feature extraction. Feature extraction means that it extract important features from image for classification, so that all pixels in images is not required. Through these layer, information of data is resampled, and refined, and go to the output node. Fully-connected (also known as FC) is used for classification.",
"_____no_output_____"
],
[
"### 2D Convolution Layer\nTo understand convolution layer, we need to see the shape of image and some elements to extract features called filters(also called kernel).\n\n\n\nIn Fashion-MNIST example, the image containing dataset is grayscale. So the range of pixel value is from 0 to 255. But most of real-world image and all the things we see now is colorized. Technically, it can expressed with 3 base color, Red, Green, Blue. The color system which can express these 3 colors are called **RGB**, and each range of pixel value is also from 0 to 255, same as grayscaled pixel.\n\n> Note: There are other color systems like RGBA (Red-Green-Blue-Alpha) or HSV (Hue-Saturation-Value), HSL (Hue-Saturation-Lightness), etc. In this post, we just assume that image has 3 channels (RGB)\n\nSo In the figure, this image has shape of 32x32 and 3 channels (RGB).\n\nAnd Next one is the filter, which shape has 5x5x3. The number we need to focus on **3**. Filter always extend the full channel of the input volume. So it requires to set same number of channels in input nodes and filters. \n\nAs you can see, filter has same channel with input image. And let's assume the notation of filter, $w$. Next thing we need to do is just slide th filter over the image spatially, and compute dot products, same as MLP operation (This operation is called **convolution**, and it's the reason why this layer is called Convolution layer). Simply speaking, we just focus on the range covered in filter and dot product like this,\n\n$$ w^T x + b $$\n\nOf course, we move the filter until it can operate convolution.\n\n\n*Fig 3. Animation of convolution operation\n\nAfter that, (Assume we don't apply padding, and stride is 1), we can get output volume which shape has 28x28x1. There is formula to calculate the shape of output volume when the stride and filter is defined,\n\n$$ \\frac{(\\text{Height of input} - \\text{Height of filter})}{\\text{Stride}} + 1 $$\n\nWe can substitute the number in this formula, then we can conclude the height of output volume is 28. The output the process convolution is called **Feature Map** (or Activation Map). One feature map gather from one filter, we can apply several filters in one input image. If we use 6 5x5 filters, we can get 6 separate feature maps. We can stack them into one object, then we get \"new image\" of size 28x28x6.\n\n\n<p>\n <em>Fig 4. stacked output of convolution</em>\n</p>\n\nHere, one number is need to focus, **6**. 6 means the number of filters we apply in this convolution layer.\n\nYou may be curious about what filter looks like. Actually, filter was widely used from classic computer vision. For example, to extract the edge of object in image, we can apply the *canny edge filer*, and there is edge detection filter named *sobel filter*, and so on. We can bring the concept of filter in CNN here. In short, filter extract the feature like where the edge is, and convolution layer refine its feature.\n\n\n*Fig 5. Applying filter for each pixel*\n\nHere, we just consider the one channel of image and apply it with 6 filters. And we can extend it in multi-channel, and lots of filters.\n\n\n*Fig 6. Convolution operation in many channels and many filters*",
"_____no_output_____"
],
[
"### Options of Convolution\n\nSo what parameteter may affect operation in convolution layer? We can introduce three main parameters for convolution layer: stride, zero-padding, and activation function.\n\n**Stride** is the size of step that how far to go to the right or the bottom to perform the next convolution. It is important that it defines the output model's size. Review the formula of calculating the feature map size,\n\n$$ \\frac{(\\text{Height of input} - \\text{Height of filter})}{\\text{Stride}} + 1 $$\n\nWe can define any size of strides unless its value is smaller than original model size. As we increase the stride, then the size of feature map will be small, and it means that feature will also be small. Think about that the picture is summarized by just a small dot.\n\n**Zero-padding** is another factor that affect the convolution layer. The meaning is contained in its name. A few numbers of zeros surrounds the original image, then process the convolution operation. As we can see from previous process, if the layer is deeper and deeper, then the information will be smaller the original one due to the convolution operation. To preserve the size of original image during process, zero-padding is required. If the filter size is FxF and stride is 1, then we can also calculate the zero-padding size,\n\n$$ \\frac{F - 1}{2} $$\n\nYou may see the **Activation Function** in MLP. It can also apply in the Convolution layer.\nFor example, when we apply Rectified Linear Unit (ReLU) in feature map, we can remove the value that lower than 0,",
"_____no_output_____"
],
[
"### Convolution Layer in Tensorflow\nLet's look at how we can use Convolution layer in tensorflow. Actually, in Tensorflow v2.x, Convolution layer is implemented in keras as an high-level class, so all we need to do is just defineing the parameters correctly. Here is the `__init__` of `tf.keras.layers.Conv2D`.\n\n```python\ntf.keras.layers.Conv2D(\n filters, kernel_size, strides=(1, 1), padding='valid', data_format=None,\n dilation_rate=(1, 1), groups=1, activation=None, use_bias=True,\n kernel_initializer='glorot_uniform', bias_initializer='zeros',\n kernel_regularizer=None, bias_regularizer=None, activity_regularizer=None,\n kernel_constraint=None, bias_constraint=None, **kwargs\n)\n```\n\nWe already covered filters, kernel_size (same as filter_size), strides, and so on. Here is brief description of arguments:\n\n| arguments | |\n| --- | --- |\n| filters | Integer, the dimensionality of the output space (i.e. the number of output filters in the convolution). |\n| kernel_size | An integer or tuple/list of 2 integers, specifying the height and width of the 2D convolution window. Can be a single integer to specify the same value for all spatial dimensions. |\n| strides | An integer or tuple/list of 2 integers, specifying the strides of the convolution along the height and width. Can be a single integer to specify the same value for all spatial dimensions. Specifying any stride value != 1 is incompatible with specifying any dilation_rate value != 1. |\n| padding | one of \"valid\" or \"same\" (case-insensitive). |\n| data_format | A string, one of channels_last (default) or channels_first. The ordering of the dimensions in the inputs. channels_last corresponds to inputs with shape (batch_size, height, width, channels) while channels_first corresponds to inputs with shape (batch_size, channels,height, width). It defaults to the image_data_format value found in your Keras config file at ~/.keras/keras.json. If you never set it, then it will be channels_last. |\n| activation | Activation function to use. If you don't specify anything, no activation is applied (see [keras.activations](https://www.tensorflow.org/api_docs/python/tf/keras/activations)). |\n| use_bias | Boolean, whether the layer uses a bias vector. |\n| kernel_initializer | Initializer for the kernel weights matrix |\n| bias_initializer | Initializer for the bias vector |\n\nWe need to focus on the `data_format`. The description said that the default value of data_format is 'channels_last'. It means that all data in this layer must follow in this format order: `(batch, height, width, channels)`\n\nAnd `padding` argument can accept `valid` and `same`. `same` means no zero-padding. In this case, it drops the last convolution if the dimensions do not match (or fraction). If you want to use zero-padding, then we need to define `padding` argument as `same`. In this case, it pads such that feature map size has same as original one.(That's why we call the `same`) Usually it is also called 'half' padding.",
"_____no_output_____"
],
[
"### Example with Toy Image\nLet's use the convolution layer. For the simplicity, we will use a simple toy image, then see the output.",
"_____no_output_____"
]
],
[
[
"image = tf.constant([[[[1], [2], [3]], \n [[4], [5], [6]],\n [[7], [8], [9]]]], dtype=np.float32)\nfig, ax = plt.subplots()\nax.imshow(image.numpy().reshape(3, 3), cmap='gray')\n\nfor (j, i), label in np.ndenumerate(image.numpy().reshape(3, 3)):\n if label < image.numpy().mean():\n ax.text(i, j, label, ha='center', va='center', color='white')\n else:\n ax.text(i, j, label, ha='center', va='center', color='k')\nplt.show()",
"_____no_output_____"
],
[
"print(image.shape)",
"(1, 3, 3, 1)\n"
]
],
[
[
"We made a simple image that has size of 3x3. Rememeber that the order of data should be `(batch, height, width, channel)`. In this case, batch size is 1, and currently generates the grayscaled image, so the channel should be 1.\n\nThen, we need to define filter and kernel_size, and padding method. We will use one filter with all-one weight that has 2x2 shape.\n\nNote that we need to set the same format in image shape and filter. If not, following error will be occurred,\n\n```python\nValueError: setting an array element with a sequence.\n```",
"_____no_output_____"
]
],
[
[
"# Weight Initialization\nweight = np.array([[[[1.]], [[1.]]],\n [[[1.]], [[1.]]]])\nweight_init = tf.constant_initializer(weight)\nprint(\"weight.shape: {}\".format(weight.shape))\n\n# Convolution layer\nlayer = tf.keras.layers.Conv2D(filters=1, kernel_size=(2, 2), padding='VALID', kernel_initializer=weight_init)\noutput = layer(image)",
"weight.shape: (2, 2, 1, 1)\n"
],
[
"# Check the result\nfig, ax = plt.subplots()\nax.imshow(output.numpy().reshape(2, 2), cmap='gray')\nfor (j, i), label in np.ndenumerate(output.numpy().reshape(2, 2)):\n if label < output.numpy().mean():\n ax.text(i, j, label, ha='center', va='center', color='white')\n else:\n ax.text(i, j, label, ha='center', va='center', color='k')\n\nplt.show()",
"_____no_output_____"
]
],
[
[
"See? This is the output of convolution layer with toy image. In this time, change the padding argument from `VALID` to `SAME` and see the result. In this case, zero padding is added ('half' padding), so the output shape will be also changed.",
"_____no_output_____"
]
],
[
[
"# Convolution layer with half padding\nlayer = tf.keras.layers.Conv2D(filters=1, kernel_size=(2, 2), padding='SAME', kernel_initializer=weight_init)\noutput2 = layer(image)",
"_____no_output_____"
],
[
"# Check the result\nfig, ax = plt.subplots()\nax.imshow(output2.numpy().reshape(3, 3), cmap='gray')\nfor (j, i), label in np.ndenumerate(output2.numpy().reshape(3, 3)):\n if label < output2.numpy().mean():\n ax.text(i, j, label, ha='center', va='center', color='white')\n else:\n ax.text(i, j, label, ha='center', va='center', color='k')\nplt.show()",
"_____no_output_____"
]
],
[
[
"And what if we apply 3 filters in here?",
"_____no_output_____"
]
],
[
[
"# Weight initialization\nweight = np.array([[[[1., 10., -1.]], [[1., 10., -1.]]],\n [[[1., 10., -1.]], [[1., 10., -1.]]]])\nweight_init = tf.constant_initializer(weight)\nprint(\"Weight shape: {}\".format(weight.shape))\n\n# Convolution layer\nlayer = tf.keras.layers.Conv2D(filters=3, kernel_size=(2, 2), padding='SAME', kernel_initializer=weight_init)\noutput = layer(image)\n\n## Check output\nfeature_maps = np.swapaxes(output, 0, 3)\n\nfig, ax = plt.subplots(1, 3)\nfor x, feature_map in enumerate(feature_maps):\n ax[x].imshow(feature_map.reshape(3, 3), cmap='gray')\n for (j, i), label in np.ndenumerate(feature_map.reshape(3, 3)):\n if label < feature_map.mean():\n ax[x].text(i, j, label, ha='center', va='center', color='white')\n else:\n ax[x].text(i, j, label, ha='center', va='center', color='k')",
"Weight shape: (2, 2, 1, 3)\n"
]
],
[
[
"### Pooling Layer\nAfter passing Activation function, the output may be changed. We can summarize the output with some rules, for example, find the maximum pixel value in specific filter that assume to represent that field.\n\n\n*Fig 7, Max-Pooling*\n\nIn the figure, we use 2x2 filter for pixel handling. When the filter is slided by stride 2, filter find the maximum pixel value, and re-define it as an output.\n\nOr we can find the average pixel value in specific filter that assume to represent that field. Prior one is called **Max-Pooling**, and latter is called **Average-Pooling**. Usually, this kind of process is called **Sub-sampling**, since this process extract the important pixel(Max or Average) from the image, and the output size is reduced by half.",
"_____no_output_____"
],
[
"#### Max Pooling Layer in Tensorflow\nSame as Convolution Layer, Max Pooling Layer is also defined in Tensorflow-keras as a high level class. Here is the `__init__` of `tf.keras.layers.MaxPool2D`. (you can also check [AveragePooling2D](https://www.tensorflow.org/api_docs/python/tf/keras/layers/AveragePooling2D) in documentation)\n\n```python\ntf.keras.layers.MaxPool2D(\n pool_size=(2, 2), strides=None, padding='valid', data_format=None, **kwargs\n)\n```\n\nActually, it is almost same as that of convolution layer. `pool_size` argument is similar with `filter_size` in convolution layer, and it will define the range to extract the maximum value. Here is brief description of arguments:\n\n| arguments | |\n| --- | --- |\n| pool_size | integer or tuple of 2 integers, factors by which to downscale (vertical, horizontal). (2, 2) will halve the input in both spatial dimension. If only one integer is specified, the same window length will be used for both dimensions.\n |\n| strides | Integer, tuple of 2 integers, or None. Strides values. If None, it will default to pool_size.\n |\n| padding | One of \"valid\" or \"same\" (case-insensitive). |\n| data_format | A string, one of channels_last (default) or channels_first. The ordering of the dimensions in the inputs. channels_last corresponds to inputs with shape (batch_size, height, width, channels) while channels_first corresponds to inputs with shape (batch_size, channels,height, width). It defaults to the image_data_format value found in your Keras config file at ~/.keras/keras.json. If you never set it, then it will be channels_last. |",
"_____no_output_____"
],
[
"### Example with Toy Image\nHere, we will check the Maxpooling operation with toy image.",
"_____no_output_____"
]
],
[
[
"# Sample image\nimage = tf.constant([[[[4.], [3.]],\n [[2.], [1.]]]], dtype=np.float32)",
"_____no_output_____"
],
[
"# Max Pooling layer\nlayer = tf.keras.layers.MaxPool2D(pool_size=(2, 2), strides=1, padding='VALID')\noutput = layer(image)\n\n# Check the output\nprint(output.numpy())",
"[[[[4.]]]]\n"
]
],
[
[
"After that, we found out that the output of this image is just 4, the maximum value. How about the case with `SAME` padding?",
"_____no_output_____"
]
],
[
[
"layer = tf.keras.layers.MaxPool2D(pool_size=(2, 2), strides=1, padding='SAME')\noutput = layer(image)\n\nprint(output.numpy())",
"[[[[4.]\n [3.]]\n\n [[2.]\n [1.]]]]\n"
]
],
[
[
"You may see that the output is different compared with previous one. That's because, while max pooling operation is held, zero-padding is also considered as an one pixel. So the 4 max-pooling operation is occurred.\n\n",
"_____no_output_____"
],
[
"## Convolution/MaxPooling in MNIST\nIn this case, we apply the convolution/MaxPooling operation in more specific dataset, the MNIST dataset. First, load the data. and normalize it. And see the sampled data.",
"_____no_output_____"
]
],
[
[
"(X_train, y_train), (X_test, y_test) = tf.keras.datasets.mnist.load_data()\n\n# Normalization\nX_train = X_train.astype(np.float32) / 255.\nX_test = X_test.astype(np.float32) / 255.\n\nimage = X_train[0]\nplt.imshow(image, cmap='gray')\nplt.show()",
"_____no_output_____"
]
],
[
[
"To handle this image in tensorflow, we need to convert it from 2d numpy array to 4D Tensor. There are several approaches to convert 4D tensor. One of approaches in Tensorflow is add `tf.newaxis` like this,",
"_____no_output_____"
]
],
[
[
"print(\"Dimension: {}\".format(image.shape))\nimage = image[tf.newaxis, ..., tf.newaxis]\nprint(\"Dimension: {}\".format(image.shape))\n\n# Convert it to tensor\nimage = tf.convert_to_tensor(image)",
"Dimension: (28, 28)\nDimension: (1, 28, 28, 1)\n"
]
],
[
[
"Same as before, we initialize the filter weight and apply it to convolution layer. In this case, we use 5 filters and (3, 3) filter size and stride to (2, 2), and padding is `SAME`.",
"_____no_output_____"
]
],
[
[
"weight_init = tf.keras.initializers.RandomNormal(stddev=0.01)\nlayer_conv = tf.keras.layers.Conv2D(filters=5, kernel_size=(3, 3), strides=(2, 2), padding='SAME',\n kernel_initializer=weight_init)\noutput = layer_conv(image)\nprint(output.shape)",
"(1, 14, 14, 5)\n"
],
[
"feature_maps = np.swapaxes(output, 0, 3)\n\nfig, ax = plt.subplots(1, 5)\nfor i, feature_map in enumerate(feature_maps):\n ax[i].imshow(feature_map.reshape(14, 14), cmap='gray')\nplt.tight_layout()\nplt.show()",
"_____no_output_____"
]
],
[
[
"After that, we use this output to push max-pooling layer as an input.",
"_____no_output_____"
]
],
[
[
"layer_pool = tf.keras.layers.MaxPool2D(pool_size=(2, 2), strides=(2, 2), padding='SAME')\noutput2 = layer_pool(output)\nprint(output2.shape)",
"(1, 7, 7, 5)\n"
],
[
"feature_maps = np.swapaxes(output2, 0, 3)\n\nfig, ax = plt.subplots(1, 5)\nfor i, feature_map in enumerate(feature_maps):\n ax[i].imshow(feature_map.reshape(7, 7), cmap='gray')\nplt.tight_layout()\nplt.show()",
"_____no_output_____"
]
],
[
[
"Using this, we extract the feature of input. Then we can use Fully-Connected Layer with softmax activation function for classification. (Actually, We already covered Fully-Connected layer with softmax activation function in previous post).",
"_____no_output_____"
],
[
"## Summary\nIn this post, We covered the definition of Convolutional Neural Network, and its basic opeartions, convolution and max-pooling. Unlike Artificial Neural Network, The purpose of these operation is actually to extract the feature and handle it efficiently without whole informations. We can use it in various ways, and of course, we can re-do the image classification with CNN, same as MLP.",
"_____no_output_____"
]
]
] | [
"markdown",
"code",
"markdown",
"code",
"markdown",
"code",
"markdown",
"code",
"markdown",
"code",
"markdown",
"code",
"markdown",
"code",
"markdown",
"code",
"markdown",
"code",
"markdown",
"code",
"markdown",
"code",
"markdown"
] | [
[
"markdown"
],
[
"code"
],
[
"markdown",
"markdown",
"markdown",
"markdown",
"markdown"
],
[
"code",
"code"
],
[
"markdown"
],
[
"code",
"code"
],
[
"markdown"
],
[
"code",
"code"
],
[
"markdown"
],
[
"code"
],
[
"markdown",
"markdown",
"markdown"
],
[
"code",
"code"
],
[
"markdown"
],
[
"code"
],
[
"markdown",
"markdown"
],
[
"code"
],
[
"markdown"
],
[
"code"
],
[
"markdown"
],
[
"code",
"code"
],
[
"markdown"
],
[
"code",
"code"
],
[
"markdown",
"markdown"
]
] |
e776c49ea19b7652edab026236bbafc410dbd7c1 | 82,048 | ipynb | Jupyter Notebook | store_sales_prediction_1.ipynb | mariosotper/Predict-Time--Series-Test | da78762cf6449726fd1d74013b775256b4fb0bdd | [
"MIT"
] | null | null | null | store_sales_prediction_1.ipynb | mariosotper/Predict-Time--Series-Test | da78762cf6449726fd1d74013b775256b4fb0bdd | [
"MIT"
] | null | null | null | store_sales_prediction_1.ipynb | mariosotper/Predict-Time--Series-Test | da78762cf6449726fd1d74013b775256b4fb0bdd | [
"MIT"
] | null | null | null | 85.288981 | 35,804 | 0.778191 | [
[
[
" # 0.0. IMPORTS",
"_____no_output_____"
]
],
[
[
"import math\nimport numpy as np\nimport pandas as pd\nimport inflection\n\nimport seaborn as sns\n\nfrom matplotlib import pyplot as plt\nfrom IPython.core.display import HTML",
"_____no_output_____"
]
],
[
[
"## 0.1. Helper Functions",
"_____no_output_____"
]
],
[
[
"def jupyter_settings():\n %matplotlib inline\n %pylab inline\n \n plt.style.use( 'bmh' )\n plt.rcParams['figure.figsize'] = [25, 12]\n plt.rcParams['font.size'] = 24\n \n display( HTML( '<style>.container { width:100% !important; }</style>') )\n pd.options.display.max_columns = None\n pd.options.display.max_rows = None\n pd.set_option( 'display.expand_frame_repr', False )\n \n sns.set()",
"_____no_output_____"
],
[
"jupyter_settings()",
"Populating the interactive namespace from numpy and matplotlib\n"
]
],
[
[
"## 0.2. Loading data",
"_____no_output_____"
]
],
[
[
"df_sales_raw = pd.read_csv( 'data/train.csv', low_memory=False )\ndf_store_raw = pd.read_csv( 'data/store.csv', low_memory=False )\n\n# merge\ndf_raw = pd.merge( df_sales_raw, df_store_raw, how='left', on='Store' )",
"_____no_output_____"
]
],
[
[
"# 1.0 DESCRIPCIÓN DE LOS DATOS",
"_____no_output_____"
]
],
[
[
"df1 = df_raw.copy()",
"_____no_output_____"
]
],
[
[
"## 1.1. Rename Columns",
"_____no_output_____"
]
],
[
[
"cols_old = ['Store', 'DayOfWeek', 'Date', 'Sales', 'Customers', 'Open', 'Promo', 'StateHoliday', 'SchoolHoliday', \n 'StoreType', 'Assortment', 'CompetitionDistance', 'CompetitionOpenSinceMonth',\n 'CompetitionOpenSinceYear', 'Promo2', 'Promo2SinceWeek', 'Promo2SinceYear', 'PromoInterval']\n\nsnakecase = lambda x: inflection.underscore( x )\n\ncols_new = list( map( snakecase, cols_old ) )\n\n# rename\ndf1.columns = cols_new",
"_____no_output_____"
]
],
[
[
"## 1.2. Data Dimensions",
"_____no_output_____"
]
],
[
[
"print( 'Number of Rows: {}'.format( df1.shape[0] ) )\nprint( 'Number of Cols: {}'.format( df1.shape[1] ) )\n\n",
"Number of Rows: 1017209\nNumber of Cols: 18\n"
]
],
[
[
"## 1.3. Data Types",
"_____no_output_____"
]
],
[
[
"df1['date'] = pd.to_datetime( df1['date'] )\ndf1.dtypes",
"_____no_output_____"
]
],
[
[
"## 1.4. Check NA",
"_____no_output_____"
]
],
[
[
"df1.isna().sum()",
"_____no_output_____"
]
],
[
[
"## 1.5. Fillout NA",
"_____no_output_____"
]
],
[
[
"df1.sample()",
"_____no_output_____"
],
[
"#competition_distance \ndf1['competition_distance'] = df1['competition_distance'].apply( lambda x: 200000.0 if math.isnan( x ) else x )\n\n#competition_open_since_month\ndf1['competition_open_since_month'] = df1.apply( lambda x: x['date'].month if math.isnan( x['competition_open_since_month'] ) else x['competition_open_since_month'], axis=1 )\n\n#competition_open_since_year \ndf1['competition_open_since_year'] = df1.apply( lambda x: x['date'].year if math.isnan( x['competition_open_since_year'] ) else x['competition_open_since_year'], axis=1 )\n\n#promo2_since_week \ndf1['promo2_since_week'] = df1.apply( lambda x: x['date'].week if math.isnan( x['promo2_since_week'] ) else x['promo2_since_week'], axis=1 )\n\n#promo2_since_year \ndf1['promo2_since_year'] = df1.apply( lambda x: x['date'].year if math.isnan( x['promo2_since_year'] ) else x['promo2_since_year'], axis=1 )\n\n#promo_interval \nmonth_map = {1: 'Jan', 2: 'Fev', 3: 'Mar', 4: 'Apr', 5: 'May', 6: 'Jun', 7: 'Jul', 8: 'Aug', 9: 'Sep', 10: 'Oct', 11: 'Nov', 12: 'Dec'}\n\ndf1['promo_interval'].fillna(0, inplace=True )\n\ndf1['month_map'] = df1['date'].dt.month.map( month_map )\n\ndf1['is_promo'] = df1[['promo_interval', 'month_map']].apply( lambda x: 0 if x['promo_interval'] == 0 else 1 if x['month_map'] in x['promo_interval'].split( ',' ) else 0, axis=1 )\n",
"_____no_output_____"
],
[
"df1.isna().sum()",
"_____no_output_____"
]
],
[
[
"## 1.6. Change Data Types",
"_____no_output_____"
]
],
[
[
"# competiton\ndf1['competition_open_since_month'] = df1['competition_open_since_month'].astype( int )\ndf1['competition_open_since_year'] = df1['competition_open_since_year'].astype( int )\n \n# promo2\ndf1['promo2_since_week'] = df1['promo2_since_week'].astype( int )\ndf1['promo2_since_year'] = df1['promo2_since_year'].astype( int )",
"_____no_output_____"
]
],
[
[
"## 1.7. Descriptive Statistics",
"_____no_output_____"
]
],
[
[
"num_attributes = df1.select_dtypes( include=['int64', 'float64'] )\ncat_attributes = df1.select_dtypes( exclude=['int64', 'float64', 'datetime64[ns]'] )",
"_____no_output_____"
]
],
[
[
"### 1.7.1. Numerical Atributes",
"_____no_output_____"
]
],
[
[
"# Central Tendency - mean, meadina \nct1 = pd.DataFrame( num_attributes.apply( np.mean ) ).T\nct2 = pd.DataFrame( num_attributes.apply( np.median ) ).T\n\n# dispersion - std, min, max, range, skew, kurtosis\nd1 = pd.DataFrame( num_attributes.apply( np.std ) ).T \nd2 = pd.DataFrame( num_attributes.apply( min ) ).T \nd3 = pd.DataFrame( num_attributes.apply( max ) ).T \nd4 = pd.DataFrame( num_attributes.apply( lambda x: x.max() - x.min() ) ).T \nd5 = pd.DataFrame( num_attributes.apply( lambda x: x.skew() ) ).T \nd6 = pd.DataFrame( num_attributes.apply( lambda x: x.kurtosis() ) ).T \n\n# concatenar\nm = pd.concat( [d2, d3, d4, ct1, ct2, d1, d5, d6] ).T.reset_index()\nm.columns = ['attributes', 'min', 'max', 'range', 'mean', 'median', 'std', 'skew', 'kurtosis']\nm",
"_____no_output_____"
],
[
"sns.distplot( df1['competition_distance'], kde=False )",
"_____no_output_____"
]
],
[
[
"### 1.7.2. Categorical Atributes",
"_____no_output_____"
]
],
[
[
"cat_attributes.apply( lambda x: x.unique().shape[0] )",
"_____no_output_____"
],
[
"aux = df1[(df1['state_holiday'] != '0') & (df1['sales'] > 0)]\n\nplt.subplot( 1, 3, 1 )\nsns.boxplot( x='state_holiday', y='sales', data=aux )\n\nplt.subplot( 1, 3, 2 )\nsns.boxplot( x='store_type', y='sales', data=aux )\n\nplt.subplot( 1, 3, 3 )\nsns.boxplot( x='assortment', y='sales', data=aux )",
"_____no_output_____"
]
]
] | [
"markdown",
"code",
"markdown",
"code",
"markdown",
"code",
"markdown",
"code",
"markdown",
"code",
"markdown",
"code",
"markdown",
"code",
"markdown",
"code",
"markdown",
"code",
"markdown",
"code",
"markdown",
"code",
"markdown",
"code",
"markdown",
"code"
] | [
[
"markdown"
],
[
"code"
],
[
"markdown"
],
[
"code",
"code"
],
[
"markdown"
],
[
"code"
],
[
"markdown"
],
[
"code"
],
[
"markdown"
],
[
"code"
],
[
"markdown"
],
[
"code"
],
[
"markdown"
],
[
"code"
],
[
"markdown"
],
[
"code"
],
[
"markdown"
],
[
"code",
"code",
"code"
],
[
"markdown"
],
[
"code"
],
[
"markdown"
],
[
"code"
],
[
"markdown"
],
[
"code",
"code"
],
[
"markdown"
],
[
"code",
"code"
]
] |
e776d8effa8cb6f8486a397af2613165ebee1c1a | 584,885 | ipynb | Jupyter Notebook | Chapter 8 - Modeling Continuous Variables.ipynb | Suraj-617/sas-viya-python | fc57ee9c5c0adc25dfede124874876cfdca1f461 | [
"Apache-2.0"
] | 19 | 2017-01-11T21:17:31.000Z | 2021-11-02T17:36:58.000Z | Chapter 8 - Modeling Continuous Variables.ipynb | Suraj-617/sas-viya-python | fc57ee9c5c0adc25dfede124874876cfdca1f461 | [
"Apache-2.0"
] | null | null | null | Chapter 8 - Modeling Continuous Variables.ipynb | Suraj-617/sas-viya-python | fc57ee9c5c0adc25dfede124874876cfdca1f461 | [
"Apache-2.0"
] | 15 | 2017-01-13T02:37:38.000Z | 2021-12-06T22:00:56.000Z | 88.005567 | 140,518 | 0.539198 | [
[
[
"# Chapter 8: Modeling Continuous Variables",
"_____no_output_____"
]
],
[
[
"import swat\n\nconn = swat.CAS('server-name.mycomany.com', 5570, 'username', 'password')",
"_____no_output_____"
],
[
"cars = conn.upload_file('https://raw.githubusercontent.com/sassoftware/sas-viya-programming/master/data/cars.csv',\n casout=dict(name='cars', replace=True))",
"NOTE: Cloud Analytic Services made the uploaded file available as table CARS in caslib CASUSER(username).\nNOTE: The table CARS has been created in caslib CASUSER(username) from binary data uploaded to Cloud Analytic Services.\n"
],
[
"cars.tableinfo()",
"_____no_output_____"
],
[
"cars.columninfo()",
"_____no_output_____"
]
],
[
[
"## Linear Regressions",
"_____no_output_____"
]
],
[
[
"conn.loadactionset('regression')\nconn.help(actionset='regression')",
"NOTE: Added action set 'regression'.\nNOTE: Information for action set 'regression':\nNOTE: regression\nNOTE: glm - Fits linear regression models using the method of least squares\nNOTE: genmod - Fits generalized linear regression models\nNOTE: logistic - Fits logistic regression models\n"
]
],
[
[
"Simple linear regression",
"_____no_output_____"
]
],
[
[
"cars.glm( \n target='MSRP',\n inputs=['MPG_City']\n)",
"_____no_output_____"
]
],
[
[
"Another way to define a model ",
"_____no_output_____"
]
],
[
[
"linear1 = cars.Glm()\nlinear1.target = 'MSRP'\nlinear1.inputs = ['MPG_City']\nlinear1()",
"_____no_output_____"
],
[
"linear1.display.names = ['ParameterEstimates']\nlinear1()",
"_____no_output_____"
]
],
[
[
"Scoring",
"_____no_output_____"
]
],
[
[
"del linear1.display.names\n\nresult1 = conn.CASTable('MSRPPrediction')\nresult1.replace = True\nlinear1.output.casout = result1\nlinear1.output.copyVars = 'ALL';\nlinear1()",
"_____no_output_____"
],
[
"result1[['pred']].summary()",
"_____no_output_____"
]
],
[
[
"Output more information in the score table",
"_____no_output_____"
]
],
[
[
"result2 = conn.CASTable('MSRPPrediction3')\nresult2.replace = True\nlinear1.output.casout = result2\nlinear1.output.pred = 'Predicted_MSRP'\nlinear1.output.resid = 'Presidual_MSRP'\nlinear1.output.lcl = 'LCL_MSRP'\nlinear1.output.ucl = 'UCL_MSRP'\nlinear1()",
"_____no_output_____"
]
],
[
[
"Use scatter plot of predicted values and residuals to check the model fitting",
"_____no_output_____"
]
],
[
[
"from bokeh.charts import Scatter, output_file, output_notebook, show\n\nout1 = result2.to_frame()\n\np = Scatter(out1, x='Predicted_MSRP', y='Residual_MSRP', color='Origin', marker='Origin')\noutput_notebook()\n#output_file('scatter.html')\nshow(p)",
"_____no_output_____"
]
],
[
[
"Investigate which observations have negative predicted MSRP values",
"_____no_output_____"
]
],
[
[
"result2[['Predicted_MSRP', 'MSRP', 'MPG_City','Make','Model']].query('Predicted_MSRP < 0').to_frame()",
"_____no_output_____"
],
[
"p = Scatter(out1, x='MPG_City', y='MSRP', color='Origin', marker='Origin')\noutput_notebook()\n#output_file('scatter.html')\nshow(p)",
"_____no_output_____"
]
],
[
[
"Remove outliers",
"_____no_output_____"
]
],
[
[
"cars.where = 'MSRP < 100000 and MPG_City < 40'\n\nresult2 = conn.CASTable('cas.MSRPPrediction2')\nresult2.replace = True\n\nlinear2 = cars.Glm()\nlinear2 = cars.query('MSRP < 100000 and MPG_City < 40').glm\nlinear2.target = 'MSRP'\nlinear2.inputs = ['MPG_City']\nlinear2.output.casout = result2\nlinear2.output.copyVars = 'ALL';\nlinear2.output.pred = 'Predicted_MSRP'\nlinear2.output.resid = 'Residual_MSRP'\nlinear2.output.lcl = 'LCL_MSRP'\nlinear2.output.ucl = 'UCL_MSRP'\nlinear2()",
"_____no_output_____"
]
],
[
[
"Check the model fitting again",
"_____no_output_____"
]
],
[
[
"out2 = result2.to_frame()\np = Scatter(out2, x='Predicted_MSRP', y='Residual_MSRP', color='Origin', marker='Origin')\noutput_notebook()\n#output_file('scatter.html')\nshow(p)",
"_____no_output_____"
]
],
[
[
"Adding more predictors",
"_____no_output_____"
]
],
[
[
"nomList = ['Origin','Type','DriveTrain']\ncontList = ['MPG_City','Weight','Length']\n\nlinear3 = conn.CASTable('cars').Glm()\nlinear3.target = 'MSRP'\nlinear3.inputs = nomList + contList\nlinear3.nominals = nomList\nlinear3.display.names = ['FitStatistics','ParameterEstimates']\nlinear3()",
"_____no_output_____"
]
],
[
[
"Groupby regression ",
"_____no_output_____"
]
],
[
[
"cars = conn.CASTable('cars')\nout = cars.groupby('Origin')[['MSRP']].summary().concat_bygroups()\nout['Summary'][['Column','Mean','Var','Std']]",
"_____no_output_____"
],
[
"cars = conn.CASTable('cars')\ncars.groupby=['Origin']\ncars.where = 'MSRP < 100000 and MPG_City < 40'\nnomList = ['Type','DriveTrain']\ncontList = ['MPG_City','Weight','Length']\ngroupBYResult =conn.CASTable('MSRPPredictionGroupBy')\n\nlinear4 = cars.glm\nlinear4.target = 'MSRP'\nlinear4.inputs = nomList + contList\nlinear4.nominals = nomList\nlinear4.display.names = ['FitStatistics','ParameterEstimates']\nlinear4.output.casout = groupBYResult\nlinear4.output.copyVars = 'ALL';\nlinear4.output.pred = 'Predicted_MSRP'\nlinear4.output.resid = 'Residual_MSRP'\nlinear4.output.lcl = 'LCL_MSRP'\nlinear4.output.ucl = 'UCL_MSRP'\nlinear4()",
"_____no_output_____"
],
[
"out = groupBYResult.to_frame()\np = Scatter(out, x='Predicted_MSRP', y='Residual_MSRP', color='Origin', marker='Origin')\noutput_notebook()\n#output_file('scatter.html')\nshow(p)",
"_____no_output_____"
]
],
[
[
"## Extensions of Ordinary Linear Regression",
"_____no_output_____"
],
[
"### Generalized Linear Models",
"_____no_output_____"
],
[
"Gamma Regression",
"_____no_output_____"
]
],
[
[
"cars = conn.CASTable('cars')\ngenmodModel1 = cars.Genmod()\ngenmodModel1.model.depvars = 'MSRP'\ngenmodModel1.model.effects = ['MPG_City']\ngenmodModel1.model.dist = 'gamma'\ngenmodModel1.model.link = 'log'\ngenmodModel1()",
"NOTE: Convergence criterion (GCONV=1E-8) satisfied.\n"
]
],
[
[
"Multinomial Regression",
"_____no_output_____"
]
],
[
[
"genmodModel1.model.depvars = 'Cylinders'\ngenmodModel1.model.dist = 'multinomial'\ngenmodModel1.model.link = 'logit'\ngenmodModel1.model.effects = ['MPG_City']\ngenmodModel1.display.names = ['ModelInfo', 'ParameterEstimates']\ngenmodModel1()",
"NOTE: Convergence criterion (GCONV=1E-8) satisfied.\n"
]
],
[
[
"Score the input table",
"_____no_output_____"
]
],
[
[
"genmodResult = conn.CASTable('CylinderPredicted', replace=True)\ngenmodModel1.output.casout = genmodResult\ngenmodModel1.output.copyVars = 'ALL';\ngenmodModel1.output.pred = 'Prob_Cylinders'\ngenmodModel1()\ngenmodResult[['Prob_Cylinders','_level_','Cylinders','MPG_City']].head(24)",
"NOTE: Convergence criterion (GCONV=1E-8) satisfied.\n"
]
],
[
[
"### Regression Trees",
"_____no_output_____"
]
],
[
[
"conn.loadactionset('decisiontree')\nconn.help(actionset='decisiontree')",
"NOTE: Added action set 'decisiontree'.\nNOTE: Information for action set 'decisiontree':\nNOTE: decisionTree\nNOTE: dtreeTrain - Train a decision tree\nNOTE: dtreeScore - Score a table using a decision tree model\nNOTE: dtreeSplit - Split decision tree nodes\nNOTE: dtreePrune - Prune a decision tree\nNOTE: dtreeMerge - Merge decision tree nodes\nNOTE: dtreeCode - Generate DATA step scoring code from a decision tree model\nNOTE: forestTrain - Train a forest\nNOTE: forestScore - Score a table using a forest model\nNOTE: forestCode - Generate DATA step scoring code from a forest model\nNOTE: gbtreeTrain - Train a gradient boosting tree\nNOTE: gbtreeScore - Score a table using a gradient boosting tree model\nNOTE: gbtreecode - Generate DATA step scoring code from a gradient boosting tree model\n"
],
[
"cars = conn.CASTable('cars')\n\noutput1 = conn.CASTable('treeModel1')\noutput1.replace = True;\n\ntree1 = cars.dtreetrain\ntree1.target = 'MSRP'\ntree1.inputs = ['MPG_City']\ntree1.casout = output1\ntree1.maxlevel = 2\ntree1()",
"_____no_output_____"
],
[
"output1[['_NodeID_', '_Parent_','_Mean_','_NodeName_','_PBLower0_','_PBUpper0_']].fetch()",
"_____no_output_____"
],
[
"conn.close()",
"_____no_output_____"
]
]
] | [
"markdown",
"code",
"markdown",
"code",
"markdown",
"code",
"markdown",
"code",
"markdown",
"code",
"markdown",
"code",
"markdown",
"code",
"markdown",
"code",
"markdown",
"code",
"markdown",
"code",
"markdown",
"code",
"markdown",
"code",
"markdown",
"code",
"markdown",
"code",
"markdown",
"code",
"markdown",
"code"
] | [
[
"markdown"
],
[
"code",
"code",
"code",
"code"
],
[
"markdown"
],
[
"code"
],
[
"markdown"
],
[
"code"
],
[
"markdown"
],
[
"code",
"code"
],
[
"markdown"
],
[
"code",
"code"
],
[
"markdown"
],
[
"code"
],
[
"markdown"
],
[
"code"
],
[
"markdown"
],
[
"code",
"code"
],
[
"markdown"
],
[
"code"
],
[
"markdown"
],
[
"code"
],
[
"markdown"
],
[
"code"
],
[
"markdown"
],
[
"code",
"code",
"code"
],
[
"markdown",
"markdown",
"markdown"
],
[
"code"
],
[
"markdown"
],
[
"code"
],
[
"markdown"
],
[
"code"
],
[
"markdown"
],
[
"code",
"code",
"code",
"code"
]
] |
e776dab7f5e812d5528f0287e3863bd79cc9fffe | 111,507 | ipynb | Jupyter Notebook | sagemaker/07_tensorflow_distributed_training_data_parallelism/sagemaker-notebook.ipynb | Shamik-07/notebooks | 1783fb76ebbf697856863d83cf0ca0dfaf6c232e | [
"Apache-2.0"
] | 765 | 2020-06-15T14:09:03.000Z | 2022-03-31T19:37:11.000Z | sagemaker/07_tensorflow_distributed_training_data_parallelism/sagemaker-notebook.ipynb | Shamik-07/notebooks | 1783fb76ebbf697856863d83cf0ca0dfaf6c232e | [
"Apache-2.0"
] | 105 | 2020-07-24T11:01:46.000Z | 2022-03-29T16:15:04.000Z | sagemaker/07_tensorflow_distributed_training_data_parallelism/sagemaker-notebook.ipynb | Shamik-07/notebooks | 1783fb76ebbf697856863d83cf0ca0dfaf6c232e | [
"Apache-2.0"
] | 433 | 2020-06-15T19:31:21.000Z | 2022-03-31T10:08:58.000Z | 177.842105 | 78,504 | 0.870726 | [
[
[
"# Huggingface Sagemaker-sdk - Distributed Training Demo for `TensorFlow`\n### Distributed Data Parallelism with `transformers` and `tensorflow`",
"_____no_output_____"
],
[
"1. [Introduction](#Introduction) \n2. [Development Environment and Permissions](#Development-Environment-and-Permissions)\n 1. [Installation](#Installation) \n 2. [Development environment](#Development-environment) \n 3. [Permissions](#Permissions)\n3. [Processing](#Preprocessing) \n 1. [Tokenization](#Tokenization) \n 2. [Uploading data to sagemaker_session_bucket](#Uploading-data-to-sagemaker_session_bucket) \n4. [Fine-tuning & starting Sagemaker Training Job](#Fine-tuning-\\&-starting-Sagemaker-Training-Job) \n 1. [Creating an Estimator and start a training job](#Creating-an-Estimator-and-start-a-training-job) \n 2. [Estimator Parameters](#Estimator-Parameters) \n 3. [Download fine-tuned model from s3](#Download-fine-tuned-model-from-s3)\n 3. [Attach to old training job to an estimator ](#Attach-to-old-training-job-to-an-estimator) \n5. [_Coming soon_:Push model to the Hugging Face hub](#Push-model-to-the-Hugging-Face-hub)",
"_____no_output_____"
],
[
"# Introduction\n\nWelcome to our distributed end-to-end binary Text-Classification example. In this demo, we will use the Hugging Faces `transformers` and `datasets` library together with a custom Amazon sagemaker-sdk extension to fine-tune a pre-trained transformer on binary text classification. In particular, the pre-trained model will be fine-tuned using the `imdb` dataset. To speed upload Training we are going to use SageMaker distributed Data Parallel library to run our training distributed across multiple gpus. To get started, we need to set up the environment with a few prerequisite steps, for permissions, configurations, and so on. \n\n\n\n_**NOTE: You can run this demo in Sagemaker Studio, your local machine or Sagemaker Notebook Instances**_",
"_____no_output_____"
],
[
"# Development Environment and Permissions ",
"_____no_output_____"
],
[
"## Installation\n\n_*Note:* we only install the required libraries from Hugging Face and AWS. You also need PyTorch or Tensorflow, if you haven´t it installed_",
"_____no_output_____"
]
],
[
[
"!pip install \"sagemaker>=2.48.0\" --upgrade",
"_____no_output_____"
]
],
[
[
"## Development environment ",
"_____no_output_____"
],
[
"**upgrade ipywidgets for `datasets` library and restart kernel, only needed when prerpocessing is done in the notebook**",
"_____no_output_____"
]
],
[
[
"%%capture\nimport IPython\n!conda install -c conda-forge ipywidgets -y\nIPython.Application.instance().kernel.do_shutdown(True) # has to restart kernel so changes are used",
"_____no_output_____"
],
[
"import sagemaker.huggingface",
"_____no_output_____"
]
],
[
[
"## Permissions",
"_____no_output_____"
],
[
"_If you are going to use Sagemaker in a local environment. You need access to an IAM Role with the required permissions for Sagemaker. You can find [here](https://docs.aws.amazon.com/sagemaker/latest/dg/sagemaker-roles.html) more about it._",
"_____no_output_____"
]
],
[
[
"import sagemaker\n\nsess = sagemaker.Session()\n# sagemaker session bucket -> used for uploading data, models and logs\n# sagemaker will automatically create this bucket if it not exists\nsagemaker_session_bucket=None\nif sagemaker_session_bucket is None and sess is not None:\n # set to default bucket if a bucket name is not given\n sagemaker_session_bucket = sess.default_bucket()\n\nrole = sagemaker.get_execution_role()\nsess = sagemaker.Session(default_bucket=sagemaker_session_bucket)\n\nprint(f\"sagemaker role arn: {role}\")\nprint(f\"sagemaker bucket: {sess.default_bucket()}\")\nprint(f\"sagemaker session region: {sess.boto_region_name}\")",
"_____no_output_____"
]
],
[
[
"# Preprocessing\n\nIn this example the preproccsing will be done in the `train.py` when executing the script. You could also move the `preprocessing` outside of the script and upload the data to s3 and pass it into it.",
"_____no_output_____"
],
[
"# Fine-tuning & starting Sagemaker Training Job\n\nIn order to create a sagemaker training job we need an `HuggingFace` Estimator. The Estimator handles end-to-end Amazon SageMaker training and deployment tasks. In a Estimator we define, which fine-tuning script should be used as `entry_point`, which `instance_type` should be used, which `hyperparameters` are passed in .....\n\n\n\n```python\nhuggingface_estimator = HuggingFace(entry_point='train.py',\n source_dir='./scripts',\n base_job_name='huggingface-sdk-extension',\n instance_type='ml.p3.2xlarge',\n instance_count=1,\n transformers_version='4.4',\n pytorch_version='1.6',\n py_version='py37',\n role=role,\n hyperparameters = {'epochs': 1,\n 'train_batch_size': 32,\n 'model_name':'distilbert-base-uncased'\n })\n```\n\nWhen we create a SageMaker training job, SageMaker takes care of starting and managing all the required ec2 instances for us with the `huggingface` container, uploads the provided fine-tuning script `train.py` and downloads the data from our `sagemaker_session_bucket` into the container at `/opt/ml/input/data`. Then, it starts the training job by running. \n\n```python\n/opt/conda/bin/python train.py --epochs 1 --model_name distilbert-base-uncased --train_batch_size 32\n```\n\nThe `hyperparameters` you define in the `HuggingFace` estimator are passed in as named arguments. \n\nSagemaker is providing useful properties about the training environment through various environment variables, including the following:\n\n* `SM_MODEL_DIR`: A string that represents the path where the training job writes the model artifacts to. After training, artifacts in this directory are uploaded to S3 for model hosting.\n\n* `SM_NUM_GPUS`: An integer representing the number of GPUs available to the host.\n\n* `SM_CHANNEL_XXXX:` A string that represents the path to the directory that contains the input data for the specified channel. For example, if you specify two input channels in the HuggingFace estimator’s fit call, named `train` and `test`, the environment variables `SM_CHANNEL_TRAIN` and `SM_CHANNEL_TEST` are set.\n\n\nTo run your training job locally you can define `instance_type='local'` or `instance_type='local_gpu'` for gpu usage. _Note: this does not working within SageMaker Studio_\n",
"_____no_output_____"
]
],
[
[
"!pygmentize ./scripts/train.py",
"\u001b[34mimport\u001b[39;49;00m \u001b[04m\u001b[36margparse\u001b[39;49;00m\r\n\u001b[34mimport\u001b[39;49;00m \u001b[04m\u001b[36mlogging\u001b[39;49;00m\r\n\u001b[34mimport\u001b[39;49;00m \u001b[04m\u001b[36mos\u001b[39;49;00m\r\n\u001b[34mimport\u001b[39;49;00m \u001b[04m\u001b[36msys\u001b[39;49;00m\r\n\r\n\u001b[34mimport\u001b[39;49;00m \u001b[04m\u001b[36mtensorflow\u001b[39;49;00m \u001b[34mas\u001b[39;49;00m \u001b[04m\u001b[36mtf\u001b[39;49;00m\r\n\u001b[34mfrom\u001b[39;49;00m \u001b[04m\u001b[36mdatasets\u001b[39;49;00m \u001b[34mimport\u001b[39;49;00m load_dataset\r\n\u001b[34mfrom\u001b[39;49;00m \u001b[04m\u001b[36mtqdm\u001b[39;49;00m \u001b[34mimport\u001b[39;49;00m tqdm\r\n\u001b[34mfrom\u001b[39;49;00m \u001b[04m\u001b[36mtransformers\u001b[39;49;00m \u001b[34mimport\u001b[39;49;00m AutoTokenizer, TFAutoModelForSequenceClassification\r\n\u001b[34mfrom\u001b[39;49;00m \u001b[04m\u001b[36mtransformers\u001b[39;49;00m\u001b[04m\u001b[36m.\u001b[39;49;00m\u001b[04m\u001b[36mfile_utils\u001b[39;49;00m \u001b[34mimport\u001b[39;49;00m is_sagemaker_distributed_available\r\n\r\n\u001b[34mif\u001b[39;49;00m os.environ.get(\u001b[33m\"\u001b[39;49;00m\u001b[33mSDP_ENABLED\u001b[39;49;00m\u001b[33m\"\u001b[39;49;00m) \u001b[35mor\u001b[39;49;00m is_sagemaker_distributed_available():\r\n SDP_ENABLED = \u001b[34mTrue\u001b[39;49;00m\r\n os.environ[\u001b[33m\"\u001b[39;49;00m\u001b[33mSAGEMAKER_INSTANCE_TYPE\u001b[39;49;00m\u001b[33m\"\u001b[39;49;00m] = \u001b[33m\"\u001b[39;49;00m\u001b[33mp3dn.24xlarge\u001b[39;49;00m\u001b[33m\"\u001b[39;49;00m\r\n \u001b[34mimport\u001b[39;49;00m \u001b[04m\u001b[36msmdistributed\u001b[39;49;00m\u001b[04m\u001b[36m.\u001b[39;49;00m\u001b[04m\u001b[36mdataparallel\u001b[39;49;00m\u001b[04m\u001b[36m.\u001b[39;49;00m\u001b[04m\u001b[36mtensorflow\u001b[39;49;00m \u001b[34mas\u001b[39;49;00m \u001b[04m\u001b[36msdp\u001b[39;49;00m\r\n\u001b[34melse\u001b[39;49;00m:\r\n SDP_ENABLED = \u001b[34mFalse\u001b[39;49;00m\r\n\r\n\r\n\u001b[34mdef\u001b[39;49;00m \u001b[32mfit\u001b[39;49;00m(model, loss, opt, train_dataset, epochs, train_batch_size, max_steps=\u001b[34mNone\u001b[39;49;00m):\r\n pbar = tqdm(train_dataset)\r\n \u001b[34mfor\u001b[39;49;00m i, batch \u001b[35min\u001b[39;49;00m \u001b[36menumerate\u001b[39;49;00m(pbar):\r\n \u001b[34mwith\u001b[39;49;00m tf.GradientTape() \u001b[34mas\u001b[39;49;00m tape:\r\n inputs, targets = batch\r\n outputs = model(batch)\r\n loss_value = loss(targets, outputs.logits)\r\n\r\n \u001b[34mif\u001b[39;49;00m SDP_ENABLED:\r\n tape = sdp.DistributedGradientTape(tape, sparse_as_dense=\u001b[34mTrue\u001b[39;49;00m)\r\n\r\n grads = tape.gradient(loss_value, model.trainable_variables)\r\n opt.apply_gradients(\u001b[36mzip\u001b[39;49;00m(grads, model.trainable_variables))\r\n\r\n pbar.set_description(\u001b[33mf\u001b[39;49;00m\u001b[33m\"\u001b[39;49;00m\u001b[33mLoss: \u001b[39;49;00m\u001b[33m{\u001b[39;49;00mloss_value\u001b[33m:\u001b[39;49;00m\u001b[33m.4f\u001b[39;49;00m\u001b[33m}\u001b[39;49;00m\u001b[33m\"\u001b[39;49;00m)\r\n\r\n \u001b[34mif\u001b[39;49;00m SDP_ENABLED:\r\n \u001b[34mif\u001b[39;49;00m i == \u001b[34m0\u001b[39;49;00m:\r\n sdp.broadcast_variables(model.variables, root_rank=\u001b[34m0\u001b[39;49;00m)\r\n sdp.broadcast_variables(opt.variables(), root_rank=\u001b[34m0\u001b[39;49;00m)\r\n first_batch = \u001b[34mFalse\u001b[39;49;00m\r\n\r\n \u001b[34mif\u001b[39;49;00m max_steps \u001b[35mand\u001b[39;49;00m i >= max_steps:\r\n \u001b[34mbreak\u001b[39;49;00m\r\n\r\n train_results = {\u001b[33m\"\u001b[39;49;00m\u001b[33mloss\u001b[39;49;00m\u001b[33m\"\u001b[39;49;00m: loss_value.numpy()}\r\n \u001b[34mreturn\u001b[39;49;00m train_results\r\n\r\n\r\n\u001b[34mdef\u001b[39;49;00m \u001b[32mget_datasets\u001b[39;49;00m():\r\n \u001b[37m# Load dataset\u001b[39;49;00m\r\n train_dataset, test_dataset = load_dataset(\u001b[33m\"\u001b[39;49;00m\u001b[33mimdb\u001b[39;49;00m\u001b[33m\"\u001b[39;49;00m, split=[\u001b[33m\"\u001b[39;49;00m\u001b[33mtrain\u001b[39;49;00m\u001b[33m\"\u001b[39;49;00m, \u001b[33m\"\u001b[39;49;00m\u001b[33mtest\u001b[39;49;00m\u001b[33m\"\u001b[39;49;00m])\r\n\r\n \u001b[37m# Preprocess train dataset\u001b[39;49;00m\r\n train_dataset = train_dataset.map(\r\n \u001b[34mlambda\u001b[39;49;00m e: tokenizer(e[\u001b[33m\"\u001b[39;49;00m\u001b[33mtext\u001b[39;49;00m\u001b[33m\"\u001b[39;49;00m], truncation=\u001b[34mTrue\u001b[39;49;00m, padding=\u001b[33m\"\u001b[39;49;00m\u001b[33mmax_length\u001b[39;49;00m\u001b[33m\"\u001b[39;49;00m), batched=\u001b[34mTrue\u001b[39;49;00m\r\n )\r\n train_dataset.set_format(\u001b[36mtype\u001b[39;49;00m=\u001b[33m\"\u001b[39;49;00m\u001b[33mtensorflow\u001b[39;49;00m\u001b[33m\"\u001b[39;49;00m, columns=[\u001b[33m\"\u001b[39;49;00m\u001b[33minput_ids\u001b[39;49;00m\u001b[33m\"\u001b[39;49;00m, \u001b[33m\"\u001b[39;49;00m\u001b[33mattention_mask\u001b[39;49;00m\u001b[33m\"\u001b[39;49;00m, \u001b[33m\"\u001b[39;49;00m\u001b[33mlabel\u001b[39;49;00m\u001b[33m\"\u001b[39;49;00m])\r\n\r\n train_features = {\r\n x: train_dataset[x].to_tensor(default_value=\u001b[34m0\u001b[39;49;00m, shape=[\u001b[34mNone\u001b[39;49;00m, tokenizer.model_max_length])\r\n \u001b[34mfor\u001b[39;49;00m x \u001b[35min\u001b[39;49;00m [\u001b[33m\"\u001b[39;49;00m\u001b[33minput_ids\u001b[39;49;00m\u001b[33m\"\u001b[39;49;00m, \u001b[33m\"\u001b[39;49;00m\u001b[33mattention_mask\u001b[39;49;00m\u001b[33m\"\u001b[39;49;00m]\r\n }\r\n tf_train_dataset = tf.data.Dataset.from_tensor_slices((train_features, train_dataset[\u001b[33m\"\u001b[39;49;00m\u001b[33mlabel\u001b[39;49;00m\u001b[33m\"\u001b[39;49;00m]))\r\n\r\n \u001b[37m# Preprocess test dataset\u001b[39;49;00m\r\n test_dataset = test_dataset.map(\r\n \u001b[34mlambda\u001b[39;49;00m e: tokenizer(e[\u001b[33m\"\u001b[39;49;00m\u001b[33mtext\u001b[39;49;00m\u001b[33m\"\u001b[39;49;00m], truncation=\u001b[34mTrue\u001b[39;49;00m, padding=\u001b[33m\"\u001b[39;49;00m\u001b[33mmax_length\u001b[39;49;00m\u001b[33m\"\u001b[39;49;00m), batched=\u001b[34mTrue\u001b[39;49;00m\r\n )\r\n test_dataset.set_format(\u001b[36mtype\u001b[39;49;00m=\u001b[33m\"\u001b[39;49;00m\u001b[33mtensorflow\u001b[39;49;00m\u001b[33m\"\u001b[39;49;00m, columns=[\u001b[33m\"\u001b[39;49;00m\u001b[33minput_ids\u001b[39;49;00m\u001b[33m\"\u001b[39;49;00m, \u001b[33m\"\u001b[39;49;00m\u001b[33mattention_mask\u001b[39;49;00m\u001b[33m\"\u001b[39;49;00m, \u001b[33m\"\u001b[39;49;00m\u001b[33mlabel\u001b[39;49;00m\u001b[33m\"\u001b[39;49;00m])\r\n\r\n test_features = {\r\n x: test_dataset[x].to_tensor(default_value=\u001b[34m0\u001b[39;49;00m, shape=[\u001b[34mNone\u001b[39;49;00m, tokenizer.model_max_length])\r\n \u001b[34mfor\u001b[39;49;00m x \u001b[35min\u001b[39;49;00m [\u001b[33m\"\u001b[39;49;00m\u001b[33minput_ids\u001b[39;49;00m\u001b[33m\"\u001b[39;49;00m, \u001b[33m\"\u001b[39;49;00m\u001b[33mattention_mask\u001b[39;49;00m\u001b[33m\"\u001b[39;49;00m]\r\n }\r\n tf_test_dataset = tf.data.Dataset.from_tensor_slices((test_features, test_dataset[\u001b[33m\"\u001b[39;49;00m\u001b[33mlabel\u001b[39;49;00m\u001b[33m\"\u001b[39;49;00m]))\r\n\r\n \u001b[34mif\u001b[39;49;00m SDP_ENABLED:\r\n tf_train_dataset = tf_train_dataset.shard(sdp.size(), sdp.rank())\r\n tf_test_dataset = tf_test_dataset.shard(sdp.size(), sdp.rank())\r\n tf_train_dataset = tf_train_dataset.batch(args.train_batch_size, drop_remainder=\u001b[34mTrue\u001b[39;49;00m)\r\n tf_test_dataset = tf_test_dataset.batch(args.eval_batch_size, drop_remainder=\u001b[34mTrue\u001b[39;49;00m)\r\n\r\n \u001b[34mreturn\u001b[39;49;00m tf_train_dataset, tf_test_dataset\r\n\r\n\r\n\u001b[34mif\u001b[39;49;00m \u001b[31m__name__\u001b[39;49;00m == \u001b[33m\"\u001b[39;49;00m\u001b[33m__main__\u001b[39;49;00m\u001b[33m\"\u001b[39;49;00m:\r\n\r\n parser = argparse.ArgumentParser()\r\n\r\n \u001b[37m# Hyperparameters sent by the client are passed as command-line arguments to the script.\u001b[39;49;00m\r\n parser.add_argument(\u001b[33m\"\u001b[39;49;00m\u001b[33m--epochs\u001b[39;49;00m\u001b[33m\"\u001b[39;49;00m, \u001b[36mtype\u001b[39;49;00m=\u001b[36mint\u001b[39;49;00m, default=\u001b[34m3\u001b[39;49;00m)\r\n parser.add_argument(\u001b[33m\"\u001b[39;49;00m\u001b[33m--train-batch-size\u001b[39;49;00m\u001b[33m\"\u001b[39;49;00m, \u001b[36mtype\u001b[39;49;00m=\u001b[36mint\u001b[39;49;00m, default=\u001b[34m16\u001b[39;49;00m)\r\n parser.add_argument(\u001b[33m\"\u001b[39;49;00m\u001b[33m--eval-batch-size\u001b[39;49;00m\u001b[33m\"\u001b[39;49;00m, \u001b[36mtype\u001b[39;49;00m=\u001b[36mint\u001b[39;49;00m, default=\u001b[34m8\u001b[39;49;00m)\r\n parser.add_argument(\u001b[33m\"\u001b[39;49;00m\u001b[33m--model_name\u001b[39;49;00m\u001b[33m\"\u001b[39;49;00m, \u001b[36mtype\u001b[39;49;00m=\u001b[36mstr\u001b[39;49;00m)\r\n parser.add_argument(\u001b[33m\"\u001b[39;49;00m\u001b[33m--learning_rate\u001b[39;49;00m\u001b[33m\"\u001b[39;49;00m, \u001b[36mtype\u001b[39;49;00m=\u001b[36mstr\u001b[39;49;00m, default=\u001b[34m5e-5\u001b[39;49;00m)\r\n parser.add_argument(\u001b[33m\"\u001b[39;49;00m\u001b[33m--do_train\u001b[39;49;00m\u001b[33m\"\u001b[39;49;00m, \u001b[36mtype\u001b[39;49;00m=\u001b[36mbool\u001b[39;49;00m, default=\u001b[34mTrue\u001b[39;49;00m)\r\n parser.add_argument(\u001b[33m\"\u001b[39;49;00m\u001b[33m--do_eval\u001b[39;49;00m\u001b[33m\"\u001b[39;49;00m, \u001b[36mtype\u001b[39;49;00m=\u001b[36mbool\u001b[39;49;00m, default=\u001b[34mTrue\u001b[39;49;00m)\r\n\r\n \u001b[37m# Data, model, and output directories\u001b[39;49;00m\r\n parser.add_argument(\u001b[33m\"\u001b[39;49;00m\u001b[33m--output_data_dir\u001b[39;49;00m\u001b[33m\"\u001b[39;49;00m, \u001b[36mtype\u001b[39;49;00m=\u001b[36mstr\u001b[39;49;00m, default=os.environ[\u001b[33m\"\u001b[39;49;00m\u001b[33mSM_OUTPUT_DATA_DIR\u001b[39;49;00m\u001b[33m\"\u001b[39;49;00m])\r\n parser.add_argument(\u001b[33m\"\u001b[39;49;00m\u001b[33m--model_dir\u001b[39;49;00m\u001b[33m\"\u001b[39;49;00m, \u001b[36mtype\u001b[39;49;00m=\u001b[36mstr\u001b[39;49;00m, default=os.environ[\u001b[33m\"\u001b[39;49;00m\u001b[33mSM_MODEL_DIR\u001b[39;49;00m\u001b[33m\"\u001b[39;49;00m])\r\n parser.add_argument(\u001b[33m\"\u001b[39;49;00m\u001b[33m--n_gpus\u001b[39;49;00m\u001b[33m\"\u001b[39;49;00m, \u001b[36mtype\u001b[39;49;00m=\u001b[36mstr\u001b[39;49;00m, default=os.environ[\u001b[33m\"\u001b[39;49;00m\u001b[33mSM_NUM_GPUS\u001b[39;49;00m\u001b[33m\"\u001b[39;49;00m])\r\n\r\n args, _ = parser.parse_known_args()\r\n\r\n \u001b[37m# Set up logging\u001b[39;49;00m\r\n logger = logging.getLogger(\u001b[31m__name__\u001b[39;49;00m)\r\n\r\n logging.basicConfig(\r\n level=logging.getLevelName(\u001b[33m\"\u001b[39;49;00m\u001b[33mINFO\u001b[39;49;00m\u001b[33m\"\u001b[39;49;00m),\r\n handlers=[logging.StreamHandler(sys.stdout)],\r\n \u001b[36mformat\u001b[39;49;00m=\u001b[33m\"\u001b[39;49;00m\u001b[33m%(asctime)s\u001b[39;49;00m\u001b[33m - \u001b[39;49;00m\u001b[33m%(name)s\u001b[39;49;00m\u001b[33m - \u001b[39;49;00m\u001b[33m%(levelname)s\u001b[39;49;00m\u001b[33m - \u001b[39;49;00m\u001b[33m%(message)s\u001b[39;49;00m\u001b[33m\"\u001b[39;49;00m,\r\n )\r\n\r\n \u001b[34mif\u001b[39;49;00m SDP_ENABLED:\r\n sdp.init()\r\n\r\n gpus = tf.config.experimental.list_physical_devices(\u001b[33m\"\u001b[39;49;00m\u001b[33mGPU\u001b[39;49;00m\u001b[33m\"\u001b[39;49;00m)\r\n \u001b[34mfor\u001b[39;49;00m gpu \u001b[35min\u001b[39;49;00m gpus:\r\n tf.config.experimental.set_memory_growth(gpu, \u001b[34mTrue\u001b[39;49;00m)\r\n \u001b[34mif\u001b[39;49;00m gpus:\r\n tf.config.experimental.set_visible_devices(gpus[sdp.local_rank()], \u001b[33m\"\u001b[39;49;00m\u001b[33mGPU\u001b[39;49;00m\u001b[33m\"\u001b[39;49;00m)\r\n\r\n \u001b[37m# Load model and tokenizer\u001b[39;49;00m\r\n model = TFAutoModelForSequenceClassification.from_pretrained(args.model_name)\r\n tokenizer = AutoTokenizer.from_pretrained(args.model_name)\r\n\r\n \u001b[37m# get datasets\u001b[39;49;00m\r\n tf_train_dataset, tf_test_dataset = get_datasets()\r\n\r\n \u001b[37m# fine optimizer and loss\u001b[39;49;00m\r\n optimizer = tf.keras.optimizers.Adam(learning_rate=args.learning_rate)\r\n loss = tf.keras.losses.SparseCategoricalCrossentropy(from_logits=\u001b[34mTrue\u001b[39;49;00m)\r\n metrics = [tf.keras.metrics.SparseCategoricalAccuracy()]\r\n model.compile(optimizer=optimizer, loss=loss, metrics=metrics)\r\n\r\n \u001b[37m# Training\u001b[39;49;00m\r\n \u001b[34mif\u001b[39;49;00m args.do_train:\r\n\r\n \u001b[37m# train_results = model.fit(tf_train_dataset, epochs=args.epochs, batch_size=args.train_batch_size)\u001b[39;49;00m\r\n train_results = fit(\r\n model, loss, optimizer, tf_train_dataset, args.epochs, args.train_batch_size, max_steps=\u001b[34mNone\u001b[39;49;00m\r\n )\r\n logger.info(\u001b[33m\"\u001b[39;49;00m\u001b[33m*** Train ***\u001b[39;49;00m\u001b[33m\"\u001b[39;49;00m)\r\n\r\n output_eval_file = os.path.join(args.output_data_dir, \u001b[33m\"\u001b[39;49;00m\u001b[33mtrain_results.txt\u001b[39;49;00m\u001b[33m\"\u001b[39;49;00m)\r\n\r\n \u001b[34mif\u001b[39;49;00m \u001b[35mnot\u001b[39;49;00m SDP_ENABLED \u001b[35mor\u001b[39;49;00m sdp.rank() == \u001b[34m0\u001b[39;49;00m:\r\n \u001b[34mwith\u001b[39;49;00m \u001b[36mopen\u001b[39;49;00m(output_eval_file, \u001b[33m\"\u001b[39;49;00m\u001b[33mw\u001b[39;49;00m\u001b[33m\"\u001b[39;49;00m) \u001b[34mas\u001b[39;49;00m writer:\r\n logger.info(\u001b[33m\"\u001b[39;49;00m\u001b[33m***** Train results *****\u001b[39;49;00m\u001b[33m\"\u001b[39;49;00m)\r\n logger.info(train_results)\r\n \u001b[34mfor\u001b[39;49;00m key, value \u001b[35min\u001b[39;49;00m train_results.items():\r\n logger.info(\u001b[33m\"\u001b[39;49;00m\u001b[33m \u001b[39;49;00m\u001b[33m%s\u001b[39;49;00m\u001b[33m = \u001b[39;49;00m\u001b[33m%s\u001b[39;49;00m\u001b[33m\"\u001b[39;49;00m, key, value)\r\n writer.write(\u001b[33m\"\u001b[39;49;00m\u001b[33m%s\u001b[39;49;00m\u001b[33m = \u001b[39;49;00m\u001b[33m%s\u001b[39;49;00m\u001b[33m\\n\u001b[39;49;00m\u001b[33m\"\u001b[39;49;00m % (key, value))\r\n\r\n \u001b[37m# Evaluation\u001b[39;49;00m\r\n \u001b[34mif\u001b[39;49;00m args.do_eval \u001b[35mand\u001b[39;49;00m (\u001b[35mnot\u001b[39;49;00m SDP_ENABLED \u001b[35mor\u001b[39;49;00m sdp.rank() == \u001b[34m0\u001b[39;49;00m):\r\n\r\n result = model.evaluate(tf_test_dataset, batch_size=args.eval_batch_size, return_dict=\u001b[34mTrue\u001b[39;49;00m)\r\n logger.info(\u001b[33m\"\u001b[39;49;00m\u001b[33m*** Evaluate ***\u001b[39;49;00m\u001b[33m\"\u001b[39;49;00m)\r\n\r\n output_eval_file = os.path.join(args.output_data_dir, \u001b[33m\"\u001b[39;49;00m\u001b[33meval_results.txt\u001b[39;49;00m\u001b[33m\"\u001b[39;49;00m)\r\n\r\n \u001b[34mwith\u001b[39;49;00m \u001b[36mopen\u001b[39;49;00m(output_eval_file, \u001b[33m\"\u001b[39;49;00m\u001b[33mw\u001b[39;49;00m\u001b[33m\"\u001b[39;49;00m) \u001b[34mas\u001b[39;49;00m writer:\r\n logger.info(\u001b[33m\"\u001b[39;49;00m\u001b[33m***** Eval results *****\u001b[39;49;00m\u001b[33m\"\u001b[39;49;00m)\r\n logger.info(result)\r\n \u001b[34mfor\u001b[39;49;00m key, value \u001b[35min\u001b[39;49;00m result.items():\r\n logger.info(\u001b[33m\"\u001b[39;49;00m\u001b[33m \u001b[39;49;00m\u001b[33m%s\u001b[39;49;00m\u001b[33m = \u001b[39;49;00m\u001b[33m%s\u001b[39;49;00m\u001b[33m\"\u001b[39;49;00m, key, value)\r\n writer.write(\u001b[33m\"\u001b[39;49;00m\u001b[33m%s\u001b[39;49;00m\u001b[33m = \u001b[39;49;00m\u001b[33m%s\u001b[39;49;00m\u001b[33m\\n\u001b[39;49;00m\u001b[33m\"\u001b[39;49;00m % (key, value))\r\n\r\n \u001b[37m# Save result\u001b[39;49;00m\r\n \u001b[34mif\u001b[39;49;00m SDP_ENABLED:\r\n \u001b[34mif\u001b[39;49;00m sdp.rank() == \u001b[34m0\u001b[39;49;00m:\r\n model.save_pretrained(args.model_dir)\r\n tokenizer.save_pretrained(args.model_dir)\r\n \u001b[34melse\u001b[39;49;00m:\r\n model.save_pretrained(args.model_dir)\r\n tokenizer.save_pretrained(args.model_dir)\r\n"
]
],
[
[
"## Creating an Estimator and start a training job",
"_____no_output_____"
]
],
[
[
"from sagemaker.huggingface import HuggingFace\n\n# hyperparameters, which are passed into the training job\nhyperparameters={\n 'epochs': 1,\n 'train_batch_size': 16,\n 'model_name':'distilbert-base-uncased',\n}\n\n# configuration for running training on smdistributed Data Parallel\ndistribution = {'smdistributed':{'dataparallel':{ 'enabled': True }}}\n\n# instance configurations\ninstance_type='ml.p3dn.24xlarge'\ninstance_count=2\nvolume_size=200",
"nieljare-hf-tf24-droplast-nodebugger-3node-sdp\nLaunched job nieljare-hf-tf24-droplast-nodebugger-3node-sdp\n"
],
[
"huggingface_estimator = HuggingFace(\n entry_point='train.py',\n source_dir='./scripts',\n instance_type=instance_type,\n instance_count=instance_count,\n role=role,\n transformers_version='4.6',\n tensorflow_version='2.4',\n py_version='py37',\n distribution=distribution,\n hyperparameters=hyperparameters,\n debugger_hook_config=False, # currently needed\n)",
"_____no_output_____"
],
[
"huggingface_estimator.fit()",
"_____no_output_____"
]
],
[
[
"## Deploying the endpoint\n\nTo deploy our endpoint, we call `deploy()` on our HuggingFace estimator object, passing in our desired number of instances and instance type.",
"_____no_output_____"
]
],
[
[
"predictor = huggingface_estimator.deploy(1,\"ml.g4dn.xlarge\")",
"_____no_output_____"
]
],
[
[
"Then, we use the returned predictor object to call the endpoint.",
"_____no_output_____"
]
],
[
[
"sentiment_input= {\"inputs\":\"I love using the new Inference DLC.\"}\n\npredictor.predict(sentiment_input)",
"_____no_output_____"
]
],
[
[
"Finally, we delete the endpoint again.",
"_____no_output_____"
]
],
[
[
"predictor.delete_endpoint()",
"_____no_output_____"
]
],
[
[
"# Extras",
"_____no_output_____"
],
[
"## Estimator Parameters",
"_____no_output_____"
]
],
[
[
"# container image used for training job\nprint(f\"container image used for training job: \\n{huggingface_estimator.image_uri}\\n\")\n\n# s3 uri where the trained model is located\nprint(f\"s3 uri where the trained model is located: \\n{huggingface_estimator.model_data}\\n\")\n\n# latest training job name for this estimator\nprint(f\"latest training job name for this estimator: \\n{huggingface_estimator.latest_training_job.name}\\n\")\n",
"container image used for training job: \n763104351884.dkr.ecr.us-east-1.amazonaws.com/huggingface-tensorflow-training:2.4.1-transformers4.5.0-gpu-py37-cu110-ubuntu18.04\n\ns3 uri where the trained model is located: \ns3://sagemaker-us-east-1-558105141721/huggingface-training-2021-02-16-11-57-47-658/output/model.tar.gz\n\nlatest training job name for this estimator: \nhuggingface-training-2021-02-16-11-57-47-658\n\n"
],
[
"# access the logs of the training job\nhuggingface_estimator.sagemaker_session.logs_for_job(huggingface_estimator.latest_training_job.name)",
"_____no_output_____"
]
],
[
[
"## Attach to old training job to an estimator \n\nIn Sagemaker you can attach an old training job to an estimator to continue training, get results etc..",
"_____no_output_____"
]
],
[
[
"from sagemaker.estimator import Estimator\n\n# job which is going to be attached to the estimator\nold_training_job_name=''",
"_____no_output_____"
],
[
"# attach old training job\nhuggingface_estimator_loaded = Estimator.attach(old_training_job_name)\n\n# get model output s3 from training job\nhuggingface_estimator_loaded.model_data",
"_____no_output_____"
]
]
] | [
"markdown",
"code",
"markdown",
"code",
"markdown",
"code",
"markdown",
"code",
"markdown",
"code",
"markdown",
"code",
"markdown",
"code",
"markdown",
"code",
"markdown",
"code",
"markdown",
"code"
] | [
[
"markdown",
"markdown",
"markdown",
"markdown",
"markdown"
],
[
"code"
],
[
"markdown",
"markdown"
],
[
"code",
"code"
],
[
"markdown",
"markdown"
],
[
"code"
],
[
"markdown",
"markdown"
],
[
"code"
],
[
"markdown"
],
[
"code",
"code",
"code"
],
[
"markdown"
],
[
"code"
],
[
"markdown"
],
[
"code"
],
[
"markdown"
],
[
"code"
],
[
"markdown",
"markdown"
],
[
"code",
"code"
],
[
"markdown"
],
[
"code",
"code"
]
] |
e776df220bb7af5db23d62dff76d0c74a4541357 | 16,321 | ipynb | Jupyter Notebook | misc/6 - ResNet - Dogs vs Cats.ipynb | oney/pytorch-image-classification | 11471078145609923b5e1fdba6ca6680bd4bbbbb | [
"MIT"
] | 568 | 2019-01-11T16:45:40.000Z | 2022-03-30T17:13:03.000Z | misc/6 - ResNet - Dogs vs Cats.ipynb | mayurgpt07/pytorch-image-classification | b2e971a52d1478c8d56cb454fc147a3c343eb882 | [
"MIT"
] | 14 | 2019-04-08T01:28:42.000Z | 2021-12-28T22:42:12.000Z | misc/6 - ResNet - Dogs vs Cats.ipynb | mayurgpt07/pytorch-image-classification | b2e971a52d1478c8d56cb454fc147a3c343eb882 | [
"MIT"
] | 157 | 2019-03-31T23:46:28.000Z | 2022-03-31T21:04:38.000Z | 34.725532 | 175 | 0.523804 | [
[
[
"import torch\nimport torch.nn as nn\nimport torch.nn.functional as F\nimport torch.optim as optim\nimport torchvision\nimport torchvision.transforms as transforms\nimport torchvision.datasets as datasets\n\nimport os\nimport random\nimport numpy as np",
"_____no_output_____"
],
[
"SEED = 1234\n\nrandom.seed(SEED)\nnp.random.seed(SEED)\ntorch.manual_seed(SEED)\ntorch.cuda.manual_seed(SEED)\ntorch.backends.cudnn.deterministic = True",
"_____no_output_____"
],
[
"train_transforms = transforms.Compose([\n transforms.RandomHorizontalFlip(),\n transforms.RandomRotation(10),\n transforms.RandomCrop((224, 224), pad_if_needed=True),\n transforms.ToTensor(),\n transforms.Normalize((0.485, 0.456, 0.406),(0.229, 0.224, 0.225))\n ])\n\ntest_transforms = transforms.Compose([\n transforms.CenterCrop((224, 224)),\n transforms.ToTensor(),\n transforms.Normalize((0.485, 0.456, 0.406),(0.229, 0.224, 0.225))\n ])",
"_____no_output_____"
],
[
"train_data = datasets.ImageFolder('data/dogs-vs-cats/train', train_transforms)\nvalid_data = datasets.ImageFolder('data/dogs-vs-cats/valid', test_transforms)\ntest_data = datasets.ImageFolder('data/dogs-vs-cats/test', test_transforms)\n\n#import os\n\n#print(len(os.listdir('data/dogs-vs-cats/train')))\n\n#n_train_examples = int(len(train_data)*0.9)\n#n_valid_examples = n_test_examples = len(train_data) - n_train_examples\n\n#train_data, valid_data = torch.utils.data.random_split(train_data, [n_train_examples, n_valid_examples])\n#train_data, test_data = torch.utils.data.random_split(train_data, [n_train_examples-n_valid_examples, n_test_examples])",
"_____no_output_____"
]
],
[
[
"https://github.com/facebook/fb.resnet.torch/issues/180\nhttps://github.com/bamos/densenet.pytorch/blob/master/compute-cifar10-mean.py",
"_____no_output_____"
]
],
[
[
"print(f'Number of training examples: {len(train_data)}')\nprint(f'Number of validation examples: {len(valid_data)}')\nprint(f'Number of testing examples: {len(test_data)}')",
"Number of training examples: 20000\nNumber of validation examples: 2500\nNumber of testing examples: 2500\n"
],
[
"BATCH_SIZE = 64\n\ntrain_iterator = torch.utils.data.DataLoader(train_data, shuffle=True, batch_size=BATCH_SIZE)\nvalid_iterator = torch.utils.data.DataLoader(valid_data, batch_size=BATCH_SIZE)\ntest_iterator = torch.utils.data.DataLoader(test_data, batch_size=BATCH_SIZE)",
"_____no_output_____"
]
],
[
[
"https://discuss.pytorch.org/t/why-does-the-resnet-model-given-by-pytorch-omit-biases-from-the-convolutional-layer/10990/4\nhttps://github.com/kuangliu/pytorch-cifar/blob/master/models/resnet.py",
"_____no_output_____"
]
],
[
[
"device = torch.device('cuda')",
"_____no_output_____"
],
[
"import torchvision.models as models\n\nmodel = models.resnet18(pretrained=True).to(device)",
"_____no_output_____"
],
[
"print(model)",
"ResNet(\n (conv1): Conv2d(3, 64, kernel_size=(7, 7), stride=(2, 2), padding=(3, 3), bias=False)\n (bn1): BatchNorm2d(64, eps=1e-05, momentum=0.1, affine=True, track_running_stats=True)\n (relu): ReLU(inplace)\n (maxpool): MaxPool2d(kernel_size=3, stride=2, padding=1, dilation=1, ceil_mode=False)\n (layer1): Sequential(\n (0): BasicBlock(\n (conv1): Conv2d(64, 64, kernel_size=(3, 3), stride=(1, 1), padding=(1, 1), bias=False)\n (bn1): BatchNorm2d(64, eps=1e-05, momentum=0.1, affine=True, track_running_stats=True)\n (relu): ReLU(inplace)\n (conv2): Conv2d(64, 64, kernel_size=(3, 3), stride=(1, 1), padding=(1, 1), bias=False)\n (bn2): BatchNorm2d(64, eps=1e-05, momentum=0.1, affine=True, track_running_stats=True)\n )\n (1): BasicBlock(\n (conv1): Conv2d(64, 64, kernel_size=(3, 3), stride=(1, 1), padding=(1, 1), bias=False)\n (bn1): BatchNorm2d(64, eps=1e-05, momentum=0.1, affine=True, track_running_stats=True)\n (relu): ReLU(inplace)\n (conv2): Conv2d(64, 64, kernel_size=(3, 3), stride=(1, 1), padding=(1, 1), bias=False)\n (bn2): BatchNorm2d(64, eps=1e-05, momentum=0.1, affine=True, track_running_stats=True)\n )\n )\n (layer2): Sequential(\n (0): BasicBlock(\n (conv1): Conv2d(64, 128, kernel_size=(3, 3), stride=(2, 2), padding=(1, 1), bias=False)\n (bn1): BatchNorm2d(128, eps=1e-05, momentum=0.1, affine=True, track_running_stats=True)\n (relu): ReLU(inplace)\n (conv2): Conv2d(128, 128, kernel_size=(3, 3), stride=(1, 1), padding=(1, 1), bias=False)\n (bn2): BatchNorm2d(128, eps=1e-05, momentum=0.1, affine=True, track_running_stats=True)\n (downsample): Sequential(\n (0): Conv2d(64, 128, kernel_size=(1, 1), stride=(2, 2), bias=False)\n (1): BatchNorm2d(128, eps=1e-05, momentum=0.1, affine=True, track_running_stats=True)\n )\n )\n (1): BasicBlock(\n (conv1): Conv2d(128, 128, kernel_size=(3, 3), stride=(1, 1), padding=(1, 1), bias=False)\n (bn1): BatchNorm2d(128, eps=1e-05, momentum=0.1, affine=True, track_running_stats=True)\n (relu): ReLU(inplace)\n (conv2): Conv2d(128, 128, kernel_size=(3, 3), stride=(1, 1), padding=(1, 1), bias=False)\n (bn2): BatchNorm2d(128, eps=1e-05, momentum=0.1, affine=True, track_running_stats=True)\n )\n )\n (layer3): Sequential(\n (0): BasicBlock(\n (conv1): Conv2d(128, 256, kernel_size=(3, 3), stride=(2, 2), padding=(1, 1), bias=False)\n (bn1): BatchNorm2d(256, eps=1e-05, momentum=0.1, affine=True, track_running_stats=True)\n (relu): ReLU(inplace)\n (conv2): Conv2d(256, 256, kernel_size=(3, 3), stride=(1, 1), padding=(1, 1), bias=False)\n (bn2): BatchNorm2d(256, eps=1e-05, momentum=0.1, affine=True, track_running_stats=True)\n (downsample): Sequential(\n (0): Conv2d(128, 256, kernel_size=(1, 1), stride=(2, 2), bias=False)\n (1): BatchNorm2d(256, eps=1e-05, momentum=0.1, affine=True, track_running_stats=True)\n )\n )\n (1): BasicBlock(\n (conv1): Conv2d(256, 256, kernel_size=(3, 3), stride=(1, 1), padding=(1, 1), bias=False)\n (bn1): BatchNorm2d(256, eps=1e-05, momentum=0.1, affine=True, track_running_stats=True)\n (relu): ReLU(inplace)\n (conv2): Conv2d(256, 256, kernel_size=(3, 3), stride=(1, 1), padding=(1, 1), bias=False)\n (bn2): BatchNorm2d(256, eps=1e-05, momentum=0.1, affine=True, track_running_stats=True)\n )\n )\n (layer4): Sequential(\n (0): BasicBlock(\n (conv1): Conv2d(256, 512, kernel_size=(3, 3), stride=(2, 2), padding=(1, 1), bias=False)\n (bn1): BatchNorm2d(512, eps=1e-05, momentum=0.1, affine=True, track_running_stats=True)\n (relu): ReLU(inplace)\n (conv2): Conv2d(512, 512, kernel_size=(3, 3), stride=(1, 1), padding=(1, 1), bias=False)\n (bn2): BatchNorm2d(512, eps=1e-05, momentum=0.1, affine=True, track_running_stats=True)\n (downsample): Sequential(\n (0): Conv2d(256, 512, kernel_size=(1, 1), stride=(2, 2), bias=False)\n (1): BatchNorm2d(512, eps=1e-05, momentum=0.1, affine=True, track_running_stats=True)\n )\n )\n (1): BasicBlock(\n (conv1): Conv2d(512, 512, kernel_size=(3, 3), stride=(1, 1), padding=(1, 1), bias=False)\n (bn1): BatchNorm2d(512, eps=1e-05, momentum=0.1, affine=True, track_running_stats=True)\n (relu): ReLU(inplace)\n (conv2): Conv2d(512, 512, kernel_size=(3, 3), stride=(1, 1), padding=(1, 1), bias=False)\n (bn2): BatchNorm2d(512, eps=1e-05, momentum=0.1, affine=True, track_running_stats=True)\n )\n )\n (avgpool): AvgPool2d(kernel_size=7, stride=1, padding=0)\n (fc): Linear(in_features=512, out_features=1000, bias=True)\n)\n"
],
[
"for param in model.parameters():\n param.requires_grad = False",
"_____no_output_____"
],
[
"print(model.fc)",
"Linear(in_features=512, out_features=1000, bias=True)\n"
],
[
"model.fc = nn.Linear(in_features=512, out_features=2).to(device)",
"_____no_output_____"
],
[
"optimizer = optim.Adam(model.parameters())",
"_____no_output_____"
],
[
"criterion = nn.CrossEntropyLoss()",
"_____no_output_____"
],
[
"def calculate_accuracy(fx, y):\n preds = fx.max(1, keepdim=True)[1]\n correct = preds.eq(y.view_as(preds)).sum()\n acc = correct.float()/preds.shape[0]\n return acc",
"_____no_output_____"
],
[
"def train(model, device, iterator, optimizer, criterion):\n \n epoch_loss = 0\n epoch_acc = 0\n \n model.train()\n \n for (x, y) in iterator:\n \n x = x.to(device)\n y = y.to(device)\n \n optimizer.zero_grad()\n \n fx = model(x)\n \n loss = criterion(fx, y)\n \n acc = calculate_accuracy(fx, y)\n \n loss.backward()\n \n optimizer.step()\n \n epoch_loss += loss.item()\n epoch_acc += acc.item()\n \n return epoch_loss / len(iterator), epoch_acc / len(iterator)",
"_____no_output_____"
],
[
"def evaluate(model, device, iterator, criterion):\n \n epoch_loss = 0\n epoch_acc = 0\n \n model.eval()\n \n with torch.no_grad():\n for (x, y) in iterator:\n\n x = x.to(device)\n y = y.to(device)\n\n fx = model(x)\n\n loss = criterion(fx, y)\n\n acc = calculate_accuracy(fx, y)\n\n epoch_loss += loss.item()\n epoch_acc += acc.item()\n \n return epoch_loss / len(iterator), epoch_acc / len(iterator)",
"_____no_output_____"
],
[
"EPOCHS = 10\nSAVE_DIR = 'models'\nMODEL_SAVE_PATH = os.path.join(SAVE_DIR, 'resnet18-dogs-vs-cats.pt')\n\nbest_valid_loss = float('inf')\n\nif not os.path.isdir(f'{SAVE_DIR}'):\n os.makedirs(f'{SAVE_DIR}')\n\nfor epoch in range(EPOCHS):\n train_loss, train_acc = train(model, device, train_iterator, optimizer, criterion)\n valid_loss, valid_acc = evaluate(model, device, valid_iterator, criterion)\n \n if valid_loss < best_valid_loss:\n best_valid_loss = valid_loss\n torch.save(model.state_dict(), MODEL_SAVE_PATH)\n \n print(f'| Epoch: {epoch+1:02} | Train Loss: {train_loss:.3f} | Train Acc: {train_acc*100:05.2f}% | Val. Loss: {valid_loss:.3f} | Val. Acc: {valid_acc*100:05.2f}% |')",
"| Epoch: 01 | Train Loss: 0.198 | Train Acc: 91.95% | Val. Loss: 0.089 | Val. Acc: 96.80% |\n| Epoch: 02 | Train Loss: 0.136 | Train Acc: 94.40% | Val. Loss: 0.069 | Val. Acc: 97.50% |\n| Epoch: 03 | Train Loss: 0.128 | Train Acc: 94.68% | Val. Loss: 0.059 | Val. Acc: 97.70% |\n| Epoch: 04 | Train Loss: 0.119 | Train Acc: 95.03% | Val. Loss: 0.070 | Val. Acc: 97.30% |\n| Epoch: 05 | Train Loss: 0.118 | Train Acc: 94.95% | Val. Loss: 0.057 | Val. Acc: 97.73% |\n| Epoch: 06 | Train Loss: 0.121 | Train Acc: 94.95% | Val. Loss: 0.056 | Val. Acc: 97.70% |\n| Epoch: 07 | Train Loss: 0.117 | Train Acc: 95.11% | Val. Loss: 0.063 | Val. Acc: 97.46% |\n| Epoch: 08 | Train Loss: 0.110 | Train Acc: 95.44% | Val. Loss: 0.052 | Val. Acc: 97.93% |\n| Epoch: 09 | Train Loss: 0.116 | Train Acc: 95.14% | Val. Loss: 0.056 | Val. Acc: 97.77% |\n| Epoch: 10 | Train Loss: 0.114 | Train Acc: 95.36% | Val. Loss: 0.063 | Val. Acc: 97.46% |\n"
],
[
"model.load_state_dict(torch.load(MODEL_SAVE_PATH))\n\ntest_loss, test_acc = evaluate(model, device, valid_iterator, criterion)\n\nprint(f'| Test Loss: {test_loss:.3f} | Test Acc: {test_acc*100:05.2f}% |')",
"| Test Loss: 0.052 | Test Acc: 97.93% |\n"
]
]
] | [
"code",
"markdown",
"code",
"markdown",
"code"
] | [
[
"code",
"code",
"code",
"code"
],
[
"markdown"
],
[
"code",
"code"
],
[
"markdown"
],
[
"code",
"code",
"code",
"code",
"code",
"code",
"code",
"code",
"code",
"code",
"code",
"code",
"code"
]
] |
e776f2adce6ef3c3017fcfb789e0c660a89c38eb | 741,280 | ipynb | Jupyter Notebook | examples/mex_hrsc_isis_cmp.ipynb | Kelvinrr/knoten | 8bc3831ed4669940ddfc4557615f7718a5891eef | [
"Unlicense"
] | null | null | null | examples/mex_hrsc_isis_cmp.ipynb | Kelvinrr/knoten | 8bc3831ed4669940ddfc4557615f7718a5891eef | [
"Unlicense"
] | null | null | null | examples/mex_hrsc_isis_cmp.ipynb | Kelvinrr/knoten | 8bc3831ed4669940ddfc4557615f7718a5891eef | [
"Unlicense"
] | null | null | null | 1,029.555556 | 155,472 | 0.952432 | [
[
[
"import os \n\nos.environ[\"ISISROOT\"] = \"/usgs/cpkgs/anaconda3_linux/envs/isis3.7.0\"\n\nimport pysis\nfrom pysis import isis\nfrom pysis.exceptions import ProcessError\n\nimport knoten\nfrom knoten import vis\n\nimport json\n\nimport ale\nale.config['mex'] = '/home/acpaquette/5270/'\nfrom ale import util\nfrom ale.drivers.mex_drivers import MexHrscPds3NaifSpiceDriver\nfrom ale.formatters.usgscsm_formatter import to_usgscsm\n\nfrom IPython.display import Image\n\nimport plotly\nplotly.offline.init_notebook_mode(connected=True)",
"_____no_output_____"
],
[
"# url = 'https://pds-imaging.jpl.nasa.gov/data/mro/mars_reconnaissance_orbiter/ctx/mrox_3365/data/K11_057871_1602_XN_19S163W.IMG'\n\n# !wget -N -P data/ {url}\n# fileName = 'data/' + os.path.split(url)[1]\nfileName = '/home/acpaquette/5270/h5270_0000_nd2.img'\ncamera = knoten.csm.create_csm(fileName)",
"_____no_output_____"
],
[
"# Set the output location of the resulting .cub\ncub_loc = os.path.splitext(fileName)[0] + '.cub'\n\ntry: \n isis.hrsc2isis(from_=fileName, to=cub_loc)\nexcept ProcessError as e:\n print(e.stderr)\n\ntry:\n isis.spiceinit(from_=cub_loc, shape='ellipsoid', ckpredicted=True, spkpredicted=True)\nexcept ProcessError as e:\n print(e.stderr)\n\n# driver = MexHrscPds3NaifSpiceDriver(fileName)\n# print(driver.metakernel)\n \n# with MexHrscPds3NaifSpiceDriver(fileName) as driver:\n# usgscsmString = to_usgscsm(driver)\n# usgscsm_dict = json.loads(usgscsmString)\n \n# csm_isd = os.path.splitext(fileName)[0] + '.json'\n# json.dump(usgscsm_dict, open(csm_isd, 'w'))",
"_____no_output_____"
],
[
"csm_isd = os.path.splitext(fileName)[0] + '.json'\n\ncsmisis_diff_lv_plot, csmisis_diff_ephem_plot, external_orientation_data = vis.external_orientation_diff(csm_isd, cub_loc, 10, 50, 600, 600)",
"_____no_output_____"
],
[
"csmisis_diff_lv_plot_bytes = csmisis_diff_lv_plot.to_image(format=\"png\")\ncsmisis_diff_ephem_plot_bytes = csmisis_diff_ephem_plot.to_image(format=\"png\")\nImage(csmisis_diff_lv_plot_bytes)",
"_____no_output_____"
],
[
"Image(csmisis_diff_ephem_plot_bytes)",
"_____no_output_____"
],
[
"external_orientation_data[['diffx', 'diffy', 'diffz', 'diffu', 'diffv', 'diffw']].describe()",
"_____no_output_____"
],
[
"isis2csm_plot, csm2isis_plot, isiscsm_plotlatlon, isiscsm_plotbf, isis2csm_data, csm2isis_data, isiscsm_latlondata, isiscsm_bfdata = vis.reprojection_diff(csm_isd, cub_loc, 10, 50, 500, 500)",
"_____no_output_____"
],
[
"Image(isis2csm_plot.to_image())",
"_____no_output_____"
],
[
"isis2csm_data[['diff sample', 'diff line']].describe()",
"_____no_output_____"
],
[
"Image(csm2isis_plot.to_image())",
"_____no_output_____"
],
[
"csm2isis_data[['diff sample', 'diff line']].describe()",
"_____no_output_____"
],
[
"Image(isiscsm_plotlatlon.to_image())",
"_____no_output_____"
],
[
"Image(isiscsm_plotbf.to_image())",
"_____no_output_____"
],
[
"isiscsm_bfdata[['diffx', 'diffy', 'diffz']].describe()",
"_____no_output_____"
]
]
] | [
"code"
] | [
[
"code",
"code",
"code",
"code",
"code",
"code",
"code",
"code",
"code",
"code",
"code",
"code",
"code",
"code",
"code"
]
] |
e77703e1b1c92ca82c52fefc623a668b0906c88f | 142,115 | ipynb | Jupyter Notebook | Bengali.Ai classification challenge/pytorch-predict.ipynb | yoviny/Kaggle-Competitions | b91efeb2d1ecdebfb8b30f144a4327daf8d1fe9a | [
"MIT"
] | null | null | null | Bengali.Ai classification challenge/pytorch-predict.ipynb | yoviny/Kaggle-Competitions | b91efeb2d1ecdebfb8b30f144a4327daf8d1fe9a | [
"MIT"
] | null | null | null | Bengali.Ai classification challenge/pytorch-predict.ipynb | yoviny/Kaggle-Competitions | b91efeb2d1ecdebfb8b30f144a4327daf8d1fe9a | [
"MIT"
] | null | null | null | 72.176232 | 44,720 | 0.756599 | [
[
[
"# Inference code for running on kaggle server",
"_____no_output_____"
]
],
[
[
"!pip install ../input/pretrainedmodels/pretrainedmodels-0.7.4/pretrainedmodels-0.7.4/ > /dev/null # no output",
"_____no_output_____"
],
[
"import gc\nimport os\nimport random\nimport sys\nimport six\nimport math\nfrom pathlib import Path\nfrom tqdm import tqdm_notebook as tqdm\nfrom IPython.core.display import display, HTML\nfrom typing import List\n\nimport plotly.offline as py\nimport plotly.graph_objs as go\nimport plotly.express as px\nimport plotly.figure_factory as ff\nfrom plotly import tools, subplots\npy.init_notebook_mode(connected=True)\n\nimport numpy\nimport numpy as np\nimport pandas as pd \nimport matplotlib.pyplot as plt\nimport seaborn as sns\nimport albumentations as A\nimport cv2\nfrom sklearn import preprocessing\nfrom sklearn.model_selection import KFold\nfrom skimage.transform import AffineTransform, warp\n\nimport torch\nimport torch.nn.functional as F\nfrom torch import nn\nfrom torch.nn import init, Sequential\nfrom torch.nn.parameter import Parameter\nfrom torch.utils.data.dataset import Dataset\nfrom torch.utils.data.dataloader import DataLoader\n\nimport pretrainedmodels\n\n# --- setup ---\npd.set_option('max_columns', 50)",
"_____no_output_____"
],
[
"debug=False\nsubmission=True\nbatch_size=32\ndevice='cuda:0'\nout='.'\n#load_model_path='/kaggle/input/pytorch-mixup1/model_097889.pt'\nload_model_path='.'\nimage_size=128\nthreshold=40.#20\nmodel_name='se_resnext50_32x4d'",
"_____no_output_____"
],
[
"datadir = Path('/kaggle/input/bengaliai-cv19')\nfeatherdir = Path('/kaggle/input/bengaliaicv19feather')\noutdir = Path('.')",
"_____no_output_____"
],
[
"# Read in the data CSV files\n# train = pd.read_csv(datadir/'train.csv')\n# test = pd.read_csv(datadir/'test.csv')\n# sample_submission = pd.read_csv(datadir/'sample_submission.csv')\n# class_map = pd.read_csv(datadir/'class_map.csv')",
"_____no_output_____"
]
],
[
[
"## Dataset",
"_____no_output_____"
]
],
[
[
"\"\"\"\nReferenced `chainer.dataset.DatasetMixin` to work with pytorch Dataset.\n\"\"\"\n\nclass DatasetMixin(Dataset):\n\n def __init__(self, transform=None):\n self.transform = transform\n\n def __getitem__(self, index):\n \"\"\"Returns an example or a sequence of examples.\"\"\"\n if torch.is_tensor(index):\n index = index.tolist()\n if isinstance(index, slice):\n current, stop, step = index.indices(len(self))\n return [self.get_example_wrapper(i) for i in\n six.moves.range(current, stop, step)]\n elif isinstance(index, list) or isinstance(index, numpy.ndarray):\n return [self.get_example_wrapper(i) for i in index]\n else:\n return self.get_example_wrapper(index)\n\n def __len__(self):\n \"\"\"Returns the number of data points.\"\"\"\n raise NotImplementedError\n\n def get_example_wrapper(self, i):\n \"\"\"Wrapper of `get_example`, to apply `transform` if necessary\"\"\"\n example = self.get_example(i)\n if self.transform:\n example = self.transform(example)\n return example\n\n def get_example(self, i):\n \"\"\"Returns the i-th example.\n\n Implementations should override it. It should raise :class:`IndexError`\n if the index is invalid.\n\n Args:\n i (int): The index of the example.\n\n Returns:\n The i-th example.\n\n \"\"\"\n raise NotImplementedError\n",
"_____no_output_____"
],
[
"class BengaliAIDataset(DatasetMixin):\n def __init__(self, images, labels=None, transform=None, indices=None):\n super(BengaliAIDataset, self).__init__(transform=transform)\n self.images = images\n self.labels = labels\n if indices is None:\n indices = np.arange(len(images))\n self.indices = indices\n self.train = labels is not None\n\n def __len__(self):\n \"\"\"return length of this dataset\"\"\"\n return len(self.indices)\n\n def get_example(self, i):\n \"\"\"Return i-th data\"\"\"\n i = self.indices[i]\n x = self.images[i]\n x = (255 - x).astype(np.float32) / 255.\n if self.train:\n y = self.labels[i]\n return x, y\n else:\n return x\n",
"_____no_output_____"
]
],
[
[
"### Data augmentation/processing",
"_____no_output_____"
]
],
[
[
"\"\"\"\nFrom https://www.kaggle.com/corochann/deep-learning-cnn-with-chainer-lb-0-99700\n\"\"\"\n\ndef affine_image(img):\n \"\"\"\n\n Args:\n img: (h, w) or (1, h, w)\n\n Returns:\n img: (h, w)\n \"\"\"\n # ch, h, w = img.shape\n # img = img / 255.\n if img.ndim == 3:\n img = img[0]\n\n # --- scale ---\n min_scale = 0.8\n max_scale = 1.2\n sx = np.random.uniform(min_scale, max_scale)\n sy = np.random.uniform(min_scale, max_scale)\n\n # --- rotation ---\n max_rot_angle = 7\n rot_angle = np.random.uniform(-max_rot_angle, max_rot_angle) * np.pi / 180.\n\n # --- shear ---\n max_shear_angle = 10\n shear_angle = np.random.uniform(-max_shear_angle, max_shear_angle) * np.pi / 180.\n\n # --- translation ---\n max_translation = 4\n tx = np.random.randint(-max_translation, max_translation)\n ty = np.random.randint(-max_translation, max_translation)\n\n tform = AffineTransform(scale=(sx, sy), rotation=rot_angle, shear=shear_angle,\n translation=(tx, ty))\n transformed_image = warp(img, tform)\n assert transformed_image.ndim == 2\n return transformed_image\n\n\ndef crop_char_image(image, threshold=40./255.):\n assert image.ndim == 2\n is_black = image > threshold\n\n is_black_vertical = np.sum(is_black, axis=0) > 0\n is_black_horizontal = np.sum(is_black, axis=1) > 0\n left = np.argmax(is_black_horizontal)\n right = np.argmax(is_black_horizontal[::-1])\n top = np.argmax(is_black_vertical)\n bottom = np.argmax(is_black_vertical[::-1])\n height, width = image.shape\n cropped_image = image[left:height - right, top:width - bottom]\n return cropped_image\n\n\ndef resize(image, size=(224, 224)):\n return cv2.resize(image, size, interpolation=cv2.INTER_AREA)",
"_____no_output_____"
],
[
"def add_gaussian_noise(x, sigma):\n x += np.random.randn(*x.shape) * sigma\n x = np.clip(x, 0., 1.)\n return x\n\ndef _evaluate_ratio(ratio):\n if ratio <= 0.:\n return False\n return np.random.uniform() < ratio\n\n\ndef apply_aug(aug, image):\n return aug(image=image)['image']\n\nclass Transform:\n def __init__(self, affine=True, crop=False, size=(224, 224),\n normalize=True, train=True, threshold=40.,\n sigma=-1., ssr_ratio=0.):\n self.affine = affine\n self.crop = crop\n self.size = size\n self.normalize = normalize\n self.train = train\n self.threshold = threshold / 255.\n self.sigma = sigma / 255.\n self.ssr_ratio = ssr_ratio\n\n def __call__(self, example):\n if self.train:\n x, y = example\n else:\n x = example\n # --- Augmentation ---\n if self.affine:\n x = affine_image(x)\n\n # --- Train/Test common preprocessing ---\n if self.crop:\n x = crop_char_image(x, threshold=self.threshold)\n if self.size is not None:\n x = resize(x, size=self.size)\n if self.sigma > 0.:\n x = add_gaussian_noise(x, sigma=self.sigma)\n if _evaluate_ratio(self.ssr_ratio):\n x = apply_aug(A.ShiftScaleRotate(\n shift_limit=0.0625,\n scale_limit=0.1,\n rotate_limit=15,\n p=1.0), x) \n if self.normalize:\n x = (x.astype(np.float32) - 0.0692) / 0.2051\n if x.ndim == 2:\n x = x[None, :, :]\n x = x.astype(np.float32)\n if self.train:\n y = y.astype(np.int64)\n return x, y\n else:\n return x",
"_____no_output_____"
],
[
"def residual_add(lhs, rhs):\n lhs_ch, rhs_ch = lhs.shape[1], rhs.shape[1]\n if lhs_ch < rhs_ch:\n out = lhs + rhs[:, :lhs_ch]\n elif lhs_ch > rhs_ch:\n out = torch.cat([lhs[:, :rhs_ch] + rhs, lhs[:, rhs_ch:]], dim=1)\n else:\n out = lhs + rhs\n return out\n",
"_____no_output_____"
],
[
"class LazyLoadModule(nn.Module):\n \"\"\"Lazy buffer/parameter loading using load_state_dict_pre_hook\n\n Define all buffer/parameter in `_lazy_buffer_keys`/`_lazy_parameter_keys` and\n save buffer with `register_buffer`/`register_parameter`\n method, which can be outside of __init__ method.\n Then this module can load any shape of Tensor during de-serializing.\n\n Note that default value of lazy buffer is torch.Tensor([]), while lazy parameter is None.\n \"\"\"\n _lazy_buffer_keys: List[str] = [] # It needs to be override to register lazy buffer\n _lazy_parameter_keys: List[str] = [] # It needs to be override to register lazy parameter\n\n def __init__(self):\n super(LazyLoadModule, self).__init__()\n for k in self._lazy_buffer_keys:\n self.register_buffer(k, torch.tensor([]))\n for k in self._lazy_parameter_keys:\n self.register_parameter(k, None)\n self._register_load_state_dict_pre_hook(self._hook)\n\n def _hook(self, state_dict, prefix, local_metadata, strict, missing_keys,\n unexpected_keys, error_msgs):\n for key in self._lazy_buffer_keys:\n self.register_buffer(key, state_dict[prefix + key])\n\n for key in self._lazy_parameter_keys:\n self.register_parameter(key, Parameter(state_dict[prefix + key]))\n",
"_____no_output_____"
],
[
"class LazyLinear(LazyLoadModule):\n \"\"\"Linear module with lazy input inference\n\n `in_features` can be `None`, and it is determined at the first time of forward step dynamically.\n \"\"\"\n\n __constants__ = ['bias', 'in_features', 'out_features']\n _lazy_parameter_keys = ['weight']\n\n def __init__(self, in_features, out_features, bias=True):\n super(LazyLinear, self).__init__()\n self.in_features = in_features\n self.out_features = out_features\n if bias:\n self.bias = Parameter(torch.Tensor(out_features))\n else:\n self.register_parameter('bias', None)\n\n if in_features is not None:\n self.weight = Parameter(torch.Tensor(out_features, in_features))\n self.reset_parameters()\n\n def reset_parameters(self):\n init.kaiming_uniform_(self.weight, a=math.sqrt(5))\n if self.bias is not None:\n fan_in, _ = init._calculate_fan_in_and_fan_out(self.weight)\n bound = 1 / math.sqrt(fan_in)\n init.uniform_(self.bias, -bound, bound)\n\n def forward(self, input):\n if self.weight is None:\n self.in_features = input.shape[-1]\n self.weight = Parameter(torch.Tensor(self.out_features, self.in_features))\n self.reset_parameters()\n\n # Need to send lazy defined parameter to device...\n self.to(input.device)\n return F.linear(input, self.weight, self.bias)\n\n def extra_repr(self):\n return 'in_features={}, out_features={}, bias={}'.format(\n self.in_features, self.out_features, self.bias is not None\n )\n",
"_____no_output_____"
],
[
"class LinearBlock(nn.Module):\n\n def __init__(self, in_features, out_features, bias=True,\n use_bn=True, activation=F.relu, dropout_ratio=-1, residual=False,):\n super(LinearBlock, self).__init__()\n if in_features is None:\n self.linear = LazyLinear(in_features, out_features, bias=bias)\n else:\n self.linear = nn.Linear(in_features, out_features, bias=bias)\n if use_bn:\n self.bn = nn.BatchNorm1d(out_features)\n if dropout_ratio > 0.:\n self.dropout = nn.Dropout(p=dropout_ratio)\n else:\n self.dropout = None\n self.activation = activation\n self.use_bn = use_bn\n self.dropout_ratio = dropout_ratio\n self.residual = residual\n\n def __call__(self, x):\n h = self.linear(x)\n if self.use_bn:\n h = self.bn(h)\n if self.activation is not None:\n h = self.activation(h)\n if self.residual:\n h = residual_add(h, x)\n if self.dropout_ratio > 0:\n h = self.dropout(h)\n return h",
"_____no_output_____"
],
[
"class PretrainedCNN(nn.Module):\n def __init__(self, model_name='se_resnext50_32x4d',\n in_channels=1, out_dim=10, use_bn=True,\n pretrained='imagenet'):\n super(PretrainedCNN, self).__init__()\n self.conv0 = nn.Conv2d(\n in_channels, 3, kernel_size=3, stride=1, padding=1, bias=True)\n self.base_model = pretrainedmodels.__dict__[model_name](pretrained=pretrained)\n activation = F.leaky_relu\n self.do_pooling = True\n if self.do_pooling:\n inch = self.base_model.last_linear.in_features\n else:\n inch = None\n hdim = 512\n lin1 = LinearBlock(inch, hdim, use_bn=use_bn, activation=activation, residual=False)\n lin2 = LinearBlock(hdim, out_dim, use_bn=use_bn, activation=None, residual=False)\n self.lin_layers = Sequential(lin1, lin2)\n\n def forward(self, x):\n #h = self.conv0(x)\n h = x.repeat(1,3,1,1)\n h = self.base_model.features(h)\n\n if self.do_pooling:\n h = torch.sum(h, dim=(-1, -2))\n else:\n bs, ch, height, width = h.shape\n h = h.view(bs, ch*height*width)\n for layer in self.lin_layers:\n h = layer(h)\n return h",
"_____no_output_____"
]
],
[
[
"## Classifier",
"_____no_output_____"
]
],
[
[
"def accuracy(y, t):\n pred_label = torch.argmax(y, dim=1)\n count = pred_label.shape[0]\n correct = (pred_label == t).sum().type(torch.float32)\n acc = correct / count\n return acc\n\n\nclass BengaliClassifier(nn.Module):\n def __init__(self, predictor, n_grapheme=168, n_vowel=11, n_consonant=7):\n super(BengaliClassifier, self).__init__()\n self.n_grapheme = n_grapheme\n self.n_vowel = n_vowel\n self.n_consonant = n_consonant\n self.n_total_class = self.n_grapheme + self.n_vowel + self.n_consonant\n self.predictor = predictor\n\n self.metrics_keys = [\n 'loss', 'loss_grapheme', 'loss_vowel', 'loss_consonant',\n 'acc_grapheme', 'acc_vowel', 'acc_consonant']\n\n def forward(self, x, y=None):\n pred = self.predictor(x)\n if isinstance(pred, tuple):\n assert len(pred) == 3\n preds = pred\n else:\n assert pred.shape[1] == self.n_total_class\n preds = torch.split(pred, [self.n_grapheme, self.n_vowel, self.n_consonant], dim=1)\n loss_grapheme = F.cross_entropy(preds[0], y[:, 0])\n loss_vowel = F.cross_entropy(preds[1], y[:, 1])\n loss_consonant = F.cross_entropy(preds[2], y[:, 2])\n loss = loss_grapheme + loss_vowel + loss_consonant\n metrics = {\n 'loss': loss.item(),\n 'loss_grapheme': loss_grapheme.item(),\n 'loss_vowel': loss_vowel.item(),\n 'loss_consonant': loss_consonant.item(),\n 'acc_grapheme': accuracy(preds[0], y[:, 0]),\n 'acc_vowel': accuracy(preds[1], y[:, 1]),\n 'acc_consonant': accuracy(preds[2], y[:, 2]),\n }\n return loss, metrics, pred\n\n def calc(self, data_loader):\n device: torch.device = next(self.parameters()).device\n self.eval()\n output_list = []\n with torch.no_grad():\n for batch in tqdm(data_loader):\n batch = batch.to(device)\n pred = self.predictor(batch)\n output_list.append(pred)\n output = torch.cat(output_list, dim=0)\n preds = torch.split(output, [self.n_grapheme, self.n_vowel, self.n_consonant], dim=1)\n return preds\n\n def predict_proba(self, data_loader):\n preds = self.calc(data_loader)\n return [F.softmax(p, dim=1) for p in preds]\n\n def predict(self, data_loader):\n preds = self.calc(data_loader)\n pred_labels = [torch.argmax(p, dim=1) for p in preds]\n return pred_labels\n",
"_____no_output_____"
],
[
"def prepare_image(datadir, featherdir, data_type='train',\n submission=False, indices=[0, 1, 2, 3]):\n assert data_type in ['train', 'test']\n if submission:\n image_df_list = [pd.read_parquet(datadir / f'{data_type}_image_data_{i}.parquet')\n for i in indices]\n else:\n image_df_list = [pd.read_feather(featherdir / f'{data_type}_image_data_{i}.feather')\n for i in indices]\n\n print('image_df_list', len(image_df_list))\n HEIGHT = 137\n WIDTH = 236\n images = [df.iloc[:, 1:].values.reshape(-1, HEIGHT, WIDTH) for df in image_df_list]\n images = np.concatenate(images, axis=0)\n return images\n",
"_____no_output_____"
],
[
"# --- Model ---\ndevice = torch.device(device)\nn_grapheme = 168\nn_vowel = 11\nn_consonant = 7\nn_total = n_grapheme + n_vowel + n_consonant\nprint('n_total', n_total)\n#predictor = PretrainedCNN(in_channels=1, out_dim=n_total, model_name=model_name, pretrained=None)\n#print('predictor', type(predictor))\n\n#classifier = BengaliClassifier(predictor)",
"n_total 186\n"
],
[
"class WrappedModel(nn.Module):\n\tdef __init__(self, module):\n\t\tsuper(WrappedModel, self).__init__()\n\t\tself.module = module \n\tdef forward(self, x):\n\t\treturn self.module(x)",
"_____no_output_____"
],
[
"def build_predictor():\n predictor = PretrainedCNN(in_channels=3, out_dim=n_total, model_name=model_name, pretrained=None)\n return predictor\n\ndef build_classifier(arch, load_model_path, n_total, model_name='', device='cuda:0'):\n if isinstance(device, str):\n device = torch.device(device)\n predictor = build_predictor()\n predictor = WrappedModel(predictor)\n print('predictor', type(predictor))\n classifier = BengaliClassifier(predictor)\n if load_model_path:\n predictor.load_state_dict(torch.load(load_model_path))\n else:\n print(\"[WARNING] Unexpected value load_model_path={}\"\n .format(load_model_path))\n classifier.to(device)\n return classifier",
"_____no_output_____"
],
[
"def predict_core(test_images, image_size, threshold,\n arch, n_total, model_name, load_model_path, batch_size=512, device='cuda:0', **kwargs):\n classifier = build_classifier(arch, load_model_path, n_total, model_name, device=device)\n test_dataset = BengaliAIDataset(\n test_images, None,\n transform=Transform(affine=False, crop=False, size=(224, 224),\n threshold=threshold, train=False, ssr_ratio=0.0))\n print('test_dataset', len(test_dataset))\n test_loader = DataLoader(test_dataset, batch_size=batch_size, shuffle=False)\n test_pred_proba = classifier.predict_proba(test_loader)\n return test_pred_proba",
"_____no_output_____"
],
[
"'''\nfrom torch.utils.data.dataloader import DataLoader\n\n# --- Prediction ---\ndata_type = 'test'\ntest_preds_list = []\nfor i in range(4):\n # --- prepare data ---\n indices = [i]\n test_images = prepare_image(\n datadir, featherdir, data_type=data_type, submission=submission, indices=indices)\n n_dataset = len(test_images)\n print(f'i={i}, n_dataset={n_dataset}')\n # test_data_size = 200 if debug else int(n_dataset * 0.9)\n test_dataset = BengaliAIDataset(\n test_images, None,\n transform=Transform(affine=False, crop=True, size=(image_size, image_size),\n threshold=threshold, train=False))\n print('test_dataset', len(test_dataset))\n test_loader = DataLoader(test_dataset, batch_size=batch_size, shuffle=False)\n test_preds = classifier.predict(test_loader)\n test_preds_list.append(test_preds)\n del test_images\n gc.collect()\n if debug:\n break\n ''' ",
"_____no_output_____"
],
[
"model_dir = '/kaggle/input/pytorch-cutmix-46/'\n\nfilenames = []\nfor filename in os.listdir(model_dir):\n if filename.endswith(\".pt\"): \n print(os.path.join(model_dir, filename))\n filenames.append(filename)\n\ntrain_args_dict={\n 'load_model_path': filename,\n 'device': device,\n 'batch_size': batch_size,\n 'debug': debug,\n 'image_size': (224, 224),\n 'model_name': model_name,\n 'threshold': 40.,\n 'arch': None,}\n \n\n# --- Prediction ---\ndata_type = 'test'\ntest_preds_list = []\nfor i in range(4):\n # --- prepare data ---\n indices = [i]\n test_images = prepare_image(\n datadir, featherdir, data_type=data_type, submission=submission, indices=indices)\n n_dataset = len(test_images)\n print(f'i={i}, n_dataset={n_dataset}')\n # test_data_size = 200 if debug else int(n_dataset * 0.9)\n model_preds_list = []\n for j in range(6):\n train_args_dict.update({\n 'load_model_path': os.path.join(model_dir, filenames[j]),\n 'device': device,\n 'batch_size': batch_size,\n 'debug': debug,\n })\n print(f'j {j} updated train_args_dict {train_args_dict}')\n test_preds = predict_core(\n test_images=test_images, n_total=n_total,\n **train_args_dict)\n\n model_preds_list.append(test_preds)\n \n # --- ensemble ---\n proba0 = torch.mean(torch.stack([test_preds[0] for test_preds in model_preds_list], dim=0), dim=0)\n proba1 = torch.mean(torch.stack([test_preds[1] for test_preds in model_preds_list], dim=0), dim=0)\n proba2 = torch.mean(torch.stack([test_preds[2] for test_preds in model_preds_list], dim=0), dim=0)\n p0 = torch.argmax(proba0, dim=1).cpu().numpy()\n p1 = torch.argmax(proba1, dim=1).cpu().numpy()\n p2 = torch.argmax(proba2, dim=1).cpu().numpy()\n print('p0', p0.shape, 'p1', p1.shape, 'p2', p2.shape)\n\n test_preds_list.append([p0, p1, p2])\n if debug:\n break\n del test_images\n gc.collect()\n '''\n test_preds_list.append(test_preds)\n del test_images\n gc.collect()\n if debug:\n break\n ''' ",
"/kaggle/input/pytorch-cutmix-46/model_079.pt\n/kaggle/input/pytorch-cutmix-46/model_109.pt\n/kaggle/input/pytorch-cutmix-46/model_073.pt\n/kaggle/input/pytorch-cutmix-46/model_111.pt\n/kaggle/input/pytorch-cutmix-46/model_100.pt\n/kaggle/input/pytorch-cutmix-46/model_098.pt\nimage_df_list 1\ni=0, n_dataset=3\nj 0 updated train_args_dict {'load_model_path': '/kaggle/input/pytorch-cutmix-46/model_079.pt', 'device': device(type='cuda', index=0), 'batch_size': 32, 'debug': False, 'image_size': (224, 224), 'model_name': 'se_resnext50_32x4d', 'threshold': 40.0, 'arch': None}\npredictor <class '__main__.WrappedModel'>\n"
],
[
"\np0 = np.concatenate([test_preds[0] for test_preds in test_preds_list], axis=0)\np1 = np.concatenate([test_preds[1] for test_preds in test_preds_list], axis=0)\np2 = np.concatenate([test_preds[2] for test_preds in test_preds_list], axis=0)\nprint('concat:', 'p0', p0.shape, 'p1', p1.shape, 'p2', p2.shape)\n\nrow_id = []\ntarget = []\nfor i in tqdm(range(len(p0))):\n row_id += [f'Test_{i}_grapheme_root', f'Test_{i}_vowel_diacritic',\n f'Test_{i}_consonant_diacritic']\n target += [p0[i], p1[i], p2[i]]\nsubmission_df = pd.DataFrame({'row_id': row_id, 'target': target})\nsubmission_df.to_csv('submission.csv', index=False)",
"100%|██████████| 12/12 [00:00<00:00, 19365.77it/s]"
],
[
"'''\np0 = np.concatenate([test_preds[0].cpu().numpy() for test_preds in test_preds_list], axis=0)\np1 = np.concatenate([test_preds[1].cpu().numpy() for test_preds in test_preds_list], axis=0)\np2 = np.concatenate([test_preds[2].cpu().numpy() for test_preds in test_preds_list], axis=0)\nprint('p0', p0.shape, 'p1', p1.shape, 'p2', p2.shape)\n\nrow_id = []\ntarget = []\nfor i in tqdm(range(len(p0))):\n row_id += [f'Test_{i}_grapheme_root', f'Test_{i}_vowel_diacritic',\n f'Test_{i}_consonant_diacritic']\n target += [p0[i], p1[i], p2[i]]\nsubmission_df = pd.DataFrame({'row_id': row_id, 'target': target})\nsubmission_df.to_csv('submission.csv', index=False)\n'''",
"_____no_output_____"
],
[
"submission_df",
"_____no_output_____"
]
],
[
[
"# Check prediction",
"_____no_output_____"
]
],
[
[
"train = pd.read_csv(datadir/'train.csv')",
"_____no_output_____"
],
[
"pred_df = pd.DataFrame({\n 'grapheme_root': p0,\n 'vowel_diacritic': p1,\n 'consonant_diacritic': p2\n})",
"_____no_output_____"
],
[
"fig, axes = plt.subplots(2, 3, figsize=(22, 6))\nplt.title('Label Count')\nsns.countplot(x=\"grapheme_root\",data=train, ax=axes[0, 0])\nsns.countplot(x=\"vowel_diacritic\",data=train, ax=axes[0, 1])\nsns.countplot(x=\"consonant_diacritic\",data=train, ax=axes[0, 2])\nsns.countplot(x=\"grapheme_root\",data=pred_df, ax=axes[1, 0])\nsns.countplot(x=\"vowel_diacritic\",data=pred_df, ax=axes[1, 1])\nsns.countplot(x=\"consonant_diacritic\",data=pred_df, ax=axes[1, 2])\nplt.tight_layout()\nplt.show()",
"_____no_output_____"
],
[
"train_labels = train[['grapheme_root', 'vowel_diacritic', 'consonant_diacritic']].values",
"_____no_output_____"
],
[
"fig, axes = plt.subplots(1, 3, figsize=(22, 6))\nsns.distplot(train_labels[:, 0], ax=axes[0], color='green', kde=False, label='train grapheme')\nsns.distplot(train_labels[:, 1], ax=axes[1], color='green', kde=False, label='train vowel')\nsns.distplot(train_labels[:, 2], ax=axes[2], color='green', kde=False, label='train consonant')\nplt.tight_layout()",
"_____no_output_____"
],
[
"fig, axes = plt.subplots(1, 3, figsize=(22, 6))\nsns.distplot(p0, ax=axes[0], color='orange', kde=False, label='test grapheme')\nsns.distplot(p1, ax=axes[1], color='orange', kde=False, label='test vowel')\nsns.distplot(p2, ax=axes[2], color='orange', kde=False, label='test consonant')\nplt.legend()\nplt.tight_layout()\n",
"_____no_output_____"
]
]
] | [
"markdown",
"code",
"markdown",
"code",
"markdown",
"code",
"markdown",
"code",
"markdown",
"code"
] | [
[
"markdown"
],
[
"code",
"code",
"code",
"code",
"code"
],
[
"markdown"
],
[
"code",
"code"
],
[
"markdown"
],
[
"code",
"code",
"code",
"code",
"code",
"code",
"code"
],
[
"markdown"
],
[
"code",
"code",
"code",
"code",
"code",
"code",
"code",
"code",
"code",
"code",
"code"
],
[
"markdown"
],
[
"code",
"code",
"code",
"code",
"code",
"code"
]
] |
e777357aa55c8f860f25ad95701ff56673515bc3 | 15,206 | ipynb | Jupyter Notebook | ml/kmeans.ipynb | lizhaoliu/RandomCollection | eca070c2723f9cf4e41a36930bfb805df623f853 | [
"MIT"
] | 1 | 2021-09-10T03:10:57.000Z | 2021-09-10T03:10:57.000Z | ml/kmeans.ipynb | lizhaoliu/RandomCollection | eca070c2723f9cf4e41a36930bfb805df623f853 | [
"MIT"
] | null | null | null | ml/kmeans.ipynb | lizhaoliu/RandomCollection | eca070c2723f9cf4e41a36930bfb805df623f853 | [
"MIT"
] | null | null | null | 127.781513 | 11,259 | 0.845193 | [
[
[
"import matplotlib.pyplot as plt\nimport numpy as np\n\ndef kmeans(points: np.ndarray, num_clusters: int, num_iterations: int) -> (np.ndarray, np.ndarray):\n \"\"\"Calculates the KMeans clusters using Euclidean distance for input points.\"\"\"\n \n num_points, point_dim = points.shape[0], points.shape[-1]\n \n # Initializes centroids as K random points from the input points, with shape [num_clusters, point_dim].\n centroids = points[np.random.choice(num_points, [num_clusters], replace=False)]\n # Augments the input points to shape [num_points, num_clusters, point_dim], for example:\n # [['x1' 'y1'] ['x2' 'y2] ['x3' 'y3']]\n # ==>\n # [[['x1' 'y1'] [['x2' 'y2'] [['x3' 'y3']\n # ['x1' 'y1'] ['x2' 'y2'] ['x3' 'y3']\n # ['x1' 'y1']] ['x2' 'y2']] ['x3' 'y3']]]\n # Such that we can take advantage of the vectorized subtraction later.\n points_augmented = np.tile(points.reshape([num_points, 1, point_dim]), [1, num_clusters, 1])\n \n for _ in range(num_iterations):\n # Calculates the element-wise diff of each point to each centroid, for example:\n # [[['x1' 'y1'] [['x2' 'y2'] [['x3' 'y3'] [[['c1x' 'c1y'] [['c1x' 'c1y'] [['c1x' 'c1y']\n # ['x1' 'y1'] ['x2' 'y2'] ['x3' 'y3'] - ['c2x' 'c2y'] ['c2x' 'c2y'] ['c2x' 'c2y']\n # ['x1' 'y1']] ['x2' 'y2']] ['x3' 'y3']]] ['c3x' 'c3y']] ['c3x' 'c3y']] ['c3x' 'c3y']]]\n diff = points_augmented - centroids\n # Calculates the Euclidean distance for each point to all centroids.\n dists = np.sqrt(np.sum(diff ** 2, axis=2))\n # Assigns the index of the closest centroid to each point.\n points_cluster = dists.argmin(axis=1)\n \n for k in range(num_clusters):\n # Updates each centroid to be the mean of all points that belong to this cluster.\n centroids[k] = points[points_cluster == k].mean(axis=0)\n \n return points_cluster, centroids\n ",
"_____no_output_____"
],
[
"x, k = np.random.normal(size=[200, 2]), 5\nclusters, centroids = kmeans(x, k, 10)\ncolors = ['b', 'g', 'r', 'c', 'm', 'y', 'k']\nfor i in range(k):\n pts = x[clusters == i]\n # Plots the clustered points.\n plt.scatter(pts[:, 0], pts[:, 1], c=colors[i], marker='.')\n # Plots the centroid.\n plt.scatter(centroids[i, 0], centroids[i, 1], c=colors[i], marker='x')\nplt.show()",
"_____no_output_____"
]
]
] | [
"code"
] | [
[
"code",
"code"
]
] |
e777381ae151a18a0c42552dd9e4959a21afcd79 | 58,428 | ipynb | Jupyter Notebook | tutorials/W2D4_DynamicNetworks/student/W2D4_Tutorial1.ipynb | carsen-stringer/course-content | e4bdc0ad9a4e846d7f2e5047c233019d0e84d2e4 | [
"CC-BY-4.0",
"BSD-3-Clause"
] | 1 | 2022-03-03T05:19:09.000Z | 2022-03-03T05:19:09.000Z | tutorials/W2D4_DynamicNetworks/student/W2D4_Tutorial1.ipynb | NiloofarT/course-content | ba1293fe831dc9b6ea9c0edac046767381ffafc6 | [
"CC-BY-4.0",
"BSD-3-Clause"
] | null | null | null | tutorials/W2D4_DynamicNetworks/student/W2D4_Tutorial1.ipynb | NiloofarT/course-content | ba1293fe831dc9b6ea9c0edac046767381ffafc6 | [
"CC-BY-4.0",
"BSD-3-Clause"
] | 1 | 2021-11-26T17:23:48.000Z | 2021-11-26T17:23:48.000Z | 37.239006 | 535 | 0.576008 | [
[
[
"<a href=\"https://colab.research.google.com/github/NeuromatchAcademy/course-content/blob/master/tutorials/W2D4_DynamicNetworks/student/W2D4_Tutorial1.ipynb\" target=\"_parent\"><img src=\"https://colab.research.google.com/assets/colab-badge.svg\" alt=\"Open In Colab\"/></a>",
"_____no_output_____"
],
[
"# Tutorial 1: Neural Rate Models\n**Week 2, Day 4: Dynamic Networks**\n\n**By Neuromatch Academy**\n\n__Content creators:__ Qinglong Gu, Songtin Li, Arvind Kumar, John Murray, Julijana Gjorgjieva \n\n__Content reviewers:__ Maryam Vaziri-Pashkam, Ella Batty, Lorenzo Fontolan, Richard Gao, Spiros Chavlis, Michael Waskom\n",
"_____no_output_____"
],
[
"**Our 2021 Sponsors, including Presenting Sponsor Facebook Reality Labs**\n\n<p align='center'><img src='https://github.com/NeuromatchAcademy/widgets/blob/master/sponsors.png?raw=True'/></p>",
"_____no_output_____"
],
[
"---\n# Tutorial Objectives\n\nThe brain is a complex system, not because it is composed of a large number of diverse types of neurons, but mainly because of how neurons are connected to each other. The brain is indeed a network of highly specialized neuronal networks. \n\nThe activity of a neural network constantly evolves in time. For this reason, neurons can be modeled as dynamical systems. The dynamical system approach is only one of the many modeling approaches that computational neuroscientists have developed (other points of view include information processing, statistical models, etc.). \n\nHow the dynamics of neuronal networks affect the representation and processing of information in the brain is an open question. However, signatures of altered brain dynamics present in many brain diseases (e.g., in epilepsy or Parkinson's disease) tell us that it is crucial to study network activity dynamics if we want to understand the brain.\n\nIn this tutorial, we will simulate and study one of the simplest models of biological neuronal networks. Instead of modeling and simulating individual excitatory neurons (e.g., LIF models that you implemented yesterday), we will treat them as a single homogeneous population and approximate their dynamics using a single one-dimensional equation describing the evolution of their average spiking rate in time.\n\nIn this tutorial, we will learn how to build a firing rate model of a single population of excitatory neurons. \n\n**Steps:**\n- Write the equation for the firing rate dynamics of a 1D excitatory population.\n- Visualize the response of the population as a function of parameters such as threshold level and gain, using the frequency-current (F-I) curve.\n- Numerically simulate the dynamics of the excitatory population and find the fixed points of the system. \n- Investigate the stability of the fixed points by linearizing the dynamics around them.\n \n",
"_____no_output_____"
],
[
"---\n# Setup",
"_____no_output_____"
]
],
[
[
"# Imports\nimport matplotlib.pyplot as plt\nimport numpy as np\nimport scipy.optimize as opt # root-finding algorithm",
"_____no_output_____"
],
[
"# @title Figure Settings\nimport ipywidgets as widgets # interactive display\n%config InlineBackend.figure_format = 'retina'\nplt.style.use(\"https://raw.githubusercontent.com/NeuromatchAcademy/course-content/master/nma.mplstyle\")",
"_____no_output_____"
],
[
"# @title Helper functions\n\n\ndef plot_fI(x, f):\n plt.figure(figsize=(6, 4)) # plot the figure\n plt.plot(x, f, 'k')\n plt.xlabel('x (a.u.)', fontsize=14)\n plt.ylabel('F(x)', fontsize=14)\n plt.show()\n\n\ndef plot_dr_r(r, drdt, x_fps=None):\n plt.figure()\n plt.plot(r, drdt, 'k')\n plt.plot(r, 0. * r, 'k--')\n if x_fps is not None:\n plt.plot(x_fps, np.zeros_like(x_fps), \"ko\", ms=12)\n plt.xlabel(r'$r$')\n plt.ylabel(r'$\\frac{dr}{dt}$', fontsize=20)\n plt.ylim(-0.1, 0.1)\n\n\ndef plot_dFdt(x, dFdt):\n plt.figure()\n plt.plot(x, dFdt, 'r')\n plt.xlabel('x (a.u.)', fontsize=14)\n plt.ylabel('dF(x)', fontsize=14)\n plt.show()",
"_____no_output_____"
]
],
[
[
"---\n# Section 1: Neuronal network dynamics",
"_____no_output_____"
]
],
[
[
"# @title Video 1: Dynamic networks\nfrom ipywidgets import widgets\n\nout2 = widgets.Output()\nwith out2:\n from IPython.display import IFrame\n class BiliVideo(IFrame):\n def __init__(self, id, page=1, width=400, height=300, **kwargs):\n self.id=id\n src = 'https://player.bilibili.com/player.html?bvid={0}&page={1}'.format(id, page)\n super(BiliVideo, self).__init__(src, width, height, **kwargs)\n\n video = BiliVideo(id=\"\", width=854, height=480, fs=1)\n print('Video available at https://www.bilibili.com/video/{0}'.format(video.id))\n display(video)\n\nout1 = widgets.Output()\nwith out1:\n from IPython.display import YouTubeVideo\n video = YouTubeVideo(id=\"p848349hPyw\", width=854, height=480, fs=1, rel=0)\n print('Video available at https://youtube.com/watch?v=' + video.id)\n display(video)\n\nout = widgets.Tab([out1, out2])\nout.set_title(0, 'Youtube')\nout.set_title(1, 'Bilibili')\n\ndisplay(out)",
"_____no_output_____"
]
],
[
[
"## Section 1.1: Dynamics of a single excitatory population\n\nIndividual neurons respond by spiking. When we average the spikes of neurons in a population, we can define the average firing activity of the population. In this model, we are interested in how the population-averaged firing varies as a function of time and network parameters. Mathematically, we can describe the firing rate dynamic as:\n\n\\begin{align}\n\\tau \\frac{dr}{dt} &= -r + F(w\\cdot r + I_{\\text{ext}}) \\quad\\qquad (1)\n\\end{align}\n\n$r(t)$ represents the average firing rate of the excitatory population at time $t$, $\\tau$ controls the timescale of the evolution of the average firing rate, $w$ denotes the strength (synaptic weight) of the recurrent input to the population, $I_{\\text{ext}}$ represents the external input, and the transfer function $F(\\cdot)$ (which can be related to f-I curve of individual neurons described in the next sections) represents the population activation function in response to all received inputs.\n\nTo start building the model, please execute the cell below to initialize the simulation parameters.",
"_____no_output_____"
]
],
[
[
"# @markdown *Execute this cell to set default parameters for a single excitatory population model*\n\n\ndef default_pars_single(**kwargs):\n pars = {}\n\n # Excitatory parameters\n pars['tau'] = 1. # Timescale of the E population [ms]\n pars['a'] = 1.2 # Gain of the E population\n pars['theta'] = 2.8 # Threshold of the E population\n\n # Connection strength\n pars['w'] = 0. # E to E, we first set it to 0\n\n # External input\n pars['I_ext'] = 0.\n\n # simulation parameters\n pars['T'] = 20. # Total duration of simulation [ms]\n pars['dt'] = .1 # Simulation time step [ms]\n pars['r_init'] = 0.2 # Initial value of E\n\n # External parameters if any\n pars.update(kwargs)\n\n # Vector of discretized time points [ms]\n pars['range_t'] = np.arange(0, pars['T'], pars['dt'])\n\n return pars",
"_____no_output_____"
]
],
[
[
"You can now use:\n- `pars = default_pars_single()` to get all the parameters, and then you can execute `print(pars)` to check these parameters. \n- `pars = default_pars_single(T=T_sim, dt=time_step)` to set new simulation time and time step\n- To update an existing parameter dictionary, use `pars['New_para'] = value`\n\nBecause `pars` is a dictionary, it can be passed to a function that requires individual parameters as arguments using `my_func(**pars)` syntax.",
"_____no_output_____"
],
[
"## Section 1.2: F-I curves\nIn electrophysiology, a neuron is often characterized by its spike rate output in response to input currents. This is often called the **F-I** curve, denoting the output spike frequency (**F**) in response to different injected currents (**I**). We estimated this for an LIF neuron in yesterday's tutorial.\n\nThe transfer function $F(\\cdot)$ in Equation $1$ represents the gain of the population as a function of the total input. The gain is often modeled as a sigmoidal function, i.e., more input drive leads to a nonlinear increase in the population firing rate. The output firing rate will eventually saturate for high input values. \n\nA sigmoidal $F(\\cdot)$ is parameterized by its gain $a$ and threshold $\\theta$.\n\n$$ F(x;a,\\theta) = \\frac{1}{1+\\text{e}^{-a(x-\\theta)}} - \\frac{1}{1+\\text{e}^{a\\theta}} \\quad(2)$$\n\nThe argument $x$ represents the input to the population. Note that the second term is chosen so that $F(0;a,\\theta)=0$.\n\nMany other transfer functions (generally monotonic) can be also used. Examples are the rectified linear function $ReLU(x)$ or the hyperbolic tangent $tanh(x)$.",
"_____no_output_____"
],
[
"### Exercise 1: Implement F-I curve \n\nLet's first investigate the activation functions before simulating the dynamics of the entire population. \n\nIn this exercise, you will implement a sigmoidal **F-I** curve or transfer function $F(x)$, with gain $a$ and threshold level $\\theta$ as parameters.",
"_____no_output_____"
]
],
[
[
"def F(x, a, theta):\n \"\"\"\n Population activation function.\n\n Args:\n x (float): the population input\n a (float): the gain of the function\n theta (float): the threshold of the function\n\n Returns:\n float: the population activation response F(x) for input x\n \"\"\"\n #################################################\n ## TODO for students: compute f = F(x) ##\n # Fill out function and remove\n raise NotImplementedError(\"Student excercise: implement the f-I function\")\n #################################################\n\n # Define the sigmoidal transfer function f = F(x)\n f = ...\n\n return f\n\n\npars = default_pars_single() # get default parameters\nx = np.arange(0, 10, .1) # set the range of input\n\n# Uncomment below to test your function\n# f = F(x, pars['a'], pars['theta'])\n# plot_fI(x, f)",
"_____no_output_____"
]
],
[
[
"[*Click for solution*](https://github.com/NeuromatchAcademy/course-content/tree/master//tutorials/W2D4_DynamicNetworks/solutions/W2D4_Tutorial1_Solution_45ddc05f.py)\n\n*Example output:*\n\n<img alt='Solution hint' align='left' width=416 height=272 src=https://raw.githubusercontent.com/NeuromatchAcademy/course-content/master/tutorials/W2D4_DynamicNetworks/static/W2D4_Tutorial1_Solution_45ddc05f_0.png>\n\n",
"_____no_output_____"
],
[
"### Interactive Demo: Parameter exploration of F-I curve\nHere's an interactive demo that shows how the F-I curve changes for different values of the gain and threshold parameters. How do the gain and threshold parameters affect the F-I curve?",
"_____no_output_____"
]
],
[
[
"# @title\n\n# @markdown Make sure you execute this cell to enable the widget!\n\n\ndef interactive_plot_FI(a, theta):\n \"\"\"\n Population activation function.\n\n Expecxts:\n a : the gain of the function\n theta : the threshold of the function\n\n Returns:\n plot the F-I curve with give parameters\n \"\"\"\n\n # set the range of input\n x = np.arange(0, 10, .1)\n plt.figure()\n plt.plot(x, F(x, a, theta), 'k')\n plt.xlabel('x (a.u.)', fontsize=14)\n plt.ylabel('F(x)', fontsize=14)\n plt.show()\n\n\n_ = widgets.interact(interactive_plot_FI, a=(0.3, 3, 0.3), theta=(2, 4, 0.2))",
"_____no_output_____"
]
],
[
[
"[*Click for solution*](https://github.com/NeuromatchAcademy/course-content/tree/master//tutorials/W2D4_DynamicNetworks/solutions/W2D4_Tutorial1_Solution_1c0165d7.py)\n\n",
"_____no_output_____"
],
[
"## Section 1.3: Simulation scheme of E dynamics\n\nBecause $F(\\cdot)$ is a nonlinear function, the exact solution of Equation $1$ can not be determined via analytical methods. Therefore, numerical methods must be used to find the solution. In practice, the derivative on the left-hand side of Equation $1$ can be approximated using the Euler method on a time-grid of stepsize $\\Delta t$:\n\n\\begin{align}\n&\\frac{dr}{dt} \\approx \\frac{r[k+1]-r[k]}{\\Delta t} \n\\end{align}\nwhere $r[k] = r(k\\Delta t)$. \n\nThus,\n\n$$\\Delta r[k] = \\frac{\\Delta t}{\\tau}[-r[k] + F(w\\cdot r[k] + I_{\\text{ext}}[k];a,\\theta)]$$\n\n\nHence, Equation (1) is updated at each time step by:\n\n$$r[k+1] = r[k] + \\Delta r[k]$$\n",
"_____no_output_____"
]
],
[
[
"# @markdown *Execute this cell to enable the single population rate model simulator: `simulate_single`*\n\n\ndef simulate_single(pars):\n \"\"\"\n Simulate an excitatory population of neurons\n\n Args:\n pars : Parameter dictionary\n\n Returns:\n rE : Activity of excitatory population (array)\n\n Example:\n pars = default_pars_single()\n r = simulate_single(pars)\n \"\"\"\n\n # Set parameters\n tau, a, theta = pars['tau'], pars['a'], pars['theta']\n w = pars['w']\n I_ext = pars['I_ext']\n r_init = pars['r_init']\n dt, range_t = pars['dt'], pars['range_t']\n Lt = range_t.size\n\n # Initialize activity\n r = np.zeros(Lt)\n r[0] = r_init\n I_ext = I_ext * np.ones(Lt)\n\n # Update the E activity\n for k in range(Lt - 1):\n dr = dt / tau * (-r[k] + F(w * r[k] + I_ext[k], a, theta))\n r[k+1] = r[k] + dr\n\n return r\n\nhelp(simulate_single)",
"_____no_output_____"
]
],
[
[
"### Interactive Demo: Parameter Exploration of single population dynamics\n\nNote that $w=0$, as in the default setting, means no recurrent input to the neuron population in Equation (1). Hence, the dynamics are entirely determined by the external input $I_{\\text{ext}}$. Explore these dynamics in this interactive demo.\n\nHow does $r_{\\text{sim}}(t)$ change with different $I_{\\text{ext}}$ values? How does it change with different $\\tau$ values? Investigate the relationship between $F(I_{\\text{ext}}; a, \\theta)$ and the steady value of $r(t)$. \n\nNote that, $r_{\\rm ana}(t)$ denotes the analytical solution - you will learn how this is computed in the next section.",
"_____no_output_____"
]
],
[
[
"# @title\n\n# @markdown Make sure you execute this cell to enable the widget!\n\n# get default parameters\npars = default_pars_single(T=20.)\n\n\ndef Myplot_E_diffI_difftau(I_ext, tau):\n # set external input and time constant\n pars['I_ext'] = I_ext\n pars['tau'] = tau\n\n # simulation\n r = simulate_single(pars)\n\n # Analytical Solution\n r_ana = (pars['r_init']\n + (F(I_ext, pars['a'], pars['theta'])\n - pars['r_init']) * (1. - np.exp(-pars['range_t'] / pars['tau'])))\n\n # plot\n plt.figure()\n plt.plot(pars['range_t'], r, 'b', label=r'$r_{\\mathrm{sim}}$(t)', alpha=0.5,\n zorder=1)\n plt.plot(pars['range_t'], r_ana, 'b--', lw=5, dashes=(2, 2),\n label=r'$r_{\\mathrm{ana}}$(t)', zorder=2)\n plt.plot(pars['range_t'],\n F(I_ext, pars['a'], pars['theta']) * np.ones(pars['range_t'].size),\n 'k--', label=r'$F(I_{\\mathrm{ext}})$')\n plt.xlabel('t (ms)', fontsize=16.)\n plt.ylabel('Activity r(t)', fontsize=16.)\n plt.legend(loc='best', fontsize=14.)\n plt.show()\n\n\n_ = widgets.interact(Myplot_E_diffI_difftau, I_ext=(0.0, 10., 1.),\n tau=(1., 5., 0.2))",
"_____no_output_____"
]
],
[
[
"[*Click for solution*](https://github.com/NeuromatchAcademy/course-content/tree/master//tutorials/W2D4_DynamicNetworks/solutions/W2D4_Tutorial1_Solution_65dee3e7.py)\n\n",
"_____no_output_____"
],
[
"## Think!\nAbove, we have numerically solved a system driven by a positive input. Yet, $r_E(t)$ either decays to zero or reaches a fixed non-zero value.\n- Why doesn't the solution of the system \"explode\" in a finite time? In other words, what guarantees that $r_E$(t) stays finite? \n- Which parameter would you change in order to increase the maximum value of the response? ",
"_____no_output_____"
],
[
"[*Click for solution*](https://github.com/NeuromatchAcademy/course-content/tree/master//tutorials/W2D4_DynamicNetworks/solutions/W2D4_Tutorial1_Solution_5a95a98e.py)\n\n",
"_____no_output_____"
],
[
"---\n# Section 2: Fixed points of the single population system\n",
"_____no_output_____"
]
],
[
[
"# @title Video 2: Fixed point\nfrom ipywidgets import widgets\n\nout2 = widgets.Output()\nwith out2:\n from IPython.display import IFrame\n class BiliVideo(IFrame):\n def __init__(self, id, page=1, width=400, height=300, **kwargs):\n self.id=id\n src = 'https://player.bilibili.com/player.html?bvid={0}&page={1}'.format(id, page)\n super(BiliVideo, self).__init__(src, width, height, **kwargs)\n\n video = BiliVideo(id=\"\", width=854, height=480, fs=1)\n print('Video available at https://www.bilibili.com/video/{0}'.format(video.id))\n display(video)\n\nout1 = widgets.Output()\nwith out1:\n from IPython.display import YouTubeVideo\n video = YouTubeVideo(id=\"Ox3ELd1UFyo\", width=854, height=480, fs=1, rel=0)\n print('Video available at https://youtube.com/watch?v=' + video.id)\n display(video)\n\nout = widgets.Tab([out1, out2])\nout.set_title(0, 'Youtube')\nout.set_title(1, 'Bilibili')\n\ndisplay(out)",
"_____no_output_____"
]
],
[
[
"As you varied the two parameters in the last Interactive Demo, you noticed that, while at first the system output quickly changes, with time, it reaches its maximum/minimum value and does not change anymore. The value eventually reached by the system is called the **steady state** of the system, or the **fixed point**. Essentially, in the steady states the derivative with respect to time of the activity ($r$) is zero, i.e. $\\displaystyle \\frac{dr}{dt}=0$. \n\nWe can find that the steady state of the Equation. (1) by setting $\\displaystyle{\\frac{dr}{dt}=0}$ and solve for $r$:\n\n$$-r_{\\text{steady}} + F(w\\cdot r_{\\text{steady}} + I_{\\text{ext}};a,\\theta) = 0, \\qquad (3)$$\n\nWhen it exists, the solution of Equation. (3) defines a **fixed point** of the dynamical system in Equation (1). Note that if $F(x)$ is nonlinear, it is not always possible to find an analytical solution, but the solution can be found via numerical simulations, as we will do later.\n\nFrom the Interactive Demo, one could also notice that the value of $\\tau$ influences how quickly the activity will converge to the steady state from its initial value. \n\nIn the specific case of $w=0$, we can also analytically compute the solution of Equation (1) (i.e., the thick blue dashed line) and deduce the role of $\\tau$ in determining the convergence to the fixed point: \n\n$$\\displaystyle{r(t) = \\big{[}F(I_{\\text{ext}};a,\\theta) -r(t=0)\\big{]} (1-\\text{e}^{-\\frac{t}{\\tau}})} + r(t=0)$$ \\\\\n\nWe can now numerically calculate the fixed point with a root finding algorithm.",
"_____no_output_____"
],
[
"## Exercise 2: Visualization of the fixed points\n\nWhen it is not possible to find the solution for Equation (3) analytically, a graphical approach can be taken. To that end, it is useful to plot $\\displaystyle{\\frac{dr}{dt}}$ as a function of $r$. The values of $r$ for which the plotted function crosses zero on the y axis correspond to fixed points. \n\nHere, let us, for example, set $w=5.0$ and $I^{\\text{ext}}=0.5$. From Equation (1), you can obtain\n\n$$\\frac{dr}{dt} = [-r + F(w\\cdot r + I^{\\text{ext}})]\\,/\\,\\tau $$\n\nThen, plot the $dr/dt$ as a function of $r$, and check for the presence of fixed points. ",
"_____no_output_____"
]
],
[
[
"def compute_drdt(r, I_ext, w, a, theta, tau, **other_pars):\n \"\"\"Given parameters, compute dr/dt as a function of r.\n\n Args:\n r (1D array) : Average firing rate of the excitatory population\n I_ext, w, a, theta, tau (numbers): Simulation parameters to use\n other_pars : Other simulation parameters are unused by this function\n\n Returns\n drdt function for each value of r\n \"\"\"\n #########################################################################\n # TODO compute drdt and disable the error\n raise NotImplementedError(\"Finish the compute_drdt function\")\n #########################################################################\n\n # Calculate drdt\n drdt = ...\n\n return drdt\n\n\n# Define a vector of r values and the simulation parameters\nr = np.linspace(0, 1, 1000)\npars = default_pars_single(I_ext=0.5, w=5)\n\n# Uncomment to test your function\n# drdt = compute_drdt(r, **pars)\n# plot_dr_r(r, drdt)",
"_____no_output_____"
]
],
[
[
"[*Click for solution*](https://github.com/NeuromatchAcademy/course-content/tree/master//tutorials/W2D4_DynamicNetworks/solutions/W2D4_Tutorial1_Solution_c5280901.py)\n\n*Example output:*\n\n<img alt='Solution hint' align='left' width=558 height=413 src=https://raw.githubusercontent.com/NeuromatchAcademy/course-content/master/tutorials/W2D4_DynamicNetworks/static/W2D4_Tutorial1_Solution_c5280901_0.png>\n\n",
"_____no_output_____"
],
[
"## Exercise 3: Fixed point calculation\n\nWe will now find the fixed points numerically. To do so, we need to specif initial values ($r_{\\text{guess}}$) for the root-finding algorithm to start from. From the line $\\displaystyle{\\frac{dr}{dt}}$ plotted above in Exercise 2, initial values can be chosen as a set of values close to where the line crosses zero on the y axis (real fixed point).\n\nThe next cell defines three helper functions that we will use:\n\n- `my_fp_single(r_guess, **pars)` uses a root-finding algorithm to locate a fixed point near a given initial value\n- `check_fp_single(x_fp, **pars)`, verifies that the values of $r_{\\rm fp}$ for which $\\displaystyle{\\frac{dr}{dt}} = 0$ are the true fixed points\n- `my_fp_finder(r_guess_vector, **pars)` accepts an array of initial values and finds the same number of fixed points, using the above two functions",
"_____no_output_____"
]
],
[
[
"# @markdown *Execute this cell to enable the fixed point functions*\n\ndef my_fp_single(r_guess, a, theta, w, I_ext, **other_pars):\n \"\"\"\n Calculate the fixed point through drE/dt=0\n\n Args:\n r_guess : Initial value used for scipy.optimize function\n a, theta, w, I_ext : simulation parameters\n\n Returns:\n x_fp : value of fixed point\n \"\"\"\n # define the right hand of E dynamics\n def my_WCr(x):\n r = x\n drdt = (-r + F(w * r + I_ext, a, theta))\n y = np.array(drdt)\n\n return y\n\n x0 = np.array(r_guess)\n x_fp = opt.root(my_WCr, x0).x.item()\n\n return x_fp\n\n\ndef check_fp_single(x_fp, a, theta, w, I_ext, mytol=1e-4, **other_pars):\n \"\"\"\n Verify |dr/dt| < mytol\n\n Args:\n fp : value of fixed point\n a, theta, w, I_ext: simulation parameters\n mytol : tolerance, default as 10^{-4}\n\n Returns :\n Whether it is a correct fixed point: True/False\n \"\"\"\n # calculate Equation(3)\n y = x_fp - F(w * x_fp + I_ext, a, theta)\n\n # Here we set tolerance as 10^{-4}\n return np.abs(y) < mytol\n\n\ndef my_fp_finder(pars, r_guess_vector, mytol=1e-4):\n \"\"\"\n Calculate the fixed point(s) through drE/dt=0\n\n Args:\n pars : Parameter dictionary\n r_guess_vector : Initial values used for scipy.optimize function\n mytol : tolerance for checking fixed point, default as 10^{-4}\n\n Returns:\n x_fps : values of fixed points\n\n \"\"\"\n x_fps = []\n correct_fps = []\n for r_guess in r_guess_vector:\n x_fp = my_fp_single(r_guess, **pars)\n if check_fp_single(x_fp, **pars, mytol=mytol):\n x_fps.append(x_fp)\n\n return x_fps\n\nhelp(my_fp_finder)",
"_____no_output_____"
],
[
"r = np.linspace(0, 1, 1000)\npars = default_pars_single(I_ext=0.5, w=5)\ndrdt = compute_drdt(r, **pars)\n\n#############################################################################\n# TODO for students:\n# Define initial values close to the intersections of drdt and y=0\n# (How many initial values? Hint: How many times do the two lines intersect?)\n# Calculate the fixed point with these initial values and plot them\n#############################################################################\nr_guess_vector = [...]\n\n# Uncomment to test your values\n# x_fps = my_fp_finder(pars, r_guess_vector)\n# plot_dr_r(r, drdt, x_fps)",
"_____no_output_____"
]
],
[
[
"[*Click for solution*](https://github.com/NeuromatchAcademy/course-content/tree/master//tutorials/W2D4_DynamicNetworks/solutions/W2D4_Tutorial1_Solution_0637b6bf.py)\n\n*Example output:*\n\n<img alt='Solution hint' align='left' width=558 height=413 src=https://raw.githubusercontent.com/NeuromatchAcademy/course-content/master/tutorials/W2D4_DynamicNetworks/static/W2D4_Tutorial1_Solution_0637b6bf_0.png>\n\n",
"_____no_output_____"
],
[
"## Interactive Demo: fixed points as a function of recurrent and external inputs.\n\nYou can now explore how the previous plot changes when the recurrent coupling $w$ and the external input $I_{\\text{ext}}$ take different values. How does the number of fixed points change?",
"_____no_output_____"
]
],
[
[
"# @title\n\n# @markdown Make sure you execute this cell to enable the widget!\n\n\ndef plot_intersection_single(w, I_ext):\n # set your parameters\n pars = default_pars_single(w=w, I_ext=I_ext)\n\n # find fixed points\n r_init_vector = [0, .4, .9]\n x_fps = my_fp_finder(pars, r_init_vector)\n\n # plot\n r = np.linspace(0, 1., 1000)\n drdt = (-r + F(w * r + I_ext, pars['a'], pars['theta'])) / pars['tau']\n\n plot_dr_r(r, drdt, x_fps)\n\n_ = widgets.interact(plot_intersection_single, w=(1, 7, 0.2),\n I_ext=(0, 3, 0.1))",
"_____no_output_____"
]
],
[
[
"[*Click for solution*](https://github.com/NeuromatchAcademy/course-content/tree/master//tutorials/W2D4_DynamicNetworks/solutions/W2D4_Tutorial1_Solution_20486792.py)\n\n",
"_____no_output_____"
],
[
"---\n# Summary\n\nIn this tutorial, we have investigated the dynamics of a rate-based single population of neurons.\n\nWe learned about:\n- The effect of the input parameters and the time constant of the network on the dynamics of the population.\n- How to find the fixed point(s) of the system.\n\nNext, we have two Bonus, but important concepts in dynamical system analysis and simulation. If you have time left, watch the next video and proceed to solve the exercises. You will learn:\n\n- How to determine the stability of a fixed point by linearizing the system.\n- How to add realistic inputs to our model.",
"_____no_output_____"
],
[
"---\n# Bonus 1: Stability of a fixed point",
"_____no_output_____"
]
],
[
[
"# @title Video 3: Stability of fixed points\nfrom ipywidgets import widgets\n\nout2 = widgets.Output()\nwith out2:\n from IPython.display import IFrame\n class BiliVideo(IFrame):\n def __init__(self, id, page=1, width=400, height=300, **kwargs):\n self.id=id\n src = 'https://player.bilibili.com/player.html?bvid={0}&page={1}'.format(id, page)\n super(BiliVideo, self).__init__(src, width, height, **kwargs)\n\n video = BiliVideo(id=\"\", width=854, height=480, fs=1)\n print('Video available at https://www.bilibili.com/video/{0}'.format(video.id))\n display(video)\n\nout1 = widgets.Output()\nwith out1:\n from IPython.display import YouTubeVideo\n video = YouTubeVideo(id=\"KKMlWWU83Jg\", width=854, height=480, fs=1, rel=0)\n print('Video available at https://youtube.com/watch?v=' + video.id)\n display(video)\n\nout = widgets.Tab([out1, out2])\nout.set_title(0, 'Youtube')\nout.set_title(1, 'Bilibili')\n\ndisplay(out)",
"_____no_output_____"
]
],
[
[
"#### Initial values and trajectories\n\nHere, let us first set $w=5.0$ and $I_{\\text{ext}}=0.5$, and investigate the dynamics of $r(t)$ starting with different initial values $r(0) \\equiv r_{\\text{init}}$. We will plot the trajectories of $r(t)$ with $r_{\\text{init}} = 0.0, 0.1, 0.2,..., 0.9$.",
"_____no_output_____"
]
],
[
[
"# @markdown Execute this cell to see the trajectories!\n\npars = default_pars_single()\npars['w'] = 5.0\npars['I_ext'] = 0.5\n\nplt.figure(figsize=(8, 5))\nfor ie in range(10):\n pars['r_init'] = 0.1 * ie # set the initial value\n r = simulate_single(pars) # run the simulation\n\n # plot the activity with given initial\n plt.plot(pars['range_t'], r, 'b', alpha=0.1 + 0.1 * ie,\n label=r'r$_{\\mathrm{init}}$=%.1f' % (0.1 * ie))\n\nplt.xlabel('t (ms)')\nplt.title('Two steady states?')\nplt.ylabel(r'$r$(t)')\nplt.legend(loc=[1.01, -0.06], fontsize=14)\nplt.show()",
"_____no_output_____"
]
],
[
[
"## Interactive Demo: dynamics as a function of the initial value\n\nLet's now set $r_{\\rm init}$ to a value of your choice in this demo. How does the solution change? What do you observe?",
"_____no_output_____"
]
],
[
[
"# @title\n\n# @markdown Make sure you execute this cell to enable the widget!\n\npars = default_pars_single(w=5.0, I_ext=0.5)\n\ndef plot_single_diffEinit(r_init):\n pars['r_init'] = r_init\n r = simulate_single(pars)\n\n plt.figure()\n plt.plot(pars['range_t'], r, 'b', zorder=1)\n plt.plot(0, r[0], 'bo', alpha=0.7, zorder=2)\n plt.xlabel('t (ms)', fontsize=16)\n plt.ylabel(r'$r(t)$', fontsize=16)\n plt.ylim(0, 1.0)\n plt.show()\n\n\n_ = widgets.interact(plot_single_diffEinit, r_init=(0, 1, 0.02))",
"_____no_output_____"
]
],
[
[
"[*Click for solution*](https://github.com/NeuromatchAcademy/course-content/tree/master//tutorials/W2D4_DynamicNetworks/solutions/W2D4_Tutorial1_Solution_4d2de6a0.py)\n\n",
"_____no_output_____"
],
[
"### Stability analysis via linearization of the dynamics\n\nJust like Equation $1$ in the case ($w=0$) discussed above, a generic linear system \n$$\\frac{dx}{dt} = \\lambda (x - b),$$ \nhas a fixed point for $x=b$. The analytical solution of such a system can be found to be:\n$$x(t) = b + \\big{(} x(0) - b \\big{)} \\text{e}^{\\lambda t}.$$ \nNow consider a small perturbation of the activity around the fixed point: $x(0) = b+ \\epsilon$, where $|\\epsilon| \\ll 1$. Will the perturbation $\\epsilon(t)$ grow with time or will it decay to the fixed point? The evolution of the perturbation with time can be written, using the analytical solution for $x(t)$, as:\n $$\\epsilon (t) = x(t) - b = \\epsilon \\text{e}^{\\lambda t}$$\n\n- if $\\lambda < 0$, $\\epsilon(t)$ decays to zero, $x(t)$ will still converge to $b$ and the fixed point is \"**stable**\".\n\n- if $\\lambda > 0$, $\\epsilon(t)$ grows with time, $x(t)$ will leave the fixed point $b$ exponentially, and the fixed point is, therefore, \"**unstable**\" .",
"_____no_output_____"
],
[
"### Compute the stability of Equation $1$\n\nSimilar to what we did in the linear system above, in order to determine the stability of a fixed point $r^{*}$ of the excitatory population dynamics, we perturb Equation (1) around $r^{*}$ by $\\epsilon$, i.e. $r = r^{*} + \\epsilon$. We can plug in Equation (1) and obtain the equation determining the time evolution of the perturbation $\\epsilon(t)$:\n\n\\begin{align}\n\\tau \\frac{d\\epsilon}{dt} \\approx -\\epsilon + w F'(w\\cdot r^{*} + I_{\\text{ext}};a,\\theta) \\epsilon \n\\end{align}\n\nwhere $F'(\\cdot)$ is the derivative of the transfer function $F(\\cdot)$. We can rewrite the above equation as:\n\n\\begin{align}\n\\frac{d\\epsilon}{dt} \\approx \\frac{\\epsilon}{\\tau }[-1 + w F'(w\\cdot r^* + I_{\\text{ext}};a,\\theta)] \n\\end{align}\n\nThat is, as in the linear system above, the value of\n\n$$\\lambda = [-1+ wF'(w\\cdot r^* + I_{\\text{ext}};a,\\theta)]/\\tau \\qquad (4)$$\n\ndetermines whether the perturbation will grow or decay to zero, i.e., $\\lambda$ defines the stability of the fixed point. This value is called the **eigenvalue** of the dynamical system.",
"_____no_output_____"
],
[
"## Exercise 4: Compute $dF$\n\nThe derivative of the sigmoid transfer function is:\n\\begin{align} \n\\frac{dF}{dx} & = \\frac{d}{dx} (1+\\exp\\{-a(x-\\theta)\\})^{-1} \\\\\n& = a\\exp\\{-a(x-\\theta)\\} (1+\\exp\\{-a(x-\\theta)\\})^{-2}. \\qquad (5)\n\\end{align}\n\nLet's now find the expression for the derivative $\\displaystyle{\\frac{dF}{dx}}$ in the following cell and plot it.",
"_____no_output_____"
]
],
[
[
"def dF(x, a, theta):\n \"\"\"\n Population activation function.\n\n Args:\n x : the population input\n a : the gain of the function\n theta : the threshold of the function\n\n Returns:\n dFdx : the population activation response F(x) for input x\n \"\"\"\n\n ###########################################################################\n # TODO for students: compute dFdx ##\n raise NotImplementedError(\"Student excercise: compute the deravitive of F\")\n ###########################################################################\n\n # Calculate the population activation\n dFdx = ...\n\n return dFdx\n\n\npars = default_pars_single() # get default parameters\nx = np.arange(0, 10, .1) # set the range of input\n\n# Uncomment below to test your function\n# df = dF(x, pars['a'], pars['theta'])\n# plot_dFdt(x, df)",
"_____no_output_____"
]
],
[
[
"[*Click for solution*](https://github.com/NeuromatchAcademy/course-content/tree/master//tutorials/W2D4_DynamicNetworks/solutions/W2D4_Tutorial1_Solution_ce2e3bc5.py)\n\n*Example output:*\n\n<img alt='Solution hint' align='left' width=560 height=416 src=https://raw.githubusercontent.com/NeuromatchAcademy/course-content/master/tutorials/W2D4_DynamicNetworks/static/W2D4_Tutorial1_Solution_ce2e3bc5_0.png>\n\n",
"_____no_output_____"
],
[
"## Exercise 5: Compute eigenvalues\n\nAs discussed above, for the case with $w=5.0$ and $I_{\\text{ext}}=0.5$, the system displays **three** fixed points. However, when we simulated the dynamics and varied the initial conditions $r_{\\rm init}$, we could only obtain **two** steady states. In this exercise, we will now check the stability of each of the three fixed points by calculating the corresponding eigenvalues with the function `eig_single`. Check the sign of each eigenvalue (i.e., stability of each fixed point). How many of the fixed points are stable?\n\nNote that the expression of the eigenvalue at fixed point $r^*$\n$$\\lambda = [-1+ wF'(w\\cdot r^* + I_{\\text{ext}};a,\\theta)]/\\tau$$",
"_____no_output_____"
]
],
[
[
"def eig_single(fp, tau, a, theta, w, I_ext, **other_pars):\n \"\"\"\n Args:\n fp : fixed point r_fp\n tau, a, theta, w, I_ext : Simulation parameters\n\n Returns:\n eig : eigevalue of the linearized system\n \"\"\"\n #####################################################################\n ## TODO for students: compute eigenvalue and disable the error\n raise NotImplementedError(\"Student excercise: compute the eigenvalue\")\n ######################################################################\n # Compute the eigenvalue\n eig = ...\n\n return eig\n\n\n# Find the eigenvalues for all fixed points of Exercise 2\npars = default_pars_single(w=5, I_ext=.5)\nr_guess_vector = [0, .4, .9]\nx_fp = my_fp_finder(pars, r_guess_vector)\n\n# Uncomment below lines after completing the eig_single function.\n\n# for fp in x_fp:\n# eig_fp = eig_single(fp, **pars)\n# print(f'Fixed point1 at {fp:.3f} with Eigenvalue={eig_fp:.3f}')",
"_____no_output_____"
]
],
[
[
"**SAMPLE OUTPUT**\n\n```\nFixed point1 at 0.042 with Eigenvalue=-0.583\nFixed point2 at 0.447 with Eigenvalue=0.498\nFixed point3 at 0.900 with Eigenvalue=-0.626\n```",
"_____no_output_____"
],
[
"[*Click for solution*](https://github.com/NeuromatchAcademy/course-content/tree/master//tutorials/W2D4_DynamicNetworks/solutions/W2D4_Tutorial1_Solution_e285f60d.py)\n\n",
"_____no_output_____"
],
[
"## Think! \nThroughout the tutorial, we have assumed $w> 0 $, i.e., we considered a single population of **excitatory** neurons. What do you think will be the behavior of a population of inhibitory neurons, i.e., where $w> 0$ is replaced by $w< 0$? ",
"_____no_output_____"
],
[
"[*Click for solution*](https://github.com/NeuromatchAcademy/course-content/tree/master//tutorials/W2D4_DynamicNetworks/solutions/W2D4_Tutorial1_Solution_579bc9c9.py)\n\n",
"_____no_output_____"
],
[
"---\n# Bonus 2: Noisy input drives the transition between two stable states\n\n",
"_____no_output_____"
],
[
"## Ornstein-Uhlenbeck (OU) process\n\nAs discussed in several previous tutorials, the OU process is usually used to generate a noisy input into the neuron. The OU input $\\eta(t)$ follows: \n\n$$\\tau_\\eta \\frac{d}{dt}\\eta(t) = -\\eta (t) + \\sigma_\\eta\\sqrt{2\\tau_\\eta}\\xi(t)$$\n\nExecute the following function `my_OU(pars, sig, myseed=False)` to generate an OU process.",
"_____no_output_____"
]
],
[
[
"# @title OU process `my_OU(pars, sig, myseed=False)`\n\n# @markdown Make sure you execute this cell to visualize the noise!\n\n\ndef my_OU(pars, sig, myseed=False):\n \"\"\"\n A functions that generates Ornstein-Uhlenback process\n\n Args:\n pars : parameter dictionary\n sig : noise amplitute\n myseed : random seed. int or boolean\n\n Returns:\n I : Ornstein-Uhlenbeck input current\n \"\"\"\n\n # Retrieve simulation parameters\n dt, range_t = pars['dt'], pars['range_t']\n Lt = range_t.size\n tau_ou = pars['tau_ou'] # [ms]\n\n # set random seed\n if myseed:\n np.random.seed(seed=myseed)\n else:\n np.random.seed()\n\n # Initialize\n noise = np.random.randn(Lt)\n I_ou = np.zeros(Lt)\n I_ou[0] = noise[0] * sig\n\n # generate OU\n for it in range(Lt - 1):\n I_ou[it + 1] = (I_ou[it]\n + dt / tau_ou * (0. - I_ou[it])\n + np.sqrt(2 * dt / tau_ou) * sig * noise[it + 1])\n\n return I_ou\n\n\npars = default_pars_single(T=100)\npars['tau_ou'] = 1. # [ms]\nsig_ou = 0.1\nI_ou = my_OU(pars, sig=sig_ou, myseed=2020)\nplt.figure(figsize=(10, 4))\nplt.plot(pars['range_t'], I_ou, 'r')\nplt.xlabel('t (ms)')\nplt.ylabel(r'$I_{\\mathrm{OU}}$')\nplt.show()",
"_____no_output_____"
]
],
[
[
"## Example: Up-Down transition\n\nIn the presence of two or more fixed points, noisy inputs can drive a transition between the fixed points! Here, we stimulate an E population for 1,000 ms applying OU inputs.",
"_____no_output_____"
]
],
[
[
"# @title Simulation of an E population with OU inputs\n\n# @markdown Make sure you execute this cell to spot the Up-Down states!\n\npars = default_pars_single(T=1000)\npars['w'] = 5.0\nsig_ou = 0.7\npars['tau_ou'] = 1. # [ms]\npars['I_ext'] = 0.56 + my_OU(pars, sig=sig_ou, myseed=2020)\n\nr = simulate_single(pars)\n\nplt.figure(figsize=(10, 4))\nplt.plot(pars['range_t'], r, 'b', alpha=0.8)\nplt.xlabel('t (ms)')\nplt.ylabel(r'$r(t)$')\nplt.show()",
"_____no_output_____"
]
]
] | [
"markdown",
"code",
"markdown",
"code",
"markdown",
"code",
"markdown",
"code",
"markdown",
"code",
"markdown",
"code",
"markdown",
"code",
"markdown",
"code",
"markdown",
"code",
"markdown",
"code",
"markdown",
"code",
"markdown",
"code",
"markdown",
"code",
"markdown",
"code",
"markdown",
"code",
"markdown",
"code",
"markdown",
"code",
"markdown",
"code"
] | [
[
"markdown",
"markdown",
"markdown",
"markdown",
"markdown"
],
[
"code",
"code",
"code"
],
[
"markdown"
],
[
"code"
],
[
"markdown"
],
[
"code"
],
[
"markdown",
"markdown",
"markdown"
],
[
"code"
],
[
"markdown",
"markdown"
],
[
"code"
],
[
"markdown",
"markdown"
],
[
"code"
],
[
"markdown"
],
[
"code"
],
[
"markdown",
"markdown",
"markdown",
"markdown"
],
[
"code"
],
[
"markdown",
"markdown"
],
[
"code"
],
[
"markdown",
"markdown"
],
[
"code",
"code"
],
[
"markdown",
"markdown"
],
[
"code"
],
[
"markdown",
"markdown",
"markdown"
],
[
"code"
],
[
"markdown"
],
[
"code"
],
[
"markdown"
],
[
"code"
],
[
"markdown",
"markdown",
"markdown",
"markdown"
],
[
"code"
],
[
"markdown",
"markdown"
],
[
"code"
],
[
"markdown",
"markdown",
"markdown",
"markdown",
"markdown",
"markdown"
],
[
"code"
],
[
"markdown"
],
[
"code"
]
] |
e77755c0b53078f0d1cd03a0ac08114b4059bfad | 9,622 | ipynb | Jupyter Notebook | chapter-1-hands_on_deep_learning/1-1-overview_of_deep_learning.ipynb | lcsss1995/tutorials | 04fc31f950cc55451d8454ffaaed65e8d65f021d | [
"Apache-2.0"
] | null | null | null | chapter-1-hands_on_deep_learning/1-1-overview_of_deep_learning.ipynb | lcsss1995/tutorials | 04fc31f950cc55451d8454ffaaed65e8d65f021d | [
"Apache-2.0"
] | null | null | null | chapter-1-hands_on_deep_learning/1-1-overview_of_deep_learning.ipynb | lcsss1995/tutorials | 04fc31f950cc55451d8454ffaaed65e8d65f021d | [
"Apache-2.0"
] | null | null | null | 48.59596 | 497 | 0.701206 | [
[
[
"# 人工智能\机器学习和深度学习的概念及关系\n\n人工智能、机器学习和深度学习的概念在近些年十分火热,但很多从业者也难以说清它们之间的关系,外行人更是雾里看花。学习深度学习,需要先从三个概念的正本清源开始。\n\n三者覆盖的技术范畴是逐层递减的,人工智能是最宽泛的概念,机器学习则是实现人工智能的一种方式,也是目前较有效的方式。深度学习是机器学习算法中最热的一个分支,在近些年取得了显著的进展,并代替了多数传统机器学习算法。所以,三者的关系可用下图表示,人工智能 > 机器学习 > 深度学习。\n\n\n\n<center>\n \n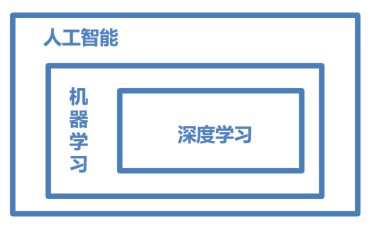\n \n</center>\n<center>图1:人工智能、机器学习和深度学习三者之间的概念范围</center>\n<br/>\n\n如字面含义,人工智能是研究、开发用于模拟、延伸和扩展人的智能的理论、方法、技术及应用系统的科学。由于这个定义只阐述了目标,而没限定方法。所以,实现人工智能存在的诸多方法和分支,导致其变成一个“大杂烩”式的学科。\n\n与此不同,机器学习,尤其是监督学习则有更加明确的指代。机器学习是专门研究计算机怎样模拟或实现人类的学习行为,以获取新的知识或技能,重新组织已有的知识结构使之不断改善自身的性能。这句话有点“云山雾罩”的感觉,让人不知所云。\n\n机器学习的实现步骤可以分成两步,训练和预测。这两个专业名词类似于归纳和演绎的含义。归纳是从具体案例中抽象一般规律,机器学习中的“训练”亦是如此。从一定数量的样本(已知模型输入$X$和模型输出$Y$)中,学习出输出$Y$与输入$X$的关系(可以想象成是某种表达式)。演绎则是从一般规律推导出具体案例的结果,机器学习中的预测亦是如此。基于训练得到的$Y$与$X$之间的关系,遇到新出现的输入$X$,计算出输出$Y$。在多数时候,通过模型计算得到的输出,如果和真实场景中的输出一致,说明模型是有效的。\n\n下面以“机器从牛顿第二定律实验中学习知识”为案例,让读者深入理解下机器学习(监督学习)到底是怎样的一种技术方法。机器学习的方法论和人类科研的过程有异曲同工之妙。下牛顿第二定律的常见表述:物体加速度的大小跟作用力成正比,跟物体的质量成反比,且与物体质量的倒数成正比。该定律是由艾萨克·牛顿在1687年于《自然哲学的数学原理》一书中提出的。牛顿第二运动定律和第一、第三定律共同组成了牛顿运动定律,阐述了经典力学中基本的运动规律。\n\n在中学课本中,牛顿第二定律有两种设计实验的方法:倾斜滑动法和水平拉线法。 \n\n\n<center>\n \n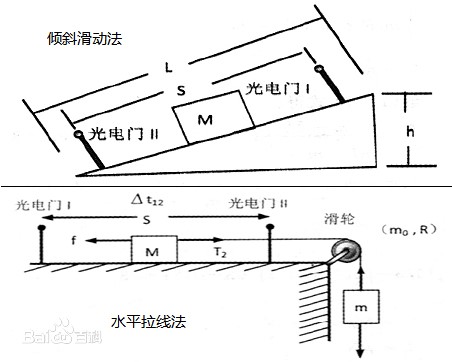\n\n</center>\n<center>图2:牛顿第二定律实验的两种方法</center>\n<br/>\n\n相信很多读者均有摆弄滑轮和小木块做实验的青涩年代和美好回忆。基于多次实验,统计到不同的作用力下木块加速度如下表所示。\n\n<br/>\n\n<center>表1:实验获取的大量数据样本和作图观测结果</center>\n\n<center>\n\n| 项目 | 作用力X | 加速度Y |\n| -------- | -----: | :----: |\n| 第1次 | 4 | 2 |\n| 第2次 | 5 | 2.5 |\n| ... | ... | ... |\n| 第n次 | 6 | 3 |\n\n</center>\n\n观察上述实验数据,不难猜测物体的加速度$a$和作用力之间的关系应该是线性关系。所以,我们提出假设 $a = w * F$,$a$代表加速度,$F$代表作用力,$w$是待确定的参数。\n\n通过大量实验数据的训练,确定参数$w$是物体质量的倒数$(1/m)$,即得到完整的模型公式$a = w * (1/m)$。当已知作用到某个物体的力时,基于模型可以方便的预测物体的加速度。例如燃料对火箭的推力$F$=10,火箭的质量$m$=2,求得火箭的加速度$a$=5。\n\n这个有趣的案例演示机器学习的基本过程,但其中有一个关键点的实现尚不清晰,即怎样确定模型的参数$(w=1/m)$?\n\n学习确定参数的过程与科学家提出假说的方式类似,合理的假说至少能够解释所有已有观测。如果在未来观测到不符合理论假说的新数据,人们会尝试提出新的假说。如天文史上,使用大圆和小圆组合的方式计算天体运行在中世纪是可以拟合观测数据的。但随着欧洲机械工业的进步,天文观测设备逐渐强大,越来越多的观测数据无法套用已有的理论。这促进了使用椭圆计算天体运行的理论假说出现。所以,模型有效的基本条件是能够拟合已知的样本,这给我们提供了学习有效模型的实现方案。以$H$为模型的假设,它是一个关于参数$\\theta$和输入$X$的函数,用$H(\\theta, X)$ 表示。模型的优化目标是使得$H(\\theta, X)$的输出与真实输入$Y$尽量一致,即两者相差程度即是模型效果的评价函数(相差越小越好)。那么,学习参数的过程就是在已知的样本上,不断减小该评价函数($H(\\theta, X)$ 和$Y$相差)的过程,直到学习到一个参数$\\theta$使得评价函数的取值最小。这个衡量模型预测值和真实值差距的评价函数也被称为损失函数(损失 Loss)。上述优化参数的过程如下图公式所示。\n\n<center>\n\n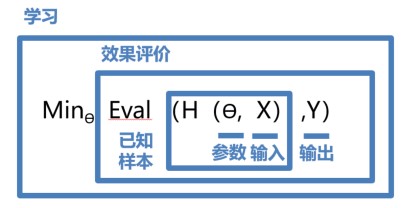\n\n</center>\n<center>图3:学习确定参数的方法</center>\n<br/>\n\n\n举例类比,机器如一个机械的学生一样,只能通过尝试答对(最小化损失)大量的习题(已知样本)来学习知识(模型参数w),期望用学习到的知识w组成完整的模型$H(\\theta, X)$,能回答不知道答案的考试题(未知样本)。最小化损失是模型的优化目标,实现损失最小化的方法称为优化算法,也称为寻解算法(找到使得损失函数最小的参数解)。参数$\\theta$和输入$X$组成公式的基本结构称为假设。在牛顿第二定律的案例中,基于对数据的观测,我们提出的是线性假设,即作用力和加速度是线性关系,用线性方程表示。由此可见,模型假设,评价函数(损失/优化目标)和优化算法是构成一个模型的三个部分。\n\n机器学习算法理论在上个世纪90年代发展成熟,在诸多领域也取得了应用效果。但平静的日子过到2010年左右,深度学习模型的异军突起,极大改变了机器学习的应用格局。在今天,多数机器学习任务均可以使用深度学习模型解决。在语音,计算机视觉和自然语言处理等领域,深度学习模型的效果比传统机器学习算法有显著提升。\n\n那么,深度学习又怎样对机器学习的算法结构提出了改进呢?其实两者的理论结构是一致的,也存在模型假设,评价函数和优化算法,最根本的差别在于假设的复杂度上。\n\n\n\n<center>\n \n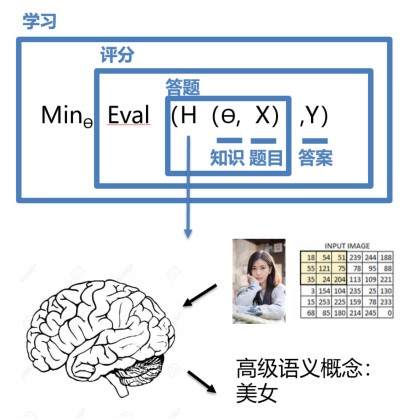\n\n</center>\n<center> 图4:从原始图片像素到高级语义概念“美女”的函数转换的复杂度难以想象!</center>\n<br/>\n\n如上图所示,不是所有的任务均如牛顿第二定律那样简单直观。对于一张图片,人脑接收到五颜六色的光学信号,计算机则接收到一个数字矩阵。人脑以极快的速度反应出这张图片是一位美女,而且是程序员喜欢的类型。这个结果是一个非常高级的语义概念,从像素到高级语义概念中间要经历怎样复杂的信息变换是难以想象的!这种变换已经复杂到无法用数学公式表达,所以研究者们借鉴了人脑神经元的结构,设计出神经网络的模型。\n\n人工神经网络包括多个神经网络层(卷积层、全连接层、LSTM等),每一层又包括很多神经元,超过三层的非线性神经网络都可以被成为深度神经网络。通俗的讲,深度学习的模型可以视为是输入到输出的映射函数,比如中文到英文的映射,足够深的神经网络理论上可以拟合任何复杂的函数,因此,神经网络非常适合学习样本数据的内在规律和表示层次,对文字\图像和声音任务有很好的适用性,因为这几个领域的任务是人工智能的基础模块,所以深度学习被称为实现人工智能的基础也就不足为奇了\n\n\n# 深度学习的历史和发展\n\n究竟神经网络是怎样的设计?先不用着急,在下一章会以一个“房价预测”的案例,演示使用Python实现神经网络模型的细节。在进入实现细节之前,让我们回顾下深度学习的悠久的历史和今日的蓬勃发展。\n\n神经网络思想的提出已经是75年前的事情了,现今的神经网络和深度学习的设计理论是一步步的完善的。在这漫长的发展岁月中,有一些取得关键突破的闪光时刻。其中有1960年代,基本网络结构设计完善后的黄金时代,也有在1969年异或问题被提出后(人们惊奇的发现神经网络模型连简单的异或问题也无法解决),神经网络模型被束之高阁的黑暗时代。虽然在1986年,新提出的多层的神经网络解决了异或问题,但随着90年代后理论更完备并且实践效果更好的SVM等机器学习模型的兴起,神经网络并未得到重视。真正的兴起是在2010年左右,基于神经网络模型改进的技术在语音和计算机视觉任务上大放异彩,也逐渐被证明在更多的任务(自然语言处理以及海量数据的任务)上有效。至此,神经网络模型重新焕发生机,并有了一个更加响亮的名字:深度学习。\n\n\n<center>\n \n<img src=\"https://ai-studio-static-online.cdn.bcebos.com/3ffdc18ef6384f4b93fcba9eff5360b926e7822eb0f5452ea85163281f31b3a2\" width = \"1000\" height = \"600\" alt=\"深度学习有悠久的发展历史,但在2010年后才逐渐成熟\" align=center />\n\n</center>\n<center> 图5:深度学习有悠久的发展历史,但在2010年后才逐渐成熟 </center>\n<br/>\n\n\n为何神经网络到2010年后才焕发生机,这与深度学习成功所依赖的先决条件有关。\n\n1. 大数据是它有效的前提。神经网络和深度学习是非常强大的模型,但也需要足够量级的训练数据。时至今日,很多传统机器学习算法和人工特征依然是足够有效的方案,原因在于很多场景下没有足够的标记数据来支撑深度学习这样强大的模型。深度学习的能力特别像科学家托罗密的豪言壮语:“给我一根足够长的杠杆,我能撬动地球!”,它也可以发出类似的豪言:“给我足够多的数据,我能够学习任何复杂的关系”。但在现实中,足够长的杠杆与足够多的数据一样,往往只能是一种美好的愿景。直到近些年,各行业IT化程度提高,累积的数据量爆发式的增长,才使得应用深度学习模型成为可能。\n2. 依靠硬件的发展和算法的优化。现阶段依靠更强大的计算机,GPU,Autoencoder预训练和并行计算等技术,深度网络在训练上的困难已经被逐渐克服。其中,数据量和硬件是更主要的原因。没有前两者,科学家们想优化算法都无从进行。\n\n 早在1998年,一些科学家就已经使用神经网络模型识别手写字母图像了。但深度学习在计算机视觉应用上的兴起,还是在2012年ImageNet比赛上,使用AlexNet做图像分类。如果比较下98年和12年的模型,会发现两者在网络结构上非常类似,仅在一些细节上有所优化。在这十四年间计算性能的大幅提升和数据量的爆发式增长,促使模型完成了从“简单的字母识别”到“复杂的图像分类”的跨越。\n\n虽然历史悠久,但深度学习在今天依然在蓬勃发展,一方面基础研究快速进展,另一方面工业实践层出不穷。\n\n如下图所示,基于深度学习的顶级会议ICLR(international conference on learning representations)统计,深度学习相关的论文数量呈逐年递增的状态。同时,不仅仅是深度学习会议,与数据和模型技术相关的会议ICML和KDD,专注视觉的CVPR和专注自然语言处理的EMNLP等国际会议的大量论文均涉及着深度学习技术。该领域和相关领域的研究方兴未艾,技术仍在不断创新突破中。\n\n<center>\n\n<img src=\"https://ai-studio-static-online.cdn.bcebos.com/098749c66df347c8b99e61156718a28ff469d69f312442638fd9e88ad93bb982\" width = \"600\" height = \"400\" alt=\"与深度学习相关的论文数量逐年攀升\" align=center />\n\n</center>\n<center>图6:与深度学习相关的论文数量逐年攀升</center>\n<br/>\n\n另一方面,以深度学习为基础的人工智能技术在升级改造众多的传统行业,存在极其广阔的应用场景。下图选自艾瑞咨询的研究报告,人工智能技术不仅可在众多行业中落地应用(广度),在部分行业(如安防)已经实现了市场化变现和高速增长(深度)。\n\n<center>\n\n<img src=\"https://ai-studio-static-online.cdn.bcebos.com/6060c5cd5f47431abb54faf47f4c5e6c4a33a210bcff470c91a47dfea5904539\" width = \"900\" height = \"700\" alt=\"以深度学习为基础的AI技术在各行业广泛应用,产生巨大经济价值\" align=center />\n\n</center>\n<center>图7:以深度学习为基础的AI技术在各行业广泛应用,产生巨大经济价值</center>\n<br/>\n\n除了应用广泛的特点外,深度学习还推动人工智能进入工业大生产阶段,算法的通用性导致标准化、自动化和模块化的框架产生。此前,不同流派的机器学习算法理论和实现均不同,导致每个算法均要独立实现,例如随机森林和支撑向量机(SVM)。但在深度学习框架下的诸多算法结构有较大的通用性,例如常用与计算机视觉的卷积神经网络模型(CNN)和常用于自然语言处理的长期短期记忆模型(LSTM),均可以分为组网模块,梯度下降的优化模块,预测模块等。这使得抽象出统一的框架成为了可能,并大大降低了编写建模代码的成本。一些相对通用的模块,如网络基础算子的实现,各种优化算法等均可以由框架实现。建模者只需要关注数据处理,配置组网的方式,以及用少量代码串起训练和预测的流程即可。\n\n<center>\n\n\n \n</center>\n<center>图8:深度学习模型具有通用性特点,可以标准化、自动化和模块化 </center>\n<br/>\n\n在深度学习框架出现之前,机器学习工程师处于手工业作坊生产的时代。为了完成建模,工程师需要储备大量数学知识,并为特征工程工作积累大量行业知识。每个模型是极其个性化的,建模者如同手工业者一样,将自己的积累形成模型的“个性化签名”。而今,“深度学习工程师”进入了工业化大生产时代。只要掌握深度学习必要但少量的理论知识,掌握Python编程即可以在深度学习框架实现极其有效的模型,甚至与该领域最领先的实现模型不相上下。建模这个被“老科学家”们长期把持的建模领域面临着颠覆,也是新入行者的机遇。\n\n<center>\n\n<img src=\"https://ai-studio-static-online.cdn.bcebos.com/09c968080e83481cb44a5aac3bdfb9a111fcac98bb254793b975f5eb3f23ba70\" width = \"800\" height = \"600\" alt=\"深度学习工程师处于工业化大生产时代,“老科学家”长期积累的优势不再牢固\" align=center />\n\n</center>\n\n<br/>\n<center>图9:深度学习工程师处于工业化大生产时代,“老科学家”长期积累的优势不再牢固</center>\n<br/>\n\n每个人的生命都是宝贵的,我们经常说要将有限的时间浪费在有价值的事情上。为何要学习深度学习技术,以及通过这本书来学习呢?一方面,深度学习的应用前景广阔,是极好的发展方向和职业选择。另一方面,本书会使用国产的深度学习框架飞桨(PaddlePaddle)来编写实践案例,基于框架的编程让深度学习变得易学易用。\n\n下面让我们尽快开始第一个实践案例:基于Python编写完成房价预测任务的神经网络模型,并在这个过程中亲身设计一个神经网络模型。\n\n### 思考题\n1. 类比牛顿第二定律的案例,在你的工作和生活中还有哪些问题可以用监督学习的框架来解决?模型假设和参数是什么?评价函数(损失)是什么?\n2. 为什么说深度学习工程师有发展前景?怎样从经济学(市场供需)的角度做出解读?",
"_____no_output_____"
]
]
] | [
"markdown"
] | [
[
"markdown"
]
] |
e77759e71608cdee2c0bb851cb90b0256dcb9c38 | 4,743 | ipynb | Jupyter Notebook | Tutorial/.ipynb_checkpoints/c5_optimizers-checkpoint.ipynb | danhtaihoang/pytorch-deeplearning | 258dd316894c8fcac2d0b7be1be3c18714164e49 | [
"MIT"
] | null | null | null | Tutorial/.ipynb_checkpoints/c5_optimizers-checkpoint.ipynb | danhtaihoang/pytorch-deeplearning | 258dd316894c8fcac2d0b7be1be3c18714164e49 | [
"MIT"
] | null | null | null | Tutorial/.ipynb_checkpoints/c5_optimizers-checkpoint.ipynb | danhtaihoang/pytorch-deeplearning | 258dd316894c8fcac2d0b7be1be3c18714164e49 | [
"MIT"
] | null | null | null | 21.461538 | 79 | 0.45351 | [
[
[
"Refs:\n\nhttps://github.com/deep-learning-with-pytorch/dlwpt-code",
"_____no_output_____"
]
],
[
[
"import numpy as np\nimport torch",
"_____no_output_____"
]
],
[
[
"### Optimizers",
"_____no_output_____"
]
],
[
[
"x = [35.7, 55.9, 58.2, 81.9, 56.3, 48.9, 33.9, 21.8, 48.4, 60.4, 68.4]\ny = [0.5, 14.0, 15.0, 28.0, 11.0, 8.0, 3.0, -4.0, 6.0, 13.0, 21.0]\n\nx = torch.tensor(x)\ny = torch.tensor(y)\n\n#x = 0.1*x # normalize",
"_____no_output_____"
],
[
"x_norm = 0.1*x",
"_____no_output_____"
],
[
"def model(x, w, b):\n return w * x + b",
"_____no_output_____"
],
[
"def loss_fn(y_p, y):\n squared_diffs = (y_p - y)**2\n return squared_diffs.mean()",
"_____no_output_____"
],
[
"import torch.optim as optim",
"_____no_output_____"
],
[
"dir(optim)",
"_____no_output_____"
],
[
"def training_loop(n_epochs, optimizer, params, x, y):\n \n for epoch in range(1, n_epochs + 1):\n y_p = model(x, *params) \n loss = loss_fn(y_p, y)\n \n ## reset gradients to zero\n optimizer.zero_grad()\n \n ## calculate gradients\n loss.backward() \n \n ## update params: params -= learning_rate * params.grad\n optimizer.step() \n\n if epoch % 500 == 0:\n print('Epoch %d, Loss %f' % (epoch, float(loss)))\n \n return params",
"_____no_output_____"
],
[
"params = torch.tensor([1.0, 0.0], requires_grad=True)\n\nlearning_rate = 1e-2\noptimizer = optim.SGD([params], lr=learning_rate)\n\ntraining_loop(n_epochs = 5000, params = params,\n optimizer = optimizer, x = x_norm, y = y)",
"Epoch 500, Loss 7.860115\nEpoch 1000, Loss 3.828538\nEpoch 1500, Loss 3.092191\nEpoch 2000, Loss 2.957698\nEpoch 2500, Loss 2.933134\nEpoch 3000, Loss 2.928648\nEpoch 3500, Loss 2.927830\nEpoch 4000, Loss 2.927679\nEpoch 4500, Loss 2.927652\nEpoch 5000, Loss 2.927647\n"
]
]
] | [
"markdown",
"code",
"markdown",
"code"
] | [
[
"markdown"
],
[
"code"
],
[
"markdown"
],
[
"code",
"code",
"code",
"code",
"code",
"code",
"code",
"code"
]
] |
e777755583daac30e464a04427934b66f16a0257 | 11,397 | ipynb | Jupyter Notebook | 1-output.ipynb | fndari/nbpy-top-tweeters | 8203b4d1c2472d8a0c1d08aeafe9de51a6868813 | [
"MIT"
] | null | null | null | 1-output.ipynb | fndari/nbpy-top-tweeters | 8203b4d1c2472d8a0c1d08aeafe9de51a6868813 | [
"MIT"
] | null | null | null | 1-output.ipynb | fndari/nbpy-top-tweeters | 8203b4d1c2472d8a0c1d08aeafe9de51a6868813 | [
"MIT"
] | null | null | null | 20.873626 | 227 | 0.521541 | [
[
[
"# The basic nbpy_top_tweeters app",
"_____no_output_____"
],
[
"First, let's get connected with the Twitter API:",
"_____no_output_____"
]
],
[
[
"import os\nimport tweepy",
"_____no_output_____"
],
[
"auth = tweepy.AppAuthHandler(\n os.environ['TWITTER_API_TOKEN'],\n os.environ['TWITTER_API_SECRET']\n)\n\napi = tweepy.API(auth)\napi",
"_____no_output_____"
],
[
"# import requests_cache\n# requests_cache.install_cache()",
"_____no_output_____"
]
],
[
[
"At this point, we use the `search()` method to get a list of tweets matching the search term:",
"_____no_output_____"
]
],
[
[
"nbpy_tweets = api.search('#nbpy', count=100)",
"_____no_output_____"
],
[
"len(nbpy_tweets)",
"_____no_output_____"
]
],
[
[
"From the iterable of tweets we get the number of tweets per user by using a `collections.Counter` object:",
"_____no_output_____"
]
],
[
[
"from collections import Counter\n\ntweet_count_by_username = Counter(tweet.user.screen_name for tweet in nbpy_tweets)",
"_____no_output_____"
],
[
"tweet_count_by_username",
"_____no_output_____"
]
],
[
[
"At this point, we can calculate the top $n$ tweeters:",
"_____no_output_____"
]
],
[
[
"top_tweeters = tweet_count_by_username.most_common(20)\ntop_tweeters",
"_____no_output_____"
]
],
[
[
"And show a scoreboard with the winners:",
"_____no_output_____"
]
],
[
[
"for username, tweet_count in top_tweeters:\n print(f'@{username:20}{tweet_count:2d}')",
"_____no_output_____"
]
],
[
[
"- We can see that, already with the \"vanilla\" notebook, we have some degree of interactivity simply by editing and running the code cell-by-cell rather than in one go",
"_____no_output_____"
],
[
"---",
"_____no_output_____"
],
[
"# From `repr()` output to rich output with `IPython.display`",
"_____no_output_____"
]
],
[
[
"import random\n\ntweet = random.choice(nbpy_tweets)\ntweet",
"_____no_output_____"
]
],
[
[
"- The repr of these objects are rich in information, but not very easy to explore",
"_____no_output_____"
]
],
[
[
"tweet.user",
"_____no_output_____"
]
],
[
[
"The `IPython.display` module contains several classes that render rich output from objects in a cell's output",
"_____no_output_____"
]
],
[
[
"from IPython.display import *",
"_____no_output_____"
]
],
[
[
"- `JSON` turns any JSON-able `dict` into an expandable, filterable widget",
"_____no_output_____"
]
],
[
[
"tweet._json",
"_____no_output_____"
],
[
"JSON(tweet._json)",
"_____no_output_____"
]
],
[
[
"- `Image` generates an image from raw PNG data, a file path, or a URL",
"_____no_output_____"
]
],
[
[
"Image(tweet.user.profile_image_url)",
"_____no_output_____"
]
],
[
[
"- `Markdown` can be used to generate rich text programmatically in a cell's output\n",
"_____no_output_____"
]
],
[
[
"Markdown(f\"\"\"\n*{tweet.user.name}* (`@{tweet.user.screen_name}`) is tweeting about **North Bay Python**!\n\"\"\")",
"_____no_output_____"
]
],
[
[
"- `HTML` is able to render arbitrary HTML code",
"_____no_output_____"
]
],
[
[
"HTML('<a class=\"twitter-timeline\" href=\"https://twitter.com/northbaypython?ref_src=twsrc%5Etfw\">Tweets by northbaypython</a> <script async src=\"https://platform.twitter.com/widgets.js\" charset=\"utf-8\"></script>');",
"_____no_output_____"
]
],
[
[
"- `FileLink` generates a \"smart\" link to a file, relative to the notebook's working directory",
"_____no_output_____"
]
],
[
[
"FileLink('hey-nbpy.md')",
"_____no_output_____"
]
],
[
[
"---",
"_____no_output_____"
],
[
"We can use these building block to create rich representations and associate them with any object\n\n- Strategy #1: Register custom formatters for object types",
"_____no_output_____"
]
],
[
[
"def tweet_as_markdown(tweet):\n quoted_text = '\\n'.join(f'> {line}' for line in tweet.text.split('\\n'))\n author = f'--*{tweet.user.name}* (`@{tweet.user.screen_name}`) on {tweet.created_at}'\n return quoted_text + '\\n\\n' + author",
"_____no_output_____"
],
[
"formatters = get_ipython().display_formatter.formatters\nformatters['text/markdown'].for_type(tweepy.Status, tweet_as_markdown)",
"_____no_output_____"
],
[
"tweet",
"_____no_output_____"
]
],
[
[
"- Strategy #2: Implement `_repr_*_()` methods for custom classes",
"_____no_output_____"
],
[
"Notes:\n\n- Let's say we want to move the tweet-counting code to its own class\n",
"_____no_output_____"
]
],
[
[
"class ScoreBoard:\n \n def __init__(self, items, display_top=5):\n self._items = items\n self.display_top = display_top\n \n @property\n def counts_by_name(self):\n return Counter(self._items)\n \n @property\n def to_display(self):\n return self.counts_by_name.most_common(self.display_top)\n\n def _repr_markdown_(self):\n # effectively we're using this \n lines = [\n f'# [North Bay Python 2019](https://2019.northbaypython.org) Top {self.display_top} Tweeters',\n '| name | # tweets |',\n '|-|-|',\n ]\n\n for name, count in self.to_display:\n lines.append(f'| {name} | {count} |')\n\n return '\\n'.join(lines)\n ",
"_____no_output_____"
],
[
"ScoreBoard(tweet_count_by_username, display_top=10)",
"_____no_output_____"
]
],
[
[
"- Rich output is rendered automatically when the object is the return value of a cell\n - Tip: use a `;` at the end of the last line in the cell to render nothing instead\n- Use the `display()` function to show rich output from anywhere in a cell (e.g. in a loop)\n - `display()` is versatile; falls back to text repr in a console",
"_____no_output_____"
]
],
[
[
"for tweet in nbpy_tweets[:10]:\n display(tweet)",
"_____no_output_____"
],
[
"nbpy_tweets = api.search('#nbpy', count=100000)",
"_____no_output_____"
],
[
"import pickle\n\nwith open('nbpy_tweets.pkl', 'wb') as f:\n pickle.dump(nbpy_tweets, f)",
"_____no_output_____"
],
[
"with open('nbpy_tweets.pkl', 'rb') as f:\n unpickled = pickle.load(f)\n \nlen(unpickled)",
"_____no_output_____"
]
]
] | [
"markdown",
"code",
"markdown",
"code",
"markdown",
"code",
"markdown",
"code",
"markdown",
"code",
"markdown",
"code",
"markdown",
"code",
"markdown",
"code",
"markdown",
"code",
"markdown",
"code",
"markdown",
"code",
"markdown",
"code",
"markdown",
"code",
"markdown",
"code",
"markdown",
"code",
"markdown",
"code"
] | [
[
"markdown",
"markdown"
],
[
"code",
"code",
"code"
],
[
"markdown"
],
[
"code",
"code"
],
[
"markdown"
],
[
"code",
"code"
],
[
"markdown"
],
[
"code"
],
[
"markdown"
],
[
"code"
],
[
"markdown",
"markdown",
"markdown"
],
[
"code"
],
[
"markdown"
],
[
"code"
],
[
"markdown"
],
[
"code"
],
[
"markdown"
],
[
"code",
"code"
],
[
"markdown"
],
[
"code"
],
[
"markdown"
],
[
"code"
],
[
"markdown"
],
[
"code"
],
[
"markdown"
],
[
"code"
],
[
"markdown",
"markdown"
],
[
"code",
"code",
"code"
],
[
"markdown",
"markdown"
],
[
"code",
"code"
],
[
"markdown"
],
[
"code",
"code",
"code",
"code"
]
] |
e7777dea6cecb622ce3264cf7bc511a09ff36749 | 12,016 | ipynb | Jupyter Notebook | example/MXNetTutorialTemplate.ipynb | simonmaurer/BMXNet-v2 | 91fcc480d66415c9c70254e00114b839e5229f10 | [
"Apache-2.0"
] | null | null | null | example/MXNetTutorialTemplate.ipynb | simonmaurer/BMXNet-v2 | 91fcc480d66415c9c70254e00114b839e5229f10 | [
"Apache-2.0"
] | null | null | null | example/MXNetTutorialTemplate.ipynb | simonmaurer/BMXNet-v2 | 91fcc480d66415c9c70254e00114b839e5229f10 | [
"Apache-2.0"
] | null | null | null | 27.43379 | 575 | 0.601698 | [
[
[
"# Tutorial Title",
"_____no_output_____"
],
[
"A brief introduction to the tutorial that describes:\n\n- The problem that that the tutorial addresses\n- Who the intended audience is\n- The expected experience level of that audience with a concept or tool \n- Which environment/language it runs in \n\nIf there is another similar tutorial that's more appropriate for another audience, direct the reader there with a linked reference.",
"_____no_output_____"
],
[
"## How to Use This Tutorial",
"_____no_output_____"
],
[
"A brief explanation of how the reader can use the tutorial. Can the reader copy each code snippet into a Python or other environment? Or can the reader run `<filename>` before or after reading through the explanations to understand how the code works?",
"_____no_output_____"
],
[
"You can use this tutorial by *insert method(s) here*. \n\nA bulleted list of the tasks the reader will accomplish and skills he or she will learn. Begin each list item with a noun (Learn, Create, Use, etc.).\n\nYou will accomplish the following:\n\n- First task or skill\n- Second task or skill\n- X task or skill",
"_____no_output_____"
],
[
"## Prerequisites",
"_____no_output_____"
],
[
"Provide a *complete* list of the software, hardware, knowledge, and skills required to be successful using the tutorial. For each item, link the item to installation instructions, specs, or skill development tools, as appropriate. If good installation instructions aren't available for required software, start the tutorial with instructions for installing it.",
"_____no_output_____"
],
[
"To complete this tutorial, you need:\n\n- [MXNet](https://mxnet.incubator.apache.org/install/#overview)\n- [Language](https://mxnet.incubator.apache.org/tutorials/)\n- [Tool](https://mxnet.incubator.apache.org/api/python/index.html)\n- [Familiarity with concept or tool](https://gluon.mxnet.io/)\n",
"_____no_output_____"
],
[
"## The Data",
"_____no_output_____"
],
[
"Provide a link to where the data is hosted and explain how to download it. If it requires more than two steps, use a numbered list.",
"_____no_output_____"
],
[
"You can download the data used in this tutorial from the [Site Name](http://) site. To download the data:\n\n1. At the `<language>` prompt, type:\n\n `<command>`\n2. Second task.\n\n3. Last task.",
"_____no_output_____"
],
[
"Briefly describe key aspects of the data. If there are two or more aspects of the data that require involved discussion, use subheads (### `<Concept or Sub-component Name>`). To include a graphic, introduce it with a brief description and use the image linking tool to include it. Store the graphic in GitHub and use the following format: <img width=\"517\" alt=\"screen shot 2016-05-06 at 10 13 16 pm\" src=\"https://cloud.githubusercontent.com/assets/5545640/15089697/d6f4fca0-13d7-11e6-9331-7f94fcc7b4c6.png\">. You do not need to provide a title for your graphics. ",
"_____no_output_____"
],
[
"The data *add description here. (optional)*",
"_____no_output_____"
],
[
"## (Optional) Concept or Component Name",
"_____no_output_____"
],
[
"If concepts or components need further introduction, include this section. If there are two or more aspects of the concept or component that require involved discussion, use subheads (### Concept or Sub-component Name).",
"_____no_output_____"
]
],
[
[
"## Prepare the Data",
"_____no_output_____"
]
],
[
[
"If appropriate, summarize the tasks required to prepare the data, defining and explaining key concepts.",
"_____no_output_____"
],
[
"To prepare the data, *provide explanation here.*",
"_____no_output_____"
],
[
"Use a numbered procedure to explain how to prepare the data. Add code snippets or blocks that show the code that the user must type or that is used for this task in the Jupyter Notebook. To include code snippets, precede each line of code with four spaces and two tick marks. Always introduce input or output with a description or context or result, followed by a colon.",
"_____no_output_____"
],
[
"To prepare the data:\n\n1.\n\n2.\n\n3.",
"_____no_output_____"
],
[
"If there are any aspects of data preparation that require elaboration, add it here.",
"_____no_output_____"
],
[
"## Create the Model",
"_____no_output_____"
],
[
"If appropriate, summarize the tasks required to create the model, defining and explaining key concepts.",
"_____no_output_____"
],
[
"To create the model, *provide explanation here.*",
"_____no_output_____"
],
[
"Use a numbered procedure to explain how to create the data. Add code snippets or blocks that show the code that the user must type or that is used for this task in the Jupyter Notebook. To include code snippets, precede each line of code with four spaces and two tick marks. Always introduce input or output with a description or context or result, followed by a colon.",
"_____no_output_____"
],
[
"To create the model:\n\n1.\n\n2.\n\n3.",
"_____no_output_____"
],
[
"If there are any aspects of model creation that require elaboration, add it here.",
"_____no_output_____"
],
[
"## Fit the Model",
"_____no_output_____"
],
[
"If appropriate, summarize the tasks required to create the model, defining and explaining key concepts.",
"_____no_output_____"
],
[
"To fit the model, *provide explanation here.*",
"_____no_output_____"
],
[
"Use a numbered procedure to explain how to fit the model. Add code snippets or blocks that show the code that the user must type or that is used for this task in the Jupyter Notebook. To include code snippets, precede each line of code with four spaces and two tick marks. Always introduce input or output with a description or context or result, followed by a colon.",
"_____no_output_____"
],
[
"To fit the model:\n\n1.\n\n2.\n\n3.",
"_____no_output_____"
],
[
"If there are any aspects of model fitting that require elaboration, add it here.",
"_____no_output_____"
],
[
"## Evaluate the Model",
"_____no_output_____"
],
[
"If appropriate, summarize the tasks required to evaluate the model, defining and explaining key concepts.",
"_____no_output_____"
],
[
"To evaluate the model, *provide explanation here.*",
"_____no_output_____"
],
[
"Use a numbered procedure to explain how to evaluate the model. Add code snippets or blocks that show the code that the user must type or that is used for this task in the Jupyter Notebook. To include code snippets, precede each line of code with four spaces and two tick marks. Always introduce input or output with a description or context or result, followed by a colon.",
"_____no_output_____"
],
[
"To evaluate the model:\n\n1.\n\n2.\n\n3.",
"_____no_output_____"
],
[
"If there are any aspects of model evaluation that require elaboration, add it here.",
"_____no_output_____"
],
[
"## (Optional) Additional Tasks",
"_____no_output_____"
],
[
"If appropriate, summarize the tasks required to perform the task, defining and explaining key concepts.",
"_____no_output_____"
],
[
"To *fperform the task*, *provide explanation here.*",
"_____no_output_____"
],
[
"Use a numbered procedure to explain how to perform the task. Add code snippets or blocks that show the code that the user must type or that is used for this task in the Jupyter Notebook. To include code snippets, precede each line of code with four spaces and two tick marks. Always introduce input or output with a description or context or result, followed by a colon.",
"_____no_output_____"
],
[
"To *perform the task*:\n\n1.\n\n2.\n\n3.",
"_____no_output_____"
],
[
"If there are any aspects of model evaluation that require elaboration, add it here.",
"_____no_output_____"
],
[
"## Summary",
"_____no_output_____"
],
[
"Briefly describe the end result of the tutorial and how the user can use it or modify it to customize it.",
"_____no_output_____"
],
[
"## Next Steps",
"_____no_output_____"
],
[
"Provide a bulleted list of other documents, tools, or tutorials that further explain the concepts discussed in this tutorial or build on this tutorial. Start each list item with a brief description of a user task followed by the title of the destination site or topic that is formatted as a link.",
"_____no_output_____"
],
[
"- For more information on *topic*, see [Site Name](http://).\n- To learn more about using *tool or task*, see [Topic Title](http://).\n- To experiment with *service*, *tool*, or *object*, see [Site Name](http://).\n- For a more advanced tutorial on *subject*, see [Tutorial Title](http://).",
"_____no_output_____"
]
]
] | [
"markdown",
"code",
"markdown"
] | [
[
"markdown",
"markdown",
"markdown",
"markdown",
"markdown",
"markdown",
"markdown",
"markdown",
"markdown",
"markdown",
"markdown",
"markdown",
"markdown",
"markdown",
"markdown"
],
[
"code"
],
[
"markdown",
"markdown",
"markdown",
"markdown",
"markdown",
"markdown",
"markdown",
"markdown",
"markdown",
"markdown",
"markdown",
"markdown",
"markdown",
"markdown",
"markdown",
"markdown",
"markdown",
"markdown",
"markdown",
"markdown",
"markdown",
"markdown",
"markdown",
"markdown",
"markdown",
"markdown",
"markdown",
"markdown",
"markdown",
"markdown",
"markdown",
"markdown",
"markdown",
"markdown"
]
] |
e77782d7c950d0d4c1326809d746b2cfc1f4bee9 | 15,693 | ipynb | Jupyter Notebook | doc/examples/navigation/0-Introduction.ipynb | flowmatters/veneer-py | af551b49038f5f93358b510fb893015c590bf6d4 | [
"0BSD"
] | 7 | 2016-11-14T13:06:40.000Z | 2020-10-13T06:13:51.000Z | doc/examples/navigation/0-Introduction.ipynb | ConnectedSystems/veneer-py | 3200c597e133255724d1355e61096ef62555a55c | [
"ISC"
] | 3 | 2016-11-06T10:22:07.000Z | 2019-05-09T09:55:14.000Z | doc/examples/navigation/0-Introduction.ipynb | ConnectedSystems/veneer-py | 3200c597e133255724d1355e61096ef62555a55c | [
"ISC"
] | 4 | 2016-11-02T00:46:32.000Z | 2020-07-30T03:24:35.000Z | 23.885845 | 314 | 0.554132 | [
[
[
"# Navigation functionality\n\nThe Navigation functionality (`veneer.navigation`) lets you query and modify the current Source model using normal Python object notation. For example:\n\n```\nscenario.Network.Nodes[0].Name = 'Renamed node'\n```\n\nThis notebook introduces the navigation functionality, including how it ties into the existing functionality in `v.model` as well as current limitations.",
"_____no_output_____"
],
[
"## Setup\n\nStart up Veneer as per usual...",
"_____no_output_____"
]
],
[
[
"import veneer\nv = veneer.Veneer(19876)",
"_____no_output_____"
],
[
"v.status()",
"_____no_output_____"
]
],
[
[
"## Initialise a Queryable object\n\n`Queryable` is the main component in `veneer.navigate`. By default, a Queryable points to the scenario.",
"_____no_output_____"
]
],
[
[
"from veneer.navigate import Queryable",
"_____no_output_____"
],
[
"scenario = Queryable(v)",
"_____no_output_____"
],
[
"scenario.Name",
"_____no_output_____"
]
],
[
[
"## Tab completion\n\n`Queryable` objects work with the tab completion in IPython/Jupyter, including, in many cases, for nested objects:\n\n",
"_____no_output_____"
]
],
[
[
"scenario.Network.nodes.Count",
"_____no_output_____"
]
],
[
[
"... However this won't work after indexing into a list or dictionary:\n\n\n\nYou can still access the properties of the list item, if you know what they're called:",
"_____no_output_____"
]
],
[
[
"scenario.Network.nodes[0].Name",
"_____no_output_____"
]
],
[
[
"... But if you want tab completion, create a variable **and run the cell that creates it**. Then tab into the new variable:\n\n",
"_____no_output_____"
]
],
[
[
"node = scenario.Network.nodes[0]\n# node.<tab> WON'T WORK YET. You need to run this cell first",
"_____no_output_____"
],
[
"# Now tab completion should work should work\nnode.Name",
"_____no_output_____"
]
],
[
[
"## Accessing a particular node/link/fu/etc\n\nThe above examples start from the scenario - which works, but is tedious when you need a particular node or link (or all water users, etc).\n\nHere, the existing functionality under `v.model` is has been expanded to return Queryable objects that can be used for object navigation.\n\nAll of the relevant `v.model` areas (link, node, catchment, etc) now have a `nav_first` method, which accepts the same query parameters as the other operations. For example, `v.model.node.nav_first` accepts the `nodes` and `node_types` query parameters, in the same way as `v.model.node.get_param_values`.\n\nAs always, the query parameters are available in the help, one level up:\n",
"_____no_output_____"
]
],
[
[
"v.model.node?",
"_____no_output_____"
]
],
[
[
"... So we can get a particular node, for navigation as follows.",
"_____no_output_____"
]
],
[
[
"callide = v.model.node.nav_first(nodes='Callide Dam')",
"_____no_output_____"
]
],
[
[
"**Note:** The function is called `nav_first` to emphasise that you will receive the **first** match for the query. So, if you query `node_types='WaterUser'`, you'll get the first Water User matched.\n\nIt's likely that we'll add a more generic `nav` method at some point that allows you to get a Queryable capable of bulk operations.",
"_____no_output_____"
],
[
"## Working with the navigation object\n\nFrom the examples above, it looks like, you can work with the `Queryable` object as a normal Python object. In *some* cases you can, but not always.\n\nFor changing values in the object (ie changing the relevant property in the Source model), you can indeed set the value directly:",
"_____no_output_____"
]
],
[
[
"callide.Name",
"_____no_output_____"
],
[
"callide.fullSupplyLevel",
"_____no_output_____"
],
[
"callide.fullSupplyVolume",
"_____no_output_____"
],
[
"callide.fullSupplyVolume = 136300000",
"_____no_output_____"
],
[
"callide.fullSupplyVolume",
"_____no_output_____"
]
],
[
[
"**Note:** Changing one thing may have a side effect, impacting another property:",
"_____no_output_____"
]
],
[
[
"callide.fullSupplyLevel",
"_____no_output_____"
]
],
[
[
"If a property can't be set this way, it *should* tell you:",
"_____no_output_____"
]
],
[
[
"# CAUSES EXCEPTION\n#callide.fullSupplyLevel = 216",
"_____no_output_____"
]
],
[
[
"### Things aren't necessarily as they seem!\n\nThe above examples suggest that the following would work:",
"_____no_output_____"
]
],
[
[
"# Would be nice, but doesn't work...\n#callide.fullSupplyVolume = 1.1 * callide.fullSupplyVolume",
"_____no_output_____"
]
],
[
[
"The reason is, `callide.fullSupplyVolume` is, itself, a `Queryable` object:",
"_____no_output_____"
]
],
[
[
"callide.fullSupplyVolume.__class__",
"_____no_output_____"
]
],
[
[
"So, although it prints out as a number, it is in fact a reference to the value within the model.\n\nIf you want to actually use the *value* in an expression (eg to set another property), you'll need to use the `",
"_____no_output_____"
]
],
[
[
"callide.fullSupplyVolume = 1.1 * callide.fullSupplyVolume._eval_()",
"_____no_output_____"
],
[
"callide.fullSupplyVolume",
"_____no_output_____"
],
[
"callide.fullSupplyVolume = callide.fullSupplyVolume._eval_() / 1.1",
"_____no_output_____"
],
[
"callide.fullSupplyVolume",
"_____no_output_____"
]
],
[
[
"## Evaluating a Queryable\n\nIt's not ideal to need to call `._eval_()` and we plan to improve this over time.\n\nAlso, the `_eval_()` workaround only works for simple types - numbers, strings, booleans, etc. It doesn't work for complex objects, such as Nodes, Links and model instances.\n\nFor example you CANNOT do this at the moment:\n\n```\nstorage = v.model.node.nav_first(nodes='my storage')\nlink = v.model.link.nav_first(links='some link')\n\n# Try to set the outlet link for the storage...\nstorage.OutletPaths[0] = link # WILL NOT WORK!\n```\n",
"_____no_output_____"
],
[
"## Bulk changes\n\nWhen you use the model configuration functionality under `v.model` every operation is a **bulk** operation by default - you use query parameters to limit the application:\n\nFor example, the following would retrieve Easting and Northing (really, just the node coordinates) for every node:\n\n```\neastings = v.model.node.get_param_values('Node.location.E')\nnorthings = v.model.node.get_param_values('Node.location.N')\n```\n\nwhile the following would do the same for only storages, by using query parameters:\n\n```\neastings = v.model.node.get_param_values('Node.location.E',node_types='Storage')\nnorthings = v.model.node.get_param_values('Node.location.N',node_types='Storage')\n```\n\nWhen using `Queryable`, everything is, currently, an operation on a single object (eg a single node):",
"_____no_output_____"
]
],
[
[
"callide.Node.location.E",
"_____no_output_____"
]
],
[
[
"However... one of the big benefits of the navigable functionality is the ability to discover the parameter name (often nested) that you need to use in a bulk operation.\n\n**When stuck for a parameter name, use a Queryable to discover it, through tab-completion and then plug the resulting path back into the `v.model.X.get_param_values()` call**",
"_____no_output_____"
],
[
"## TODO\n\nThe Queryable approach is new and will expand.\n\nThings on the list are:\n\n* Ability to call methods on Queryable objects\n* Ability to use Queryable objects in expressions, assignments to other Queryables and as method parameters\n* Ability to perform bulk operations using Queryables\n* Ability to write Python loops / list comprehensions that iterate over Source collections\n\nOther related functionality that would be nice:\n\n* Ability to identify functions, data sources, etc that point at a given variable. Eg instead of seeing `node.Inflow = 0`, see the data source that is connected.\n",
"_____no_output_____"
]
]
] | [
"markdown",
"code",
"markdown",
"code",
"markdown",
"code",
"markdown",
"code",
"markdown",
"code",
"markdown",
"code",
"markdown",
"code",
"markdown",
"code",
"markdown",
"code",
"markdown",
"code",
"markdown",
"code",
"markdown",
"code",
"markdown",
"code",
"markdown",
"code",
"markdown"
] | [
[
"markdown",
"markdown"
],
[
"code",
"code"
],
[
"markdown"
],
[
"code",
"code",
"code"
],
[
"markdown"
],
[
"code"
],
[
"markdown"
],
[
"code"
],
[
"markdown"
],
[
"code",
"code"
],
[
"markdown"
],
[
"code"
],
[
"markdown"
],
[
"code"
],
[
"markdown",
"markdown"
],
[
"code",
"code",
"code",
"code",
"code"
],
[
"markdown"
],
[
"code"
],
[
"markdown"
],
[
"code"
],
[
"markdown"
],
[
"code"
],
[
"markdown"
],
[
"code"
],
[
"markdown"
],
[
"code",
"code",
"code",
"code"
],
[
"markdown",
"markdown"
],
[
"code"
],
[
"markdown",
"markdown"
]
] |
e7778b242e19eaf9e136dfaddf34b586f6601073 | 22,665 | ipynb | Jupyter Notebook | notebooks/swath_generation.ipynb | CNES/swot_simulator | 92d0bb4a274ec9923265567968beea3be4283e61 | [
"BSD-3-Clause"
] | 17 | 2020-05-28T08:20:11.000Z | 2022-03-25T07:40:48.000Z | notebooks/swath_generation.ipynb | CNES/swot_simulator | 92d0bb4a274ec9923265567968beea3be4283e61 | [
"BSD-3-Clause"
] | 7 | 2021-07-21T02:15:52.000Z | 2021-11-14T10:46:41.000Z | notebooks/swath_generation.ipynb | CNES/swot_simulator | 92d0bb4a274ec9923265567968beea3be4283e61 | [
"BSD-3-Clause"
] | 8 | 2020-05-17T13:53:43.000Z | 2022-03-25T07:40:58.000Z | 34.445289 | 525 | 0.562453 | [
[
[
"## Generate a SWOT swath.\n\nThis example lets us understand how to initialize the simulation parameters (error, SSH interpolation, orbit), generate an orbit, generate a swath, interpolate the SSH, and simulate the measurement errors. Finally, we visualize the simulated data.\n\n### Simulation setup\n\nThe configuration is defined using an associative dictionary between the expected parameters and the values of its parameters. The description of the parameters is available on the [online help](https://swot-simulator.readthedocs.io/en/latest/generated/swot_simulator.settings.Parameters.html#swot_simulator.settings.Parameters).\n\nThis array can be loaded from a Python file using the [eval_config_file](https://swot-simulator.readthedocs.io/en/latest/generated/swot_simulator.settings.eval_config_file.html#swot_simulator.settings.eval_config_file) method. But it can also be declared directly in Python code.",
"_____no_output_____"
]
],
[
[
"import swot_simulator",
"_____no_output_____"
],
[
"configuration = dict(\n # The swath contains in its centre a central pixel divided in two by the\n # reference ground track.\n central_pixel=True,\n # Distance, in km, between two points along track direction.\n delta_al=2.0,\n # Distance, in km, between two points across track direction.\n delta_ac=2.0,\n # Distance, in km, between the nadir and the center of the first pixel of the\n # swath\n half_gap=2.0,\n # Distance, in km, between the nadir and the center of the last pixel of the\n # swath\n half_swath=70.0,\n # Limits of SWOT swath requirements. Measurements outside the span will be\n # set with fill values.\n requirement_bounds=[10, 60],\n # Ephemeris file to read containing the satellite's orbit.\n ephemeris=swot_simulator.DATA.joinpath(\n \"ephemeris_calval_june2015_ell.txt\"),\n # Generation of measurement noise.\n noise=[\n 'altimeter',\n 'baseline_dilation',\n 'karin',\n 'roll_phase',\n #'orbital', (This generator consumes too much memory to run with binder)\n 'timing',\n 'wet_troposphere',\n ],\n # File containing spectrum of instrument error\n error_spectrum=swot_simulator.DATA.joinpath(\"error_spectrum.nc\"),\n # KaRIN file containing spectrum for several SWH\n karin_noise=swot_simulator.DATA.joinpath(\"karin_noise_v2.nc\"),\n # The plug-in handling the SSH interpolation under the satellite swath.\n #ssh_plugin = TODO\n)",
"_____no_output_____"
]
],
[
[
"We create the parameter object for our simulation.",
"_____no_output_____"
]
],
[
[
"import swot_simulator.settings\n\nparameters = swot_simulator.settings.Parameters(configuration)",
"_____no_output_____"
]
],
[
[
"---\n**Note**\n\nThe [Parameter](https://swot-simulator.readthedocs.io/en/latest/generated/swot_simulator.settings.Parameters.html#swot_simulator.settings.Parameters) class exposes the [load_default](https://swot-simulator.readthedocs.io/en/latest/generated/swot_simulator.settings.Parameters.load_default.html#swot_simulator.settings.Parameters.load_default) method returning the default parameters of the simulation:\n\n```python\nparameters = swot_simulator.settings.Parameters.load_default()\n```\n\nIt is also possible to [automatically load the\ndictionary](https://swot-simulator.readthedocs.io/en/latest/generated/swot_simulator.settings.template.html)\ncontaining the simulation parameters to adapt them to your needs and after all\ncreate the parameters of your simulation.\n\n```python\nconfiguration = swot_simulator.settings.template(python=True)\nparameters = swot_simulator.settings.Parameters(configuration)\n```\n---",
"_____no_output_____"
],
[
"### SSH interpolation\n\nThe written configuration allows us to simulate a swath. However, the interpolation of the SSH under the satellite swath remains undefined. If you don't need this parameter, you can skip this setting.\n\nFor our example, we use the SSH of the CMEMS grids provided on the Pangeo site.",
"_____no_output_____"
]
],
[
[
"import intake\n\ncat = intake.open_catalog(\"https://raw.githubusercontent.com/pangeo-data/\"\n \"pangeo-datastore/master/intake-catalogs/master.yaml\")",
"_____no_output_____"
],
[
"ds = cat.ocean.sea_surface_height.to_dask()\nds",
"_____no_output_____"
]
],
[
[
"To interpolate SSH, we need to implement a class that must define a method to\ninterpolate the data under the swath. This class must be derived from the\n[CartesianGridHandler](https://swot-simulator.readthedocs.io/en/latest/generated/swot_simulator.plugins.data_handler.CartesianGridHandler.html)\nclass to be correctly taken into account by the class managing the parameters.",
"_____no_output_____"
]
],
[
[
"import pyinterp.backends.xarray\nimport numpy\nimport xarray\n#\nimport swot_simulator.plugins.data_handler\n\n\nclass CMEMS(swot_simulator.plugins.data_handler.CartesianGridHandler):\n \"\"\"\n Interpolation of the SSH AVISO (CMEMS L4 products).\n \"\"\"\n def __init__(self, adt):\n self.adt = adt\n ts = adt.time.data\n\n assert numpy.all(ts[:-1] <= ts[1:])\n\n # The frequency between the grids must be constant.\n frequency = set(numpy.diff(ts.astype(\"datetime64[s]\").astype(\"int64\")))\n if len(frequency) != 1:\n raise RuntimeError(\n \"Time series does not have a constant step between two \"\n f\"grids: {frequency} seconds\")\n\n # The frequency is stored in order to load the grids required to\n # interpolate the SSH.\n self.dt = numpy.timedelta64(frequency.pop(), 'ns')\n\n def load_dataset(self, first_date, last_date):\n \"\"\"Loads the 3D cube describing the SSH in time and space.\"\"\"\n if first_date < self.adt.time[0] or last_date > self.adt.time[-1]:\n raise IndexError(\n f\"period [{first_date}, {last_date}] is out of range: \"\n f\"[{self.adt.time[0]}, {self.adt.time[-1]}]\")\n first_date = self.adt.time.sel(time=first_date, method='pad')\n last_date = self.adt.time.sel(time=last_date, method='backfill')\n selected = self.adt.loc[dict(time=slice(first_date, last_date))]\n selected = selected.compute()\n return pyinterp.backends.xarray.Grid3D(selected.adt)\n\n def interpolate(self, lon, lat, time):\n \"\"\"Interpolate the SSH to the required coordinates\"\"\"\n interpolator = self.load_dataset(time.min(), time.max())\n ssh = interpolator.trivariate(dict(longitude=lon,\n latitude=lat,\n time=time),\n interpolator='bilinear')\n return ssh",
"_____no_output_____"
]
],
[
[
"Now we can update our parameters.",
"_____no_output_____"
]
],
[
[
"parameters.ssh_plugin = CMEMS(ds)",
"_____no_output_____"
]
],
[
[
"### Initiating orbit propagator.\n\nInitialization is simply done by [loading](https://swot-simulator.readthedocs.io/en/latest/generated/swot_simulator.orbit_propagator.load_ephemeris.html#swot_simulator.orbit_propagator.load_ephemeris) the ephemeris file. The satellite's one-day pass is taken into account in this case.",
"_____no_output_____"
]
],
[
[
"import swot_simulator.orbit_propagator\n\n\nwith open(parameters.ephemeris, \"r\") as stream:\n orbit = swot_simulator.orbit_propagator.calculate_orbit(parameters, stream)",
"_____no_output_____"
]
],
[
[
"### Iterate on the half-orbits of a period.\n\nTo iterate over all the half-orbits of a period, call the method [iterate](https://swot-simulator.readthedocs.io/en/latest/generated/swot_simulator.orbit_propagator.Orbit.iterate.html#swot_simulator.orbit_propagator.Orbit.iterate). This method returns all cycle numbers, trace numbers, and start dates of the half orbits within the period. If the start date remains not set, the method uses the current date. If the end date remains undefined, the method sets the end date to the start date plus the cycle duration.\n\nIn our case, we generate a cycle from January 1, 2000.",
"_____no_output_____"
]
],
[
[
"first_date = numpy.datetime64(\"2000-01-01\")\niterator = orbit.iterate(first_date)\ncycle_number, pass_number, date = next(iterator)\ncycle_number, pass_number, date",
"_____no_output_____"
]
],
[
[
"### Initialization of measurement error generators\n\nError initialization is done simply by calling the appropriate [class](https://swot-simulator.readthedocs.io/en/latest/generated/swot_simulator.error.generator.Generator.html#swot_simulator.error.generator.Generator). The initialization of the wet troposphere error generator takes a little time (about 40 seconds), which explains the processing time for the next cell.",
"_____no_output_____"
]
],
[
[
"import swot_simulator.error.generator\n\nerror_generator = swot_simulator.error.generator.Generator(\n parameters, first_date, orbit.orbit_duration())",
"_____no_output_____"
]
],
[
[
"### Generate the positions under the swath.\n\nTo perform this task, the following function is implemented.\n\n> If the position of the pass is outside the area of interest (`parameters.area`),\n> the generation of the pass can return `None`.",
"_____no_output_____"
]
],
[
[
"def generate_one_track(pass_number, date, orbit):\n # Compute the spatial/temporal position of the satellite\n track = swot_simulator.orbit_propagator.calculate_pass(\n pass_number, orbit, parameters)\n\n # If the pass is not located in the area of interest (parameter.area)\n # the result of the generation can be null.\n if track is None:\n return None\n\n # Set the simulated date\n track.set_simulated_date(date)\n\n return track",
"_____no_output_____"
]
],
[
[
"### Interpolate SSH\n\nInterpolation of the SSH for the space-time coordinates generated by the simulator.",
"_____no_output_____"
]
],
[
[
"def interpolate_ssh(parameters, track):\n swath_time = numpy.repeat(track.time, track.lon.shape[1]).reshape(track.lon.shape)\n ssh = parameters.ssh_plugin.interpolate(track.lon.flatten(),\n track.lat.flatten(),\n swath_time.flatten())\n return ssh.reshape(track.lon.shape)",
"_____no_output_____"
]
],
[
[
"### Calculation of instrumental errors\n\nSimulation of instrumental errors. \n\n> Karin's instrumental noise can be modulated by wave heights.\n> The parameter SWH takes either a constant or a matrix defining\n> the SWH for the swath positions. ",
"_____no_output_____"
]
],
[
[
"def generate_instrumental_errors(error_generator, cycle_number, pass_number,\n orbit, track):\n return error_generator.generate(cycle_number,\n pass_number,\n orbit.curvilinear_distance,\n track.time,\n track.x_al,\n track.x_ac,\n swh=2.0)",
"_____no_output_____"
]
],
[
[
"### Calculates the sum of the simulated errors.",
"_____no_output_____"
]
],
[
[
"def sum_error(errors, swath=True):\n \"\"\"Calculate the sum of errors\"\"\"\n dims = 2 if swath else 1\n return numpy.add.reduce(\n [item for item in errors.values() if len(item.shape) == dims])",
"_____no_output_____"
]
],
[
[
" ### Create the swath dataset\n \n Generation of the simulated swath. The function returns an xarray dataset for the half-orbit generated.",
"_____no_output_____"
]
],
[
[
"import swot_simulator.netcdf\n\n\ndef generate_dataset(cycle_number,\n pass_number,\n track,\n ssh,\n noise_errors,\n complete_product=False):\n product = swot_simulator.netcdf.Swath(track, central_pixel=True)\n # Mask to set the measurements outside the requirements of the mission to\n # NaN.\n mask = track.mask()\n ssh *= mask\n product.ssh(ssh + sum_error(noise_errors))\n product.simulated_true_ssh(ssh)\n for error in noise_errors.values():\n # Only the swaths must be masked\n if len(error.shape) == 2:\n error *= mask\n product.update_noise_errors(noise_errors)\n return product.to_xarray(cycle_number, pass_number, complete_product)",
"_____no_output_____"
]
],
[
[
"### Swath generation.\n\nNow we can combine the different components to generate the swath.",
"_____no_output_____"
]
],
[
[
"import dask\nimport dask.distributed\n\n\ndef generate_swath(cycle_number, pass_number, date, parameters,\n error_generator, orbit):\n client = dask.distributed.get_client()\n # Scatter big data\n orbit_ = client.scatter(orbit)\n error_generator_ = client.scatter(error_generator)\n # Compute swath positions\n track = dask.delayed(generate_one_track)(pass_number, date, orbit_)\n # Interpolate SSH\n ssh = dask.delayed(interpolate_ssh)(parameters, track)\n # Simulate instrumental errors\n noise_errors = dask.delayed(generate_instrumental_errors)(error_generator_,\n cycle_number,\n pass_number,\n orbit_, track)\n # Finally generate the dataset\n return dask.delayed(generate_dataset)(\n cycle_number, pass_number, track, ssh, noise_errors,\n parameters.complete_product).compute()",
"_____no_output_____"
]
],
[
[
"The simulator calculation can be distributed on a Dask cluster.",
"_____no_output_____"
]
],
[
[
"import dask.distributed\n\n# A local cluster is used here.\ncluster = dask.distributed.LocalCluster()\nclient = dask.distributed.Client(cluster)\nclient",
"_____no_output_____"
],
[
"error_generator_ = client.scatter(error_generator)\nparameters_ = client.scatter(parameters)\norbit_ = client.scatter(orbit)",
"_____no_output_____"
],
[
"future = client.submit(generate_swath, cycle_number, pass_number, date,\n parameters_, error_generator_, orbit_)\nds = client.gather(future)\nds",
"_____no_output_____"
]
],
[
[
"To calculate a trace set you can use the following code\n\n futures = []\n for cycle_number, pass_number, date in iterator:\n futures.append(client.submit(generate_swath,\n cycle_number,\n pass_number,\n date,\n error_generator_,\n orbit_,\n parameters))\n client.gather(futures)\n \n### Visualization",
"_____no_output_____"
]
],
[
[
"import matplotlib.pyplot\nimport cartopy.crs\nimport cartopy.feature\n%matplotlib inline",
"_____no_output_____"
]
],
[
[
"Selection of a reduced geographical area for visualization.",
"_____no_output_____"
]
],
[
[
"selected = ds.where((ds.latitude > -50) & (ds.latitude < -40), drop=True)",
"_____no_output_____"
]
],
[
[
"Simulated SSH measurements (Interpolated SSH and simulated instrumental errors).",
"_____no_output_____"
]
],
[
[
"fig = matplotlib.pyplot.figure(figsize=(24, 12))\nax = fig.add_subplot(1, 1, 1, projection=cartopy.crs.PlateCarree())\ncontourf = ax.contourf(selected.longitude,\n selected.latitude,\n selected.ssh_karin,\n transform=cartopy.crs.PlateCarree(),\n levels=255,\n cmap='jet')\nfig.colorbar(contourf, orientation='vertical')\nax.set_extent([60, 69, -50, -40], crs=cartopy.crs.PlateCarree())\nax.gridlines(draw_labels=True, dms=True, x_inline=False, y_inline=False)\nax.coastlines()",
"_____no_output_____"
]
],
[
[
"Simulated KaRIN instrumental noise.",
"_____no_output_____"
]
],
[
[
"for item in selected.variables:\n if item.startswith(\"simulated_error\"):\n variable = selected.variables[item]\n fig = matplotlib.pyplot.figure(figsize=(18, 8))\n ax = fig.add_subplot(1, 1, 1)\n image = ax.imshow(variable.T,\n extent=[0, len(selected.num_lines), -70, 70],\n cmap='jet')\n ax.set_title(variable.attrs['long_name'] + \"(\" +\n variable.attrs['units'] + \")\")\n ax.set_xlabel(\"num_lines\")\n ax.set_ylabel(\"num_pixels\")\n fig.colorbar(image,\n orientation='vertical',\n fraction=0.046 * 70 / 250,\n pad=0.04)",
"_____no_output_____"
]
]
] | [
"markdown",
"code",
"markdown",
"code",
"markdown",
"code",
"markdown",
"code",
"markdown",
"code",
"markdown",
"code",
"markdown",
"code",
"markdown",
"code",
"markdown",
"code",
"markdown",
"code",
"markdown",
"code",
"markdown",
"code",
"markdown",
"code",
"markdown",
"code",
"markdown",
"code",
"markdown",
"code",
"markdown",
"code",
"markdown",
"code",
"markdown",
"code"
] | [
[
"markdown"
],
[
"code",
"code"
],
[
"markdown"
],
[
"code"
],
[
"markdown",
"markdown"
],
[
"code",
"code"
],
[
"markdown"
],
[
"code"
],
[
"markdown"
],
[
"code"
],
[
"markdown"
],
[
"code"
],
[
"markdown"
],
[
"code"
],
[
"markdown"
],
[
"code"
],
[
"markdown"
],
[
"code"
],
[
"markdown"
],
[
"code"
],
[
"markdown"
],
[
"code"
],
[
"markdown"
],
[
"code"
],
[
"markdown"
],
[
"code"
],
[
"markdown"
],
[
"code"
],
[
"markdown"
],
[
"code",
"code",
"code"
],
[
"markdown"
],
[
"code"
],
[
"markdown"
],
[
"code"
],
[
"markdown"
],
[
"code"
],
[
"markdown"
],
[
"code"
]
] |
e7778e32a639ba9e6b7f8513946313006b2ad666 | 11,219 | ipynb | Jupyter Notebook | notebooks/intro.ipynb | codingalzi/pyfast | bba69b83e554dde39869b91565807d69ce3d2e11 | [
"MIT"
] | null | null | null | notebooks/intro.ipynb | codingalzi/pyfast | bba69b83e554dde39869b91565807d69ce3d2e11 | [
"MIT"
] | null | null | null | notebooks/intro.ipynb | codingalzi/pyfast | bba69b83e554dde39869b91565807d69ce3d2e11 | [
"MIT"
] | null | null | null | 24.231102 | 180 | 0.493538 | [
[
[
"# 데이터 과학과 파이썬",
"_____no_output_____"
],
[
"**감사의 말**: [SciPy Lecture Notes](https://scipy-lectures.org/_downloads/ScipyLectures-simple.pdf)의 1장 내용을 참고합니다. ",
"_____no_output_____"
],
[
"## 파이썬",
"_____no_output_____"
],
[
"데이터 과학자는 보통 다음 세 가지를 필요로 한다.\n\n* 데이터 구하기: 모의 실험\n* 데이터 처리: 데이터로부터 정보 얻기\n* 데이터 시각화: 얻어진 정보 이해 및 전달",
"_____no_output_____"
],
[
"파이썬의 장점은 다음과 같다.\n\n* 수치 계산, 데이터 처리 및 시각화와 관련된 많고 다양한 툴을 제공한다.\n* 배우고 사용하기 쉽다.\n* 문법이 단순하다.\n* 효율적인 코드를 작성하기 쉽다.\n* 데이터 분석 이외에 프로그래밍 관련 모든 분야에서 사용된다.",
"_____no_output_____"
],
[
"## 파이썬 대 기타 언어",
"_____no_output_____"
],
[
"**컴파일 언어**\n\nC, C++, C#, 자바 등 작성된 코드를 컴파일<font size=\"2\">compile</font>한 후에 실행하도록 하는 \n컴파일 언어<font size=\"2\">compiled language</font>의\n장점과 단점은 다음과 같다.\n\n* 장점\n * 실행이 매우 빠르다. 따라서 매우 많은 양의 계산에 적합하다.\n* 단점\n * 사용하기 어렵다. 메모리 수동 관리, 복잡한 구문 등 일반 사용자가 접근하기에는 \n 조금 어려운 프로그래밍 언어이다.",
"_____no_output_____"
],
[
"**매트랩**<font size=\"2\">Matlab</font>\n\n* 장점\n * 다양한 분야에서 활용될 수 있는 효율적인 수치 계산용 알고리즘을 포함하는 라이브러리를 제공한다.\n * 편리한 개발환경을 제공한다.\n * (유료) 기술 지원이 제공된다.\n* 단점\n * 언어 자체가 고수준의 기능을 지원하지 않는다.\n * 유료.",
"_____no_output_____"
],
[
"**R**\n\n* 장점\n * 오픈 소스<font size=\"2\">open source</font>이며 무료.\n * 통계 관련 고수준의 기능을 지원한다.\n \n* 단점\n * 매트랩보다 지원되는 알고리즘이 적으며, 언어 자체도 고수준의 기능을 제대로 지원하지 않는다.\n * 지원되는 기능도 제한적인 영역에서만 활용되어 보편성이 떨어진다.",
"_____no_output_____"
],
[
"**파이썬**\n\n* 장점\n * 계산 관련 라이브러리가 풍부하다.\n * 체계적이며 가독성이 높은 코드 작성을 지원한다.\n * 웹서버 등 계산 과학 이외의 기타 분야에서도 활용되는 라이브러리를 지원한다.\n * 오픈 소스이자 무료이고 많은 사용자 그룹이 존재한다.\n * 다양한 개발환경이 지원된다.\n * [IPython](http://ipython.readthedocs.io/en/stable/)\n * [Spyder](https://www.spyder-ide.org/)\n * [Jupyter notebooks](http://jupyter.org/)\n * [Visual Studio Code](https://code.visualstudio.com/docs/languages/python)\n \n* 단점\n * 컴파일 언어로 작성된 프로그램에 비해 상대적으로 느리게 작동한다.\n * 시스템 개발 등에 사용되기에는 제한적이다.",
"_____no_output_____"
],
[
"## 데이터 과학용 파이썬 생태계",
"_____no_output_____"
],
[
"매트랩과 R은 수치계산 또는 통계 분석에 특화된 프로그래밍 언어이다.\n반면에 파이썬은 모든 영역의 문제를 해결하는 데에 사용되는 \n범용<font size=\"2\">universal</font> 프로그래밍 언어이다.\n데이터 과학용으로 파이썬을 사용할 때 사용되는 기본 구성요소는 다음과 같다.",
"_____no_output_____"
],
[
"**파이썬 기본 패키지**\n\n- 파이썬 프로그래밍 언어 기본: 변수, 표현식, 함수, 클래스, 모듈, 명령문 등등\n- 기본 자료구조: 정수(`int`), 부동소수점(`float`), 문자열(`string`), \n 리스트(`list`), 튜플(`tuple`), 사전(`dict`), 집합(`set`) 등등\n- 웹 프로그래밍, 계산 과학 등 다양한 분야에 특화된 모듈 및 라이브러리\n- 테스트 자동화, 문서 생성 등의 개발툴",
"_____no_output_____"
],
[
"**NumPy(넘파이) 패키지**\n\n- 수치 계산 전용 라이브러리, 특히 행렬 연산에 효율적임. \n- 참고: [http://www.numpy.org/](http://www.numpy.org/)",
"_____no_output_____"
],
[
"**Scipy(싸이파이) 패키지**\n\n- 최적화<font size=\"2\">optimization</font>, \n회귀 분석<font size=\"2\">regression analysis</font>, \n보간법<font size=\"2\">interpolation</font> 등등과 관련된 고수준 라이브러리\n- 참고: [http://www.scipy.org/](http://www.scipy.org/)",
"_____no_output_____"
],
[
"**Matplotlib(맷플롯립) 패키지**\n\n- 2D 시각화\n- 참고: [http://matplotlib.org/](http://matplotlib.org/)\n <br>\n\n<div align=\"center\"><img src=\"https://github.com/codingalzi/pyfast/blob/master/notebooks/images/random_c.jpg?raw=true\" width=\"60%\"></div>",
"_____no_output_____"
],
[
"**대화식<font size=\"2\">interactive</font> 개발환경**\n\n* **IPython**: 개선된 파이썬 콘솔([http://ipython.org/](http://ipython.org/))\n <br>\n\n <div align=\"center\"><img src=\"https://github.com/codingalzi/pyfast/blob/master/notebooks/images/snapshot_ipython.png?raw=true\" width=\"50%\"></div>",
"_____no_output_____"
],
[
"* **Jupyter notebooks**: 웹 브라우저 활용 파이썬 프로그래밍 인터페이스([http://jupyter.org/](http://jupyter.org/))\n <br>\n\n <div align=\"center\"><img src=\"https://jupyter.org/assets/homepage/jupyterpreview.webp\" width=\"50%\"></div>",
"_____no_output_____"
],
[
"**도메인 특화 패키지**",
"_____no_output_____"
],
[
"* **pandas**, **statsmodels**, **seaborn**: 통계",
"_____no_output_____"
],
[
"* **sympy**: 기호 계산<font size=\"2\">symbolic computing</font>",
"_____no_output_____"
],
[
"* **scikit-image**: 이미지 처리",
"_____no_output_____"
],
[
"* **scikit-learn**: 머신러닝",
"_____no_output_____"
],
[
"* **tensorflow**, **pytorch**: 딥러닝",
"_____no_output_____"
],
[
"* **mayavi**: 3D 시각화\n\n <div align=\"center\"><img src=\"https://github.com/codingalzi/pyfast/blob/master/notebooks/images/example_surface_from_irregular_data.jpg?raw=true\" width=\"50%\"></div>",
"_____no_output_____"
],
[
"## 데이터 과학용 파이썬 설치 및 개발환경",
"_____no_output_____"
],
[
"### 파이썬 설치",
"_____no_output_____"
],
[
"앞서 언급된 라이브러리 중에 mayavi를 제외한 모든 것을\n한 번에 설치하는 패키지로 **아나콘다**<font size=\"2\">Anaconda</font>를 추천한다.",
"_____no_output_____"
],
[
"### 개발 환경",
"_____no_output_____"
],
[
"**대화식 프로그래밍**\n\n간단한 파이썬 코딩 설명을 위해서 대화식 프로그래밍<font size=\"2\">interactive programming</font>을\n지원하는 \n주피터 노트북<font size=\"2\">Jupyter notebook</font>을 기본적으로 사용한다.\n주피터 노트북은 \n파이썬의 기본 콘솔의 기능을 확장한 IPython 콘솔을 포함한다.\n\n파이썬 기본 콘솔은 다음 모양의 대화식 코딩을 지원하는다.\n\n```python\n>>> print('Hello world')\nHello world\n```\n\n반면에 IPython 콘솔의 대화식 코딩은 다음과 같다.\n\n```python\nIn [1]: print('Hello world')\nHello world\n```\n\nIPython 콘솔은 이외에 아래에서 설명하는 매직 커맨드 보다\n유용한 기능을 지원한다.",
"_____no_output_____"
],
[
":::{admonition} 아나콘다 설치와 주피터 노트북 사용법\n:class: tip\n\n[나도코딩의 동영상](https://www.youtube.com/watch?v=dJfq-eCi7KI&t=2298s)에서 아나콘다의 설치 과정과\n주피터 노트북의 기초 사용법에 대한 상세한 설명을 들을 수 있다.\n:::\n",
"_____no_output_____"
],
[
"**IDE(통합 개발 환경)**",
"_____no_output_____"
],
[
"보다 복잡한 파이썬 코딩을 위해서는 코드 편집과 실행, 디버깅 등 \n프로그래밍 관련 모든 작업을 통합해서 지원하는 \n통합 개발 환경을 사용한다.\n\n일명 **IDE**<font size=\"2\">Interactive Development Environment</font>라고 불리는\n통합 개발 환경을 지원하는 다양한 툴이 존재하며, \n대표적으로 다음과 같다.\n\n* [Spyder](https://www.spyderide.org/): 아나콘다 패키지에 포함되어 있다.\n* [PyCharm](https://www.jetbrains.com/pycharm): 가장 인기있는 상용 프로그램이다.\n Pro 와 Community 두 버전을 지원하며 무료 버전인 Community 만으로도 입문용으로 충분하다.\n* [Visual Studio Code](https://code.visualstudio.com/docs/languages/python): \n 마이크로소프트에서 지원하며, 현재 가장 인기 있는 무료 프로그램이다. \n 파이썬 뿐만 아니라 거의 모든 프로그래밍 언어를 위한 통합 개발 환경을 지원한다.\n* [Atom](https://atom.io): 소스코드 저장소로 가장 유명한 \n [GitHub](https://github.com/)에서 개발한 IDE. \n Visual Studio Code 처럼 거의 모든 프로그래밍 언어를 지원한다.",
"_____no_output_____"
],
[
"여기서는 일명 **VS Code**라 불리는 \n비주얼 스튜디오 코드<font size=\"2\">Visual Studio Code</font>를 사용할 것을 추천한다.",
"_____no_output_____"
],
[
":::{admonition} VS Code 설치와 사용법\n:class: tip\n\n[생활코딩의 동영상](https://www.youtube.com/watch?v=K8qVH8V0VvY&t=337s)에서 \nVS Code의 설치와 사용법에 대한 상세한 설명을 들을 수 있다.\n:::\n",
"_____no_output_____"
],
[
":::{prf:example}\n:label: my_file\n\n아래 내용을 담은 *my_file.py* 파일을 작성하고 저장하라.\n여기서는 *codes* 라는 하위 디렉터리(폴더)에 저장되어 있다고 가정한다.\n\n```\ns = 'Hello world'\nprint(s)\n```\n\n이제 주피터 노트북의 코드 셀에서 아래 명령문을 실행해보자.\n\n```python\nIn [1]: %run codes/my_file.py\n파이썬 안녕\n```\n\n`%run my_file.py`은 IPython에서 *my_file.py* 파일에 포함된 모든 코드를 실행한다.\n그리고 이제는 코드에서 정의된 변수와 변수가 가리키는 값을 확인할 수 있다.\n\n```python\nIn [2]: s\nOut[2]: 'Hello world'\n```\n\n`%whos`는 선언된 변수에 대한 정보를 보여준다.\n여기서는 변수 `s`는 `'파이썬 안녕'` 이라는 문자열(`str`)을 가리키고 있음을 확인해준다. \n\n```python\nIn [3]: %whos\nVariable Type Data/Info\n----------------------------\ns str 파이썬 안녕\n```\n:::",
"_____no_output_____"
],
[
":::{admonition} 매직 커맨드\n:class: info\n\n퍼센트 기호 `%`로 시작하는 명령어는 파이썬 명령어가 아니며,\n파이썬 코드 파일 및 디렉터리 경로 관리 등에 사용하는 IPython 전용 명령어이다.\n**매직 커맨드**<font size=\"2\">magic command</font>라 불리며,\n앞으로 기회될 때마다 하나씩 소개될 것이다.\n매직 커맨드에 자세한 정보는 [IPython 공식 문서](https://ipython.readthedocs.io/en/stable/interactive/magics.html)에서 확인할 수 있다.\n:::",
"_____no_output_____"
]
]
] | [
"markdown"
] | [
[
"markdown",
"markdown",
"markdown",
"markdown",
"markdown",
"markdown",
"markdown",
"markdown",
"markdown",
"markdown",
"markdown",
"markdown",
"markdown",
"markdown",
"markdown",
"markdown",
"markdown",
"markdown",
"markdown",
"markdown",
"markdown",
"markdown",
"markdown",
"markdown",
"markdown",
"markdown",
"markdown",
"markdown",
"markdown",
"markdown",
"markdown",
"markdown",
"markdown",
"markdown",
"markdown",
"markdown",
"markdown"
]
] |
e777a5b2b0d4a13a4ca83b906a057e325cc79986 | 5,441 | ipynb | Jupyter Notebook | L01_Research_computing_with_Python.ipynb | Olaolutosin/phythosin | 3e33952831f64e0b7a7b2e6ba122aeb666cbac79 | [
"Unlicense"
] | 11 | 2019-01-23T09:37:35.000Z | 2021-07-06T11:51:15.000Z | L01_Research_computing_with_Python.ipynb | Olaolutosin/phythosin | 3e33952831f64e0b7a7b2e6ba122aeb666cbac79 | [
"Unlicense"
] | null | null | null | L01_Research_computing_with_Python.ipynb | Olaolutosin/phythosin | 3e33952831f64e0b7a7b2e6ba122aeb666cbac79 | [
"Unlicense"
] | 6 | 2018-09-27T11:05:47.000Z | 2021-04-29T23:11:14.000Z | 46.504274 | 326 | 0.645286 | [
[
[
"<img align=\"left\" src=\"./img/lu.png\" hspace=\"20\"/> <img align=\"right\" src=\"./img/midlands+.png\"/>\n<br/><br/><br/><br/><br/><br/><br/>\n\n## Research computing with Python\n\n------\n\n### Workflow of research computing \n* Literature review\n* Ideas\n\t- Hypothesis formulation\n\t- Methodology\n* Data generation\n\t- Simulation - coding by yourself or use existing models (such as [LAMMPS](http://lammps.sandia.gov/doc/Manual.html) and [OpenFOAM](https://openfoam.org/))\n\t- Experiment control \n* Data analysis\n\t- Tools for data post-processing\n\t- Implementation of algorithm\n\t- Visualisation \n* Publishing your results\n\t- Manuscripts\n\t- Figures/tables/animations\n\t- Your research data and source codes might be required by reviewers so that they can reproduce and validate your published results\n\n------\n\n### Requirements in computational-based research\n_\"Just make it work\"_ is NOT enough.\n\n* Minimum standard: __reproducibility__ - both the author and other researchers are able to rerun the simulations and reproduce the same results using the data and computer code that are used to generate the published results by the author\n\n* Ultimate standard: __replicability__ - research findings/claims that invlove numerical simulations are able to be replicated by other researchers using independent methods and data (e.g. can your simulation results be validated against that of experiments?) \n\nTherefore reproducible may _NOT_ be replicible. \n\nSee: [Peng, 2011. Reproducible Research in Computational Science. DOI: 10.1126/science.1213847](http://science.sciencemag.org/content/334/6060/1226.full) \n\n------\n\n### Why Python\nPython is a modern, fully-featured, general-purpose, high-level interpreted language, and supports multiple programming paradigms, e.g. object-oriented and functional programming.\n\n* Easy to learn and quick to program in - more time on scientific thinking, less time on programming. Note that our main work is research.\n* Dynamically-typed and automatic memory management - No need to define types for variables and functions. No need to manually allocate and deallocate memory for data arrays \n* Expressive - not only readable, but also concise ",
"_____no_output_____"
]
],
[
[
"persons = [name for name in [\"Adam\", \"James\", \"Dan\", \"Smith\"] if name.startswith(\"A\") or name.endswith(\"s\")]\nprint(persons)",
"['Adam', 'James']\n"
]
],
[
[
"* Very rich collection of numerical libraries, scientifc packages and visualisation functionalities - [Numpy](http://www.numpy.org/), [Scipy](https://www.scipy.org/scipylib/index.html), [SymPy](http://www.sympy.org/en/index.html), [Matplotlib](https://matplotlib.org/), [PyQtGraph](http://www.pyqtgraph.org/) and etc.\n* A variety of development environments\n\t- Interactive: [IPython](https://ipython.org/) and [Jupyter notebooks](https://jupyter.org/). \n\t- IDE: [PyCharm](https://www.jetbrains.com/pycharm/), [Spyder](https://pythonhosted.org/spyder/) and [Eclipse](http://www.eclipse.org/) with [PyDev](http://www.pydev.org/) plugin. Note that PyCharm also supports IPython and Jupyter notebooks.\n* Interfacing with C/C++/Fortran - leave heavy computations with C/C++/Fortran and use Python as \"glue\" or \"wapper\". F2py (included in Numpy) for Fortran and [Cython](http://cython.org/)/[SWIG](http://www.swig.org/) for C/C++\n* Good support for parallel computing - e.g. [Multiprocessing](https://docs.python.org/2/library/multiprocessing.html) for shared memory system, MPI based [MPI4Py](http://mpi4py.readthedocs.io/en/stable/) for distributed memory system and [PyCUDA](https://mathema.tician.de/software/pycuda/) for GPU computing \n* Free, open source and large user community\n\nUseful links:\n>Python documentation official site: [https://www.python.org/doc/](https://www.python.org/doc/)<br/>\n>Python scientific computing ecosystem: [https://www.scipy.org/](https://www.scipy.org/); [http://www.scipy-lectures.org/intro/intro.html](http://www.scipy-lectures.org/intro/intro.html)<br/>\n>Think Python: [http://greenteapress.com/thinkpython/thinkpython.pdf](http://greenteapress.com/thinkpython/thinkpython.pdf)",
"_____no_output_____"
]
]
] | [
"markdown",
"code",
"markdown"
] | [
[
"markdown"
],
[
"code"
],
[
"markdown"
]
] |
e777be4b5924281fdfa90a1b3529bcd45d8227f0 | 40,364 | ipynb | Jupyter Notebook | notebooks/gold standard/.ipynb_checkpoints/2. recon_tsr_strikes_normalVol-checkpoint.ipynb | nkapchenko/TSR | 3c654ad0fcc10c36d64da238fcc60acf0610c8d5 | [
"MIT"
] | null | null | null | notebooks/gold standard/.ipynb_checkpoints/2. recon_tsr_strikes_normalVol-checkpoint.ipynb | nkapchenko/TSR | 3c654ad0fcc10c36d64da238fcc60acf0610c8d5 | [
"MIT"
] | null | null | null | notebooks/gold standard/.ipynb_checkpoints/2. recon_tsr_strikes_normalVol-checkpoint.ipynb | nkapchenko/TSR | 3c654ad0fcc10c36d64da238fcc60acf0610c8d5 | [
"MIT"
] | null | null | null | 87.179266 | 14,252 | 0.828164 | [
[
[
"import numpy as np\nimport pandas as pd\nimport math\nfrom numpy import array, median\nfrom hw import Jamshidian as jamsh\nfrom hw import Henrard as henr\nfrom hw import calibration as hw_calib\nfrom hw.const import *\nfrom fox_toolbox.utils import rates\nfrom fox_toolbox.utils import volatility\nfrom fox_toolbox.utils import xml_parser\n# from fox_toolbox.utils \nfrom tsr import csv_parser, tsr, linear\nfrom random import choice\nimport matplotlib.pyplot as plt\nfrom termcolor import colored\n\nnp.set_printoptions(precision=4)\n\n%load_ext autoreload\n%autoreload 2",
"The autoreload extension is already loaded. To reload it, use:\n %reload_ext autoreload\n"
]
],
[
[
"### Read IRSM FORM",
"_____no_output_____"
]
],
[
[
"cms_xml = xml_parser.get_files('irsmform xml', folder = 'linear TSR logs')\ncms_xml_out = xml_parser.get_files('out xml', folder = 'linear TSR logs')\ncms_csv = xml_parser.get_files('CMS 10y csv', folder = 'linear TSR logs')\n\ncms_replic_basket = csv_parser.parse_csv(cms_csv)\ncal_basket = list(xml_parser.get_calib_basket(cms_xml_out))",
"Parsing CmsFlow csv log\n"
],
[
"main_curve, sprds = xml_parser.get_rate_curves(cms_xml)\ndsc_curve = main_curve\n\ntry:\n estim_curve = sprds[0]\nexcept TypeError:\n estim_curve = main_curve",
"_____no_output_____"
]
],
[
[
"### Kmin & Kmax reconciliation",
"_____no_output_____"
]
],
[
[
"maxe = 0\ntsr_fwds, xml_fwds = [], []\nfor (caplet, floorlet, swaplet), swo in zip(cms_replic_basket, cal_basket):\n tsr_fwds.append(caplet.fwd)\n xml_fwds.append(swo.get_swap_rate(dsc_curve, estim_curve))\n \n kmax = tsr.minmax_strikes(swo.vol, swo.expiry, swo.get_swap_rate(dsc_curve, estim_curve), caplet.n).kmax \n kmin = tsr.minmax_strikes(swo.vol, swo.expiry, swo.get_swap_rate(dsc_curve, estim_curve), floorlet.n).kmin\n maxe = max(maxe, kmax - caplet.strike_max)\n# print('Tf', int(caplet.fixing_date),'kmax diff: ',kmax - caplet.strike_max,'kmin diff: ',kmin - floorlet.strike_min)\n \nprint(colored('hello', 'red') if maxe > 1e-09 else colored('OK', 'green'))",
"\u001b[32mOK\u001b[0m\n"
],
[
"array(xml_fwds) - array(tsr_fwds)",
"_____no_output_____"
],
[
"print(colored('hello', 'red') if max(array(xml_fwds) - array(tsr_fwds)) > 1e-09 else colored('OK', 'green'))",
"\u001b[32mOK\u001b[0m\n"
]
],
[
[
"### Strike ladder recon",
"_____no_output_____"
]
],
[
[
"swo = cal_basket[-1]\ncaplet, floorlet, swaplet = cms_replic_basket[-1]\n\nfwd = swo.get_swap_rate(dsc_curve, estim_curve)\nnref = caplet.n\n\nminmax_strikes = tsr.minmax_strikes(swo.vol, swo.expiry, fwd, nref)\n\nneff_capl = math.ceil((minmax_strikes.kmax - minmax_strikes.fwd)/minmax_strikes.kstep) + 1\nneff_floo = math.floor((minmax_strikes.fwd - minmax_strikes.kmin)/minmax_strikes.kstep) + 1\n\nstrikes_ladders = tsr.build_strike_ladders(minmax_strikes, neff_capl, neff_floo)\n\n# print(caplet.calib_basket.Strike.values - strikes_ladders.caplet_ladder)\nmax(caplet.calib_basket.Strike.values - strikes_ladders.caplet_ladder)\nprint(colored('hello', 'red') if max(caplet.calib_basket.Strike.values - strikes_ladders.caplet_ladder) > 1e-09 else\\\n colored('OK', 'green'))",
"\u001b[32mOK\u001b[0m\n"
],
[
"# print(floorlet.calib_basket.Strike.values - strikes_ladders.floorlet_ladder)\n\nprint(colored('hello', 'red') if max(floorlet.calib_basket.Strike.values - strikes_ladders.floorlet_ladder) > 1e-09\\\n else colored('OK', 'green'))",
"\u001b[32mOK\u001b[0m\n"
]
],
[
[
"### Weights recon",
"_____no_output_____"
]
],
[
[
"mr = xml_parser.get_tsr_params(cms_xml_out).meanRevTSRSwapRate(caplet.fixing_date)\ntsr_coeff = linear.get_coeff(caplet.pmnt_date, dsc_curve, swo, mr, estim_curve)\ntsr_weights = tsr.build_weights(minmax_strikes, neff_capl, neff_floo, tsr_coeff)\n\n# print(caplet.calib_basket.Weights.values - tsr_weights.capletWeights)\n\nprint(colored('ALARM', 'red') if max(abs(caplet.calib_basket.Weights.values - tsr_weights.capletWeights)) > 3e-09\\\n else colored('OK', 'green'))",
"\u001b[32mOK\u001b[0m\n"
],
[
"# print(floorlet.calib_basket.Weights.values - tsr_weights.floorletWeights)\n\nprint(colored('ALARM', 'red') if max(abs(floorlet.calib_basket.Weights.values - tsr_weights.floorletWeights)) > 3e-09\\\n else colored('OK', 'green'))",
"\u001b[32mOK\u001b[0m\n"
]
],
[
[
"### Disc(Tf, Tp) / Annuity",
"_____no_output_____"
]
],
[
[
"print(caplet.calib_basket['Disc/A'].values - tsr.get_DiscOverAnnuity(strikes_ladders.caplet_ladder, tsr_coeff))\n\nprint(colored('ALARM', 'red') if max(abs(caplet.calib_basket['Disc/A'].values -\\\n tsr.get_DiscOverAnnuity(strikes_ladders.caplet_ladder, tsr_coeff))) > 3e-09 else colored('OK', 'green'))",
"[2.1060e-09 2.0325e-09 1.9589e-09 1.8852e-09 1.8117e-09 1.7381e-09\n 1.6646e-09 1.5909e-09 1.5174e-09 1.4438e-09 1.3702e-09 1.2966e-09\n 1.2230e-09 1.1495e-09 1.0758e-09 1.0023e-09 9.2870e-10 8.5514e-10\n 7.8148e-10 7.0793e-10 6.3437e-10 5.6071e-10 4.8715e-10 4.1360e-10\n 3.4004e-10]\n\u001b[32mOK\u001b[0m\n"
],
[
"# print(floorlet.calib_basket['Disc/A'].values - tsr.get_DiscOverAnnuity(strikes_ladders.floorlet_ladder, tsr_coeff))\n\n\nprint(colored('ALARM', 'red') if max(abs(floorlet.calib_basket['Disc/A'].values - \\\n tsr.get_DiscOverAnnuity(strikes_ladders.floorlet_ladder, tsr_coeff))) > 4e-09 else colored('OK', 'green'))",
"\u001b[32mOK\u001b[0m\n"
],
[
"myBachelierCaplet = array([volatility.BachelierPrice(F=swo.get_swap_rate(dsc_curve, estim_curve), \n K=strike, \n v=swo.vol.value*np.sqrt(swo.expiry)) \n * swo.get_annuity(dsc_curve) / dsc_curve.get_dsc(swo.start_date)\n for strike in strikes_ladders.caplet_ladder])\n\nplt.plot(myBachelierCaplet, label='Bachelier')\n\nplt.plot(caplet.calib_basket.SwoPrice.values, label='Swaption', ls='--')\nplt.legend()\n\nmax(myBachelierCaplet - caplet.calib_basket.SwoPrice.values)\n\nprint(colored('ALARM', 'red') if max(myBachelierCaplet - caplet.calib_basket.SwoPrice.values) > 2e-05 else colored('OK e-05', 'green'))",
"\u001b[32mOK e-05\u001b[0m\n"
],
[
"(myBachelierCaplet - caplet.calib_basket.SwoPrice.values)",
"_____no_output_____"
],
[
"myBachelierFloorlet = array([volatility.BachelierPrice(\n F=swo.get_swap_rate(dsc_curve, estim_curve), \n K=strike, \n v=swo.vol.value*np.sqrt(swo.expiry), w=-1) \n * swo.get_annuity(dsc_curve) / dsc_curve.get_dsc(swo.start_date)\n for strike in strikes_ladders.floorlet_ladder])\n\nplt.plot(myBachelierFloorlet, label='Bachelier')\n\nplt.plot(floorlet.calib_basket.SwoPrice.values, label='Swaption')\nplt.legend()\n\nprint(colored('ALARM', 'red') if max(myBachelierFloorlet - floorlet.calib_basket.SwoPrice.values) > 2e-05 else colored('OK e-05', 'green'))",
"\u001b[32mOK e-05\u001b[0m\n"
]
]
] | [
"code",
"markdown",
"code",
"markdown",
"code",
"markdown",
"code",
"markdown",
"code",
"markdown",
"code"
] | [
[
"code"
],
[
"markdown"
],
[
"code",
"code"
],
[
"markdown"
],
[
"code",
"code",
"code"
],
[
"markdown"
],
[
"code",
"code"
],
[
"markdown"
],
[
"code",
"code"
],
[
"markdown"
],
[
"code",
"code",
"code",
"code",
"code"
]
] |
e777bee50eb2328b9797e1e31f606a67662a7552 | 7,537 | ipynb | Jupyter Notebook | project_euler/061_Cyclical figurate numbers_incomplete.ipynb | Sabihxh/projectEuler | 4b0d4bc56598dd2a32c1f00b41bfcadbb495a88b | [
"MIT"
] | 1 | 2018-03-20T12:04:06.000Z | 2018-03-20T12:04:06.000Z | project_euler/061_Cyclical figurate numbers_incomplete.ipynb | Sabihxh/projectEuler | 4b0d4bc56598dd2a32c1f00b41bfcadbb495a88b | [
"MIT"
] | null | null | null | project_euler/061_Cyclical figurate numbers_incomplete.ipynb | Sabihxh/projectEuler | 4b0d4bc56598dd2a32c1f00b41bfcadbb495a88b | [
"MIT"
] | null | null | null | 26.353147 | 234 | 0.490115 | [
[
[
"### Cyclical figurate numbers\nProblem 61\n\nhttps://projecteuler.net/minimal=61",
"_____no_output_____"
],
[
"<p>Triangle, square, pentagonal, hexagonal, heptagonal, and octagonal numbers are all figurate (polygonal) numbers and are generated by the following formulae:</p>\n<table><tr><td>Triangle</td>\n<td> </td>\n<td>P<sub>3,<i>n</i></sub>=<i>n</i>(<i>n</i>+1)/2</td>\n<td> </td>\n<td>1, 3, 6, 10, 15, ...</td>\n</tr><tr><td>Square</td>\n<td> </td>\n<td>P<sub>4,<i>n</i></sub>=<i>n</i><sup>2</sup></td>\n<td> </td>\n<td>1, 4, 9, 16, 25, ...</td>\n</tr><tr><td>Pentagonal</td>\n<td> </td>\n<td>P<sub>5,<i>n</i></sub>=<i>n</i>(3<i>n</i>−1)/2</td>\n<td> </td>\n<td>1, 5, 12, 22, 35, ...</td>\n</tr><tr><td>Hexagonal</td>\n<td> </td>\n<td>P<sub>6,<i>n</i></sub>=<i>n</i>(2<i>n</i>−1)</td>\n<td> </td>\n<td>1, 6, 15, 28, 45, ...</td>\n</tr><tr><td>Heptagonal</td>\n<td> </td>\n<td>P<sub>7,<i>n</i></sub>=<i>n</i>(5<i>n</i>−3)/2</td>\n<td> </td>\n<td>1, 7, 18, 34, 55, ...</td>\n</tr><tr><td>Octagonal</td>\n<td> </td>\n<td>P<sub>8,<i>n</i></sub>=<i>n</i>(3<i>n</i>−2)</td>\n<td> </td>\n<td>1, 8, 21, 40, 65, ...</td>\n</tr></table><p>The ordered set of three 4-digit numbers: 8128, 2882, 8281, has three interesting properties.</p>\n<ol><li>The set is cyclic, in that the last two digits of each number is the first two digits of the next number (including the last number with the first).</li>\n<li>Each polygonal type: triangle (P<sub>3,127</sub>=8128), square (P<sub>4,91</sub>=8281), and pentagonal (P<sub>5,44</sub>=2882), is represented by a different number in the set.</li>\n<li>This is the only set of 4-digit numbers with this property.</li>\n</ol><p>Find the sum of the only ordered set of six cyclic 4-digit numbers for which each polygonal type: triangle, square, pentagonal, hexagonal, heptagonal, and octagonal, is represented by a different number in the set.</p>\n",
"_____no_output_____"
]
],
[
[
"triangle = lambda n: int( (n*(n+1))/2 )\nsquare = lambda n: int( n**2 )\npentagonal = lambda n: int( (n*(3*n-1))/2 )\nhexagonal = lambda n: ( n*(2*n-1) )\nheptagonal = lambda n: int( (n*(5*n-3))/2 )\noctagonal = lambda n: int( n*(3*n-2) )\n\n\n# Tests\nassert [triangle(x) for x in range(1, 6)] == [1,3,6,10,15]\nassert [square(x) for x in range(1, 6)] == [1,4,9,16,25]\nassert [pentagonal(x) for x in range(1, 6)] == [1,5,12,22,35]\nassert [hexagonal(x) for x in range(1, 6)] == [1,6,15,28,45]\nassert [heptagonal(x) for x in range(1, 6)] == [1,7,18,34,55]\nassert [octagonal(x) for x in range(1, 6)] == [1,8,21,40,65]\n\n\ndef get_q_r(x):\n return (x // 100, x % 100)\n\n\ndef generate_polygonal_numbers(func):\n \"\"\"\n Returns list of numbers between 1010 and 9999 given a function.\n \n \"\"\"\n result = []\n \n for n in range(1, 150):\n p = func(n)\n q, r = get_q_r(p)\n if (1010 <= p <= 9999) & (r >= 10):\n result.append(p)\n if p >= 9999:\n break\n\n return result\n\ntriangle_nums = generate_polygonal_numbers(triangle)\nsquare_nums = generate_polygonal_numbers(square)\npentagonal_nums = generate_polygonal_numbers(pentagonal)\nhexagonal_nums = generate_polygonal_numbers(hexagonal)\nheptagonal_nums = generate_polygonal_numbers(heptagonal)\noctagonal_nums = generate_polygonal_numbers(octagonal)\n\npolygonal_sets = [triangle_nums, square_nums, pentagonal_nums, hexagonal_nums, heptagonal_nums, octagonal_nums]\n\n",
"_____no_output_____"
],
[
"for p3 in triangle_nums:\n for p4 in square_nums:\n for p5 in pentagonal_nums:\n if len(set((p3, p4, p5))) < 3:\n continue\n q3, r3 = get_q_r(p3)\n q4, r4 = get_q_r(p4)\n q5, r5 = get_q_r(p5)\n if (r3 == q4) & (r4 == q5) & (r5 == q3):\n print(p3, p4, p5)\n elif (r3 == q5) & (r5 == q4) & (r4 == q3):\n print(p3, p4, p5)\n",
"8128 8281 2882\n"
],
[
"1024**0.5",
"_____no_output_____"
]
]
] | [
"markdown",
"code"
] | [
[
"markdown",
"markdown"
],
[
"code",
"code",
"code"
]
] |
e777e7d79b325531cdd496591b541add8400698e | 14,752 | ipynb | Jupyter Notebook | xgboostml.ipynb | LeadingIndiaAI/Review-On-Machine-Learning-Algorithms-For-Dengue-Disease-Spread-Prediction | a162be03ac6c4551c18ca9b4f3ad2af5204ed1e1 | [
"MIT"
] | 1 | 2019-11-19T04:33:57.000Z | 2019-11-19T04:33:57.000Z | xgboostml.ipynb | LeadingIndiaAI/Review-On-Machine-Learning-Algorithms-For-Dengue-Disease-Spread-Prediction | a162be03ac6c4551c18ca9b4f3ad2af5204ed1e1 | [
"MIT"
] | null | null | null | xgboostml.ipynb | LeadingIndiaAI/Review-On-Machine-Learning-Algorithms-For-Dengue-Disease-Spread-Prediction | a162be03ac6c4551c18ca9b4f3ad2af5204ed1e1 | [
"MIT"
] | 1 | 2021-01-29T19:37:52.000Z | 2021-01-29T19:37:52.000Z | 32.855234 | 235 | 0.43872 | [
[
[
"import numpy as np\nimport pandas as pd\nimport matplotlib.pyplot as plt\nimport seaborn as sns\n%matplotlib inline",
"_____no_output_____"
],
[
"x=pd.read_csv('https://s3.amazonaws.com/drivendata/data/44/public/dengue_features_train.csv')\ny=pd.read_csv('https://s3.amazonaws.com/drivendata/data/44/public/dengue_labels_train.csv')\nx_test=pd.read_csv('https://s3.amazonaws.com/drivendata/data/44/public/dengue_features_test.csv')\nsubmission_file=pd.read_csv('https://s3.amazonaws.com/drivendata/data/44/public/submission_format.csv')",
"_____no_output_____"
],
[
"x.fillna(method='ffill', inplace=True)\nx_test.fillna(method='ffill', inplace=True)",
"_____no_output_____"
],
[
"features=['ndvi_ne','year','weekofyear','ndvi_se','ndvi_nw','reanalysis_air_temp_k','precipitation_amt_mm']\nfe=['year','weekofyear','station_avg_temp_c','reanalysis_dew_point_temp_k','ndvi_ne','reanalysis_tdtr_k']",
"_____no_output_____"
],
[
"X=x[fe]\nX.info()",
"<class 'pandas.core.frame.DataFrame'>\nRangeIndex: 1456 entries, 0 to 1455\nData columns (total 6 columns):\nyear 1456 non-null int64\nweekofyear 1456 non-null int64\nstation_avg_temp_c 1456 non-null float64\nreanalysis_dew_point_temp_k 1456 non-null float64\nndvi_ne 1456 non-null float64\nreanalysis_tdtr_k 1456 non-null float64\ndtypes: float64(4), int64(2)\nmemory usage: 68.3 KB\n"
],
[
"y.drop(columns='city',inplace=True)\ny.drop(columns='year',inplace=True)\ny.drop(columns='weekofyear',inplace=True)",
"_____no_output_____"
],
[
"from sklearn.model_selection import train_test_split\nseed = 0\ntest_size = 0.2\nX_train, X_test, y_train, y_test = train_test_split(X, y, test_size=test_size, random_state=seed)",
"_____no_output_____"
],
[
"from xgboost import XGBRegressor\nmy_model = XGBRegressor(learning_rate=0.009,n_estimators=1500,subsample=1,max_depth=7,cosample_bytree=1,gamma=1)\nmy_model.fit(X_train, y_train)",
"[21:15:20] WARNING: /workspace/src/objective/regression_obj.cu:152: reg:linear is now deprecated in favor of reg:squarederror.\n"
],
[
"from sklearn.metrics import mean_absolute_error\npredictions = my_model.predict(X_test)\n\nprint(\"Mean Absolute Error: \" + str(mean_absolute_error(predictions, y_test)))",
"Mean Absolute Error: 9.662770768142726\n"
],
[
"model_full=XGBRegressor(learning_rate=0.009,n_estimators=1500,subsample=1,max_depth=7,cosample_bytree=1,gamma=1)\nmodel_full.fit(X,y)\nx_test=x_test[fe]\nx.head()\nx_test.head()",
"[21:15:23] WARNING: /workspace/src/objective/regression_obj.cu:152: reg:linear is now deprecated in favor of reg:squarederror.\n"
],
[
"#x_test['city1']=np.where(x_test['city']=='sj',1,-1)\n#x_test.drop(columns='city',inplace=True)",
"_____no_output_____"
],
[
"test_pred=model_full.predict(x_test).astype(int)\nsubmission=pd.read_csv('https://s3.amazonaws.com/drivendata/data/44/public/submission_format.csv',index_col=[0,1,2])\nsubmission.total_cases=test_pred\nsubmission.to_csv('value.csv')",
"_____no_output_____"
],
[
"from sklearn.ensemble import RandomForestRegressor\nrf=RandomForestRegressor()\nrf.fit(X_train,y_train)\n",
"/usr/local/lib/python3.6/dist-packages/sklearn/ensemble/forest.py:245: FutureWarning: The default value of n_estimators will change from 10 in version 0.20 to 100 in 0.22.\n \"10 in version 0.20 to 100 in 0.22.\", FutureWarning)\n/usr/local/lib/python3.6/dist-packages/ipykernel_launcher.py:3: DataConversionWarning: A column-vector y was passed when a 1d array was expected. Please change the shape of y to (n_samples,), for example using ravel().\n This is separate from the ipykernel package so we can avoid doing imports until\n"
],
[
"from sklearn.metrics import mean_absolute_error\npredictions = rf.predict(X_test)\nprint(\"Mean Absolute Error: \" + str(mean_absolute_error(predictions, y_test)))",
"Mean Absolute Error: 10.837328767123287\n"
]
]
] | [
"code"
] | [
[
"code",
"code",
"code",
"code",
"code",
"code",
"code",
"code",
"code",
"code",
"code",
"code",
"code",
"code"
]
] |
e777ea639fc190324c9e97de54af5682dd170482 | 45,857 | ipynb | Jupyter Notebook | GetFromHypeAuditor.ipynb | santiagosilas/GetOpenDataFromHA | 89ec324e29154fe6e5ce3aab59f71f9edfa9b5d2 | [
"MIT"
] | null | null | null | GetFromHypeAuditor.ipynb | santiagosilas/GetOpenDataFromHA | 89ec324e29154fe6e5ce3aab59f71f9edfa9b5d2 | [
"MIT"
] | null | null | null | GetFromHypeAuditor.ipynb | santiagosilas/GetOpenDataFromHA | 89ec324e29154fe6e5ce3aab59f71f9edfa9b5d2 | [
"MIT"
] | null | null | null | 33.496713 | 101 | 0.338204 | [
[
[
"import requests\nfrom bs4 import BeautifulSoup\nimport pandas as pd",
"_____no_output_____"
],
[
"data = list()\nfor i in list(range(1, 21)):\n page = i\n url = \"https://hypeauditor.com/top-instagram-all-brazil/?p={}\".format(page)\n texto = requests.get(url).text\n soup = BeautifulSoup(texto, 'lxml')\n _table = soup.find('table',{'class':'kyb-table bloggers-top-table'})\n tbody = _table.find('tbody')\n trs = tbody.findAll('tr')\n # loop\n for tr in trs:\n tds = tr.findAll('td')\n name = tds[2].find('h4').get_text()\n username = tds[2].find('a').get_text()\n topics = [span.get_text() for span in tds[3].findAll('span')]\n topics = str(topics).replace(\"'\", '').replace(\"[\", '').replace(\"]\", '')\n from_brazil = tds[4].get_text().replace(' ', '')\n if 'M' in from_brazil:\n from_brazil = float(from_brazil.replace('M', '')) * 1000000\n elif 'K' in from_brazil:\n from_brazil = float(from_brazil.replace('K', '')) * 1000\n elif 'N/A' in from_brazil:\n from_brazil = 0.0\n else:\n from_brazil = float(from_brazil) \n engagement = tds[6].get_text().replace('\\n', '').replace(' ', '')\n data.append([name, username, topics, from_brazil, engagement])",
"_____no_output_____"
],
[
"df = pd.DataFrame(data, columns = ['name', 'username', 'topics', 'followers', 'engagement'])\ndf.head()",
"_____no_output_____"
],
[
"df.shape",
"_____no_output_____"
],
[
"ordered = df.sort_values('followers', ascending=True)",
"_____no_output_____"
],
[
"ordered.to_csv('instagram.csv')",
"_____no_output_____"
],
[
"topics = [value[0] for value in ordered[['topics']].values]\ntopics_set = list()\nfor t in topics:\n if t == '':\n continue\n else:\n ts = t.split(',')\n for item in ts:\n topics_set.append(item)",
"_____no_output_____"
],
[
"topics_set = set(topics_set)",
"_____no_output_____"
],
[
"pd.DataFrame(data = list(topics_set), columns=['topics'])",
"_____no_output_____"
],
[
"ordered[ordered.topics.str.contains('Music')]",
"_____no_output_____"
],
[
"import os\nos.system('instagram-scraper @kicky -u aurevoirstuffs -p Ea@901facebook')",
"_____no_output_____"
],
[
"!pwd",
"/f/repositories/skhb\n"
],
[
"import requests\nimport urllib.request\nimport urllib.parse\nimport urllib.error\nfrom bs4 import BeautifulSoup\nimport ssl\nimport json",
"_____no_output_____"
],
[
"class Insta_Info_Scraper:\n\n def getinfo(self, url):\n self.ctx = ssl.create_default_context()\n html = urllib.request.urlopen(url, context=self.ctx).read()\n soup = BeautifulSoup(html, 'html.parser')\n data = soup.find_all('meta', attrs={'property': 'og:description'\n })\n text = data[0].get('content').split()\n user = '%s %s %s' % (text[-3], text[-2], text[-1])\n followers = text[0]\n following = text[2]\n posts = text[4]\n print ('User:', user)\n print ('Followers:', followers)\n print ('Following:', following)\n print ('Posts:', posts)\n print ('---------------------------')",
"_____no_output_____"
],
[
"obj = Insta_Info_Scraper()\nobj.getinfo('https://www.instagram.com/maisa/')",
"User: from +A (@maisa)\nFollowers: 23.3m\nFollowing: 2,869\nPosts: 2,061\n---------------------------\n"
]
]
] | [
"code"
] | [
[
"code",
"code",
"code",
"code",
"code",
"code",
"code",
"code",
"code",
"code",
"code",
"code",
"code",
"code",
"code"
]
] |
e777f99a5c3c59edf97c6a7be916c66f3bd0033f | 773,236 | ipynb | Jupyter Notebook | stats-deces.ipynb | SteamFred/data-analysis | c680147c9bd0cadcc3b389b139a666d8f350fed1 | [
"MIT"
] | null | null | null | stats-deces.ipynb | SteamFred/data-analysis | c680147c9bd0cadcc3b389b139a666d8f350fed1 | [
"MIT"
] | null | null | null | stats-deces.ipynb | SteamFred/data-analysis | c680147c9bd0cadcc3b389b139a666d8f350fed1 | [
"MIT"
] | null | null | null | 321.244703 | 206,376 | 0.910375 | [
[
[
"# Analyse des décès en France (toutes causes confondues)\nCe notebook a pour but de mettre les chiffres de décès de ces dernières années en France en perspective afin d'en tirer des conclusions éclairées.",
"_____no_output_____"
]
],
[
[
"# Bibliothèque d'analyse et de traitement de données\nimport pandas as pd\nfrom pandas.api.types import CategoricalDtype\nprint(\"Pandas version :\", pd.__version__)\nimport numpy as np\nfrom datetime import datetime\n# Bibliothèques de gestion des graphiques\nimport matplotlib.pyplot as plt\n# Bibliothèques de gestion des fichiers\nimport glob\nimport os.path\n# Bibliothèque de gestion du format csv pour écrire les fichiers cache\nimport csv\n# \"Constantes\" ou variables globales\ncsv_delimiter = ';'\nprint ('Imports des bibliothèques terminés !')",
"Pandas version : 1.1.3\nImports des bibliothèques terminés !\n"
]
],
[
[
"# Données sources\nLes données sources sont issues du __[Fichier des personnes décédées](https://www.data.gouv.fr/fr/datasets/fichier-des-personnes-decedees/)__ fournies en données ouvertes sur [data.gouv.fr](https://www.data.gouv.fr/fr/).\n\nLes fichiers `deces-*.txt` doivent être téléchargés dans le dossier de travail du notebook.\nPour un traitement rapide, commencer par un petit nombre de fichiers, ex: 2002 et 2003.\n\n**note** : Certains fichiers portent parfois quelques \"caractères non imprimables\" qui font échouer sa lecture et son interprétation. Dans ce cas, il faut l'éditer pour rechercher et retirer ces caractères. Je ne sais pas si c'est un problème du fichier d'origine ou si c'est dû à un parasitage du téléchargement, je n'ai pas investigué.\n\n## Analyse et extraction\nPour accélérer le travail, nous alons lire les fichiers `deces-*.txt` qui ont un format \"en largeur fixe\" et en extraire les informations essentielles suivantes :\n - date de décès\n - date de naissance\n \nCes informations seront écrites dans un fichier cache au format CSV qui permettra plusieurs choses :\n 1. Accélérer une éventuelle relecture des données sources : au lieu de lire pour chaque année un fichier de plus de 100 Mo, on ne lit que quelques Mo.\n 1. Manipuler ces données dans un tableur indépendamment de cet exercice à des fins de recoupement, par exemple.\n 1. Porter directement l'âge du décès par soustraction plutôt que la date de naissance pour éviter de refaire le calcul à chaque utilisation de la donnée.\n \nPour que le code soit compréhensible, je laisse un certain nombre de variables intermédiaires.",
"_____no_output_____"
]
],
[
[
"# Lister tous les fichiers qui commencent par \"deces\" et sont au format texte (finissent par \".txt\")\nsourcelist = [f for f in glob.glob(\"deces*.txt\")]\n# Pour chaque fichier de cette liste...\nfor source in sourcelist:\n # on va créer un pendant au même nom, mais se terminant par .csv\n cachefile = source[0:-4] + '.csv'\n # Par contre, on ne crée ce fichier que s'il n'existe pas ou s'il est plus vieux que le fichier de données\n if not(os.path.exists(cachefile)) or os.path.getmtime(source) > os.path.getmtime(cachefile):\n if os.path.exists(cachefile):\n os.remove(cachefile)\n with open(cachefile, 'w', newline='') as cache:\n cache_writer = csv.writer(cache, delimiter = csv_delimiter, quotechar='\"', quoting=csv.QUOTE_MINIMAL)\n print ('Génération du cache CSV pour le fichier : ' + source)\n # On crée une première ligne portant le nom des 2 colonnes\n cache_writer.writerow(['date', 'age'])\n # On ouvre ensuite le fichier source pour en lire toutes ses lignes en gérant les caractères accentués\n with open(source,'r', errors='replace') as f:\n lines = f.readlines()\n # Pour chaque ligne du fichier source...\n for l in lines:\n # ...on interprète la date de décès aux caractères 154 à 161 inclus (donc 162 exclus)\n txt_date_d = l[154:162]\n try:\n date_d = datetime.strptime(txt_date_d, \"%Y%m%d\")\n except ValueError:\n # Si la date n'est pas interprétable, alors on met zero\n date_d = 0\n continue\n # ...on interprète la date de naissance aux caractères 81 à 88\n try:\n date_n = datetime.strptime(l[81:89], \"%Y%m%d\")\n except ValueError:\n # Si la date n'est pas interprétable, alors on met zero\n date_n = 0\n continue\n # Ici, on peut choisir de filtrer.\n # Par exemple, on peut choisir de ne conserver que les années 20xx et 199x\n # Mais surtout, on ignore les dates non reconnues\n if date_d != 0 and date_n != 0 and (txt_date_d[0:2] == '20' or txt_date_d[0:3] == '199'):\n # Puis on calcule l'âge qu'on écrit avec la date de décès dans le fichier csv\n age = date_d.year - date_n.year - ((date_d.month, date_d.day) < (date_n.month, date_n.day))\n cache_writer.writerow([txt_date_d[0:4]+'/'+txt_date_d[4:6]+'/'+txt_date_d[6:8],age])\nprint ('(Ré)génération des caches CSV terminée !')",
"(Ré)génération des caches CSV terminée !\n"
]
],
[
[
"## Relecture du cache\nCi-après, on lit tous les fichiers cache pour les concaténer en mémoire afin de faire notre analyse.\n\nPour réinitialiser toutes les données de la \"dataframe\" df, c'est cette cellule qu'il faut réexécuter.",
"_____no_output_____"
]
],
[
[
"print ('Lecture des fichiers cache...')\n# Créer une liste de tous les csv\nchunks = []\ncachelist = [f for f in glob.glob(\"deces*.csv\")]\nfor cache in cachelist:\n # print ('Lecture de :', cache)\n chunks.append(pd.read_csv(cache,\n sep=';',\n encoding='latin_1', # Spécifie l'encodage du fichier à lire\n dtype={'age':np.int8}, # Stocke l'age dans un format court pour la performance\n index_col=['date'], # Crée un indexe sur la colonne date\n parse_dates=True)) # Interprète automatiquement ce qui ressemble à une date\n# Concatène le tout\ndf = pd.concat(chunks, axis=0, ignore_index=False)\n# Affiche les premières lignes pour vérifier que tout s'est bien passé\ndf.tail()\n# Exemples d'utilisation :\n# * Sélection de tous les décès à moins de 50 ans : df[df.age < 50]\n# * Sélection de tous les décès du mois de février 2020 : df.loc['2020-02']",
"Lecture des fichiers cache...\n"
]
],
[
[
"## Classification\nPour se simplifier l'interprétation graphique, on va classer chaque décès dans une catégorie d'âge.\n\nJ'ai découpé en tranches plus ou moins fines...",
"_____no_output_____"
]
],
[
[
"df['classe'] = pd.cut(df.age, bins=[0, 18, 25, 45, 55, 65, 75, 85, 150],\n labels=['mineur', '18-25 ans', '26-45 ans', '46-55 ans', '56-65 ans', '66-75 ans', '76-85 ans', 'plus de 86 ans'])\ndf.tail()",
"_____no_output_____"
]
],
[
[
"## Nombre de décès par date par catégorie\nA partir de la dataframe contenant les catégories, on crée une matrice pour compter le nombre de décès par date par catégorie. ",
"_____no_output_____"
]
],
[
[
"ages_df = df.groupby(['date', 'classe']).age.count().astype(np.int16).unstack()\nages_df.tail()",
"_____no_output_____"
]
],
[
[
"# Analyses graphiques\n## Analyse graphique par classe d'âge durant le confinement en France\nAfin de rendre la courbe plus lisible (la débruiter), on utilise une fonction de lissage ewm moyenné avec un facteur alpha assez faible.\nAnalysons la période du confinement élargie de mars à mai.\nConstate-t-on que pour la population au dessous de 65 ans, ne nombre de décès n'a pas varié de façon notable ?",
"_____no_output_____"
]
],
[
[
"# Pour supprimer le lissage, mettre alpha=1\n# _= est une petite astuce de Romain WaaY pour ne pas afficher de message parasite avec le graphique\n_=ages_df.ewm(alpha=0.2).mean().loc['2020-03':'2020-05'].plot(figsize=(18,10), legend='reverse')",
"_____no_output_____"
]
],
[
[
"## Analyse graphique sur les 20 dernières années\nMettons maintenant en perspective la surmortalité du printemps 2020 par rapport aux 20 dernières années, toutes classes d'âge confondues.\n\nD'une manière générale, on constate des pics de décès de plus en plus hauts tous les 2 à 4 ans.\n\nLe pic de 2020 est élevé, mais semble rester dans la tendance haussière générale...",
"_____no_output_____"
]
],
[
[
"_=df.loc['2000-09':'2020-11-25'].classe.groupby('date').count().plot(figsize=(18,8))\n# Calcul des moyennes annuelles\nan_debut = 2001\nan_fin = 2021\nmoyennes = pd.Series([int(ages_df.loc[str(an)].mean().sum()) for an in range(an_debut,an_fin)],\n index=[datetime.strptime(str(an), \"%Y\") for an in range(an_debut,an_fin)])\nmoyennes.plot()",
"_____no_output_____"
],
[
"#Todo: superposer les courbes\n#plt.figure(figsize=(18,8))\n#df.loc['2009-09'].classe.groupby('date').count().plot()\n#df.loc['2010-09'].classe.groupby('date').count().plot()",
"_____no_output_____"
],
[
"ddf = pd.DataFrame(df.classe.groupby('date').count())\nddf.tail()",
"_____no_output_____"
],
[
"#Todo: superposer les courbes\nplt.figure(figsize=(18,8))\n#df.loc['2019-09-01':'2019-10-26'].classe.groupby('date').count().ewm(alpha=0.2).mean().plot()\ndf.loc['2019-10-01':].classe.groupby('date').count().ewm(alpha=0.2).mean().plot()",
"_____no_output_____"
],
[
"ddf['annee'] = ddf.index.year\nddf['doy'] = ddf.index.dayofyear\nddf.tail()",
"_____no_output_____"
],
[
"ddf.reset_index(inplace=True)\nddf.set_index(['annee', 'doy'], inplace=True)\nddf.loc[2020].classe.plot(x=ddf.date)",
"_____no_output_____"
]
],
[
[
"INSEE.fr : [Nombre de décès quotidiensFrance, régions et départements](https://www.insee.fr/fr/statistiques/4487988?sommaire=4487854), Fichier individuel comportant des informations sur chaque décès - 20 novembre 2020",
"_____no_output_____"
]
],
[
[
"ds2020 = pd.read_csv('DC_20202021_det.csv', sep=';', encoding='utf-8')\nds2020 = ds2020.drop(['COMDEC', 'DEPDEC', 'SEXE', 'COMDOM', 'LIEUDEC2'], axis=1)\nnb_lignes_brut = ds2020.shape[0]\nds2020 = ds2020.dropna(axis=0)\nprint (\"Nombre de lignes incomplètes retirées :\", str(nb_lignes_brut - ds2020.shape[0]))\nds2020.tail()",
"Nombre de lignes incomplètes retirées : 9208\n"
],
[
"ds2020['MNAIS'] = ds2020['MNAIS'].astype('int64')\nds2020['JNAIS'] = ds2020['JNAIS'].astype('int64')\nds2020['age'] = ds2020['ADEC'] - ds2020['ANAIS'] - (ds2020['MDEC'] < ds2020['MNAIS'])\nds2020.drop(['ANAIS', 'MNAIS', 'JNAIS'], axis=1, inplace=True)\nds2020.tail()",
"_____no_output_____"
],
[
"ds2020['classe'] = pd.cut(ds2020.age, bins=[0, 18, 25, 45, 55, 65, 75, 85, 150],\n labels=['mineur', '18-25 ans', '26-45 ans', '46-55 ans', '56-65 ans', '66-75 ans', '76-85 ans', 'plus de 86 ans'])\n#ds2020['date'] = pd.to_datetime(str(ds2020['ADEC'])+'-'+str(ds2020['MDEC'])+'-'+str(ds2020['JDEC']), format='%y-%m-%d')\nds2020.rename(columns={\"ADEC\": \"year\", \"MDEC\": \"month\", \"JDEC\": \"day\"}, inplace = True)\nds2020['date'] = pd.to_datetime(ds2020.loc[:,['year', 'month', 'day']])\nds2020.tail()",
"_____no_output_____"
],
[
"ds2020.drop(['month', 'day', 'age'], axis=1, inplace = True)\nds2020.rename(columns={\"year\": \"annee\"}, inplace = True)\nds2020.set_index('date', inplace=True)\nds2020.tail()",
"_____no_output_____"
],
[
"ages_ds = ds2020.groupby(['date', 'classe']).annee.count().astype(np.int16).unstack()\nages_ds.tail()",
"_____no_output_____"
],
[
"_=ages_ds.ewm(alpha=0.1).mean().loc['2020-09':].plot(figsize=(18,10), legend='reverse')",
"_____no_output_____"
],
[
"dds = pd.DataFrame(ds2020.classe.groupby('date').count())\ndds.tail()\ndds['annee'] = dds.index.year\ndds['doy'] = dds.index.dayofyear\ndds.reset_index(inplace=True)\ndds.set_index(['annee', 'doy'], inplace=True)\ndds.tail()",
"_____no_output_____"
]
],
[
[
"Sources des données hospitalières : https://www.data.gouv.fr/fr/datasets/donnees-hospitalieres-relatives-a-lepidemie-de-covid-19/ ",
"_____no_output_____"
]
],
[
[
"dcc = pd.read_csv('donnees-hospitalieres-classe-age-covid19-2021-01-09-19h03.csv', sep=';', encoding='utf-8',\n index_col=['jour'], # Crée un indexe sur la colonne date\n parse_dates=True)\ndcc = dcc.drop(['reg', 'hosp', 'rea', 'rad'], axis=1)\nnb_lignes_brut = dcc.shape[0]\ndcc = dcc.dropna(axis=0)\nprint (\"Nombre de lignes incomplètes retirées :\", str(nb_lignes_brut - dcc.shape[0]))\ndcc.tail(20)",
"Nombre de lignes incomplètes retirées : 0\n"
],
[
"ages_dcc = dcc.groupby(['jour', 'cl_age90'])['dc'].sum().unstack()\n#.drop([0], axis=1)\nages_dcc.tail(20)",
"_____no_output_____"
],
[
"total_dcc = pd.DataFrame(ages_dcc[0])\nidx = pd.IndexSlice\ntotal_dcc['delta'] = total_dcc[0]\ndelta = 0\ny_prev = 0\nx_prev = 0\nfor x, y in total_dcc.iterrows():\n if x_prev != 0:\n delta = y[0] - y_prev\n total_dcc.loc[idx[x]]['delta'] = delta\n else:\n total_dcc.loc[idx[x]]['delta'] = y[0]\n y_prev = y[0]\n x_prev = x\ntotal_dcc.head()",
"_____no_output_____"
],
[
"_=total_dcc['2020']['delta'].ewm(alpha=0.3).mean().plot(figsize=(18,10))",
"_____no_output_____"
],
[
"# _=total_dcc['2021']['delta'].ewm(alpha=0.3).mean().plot(figsize=(18,10))",
"_____no_output_____"
],
[
"total_dcc['annee'] = total_dcc.index.year\ntotal_dcc['doy'] = total_dcc.index.dayofyear\ntotal_dcc.reset_index(inplace=True)\ntotal_dcc.set_index(['annee', 'doy'], inplace=True)\ntotal_dcc.tail()",
"_____no_output_____"
],
[
"_=ages_dcc.ewm(alpha=0.3).mean().plot(figsize=(18,10), legend='reverse')",
"_____no_output_____"
],
[
"#Essayons de mettre des légendes sur le graphe...\nfig, ax = plt.subplots(figsize=(18,10))\nax.set_ylim(bottom=0, top=3000)\ndoy = range(1,367)\nc2017 = ax.plot(ddf.loc[2017].classe)\nc2018 = ax.plot(ddf.loc[2018].classe)\nc2019 = ax.plot(ddf.loc[2019].classe)\nc2020 = ax.plot(dds.loc[2020].classe)\nc2021 = ax.plot(dds.loc[2021].classe)\nd2020 = ax.plot(total_dcc.loc[2020].delta)\n#ax.legend((c2019, c2020), ('2019', '2020'), loc='upper right')\nax.set_xlabel('jour de l''année')\nax.set_ylabel('nombre de décès par jour')\nax.set_title('Courbes annuelles de décès en France')",
"_____no_output_____"
],
[
"total_dcc['surmortalite'] = dds.loc[2020].classe - total_dcc.loc[2020].delta",
"_____no_output_____"
],
[
"total_dcc['surmortalite']",
"_____no_output_____"
]
]
] | [
"markdown",
"code",
"markdown",
"code",
"markdown",
"code",
"markdown",
"code",
"markdown",
"code",
"markdown",
"code",
"markdown",
"code",
"markdown",
"code",
"markdown",
"code"
] | [
[
"markdown"
],
[
"code"
],
[
"markdown"
],
[
"code"
],
[
"markdown"
],
[
"code"
],
[
"markdown"
],
[
"code"
],
[
"markdown"
],
[
"code"
],
[
"markdown"
],
[
"code"
],
[
"markdown"
],
[
"code",
"code",
"code",
"code",
"code",
"code"
],
[
"markdown"
],
[
"code",
"code",
"code",
"code",
"code",
"code",
"code"
],
[
"markdown"
],
[
"code",
"code",
"code",
"code",
"code",
"code",
"code",
"code",
"code",
"code"
]
] |
e77819794590d834d2c15b5b783389022fbde8ad | 11,968 | ipynb | Jupyter Notebook | Notebook/7/7.3.NiN.ipynb | zihan987/d2l-PaddlePaddle | 6059936633b51e66ed2ab89faddc30dca32366fa | [
"MIT"
] | 4 | 2021-07-01T10:07:21.000Z | 2022-01-17T14:11:30.000Z | Notebook/7/7.3.NiN.ipynb | zihan987/d2l-PaddlePaddle | 6059936633b51e66ed2ab89faddc30dca32366fa | [
"MIT"
] | null | null | null | Notebook/7/7.3.NiN.ipynb | zihan987/d2l-PaddlePaddle | 6059936633b51e66ed2ab89faddc30dca32366fa | [
"MIT"
] | 2 | 2021-07-01T11:05:49.000Z | 2021-07-26T10:47:32.000Z | 35.513353 | 160 | 0.471257 | [
[
[
"The following additional libraries are needed to run this\nnotebook. Note that running on Colab is experimental, please report a Github\nissue if you have any problem.",
"_____no_output_____"
]
],
[
[
"!pip install git+https://github.com/d2l-ai/d2l-zh@release # installing d2l\n",
"_____no_output_____"
]
],
[
[
"# 7.3. 网络中的网络(NiN)\n:label:`sec_nin`\n\nLeNet、AlexNet 和 VGG 都有一个共同的设计模式:通过一系列的卷积层与池化层来提取空间结构特征;然后通过全连接层对特征的表征进行处理。\nAlexNet 和 VGG 对 LeNet 的改进主要在于如何扩大和加深这两个模块。\n或者,可以想象在这个过程的早期使用全连接层。\n然而,如果使用稠密层了,可能会完全放弃表征的空间结构。\n*网络中的网络* (*NiN*) 提供了一个非常简单的解决方案:在每个像素的通道上分别使用多层感知机 :cite:`Lin.Chen.Yan.2013`\n\n## (**7.3.1. NiN块**)\n\n回想一下,卷积层的输入和输出由四维张量组成,张量的每个轴分别对应样本、通道、高度和宽度。\n另外,全连接层的输入和输出通常是分别对应于样本和特征的二维张量。\nNiN 的想法是在每个像素位置(针对每个高度和宽度)应用一个全连接层。\n如果我们将权重连接到每个空间位置,我们可以将其视为 $1\\times 1$ 卷积层(如 :numref:`sec_channels` 中所述),或作为在每个像素位置上独立作用的全连接层。\n从另一个角度看,即将空间维度中的每个像素视为单个样本,将通道维度视为不同特征(feature)。\n\n:numref:`fig_nin` 说明了 VGG 和 NiN 及它们的块之间主要结构差异。\nNiN 块以一个普通卷积层开始,后面是两个 $1\\times 1$ 的卷积层。这两个$1\\times 1$ 卷积层充当带有 ReLU 激活函数的逐像素全连接层。\n第一层的卷积窗口形状通常由用户设置。\n随后的卷积窗口形状固定为 $1 \\times 1$。\n\n\n:width:`600px`\n:label:`fig_nin`\n",
"_____no_output_____"
]
],
[
[
"import paddle\nimport paddle.nn as nn\nimport numpy as np\n\nclass Nin(nn.Layer):\n def __init__(self, num_channels, num_filters, kernel_size, strides, padding):\n super(Nin, self).__init__()\n model = [\n nn.Conv2D(num_channels, num_filters, kernel_size, stride=strides, padding=padding),\n nn.ReLU(),\n nn.Conv2D(num_filters, num_filters, 1),\n nn.ReLU(),\n nn.Conv2D(num_filters, num_filters, 1),\n nn.ReLU()\n ]\n self.model = nn.Sequential(*model)\n def forward(self, X):\n return self.model(X)",
"_____no_output_____"
]
],
[
[
"## [**7.3.2. NiN模型**]\n\n最初的 NiN 网络是在 AlexNet 后不久提出的,显然从中得到了一些启示。\nNiN使用窗口形状为 $11\\times 11$、$5\\times 5$ 和 $3\\times 3$的卷积层,输出通道数量与 AlexNet 中的相同。\n每个 NiN 块后有一个最大池化层,池化窗口形状为 $3\\times 3$,步幅为 2。\n\nNiN 和 AlexNet 之间的一个显著区别是 NiN 完全取消了全连接层。\n相反,NiN 使用一个 NiN块,其输出通道数等于标签类别的数量。最后放一个 *全局平均池化层*(global average pooling layer),生成一个多元逻辑向量(logits)。NiN 设计的一个优点是,它显著减少了模型所需参数的数量。然而,在实践中,这种设计有时会增加训练模型的时间。\n",
"_____no_output_____"
]
],
[
[
"class Net(nn.Layer):\n def __init__(self, num_channels, class_dim):\n super(Net, self).__init__()\n model = [\n Nin(num_channels, 96, 11, strides=4, padding=0),\n nn.MaxPool2D(kernel_size=3, stride=2),\n Nin(96, 256, 5, strides=1, padding=2),\n nn.MaxPool2D(kernel_size=3, stride=2),\n # Nin(256, 384, 3, strides=1, padding=1),\n # nn.MaxPool2D(kernel_size=3, stride=2),\n nn.Dropout(),\n # 标签类别数是10\n # Nin(384, 10, 3, strides=1, padding=1),\n Nin(256, 10, 3, strides=1, padding=1),\n paddle.fluid.dygraph.Pool2D(pool_type='max', global_pooling=True)\n ]\n self.model = nn.Sequential(*model)\n def forward(self, X):\n Y = self.model(X)\n Y = paddle.flatten(Y, start_axis=1)\n return Y\n\nwith paddle.fluid.dygraph.guard():\n net = Net(3, 10)\n X = paddle.to_tensor(np.random.uniform(-1., 1., [5, 3, 28, 28]).astype('float32'))\n Y = net(X)\n print(Y.shape)",
"[5, 10]\n"
]
],
[
[
"我们创建一个数据样本来[**查看每个块的输出形状**]。\n",
"_____no_output_____"
]
],
[
[
"with paddle.fluid.dygraph.guard():\n net = Net(1, 10)\n param_info = paddle.summary(net, (1, 1, 28, 28))\n print(param_info)",
"---------------------------------------------------------------------------\n Layer (type) Input Shape Output Shape Param # \n===========================================================================\n Conv2D-1 [[1, 1, 28, 28]] [1, 96, 5, 5] 11,712 \n ReLU-1 [[1, 96, 5, 5]] [1, 96, 5, 5] 0 \n Conv2D-2 [[1, 96, 5, 5]] [1, 96, 5, 5] 9,312 \n ReLU-2 [[1, 96, 5, 5]] [1, 96, 5, 5] 0 \n Conv2D-3 [[1, 96, 5, 5]] [1, 96, 5, 5] 9,312 \n ReLU-3 [[1, 96, 5, 5]] [1, 96, 5, 5] 0 \n Nin-1 [[1, 1, 28, 28]] [1, 96, 5, 5] 0 \n MaxPool2D-1 [[1, 96, 5, 5]] [1, 96, 2, 2] 0 \n Conv2D-4 [[1, 96, 2, 2]] [1, 256, 2, 2] 614,656 \n ReLU-4 [[1, 256, 2, 2]] [1, 256, 2, 2] 0 \n Conv2D-5 [[1, 256, 2, 2]] [1, 256, 2, 2] 65,792 \n ReLU-5 [[1, 256, 2, 2]] [1, 256, 2, 2] 0 \n Conv2D-6 [[1, 256, 2, 2]] [1, 256, 2, 2] 65,792 \n ReLU-6 [[1, 256, 2, 2]] [1, 256, 2, 2] 0 \n Nin-2 [[1, 96, 2, 2]] [1, 256, 2, 2] 0 \n MaxPool2D-2 [[1, 256, 2, 2]] [1, 256, 1, 1] 0 \n Dropout-1 [[1, 256, 1, 1]] [1, 256, 1, 1] 0 \n Conv2D-7 [[1, 256, 1, 1]] [1, 10, 1, 1] 23,050 \n ReLU-7 [[1, 10, 1, 1]] [1, 10, 1, 1] 0 \n Conv2D-8 [[1, 10, 1, 1]] [1, 10, 1, 1] 110 \n ReLU-8 [[1, 10, 1, 1]] [1, 10, 1, 1] 0 \n Conv2D-9 [[1, 10, 1, 1]] [1, 10, 1, 1] 110 \n ReLU-9 [[1, 10, 1, 1]] [1, 10, 1, 1] 0 \n Nin-3 [[1, 256, 1, 1]] [1, 10, 1, 1] 0 \n Pool2D-1 [[1, 10, 1, 1]] [1, 10, 1, 1] 0 \n===========================================================================\nTotal params: 799,846\nTrainable params: 799,846\nNon-trainable params: 0\n---------------------------------------------------------------------------\nInput size (MB): 0.00\nForward/backward pass size (MB): 0.19\nParams size (MB): 3.05\nEstimated Total Size (MB): 3.24\n---------------------------------------------------------------------------\n\n{'total_params': 799846, 'trainable_params': 799846}\n"
]
],
[
[
"## [**7.3.3训练模型**]\n\n和以前一样,我们使用 Fashion-MNIST 来训练模型。训练 NiN 与训练 AlexNet、VGG时相似。\n",
"_____no_output_____"
]
],
[
[
"import paddle\nimport paddle.vision.transforms as T\nfrom paddle.vision.datasets import FashionMNIST\n\n# 数据集处理\ntransform = T.Compose([\n T.Resize(64),\n T.Transpose(),\n T.Normalize([127.5], [127.5]),\n])\ntrain_dataset = FashionMNIST(mode='train', transform=transform)\nval_dataset = FashionMNIST(mode='test', transform=transform)\n# 模型定义\nmodel = paddle.Model(Net(1, 10))\n# 设置训练模型所需的optimizer, loss, metric\nmodel.prepare(\n paddle.optimizer.Adam(learning_rate=0.001, parameters=model.parameters()),\n paddle.nn.CrossEntropyLoss(),\n paddle.metric.Accuracy(topk=(1, 5)))\n# 启动训练、评估\nmodel.fit(train_dataset, val_dataset, epochs=2, batch_size=64, log_freq=100)",
"The loss value printed in the log is the current step, and the metric is the average value of previous steps.\nEpoch 1/2\nstep 100/938 - loss: 1.7510 - acc_top1: 0.3219 - acc_top5: 0.6873 - 13ms/step\nstep 200/938 - loss: 1.2018 - acc_top1: 0.3822 - acc_top5: 0.7639 - 13ms/step\nstep 300/938 - loss: 0.8502 - acc_top1: 0.4805 - acc_top5: 0.8007 - 13ms/step\nstep 400/938 - loss: 0.8844 - acc_top1: 0.5520 - acc_top5: 0.8247 - 13ms/step\nstep 500/938 - loss: 0.7246 - acc_top1: 0.5986 - acc_top5: 0.8372 - 13ms/step\nstep 600/938 - loss: 0.5191 - acc_top1: 0.6321 - acc_top5: 0.8473 - 13ms/step\nstep 700/938 - loss: 0.6790 - acc_top1: 0.6568 - acc_top5: 0.8539 - 13ms/step\nstep 800/938 - loss: 0.6095 - acc_top1: 0.6762 - acc_top5: 0.8587 - 13ms/step\nstep 900/938 - loss: 0.6649 - acc_top1: 0.6910 - acc_top5: 0.8624 - 13ms/step\nstep 938/938 - loss: 0.6247 - acc_top1: 0.6953 - acc_top5: 0.8635 - 13ms/step\nEval begin...\nstep 100/157 - loss: 0.5220 - acc_top1: 0.8167 - acc_top5: 0.8983 - 11ms/step\n"
]
],
[
[
"## 7.3.4. 小结\n\n* NiN使用由一个卷积层和多个 $1\\times 1$ 卷积层组成的块。该块可以在卷积神经网络中使用,以允许更多的每像素非线性。\n* NiN去除了容易造成过拟合的全连接层,将它们替换为全局平均池化层(即在所有位置上进行求和)。该池化层通道数量为所需的输出数量(例如,Fashion-MNIST的输出为10)。\n* 移除全连接层可减少过拟合,同时显著减少NiN的参数。\n* NiN的设计影响了许多后续卷积神经网络的设计。\n\n## 7.3.5.练习\n\n1. 调整NiN的超参数,以提高分类准确性。\n1. 为什么NiN块中有两个 $1\\times 1$ 卷积层?删除其中一个,然后观察和分析实验现象。\n1. 计算NiN的资源使用情况。\n 1. 参数的数量是多少?\n 1. 计算量是多少?\n 1. 训练期间需要多少显存?\n 1. 预测期间需要多少显存?\n1. 一次性直接将 $384 \\times 5 \\times 5$ 的表示缩减为 $10 \\times 5 \\times 5$ 的表示,会存在哪些问题?\n",
"_____no_output_____"
],
[
"[Discussions](https://discuss.d2l.ai/t/1869)\n",
"_____no_output_____"
]
]
] | [
"markdown",
"code",
"markdown",
"code",
"markdown",
"code",
"markdown",
"code",
"markdown",
"code",
"markdown"
] | [
[
"markdown"
],
[
"code"
],
[
"markdown"
],
[
"code"
],
[
"markdown"
],
[
"code"
],
[
"markdown"
],
[
"code"
],
[
"markdown"
],
[
"code"
],
[
"markdown",
"markdown"
]
] |
e7782a76160c0cc9a859a378171cd291c77fb269 | 99,062 | ipynb | Jupyter Notebook | Project_Plagiarism_Detection/2_Plagiarism_Feature_Engineering.ipynb | pooja63/Plagiarism-detection | 30f5bd7e3b051c06da2e898972d5625508a159a8 | [
"MIT"
] | null | null | null | Project_Plagiarism_Detection/2_Plagiarism_Feature_Engineering.ipynb | pooja63/Plagiarism-detection | 30f5bd7e3b051c06da2e898972d5625508a159a8 | [
"MIT"
] | null | null | null | Project_Plagiarism_Detection/2_Plagiarism_Feature_Engineering.ipynb | pooja63/Plagiarism-detection | 30f5bd7e3b051c06da2e898972d5625508a159a8 | [
"MIT"
] | null | null | null | 41.868977 | 1,359 | 0.55012 | [
[
[
"# Plagiarism Detection, Feature Engineering\n\nIn this project, you will be tasked with building a plagiarism detector that examines an answer text file and performs binary classification; labeling that file as either plagiarized or not, depending on how similar that text file is to a provided, source text. \n\nYour first task will be to create some features that can then be used to train a classification model. This task will be broken down into a few discrete steps:\n\n* Clean and pre-process the data.\n* Define features for comparing the similarity of an answer text and a source text, and extract similarity features.\n* Select \"good\" features, by analyzing the correlations between different features.\n* Create train/test `.csv` files that hold the relevant features and class labels for train/test data points.\n\nIn the _next_ notebook, Notebook 3, you'll use the features and `.csv` files you create in _this_ notebook to train a binary classification model in a SageMaker notebook instance.\n\nYou'll be defining a few different similarity features, as outlined in [this paper](https://s3.amazonaws.com/video.udacity-data.com/topher/2019/January/5c412841_developing-a-corpus-of-plagiarised-short-answers/developing-a-corpus-of-plagiarised-short-answers.pdf), which should help you build a robust plagiarism detector!\n\nTo complete this notebook, you'll have to complete all given exercises and answer all the questions in this notebook.\n> All your tasks will be clearly labeled **EXERCISE** and questions as **QUESTION**.\n\nIt will be up to you to decide on the features to include in your final training and test data.\n\n---",
"_____no_output_____"
],
[
"## Read in the Data\n\nThe cell below will download the necessary, project data and extract the files into the folder `data/`.\n\nThis data is a slightly modified version of a dataset created by Paul Clough (Information Studies) and Mark Stevenson (Computer Science), at the University of Sheffield. You can read all about the data collection and corpus, at [their university webpage](https://ir.shef.ac.uk/cloughie/resources/plagiarism_corpus.html). \n\n> **Citation for data**: Clough, P. and Stevenson, M. Developing A Corpus of Plagiarised Short Answers, Language Resources and Evaluation: Special Issue on Plagiarism and Authorship Analysis, In Press. [Download]",
"_____no_output_____"
]
],
[
[
"# NOTE:\n# you only need to run this cell if you have not yet downloaded the data\n# otherwise you may skip this cell or comment it out\n\n!wget https://s3.amazonaws.com/video.udacity-data.com/topher/2019/January/5c4147f9_data/data.zip\n!unzip data",
"--2019-12-01 11:39:14-- https://s3.amazonaws.com/video.udacity-data.com/topher/2019/January/5c4147f9_data/data.zip\nResolving s3.amazonaws.com (s3.amazonaws.com)... 52.216.98.85\nConnecting to s3.amazonaws.com (s3.amazonaws.com)|52.216.98.85|:443... connected.\nHTTP request sent, awaiting response... 200 OK\nLength: 113826 (111K) [application/zip]\nSaving to: ‘data.zip’\n\ndata.zip 100%[===================>] 111.16K --.-KB/s in 0.02s \n\n2019-12-01 11:39:14 (4.99 MB/s) - ‘data.zip’ saved [113826/113826]\n\nArchive: data.zip\n creating: data/\n inflating: data/.DS_Store \n creating: __MACOSX/\n creating: __MACOSX/data/\n inflating: __MACOSX/data/._.DS_Store \n inflating: data/file_information.csv \n inflating: __MACOSX/data/._file_information.csv \n inflating: data/g0pA_taska.txt \n inflating: __MACOSX/data/._g0pA_taska.txt \n inflating: data/g0pA_taskb.txt \n inflating: __MACOSX/data/._g0pA_taskb.txt \n inflating: data/g0pA_taskc.txt \n inflating: __MACOSX/data/._g0pA_taskc.txt \n inflating: data/g0pA_taskd.txt \n inflating: __MACOSX/data/._g0pA_taskd.txt \n inflating: data/g0pA_taske.txt \n inflating: __MACOSX/data/._g0pA_taske.txt \n inflating: data/g0pB_taska.txt \n inflating: __MACOSX/data/._g0pB_taska.txt \n inflating: data/g0pB_taskb.txt \n inflating: __MACOSX/data/._g0pB_taskb.txt \n inflating: data/g0pB_taskc.txt \n inflating: __MACOSX/data/._g0pB_taskc.txt \n inflating: data/g0pB_taskd.txt \n inflating: __MACOSX/data/._g0pB_taskd.txt \n inflating: data/g0pB_taske.txt \n inflating: __MACOSX/data/._g0pB_taske.txt \n inflating: data/g0pC_taska.txt \n inflating: __MACOSX/data/._g0pC_taska.txt \n inflating: data/g0pC_taskb.txt \n inflating: __MACOSX/data/._g0pC_taskb.txt \n inflating: data/g0pC_taskc.txt \n inflating: __MACOSX/data/._g0pC_taskc.txt \n inflating: data/g0pC_taskd.txt \n inflating: __MACOSX/data/._g0pC_taskd.txt \n inflating: data/g0pC_taske.txt \n inflating: __MACOSX/data/._g0pC_taske.txt \n inflating: data/g0pD_taska.txt \n inflating: __MACOSX/data/._g0pD_taska.txt \n inflating: data/g0pD_taskb.txt \n inflating: __MACOSX/data/._g0pD_taskb.txt \n inflating: data/g0pD_taskc.txt \n inflating: __MACOSX/data/._g0pD_taskc.txt \n inflating: data/g0pD_taskd.txt \n inflating: __MACOSX/data/._g0pD_taskd.txt \n inflating: data/g0pD_taske.txt \n inflating: __MACOSX/data/._g0pD_taske.txt \n inflating: data/g0pE_taska.txt \n inflating: __MACOSX/data/._g0pE_taska.txt \n inflating: data/g0pE_taskb.txt \n inflating: __MACOSX/data/._g0pE_taskb.txt \n inflating: data/g0pE_taskc.txt \n inflating: __MACOSX/data/._g0pE_taskc.txt \n inflating: data/g0pE_taskd.txt \n inflating: __MACOSX/data/._g0pE_taskd.txt \n inflating: data/g0pE_taske.txt \n inflating: __MACOSX/data/._g0pE_taske.txt \n inflating: data/g1pA_taska.txt \n inflating: __MACOSX/data/._g1pA_taska.txt \n inflating: data/g1pA_taskb.txt \n inflating: __MACOSX/data/._g1pA_taskb.txt \n inflating: data/g1pA_taskc.txt \n inflating: __MACOSX/data/._g1pA_taskc.txt \n inflating: data/g1pA_taskd.txt \n inflating: __MACOSX/data/._g1pA_taskd.txt \n inflating: data/g1pA_taske.txt \n inflating: __MACOSX/data/._g1pA_taske.txt \n inflating: data/g1pB_taska.txt \n inflating: __MACOSX/data/._g1pB_taska.txt \n inflating: data/g1pB_taskb.txt \n inflating: __MACOSX/data/._g1pB_taskb.txt \n inflating: data/g1pB_taskc.txt \n inflating: __MACOSX/data/._g1pB_taskc.txt \n inflating: data/g1pB_taskd.txt \n inflating: __MACOSX/data/._g1pB_taskd.txt \n inflating: data/g1pB_taske.txt \n inflating: __MACOSX/data/._g1pB_taske.txt \n inflating: data/g1pD_taska.txt \n inflating: __MACOSX/data/._g1pD_taska.txt \n inflating: data/g1pD_taskb.txt \n inflating: __MACOSX/data/._g1pD_taskb.txt \n inflating: data/g1pD_taskc.txt \n inflating: __MACOSX/data/._g1pD_taskc.txt \n inflating: data/g1pD_taskd.txt \n inflating: __MACOSX/data/._g1pD_taskd.txt \n inflating: data/g1pD_taske.txt \n inflating: __MACOSX/data/._g1pD_taske.txt \n inflating: data/g2pA_taska.txt \n inflating: __MACOSX/data/._g2pA_taska.txt \n inflating: data/g2pA_taskb.txt \n inflating: __MACOSX/data/._g2pA_taskb.txt \n inflating: data/g2pA_taskc.txt \n inflating: __MACOSX/data/._g2pA_taskc.txt \n inflating: data/g2pA_taskd.txt \n inflating: __MACOSX/data/._g2pA_taskd.txt \n inflating: data/g2pA_taske.txt \n inflating: __MACOSX/data/._g2pA_taske.txt \n inflating: data/g2pB_taska.txt \n inflating: __MACOSX/data/._g2pB_taska.txt \n inflating: data/g2pB_taskb.txt \n inflating: __MACOSX/data/._g2pB_taskb.txt \n inflating: data/g2pB_taskc.txt \n inflating: __MACOSX/data/._g2pB_taskc.txt \n inflating: data/g2pB_taskd.txt \n inflating: __MACOSX/data/._g2pB_taskd.txt \n inflating: data/g2pB_taske.txt \n inflating: __MACOSX/data/._g2pB_taske.txt \n inflating: data/g2pC_taska.txt \n inflating: __MACOSX/data/._g2pC_taska.txt \n inflating: data/g2pC_taskb.txt \n inflating: __MACOSX/data/._g2pC_taskb.txt \n inflating: data/g2pC_taskc.txt \n inflating: __MACOSX/data/._g2pC_taskc.txt \n inflating: data/g2pC_taskd.txt \n inflating: __MACOSX/data/._g2pC_taskd.txt \n inflating: data/g2pC_taske.txt \n inflating: __MACOSX/data/._g2pC_taske.txt \n inflating: data/g2pE_taska.txt \n inflating: __MACOSX/data/._g2pE_taska.txt \n inflating: data/g2pE_taskb.txt \n inflating: __MACOSX/data/._g2pE_taskb.txt \n inflating: data/g2pE_taskc.txt \n inflating: __MACOSX/data/._g2pE_taskc.txt \n inflating: data/g2pE_taskd.txt \n inflating: __MACOSX/data/._g2pE_taskd.txt \n inflating: data/g2pE_taske.txt \n inflating: __MACOSX/data/._g2pE_taske.txt \n inflating: data/g3pA_taska.txt \n inflating: __MACOSX/data/._g3pA_taska.txt \n inflating: data/g3pA_taskb.txt \n inflating: __MACOSX/data/._g3pA_taskb.txt \n inflating: data/g3pA_taskc.txt \n inflating: __MACOSX/data/._g3pA_taskc.txt \n inflating: data/g3pA_taskd.txt \n inflating: __MACOSX/data/._g3pA_taskd.txt \n inflating: data/g3pA_taske.txt \n inflating: __MACOSX/data/._g3pA_taske.txt \n inflating: data/g3pB_taska.txt \n inflating: __MACOSX/data/._g3pB_taska.txt \n inflating: data/g3pB_taskb.txt \n inflating: __MACOSX/data/._g3pB_taskb.txt \n inflating: data/g3pB_taskc.txt \n inflating: __MACOSX/data/._g3pB_taskc.txt \n inflating: data/g3pB_taskd.txt \n inflating: __MACOSX/data/._g3pB_taskd.txt \n inflating: data/g3pB_taske.txt \n inflating: __MACOSX/data/._g3pB_taske.txt \n inflating: data/g3pC_taska.txt \n inflating: __MACOSX/data/._g3pC_taska.txt \n inflating: data/g3pC_taskb.txt \n inflating: __MACOSX/data/._g3pC_taskb.txt \n inflating: data/g3pC_taskc.txt \n inflating: __MACOSX/data/._g3pC_taskc.txt \n inflating: data/g3pC_taskd.txt \n inflating: __MACOSX/data/._g3pC_taskd.txt \n inflating: data/g3pC_taske.txt \n inflating: __MACOSX/data/._g3pC_taske.txt \n inflating: data/g4pB_taska.txt \n inflating: __MACOSX/data/._g4pB_taska.txt \n inflating: data/g4pB_taskb.txt \n inflating: __MACOSX/data/._g4pB_taskb.txt \n inflating: data/g4pB_taskc.txt \n inflating: __MACOSX/data/._g4pB_taskc.txt \n inflating: data/g4pB_taskd.txt \n inflating: __MACOSX/data/._g4pB_taskd.txt \n inflating: data/g4pB_taske.txt \n inflating: __MACOSX/data/._g4pB_taske.txt \n inflating: data/g4pC_taska.txt \n inflating: __MACOSX/data/._g4pC_taska.txt \n inflating: data/g4pC_taskb.txt \n inflating: __MACOSX/data/._g4pC_taskb.txt \n inflating: data/g4pC_taskc.txt \n inflating: __MACOSX/data/._g4pC_taskc.txt \n inflating: data/g4pC_taskd.txt \n inflating: __MACOSX/data/._g4pC_taskd.txt \n inflating: data/g4pC_taske.txt \n inflating: __MACOSX/data/._g4pC_taske.txt \n inflating: data/g4pD_taska.txt \n inflating: __MACOSX/data/._g4pD_taska.txt \n inflating: data/g4pD_taskb.txt \n inflating: __MACOSX/data/._g4pD_taskb.txt \n inflating: data/g4pD_taskc.txt \n inflating: __MACOSX/data/._g4pD_taskc.txt \n inflating: data/g4pD_taskd.txt \n inflating: __MACOSX/data/._g4pD_taskd.txt \n inflating: data/g4pD_taske.txt \n inflating: __MACOSX/data/._g4pD_taske.txt \n inflating: data/g4pE_taska.txt \n inflating: __MACOSX/data/._g4pE_taska.txt \n inflating: data/g4pE_taskb.txt \n inflating: __MACOSX/data/._g4pE_taskb.txt \n inflating: data/g4pE_taskc.txt \n inflating: __MACOSX/data/._g4pE_taskc.txt \n inflating: data/g4pE_taskd.txt \n inflating: __MACOSX/data/._g4pE_taskd.txt \n inflating: data/g4pE_taske.txt \n inflating: __MACOSX/data/._g4pE_taske.txt \n inflating: data/orig_taska.txt \n inflating: __MACOSX/data/._orig_taska.txt \n inflating: data/orig_taskb.txt \n inflating: data/orig_taskc.txt \n inflating: __MACOSX/data/._orig_taskc.txt \n inflating: data/orig_taskd.txt \n inflating: __MACOSX/data/._orig_taskd.txt \n inflating: data/orig_taske.txt \n inflating: __MACOSX/data/._orig_taske.txt \n inflating: data/test_info.csv \n inflating: __MACOSX/data/._test_info.csv \n inflating: __MACOSX/._data \n"
],
[
"# import libraries\nimport pandas as pd\nimport numpy as np\nimport os",
"_____no_output_____"
]
],
[
[
"This plagiarism dataset is made of multiple text files; each of these files has characteristics that are is summarized in a `.csv` file named `file_information.csv`, which we can read in using `pandas`.",
"_____no_output_____"
]
],
[
[
"csv_file = 'data/file_information.csv'\nplagiarism_df = pd.read_csv(csv_file)\n\n# print out the first few rows of data info\nplagiarism_df.head()",
"_____no_output_____"
]
],
[
[
"## Types of Plagiarism\n\nEach text file is associated with one **Task** (task A-E) and one **Category** of plagiarism, which you can see in the above DataFrame.\n\n### Tasks, A-E\n\nEach text file contains an answer to one short question; these questions are labeled as tasks A-E. For example, Task A asks the question: \"What is inheritance in object oriented programming?\"\n\n### Categories of plagiarism \n\nEach text file has an associated plagiarism label/category:\n\n**1. Plagiarized categories: `cut`, `light`, and `heavy`.**\n* These categories represent different levels of plagiarized answer texts. `cut` answers copy directly from a source text, `light` answers are based on the source text but include some light rephrasing, and `heavy` answers are based on the source text, but *heavily* rephrased (and will likely be the most challenging kind of plagiarism to detect).\n \n**2. Non-plagiarized category: `non`.** \n* `non` indicates that an answer is not plagiarized; the Wikipedia source text is not used to create this answer.\n \n**3. Special, source text category: `orig`.**\n* This is a specific category for the original, Wikipedia source text. We will use these files only for comparison purposes.",
"_____no_output_____"
],
[
"---\n## Pre-Process the Data\n\nIn the next few cells, you'll be tasked with creating a new DataFrame of desired information about all of the files in the `data/` directory. This will prepare the data for feature extraction and for training a binary, plagiarism classifier.",
"_____no_output_____"
],
[
"### EXERCISE: Convert categorical to numerical data\n\nYou'll notice that the `Category` column in the data, contains string or categorical values, and to prepare these for feature extraction, we'll want to convert these into numerical values. Additionally, our goal is to create a binary classifier and so we'll need a binary class label that indicates whether an answer text is plagiarized (1) or not (0). Complete the below function `numerical_dataframe` that reads in a `file_information.csv` file by name, and returns a *new* DataFrame with a numerical `Category` column and a new `Class` column that labels each answer as plagiarized or not. \n\nYour function should return a new DataFrame with the following properties:\n\n* 4 columns: `File`, `Task`, `Category`, `Class`. The `File` and `Task` columns can remain unchanged from the original `.csv` file.\n* Convert all `Category` labels to numerical labels according to the following rules (a higher value indicates a higher degree of plagiarism):\n * 0 = `non`\n * 1 = `heavy`\n * 2 = `light`\n * 3 = `cut`\n * -1 = `orig`, this is a special value that indicates an original file.\n* For the new `Class` column\n * Any answer text that is not plagiarized (`non`) should have the class label `0`. \n * Any plagiarized answer texts should have the class label `1`. \n * And any `orig` texts will have a special label `-1`. \n\n### Expected output\n\nAfter running your function, you should get a DataFrame with rows that looks like the following: \n```\n\n File\t Task Category Class\n0\tg0pA_taska.txt\ta\t 0 \t0\n1\tg0pA_taskb.txt\tb\t 3 \t1\n2\tg0pA_taskc.txt\tc\t 2 \t1\n3\tg0pA_taskd.txt\td\t 1 \t1\n4\tg0pA_taske.txt\te\t 0\t 0\n...\n...\n99 orig_taske.txt e -1 -1\n\n```",
"_____no_output_____"
]
],
[
[
"# Read in a csv file and return a transformed dataframe\ndef numerical_dataframe(csv_file='data/file_information.csv'):\n '''Reads in a csv file which is assumed to have `File`, `Category` and `Task` columns.\n This function does two things: \n 1) converts `Category` column values to numerical values \n 2) Adds a new, numerical `Class` label column.\n The `Class` column will label plagiarized answers as 1 and non-plagiarized as 0.\n Source texts have a special label, -1.\n :param csv_file: The directory for the file_information.csv file\n :return: A dataframe with numerical categories and a new `Class` label column'''\n \n # your code here\n df = pd.read_csv(csv_file)\n df['Class'] = df[\"Category\"].map({'non':0,'heavy':1,'light':1,'cut':1,'orig':-1})\n\n df['Category'] = df['Category'].map({'non':0,'heavy':1,'light':2,'cut':3,'orig':-1})\n return df\n",
"_____no_output_____"
]
],
[
[
"### Test cells\n\nBelow are a couple of test cells. The first is an informal test where you can check that your code is working as expected by calling your function and printing out the returned result.\n\nThe **second** cell below is a more rigorous test cell. The goal of a cell like this is to ensure that your code is working as expected, and to form any variables that might be used in _later_ tests/code, in this case, the data frame, `transformed_df`.\n\n> The cells in this notebook should be run in chronological order (the order they appear in the notebook). This is especially important for test cells.\n\nOften, later cells rely on the functions, imports, or variables defined in earlier cells. For example, some tests rely on previous tests to work.\n\nThese tests do not test all cases, but they are a great way to check that you are on the right track!",
"_____no_output_____"
]
],
[
[
"# informal testing, print out the results of a called function\n# create new `transformed_df`\ntransformed_df = numerical_dataframe(csv_file ='data/file_information.csv')\n\n# check work\n# check that all categories of plagiarism have a class label = 1\ntransformed_df.head(10)",
"_____no_output_____"
],
[
"# test cell that creates `transformed_df`, if tests are passed\n\n\"\"\"\nDON'T MODIFY ANYTHING IN THIS CELL THAT IS BELOW THIS LINE\n\"\"\"\n\n# importing tests\nimport problem_unittests as tests\n\n# test numerical_dataframe function\ntests.test_numerical_df(numerical_dataframe)\n\n# if above test is passed, create NEW `transformed_df`\ntransformed_df = numerical_dataframe(csv_file ='data/file_information.csv')\n\n# check work\nprint('\\nExample data: ')\ntransformed_df.head()",
"Tests Passed!\n\nExample data: \n"
]
],
[
[
"## Text Processing & Splitting Data\n\nRecall that the goal of this project is to build a plagiarism classifier. At it's heart, this task is a comparison text; one that looks at a given answer and a source text, compares them and predicts whether an answer has plagiarized from the source. To effectively do this comparison, and train a classifier we'll need to do a few more things: pre-process all of our text data and prepare the text files (in this case, the 95 answer files and 5 original source files) to be easily compared, and split our data into a `train` and `test` set that can be used to train a classifier and evaluate it, respectively. \n\nTo this end, you've been provided code that adds additional information to your `transformed_df` from above. The next two cells need not be changed; they add two additional columns to the `transformed_df`:\n\n1. A `Text` column; this holds all the lowercase text for a `File`, with extraneous punctuation removed.\n2. A `Datatype` column; this is a string value `train`, `test`, or `orig` that labels a data point as part of our train or test set\n\nThe details of how these additional columns are created can be found in the `helpers.py` file in the project directory. You're encouraged to read through that file to see exactly how text is processed and how data is split.\n\nRun the cells below to get a `complete_df` that has all the information you need to proceed with plagiarism detection and feature engineering.",
"_____no_output_____"
]
],
[
[
"\"\"\"\nDON'T MODIFY ANYTHING IN THIS CELL THAT IS BELOW THIS LINE\n\"\"\"\nimport helpers \n\n# create a text column \ntext_df = helpers.create_text_column(transformed_df)\ntext_df.head()",
"_____no_output_____"
],
[
"# after running the cell above\n# check out the processed text for a single file, by row index\nrow_idx = 0 # feel free to change this index\n\nsample_text = text_df.iloc[0]['Text']\n\nprint('Sample processed text:\\n\\n', sample_text)",
"Sample processed text:\n\n inheritance is a basic concept of object oriented programming where the basic idea is to create new classes that add extra detail to existing classes this is done by allowing the new classes to reuse the methods and variables of the existing classes and new methods and classes are added to specialise the new class inheritance models the is kind of relationship between entities or objects for example postgraduates and undergraduates are both kinds of student this kind of relationship can be visualised as a tree structure where student would be the more general root node and both postgraduate and undergraduate would be more specialised extensions of the student node or the child nodes in this relationship student would be known as the superclass or parent class whereas postgraduate would be known as the subclass or child class because the postgraduate class extends the student class inheritance can occur on several layers where if visualised would display a larger tree structure for example we could further extend the postgraduate node by adding two extra extended classes to it called msc student and phd student as both these types of student are kinds of postgraduate student this would mean that both the msc student and phd student classes would inherit methods and variables from both the postgraduate and student classes \n"
]
],
[
[
"## Split data into training and test sets\n\nThe next cell will add a `Datatype` column to a given DataFrame to indicate if the record is: \n* `train` - Training data, for model training.\n* `test` - Testing data, for model evaluation.\n* `orig` - The task's original answer from wikipedia.\n\n### Stratified sampling\n\nThe given code uses a helper function which you can view in the `helpers.py` file in the main project directory. This implements [stratified random sampling](https://en.wikipedia.org/wiki/Stratified_sampling) to randomly split data by task & plagiarism amount. Stratified sampling ensures that we get training and test data that is fairly evenly distributed across task & plagiarism combinations. Approximately 26% of the data is held out for testing and 74% of the data is used for training.\n\nThe function **train_test_dataframe** takes in a DataFrame that it assumes has `Task` and `Category` columns, and, returns a modified frame that indicates which `Datatype` (train, test, or orig) a file falls into. This sampling will change slightly based on a passed in *random_seed*. Due to a small sample size, this stratified random sampling will provide more stable results for a binary plagiarism classifier. Stability here is smaller *variance* in the accuracy of classifier, given a random seed.",
"_____no_output_____"
]
],
[
[
"random_seed = 1 # can change; set for reproducibility\n\n\"\"\"\nDON'T MODIFY ANYTHING IN THIS CELL THAT IS BELOW THIS LINE\n\"\"\"\nimport helpers\n\n# create new df with Datatype (train, test, orig) column\n# pass in `text_df` from above to create a complete dataframe, with all the information you need\ncomplete_df = helpers.train_test_dataframe(text_df, random_seed=random_seed)\n\n# check results\ncomplete_df.head(10)",
"_____no_output_____"
]
],
[
[
"# Determining Plagiarism\n\nNow that you've prepared this data and created a `complete_df` of information, including the text and class associated with each file, you can move on to the task of extracting similarity features that will be useful for plagiarism classification. \n\n> Note: The following code exercises, assume that the `complete_df` as it exists now, will **not** have its existing columns modified. \n\nThe `complete_df` should always include the columns: `['File', 'Task', 'Category', 'Class', 'Text', 'Datatype']`. You can add additional columns, and you can create any new DataFrames you need by copying the parts of the `complete_df` as long as you do not modify the existing values, directly.\n\n---",
"_____no_output_____"
],
[
"\n# Similarity Features \n\nOne of the ways we might go about detecting plagiarism, is by computing **similarity features** that measure how similar a given answer text is as compared to the original wikipedia source text (for a specific task, a-e). The similarity features you will use are informed by [this paper on plagiarism detection](https://s3.amazonaws.com/video.udacity-data.com/topher/2019/January/5c412841_developing-a-corpus-of-plagiarised-short-answers/developing-a-corpus-of-plagiarised-short-answers.pdf). \n> In this paper, researchers created features called **containment** and **longest common subsequence**. \n\nUsing these features as input, you will train a model to distinguish between plagiarized and not-plagiarized text files.\n\n## Feature Engineering\n\nLet's talk a bit more about the features we want to include in a plagiarism detection model and how to calculate such features. In the following explanations, I'll refer to a submitted text file as a **Student Answer Text (A)** and the original, wikipedia source file (that we want to compare that answer to) as the **Wikipedia Source Text (S)**.\n\n### Containment\n\nYour first task will be to create **containment features**. To understand containment, let's first revisit a definition of [n-grams](https://en.wikipedia.org/wiki/N-gram). An *n-gram* is a sequential word grouping. For example, in a line like \"bayes rule gives us a way to combine prior knowledge with new information,\" a 1-gram is just one word, like \"bayes.\" A 2-gram might be \"bayes rule\" and a 3-gram might be \"combine prior knowledge.\"\n\n> Containment is defined as the **intersection** of the n-gram word count of the Wikipedia Source Text (S) with the n-gram word count of the Student Answer Text (S) *divided* by the n-gram word count of the Student Answer Text.\n\n$$ \\frac{\\sum{count(\\text{ngram}_{A}) \\cap count(\\text{ngram}_{S})}}{\\sum{count(\\text{ngram}_{A})}} $$\n\nIf the two texts have no n-grams in common, the containment will be 0, but if _all_ their n-grams intersect then the containment will be 1. Intuitively, you can see how having longer n-gram's in common, might be an indication of cut-and-paste plagiarism. In this project, it will be up to you to decide on the appropriate `n` or several `n`'s to use in your final model.\n\n### EXERCISE: Create containment features\n\nGiven the `complete_df` that you've created, you should have all the information you need to compare any Student Answer Text (A) with its appropriate Wikipedia Source Text (S). An answer for task A should be compared to the source text for task A, just as answers to tasks B, C, D, and E should be compared to the corresponding original source text.\n\nIn this exercise, you'll complete the function, `calculate_containment` which calculates containment based upon the following parameters:\n* A given DataFrame, `df` (which is assumed to be the `complete_df` from above)\n* An `answer_filename`, such as 'g0pB_taskd.txt' \n* An n-gram length, `n`\n\n### Containment calculation\n\nThe general steps to complete this function are as follows:\n1. From *all* of the text files in a given `df`, create an array of n-gram counts; it is suggested that you use a [CountVectorizer](https://scikit-learn.org/stable/modules/generated/sklearn.feature_extraction.text.CountVectorizer.html) for this purpose.\n2. Get the processed answer and source texts for the given `answer_filename`.\n3. Calculate the containment between an answer and source text according to the following equation.\n\n >$$ \\frac{\\sum{count(\\text{ngram}_{A}) \\cap count(\\text{ngram}_{S})}}{\\sum{count(\\text{ngram}_{A})}} $$\n \n4. Return that containment value.\n\nYou are encouraged to write any helper functions that you need to complete the function below.",
"_____no_output_____"
]
],
[
[
"# Calculate the ngram containment for one answer file/source file pair in a df\nfrom sklearn.feature_extraction.text import CountVectorizer\ndef calculate_containment(df, n, answer_filename):\n '''Calculates the containment between a given answer text and its associated source text.\n This function creates a count of ngrams (of a size, n) for each text file in our data.\n Then calculates the containment by finding the ngram count for a given answer text, \n and its associated source text, and calculating the normalized intersection of those counts.\n :param df: A dataframe with columns,\n 'File', 'Task', 'Category', 'Class', 'Text', and 'Datatype'\n :param n: An integer that defines the ngram size\n :param answer_filename: A filename for an answer text in the df, ex. 'g0pB_taskd.txt'\n :return: A single containment value that represents the similarity\n between an answer text and its source text.\n '''\n \n # your code here\n df_answer = df.loc[df['File']==answer_filename]\n task = df_answer['Task'].values[0]\n answer_text = df_answer['Text'].values[0]\n source_text = df.loc[(df['Task']==task) & (df['Datatype']=='orig')]['Text'].values[0]\n counts = CountVectorizer(analyzer='word', ngram_range=(n,n))\n ngrams = counts.fit_transform([answer_text, source_text])\n \n ngram_array = ngrams.toarray()\n answer_ngram = ngram_array[0]\n source_ngram = ngram_array[1]\n #print(answer_ngram, source_ngram)\n containment = 0\n for i,j in zip(answer_ngram, source_ngram):\n containment += min(i,j)\n \n return containment/sum(answer_ngram)\n",
"_____no_output_____"
]
],
[
[
"### Test cells\n\nAfter you've implemented the containment function, you can test out its behavior. \n\nThe cell below iterates through the first few files, and calculates the original category _and_ containment values for a specified n and file.\n\n>If you've implemented this correctly, you should see that the non-plagiarized have low or close to 0 containment values and that plagiarized examples have higher containment values, closer to 1.\n\nNote what happens when you change the value of n. I recommend applying your code to multiple files and comparing the resultant containment values. You should see that the highest containment values correspond to files with the highest category (`cut`) of plagiarism level.",
"_____no_output_____"
]
],
[
[
"# select a value for n\nn = 3\n\n# indices for first few files\ntest_indices = range(5)\n\n# iterate through files and calculate containment\ncategory_vals = []\ncontainment_vals = []\nfor i in test_indices:\n # get level of plagiarism for a given file index\n category_vals.append(complete_df.loc[i, 'Category'])\n # calculate containment for given file and n\n filename = complete_df.loc[i, 'File']\n c = calculate_containment(complete_df, n, filename)\n containment_vals.append(c)\n\n# print out result, does it make sense?\nprint('Original category values: \\n', category_vals)\nprint()\nprint(str(n)+'-gram containment values: \\n', containment_vals)",
"Original category values: \n [0, 3, 2, 1, 0]\n\n3-gram containment values: \n [0.009345794392523364, 0.9641025641025641, 0.6136363636363636, 0.15675675675675677, 0.031746031746031744]\n"
],
[
"# run this test cell\n\"\"\"\nDON'T MODIFY ANYTHING IN THIS CELL THAT IS BELOW THIS LINE\n\"\"\"\n# test containment calculation\n# params: complete_df from before, and containment function\ntests.test_containment(complete_df, calculate_containment)",
"Tests Passed!\n"
]
],
[
[
"### QUESTION 1: Why can we calculate containment features across *all* data (training & test), prior to splitting the DataFrame for modeling? That is, what about the containment calculation means that the test and training data do not influence each other?",
"_____no_output_____"
],
[
"**Answer:**\nTesting and training data need to be split while training a model. Since containment calculation does not involve training a model but just preprocessing of data that is required for both testing and training data, it doesnot matter that the data is not split.",
"_____no_output_____"
],
[
"---\n## Longest Common Subsequence\n\nContainment a good way to find overlap in word usage between two documents; it may help identify cases of cut-and-paste as well as paraphrased levels of plagiarism. Since plagiarism is a fairly complex task with varying levels, it's often useful to include other measures of similarity. The paper also discusses a feature called **longest common subsequence**.\n\n> The longest common subsequence is the longest string of words (or letters) that are *the same* between the Wikipedia Source Text (S) and the Student Answer Text (A). This value is also normalized by dividing by the total number of words (or letters) in the Student Answer Text. \n\nIn this exercise, we'll ask you to calculate the longest common subsequence of words between two texts.\n\n### EXERCISE: Calculate the longest common subsequence\n\nComplete the function `lcs_norm_word`; this should calculate the *longest common subsequence* of words between a Student Answer Text and corresponding Wikipedia Source Text. \n\nIt may be helpful to think of this in a concrete example. A Longest Common Subsequence (LCS) problem may look as follows:\n* Given two texts: text A (answer text) of length n, and string S (original source text) of length m. Our goal is to produce their longest common subsequence of words: the longest sequence of words that appear left-to-right in both texts (though the words don't have to be in continuous order).\n* Consider:\n * A = \"i think pagerank is a link analysis algorithm used by google that uses a system of weights attached to each element of a hyperlinked set of documents\"\n * S = \"pagerank is a link analysis algorithm used by the google internet search engine that assigns a numerical weighting to each element of a hyperlinked set of documents\"\n\n* In this case, we can see that the start of each sentence of fairly similar, having overlap in the sequence of words, \"pagerank is a link analysis algorithm used by\" before diverging slightly. Then we **continue moving left -to-right along both texts** until we see the next common sequence; in this case it is only one word, \"google\". Next we find \"that\" and \"a\" and finally the same ending \"to each element of a hyperlinked set of documents\".\n* Below, is a clear visual of how these sequences were found, sequentially, in each text.\n\n<img src='notebook_ims/common_subseq_words.png' width=40% />\n\n* Now, those words appear in left-to-right order in each document, sequentially, and even though there are some words in between, we count this as the longest common subsequence between the two texts. \n* If I count up each word that I found in common I get the value 20. **So, LCS has length 20**. \n* Next, to normalize this value, divide by the total length of the student answer; in this example that length is only 27. **So, the function `lcs_norm_word` should return the value `20/27` or about `0.7408`.**\n\nIn this way, LCS is a great indicator of cut-and-paste plagiarism or if someone has referenced the same source text multiple times in an answer.",
"_____no_output_____"
],
[
"### LCS, dynamic programming\n\nIf you read through the scenario above, you can see that this algorithm depends on looking at two texts and comparing them word by word. You can solve this problem in multiple ways. First, it may be useful to `.split()` each text into lists of comma separated words to compare. Then, you can iterate through each word in the texts and compare them, adding to your value for LCS as you go. \n\nThe method I recommend for implementing an efficient LCS algorithm is: using a matrix and dynamic programming. **Dynamic programming** is all about breaking a larger problem into a smaller set of subproblems, and building up a complete result without having to repeat any subproblems. \n\nThis approach assumes that you can split up a large LCS task into a combination of smaller LCS tasks. Let's look at a simple example that compares letters:\n\n* A = \"ABCD\"\n* S = \"BD\"\n\nWe can see right away that the longest subsequence of _letters_ here is 2 (B and D are in sequence in both strings). And we can calculate this by looking at relationships between each letter in the two strings, A and S.\n\nHere, I have a matrix with the letters of A on top and the letters of S on the left side:\n\n<img src='notebook_ims/matrix_1.png' width=40% />\n\nThis starts out as a matrix that has as many columns and rows as letters in the strings S and O **+1** additional row and column, filled with zeros on the top and left sides. So, in this case, instead of a 2x4 matrix it is a 3x5.\n\nNow, we can fill this matrix up by breaking it into smaller LCS problems. For example, let's first look at the shortest substrings: the starting letter of A and S. We'll first ask, what is the Longest Common Subsequence between these two letters \"A\" and \"B\"? \n\n**Here, the answer is zero and we fill in the corresponding grid cell with that value.**\n\n<img src='notebook_ims/matrix_2.png' width=30% />\n\nThen, we ask the next question, what is the LCS between \"AB\" and \"B\"?\n\n**Here, we have a match, and can fill in the appropriate value 1**.\n\n<img src='notebook_ims/matrix_3_match.png' width=25% />\n\nIf we continue, we get to a final matrix that looks as follows, with a **2** in the bottom right corner.\n\n<img src='notebook_ims/matrix_6_complete.png' width=25% />\n\nThe final LCS will be that value **2** *normalized* by the number of n-grams in A. So, our normalized value is 2/4 = **0.5**.\n\n### The matrix rules\n\nOne thing to notice here is that, you can efficiently fill up this matrix one cell at a time. Each grid cell only depends on the values in the grid cells that are directly on top and to the left of it, or on the diagonal/top-left. The rules are as follows:\n* Start with a matrix that has one extra row and column of zeros.\n* As you traverse your string:\n * If there is a match, fill that grid cell with the value to the top-left of that cell *plus* one. So, in our case, when we found a matching B-B, we added +1 to the value in the top-left of the matching cell, 0.\n * If there is not a match, take the *maximum* value from either directly to the left or the top cell, and carry that value over to the non-match cell.\n\n<img src='notebook_ims/matrix_rules.png' width=50% />\n\nAfter completely filling the matrix, **the bottom-right cell will hold the non-normalized LCS value**.\n\nThis matrix treatment can be applied to a set of words instead of letters. Your function should apply this to the words in two texts and return the normalized LCS value.",
"_____no_output_____"
]
],
[
[
"# Compute the normalized LCS given an answer text and a source text\ndef lcs_norm_word(answer_text, source_text):\n '''Computes the longest common subsequence of words in two texts; returns a normalized value.\n :param answer_text: The pre-processed text for an answer text\n :param source_text: The pre-processed text for an answer's associated source text\n :return: A normalized LCS value'''\n \n # your code here\n ans_words = answer_text.split()\n src_words = source_text.split()\n ans_length = len(ans_words)\n src_length = len(src_words)\n lcs = np.zeros((ans_length + 1, src_length+1),dtype=int)\n for i, ans in enumerate(ans_words,1):\n for j, src in enumerate(src_words,1):\n if ans == src:\n lcs[i][j] = lcs[i-1][j-1] +1\n else:\n lcs[i][j] = max(lcs[i-1][j], lcs[i][j-1])\n lcs_res = lcs[i][j]/i\n return lcs_res",
"_____no_output_____"
]
],
[
[
"### Test cells\n\nLet's start by testing out your code on the example given in the initial description.\n\nIn the below cell, we have specified strings A (answer text) and S (original source text). We know that these texts have 20 words in common and the submitted answer is 27 words long, so the normalized, longest common subsequence should be 20/27.\n",
"_____no_output_____"
]
],
[
[
"# Run the test scenario from above\n# does your function return the expected value?\n\nA = \"i think pagerank is a link analysis algorithm used by google that uses a system of weights attached to each element of a hyperlinked set of documents\"\nS = \"pagerank is a link analysis algorithm used by the google internet search engine that assigns a numerical weighting to each element of a hyperlinked set of documents\"\n\n# calculate LCS\nlcs = lcs_norm_word(A, S)\nprint('LCS = ', lcs)\n\n\n# expected value test\nassert lcs==20/27., \"Incorrect LCS value, expected about 0.7408, got \"+str(lcs)\n\nprint('Test passed!')",
"LCS = 0.7407407407407407\nTest passed!\n"
]
],
[
[
"This next cell runs a more rigorous test.",
"_____no_output_____"
]
],
[
[
"# run test cell\n\"\"\"\nDON'T MODIFY ANYTHING IN THIS CELL THAT IS BELOW THIS LINE\n\"\"\"\n# test lcs implementation\n# params: complete_df from before, and lcs_norm_word function\ntests.test_lcs(complete_df, lcs_norm_word)",
"Tests Passed!\n"
]
],
[
[
"Finally, take a look at a few resultant values for `lcs_norm_word`. Just like before, you should see that higher values correspond to higher levels of plagiarism.",
"_____no_output_____"
]
],
[
[
"# test on your own\ntest_indices = range(5) # look at first few files\n\ncategory_vals = []\nlcs_norm_vals = []\n# iterate through first few docs and calculate LCS\nfor i in test_indices:\n category_vals.append(complete_df.loc[i, 'Category'])\n # get texts to compare\n answer_text = complete_df.loc[i, 'Text'] \n task = complete_df.loc[i, 'Task']\n # we know that source texts have Class = -1\n orig_rows = complete_df[(complete_df['Class'] == -1)]\n orig_row = orig_rows[(orig_rows['Task'] == task)]\n source_text = orig_row['Text'].values[0]\n \n # calculate lcs\n lcs_val = lcs_norm_word(answer_text, source_text)\n lcs_norm_vals.append(lcs_val)\n\n# print out result, does it make sense?\nprint('Original category values: \\n', category_vals)\nprint()\nprint('Normalized LCS values: \\n', lcs_norm_vals)",
"Original category values: \n [0, 3, 2, 1, 0]\n\nNormalized LCS values: \n [0.1917808219178082, 0.8207547169811321, 0.8464912280701754, 0.3160621761658031, 0.24257425742574257]\n"
]
],
[
[
"---\n# Create All Features\n\nNow that you've completed the feature calculation functions, it's time to actually create multiple features and decide on which ones to use in your final model! In the below cells, you're provided two helper functions to help you create multiple features and store those in a DataFrame, `features_df`.\n\n### Creating multiple containment features\n\nYour completed `calculate_containment` function will be called in the next cell, which defines the helper function `create_containment_features`. \n\n> This function returns a list of containment features, calculated for a given `n` and for *all* files in a df (assumed to the the `complete_df`).\n\nFor our original files, the containment value is set to a special value, -1.\n\nThis function gives you the ability to easily create several containment features, of different n-gram lengths, for each of our text files.",
"_____no_output_____"
]
],
[
[
"\"\"\"\nDON'T MODIFY ANYTHING IN THIS CELL THAT IS BELOW THIS LINE\n\"\"\"\n# Function returns a list of containment features, calculated for a given n \n# Should return a list of length 100 for all files in a complete_df\ndef create_containment_features(df, n, column_name=None):\n \n containment_values = []\n \n if(column_name==None):\n column_name = 'c_'+str(n) # c_1, c_2, .. c_n\n \n # iterates through dataframe rows\n for i in df.index:\n file = df.loc[i, 'File']\n # Computes features using calculate_containment function\n if df.loc[i,'Category'] > -1:\n c = calculate_containment(df, n, file)\n containment_values.append(c)\n # Sets value to -1 for original tasks \n else:\n containment_values.append(-1)\n \n print(str(n)+'-gram containment features created!')\n return containment_values\n",
"_____no_output_____"
]
],
[
[
"### Creating LCS features\n\nBelow, your complete `lcs_norm_word` function is used to create a list of LCS features for all the answer files in a given DataFrame (again, this assumes you are passing in the `complete_df`. It assigns a special value for our original, source files, -1.\n",
"_____no_output_____"
]
],
[
[
"\"\"\"\nDON'T MODIFY ANYTHING IN THIS CELL THAT IS BELOW THIS LINE\n\"\"\"\n# Function creates lcs feature and add it to the dataframe\ndef create_lcs_features(df, column_name='lcs_word'):\n \n lcs_values = []\n \n # iterate through files in dataframe\n for i in df.index:\n # Computes LCS_norm words feature using function above for answer tasks\n if df.loc[i,'Category'] > -1:\n # get texts to compare\n answer_text = df.loc[i, 'Text'] \n task = df.loc[i, 'Task']\n # we know that source texts have Class = -1\n orig_rows = df[(df['Class'] == -1)]\n orig_row = orig_rows[(orig_rows['Task'] == task)]\n source_text = orig_row['Text'].values[0]\n\n # calculate lcs\n lcs = lcs_norm_word(answer_text, source_text)\n lcs_values.append(lcs)\n # Sets to -1 for original tasks \n else:\n lcs_values.append(-1)\n\n print('LCS features created!')\n return lcs_values\n ",
"_____no_output_____"
]
],
[
[
"## EXERCISE: Create a features DataFrame by selecting an `ngram_range`\n\nThe paper suggests calculating the following features: containment *1-gram to 5-gram* and *longest common subsequence*. \n> In this exercise, you can choose to create even more features, for example from *1-gram to 7-gram* containment features and *longest common subsequence*. \n\nYou'll want to create at least 6 features to choose from as you think about which to give to your final, classification model. Defining and comparing at least 6 different features allows you to discard any features that seem redundant, and choose to use the best features for your final model!\n\nIn the below cell **define an n-gram range**; these will be the n's you use to create n-gram containment features. The rest of the feature creation code is provided.",
"_____no_output_____"
]
],
[
[
"# Define an ngram range\nngram_range = range(1,7)\n\n\n# The following code may take a minute to run, depending on your ngram_range\n\"\"\"\nDON'T MODIFY ANYTHING IN THIS CELL THAT IS BELOW THIS LINE\n\"\"\"\nfeatures_list = []\n\n# Create features in a features_df\nall_features = np.zeros((len(ngram_range)+1, len(complete_df)))\n\n# Calculate features for containment for ngrams in range\ni=0\nfor n in ngram_range:\n column_name = 'c_'+str(n)\n features_list.append(column_name)\n # create containment features\n all_features[i]=np.squeeze(create_containment_features(complete_df, n))\n i+=1\n\n# Calculate features for LCS_Norm Words \nfeatures_list.append('lcs_word')\nall_features[i]= np.squeeze(create_lcs_features(complete_df))\n\n# create a features dataframe\nfeatures_df = pd.DataFrame(np.transpose(all_features), columns=features_list)\n\n# Print all features/columns\nprint()\nprint('Features: ', features_list)\nprint()",
"1-gram containment features created!\n2-gram containment features created!\n3-gram containment features created!\n4-gram containment features created!\n5-gram containment features created!\n6-gram containment features created!\nLCS features created!\n\nFeatures: ['c_1', 'c_2', 'c_3', 'c_4', 'c_5', 'c_6', 'lcs_word']\n\n"
],
[
"# print some results \nfeatures_df.head(10)",
"_____no_output_____"
]
],
[
[
"## Correlated Features\n\nYou should use feature correlation across the *entire* dataset to determine which features are ***too*** **highly-correlated** with each other to include both features in a single model. For this analysis, you can use the *entire* dataset due to the small sample size we have. \n\nAll of our features try to measure the similarity between two texts. Since our features are designed to measure similarity, it is expected that these features will be highly-correlated. Many classification models, for example a Naive Bayes classifier, rely on the assumption that features are *not* highly correlated; highly-correlated features may over-inflate the importance of a single feature. \n\nSo, you'll want to choose your features based on which pairings have the lowest correlation. These correlation values range between 0 and 1; from low to high correlation, and are displayed in a [correlation matrix](https://www.displayr.com/what-is-a-correlation-matrix/), below.",
"_____no_output_____"
]
],
[
[
"\"\"\"\nDON'T MODIFY ANYTHING IN THIS CELL THAT IS BELOW THIS LINE\n\"\"\"\n# Create correlation matrix for just Features to determine different models to test\ncorr_matrix = features_df.corr().abs().round(2)\n\n# display shows all of a dataframe\ndisplay(corr_matrix)",
"_____no_output_____"
]
],
[
[
"## EXERCISE: Create selected train/test data\n\nComplete the `train_test_data` function below. This function should take in the following parameters:\n* `complete_df`: A DataFrame that contains all of our processed text data, file info, datatypes, and class labels\n* `features_df`: A DataFrame of all calculated features, such as containment for ngrams, n= 1-5, and lcs values for each text file listed in the `complete_df` (this was created in the above cells)\n* `selected_features`: A list of feature column names, ex. `['c_1', 'lcs_word']`, which will be used to select the final features in creating train/test sets of data.\n\nIt should return two tuples:\n* `(train_x, train_y)`, selected training features and their corresponding class labels (0/1)\n* `(test_x, test_y)`, selected training features and their corresponding class labels (0/1)\n\n** Note: x and y should be arrays of feature values and numerical class labels, respectively; not DataFrames.**\n\nLooking at the above correlation matrix, you should decide on a **cutoff** correlation value, less than 1.0, to determine which sets of features are *too* highly-correlated to be included in the final training and test data. If you cannot find features that are less correlated than some cutoff value, it is suggested that you increase the number of features (longer n-grams) to choose from or use *only one or two* features in your final model to avoid introducing highly-correlated features.\n\nRecall that the `complete_df` has a `Datatype` column that indicates whether data should be `train` or `test` data; this should help you split the data appropriately.",
"_____no_output_____"
]
],
[
[
"# Takes in dataframes and a list of selected features (column names) \n# and returns (train_x, train_y), (test_x, test_y)\ndef train_test_data(complete_df, features_df, selected_features):\n '''Gets selected training and test features from given dataframes, and \n returns tuples for training and test features and their corresponding class labels.\n :param complete_df: A dataframe with all of our processed text data, datatypes, and labels\n :param features_df: A dataframe of all computed, similarity features\n :param selected_features: An array of selected features that correspond to certain columns in `features_df`\n :return: training and test features and labels: (train_x, train_y), (test_x, test_y)'''\n \n df = pd.concat([complete_df, features_df[selected_features]], axis=1)\n df_train = df[df['Datatype']=='train']\n df_test = df[df['Datatype']=='test']\n # get the training features\n train_x = df_train[selected_features].values\n # And training class labels (0 or 1)\n train_y = df_train['Class'].values\n \n # get the test features and labels\n test_x = df_test[selected_features].values\n test_y = df_test['Class'].values\n \n return (train_x, train_y), (test_x, test_y)\n\n ",
"_____no_output_____"
]
],
[
[
"### Test cells\n\nBelow, test out your implementation and create the final train/test data.",
"_____no_output_____"
]
],
[
[
"\"\"\"\nDON'T MODIFY ANYTHING IN THIS CELL THAT IS BELOW THIS LINE\n\"\"\"\ntest_selection = list(features_df)[:2] # first couple columns as a test\n# test that the correct train/test data is created\n(train_x, train_y), (test_x, test_y) = train_test_data(complete_df, features_df, test_selection)\n\n# params: generated train/test data\ntests.test_data_split(train_x, train_y, test_x, test_y)",
"Tests Passed!\n"
]
],
[
[
"## EXERCISE: Select \"good\" features\n\nIf you passed the test above, you can create your own train/test data, below. \n\nDefine a list of features you'd like to include in your final mode, `selected_features`; this is a list of the features names you want to include.",
"_____no_output_____"
]
],
[
[
"# Select your list of features, this should be column names from features_df\n# ex. ['c_1', 'lcs_word']\nselected_features = ['c_1', 'c_5', 'lcs_word']\n\n\n\"\"\"\nDON'T MODIFY ANYTHING IN THIS CELL THAT IS BELOW THIS LINE\n\"\"\"\n\n(train_x, train_y), (test_x, test_y) = train_test_data(complete_df, features_df, selected_features)\n\n# check that division of samples seems correct\n# these should add up to 95 (100 - 5 original files)\nprint('Training size: ', len(train_x))\nprint('Test size: ', len(test_x))\nprint()\nprint('Training df sample: \\n', train_x[:10])",
"Training size: 70\nTest size: 25\n\nTraining df sample: \n [[0.39814815 0. 0.19178082]\n [0.86936937 0.44954128 0.84649123]\n [0.59358289 0.08196721 0.31606218]\n [0.54450262 0. 0.24257426]\n [0.32950192 0. 0.16117216]\n [0.59030837 0. 0.30165289]\n [0.75977654 0.24571429 0.48430493]\n [0.51612903 0. 0.27083333]\n [0.44086022 0. 0.22395833]\n [0.97945205 0.78873239 0.9 ]]\n"
]
],
[
[
"### Question 2: How did you decide on which features to include in your final model? ",
"_____no_output_____"
],
[
"**Answer:**\nlcs is one of the most important features so I have selected that. Also, its important to assess the number of words in common, so I have selected c_1 and c_5 because the correlation factor is 1 with c_4 and c_6 and also close to 1 for c_2 and c_3",
"_____no_output_____"
],
[
"---\n## Creating Final Data Files\n\nNow, you are almost ready to move on to training a model in SageMaker!\n\nYou'll want to access your train and test data in SageMaker and upload it to S3. In this project, SageMaker will expect the following format for your train/test data:\n* Training and test data should be saved in one `.csv` file each, ex `train.csv` and `test.csv`\n* These files should have class labels in the first column and features in the rest of the columns\n\nThis format follows the practice, outlined in the [SageMaker documentation](https://docs.aws.amazon.com/sagemaker/latest/dg/cdf-training.html), which reads: \"Amazon SageMaker requires that a CSV file doesn't have a header record and that the target variable [class label] is in the first column.\"\n\n## EXERCISE: Create csv files\n\nDefine a function that takes in x (features) and y (labels) and saves them to one `.csv` file at the path `data_dir/filename`.\n\nIt may be useful to use pandas to merge your features and labels into one DataFrame and then convert that into a csv file. You can make sure to get rid of any incomplete rows, in a DataFrame, by using `dropna`.",
"_____no_output_____"
]
],
[
[
"def make_csv(x, y, filename, data_dir):\n '''Merges features and labels and converts them into one csv file with labels in the first column.\n :param x: Data features\n :param y: Data labels\n :param file_name: Name of csv file, ex. 'train.csv'\n :param data_dir: The directory where files will be saved\n '''\n # make data dir, if it does not exist\n if not os.path.exists(data_dir):\n os.makedirs(data_dir)\n \n \n # your code here\n \n pd.concat([pd.DataFrame(y), pd.DataFrame(x)], axis=1).dropna().to_csv(os.path.join(data_dir, filename), header=False, index=False)\n\n # nothing is returned, but a print statement indicates that the function has run\n print('Path created: '+str(data_dir)+'/'+str(filename))",
"_____no_output_____"
]
],
[
[
"### Test cells\n\nTest that your code produces the correct format for a `.csv` file, given some text features and labels.",
"_____no_output_____"
]
],
[
[
"\"\"\"\nDON'T MODIFY ANYTHING IN THIS CELL THAT IS BELOW THIS LINE\n\"\"\"\nfake_x = [ [0.39814815, 0.0001, 0.19178082], \n [0.86936937, 0.44954128, 0.84649123], \n [0.44086022, 0., 0.22395833] ]\n\nfake_y = [0, 1, 1]\n\nmake_csv(fake_x, fake_y, filename='to_delete.csv', data_dir='test_csv')\n\n# read in and test dimensions\nfake_df = pd.read_csv('test_csv/to_delete.csv', header=None)\n\n# check shape\nassert fake_df.shape==(3, 4), \\\n 'The file should have as many rows as data_points and as many columns as features+1 (for indices).'\n# check that first column = labels\nassert np.all(fake_df.iloc[:,0].values==fake_y), 'First column is not equal to the labels, fake_y.'\nprint('Tests passed!')",
"Path created: test_csv/to_delete.csv\nTests passed!\n"
],
[
"# delete the test csv file, generated above\n! rm -rf test_csv",
"_____no_output_____"
]
],
[
[
"If you've passed the tests above, run the following cell to create `train.csv` and `test.csv` files in a directory that you specify! This will save the data in a local directory. Remember the name of this directory because you will reference it again when uploading this data to S3.",
"_____no_output_____"
]
],
[
[
"# can change directory, if you want\ndata_dir = 'plagiarism_data'\n\n\"\"\"\nDON'T MODIFY ANYTHING IN THIS CELL THAT IS BELOW THIS LINE\n\"\"\"\n\nmake_csv(train_x, train_y, filename='train.csv', data_dir=data_dir)\nmake_csv(test_x, test_y, filename='test.csv', data_dir=data_dir)",
"Path created: plagiarism_data/train.csv\nPath created: plagiarism_data/test.csv\n"
]
],
[
[
"## Up Next\n\nNow that you've done some feature engineering and created some training and test data, you are ready to train and deploy a plagiarism classification model. The next notebook will utilize SageMaker resources to train and test a model that you design.",
"_____no_output_____"
]
]
] | [
"markdown",
"code",
"markdown",
"code",
"markdown",
"code",
"markdown",
"code",
"markdown",
"code",
"markdown",
"code",
"markdown",
"code",
"markdown",
"code",
"markdown",
"code",
"markdown",
"code",
"markdown",
"code",
"markdown",
"code",
"markdown",
"code",
"markdown",
"code",
"markdown",
"code",
"markdown",
"code",
"markdown",
"code",
"markdown",
"code",
"markdown",
"code",
"markdown",
"code",
"markdown",
"code",
"markdown",
"code",
"markdown"
] | [
[
"markdown",
"markdown"
],
[
"code",
"code"
],
[
"markdown"
],
[
"code"
],
[
"markdown",
"markdown",
"markdown"
],
[
"code"
],
[
"markdown"
],
[
"code",
"code"
],
[
"markdown"
],
[
"code",
"code"
],
[
"markdown"
],
[
"code"
],
[
"markdown",
"markdown"
],
[
"code"
],
[
"markdown"
],
[
"code",
"code"
],
[
"markdown",
"markdown",
"markdown",
"markdown"
],
[
"code"
],
[
"markdown"
],
[
"code"
],
[
"markdown"
],
[
"code"
],
[
"markdown"
],
[
"code"
],
[
"markdown"
],
[
"code"
],
[
"markdown"
],
[
"code"
],
[
"markdown"
],
[
"code",
"code"
],
[
"markdown"
],
[
"code"
],
[
"markdown"
],
[
"code"
],
[
"markdown"
],
[
"code"
],
[
"markdown"
],
[
"code"
],
[
"markdown",
"markdown",
"markdown"
],
[
"code"
],
[
"markdown"
],
[
"code",
"code"
],
[
"markdown"
],
[
"code"
],
[
"markdown"
]
] |
e7782b71b88fc70bc798e205e0c16845e2d32a0a | 28,864 | ipynb | Jupyter Notebook | notebooks/Behaviour.ipynb | CallumJHays/g26-egb320-2019 | 6dde6b5d2f72fac3928c5042a27dc50e978c3425 | [
"MIT"
] | null | null | null | notebooks/Behaviour.ipynb | CallumJHays/g26-egb320-2019 | 6dde6b5d2f72fac3928c5042a27dc50e978c3425 | [
"MIT"
] | null | null | null | notebooks/Behaviour.ipynb | CallumJHays/g26-egb320-2019 | 6dde6b5d2f72fac3928c5042a27dc50e978c3425 | [
"MIT"
] | null | null | null | 80.177778 | 1,964 | 0.661724 | [
[
[
"import sys\nsys.path.append('..')",
"_____no_output_____"
],
[
"from VisionSystem import VisionSystem, VideoStream, VisualObject\nfrom VisionSystem.DetectionModel import ThreshBlob\nfrom DisplayPane import DisplayPane\nfrom KickerSystem import KickerSystem\nfrom DriveSystem import DriveSystem",
"before {}\nafter {13: ('/home/pi/SoccerRobot/DriveSystem/DriveSystem.py, line 10', <gpiozero.LED object on pin GPIO13, active_high=True, is_active=False>), 26: ('/home/pi/SoccerRobot/DriveSystem/DriveSystem.py, line 12', <gpiozero.LED object on pin GPIO26, active_high=True, is_active=False>)}\nbefore {13: ('/home/pi/SoccerRobot/DriveSystem/DriveSystem.py, line 10', <gpiozero.LED object on pin GPIO13, active_high=True, is_active=False>), 26: ('/home/pi/SoccerRobot/DriveSystem/DriveSystem.py, line 12', <gpiozero.LED object on pin GPIO26, active_high=True, is_active=False>)}\nafter {13: ('/home/pi/SoccerRobot/DriveSystem/DriveSystem.py, line 10', <gpiozero.LED object on pin GPIO13, active_high=True, is_active=False>), 26: ('/home/pi/SoccerRobot/DriveSystem/DriveSystem.py, line 12', <gpiozero.LED object on pin GPIO26, active_high=True, is_active=False>), 5: ('/home/pi/SoccerRobot/DriveSystem/DriveSystem.py, line 10', <gpiozero.LED object on pin GPIO5, active_high=True, is_active=False>), 12: ('/home/pi/SoccerRobot/DriveSystem/DriveSystem.py, line 12', <gpiozero.LED object on pin GPIO12, active_high=True, is_active=False>)}\nbefore {13: ('/home/pi/SoccerRobot/DriveSystem/DriveSystem.py, line 10', <gpiozero.LED object on pin GPIO13, active_high=True, is_active=False>), 26: ('/home/pi/SoccerRobot/DriveSystem/DriveSystem.py, line 12', <gpiozero.LED object on pin GPIO26, active_high=True, is_active=False>), 5: ('/home/pi/SoccerRobot/DriveSystem/DriveSystem.py, line 10', <gpiozero.LED object on pin GPIO5, active_high=True, is_active=False>), 12: ('/home/pi/SoccerRobot/DriveSystem/DriveSystem.py, line 12', <gpiozero.LED object on pin GPIO12, active_high=True, is_active=False>)}\nafter {13: ('/home/pi/SoccerRobot/DriveSystem/DriveSystem.py, line 10', <gpiozero.LED object on pin GPIO13, active_high=True, is_active=False>), 26: ('/home/pi/SoccerRobot/DriveSystem/DriveSystem.py, line 12', <gpiozero.LED object on pin GPIO26, active_high=True, is_active=False>), 5: ('/home/pi/SoccerRobot/DriveSystem/DriveSystem.py, line 10', <gpiozero.LED object on pin GPIO5, active_high=True, is_active=False>), 12: ('/home/pi/SoccerRobot/DriveSystem/DriveSystem.py, line 12', <gpiozero.LED object on pin GPIO12, active_high=True, is_active=False>), 21: ('/home/pi/SoccerRobot/DriveSystem/DriveSystem.py, line 10', <gpiozero.LED object on pin GPIO21, active_high=True, is_active=False>), 16: ('/home/pi/SoccerRobot/DriveSystem/DriveSystem.py, line 12', <gpiozero.LED object on pin GPIO16, active_high=True, is_active=False>)}\n"
],
[
"drive = DriveSystem()\nkicker = KickerSystem()\nkicker.setup() # dont ask",
"_____no_output_____"
],
[
"def load_or_create_new_threshblob(path):\n try:\n model = ThreshBlob.load(path)\n print(\"Loaded \" + path)\n except Exception:\n model = ThreshBlob()\n finally:\n return model\n \n\ndef setup_vision_system(resolution):\n objects_to_size_and_result_limit = [\n (\"ball\", (0.043, 0.043, 0.043), 1),\n (\"obstacle\", (0.18, 0.18, 0.2), None),\n # 30 centimetres long, 10 cm high? i guess\n (\"blue_goal\", (0, 0, 0.1), 1),\n (\"yellow_goal\", (0, 0, 0.1), 1)\n ]\n\n return VisionSystem(\n resolution=resolution,\n objects_to_track={\n name: VisualObject(\n real_size=size,\n detection_model=load_or_create_new_threshblob(f\"../models/{name}.threshblob.pkl\"),\n result_limit=result_limit\n ) for name, size, result_limit in objects_to_size_and_result_limit\n }\n )\n\nvideo = VideoStream(downsample_scale=5, crop=((0.13, 0), (.9, 1)))\nvision = setup_vision_system(video.resolution)",
"_____no_output_____"
],
[
"from DisplayPane.Interactor.VisionSystemTuner import VisionSystemTuner\n\nDisplayPane(vision_system=vision, video_stream=video, interactors=[VisionSystemTuner(vision)])",
"_____no_output_____"
],
[
"# RUN THIS CELL TO SAVE THE MODELs tinkered with\nfor name, obj in vision.objects_to_track.items():\n obj.detection_model.save(f\"../models/{name}.threshblob.pkl\")",
"_____no_output_____"
],
[
"def get_vision_results_vrep_format():\n objs = vision.objects_to_track # for shorthand\n\n def vrep_format(bearings_distances, multi=False):\n if any(bearings_distances):\n if multi:\n bds = bearings_distances[::-1]\n for idx, (bear, dist) in enumerate(bds):\n bds[idx] = (np.pi - bear), dist\n return bds\n else:\n bear, dist = bearings_distances[0][::-1]\n return (np.pi - bear), dist\n else:\n return None\n\n return (\n vrep_format(objs[\"ball\"].bearings_distances),\n vrep_format(objs[\"blue_goal\"].bearings_distances),\n vrep_format(objs[\"yellow_goal\"].bearings_distances),\n vrep_format(objs[\"obstacle\"].bearings_distances, multi=True),\n )",
"_____no_output_____"
],
[
"from math import *\nfrom time import sleep\n\nT_SPEED = 0.03\nR_SPEED = 0.02\n\nfor frame in video:\n vision.update_with_frame(frame)\n \n ball_br, blue_br, yellow_br, obstacle_brs = get_vision_results_vrep_format()\n \n if ball_br is not None:\n ball_bear, ball_range = (-pi / 2, 1)\n print(\"BALL\", ball_br)\n t_speed = T_SPEED if ball_range > 0.3 else ball_range / 0.3 * T_SPEED\n r_speed = R_SPEED if ball_bear > 0.5 else ball_bear / 0.5 * R_SPEED\n \n drive.set_desired_motion(\n t_speed * cos(ball_bear),\n t_speed * sin(ball_bear),\n r_speed * ball_bear)\n \n sleep(0.2)\n \n drive.set_desired_motion(0,0,0)\n sleep(0.1)\n ",
"../VisionSystem/DetectionModel/DetectionResult.py:29: RuntimeWarning: overflow encountered in long_scalars\n return 0.5*np.abs(np.dot(x, np.roll(y, 1))-np.dot(y, np.roll(x, 1)))\n"
],
[
"drive.set_desired_motion(0,0,0)",
"set desired motion 0 0 0\ndriving left, right, back 0.0 0.0 0.0\n"
],
[
"# run the kicker\n",
"_____no_output_____"
]
]
] | [
"code"
] | [
[
"code",
"code",
"code",
"code",
"code",
"code",
"code",
"code",
"code",
"code"
]
] |
e778452ad5a04585ef4ec4b7155d1891b63020a9 | 486,812 | ipynb | Jupyter Notebook | GBDA_exercise_5.ipynb | giorgosouz/HSI-supervised-classification | 9e70cbb7c93e24201c94e43adbb1c04378289476 | [
"MIT"
] | null | null | null | GBDA_exercise_5.ipynb | giorgosouz/HSI-supervised-classification | 9e70cbb7c93e24201c94e43adbb1c04378289476 | [
"MIT"
] | null | null | null | GBDA_exercise_5.ipynb | giorgosouz/HSI-supervised-classification | 9e70cbb7c93e24201c94e43adbb1c04378289476 | [
"MIT"
] | null | null | null | 502.385965 | 64,448 | 0.933383 | [
[
[
"import numpy as np\nimport pandas as pd\nimport gdal\nimport matplotlib.pyplot as plt\n\nfrom sklearn.model_selection import train_test_split\nfrom sklearn.naive_bayes import GaussianNB\nfrom sklearn.neighbors import KNeighborsClassifier\nfrom sklearn.linear_model import Perceptron\n\nfrom sklearn.svm import SVC\nfrom sklearn.ensemble import RandomForestClassifier\nfrom sklearn.neural_network import MLPClassifier\n\nfrom sklearn.metrics import confusion_matrix\nfrom sklearn.metrics import precision_recall_fscore_support\nfrom sklearn.metrics import plot_confusion_matrix\n\nfrom sklearn.preprocessing import StandardScaler,MinMaxScaler\n\nfrom rasterio import plot\n\nimport seaborn as sn\nimport pandas as pd\n",
"_____no_output_____"
]
],
[
[
"# Μέρος Α",
"_____no_output_____"
]
],
[
[
"green_tif = gdal.Open(\"./GBDA2020_ML1/partA/green.tif\")\ngreen_data = green_tif.ReadAsArray().flatten()\n\nnir_tif = gdal.Open(\"./GBDA2020_ML1/partA/nir.tif\")\nnir_data = nir_tif.ReadAsArray().flatten()\n\nlabels_tif = gdal.Open(\"./GBDA2020_ML1/partA/gt.tif\")\nlabels_data = labels_tif.ReadAsArray().flatten()\nlabels_data = np.where(labels_data==255,1,labels_data)\n\ndata = np.concatenate((np.expand_dims(green_data,axis=1),np.expand_dims(nir_data,axis=1)),axis=1)\n",
"_____no_output_____"
],
[
"# AM: 03400121\n# train-test set, 70-30 %\nX_train, X_test, y_train, y_test = train_test_split(data, labels_data, test_size=0.3, random_state=121)",
"_____no_output_____"
]
],
[
[
"Αρχικοποιώ τα μοντέλα του πρώτου ερωτήματος, τα εκπαιδεύω και εμφανίζω τις απαιτούμενες μετρικές αξιολόγησης.",
"_____no_output_____"
]
],
[
[
"my_clfs = { \n \"Gaussian Naive Bayes\" : GaussianNB(),\n \"K-Nearest Neighbors\" : KNeighborsClassifier(n_neighbors=3,n_jobs=-1),\n \"Simple Perceptron\" : Perceptron(tol=1e-3, random_state=121,n_jobs=-1)\n}\nfor title, clf in my_clfs.items():\n print(title)\n clf.fit(X_train,y_train)\n \n pred = clf.predict(X_test)\n print(\"Accuracy :\", clf.score(X_test, y_test))\n tn, fp, fn, tp = confusion_matrix(y_test, pred).ravel()\n prec, rec, f1, sup = precision_recall_fscore_support(y_test, pred,average='binary')\n \n print(\"Precision :\", prec)\n print(\"Recall :\", rec)\n print(\"F1 Score :\", f1)\n \n print(\"True Positives :\", tp)\n print(\"True Negatives :\", tn)\n print(\"False Positives :\",fp)\n print(\"False Negatives :\",fn)\n \n fig, ax = plt.subplots(figsize=(12, 6))\n ax.set_title(title)\n plot_confusion_matrix(clf, X_test, y_test, display_labels=[\"Land\",\"Water\"],ax=ax) \n plt.show()\n \n \n ",
"Gaussian Naive Bayes\nAccuracy : 0.9980966666666666\nPrecision : 0.9997233383835057\nRecall : 0.9928042494177983\nF1 Score : 0.9962517805683377\nTrue Positives : 75884\nTrue Negatives : 223545\nFalse Positives : 21\nFalse Negatives : 550\n"
]
],
[
[
"Με βάση τα αποτελέσματα που δίνουν οι αλγόριθμοι είναι εμφανές πως τα δεδομένα μας είναι καλώς ορισμένα και μπορούν να διαχωρίσουν τις δυο κλάσεις τέλεια. Τα αποτελέσματα είναι τέλεια με μόνο τον Gaussian classifier να πραγματοποιεί ελάχιστα λάθη. Το πρόβλημα που καλούνται να λύσουν οι αλγόριθμοι είναι σχετικά απλό, ενώ η παρουσία μεγάλου αριθμού δεδομένων για την εκπαίδευση καθιστά δυνατά αυτά τα ποσοστά επιτυχίας.",
"_____no_output_____"
],
[
"# Μέρος Β",
"_____no_output_____"
],
[
"Φορτώνω και επεξεργάζομαι τα δεδομένα του δεύτερου ερωτήματος. Αφαιρείται η κλάση 0 από τα data και τα labels, και οι κλάσεις για λόγους ευκολίας στη συνέχεια μετατρέπονται από 1-16 σε 0-15.",
"_____no_output_____"
]
],
[
[
"data_b = np.load(\"./GBDA2020_ML1/partB/indianpinearray.npy\").transpose().reshape((200,-1)).transpose().astype(np.int16)\nlabels_b = np.load(\"./GBDA2020_ML1/partB/IPgt.npy\").flatten().astype(np.int16)\n\nclean_data = np.delete(data_b,np.where(labels_b==0),0)\nclean_labels = np.delete(labels_b,np.where(labels_b==0),0)\nclean_labels = clean_labels-1",
"_____no_output_____"
]
],
[
[
"Για τα μοντέλα που θα ζητούνται να εξεταστούν είναι απαραίτητη η προεπεξέργασία των δεδομένων. Συγκεκριμένα γίνεται κανονικοποίηση των δεδομένων. Δύο επιλογές εξετάστηκαν:\n\n1. Κανονικοποίηση Max-Min δίνονται νέες τιμές στα δεδομένα στο διάστημα [0,1]\n2. Κανονικοποίηση χρησιμοποιώντας τη μέση τιμή και τη διασπορά του κάθε χαρακτηριστικού ώστε να ακολουθούν τα δεδομένα την κανονική κατανομή ανά χαρακτηριστικό.\n\nΕπιλέχθηκε η δεύτερη προσέγγιση καθώς έδωσε καλύτερα αποτελέσματα.",
"_____no_output_____"
]
],
[
[
"# scaler = MinMaxScaler()\nscaler = StandardScaler()\nscaler.fit(clean_data)\nscaled_data = scaler.transform(clean_data)",
"_____no_output_____"
]
],
[
[
"Για τα μοντέλα MLP χρειάστηκε και ένα validation set. Για να είναι δυνατή η σύγκριση μεταξύ όλων των εξεταζόμενων μοντέλων, χρησιμοποιήθηκαν τα ίδια δεδομένα εκπαίδευσης και ελέγχου σε όλες τις περιπτώσεις.",
"_____no_output_____"
]
],
[
[
"# AM: 03400121\n# train-val-test 60-10-30 , \nX_train, X_test, y_train, y_test = train_test_split(scaled_data, clean_labels, test_size=0.3, random_state=121,stratify=clean_labels)\nX_train, X_val, y_train, y_val = train_test_split(X_train, y_train, test_size=0.143, random_state=121,stratify=y_train) #1/7 of train set or 1/10 of total data",
"_____no_output_____"
]
],
[
[
"Αρχικοποιώ τα μοντέλα των SVM και RF, τα εκπαιδεύω και εμφανίζω τις απαιτούμενες μετρικές.",
"_____no_output_____"
]
],
[
[
"my_clfs_b = { \n \"Support Vector Machine\" : SVC(C=50),\n \"Random Forest\" : RandomForestClassifier(criterion=\"entropy\",max_features=\"sqrt\",random_state=121,n_jobs=12),\n}\n\nfor title, clf in my_clfs_b.items():\n print(title)\n clf.fit(X_train,y_train)\n \n pred = clf.predict(X_test)\n print(\"Accuracy :\", clf.score(X_test, y_test))\n prec, rec, f1, sup = precision_recall_fscore_support(y_test, pred)\n \n print(\"Precision :\", prec)\n print(\"Recall :\", rec)\n print(\"F1 Score :\", f1)\n \n prec_micro, rec_micro, f1_micro, sup_micro = precision_recall_fscore_support(y_test, pred,average='micro')\n \n print(\"Precision micro:\", prec_micro)\n print(\"Recall micro:\", rec_micro)\n print(\"F1 Score micro:\", f1_micro)\n\n \n fig, ax = plt.subplots(figsize=(12, 6))\n ax.set_title(title)\n plot_confusion_matrix(clf, X_test, y_test, ax=ax) \n plt.show()\n \n print(\"----------------------------------------------------------------------\")\n# break\n\n",
"Support Vector Machine\nAccuracy : 0.6386991869918699\nPrecision : [0.27777778 0.61571125 0.55597015 0.80519481 0.5112782 0.52763819\n 0.5 0.7 0.5 0.6294964 0.68970013 0.53157895\n 0.54054054 0.74185464 0.68918919 0.72727273]\nRecall : [0.35714286 0.67757009 0.59839357 0.87323944 0.46896552 0.47945205\n 0.375 0.63636364 0.5 0.59931507 0.71777476 0.56741573\n 0.32786885 0.77894737 0.43965517 0.57142857]\nF1 Score : [0.3125 0.64516129 0.57640232 0.83783784 0.48920863 0.50239234\n 0.42857143 0.66666667 0.5 0.61403509 0.70345745 0.54891304\n 0.40816327 0.75994865 0.53684211 0.64 ]\nPrecision micro: 0.6386991869918699\nRecall micro: 0.6386991869918699\nF1 Score micro: 0.6386991869918699\n"
]
],
[
[
"Για τα μονέλα MLP δημιουργήθηκαν δυο απλά δίκτυα. Το πρώτο αποτελείται από ένα shallow network χωρίς κανένα κρυφό επίπεδο, το οποίο προβάλει την είσοδο των 200 χαρακηριστικών στις 16 κατηγορίες εξόδου. Ως δευτερη αρχιτεκτονική για τα MLP δημιουργήθηκε ένα δίκτυο με 3 κρυφά επίπεδα με 100,50,25 νευρώνες αντίστοιχα. Στο δεύτερο δίκτυο παρατηρήθηκε overfitting γι αυτό χρησιμοποιήθηκε η τεχνική του dropout για να μειωθεί η επίδρασή του. Προς το τέλος του notebook υπάρχουν διαγράμματα για την εκπαίδευση των μοντέλων όπου γίνεται εμφανές το overfitting.",
"_____no_output_____"
]
],
[
[
"import torch\nfrom torch import nn\nfrom torch import optim\nimport torch.nn.functional as F\n\nimport numpy as np\nfrom torch.utils.data import TensorDataset, DataLoader\n\n \nclass my_MLP_V1(nn.Module):\n def __init__(self):\n super().__init__()\n \n# self.fc1 = nn.Linear(200,50)\n# self.fc2 = nn.Linear(50,25)\n self.fc3 = nn.Linear(200,16)\n\n \n \n def forward(self,x):\n \n# x = F.relu(self.fc1(x))\n# x = F.relu(self.fc2(x))\n x = F.log_softmax(self.fc3(x),dim=1)\n \n return x\n\n \nclass my_MLP_V2(nn.Module):\n def __init__(self):\n super().__init__()\n \n self.fc1 = nn.Linear(200,100)\n self.fc2 = nn.Linear(100,50)\n self.fc3 = nn.Linear(50,25)\n self.fc4 = nn.Linear(25,16)\n \n self.dropout = nn.Dropout(p=0.2)\n \n \n def forward(self,x):\n \n# x = F.relu(self.fc1(x))\n# x = F.relu(self.fc2(x))\n# x = F.relu(self.fc3(x))\n \n x = self.dropout(F.relu(self.fc1(x)))\n x = self.dropout(F.relu(self.fc2(x)))\n x = self.dropout(F.relu(self.fc3(x)))\n x = F.log_softmax(self.fc4(x),dim=1)\n \n return x\n\n\n# transform to torch tensors\ntensor_x_train = torch.from_numpy(X_train).type(torch.FloatTensor) \ntensor_y_train = torch.from_numpy(y_train).type(torch.LongTensor)\n\ntensor_x_val = torch.from_numpy(X_val).type(torch.FloatTensor) \ntensor_y_val = torch.from_numpy(y_val).type(torch.LongTensor)\n\ntensor_x_test = torch.from_numpy(X_test).type(torch.FloatTensor) \ntensor_y_test = torch.from_numpy(y_test).type(torch.LongTensor)\n\n\n# create train, validation, test datsets and create dataloaders\nmy_dataset_train = TensorDataset(tensor_x_train,tensor_y_train) \nmy_dataloader_train = DataLoader(my_dataset_train,batch_size=64, shuffle=True) \n\nmy_dataset_val = TensorDataset(tensor_x_val,tensor_y_val) \nmy_dataloader_val = DataLoader(my_dataset_val,batch_size=64, shuffle=True)\n\nmy_dataset_test = TensorDataset(tensor_x_test,tensor_y_test) \nmy_dataloader_test = DataLoader(my_dataset_test,batch_size=64, shuffle=True)",
"_____no_output_____"
],
[
"# mlp train function\ndef MLP_train_model(model,epochs=300):\n \n optimizer = optim.Adam(model.parameters(), lr=0.001)\n criterion = nn.NLLLoss()\n \n train_losses, val_losses = [], []\n for e in range(epochs):\n tot_train_loss = 0\n for images, labels in my_dataloader_train:\n optimizer.zero_grad()\n log_ps = model(images)\n loss = criterion(log_ps, labels)\n \n\n loss.backward()\n optimizer.step()\n tot_train_loss += loss.item()\n else:\n tot_val_loss = 0\n # Number of correct predictions on the validation set\n val_correct = 0 \n\n # Turn off gradients for validation and put model on evaluation mode\n with torch.no_grad():\n model.eval()\n for images, labels in my_dataloader_val:\n log_ps = model(images)\n loss = criterion(log_ps, labels)\n tot_val_loss += loss.item()\n\n ps = torch.exp(log_ps)\n top_p, top_class = ps.topk(1, dim=1)\n equals = top_class == labels.view(*top_class.shape)\n val_correct += equals.sum().item()\n \n # Put model on training mode again\n model.train()\n\n # Get mean loss to enable comparison between train and test sets\n train_loss = tot_train_loss / len(my_dataloader_train.dataset)\n val_loss = tot_val_loss / len(my_dataloader_val.dataset)\n\n # At completion of epoch\n train_losses.append(train_loss)\n val_losses.append(val_loss)\n\n if (e)%10==9 or e==0:\n print(\"Epoch: {}/{}.. \".format(e+1, epochs),\n \"Training Loss: {:.3f}.. \".format(train_loss),\n \"Validation Loss: {:.3f}.. \".format(val_loss),\n \"Validation Accuracy: {:.3f}\".format(val_correct / len(my_dataloader_val.dataset)))\n\n \n plt.figure(figsize=(14,7))\n plt.plot(train_losses, label='Training loss')\n plt.plot(val_losses, label='Validation loss')\n plt.legend(frameon=False)\n \n return\n",
"_____no_output_____"
],
[
"model1 = my_MLP_V1()\nMLP_train_model(model1)",
"Epoch: 1/300.. Training Loss: 0.040.. Validation Loss: 0.041.. Validation Accuracy: 0.229\nEpoch: 10/300.. Training Loss: 0.030.. Validation Loss: 0.033.. Validation Accuracy: 0.349\nEpoch: 20/300.. Training Loss: 0.027.. Validation Loss: 0.031.. Validation Accuracy: 0.405\nEpoch: 30/300.. Training Loss: 0.026.. Validation Loss: 0.030.. Validation Accuracy: 0.395\nEpoch: 40/300.. Training Loss: 0.025.. Validation Loss: 0.027.. Validation Accuracy: 0.434\nEpoch: 50/300.. Training Loss: 0.025.. Validation Loss: 0.026.. Validation Accuracy: 0.426\nEpoch: 60/300.. Training Loss: 0.024.. Validation Loss: 0.026.. Validation Accuracy: 0.439\nEpoch: 70/300.. Training Loss: 0.024.. Validation Loss: 0.026.. Validation Accuracy: 0.446\nEpoch: 80/300.. Training Loss: 0.024.. Validation Loss: 0.026.. Validation Accuracy: 0.446\nEpoch: 90/300.. Training Loss: 0.023.. Validation Loss: 0.027.. Validation Accuracy: 0.446\nEpoch: 100/300.. Training Loss: 0.023.. Validation Loss: 0.026.. Validation Accuracy: 0.448\nEpoch: 110/300.. Training Loss: 0.023.. Validation Loss: 0.027.. Validation Accuracy: 0.456\nEpoch: 120/300.. Training Loss: 0.023.. Validation Loss: 0.025.. Validation Accuracy: 0.467\nEpoch: 130/300.. Training Loss: 0.022.. Validation Loss: 0.026.. Validation Accuracy: 0.459\nEpoch: 140/300.. Training Loss: 0.022.. Validation Loss: 0.027.. Validation Accuracy: 0.463\nEpoch: 150/300.. Training Loss: 0.022.. Validation Loss: 0.026.. Validation Accuracy: 0.455\nEpoch: 160/300.. Training Loss: 0.022.. Validation Loss: 0.025.. Validation Accuracy: 0.467\nEpoch: 170/300.. Training Loss: 0.022.. Validation Loss: 0.025.. Validation Accuracy: 0.460\nEpoch: 180/300.. Training Loss: 0.022.. Validation Loss: 0.027.. Validation Accuracy: 0.466\nEpoch: 190/300.. Training Loss: 0.022.. Validation Loss: 0.026.. Validation Accuracy: 0.470\nEpoch: 200/300.. Training Loss: 0.022.. Validation Loss: 0.024.. Validation Accuracy: 0.461\nEpoch: 210/300.. Training Loss: 0.022.. Validation Loss: 0.025.. Validation Accuracy: 0.468\nEpoch: 220/300.. Training Loss: 0.022.. Validation Loss: 0.026.. Validation Accuracy: 0.474\nEpoch: 230/300.. Training Loss: 0.021.. Validation Loss: 0.025.. Validation Accuracy: 0.477\nEpoch: 240/300.. Training Loss: 0.021.. Validation Loss: 0.024.. Validation Accuracy: 0.484\nEpoch: 250/300.. Training Loss: 0.021.. Validation Loss: 0.024.. Validation Accuracy: 0.473\nEpoch: 260/300.. Training Loss: 0.021.. Validation Loss: 0.026.. Validation Accuracy: 0.467\nEpoch: 270/300.. Training Loss: 0.021.. Validation Loss: 0.025.. Validation Accuracy: 0.467\nEpoch: 280/300.. Training Loss: 0.021.. Validation Loss: 0.024.. Validation Accuracy: 0.481\nEpoch: 290/300.. Training Loss: 0.021.. Validation Loss: 0.024.. Validation Accuracy: 0.478\nEpoch: 300/300.. Training Loss: 0.021.. Validation Loss: 0.025.. Validation Accuracy: 0.474\n"
],
[
"# without dropout layers we get overfitting\nmodel2 = my_MLP_V2()\nMLP_train_model(model2)",
"Epoch: 1/300.. Training Loss: 0.036.. Validation Loss: 0.036.. Validation Accuracy: 0.279\nEpoch: 10/300.. Training Loss: 0.023.. Validation Loss: 0.027.. Validation Accuracy: 0.449\nEpoch: 20/300.. Training Loss: 0.019.. Validation Loss: 0.023.. Validation Accuracy: 0.527\nEpoch: 30/300.. Training Loss: 0.017.. Validation Loss: 0.021.. Validation Accuracy: 0.555\nEpoch: 40/300.. Training Loss: 0.015.. Validation Loss: 0.022.. Validation Accuracy: 0.574\nEpoch: 50/300.. Training Loss: 0.014.. Validation Loss: 0.019.. Validation Accuracy: 0.566\nEpoch: 60/300.. Training Loss: 0.013.. Validation Loss: 0.019.. Validation Accuracy: 0.585\nEpoch: 70/300.. Training Loss: 0.011.. Validation Loss: 0.020.. Validation Accuracy: 0.573\nEpoch: 80/300.. Training Loss: 0.010.. Validation Loss: 0.020.. Validation Accuracy: 0.605\nEpoch: 90/300.. Training Loss: 0.010.. Validation Loss: 0.020.. Validation Accuracy: 0.598\nEpoch: 100/300.. Training Loss: 0.009.. Validation Loss: 0.022.. Validation Accuracy: 0.580\nEpoch: 110/300.. Training Loss: 0.009.. Validation Loss: 0.021.. Validation Accuracy: 0.592\nEpoch: 120/300.. Training Loss: 0.007.. Validation Loss: 0.023.. Validation Accuracy: 0.621\nEpoch: 130/300.. Training Loss: 0.008.. Validation Loss: 0.023.. Validation Accuracy: 0.605\nEpoch: 140/300.. Training Loss: 0.006.. Validation Loss: 0.028.. Validation Accuracy: 0.587\nEpoch: 150/300.. Training Loss: 0.006.. Validation Loss: 0.024.. Validation Accuracy: 0.611\nEpoch: 160/300.. Training Loss: 0.005.. Validation Loss: 0.025.. Validation Accuracy: 0.611\nEpoch: 170/300.. Training Loss: 0.005.. Validation Loss: 0.026.. Validation Accuracy: 0.600\nEpoch: 180/300.. Training Loss: 0.004.. Validation Loss: 0.028.. Validation Accuracy: 0.606\nEpoch: 190/300.. Training Loss: 0.004.. Validation Loss: 0.029.. Validation Accuracy: 0.602\nEpoch: 200/300.. Training Loss: 0.004.. Validation Loss: 0.037.. Validation Accuracy: 0.583\nEpoch: 210/300.. Training Loss: 0.004.. Validation Loss: 0.032.. Validation Accuracy: 0.590\nEpoch: 220/300.. Training Loss: 0.004.. Validation Loss: 0.036.. Validation Accuracy: 0.591\nEpoch: 230/300.. Training Loss: 0.004.. Validation Loss: 0.033.. Validation Accuracy: 0.603\nEpoch: 240/300.. Training Loss: 0.003.. Validation Loss: 0.040.. Validation Accuracy: 0.596\nEpoch: 250/300.. Training Loss: 0.003.. Validation Loss: 0.043.. Validation Accuracy: 0.585\nEpoch: 260/300.. Training Loss: 0.002.. Validation Loss: 0.043.. Validation Accuracy: 0.605\nEpoch: 270/300.. Training Loss: 0.003.. Validation Loss: 0.048.. Validation Accuracy: 0.605\nEpoch: 280/300.. Training Loss: 0.001.. Validation Loss: 0.044.. Validation Accuracy: 0.601\nEpoch: 290/300.. Training Loss: 0.002.. Validation Loss: 0.042.. Validation Accuracy: 0.593\nEpoch: 300/300.. Training Loss: 0.001.. Validation Loss: 0.048.. Validation Accuracy: 0.608\n"
],
[
"# with dropout to counter overfitting\nmodel2 = my_MLP_V2()\nMLP_train_model(model2)",
"Epoch: 1/300.. Training Loss: 0.038.. Validation Loss: 0.035.. Validation Accuracy: 0.283\nEpoch: 10/300.. Training Loss: 0.027.. Validation Loss: 0.027.. Validation Accuracy: 0.444\nEpoch: 20/300.. Training Loss: 0.023.. Validation Loss: 0.024.. Validation Accuracy: 0.504\nEpoch: 30/300.. Training Loss: 0.022.. Validation Loss: 0.021.. Validation Accuracy: 0.514\nEpoch: 40/300.. Training Loss: 0.021.. Validation Loss: 0.020.. Validation Accuracy: 0.545\nEpoch: 50/300.. Training Loss: 0.020.. Validation Loss: 0.022.. Validation Accuracy: 0.543\nEpoch: 60/300.. Training Loss: 0.020.. Validation Loss: 0.020.. Validation Accuracy: 0.580\nEpoch: 70/300.. Training Loss: 0.019.. Validation Loss: 0.021.. Validation Accuracy: 0.578\nEpoch: 80/300.. Training Loss: 0.018.. Validation Loss: 0.018.. Validation Accuracy: 0.575\nEpoch: 90/300.. Training Loss: 0.018.. Validation Loss: 0.019.. Validation Accuracy: 0.583\nEpoch: 100/300.. Training Loss: 0.018.. Validation Loss: 0.019.. Validation Accuracy: 0.585\nEpoch: 110/300.. Training Loss: 0.017.. Validation Loss: 0.018.. Validation Accuracy: 0.602\nEpoch: 120/300.. Training Loss: 0.017.. Validation Loss: 0.018.. Validation Accuracy: 0.588\nEpoch: 130/300.. Training Loss: 0.017.. Validation Loss: 0.018.. Validation Accuracy: 0.592\nEpoch: 140/300.. Training Loss: 0.017.. Validation Loss: 0.020.. Validation Accuracy: 0.587\nEpoch: 150/300.. Training Loss: 0.016.. Validation Loss: 0.018.. Validation Accuracy: 0.609\nEpoch: 160/300.. Training Loss: 0.016.. Validation Loss: 0.017.. Validation Accuracy: 0.601\nEpoch: 170/300.. Training Loss: 0.015.. Validation Loss: 0.018.. Validation Accuracy: 0.602\nEpoch: 180/300.. Training Loss: 0.015.. Validation Loss: 0.019.. Validation Accuracy: 0.607\nEpoch: 190/300.. Training Loss: 0.015.. Validation Loss: 0.019.. Validation Accuracy: 0.599\nEpoch: 200/300.. Training Loss: 0.016.. Validation Loss: 0.017.. Validation Accuracy: 0.614\nEpoch: 210/300.. Training Loss: 0.015.. Validation Loss: 0.017.. Validation Accuracy: 0.627\nEpoch: 220/300.. Training Loss: 0.015.. Validation Loss: 0.018.. Validation Accuracy: 0.604\nEpoch: 230/300.. Training Loss: 0.015.. Validation Loss: 0.018.. Validation Accuracy: 0.609\nEpoch: 240/300.. Training Loss: 0.014.. Validation Loss: 0.018.. Validation Accuracy: 0.621\nEpoch: 250/300.. Training Loss: 0.014.. Validation Loss: 0.018.. Validation Accuracy: 0.621\nEpoch: 260/300.. Training Loss: 0.014.. Validation Loss: 0.017.. Validation Accuracy: 0.619\nEpoch: 270/300.. Training Loss: 0.014.. Validation Loss: 0.018.. Validation Accuracy: 0.622\nEpoch: 280/300.. Training Loss: 0.014.. Validation Loss: 0.018.. Validation Accuracy: 0.621\nEpoch: 290/300.. Training Loss: 0.015.. Validation Loss: 0.018.. Validation Accuracy: 0.596\nEpoch: 300/300.. Training Loss: 0.014.. Validation Loss: 0.018.. Validation Accuracy: 0.614\n"
],
[
"# mlp test function and resutls\ndef MLP_test_results(model):\n tot_test_loss = 0\n test_correct = 0 \n all_predictions = []\n all_labels = []\n \n optimizer = optim.Adam(model.parameters(), lr=0.001)\n criterion = nn.NLLLoss()\n \n with torch.no_grad():\n model.eval()\n for images, labels in my_dataloader_test:\n log_ps = model(images)\n loss = criterion(log_ps, labels)\n tot_test_loss += loss.item()\n\n ps = torch.exp(log_ps)\n top_p, top_class = ps.topk(1, dim=1)\n all_predictions.append(list(top_class.numpy().squeeze()))\n all_labels.append(list(labels.numpy().squeeze()))\n equals = top_class == labels.view(*top_class.shape)\n test_correct += equals.sum().item()\n\n test_loss = tot_test_loss / len(my_dataloader_test.dataset)\n\n print(\"Test Loss: {:.3f}.. \".format(test_loss),\"Test Accuracy: {:.3f}\".format(test_correct / len(my_dataloader_test.dataset)))\n\n pred_list = [item for sublist in all_predictions for item in sublist]\n labels_list = [item for sublist in all_labels for item in sublist]\n \n prec, rec, f1, sup = precision_recall_fscore_support(labels_list, pred_list)\n \n print(\"Precision :\", prec)\n print(\"Recall :\", rec)\n print(\"F1 Score :\", f1)\n \n \n \n prec_micro, rec_micro, f1_micro, sup_micro = precision_recall_fscore_support(labels_list, pred_list,average='micro')\n \n print(\"Precision micro:\", prec_micro)\n print(\"Recall micro:\", rec_micro)\n print(\"F1 Score micro:\", f1_micro)\n \n cm=confusion_matrix(labels_list,pred_list)\n\n df_cm = pd.DataFrame(cm, index = range(1,17),\n columns = range(1,17))\n plt.figure(figsize = (10,8)) \n sn.heatmap(df_cm, annot=True,fmt='d')\n t = plt.yticks(rotation=0)\n \n return",
"_____no_output_____"
],
[
"MLP_test_results(model1)",
"Test Loss: 0.023.. Test Accuracy: 0.498\nPrecision : [1. 0.46131805 0.45454545 0.625 0.41353383 0.34782609\n 0.66666667 0.54716981 0.2 0.46258503 0.51896208 0.39072848\n 0.35 0.59396752 0.61538462 0.69230769]\nRecall : [0.07142857 0.37616822 0.46184739 0.77464789 0.37931034 0.25570776\n 0.25 0.40559441 0.16666667 0.46575342 0.70556309 0.33146067\n 0.1147541 0.67368421 0.34482759 0.32142857]\nF1 Score : [0.13333333 0.41441441 0.45816733 0.6918239 0.39568345 0.29473684\n 0.36363636 0.46586345 0.18181818 0.46416382 0.59804485 0.35866261\n 0.17283951 0.63131936 0.44198895 0.43902439]\nPrecision micro: 0.4978861788617886\nRecall micro: 0.4978861788617886\nF1 Score micro: 0.4978861788617886\n"
],
[
"MLP_test_results(model2)",
"Test Loss: 0.038.. Test Accuracy: 0.631\nPrecision : [0.33333333 0.64332604 0.546875 0.78666667 0.56557377 0.50490196\n 0.33333333 0.57228916 0.5 0.63773585 0.69559413 0.56424581\n 0.33870968 0.75193798 0.52777778 0.51851852]\nRecall : [0.21428571 0.68691589 0.562249 0.83098592 0.47586207 0.47031963\n 0.125 0.66433566 0.5 0.57876712 0.70691995 0.56741573\n 0.3442623 0.76578947 0.49137931 0.5 ]\nF1 Score : [0.26086957 0.66440678 0.55445545 0.80821918 0.51685393 0.48699764\n 0.18181818 0.61488673 0.5 0.60682226 0.70121131 0.56582633\n 0.34146341 0.75880052 0.50892857 0.50909091]\nPrecision micro: 0.631219512195122\nRecall micro: 0.631219512195122\nF1 Score micro: 0.631219512195122\n"
]
],
[
[
"## Σχολιασμός αποτελεσμάτων\n\n### Β1\nΠαρατηρούμε πως όλοι οι αλγόριθμοι δίνουν σχετικά κοντινά αποτελέσματα γύρω από το 60% εκτός του πρώτου mlp που κινείται κάτω από το 50%. Συγκεκριμένα τα αποτελέσματά του accuracy τους είναι:\n- SVM: 63.8%\n- RF: 58.8%\n- MLP v1: 48.5%\n- MLP v2: 63.0%\n\nΓια τα απλα μοντέλα που εξετάζουμε και τα imbalanced δεδομένα που έχουμε στη διάθεση μας το ποσοστό επιτυχίας είναι αποδεκτό. Αν οι αλγοριθμοι αποτύγχαναν τελείως θα έδιναν τυχαίες προβλέψεις για κάθε κλαση με ποσοστό επιτυχίας περίπου 1/16=6.25%, ενώ εμείς λαμβάνουμε ποσοστό επιτυχίας 10 φορές μεγαλύτερο που σημαίνει πως τα μοντέλα μας καταφέρνουν ως ενα βαθμό να διαχωρίσουν τις κλάσεις. Στα χαμηλά αυτά ποσοστά συμβάλει και το γεγονός ομοιότητας που υπάρχει στα δεδομένα μας. Οι φασματικές υπογραφές των φυτών μοιάζουν αρκετά με αποτέλεσμα τα μοντέλα να μπερδεύονται. Αν συγκρίναμε φυτά με άλλα υλικά όπως χώμα, νερό ή τεχνητό ανρθώπινο περιβάλλον τότε τα φάσματα θα ήταν πολύ διαφορετικά και ο διαχωρισμός των κλάσεων πολύ πιο εύκολος. Αυτό συμβαίνει στο πρώτο μέρος της με αρκετά πιο απλοϊκό τρόπο καθώς έχουμε να συγκρίνουμε στεριά με θάλασσα, τα οποία παρουσιάζουν μεγάλες διαφορές στις επιλεγμένες συχνότητες.\n\n### Β2\n- Ο αλγόριθμος SVM σημείωσε σημαντική βελτίωση όταν η υπερπαράμετρός του C επιλέχθηκε να έχει μεγαλύτερη τιμή (C=50), η default τιμή της ήταν αρκετά μικρή για το συγκεκριμένο πρόβλημα (C=1). Η υπερπαράμετρος αυτή δείχνει πόσο αυστηρό θα είναι το μοντέλο στις περιπτώσεις missclasification. Όσο μεγαλύτερη είναι τόσο λιγότερη ανοχή δείχνει στα λανθασμένα δείγματα και προσπαθεί να αναπροσαρμώσει τις τιμές του ώστε να τα ταξινομέι σωστά. Εξεταστήκανε και άλλοι διαθέσιμοι kernels αλλά ο rbf που υπάρχει by default έδωσε τα καλύτερα αποτελέσματα.\n- Ο αλγόριθμός του Random Forest παρουσίασε μικρή βελτίωση όταν επιλέχθηκε το κριτήριο της εντροπίας σε σχέση με αυτό του gini, ενώ άλλες αλλαγές στις υπερπαραμέτρους δεν επέφεραν σημαντικές αλλαγές στα αποτελέσματα του. Συγκεκριμένα η αύξηση του αριθμού των παραγώμενων δέντρων δεν οδήγησε σε βελτίωση των αποτελεσμάτων ενώ η μείωση τους επέφερε χειρότερα αποτελέσματα.\n- Στα δίκτυα των MLP παίζει σημαντικό ρόλο η αρχιτεκτονική του δικτύου. Για το απλό MLP, στο validtation set το accuracy φτάνει περίπου στο 0.475 με vallidation loss 0.025. Από το διάγραμμα των losses κατά την εκπαίδευση μπορούμε να δούμε πως υπάρχει overfitting αφού το training loss συνεχίζει να πέφτει ενώ το validation loss ταλαντώνεται γύρω από την τιμή του 0.026. Στο βαθύτερο mlp το accuracy\n\n\n### Β3\n1. Βλέποντας τις μετρικές για την κάθε κατηγορία σε όλα τα μοντέλα μας βλέπουμε διακυμάνσεις. Κάποιες κατηγορίες έχουν καλύτερα αποτελέσματα καθώς υπάρχουν περισσότερα δείγματα για να εκπαιδευτεί το μοντέλο μας πάνω σε αυτές και να τις αναγνωρίζει πιο εύκολα. Επίσης, μερικές κατηγορίες έχουν ίδια χαρακτηριστικά. Για παράδειγμα μπορούμε να δούμε πως οι κατηγορίες 2 και 11 (Corn-notill και Soybean-mintill αντίστοιχα) μπερδεύουν αρκετά το μοντέλο μας. Αυτό πιθανώς οφείλεται στο γεγονός πως τα δύο αυτά φυτά παρουσιάζουν παρόμοια υπερφασματική ταυτότητα στο συγκεκριμένο data cube που έχουμε στη διάθεσή μας.\n2. Στα mlp όπως προαναφέρθηκε υπάρχουν φαινόμενα overfitting. Αυτό φαίνεται και από τα διαγράμματα των εκπαιδεύσεων όπου το training loss συνεχίζει να πέφτει ενώ το validation loss αυξάνεται. Αυτό συμβαίνει γιατί έχουμε λίγα δεδομένα στη διάθεσή μας για εκπαίδευση με αποτέλεσμα να πρέπει να τα περάσουμε πολλές φορές από το μοντέλο το οποίο μετά από ένα σημειό αρχίζει να τα απομνημονεύει. Στην περίπτωση του δεύτερου mlp είναι πιο έντονο το φαινόμενο και για αυτό χρησιμοποιείται το dropout για να αντιμετωπιστεί το φαινόμενο αυτό.\n3. Έγιναν δοκιμες για το learning rate και σε 0.01 και σε 0.0001. Την πρωτη φορα το μοντέλο έκανε ταλάντωση ενώ την δεύτερη είχε πολύ αργή πρόοδο. Το 0.001 που είναι by default έδωσε τα καλύτερα αποτελέσματα σε λογικό αριθμό εποχών.\n4. Και για τις δύο αρχιτεκτονικές (το δεύτερο mlp με dropout) μπορούμε να δούμε πως το test loss δεν μειώνεται περαιτέρω μετά από έναν συγκεκριμένο αριθμό εποχών. Και στις δύο περιπτώσεις μπορούμε να πούμε με ασφάλεια πως οι 100 εποχές είναι αρκετές για να εκπαιδευτέι πλήρως το μοντέλο.",
"_____no_output_____"
]
]
] | [
"code",
"markdown",
"code",
"markdown",
"code",
"markdown",
"code",
"markdown",
"code",
"markdown",
"code",
"markdown",
"code",
"markdown",
"code",
"markdown"
] | [
[
"code"
],
[
"markdown"
],
[
"code",
"code"
],
[
"markdown"
],
[
"code"
],
[
"markdown",
"markdown",
"markdown"
],
[
"code"
],
[
"markdown"
],
[
"code"
],
[
"markdown"
],
[
"code"
],
[
"markdown"
],
[
"code"
],
[
"markdown"
],
[
"code",
"code",
"code",
"code",
"code",
"code",
"code",
"code"
],
[
"markdown"
]
] |
e7785729b31c99bb435d286fbf3f598394dc2796 | 358,659 | ipynb | Jupyter Notebook | example_vis_arctic.ipynb | bmorris3/stsp_osg_results | 655ccf972193941ef148b62f861cbe5e58650f6b | [
"MIT"
] | null | null | null | example_vis_arctic.ipynb | bmorris3/stsp_osg_results | 655ccf972193941ef148b62f861cbe5e58650f6b | [
"MIT"
] | null | null | null | example_vis_arctic.ipynb | bmorris3/stsp_osg_results | 655ccf972193941ef148b62f861cbe5e58650f6b | [
"MIT"
] | null | null | null | 990.770718 | 143,638 | 0.943088 | [
[
[
"# MCMC visualizations",
"_____no_output_____"
]
],
[
[
"%matplotlib inline\nimport numpy as np\nimport matplotlib.pyplot as plt\nfrom results import MCMCResults\nimport sys\nsys.path.insert(0, '/Users/bmmorris/git/friedrich')\nfrom friedrich.lightcurve import hat11_params_morris\ntransit_params = hat11_params_morris()\n\n\nfrom corner import corner",
"_____no_output_____"
],
[
"transit_params = hat11_params_morris()\n\nm1 = MCMCResults.from_stsp_local('/Users/bmmorris/git/friedrich/hat11/arctic/first_half_mcmc.txt', \n '/Users/bmmorris/git/friedrich/hat11/arctic/first_half.dat', \n transit_params=hat11_params_morris())\n\nm2 = MCMCResults.from_stsp_local('/Users/bmmorris/git/friedrich/hat11/arctic/second_half_mcmc.txt', \n '/Users/bmmorris/git/friedrich/hat11/arctic/second_half.dat', \n transit_params=hat11_params_morris())",
"_____no_output_____"
],
[
"# fig, ax = m.light_curve.plot_transit()",
"_____no_output_____"
]
],
[
[
"Identify the burn-in period",
"_____no_output_____"
]
],
[
[
"burn_in_step = 2000\nfor i in range(len(m.chi2_chains)):\n plt.plot(np.log(m.chi2_chains[i]), '.', color='k', alpha=0.005)\nplt.xlabel('step')\nplt.ylabel('$\\log\\chi^2$')\n#plt.axvline(burn_in_step, color='k', lw=2)\nplt.show()",
"_____no_output_____"
],
[
"flat_radius = []\nflat_phi = []\nflat_theta = []\nfor i in range(len(m.chi2_chains)):\n flat_radius.append(m.radius_chains[i][burn_in_step:, :])\n flat_phi.append(m.phi_chains[i][burn_in_step:, :])\n flat_theta.append(m.theta_chains[i][burn_in_step:, :])\nflat_radius = np.vstack(flat_radius)\nflat_phi = np.vstack(flat_phi)\nflat_theta = np.vstack(flat_theta)",
"_____no_output_____"
],
[
"from astropy.time import Time\nerrorbar_color = '#b3b3b3'\nfontsize = 16\n\nfig, ax = plt.subplots(1, figsize=(8, 5))\nax.errorbar(m1.light_curve.kepler_lc.times.plot_date, m1.light_curve.fluxes_kepler,\n m1.light_curve.kepler_lc.errors, fmt='.',\n color='k', ecolor=errorbar_color, capsize=0, label='Kepler')\nax.plot(m1.light_curve.model_lc.times.plot_date, m1.light_curve.fluxes_model, 'r', label='STSP', lw=2)\n\nax.errorbar(m2.light_curve.kepler_lc.times.plot_date, m2.light_curve.fluxes_kepler,\n m2.light_curve.kepler_lc.errors, fmt='.',\n color='k', ecolor=errorbar_color, capsize=0, label='Kepler')\nax.plot(m2.light_curve.model_lc.times.plot_date, m2.light_curve.fluxes_model, 'r', label='STSP', lw=2)\n\nlabel_times = Time(ax.get_xticks(), format='plot_date')\nax.set_xticklabels([lt.strftime(\"%H:%M\") for lt in label_times.datetime])\n\nax.set_xlabel('Time on {0} UTC'.format(label_times[0].datetime.date()),\n fontsize=fontsize)\nax.set_ylabel('Flux', fontsize=fontsize)\n\nax.axvline(m2.light_curve.kepler_lc.times.plot_date[0])\n\n# ax.set_xlim([m.light_curve.kepler_lc.times.plot_date.min(),\n# m.light_curve.kepler_lc.times.plot_date.max()])\nfig.savefig('plots/transit_{0:03d}.png'.format(m.window_ind), bbox_inches='tight', dpi=200)",
"_____no_output_____"
],
[
"\n!mkdir arctic_outputs",
"_____no_output_____"
],
[
"\nfor m in [m1, m2]:\n corner(np.vstack([m.radius.ravel(), m.theta.ravel(), m.phi.ravel()]).T,\n labels=['$r$', r'$\\theta$', r'$\\phi$'])",
"_____no_output_____"
],
[
"attrs = ['radius', 'theta', 'phi']\ntrans = [lambda r: r, \n lambda t: np.degrees(np.pi/2 - t), \n lambda p: np.degrees(p)]\n\nspots_rad = []\nspots_deg = []\n\nfor m in [m1, m2]:\n measurements_rad = []\n measurements_deg = []\n for attr, transformation in zip(attrs, trans):\n l, med, u = np.percentile(getattr(m, attr).ravel(), [16, 50, 84])\n measurements_rad.append(dict(attr=attr, lower=med-l, best=med, upper=med+l))\n\n l, med, u = np.percentile(transformation(getattr(m, attr).ravel()), [16, 50, 84])\n measurements_deg.append(dict(attr=attr, lower=med-l, best=med, upper=med+l))\n \n spots_rad.append(measurements_rad)\n spots_deg.append(measurements_deg)",
"_____no_output_____"
],
[
"from astropy.table import Table\n\nfor measurements_deg in spots_deg:\n print(Table(rows=measurements_deg))",
" attr best lower upper \n------ -------------- ------------- --------------\nradius 0.512683 0.39675188 0.62861412\n theta -8.37756962121 20.3741626168 -37.1293018592\n phi 253.980330355 163.959035049 344.001625661\n attr best lower upper \n------ ------------- ------------- -------------\nradius 0.2676935 0.08163682 0.45375018\n theta 15.4532920643 9.15533271544 21.7512514132\n phi 278.051633779 136.452673172 419.650594387\n"
],
[
"skip = 100\n#plt.subplot(111, projection='hammer')\nplt.scatter(m1.theta.ravel()[::skip], m1.phi.ravel()[::skip], s=4, alpha=0.2)\nplt.scatter(m2.theta.ravel()[::skip], m2.phi.ravel()[::skip], s=4, alpha=0.2)",
"_____no_output_____"
]
]
] | [
"markdown",
"code",
"markdown",
"code"
] | [
[
"markdown"
],
[
"code",
"code",
"code"
],
[
"markdown"
],
[
"code",
"code",
"code",
"code",
"code",
"code",
"code",
"code"
]
] |
e7785de96b06afd0e8eb9cc0342baacf7233edf2 | 3,473 | ipynb | Jupyter Notebook | KonputaziorakoSarrera-MAT/Gardenkiak/Kontrol egiturak.ipynb | mpenagar/Irakaskuntza-Docencia-2019-2020 | ac259c5a9949f13edb491a609e5cc6c7543344e9 | [
"MIT"
] | null | null | null | KonputaziorakoSarrera-MAT/Gardenkiak/Kontrol egiturak.ipynb | mpenagar/Irakaskuntza-Docencia-2019-2020 | ac259c5a9949f13edb491a609e5cc6c7543344e9 | [
"MIT"
] | null | null | null | KonputaziorakoSarrera-MAT/Gardenkiak/Kontrol egiturak.ipynb | mpenagar/Irakaskuntza-Docencia-2019-2020 | ac259c5a9949f13edb491a609e5cc6c7543344e9 | [
"MIT"
] | null | null | null | 25.166667 | 239 | 0.543334 | [
[
[
"# Kontrol egiturak\n\nKontrol egiturek exekuzioaren fluxua adierazteko balio dute. Defektuz, ezekuzioa sekuentziala izango da, hau da, aginduak bata bestearen atzetik exekutatuko dira adieraziak dauden ordenean:",
"_____no_output_____"
]
],
[
[
"print(1)\nprint(2)\nprint(3)\nprint(4)",
"1\n2\n3\n4\n"
]
],
[
[
"Kontrol egiturak bi taldetan sailkatu daitezke eratzen duten fluxu motaren arabera:\n\n 1. **Baldintzazko kontrol egiturak** → exekutatua izango den sententzia multzoa zein izango den baldintzen arabera adierazteko aukera eskeintzen dute.\n * `IF`\n 1. **Kontrol egitura errepikakorrak** → sententzi multzo bat behin eta berriro exekutatzeko aukera eskeintzen dute.\n * `WHILE`\n * `FOR`",
"_____no_output_____"
],
[
"## Egiturak eta sententzi multzoak (kode blokeak)\n\nPythonek *indentazioa* erabiltzen du sententzi multzoak edo kode blokeak adierazteko. Generalean, *egitura* baten definizioa `:` zeinuaz hasten da eta barnean dauden sententzi guztiak **4 hutsunetako** indentazioa izan behar dute.\n\n```\negitura bat :\n| sententzia\n| sententzia\n| beste egitura bat :\n| | sententzia\n| | sententzia\n| | sententzia\n| | azken egitura :\n| | | sententzia\n| | | sententzia\n| | | sententzia\n| | sententzia\n| | sententzia\n| sentenztia\n| sentenztia\n```\n\nOrain kontrol egiturekin ari bagara ere, eredu hau beste egitura guztietan ematen da: funtzioak, klaseak, etab.",
"_____no_output_____"
],
[
"<table border=\"0\" width=\"100%\" style=\"margin: 0px;\">\n<tr> \n <td style=\"text-align:left\"><a href=\"Oinarrizko datu sarrera eta irteera.ipynb\">< < Oinarrizko datu sarrera eta irteera < <</a></td>\n <td style=\"text-align:right\"><a href=\"if sententzia.ipynb\">> > if sententzia > ></a></td>\n</tr>\n</table>",
"_____no_output_____"
]
]
] | [
"markdown",
"code",
"markdown"
] | [
[
"markdown"
],
[
"code"
],
[
"markdown",
"markdown",
"markdown"
]
] |
e778622cda03bd8ecae4b1e371c068f06481fab7 | 951,773 | ipynb | Jupyter Notebook | .ipynb_checkpoints/20170622 Experiment-checkpoint.ipynb | JustWon/DCGAN-Experiment | f077ca3899ea169d0ab841e59546d4f833b60a74 | [
"MIT"
] | null | null | null | .ipynb_checkpoints/20170622 Experiment-checkpoint.ipynb | JustWon/DCGAN-Experiment | f077ca3899ea169d0ab841e59546d4f833b60a74 | [
"MIT"
] | null | null | null | .ipynb_checkpoints/20170622 Experiment-checkpoint.ipynb | JustWon/DCGAN-Experiment | f077ca3899ea169d0ab841e59546d4f833b60a74 | [
"MIT"
] | null | null | null | 1,407.948225 | 157,020 | 0.945248 | [
[
[
"import os\nimport scipy.misc\nimport numpy as np\nfrom sklearn.decomposition import PCA\n\nfrom model import DCGAN\nfrom utils import pp, visualize, to_json, show_all_variables\n\nimport tensorflow as tf\n\nfrom glob import glob\n\nimport sys\n\nflags = tf.app.flags\nflags.DEFINE_integer(\"epoch\", 25, \"Epoch to train [25]\")\nflags.DEFINE_float(\"learning_rate\", 0.0002, \"Learning rate of for adam [0.0002]\")\nflags.DEFINE_float(\"beta1\", 0.5, \"Momentum term of adam [0.5]\")\nflags.DEFINE_integer(\"train_size\", np.inf, \"The size of train images [np.inf]\")\nflags.DEFINE_integer(\"batch_size\", 64, \"The size of batch images [64]\")\nflags.DEFINE_integer(\"input_height\", 64, \"The size of image to use (will be center cropped). [108]\")\nflags.DEFINE_integer(\"input_width\", None,\n \"The size of image to use (will be center cropped). If None, same value as input_height [None]\")\nflags.DEFINE_integer(\"output_height\", 64, \"The size of the output images to produce [64]\")\nflags.DEFINE_integer(\"output_width\", None,\n \"The size of the output images to produce. If None, same value as output_height [None]\")\nflags.DEFINE_string(\"dataset\", \"PatchofPlaces\", \"The name of dataset [celebA, mnist, lsun]\")\nflags.DEFINE_string(\"input_fname_pattern\", \"*.jpg\", \"Glob pattern of filename of input images [*]\")\nflags.DEFINE_string(\"checkpoint_dir\", \"checkpoint\", \"Directory name to save the checkpoints [checkpoint]\")\nflags.DEFINE_string(\"sample_dir\", \"samples\", \"Directory name to save the image samples [samples]\")\nflags.DEFINE_boolean(\"train\", False, \"True for training, False for testing [False]\")\nflags.DEFINE_boolean(\"crop\", False, \"True for training, False for testing [False]\")\nflags.DEFINE_boolean(\"visualize\", False, \"True for visualizing, False for nothing [False]\")\nFLAGS = flags.FLAGS\n\n\n\npp.pprint(flags.FLAGS.__flags)\n\nif FLAGS.input_width is None:\n FLAGS.input_width = FLAGS.input_height\nif FLAGS.output_width is None:\n FLAGS.output_width = FLAGS.output_height\n\nif not os.path.exists(FLAGS.checkpoint_dir):\n os.makedirs(FLAGS.checkpoint_dir)\nif not os.path.exists(FLAGS.sample_dir):\n os.makedirs(FLAGS.sample_dir)\n\n# gpu_options = tf.GPUOptions(per_process_gpu_memory_fraction=0.333)\nrun_config = tf.ConfigProto()\nrun_config.gpu_options.allow_growth = True\n\n\nsess = tf.Session(config=run_config)\n\ndcgan = DCGAN(\n sess,\n input_width=FLAGS.input_width,\n input_height=FLAGS.input_height,\n output_width=FLAGS.output_width,\n output_height=FLAGS.output_height,\n batch_size=FLAGS.batch_size,\n sample_num=FLAGS.batch_size,\n dataset_name=FLAGS.dataset,\n input_fname_pattern=FLAGS.input_fname_pattern,\n crop=FLAGS.crop,\n checkpoint_dir=FLAGS.checkpoint_dir,\n sample_dir=FLAGS.sample_dir)\n\nshow_all_variables()\n\nif not dcgan.load(FLAGS.checkpoint_dir)[0]:\n raise Exception(\"[!] Train a model first, then run test mode\")",
"{}\n---------\nVariables: name (type shape) [size]\n---------\ngenerator/g_h0_lin/Matrix:0 (float32_ref 100x8192) [819200, bytes: 3276800]\ngenerator/g_h0_lin/bias:0 (float32_ref 8192) [8192, bytes: 32768]\ngenerator/g_bn0/beta:0 (float32_ref 512) [512, bytes: 2048]\ngenerator/g_bn0/gamma:0 (float32_ref 512) [512, bytes: 2048]\ngenerator/g_h1/w:0 (float32_ref 5x5x256x512) [3276800, bytes: 13107200]\ngenerator/g_h1/biases:0 (float32_ref 256) [256, bytes: 1024]\ngenerator/g_bn1/beta:0 (float32_ref 256) [256, bytes: 1024]\ngenerator/g_bn1/gamma:0 (float32_ref 256) [256, bytes: 1024]\ngenerator/g_h2/w:0 (float32_ref 5x5x128x256) [819200, bytes: 3276800]\ngenerator/g_h2/biases:0 (float32_ref 128) [128, bytes: 512]\ngenerator/g_bn2/beta:0 (float32_ref 128) [128, bytes: 512]\ngenerator/g_bn2/gamma:0 (float32_ref 128) [128, bytes: 512]\ngenerator/g_h3/w:0 (float32_ref 5x5x64x128) [204800, bytes: 819200]\ngenerator/g_h3/biases:0 (float32_ref 64) [64, bytes: 256]\ngenerator/g_bn3/beta:0 (float32_ref 64) [64, bytes: 256]\ngenerator/g_bn3/gamma:0 (float32_ref 64) [64, bytes: 256]\ngenerator/g_h4/w:0 (float32_ref 5x5x3x64) [4800, bytes: 19200]\ngenerator/g_h4/biases:0 (float32_ref 3) [3, bytes: 12]\ndiscriminator/d_h0_conv/w:0 (float32_ref 5x5x3x64) [4800, bytes: 19200]\ndiscriminator/d_h0_conv/biases:0 (float32_ref 64) [64, bytes: 256]\ndiscriminator/d_h1_conv/w:0 (float32_ref 5x5x64x128) [204800, bytes: 819200]\ndiscriminator/d_h1_conv/biases:0 (float32_ref 128) [128, bytes: 512]\ndiscriminator/d_bn1/beta:0 (float32_ref 128) [128, bytes: 512]\ndiscriminator/d_bn1/gamma:0 (float32_ref 128) [128, bytes: 512]\ndiscriminator/d_h2_conv/w:0 (float32_ref 5x5x128x256) [819200, bytes: 3276800]\ndiscriminator/d_h2_conv/biases:0 (float32_ref 256) [256, bytes: 1024]\ndiscriminator/d_bn2/beta:0 (float32_ref 256) [256, bytes: 1024]\ndiscriminator/d_bn2/gamma:0 (float32_ref 256) [256, bytes: 1024]\ndiscriminator/d_h3_conv/w:0 (float32_ref 5x5x256x512) [3276800, bytes: 13107200]\ndiscriminator/d_h3_conv/biases:0 (float32_ref 512) [512, bytes: 2048]\ndiscriminator/d_bn3/beta:0 (float32_ref 512) [512, bytes: 2048]\ndiscriminator/d_bn3/gamma:0 (float32_ref 512) [512, bytes: 2048]\ndiscriminator/d_h3_lin/Matrix:0 (float32_ref 8192x1) [8192, bytes: 32768]\ndiscriminator/d_h3_lin/bias:0 (float32_ref 1) [1, bytes: 4]\nTotal size of variables: 9451908\nTotal bytes of variables: 37807632\n [*] Reading checkpoints...\nINFO:tensorflow:Restoring parameters from checkpoint/PatchofPlaces_64_64_64/DCGAN.model-150002\n [*] Success to read DCGAN.model-150002\n"
]
],
[
[
"## Utility functions",
"_____no_output_____"
]
],
[
[
"def layer_extraction(dcgan, file_names):\n return dcgan.get_feature(FLAGS, file_names)\n \ndef maxpooling(disc):\n kernel_stride_size = 4\n maxpooling = [\n tf.nn.max_pool(disc[i],ksize=[1,2**(4-i),2**(4-i),1],\n strides=[1,2**(4-i),2**(4-i),1],padding='SAME')\n for i in range(4)\n ]\n\n# tf.global_variables_initializer().run()\n maxpool_result = sess.run(maxpooling)\n\n# for idx in range(4):\n# print(idx, maxpool_result[idx].shape)\n\n return maxpool_result\n\ndef flatten(disc):\n flatten = [\n tf.reshape(disc[i],[64, -1])\n for i in range(4)\n ]\n# tf.global_variables_initializer().run()\n flatten_result = sess.run(flatten)\n \n return flatten_result\n\ndef concat(disc):\n concat = tf.concat(disc,1)\n\n# tf.global_variables_initializer().run()\n concat_result = sess.run(concat)\n \n return concat_result\n\ndef feature_ext_GAN(file_names):\n \n ret = layer_extraction(dcgan, file_names)\n ret = maxpooling(ret)\n ret = flatten(ret)\n ret = concat(ret)\n \n return ret\n",
"_____no_output_____"
]
],
[
[
"# Integration",
"_____no_output_____"
]
],
[
[
"for term in range(11,15):\n print('%d ~ %d' % (50*term,50*(term+1)))\n \n disc_list = []\n batch_list = []\n file_names = []\n \n for idx in range(50*term,50*(term+1)):\n patch_path =\"/media/dongwonshin/Ubuntu Data/Datasets/FAB-MAP/Image Data/City Centre/patches/#300/\"\n data = sorted(glob(\"%s/%04d/*.jpg\" % (patch_path, idx)))\n# patch_path =\"/media/dongwonshin/Ubuntu Data/Datasets/Places365/Large_images/val_large/patches\"\n# data = glob(\"%s/Places365_val_%08d/*.jpg\" % (patch_path, idx))\n file_names.append(data)\n\n file_names=np.concatenate(file_names)\n print('total:',len(file_names))\n# print(file_names)\n\n for idx in range(0, len(file_names)-64,64):\n batch_files = file_names[idx: idx+64]\n\n disc = feature_ext_GAN(batch_files)\n\n disc_list.append(disc)\n batch_list.append(batch_files)\n sys.stdout.write('.')\n\n final_disc_list = np.concatenate(disc_list)\n final_batch_list = np.concatenate(batch_list)\n \n X = np.array(final_disc_list)\n pca = PCA(n_components = 128)\n pca.fit(X)\n final_disc_list = pca.transform(X)\n \n for idx, name in enumerate(final_batch_list):\n# output_filename = '/media/dongwonshin/Ubuntu Data/Datasets/Places365/Large_images/val_large/descs/128dim/' + (name.split('/')[-2])+'.desc'\n output_filename = '/media/dongwonshin/Ubuntu Data/Datasets/FAB-MAP/Image Data/City Centre/descs/128dim/' + (name.split('/')[-2])+'.desc'\n\n with open(output_filename,'at') as fp:\n for v in final_disc_list[idx]:\n fp.write('%f ' % v)\n fp.write('\\n')",
"550 ~ 600\ntotal: 14927\n.........................................................................................................................................................................................................................................600 ~ 650\ntotal: 14647\n....................................................................................................................................................................................................................................650 ~ 700\ntotal: 15000\n..........................................................................................................................................................................................................................................700 ~ 750\ntotal: 15000\n...."
],
[
"desc_path =\"/media/dongwonshin/Ubuntu Data/Datasets/FAB-MAP/Image Data/City Centre/descs\"\ndesc_name = glob(\"%s/*.desc\" % (desc_path))\ndesc_name.sort()\nfor i, d in enumerate(desc_name):\n if (i+1 != int(d[77:81])):\n print(i+1)\n break\n",
"1020\n"
]
],
[
[
"# Descriptor Save",
"_____no_output_____"
]
],
[
[
"for idx, name in enumerate(final_batch_list):\n output_filename = '/media/dongwonshin/Ubuntu Data/Datasets/FAB-MAP/Image Data/City Centre/descs/' + (name.split('/')[-2])+'.desc'\n \n with open(output_filename,'at') as fp:\n for v in final_disc_list[idx]:\n fp.write('%f ' % v)\n fp.write('\\n')",
"_____no_output_____"
]
],
[
[
"# Result Analysis",
"_____no_output_____"
]
],
[
[
"# import cv2 \nimport numpy as np\nimport matplotlib.pyplot as plt\nimport matplotlib.image as mpimg\n\nSURF_result_text = '/home/dongwonshin/Desktop/LC_text_results/20170622_SURF_result_2.txt'\nDCGAN_result_text = '/home/dongwonshin/Desktop/LC_text_results/20170622_DCGAN_result_128dim_2.txt'\nhow_many = 199\n\nwith open(SURF_result_text) as fp:\n \n SURF_current_idx = []\n SURF_most_related_idx = []\n \n lines = fp.readlines()\n for line in lines:\n ele = line.strip().split(',')\n SURF_current_idx.append(ele[0].split('=')[1])\n SURF_most_related_idx.append(ele[2].split('=')[1])\n\nwith open(DCGAN_result_text) as fp:\n \n DCGAN_current_idx = []\n DCGAN_most_related_idx = []\n \n lines = fp.readlines()\n for line in lines:\n ele = line.strip().split(',')\n DCGAN_current_idx.append(ele[0].split('=')[1])\n DCGAN_most_related_idx.append(ele[2].split('=')[1])\n \ncnt = 0\nLC_cs_cnt = 0\nLC_cd_cnt = 0\nfor c, s, d in zip(SURF_current_idx, SURF_most_related_idx, DCGAN_most_related_idx):\n\n gps_c = np.array(GPS_info_list[int(c)])\n gps_s = np.array(GPS_info_list[int(s)])\n gps_d = np.array(GPS_info_list[int(d)])\n \n gps_cs = np.linalg.norm(gps_c-gps_s)\n gps_cd = np.linalg.norm(gps_c-gps_d)\n \n threshold = 5\n if (gps_cs < threshold):\n LC_cs = 'true'\n LC_cs_cnt += 1\n else:\n LC_cs = 'false'\n \n if (gps_cd < threshold):\n LC_cd = 'true'\n LC_cd_cnt += 1\n else:\n LC_cd = 'false'\n \n# print('%4d' % int(c), gps_c)\n# print('%4d' % int(s), gps_s, gps_cs, LC_cs)\n# print('%4d' % int(d), gps_d, gps_cd, LC_cd)\n# print()\n \n# cur_path = '/media/dongwonshin/Ubuntu Data/Datasets/FAB-MAP/Image Data/City Centre/images/%04d.jpg' % int(c)\n# surf_path = '/media/dongwonshin/Ubuntu Data/Datasets/FAB-MAP/Image Data/City Centre/images/%04d.jpg' % int(s)\n# dcgan_path = '/media/dongwonshin/Ubuntu Data/Datasets/FAB-MAP/Image Data/City Centre/images/%04d.jpg' % int(d)\n \n# print(cur_path)\n# print(surf_path)\n# print(dcgan_path)\n \n# cur_img = mpimg.imread(cur_path)\n# surf_img = mpimg.imread(surf_path)\n# dcgan_img = mpimg.imread(dcgan_path)\n \n# one_img = np.hstack([cur_img, surf_img, dcgan_img])\n# plt.imshow(one_img)\n# plt.show()\n \n if (cnt > how_many):\n break\n else:\n cnt += 1\n\nprint('LC_cs_cnt = %d, LC_cd_cnt = %d' % (LC_cs_cnt, LC_cd_cnt))\n ",
"LC_cs_cnt = 82, LC_cd_cnt = 150\n"
]
],
[
[
"# Loop Closure GroundTruth Text Handling",
"_____no_output_____"
]
],
[
[
"LC_corr_list = []\nwith open('/media/dongwonshin/Ubuntu Data/Datasets/FAB-MAP/GroundTruth Text/CityCentreGroundTruth.txt') as fp:\n row = 1\n for line in fp:\n row_ele = line.strip().split(',')\n if ('1' in row_ele):\n \n col = 1\n for r in row_ele:\n if (r == '1'):\n# print('(row, col) (%d, %d)' % (row, col))\n LC_corr_list.append([row,col])\n col+=1\n \n row += 1 \n else:\n print('eof')",
"eof\n"
],
[
"GPS_info_list = [[0,0]] # dummy for a start index 1\nwith open('/media/dongwonshin/Ubuntu Data/Datasets/FAB-MAP/GroundTruth Text/CityCentreGPSData.txt') as fp:\n for line in fp:\n GPS_info_list.append(\n [float(line.strip().split(' ')[1]) , float(line.strip().split(' ')[2])]\n )\n else:\n print('eof')",
"eof\n"
],
[
"def isOdd(val):\n return not (val%2==0)\n\ndef isEven(val):\n return (val%2==0)\n\nfor i, corr in enumerate(LC_corr_list):\n if (isOdd(corr[0]) and isEven(corr[1])):\n continue\n if (isEven(corr[0]) and isOdd(corr[1])):\n continue\n \n img_i_path = ('/media/dongwonshin/Ubuntu Data/Datasets/FAB-MAP/Image Data/City Centre/images/%04d.jpg' % corr[0])\n img_j_path = ('/media/dongwonshin/Ubuntu Data/Datasets/FAB-MAP/Image Data/City Centre/images/%04d.jpg' % corr[1])\n print(corr[0], GPS_info_list[corr[0]])\n print(corr[1], GPS_info_list[corr[1]])\n \n img_i = mpimg.imread(img_i_path)\n img_j = mpimg.imread(img_j_path)\n \n merge_img = np.hstack([img_i, img_j])\n plt.imshow(merge_img)\n plt.show()\n \n if i > 10:\n break",
"1353 [201.13763, -174.712228]\n305 [196.393236, -168.938331]\n"
]
]
] | [
"code",
"markdown",
"code",
"markdown",
"code",
"markdown",
"code",
"markdown",
"code",
"markdown",
"code"
] | [
[
"code"
],
[
"markdown"
],
[
"code"
],
[
"markdown"
],
[
"code",
"code"
],
[
"markdown"
],
[
"code"
],
[
"markdown"
],
[
"code"
],
[
"markdown"
],
[
"code",
"code",
"code"
]
] |
e7786cb1a5ed7e8c1b9b1ebfd4eb346210048c2a | 80,580 | ipynb | Jupyter Notebook | microsoft-ml/Microsoft ML Tutorial/Microsoft ML Tutorial Notebooks/Microsoft ML Twitter Sentiment Analysis Tutorial.ipynb | Microsoft/microsoft-r | a6927bbf51b748a61ad4074434b811bcfb4d6790 | [
"MIT"
] | 87 | 2017-02-08T16:14:29.000Z | 2019-04-09T15:13:07.000Z | microsoft-ml/Microsoft ML Tutorial/Microsoft ML Tutorial Notebooks/Microsoft ML Twitter Sentiment Analysis Tutorial.ipynb | microsoft/microsoft-r | c42fa71a37da921807839105b63f96282ca5b7c0 | [
"MIT"
] | 19 | 2017-04-21T04:01:22.000Z | 2019-04-18T15:58:50.000Z | microsoft-ml/Microsoft ML Tutorial/Microsoft ML Tutorial Notebooks/Microsoft ML Twitter Sentiment Analysis Tutorial.ipynb | Microsoft/microsoft-r | a6927bbf51b748a61ad4074434b811bcfb4d6790 | [
"MIT"
] | 73 | 2017-01-19T00:27:26.000Z | 2019-04-18T08:47:26.000Z | 72.464029 | 26,966 | 0.670985 | [
[
[
"# Microsoft ML Twitter Sentiment Analysis Tutorial\n\n1. [Overview](#Overview)\n2. [Fitting Models That Identify Sentiment](#TwitterSentiment)\n3. [What's Next?](#Next)",
"_____no_output_____"
],
[
"## <a id=\"Overview\">1. OVERVIEW</a>\n\nMicrosoft Machine Learning, or Microsoft ML for short, is an R package within Microsoft R Services that includes powerful machine learning algorithms and associated tools. The tutorial is an introduction to Microsoft ML for data scientists who want to take advantage of its unique capabilities. It is intended primarily for those who are comfortable with using Microsoft R Services for data science, and want to see an end-to-end example that uses Microsoft ML to carry out common data science tasks.",
"_____no_output_____"
],
[
"## <a id=\"TwitterSentiment\">2. FITTING MODELS THAT IDENTIFY SENTIMENT</a>\n\nThe tutorial steps through the fitting of a model for identifying sentiment from Twitter text. Identifying how people feel about a product or an event is important to sales. We will focus on identifying which tweets identify happiness or sadness.\n\nThe tutorial begins from data imported from a twitter database. In this tutorial, the features are automatically extracted from the text using the featurizeText Microsoft ML transform. Then, a model is fit by multiple learning algorithms, and the performance of these fit models is compared to select the best one. The initial and final steps in this process will be familiar to Microsoft R Services users, while the model fitting and performance evaluation steps will involve new Microsoft ML commands.",
"_____no_output_____"
],
[
"### <a id=\"Packages\">2.1. LOADING THE PACKAGES</a>\n\nThe tutorial is broken into steps, the first being loading the Microsoft ML package. When you execute the first step, there should be no output.",
"_____no_output_____"
]
],
[
[
"#-----------------------------------------------------------------------\n# 1. Load packages.\n#-----------------------------------------------------------------------\nif (!suppressPackageStartupMessages(require(\"MicrosoftML\",\n quietly = TRUE,\n warn.conflicts = FALSE))) {\n stop(\"The MicrosoftML package does not seem to be installed, so this\\n\",\n \"script cannot be run. If Microsoft R Server with MML is installed,\\n\",\n \"you may need to switch the R engine option. In R Tools for Visual\\n\",\n \"Studio, this option is under:\\n\",\n \"\\tR Tools -> Options -> R Engine.\\n\",\n \"If Microsoft R Server with MML is not installed, you can download it\\n\",\n \"from https://microsoft.sharepoint.com/teams/TLC/SitePages/MicrosoftML.aspx\\n\")\n}",
"_____no_output_____"
]
],
[
[
"### <a id=\"Import\">2.2. IMPORT DATA</a>\n\nThe second step consists of importing the data we will use to fit a model. There is only one table of data: the HappyOrSad table. This section imports that table into an Xdf. These Xdfs are an efficient way of working with large amounts of data. They are files in which the rows are grouped in blocks whose size is specified by the parameter rowsPerBlock.",
"_____no_output_____"
]
],
[
[
"#-----------------------------------------------------------------------\n# 2. Import data.\n#-----------------------------------------------------------------------\n\n# The directory containing data files.\ndataDir <- file.path(\"Data\")\n\n# Verify that the data file exists.\nif (!file.exists(file.path(dataDir, \"HappyOrSad.csv\"))) {\n stop(\"The data files needed for running this script cannot be found.\\n\",\n \"You may need to set R's working directory to the location of the Data\\n\",\n \"directory.\")\n}\n\n# The data chunk size.\nrowsPerBlock <- 1000000",
"_____no_output_____"
]
],
[
[
"The HappyOrSad table has one row per tweet, and three columns: id_nfpu, features, and label. Because the id_nfpu column uniquely identifies each tweet, it is ignored. The two remaining columns are text, and they are respectively renamed Text and sentiment. As part of the importing process, we create Label, a logical variable that is TRUE when the sentiment is happiness, and false otherwise.",
"_____no_output_____"
]
],
[
[
"# The data source has three columns. Keep the tweet text and sentiment.\ndatasetSource <-\n RxTextData(file.path(dataDir, \"HappyOrSad.csv\"),\n varsToKeep = c(\"features\", \"label\"),\n colInfo = list(features = list(type = \"character\",\n newName = \"Text\"),\n label = list(type = \"character\",\n newName = \"sentiment\")),\n quotedDelimiters = TRUE)\n\n# Import the data. Define Label.\ndataset <-\n rxImport(datasetSource,\n transforms = list(Label = sentiment == \"happiness\"),\n outFile = tempfile(fileext = \".xdf\"),\n rowsPerRead = rowsPerBlock)",
"Rows Read: 10362, Total Rows Processed: 10362, Total Chunk Time: 0.152 seconds \n"
]
],
[
[
"We can see from the output that the activity table has 252,204 rows, and its first few rows are",
"_____no_output_____"
]
],
[
[
"head(dataset)",
"_____no_output_____"
]
],
[
[
"### <a id=\"Split\">2.3. SPLIT THE DATASET INTO TRAIN AND TEST</a>\n\nTo create train and test sets, the data are randomly split by tweet into two datasets. The training data tweets will be used by the learners to fit models, while the test data tweets will be used as a fair measure the performance of the fit models. Because the split is randomized, we first set the random seed used by the randomizer to guarantee we will be able to reproduce our results at a later date.",
"_____no_output_____"
]
],
[
[
"#-----------------------------------------------------------------------\n# 3. Split the dataset into train and test data.\n#-----------------------------------------------------------------------\n# Set the random seed for reproducibility of randomness.\nset.seed(2345, \"L'Ecuyer-CMRG\")\n# Randomly split the data 80-20 between train and test sets.\ndataProb <- c(Train = 0.8, Test = 0.2)\ndataSplit <-\n rxSplit(dataset,\n splitByFactor = \"splitVar\",\n transforms = list(splitVar =\n sample(dataFactor,\n size = .rxNumRows,\n replace = TRUE,\n prob = dataProb)),\n transformObjects =\n list(dataProb = dataProb,\n dataFactor = factor(names(dataProb),\n levels = names(dataProb))),\n outFilesBase = tempfile())\n\n# Name the train and test datasets.\ndataTrain <- dataSplit[[1]]\ndataTest <- dataSplit[[2]]",
"Rows Read: 10362, Total Rows Processed: 10362Rows Read: 8279, Total Rows Processed: 8279, Total Chunk Time: 0.020 seconds \nRows Read: 2083, Total Rows Processed: 2083, Total Chunk Time: 0.006 seconds \n, Total Chunk Time: 0.219 seconds \n"
]
],
[
[
"We can explore the distribution of \"Label\" in the train and test sets.",
"_____no_output_____"
]
],
[
[
"rxSummary(~ Label, dataTrain)$sDataFrame\nrxSummary(~ Label, dataTest)$sDataFrame",
"Rows Read: 8279, Total Rows Processed: 8279, Total Chunk Time: Less than .001 seconds \nComputation time: 0.002 seconds.\n"
]
],
[
[
"We read from the output that train has 8,279 rows while test has 2,083 rows. Because Label is a boolean, its mean shows the proportion of happy tweets in the data. We see that train has more than 50% happy tweets and that test has almost 49% happy tweets, which is a reasonable split.",
"_____no_output_____"
],
[
"### <a id=\"Model\">2.4. DEFINE THE MODEL</a>\n\nThe model is a formula that describes what column has the label, and what columns are to be used to rxPredict the label. We will be using as rxPredictors features that will be automatically obtained from the Text column. Then we create a formula that says that Label is to be rxPredicted by these features.",
"_____no_output_____"
]
],
[
[
"#-----------------------------------------------------------------------\n# 4. Define the model to be fit.\n#-----------------------------------------------------------------------\n# The model is a formula that says that sentiments are to be identified\n# using Features, a stand-in for variables created on-the-fly from text\n# by the text transform.\n(model <- Label ~ Features)",
"_____no_output_____"
]
],
[
[
"The left-hand side of the formula is the label, while the right-hand side lists the source of the rxPredictors.",
"_____no_output_____"
],
[
"### <a id=\"Fit\">2.5. FIT THE MODEL</a>\n\nThe model will be fit by learners that can rxPredict class data: rxLogisticRegression, rxFastLinear, rxFastTrees, rxFastForest, and rxNeuralNet. In the next section, each fit will be used to score the test data. The comments in this section give a glimpse of the kind of work done by each learner.\n\nEach command has two mlTransforms. The featurizeText transform automatically creates text-based features from the tweets, while the selectFeatures transform sorts through the created features to include in the model those that are most informative about each tweet's label.",
"_____no_output_____"
]
],
[
[
"#-----------------------------------------------------------------------\n# 5. Fit the model using different learners.\n#-----------------------------------------------------------------------\n# Fit the model with logistic regression. This finds the variable\n# weights that are most useful for rxPredicting sentiment. The\n# rxLogisticRegression learner automatically adjusts the weights to select\n# those variables that are most useful for making rxPredictions.\nrxLogisticRegressionFit <-\n rxLogisticRegression(model, data = dataTrain,\n mlTransforms =\n list(featurizeText(vars = c(Features = \"Text\")),\n selectFeatures(model, mutualInformation())))",
"Beginning read for block: 1\nRows Read: 8279, Read Time: 0.007, Transform Time: 0\nBeginning read for block: 2\nNo rows remaining. Finished reading data set. \nBeginning read for block: 1\nRows Read: 8279, Read Time: 0.007, Transform Time: 0\nBeginning read for block: 2\nNo rows remaining. Finished reading data set. \nComputing mutual information\nBeginning read for block: 1\nRows Read: 8279, Read Time: 0.007, Transform Time: 0\nBeginning read for block: 2\nNo rows remaining. Finished reading data set. \nBeginning read for block: 1\nRows Read: 8279, Read Time: 0.009, Transform Time: 0\nBeginning read for block: 2\nNo rows remaining. Finished reading data set. \nBeginning read for block: 1\nRows Read: 8279, Read Time: 0.006, Transform Time: 0\nBeginning read for block: 2\nNo rows remaining. Finished reading data set. \nFinished mutual information computation in 00:00:01.4135176\nSelecting features to drop\nSelected 1000 slots out of 15588 in column 'Features'\nTotal number of slots selected: 1000\nNot adding a normalizer.\nBeginning read for block: 1\nRows Read: 8279, Read Time: 0.007, Transform Time: 0\nBeginning read for block: 2\nNo rows remaining. Finished reading data set. \nBeginning read for block: 1\nRows Read: 8279, Read Time: 0.006, Transform Time: 0\nBeginning read for block: 2\nNo rows remaining. Finished reading data set. \nWrote 8279 rows across 2 columns in 00:00:00.1258488\nLBFGS multi-threading will attempt to load dataset into memory. In case of out-of-memory issues, turn off multi-threading by setting trainThreads to 1.\nBeginning optimization\nnum vars: 1001\nimprovement criterion: Mean Improvement\nL1 regularization selected 346 of 1001 weights.\nNot training a calibrator because it is not needed.\nElapsed time: 00:00:02.6625234\nElapsed time: 00:00:00.2480366\n"
],
[
"#-----------------------------------------------------------------------\n# Fit the model with linear regression. This finds the variable\n# weights that are most useful for rxPredicting sentiment. The\n# rxFastLinear learner automatically adjusts the weights to select\n# those variables that are most useful for making rxPredictions.\nrxFastLinearFit <-\n rxFastLinear(model, data = dataTrain,\n mlTransforms =\n list(featurizeText(vars = c(Features = \"Text\")),\n selectFeatures(model, mutualInformation())))",
"Beginning read for block: 1\nRows Read: 8279, Read Time: 0.006, Transform Time: 0\nBeginning read for block: 2\nNo rows remaining. Finished reading data set. \nBeginning read for block: 1\nRows Read: 8279, Read Time: 0.006, Transform Time: 0\nBeginning read for block: 2\nNo rows remaining. Finished reading data set. \nComputing mutual information\nBeginning read for block: 1\nRows Read: 8279, Read Time: 0.006, Transform Time: 0\nBeginning read for block: 2\nNo rows remaining. Finished reading data set. \nBeginning read for block: 1\nRows Read: 8279, Read Time: 0.007, Transform Time: 0\nBeginning read for block: 2\nNo rows remaining. Finished reading data set. \nBeginning read for block: 1\nRows Read: 8279, Read Time: 0.005, Transform Time: 0\nBeginning read for block: 2\nNo rows remaining. Finished reading data set. \nFinished mutual information computation in 00:00:01.2399867\nSelecting features to drop\nSelected 1000 slots out of 15588 in column 'Features'\nTotal number of slots selected: 1000\nNot adding a normalizer.\nBeginning read for block: 1\nRows Read: 8279, Read Time: 0.007, Transform Time: 0\nBeginning read for block: 2\nNo rows remaining. Finished reading data set. \nBeginning read for block: 1\nRows Read: 8279, Read Time: 0.005, Transform Time: 0\nBeginning read for block: 2\nNo rows remaining. Finished reading data set. \nWrote 8279 rows across 2 columns in 00:00:00.1051141\nUsing 2 threads to train.\nAutomatically choosing a check frequency of 2.\nAuto-tuning parameters: maxIterations = 180.\nAuto-tuning parameters: L2 = 2.684168E-05.\nAuto-tuning parameters: L1Threshold (L1/L2) = 0.5.\nUsing best model from iteration 178.\nNot training a calibrator because it is not needed.\nElapsed time: 00:00:03.3116258\nElapsed time: 00:00:00.1146629\n"
],
[
"#-----------------------------------------------------------------------\n# Fit the model with boosted trees. This finds the combinations of\n# variables and threshold values that are useful for rxPredicting sentiment.\n# The rxFastTrees learner automatically builds a sequence of trees so that\n# trees later in the sequence repair errors made by trees earlier in the\n# sequence.\nrxFastTreesFit <-\n rxFastTrees(model, data = dataTrain,\n mlTransforms =\n list(featurizeText(vars = c(Features = \"Text\")),\n selectFeatures(model, mutualInformation())),\n randomSeed = 23648)",
"Beginning read for block: 1\nRows Read: 8279, Read Time: 0.008, Transform Time: 0\nBeginning read for block: 2\nNo rows remaining. Finished reading data set. \nBeginning read for block: 1\nRows Read: 8279, Read Time: 0.007, Transform Time: 0\nBeginning read for block: 2\nNo rows remaining. Finished reading data set. \nComputing mutual information\nBeginning read for block: 1\nRows Read: 8279, Read Time: 0.007, Transform Time: 0\nBeginning read for block: 2\nNo rows remaining. Finished reading data set. \nBeginning read for block: 1\nRows Read: 8279, Read Time: 0.008, Transform Time: 0\nBeginning read for block: 2\nNo rows remaining. Finished reading data set. \nBeginning read for block: 1\nRows Read: 8279, Read Time: 0.006, Transform Time: 0\nBeginning read for block: 2\nNo rows remaining. Finished reading data set. \nFinished mutual information computation in 00:00:01.3049749\nSelecting features to drop\nSelected 1000 slots out of 15588 in column 'Features'\nTotal number of slots selected: 1000\nNot adding a normalizer.\nMaking per-feature arrays\nChanging data from row-wise to column-wise\nBeginning read for block: 1\nRows Read: 8279, Read Time: 0.006, Transform Time: 0\nBeginning read for block: 2\nNo rows remaining. Finished reading data set. \nProcessed 8279 instances\nBinning and forming Feature objects\nReserved memory for tree learner: 4848064 bytes\nStarting to train ...\nNot training a calibrator because it is not needed.\nElapsed time: 00:00:03.7108232\n"
],
[
"#-----------------------------------------------------------------------\n# Fit the model with random forest. This finds the combinations of\n# variables and threshold values that are useful for rxPredicting sentiment.\n# The rxFastForest learner automatically builds a set of trees whose\n# combined rxPredictions are better than the rxPredictions of any one of the\n# trees.\nrxFastForestFit <-\n rxFastForest(model, data = dataTrain,\n mlTransforms =\n list(featurizeText(vars = c(Features = \"Text\")),\n selectFeatures(model, mutualInformation())),\n randomSeed = 23648)",
"Beginning read for block: 1\nRows Read: 8279, Read Time: 0.005, Transform Time: 0\nBeginning read for block: 2\nNo rows remaining. Finished reading data set. \nBeginning read for block: 1\nRows Read: 8279, Read Time: 0.006, Transform Time: 0\nBeginning read for block: 2\nNo rows remaining. Finished reading data set. \nComputing mutual information\nBeginning read for block: 1\nRows Read: 8279, Read Time: 0.006, Transform Time: 0\nBeginning read for block: 2\nNo rows remaining. Finished reading data set. \nBeginning read for block: 1\nRows Read: 8279, Read Time: 0.009, Transform Time: 0\nBeginning read for block: 2\nNo rows remaining. Finished reading data set. \nBeginning read for block: 1\nRows Read: 8279, Read Time: 0.005, Transform Time: 0\nBeginning read for block: 2\nNo rows remaining. Finished reading data set. \nFinished mutual information computation in 00:00:01.2482423\nSelecting features to drop\nSelected 1000 slots out of 15588 in column 'Features'\nTotal number of slots selected: 1000\nNot adding a normalizer.\nMaking per-feature arrays\nChanging data from row-wise to column-wise\nBeginning read for block: 1\nRows Read: 8279, Read Time: 0.006, Transform Time: 0\nBeginning read for block: 2\nNo rows remaining. Finished reading data set. \nProcessed 8279 instances\nBinning and forming Feature objects\nReserved memory for tree learner: 4848064 bytes\nStarting to train ...\nTraining calibrator.\nBeginning read for block: 1\nRows Read: 8279, Read Time: 0.006, Transform Time: 0\nBeginning read for block: 2\nNo rows remaining. Finished reading data set. \nElapsed time: 00:00:03.2268725\n"
],
[
"#-----------------------------------------------------------------------\n# Fit the model with neural net. This finds the variable weights that\n# are most useful for rxPredicting sentiment. Neural net can excel when\n# dealing with non-linear relationships between the variables.\nrxNeuralNetFit <-\n rxNeuralNet(model, data = dataTrain,\n mlTransforms =\n list(featurizeText(vars = c(Features = \"Text\")),\n selectFeatures(model, mutualInformation())))",
"Beginning read for block: 1\nRows Read: 8279, Read Time: 0.007, Transform Time: 0\nBeginning read for block: 2\nNo rows remaining. Finished reading data set. \nBeginning read for block: 1\nRows Read: 8279, Read Time: 0.005, Transform Time: 0\nBeginning read for block: 2\nNo rows remaining. Finished reading data set. \nComputing mutual information\nBeginning read for block: 1\nRows Read: 8279, Read Time: 0.009, Transform Time: 0\nBeginning read for block: 2\nNo rows remaining. Finished reading data set. \nBeginning read for block: 1\nRows Read: 8279, Read Time: 0.008, Transform Time: 0\nBeginning read for block: 2\nNo rows remaining. Finished reading data set. \nBeginning read for block: 1\nRows Read: 8279, Read Time: 0.006, Transform Time: 0\nBeginning read for block: 2\nNo rows remaining. Finished reading data set. \nFinished mutual information computation in 00:00:01.2677761\nSelecting features to drop\nSelected 1000 slots out of 15588 in column 'Features'\nTotal number of slots selected: 1000\nNot adding a normalizer.\nBeginning read for block: 1\nRows Read: 8279, Read Time: 0.005, Transform Time: 0\nBeginning read for block: 2\nNo rows remaining. Finished reading data set. \nBeginning read for block: 1\nRows Read: 8279, Read Time: 0.006, Transform Time: 0\nBeginning read for block: 2\nNo rows remaining. Finished reading data set. \nWrote 8279 rows across 2 columns in 00:00:00.1013217\nUsing: SSE Math\n\r\n***** Net definition *****\r\n input Data [1000];\r\n hidden H [100] sigmoid { // Depth 1\r\n from Data all;\r\n }\r\n output Result [1] sigmoid { // Depth 0\r\n from H all;\r\n }\r\n***** End net definition *****\r\nInput count: 1000\nOutput count: 1\nOutput Function: Sigmoid\nLoss Function: CrossEntropy\nPreTrainer: NoPreTrainer\n___________________________________________________________________\nStarting training...\nLearning rate: 0.001000\nMomentum: 0.000000\nInitWtsDiameter: 0.100000\n___________________________________________________________________\nInitializing 1 Hidden Layers, 100201 Weights...\nEstimated Pre-training MeanError = 0.698938\nIter:1/100, MeanErr=0.694743(-0.60%%), 15065.45M WeightUpdates/sec\nIter:2/100, MeanErr=0.694840(0.01%%), 18442.02M WeightUpdates/sec\nIter:3/100, MeanErr=0.694486(-0.05%%), 17963.72M WeightUpdates/sec\nIter:4/100, MeanErr=0.694071(-0.06%%), 18966.84M WeightUpdates/sec\nIter:5/100, MeanErr=0.694328(0.04%%), 14103.88M WeightUpdates/sec\nIter:6/100, MeanErr=0.694218(-0.02%%), 17415.57M WeightUpdates/sec\nIter:7/100, MeanErr=0.693784(-0.06%%), 18259.03M WeightUpdates/sec\nIter:8/100, MeanErr=0.693539(-0.04%%), 19027.81M WeightUpdates/sec\nIter:9/100, MeanErr=0.693908(0.05%%), 18918.35M WeightUpdates/sec\nIter:10/100, MeanErr=0.693858(-0.01%%), 17816.30M WeightUpdates/sec\nIter:11/100, MeanErr=0.693686(-0.02%%), 18904.39M WeightUpdates/sec\nIter:12/100, MeanErr=0.692638(-0.15%%), 18432.16M WeightUpdates/sec\nIter:13/100, MeanErr=0.693043(0.06%%), 14960.45M WeightUpdates/sec\nIter:14/100, MeanErr=0.692614(-0.06%%), 18872.47M WeightUpdates/sec\nIter:15/100, MeanErr=0.692331(-0.04%%), 18877.39M WeightUpdates/sec\nIter:16/100, MeanErr=0.691354(-0.14%%), 18753.40M WeightUpdates/sec\nIter:17/100, MeanErr=0.690383(-0.14%%), 18039.59M WeightUpdates/sec\nIter:18/100, MeanErr=0.690110(-0.04%%), 18911.54M WeightUpdates/sec\nIter:19/100, MeanErr=0.690243(0.02%%), 18956.40M WeightUpdates/sec\nIter:20/100, MeanErr=0.689222(-0.15%%), 16790.78M WeightUpdates/sec\nIter:21/100, MeanErr=0.688592(-0.09%%), 18898.61M WeightUpdates/sec\nIter:22/100, MeanErr=0.687258(-0.19%%), 18102.53M WeightUpdates/sec\nIter:23/100, MeanErr=0.686780(-0.07%%), 18572.74M WeightUpdates/sec\nIter:24/100, MeanErr=0.685722(-0.15%%), 17177.13M WeightUpdates/sec\nIter:25/100, MeanErr=0.684179(-0.23%%), 19023.85M WeightUpdates/sec\nIter:26/100, MeanErr=0.683205(-0.14%%), 18716.99M WeightUpdates/sec\nIter:27/100, MeanErr=0.680451(-0.40%%), 16627.90M WeightUpdates/sec\nIter:28/100, MeanErr=0.679039(-0.21%%), 19057.83M WeightUpdates/sec\nIter:29/100, MeanErr=0.677644(-0.21%%), 19033.67M WeightUpdates/sec\nIter:30/100, MeanErr=0.675449(-0.32%%), 17345.71M WeightUpdates/sec\nIter:31/100, MeanErr=0.673144(-0.34%%), 18844.55M WeightUpdates/sec\nIter:32/100, MeanErr=0.671218(-0.29%%), 18907.28M WeightUpdates/sec\nIter:33/100, MeanErr=0.668102(-0.46%%), 18898.61M WeightUpdates/sec\nIter:34/100, MeanErr=0.665372(-0.41%%), 15378.79M WeightUpdates/sec\nIter:35/100, MeanErr=0.662624(-0.41%%), 19117.47M WeightUpdates/sec\nIter:36/100, MeanErr=0.659697(-0.44%%), 17391.65M WeightUpdates/sec\nIter:37/100, MeanErr=0.656194(-0.53%%), 17776.21M WeightUpdates/sec\nIter:38/100, MeanErr=0.653975(-0.34%%), 19070.63M WeightUpdates/sec\nIter:39/100, MeanErr=0.650510(-0.53%%), 19058.01M WeightUpdates/sec\nIter:40/100, MeanErr=0.647118(-0.52%%), 19036.25M WeightUpdates/sec\nIter:41/100, MeanErr=0.644360(-0.43%%), 18140.19M WeightUpdates/sec\nIter:42/100, MeanErr=0.641092(-0.51%%), 18903.37M WeightUpdates/sec\nIter:43/100, MeanErr=0.638272(-0.44%%), 18605.30M WeightUpdates/sec\nIter:44/100, MeanErr=0.634489(-0.59%%), 15621.61M WeightUpdates/sec\nIter:45/100, MeanErr=0.630816(-0.58%%), 18980.20M WeightUpdates/sec\nIter:46/100, MeanErr=0.629236(-0.25%%), 19120.26M WeightUpdates/sec\nIter:47/100, MeanErr=0.626434(-0.45%%), 16547.63M WeightUpdates/sec\nIter:48/100, MeanErr=0.623489(-0.47%%), 18871.97M WeightUpdates/sec\nIter:49/100, MeanErr=0.620743(-0.44%%), 18923.29M WeightUpdates/sec\nIter:50/100, MeanErr=0.618342(-0.39%%), 19074.44M WeightUpdates/sec\nIter:51/100, MeanErr=0.615784(-0.41%%), 17540.29M WeightUpdates/sec\nIter:52/100, MeanErr=0.613617(-0.35%%), 18947.35M WeightUpdates/sec\nIter:53/100, MeanErr=0.610573(-0.50%%), 18574.22M WeightUpdates/sec\nIter:54/100, MeanErr=0.608551(-0.33%%), 17783.28M WeightUpdates/sec\nIter:55/100, MeanErr=0.606447(-0.35%%), 18357.44M WeightUpdates/sec\nIter:56/100, MeanErr=0.604080(-0.39%%), 18889.78M WeightUpdates/sec\nIter:57/100, MeanErr=0.601244(-0.47%%), 18894.53M WeightUpdates/sec\nIter:58/100, MeanErr=0.599538(-0.28%%), 14938.23M WeightUpdates/sec\nIter:59/100, MeanErr=0.597053(-0.41%%), 17991.71M WeightUpdates/sec\nIter:60/100, MeanErr=0.594561(-0.42%%), 18999.59M WeightUpdates/sec\nIter:61/100, MeanErr=0.592751(-0.30%%), 17431.61M WeightUpdates/sec\nIter:62/100, MeanErr=0.590446(-0.39%%), 18461.63M WeightUpdates/sec\nIter:63/100, MeanErr=0.588331(-0.36%%), 18935.56M WeightUpdates/sec\nIter:64/100, MeanErr=0.585737(-0.44%%), 19117.82M WeightUpdates/sec\nIter:65/100, MeanErr=0.583760(-0.34%%), 17786.29M WeightUpdates/sec\nIter:66/100, MeanErr=0.581722(-0.35%%), 18881.12M WeightUpdates/sec\nIter:67/100, MeanErr=0.579230(-0.43%%), 18102.06M WeightUpdates/sec\nIter:68/100, MeanErr=0.576833(-0.41%%), 15406.07M WeightUpdates/sec\nIter:69/100, MeanErr=0.574782(-0.36%%), 18947.00M WeightUpdates/sec\nIter:70/100, MeanErr=0.572724(-0.36%%), 17429.73M WeightUpdates/sec\nIter:71/100, MeanErr=0.570328(-0.42%%), 17659.24M WeightUpdates/sec\nIter:72/100, MeanErr=0.568012(-0.41%%), 18887.06M WeightUpdates/sec\nIter:73/100, MeanErr=0.565969(-0.36%%), 19215.89M WeightUpdates/sec\nIter:74/100, MeanErr=0.563822(-0.38%%), 19148.83M WeightUpdates/sec\nIter:75/100, MeanErr=0.561578(-0.40%%), 18060.21M WeightUpdates/sec\nIter:76/100, MeanErr=0.558682(-0.52%%), 18741.03M WeightUpdates/sec\nIter:77/100, MeanErr=0.557239(-0.26%%), 18885.36M WeightUpdates/sec\nIter:78/100, MeanErr=0.554676(-0.46%%), 15681.76M WeightUpdates/sec\nIter:79/100, MeanErr=0.552723(-0.35%%), 18717.49M WeightUpdates/sec\nIter:80/100, MeanErr=0.549682(-0.55%%), 18302.29M WeightUpdates/sec\nIter:81/100, MeanErr=0.547993(-0.31%%), 18738.52M WeightUpdates/sec\nIter:82/100, MeanErr=0.545911(-0.38%%), 16833.96M WeightUpdates/sec\nIter:83/100, MeanErr=0.542927(-0.55%%), 18391.18M WeightUpdates/sec\nIter:84/100, MeanErr=0.540912(-0.37%%), 18538.83M WeightUpdates/sec\nIter:85/100, MeanErr=0.538768(-0.40%%), 18926.36M WeightUpdates/sec\nIter:86/100, MeanErr=0.537217(-0.29%%), 17828.54M WeightUpdates/sec\nIter:87/100, MeanErr=0.534463(-0.51%%), 18861.63M WeightUpdates/sec\nIter:88/100, MeanErr=0.532809(-0.31%%), 18692.18M WeightUpdates/sec\nIter:89/100, MeanErr=0.530901(-0.36%%), 18734.68M WeightUpdates/sec\nIter:90/100, MeanErr=0.528513(-0.45%%), 17563.02M WeightUpdates/sec\nIter:91/100, MeanErr=0.526226(-0.43%%), 18897.25M WeightUpdates/sec\nIter:92/100, MeanErr=0.524161(-0.39%%), 18779.38M WeightUpdates/sec\nIter:93/100, MeanErr=0.522293(-0.36%%), 16593.89M WeightUpdates/sec\nIter:94/100, MeanErr=0.520065(-0.43%%), 18697.51M WeightUpdates/sec\nIter:95/100, MeanErr=0.518003(-0.40%%), 17215.55M WeightUpdates/sec\nIter:96/100, MeanErr=0.515826(-0.42%%), 18950.25M WeightUpdates/sec\nIter:97/100, MeanErr=0.513923(-0.37%%), 18572.91M WeightUpdates/sec\nIter:98/100, MeanErr=0.511994(-0.38%%), 17661.77M WeightUpdates/sec\nIter:99/100, MeanErr=0.510253(-0.34%%), 19049.54M WeightUpdates/sec\nIter:100/100, MeanErr=0.508042(-0.43%%), 18888.93M WeightUpdates/sec\nDone!\nEstimated Post-training MeanError = 0.505545\n___________________________________________________________________\nNot training a calibrator because it is not needed.\nElapsed time: 00:00:06.6045457\n"
]
],
[
[
"### <a id=\"Score\">2.6. SCORE THE TEST DATA</a>\n\nEach fit will be used to score the test data. In order to plot together each fit's performance for convenient side-by-side comparison, we append each rxPrediction column to the test dataset. This will also conveniently include the Label column with the rxPredictions, so that the rxPrediction performance can be computed. When the test data are huge, scoring in this manner may not be possible. In that case, each rxPrediction set will have to be computed separately, and then merged into one data table.",
"_____no_output_____"
]
],
[
[
"#-----------------------------------------------------------------------\n# 6. Score the held-aside test data with the fit models.\n#-----------------------------------------------------------------------\n# The scores are each test record's probability of being a sentiment.\n# This combines each fit model's rxPredictions and the label into one\n# table for side-by-side plotting and comparison.\nfitScores <-\n rxPredict(rxLogisticRegressionFit, dataTest, suffix = \".rxLogisticRegression\",\n extraVarsToWrite = names(dataTest),\n outData = tempfile(fileext = \".xdf\"))\nfitScores <-\n rxPredict(rxFastLinearFit, fitScores, suffix = \".rxFastLinear\",\n extraVarsToWrite = names(fitScores),\n outData = tempfile(fileext = \".xdf\"))\nfitScores <-\n rxPredict(rxFastTreesFit, fitScores, suffix = \".rxFastTrees\",\n extraVarsToWrite = names(fitScores),\n outData = tempfile(fileext = \".xdf\"))\nfitScores <-\n rxPredict(rxFastForestFit, fitScores, suffix = \".rxFastForest\",\n extraVarsToWrite = names(fitScores),\n outData = tempfile(fileext = \".xdf\"))\nfitScores <-\n rxPredict(rxNeuralNetFit, fitScores, suffix = \".rxNeuralNet\",\n extraVarsToWrite = names(fitScores),\n outData = tempfile(fileext = \".xdf\"))",
"Beginning read for block: 1\nRows Read: 2083, Read Time: 0.002, Transform Time: 0\nBeginning read for block: 2\nNo rows remaining. Finished reading data set. \nElapsed time: 00:00:00.3493465\nFinished writing 2083 rows.\nWriting completed.\nBeginning read for block: 1\nRows Read: 2083, Read Time: 0.002, Transform Time: 0\nBeginning read for block: 2\nNo rows remaining. Finished reading data set. \nElapsed time: 00:00:00.2835029\nFinished writing 2083 rows.\nWriting completed.\nBeginning read for block: 1\nRows Read: 2083, Read Time: 0.002, Transform Time: 0\nBeginning read for block: 2\nNo rows remaining. Finished reading data set. \nElapsed time: 00:00:00.4074182\nFinished writing 2083 rows.\nWriting completed.\nBeginning read for block: 1\nRows Read: 2083, Read Time: 0.002, Transform Time: 0\nBeginning read for block: 2\nNo rows remaining. Finished reading data set. \nElapsed time: 00:00:00.3653417\nFinished writing 2083 rows.\nWriting completed.\nBeginning read for block: 1\nRows Read: 2083, Read Time: 0.002, Transform Time: 0\nBeginning read for block: 2\nNo rows remaining. Finished reading data set. \nElapsed time: 00:00:00.3158979\nFinished writing 2083 rows.\nWriting completed.\n"
]
],
[
[
"We see in the output of the command that the number of rows in the results is the same as the number of rows in the test data.",
"_____no_output_____"
],
[
"### <a id=\"Compare\">2.7. COMPARE THE FIT MODEL PERFORMANCE</a>\n\nFor each fit model, its rxPredictions and the Label are used to compute an ROC curve for that fit. The curves will then be plotted side-by-side in a graph.",
"_____no_output_____"
]
],
[
[
"#-----------------------------------------------------------------------\n# 7. Compare the performance of fit models.\n#-----------------------------------------------------------------------\n# Compute the fit models's ROC curves.\nfitRoc <-\n rxRoc(\"Label\",\n paste(\"Probability\",\n c(\"rxLogisticRegression\", \"rxFastLinear\", \"rxFastTrees\",\n \"rxFastForest\", \"rxNeuralNet\"),\n sep = \".\"),\n fitScores)\n# Plot the ROC curves and report their AUCs.\nplot(fitRoc)",
"_____no_output_____"
]
],
[
[
"The fit models are then used to compute the fit model AUCs, and these are used to select the best model.",
"_____no_output_____"
]
],
[
[
"# Create a named list of the fit models.\nfitList <-\n list(rxLogisticRegression = rxLogisticRegressionFit,\n rxFastLinear = rxFastLinearFit,\n rxFastTrees = rxFastTreesFit,\n rxFastForest = rxFastForestFit,\n rxNeuralNet = rxNeuralNetFit)\n\n# Compute the fit models's AUCs.\nfitAuc <- rxAuc(fitRoc)\nnames(fitAuc) <- substring(names(fitAuc), nchar(\"Probability.\") + 1)\n\n# Find the name of the fit with the largest AUC.\nbestFitName <- names(which.max(fitAuc))\n\n# Select the fit model with the largest AUC.\nbestFit <- fitList[[bestFitName]]\n\n# Report the fit AUCs.\ncat(\"Fit model AUCs:\\n\")\nprint(fitAuc, digits = 2)\n\n# Report the best fit.\ncat(paste0(\"Best fit model with \", bestFitName,\n \", AUC = \", signif(fitAuc[[bestFitName]], digits = 2),\n \".\\n\"))",
"Fit model AUCs:\n rxFastForest rxFastLinear rxFastTrees \n 0.80 0.87 0.88 \nrxLogisticRegression rxNeuralNet \n 0.85 0.82 \nBest fit model with rxFastTrees, AUC = 0.88.\n"
]
],
[
[
"In the end, the best fit model is stored in variable bestFit.",
"_____no_output_____"
],
[
"## <a id=\"Next\">3. WHAT'S NEXT?</a>\n\nIn the Microsoft ML samples directory, the script TwitterSentiment.R contains all the R code used in this tutorial. It also contains other scripts formatted to follow the steps used for retail churn. Try them out interactively, and learn about other Microsoft ML capabilities.",
"_____no_output_____"
]
]
] | [
"markdown",
"code",
"markdown",
"code",
"markdown",
"code",
"markdown",
"code",
"markdown",
"code",
"markdown",
"code",
"markdown",
"code",
"markdown",
"code",
"markdown",
"code",
"markdown",
"code",
"markdown",
"code",
"markdown"
] | [
[
"markdown",
"markdown",
"markdown",
"markdown"
],
[
"code"
],
[
"markdown"
],
[
"code"
],
[
"markdown"
],
[
"code"
],
[
"markdown"
],
[
"code"
],
[
"markdown"
],
[
"code"
],
[
"markdown"
],
[
"code"
],
[
"markdown",
"markdown"
],
[
"code"
],
[
"markdown",
"markdown"
],
[
"code",
"code",
"code",
"code",
"code"
],
[
"markdown"
],
[
"code"
],
[
"markdown",
"markdown"
],
[
"code"
],
[
"markdown"
],
[
"code"
],
[
"markdown",
"markdown"
]
] |
e7788413279a7e9d85170bbe9109e1319704205a | 28,048 | ipynb | Jupyter Notebook | protopipe/benchmarks/notebooks/DL2/benchmarks_DL2_direction-reconstruction.ipynb | Iburelli/protopipe | 1f392ea5abca6b09b684fca1e4ff3b138faa0b5a | [
"CECILL-B"
] | null | null | null | protopipe/benchmarks/notebooks/DL2/benchmarks_DL2_direction-reconstruction.ipynb | Iburelli/protopipe | 1f392ea5abca6b09b684fca1e4ff3b138faa0b5a | [
"CECILL-B"
] | null | null | null | protopipe/benchmarks/notebooks/DL2/benchmarks_DL2_direction-reconstruction.ipynb | Iburelli/protopipe | 1f392ea5abca6b09b684fca1e4ff3b138faa0b5a | [
"CECILL-B"
] | null | null | null | 34.541872 | 186 | 0.56004 | [
[
[
"# Remove input cells at runtime (nbsphinx)\nimport IPython.core.display as d\nd.display_html('<script>jQuery(function() {if (jQuery(\"body.notebook_app\").length == 0) { jQuery(\".input_area\").toggle(); jQuery(\".prompt\").toggle();}});</script>', raw=True)",
"_____no_output_____"
]
],
[
[
"# Direction recontruction (DL2)",
"_____no_output_____"
],
[
"**Recommended datasample(s):**\nDatasets of fully-analyzed showers used to obtain Instrument Response Functions, which in the default pipeline workflow are called ``gamma3``, ``proton2`` and ``electron``.\n\n**Data level(s):** DL2 (shower geometry + estimated energy + estimated particle classification)\n\n**Description:**\n\nThis notebook contains benchmarks for the _protopipe_ pipeline regarding the shower geometry of events which have been completely analyzed.\n\n**Requirements and steps to reproduce:**\n\n- get a TRAINING file generated using ``protopipe-DL2`` or the equivalent command from the DIRAC Grid interface\n\n- execute the notebook with ``protopipe-BENCHMARK``,\n\n``protopipe-BENCHMARK launch --config_file configs/benchmarks.yaml -n DL2/benchmarks_DL2_direction-reconstruction``\n\nTo obtain the list of all available parameters add ``--help-notebook``.\n\n**Development and testing:** \n \nAs with any other part of _protopipe_ and being part of the official repository, this notebook can be further developed by any interested contributor. \nThe execution of this notebook is not currently automatic, it must be done locally by the user _before_ pushing a pull-request. \nPlease, strip the output before pushing.\n\n**TODO:**\n\n* ...",
"_____no_output_____"
],
[
"## Table of contents\n - [Energy-dependent offset distribution](#Energy-dependent-offset-distribution)\n - [Angular-resolution-as-a-function-of-telescope-multiplicity](#Angular-resolution-as-a-function-of-telescope-multiplicity)\n - [Angular resolution for different containment radii and fixed signal efficiency](Angular-resolution-for-different-containment-radii-and-fixed-signal-efficiency)\n - [PSF asymmetry](#PSF-asymmetry)\n - [True energy distributions](#True-energy-distributions)",
"_____no_output_____"
],
[
"## Imports",
"_____no_output_____"
]
],
[
[
"import os\nfrom pathlib import Path\n\n%matplotlib inline\nimport matplotlib.pyplot as plt\nfrom matplotlib.colors import LogNorm\nfrom matplotlib.pyplot import rc\nimport matplotlib.style as style\nfrom cycler import cycler\n\nimport numpy as np\nimport pandas as pd\n\nimport astropy.units as u\nfrom astropy.coordinates import SkyCoord\nfrom ctapipe.coordinates import NominalFrame\nfrom pyirf.binning import (\n add_overflow_bins,\n create_bins_per_decade\n)\n\nfrom protopipe.benchmarks.utils import get_fig_size, string_to_boolean, get_fig_size\nfrom protopipe.benchmarks.operations import compute_psf\nfrom protopipe.benchmarks.plot import plot_psf",
"_____no_output_____"
]
],
[
[
"## Load data",
"_____no_output_____"
]
],
[
[
"# Parametrized cell\nanalyses_directory = None\noutput_directory = Path.cwd() # default output directory for plots\nanalysis_name = None\nanalysis_name_2 = None\ngammas_infile_name = \"DL2_tail_gamma_merged.h5\"\nprotons_infile_name = \"DL2_tail_proton_merged.h5\"\nelectrons_infile_name = \"DL2_tail_electron_merged.h5\"\nefficiency_cut = 0.9\nexport_data = False\nsuperimpose_analysis_2 = False\nuse_seaborn = True\nplots_scale = None",
"_____no_output_____"
],
[
"# Handle boolean variables (papermill reads them as strings)\n[use_seaborn,\n export_data,\n superimpose_analysis_2] = string_to_boolean([use_seaborn,\n export_data,\n superimpose_analysis_2])",
"_____no_output_____"
],
[
"input_directory = Path(analyses_directory) / analysis_name / Path(\"data/DL2\")\ngammas = pd.read_hdf(os.path.join(input_directory, \"gamma\", gammas_infile_name), \"/reco_events\")\nprotons = pd.read_hdf(os.path.join(input_directory, \"proton\", protons_infile_name), \"/reco_events\")\nelectrons = pd.read_hdf(os.path.join(input_directory, \"electron\", electrons_infile_name), \"/reco_events\")",
"_____no_output_____"
],
[
"basic_selection_cut = (gammas[\"is_valid\"]==True) & (gammas[\"NTels_reco\"] >= 2)\nselected_gammaness = gammas[basic_selection_cut][\"gammaness\"]\ngammaness_cut = np.quantile(selected_gammaness, efficiency_cut)\nselected_gammas = gammas[basic_selection_cut & (gammas[\"gammaness\"] >= gammaness_cut)]",
"_____no_output_____"
],
[
"#selected_gammas = gammas[(gammas[\"is_valid\"]==True) & (gammas[\"NTels_reco\"] >= 2) & (gammas[\"gammaness\"] >= 0.90)]",
"_____no_output_____"
],
[
"# First we check if a _plots_ folder exists already. \n# If not, we create it.\nplots_folder = Path(output_directory) / \"plots\"\nplots_folder.mkdir(parents=True, exist_ok=True)\n\n# Next we check if a _data_ folder exists already. \n# If not, we create it.\ndata_folder = Path(output_directory) / \"data\"\ndata_folder.mkdir(parents=True, exist_ok=True)\n\ninput_directory_data_2 = Path(analyses_directory) / analysis_name_2/ \"benchmarks_results/TRAINING\"",
"_____no_output_____"
],
[
"# Plot aesthetics settings\n\nscale = matplotlib_settings[\"scale\"] if plots_scale is None else float(plots_scale)\nstyle.use(matplotlib_settings[\"style\"])\ncmap = matplotlib_settings[\"cmap\"]\n\nif matplotlib_settings[\"style\"] == \"seaborn-colorblind\":\n \n colors_order = ['#0072B2', '#D55E00', '#F0E442', '#009E73', '#CC79A7', '#56B4E9']\n rc('axes', prop_cycle=cycler(color=colors_order))\n\nif use_seaborn:\n import seaborn as sns\n\n sns.set_theme(context=seaborn_settings[\"theme\"][\"context\"] if \"context\" in seaborn_settings[\"theme\"] else \"talk\",\n style=seaborn_settings[\"theme\"][\"style\"] if \"style\" in seaborn_settings[\"theme\"] else \"whitegrid\",\n palette=seaborn_settings[\"theme\"][\"palette\"] if \"palette\" in seaborn_settings[\"theme\"] else None,\n font=seaborn_settings[\"theme\"][\"font\"] if \"font\" in seaborn_settings[\"theme\"] else \"Fira Sans\",\n font_scale=seaborn_settings[\"theme\"][\"font_scale\"] if \"font_scale\" in seaborn_settings[\"theme\"] else 1.0,\n color_codes=seaborn_settings[\"theme\"][\"color_codes\"] if \"color_codes\" in seaborn_settings[\"theme\"] else True\n )\n \n sns.set_style(seaborn_settings[\"theme\"][\"style\"], rc=seaborn_settings[\"rc_style\"])\n sns.set_context(seaborn_settings[\"theme\"][\"context\"],\n font_scale=seaborn_settings[\"theme\"][\"font_scale\"] if \"font_scale\" in seaborn_settings[\"theme\"] else 1.0)",
"_____no_output_____"
]
],
[
[
"## Benchmarks",
"_____no_output_____"
],
[
"Here we use events with the following cuts:\n- valid reconstructed events\n- at least 2 reconstructed images, regardless of the camera (on top of any other hardware trigger)\n- gammaness > 0.75 (mostly a conservative choice)",
"_____no_output_____"
]
],
[
[
"min_true_energy = 0.006\nmax_true_energy = 660",
"_____no_output_____"
]
],
[
[
"### Energy-dependent offset distribution\n[back to top](#Table-of-contents)",
"_____no_output_____"
]
],
[
[
"n_bins = 4\ntrue_energy_bin_edges = np.logspace(np.log10(min_true_energy),\n np.log10(max_true_energy), n_bins + 1)\n\nplt.figure(figsize=get_fig_size(ratio=4./3., scale=scale))\n\nplt.xlabel(\"Offset [deg]\")\nplt.ylabel(\"Number of events\")\n\n\nfor i in range(len(true_energy_bin_edges)-1):\n \n low_E = true_energy_bin_edges[i]\n high_E = true_energy_bin_edges[i+1]\n \n selected_events = selected_gammas[(selected_gammas[\"true_energy\"]>low_E) & (selected_gammas[\"true_energy\"]<high_E)]\n \n plt.hist(selected_events[\"offset\"], \n bins=100,\n #range = [0,10],\n label=f\"{low_E:.2f} < E_true [TeV] < {high_E:.2f}\",\n histtype=\"step\",\n linewidth=2)\n\nplt.yscale(\"log\")\nplt.legend(loc=\"best\")\nplt.grid(which=\"both\")\n\nplt.savefig(plots_folder / f\"DL2_offsets_{analysis_name}.png\")\n\nplt.show()",
"_____no_output_____"
],
[
"min_true_energy = [0.02, 0.2, 2, 20]\nmax_true_energy = [0.2, 2, 20, 200]\n\nplt.figure(figsize=(10,5))\nplt.xlabel(\"Offset [deg]\")\nplt.ylabel(\"Number of events\")\n\nfor low_E, high_E in zip(min_true_energy, max_true_energy):\n \n selected_events = selected_gammas[(selected_gammas[\"true_energy\"]>low_E) & (selected_gammas[\"true_energy\"]<high_E)]\n \n plt.hist(selected_events[\"offset\"], \n bins=100,\n range = [0,10],\n label=f\"{low_E} < E_true [TeV] < {high_E}\",\n histtype=\"step\",\n linewidth=2)\n\nplt.yscale(\"log\")\nplt.legend(loc=\"best\")\nplt.grid(which=\"both\")\n\nplt.savefig(plots_folder / f\"DL2_offsets_{analysis_name}.png\")\n\nplt.show()",
"_____no_output_____"
],
[
"min_true_energy = [0.02, 0.2, 2, 20]\nmax_true_energy = [0.2, 2, 20, 200]\n\nplt.figure(figsize=(10,5))\nplt.xlabel(\"Offset [deg]\")\nplt.ylabel(\"Number of events\")\n\nfor low_E, high_E in zip(min_true_energy, max_true_energy):\n \n selected_events = selected_gammas[(selected_gammas[\"true_energy\"]>low_E) & (selected_gammas[\"true_energy\"]<high_E)]\n \n plt.hist(selected_events[\"offset\"], \n bins=100,\n range = [0,10],\n label=f\"{low_E} < E_true [TeV] < {high_E}\",\n histtype=\"step\",\n linewidth=2)\n\nplt.yscale(\"log\")\nplt.legend(loc=\"best\")\nplt.grid(which=\"both\")\n\nplt.savefig(plots_folder / f\"DL2_offsets_{analysis_name}.png\")\n\nplt.show()",
"_____no_output_____"
]
],
[
[
"### Angular resolution as a function of telescope multiplicity\n[back to top](#Table-of-contents)",
"_____no_output_____"
],
[
"Here we compare how the multiplicity influences the performance of reconstructed events with a 90% gamma efficiency within a 68% containment radius.",
"_____no_output_____"
]
],
[
[
"r_containment = 68\n\nmin_true_energy = 0.003\nmax_true_energy = 330\nn_true_energy_bins = 21\ntrue_energy_bin_edges = np.logspace(np.log10(min_true_energy),\n np.log10(max_true_energy),\n n_true_energy_bins)\ntrue_energy_bin_centers = 0.5 * (true_energy_bin_edges[:-1]+true_energy_bin_edges[1:])\n\nmultiplicity_cuts = ['NTels_reco == 2',\n 'NTels_reco == 3',\n 'NTels_reco == 4',\n 'NTels_reco >= 2']\n\nfig, axes = plt.subplots(nrows=1, ncols=2, figsize=(16, 8))\naxes = axes.flatten()\n\nfor cut_idx, cut in enumerate(multiplicity_cuts):\n data_mult = selected_gammas.query(cut)\n psf, err_psf = compute_psf(data_mult, true_energy_bin_edges, 68)\n plot_psf(axes[0], true_energy_bin_centers, psf, err_psf, label=multiplicity_cuts[cut_idx])\n \n y, tmp = np.histogram(data_mult['true_energy'], bins=true_energy_bin_edges)\n weights = np.ones_like(y)\n #weights = weights / float(np.sum(y))\n yerr = np.sqrt(y) * weights\n width = np.diff(true_energy_bin_edges)\n axes[1].bar(true_energy_bin_centers, y * weights, width=width, yerr=yerr, **{'label': multiplicity_cuts[cut_idx], 'lw': 2, 'fill': False})\n axes[1].set_ylabel('Number of events')\n \nfor ax in axes:\n #ax.set_xlim(limit)\n ax.set_xscale('log')\n ax.legend(loc='best')\n ax.grid(which='both', visible=True)\n ax.set_xlabel('True energy [TeV]')\n\nplt.tight_layout()\n\nfig.savefig(plots_folder / f\"DL2_PSF_{analysis_name}.png\")",
"_____no_output_____"
]
],
[
[
"### Angular resolution for different containment radii and fixed signal efficiency\n[back to top](#Table-of-contents)",
"_____no_output_____"
],
[
"Apply fixed signal efficiency cut (requires well defined ML separator and ML train-ing)\nCalculate angular resolution for 68%, 80%, and 95% containment radius.",
"_____no_output_____"
]
],
[
[
"scale=0.75\nplt.figure(figsize=(16*scale,9*scale))\n\ntrue_energy_bins = create_bins_per_decade(10**-1.9 * u.TeV, 10**2.31 * u.TeV, 5).value\n\ngamma_efficiency = 0.9\nreconstructed_gammas = gammas.query(\"is_valid == True\")\ngammaness = reconstructed_gammas[\"gammaness\"]\ngammaness_cut = np.quantile(gammaness, gamma_efficiency)\nselected_events = reconstructed_gammas.query(f\"gammaness > {gammaness_cut}\")\n\nax = plt.gca()\n\ndef angular_resolution_vs_true_energy(ax, events, true_energy_bins, containment):\n \n ang_res = []\n for i in range(len(true_energy_bins)-1):\n true_energy_mask = f\"true_energy > {true_energy_bins[i]} & true_energy < {true_energy_bins[i+1]}\"\n selected_offsets = events.query(true_energy_mask)[\"offset\"]\n if len(selected_offsets)==0:\n ang_res.append(np.nan)\n else:\n ang_res.append(np.quantile(selected_offsets, containment/100.))\n\n ax.errorbar(\n 0.5 * (true_energy_bins[:-1] + true_energy_bins[1:]),\n ang_res,\n xerr=0.5 * (true_energy_bins[:-1] - true_energy_bins[1:]),\n label=f'{containment}% containment radius',\n fmt='o',\n )\n \n return ax\n\nangular_resolution_vs_true_energy(ax, selected_events, true_energy_bins, 68)\nangular_resolution_vs_true_energy(ax, selected_events, true_energy_bins, 80)\nangular_resolution_vs_true_energy(ax, selected_events, true_energy_bins, 95)\n\nplt.xlabel(\"True energy [TeV]\")\nplt.xscale(\"log\")\nplt.ylabel(\"Angular resolution [deg]\")\nplt.legend()\nplt.title(f\"Reconstructed gammas with {gamma_efficiency*100}% signal efficiency\")\nplt.grid()",
"_____no_output_____"
]
],
[
[
"### H_max as a function of energy for gammas and protons",
"_____no_output_____"
],
[
"Fixed gamma efficiency at 90%",
"_____no_output_____"
]
],
[
[
"reconstructed_gammas = gammas.query(\"is_valid == True\")\nreconstructed_protons = protons.query(\"is_valid == True\")\n\nplt.figure(figsize=(12,6))\n\nmask_gammaness = f\"gammaness > 0.9\"\n\nplt.subplot(1, 2, 1)\n\nhist_opt = {\"bins\":[100,100],\n \"range\": [[0.003, 300],[1,8]],\n \"norm\": LogNorm(vmin=1,vmax=1.e6),\n \"cmap\": cmap}\n\nplt.hist2d(reconstructed_gammas.query(mask_gammaness)[\"reco_energy\"],\n np.log10(reconstructed_gammas.query(mask_gammaness)[\"h_max\"]),\n **hist_opt\n )\nplt.xlabel(\"Reconstructed energy [TeV]\")\nplt.ylabel(\"log10(H max)\")\nplt.colorbar()\nplt.title(\"DL2 gammas\")\n\nplt.subplot(1, 2, 2)\n\nplt.hist2d(reconstructed_protons.query(mask_gammaness)[\"reco_energy\"],\n np.log10(reconstructed_protons.query(mask_gammaness)[\"h_max\"]),\n **hist_opt\n )\nplt.xlabel(\"Reconstructed energy [TeV]\")\nplt.ylabel(\"log10(H max)\")\nplt.colorbar()\nplt.title(\"DL2 protons\")\nNone",
"_____no_output_____"
]
],
[
[
"### PSF asymmetry\n[back to top](#Table-of-contents)",
"_____no_output_____"
]
],
[
[
"reco_alt = selected_gammas.reco_alt\nreco_az = selected_gammas.reco_az\n\n# right now all reco_az for a 180° deg simualtion turn out to be all around -180°\n#if ~np.count_nonzero(np.sign(reco_az) + 1):\nreco_az = np.abs(reco_az)\n\n# this is needed for projecting the angle onto the sky\nreco_az_corr = reco_az * np.cos(np.deg2rad(selected_gammas.reco_alt))\n\ntrue_alt = selected_gammas.iloc[0].true_alt\ntrue_az = selected_gammas.iloc[0].true_az\n\ndaz = reco_az - true_az\ndaz_corr = daz * np.cos(np.deg2rad(reco_alt))\ndalt = reco_alt - true_alt\n\nplt.figure(figsize=(5, 5))\n\nplt.xlabel(\"Mis-recontruction [deg]\")\nplt.ylabel(\"Number of events\")\n\nplt.hist(daz_corr, bins=100, alpha=0.5, label = \"azimuth\")\nplt.hist(dalt, bins=100, alpha=0.5, label = \"altitude\")\n\nplt.legend()\nplt.yscale(\"log\")\nplt.grid()\n\nprint(\"Mean and STDs of sky-projected mis-reconstruction axes\")\nprint('daz = {:.4f} +/- {:.4f} deg'.format(daz_corr.mean(), daz_corr.std()))\nprint('dalt = {:.4f} +/- {:.4f} deg'.format(dalt.mean(), dalt.std()))\n\nplt.show()",
"_____no_output_____"
]
],
[
[
"2D representation with **orange** events being those with **offset < 1 deg** and **E_true > 20 TeV**",
"_____no_output_____"
]
],
[
[
"angcut = (selected_gammas['offset'] < 1) & (selected_gammas['true_energy'] > 20)\n\nplt.figure(figsize=(5,5))\nax = plt.gca()\nFOV_size = 2.5 # deg\n\nax.scatter(daz_corr, dalt, alpha=0.1, s=1, label='no angular cut')\nax.scatter(daz_corr[angcut], dalt[angcut], alpha=0.05, s=1, label='offset < 1 deg & E_true > 20 TeV')\n\nax.set_aspect('equal')\nax.set_xlabel('cent. Az [deg]')\nax.set_ylabel('cent. Alt [deg]')\nax.set_xlim(-FOV_size,FOV_size)\nax.set_ylim(-FOV_size,FOV_size)\nplt.tight_layout()\nplt.grid(which=\"both\")\n\nfig.savefig(plots_folder / f\"PSFasymmetry_2D_altaz_{analysis_name}.png\")",
"_____no_output_____"
]
],
[
[
"### True energy distributions\n[back to top](#Table-of-contents)",
"_____no_output_____"
]
],
[
[
"#min_true_energy = 0.003\n#max_true_energy = 330\ntrue_energy_bins_edges = np.logspace(np.log10(min_true_energy), np.log10(max_true_energy), 6 + 1)\n\nif len(np.unique(gammas[\"true_az\"]))==1:\n true_az = np.unique(gammas[\"true_az\"]) * u.deg\n true_alt = np.unique(gammas[\"true_alt\"]) * u.deg\nelse:\n print(\"WARNING: diffuse simulations not yet supported.\")\n\nprint(f\"true AZ = {true_az}\")\nprint(f\"true ALT = {true_alt}\")",
"_____no_output_____"
],
[
"get_fig_size(ratio=(9/16), scale=None)\nplt.subplots_adjust(wspace=0.5, hspace=0.3)\n\ncenter = SkyCoord(az=true_az, alt=true_alt, frame=\"altaz\")\nnominal_frame = NominalFrame(origin=center)\n\nfor i in range(len(true_energy_bins_edges)-1):\n \n plt.subplot(3,2,i+1)\n \n ax = plt.gca()\n ax.set_aspect(\"equal\")\n\n reconstruction_mask = \"is_valid == True and \"\n true_energy_mask = f\"true_energy > {true_energy_bins_edges[i]} and true_energy < {true_energy_bins_edges[i+1]}\"\n selected_gammas = gammas.query(reconstruction_mask + true_energy_mask)\n\n reconstructed_coordinates = SkyCoord(az=selected_gammas.reco_az.values * u.degree,\n alt=selected_gammas.reco_alt.values * u.degree,\n frame=\"altaz\")\n\n reconstructed_coordinates_nominal_frame = reconstructed_coordinates.transform_to(nominal_frame)\n\n hist_opt = {\"bins\":[100,100],\n \"range\":[[-10, 10], [-10, 10]],\n \"norm\":LogNorm(),\n \"cmap\":cmap}\n plt.hist2d(reconstructed_coordinates_nominal_frame.fov_lon.value,\n reconstructed_coordinates_nominal_frame.fov_lat.value,\n **hist_opt)\n plt.plot(0, 0, \"*\", markersize=15, color='#D55E00')\n plt.colorbar()\n plt.xlabel(\"FOV Longitude [deg]\")\n plt.ylabel(\"FOV Latitude [deg]\")\n plt.title(f\"{true_energy_bins_edges[i]:.2f} TeV < True energy < {true_energy_bins_edges[i+1]:.2f} TeV\")\nNone",
"_____no_output_____"
]
],
[
[
"Same, but with a fixed gamma efficiency cut of 90%",
"_____no_output_____"
]
],
[
[
"plt.figure(figsize=(15,15))\nplt.subplots_adjust(wspace=0.3, hspace=0.3)\n\ncenter = SkyCoord(az=true_az, alt=true_alt, frame=\"altaz\")\nnominal_frame = NominalFrame(origin=center)\n\nfor i in range(len(true_energy_bins_edges)-1):\n \n plt.subplot(3,2,i+1)\n \n ax = plt.gca()\n ax.set_aspect(\"equal\")\n\n reconstruction_mask = \"is_valid == True and \"\n true_energy_mask = f\"true_energy > {true_energy_bins_edges[i]} and true_energy < {true_energy_bins_edges[i+1]}\"\n reconstructed_gammas_per_true_energy = gammas.query(reconstruction_mask + true_energy_mask)\n gammaness = reconstructed_gammas_per_true_energy[\"gammaness\"]\n gammaness_cut = np.quantile(gammaness, gamma_efficiency)\n selected_gammas = reconstructed_gammas_per_true_energy.query(f\"gammaness > {gammaness_cut}\")\n \n selected_gammas\n\n reconstructed_coordinates = SkyCoord(az=selected_gammas.reco_az.values * u.degree,\n alt=selected_gammas.reco_alt.values * u.degree,\n frame=\"altaz\")\n\n reconstructed_coordinates_nominal_frame = reconstructed_coordinates.transform_to(nominal_frame)\n\n hist_opt = {\"bins\":[100,100],\n \"range\":[[-10, 10], [-10, 10]],\n \"norm\":LogNorm(),\n \"cmap\":cmap}\n plt.hist2d(reconstructed_coordinates_nominal_frame.fov_lon.value,\n reconstructed_coordinates_nominal_frame.fov_lat.value,\n **hist_opt)\n plt.plot(0, 0, \"*\", markersize=20, color='#D55E00')\n plt.colorbar()\n plt.xlabel(\"FOV Longitude [deg]\")\n plt.ylabel(\"FOV Latitude [deg]\")\n plt.title(f\"{true_energy_bins_edges[i]:.2f} TeV < True energy < {true_energy_bins_edges[i+1]:.2f} TeV\")\nNone",
"_____no_output_____"
]
]
] | [
"code",
"markdown",
"code",
"markdown",
"code",
"markdown",
"code",
"markdown",
"code",
"markdown",
"code",
"markdown",
"code",
"markdown",
"code",
"markdown",
"code",
"markdown",
"code",
"markdown",
"code",
"markdown",
"code"
] | [
[
"code"
],
[
"markdown",
"markdown",
"markdown",
"markdown"
],
[
"code"
],
[
"markdown"
],
[
"code",
"code",
"code",
"code",
"code",
"code",
"code"
],
[
"markdown",
"markdown"
],
[
"code"
],
[
"markdown"
],
[
"code",
"code",
"code"
],
[
"markdown",
"markdown"
],
[
"code"
],
[
"markdown",
"markdown"
],
[
"code"
],
[
"markdown",
"markdown"
],
[
"code"
],
[
"markdown"
],
[
"code"
],
[
"markdown"
],
[
"code"
],
[
"markdown"
],
[
"code",
"code"
],
[
"markdown"
],
[
"code"
]
] |
e77889eaafc0a2dcff4820c85ec044cf81f03417 | 15,618 | ipynb | Jupyter Notebook | 3_Data_Cube_Basics/notebooks/02_xarrayI_data_structure.ipynb | eo2cube/datacube_eagles | f0b1669a5010aef07b7b9f3155edc86dfb3e9cd2 | [
"MIT"
] | 1 | 2022-03-20T20:04:39.000Z | 2022-03-20T20:04:39.000Z | 3_Data_Cube_Basics/notebooks/02_xarrayI_data_structure.ipynb | eo2cube/datacube_eagles | f0b1669a5010aef07b7b9f3155edc86dfb3e9cd2 | [
"MIT"
] | null | null | null | 3_Data_Cube_Basics/notebooks/02_xarrayI_data_structure.ipynb | eo2cube/datacube_eagles | f0b1669a5010aef07b7b9f3155edc86dfb3e9cd2 | [
"MIT"
] | null | null | null | 33.659483 | 455 | 0.609105 | [
[
[
"<img align=\"right\" src=\"../../additional_data/banner_siegel.png\" style=\"width:1000px;\">",
"_____no_output_____"
],
[
"# Xarray-I: Data Structure \n\n* [**Sign up to the JupyterHub**](https://www.phenocube.org/) to run this notebook interactively from your browser\n* **Compatibility:** Notebook currently compatible with the Open Data Cube environments of the University of Wuerzburg\n* **Prerequisites**: There is no prerequisite learning required.\n",
"_____no_output_____"
],
[
"## Background\n\nIn the previous notebook, we experienced that the data we wanna access are loaded in a form called **`xarray.dataset`**. This is the form in which earth observation data are usually stored in a datacube.\n\n**`xarray`** is an open source project and Python package which offers a toolkit for working with ***multi-dimensional arrays*** of data. **`xarray.dataset`** is an in-memory representation of a netCDF (network Common Data Form) file. Understanding the structure of a **`xarray.dataset`** is the key to enable us work with these data. Thus, in this notebook, we are mainly dedicated to helping users of our datacube understand its data structure.\n\nFirstly let's come to the end stage of the previous notebook, where we have loaded a data product. The data product \"s2_l2a_bavaria\" is used as example in this notebook.",
"_____no_output_____"
],
[
"## Description\n\nThe following topics are convered in this notebook:\n* **What is inside a `xrray.dataset` (the structure)?**\n* **(Basic) Subset Dataset / DataArray**\n* **Reshape a Dataset**",
"_____no_output_____"
]
],
[
[
"import datacube\nimport pandas as pd\nfrom odc.ui import DcViewer \nfrom odc.ui import with_ui_cbk\nimport xarray as xr\nimport matplotlib.pyplot as plt\n\n# Set config for displaying tables nicely\n# !! USEFUL !! otherwise parts of longer infos won't be displayed in tables\npd.set_option(\"display.max_colwidth\", 200)\npd.set_option(\"display.max_rows\", None)\n\n# Connect to DataCube\n# argument \"app\" --- user defined name for a session (e.g. choose one matching the purpose of this notebook)\ndc = datacube.Datacube(app = \"nb_understand_ndArrays\")",
"_____no_output_____"
],
[
"# Load Data Product\nds = dc.load(product= \"s2_l2a\",\n x= [12.94 ,13.05],\n y= [53.88,53.94],\n output_crs = \"EPSG:32632\",\n time = (\"2020-10-01\", \"2020-12-31\"),\n measurements= [\"blue\", \"green\", \"red\",\"nir\"],\n resolution = (-10,10),\n group_by = \"solar_day\",\n progress_cbk=with_ui_cbk())\n\nds",
"_____no_output_____"
],
[
"#da = ds.to_array().rename({\"variable\":\"band\"})\n#print(da)",
"_____no_output_____"
],
[
"#ds2 = da.to_dataset(dim=\"time\")\n#ds2",
"_____no_output_____"
]
],
[
[
"## **What is inside a `xarray.dataset`?**\nThe figure below is a diagramm depicting the structure of the **`xarray.dataset`** we've just loaded. Combined with the diagramm, we hope you may better interpret the texts below explaining the data strucutre of a **`xarray.dataset`**.\n\n",
"_____no_output_____"
],
[
"As read from the output block, this dataset has three ***Data Variables*** , \"blue\", \"green\" and \"red\" (shown with colors in the diagramm), referring to individual spectral band.\n\nEach data variable can be regarded as a **multi-dimensional *Data Array*** of same structure; in this case, it is a **three-dimensional array** (shown as 3D Cube in the diagramm) where `time`, `x` and `y` are its ***Dimensions*** (shown as axis along each cube in the diagramm).\n\nIn this dataset, there are 35 ***Coordinates*** under `time` dimension, which means there are 35 time steps along the `time` axis. There are 164 coordinates under `x` dimension and 82 coordinates under `y` dimension, indicating that there are 164 pixels along `x` axis and 82 pixels along `y` axis.\n\nAs for the term ***Dataset***, it is like a *Container* holding all the multi-dimensional arrays of same structure (shown as the red-lined box holding all 3D Cubes in the diagramm).\n\nSo this instance dataset has a spatial extent of 164 by 82 pixels at given lon/lat locations, spans over 35 time stamps and 3 spectral band.\n\n**In summary, *`xarray.dataset`* is substantially a container for high-dimensional *`DataArray`* with common attributes (e.g. crs) attached**, :\n* **Data Variables (`values`)**: **it's generally the first/highest dimension to subset from a high dimensional array.** Each `data variable` contains a multi-dimensional array of all other dimensions.\n* **Dimensions (`dims`)**: other dimensions arranged in hierachical order *(e.g. 'time', 'y', 'x')*.\n* **Coordinates (`coords`)**: Coordinates along each `Dimension` *(e.g. timesteps along 'time' dimension, latitudes along 'y' dimension, longitudes along 'x' dimension)*\n* **Attributes (`attrs`)**: A dictionary(`dict`) containing Metadata.",
"_____no_output_____"
],
[
"Now let's deconstruct the dataset we have just loaded a bit further to have things more clarified!:D",
"_____no_output_____"
],
[
"* **To check existing dimensions of a dataset**",
"_____no_output_____"
]
],
[
[
"ds.dims",
"_____no_output_____"
]
],
[
[
"* **To check the coordinates of a dataset**",
"_____no_output_____"
]
],
[
[
"ds.coords#['time']",
"_____no_output_____"
]
],
[
[
"* **To check all coordinates along a specific dimension**\n<br>\n<img src=https://live.staticflickr.com/65535/51115452191_ec160d4514_o.png, width=\"450\">",
"_____no_output_____"
]
],
[
[
"ds.time\n# OR\n#ds.coords['time']",
"_____no_output_____"
]
],
[
[
"* **To check attributes of the dataset**",
"_____no_output_____"
]
],
[
[
"ds.attrs",
"_____no_output_____"
]
],
[
[
"## **Subset Dataset / DataArray**\n\n* **To select all data of \"blue\" band**\n<br>\n<img src=https://live.staticflickr.com/65535/51115092614_366cb774a8_o.png, width=\"350\">",
"_____no_output_____"
]
],
[
[
"ds.blue\n# OR\n#ds['blue']",
"_____no_output_____"
],
[
"# Only print pixel values\nds.blue.values",
"_____no_output_____"
]
],
[
[
"* **To select blue band data at the first time stamp**\n<br>\n<img src=https://live.staticflickr.com/65535/51116131265_8464728bc1_o.png, width=\"350\">",
"_____no_output_____"
]
],
[
[
"ds.blue[0]",
"_____no_output_____"
]
],
[
[
"* **To select blue band data at the first time stamp while the latitude is the largest in the defined spatial extent**\n<img src=https://live.staticflickr.com/65535/51115337046_aeb75d0d03_o.png, width=\"350\">",
"_____no_output_____"
]
],
[
[
"ds.blue[0][0]",
"_____no_output_____"
]
],
[
[
"* **To select the upper-left corner pixel**\n<br>\n<img src=https://live.staticflickr.com/65535/51116131235_b0cca9589f_o.png, width=\"350\">",
"_____no_output_____"
]
],
[
[
"ds.blue[0][0][0]",
"_____no_output_____"
]
],
[
[
"### **subset dataset with `isel` vs. `sel`**\n* Use `isel` when subsetting with **index**\n* Use `sel` when subsetting with **labels**",
"_____no_output_____"
],
[
"* **To select data of all spectral bands at the first time stamp**\n<br>\n<img src=https://live.staticflickr.com/65535/51114879732_7d62db54f4_o.png, width=\"750\">",
"_____no_output_____"
]
],
[
[
"ds.isel(time=[0])",
"_____no_output_____"
]
],
[
[
"* **To select data of all spectral bands of year 2020** \n<br>\n<img src=https://live.staticflickr.com/65535/51116281070_75f1b46a9c_o.png, width=\"750\">",
"_____no_output_____"
]
],
[
[
"ds.sel(time='2020-12')\n#print(ds.sel(time='2019'))",
"_____no_output_____"
]
],
[
[
"***Tip: More about indexing and sebsetting Dataset or DataArray is presented in the [Notebook_05](https://github.com/eo2cube/eo2cube_notebooks/blob/main/get_started/intro_to_eo2cube/05_xarrayII.ipynb).***",
"_____no_output_____"
],
[
"## **Reshape Dataset**\n\n* **Convert the Dataset (subset to 2019) to a *4-dimension* DataArray**",
"_____no_output_____"
]
],
[
[
"da = ds.sel(time='2020-12').to_array().rename({\"variable\":\"band\"})\nda",
"_____no_output_____"
]
],
[
[
"* **Convert the *4-dimension* DataArray back to a Dataset by setting the \"time\" as DataVariable (reshaped)**\n\n",
"_____no_output_____"
]
],
[
[
"ds_reshp = da.to_dataset(dim=\"time\")\nprint(ds_reshp)",
"_____no_output_____"
]
],
[
[
"## Recommended next steps\n\nIf you now understand the **data structure** of `xarray.dataset` and **basic indexing** methods illustrated in this notebook, you are ready to move on to the next notebook where you will learn more about **advanced indexing** and calculating some **basic statistical parameters** of the n-dimensional arrays!:D\n\nIn case you are gaining interest in exploring the world of **xarrays**, you may lay yourself into the [Xarray user guide](http://xarray.pydata.org/en/stable/index.html).\n\n<br>\nTo continue working through the notebooks in this beginner's guide, the following notebooks are designed to be worked through in the following order:\n\n1. [Jupyter Notebooks](https://github.com/eo2cube/eo2cube_notebooks/blob/main/get_started/intro_to_eo2cube/01_jupyter_introduction.ipynb)\n2. [eo2cube](https://github.com/eo2cube/eo2cube_notebooks/blob/main/get_started/intro_to_eo2cube/02_eo2cube_introduction.ipynb)\n3. [Loading Data](https://github.com/eo2cube/eo2cube_notebooks/blob/main/get_started/intro_to_eo2cube/03_data_lookup_and_loading.ipynb)\n4. ***Xarray I: Data Structure (this notebook)***\n5. [Xarray II: Index and Statistics](https://github.com/eo2cube/eo2cube_notebooks/blob/main/get_started/intro_to_eo2cube/05_xarrayII.ipynb)\n6. [Plotting data](https://github.com/eo2cube/eo2cube_notebooks/blob/main/get_started/intro_to_eo2cube/06_plotting_basics.ipynb)\n7. [Spatial analysis](https://github.com/eo2cube/eo2cube_notebooks/blob/main/get_started/intro_to_eo2cube/07_basic_analysis.ipynb)\n8. [Parallel processing with Dask](https://github.com/eo2cube/eo2cube_notebooks/blob/main/get_started/intro_to_eo2cube/08_parallel_processing_with_dask.ipynb)\n\nThe additional notebooks are designed for users to build up both basic and advanced skills which are not covered by the beginner's guide. Self-motivated users can go through them according to their own needs. They act as complements for the guide:\n<br>\n\n1. [Python's file management tools](https://github.com/eo2cube/eo2cube_notebooks/blob/main/get_started/intro_to_eo2cube/I_file_management.ipynb)\n2. [Image Processing basics using NumPy and Matplotlib](https://github.com/eo2cube/eo2cube_notebooks/blob/main/get_started/intro_to_eo2cube/II_numpy_image_processing.ipynb)\n3. [Vector Processing](https://github.com/eo2cube/eo2cube_notebooks/blob/main/get_started/intro_to_eo2cube/III_process_vector_data.ipynb)\n4. [Advanced Plotting](https://github.com/eo2cube/eo2cube_notebooks/blob/main/get_started/intro_to_eo2cube/IV_advanced_plotting.ipynb)",
"_____no_output_____"
],
[
"***\n## Additional information\n\nThis notebook is for the usage of Jupyter Notebook of the [Department of Remote Sensing](http://remote-sensing.org/), [University of Wuerzburg](https://www.uni-wuerzburg.de/startseite/).\n\n**License:** The code in this notebook is licensed under the [Apache License, Version 2.0](https://www.apache.org/licenses/LICENSE-2.0). \n\n\n**Contact:** If you would like to report an issue with this notebook, you can file one on [Github](https://github.com).\n\n**Last modified:** April 2021",
"_____no_output_____"
]
]
] | [
"markdown",
"code",
"markdown",
"code",
"markdown",
"code",
"markdown",
"code",
"markdown",
"code",
"markdown",
"code",
"markdown",
"code",
"markdown",
"code",
"markdown",
"code",
"markdown",
"code",
"markdown",
"code",
"markdown",
"code",
"markdown",
"code",
"markdown"
] | [
[
"markdown",
"markdown",
"markdown",
"markdown"
],
[
"code",
"code",
"code",
"code"
],
[
"markdown",
"markdown",
"markdown",
"markdown"
],
[
"code"
],
[
"markdown"
],
[
"code"
],
[
"markdown"
],
[
"code"
],
[
"markdown"
],
[
"code"
],
[
"markdown"
],
[
"code",
"code"
],
[
"markdown"
],
[
"code"
],
[
"markdown"
],
[
"code"
],
[
"markdown"
],
[
"code"
],
[
"markdown",
"markdown"
],
[
"code"
],
[
"markdown"
],
[
"code"
],
[
"markdown",
"markdown"
],
[
"code"
],
[
"markdown"
],
[
"code"
],
[
"markdown",
"markdown"
]
] |
e7788c19416e83700f9d091d6e9cae3490b67d16 | 3,131 | ipynb | Jupyter Notebook | Untitled4.ipynb | bmb29/Leap_Frog_Code | 6617b763f7311e6465f9c37901e010cedceb3242 | [
"BSD-3-Clause"
] | null | null | null | Untitled4.ipynb | bmb29/Leap_Frog_Code | 6617b763f7311e6465f9c37901e010cedceb3242 | [
"BSD-3-Clause"
] | null | null | null | Untitled4.ipynb | bmb29/Leap_Frog_Code | 6617b763f7311e6465f9c37901e010cedceb3242 | [
"BSD-3-Clause"
] | null | null | null | 20.2 | 81 | 0.508783 | [
[
[
"empty"
]
]
] | [
"empty"
] | [
[
"empty"
]
] |
e778973436b36e7fbf50d3a01ea913228bb66e05 | 12,078 | ipynb | Jupyter Notebook | ml masters assignments/LinearRegressionAssignment.ipynb | bhanuteja2001/ML-Masters-with-Deployment | a68080e2bc8698f682f1e7c452c4e4863a1984ce | [
"MIT"
] | null | null | null | ml masters assignments/LinearRegressionAssignment.ipynb | bhanuteja2001/ML-Masters-with-Deployment | a68080e2bc8698f682f1e7c452c4e4863a1984ce | [
"MIT"
] | null | null | null | ml masters assignments/LinearRegressionAssignment.ipynb | bhanuteja2001/ML-Masters-with-Deployment | a68080e2bc8698f682f1e7c452c4e4863a1984ce | [
"MIT"
] | null | null | null | 23.54386 | 232 | 0.586521 | [
[
[
"1.\tWhat is Regression?",
"_____no_output_____"
],
[
"It is the relation between the dependent features and target feature.",
"_____no_output_____"
],
[
"2.\tWhat is Linear Regression?",
"_____no_output_____"
],
[
"It is the fundamental and basic ML algorithm which finds the best fit line and finds the output.\n\nIf the relationship between the features and target column is linear",
"_____no_output_____"
],
[
"3.\tWhen to use Linear Regression? Explain the equation of a straight line.",
"_____no_output_____"
],
[
"Linear Regression can be used\n1. When the variables are independent.\n2. When the variance in the dataset is constant.\n3. The Residuals are normally distributed.\n\nIn the Linear Regression Equation,\nThe line is the best fit line.\nthe term y is the dependent variable and the term x is the independent varible/variables(if multi-linear regression)\nand the term c is the intercept of the line and the term m is the slope of the line.",
"_____no_output_____"
],
[
"4.\tWhat kind of plots will you use to showcase the relationship amongst the columns?",
"_____no_output_____"
],
[
"Correlation heatmap can be used to find the correlation if there is any\n\nwe can use scatter plot to find the relationship between features and the target column",
"_____no_output_____"
],
[
"5.\tHow is the best fit line chosen?",
"_____no_output_____"
],
[
"The Best fit line is the line that gives the least residual value and the residual value should be constant",
"_____no_output_____"
],
[
"6.\tWhat is gradient descent, and why is it used? Explain the maths.",
"_____no_output_____"
],
[
"It is a ML algorithm which helps to find the minima.\nGradient meaning 'an inclined part' and descending means decreasing.\nAs for finding the minimum residual value, we square in order to get only positive values.\nWhen plotted it results in a Paraboliod and the best values for m and b can be found by finding the minima of the plot.\nThere by we use gradient-descend",
"_____no_output_____"
],
[
"7.\tWhat are residuals?",
"_____no_output_____"
],
[
"The difference between the actual value and the predicted value",
"_____no_output_____"
],
[
"8.\tWhat is correlation?",
"_____no_output_____"
],
[
"If there exists a relationship between the features then they are said to be correlated",
"_____no_output_____"
],
[
"9.\tWhat is multicollinearity?",
"_____no_output_____"
],
[
"If two or more features are highly correlated",
"_____no_output_____"
],
[
"10.\tHow to detect multicollinearity?",
"_____no_output_____"
],
[
"1. Using correlation matrix\n2. By finding VIF value",
"_____no_output_____"
],
[
"11.\tWhat are the remedies for multicollinearity?",
"_____no_output_____"
],
[
"1. PCA\n2. Do nothing\n3. Removing the feature\n4. Create a new feature",
"_____no_output_____"
],
[
"12.\tWhat is the R-Squared Statistics?",
"_____no_output_____"
],
[
"It is a measure of fit",
"_____no_output_____"
],
[
"13.\tWhat is an adjusted R-Squared Statistics?",
"_____no_output_____"
],
[
"As the number of features are increased we use R-Squared method",
"_____no_output_____"
],
[
"14.\tWhy do we use adj R-squared?",
"_____no_output_____"
],
[
"As we increase the features the value of R-Squared value also increases, so to rectify this isssue we use adj R-Squared",
"_____no_output_____"
],
[
"15.\tWhy adj R-squared decreases when we use incompetent variables?\n\n",
"_____no_output_____"
],
[
"As per the denomintor of the adj R-squared formula, if there are not many useful features the value of the denomintor increase and hence the value of adj R-squared increases",
"_____no_output_____"
],
[
"16.\tHow to interpret a Linear Regression model?",
"_____no_output_____"
],
[
"With the coefficients of the model",
"_____no_output_____"
],
[
"17.\tWhat is the difference between fit, fit_transform and predict methods?",
"_____no_output_____"
],
[
"fit() - This basically fits it to model without any change\n\nfit_transform() - scales the x and y values and fits them\n\npredict() - Uses the model and makes the predictions.",
"_____no_output_____"
],
[
"18.\tHow do you plot the least squared line?",
"_____no_output_____"
],
[
"1. First find the minimum and maximum values\n2. Then make their predictions.\n3. Then plot the observed data as scatter plot and this plot is the best fit line",
"_____no_output_____"
],
[
"19.\tWhat are Bias and Variance? What is Bias Variance Trade-off?",
"_____no_output_____"
],
[
"Bias is basically the error value and Variance is the spread.\nWe do regularization to reduce the variance, and it also results in slight increase in bias.\nA best fit line should be selected which cand give less variance and less bias line.",
"_____no_output_____"
],
[
"20.\tWhat is the null and alternate hypothesis?",
"_____no_output_____"
],
[
"Null hypothesis is something we assume to be correct and we do hypothesis testing to prove that it is incorrect.If we are able to prove then we go for alternate hypothesis(which is opposite to Null hypothesis) else vice-versa.",
"_____no_output_____"
],
[
"21.\tWhat is multiple linear regression?",
"_____no_output_____"
],
[
"linear regression with multiple features and a target variable.",
"_____no_output_____"
],
[
"22.\tWhat is the OLS method? Derive the formulae used in the OLS method.",
"_____no_output_____"
],
[
"The OLS method corresponds to minimizing the sum of square differences between the observed and predicted values.\nUsing RSS formula and by substituting the y value in the RSS formula we obtain that cost function.",
"_____no_output_____"
],
[
"23.\tWhat is the p-value? How does it help in feature selection?",
"_____no_output_____"
],
[
"If the p-value is a metric in hypothesis testing.\n\nIf in an example with CI 95% if the zero lies in the CI and p-value is less than 5% we reject the null hypothesis and we say that there is a relationship b/w the feature and the target column",
"_____no_output_____"
],
[
"24.\tHow to handle categorical values in the data?",
"_____no_output_____"
],
[
"Using encoding methods,dummy variables method.",
"_____no_output_____"
],
[
"25.\tWhat is regularization, and why do we need it?",
"_____no_output_____"
],
[
"Regularization helps when there is overfitting condition in the model.It reduces the variance and restricts the degree of freedom of a given equation. ",
"_____no_output_____"
],
[
"26.\tExplain Ridge Regression.",
"_____no_output_____"
],
[
"Ridge regression penalizes the model based on the sum of squares of magnitude of the coefficients.\nRidge regression shrinks the coefficients and it helps to reduce the model complexity and multi-collinearity",
"_____no_output_____"
],
[
"27.\tExplain Lasso Regression.",
"_____no_output_____"
],
[
"LASSO regression penalizes the model based on the sum of magnitude of the coefficients.",
"_____no_output_____"
],
[
"28.\tExplain Elastic Net.",
"_____no_output_____"
],
[
"elastic Net is a middle ground between Ridge Regression and Lasso Regression",
"_____no_output_____"
],
[
"29.\tWhy do we do a train test split?",
"_____no_output_____"
],
[
"Train test split is usually done if there are sufficient number of entries.\nTrain data set helps us to train the model and test data set helps us to test the model that we created using training data set.\n",
"_____no_output_____"
],
[
"30.\tWhat is polynomial regression? When to use it?",
"_____no_output_____"
],
[
"If the relationship between the independent and dependent variables doesn't turn out to be linear then we use polynomial regression.",
"_____no_output_____"
],
[
"31.\tExplain the steps for GCP deployment.",
"_____no_output_____"
],
[
"1. Go to GCP console\n2. Create a new project\n3. Download and install Google cloud SDK\n4. Click the correct project name in the console\n5. Create a yaml and procfile for the flask app\n6. using the SDK, and necessary commands deploy your app",
"_____no_output_____"
],
[
"32. What difficulties did you face in cloud deployment?",
"_____no_output_____"
],
[
"None",
"_____no_output_____"
]
]
] | [
"markdown"
] | [
[
"markdown",
"markdown",
"markdown",
"markdown",
"markdown",
"markdown",
"markdown",
"markdown",
"markdown",
"markdown",
"markdown",
"markdown",
"markdown",
"markdown",
"markdown",
"markdown",
"markdown",
"markdown",
"markdown",
"markdown",
"markdown",
"markdown",
"markdown",
"markdown",
"markdown",
"markdown",
"markdown",
"markdown",
"markdown",
"markdown",
"markdown",
"markdown",
"markdown",
"markdown",
"markdown",
"markdown",
"markdown",
"markdown",
"markdown",
"markdown",
"markdown",
"markdown",
"markdown",
"markdown",
"markdown",
"markdown",
"markdown",
"markdown",
"markdown",
"markdown",
"markdown",
"markdown",
"markdown",
"markdown",
"markdown",
"markdown",
"markdown",
"markdown",
"markdown",
"markdown",
"markdown",
"markdown",
"markdown",
"markdown"
]
] |
e7789dc39fb7889ed5ab5735c120c8ddc2dd7324 | 154,831 | ipynb | Jupyter Notebook | 3-Visualization/2-Seaborn/7-Seaborn Uebung - Aufgabe.ipynb | Klaynie/Jupyter-Test | 8d4d165568527bdd789a1ca9a8f1a56efade9bf8 | [
"BSD-3-Clause"
] | null | null | null | 3-Visualization/2-Seaborn/7-Seaborn Uebung - Aufgabe.ipynb | Klaynie/Jupyter-Test | 8d4d165568527bdd789a1ca9a8f1a56efade9bf8 | [
"BSD-3-Clause"
] | null | null | null | 3-Visualization/2-Seaborn/7-Seaborn Uebung - Aufgabe.ipynb | Klaynie/Jupyter-Test | 8d4d165568527bdd789a1ca9a8f1a56efade9bf8 | [
"BSD-3-Clause"
] | null | null | null | 300.060078 | 68,150 | 0.915333 | [
[
[
"# Seaborn Übung - Aufgabe\nZeit unsere neu gelernten Seaborn Fähigkeiten anzuwenden! Versucht die gezeigten Diagramme selbst nachzustellen. Dabei ist die Farbgebung nicht entscheidend, es geht um die Inhalte.\n## Die Daten\nWir werde dazu ein berühmten Datensatz zur Titanic benutzen. Später im Machine Learning Teil des Kurses kommen wir auf diesen Datensatz zurück, um Überlebenswahrscheinlichkeiten zu berechnen.",
"_____no_output_____"
]
],
[
[
"import seaborn as sns\nimport matplotlib.pyplot as plt\n%matplotlib inline",
"_____no_output_____"
],
[
"titanic = sns.load_dataset('titanic')",
"_____no_output_____"
],
[
"titanic.head()",
"_____no_output_____"
]
],
[
[
"## Übung\nVersucht die gezeigten Diagramme selbst nachzustellen. Dabei ist die Farbgebung nicht entscheidend, es geht um die Inhalte.\n\nAchtet außerdem darauf, die Zelle direkt über dem Diagramm nicht zu verwenden. So könnt ihr verhindern, dass das Diagramm verloren geht. Wir haben eine extra Zelle zum Coden eingebaut.",
"_____no_output_____"
]
],
[
[
"# Hier eigenen Code schreiben\n# Nächste Zelle nicht nutzen",
"_____no_output_____"
],
[
"# Hier eigenen Code schreiben\n# Nächste Zelle nicht nutzen",
"_____no_output_____"
],
[
"# Hier eigenen Code schreiben\n# Nächste Zelle nicht nutzen",
"_____no_output_____"
],
[
"# Hier eigenen Code schreiben\n# Nächste Zelle nicht nutzen",
"_____no_output_____"
],
[
"sns.swarmplot(x='class', y='age', data=titanic, palette='Set2')",
"_____no_output_____"
],
[
"# Hier eigenen Code schreiben\n# Nächste Zelle nicht nutzen",
"_____no_output_____"
],
[
"# Hier eigenen Code schreiben\n# Nächste Zelle nicht nutzen",
"_____no_output_____"
],
[
"# Hier eigenen Code schreiben\n# Nächste Zelle nicht nutzen",
"_____no_output_____"
],
[
"g = sns.FacetGrid(data=titanic, col='sex')\ng.map(sns.distplot,'age')",
"_____no_output_____"
]
],
[
[
"# Gut gemacht!\n\nDas sei soweit genug. Wir werden Seaborn noch viel mehr verwenden, sobald wir zum Machine Learning Teil des Kurses gekommen sind!",
"_____no_output_____"
]
]
] | [
"markdown",
"code",
"markdown",
"code",
"markdown"
] | [
[
"markdown"
],
[
"code",
"code",
"code"
],
[
"markdown"
],
[
"code",
"code",
"code",
"code",
"code",
"code",
"code",
"code",
"code"
],
[
"markdown"
]
] |
e778b102d5d6429a75ddba237ebe3bf139fcf651 | 22,366 | ipynb | Jupyter Notebook | DataFrame row.column.ipynb | ghdtjf/Beakjon | 5e2e05540c53f277397a64a367294c6ba4dbeb43 | [
"MIT"
] | null | null | null | DataFrame row.column.ipynb | ghdtjf/Beakjon | 5e2e05540c53f277397a64a367294c6ba4dbeb43 | [
"MIT"
] | null | null | null | DataFrame row.column.ipynb | ghdtjf/Beakjon | 5e2e05540c53f277397a64a367294c6ba4dbeb43 | [
"MIT"
] | null | null | null | 23.793617 | 57 | 0.344675 | [
[
[
"import pandas as pd",
"_____no_output_____"
],
[
"friend_list = [\n ['name',['john', 'jenny', 'Nate']],\n ['age',[20, 30, 30]],\n ['job', ['student', 'developer', 'teacher']]\n]\ndf = pd.DataFrame.from_dict(dict(friend_list))",
"_____no_output_____"
],
[
"df",
"_____no_output_____"
],
[
"df[1:3]",
"_____no_output_____"
],
[
"df",
"_____no_output_____"
],
[
"df.loc[[0,2]]",
"_____no_output_____"
],
[
"df = df.loc[[0,2]]",
"_____no_output_____"
],
[
"df",
"_____no_output_____"
],
[
"friend_list = [\n ['name',['john', 'jenny', 'Nate']],\n ['age',[20, 30, 30]],\n ['job', ['student', 'developer', 'teacher']]\n]\ndf = pd.DataFrame.from_dict(dict(friend_list))",
"_____no_output_____"
],
[
"df",
"_____no_output_____"
],
[
"# by column condition",
"_____no_output_____"
],
[
"df[df.age > 25]",
"_____no_output_____"
],
[
"df.query('age>25')",
"_____no_output_____"
],
[
"df",
"_____no_output_____"
],
[
"df[(df.age>25 & (df.name == 'Nate'))]",
"_____no_output_____"
],
[
"#Filter Column",
"_____no_output_____"
],
[
"# by index",
"_____no_output_____"
],
[
"import pandas as pd\nfriend_list = [\n ['John', 20, 'student'],\n ['Jenny', 30, 'developer'],\n ['Nate', 30, 'teacher']\n]\ndf = pd.DataFrame.from_records(friend_list)\ndf",
"_____no_output_____"
],
[
"df.iloc[0:2,0:2]",
"_____no_output_____"
],
[
"##by column name",
"_____no_output_____"
],
[
"df = ",
"_____no_output_____"
]
]
] | [
"code"
] | [
[
"code",
"code",
"code",
"code",
"code",
"code",
"code",
"code",
"code",
"code",
"code",
"code",
"code",
"code",
"code",
"code",
"code",
"code",
"code",
"code",
"code"
]
] |
e778bbf52b3f88b0a19929b44da14b04fa08fe8e | 177,456 | ipynb | Jupyter Notebook | Semana3/Clase4_NumpyFundamentos.ipynb | mr1023/prope_programacion_2021 | 4ce7b2fc6533484ae96e3819b54e32a0d619da9c | [
"MIT"
] | 3 | 2021-06-08T01:14:27.000Z | 2021-06-15T02:42:45.000Z | Semana3/Clase4_NumpyFundamentos.ipynb | mr1023/prope_programacion_2021 | 4ce7b2fc6533484ae96e3819b54e32a0d619da9c | [
"MIT"
] | null | null | null | Semana3/Clase4_NumpyFundamentos.ipynb | mr1023/prope_programacion_2021 | 4ce7b2fc6533484ae96e3819b54e32a0d619da9c | [
"MIT"
] | 13 | 2021-06-08T01:13:39.000Z | 2022-03-03T05:15:03.000Z | 32.049124 | 380 | 0.436604 | [
[
[
"# Fundamentos de Numpy\n\n<img style=\"float: right; margin: 0px 0px 15px 15px;\" src=\"https://upload.wikimedia.org/wikipedia/commons/1/1a/NumPy_logo.svg\" width=\"400px\" height=\"400px\" />\n\n> Hasta ahora sólo hemos hablado acerca de tipos (clases) de variables y funciones que vienen por defecto en Python.\n\n> Sin embargo, una de las mejores cosas de Python (especialmente si eres o te preparas para ser un científico de datos) es la gran cantidad de librerías de alto nivel que se encuentran disponibles.\n\n> Algunas de estas librerías se encuentran en la librería estándar, es decir, se pueden encontrar donde sea que esté Python. Otras librerías se pueden añadir fácilmente.\n\n> La primer librería externa que cubriremos en este curso es NumPy (Numerical Python).\n\n\nReferencias:\n- https://www.numpy.org/\n- https://towardsdatascience.com/first-step-in-data-science-with-python-numpy-5e99d6821953\n___",
"_____no_output_____"
],
[
"# 0. Motivación \n\n¿Recuerdan algo de álgebra lineal? Por ejemplo:\n- vectores;\n- suma de vectores;\n- producto por un escalar ...\n\n¿Cómo se les ocurre que podríamos manejar lo anterior en Python?",
"_____no_output_____"
]
],
[
[
"# Crear dos vectores\nx = [4, 5, 8, -2, 3]\ny = [3, 1, -7, -9, 5]",
"_____no_output_____"
],
[
"# Suma de vectores\nx + y",
"_____no_output_____"
],
[
"# ¿con ciclos quizá?\nsum_ = [x[i] + y[i] for i in range(len(x))]\nsum_",
"_____no_output_____"
],
[
"# Producto por escalar\n3 * x",
"_____no_output_____"
],
[
"# ¿con ciclos quizá?\nprod_ = [3 * x[i] for i in range(len(x))]\nprod_",
"_____no_output_____"
]
],
[
[
"### Solución: NumPy\n\nNumPy es la librería fundamental para computación científica con Python. Contiene, entre otros:\n- una clase de objetos tipo arreglo N-dimensional muy poderso;\n- funciones matemáticas sofisticadas;\n- herramientas matemáticas útiles de álgebra lineal, transformada de Fourier y números aleatorios.",
"_____no_output_____"
],
[
"Aparte de sus usos científicos, NumPy puede ser usada como un contenedor eficiente de datos multidimensional, lo que le otorga a NumPy una capacidad impresionante de integración con bases de datos.\n\nPor otra parte, casi todas las librerías de Python relacionadas con ciencia de datos y machine learning tales como SciPy (Scientific Python), Mat-plotlib (librería de gráficos), Scikit-learn, dependen de NumPy razonablemente.",
"_____no_output_____"
],
[
"Para nuestra fortuna, NumPy ya viene instalado por defecto en la instalación de Anaconda.\n\nAsí que si queremos empezar a utilizarlo, lo único que debemos hacer es importarlo:",
"_____no_output_____"
]
],
[
[
"# Importar numpy\nimport numpy as np",
"_____no_output_____"
],
[
"np.sin(np.pi / 2)",
"_____no_output_____"
]
],
[
[
"Lo que acabamos de hacer es un procedimiento genérico para importar librerías:\n- se comienza con la palabra clave `import`;\n- a continuación el nombre de la librería, en este caso `numpy`;\n- opcionalmente se puede incluir una cláusula `as` y una abreviación del nombre de la librería. Para el caso de NumPy, la comunidad comúmente usa la abreviación `np`.",
"_____no_output_____"
],
[
"Ahora, intentemos hacer lo mismo que que antes, pero con el arreglo n-dimensional que provee NumPy como vector:",
"_____no_output_____"
]
],
[
[
"# Ayuda sobre arreglo N-dimensional\nhelp(np.array)",
"Help on built-in function array in module numpy:\n\narray(...)\n array(object, dtype=None, copy=True, order='K', subok=False, ndmin=0)\n \n Create an array.\n \n Parameters\n ----------\n object : array_like\n An array, any object exposing the array interface, an object whose\n __array__ method returns an array, or any (nested) sequence.\n dtype : data-type, optional\n The desired data-type for the array. If not given, then the type will\n be determined as the minimum type required to hold the objects in the\n sequence.\n copy : bool, optional\n If true (default), then the object is copied. Otherwise, a copy will\n only be made if __array__ returns a copy, if obj is a nested sequence,\n or if a copy is needed to satisfy any of the other requirements\n (`dtype`, `order`, etc.).\n order : {'K', 'A', 'C', 'F'}, optional\n Specify the memory layout of the array. If object is not an array, the\n newly created array will be in C order (row major) unless 'F' is\n specified, in which case it will be in Fortran order (column major).\n If object is an array the following holds.\n \n ===== ========= ===================================================\n order no copy copy=True\n ===== ========= ===================================================\n 'K' unchanged F & C order preserved, otherwise most similar order\n 'A' unchanged F order if input is F and not C, otherwise C order\n 'C' C order C order\n 'F' F order F order\n ===== ========= ===================================================\n \n When ``copy=False`` and a copy is made for other reasons, the result is\n the same as if ``copy=True``, with some exceptions for `A`, see the\n Notes section. The default order is 'K'.\n subok : bool, optional\n If True, then sub-classes will be passed-through, otherwise\n the returned array will be forced to be a base-class array (default).\n ndmin : int, optional\n Specifies the minimum number of dimensions that the resulting\n array should have. Ones will be pre-pended to the shape as\n needed to meet this requirement.\n \n Returns\n -------\n out : ndarray\n An array object satisfying the specified requirements.\n \n See Also\n --------\n empty_like : Return an empty array with shape and type of input.\n ones_like : Return an array of ones with shape and type of input.\n zeros_like : Return an array of zeros with shape and type of input.\n full_like : Return a new array with shape of input filled with value.\n empty : Return a new uninitialized array.\n ones : Return a new array setting values to one.\n zeros : Return a new array setting values to zero.\n full : Return a new array of given shape filled with value.\n \n \n Notes\n -----\n When order is 'A' and `object` is an array in neither 'C' nor 'F' order,\n and a copy is forced by a change in dtype, then the order of the result is\n not necessarily 'C' as expected. This is likely a bug.\n \n Examples\n --------\n >>> np.array([1, 2, 3])\n array([1, 2, 3])\n \n Upcasting:\n \n >>> np.array([1, 2, 3.0])\n array([ 1., 2., 3.])\n \n More than one dimension:\n \n >>> np.array([[1, 2], [3, 4]])\n array([[1, 2],\n [3, 4]])\n \n Minimum dimensions 2:\n \n >>> np.array([1, 2, 3], ndmin=2)\n array([[1, 2, 3]])\n \n Type provided:\n \n >>> np.array([1, 2, 3], dtype=complex)\n array([ 1.+0.j, 2.+0.j, 3.+0.j])\n \n Data-type consisting of more than one element:\n \n >>> x = np.array([(1,2),(3,4)],dtype=[('a','<i4'),('b','<i4')])\n >>> x['a']\n array([1, 3])\n \n Creating an array from sub-classes:\n \n >>> np.array(np.mat('1 2; 3 4'))\n array([[1, 2],\n [3, 4]])\n \n >>> np.array(np.mat('1 2; 3 4'), subok=True)\n matrix([[1, 2],\n [3, 4]])\n\n"
],
[
"a = [5, 6]\na",
"_____no_output_____"
],
[
"import copy",
"_____no_output_____"
],
[
"b = copy.copy(a)",
"_____no_output_____"
],
[
"b",
"_____no_output_____"
],
[
"a[1] = 8",
"_____no_output_____"
],
[
"b",
"_____no_output_____"
],
[
"# Crear dos vectores\nx = np.array([4, 5, 8, -2, 3])\ny = np.array([3, 1, -7, -9, 5])\nx, y",
"_____no_output_____"
],
[
"# Tipo\ntype(x)",
"_____no_output_____"
],
[
"# Suma de vectores\nx + y",
"_____no_output_____"
],
[
"# Producto interno\n7 * x",
"_____no_output_____"
],
[
"x.dtype",
"_____no_output_____"
],
[
"np.array([5], dtype=\"float64\")**np.array([28], dtype=\"float64\") # == 5**28",
"_____no_output_____"
],
[
"5**28",
"_____no_output_____"
]
],
[
[
"### Diferencias fundamentales entre Listas de Python y Arreglos de NumPy\n\nMientras que las listas y los arreglos tienen algunas similaridades (ambos son colecciones ordenadas de valores), existen ciertas diferencias abismales entre este tipo de estructuras de datos:\n\n- A diferencia de las listas, todos los elementos en un arreglo de NumPy deben ser del mismo tipo de datos (esto es, todos enteros, o flotantes, o strings, etc).\n\n- Por lo anterior, los arreglos de NumPy soportan operaciones aritméticas y otras funciones matemáticas que se ejecutan en cada elemento del arreglo. Las listas no soportan estos cálculos.\n\n- Los arreglos de NumPy tienen dimensionalidad.",
"_____no_output_____"
]
],
[
[
"np.array([6, 'hola', help])",
"_____no_output_____"
]
],
[
[
"# 1. ¿Qué podemos hacer en NumPy?\n\nYa vimos como crear arreglos básicos en NumPy, con el comando `np.array()`",
"_____no_output_____"
]
],
[
[
"x",
"_____no_output_____"
]
],
[
[
"¿Cuál es el tipo de estos arreglos?",
"_____no_output_____"
]
],
[
[
"type(x)",
"_____no_output_____"
],
[
"len(x)",
"_____no_output_____"
],
[
"x.shape",
"_____no_output_____"
],
[
"x.size",
"_____no_output_____"
],
[
"x.ndim",
"_____no_output_____"
]
],
[
[
"También podemos crear arreglos multidimensionales:",
"_____no_output_____"
]
],
[
[
"# Matriz 4x5\nA = np.array([[1, 2, 0, 5, -2],\n [9, -7, 5, 3, 0],\n [2, 1, 1, 1, -3],\n [4, 8, -3, 2, 1]])",
"_____no_output_____"
],
[
"len([[1, 2, 0, 5, -2],\n [9, -7, 5, 3, 0],\n [2, 1, 1, 1, -3],\n [4, 8, -3, 2, 1]])",
"_____no_output_____"
],
[
"# Tipo\ntype(A)",
"_____no_output_____"
],
[
"# Atributos\nA.shape",
"_____no_output_____"
],
[
"A.size",
"_____no_output_____"
],
[
"A.ndim",
"_____no_output_____"
],
[
"len(A)",
"_____no_output_____"
]
],
[
[
"## 1.1 Funciones de NumPy",
"_____no_output_____"
],
[
"Seguiremos nuestra introducción a NumPy mediante la resolución del siguiente problema:",
"_____no_output_____"
],
[
"### Problema 1\n\n> Dados cinco (5) contenedores cilíndricos con diferentes radios y alturas que pueden variar entre 5 y 25 cm, encontrar:\n> 1. El volumen del agua que puede almacenar cada contenedor;\n> 2. El volumen total del agua que pueden almacenar todos los contenedores juntos;\n> 3. Cual contenedor puede almacenar más volumen, y cuanto;\n> 4. Cual contenedor puede almacenar menos volumen, y cuanto;\n> 5. Obtener la media, la mediana y la desviación estándar de los volúmenes de agua que pueden ser almacenados en los contenedores.",
"_____no_output_____"
],
[
"Antes que nada, definamos las variables que nos dan:",
"_____no_output_____"
]
],
[
[
"# Definir numero de contenedores, medida minima y medida maxima\nn_contenedores = 5\nmedida_min = 5\nmedida_max = 25",
"_____no_output_____"
]
],
[
[
"A continuación, generaremos un arreglo de números enteros aleatorios entre 5 y 25 cm que representarán los radios y las alturas de los cilindros:",
"_____no_output_____"
]
],
[
[
"# Ayuda de np.random.randint()\nhelp(np.random.randint)",
"Help on built-in function randint:\n\nrandint(...) method of numpy.random.mtrand.RandomState instance\n randint(low, high=None, size=None, dtype='l')\n \n Return random integers from `low` (inclusive) to `high` (exclusive).\n \n Return random integers from the \"discrete uniform\" distribution of\n the specified dtype in the \"half-open\" interval [`low`, `high`). If\n `high` is None (the default), then results are from [0, `low`).\n \n .. note::\n New code should use the ``integers`` method of a ``default_rng()``\n instance instead; see `random-quick-start`.\n \n Parameters\n ----------\n low : int or array-like of ints\n Lowest (signed) integers to be drawn from the distribution (unless\n ``high=None``, in which case this parameter is one above the\n *highest* such integer).\n high : int or array-like of ints, optional\n If provided, one above the largest (signed) integer to be drawn\n from the distribution (see above for behavior if ``high=None``).\n If array-like, must contain integer values\n size : int or tuple of ints, optional\n Output shape. If the given shape is, e.g., ``(m, n, k)``, then\n ``m * n * k`` samples are drawn. Default is None, in which case a\n single value is returned.\n dtype : dtype, optional\n Desired dtype of the result. All dtypes are determined by their\n name, i.e., 'int64', 'int', etc, so byteorder is not available\n and a specific precision may have different C types depending\n on the platform. The default value is `np.int_`.\n \n .. versionadded:: 1.11.0\n \n Returns\n -------\n out : int or ndarray of ints\n `size`-shaped array of random integers from the appropriate\n distribution, or a single such random int if `size` not provided.\n \n See Also\n --------\n random_integers : similar to `randint`, only for the closed\n interval [`low`, `high`], and 1 is the lowest value if `high` is\n omitted.\n Generator.integers: which should be used for new code.\n \n Examples\n --------\n >>> np.random.randint(2, size=10)\n array([1, 0, 0, 0, 1, 1, 0, 0, 1, 0]) # random\n >>> np.random.randint(1, size=10)\n array([0, 0, 0, 0, 0, 0, 0, 0, 0, 0])\n \n Generate a 2 x 4 array of ints between 0 and 4, inclusive:\n \n >>> np.random.randint(5, size=(2, 4))\n array([[4, 0, 2, 1], # random\n [3, 2, 2, 0]])\n \n Generate a 1 x 3 array with 3 different upper bounds\n \n >>> np.random.randint(1, [3, 5, 10])\n array([2, 2, 9]) # random\n \n Generate a 1 by 3 array with 3 different lower bounds\n \n >>> np.random.randint([1, 5, 7], 10)\n array([9, 8, 7]) # random\n \n Generate a 2 by 4 array using broadcasting with dtype of uint8\n \n >>> np.random.randint([1, 3, 5, 7], [[10], [20]], dtype=np.uint8)\n array([[ 8, 6, 9, 7], # random\n [ 1, 16, 9, 12]], dtype=uint8)\n\n"
],
[
"help(np.random.seed)",
"Help on built-in function seed:\n\nseed(...) method of numpy.random.mtrand.RandomState instance\n seed(self, seed=None)\n \n Reseed a legacy MT19937 BitGenerator\n \n Notes\n -----\n This is a convenience, legacy function.\n \n The best practice is to **not** reseed a BitGenerator, rather to\n recreate a new one. This method is here for legacy reasons.\n This example demonstrates best practice.\n \n >>> from numpy.random import MT19937\n >>> from numpy.random import RandomState, SeedSequence\n >>> rs = RandomState(MT19937(SeedSequence(123456789)))\n # Later, you want to restart the stream\n >>> rs = RandomState(MT19937(SeedSequence(987654321)))\n\n"
],
[
"import numpy as np",
"_____no_output_____"
],
[
"# Números aleatorios que representan radios y alturas.\n# Inicializar la semilla\nnp.random.seed(1001)\nmedidas = np.random.randint(medida_min, medida_max, size=(10,))",
"_____no_output_____"
],
[
"# Ver valores\nmedidas",
"_____no_output_____"
],
[
"help(medidas.reshape)",
"Help on built-in function reshape:\n\nreshape(...) method of numpy.ndarray instance\n a.reshape(shape, order='C')\n \n Returns an array containing the same data with a new shape.\n \n Refer to `numpy.reshape` for full documentation.\n \n See Also\n --------\n numpy.reshape : equivalent function\n \n Notes\n -----\n Unlike the free function `numpy.reshape`, this method on `ndarray` allows\n the elements of the shape parameter to be passed in as separate arguments.\n For example, ``a.reshape(10, 11)`` is equivalent to\n ``a.reshape((10, 11))``.\n\n"
],
[
"# array.reshape\nmedidas = medidas.reshape((2, 5))",
"_____no_output_____"
],
[
"medidas",
"_____no_output_____"
]
],
[
[
"De los números generados, separemos los que corresponden a los radios, y los que corresponden a las alturas:",
"_____no_output_____"
]
],
[
[
"# Radios\nradios = medidas[0, :]\nradios",
"_____no_output_____"
],
[
"medidas[:, 3:5]",
"_____no_output_____"
],
[
"medidas[:, ::2]",
"_____no_output_____"
],
[
"# Alturas\nalturas = medidas[1, :]\nalturas",
"_____no_output_____"
]
],
[
[
"1. Con lo anterior, calculemos cada uno los volúmenes:",
"_____no_output_____"
]
],
[
[
"radios",
"_____no_output_____"
],
[
"radios**2",
"_____no_output_____"
],
[
"alturas",
"_____no_output_____"
],
[
"# Volúmenes de los contenedores\nvolumenes = (np.pi * radios**2) * alturas\nvolumenes",
"_____no_output_____"
]
],
[
[
"<img style=\"float: right; margin: 0px 0px 15px 15px;\" src=\"https://upload.wikimedia.org/wikipedia/commons/b/b3/Symbol_great.svg\" width=\"400px\" height=\"400px\" />\n\n### ¡Excelente!\n\nCon esta línea de código tan sencilla, pudimos obtener de un solo jalón todos los volúmenes de nuestros contenedores.\n\nEsta es la potencia que nos ofrece NumPy. Podemos operar los arreglos de forma rápida, sencilla, y muy eficiente.",
"_____no_output_____"
],
[
"2. Ahora, el volumen total",
"_____no_output_____"
]
],
[
[
"# Volumen total\nvolumenes.sum()",
"_____no_output_____"
]
],
[
[
"3. ¿Cuál contenedor puede almacenar más volumen? ¿Cuánto?",
"_____no_output_____"
]
],
[
[
"volumenes",
"_____no_output_____"
],
[
"# Contenedor que puede almacenar más volumen\nvolumenes.argmax()",
"_____no_output_____"
],
[
"# Volumen máximo\nvolumenes.max()",
"_____no_output_____"
],
[
"# También se puede, pero no es recomendable. Ver comparación de tiempos\nmax(volumenes)",
"_____no_output_____"
],
[
"random_vector = np.random.randint(0, 1000, size=(1000,))",
"_____no_output_____"
],
[
"%timeit random_vector.max()",
"5.22 µs ± 58.8 ns per loop (mean ± std. dev. of 7 runs, 100000 loops each)\n"
],
[
"%timeit np.max(random_vector)",
"7.95 µs ± 116 ns per loop (mean ± std. dev. of 7 runs, 100000 loops each)\n"
],
[
"%timeit max(random_vector)",
"181 µs ± 4.44 µs per loop (mean ± std. dev. of 7 runs, 1000 loops each)\n"
]
],
[
[
"4. ¿Cuál contenedor puede almacenar menos volumen? ¿Cuánto?",
"_____no_output_____"
]
],
[
[
"# Contenedor que puede almacenar menos volumen\nvolumenes.argmin()",
"_____no_output_____"
],
[
"# Volumen mínimo\nvolumenes.min()",
"_____no_output_____"
]
],
[
[
"5. Media, mediana y desviación estándar de los volúmenes",
"_____no_output_____"
]
],
[
[
"# Media, mediana y desviación estándar\nvolumenes.mean(), volumenes.std()",
"_____no_output_____"
],
[
"np.median(volumenes)",
"_____no_output_____"
],
[
"# Atributos shape y dtype\nvolumenes.shape",
"_____no_output_____"
],
[
"volumenes.dtype",
"_____no_output_____"
],
[
"A",
"_____no_output_____"
],
[
"A.shape",
"_____no_output_____"
],
[
"A.size",
"_____no_output_____"
]
],
[
[
"## 1.2 Trabajando con matrices",
"_____no_output_____"
],
[
"### Problema 2\n\n> 25 cartas numeradas de la 1 a la 25 se distribuyen aleatoriamente y en partes iguales a 5 personas. Encuentre la suma de cartas para cada persona tal que: \n> - para la primera persona, la suma es el valor de la primera carta menos la suma del resto de las cartas;\n> - para la segunda persona, la suma es el valor de la segunda carta menos la suma del resto de las cartas;\n> - y así sucesivamente ...\n\n> La persona para la cual la suma sea mayor, será el ganador. Encontrar el ganador.",
"_____no_output_____"
],
[
"Lo primero será generar los números del 1 al 25. ¿Cómo podemos hacer esto?",
"_____no_output_____"
],
[
"np.arange = np.array(range)",
"_____no_output_____"
]
],
[
[
"# Ayuda en la función np.arange()\nhelp(np.arange)",
"Help on built-in function arange in module numpy:\n\narange(...)\n arange([start,] stop[, step,], dtype=None)\n \n Return evenly spaced values within a given interval.\n \n Values are generated within the half-open interval ``[start, stop)``\n (in other words, the interval including `start` but excluding `stop`).\n For integer arguments the function is equivalent to the Python built-in\n `range` function, but returns an ndarray rather than a list.\n \n When using a non-integer step, such as 0.1, the results will often not\n be consistent. It is better to use `numpy.linspace` for these cases.\n \n Parameters\n ----------\n start : number, optional\n Start of interval. The interval includes this value. The default\n start value is 0.\n stop : number\n End of interval. The interval does not include this value, except\n in some cases where `step` is not an integer and floating point\n round-off affects the length of `out`.\n step : number, optional\n Spacing between values. For any output `out`, this is the distance\n between two adjacent values, ``out[i+1] - out[i]``. The default\n step size is 1. If `step` is specified as a position argument,\n `start` must also be given.\n dtype : dtype\n The type of the output array. If `dtype` is not given, infer the data\n type from the other input arguments.\n \n Returns\n -------\n arange : ndarray\n Array of evenly spaced values.\n \n For floating point arguments, the length of the result is\n ``ceil((stop - start)/step)``. Because of floating point overflow,\n this rule may result in the last element of `out` being greater\n than `stop`.\n \n See Also\n --------\n numpy.linspace : Evenly spaced numbers with careful handling of endpoints.\n numpy.ogrid: Arrays of evenly spaced numbers in N-dimensions.\n numpy.mgrid: Grid-shaped arrays of evenly spaced numbers in N-dimensions.\n \n Examples\n --------\n >>> np.arange(3)\n array([0, 1, 2])\n >>> np.arange(3.0)\n array([ 0., 1., 2.])\n >>> np.arange(3,7)\n array([3, 4, 5, 6])\n >>> np.arange(3,7,2)\n array([3, 5])\n\n"
],
[
"# Números del 1 al 25\ncartas = np.arange(1, 26)\ncartas",
"_____no_output_____"
]
],
[
[
"Luego, tal y como en un juego de cartas, deberíamos barajarlos, antes de repartirlos:",
"_____no_output_____"
]
],
[
[
"# Ayuda en la función np.random.shuffle()\nhelp(np.random.shuffle)",
"Help on built-in function shuffle:\n\nshuffle(...) method of numpy.random.mtrand.RandomState instance\n shuffle(x)\n \n Modify a sequence in-place by shuffling its contents.\n \n This function only shuffles the array along the first axis of a\n multi-dimensional array. The order of sub-arrays is changed but\n their contents remains the same.\n \n .. note::\n New code should use the ``shuffle`` method of a ``default_rng()``\n instance instead; see `random-quick-start`.\n \n Parameters\n ----------\n x : array_like\n The array or list to be shuffled.\n \n Returns\n -------\n None\n \n See Also\n --------\n Generator.shuffle: which should be used for new code.\n \n Examples\n --------\n >>> arr = np.arange(10)\n >>> np.random.shuffle(arr)\n >>> arr\n [1 7 5 2 9 4 3 6 0 8] # random\n \n Multi-dimensional arrays are only shuffled along the first axis:\n \n >>> arr = np.arange(9).reshape((3, 3))\n >>> np.random.shuffle(arr)\n >>> arr\n array([[3, 4, 5], # random\n [6, 7, 8],\n [0, 1, 2]])\n\n"
],
[
"# Barajar\nnp.random.shuffle(cartas)",
"_____no_output_____"
],
[
"# Ver valores\ncartas",
"_____no_output_____"
]
],
[
[
"Bien. Ahora, deberíamos distribuir las cartas. Podemos imaginarnos la distribución como una matriz 5x5:",
"_____no_output_____"
]
],
[
[
"# Repartir cartas\ncartas = cartas.reshape((5, 5))",
"_____no_output_____"
],
[
"# Ver valores\ncartas",
"_____no_output_____"
]
],
[
[
"Entonces, tenemos 5 cartas para cada una de las 5 personas, visualizadas como una matriz 5x5.\n\nLo único que nos falta es encontrar la suma para cada uno, es decir, sumar el elemento de la diagonal principal y restar las demás entradas de la fila (o columna).\n\n¿Cómo hacemos esto?",
"_____no_output_____"
]
],
[
[
"# Ayuda en la función np.eye()\nhelp(np.eye)",
"Help on function eye in module numpy:\n\neye(N, M=None, k=0, dtype=<class 'float'>, order='C')\n Return a 2-D array with ones on the diagonal and zeros elsewhere.\n \n Parameters\n ----------\n N : int\n Number of rows in the output.\n M : int, optional\n Number of columns in the output. If None, defaults to `N`.\n k : int, optional\n Index of the diagonal: 0 (the default) refers to the main diagonal,\n a positive value refers to an upper diagonal, and a negative value\n to a lower diagonal.\n dtype : data-type, optional\n Data-type of the returned array.\n order : {'C', 'F'}, optional\n Whether the output should be stored in row-major (C-style) or\n column-major (Fortran-style) order in memory.\n \n .. versionadded:: 1.14.0\n \n Returns\n -------\n I : ndarray of shape (N,M)\n An array where all elements are equal to zero, except for the `k`-th\n diagonal, whose values are equal to one.\n \n See Also\n --------\n identity : (almost) equivalent function\n diag : diagonal 2-D array from a 1-D array specified by the user.\n \n Examples\n --------\n >>> np.eye(2, dtype=int)\n array([[1, 0],\n [0, 1]])\n >>> np.eye(3, k=1)\n array([[0., 1., 0.],\n [0., 0., 1.],\n [0., 0., 0.]])\n\n"
],
[
"# Matriz con la diagonal principal\nI5 = np.eye(5)",
"_____no_output_____"
],
[
"I5",
"_____no_output_____"
],
[
"I5 * cartas",
"_____no_output_____"
],
[
"# Ayuda en la función np.ones()\nhelp(np.ones)",
"Help on function ones in module numpy:\n\nones(shape, dtype=None, order='C')\n Return a new array of given shape and type, filled with ones.\n \n Parameters\n ----------\n shape : int or sequence of ints\n Shape of the new array, e.g., ``(2, 3)`` or ``2``.\n dtype : data-type, optional\n The desired data-type for the array, e.g., `numpy.int8`. Default is\n `numpy.float64`.\n order : {'C', 'F'}, optional, default: C\n Whether to store multi-dimensional data in row-major\n (C-style) or column-major (Fortran-style) order in\n memory.\n \n Returns\n -------\n out : ndarray\n Array of ones with the given shape, dtype, and order.\n \n See Also\n --------\n ones_like : Return an array of ones with shape and type of input.\n empty : Return a new uninitialized array.\n zeros : Return a new array setting values to zero.\n full : Return a new array of given shape filled with value.\n \n \n Examples\n --------\n >>> np.ones(5)\n array([1., 1., 1., 1., 1.])\n \n >>> np.ones((5,), dtype=int)\n array([1, 1, 1, 1, 1])\n \n >>> np.ones((2, 1))\n array([[1.],\n [1.]])\n \n >>> s = (2,2)\n >>> np.ones(s)\n array([[1., 1.],\n [1., 1.]])\n\n"
],
[
"# Matriz con los elementos fuera de la diagonal negativos\ncomplement = np.ones((5, 5)) - I5",
"_____no_output_____"
],
[
"complement",
"_____no_output_____"
],
[
"complement * cartas",
"_____no_output_____"
],
[
"# Matriz completa\nmatriz_para_suma = I5 * cartas - complement * cartas",
"_____no_output_____"
],
[
"matriz_para_suma",
"_____no_output_____"
],
[
"# Sumar por filas\nsuma = matriz_para_suma.sum(axis=0)\nsuma",
"_____no_output_____"
]
],
[
[
"¿Quién es el ganador?",
"_____no_output_____"
]
],
[
[
"suma.argmax()",
"_____no_output_____"
]
],
[
[
"# 2. Algo de álgebra lineal con NumPy\n\nBueno, ya hemos utilizado NumPy para resolver algunos problemas de juguete. A través de estos problemas, hemos introducido el tipo de objetos que podemos manipular con NumPy, además de varias funcionalidades que podemos utilizar.\n\nPues bien, este tipo de objetos nos sirven perfectamente para representar vectores y matrices con entradas reales o complejas... si, de las que estudiamos en algún momento en álgebra lineal.\n\nMejor aún, NumPy nos ofrece un módulo de álgebra lineal para efectuar las operaciones básicas que podríamos necesitar.",
"_____no_output_____"
],
[
"Consideremos la siguiente matriz:",
"_____no_output_____"
]
],
[
[
"A = np.array([[1, 0, 1],\n [-1, 2, 4],\n [2, 1, 1]])",
"_____no_output_____"
],
[
"A",
"_____no_output_____"
]
],
[
[
"Podemos obtener varios cálculos útiles alrededor de la matriz A:",
"_____no_output_____"
]
],
[
[
"# Rango de la matriz A\nnp.linalg.matrix_rank(A)",
"_____no_output_____"
],
[
"# Determinante de la matriz A\nnp.linalg.det(A)",
"_____no_output_____"
],
[
"# Inversa de la matriz A\nnp.linalg.inv(A)",
"_____no_output_____"
],
[
"A.dot(np.linalg.inv(A))",
"_____no_output_____"
],
[
"np.linalg.inv(A).dot(A)",
"_____no_output_____"
],
[
"np.dot(A, np.linalg.inv(A))",
"_____no_output_____"
],
[
"np.dot(np.linalg.inv(A), A)",
"_____no_output_____"
],
[
"# Potencia de la matriz A\n# A.dot(A).dot(A).dot(A).dot(A)\nnp.linalg.matrix_power(A, 5)",
"_____no_output_____"
],
[
"A.dot(A).dot(A).dot(A).dot(A)",
"_____no_output_____"
],
[
"A**5",
"_____no_output_____"
],
[
"A",
"_____no_output_____"
],
[
"# Eigenvalores y eigenvectores de la matriz A\nl, v = np.linalg.eig(A)",
"_____no_output_____"
],
[
"l",
"_____no_output_____"
],
[
"v",
"_____no_output_____"
]
],
[
[
"Por otra parte, si tenemos dos vectores:",
"_____no_output_____"
]
],
[
[
"x, y",
"_____no_output_____"
]
],
[
[
"podemos calcular su producto interno (producto punto)",
"_____no_output_____"
]
],
[
[
"x.dot(y)",
"_____no_output_____"
],
[
"(x * y).sum()",
"_____no_output_____"
],
[
"x[:3], y[:3]",
"_____no_output_____"
],
[
"np.cross(x[:3], y[:3])",
"_____no_output_____"
]
],
[
[
"De la misma manera, podemos calcular la multiplicación de la matriz A por un vector",
"_____no_output_____"
]
],
[
[
"A",
"_____no_output_____"
],
[
"z = np.array([1, 0, 1])",
"_____no_output_____"
],
[
"A.dot(z)",
"_____no_output_____"
]
],
[
[
"$$\nA x = z\n$$",
"_____no_output_____"
]
],
[
[
"np.linalg.inv(A).dot(z)",
"_____no_output_____"
],
[
"np.linalg.solve(A, z)",
"_____no_output_____"
],
[
"help(np.linalg.cross)",
"_____no_output_____"
],
[
"help(np.linalg.svd)",
"Help on function svd in module numpy.linalg:\n\nsvd(a, full_matrices=True, compute_uv=True, hermitian=False)\n Singular Value Decomposition.\n \n When `a` is a 2D array, it is factorized as ``u @ np.diag(s) @ vh\n = (u * s) @ vh``, where `u` and `vh` are 2D unitary arrays and `s` is a 1D\n array of `a`'s singular values. When `a` is higher-dimensional, SVD is\n applied in stacked mode as explained below.\n \n Parameters\n ----------\n a : (..., M, N) array_like\n A real or complex array with ``a.ndim >= 2``.\n full_matrices : bool, optional\n If True (default), `u` and `vh` have the shapes ``(..., M, M)`` and\n ``(..., N, N)``, respectively. Otherwise, the shapes are\n ``(..., M, K)`` and ``(..., K, N)``, respectively, where\n ``K = min(M, N)``.\n compute_uv : bool, optional\n Whether or not to compute `u` and `vh` in addition to `s`. True\n by default.\n hermitian : bool, optional\n If True, `a` is assumed to be Hermitian (symmetric if real-valued),\n enabling a more efficient method for finding singular values.\n Defaults to False.\n \n .. versionadded:: 1.17.0\n \n Returns\n -------\n u : { (..., M, M), (..., M, K) } array\n Unitary array(s). The first ``a.ndim - 2`` dimensions have the same\n size as those of the input `a`. The size of the last two dimensions\n depends on the value of `full_matrices`. Only returned when\n `compute_uv` is True.\n s : (..., K) array\n Vector(s) with the singular values, within each vector sorted in\n descending order. The first ``a.ndim - 2`` dimensions have the same\n size as those of the input `a`.\n vh : { (..., N, N), (..., K, N) } array\n Unitary array(s). The first ``a.ndim - 2`` dimensions have the same\n size as those of the input `a`. The size of the last two dimensions\n depends on the value of `full_matrices`. Only returned when\n `compute_uv` is True.\n \n Raises\n ------\n LinAlgError\n If SVD computation does not converge.\n \n Notes\n -----\n \n .. versionchanged:: 1.8.0\n Broadcasting rules apply, see the `numpy.linalg` documentation for\n details.\n \n The decomposition is performed using LAPACK routine ``_gesdd``.\n \n SVD is usually described for the factorization of a 2D matrix :math:`A`.\n The higher-dimensional case will be discussed below. In the 2D case, SVD is\n written as :math:`A = U S V^H`, where :math:`A = a`, :math:`U= u`,\n :math:`S= \\mathtt{np.diag}(s)` and :math:`V^H = vh`. The 1D array `s`\n contains the singular values of `a` and `u` and `vh` are unitary. The rows\n of `vh` are the eigenvectors of :math:`A^H A` and the columns of `u` are\n the eigenvectors of :math:`A A^H`. In both cases the corresponding\n (possibly non-zero) eigenvalues are given by ``s**2``.\n \n If `a` has more than two dimensions, then broadcasting rules apply, as\n explained in :ref:`routines.linalg-broadcasting`. This means that SVD is\n working in \"stacked\" mode: it iterates over all indices of the first\n ``a.ndim - 2`` dimensions and for each combination SVD is applied to the\n last two indices. The matrix `a` can be reconstructed from the\n decomposition with either ``(u * s[..., None, :]) @ vh`` or\n ``u @ (s[..., None] * vh)``. (The ``@`` operator can be replaced by the\n function ``np.matmul`` for python versions below 3.5.)\n \n If `a` is a ``matrix`` object (as opposed to an ``ndarray``), then so are\n all the return values.\n \n Examples\n --------\n >>> a = np.random.randn(9, 6) + 1j*np.random.randn(9, 6)\n >>> b = np.random.randn(2, 7, 8, 3) + 1j*np.random.randn(2, 7, 8, 3)\n \n Reconstruction based on full SVD, 2D case:\n \n >>> u, s, vh = np.linalg.svd(a, full_matrices=True)\n >>> u.shape, s.shape, vh.shape\n ((9, 9), (6,), (6, 6))\n >>> np.allclose(a, np.dot(u[:, :6] * s, vh))\n True\n >>> smat = np.zeros((9, 6), dtype=complex)\n >>> smat[:6, :6] = np.diag(s)\n >>> np.allclose(a, np.dot(u, np.dot(smat, vh)))\n True\n \n Reconstruction based on reduced SVD, 2D case:\n \n >>> u, s, vh = np.linalg.svd(a, full_matrices=False)\n >>> u.shape, s.shape, vh.shape\n ((9, 6), (6,), (6, 6))\n >>> np.allclose(a, np.dot(u * s, vh))\n True\n >>> smat = np.diag(s)\n >>> np.allclose(a, np.dot(u, np.dot(smat, vh)))\n True\n \n Reconstruction based on full SVD, 4D case:\n \n >>> u, s, vh = np.linalg.svd(b, full_matrices=True)\n >>> u.shape, s.shape, vh.shape\n ((2, 7, 8, 8), (2, 7, 3), (2, 7, 3, 3))\n >>> np.allclose(b, np.matmul(u[..., :3] * s[..., None, :], vh))\n True\n >>> np.allclose(b, np.matmul(u[..., :3], s[..., None] * vh))\n True\n \n Reconstruction based on reduced SVD, 4D case:\n \n >>> u, s, vh = np.linalg.svd(b, full_matrices=False)\n >>> u.shape, s.shape, vh.shape\n ((2, 7, 8, 3), (2, 7, 3), (2, 7, 3, 3))\n >>> np.allclose(b, np.matmul(u * s[..., None, :], vh))\n True\n >>> np.allclose(b, np.matmul(u, s[..., None] * vh))\n True\n\n"
],
[
"a = np.arange(25).reshape(5,5)\na",
"_____no_output_____"
],
[
"np.einsum('ii', a)",
"_____no_output_____"
],
[
"help(a)",
"Help on ndarray object:\n\nclass ndarray(builtins.object)\n | ndarray(shape, dtype=float, buffer=None, offset=0,\n | strides=None, order=None)\n | \n | An array object represents a multidimensional, homogeneous array\n | of fixed-size items. An associated data-type object describes the\n | format of each element in the array (its byte-order, how many bytes it\n | occupies in memory, whether it is an integer, a floating point number,\n | or something else, etc.)\n | \n | Arrays should be constructed using `array`, `zeros` or `empty` (refer\n | to the See Also section below). The parameters given here refer to\n | a low-level method (`ndarray(...)`) for instantiating an array.\n | \n | For more information, refer to the `numpy` module and examine the\n | methods and attributes of an array.\n | \n | Parameters\n | ----------\n | (for the __new__ method; see Notes below)\n | \n | shape : tuple of ints\n | Shape of created array.\n | dtype : data-type, optional\n | Any object that can be interpreted as a numpy data type.\n | buffer : object exposing buffer interface, optional\n | Used to fill the array with data.\n | offset : int, optional\n | Offset of array data in buffer.\n | strides : tuple of ints, optional\n | Strides of data in memory.\n | order : {'C', 'F'}, optional\n | Row-major (C-style) or column-major (Fortran-style) order.\n | \n | Attributes\n | ----------\n | T : ndarray\n | Transpose of the array.\n | data : buffer\n | The array's elements, in memory.\n | dtype : dtype object\n | Describes the format of the elements in the array.\n | flags : dict\n | Dictionary containing information related to memory use, e.g.,\n | 'C_CONTIGUOUS', 'OWNDATA', 'WRITEABLE', etc.\n | flat : numpy.flatiter object\n | Flattened version of the array as an iterator. The iterator\n | allows assignments, e.g., ``x.flat = 3`` (See `ndarray.flat` for\n | assignment examples; TODO).\n | imag : ndarray\n | Imaginary part of the array.\n | real : ndarray\n | Real part of the array.\n | size : int\n | Number of elements in the array.\n | itemsize : int\n | The memory use of each array element in bytes.\n | nbytes : int\n | The total number of bytes required to store the array data,\n | i.e., ``itemsize * size``.\n | ndim : int\n | The array's number of dimensions.\n | shape : tuple of ints\n | Shape of the array.\n | strides : tuple of ints\n | The step-size required to move from one element to the next in\n | memory. For example, a contiguous ``(3, 4)`` array of type\n | ``int16`` in C-order has strides ``(8, 2)``. This implies that\n | to move from element to element in memory requires jumps of 2 bytes.\n | To move from row-to-row, one needs to jump 8 bytes at a time\n | (``2 * 4``).\n | ctypes : ctypes object\n | Class containing properties of the array needed for interaction\n | with ctypes.\n | base : ndarray\n | If the array is a view into another array, that array is its `base`\n | (unless that array is also a view). The `base` array is where the\n | array data is actually stored.\n | \n | See Also\n | --------\n | array : Construct an array.\n | zeros : Create an array, each element of which is zero.\n | empty : Create an array, but leave its allocated memory unchanged (i.e.,\n | it contains \"garbage\").\n | dtype : Create a data-type.\n | \n | Notes\n | -----\n | There are two modes of creating an array using ``__new__``:\n | \n | 1. If `buffer` is None, then only `shape`, `dtype`, and `order`\n | are used.\n | 2. If `buffer` is an object exposing the buffer interface, then\n | all keywords are interpreted.\n | \n | No ``__init__`` method is needed because the array is fully initialized\n | after the ``__new__`` method.\n | \n | Examples\n | --------\n | These examples illustrate the low-level `ndarray` constructor. Refer\n | to the `See Also` section above for easier ways of constructing an\n | ndarray.\n | \n | First mode, `buffer` is None:\n | \n | >>> np.ndarray(shape=(2,2), dtype=float, order='F')\n | array([[0.0e+000, 0.0e+000], # random\n | [ nan, 2.5e-323]])\n | \n | Second mode:\n | \n | >>> np.ndarray((2,), buffer=np.array([1,2,3]),\n | ... offset=np.int_().itemsize,\n | ... dtype=int) # offset = 1*itemsize, i.e. skip first element\n | array([2, 3])\n | \n | Methods defined here:\n | \n | __abs__(self, /)\n | abs(self)\n | \n | __add__(self, value, /)\n | Return self+value.\n | \n | __and__(self, value, /)\n | Return self&value.\n | \n | __array__(...)\n | a.__array__(|dtype) -> reference if type unchanged, copy otherwise.\n | \n | Returns either a new reference to self if dtype is not given or a new array\n | of provided data type if dtype is different from the current dtype of the\n | array.\n | \n | __array_function__(...)\n | \n | __array_prepare__(...)\n | a.__array_prepare__(obj) -> Object of same type as ndarray object obj.\n | \n | __array_ufunc__(...)\n | \n | __array_wrap__(...)\n | a.__array_wrap__(obj) -> Object of same type as ndarray object a.\n | \n | __bool__(self, /)\n | self != 0\n | \n | __complex__(...)\n | \n | __contains__(self, key, /)\n | Return key in self.\n | \n | __copy__(...)\n | a.__copy__()\n | \n | Used if :func:`copy.copy` is called on an array. Returns a copy of the array.\n | \n | Equivalent to ``a.copy(order='K')``.\n | \n | __deepcopy__(...)\n | a.__deepcopy__(memo, /) -> Deep copy of array.\n | \n | Used if :func:`copy.deepcopy` is called on an array.\n | \n | __delitem__(self, key, /)\n | Delete self[key].\n | \n | __divmod__(self, value, /)\n | Return divmod(self, value).\n | \n | __eq__(self, value, /)\n | Return self==value.\n | \n | __float__(self, /)\n | float(self)\n | \n | __floordiv__(self, value, /)\n | Return self//value.\n | \n | __format__(...)\n | Default object formatter.\n | \n | __ge__(self, value, /)\n | Return self>=value.\n | \n | __getitem__(self, key, /)\n | Return self[key].\n | \n | __gt__(self, value, /)\n | Return self>value.\n | \n | __iadd__(self, value, /)\n | Return self+=value.\n | \n | __iand__(self, value, /)\n | Return self&=value.\n | \n | __ifloordiv__(self, value, /)\n | Return self//=value.\n | \n | __ilshift__(self, value, /)\n | Return self<<=value.\n | \n | __imatmul__(self, value, /)\n | Return self@=value.\n | \n | __imod__(self, value, /)\n | Return self%=value.\n | \n | __imul__(self, value, /)\n | Return self*=value.\n | \n | __index__(self, /)\n | Return self converted to an integer, if self is suitable for use as an index into a list.\n | \n | __int__(self, /)\n | int(self)\n | \n | __invert__(self, /)\n | ~self\n | \n | __ior__(self, value, /)\n | Return self|=value.\n | \n | __ipow__(self, value, /)\n | Return self**=value.\n | \n | __irshift__(self, value, /)\n | Return self>>=value.\n | \n | __isub__(self, value, /)\n | Return self-=value.\n | \n | __iter__(self, /)\n | Implement iter(self).\n | \n | __itruediv__(self, value, /)\n | Return self/=value.\n | \n | __ixor__(self, value, /)\n | Return self^=value.\n | \n | __le__(self, value, /)\n | Return self<=value.\n | \n | __len__(self, /)\n | Return len(self).\n | \n | __lshift__(self, value, /)\n | Return self<<value.\n | \n | __lt__(self, value, /)\n | Return self<value.\n | \n | __matmul__(self, value, /)\n | Return self@value.\n | \n | __mod__(self, value, /)\n | Return self%value.\n | \n | __mul__(self, value, /)\n | Return self*value.\n | \n | __ne__(self, value, /)\n | Return self!=value.\n | \n | __neg__(self, /)\n | -self\n | \n | __or__(self, value, /)\n | Return self|value.\n | \n | __pos__(self, /)\n | +self\n | \n | __pow__(self, value, mod=None, /)\n | Return pow(self, value, mod).\n | \n | __radd__(self, value, /)\n | Return value+self.\n | \n | __rand__(self, value, /)\n | Return value&self.\n | \n | __rdivmod__(self, value, /)\n | Return divmod(value, self).\n | \n | __reduce__(...)\n | a.__reduce__()\n | \n | For pickling.\n | \n | __reduce_ex__(...)\n | Helper for pickle.\n | \n | __repr__(self, /)\n | Return repr(self).\n | \n | __rfloordiv__(self, value, /)\n | Return value//self.\n | \n | __rlshift__(self, value, /)\n | Return value<<self.\n | \n | __rmatmul__(self, value, /)\n | Return value@self.\n | \n | __rmod__(self, value, /)\n | Return value%self.\n | \n | __rmul__(self, value, /)\n | Return value*self.\n | \n | __ror__(self, value, /)\n | Return value|self.\n | \n | __rpow__(self, value, mod=None, /)\n | Return pow(value, self, mod).\n | \n | __rrshift__(self, value, /)\n | Return value>>self.\n | \n | __rshift__(self, value, /)\n | Return self>>value.\n | \n | __rsub__(self, value, /)\n | Return value-self.\n | \n | __rtruediv__(self, value, /)\n | Return value/self.\n | \n | __rxor__(self, value, /)\n | Return value^self.\n | \n | __setitem__(self, key, value, /)\n | Set self[key] to value.\n | \n | __setstate__(...)\n | a.__setstate__(state, /)\n | \n | For unpickling.\n | \n | The `state` argument must be a sequence that contains the following\n | elements:\n | \n | Parameters\n | ----------\n | version : int\n | optional pickle version. If omitted defaults to 0.\n | shape : tuple\n | dtype : data-type\n | isFortran : bool\n | rawdata : string or list\n | a binary string with the data (or a list if 'a' is an object array)\n | \n | __sizeof__(...)\n | Size of object in memory, in bytes.\n | \n | __str__(self, /)\n | Return str(self).\n | \n | __sub__(self, value, /)\n | Return self-value.\n | \n | __truediv__(self, value, /)\n | Return self/value.\n | \n | __xor__(self, value, /)\n | Return self^value.\n | \n | all(...)\n | a.all(axis=None, out=None, keepdims=False)\n | \n | Returns True if all elements evaluate to True.\n | \n | Refer to `numpy.all` for full documentation.\n | \n | See Also\n | --------\n | numpy.all : equivalent function\n | \n | any(...)\n | a.any(axis=None, out=None, keepdims=False)\n | \n | Returns True if any of the elements of `a` evaluate to True.\n | \n | Refer to `numpy.any` for full documentation.\n | \n | See Also\n | --------\n | numpy.any : equivalent function\n | \n | argmax(...)\n | a.argmax(axis=None, out=None)\n | \n | Return indices of the maximum values along the given axis.\n | \n | Refer to `numpy.argmax` for full documentation.\n | \n | See Also\n | --------\n | numpy.argmax : equivalent function\n | \n | argmin(...)\n | a.argmin(axis=None, out=None)\n | \n | Return indices of the minimum values along the given axis of `a`.\n | \n | Refer to `numpy.argmin` for detailed documentation.\n | \n | See Also\n | --------\n | numpy.argmin : equivalent function\n | \n | argpartition(...)\n | a.argpartition(kth, axis=-1, kind='introselect', order=None)\n | \n | Returns the indices that would partition this array.\n | \n | Refer to `numpy.argpartition` for full documentation.\n | \n | .. versionadded:: 1.8.0\n | \n | See Also\n | --------\n | numpy.argpartition : equivalent function\n | \n | argsort(...)\n | a.argsort(axis=-1, kind=None, order=None)\n | \n | Returns the indices that would sort this array.\n | \n | Refer to `numpy.argsort` for full documentation.\n | \n | See Also\n | --------\n | numpy.argsort : equivalent function\n | \n | astype(...)\n | a.astype(dtype, order='K', casting='unsafe', subok=True, copy=True)\n | \n | Copy of the array, cast to a specified type.\n | \n | Parameters\n | ----------\n | dtype : str or dtype\n | Typecode or data-type to which the array is cast.\n | order : {'C', 'F', 'A', 'K'}, optional\n | Controls the memory layout order of the result.\n | 'C' means C order, 'F' means Fortran order, 'A'\n | means 'F' order if all the arrays are Fortran contiguous,\n | 'C' order otherwise, and 'K' means as close to the\n | order the array elements appear in memory as possible.\n | Default is 'K'.\n | casting : {'no', 'equiv', 'safe', 'same_kind', 'unsafe'}, optional\n | Controls what kind of data casting may occur. Defaults to 'unsafe'\n | for backwards compatibility.\n | \n | * 'no' means the data types should not be cast at all.\n | * 'equiv' means only byte-order changes are allowed.\n | * 'safe' means only casts which can preserve values are allowed.\n | * 'same_kind' means only safe casts or casts within a kind,\n | like float64 to float32, are allowed.\n | * 'unsafe' means any data conversions may be done.\n | subok : bool, optional\n | If True, then sub-classes will be passed-through (default), otherwise\n | the returned array will be forced to be a base-class array.\n | copy : bool, optional\n | By default, astype always returns a newly allocated array. If this\n | is set to false, and the `dtype`, `order`, and `subok`\n | requirements are satisfied, the input array is returned instead\n | of a copy.\n | \n | Returns\n | -------\n | arr_t : ndarray\n | Unless `copy` is False and the other conditions for returning the input\n | array are satisfied (see description for `copy` input parameter), `arr_t`\n | is a new array of the same shape as the input array, with dtype, order\n | given by `dtype`, `order`.\n | \n | Notes\n | -----\n | .. versionchanged:: 1.17.0\n | Casting between a simple data type and a structured one is possible only\n | for \"unsafe\" casting. Casting to multiple fields is allowed, but\n | casting from multiple fields is not.\n | \n | .. versionchanged:: 1.9.0\n | Casting from numeric to string types in 'safe' casting mode requires\n | that the string dtype length is long enough to store the max\n | integer/float value converted.\n | \n | Raises\n | ------\n | ComplexWarning\n | When casting from complex to float or int. To avoid this,\n | one should use ``a.real.astype(t)``.\n | \n | Examples\n | --------\n | >>> x = np.array([1, 2, 2.5])\n | >>> x\n | array([1. , 2. , 2.5])\n | \n | >>> x.astype(int)\n | array([1, 2, 2])\n | \n | byteswap(...)\n | a.byteswap(inplace=False)\n | \n | Swap the bytes of the array elements\n | \n | Toggle between low-endian and big-endian data representation by\n | returning a byteswapped array, optionally swapped in-place.\n | Arrays of byte-strings are not swapped. The real and imaginary\n | parts of a complex number are swapped individually.\n | \n | Parameters\n | ----------\n | inplace : bool, optional\n | If ``True``, swap bytes in-place, default is ``False``.\n | \n | Returns\n | -------\n | out : ndarray\n | The byteswapped array. If `inplace` is ``True``, this is\n | a view to self.\n | \n | Examples\n | --------\n | >>> A = np.array([1, 256, 8755], dtype=np.int16)\n | >>> list(map(hex, A))\n | ['0x1', '0x100', '0x2233']\n | >>> A.byteswap(inplace=True)\n | array([ 256, 1, 13090], dtype=int16)\n | >>> list(map(hex, A))\n | ['0x100', '0x1', '0x3322']\n | \n | Arrays of byte-strings are not swapped\n | \n | >>> A = np.array([b'ceg', b'fac'])\n | >>> A.byteswap()\n | array([b'ceg', b'fac'], dtype='|S3')\n | \n | ``A.newbyteorder().byteswap()`` produces an array with the same values\n | but different representation in memory\n | \n | >>> A = np.array([1, 2, 3])\n | >>> A.view(np.uint8)\n | array([1, 0, 0, 0, 0, 0, 0, 0, 2, 0, 0, 0, 0, 0, 0, 0, 3, 0, 0, 0, 0, 0,\n | 0, 0], dtype=uint8)\n | >>> A.newbyteorder().byteswap(inplace=True)\n | array([1, 2, 3])\n | >>> A.view(np.uint8)\n | array([0, 0, 0, 0, 0, 0, 0, 1, 0, 0, 0, 0, 0, 0, 0, 2, 0, 0, 0, 0, 0, 0,\n | 0, 3], dtype=uint8)\n | \n | choose(...)\n | a.choose(choices, out=None, mode='raise')\n | \n | Use an index array to construct a new array from a set of choices.\n | \n | Refer to `numpy.choose` for full documentation.\n | \n | See Also\n | --------\n | numpy.choose : equivalent function\n | \n | clip(...)\n | a.clip(min=None, max=None, out=None, **kwargs)\n | \n | Return an array whose values are limited to ``[min, max]``.\n | One of max or min must be given.\n | \n | Refer to `numpy.clip` for full documentation.\n | \n | See Also\n | --------\n | numpy.clip : equivalent function\n | \n | compress(...)\n | a.compress(condition, axis=None, out=None)\n | \n | Return selected slices of this array along given axis.\n | \n | Refer to `numpy.compress` for full documentation.\n | \n | See Also\n | --------\n | numpy.compress : equivalent function\n | \n | conj(...)\n | a.conj()\n | \n | Complex-conjugate all elements.\n | \n | Refer to `numpy.conjugate` for full documentation.\n | \n | See Also\n | --------\n | numpy.conjugate : equivalent function\n | \n | conjugate(...)\n | a.conjugate()\n | \n | Return the complex conjugate, element-wise.\n | \n | Refer to `numpy.conjugate` for full documentation.\n | \n | See Also\n | --------\n | numpy.conjugate : equivalent function\n | \n | copy(...)\n | a.copy(order='C')\n | \n | Return a copy of the array.\n | \n | Parameters\n | ----------\n | order : {'C', 'F', 'A', 'K'}, optional\n | Controls the memory layout of the copy. 'C' means C-order,\n | 'F' means F-order, 'A' means 'F' if `a` is Fortran contiguous,\n | 'C' otherwise. 'K' means match the layout of `a` as closely\n | as possible. (Note that this function and :func:`numpy.copy` are very\n | similar, but have different default values for their order=\n | arguments.)\n | \n | See also\n | --------\n | numpy.copy\n | numpy.copyto\n | \n | Examples\n | --------\n | >>> x = np.array([[1,2,3],[4,5,6]], order='F')\n | \n | >>> y = x.copy()\n | \n | >>> x.fill(0)\n | \n | >>> x\n | array([[0, 0, 0],\n | [0, 0, 0]])\n | \n | >>> y\n | array([[1, 2, 3],\n | [4, 5, 6]])\n | \n | >>> y.flags['C_CONTIGUOUS']\n | True\n | \n | cumprod(...)\n | a.cumprod(axis=None, dtype=None, out=None)\n | \n | Return the cumulative product of the elements along the given axis.\n | \n | Refer to `numpy.cumprod` for full documentation.\n | \n | See Also\n | --------\n | numpy.cumprod : equivalent function\n | \n | cumsum(...)\n | a.cumsum(axis=None, dtype=None, out=None)\n | \n | Return the cumulative sum of the elements along the given axis.\n | \n | Refer to `numpy.cumsum` for full documentation.\n | \n | See Also\n | --------\n | numpy.cumsum : equivalent function\n | \n | diagonal(...)\n | a.diagonal(offset=0, axis1=0, axis2=1)\n | \n | Return specified diagonals. In NumPy 1.9 the returned array is a\n | read-only view instead of a copy as in previous NumPy versions. In\n | a future version the read-only restriction will be removed.\n | \n | Refer to :func:`numpy.diagonal` for full documentation.\n | \n | See Also\n | --------\n | numpy.diagonal : equivalent function\n | \n | dot(...)\n | a.dot(b, out=None)\n | \n | Dot product of two arrays.\n | \n | Refer to `numpy.dot` for full documentation.\n | \n | See Also\n | --------\n | numpy.dot : equivalent function\n | \n | Examples\n | --------\n | >>> a = np.eye(2)\n | >>> b = np.ones((2, 2)) * 2\n | >>> a.dot(b)\n | array([[2., 2.],\n | [2., 2.]])\n | \n | This array method can be conveniently chained:\n | \n | >>> a.dot(b).dot(b)\n | array([[8., 8.],\n | [8., 8.]])\n | \n | dump(...)\n | a.dump(file)\n | \n | Dump a pickle of the array to the specified file.\n | The array can be read back with pickle.load or numpy.load.\n | \n | Parameters\n | ----------\n | file : str or Path\n | A string naming the dump file.\n | \n | .. versionchanged:: 1.17.0\n | `pathlib.Path` objects are now accepted.\n | \n | dumps(...)\n | a.dumps()\n | \n | Returns the pickle of the array as a string.\n | pickle.loads or numpy.loads will convert the string back to an array.\n | \n | Parameters\n | ----------\n | None\n | \n | fill(...)\n | a.fill(value)\n | \n | Fill the array with a scalar value.\n | \n | Parameters\n | ----------\n | value : scalar\n | All elements of `a` will be assigned this value.\n | \n | Examples\n | --------\n | >>> a = np.array([1, 2])\n | >>> a.fill(0)\n | >>> a\n | array([0, 0])\n | >>> a = np.empty(2)\n | >>> a.fill(1)\n | >>> a\n | array([1., 1.])\n | \n | flatten(...)\n | a.flatten(order='C')\n | \n | Return a copy of the array collapsed into one dimension.\n | \n | Parameters\n | ----------\n | order : {'C', 'F', 'A', 'K'}, optional\n | 'C' means to flatten in row-major (C-style) order.\n | 'F' means to flatten in column-major (Fortran-\n | style) order. 'A' means to flatten in column-major\n | order if `a` is Fortran *contiguous* in memory,\n | row-major order otherwise. 'K' means to flatten\n | `a` in the order the elements occur in memory.\n | The default is 'C'.\n | \n | Returns\n | -------\n | y : ndarray\n | A copy of the input array, flattened to one dimension.\n | \n | See Also\n | --------\n | ravel : Return a flattened array.\n | flat : A 1-D flat iterator over the array.\n | \n | Examples\n | --------\n | >>> a = np.array([[1,2], [3,4]])\n | >>> a.flatten()\n | array([1, 2, 3, 4])\n | >>> a.flatten('F')\n | array([1, 3, 2, 4])\n | \n | getfield(...)\n | a.getfield(dtype, offset=0)\n | \n | Returns a field of the given array as a certain type.\n | \n | A field is a view of the array data with a given data-type. The values in\n | the view are determined by the given type and the offset into the current\n | array in bytes. The offset needs to be such that the view dtype fits in the\n | array dtype; for example an array of dtype complex128 has 16-byte elements.\n | If taking a view with a 32-bit integer (4 bytes), the offset needs to be\n | between 0 and 12 bytes.\n | \n | Parameters\n | ----------\n | dtype : str or dtype\n | The data type of the view. The dtype size of the view can not be larger\n | than that of the array itself.\n | offset : int\n | Number of bytes to skip before beginning the element view.\n | \n | Examples\n | --------\n | >>> x = np.diag([1.+1.j]*2)\n | >>> x[1, 1] = 2 + 4.j\n | >>> x\n | array([[1.+1.j, 0.+0.j],\n | [0.+0.j, 2.+4.j]])\n | >>> x.getfield(np.float64)\n | array([[1., 0.],\n | [0., 2.]])\n | \n | By choosing an offset of 8 bytes we can select the complex part of the\n | array for our view:\n | \n | >>> x.getfield(np.float64, offset=8)\n | array([[1., 0.],\n | [0., 4.]])\n | \n | item(...)\n | a.item(*args)\n | \n | Copy an element of an array to a standard Python scalar and return it.\n | \n | Parameters\n | ----------\n | \\*args : Arguments (variable number and type)\n | \n | * none: in this case, the method only works for arrays\n | with one element (`a.size == 1`), which element is\n | copied into a standard Python scalar object and returned.\n | \n | * int_type: this argument is interpreted as a flat index into\n | the array, specifying which element to copy and return.\n | \n | * tuple of int_types: functions as does a single int_type argument,\n | except that the argument is interpreted as an nd-index into the\n | array.\n | \n | Returns\n | -------\n | z : Standard Python scalar object\n | A copy of the specified element of the array as a suitable\n | Python scalar\n | \n | Notes\n | -----\n | When the data type of `a` is longdouble or clongdouble, item() returns\n | a scalar array object because there is no available Python scalar that\n | would not lose information. Void arrays return a buffer object for item(),\n | unless fields are defined, in which case a tuple is returned.\n | \n | `item` is very similar to a[args], except, instead of an array scalar,\n | a standard Python scalar is returned. This can be useful for speeding up\n | access to elements of the array and doing arithmetic on elements of the\n | array using Python's optimized math.\n | \n | Examples\n | --------\n | >>> np.random.seed(123)\n | >>> x = np.random.randint(9, size=(3, 3))\n | >>> x\n | array([[2, 2, 6],\n | [1, 3, 6],\n | [1, 0, 1]])\n | >>> x.item(3)\n | 1\n | >>> x.item(7)\n | 0\n | >>> x.item((0, 1))\n | 2\n | >>> x.item((2, 2))\n | 1\n | \n | itemset(...)\n | a.itemset(*args)\n | \n | Insert scalar into an array (scalar is cast to array's dtype, if possible)\n | \n | There must be at least 1 argument, and define the last argument\n | as *item*. Then, ``a.itemset(*args)`` is equivalent to but faster\n | than ``a[args] = item``. The item should be a scalar value and `args`\n | must select a single item in the array `a`.\n | \n | Parameters\n | ----------\n | \\*args : Arguments\n | If one argument: a scalar, only used in case `a` is of size 1.\n | If two arguments: the last argument is the value to be set\n | and must be a scalar, the first argument specifies a single array\n | element location. It is either an int or a tuple.\n | \n | Notes\n | -----\n | Compared to indexing syntax, `itemset` provides some speed increase\n | for placing a scalar into a particular location in an `ndarray`,\n | if you must do this. However, generally this is discouraged:\n | among other problems, it complicates the appearance of the code.\n | Also, when using `itemset` (and `item`) inside a loop, be sure\n | to assign the methods to a local variable to avoid the attribute\n | look-up at each loop iteration.\n | \n | Examples\n | --------\n | >>> np.random.seed(123)\n | >>> x = np.random.randint(9, size=(3, 3))\n | >>> x\n | array([[2, 2, 6],\n | [1, 3, 6],\n | [1, 0, 1]])\n | >>> x.itemset(4, 0)\n | >>> x.itemset((2, 2), 9)\n | >>> x\n | array([[2, 2, 6],\n | [1, 0, 6],\n | [1, 0, 9]])\n | \n | max(...)\n | a.max(axis=None, out=None, keepdims=False, initial=<no value>, where=True)\n | \n | Return the maximum along a given axis.\n | \n | Refer to `numpy.amax` for full documentation.\n | \n | See Also\n | --------\n | numpy.amax : equivalent function\n | \n | mean(...)\n | a.mean(axis=None, dtype=None, out=None, keepdims=False)\n | \n | Returns the average of the array elements along given axis.\n | \n | Refer to `numpy.mean` for full documentation.\n | \n | See Also\n | --------\n | numpy.mean : equivalent function\n | \n | min(...)\n | a.min(axis=None, out=None, keepdims=False, initial=<no value>, where=True)\n | \n | Return the minimum along a given axis.\n | \n | Refer to `numpy.amin` for full documentation.\n | \n | See Also\n | --------\n | numpy.amin : equivalent function\n | \n | newbyteorder(...)\n | arr.newbyteorder(new_order='S')\n | \n | Return the array with the same data viewed with a different byte order.\n | \n | Equivalent to::\n | \n | arr.view(arr.dtype.newbytorder(new_order))\n | \n | Changes are also made in all fields and sub-arrays of the array data\n | type.\n | \n | \n | \n | Parameters\n | ----------\n | new_order : string, optional\n | Byte order to force; a value from the byte order specifications\n | below. `new_order` codes can be any of:\n | \n | * 'S' - swap dtype from current to opposite endian\n | * {'<', 'L'} - little endian\n | * {'>', 'B'} - big endian\n | * {'=', 'N'} - native order\n | * {'|', 'I'} - ignore (no change to byte order)\n | \n | The default value ('S') results in swapping the current\n | byte order. The code does a case-insensitive check on the first\n | letter of `new_order` for the alternatives above. For example,\n | any of 'B' or 'b' or 'biggish' are valid to specify big-endian.\n | \n | \n | Returns\n | -------\n | new_arr : array\n | New array object with the dtype reflecting given change to the\n | byte order.\n | \n | nonzero(...)\n | a.nonzero()\n | \n | Return the indices of the elements that are non-zero.\n | \n | Refer to `numpy.nonzero` for full documentation.\n | \n | See Also\n | --------\n | numpy.nonzero : equivalent function\n | \n | partition(...)\n | a.partition(kth, axis=-1, kind='introselect', order=None)\n | \n | Rearranges the elements in the array in such a way that the value of the\n | element in kth position is in the position it would be in a sorted array.\n | All elements smaller than the kth element are moved before this element and\n | all equal or greater are moved behind it. The ordering of the elements in\n | the two partitions is undefined.\n | \n | .. versionadded:: 1.8.0\n | \n | Parameters\n | ----------\n | kth : int or sequence of ints\n | Element index to partition by. The kth element value will be in its\n | final sorted position and all smaller elements will be moved before it\n | and all equal or greater elements behind it.\n | The order of all elements in the partitions is undefined.\n | If provided with a sequence of kth it will partition all elements\n | indexed by kth of them into their sorted position at once.\n | axis : int, optional\n | Axis along which to sort. Default is -1, which means sort along the\n | last axis.\n | kind : {'introselect'}, optional\n | Selection algorithm. Default is 'introselect'.\n | order : str or list of str, optional\n | When `a` is an array with fields defined, this argument specifies\n | which fields to compare first, second, etc. A single field can\n | be specified as a string, and not all fields need to be specified,\n | but unspecified fields will still be used, in the order in which\n | they come up in the dtype, to break ties.\n | \n | See Also\n | --------\n | numpy.partition : Return a parititioned copy of an array.\n | argpartition : Indirect partition.\n | sort : Full sort.\n | \n | Notes\n | -----\n | See ``np.partition`` for notes on the different algorithms.\n | \n | Examples\n | --------\n | >>> a = np.array([3, 4, 2, 1])\n | >>> a.partition(3)\n | >>> a\n | array([2, 1, 3, 4])\n | \n | >>> a.partition((1, 3))\n | >>> a\n | array([1, 2, 3, 4])\n | \n | prod(...)\n | a.prod(axis=None, dtype=None, out=None, keepdims=False, initial=1, where=True)\n | \n | Return the product of the array elements over the given axis\n | \n | Refer to `numpy.prod` for full documentation.\n | \n | See Also\n | --------\n | numpy.prod : equivalent function\n | \n | ptp(...)\n | a.ptp(axis=None, out=None, keepdims=False)\n | \n | Peak to peak (maximum - minimum) value along a given axis.\n | \n | Refer to `numpy.ptp` for full documentation.\n | \n | See Also\n | --------\n | numpy.ptp : equivalent function\n | \n | put(...)\n | a.put(indices, values, mode='raise')\n | \n | Set ``a.flat[n] = values[n]`` for all `n` in indices.\n | \n | Refer to `numpy.put` for full documentation.\n | \n | See Also\n | --------\n | numpy.put : equivalent function\n | \n | ravel(...)\n | a.ravel([order])\n | \n | Return a flattened array.\n | \n | Refer to `numpy.ravel` for full documentation.\n | \n | See Also\n | --------\n | numpy.ravel : equivalent function\n | \n | ndarray.flat : a flat iterator on the array.\n | \n | repeat(...)\n | a.repeat(repeats, axis=None)\n | \n | Repeat elements of an array.\n | \n | Refer to `numpy.repeat` for full documentation.\n | \n | See Also\n | --------\n | numpy.repeat : equivalent function\n | \n | reshape(...)\n | a.reshape(shape, order='C')\n | \n | Returns an array containing the same data with a new shape.\n | \n | Refer to `numpy.reshape` for full documentation.\n | \n | See Also\n | --------\n | numpy.reshape : equivalent function\n | \n | Notes\n | -----\n | Unlike the free function `numpy.reshape`, this method on `ndarray` allows\n | the elements of the shape parameter to be passed in as separate arguments.\n | For example, ``a.reshape(10, 11)`` is equivalent to\n | ``a.reshape((10, 11))``.\n | \n | resize(...)\n | a.resize(new_shape, refcheck=True)\n | \n | Change shape and size of array in-place.\n | \n | Parameters\n | ----------\n | new_shape : tuple of ints, or `n` ints\n | Shape of resized array.\n | refcheck : bool, optional\n | If False, reference count will not be checked. Default is True.\n | \n | Returns\n | -------\n | None\n | \n | Raises\n | ------\n | ValueError\n | If `a` does not own its own data or references or views to it exist,\n | and the data memory must be changed.\n | PyPy only: will always raise if the data memory must be changed, since\n | there is no reliable way to determine if references or views to it\n | exist.\n | \n | SystemError\n | If the `order` keyword argument is specified. This behaviour is a\n | bug in NumPy.\n | \n | See Also\n | --------\n | resize : Return a new array with the specified shape.\n | \n | Notes\n | -----\n | This reallocates space for the data area if necessary.\n | \n | Only contiguous arrays (data elements consecutive in memory) can be\n | resized.\n | \n | The purpose of the reference count check is to make sure you\n | do not use this array as a buffer for another Python object and then\n | reallocate the memory. However, reference counts can increase in\n | other ways so if you are sure that you have not shared the memory\n | for this array with another Python object, then you may safely set\n | `refcheck` to False.\n | \n | Examples\n | --------\n | Shrinking an array: array is flattened (in the order that the data are\n | stored in memory), resized, and reshaped:\n | \n | >>> a = np.array([[0, 1], [2, 3]], order='C')\n | >>> a.resize((2, 1))\n | >>> a\n | array([[0],\n | [1]])\n | \n | >>> a = np.array([[0, 1], [2, 3]], order='F')\n | >>> a.resize((2, 1))\n | >>> a\n | array([[0],\n | [2]])\n | \n | Enlarging an array: as above, but missing entries are filled with zeros:\n | \n | >>> b = np.array([[0, 1], [2, 3]])\n | >>> b.resize(2, 3) # new_shape parameter doesn't have to be a tuple\n | >>> b\n | array([[0, 1, 2],\n | [3, 0, 0]])\n | \n | Referencing an array prevents resizing...\n | \n | >>> c = a\n | >>> a.resize((1, 1))\n | Traceback (most recent call last):\n | ...\n | ValueError: cannot resize an array that references or is referenced ...\n | \n | Unless `refcheck` is False:\n | \n | >>> a.resize((1, 1), refcheck=False)\n | >>> a\n | array([[0]])\n | >>> c\n | array([[0]])\n | \n | round(...)\n | a.round(decimals=0, out=None)\n | \n | Return `a` with each element rounded to the given number of decimals.\n | \n | Refer to `numpy.around` for full documentation.\n | \n | See Also\n | --------\n | numpy.around : equivalent function\n | \n | searchsorted(...)\n | a.searchsorted(v, side='left', sorter=None)\n | \n | Find indices where elements of v should be inserted in a to maintain order.\n | \n | For full documentation, see `numpy.searchsorted`\n | \n | See Also\n | --------\n | numpy.searchsorted : equivalent function\n | \n | setfield(...)\n | a.setfield(val, dtype, offset=0)\n | \n | Put a value into a specified place in a field defined by a data-type.\n | \n | Place `val` into `a`'s field defined by `dtype` and beginning `offset`\n | bytes into the field.\n | \n | Parameters\n | ----------\n | val : object\n | Value to be placed in field.\n | dtype : dtype object\n | Data-type of the field in which to place `val`.\n | offset : int, optional\n | The number of bytes into the field at which to place `val`.\n | \n | Returns\n | -------\n | None\n | \n | See Also\n | --------\n | getfield\n | \n | Examples\n | --------\n | >>> x = np.eye(3)\n | >>> x.getfield(np.float64)\n | array([[1., 0., 0.],\n | [0., 1., 0.],\n | [0., 0., 1.]])\n | >>> x.setfield(3, np.int32)\n | >>> x.getfield(np.int32)\n | array([[3, 3, 3],\n | [3, 3, 3],\n | [3, 3, 3]], dtype=int32)\n | >>> x\n | array([[1.0e+000, 1.5e-323, 1.5e-323],\n | [1.5e-323, 1.0e+000, 1.5e-323],\n | [1.5e-323, 1.5e-323, 1.0e+000]])\n | >>> x.setfield(np.eye(3), np.int32)\n | >>> x\n | array([[1., 0., 0.],\n | [0., 1., 0.],\n | [0., 0., 1.]])\n | \n | setflags(...)\n | a.setflags(write=None, align=None, uic=None)\n | \n | Set array flags WRITEABLE, ALIGNED, (WRITEBACKIFCOPY and UPDATEIFCOPY),\n | respectively.\n | \n | These Boolean-valued flags affect how numpy interprets the memory\n | area used by `a` (see Notes below). The ALIGNED flag can only\n | be set to True if the data is actually aligned according to the type.\n | The WRITEBACKIFCOPY and (deprecated) UPDATEIFCOPY flags can never be set\n | to True. The flag WRITEABLE can only be set to True if the array owns its\n | own memory, or the ultimate owner of the memory exposes a writeable buffer\n | interface, or is a string. (The exception for string is made so that\n | unpickling can be done without copying memory.)\n | \n | Parameters\n | ----------\n | write : bool, optional\n | Describes whether or not `a` can be written to.\n | align : bool, optional\n | Describes whether or not `a` is aligned properly for its type.\n | uic : bool, optional\n | Describes whether or not `a` is a copy of another \"base\" array.\n | \n | Notes\n | -----\n | Array flags provide information about how the memory area used\n | for the array is to be interpreted. There are 7 Boolean flags\n | in use, only four of which can be changed by the user:\n | WRITEBACKIFCOPY, UPDATEIFCOPY, WRITEABLE, and ALIGNED.\n | \n | WRITEABLE (W) the data area can be written to;\n | \n | ALIGNED (A) the data and strides are aligned appropriately for the hardware\n | (as determined by the compiler);\n | \n | UPDATEIFCOPY (U) (deprecated), replaced by WRITEBACKIFCOPY;\n | \n | WRITEBACKIFCOPY (X) this array is a copy of some other array (referenced\n | by .base). When the C-API function PyArray_ResolveWritebackIfCopy is\n | called, the base array will be updated with the contents of this array.\n | \n | All flags can be accessed using the single (upper case) letter as well\n | as the full name.\n | \n | Examples\n | --------\n | >>> y = np.array([[3, 1, 7],\n | ... [2, 0, 0],\n | ... [8, 5, 9]])\n | >>> y\n | array([[3, 1, 7],\n | [2, 0, 0],\n | [8, 5, 9]])\n | >>> y.flags\n | C_CONTIGUOUS : True\n | F_CONTIGUOUS : False\n | OWNDATA : True\n | WRITEABLE : True\n | ALIGNED : True\n | WRITEBACKIFCOPY : False\n | UPDATEIFCOPY : False\n | >>> y.setflags(write=0, align=0)\n | >>> y.flags\n | C_CONTIGUOUS : True\n | F_CONTIGUOUS : False\n | OWNDATA : True\n | WRITEABLE : False\n | ALIGNED : False\n | WRITEBACKIFCOPY : False\n | UPDATEIFCOPY : False\n | >>> y.setflags(uic=1)\n | Traceback (most recent call last):\n | File \"<stdin>\", line 1, in <module>\n | ValueError: cannot set WRITEBACKIFCOPY flag to True\n | \n | sort(...)\n | a.sort(axis=-1, kind=None, order=None)\n | \n | Sort an array in-place. Refer to `numpy.sort` for full documentation.\n | \n | Parameters\n | ----------\n | axis : int, optional\n | Axis along which to sort. Default is -1, which means sort along the\n | last axis.\n | kind : {'quicksort', 'mergesort', 'heapsort', 'stable'}, optional\n | Sorting algorithm. The default is 'quicksort'. Note that both 'stable'\n | and 'mergesort' use timsort under the covers and, in general, the\n | actual implementation will vary with datatype. The 'mergesort' option\n | is retained for backwards compatibility.\n | \n | .. versionchanged:: 1.15.0.\n | The 'stable' option was added.\n | \n | order : str or list of str, optional\n | When `a` is an array with fields defined, this argument specifies\n | which fields to compare first, second, etc. A single field can\n | be specified as a string, and not all fields need be specified,\n | but unspecified fields will still be used, in the order in which\n | they come up in the dtype, to break ties.\n | \n | See Also\n | --------\n | numpy.sort : Return a sorted copy of an array.\n | numpy.argsort : Indirect sort.\n | numpy.lexsort : Indirect stable sort on multiple keys.\n | numpy.searchsorted : Find elements in sorted array.\n | numpy.partition: Partial sort.\n | \n | Notes\n | -----\n | See `numpy.sort` for notes on the different sorting algorithms.\n | \n | Examples\n | --------\n | >>> a = np.array([[1,4], [3,1]])\n | >>> a.sort(axis=1)\n | >>> a\n | array([[1, 4],\n | [1, 3]])\n | >>> a.sort(axis=0)\n | >>> a\n | array([[1, 3],\n | [1, 4]])\n | \n | Use the `order` keyword to specify a field to use when sorting a\n | structured array:\n | \n | >>> a = np.array([('a', 2), ('c', 1)], dtype=[('x', 'S1'), ('y', int)])\n | >>> a.sort(order='y')\n | >>> a\n | array([(b'c', 1), (b'a', 2)],\n | dtype=[('x', 'S1'), ('y', '<i8')])\n | \n | squeeze(...)\n | a.squeeze(axis=None)\n | \n | Remove single-dimensional entries from the shape of `a`.\n | \n | Refer to `numpy.squeeze` for full documentation.\n | \n | See Also\n | --------\n | numpy.squeeze : equivalent function\n | \n | std(...)\n | a.std(axis=None, dtype=None, out=None, ddof=0, keepdims=False)\n | \n | Returns the standard deviation of the array elements along given axis.\n | \n | Refer to `numpy.std` for full documentation.\n | \n | See Also\n | --------\n | numpy.std : equivalent function\n | \n | sum(...)\n | a.sum(axis=None, dtype=None, out=None, keepdims=False, initial=0, where=True)\n | \n | Return the sum of the array elements over the given axis.\n | \n | Refer to `numpy.sum` for full documentation.\n | \n | See Also\n | --------\n | numpy.sum : equivalent function\n | \n | swapaxes(...)\n | a.swapaxes(axis1, axis2)\n | \n | Return a view of the array with `axis1` and `axis2` interchanged.\n | \n | Refer to `numpy.swapaxes` for full documentation.\n | \n | See Also\n | --------\n | numpy.swapaxes : equivalent function\n | \n | take(...)\n | a.take(indices, axis=None, out=None, mode='raise')\n | \n | Return an array formed from the elements of `a` at the given indices.\n | \n | Refer to `numpy.take` for full documentation.\n | \n | See Also\n | --------\n | numpy.take : equivalent function\n | \n | tobytes(...)\n | a.tobytes(order='C')\n | \n | Construct Python bytes containing the raw data bytes in the array.\n | \n | Constructs Python bytes showing a copy of the raw contents of\n | data memory. The bytes object can be produced in either 'C' or 'Fortran',\n | or 'Any' order (the default is 'C'-order). 'Any' order means C-order\n | unless the F_CONTIGUOUS flag in the array is set, in which case it\n | means 'Fortran' order.\n | \n | .. versionadded:: 1.9.0\n | \n | Parameters\n | ----------\n | order : {'C', 'F', None}, optional\n | Order of the data for multidimensional arrays:\n | C, Fortran, or the same as for the original array.\n | \n | Returns\n | -------\n | s : bytes\n | Python bytes exhibiting a copy of `a`'s raw data.\n | \n | Examples\n | --------\n | >>> x = np.array([[0, 1], [2, 3]], dtype='<u2')\n | >>> x.tobytes()\n | b'\\x00\\x00\\x01\\x00\\x02\\x00\\x03\\x00'\n | >>> x.tobytes('C') == x.tobytes()\n | True\n | >>> x.tobytes('F')\n | b'\\x00\\x00\\x02\\x00\\x01\\x00\\x03\\x00'\n | \n | tofile(...)\n | a.tofile(fid, sep=\"\", format=\"%s\")\n | \n | Write array to a file as text or binary (default).\n | \n | Data is always written in 'C' order, independent of the order of `a`.\n | The data produced by this method can be recovered using the function\n | fromfile().\n | \n | Parameters\n | ----------\n | fid : file or str or Path\n | An open file object, or a string containing a filename.\n | \n | .. versionchanged:: 1.17.0\n | `pathlib.Path` objects are now accepted.\n | \n | sep : str\n | Separator between array items for text output.\n | If \"\" (empty), a binary file is written, equivalent to\n | ``file.write(a.tobytes())``.\n | format : str\n | Format string for text file output.\n | Each entry in the array is formatted to text by first converting\n | it to the closest Python type, and then using \"format\" % item.\n | \n | Notes\n | -----\n | This is a convenience function for quick storage of array data.\n | Information on endianness and precision is lost, so this method is not a\n | good choice for files intended to archive data or transport data between\n | machines with different endianness. Some of these problems can be overcome\n | by outputting the data as text files, at the expense of speed and file\n | size.\n | \n | When fid is a file object, array contents are directly written to the\n | file, bypassing the file object's ``write`` method. As a result, tofile\n | cannot be used with files objects supporting compression (e.g., GzipFile)\n | or file-like objects that do not support ``fileno()`` (e.g., BytesIO).\n | \n | tolist(...)\n | a.tolist()\n | \n | Return the array as an ``a.ndim``-levels deep nested list of Python scalars.\n | \n | Return a copy of the array data as a (nested) Python list.\n | Data items are converted to the nearest compatible builtin Python type, via\n | the `~numpy.ndarray.item` function.\n | \n | If ``a.ndim`` is 0, then since the depth of the nested list is 0, it will\n | not be a list at all, but a simple Python scalar.\n | \n | Parameters\n | ----------\n | none\n | \n | Returns\n | -------\n | y : object, or list of object, or list of list of object, or ...\n | The possibly nested list of array elements.\n | \n | Notes\n | -----\n | The array may be recreated via ``a = np.array(a.tolist())``, although this\n | may sometimes lose precision.\n | \n | Examples\n | --------\n | For a 1D array, ``a.tolist()`` is almost the same as ``list(a)``, \n | except that ``tolist`` changes numpy scalars to Python scalars:\n | \n | >>> a = np.uint32([1, 2])\n | >>> a_list = list(a)\n | >>> a_list\n | [1, 2]\n | >>> type(a_list[0])\n | <class 'numpy.uint32'>\n | >>> a_tolist = a.tolist()\n | >>> a_tolist\n | [1, 2]\n | >>> type(a_tolist[0])\n | <class 'int'>\n | \n | Additionally, for a 2D array, ``tolist`` applies recursively:\n | \n | >>> a = np.array([[1, 2], [3, 4]])\n | >>> list(a)\n | [array([1, 2]), array([3, 4])]\n | >>> a.tolist()\n | [[1, 2], [3, 4]]\n | \n | The base case for this recursion is a 0D array:\n | \n | >>> a = np.array(1)\n | >>> list(a)\n | Traceback (most recent call last):\n | ...\n | TypeError: iteration over a 0-d array\n | >>> a.tolist()\n | 1\n | \n | tostring(...)\n | a.tostring(order='C')\n | \n | Construct Python bytes containing the raw data bytes in the array.\n | \n | Constructs Python bytes showing a copy of the raw contents of\n | data memory. The bytes object can be produced in either 'C' or 'Fortran',\n | or 'Any' order (the default is 'C'-order). 'Any' order means C-order\n | unless the F_CONTIGUOUS flag in the array is set, in which case it\n | means 'Fortran' order.\n | \n | This function is a compatibility alias for tobytes. Despite its name it returns bytes not strings.\n | \n | Parameters\n | ----------\n | order : {'C', 'F', None}, optional\n | Order of the data for multidimensional arrays:\n | C, Fortran, or the same as for the original array.\n | \n | Returns\n | -------\n | s : bytes\n | Python bytes exhibiting a copy of `a`'s raw data.\n | \n | Examples\n | --------\n | >>> x = np.array([[0, 1], [2, 3]], dtype='<u2')\n | >>> x.tobytes()\n | b'\\x00\\x00\\x01\\x00\\x02\\x00\\x03\\x00'\n | >>> x.tobytes('C') == x.tobytes()\n | True\n | >>> x.tobytes('F')\n | b'\\x00\\x00\\x02\\x00\\x01\\x00\\x03\\x00'\n | \n | trace(...)\n | a.trace(offset=0, axis1=0, axis2=1, dtype=None, out=None)\n | \n | Return the sum along diagonals of the array.\n | \n | Refer to `numpy.trace` for full documentation.\n | \n | See Also\n | --------\n | numpy.trace : equivalent function\n | \n | transpose(...)\n | a.transpose(*axes)\n | \n | Returns a view of the array with axes transposed.\n | \n | For a 1-D array this has no effect, as a transposed vector is simply the\n | same vector. To convert a 1-D array into a 2D column vector, an additional\n | dimension must be added. `np.atleast2d(a).T` achieves this, as does\n | `a[:, np.newaxis]`.\n | For a 2-D array, this is a standard matrix transpose.\n | For an n-D array, if axes are given, their order indicates how the\n | axes are permuted (see Examples). If axes are not provided and\n | ``a.shape = (i[0], i[1], ... i[n-2], i[n-1])``, then\n | ``a.transpose().shape = (i[n-1], i[n-2], ... i[1], i[0])``.\n | \n | Parameters\n | ----------\n | axes : None, tuple of ints, or `n` ints\n | \n | * None or no argument: reverses the order of the axes.\n | \n | * tuple of ints: `i` in the `j`-th place in the tuple means `a`'s\n | `i`-th axis becomes `a.transpose()`'s `j`-th axis.\n | \n | * `n` ints: same as an n-tuple of the same ints (this form is\n | intended simply as a \"convenience\" alternative to the tuple form)\n | \n | Returns\n | -------\n | out : ndarray\n | View of `a`, with axes suitably permuted.\n | \n | See Also\n | --------\n | ndarray.T : Array property returning the array transposed.\n | ndarray.reshape : Give a new shape to an array without changing its data.\n | \n | Examples\n | --------\n | >>> a = np.array([[1, 2], [3, 4]])\n | >>> a\n | array([[1, 2],\n | [3, 4]])\n | >>> a.transpose()\n | array([[1, 3],\n | [2, 4]])\n | >>> a.transpose((1, 0))\n | array([[1, 3],\n | [2, 4]])\n | >>> a.transpose(1, 0)\n | array([[1, 3],\n | [2, 4]])\n | \n | var(...)\n | a.var(axis=None, dtype=None, out=None, ddof=0, keepdims=False)\n | \n | Returns the variance of the array elements, along given axis.\n | \n | Refer to `numpy.var` for full documentation.\n | \n | See Also\n | --------\n | numpy.var : equivalent function\n | \n | view(...)\n | a.view(dtype=None, type=None)\n | \n | New view of array with the same data.\n | \n | Parameters\n | ----------\n | dtype : data-type or ndarray sub-class, optional\n | Data-type descriptor of the returned view, e.g., float32 or int16. The\n | default, None, results in the view having the same data-type as `a`.\n | This argument can also be specified as an ndarray sub-class, which\n | then specifies the type of the returned object (this is equivalent to\n | setting the ``type`` parameter).\n | type : Python type, optional\n | Type of the returned view, e.g., ndarray or matrix. Again, the\n | default None results in type preservation.\n | \n | Notes\n | -----\n | ``a.view()`` is used two different ways:\n | \n | ``a.view(some_dtype)`` or ``a.view(dtype=some_dtype)`` constructs a view\n | of the array's memory with a different data-type. This can cause a\n | reinterpretation of the bytes of memory.\n | \n | ``a.view(ndarray_subclass)`` or ``a.view(type=ndarray_subclass)`` just\n | returns an instance of `ndarray_subclass` that looks at the same array\n | (same shape, dtype, etc.) This does not cause a reinterpretation of the\n | memory.\n | \n | For ``a.view(some_dtype)``, if ``some_dtype`` has a different number of\n | bytes per entry than the previous dtype (for example, converting a\n | regular array to a structured array), then the behavior of the view\n | cannot be predicted just from the superficial appearance of ``a`` (shown\n | by ``print(a)``). It also depends on exactly how ``a`` is stored in\n | memory. Therefore if ``a`` is C-ordered versus fortran-ordered, versus\n | defined as a slice or transpose, etc., the view may give different\n | results.\n | \n | \n | Examples\n | --------\n | >>> x = np.array([(1, 2)], dtype=[('a', np.int8), ('b', np.int8)])\n | \n | Viewing array data using a different type and dtype:\n | \n | >>> y = x.view(dtype=np.int16, type=np.matrix)\n | >>> y\n | matrix([[513]], dtype=int16)\n | >>> print(type(y))\n | <class 'numpy.matrix'>\n | \n | Creating a view on a structured array so it can be used in calculations\n | \n | >>> x = np.array([(1, 2),(3,4)], dtype=[('a', np.int8), ('b', np.int8)])\n | >>> xv = x.view(dtype=np.int8).reshape(-1,2)\n | >>> xv\n | array([[1, 2],\n | [3, 4]], dtype=int8)\n | >>> xv.mean(0)\n | array([2., 3.])\n | \n | Making changes to the view changes the underlying array\n | \n | >>> xv[0,1] = 20\n | >>> x\n | array([(1, 20), (3, 4)], dtype=[('a', 'i1'), ('b', 'i1')])\n | \n | Using a view to convert an array to a recarray:\n | \n | >>> z = x.view(np.recarray)\n | >>> z.a\n | array([1, 3], dtype=int8)\n | \n | Views share data:\n | \n | >>> x[0] = (9, 10)\n | >>> z[0]\n | (9, 10)\n | \n | Views that change the dtype size (bytes per entry) should normally be\n | avoided on arrays defined by slices, transposes, fortran-ordering, etc.:\n | \n | >>> x = np.array([[1,2,3],[4,5,6]], dtype=np.int16)\n | >>> y = x[:, 0:2]\n | >>> y\n | array([[1, 2],\n | [4, 5]], dtype=int16)\n | >>> y.view(dtype=[('width', np.int16), ('length', np.int16)])\n | Traceback (most recent call last):\n | ...\n | ValueError: To change to a dtype of a different size, the array must be C-contiguous\n | >>> z = y.copy()\n | >>> z.view(dtype=[('width', np.int16), ('length', np.int16)])\n | array([[(1, 2)],\n | [(4, 5)]], dtype=[('width', '<i2'), ('length', '<i2')])\n | \n | ----------------------------------------------------------------------\n | Static methods defined here:\n | \n | __new__(*args, **kwargs) from builtins.type\n | Create and return a new object. See help(type) for accurate signature.\n | \n | ----------------------------------------------------------------------\n | Data descriptors defined here:\n | \n | T\n | The transposed array.\n | \n | Same as ``self.transpose()``.\n | \n | Examples\n | --------\n | >>> x = np.array([[1.,2.],[3.,4.]])\n | >>> x\n | array([[ 1., 2.],\n | [ 3., 4.]])\n | >>> x.T\n | array([[ 1., 3.],\n | [ 2., 4.]])\n | >>> x = np.array([1.,2.,3.,4.])\n | >>> x\n | array([ 1., 2., 3., 4.])\n | >>> x.T\n | array([ 1., 2., 3., 4.])\n | \n | See Also\n | --------\n | transpose\n | \n | __array_finalize__\n | None.\n | \n | __array_interface__\n | Array protocol: Python side.\n | \n | __array_priority__\n | Array priority.\n | \n | __array_struct__\n | Array protocol: C-struct side.\n | \n | base\n | Base object if memory is from some other object.\n | \n | Examples\n | --------\n | The base of an array that owns its memory is None:\n | \n | >>> x = np.array([1,2,3,4])\n | >>> x.base is None\n | True\n | \n | Slicing creates a view, whose memory is shared with x:\n | \n | >>> y = x[2:]\n | >>> y.base is x\n | True\n | \n | ctypes\n | An object to simplify the interaction of the array with the ctypes\n | module.\n | \n | This attribute creates an object that makes it easier to use arrays\n | when calling shared libraries with the ctypes module. The returned\n | object has, among others, data, shape, and strides attributes (see\n | Notes below) which themselves return ctypes objects that can be used\n | as arguments to a shared library.\n | \n | Parameters\n | ----------\n | None\n | \n | Returns\n | -------\n | c : Python object\n | Possessing attributes data, shape, strides, etc.\n | \n | See Also\n | --------\n | numpy.ctypeslib\n | \n | Notes\n | -----\n | Below are the public attributes of this object which were documented\n | in \"Guide to NumPy\" (we have omitted undocumented public attributes,\n | as well as documented private attributes):\n | \n | .. autoattribute:: numpy.core._internal._ctypes.data\n | :noindex:\n | \n | .. autoattribute:: numpy.core._internal._ctypes.shape\n | :noindex:\n | \n | .. autoattribute:: numpy.core._internal._ctypes.strides\n | :noindex:\n | \n | .. automethod:: numpy.core._internal._ctypes.data_as\n | :noindex:\n | \n | .. automethod:: numpy.core._internal._ctypes.shape_as\n | :noindex:\n | \n | .. automethod:: numpy.core._internal._ctypes.strides_as\n | :noindex:\n | \n | If the ctypes module is not available, then the ctypes attribute\n | of array objects still returns something useful, but ctypes objects\n | are not returned and errors may be raised instead. In particular,\n | the object will still have the ``as_parameter`` attribute which will\n | return an integer equal to the data attribute.\n | \n | Examples\n | --------\n | >>> import ctypes\n | >>> x\n | array([[0, 1],\n | [2, 3]])\n | >>> x.ctypes.data\n | 30439712\n | >>> x.ctypes.data_as(ctypes.POINTER(ctypes.c_long))\n | <ctypes.LP_c_long object at 0x01F01300>\n | >>> x.ctypes.data_as(ctypes.POINTER(ctypes.c_long)).contents\n | c_long(0)\n | >>> x.ctypes.data_as(ctypes.POINTER(ctypes.c_longlong)).contents\n | c_longlong(4294967296L)\n | >>> x.ctypes.shape\n | <numpy.core._internal.c_long_Array_2 object at 0x01FFD580>\n | >>> x.ctypes.shape_as(ctypes.c_long)\n | <numpy.core._internal.c_long_Array_2 object at 0x01FCE620>\n | >>> x.ctypes.strides\n | <numpy.core._internal.c_long_Array_2 object at 0x01FCE620>\n | >>> x.ctypes.strides_as(ctypes.c_longlong)\n | <numpy.core._internal.c_longlong_Array_2 object at 0x01F01300>\n | \n | data\n | Python buffer object pointing to the start of the array's data.\n | \n | dtype\n | Data-type of the array's elements.\n | \n | Parameters\n | ----------\n | None\n | \n | Returns\n | -------\n | d : numpy dtype object\n | \n | See Also\n | --------\n | numpy.dtype\n | \n | Examples\n | --------\n | >>> x\n | array([[0, 1],\n | [2, 3]])\n | >>> x.dtype\n | dtype('int32')\n | >>> type(x.dtype)\n | <type 'numpy.dtype'>\n | \n | flags\n | Information about the memory layout of the array.\n | \n | Attributes\n | ----------\n | C_CONTIGUOUS (C)\n | The data is in a single, C-style contiguous segment.\n | F_CONTIGUOUS (F)\n | The data is in a single, Fortran-style contiguous segment.\n | OWNDATA (O)\n | The array owns the memory it uses or borrows it from another object.\n | WRITEABLE (W)\n | The data area can be written to. Setting this to False locks\n | the data, making it read-only. A view (slice, etc.) inherits WRITEABLE\n | from its base array at creation time, but a view of a writeable\n | array may be subsequently locked while the base array remains writeable.\n | (The opposite is not true, in that a view of a locked array may not\n | be made writeable. However, currently, locking a base object does not\n | lock any views that already reference it, so under that circumstance it\n | is possible to alter the contents of a locked array via a previously\n | created writeable view onto it.) Attempting to change a non-writeable\n | array raises a RuntimeError exception.\n | ALIGNED (A)\n | The data and all elements are aligned appropriately for the hardware.\n | WRITEBACKIFCOPY (X)\n | This array is a copy of some other array. The C-API function\n | PyArray_ResolveWritebackIfCopy must be called before deallocating\n | to the base array will be updated with the contents of this array.\n | UPDATEIFCOPY (U)\n | (Deprecated, use WRITEBACKIFCOPY) This array is a copy of some other array.\n | When this array is\n | deallocated, the base array will be updated with the contents of\n | this array.\n | FNC\n | F_CONTIGUOUS and not C_CONTIGUOUS.\n | FORC\n | F_CONTIGUOUS or C_CONTIGUOUS (one-segment test).\n | BEHAVED (B)\n | ALIGNED and WRITEABLE.\n | CARRAY (CA)\n | BEHAVED and C_CONTIGUOUS.\n | FARRAY (FA)\n | BEHAVED and F_CONTIGUOUS and not C_CONTIGUOUS.\n | \n | Notes\n | -----\n | The `flags` object can be accessed dictionary-like (as in ``a.flags['WRITEABLE']``),\n | or by using lowercased attribute names (as in ``a.flags.writeable``). Short flag\n | names are only supported in dictionary access.\n | \n | Only the WRITEBACKIFCOPY, UPDATEIFCOPY, WRITEABLE, and ALIGNED flags can be\n | changed by the user, via direct assignment to the attribute or dictionary\n | entry, or by calling `ndarray.setflags`.\n | \n | The array flags cannot be set arbitrarily:\n | \n | - UPDATEIFCOPY can only be set ``False``.\n | - WRITEBACKIFCOPY can only be set ``False``.\n | - ALIGNED can only be set ``True`` if the data is truly aligned.\n | - WRITEABLE can only be set ``True`` if the array owns its own memory\n | or the ultimate owner of the memory exposes a writeable buffer\n | interface or is a string.\n | \n | Arrays can be both C-style and Fortran-style contiguous simultaneously.\n | This is clear for 1-dimensional arrays, but can also be true for higher\n | dimensional arrays.\n | \n | Even for contiguous arrays a stride for a given dimension\n | ``arr.strides[dim]`` may be *arbitrary* if ``arr.shape[dim] == 1``\n | or the array has no elements.\n | It does *not* generally hold that ``self.strides[-1] == self.itemsize``\n | for C-style contiguous arrays or ``self.strides[0] == self.itemsize`` for\n | Fortran-style contiguous arrays is true.\n | \n | flat\n | A 1-D iterator over the array.\n | \n | This is a `numpy.flatiter` instance, which acts similarly to, but is not\n | a subclass of, Python's built-in iterator object.\n | \n | See Also\n | --------\n | flatten : Return a copy of the array collapsed into one dimension.\n | \n | flatiter\n | \n | Examples\n | --------\n | >>> x = np.arange(1, 7).reshape(2, 3)\n | >>> x\n | array([[1, 2, 3],\n | [4, 5, 6]])\n | >>> x.flat[3]\n | 4\n | >>> x.T\n | array([[1, 4],\n | [2, 5],\n | [3, 6]])\n | >>> x.T.flat[3]\n | 5\n | >>> type(x.flat)\n | <class 'numpy.flatiter'>\n | \n | An assignment example:\n | \n | >>> x.flat = 3; x\n | array([[3, 3, 3],\n | [3, 3, 3]])\n | >>> x.flat[[1,4]] = 1; x\n | array([[3, 1, 3],\n | [3, 1, 3]])\n | \n | imag\n | The imaginary part of the array.\n | \n | Examples\n | --------\n | >>> x = np.sqrt([1+0j, 0+1j])\n | >>> x.imag\n | array([ 0. , 0.70710678])\n | >>> x.imag.dtype\n | dtype('float64')\n | \n | itemsize\n | Length of one array element in bytes.\n | \n | Examples\n | --------\n | >>> x = np.array([1,2,3], dtype=np.float64)\n | >>> x.itemsize\n | 8\n | >>> x = np.array([1,2,3], dtype=np.complex128)\n | >>> x.itemsize\n | 16\n | \n | nbytes\n | Total bytes consumed by the elements of the array.\n | \n | Notes\n | -----\n | Does not include memory consumed by non-element attributes of the\n | array object.\n | \n | Examples\n | --------\n | >>> x = np.zeros((3,5,2), dtype=np.complex128)\n | >>> x.nbytes\n | 480\n | >>> np.prod(x.shape) * x.itemsize\n | 480\n | \n | ndim\n | Number of array dimensions.\n | \n | Examples\n | --------\n | >>> x = np.array([1, 2, 3])\n | >>> x.ndim\n | 1\n | >>> y = np.zeros((2, 3, 4))\n | >>> y.ndim\n | 3\n | \n | real\n | The real part of the array.\n | \n | Examples\n | --------\n | >>> x = np.sqrt([1+0j, 0+1j])\n | >>> x.real\n | array([ 1. , 0.70710678])\n | >>> x.real.dtype\n | dtype('float64')\n | \n | See Also\n | --------\n | numpy.real : equivalent function\n | \n | shape\n | Tuple of array dimensions.\n | \n | The shape property is usually used to get the current shape of an array,\n | but may also be used to reshape the array in-place by assigning a tuple of\n | array dimensions to it. As with `numpy.reshape`, one of the new shape\n | dimensions can be -1, in which case its value is inferred from the size of\n | the array and the remaining dimensions. Reshaping an array in-place will\n | fail if a copy is required.\n | \n | Examples\n | --------\n | >>> x = np.array([1, 2, 3, 4])\n | >>> x.shape\n | (4,)\n | >>> y = np.zeros((2, 3, 4))\n | >>> y.shape\n | (2, 3, 4)\n | >>> y.shape = (3, 8)\n | >>> y\n | array([[ 0., 0., 0., 0., 0., 0., 0., 0.],\n | [ 0., 0., 0., 0., 0., 0., 0., 0.],\n | [ 0., 0., 0., 0., 0., 0., 0., 0.]])\n | >>> y.shape = (3, 6)\n | Traceback (most recent call last):\n | File \"<stdin>\", line 1, in <module>\n | ValueError: total size of new array must be unchanged\n | >>> np.zeros((4,2))[::2].shape = (-1,)\n | Traceback (most recent call last):\n | File \"<stdin>\", line 1, in <module>\n | AttributeError: incompatible shape for a non-contiguous array\n | \n | See Also\n | --------\n | numpy.reshape : similar function\n | ndarray.reshape : similar method\n | \n | size\n | Number of elements in the array.\n | \n | Equal to ``np.prod(a.shape)``, i.e., the product of the array's\n | dimensions.\n | \n | Notes\n | -----\n | `a.size` returns a standard arbitrary precision Python integer. This\n | may not be the case with other methods of obtaining the same value\n | (like the suggested ``np.prod(a.shape)``, which returns an instance\n | of ``np.int_``), and may be relevant if the value is used further in\n | calculations that may overflow a fixed size integer type.\n | \n | Examples\n | --------\n | >>> x = np.zeros((3, 5, 2), dtype=np.complex128)\n | >>> x.size\n | 30\n | >>> np.prod(x.shape)\n | 30\n | \n | strides\n | Tuple of bytes to step in each dimension when traversing an array.\n | \n | The byte offset of element ``(i[0], i[1], ..., i[n])`` in an array `a`\n | is::\n | \n | offset = sum(np.array(i) * a.strides)\n | \n | A more detailed explanation of strides can be found in the\n | \"ndarray.rst\" file in the NumPy reference guide.\n | \n | Notes\n | -----\n | Imagine an array of 32-bit integers (each 4 bytes)::\n | \n | x = np.array([[0, 1, 2, 3, 4],\n | [5, 6, 7, 8, 9]], dtype=np.int32)\n | \n | This array is stored in memory as 40 bytes, one after the other\n | (known as a contiguous block of memory). The strides of an array tell\n | us how many bytes we have to skip in memory to move to the next position\n | along a certain axis. For example, we have to skip 4 bytes (1 value) to\n | move to the next column, but 20 bytes (5 values) to get to the same\n | position in the next row. As such, the strides for the array `x` will be\n | ``(20, 4)``.\n | \n | See Also\n | --------\n | numpy.lib.stride_tricks.as_strided\n | \n | Examples\n | --------\n | >>> y = np.reshape(np.arange(2*3*4), (2,3,4))\n | >>> y\n | array([[[ 0, 1, 2, 3],\n | [ 4, 5, 6, 7],\n | [ 8, 9, 10, 11]],\n | [[12, 13, 14, 15],\n | [16, 17, 18, 19],\n | [20, 21, 22, 23]]])\n | >>> y.strides\n | (48, 16, 4)\n | >>> y[1,1,1]\n | 17\n | >>> offset=sum(y.strides * np.array((1,1,1)))\n | >>> offset/y.itemsize\n | 17\n | \n | >>> x = np.reshape(np.arange(5*6*7*8), (5,6,7,8)).transpose(2,3,1,0)\n | >>> x.strides\n | (32, 4, 224, 1344)\n | >>> i = np.array([3,5,2,2])\n | >>> offset = sum(i * x.strides)\n | >>> x[3,5,2,2]\n | 813\n | >>> offset / x.itemsize\n | 813\n | \n | ----------------------------------------------------------------------\n | Data and other attributes defined here:\n | \n | __hash__ = None\n\n"
]
],
[
[
"**Recomendado el siguiente [tutorial](https://www.numpy.org/devdocs/user/quickstart.html) para que profundicen más en todo lo que pueden hacer con NumPy**",
"_____no_output_____"
],
[
"<script>\n $(document).ready(function(){\n $('div.prompt').hide();\n $('div.back-to-top').hide();\n $('nav#menubar').hide();\n $('.breadcrumb').hide();\n $('.hidden-print').hide();\n });\n</script>\n\n<footer id=\"attribution\" style=\"float:right; color:#808080; background:#fff;\">\nCreated with Jupyter by Esteban Jiménez Rodríguez.\n</footer>",
"_____no_output_____"
]
]
] | [
"markdown",
"code",
"markdown",
"code",
"markdown",
"code",
"markdown",
"code",
"markdown",
"code",
"markdown",
"code",
"markdown",
"code",
"markdown",
"code",
"markdown",
"code",
"markdown",
"code",
"markdown",
"code",
"markdown",
"code",
"markdown",
"code",
"markdown",
"code",
"markdown",
"code",
"markdown",
"code",
"markdown",
"code",
"markdown",
"code",
"markdown",
"code",
"markdown",
"code",
"markdown",
"code",
"markdown",
"code",
"markdown",
"code",
"markdown",
"code",
"markdown",
"code",
"markdown",
"code",
"markdown"
] | [
[
"markdown",
"markdown"
],
[
"code",
"code",
"code",
"code",
"code"
],
[
"markdown",
"markdown",
"markdown"
],
[
"code",
"code"
],
[
"markdown",
"markdown"
],
[
"code",
"code",
"code",
"code",
"code",
"code",
"code",
"code",
"code",
"code",
"code",
"code",
"code",
"code"
],
[
"markdown"
],
[
"code"
],
[
"markdown"
],
[
"code"
],
[
"markdown"
],
[
"code",
"code",
"code",
"code",
"code"
],
[
"markdown"
],
[
"code",
"code",
"code",
"code",
"code",
"code",
"code"
],
[
"markdown",
"markdown",
"markdown",
"markdown"
],
[
"code"
],
[
"markdown"
],
[
"code",
"code",
"code",
"code",
"code",
"code",
"code",
"code"
],
[
"markdown"
],
[
"code",
"code",
"code",
"code"
],
[
"markdown"
],
[
"code",
"code",
"code",
"code"
],
[
"markdown",
"markdown"
],
[
"code"
],
[
"markdown"
],
[
"code",
"code",
"code",
"code",
"code",
"code",
"code",
"code"
],
[
"markdown"
],
[
"code",
"code"
],
[
"markdown"
],
[
"code",
"code",
"code",
"code",
"code",
"code",
"code"
],
[
"markdown",
"markdown",
"markdown",
"markdown"
],
[
"code",
"code"
],
[
"markdown"
],
[
"code",
"code",
"code"
],
[
"markdown"
],
[
"code",
"code"
],
[
"markdown"
],
[
"code",
"code",
"code",
"code",
"code",
"code",
"code",
"code",
"code",
"code",
"code"
],
[
"markdown"
],
[
"code"
],
[
"markdown",
"markdown"
],
[
"code",
"code"
],
[
"markdown"
],
[
"code",
"code",
"code",
"code",
"code",
"code",
"code",
"code",
"code",
"code",
"code",
"code",
"code",
"code"
],
[
"markdown"
],
[
"code"
],
[
"markdown"
],
[
"code",
"code",
"code",
"code"
],
[
"markdown"
],
[
"code",
"code",
"code"
],
[
"markdown"
],
[
"code",
"code",
"code",
"code",
"code",
"code",
"code"
],
[
"markdown",
"markdown"
]
] |
e778bf31b6d71df7f1fd5e487015991eceffc4a4 | 44,542 | ipynb | Jupyter Notebook | solutions by participants/ex5/ex5-AnurananDas-3cnot-2.339767mHa-32params.ipynb | fazliberkordek/ibm-quantum-challenge-2021 | 2206a364e354965b749dcda7c5d62631f571d718 | [
"Apache-2.0"
] | 136 | 2021-05-20T14:07:53.000Z | 2022-03-19T17:19:31.000Z | solutions by participants/ex5/ex5-AnurananDas-3cnot-2.339767mHa-32params.ipynb | fazliberkordek/ibm-quantum-challenge-2021 | 2206a364e354965b749dcda7c5d62631f571d718 | [
"Apache-2.0"
] | 106 | 2021-05-21T15:41:13.000Z | 2021-11-08T08:29:25.000Z | solutions by participants/ex5/ex5-AnurananDas-3cnot-2.339767mHa-32params.ipynb | fazliberkordek/ibm-quantum-challenge-2021 | 2206a364e354965b749dcda7c5d62631f571d718 | [
"Apache-2.0"
] | 190 | 2021-05-20T14:02:09.000Z | 2022-03-27T16:31:20.000Z | 61.100137 | 15,860 | 0.684253 | [
[
[
"### Reducing the problem size\n\nI reduced the number of qubits for my simulation considering the following:\n- I froze the core electrons that do not contribute significantly to chemistry and considered only the valence electrons. Qiskit already has this functionality implemented. So inspected the different transformers in `qiskit_nature.transformers` and find the one that performs the freeze core approximation. Still for further optimization, I removed two orbitals(indexed 3 and 4) which was also less contributing to these cases.\n- Used `ParityMapper` with `two_qubit_reduction=True` to eliminate 2 qubits, motive was to reduce the number of qubits used\n- There weren't any symmetry left after implementing the above.",
"_____no_output_____"
]
],
[
[
"from qiskit_nature.drivers import PySCFDriver\nfrom qiskit_nature.transformers import FreezeCoreTransformer, ActiveSpaceTransformer\nfrom qiskit.visualization import array_to_latex\nmolecule = 'Li 0.0 0.0 0.0; H 0.0 0.0 1.5474'\nfreezeCoreTransfomer = FreezeCoreTransformer(True, [3,4])\ndriver = PySCFDriver(atom=molecule)\nqmolecule = driver.run()\nqmolecule = freezeCoreTransfomer.transform(qmolecule)\nprint(qmolecule.energy_shift)\narray_to_latex(qmolecule.mo_coeff)\n",
"{'FreezeCoreTransformer': -7.818690295581144}\n"
],
[
"# WRITE YOUR CODE BETWEEN THESE LINES - START\nprint(\"Total number of electrons is {}\".format(qmolecule.num_alpha + qmolecule.num_beta))\nprint(\"Total number of molecular orbitals is {}\".format(qmolecule.num_molecular_orbitals))\nprint(\"Total number of spin orbitals is {}\".format(2 * qmolecule.num_molecular_orbitals))\nprint(\"qubits you need to simulate this molecule with Jordan-Wigner mapping is {}\".format(2 * qmolecule.num_molecular_orbitals))\nprint(\"The value of the nuclear repulsion energy is {}\".format(qmolecule.nuclear_repulsion_energy))\n# WRITE YOUR CODE BETWEEN THESE LINES - END",
"Total number of electrons is 2\nTotal number of molecular orbitals is 3\nTotal number of spin orbitals is 6\nqubits you need to simulate this molecule with Jordan-Wigner mapping is 6\nThe value of the nuclear repulsion energy is 1.0259348796432726\n"
]
],
[
[
"#### 2. Electronic structure problem\n\nCreated an `ElectronicStructureProblem` that can produce the list of fermionic operators before mapping them to qubits (Pauli strings), included the 'freezecore' parameter.",
"_____no_output_____"
]
],
[
[
"from qiskit_nature.problems.second_quantization.electronic import ElectronicStructureProblem\nproblem = ElectronicStructureProblem(driver, [freezeCoreTransfomer])\n\n# Generate the second-quantized operators\nsecond_q_ops = problem.second_q_ops()\n\n# Hamiltonian\nmain_op = second_q_ops[0]\nprint(problem.__dict__)",
"{'driver': <qiskit_nature.drivers.pyscfd.pyscfdriver.PySCFDriver object at 0x7fe3a68f8e50>, 'transformers': [<qiskit_nature.transformers.freeze_core_transformer.FreezeCoreTransformer object at 0x7fe3a2965850>], '_molecule_data': <qiskit_nature.drivers.qmolecule.QMolecule object at 0x7fe398925250>, '_molecule_data_transformed': <qiskit_nature.drivers.qmolecule.QMolecule object at 0x7fe39d6db9d0>}\n"
]
],
[
[
"#### 3. QubitConverter\n\nMapping defined as `ParityMapper` with ``two_qubit_reduction=True``",
"_____no_output_____"
]
],
[
[
"from qiskit_nature.mappers.second_quantization import ParityMapper, BravyiKitaevMapper, JordanWignerMapper\nfrom qiskit_nature.converters.second_quantization.qubit_converter import QubitConverter\n\n# Setup the mapper and qubit converter\nmapper_type = 'ParityMapper'\n\nif mapper_type == 'ParityMapper':\n mapper = ParityMapper()\nelif mapper_type == 'JordanWignerMapper':\n mapper = JordanWignerMapper()\nelif mapper_type == 'BravyiKitaevMapper':\n mapper = BravyiKitaevMapper()\n\nconverter = QubitConverter(mapper=mapper, two_qubit_reduction=True, z2symmetry_reduction=None)\n\n# The fermionic operators are mapped to qubit operators\nnum_particles = (problem.molecule_data_transformed.num_alpha,\n problem.molecule_data_transformed.num_beta)\nqubit_op = converter.convert(main_op, num_particles=num_particles)\nprint(converter.z2symmetries)",
"Z2 symmetries:\nSymmetries:\nSingle-Qubit Pauli X:\nCliffords:\nQubit index:\n[]\nTapering values:\n - Possible values: []\n"
]
],
[
[
"#### 4. Initial state\nAs we described in the Theory section, a good initial state in chemistry is the HF state (i.e. $|\\Psi_{HF} \\rangle = |0101 \\rangle$). I initialize it as follows:",
"_____no_output_____"
]
],
[
[
"from qiskit_nature.circuit.library import HartreeFock\n\nnum_particles = (problem.molecule_data_transformed.num_alpha,\n problem.molecule_data_transformed.num_beta)\nnum_spin_orbitals = 2 * problem.molecule_data_transformed.num_molecular_orbitals\ninit_state = HartreeFock(num_spin_orbitals, num_particles, converter)\nprint(init_state)",
" ┌───┐\nq_0: ┤ X ├\n ├───┤\nq_1: ┤ X ├\n └───┘\nq_2: ─────\n \nq_3: ─────\n \n"
]
],
[
[
"#### 5. Ansatz\nThe ansatz used was `TwoLocal`, with rotation layers as `['ry', 'rx', 'ry', 'rx']`, entanglement gate was only `cx`, `linear` type of entanglement, `repetitions` set to `1`. Idea was to get maximum entanglement with minimum circuit depth, all the while satisfying the costs.",
"_____no_output_____"
]
],
[
[
"from qiskit.circuit.library import TwoLocal\nfrom qiskit_nature.circuit.library import UCCSD, PUCCD, SUCCD\n\n# Choose the ansatz\nansatz_type = \"TwoLocal\"\n\n# Parameters for q-UCC antatze\nnum_particles = (problem.molecule_data_transformed.num_alpha,\n problem.molecule_data_transformed.num_beta)\nnum_spin_orbitals = 2 * problem.molecule_data_transformed.num_molecular_orbitals\n\n# Put arguments for twolocal\nif ansatz_type == \"TwoLocal\":\n # Single qubit rotations that are placed on all qubits with independent parameters\n rotation_blocks = ['ry', 'rx', 'ry', 'rx']\n # Entangling gates\n entanglement_blocks = ['cx']\n # How the qubits are entangled \n entanglement = \"linear\"\n # Repetitions of rotation_blocks + entanglement_blocks with independent parameters\n repetitions = 1\n # Skip the final rotation_blocks layer\n skip_final_rotation_layer = False\n ansatz = TwoLocal(qubit_op.num_qubits, rotation_blocks, entanglement_blocks, reps=repetitions, \n entanglement=entanglement, skip_final_rotation_layer=skip_final_rotation_layer)\n # Add the initial state\n ansatz.compose(init_state, front=True, inplace=True)\nelif ansatz_type == \"UCCSD\":\n ansatz = UCCSD(converter,num_particles,num_spin_orbitals,initial_state = init_state)\nelif ansatz_type == \"PUCCD\":\n ansatz = PUCCD(converter,num_particles,num_spin_orbitals,initial_state = init_state)\nelif ansatz_type == \"SUCCD\":\n ansatz = SUCCD(converter,num_particles,num_spin_orbitals,initial_state = init_state)\nelif ansatz_type == \"Custom\":\n # Example of how to write your own circuit\n from qiskit.circuit import Parameter, QuantumCircuit, QuantumRegister\n # Define the variational parameter\n thetas = []\n \n n = qubit_op.num_qubits\n # Make an empty quantum circuit\n qc = QuantumCircuit(qubit_op.num_qubits)\n qubit_label = 0\n # Place a Hadamard gate\n# qc.h(qubit_label)\n# qc.rz(theta_z, range(n))\n qc.rx(theta_x, range(n))\n qc.ry(theta_y, range(n))\n # Place a CNOT ladder\n for i in range(n-1):\n qc.cx(i, i+1)\n # Visual separator\n# qc.barrier()\n # rz rotations on all qubits\n# qc.rz(theta_z, range(n))\n# qc.ry(theta_x, range(n))\n# qc.rx(theta_y, range(n))\n ansatz = qc\n ansatz.compose(init_state, front=True, inplace=True)\n\nprint(ansatz)",
" ┌───┐ ┌──────────┐ ┌──────────┐ ┌──────────┐┌───────────┐ »\nq_0: ───┤ X ├────┤ RY(θ[0]) ├─┤ RX(θ[4]) ├─┤ RY(θ[8]) ├┤ RX(θ[12]) ├──■──»\n ├───┤ ├──────────┤ ├──────────┤ ├──────────┤├───────────┤┌─┴─┐»\nq_1: ───┤ X ├────┤ RY(θ[1]) ├─┤ RX(θ[5]) ├─┤ RY(θ[9]) ├┤ RX(θ[13]) ├┤ X ├»\n ┌──┴───┴───┐├──────────┤┌┴──────────┤┌┴──────────┤└───────────┘└───┘»\nq_2: ┤ RY(θ[2]) ├┤ RX(θ[6]) ├┤ RY(θ[10]) ├┤ RX(θ[14]) ├──────────────────»\n ├──────────┤├──────────┤├───────────┤├───────────┤ »\nq_3: ┤ RY(θ[3]) ├┤ RX(θ[7]) ├┤ RY(θ[11]) ├┤ RX(θ[15]) ├──────────────────»\n └──────────┘└──────────┘└───────────┘└───────────┘ »\n« ┌───────────┐┌───────────┐┌───────────┐┌───────────┐ »\n«q_0: ┤ RY(θ[16]) ├┤ RX(θ[20]) ├┤ RY(θ[24]) ├┤ RX(θ[28]) ├─────────────»\n« └───────────┘├───────────┤├───────────┤├───────────┤┌───────────┐»\n«q_1: ──────■──────┤ RY(θ[17]) ├┤ RX(θ[21]) ├┤ RY(θ[25]) ├┤ RX(θ[29]) ├»\n« ┌─┴─┐ └───────────┘├───────────┤├───────────┤├───────────┤»\n«q_2: ────┤ X ├──────────■──────┤ RY(θ[18]) ├┤ RX(θ[22]) ├┤ RY(θ[26]) ├»\n« └───┘ ┌─┴─┐ ├───────────┤├───────────┤├───────────┤»\n«q_3: ─────────────────┤ X ├────┤ RY(θ[19]) ├┤ RX(θ[23]) ├┤ RY(θ[27]) ├»\n« └───┘ └───────────┘└───────────┘└───────────┘»\n« \n«q_0: ─────────────\n« \n«q_1: ─────────────\n« ┌───────────┐\n«q_2: ┤ RX(θ[30]) ├\n« ├───────────┤\n«q_3: ┤ RX(θ[31]) ├\n« └───────────┘\n"
],
[
"from qiskit import Aer\nbackend = Aer.get_backend('statevector_simulator')",
"_____no_output_____"
]
],
[
[
"#### 7. Optimizer\n\nThe optimizer guides the evolution of the parameters of the ansatz so it is very important to investigate the energy convergence as it would define the number of measurements that have to be performed on the QPU. Here it was set to `COBYLA` with sufficient amount of maximum iterations possible before convergence\n",
"_____no_output_____"
]
],
[
[
"from qiskit.algorithms.optimizers import COBYLA, L_BFGS_B, SPSA, SLSQP\n\noptimizer_type = 'COBYLA'\n\n# You may want to tune the parameters \n# of each optimizer, here the defaults are used\nif optimizer_type == 'COBYLA':\n optimizer = COBYLA(maxiter=30000)\nelif optimizer_type == 'L_BFGS_B':\n optimizer = L_BFGS_B(maxfun=60000)\nelif optimizer_type == 'SPSA':\n optimizer = SPSA(maxiter=50000)\nelif optimizer_type == 'SLSQP':\n optimizer = SLSQP(maxiter=3000)",
"_____no_output_____"
]
],
[
[
"#### 8. Exact eigensolver",
"_____no_output_____"
]
],
[
[
"from qiskit_nature.algorithms.ground_state_solvers.minimum_eigensolver_factories import NumPyMinimumEigensolverFactory\nfrom qiskit_nature.algorithms.ground_state_solvers import GroundStateEigensolver\nimport numpy as np \n\ndef exact_diagonalizer(problem, converter):\n solver = NumPyMinimumEigensolverFactory()\n calc = GroundStateEigensolver(converter, solver)\n result = calc.solve(problem)\n return result\n\nresult_exact = exact_diagonalizer(problem, converter)\nexact_energy = np.real(result_exact.eigenenergies[0])\nprint(\"Exact electronic energy after freezing core, for the valence electrons is\", exact_energy)\n# print(result_exact)",
"Exact electronic energy after freezing core, for the valence electrons is -1.0887060157347412\n"
]
],
[
[
"#### 9. VQE and initial parameters for the ansatz\nNow we can import the VQE class and run the algorithm.",
"_____no_output_____"
]
],
[
[
"from qiskit.algorithms import VQE\nfrom IPython.display import display, clear_output\n\n# Print and save the data in lists\ndef callback(eval_count, parameters, mean, std): \n # Overwrites the same line when printing\n display(\"Evaluation: {}, Energy: {}, Std: {}\".format(eval_count, mean, std))\n clear_output(wait=True)\n counts.append(eval_count)\n values.append(mean)\n params.append(parameters)\n deviation.append(std)\n\ncounts = []\nvalues = []\nparams = []\ndeviation = []\n\n# Set initial parameters of the ansatz\n# We choose a fixed small displacement \n# So all participants start from similar starting point\ntry:\n initial_point = [0.01] * len(ansatz.ordered_parameters)\nexcept:\n initial_point = [0.01] * ansatz.num_parameters\n\nalgorithm = VQE(ansatz,\n optimizer=optimizer,\n quantum_instance=backend,\n callback=callback,\n initial_point=initial_point)\n\nresult = algorithm.compute_minimum_eigenvalue(qubit_op)\n\nprint(result)",
"{ 'aux_operator_eigenvalues': None,\n 'cost_function_evals': 9660,\n 'eigenstate': array([ 1.42330541e-04+1.43431962e-03j, -4.23490958e-04-4.89065970e-03j,\n 2.23678404e-03+2.63113429e-02j, -8.30964184e-02-9.87905676e-01j,\n -3.50684854e-03-5.38647331e-02j, -1.74375790e-05-3.17583535e-04j,\n 4.88898409e-05+9.08366879e-04j, -1.18573222e-03-2.40722890e-02j,\n -3.32766414e-04-2.74166551e-03j, -5.66367067e-07-8.21282984e-06j,\n -1.45660908e-06+3.46419632e-06j, 1.14985281e-04+3.80322133e-04j,\n 9.77945785e-03+1.13200984e-01j, 3.25326673e-05+4.16855646e-04j,\n -4.70472273e-05-5.61752304e-04j, -1.69759079e-06+1.89927731e-05j]),\n 'eigenvalue': -1.08636624859473,\n 'optimal_parameters': { ParameterVectorElement(θ[16]): 0.6023040974600175,\n ParameterVectorElement(θ[2]): 0.2754752513533806,\n ParameterVectorElement(θ[11]): 0.08498065538345674,\n ParameterVectorElement(θ[12]): 0.49405851441676,\n ParameterVectorElement(θ[14]): -0.13158130545342372,\n ParameterVectorElement(θ[6]): 0.1367791221567639,\n ParameterVectorElement(θ[13]): 0.17919625235084016,\n ParameterVectorElement(θ[31]): -0.4430577995088451,\n ParameterVectorElement(θ[3]): -0.5287436933434927,\n ParameterVectorElement(θ[0]): 0.9194205453662906,\n ParameterVectorElement(θ[10]): -0.2779671919120382,\n ParameterVectorElement(θ[15]): 0.27088658149510303,\n ParameterVectorElement(θ[5]): -0.1821021597121472,\n ParameterVectorElement(θ[4]): -0.6817850823310849,\n ParameterVectorElement(θ[25]): 0.026278322889119826,\n ParameterVectorElement(θ[26]): 0.173200889411082,\n ParameterVectorElement(θ[24]): -0.6514974023548069,\n ParameterVectorElement(θ[27]): -0.70010160265166,\n ParameterVectorElement(θ[23]): 0.18593894311708528,\n ParameterVectorElement(θ[30]): 0.3535595958735285,\n ParameterVectorElement(θ[1]): 0.18118039040665185,\n ParameterVectorElement(θ[9]): -0.18998201653013846,\n ParameterVectorElement(θ[18]): -0.11380267575601392,\n ParameterVectorElement(θ[19]): 1.1198256842268843,\n ParameterVectorElement(θ[21]): 1.998490447687628,\n ParameterVectorElement(θ[20]): 0.6493468545437504,\n ParameterVectorElement(θ[28]): -0.5224124263847214,\n ParameterVectorElement(θ[29]): 1.1427592303144563,\n ParameterVectorElement(θ[17]): 0.020817230460885735,\n ParameterVectorElement(θ[8]): -0.7806273236070762,\n ParameterVectorElement(θ[22]): -0.35721805043525556,\n ParameterVectorElement(θ[7]): 0.06268198714828736},\n 'optimal_point': array([ 0.91942055, -0.27796719, 0.08498066, 0.49405851, 0.17919625,\n -0.13158131, 0.27088658, 0.6023041 , 0.02081723, -0.11380268,\n 1.11982568, 0.18118039, 0.64934685, 1.99849045, -0.35721805,\n 0.18593894, -0.6514974 , 0.02627832, 0.17320089, -0.7001016 ,\n -0.52241243, 1.14275923, 0.27547525, 0.3535596 , -0.4430578 ,\n -0.52874369, -0.68178508, -0.18210216, 0.13677912, 0.06268199,\n -0.78062732, -0.18998202]),\n 'optimal_value': -1.08636624859473,\n 'optimizer_evals': 9660,\n 'optimizer_time': 75.38813042640686}\n"
]
],
[
[
"#### 9. Scoring function \nThe following was the simple scoring function:\n\n$$ score = N_{CNOT}$$\n\nwhere $N_{CNOT}$ is the number of CNOTs. \nWe had to reach the chemical accuracy which is $\\delta E_{chem} = 0.004$ Ha $= 4$ mHa.\n\nThe lower the score the better!",
"_____no_output_____"
]
],
[
[
"# Store results in a dictionary\nfrom qiskit.transpiler import PassManager\nfrom qiskit.transpiler.passes import Unroller\n\n# Unroller transpile your circuit into CNOTs and U gates\npass_ = Unroller(['u', 'cx'])\npm = PassManager(pass_)\nansatz_tp = pm.run(ansatz)\ncnots = ansatz_tp.count_ops()['cx']\nscore = cnots\n\naccuracy_threshold = 4.0 # in mHa\nenergy = result.optimal_value\n\nif ansatz_type == \"TwoLocal\":\n result_dict = {\n 'optimizer': optimizer.__class__.__name__,\n 'mapping': converter.mapper.__class__.__name__,\n 'ansatz': ansatz.__class__.__name__,\n 'rotation blocks': rotation_blocks,\n 'entanglement_blocks': entanglement_blocks,\n 'entanglement': entanglement,\n 'repetitions': repetitions,\n 'skip_final_rotation_layer': skip_final_rotation_layer,\n 'energy (Ha)': energy,\n 'error (mHa)': (energy-exact_energy)*1000,\n 'pass': (energy-exact_energy)*1000 <= accuracy_threshold,\n '# of parameters': len(result.optimal_point),\n 'final parameters': result.optimal_point,\n '# of evaluations': result.optimizer_evals,\n 'optimizer time': result.optimizer_time,\n '# of qubits': int(qubit_op.num_qubits),\n '# of CNOTs': cnots,\n 'score': score}\nelse:\n result_dict = {\n 'optimizer': optimizer.__class__.__name__,\n 'mapping': converter.mapper.__class__.__name__,\n 'ansatz': ansatz.__class__.__name__,\n 'rotation blocks': None,\n 'entanglement_blocks': None,\n 'entanglement': None,\n 'repetitions': None,\n 'skip_final_rotation_layer': None,\n 'energy (Ha)': energy,\n 'error (mHa)': (energy-exact_energy)*1000,\n 'pass': (energy-exact_energy)*1000 <= accuracy_threshold,\n '# of parameters': len(result.optimal_point),\n 'final parameters': result.optimal_point,\n '# of evaluations': result.optimizer_evals,\n 'optimizer time': result.optimizer_time,\n '# of qubits': int(qubit_op.num_qubits),\n '# of CNOTs': cnots,\n 'score': score}\n\n# Plot the results\nimport matplotlib.pyplot as plt\n\nfig, ax = plt.subplots(1, 1)\nax.set_xlabel('Iterations')\nax.set_ylabel('Energy')\nax.grid()\nfig.text(0.7, 0.75, f'Energy: {result.optimal_value:.3f}\\nScore: {score:.0f}')\nplt.title(f\"{result_dict['optimizer']}-{result_dict['mapping']}\\n{result_dict['ansatz']}\")\nax.plot(counts, values)\nax.axhline(exact_energy, linestyle='--')\nfig_title = f\"\\\n{result_dict['optimizer']}-\\\n{result_dict['mapping']}-\\\n{result_dict['ansatz']}-\\\nEnergy({result_dict['energy (Ha)']:.3f})-\\\nScore({result_dict['score']:.0f})\\\n.png\"\nfig.savefig(fig_title, dpi=300)\n\n# Display and save the data\nimport pandas as pd\nimport os.path\nfilename = 'results_h2.csv'\nif os.path.isfile(filename):\n result_df = pd.read_csv(filename)\n result_df = result_df.append([result_dict])\nelse:\n result_df = pd.DataFrame.from_dict([result_dict])\nresult_df.to_csv(filename)\nresult_df[['optimizer','ansatz', '# of qubits', '# of parameters','rotation blocks', 'entanglement_blocks',\n 'entanglement', 'repetitions', 'error (mHa)', 'pass', 'score']]",
"_____no_output_____"
]
]
] | [
"markdown",
"code",
"markdown",
"code",
"markdown",
"code",
"markdown",
"code",
"markdown",
"code",
"markdown",
"code",
"markdown",
"code",
"markdown",
"code",
"markdown",
"code"
] | [
[
"markdown"
],
[
"code",
"code"
],
[
"markdown"
],
[
"code"
],
[
"markdown"
],
[
"code"
],
[
"markdown"
],
[
"code"
],
[
"markdown"
],
[
"code",
"code"
],
[
"markdown"
],
[
"code"
],
[
"markdown"
],
[
"code"
],
[
"markdown"
],
[
"code"
],
[
"markdown"
],
[
"code"
]
] |
e778c8c0a9e0eb1a3b518b2cdbf7e439947c8d71 | 555,087 | ipynb | Jupyter Notebook | notebooks/1.0-mayab-data-munging.ipynb | mayabenowitz/neuralcraft | 00622818e6a078d906399d2be6d641acf040186c | [
"MIT"
] | 1 | 2020-09-28T21:24:25.000Z | 2020-09-28T21:24:25.000Z | notebooks/1.0-mayab-data-munging.ipynb | mayabenowitz/neuralcraft | 00622818e6a078d906399d2be6d641acf040186c | [
"MIT"
] | null | null | null | notebooks/1.0-mayab-data-munging.ipynb | mayabenowitz/neuralcraft | 00622818e6a078d906399d2be6d641acf040186c | [
"MIT"
] | null | null | null | 267.125602 | 105,144 | 0.898556 | [
[
[
"import os\nimport sys\nROOT_DIR = os.path.dirname(os.path.abspath('..'))\nsys.path.insert(0, os.path.abspath(ROOT_DIR))\n\n%load_ext autoreload\n%autoreload 2\n\nfrom IPython.core.display import display, HTML\ndisplay(HTML(\"<style>.container { width:100% !important; }</style>\"))\n\nimport pandas as pd\nimport numpy as np\nimport matplotlib.pyplot as plt\nfrom jupyterthemes import jtplot\njtplot.style()\nimport seaborn as sns\nimport statsmodels\nimport sklearn",
"_____no_output_____"
],
[
"df = pd.read_csv('../data/raw/productivity_growth.csv')\ndf.info()",
"<class 'pandas.core.frame.DataFrame'>\nRangeIndex: 24442 entries, 0 to 24441\nData columns (total 17 columns):\nLOCATION 24442 non-null object\nCountry 24442 non-null object\nSUBJECT 24442 non-null object\nSubject 24442 non-null object\nMEASURE 24442 non-null object\nMeasure 24442 non-null object\nTIME 24442 non-null int64\nTime 24442 non-null int64\nUnit Code 24442 non-null object\nUnit 24442 non-null object\nPowerCode Code 24442 non-null int64\nPowerCode 24442 non-null object\nReference Period Code 0 non-null float64\nReference Period 0 non-null float64\nValue 24442 non-null float64\nFlag Codes 150 non-null object\nFlags 150 non-null object\ndtypes: float64(3), int64(3), object(11)\nmemory usage: 3.2+ MB\n"
],
[
"df.head()",
"_____no_output_____"
],
[
"df['Subject'].unique()",
"_____no_output_____"
],
[
"df['Measure'].unique()",
"_____no_output_____"
],
[
"df['Time'].unique()",
"_____no_output_____"
],
[
"countries = df['Country'].unique()\ncountries",
"_____no_output_____"
],
[
"df1 = pd.read_csv('../data/raw/productivity_growth_by_industry.csv')\ndf1.head()",
"_____no_output_____"
],
[
"df1.drop(\n columns=[\n 'LOCATION',\n 'SUBJECT',\n 'MEASURE',\n 'ACTIVITY',\n 'TIME',\n 'Unit Code',\n 'PowerCode Code',\n 'PowerCode',\n 'Reference Period Code',\n 'Reference Period',\n 'Flag Codes',\n 'Flags'\n ],\n inplace=True\n)\ndf1.head()",
"_____no_output_____"
],
[
"df1['Subject'] = df1['Subject'].map(lambda s: s.strip(' '))\ndf1['Activity'] = df1['Activity'].map(lambda s: s.strip(' '))",
"_____no_output_____"
],
[
"df1['Subject'].unique()",
"_____no_output_____"
],
[
"df1['Activity'].unique()",
"_____no_output_____"
],
[
"set(df1['Country'].unique()) == set(countries)",
"_____no_output_____"
],
[
"countries2 = df1['Country'].unique()\ncountries2",
"_____no_output_____"
],
[
"df1['Subject'].unique()",
"_____no_output_____"
],
[
"df1['Activity'].unique()",
"_____no_output_____"
],
[
"df2 = pd.read_csv('../data/raw/lpc_by_industry.csv', low_memory=False)\ndf2.head()",
"_____no_output_____"
],
[
"df2.columns = df2.iloc[0]\ndf2.drop(df2.index[0], inplace=True)\ndf2.head()",
"_____no_output_____"
],
[
"# {col:type(col) for col in df2.columns}\nids = df2.iloc[:, 0:5].columns.tolist()\nvalues = df2.iloc[:, 5:38].columns.tolist()\nvalues = [str(int(i)) for i in values]\ndf2.columns = ids + values",
"_____no_output_____"
],
[
"df2 = pd.melt(df2, id_vars=ids, value_vars=values, var_name='Year')\ndf2.head()",
"_____no_output_____"
],
[
"df2.info()",
"<class 'pandas.core.frame.DataFrame'>\nRangeIndex: 589842 entries, 0 to 589841\nData columns (total 7 columns):\nIndustry 525987 non-null object\nIndustry Sector 525987 non-null object\nIndustry Digit 525987 non-null object\nMeasure 525987 non-null object\nUnits 525987 non-null object\nYear 589842 non-null object\nvalue 525987 non-null object\ndtypes: object(7)\nmemory usage: 31.5+ MB\n"
],
[
"df2.dropna(inplace=True)\ndf2.drop(columns=['Industry Digit'], inplace=True)\ndf2['Industry'] = df2['Industry'].str.replace('-', '').map(lambda s: ''.join([i for i in s if not i.isdigit()]))\ndf2['Industry Sector'] = df2['Industry Sector'].str.replace('-', '').map(lambda s: ''.join([i for i in s if not i.isdigit()]))",
"_____no_output_____"
],
[
"df2['value'] = df2['value'].replace('n.a.', np.nan)",
"_____no_output_____"
],
[
"df2['Industry'].unique()",
"_____no_output_____"
],
[
"df2['Industry Sector'].unique()",
"_____no_output_____"
],
[
"df2['Industry'] = df2['Industry'].map(lambda s: s.lstrip(' '))\ndf2['Industry Sector'] = df2['Industry Sector'].map(lambda s: s.lstrip(' '))",
"_____no_output_____"
],
[
"df2['Industry Sector'] = df2['Industry Sector'].map(lambda s: s.lstrip(', '))",
"_____no_output_____"
],
[
"df2['Units'].unique()",
"_____no_output_____"
],
[
"def _regplot(df, country, subject):\n fig, ax = plt.subplots(figsize=(20,10))\n # locally weighted linear regression\n sns.regplot(x='Time', y='Value', lowess=True, scatter=False, data=df)\n # linear regression\n sns.regplot(x='Time', y='Value', scatter=False, data=df)\n sns.lineplot(x='Time', y='Value', data=df)\n plt.title(f'{country} Productivity Growth')\n plt.ylabel(f'Change in {subject}')",
"_____no_output_____"
],
[
"def collect_data(df, subject, activity=None) -> dict:\n countries = df['Country'].unique().tolist()\n df_cdict={}\n if activity is None:\n for c in countries:\n df_cdict[c] = df[\n (df['Country'] == c) & (df['Subject'] == subject) \n ]\n return df_cdict\n else:\n for c in countries:\n df_cdict[c] = df[\n (df['Country'] == c) & (df['Subject'] == subject) & (df['Activity'] == activity)\n ]\n return df_cdict",
"_____no_output_____"
],
[
"df1[df1['Country'] == 'United States']['Activity'].unique()",
"_____no_output_____"
],
[
"df1[df1['Country'] == 'United States']['Subject'].unique()",
"_____no_output_____"
],
[
"c='United Kingdom'\nsubject='Gross value added per hour worked, constant prices'\nactivity='Information and communication'\nt=df1[(df1['Country'] == c) & (df1['Subject'] == subject)]",
"_____no_output_____"
],
[
"def regplot(df, country, subject, activity=None):\n if activity is None:\n d = collect_data(df, subject)\n return _regplot(d[country], country, subject)\n else:\n d = collect_data(df, subject, activity)\n return _regplot(d[country], country, subject)",
"_____no_output_____"
],
[
"regplot(\n df1,\n country='European Union (28 countries)',\n subject='Gross value added per hour worked, constant prices',\n activity='Information and communication',\n)",
"_____no_output_____"
],
[
"regplot(\n df, \n country='United States', \n subject='GDP per hour worked, constant prices '\n)",
"_____no_output_____"
],
[
"regplot(\n df, \n country='United States', \n subject='GDP per capita, constant prices '\n)",
"_____no_output_____"
],
[
"regplot(\n df, \n country='G7', \n subject='GDP per hour worked, constant prices '\n)",
"_____no_output_____"
],
[
"regplot(\n df, \n country='G7', \n subject='GDP per capita, constant prices '\n)",
"_____no_output_____"
]
]
] | [
"code"
] | [
[
"code",
"code",
"code",
"code",
"code",
"code",
"code",
"code",
"code",
"code",
"code",
"code",
"code",
"code",
"code",
"code",
"code",
"code",
"code",
"code",
"code",
"code",
"code",
"code",
"code",
"code",
"code",
"code",
"code",
"code",
"code",
"code",
"code",
"code",
"code",
"code",
"code",
"code",
"code"
]
] |
e778d3cbefb70da26e044594f28b7aebc59bb357 | 648,835 | ipynb | Jupyter Notebook | Starbucks_Capstone_notebook.ipynb | binh-bk/Starbucks-Promotion-Recommender | a82f4384380ec71d30b722e34e1d2cd36dccfa62 | [
"MIT"
] | null | null | null | Starbucks_Capstone_notebook.ipynb | binh-bk/Starbucks-Promotion-Recommender | a82f4384380ec71d30b722e34e1d2cd36dccfa62 | [
"MIT"
] | null | null | null | Starbucks_Capstone_notebook.ipynb | binh-bk/Starbucks-Promotion-Recommender | a82f4384380ec71d30b722e34e1d2cd36dccfa62 | [
"MIT"
] | null | null | null | 95.670156 | 56,344 | 0.801888 | [
[
[
"# Starbucks Capstone Challenge\n\n### Introduction\n\nThis data set contains simulated data that mimics customer behavior on the Starbucks rewards mobile app. Once every few days, Starbucks sends out an offer to users of the mobile app. An offer can be merely an advertisement for a drink or an actual offer such as a discount or BOGO (buy one get one free). Some users might not receive any offer during certain weeks. \n\nNot all users receive the same offer, and that is the challenge to solve with this data set.\n\nYour task is to combine transaction, demographic and offer data to determine which demographic groups respond best to which offer type. This data set is a simplified version of the real Starbucks app because the underlying simulator only has one product whereas Starbucks actually sells dozens of products.\n\nEvery offer has a validity period before the offer expires. As an example, a BOGO offer might be valid for only 5 days. You'll see in the data set that informational offers have a validity period even though these ads are merely providing information about a product; for example, if an informational offer has 7 days of validity, you can assume the customer is feeling the influence of the offer for 7 days after receiving the advertisement.\n\nYou'll be given transactional data showing user purchases made on the app including the timestamp of purchase and the amount of money spent on a purchase. This transactional data also has a record for each offer that a user receives as well as a record for when a user actually views the offer. There are also records for when a user completes an offer. \n\nKeep in mind as well that someone using the app might make a purchase through the app without having received an offer or seen an offer.\n\n### Example\n\nTo give an example, a user could receive a discount offer buy 10 dollars get 2 off on Monday. The offer is valid for 10 days from receipt. If the customer accumulates at least 10 dollars in purchases during the validity period, the customer completes the offer.\n\nHowever, there are a few things to watch out for in this data set. Customers do not opt into the offers that they receive; in other words, a user can receive an offer, never actually view the offer, and still complete the offer. For example, a user might receive the \"buy 10 dollars get 2 dollars off offer\", but the user never opens the offer during the 10 day validity period. The customer spends 15 dollars during those ten days. There will be an offer completion record in the data set; however, the customer was not influenced by the offer because the customer never viewed the offer.\n\n### Cleaning\n\nThis makes data cleaning especially important and tricky.\n\nYou'll also want to take into account that some demographic groups will make purchases even if they don't receive an offer. From a business perspective, if a customer is going to make a 10 dollar purchase without an offer anyway, you wouldn't want to send a buy 10 dollars get 2 dollars off offer. You'll want to try to assess what a certain demographic group will buy when not receiving any offers.\n\n### Final Advice\n\nBecause this is a capstone project, you are free to analyze the data any way you see fit. For example, you could build a machine learning model that predicts how much someone will spend based on demographics and offer type. Or you could build a model that predicts whether or not someone will respond to an offer. Or, you don't need to build a machine learning model at all. You could develop a set of heuristics that determine what offer you should send to each customer (i.e., 75 percent of women customers who were 35 years old responded to offer A vs 40 percent from the same demographic to offer B, so send offer A).",
"_____no_output_____"
],
[
"# Data Sets\n\nThe data is contained in three files:\n\n* portfolio.json - containing offer ids and meta data about each offer (duration, type, etc.)\n* profile.json - demographic data for each customer\n* transcript.json - records for transactions, offers received, offers viewed, and offers completed\n\nHere is the schema and explanation of each variable in the files:\n\n**portfolio.json**\n* id (string) - offer id\n* offer_type (string) - type of offer ie BOGO, discount, informational\n* difficulty (int) - minimum required spend to complete an offer\n* reward (int) - reward given for completing an offer\n* duration (int) - time for offer to be open, in days\n* channels (list of strings)\n\n**profile.json**\n* age (int) - age of the customer \n* became_member_on (int) - date when customer created an app account\n* gender (str) - gender of the customer (note some entries contain 'O' for other rather than M or F)\n* id (str) - customer id\n* income (float) - customer's income\n\n**transcript.json**\n* event (str) - record description (ie transaction, offer received, offer viewed, etc.)\n* person (str) - customer id\n* time (int) - time in hours since start of test. The data begins at time t=0\n* value - (dict of strings) - either an offer id or transaction amount depending on the record\n\n**Note:** If you are using the workspace, you will need to go to the terminal and run the command `conda update pandas` before reading in the files. This is because the version of pandas in the workspace cannot read in the transcript.json file correctly, but the newest version of pandas can. You can access the termnal from the orange icon in the top left of this notebook. ",
"_____no_output_____"
],
[
"# Problem statement and Approach",
"_____no_output_____"
],
[
"In scope of this program and with the data presented, we will looking at the association of promotion types, customer demographics and transaction records. The maingoals are:\n\n**1. Which promotion would return the best outcome?**\n\nThe \"best\" outcome measured by the reach to audience and amount spending on each promotion. This is comprarison of one promotion versus the other over the sampling of customers (all customers/users in dataset)\n\n**2. Which group of customer would enjoy the promotion or which promotion is prefer?**\n\nThis measured by comparative data from other group similar to a the same promotion. This is subset data, in which one group of users are compared to other or the rest of users to a certain promotion or offer\n\n**3. Could we recommend a user a certain promotion, knowing that there is similarity of this user vs. other similar users in the dataset?**\n\nEssentially, for \\#3 we can build a recommendation system having following concepts:\n- if user is in the data base, we can check the performance on received offers vs. the most similar n users. We then recommend the ranked offer that the group with n users responded in the dataset. The performance could be measured by completion records or actually dollars purchased\n- if we don't have anything information of the user, we could send the most successful promotion on the step 1, measured by completion response or by dollars purchased.",
"_____no_output_____"
],
[
"# Data Exploration",
"_____no_output_____"
]
],
[
[
"import warnings\nwarnings.filterwarnings('ignore') # make the output cleaner",
"_____no_output_____"
],
[
"import seaborn as sns\nimport matplotlib.pyplot as plt\n%matplotlib inline\nplt.style.use('seaborn-white')\n\nplt.rcParams['figure.figsize'] = (10,6)\nplt.rcParams['font.size'] = 13\nplt.rcParams['font.sans-serif'] = 'Open Sans'\nplt.rcParams['font.family'] = 'sans-serif'\nplt.rcParams['text.color'] = '#4c4c4c'\nplt.rcParams['axes.labelcolor']= '#4c4c4c'\nplt.rcParams['xtick.color'] = '#4c4c4c'\nplt.rcParams['ytick.color'] = '#4c4c4c'",
"_____no_output_____"
],
[
"import pandas as pd\nimport numpy as np\nimport math\nimport json",
"_____no_output_____"
],
[
"# one analysis could ran for 20 minutes, the LOAD_JSON option here let us load file from a json file\n# else the actually iteration will take place\nLOAD_JSON = True",
"_____no_output_____"
]
],
[
[
"## Portfolio",
"_____no_output_____"
]
],
[
[
"# read in the json files\nportfolio = pd.read_json('data/portfolio.json', orient='records', lines=True)\nportfolio",
"_____no_output_____"
]
],
[
[
"three types of promotion:\n\n- **BOGO (Buy One Get One free)**: customer received this offer, customer can get two similar drinks and pay for one, essentially 50% except customer now have two drinks, works better for customer who has a friend or a colleague to join that drink\n- **Discount**: after certain dollars purchased for example \\\\$10, then a reward of \\\\$5 is added to buyers' Starbucks account\n- **Informational**: a notice or email informing a new product, or a new type of service. Customer is considered under *influence* during valid period if customer **viewed** the offer\n\nThere are four types of BOGO, four types of discounts, and two types of informational included in this campaign. We will comback with this **portfolio** data after seeing more date in **transcript**",
"_____no_output_____"
],
[
"## Profile",
"_____no_output_____"
]
],
[
[
"# load data in show first 5 lines\nprofile = pd.read_json('data/profile.json', orient='records', lines=True)\nprofile.head()",
"_____no_output_____"
],
[
"# shape: rows by columns\nprofile.shape",
"_____no_output_____"
],
[
"profile.info(verbose=True)",
"<class 'pandas.core.frame.DataFrame'>\nRangeIndex: 17000 entries, 0 to 16999\nData columns (total 5 columns):\n # Column Non-Null Count Dtype \n--- ------ -------------- ----- \n 0 gender 14825 non-null object \n 1 age 17000 non-null int64 \n 2 id 17000 non-null object \n 3 became_member_on 17000 non-null int64 \n 4 income 14825 non-null float64\ndtypes: float64(1), int64(2), object(2)\nmemory usage: 664.2+ KB\n"
],
[
"# genders\nprofile.gender.value_counts()",
"_____no_output_____"
],
[
"profile.gender.value_counts().plot(kind='bar');",
"_____no_output_____"
],
[
"# age distribution, we saw some discrepancy with 118 (years old)\nprofile.age.value_counts()",
"_____no_output_____"
],
[
"# abnormal with 118 value, setting by NaN\nprofile.loc[profile['age']==118, 'age'] = np.NaN",
"_____no_output_____"
],
[
"# all genders\nprofile.age.plot.hist();",
"_____no_output_____"
],
[
"# distribution of age by gender\nprofile.query('gender==\"M\"')['age'].hist()\nprofile.query('gender==\"F\"')['age'].hist();",
"_____no_output_____"
]
],
[
[
"- clear more young male members than female members in the dataset. It makes sense since Starbuck sells coffee as the main drink, ",
"_____no_output_____"
]
],
[
[
"# let group genders and age \ngender = profile.groupby(['age', 'gender']).count()['id'].unstack()\ngender",
"_____no_output_____"
],
[
"gender['M/F'] = gender['M']/gender['F']",
"_____no_output_____"
],
[
"plt.title('Ratio of Male/Female of Starbucks customers over age')\nplt.ylabel('Male/Female')\nplt.xlabel('age')\nplt.grid()\nplt.plot(gender.index, gender['M/F']);",
"_____no_output_____"
]
],
[
[
"- more male customers under 40, and female customers are increased propotionally with age\n- over 80 years old, more female than male using Starbucks products (or as Starbucks customer)",
"_____no_output_____"
]
],
[
[
"# explore member join \nprofile['became_member_on'] = pd.to_datetime(profile.became_member_on, format='%Y%m%d')",
"_____no_output_____"
],
[
"latest_ts = profile.became_member_on.max()\nprofile.became_member_on.apply(lambda x: (latest_ts - x).total_seconds()/\n (3600*24*365)).plot(kind='hist', bins=20);",
"_____no_output_____"
],
[
"profile['membership_age'] = profile.became_member_on.apply(lambda x: int((latest_ts - x).total_seconds()/\n (3600*24*365)))",
"_____no_output_____"
],
[
"# portion with nans in incomes\nprofile.income.isna().sum()/profile.shape[0]",
"_____no_output_____"
],
[
"profile.income.plot(kind='hist', bins=20);",
"_____no_output_____"
],
[
"profile.head()",
"_____no_output_____"
],
[
"# make a combined graph\nfig, axs = plt.subplots(2,2, figsize=(14,8))\nfig.suptitle('Customer demographics', fontsize=20)\ndf_ = profile.gender.value_counts()\naxs[0,0].set_title('Gender')\naxs[0,0].bar(x = df_.index, height=df_.values)\naxs[0,1].set_title('Age')\naxs[0,1].hist(profile.age)\naxs[1,1].set_title('Income, $')\naxs[1,1].hist(profile.income)\ndf_ = profile.membership_age.value_counts()\naxs[1,0].set_title('Membership in year')\naxs[1,0].bar(x=df_.index, height=df_.values)\nfig.tight_layout()\nfig.savefig('img/profile.png', optimize=True, dpi=200);",
"_____no_output_____"
],
[
"fig, axs = plt.subplots(1,2, figsize=(12,5))\nfig.subplots_adjust(wspace=0.5)\nfig.suptitle('Customers\\' gender distribution over age', fontsize=20)\nm = profile.query('gender==\"M\"')['age']\nfm = profile.query('gender==\"F\"')['age']\n\naxs[0,].set_title('Age distribution by gender')\naxs[0,].hist(m.values, alpha=0.5, label='M')\naxs[0,].hist(fm.values, alpha=0.5, label='F')\naxs[0].legend()\n\naxs[1,].set_title('M/F ratio over age')\naxs[1,].set_ylabel('M/F')\naxs[1,].plot(gender.index, gender['M/F']);\naxs[1,].grid()\n# fig.legend()\nfig.tight_layout()\nfig.savefig('img/age_dist.png', optimize=True, dpi=200);",
"_____no_output_____"
]
],
[
[
"### encoded profile",
"_____no_output_____"
]
],
[
[
"# for columns with a few categorical values, we can get encode them direcly such as gender, became_member_on\n# other need to be cut into a larger bin and then encoded by pd.get_dummies\n# will we find a similar customer based on characteristics from profile\nprofile.columns",
"_____no_output_____"
],
[
"# membership age\nprofile['membership_age'] = profile.became_member_on.apply(lambda x: int((latest_ts - x).total_seconds()/\n (3600*24*365)))\ndf_m_age = pd.get_dummies(profile.membership_age, prefix=\"m_age\", dummy_na=True)",
"_____no_output_____"
],
[
"# genders\ndf_gender = pd.get_dummies(profile.gender, prefix=\"gender\", dummy_na=True)",
"_____no_output_____"
],
[
"# for age columns, we have 84 members, which is is too large to encoded for each year\nprofile.age.value_counts()",
"_____no_output_____"
],
[
"# instead we gather in to a bin of 10 years\nmin_, max_ = profile.age.describe().loc['min'], profile.age.describe().loc['max']\nmin_, max_",
"_____no_output_____"
],
[
"age_bins = np.arange(min_, max_+1, 10)\nage_labels = [int((age_bins[i]+age_bins[i+1])/2) for i in range(0, len(age_bins)-1)]\nage_labels # average values",
"_____no_output_____"
],
[
"df_age = pd.get_dummies(pd.cut(profile.age, bins=age_bins, \n labels = age_labels), prefix=\"age\", dummy_na=True)",
"_____no_output_____"
],
[
"# and finally income\nprofile.income.describe()",
"_____no_output_____"
],
[
"# similar to age, we bins income to smaller groups\nmin_, max_ = profile.income.describe().loc['min'], profile.income.describe().loc['max']\nincome_bins = np.arange(min_, max_+1, 10_000)\nincome_labels = [int((income_bins[i]+income_bins[i+1])/2) for i in range(0, len(income_bins)-1) ]\nincome_labels # average values",
"_____no_output_____"
],
[
"df_income = pd.get_dummies(pd.cut(profile.income, bins=income_bins, \n labels = income_labels), prefix=\"income\", dummy_na=True)",
"_____no_output_____"
],
[
"# then we concat all users with encoded columns\nprofile_encoded = pd.concat([profile.id, df_gender, df_age, df_income, df_m_age], axis=1)\nprofile_encoded.set_index('id', inplace=True)\nprofile_encoded.shape",
"_____no_output_____"
],
[
"# see what np.dot production looks like\nnp.dot(profile_encoded.iloc[1], profile_encoded.iloc[1])",
"_____no_output_____"
],
[
"def encoding_profile(df=None):\n '''encode values of columns in user profile.\n \n INPUT: user profile dataframe\n \n OUTPUT: a dataframe with value encoded\n '''\n \n # membership age\n df['membership_age'] = df.became_member_on.apply(\n lambda x: int((latest_ts - x).total_seconds()/(3600*24*365)))\n df_m_age = pd.get_dummies(df.membership_age, prefix=\"m_age\", dummy_na=True)\n \n # gender\n df_gender = pd.get_dummies(df.gender, prefix=\"gender\", dummy_na=True)\n min_, max_ = df.age.describe().loc['min'], df.age.describe().loc['max']\n \n # user age\n age_bins = np.arange(min_, max_+1, 10)\n age_labels = [int((age_bins[i]+age_bins[i+1])/2) for i in range(0, len(age_bins)-1)]\n df_age = pd.get_dummies(pd.cut(df.age, bins=age_bins, \n labels = age_labels), prefix=\"age\", dummy_na=True)\n \n # user income\n min_, max_ = df.income.describe().loc['min'], df.income.describe().loc['max']\n income_bins = np.arange(min_, max_+1, 10_000)\n income_labels = [int((income_bins[i]+income_bins[i+1])/2) for i in range(0, len(income_bins)-1)]\n df_income = pd.get_dummies(pd.cut(df.income, bins=income_bins, \n labels = income_labels), prefix=\"income\", dummy_na=True)\n # concatinate\n profile_encoded = pd.concat([df.id, df_gender, df_age, df_income, df_m_age], axis=1)\n profile_encoded.set_index('id', inplace=True)\n return profile_encoded",
"_____no_output_____"
],
[
"def find_similar_users(user_id, df=None, n_top=100):\n '''find n_top similars to user_id based np.dot product\n \n INPUT:\n user_id: a select user id \n df: a dataframe contains encoded columns characterize each user\n n_top: number of top users would be return\n \n OUTPUT:\n a dictionary contain a list of user_id and similar score \n '''\n # select all users except the user_id\n users = df.index.drop(user_id)\n \n # find similarity \n scores = [{'user': user, 'score':np.dot(df.loc[user_id], \n df.loc[user])} for user in users]\n # sort from top score\n scores = sorted(scores, key=lambda k: k['score'], reverse=True)\n return scores[:n_top]",
"_____no_output_____"
],
[
"# select a user based on index\nuser_id = profile_encoded.index[100]\nuser_id",
"_____no_output_____"
],
[
"profile_encoded = encoding_profile(df=profile)",
"_____no_output_____"
],
[
"profile_encoded.head()",
"_____no_output_____"
],
[
"find_similar_users(user_id, df=profile_encoded)",
"_____no_output_____"
]
],
[
[
"## Transcript",
"_____no_output_____"
]
],
[
[
"# transaction record\ntranscript = pd.read_json('data/transcript.json', orient='records', lines=True)\ntranscript.head()",
"_____no_output_____"
],
[
"transcript.info()",
"<class 'pandas.core.frame.DataFrame'>\nRangeIndex: 306534 entries, 0 to 306533\nData columns (total 4 columns):\n # Column Non-Null Count Dtype \n--- ------ -------------- ----- \n 0 person 306534 non-null object\n 1 event 306534 non-null object\n 2 value 306534 non-null object\n 3 time 306534 non-null int64 \ndtypes: int64(1), object(3)\nmemory usage: 9.4+ MB\n"
],
[
"# long table\ntranscript.shape",
"_____no_output_____"
],
[
"# average traffic per user\ntranscript.shape[0]/transcript.person.nunique()",
"_____no_output_____"
],
[
"# transaction by timestamp (hours)\ntranscript.time.plot(kind='hist');",
"_____no_output_____"
],
[
"# transaction categories\ntranscript.event.value_counts()",
"_____no_output_____"
],
[
"transcript.event.value_counts().iloc[1:].plot(kind='bar')\nplt.xticks(rotation=0);\nplt.ylabel('Count')\nplt.title('Overall transaction summary of dataset');\n",
"_____no_output_____"
]
],
[
[
"- over the all transaction, about 44% promotion received gets completed\n- 75% of promotions received gets viewed\n- 58% of promotions viewed gets completed\n- two informational promotions don't have \"completed\" record",
"_____no_output_____"
],
[
"### user-offer-matrix",
"_____no_output_____"
]
],
[
[
"transcript.head()",
"_____no_output_____"
],
[
"# get content of value columns, unpack\ntranscript['amount'] = transcript['value'].apply(lambda x: list(x.values())[0] \n if 'amount' in list(x.keys())[0] else np.NaN)\ntranscript['offer'] = transcript['value'].apply(lambda x: list(x.values())[0] \n if 'offer' in list(x.keys())[0] else np.NaN)",
"_____no_output_____"
],
[
"transcript.drop(columns='value', inplace=True)\ntranscript.shape",
"_____no_output_____"
],
[
"# let see total purchase in $M\ntranscript.amount.sum()/1_000_000",
"_____no_output_____"
],
[
"# on average, customer make a $12.77, and 50% customers make $8.9 or more\ntranscript.amount.describe()",
"_____no_output_____"
],
[
"transcript.amount.plot(kind='hist', bins=100, logy=True);",
"_____no_output_____"
],
[
"# let see who make a $100 or more\ntranscript[transcript.amount > 100]",
"_____no_output_____"
],
[
"# look like we have some office parties, let see if we can filter out transaction outside 1.5IRQ\nirq = transcript.amount.describe().loc['75%'] - transcript.amount.describe().loc['25%']\nirq",
"_____no_output_____"
],
[
"upper_limit = transcript.amount.describe().loc['75%'] + 1.5*irq\nupper_limit",
"_____no_output_____"
],
[
"transcript.shape",
"_____no_output_____"
],
[
"# seem we are losing more than 50% of transaction if we filter out\n# we will proceed WITHOUT filter out,\ntranscript[transcript.amount < upper_limit].shape",
"_____no_output_____"
],
[
"# moving on to unique users\ntranscript.person.nunique()",
"_____no_output_____"
],
[
"# first let tackle the promo with BOGO or discount\ndiscount_bogo_ids = portfolio[portfolio['offer_type'] != 'informational'].id.values\ndiscount_bogo_ids",
"_____no_output_____"
],
[
"# informational offer id\ninfo_ids = portfolio[portfolio['offer_type'] == 'informational'].id.values\ninfo_ids",
"_____no_output_____"
]
],
[
[
"- We need to rate how a promotion is sucess or not, for discout or BOGO, a **success case** this should be: \n\n `offer received >> offer viewed >> offer completed`\n- A **failed** promotion is:\n\n `offer received >> no view on offer >> offer completed`\nbasically, an offer made, customer did not know about an offer but still purchase goods to the minimum amount required by an offer. From pretext of the problem, it is not desirable since the marketing campaign had no influence on customer's purchase\n\n- Another failed case: \n\n `offer received >> offer viewed >> offer not completed` before time expired\n- and another case: \n\n `offer received >> no view on offer >> offer not completed` before time expired\n\nthe last one represents a case that is more complicated. We do not know offer not completed because they are not aware of a promotion or the promotion is not appeal enough to get it completed.\n\nLet tackle problem by trying a simpler approach: rating a success/fail offer by looking in `viewed, completed` records. This approach is only applicable for 8 discounts or BOGO promotions. For informational ones, we could simplify by looking only to `viewed` records",
"_____no_output_____"
],
[
"## Simple rating based on records of viewed, completed",
"_____no_output_____"
]
],
[
[
"def rate_offer_discount_bogo(offer_id, df=None):\n '''\n rate a offer based on average number of viewed to number of completed \n and total offer received.\n \n score = number of completed / number of received (promotion)\n For example: \n - if a customer received two offers, viewed two and completed two, the score is 1\n - if a customer received two, one viewed, completed 2, score is 2/2 = 1\n - if a customer received two, one viewed, completed 0, score is 0/2 = 0\n \n INPUT:\n offer_id: id of offer of discount or BOGO\n df: dataframe with promoting events (filter out transacion)\n \n OUTPUT: a dataframe with index as the users received the offer and the column name as the offer id\n '''\n \n df_group = df.query(f'offer==@offer_id').groupby(\n ['person', 'event']).count()['time'].unstack(fill_value=0)\n df_group = df_group.apply(lambda row: \n row['offer completed']/row['offer received']\n if row['offer received']>0 else np.NaN, axis=1)\n return df_group.rename(offer_id).to_frame()",
"_____no_output_____"
],
[
"# for informational events, it is harder to evaluate influence of seeing the offer and the follow up transaction\n# for a simple case, I will rate them based on viewed/received ratio\ndef rate_offer_info(offer_id, df=None):\n '''rate informational offer based number of viewed and received.\n rate = number of viewed/ number of received\n \n For example:\n - if all offers were viewed, the rate = 1\n - if none of offers were viewed, teh rate = 0\n \n INPUT: offer_id - id for the offer\n OUTPUT: a dataframe with promoting events (filter out transacion)\n '''\n \n df_group = df.query('offer==@offer_id').groupby(\n ['person', 'event']).count()['time'].unstack(fill_value=0)\n df_group = df_group.apply(lambda row: row['offer viewed'] /row['offer received']\n if row['offer received']>0 else np.NaN, axis=1)\n return df_group.rename(offer_id).to_frame()",
"_____no_output_____"
],
[
"dfs = list()\nfor offer_id in discount_bogo_ids:\n dft = rate_offer_discount_bogo(offer_id, df=transcript)\n dfs.append(dft)\n# append to a list of dataframe on the previous step\nfor offer_id in info_ids:\n dft = rate_offer_info(offer_id, df=transcript)\n dfs.append(dft)",
"_____no_output_____"
],
[
"for df in dfs:\n# print(df.info())\n print(df.shape)",
"(6374, 1)\n(6330, 1)\n(6355, 1)\n(6374, 1)\n(6325, 1)\n(6332, 1)\n(6262, 1)\n(6285, 1)\n(6331, 1)\n(6320, 1)\n"
],
[
"# check set of users received all offers\ncommon_users = set(dfs[0].index)\nfor df in dfs[1:]:\n users = df.index\n common_users = common_users.intersection(users)\n print(len(common_users))\n# none of user received all offers ",
"2118\n604\n127\n36\n4\n0\n0\n0\n0\n"
],
[
"# pd.concat is easier for applying, but only along columns or a along the rows which is less flexible\ndf = dfs[0]\nfor df_ in dfs[1:]:\n df = df.merge(df_, on='person', how='outer')\n print(df.shape)",
"(10586, 2)\n(13319, 3)\n(15016, 4)\n(16013, 5)\n(16549, 6)\n(16805, 7)\n(16928, 8)\n(16972, 9)\n(16994, 10)\n"
],
[
"# there is 6 person is missing between transcript and this df\ntranscript.person.nunique()",
"_____no_output_____"
],
[
"df.shape",
"_____no_output_____"
],
[
"# let see which ids are not have any record on transaction\nno_record_users = set(np.setdiff1d(transcript.person.to_list(), df.index.to_list()))\nno_record_users",
"_____no_output_____"
],
[
"# look normal, I want to make sure the data is still there\nprofile.set_index('id').loc[no_record_users]",
"_____no_output_____"
]
],
[
[
"### Apply FunkSVD",
"_____no_output_____"
]
],
[
[
"# we get about 2/3 matrix is null values\nsum(df.isnull().sum())/(df.shape[0]*df.shape[1])",
"_____no_output_____"
],
[
"# is any user with no promotional available?\n# user will get at least 4 offers, and maximum 9 offers\ndf.isnull().sum(axis=1).sort_values(ascending=False).value_counts().sort_values()",
"_____no_output_____"
],
[
"# adopted from Udacity's exercise\ndef FunkSVD(user_offer_mat, latent_features=10, learning_rate=0.0001, iters=100):\n '''\n This function performs matrix factorization using a basic form of FunkSVD with no regularization\n \n INPUT:\n ratings_mat - (numpy array) a matrix with users as rows, promotion_id as columns, and ratings as values\n latent_features - (int) the number of latent features used\n learning_rate - (float) the learning rate \n iters - (int) the number of iterations\n \n OUTPUT:\n user_mat - (numpy array) a user by latent feature matrix\n movie_mat - (numpy array) a latent feature by promotion_id matrix\n '''\n \n # Set up useful values to be used through the rest of the function\n n_users = user_offer_mat.shape[0]\n n_offers = user_offer_mat.shape[1]\n num_ratings = np.count_nonzero(~np.isnan(user_offer_mat))\n \n # initialize the user and promotion matrices with random values\n user_mat = np.random.rand(n_users, latent_features)\n offer_mat = np.random.rand(latent_features, n_offers)\n \n # initialize sse at 0 for first iteration\n sse_accum = 0\n \n # header for running results\n print(\"Optimizaiton Statistics\")\n print(\"Iterations | Mean Squared Error \")\n \n # for each iteration\n for iteration in range(iters):\n\n # update our sse\n old_sse = sse_accum\n sse_accum = 0\n \n # For each user-promotion pair\n for i in range(n_users):\n for j in range(n_offers):\n \n # if the rating exists\n if user_offer_mat[i, j] > 0:\n \n # compute the error as the actual minus the dot product of the user and promotion latent features\n diff = user_offer_mat[i, j] - np.dot(user_mat[i, :], offer_mat[:, j])\n \n # Keep track of the sum of squared errors for the matrix\n sse_accum += diff**2\n \n # update the values in each matrix in the direction of the gradient\n for k in range(latent_features):\n user_mat[i, k] += learning_rate * (2*diff*offer_mat[k, j])\n offer_mat[k, j] += learning_rate * (2*diff*user_mat[i, k])\n\n # print results for iteration\n print(\"%d \\t\\t %f\" % (iteration+1, sse_accum / num_ratings))\n \n return user_mat, offer_mat ",
"_____no_output_____"
],
[
"# this is sparse matrix, let see how FunkSVD algorithm performs on this set\ndf_ = df.to_numpy()\nuser_mat, offer_mat = FunkSVD(df_, latent_features=10, learning_rate=0.005, iters=10)",
"Optimizaiton Statistics\nIterations | Mean Squared Error \n1 \t\t 0.042280\n2 \t\t 0.028292\n3 \t\t 0.027818\n4 \t\t 0.027364\n5 \t\t 0.026920\n6 \t\t 0.026486\n7 \t\t 0.026063\n8 \t\t 0.025649\n9 \t\t 0.025245\n10 \t\t 0.024850\n"
]
],
[
[
"- small error, but is this a good approximation?",
"_____no_output_____"
]
],
[
[
"# reconstruct user-item matrix based on decomposed matrices\npred_mat = np.dot(user_mat, offer_mat)",
"_____no_output_____"
],
[
"# check average value of each columns\ndf.mean(axis=1)",
"_____no_output_____"
],
[
"# a quick check on the mean value is not promissing, we are looking value between -1 to 1\npred_mat.mean(axis=1)",
"_____no_output_____"
]
],
[
[
"- this approximation using FunkSVD seems NOT working well with our dataset",
"_____no_output_____"
],
[
"### How FunkSVD with a denser matrix?",
"_____no_output_____"
]
],
[
[
"# select matrix have 5 or less cells as null values\ndf2 = df[df.isnull().sum(axis=1) <= 5]\ndf_ = df2.to_numpy()\nuser_mat, offer_mat = FunkSVD(df_, latent_features=20, learning_rate=0.005, iters=10)",
"Optimizaiton Statistics\nIterations | Mean Squared Error \n1 \t\t 0.141795\n2 \t\t 0.020232\n3 \t\t 0.015170\n4 \t\t 0.014100\n5 \t\t 0.013749\n6 \t\t 0.013549\n7 \t\t 0.013384\n8 \t\t 0.013231\n9 \t\t 0.013082\n10 \t\t 0.012937\n"
],
[
"pred_mat = np.dot(user_mat, offer_mat)",
"_____no_output_____"
],
[
"# still. something is not working right\npred_mat.mean(axis=1)",
"_____no_output_____"
],
[
"pred_mat.max(axis=1)",
"_____no_output_____"
],
[
"diff_sqr = np.nansum((pred_mat - df_)**2)",
"_____no_output_____"
],
[
"# mean squared root error. It is almost a guess work. \nnp.sqrt(diff_sqr/(pred_mat.shape[0]*pred_mat.shape[1]))",
"_____no_output_____"
]
],
[
[
"### Surprise SVD ",
"_____no_output_____"
]
],
[
[
"# let try out another FunkSVD\n# https://surprise.readthedocs.io/en/stable/getting_started.html\nfrom surprise import SVD\nfrom surprise import Reader\nfrom surprise import Dataset\nfrom surprise.model_selection import cross_validate\nfrom surprise.model_selection import KFold\nfrom surprise import accuracy",
"_____no_output_____"
],
[
"reader = Reader(rating_scale=(0, 1))",
"_____no_output_____"
],
[
"# transfer from wide table to long table\ndf3 = pd.melt(df.reset_index(), id_vars=['person'], value_name='rating')\ndf3.columns = ['user', 'offer', 'rating']\nprint(df3.shape)\ndf3.head()",
"(169940, 3)\n"
],
[
"# load data from dataframe\ndata = Dataset.load_from_df(df3[['user', 'offer', 'rating']], reader)",
"_____no_output_____"
],
[
"kf = KFold(n_splits=3)\nalgo = SVD()",
"_____no_output_____"
],
[
"for trainset, testset in kf.split(data):\n\n # train and test algorithm.\n algo.fit(trainset)\n predictions = algo.test(testset)\n\n # Compute and print Root Mean Squared Error\n accuracy.rmse(predictions, verbose=True)",
"RMSE: nan\nRMSE: nan\nRMSE: nan\n"
]
],
[
[
"- it appeared that SVD algorithm is not converged. It is consistent with the RMSE error of 0.5 as above for value between 0 and zero",
"_____no_output_____"
],
[
"# Deeper dive into `Transcript`",
"_____no_output_____"
],
[
"## Rate transaction by completion",
"_____no_output_____"
]
],
[
[
"# let see transaction records again\ntranscript.head()",
"_____no_output_____"
],
[
"# and make a wide table by counting records\ntranscript.groupby(['offer', 'event']).count()['person'].unstack()",
"_____no_output_____"
],
[
"df_offer = transcript.groupby(['offer', 'event']).count()['person'].unstack()\ndf_offer.index.set_names(names='id', inplace=True)",
"_____no_output_____"
],
[
"# and calculate some ratios\ndf_offer['view/receive'] = df_offer.apply(lambda row: row['offer viewed']/row['offer received'], axis=1)\ndf_offer['comp/receive'] = df_offer.apply(lambda row: row['offer completed']/row['offer received'], axis=1)\ndf_offer['comp/view'] = df_offer.apply(lambda row: row['offer completed']/row['offer viewed'], axis=1)\ndf_offer",
"_____no_output_____"
],
[
"# checking column on portfolio dataset\nportfolio.columns",
"_____no_output_____"
],
[
"# join promotion profile and promotion records\nportfolio_extra = portfolio.merge(df_offer, how='right', left_on='id', right_on='id')",
"_____no_output_____"
],
[
"# list comprehension over 2D array, cast to a set to get unique element\nchannels = set([channel for row in portfolio_extra.channels.values for channel in row])",
"_____no_output_____"
],
[
"# encode channels\nfor channel in channels:\n portfolio_extra[channel] = portfolio_extra.channels.apply(lambda cell: 1 if channel in cell else 0)",
"_____no_output_____"
],
[
"portfolio_extra.head()",
"_____no_output_____"
],
[
"# or a cleaner view\nportfolio_extra[['id', 'channels', 'difficulty', 'duration', 'offer_type',\n 'view/receive', 'comp/receive', 'comp/view']]",
"_____no_output_____"
],
[
"# ratio of views and advertising channels\nplt.figure(figsize=(8,5))\nplt.plot(portfolio_extra[['email', 'mobile', 'web', 'social']].sum(axis=1),\n portfolio_extra['view/receive'],\n ls='', marker='o', markersize=20)\nplt.title('Marketing channels vs. view rate')\nplt.ylabel('#view/#receive per promotion')\nplt.xlabel('#channel')\nplt.tight_layout()\nplt.savefig('img/view_rate.png', optimize=True, dpi=120)",
"_____no_output_____"
]
],
[
[
"summary:\n- more marketing channels resulted a higher view rate\n- all promotion has email as one of the channel\n- view rate is not linear over each channel, \n - without social marketing, the view rate is about 0.54 (or 54% customers saw their promotion)\n - without social plus mobile, the view rate drops to 0.35\n - without web marketing, the view rate is 0.87, which is the least influence channels\n - all promotion sent via email so we are not able to draw any relation on this channel",
"_____no_output_____"
]
],
[
[
"# let see all the rates with each promotion id\ncategories = ['view/receive','comp/receive', 'comp/view']\nportfolio_extra[categories].plot(kind='bar', subplots=True);",
"_____no_output_____"
]
],
[
[
"**Successful rate of completion**\n- we will define a term to quantify a successful transaction by:\n s_rate = 1 - (#completed/ #received)*(#completed/ #viewed)\n\nfor each transaction or overall users in this dataset. \n- in a perfect case, an offer was received, viewed, then completed, `s_rate = 0`\n- if a customer received an offer, NOT see the offer, but still completed the offer, the `s_rate = -infinity`\n- if a customer received an offer, saw the offer, but not completed the offer, the `s_rate = 1`\n- if we want to find the most successful transaction, we can use the absolute value (`abs(s_rate)`) of s_rate, in which s_rate close to zero is more sucess than larger (a far away) from zero.\n",
"_____no_output_____"
]
],
[
[
"# for a simpler approach, I will use the ratio of (completed/received)*(completed/viewed)\n# as one rating for how succesful the promotion is. This is only applicable for discount for BOGO\ncategories = ['view/receive','comp/receive', 'comp/view']\nportfolio_extra['s_rate'] = 1 - portfolio_extra['comp/receive']*portfolio_extra['comp/view']",
"_____no_output_____"
],
[
"portfolio_extra.columns",
"_____no_output_____"
],
[
"portfolio_extra[['id', 'offer_type', 'difficulty', 'duration', 's_rate']].\\\nsort_values(by='s_rate', ascending=True)",
"_____no_output_____"
],
[
"def find_pop_offers(transcript, portfolio):\n '''find offer that has a high successful rate\n \n INPUT:\n transcript: dataframe of records of transaction of customers with promo offered\n portfolio: information of promotion including type, duration, difficulty\n \n OUTPUT:\n a sorted offers which show the highest successful rate `s_rate`.\n s_rate = (number of completed/number of received)*(number of viewed/number of received)\n for each promotion\n '''\n \n df = transcript.groupby(['offer', 'event']).count()['person'].unstack()\n df.index.set_names(names='id', inplace=True)\n \n df['view/receive'] = df.apply(lambda row: row['offer viewed']/row['offer received'], axis=1)\n df['comp/receive'] = df.apply(lambda row: row['offer completed']/row['offer received'], axis=1)\n df['comp/view'] = df.apply(lambda row: row['offer completed']/row['offer viewed'], axis=1)\n \n df = portfolio.merge(df, how='right', left_on='id', right_on='id')\n \n # define an `s_rate` as sucessful rate based on completion\n df['s_rate'] = abs(1-df['comp/receive']*df['comp/view'])\n offers = df[['id', 'offer_type', 'difficulty', 'duration',\n 's_rate']].sort_values(by='s_rate', ascending=True)\n return offers",
"_____no_output_____"
],
[
"ranked_offers = find_pop_offers(transcript, portfolio)\nranked_offers",
"_____no_output_____"
],
[
"labels = ranked_offers[['id', 'offer_type']].apply(\n lambda row: row['offer_type']+'_' + row['id'][-5:], axis=1).values\nlabels",
"_____no_output_____"
],
[
"plt.figure(figsize=(12,6))\nplt.bar(x=labels, height=ranked_offers['s_rate'], width=0.4)\nplt.xticks(rotation=0)\nplt.title('Successful rate by promotion id (lower is more completion)')\nplt.tight_layout()\nplt.grid()\nplt.savefig('img/s_rate.png', optimize=True, dpi=120);\n",
"_____no_output_____"
],
[
"transcript.amount.describe()",
"_____no_output_____"
],
[
"plt.figure(figsize=(12,8))\n# plt.subplot_adjust(h_space=0.5)\nplt.suptitle('Transaction records')\n\nax1 = plt.subplot(221)\nax1.set_title('Overall ')\ndf_ = transcript.event.value_counts()\nshort_labels = [label.split(' ')[-1] for label in df_.index]\nax1.bar(x=short_labels, height=df_.values, width=0.3)\n\nax2 = plt.subplot(222)\nax2.set_title('Amount per transaction, $')\nax2.hist(transcript.amount, log=True, bins=21)\n\nax3 = plt.subplot(212)\nax3.set_title('Number of events by promotion id')\ndf_ = transcript.groupby(['offer', 'event']).count()['time'].unstack()\nsort_labels = [label[-5:] for label in df_.index]\ndf_[['offer received', 'offer viewed', 'offer completed']].plot(kind='bar', ax=ax3)\nax3.set_xticklabels(sort_labels, rotation=0)\nax3.legend(loc='best', ncol=3, bbox_to_anchor=(0.2, 0., 0.5, -0.15))\nax3.set_xlabel('')\n\n# # axs[1,0].bar(df_.index, df_.values)\nplt.tight_layout()\nplt.savefig('img/transaction.png', optimize=True, dpi=120)",
"_____no_output_____"
]
],
[
[
"## Grouping transaction by users",
"_____no_output_____"
]
],
[
[
"def analyze_df(df, offer_id, info_offer=False): \n '''summary a transaction if it was viewed, other offer, and amount spent.\n \n INPUT:\n df: a dataframe containing transaction records\n offer_id: an promotion id about an offer\n \n OUPUT:\n a dictionary containing summary\n '''\n# print(df)\n start_idx = df.head(1).index[0]\n result = {'viewed': False,\n 'completed': False,\n 'other offer viewed': False,\n 'amount': 0}\n count_viewed_offers = df.query('event==\"offer viewed\"')['offer'].value_counts().index\n if offer_id in count_viewed_offers:\n result['viewed'] = True\n if len(count_viewed_offers) > 1:\n result['other offer viewed'] = True\n amount = df['amount'].sum()\n result['amount'] = amount\n \n if info_offer:\n # for informational offer, if we saw at least one transaction, we marked it as a completed one\n if len(df['offer']=='transaction') >0:\n result['completed'] = True\n else:\n # for discount for BOGO offers, we look for \"offer completed\" in event columns\n if offer_id in df.query('event==\"offer completed\"')['offer'].values:\n result['completed'] = True\n return {start_idx: result}",
"_____no_output_____"
],
[
"def slice_df(df, start_point, valid_hours):\n '''slice a dataframe based on duration of offer since it's received.\n \n INPUT:\n df: a larger dataframe contains all transaction records\n start_point: starting index, usually when offer is received\n valid_hours: duration of offer to be valid\n OUPUT:\n a sliced dataframe \n '''\n \n time_track = df.loc[start_point]['time']\n time_expired = time_track + valid_hours\n \n # \n for idx, row in df[['offer', 'event', 'time', 'amount']].loc[start_point:,].iterrows():\n time_expired = time_track + valid_hours\n if row['time'] > time_expired:\n return df.loc[start_point:idx]\n else:\n return df.loc[start_point:]",
"_____no_output_____"
],
[
"portfolio.query('offer_type==\"informational\"').id.values",
"_____no_output_____"
],
[
"transcript.groupby('event').count()",
"_____no_output_____"
],
[
"transcript.head()",
"_____no_output_____"
],
[
"def info_offer(user, transcript, portfolio):\n '''summary of offers for each user by itering through each promotion and count numbers \n of received, viewed, and completed transaction.\n \n INPUT:\n person: an id associated with an user\n portfolio: a dataframe containg promotion id and valid hours\n \n OUTPUT:\n a list of dictionary with person_id as the key and summary stat as the value\n '''\n # slice the main dataset to each user/person with 4 columns\n df = transcript.query('person==@user')[['time', 'offer', 'event', 'amount']]\n res = dict()\n \n for offer in portfolio['id'].values:\n # if informational offer, we count one transaction for completion\n if offer in portfolio.query('offer_type==\"informational\"').id.values:\n info_offer = True\n else:\n info_offer = False\n num_offer = df.query(f'offer==\"{offer}\" & event==\"offer received\"').index\n valid_hours = portfolio.query(f'id==\"{offer}\"')['duration'].values[0]*24\n offer_stats = []\n for segment in num_offer: \n dft = slice_df(df, segment, valid_hours)\n result = analyze_df(dft, offer, info_offer=info_offer)\n offer_stats.append(result)\n \n \n # summary over each offer\n result = {\n 'viewed': 0,\n 'completed': 0,\n 'other offer viewed': 0,\n 'amount': 0}\n \n # counting all offer for each promotion id\n result['received'] = len(num_offer)\n result['info'] = info_offer\n for stat in offer_stats:\n stat_v = list(stat.values())[0]\n result['viewed'] += stat_v['viewed']\n result['completed'] += stat_v['completed']\n result['other offer viewed'] += stat_v['other offer viewed']\n result['amount'] += stat_v['amount'] \n \n res[offer] = result\n \n return res",
"_____no_output_____"
],
[
"# iterate through all users and make a summary of transaction based on offer id\n# the would take 20 minutes to complete\ndef user_transaction(transcript=None, portfolio=None, save_file=True):\n info_res = dict()\n max_value = transcript['person'].nunique()\n i= 0\n with progressbar.ProgressBar(max_value=max_value) as bar:\n for person in transcript['person'].unique():\n person_ = info_offer(person, transcript, portfolio)\n info_res[person] = person_\n i +=1\n bar.update(i)\n df_info = pd.DataFrame.from_dict(data=info_res, orient='index')\n if save_file:\n# save to file, and save 20 minutes if we need to load them \n df_info.to_json('data/offer_summary.json')\n return df_info",
"_____no_output_____"
]
],
[
[
"If you want to test the grouping transaction with with person, this is a screenshot. Otherwise, change `LOAD_JSON` from `True` to `False` at the beginning of the file\n",
"_____no_output_____"
]
],
[
[
"# LOAD_JSON = False\nif not LOAD_JSON: \n import progressbar\n df_info = user_transaction(transcript=transcript, portfolio=portfolio)\n df_info\nelse:\n df_info = pd.read_json('data/offer_summary.json')",
"_____no_output_____"
],
[
"# make a short label from portfolio dataframe\nlabels = portfolio[['id', 'offer_type']].apply(\n lambda row: row['offer_type'][:4]+'_' + row['id'][-5:], axis=1).values\nlabels",
"_____no_output_____"
],
[
"# compare the total dollars spent for each transaction\ndf_amount = pd.DataFrame()\namounts = dict()\ndf_info.columns = labels\n\nfor offer in df_info.columns:\n df_amount[offer] = df_info[offer].apply(lambda x: x['amount']/x['received'] if x['received']>0 else np.NaN)\n\n# save average values to a dictionary\namounts['received'] = df_amount.describe().loc['mean'].to_dict()",
"_____no_output_____"
],
[
"# for viewed event\ndf_amount = pd.DataFrame()\n\n# average spending per transaction if offer NOT viewed\nfor offer in df_info.columns:\n df_amount[offer] = df_info[offer].apply(lambda x: x['amount'] if x['viewed']==0 else np.NaN)\namounts['not_viewed'] = df_amount.describe().loc['mean'].to_dict()\n\n# if offer was viewed\nfor offer in df_info.columns:\n df_amount[offer] = df_info[offer].apply(lambda x: x['amount']/x['viewed'] if x['viewed']>0 else np.NaN)\n\namounts['viewed'] = df_amount.describe().loc['mean'].to_dict()",
"_____no_output_____"
],
[
"# for transaction not completed\ndf_amount = pd.DataFrame()\n\nfor offer in df_info.columns:\n df_amount[offer] = df_info[offer].apply(lambda x: x['amount'] if x['completed']==0 else np.NaN)\namounts['not_completed'] = df_amount.describe().loc['mean'].to_dict()\n\n# and completed transaction\nfor offer in df_info.columns:\n df_amount[offer] = df_info[offer].apply(lambda x: x['amount']/x['completed'] if x['completed']>0 else np.NaN)\namounts['completed'] = df_amount.describe().loc['mean'].to_dict()",
"_____no_output_____"
],
[
"df_amount = pd.DataFrame().from_dict(amounts, orient='index')\ndf_amount",
"_____no_output_____"
],
[
"fig, ax = plt.subplots(figsize=(12,6))\ndf_amount.transpose().plot(kind='bar', ax=ax)\nax.legend(ncol=5)\nax.set_title('Amount purchased by offer id')\nax.set_ylabel('Average amount per offer, $')\nax.set_xticklabels(labels=df_amount.columns, rotation=0)\nax.grid()\nfig.tight_layout()\nfig.savefig('img/count_event.png', optimize=True, dpi=120)",
"_____no_output_____"
],
[
"df_amount.loc['viewed']/df_amount.loc['not_viewed']",
"_____no_output_____"
]
],
[
[
"remarks:\n - one transaction marked completion of informational offer is not sufficient to distinguish. All received offers were completed based on this assumption, which is minimal.\n - when a BOGO and discount offer was viewed, the total transaction during the offer was valid make a huge different to the offer not viewed. Similar observation with completed event on offer\n - the total dollar spent was 7 to 100 times more with offer that user saw. The precaution is that this is confounding with activeness of users. If users used the Starbucks product more, they should checked the app or promotion more offen, made more purchase in general. ",
"_____no_output_____"
],
[
"# Recommendation system\n\n## Approach\n\n\n- if a user is on the database and have completed of a few offesr, combining promotion by user history purchase and user similarity purchase\n- if a user is totally new to the app, recommend the top promotion by overall population",
"_____no_output_____"
]
],
[
[
"# load group transaction from json file\ndf_info = pd.read_json('data/offer_summary.json')\nencoded_profile = encoding_profile(df=profile)",
"_____no_output_____"
],
[
"def evaluate_similar_users(user_id, profile_df=None, \n info_df=None, sort_amount=False, n_top=100):\n '''evaluate similar users responded to promotions\n \n INPUT: a dataframe containing similar users responses to promotion\n \n OUTPUT: a dataframe sorted by sucessful rate of view, complete \n and average amount of purchase \n '''\n users = find_similar_users(user_id, df=profile_df, n_top=n_top)\n users = [item['user'] for item in users]\n df_users = info_df.loc[users]\n\n cols = df_users.columns\n ranking = dict()\n for col in cols:\n receives = df_users[col].apply(lambda x: x['received']).sum()\n completes = df_users[col].apply(lambda x: x['completed']).sum()\n views = df_users[col].apply(lambda x: x['viewed']).sum()\n amount_avg = df_users[col].apply(lambda x: x['amount']).mean()\n \n # rank metrics\n ranking[col] = {'rank': abs(1-completes**2/(views*receives)),\n 'amount': amount_avg}\n ranks = pd.DataFrame().from_dict(ranking,orient='index')\n \n if not sort_amount: # sort by completion rate first then amount\n ranks = ranks.sort_values(['rank', 'amount'], ascending=[True, False])\n else: # by amount first, then rank\n ranks = ranks.sort_values(['amount', 'rank'], ascending=[False, True])\n ranks = {k:round(v,2) for k,v in ranks['amount'].to_dict().items()} \n return ranks",
"_____no_output_____"
],
[
"def evaluate_user_history(user_id, df=None):\n '''evaluate user history preference by evaluating number of view and completion\n on each promotion\n \n INPUT: a user_id - a string encoded for each user\n df: a dataframe encoded user demographic\n \n OUTPUT: a ranking dictionary containing promotion id and amount of \n dollar purchased on offers that have number of views equals to number\n of completion\n '''\n \n dft = df.loc[user_id]\n user_hist = dict()\n for promotion in dft.index:\n dft_ = dft.loc[promotion]\n if dft_['viewed'] == dft_['completed'] > 0:\n user_hist[promotion] = dft_['amount']\n# print(dft.loc[promotion])\n user_hist = {k:v for k,v in sorted(user_hist.items(), \n key=lambda item: item[1],\n reverse=True)}\n return user_hist",
"_____no_output_____"
],
[
"def recommend_offers(user_id, profile_df=encoded_profile, info_df=df_info, sort_amount=False):\n '''recommend a few promotions for a user based on user history preference,\n group similarity, overall \n \n INPUT: user_id (str) - user identification on dataset\n \n OUTPUT: a dictionary of top three promotions with expected dollar spent\n '''\n \n # existing users:\n if user_id in profile_df.index:\n offers = evaluate_similar_users(user_id, profile_df=profile_df, \n info_df = info_df, sort_amount=sort_amount)\n user_pref = evaluate_user_history(user_id, df=info_df)\n \n # if user make a large dollar amount, the group preference will be updated\n for k,v in user_pref.items():\n if v > offers[k]:\n offers[k] = v\n if sort_amount:\n offers = {k:v for k,v in sorted(offers.items(), \n key=lambda item: item[1],\n reverse=True)}\n else: # new user\n offers = find_pop_offers(transcript, portfolio).set_index('id')['s_rate'].to_dict()\n \n offers = {k: round(v,2) for k,v in offers.items()}\n return offers",
"_____no_output_____"
]
],
[
[
"## Test recommendation",
"_____no_output_____"
]
],
[
[
"import random\nrandom.seed(2021)\nmax_idx = len(profile)",
"_____no_output_____"
],
[
"user_idx = random.randint(0, max_idx)\nuser_id = profile.iloc[user_idx].id\nuser_id\n# '3713b8ef49c541beaa07ed83ed0136d5'",
"_____no_output_____"
],
[
"# group preference\ngroup = evaluate_similar_users(user_id, profile_df=encoded_profile, \n info_df=df_info, sort_amount=True)\ngroup",
"_____no_output_____"
],
[
"# user preference\nuser = evaluate_user_history(user_id, df=df_info)\nuser",
"_____no_output_____"
],
[
"# based on both the user and similar users preference\nrecommend_offers(user_id=user_id, profile_df=encoded_profile, \n info_df=df_info, sort_amount=False)",
"_____no_output_____"
],
[
"recommend_offers(user_id=user_id, profile_df=encoded_profile, \n info_df=df_info, sort_amount=True)",
"_____no_output_____"
],
[
"# new user, this show the rate of completion \nrecommend_offers(user_id='new_user')",
"_____no_output_____"
]
],
[
[
"# Summary",
"_____no_output_____"
],
[
"- In this *simulated* dataset featuring Starbucks promotion. The dataset including three `json` files:\n\n - **portfolio**: a table of 10 rows by 6 columns listed 10 promotion id, four of them are discounts, four of them are BOGO (Buy One Get One free), and two are informational which is informing customer a new product or service\n - **profile**: a table of 17000 rows by 6 columns listed user demographic data such age, income, year of membership, user_id, gender\n - **transcript**: a table of 306 534 rows by 5 columns listed all transaction during simulating periods. This a main file with a long-table format that records when promotion is received, viewed or completed with a mount of dollar in each transaction\n\n\n- Data Exploration and Analysis: \n - this include exploratory steps on **portfolio** table. The portfolio data is important to refer to characteristics of each promotion. \n - analysis on **profile** were mainly on distribution by histogram using cleaning out abnormal values (age). The most important function built out of profile data is `find_similar_users` by ranking `nump.dot` of *encoded* user profile. This function is used to finding a similar group of users given a particular `user_id`.\n - high level summary on **transcript** is available without changing structure of the table such as the number of received, viewed, and completed events on each promotion. Similar to **movie recommender**, the transcript table contains information to build a `user-offer-matrix`\n\n\n- Attempt with Single Value Decomposition (SVD) for collaborative filtering\n - the `user-offer` matrix is the first step to reconstruct a **user-latent features** and **offer-latent features** to calculate **user preference's similarity** \n - I attempted `FunkSVD` and `Surprise SVD` to decompose `user-offer` matrix, but I failed to get reliable matrices. In fact, the algorithms did not able to \"converged\" resulted in a biased RMS or a NaN values\n\n\n- Grouping transaction by user\n - this is an alternative approach, in which each offer for each person was summarized based on *received, viewed, completed* events and transaction amount. The summary of each offer was then aggregated to `person-offer-stats` frame. We can use this dataframe to pull out amount of each transaction by each user, the completed rate on each transaction by user\n - one big caveat of this approach is a biased ranking. I ranked each transaction by two metrics:\n - rate of completion = 1- (\\#completed)**2)/(\\#viewed*\\#received)\n - and amount of dollar spent\n \n which is subjective to my selection. However, with the context of effectiveness of promotion, the first metrics seems resonable, and the second metrics would be find for business.\n \n\n- Recommendation system: a short summary, the function returns ranked dictionary from a mixed of **similar users** on **rate of completion** and **dolar spent** and **user history preference** if that user is **existed** in database; otherwise, the function returns a ranked dictionary based on `find_pop_offers` which is ranked **completion rate** based on the average of all users in this dataset. ",
"_____no_output_____"
]
]
] | [
"markdown",
"code",
"markdown",
"code",
"markdown",
"code",
"markdown",
"code",
"markdown",
"code",
"markdown",
"code",
"markdown",
"code",
"markdown",
"code",
"markdown",
"code",
"markdown",
"code",
"markdown",
"code",
"markdown",
"code",
"markdown",
"code",
"markdown",
"code",
"markdown",
"code",
"markdown",
"code",
"markdown",
"code",
"markdown",
"code",
"markdown",
"code",
"markdown",
"code",
"markdown"
] | [
[
"markdown",
"markdown",
"markdown",
"markdown",
"markdown"
],
[
"code",
"code",
"code",
"code"
],
[
"markdown"
],
[
"code"
],
[
"markdown",
"markdown"
],
[
"code",
"code",
"code",
"code",
"code",
"code",
"code",
"code",
"code"
],
[
"markdown"
],
[
"code",
"code",
"code"
],
[
"markdown"
],
[
"code",
"code",
"code",
"code",
"code",
"code",
"code",
"code"
],
[
"markdown"
],
[
"code",
"code",
"code",
"code",
"code",
"code",
"code",
"code",
"code",
"code",
"code",
"code",
"code",
"code",
"code",
"code",
"code",
"code"
],
[
"markdown"
],
[
"code",
"code",
"code",
"code",
"code",
"code",
"code"
],
[
"markdown",
"markdown"
],
[
"code",
"code",
"code",
"code",
"code",
"code",
"code",
"code",
"code",
"code",
"code",
"code",
"code",
"code"
],
[
"markdown",
"markdown"
],
[
"code",
"code",
"code",
"code",
"code",
"code",
"code",
"code",
"code",
"code"
],
[
"markdown"
],
[
"code",
"code",
"code",
"code"
],
[
"markdown"
],
[
"code",
"code",
"code"
],
[
"markdown",
"markdown"
],
[
"code",
"code",
"code",
"code",
"code",
"code"
],
[
"markdown"
],
[
"code",
"code",
"code",
"code",
"code",
"code"
],
[
"markdown",
"markdown",
"markdown"
],
[
"code",
"code",
"code",
"code",
"code",
"code",
"code",
"code",
"code",
"code",
"code"
],
[
"markdown"
],
[
"code"
],
[
"markdown"
],
[
"code",
"code",
"code",
"code",
"code",
"code",
"code",
"code",
"code"
],
[
"markdown"
],
[
"code",
"code",
"code",
"code",
"code",
"code",
"code"
],
[
"markdown"
],
[
"code",
"code",
"code",
"code",
"code",
"code",
"code",
"code"
],
[
"markdown",
"markdown"
],
[
"code",
"code",
"code",
"code"
],
[
"markdown"
],
[
"code",
"code",
"code",
"code",
"code",
"code",
"code"
],
[
"markdown",
"markdown"
]
] |
e778d52bbbd7376445a7bce18b81a8579fe7d273 | 11,401 | ipynb | Jupyter Notebook | Untitled.ipynb | Pomidorka1234/covid-19 | 2f2258c4747e8821340d526af0611e2d2e9f230c | [
"MIT"
] | null | null | null | Untitled.ipynb | Pomidorka1234/covid-19 | 2f2258c4747e8821340d526af0611e2d2e9f230c | [
"MIT"
] | null | null | null | Untitled.ipynb | Pomidorka1234/covid-19 | 2f2258c4747e8821340d526af0611e2d2e9f230c | [
"MIT"
] | null | null | null | 32.025281 | 117 | 0.379177 | [
[
[
"import pandas as pd\nimport plotly.graph_objects as go\nfrom datetime import datetime\nimport plotly\nimport france_data_management as data",
"_____no_output_____"
],
[
"df_capa = pd.read_csv(\"/Users/guillaumerozier/Downloads/sp-capa-quot-fra-2020-10-18-19h15.csv\", sep=\";\")",
"_____no_output_____"
],
[
"df, df_confirmed, dates, df_new, df_tests, df_deconf, df_sursaud, df_incid, df_tests_viros = data.import_data()",
"\n 0%| | 0/4 [00:00<?, ?it/s]\u001b[A\n 75%|███████▌ | 3/4 [00:01<00:00, 2.39it/s]\u001b[A"
],
[
"df_tests_viros = df_tests_viros.groupby([\"jour\"]).sum().reset_index()\ndf_tests = df_tests.groupby([\"jour\"]).sum().reset_index()",
"_____no_output_____"
],
[
"df_tests",
"_____no_output_____"
],
[
"title = \"<b>Estimations des cas</b> du Covid19 à partir des décès<br>\"\nsub = \"Hypothèses : taux de mortalité de 0,5 % ; décalage de 21 j. entre cas et décès\"\n\nfig = go.Figure()\n\n#estimated_rolling = df_france.diff().rolling(window=7).mean().shift(-21).dropna()/0.005\n#confirmed_rolling = df_france.diff().rolling(window=7, center=True).mean()\n\nfig.add_trace(go.Scatter(\n x = df_tests_viros[\"jour\"],\n y = df_tests_viros[\"T\"],\n name = \"Est.\",\n marker_color='black',\n line_width=6,\n opacity=0.6,\n fill='tozeroy',\n fillcolor=\"rgba(0,0,0,0.3)\",\n showlegend=False\n))\n\nfig.add_trace(go.Scatter(\n x = df_tests[\"jour\"],\n y = df_tests[\"nb_test\"],\n name = \"Conf\",\n marker_color='red',\n line_width=4,\n opacity=0.8,\n fill='tozeroy',\n fillcolor=\"rgba(201, 4, 4,0.3)\",\n showlegend=False\n))\n\nfig.update_yaxes(zerolinecolor='Grey', tickfont=dict(size=18))\nfig.update_xaxes(nticks=10, ticks='inside', tickangle=0, tickfont=dict(size=18))\n\n# Here we modify the tickangle of the xaxis, resulting in rotated labels.\nfig.update_layout(\n margin=dict(\n l=50,\n r=0,\n b=50,\n t=70,\n pad=0\n ),\n legend_orientation=\"h\",\n barmode='group',\n title={\n 'text': title,\n 'y':0.95,\n 'x':0.5,\n 'xanchor': 'center',\n 'yanchor': 'top'},\n titlefont = dict(\n size=30),\n xaxis=dict(\n title='',\n tickformat='%d/%m'),\n\n )\n\nfig.write_image(\"images/charts/france/{}.jpeg\".format(\"test_asuppr\"), scale=2, width=900, height=600)\n\n#plotly.offline.plot(fig, filename = 'images/html_exports/france/{}.html'.format(t), auto_open=False)\nprint(\"> \" + \"name_fig\")\n",
"> name_fig\n"
]
]
] | [
"code"
] | [
[
"code",
"code",
"code",
"code",
"code",
"code"
]
] |
e778e5a767360641edf3fa0b556abfea890fc80d | 57,653 | ipynb | Jupyter Notebook | simulation/portfolio-optimization.ipynb | juntaoduan/Stock-Prediction-Models | 0e963b4c599dc86bb4b88706e46971948a3b87f6 | [
"Apache-2.0"
] | null | null | null | simulation/portfolio-optimization.ipynb | juntaoduan/Stock-Prediction-Models | 0e963b4c599dc86bb4b88706e46971948a3b87f6 | [
"Apache-2.0"
] | null | null | null | simulation/portfolio-optimization.ipynb | juntaoduan/Stock-Prediction-Models | 0e963b4c599dc86bb4b88706e46971948a3b87f6 | [
"Apache-2.0"
] | 1 | 2021-06-15T16:53:20.000Z | 2021-06-15T16:53:20.000Z | 165.194842 | 48,380 | 0.881064 | [
[
[
"import numpy as np\nimport pandas as pd\nimport matplotlib.pyplot as plt\nimport seaborn as sns\nsns.set()",
"_____no_output_____"
],
[
"directory = '../dataset/'\nstocks = ['AMD.csv', 'FB.csv', 'TSLA.csv', 'TWTR.csv', 'MONDY.csv']\nstocks = [directory + s for s in stocks]\nstocks",
"_____no_output_____"
],
[
"dfs = [pd.read_csv(s)[['Date', 'Close']] for s in stocks]",
"_____no_output_____"
],
[
"from functools import reduce\ndata = reduce(lambda left,right: pd.merge(left,right,on='Date'), dfs).iloc[:, 1:]\ndata.head()",
"_____no_output_____"
],
[
"returns = data.pct_change()\nmean_daily_returns = returns.mean()\ncov_matrix = returns.cov()",
"_____no_output_____"
],
[
"cov_matrix",
"_____no_output_____"
],
[
"num_portfolios = 25000\nresults = np.zeros((3,num_portfolios))",
"_____no_output_____"
],
[
"for i in range(num_portfolios):\n weights = np.random.random(cov_matrix.shape[0])\n weights /= np.sum(weights)\n portfolio_return = np.sum(mean_daily_returns * weights) * 252\n portfolio_std_dev = np.sqrt(np.dot(weights.T,np.dot(cov_matrix, weights))) * np.sqrt(252)\n results[0,i] = portfolio_return\n results[1,i] = portfolio_std_dev\n results[2,i] = results[0,i] / results[1,i]",
"_____no_output_____"
],
[
"results_frame = pd.DataFrame(results.T,columns=['ret','stdev','sharpe'])",
"_____no_output_____"
],
[
"plt.figure(figsize = (7, 5))\nplt.scatter(results_frame.stdev,results_frame.ret,c=results_frame.sharpe,cmap='RdYlBu')\nplt.colorbar()\nplt.xlabel('volatility')\nplt.ylabel('returns')\nplt.show()",
"_____no_output_____"
]
]
] | [
"code"
] | [
[
"code",
"code",
"code",
"code",
"code",
"code",
"code",
"code",
"code",
"code"
]
] |
e778f3c6f8f25f2fe8ade443fc37ef839d2fd477 | 18,830 | ipynb | Jupyter Notebook | day3/aws.ipynb | NBISweden/workshop-advanced-python | 44c2b413389ed50e1bc6bbe501d77f952de1dd84 | [
"CC0-1.0"
] | 1 | 2021-11-07T16:09:39.000Z | 2021-11-07T16:09:39.000Z | day3/aws.ipynb | NBISweden/workshop-advanced-python | 44c2b413389ed50e1bc6bbe501d77f952de1dd84 | [
"CC0-1.0"
] | null | null | null | day3/aws.ipynb | NBISweden/workshop-advanced-python | 44c2b413389ed50e1bc6bbe501d77f952de1dd84 | [
"CC0-1.0"
] | 2 | 2021-04-27T07:01:59.000Z | 2021-12-01T21:40:34.000Z | 39.642105 | 455 | 0.621402 | [
[
[
"# Amazon web services (AWS)\n\n- [Loading data into S3 buckets](#Loading-data-into-S3-buckets)\n - via Console, CLI, Boto3\n- [Setting up an EC2 reserved instance](#Setting-up-a-reserved-instance)\n - via Console, CLI, Boto3\n- [Spin up containers via Docker Machine](#Spin-up-containers-via-Docker-Machine)\n- [Instance types](#Instance-types)\n- [ECS clusters and Docker Cloud](#ECS-clusters-and-Docker-Cloud)\n\nTODO:\n- (Make task) Getting Spark Python and Jupyter notebook running on Amazon EC2\n- https://medium.com/@josemarcialportilla/getting-spark-python-and-jupyter-notebook-running-on-amazon-ec2-dec599e1c297\n",
"_____no_output_____"
],
[
"## Introduction\n\nThere is not special reason to study AWS compared to Google Cloud, Azure, Digital Ocean etc. Amazon Cloud is probably the most popular today, so it offers a nice parralel to Python. AWS is the web-based gateway to the Amazon Cloud computing resources.\n\nOf note, AWS [will deploy a region in Sweden](https://aws.amazon.com/blogs/aws/coming-in-2018-new-aws-region-in-sweden/) this year, which will make it interesting for genomics research, especially since it will be made GDPR compliant. Currently no Swedish patient data can be processed on premises outside of Sweden, but the cloud is a player in general non-clinical research.\n\n[AWS](https://aws.amazon.com/) is an umbrella for a large number of computing resources, starting from storage and ending with the management of the remote computing infrastructure. To be practical, our focus is on loading data into a bucket, setting up a cloud instance, and later using Docker to remotely spin up cloud instances. We will also learn how to manage these resources via Python.",
"_____no_output_____"
],
[
"## Loading data into S3 buckets\n\nLet us start with loading data. This is a common operation when you want to share your research result with someone, but it can also be useful for yourself as a way to backup your data. Clouds use the concept of 'buckets' to hold data. The 'objects' stored in a bucket can have any encoding, from text to film. There used to be severe penalties on loading super massive objects. Today however, the maximum size for an object is 5TB (on AWS).\n\nWe will learn how to do this via the web console, via the command line interface and via Python. Note that even thogh these options seem like separated, they are actually using the same API.\n\n### Web Console\n\nTask:\n- Use the console to load a test file onto a S3 bucket\n- Follow this doc link: https://docs.aws.amazon.com/AmazonS3/latest/user-guide/upload-objects.html\n- Use the following shell command to generate some test data, or use your own:\n```\n$ for i in {1..5}; do echo \"l$i\">f$i.txt && gzip f$i.txt; done && \\\nzcat f*.txt.gz| gzip > f.gz\n```\n- Figure out how much your bucket would cost (tip: it is free up to a threshold)!\n\n### Amazon CLI\n\nNow let's repeat those steps using the command line interface. But first, we must install it.\n\nLinks: \n- https://docs.aws.amazon.com/cli/latest/userguide/using-s3-commands.html\n- https://aws.amazon.com/getting-started/tutorials/backup-to-s3-cli/\n\n```\n$ sudo apt install awscli\n$ aws configure\nAWS Access Key ID [None]: \nAWS Secret Access Key [None]:\n(also used eu-central-1 for region, and json as format)\n```\n\nThe above commang needs SSL certificates. To generate the aws keys:\n- https://docs.aws.amazon.com/AWSEC2/latest/UserGuide/set-up-ami-tools.html?icmpid=docs_iam_console#ami-tools-managing-certs\n\n```\n$ openssl genrsa 2048 > aws-private.pem\n$ openssl req -new -x509 -nodes -sha256 -days 365 -key aws-private.pem -outform PEM -out aws-certificate.pem\n\n# if in dire need for security use:\n$ sudo apt-get install xclip\n$ xclip -sel clip < ~/.ssh/aws-private.pem\n```\n\nNow that you installed the CLI, here are the main bucket related activities:\n\n```\naws s3 mb s3://my-first-backup-bucket\nupload:\naws s3 cp “C:\\users\\my first backup.bak” s3://my-first-backup-bucket/\ndownload:\naws s3 cp s3://my-first-backup-bucket/my-first-backup.bak ./\ndelete:\naws s3 rm s3://my-first-backup-bucket/my-first-backup.bak\n```\n\nData can also be streamed towards a bucket. This can be useful to avoid unnecesary space waste onto the local cloud or PC, but it can be just as useful when it comes to using bucket data without storing all that data locally. It can be done via piping, or proccess substitution:\n\n```\n$ aws s3 mb s3://siofuysni78\n$ zcat f*.txt.gz| gzip | aws s3 cp - s3://siofuysni78/f.gz\n$ aws s3 rm s3://siofuysni78/f.gz\n$ aws s3 rb s3://siofuysni78 --force\n```\n\nWhy did I use such a weird name? It is because Amazon indexes all buckets by their name, thus a name such as \"test123\" will never fly. Here is how to stream from S3 to your computing resource (it can be a cloud instance, you local machine or a remore server)\n\n```\n$ aws s3 mb s3://siofuysni78\n$ zcat f*.txt.gz| gzip | aws s3 cp - s3://siofuysni78/f.gz\n$ aws s3 cp s3://siofuysni78/f.gz - | gunzip | grep 1\nl1\n```\n\n### Boto3\n\n\nLinks:\n- http://boto3.readthedocs.io/en/latest/guide/migration.html#installation-configuration\n- https://boto3.readthedocs.io/en/latest/guide/s3-example-creating-buckets.html\n- http://boto3.readthedocs.io/en/latest/reference/services/s3.html\n\n\n```\nconda install -c anaconda boto3\npip install boto3\n```",
"_____no_output_____"
]
],
[
[
"import boto3\n\n# initialize the S3 service\ns3 = boto3.client('s3')\n\n# create a test bucket (tip: use a different name!)\ns3.create_bucket(Bucket='jo8a7fn8sfn8', CreateBucketConfiguration={'LocationConstraint': 'eu-central-1'})\n\n# Call S3 to list current buckets\nresponse = s3.list_buckets()\n\n# Get a list of all bucket names from the response\nbuckets = [bucket['Name'] for bucket in response['Buckets']]\n\n# Print out the bucket list\nprint(\"Bucket List: %s\" % buckets)",
"Bucket List: ['crasstestdummy', 'jo8a7fn8sfn8', 'siofuysni78', 'snlmocombined']\n"
],
[
"import boto3\n\n# Create an S3 client\ns3 = boto3.client('s3')\n\nfilename = '/path/to/test/file'\nbucket_name = 'jo8a7fn8sfn8'\n\n# Uploads the given file using a managed uploader, which will split up large\n# files automatically and upload parts in parallel.\ns3.upload_file(filename, bucket_name, filename)\n\n# or\n# s3.Object('mybucket', 'hello.txt').put(Body=open('/tmp/hello.txt', 'rb'))",
"_____no_output_____"
],
[
"# https://boto3.readthedocs.io/en/latest/guide/migrations3.html#deleting-a-bucket\nimport boto3\nimport botocore\n\ns3 = boto3.resource('s3')\nbucket = s3.Bucket('jo8a7fn8sfn8')\n\nfor key in bucket.objects.all():\n key.delete()\nbucket.delete()",
"_____no_output_____"
]
],
[
[
"\n\n\n\nNow I want to test using the buchet without local file storage.\n\n",
"_____no_output_____"
],
[
"## Setting up a reserved instance\n\nAmazon names their most popular instances Elastic Compute Cloud (EC2).\n- https://aws.amazon.com/ec2/\n- https://docs.aws.amazon.com/efs/latest/ug/gs-step-one-create-ec2-resources.html\n- https://docs.aws.amazon.com/AWSEC2/latest/UserGuide/get-set-up-for-amazon-ec2.html\n\nProbably the most basic level of access to the Amazon computing infrastructure is setting up a free tier reserved instance. \n\n### Web Console\n\nTask:\n- Setup an AWS instance using the Free Tier (don't forget to close it!).\n- [https://aws.amazon.com/console/](https://aws.amazon.com/console/)\n\n\n### Amazon CLI\n\n\n```\naws ec2 run-instances --image-id ami-xxxxxxxx --count 1 --instance-type t1.micro --key-name MyKeyPair --security-groups my-sg\n```\n\n \n### Boto3\n\n\n- http://boto3.readthedocs.io/en/latest/reference/services/ec2.html#instance\n- http://boto3.readthedocs.io/en/latest/guide/migrationec2.html#launching-new-instances\n\nTask:\n- A larger task is to create an instance with Boto3, install an SSH client such as Paramaiko and run commands on the remote client.\n\nHelpful code:",
"_____no_output_____"
]
],
[
[
"\nimport boto3\nimport botocore\nimport paramiko\n\n\nec2 = boto3.resource('ec2')\ninstance = ec2.Instance('id')\nec2.create_instances(ImageId='<ami-image-id>', MinCount=1, MaxCount=5)\n\nkey = paramiko.RSAKey.from_private_key_file(path/to/mykey.pem)\nclient = paramiko.SSHClient()\nclient.set_missing_host_key_policy(paramiko.AutoAddPolicy())\n\n# Connect/ssh to an instance\ntry:\n # Here 'ubuntu' is user name and 'instance_ip' is public IP of EC2\n client.connect(hostname=instance_ip, username=\"ubuntu\", pkey=key)\n\n # Execute a command(cmd) after connecting/ssh to an instance\n stdin, stdout, stderr = client.exec_command(cmd)\n print stdout.read()\n\n # close the client connection once the job is done\n client.close()\n break\n\nexcept Exception, e:\n print e",
"_____no_output_____"
]
],
[
[
"## Spin up containers via Docker Machine\n\nMy preferred way is to use docker machine in order to manage cloud instances that are already set-up with Docker. Then you can pull your intended container from the Docker Hub and run it on the instance. An alternative is usign AWS services to create your instance, which has its own benefits (basically most benefits except for time). Another alternative is usign Docker Cloud or Kubernetes, which is the way to go for multiple instances.\n\n\n```\n# install docker machine\n$ base=https://github.com/docker/machine/releases/download/v0.14.0 && \\\ncurl -L $base/docker-machine-$(uname -s)-$(uname -m) >/tmp/docker-machine && \\\nsudo install /tmp/docker-machine /usr/local/bin/docker-machine\n\n# setup a cloud instance\n$ export SECRET_KEY=\"...\"\n$ docker-machine create --driver amazonec2 --amazonec2-region eu-central-1 \\\n--amazonec2-access-key AKIAJPBEKSXQ7NJGSL3A \\\n--amazonec2-secret-key $SECRET_KEY \\\naws-test\n\n# ssh and delete\ndocker-machine ssh aws-test\ndocker-machine rm aws-test\n\n# for other options: --amazonec2-instance-type \"t2.2xlarge\"\ndocker-machine create --driver amazonec2\n```\n\n\n**Further read**\n\n- On Docker Machine:\n - https://docs.docker.com/machine/examples/aws/\n - https://docker-curriculum.com/\n - https://sreeninet.wordpress.com/2016/09/03/docker-machine-for-aws/\n - http://blog.wimwauters.com/docker-getting-started-with-docker-machine-aws/\n - http://docker-k8s-lab.readthedocs.io/en/latest/docker/docker-machine-aws.html\n- Using AWS alternative:\n - https://aws.amazon.com/getting-started/tutorials/deploy-docker-containers/\n - https://docs.aws.amazon.com/AmazonECS/latest/developerguide/docker-basics.html#docker-basics-create-image\n - https://docs.aws.amazon.com/AmazonECR/latest/userguide/docker-push-ecr-image.html\n- Or the more simplified use of Docker cloud, by linking to AWS. There are other, such as Kubernetes, but it is too complex (we only need one instance):\n - https://docs.docker.com/docker-cloud/infrastructure/link-aws/\n - https://docs.docker.com/docker-cloud/getting-started/your_first_node/\n\n",
"_____no_output_____"
],
[
"### Pull the docker hub container on the EC2 instance, open shell and run test\n\n```\n# run inside the EC2 instance\nexport DOCKER_ID_USER=\"grokkaine\"\ndocker login\ndocker pull $DOCKER_ID_USER/awstest\ndocker run -ti $DOCKER_ID_USER/awstest /bin/bash\n\n# now run your commands inside the container\n```\n\nThis works only if you must run short tasks, because once you log out from the container, the container will end. What you need is to be able to run long jobs. So we must create a detached container, then attach to it during and after the execution of the program with a shell in order to check the logs and save data.\n\n```\n# run inside the EC2 instance\nexport DOCKER_ID_USER=\"\"\nsudo docker login\nsudo docker pull $DOCKER_ID_USER/awscrass\nsudo docker run -w /home/ -tid $DOCKER_ID_USER/awscrass /bin/bash\n\n# exit container, start it\nsudo docker ps\n# run a command in detached mode\n#sudo docker exec -d containerid bash -c \"your command line\"\n\n#alternative is to log into the container and run the command there\nsudo docker exec -it containerid bash\n\n# start, attach\ndocker start containerid\ndocker attach containerid\n\n```\n\n",
"_____no_output_____"
],
[
"# Pricing\n\n- storage on S3 buckets:\n150 GB * 0.022$ /month = 3$\n- transfer:\n150 GB * 0.09$ /month = 13$\n- compute using m5.4xlarge (64GiB RAM) on demand instances:\n20 days * 0.9$/hour = 432$\n- compute using t2.2xlarge (32GiB RAM)\n20 days * 0.42$/hour = 201$\n\nFurther read:\n\n- autoscaling? https://aws.amazon.com/autoscaling/\n- https://docs.aws.amazon.com/AWSEC2/latest/UserGuide/EC2_GetStarted.html\n- https://docs.aws.amazon.com/AWSEC2/latest/UserGuide/Stop_Start.html\n- https://docs.aws.amazon.com/AWSEC2/latest/UserGuide/ec2-instance-resize.html",
"_____no_output_____"
],
[
"## Task\n\n\nYour task will be to create a Docker container, push it to Docker Hub, start an EC2 instance and remotely run your container, log out from the instance, then logging back and checking that the output is preserved.\n",
"_____no_output_____"
],
[
"## Instance Types\n\nOn AWS you can opt for different types of instances, and you can also upgrade or downgrade your instance to meet your need for resources. One can opt for example for instances that have a lot of RAM assigned when using a RAM intensive computation such as sequence alignment, or GPU instances when needing deep learning or other forms of GPU accelerated computing. More here: https://docs.aws.amazon.com/AWSEC2/latest/UserGuide/instance-types.html\n\nFrom the purchasing point of view there are however several major classes of instances, most notably:\n- On demand instances. Such instances are available when you request them and will be held up until you close them. - Spot instances. A Spot Instance is an unused EC2 instance that is available for less than the On-Demand price. Your Spot Instance runs whenever capacity is available and the maximum price per hour for your request exceeds the Spot price. \n- read about the other types here: https://docs.aws.amazon.com/AWSEC2/latest/UserGuide/instance-purchasing-options.html\n\n\n",
"_____no_output_____"
],
[
"## ECS clusters and Docker Cloud\n\n\nYou can run containerized clusters of EC2 instances using another AWS web service called ECS clusters. More information here: https://docs.aws.amazon.com/AmazonECS/latest/developerguide/ECS_clusters.html\n\nAnother popular option is to use Docker Cloud, allowing you to define and store images and build mechanisms of continuous integration then run tests or production clusters on AWS.\n\nTask:\n- Learn how to deply an Elastic HPC cluster: https://aws.amazon.com/getting-started/projects/deploy-elastic-hpc-cluster/\n\n",
"_____no_output_____"
]
]
] | [
"markdown",
"code",
"markdown",
"code",
"markdown"
] | [
[
"markdown",
"markdown",
"markdown"
],
[
"code",
"code",
"code"
],
[
"markdown",
"markdown"
],
[
"code"
],
[
"markdown",
"markdown",
"markdown",
"markdown",
"markdown",
"markdown"
]
] |
e77902794d691876ec0ae14ca37ecd31bdac3727 | 9,989 | ipynb | Jupyter Notebook | NLTK_korean.ipynb | creamcheesesteak/test_deeplearning | 141d7371d7d4257468d6db33f084357f83c0c85a | [
"Apache-2.0"
] | null | null | null | NLTK_korean.ipynb | creamcheesesteak/test_deeplearning | 141d7371d7d4257468d6db33f084357f83c0c85a | [
"Apache-2.0"
] | null | null | null | NLTK_korean.ipynb | creamcheesesteak/test_deeplearning | 141d7371d7d4257468d6db33f084357f83c0c85a | [
"Apache-2.0"
] | null | null | null | 36.724265 | 403 | 0.533287 | [
[
[
"<a href=\"https://colab.research.google.com/github/creamcheesesteak/test_deeplearning/blob/master/NLTK_korean.ipynb\" target=\"_parent\"><img src=\"https://colab.research.google.com/assets/colab-badge.svg\" alt=\"Open In Colab\"/></a>",
"_____no_output_____"
]
],
[
[
"import pandas as pd",
"_____no_output_____"
],
[
"!ls ./drive/MyDrive/Test01",
" boston_housing_withregression.ipynb NLTK_korean.ipynb\n CEO3.csv\t\t\t reuters_embeddinglayer_9.ipynb\n dff_sigmoid_softmax.ipynb\t reuters_LSTM.ipynb\n embeddinglayer.ipynb\t\t roc_curve.ipynb\n imdb_embedding.ipynb\t\t single_perceptron.ipynb\n imdb_LSTM_9.ipynb\t\t smtph_total.csv\n iris_classification.ipynb\t Untitled0.ipynb\n mnist_classification.ipynb\t Untitled1.ipynb\n multi_perceptron.ipynb\t\t Untitled2.ipynb\n'NLTK_english (1).ipynb'\t Untitled3.ipynb\n NLTK_english.ipynb\t\t win_deeplearning_practice.ipynb\n"
],
[
"!ls ./smtphP",
"_____no_output_____"
],
[
"df = pd.read_csv('./drive/MyDrive/Test01/smtph_total.csv')\ndf.head(5)",
"_____no_output_____"
],
[
"posts = df['Description']\nposts",
"_____no_output_____"
],
[
"!python -m pip install konlpy",
"_____no_output_____"
],
[
"!python -m pip install eunjeon",
"Collecting eunjeon\n Downloading eunjeon-0.4.0.tar.gz (34.7 MB)\n\u001b[K |████████████████████████████████| 34.7 MB 69 kB/s \n\u001b[33mWARNING: Discarding https://files.pythonhosted.org/packages/68/90/3232725f974abf6d38f1e2cfd7a6b958337133b3fdc5b3e8994e03d7c2d3/eunjeon-0.4.0.tar.gz#sha256=60865fbe28537e820ab864cd135467ea277c8182908e2bc364e83e5fd29ef07f (from https://pypi.org/simple/eunjeon/). Command errored out with exit status 1: python setup.py egg_info Check the logs for full command output.\u001b[0m\n\u001b[?25h Downloading eunjeon-0.3.9.6.tar.gz (34.7 MB)\n\u001b[K |████████████████████████████████| 34.7 MB 53 kB/s \n\u001b[33mWARNING: Discarding https://files.pythonhosted.org/packages/64/d3/743807b1ec82a99872ad785c5c244a6806ecbc3ba369cf09fdc1c4767509/eunjeon-0.3.9.6.tar.gz#sha256=d088714a537d999e16afb1cd3c1375c81fd1f03a169c47c95250ee5b704e1bd5 (from https://pypi.org/simple/eunjeon/). Command errored out with exit status 1: python setup.py egg_info Check the logs for full command output.\u001b[0m\n\u001b[?25h Downloading eunjeon-0.3.9.5.tar.gz (34.1 MB)\n\u001b[K |████████████████████████████████| 34.1 MB 75 kB/s \n\u001b[33mWARNING: Discarding https://files.pythonhosted.org/packages/aa/8e/909ee5bdb2e6e4255f5f9c80a8cf180adcc002bd319bde1aa9141416cf6e/eunjeon-0.3.9.5.tar.gz#sha256=556d99ce96f39dedd3e0f2470e6c45343740ba85a27ef34c2b6d8c61f57c7388 (from https://pypi.org/simple/eunjeon/). Command errored out with exit status 1: python setup.py egg_info Check the logs for full command output.\u001b[0m\n\u001b[?25h Downloading eunjeon-0.3.9.4.tar.gz (33.2 MB)\n\u001b[K |████████████████████████████████| 33.2 MB 45 kB/s \n\u001b[33mWARNING: Discarding https://files.pythonhosted.org/packages/16/d1/804f0322c6b2715ddb0c5f47d78c03e5fcdbe252a0d5c4f4b0936c2bdf46/eunjeon-0.3.9.4.tar.gz#sha256=9032fe0860fb4d4edec4113a4258f2599fea5df46e0e86413d7ffdf8c6adbee9 (from https://pypi.org/simple/eunjeon/). Command errored out with exit status 1: python setup.py egg_info Check the logs for full command output.\u001b[0m\n\u001b[?25h Downloading eunjeon-0.3.9.3.tar.gz (33.2 MB)\n\u001b[K |████████████████████████████████| 33.2 MB 45 kB/s \n\u001b[33mWARNING: Discarding https://files.pythonhosted.org/packages/e3/24/c1c6bf9d0adfcf5a49ed1adb3d3a45fd014c6d9621b5ac7bec1fde4d4666/eunjeon-0.3.9.3.tar.gz#sha256=4004da49581b44b14746521e20a69e1a08acec1778a1ccfa59b7442c16a86490 (from https://pypi.org/simple/eunjeon/). Command errored out with exit status 1: python setup.py egg_info Check the logs for full command output.\u001b[0m\n\u001b[?25h Downloading eunjeon-0.3.9.0.tar.gz (33.2 MB)\n\u001b[K |████████████████████████████████| 33.2 MB 57 kB/s \n\u001b[33mWARNING: Discarding https://files.pythonhosted.org/packages/08/df/7981c91ccea41cbabded1a28cb36442b8ff75a355aa77a2ef65272fa1472/eunjeon-0.3.9.0.tar.gz#sha256=46c96b1797211e179e3e61d90c7a1aa86a34cdba825a0979b8f83480a90adf21 (from https://pypi.org/simple/eunjeon/). Command errored out with exit status 1: python setup.py egg_info Check the logs for full command output.\u001b[0m\n\u001b[31mERROR: Could not find a version that satisfies the requirement eunjeon (from versions: 0.3.9.0, 0.3.9.3, 0.3.9.4, 0.3.9.5, 0.3.9.6, 0.4.0)\u001b[0m\n\u001b[31mERROR: No matching distribution found for eunjeon\u001b[0m\n\u001b[?25h"
],
[
"from konlpy.tag import Mecab\ntagger = Mecab()",
"_____no_output_____"
],
[
"",
"_____no_output_____"
],
[
"",
"_____no_output_____"
],
[
"",
"_____no_output_____"
],
[
"",
"_____no_output_____"
],
[
"",
"_____no_output_____"
],
[
"",
"_____no_output_____"
]
]
] | [
"markdown",
"code"
] | [
[
"markdown"
],
[
"code",
"code",
"code",
"code",
"code",
"code",
"code",
"code",
"code",
"code",
"code",
"code",
"code",
"code"
]
] |
e77924d47d02b6b3260c29996692c83fd95e66e1 | 959,338 | ipynb | Jupyter Notebook | Python Analysis/Visualisations/Code/Visualisation_TFR_and_FLPR_of_Highest_and_Lowest_HDI_countries.ipynb | ChangHuaHua/QM2-Group-12 | fdad787087c403236243fdead96345a7a80df873 | [
"MIT"
] | null | null | null | Python Analysis/Visualisations/Code/Visualisation_TFR_and_FLPR_of_Highest_and_Lowest_HDI_countries.ipynb | ChangHuaHua/QM2-Group-12 | fdad787087c403236243fdead96345a7a80df873 | [
"MIT"
] | null | null | null | Python Analysis/Visualisations/Code/Visualisation_TFR_and_FLPR_of_Highest_and_Lowest_HDI_countries.ipynb | ChangHuaHua/QM2-Group-12 | fdad787087c403236243fdead96345a7a80df873 | [
"MIT"
] | null | null | null | 176.934342 | 46,206 | 0.828198 | [
[
[
"<a href=\"https://colab.research.google.com/github/ChangHuaHua/QM2-Group-12/blob/main/Visualisation_TFR_and_FLPR_of_Highest_and_Lowest_HDI_countries.ipynb\" target=\"_parent\"><img src=\"https://colab.research.google.com/assets/colab-badge.svg\" alt=\"Open In Colab\"/></a>",
"_____no_output_____"
],
[
"Creating two graphs including the trends of TFR and FLPR. One graph includes 3 countries with the highest current HDI and the other graph includes 3 with the lowest current HDI.",
"_____no_output_____"
],
[
"**Importing Libraries and Uploading Files**",
"_____no_output_____"
]
],
[
[
"import matplotlib.pyplot as plt\r\nimport pandas as pd\r\nimport pylab\r\n%matplotlib inline\r\npylab.rcParams['figure.figsize'] = (10., 8.)",
"_____no_output_____"
],
[
"from google.colab import files\r\nuploaded = files.upload()\r\n#upload Africa_FULL_MERGE.csv file",
"_____no_output_____"
],
[
"data = 'Africa_FULL_MERGE.csv'\r\nafrica = pd.read_csv(data)\r\nafrica",
"_____no_output_____"
],
[
"africa['HDI'] = pd.to_numeric(africa['HDI'], errors = 'coerce')\r\nafrica['HDI Rank (2018)'] = pd.to_numeric(africa['HDI Rank (2018)'], errors = 'coerce')",
"_____no_output_____"
]
],
[
[
"**3 Highest and Lowest HDI**",
"_____no_output_____"
]
],
[
[
"africa_2018 = africa[africa['Year'] == 2018]\r\nafrica_2018 = africa_2018.reset_index(drop=True)\r\nafrica_2018",
"_____no_output_____"
],
[
"africa_string = africa_2018['HDI'].astype(float)\r\nafrica_string.nlargest(3)",
"_____no_output_____"
],
[
"# corresponding countries to index numbers 43, 35, and 3 are:\r\n# 43 Seychelles 0.801\r\n# 35 Mauritius 0.796\r\n# 3 Algeria 0.759",
"_____no_output_____"
],
[
"africa_string.nsmallest(3)",
"_____no_output_____"
],
[
"# corresponding countries to index numbers 38, 13, and 14 are:\r\n# 38 Niger 0.377\r\n# 13 Central African Republic 0.381\r\n# 14 Chad 0.401",
"_____no_output_____"
]
],
[
[
"**Segregating Dataframe**",
"_____no_output_____"
]
],
[
[
"africa_seychelles = africa[africa['Country'] == 'Seychelles']\r\nafrica_mauritius = africa[africa['Country'] == 'Mauritius']\r\nafrica_algeria = africa[africa['Country'] == 'Algeria']\r\nafrica_niger = africa[africa['Country'] == 'Niger']\r\nafrica_CAR = africa[africa['Country'] == 'Central African Republic']\r\nafrica_chad = africa[africa['Country'] == 'Chad']",
"_____no_output_____"
],
[
"frames = [africa_seychelles, africa_mauritius, africa_algeria]\r\nafrica_high = pd.concat(frames)\r\nafrica_high.reset_index(drop=True)",
"_____no_output_____"
],
[
"frames_2 = [africa_niger, africa_CAR, africa_chad]\r\nafrica_low = pd.concat(frames_2)\r\nafrica_low.reset_index(drop=True)",
"_____no_output_____"
]
],
[
[
"**Getting Values for Analysis**",
"_____no_output_____"
]
],
[
[
"africa_algeria[africa_algeria['Year']==1960]",
"_____no_output_____"
],
[
"africa_algeria[africa_algeria['Year']==1990]",
"_____no_output_____"
],
[
"africa_algeria[africa_algeria['Year']==2001]",
"_____no_output_____"
],
[
"africa_algeria[africa_algeria['Year']==2002]",
"_____no_output_____"
],
[
"africa_algeria[africa_algeria['Year']==2003]",
"_____no_output_____"
],
[
"africa_algeria[africa_algeria['Year']==2017]",
"_____no_output_____"
],
[
"africa_algeria[africa_algeria['Year']==2018]",
"_____no_output_____"
],
[
"africa_mauritius[africa_mauritius['Year']==1960]",
"_____no_output_____"
],
[
"africa_mauritius[africa_mauritius['Year']==1990]",
"_____no_output_____"
],
[
"africa_mauritius[africa_mauritius['Year']==1985]",
"_____no_output_____"
],
[
"africa_mauritius[africa_mauritius['Year']==1986]",
"_____no_output_____"
],
[
"africa_mauritius[africa_mauritius['Year']==1987]",
"_____no_output_____"
],
[
"africa_mauritius[africa_mauritius['Year']==1989]",
"_____no_output_____"
],
[
"africa_mauritius[africa_mauritius['Year']==2017]",
"_____no_output_____"
],
[
"africa_mauritius[africa_mauritius['Year']==2018]",
"_____no_output_____"
],
[
"africa_seychelles[africa_seychelles['Year']==1990]",
"_____no_output_____"
],
[
"africa_seychelles[africa_seychelles['Year']==2018]",
"_____no_output_____"
],
[
"africa_CAR[africa_CAR['Year']==1990]",
"_____no_output_____"
],
[
"africa_CAR[africa_CAR['Year']==1998]",
"_____no_output_____"
],
[
"africa_CAR[africa_CAR['Year']==1999]",
"_____no_output_____"
],
[
"africa_CAR[africa_CAR['Year']==2000]",
"_____no_output_____"
],
[
"africa_CAR[africa_CAR['Year']==2018]",
"_____no_output_____"
],
[
"africa_chad[africa_chad['Year']==1990]",
"_____no_output_____"
],
[
"africa_chad[africa_chad['Year']==1995]",
"_____no_output_____"
],
[
"africa_chad[africa_chad['Year']==1996]",
"_____no_output_____"
],
[
"africa_chad[africa_chad['Year']==1997]",
"_____no_output_____"
],
[
"africa_chad[africa_chad['Year']==1998]",
"_____no_output_____"
],
[
"africa_chad[africa_chad['Year']==1999]",
"_____no_output_____"
],
[
"africa_chad[africa_chad['Year']==2000]",
"_____no_output_____"
],
[
"africa_chad[africa_chad['Year']==2018]",
"_____no_output_____"
],
[
"africa_niger[africa_niger['Year']==1990]",
"_____no_output_____"
],
[
"africa_niger[africa_niger['Year']==2018]",
"_____no_output_____"
]
],
[
[
"**Creating Visualisations**\r\n\r\nThe darker the colour, the higher the HDI\r\nRed = highest HDI countries\r\nBlue = lowest HDI countries",
"_____no_output_____"
],
[
"3 Highest HDI Countries",
"_____no_output_____"
]
],
[
[
"ax = africa_high.loc[africa_high['Country']=='Seychelles'].plot(x='Year', y= 'TFR', kind='scatter', c = 'maroon', label = 'Seychelles')\r\nafrica_high.loc[africa_high['Country']=='Mauritius'].plot(x='Year', y= 'TFR', kind='scatter', c = 'red', ax=ax, label = 'Mauritius')\r\nafrica_high.loc[africa_high['Country']=='Algeria'].plot(x='Year', y= 'TFR', kind='scatter', c = 'lightcoral', ax=ax, label = 'Algeria')\r\nax.legend(loc='upper right')\r\nax.set_title('3 Highest HDI Countries: TFR 1960-2018')\r\nax.figure.savefig('Scatter Plot 3 Highest HDI Countries: TFR 1960-2018.png')\r\n#TFR of 3 highest HDI countries",
"_____no_output_____"
],
[
"ax = africa_high.loc[africa_high['Country']=='Seychelles'].plot(x='Year', y='TFR', kind='line', c = 'maroon', label = 'Seychelles')\r\nafrica_high.loc[africa_high['Country']=='Mauritius'].plot(x='Year', y='TFR', kind='line', c = 'red', ax=ax, label = 'Mauritius')\r\nafrica_high.loc[africa_high['Country']=='Algeria'].plot(x='Year', y='TFR', kind='line', c = 'lightcoral', ax=ax, label = 'Algeria')\r\nax.set_ylabel('TFR')\r\nax.legend(loc='upper right')\r\nax.set_title('3 Highest HDI Countries: TFR 1960-2020')\r\nax.figure.savefig('Line Plot 3 Highest HDI Countries: TFR 1960-2018.png')\r\n#TFR of 3 highest HDI countries",
"_____no_output_____"
],
[
"ax = africa_high.loc[africa_high['Country']=='Seychelles'].plot(x='Year', y= 'Labor force participation rate, female (% of female population ages 15+) (modeled ILO estimate)', kind='scatter', c = 'maroon', label = 'Seychelles')\r\nafrica_high.loc[africa_high['Country']=='Mauritius'].plot(x='Year', y= 'Labor force participation rate, female (% of female population ages 15+) (modeled ILO estimate)', kind='scatter', c = 'red', ax=ax, label = 'Mauritius')\r\nafrica_high.loc[africa_high['Country']=='Algeria'].plot(x='Year', y= 'Labor force participation rate, female (% of female population ages 15+) (modeled ILO estimate)', kind='scatter', c = 'lightcoral', ax=ax, label = 'Algeria')\r\nax.legend(loc='best')\r\nax.set_title('3 Highest HDI Countries: FLPR 1990-2017')\r\nax.figure.savefig('Scatter Plot 3 Highest HDI Countries: FLPR 1990-2017.png')\r\n#FLPR of 3 highest HDI countries",
"_____no_output_____"
],
[
"ax = africa_high.loc[africa_high['Country']=='Seychelles'].plot(x='Year', y= 'Labor force participation rate, female (% of female population ages 15+) (modeled ILO estimate)', kind='line', c = 'maroon', label = 'Seychelles')\r\nafrica_high.loc[africa_high['Country']=='Mauritius'].plot(x='Year', y= 'Labor force participation rate, female (% of female population ages 15+) (modeled ILO estimate)', kind='line', c = 'red', ax=ax, label = 'Mauritius')\r\nafrica_high.loc[africa_high['Country']=='Algeria'].plot(x='Year', y= 'Labor force participation rate, female (% of female population ages 15+) (modeled ILO estimate)', kind='line', c = 'lightcoral', ax=ax, label = 'Algeria')\r\nax.set_ylabel('Labor force participation rate, female (% of female population ages 15+) (modeled ILO estimate)')\r\nax.legend(loc='best')\r\nax.set_title('3 Highest HDI Countries: FLPR 1990-2017')\r\nax.figure.savefig('Line Plot 3 Highest HDI Countries: FLPR 1990-2017.png')\r\n#FLPR of 3 highest HDI countries",
"_____no_output_____"
],
[
"ax = africa_high.loc[africa_high['Country']=='Seychelles'].plot(x='Year', y= 'HDI', kind='scatter', c = 'maroon', label = 'Seychelles')\r\nafrica_high.loc[africa_high['Country']=='Mauritius'].plot(x='Year', y= 'HDI', kind='scatter', c = 'red', ax=ax, label = 'Mauritius')\r\nafrica_high.loc[africa_high['Country']=='Algeria'].plot(x='Year', y= 'HDI', kind='scatter', c = 'lightcoral', ax=ax, label = 'Algeria')\r\nax.legend(loc='best')\r\nax.set_title('3 Highest HDI Countries: HDI 1990-2018')\r\nax.figure.savefig('Scatter Plot 3 Highest HDI Countries: HDI 1990-2018.png')\r\n#HDI of 3 highest HDI countries",
"_____no_output_____"
],
[
"ax = africa_high.loc[africa_high['Country']=='Seychelles'].plot(x='Year', y= 'HDI', kind='line', c = 'maroon', label = 'Seychelles')\r\nafrica_high.loc[africa_high['Country']=='Mauritius'].plot(x='Year', y= 'HDI', kind='line', c = 'red', ax=ax, label = 'Mauritius')\r\nafrica_high.loc[africa_high['Country']=='Algeria'].plot(x='Year', y= 'HDI', kind='line', c = 'lightcoral', ax=ax, label = 'Algeria')\r\nax.set_ylabel('HDI')\r\nax.legend(loc='best')\r\nax.set_title('3 Highest HDI Countries: HDI 1990-2018')\r\nax.figure.savefig('Line Plot 3 Highest HDI Countries: HDI 1990-2018.png')\r\n#HDI of 3 highest HDI countries",
"_____no_output_____"
],
[
"ax = africa_high.loc[africa_high['Country']=='Seychelles'].plot(x='HDI', y= 'TFR', kind='scatter', c = 'maroon', label = 'Seychelles')\r\nafrica_high.loc[africa_high['Country']=='Mauritius'].plot(x='HDI', y= 'TFR', kind='scatter', c = 'red', ax=ax, label = 'Mauritius')\r\nafrica_high.loc[africa_high['Country']=='Algeria'].plot(x='HDI', y= 'TFR', kind='scatter', c = 'lightcoral', ax=ax, label = 'Algeria')\r\nax.legend(loc='best')\r\nax.set_title('3 Highest HDI Countries: HDI vs TFR')\r\nax.figure.savefig('Scatter Plot 3 Highest HDI Countries: HDI vs TFR.png')\r\n#HDI vs TFR of 3 highest HDI countries",
"_____no_output_____"
],
[
"ax = africa_high.loc[africa_high['Country']=='Seychelles'].plot(x='HDI', y= 'TFR', kind='line', c = 'maroon', label = 'Seychelles')\r\nafrica_high.loc[africa_high['Country']=='Mauritius'].plot(x='HDI', y= 'TFR', kind='line', c = 'red', ax=ax, label = 'Mauritius')\r\nafrica_high.loc[africa_high['Country']=='Algeria'].plot(x='HDI', y= 'TFR', kind='line', c = 'lightcoral', ax=ax, label = 'Algeria')\r\nax.set_ylabel('TFR')\r\nax.legend(loc='best')\r\nax.set_title('3 Highest HDI Countries: HDI vs TFR')\r\nax.figure.savefig('Line Plot 3 Highest HDI Countries: HDI vs TFR.png')\r\n#HDI vs TFR of 3 highest HDI countries",
"_____no_output_____"
],
[
"ax = africa_high.loc[africa_high['Country']=='Seychelles'].plot(x='HDI', y= 'Labor force participation rate, female (% of female population ages 15+) (modeled ILO estimate)', kind='scatter', c = 'maroon', label = 'Seychelles')\r\nafrica_high.loc[africa_high['Country']=='Mauritius'].plot(x='HDI', y= 'Labor force participation rate, female (% of female population ages 15+) (modeled ILO estimate)', kind='scatter', c = 'red', ax=ax, label = 'Mauritius')\r\nafrica_high.loc[africa_high['Country']=='Algeria'].plot(x='HDI', y= 'Labor force participation rate, female (% of female population ages 15+) (modeled ILO estimate)', kind='scatter', c = 'lightcoral', ax=ax, label = 'Algeria')\r\nax.legend(loc='best')\r\nax.set_title('3 Highest HDI Countries: HDI vs FLPR')\r\nax.figure.savefig('Scatter Plot 3 Highest HDI Countries: HDI vs FLPR.png')\r\n#HDI vs FLPR of 3 highest HDI countries",
"_____no_output_____"
],
[
"ax = africa_high.loc[africa_high['Country']=='Seychelles'].plot(x='HDI', y= 'Labor force participation rate, female (% of female population ages 15+) (modeled ILO estimate)', kind='line', c = 'maroon', label = 'Seychelles')\r\nafrica_high.loc[africa_high['Country']=='Mauritius'].plot(x='HDI', y= 'Labor force participation rate, female (% of female population ages 15+) (modeled ILO estimate)', kind='line', c = 'red', ax=ax, label = 'Mauritius')\r\nafrica_high.loc[africa_high['Country']=='Algeria'].plot(x='HDI', y= 'Labor force participation rate, female (% of female population ages 15+) (modeled ILO estimate)', kind='line', c = 'lightcoral', ax=ax, label = 'Algeria')\r\nax.set_ylabel('Labor force participation rate, female (% of female population ages 15+) (modeled ILO estimate)')\r\nax.legend(loc='best')\r\nax.set_title('3 Highest HDI Countries: HDI vs FLPR')\r\nax.figure.savefig('Line Plot 3 Highest HDI Countries: HDI vs FLPR.png')\r\n#HDI vs FLPR of 3 highest HDI countries",
"_____no_output_____"
],
[
"ax = africa_high.loc[africa_high['Country']=='Seychelles'].plot(x='TFR', y= 'Labor force participation rate, female (% of female population ages 15+) (modeled ILO estimate)', kind='scatter', c = 'maroon', label = 'Seychelles')\r\nafrica_high.loc[africa_high['Country']=='Mauritius'].plot(x='TFR', y= 'Labor force participation rate, female (% of female population ages 15+) (modeled ILO estimate)', kind='scatter', c = 'red', ax=ax, label = 'Mauritius')\r\nafrica_high.loc[africa_high['Country']=='Algeria'].plot(x='TFR', y= 'Labor force participation rate, female (% of female population ages 15+) (modeled ILO estimate)', kind='scatter', c = 'lightcoral', ax=ax, label = 'Algeria')\r\nax.legend(loc='best')\r\nax.set_title('3 Highest HDI Countries: TFR vs FLPR')\r\nax.figure.savefig('Scatter Plot 3 Highest HDI Countries: TFR vs FLPR.png')\r\n#TFR vs FLPR of 3 highest HDI countries",
"_____no_output_____"
],
[
"ax = africa_high.loc[africa_high['Country']=='Seychelles'].plot(x='TFR', y= 'Labor force participation rate, female (% of female population ages 15+) (modeled ILO estimate)', kind='line', c = 'maroon', label = 'Seychelles')\r\nafrica_high.loc[africa_high['Country']=='Mauritius'].plot(x='TFR', y= 'Labor force participation rate, female (% of female population ages 15+) (modeled ILO estimate)', kind='line', c = 'red', ax=ax, label = 'Mauritius')\r\nafrica_high.loc[africa_high['Country']=='Algeria'].plot(x='TFR', y= 'Labor force participation rate, female (% of female population ages 15+) (modeled ILO estimate)', kind='line', c = 'lightcoral', ax=ax, label = 'Algeria')\r\nax.set_ylabel('Labor force participation rate, female (% of female population ages 15+) (modeled ILO estimate)')\r\nax.legend(loc='best')\r\nax.set_title('3 Highest HDI Countries: TFR vs FLPR')\r\nax.figure.savefig('Line Plot 3 Highest HDI Countries: TFR vs FLPR.png')\r\n#TFR vs FLPR of 3 highest HDI countries",
"_____no_output_____"
]
],
[
[
"3 Lowest HDI Countries",
"_____no_output_____"
]
],
[
[
"ax = africa_low.loc[africa_low['Country']=='Niger'].plot(x='Year', y= 'TFR', kind='scatter', c = 'cornflowerblue', label = 'Niger')\r\nafrica_low.loc[africa_low['Country']=='Central African Republic'].plot(x='Year', y= 'TFR', kind='scatter', c = 'mediumblue', ax=ax, label = 'Central African Republic')\r\nafrica_low.loc[africa_low['Country']=='Chad'].plot(x='Year', y= 'TFR', kind='scatter', c = 'navy', ax=ax, label = 'Chad')\r\nax.legend(loc='best')\r\nax.set_title('3 Lowest HDI Countries: TFR 1960-2018')\r\nax.figure.savefig('Scatter Plot 3 Lowest HDI Countries: TFR 1960-2018.png')\r\n#TFR of 3 lowest HDI countries",
"_____no_output_____"
],
[
"ax = africa_low.loc[africa_low['Country']=='Niger'].plot(x='Year', y= 'TFR', kind='line', c = 'cornflowerblue', label = 'Niger')\r\nafrica_low.loc[africa_low['Country']=='Central African Republic'].plot(x='Year', y= 'TFR', kind='line', c = 'mediumblue', ax=ax, label = 'Central African Republic')\r\nafrica_low.loc[africa_low['Country']=='Chad'].plot(x='Year', y= 'TFR', kind='line', c = 'navy', ax=ax, label = 'Chad')\r\nax.set_ylabel('TFR')\r\nax.legend(loc='best')\r\nax.set_title('3 Lowest HDI Countries: TFR 1960-2018')\r\nax.figure.savefig('Line Plot 3 Lowest HDI Countries: TFR 1960-2018.png')\r\n#TFR of 3 lowest HDI countries",
"_____no_output_____"
],
[
"ax = africa_low.loc[africa_low['Country']=='Niger'].plot(x='Year', y= 'Labor force participation rate, female (% of female population ages 15+) (modeled ILO estimate)', kind='scatter', c = 'cornflowerblue', label = 'Niger')\r\nafrica_low.loc[africa_low['Country']=='Central African Republic'].plot(x='Year', y= 'Labor force participation rate, female (% of female population ages 15+) (modeled ILO estimate)', kind='scatter', c = 'mediumblue', ax=ax, label = 'Central African Republic')\r\nafrica_low.loc[africa_low['Country']=='Chad'].plot(x='Year', y= 'Labor force participation rate, female (% of female population ages 15+) (modeled ILO estimate)', kind='scatter', c = 'navy', ax=ax, label = 'Chad')\r\nax.legend(loc='best')\r\nax.set_title('3 Lowest HDI Countries: FLPR 1990-2017')\r\nax.figure.savefig('Scatter Plot 3 Lowest HDI Countries: FLPR 1990-2017.png')\r\n#FLPR of 3 lowest HDI countries",
"_____no_output_____"
],
[
"ax = africa_low.loc[africa_low['Country']=='Niger'].plot(x='Year', y= 'Labor force participation rate, female (% of female population ages 15+) (modeled ILO estimate)', kind='line', c = 'cornflowerblue', label = 'Niger')\r\nafrica_low.loc[africa_low['Country']=='Central African Republic'].plot(x='Year', y= 'Labor force participation rate, female (% of female population ages 15+) (modeled ILO estimate)', kind='line', c = 'mediumblue', ax=ax, label = 'Central African Republic')\r\nafrica_low.loc[africa_low['Country']=='Chad'].plot(x='Year', y= 'Labor force participation rate, female (% of female population ages 15+) (modeled ILO estimate)', kind='line', c = 'navy', ax=ax, label = 'Chad')\r\nax.set_ylabel('Labor force participation rate, female (% of female population ages 15+) (modeled ILO estimate)')\r\nax.legend(loc='best')\r\nax.set_title('3 Lowest HDI Countries: FLPR 1990-2017')\r\nax.figure.savefig('Line Plot 3 Lowest HDI Countries: FLPR 1990-2017.png')\r\n#FLPR of 3 lowest HDI countries",
"_____no_output_____"
],
[
"ax = africa_low.loc[africa_low['Country']=='Niger'].plot(x='Year', y= 'HDI', kind='scatter', c = 'cornflowerblue', label = 'Niger')\r\nafrica_low.loc[africa_low['Country']=='Central African Republic'].plot(x='Year', y= 'HDI', kind='scatter', c = 'mediumblue', ax=ax, label = 'Central African Republic')\r\nafrica_low.loc[africa_low['Country']=='Chad'].plot(x='Year', y= 'HDI', kind='scatter', c = 'navy', ax=ax, label = 'Chad')\r\nax.set_ylabel('HDI')\r\nax.legend(loc='lower right')\r\nax.set_title('3 Lowest HDI Countries: HDI 1990-2018')\r\nax.figure.savefig('Scatter Plot 3 Lowest HDI Countries: HDI 1990-2018.png')\r\n#HDI of 3 lowest HDI countries",
"_____no_output_____"
],
[
"ax = africa_low.loc[africa_low['Country']=='Niger'].plot(x='Year', y= 'HDI', kind='line', c = 'cornflowerblue', label = 'Niger')\r\nafrica_low.loc[africa_low['Country']=='Central African Republic'].plot(x='Year', y= 'HDI', kind='line', c = 'mediumblue', ax=ax, label = 'Central African Republic')\r\nafrica_low.loc[africa_low['Country']=='Chad'].plot(x='Year', y= 'HDI', kind='line', c = 'navy', ax=ax, label = 'Chad')\r\nax.set_ylabel('HDI')\r\nax.legend(loc='lower right')\r\nax.set_title('3 Lowest HDI Countries: HDI 1990-2018')\r\nax.figure.savefig('Line Plot 3 Lowest HDI Countries: HDI 1990-2018.png')\r\n#HDI of 3 lowest HDI countries",
"_____no_output_____"
],
[
"ax = africa_low.loc[africa_low['Country']=='Niger'].plot(x='HDI', y= 'TFR', kind='scatter', c = 'cornflowerblue', label = 'Niger')\r\nafrica_low.loc[africa_low['Country']=='Central African Republic'].plot(x='HDI', y= 'TFR', kind='scatter', c = 'mediumblue', ax=ax, label = 'Central African Republic')\r\nafrica_low.loc[africa_low['Country']=='Chad'].plot(x='HDI', y= 'TFR', kind='scatter', c = 'navy', ax=ax, label = 'Chad')\r\nax.legend(loc='best')\r\nax.set_title('3 Lowest HDI Countries: HDI vs TFR')\r\nax.figure.savefig('Scatter Plot 3 Lowest HDI Countries: HDI vs TFR.png')\r\n#HDI vs TFR of 3 lowest HDI countries",
"_____no_output_____"
],
[
"ax = africa_low.loc[africa_low['Country']=='Niger'].plot(x='HDI', y= 'TFR', kind='line', c = 'cornflowerblue', label = 'Niger')\r\nafrica_low.loc[africa_low['Country']=='Central African Republic'].plot(x='HDI', y= 'TFR', kind='line', c = 'mediumblue', ax=ax, label = 'Central African Republic')\r\nafrica_low.loc[africa_low['Country']=='Chad'].plot(x='HDI', y= 'TFR', kind='line', c = 'navy', ax=ax, label = 'Chad')\r\nax.set_ylabel('TFR')\r\nax.legend(loc='best')\r\nax.set_title('3 Lowest HDI Countries: HDI vs TFR')\r\nax.figure.savefig('Line Plot 3 Lowest HDI Countries: TFR vs HDI.png')\r\n#HDI vs TFR of 3 lowest HDI countries",
"_____no_output_____"
],
[
"ax = africa_low.loc[africa_low['Country']=='Niger'].plot(x='HDI', y= 'Labor force participation rate, female (% of female population ages 15+) (modeled ILO estimate)', kind='scatter', c = 'cornflowerblue', label = 'Niger')\r\nafrica_low.loc[africa_low['Country']=='Central African Republic'].plot(x='HDI', y= 'Labor force participation rate, female (% of female population ages 15+) (modeled ILO estimate)', kind='scatter', c = 'mediumblue', ax=ax, label = 'Central African Republic')\r\nafrica_low.loc[africa_low['Country']=='Chad'].plot(x='HDI', y= 'Labor force participation rate, female (% of female population ages 15+) (modeled ILO estimate)', kind='scatter', c = 'navy', ax=ax, label = 'Chad')\r\nax.legend(loc='best')\r\nax.set_title('3 Lowest HDI Countries: HDI vs FLPR')\r\nax.figure.savefig('Scatter Plot 3 Lowest HDI Countries: HDI vs FLPR.png')\r\n#HDI vs FLPR of 3 lowest HDI countries",
"_____no_output_____"
],
[
"ax = africa_low.loc[africa_low['Country']=='Niger'].plot(x='HDI', y= 'Labor force participation rate, female (% of female population ages 15+) (modeled ILO estimate)', kind='line', c = 'cornflowerblue', label = 'Niger')\r\nafrica_low.loc[africa_low['Country']=='Central African Republic'].plot(x='HDI', y= 'Labor force participation rate, female (% of female population ages 15+) (modeled ILO estimate)', kind='line', c = 'mediumblue', ax=ax, label = 'Central African Republic')\r\nafrica_low.loc[africa_low['Country']=='Chad'].plot(x='HDI', y= 'Labor force participation rate, female (% of female population ages 15+) (modeled ILO estimate)', kind='line', c = 'navy', ax=ax, label = 'Chad')\r\nax.set_ylabel('Labor force participation rate, female (% of female population ages 15+) (modeled ILO estimate)')\r\nax.legend(loc='best')\r\nax.set_title('3 Lowest HDI Countries: HDI vs FLPR')\r\nax.figure.savefig('Line Plot 3 Lowest HDI Countries: HDI vs FLPR.png')\r\n#HDI vs FLPR of 3 lowest HDI countries",
"_____no_output_____"
],
[
"ax = africa_low.loc[africa_low['Country']=='Niger'].plot(x='TFR', y= 'Labor force participation rate, female (% of female population ages 15+) (modeled ILO estimate)', kind='scatter', c = 'cornflowerblue', label = 'Niger')\r\nafrica_low.loc[africa_low['Country']=='Central African Republic'].plot(x='TFR', y= 'Labor force participation rate, female (% of female population ages 15+) (modeled ILO estimate)', kind='scatter', c = 'mediumblue', ax=ax, label = 'Central African Republic')\r\nafrica_low.loc[africa_low['Country']=='Chad'].plot(x='TFR', y= 'Labor force participation rate, female (% of female population ages 15+) (modeled ILO estimate)', kind='scatter', c = 'navy', ax=ax, label = 'Chad')\r\nax.legend(loc='lower right')\r\nax.set_title('3 Lowest HDI Countries: TFR vs FLPR')\r\nax.figure.savefig('Scatter Plot 3 Lowest HDI Countries: TFR vs FLPR.png')\r\n#TFR vs FLPR of 3 lowest HDI countries",
"_____no_output_____"
],
[
"ax = africa_low.loc[africa_low['Country']=='Niger'].plot(x='TFR', y= 'Labor force participation rate, female (% of female population ages 15+) (modeled ILO estimate)', kind='line', c = 'cornflowerblue', label = 'Niger')\r\nafrica_low.loc[africa_low['Country']=='Central African Republic'].plot(x='TFR', y= 'Labor force participation rate, female (% of female population ages 15+) (modeled ILO estimate)', kind='line', c = 'mediumblue', ax=ax, label = 'Central African Republic')\r\nafrica_low.loc[africa_low['Country']=='Chad'].plot(x='TFR', y= 'Labor force participation rate, female (% of female population ages 15+) (modeled ILO estimate)', kind='line', c = 'navy', ax=ax, label = 'Chad')\r\nax.set_ylabel('Labor force participation rate, female (% of female population ages 15+) (modeled ILO estimate)')\r\nax.legend(loc='lower right')\r\nax.set_title('3 Lowest HDI Countries: TFR vs FLPR')\r\nax.figure.savefig('Line Plot 3 Lowest HDI Countries: TFR vs FLPR.png')\r\n#TFR vs FLPR of 3 lowest HDI countries",
"_____no_output_____"
]
]
] | [
"markdown",
"code",
"markdown",
"code",
"markdown",
"code",
"markdown",
"code",
"markdown",
"code",
"markdown",
"code"
] | [
[
"markdown",
"markdown",
"markdown"
],
[
"code",
"code",
"code",
"code"
],
[
"markdown"
],
[
"code",
"code",
"code",
"code",
"code"
],
[
"markdown"
],
[
"code",
"code",
"code"
],
[
"markdown"
],
[
"code",
"code",
"code",
"code",
"code",
"code",
"code",
"code",
"code",
"code",
"code",
"code",
"code",
"code",
"code",
"code",
"code",
"code",
"code",
"code",
"code",
"code",
"code",
"code",
"code",
"code",
"code",
"code",
"code",
"code",
"code",
"code"
],
[
"markdown",
"markdown"
],
[
"code",
"code",
"code",
"code",
"code",
"code",
"code",
"code",
"code",
"code",
"code",
"code"
],
[
"markdown"
],
[
"code",
"code",
"code",
"code",
"code",
"code",
"code",
"code",
"code",
"code",
"code",
"code"
]
] |
e7792bc2d54ad43237deff712b2614a4975ccaed | 10,124 | ipynb | Jupyter Notebook | sagemaker-python-sdk/dgl_kge/kge_pytorch_hypertune.ipynb | P15241328/amazon-sagemaker-examples | 00cba545be0822474f070321a62d22865187e09b | [
"Apache-2.0"
] | 5 | 2019-01-19T23:53:35.000Z | 2022-01-29T14:04:31.000Z | sagemaker-python-sdk/dgl_kge/kge_pytorch_hypertune.ipynb | P15241328/amazon-sagemaker-examples | 00cba545be0822474f070321a62d22865187e09b | [
"Apache-2.0"
] | 4 | 2020-09-26T01:30:01.000Z | 2022-02-10T02:20:35.000Z | sagemaker-python-sdk/dgl_kge/kge_pytorch_hypertune.ipynb | P15241328/amazon-sagemaker-examples | 00cba545be0822474f070321a62d22865187e09b | [
"Apache-2.0"
] | 7 | 2020-03-04T22:23:51.000Z | 2021-07-13T14:05:46.000Z | 37.776119 | 606 | 0.615567 | [
[
[
"# Hyperparameter tuning with Amazon SageMaker and Deep Graph Library with PyTorch backend\n_**Creating a Hyperparameter tuning job for a DGL network**_\n___\n___\n\n\n## Contents\n1. [Background](#Background) \n2. [Setup](#Setup) \n3. [Tune](#Train) \n4. [Wrap-up](#Wrap-up) \n\n## Background\nThis example notebook shows how to generate knowledge graph embedding using the DMLC DGL API and FB15k dataset. It uses the Amazon SageMaker hyperparameter tuning to start multiple training jobs with different hyperparameter combinations. This helps you find the set with best model performance. This is an important step in the machine learning process as hyperparameter settings can have a large effect on model accuracy. In this example, you use the [Amazon SageMaker Python SDK](https://github.com/aws/sagemaker-python-sdk) to create a hyperparameter tuning job for an Amazon SageMaker estimator.",
"_____no_output_____"
],
[
"## Setup\nThis notebook was created and tested on an ml.p3.2xlarge notebook instance.\n\nPrerequisites\n * You can successfully run the kge_pytorch example (see kge_pytorch.ipynb).\n * You have an S3 bucket and prefix that you want to use for training and model data. This should be within the same Region as the notebook instance, training, and hosting.\n * You have the IAM role ARN used to give training and hosting access to your data. See the documentation for more details on creating these. If a role not associated with the current notebook instance, or more than one role, is required for training or hosting, replace sagemaker.get_execution_role() with the appropriate full IAM role ARN strings.\n",
"_____no_output_____"
]
],
[
[
"import sagemaker\nfrom sagemaker import get_execution_role\nfrom sagemaker.session import Session\n\n# Setup session\nsess = sagemaker.Session()\n\n# S3 bucket for saving code and model artifacts.\n# Feel free to specify a different bucket here if you wish.\nbucket = sess.default_bucket()\n\n# Location to put your custom code.\ncustom_code_upload_location = 'customcode'\n\n# IAM execution role that gives Amazon SageMaker access to resources in your AWS account.\n# You can use the Amazon SageMaker Python SDK to get the role from the notebook environment. \nrole = get_execution_role()",
"_____no_output_____"
]
],
[
[
"Now we'll import the Python libraries we'll need.",
"_____no_output_____"
]
],
[
[
"import boto3\nfrom sagemaker.tuner import IntegerParameter, CategoricalParameter, ContinuousParameter, HyperparameterTuner",
"_____no_output_____"
]
],
[
[
"## Tune\nSimilar to training a single training job in Amazon SageMaker, you define the training estimator passing in the code scripts, IAM role, (per job) hardware configuration, and any hyperparameters you're not tuning.",
"_____no_output_____"
]
],
[
[
"from sagemaker.pytorch import PyTorch\n\nENTRY_POINT = 'train.py'\nCODE_PATH = './'\n\naccount = sess.boto_session.client('sts').get_caller_identity()['Account']\nregion = sess.boto_session.region_name\n\nparams = {}\nparams['dataset'] = 'FB15k'\nparams['model'] = 'DistMult'\nparams['batch_size'] = 1024\nparams['neg_sample_size'] = 256\nparams['hidden_dim'] = 2000\nparams['max_step'] = 100000\nparams['batch_size_eval'] = 16\nparams['valid'] = True\nparams['test'] = True\nparams['neg_adversarial_sampling'] = True\n\nestimator = PyTorch(entry_point=ENTRY_POINT,\n source_dir=CODE_PATH,\n role=role,\n train_instance_count=1,\n train_instance_type='ml.p3.2xlarge',\n framework_version=\"1.3.1\",\n py_version='py3',\n debugger_hook_config=False,\n hyperparameters=params,\n sagemaker_session=sess)",
"_____no_output_____"
]
],
[
[
"After you define your estimator, specify the hyperparameters you want to tune and their possible values. You have three different types of hyperparameters.\n * Categorical parameters need to take one value from a discrete set. Define this by passing the list of possible values to CategoricalParameter(list)\n * Continuous parameters can take any real number value between the minimum and maximum value, defined by ContinuousParameter(min, max)\n * Integer parameters can take any integer value between the minimum and maximum value, defined by IntegerParameter(min, max)\n \nIf possible, it's almost always best to specify a value as the least restrictive type. For example, tuning threshold as a continuous value between 0.01 and 0.2 is likely to yield a better result than tuning as a categorical parameter with possible values of 0.01, 0.1, 0.15, or 0.2.",
"_____no_output_____"
]
],
[
[
"hyperparameter_ranges = {'lr': ContinuousParameter(0.01, 0.1),\n 'gamma': ContinuousParameter(400, 600)}",
"_____no_output_____"
]
],
[
[
"Next, specify the objective metric that you want to tune and its definition. This includes the regular expression needed to extract that metric from the Amazon CloudWatch logs of the training job.\n\nYou can capture evalution results such as MR, MRR and Hit10.",
"_____no_output_____"
]
],
[
[
"metric = []\nmr_metric = {'Name': 'final_MR', 'Regex':\"Test average MR at \\[\\S*\\]: (\\S*)\"}\nmrr_metric = {'Name': 'final_MRR', 'Regex':\"Test average MRR at \\[\\S*\\]: (\\S*)\"}\nhit10_metric = {'Name': 'final_Hit10', 'Regex':\"Test average HITS@10 at \\[\\S*\\]: (\\S*)\"}\nmetric.append(mr_metric)\nmetric.append(mrr_metric)\nmetric.append(hit10_metric)",
"_____no_output_____"
]
],
[
[
"Now, create a HyperparameterTuner object, which you pass.\n\n * The training estimator you created above\n * The hyperparameter ranges\n * Objective metric name and definition\n * Number of training jobs to run in-total and how many training jobs should be run simultaneously. More parallel jobs will finish tuning sooner, but may sacrifice accuracy. We recommend that you set the parallel jobs value to less than 10 percent of the total number of training jobs It's set it higher in this example to keep it short.\n * Whether you should maximize or minimize the objective metric. You choose 'Minimize' in this example, which is what you want for the MR result.\n\nYou can also add a task_tag with value 'DGL' to help tracking the hyperparameter tuning task.",
"_____no_output_____"
]
],
[
[
"task_tags = [{'Key':'ML Task', 'Value':'DGL'}]\ntuner = HyperparameterTuner(estimator,\n objective_metric_name='final_MR',\n objective_type='Minimize',\n hyperparameter_ranges=hyperparameter_ranges,\n metric_definitions=metric,\n tags=task_tags,\n max_jobs=6,\n max_parallel_jobs=2)",
"_____no_output_____"
]
],
[
[
"And finally, you can start the tuning job by calling .fit().",
"_____no_output_____"
]
],
[
[
"tuner.fit()",
"_____no_output_____"
]
],
[
[
"Run a quick check of the hyperparameter tuning jobs status to make sure it started successfully and is InProgress.",
"_____no_output_____"
]
],
[
[
"boto3.client('sagemaker').describe_hyper_parameter_tuning_job(\n HyperParameterTuningJobName=tuner.latest_tuning_job.job_name)['HyperParameterTuningJobStatus']",
"_____no_output_____"
]
],
[
[
"## Wrap-up\nNow that we've started the hyperparameter tuning job, it will run in the background. You can close this notebook. When it's finished, you can go to console to analyze the result.\n\nFor more information about Amazon SageMaker's Hyperparameter Tuning, see the AWS documentation.",
"_____no_output_____"
]
]
] | [
"markdown",
"code",
"markdown",
"code",
"markdown",
"code",
"markdown",
"code",
"markdown",
"code",
"markdown",
"code",
"markdown",
"code",
"markdown",
"code",
"markdown"
] | [
[
"markdown",
"markdown"
],
[
"code"
],
[
"markdown"
],
[
"code"
],
[
"markdown"
],
[
"code"
],
[
"markdown"
],
[
"code"
],
[
"markdown"
],
[
"code"
],
[
"markdown"
],
[
"code"
],
[
"markdown"
],
[
"code"
],
[
"markdown"
],
[
"code"
],
[
"markdown"
]
] |
e7792ccd1ef5a90a7f96cc8688ac32008e60ee56 | 311,103 | ipynb | Jupyter Notebook | examples/classification_tutorial.ipynb | ayeffkay/DeepPavlov | f5117cd9ad1e64f6c2d970ecaa42fc09ccb23144 | [
"Apache-2.0"
] | 5,893 | 2018-02-01T18:13:20.000Z | 2022-03-31T19:22:21.000Z | examples/classification_tutorial.ipynb | Aniket27100709/DeepPavlov | d73f45733d6b23347871aa293309730303b64450 | [
"Apache-2.0"
] | 749 | 2018-01-31T11:36:02.000Z | 2022-03-30T07:24:22.000Z | examples/classification_tutorial.ipynb | Aniket27100709/DeepPavlov | d73f45733d6b23347871aa293309730303b64450 | [
"Apache-2.0"
] | 1,155 | 2018-02-01T10:52:15.000Z | 2022-03-29T02:12:15.000Z | 105.031398 | 9,056 | 0.612559 | [
[
[
"## You can also run the notebook in [COLAB](https://colab.research.google.com/github/deepmipt/DeepPavlov/blob/master/examples/classification_tutorial.ipynb).",
"_____no_output_____"
]
],
[
[
"!pip3 install deeppavlov",
"_____no_output_____"
]
],
[
[
"# Classification on DeepPavlov",
"_____no_output_____"
],
[
"**Task**:\nIntent recognition on SNIPS dataset: https://github.com/snipsco/nlu-benchmark/tree/master/2017-06-custom-intent-engines that has already been recomposed to `csv` format and can be downloaded from http://files.deeppavlov.ai/datasets/snips_intents/train.csv\n\nFastText English word embeddings ~8Gb: http://files.deeppavlov.ai/deeppavlov_data/embeddings/wiki.en.bin",
"_____no_output_____"
],
[
"## Plan of the notebook with documentation links:\n\n1. [Data aggregation](#Data-aggregation)\n * [DatasetReader](#DatasetReader): [docs link](https://deeppavlov.readthedocs.io/en/latest/apiref/dataset_readers.html)\n * [DatasetIterator](#DatasetIterator): [docs link](https://deeppavlov.readthedocs.io/en/latest/apiref/dataset_iterators.html)\n2. [Data preprocessing](#Data-preprocessing): [docs link](https://deeppavlov.readthedocs.io/en/latest/components/data_processors.html)\n * [Lowercasing](#Lowercasing)\n * [Tokenization](#Tokenization)\n * [Vocabulary](#Vocabulary)\n3. [Featurization](#Featurization): [docs link](https://deeppavlov.readthedocs.io/en/latest/components/data_processors.html), [pre-trained embeddings link](https://deeppavlov.readthedocs.io/en/latest/intro/pretrained_vectors.html)\n * [Bag-of-words embedder](#Bag-of-words)\n * [TF-IDF vectorizer](#TF-IDF-Vectorizer)\n * [GloVe embedder](#GloVe-embedder)\n * [Mean GloVe embedder](#Mean-GloVe-embedder)\n * [GloVe weighted by TF-IDF embedder](#GloVe-weighted-by-TF-IDF-embedder)\n4. [Models](#Models): [docs link](https://deeppavlov.readthedocs.io/en/latest/components/classifiers.html)\n * [Building models in python](#Models-in-python)\n - [Sklearn component classifiers](#SklearnComponent-classifier-on-Tfidf-features-in-python)\n - [Keras classification model on GloVe emb](#KerasClassificationModel-on-GloVe-embeddings-in-python)\n - [Sklearn component classifier on GloVe weighted emb](#SklearnComponent-classifier-on-GloVe-weighted-by-TF-IDF-embeddings-in-python)\n * [Building models from configs](#Models-from-configs)\n - [Sklearn component classifiers](#SklearnComponent-classifier-on-Tfidf-features-from-config)\n - [Keras classification model](#KerasClassificationModel-on-fastText-embeddings-from-config)\n - [Sklearn component classifier on GloVe weighted emb](#SklearnComponent-classifier-on-GloVe-weighted-by-TF-IDF-embeddings-from-config)\n * [Bonus: pre-trained CNN model in DeepPavlov](#Bonus:-pre-trained-CNN-model-in-DeepPavlov)",
"_____no_output_____"
],
[
"## Data aggregation",
"_____no_output_____"
],
[
"First of all, let's download and look into data we will work with.",
"_____no_output_____"
]
],
[
[
"from deeppavlov.core.data.utils import simple_download\n\n#download train data file for SNIPS\nsimple_download(url=\"http://files.deeppavlov.ai/datasets/snips_intents/train.csv\", \n destination=\"./snips/train.csv\")",
"2019-02-12 12:14:21.101 INFO in 'deeppavlov.core.data.utils'['utils'] at line 63: Downloading from http://files.deeppavlov.ai/datasets/snips_intents/train.csv to snips/train.csv\n100%|██████████| 981k/981k [00:00<00:00, 63.5MB/s]\n"
],
[
"! head -n 15 snips/train.csv",
"text,intents\r\nAdd another song to the Cita RomГЎntica playlist. ,AddToPlaylist\r\nadd clem burke in my playlist Pre-Party R&B Jams,AddToPlaylist\r\nAdd Live from Aragon Ballroom to Trapeo,AddToPlaylist\r\nadd Unite and Win to my night out,AddToPlaylist\r\nAdd track to my Digster Future Hits,AddToPlaylist\r\nadd the piano bar to my Cindy Wilson,AddToPlaylist\r\nAdd Spanish Harlem Incident to cleaning the house,AddToPlaylist\r\nadd The Greyest of Blue Skies in Indie EspaГ±ol my playlist,AddToPlaylist\r\nAdd the name kids in the street to the plylist New Indie Mix,AddToPlaylist\r\nadd album radar latino,AddToPlaylist\r\nAdd Tranquility to the Latin Pop Rising playlist. ,AddToPlaylist\r\nAdd d flame to the Dcode2016 playlist.,AddToPlaylist\r\nAdd album to my fairy tales,AddToPlaylist\r\nI need another artist in the New Indie Mix playlist. ,AddToPlaylist\r\n"
]
],
[
[
"### DatasetReader\n\nRead data using `BasicClassificationDatasetReader` из DeepPavlov",
"_____no_output_____"
]
],
[
[
"from deeppavlov.dataset_readers.basic_classification_reader import BasicClassificationDatasetReader",
"_____no_output_____"
],
[
"# read data from particular columns of `.csv` file\ndr = BasicClassificationDatasetReader().read(\n data_path='./snips/',\n train='train.csv',\n x = 'text',\n y = 'intents'\n)",
"2019-02-12 12:14:23.376 WARNING in 'deeppavlov.dataset_readers.basic_classification_reader'['basic_classification_reader'] at line 96: Cannot find snips/valid.csv file\n2019-02-12 12:14:23.376 WARNING in 'deeppavlov.dataset_readers.basic_classification_reader'['basic_classification_reader'] at line 96: Cannot find snips/test.csv file\n"
]
],
[
[
"We don't have a ready train/valid/test split.",
"_____no_output_____"
]
],
[
[
"# check train/valid/test sizes\n[(k, len(dr[k])) for k in dr.keys()]",
"_____no_output_____"
]
],
[
[
"### DatasetIterator\n\nUse `BasicClassificationDatasetIterator` to split `train` on `train` and `valid` and to generate batches of samples.",
"_____no_output_____"
]
],
[
[
"from deeppavlov.dataset_iterators.basic_classification_iterator import BasicClassificationDatasetIterator",
"_____no_output_____"
],
[
"# initialize data iterator splitting `train` field to `train` and `valid` in proportion 0.8/0.2\ntrain_iterator = BasicClassificationDatasetIterator(\n data=dr,\n field_to_split='train', # field that will be splitted\n split_fields=['train', 'valid'], # fields to which the fiald above will be splitted\n split_proportions=[0.8, 0.2], #proportions for splitting\n split_seed=23, # seed for splitting dataset\n seed=42) # seed for iteration over dataset",
"2019-02-12 12:14:23.557 INFO in 'deeppavlov.dataset_iterators.basic_classification_iterator'['basic_classification_iterator'] at line 73: Splitting field <<train>> to new fields <<['train', 'valid']>>\n"
]
],
[
[
"Let's look into training samples. ",
"_____no_output_____"
]
],
[
[
"# one can get train instances (or any other data type including `all`)\nx_train, y_train = train_iterator.get_instances(data_type='train')\nfor x, y in list(zip(x_train, y_train))[:5]:\n print('x:', x)\n print('y:', y)\n print('=================')",
"x: Is it freezing in Offerman, California?\ny: ['GetWeather']\n=================\nx: put this song in the playlist Trap Land\ny: ['AddToPlaylist']\n=================\nx: show me a textbook with a rating of 2 and a maximum rating of 6 that is current\ny: ['RateBook']\n=================\nx: Will the weather be okay in Northern Luzon Heroes Hill National Park 4 and a half months from now?\ny: ['GetWeather']\n=================\nx: Rate the current album a four\ny: ['RateBook']\n=================\n"
]
],
[
[
"## Data preprocessing",
"_____no_output_____"
],
[
"We will be using lowercasing and tokenization as data preparation. \n\nDeepPavlov also contains several other preprocessors and tokenizers.",
"_____no_output_____"
],
[
"### Lowercasing",
"_____no_output_____"
],
[
"`str_lower` lowercases texts.",
"_____no_output_____"
]
],
[
[
"from deeppavlov.models.preprocessors.str_lower import str_lower",
"[nltk_data] Downloading package punkt to /home/vimary/nltk_data...\n[nltk_data] Package punkt is already up-to-date!\n[nltk_data] Downloading package stopwords to /home/vimary/nltk_data...\n[nltk_data] Package stopwords is already up-to-date!\n[nltk_data] Downloading package perluniprops to\n[nltk_data] /home/vimary/nltk_data...\n[nltk_data] Package perluniprops is already up-to-date!\n[nltk_data] Downloading package nonbreaking_prefixes to\n[nltk_data] /home/vimary/nltk_data...\n[nltk_data] Package nonbreaking_prefixes is already up-to-date!\n"
],
[
"str_lower(['Is it freezing in Offerman, California?'])",
"_____no_output_____"
]
],
[
[
"### Tokenization\n\n`NLTKTokenizer` can split string to tokens.",
"_____no_output_____"
]
],
[
[
"from deeppavlov.models.tokenizers.nltk_moses_tokenizer import NLTKMosesTokenizer",
"_____no_output_____"
],
[
"tokenizer = NLTKMosesTokenizer()\ntokenizer(['Is it freezing in Offerman, California?'])",
"_____no_output_____"
]
],
[
[
"Let's preprocess all `train` part of the dataset.",
"_____no_output_____"
]
],
[
[
"train_x_lower_tokenized = str_lower(tokenizer(train_iterator.get_instances(data_type='train')[0]))",
"_____no_output_____"
]
],
[
[
"### Vocabulary\n\nNow we are ready to use `vocab`. They are very usefull for:\n* extracting class labels and converting labels to indices and vice versa,\n* building of characters or tokens vocabularies.",
"_____no_output_____"
]
],
[
[
"from deeppavlov.core.data.simple_vocab import SimpleVocabulary",
"_____no_output_____"
],
[
"# initialize simple vocabulary to collect all appeared in the dataset classes\nclasses_vocab = SimpleVocabulary(\n save_path='./snips/classes.dict',\n load_path='./snips/classes.dict')",
"_____no_output_____"
],
[
"classes_vocab.fit((train_iterator.get_instances(data_type='train')[1]))\nclasses_vocab.save()",
"2019-02-12 12:14:25.35 INFO in 'deeppavlov.core.data.simple_vocab'['simple_vocab'] at line 89: [saving vocabulary to /home/vimary/ipavlov/Pilot/examples/tutorials/snips/classes.dict]\n"
]
],
[
[
"Let's see what classes the dataset contains and their indices in the vocabulary.",
"_____no_output_____"
]
],
[
[
"list(classes_vocab.items())",
"_____no_output_____"
],
[
"# also one can collect vocabulary of textual tokens appeared 2 and more times in the dataset\ntoken_vocab = SimpleVocabulary(\n save_path='./snips/tokens.dict',\n load_path='./snips/tokens.dict',\n min_freq=2,\n special_tokens=('<PAD>', '<UNK>',),\n unk_token='<UNK>')",
"_____no_output_____"
],
[
"token_vocab.fit(train_x_lower_tokenized)\ntoken_vocab.save()",
"2019-02-12 12:14:25.157 INFO in 'deeppavlov.core.data.simple_vocab'['simple_vocab'] at line 89: [saving vocabulary to /home/vimary/ipavlov/Pilot/examples/tutorials/snips/tokens.dict]\n"
],
[
"# number of tokens in dictionary\nlen(token_vocab)",
"_____no_output_____"
],
[
"# 10 most common words and number of times their appeared\ntoken_vocab.freqs.most_common()[:10]",
"_____no_output_____"
],
[
"token_ids = token_vocab(str_lower(tokenizer(['Is it freezing in Offerman, California?'])))\ntoken_ids",
"_____no_output_____"
],
[
"tokenizer(token_vocab(token_ids))",
"_____no_output_____"
]
],
[
[
"## Featurization\n\nThis part contains several possible ways of featurization of text samples. One can chose any appropriate vectorizer/embedder according to available resources and given task.\n\nBag-of-words (BoW) and TF-IDF vectorizers converts text samples to vectors (one vector per sample) while fastText, GloVe, fastText weighted by TF-IDF embedders either produce an embedding vector per token or an embedding vector per text sample (if `mean` set to True).",
"_____no_output_____"
],
[
"### Bag-of-words\n\nMatches a vector to each text sample indicating which words appeared in the given sample: text -> binary vector $v$: \\[0, 1, 0, 0, 0, 1, ..., ...1, 0, 1\\]. \n\nDimensionality of vector $v$ is equal to vocabulary size.\n\n$v_i$ == 1, if word $i$ is in the text,\n\n$v_i$ == 0, else.",
"_____no_output_____"
]
],
[
[
"import numpy as np\nfrom deeppavlov.models.embedders.bow_embedder import BoWEmbedder",
"_____no_output_____"
],
[
"# initialize bag-of-words embedder giving total number of tokens\nbow = BoWEmbedder(depth=token_vocab.len)\n# it assumes indexed tokenized samples\nbow(token_vocab(str_lower(tokenizer(['Is it freezing in Offerman, California?']))))",
"_____no_output_____"
],
[
"# all 8 tokens are in the vocabulary\nsum(bow(token_vocab(str_lower(tokenizer(['Is it freezing in Offerman, California?']))))[0])",
"_____no_output_____"
]
],
[
[
"### TF-IDF Vectorizer\n\nMatches a vector to each text sample: text -> vector $v$ from $R^N$ where $N$ is a vocabulary size.\n\n$TF-IDF(token, document) = TF(token, document) * IDF(token, document)$\n\n$TF$ is a term frequency:\n\n$TF(token, document) = \\frac{n_{token}}{\\sum_{k}n_k}.$\n\n$IDF$ is a inverse document frequency:\n\n$IDF(token, all\\_documents) = \\frac{Total\\ number\\ of\\ documents}{number\\ of\\ documents\\ where\\ token\\ appeared}.$",
"_____no_output_____"
],
[
"`SklearnComponent` in DeepPavlov is a universal wrapper for any vecotirzer/estimator from `sklearn` package. The only requirement to specify component usage is following: model class and name of infer method should be passed as parameters.",
"_____no_output_____"
]
],
[
[
"from deeppavlov.models.sklearn import SklearnComponent",
"_____no_output_____"
],
[
"# initialize TF-IDF vectorizer sklearn component with `transform` as infer method\ntfidf = SklearnComponent(\n model_class=\"sklearn.feature_extraction.text:TfidfVectorizer\",\n infer_method=\"transform\",\n save_path='./tfidf_v0.pkl',\n load_path='./tfidf_v0.pkl',\n mode='train')",
"2019-02-12 12:14:25.268 WARNING in 'deeppavlov.models.sklearn.sklearn_component'['sklearn_component'] at line 218: Cannot load model from /home/vimary/ipavlov/Pilot/examples/tutorials/tfidf_v0.pkl\n2019-02-12 12:14:25.269 INFO in 'deeppavlov.models.sklearn.sklearn_component'['sklearn_component'] at line 165: Initializing model sklearn.feature_extraction.text:TfidfVectorizer from scratch\n"
],
[
"# fit on textual train instances and save it\ntfidf.fit(str_lower(train_iterator.get_instances(data_type='train')[0]))\ntfidf.save()",
"2019-02-12 12:14:25.296 INFO in 'deeppavlov.models.sklearn.sklearn_component'['sklearn_component'] at line 108: Fitting model sklearn.feature_extraction.text:TfidfVectorizer\n2019-02-12 12:14:25.395 INFO in 'deeppavlov.models.sklearn.sklearn_component'['sklearn_component'] at line 240: Saving model to /home/vimary/ipavlov/Pilot/examples/tutorials/tfidf_v0.pkl\n"
],
[
"tfidf(str_lower(['Is it freezing in Offerman, California?']))",
"_____no_output_____"
],
[
"# number of tokens in the TF-IDF vocabulary\nlen(tfidf.model.vocabulary_)",
"_____no_output_____"
]
],
[
[
"### GloVe embedder\n\n[GloVe](https://nlp.stanford.edu/projects/glove/) is an unsupervised learning algorithm for obtaining vector representations for words. Training is performed on aggregated global word-word co-occurrence statistics from a corpus, and the resulting representations showcase interesting linear substructures of the word vector space.",
"_____no_output_____"
]
],
[
[
"from deeppavlov.models.embedders.glove_embedder import GloVeEmbedder",
"Using TensorFlow backend.\n"
]
],
[
[
"Let's download GloVe embedding file",
"_____no_output_____"
]
],
[
[
"simple_download(url=\"http://files.deeppavlov.ai/embeddings/glove.6B.100d.txt\", \n destination=\"./glove.6B.100d.txt\")",
"2019-02-12 12:14:26.153 INFO in 'deeppavlov.core.data.utils'['utils'] at line 63: Downloading from http://files.deeppavlov.ai/embeddings/glove.6B.100d.txt to glove.6B.100d.txt\n347MB [00:06, 50.0MB/s] \n"
],
[
"embedder = GloVeEmbedder(load_path='./glove.6B.100d.txt',\n dim=100, pad_zero=True)",
"2019-02-12 12:14:33.99 INFO in 'deeppavlov.models.embedders.glove_embedder'['glove_embedder'] at line 52: [loading GloVe embeddings from `/home/vimary/ipavlov/Pilot/examples/tutorials/glove.6B.100d.txt`]\n"
],
[
"# output shape is (batch_size x max_num_tokens_in_the_batch x embedding_dim)\nembedded_batch = embedder(str_lower(tokenizer(['Is it freezing in Offerman, California?']))) \nlen(embedded_batch), len(embedded_batch[0]), embedded_batch[0][0].shape",
"_____no_output_____"
]
],
[
[
"### Mean GloVe embedder",
"_____no_output_____"
],
[
"Embedder returns a vector per token while we want to get a vector per text sample. Therefore, let's calculate mean vector of embeddings of tokens. \nFor that we can either init `GloVeEmbedder` with `mean=True` parameter (`mean=false` by default), or pass `mean=true` while calling function (this way `mean` value is assigned only for this call).",
"_____no_output_____"
]
],
[
[
"# output shape is (batch_size x embedding_dim)\nembedded_batch = embedder(str_lower(tokenizer(['Is it freezing in Offerman, California?'])), mean=True) \nlen(embedded_batch), embedded_batch[0].shape",
"_____no_output_____"
]
],
[
[
"### GloVe weighted by TF-IDF embedder\n\nOne of the possible ways to combine TF-IDF vectorizer and any token embedder is to weigh token embeddings by TF-IDF coefficients (therefore, `mean` set to True is obligatory to obtain embeddings of interest while it still **by default** returns embeddings of tokens.",
"_____no_output_____"
]
],
[
[
"from deeppavlov.models.embedders.tfidf_weighted_embedder import TfidfWeightedEmbedder",
"_____no_output_____"
],
[
"weighted_embedder = TfidfWeightedEmbedder(\n embedder=embedder, # our GloVe embedder instance\n tokenizer=tokenizer, # our tokenizer instance\n mean=True, # to return one vector per sample\n vectorizer=tfidf # our TF-IDF vectorizer\n)",
"_____no_output_____"
],
[
"# output shape is (batch_size x embedding_dim)\nembedded_batch = weighted_embedder(str_lower(tokenizer(['Is it freezing in Offerman, California?']))) \nlen(embedded_batch), embedded_batch[0].shape",
"_____no_output_____"
]
],
[
[
"## Models",
"_____no_output_____"
]
],
[
[
"from deeppavlov.metrics.accuracy import sets_accuracy",
"_____no_output_____"
],
[
"# get all train and valid data from iterator\nx_train, y_train = train_iterator.get_instances(data_type=\"train\")\nx_valid, y_valid = train_iterator.get_instances(data_type=\"valid\")",
"_____no_output_____"
]
],
[
[
"### Models in python",
"_____no_output_____"
],
[
"#### SklearnComponent classifier on Tfidf-features in python",
"_____no_output_____"
]
],
[
[
"# initialize sklearn classifier, all parameters for classifier could be passed\ncls = SklearnComponent(\n model_class=\"sklearn.linear_model:LogisticRegression\",\n infer_method=\"predict\",\n save_path='./logreg_v0.pkl',\n load_path='./logreg_v0.pkl',\n C=1,\n mode='train')",
"2019-02-12 12:14:53.75 WARNING in 'deeppavlov.models.sklearn.sklearn_component'['sklearn_component'] at line 218: Cannot load model from /home/vimary/ipavlov/Pilot/examples/tutorials/logreg_v0.pkl\n2019-02-12 12:14:53.75 INFO in 'deeppavlov.models.sklearn.sklearn_component'['sklearn_component'] at line 165: Initializing model sklearn.linear_model:LogisticRegression from scratch\n"
],
[
"# fit sklearn classifier and save it\ncls.fit(tfidf(x_train), y_train)\ncls.save()",
"2019-02-12 12:14:53.591 INFO in 'deeppavlov.models.sklearn.sklearn_component'['sklearn_component'] at line 108: Fitting model sklearn.linear_model:LogisticRegression\n2019-02-12 12:14:53.756 INFO in 'deeppavlov.models.sklearn.sklearn_component'['sklearn_component'] at line 240: Saving model to /home/vimary/ipavlov/Pilot/examples/tutorials/logreg_v0.pkl\n"
],
[
"y_valid_pred = cls(tfidf(x_valid))",
"_____no_output_____"
],
[
"# Let's look into obtained result\nprint(\"Text sample: {}\".format(x_valid[0]))\nprint(\"True label: {}\".format(y_valid[0]))\nprint(\"Predicted label: {}\".format(y_valid_pred[0]))",
"Text sample: I need seating at Floating restaurant in Tennessee for a group of 9\nTrue label: ['BookRestaurant']\nPredicted label: BookRestaurant\n"
],
[
"# let's calculate sets accuracy (because each element is a list of labels)\nsets_accuracy(np.squeeze(y_valid), y_valid_pred)",
"_____no_output_____"
]
],
[
[
"#### KerasClassificationModel on GloVe embeddings in python",
"_____no_output_____"
]
],
[
[
"from deeppavlov.models.classifiers.keras_classification_model import KerasClassificationModel\nfrom deeppavlov.models.preprocessors.one_hotter import OneHotter\nfrom deeppavlov.models.classifiers.proba2labels import Proba2Labels",
"_____no_output_____"
],
[
"# Intialize `KerasClassificationModel` that composes CNN shallow-and-wide network \n# (name here as`cnn_model`)\ncls = KerasClassificationModel(save_path=\"./cnn_model_v0\", \n load_path=\"./cnn_model_v0\", \n embedding_size=embedder.dim,\n n_classes=classes_vocab.len,\n model_name=\"cnn_model\",\n text_size=15, # number of tokens\n kernel_sizes_cnn=[3, 5, 7],\n filters_cnn=128,\n dense_size=100,\n optimizer=\"Adam\",\n learning_rate=0.1,\n learning_rate_decay=0.01,\n loss=\"categorical_crossentropy\")",
"2019-02-12 12:14:54.421 INFO in 'deeppavlov.models.classifiers.keras_classification_model'['keras_classification_model'] at line 272: [initializing `KerasClassificationModel` from scratch as cnn_model]\n2019-02-12 12:14:54.818 INFO in 'deeppavlov.models.classifiers.keras_classification_model'['keras_classification_model'] at line 136: Model was successfully initialized!\nModel summary:\n__________________________________________________________________________________________________\nLayer (type) Output Shape Param # Connected to \n==================================================================================================\ninput_1 (InputLayer) (None, 15, 100) 0 \n__________________________________________________________________________________________________\nconv1d_1 (Conv1D) (None, 15, 128) 38528 input_1[0][0] \n__________________________________________________________________________________________________\nconv1d_2 (Conv1D) (None, 15, 128) 64128 input_1[0][0] \n__________________________________________________________________________________________________\nconv1d_3 (Conv1D) (None, 15, 128) 89728 input_1[0][0] \n__________________________________________________________________________________________________\nbatch_normalization_1 (BatchNor (None, 15, 128) 512 conv1d_1[0][0] \n__________________________________________________________________________________________________\nbatch_normalization_2 (BatchNor (None, 15, 128) 512 conv1d_2[0][0] \n__________________________________________________________________________________________________\nbatch_normalization_3 (BatchNor (None, 15, 128) 512 conv1d_3[0][0] \n__________________________________________________________________________________________________\nactivation_1 (Activation) (None, 15, 128) 0 batch_normalization_1[0][0] \n__________________________________________________________________________________________________\nactivation_2 (Activation) (None, 15, 128) 0 batch_normalization_2[0][0] \n__________________________________________________________________________________________________\nactivation_3 (Activation) (None, 15, 128) 0 batch_normalization_3[0][0] \n__________________________________________________________________________________________________\nglobal_max_pooling1d_1 (GlobalM (None, 128) 0 activation_1[0][0] \n__________________________________________________________________________________________________\nglobal_max_pooling1d_2 (GlobalM (None, 128) 0 activation_2[0][0] \n__________________________________________________________________________________________________\nglobal_max_pooling1d_3 (GlobalM (None, 128) 0 activation_3[0][0] \n__________________________________________________________________________________________________\nconcatenate_1 (Concatenate) (None, 384) 0 global_max_pooling1d_1[0][0] \n global_max_pooling1d_2[0][0] \n global_max_pooling1d_3[0][0] \n__________________________________________________________________________________________________\ndropout_1 (Dropout) (None, 384) 0 concatenate_1[0][0] \n__________________________________________________________________________________________________\ndense_1 (Dense) (None, 100) 38500 dropout_1[0][0] \n__________________________________________________________________________________________________\nbatch_normalization_4 (BatchNor (None, 100) 400 dense_1[0][0] \n__________________________________________________________________________________________________\nactivation_4 (Activation) (None, 100) 0 batch_normalization_4[0][0] \n__________________________________________________________________________________________________\ndropout_2 (Dropout) (None, 100) 0 activation_4[0][0] \n__________________________________________________________________________________________________\ndense_2 (Dense) (None, 7) 707 dropout_2[0][0] \n__________________________________________________________________________________________________\nbatch_normalization_5 (BatchNor (None, 7) 28 dense_2[0][0] \n__________________________________________________________________________________________________\nactivation_5 (Activation) (None, 7) 0 batch_normalization_5[0][0] \n==================================================================================================\nTotal params: 233,555\nTrainable params: 232,573\nNon-trainable params: 982\n__________________________________________________________________________________________________\n"
],
[
"# `KerasClassificationModel` assumes one-hotted distribution of classes per sample.\n# `OneHotter` converts indices to one-hot vectors representation.\n# To obtain indices we can use our `classes_vocab` intialized and fitted above\nonehotter = OneHotter(depth=classes_vocab.len, single_vector=True)",
"_____no_output_____"
],
[
"# Train for 10 epochs\nfor ep in range(10):\n for x, y in train_iterator.gen_batches(batch_size=64, \n data_type=\"train\"):\n x_embed = embedder(tokenizer(str_lower(x)))\n y_onehot = onehotter(classes_vocab(y))\n cls.train_on_batch(x_embed, y_onehot)",
"_____no_output_____"
],
[
"# Save model weights and parameters\ncls.save()",
"2019-02-12 12:15:22.184 INFO in 'deeppavlov.models.classifiers.keras_classification_model'['keras_classification_model'] at line 386: [saving model to /home/vimary/ipavlov/Pilot/examples/tutorials/cnn_model_v0_opt.json]\n"
],
[
"# Infering on validation data we get probability distribution on given data.\ny_valid_pred = cls(embedder(tokenizer(str_lower(x_valid))))",
"_____no_output_____"
],
[
"# To convert probability distribution to labels, \n# we first need to convert probabilities to indices,\n# and then using vocabulary `classes_vocab` convert indices to labels.\n# \n# `Proba2Labels` converts probabilities to indices and supports three different modes:\n# if `max_proba` is true, returns indices of the highest probabilities\n# if `confidence_threshold` is given, returns indices with probabiltiies higher than threshold\n# if `top_n` is given, returns `top_n` indices with highest probabilities\nprob2labels = Proba2Labels(max_proba=True)",
"_____no_output_____"
],
[
"# Let's look into obtained result\nprint(\"Text sample: {}\".format(x_valid[0]))\nprint(\"True label: {}\".format(y_valid[0]))\nprint(\"Predicted probability distribution: {}\".format(dict(zip(classes_vocab.keys(), \n y_valid_pred[0]))))\nprint(\"Predicted label: {}\".format(classes_vocab(prob2labels(y_valid_pred))[0]))",
"Text sample: I need seating at Floating restaurant in Tennessee for a group of 9\nTrue label: ['BookRestaurant']\nPredicted probability distribution: {'GetWeather': 4.443174475454725e-05, 'PlayMusic': 0.0002085473679471761, 'SearchScreeningEvent': 6.492184911621734e-05, 'BookRestaurant': 0.9995043277740479, 'RateBook': 0.00021818796813022345, 'SearchCreativeWork': 0.0013526129769161344, 'AddToPlaylist': 8.029041782720014e-05}\nPredicted label: ['BookRestaurant']\n"
],
[
"# calculate sets accuracy\nsets_accuracy(y_valid, classes_vocab(prob2labels(y_valid_pred)))",
"_____no_output_____"
]
],
[
[
"#### SklearnComponent classifier on GloVe weighted by TF-IDF embeddings in python",
"_____no_output_____"
]
],
[
[
"# initialize sklearn classifier, all parameters for classifier could be passed\ncls = SklearnComponent(\n model_class=\"sklearn.linear_model:LogisticRegression\",\n infer_method=\"predict\",\n save_path='./logreg_v1.pkl',\n load_path='./logreg_v1.pkl',\n C=1,\n mode='train')",
"2019-02-12 12:15:22.961 WARNING in 'deeppavlov.models.sklearn.sklearn_component'['sklearn_component'] at line 218: Cannot load model from /home/vimary/ipavlov/Pilot/examples/tutorials/logreg_v1.pkl\n2019-02-12 12:15:22.962 INFO in 'deeppavlov.models.sklearn.sklearn_component'['sklearn_component'] at line 165: Initializing model sklearn.linear_model:LogisticRegression from scratch\n"
],
[
"# fit sklearn classifier and save it\ncls.fit(weighted_embedder(str_lower(tokenizer(x_train))), y_train)\ncls.save()",
"2019-02-12 12:15:44.521 INFO in 'deeppavlov.models.sklearn.sklearn_component'['sklearn_component'] at line 108: Fitting model sklearn.linear_model:LogisticRegression\n2019-02-12 12:15:46.59 INFO in 'deeppavlov.models.sklearn.sklearn_component'['sklearn_component'] at line 240: Saving model to /home/vimary/ipavlov/Pilot/examples/tutorials/logreg_v1.pkl\n"
],
[
"y_valid_pred = cls(weighted_embedder(str_lower(tokenizer(x_valid))))",
"_____no_output_____"
],
[
"# Let's look into obtained result\nprint(\"Text sample: {}\".format(x_valid[0]))\nprint(\"True label: {}\".format(y_valid[0]))\nprint(\"Predicted label: {}\".format(y_valid_pred[0]))",
"Text sample: I need seating at Floating restaurant in Tennessee for a group of 9\nTrue label: ['BookRestaurant']\nPredicted label: BookRestaurant\n"
],
[
"# let's calculate sets accuracy (because each element is a list of labels)\nsets_accuracy(np.squeeze(y_valid), y_valid_pred)",
"_____no_output_____"
]
],
[
[
"### Let's free our memory from embeddings and models",
"_____no_output_____"
]
],
[
[
"embedder.reset()\ncls.reset()",
"_____no_output_____"
]
],
[
[
"### Models from configs",
"_____no_output_____"
]
],
[
[
"from deeppavlov import build_model\nfrom deeppavlov import train_model",
"_____no_output_____"
]
],
[
[
"#### SklearnComponent classifier on Tfidf-features from config",
"_____no_output_____"
]
],
[
[
"logreg_config = {\n \"dataset_reader\": {\n \"class_name\": \"basic_classification_reader\",\n \"x\": \"text\",\n \"y\": \"intents\",\n \"data_path\": \"./snips\"\n },\n \"dataset_iterator\": {\n \"class_name\": \"basic_classification_iterator\",\n \"seed\": 42,\n \"split_seed\": 23,\n \"field_to_split\": \"train\",\n \"split_fields\": [\n \"train\",\n \"valid\"\n ],\n \"split_proportions\": [\n 0.9,\n 0.1\n ]\n },\n \"chainer\": {\n \"in\": [\n \"x\"\n ],\n \"in_y\": [\n \"y\"\n ],\n \"pipe\": [\n {\n \"id\": \"classes_vocab\",\n \"class_name\": \"simple_vocab\",\n \"fit_on\": [\n \"y\"\n ],\n \"save_path\": \"./snips/classes.dict\",\n \"load_path\": \"./snips/classes.dict\",\n \"in\": \"y\",\n \"out\": \"y_ids\"\n },\n {\n \"in\": [\n \"x\"\n ],\n \"out\": [\n \"x_vec\"\n ],\n \"fit_on\": [\n \"x\",\n \"y_ids\"\n ],\n \"id\": \"tfidf_vec\",\n \"class_name\": \"sklearn_component\",\n \"save_path\": \"tfidf_v1.pkl\",\n \"load_path\": \"tfidf_v1.pkl\",\n \"model_class\": \"sklearn.feature_extraction.text:TfidfVectorizer\",\n \"infer_method\": \"transform\"\n },\n {\n \"in\": \"x\",\n \"out\": \"x_tok\",\n \"id\": \"my_tokenizer\",\n \"class_name\": \"nltk_moses_tokenizer\",\n \"tokenizer\": \"wordpunct_tokenize\"\n },\n {\n \"in\": [\n \"x_vec\"\n ],\n \"out\": [\n \"y_pred\"\n ],\n \"fit_on\": [\n \"x_vec\",\n \"y\"\n ],\n \"class_name\": \"sklearn_component\",\n \"main\": True,\n \"save_path\": \"logreg_v2.pkl\",\n \"load_path\": \"logreg_v2.pkl\",\n \"model_class\": \"sklearn.linear_model:LogisticRegression\",\n \"infer_method\": \"predict\",\n \"ensure_list_output\": True\n }\n ],\n \"out\": [\n \"y_pred\"\n ]\n },\n \"train\": {\n \"batch_size\": 64,\n \"metrics\": [\n \"accuracy\"\n ],\n \"validate_best\": True,\n \"test_best\": False\n }\n}\n",
"_____no_output_____"
],
[
"# we can train and evaluate model from config\nm = train_model(logreg_config)",
"2019-02-12 12:15:52.310 WARNING in 'deeppavlov.dataset_readers.basic_classification_reader'['basic_classification_reader'] at line 96: Cannot find /home/vimary/ipavlov/Pilot/examples/tutorials/snips/valid.csv file\n2019-02-12 12:15:52.310 WARNING in 'deeppavlov.dataset_readers.basic_classification_reader'['basic_classification_reader'] at line 96: Cannot find /home/vimary/ipavlov/Pilot/examples/tutorials/snips/test.csv file\n2019-02-12 12:15:52.311 INFO in 'deeppavlov.dataset_iterators.basic_classification_iterator'['basic_classification_iterator'] at line 73: Splitting field <<train>> to new fields <<['train', 'valid']>>\n2019-02-12 12:15:52.314 WARNING in 'deeppavlov.core.commands.train'['train'] at line 108: \"validate_best\" and \"test_best\" parameters are deprecated. Please, use \"evaluation_targets\" list instead\n2019-02-12 12:15:52.322 INFO in 'deeppavlov.core.data.simple_vocab'['simple_vocab'] at line 103: [loading vocabulary from /home/vimary/ipavlov/Pilot/examples/tutorials/snips/classes.dict]\n2019-02-12 12:15:52.339 INFO in 'deeppavlov.core.data.simple_vocab'['simple_vocab'] at line 89: [saving vocabulary to /home/vimary/ipavlov/Pilot/examples/tutorials/snips/classes.dict]\n2019-02-12 12:15:52.340 WARNING in 'deeppavlov.models.sklearn.sklearn_component'['sklearn_component'] at line 218: Cannot load model from /home/vimary/ipavlov/Pilot/examples/tutorials/tfidf_v1.pkl\n2019-02-12 12:15:52.341 INFO in 'deeppavlov.models.sklearn.sklearn_component'['sklearn_component'] at line 165: Initializing model sklearn.feature_extraction.text:TfidfVectorizer from scratch\n2019-02-12 12:15:52.389 INFO in 'deeppavlov.models.sklearn.sklearn_component'['sklearn_component'] at line 108: Fitting model sklearn.feature_extraction.text:TfidfVectorizer\n2019-02-12 12:15:52.493 INFO in 'deeppavlov.models.sklearn.sklearn_component'['sklearn_component'] at line 240: Saving model to /home/vimary/ipavlov/Pilot/examples/tutorials/tfidf_v1.pkl\n2019-02-12 12:15:52.510 WARNING in 'deeppavlov.models.sklearn.sklearn_component'['sklearn_component'] at line 218: Cannot load model from /home/vimary/ipavlov/Pilot/examples/tutorials/logreg_v2.pkl\n2019-02-12 12:15:52.510 INFO in 'deeppavlov.models.sklearn.sklearn_component'['sklearn_component'] at line 165: Initializing model sklearn.linear_model:LogisticRegression from scratch\n2019-02-12 12:15:53.67 INFO in 'deeppavlov.models.sklearn.sklearn_component'['sklearn_component'] at line 108: Fitting model sklearn.linear_model:LogisticRegression\n2019-02-12 12:15:53.254 INFO in 'deeppavlov.models.sklearn.sklearn_component'['sklearn_component'] at line 240: Saving model to /home/vimary/ipavlov/Pilot/examples/tutorials/logreg_v2.pkl\n2019-02-12 12:15:53.255 WARNING in 'deeppavlov.core.trainers.nn_trainer'['nn_trainer'] at line 295: Using NNTrainer for a pipeline without batched training\n2019-02-12 12:15:53.256 INFO in 'deeppavlov.models.sklearn.sklearn_component'['sklearn_component'] at line 240: Saving model to /home/vimary/ipavlov/Pilot/examples/tutorials/logreg_v2.pkl\n2019-02-12 12:15:53.257 INFO in 'deeppavlov.core.data.simple_vocab'['simple_vocab'] at line 103: [loading vocabulary from /home/vimary/ipavlov/Pilot/examples/tutorials/snips/classes.dict]\n2019-02-12 12:15:53.258 INFO in 'deeppavlov.models.sklearn.sklearn_component'['sklearn_component'] at line 202: Loading model sklearn.feature_extraction.text:TfidfVectorizer from /home/vimary/ipavlov/Pilot/examples/tutorials/tfidf_v1.pkl\n2019-02-12 12:15:53.263 INFO in 'deeppavlov.models.sklearn.sklearn_component'['sklearn_component'] at line 209: Model sklearn.feature_extraction.textTfidfVectorizer loaded with parameters\n2019-02-12 12:15:53.264 WARNING in 'deeppavlov.models.sklearn.sklearn_component'['sklearn_component'] at line 215: Fitting of loaded model can not be continued. Model can be fitted from scratch.If one needs to continue fitting, please, look at `warm_start` parameter\n2019-02-12 12:15:53.266 INFO in 'deeppavlov.models.sklearn.sklearn_component'['sklearn_component'] at line 202: Loading model sklearn.linear_model:LogisticRegression from /home/vimary/ipavlov/Pilot/examples/tutorials/logreg_v2.pkl\n2019-02-12 12:15:53.266 INFO in 'deeppavlov.models.sklearn.sklearn_component'['sklearn_component'] at line 209: Model sklearn.linear_model.logisticLogisticRegression loaded with parameters\n2019-02-12 12:15:53.267 WARNING in 'deeppavlov.models.sklearn.sklearn_component'['sklearn_component'] at line 215: Fitting of loaded model can not be continued. Model can be fitted from scratch.If one needs to continue fitting, please, look at `warm_start` parameter\n2019-02-12 12:15:53.346 INFO in 'deeppavlov.core.data.simple_vocab'['simple_vocab'] at line 103: [loading vocabulary from /home/vimary/ipavlov/Pilot/examples/tutorials/snips/classes.dict]\n2019-02-12 12:15:53.347 INFO in 'deeppavlov.models.sklearn.sklearn_component'['sklearn_component'] at line 202: Loading model sklearn.feature_extraction.text:TfidfVectorizer from /home/vimary/ipavlov/Pilot/examples/tutorials/tfidf_v1.pkl\n2019-02-12 12:15:53.352 INFO in 'deeppavlov.models.sklearn.sklearn_component'['sklearn_component'] at line 209: Model sklearn.feature_extraction.textTfidfVectorizer loaded with parameters\n2019-02-12 12:15:53.352 WARNING in 'deeppavlov.models.sklearn.sklearn_component'['sklearn_component'] at line 215: Fitting of loaded model can not be continued. Model can be fitted from scratch.If one needs to continue fitting, please, look at `warm_start` parameter\n2019-02-12 12:15:53.354 INFO in 'deeppavlov.models.sklearn.sklearn_component'['sklearn_component'] at line 202: Loading model sklearn.linear_model:LogisticRegression from /home/vimary/ipavlov/Pilot/examples/tutorials/logreg_v2.pkl\n2019-02-12 12:15:53.354 INFO in 'deeppavlov.models.sklearn.sklearn_component'['sklearn_component'] at line 209: Model sklearn.linear_model.logisticLogisticRegression loaded with parameters\n2019-02-12 12:15:53.355 WARNING in 'deeppavlov.models.sklearn.sklearn_component'['sklearn_component'] at line 215: Fitting of loaded model can not be continued. Model can be fitted from scratch.If one needs to continue fitting, please, look at `warm_start` parameter\n"
],
[
"# or we can just load pre-trained model (conicides with what we did above)\nm = build_model(logreg_config)",
"2019-02-12 12:15:53.359 INFO in 'deeppavlov.core.data.simple_vocab'['simple_vocab'] at line 103: [loading vocabulary from /home/vimary/ipavlov/Pilot/examples/tutorials/snips/classes.dict]\n2019-02-12 12:15:53.360 INFO in 'deeppavlov.models.sklearn.sklearn_component'['sklearn_component'] at line 202: Loading model sklearn.feature_extraction.text:TfidfVectorizer from /home/vimary/ipavlov/Pilot/examples/tutorials/tfidf_v1.pkl\n2019-02-12 12:15:53.366 INFO in 'deeppavlov.models.sklearn.sklearn_component'['sklearn_component'] at line 209: Model sklearn.feature_extraction.textTfidfVectorizer loaded with parameters\n2019-02-12 12:15:53.367 WARNING in 'deeppavlov.models.sklearn.sklearn_component'['sklearn_component'] at line 215: Fitting of loaded model can not be continued. Model can be fitted from scratch.If one needs to continue fitting, please, look at `warm_start` parameter\n2019-02-12 12:15:53.368 INFO in 'deeppavlov.models.sklearn.sklearn_component'['sklearn_component'] at line 202: Loading model sklearn.linear_model:LogisticRegression from /home/vimary/ipavlov/Pilot/examples/tutorials/logreg_v2.pkl\n2019-02-12 12:15:53.369 INFO in 'deeppavlov.models.sklearn.sklearn_component'['sklearn_component'] at line 209: Model sklearn.linear_model.logisticLogisticRegression loaded with parameters\n2019-02-12 12:15:53.369 WARNING in 'deeppavlov.models.sklearn.sklearn_component'['sklearn_component'] at line 215: Fitting of loaded model can not be continued. Model can be fitted from scratch.If one needs to continue fitting, please, look at `warm_start` parameter\n"
],
[
"m([\"Is it freezing in Offerman, California?\"])",
"_____no_output_____"
]
],
[
[
"#### KerasClassificationModel on GloVe embeddings from config",
"_____no_output_____"
]
],
[
[
"cnn_config = {\n \"dataset_reader\": {\n \"class_name\": \"basic_classification_reader\",\n \"x\": \"text\",\n \"y\": \"intents\",\n \"data_path\": \"snips\"\n },\n \"dataset_iterator\": {\n \"class_name\": \"basic_classification_iterator\",\n \"seed\": 42,\n \"split_seed\": 23,\n \"field_to_split\": \"train\",\n \"split_fields\": [\n \"train\",\n \"valid\"\n ],\n \"split_proportions\": [\n 0.9,\n 0.1\n ]\n },\n \"chainer\": {\n \"in\": [\n \"x\"\n ],\n \"in_y\": [\n \"y\"\n ],\n \"pipe\": [\n {\n \"id\": \"classes_vocab\",\n \"class_name\": \"simple_vocab\",\n \"fit_on\": [\n \"y\"\n ],\n \"level\": \"token\",\n \"save_path\": \"./snips/classes.dict\",\n \"load_path\": \"./snips/classes.dict\",\n \"in\": \"y\",\n \"out\": \"y_ids\"\n },\n {\n \"in\": \"x\",\n \"out\": \"x_tok\",\n \"id\": \"my_tokenizer\",\n \"class_name\": \"nltk_tokenizer\",\n \"tokenizer\": \"wordpunct_tokenize\"\n },\n {\n \"in\": \"x_tok\",\n \"out\": \"x_emb\",\n \"id\": \"my_embedder\",\n \"class_name\": \"glove\",\n \"load_path\": \"./glove.6B.100d.txt\",\n \"dim\": 100,\n \"pad_zero\": True\n },\n {\n \"in\": \"y_ids\",\n \"out\": \"y_onehot\",\n \"class_name\": \"one_hotter\",\n \"depth\": \"#classes_vocab.len\",\n \"single_vector\": True\n },\n {\n \"in\": [\n \"x_emb\"\n ],\n \"in_y\": [\n \"y_onehot\"\n ],\n \"out\": [\n \"y_pred_probas\"\n ],\n \"main\": True,\n \"class_name\": \"keras_classification_model\",\n \"save_path\": \"./cnn_model_v1\",\n \"load_path\": \"./cnn_model_v1\",\n \"embedding_size\": \"#my_embedder.dim\",\n \"n_classes\": \"#classes_vocab.len\",\n \"kernel_sizes_cnn\": [\n 1,\n 2,\n 3\n ],\n \"filters_cnn\": 256,\n \"optimizer\": \"Adam\",\n \"learning_rate\": 0.01,\n \"learning_rate_decay\": 0.1,\n \"loss\": \"categorical_crossentropy\",\n \"coef_reg_cnn\": 1e-4,\n \"coef_reg_den\": 1e-4,\n \"dropout_rate\": 0.5,\n \"dense_size\": 100,\n \"model_name\": \"cnn_model\"\n },\n {\n \"in\": \"y_pred_probas\",\n \"out\": \"y_pred_ids\",\n \"class_name\": \"proba2labels\",\n \"max_proba\": True\n },\n {\n \"in\": \"y_pred_ids\",\n \"out\": \"y_pred_labels\",\n \"ref\": \"classes_vocab\"\n }\n ],\n \"out\": [\n \"y_pred_labels\"\n ]\n },\n \"train\": {\n \"epochs\": 10,\n \"batch_size\": 64,\n \"metrics\": [\n \"sets_accuracy\",\n \"f1_macro\",\n {\n \"name\": \"roc_auc\",\n \"inputs\": [\"y_onehot\", \"y_pred_probas\"]\n }\n ],\n \"validation_patience\": 5,\n \"val_every_n_epochs\": 1,\n \"log_every_n_epochs\": 1,\n \"show_examples\": True,\n \"validate_best\": True,\n \"test_best\": False\n }\n}\n",
"_____no_output_____"
],
[
"# we can train and evaluate model from config\nm = train_model(cnn_config)",
"2019-02-12 12:15:54.311 WARNING in 'deeppavlov.dataset_readers.basic_classification_reader'['basic_classification_reader'] at line 96: Cannot find /home/vimary/ipavlov/Pilot/examples/tutorials/snips/valid.csv file\n2019-02-12 12:15:54.312 WARNING in 'deeppavlov.dataset_readers.basic_classification_reader'['basic_classification_reader'] at line 96: Cannot find /home/vimary/ipavlov/Pilot/examples/tutorials/snips/test.csv file\n2019-02-12 12:15:54.313 INFO in 'deeppavlov.dataset_iterators.basic_classification_iterator'['basic_classification_iterator'] at line 73: Splitting field <<train>> to new fields <<['train', 'valid']>>\n2019-02-12 12:15:54.316 WARNING in 'deeppavlov.core.commands.train'['train'] at line 108: \"validate_best\" and \"test_best\" parameters are deprecated. Please, use \"evaluation_targets\" list instead\n2019-02-12 12:15:54.319 INFO in 'deeppavlov.core.data.simple_vocab'['simple_vocab'] at line 103: [loading vocabulary from /home/vimary/ipavlov/Pilot/examples/tutorials/snips/classes.dict]\n2019-02-12 12:15:54.335 INFO in 'deeppavlov.core.data.simple_vocab'['simple_vocab'] at line 89: [saving vocabulary to /home/vimary/ipavlov/Pilot/examples/tutorials/snips/classes.dict]\n2019-02-12 12:15:54.337 INFO in 'deeppavlov.models.embedders.glove_embedder'['glove_embedder'] at line 52: [loading GloVe embeddings from `/home/vimary/ipavlov/Pilot/examples/tutorials/glove.6B.100d.txt`]\n2019-02-12 12:16:14.207 INFO in 'deeppavlov.models.classifiers.keras_classification_model'['keras_classification_model'] at line 272: [initializing `KerasClassificationModel` from scratch as cnn_model]\n2019-02-12 12:16:14.548 INFO in 'deeppavlov.models.classifiers.keras_classification_model'['keras_classification_model'] at line 136: Model was successfully initialized!\nModel summary:\n__________________________________________________________________________________________________\nLayer (type) Output Shape Param # Connected to \n==================================================================================================\ninput_1 (InputLayer) (None, None, 100) 0 \n__________________________________________________________________________________________________\nconv1d_1 (Conv1D) (None, None, 256) 25856 input_1[0][0] \n__________________________________________________________________________________________________\nconv1d_2 (Conv1D) (None, None, 256) 51456 input_1[0][0] \n__________________________________________________________________________________________________\nconv1d_3 (Conv1D) (None, None, 256) 77056 input_1[0][0] \n__________________________________________________________________________________________________\nbatch_normalization_1 (BatchNor (None, None, 256) 1024 conv1d_1[0][0] \n__________________________________________________________________________________________________\nbatch_normalization_2 (BatchNor (None, None, 256) 1024 conv1d_2[0][0] \n__________________________________________________________________________________________________\nbatch_normalization_3 (BatchNor (None, None, 256) 1024 conv1d_3[0][0] \n__________________________________________________________________________________________________\nactivation_1 (Activation) (None, None, 256) 0 batch_normalization_1[0][0] \n__________________________________________________________________________________________________\nactivation_2 (Activation) (None, None, 256) 0 batch_normalization_2[0][0] \n__________________________________________________________________________________________________\nactivation_3 (Activation) (None, None, 256) 0 batch_normalization_3[0][0] \n__________________________________________________________________________________________________\nglobal_max_pooling1d_1 (GlobalM (None, 256) 0 activation_1[0][0] \n__________________________________________________________________________________________________\nglobal_max_pooling1d_2 (GlobalM (None, 256) 0 activation_2[0][0] \n__________________________________________________________________________________________________\nglobal_max_pooling1d_3 (GlobalM (None, 256) 0 activation_3[0][0] \n__________________________________________________________________________________________________\nconcatenate_1 (Concatenate) (None, 768) 0 global_max_pooling1d_1[0][0] \n global_max_pooling1d_2[0][0] \n global_max_pooling1d_3[0][0] \n__________________________________________________________________________________________________\ndropout_1 (Dropout) (None, 768) 0 concatenate_1[0][0] \n__________________________________________________________________________________________________\ndense_1 (Dense) (None, 100) 76900 dropout_1[0][0] \n__________________________________________________________________________________________________\nbatch_normalization_4 (BatchNor (None, 100) 400 dense_1[0][0] \n__________________________________________________________________________________________________\nactivation_4 (Activation) (None, 100) 0 batch_normalization_4[0][0] \n__________________________________________________________________________________________________\ndropout_2 (Dropout) (None, 100) 0 activation_4[0][0] \n__________________________________________________________________________________________________\ndense_2 (Dense) (None, 7) 707 dropout_2[0][0] \n__________________________________________________________________________________________________\nbatch_normalization_5 (BatchNor (None, 7) 28 dense_2[0][0] \n__________________________________________________________________________________________________\nactivation_5 (Activation) (None, 7) 0 batch_normalization_5[0][0] \n==================================================================================================\nTotal params: 235,475\nTrainable params: 233,725\nNon-trainable params: 1,750\n__________________________________________________________________________________________________\n/home/vimary/tensorflow/lib/python3.6/site-packages/sklearn/metrics/classification.py:1135: UndefinedMetricWarning: F-score is ill-defined and being set to 0.0 in labels with no predicted samples.\n 'precision', 'predicted', average, warn_for)\n2019-02-12 12:16:14.932 INFO in 'deeppavlov.core.trainers.nn_trainer'['nn_trainer'] at line 163: New best sets_accuracy of 0.1479\n2019-02-12 12:16:14.932 INFO in 'deeppavlov.core.trainers.nn_trainer'['nn_trainer'] at line 165: Saving model\n2019-02-12 12:16:14.933 INFO in 'deeppavlov.models.classifiers.keras_classification_model'['keras_classification_model'] at line 386: [saving model to /home/vimary/ipavlov/Pilot/examples/tutorials/cnn_model_v1_opt.json]\n"
],
[
"# or we can just load pre-trained model (conicides with what we did above)\nm = build_model(cnn_config)",
"2019-02-12 12:17:21.914 INFO in 'deeppavlov.core.data.simple_vocab'['simple_vocab'] at line 103: [loading vocabulary from /home/vimary/ipavlov/Pilot/examples/tutorials/snips/classes.dict]\n2019-02-12 12:17:21.915 INFO in 'deeppavlov.models.embedders.glove_embedder'['glove_embedder'] at line 52: [loading GloVe embeddings from `/home/vimary/ipavlov/Pilot/examples/tutorials/glove.6B.100d.txt`]\n2019-02-12 12:17:42.89 INFO in 'deeppavlov.models.classifiers.keras_classification_model'['keras_classification_model'] at line 302: [initializing `KerasClassificationModel` from saved]\n2019-02-12 12:17:42.406 INFO in 'deeppavlov.models.classifiers.keras_classification_model'['keras_classification_model'] at line 312: [loading weights from cnn_model_v1.h5]\n2019-02-12 12:17:42.569 INFO in 'deeppavlov.models.classifiers.keras_classification_model'['keras_classification_model'] at line 136: Model was successfully initialized!\nModel summary:\n__________________________________________________________________________________________________\nLayer (type) Output Shape Param # Connected to \n==================================================================================================\ninput_1 (InputLayer) (None, None, 100) 0 \n__________________________________________________________________________________________________\nconv1d_1 (Conv1D) (None, None, 256) 25856 input_1[0][0] \n__________________________________________________________________________________________________\nconv1d_2 (Conv1D) (None, None, 256) 51456 input_1[0][0] \n__________________________________________________________________________________________________\nconv1d_3 (Conv1D) (None, None, 256) 77056 input_1[0][0] \n__________________________________________________________________________________________________\nbatch_normalization_1 (BatchNor (None, None, 256) 1024 conv1d_1[0][0] \n__________________________________________________________________________________________________\nbatch_normalization_2 (BatchNor (None, None, 256) 1024 conv1d_2[0][0] \n__________________________________________________________________________________________________\nbatch_normalization_3 (BatchNor (None, None, 256) 1024 conv1d_3[0][0] \n__________________________________________________________________________________________________\nactivation_1 (Activation) (None, None, 256) 0 batch_normalization_1[0][0] \n__________________________________________________________________________________________________\nactivation_2 (Activation) (None, None, 256) 0 batch_normalization_2[0][0] \n__________________________________________________________________________________________________\nactivation_3 (Activation) (None, None, 256) 0 batch_normalization_3[0][0] \n__________________________________________________________________________________________________\nglobal_max_pooling1d_1 (GlobalM (None, 256) 0 activation_1[0][0] \n__________________________________________________________________________________________________\nglobal_max_pooling1d_2 (GlobalM (None, 256) 0 activation_2[0][0] \n__________________________________________________________________________________________________\nglobal_max_pooling1d_3 (GlobalM (None, 256) 0 activation_3[0][0] \n__________________________________________________________________________________________________\nconcatenate_1 (Concatenate) (None, 768) 0 global_max_pooling1d_1[0][0] \n global_max_pooling1d_2[0][0] \n global_max_pooling1d_3[0][0] \n__________________________________________________________________________________________________\ndropout_1 (Dropout) (None, 768) 0 concatenate_1[0][0] \n__________________________________________________________________________________________________\ndense_1 (Dense) (None, 100) 76900 dropout_1[0][0] \n__________________________________________________________________________________________________\nbatch_normalization_4 (BatchNor (None, 100) 400 dense_1[0][0] \n__________________________________________________________________________________________________\nactivation_4 (Activation) (None, 100) 0 batch_normalization_4[0][0] \n__________________________________________________________________________________________________\ndropout_2 (Dropout) (None, 100) 0 activation_4[0][0] \n__________________________________________________________________________________________________\ndense_2 (Dense) (None, 7) 707 dropout_2[0][0] \n__________________________________________________________________________________________________\nbatch_normalization_5 (BatchNor (None, 7) 28 dense_2[0][0] \n__________________________________________________________________________________________________\nactivation_5 (Activation) (None, 7) 0 batch_normalization_5[0][0] \n==================================================================================================\nTotal params: 235,475\nTrainable params: 233,725\nNon-trainable params: 1,750\n__________________________________________________________________________________________________\n"
],
[
"m([\"Is it freezing in Offerman, California?\"])",
"_____no_output_____"
]
],
[
[
"#### SklearnComponent classifier on GloVe weighted by TF-IDF embeddings from config",
"_____no_output_____"
]
],
[
[
"logreg_config = {\n \"dataset_reader\": {\n \"class_name\": \"basic_classification_reader\",\n \"x\": \"text\",\n \"y\": \"intents\",\n \"data_path\": \"snips\"\n },\n \"dataset_iterator\": {\n \"class_name\": \"basic_classification_iterator\",\n \"seed\": 42,\n \"split_seed\": 23,\n \"field_to_split\": \"train\",\n \"split_fields\": [\n \"train\",\n \"valid\"\n ],\n \"split_proportions\": [\n 0.9,\n 0.1\n ]\n },\n \"chainer\": {\n \"in\": [\n \"x\"\n ],\n \"in_y\": [\n \"y\"\n ],\n \"pipe\": [\n {\n \"id\": \"classes_vocab\",\n \"class_name\": \"simple_vocab\",\n \"fit_on\": [\n \"y\"\n ],\n \"save_path\": \"./snips/classes.dict\",\n \"load_path\": \"./snips/classes.dict\",\n \"in\": \"y\",\n \"out\": \"y_ids\"\n },\n {\n \"in\": [\n \"x\"\n ],\n \"out\": [\n \"x_vec\"\n ],\n \"fit_on\": [\n \"x\",\n \"y_ids\"\n ],\n \"id\": \"my_tfidf_vectorizer\",\n \"class_name\": \"sklearn_component\",\n \"save_path\": \"tfidf_v2.pkl\",\n \"load_path\": \"tfidf_v2.pkl\",\n \"model_class\": \"sklearn.feature_extraction.text:TfidfVectorizer\",\n \"infer_method\": \"transform\"\n },\n {\n \"in\": \"x\",\n \"out\": \"x_tok\",\n \"id\": \"my_tokenizer\",\n \"class_name\": \"nltk_moses_tokenizer\"\n },\n {\n \"in\": \"x_tok\",\n \"out\": \"x_emb\",\n \"id\": \"my_embedder\",\n \"class_name\": \"glove\",\n \"save_path\": \"./glove.6B.100d.txt\",\n \"load_path\": \"./glove.6B.100d.txt\",\n \"dim\": 100,\n \"pad_zero\": True\n },\n {\n \"class_name\": \"one_hotter\",\n \"id\": \"my_onehotter\",\n \"depth\": \"#classes_vocab.len\",\n \"in\": \"y_ids\",\n \"out\": \"y_onehot\",\n \"single_vector\": True\n },\n {\n \"in\": \"x_tok\",\n \"out\": \"x_weighted_emb\",\n \"class_name\": \"tfidf_weighted\",\n \"id\": \"my_weighted_embedder\",\n \"embedder\": \"#my_embedder\",\n \"tokenizer\": \"#my_tokenizer\",\n \"vectorizer\": \"#my_tfidf_vectorizer\",\n \"mean\": True\n },\n {\n \"in\": [\n \"x_weighted_emb\"\n ],\n \"out\": [\n \"y_pred\"\n ],\n \"fit_on\": [\n \"x_weighted_emb\",\n \"y\"\n ],\n \"class_name\": \"sklearn_component\",\n \"main\": True,\n \"save_path\": \"logreg_v3.pkl\",\n \"load_path\": \"logreg_v3.pkl\",\n \"model_class\": \"sklearn.linear_model:LogisticRegression\",\n \"infer_method\": \"predict\",\n \"ensure_list_output\": True\n }\n ],\n \"out\": [\n \"y_pred\"\n ]\n },\n \"train\": {\n \"epochs\": 10,\n \"batch_size\": 64,\n \"metrics\": [\n \"sets_accuracy\"\n ],\n \"show_examples\": False,\n \"validate_best\": True,\n \"test_best\": False\n }\n}\n",
"_____no_output_____"
],
[
"# we can train and evaluate model from config\nm = train_model(logreg_config)",
"2019-02-12 12:32:01.417 WARNING in 'deeppavlov.dataset_readers.basic_classification_reader'['basic_classification_reader'] at line 96: Cannot find /home/vimary/ipavlov/Pilot/examples/tutorials/snips/valid.csv file\n2019-02-12 12:32:01.417 WARNING in 'deeppavlov.dataset_readers.basic_classification_reader'['basic_classification_reader'] at line 96: Cannot find /home/vimary/ipavlov/Pilot/examples/tutorials/snips/test.csv file\n2019-02-12 12:32:01.418 INFO in 'deeppavlov.dataset_iterators.basic_classification_iterator'['basic_classification_iterator'] at line 73: Splitting field <<train>> to new fields <<['train', 'valid']>>\n2019-02-12 12:32:01.420 WARNING in 'deeppavlov.core.commands.train'['train'] at line 108: \"validate_best\" and \"test_best\" parameters are deprecated. Please, use \"evaluation_targets\" list instead\n2019-02-12 12:32:01.421 INFO in 'deeppavlov.core.data.simple_vocab'['simple_vocab'] at line 103: [loading vocabulary from /home/vimary/ipavlov/Pilot/examples/tutorials/snips/classes.dict]\n2019-02-12 12:32:01.439 INFO in 'deeppavlov.core.data.simple_vocab'['simple_vocab'] at line 89: [saving vocabulary to /home/vimary/ipavlov/Pilot/examples/tutorials/snips/classes.dict]\n2019-02-12 12:32:01.440 WARNING in 'deeppavlov.models.sklearn.sklearn_component'['sklearn_component'] at line 218: Cannot load model from /home/vimary/ipavlov/Pilot/examples/tutorials/tfidf_v2.pkl\n2019-02-12 12:32:01.441 INFO in 'deeppavlov.models.sklearn.sklearn_component'['sklearn_component'] at line 165: Initializing model sklearn.feature_extraction.text:TfidfVectorizer from scratch\n2019-02-12 12:32:01.486 INFO in 'deeppavlov.models.sklearn.sklearn_component'['sklearn_component'] at line 108: Fitting model sklearn.feature_extraction.text:TfidfVectorizer\n2019-02-12 12:32:01.587 INFO in 'deeppavlov.models.sklearn.sklearn_component'['sklearn_component'] at line 240: Saving model to /home/vimary/ipavlov/Pilot/examples/tutorials/tfidf_v2.pkl\n2019-02-12 12:32:01.603 INFO in 'deeppavlov.models.embedders.glove_embedder'['glove_embedder'] at line 52: [loading GloVe embeddings from `/home/vimary/ipavlov/Pilot/examples/tutorials/glove.6B.100d.txt`]\n2019-02-12 12:32:21.226 WARNING in 'deeppavlov.models.sklearn.sklearn_component'['sklearn_component'] at line 218: Cannot load model from /home/vimary/ipavlov/Pilot/examples/tutorials/logreg_v3.pkl\n2019-02-12 12:32:21.227 INFO in 'deeppavlov.models.sklearn.sklearn_component'['sklearn_component'] at line 165: Initializing model sklearn.linear_model:LogisticRegression from scratch\n2019-02-12 12:32:43.431 INFO in 'deeppavlov.models.sklearn.sklearn_component'['sklearn_component'] at line 108: Fitting model sklearn.linear_model:LogisticRegression\n2019-02-12 12:32:45.621 INFO in 'deeppavlov.models.sklearn.sklearn_component'['sklearn_component'] at line 240: Saving model to /home/vimary/ipavlov/Pilot/examples/tutorials/logreg_v3.pkl\n2019-02-12 12:32:45.626 WARNING in 'deeppavlov.core.trainers.nn_trainer'['nn_trainer'] at line 295: Using NNTrainer for a pipeline without batched training\n2019-02-12 12:32:45.626 INFO in 'deeppavlov.models.sklearn.sklearn_component'['sklearn_component'] at line 240: Saving model to /home/vimary/ipavlov/Pilot/examples/tutorials/logreg_v3.pkl\n2019-02-12 12:32:45.658 INFO in 'deeppavlov.core.data.simple_vocab'['simple_vocab'] at line 103: [loading vocabulary from /home/vimary/ipavlov/Pilot/examples/tutorials/snips/classes.dict]\n2019-02-12 12:32:45.659 INFO in 'deeppavlov.models.sklearn.sklearn_component'['sklearn_component'] at line 202: Loading model sklearn.feature_extraction.text:TfidfVectorizer from /home/vimary/ipavlov/Pilot/examples/tutorials/tfidf_v2.pkl\n2019-02-12 12:32:45.664 INFO in 'deeppavlov.models.sklearn.sklearn_component'['sklearn_component'] at line 209: Model sklearn.feature_extraction.textTfidfVectorizer loaded with parameters\n2019-02-12 12:32:45.665 WARNING in 'deeppavlov.models.sklearn.sklearn_component'['sklearn_component'] at line 215: Fitting of loaded model can not be continued. Model can be fitted from scratch.If one needs to continue fitting, please, look at `warm_start` parameter\n2019-02-12 12:32:45.666 INFO in 'deeppavlov.models.embedders.glove_embedder'['glove_embedder'] at line 52: [loading GloVe embeddings from `/home/vimary/ipavlov/Pilot/examples/tutorials/glove.6B.100d.txt`]\n2019-02-12 12:33:05.258 INFO in 'deeppavlov.models.sklearn.sklearn_component'['sklearn_component'] at line 202: Loading model sklearn.linear_model:LogisticRegression from /home/vimary/ipavlov/Pilot/examples/tutorials/logreg_v3.pkl\n2019-02-12 12:33:05.259 INFO in 'deeppavlov.models.sklearn.sklearn_component'['sklearn_component'] at line 209: Model sklearn.linear_model.logisticLogisticRegression loaded with parameters\n2019-02-12 12:33:05.259 WARNING in 'deeppavlov.models.sklearn.sklearn_component'['sklearn_component'] at line 215: Fitting of loaded model can not be continued. Model can be fitted from scratch.If one needs to continue fitting, please, look at `warm_start` parameter\n2019-02-12 12:33:07.749 INFO in 'deeppavlov.core.data.simple_vocab'['simple_vocab'] at line 103: [loading vocabulary from /home/vimary/ipavlov/Pilot/examples/tutorials/snips/classes.dict]\n2019-02-12 12:33:07.750 INFO in 'deeppavlov.models.sklearn.sklearn_component'['sklearn_component'] at line 202: Loading model sklearn.feature_extraction.text:TfidfVectorizer from /home/vimary/ipavlov/Pilot/examples/tutorials/tfidf_v2.pkl\n2019-02-12 12:33:07.755 INFO in 'deeppavlov.models.sklearn.sklearn_component'['sklearn_component'] at line 209: Model sklearn.feature_extraction.textTfidfVectorizer loaded with parameters\n2019-02-12 12:33:07.755 WARNING in 'deeppavlov.models.sklearn.sklearn_component'['sklearn_component'] at line 215: Fitting of loaded model can not be continued. Model can be fitted from scratch.If one needs to continue fitting, please, look at `warm_start` parameter\n2019-02-12 12:33:07.756 INFO in 'deeppavlov.models.embedders.glove_embedder'['glove_embedder'] at line 52: [loading GloVe embeddings from `/home/vimary/ipavlov/Pilot/examples/tutorials/glove.6B.100d.txt`]\n"
],
[
"# or we can just load pre-trained model (conicides with what we did above)\nm = build_model(logreg_config)",
"2019-02-12 12:33:27.742 INFO in 'deeppavlov.core.data.simple_vocab'['simple_vocab'] at line 103: [loading vocabulary from /home/vimary/ipavlov/Pilot/examples/tutorials/snips/classes.dict]\n2019-02-12 12:33:27.743 INFO in 'deeppavlov.models.sklearn.sklearn_component'['sklearn_component'] at line 202: Loading model sklearn.feature_extraction.text:TfidfVectorizer from /home/vimary/ipavlov/Pilot/examples/tutorials/tfidf_v2.pkl\n2019-02-12 12:33:27.748 INFO in 'deeppavlov.models.sklearn.sklearn_component'['sklearn_component'] at line 209: Model sklearn.feature_extraction.textTfidfVectorizer loaded with parameters\n2019-02-12 12:33:27.749 WARNING in 'deeppavlov.models.sklearn.sklearn_component'['sklearn_component'] at line 215: Fitting of loaded model can not be continued. Model can be fitted from scratch.If one needs to continue fitting, please, look at `warm_start` parameter\n2019-02-12 12:33:27.750 INFO in 'deeppavlov.models.embedders.glove_embedder'['glove_embedder'] at line 52: [loading GloVe embeddings from `/home/vimary/ipavlov/Pilot/examples/tutorials/glove.6B.100d.txt`]\n2019-02-12 12:33:47.483 INFO in 'deeppavlov.models.sklearn.sklearn_component'['sklearn_component'] at line 202: Loading model sklearn.linear_model:LogisticRegression from /home/vimary/ipavlov/Pilot/examples/tutorials/logreg_v3.pkl\n2019-02-12 12:33:47.484 INFO in 'deeppavlov.models.sklearn.sklearn_component'['sklearn_component'] at line 209: Model sklearn.linear_model.logisticLogisticRegression loaded with parameters\n2019-02-12 12:33:47.484 WARNING in 'deeppavlov.models.sklearn.sklearn_component'['sklearn_component'] at line 215: Fitting of loaded model can not be continued. Model can be fitted from scratch.If one needs to continue fitting, please, look at `warm_start` parameter\n"
],
[
"m([\"Is it freezing in Offerman, California?\"])",
"_____no_output_____"
],
[
"# let's free memory\ndel m",
"_____no_output_____"
]
],
[
[
"## Bonus: pre-trained CNN model in DeepPavlov",
"_____no_output_____"
],
[
"Download model files (`wiki.en.bin` 8Gb embeddings):",
"_____no_output_____"
],
[
"! python -m deeppavlov download intents_snips_big",
"_____no_output_____"
],
[
"Evaluate metrics on validation set (no test set provided):",
"_____no_output_____"
],
[
"! python -m deeppavlov evaluate intents_snips_big",
"_____no_output_____"
],
[
"Or one can use model from python code:",
"_____no_output_____"
]
],
[
[
"from pathlib import Path\n\nimport deeppavlov\nfrom deeppavlov import build_model, evaluate_model\nfrom deeppavlov.download import deep_download\n\nconfig_path = Path(deeppavlov.__file__).parent.joinpath('configs/classifiers/intents_snips_big.json')",
"_____no_output_____"
],
[
"# let's download all the required data - model files, embeddings, vocabularies\ndeep_download(config_path)",
"2018-12-13 18:44:55.284 DEBUG in 'urllib3.connectionpool'['connectionpool'] at line 205: Starting new HTTP connection (1): files.deeppavlov.ai:80\n2018-12-13 18:44:55.341 DEBUG in 'urllib3.connectionpool'['connectionpool'] at line 393: http://files.deeppavlov.ai:80 \"GET /datasets/snips_intents/train.csv.md5 HTTP/1.1\" 200 44\n2018-12-13 18:44:55.346 INFO in 'deeppavlov.download'['download'] at line 115: Skipped http://files.deeppavlov.ai/datasets/snips_intents/train.csv download because of matching hashes\n2018-12-13 18:44:55.348 DEBUG in 'urllib3.connectionpool'['connectionpool'] at line 205: Starting new HTTP connection (1): files.deeppavlov.ai:80\n2018-12-13 18:44:55.540 DEBUG in 'urllib3.connectionpool'['connectionpool'] at line 393: http://files.deeppavlov.ai:80 \"GET /deeppavlov_data/classifiers/intents_snips_v10.tar.gz.md5 HTTP/1.1\" 200 193\n2018-12-13 18:44:55.589 INFO in 'deeppavlov.download'['download'] at line 115: Skipped http://files.deeppavlov.ai/deeppavlov_data/classifiers/intents_snips_v10.tar.gz download because of matching hashes\n2018-12-13 18:44:55.593 DEBUG in 'urllib3.connectionpool'['connectionpool'] at line 205: Starting new HTTP connection (1): files.deeppavlov.ai:80\n2018-12-13 18:44:55.629 DEBUG in 'urllib3.connectionpool'['connectionpool'] at line 393: http://files.deeppavlov.ai:80 \"GET /deeppavlov_data/embeddings/wiki.en.bin.md5 HTTP/1.1\" 200 46\n2018-12-13 18:45:11.617 INFO in 'deeppavlov.download'['download'] at line 115: Skipped http://files.deeppavlov.ai/deeppavlov_data/embeddings/wiki.en.bin download because of matching hashes\n"
],
[
"# now one can initialize model\nm = build_model(config_path)",
"2018-12-13 18:45:11.621 INFO in 'deeppavlov.core.data.simple_vocab'['simple_vocab'] at line 100: [loading vocabulary from /home/dilyara/.deeppavlov/models/classifiers/intents_snips_v10/classes.dict]\n2018-12-13 18:45:11.632 INFO in 'deeppavlov.models.embedders.fasttext_embedder'['fasttext_embedder'] at line 52: [loading fastText embeddings from `/home/dilyara/.deeppavlov/downloads/embeddings/wiki.en.bin`]\n2018-12-13 18:45:32.229 INFO in 'deeppavlov.models.classifiers.keras_classification_model'['keras_classification_model'] at line 287: [initializing `KerasClassificationModel` from saved]\n2018-12-13 18:45:32.554 INFO in 'deeppavlov.models.classifiers.keras_classification_model'['keras_classification_model'] at line 297: [loading weights from model.h5]\n2018-12-13 18:45:32.772 INFO in 'deeppavlov.models.classifiers.keras_classification_model'['keras_classification_model'] at line 137: Model was successfully initialized!\nModel summary:\n__________________________________________________________________________________________________\nLayer (type) Output Shape Param # Connected to \n==================================================================================================\ninput_1 (InputLayer) (None, None, 300) 0 \n__________________________________________________________________________________________________\nconv1d_1 (Conv1D) (None, None, 256) 230656 input_1[0][0] \n__________________________________________________________________________________________________\nconv1d_2 (Conv1D) (None, None, 256) 384256 input_1[0][0] \n__________________________________________________________________________________________________\nconv1d_3 (Conv1D) (None, None, 256) 537856 input_1[0][0] \n__________________________________________________________________________________________________\nbatch_normalization_1 (BatchNor (None, None, 256) 1024 conv1d_1[0][0] \n__________________________________________________________________________________________________\nbatch_normalization_2 (BatchNor (None, None, 256) 1024 conv1d_2[0][0] \n__________________________________________________________________________________________________\nbatch_normalization_3 (BatchNor (None, None, 256) 1024 conv1d_3[0][0] \n__________________________________________________________________________________________________\nactivation_1 (Activation) (None, None, 256) 0 batch_normalization_1[0][0] \n__________________________________________________________________________________________________\nactivation_2 (Activation) (None, None, 256) 0 batch_normalization_2[0][0] \n__________________________________________________________________________________________________\nactivation_3 (Activation) (None, None, 256) 0 batch_normalization_3[0][0] \n__________________________________________________________________________________________________\nglobal_max_pooling1d_1 (GlobalM (None, 256) 0 activation_1[0][0] \n__________________________________________________________________________________________________\nglobal_max_pooling1d_2 (GlobalM (None, 256) 0 activation_2[0][0] \n__________________________________________________________________________________________________\nglobal_max_pooling1d_3 (GlobalM (None, 256) 0 activation_3[0][0] \n__________________________________________________________________________________________________\nconcatenate_1 (Concatenate) (None, 768) 0 global_max_pooling1d_1[0][0] \n global_max_pooling1d_2[0][0] \n global_max_pooling1d_3[0][0] \n__________________________________________________________________________________________________\ndropout_1 (Dropout) (None, 768) 0 concatenate_1[0][0] \n__________________________________________________________________________________________________\ndense_1 (Dense) (None, 100) 76900 dropout_1[0][0] \n__________________________________________________________________________________________________\nbatch_normalization_4 (BatchNor (None, 100) 400 dense_1[0][0] \n__________________________________________________________________________________________________\nactivation_4 (Activation) (None, 100) 0 batch_normalization_4[0][0] \n__________________________________________________________________________________________________\ndropout_2 (Dropout) (None, 100) 0 activation_4[0][0] \n__________________________________________________________________________________________________\ndense_2 (Dense) (None, 7) 707 dropout_2[0][0] \n__________________________________________________________________________________________________\nbatch_normalization_5 (BatchNor (None, 7) 28 dense_2[0][0] \n__________________________________________________________________________________________________\nactivation_5 (Activation) (None, 7) 0 batch_normalization_5[0][0] \n==================================================================================================\nTotal params: 1,233,875\nTrainable params: 1,232,125\nNon-trainable params: 1,750\n__________________________________________________________________________________________________\n"
],
[
"m([\"Is it freezing in Offerman, California?\"])",
"_____no_output_____"
],
[
"# let's free memory\ndel m",
"_____no_output_____"
],
[
"# or one can evaluate model WITHOUT training\nevaluate_model(config_path)",
"2018-12-13 18:45:33.675 WARNING in 'deeppavlov.dataset_readers.basic_classification_reader'['basic_classification_reader'] at line 97: Cannot find /home/dilyara/.deeppavlov/downloads/snips/valid.csv file\n2018-12-13 18:45:33.675 WARNING in 'deeppavlov.dataset_readers.basic_classification_reader'['basic_classification_reader'] at line 97: Cannot find /home/dilyara/.deeppavlov/downloads/snips/test.csv file\n2018-12-13 18:45:33.676 INFO in 'deeppavlov.dataset_iterators.basic_classification_iterator'['basic_classification_iterator'] at line 73: Splitting field <<train>> to new fields <<['train', 'valid']>>\n2018-12-13 18:45:33.679 INFO in 'deeppavlov.core.data.simple_vocab'['simple_vocab'] at line 100: [loading vocabulary from /home/dilyara/.deeppavlov/models/classifiers/intents_snips_v10/classes.dict]\n2018-12-13 18:45:33.680 INFO in 'deeppavlov.models.embedders.fasttext_embedder'['fasttext_embedder'] at line 52: [loading fastText embeddings from `/home/dilyara/.deeppavlov/downloads/embeddings/wiki.en.bin`]\n2018-12-13 18:45:54.568 INFO in 'deeppavlov.models.classifiers.keras_classification_model'['keras_classification_model'] at line 287: [initializing `KerasClassificationModel` from saved]\n2018-12-13 18:45:54.913 INFO in 'deeppavlov.models.classifiers.keras_classification_model'['keras_classification_model'] at line 297: [loading weights from model.h5]\n2018-12-13 18:45:55.112 INFO in 'deeppavlov.models.classifiers.keras_classification_model'['keras_classification_model'] at line 137: Model was successfully initialized!\nModel summary:\n__________________________________________________________________________________________________\nLayer (type) Output Shape Param # Connected to \n==================================================================================================\ninput_1 (InputLayer) (None, None, 300) 0 \n__________________________________________________________________________________________________\nconv1d_1 (Conv1D) (None, None, 256) 230656 input_1[0][0] \n__________________________________________________________________________________________________\nconv1d_2 (Conv1D) (None, None, 256) 384256 input_1[0][0] \n__________________________________________________________________________________________________\nconv1d_3 (Conv1D) (None, None, 256) 537856 input_1[0][0] \n__________________________________________________________________________________________________\nbatch_normalization_1 (BatchNor (None, None, 256) 1024 conv1d_1[0][0] \n__________________________________________________________________________________________________\nbatch_normalization_2 (BatchNor (None, None, 256) 1024 conv1d_2[0][0] \n__________________________________________________________________________________________________\nbatch_normalization_3 (BatchNor (None, None, 256) 1024 conv1d_3[0][0] \n__________________________________________________________________________________________________\nactivation_1 (Activation) (None, None, 256) 0 batch_normalization_1[0][0] \n__________________________________________________________________________________________________\nactivation_2 (Activation) (None, None, 256) 0 batch_normalization_2[0][0] \n__________________________________________________________________________________________________\nactivation_3 (Activation) (None, None, 256) 0 batch_normalization_3[0][0] \n__________________________________________________________________________________________________\nglobal_max_pooling1d_1 (GlobalM (None, 256) 0 activation_1[0][0] \n__________________________________________________________________________________________________\nglobal_max_pooling1d_2 (GlobalM (None, 256) 0 activation_2[0][0] \n__________________________________________________________________________________________________\nglobal_max_pooling1d_3 (GlobalM (None, 256) 0 activation_3[0][0] \n__________________________________________________________________________________________________\nconcatenate_1 (Concatenate) (None, 768) 0 global_max_pooling1d_1[0][0] \n global_max_pooling1d_2[0][0] \n global_max_pooling1d_3[0][0] \n__________________________________________________________________________________________________\ndropout_1 (Dropout) (None, 768) 0 concatenate_1[0][0] \n__________________________________________________________________________________________________\ndense_1 (Dense) (None, 100) 76900 dropout_1[0][0] \n__________________________________________________________________________________________________\nbatch_normalization_4 (BatchNor (None, 100) 400 dense_1[0][0] \n__________________________________________________________________________________________________\nactivation_4 (Activation) (None, 100) 0 batch_normalization_4[0][0] \n__________________________________________________________________________________________________\ndropout_2 (Dropout) (None, 100) 0 activation_4[0][0] \n__________________________________________________________________________________________________\ndense_2 (Dense) (None, 7) 707 dropout_2[0][0] \n__________________________________________________________________________________________________\nbatch_normalization_5 (BatchNor (None, 7) 28 dense_2[0][0] \n__________________________________________________________________________________________________\nactivation_5 (Activation) (None, 7) 0 batch_normalization_5[0][0] \n==================================================================================================\nTotal params: 1,233,875\nTrainable params: 1,232,125\nNon-trainable params: 1,750\n__________________________________________________________________________________________________\n2018-12-13 18:45:55.113 INFO in 'deeppavlov.core.commands.train'['train'] at line 207: Testing the best saved model\n"
]
]
] | [
"markdown",
"code",
"markdown",
"code",
"markdown",
"code",
"markdown",
"code",
"markdown",
"code",
"markdown",
"code",
"markdown",
"code",
"markdown",
"code",
"markdown",
"code",
"markdown",
"code",
"markdown",
"code",
"markdown",
"code",
"markdown",
"code",
"markdown",
"code",
"markdown",
"code",
"markdown",
"code",
"markdown",
"code",
"markdown",
"code",
"markdown",
"code",
"markdown",
"code",
"markdown",
"code",
"markdown",
"code",
"markdown",
"code",
"markdown",
"code",
"markdown",
"code",
"markdown",
"code",
"markdown",
"code"
] | [
[
"markdown"
],
[
"code"
],
[
"markdown",
"markdown",
"markdown",
"markdown",
"markdown"
],
[
"code",
"code"
],
[
"markdown"
],
[
"code",
"code"
],
[
"markdown"
],
[
"code"
],
[
"markdown"
],
[
"code",
"code"
],
[
"markdown"
],
[
"code"
],
[
"markdown",
"markdown",
"markdown",
"markdown"
],
[
"code",
"code"
],
[
"markdown"
],
[
"code",
"code"
],
[
"markdown"
],
[
"code"
],
[
"markdown"
],
[
"code",
"code",
"code"
],
[
"markdown"
],
[
"code",
"code",
"code",
"code",
"code",
"code",
"code"
],
[
"markdown",
"markdown"
],
[
"code",
"code",
"code"
],
[
"markdown",
"markdown"
],
[
"code",
"code",
"code",
"code",
"code"
],
[
"markdown"
],
[
"code"
],
[
"markdown"
],
[
"code",
"code",
"code"
],
[
"markdown",
"markdown"
],
[
"code"
],
[
"markdown"
],
[
"code",
"code",
"code"
],
[
"markdown"
],
[
"code",
"code"
],
[
"markdown",
"markdown"
],
[
"code",
"code",
"code",
"code",
"code"
],
[
"markdown"
],
[
"code",
"code",
"code",
"code",
"code",
"code",
"code",
"code",
"code"
],
[
"markdown"
],
[
"code",
"code",
"code",
"code",
"code"
],
[
"markdown"
],
[
"code"
],
[
"markdown"
],
[
"code"
],
[
"markdown"
],
[
"code",
"code",
"code",
"code"
],
[
"markdown"
],
[
"code",
"code",
"code",
"code"
],
[
"markdown"
],
[
"code",
"code",
"code",
"code",
"code"
],
[
"markdown",
"markdown",
"markdown",
"markdown",
"markdown",
"markdown"
],
[
"code",
"code",
"code",
"code",
"code",
"code"
]
] |
e779352ed3a42a5ff595712bc7bb8a1a228f346c | 100,992 | ipynb | Jupyter Notebook | notebooks/trunk/regression-v2.ipynb | mehdirezaie/LSSutils | aa0505b4d711e591f8a54121ea103ca3e72bdfc8 | [
"MIT"
] | 1 | 2021-12-15T22:38:31.000Z | 2021-12-15T22:38:31.000Z | notebooks/trunk/regression-v2.ipynb | mehdirezaie/LSSutils | aa0505b4d711e591f8a54121ea103ca3e72bdfc8 | [
"MIT"
] | 3 | 2019-08-19T21:47:47.000Z | 2020-08-25T17:57:19.000Z | notebooks/trunk/regression-v2.ipynb | mehdirezaie/LSSutils | aa0505b4d711e591f8a54121ea103ca3e72bdfc8 | [
"MIT"
] | null | null | null | 106.195584 | 57,988 | 0.807381 | [
[
[
"import pandas as pd\nimport sys\nsys.path.append('/Users/mehdi/github/LSSutils')\nimport LSSutils.nn.nnutils as utils\nimport LSSutils.nn.ffnn as ffnn",
"_____no_output_____"
]
],
[
[
"# Auto MPG data",
"_____no_output_____"
]
],
[
[
"dataset_path = '/Users/mehdi/.keras/datasets/auto-mpg.data'",
"_____no_output_____"
],
[
"# read using pandas\ncolumn_names = ['MPG','Cylinders','Displacement','Horsepower','Weight',\n 'Acceleration', 'Model Year', 'Origin']\nraw_dataset = pd.read_csv(dataset_path, names=column_names,\n na_values = \"?\", comment='\\t',\n sep=\" \", skipinitialspace=True)\ndataset = raw_dataset.copy()\ndataset.tail()",
"_____no_output_____"
],
[
"dataset = dataset.dropna()\norigin = dataset.pop('Origin')\ndataset['USA'] = (origin == 1)*1.0\ndataset['Europe'] = (origin == 2)*1.0\ndataset['Japan'] = (origin == 3)*1.0",
"_____no_output_____"
],
[
"dataset.shape",
"_____no_output_____"
],
[
"t_dataset = dataset.sample(frac=0.8,random_state=0)\ntest_dataset = dataset.drop(t_dataset.index)\nval_dataset = t_dataset.sample(frac=0.25, random_state=0)\ntrain_dataset = t_dataset.drop(val_dataset.index)\ntrain_dataset.shape, val_dataset.shape, test_dataset.shape",
"_____no_output_____"
],
[
"t_dataset.shape, dataset.shape",
"_____no_output_____"
],
[
"train_stats = train_dataset.describe()\ntrain_stats.pop(\"MPG\")\ntrain_stats = train_stats.transpose()\ntrain_stats",
"_____no_output_____"
],
[
"train_labels = train_dataset.pop('MPG')\ntest_labels = test_dataset.pop('MPG')\nval_labels = val_dataset.pop('MPG')",
"_____no_output_____"
],
[
"def norm(x):\n return (x - train_stats['mean']) / train_stats['std']\n\nnormed_train_data = norm(train_dataset)\nnormed_test_data = norm(test_dataset)\nnormed_val_data = norm(val_dataset)",
"_____no_output_____"
],
[
"import numpy as np",
"_____no_output_____"
],
[
"class DATA(object): \n def __init__(self, x, y, w=1.0):\n self.X = x\n self.Y = y\n self.W = w*np.ones_like(y)",
"_____no_output_____"
],
[
"train = DATA(normed_train_data, train_labels)\ntest = DATA(normed_test_data, test_labels)\nval = DATA(normed_val_data, val_labels)",
"_____no_output_____"
],
[
"kw = dict(nfeature=9)\nmodel0 = ffnn.run_model(9, train, val, test, units=[0], batch_size=20)\nmodel1 = ffnn.run_model(9, train, val, test, units=[10, 10], batch_size=20)\nmodel2 = ffnn.run_model(9, train, val, test, units=[50, 50], batch_size=20)\nmodel3 = ffnn.run_model(9, train, val, test, units=[100, 100], batch_size=20)",
"run linear model\n\n....................................................................................................done in 1.8 secs\nrun with two hidden layer\n\n....................................................................................................done in 2.1 secs\nrun with two hidden layer\n\n............................................................................done in 1.9 secs\nrun with two hidden layer\n\n...............................................done in 1.3 secs\n"
],
[
"import matplotlib.pyplot as plt",
"_____no_output_____"
],
[
"utils.plot_history([model0['history'], \n model1['history'], \n model2['history'], \n model3['history']],\n ['linear', \n '2 layers of 10',\n '2 layers of 50',\n '2 layers of 100'])\nplt.show()",
"_____no_output_____"
],
[
"import pandas as pd",
"_____no_output_____"
],
[
"pd.DataFrame(model0['history'].history).tail().T",
"_____no_output_____"
],
[
"pd.DataFrame(model0['history'].history).tail().T",
"_____no_output_____"
],
[
"utils.plot_prederr(test.Y, model1['Ypred'].flatten())",
"_____no_output_____"
],
[
"model2['history'].model.get_config()",
"_____no_output_____"
],
[
"sys.path.append('/Users/mehdi/github/SYSNet/src')\nimport NN",
"_____no_output_____"
],
[
"help(NN.Netregression)",
"Help on class Netregression in module NN:\n\nclass Netregression(builtins.object)\n | Netregression(train, valid, test, axfit=None)\n | \n | class for a general regression\n | \n | Methods defined here:\n | \n | __init__(self, train, valid, test, axfit=None)\n | Initialize self. See help(type(self)) for accurate signature.\n | \n | savez(self, indir='./', name='regression_2hl_5chain_10epoch')\n | \n | train_evaluate(self, learning_rate=0.001, batchsize=100, nepoch=10, nchain=5, Units=[10, 10], tol=1e-05, scale=0.0, actfunc=<function relu at 0x12d9c4bf8>, patience=10)\n | \n | ----------------------------------------------------------------------\n | Data descriptors defined here:\n | \n | __dict__\n | dictionary for instance variables (if defined)\n | \n | __weakref__\n | list of weak references to the object (if defined)\n\n"
],
[
"NN.Netregression?",
"_____no_output_____"
]
]
] | [
"code",
"markdown",
"code"
] | [
[
"code"
],
[
"markdown"
],
[
"code",
"code",
"code",
"code",
"code",
"code",
"code",
"code",
"code",
"code",
"code",
"code",
"code",
"code",
"code",
"code",
"code",
"code",
"code",
"code",
"code",
"code",
"code"
]
] |
e779428e79dd27231f63eda9b6b51bd736763dbf | 24,968 | ipynb | Jupyter Notebook | chatbot/universal transformer chatbot.ipynb | flynougat/ML | b159a5f93dd2d3e43005d1b298d61da0b5f84e7f | [
"MIT"
] | null | null | null | chatbot/universal transformer chatbot.ipynb | flynougat/ML | b159a5f93dd2d3e43005d1b298d61da0b5f84e7f | [
"MIT"
] | 1 | 2021-09-18T10:21:54.000Z | 2021-09-18T10:21:54.000Z | chatbot/universal transformer chatbot.ipynb | flynougat/ML | b159a5f93dd2d3e43005d1b298d61da0b5f84e7f | [
"MIT"
] | null | null | null | 35.365439 | 140 | 0.526354 | [
[
[
"from collections import Counter\nimport json\nimport torch\nimport torch.nn as nn\nfrom torch.utils.data import Dataset\nimport torch.utils.data\nimport math\nimport torch.nn.functional as F",
"_____no_output_____"
],
[
"corpus_movie_conv = 'cornell movie-dialogs corpus/movie_conversations.txt'\ncorpus_movie_lines = 'cornell movie-dialogs corpus/movie_lines.txt'\nmax_len = 25",
"_____no_output_____"
],
[
"with open(corpus_movie_conv, 'r') as c:\n conv = c.readlines()",
"_____no_output_____"
],
[
"with open(corpus_movie_lines, 'r') as l:\n lines = l.readlines()",
"_____no_output_____"
],
[
"lines_dic = {}\nfor line in lines:\n objects = line.split(\" +++$+++ \")\n lines_dic[objects[0]] = objects[-1]",
"_____no_output_____"
],
[
"def remove_punc(string):\n punctuations = '''!()-[]{};:'\"\\,<>./?@#$%^&*_~'''\n no_punct = \"\"\n for char in string:\n if char not in punctuations:\n no_punct = no_punct + char # space is also a character\n return no_punct.lower()",
"_____no_output_____"
],
[
"pairs = []\nfor con in conv:\n ids = eval(con.split(\" +++$+++ \")[-1])\n for i in range(len(ids)):\n qa_pairs = []\n \n if i==len(ids)-1:\n break\n \n first = remove_punc(lines_dic[ids[i]].strip()) \n second = remove_punc(lines_dic[ids[i+1]].strip())\n qa_pairs.append(first.split()[:max_len])\n qa_pairs.append(second.split()[:max_len])\n pairs.append(qa_pairs)",
"_____no_output_____"
],
[
"word_freq = Counter()\nfor pair in pairs:\n word_freq.update(pair[0])\n word_freq.update(pair[1])",
"_____no_output_____"
],
[
"min_word_freq = 5\nwords = [w for w in word_freq.keys() if word_freq[w] > min_word_freq]\nword_map = {k: v + 1 for v, k in enumerate(words)}\nword_map['<unk>'] = len(word_map) + 1\nword_map['<start>'] = len(word_map) + 1\nword_map['<end>'] = len(word_map) + 1\nword_map['<pad>'] = 0",
"_____no_output_____"
],
[
"print(\"Total words are: {}\".format(len(word_map)))",
"_____no_output_____"
],
[
"with open('WORDMAP_corpus.json', 'w') as j:\n json.dump(word_map, j)",
"_____no_output_____"
],
[
"def encode_question(words, word_map):\n enc_c = [word_map.get(word, word_map['<unk>']) for word in words] + [word_map['<pad>']] * (max_len - len(words))\n return enc_c",
"_____no_output_____"
],
[
"def encode_reply(words, word_map):\n enc_c = [word_map['<start>']] + [word_map.get(word, word_map['<unk>']) for word in words] + \\\n [word_map['<end>']] + [word_map['<pad>']] * (max_len - len(words))\n return enc_c",
"_____no_output_____"
],
[
"pairs_encoded = []\nfor pair in pairs:\n qus = encode_question(pair[0], word_map)\n ans = encode_reply(pair[1], word_map)\n pairs_encoded.append([qus, ans])",
"_____no_output_____"
],
[
"with open('pairs_encoded.json', 'w') as p:\n json.dump(pairs_encoded, p)",
"_____no_output_____"
],
[
"# rev_word_map = {v: k for k, v in word_map.items()}\n# ' '.join([rev_word_map[v] for v in pairs_encoded[1][0]])",
"_____no_output_____"
],
[
"class Dataset(Dataset):\n\n def __init__(self):\n\n self.pairs = json.load(open('pairs_encoded.json'))\n self.dataset_size = len(self.pairs)\n\n def __getitem__(self, i):\n \n question = torch.LongTensor(self.pairs[i][0])\n reply = torch.LongTensor(self.pairs[i][1])\n \n return question, reply\n\n def __len__(self):\n return self.dataset_size",
"_____no_output_____"
],
[
"train_loader = torch.utils.data.DataLoader(Dataset(),\n batch_size = 100, \n shuffle=True, \n pin_memory=True)",
"_____no_output_____"
],
[
"# question, reply = next(iter(train_loader))",
"_____no_output_____"
],
[
"def create_masks(question, reply_input, reply_target):\n \n def subsequent_mask(size):\n mask = torch.triu(torch.ones(size, size)).transpose(0, 1).type(dtype=torch.uint8)\n return mask.unsqueeze(0)\n \n question_mask = question!=0\n question_mask = question_mask.to(device)\n question_mask = question_mask.unsqueeze(1).unsqueeze(1) # (batch_size, 1, 1, max_words)\n \n reply_input_mask = reply_input!=0\n reply_input_mask = reply_input_mask.unsqueeze(1) # (batch_size, 1, max_words)\n reply_input_mask = reply_input_mask & subsequent_mask(reply_input.size(-1)).type_as(reply_input_mask.data) \n reply_input_mask = reply_input_mask.unsqueeze(1) # (batch_size, 1, max_words, max_words)\n reply_target_mask = reply_target!=0 # (batch_size, max_words)\n \n return question_mask, reply_input_mask, reply_target_mask",
"_____no_output_____"
],
[
"class Embeddings(nn.Module):\n \"\"\"\n Implements embeddings of the words and adds their positional encodings. \n \"\"\"\n def __init__(self, vocab_size, d_model, max_len = 50, num_layers = 6):\n super(Embeddings, self).__init__()\n self.d_model = d_model\n self.dropout = nn.Dropout(0.1)\n self.embed = nn.Embedding(vocab_size, d_model)\n self.pe = self.create_positinal_encoding(max_len, self.d_model) # (1, max_len, d_model)\n self.te = self.create_positinal_encoding(num_layers, self.d_model) # (1, num_layers, d_model)\n self.dropout = nn.Dropout(0.1)\n \n def create_positinal_encoding(self, max_len, d_model):\n pe = torch.zeros(max_len, d_model).to(device)\n for pos in range(max_len): # for each position of the word\n for i in range(0, d_model, 2): # for each dimension of the each position\n pe[pos, i] = math.sin(pos / (10000 ** ((2 * i)/d_model)))\n pe[pos, i + 1] = math.cos(pos / (10000 ** ((2 * (i + 1))/d_model)))\n pe = pe.unsqueeze(0) # include the batch size\n return pe\n \n def forward(self, embedding, layer_idx):\n if layer_idx == 0:\n embedding = self.embed(embedding) * math.sqrt(self.d_model)\n embedding += self.pe[:, :embedding.size(1)] # pe will automatically be expanded with the same batch size as encoded_words\n # embedding: (batch_size, max_len, d_model), te: (batch_size, 1, d_model)\n embedding += self.te[:, layer_idx, :].unsqueeze(1).repeat(1, embedding.size(1), 1)\n embedding = self.dropout(embedding)\n return embedding",
"_____no_output_____"
],
[
"class MultiHeadAttention(nn.Module):\n \n def __init__(self, heads, d_model):\n \n super(MultiHeadAttention, self).__init__()\n assert d_model % heads == 0\n self.d_k = d_model // heads\n self.heads = heads\n self.dropout = nn.Dropout(0.1)\n self.query = nn.Linear(d_model, d_model)\n self.key = nn.Linear(d_model, d_model)\n self.value = nn.Linear(d_model, d_model)\n self.concat = nn.Linear(d_model, d_model)\n \n def forward(self, query, key, value, mask):\n \"\"\"\n query, key, value of shape: (batch_size, max_len, 512)\n mask of shape: (batch_size, 1, 1, max_words)\n \"\"\"\n # (batch_size, max_len, 512)\n query = self.query(query)\n key = self.key(key) \n value = self.value(value) \n \n # (batch_size, max_len, 512) --> (batch_size, max_len, h, d_k) --> (batch_size, h, max_len, d_k)\n query = query.view(query.shape[0], -1, self.heads, self.d_k).permute(0, 2, 1, 3) \n key = key.view(key.shape[0], -1, self.heads, self.d_k).permute(0, 2, 1, 3) \n value = value.view(value.shape[0], -1, self.heads, self.d_k).permute(0, 2, 1, 3) \n \n # (batch_size, h, max_len, d_k) matmul (batch_size, h, d_k, max_len) --> (batch_size, h, max_len, max_len)\n scores = torch.matmul(query, key.permute(0,1,3,2)) / math.sqrt(query.size(-1))\n scores = scores.masked_fill(mask == 0, -1e9) # (batch_size, h, max_len, max_len)\n weights = F.softmax(scores, dim = -1) # (batch_size, h, max_len, max_len)\n weights = self.dropout(weights)\n # (batch_size, h, max_len, max_len) matmul (batch_size, h, max_len, d_k) --> (batch_size, h, max_len, d_k)\n context = torch.matmul(weights, value)\n # (batch_size, h, max_len, d_k) --> (batch_size, max_len, h, d_k) --> (batch_size, max_len, h * d_k)\n context = context.permute(0,2,1,3).contiguous().view(context.shape[0], -1, self.heads * self.d_k)\n # (batch_size, max_len, h * d_k)\n interacted = self.concat(context)\n return interacted ",
"_____no_output_____"
],
[
"class FeedForward(nn.Module):\n\n def __init__(self, d_model, middle_dim = 2048):\n super(FeedForward, self).__init__()\n \n self.fc1 = nn.Linear(d_model, middle_dim)\n self.fc2 = nn.Linear(middle_dim, d_model)\n self.dropout = nn.Dropout(0.1)\n\n def forward(self, x):\n out = F.relu(self.fc1(x))\n out = self.fc2(self.dropout(out))\n return out",
"_____no_output_____"
],
[
"class EncoderLayer(nn.Module):\n\n def __init__(self, d_model, heads):\n super(EncoderLayer, self).__init__()\n self.layernorm = nn.LayerNorm(d_model)\n self.self_multihead = MultiHeadAttention(heads, d_model)\n self.feed_forward = FeedForward(d_model)\n self.dropout = nn.Dropout(0.1)\n\n def forward(self, embeddings, mask):\n interacted = self.dropout(self.self_multihead(embeddings, embeddings, embeddings, mask))\n interacted = self.layernorm(interacted + embeddings)\n feed_forward_out = self.dropout(self.feed_forward(interacted))\n encoded = self.layernorm(feed_forward_out + interacted)\n return encoded",
"_____no_output_____"
],
[
"class DecoderLayer(nn.Module):\n \n def __init__(self, d_model, heads):\n super(DecoderLayer, self).__init__()\n self.layernorm = nn.LayerNorm(d_model)\n self.self_multihead = MultiHeadAttention(heads, d_model)\n self.src_multihead = MultiHeadAttention(heads, d_model)\n self.feed_forward = FeedForward(d_model)\n self.dropout = nn.Dropout(0.1)\n \n def forward(self, embeddings, encoded, src_mask, target_mask):\n query = self.dropout(self.self_multihead(embeddings, embeddings, embeddings, target_mask))\n query = self.layernorm(query + embeddings)\n interacted = self.dropout(self.src_multihead(query, encoded, encoded, src_mask))\n interacted = self.layernorm(interacted + query)\n feed_forward_out = self.dropout(self.feed_forward(interacted))\n decoded = self.layernorm(feed_forward_out + interacted)\n return decoded",
"_____no_output_____"
],
[
"class Transformer(nn.Module):\n \n def __init__(self, d_model, heads, num_layers, word_map):\n super(Transformer, self).__init__()\n \n self.d_model = d_model\n self.num_layers = num_layers\n self.vocab_size = len(word_map)\n self.embed = Embeddings(self.vocab_size, d_model, num_layers = num_layers)\n self.encoder = EncoderLayer(d_model, heads) \n self.decoder = DecoderLayer(d_model, heads)\n self.logit = nn.Linear(d_model, self.vocab_size)\n \n def encode(self, src_embeddings, src_mask):\n for i in range(self.num_layers):\n src_embeddings = self.embed(src_embeddings, i)\n src_embeddings = self.encoder(src_embeddings, src_mask)\n return src_embeddings\n \n def decode(self, tgt_embeddings, target_mask, src_embeddings, src_mask):\n for i in range(self.num_layers):\n tgt_embeddings = self.embed(tgt_embeddings, i)\n tgt_embeddings = self.decoder(tgt_embeddings, src_embeddings, src_mask, target_mask)\n return tgt_embeddings\n \n def forward(self, src_words, src_mask, target_words, target_mask):\n encoded = self.encode(src_words, src_mask)\n decoded = self.decode(target_words, target_mask, encoded, src_mask)\n out = F.log_softmax(self.logit(decoded), dim = 2)\n return out",
"_____no_output_____"
],
[
"class AdamWarmup:\n \n def __init__(self, model_size, warmup_steps, optimizer):\n \n self.model_size = model_size\n self.warmup_steps = warmup_steps\n self.optimizer = optimizer\n self.current_step = 0\n self.lr = 0\n \n def get_lr(self):\n return self.model_size ** (-0.5) * min(self.current_step ** (-0.5), self.current_step * self.warmup_steps ** (-1.5))\n \n def step(self):\n # Increment the number of steps each time we call the step function\n self.current_step += 1\n lr = self.get_lr()\n for param_group in self.optimizer.param_groups:\n param_group['lr'] = lr\n # update the learning rate\n self.lr = lr\n self.optimizer.step() ",
"_____no_output_____"
],
[
"class LossWithLS(nn.Module):\n\n def __init__(self, size, smooth):\n super(LossWithLS, self).__init__()\n self.criterion = nn.KLDivLoss(size_average=False, reduce=False)\n self.confidence = 1.0 - smooth\n self.smooth = smooth\n self.size = size\n \n def forward(self, prediction, target, mask):\n \"\"\"\n prediction of shape: (batch_size, max_words, vocab_size)\n target and mask of shape: (batch_size, max_words)\n \"\"\"\n prediction = prediction.view(-1, prediction.size(-1)) # (batch_size * max_words, vocab_size)\n target = target.contiguous().view(-1) # (batch_size * max_words)\n mask = mask.float()\n mask = mask.view(-1) # (batch_size * max_words)\n labels = prediction.data.clone()\n labels.fill_(self.smooth / (self.size - 1))\n labels.scatter_(1, target.data.unsqueeze(1), self.confidence)\n loss = self.criterion(prediction, labels) # (batch_size * max_words, vocab_size)\n loss = (loss.sum(1) * mask).sum() / mask.sum()\n return loss",
"_____no_output_____"
],
[
"d_model = 512\nheads = 8\nnum_layers = 1\ndevice = torch.device(\"cuda\" if torch.cuda.is_available() else \"cpu\")\nepochs = 10\n\nwith open('WORDMAP_corpus.json', 'r') as j:\n word_map = json.load(j)\n \ntransformer = Transformer(d_model = d_model, heads = heads, num_layers = num_layers, word_map = word_map)\ntransformer = transformer.to(device)\nadam_optimizer = torch.optim.Adam(transformer.parameters(), lr=0, betas=(0.9, 0.98), eps=1e-9)\ntransformer_optimizer = AdamWarmup(model_size = d_model, warmup_steps = 4000, optimizer = adam_optimizer)\ncriterion = LossWithLS(len(word_map), 0.2)",
"_____no_output_____"
],
[
"def train(train_loader, transformer, criterion, epoch):\n \n transformer.train()\n sum_loss = 0\n count = 0\n\n for i, (question, reply) in enumerate(train_loader):\n \n samples = question.shape[0]\n\n # Move to device\n question = question.to(device)\n reply = reply.to(device)\n\n # Prepare Target Data\n reply_input = reply[:, :-1]\n reply_target = reply[:, 1:]\n\n # Create mask and add dimensions\n question_mask, reply_input_mask, reply_target_mask = create_masks(question, reply_input, reply_target)\n\n # Get the transformer outputs\n out = transformer(question, question_mask, reply_input, reply_input_mask)\n\n # Compute the loss\n loss = criterion(out, reply_target, reply_target_mask)\n \n # Backprop\n transformer_optimizer.optimizer.zero_grad()\n loss.backward()\n transformer_optimizer.step()\n \n sum_loss += loss.item() * samples\n count += samples\n \n if i % 100 == 0:\n print(\"Epoch [{}][{}/{}]\\tLoss: {:.3f}\".format(epoch, i, len(train_loader), sum_loss/count))",
"_____no_output_____"
],
[
"def evaluate(transformer, question, question_mask, max_len, word_map):\n \"\"\"\n Performs Greedy Decoding with a batch size of 1\n \"\"\"\n rev_word_map = {v: k for k, v in word_map.items()}\n transformer.eval()\n start_token = word_map['<start>']\n encoded = transformer.encode(question, question_mask)\n words = torch.LongTensor([[start_token]]).to(device)\n \n for step in range(max_len - 1):\n size = words.shape[1]\n target_mask = torch.triu(torch.ones(size, size)).transpose(0, 1).type(dtype=torch.uint8)\n target_mask = target_mask.to(device).unsqueeze(0).unsqueeze(0)\n decoded = transformer.decode(words, target_mask, encoded, question_mask)\n predictions = transformer.logit(decoded[:, -1])\n _, next_word = torch.max(predictions, dim = 1)\n next_word = next_word.item()\n if next_word == word_map['<end>']:\n break\n words = torch.cat([words, torch.LongTensor([[next_word]]).to(device)], dim = 1) # (1,step+2)\n \n # Construct Sentence\n if words.dim() == 2:\n words = words.squeeze(0)\n words = words.tolist()\n \n sen_idx = [w for w in words if w not in {word_map['<start>']}]\n sentence = ' '.join([rev_word_map[sen_idx[k]] for k in range(len(sen_idx))])\n \n return sentence",
"_____no_output_____"
],
[
"for epoch in range(epochs):\n \n train(train_loader, transformer, criterion, epoch)\n \n state = {'epoch': epoch, 'transformer': transformer, 'transformer_optimizer': transformer_optimizer}\n torch.save(state, 'checkpoint_' + str(epoch) + '.pth.tar')",
"_____no_output_____"
],
[
"checkpoint = torch.load('checkpoint_0.pth.tar')\ntransformer = checkpoint['transformer']",
"_____no_output_____"
],
[
"while(1):\n question = input(\"Question: \") \n if question == 'quit':\n break\n max_len = input(\"Maximum Reply Length: \")\n enc_qus = [word_map.get(word, word_map['<unk>']) for word in question.split()]\n question = torch.LongTensor(enc_qus).to(device).unsqueeze(0)\n question_mask = (question!=0).to(device).unsqueeze(1).unsqueeze(1) \n sentence = evaluate(transformer, question, question_mask, int(max_len), word_map)\n print(sentence)",
"_____no_output_____"
]
]
] | [
"code"
] | [
[
"code",
"code",
"code",
"code",
"code",
"code",
"code",
"code",
"code",
"code",
"code",
"code",
"code",
"code",
"code",
"code",
"code",
"code",
"code",
"code",
"code",
"code",
"code",
"code",
"code",
"code",
"code",
"code",
"code",
"code",
"code",
"code",
"code",
"code"
]
] |
e77944932cf2add8d1c37d363b567100bc260041 | 1,460 | ipynb | Jupyter Notebook | _notebooks/Daily Summary (In Progress).ipynb | SamCurtis111/attunga | bea6cb896e9ab63d99d6c207f9acbcad10d60692 | [
"Apache-2.0"
] | 1 | 2021-03-13T01:51:56.000Z | 2021-03-13T01:51:56.000Z | _notebooks/Daily Summary (In Progress).ipynb | SamCurtis111/attunga | bea6cb896e9ab63d99d6c207f9acbcad10d60692 | [
"Apache-2.0"
] | null | null | null | _notebooks/Daily Summary (In Progress).ipynb | SamCurtis111/attunga | bea6cb896e9ab63d99d6c207f9acbcad10d60692 | [
"Apache-2.0"
] | null | null | null | 21.15942 | 69 | 0.507534 | [
[
[
"# Morning Review\n(not in production) \nneed to put in appropriate formatting prior to production",
"_____no_output_____"
],
[
"## Rough Format\n\n#### NEM Summary\n* Spot market summary:\n * Yest spot prices\n * PD 7 Day spoot prices\n * Average 7 day price table (incl. caps)\n \n* Supply / Demand / Reserve\n * IC flows / constraints\n \n* Generation\n * Fuel mix\n * By DUID - outages / trips\n * Bid stacks (some of these might not be worth the work)\n \n#### ASX Summary\n* Yesterday biggest movers",
"_____no_output_____"
]
]
] | [
"markdown"
] | [
[
"markdown",
"markdown"
]
] |
e7794eb63c153f8960c9347c0b99755d0c285c71 | 112,704 | ipynb | Jupyter Notebook | notebooks/EDA - Incident data from CSR vs Ongevallen from RWS.ipynb | G-Simeone/Learning_Accident_Occurence_on_Dutch_Highways | 1f3992a529fed70fd488811d68128a1e255fac5f | [
"MIT"
] | 4 | 2018-11-09T16:18:28.000Z | 2019-04-09T11:19:23.000Z | notebooks/EDA - Incident data from CSR vs Ongevallen from RWS.ipynb | G-Simeone/Learning_Accident_Occurence_on_Dutch_Highways | 1f3992a529fed70fd488811d68128a1e255fac5f | [
"MIT"
] | null | null | null | notebooks/EDA - Incident data from CSR vs Ongevallen from RWS.ipynb | G-Simeone/Learning_Accident_Occurence_on_Dutch_Highways | 1f3992a529fed70fd488811d68128a1e255fac5f | [
"MIT"
] | 1 | 2020-05-28T18:48:17.000Z | 2020-05-28T18:48:17.000Z | 38.769866 | 107 | 0.36028 | [
[
[
"import pandas as pd\nimport sys",
"_____no_output_____"
],
[
"sys.path.append('..')",
"_____no_output_____"
],
[
"from scripts import util",
"_____no_output_____"
],
[
"conn = util.connect_rds()",
"_____no_output_____"
]
],
[
[
"The aim of this notebook is to explore the following questions:\n - [ ] Does CSR ongevellan have similar numbers as the incident data that has been provided by RWS\n - [ ] Is there a common key between the 2 datasets such that we can beef up RWS using Ongavellen.",
"_____no_output_____"
]
],
[
[
"rws = pd.read_sql('select * from rws_schema.ongevallen_raw;', con=conn)",
"_____no_output_____"
],
[
"csr = pd.read_sql('select * from rws_schema.incidents;', con=conn)",
"_____no_output_____"
],
[
"csr.head()",
"_____no_output_____"
],
[
"rws.columns",
"_____no_output_____"
],
[
"csr.columns",
"_____no_output_____"
],
[
"csr.inc_type.value_counts(normalize=True)",
"_____no_output_____"
],
[
"csr.loc[:,'inc_start'] = pd.to_datetime(csr.inc_start)\n",
"_____no_output_____"
],
[
"csr.loc[:,'date'] = csr.inc_start.apply(lambda x: x.date())\ncsr.loc[:,'year'] = csr.inc_start.apply(lambda x: x.year)",
"_____no_output_____"
],
[
"csr.loc[:,'accident'] = 1",
"_____no_output_____"
],
[
"d = csr.pivot_table(index='inc_type',columns='year', values='accident', aggfunc=sum)",
"_____no_output_____"
],
[
"d.loc['Ongeval',:]",
"_____no_output_____"
],
[
"rws.jaar.value_counts().sort_index()",
"_____no_output_____"
],
[
"csr.head(1).transpose()",
"_____no_output_____"
]
],
[
[
"## Do they have a common key?",
"_____no_output_____"
]
],
[
[
"# what are the common columns",
"_____no_output_____"
],
[
"c = set(csr.columns)\nr = set(rws.columns)",
"_____no_output_____"
],
[
"c.intersection(r)",
"_____no_output_____"
],
[
"r.intersection(c)",
"_____no_output_____"
]
],
[
[
"Because column names have been edited in english, so there is no direct intersection",
"_____no_output_____"
]
],
[
[
"csr.loc[csr.inc_type=='Ongeval']",
"_____no_output_____"
],
[
"rws.head()",
"_____no_output_____"
],
[
"csr.shape",
"_____no_output_____"
],
[
"pd.to_numeric(rws.id_jaar.map(lambda x: x.split('.')[0])).describe()",
"_____no_output_____"
]
]
] | [
"code",
"markdown",
"code",
"markdown",
"code",
"markdown",
"code"
] | [
[
"code",
"code",
"code",
"code"
],
[
"markdown"
],
[
"code",
"code",
"code",
"code",
"code",
"code",
"code",
"code",
"code",
"code",
"code",
"code",
"code"
],
[
"markdown"
],
[
"code",
"code",
"code",
"code"
],
[
"markdown"
],
[
"code",
"code",
"code",
"code"
]
] |
e7795b541d127aa2f849a61079ec612c732651c2 | 32,355 | ipynb | Jupyter Notebook | Binary-Classification/it will rain tomorrow/notebooks/Binary Classification-Random Forest.ipynb | mamonteiro-brg/Lisbon-Data-Science-Academy | 29cbabb3ae65b6e731af6702518b0bccc3dec162 | [
"MIT"
] | null | null | null | Binary-Classification/it will rain tomorrow/notebooks/Binary Classification-Random Forest.ipynb | mamonteiro-brg/Lisbon-Data-Science-Academy | 29cbabb3ae65b6e731af6702518b0bccc3dec162 | [
"MIT"
] | null | null | null | Binary-Classification/it will rain tomorrow/notebooks/Binary Classification-Random Forest.ipynb | mamonteiro-brg/Lisbon-Data-Science-Academy | 29cbabb3ae65b6e731af6702518b0bccc3dec162 | [
"MIT"
] | null | null | null | 28.506608 | 202 | 0.39218 | [
[
[
"%load_ext autoreload",
"_____no_output_____"
],
[
"from utils import basic_data_wrangling\nimport pandas as pd\nimport numpy as np\nfrom scipy.stats import randint\n\n\nimport seaborn as sns\nimport matplotlib\nimport matplotlib.pyplot as plt\n%matplotlib inline\n\n#Import stuff to scale data\nfrom sklearn.preprocessing import MinMaxScaler\nfrom sklearn.preprocessing import StandardScaler\n\n\n# Import the classifiers we will be using\nfrom sklearn.neighbors import KNeighborsClassifier\nfrom sklearn.naive_bayes import GaussianNB\nfrom sklearn.tree import DecisionTreeClassifier\nfrom sklearn.ensemble import RandomForestClassifier, VotingClassifier\nfrom sklearn.model_selection import RandomizedSearchCV\nfrom sklearn.linear_model import LogisticRegression\n\n\n# Import ROC AUC scoring function\nfrom sklearn.metrics import roc_auc_score\n\n# Import train/test split function\nfrom sklearn.model_selection import train_test_split\n\n\n%autoreload 2",
"_____no_output_____"
],
[
"#Import data\ntrain = pd.read_csv(\"../data/train.csv\", index_col='ID')\ntest = pd.read_csv(\"../data/test.csv\", index_col='ID')",
"_____no_output_____"
]
],
[
[
"#Non Numerical data\nnon_numerical_data = train.select_dtypes(include=\"object\")\nnon_numerical_data.head(3)\ntrain.head()",
"_____no_output_____"
]
],
[
[
"#Numerical data\nnumerical_data = train.select_dtypes(exclude=\"object\")\nnumerical_data.head(3)\ntrain.head()",
"_____no_output_____"
],
[
"#Sub every empty postion with smtg\nnumericals = train.select_dtypes(include=[np.number]).columns.tolist()\nnumericals.remove(\"TomorrowRainForecast\")\n#Get categoricals\ncategoricals = train.select_dtypes(exclude=[np.number]).columns.tolist() \n\n#Clen data\ntrain = basic_data_wrangling(train) \nX_final_test = basic_data_wrangling(test)\ntrain.head()",
"_____no_output_____"
],
[
"#Ready data\nlabel = train.TomorrowRainForecast\nfeatures = train.drop('TomorrowRainForecast', axis=1)\nX_train, X_test, y_train, y_test = train_test_split(features, label, test_size=0.33, random_state=0)",
"_____no_output_____"
],
[
"#Get rid of every feature with a direction\n\nold_X_train = X_train\n\nfor col_name in X_train:\n \n if col_name.find('Dir') != -1 and col_name.find('StrongWind')!= -1 :\n\n X_train = X_train.drop(col_name,axis=1)\n\n X_test = X_test.drop(col_name,axis=1)\n ",
"_____no_output_____"
],
[
"\"\"\"\n\nrange_min=0\n\nrange_max=1\n\nmin_max_scaler = MinMaxScaler(feature_range=(range_min, range_max))\n\nX_train = min_max_scaler.fit_transform(X_train)\n\npd.DataFrame( X_train)\n\"\"\"",
"_____no_output_____"
],
[
"#Classifier \n\n# Choose the model\nrandom_forest = RandomForestClassifier(random_state=10, n_estimators=500) #, n_estimators = 500) # max_depth=10\n\n'''\nrandom_forest = RandomForestClassifier(bootstrap=True, class_weight=None, criterion='gini',\n max_depth=6, max_features=45, max_leaf_nodes=None,\n min_impurity_decrease=0.0, min_impurity_split=None,\n min_samples_leaf=1, min_samples_split=2,\n min_weight_fraction_leaf=0.0, n_estimators=500, n_jobs=1,\n oob_score=False, random_state=10, verbose=0,\n warm_start=False)\n'''\n\n\n# Fit the model\nrandom_forest.fit(X_train, y_train)\n\n# Make the predictions\nrandom_forest_preds = random_forest.predict_proba(X_test)\n\ncols = X_train.columns.tolist()\nsubmit_preds = random_forest.predict_proba(X_final_test[cols])\n\n# Score the predictions\nscore_frst = roc_auc_score(y_test, random_forest_preds[:,1])\n\nprint(\"ROC AUC: %f\" % score_frst)",
"ROC AUC: 0.870390\n"
],
[
"X_train.shape",
"_____no_output_____"
],
[
"y_train.shape",
"_____no_output_____"
],
[
"X_final_test[cols].shape",
"_____no_output_____"
],
[
"#Classifier gaussian naive bayes# Choose the model\n\nnaive_bayes = GaussianNB()# Fit the model\n\nnaive_bayes = naive_bayes.fit(X_train, y_train)# Make the predictions\n\nnaive_bayes_preds = naive_bayes.predict_proba( X_final_test[cols])\nnaive_bayes_preds2 = naive_bayes.predict_proba( X_test)\n# Score the predictions\n\nscore_gaus = roc_auc_score(y_test, naive_bayes_preds2[:,1])\n#print(\"ROC AUC: %f\" % score_gaus)\n",
"_____no_output_____"
],
[
"logReg = LogisticRegression(random_state=10)\n",
"_____no_output_____"
],
[
"#Voting classifier\n\n#total = score_frst + score_gaus\n\n#weights = [score_frst/total ,score_gaus/total ]\n\n#eclf1 = VotingClassifier(estimators=[('rand_frst', random_forest), ('naive_bayes', naive_bayes)], voting='soft', weights = weights )\neclf1 = VotingClassifier(estimators=[('rand_frst', random_forest), ('naive_bayes', naive_bayes),('logreg',logReg)], voting='soft')\n\neclf1 = eclf1.fit(X_train,y_train)\n\nresults=eclf1.predict_proba(X_final_test[cols])[:,1]\n\nprint(results)",
"[0.8812981 0.01354855 0.17339592 ... 0.03721223 0.07927122 0.05134079]\n"
],
[
"X_train.head()",
"_____no_output_____"
],
[
"from sklearn.model_selection import cross_val_score, cross_validate\ngrid_results = cross_validate(random_forest, X_test, y_test, scoring=\"roc_auc\", \n return_train_score=True, cv=5)\n\n",
"_____no_output_____"
],
[
"grid_results\npd.DataFrame(grid_results).mean()\n",
"_____no_output_____"
],
[
"X_test.head()",
"_____no_output_____"
],
[
"independent_variables = numericals\nestimator=DecisionTreeClassifier()\n\nrandom_search_parameter_space_dist = {\n \"max_depth\": randint(1, 100),\n \"max_features\": randint(1, len(independent_variables)),\n \"class_weight\": [\"balanced\", None]\n }\n\nrandomized_search = RandomizedSearchCV(\n estimator, \n random_search_parameter_space_dist,\n cv=5, n_iter=250,\n random_state=42,\n return_train_score=True, \n n_jobs = 10 )",
"_____no_output_____"
],
[
"%%timeit -n 1 -r 1\n\nrandomized_search.fit(X_train, y_train)",
"_____no_output_____"
],
[
"randomized_search.best_estimator_",
"_____no_output_____"
],
[
"randomized_search.best_score_",
"_____no_output_____"
],
[
"randomized_search = RandomizedSearchCV(\n RandomForestClassifier(), \n random_search_parameter_space_dist,\n cv=5, n_iter=250,\n random_state=42,\n return_train_score=True, \n n_jobs = 10 )",
"_____no_output_____"
],
[
"%%timeit -n 1 -r 1\n\nrandomized_search.fit(X_train, y_train)",
"_____no_output_____"
],
[
"randomized_search.best_estimator_",
"_____no_output_____"
],
[
"randomized_search.best_score_",
"_____no_output_____"
],
[
"#results = submit_preds[:,1]\nprint(len(results))\npredictions = pd.DataFrame({'ID': X_final_test.index,'TomorrowRainForecast':results})\n#Output\n\npredictions.to_csv('predictions_vote_2.csv', index=False)\n",
"3000\n"
],
[
"#Output\n\npredictions.to_csv('predictions.csv', index=False)",
"_____no_output_____"
],
[
"#Plot\n\"\"\"\nfeature_importances = random_forest.feature_importances_\nfeature_importances = pd.Series(feature_importances, index=X_train.columns, name=\"feature_importance_value\")\nmatplotlib.rcParams[\"figure.figsize\"] = [18, 18]\nfeature_importances.plot.barh();\"\"\"",
"_____no_output_____"
],
[
"random_forest_preds",
"_____no_output_____"
]
]
] | [
"code",
"raw",
"code"
] | [
[
"code",
"code",
"code"
],
[
"raw"
],
[
"code",
"code",
"code",
"code",
"code",
"code",
"code",
"code",
"code",
"code",
"code",
"code",
"code",
"code",
"code",
"code",
"code",
"code",
"code",
"code",
"code",
"code",
"code",
"code",
"code",
"code",
"code",
"code"
]
] |
e77961d23abec30f16c69cb067b7d7391874c38e | 966,693 | ipynb | Jupyter Notebook | notebooks/executed/037_afox_RunParcels_TS_MXL_Multiline_Randomvel_Papermill_executed_2019-10-20.ipynb | alanfox/spg_fresh_blob_202104 | e0221e31d690b08585c1d75b34398610f91fc2ba | [
"MIT"
] | null | null | null | notebooks/executed/037_afox_RunParcels_TS_MXL_Multiline_Randomvel_Papermill_executed_2019-10-20.ipynb | alanfox/spg_fresh_blob_202104 | e0221e31d690b08585c1d75b34398610f91fc2ba | [
"MIT"
] | null | null | null | notebooks/executed/037_afox_RunParcels_TS_MXL_Multiline_Randomvel_Papermill_executed_2019-10-20.ipynb | alanfox/spg_fresh_blob_202104 | e0221e31d690b08585c1d75b34398610f91fc2ba | [
"MIT"
] | null | null | null | 78.554608 | 237,332 | 0.790989 | [
[
[
"# Parcels Experiment:<br><br>Expanding the polyline code to release particles at density based on local velocity normal to section.\n\n_(Based on an experiment originally designed by Christina Schmidt.)_\n\n_(Runs on GEOMAR Jupyter Server at https://schulung3.geomar.de/user/workshop007/lab)_",
"_____no_output_____"
],
[
"## To do\n\n- Check/ask how OceanParcels deals with partial cells, if it does.\n - It doesn't. Does it matter?",
"_____no_output_____"
],
[
"## Technical preamble",
"_____no_output_____"
]
],
[
[
"%matplotlib inline\n\nfrom parcels import (\n AdvectionRK4_3D,\n ErrorCode,\n FieldSet,\n JITParticle,\n ParticleSet,\n Variable\n)\n\n# from operator import attrgetter\nfrom datetime import datetime, timedelta\nimport numpy as np\nfrom pathlib import Path\nimport matplotlib.pyplot as plt\nimport cmocean as co\nimport pandas as pd\nimport xarray as xr\n# import dask as dask\n",
"INFO: Compiled ParcelsRandom ==> /tmp/parcels-62665/libparcels_random_657e0035-5181-471b-9b3b-09640069ddf8.so\n"
]
],
[
[
"## Experiment settings (user input)",
"_____no_output_____"
],
[
"### Parameters\nThese can be set in papermill",
"_____no_output_____"
]
],
[
[
"# OSNAP multiline details\nsectionPathname = '../data/external/'\nsectionFilename = 'osnap_pos_wp.txt'\nsectionname = 'osnap'\n# location of input data\npath_name = '/data/iAtlantic/ocean-only/VIKING20X.L46-KKG36107B/nemo/output/'\nexperiment_name = 'VIKING20X.L46-KKG36107B' \ndata_resolution = '1m'\nw_name_extension = '_repaire_depthw_time'\n\n# location of mask data\nmask_path_name = '/data/iAtlantic/ocean-only/VIKING20X.L46-KKG36107B/nemo/suppl/'\nmesh_mask_filename = '1_mesh_mask.nc_notime_depthw'\n# location of output data\noutpath_name = '../data/raw/'\n\nyear_prefix = 201 # this does from 2000 onwards\n\n# set line segment to use\nstart_vertex = 4\nend_vertex = 12\n\n# experiment duration etc\nruntime_in_days = 10\ndt_in_minutes = -10\n# repeatdt = timedelta(days=3)\n\n# number of particles to track\ncreate_number_particles = 200000 # many will not be ocean points\nuse_number_particles = 200000\n\nmin_release_depth = 0\nmax_release_depth = 1_000 \n\n# max current speed for particle selection\nmax_current = 1.0\n\n# set base release date and time\nt_0_str = '2010-01-16T12:00:00'\nt_start_str = '2016-01-16T12:00:00'\n\n# particle positions are stored every x hours \noutputdt_in_hours = 120\n\n# select subdomain (to decrease needed resources) comment out to use whole domain\n# sd_i1, sd_i2 = 0, 2404 # western/eastern limit (indices not coordinates)\n# sd_j1, sd_j2 = 1200, 2499 # southern/northern limit (indices not coordinates)\n# sd_z1, sd_z2 = 0, 46\n\n# how to initialize the random number generator\n# --> is set in next cell\n# RNG_seed = 123\n\nuse_dask_chunks = True",
"_____no_output_____"
],
[
"# Parameters\npath_name = \"/gxfs_work1/geomar/smomw355/model_data/ocean-only/VIKING20X.L46-KKG36107B/nemo/output/\"\ndata_resolution = \"5d\"\nw_name_extension = \"\"\nmask_path_name = \"/gxfs_work1/geomar/smomw355/model_data/ocean-only/VIKING20X.L46-KKG36107B/nemo/suppl/\"\nmesh_mask_filename = \"1_mesh_mask.nc\"\nyear_prefix = \"\"\nruntime_in_days = 3650\ncreate_number_particles = 4000000\nuse_number_particles = 4000000\nmax_release_depth = 1000\nmax_current = 2.0\nt_0_str = \"1980-01-03T12:00:00\"\nt_start_str = \"2019-10-20T12:00:00\"\nuse_dask_chunks = False\n",
"_____no_output_____"
]
],
[
[
"### Derived variables",
"_____no_output_____"
]
],
[
[
"# times\nt_0 = datetime.fromisoformat(t_0_str) # using monthly mean fields. Check dates.\nt_start = datetime.fromisoformat(t_start_str)\n# RNG seed based on release day (days since 1980-01-03)\nRNG_seed = int((t_start - t_0).total_seconds() / (60*60*24))\n\n# names of files to load \nfname_U = f'1_{experiment_name}_{data_resolution}_{year_prefix}*_grid_U.nc' \nfname_V = f'1_{experiment_name}_{data_resolution}_{year_prefix}*_grid_V.nc'\nfname_T = f'1_{experiment_name}_{data_resolution}_{year_prefix}*_grid_T.nc'\nfname_W = f'1_{experiment_name}_{data_resolution}_{year_prefix}*_grid_W.nc{w_name_extension}'\n\nsectionPath = Path(sectionPathname)\n\ndata_path = Path(path_name)\n\nmask_path = Path(mask_path_name)\n\noutpath = Path(outpath_name)\n\ndisplay(t_0)\ndisplay(t_start)",
"_____no_output_____"
],
[
"if dt_in_minutes > 0:\n direction = '_forwards_'\nelse:\n direction = '_backward_'\n\nyear_str = str(t_start.year)\nmonth_str = str(t_start.month).zfill(2)\nday_str = str(t_start.day).zfill(2)\ndays = str(runtime_in_days)\nseed = str(RNG_seed)\nnpart= str(use_number_particles)\n\n",
"_____no_output_____"
],
[
"degree2km = 1.852*60.0\n",
"_____no_output_____"
]
],
[
[
"## Construct input / output paths etc.",
"_____no_output_____"
]
],
[
[
"mesh_mask = mask_path / mesh_mask_filename\n",
"_____no_output_____"
]
],
[
[
"## Load input datasets",
"_____no_output_____"
]
],
[
[
"def fieldset_defintions(\n list_of_filenames_U, list_of_filenames_V,\n list_of_filenames_W, list_of_filenames_T,\n mesh_mask\n):\n ds_mask = xr.open_dataset(mesh_mask)\n \n filenames = {'U': {'lon': (mesh_mask),\n 'lat': (mesh_mask),\n 'depth': list_of_filenames_W[0],\n 'data': list_of_filenames_U},\n 'V': {'lon': (mesh_mask),\n 'lat': (mesh_mask),\n 'depth': list_of_filenames_W[0],\n 'data': list_of_filenames_V},\n 'W': {'lon': (mesh_mask),\n 'lat': (mesh_mask),\n 'depth': list_of_filenames_W[0],\n 'data': list_of_filenames_W},\n 'T': {'lon': (mesh_mask),\n 'lat': (mesh_mask),\n 'depth': list_of_filenames_W[0],\n 'data': list_of_filenames_T},\n 'S': {'lon': (mesh_mask),\n 'lat': (mesh_mask),\n 'depth': list_of_filenames_W[0],\n 'data': list_of_filenames_T},\n 'MXL': {'lon': (mesh_mask),\n 'lat': (mesh_mask),\n 'data': list_of_filenames_T}\n }\n \n variables = {'U': 'vozocrtx',\n 'V': 'vomecrty',\n 'W': 'vovecrtz',\n 'T': 'votemper',\n 'S': 'vosaline',\n 'MXL':'somxl010'\n }\n \n dimensions = {'U': {'lon': 'glamf', 'lat': 'gphif', 'depth': 'depthw',\n 'time': 'time_counter'}, # needs to be on f-nodes\n 'V': {'lon': 'glamf', 'lat': 'gphif', 'depth': 'depthw',\n 'time': 'time_counter'}, # needs to be on f-nodes\n 'W': {'lon': 'glamf', 'lat': 'gphif', 'depth': 'depthw',\n 'time': 'time_counter'}, # needs to be on f-nodes\n 'T': {'lon': 'glamf', 'lat': 'gphif', 'depth': 'depthw',\n 'time': 'time_counter'}, # needs to be on t-nodes\n 'S': {'lon': 'glamf', 'lat': 'gphif', 'depth': 'depthw',\n 'time': 'time_counter'}, # needs to be on t-nodes\n 'MXL': {'lon': 'glamf', 'lat': 'gphif',\n 'time': 'time_counter'}, # needs to be on t-nodes\n }\n \n # exclude the two grid cells at the edges of the nest as they contain 0\n # and everything south of 20N\n indices = {'lon': range(2, ds_mask.x.size-2), 'lat': range(1132, ds_mask.y.size-2)}\n \n# indices = {\n# 'U': {'depth': range(sd_z1, sd_z2), 'lon': range(sd_i1, sd_i2), 'lat': range(sd_j1, sd_j2)},\n# 'V': {'depth': range(sd_z1, sd_z2), 'lon': range(sd_i1, sd_i2), 'lat': range(sd_j1, sd_j2)},\n# 'W': {'depth': range(sd_z1, sd_z2), 'lon': range(sd_i1, sd_i2), 'lat':range(sd_j1, sd_j2)},\n# 'T': {'depth': range(sd_z1, sd_z2), 'lon': range(sd_i1, sd_i2), 'lat':range(sd_j1, sd_j2)},\n# 'S': {'depth': range(sd_z1, sd_z2), 'lon': range(sd_i1, sd_i2), 'lat':range(sd_j1, sd_j2)}\n# } \n \n if use_dask_chunks:\n field_chunksizes = {'U': {'lon':('x', 1024), 'lat':('y',128), 'depth': ('depthw', 64),\n 'time': ('time_counter',3)}, # needs to be on f-nodes\n 'V': {'lon':('x', 1024), 'lat':('y',128), 'depth': ('depthw', 64),\n 'time': ('time_counter',3)}, # needs to be on f-nodes\n 'W': {'lon':('x', 1024), 'lat':('y',128), 'depth': ('depthw', 64),\n 'time': ('time_counter',3)}, # needs to be on f-nodes\n 'T': {'lon':('x', 1024), 'lat':('y',128), 'depth': ('depthw', 64),\n 'time': ('time_counter',3)}, # needs to be on t-nodes\n 'S': {'lon':('x', 1024), 'lat':('y',128), 'depth': ('depthw', 64),\n 'time': ('time_counter',3)}, # needs to be on t-nodes\n 'MXL': {'lon':('x', 1024), 'lat':('y',128),\n 'time': ('time_counter',3)}, # needs to be on t-nodes\n }\n else:\n field_chunksizes = None\n\n return FieldSet.from_nemo(\n filenames, variables, dimensions, \n indices=indices,\n chunksize=field_chunksizes, # = None for no chunking\n mesh='spherical',\n tracer_interp_method='cgrid_tracer'\n# ,time_periodic=time_loop_period\n# ,allow_time_extrapolation=True\n )",
"_____no_output_____"
],
[
"def create_fieldset(\n data_path=data_path, experiment_name=experiment_name,\n fname_U=fname_U, fname_V=fname_V, fname_W=fname_W, fname_T=fname_T,\n mesh_mask = mesh_mask\n):\n \n files_U = list(sorted((data_path).glob(fname_U)))\n files_V = list(sorted((data_path).glob(fname_V)))\n files_W = list(sorted((data_path).glob(fname_W)))\n files_T = list(sorted((data_path).glob(fname_T)))\n \n print(files_U)\n \n fieldset = fieldset_defintions(\n files_U, files_V,\n files_W, files_T, mesh_mask)\n\n return fieldset",
"_____no_output_____"
],
[
"fieldset = create_fieldset()",
"[PosixPath('/gxfs_work1/geomar/smomw355/model_data/ocean-only/VIKING20X.L46-KKG36107B/nemo/output/1_VIKING20X.L46-KKG36107B_5d_19800101_19801231_grid_U.nc'), PosixPath('/gxfs_work1/geomar/smomw355/model_data/ocean-only/VIKING20X.L46-KKG36107B/nemo/output/1_VIKING20X.L46-KKG36107B_5d_19810101_19811231_grid_U.nc'), PosixPath('/gxfs_work1/geomar/smomw355/model_data/ocean-only/VIKING20X.L46-KKG36107B/nemo/output/1_VIKING20X.L46-KKG36107B_5d_19820101_19821231_grid_U.nc'), PosixPath('/gxfs_work1/geomar/smomw355/model_data/ocean-only/VIKING20X.L46-KKG36107B/nemo/output/1_VIKING20X.L46-KKG36107B_5d_19830101_19831231_grid_U.nc'), PosixPath('/gxfs_work1/geomar/smomw355/model_data/ocean-only/VIKING20X.L46-KKG36107B/nemo/output/1_VIKING20X.L46-KKG36107B_5d_19840101_19841231_grid_U.nc'), PosixPath('/gxfs_work1/geomar/smomw355/model_data/ocean-only/VIKING20X.L46-KKG36107B/nemo/output/1_VIKING20X.L46-KKG36107B_5d_19850101_19851231_grid_U.nc'), PosixPath('/gxfs_work1/geomar/smomw355/model_data/ocean-only/VIKING20X.L46-KKG36107B/nemo/output/1_VIKING20X.L46-KKG36107B_5d_19860101_19861231_grid_U.nc'), PosixPath('/gxfs_work1/geomar/smomw355/model_data/ocean-only/VIKING20X.L46-KKG36107B/nemo/output/1_VIKING20X.L46-KKG36107B_5d_19870101_19871231_grid_U.nc'), PosixPath('/gxfs_work1/geomar/smomw355/model_data/ocean-only/VIKING20X.L46-KKG36107B/nemo/output/1_VIKING20X.L46-KKG36107B_5d_19880101_19881231_grid_U.nc'), PosixPath('/gxfs_work1/geomar/smomw355/model_data/ocean-only/VIKING20X.L46-KKG36107B/nemo/output/1_VIKING20X.L46-KKG36107B_5d_19890101_19891231_grid_U.nc'), PosixPath('/gxfs_work1/geomar/smomw355/model_data/ocean-only/VIKING20X.L46-KKG36107B/nemo/output/1_VIKING20X.L46-KKG36107B_5d_19900101_19901231_grid_U.nc'), PosixPath('/gxfs_work1/geomar/smomw355/model_data/ocean-only/VIKING20X.L46-KKG36107B/nemo/output/1_VIKING20X.L46-KKG36107B_5d_19910101_19911231_grid_U.nc'), PosixPath('/gxfs_work1/geomar/smomw355/model_data/ocean-only/VIKING20X.L46-KKG36107B/nemo/output/1_VIKING20X.L46-KKG36107B_5d_19920101_19921231_grid_U.nc'), PosixPath('/gxfs_work1/geomar/smomw355/model_data/ocean-only/VIKING20X.L46-KKG36107B/nemo/output/1_VIKING20X.L46-KKG36107B_5d_19930101_19931231_grid_U.nc'), PosixPath('/gxfs_work1/geomar/smomw355/model_data/ocean-only/VIKING20X.L46-KKG36107B/nemo/output/1_VIKING20X.L46-KKG36107B_5d_19940101_19941231_grid_U.nc'), PosixPath('/gxfs_work1/geomar/smomw355/model_data/ocean-only/VIKING20X.L46-KKG36107B/nemo/output/1_VIKING20X.L46-KKG36107B_5d_19950101_19951231_grid_U.nc'), PosixPath('/gxfs_work1/geomar/smomw355/model_data/ocean-only/VIKING20X.L46-KKG36107B/nemo/output/1_VIKING20X.L46-KKG36107B_5d_19960101_19961231_grid_U.nc'), PosixPath('/gxfs_work1/geomar/smomw355/model_data/ocean-only/VIKING20X.L46-KKG36107B/nemo/output/1_VIKING20X.L46-KKG36107B_5d_19970101_19971231_grid_U.nc'), PosixPath('/gxfs_work1/geomar/smomw355/model_data/ocean-only/VIKING20X.L46-KKG36107B/nemo/output/1_VIKING20X.L46-KKG36107B_5d_19980101_19981231_grid_U.nc'), PosixPath('/gxfs_work1/geomar/smomw355/model_data/ocean-only/VIKING20X.L46-KKG36107B/nemo/output/1_VIKING20X.L46-KKG36107B_5d_19990101_19991231_grid_U.nc'), PosixPath('/gxfs_work1/geomar/smomw355/model_data/ocean-only/VIKING20X.L46-KKG36107B/nemo/output/1_VIKING20X.L46-KKG36107B_5d_20000101_20001231_grid_U.nc'), PosixPath('/gxfs_work1/geomar/smomw355/model_data/ocean-only/VIKING20X.L46-KKG36107B/nemo/output/1_VIKING20X.L46-KKG36107B_5d_20010101_20011231_grid_U.nc'), PosixPath('/gxfs_work1/geomar/smomw355/model_data/ocean-only/VIKING20X.L46-KKG36107B/nemo/output/1_VIKING20X.L46-KKG36107B_5d_20020101_20021231_grid_U.nc'), PosixPath('/gxfs_work1/geomar/smomw355/model_data/ocean-only/VIKING20X.L46-KKG36107B/nemo/output/1_VIKING20X.L46-KKG36107B_5d_20030101_20031231_grid_U.nc'), PosixPath('/gxfs_work1/geomar/smomw355/model_data/ocean-only/VIKING20X.L46-KKG36107B/nemo/output/1_VIKING20X.L46-KKG36107B_5d_20040101_20041231_grid_U.nc'), PosixPath('/gxfs_work1/geomar/smomw355/model_data/ocean-only/VIKING20X.L46-KKG36107B/nemo/output/1_VIKING20X.L46-KKG36107B_5d_20050101_20051231_grid_U.nc'), PosixPath('/gxfs_work1/geomar/smomw355/model_data/ocean-only/VIKING20X.L46-KKG36107B/nemo/output/1_VIKING20X.L46-KKG36107B_5d_20060101_20061231_grid_U.nc'), PosixPath('/gxfs_work1/geomar/smomw355/model_data/ocean-only/VIKING20X.L46-KKG36107B/nemo/output/1_VIKING20X.L46-KKG36107B_5d_20070101_20071231_grid_U.nc'), PosixPath('/gxfs_work1/geomar/smomw355/model_data/ocean-only/VIKING20X.L46-KKG36107B/nemo/output/1_VIKING20X.L46-KKG36107B_5d_20080101_20081231_grid_U.nc'), PosixPath('/gxfs_work1/geomar/smomw355/model_data/ocean-only/VIKING20X.L46-KKG36107B/nemo/output/1_VIKING20X.L46-KKG36107B_5d_20090101_20091231_grid_U.nc'), PosixPath('/gxfs_work1/geomar/smomw355/model_data/ocean-only/VIKING20X.L46-KKG36107B/nemo/output/1_VIKING20X.L46-KKG36107B_5d_20100101_20101231_grid_U.nc'), PosixPath('/gxfs_work1/geomar/smomw355/model_data/ocean-only/VIKING20X.L46-KKG36107B/nemo/output/1_VIKING20X.L46-KKG36107B_5d_20110101_20111231_grid_U.nc'), PosixPath('/gxfs_work1/geomar/smomw355/model_data/ocean-only/VIKING20X.L46-KKG36107B/nemo/output/1_VIKING20X.L46-KKG36107B_5d_20120101_20121231_grid_U.nc'), PosixPath('/gxfs_work1/geomar/smomw355/model_data/ocean-only/VIKING20X.L46-KKG36107B/nemo/output/1_VIKING20X.L46-KKG36107B_5d_20130101_20131231_grid_U.nc'), PosixPath('/gxfs_work1/geomar/smomw355/model_data/ocean-only/VIKING20X.L46-KKG36107B/nemo/output/1_VIKING20X.L46-KKG36107B_5d_20140101_20141231_grid_U.nc'), PosixPath('/gxfs_work1/geomar/smomw355/model_data/ocean-only/VIKING20X.L46-KKG36107B/nemo/output/1_VIKING20X.L46-KKG36107B_5d_20150101_20151231_grid_U.nc'), PosixPath('/gxfs_work1/geomar/smomw355/model_data/ocean-only/VIKING20X.L46-KKG36107B/nemo/output/1_VIKING20X.L46-KKG36107B_5d_20160101_20161231_grid_U.nc'), PosixPath('/gxfs_work1/geomar/smomw355/model_data/ocean-only/VIKING20X.L46-KKG36107B/nemo/output/1_VIKING20X.L46-KKG36107B_5d_20170101_20171231_grid_U.nc'), PosixPath('/gxfs_work1/geomar/smomw355/model_data/ocean-only/VIKING20X.L46-KKG36107B/nemo/output/1_VIKING20X.L46-KKG36107B_5d_20180101_20181231_grid_U.nc'), PosixPath('/gxfs_work1/geomar/smomw355/model_data/ocean-only/VIKING20X.L46-KKG36107B/nemo/output/1_VIKING20X.L46-KKG36107B_5d_20190101_20191231_grid_U.nc')]\n"
]
],
[
[
"## Create Virtual Particles",
"_____no_output_____"
],
[
"#### add a couple of simple plotting routines",
"_____no_output_____"
]
],
[
[
"def plot_section_sdist():\n plt.figure(figsize=(10,5))\n\n u = np.array([p.uvel for p in pset]) * degree2km * 1000.0 * np.cos(np.radians(pset.lat))\n v = np.array([p.vvel for p in pset]) * degree2km * 1000.0\n section_index = np.searchsorted(lonlat.lon,pset.lon)-1\n u_normal = v * lonlatdiff.costheta[section_index].data - u * lonlatdiff.sintheta[section_index].data\n y = (pset.lat - lonlat.lat[section_index]) * degree2km\n x = (pset.lon - lonlat.lon[section_index]) * degree2km*np.cos(np.radians(lonlat2mean.lat[section_index+1].data))\n dist = np.sqrt(x**2 + y**2) + lonlatdiff.length_west[section_index].data\n \n \n plt.scatter(\n dist,\n [p.depth for p in pset],\n 1,\n u_normal,\n cmap=co.cm.balance,vmin=-0.3,vmax=0.3\n )\n plt.ylim(1200,0)\n plt.colorbar(label = r'normal velocity [$\\mathrm{m\\ s}^{-1}$]')\n plt.xlabel('distance [km]')\n plt.ylabel('depth [m]')\n return\n",
"_____no_output_____"
],
[
"def plot_section_lon():\n plt.figure(figsize=(10,5))\n\n u = np.array([p.uvel for p in pset]) * degree2km * 1000.0 * np.cos(np.radians(pset.lat))\n v = np.array([p.vvel for p in pset]) * degree2km * 1000.0\n section_index = np.searchsorted(lonlat.lon,pset.lon)-1\n u_normal = v * lonlatdiff.costheta[section_index].data - u * lonlatdiff.sintheta[section_index].data\n \n plt.scatter(\n [p.lon for p in pset],\n [p.depth for p in pset],\n 1,\n u_normal,\n cmap=co.cm.balance,vmin=-0.3,vmax=0.3\n )\n plt.ylim(1200,0)\n plt.colorbar(label = r'normal velocity [$\\mathrm{m\\ s}^{-1}$]');\n plt.xlabel('longitude [$\\degree$E]')\n plt.ylabel('depth [m]')\n return\n",
"_____no_output_____"
],
[
"class SampleParticle(JITParticle):\n \"\"\"Add variables to the standard particle class.\n \n Particles will sample temperature and track the age of the particle.\n Particles also have a flag `alive` that is 1 if the particle is alive and 0 otherwise.\n Furthermore, we have a `speed_param` that scales the velocity with which particles can\n swim towards the surface.\n\n Note that we don't initialize temp from the actual data.\n This speeds up particle creation, but might render initial data point less useful.\n \"\"\"\n mxl = Variable('mxl', dtype=np.float32, initial=-100)\n temp = Variable('temp', dtype=np.float32, initial=-100)\n salt = Variable('salt', dtype=np.float32, initial=-100)\n uvel = Variable('uvel', dtype=np.float32, initial=0)\n vvel = Variable('vvel', dtype=np.float32, initial=0)\n# wvel = Variable('wvel', dtype=np.float32, initial=0)\n# alive = Variable('alive', dtype=np.int32, initial=1)\n# speed_param = Variable('speed_param', dtype=np.float32, initial=1)\n# age = Variable('age', dtype=np.int32, initial=0, to_write=True)",
"_____no_output_____"
]
],
[
[
"## Create a set of particles with random initial positions\n\nWe seed the RNG to be reproducible (and to be able to quickly create a second equivalent experiment with differently chosen compatible initial positions), and create arrays of random starting times, lats, lons, depths, and speed parameters (see kernel definitions below for details).\n\nInitially create points on 'rectangle'. Land points are removed later in a OceanParcels 'run' with runtime and timedelta zero.",
"_____no_output_____"
],
[
"### First set up the piecewise section",
"_____no_output_____"
]
],
[
[
"lonlat = xr.Dataset(pd.read_csv(sectionPath / sectionFilename,delim_whitespace=True))",
"_____no_output_____"
],
[
"lonlat.lon.attrs['long_name']='Longitude'\nlonlat.lat.attrs['long_name']='Latitude'\nlonlat.lon.attrs['standard_name']='longitude'\nlonlat.lat.attrs['standard_name']='latitude'\nlonlat.lon.attrs['units']='degrees_east'\nlonlat.lat.attrs['units']='degrees_north'\n\nlonlatdiff = lonlat.diff('dim_0')\nlonlat2mean= lonlat.rolling({'dim_0':2}).mean()\n\nlonlat.plot.scatter(x='lon',y='lat')\nlonlat2mean.plot.scatter(x='lon',y='lat')\n\nlonlatdiff = lonlatdiff.assign({'y':lonlatdiff['lat']*degree2km})\nlonlatdiff = lonlatdiff.assign({'x':lonlatdiff['lon']*degree2km*np.cos(np.radians(lonlat2mean.lat.data[1:]))})\nlonlatdiff=lonlatdiff.assign({'length':np.sqrt(lonlatdiff['x']**2+lonlatdiff['y']**2)})\nlonlatdiff=lonlatdiff.assign({'length_west':lonlatdiff.length.sum() - np.cumsum(lonlatdiff.length[::-1])[::-1]})\nlonlatdiff=lonlatdiff.assign({'costheta':lonlatdiff['x']/lonlatdiff['length']})\nlonlatdiff=lonlatdiff.assign({'sintheta':lonlatdiff['y']/lonlatdiff['length']})\n\ntotal_length = lonlatdiff.length.sum().data\nprint(total_length)",
"3594.572878394652\n"
],
[
"lonlatdiff.length.shape[0]",
"_____no_output_____"
]
],
[
[
"### Seed particles uniform random along OSNAP section",
"_____no_output_____"
]
],
[
[
"np.random.seed(RNG_seed)\n\n# define time of release for each particle relative to t0\n# can start each particle at a different time if required\n# here all start at time t_start.\ntimes = []\nlons = []\nlats = []\ndepths = []\n\n# for subsect in range(lonlatdiff.length.shape[0]):\nfor subsect in range(start_vertex,end_vertex):\n \n number_particles = int(create_number_particles*lonlatdiff.length[subsect]/total_length)\n time = np.zeros(number_particles)\n time += (t_start - t_0).total_seconds()\n\n # start along a line from west to east\n\n west_lat = lonlat.lat[subsect].data\n west_lon = lonlat.lon[subsect].data\n east_lat = lonlat.lat[subsect+1].data\n east_lon = lonlat.lon[subsect+1].data\n \n lon = np.random.uniform(\n low=west_lon, high = east_lon,\n size=time.shape\n )\n lat = west_lat + ((lon - west_lon) * (east_lat - west_lat)/ (east_lon - west_lon))\n\n # at depths from surface to max_release_depth\n\n depth = np.random.uniform(\n low=min_release_depth, high=max_release_depth,\n size=time.shape\n )\n times.append(time)\n lons.append(lon)\n lats.append(lat)\n depths.append(depth)\n\n \n \ntime = np.concatenate(times)\nlon = np.concatenate(lons)\nlat = np.concatenate(lats)\ndepth = np.concatenate(depths)\n",
"_____no_output_____"
]
],
[
[
"### Build particle set",
"_____no_output_____"
]
],
[
[
"%%time\n\npset = ParticleSet(\n fieldset=fieldset,\n pclass=SampleParticle,\n lat=lat,\n lon=lon,\n# speed_param=speed_param,\n depth=depth,\n time=time\n# repeatdt = repeatdt\n)\n",
"CPU times: user 745 ms, sys: 193 ms, total: 937 ms\nWall time: 942 ms\n"
],
[
"print(f\"Created {len(pset)} particles.\")\n# display(pset[:5])\n# display(pset[-5:])",
"Created 2643886 particles.\n"
]
],
[
[
"## Compose custom kernel\n\nWe'll create three additional kernels:\n- One Kernel adds velocity sampling\n- One Kernel adds temperature sampling\n- One kernel adds salinity sampling\n\nThen, we combine the builtin `AdvectionRK4_3D` kernel with these additional kernels.",
"_____no_output_____"
]
],
[
[
"def velocity_sampling(particle, fieldset, time):\n '''Sample velocity.'''\n \n (particle.uvel,particle.vvel) = fieldset.UV[time, particle.depth, particle.lat, particle.lon]\n ",
"_____no_output_____"
],
[
"def temperature_sampling(particle, fieldset, time):\n '''Sample temperature.'''\n \n particle.temp = fieldset.T[time, particle.depth, particle.lat, particle.lon]",
"_____no_output_____"
],
[
"def salinity_sampling(particle, fieldset, time):\n '''Sample salinity.'''\n \n particle.salt = fieldset.S[time, particle.depth, particle.lat, particle.lon]",
"_____no_output_____"
],
[
"def mxl_sampling(particle, fieldset, time):\n '''Sample mixed layer depth.'''\n \n particle.mxl = fieldset.MXL[time, particle.depth, particle.lat, particle.lon]",
"_____no_output_____"
],
[
"custom_kernel = (\n pset.Kernel(AdvectionRK4_3D)\n# + pset.Kernel(temperature_sensitivity)\n + pset.Kernel(temperature_sampling)\n + pset.Kernel(salinity_sampling)\n + pset.Kernel(velocity_sampling)\n + pset.Kernel(mxl_sampling)\n)",
"_____no_output_____"
]
],
[
[
"## Be able to handle errors during integration\n\nWe have restricted our domain so in principle, particles could reach undefined positions.\nIn that case, we want to just delete the particle (without forgetting its history).",
"_____no_output_____"
]
],
[
[
"def DeleteParticle(particle, fieldset, time):\n particle.delete()\n\n\nrecovery_cases = {\n ErrorCode.ErrorOutOfBounds: DeleteParticle,\n ErrorCode.Error: DeleteParticle,\n ErrorCode.ErrorInterpolation: DeleteParticle\n}",
"_____no_output_____"
]
],
[
[
"## Run with runtime=0 to initialise fields",
"_____no_output_____"
]
],
[
[
"%%time\n\n# with dask.config.set(**{'array.slicing.split_large_chunks': False}):\npset.execute(\n custom_kernel,\n runtime=0, \n# dt=timedelta(minutes=0), \n# output_file=outputfile,\n recovery=recovery_cases\n)",
"INFO: Compiled SampleParticleAdvectionRK4_3Dtemperature_samplingsalinity_samplingvelocity_samplingmxl_sampling ==> /tmp/parcels-62665/f1203dc1d27ed108049fd0163c6550ba_0.so\n"
],
[
"plot_section_sdist()",
"_____no_output_____"
]
],
[
[
"## Trim unwanted points from ParticleSet\n\nUse initialised fields to remove land points. We test `temp == 0.0` (the mask value over land).\n",
"_____no_output_____"
]
],
[
[
"t = np.array([p.temp for p in pset])\n# u = np.array([p.uvel for p in pset])\n# v = np.array([p.vvel for p in pset])\npset.remove_indices(np.argwhere(t == 0).flatten())\n# pset.remove(np.argwhere(x * y * z == 0).flatten())\nprint(len(pset))",
"2099868\n"
],
[
"plot_section_sdist()",
"_____no_output_____"
]
],
[
[
"### Test velocity normal to section",
"_____no_output_____"
],
[
"#### Velocity conversions from degrees lat/lon per second to m/s",
"_____no_output_____"
]
],
[
[
"u = np.array([p.uvel for p in pset])\nv = np.array([p.vvel for p in pset])\n",
"_____no_output_____"
],
[
"u=u * degree2km * 1000.0 * np.cos(np.radians(pset.lat))\nv=v * degree2km * 1000.0",
"_____no_output_____"
]
],
[
[
"#### normal velocities",
"_____no_output_____"
]
],
[
[
"section_index = np.searchsorted(lonlat.lon,pset.lon)-1\nu_normal = v * lonlatdiff.costheta[section_index].data - u * lonlatdiff.sintheta[section_index].data",
"_____no_output_____"
],
[
"abs(u_normal).max()",
"_____no_output_____"
]
],
[
[
"#### remove particles randomly with probability proportional to normal speed",
"_____no_output_____"
]
],
[
[
"u_random = np.random.rand(len(u_normal))*max_current\npset.remove_indices(np.argwhere(abs(u_normal) < u_random).flatten())\n\nprint(len(pset))",
"59894\n"
],
[
"plot_section_sdist()",
"_____no_output_____"
]
],
[
[
"## Prepare output\n\nWe define an output file and specify the desired output frequency.",
"_____no_output_____"
]
],
[
[
"# output_filename = 'Parcels_IFFForwards_1m_June2016_2000.nc'\nnpart = str(len(pset))\noutput_filename = 'tracks_randomvel_mxl_'+sectionname+direction+year_str+month_str+day_str+'_N'+npart+'_D'+days+'_Rnd'+ seed+'.nc'\noutfile = outpath / output_filename\n\nprint(outfile)\noutputfile = pset.ParticleFile(\n name=outfile,\n outputdt=timedelta(hours=outputdt_in_hours)\n)",
"../data/raw/tracks_randomvel_mxl_osnap_backward_20191020_N59894_D3650_Rnd14535.nc\n"
]
],
[
[
"## Execute the experiment\n\nWe'll evolve particles, log their positions and variables to the output buffer and finally export the output to a the file.",
"_____no_output_____"
],
[
"### Run the experiment",
"_____no_output_____"
]
],
[
[
"%%time\n\n# with dask.config.set(**{'array.slicing.split_large_chunks': False}):\npset.execute(\n custom_kernel,\n runtime=timedelta(days=runtime_in_days), \n dt=timedelta(minutes=dt_in_minutes), \n output_file=outputfile,\n recovery=recovery_cases\n)",
"INFO: Temporary output files are stored in ../data/raw/out-BLRXXCGD.\n"
],
[
"# outputfile.export()",
"_____no_output_____"
],
[
"outputfile.close()",
"_____no_output_____"
],
[
"conda list\n",
"# packages in environment at /opt/conda:\r\n#\r\n# Name Version Build Channel\r\n_libgcc_mutex 0.1 conda_forge conda-forge\r\n_openmp_mutex 4.5 1_gnu conda-forge\r\nalembic 1.5.5 pyhd8ed1ab_0 conda-forge\r\nansiwrap 0.8.4 py_0 conda-forge\r\nanyio 2.2.0 py38h578d9bd_0 conda-forge\r\nappdirs 1.4.4 pyh9f0ad1d_0 conda-forge\r\nargon2-cffi 20.1.0 py38h497a2fe_2 conda-forge\r\nasciitree 0.3.3 py_2 conda-forge\r\nasync_generator 1.10 py_0 conda-forge\r\nattrs 20.3.0 pyhd3deb0d_0 conda-forge\r\nbabel 2.9.0 pyhd3deb0d_0 conda-forge\r\nbackcall 0.2.0 pyh9f0ad1d_0 conda-forge\r\nbackports 1.0 py_2 conda-forge\r\nbackports.functools_lru_cache 1.6.1 py_0 conda-forge\r\nbasemap 1.2.2 py38h85717e3_3 conda-forge\r\nbinutils_impl_linux-64 2.35.1 h193b22a_2 conda-forge\r\nbinutils_linux-64 2.35 h67ddf6f_30 conda-forge\r\nblack 20.8b1 py_1 conda-forge\r\nbleach 3.3.0 pyh44b312d_0 conda-forge\r\nblinker 1.4 py_1 conda-forge\r\nblosc 1.21.0 h9c3ff4c_0 conda-forge\r\nbokeh 2.3.0 py38h578d9bd_0 conda-forge\r\nboost-cpp 1.74.0 hc6e9bd1_2 conda-forge\r\nbottleneck 1.3.2 py38h5c078b8_3 conda-forge\r\nbrotlipy 0.7.0 py38h497a2fe_1001 conda-forge\r\nbzip2 1.0.8 h7f98852_4 conda-forge\r\nc-ares 1.17.1 h36c2ea0_0 conda-forge\r\nca-certificates 2020.12.5 ha878542_0 conda-forge\r\ncached-property 1.5.2 hd8ed1ab_1 conda-forge\r\ncached_property 1.5.2 pyha770c72_1 conda-forge\r\ncachetools 4.2.1 pyhd8ed1ab_0 conda-forge\r\ncairo 1.16.0 h7979940_1007 conda-forge\r\ncartopy 0.18.0 py38h9b98833_8 conda-forge\r\ncertifi 2020.12.5 py38h578d9bd_1 conda-forge\r\ncertipy 0.1.3 py_0 conda-forge\r\ncffi 1.14.5 py38ha65f79e_0 conda-forge\r\ncfitsio 3.470 hb418390_7 conda-forge\r\ncftime 1.4.1 py38h5c078b8_0 conda-forge\r\ncgen 2020.1 py_0 conda-forge\r\nchardet 4.0.0 py38h578d9bd_1 conda-forge\r\nclick 7.1.2 pyh9f0ad1d_0 conda-forge\r\nclick-plugins 1.1.1 py_0 conda-forge\r\ncligj 0.7.1 pyhd8ed1ab_0 conda-forge\r\ncloudpickle 1.6.0 py_0 conda-forge\r\ncmocean 2.0 py_3 conda-forge\r\ncolorcet 2.0.6 pyhd8ed1ab_0 conda-forge\r\ncolorspacious 1.1.2 pyh24bf2e0_0 conda-forge\r\nconda 4.9.2 py38h578d9bd_0 conda-forge\r\nconda-package-handling 1.7.2 py38h8df0ef7_0 conda-forge\r\nconfigurable-http-proxy 4.2.3 node15_he6ea98c_0 conda-forge\r\ncryptography 3.4.4 py38h3e25421_0 conda-forge\r\ncurl 7.71.1 he644dc0_8 conda-forge\r\ncycler 0.10.0 py_2 conda-forge\r\ncytoolz 0.11.0 py38h497a2fe_3 conda-forge\r\ndask 2021.2.0 pyhd8ed1ab_0 conda-forge\r\ndask-core 2021.2.0 pyhd8ed1ab_0 conda-forge\r\ndataclasses 0.8 pyhc8e2a94_1 conda-forge\r\ndatashader 0.12.0 pyhd3deb0d_0 conda-forge\r\ndatashape 0.5.4 py_1 conda-forge\r\ndbus 1.13.6 hfdff14a_1 conda-forge\r\ndecorator 4.4.2 py_0 conda-forge\r\ndefusedxml 0.6.0 py_0 conda-forge\r\ndistributed 2021.2.0 py38h578d9bd_0 conda-forge\r\nentrypoints 0.3 pyhd8ed1ab_1003 conda-forge\r\nexpat 2.2.10 h9c3ff4c_0 conda-forge\r\nfasteners 0.14.1 py_3 conda-forge\r\nffmpeg 4.3.1 hca11adc_2 conda-forge\r\nfiona 1.8.18 py38h58f84aa_1 conda-forge\r\nfontconfig 2.13.1 hba837de_1004 conda-forge\r\nfreetype 2.10.4 h0708190_1 conda-forge\r\nfreexl 1.0.6 h7f98852_0 conda-forge\r\nfsspec 0.8.7 pyhd8ed1ab_0 conda-forge\r\ngcc_impl_linux-64 9.3.0 h70c0ae5_18 conda-forge\r\ngcc_linux-64 9.3.0 hf25ea35_30 conda-forge\r\ngdal 3.2.1 py38hc0b2d6b_3 conda-forge\r\ngeopandas 0.9.0 pyhd8ed1ab_0 conda-forge\r\ngeos 3.8.1 he1b5a44_0 conda-forge\r\ngeotiff 1.6.0 h2b14fbe_4 conda-forge\r\ngeoviews 1.9.1 pyhd8ed1ab_0 conda-forge\r\ngeoviews-core 1.9.1 pyha770c72_0 conda-forge\r\ngettext 0.19.8.1 h0b5b191_1005 conda-forge\r\ngiflib 5.2.1 h36c2ea0_2 conda-forge\r\nglib 2.66.7 h9c3ff4c_1 conda-forge\r\nglib-tools 2.66.7 h9c3ff4c_1 conda-forge\r\ngmp 6.2.1 h58526e2_0 conda-forge\r\ngnutls 3.6.13 h85f3911_1 conda-forge\r\ngst-plugins-base 1.14.5 h0935bb2_2 conda-forge\r\ngstreamer 1.18.4 h76c114f_0 conda-forge\r\ngsw 3.4.0 py38h5c078b8_1 conda-forge\r\nh5netcdf 0.10.0 pyhd8ed1ab_0 conda-forge\r\nh5py 3.1.0 nompi_py38hafa665b_100 conda-forge\r\nhdf4 4.2.13 h10796ff_1004 conda-forge\r\nhdf5 1.10.6 nompi_h6a2412b_1114 conda-forge\r\nheapdict 1.0.1 py_0 conda-forge\r\nholoviews 1.14.2 pyhd8ed1ab_0 conda-forge\r\nhvplot 0.7.1 pyh44b312d_0 conda-forge\r\nicu 68.1 h58526e2_0 conda-forge\r\nidna 2.10 pyh9f0ad1d_0 conda-forge\r\nimportlib-metadata 3.7.0 py38h578d9bd_0 conda-forge\r\nipykernel 5.5.0 py38h81c977d_1 conda-forge\r\nipython 7.21.0 py38h81c977d_0 conda-forge\r\nipython_genutils 0.2.0 py_1 conda-forge\r\njedi 0.18.0 py38h578d9bd_2 conda-forge\r\njinja2 2.11.3 pyh44b312d_0 conda-forge\r\njoblib 1.0.1 pyhd8ed1ab_0 conda-forge\r\njpeg 9d h36c2ea0_0 conda-forge\r\njson-c 0.13.1 hbfbb72e_1002 conda-forge\r\njson5 0.9.5 pyh9f0ad1d_0 conda-forge\r\njsonschema 3.2.0 pyhd8ed1ab_3 conda-forge\r\njupyter-packaging 0.7.12 pyhd8ed1ab_0 conda-forge\r\njupyter_client 6.1.11 pyhd8ed1ab_1 conda-forge\r\njupyter_core 4.7.1 py38h578d9bd_0 conda-forge\r\njupyter_server 1.4.1 py38h578d9bd_0 conda-forge\r\njupyter_telemetry 0.1.0 pyhd8ed1ab_1 conda-forge\r\njupyterhub 1.3.0 py38h578d9bd_1 conda-forge\r\njupyterhub-base 1.3.0 py38h578d9bd_1 conda-forge\r\njupyterlab 3.0.9 pyhd8ed1ab_0 conda-forge\r\njupyterlab_pygments 0.1.2 pyh9f0ad1d_0 conda-forge\r\njupyterlab_server 2.3.0 pyhd8ed1ab_0 conda-forge\r\nkealib 1.4.14 hcc255d8_2 conda-forge\r\nkernel-headers_linux-64 2.6.32 h77966d4_13 conda-forge\r\nkiwisolver 1.3.1 py38h1fd1430_1 conda-forge\r\nkrb5 1.17.2 h926e7f8_0 conda-forge\r\nlame 3.100 h7f98852_1001 conda-forge\r\nlcms2 2.12 hddcbb42_0 conda-forge\r\nld_impl_linux-64 2.35.1 hea4e1c9_2 conda-forge\r\nlibarchive 3.5.1 h3f442fb_1 conda-forge\r\nlibblas 3.9.0 3_h92ddd45_netlib conda-forge\r\nlibcblas 3.9.0 3_h92ddd45_netlib conda-forge\r\nlibclang 11.1.0 default_ha53f305_0 conda-forge\r\nlibcurl 7.71.1 hcdd3856_8 conda-forge\r\nlibdap4 3.20.6 hd7c4107_1 conda-forge\r\nlibedit 3.1.20191231 he28a2e2_2 conda-forge\r\nlibev 4.33 h516909a_1 conda-forge\r\nlibevent 2.1.10 hcdb4288_3 conda-forge\r\nlibffi 3.3 h58526e2_2 conda-forge\r\nlibgcc-devel_linux-64 9.3.0 h7864c58_18 conda-forge\r\nlibgcc-ng 9.3.0 h2828fa1_18 conda-forge\r\nlibgdal 3.2.1 h744752d_3 conda-forge\r\nlibgfortran-ng 9.3.0 hff62375_18 conda-forge\r\nlibgfortran5 9.3.0 hff62375_18 conda-forge\r\nlibglib 2.66.7 h3e27bee_1 conda-forge\r\nlibgomp 9.3.0 h2828fa1_18 conda-forge\r\nlibiconv 1.16 h516909a_0 conda-forge\r\nlibkml 1.3.0 h02e6976_1012 conda-forge\r\nliblapack 3.9.0 3_h92ddd45_netlib conda-forge\r\nlibllvm10 10.0.1 he513fc3_3 conda-forge\r\nlibllvm11 11.1.0 hf817b99_0 conda-forge\r\nlibnetcdf 4.7.4 nompi_h56d31a8_107 conda-forge\r\nlibnghttp2 1.43.0 h812cca2_0 conda-forge\r\nlibopenblas 0.3.13 pthreads_h8fe5266_0 conda-forge\r\nlibpng 1.6.37 h21135ba_2 conda-forge\r\nlibpq 12.3 h255efa7_3 conda-forge\r\nlibrttopo 1.1.0 hb271727_4 conda-forge\r\nlibsodium 1.0.18 h36c2ea0_1 conda-forge\r\nlibsolv 0.7.17 h780b84a_0 conda-forge\r\nlibspatialindex 1.9.3 h9c3ff4c_3 conda-forge\r\nlibspatialite 5.0.1 h4e7eb2b_1 conda-forge\r\nlibssh2 1.9.0 hab1572f_5 conda-forge\r\nlibstdcxx-ng 9.3.0 h6de172a_18 conda-forge\r\nlibtiff 4.2.0 hdc55705_0 conda-forge\r\nlibuuid 2.32.1 h7f98852_1000 conda-forge\r\nlibuv 1.41.0 h7f98852_0 conda-forge\r\nlibwebp-base 1.2.0 h7f98852_2 conda-forge\r\nlibxcb 1.13 h7f98852_1003 conda-forge\r\nlibxkbcommon 1.0.3 he3ba5ed_0 conda-forge\r\nlibxml2 2.9.10 h72842e0_3 conda-forge\r\nllvmlite 0.36.0 py38h4630a5e_0 conda-forge\r\nlocket 0.2.0 py_2 conda-forge\r\nlz4-c 1.9.3 h9c3ff4c_0 conda-forge\r\nlzo 2.10 h516909a_1000 conda-forge\r\nmako 1.1.4 pyh44b312d_0 conda-forge\r\nmamba 0.7.14 py38h2aa5da1_0 conda-forge\r\nmarkdown 3.3.4 pyhd8ed1ab_0 conda-forge\r\nmarkupsafe 1.1.1 py38h497a2fe_3 conda-forge\r\nmatplotlib 3.3.4 py38h578d9bd_0 conda-forge\r\nmatplotlib-base 3.3.4 py38h0efea84_0 conda-forge\r\nmistune 0.8.4 py38h497a2fe_1003 conda-forge\r\nmonotonic 1.5 py_0 conda-forge\r\nmsgpack-python 1.0.2 py38h1fd1430_1 conda-forge\r\nmultipledispatch 0.6.0 py_0 conda-forge\r\nmunch 2.5.0 py_0 conda-forge\r\nmypy_extensions 0.4.3 py38h578d9bd_3 conda-forge\r\nmysql-common 8.0.22 ha770c72_3 conda-forge\r\nmysql-libs 8.0.22 h935591d_3 conda-forge\r\nnbclassic 0.2.6 pyhd8ed1ab_0 conda-forge\r\nnbclient 0.5.3 pyhd8ed1ab_0 conda-forge\r\nnbconvert 6.0.7 py38h578d9bd_3 conda-forge\r\nnbformat 5.1.2 pyhd8ed1ab_1 conda-forge\r\nncurses 6.2 h58526e2_4 conda-forge\r\nnest-asyncio 1.4.3 pyhd8ed1ab_0 conda-forge\r\nnetcdf4 1.5.6 nompi_py38h1cdf482_100 conda-forge\r\nnettle 3.6 he412f7d_0 conda-forge\r\nnodejs 15.11.0 h92b4a50_0 conda-forge\r\nnotebook 6.2.0 py38h578d9bd_0 conda-forge\r\nnspr 4.30 h9c3ff4c_0 conda-forge\r\nnss 3.62 hb5efdd6_0 conda-forge\r\nnumba 0.53.0 py38h5e62926_0 conda-forge\r\nnumcodecs 0.7.3 py38h709712a_0 conda-forge\r\nnumpy 1.20.1 py38h18fd61f_0 conda-forge\r\noauthlib 3.0.1 py_0 conda-forge\r\nolefile 0.46 pyh9f0ad1d_1 conda-forge\r\nopenblas 0.3.13 pthreads_h4748800_0 conda-forge\r\nopenh264 2.1.1 h780b84a_0 conda-forge\r\nopenjpeg 2.4.0 hf7af979_0 conda-forge\r\nopenssl 1.1.1j h7f98852_0 conda-forge\r\npackaging 20.9 pyh44b312d_0 conda-forge\r\npamela 1.0.0 py_0 conda-forge\r\npandas 1.2.3 py38h51da96c_0 conda-forge\r\npandoc 2.11.4 h7f98852_0 conda-forge\r\npandocfilters 1.4.2 py_1 conda-forge\r\npanel 0.11.0 pyhd8ed1ab_0 conda-forge\r\npapermill 2.3.3 pyhd8ed1ab_0 conda-forge\r\nparam 1.10.1 pyhd3deb0d_0 conda-forge\r\nparcels 2.2.2 py38h578d9bd_0 conda-forge\r\nparso 0.8.1 pyhd8ed1ab_0 conda-forge\r\npartd 1.1.0 py_0 conda-forge\r\npathspec 0.8.1 pyhd3deb0d_0 conda-forge\r\npatsy 0.5.1 py_0 conda-forge\r\npcre 8.44 he1b5a44_0 conda-forge\r\npexpect 4.8.0 pyh9f0ad1d_2 conda-forge\r\npickleshare 0.7.5 py_1003 conda-forge\r\npillow 8.1.2 py38ha0e1e83_0 conda-forge\r\npip 21.0.1 pyhd8ed1ab_0 conda-forge\r\npixman 0.40.0 h36c2ea0_0 conda-forge\r\npoppler 0.89.0 h2de54a5_5 conda-forge\r\npoppler-data 0.4.10 0 conda-forge\r\npostgresql 12.3 hc2f5b80_3 conda-forge\r\nprogressbar2 3.53.1 pyh9f0ad1d_0 conda-forge\r\nproj 7.2.0 h277dcde_2 conda-forge\r\nprometheus_client 0.9.0 pyhd3deb0d_0 conda-forge\r\nprompt-toolkit 3.0.16 pyha770c72_0 conda-forge\r\npsutil 5.8.0 py38h497a2fe_1 conda-forge\r\npthread-stubs 0.4 h36c2ea0_1001 conda-forge\r\nptyprocess 0.7.0 pyhd3deb0d_0 conda-forge\r\npycosat 0.6.3 py38h497a2fe_1006 conda-forge\r\npycparser 2.20 pyh9f0ad1d_2 conda-forge\r\npyct 0.4.6 py_0 conda-forge\r\npyct-core 0.4.6 py_0 conda-forge\r\npycurl 7.43.0.6 py38h996a351_1 conda-forge\r\npygments 2.8.0 pyhd8ed1ab_0 conda-forge\r\npyjwt 2.0.1 pyhd8ed1ab_0 conda-forge\r\npymbolic 2020.1 pyh9f0ad1d_0 conda-forge\r\npyopenssl 20.0.1 pyhd8ed1ab_0 conda-forge\r\npyparsing 2.4.7 pyh9f0ad1d_0 conda-forge\r\npyproj 3.0.1 py38h16ecdd7_0 conda-forge\r\npyqt 5.12.3 py38h578d9bd_7 conda-forge\r\npyqt-impl 5.12.3 py38h7400c14_7 conda-forge\r\npyqt5-sip 4.19.18 py38h709712a_7 conda-forge\r\npyqtchart 5.12 py38h7400c14_7 conda-forge\r\npyqtwebengine 5.12.1 py38h7400c14_7 conda-forge\r\npyrsistent 0.17.3 py38h497a2fe_2 conda-forge\r\npyshp 2.1.3 pyh44b312d_0 conda-forge\r\npysocks 1.7.1 py38h578d9bd_3 conda-forge\r\npython 3.8.8 hffdb5ce_0_cpython conda-forge\r\npython-blosc 1.10.2 py38h51da96c_0 conda-forge\r\npython-dateutil 2.8.1 py_0 conda-forge\r\npython-editor 1.0.4 py_0 conda-forge\r\npython-json-logger 2.0.1 pyh9f0ad1d_0 conda-forge\r\npython-utils 2.5.5 pyh44b312d_0 conda-forge\r\npython_abi 3.8 1_cp38 conda-forge\r\npytools 2021.2 pyhd8ed1ab_0 conda-forge\r\npytz 2021.1 pyhd8ed1ab_0 conda-forge\r\npyviz_comms 2.0.1 pyhd3deb0d_0 conda-forge\r\npyyaml 5.4.1 py38h497a2fe_0 conda-forge\r\npyzmq 22.0.3 py38h2035c66_1 conda-forge\r\nqt 5.12.9 h9d6b050_2 conda-forge\r\nreadline 8.0 he28a2e2_2 conda-forge\r\nregex 2020.11.13 py38h497a2fe_1 conda-forge\r\nreproc 14.2.1 h36c2ea0_0 conda-forge\r\nreproc-cpp 14.2.1 h58526e2_0 conda-forge\r\nrequests 2.25.1 pyhd3deb0d_0 conda-forge\r\nrtree 0.9.7 py38h02d302b_1 conda-forge\r\nruamel.yaml 0.16.12 py38h497a2fe_2 conda-forge\r\nruamel.yaml.clib 0.2.2 py38h497a2fe_2 conda-forge\r\nruamel_yaml 0.15.80 py38h497a2fe_1004 conda-forge\r\nscikit-learn 0.24.1 py38h658cfdd_0 conda-forge\r\nscipy 1.6.1 py38hb2138dd_0 conda-forge\r\nseaborn 0.11.1 hd8ed1ab_1 conda-forge\r\nseaborn-base 0.11.1 pyhd8ed1ab_1 conda-forge\r\nseawater 3.3.4 py_1 conda-forge\r\nsend2trash 1.5.0 py_0 conda-forge\r\nsetuptools 49.6.0 py38h578d9bd_3 conda-forge\r\nshapely 1.7.1 py38ha11d057_1 conda-forge\r\nsix 1.15.0 pyh9f0ad1d_0 conda-forge\r\nsniffio 1.2.0 py38h578d9bd_1 conda-forge\r\nsortedcontainers 2.3.0 pyhd8ed1ab_0 conda-forge\r\nsparse 0.11.2 py_0 conda-forge\r\nsqlalchemy 1.3.23 py38h497a2fe_0 conda-forge\r\nsqlite 3.34.0 h74cdb3f_0 conda-forge\r\nstatsmodels 0.12.2 py38h5c078b8_0 conda-forge\r\nsysroot_linux-64 2.12 h77966d4_13 conda-forge\r\ntblib 1.6.0 py_0 conda-forge\r\ntenacity 7.0.0 pyhd8ed1ab_0 conda-forge\r\nterminado 0.9.2 py38h578d9bd_0 conda-forge\r\ntestpath 0.4.4 py_0 conda-forge\r\ntextwrap3 0.9.2 py_0 conda-forge\r\nthreadpoolctl 2.1.0 pyh5ca1d4c_0 conda-forge\r\ntiledb 2.2.5 h91fcb0e_0 conda-forge\r\ntini 0.18.0 h14c3975_1001 conda-forge\r\ntk 8.6.10 h21135ba_1 conda-forge\r\ntoml 0.10.2 pyhd8ed1ab_0 conda-forge\r\ntoolz 0.11.1 py_0 conda-forge\r\ntornado 6.1 py38h497a2fe_1 conda-forge\r\ntqdm 4.58.0 pyhd8ed1ab_0 conda-forge\r\ntraitlets 5.0.5 py_0 conda-forge\r\ntyped-ast 1.4.2 py38h497a2fe_0 conda-forge\r\ntyping_extensions 3.7.4.3 py_0 conda-forge\r\ntzcode 2021a h7f98852_1 conda-forge\r\nurllib3 1.26.3 pyhd8ed1ab_0 conda-forge\r\nwcwidth 0.2.5 pyh9f0ad1d_2 conda-forge\r\nwebencodings 0.5.1 py_1 conda-forge\r\nwheel 0.36.2 pyhd3deb0d_0 conda-forge\r\nx264 1!161.3030 h7f98852_0 conda-forge\r\nxarray 0.17.0 pyhd8ed1ab_0 conda-forge\r\nxerces-c 3.2.3 h9d8b166_2 conda-forge\r\nxhistogram 0.1.2 pyhd8ed1ab_0 conda-forge\r\nxorg-kbproto 1.0.7 h7f98852_1002 conda-forge\r\nxorg-libice 1.0.10 h7f98852_0 conda-forge\r\nxorg-libsm 1.2.3 hd9c2040_1000 conda-forge\r\nxorg-libx11 1.7.0 h7f98852_0 conda-forge\r\nxorg-libxau 1.0.9 h7f98852_0 conda-forge\r\nxorg-libxdmcp 1.1.3 h7f98852_0 conda-forge\r\nxorg-libxext 1.3.4 h7f98852_1 conda-forge\r\nxorg-libxrender 0.9.10 h7f98852_1003 conda-forge\r\nxorg-renderproto 0.11.1 h7f98852_1002 conda-forge\r\nxorg-xextproto 7.3.0 h7f98852_1002 conda-forge\r\nxorg-xproto 7.0.31 h7f98852_1007 conda-forge\r\nxz 5.2.5 h516909a_1 conda-forge\r\nyaml 0.2.5 h516909a_0 conda-forge\r\nzarr 2.6.1 pyhd8ed1ab_0 conda-forge\r\nzeromq 4.3.4 h9c3ff4c_0 conda-forge\r\nzict 2.0.0 py_0 conda-forge\r\nzipp 3.4.0 py_0 conda-forge\r\nzlib 1.2.11 h516909a_1010 conda-forge\r\nzstd 1.4.9 ha95c52a_0 conda-forge\r\n"
],
[
"pip list\n",
"Package Version\r\n----------------------------- --------------------------\r\nalembic 1.5.5\r\nansiwrap 0.8.4\r\nanyio 2.2.0\r\nappdirs 1.4.4\r\nargon2-cffi 20.1.0\r\nasciitree 0.3.3\r\nasync-generator 1.10\r\nattrs 20.3.0\r\nBabel 2.9.0\r\nbackcall 0.2.0\r\nbackports.functools-lru-cache 1.6.1\r\nbasemap 1.2.1\r\nblack 20.8b1\r\nbleach 3.3.0\r\nblinker 1.4\r\nblosc 1.10.2\r\nbokeh 2.3.0\r\nBottleneck 1.3.2\r\nbrotlipy 0.7.0\r\ncached-property 1.5.2\r\ncachetools 4.2.1\r\nCartopy 0.18.0\r\ncertifi 2020.12.5\r\ncertipy 0.1.3\r\ncffi 1.14.5\r\ncftime 1.4.1\r\ncgen 2020.1\r\nchardet 4.0.0\r\nclick 7.1.2\r\nclick-plugins 1.1.1\r\ncligj 0.7.1\r\ncloudpickle 1.6.0\r\ncmocean 2.0\r\ncolorcet 2.0.6\r\ncolorspacious 1.1.2\r\nconda 4.9.2\r\nconda-package-handling 1.7.2\r\ncryptography 3.4.4\r\ncycler 0.10.0\r\ncytoolz 0.11.0\r\ndask 2021.2.0\r\ndatashader 0.12.0\r\ndatashape 0.5.4\r\ndecorator 4.4.2\r\ndefusedxml 0.6.0\r\ndistributed 2021.2.0\r\nentrypoints 0.3\r\nfasteners 0.14.1\r\nFiona 1.8.18\r\nfsspec 0.8.7\r\nGDAL 3.2.1\r\ngeopandas 0.9.0\r\ngeoviews 0.0.0+g33876c88.gitarchive\r\ngsw 3.4.0\r\nh5netcdf 0.10.0\r\nh5py 3.1.0\r\nHeapDict 1.0.1\r\nholoviews 1.14.2\r\nhvplot 0.7.1\r\nidna 2.10\r\nimportlib-metadata 3.7.0\r\nipykernel 5.5.0\r\nipython 7.21.0\r\nipython-genutils 0.2.0\r\njedi 0.18.0\r\nJinja2 2.11.3\r\njoblib 1.0.1\r\njson5 0.9.5\r\njsonschema 3.2.0\r\njupyter-client 6.1.11\r\njupyter-core 4.7.1\r\njupyter-packaging 0.7.12\r\njupyter-server 1.4.1\r\njupyter-telemetry 0.1.0\r\njupyterhub 1.3.0\r\njupyterlab 3.0.9\r\njupyterlab-pygments 0.1.2\r\njupyterlab-server 2.3.0\r\nkiwisolver 1.3.1\r\nllvmlite 0.36.0\r\nlocket 0.2.0\r\nMako 1.1.4\r\nmamba 0.7.14\r\nMarkdown 3.3.4\r\nMarkupSafe 1.1.1\r\nmatplotlib 3.3.4\r\nmistune 0.8.4\r\nmonotonic 1.5\r\nmsgpack 1.0.2\r\nmultipledispatch 0.6.0\r\nmunch 2.5.0\r\nmypy-extensions 0.4.3\r\nnbclassic 0.2.6\r\nnbclient 0.5.3\r\nnbconvert 6.0.7\r\nnbformat 5.1.2\r\nnest-asyncio 1.4.3\r\nnetCDF4 1.5.6\r\nnotebook 6.2.0\r\nnumba 0.53.0\r\nnumcodecs 0.7.3\r\nnumpy 1.20.1\r\noauthlib 3.0.1\r\nolefile 0.46\r\npackaging 20.9\r\npamela 1.0.0\r\npandas 1.2.3\r\npandocfilters 1.4.2\r\npanel 0.11.0\r\npapermill 2.3.3\r\nparam 1.10.1\r\nparcels 2.2.2\r\nparso 0.8.1\r\npartd 1.1.0\r\npathspec 0.8.1\r\npatsy 0.5.1\r\npexpect 4.8.0\r\npickleshare 0.7.5\r\nPillow 8.1.2\r\npip 21.0.1\r\nprogressbar2 3.53.1\r\nprometheus-client 0.9.0\r\nprompt-toolkit 3.0.16\r\npsutil 5.8.0\r\nptyprocess 0.7.0\r\npycosat 0.6.3\r\npycparser 2.20\r\npyct 0.4.6\r\npycurl 7.43.0.6\r\nPygments 2.8.0\r\nPyJWT 2.0.1\r\npymbolic 2020.1\r\npyOpenSSL 20.0.1\r\npyparsing 2.4.7\r\npyproj 3.0.1\r\nPyQt5 5.12.3\r\nPyQt5-sip 4.19.18\r\nPyQtChart 5.12\r\nPyQtWebEngine 5.12.1\r\npyrsistent 0.17.3\r\npyshp 2.1.3\r\nPySocks 1.7.1\r\npython-dateutil 2.8.1\r\npython-editor 1.0.4\r\npython-json-logger 2.0.1\r\npython-utils 2.5.5\r\npytools 2021.2\r\npytz 2021.1\r\npyviz-comms 2.0.1\r\nPyYAML 5.4.1\r\npyzmq 22.0.3\r\nregex 2020.11.13\r\nrequests 2.25.1\r\nRtree 0.9.7\r\nruamel-yaml-conda 0.15.80\r\nruamel.yaml 0.16.12\r\nruamel.yaml.clib 0.2.2\r\nscikit-learn 0.24.1\r\nscipy 1.6.1\r\nseaborn 0.11.1\r\nseawater 3.3.4\r\nSend2Trash 1.5.0\r\nsetuptools 49.6.0.post20210108\r\nShapely 1.7.1\r\nsix 1.15.0\r\nsniffio 1.2.0\r\nsortedcontainers 2.3.0\r\nsparse 0.11.2\r\nSQLAlchemy 1.3.23\r\nstatsmodels 0.12.2\r\ntblib 1.6.0\r\ntenacity 7.0.0\r\nterminado 0.9.2\r\ntestpath 0.4.4\r\ntextwrap3 0.9.2\r\nthreadpoolctl 2.1.0\r\ntoml 0.10.2\r\ntoolz 0.11.1\r\ntornado 6.1\r\ntqdm 4.58.0\r\ntraitlets 5.0.5\r\ntyped-ast 1.4.2\r\ntyping-extensions 3.7.4.3\r\nurllib3 1.26.3\r\nwcwidth 0.2.5\r\nwebencodings 0.5.1\r\nwheel 0.36.2\r\nxarray 0.17.0\r\nxhistogram 0.1.2\r\nzarr 2.6.1\r\nzict 2.0.0\r\nzipp 3.4.0\r\n"
]
]
] | [
"markdown",
"code",
"markdown",
"code",
"markdown",
"code",
"markdown",
"code",
"markdown",
"code",
"markdown",
"code",
"markdown",
"code",
"markdown",
"code",
"markdown",
"code",
"markdown",
"code",
"markdown",
"code",
"markdown",
"code",
"markdown",
"code",
"markdown",
"code",
"markdown",
"code",
"markdown",
"code",
"markdown",
"code",
"markdown",
"code"
] | [
[
"markdown",
"markdown",
"markdown"
],
[
"code"
],
[
"markdown",
"markdown"
],
[
"code",
"code"
],
[
"markdown"
],
[
"code",
"code",
"code"
],
[
"markdown"
],
[
"code"
],
[
"markdown"
],
[
"code",
"code",
"code"
],
[
"markdown",
"markdown"
],
[
"code",
"code",
"code"
],
[
"markdown",
"markdown"
],
[
"code",
"code",
"code"
],
[
"markdown"
],
[
"code"
],
[
"markdown"
],
[
"code",
"code"
],
[
"markdown"
],
[
"code",
"code",
"code",
"code",
"code"
],
[
"markdown"
],
[
"code"
],
[
"markdown"
],
[
"code",
"code"
],
[
"markdown"
],
[
"code",
"code"
],
[
"markdown",
"markdown"
],
[
"code",
"code"
],
[
"markdown"
],
[
"code",
"code"
],
[
"markdown"
],
[
"code",
"code"
],
[
"markdown"
],
[
"code"
],
[
"markdown",
"markdown"
],
[
"code",
"code",
"code",
"code",
"code"
]
] |
e77964f41f8c474fe7b171d6d80e6aa67e4497e9 | 3,632 | ipynb | Jupyter Notebook | Plotly/Create Candlestick chart.ipynb | vivard/awesome-notebooks | 899558bcc2165bb2155f5ab69ac922c6458e1799 | [
"BSD-3-Clause"
] | null | null | null | Plotly/Create Candlestick chart.ipynb | vivard/awesome-notebooks | 899558bcc2165bb2155f5ab69ac922c6458e1799 | [
"BSD-3-Clause"
] | null | null | null | Plotly/Create Candlestick chart.ipynb | vivard/awesome-notebooks | 899558bcc2165bb2155f5ab69ac922c6458e1799 | [
"BSD-3-Clause"
] | null | null | null | 20.290503 | 289 | 0.504681 | [
[
[
"<img width=\"10%\" alt=\"Naas\" src=\"https://landen.imgix.net/jtci2pxwjczr/assets/5ice39g4.png?w=160\"/>\n",
"_____no_output_____"
],
[
"# Plotly - Create Candlestick chart\n<a href=\"https://app.naas.ai/user-redirect/naas/downloader?url=https://raw.githubusercontent.com/jupyter-naas/awesome-notebooks/master/Plotly/Create%20Candlestick%20chart.ipynb\" target=\"_parent\"><img src=\"https://naasai-public.s3.eu-west-3.amazonaws.com/open_in_naas.svg\"/></a>",
"_____no_output_____"
],
[
"**Tags:** #plotly #chart #candlestick #trading #dataviz",
"_____no_output_____"
],
[
"## Input",
"_____no_output_____"
],
[
"### Import libraries",
"_____no_output_____"
]
],
[
[
"import plotly.graph_objects as go\n\nimport pandas as pd\nfrom datetime import datetime",
"_____no_output_____"
]
],
[
[
"## Model",
"_____no_output_____"
],
[
"### Read a csv and map the plot",
"_____no_output_____"
]
],
[
[
"df = pd.read_csv('https://raw.githubusercontent.com/plotly/datasets/master/finance-charts-apple.csv')\n\nfig = go.Figure(data=[go.Candlestick(x=df['Date'],\n open=df['AAPL.Open'],\n high=df['AAPL.High'],\n low=df['AAPL.Low'],\n close=df['AAPL.Close'])])",
"_____no_output_____"
]
],
[
[
"## Output",
"_____no_output_____"
],
[
"### Display result",
"_____no_output_____"
]
],
[
[
"fig.show()",
"_____no_output_____"
]
]
] | [
"markdown",
"code",
"markdown",
"code",
"markdown",
"code"
] | [
[
"markdown",
"markdown",
"markdown",
"markdown",
"markdown"
],
[
"code"
],
[
"markdown",
"markdown"
],
[
"code"
],
[
"markdown",
"markdown"
],
[
"code"
]
] |
e7796d9b9a0409a53afe57fde3bc5e56d1a65ea1 | 1,080 | ipynb | Jupyter Notebook | Section2/while.ipynb | BartlomiejSkwira/udemy_python-az-for-data-science | e164ae734679ae8d5365d04beccef2709df38810 | [
"MIT"
] | null | null | null | Section2/while.ipynb | BartlomiejSkwira/udemy_python-az-for-data-science | e164ae734679ae8d5365d04beccef2709df38810 | [
"MIT"
] | null | null | null | Section2/while.ipynb | BartlomiejSkwira/udemy_python-az-for-data-science | e164ae734679ae8d5365d04beccef2709df38810 | [
"MIT"
] | null | null | null | 16.615385 | 34 | 0.469444 | [
[
[
"while False:\n print(\"not gonna run\")",
"_____no_output_____"
],
[
"counter = 0\nwhile counter < 3:\n print(counter)\n counter = counter + 1",
"0\n1\n2\n"
]
]
] | [
"code"
] | [
[
"code",
"code"
]
] |
e779757446624e034dcfafffa77e303dc3e06843 | 383,744 | ipynb | Jupyter Notebook | RANDOM PATCHES WITH NON-UNIFORM SAMPLING.ipynb | kbogas/Cascada | e5c614bd9251c73c95fbf8d7634b96166cf5234e | [
"MIT"
] | null | null | null | RANDOM PATCHES WITH NON-UNIFORM SAMPLING.ipynb | kbogas/Cascada | e5c614bd9251c73c95fbf8d7634b96166cf5234e | [
"MIT"
] | null | null | null | RANDOM PATCHES WITH NON-UNIFORM SAMPLING.ipynb | kbogas/Cascada | e5c614bd9251c73c95fbf8d7634b96166cf5234e | [
"MIT"
] | null | null | null | 119.101179 | 164,424 | 0.773344 | [
[
[
"The idea is to do random patches but try out different methodologies regarding the sampling procedure. First, in the form of weighted samples where ideas from Breiman's Paper (pasting) and Adaboost can be used.\nSecond, in the form of weighted features with respect to correlation (chi square, best of k?) between the selected samples?\n\n- [Link to breiman Paper](https://link.springer.com/content/pdf/10.1023%2FA%3A1007563306331.pdf)\n- [Link to Louppe Paper](https://orbi.uliege.be/bitstream/2268/130099/1/glouppe12.pdf)",
"_____no_output_____"
],
[
"Some more similar ideas:\n- use for each new estimator the n1 closest from the same class and the n2 closest from other classes. if the average distance between the sample and the same-class samples is bigger than the avg.distance with the other-class samples, do not take it into account and say it's an outlier? n1 and n2 should be trained probably?\n- Based on the above, maybe pick the largest possible linearly separable dataset for this sample?\n- idea of linearly separable classifiers? do we need one good and create multiple linear for the misclassifier samples or go directly for multiple classifiers?",
"_____no_output_____"
]
],
[
[
"from sklearn.cross_validation import cross_val_score\nfrom sklearn.ensemble import BaggingClassifier\nfrom sklearn.neighbors import KNeighborsClassifier\n\n\nimport numpy as np\nimport matplotlib.pyplot as plt\nimport matplotlib.gridspec as gridspec\nimport itertools\n\nimport sklearn\n\nfrom sklearn.linear_model import LogisticRegression\nfrom sklearn.svm import SVC\nfrom sklearn.ensemble import RandomForestClassifier\n\nfrom brew.base import Ensemble, EnsembleClassifier\nfrom brew.stacking.stacker import EnsembleStack, EnsembleStackClassifier\nfrom brew.combination.combiner import Combiner\nfrom sklearn.metrics import accuracy_score, confusion_matrix, classification_report\n\n\nfrom mlxtend.data import wine_data, iris_data\n\nfrom mlxtend.plotting import plot_decision_regions\n\n\nfrom sklearn.base import TransformerMixin, BaseEstimator\nfrom sklearn.ensemble import ExtraTreesClassifier\nfrom itertools import combinations\nimport random\nrandom.seed(10)\n\n\n\n\nX, y = wine_data()\n\n\nknn = KNeighborsClassifier()\nbagging = BaggingClassifier(knn, max_samples=0.5, max_features=0.5)\n\nprint(\"KNN Score:\")\nprint(cross_val_score(knn, X, y, cv=5, n_jobs=-1).mean())\nprint(\"Bagging Score:\")\nprint(cross_val_score(bagging, X, y, cv=5, n_jobs=-1).mean())\n\n\n",
"/usr/local/lib/python2.7/dist-packages/sklearn/cross_validation.py:41: DeprecationWarning: This module was deprecated in version 0.18 in favor of the model_selection module into which all the refactored classes and functions are moved. Also note that the interface of the new CV iterators are different from that of this module. This module will be removed in 0.20.\n \"This module will be removed in 0.20.\", DeprecationWarning)\n"
],
[
"import matplotlib.pyplot as plt\nimport numpy\n\nx = numpy.linspace(0,1,100) # 100 linearly spaced numbers\nyy = np.exp(0.5*np.log((1-x)/x)) # computing the values of sin(x)/x\n\n# compose plot\nplt.plot(x,yy) # sin(x)/x\n#plt.plot(x,yy,'co') # same function with cyan dots\nplt.show() # show the plot",
"/usr/local/lib/python2.7/dist-packages/ipykernel_launcher.py:5: RuntimeWarning: divide by zero encountered in divide\n \"\"\"\n/usr/local/lib/python2.7/dist-packages/ipykernel_launcher.py:5: RuntimeWarning: divide by zero encountered in log\n \"\"\"\n"
],
[
"print(a)\nprint(signs[0])\nprint(np.exp(-a*signs[0]))\nprint(signs[4])\nprint(np.exp(-a*signs[4]))",
"_____no_output_____"
],
[
"knn.fit(X,y)\npreds2 = knn.predict(X)\nsc = 1-accuracy_score(y,preds2, normalize=True)\nprint(sc)\na = 0.5*np.log((1-sc)/float(sc))\nprint(a)\nsigns = sign(y, preds2)\n#print(signs)\ntemp =np.array([1/float(len(y)) for i in y])*np.exp(-a*signs)\nprint(temp)\nprint(temp/sum(temp))\nfor i, sign_ in enumerate(signs):\n if sign_ <0:\n pass\n #print(\"DIFFERENT\")\n #print(y[i], preds2[i])\n #print(np.mean(temp), temp[i])\n elif sign_ >0:\n pass\n #print(\"SAME\")\n #print(y[i], preds2[i])\n #print(np.mean(temp), temp[i])",
"0.2134831460674157\n0.6520281314414593\n"
],
[
"ssign = np.ones_like(preds)\nssign[np.where(preds!=y)] = -1\nssign",
"_____no_output_____"
],
[
"#rp2.fit(X,y)\npreds = rp2.predict(X)\nrp2.samples_weights[-1][np.argsort(rp2.samples_weights[-1]).flatten()]\n#for i, y_ in enumerate(preds):\n #print(\"%d -- %d\" % (y_, y[i]))\n #print(rp2.samples_weights[-1][i])",
"_____no_output_____"
],
[
"from sklearn.base import BaseEstimator, ClassifierMixin, clone\nfrom sklearn.neighbors import KNeighborsClassifier\nfrom sklearn.utils import check_X_y, column_or_1d, check_random_state\nfrom sklearn.utils.multiclass import check_classification_targets\nfrom sklearn.model_selection import train_test_split\nfrom sklearn.utils import check_random_state, check_X_y, check_array, column_or_1d\nfrom sklearn.utils.random import sample_without_replacement\nfrom sklearn.utils.validation import has_fit_parameter, check_is_fitted\nfrom sklearn.utils import indices_to_mask, check_consistent_length\nfrom sklearn.utils.metaestimators import if_delegate_has_method\nfrom sklearn.utils.multiclass import check_classification_targets\n\n\n\ndef _generate_indices(random_state, bootstrap, n_population, n_samples, prob= None):\n \"\"\"Draw randomly sampled indices.\"\"\"\n # Draw sample indices\n if np.all(prob)!=None:\n #print(prob.shape)\n #print(prob)\n indices = random_state.choice([i for i in xrange(0, n_population)], n_samples, p=prob)\n else:\n if bootstrap:\n #print(random_state)\n #print(n_population, n_samples)\n indices = random_state.randint(0, n_population, n_samples)\n else:\n indices = sample_without_replacement(n_population, n_samples,\n random_state=random_state)\n\n return indices\n\n\ndef generate_bagging_indices(random_state, bootstrap_features,\n bootstrap_samples, n_features, n_samples,\n max_features, max_samples, samples_weights):\n \"\"\"Randomly draw feature and sample indices.\"\"\"\n # Get valid random state\n random_state = check_random_state(random_state)\n\n # Draw indices\n #print(random_state, type(random_state))\n \n feature_indices = _generate_indices(random_state, bootstrap_features,\n n_features, max_features)\n #print(bootstrap_samples, n_samples, max_samples, samples_weights)\n sample_indices = _generate_indices(random_state, bootstrap_samples,\n n_samples, max_samples, prob=samples_weights)\n\n return feature_indices, sample_indices\n\n\n\ndef sign(true, preds):\n ssign = np.ones_like(true)\n ssign[np.where(preds!=true)] = -1\n return ssign\n\nclass Vanilla_RP(BaseEstimator, ClassifierMixin):\n \n def __init__(self, \n base_estimator_=KNeighborsClassifier(),\n n_estimators=10,\n max_samples=1.0,\n max_features=1.0,\n bootstrap_samples=True,\n bootstrap_features = False,\n patcher='random',\n dev_set=0.1,\n random_state=42):\n self.base_estimator_ = base_estimator_\n self.n_estimators= n_estimators\n self.max_samples = max_samples\n self.max_features = max_features\n self.bootstrap_samples = bootstrap_samples\n self.bootstrap_features = bootstrap_features\n self.patcher = patcher\n self.dev_set = 0.1\n self.random_state = check_random_state(random_state)\n self.ensemble = []\n self.prev_samples_indices = []\n self.estimators_features = []\n self.samples_weights = []\n self.samples_times_selected = None\n self.scores = []\n self.a = 0\n self.X_dev = None\n self.y_dev = None\n\n \n def fit(self, X, y):\n \n X, y = check_X_y(\n X, y, ['csr', 'csc'], dtype=None, force_all_finite=False,\n multi_output=True)\n #print(y)\n #print(type(y), y.shape)\n #print(X.shape)\n #X, self.X_dev, y, self.y_dev = train_test_split(X, y, test_size=self.dev_set, stratify=y)\n n_samples, self.n_features_ = X.shape\n self._n_samples = n_samples\n self.samples_times_selected = np.zeros_like(y)\n self.default_sample_weight = 1/float(X.shape[0])\n self.max_samples = int(self.max_samples*self._n_samples)\n self.max_features = int(self.max_features*self.n_features_)\n \n y = self._validate_y(y)\n for i_est in xrange(self.n_estimators):\n estimator = clone(self.base_estimator_)\n if self.patcher == 'random':\n features_indices, samples_indices = generate_bagging_indices(\n self.random_state, self.bootstrap_features,\n self.bootstrap_samples, X.shape[1], X.shape[0],\n self.max_features, self.max_samples, [None])\n elif self.patcher == 'weighted':\n# X_train, X_dev, y_train, y_dev = train_test_split(X, y, stratify = True,\n# test_size=self.dev_set, \n# random_state=self.random_state)\n if i_est==0:\n self.samples_weights.append(np.array([self.default_sample_weight for i in xrange(X.shape[0])]))\n self.scores.append(1)\n signs = np.ones_like(y)\n else:\n signs = self.update_weights(X, y)\n #print(\"ROUND %d\"%i_est)\n #print(self.samples_weights[i_est])\n #print(\"INDEX %d\" % i)\n #print(self.samples_weights[i_est])\n features_indices, samples_indices = generate_bagging_indices(\n self.random_state, self.bootstrap_features,\n self.bootstrap_samples, X.shape[1], X.shape[0],\n self.max_features, self.max_samples, self.samples_weights[i_est])\n # print(\"ROUND %d\" % i_est)\n # print(\"SCORE %0.3f\"%(self.scores[-1]))\n if i_est != 0:\n accs = []\n accs_last = []\n overlaps = []\n accs_total = []\n for jj, ens in enumerate(self.ensemble):\n # print(len(self.prev_samples_indices), len(self.estimators_features))\n p_pred = ens.predict(X[self.prev_samples_indices[jj]][:, self.estimators_features[jj]])\n last_pred = ens.predict(X[self.prev_samples_indices[-1]][:, self.estimators_features[jj]])\n total_pred = self.predict(X[self.prev_samples_indices[jj]])\n accs.append(1-accuracy_score(y[self.prev_samples_indices[jj]], p_pred, normalize=True))\n accs_last.append(1-accuracy_score(y[self.prev_samples_indices[-1]], last_pred, normalize=True))\n accs_total.append(1-accuracy_score(y[self.prev_samples_indices[jj]], total_pred, normalize=True))\n #try:\n # self.ensemble[jj+1]\n #except IndexError:\n # print(\"CURRENT\")\n # print(self.prev_samples_indices[jj])\n # print(\"LAST\")\n # print(self.prev_samples_indices[-1])\n # print(np.array_equal(self.prev_samples_indices[jj], self.prev_samples_indices[-1]))\n # print(np.intersect1d(self.prev_samples_indices[jj], self.prev_samples_indices[-1]).shape[0], self.prev_samples_indices[-1].shape[0], self.prev_samples_indices[jj].shape[0])\n overlaps.append(np.intersect1d(self.prev_samples_indices[jj], self.prev_samples_indices[-1]).shape[0]/float(np.unique(self.prev_samples_indices[jj]).shape[0]))\n accs = np.round(100*np.array(accs),3)\n accs_last = np.round(100*np.array(accs_last),3)\n overlaps = np.round(100*np.array(overlaps),3)\n accs_total = np.round(100*np.array(accs_total),3)\n# print(\"PREVIOUS ERRORS ON CORRESPONDING DATA\")\n# print(accs)\n# print(\"ERRORS OF THE ENSEMBLE ON CORRESPONDING DATA\")\n# print(accs_total)\n# print(\"PREVIOUS ERRORS ON LAST SELECTED DATA\")\n# print(accs_last)\n# print(\"OVERLAPS\")\n# print(overlaps)\n# print(\"a: %0.3f\"%self.a)\n# print(\"WEIGHTS\")\n# print(np.min(self.samples_weights[-1]), \n# np.mean(self.samples_weights[-1]), \n# np.max(self.samples_weights[-1]))\n# #print(self.samples_weights[-1])\n# print(\"SAMPLED INSTANCES\")\n# print(self.samples_weights[-1][samples_indices])\n# print(signs[samples_indices])\n# print(y[samples_indices])\n# print(\"~\"*50)\n else:\n print(\"UNSUPPORTED WAY OF PATCHING: %s !\" % self.patcher)\n # minor fix for when one class is not represented during sampling\n #print(samples_indices.shape)\n samples_indices = self.fix_class_indices(y, samples_indices)\n #print(\"AFTER\")\n #print(samples_indices.shape)\n estimator.fit(X[samples_indices][:, features_indices], y[samples_indices])\n self.prev_samples_indices.append(samples_indices)\n self.estimators_features.append(features_indices)\n self.ensemble.append(estimator)\n self.samples_times_selected[samples_indices] += 1\n return self\n \n def fix_class_indices(self, y, samples_indices):\n in_set = set(y[samples_indices])\n a = set(y).difference(in_set)\n for item in a:\n samples_indices= np.append(samples_indices, [np.where(y==item)[0][0]])\n return samples_indices\n \n def update_weights(self, X, y): \n #print(\"REMOVED %d\" % np.where(self.samples_times_selected > 3)[0].shape[0])\n #self.samples_weights[-1][np.where(self.samples_times_selected > 3)] = self.default_sample_weight\n #self.samples_times_selected[np.where(self.samples_times_selected > 3)] = 0\n preds = self.predict(X)\n self.scores.append(1-accuracy_score(y, preds, normalize=True)+0.0001)\n self.a = 0.5*np.log((1-self.scores[-1])/float(self.scores[-1]))\n #self.a = 9\n #self.a = 1\n signs = sign(y, preds)\n temp = self.samples_weights[-1]*np.exp(-self.a*signs)\n \n #print(\"MEAN\")\n #print(np.mean(temp))\n #print(temp[np.where(signs<0)])\n self.samples_weights.append(temp/np.sum(temp))\n preds = np.ones((X.shape[0], len(self.ensemble)))\n for ii, est in enumerate(self.ensemble):\n sc = accuracy_score(y, est.predict(X[:, self.estimators_features[ii]]), normalize=True)\n preds[:, ii] = sc*sign(y, est.predict(X[:, self.estimators_features[ii]]))\n #print(\"INITIAL\")\n #print(preds)\n #print(np.min(preds))\n #print(\"AFTER COLLAPSE\")\n preds = np.sum(preds, axis=1)\n #print(preds)\n #print(\"AFTER RESHAPE\")\n preds = preds.reshape(-1,)\n #print(preds)\n if np.any(preds<0):\n min_ = np.min(preds)\n if min_ < 0:\n min_ = -1*min_\n #print(\"MIN\")\n #print(min_)\n preds = preds + min_ + 0.001\n #else:\n #preds = preds - np.min(preds) + 0.001\n #print(\"AFTER_MIN\")\n #print(preds)\n #print(\"AFTER NORMALIZATION\")\n preds /= np.sum(preds)\n #print(preds)\n #print(preds)\n #print(np.sum(preds))\n self.samples_weights.append(preds)\n return signs\n \n def _validate_y(self, y):\n y = column_or_1d(y, warn=True)\n check_classification_targets(y)\n self.classes_, y = np.unique(y, return_inverse=True)\n self.n_classes_ = len(self.classes_)\n return y\n \n def predict(self, X):\n \"\"\"Predict class for X.\n The predicted class of an input sample is computed as the class with\n the highest mean predicted probability. If base estimators do not\n implement a ``predict_proba`` method, then it resorts to voting.\n Parameters\n ----------\n X : {array-like, sparse matrix} of shape = [n_samples, n_features]\n The training input samples. Sparse matrices are accepted only if\n they are supported by the base estimator.\n Returns\n -------\n y : array of shape = [n_samples]\n The predicted classes.\n \"\"\"\n \n if hasattr(self.base_estimator_, \"predict_proba\"):\n predicted_probability = self.predict_proba(X)\n return self.classes_.take((np.argmax(predicted_probability, axis=1)),\n axis=0)\n else:\n predicted_probability = np.zeros((X.shape[0],1))\n for i, ens in enumerate(self.ensemble):\n predicted_probability = np.hstack((predicted_probability, ens.predict(X[:, self.estimators_features[i]]).reshape(-1,1)))\n predicted_probability = np.delete(predicted_probability,0,axis=1)\n final_pred = []\n for sample in xrange(X.shape[0]):\n final_pred.append(most_common(predicted_probability[sample,:]))\n #votes = []\n #for i, mod_vote in predictions[sample,:]:\n # votes.extend([predictions[sample, i] for j in xrange(int(self.acc[i]))])\n #final_pred = most_common(votes)\n return np.array(final_pred) \n \n\n\n def predict_proba(self, X):\n \"\"\"Predict class probabilities for X.\n The predicted class probabilities of an input sample is computed as\n the mean predicted class probabilities of the base estimators in the\n ensemble. If base estimators do not implement a ``predict_proba``\n method, then it resorts to voting and the predicted class probabilities\n of an input sample represents the proportion of estimators predicting\n each class.\n Parameters\n ----------\n X : {array-like, sparse matrix} of shape = [n_samples, n_features]\n The training input samples. Sparse matrices are accepted only if\n they are supported by the base estimator.\n Returns\n -------\n p : array of shape = [n_samples, n_classes]\n The class probabilities of the input samples. The order of the\n classes corresponds to that in the attribute `classes_`.\n \"\"\"\n check_is_fitted(self, \"classes_\")\n # Check data\n X = check_array(\n X, accept_sparse=['csr', 'csc'], dtype=None,\n force_all_finite=False\n )\n\n if self.n_features_ != X.shape[1]:\n raise ValueError(\"Number of features of the model must \"\n \"match the input. Model n_features is {0} and \"\n \"input n_features is {1}.\"\n \"\".format(self.n_features_, X.shape[1]))\n\n all_proba = np.zeros((X.shape[0], self.n_classes_))\n for i, ens in enumerate(self.ensemble):\n all_proba += ens.predict_proba(X[:, self.estimators_features[i]])\n all_proba /= self.n_estimators\n #print(all_proba.shape)\n #print(all_proba)\n #proba = np.sum(all_proba, axis=0) / self.n_estimators\n #print(proba.shape)\n #print(proba)\n return all_proba\n\n\n @if_delegate_has_method(delegate='base_estimator')\n def decision_function(self, X):\n \"\"\"Average of the decision functions of the base classifiers.\n Parameters\n ----------\n X : {array-like, sparse matrix} of shape = [n_samples, n_features]\n The training input samples. Sparse matrices are accepted only if\n they are supported by the base estimator.\n Returns\n -------\n score : array, shape = [n_samples, k]\n The decision function of the input samples. The columns correspond\n to the classes in sorted order, as they appear in the attribute\n ``classes_``. Regression and binary classification are special\n cases with ``k == 1``, otherwise ``k==n_classes``.\n \"\"\"\n check_is_fitted(self, \"classes_\")\n\n # Check data\n X = check_array(\n X, accept_sparse=['csr', 'csc'], dtype=None,\n force_all_finite=False\n )\n\n if self.n_features_ != X.shape[1]:\n raise ValueError(\"Number of features of the model must \"\n \"match the input. Model n_features is {0} and \"\n \"input n_features is {1} \"\n \"\".format(self.n_features_, X.shape[1]))\n all_decisions = np.zeros((X.shape[0], self.n_classes_))\n for i, ens in enumerate(self.ensemble):\n all_decisions += ens.predict_proba(X[:, self.estimators_features[i]]) \n decisions = sum(all_decisions) / self.n_estimators\n\n return decisions\n\n \n \n\n \n \ndef most_common(lst):\n if isinstance(lst, np.ndarray):\n lst = lst.tolist()\n #print(lst, max(set(lst), key=lst.count) )\n return max(set(lst), key=lst.count) \n \n ",
"_____no_output_____"
],
[
"min(min(np.array([[1,2]])))",
"_____no_output_____"
],
[
"#X, y = wine_data()\nrp2 = Vanilla_RP(knn,max_samples=0.5, max_features=0.5, patcher='weighted')\nrp2.fit(X,y)\n\n#train_test_split(X,y,test_size=0.2, stratify=y)",
"_____no_output_____"
],
[
"knn = KNeighborsClassifier()\nbagging = BaggingClassifier(knn, max_samples=0.5, max_features=0.5)\nrp = Vanilla_RP(knn,max_samples=0.5, max_features=0.5)\nrp2 = Vanilla_RP(knn,max_samples=0.5, max_features=0.5, patcher='weighted')\ncv = 10\nprint(\"KNN Score:\")\nprint(cross_val_score(knn, X, y, cv=cv, n_jobs=-1).mean())\nprint(\"Bagging Score:\")\nprint(cross_val_score(bagging, X, y, cv=cv, n_jobs=-1).mean())\nprint(\"RP Score:\")\nprint(cross_val_score(rp, X, y, cv=cv, n_jobs=-1).mean())\nprint(\"RP-WEIGHTED Score:\")\nprint(cross_val_score(rp2, X, y, cv=cv, n_jobs=-1).mean())\n",
"KNN Score:\n0.6757739938080495\nBagging Score:\n0.9442251461988305\nRP Score:\n0.9400584795321638\nRP-WEIGHTED Score:\n0.9213837289301685\n"
],
[
"\n\"\"\"Bagging meta-estimator.\"\"\"\n\n# Author: Gilles Louppe <[email protected]>\n# License: BSD 3 clause\n\nfrom __future__ import division\n\nimport itertools\nimport numbers\nimport numpy as np\nfrom warnings import warn\nfrom abc import ABCMeta, abstractmethod\n\nfrom sklearn.base import ClassifierMixin, RegressorMixin\nfrom sklearn.externals.joblib import Parallel, delayed\nfrom sklearn.externals.six import with_metaclass\nfrom sklearn.externals.six.moves import zip\nfrom sklearn.metrics import r2_score, accuracy_score\nfrom sklearn.tree import DecisionTreeClassifier, DecisionTreeRegressor\nfrom sklearn.utils import check_random_state, check_X_y, check_array, column_or_1d\nfrom sklearn.utils.random import sample_without_replacement\nfrom sklearn.utils.validation import has_fit_parameter, check_is_fitted\nfrom sklearn.utils import indices_to_mask, check_consistent_length\nfrom sklearn.utils.metaestimators import if_delegate_has_method\nfrom sklearn.utils.multiclass import check_classification_targets\n\nfrom sklearn.base import BaseEnsemble, _partition_estimators\n\n\n__all__ = [\"BaggingClassifier\",\n \"BaggingRegressor\"]\n\nMAX_INT = np.iinfo(np.int32).max\n\n\ndef _generate_indices(random_state, bootstrap, n_population, n_samples, prob= None):\n \"\"\"Draw randomly sampled indices.\"\"\"\n # Draw sample indices\n if prob:\n indices = random_state.choice([i for i in xrange(0, n_population)], n_samples, p=prob)\n else:\n if bootstrap:\n indices = random_state.randint(0, n_population, n_samples)\n else:\n indices = sample_without_replacement(n_population, n_samples,\n random_state=random_state)\n\n return indices\n\n\ndef _generate_bagging_indices(random_state, bootstrap_features,\n bootstrap_samples, n_features, n_samples,\n max_features, max_samples):\n \"\"\"Randomly draw feature and sample indices.\"\"\"\n # Get valid random state\n random_state = check_random_state(random_state)\n\n # Draw indices\n feature_indices = _generate_indices(random_state, bootstrap_features,\n n_features, max_features)\n sample_indices = _generate_indices(random_state, bootstrap_samples,\n n_samples, max_samples)\n\n return feature_indices, sample_indices\n\ndef sign_()\n\ndef _parallel_build_estimators(n_estimators, ensemble, X, y, sample_weight,\n seeds, total_n_estimators, verbose, sample_weights):\n \"\"\"Private function used to build a batch of estimators within a job.\"\"\"\n # Retrieve settings\n n_samples, n_features = X.shape\n max_features = ensemble._max_features\n max_samples = ensemble._max_samples\n bootstrap = ensemble.bootstrap\n bootstrap_features = ensemble.bootstrap_features\n support_sample_weight = has_fit_parameter(ensemble.base_estimator_,\n \"sample_weight\")\n if not support_sample_weight and sample_weight is not None:\n raise ValueError(\"The base estimator doesn't support sample weight\")\n\n # Build estimators\n estimators = []\n estimators_features = []\n\n for i in range(n_estimators):\n if verbose > 1:\n print(\"Building estimator %d of %d for this parallel run \"\n \"(total %d)...\" % (i + 1, n_estimators, total_n_estimators))\n\n random_state = np.random.RandomState(seeds[i])\n estimator = ensemble._make_estimator(append=False,\n random_state=random_state)\n\n # Draw random feature, sample indices\n a = 0.5*np.log((1-self.oob_score_)/self.oob_score_)\n sample_weights =\n features, indices = _generate_bagging_indices(random_state,\n bootstrap_features,\n bootstrap, n_features,\n n_samples, max_features,\n max_samples, prob=sample_weights)\n\n # Draw samples, using sample weights, and then fit\n if support_sample_weight:\n if sample_weight is None:\n curr_sample_weight = np.ones((n_samples,))\n else:\n curr_sample_weight = sample_weight.copy()\n\n if bootstrap:\n sample_counts = np.bincount(indices, minlength=n_samples)\n curr_sample_weight *= sample_counts\n else:\n not_indices_mask = ~indices_to_mask(indices, n_samples)\n curr_sample_weight[not_indices_mask] = 0\n\n estimator.fit(X[:, features], y, sample_weight=curr_sample_weight)\n\n # Draw samples, using a mask, and then fit\n else:\n estimator.fit((X[indices])[:, features], y[indices])\n\n estimators.append(estimator)\n estimators_features.append(features)\n\n return estimators, estimators_features\n\n\ndef _parallel_predict_proba(estimators, estimators_features, X, n_classes):\n \"\"\"Private function used to compute (proba-)predictions within a job.\"\"\"\n n_samples = X.shape[0]\n p_parallel_predict_probaroba = np.zeros((n_samples, n_classes))\n\n for estimator, features in zip(estimators, estimators_features):\n if hasattr(estimator, \"predict_proba\"):\n proba_estimator = estimator.predict_proba(X[:, features])\n\n if n_classes == len(estimator.classes_):\n proba += proba_estimator\n\n else:\n proba[:, estimator.classes_] += \\\n proba_estimator[:, range(len(estimator.classes_))]\n\n else:\n # Resort to voting\n predictions = estimator.predict(X[:, features])\n\n for i in range(n_samples):\n proba[i, predictions[i]] += 1\n\n return proba\n\n\ndef _parallel_predict_log_proba(estimators, estimators_features, X, n_classes):\n \"\"\"Private function used to compute log probabilities within a job.\"\"\"\n n_samples = X.shape[0]\n log_proba = np.empty((n_samples, n_classes))\n log_proba.fill(-np.inf)\n all_classes = np.arange(n_classes, dtype=np.int)\n\n for estimator, features in zip(estimators, estimators_features):\n log_proba_estimator = estimator.predict_log_proba(X[:, features])\n\n if n_classes == len(estimator.classes_):\n log_proba = np.logaddexp(log_proba, log_proba_estimator)\n\n else:\n log_proba[:, estimator.classes_] = np.logaddexp(\n log_proba[:, estimator.classes_],\n log_proba_estimator[:, range(len(estimator.classes_))])\n\n missing = np.setdiff1d(all_classes, estimator.classes_)\n log_proba[:, missing] = np.logaddexp(log_proba[:, missing],\n -np.inf)\n\n return log_proba\n\n\ndef _parallel_decision_function(estimators, estimators_features, X):\n \"\"\"Private function used to compute decisions within a job.\"\"\"\n return sum(estimator.decision_function(X[:, features])\n for estimator, features in zip(estimators,\n estimators_features))\n\n\ndef _parallel_predict_regression(estimators, estimators_features, X):\n \"\"\"Private function used to compute predictions within a job.\"\"\"\n return sum(estimator.predict(X[:, features])\n for estimator, features in zip(estimators,\n estimators_features))\n\n\nclass BaseBagging(with_metaclass(ABCMeta, BaseEnsemble)):\n \"\"\"Base class for Bagging meta-estimator.\n Warning: This class should not be used directly. Use derived classes\n instead.\n \"\"\"\n\n @abstractmethod\n def __init__(self,\n base_estimator=None,\n n_estimators=10,\n max_samples=1.0,\n max_features=1.0,\n bootstrap=True,\n bootstrap_features=False,\n oob_score=False,\n warm_start=False,\n n_jobs=1,\n random_state=None,\n verbose=0):\n super(BaseBagging, self).__init__(\n base_estimator=base_estimator,\n n_estimators=n_estimators)\n\n self.max_samples = max_samples\n self.max_features = max_features\n self.bootstrap = bootstrap\n self.bootstrap_features = bootstrap_features\n self.oob_score = oob_score\n self.warm_start = warm_start\n self.n_jobs = n_jobs\n self.random_state = random_state\n self.verbose = verbose\n self.samples_weights = None \n\n def fit(self, X, y, sample_weight=None):\n \"\"\"Build a Bagging ensemble of estimators from the training\n set (X, y).\n Parameters\n ----------\n X : {array-like, sparse matrix} of shape = [n_samples, n_features]\n The training input samples. Sparse matrices are accepted only if\n they are supported by the base estimator.\n y : array-like, shape = [n_samples]\n The target values (class labels in classification, real numbers in\n regression).\n sample_weight : array-like, shape = [n_samples] or None\n Sample weights. If None, then samples are equally weighted.\n Note that this is supported only if the base estimator supports\n sample weighting.\n Returns\n -------\n self : object\n Returns self.\n \"\"\"\n return self._fit(X, y, self.max_samples, sample_weight=sample_weight)\n\n def _fit(self, X, y, max_samples=None, max_depth=None, sample_weight=None):\n \"\"\"Build a Bagging ensemble of estimators from the training\n set (X, y).\n Parameters\n ----------\n X : {array-like, sparse matrix} of shape = [n_samples, n_features]\n The training input samples. Sparse matrices are accepted only if\n they are supported by the base estimator.\n y : array-like, shape = [n_samples]\n The target values (class labels in classification, real numbers in\n regression).\n max_samples : int or float, optional (default=None)\n Argument to use instead of self.max_samples.\n max_depth : int, optional (default=None)\n Override value used when constructing base estimator. Only\n supported if the base estimator has a max_depth parameter.\n sample_weight : array-like, shape = [n_samples] or None\n Sample weights. If None, then samples are equally weighted.\n Note that this is supported only if the base estimator supports\n sample weighting.\n Returns\n -------\n self : object\n Returns self.\n \"\"\"\n random_state = check_random_state(self.random_state)\n\n # Convert data\n X, y = check_X_y(X, y, ['csr', 'csc'])\n if sample_weight is not None:\n sample_weight = check_array(sample_weight, ensure_2d=False)\n check_consistent_length(y, sample_weight)\n\n # Remap output\n n_samples, self.n_features_ = X.shape\n self._n_samples = n_samples\n self.samples_weights = np.array([1/float(n_samples) for i in xrange(n_samples)])\n y = self._validate_y(y)\n\n # Check parameters\n self._validate_estimator()\n\n if max_depth is not None:\n self.base_estimator_.max_depth = max_depth\n\n # Validate max_samples\n if max_samples is None:\n max_samples = self.max_samples\n elif not isinstance(max_samples, (numbers.Integral, np.integer)):\n max_samples = int(max_samples * X.shape[0])\n\n if not (0 < max_samples <= X.shape[0]):\n raise ValueError(\"max_samples must be in (0, n_samples]\")\n\n # Store validated integer row sampling value\n self._max_samples = max_samples\n\n # Validate max_features\n if isinstance(self.max_features, (numbers.Integral, np.integer)):\n max_features = self.max_features\n else: # float\n max_features = int(self.max_features * self.n_features_)\n\n if not (0 < max_features <= self.n_features_):\n raise ValueError(\"max_features must be in (0, n_features]\")\n\n # Store validated integer feature sampling value\n self._max_features = max_features\n\n # Other checks\n if not self.bootstrap and self.oob_score:\n raise ValueError(\"Out of bag estimation only available\"\n \" if bootstrap=True\")\n\n if self.warm_start and self.oob_score:\n raise ValueError(\"Out of bag estimate only available\"\n \" if warm_start=False\")\n\n if hasattr(self, \"oob_score_\") and self.warm_start:\n del self.oob_score_\n\n if not self.warm_start or not hasattr(self, 'estimators_'):\n # Free allocated memory, if any\n self.estimators_ = []\n self.estimators_features_ = []\n\n n_more_estimators = self.n_estimators - len(self.estimators_)\n\n if n_more_estimators < 0:\n raise ValueError('n_estimators=%d must be larger or equal to '\n 'len(estimators_)=%d when warm_start==True'\n % (self.n_estimators, len(self.estimators_)))\n\n elif n_more_estimators == 0:\n warn(\"Warm-start fitting without increasing n_estimators does not \"\n \"fit new trees.\")\n return self\n\n # Parallel loop\n n_jobs, n_estimators, starts = _partition_estimators(n_more_estimators,\n self.n_jobs)\n total_n_estimators = sum(n_estimators)\n\n # Advance random state to state after training\n # the first n_estimators\n if self.warm_start and len(self.estimators_) > 0:\n random_state.randint(MAX_INT, size=len(self.estimators_))\n\n seeds = random_state.randint(MAX_INT, size=n_more_estimators)\n self._seeds = seeds\n\n all_results = Parallel(n_jobs=n_jobs, verbose=self.verbose)(\n delayed(_parallel_build_estiself.samples_weights = np.array([1/float(n_samples) for i in xrange(n_samples)])mators)(\n n_estimators[i],\n self,\n X,\n y,\n sample_weight,\n seeds[starts[i]:starts[i + 1]],\n total_n_estimators,\n verbose=self.verbose,\n sample_weights = self.samples_weights)\n for i in range(n_jobs))\n\n # Reduce\n self.estimators_ += list(itertools.chain.from_iterable(\n t[0] for t in all_results))\n self.estimators_features_ += list(itertools.chain.from_iterable(\n t[1] for t in all_results))\n\n if self.oob_score:\n self._set_oob_score(X, y)\n\n return self\n\n @abstractmethod\n def _set_oob_score(self, X, y):\n \"\"\"Calculate out of bag predictions and score.\"\"\"\n\n def _validate_y(self, y):\n # Default implementation\n return column_or_1d(y, warn=True)\n\n def _get_estimators_indices(self):\n # Get drawn indices along both sample and feature axes\n for seed in self._seeds:\n # Operations accessing random_state must be performed identically\n # to those in `_parallel_build_estimators()`\n random_state = np.random.RandomState(seed)\n feature_indices, sample_indices = _generate_bagging_indices(\n random_state, self.bootstrap_features, self.bootstrap,\n self.n_features_, self._n_samples, self._max_features,\n self._max_samples)\n\n yield feature_indices, sample_indices\n\n @property\n def estimators_samples_(self):\n \"\"\"The subset of drawn samples for each base estimator.\n Returns a dynamically generated list of boolean masks identifying\n the samples used for fitting each member of the ensemble, i.e.,\n the in-bag samples.\n Note: the list is re-created at each call to the property in order\n to reduce the object memory footprint by not storing the sampling\n data. Thus fetching the property may be slower than expected.\n \"\"\"\n sample_masks = []\n for _, sample_indices in self._get_estimators_indices():\n mask = indices_to_mask(sample_indices, self._n_samples)\n sample_masks.append(mask)\n\n return sample_masks\n\n\nclass BaggingClassifier2(BaseBagging, ClassifierMixin):\n \"\"\"A Bagging classifier.\n A Bagging classifier is an ensemble meta-estimator that fits base\n classifiers each on random subsets of the original dataset and then\n aggregate their individual predictions (either by voting or by averaging)\n to form a final prediction. Such a meta-estimator can typically be used as\n a way to reduce the variance of a black-box estimator (e.g., a decision\n tree), by introducing randomization into its construction procedure and\n then making an ensemble out of it.\n This algorithm encompasses several works from the literature. When random\n subsets of the dataset are drawn as random subsets of the samples, then\n this algorithm is known as Pasting [1]_. If samples are drawn with\n replacement, then the method is known as Bagging [2]_. When random subsets\n of the dataset are drawn as random subsets of the features, then the method\n is known as Random Subspaces [3]_. Finally, when base estimators are built\n on subsets of both samples and features, then the method is known as\n Random Patches [4]_.\n Read more in the :ref:`User Guide <bagging>`.\n Parameters\n ----------\n base_estimator : object or None, optional (default=None)\n The base estimator to fit on random subsets of the dataset.\n If None, then the base estimator is a decision tree.\n n_estimators : int, optional (default=10)\n The number of base estimators in the ensemble.\n max_samples : int or float, optional (default=1.0)\n The number of samples to draw from X to train each base estimator.\n - If int, then draw `max_samples` samples.\n - If float, then draw `max_samples * X.shape[0]` samples.\n max_features : int or float, optional (default=1.0)\n The number of features to draw from X to train each base estimator.\n - If int, then draw `max_features` features.\n - If float, then draw `max_features * X.shape[1]` features.\n bootstrap : boolean, optional (default=True)\n Whether samples are drawn with replacement.\n bootstrap_features : boolean, optional (default=False)\n Whether features are drawn with replacement.\n oob_score : bool\n Whether to use out-of-bag samples to estimate\n the generalization error.\n warm_start : bool, optional (default=False)\n When set to True, reuse the solution of the previous call to fit\n and add more estimators to the ensemble, otherwise, just fit\n a whole new ensemble.\n .. versionadded:: 0.17\n *warm_start* constructor parameter.\n n_jobs : int, optional (default=1)\n The number of jobs to run in parallel for both `fit` and `predict`.\n If -1, then the number of jobs is set to the number of cores.\n random_state : int, RandomState instance or None, optional (default=None)\n If int, random_state is the seed used by the random number generator;\n If RandomState instance, random_state is the random number generator;\n If None, the random number generator is the RandomState instance used\n by `np.random`.\n verbose : int, optional (default=0)\n Controls the verbosity of the building process.\n Attributes\n ----------\n base_estimator_ : estimator\n The base estimator from which the ensemble is grown.\n estimators_ : list of estimators\n The collection of fitted base estimators.\n estimators_samples_ : list of arrays\n The subset of drawn samples (i.e., the in-bag samples) for each base\n estimator. Each subset is defined by a boolean mask.\n estimators_features_ : list of arrays\n The subset of drawn features for each base estimator.\n classes_ : array of shape = [n_classes]\n The classes labels.\n n_classes_ : int or list\n The number of classes.\n oob_score_ : float\n Score of the training dataset obtained using an out-of-bag estimate.\n oob_decision_function_ : array of shape = [n_samples, n_classes]\n Decision function computed with out-of-bag estimate on the training\n set. If n_estimators is small it might be possible that a data point\n was never left out during the bootstrap. In this case,\n `oob_decision_function_` might contain NaN.\n References\n ----------\n .. [1] L. Breiman, \"Pasting small votes for classification in large\n databases and on-line\", Machine Learning, 36(1), 85-103, 1999.\n .. [2] L. Breiman, \"Bagging predictors\", Machine Learning, 24(2), 123-140,\n 1996.\n .. [3] T. Ho, \"The random subspace method for constructing decision\n forests\", Pattern Analysis and Machine Intelligence, 20(8), 832-844,\n 1998.\n .. [4] G. Louppe and P. Geurts, \"Ensembles on Random Patches\", Machine\n Learning and Knowledge Discovery in Databases, 346-361, 2012.\n \"\"\"\n def __init__(self,\n base_estimator=None,\n n_estimators=10,\n max_samples=1.0,\n max_features=1.0,\n bootstrap=True,\n bootstrap_features=False,\n oob_score=False,\n warm_start=False,\n n_jobs=1,\n random_state=None,\n verbose=0):\n\n super(BaggingClassifier, self).__init__(\n base_estimator,\n n_estimators=n_estimators,\n max_samples=max_samples,\n max_features=max_features,\n bootstrap=bootstrap,\n bootstrap_features=bootstrap_features,\n oob_score=oob_score,\n warm_start=warm_start,\n n_jobs=n_jobs,\n random_state=random_state,\n verbose=verbose)\n\n def _validate_estimator(self):\n \"\"\"Check the estimator and set the base_estimator_ attribute.\"\"\"\n super(BaggingClassifier, self)._validate_estimator(\n default=DecisionTreeClassifier())\n\n def _set_oob_score(self, X, y):\n n_samples = y.shape[0]\n n_classes_ = self.n_classes_\n classes_ = self.classes_\n\n predictions = np.zeros((n_samples, n_classes_))\n\n for estimator, samples, features in zip(self.estimators_,\n self.estimators_samples_,\n self.estimators_features_):\n # Create mask for OOB samples\n mask = ~samples\n\n if hasattr(estimator, \"predict_proba\"):\n predictions[mask, :] += estimator.predict_proba(\n (X[mask, :])[:, features])\n\n else:\n p = estimator.predict((X[mask, :])[:, features])\n j = 0\n\n for i in range(n_samples):\n if mask[i]:\n predictions[i, p[j]] += 1\n j += 1\n\n if (predictions.sum(axis=1) == 0).any():\n warn(\"Some inputs do not have OOB scores. \"\n \"This probably means too few estimators were used \"\n \"to compute any reliable oob estimates.\")\n\n oob_decision_function = (predictions /\n predictions.sum(axis=1)[:, np.newaxis])\n oob_score = accuracy_score(y, np.argmax(predictions, axis=1))\n\n self.oob_decision_function_ = oob_decision_function\n self.oob_score_ = oob_score\n\n def _validate_y(self, y):\n y = column_or_1d(y, warn=True)\n check_classification_targets(y)\n self.classes_, y = np.unique(y, return_inverse=True)\n self.n_classes_ = len(self.classes_)\n\n return y\n\n def predict(self, X):\n \"\"\"Predict class for X.\n The predicted class of an input sample is computed as the class with\n the highest mean predicted probability. If base estimators do not\n implement a ``predict_proba`` method, then it resorts to voting.\n Parameters\n ----------\n X : {array-like, sparse matrix} of shape = [n_samples, n_features]\n The training input samples. Sparse matrices are accepted only if\n they are supported by the base estimator.\n Returns\n -------\n y : array of shape = [n_samples]\n The predicted classes.\n \"\"\"\n predicted_probabilitiy = self.predict_proba(X)\n return self.classes_.take((np.argmax(predicted_probabilitiy, axis=1)),\n axis=0)\n\n def predict_proba(self, X):\n \"\"\"Predict class probabilities for X.\n The predicted class probabilities of an input sample is computed as\n the mean predicted class probabilities of the base estimators in the\n ensemble. If base estimators do not implement a ``predict_proba``\n method, then it resorts to voting and the predicted class probabilities\n of an input sample represents the proportion of estimators predicting\n each class.\n Parameters\n ----------\n X : {array-like, sparse matrix} of shape = [n_samples, n_features]\n The training input samples. Sparse matrices are accepted only if\n they are supported by the base estimator.\n Returns\n -------\n p : array of shape = [n_samples, n_classes]\n The class probabilities of the input samples. The order of the\n classes corresponds to that in the attribute `classes_`.\n \"\"\"\n check_is_fitted(self, \"classes_\")\n # Check data\n X = check_array(X, accept_sparse=['csr', 'csc'])\n\n if self.n_features_ != X.shape[1]:\n raise ValueError(\"Number of features of the model must \"\n \"match the input. Model n_features is {0} and \"\n \"input n_features is {1}.\"\n \"\".format(self.n_features_, X.shape[1]))\n\n # Parallel loop\n n_jobs, n_estimators, starts = _partition_estimators(self.n_estimators,\n self.n_jobs)\n\n all_proba = Parallel(n_jobs=n_jobs, verbose=self.verbose)(\n delayed(_parallel_predict_proba)(\n self.estimators_[starts[i]:starts[i + 1]],\n self.estimators_features_[starts[i]:starts[i + 1]],\n X,\n self.n_classes_)\n for i in range(n_jobs))\n\n # Reduce\n proba = sum(all_proba) / self.n_estimators\n\n return proba\n\n def predict_log_proba(self, X):\n \"\"\"Predict class log-probabilities for X.\n The predicted class log-probabilities of an input sample is computed as\n the log of the mean predicted class probabilities of the base\n estimators in the ensemble.\n Parameters\n ----------\n X : {array-like, sparse matrix} of shape = [n_samples, n_features]\n The training input samples. Sparse matrices are accepted only if\n they are supported by the base estimator.\n Returns\n -------\n p : array of shape = [n_samples, n_classes]\n The class log-probabilities of the input samples. The order of the\n classes corresponds to that in the attribute `classes_`.\n \"\"\"\n check_is_fitted(self, \"classes_\")\n if hasattr(self.base_estimator_, \"predict_log_proba\"):\n # Check data\n X = check_array(X, accept_sparse=['csr', 'csc'])\n\n if self.n_features_ != X.shape[1]:\n raise ValueError(\"Number of features of the model must \"\n \"match the input. Model n_features is {0} \"\n \"and input n_features is {1} \"\n \"\".format(self.n_features_, X.shape[1]))\n\n # Parallel loop\n n_jobs, n_estimators, starts = _partition_estimators(\n self.n_estimators, self.n_jobs)\n\n all_log_proba = Parallel(n_jobs=n_jobs, verbose=self.verbose)(\n delayed(_parallel_predict_log_proba)(\n self.estimators_[starts[i]:starts[i + 1]],\n self.estimators_features_[starts[i]:starts[i + 1]],\n X,\n self.n_classes_)\n for i in range(n_jobs))\n\n # Reduce\n log_proba = all_log_proba[0]\n\n for j in range(1, len(all_log_proba)):\n log_proba = np.logaddexp(log_proba, all_log_proba[j])\n\n log_proba -= np.log(self.n_estimators)\n\n return log_proba\n\n else:\n return np.log(self.predict_proba(X))\n\n @if_delegate_has_method(delegate='base_estimator')\n def decision_function(self, X):\n \"\"\"Average of the decision functions of the base classifiers.\n Parameters\n ----------\n X : {array-like, sparse matrix} of shape = [n_samples, n_features]\n The training input samples. Sparse matrices are accepted only if\n they are supported by the base estimator.\n Returns\n -------\n score : array, shape = [n_samples, k]\n The decision function of the input samples. The columns correspond\n to the classes in sorted order, as they appear in the attribute\n ``classes_``. Regression and binary classification are special\n cases with ``k == 1``, otherwise ``k==n_classes``.\n \"\"\"\n check_is_fitted(self, \"classes_\")\n\n # Check data\n X = check_array(X, accept_sparse=['csr', 'csc'])\n\n if self.n_features_ != X.shape[1]:\n raise ValueError(\"Number of features of the model must \"\n \"match the input. Model n_features is {0} and \"\n \"input n_features is {1} \"\n \"\".format(self.n_features_, X.shape[1]))\n\n # Parallel loop\n n_jobs, n_estimators, starts = _partition_estimators(self.n_estimators,\n self.n_jobs)\n\n all_decisions = Parallel(n_jobs=n_jobs, verbose=self.verbose)(\n delayed(_parallel_decision_function)(\n self.estimators_[starts[i]:starts[i + 1]],\n self.estimators_features_[starts[i]:starts[i + 1]],\n X)\n for i in range(n_jobs))\n\n # Reduce\n decisions = sum(all_decisions) / self.n_estimators\n\n return decisions\n\n",
"_____no_output_____"
],
[
"\n #print(y)\n #print(type(y), y.shape)\n #print(X.shape)\n #X, self.X_dev, y, self.y_dev = train_test_split(X, y, test_size=self.dev_set, stratify=y)\n n_samples, self.n_features_ = X.shape\n self._n_samples = n_samples\n self.samples_times_selected = np.zeros_like(y)\n self.default_sample_weight = 1/float(X.shape[0])\n self.max_samples = int(self.max_samples*self._n_samples)\n self.max_features = int(self.max_features*self.n_features_)\n \n y = self._validate_y(y)\n for i_est in xrange(self.n_estimators):\n estimator = clone(self.base_estimator_)\n if self.patcher == 'random':\n features_indices, samples_indices = generate_bagging_indices(\n self.random_state, self.bootstrap_features,\n self.bootstrap_samples, X.shape[1], X.shape[0],\n self.max_features, self.max_samples, [None])\n elif self.patcher == 'weighted':\n# X_train, X_dev, y_train, y_dev = train_test_split(X, y, stratify = True,\n# test_size=self.dev_set, \n# random_state=self.random_state)\n if i_est==0:\n self.samples_weights.append(np.array([self.default_sample_weight for i in xrange(X.shape[0])]))\n self.scores.append(1)\n signs = np.ones_like(y)\n else:\n signs = self.update_weights(X, y)\n #print(\"ROUND %d\"%i_est)\n #print(self.samples_weights[i_est])\n #print(\"INDEX %d\" % i)\n #print(self.samples_weights[i_est])\n features_indices, samples_indices = generate_bagging_indices(\n self.random_state, self.bootstrap_features,\n self.bootstrap_samples, X.shape[1], X.shape[0],\n self.max_features, self.max_samples, self.samples_weights[i_est])\n print(\"ROUND %d\" % i_est)\n print(\"SCORE %0.3f\"%(self.scores[-1]))\n if i_est != 0:\n accs = []\n accs_last = []\n overlaps = []\n accs_total = []\n for jj, ens in enumerate(self.ensemble):\n print(len(self.prev_samples_indices), len(self.estimators_features))\n p_pred = ens.predict(X[self.prev_samples_indices[jj]][:, self.estimators_features[jj]])\n last_pred = ens.predict(X[self.prev_samples_indices[-1]][:, self.estimators_features[jj]])\n total_pred = self.predict(X[self.prev_samples_indices[jj]])\n accs.append(1-accuracy_score(y[self.prev_samples_indices[jj]], p_pred, normalize=True))\n accs_last.append(1-accuracy_score(y[self.prev_samples_indices[-1]], last_pred, normalize=True))\n accs_total.append(1-accuracy_score(y[self.prev_samples_indices[jj]], total_pred, normalize=True))\n #try:\n # self.ensemble[jj+1]\n #except IndexError:\n # print(\"CURRENT\")\n # print(self.prev_samples_indices[jj])\n # print(\"LAST\")\n # print(self.prev_samples_indices[-1])\n # print(np.array_equal(self.prev_samples_indices[jj], self.prev_samples_indices[-1]))\n # print(np.intersect1d(self.prev_samples_indices[jj], self.prev_samples_indices[-1]).shape[0], self.prev_samples_indices[-1].shape[0], self.prev_samples_indices[jj].shape[0])\n overlaps.append(np.intersect1d(self.prev_samples_indices[jj], self.prev_samples_indices[-1]).shape[0]/float(np.unique(self.prev_samples_indices[jj]).shape[0]))\n accs = np.round(100*np.array(accs),3)\n accs_last = np.round(100*np.array(accs_last),3)\n overlaps = np.round(100*np.array(overlaps),3)\n accs_total = np.round(100*np.array(accs_total),3)\n print(\"PREVIOUS ERRORS ON CORRESPONDING DATA\")\n print(accs)\n print(\"ERRORS OF THE ENSEMBLE ON CORRESPONDING DATA\")\n print(accs_total)\n print(\"PREVIOUS ERRORS ON LAST SELECTED DATA\")\n print(accs_last)\n print(\"OVERLAPS\")\n print(overlaps)\n print(\"a: %0.3f\"%self.a)\n print(\"WEIGHTS\")\n print(np.min(self.samples_weights[-1]), \n np.mean(self.samples_weights[-1]), \n np.max(self.samples_weights[-1]))\n #print(self.samples_weights[-1])\n print(\"SAMPLED INSTANCES\")\n print(self.samples_weights[-1][samples_indices])\n print(signs[samples_indices])\n print(y[samples_indices])\n print(\"~\"*50)\n else:\n print(\"UNSUPPORTED WAY OF PATCHING: %s !\" % self.patcher)\n # minor fix for when one class is not represented during sampling\n #print(samples_indices.shape)\n samples_indices = self.fix_class_indices(y, samples_indices)\n #print(\"AFTER\")\n #print(samples_indices.shape)\n estimator.fit(X[samples_indices][:, features_indices], y[samples_indices])\n self.prev_samples_indices.append(samples_indices)\n self.estimators_features.append(features_indices)\n self.ensemble.append(estimator)\n self.samples_times_selected[samples_indices] += 1\n return\n \n def fix_class_indices(self, y, samples_indices):\n in_set = set(y[samples_indices])\n a = set(y).difference(in_set)\n for item in a:\n samples_indices= np.append(samples_indices, [np.where(y==item)[0][0]])\n return samples_indices\n \n def update_weights(self, X, y): \n #print(\"REMOVED %d\" % np.where(self.samples_times_selected > 3)[0].shape[0])\n #self.samples_weights[-1][np.where(self.samples_times_selected > 3)] = self.default_sample_weight\n #self.samples_times_selected[np.where(self.samples_times_selected > 3)] = 0\n preds = self.predict(X)\n self.scores.append(1-accuracy_score(y, preds, normalize=True)+0.0001)\n self.a = 0.5*np.log((1-self.scores[-1])/float(self.scores[-1]))\n #self.a = 9\n #self.a = 1\n signs = sign(y, preds)\n temp = self.samples_weights[-1]*np.exp(-self.a*signs)\n \n #print(\"MEAN\")\n #print(np.mean(temp))\n #print(temp[np.where(signs<0)])\n self.samples_weights.append(temp/np.sum(temp))\n preds = np.ones((X.shape[0], len(self.ensemble)))\n for ii, est in enumerate(self.ensemble):\n sc = accuracy_score(y, est.predict(X[:, self.estimators_features[ii]]), normalize=True)\n preds[:, ii] = sc*sign(y, est.predict(X[:, self.estimators_features[ii]]))\n #print(\"INITIAL\")\n #print(preds)\n #print(np.min(preds))\n #print(\"AFTER COLLAPSE\")\n preds = np.sum(preds, axis=1)\n #print(preds)\n #print(\"AFTER RESHAPE\")\n preds = preds.reshape(-1,)\n #print(preds)\n if np.any(preds<0):\n min_ = np.min(preds)\n if min_ < 0:\n min_ = -1*min_\n #print(\"MIN\")\n #print(min_)\n preds = preds + min_ + 0.001\n #print(\"AFTER_MIN\")\n #print(preds)\n #print(\"AFTER NORMALIZATION\")\n preds /= np.sum(preds)\n #print(preds)\n #print(preds)\n #print(np.sum(preds))\n self.samples_weights.append(preds)\n return signs\n \n",
"_____no_output_____"
],
[
"from sklearn.utils import check_random_state, check_X_y, column_or_1d\nfrom sklearn.utils.multiclass import check_classification_targets\nfrom sklearn.base import BaseEstimator, ClassifierMixin, clone\nfrom sklearn.neighbors import KNeighborsClassifier\nfrom sklearn.metrics import pairwise_distances\nfrom sklearn.metrics import accuracy_score, get_scorer\nimport copy\n\nclass Adversarial_Cascade(BaseEstimator, ClassifierMixin):\n \n def __init__(self, base_estimator=KNeighborsClassifier(), n_estimators=10, acc_target=0.99,\n num_adversaries_per_instance=4, way = 'prob', \n random_state=42, optim=False, parameters=None, metric='accuracy', oob=False, oob_size=0.1):\n self.base_estimator = base_estimator\n self.n_estimators = n_estimators\n self.acc_target = acc_target\n self.num_adversaries_per_instance = num_adversaries_per_instance\n self.way = way\n self.random_state = check_random_state(random_state)\n self.optim = optim\n self.oob = oob\n self.oob_size = 0.1\n self.X_oob = None\n self.y_oob = None\n if self.optim:\n self.parameters = parameters\n else:\n self.parameters = None\n self.scoring = get_scorer(metric)\n self.acc = 0\n self.ensemble = []\n self.selected_indices = []\n \n def fit(self, X, y):\n return self._fit(X, y)\n \n def _fit(self,X,y):\n X, y = check_X_y(\n X, y, ['csr', 'csc'], dtype=None, force_all_finite=False,\n multi_output=True)\n y = self._validate_y(y)\n if self.oob:\n X, self.X_oob, y, self.y_oob = train_test_split(X,y,test_size=0.1,stratify=y)\n n_samples, self.n_features_ = X.shape\n cur_X, cur_y = X, y\n self.selected_indices.append([i for i in xrange(X.shape[0])])\n flag_target = False\n for i_est in xrange(self.n_estimators):\n cur_mod = clone(self.base_estimator)\n if self.optim:\n grid_search = GridSearchCV(cur_mod, self.parameters, n_jobs=-1, verbose=1, refit=True)\n grid_search.fit(cur_X, cur_y)\n cur_mod = grid_search.best_estimator_\n else:\n cur_mod.fit(cur_X, cur_y)\n self.ensemble.append(cur_mod)\n cur_X, cur_y, flag_target = self._create_next_batch(X, y)\n if flag_target:\n break\n #print(cur_X.shape, cur_y.shape)\n print(\"%d ESTIMATORS -- %0.3f\" % (len(self.ensemble), 100*accuracy_score(y, self.predict(X), normalize=True)))\n return self\n \n def _create_next_batch(self, X, y):\n if self.oob:\n preds = self.predict(self.X_oob)\n centroids = self.X_oob[preds != self.y_oob]\n centroids_ind = np.argwhere(preds != self.y_oob).reshape(-1,)\n cur_X = copy.deepcopy(self.X_oob[centroids_ind,:])\n cur_y = copy.deepcopy(self.y_oob[centroids_ind])\n str_target = \"OOB SAMPLE\"\n self.acc = accuracy_score(self.y_oob, preds, normalize=True)\n #acc = (1-(centroids.shape[0])/float(self.X_oob.shape[0]))\n else:\n preds = self.predict(X)\n centroids = X[preds != y]\n centroids_ind = np.argwhere(preds!=y).reshape(-1,)\n cur_X = copy.deepcopy(X[centroids_ind,:])\n cur_y = copy.deepcopy(y[centroids_ind])\n str_target = \"TRAIN SAMPLE\"\n self.acc = accuracy_score(y, preds, normalize=True)\n #acc = (1-(centroids.shape[0])/float(X.shape[0]))\n if self.acc > self.acc_target:\n #return X, y, False\n #print(\"ACCURACY ON THE %s IS %0.3f\" % (str_target, 100*(1-(centroids.shape[0])/float(X.shape[0]))))\n #print(\"STOPPING WITH %d BASE MODELS\" % len(self.ensemble))\n return _, _,True\n probas = pairwise_distances(centroids, X)\n probas /= np.sum(probas, axis=1).reshape(-1,1)\n\n \n for i_centr in xrange(probas.shape[0]):\n if self.way == 'prob':\n indices = self.random_state.choice([i for i in xrange(0, probas.shape[1])],\n self.num_adversaries_per_instance, p=probas[i_centr,:])\n if self.way == 'furthest':\n indices = np.argsort(probas[i_centr,:])[::-1][:self.num_adversaries_per_instance]\n indices = self._fix_class_indices(y, indices)\n #print(cur_X.shape, X[indices,:].shape)\n cur_X = np.vstack((cur_X, X[indices,:]))\n cur_y = np.append(cur_y, y[indices])\n #cur_y.extend(indices)\n\n #cur_X = np.delete(cur_X, 0, axis=0)\n #cur_y = y[cur_y]\n return cur_X, cur_y, False\n \n def _fix_class_indices(self, y, samples_indices):\n in_set = set(y[samples_indices])\n a = set(y).difference(in_set)\n for item in a:\n samples_indices= np.append(samples_indices, [np.where(y==item)[0][0]])\n return samples_indices \n \n def _validate_y(self, y):\n y = column_or_1d(y, warn=True)\n check_classification_targets(y)\n self.classes_, y = np.unique(y, return_inverse=True)\n self.n_classes_ = len(self.classes_)\n return y\n \n def predict(self, X):\n \"\"\"Predict class for X.\n The predicted class of an input sample is computed as the class with\n the highest mean predicted probability. If base estimators do not\n implement a ``predict_proba`` method, then it resorts to voting.\n Parameters\n ----------\n X : {array-like, sparse matrix} of shape = [n_samples, n_features]\n The training input samples. Sparse matrices are accepted only if\n they are supported by the base estimator.\n Returns\n -------\n y : array of shape = [n_samples]\n The predicted classes.\n \"\"\"\n \n if hasattr(self.base_estimator, \"predict_proba\"):\n predicted_probability = self.predict_proba(X)\n return self.classes_.take((np.argmax(predicted_probability, axis=1)),\n axis=0)\n else:\n predicted_probability = np.zeros((X.shape[0],1), dtype=int)\n for i, ens in enumerate(self.ensemble):\n predicted_probability = np.hstack((predicted_probability, ens.predict(X).reshape(-1,1)))\n predicted_probability = np.delete(predicted_probability,0,axis=1)\n final_pred = []\n for sample in xrange(X.shape[0]):\n final_pred.append(most_common(predicted_probability[sample,:]))\n #votes = []\n #for i, mod_vote in predictions[sample,:]:\n # votes.extend([predictions[sample, i] for j in xrange(int(self.acc[i]))])\n #final_pred = most_common(votes)\n return np.array(final_pred) \n\n\n def predict_proba(self, X):\n \"\"\"Predict class probabilities for X.\n The predicted class probabilities of an input sample is computed as\n the mean predicted class probabilities of the base estimators in the\n ensemble. If base estimators do not implement a ``predict_proba``\n method, then it resorts to voting and the predicted class probabilities\n of an input sample represents the proportion of estimators predicting\n each class.\n Parameters\n ----------\n X : {array-like, sparse matrix} of shape = [n_samples, n_features]\n The training input samples. Sparse matrices are accepted only if\n they are supported by the base estimator.\n Returns\n -------\n p : array of shape = [n_samples, n_classes]\n The class probabilities of the input samples. The order of the\n classes corresponds to that in the attribute `classes_`.\n \"\"\"\n check_is_fitted(self, \"classes_\")\n # Check data\n X = check_array(\n X, accept_sparse=['csr', 'csc'], dtype=None,\n force_all_finite=False\n )\n\n if self.n_features_ != X.shape[1]:\n raise ValueError(\"Number of features of the model must \"\n \"match the input. Model n_features is {0} and \"\n \"input n_features is {1}.\"\n \"\".format(self.n_features_, X.shape[1]))\n\n all_proba = np.zeros((X.shape[0], self.n_classes_))\n for i, ens in enumerate(self.ensemble):\n all_proba += ens.predict_proba(X)\n all_proba /= self.n_estimators\n #print(all_proba.shape)\n #print(all_proba)\n #proba = np.sum(all_proba, axis=0) / self.n_estimators\n #print(proba.shape)\n #print(proba)\n return all_proba\n\n\n @if_delegate_has_method(delegate='base_estimator')\n def decision_function(self, X):\n \"\"\"Average of the decision functions of the base classifiers.\n Parameters\n ----------\n X : {array-like, sparse matrix} of shape = [n_samples, n_features]\n The training input samples. Sparse matrices are accepted only if\n they are supported by the base estimator.\n Returns\n -------\n score : array, shape = [n_samples, k]\n The decision function of the input samples. The columns correspond\n to the classes in sorted order, as they appear in the attribute\n ``classes_``. Regression and binary classification are special\n cases with ``k == 1``, otherwise ``k==n_classes``.\n \"\"\"\n check_is_fitted(self, \"classes_\")\n\n # Check data\n X = check_array(\n X, accept_sparse=['csr', 'csc'], dtype=None,\n force_all_finite=False\n )\n\n if self.n_features_ != X.shape[1]:\n raise ValueError(\"Number of features of the model must \"\n \"match the input. Model n_features is {0} and \"\n \"input n_features is {1} \"\n \"\".format(self.n_features_, X.shape[1]))\n all_decisions = np.zeros((X.shape[0], self.n_classes_))\n for i, ens in enumerate(self.ensemble):\n all_decisions += ens.predict_proba(X) \n decisions = sum(all_decisions) / self.n_estimators\n\n return decisions\n\ndef most_common(lst):\n if isinstance(lst, np.ndarray):\n lst = lst.tolist()\n #print(lst, max(set(lst), key=lst.count) )\n return max(set(lst), key=lst.count) ",
"_____no_output_____"
],
[
"ac = Adversarial_Cascade(base_estimator=knn, oob=False, num_adversaries_per_instance=10)\nac.fit(X,y)\npreds = ac.predict(X)\nprint(accuracy_score(y,preds,normalize=True))\n#ac.selected_indices",
"10 ESTIMATORS -- 86.517\n0.8651685393258427\n"
],
[
"X_train, X_dev, y_train, y_dev = train_test_split(X,y,stratify=y,test_size=0.1, random_state=42)\nac = Adversarial_Cascade(base_estimator=KNeighborsClassifier(), \n oob=False, \n num_adversaries_per_instance=5, way='furthest')\nac.fit(X_train,y_train)\npreds = ac.predict(X_dev)\nprint(accuracy_score(y_dev,preds,normalize=True))",
"10 ESTIMATORS -- 76.250\n0.7222222222222222\n"
],
[
"ac = Adversarial_Cascade(base_estimator=base, optim=True, parameters=parameters, oob=True, random_state=i)\nprint(cross_val_score(ac, X, y, cv=5, n_jobs=-1).mean())",
"_____no_output_____"
],
[
"knn.fit(X,y)\npreds = knn.predict(X)\ncentroids = X[preds!=y]",
"_____no_output_____"
],
[
"knn.fit(X,y)\nlist(knn.predict(X))",
"_____no_output_____"
],
[
"probas = pairwise_distances(centroids, X)\nprobas /= np.sum(probas, axis=1).reshape(-1,1)\ncheck_random_state(42).rand",
"_____no_output_____"
],
[
"from sklearn.preprocessing import StandardScaler\nfrom sklearn.linear_model import SGDClassifier\nfrom sklearn.model_selection import GridSearchCV\nfrom sklearn.pipeline import Pipeline\n\n\nparameters = {\n 'clf__alpha': (0.00001, 0.000001),\n 'clf__penalty': ('l2', 'elasticnet'),\n 'clf__max_iter': (10, 50, 80, 150),\n}\n\npipeline = Pipeline([ ('std', StandardScaler()), ('clf', SGDClassifier())])\ngrid_search = GridSearchCV(pipeline, parameters, n_jobs=-1, verbose=1)\n",
"_____no_output_____"
],
[
"model_names = ['BASE', \"BAGGING\", \"RANDOM_PATCHES\", \"WEIGHTED_RP\", \"AC\"]\nbase = pipeline\nmodels = [\n base,\n BaggingClassifier(base, max_samples=0.5, max_features=0.5),\n Vanilla_RP(base,max_samples=0.5, max_features=0.5),\n Vanilla_RP(base, max_samples=0.5, max_features=0.5, patcher='weighted'),\n Adversarial_Cascade(base_estimator=base, optim=False, parameters=parameters, oob=True)\n]\nscores = {}\nfor m in model_names:\n scores[m] = []\nfor i in xrange(10):\n print(\"ROUND %d\" % i)\n for m_i in xrange(len(model_names)):\n try:\n models[m_i].set_params(**{'clf__random_state':i})\n except ValueError:\n models[m_i].set_params(**{'random_state':i})\n print(models[m_i])\n sc = cross_val_score(models[m_i], X, y, cv=5, n_jobs=-1).mean()\n print(\"MODEL %s -- %0.3f\" %(model_names[m_i], 100*sc))\n scores[model_names[m_i]].append(sc)\n",
"ROUND 0\nPipeline(memory=None,\n steps=[('std', StandardScaler(copy=True, with_mean=True, with_std=True)), ('clf', SGDClassifier(alpha=0.0001, average=False, class_weight=None, epsilon=0.1,\n eta0=0.0, fit_intercept=True, l1_ratio=0.15,\n learning_rate='optimal', loss='hinge', max_iter=None, n_iter=None,\n n_jobs=1, penalty='l2', power_t=0.5, random_state=0, shuffle=True,\n tol=None, verbose=0, warm_start=False))])\n"
],
[
"base = KNeighborsClassifier()\nbagging = BaggingClassifier(base, max_samples=0.5, max_features=0.5, random_state=42)\nrp = Vanilla_RP(base,max_samples=0.5, max_features=0.5, random_state=42)\nrp_w = Vanilla_RP(base, max_samples=0.5, max_features=0.5, patcher='weighted', random_state=42)\nac = Adversarial_Cascade(base_estimator=base, num_adversaries_per_instance=10,\n optim=False, \n parameters=parameters, \n oob=False, random_state=42)\nprint(\"KNN Score:\")\nprint(cross_val_score(knn, X, y, cv=5, n_jobs=-1).mean())\nprint(\"Bagging Score:\")\nprint(cross_val_score(bagging, X, y, cv=5, n_jobs=-1).mean())\nprint(\"RP Score:\")\nprint(cross_val_score(rp, X, y, cv=5, n_jobs=-1).mean())\nprint(\"RP-WEIGHTED Score:\")\nprint(cross_val_score(rp_w, X, y, cv=5, n_jobs=-1).mean())\nprint(\"AC\")\nprint(cross_val_score(ac, X, y, cv=5, n_jobs=-1).mean())\nprint(\"~\"*50)",
"KNN Score:\n0.6860213995508112\nBagging Score:\n0.8260564766447119\nRP Score:\n0.9226555126555127\nRP-WEIGHTED Score:\n0.9334039922275217\nAC\n10 ESTIMATORS -- 86.713\n10 ESTIMATORS -- 84.397\n10 ESTIMATORS -- 84.507\n10 ESTIMATORS -- 85.915\n10 ESTIMATORS -- 82.639\n0.7325760213995508\n~~~~~~~~~~~~~~~~~~~~~~~~~~~~~~~~~~~~~~~~~~~~~~~~~~\n"
],
[
"for i in xrange(10):\n print(\"ROUND %d\" % i)\n base = pipeline\n bagging = BaggingClassifier(base, max_samples=0.5, max_features=0.5, random_state=i)\n rp = Vanilla_RP(base,max_samples=0.5, max_features=0.5, random_state=i)\n rp_w = Vanilla_RP(base, max_samples=0.5, max_features=0.5, patcher='weighted', random_state=i)\n ac = Adversarial_Cascade(base_estimator=base, optim=True, parameters=parameters, oob=True, random_state=i)\n print(\"KNN Score:\")\n print(cross_val_score(knn, X, y, cv=5, n_jobs=-1).mean())\n print(\"Bagging Score:\")\n print(cross_val_score(bagging, X, y, cv=5, n_jobs=-1).mean())\n print(\"RP Score:\")\n print(cross_val_score(rp, X, y, cv=5, n_jobs=-1).mean())\n print(\"RP-WEIGHTED Score:\")\n print(cross_val_score(rp_w, X, y, cv=5, n_jobs=-1).mean())\n print(\"AC\")\n print(cross_val_score(ac, X, y, cv=5, n_jobs=-1).mean())\n print(\"~\"*50)",
"_____no_output_____"
],
[
"print(__doc__)\n\nimport numpy as np\nimport matplotlib.pyplot as plt\nfrom sklearn import svm, datasets\n\n\ndef make_meshgrid(x, y, h=.02):\n \"\"\"Create a mesh of points to plot in\n\n Parameters\n ----------\n x: data to base x-axis meshgrid on\n y: data to base y-axis meshgrid on\n h: stepsize for meshgrid, optional\n\n Returns\n -------\n xx, yy : ndarray\n \"\"\"\n x_min, x_max = x.min() - 1, x.max() + 1\n y_min, y_max = y.min() - 1, y.max() + 1\n xx, yy = np.meshgrid(np.arange(x_min, x_max, h),\n np.arange(y_min, y_max, h))\n return xx, yy\n\n\ndef plot_contours(ax, clf, xx, yy, **params):\n \"\"\"Plot the decision boundaries for a classifier.\n\n Parameters\n ----------\n ax: matplotlib axes object\n clf: a classifier\n xx: meshgrid ndarray\n yy: meshgrid ndarray\n params: dictionary of params to pass to contourf, optional\n \"\"\"\n #print(clf)\n Z = clf.predict(np.c_[xx.ravel(), yy.ravel()])\n Z = Z.reshape(xx.shape)\n out = ax.contourf(xx, yy, Z, **params)\n return out\n\n\n# import some data to play with\niris = datasets.load_iris()\n# Take the first two features. We could avoid this by using a two-dim dataset\nX = iris.data[:, :2]\ny = iris.target\n\nfrom sklearn.tree import DecisionTreeClassifier, ExtraTreeClassifier\nfrom sklearn.ensemble import RandomForestClassifier, AdaBoostClassifier, GradientBoostingClassifier\n\nC = 1.0 # SVM regularization parameter\nbase = AdaBoostClassifier()\n #KNeighborsClassifier() \n #ExtraTreeClassifier()\n #DecisionTreeClassifier()\n #SGDClassifier() \n #svm.SVC(kernel='linear', C=C, probability=True)\nbagging = BaggingClassifier(base, max_samples=0.5, max_features=0.5, random_state=42)\nrp = Vanilla_RP(base,max_samples=0.5, max_features=0.5, random_state=42)\nrp_w = Vanilla_RP(base, max_samples=0.5, max_features=0.5, patcher='weighted', random_state=42)\nac = Adversarial_Cascade(base_estimator=base, num_adversaries_per_instance=10,\n optim=False, \n parameters=None, oob=True, way='furthest')\n\n# we create an instance of SVM and fit out data. We do not scale our\n# data since we want to plot the support vectors\n\nmodels = (svm.SVC(kernel='linear', C=C),\n svm.SVC(kernel='rbf', gamma=0.7, C=C), \n KNeighborsClassifier(),\n pipeline,\n bagging,\n rp,\n rp_w,\n ac)\n\nmodels = (clf.fit(X, y) for clf in models)\n\n# title for the plots\ntitles = ('SVC with linear kernel',\n 'SVC with RBF kernel',\n 'KNN',\n 'SGD', \n \"Bagging\",\n \"RP\",\n \"RP-W\",\n 'AC')\n\n# Set-up 2x2 grid for plotting.\nfig, sub = plt.subplots(2, 4)\nplt.subplots_adjust(wspace=0.4, hspace=0.4)\n\nX0, X1 = X[:, 0], X[:, 1]\nxx, yy = make_meshgrid(X0, X1)\n\nfor clf, title, ax in zip(models, titles, sub.flatten()):\n \n print(\"%s : %0.3f\"% (title, 100*accuracy_score(y, clf.predict(X))))\n plot_contours(ax, clf, xx, yy,\n cmap=plt.cm.coolwarm, alpha=0.8)\n ax.scatter(X0, X1, c=y, cmap=plt.cm.coolwarm, s=20, edgecolors='k')\n ax.set_xlim(xx.min(), xx.max())\n ax.set_ylim(yy.min(), yy.max())\n ax.set_xlabel('Sepal length')\n ax.set_ylabel('Sepal width')\n ax.set_xticks(())\n ax.set_yticks(())\n ax.set_title(title)\nfig.set_figwidth(20)\nfig.set_figheight(10)\nplt.show()",
"Automatically created module for IPython interactive environment\n"
],
[
"class Adversarial_Cascade(BaseEstimator, ClassifierMixin):\n \n def __init__(self, base_estimator=KNeighborsClassifier(), n_estimators=10, acc_target=0.99,\n num_adversaries_per_instance=4, way = 'prob', \n random_state=42, optim=False, parameters=None, metric='accuracy', oob=False, oob_size=0.1):\n self.base_estimator = base_estimator\n self.n_estimators = n_estimators\n self.acc_target = acc_target\n self.num_adversaries_per_instance = num_adversaries_per_instance\n self.way = way\n self.random_state = check_random_state(random_state)\n self.optim = optim\n self.oob = oob\n self.oob_size = 0.1\n self.X_oob = None\n self.y_oob = None\n if self.optim:\n self.parameters = parameters\n else:\n self.parameters = None\n self.scoring = get_scorer(metric)\n self.acc = 0\n self.ensemble = []\n self.selected_indices = []\n \n def fit(self, X, y):\n return self._fit(X, y)\n \n def _fit(self,X,y):\n X, y = check_X_y(\n X, y, ['csr', 'csc'], dtype=None, force_all_finite=False,\n multi_output=True)\n y = self._validate_y(y)\n if self.oob:\n X, self.X_oob, y, self.y_oob = train_test_split(X,y,test_size=0.1,stratify=y)\n n_samples, self.n_features_ = X.shape\n cur_X, cur_y = X, y\n self.selected_indices.append([i for i in xrange(X.shape[0])])\n flag_target = False\n for i_est in xrange(self.n_estimators):\n cur_mod = clone(self.base_estimator)\n if self.optim:\n grid_search = GridSearchCV(cur_mod, self.parameters, n_jobs=-1, verbose=1, refit=True)\n grid_search.fit(cur_X, cur_y)\n cur_mod = grid_search.best_estimator_\n else:\n cur_mod.fit(cur_X, cur_y)\n self.ensemble.append(cur_mod)\n cur_X, cur_y, flag_target = self._create_next_batch(X, y)\n if flag_target:\n break\n #print(cur_X.shape, cur_y.shape)\n print(\"%d ESTIMATORS -- %0.3f\" % (len(self.ensemble), 100*accuracy_score(y, self.predict(X), normalize=True)))\n return self\n \n def _create_next_batch(self, X, y):\n if self.oob:\n preds = self.predict(self.X_oob)\n centroids = self.X_oob[preds != self.y_oob]\n centroids_ind = np.argwhere(preds != self.y_oob).reshape(-1,)\n cur_X = copy.deepcopy(self.X_oob[centroids_ind,:])\n cur_y = copy.deepcopy(self.y_oob[centroids_ind])\n str_target = \"OOB SAMPLE\"\n self.acc = accuracy_score(self.y_oob, preds, normalize=True)\n #acc = (1-(centroids.shape[0])/float(self.X_oob.shape[0]))\n else:\n preds = self.predict(X)\n centroids = X[preds != y]\n centroids_ind = np.argwhere(preds!=y).reshape(-1,)\n cur_X = copy.deepcopy(X[centroids_ind,:])\n cur_y = copy.deepcopy(y[centroids_ind])\n str_target = \"TRAIN SAMPLE\"\n self.acc = accuracy_score(y, preds, normalize=True)\n #acc = (1-(centroids.shape[0])/float(X.shape[0]))\n if self.acc > self.acc_target:\n #return X, y, False\n #print(\"ACCURACY ON THE %s IS %0.3f\" % (str_target, 100*(1-(centroids.shape[0])/float(X.shape[0]))))\n #print(\"STOPPING WITH %d BASE MODELS\" % len(self.ensemble))\n return _, _,True\n probas = pairwise_distances(centroids, X)\n probas /= np.sum(probas, axis=1).reshape(-1,1)\n for i_centr in xrange(probas.shape[0]):\n # Make zero the probability that a same-class sample is picked\n cur_prob = copy.deepcopy(probas[i_centr,:])\n cur_prob[y[centroids_ind[i_centr]]==y]=0\n print(cur_prob.shape, np.sum(cur_prob))\n cur_prob /= np.sum(cur_prob)\n if self.way == 'prob':\n indices = self.random_state.choice([i for i in xrange(0, probas.shape[1])],\n self.num_adversaries_per_instance, p=cur_prob)\n if self.way == 'furthest':\n indices = np.argsort(cur_prob)[::-1][:self.num_adversaries_per_instance]\n if self.way == 'closest':\n cur_prob[y[centroids_ind[i_centr]]==y]=1\n indices = np.argsort(cur_prob)[:self.num_adversaries_per_instance]\n indices = self._fix_class_indices(y, indices)\n #print(cur_X.shape, X[indices,:].shape)\n cur_X = np.vstack((cur_X, X[indices,:]))\n cur_y = np.append(cur_y, y[indices])\n #cur_y.extend(indices)\n\n #cur_X = np.delete(cur_X, 0, axis=0)\n #cur_y = y[cur_y]\n return cur_X, cur_y, False\n \n def _fix_class_indices(self, y, samples_indices):\n in_set = set(y[samples_indices])\n a = set(y).difference(in_set)\n for item in a:\n samples_indices= np.append(samples_indices, [np.where(y==item)[0][0]])\n return samples_indices \n \n def _validate_y(self, y):\n y = column_or_1d(y, warn=True)\n check_classification_targets(y)\n self.classes_, y = np.unique(y, return_inverse=True)\n self.n_classes_ = len(self.classes_)\n return y\n \n def predict(self, X):\n \"\"\"Predict class for X.\n The predicted class of an input sample is computed as the class with\n the highest mean predicted probability. If base estimators do not\n implement a ``predict_proba`` method, then it resorts to voting.\n Parameters\n ----------\n X : {array-like, sparse matrix} of shape = [n_samples, n_features]\n The training input samples. Sparse matrices are accepted only if\n they are supported by the base estimator.\n Returns\n -------\n y : array of shape = [n_samples]\n The predicted classes.\n \"\"\"\n \n if hasattr(self.base_estimator, \"predict_proba\"):\n predicted_probability = self.predict_proba(X)\n return self.classes_.take((np.argmax(predicted_probability, axis=1)),\n axis=0)\n else:\n predicted_probability = np.zeros((X.shape[0],1), dtype=int)\n for i, ens in enumerate(self.ensemble):\n predicted_probability = np.hstack((predicted_probability, ens.predict(X).reshape(-1,1)))\n predicted_probability = np.delete(predicted_probability,0,axis=1)\n final_pred = []\n for sample in xrange(X.shape[0]):\n final_pred.append(most_common(predicted_probability[sample,:]))\n #votes = []\n #for i, mod_vote in predictions[sample,:]:\n # votes.extend([predictions[sample, i] for j in xrange(int(self.acc[i]))])\n #final_pred = most_common(votes)\n return np.array(final_pred) \n\n\n def predict_proba(self, X):\n \"\"\"Predict class probabilities for X.\n The predicted class probabilities of an input sample is computed as\n the mean predicted class probabilities of the base estimators in the\n ensemble. If base estimators do not implement a ``predict_proba``\n method, then it resorts to voting and the predicted class probabilities\n of an input sample represents the proportion of estimators predicting\n each class.\n Parameters\n ----------\n X : {array-like, sparse matrix} of shape = [n_samples, n_features]\n The training input samples. Sparse matrices are accepted only if\n they are supported by the base estimator.\n Returns\n -------\n p : array of shape = [n_samples, n_classes]\n The class probabilities of the input samples. The order of the\n classes corresponds to that in the attribute `classes_`.\n \"\"\"\n check_is_fitted(self, \"classes_\")\n # Check data\n X = check_array(\n X, accept_sparse=['csr', 'csc'], dtype=None,\n force_all_finite=False\n )\n\n if self.n_features_ != X.shape[1]:\n raise ValueError(\"Number of features of the model must \"\n \"match the input. Model n_features is {0} and \"\n \"input n_features is {1}.\"\n \"\".format(self.n_features_, X.shape[1]))\n\n all_proba = np.zeros((X.shape[0], self.n_classes_))\n for i, ens in enumerate(self.ensemble):\n all_proba += ens.predict_proba(X)\n all_proba /= self.n_estimators\n #print(all_proba.shape)\n #print(all_proba)\n #proba = np.sum(all_proba, axis=0) / self.n_estimators\n #print(proba.shape)\n #print(proba)\n return all_proba\n\n\n @if_delegate_has_method(delegate='base_estimator')\n def decision_function(self, X):\n \"\"\"Average of the decision functions of the base classifiers.\n Parameters\n ----------\n X : {array-like, sparse matrix} of shape = [n_samples, n_features]\n The training input samples. Sparse matrices are accepted only if\n they are supported by the base estimator.\n Returns\n -------\n score : array, shape = [n_samples, k]\n The decision function of the input samples. The columns correspond\n to the classes in sorted order, as they appear in the attribute\n ``classes_``. Regression and binary classification are special\n cases with ``k == 1``, otherwise ``k==n_classes``.\n \"\"\"\n check_is_fitted(self, \"classes_\")\n\n # Check data\n X = check_array(\n X, accept_sparse=['csr', 'csc'], dtype=None,\n force_all_finite=False\n )\n\n if self.n_features_ != X.shape[1]:\n raise ValueError(\"Number of features of the model must \"\n \"match the input. Model n_features is {0} and \"\n \"input n_features is {1} \"\n \"\".format(self.n_features_, X.shape[1]))\n all_decisions = np.zeros((X.shape[0], self.n_classes_))\n for i, ens in enumerate(self.ensemble):\n all_decisions += ens.predict_proba(X) \n decisions = sum(all_decisions) / self.n_estimators\n\n return decisions\n \n def viz_fit(self, X, y):\n X, y = check_X_y(\n X, y, ['csr', 'csc'], dtype=None, force_all_finite=False,\n multi_output=True)\n y = self._validate_y(y)\n if self.oob:\n X, self.X_oob, y, self.y_oob = train_test_split(X,y,test_size=0.1,stratify=y)\n n_samples, self.n_features_ = X.shape\n cur_X, cur_y = X, y\n self.selected_indices.append([i for i in xrange(X.shape[0])])\n flag_target = False\n for i_est in xrange(self.n_estimators):\n cur_mod = clone(self.base_estimator)\n if self.optim:\n grid_search = GridSearchCV(cur_mod, self.parameters, n_jobs=-1, verbose=1, refit=True)\n grid_search.fit(cur_X, cur_y)\n cur_mod = grid_search.best_estimator_\n else:\n cur_mod.fit(cur_X, cur_y)\n self.ensemble.append(cur_mod)\n cur_X, cur_y, flag_target = self.viz_create_next_batch(X, y)\n if flag_target:\n break\n #print(cur_X.shape, cur_y.shape)\n print(\"%d ESTIMATORS -- %0.3f\" % (len(self.ensemble), 100*accuracy_score(y, self.predict(X), normalize=True)))\n return self\n \n def viz_create_next_batch(self, X, y):\n if self.oob:\n preds = self.predict(self.X_oob)\n centroids = self.X_oob[preds != self.y_oob]\n centroids_ind = np.argwhere(preds != self.y_oob).reshape(-1,)\n cur_X = copy.deepcopy(self.X_oob[centroids_ind,:])\n cur_y = copy.deepcopy(self.y_oob[centroids_ind])\n str_target = \"OOB SAMPLE\"\n self.acc = accuracy_score(self.y_oob, preds, normalize=True)\n #acc = (1-(centroids.shape[0])/float(self.X_oob.shape[0]))\n else:\n preds = self.predict(X)\n centroids = X[preds != y]\n centroids_ind = np.argwhere(preds!=y).reshape(-1,)\n cur_X = copy.deepcopy(X[centroids_ind,:])\n cur_y = copy.deepcopy(y[centroids_ind])\n str_target = \"TRAIN SAMPLE\"\n self.acc = accuracy_score(y, preds, normalize=True)\n #acc = (1-(centroids.shape[0])/float(X.shape[0]))\n if self.acc > self.acc_target:\n #return X, y, False\n #print(\"ACCURACY ON THE %s IS %0.3f\" % (str_target, 100*(1-(centroids.shape[0])/float(X.shape[0]))))\n #print(\"STOPPING WITH %d BASE MODELS\" % len(self.ensemble))\n return _, _,True\n probas = pairwise_distances(centroids, X)\n probas /= np.sum(probas, axis=1).reshape(-1,1)\n for i_centr in xrange(probas.shape[0]):\n # Make zero the probability that a same-class sample is picked\n cur_prob = copy.deepcopy(probas[i_centr,:])\n cur_prob[y[centroids_ind[i_centr]]==y]=0\n print(cur_prob.shape, np.sum(cur_prob))\n cur_prob /= np.sum(cur_prob)\n if self.way == 'prob':\n indices = self.random_state.choice([i for i in xrange(0, probas.shape[1])],\n self.num_adversaries_per_instance, p=cur_prob)\n if self.way == 'furthest':\n indices = np.argsort(cur_prob)[::-1][:self.num_adversaries_per_instance]\n if self.way == 'closest':\n cur_prob[y[centroids_ind[i_centr]]==y]=1\n indices = np.argsort(cur_prob)[:self.num_adversaries_per_instance]\n indices = self._fix_class_indices(y, indices)\n \n #print(cur_X.shape, X[indices,:].shape)\n cur_X = np.vstack((cur_X, X[indices,:]))\n cur_y = np.append(cur_y, y[indices])\n #cur_y.extend(indices)\n\n #cur_X = np.delete(cur_X, 0, axis=0)\n #cur_y = y[cur_y]\n plot_selected_points(self, X,y, centroids_ind[i_centr], indices)\n cc = raw_input()\n if cc == 'q':\n exit\n return cur_X, cur_y, False\n \n\ndef most_common(lst):\n if isinstance(lst, np.ndarray):\n lst = lst.tolist()\n #print(lst, max(set(lst), key=lst.count) )\n return max(set(lst), key=lst.count)",
"_____no_output_____"
],
[
"ac = Adversarial_Cascade(way='closest')\nac.viz_fit(X,y)",
"((150,), 0.749618053103047)\n((150,), 0.7030413831294353)\n((150,), 0.7333781004411971)\n((150,), 0.7136448918796773)\n((150,), 0.7601507426572564)\n((150,), 0.7248006287638418)\n((150,), 0.7984514622437389)\n((150,), 0.7788390737899221)\n((150,), 0.7627554359400545)\n((150,), 0.7413436854865884)\n((150,), 0.7984514622437389)\n((150,), 0.7116112378255705)\n((150,), 0.7778260248930959)\n((150,), 0.5429528319909134)\n((150,), 0.787109573424457)\n((150,), 0.6303892204279558)\n((150,), 0.6416119464106853)\n((150,), 0.6886400659980525)\n((150,), 0.5914062113872718)\n((150,), 0.7464179471235344)\n((150,), 0.7194995176572122)\n((150,), 0.7181016216618819)\n((150,), 0.8137265836816736)\n((150,), 0.8150894085124069)\n((150,), 0.6627973573931975)\n"
],
[
"def plot_selected_points(clf, X, y, center_id, indices, s=100):\n fig = plt.figure(figsize=(10,10))\n ax = plt.gca()\n plt.subplots_adjust(wspace=0.4, hspace=0.4)\n X0, X1 = X[:, 0], X[:, 1]\n xx, yy = make_meshgrid(X0, X1)\n plot_contours(ax, clf, xx, yy, cmap=plt.cm.coolwarm, alpha=0.8)\n ax.scatter(X0, X1, c=y, cmap=plt.cm.coolwarm, s=s, edgecolors='grey')\n ax.scatter(X[center_id,0], X[center_id,1], s=s, edgecolors='green', facecolors='none')\n ax.scatter(X[indices,0], X[indices, 1], s=s, edgecolors='black', facecolors='none')\n ax.set_xlim(xx.min(), xx.max())\n ax.set_ylim(yy.min(), yy.max())\n ax.set_xlabel('Sepal length')\n ax.set_ylabel('Sepal width')\n ax.set_xticks(())\n ax.set_yticks(())\n ax.set_title(title)\n plt.show()\n ",
"_____no_output_____"
],
[
"fig = plt.figure(figsize=(10,10))\nax = plt.gca()\nplt.subplots_adjust(wspace=0.4, hspace=0.4)\n\nX0, X1 = X[:, 0], X[:, 1]\nxx, yy = make_meshgrid(X0, X1)\n \nprint(\"%s : %0.3f\"% (title, 100*accuracy_score(y, clf.predict(X))))\nplot_contours(ax, clf, xx, yy,\n cmap=plt.cm.coolwarm, alpha=0.8)\nax.scatter(X0, X1, c=y, cmap=plt.cm.coolwarm, s=80, edgecolors='grey', facecolors='none')\nax.set_xlim(xx.min(), xx.max())\nax.set_ylim(yy.min(), yy.max())\nax.set_xlabel('Sepal length')\nax.set_ylabel('Sepal width')\nax.set_xticks(())\nax.set_yticks(())\nax.set_title(title)\nplt.show()",
"AC : 64.000\n"
],
[
"class Linear_Classifiers(BaseEstimator, ClassifierMixin):\n \n def __init__(self, base_estimator=KNeighborsClassifier(), n_estimators=10, acc_target=0.99,\n num_adversaries_per_instance=4, way = 'prob', \n random_state=42, optim=False, parameters=None, metric='accuracy', oob=False, oob_size=0.1):\n self.base_estimator = base_estimator\n self.n_estimators = n_estimators\n self.acc_target = acc_target\n self.num_adversaries_per_instance = num_adversaries_per_instance\n self.way = way\n self.random_state = check_random_state(random_state)\n self.optim = optim\n self.oob = oob\n self.oob_size = 0.1\n self.X_oob = None\n self.y_oob = None\n if self.optim:\n self.parameters = parameters\n else:\n self.parameters = None\n self.scoring = get_scorer(metric)\n self.acc = 0\n self.ensemble = []\n self.selected_indices = []\n \n def fit(self, X, y):\n return self._fit(X, y)\n \n def _fit(self,X,y):\n X, y = check_X_y(\n X, y, ['csr', 'csc'], dtype=None, force_all_finite=False,\n multi_output=True)\n y = self._validate_y(y)\n if self.oob:\n X, self.X_oob, y, self.y_oob = train_test_split(X,y,test_size=0.1,stratify=y)\n n_samples, self.n_features_ = X.shape\n cur_X, cur_y = X, y\n self.selected_indices.append([i for i in xrange(X.shape[0])])\n flag_target = False\n for i_est in xrange(self.n_estimators):\n cur_mod = clone(self.base_estimator)\n if self.optim:\n grid_search = GridSearchCV(cur_mod, self.parameters, n_jobs=-1, verbose=1, refit=True)\n grid_search.fit(cur_X, cur_y)\n cur_mod = grid_search.best_estimator_\n else:\n cur_mod.fit(cur_X, cur_y)\n self.ensemble.append(cur_mod)\n cur_X, cur_y, flag_target = self._create_next_batch(X, y)\n if flag_target:\n break\n #print(cur_X.shape, cur_y.shape)\n print(\"%d ESTIMATORS -- %0.3f\" % (len(self.ensemble), 100*accuracy_score(y, self.predict(X), normalize=True)))\n return self\n \n def _create_next_batch(self, X, y):\n if self.oob:\n preds = self.predict(self.X_oob)\n centroids = self.X_oob[preds != self.y_oob]\n centroids_ind = np.argwhere(preds != self.y_oob).reshape(-1,)\n cur_X = copy.deepcopy(self.X_oob[centroids_ind,:])\n cur_y = copy.deepcopy(self.y_oob[centroids_ind])\n str_target = \"OOB SAMPLE\"\n self.acc = accuracy_score(self.y_oob, preds, normalize=True)\n #acc = (1-(centroids.shape[0])/float(self.X_oob.shape[0]))\n else:\n preds = self.predict(X)\n centroids = X[preds != y]\n centroids_ind = np.argwhere(preds!=y).reshape(-1,)\n cur_X = copy.deepcopy(X[centroids_ind,:])\n cur_y = copy.deepcopy(y[centroids_ind])\n str_target = \"TRAIN SAMPLE\"\n self.acc = accuracy_score(y, preds, normalize=True)\n #acc = (1-(centroids.shape[0])/float(X.shape[0]))\n if self.acc > self.acc_target:\n #return X, y, False\n #print(\"ACCURACY ON THE %s IS %0.3f\" % (str_target, 100*(1-(centroids.shape[0])/float(X.shape[0]))))\n #print(\"STOPPING WITH %d BASE MODELS\" % len(self.ensemble))\n return _, _,True\n probas = pairwise_distances(centroids, X)\n probas /= np.sum(probas, axis=1).reshape(-1,1)\n for i_centr in xrange(probas.shape[0]):\n # Make zero the probability that a same-class sample is picked\n cur_prob = copy.deepcopy(probas[i_centr,:])\n cur_prob[y[centroids_ind[i_centr]]==y]=0\n print(cur_prob.shape, np.sum(cur_prob))\n cur_prob /= np.sum(cur_prob)\n if self.way == 'prob':\n indices = self.random_state.choice([i for i in xrange(0, probas.shape[1])],\n self.num_adversaries_per_instance, p=cur_prob)\n if self.way == 'furthest':\n indices = np.argsort(cur_prob)[::-1][:self.num_adversaries_per_instance]\n if self.way == 'closest':\n cur_prob[y[centroids_ind[i_centr]]==y]=1\n indices = np.argsort(cur_prob)[:self.num_adversaries_per_instance]\n indices = self._fix_class_indices(y, indices)\n #print(cur_X.shape, X[indices,:].shape)\n cur_X = np.vstack((cur_X, X[indices,:]))\n cur_y = np.append(cur_y, y[indices])\n #cur_y.extend(indices)\n\n #cur_X = np.delete(cur_X, 0, axis=0)\n #cur_y = y[cur_y]\n return cur_X, cur_y, False\n \n def _fix_class_indices(self, y, samples_indices):\n in_set = set(y[samples_indices])\n a = set(y).difference(in_set)\n for item in a:\n samples_indices= np.append(samples_indices, [np.where(y==item)[0][0]])\n return samples_indices \n \n def _validate_y(self, y):\n y = column_or_1d(y, warn=True)\n check_classification_targets(y)\n self.classes_, y = np.unique(y, return_inverse=True)\n self.n_classes_ = len(self.classes_)\n return y\n \n def predict(self, X):\n \"\"\"Predict class for X.\n The predicted class of an input sample is computed as the class with\n the highest mean predicted probability. If base estimators do not\n implement a ``predict_proba`` method, then it resorts to voting.\n Parameters\n ----------\n X : {array-like, sparse matrix} of shape = [n_samples, n_features]\n The training input samples. Sparse matrices are accepted only if\n they are supported by the base estimator.\n Returns\n -------\n y : array of shape = [n_samples]\n The predicted classes.\n \"\"\"\n \n if hasattr(self.base_estimator, \"predict_proba\"):\n predicted_probability = self.predict_proba(X)\n return self.classes_.take((np.argmax(predicted_probability, axis=1)),\n axis=0)\n else:\n predicted_probability = np.zeros((X.shape[0],1), dtype=int)\n for i, ens in enumerate(self.ensemble):\n predicted_probability = np.hstack((predicted_probability, ens.predict(X).reshape(-1,1)))\n predicted_probability = np.delete(predicted_probability,0,axis=1)\n final_pred = []\n for sample in xrange(X.shape[0]):\n final_pred.append(most_common(predicted_probability[sample,:]))\n #votes = []\n #for i, mod_vote in predictions[sample,:]:\n # votes.extend([predictions[sample, i] for j in xrange(int(self.acc[i]))])\n #final_pred = most_common(votes)\n return np.array(final_pred) \n\n\n def predict_proba(self, X):\n \"\"\"Predict class probabilities for X.\n The predicted class probabilities of an input sample is computed as\n the mean predicted class probabilities of the base estimators in the\n ensemble. If base estimators do not implement a ``predict_proba``\n method, then it resorts to voting and the predicted class probabilities\n of an input sample represents the proportion of estimators predicting\n each class.\n Parameters\n ----------\n X : {array-like, sparse matrix} of shape = [n_samples, n_features]\n The training input samples. Sparse matrices are accepted only if\n they are supported by the base estimator.\n Returns\n -------\n p : array of shape = [n_samples, n_classes]\n The class probabilities of the input samples. The order of the\n classes corresponds to that in the attribute `classes_`.\n \"\"\"\n check_is_fitted(self, \"classes_\")\n # Check data\n X = check_array(\n X, accept_sparse=['csr', 'csc'], dtype=None,\n force_all_finite=False\n )\n\n if self.n_features_ != X.shape[1]:\n raise ValueError(\"Number of features of the model must \"\n \"match the input. Model n_features is {0} and \"\n \"input n_features is {1}.\"\n \"\".format(self.n_features_, X.shape[1]))\n\n all_proba = np.zeros((X.shape[0], self.n_classes_))\n for i, ens in enumerate(self.ensemble):\n all_proba += ens.predict_proba(X)\n all_proba /= self.n_estimators\n #print(all_proba.shape)\n #print(all_proba)\n #proba = np.sum(all_proba, axis=0) / self.n_estimators\n #print(proba.shape)\n #print(proba)\n return all_proba\n\n\n @if_delegate_has_method(delegate='base_estimator')\n def decision_function(self, X):\n \"\"\"Average of the decision functions of the base classifiers.\n Parameters\n ----------\n X : {array-like, sparse matrix} of shape = [n_samples, n_features]\n The training input samples. Sparse matrices are accepted only if\n they are supported by the base estimator.\n Returns\n -------\n score : array, shape = [n_samples, k]\n The decision function of the input samples. The columns correspond\n to the classes in sorted order, as they appear in the attribute\n ``classes_``. Regression and binary classification are special\n cases with ``k == 1``, otherwise ``k==n_classes``.\n \"\"\"\n check_is_fitted(self, \"classes_\")\n\n # Check data\n X = check_array(\n X, accept_sparse=['csr', 'csc'], dtype=None,\n force_all_finite=False\n )\n\n if self.n_features_ != X.shape[1]:\n raise ValueError(\"Number of features of the model must \"\n \"match the input. Model n_features is {0} and \"\n \"input n_features is {1} \"\n \"\".format(self.n_features_, X.shape[1]))\n all_decisions = np.zeros((X.shape[0], self.n_classes_))\n for i, ens in enumerate(self.ensemble):\n all_decisions += ens.predict_proba(X) \n decisions = sum(all_decisions) / self.n_estimators\n\n return decisions\n \n def viz_fit(self, X, y):\n X, y = check_X_y(\n X, y, ['csr', 'csc'], dtype=None, force_all_finite=False,\n multi_output=True)\n y = self._validate_y(y)\n if self.oob:\n X, self.X_oob, y, self.y_oob = train_test_split(X,y,test_size=0.1,stratify=y)\n n_samples, self.n_features_ = X.shape\n cur_X, cur_y = X, y\n self.selected_indices.append([i for i in xrange(X.shape[0])])\n flag_target = False\n for i_est in xrange(self.n_estimators):\n cur_mod = clone(self.base_estimator)\n if self.optim:\n grid_search = GridSearchCV(cur_mod, self.parameters, n_jobs=-1, verbose=1, refit=True)\n grid_search.fit(cur_X, cur_y)\n cur_mod = grid_search.best_estimator_\n else:\n cur_mod.fit(cur_X, cur_y)\n self.ensemble.append(cur_mod)\n cur_X, cur_y, flag_target = self.viz_create_next_batch(X, y)\n if flag_target:\n break\n #print(cur_X.shape, cur_y.shape)\n print(\"%d ESTIMATORS -- %0.3f\" % (len(self.ensemble), 100*accuracy_score(y, self.predict(X), normalize=True)))\n return self\n \n def viz_create_next_batch(self, X, y):\n if self.oob:\n preds = self.predict(self.X_oob)\n centroids = self.X_oob[preds != self.y_oob]\n centroids_ind = np.argwhere(preds != self.y_oob).reshape(-1,)\n cur_X = copy.deepcopy(self.X_oob[centroids_ind,:])\n cur_y = copy.deepcopy(self.y_oob[centroids_ind])\n str_target = \"OOB SAMPLE\"\n self.acc = accuracy_score(self.y_oob, preds, normalize=True)\n #acc = (1-(centroids.shape[0])/float(self.X_oob.shape[0]))\n else:\n preds = self.predict(X)\n centroids = X[preds != y]\n centroids_ind = np.argwhere(preds!=y).reshape(-1,)\n cur_X = copy.deepcopy(X[centroids_ind,:])\n cur_y = copy.deepcopy(y[centroids_ind])\n str_target = \"TRAIN SAMPLE\"\n self.acc = accuracy_score(y, preds, normalize=True)\n #acc = (1-(centroids.shape[0])/float(X.shape[0]))\n if self.acc > self.acc_target:\n #return X, y, False\n #print(\"ACCURACY ON THE %s IS %0.3f\" % (str_target, 100*(1-(centroids.shape[0])/float(X.shape[0]))))\n #print(\"STOPPING WITH %d BASE MODELS\" % len(self.ensemble))\n return _, _,True\n probas = pairwise_distances(centroids, X)\n probas /= np.sum(probas, axis=1).reshape(-1,1)\n for i_centr in xrange(probas.shape[0]):\n # Make zero the probability that a same-class sample is picked\n cur_prob = copy.deepcopy(probas[i_centr,:])\n cur_prob[y[centroids_ind[i_centr]]==y]=0\n print(cur_prob.shape, np.sum(cur_prob))\n cur_prob /= np.sum(cur_prob)\n if self.way == 'prob':\n indices = self.random_state.choice([i for i in xrange(0, probas.shape[1])],\n self.num_adversaries_per_instance, p=cur_prob)\n if self.way == 'furthest':\n indices = np.argsort(cur_prob)[::-1][:self.num_adversaries_per_instance]\n if self.way == 'closest':\n cur_prob[y[centroids_ind[i_centr]]==y]=1\n indices = np.argsort(cur_prob)[:self.num_adversaries_per_instance]\n indices = self._fix_class_indices(y, indices)\n \n #print(cur_X.shape, X[indices,:].shape)\n cur_X = np.vstack((cur_X, X[indices,:]))\n cur_y = np.append(cur_y, y[indices])\n #cur_y.extend(indices)\n\n #cur_X = np.delete(cur_X, 0, axis=0)\n #cur_y = y[cur_y]\n plot_selected_points(self, X,y, centroids_ind[i_centr], indices)\n cc = raw_input()\n if cc == 'q':\n exit\n return cur_X, cur_y, False\n \n\ndef most_common(lst):\n if isinstance(lst, np.ndarray):\n lst = lst.tolist()\n #print(lst, max(set(lst), key=lst.count) )\n return max(set(lst), key=lst.count)",
"_____no_output_____"
]
]
] | [
"markdown",
"code"
] | [
[
"markdown",
"markdown"
],
[
"code",
"code",
"code",
"code",
"code",
"code",
"code",
"code",
"code",
"code",
"code",
"code",
"code",
"code",
"code",
"code",
"code",
"code",
"code",
"code",
"code",
"code",
"code",
"code",
"code",
"code",
"code",
"code",
"code"
]
] |
e7799608ba0345471743bb0652e06a3f90140fad | 508,442 | ipynb | Jupyter Notebook | nbs/74_callback.cutmix.ipynb | hanshin-back/fastai | eb98c4a490c319f8136be92cfc1628b5de3f33e2 | [
"Apache-2.0"
] | 1 | 2020-11-28T20:01:39.000Z | 2020-11-28T20:01:39.000Z | nbs/74_callback.cutmix.ipynb | hanshin-back/fastai | eb98c4a490c319f8136be92cfc1628b5de3f33e2 | [
"Apache-2.0"
] | null | null | null | nbs/74_callback.cutmix.ipynb | hanshin-back/fastai | eb98c4a490c319f8136be92cfc1628b5de3f33e2 | [
"Apache-2.0"
] | null | null | null | 1,499.828909 | 496,960 | 0.957053 | [
[
[
"#hide\n#skip\n! [ -e /content ] && pip install -Uqq fastai # upgrade fastai on colab",
"_____no_output_____"
],
[
"#default_exp callback.cutmix",
"_____no_output_____"
],
[
"#export\nfrom torch.distributions.beta import Beta\nfrom fastai.vision.all import *",
"_____no_output_____"
]
],
[
[
"# CutMix Callback\n> Callback to apply [CutMix](https://arxiv.org/pdf/1905.04899.pdf) data augmentation technique to the training data.",
"_____no_output_____"
],
[
"From the [research paper](https://arxiv.org/pdf/1905.04899.pdf), `CutMix` is a way to combine two images. It comes from `MixUp` and `Cutout`. In this data augmentation technique:\n> patches are cut and pasted among training images where the ground truth labels are also mixed proportionally to the area of the patches\n\nAlso, from the paper: \n> By making efficient use of training pixels and retaining the regularization effect of regional dropout, CutMix consistently outperforms the state-of-the-art augmentation strategies on CIFAR and ImageNet classification tasks, as well as on the ImageNet weakly-supervised localization task. Moreover, unlike previous augmentation methods, our CutMix-trained ImageNet classifier, when used as a pretrained model, results in consistent performance gains in Pascal detection and MS-COCO image captioning benchmarks. We also show that CutMix improves the model robustness against input corruptions and its out-of-distribution detection performances. ",
"_____no_output_____"
]
],
[
[
"#export\nclass CutMix(Callback):\n \"Implementation of `https://arxiv.org/abs/1905.04899`\"\n run_after,run_valid = [Normalize],False\n def __init__(self, alpha=1.): self.distrib = Beta(tensor(alpha), tensor(alpha))\n def before_fit(self):\n self.stack_y = getattr(self.learn.loss_func, 'y_int', False)\n if self.stack_y: self.old_lf,self.learn.loss_func = self.learn.loss_func,self.lf\n\n def after_fit(self):\n if self.stack_y: self.learn.loss_func = self.old_lf\n\n def before_batch(self):\n W, H = self.xb[0].size(3), self.xb[0].size(2)\n lam = self.distrib.sample((1,)).squeeze().to(self.x.device)\n lam = torch.stack([lam, 1-lam])\n self.lam = lam.max()\n shuffle = torch.randperm(self.y.size(0)).to(self.x.device)\n xb1,self.yb1 = tuple(L(self.xb).itemgot(shuffle)),tuple(L(self.yb).itemgot(shuffle))\n nx_dims = len(self.x.size())\n x1, y1, x2, y2 = self.rand_bbox(W, H, self.lam)\n self.learn.xb[0][:, :, x1:x2, y1:y2] = xb1[0][:, :, x1:x2, y1:y2]\n self.lam = (1 - ((x2-x1)*(y2-y1))/float(W*H)).item()\n\n if not self.stack_y:\n ny_dims = len(self.y.size())\n self.learn.yb = tuple(L(self.yb1,self.yb).map_zip(torch.lerp,weight=unsqueeze(self.lam, n=ny_dims-1)))\n\n def lf(self, pred, *yb):\n if not self.training: return self.old_lf(pred, *yb)\n with NoneReduce(self.old_lf) as lf:\n loss = torch.lerp(lf(pred,*self.yb1), lf(pred,*yb), self.lam)\n return reduce_loss(loss, getattr(self.old_lf, 'reduction', 'mean'))\n\n def rand_bbox(self, W, H, lam):\n cut_rat = torch.sqrt(1. - lam)\n cut_w = (W * cut_rat).type(torch.long)\n cut_h = (H * cut_rat).type(torch.long)\n # uniform\n cx = torch.randint(0, W, (1,)).to(self.x.device)\n cy = torch.randint(0, H, (1,)).to(self.x.device)\n x1 = torch.clamp(cx - cut_w // 2, 0, W)\n y1 = torch.clamp(cy - cut_h // 2, 0, H)\n x2 = torch.clamp(cx + cut_w // 2, 0, W)\n y2 = torch.clamp(cy + cut_h // 2, 0, H)\n return x1, y1, x2, y2",
"_____no_output_____"
]
],
[
[
"## How does the batch with `CutMix` data augmentation technique look like?",
"_____no_output_____"
],
[
"First, let's quickly create the `dls` using `ImageDataLoaders.from_name_re` DataBlocks API.",
"_____no_output_____"
]
],
[
[
"path = untar_data(URLs.PETS)\npat = r'([^/]+)_\\d+.*$'\nfnames = get_image_files(path/'images')\nitem_tfms = [Resize(256, method='crop')]\nbatch_tfms = [*aug_transforms(size=224), Normalize.from_stats(*imagenet_stats)]\ndls = ImageDataLoaders.from_name_re(path, fnames, pat, bs=64, item_tfms=item_tfms, \n batch_tfms=batch_tfms)",
"_____no_output_____"
]
],
[
[
"Next, let's initialize the callback `CutMix`, create a learner, do one batch and display the images with the labels. `CutMix` inside updates the loss function based on the ratio of the cutout bbox to the complete image.",
"_____no_output_____"
]
],
[
[
"cutmix = CutMix(alpha=1.)",
"_____no_output_____"
],
[
"with Learner(dls, resnet18(), loss_func=CrossEntropyLossFlat(), cbs=cutmix) as learn:\n learn.epoch,learn.training = 0,True\n learn.dl = dls.train\n b = dls.one_batch()\n learn._split(b)\n learn('before_batch')\n\n_,axs = plt.subplots(3,3, figsize=(9,9))\ndls.show_batch(b=(cutmix.x,cutmix.y), ctxs=axs.flatten())",
"_____no_output_____"
]
],
[
[
"## Using `CutMix` in Training",
"_____no_output_____"
]
],
[
[
"learn = cnn_learner(dls, resnet18, loss_func=CrossEntropyLossFlat(), cbs=cutmix, metrics=[accuracy, error_rate])\n# learn.fit_one_cycle(1)",
"_____no_output_____"
]
],
[
[
"\n## Export -",
"_____no_output_____"
]
],
[
[
"#hide\nfrom nbdev.export import notebook2script\nnotebook2script()",
"Converted 00_torch_core.ipynb.\nConverted 01_layers.ipynb.\nConverted 01a_losses.ipynb.\nConverted 02_data.load.ipynb.\nConverted 03_data.core.ipynb.\nConverted 04_data.external.ipynb.\nConverted 05_data.transforms.ipynb.\nConverted 06_data.block.ipynb.\nConverted 07_vision.core.ipynb.\nConverted 08_vision.data.ipynb.\nConverted 09_vision.augment.ipynb.\nConverted 09b_vision.utils.ipynb.\nConverted 09c_vision.widgets.ipynb.\nConverted 10_tutorial.pets.ipynb.\nConverted 10b_tutorial.albumentations.ipynb.\nConverted 11_vision.models.xresnet.ipynb.\nConverted 12_optimizer.ipynb.\nConverted 13_callback.core.ipynb.\nConverted 13a_learner.ipynb.\nConverted 13b_metrics.ipynb.\nConverted 14_callback.schedule.ipynb.\nConverted 14a_callback.data.ipynb.\nConverted 15_callback.hook.ipynb.\nConverted 15a_vision.models.unet.ipynb.\nConverted 16_callback.progress.ipynb.\nConverted 17_callback.tracker.ipynb.\nConverted 18_callback.fp16.ipynb.\nConverted 18a_callback.training.ipynb.\nConverted 18b_callback.preds.ipynb.\nConverted 19_callback.mixup.ipynb.\nConverted 20_interpret.ipynb.\nConverted 20a_distributed.ipynb.\nConverted 21_vision.learner.ipynb.\nConverted 22_tutorial.imagenette.ipynb.\nConverted 23_tutorial.vision.ipynb.\nConverted 24_tutorial.siamese.ipynb.\nConverted 24_vision.gan.ipynb.\nConverted 30_text.core.ipynb.\nConverted 31_text.data.ipynb.\nConverted 32_text.models.awdlstm.ipynb.\nConverted 33_text.models.core.ipynb.\nConverted 34_callback.rnn.ipynb.\nConverted 35_tutorial.wikitext.ipynb.\nConverted 36_text.models.qrnn.ipynb.\nConverted 37_text.learner.ipynb.\nConverted 38_tutorial.text.ipynb.\nConverted 39_tutorial.transformers.ipynb.\nConverted 40_tabular.core.ipynb.\nConverted 41_tabular.data.ipynb.\nConverted 42_tabular.model.ipynb.\nConverted 43_tabular.learner.ipynb.\nConverted 44_tutorial.tabular.ipynb.\nConverted 45_collab.ipynb.\nConverted 46_tutorial.collab.ipynb.\nConverted 50_tutorial.datablock.ipynb.\nConverted 60_medical.imaging.ipynb.\nConverted 61_tutorial.medical_imaging.ipynb.\nConverted 65_medical.text.ipynb.\nConverted 70_callback.wandb.ipynb.\nConverted 71_callback.tensorboard.ipynb.\nConverted 72_callback.neptune.ipynb.\nConverted 73_callback.captum.ipynb.\nConverted 74_callback.cutmix.ipynb.\nConverted 97_test_utils.ipynb.\nConverted 99_pytorch_doc.ipynb.\nConverted dev-setup.ipynb.\nConverted index.ipynb.\nConverted quick_start.ipynb.\nConverted tutorial.ipynb.\n"
]
]
] | [
"code",
"markdown",
"code",
"markdown",
"code",
"markdown",
"code",
"markdown",
"code",
"markdown",
"code"
] | [
[
"code",
"code",
"code"
],
[
"markdown",
"markdown"
],
[
"code"
],
[
"markdown",
"markdown"
],
[
"code"
],
[
"markdown"
],
[
"code",
"code"
],
[
"markdown"
],
[
"code"
],
[
"markdown"
],
[
"code"
]
] |
e779a18d2490d0f83dbc91124253f53e6e3d34db | 9,355 | ipynb | Jupyter Notebook | 3_VectorAssembler_example.ipynb | edsonlourenco/pyspark_ml_examples | 995045ebdc6648f9ceabe91c233f8303d5978534 | [
"MIT"
] | null | null | null | 3_VectorAssembler_example.ipynb | edsonlourenco/pyspark_ml_examples | 995045ebdc6648f9ceabe91c233f8303d5978534 | [
"MIT"
] | null | null | null | 3_VectorAssembler_example.ipynb | edsonlourenco/pyspark_ml_examples | 995045ebdc6648f9ceabe91c233f8303d5978534 | [
"MIT"
] | null | null | null | 34.393382 | 121 | 0.330305 | [
[
[
"# Installing required packages",
"_____no_output_____"
]
],
[
[
"from IPython.display import clear_output\n\n!pip install --upgrade pip\n!pip install findspark\n!pip install pyspark\n\nclear_output(wait=False)",
"_____no_output_____"
]
],
[
[
"# Importing global objects",
"_____no_output_____"
]
],
[
[
"import findspark, pyspark\nfrom pyspark.sql import SparkSession\nfrom pyspark import SparkFiles",
"_____no_output_____"
]
],
[
[
"# Global Settings\nNeeded for environments not Databricks",
"_____no_output_____"
]
],
[
[
"findspark.init()\nspark = SparkSession.builder.getOrCreate()",
"_____no_output_____"
]
],
[
[
"# Reading data source",
"_____no_output_____"
]
],
[
[
"url = 'https://raw.githubusercontent.com/edsonlourenco/public_datasets/main/Carros.csv'\nspark.sparkContext.addFile(url)\ncsv_cars = SparkFiles.get(\"Carros.csv\")\ndf_cars = spark.read.csv(csv_cars, header=True, inferSchema=True, sep=';')",
"_____no_output_____"
]
],
[
[
"# Checking **data**",
"_____no_output_____"
]
],
[
[
"df_cars.orderBy('Consumo').show(truncate=False)",
"+-------+---------+-----------+---------------+----+-----+---------+-----------+-------+-----------+---+\n|Consumo|Cilindros|Cilindradas|RelEixoTraseiro|Peso|Tempo|TipoMotor|Transmissao|Marchas|Carburadors|HP |\n+-------+---------+-----------+---------------+----+-----+---------+-----------+-------+-----------+---+\n|15 |8 |301 |354 |357 |146 |0 |1 |5 |8 |335|\n|21 |6 |160 |39 |2875|1702 |0 |1 |4 |4 |110|\n|21 |6 |160 |39 |262 |1646 |0 |1 |4 |4 |110|\n|26 |4 |1203 |443 |214 |167 |0 |1 |5 |2 |91 |\n|104 |8 |472 |293 |525 |1798 |0 |0 |3 |4 |205|\n|104 |8 |460 |3 |5424|1782 |0 |0 |3 |4 |215|\n|133 |8 |350 |373 |384 |1541 |0 |0 |3 |4 |245|\n|143 |8 |360 |321 |357 |1584 |0 |0 |3 |4 |245|\n|147 |8 |440 |323 |5345|1742 |0 |0 |3 |4 |230|\n|152 |8 |2758 |307 |378 |18 |0 |0 |3 |3 |180|\n|152 |8 |304 |315 |3435|173 |0 |0 |3 |2 |150|\n|155 |8 |318 |276 |352 |1687 |0 |0 |3 |2 |150|\n|158 |8 |351 |422 |317 |145 |0 |1 |5 |4 |264|\n|164 |8 |2758 |307 |407 |174 |0 |0 |3 |3 |180|\n|173 |8 |2758 |307 |373 |176 |0 |0 |3 |3 |180|\n|178 |6 |1676 |392 |344 |189 |1 |0 |4 |4 |123|\n|181 |6 |225 |276 |346 |2022 |1 |0 |3 |1 |105|\n|187 |8 |360 |315 |344 |1702 |0 |0 |3 |2 |175|\n|192 |6 |1676 |392 |344 |183 |1 |0 |4 |4 |123|\n|192 |8 |400 |308 |3845|1705 |0 |0 |3 |2 |175|\n+-------+---------+-----------+---------------+----+-----+---------+-----------+-------+-----------+---+\nonly showing top 20 rows\n\n"
]
],
[
[
"## Transform VectorAssembler",
"_____no_output_____"
],
[
"### Importing **VectorAssembler** class",
"_____no_output_____"
]
],
[
[
"from pyspark.ml.feature import VectorAssembler",
"_____no_output_____"
]
],
[
[
"### Doing transformation and creating features column",
"_____no_output_____"
]
],
[
[
"vectas = VectorAssembler(inputCols=[\n \"Consumo\",\n \"Cilindros\",\n \"Cilindradas\",\n \"RelEixoTraseiro\",\n \"Peso\",\n \"Tempo\",\n \"TipoMotor\",\n \"Transmissao\",\n \"Marchas\",\n \"Carburadors\"\n ],\n outputCol=\"features\")\n\ndf_cars_vet = vectas.transform(df_cars)\ndf_cars_vet.orderBy('Consumo').select('features').show(truncate=True) #('caracteristicas').display()",
"+--------------------+\n| features|\n+--------------------+\n|[15.0,8.0,301.0,3...|\n|[21.0,6.0,160.0,3...|\n|[21.0,6.0,160.0,3...|\n|[26.0,4.0,1203.0,...|\n|[104.0,8.0,472.0,...|\n|[104.0,8.0,460.0,...|\n|[133.0,8.0,350.0,...|\n|[143.0,8.0,360.0,...|\n|[147.0,8.0,440.0,...|\n|[152.0,8.0,2758.0...|\n|[152.0,8.0,304.0,...|\n|[155.0,8.0,318.0,...|\n|[158.0,8.0,351.0,...|\n|[164.0,8.0,2758.0...|\n|[173.0,8.0,2758.0...|\n|[178.0,6.0,1676.0...|\n|[181.0,6.0,225.0,...|\n|[187.0,8.0,360.0,...|\n|[192.0,6.0,1676.0...|\n|[192.0,8.0,400.0,...|\n+--------------------+\nonly showing top 20 rows\n\n"
]
]
] | [
"markdown",
"code",
"markdown",
"code",
"markdown",
"code",
"markdown",
"code",
"markdown",
"code",
"markdown",
"code",
"markdown",
"code"
] | [
[
"markdown"
],
[
"code"
],
[
"markdown"
],
[
"code"
],
[
"markdown"
],
[
"code"
],
[
"markdown"
],
[
"code"
],
[
"markdown"
],
[
"code"
],
[
"markdown",
"markdown"
],
[
"code"
],
[
"markdown"
],
[
"code"
]
] |
e779b6938583f74b8c5495a3810325b6c9a7b144 | 2,563 | ipynb | Jupyter Notebook | templ.ipynb | mirkoklukas/nbx | 2181c7a5fb49a167eae07a73ffc3d95c4ee953c8 | [
"Apache-2.0"
] | null | null | null | templ.ipynb | mirkoklukas/nbx | 2181c7a5fb49a167eae07a73ffc3d95c4ee953c8 | [
"Apache-2.0"
] | 4 | 2021-01-25T20:23:14.000Z | 2022-02-26T06:21:38.000Z | templ.ipynb | mirkoklukas/nbx | 2181c7a5fb49a167eae07a73ffc3d95c4ee953c8 | [
"Apache-2.0"
] | null | null | null | 23.09009 | 85 | 0.525556 | [
[
[
"#export\nfrom pathlib import Path\nimport jinja2, jinja2.meta as meta\n\ndef get_templ_args(path):\n path = Path(path)\n loader = jinja2.FileSystemLoader(searchpath=str(path.parent))\n env = jinja2.Environment(loader=loader)\n\n src = env.loader.get_source(env, path.name)[0]\n parsed = env.parse(src)\n args = meta.find_undeclared_variables(parsed)\n\n return args\n\ndef render_templ(path, vars):\n path = Path(path)\n loader = jinja2.FileSystemLoader(searchpath=str(path.parent))\n env = jinja2.Environment(loader=loader)\n\n template = env.get_template(path.name)\n text = template.render(vars) \n return text\n\n\ndef create_file_from_template(tpath, fpath, vars):\n script_src = render_templ(tpath, vars)\n\n with open(fpath, \"w\", newline=\"\\n\") as f:\n f.write(script_src)\n\ndef render_template_from_string(s, vars):\n template = jinja2.Environment(loader=jinja2.BaseLoader()).from_string(s)\n return template.render(vars)\n",
"_____no_output_____"
],
[
"get_templ_args(\"nbx/templates/experiment.tpl\")",
"_____no_output_____"
]
],
[
[
"# Export",
"_____no_output_____"
]
],
[
[
"#default_exp templ\nfrom nbdev.export import notebook2script\nnotebook2script()",
"Converted om.ipynb.\nConverted pspace.ipynb.\nConverted templ.ipynb.\n"
]
]
] | [
"code",
"markdown",
"code"
] | [
[
"code",
"code"
],
[
"markdown"
],
[
"code"
]
] |
e779bc64e2d933ad06d3fe675c9c19e213e50ab9 | 13,674 | ipynb | Jupyter Notebook | test/cpp/Priestlytaylor.ipynb | AgriculturalModelExchangeInitiative/SQ_Energy_Balance | 928190fa117779626cf55597dbff451e31237f41 | [
"BSD-3-Clause"
] | 1 | 2018-12-06T07:54:31.000Z | 2018-12-06T07:54:31.000Z | test/cpp/Priestlytaylor.ipynb | AgriculturalModelExchangeInitiative/SQ_Energy_Balance | 928190fa117779626cf55597dbff451e31237f41 | [
"BSD-3-Clause"
] | 2 | 2018-12-07T17:00:22.000Z | 2020-11-13T06:45:51.000Z | test/cpp/Priestlytaylor.ipynb | AgriculturalModelExchangeInitiative/SQ_Energy_Balance | 928190fa117779626cf55597dbff451e31237f41 | [
"BSD-3-Clause"
] | 7 | 2019-01-15T04:33:23.000Z | 2021-12-09T07:29:46.000Z | 53.834646 | 199 | 0.599678 | [
[
[
"empty"
]
]
] | [
"empty"
] | [
[
"empty"
]
] |
e779c46b6292d1890cf4266731f523afc56036ff | 59,450 | ipynb | Jupyter Notebook | tutorials/jupyter/8- Sarcasm Classifiers (GloVe and CNN).ipynb | nabinkhadka/spark-nlp-workshop | d2d47b4320a0a7bd58d5f6b27724f5ff80435697 | [
"Apache-2.0"
] | 3 | 2020-04-18T20:21:11.000Z | 2022-02-08T23:57:46.000Z | tutorials/jupyter/8- Sarcasm Classifiers (GloVe and CNN).ipynb | nabinkhadka/spark-nlp-workshop | d2d47b4320a0a7bd58d5f6b27724f5ff80435697 | [
"Apache-2.0"
] | null | null | null | tutorials/jupyter/8- Sarcasm Classifiers (GloVe and CNN).ipynb | nabinkhadka/spark-nlp-workshop | d2d47b4320a0a7bd58d5f6b27724f5ff80435697 | [
"Apache-2.0"
] | 1 | 2021-01-23T15:24:52.000Z | 2021-01-23T15:24:52.000Z | 91.885626 | 35,560 | 0.764407 | [
[
[
"! wget -N https://s3.amazonaws.com/auxdata.johnsnowlabs.com/public/resources/en/sarcasm/train-balanced-sarcasm.csv -P /tmp",
"--2020-02-10 14:42:31-- https://s3.amazonaws.com/auxdata.johnsnowlabs.com/public/resources/en/sarcasm/train-balanced-sarcasm.csv\nLoaded CA certificate '/etc/ssl/certs/ca-certificates.crt'\nResolving s3.amazonaws.com (s3.amazonaws.com)... 52.217.15.126\nConnecting to s3.amazonaws.com (s3.amazonaws.com)|52.217.15.126|:443... connected.\nHTTP request sent, awaiting response... 304 Not Modified\nFile ‘/tmp/train-balanced-sarcasm.csv’ not modified on server. Omitting download.\n\n"
]
],
[
[
"# Additional dependencies\nYou will need to have tensorflow keras pydot and graphviz in your OS installed and added to the path \n```bash\npython -m pip install pydot\n```\n\n```bash\nyay graphviz \n\n```bash\nsudo apt install python-pydot python-pydot-ng graphviz\n```",
"_____no_output_____"
]
],
[
[
"import os\nimport sys\nimport time\nimport warnings\n\nwarnings.filterwarnings(\"ignore\")\nos.environ['TF_CPP_MIN_LOG_LEVEL'] = '2' \n\nimport numpy as np\nimport pandas as pd\n\nfrom pyspark.sql import SparkSession\npackages = [\n 'JohnSnowLabs:spark-nlp: 2.4.2'\n]\nspark = SparkSession \\\n .builder \\\n .appName(\"ML SQL session\") \\\n .config('spark.jars.packages', ','.join(packages)) \\\n .config(\"spark.driver.memory\",\"2g\") \\\n .getOrCreate()",
"_____no_output_____"
],
[
"import sparknlp\n\nprint(\"Spark NLP version: \", sparknlp.version())\nprint(\"Apache Spark version: \", spark.version)",
"Spark NLP version: 2.4.2\nApache Spark version: 2.4.4\n"
],
[
"from pyspark.sql import SQLContext\n\nsql = SQLContext(spark)\n\ntrainBalancedSarcasmDF = spark.read.option(\"header\", True).option(\"inferSchema\", True) \\\n .csv(\"/tmp/train-balanced-sarcasm.csv\")\ntrainBalancedSarcasmDF.printSchema()\n\n# Let's create a temp view (table) for our SQL queries\ntrainBalancedSarcasmDF.createOrReplaceTempView('sarcasm')\n\nsql.sql('SELECT COUNT(*) FROM sarcasm').collect()",
"root\n |-- label: integer (nullable = true)\n |-- comment: string (nullable = true)\n |-- author: string (nullable = true)\n |-- subreddit: string (nullable = true)\n |-- score: string (nullable = true)\n |-- ups: string (nullable = true)\n |-- downs: string (nullable = true)\n |-- date: string (nullable = true)\n |-- created_utc: string (nullable = true)\n |-- parent_comment: string (nullable = true)\n\n"
],
[
"df = sql.sql('''\nselect label, concat(parent_comment,\"\\n\",comment) as comment \nfrom sarcasm \nwhere comment is not null and parent_comment is not null limit 100000''')\nprint(type(df))\ndf.printSchema()\nprint('rows', df.count())\ndf = df.limit(2000) #minimize dataset if you are not running on a cluster\ndf.show()\n",
"<class 'pyspark.sql.dataframe.DataFrame'>\nroot\n |-- label: integer (nullable = true)\n |-- comment: string (nullable = true)\n\nrows 100000\n+-----+--------------------+\n|label| comment|\n+-----+--------------------+\n| 0|Yeah, I get that ...|\n| 0|The blazers and M...|\n| 0|They're favored t...|\n| 0|deadass don't kil...|\n| 0|Yep can confirm I...|\n| 0|do you find arian...|\n| 0|What's your weird...|\n| 0|Probably Sephirot...|\n| 0|What to upgrade? ...|\n| 0|Probably count Ka...|\n| 0|I bet if that mon...|\n| 0|James Shields Wil...|\n| 0|There's no time t...|\n| 0|Team Specific Thr...|\n| 0|Ill give you a hi...|\n| 0|Star Wars, easy. ...|\n| 0|You're adorable.\n...|\n| 0|He actually acts ...|\n| 0|Clinton struggles...|\n| 0|Is that the Older...|\n+-----+--------------------+\nonly showing top 20 rows\n\n"
],
[
"from sparknlp.annotator import *\nfrom sparknlp.common import *\nfrom sparknlp.base import *\n\nfrom pyspark.ml import Pipeline\n\ndocument_assembler = DocumentAssembler() \\\n .setInputCol(\"comment\") \\\n .setOutputCol(\"document\")\n \nsentence_detector = SentenceDetector() \\\n .setInputCols([\"document\"]) \\\n .setOutputCol(\"sentence\") \\\n .setUseAbbreviations(True)\n \ntokenizer = Tokenizer() \\\n .setInputCols([\"sentence\"]) \\\n .setOutputCol(\"token\")\n\nnlp_pipeline = Pipeline(stages=[document_assembler, sentence_detector, tokenizer])\nnlp_model = nlp_pipeline.fit(df)\n\nprocessed = nlp_model.transform(df)\nprocessed.show()\n\ntrain, test = processed.randomSplit(weights=[0.7, 0.3], seed=123)\n\nprint(train.count())\nprint(test.count())",
"+-----+--------------------+--------------------+--------------------+--------------------+\n|label| comment| document| sentence| token|\n+-----+--------------------+--------------------+--------------------+--------------------+\n| 0|Yeah, I get that ...|[[document, 0, 90...|[[document, 0, 25...|[[token, 0, 3, Ye...|\n| 0|The blazers and M...|[[document, 0, 20...|[[document, 0, 13...|[[token, 0, 2, Th...|\n| 0|They're favored t...|[[document, 0, 14...|[[document, 0, 22...|[[token, 0, 6, Th...|\n| 0|deadass don't kil...|[[document, 0, 90...|[[document, 0, 90...|[[token, 0, 6, de...|\n| 0|Yep can confirm I...|[[document, 0, 11...|[[document, 0, 48...|[[token, 0, 2, Ye...|\n| 0|do you find arian...|[[document, 0, 14...|[[document, 0, 14...|[[token, 0, 1, do...|\n| 0|What's your weird...|[[document, 0, 98...|[[document, 0, 52...|[[token, 0, 5, Wh...|\n| 0|Probably Sephirot...|[[document, 0, 14...|[[document, 0, 18...|[[token, 0, 7, Pr...|\n| 0|What to upgrade? ...|[[document, 0, 77...|[[document, 0, 15...|[[token, 0, 3, Wh...|\n| 0|Probably count Ka...|[[document, 0, 33...|[[document, 0, 93...|[[token, 0, 7, Pr...|\n| 0|I bet if that mon...|[[document, 0, 20...|[[document, 0, 11...|[[token, 0, 0, I,...|\n| 0|James Shields Wil...|[[document, 0, 76...|[[document, 0, 76...|[[token, 0, 4, Ja...|\n| 0|There's no time t...|[[document, 0, 99...|[[document, 0, 78...|[[token, 0, 6, Th...|\n| 0|Team Specific Thr...|[[document, 0, 51...|[[document, 0, 15...|[[token, 0, 3, Te...|\n| 0|Ill give you a hi...|[[document, 0, 10...|[[document, 0, 19...|[[token, 0, 2, Il...|\n| 0|Star Wars, easy. ...|[[document, 0, 75...|[[document, 0, 15...|[[token, 0, 3, St...|\n| 0|You're adorable.\n...|[[document, 0, 22...|[[document, 0, 15...|[[token, 0, 5, Yo...|\n| 0|He actually acts ...|[[document, 0, 27...|[[document, 0, 49...|[[token, 0, 1, He...|\n| 0|Clinton struggles...|[[document, 0, 13...|[[document, 0, 13...|[[token, 0, 6, Cl...|\n| 0|Is that the Older...|[[document, 0, 69...|[[document, 0, 38...|[[token, 0, 1, Is...|\n+-----+--------------------+--------------------+--------------------+--------------------+\nonly showing top 20 rows\n\n709\n291\n"
],
[
"glove = WordEmbeddingsModel.pretrained()\ntrain_featurized = glove.transform(train)\ntrain_featurized.show()",
"glove_100d download started this may take some time.\nApproximate size to download 145.3 MB\n[OK!]\n+-----+--------------------+--------------------+--------------------+--------------------+--------------------+\n|label| comment| document| sentence| token| embeddings|\n+-----+--------------------+--------------------+--------------------+--------------------+--------------------+\n| 0|\"\"\"Agreed. I thin...|[[document, 0, 13...|[[document, 0, 9,...|[[token, 0, 2, \"\"...|[[word_embeddings...|\n| 0|\"\"\"It's kind of h...|[[document, 0, 11...|[[document, 0, 11...|[[token, 0, 2, \"\"...|[[word_embeddings...|\n| 0|\"\"\"People\"\"\nUmm, ...|[[document, 0, 54...|[[document, 0, 54...|[[token, 0, 2, \"\"...|[[word_embeddings...|\n| 0|\"\"\"QR Code\"\"\n\"For...|[[document, 0, 73...|[[document, 0, 73...|[[token, 0, 2, \"\"...|[[word_embeddings...|\n| 0|\"\"\"You are like t...|[[document, 0, 71...|[[document, 0, 71...|[[token, 0, 2, \"\"...|[[word_embeddings...|\n| 0|\"*Guilt roles ove...|[[document, 0, 19...|[[document, 0, 88...|[[token, 0, 1, \"*...|[[word_embeddings...|\n| 0|\"A quick google s...|[[document, 0, 44...|[[document, 0, 44...|[[token, 0, 0, \",...|[[word_embeddings...|\n| 0|\"BREAKING: Trump ...|[[document, 0, 13...|[[document, 0, 60...|[[token, 0, 0, \",...|[[word_embeddings...|\n| 0|\"BU only follows ...|[[document, 0, 16...|[[document, 0, 16...|[[token, 0, 0, \",...|[[word_embeddings...|\n| 0|\"Bug/Feature? Ver...|[[document, 0, 29...|[[document, 0, 12...|[[token, 0, 0, \",...|[[word_embeddings...|\n| 0|\"Electrical Engin...|[[document, 0, 20...|[[document, 0, 20...|[[token, 0, 0, \",...|[[word_embeddings...|\n| 0|\"Has Persona 5 ma...|[[document, 0, 75...|[[document, 0, 55...|[[token, 0, 0, \",...|[[word_embeddings...|\n| 0|\"Hey man, no need...|[[document, 0, 22...|[[document, 0, 22...|[[token, 0, 0, \",...|[[word_embeddings...|\n| 0|\"Hey... Don't you...|[[document, 0, 29...|[[document, 0, 6,...|[[token, 0, 0, \",...|[[word_embeddings...|\n| 0|\"I dunno, doesnt ...|[[document, 0, 40...|[[document, 0, 44...|[[token, 0, 0, \",...|[[word_embeddings...|\n| 0|\"I just worry (a ...|[[document, 0, 29...|[[document, 0, 67...|[[token, 0, 0, \",...|[[word_embeddings...|\n| 0|\"I love it becaus...|[[document, 0, 25...|[[document, 0, 12...|[[token, 0, 0, \",...|[[word_embeddings...|\n| 0|\"I still doubt th...|[[document, 0, 32...|[[document, 0, 73...|[[token, 0, 0, \",...|[[word_embeddings...|\n| 0|\"If your employee...|[[document, 0, 15...|[[document, 0, 11...|[[token, 0, 0, \",...|[[word_embeddings...|\n| 0|\"Irrelevant. You ...|[[document, 0, 22...|[[document, 0, 32...|[[token, 0, 0, \",...|[[word_embeddings...|\n+-----+--------------------+--------------------+--------------------+--------------------+--------------------+\nonly showing top 20 rows\n\n"
],
[
"test_featurized = glove.transform(test)\ntest_featurized.show()",
"+-----+--------------------+--------------------+--------------------+--------------------+--------------------+\n|label| comment| document| sentence| token| embeddings|\n+-----+--------------------+--------------------+--------------------+--------------------+--------------------+\n| 0|\"\"\"Did Hillary Cl...|[[document, 0, 24...|[[document, 0, 10...|[[token, 0, 2, \"\"...|[[word_embeddings...|\n| 0|\"\"\"Gingrich\nAnd C...|[[document, 0, 98...|[[document, 0, 98...|[[token, 0, 2, \"\"...|[[word_embeddings...|\n| 0|\"\"\"Hey you wanna ...|[[document, 0, 77...|[[document, 0, 74...|[[token, 0, 2, \"\"...|[[word_embeddings...|\n| 0|\"*Danny reaches f...|[[document, 0, 10...|[[document, 0, 63...|[[token, 0, 1, \"*...|[[word_embeddings...|\n| 0|\"@Senator_Assange...|[[document, 0, 18...|[[document, 0, 13...|[[token, 0, 0, \",...|[[word_embeddings...|\n| 0|\"Can we have a \"\"...|[[document, 0, 18...|[[document, 0, 56...|[[token, 0, 0, \",...|[[word_embeddings...|\n| 0|\"Dem Strategist M...|[[document, 0, 13...|[[document, 0, 13...|[[token, 0, 0, \",...|[[word_embeddings...|\n| 0|\"Dogs would sell ...|[[document, 0, 11...|[[document, 0, 11...|[[token, 0, 0, \",...|[[word_embeddings...|\n| 0|\"I like the one w...|[[document, 0, 12...|[[document, 0, 10...|[[token, 0, 0, \",...|[[word_embeddings...|\n| 0|\"If you were a Tr...|[[document, 0, 47...|[[document, 0, 59...|[[token, 0, 0, \",...|[[word_embeddings...|\n| 0|\"Im replying to h...|[[document, 0, 15...|[[document, 0, 10...|[[token, 0, 0, \",...|[[word_embeddings...|\n| 0|\"It would depend ...|[[document, 0, 59...|[[document, 0, 46...|[[token, 0, 0, \",...|[[word_embeddings...|\n| 0|\"Live Discussion ...|[[document, 0, 68...|[[document, 0, 10...|[[token, 0, 0, \",...|[[word_embeddings...|\n| 0|\"Most likely it w...|[[document, 0, 22...|[[document, 0, 22...|[[token, 0, 0, \",...|[[word_embeddings...|\n| 0|\"My roommate woul...|[[document, 0, 89...|[[document, 0, 12...|[[token, 0, 0, \",...|[[word_embeddings...|\n| 0|\"Next expansion, ...|[[document, 0, 94...|[[document, 0, 94...|[[token, 0, 0, \",...|[[word_embeddings...|\n| 0|\"Show me where I ...|[[document, 0, 27...|[[document, 0, 73...|[[token, 0, 0, \",...|[[word_embeddings...|\n| 0|\"Something that g...|[[document, 0, 31...|[[document, 0, 67...|[[token, 0, 0, \",...|[[word_embeddings...|\n| 0|\"That's funny. Al...|[[document, 0, 96...|[[document, 0, 13...|[[token, 0, 0, \",...|[[word_embeddings...|\n| 0|\"Those \"\"cuts\"\" i...|[[document, 0, 83...|[[document, 0, 83...|[[token, 0, 0, \",...|[[word_embeddings...|\n+-----+--------------------+--------------------+--------------------+--------------------+--------------------+\nonly showing top 20 rows\n\n"
],
[
"def get_features(row):\n result = []\n for tk in row:\n result.append(tk['embeddings'])\n return np.array(result)\n\ndef build_data(df, chunks=10):\n x_train = []\n y_train = []\n\n row_count = df.count()\n i = 0\n \n chunks = df.randomSplit(weights=[1/chunks] * chunks)\n\n for chunk in chunks:\n rows = chunk.collect()\n for row in rows:\n if i % 1000 == 0:\n print('row {} / {} ({:.1f} %)'.format(i, row_count, 100 * i / row_count))\n embeddings = get_features(row['embeddings'])\n label = row['label']\n x_train.append(embeddings)\n y_train.append(label)\n i += 1\n\n x_train = np.array(x_train)\n y_train = np.array(y_train)\n return x_train, y_train",
"_____no_output_____"
],
[
"x_train, y_train = build_data(train_featurized)",
"row 0 / 709 (0.0 %)\n"
],
[
"x_test, y_test = build_data(test_featurized)",
"row 0 / 291 (0.0 %)\n"
],
[
"spark.stop()",
"_____no_output_____"
],
[
"print('Train Labels:\\n', pd.Series(y_train).value_counts())\nprint('Test Labels:\\n', pd.Series(y_test).value_counts())",
"Train Labels:\n 0 663\n1 46\ndtype: int64\nTest Labels:\n 0 277\n1 14\ndtype: int64\n"
],
[
"from keras.preprocessing import sequence\nfrom keras.models import Sequential\nfrom keras.layers import Dense, Dropout, Activation\nfrom keras.layers import Embedding\nfrom keras.layers import Conv1D, GlobalMaxPooling1D\n\n# set parameters for our model:\nmaxlen = 100 #max 50 words per article\nbatch_size = 32 #size of the batch \nfilters = 50 #dimension of filters for the convolutional layer\nkernel_size = 3 #size of the kernel used in the convolutional layer\nhidden_dims = 250 #dimension of the hidden layer\nepochs = 5 #number of training epochs\n\nx_train = sequence.pad_sequences(x_train, maxlen=maxlen)\nx_test = sequence.pad_sequences(x_test, maxlen=maxlen)",
"Using TensorFlow backend.\n"
],
[
"print('Build model...')\nmodel = Sequential()\n\n# we add a Convolution1D, which will learn filters\n# word group filters of size filter_length:\nmodel.add(Conv1D(filters,\n kernel_size,\n padding='valid',\n activation='relu',\n strides=1))\n# we use max pooling:\nmodel.add(GlobalMaxPooling1D())\n\n# We add a vanilla hidden layer:\nmodel.add(Dense(hidden_dims))\nmodel.add(Dropout(0.2))\nmodel.add(Activation('relu'))\n\n# We project onto a single unit output layer, and squash it with a sigmoid:\nmodel.add(Dense(1))\nmodel.add(Activation('sigmoid'))\n\nfrom keras import backend as K\n\n\nmodel.compile(loss='binary_crossentropy',\n optimizer='adam',\n metrics=['accuracy','mae'])",
"Build model...\n"
],
[
"model.fit(x_train, y_train,\n batch_size=batch_size,\n epochs=epochs,\n validation_data=(x_test, y_test))",
"Train on 709 samples, validate on 291 samples\nEpoch 1/5\n709/709 [==============================] - 2s 2ms/step - loss: 0.3482 - accuracy: 0.8942 - mae: 0.1879 - val_loss: 0.1974 - val_accuracy: 0.9519 - val_mae: 0.0865\nEpoch 2/5\n709/709 [==============================] - 0s 325us/step - loss: 0.2448 - accuracy: 0.9351 - mae: 0.1161 - val_loss: 0.2031 - val_accuracy: 0.9519 - val_mae: 0.1207\nEpoch 3/5\n709/709 [==============================] - 0s 327us/step - loss: 0.2370 - accuracy: 0.9351 - mae: 0.1306 - val_loss: 0.1979 - val_accuracy: 0.9519 - val_mae: 0.1036\nEpoch 4/5\n709/709 [==============================] - 0s 324us/step - loss: 0.2315 - accuracy: 0.9351 - mae: 0.1147 - val_loss: 0.2000 - val_accuracy: 0.9519 - val_mae: 0.1096\nEpoch 5/5\n709/709 [==============================] - 0s 338us/step - loss: 0.2221 - accuracy: 0.9351 - mae: 0.1145 - val_loss: 0.2047 - val_accuracy: 0.9519 - val_mae: 0.1199\n"
],
[
"from IPython.display import Image\nfrom keras.utils.vis_utils import model_to_dot\ndot = model_to_dot(model)\nImage(dot.create_png())",
"_____no_output_____"
]
]
] | [
"code",
"markdown",
"code"
] | [
[
"code"
],
[
"markdown"
],
[
"code",
"code",
"code",
"code",
"code",
"code",
"code",
"code",
"code",
"code",
"code",
"code",
"code",
"code",
"code",
"code"
]
] |
e779d01eba76d7ab82478776b6b560088a06d18c | 17,184 | ipynb | Jupyter Notebook | trainUnet.ipynb | chitrakumarsai/Semantic-segmentation---Unet- | fdef7ce48ee661171436a9b9f8c78dbbb4c321e6 | [
"MIT"
] | null | null | null | trainUnet.ipynb | chitrakumarsai/Semantic-segmentation---Unet- | fdef7ce48ee661171436a9b9f8c78dbbb4c321e6 | [
"MIT"
] | null | null | null | trainUnet.ipynb | chitrakumarsai/Semantic-segmentation---Unet- | fdef7ce48ee661171436a9b9f8c78dbbb4c321e6 | [
"MIT"
] | null | null | null | 86.351759 | 1,682 | 0.670158 | [
[
[
"from model import *\nfrom data import *",
"Using TensorFlow backend.\n"
]
],
[
[
"## Train your Unet with membrane data\nmembrane data is in folder membrane/, it is a binary classification task.\n\nThe input shape of image and mask are the same :(batch_size,rows,cols,channel = 1)",
"_____no_output_____"
],
[
"### Train with data generator",
"_____no_output_____"
]
],
[
[
"data_gen_args = dict(rotation_range=0.2,\n width_shift_range=0.05,\n height_shift_range=0.05,\n shear_range=0.05,\n zoom_range=0.05,\n horizontal_flip=True,\n fill_mode='nearest')\nmyGene = trainGenerator(2,'data/membrane/train','image','label',data_gen_args,save_to_dir = None)\nmodel = unet()\nmodel_checkpoint = ModelCheckpoint('unet_membrane.hdf5', monitor='loss',verbose=1, save_best_only=True)\nmodel.fit_generator(myGene,steps_per_epoch=2000,epochs=5,callbacks=[model_checkpoint])",
"_____no_output_____"
]
],
[
[
"### Train with npy file",
"_____no_output_____"
]
],
[
[
"#imgs_train,imgs_mask_train = geneTrainNpy(\"data/membrane/train/aug/\",\"data/membrane/train/aug/\")\n#model.fit(imgs_train, imgs_mask_train, batch_size=2, nb_epoch=10, verbose=1,validation_split=0.2, shuffle=True, callbacks=[model_checkpoint])",
"_____no_output_____"
]
],
[
[
"### test your model and save predicted results",
"_____no_output_____"
]
],
[
[
"testGene = testGenerator(\"data/membrane/test\")\nmodel = unet()\nmodel.load_weights(\"unet_membrane.hdf5\")\nresults = model.predict_generator(testGene,30,verbose=1)\nsaveResult(\"data/membrane/test\",results)",
"C:\\Users\\xuhaozhi\\Documents\\Study\\unet\\model.py:34: UserWarning: The `merge` function is deprecated and will be removed after 08/2017. Use instead layers from `keras.layers.merge`, e.g. `add`, `concatenate`, etc.\n merge6 = merge([drop4,up6], mode = 'concat', concat_axis = 3)\nC:\\SoftWare\\Anaconda2\\envs\\python3\\lib\\site-packages\\keras\\legacy\\layers.py:465: UserWarning: The `Merge` layer is deprecated and will be removed after 08/2017. Use instead layers from `keras.layers.merge`, e.g. `add`, `concatenate`, etc.\n name=name)\nC:\\Users\\xuhaozhi\\Documents\\Study\\unet\\model.py:39: UserWarning: The `merge` function is deprecated and will be removed after 08/2017. Use instead layers from `keras.layers.merge`, e.g. `add`, `concatenate`, etc.\n merge7 = merge([conv3,up7], mode = 'concat', concat_axis = 3)\nC:\\Users\\xuhaozhi\\Documents\\Study\\unet\\model.py:44: UserWarning: The `merge` function is deprecated and will be removed after 08/2017. Use instead layers from `keras.layers.merge`, e.g. `add`, `concatenate`, etc.\n merge8 = merge([conv2,up8], mode = 'concat', concat_axis = 3)\nC:\\Users\\xuhaozhi\\Documents\\Study\\unet\\model.py:49: UserWarning: The `merge` function is deprecated and will be removed after 08/2017. Use instead layers from `keras.layers.merge`, e.g. `add`, `concatenate`, etc.\n merge9 = merge([conv1,up9], mode = 'concat', concat_axis = 3)\nC:\\Users\\xuhaozhi\\Documents\\Study\\unet\\model.py:55: UserWarning: Update your `Model` call to the Keras 2 API: `Model(inputs=Tensor(\"in..., outputs=Tensor(\"co...)`\n model = Model(input = inputs, output = conv10)\n"
]
]
] | [
"code",
"markdown",
"code",
"markdown",
"code",
"markdown",
"code"
] | [
[
"code"
],
[
"markdown",
"markdown"
],
[
"code"
],
[
"markdown"
],
[
"code"
],
[
"markdown"
],
[
"code"
]
] |
Subsets and Splits
No community queries yet
The top public SQL queries from the community will appear here once available.