hexsha
stringlengths 40
40
| size
int64 6
14.9M
| ext
stringclasses 1
value | lang
stringclasses 1
value | max_stars_repo_path
stringlengths 6
260
| max_stars_repo_name
stringlengths 6
119
| max_stars_repo_head_hexsha
stringlengths 40
41
| max_stars_repo_licenses
sequence | max_stars_count
int64 1
191k
⌀ | max_stars_repo_stars_event_min_datetime
stringlengths 24
24
⌀ | max_stars_repo_stars_event_max_datetime
stringlengths 24
24
⌀ | max_issues_repo_path
stringlengths 6
260
| max_issues_repo_name
stringlengths 6
119
| max_issues_repo_head_hexsha
stringlengths 40
41
| max_issues_repo_licenses
sequence | max_issues_count
int64 1
67k
⌀ | max_issues_repo_issues_event_min_datetime
stringlengths 24
24
⌀ | max_issues_repo_issues_event_max_datetime
stringlengths 24
24
⌀ | max_forks_repo_path
stringlengths 6
260
| max_forks_repo_name
stringlengths 6
119
| max_forks_repo_head_hexsha
stringlengths 40
41
| max_forks_repo_licenses
sequence | max_forks_count
int64 1
105k
⌀ | max_forks_repo_forks_event_min_datetime
stringlengths 24
24
⌀ | max_forks_repo_forks_event_max_datetime
stringlengths 24
24
⌀ | avg_line_length
float64 2
1.04M
| max_line_length
int64 2
11.2M
| alphanum_fraction
float64 0
1
| cells
sequence | cell_types
sequence | cell_type_groups
sequence |
---|---|---|---|---|---|---|---|---|---|---|---|---|---|---|---|---|---|---|---|---|---|---|---|---|---|---|---|---|---|---|
e77e119348d3c2c200541b9dc67ae7125c3a15fb | 258,489 | ipynb | Jupyter Notebook | examples/_debug/legend.ipynb | CristianPachacama/cartoframes | 3dc4e10d175069a7d7b734db3d9526127aad9dec | [
"BSD-3-Clause"
] | 1 | 2020-11-23T23:44:32.000Z | 2020-11-23T23:44:32.000Z | examples/_debug/legend.ipynb | CristianPachacama/cartoframes | 3dc4e10d175069a7d7b734db3d9526127aad9dec | [
"BSD-3-Clause"
] | null | null | null | examples/_debug/legend.ipynb | CristianPachacama/cartoframes | 3dc4e10d175069a7d7b734db3d9526127aad9dec | [
"BSD-3-Clause"
] | null | null | null | 40.636535 | 867 | 0.424146 | [
[
[
"from cartoframes.auth import set_default_credentials\nfrom cartoframes.viz import Map, Layer, Source, Style, Legend\n\nset_default_credentials('cartovl')",
"_____no_output_____"
],
[
"# Legend color\nmap = Map(\n Layer(\n Source('populated_places'),\n Style('color: ramp(top($adm0name, 5), bold)'),\n legend=Legend({\n 'type': 'color-category',\n 'title': '[TITLE]',\n 'description': '[description]',\n 'footer': '[footer]'\n })\n )\n)\n\n# Legend color + sugar\nMap(\n Layer(\n 'populated_places',\n 'color: ramp(top($adm0name, 5), bold)',\n legend={\n 'type': 'color-category',\n 'title': '[TITLE]',\n 'description': '[description]',\n 'footer': '[footer]'\n }\n )\n)",
"_____no_output_____"
],
[
"# Legend strokeColor\nMap(\n Layer(\n 'populated_places',\n 'color: transparent strokeWidth: 1 strokeColor: ramp(top($adm0name, 5), bold)',\n legend={\n 'type': 'color-category',\n 'prop': 'strokeColor',\n 'title': '[TITLE]',\n 'description': '[description]'\n }\n )\n)",
"_____no_output_____"
],
[
"# Legend: only info\nMap(\n Layer(\n 'populated_places',\n legend={\n 'title': '[TITLE]',\n 'description': '[description]',\n 'footer': '[footer]'\n }\n )\n)",
"_____no_output_____"
],
[
"# Legend: color\nMap(\n Layer(\n 'populated_places',\n legend={\n 'type': 'color-category'\n }\n )\n)",
"_____no_output_____"
],
[
"# Legend: size\nMap(\n Layer(\n 'populated_places',\n '''\n width: ramp($pop_max, [0, 50])\n strokeWidth: 1\n strokeColor: opacity(white, 0.4)\n ''',\n legend={\n 'type': 'size-bins'\n }\n )\n)",
"_____no_output_____"
],
[
"# Legend: complete\nMap(\n Layer(\n 'SELECT * FROM populated_places WHERE adm0name = \\'Spain\\'',\n 'color: ramp(globalQuantiles($pop_max, 5), reverse(purpor))',\n legend={\n 'type': 'color-category',\n 'title': 'Population'\n }\n )\n)",
"_____no_output_____"
],
[
"Map(\n Layer('populated_places'),\n default_legend=True\n)",
"_____no_output_____"
]
]
] | [
"code"
] | [
[
"code",
"code",
"code",
"code",
"code",
"code",
"code",
"code"
]
] |
e77e1242b13ce4bf21960fadb30c638e647c9249 | 17,175 | ipynb | Jupyter Notebook | Color_Ai/Learner_Color_Ai/Color AI.ipynb | xxmeowxx/AI-s | 9f062b08765314142791a2342d25c1f7a7498863 | [
"MIT"
] | 1 | 2020-08-27T08:07:33.000Z | 2020-08-27T08:07:33.000Z | Color_Ai/Learner_Color_Ai/Color AI.ipynb | xxmeowxx/AI-s | 9f062b08765314142791a2342d25c1f7a7498863 | [
"MIT"
] | null | null | null | Color_Ai/Learner_Color_Ai/Color AI.ipynb | xxmeowxx/AI-s | 9f062b08765314142791a2342d25c1f7a7498863 | [
"MIT"
] | null | null | null | 37.997788 | 467 | 0.441572 | [
[
[
"<h1><p style=\"text-align:center\">ColorAI</p></h1>\n<p style=\"text-align:center\">By: Mark John A. Velmonte</p>\n\n<p style=\"text-align: justify;\">ColorAi is a type of <span style=\"font-weight: bold;\">simple</span> supervised classification machine learning AI. It can classify what shade of color the given rgb is and can also learn new color base on what the teacher teach it. The performance of this AI will depend on what you teach it. It uses <span style=\"font-weight: bold;\">KNN (K-nearest neighbor) and Random Forest algorithm's </span> to calculate inputs</p>\n\n\n###### dependencies\n1. python3\n1. pandas\n1. numpy\n1. sklearn\n1. matplotlib\n\n\n<br><br><br>\n\n\n### Class\n\n##### ColorAI(n_neighbors):\n parameters:\n> n_neigbors : default value 15\n> set number of neigbors for KNN algorithm\n\n\n\n<br><br><br>\n\n\n### Methods\n\n###### showMethods()\n parameter:\n> Accept no parameter\n<br>\n> Print all availble methods \n\n<br><br>\n###### showDataMemory()\n parameter:\n> Accept no parameter\n<br>\n> Print out all the data in datasets\n\n<br><br>\n###### accuracyTest()\n parameter:\n> Accept no parameter\n<br>\n> Print out the accuracy of the data set being use\n\n<br><br>\n###### getColor(color_inp, data_ref, ret_val, show_predicted)\n parameter:\n> color_inp = list of 3 intiger.\n<br>\n> data_ref = A read csv file\n<br>\n> ret_val = bool, if True it will return a value of a color name. default value False\n<br>\n> show_predicted = bool, if True will print a value of a color name. default value True\n\n<br><br>\n##### teach(save_count)\nThis method will ask for a rgb value of color and will try to predict that color. \n<br>\n parameter\n> save_count = int, number of times the new data will added to the data sets, default value 1\n\n<br><br>\n###### getColorFromImage(show_plot, show_info, read_img ):\n parameter\n> show_plot = bool, if True will plot the image, default value False\n<br>\n> show_info = bool, if True will print all the information about the image, default value False\n<br>\n> read_img = string either (\"strips\", \"full\"), \"strips\" value will proccess the image by slicing it on top bottom and middle to find a prominent color on image, \"full\" value will process the whole image (This method takes longer to process)",
"_____no_output_____"
]
],
[
[
"import pandas as pd\nimport matplotlib.pyplot as plt\nimport numpy as np\nimport re\nfrom datetime import datetime\nfrom sklearn import metrics\nfrom sklearn.model_selection import train_test_split\nimport matplotlib.image as mpimg\nfrom sklearn.neighbors import KNeighborsClassifier\nfrom sklearn.ensemble import RandomForestClassifier\nfrom PIL import Image",
"_____no_output_____"
],
[
"class ColorAI():\n def __init__(self, n_neighbors = 15):\n self.trained_data = pd.read_csv(\"learned_color_data.csv\")\n self.number_of_neighbors = n_neighbors\n \n \n def showMethods(self):\n print(\"showDataMemory, accuracyTest, getColor, showDataFrame, teach, getColorFromImage\")\n \n\n def showDataMemory(self):\n color_name_guide = self.trained_data[\"Color name\"]\n result_color_name = color_name_guide.drop_duplicates()\n\n color_id = self.trained_data[\"Id\"]\n result_color_id = color_id.drop_duplicates()\n user_guide = pd.DataFrame({\"Color family\" : result_color_name, \"ID\" : result_color_id})\n\n print(user_guide)\n \n \n def accuracyTest(self):\n test_data = self.trained_data\n\n X = test_data.iloc[:, :-2].values\n y = test_data[\"Id\"]\n\n\n X_train, X_test, y_train, y_test = train_test_split(X, y, test_size=0.3)\n\n knn = KNeighborsClassifier(n_neighbors = self.number_of_neighbors)\n knn.fit(X_train, y_train)\n\n y_pred = knn.predict(X_test)\n \n print(y_pred)\n print(\"Accuracy:\",metrics.accuracy_score(y_test, y_pred))\n \n \n def getColor(self, color_inp, data_ref, ret_val = \"False\", show_predicted = True):\n self.data = data_ref\n\n R = self.data[\"R\"]\n G = self.data[\"G\"]\n B = self.data[\"B\"]\n\n X = self.data.iloc[:, :-2].values\n y = self.data[\"Id\"]\n\n\n model = KNeighborsClassifier(n_neighbors = self.number_of_neighbors)\n model.fit(X, y)\n\n\n u_input = color_inp\n\n\n prediction = model.predict([u_input])\n\n self.prediction_index = np.where(self.data == prediction[0])[0][0]\n \n if show_predicted == True:\n print(\"prediction:\", self.data[\"Color name\"][self.prediction_index])\n \n elif show_predicted == False:\n pass\n \n else:\n print(\"no such parameter\")\n \n \n if ret_val == True:\n return prediction\n\n \n def showDataFrame(self):\n pd.set_option(\"display.max_rows\", 10000)\n print(self.trained_data)\n \n \n \n def teach(self, save_count = 1):\n teach_status = \"T\"\n n_test = 0\n n_correct = 0\n n_wrong = 0\n \n while teach_status == \"T\":\n print(\"-\" * 10 + str(n_test) +\"-\" * 10)\n \n n_test += 1\n \n uinp = input(\"color:\")\n uinp_enc = re.split(\",\", uinp)\n print(\"inp\", uinp_enc)\n RGB = []\n \n for num in uinp_enc:\n print(num)\n RGB.append(int(num))\n \n print(\"rgb\", type(RGB[1]))\n\n self.getColor(RGB, self.trained_data)\n\n answer_status = input(\"answer status C/W:\")\n\n if answer_status == \"C\":\n n_correct += 1\n \n R = RGB[0]\n G = RGB[1]\n B = RGB[2]\n \n print(R, G, B)\n save_count = save_count\n while save_count > 0:\n shade_fam = self.data[\"Color name\"][self.prediction_index]\n data_id = self.data[\"Id\"][self.prediction_index]\n new_data = pd.DataFrame({\"R\":R, \"G\":G, \"B\":B, \"Color name\":shade_fam, \"Id\":data_id}, index = [0])\n\n self.trained_data = pd.concat([new_data, self.trained_data]).reset_index(drop = True)\n self.trained_data.to_csv(\"learned_color_data.csv\", index=False)\n \n save_count -= 1\n if save_count == 0: break\n \n \n\n elif answer_status == \"W\":\n R = RGB[0]\n G = RGB[1]\n B = RGB[2]\n \n n_wrong += 1\n\n add_learnings = input(\"Add New Lesson? Y/N :\")\n \n if add_learnings == \"Y\":\n \n \n self.showDataMemory()\n \n shade_fam = input(\"shader family:\")\n data_id = int(input(\"new data id:\"))\n \n save_count = save_count\n \n while save_count > 0:\n new_data = pd.DataFrame({\"R\":R, \"G\":G, \"B\":B, \"Color name\":shade_fam, \"Id\":data_id}, index = [0])\n\n self.trained_data = pd.concat([new_data, self.trained_data]).reset_index(drop = True)\n self.trained_data.to_csv(\"learned_color_data.csv\", index=False)\n \n save_count -= 1\n if save_count == 0: break\n \n else:\n print(\"input error\")\n break\n \n \n if teach_status == \"F\":\n print(\"-\" * 10 + \"teaching ended\" + \"-\" * 10)\n print(\"number of tests : \", n_test)\n print(\"correct answer : \", n_correct)\n print(\"wrong answer : \", n_wrong)\n break\n \n teach_status = input(\"teaching status T/F:\")\n \n \n \n \n def getColorFromImage(self, show_plot = False, show_info = False, read_img = \"strips\"):\n uinp = input(\"image:\")\n \n if read_img == \"strips\":\n print(\"analizyng image\")\n \n try:\n image_inp = mpimg.imread(uinp)\n except Exception as error:\n print(error)\n \n\n image_size = np.array(image_inp)\n image_total_pixel = int((image_inp.shape[2] * image_inp.shape[1] * image_inp.shape[0]))\n\n dim1 = int(image_total_pixel / 3)\n\n image_data = image_size.reshape(dim1, 3)\n\n seq_shape = int((image_inp[0:50].shape[2] * image_inp[0:50].shape[1] * image_inp[0:50].shape[0]) / 3)\n\n sequence_1 = np.array(image_inp[0:50]).reshape(seq_shape, 3)\n sequence_2 = np.array(image_inp[ int(image_inp.shape[0] / 2): int((image_inp.shape[0] / 2) + 50)]).reshape(seq_shape, 3)\n sequence_3 = np.array(image_inp[ int(image_inp.shape[0] - 50 ): int(image_inp.shape[0])]).reshape(seq_shape, 3)\n\n\n print(\"---\" * 15 + \"---\" * 15 )\n\n if show_plot == True:\n fig, axs = plt.subplots(3)\n\n\n axs[0].imshow(image_inp[0:50])\n axs[1].imshow(image_inp[ int(image_inp.shape[0] / 2): int((image_inp.shape[0] / 2) + 50)])\n axs[2].imshow(image_inp[ int(image_inp.shape[0] - 50 ): int(image_inp.shape[0])])\n\n\n\n readings = np.array([sequence_1, sequence_2, sequence_3])\n tota_pixels = readings.shape[2]* readings.shape[1] * readings.shape[0]\n\n enc_reading = readings.reshape(int(tota_pixels / 3), 3)\n\n\n data = pd.read_csv(\"learned_color_data.csv\")\n\n Red_pixel = data[\"R\"]\n Green_pixel = data[\"G\"]\n Blue_pixel = data[\"B\"]\n\n feat = np.array([Red_pixel, Green_pixel, Blue_pixel])\n\n\n X = data.iloc[:, :-2].values\n y = data[\"Id\"]\n\n \n model = RandomForestClassifier(max_depth=100, random_state=0)\n model.fit(X, y)\n\n prediction = model.predict(enc_reading)\n\n\n result_color_name = pd.DataFrame({\"answers\" : prediction}).drop_duplicates()\n\n answers = np.array(result_color_name[\"answers\"])\n\n\n if show_info == True:\n print(\"INFORMATION:\" + \"\\n\")\n print(\"colors found\", answers)\n self.showDataMemory()\n\n\n turn = 0\n n_total = 0\n ans_arr = []\n\n for index in answers:\n for pixel in prediction:\n if pixel == answers[turn]:\n n_total += 1\n\n turn += 1\n ans_arr.append(n_total)\n n_total -= n_total\n\n if turn >= answers.shape[0]:\n break\n\n superior = np.max(ans_arr)\n answer_index = ans_arr.index(superior)\n\n final_answer_index = answers[answer_index]\n final_answer = np.where(data[\"Id\"] == final_answer_index)[0][0]\n\n print(\"\\n\" + \"Prominent Color:\", data[\"Color name\"].iloc[final_answer])\n \n \n if read_img == \"full\":\n print(\"analyzing image. It will take time depending on the size of the image and tour proccesssing power\")\n \n res_img = Image.open(uinp)\n \n img_height = res_img.size[1]\n img_width = res_img.size[0]\n\n res_img = res_img.resize((int(img_width / 2), int(img_height / 2)),Image.ANTIALIAS)\n res_img.save(\"images/res_image.jpg\",optimize=True,quality=100)\n \n res_img = mpimg.imread(\"images/res_image.jpg\")\n \n img_array = np.array(res_img)\n \n print(img_array.shape)\n \n dimension = img_array.shape[0] * img_array.shape[1]\n img_array = img_array.reshape(dimension, 3)\n \n print(img_array.shape)\n \n color_found = []\n \n data = pd.read_csv(\"learned_color_data.csv\")\n \n count = 0\n for color in img_array: \n color_found.append(self.getColor(color, data, ret_val = True, show_predicted = False)[0])\n print(color_found)\n count += 1\n \n if count >= 20:\n break\n \n \n result_color_name = pd.DataFrame({\"answers\" : color_found}).drop_duplicates()\n answers = np.array(result_color_name[\"answers\"])\n \n self.showDataMemory()\n print(\"found colors:\", answers)",
"_____no_output_____"
]
]
] | [
"markdown",
"code"
] | [
[
"markdown"
],
[
"code",
"code"
]
] |
e77e4136d192821f3db165e845b2cf12010f03bb | 94,530 | ipynb | Jupyter Notebook | Chapter4-3.ipynb | huiselilun/LBM_Applications | 2af107683d63995e5cc960e029c1dc508ee3f6ea | [
"MIT"
] | 3 | 2020-06-30T03:11:47.000Z | 2021-01-16T07:01:17.000Z | Chapter4-3.ipynb | huiselilun/LBM_Applications | 2af107683d63995e5cc960e029c1dc508ee3f6ea | [
"MIT"
] | null | null | null | Chapter4-3.ipynb | huiselilun/LBM_Applications | 2af107683d63995e5cc960e029c1dc508ee3f6ea | [
"MIT"
] | 1 | 2021-01-16T07:01:23.000Z | 2021-01-16T07:01:23.000Z | 484.769231 | 88,800 | 0.930382 | [
[
[
"# A.2.5 The LBM Code (D2Q9)",
"_____no_output_____"
]
],
[
[
"# LBM advection-diffusion D2Q9\n\nimport numpy as np\nimport matplotlib.pyplot as plt\n% matplotlib inline\n\nn = 100\nm = 100\nf = np.zeros((9,n+1,m+1), dtype=float)\nfeq = np.zeros(9,dtype=float)\nrho = np.zeros((n+1,m+1), dtype=float)\nx = np.zeros(n+1, dtype=float)\ny = np.zeros(m+1,dtype=float)\nw = np.zeros(9,dtype=float)\n\nu = 1.0\nv = 0.4\ndt = 1.0\ndx = 1.0\ndy = 1.0\nfor i in range(1, n+1):\n x[i] = x[i-1] + dx\nfor j in range(1, m+1):\n y[i] = y[i-1] + dy\n \ntw = 1.0\nalpha = 1.0\nck = dx/dt\ncsq = ck*ck\nomega = 1.0/(3.*alpha/(csq*dt) + 0.5)\nmstep = 400\nw = [4/9,1/9, 1/9, 1/9, 1/9, 1/36, 1/36, 1/36, 1/36]\ndensity = 0.\nfor j in range(0,m+1):\n for i in range(0,n+1):\n for k in range(0,9):\n f[k,i,j] = w[k] * density\n if(i == 0) :\n f[k,i,j] = w[k] * tw\n \n \nfor kk in range(1,mstep+1):\n for j in range(0,m+1):\n for i in range(0,n+1):\n sum = 0.0\n for k in range(0,9):\n sum += f[k,i,j] \n rho[i,j] = sum\n \n for j in range(0,m+1):\n for i in range(0,n+1):\n feq[0] = w[0]*rho[i,j]\n feq[1] = w[1]*rho[i,j]*(1. + 3.*u/ck)\n feq[2] = w[2]*rho[i,j]*(1. + 3.*v/ck)\n feq[3] = w[3]*rho[i,j]*(1. - 3.*u/ck)\n feq[4] = w[4]*rho[i,j]*(1. - 3.*v/ck)\n feq[5] = w[5]*rho[i,j]*(1. + 3.*(u+v)/ck)\n feq[6] = w[6]*rho[i,j]*(1. + 3.*(-u+v)/ck)\n feq[7] = w[7]*rho[i,j]*(1. + 3.*(-u-v)/ck)\n feq[8] = w[8]*rho[i,j]*(1. + 3.*(u-v)/ck)\n for k in range(0,9):\n f[k,i,j] = omega*feq[k] + (1.-omega)*f[k,i,j]\n \n # streaming\n for j in range(m,-1,-1):\n for i in range(0,n):\n f[2,i,j] = f[2,i,j-1]\n f[6,i,j] = f[6,i+1,j-1]\n \n for j in range(m,-1,-1):\n for i in range(n,0,-1):\n f[1,i,j] = f[1,i-1,j]\n f[5,i,j] = f[5,i-1,j-1]\n \n for j in range(0,m):\n for i in range(n,0,-1):\n f[4,i,j] = f[4,i,j+1]\n f[8,i,j] = f[8,i-1,j+1]\n \n for j in range(0,m):\n for i in range(0,n):\n f[3,i,j] = f[3,i+1,j]\n f[7,i,j] = f[7,i+1,j+1]\n \n # boundary condition\n # left boundary condition ,the temperature is given,tw\n for j in range(0,m+1):\n f[1,0,j] = w[1]*tw + w[3]*tw - f[3,0,j]\n f[5,0,j] = w[5]*tw + w[7]*tw - f[7,0,j]\n f[8,0,j] = w[8]*tw + w[6]*tw - f[6,0,j]\n \n # right boundary condition, T = 0\n for j in range(0,m+1):\n f[6,n,j] = -f[8,n,j]\n f[3,n,j] = -f[1,n,j]\n f[7,n,j] = -f[5,n,j]\n f[2,n,j] = -f[4,n,j]\n f[0,n,j] = 0.0\n \n # top boundary condition, T = 0.0\n for i in range(0,n+1):\n f[8,i,m] = -f[6,i,m]\n f[7,i,m] = -f[5,i,m]\n f[4,i,m] = -f[2,i,m]\n f[1,i,m] = -f[3,i,m]\n f[0,i,m] = 0.0\n \n # bottom boundary condition, T = 0.0\n for i in range(0,n+1):\n f[2,i,0] = -f[4,i,0]\n f[6,i,0] = -f[8,i,0]\n f[5,i,0] = -f[7,i,0]\n f[1,i,0] = -f[3,i,0]\n f[0,i,0] = 0.0\n \nfor j in range(0,m+1):\n for i in range(0,n+1):\n sum = 0.0\n for k in range(0,9):\n sum += f[k,i,j] \n rho[i,j] = sum",
"_____no_output_____"
],
[
"temp = rho[:,50]\nfig = plt.figure(figsize=(15, 5))\nplt.subplot(1, 3, 3)\nplt.plot(temp)\n\nplt.subplot(1, 3, 2)\nplt.contour(rho,16,linewidths=0.5)\nplt.colorbar()\n\nplt.subplot(1, 3, 1)\nplt.imshow(rho, interpolation='nearest', origin='lower')\nplt.colorbar()\n\nplt.show()",
"_____no_output_____"
]
]
] | [
"markdown",
"code"
] | [
[
"markdown"
],
[
"code",
"code"
]
] |
e77e479f643cf6c3834d5f54b78bb4c2ff3104f4 | 31,359 | ipynb | Jupyter Notebook | tables/automl/notebooks/music_recommendation/music_recommendation.ipynb | CodingFanSteve/python-docs-samples | c438ba15fbd1c4288d7a243ad597bce1d8ac075d | [
"Apache-2.0"
] | 1 | 2019-09-26T07:09:48.000Z | 2019-09-26T07:09:48.000Z | tables/automl/notebooks/music_recommendation/music_recommendation.ipynb | CodingFanSteve/python-docs-samples | c438ba15fbd1c4288d7a243ad597bce1d8ac075d | [
"Apache-2.0"
] | 320 | 2020-11-08T21:02:43.000Z | 2022-02-10T10:43:29.000Z | tables/automl/notebooks/music_recommendation/music_recommendation.ipynb | CodingFanSteve/python-docs-samples | c438ba15fbd1c4288d7a243ad597bce1d8ac075d | [
"Apache-2.0"
] | null | null | null | 36.720141 | 612 | 0.602857 | [
[
[
"# Copyright 2019 Google LLC\n#\n# Licensed under the Apache License, Version 2.0 (the \"License\");\n# you may not use this file except in compliance with the License.\n# You may obtain a copy of the License at\n#\n# https://www.apache.org/licenses/LICENSE-2.0\n#\n# Unless required by applicable law or agreed to in writing, software\n# distributed under the License is distributed on an \"AS IS\" BASIS,\n# WITHOUT WARRANTIES OR CONDITIONS OF ANY KIND, either express or implied.\n# See the License for the specific language governing permissions and\n# limitations under the License.",
"_____no_output_____"
]
],
[
[
"# Music Recommendation using AutoML Tables\n\n## Overview\nIn this notebook we will see how [AutoML Tables](https://cloud.google.com/automl-tables/) can be used to make music recommendations to users. AutoML Tables is a supervised learning service for structured data that can vastly simplify the model building process.\n\n### Dataset\nAutoML Tables allows data to be imported from either GCS or BigQuery. This tutorial uses the [ListenBrainz](https://console.cloud.google.com/marketplace/details/metabrainz/listenbrainz) dataset from [Cloud Marketplace](https://console.cloud.google.com/marketplace), hosted in BigQuery.\n\nThe ListenBrainz dataset is a log of songs played by users, some notable pieces of the schema include:\n - **user_name:** a user id.\n - **track_name:** a song id.\n - **artist_name:** the artist of the song.\n - **release_name:** the album of the song.\n - **tags:** the genres of the song.\n\n### Objective\nThe goal of this notebook is to demonstrate how to create a lookup table in BigQuery of songs to recommend to users using a log of user-song listens and AutoML Tables. This will be done by training a binary classification model to predict whether or not a `user` will like a given `song`. In the training data, liking a song was defined as having listened to a song more than twice. **Using the predictions for every `(user, song)` pair to generate a ranking of the most similar songs for each user.**\n\nAs the number of `(user, song)` pairs grows exponentially with the number of unique users and songs, this approach may not be optimal for extremely large datasets. One workaround would be to train a model that learns to embed users and songs in the same embedding space, and use a nearest-neighbors algorithm to get recommendations for users. Unfortunately, AutoML Tables does not expose any feature for training and using embeddings, so a [custom ML model](https://github.com/GoogleCloudPlatform/professional-services/tree/master/examples/cloudml-collaborative-filtering) would need to be used instead.\n\nAnother recommendation approach that is worth mentioning is [using extreme multiclass classification](https://ai.google/research/pubs/pub45530), as that also circumvents storing every possible pair of users and songs. Unfortunately, AutoML Tables does not support the multiclass classification of more than [100 classes](https://cloud.google.com/automl-tables/docs/prepare#target-requirements).\n\n### Costs\nThis tutorial uses billable components of Google Cloud Platform (GCP):\n- Cloud AutoML Tables\n\nLearn about [AutoML Tables pricing](https://cloud.google.com/automl-tables/pricing), and use the [Pricing Calculator](https://cloud.google.com/products/calculator/) to generate a cost estimate based on your projected usage.",
"_____no_output_____"
],
[
"## 1. Setup",
"_____no_output_____"
],
[
"Follow the [AutoML Tables documentation](https://cloud.google.com/automl-tables/docs/) to\n* [Enable billing](https://cloud.google.com/billing/docs/how-to/modify-project).\n* [Enable AutoML API](https://console.cloud.google.com/apis/library/automl.googleapis.com?q=automl)",
"_____no_output_____"
],
[
"### 1.1 PIP Install Packages and dependencies\nInstall addional dependencies not installed in the notebook environment.",
"_____no_output_____"
]
],
[
[
"! pip install --upgrade --quiet google-cloud-automl google-cloud-bigquery",
"_____no_output_____"
]
],
[
[
"Restart the kernel to allow `automl_v1beta1` to be imported. The following cell should succeed after a kernel restart:",
"_____no_output_____"
]
],
[
[
"from google.cloud import automl_v1beta1",
"_____no_output_____"
]
],
[
[
"### 1.2 Import libraries and define constants",
"_____no_output_____"
],
[
"Populate the following cell with the necessary constants and run it to initialize constants and create clients for BigQuery and AutoML Tables.",
"_____no_output_____"
]
],
[
[
"# The GCP project id.\nPROJECT_ID = \"\"\n# The region to use for compute resources (AutoML isn't supported in some regions).\nLOCATION = \"us-central1\"\n# A name for the AutoML tables Dataset to create.\nDATASET_DISPLAY_NAME = \"\"\n# The BigQuery dataset to import data from (doesn't need to exist).\nINPUT_BQ_DATASET = \"\"\n# The BigQuery table to import data from (doesn't need to exist).\nINPUT_BQ_TABLE = \"\"\n# A name for the AutoML tables model to create.\nMODEL_DISPLAY_NAME = \"\"\n# The number of hours to train the model.\nMODEL_TRAIN_HOURS = 0\n\nassert all([\n PROJECT_ID,\n LOCATION,\n DATASET_DISPLAY_NAME,\n INPUT_BQ_DATASET,\n INPUT_BQ_TABLE,\n MODEL_DISPLAY_NAME,\n MODEL_TRAIN_HOURS,\n])",
"_____no_output_____"
]
],
[
[
"Import relevant packages and initialize clients for BigQuery and AutoML Tables.",
"_____no_output_____"
]
],
[
[
"from __future__ import absolute_import\nfrom __future__ import division\nfrom __future__ import print_function\n\nfrom google.cloud import automl_v1beta1\nfrom google.cloud import bigquery\nfrom google.cloud import exceptions\nimport seaborn as sns\n\n%matplotlib inline\n\n\ntables_client = automl_v1beta1.TablesClient(project=PROJECT_ID, region=LOCATION)\nbq_client = bigquery.Client()",
"_____no_output_____"
]
],
[
[
"## 2. Create a Dataset",
"_____no_output_____"
],
[
"In order to train a model, a structured dataset must be injested into AutoML tables from either BigQuery or Google Cloud Storage. Once injested, the user will be able to cherry pick columns to use as features, labels, or weights and configure the loss function.",
"_____no_output_____"
],
[
"### 2.1 Create BigQuery table",
"_____no_output_____"
],
[
"First, do some feature engineering on the original ListenBrainz dataset to turn it into a dataset for training and export it into a seperate BigQuery table:\n\n 1. Make each sample a unique `(user, song)` pair.\n 2. For features, use the user's top 10 songs ever played and the song's number of albums, artist, and genres.\n 3. For a label, use the number of times the user has listened to the song, normalized by dividing by the maximum number of times that user has listened to any song. Normalizing the listen counts ensures active users don't have disproportionate effect on the model error.\n 4. Add a weight equal to the label to give songs more popular with the user higher weights. This is to help account for the skew in the label distribution.",
"_____no_output_____"
]
],
[
[
"query = \"\"\"\n WITH\n songs AS (\n SELECT CONCAT(track_name, \" by \", artist_name) AS song,\n MAX(tags) as tags\n FROM `listenbrainz.listenbrainz.listen`\n GROUP BY song\n HAVING tags != \"\"\n ORDER BY COUNT(*) DESC\n LIMIT 10000\n ),\n user_songs AS (\n SELECT user_name AS user, ANY_VALUE(artist_name) AS artist,\n CONCAT(track_name, \" by \", artist_name) AS song,\n SPLIT(ANY_VALUE(songs.tags), \",\") AS tags,\n COUNT(*) AS user_song_listens\n FROM `listenbrainz.listenbrainz.listen`\n JOIN songs ON songs.song = CONCAT(track_name, \" by \", artist_name)\n GROUP BY user_name, song\n ),\n user_tags AS (\n SELECT user, tag, COUNT(*) AS COUNT\n FROM user_songs,\n UNNEST(tags) tag\n WHERE tag != \"\"\n GROUP BY user, tag\n ),\n top_tags AS (\n SELECT tag\n FROM user_tags\n GROUP BY tag\n ORDER BY SUM(count) DESC\n LIMIT 20\n ),\n tag_table AS (\n SELECT user, b.tag\n FROM user_tags a, top_tags b\n GROUP BY user, b.tag\n ),\n user_tag_features AS (\n SELECT user,\n ARRAY_AGG(IFNULL(count, 0) ORDER BY tag) as user_tags,\n SUM(count) as tag_count\n FROM tag_table\n LEFT JOIN user_tags USING (user, tag)\n GROUP BY user\n ), user_features AS (\n SELECT user, MAX(user_song_listens) AS user_max_listen,\n ANY_VALUE(user_tags)[OFFSET(0)]/ANY_VALUE(tag_count) as user_tags0,\n ANY_VALUE(user_tags)[OFFSET(1)]/ANY_VALUE(tag_count) as user_tags1,\n ANY_VALUE(user_tags)[OFFSET(2)]/ANY_VALUE(tag_count) as user_tags2,\n ANY_VALUE(user_tags)[OFFSET(3)]/ANY_VALUE(tag_count) as user_tags3,\n ANY_VALUE(user_tags)[OFFSET(4)]/ANY_VALUE(tag_count) as user_tags4,\n ANY_VALUE(user_tags)[OFFSET(5)]/ANY_VALUE(tag_count) as user_tags5,\n ANY_VALUE(user_tags)[OFFSET(6)]/ANY_VALUE(tag_count) as user_tags6,\n ANY_VALUE(user_tags)[OFFSET(7)]/ANY_VALUE(tag_count) as user_tags7,\n ANY_VALUE(user_tags)[OFFSET(8)]/ANY_VALUE(tag_count) as user_tags8,\n ANY_VALUE(user_tags)[OFFSET(9)]/ANY_VALUE(tag_count) as user_tags9,\n ANY_VALUE(user_tags)[OFFSET(10)]/ANY_VALUE(tag_count) as user_tags10,\n ANY_VALUE(user_tags)[OFFSET(11)]/ANY_VALUE(tag_count) as user_tags11,\n ANY_VALUE(user_tags)[OFFSET(12)]/ANY_VALUE(tag_count) as user_tags12,\n ANY_VALUE(user_tags)[OFFSET(13)]/ANY_VALUE(tag_count) as user_tags13,\n ANY_VALUE(user_tags)[OFFSET(14)]/ANY_VALUE(tag_count) as user_tags14,\n ANY_VALUE(user_tags)[OFFSET(15)]/ANY_VALUE(tag_count) as user_tags15,\n ANY_VALUE(user_tags)[OFFSET(16)]/ANY_VALUE(tag_count) as user_tags16,\n ANY_VALUE(user_tags)[OFFSET(17)]/ANY_VALUE(tag_count) as user_tags17,\n ANY_VALUE(user_tags)[OFFSET(18)]/ANY_VALUE(tag_count) as user_tags18,\n ANY_VALUE(user_tags)[OFFSET(19)]/ANY_VALUE(tag_count) as user_tags19\n FROM user_songs\n LEFT JOIN user_tag_features USING (user)\n GROUP BY user\n HAVING COUNT(*) < 5000 AND user_max_listen > 2\n ),\n item_features AS (\n SELECT CONCAT(track_name, \" by \", artist_name) AS song,\n COUNT(DISTINCT(release_name)) AS albums\n FROM `listenbrainz.listenbrainz.listen`\n WHERE track_name != \"\"\n GROUP BY song\n )\n SELECT user, song, artist, tags, albums,\n user_tags0,\n user_tags1,\n user_tags2,\n user_tags3,\n user_tags4,\n user_tags5,\n user_tags6,\n user_tags7,\n user_tags8,\n user_tags9,\n user_tags10,\n user_tags11,\n user_tags12,\n user_tags13,\n user_tags14,\n user_tags15,\n user_tags16,\n user_tags17,\n user_tags18,\n user_tags19,\n IF(user_song_listens > 2, \n SQRT(user_song_listens/user_max_listen), \n .5/user_song_listens) AS weight,\n IF(user_song_listens > 2, 1, 0) as label\n FROM user_songs\n JOIN user_features USING(user)\n JOIN item_features USING(song)\n\"\"\"",
"_____no_output_____"
],
[
"def create_table_from_query(query, table):\n \"\"\"Creates a new table using the results from the given query.\n \n Args:\n query: a query string.\n table: a name to give the new table.\n \"\"\"\n job_config = bigquery.QueryJobConfig()\n bq_dataset = bigquery.Dataset(\"{0}.{1}\".format(PROJECT_ID, INPUT_BQ_DATASET))\n bq_dataset.location = \"US\"\n\n try:\n bq_dataset = bq_client.create_dataset(bq_dataset)\n except exceptions.Conflict:\n pass\n\n table_ref = bq_client.dataset(INPUT_BQ_DATASET).table(table)\n job_config.destination = table_ref\n\n query_job = bq_client.query(query,\n location=bq_dataset.location,\n job_config=job_config)\n\n query_job.result()\n print('Query results loaded to table {}'.format(table_ref.path))",
"_____no_output_____"
],
[
"create_table_from_query(query, INPUT_BQ_TABLE)",
"_____no_output_____"
]
],
[
[
"### 2.2 Create AutoML Dataset",
"_____no_output_____"
],
[
"Create a Dataset by importing the BigQuery table that was just created. Importing data may take a few minutes or hours depending on the size of your data.",
"_____no_output_____"
]
],
[
[
"dataset = tables_client.create_dataset(\n dataset_display_name=DATASET_DISPLAY_NAME)\n\ndataset_bq_input_uri = 'bq://{0}.{1}.{2}'.format(\n PROJECT_ID, INPUT_BQ_DATASET, INPUT_BQ_TABLE)\nimport_data_response = tables_client.import_data(\n dataset=dataset, bigquery_input_uri=dataset_bq_input_uri)\nimport_data_result = import_data_response.result()\nimport_data_result",
"_____no_output_____"
]
],
[
[
"Inspect the datatypes assigned to each column. In this case, the `song` and `artist` should be categorical, not textual.",
"_____no_output_____"
]
],
[
[
"list_column_specs_response = tables_client.list_column_specs(\n dataset_display_name=DATASET_DISPLAY_NAME)\ncolumn_specs = {s.display_name: s for s in list_column_specs_response}\n\ndef print_column_specs(column_specs):\n \"\"\"Parses the given specs and prints each column and column type.\"\"\"\n data_types = automl_v1beta1.proto.data_types_pb2\n return [(x, data_types.TypeCode.Name(\n column_specs[x].data_type.type_code)) for x in column_specs.keys()]\n\nprint_column_specs(column_specs)",
"_____no_output_____"
]
],
[
[
"### 2.3 Update Dataset params",
"_____no_output_____"
],
[
"Sometimes, the types AutoML Tables automatically assigns each column will be off from that they were intended to be. When that happens, we need to update Tables with different types for certain columns.\n\nIn this case, set the `song` and `artist` column types to `CATEGORY`.",
"_____no_output_____"
]
],
[
[
"for col in [\"song\", \"artist\"]:\n tables_client.update_column_spec(dataset_display_name=DATASET_DISPLAY_NAME,\n column_spec_display_name=col,\n type_code=\"CATEGORY\")\n\nlist_column_specs_response = tables_client.list_column_specs(\n dataset_display_name=DATASET_DISPLAY_NAME)\ncolumn_specs = {s.display_name: s for s in list_column_specs_response}\nprint_column_specs(column_specs)",
"_____no_output_____"
]
],
[
[
"Not all columns are feature columns, in order to train a model, we need to tell Tables which column should be used as the target variable and, optionally, which column should be used as sample weights.",
"_____no_output_____"
]
],
[
[
"tables_client.set_target_column(dataset_display_name=DATASET_DISPLAY_NAME,\n column_spec_display_name=\"label\")\n\ntables_client.set_weight_column(dataset_display_name=DATASET_DISPLAY_NAME,\n column_spec_display_name=\"weight\")",
"_____no_output_____"
]
],
[
[
"## 3. Create a Model",
"_____no_output_____"
],
[
"Once the Dataset has been configured correctly, we can tell AutoML Tables to train a new model. The amount of resources spent to train this model can be adjusted using a parameter called `train_budget_milli_node_hours`. As the name implies, this puts a maximum budget on how many resources a training job can use up before exporting a servable model.\n\nEven with a budget of 1 node hour (the minimum possible budget), training a model can take several hours.",
"_____no_output_____"
]
],
[
[
"tables_client.create_model(\n model_display_name=MODEL_DISPLAY_NAME,\n dataset_display_name=DATASET_DISPLAY_NAME,\n train_budget_milli_node_hours= MODEL_TRAIN_HOURS * 1000).result()",
"_____no_output_____"
]
],
[
[
"## 4. Model Evaluation",
"_____no_output_____"
],
[
"Because we are optimizing a surrogate problem (predicting the similarity between `(user, song)` pairs) in order to achieve our final objective of producing a list of recommended songs for a user, it's difficult to tell how well the model performs by looking only at the final loss function. Instead, an evaluation metric we can use for our model is `recall@n` for the top `m` most listened to songs for each user. This metric will give us the probability that one of a user's top `m` most listened to songs will appear in the top `n` recommendations we make.\n\nIn order to get the top recommendations for each user, we need to create a batch job to predict similarity scores between each user and item pair. These similarity scores would then be sorted per user to produce an ordered list of recommended songs.",
"_____no_output_____"
],
[
"### 4.1 Create an evaluation table",
"_____no_output_____"
],
[
"Instead of creating a lookup table for all users, let's just focus on the performance for a few users for this demo. We will focus especially on recommendations for the user `rob`, and demonstrate how the others can be included in an overall evaluation metric for the model. We start by creatings a dataset for prediction to feed into the trained model; this is a table of every possible `(user, song)` pair containing the users and corresponding features.",
"_____no_output_____"
]
],
[
[
"users = [\"rob\", \"fiveofoh\", \"Aerion\"]\ntraining_table = \"{}.{}.{}\".format(PROJECT_ID, INPUT_BQ_DATASET, INPUT_BQ_TABLE)\nquery = \"\"\"\n WITH user as (\n SELECT user, \n user_tags0, user_tags1, user_tags2, user_tags3, user_tags4,\n user_tags5, user_tags6, user_tags7, user_tags8, user_tags9,\n user_tags10,user_tags11, user_tags12, user_tags13, user_tags14,\n user_tags15, user_tags16, user_tags17, user_tags18, user_tags19, label\n FROM `{0}`\n WHERE user in ({1})\n )\n SELECT ANY_VALUE(a).*, song, ANY_VALUE(artist) as artist,\n ANY_VALUE(tags) as tags, ANY_VALUE(albums) as albums\n FROM `{0}`, user a\n GROUP BY song\n\"\"\".format(training_table, \",\".join([\"\\\"{}\\\"\".format(x) for x in users]))",
"_____no_output_____"
],
[
"eval_table = \"{}_example\".format(INPUT_BQ_TABLE)\ncreate_table_from_query(query, eval_table)",
"_____no_output_____"
]
],
[
[
"### 4.2 Make predictions",
"_____no_output_____"
],
[
"Once the prediction table is created, start a batch prediction job. This may take a few minutes.",
"_____no_output_____"
]
],
[
[
"preds_bq_input_uri = \"bq://{}.{}.{}\".format(PROJECT_ID, INPUT_BQ_DATASET, eval_table)\npreds_bq_output_uri = \"bq://{}\".format(PROJECT_ID)\nresponse = tables_client.batch_predict(model_display_name=MODEL_DISPLAY_NAME,\n bigquery_input_uri=preds_bq_input_uri,\n bigquery_output_uri=preds_bq_output_uri)\nresponse.result()\noutput_uri = response.metadata.batch_predict_details.output_info.bigquery_output_dataset",
"_____no_output_____"
]
],
[
[
"With the similarity predictions for `rob`, we can order by the predictions to get a ranked list of songs to recommend to `rob`.",
"_____no_output_____"
]
],
[
[
"n = 10\nquery = \"\"\"\n SELECT user, song, tables.score as score, a.label as pred_label,\n b.label as true_label\n FROM `{}.predictions` a, UNNEST(predicted_label)\n LEFT JOIN `{}` b USING(user, song)\n WHERE user = \"{}\" AND CAST(tables.value AS INT64) = 1\n ORDER BY score DESC\n LIMIT {}\n\"\"\".format(output_uri[5:].replace(\":\", \".\"), training_table, user, n)\nquery_job = bq_client.query(query)\n\nprint(\"Top {} song recommended for {}:\".format(n, user))\nfor idx, row in enumerate(query_job):\n print(\"{}.\".format(idx + 1), row[\"song\"])",
"_____no_output_____"
]
],
[
[
"### 4.3 Evaluate predictions",
"_____no_output_____"
],
[
"#### Precision@k and Recall@k\n\nTo evaluate the recommendations, we can look at the precision@k and recall@k of our predictions for `rob`. Run the cells below to load the recommendations into a pandas dataframe and plot the precisions and recalls at various top-k recommendations. ",
"_____no_output_____"
]
],
[
[
"query = \"\"\"\n WITH \n top_k AS (\n SELECT user, song, label,\n ROW_NUMBER() OVER (PARTITION BY user ORDER BY label + weight DESC) as user_rank\n FROM `{0}`\n )\n SELECT user, song, tables.score as score, b.label,\n ROW_NUMBER() OVER (ORDER BY tables.score DESC) as rank, user_rank\n FROM `{1}.predictions` a, UNNEST(predicted_label)\n LEFT JOIN top_k b USING(user, song)\n WHERE CAST(tables.value AS INT64) = 1\n ORDER BY score DESC\n\"\"\".format(training_table, output_uri[5:].replace(\":\", \".\"))\n\ndf = bq_client.query(query).result().to_dataframe()\ndf.head()",
"_____no_output_____"
],
[
"precision_at_k = {}\nrecall_at_k = {}\n\nfor user in users:\n precision_at_k[user] = []\n recall_at_k[user] = []\n for k in range(1, 1000):\n precision = df[\"label\"][:k].sum() / k\n recall = df[\"label\"][:k].sum() / df[\"label\"].sum()\n precision_at_k[user].append(precision)\n recall_at_k[user].append(recall)\n\n# plot the precision-recall curve\nax = sns.lineplot(recall_at_k[users[0]], precision_at_k[users[0]])\nax.set_title(\"precision-recall curve for varying k\")\nax.set_xlabel(\"recall@k\")\nax.set_ylabel(\"precision@k\")",
"_____no_output_____"
]
],
[
[
"Achieving a high precision@k means a large proportion of top-k recommended items are relevant to the user. Recall@k shows what proportion of all relevant items appeared in the top-k recommendations.",
"_____no_output_____"
],
[
"#### Mean Average Precision (MAP)\n\nPrecision@k is a good metric for understanding how many relevant recommendations we might make at each top-k. However, we would prefer relevant items to be recommended first when possible and should encode that into our evaluation metric. __Average Precision (AP)__ is a running average of precision@k, rewarding recommendations where the revelant items are seen earlier rather than later. When the averaged across all users for some k, the AP metric is called MAP.",
"_____no_output_____"
]
],
[
[
"def calculate_ap(precision):\n ap = [precision[0]]\n for p in precision[1:]:\n ap.append(ap[-1] + p)\n ap = [x / (n + 1) for x, n in zip(ap, range(len(ap)))]\n return ap\n\nap_at_k = {user: calculate_ap(pk)\n for user, pk in precision_at_k.items()}\n\nnum_k = 500\nmap_at_k = [sum([ap_at_k[user][k] for user in users]) / len(users)\n for k in range(num_k)]\nprint(\"MAP@50: {}\".format(map_at_k[49]))\n\n# plot average precision\nax = sns.lineplot(range(num_k), map_at_k)\nax.set_title(\"MAP@k for varying k\")\nax.set_xlabel(\"k\")\nax.set_ylabel(\"MAP\")",
"_____no_output_____"
]
],
[
[
"## 5. Cleanup",
"_____no_output_____"
],
[
"The following cells clean up the BigQuery tables and AutoML Table Datasets that were created with this notebook to avoid additional charges for storage.",
"_____no_output_____"
],
[
"### 5.1 Delete the Model and Dataset",
"_____no_output_____"
]
],
[
[
"tables_client.delete_model(model_display_name=MODEL_DISPLAY_NAME)\n\ntables_client.delete_dataset(dataset_display_name=DATASET_DISPLAY_NAME)",
"_____no_output_____"
]
],
[
[
"### 5.2 Delete BigQuery datasets",
"_____no_output_____"
],
[
"In order to delete BigQuery tables, make sure the service account linked to this notebook has a role with the `bigquery.tables.delete` permission such as `Big Query Data Owner`. The following command displays the current service account.\n\nIAM permissions can be adjusted [here](https://console.cloud.google.com/iam-admin/iam).",
"_____no_output_____"
]
],
[
[
"!gcloud config list account --format \"value(core.account)\"",
"_____no_output_____"
]
],
[
[
"Clean up the BigQuery tables created by this notebook.",
"_____no_output_____"
]
],
[
[
"# Delete the prediction dataset.\ndataset_id = str(output_uri[5:].replace(\":\", \".\"))\nbq_client.delete_dataset(dataset_id, delete_contents=True, not_found_ok=True)\n\n# Delete the training dataset.\ndataset_id = \"{0}.{1}\".format(PROJECT_ID, INPUT_BQ_DATASET)\nbq_client.delete_dataset(dataset_id, delete_contents=True, not_found_ok=True)",
"_____no_output_____"
]
]
] | [
"code",
"markdown",
"code",
"markdown",
"code",
"markdown",
"code",
"markdown",
"code",
"markdown",
"code",
"markdown",
"code",
"markdown",
"code",
"markdown",
"code",
"markdown",
"code",
"markdown",
"code",
"markdown",
"code",
"markdown",
"code",
"markdown",
"code",
"markdown",
"code",
"markdown",
"code",
"markdown",
"code",
"markdown",
"code",
"markdown",
"code"
] | [
[
"code"
],
[
"markdown",
"markdown",
"markdown",
"markdown"
],
[
"code"
],
[
"markdown"
],
[
"code"
],
[
"markdown",
"markdown"
],
[
"code"
],
[
"markdown"
],
[
"code"
],
[
"markdown",
"markdown",
"markdown",
"markdown"
],
[
"code",
"code",
"code"
],
[
"markdown",
"markdown"
],
[
"code"
],
[
"markdown"
],
[
"code"
],
[
"markdown",
"markdown"
],
[
"code"
],
[
"markdown"
],
[
"code"
],
[
"markdown",
"markdown"
],
[
"code"
],
[
"markdown",
"markdown",
"markdown",
"markdown"
],
[
"code",
"code"
],
[
"markdown",
"markdown"
],
[
"code"
],
[
"markdown"
],
[
"code"
],
[
"markdown",
"markdown"
],
[
"code",
"code"
],
[
"markdown",
"markdown"
],
[
"code"
],
[
"markdown",
"markdown",
"markdown"
],
[
"code"
],
[
"markdown",
"markdown"
],
[
"code"
],
[
"markdown"
],
[
"code"
]
] |
e77e4e2df83b2e428f3cba758469351da23e4f68 | 21,334 | ipynb | Jupyter Notebook | Deutsch-Jozsa-Algorithmus.ipynb | Gruschtel/Quantum_Computing | 3f12395e26e45fcc7268806c28c5ca3428411193 | [
"MIT"
] | null | null | null | Deutsch-Jozsa-Algorithmus.ipynb | Gruschtel/Quantum_Computing | 3f12395e26e45fcc7268806c28c5ca3428411193 | [
"MIT"
] | null | null | null | Deutsch-Jozsa-Algorithmus.ipynb | Gruschtel/Quantum_Computing | 3f12395e26e45fcc7268806c28c5ca3428411193 | [
"MIT"
] | null | null | null | 38.859745 | 794 | 0.563654 | [
[
[
"empty"
]
]
] | [
"empty"
] | [
[
"empty"
]
] |
e77e69116e79e7be02cb77f3d6aa661d2ea76ca3 | 107,436 | ipynb | Jupyter Notebook | Pandas/.ipynb_checkpoints/top_25_pandas_tricks-checkpoint.ipynb | piszewc/python-deep-learning-data-science | 789b4796a41830040143aef073aa3f818d577b6c | [
"MIT",
"Unlicense"
] | 1 | 2020-11-21T17:06:08.000Z | 2020-11-21T17:06:08.000Z | Pandas/.ipynb_checkpoints/top_25_pandas_tricks-checkpoint.ipynb | piszewc/python-deep-learning-data-science | 789b4796a41830040143aef073aa3f818d577b6c | [
"MIT",
"Unlicense"
] | null | null | null | Pandas/.ipynb_checkpoints/top_25_pandas_tricks-checkpoint.ipynb | piszewc/python-deep-learning-data-science | 789b4796a41830040143aef073aa3f818d577b6c | [
"MIT",
"Unlicense"
] | 2 | 2020-06-06T15:40:34.000Z | 2021-05-04T10:44:20.000Z | 28.168852 | 1,490 | 0.479867 | [
[
[
"# Data School's top 25 pandas tricks ([video](https://www.youtube.com/watch?v=RlIiVeig3hc))\n\n- Watch the [complete pandas video series](https://www.dataschool.io/easier-data-analysis-with-pandas/)\n- Connect on [Twitter](https://twitter.com/justmarkham), [Facebook](https://www.facebook.com/DataScienceSchool/), and [LinkedIn](https://www.linkedin.com/in/justmarkham/)\n- Subscribe on [YouTube](https://www.youtube.com/dataschool?sub_confirmation=1)\n- Join the [email newsletter](https://www.dataschool.io/subscribe/)",
"_____no_output_____"
],
[
"## Table of contents\n\n1. <a href=\"#1.-Show-installed-versions\">Show installed versions</a>\n2. <a href=\"#2.-Create-an-example-DataFrame\">Create an example DataFrame</a>\n3. <a href=\"#3.-Rename-columns\">Rename columns</a>\n4. <a href=\"#4.-Reverse-row-order\">Reverse row order</a>\n5. <a href=\"#5.-Reverse-column-order\">Reverse column order</a>\n6. <a href=\"#6.-Select-columns-by-data-type\">Select columns by data type</a>\n7. <a href=\"#7.-Convert-strings-to-numbers\">Convert strings to numbers</a>\n8. <a href=\"#8.-Reduce-DataFrame-size\">Reduce DataFrame size</a>\n9. <a href=\"#9.-Build-a-DataFrame-from-multiple-files-(row-wise)\">Build a DataFrame from multiple files (row-wise)</a>\n10. <a href=\"#10.-Build-a-DataFrame-from-multiple-files-(column-wise)\">Build a DataFrame from multiple files (column-wise)</a>\n11. <a href=\"#11.-Create-a-DataFrame-from-the-clipboard\">Create a DataFrame from the clipboard</a>\n12. <a href=\"#12.-Split-a-DataFrame-into-two-random-subsets\">Split a DataFrame into two random subsets</a>\n13. <a href=\"#13.-Filter-a-DataFrame-by-multiple-categories\">Filter a DataFrame by multiple categories</a>\n14. <a href=\"#14.-Filter-a-DataFrame-by-largest-categories\">Filter a DataFrame by largest categories</a>\n15. <a href=\"#15.-Handle-missing-values\">Handle missing values</a>\n16. <a href=\"#16.-Split-a-string-into-multiple-columns\">Split a string into multiple columns</a>\n17. <a href=\"#17.-Expand-a-Series-of-lists-into-a-DataFrame\">Expand a Series of lists into a DataFrame</a>\n18. <a href=\"#18.-Aggregate-by-multiple-functions\">Aggregate by multiple functions</a>\n19. <a href=\"#19.-Combine-the-output-of-an-aggregation-with-a-DataFrame\">Combine the output of an aggregation with a DataFrame</a>\n20. <a href=\"#20.-Select-a-slice-of-rows-and-columns\">Select a slice of rows and columns</a>\n21. <a href=\"#21.-Reshape-a-MultiIndexed-Series\">Reshape a MultiIndexed Series</a>\n22. <a href=\"#22.-Create-a-pivot-table\">Create a pivot table</a>\n23. <a href=\"#23.-Convert-continuous-data-into-categorical-data\">Convert continuous data into categorical data</a>\n24. <a href=\"#24.-Change-display-options\">Change display options</a>\n25. <a href=\"#25.-Style-a-DataFrame\">Style a DataFrame</a>\n26. <a href=\"#Bonus:-Profile-a-DataFrame\">Bonus trick: Profile a DataFrame</a>",
"_____no_output_____"
],
[
"## Load example datasets",
"_____no_output_____"
]
],
[
[
"import pandas as pd\nimport numpy as np",
"_____no_output_____"
],
[
"drinks = pd.read_csv('http://bit.ly/drinksbycountry')\nmovies = pd.read_csv('http://bit.ly/imdbratings')\norders = pd.read_csv('http://bit.ly/chiporders', sep='\\t')\norders['item_price'] = orders.item_price.str.replace('$', '').astype('float')\nstocks = pd.read_csv('http://bit.ly/smallstocks', parse_dates=['Date'])\ntitanic = pd.read_csv('http://bit.ly/kaggletrain')\nufo = pd.read_csv('http://bit.ly/uforeports', parse_dates=['Time'])",
"_____no_output_____"
]
],
[
[
"## 1. Show installed versions",
"_____no_output_____"
],
[
"Sometimes you need to know the pandas version you're using, especially when reading the pandas documentation. You can show the pandas version by typing:",
"_____no_output_____"
]
],
[
[
"pd.__version__",
"_____no_output_____"
]
],
[
[
"But if you also need to know the versions of pandas' dependencies, you can use the `show_versions()` function:",
"_____no_output_____"
]
],
[
[
"pd.show_versions()",
"\nINSTALLED VERSIONS\n------------------\ncommit: None\npython: 3.7.1.final.0\npython-bits: 64\nOS: Windows\nOS-release: 10\nmachine: AMD64\nprocessor: Intel64 Family 6 Model 60 Stepping 3, GenuineIntel\nbyteorder: little\nLC_ALL: None\nLANG: None\nLOCALE: None.None\n\npandas: 0.23.4\npytest: 4.0.2\npip: 18.1\nsetuptools: 40.6.3\nCython: 0.29.2\nnumpy: 1.15.4\nscipy: 1.1.0\npyarrow: None\nxarray: None\nIPython: 7.2.0\nsphinx: 1.8.2\npatsy: 0.5.1\ndateutil: 2.7.5\npytz: 2018.7\nblosc: None\nbottleneck: 1.2.1\ntables: 3.4.4\nnumexpr: 2.6.8\nfeather: None\nmatplotlib: 3.0.2\nopenpyxl: 2.5.12\nxlrd: 1.2.0\nxlwt: 1.3.0\nxlsxwriter: 1.1.2\nlxml: 4.2.5\nbs4: 4.6.3\nhtml5lib: 1.0.1\nsqlalchemy: 1.2.15\npymysql: None\npsycopg2: None\njinja2: 2.10\ns3fs: None\nfastparquet: None\npandas_gbq: None\npandas_datareader: None\n"
]
],
[
[
"You can see the versions of Python, pandas, NumPy, matplotlib, and more.",
"_____no_output_____"
],
[
"## 2. Create an example DataFrame",
"_____no_output_____"
],
[
"Let's say that you want to demonstrate some pandas code. You need an example DataFrame to work with.\n\nThere are many ways to do this, but my favorite way is to pass a dictionary to the DataFrame constructor, in which the dictionary keys are the column names and the dictionary values are lists of column values:",
"_____no_output_____"
]
],
[
[
"df = pd.DataFrame({'col one':[100, 200], 'col two':[300, 400]})\ndf",
"_____no_output_____"
]
],
[
[
"Now if you need a much larger DataFrame, the above method will require way too much typing. In that case, you can use NumPy's `random.rand()` function, tell it the number of rows and columns, and pass that to the DataFrame constructor:",
"_____no_output_____"
]
],
[
[
"pd.DataFrame(np.random.rand(4, 8))",
"_____no_output_____"
]
],
[
[
"That's pretty good, but if you also want non-numeric column names, you can coerce a string of letters to a list and then pass that list to the columns parameter:",
"_____no_output_____"
]
],
[
[
"pd.DataFrame(np.random.rand(4, 8), columns=list('abcdefgh'))",
"_____no_output_____"
]
],
[
[
"As you might guess, your string will need to have the same number of characters as there are columns.",
"_____no_output_____"
],
[
"## 3. Rename columns",
"_____no_output_____"
],
[
"Let's take a look at the example DataFrame we created in the last trick:",
"_____no_output_____"
]
],
[
[
"df",
"_____no_output_____"
]
],
[
[
"I prefer to use dot notation to select pandas columns, but that won't work since the column names have spaces. Let's fix this.\n\nThe most flexible method for renaming columns is the `rename()` method. You pass it a dictionary in which the keys are the old names and the values are the new names, and you also specify the axis:",
"_____no_output_____"
]
],
[
[
"df = df.rename({'col one':'col_one', 'col two':'col_two'}, axis='columns')",
"_____no_output_____"
]
],
[
[
"The best thing about this method is that you can use it to rename any number of columns, whether it be just one column or all columns.\n\nNow if you're going to rename all of the columns at once, a simpler method is just to overwrite the columns attribute of the DataFrame:",
"_____no_output_____"
]
],
[
[
"df.columns = ['col_one', 'col_two']",
"_____no_output_____"
]
],
[
[
"Now if the only thing you're doing is replacing spaces with underscores, an even better method is to use the `str.replace()` method, since you don't have to type out all of the column names:",
"_____no_output_____"
]
],
[
[
"df.columns = df.columns.str.replace(' ', '_')",
"_____no_output_____"
]
],
[
[
"All three of these methods have the same result, which is to rename the columns so that they don't have any spaces:",
"_____no_output_____"
]
],
[
[
"df",
"_____no_output_____"
]
],
[
[
"Finally, if you just need to add a prefix or suffix to all of your column names, you can use the `add_prefix()` method...",
"_____no_output_____"
]
],
[
[
"df.add_prefix('X_')",
"_____no_output_____"
]
],
[
[
"...or the `add_suffix()` method:",
"_____no_output_____"
]
],
[
[
"df.add_suffix('_Y')",
"_____no_output_____"
]
],
[
[
"## 4. Reverse row order",
"_____no_output_____"
],
[
"Let's take a look at the drinks DataFrame:",
"_____no_output_____"
]
],
[
[
"drinks.head()",
"_____no_output_____"
]
],
[
[
"This is a dataset of average alcohol consumption by country. What if you wanted to reverse the order of the rows?\n\nThe most straightforward method is to use the `loc` accessor and pass it `::-1`, which is the same slicing notation used to reverse a Python list:",
"_____no_output_____"
]
],
[
[
"drinks.loc[::-1].head()",
"_____no_output_____"
]
],
[
[
"What if you also wanted to reset the index so that it starts at zero?\n\nYou would use the `reset_index()` method and tell it to drop the old index entirely:",
"_____no_output_____"
]
],
[
[
"drinks.loc[::-1].reset_index(drop=True).head()",
"_____no_output_____"
]
],
[
[
"As you can see, the rows are in reverse order but the index has been reset to the default integer index.",
"_____no_output_____"
],
[
"## 5. Reverse column order",
"_____no_output_____"
],
[
"Similar to the previous trick, you can also use `loc` to reverse the left-to-right order of your columns:",
"_____no_output_____"
]
],
[
[
"drinks.loc[:, ::-1].head()",
"_____no_output_____"
]
],
[
[
"The colon before the comma means \"select all rows\", and the `::-1` after the comma means \"reverse the columns\", which is why \"country\" is now on the right side.",
"_____no_output_____"
],
[
"## 6. Select columns by data type",
"_____no_output_____"
],
[
"Here are the data types of the drinks DataFrame:",
"_____no_output_____"
]
],
[
[
"drinks.dtypes",
"_____no_output_____"
]
],
[
[
"Let's say you need to select only the numeric columns. You can use the `select_dtypes()` method:",
"_____no_output_____"
]
],
[
[
"drinks.select_dtypes(include='number').head()",
"_____no_output_____"
]
],
[
[
"This includes both int and float columns.\n\nYou could also use this method to select just the object columns:",
"_____no_output_____"
]
],
[
[
"drinks.select_dtypes(include='object').head()",
"_____no_output_____"
]
],
[
[
"You can tell it to include multiple data types by passing a list:",
"_____no_output_____"
]
],
[
[
"drinks.select_dtypes(include=['number', 'object', 'category', 'datetime']).head()",
"_____no_output_____"
]
],
[
[
"You can also tell it to exclude certain data types:",
"_____no_output_____"
]
],
[
[
"drinks.select_dtypes(exclude='number').head()",
"_____no_output_____"
]
],
[
[
"## 7. Convert strings to numbers",
"_____no_output_____"
],
[
"Let's create another example DataFrame:",
"_____no_output_____"
]
],
[
[
"df = pd.DataFrame({'col_one':['1.1', '2.2', '3.3'],\n 'col_two':['4.4', '5.5', '6.6'],\n 'col_three':['7.7', '8.8', '-']})\ndf",
"_____no_output_____"
]
],
[
[
"These numbers are actually stored as strings, which results in object columns:",
"_____no_output_____"
]
],
[
[
"df.dtypes",
"_____no_output_____"
]
],
[
[
"In order to do mathematical operations on these columns, we need to convert the data types to numeric. You can use the `astype()` method on the first two columns:",
"_____no_output_____"
]
],
[
[
"df.astype({'col_one':'float', 'col_two':'float'}).dtypes",
"_____no_output_____"
]
],
[
[
"However, this would have resulted in an error if you tried to use it on the third column, because that column contains a dash to represent zero and pandas doesn't understand how to handle it.\n\nInstead, you can use the `to_numeric()` function on the third column and tell it to convert any invalid input into `NaN` values:",
"_____no_output_____"
]
],
[
[
"pd.to_numeric(df.col_three, errors='coerce')",
"_____no_output_____"
]
],
[
[
"If you know that the `NaN` values actually represent zeros, you can fill them with zeros using the `fillna()` method:",
"_____no_output_____"
]
],
[
[
"pd.to_numeric(df.col_three, errors='coerce').fillna(0)",
"_____no_output_____"
]
],
[
[
"Finally, you can apply this function to the entire DataFrame all at once by using the `apply()` method:",
"_____no_output_____"
]
],
[
[
"df = df.apply(pd.to_numeric, errors='coerce').fillna(0)\ndf",
"_____no_output_____"
]
],
[
[
"This one line of code accomplishes our goal, because all of the data types have now been converted to float:",
"_____no_output_____"
]
],
[
[
"df.dtypes",
"_____no_output_____"
]
],
[
[
"## 8. Reduce DataFrame size",
"_____no_output_____"
],
[
"pandas DataFrames are designed to fit into memory, and so sometimes you need to reduce the DataFrame size in order to work with it on your system.\n\nHere's the size of the drinks DataFrame:",
"_____no_output_____"
]
],
[
[
"drinks.info(memory_usage='deep')",
"<class 'pandas.core.frame.DataFrame'>\nRangeIndex: 193 entries, 0 to 192\nData columns (total 6 columns):\ncountry 193 non-null object\nbeer_servings 193 non-null int64\nspirit_servings 193 non-null int64\nwine_servings 193 non-null int64\ntotal_litres_of_pure_alcohol 193 non-null float64\ncontinent 193 non-null object\ndtypes: float64(1), int64(3), object(2)\nmemory usage: 30.4 KB\n"
]
],
[
[
"You can see that it currently uses 30.4 KB.\n\nIf you're having performance problems with your DataFrame, or you can't even read it into memory, there are two easy steps you can take during the file reading process to reduce the DataFrame size.\n\nThe first step is to only read in the columns that you actually need, which we specify with the \"usecols\" parameter:",
"_____no_output_____"
]
],
[
[
"cols = ['beer_servings', 'continent']\nsmall_drinks = pd.read_csv('http://bit.ly/drinksbycountry', usecols=cols)\nsmall_drinks.info(memory_usage='deep')",
"<class 'pandas.core.frame.DataFrame'>\nRangeIndex: 193 entries, 0 to 192\nData columns (total 2 columns):\nbeer_servings 193 non-null int64\ncontinent 193 non-null object\ndtypes: int64(1), object(1)\nmemory usage: 13.6 KB\n"
]
],
[
[
"By only reading in these two columns, we've reduced the DataFrame size to 13.6 KB.\n\nThe second step is to convert any object columns containing categorical data to the category data type, which we specify with the \"dtype\" parameter:",
"_____no_output_____"
]
],
[
[
"dtypes = {'continent':'category'}\nsmaller_drinks = pd.read_csv('http://bit.ly/drinksbycountry', usecols=cols, dtype=dtypes)\nsmaller_drinks.info(memory_usage='deep')",
"<class 'pandas.core.frame.DataFrame'>\nRangeIndex: 193 entries, 0 to 192\nData columns (total 2 columns):\nbeer_servings 193 non-null int64\ncontinent 193 non-null category\ndtypes: category(1), int64(1)\nmemory usage: 2.3 KB\n"
]
],
[
[
"By reading in the continent column as the category data type, we've further reduced the DataFrame size to 2.3 KB.\n\nKeep in mind that the category data type will only reduce memory usage if you have a small number of categories relative to the number of rows.",
"_____no_output_____"
],
[
"## 9. Build a DataFrame from multiple files (row-wise)",
"_____no_output_____"
],
[
"Let's say that your dataset is spread across multiple files, but you want to read the dataset into a single DataFrame.\n\nFor example, I have a small dataset of stock data in which each CSV file only includes a single day. Here's the first day:",
"_____no_output_____"
]
],
[
[
"pd.read_csv('data/stocks1.csv')",
"_____no_output_____"
]
],
[
[
"Here's the second day:",
"_____no_output_____"
]
],
[
[
"pd.read_csv('data/stocks2.csv')",
"_____no_output_____"
]
],
[
[
"And here's the third day:",
"_____no_output_____"
]
],
[
[
"pd.read_csv('data/stocks3.csv')",
"_____no_output_____"
]
],
[
[
"You could read each CSV file into its own DataFrame, combine them together, and then delete the original DataFrames, but that would be memory inefficient and require a lot of code.\n\nA better solution is to use the built-in glob module:",
"_____no_output_____"
]
],
[
[
"from glob import glob",
"_____no_output_____"
]
],
[
[
"You can pass a pattern to `glob()`, including wildcard characters, and it will return a list of all files that match that pattern.\n\nIn this case, glob is looking in the \"data\" subdirectory for all CSV files that start with the word \"stocks\":",
"_____no_output_____"
]
],
[
[
"stock_files = sorted(glob('data/stocks*.csv'))\nstock_files",
"_____no_output_____"
]
],
[
[
"glob returns filenames in an arbitrary order, which is why we sorted the list using Python's built-in `sorted()` function.\n\nWe can then use a generator expression to read each of the files using `read_csv()` and pass the results to the `concat()` function, which will concatenate the rows into a single DataFrame:",
"_____no_output_____"
]
],
[
[
"pd.concat((pd.read_csv(file) for file in stock_files))",
"_____no_output_____"
]
],
[
[
"Unfortunately, there are now duplicate values in the index. To avoid that, we can tell the `concat()` function to ignore the index and instead use the default integer index:",
"_____no_output_____"
]
],
[
[
"pd.concat((pd.read_csv(file) for file in stock_files), ignore_index=True)",
"_____no_output_____"
]
],
[
[
"## 10. Build a DataFrame from multiple files (column-wise)",
"_____no_output_____"
],
[
"The previous trick is useful when each file contains rows from your dataset. But what if each file instead contains columns from your dataset?\n\nHere's an example in which the drinks dataset has been split into two CSV files, and each file contains three columns:",
"_____no_output_____"
]
],
[
[
"pd.read_csv('data/drinks1.csv').head()",
"_____no_output_____"
],
[
"pd.read_csv('data/drinks2.csv').head()",
"_____no_output_____"
]
],
[
[
"Similar to the previous trick, we'll start by using `glob()`:",
"_____no_output_____"
]
],
[
[
"drink_files = sorted(glob('data/drinks*.csv'))",
"_____no_output_____"
]
],
[
[
"And this time, we'll tell the `concat()` function to concatenate along the columns axis:",
"_____no_output_____"
]
],
[
[
"pd.concat((pd.read_csv(file) for file in drink_files), axis='columns').head()",
"_____no_output_____"
]
],
[
[
"Now our DataFrame has all six columns.",
"_____no_output_____"
],
[
"## 11. Create a DataFrame from the clipboard",
"_____no_output_____"
],
[
"Let's say that you have some data stored in an Excel spreadsheet or a [Google Sheet](https://docs.google.com/spreadsheets/d/1ipv_HAykbky8OXUubs9eLL-LQ1rAkexXG61-B4jd0Rc/edit?usp=sharing), and you want to get it into a DataFrame as quickly as possible.\n\nJust select the data and copy it to the clipboard. Then, you can use the `read_clipboard()` function to read it into a DataFrame:",
"_____no_output_____"
]
],
[
[
"df = pd.read_clipboard()\ndf",
"_____no_output_____"
]
],
[
[
"Just like the `read_csv()` function, `read_clipboard()` automatically detects the correct data type for each column:",
"_____no_output_____"
]
],
[
[
"df.dtypes",
"_____no_output_____"
]
],
[
[
"Let's copy one other dataset to the clipboard:",
"_____no_output_____"
]
],
[
[
"df = pd.read_clipboard()\ndf",
"_____no_output_____"
]
],
[
[
"Amazingly, pandas has even identified the first column as the index:",
"_____no_output_____"
]
],
[
[
"df.index",
"_____no_output_____"
]
],
[
[
"Keep in mind that if you want your work to be reproducible in the future, `read_clipboard()` is not the recommended approach.",
"_____no_output_____"
],
[
"## 12. Split a DataFrame into two random subsets",
"_____no_output_____"
],
[
"Let's say that you want to split a DataFrame into two parts, randomly assigning 75% of the rows to one DataFrame and the other 25% to a second DataFrame.\n\nFor example, we have a DataFrame of movie ratings with 979 rows:",
"_____no_output_____"
]
],
[
[
"len(movies)",
"_____no_output_____"
]
],
[
[
"We can use the `sample()` method to randomly select 75% of the rows and assign them to the \"movies_1\" DataFrame:",
"_____no_output_____"
]
],
[
[
"movies_1 = movies.sample(frac=0.75, random_state=1234)",
"_____no_output_____"
]
],
[
[
"Then we can use the `drop()` method to drop all rows that are in \"movies_1\" and assign the remaining rows to \"movies_2\":",
"_____no_output_____"
]
],
[
[
"movies_2 = movies.drop(movies_1.index)",
"_____no_output_____"
]
],
[
[
"You can see that the total number of rows is correct:",
"_____no_output_____"
]
],
[
[
"len(movies_1) + len(movies_2)",
"_____no_output_____"
]
],
[
[
"And you can see from the index that every movie is in either \"movies_1\":",
"_____no_output_____"
]
],
[
[
"movies_1.index.sort_values()",
"_____no_output_____"
]
],
[
[
"...or \"movies_2\":",
"_____no_output_____"
]
],
[
[
"movies_2.index.sort_values()",
"_____no_output_____"
]
],
[
[
"Keep in mind that this approach will not work if your index values are not unique.",
"_____no_output_____"
],
[
"## 13. Filter a DataFrame by multiple categories",
"_____no_output_____"
],
[
"Let's take a look at the movies DataFrame:",
"_____no_output_____"
]
],
[
[
"movies.head()",
"_____no_output_____"
]
],
[
[
"One of the columns is genre:",
"_____no_output_____"
]
],
[
[
"movies.genre.unique()",
"_____no_output_____"
]
],
[
[
"If we wanted to filter the DataFrame to only show movies with the genre Action or Drama or Western, we could use multiple conditions separated by the \"or\" operator:",
"_____no_output_____"
]
],
[
[
"movies[(movies.genre == 'Action') |\n (movies.genre == 'Drama') |\n (movies.genre == 'Western')].head()",
"_____no_output_____"
]
],
[
[
"However, you can actually rewrite this code more clearly by using the `isin()` method and passing it a list of genres:",
"_____no_output_____"
]
],
[
[
"movies[movies.genre.isin(['Action', 'Drama', 'Western'])].head()",
"_____no_output_____"
]
],
[
[
"And if you want to reverse this filter, so that you are excluding (rather than including) those three genres, you can put a tilde in front of the condition:",
"_____no_output_____"
]
],
[
[
"movies[~movies.genre.isin(['Action', 'Drama', 'Western'])].head()",
"_____no_output_____"
]
],
[
[
"This works because tilde is the \"not\" operator in Python.",
"_____no_output_____"
],
[
"## 14. Filter a DataFrame by largest categories",
"_____no_output_____"
],
[
"Let's say that you needed to filter the movies DataFrame by genre, but only include the 3 largest genres.\n\nWe'll start by taking the `value_counts()` of genre and saving it as a Series called counts:",
"_____no_output_____"
]
],
[
[
"counts = movies.genre.value_counts()\ncounts",
"_____no_output_____"
]
],
[
[
"The Series method `nlargest()` makes it easy to select the 3 largest values in this Series:",
"_____no_output_____"
]
],
[
[
"counts.nlargest(3)",
"_____no_output_____"
]
],
[
[
"And all we actually need from this Series is the index:",
"_____no_output_____"
]
],
[
[
"counts.nlargest(3).index",
"_____no_output_____"
]
],
[
[
"Finally, we can pass the index object to `isin()`, and it will be treated like a list of genres:",
"_____no_output_____"
]
],
[
[
"movies[movies.genre.isin(counts.nlargest(3).index)].head()",
"_____no_output_____"
]
],
[
[
"Thus, only Drama and Comedy and Action movies remain in the DataFrame.",
"_____no_output_____"
],
[
"## 15. Handle missing values",
"_____no_output_____"
],
[
"Let's look at a dataset of UFO sightings:",
"_____no_output_____"
]
],
[
[
"ufo.head()",
"_____no_output_____"
]
],
[
[
"You'll notice that some of the values are missing.\n\nTo find out how many values are missing in each column, you can use the `isna()` method and then take the `sum()`:",
"_____no_output_____"
]
],
[
[
"ufo.isna().sum()",
"_____no_output_____"
]
],
[
[
"`isna()` generated a DataFrame of True and False values, and `sum()` converted all of the True values to 1 and added them up.\n\nSimilarly, you can find out the percentage of values that are missing by taking the `mean()` of `isna()`:",
"_____no_output_____"
]
],
[
[
"ufo.isna().mean()",
"_____no_output_____"
]
],
[
[
"If you want to drop the columns that have any missing values, you can use the `dropna()` method:",
"_____no_output_____"
]
],
[
[
"ufo.dropna(axis='columns').head()",
"_____no_output_____"
]
],
[
[
"Or if you want to drop columns in which more than 10% of the values are missing, you can set a threshold for `dropna()`:",
"_____no_output_____"
]
],
[
[
"ufo.dropna(thresh=len(ufo)*0.9, axis='columns').head()",
"_____no_output_____"
]
],
[
[
"`len(ufo)` returns the total number of rows, and then we multiply that by 0.9 to tell pandas to only keep columns in which at least 90% of the values are not missing.",
"_____no_output_____"
],
[
"## 16. Split a string into multiple columns",
"_____no_output_____"
],
[
"Let's create another example DataFrame:",
"_____no_output_____"
]
],
[
[
"df = pd.DataFrame({'name':['John Arthur Doe', 'Jane Ann Smith'],\n 'location':['Los Angeles, CA', 'Washington, DC']})\ndf",
"_____no_output_____"
]
],
[
[
"What if we wanted to split the \"name\" column into three separate columns, for first, middle, and last name? We would use the `str.split()` method and tell it to split on a space character and expand the results into a DataFrame:",
"_____no_output_____"
]
],
[
[
"df.name.str.split(' ', expand=True)",
"_____no_output_____"
]
],
[
[
"These three columns can actually be saved to the original DataFrame in a single assignment statement:",
"_____no_output_____"
]
],
[
[
"df[['first', 'middle', 'last']] = df.name.str.split(' ', expand=True)\ndf",
"_____no_output_____"
]
],
[
[
"What if we wanted to split a string, but only keep one of the resulting columns? For example, let's split the location column on \"comma space\":",
"_____no_output_____"
]
],
[
[
"df.location.str.split(', ', expand=True)",
"_____no_output_____"
]
],
[
[
"If we only cared about saving the city name in column 0, we can just select that column and save it to the DataFrame:",
"_____no_output_____"
]
],
[
[
"df['city'] = df.location.str.split(', ', expand=True)[0]\ndf",
"_____no_output_____"
]
],
[
[
"## 17. Expand a Series of lists into a DataFrame",
"_____no_output_____"
],
[
"Let's create another example DataFrame:",
"_____no_output_____"
]
],
[
[
"df = pd.DataFrame({'col_one':['a', 'b', 'c'], 'col_two':[[10, 40], [20, 50], [30, 60]]})\ndf",
"_____no_output_____"
]
],
[
[
"There are two columns, and the second column contains regular Python lists of integers.\n\nIf we wanted to expand the second column into its own DataFrame, we can use the `apply()` method on that column and pass it the Series constructor:",
"_____no_output_____"
]
],
[
[
"df_new = df.col_two.apply(pd.Series)\ndf_new",
"_____no_output_____"
]
],
[
[
"And by using the `concat()` function, you can combine the original DataFrame with the new DataFrame:",
"_____no_output_____"
]
],
[
[
"pd.concat([df, df_new], axis='columns')",
"_____no_output_____"
]
],
[
[
"## 18. Aggregate by multiple functions",
"_____no_output_____"
],
[
"Let's look at a DataFrame of orders from the Chipotle restaurant chain:",
"_____no_output_____"
]
],
[
[
"orders.head(10)",
"_____no_output_____"
]
],
[
[
"Each order has an order_id and consists of one or more rows. To figure out the total price of an order, you sum the item_price for that order_id. For example, here's the total price of order number 1:",
"_____no_output_____"
]
],
[
[
"orders[orders.order_id == 1].item_price.sum()",
"_____no_output_____"
]
],
[
[
"If you wanted to calculate the total price of every order, you would `groupby()` order_id and then take the sum of item_price for each group:",
"_____no_output_____"
]
],
[
[
"orders.groupby('order_id').item_price.sum().head()",
"_____no_output_____"
]
],
[
[
"However, you're not actually limited to aggregating by a single function such as `sum()`. To aggregate by multiple functions, you use the `agg()` method and pass it a list of functions such as `sum()` and `count()`:",
"_____no_output_____"
]
],
[
[
"orders.groupby('order_id').item_price.agg(['sum', 'count']).head()",
"_____no_output_____"
]
],
[
[
"That gives us the total price of each order as well as the number of items in each order.",
"_____no_output_____"
],
[
"## 19. Combine the output of an aggregation with a DataFrame",
"_____no_output_____"
],
[
"Let's take another look at the orders DataFrame:",
"_____no_output_____"
]
],
[
[
"orders.head(10)",
"_____no_output_____"
]
],
[
[
"What if we wanted to create a new column listing the total price of each order? Recall that we calculated the total price using the `sum()` method:",
"_____no_output_____"
]
],
[
[
"orders.groupby('order_id').item_price.sum().head()",
"_____no_output_____"
]
],
[
[
"`sum()` is an aggregation function, which means that it returns a reduced version of the input data.\n\nIn other words, the output of the `sum()` function:",
"_____no_output_____"
]
],
[
[
"len(orders.groupby('order_id').item_price.sum())",
"_____no_output_____"
]
],
[
[
"...is smaller than the input to the function:",
"_____no_output_____"
]
],
[
[
"len(orders.item_price)",
"_____no_output_____"
]
],
[
[
"The solution is to use the `transform()` method, which performs the same calculation but returns output data that is the same shape as the input data:",
"_____no_output_____"
]
],
[
[
"total_price = orders.groupby('order_id').item_price.transform('sum')\nlen(total_price)",
"_____no_output_____"
]
],
[
[
"We'll store the results in a new DataFrame column called total_price:",
"_____no_output_____"
]
],
[
[
"orders['total_price'] = total_price\norders.head(10)",
"_____no_output_____"
]
],
[
[
"As you can see, the total price of each order is now listed on every single line.\n\nThat makes it easy to calculate the percentage of the total order price that each line represents:",
"_____no_output_____"
]
],
[
[
"orders['percent_of_total'] = orders.item_price / orders.total_price\norders.head(10)",
"_____no_output_____"
]
],
[
[
"## 20. Select a slice of rows and columns",
"_____no_output_____"
],
[
"Let's take a look at another dataset:",
"_____no_output_____"
]
],
[
[
"titanic.head()",
"_____no_output_____"
]
],
[
[
"This is the famous Titanic dataset, which shows information about passengers on the Titanic and whether or not they survived.\n\nIf you wanted a numerical summary of the dataset, you would use the `describe()` method:",
"_____no_output_____"
]
],
[
[
"titanic.describe()",
"_____no_output_____"
]
],
[
[
"However, the resulting DataFrame might be displaying more information than you need.\n\nIf you wanted to filter it to only show the \"five-number summary\", you can use the `loc` accessor and pass it a slice of the \"min\" through the \"max\" row labels:",
"_____no_output_____"
]
],
[
[
"titanic.describe().loc['min':'max']",
"_____no_output_____"
]
],
[
[
"And if you're not interested in all of the columns, you can also pass it a slice of column labels:",
"_____no_output_____"
]
],
[
[
"titanic.describe().loc['min':'max', 'Pclass':'Parch']",
"_____no_output_____"
]
],
[
[
"## 21. Reshape a MultiIndexed Series",
"_____no_output_____"
],
[
"The Titanic dataset has a \"Survived\" column made up of ones and zeros, so you can calculate the overall survival rate by taking a mean of that column:",
"_____no_output_____"
]
],
[
[
"titanic.Survived.mean()",
"_____no_output_____"
]
],
[
[
"If you wanted to calculate the survival rate by a single category such as \"Sex\", you would use a `groupby()`:",
"_____no_output_____"
]
],
[
[
"titanic.groupby('Sex').Survived.mean()",
"_____no_output_____"
]
],
[
[
"And if you wanted to calculate the survival rate across two different categories at once, you would `groupby()` both of those categories:",
"_____no_output_____"
]
],
[
[
"titanic.groupby(['Sex', 'Pclass']).Survived.mean()",
"_____no_output_____"
]
],
[
[
"This shows the survival rate for every combination of Sex and Passenger Class. It's stored as a MultiIndexed Series, meaning that it has multiple index levels to the left of the actual data.\n\nIt can be hard to read and interact with data in this format, so it's often more convenient to reshape a MultiIndexed Series into a DataFrame by using the `unstack()` method:",
"_____no_output_____"
]
],
[
[
"titanic.groupby(['Sex', 'Pclass']).Survived.mean().unstack()",
"_____no_output_____"
]
],
[
[
"This DataFrame contains the same exact data as the MultiIndexed Series, except that now you can interact with it using familiar DataFrame methods.",
"_____no_output_____"
],
[
"## 22. Create a pivot table",
"_____no_output_____"
],
[
"If you often create DataFrames like the one above, you might find it more convenient to use the `pivot_table()` method instead:",
"_____no_output_____"
]
],
[
[
"titanic.pivot_table(index='Sex', columns='Pclass', values='Survived', aggfunc='mean')",
"_____no_output_____"
]
],
[
[
"With a pivot table, you directly specify the index, the columns, the values, and the aggregation function.\n\nAn added benefit of a pivot table is that you can easily add row and column totals by setting `margins=True`:",
"_____no_output_____"
]
],
[
[
"titanic.pivot_table(index='Sex', columns='Pclass', values='Survived', aggfunc='mean',\n margins=True)",
"_____no_output_____"
]
],
[
[
"This shows the overall survival rate as well as the survival rate by Sex and Passenger Class.\n\nFinally, you can create a cross-tabulation just by changing the aggregation function from \"mean\" to \"count\":",
"_____no_output_____"
]
],
[
[
"titanic.pivot_table(index='Sex', columns='Pclass', values='Survived', aggfunc='count',\n margins=True)",
"_____no_output_____"
]
],
[
[
"This shows the number of records that appear in each combination of categories.",
"_____no_output_____"
],
[
"## 23. Convert continuous data into categorical data",
"_____no_output_____"
],
[
"Let's take a look at the Age column from the Titanic dataset:",
"_____no_output_____"
]
],
[
[
"titanic.Age.head(10)",
"_____no_output_____"
]
],
[
[
"It's currently continuous data, but what if you wanted to convert it into categorical data?\n\nOne solution would be to label the age ranges, such as \"child\", \"young adult\", and \"adult\". The best way to do this is by using the `cut()` function:",
"_____no_output_____"
]
],
[
[
"pd.cut(titanic.Age, bins=[0, 18, 25, 99], labels=['child', 'young adult', 'adult']).head(10)",
"_____no_output_____"
]
],
[
[
"This assigned each value to a bin with a label. Ages 0 to 18 were assigned the label \"child\", ages 18 to 25 were assigned the label \"young adult\", and ages 25 to 99 were assigned the label \"adult\".\n\nNotice that the data type is now \"category\", and the categories are automatically ordered.",
"_____no_output_____"
],
[
"## 24. Change display options",
"_____no_output_____"
],
[
"Let's take another look at the Titanic dataset:",
"_____no_output_____"
]
],
[
[
"titanic.head()",
"_____no_output_____"
]
],
[
[
"Notice that the Age column has 1 decimal place and the Fare column has 4 decimal places. What if you wanted to standardize the display to use 2 decimal places?\n\nYou can use the `set_option()` function:",
"_____no_output_____"
]
],
[
[
"pd.set_option('display.float_format', '{:.2f}'.format)",
"_____no_output_____"
]
],
[
[
"The first argument is the name of the option, and the second argument is a Python format string.",
"_____no_output_____"
]
],
[
[
"titanic.head()",
"_____no_output_____"
]
],
[
[
"You can see that Age and Fare are now using 2 decimal places. Note that this did not change the underlying data, only the display of the data.\n\nYou can also reset any option back to its default:",
"_____no_output_____"
]
],
[
[
"pd.reset_option('display.float_format')",
"_____no_output_____"
]
],
[
[
"There are many more options you can specify is a similar way.",
"_____no_output_____"
],
[
"## 25. Style a DataFrame",
"_____no_output_____"
],
[
"The previous trick is useful if you want to change the display of your entire notebook. However, a more flexible and powerful approach is to define the style of a particular DataFrame.\n\nLet's return to the stocks DataFrame:",
"_____no_output_____"
]
],
[
[
"stocks",
"_____no_output_____"
]
],
[
[
"We can create a dictionary of format strings that specifies how each column should be formatted:",
"_____no_output_____"
]
],
[
[
"format_dict = {'Date':'{:%m/%d/%y}', 'Close':'${:.2f}', 'Volume':'{:,}'}",
"_____no_output_____"
]
],
[
[
"And then we can pass it to the DataFrame's `style.format()` method:",
"_____no_output_____"
]
],
[
[
"stocks.style.format(format_dict)",
"_____no_output_____"
]
],
[
[
"Notice that the Date is now in month-day-year format, the closing price has a dollar sign, and the Volume has commas.\n\nWe can apply more styling by chaining additional methods:",
"_____no_output_____"
]
],
[
[
"(stocks.style.format(format_dict)\n .hide_index()\n .highlight_min('Close', color='red')\n .highlight_max('Close', color='lightgreen')\n)",
"_____no_output_____"
]
],
[
[
"We've now hidden the index, highlighted the minimum Close value in red, and highlighted the maximum Close value in green.\n\nHere's another example of DataFrame styling:",
"_____no_output_____"
]
],
[
[
"(stocks.style.format(format_dict)\n .hide_index()\n .background_gradient(subset='Volume', cmap='Blues')\n)",
"_____no_output_____"
]
],
[
[
"The Volume column now has a background gradient to help you easily identify high and low values.\n\nAnd here's one final example:",
"_____no_output_____"
]
],
[
[
"(stocks.style.format(format_dict)\n .hide_index()\n .bar('Volume', color='lightblue', align='zero')\n .set_caption('Stock Prices from October 2016')\n)",
"_____no_output_____"
]
],
[
[
"There's now a bar chart within the Volume column and a caption above the DataFrame.\n\nNote that there are many more options for how you can style your DataFrame.",
"_____no_output_____"
],
[
"## Bonus: Profile a DataFrame",
"_____no_output_____"
],
[
"Let's say that you've got a new dataset, and you want to quickly explore it without too much work. There's a separate package called [pandas-profiling](https://github.com/pandas-profiling/pandas-profiling) that is designed for this purpose.\n\nFirst you have to install it using conda or pip. Once that's done, you import `pandas_profiling`:",
"_____no_output_____"
]
],
[
[
"import pandas_profiling",
"_____no_output_____"
]
],
[
[
"Then, simply run the `ProfileReport()` function and pass it any DataFrame. It returns an interactive HTML report:\n\n- The first section is an overview of the dataset and a list of possible issues with the data.\n- The next section gives a summary of each column. You can click \"toggle details\" for even more information.\n- The third section shows a heatmap of the correlation between columns.\n- And the fourth section shows the head of the dataset.",
"_____no_output_____"
]
],
[
[
"pandas_profiling.ProfileReport(titanic)",
"_____no_output_____"
]
]
] | [
"markdown",
"code",
"markdown",
"code",
"markdown",
"code",
"markdown",
"code",
"markdown",
"code",
"markdown",
"code",
"markdown",
"code",
"markdown",
"code",
"markdown",
"code",
"markdown",
"code",
"markdown",
"code",
"markdown",
"code",
"markdown",
"code",
"markdown",
"code",
"markdown",
"code",
"markdown",
"code",
"markdown",
"code",
"markdown",
"code",
"markdown",
"code",
"markdown",
"code",
"markdown",
"code",
"markdown",
"code",
"markdown",
"code",
"markdown",
"code",
"markdown",
"code",
"markdown",
"code",
"markdown",
"code",
"markdown",
"code",
"markdown",
"code",
"markdown",
"code",
"markdown",
"code",
"markdown",
"code",
"markdown",
"code",
"markdown",
"code",
"markdown",
"code",
"markdown",
"code",
"markdown",
"code",
"markdown",
"code",
"markdown",
"code",
"markdown",
"code",
"markdown",
"code",
"markdown",
"code",
"markdown",
"code",
"markdown",
"code",
"markdown",
"code",
"markdown",
"code",
"markdown",
"code",
"markdown",
"code",
"markdown",
"code",
"markdown",
"code",
"markdown",
"code",
"markdown",
"code",
"markdown",
"code",
"markdown",
"code",
"markdown",
"code",
"markdown",
"code",
"markdown",
"code",
"markdown",
"code",
"markdown",
"code",
"markdown",
"code",
"markdown",
"code",
"markdown",
"code",
"markdown",
"code",
"markdown",
"code",
"markdown",
"code",
"markdown",
"code",
"markdown",
"code",
"markdown",
"code",
"markdown",
"code",
"markdown",
"code",
"markdown",
"code",
"markdown",
"code",
"markdown",
"code",
"markdown",
"code",
"markdown",
"code",
"markdown",
"code",
"markdown",
"code",
"markdown",
"code",
"markdown",
"code",
"markdown",
"code",
"markdown",
"code",
"markdown",
"code",
"markdown",
"code",
"markdown",
"code",
"markdown",
"code",
"markdown",
"code",
"markdown",
"code",
"markdown",
"code",
"markdown",
"code",
"markdown",
"code",
"markdown",
"code",
"markdown",
"code",
"markdown",
"code",
"markdown",
"code",
"markdown",
"code",
"markdown",
"code",
"markdown",
"code",
"markdown",
"code",
"markdown",
"code",
"markdown",
"code",
"markdown",
"code",
"markdown",
"code",
"markdown",
"code",
"markdown",
"code",
"markdown",
"code",
"markdown",
"code",
"markdown",
"code",
"markdown",
"code",
"markdown",
"code",
"markdown",
"code"
] | [
[
"markdown",
"markdown",
"markdown"
],
[
"code",
"code"
],
[
"markdown",
"markdown"
],
[
"code"
],
[
"markdown"
],
[
"code"
],
[
"markdown",
"markdown",
"markdown"
],
[
"code"
],
[
"markdown"
],
[
"code"
],
[
"markdown"
],
[
"code"
],
[
"markdown",
"markdown",
"markdown"
],
[
"code"
],
[
"markdown"
],
[
"code"
],
[
"markdown"
],
[
"code"
],
[
"markdown"
],
[
"code"
],
[
"markdown"
],
[
"code"
],
[
"markdown"
],
[
"code"
],
[
"markdown"
],
[
"code"
],
[
"markdown",
"markdown"
],
[
"code"
],
[
"markdown"
],
[
"code"
],
[
"markdown"
],
[
"code"
],
[
"markdown",
"markdown",
"markdown"
],
[
"code"
],
[
"markdown",
"markdown",
"markdown"
],
[
"code"
],
[
"markdown"
],
[
"code"
],
[
"markdown"
],
[
"code"
],
[
"markdown"
],
[
"code"
],
[
"markdown"
],
[
"code"
],
[
"markdown",
"markdown"
],
[
"code"
],
[
"markdown"
],
[
"code"
],
[
"markdown"
],
[
"code"
],
[
"markdown"
],
[
"code"
],
[
"markdown"
],
[
"code"
],
[
"markdown"
],
[
"code"
],
[
"markdown"
],
[
"code"
],
[
"markdown",
"markdown"
],
[
"code"
],
[
"markdown"
],
[
"code"
],
[
"markdown"
],
[
"code"
],
[
"markdown",
"markdown",
"markdown"
],
[
"code"
],
[
"markdown"
],
[
"code"
],
[
"markdown"
],
[
"code"
],
[
"markdown"
],
[
"code"
],
[
"markdown"
],
[
"code"
],
[
"markdown"
],
[
"code"
],
[
"markdown"
],
[
"code"
],
[
"markdown",
"markdown"
],
[
"code",
"code"
],
[
"markdown"
],
[
"code"
],
[
"markdown"
],
[
"code"
],
[
"markdown",
"markdown",
"markdown"
],
[
"code"
],
[
"markdown"
],
[
"code"
],
[
"markdown"
],
[
"code"
],
[
"markdown"
],
[
"code"
],
[
"markdown",
"markdown",
"markdown"
],
[
"code"
],
[
"markdown"
],
[
"code"
],
[
"markdown"
],
[
"code"
],
[
"markdown"
],
[
"code"
],
[
"markdown"
],
[
"code"
],
[
"markdown"
],
[
"code"
],
[
"markdown",
"markdown",
"markdown"
],
[
"code"
],
[
"markdown"
],
[
"code"
],
[
"markdown"
],
[
"code"
],
[
"markdown"
],
[
"code"
],
[
"markdown"
],
[
"code"
],
[
"markdown",
"markdown",
"markdown"
],
[
"code"
],
[
"markdown"
],
[
"code"
],
[
"markdown"
],
[
"code"
],
[
"markdown"
],
[
"code"
],
[
"markdown",
"markdown",
"markdown"
],
[
"code"
],
[
"markdown"
],
[
"code"
],
[
"markdown"
],
[
"code"
],
[
"markdown"
],
[
"code"
],
[
"markdown"
],
[
"code"
],
[
"markdown",
"markdown",
"markdown"
],
[
"code"
],
[
"markdown"
],
[
"code"
],
[
"markdown"
],
[
"code"
],
[
"markdown"
],
[
"code"
],
[
"markdown"
],
[
"code"
],
[
"markdown",
"markdown"
],
[
"code"
],
[
"markdown"
],
[
"code"
],
[
"markdown"
],
[
"code"
],
[
"markdown",
"markdown"
],
[
"code"
],
[
"markdown"
],
[
"code"
],
[
"markdown"
],
[
"code"
],
[
"markdown"
],
[
"code"
],
[
"markdown",
"markdown",
"markdown"
],
[
"code"
],
[
"markdown"
],
[
"code"
],
[
"markdown"
],
[
"code"
],
[
"markdown"
],
[
"code"
],
[
"markdown"
],
[
"code"
],
[
"markdown"
],
[
"code"
],
[
"markdown"
],
[
"code"
],
[
"markdown",
"markdown"
],
[
"code"
],
[
"markdown"
],
[
"code"
],
[
"markdown"
],
[
"code"
],
[
"markdown"
],
[
"code"
],
[
"markdown",
"markdown"
],
[
"code"
],
[
"markdown"
],
[
"code"
],
[
"markdown"
],
[
"code"
],
[
"markdown"
],
[
"code"
],
[
"markdown",
"markdown",
"markdown"
],
[
"code"
],
[
"markdown"
],
[
"code"
],
[
"markdown"
],
[
"code"
],
[
"markdown",
"markdown",
"markdown"
],
[
"code"
],
[
"markdown"
],
[
"code"
],
[
"markdown",
"markdown",
"markdown"
],
[
"code"
],
[
"markdown"
],
[
"code"
],
[
"markdown"
],
[
"code"
],
[
"markdown"
],
[
"code"
],
[
"markdown",
"markdown",
"markdown"
],
[
"code"
],
[
"markdown"
],
[
"code"
],
[
"markdown"
],
[
"code"
],
[
"markdown"
],
[
"code"
],
[
"markdown"
],
[
"code"
],
[
"markdown"
],
[
"code"
],
[
"markdown",
"markdown",
"markdown"
],
[
"code"
],
[
"markdown"
],
[
"code"
]
] |
e77e70eaf347ecc27430bf94b2c36de37c2f3f6c | 211,369 | ipynb | Jupyter Notebook | MainProjectNotebook.ipynb | sarandara/Udacity_ISDC_Implement_Route_Planner | ce67312942a499cf7fded001f62ed6006c93df1d | [
"MIT"
] | null | null | null | MainProjectNotebook.ipynb | sarandara/Udacity_ISDC_Implement_Route_Planner | ce67312942a499cf7fded001f62ed6006c93df1d | [
"MIT"
] | null | null | null | MainProjectNotebook.ipynb | sarandara/Udacity_ISDC_Implement_Route_Planner | ce67312942a499cf7fded001f62ed6006c93df1d | [
"MIT"
] | null | null | null | 51.141786 | 15,208 | 0.640084 | [
[
[
"# Implementing a Route Planner\nIn this project you will use A\\* search to implement a \"Google-maps\" style route planning algorithm.",
"_____no_output_____"
],
[
"## The Map",
"_____no_output_____"
]
],
[
[
"# Run this cell first!\n\nfrom helpers import Map, load_map_10, load_map_40, show_map\nimport math\n\n%load_ext autoreload\n%autoreload 2",
"_____no_output_____"
]
],
[
[
"### Map Basics",
"_____no_output_____"
]
],
[
[
"map_10 = load_map_10()\nshow_map(map_10)",
"_____no_output_____"
]
],
[
[
"The map above (run the code cell if you don't see it) shows a disconnected network of 10 intersections. The two intersections on the left are connected to each other but they are not connected to the rest of the road network. This map is quite literal in its expression of distance and connectivity. On the graph above, the edge between 2 nodes(intersections) represents a literal straight road not just an abstract connection of 2 cities.\n\nThese `Map` objects have two properties you will want to use to implement A\\* search: `intersections` and `roads`\n\n**Intersections**\n\nThe `intersections` are represented as a dictionary. \n\nIn this example, there are 10 intersections, each identified by an x,y coordinate. The coordinates are listed below. You can hover over each dot in the map above to see the intersection number.",
"_____no_output_____"
]
],
[
[
"map_10.intersections\n#type(len(map_10.intersections))",
"_____no_output_____"
]
],
[
[
"**Roads**\n\nThe `roads` property is a list where `roads[i]` contains a list of the intersections that intersection `i` connects to.",
"_____no_output_____"
]
],
[
[
"# this shows that intersection 0 connects to intersections 7, 6, and 5\nmap_10.roads[0] ",
"_____no_output_____"
],
[
"# This shows the full connectivity of the map\nmap_10.roads",
"_____no_output_____"
],
[
"# map_40 is a bigger map than map_10\nmap_40 = load_map_40()\nshow_map(map_40)",
"_____no_output_____"
]
],
[
[
"### Advanced Visualizations\n\nThe map above shows a network of roads which spans 40 different intersections (labeled 0 through 39). \n\nThe `show_map` function which generated this map also takes a few optional parameters which might be useful for visualizing the output of the search algorithm you will write.\n\n* `start` - The \"start\" node for the search algorithm.\n* `goal` - The \"goal\" node.\n* `path` - An array of integers which corresponds to a valid sequence of intersection visits on the map.",
"_____no_output_____"
]
],
[
[
"# run this code, note the effect of including the optional\n# parameters in the function call.\nshow_map(map_40, start=5, goal=34, path=[5,16,37,12,34])",
"_____no_output_____"
]
],
[
[
"## The Algorithm\n### Writing your algorithm\nThe algorithm written will be responsible for generating a `path` like the one passed into `show_map` above. In fact, when called with the same map, start and goal, as above you algorithm should produce the path `[5, 16, 37, 12, 34]`. However you must complete several methods before it will work.\n\n```bash\n> PathPlanner(map_40, 5, 34).path\n[5, 16, 37, 12, 34]\n```",
"_____no_output_____"
],
[
"### PathPlanner class\n\nThe below class is already partly implemented for you - you will implement additional functions that will also get included within this class further below.\n\nLet's very briefly walk through each part below.\n\n`__init__` - We initialize our path planner with a map, M, and typically a start and goal node. If either of these are `None`, the rest of the variables here are also set to none. If you don't have both a start and a goal, there's no path to plan! The rest of these variables come from functions you will soon implement. \n- `closedSet` includes any explored/visited nodes. \n- `openSet` are any nodes on our frontier for potential future exploration. \n- `cameFrom` will hold the previous node that best reaches a given node\n- `gScore` is the `g` in our `f = g + h` equation, or the actual cost to reach our current node\n- `fScore` is the combination of `g` and `h`, i.e. the `gScore` plus a heuristic; total cost to reach the goal\n- `path` comes from the `run_search` function, which is already built for you.\n\n`reconstruct_path` - This function just rebuilds the path after search is run, going from the goal node backwards using each node's `cameFrom` information.\n\n`_reset` - Resets *most* of our initialized variables for PathPlanner. This *does not* reset the map, start or goal variables, for reasons which you may notice later, depending on your implementation.\n\n`run_search` - This does a lot of the legwork to run search once you've implemented everything else below. First, it checks whether the map, goal and start have been added to the class. Then, it will also check if the other variables, other than `path` are initialized (note that these are only needed to be re-run if the goal or start were not originally given when initializing the class, based on what we discussed above for `__init__`.\n\nFrom here, we use a function you will implement, `is_open_empty`, to check that there are still nodes to explore (you'll need to make sure to feed `openSet` the start node to make sure the algorithm doesn't immediately think there is nothing to open!). If we're at our goal, we reconstruct the path. If not, we move our current node from the frontier (`openSet`) and into explored (`closedSet`). Then, we check out the neighbors of the current node, check out their costs, and plan our next move.\n\nThis is the main idea behind A*, but none of it is going to work until you implement all the relevant parts, which will be included below after the class code.",
"_____no_output_____"
]
],
[
[
"# Do not change this cell\n# When you write your methods correctly this cell will execute\n# without problems\nclass PathPlanner():\n \"\"\"Construct a PathPlanner Object\"\"\"\n def __init__(self, M, start=None, goal=None):\n \"\"\" \"\"\"\n self.map = M\n self.start= start\n self.goal = goal\n self.closedSet = self.create_closedSet() if goal != None and start != None else None\n self.openSet = self.create_openSet() if goal != None and start != None else None\n self.cameFrom = self.create_cameFrom() if goal != None and start != None else None\n self.gScore = self.create_gScore() if goal != None and start != None else None\n self.fScore = self.create_fScore() if goal != None and start != None else None\n self.path = self.run_search() if self.map and self.start != None and self.goal != None else None\n\n \n \n def reconstruct_path(self, current):\n \"\"\" Reconstructs path after search \"\"\"\n total_path = [current]\n while current in self.cameFrom.keys():\n current = self.cameFrom[current]\n total_path.append(current)\n return total_path\n \n def _reset(self):\n \"\"\"Private method used to reset the closedSet, openSet, cameFrom, gScore, fScore, and path attributes\"\"\"\n self.closedSet = None\n self.openSet = None\n self.cameFrom = None\n self.gScore = None\n self.fScore = None\n self.path = self.run_search() if self.map and self.start and self.goal else None\n\n def run_search(self):\n \"\"\" \"\"\"\n if self.map == None:\n raise(ValueError, \"Must create map before running search. Try running PathPlanner.set_map(start_node)\")\n if self.goal == None:\n raise(ValueError, \"Must create goal node before running search. Try running PathPlanner.set_goal(start_node)\")\n if self.start == None:\n raise(ValueError, \"Must create start node before running search. Try running PathPlanner.set_start(start_node)\")\n\n self.closedSet = self.closedSet if self.closedSet != None else self.create_closedSet()\n self.openSet = self.openSet if self.openSet != None else self.create_openSet()\n self.cameFrom = self.cameFrom if self.cameFrom != None else self.create_cameFrom()\n self.gScore = self.gScore if self.gScore != None else self.create_gScore()\n self.fScore = self.fScore if self.fScore != None else self.create_fScore()\n\n while not self.is_open_empty():\n current = self.get_current_node()\n\n if current == self.goal:\n self.path = [x for x in reversed(self.reconstruct_path(current))]\n return self.path\n else:\n self.openSet.remove(current)\n self.closedSet.add(current)\n \n for neighbor in self.get_neighbors(current):\n \n if neighbor in self.closedSet:\n continue # Ignore the neighbor which is already evaluated.\n \n if not neighbor in self.openSet: # Discover a new node\n self.openSet.add(neighbor)\n\n # The distance from start to a neighbor\n #the \"dist_between\" function may vary as per the solution requirements.\n if self.get_tentative_gScore(current, neighbor) >= self.get_gScore(neighbor): \n \n continue # This is not a better path.\n\n # This path is the best until now. Record it!\n self.record_best_path_to(current, neighbor) \n print(\"No Path Found\")\n self.path = None\n return False",
"_____no_output_____"
]
],
[
[
"## Your Turn\n\nImplement the following functions to get your search algorithm running smoothly!",
"_____no_output_____"
],
[
"### Data Structures\n\nThe next few functions requre you to decide on data structures to use - lists, sets, dictionaries, etc. Make sure to think about what would work most efficiently for each of these. Some can be returned as just an empty data structure (see `create_closedSet()` for an example), while others should be initialized with one or more values within.",
"_____no_output_____"
]
],
[
[
"def create_closedSet(self):\n \"\"\" Creates and returns a data structure suitable to hold the set of nodes already evaluated\"\"\"\n # EXAMPLE: return a data structure suitable to hold the set of nodes already evaluated\n return set()",
"_____no_output_____"
],
[
"def create_openSet(self):\n \"\"\" Creates and returns a data structure suitable to hold the set of currently discovered nodes \n that are not evaluated yet. Initially, only the start node is known.\"\"\"\n if self.start != None:\n # TODO: return a data structure suitable to hold the set of currently discovered nodes \n # that are not evaluated yet. Make sure to include the start node.\n \n return set([self.start])\n \n raise(ValueError, \"Must create start node before creating an open set. Try running PathPlanner.set_start(start_node)\")",
"_____no_output_____"
],
[
"def create_cameFrom(self):\n \"\"\"Creates and returns a data structure that shows which node can most efficiently be reached from another,\n for each node.\"\"\"\n # TODO: return a data structure that shows which node can most efficiently be reached from another,\n # for each node. \n #cameFrom_Register = {}\n #cameFrom_Register[self.start] = None\n cameFrom_Register = {}\n return cameFrom_Register",
"_____no_output_____"
],
[
"def create_gScore(self):\n \"\"\"Creates and returns a data structure that holds the cost of getting from the start node to that node, \n for each node. The cost of going from start to start is zero.\"\"\"\n # TODO: return a data structure that holds the cost of getting from the start node to that node, for each node.\n # for each node. The cost of going from start to start is zero. The rest of the node's values should \n # be set to infinity.\n \n the_map = self.map\n node_data = the_map.intersections\n start = self.start\n goal = self.goal\n \n gScore_register = {}\n g0 = 0\n for node, cood in node_data.items():\n if node == start:\n gScore_register[node] = g0\n else:\n gScore_register[node] = float('Inf')\n \n \n #print(gScore_register)\n return gScore_register\n ",
"_____no_output_____"
],
[
"def create_fScore(self):\n \"\"\"Creates and returns a data structure that holds the total cost of getting from the start node to the goal\n by passing by that node, for each node. That value is partly known, partly heuristic.\n For the first node, that value is completely heuristic.\"\"\"\n # TODO: return a data structure that holds the total cost of getting from the start node to the goal\n # by passing by that node, for each node. That value is partly known, partly heuristic.\n # For the first node, that value is completely heuristic. The rest of the node's value should be \n # set to infinity.\n\n #fScore_register = {node:fscore_for_the_node}\n #fScore_register = {node_0:h, node_n:g+h}\n \n #fScore_register[0] = distance(start,goal)\n # for nodes in range(0,len(map_10.intersections))\n the_map = self.map\n node_data = the_map.intersections\n start = self.start\n #print(\"start = \",start)\n goal = self.goal\n #print(\"goal = \",goal)\n \n fScore_register = {}\n h0 = self.distance(node_1=start,node_2=goal)\n #h0 = 10\n for node, cood in node_data.items():\n #if node == 0:\n if node == start:\n fScore_register[node] = h0\n else:\n fScore_register[node] = float('Inf')\n \n #print(fScore_register)\n return fScore_register\n \n",
"_____no_output_____"
]
],
[
[
"### Set certain variables\n\nThe below functions help set certain variables if they weren't a part of initializating our `PathPlanner` class, or if they need to be changed for anothe reason.",
"_____no_output_____"
]
],
[
[
"def set_map(self, M):\n \"\"\"Method used to set map attribute \"\"\"\n self._reset(self)\n self.start = None\n self.goal = None\n # TODO: Set map to new value. \n self.map = M\n \n",
"_____no_output_____"
],
[
"def set_start(self, start):\n \"\"\"Method used to set start attribute \"\"\"\n self._reset(self)\n # TODO: Set start value. Remember to remove goal, closedSet, openSet, cameFrom, gScore, fScore, \n # and path attributes' values.\n self.start = start\n \n ",
"_____no_output_____"
],
[
"def set_goal(self, goal):\n \"\"\"Method used to set goal attribute \"\"\"\n self._reset(self)\n # TODO: Set goal value.\n self.goal = goal\n \n",
"_____no_output_____"
]
],
[
[
"### Get node information\n\nThe below functions concern grabbing certain node information. In `is_open_empty`, you are checking whether there are still nodes on the frontier to explore. In `get_current_node()`, you'll want to come up with a way to find the lowest `fScore` of the nodes on the frontier. In `get_neighbors`, you'll need to gather information from the map to find the neighbors of the current node.",
"_____no_output_____"
]
],
[
[
"def is_open_empty(self):\n \"\"\"returns True if the open set is empty. False otherwise. \"\"\"\n # TODO: Return True if the open set is empty. False otherwise.\n openSet = self.openSet\n if len(openSet) == 0:\n return True\n else:\n return False",
"_____no_output_____"
],
[
"def get_current_node(self):\n \"\"\" Returns the node in the open set with the lowest value of f(node).\"\"\"\n # TODO: Return the node in the open set with the lowest value of f(node).\n openSet = self.openSet\n fScore = self.fScore\n #print(fScore)\n openSet_fScore = {}\n for node in openSet:\n openSet_fScore[node] = fScore[node]\n #the assumption here is that fScore is a dictionary that is like {node:fScore}\n #print(\"openSet_fScore = \", openSet_fScore)\n min_openSet_fScore_node = min(openSet_fScore,key=openSet_fScore.get)\n #print(\"Min fScore of node \", min_openSet_fScore_node,\" = \",openSet_fScore[min_openSet_fScore_node])\n return min_openSet_fScore_node",
"_____no_output_____"
],
[
"def get_neighbors(self, node):\n \"\"\"Returns the neighbors of a node\"\"\"\n # TODO: Return the neighbors of a node\n the_map = self.map\n return the_map.roads[node] ",
"_____no_output_____"
]
],
[
[
"### Scores and Costs\n\nBelow, you'll get into the main part of the calculation for determining the best path - calculating the various parts of the `fScore`.",
"_____no_output_____"
]
],
[
[
"def get_gScore(self, node):\n \"\"\"Returns the g Score of a node\"\"\"\n # TODO: Return the g Score of a node\n \n # I need to go to some dictionary and enter the node number (and maybe point ot the g-score) and get the g-score\n # I will call the \"some dictionary\" node_data\n gScore_data = self.gScore\n gScore = gScore_data[node]\n return gScore\n \n \n",
"_____no_output_____"
],
[
"def distance(self, node_1, node_2):\n \"\"\" Computes the Euclidean L2 Distance\"\"\"\n # TODO: Compute and return the Euclidean L2 Distance\n #print('here')\n nodal_data = self.map.intersections\n #print(nodal_data)\n node_1_x = nodal_data[node_1][0] \n node_1_y = nodal_data[node_1][1] \n node_2_x = nodal_data[node_2][0] \n node_2_y = nodal_data[node_2][1]\n euc_dis = ((node_2_x - node_1_x)**2 + (node_2_y - node_1_y)**2)**0.5\n return euc_dis\n",
"_____no_output_____"
],
[
"def get_tentative_gScore(self, current, neighbor):\n \"\"\"Returns the tentative g Score of a node\"\"\"\n # TODO: Return the g Score of the current node \n # plus distance from the current node to it's neighbors\n \n #print(\"current node is \",current)\n current_node_g_score = self.get_gScore(current)\n #print(\"current node gscore = \", current_node_g_score)\n distance_from_current_to_neighbor = self.distance(current,neighbor)\n #print(\"distance from current to neighbor\", distance_from_current_to_neighbor)\n tentative_g_score = current_node_g_score + distance_from_current_to_neighbor\n return tentative_g_score\n",
"_____no_output_____"
],
[
"def heuristic_cost_estimate(self, node):\n \"\"\" Returns the heuristic cost estimate of a node \"\"\"\n # TODO: Return the heuristic cost estimate of a node\n goal = self.goal\n distance_from_node_to_goal = self.distance(node,goal) \n return distance_from_node_to_goal\n",
"_____no_output_____"
],
[
"def calculate_fscore(self, node):\n \"\"\"Calculate the f score of a node. \"\"\"\n # TODO: Calculate and returns the f score of a node. \n # REMEMBER F = G + H\n fscore = self.get_gScore(node) + self.heuristic_cost_estimate(node)\n ",
"_____no_output_____"
]
],
[
[
"### Recording the best path\n\nNow that you've implemented the various functions on scoring, you can record the best path to a given neighbor node from the current node!",
"_____no_output_____"
]
],
[
[
"def record_best_path_to(self, current, neighbor):\n \"\"\"Record the best path to a node \"\"\"\n # TODO: Record the best path to a node, by updating cameFrom, gScore, and fScore\n #self.cameFrom[neighbor] = current\n #self.gScore[neighbor] = self.distance(current,neighbor)\n #print(gScore)\n #fScore_register = self.fScore\n \n self.cameFrom[neighbor] = current\n self.gScore[neighbor] = self.get_tentative_gScore(current,neighbor)\n self.fScore[neighbor] = self.gScore[neighbor] + self.heuristic_cost_estimate(neighbor)\n \n \n \n",
"_____no_output_____"
]
],
[
[
"### Associating your functions with the `PathPlanner` class\n\nTo check your implementations, we want to associate all of the above functions back to the `PathPlanner` class. Python makes this easy using the dot notation (i.e. `PathPlanner.myFunction`), and setting them equal to your function implementations. Run the below code cell for this to occur.\n\n*Note*: If you need to make further updates to your functions above, you'll need to re-run this code cell to associate the newly updated function back with the `PathPlanner` class again!",
"_____no_output_____"
]
],
[
[
"# Associates implemented functions with PathPlanner class\nPathPlanner.create_closedSet = create_closedSet\nPathPlanner.create_openSet = create_openSet\nPathPlanner.create_cameFrom = create_cameFrom\nPathPlanner.create_gScore = create_gScore\nPathPlanner.create_fScore = create_fScore\nPathPlanner.set_map = set_map\nPathPlanner.set_start = set_start\nPathPlanner.set_goal = set_goal\nPathPlanner.is_open_empty = is_open_empty\nPathPlanner.get_current_node = get_current_node\nPathPlanner.get_neighbors = get_neighbors\nPathPlanner.get_gScore = get_gScore\nPathPlanner.distance = distance\nPathPlanner.get_tentative_gScore = get_tentative_gScore\nPathPlanner.heuristic_cost_estimate = heuristic_cost_estimate\nPathPlanner.calculate_fscore = calculate_fscore\nPathPlanner.record_best_path_to = record_best_path_to",
"_____no_output_____"
]
],
[
[
"### Preliminary Test\n\nThe below is the first test case, just based off of one set of inputs. If some of the functions above aren't implemented yet, or are implemented incorrectly, you likely will get an error from running this cell. Try debugging the error to help you figure out what needs further revision!",
"_____no_output_____"
]
],
[
[
"planner = PathPlanner(map_40, 5, 34)\npath = planner.path\nif path == [5, 16, 37, 12, 34]:\n print(\"great! Your code works for these inputs!\")\nelse:\n print(\"something is off, your code produced the following:\")\n print(path)",
"great! Your code works for these inputs!\n"
]
],
[
[
"#### Visualize\n\nOnce the above code worked for you, let's visualize the results of your algorithm!",
"_____no_output_____"
]
],
[
[
"# Visualize your the result of the above test! You can also change start and goal here to check other paths\nstart = 5\ngoal = 34\n\nshow_map(map_40, start=start, goal=goal, path=PathPlanner(map_40, start, goal).path)",
"_____no_output_____"
]
],
[
[
"### Testing your Code\nIf the code below produces no errors, your algorithm is behaving correctly. You are almost ready to submit! Before you submit, go through the following submission checklist:\n\n**Submission Checklist**\n\n1. Does my code pass all tests?\n2. Does my code implement `A*` search and not some other search algorithm?\n3. Do I use an **admissible heuristic** to direct search efforts towards the goal?\n4. Do I use data structures which avoid unnecessarily slow lookups?\n\nWhen you can answer \"yes\" to all of these questions, and also have answered the written questions below, submit by pressing the Submit button in the lower right!",
"_____no_output_____"
]
],
[
[
"from test import test\n\ntest(PathPlanner)",
"All tests pass! Congratulations!\n"
]
],
[
[
"## Questions\n\n**Instructions** \n\nAnswer the following questions in your own words. We do not you expect you to know all of this knowledge on the top of your head. We expect you to do research and ask question. However do not merely copy and paste the answer from a google or stackoverflow. Read the information and understand it first. Then use your own words to explain the answer.",
"_____no_output_____"
],
[
"---\nHow would you explain A-Star to a family member(layman)?\n\n**ANSWER**:\n\nA-Start figures out which path to the goal is the shortest by taking into account the current cost (up to that point), i.e. the current distance travelled, and an estimate of the distance from your current location. It runs this logic in loop until the destination has been reached.\n",
"_____no_output_____"
],
[
"---\nHow does A-Star search algorithm differ from Uniform cost search? What about Best First search?\n\n**ANSWER**:\n\nA uniform cost search will expand out in all directions, in trying to figure out where the goal is. An A-star search is more directed in the direction of the goal.\n\nA best-first search is also more directed that the uniform cost serach, but since it is always trying to reduce the distance between its location and the goal, it can run into problems if it encounters obstacles. It will still get to the destination, but at the cost of not taking the shortest route. The A-star search keeps tabs on the current cost, so if a roundabout route is being taken by the alogrithm, it will know.\n",
"_____no_output_____"
],
[
"---\nWhat is a heuristic?\n\n**ANSWER**:\n\nA heuristic is an estimated solution to a problem. E.g. the actual distance between City A and B will have to take into account the true length of the road, whilst a heuristic would be to estimate the distance beteen City A and B by drawing a straight line and measure this distance.",
"_____no_output_____"
],
[
"---\nWhat is a consistent heuristic?\n\n**ANSWER**:\n\nA consistent heuristic is one where the estimated cost from the current location to the goal is less than the summation of the actual cost and heuristic of path running through an alternate node to the goal.\n\nh(n) <= cost(n,n') + h(n')\n\nwhere:\n\nn is the current node\n\nn' is another node on the path to the goal\n\ncost(n,n') is the actual cost from n to n'\n\nh(n) is the estimated cost from the current node n to the goal\n\nh(n') is the estimated cost from the node n' to the to the goal\n\n\n(Source: https://artint.info/2e/html/ArtInt2e.Ch3.S7.SS2.html)\n ",
"_____no_output_____"
],
[
"---\nWhat is a admissible heuristic? \n\n**ANSWER**:\n\nA heuristic is admissible if the estimated cost (i.e. the heuristic) of getting to the goal from the current location is always less than or equal to actual cost of the getting to that goal (through the lowest cost path). ",
"_____no_output_____"
],
[
"---\n___ admissible heuristic are consistent.\n\n*CHOOSE ONE*\n - All \n - Some\n - None\n \n**ANSWER**:\nSome",
"_____no_output_____"
],
[
"---\n___ Consistent heuristic are admissible.\n\n*CHOOSE ONE*\n - All\n - Some\n - None\n \n**ANSWER**:\nAll",
"_____no_output_____"
],
[
"---",
"_____no_output_____"
]
]
] | [
"markdown",
"code",
"markdown",
"code",
"markdown",
"code",
"markdown",
"code",
"markdown",
"code",
"markdown",
"code",
"markdown",
"code",
"markdown",
"code",
"markdown",
"code",
"markdown",
"code",
"markdown",
"code",
"markdown",
"code",
"markdown",
"code",
"markdown",
"code",
"markdown",
"code",
"markdown"
] | [
[
"markdown",
"markdown"
],
[
"code"
],
[
"markdown"
],
[
"code"
],
[
"markdown"
],
[
"code"
],
[
"markdown"
],
[
"code",
"code",
"code"
],
[
"markdown"
],
[
"code"
],
[
"markdown",
"markdown"
],
[
"code"
],
[
"markdown",
"markdown"
],
[
"code",
"code",
"code",
"code",
"code"
],
[
"markdown"
],
[
"code",
"code",
"code"
],
[
"markdown"
],
[
"code",
"code",
"code"
],
[
"markdown"
],
[
"code",
"code",
"code",
"code",
"code"
],
[
"markdown"
],
[
"code"
],
[
"markdown"
],
[
"code"
],
[
"markdown"
],
[
"code"
],
[
"markdown"
],
[
"code"
],
[
"markdown"
],
[
"code"
],
[
"markdown",
"markdown",
"markdown",
"markdown",
"markdown",
"markdown",
"markdown",
"markdown",
"markdown"
]
] |
e77eab3059a8ba9f0fc1d2e6386c295e6c5662c3 | 130,870 | ipynb | Jupyter Notebook | fiona_nanopore/pipeline/notebook_processed/yanocomp_upsetplots.py.ipynb | bartongroup/Simpson_Davies_Barton_U6_methylation | 85b0936fd95e579a3ea6391e76253e96353f94c6 | [
"MIT"
] | null | null | null | fiona_nanopore/pipeline/notebook_processed/yanocomp_upsetplots.py.ipynb | bartongroup/Simpson_Davies_Barton_U6_methylation | 85b0936fd95e579a3ea6391e76253e96353f94c6 | [
"MIT"
] | null | null | null | fiona_nanopore/pipeline/notebook_processed/yanocomp_upsetplots.py.ipynb | bartongroup/Simpson_Davies_Barton_U6_methylation | 85b0936fd95e579a3ea6391e76253e96353f94c6 | [
"MIT"
] | null | null | null | 259.662698 | 47,348 | 0.904753 | [
[
[
"\n######## snakemake preamble start (automatically inserted, do not edit) ########\nimport sys; sys.path.extend(['/cluster/ggs_lab/mtparker/.conda/envs/snakemake6/lib/python3.10/site-packages', '/cluster/ggs_lab/mtparker/papers/fiona/fiona_nanopore/rules/notebook_templates']); import pickle; snakemake = pickle.loads(b'\\x80\\x04\\x95\\xfc\\n\\x00\\x00\\x00\\x00\\x00\\x00\\x8c\\x10snakemake.script\\x94\\x8c\\tSnakemake\\x94\\x93\\x94)\\x81\\x94}\\x94(\\x8c\\x05input\\x94\\x8c\\x0csnakemake.io\\x94\\x8c\\nInputFiles\\x94\\x93\\x94)\\x81\\x94(\\x8c\"yanocomp/fip37_vs_fio1_vs_col0.bed\\x94\\x8c\"../annotations/miclip_peaks.bed.gz\\x94\\x8cA../annotations/Araport11_GFF3_genes_transposons.201606.no_chr.gtf\\x94\\x8c:../annotations/Arabidopsis_thaliana.TAIR10.dna.toplevel.fa\\x94e}\\x94(\\x8c\\x06_names\\x94}\\x94(\\x8c\\x0eyanocomp_sites\\x94K\\x00K\\x01\\x86\\x94\\x8c\\x0cmiclip_peaks\\x94K\\x01N\\x86\\x94\\x8c\\x03gtf\\x94K\\x02N\\x86\\x94\\x8c\\x05fasta\\x94K\\x03N\\x86\\x94u\\x8c\\x12_allowed_overrides\\x94]\\x94(\\x8c\\x05index\\x94\\x8c\\x04sort\\x94eh\\x1b\\x8c\\tfunctools\\x94\\x8c\\x07partial\\x94\\x93\\x94h\\x06\\x8c\\x19Namedlist._used_attribute\\x94\\x93\\x94\\x85\\x94R\\x94(h!)}\\x94\\x8c\\x05_name\\x94h\\x1bsNt\\x94bh\\x1ch\\x1fh!\\x85\\x94R\\x94(h!)}\\x94h%h\\x1csNt\\x94bh\\x11h\\x06\\x8c\\tNamedlist\\x94\\x93\\x94)\\x81\\x94h\\na}\\x94(h\\x0f}\\x94h\\x19]\\x94(h\\x1bh\\x1ceh\\x1bh\\x1fh!\\x85\\x94R\\x94(h!)}\\x94h%h\\x1bsNt\\x94bh\\x1ch\\x1fh!\\x85\\x94R\\x94(h!)}\\x94h%h\\x1csNt\\x94bubh\\x13h\\x0bh\\x15h\\x0ch\\x17h\\rub\\x8c\\x06output\\x94h\\x06\\x8c\\x0bOutputFiles\\x94\\x93\\x94)\\x81\\x94(\\x8c\"yanocomp/fip37_vs_fio1.posthoc.bed\\x94\\x8c\"yanocomp/fip37_vs_col0.posthoc.bed\\x94\\x8c!yanocomp/fio1_vs_col0.posthoc.bed\\x94\\x8c6yanocomp/fip37_vs_fio1__not__fip37_vs_col0.posthoc.bed\\x94\\x8c5yanocomp/fip37_vs_fio1__not__fio1_vs_col0.posthoc.bed\\x94\\x8c6yanocomp/fip37_vs_col0__not__fip37_vs_fio1.posthoc.bed\\x94\\x8c5yanocomp/fip37_vs_col0__not__fio1_vs_col0.posthoc.bed\\x94\\x8c5yanocomp/fio1_vs_col0__not__fip37_vs_fio1.posthoc.bed\\x94\\x8c5yanocomp/fio1_vs_col0__not__fip37_vs_col0.posthoc.bed\\x94\\x8c(figures/yanocomp/yanocomp_site_upset.svg\\x94\\x8c.figures/yanocomp/yanocomp_site_effect_size.svg\\x94e}\\x94(h\\x0f}\\x94(\\x8c\\x05sites\\x94K\\x00K\\t\\x86\\x94\\x8c\\nsite_upset\\x94K\\tN\\x86\\x94\\x8c\\x10site_effect_size\\x94K\\nN\\x86\\x94uh\\x19]\\x94(h\\x1bh\\x1ceh\\x1bh\\x1fh!\\x85\\x94R\\x94(h!)}\\x94h%h\\x1bsNt\\x94bh\\x1ch\\x1fh!\\x85\\x94R\\x94(h!)}\\x94h%h\\x1csNt\\x94bhJh,)\\x81\\x94(h=h>h?h@hAhBhChDhEe}\\x94(h\\x0f}\\x94h\\x19]\\x94(h\\x1bh\\x1ceh\\x1bh\\x1fh!\\x85\\x94R\\x94(h!)}\\x94h%h\\x1bsNt\\x94bh\\x1ch\\x1fh!\\x85\\x94R\\x94(h!)}\\x94h%h\\x1csNt\\x94bubhLhFhNhGub\\x8c\\x06params\\x94h\\x06\\x8c\\x06Params\\x94\\x93\\x94)\\x81\\x94}\\x94(h\\x0f}\\x94h\\x19]\\x94(h\\x1bh\\x1ceh\\x1bh\\x1fh!\\x85\\x94R\\x94(h!)}\\x94h%h\\x1bsNt\\x94bh\\x1ch\\x1fh!\\x85\\x94R\\x94(h!)}\\x94h%h\\x1csNt\\x94bub\\x8c\\twildcards\\x94h\\x06\\x8c\\tWildcards\\x94\\x93\\x94)\\x81\\x94}\\x94(h\\x0f}\\x94h\\x19]\\x94(h\\x1bh\\x1ceh\\x1bh\\x1fh!\\x85\\x94R\\x94(h!)}\\x94h%h\\x1bsNt\\x94bh\\x1ch\\x1fh!\\x85\\x94R\\x94(h!)}\\x94h%h\\x1csNt\\x94bub\\x8c\\x07threads\\x94K\\x01\\x8c\\tresources\\x94h\\x06\\x8c\\tResources\\x94\\x93\\x94)\\x81\\x94(K\\x01K\\x01M\\xe8\\x03M\\xe8\\x03\\x8c\\x13/tmp/370463.1.all.q\\x94\\x8c\\x03c6*\\x94e}\\x94(h\\x0f}\\x94(\\x8c\\x06_cores\\x94K\\x00N\\x86\\x94\\x8c\\x06_nodes\\x94K\\x01N\\x86\\x94\\x8c\\x06mem_mb\\x94K\\x02N\\x86\\x94\\x8c\\x07disk_mb\\x94K\\x03N\\x86\\x94\\x8c\\x06tmpdir\\x94K\\x04N\\x86\\x94\\x8c\\x08hostname\\x94K\\x05N\\x86\\x94uh\\x19]\\x94(h\\x1bh\\x1ceh\\x1bh\\x1fh!\\x85\\x94R\\x94(h!)}\\x94h%h\\x1bsNt\\x94bh\\x1ch\\x1fh!\\x85\\x94R\\x94(h!)}\\x94h%h\\x1csNt\\x94bh\\x8cK\\x01h\\x8eK\\x01h\\x90M\\xe8\\x03h\\x92M\\xe8\\x03h\\x94h\\x88\\x8c\\x08hostname\\x94h\\x89ub\\x8c\\x03log\\x94h\\x06\\x8c\\x03Log\\x94\\x93\\x94)\\x81\\x94\\x8c/notebook_processed/yanocomp_upsetplots.py.ipynb\\x94a}\\x94(h\\x0f}\\x94\\x8c\\x08notebook\\x94K\\x00N\\x86\\x94sh\\x19]\\x94(h\\x1bh\\x1ceh\\x1bh\\x1fh!\\x85\\x94R\\x94(h!)}\\x94h%h\\x1bsNt\\x94bh\\x1ch\\x1fh!\\x85\\x94R\\x94(h!)}\\x94h%h\\x1csNt\\x94bh\\xa9h\\xa6ub\\x8c\\x06config\\x94}\\x94(\\x8c\\x16transcriptome_fasta_fn\\x94\\x8c0../annotations/Araport11_genes.201606.cdna.fasta\\x94\\x8c\\x0fgenome_fasta_fn\\x94\\x8c:../annotations/Arabidopsis_thaliana.TAIR10.dna.toplevel.fa\\x94\\x8c\\x06gtf_fn\\x94\\x8cA../annotations/Araport11_GFF3_genes_transposons.201606.no_chr.gtf\\x94\\x8c\\x0cmiclip_peaks\\x94\\x8c\"../annotations/miclip_peaks.bed.gz\\x94\\x8c\\x08flowcell\\x94\\x8c\\nFLO-MIN106\\x94\\x8c\\x03kit\\x94\\x8c\\nSQK-RNA002\\x94\\x8c\\x13minimap2_parameters\\x94}\\x94\\x8c\\x0fmax_intron_size\\x94M Ns\\x8c\\x12d3pendr_parameters\\x94}\\x94(\\x8c\\x10min_read_overlap\\x94G?\\xc9\\x99\\x99\\x99\\x99\\x99\\x9a\\x8c\\x06nboots\\x94M\\xe7\\x03\\x8c\\x0fuse_gamma_model\\x94\\x88\\x8c\\x10test_homogeneity\\x94\\x89u\\x8c\\x0eexpected_motif\\x94\\x8c\\x05NNANN\\x94\\x8c\\x0bcomparisons\\x94]\\x94(\\x8c\\rfip37_vs_col0\\x94\\x8c\\x0cfio1_vs_col0\\x94e\\x8c\\tmulticomp\\x94]\\x94\\x8c\\x15fip37_vs_fio1_vs_col0\\x94au\\x8c\\x04rule\\x94\\x8c\\x1cgenerate_yanocomp_upsetplots\\x94\\x8c\\x0fbench_iteration\\x94N\\x8c\\tscriptdir\\x94\\x8cN/cluster/ggs_lab/mtparker/papers/fiona/fiona_nanopore/rules/notebook_templates\\x94ub.'); from snakemake.logging import logger; logger.printshellcmds = True; import os; os.chdir(r'/cluster/ggs_lab/mtparker/papers/fiona/fiona_nanopore/pipeline');\n######## snakemake preamble end #########\n",
"_____no_output_____"
],
[
"import os\nfrom glob import glob\nimport random\nimport re\nimport itertools as it\nfrom collections import Counter\nimport json\nimport gzip\nfrom collections import defaultdict, Counter\nfrom snakemake.io import glob_wildcards\n\nimport numpy as np\nimport pandas as pd\nfrom scipy import stats\nfrom statsmodels.stats.multitest import fdrcorrection\nimport matplotlib.pyplot as plt\nfrom matplotlib_logo import draw_logo\nfrom upsetplot import plot as plot_upset, UpSet\nfrom matplotlib.colors import ListedColormap\nimport seaborn as sns\nfrom IPython.display import Markdown, display_markdown\n\nimport pysam\nimport pybedtools as pybt\n\n## Default plotting params\n\n%matplotlib inline\nsns.set(font='Arial')\nplt.rcParams['svg.fonttype'] = 'none'\nstyle = sns.axes_style('white')\nstyle.update(sns.axes_style('ticks'))\nstyle['xtick.major.size'] = 2\nstyle['ytick.major.size'] = 2\nsns.set(font_scale=1.2, style=style)\npal = sns.color_palette(['#0072b2', '#d55e00', '#009e73', '#f0e442', '#cc79a7', '#56b4e9', '#e69f00'])\ncmap = ListedColormap(pal.as_hex())\nsns.set_palette(pal)",
"_____no_output_____"
],
[
"def display_formatted_markdown(md, **kwargs):\n md_f = md.format(**kwargs)\n display_markdown(Markdown(md_f))\n\nMD_TEXT = open('../rules/notebook_templates/md_text/upset.md').readlines()\n\ndisplay_formatted_markdown(\n '# Intersection plots of modification sites detected in Col-0, *fip37-4* and *fio1-1*',\n)",
"_____no_output_____"
],
[
"YANOCOMP_COLUMNS = [\n 'chrom', 'start', 'end', 'gene_id', 'kmer', 'score', 'strand',\n 'log_odds', 'log_odds_lower_ci', 'log_odds_upper_ci',\n 'pval', 'fdr', 'fip37_frac_mod', 'fio1_frac_mod', 'col0_frac_mod',\n 'g_stat', 'hom_g_stat', 'fip37_vs_fio1_g_stat', 'fip37_vs_col0_g_stat',\n 'fio1_vs_col0_g_stat', 'fip37_vs_fio1_fdr', 'fip37_vs_col0_fdr',\n 'fio1_vs_col0_fdr', 'unmod_mean', 'unmod_std', 'mod_mean', 'mod_std',\n 'ks_stat'\n]",
"_____no_output_____"
],
[
"tmp_bed = os.path.join(os.environ['TMPDIR'], 'yanocomp_miclip_dist.bed')\n\npybt.BedTool(snakemake.input.yanocomp_sites[0]).closest(\n b=snakemake.input.miclip_peaks,\n s=True, D='a'\n).saveas(tmp_bed)\n\n\nMICLIP_COLUMNS = ['miclip_chrom', 'miclip_start', 'miclip_end', 'miclip_name', 'miclip_score', 'miclip_strand', 'miclip_dist']\n\nfip37_fio1_res_miclip_dist = pd.read_csv(\n tmp_bed, sep='[\\t:\\[\\],]+',\n names=YANOCOMP_COLUMNS + MICLIP_COLUMNS, engine='python'\n).drop_duplicates(['chrom', 'start', 'end', 'gene_id', 'kmer', 'strand'])",
"_____no_output_____"
],
[
"COMPS = ['fip37_vs_fio1', 'fip37_vs_col0', 'fio1_vs_col0']\n\nfor comp in COMPS:\n comp_sig = fip37_fio1_res_miclip_dist.query(f'{comp}_fdr < 0.05').iloc[:, [0, 1, 2, 3, 5, 6]]\n comp_sig.to_csv(f'yanocomp/{comp}.posthoc.bed', sep='\\t', header=False, index=False)\n\nfor comp1, comp2 in it.permutations(COMPS, r=2):\n # for difference sets only use examples with miCLIP support\n comp_sig = fip37_fio1_res_miclip_dist.query(f'{comp1}_fdr < 0.05 & {comp2}_fdr > 0.05 & abs(miclip_dist) < 5').iloc[:, [0, 1, 2, 3, 5, 6]]\n comp_sig.to_csv(f'yanocomp/{comp1}__not__{comp2}.posthoc.bed', sep='\\t', header=False, index=False)",
"_____no_output_____"
],
[
"fip37_fio1_res_miclip_dist['FIP37_dependent'] = fip37_fio1_res_miclip_dist['fip37_vs_col0_fdr'] < 0.05\nfip37_fio1_res_miclip_dist['FIO1_dependent'] = fip37_fio1_res_miclip_dist['fio1_vs_col0_fdr'] < 0.05\nfip37_fio1_res_miclip_dist['miCLIP'] = fip37_fio1_res_miclip_dist['miclip_dist'].abs() < 5\n\ndisplay_formatted_markdown(\n MD_TEXT[0],\n n_sites_total=len(fip37_fio1_res_miclip_dist),\n perc_fip=fip37_fio1_res_miclip_dist.FIP37_dependent.mean() * 100,\n num_fip=fip37_fio1_res_miclip_dist.FIP37_dependent.sum(),\n perc_fio=fip37_fio1_res_miclip_dist.FIO1_dependent.mean() * 100,\n num_fio=fip37_fio1_res_miclip_dist.FIO1_dependent.sum(),\n)\n\ndisplay_formatted_markdown(\n MD_TEXT[1],\n fip_perc_miclip=fip37_fio1_res_miclip_dist.query('FIP37_dependent').miCLIP.mean() * 100,\n fip_num_miclip=fip37_fio1_res_miclip_dist.query('FIP37_dependent').miCLIP.sum(),\n num_fio_only=len(fip37_fio1_res_miclip_dist.query('FIO1_dependent & ~FIP37_dependent')),\n fio_only_perc_miclip=fip37_fio1_res_miclip_dist.query('FIO1_dependent & ~FIP37_dependent').miCLIP.mean() * 100,\n fio_only_num_miclip=fip37_fio1_res_miclip_dist.query('FIO1_dependent & ~FIP37_dependent').miCLIP.sum(),\n)\n\nupset_data = fip37_fio1_res_miclip_dist[['FIP37_dependent', 'FIO1_dependent', 'miCLIP']]\nupset_data = upset_data.groupby(['FIP37_dependent', 'FIO1_dependent']).apply(\n lambda g: pd.Series((len(g), sum(g.miCLIP), g.miCLIP.mean() * 100), index=['total', 'miclip', 'miclip_perc']))\nupset = plot_upset(\n upset_data.total, facecolor='#0072b2', sort_by='degree', sort_categories_by=None, element_size=100,\n intersection_plot_elements=4, totals_plot_elements=1,\n)\nupset['intersections'].bar(x=np.arange(len(upset_data)), height=upset_data.miclip.values[[0, 2, 1, 3]], width=0.5, color=pal[1], zorder=10)\nupset['intersections'].legend(['False', 'True'], title='miCLIP support')\ni = 0\nfor h, _, p in upset_data.iloc[[0, 2, 1, 3]].itertuples(index=False):\n upset['intersections'].annotate(s=f'{h:.0f}\\n({p:.1f}%)', xy=(i, h), ha='center', va='bottom')\n i += 1\nplt.savefig(snakemake.output.site_upset)\nplt.show()",
"_____no_output_____"
],
[
"m = fip37_fio1_res_miclip_dist.query('FIO1_dependent')\nm = m.assign(\n fip_lod=np.abs(np.log(m.fip37_frac_mod + 1e-3) - np.log(m.col0_frac_mod + 1e-3)),\n fio_lod=np.abs(np.log(m.fio1_frac_mod + 1e-3) - np.log(m.col0_frac_mod + 1e-3)),\n)\n\ndisplay_formatted_markdown(\n MD_TEXT[2],\n fio_perc_fip=fip37_fio1_res_miclip_dist.query('FIO1_dependent').FIP37_dependent.mean() * 100,\n fio_num_fip=fip37_fio1_res_miclip_dist.query('FIO1_dependent').FIP37_dependent.sum(),\n)\n\n\nm = pd.melt(\n m, id_vars=[], value_vars=['fip_lod', 'fio_lod'],\n var_name='Genotype',\n value_name='Effect size (absolute log ratio)'\n)\n\nfig, ax = plt.subplots(figsize=(5, 5))\nsns.boxplot(\n x='Genotype',\n y='Effect size (absolute log ratio)',\n data=m, palette=[pal[2], pal[1]], showfliers=False\n)\nax.set_xticklabels(['fip37-4', 'fio1-1'])\nplt.savefig(snakemake.output.site_effect_size)\nplt.show()",
"_____no_output_____"
]
],
[
[
"## U6 m6A IP analysis",
"_____no_output_____"
]
],
[
[
"u6_ip_data = pd.read_csv('ext_data/u6_m6a_ip.tsv', sep='\\t')\nu6_ip_data = u6_ip_data.pivot_table(index=['genotype', 'bio_rep', 'gene_id'], columns='treatment', values=['ct'])\nu6_ip_data.columns = u6_ip_data.columns.droplevel(0)\nu6_ip_data = u6_ip_data.reset_index()\nu6_ip_data['dct'] = ((u6_ip_data['input'] - np.log2(2)) - u6_ip_data['IP'])\n\nu6_u2_exprs_data = u6_ip_data.pivot_table(index=['genotype', 'bio_rep'], columns='gene_id', values=['input'])\nu6_u2_exprs_data.columns = u6_u2_exprs_data.columns.droplevel(0)\nu6_u2_exprs_data = u6_u2_exprs_data.reset_index()\nu6_u2_exprs_data['dct'] = ((u6_u2_exprs_data['U2']) - u6_u2_exprs_data['U6'])\n\ndisplay_formatted_markdown(MD_TEXT[3])\n\nfig, axes = plt.subplots(figsize=(12, 5), ncols=2)\nsns.pointplot(\n x='genotype',\n y='dct',\n data=u6_u2_exprs_data,\n order=['col0', 'fio1'],\n color='#777777',\n join=False,\n errwidth=2,\n capsize=0.1,\n ci='sd',\n ax=axes[0]\n \n)\nsns.stripplot(\n x='genotype',\n y='dct',\n data=u6_u2_exprs_data,\n jitter=0.2,\n size=8,\n order=['col0', 'fio1'],\n ax=axes[0]\n)\naxes[0].set_xticklabels(['Col-0', 'fio1-1'])\naxes[0].set_ylabel('Expression relative to U2 (-ΔCt)')\naxes[0].set_xlabel('Genotype')\naxes[0].set_title('U6 expression')\n\nsns.pointplot(\n hue='genotype',\n y='dct',\n x='gene_id',\n data=u6_ip_data,\n hue_order=['col0', 'fio1'],\n order=['U6', 'U2'],\n palette=['#777777', '#777777'],\n dodge=0.5,\n join=False,\n errwidth=2,\n capsize=0.1,\n ci='sd',\n ax=axes[1]\n \n)\nsns.stripplot(\n hue='genotype',\n y='dct',\n x='gene_id',\n data=u6_ip_data,\n jitter=0.2,\n dodge=0.5,\n size=8,\n hue_order=['col0', 'fio1'],\n order=['U6', 'U2'],\n ax=axes[1]\n)\naxes[1].set_xticklabels(['U6', 'U2'])\naxes[1].set_ylabel('Enrichment over input (-ΔCt)')\naxes[1].set_xlabel('Template')\naxes[1].set_title('m6A-IP')\naxes[1].axvline(0.5, ls='--', color='#252525')\naxes[1].legend_.remove()\nh1 = axes[1].scatter([], [], color=pal[0], label='Col-0')\nh2 = axes[1].scatter([], [], color=pal[1], label='fio1-1')\naxes[1].legend([h1, h2], ['Col-0', 'fio1-1'], loc=4)\nplt.savefig('figures/u6_m6a_ip_qpcr.svg')\nplt.show()",
"_____no_output_____"
]
]
] | [
"code",
"markdown",
"code"
] | [
[
"code",
"code",
"code",
"code",
"code",
"code",
"code",
"code"
],
[
"markdown"
],
[
"code"
]
] |
e77eb662228a1cd99115dbc7b5be26d93b68d02a | 2,932 | ipynb | Jupyter Notebook | code/my first python code.ipynb | caarrick/DataAnalytics | fbaf65b9f120b50f666e8caa374ccb6538e0c933 | [
"Unlicense"
] | 3 | 2021-02-14T00:35:05.000Z | 2022-03-18T16:58:08.000Z | code/my first python code.ipynb | caarrick/DataAnalytics | fbaf65b9f120b50f666e8caa374ccb6538e0c933 | [
"Unlicense"
] | null | null | null | code/my first python code.ipynb | caarrick/DataAnalytics | fbaf65b9f120b50f666e8caa374ccb6538e0c933 | [
"Unlicense"
] | 2 | 2021-02-03T17:53:16.000Z | 2021-02-12T17:29:25.000Z | 16.288889 | 34 | 0.470327 | [
[
[
"* print(\"Welcome 2021!\")",
"_____no_output_____"
]
],
[
[
"# Welcome!\nhello=\"Welcome 2021!!!\"\nprint(hello)",
"Welcome 2021!!!\n"
],
[
"what is hello",
"_____no_output_____"
]
]
] | [
"markdown",
"code"
] | [
[
"markdown"
],
[
"code",
"code"
]
] |
e77eb72128852422d5d5d84100fe76625dd83ef9 | 11,430 | ipynb | Jupyter Notebook | scripts/poet_experiments/poet_sweep_linear16_unitcost.ipynb | hy00nc/checkmate | f3452a30dbf8d00c5ce9607712e335f39d2f6c5b | [
"Apache-2.0"
] | 91 | 2020-01-15T01:10:29.000Z | 2022-01-19T13:12:16.000Z | scripts/poet_experiments/poet_sweep_linear16_unitcost.ipynb | hy00nc/checkmate | f3452a30dbf8d00c5ce9607712e335f39d2f6c5b | [
"Apache-2.0"
] | 40 | 2020-01-15T01:37:27.000Z | 2021-06-25T23:30:45.000Z | scripts/poet_experiments/poet_sweep_linear16_unitcost.ipynb | hy00nc/checkmate | f3452a30dbf8d00c5ce9607712e335f39d2f6c5b | [
"Apache-2.0"
] | 17 | 2020-01-15T05:10:05.000Z | 2021-11-27T17:14:46.000Z | 59.53125 | 1,710 | 0.62336 | [
[
[
"%matplotlib inline\nfrom checkmate.core.graph_builder import gen_linear_graph\nfrom checkmate.core.solvers.poet_solver import extract_costs_from_dfgraph, solve_poet_cvxpy\nimport matplotlib.pyplot as plt\nimport seaborn as sns\nimport numpy as np\nfrom tqdm import tqdm\nimport logging\nimport pandas as pd\nsns.set('notebook')\nsns.set_style('dark')",
"_____no_output_____"
],
[
"!mkdir -p data/poet_sweep\ndata = []\nfor cost_factor in tqdm([0.1, 1.0, 2.0, 4.0, 5.0, 8.0, 16.0]):\n for budget in [1.0, 2.0, 3.0, 4.0]:\n try:\n g = gen_linear_graph(16)\n cpu_cost, page_in_cost, page_out_cost = extract_costs_from_dfgraph(g, cost_factor)\n solution = solve_poet_cvxpy(g, budget, cpu_cost, page_in_cost, page_out_cost, solver_override=\"GUROBI\", verbose=True)\n\n data.append(dict(cost_factor=cost_factor, budget=budget, solution=solution, dfgraph=g,\n cpu_cost=cpu_cost, page_in_cost=page_in_cost, page_out_cost=page_out_cost))\n\n plt.figure()\n fig, axarr = plt.subplots(1, 5, figsize=(25, 5))\n for arr, ax, name in zip(solution, axarr, ['R', 'S_RAM', 'S_SD', 'M_sd2ram', 'M_ram2sd']):\n ax.invert_yaxis()\n ax.pcolormesh(arr, cmap=\"Greys\", vmin=0, vmax=1)\n ax.set_title(name)\n fig.savefig('data/poet_sweep/{}_{}.png'.format(cost_factor, budget))\n except Exception as e:\n logging.exception(e)\n continue",
"_____no_output_____"
],
[
"def featurize_row(data_row):\n out_vec = dict(cost_factor=data_row['cost_factor'], budget=data_row['budget'])\n R, S_RAM, S_SD, M_sd2ram, M_ram2sd = data_row['solution']\n out_vec['total_compute_runtime'] = np.sum(R @ data_row['cpu_cost'])\n out_vec['total_page_cost'] = (np.sum(M_sd2ram @ data_row['page_out_cost']) + np.sum(M_ram2sd @ data_row['page_in_cost'])) / data_row['cost_factor']\n return out_vec\ndf = pd.DataFrame(map(featurize_row, data))",
"_____no_output_____"
],
[
"for budget in [2.0, 3.0, 4.0]:\n df[df['budget'] == budget].plot(x='cost_factor', y=['total_compute_runtime', 'total_page_cost'], kind='bar', stacked=True)",
"_____no_output_____"
],
[
"df[df['cost_factor'] == 2.0].plot(x='budget', y=['total_compute_runtime', 'total_page_cost'], kind='bar', stacked=True)",
"_____no_output_____"
],
[
"data[0]",
"_____no_output_____"
]
]
] | [
"code"
] | [
[
"code",
"code",
"code",
"code",
"code",
"code"
]
] |
e77ebf77a576f0135914b8b8b2cc82dbb8ce38f8 | 10,830 | ipynb | Jupyter Notebook | Dzien01/03-numpy-oper.ipynb | gitgeoman/PAD2022 | 49266a5f130971a8b9b7fda890f0f43e6d6d64fe | [
"MIT"
] | null | null | null | Dzien01/03-numpy-oper.ipynb | gitgeoman/PAD2022 | 49266a5f130971a8b9b7fda890f0f43e6d6d64fe | [
"MIT"
] | null | null | null | Dzien01/03-numpy-oper.ipynb | gitgeoman/PAD2022 | 49266a5f130971a8b9b7fda890f0f43e6d6d64fe | [
"MIT"
] | null | null | null | 20.988372 | 117 | 0.410988 | [
[
[
"import numpy as np",
"_____no_output_____"
],
[
"a = np.arange(10,71,10)\nb = np.arange(1,8,1)\na, b",
"_____no_output_____"
],
[
"#operacje na tabelach\n#dodawanie, odejmowanie, mnożenie, dodatawanie stałej macierzy\na+b, a-b, a*b, a+5, b**2",
"_____no_output_____"
],
[
"#mnożenie tabel\na*=2\na",
"_____no_output_____"
],
[
"#a/=3 to nie zadzaiała ponieważ w wyniku dzielenia zmienia się typ wartości wewnątrz tablicy z int na float \na=a/3\na.dtype",
"_____no_output_____"
]
],
[
[
"## Broadcasting tablic pythonowych:",
"_____no_output_____"
]
],
[
[
"#tworze tablice do przeprowadzenia bradcastowania, wyświetlam obie tablice \narr1= np.array([[1],[2],[3]])\narr2= np.array([1, 2, 3])\narr1, arr2",
"_____no_output_____"
],
[
"#to jest broadcasting mówiąc prościej jest to reshape (rozciąganie) mniejszej macierzy do większej\n#możliwe jest też dociąganie obu tablic, zwrócić uwagę należy że to nie jest operator mnożenia macieżowego\n#mnożone macieżowe jest zdefiniowane specjalną funkcją numpy\narr1*arr2",
"_____no_output_____"
]
],
[
[
"## wybieranie danych z tablicy\n\n",
"_____no_output_____"
]
],
[
[
"np.random.seed(0)\narr = np.random.randint(-10,11,(7,8))\narr",
"_____no_output_____"
],
[
"#ostatni wiersz\narr[6],arr[-1]",
"_____no_output_____"
],
[
"#wiersz 2 i 3, co 3 cia kolumna (slice danych)\narr[1:3, ::3]",
"_____no_output_____"
],
[
"#tylko wiersz pierwszy i ostatni\narr[[0,-1]]",
"_____no_output_____"
],
[
"#tylko wiersz ostatni a potem pierwszy\narr[[-1,0]]",
"_____no_output_____"
],
[
"#ostatnia kolumna\narr[:,-1]",
"_____no_output_____"
],
[
"#ostatnia kolumna\narr[:,[-1]]",
"_____no_output_____"
],
[
"#TRANSPOZYCJA \narr.transpose()[-1] #pierwsza metoda\narr.T[-1] #druga metoda",
"_____no_output_____"
],
[
"#numpy - indexing/slicing - doczytać\n\n#iterowanie - tablice mają wbudowany generator więc można względem nich interować",
"_____no_output_____"
],
[
"for row in arr:\n print (row)\n print('='*50)",
"[ 2 5 -10 -7 -7 -3 -1 9]\n==================================================\n[ 8 -6 -4 2 -9 -4 -3 4]\n==================================================\n[ 7 -5 3 -2 -1 10 9 6]\n==================================================\n[ 9 -5 5 5 -10 8 -7 7]\n==================================================\n[ 9 9 9 4 -3 -10 -9 -1]\n==================================================\n[-10 0 10 -7 1 8 -8 -10]\n==================================================\n[-10 -6 -5 -4 -2 10 7 5]\n==================================================\n"
]
],
[
[
"# Porównanie wartości między python list a numpy list",
"_____no_output_____"
]
],
[
[
"%%timeit -r 20 -n 500 \n[x**2 for x in range(1, 1001)]",
"298 µs ± 11 µs per loop (mean ± std. dev. of 20 runs, 500 loops each)\n"
],
[
"%%timeit -r 20 -n 500\nnp.arange(1, 1001)**2",
"3.59 µs ± 461 ns per loop (mean ± std. dev. of 20 runs, 500 loops each)\n"
],
[
"298/3.59",
"_____no_output_____"
]
]
] | [
"code",
"markdown",
"code",
"markdown",
"code",
"markdown",
"code"
] | [
[
"code",
"code",
"code",
"code",
"code"
],
[
"markdown"
],
[
"code",
"code"
],
[
"markdown"
],
[
"code",
"code",
"code",
"code",
"code",
"code",
"code",
"code",
"code",
"code"
],
[
"markdown"
],
[
"code",
"code",
"code"
]
] |
e77ec46fbba5947ac2999beeef9a4cccb080abce | 31,010 | ipynb | Jupyter Notebook | reinforcement_learning/rl_traveling_salesman_vehicle_routing_coach/rl_traveling_salesman_vehicle_routing_coach.ipynb | Amirosimani/amazon-sagemaker-examples | bc35e7a9da9e2258e77f98098254c2a8e308041a | [
"Apache-2.0"
] | 2,610 | 2020-10-01T14:14:53.000Z | 2022-03-31T18:02:31.000Z | reinforcement_learning/rl_traveling_salesman_vehicle_routing_coach/rl_traveling_salesman_vehicle_routing_coach.ipynb | Amirosimani/amazon-sagemaker-examples | bc35e7a9da9e2258e77f98098254c2a8e308041a | [
"Apache-2.0"
] | 1,959 | 2020-09-30T20:22:42.000Z | 2022-03-31T23:58:37.000Z | reinforcement_learning/rl_traveling_salesman_vehicle_routing_coach/rl_traveling_salesman_vehicle_routing_coach.ipynb | Amirosimani/amazon-sagemaker-examples | bc35e7a9da9e2258e77f98098254c2a8e308041a | [
"Apache-2.0"
] | 2,052 | 2020-09-30T22:11:46.000Z | 2022-03-31T23:02:51.000Z | 37.771011 | 556 | 0.615124 | [
[
[
"# Traveling Salesman Problem with Reinforcement Learning",
"_____no_output_____"
],
[
"## Description of Problem\n\nThe travelling salesman problem (TSP) is a classic algorithmic problem in the field of computer science and operations research. Given a list of cities and the distances between each pair of cities, the problem is to find the shortest possible route that visits each city and returns to the origin city.\n\nThe problem is NP-complete as the number of combinations of cities grows larger as we add more cities. \n\nIn the classic version of the problem, the salesman picks a city to start, travels through remaining cities and returns to the original city. \n\nIn this version, we have slightly modified the problem, presenting it as a restaurant order delivery problem on a 2D gridworld. The agent (driver) starts at the restaurant, a fixed point on the grid. Then, delivery orders appear elsewhere on the grid. The driver needs to visit the orders, and return to the restaurant, to obtain rewards. Rewards are proportional to the time taken to do this (equivalent to the distance, as each timestep moves one square on the grid).",
"_____no_output_____"
],
[
"## Why Reinforcement Learning?\n\nFor canonical Operations problems like this one, we're very interested about RL's potential to push the state of the art.\n\nThere are a few reasons we think RL offers some unique value for this type of problem:\n1. RL seems to perform well in high-dimensional spaces, when an approximate solution to a complex problem may be of greater value than an exact/optimal solution to a simpler problem.\n2. RL can do quite well in partially observed environments. When there are aspects of a problem we don't know about and therefore can't model, which is often the case in the real-world (and we can pretend is the case with these problems), RL's ability to deal with the messiness is valuable.\n3. RL may have things to teach us! We've seen this to be the case with Go, and Dota 2, where the RL agent came up with innovative strategies that have later been adopted by human players. What if there are clever strategies we can use to solve versions of TSP, Knapsack, Newsvendor, or extensions of any of those? RL might surprise us.",
"_____no_output_____"
],
[
"## Easy Version of TSP\nIn the Easy Version, we are on a 5x5 grid. All orders are generated at the start of the episode. Order locations are fixed, and are invariant (non-random) from episode to episode. The objective is to visit each order location, and return to the restaurant. We have a maximum time-limit of 50 steps. \n\n### States\nAt each time step, our agent is aware of the following information:\n\n1. For the Restuarant:\n 1. Location (x,y coordinates)\n \n2. For the Driver\n 1. Location (x,y coordinates)\n 2. Is driver at restaurant (yes/no)\n\n3. For each Order: \n 1. Location (x,y coordinates)\n 2. Status (Delivered or Not Delivered)\n 3. Time (Time taken to deliver reach order -- incrementing until delivered)\n4. Miscellaneous\n 1. Time since start of episode\n 2. Time remaining until end of episode (i.e. until max time)\n\n### Actions\nAt each time step, our agent can take the following steps:\n- Up - Move one step up in the map\n- Down - Move one step down in the map\n- Right - Move one step right in the map\n- Left - Move one step left in the map\n\n### Rewards\nAgent gets a reward of -1 for each time step. If an order is delivered in that timestep, it gets a positive reward inversely proportional to the time taken to deliver. If all the orders are delivered and the agent is back to the restaurant, it gets an additional reward inversely proportional to time since start of episode. \n",
"_____no_output_____"
],
[
"## Using AWS SageMaker for RL\n\nAWS SageMaker allows you to train your RL agents in cloud machines using docker containers. You do not have to worry about setting up your machines with the RL toolkits and deep learning frameworks. You can easily switch between many different machines setup for you, including powerful GPU machines that give a big speedup. You can also choose to use multiple machines in a cluster to further speedup training, often necessary for production level loads.",
"_____no_output_____"
],
[
"### Prerequisites \n\n#### Imports\n\nTo get started, we'll import the Python libraries we need, set up the environment with a few prerequisites for permissions and configurations.",
"_____no_output_____"
]
],
[
[
"import sagemaker\nimport boto3\nimport sys\nimport os\nimport glob\nimport re\nimport subprocess\nfrom IPython.display import HTML\nimport time\nfrom time import gmtime, strftime\n\nsys.path.append(\"common\")\nfrom misc import get_execution_role, wait_for_s3_object\nfrom sagemaker.rl import RLEstimator, RLToolkit, RLFramework",
"_____no_output_____"
]
],
[
[
"#### Settings\n\nYou can run this notebook from your local host or from a SageMaker notebook instance. In both of these scenarios, you can run the following in either local or SageMaker modes. The local mode uses the SageMaker Python SDK to run your code in a local container before deploying to SageMaker. This can speed up iterative testing and debugging while using the same familiar Python SDK interface. You just need to set local_mode = True.",
"_____no_output_____"
]
],
[
[
"# run in local mode?\nlocal_mode = False\n\nenv_type = \"tsp-easy\"\n\n# create unique job name\njob_name_prefix = \"rl-\" + env_type\n\n# S3 bucket\nsage_session = sagemaker.session.Session()\ns3_bucket = sage_session.default_bucket()\ns3_output_path = \"s3://{}/\".format(s3_bucket)\nprint(\"S3 bucket path: {}\".format(s3_output_path))",
"_____no_output_____"
]
],
[
[
"#### Install docker for `local` mode\n\nIn order to work in `local` mode, you need to have docker installed. When running from you local machine, please make sure that you have docker or docker-compose (for local CPU machines) and nvidia-docker (for local GPU machines) installed. Alternatively, when running from a SageMaker notebook instance, you can simply run the following script to install dependenceis.\n\nNote, you can only run a single local notebook at one time.",
"_____no_output_____"
]
],
[
[
"# only run from SageMaker notebook instance\nif local_mode:\n !/bin/bash common/setup.sh",
"_____no_output_____"
]
],
[
[
"#### Create an IAM role\nEither get the execution role when running from a SageMaker notebook `role = sagemaker.get_execution_role()` or, when running from local machine, use utils method `role = get_execution_role('role_name')` to create an execution role.",
"_____no_output_____"
]
],
[
[
"try:\n role = sagemaker.get_execution_role()\nexcept:\n role = get_execution_role()\n\nprint(\"Using IAM role arn: {}\".format(role))",
"_____no_output_____"
]
],
[
[
"#### Setup the environment\n\nThe environment is defined in a Python file called “TSP_env.py” and the file is uploaded on /src directory. \n\nThe environment also implements the init(), step(), reset() and render() functions that describe how the environment behaves. This is consistent with Open AI Gym interfaces for defining an environment. \n\n\n1. Init() - initialize the environment in a pre-defined state\n2. Step() - take an action on the environment\n3. reset()- restart the environment on a new episode\n4. render() - get a rendered image of the environment in its current state",
"_____no_output_____"
],
[
"#### Configure the presets for RL algorithm \n\nThe presets that configure the RL training jobs are defined in the “preset-tsp-easy.py” file which is also uploaded on the /src directory. Using the preset file, you can define agent parameters to select the specific agent algorithm. You can also set the environment parameters, define the schedule and visualization parameters, and define the graph manager. The schedule presets will define the number of heat up steps, periodic evaluation steps, training steps between evaluations.\n\nThese can be overridden at runtime by specifying the RLCOACH_PRESET hyperparameter. Additionally, it can be used to define custom hyperparameters. ",
"_____no_output_____"
]
],
[
[
"!pygmentize src/preset-tsp-easy.py",
"_____no_output_____"
]
],
[
[
"#### Write the Training Code \n\nThe training code is written in the file “train-coach.py” which is uploaded in the /src directory. \nFirst import the environment files and the preset files, and then define the main() function. ",
"_____no_output_____"
]
],
[
[
"!pygmentize src/train-coach.py",
"_____no_output_____"
]
],
[
[
"### Train the RL model using the Python SDK Script mode\n\nIf you are using local mode, the training will run on the notebook instance. When using SageMaker for training, you can select a GPU or CPU instance. The RLEstimator is used for training RL jobs. \n\n1. Specify the source directory where the environment, presets and training code is uploaded.\n2. Specify the entry point as the training code \n3. Specify the choice of RL toolkit and framework. This automatically resolves to the ECR path for the RL Container. \n4. Define the training parameters such as the instance count, job name, S3 path for output and job name. \n5. Specify the hyperparameters for the RL agent algorithm. The RLCOACH_PRESET or the RLRAY_PRESET can be used to specify the RL agent algorithm you want to use. \n6. Define the metrics definitions that you are interested in capturing in your logs. These can also be visualized in CloudWatch and SageMaker Notebooks. ",
"_____no_output_____"
]
],
[
[
"%%time\n\nif local_mode:\n instance_type = \"local\"\nelse:\n instance_type = \"ml.m4.4xlarge\"\n\nestimator = RLEstimator(\n entry_point=\"train-coach.py\",\n source_dir=\"src\",\n dependencies=[\"common/sagemaker_rl\"],\n toolkit=RLToolkit.COACH,\n toolkit_version=\"1.0.0\",\n framework=RLFramework.TENSORFLOW,\n role=role,\n instance_type=instance_type,\n instance_count=1,\n output_path=s3_output_path,\n base_job_name=job_name_prefix,\n hyperparameters={\n # expected run time 12 mins for TSP Easy\n \"RLCOACH_PRESET\": \"preset-\"\n + env_type,\n },\n)\n\nestimator.fit(wait=local_mode)",
"_____no_output_____"
]
],
[
[
"### Store intermediate training output and model checkpoints \n\nThe output from the training job above is stored on S3. The intermediate folder contains gifs and metadata of the training.",
"_____no_output_____"
]
],
[
[
"job_name = estimator._current_job_name\nprint(\"Job name: {}\".format(job_name))\n\ns3_url = \"s3://{}/{}\".format(s3_bucket, job_name)\n\nif local_mode:\n output_tar_key = \"{}/output.tar.gz\".format(job_name)\nelse:\n output_tar_key = \"{}/output/output.tar.gz\".format(job_name)\n\nintermediate_folder_key = \"{}/output/intermediate\".format(job_name)\noutput_url = \"s3://{}/{}\".format(s3_bucket, output_tar_key)\nintermediate_url = \"s3://{}/{}\".format(s3_bucket, intermediate_folder_key)\n\nprint(\"S3 job path: {}\".format(s3_url))\nprint(\"Output.tar.gz location: {}\".format(output_url))\nprint(\"Intermediate folder path: {}\".format(intermediate_url))\n\ntmp_dir = \"/tmp/{}\".format(job_name)\nos.system(\"mkdir {}\".format(tmp_dir))\nprint(\"Create local folder {}\".format(tmp_dir))",
"_____no_output_____"
]
],
[
[
"### Visualization",
"_____no_output_____"
],
[
"#### Comparing against a baseline policy",
"_____no_output_____"
]
],
[
[
"!pip install gym",
"_____no_output_____"
],
[
"!pip install pygame",
"_____no_output_____"
],
[
"os.chdir(\"src\")",
"_____no_output_____"
],
[
"# Get baseline reward\nfrom TSP_env import TSPEasyEnv\nfrom TSP_baseline import get_mean_baseline_reward\n\nbaseline_mean, baseline_std_dev = get_mean_baseline_reward(env=TSPEasyEnv(), num_of_episodes=1)\nprint(baseline_mean, baseline_std_dev)",
"_____no_output_____"
],
[
"os.chdir(\"../\")",
"_____no_output_____"
]
],
[
[
"#### Plot metrics for training job\nWe can pull the reward metric of the training and plot it to see the performance of the model over time.",
"_____no_output_____"
]
],
[
[
"import pandas as pd\nimport matplotlib\n\n%matplotlib inline\n\n# csv_file has all the RL training metrics\n# csv_file = \"{}/worker_0.simple_rl_graph.main_level.main_level.agent_0.csv\".format(tmp_dir)\ncsv_file_name = \"worker_0.simple_rl_graph.main_level.main_level.agent_0.csv\"\nkey = intermediate_folder_key + \"/\" + csv_file_name\nwait_for_s3_object(s3_bucket, key, tmp_dir)\n\ncsv_file = \"{}/{}\".format(tmp_dir, csv_file_name)\ndf = pd.read_csv(csv_file)\nx_axis = \"Episode #\"\ny_axis_rl = \"Training Reward\"\ny_axis_base = \"Baseline Reward\"\ndf[y_axis_rl] = df[y_axis_rl].rolling(5).mean()\ndf[y_axis_base] = baseline_mean\ny_axes = [y_axis_rl]\nax = df.plot(\n x=x_axis,\n y=[y_axis_rl, y_axis_base],\n figsize=(18, 6),\n fontsize=18,\n legend=True,\n color=[\"b\", \"r\"],\n)\nfig = ax.get_figure()\nax.set_xlabel(x_axis, fontsize=20)\n# ax.set_ylabel(y_axis,fontsize=20)\n# fig.savefig('training_reward_vs_wall_clock_time.pdf')",
"_____no_output_____"
]
],
[
[
"#### Visualize the rendered gifs\nThe latest gif file found in the gifs directory is displayed. You can replace the tmp.gif file below to visualize other files generated.",
"_____no_output_____"
]
],
[
[
"key = intermediate_folder_key + \"/gifs\"\nwait_for_s3_object(s3_bucket, key, tmp_dir)\nprint(\"Copied gifs files to {}\".format(tmp_dir))\n\nglob_pattern = os.path.join(\"{}/*.gif\".format(tmp_dir))\ngifs = [file for file in glob.iglob(glob_pattern, recursive=True)]\nextract_episode = lambda string: int(\n re.search(\".*episode-(\\d*)_.*\", string, re.IGNORECASE).group(1)\n)\ngifs.sort(key=extract_episode)\nprint(\"GIFs found:\\n{}\".format(\"\\n\".join([os.path.basename(gif) for gif in gifs])))\n\n# visualize a specific episode\ngif_index = -1 # since we want last gif\ngif_filepath = gifs[gif_index]\ngif_filename = os.path.basename(gif_filepath)\nprint(\"Selected GIF: {}\".format(gif_filename))\nos.system(\n \"mkdir -p ./src/tmp_render/ && cp {} ./src/tmp_render/{}.gif\".format(gif_filepath, gif_filename)\n)\nHTML('<img src=\"./src/tmp_render/{}.gif\">'.format(gif_filename))",
"_____no_output_____"
]
],
[
[
"### Evaluation of RL models\n\nWe use the last checkpointed model to run evaluation for the RL Agent. \n\n#### Load checkpointed model\n\nCheckpointed data from the previously trained models will be passed on for evaluation / inference in the checkpoint channel. In local mode, we can simply use the local directory, whereas in the SageMaker mode, it needs to be moved to S3 first.",
"_____no_output_____"
]
],
[
[
"%%time\n\nwait_for_s3_object(s3_bucket, output_tar_key, tmp_dir)\n\nif not os.path.isfile(\"{}/output.tar.gz\".format(tmp_dir)):\n raise FileNotFoundError(\"File output.tar.gz not found\")\nos.system(\"tar -xvzf {}/output.tar.gz -C {}\".format(tmp_dir, tmp_dir))\n\nif local_mode:\n checkpoint_dir = \"{}/data/checkpoint\".format(tmp_dir)\nelse:\n checkpoint_dir = \"{}/checkpoint\".format(tmp_dir)\n\nprint(\"Checkpoint directory {}\".format(checkpoint_dir))",
"_____no_output_____"
],
[
"%%time\n\nif local_mode:\n checkpoint_path = \"file://{}\".format(checkpoint_dir)\n print(\"Local checkpoint file path: {}\".format(checkpoint_path))\nelse:\n checkpoint_path = \"s3://{}/{}/checkpoint/\".format(s3_bucket, job_name)\n if not os.listdir(checkpoint_dir):\n raise FileNotFoundError(\"Checkpoint files not found under the path\")\n os.system(\"aws s3 cp --recursive {} {}\".format(checkpoint_dir, checkpoint_path))\n print(\"S3 checkpoint file path: {}\".format(checkpoint_path))",
"_____no_output_____"
]
],
[
[
"#### Run the evaluation step\n\nUse the checkpointed model to run the evaluation step. ",
"_____no_output_____"
]
],
[
[
"estimator_eval = RLEstimator(\n role=role,\n source_dir=\"src/\",\n dependencies=[\"common/sagemaker_rl\"],\n toolkit=RLToolkit.COACH,\n toolkit_version=\"1.0.0\",\n framework=RLFramework.TENSORFLOW,\n entry_point=\"evaluate-coach.py\",\n instance_count=1,\n instance_type=instance_type,\n base_job_name=job_name_prefix + \"-evaluation\",\n hyperparameters={\n \"RLCOACH_PRESET\": \"preset-tsp-easy\",\n \"evaluate_steps\": 200, # max 4 episodes\n },\n)\n\nestimator_eval.fit({\"checkpoint\": checkpoint_path})",
"_____no_output_____"
]
],
[
[
"## Medium version of TSP <a name=\"TSP-Medium\"></a>\nWe make the problem much harder in this version by randomizing the location of destiations each episode. Hence, RL agent has to come up with a general strategy to navigate the grid. Parameters, states, actions, and rewards are identical to the Easy version of TSP.\n\n### States\nAt each time step, our agent is aware of the following information:\n\n1. For the Restuarant:\n 1. Location (x,y coordinates)\n \n2. For the Driver\n 1. Location (x,y coordinates)\n 2. Is driver at restaurant (yes/no)\n\n3. For each Order: \n 1. Location (x,y coordinates)\n 2. Status (Delivered or Not Delivered)\n 3. Time (Time taken to deliver reach order -- incrementing until delivered)\n4. Miscellaneous\n 1. Time since start of episode\n 2. Time remaining until end of episode (i.e. until max time)\n\n\n### Actions\nAt each time step, our agent can take the following steps:\n- Up - Move one step up in the map\n- Down - Move one step down in the map\n- Right - Move one step right in the map\n- Left - Move one step left in the map\n\n### Rewards\nAgent gets a reward of -1 for each time step. If an order is delivered in that timestep, it gets a positive reward inversely proportional to the time taken to deliver. If all the orders are delivered and the agent is back to the restaurant, it gets an additional reward inversely proportional to time since start of episode.",
"_____no_output_____"
],
[
"## Using AWS SageMaker for RL <a name=\"SM-TSP-Medium\"></a>\n\n### Train the model using Python SDK/Script mode\n\nSkipping through the basic setup, assuming you did that already for the easy version. For good results, we suggest you train for at least 1,000,000 steps. You can edit this either as a hyperparameter in the cell or directly change the preset file.",
"_____no_output_____"
]
],
[
[
"%%time\n\n# run in local mode?\nlocal_mode = False\n\n# create unique job name\njob_name_prefix = \"rl-tsp-medium\"",
"_____no_output_____"
],
[
"%%time\n\nif local_mode:\n instance_type = \"local\"\nelse:\n instance_type = \"ml.m4.4xlarge\"\n\nestimator = RLEstimator(\n entry_point=\"train-coach.py\",\n source_dir=\"src\",\n dependencies=[\"common/sagemaker_rl\"],\n toolkit=RLToolkit.COACH,\n toolkit_version=\"1.0.0\",\n framework=RLFramework.TENSORFLOW,\n role=role,\n instance_type=instance_type,\n instance_count=1,\n output_path=s3_output_path,\n base_job_name=job_name_prefix,\n hyperparameters={\n \"RLCOACH_PRESET\": \"preset-tsp-medium\",\n },\n)\n\nestimator.fit(wait=local_mode)",
"_____no_output_____"
]
],
[
[
"## Visualize, Compare with Baseline and Evaluate\n\nYou can follow the same set of code used for TSP easy version.",
"_____no_output_____"
],
[
"# Vehicle Routing Problem with Reinforcement Learning <a name=\"VRP-Easy\"></a>\n\nVehicle Routing Problem (VRP) is a similar problem where the algorithm optimizes the movement of a fleet of vehicles. Our VRP formulation is a bit different, we have a delivery driver who accepts orders from customers, picks up food from a restaurant and delivers it to the customer. The driver optimizes to increase the number of successful deliveries within a time limit.\n\nKey differences from TSP: \n\n- Pathing is now automatic. Instead of choosing \"left, right, up, down\", now you just select your destination as your action. The environment will get you there in the fewest steps possible.\n- Since the routing/pathing is now taken care of, we add in complexity elsewhere...\n- There can be more than one restaurant, each with a different type of order (e.g. Pizzas vs. Burritos). Each order will have a different type, and you have to visit the correct restuarant to pick up an order before dropping it off.\n- Drivers have a limited capacity; they cannot pick up an infinite number of orders. Instead, they can only have (e.g. 5) orders in the car at any given time. This means they will have to return to the restaurant(s) in between deliveries to pick up more supply.\n- Orders now come in dynamically over time, rather than all being known at time zero. Each time step, there is some probability that an order will be generated. \n- As the driver/agent, we now have the choice to fulfill an order or not -- there's no penalty associated with not accepting an order, but a potential penalty if we accept an order and fail to deliver it before Timeout.\n\n### States\nAt each time step, our agent is aware of the following information:\n\n1. For each Restuarant:\n 1. Location (x,y coordinates)\n \n1. For the Driver\n 1. Location (x,y coordinates)\n 2. Capacity (maximum # of orders you can carry, at one time, on the driver)\n 3. Used Capacity (# of orders you currently carry on the driver)\n\n1. For each Order: \n 1. Location (x,y coordinates)\n 2. Status (Accepted, Not Accepted, Delivered, Not Delivered)\n 3. Type (Which restuarant the order belongs to, like Pizza, or Burrito)\n 4. Time (Time since order was generated)\n 5. Promise (If you deliver the order by this time, you get a bonus reward)\n 6. Timeout (If you deliver the order after this time, you get a penalty)\n\n### Actions\nAt each time step, our agent can do ONE of the following:\n- Choose a restaurant to visit (incremental step L,R,U,D, will be auto-pathed)\n- Choose an order to visit (incremental step L,R,U,D, will be auto-pathed)\n- Accept an order (no movement will occur)\n- Do nothing\n\n### Rewards\n- Driver gets a reward of -1 for each time step. \n- If driver delivers order, get a reward proportional to the time taken to deliver (extra bonus for beating Promise time)\n- If order expires (reaches Timeout), get a penalty",
"_____no_output_____"
],
[
"## Using AWS SageMaker RL <a name=\"SM-VRP-Easy\"></a>\n\n### Train the model using Python SDK/Script mode\n\nSkipping through the basic setup, assuming you did that already for the easy version. For good results, we suggest a minimum of 5,000,000 steps of training.",
"_____no_output_____"
]
],
[
[
"%%time\n\n# run in local mode?\nlocal_mode = False\n\n# create unique job name\njob_name_prefix = \"rl-vrp-easy\"",
"_____no_output_____"
],
[
"%%time\n\nif local_mode:\n instance_type = \"local\"\nelse:\n instance_type = \"ml.m4.4xlarge\"\n\nestimator = RLEstimator(\n entry_point=\"train-coach.py\",\n source_dir=\"src\",\n dependencies=[\"common/sagemaker_rl\"],\n toolkit=RLToolkit.COACH,\n toolkit_version=\"1.0.0\",\n framework=RLFramework.TENSORFLOW,\n role=role,\n instance_type=instance_type,\n instance_count=1,\n output_path=s3_output_path,\n base_job_name=job_name_prefix,\n hyperparameters={\n \"RLCOACH_PRESET\": \"preset-vrp-easy\",\n },\n)\n\nestimator.fit(wait=local_mode)",
"_____no_output_____"
]
],
[
[
"## Visualize, Compare with Baseline and Evaluate\n\nYou can follow the same set of code used for TSP easy version.",
"_____no_output_____"
]
]
] | [
"markdown",
"code",
"markdown",
"code",
"markdown",
"code",
"markdown",
"code",
"markdown",
"code",
"markdown",
"code",
"markdown",
"code",
"markdown",
"code",
"markdown",
"code",
"markdown",
"code",
"markdown",
"code",
"markdown",
"code",
"markdown",
"code",
"markdown",
"code",
"markdown",
"code",
"markdown"
] | [
[
"markdown",
"markdown",
"markdown",
"markdown",
"markdown",
"markdown"
],
[
"code"
],
[
"markdown"
],
[
"code"
],
[
"markdown"
],
[
"code"
],
[
"markdown"
],
[
"code"
],
[
"markdown",
"markdown"
],
[
"code"
],
[
"markdown"
],
[
"code"
],
[
"markdown"
],
[
"code"
],
[
"markdown"
],
[
"code"
],
[
"markdown",
"markdown"
],
[
"code",
"code",
"code",
"code",
"code"
],
[
"markdown"
],
[
"code"
],
[
"markdown"
],
[
"code"
],
[
"markdown"
],
[
"code",
"code"
],
[
"markdown"
],
[
"code"
],
[
"markdown",
"markdown"
],
[
"code",
"code"
],
[
"markdown",
"markdown",
"markdown"
],
[
"code",
"code"
],
[
"markdown"
]
] |
e77ec8bde317a14cf32aa1646cdf7613c041f716 | 1,491 | ipynb | Jupyter Notebook | Notebook - JavaScript .ipynb | luiseduardogfranca/students-perfomance | 52ae5a0d41992037a3e58b56fb910f598ba9312b | [
"MIT"
] | null | null | null | Notebook - JavaScript .ipynb | luiseduardogfranca/students-perfomance | 52ae5a0d41992037a3e58b56fb910f598ba9312b | [
"MIT"
] | 2 | 2018-12-17T12:34:34.000Z | 2018-12-17T12:36:55.000Z | Notebook - JavaScript .ipynb | luiseduardogfranca/students-perfomance | 52ae5a0d41992037a3e58b56fb910f598ba9312b | [
"MIT"
] | null | null | null | 27.611111 | 363 | 0.537223 | [
[
[
"console.log(\"Ola\");",
"_____no_output_____"
]
]
] | [
"code"
] | [
[
"code"
]
] |
e77ec8cbd92121379ec98bc220b11f8b4ace8559 | 8,769 | ipynb | Jupyter Notebook | S+P_Week_2_Lesson_1.ipynb | EgorBEremeev/SoloLearmML-coursera-deeplearning.ai | 18e84f4cef8252c9c2a429b57ffcab590bd6c2df | [
"Apache-2.0"
] | null | null | null | S+P_Week_2_Lesson_1.ipynb | EgorBEremeev/SoloLearmML-coursera-deeplearning.ai | 18e84f4cef8252c9c2a429b57ffcab590bd6c2df | [
"Apache-2.0"
] | null | null | null | S+P_Week_2_Lesson_1.ipynb | EgorBEremeev/SoloLearmML-coursera-deeplearning.ai | 18e84f4cef8252c9c2a429b57ffcab590bd6c2df | [
"Apache-2.0"
] | null | null | null | 26.572727 | 269 | 0.409283 | [
[
[
"<a href=\"https://colab.research.google.com/github/EgorBEremeev/SoloLearmML-coursera-deeplearning.ai/blob/master/S%2BP_Week_2_Lesson_1.ipynb\" target=\"_parent\"><img src=\"https://colab.research.google.com/assets/colab-badge.svg\" alt=\"Open In Colab\"/></a>",
"_____no_output_____"
]
],
[
[
"!pip install tf-nightly-2.0-preview\n\n",
"_____no_output_____"
],
[
"import tensorflow as tf\nimport numpy as np\nimport matplotlib.pyplot as plt\nprint(tf.__version__)",
"2.0.0-dev20190628\n"
],
[
"dataset = tf.data.Dataset.range(10)\nfor val in dataset:\n print(val.numpy())",
"0\n1\n2\n3\n4\n5\n6\n7\n8\n9\n"
],
[
"dataset = tf.data.Dataset.range(10)\ndataset = dataset.window(5, shift=1)\nfor window_dataset in dataset:\n for val in window_dataset:\n print(val.numpy(), end=\" \")\n print()",
"0 1 2 3 4 \n1 2 3 4 5 \n2 3 4 5 6 \n3 4 5 6 7 \n4 5 6 7 8 \n5 6 7 8 9 \n6 7 8 9 \n7 8 9 \n8 9 \n9 \n"
],
[
"dataset = tf.data.Dataset.range(10)\ndataset = dataset.window(5, shift=1, drop_remainder=True)\nfor window_dataset in dataset:\n for val in window_dataset:\n print(val.numpy(), end=\" \")\n print()",
"0 1 2 3 4 \n1 2 3 4 5 \n2 3 4 5 6 \n3 4 5 6 7 \n4 5 6 7 8 \n5 6 7 8 9 \n"
],
[
"dataset = tf.data.Dataset.range(10)\ndataset = dataset.window(5, shift=1, drop_remainder=True)\ndataset = dataset.flat_map(lambda window: window.batch(5))\nfor window in dataset:\n print(window.numpy())\n",
"[0 1 2 3 4]\n[1 2 3 4 5]\n[2 3 4 5 6]\n[3 4 5 6 7]\n[4 5 6 7 8]\n[5 6 7 8 9]\n"
],
[
"dataset = tf.data.Dataset.range(10)\ndataset = dataset.window(5, shift=1, drop_remainder=True)\ndataset = dataset.flat_map(lambda window: window.batch(5))\ndataset = dataset.map(lambda window: (window[:-1], window[-1:]))\nfor x,y in dataset:\n print(x.numpy(), y.numpy())",
"[0 1 2 3] [4]\n[1 2 3 4] [5]\n[2 3 4 5] [6]\n[3 4 5 6] [7]\n[4 5 6 7] [8]\n[5 6 7 8] [9]\n"
],
[
"dataset = tf.data.Dataset.range(10)\ndataset = dataset.window(5, shift=1, drop_remainder=True)\ndataset = dataset.flat_map(lambda window: window.batch(5))\ndataset = dataset.map(lambda window: (window[:-1], window[-1:]))\ndataset = dataset.shuffle(buffer_size=10)\nfor x,y in dataset:\n print(x.numpy(), y.numpy())\n",
"[1 2 3 4] [5]\n[3 4 5 6] [7]\n[4 5 6 7] [8]\n[5 6 7 8] [9]\n[0 1 2 3] [4]\n[2 3 4 5] [6]\n"
],
[
"dataset = tf.data.Dataset.range(10)\ndataset = dataset.window(5, shift=1, drop_remainder=True)\ndataset = dataset.flat_map(lambda window: window.batch(5))\ndataset = dataset.map(lambda window: (window[:-1], window[-1:]))\ndataset = dataset.shuffle(buffer_size=10)\ndataset = dataset.batch(2).prefetch(1)\nfor x,y in dataset:\n print(\"x = \", x.numpy())\n print(\"y = \", y.numpy())\n",
"x = [[0 1 2 3]\n [4 5 6 7]]\ny = [[4]\n [8]]\nx = [[2 3 4 5]\n [3 4 5 6]]\ny = [[6]\n [7]]\nx = [[5 6 7 8]\n [1 2 3 4]]\ny = [[9]\n [5]]\n"
]
]
] | [
"markdown",
"code"
] | [
[
"markdown"
],
[
"code",
"code",
"code",
"code",
"code",
"code",
"code",
"code",
"code"
]
] |
e77ed1054c06c063dd211abb6b009186c4e992d5 | 35,022 | ipynb | Jupyter Notebook | workshop/session_2/06_introduction_to_pandas.ipynb | MEDC0106/PythonWorkshop | 8dab8d6dd76009c889528e477374a4bd1a41841c | [
"CC-BY-4.0"
] | 1 | 2021-12-16T22:51:57.000Z | 2021-12-16T22:51:57.000Z | workshop/session_2/06_introduction_to_pandas.ipynb | MEDC0106/PythonWorkshop | 8dab8d6dd76009c889528e477374a4bd1a41841c | [
"CC-BY-4.0"
] | 1 | 2021-11-24T10:50:01.000Z | 2021-11-24T11:01:59.000Z | workshop/session_2/06_introduction_to_pandas.ipynb | MEDC0106/PythonWorkshop | 8dab8d6dd76009c889528e477374a4bd1a41841c | [
"CC-BY-4.0"
] | null | null | null | 31.896175 | 842 | 0.612672 | [
[
[
"### MEDC0106: Bioinformatics in Applied Biomedical Science\n\n<p align=\"center\">\n <img src=\"../../resources/static/Banner.png\" alt=\"MEDC0106 Banner\" width=\"90%\"/>\n <br>\n</p>\n\n---------------------------------------------------------------\n\n# 06 - Introduction to Pandas\n\n*Written by:* Oliver Scott\n\n**This notebook provides a general introduction to Pandas.**\n\nDo not be afraid to make changes to the code cells to explore how things work!\n\n### What is Pandas?\n\n**[Pandas](https://pandas.pydata.org/)** is a Python package for data analysis, providing functions for analysing, cleaning and manipulating data. Pandas is probably one of the most important tools for data scientists and is the backbone of most data science projects using Python.\n\nPandas is built on top of NumPy, hence the Numpy structure is used a lot in the Pandas interface. Data manipulation often prefaces further analysis using other Python packages such as statistical analysis using [SciPy](https://www.scipy.org/), visualisation using tools such as [Matplotlib](https://matplotlib.org/) and machine learning using [scikit-learn](https://scikit-learn.org/stable/). These tools and others make up the Python scientific stack and are essential to learn for a career in informatics or data-science. To be effective in pandas it is essential to have a good grasp of the core concepts in Python (these concepts are outlined in the first session) along with some familiarity with NumPy. If you get lost with some concepts it might be a good idea to take a look through the previous material across the sessions.\n\nIn this notebook we will learn the basics of Pandas. Pandas is a huge package and is deserving of an entire lecture series itself, so here we will learn tyhe fundamentals from which you will be able to build upon if you want to learn more.\n\n-----\n\n## Contents\n\n1. [The Basics](#The-Basics)\n2. [Creating DataFrames](#Creating-DataFrames)\n3. [Reading Data](#Reading-Data)\n4. [Essential Operations](#Essential-Operations)\n5. [Slicing and Selecting](#Slicing-and-Selecting)\n5. [Arithmetic](#Arithmetic)\n6. [Applying Functions](#Applying-Functions)\n7. [Time-Series](#Time-Series)\n8. [Plotting](#Plotting)\n\n-----\n\n#### Extra Resources:\n\n- [Pandas Getting Started Guide](https://pandas.pydata.org/pandas-docs/stable/getting_started/index.html)\n- [RealPython-01](https://realpython.com/pandas-python-explore-dataset/)\n- [RealPython-02](https://realpython.com/pandas-dataframe/)\n\n-----\n\n#### References:\n\n- [Pandas Documentation](https://pandas.pydata.org/docs/)\n- [Learn Data Science](https://www.learndatasci.com/tutorials/python-pandas-tutorial-complete-introduction-for-beginners/)\n-----\n\n## The Basics\n\nImporting Pandas is no different to any other package/module. Pandas users often use the `pd` alias to keep code clean:\n",
"_____no_output_____"
]
],
[
[
"import pandas as pd\n\ns = pd.Series([1.0, 2.0, 3.0, 5.0])\ns",
"_____no_output_____"
]
],
[
[
"### Core Components\n\nPandas has two core components, the `Series` and the `DataFrame`.\n\nThe `Series` can be imagined as a single column in a data table, whereas the `DataFrame` can be imagined as a full data table made up of multiple `Series`. Both types have a similar interface allowing a user to perform similar operations. DataFrames are similar to spreadsheets that you may have interacted with in software such as Excel. DataFrames are often faster, easier to use and more powerful than spreadsheets.\n\n<p align=\"center\">\n <img src=\"https://www.datasciencemadesimple.com/wp-content/uploads/2020/05/create-series-in-python-pandas-0.png?ezimgfmt=rs%3Adevice%2Frscb1-1\" alt=\"Pandas DataFrame\" width=\"70%\"/>\n <br>\n</p>\n\n[Image source](https://www.datasciencemadesimple.com/wp-content/uploads/2020/05/create-series-in-python-pandas-0.png?ezimgfmt=rs%3Adevice%2Frscb1-1)\n\n-----\n\n## Creating DataFrames\n\nThere are numerous ways to create a DataFrame using the Pandas package. In most cases it is likely that you will want to read in data from a paticular file, however DataFrames can also be constructed from scratch from lists, tuples, NumPy arrays or Pandas series. Probably the most simple way however is from a simple Python dictionary `dict`. Suppose we wanted to construct a table like the one below:\n\n| PatientID | Gender | Age | Outcome |\n|-----------|--------|-----|----------|\n| 556785 | M | 19 | Negative |\n| 998764 | F | 38 | Positive |\n| 477822 | M | 54 | Positive |\n| 678329 | M | 22 | Negative |\n| 675859 | F | 41 | Negative |\n\nWe can construct this using a Python dictionary where the key corresponds to the column name and the list the data present in the rows. For this we can use the default constructor `pd.DataFrame()`. Notice how there is also an unnamed column containing the numbers 0-4, this is the **index** of each row. In fact you may also specify a custom index when contructing a dataframe; (`pd.DataFrame(data, index=['Tom', 'Joanne', 'Joe', 'Xander', 'Selena'])`) In this case the index is the names of the patients.",
"_____no_output_____"
]
],
[
[
"# This is or dictionary containing the raw data\ndata = {\n 'PatientID': [556785, 998764, 477822, 678329, 675859],\n 'Gender': ['M', 'F', 'M', 'M', 'F'],\n 'Age': [19, 38, 54, 22, 41],\n 'Outcome': ['Negative', 'Poisitive', 'Positive', 'Negative', 'Negative']\n}\n\n# We can now construct a DataFrame like so:\ndf = pd.DataFrame(data)\ndf # show the data",
"_____no_output_____"
]
],
[
[
"Often you will be working with very large tables of data making it impractical to view the whole table. Pandas provides a method `.head()` to display the first few n items or `.tail()` for the last few:",
"_____no_output_____"
]
],
[
[
"# Display the first three rows\ndf.head(n=3)",
"_____no_output_____"
],
[
"# Display the last two rows\ndf.tail(n=2)",
"_____no_output_____"
]
],
[
[
"Accessing an individual column is easy using the same syntax as a Python dictionary `dict`:",
"_____no_output_____"
]
],
[
[
"gender_column = df['Gender']\ngender_column",
"_____no_output_____"
]
],
[
[
"If the column label is a string you may also use **dot-syntax** to access the column:",
"_____no_output_____"
]
],
[
[
"age_column = df.Age\nage_column",
"_____no_output_____"
]
],
[
[
"## Reading Data\n\nReading and writing data from/to files in multiple formats is an essential part of the data analysis pipeline. Pandas can read data from file including; CSV, JSON, Excel, SQL and [many more](https://pandas.pydata.org/pandas-docs/stable/reference/io.html).\n\nIn the folder `data` we have provided a dataset downloaded from the [UK government](https://coronavirus.data.gov.uk/details/cases?areaType=overview&areaName=United%20Kingdom) detailing the number of reported positive COVID-19 test results in the United Kingdom by date reported (up to Oct-31-21). The file is in the CSV format and can be read using Pandas with the function `.read_csv()`: \n",
"_____no_output_____"
]
],
[
[
"cv_data_path = './data/data_2021-Oct-31.csv' # This is the path to our data\n\ncv_data = pd.read_csv(cv_data_path)\ncv_data.head(n=10)",
"_____no_output_____"
]
],
[
[
"We could also easily write this DataFrame to a new CSV file using the method `df.to_csv()`:\n\n```python\ncv_data.to_csv('./data/coronavirus_testing_results.csv')\n```\n\nGive it a go. Maybe also saving to a different [format](https://pandas.pydata.org/pandas-docs/stable/reference/io.html)!",
"_____no_output_____"
],
[
"## Essential Operations\n\nNow that we have loaded some data into a `DataFrame` we can perform operations for performing analysis. Typically once you have loaded some data you should view your data to make sure that it looks correct and to get an idea of what values you will be dealing with. Since we have already coovered visualising the data using `.head()`/`.tail()`, the next function you should probbaly run is `.info()` which provide essential details about your dataset including the number of rows/columns the number of none-null values (None), what type of data is in each column and how much memory the data is taking up:\n",
"_____no_output_____"
]
],
[
[
"cv_data.info()",
"_____no_output_____"
]
],
[
[
"Notice that we have 6 columns of which four are of type `object` (this could be something like a string) and two that are `int64` (integers) (these types correspond the the types used in NumPy). The info also tells us that we have 2466 non-null values and no null-values in this case. Knowing the datatype of ourt columns is very important as it will determine what operations we can perform on each column (we wouldnt want to calculate the mean of a column containing strings). Just like NumPy you can also use `.shape` to see the number of (rows/columns):",
"_____no_output_____"
]
],
[
[
"cv_data.shape",
"_____no_output_____"
]
],
[
[
"#### Removing duplicate data\n\nOften input data is noisy and needs cleaning up before we do any further analysis. It is often the case that data contains duplicated rows which is not great when we are trying to do statitical analysis. Luckily Pandas has utilities for dealing with this problem easily. The data we have read does not contain any duplicated rows so we will arbritrarily create some by duplicating the data and adding it to itself:",
"_____no_output_____"
]
],
[
[
"duplicated = cv_data.append(cv_data) # here we have copied the data and added it to itself\nduplicated.shape",
"_____no_output_____"
]
],
[
[
"Notice that we have to assign the result of the `append` to a new variable. Here we have copied the data so we wont do anything to the original DataFrame. We can now easily drop the duplicates using the `.drop_duplicates()` [method](https://pandas.pydata.org/docs/reference/api/pandas.DataFrame.drop_duplicates.html). It is always a good idea to look at the [documentation](https://pandas.pydata.org/docs/reference/api/pandas.DataFrame.drop_duplicates.html) to see what other arguments these functions accept.",
"_____no_output_____"
]
],
[
[
"duplicated = duplicated.drop_duplicates()\nduplicated.shape",
"_____no_output_____"
]
],
[
[
"Notice that the shape is now the same as the original data. Also notice that again we assigned the result to a new variable (with the same name). This technique can get quite annoying so Pandas often offers an argument `inplace` which if we set to `True` allows pandas to perform the operation modifying the original data rather than a copy.\n\n```python\nduplicated.drop_duplicates(inplace=True) # no need to assign to a new variable\n```",
"_____no_output_____"
],
[
"#### Removing Null values (None)\n\nData before cleaning commonly has missing values that you will need to deal with before further analysis. Missing values are represented by `None` or `np.nan`. There is usually two options to dealing with missing values:\n\n1. Remove all rows with missing data\n2. Imputing the missing values\n\nIn this tutorial we will stick to the first case.\n\nAgain as our data is nice and clean it contains no null values so for this example we will inject a new column containing some null values, let's do this first:",
"_____no_output_____"
]
],
[
[
"import numpy as np\n\nn_rows = cv_data.shape[0]\n# p is for weighting the choice here it is more likely to choose 1 than None\nnull_containing_data = np.random.choice([None, 1], n_rows, p=[0.2, 0.8])\nnull_containing_data",
"_____no_output_____"
]
],
[
[
"Now add a row to the data containing our constructed data:",
"_____no_output_____"
]
],
[
[
"cv_data['RandomData'] = null_containing_data # make a colum called 'RandomData'\ncv_data.head(10)",
"_____no_output_____"
]
],
[
[
"We can also see now that we have null values:",
"_____no_output_____"
]
],
[
[
"cv_data.info()",
"_____no_output_____"
]
],
[
[
"We can also check the number of null values in each column using `.isnull()`. This returns a dataframe with boolean columns where `True` indicated a null value. We can then use `.sum()` to count the number of `True` values in each column:",
"_____no_output_____"
]
],
[
[
"cv_data.isnull().sum()",
"_____no_output_____"
]
],
[
[
"When performing data analysis you often have to make the choice to remove missing values or impute them in some way. Removing data is only really recommended if the number of missing data points is relatively small. To remove null values you can simply use the [method](https://pandas.pydata.org/pandas-docs/stable/reference/api/pandas.DataFrame.dropna.html) `.dropna()`. This operation will remove any row with at least 1 null value, returning a new DataFrame unless you specified inplace. Instead of dropping rows we could instead drop columns with null values by changing the axis of operation. Columns are represented by `axis=1` (The axes are defined in the same way as NumPy!):",
"_____no_output_____"
]
],
[
[
"# First lets remove rows with null values\nremove_rows = cv_data.dropna()\nremove_rows.head(10)",
"_____no_output_____"
],
[
"remove_rows.shape",
"_____no_output_____"
]
],
[
[
"Now lets change the axis and remove the colum we injected:",
"_____no_output_____"
]
],
[
[
"cv_data.dropna(axis=1, inplace=True) # We can do it inplace since we do not care about this column\ncv_data.head(10)",
"_____no_output_____"
]
],
[
[
"#### Understanding Data\n\nNow that your data is clean(er) than when we started, it is time to do some basic stats to understand the data that we have in each column. This may help inform us how to continue with our analysis and maybe how to plot the data. Pandas provides us with an easy way to get a quick summary of the distribution of our continuous variables `.describe()`:",
"_____no_output_____"
]
],
[
[
"cv_data.describe()",
"_____no_output_____"
]
],
[
[
"We can also do the same for categorical columns but we will have to do it seperately:",
"_____no_output_____"
]
],
[
[
"cv_data.areaName.describe()",
"_____no_output_____"
]
],
[
[
"This shows us that in this dataset there are four unique area names with 'England' being the most frequent with a frequency of 640. We can also check the unique values using the `.unique()` method:",
"_____no_output_____"
]
],
[
[
"cv_data.areaName.unique()",
"_____no_output_____"
]
],
[
[
"We can see that the dataset contains data for:\n\n- England\n- Northern Ireland\n- Scotland\n- Wales\n\nBut how many times are these values recorded? We can use the method `.value_counts()` to find out:",
"_____no_output_____"
]
],
[
[
"cv_data.areaName.value_counts()",
"_____no_output_____"
]
],
[
[
"## Slicing and Selecting\n\nIn the previous section we saw how to produce summaries of the entire data which is useful however, sometimes we will want to perform analysis on certain subsets of data. We have already seen how to extract a column of data using square brackets and dot-syntax `df['col'] / df.col` and now we will dive deeper into the Pandas selction language. When selecting parts of a DataFrame we may be returning either a `DataFrame` or a `Series`, it is important to know which so that yopu use the correct syntax.\n\n\n#### Selecting by Column(s)\n\nUsing the square-bracket syntax we mentioned previously will return a Pandas `Series`",
"_____no_output_____"
]
],
[
[
"type(cv_data['areaName'])",
"_____no_output_____"
]
],
[
[
"If you wish to access it as a dataframe you can supply the column name as a list:",
"_____no_output_____"
]
],
[
[
"type(cv_data[['areaName']])",
"_____no_output_____"
]
],
[
[
"Adding another column to our selection is a simple as adding another column name to the list. Obviosuly inh this case our code will return a `DataFrame`:",
"_____no_output_____"
]
],
[
[
"selection = cv_data[['areaName', 'areaCode']]\nselection.head(5)",
"_____no_output_____"
]
],
[
[
"#### Selecting by rows\n\nSelecting rows is a little trickier with two methods:\n\n- `.loc`: locate by name\n- `.iloc`: locate by numerical index\n\nConsidering that our data has a numerical index it makes sense for us to use `.iloc`. If our data has an index using strings `.loc` would be the correct solution if we want to select using the string. Of course `.iloc` will also work returning the data at the numerical position instead of the name.\n\nBoth methods are similar to indexing lists or NumPy arrays:",
"_____no_output_____"
]
],
[
[
"cv_data.loc[222] # Return the row with index 222",
"_____no_output_____"
]
],
[
[
"Since Pandas is backed by NumPy we can also use slices to select a range of data:",
"_____no_output_____"
]
],
[
[
"cv_data.loc[222:226]",
"_____no_output_____"
]
],
[
[
"#### Conditional Selections\n\nSelecting data by index can be useful, but if we do not know what dat the indexes correspond too this can be limiting. Perhaps we are only interested in the data from Wales, we can use conditional selections to make informed selections.\n\nPandas like numpy can be indexed using a boolean array/Series/DataFrame generated using a conditional expression:",
"_____no_output_____"
]
],
[
[
"ind = cv_data['areaName'] == 'Wales' # A boolean Series\nind.tail(5)",
"_____no_output_____"
]
],
[
[
"Using this boolean Series we can index the DataFrame!",
"_____no_output_____"
]
],
[
[
"wales_data = cv_data[ind]\nwales_data.head(5)",
"_____no_output_____"
]
],
[
[
"We can simplify this quite nicely into a one line expression:",
"_____no_output_____"
]
],
[
[
"wales_data = cv_data[cv_data['areaName'] == 'Wales']\nwales_data.head(5)",
"_____no_output_____"
]
],
[
[
"Of course we can apply this to numerical columns also:",
"_____no_output_____"
]
],
[
[
"# Select rows where reported positives is less than 100\ncv_data[cv_data['newCasesByPublishDate'] < 100].head(5)",
"_____no_output_____"
]
],
[
[
"Chaining conditional expressions allows us to create powerful selections. For this we can use the logical operators `|` and `&`. Remeber to put seperate conditions in brackets!",
"_____no_output_____"
]
],
[
[
"# Count dates in england with reported positive results > 10,000\ncv_data[(cv_data['areaName'] == 'England') & (cv_data['newCasesByPublishDate'] > 10000)].shape",
"_____no_output_____"
]
],
[
[
"## Arithmetic\n\nBasic arithmentic operations can be applied in the same way as NumPy arrays, so we will quickly brush over it:",
"_____no_output_____"
]
],
[
[
"cv_data.newCasesByPublishDate / 100 # divide a column by 100 return a Series",
"_____no_output_____"
]
],
[
[
"You may also perform arithmetic between columns:",
"_____no_output_____"
]
],
[
[
"cv_data.newCasesByPublishDate + cv_data.cumCasesByPublishDate",
"_____no_output_____"
]
],
[
[
"You can insert a new column with the result:",
"_____no_output_____"
]
],
[
[
"cv_data['Rubbish'] = cv_data.newCasesByPublishDate * 0.3 / cv_data.cumCasesByPublishDate\ncv_data.head(5)",
"_____no_output_____"
]
],
[
[
"Pandas also provides some handy utility functions:",
"_____no_output_____"
]
],
[
[
"print(cv_data.newCasesByPublishDate.mean())\nprint(cv_data.newCasesByPublishDate.std())",
"_____no_output_____"
]
],
[
[
"## Applying Functions\n\nWhile it is possible to iterate over a DataFrame/Series like a NumPy array, it is slow in Python so instead we can use the `.apply()` function to apply a function to each element in a column or across columns. We can also save this result to a new column. Let's create an arbritary function that we can apply to the data we have:\n\n",
"_____no_output_____"
]
],
[
[
"def categorize_cases(x):\n if x >= 10000:\n return 'High'\n elif x <= 200:\n return 'Low'\n else:\n return 'Medium'",
"_____no_output_____"
]
],
[
[
"The above function categorises a case count into arbritarty categories: 'High', 'Medium' and 'Low'. Now we can apply this to the column 'newCasesByPublishDate':",
"_____no_output_____"
]
],
[
[
"cv_data['Category'] = cv_data['newCasesByPublishDate'].apply(categorize_cases)\ncv_data.head(10)",
"_____no_output_____"
]
],
[
[
"Users often will use anonymous functions instead of defining an explicit function like above:",
"_____no_output_____"
]
],
[
[
"cv_data['newCategory'] = cv_data['newCasesByPublishDate'].apply(lambda x: 'Red' if x >= 20000 else 'Amber')\ncv_data.head(10)",
"_____no_output_____"
]
],
[
[
"## Time-Series\n\nSome of you may have notices that one of the columns contains dates as a string (object). This isnt paticularly useful to us in this form. Pandas however has a datetime type which we can use to make some more intelligent selections based on time spans. First we need to tell pandas that our column is a datetime column:",
"_____no_output_____"
]
],
[
[
"cv_data['date'] = pd.to_datetime(cv_data['date'])\ncv_data.info()",
"_____no_output_____"
]
],
[
[
"Now we have the date in this form we can make selections within time ranges using the `.between()` method:",
"_____no_output_____"
]
],
[
[
"# Lets select data between the 20th and the 30th October 2021 and restrict it to England\nselection = cv_data[(cv_data.date.between('2021-10-20','2021-10-30')) & (cv_data.areaName == 'England')]\nselection.head(10)",
"_____no_output_____"
]
],
[
[
"Working with time-series data is even more powerful if we use the time as our index. Lets first only consider 'Scotland' in our analysis",
"_____no_output_____"
]
],
[
[
"scotland_data = pd.DataFrame(cv_data[cv_data.areaName == 'Scotland']) # also copy into a new DataFrame",
"_____no_output_____"
]
],
[
[
"Now we can set the index of the 'scotland_data' DataFrame as the index:",
"_____no_output_____"
]
],
[
[
"scotland_data.set_index('date', inplace=True)\nscotland_data.head(5)",
"_____no_output_____"
]
],
[
[
"You may have noticed that the data is in time-decending order, often we we will want to reverse this ordering. Now that the index is the date we can sort it easilt using the `.sort_index()` method:",
"_____no_output_____"
]
],
[
[
"scotland_data.sort_index(inplace=True)\nscotland_data.head(5)",
"_____no_output_____"
]
],
[
[
"Also we can simply use slicing to select a data range with `.loc`!",
"_____no_output_____"
]
],
[
[
"scotland_data.loc['2021-10-20':'2021-10-30']",
"_____no_output_____"
]
],
[
[
"We can resample time-series data into different intervals and get a mean value for that interval. Below we resmaple the data into 10-day intervals and calculate the mean of 'newCasesByPublishDate':",
"_____no_output_____"
]
],
[
[
"scotland_data.resample(rule='10d')['newCasesByPublishDate'].mean()",
"_____no_output_____"
]
],
[
[
"Instead of mean you could use other functions such as `min()`, `max()`, `sum()` etc. Indeed you can also calculate a rolling statistic using `.rolling()` and a window size. Here we will calculate a rolling average using a ten day window:",
"_____no_output_____"
]
],
[
[
"scotland_data['rollingAvgTenDay'] = scotland_data.rolling(10)['newCasesByPublishDate'].mean()\nscotland_data.head(20)",
"_____no_output_____"
]
],
[
[
"## Plotting\n\nPandas allows the visualisation of data in DataFrames/Series interfacing with the plotting package [matplotlib](https://matplotlib.org/). Displaying the plots will first require that import matplotlib:\n\n",
"_____no_output_____"
]
],
[
[
"# We also add this 'Jupyter magic' to display plots in the notebook.\n%matplotlib inline\n\nimport matplotlib.pyplot as plt",
"_____no_output_____"
]
],
[
[
"Now creating a plot with pandas is as simple as calling `.plot()` on some selected data!",
"_____no_output_____"
]
],
[
[
"scotland_data.newCasesByPublishDate.plot(); # We also add the semicolon when plotting in Jupyter",
"_____no_output_____"
]
],
[
[
"We could have also achieved the same result using the syntax:\n\n```python\nscotland_data.newCasesByPublishDate.plot.line()\n```\n\nThese plotting functions also have many [arguments](https://pandas.pydata.org/docs/reference/api/pandas.DataFrame.plot.html) which you can specify to tune the look of your plots. Thes arguments are passed to the underlying matplotlib methods. We can also specify other types of plot. For example we could visualise the data as a box plot, do you notice anything unusual?:",
"_____no_output_____"
]
],
[
[
"# Select a time window (1-month)\nwindow = scotland_data['2021-09-30':'2021-10-30']\n\nwindow.newCasesByPublishDate.plot.box();",
"_____no_output_____"
]
],
[
[
"How about we plot the raw data along with the 10 day rolling average:",
"_____no_output_____"
]
],
[
[
"scotland_data.newCasesByPublishDate.plot(figsize=(12, 8)) # also specify the size!\nscotland_data.rollingAvgTenDay.plot()\nplt.legend(); # We can also add a legend using matplotlib!",
"_____no_output_____"
]
],
[
[
"We can also save figures using `.savefig()`, check the data directory!",
"_____no_output_____"
]
],
[
[
"figure = scotland_data.newCasesByPublishDate.plot(figsize=(12, 8)).get_figure()\nfigure.savefig('./data/Scotland_2021-Oct-31.png');",
"_____no_output_____"
]
],
[
[
"## Discussion\n\nCleaning, analysing, manipulating and visualizing data is an essential skill for an informatician/data-scientist. In fact 80% of a data-scientists job is cleaning data for analysis. As Pandas is such a widley used tool if you want to know more there are hundreds of resources online to help you learn.\n\nFeel free to add more code cells and experiment with the concepts you have learnt.\n\nIf you want to know more there are some extra resources from external sources linked in the beginning section. You can click the link below to go back to the top.\n\nClick [here](#Contents) to go back to the contents.",
"_____no_output_____"
]
]
] | [
"markdown",
"code",
"markdown",
"code",
"markdown",
"code",
"markdown",
"code",
"markdown",
"code",
"markdown",
"code",
"markdown",
"code",
"markdown",
"code",
"markdown",
"code",
"markdown",
"code",
"markdown",
"code",
"markdown",
"code",
"markdown",
"code",
"markdown",
"code",
"markdown",
"code",
"markdown",
"code",
"markdown",
"code",
"markdown",
"code",
"markdown",
"code",
"markdown",
"code",
"markdown",
"code",
"markdown",
"code",
"markdown",
"code",
"markdown",
"code",
"markdown",
"code",
"markdown",
"code",
"markdown",
"code",
"markdown",
"code",
"markdown",
"code",
"markdown",
"code",
"markdown",
"code",
"markdown",
"code",
"markdown",
"code",
"markdown",
"code",
"markdown",
"code",
"markdown",
"code",
"markdown",
"code",
"markdown",
"code",
"markdown",
"code",
"markdown",
"code",
"markdown",
"code",
"markdown",
"code",
"markdown",
"code",
"markdown",
"code",
"markdown",
"code",
"markdown",
"code",
"markdown",
"code",
"markdown",
"code",
"markdown",
"code",
"markdown",
"code",
"markdown"
] | [
[
"markdown"
],
[
"code"
],
[
"markdown"
],
[
"code"
],
[
"markdown"
],
[
"code",
"code"
],
[
"markdown"
],
[
"code"
],
[
"markdown"
],
[
"code"
],
[
"markdown"
],
[
"code"
],
[
"markdown",
"markdown"
],
[
"code"
],
[
"markdown"
],
[
"code"
],
[
"markdown"
],
[
"code"
],
[
"markdown"
],
[
"code"
],
[
"markdown",
"markdown"
],
[
"code"
],
[
"markdown"
],
[
"code"
],
[
"markdown"
],
[
"code"
],
[
"markdown"
],
[
"code"
],
[
"markdown"
],
[
"code",
"code"
],
[
"markdown"
],
[
"code"
],
[
"markdown"
],
[
"code"
],
[
"markdown"
],
[
"code"
],
[
"markdown"
],
[
"code"
],
[
"markdown"
],
[
"code"
],
[
"markdown"
],
[
"code"
],
[
"markdown"
],
[
"code"
],
[
"markdown"
],
[
"code"
],
[
"markdown"
],
[
"code"
],
[
"markdown"
],
[
"code"
],
[
"markdown"
],
[
"code"
],
[
"markdown"
],
[
"code"
],
[
"markdown"
],
[
"code"
],
[
"markdown"
],
[
"code"
],
[
"markdown"
],
[
"code"
],
[
"markdown"
],
[
"code"
],
[
"markdown"
],
[
"code"
],
[
"markdown"
],
[
"code"
],
[
"markdown"
],
[
"code"
],
[
"markdown"
],
[
"code"
],
[
"markdown"
],
[
"code"
],
[
"markdown"
],
[
"code"
],
[
"markdown"
],
[
"code"
],
[
"markdown"
],
[
"code"
],
[
"markdown"
],
[
"code"
],
[
"markdown"
],
[
"code"
],
[
"markdown"
],
[
"code"
],
[
"markdown"
],
[
"code"
],
[
"markdown"
],
[
"code"
],
[
"markdown"
],
[
"code"
],
[
"markdown"
],
[
"code"
],
[
"markdown"
],
[
"code"
],
[
"markdown"
],
[
"code"
],
[
"markdown"
],
[
"code"
],
[
"markdown"
],
[
"code"
],
[
"markdown"
]
] |
e77ed47c4cbc275c519e01780f53cb6368ad0dd6 | 201,377 | ipynb | Jupyter Notebook | notebooks/GalsimHubDemo.ipynb | Hbretonniere/galsim_hub | 1fb8a942f49710b02e584e05fc7d715e8ddef821 | [
"MIT"
] | 7 | 2020-08-11T06:58:28.000Z | 2021-12-20T10:54:09.000Z | notebooks/GalsimHubDemo.ipynb | Hbretonniere/galsim_hub | 1fb8a942f49710b02e584e05fc7d715e8ddef821 | [
"MIT"
] | 4 | 2020-03-11T19:36:00.000Z | 2021-05-26T14:42:58.000Z | notebooks/GalsimHubDemo.ipynb | Hbretonniere/galsim_hub | 1fb8a942f49710b02e584e05fc7d715e8ddef821 | [
"MIT"
] | 4 | 2020-06-18T12:52:22.000Z | 2021-07-27T09:20:54.000Z | 349.006932 | 183,538 | 0.92588 | [
[
[
"<a href=\"https://colab.research.google.com/github/McWilliamsCenter/galsim_hub/blob/master/notebooks/GalsimHubDemo.ipynb\" target=\"_parent\"><img src=\"https://colab.research.google.com/assets/colab-badge.svg\" alt=\"Open In Colab\"/></a>",
"_____no_output_____"
],
[
"# Introduction to GalSim Hub\n\nAuthors: [@EiffL](https://github.com/EiffL)\n\nThis notebook contains a short introduction to using GalSim-Hub for sampling\nrandomly generated galaxy light profiles, and drawing them using GalSim.\n",
"_____no_output_____"
],
[
"## Setting up the environment\n\nBesides GalSim, GalSim-Hub requires TensorFlow (version 1.15, for stability reasons), and TensorFlow Hub. In a Colab environment, TensorFlow is already installed, so we only need to install GalSim, using some conda magic.",
"_____no_output_____"
]
],
[
[
"# Activating TensorFlow v1.15 environment on Colab\n%tensorflow_version 1.x",
"_____no_output_____"
],
[
"# Installing and updating conda for Python 3.6\n!wget https://repo.continuum.io/miniconda/Miniconda3-4.5.4-Linux-x86_64.sh && bash Miniconda3-4.5.4-Linux-x86_64.sh -bfp /usr/local\n!conda install --channel defaults conda python=3.6 --yes\n!conda update --channel defaults --all --yes\n\n# Installing GalSim\n!conda install -y -q -c conda-forge galsim=2.2.4=py36hbfbe4e9_0\n\n# Adding conda packages to Python path \nimport sys\nsys.path.append('/usr/local/lib/python3.6/site-packages')",
"_____no_output_____"
]
],
[
[
"And finally, installing GalSim Hub itself:",
"_____no_output_____"
]
],
[
[
"!pip install --no-deps git+https://github.com/McWilliamsCenter/galsim_hub.git",
"_____no_output_____"
]
],
[
[
"## Loading and Using generative models from Python\n\nThe first step is to load a generative model. This is done by creating an instance of the `galsim_hub.GenerativeGalaxyModel` class, either by providing a local path to a model directory, or much more conveniently, by\nusing the `hub:xxxxxx` syntax, where `xxxxxx` is the name of a published model, hosted on the GalSim Hub repository, see [here](https://github.com/McWilliamsCenter/galsim_hub/tree/master/hub).\n\nAs an example, we will load the generative model presented in Lanusse et al. 2020:",
"_____no_output_____"
]
],
[
[
"import galsim\nimport galsim_hub\n\nmodel = galsim_hub.GenerativeGalaxyModel('hub:Lanusse2020')",
"_____no_output_____"
]
],
[
[
"Behind the scene, the generative model has been downloaded from the repository,\nand is now ready to use.\n\nModels can be conditional, i.e. generating a light profile given some particular attributes as inputs. To introspect the model, and see what inputs are expected, you can use the `quantities` attribute: ",
"_____no_output_____"
]
],
[
[
"model.quantities",
"_____no_output_____"
]
],
[
[
"We see that this model generates light profiles, given a particular magnitude, redshift, and size.\n\nOther interesting properties saved with the model (but knowledge of which is not necessary) are the native stamp size, and native pixel size at which the generative model is producing light profiles: ",
"_____no_output_____"
]
],
[
[
"# Pixel size, in arcsec\nmodel.pixel_size",
"_____no_output_____"
],
[
"# Stamp size\nmodel.stamp_size",
"_____no_output_____"
]
],
[
[
"Now that the model is loaded, and that we know what inputs it expects, we can \ncreate a catalog listing some desired input quantities:",
"_____no_output_____"
]
],
[
[
"from astropy.table import Table\ncatalog = Table([[5., 10. ,20.], [24., 24., 24.], [0.5, 0.5, 0.5] ],\n names=['flux_radius', 'mag_auto', 'zphot'])",
"_____no_output_____"
]
],
[
[
"In this example, we want 3 galaxies, all at the same i-band magnitude of 24, and redshift 0.5, but with different and increasing size.\n\n\nWe can now sample actual GalSim light profiles with those properties from the model using the `sample()` method:",
"_____no_output_____"
]
],
[
[
"# Sample light profiles for these parameters\nprofiles = model.sample(catalog)",
"_____no_output_____"
]
],
[
[
"This returns a list of 3 profiles, represented by `galsim.InterpolatedImage` objects:",
"_____no_output_____"
]
],
[
[
"profiles[0]",
"_____no_output_____"
]
],
[
[
"**These objects can now be manipulated inside GalSim as any other light profile.**\n\nFor instance, we can convolve these images with a PSF and add some observational noise:",
"_____no_output_____"
]
],
[
[
"%pylab inline\n\nPSF = galsim.Gaussian(fwhm=0.06)\n\nfigure(figsize=(10,5))\n\nfor i in range(3):\n\n # Convolving light profile with PSF\n gal = galsim.Convolve(profiles[i], PSF)\n\n # Drawing postage stamp of any desired size and pixel scale\n im = gal.drawImage(nx=96, ny=96, scale=0.03)\n\n # Adding some noise for realism\n im.addNoise(galsim.getCOSMOSNoise())\n\n # Drawing the image\n subplot(1,3,i+1)\n imshow(im.array)",
"Populating the interactive namespace from numpy and matplotlib\n"
]
],
[
[
"And voila!",
"_____no_output_____"
],
[
"## Using generative models directly from Yaml\n\nGalSim Hub also provides a driver for using generative models direclty from \na GalSim Yaml script. In this section, we will illustrate how to write such a script and execute it from the command line.\n\nLet's retrieve a script from the example folder of GalSim Hub:",
"_____no_output_____"
]
],
[
[
"!wget -q https://raw.githubusercontent.com/McWilliamsCenter/galsim_hub/master/examples/demo14.yaml\n!cat demo14.yaml",
"modules:\n - galsim_hub\n\npsf :\n type : Gaussian\n sigma : 0.06 # arcsec\n\n# Define the galaxy profile\ngal :\n type : GenerativeModelGalaxy\n flux_radius : { type : Random , min : 5, max : 10 }\n mag_auto : { type : Random , min : 24., max : 25. }\n\n# The image field specifies some other information about the image to be drawn.\nimage :\n type : Tiled\n nx_tiles : 10\n ny_tiles : 10\n\n stamp_size : 64 # pixels\n\n pixel_scale : 0.03 # arcsec / pixel\n\n noise :\n type : COSMOS\n\noutput :\n dir : output_yaml\n file_name : demo14.fits\n\n# Define the input files\ninput :\n generative_model :\n file_name : 'hub:cosmos_size_mag'\n"
]
],
[
[
"Note the following points that are directly related to generative models:\n - In the preamble of the file, we load the `galsim_hub` module\n - In the galaxy section, we use the `GenerativeModelGalaxy` type, and provide some input distributions for the input quantities used by the model\n - In the `input` section, we provide the path to the generative model, or as \n in this case, only its hub tag, so that it can be automatically downloaded.\n\nWe direct the interested reader to the GalSim documentation for further details on how to compose such a Yaml file.\n\nWe can now execute that file from the command line:",
"_____no_output_____"
]
],
[
[
"!python /galsim demo14.yaml",
"_____no_output_____"
]
],
[
[
"This should generate a fits file corresponding to the Yaml description. Unfortunately, couldn't manage to get it to work yet on this hybrid conda/google environment... Suggestions welcome :-)",
"_____no_output_____"
]
],
[
[
"",
"_____no_output_____"
]
]
] | [
"markdown",
"code",
"markdown",
"code",
"markdown",
"code",
"markdown",
"code",
"markdown",
"code",
"markdown",
"code",
"markdown",
"code",
"markdown",
"code",
"markdown",
"code",
"markdown",
"code",
"markdown",
"code",
"markdown",
"code"
] | [
[
"markdown",
"markdown",
"markdown"
],
[
"code",
"code"
],
[
"markdown"
],
[
"code"
],
[
"markdown"
],
[
"code"
],
[
"markdown"
],
[
"code"
],
[
"markdown"
],
[
"code",
"code"
],
[
"markdown"
],
[
"code"
],
[
"markdown"
],
[
"code"
],
[
"markdown"
],
[
"code"
],
[
"markdown"
],
[
"code"
],
[
"markdown",
"markdown"
],
[
"code"
],
[
"markdown"
],
[
"code"
],
[
"markdown"
],
[
"code"
]
] |
e77ed5d1bb356ff0ff9d8f3862a1e7e8694ad425 | 355,456 | ipynb | Jupyter Notebook | notebooks/CST_ALL_PTMs_Normalization.ipynb | MaayanLab/cst_drug_treatment | f091846f2eeb630002bae43a6b427a2beb04955c | [
"MIT"
] | 1 | 2019-01-10T18:23:06.000Z | 2019-01-10T18:23:06.000Z | notebooks/CST_ALL_PTMs_Normalization.ipynb | MaayanLab/cst_drug_treatment | f091846f2eeb630002bae43a6b427a2beb04955c | [
"MIT"
] | null | null | null | notebooks/CST_ALL_PTMs_Normalization.ipynb | MaayanLab/cst_drug_treatment | f091846f2eeb630002bae43a6b427a2beb04955c | [
"MIT"
] | null | null | null | 807.854545 | 76,996 | 0.944812 | [
[
[
"# CST_ALL_PTMs_Normalization\nThis notebook will make the case for normalizing the distributions of PTM levels in all cell lines. I will combine the PTM data from all cell lines and look at the average properties of all PTM distributions in all cell lines. \n\n### imports and function definitions",
"_____no_output_____"
]
],
[
[
"# imports and plotting defaults\nimport pandas as pd\nimport numpy as np\nimport matplotlib.pyplot as plt\n%matplotlib inline \nimport matplotlib\nmatplotlib.style.use('ggplot')\nfrom copy import deepcopy\n\n# use clustergrammer module to load/process (source code in clustergrammer directory)\nfrom clustergrammer import Network\n\n# load data data and export as pandas dataframe: inst_df\ndef load_data(filename):\n ''' \n load data using clustergrammer and export as pandas dataframe\n '''\n net = deepcopy(Network())\n net.load_file(filename)\n tmp_df = net.dat_to_df()\n inst_df = tmp_df['mat']\n\n \n# # simplify column names (remove categories)\n# col_names = inst_df.columns.tolist()\n# simple_col_names = []\n# for inst_name in col_names:\n# simple_col_names.append(inst_name[0])\n\n# inst_df.columns = simple_col_names\n\n print(inst_df.shape)\n \n return inst_df\n\ndef plot_cl_boxplot_with_missing_data(inst_df):\n '''\n Make a box plot of the cell lines where the cell lines are ranked based \n on their average PTM levels\n '''\n # get the order of the cell lines based on their mean \n sorter = inst_df.mean().sort_values().index.tolist()\n # reorder based on ascending mean values\n sort_df = inst_df[sorter]\n # box plot of PTM values ordered based on increasing mean \n sort_df.plot(kind='box', figsize=(10,3), rot=90, ylim=(-4,4))\n\ndef plot_cl_boxplot_no_missing_data(inst_df):\n # get the order of the cell lines based on their mean \n sorter = inst_df.mean().sort_values().index.tolist()\n # reorder based on ascending mean values\n sort_df = inst_df[sorter]\n\n # transpose to get PTMs as columns \n tmp_df = sort_df.transpose()\n\n # keep only PTMs that are measured in all cell lines\n ptm_num_meas = tmp_df.count()\n ptm_all_meas = ptm_num_meas[ptm_num_meas == 45]\n ptm_all_meas = ptm_all_meas.index.tolist()\n\n print('There are ' + str(len(ptm_all_meas)) + ' PTMs measured in all cell lines')\n \n # only keep ptms that are measured in all cell lines \n # I will call this full_df as in no missing measurements\n full_df = tmp_df[ptm_all_meas]\n\n # transpose back to PTMs as rows\n full_df = full_df.transpose()\n\n full_df.plot(kind='box', figsize=(10,3), rot=900, ylim=(-8,8))\n num_ptm_all_meas = len(ptm_all_meas) ",
"_____no_output_____"
]
],
[
[
"## Load all PTM data and combine into single dataframe",
"_____no_output_____"
]
],
[
[
"filename = '../lung_cellline_3_1_16/lung_cl_all_ptm/all_ptm_ratios.tsv'\ndf_all = load_data(filename)",
"(8468, 45)\n"
],
[
"df_all.count().sort_values().plot(kind='bar', figsize=(10,2))",
"_____no_output_____"
],
[
"plot_cl_boxplot_with_missing_data(df_all)",
"_____no_output_____"
]
],
[
[
"# Merge Plex-duplicate cell lines",
"_____no_output_____"
]
],
[
[
"filename = '../lung_cellline_3_1_16/lung_cl_all_ptm/all_ptm_ratios_uni_cl.tsv'\ndf_all = load_data(filename)",
"(8468, 42)\n"
],
[
"df_all.count().sort_values().plot(kind='bar', figsize=(10,2))",
"_____no_output_____"
],
[
"plot_cl_boxplot_with_missing_data(df_all)",
"_____no_output_____"
]
],
[
[
"# Zscore rows",
"_____no_output_____"
]
],
[
[
"df_tmp = deepcopy(df_all)\ndf_tmp = df_tmp.transpose()\nzdf_all = (df_tmp - df_tmp.mean())/df_tmp.std()\nzdf_all = zdf_all.transpose()\n\nprint(zdf_all.shape)",
"(8468, 45)\n"
],
[
"zdf_all.count().sort_values().plot(kind='bar', figsize=(10,2))",
"_____no_output_____"
],
[
"plot_cl_boxplot_with_missing_data(zdf_all)",
"_____no_output_____"
]
]
] | [
"markdown",
"code",
"markdown",
"code",
"markdown",
"code",
"markdown",
"code"
] | [
[
"markdown"
],
[
"code"
],
[
"markdown"
],
[
"code",
"code",
"code"
],
[
"markdown"
],
[
"code",
"code",
"code"
],
[
"markdown"
],
[
"code",
"code",
"code"
]
] |
e77f10cad4d10716e704a4c895d2210034f57991 | 21,592 | ipynb | Jupyter Notebook | code/build_format_cv/build-page-categories.ipynb | edwbaker/ac | f594a73fd2603ce5c2268119522f8d4454765b98 | [
"CC-BY-4.0"
] | 7 | 2018-05-01T23:36:29.000Z | 2022-02-03T18:38:04.000Z | code/build_format_cv/build-page-categories.ipynb | edwbaker/ac | f594a73fd2603ce5c2268119522f8d4454765b98 | [
"CC-BY-4.0"
] | 151 | 2015-06-02T21:03:18.000Z | 2022-02-22T13:26:54.000Z | code/build_format_cv/build-page-categories.ipynb | edwbaker/ac | f594a73fd2603ce5c2268119522f8d4454765b98 | [
"CC-BY-4.0"
] | 8 | 2018-01-26T00:45:38.000Z | 2021-11-04T16:49:42.000Z | 46.03838 | 243 | 0.524037 | [
[
[
"# Script to build Markdown pages that provide term metadata for complex vocabularies\n# Steve Baskauf 2020-06-28 CC0\n# This script merges static Markdown header and footer documents with term information tables (in Markdown) generated from data in the rs.tdwg.org repo from the TDWG Github site\n\nimport re\nimport requests # best library to manage HTTP transactions\nimport csv # library to read/write/parse CSV files\nimport json # library to convert JSON to Python data structures\nimport pandas as pd\n\n# -----------------\n# Configuration section\n# -----------------\n\n# !!!! NOTE !!!!\n# There is not currently an example of a complex vocabulary that has the column headers\n# used in the sample files. In order to test this script, it uses the Audubon Core files,\n# which have headers that differ from the samples. So throughout the code, there are\n# pairs of lines where the default header names are commented out and the Audubon Core\n# headers are not. To build a page using the sample files, you will need to reverse the\n# commenting of these pairs.\n\n# This is the base URL for raw files from the branch of the repo that has been pushed to GitHub\ngithubBaseUri = 'https://raw.githubusercontent.com/tdwg/rs.tdwg.org/master/'\n\nheaderFileName = 'termlist-header.md'\nfooterFileName = 'termlist-footer.md'\noutFileName = '../../docs/format.md'\n\n# This is a Python list of the database names of the term lists to be included in the document.\ntermLists = ['format']\n#termLists = ['pathway']\n\n# NOTE! There may be problems unless every term list is of the same vocabulary type since the number of columns will differ\n# However, there probably aren't any circumstances where mixed types will be used to generate the same page.\nvocab_type = 3 # 1 is simple vocabulary, 2 is simple controlled vocabulary, 3 is c.v. with broader hierarchy\n\n# Terms in large vocabularies like Darwin and Audubon Cores may be organized into categories using tdwgutility_organizedInClass\n# If so, those categories can be used to group terms in the generated term list document.\norganized_in_categories = True\n\n# If organized in categories, the display_order list must contain the IRIs that are values of tdwgutility_organizedInClass\n# If not organized into categories, the value is irrelevant. There just needs to be one item in the list.\ndisplay_order = ['', 'm', 'e']\ndisplay_label = ['Concept schemes', 'Media types and physical media concept scheme', 'File extensions concept scheme']\ndisplay_comments = ['','','']\ndisplay_id = ['general_types', 'media_types', 'file_extensions']\n\n#display_order = ['']\n#display_label = ['Vocabulary'] # these are the section labels for the categories in the page\n#display_comments = [''] # these are the comments about the category to be appended following the section labels\n#display_id = ['Vocabulary'] # these are the fragment identifiers for the associated sections for the categories\n\n# ---------------\n# Function definitions\n# ---------------\n\n# replace URL with link\n#\ndef createLinks(text):\n def repl(match):\n if match.group(1)[-1] == '.':\n return '<a href=\"' + match.group(1)[:-1] + '\">' + match.group(1)[:-1] + '</a>.'\n return '<a href=\"' + match.group(1) + '\">' + match.group(1) + '</a>'\n\n pattern = '(https?://[^\\s,;\\)\"<]*)'\n result = re.sub(pattern, repl, text)\n return result\n\n# 2021-08-05 Add code to convert backticks copied from the DwC QRG build script written by S. Van Hoey\ndef convert_code(text_with_backticks):\n \"\"\"Takes all back-quoted sections in a text field and converts it to\n the html tagged version of code blocks <code>...</code>\n \"\"\"\n return re.sub(r'`([^`]*)`', r'<code>\\1</code>', text_with_backticks)\n\ndef convert_link(text_with_urls):\n \"\"\"Takes all links in a text field and converts it to the html tagged\n version of the link\n \"\"\"\n def _handle_matched(inputstring):\n \"\"\"quick hack version of url handling on the current prime versions data\"\"\"\n url = inputstring.group()\n return \"<a href=\\\"{}\\\">{}</a>\".format(url, url)\n\n regx = \"(http[s]?://[\\w\\d:#@%/;$()~_?\\+-;=\\\\\\.&]*)(?<![\\)\\.,])\"\n return re.sub(regx, _handle_matched, text_with_urls)\n",
"_____no_output_____"
],
[
"term_lists_info = []\n\nframe = pd.read_csv(githubBaseUri + 'term-lists/term-lists.csv', na_filter=False)\nfor termList in termLists:\n term_list_dict = {'list_iri': termList}\n term_list_dict = {'database': termList}\n for index,row in frame.iterrows():\n if row['database'] == termList:\n term_list_dict['pref_ns_prefix'] = row['vann_preferredNamespacePrefix']\n term_list_dict['pref_ns_uri'] = row['vann_preferredNamespaceUri']\n term_list_dict['list_iri'] = row['list']\n term_lists_info.append(term_list_dict)\nprint(term_lists_info)",
"_____no_output_____"
],
[
"# Create column list\ncolumn_list = ['pref_ns_prefix', 'pref_ns_uri', 'term_localName', 'label', 'definition', 'usage', 'notes', 'examples', 'term_modified', 'term_deprecated', 'type']\nif vocab_type == 2:\n column_list += ['controlled_value_string']\nelif vocab_type == 3:\n column_list += ['controlled_value_string', 'skos_broader', 'skos_exactMatch']\nif organized_in_categories:\n column_list.append('skos_inScheme')\ncolumn_list.append('version_iri')\n\n# Create list of lists metadata table\ntable_list = []\nfor term_list in term_lists_info:\n # retrieve versions metadata for term list\n versions_url = githubBaseUri + term_list['database'] + '-versions/' + term_list['database'] + '-versions.csv'\n versions_df = pd.read_csv(versions_url, na_filter=False)\n \n # retrieve current term metadata for term list\n data_url = githubBaseUri + term_list['database'] + '/' + term_list['database'] + '.csv'\n frame = pd.read_csv(data_url, na_filter=False)\n for index,row in frame.iterrows():\n row_list = [term_list['pref_ns_prefix'], term_list['pref_ns_uri'], row['term_localName'], row['label'], row['definition'], row['usage'], row['notes'], row['examples'], row['term_modified'], row['term_deprecated'], row['type']]\n if vocab_type == 2:\n row_list += [row['controlled_value_string']]\n elif vocab_type == 3:\n if row['skos_broader'] =='':\n row_list += [row['controlled_value_string'], '']\n else:\n row_list += [row['controlled_value_string'], term_list['pref_ns_prefix'] + ':' + row['skos_broader']]\n if row['skos_exactMatch'] =='':\n row_list += ['']\n else:\n row_list += [term_list['pref_ns_prefix'] + ':' + row['skos_exactMatch']]\n if organized_in_categories:\n row_list.append(row['skos_inScheme'])\n\n # Borrowed terms really don't have implemented versions. They may be lacking values for version_status.\n # In their case, their version IRI will be omitted.\n found = False\n for vindex, vrow in versions_df.iterrows():\n if vrow['term_localName']==row['term_localName'] and vrow['version_status']=='recommended':\n found = True\n version_iri = vrow['version']\n # NOTE: the current hack for non-TDWG terms without a version is to append # to the end of the term IRI\n if version_iri[len(version_iri)-1] == '#':\n version_iri = ''\n if not found:\n version_iri = ''\n row_list.append(version_iri)\n\n table_list.append(row_list)\n\n# Turn list of lists into dataframe\nterms_df = pd.DataFrame(table_list, columns = column_list)\n\n#terms_sorted_by_label = terms_df.sort_values(by='label')\n# make case insensitive\nterms_sorted_by_label = terms_df.iloc[terms_df.label.str.lower().argsort()]\nterms_sorted_by_localname = terms_df.sort_values(by='term_localName')\nterms_sorted_by_label",
"_____no_output_____"
]
],
[
[
"Run the following cell to generate an index sorted alphabetically by lowercase term local name. Omit this index if the terms have opaque local names.",
"_____no_output_____"
]
],
[
[
"# generate the index of terms grouped by category and sorted alphabetically by lowercase term local name\n\ntext = '### 3.1 Index By Term Name\\n\\n'\ntext += '(See also [3.2 Index By Label](#32-index-by-label))\\n\\n'\nfor category in range(0,len(display_order)):\n text += '**' + display_label[category] + '**\\n'\n text += '\\n'\n if organized_in_categories:\n filtered_table = terms_sorted_by_localname[terms_sorted_by_localname['skos_inScheme']==display_order[category]]\n filtered_table.reset_index(drop=True, inplace=True)\n else:\n filtered_table = terms_sorted_by_localname\n filtered_table.reset_index(drop=True, inplace=True)\n \n for row_index,row in filtered_table.iterrows():\n curie = row['pref_ns_prefix'] + \":\" + row['term_localName']\n curie_anchor = curie.replace(':','_')\n text += '[' + row['label'] + '](#' + curie_anchor + ') |\\n'\n text = text[:len(text)-2] # remove final trailing vertical bar and newline\n text += '\\n\\n' # put back removed newline\n\nindex_by_name = text\n\nprint(index_by_name)",
"_____no_output_____"
]
],
[
[
"Run the following cell to generate an index by term label",
"_____no_output_____"
]
],
[
[
"text = '\\n\\n'\n\n# Comment out the following two lines if there is no index by local names\n#text = '### 3.2 Index By Label\\n\\n'\n#text += '(See also [3.1 Index By Term Name](#31-index-by-term-name))\\n\\n'\nfor category in range(0,len(display_order)):\n if organized_in_categories:\n text += '**' + display_label[category] + '**\\n'\n text += '\\n'\n filtered_table = terms_sorted_by_label[terms_sorted_by_label['skos_inScheme']==display_order[category]]\n filtered_table.reset_index(drop=True, inplace=True)\n else:\n filtered_table = terms_sorted_by_label\n filtered_table.reset_index(drop=True, inplace=True)\n \n for row_index,row in filtered_table.iterrows():\n if row_index == 0 or (row_index != 0 and row['label'] != filtered_table.iloc[row_index - 1].loc['label']): # this is a hack to prevent duplicate labels\n curie_anchor = row['pref_ns_prefix'] + \"_\" + row['term_localName']\n text += '[' + row['label'] + '](#' + curie_anchor + ') |\\n'\n text = text[:len(text)-2] # remove final trailing vertical bar and newline\n text += '\\n\\n' # put back removed newline\n\nindex_by_label = text\n\nprint(index_by_label)",
"_____no_output_____"
],
[
"decisions_df = pd.read_csv('https://raw.githubusercontent.com/tdwg/rs.tdwg.org/master/decisions/decisions-links.csv', na_filter=False)\n\n# generate a table for each term, with terms grouped by category\n\n# generate the Markdown for the terms table\ntext = '## 4 Vocabulary\\n'\nfor category in range(0,len(display_order)):\n if organized_in_categories:\n text += '### 4.' + str(category + 1) + ' ' + display_label[category] + '\\n'\n text += '\\n'\n text += display_comments[category] # insert the comments for the category, if any.\n filtered_table = terms_sorted_by_localname[terms_sorted_by_localname['skos_inScheme']==display_order[category]]\n filtered_table.reset_index(drop=True, inplace=True)\n else:\n filtered_table = terms_sorted_by_localname\n filtered_table.reset_index(drop=True, inplace=True)\n\n for row_index,row in filtered_table.iterrows():\n text += '<table>\\n'\n curie = row['pref_ns_prefix'] + \":\" + row['term_localName']\n curieAnchor = curie.replace(':','_')\n text += '\\t<thead>\\n'\n text += '\\t\\t<tr>\\n'\n text += '\\t\\t\\t<th colspan=\"2\"><a id=\"' + curieAnchor + '\"></a>Term Name ' + curie + '</th>\\n'\n text += '\\t\\t</tr>\\n'\n text += '\\t</thead>\\n'\n text += '\\t<tbody>\\n'\n text += '\\t\\t<tr>\\n'\n text += '\\t\\t\\t<td>Term IRI</td>\\n'\n uri = row['pref_ns_uri'] + row['term_localName']\n text += '\\t\\t\\t<td><a href=\"' + uri + '\">' + uri + '</a></td>\\n'\n text += '\\t\\t</tr>\\n'\n text += '\\t\\t<tr>\\n'\n text += '\\t\\t\\t<td>Modified</td>\\n'\n text += '\\t\\t\\t<td>' + row['term_modified'] + '</td>\\n'\n text += '\\t\\t</tr>\\n'\n\n if row['version_iri'] != '':\n text += '\\t\\t<tr>\\n'\n text += '\\t\\t\\t<td>Term version IRI</td>\\n'\n text += '\\t\\t\\t<td><a href=\"' + row['version_iri'] + '\">' + row['version_iri'] + '</a></td>\\n'\n text += '\\t\\t</tr>\\n'\n\n text += '\\t\\t<tr>\\n'\n text += '\\t\\t\\t<td>Label</td>\\n'\n text += '\\t\\t\\t<td>' + row['label'] + '</td>\\n'\n text += '\\t\\t</tr>\\n'\n\n if row['term_deprecated'] != '':\n text += '\\t\\t<tr>\\n'\n text += '\\t\\t\\t<td></td>\\n'\n text += '\\t\\t\\t<td><strong>This term is deprecated and should no longer be used.</strong></td>\\n'\n text += '\\t\\t</tr>\\n'\n\n text += '\\t\\t<tr>\\n'\n text += '\\t\\t\\t<td>Definition</td>\\n'\n text += '\\t\\t\\t<td>' + row['definition'] + '</td>\\n'\n text += '\\t\\t</tr>\\n'\n\n if row['usage'] != '':\n text += '\\t\\t<tr>\\n'\n text += '\\t\\t\\t<td>Usage</td>\\n'\n text += '\\t\\t\\t<td>' + convert_link(convert_code(row['usage'])) + '</td>\\n'\n text += '\\t\\t</tr>\\n'\n\n if row['notes'] != '':\n text += '\\t\\t<tr>\\n'\n text += '\\t\\t\\t<td>Notes</td>\\n'\n text += '\\t\\t\\t<td>' + convert_link(convert_code(row['notes'])) + '</td>\\n'\n text += '\\t\\t</tr>\\n'\n\n if row['examples'] != '':\n text += '\\t\\t<tr>\\n'\n text += '\\t\\t\\t<td>Examples</td>\\n'\n text += '\\t\\t\\t<td>' + convert_link(convert_code(row['examples'])) + '</td>\\n'\n text += '\\t\\t</tr>\\n'\n\n if (vocab_type == 2 or vocab_type == 3) and row['controlled_value_string'] != '': # controlled vocabulary\n text += '\\t\\t<tr>\\n'\n text += '\\t\\t\\t<td>Controlled value</td>\\n'\n text += '\\t\\t\\t<td>' + row['controlled_value_string'] + '</td>\\n'\n text += '\\t\\t</tr>\\n'\n\n if vocab_type == 3 and row['skos_broader'] != '': # controlled vocabulary with skos:broader relationships\n text += '\\t\\t<tr>\\n'\n text += '\\t\\t\\t<td>Has broader concept</td>\\n'\n curieAnchor = row['skos_broader'].replace(':','_')\n text += '\\t\\t\\t<td><a href=\"#' + curieAnchor + '\">' + row['skos_broader'] + '</a></td>\\n'\n text += '\\t\\t</tr>\\n'\n\n if vocab_type == 3 and row['skos_exactMatch'] != '': # controlled vocabulary with skos:exactMatch relationships\n text += '\\t\\t<tr>\\n'\n text += '\\t\\t\\t<td>Has exact match</td>\\n'\n curieAnchor = row['skos_exactMatch'].replace(':','_')\n text += '\\t\\t\\t<td><a href=\"#' + curieAnchor + '\">' + row['skos_exactMatch'] + '</a></td>\\n'\n text += '\\t\\t</tr>\\n'\n\n text += '\\t\\t<tr>\\n'\n text += '\\t\\t\\t<td>Type</td>\\n'\n if row['type'] == 'http://www.w3.org/1999/02/22-rdf-syntax-ns#Property':\n text += '\\t\\t\\t<td>Property</td>\\n'\n elif row['type'] == 'http://www.w3.org/2000/01/rdf-schema#Class':\n text += '\\t\\t\\t<td>Class</td>\\n'\n elif row['type'] == 'http://www.w3.org/2004/02/skos/core#Concept':\n text += '\\t\\t\\t<td>Concept</td>\\n'\n else:\n text += '\\t\\t\\t<td>' + row['type'] + '</td>\\n' # this should rarely happen\n text += '\\t\\t</tr>\\n'\n\n # Look up decisions related to this term\n for drow_index,drow in decisions_df.iterrows():\n if drow['linked_affected_resource'] == uri:\n text += '\\t\\t<tr>\\n'\n text += '\\t\\t\\t<td>Executive Committee decision</td>\\n'\n text += '\\t\\t\\t<td><a href=\"http://rs.tdwg.org/decisions/' + drow['decision_localName'] + '\">http://rs.tdwg.org/decisions/' + drow['decision_localName'] + '</a></td>\\n'\n text += '\\t\\t</tr>\\n' \n\n text += '\\t</tbody>\\n'\n text += '</table>\\n'\n text += '\\n'\n text += '\\n'\nterm_table = text\n\nprint(term_table)",
"_____no_output_____"
]
],
[
[
"Modify to display the indices that you want",
"_____no_output_____"
]
],
[
[
"text = index_by_label + term_table\n#text = index_by_name + index_by_label + term_table",
"_____no_output_____"
],
[
"# read in header and footer, merge with terms table, and output\n\nheaderObject = open(headerFileName, 'rt', encoding='utf-8')\nheader = headerObject.read()\nheaderObject.close()\n\nfooterObject = open(footerFileName, 'rt', encoding='utf-8')\nfooter = footerObject.read()\nfooterObject.close()\n\noutput = header + text + footer\noutputObject = open(outFileName, 'wt', encoding='utf-8')\noutputObject.write(output)\noutputObject.close()\n \nprint('done')",
"_____no_output_____"
]
]
] | [
"code",
"markdown",
"code",
"markdown",
"code",
"markdown",
"code"
] | [
[
"code",
"code",
"code"
],
[
"markdown"
],
[
"code"
],
[
"markdown"
],
[
"code",
"code"
],
[
"markdown"
],
[
"code",
"code"
]
] |
e77f20f22c645928ddd0cae9f3de40c258ab8c56 | 1,610 | ipynb | Jupyter Notebook | crawler/Untitled.ipynb | yskft04/satobot-crawler-open | 50b8b72d20b18de45671475299e0f45000cc7055 | [
"Unlicense"
] | 1 | 2021-12-19T05:40:32.000Z | 2021-12-19T05:40:32.000Z | crawler/Untitled.ipynb | yskft04/satobot-crawler-open | 50b8b72d20b18de45671475299e0f45000cc7055 | [
"Unlicense"
] | null | null | null | crawler/Untitled.ipynb | yskft04/satobot-crawler-open | 50b8b72d20b18de45671475299e0f45000cc7055 | [
"Unlicense"
] | null | null | null | 25.555556 | 129 | 0.551553 | [
[
[
"from urllib import request # urllib.requestモジュールをインポート\nfrom bs4 import BeautifulSoup # BeautifulSoupクラスをインポート\n\nurl = \"https://news.google.com/topics/CAAqJAgKIh5DQkFTRUFvS0wyMHZNSEJyWHpkNGN4SUNhbUVvQUFQAQ?hl=ja&gl=JP&ceid=JP%3Aja\"\nresponse = request.urlopen(url)\nsoup = BeautifulSoup(response)\nresponse.close()\n#soup\n\n## soup と実行すると、ソースコードを吐き出してくれる。\n## そこから、必要な要素を考える。\nlinks = soup.find_all(\"div\",class_=\"gb_Fd gb_Wd gb_Md\")\nfor li in links:\n #print(li)\n l = li.find(\"a\")\n if l != None: #liに実態があれば\n url = l.get(\"href\")\n title = li.text.replace( '\\n' , '' )#何故か改行が入ってしまってる場合がある。\n print(title)\n print(url)",
"_____no_output_____"
]
]
] | [
"code"
] | [
[
"code"
]
] |
e77f254ac01a7fc0f97d0d8ea8a249112a53fae0 | 131,325 | ipynb | Jupyter Notebook | 8-Labs/Lab14/old_src/.ipynb_checkpoints/Lab14-checkpoint.ipynb | dustykat/engr-1330-psuedo-course | 3e7e31a32a1896fcb1fd82b573daa5248e465a36 | [
"CC0-1.0"
] | null | null | null | 8-Labs/Lab14/old_src/.ipynb_checkpoints/Lab14-checkpoint.ipynb | dustykat/engr-1330-psuedo-course | 3e7e31a32a1896fcb1fd82b573daa5248e465a36 | [
"CC0-1.0"
] | null | null | null | 8-Labs/Lab14/old_src/.ipynb_checkpoints/Lab14-checkpoint.ipynb | dustykat/engr-1330-psuedo-course | 3e7e31a32a1896fcb1fd82b573daa5248e465a36 | [
"CC0-1.0"
] | null | null | null | 48.441534 | 11,944 | 0.645391 | [
[
[
"**Download** (right-click, save target as ...) this page as a jupyterlab notebook from: [Lab14](http://54.243.252.9/engr-1330-webroot/8-Labs/Lab14/Lab14.ipynb)\n\n___",
"_____no_output_____"
],
[
"# <font color=darkgreen>Laboratory 14: Causality, Simulation, and Probability </font>\n\nLAST NAME, FIRST NAME\n\nR00000000\n\nENGR 1330 Laboratory 14 - In-Lab\n",
"_____no_output_____"
]
],
[
[
"# Preamble script block to identify host, user, and kernel\nimport sys\n! hostname\n! whoami\nprint(sys.executable)\nprint(sys.version)\nprint(sys.version_info)",
"atomickitty\nsensei\n/opt/jupyterhub/bin/python3\n3.8.10 (default, Sep 28 2021, 16:10:42) \n[GCC 9.3.0]\nsys.version_info(major=3, minor=8, micro=10, releaselevel='final', serial=0)\n"
]
],
[
[
"---\n\n# <font color=purple>Python for Simulation</font>",
"_____no_output_____"
],
[
"## What is Russian roulette?\n>Russian roulette (Russian: русская рулетка, russkaya ruletka) is a lethal game of chance in which a player places a single round in a revolver, spins the cylinder, places the muzzle against their head, and pulls the trigger in hopes that the loaded chamber does not align with the primer percussion mechanism and the barrel, causing the weapon to discharge. Russian refers to the supposed country of origin, and roulette to the element of risk-taking and the spinning of the revolver's cylinder, which is reminiscent of a spinning roulette wheel. <br>\n- Wikipedia @ https://en.wikipedia.org/wiki/Russian_roulette",
"_____no_output_____"
],
[
"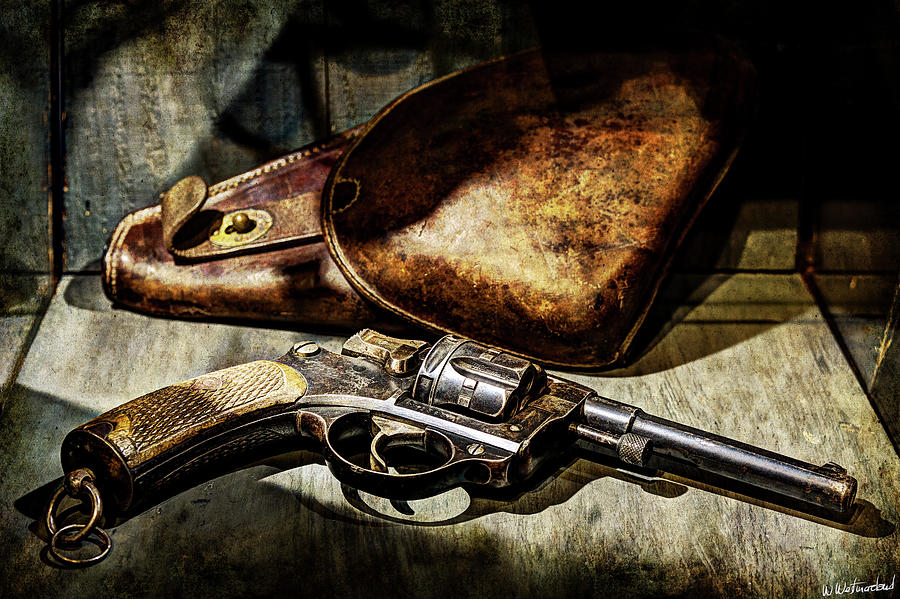",
"_____no_output_____"
],
[
">A game of dafts, a game of chance <br>\nOne where revolver's the one to dance <br>\nRounds and rounds, it goes and spins <br>\nMakes you regret all those sins <br> \\\nA game of fools, one of lethality <br>\nWith a one to six probability <br>\nThere were two guys and a gun <br>\nWith six chambers but only one... <br> \\\nCLICK, one pushed the gun <br>\nCLICK, one missed the fun <br>\nCLICK, \"that awful sound\" ... <br>\nBANG!, one had his brains all around! <br>",
"_____no_output_____"
],
[
"___\n### Example: Simulate a game of Russian Roulette:\n- For 2 rounds\n- For 5 rounds\n- For 10 rounds",
"_____no_output_____"
]
],
[
[
"import numpy as np #import numpy\nrevolver = np.array([1,0,0,0,0,0]) #create a numpy array with 1 bullet and 5 empty chambers\nprint(np.random.choice(revolver,2)) #randomly select a value from revolver - simulation",
"[0 0]\n"
],
[
"print(np.random.choice(revolver,5))",
"[0 0 1 0 0]\n"
],
[
"print(np.random.choice(revolver,10))",
"[0 0 0 1 0 0 0 0 0 0]\n"
]
],
[
[
"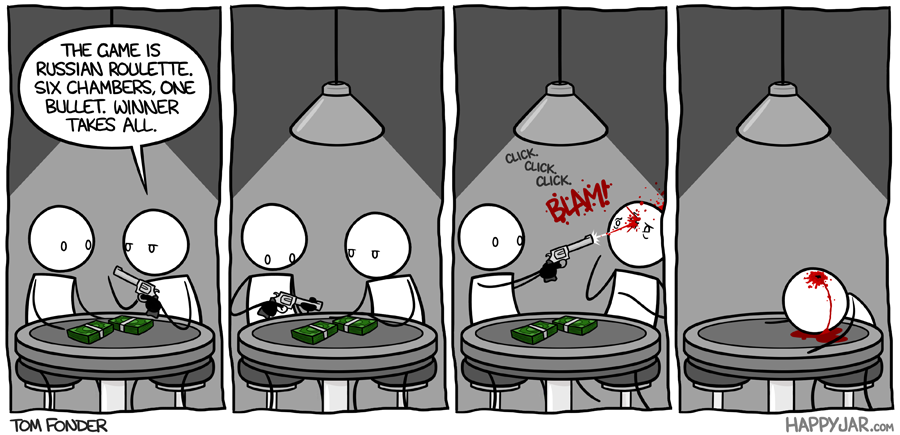",
"_____no_output_____"
],
[
"___\n### Example: Simulate the results of throwing a D6 (regular dice) for 10 times. ",
"_____no_output_____"
]
],
[
[
"import numpy as np #import numpy\ndice = np.array([1,2,3,4,5,6]) #create a numpy array with values of a D6\nnp.random.choice(dice,10) #randomly selecting a value from dice for 10 times- simulation",
"_____no_output_____"
]
],
[
[
"___\n### Example: Assume the following rules:\n\n- If the dice shows 1 or 2 spots, my net gain is -1 dollar.\n\n- If the dice shows 3 or 4 spots, my net gain is 0 dollars.\n\n- If the dice shows 5 or 6 spots, my net gain is 1 dollar.\n\n__Define a function to simulate a game with the above rules, assuming a D6, and compute the net gain of the player over any given number of rolls. <br>\nCompute the net gain for 5, 50, and 500 rolls__",
"_____no_output_____"
]
],
[
[
"def D6game(nrolls):\n import numpy as np #import numpy\n dice = np.array([1,2,3,4,5,6]) #create a numpy array with values of a D6\n rolls = np.random.choice(dice,nrolls) #randomly selecting a value from dice for nrolls times- simulation\n gainlist =[] #create an empty list for gains|losses\n for i in np.arange(len(rolls)): #Apply the rules \n if rolls[i]<=2:\n gainlist.append(-1)\n elif rolls[i]<=4:\n gainlist.append(0)\n elif rolls[i]<=6:\n gainlist.append(+1)\n return (np.sum(gainlist)) #sum up all gains|losses\n# return (gainlist,\"The net gain is equal to:\",np.sum(gainlist))\n",
"_____no_output_____"
],
[
"D6game(5)",
"_____no_output_____"
],
[
"D6game(50)",
"_____no_output_____"
],
[
"D6game(500)",
"_____no_output_____"
]
],
[
[
"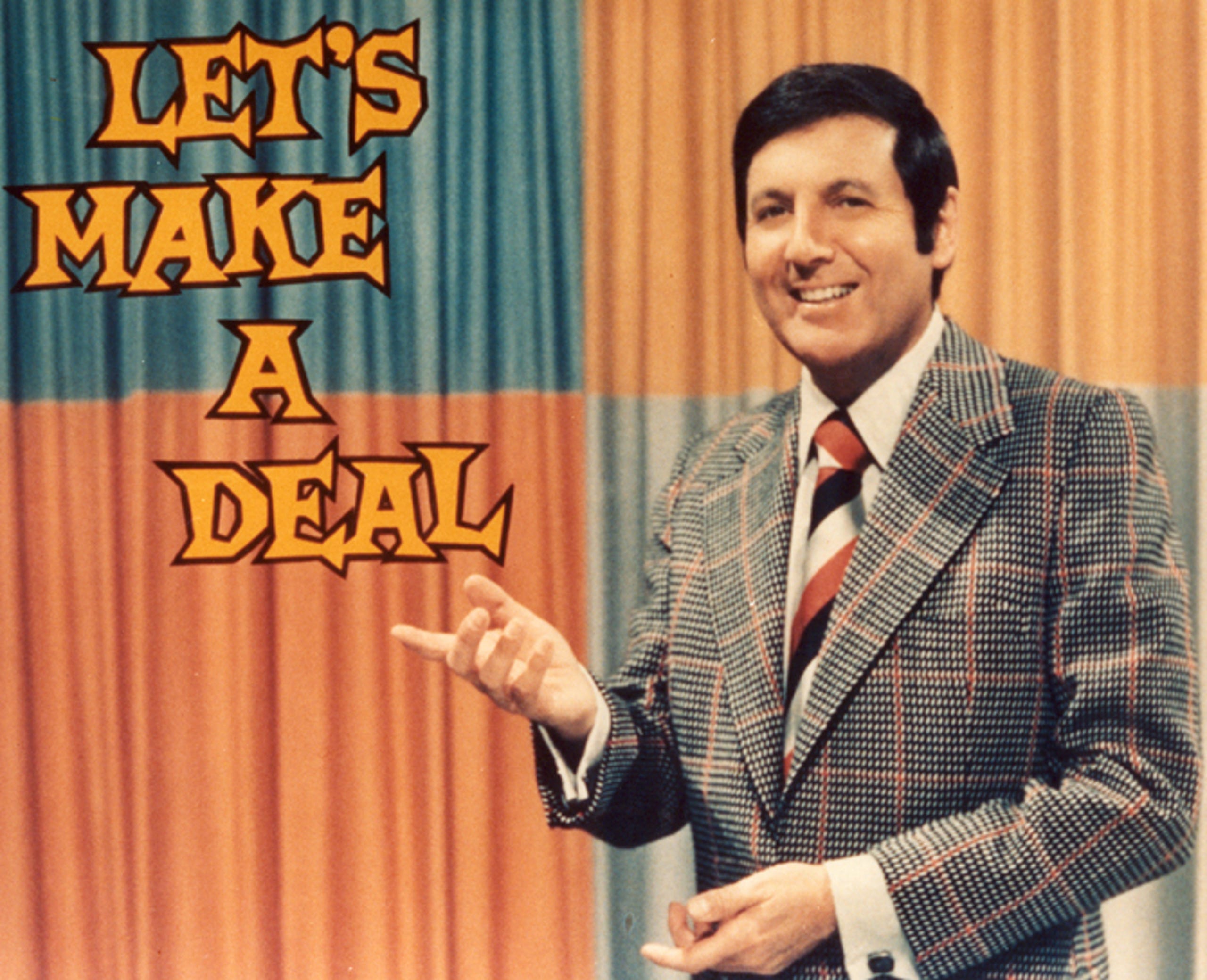",
"_____no_output_____"
],
[
"### Let's Make A Deal Game Show and Monty Hall Problem \n__The Monty Hall problem is a brain teaser, in the form of a probability puzzle, loosely based on the American television game show Let's Make a Deal and named after its original host, Monty Hall. The problem was originally posed (and solved) in a letter by Steve Selvin to the American Statistician in 1975 (Selvin 1975a), (Selvin 1975b).__\n\n>\"Suppose you're on a game show, and you're given the choice of three doors: Behind one door is a car; behind the others, goats. You pick a door, say No. 1, and the host, who knows what's behind the doors, opens another door, say No. 3, which has a goat. He then says to you, \"Do you want to pick door No. 2?\" Is it to your advantage to switch your choice?\"\n\n__*From Wikipedia: https://en.wikipedia.org/wiki/Monty_Hall_problem*__",
"_____no_output_____"
],
[
"",
"_____no_output_____"
],
[
"/data/img1.png)",
"_____no_output_____"
],
[
"",
"_____no_output_____"
],
[
"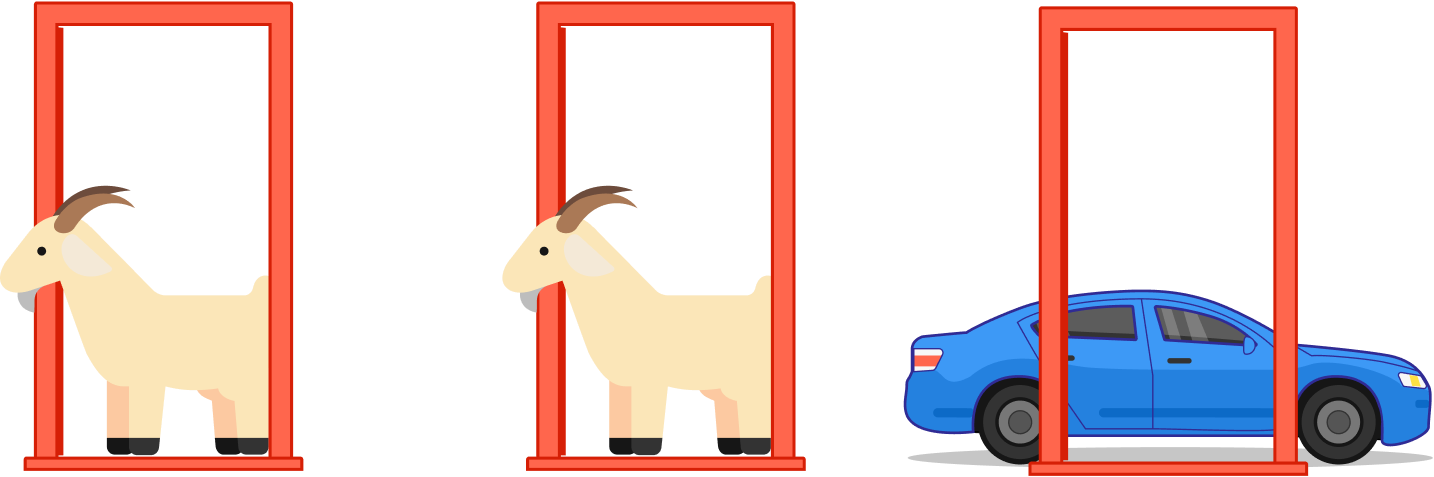",
"_____no_output_____"
],
[
"___\n### Example: Simulate Monty Hall Game for 1000 times. Use a barplot and discuss whether players are better off sticking to their initial choice, or switching doors? ",
"_____no_output_____"
]
],
[
[
"def othergoat(x): #Define a function to return \"the other goat\"!\n if x == \"Goat 1\":\n return \"Goat 2\"\n elif x == \"Goat 2\":\n return \"Goat 1\"",
"_____no_output_____"
],
[
"Doors = np.array([\"Car\",\"Goat 1\",\"Goat 2\"]) #Define a list for objects behind the doors\ngoats = np.array([\"Goat 1\" , \"Goat 2\"]) #Define a list for goats!\n\ndef MHgame():\n #Function to simulate the Monty Hall Game\n #For each guess, return [\"the guess\",\"the revealed\", \"the remaining\"]\n userguess=np.random.choice(Doors) #randomly selects a door as userguess\n if userguess == \"Goat 1\":\n return [userguess, \"Goat 2\",\"Car\"]\n if userguess == \"Goat 2\":\n return [userguess, \"Goat 1\",\"Car\"]\n if userguess == \"Car\":\n revealed = np.random.choice(goats)\n return [userguess, revealed,othergoat(revealed)]",
"_____no_output_____"
],
[
"# Check and see if the MHgame function is doing what it is supposed to do:\nfor i in np.arange(1):\n a =MHgame()\n print(a)\n print(a[0])\n print(a[1])\n print(a[2])",
"['Goat 2', 'Goat 1', 'Car']\nGoat 2\nGoat 1\nCar\n"
],
[
"c1 = [] #Create an empty list for the userguess\nc2 = [] #Create an empty list for the revealed\nc3 = [] #Create an empty list for the remaining\nfor i in np.arange(1000): #Simulate the game for 1000 rounds - or any other number of rounds you desire\n game = MHgame()\n c1.append(game[0]) #In each round, add the first element to the userguess list\n c2.append(game[1]) #In each round, add the second element to the revealed list\n c3.append(game[2]) #In each round, add the third element to the remaining list\n",
"_____no_output_____"
],
[
"import pandas as pd\n#Create a data frame (gamedf) with 3 columns (\"Guess\",\"Revealed\", \"Remaining\") and 1000 (or how many number of rounds) rows\ngamedf = pd.DataFrame({'Guess':c1,\n 'Revealed':c2,\n 'Remaining':c3})\ngamedf",
"_____no_output_____"
],
[
"# Get the count of each item in the first and 3rd column\noriginal_car =gamedf[gamedf.Guess == 'Car'].shape[0]\nremaining_car =gamedf[gamedf.Remaining == 'Car'].shape[0]\n\noriginal_g1 =gamedf[gamedf.Guess == 'Goat 1'].shape[0]\nremaining_g1 =gamedf[gamedf.Remaining == 'Goat 1'].shape[0]\n\noriginal_g2 =gamedf[gamedf.Guess == 'Goat 2'].shape[0]\nremaining_g2 =gamedf[gamedf.Remaining == 'Goat 2'].shape[0]",
"_____no_output_____"
],
[
"# Let's plot a grouped barplot\nimport matplotlib.pyplot as plt \n\n# set width of bar\nbarWidth = 0.25\n \n# set height of bar\nbars1 = [original_car,original_g1,original_g2]\nbars2 = [remaining_car,remaining_g1,remaining_g2]\n \n# Set position of bar on X axis\nr1 = np.arange(len(bars1))\nr2 = [x + barWidth for x in r1]\n \n# Make the plot\nplt.bar(r1, bars1, color='darkorange', width=barWidth, edgecolor='white', label='Original Guess')\nplt.bar(r2, bars2, color='midnightblue', width=barWidth, edgecolor='white', label='Remaining Door')\n \n# Add xticks on the middle of the group bars\nplt.xlabel('Item', fontweight='bold')\nplt.xticks([r + barWidth/2 for r in range(len(bars1))], ['Car', 'Goat 1', 'Goat 2'])\n \n# Create legend & Show graphic\nplt.legend()\nplt.show()\n",
"_____no_output_____"
]
],
[
[
"<font color=crimson>__According to the plot, it is statitically beneficial for the players to switch doors because the initial chance for being correct is only 1/3__</font>",
"_____no_output_____"
],
[
"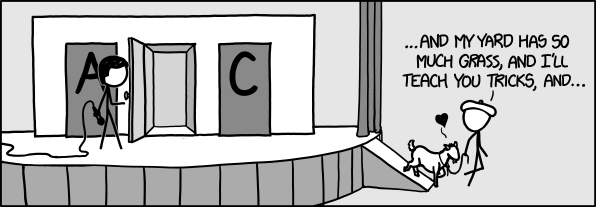",
"_____no_output_____"
],
[
"# <font color=purple>Python for Probability</font>",
"_____no_output_____"
],
[
"",
"_____no_output_____"
],
[
"### <font color=purple>Important Terminology:</font>\n \n__Experiment:__ An occurrence with an uncertain outcome that we can observe. <br>\n*For example, rolling a die.*<br>\n__Outcome:__ The result of an experiment; one particular state of the world. What Laplace calls a \"case.\"<br>\n*For example: 4.*<br>\n__Sample Space:__ The set of all possible outcomes for the experiment.<br>\n*For example, {1, 2, 3, 4, 5, 6}.*<br>\n__Event:__ A subset of possible outcomes that together have some property we are interested in.<br>\n*For example, the event \"even die roll\" is the set of outcomes {2, 4, 6}.*<br>\n__Probability:__ As Laplace said, the probability of an event with respect to a sample space is the number of favorable cases (outcomes from the sample space that are in the event) divided by the total number of cases in the sample space. (This assumes that all outcomes in the sample space are equally likely.) Since it is a ratio, probability will always be a number between 0 (representing an impossible event) and 1 (representing a certain event).<br>\n*For example, the probability of an even die roll is 3/6 = 1/2.*<br>\n\n__*From https://people.math.ethz.ch/~jteichma/probability.html*__",
"_____no_output_____"
]
],
[
[
"import numpy as np\nimport pandas as pd\nimport matplotlib.pyplot as plt ",
"_____no_output_____"
]
],
[
[
"___\n### Example: In a game of Russian Roulette, the chance of surviving each round is 5/6 which is almost 83%. Using a for loop, compute probability of surviving \n- For 2 rounds\n- For 5 rounds\n- For 10 rounds",
"_____no_output_____"
]
],
[
[
"nrounds =[]\nprobs =[]\n\nfor i in range(3):\n nrounds.append(i)\n probs.append((5/6)**i) #probability of surviving- not getting the bullet!\n\nRRDF = pd.DataFrame({\"# of Rounds\": nrounds, \"Probability of Surviving\": probs})\nRRDF",
"_____no_output_____"
],
[
"nrounds =[]\nprobs =[]\n\nfor i in range(6):\n nrounds.append(i)\n probs.append((5/6)**i) #probability of surviving- not getting the bullet!\n\nRRDF = pd.DataFrame({\"# of Rounds\": nrounds, \"Probability of Surviving\": probs})\nRRDF",
"_____no_output_____"
],
[
"nrounds =[]\nprobs =[]\n\nfor i in range(11):\n nrounds.append(i)\n probs.append((5/6)**i) #probability of surviving- not getting the bullet!\n\nRRDF = pd.DataFrame({\"# of Rounds\": nrounds, \"Probability of Surviving\": probs})\nRRDF",
"_____no_output_____"
],
[
"RRDF.plot.scatter(x=\"# of Rounds\", y=\"Probability of Surviving\",color=\"red\")",
"_____no_output_____"
]
],
[
[
"___\n### Example: What will be the probability of constantly throwing an even number with a D20 in\n- For 2 rolls\n- For 5 rolls\n- For 10 rolls\n- For 15 rolls",
"_____no_output_____"
]
],
[
[
"nrolls =[]\nprobs =[]\n\nfor i in range(1,16,1):\n nrolls.append(i)\n probs.append((1/2)**i) #probability of throwing an even number-10/20 or 1/2\n\nDRDF = pd.DataFrame({\"# of Rolls\": nrolls, \"Probability of constantly throwing an even number\": probs})\nDRDF",
"_____no_output_____"
],
[
"DRDF.plot.scatter(x=\"# of Rolls\", y=\"Probability of constantly throwing an even number\",color=\"crimson\")",
"_____no_output_____"
]
],
[
[
"___\n### Example: What will be the probability of throwing at least one 6 with a D6:\n- For 2 rolls\n- For 5 rolls\n- For 10 rolls\n- For 50 rolls - Make a scatter plot for this one!",
"_____no_output_____"
]
],
[
[
"nRolls =[]\nprobs =[]\n\nfor i in range(1,3,1):\n nRolls.append(i)\n probs.append(1-(5/6)**i) #probability of at least one 6: 1-(5/6)\n\nrollsDF = pd.DataFrame({\"# of Rolls\": nRolls, \"Probability of rolling at least one 6\": probs})\nrollsDF",
"_____no_output_____"
],
[
"nRolls =[]\nprobs =[]\n\nfor i in range(1,6,1):\n nRolls.append(i)\n probs.append(1-(5/6)**i) #probability of at least one 6: 1-(5/6)\n\nrollsDF = pd.DataFrame({\"# of Rolls\": nRolls, \"Probability of rolling at least one 6\": probs})\nrollsDF",
"_____no_output_____"
],
[
"nRolls =[]\nprobs =[]\n\nfor i in range(1,11,1):\n nRolls.append(i)\n probs.append(1-(5/6)**i) #probability of at least one 6: 1-(5/6)\n\nrollsDF = pd.DataFrame({\"# of Rolls\": nRolls, \"Probability of rolling at least one 6\": probs})\nrollsDF",
"_____no_output_____"
],
[
"nRolls =[]\nprobs =[]\n\nfor i in range(1,51,1):\n nRolls.append(i)\n probs.append(1-(5/6)**i) #probability of at least one 6: 1-(5/6)\n\nrollsDF = pd.DataFrame({\"# of Rolls\": nRolls, \"Probability of rolling at least one 6\": probs})",
"_____no_output_____"
],
[
"rollsDF.plot.scatter(x=\"# of Rolls\", y=\"Probability of rolling at least one 6\")",
"_____no_output_____"
]
],
[
[
"___\n### Example: What is the probability of drawing an ace at least once (with replacement):\n- in 2 tries\n- in 5 tries\n- in 10 tries\n- in 20 tries - make a scatter plot.\n",
"_____no_output_____"
]
],
[
[
"nDraws =[]\nprobs =[]\n\nfor i in range(1,3,1):\n nDraws.append(i)\n probs.append(1-(48/52)**i) #probability of drawing an ace least once : 1-(48/52)\n\nDrawsDF = pd.DataFrame({\"# of Draws\": nDraws, \"Probability of drawing an ace at least once\": probs})\nDrawsDF",
"_____no_output_____"
],
[
"nDraws =[]\nprobs =[]\n\nfor i in range(1,6,1):\n nDraws.append(i)\n probs.append(1-(48/52)**i) #probability of drawing an ace least once : 1-(48/52)\n\nDrawsDF = pd.DataFrame({\"# of Draws\": nDraws, \"Probability of drawing an ace at least once\": probs})\nDrawsDF",
"_____no_output_____"
],
[
"nDraws =[]\nprobs =[]\n\nfor i in range(1,11,1):\n nDraws.append(i)\n probs.append(1-(48/52)**i) #probability of drawing an ace least once : 1-(48/52)\n\nDrawsDF = pd.DataFrame({\"# of Draws\": nDraws, \"Probability of drawing an ace at least once\": probs})\nDrawsDF",
"_____no_output_____"
],
[
"nDraws =[]\nprobs =[]\n\nfor i in range(1,21,1):\n nDraws.append(i)\n probs.append(1-(48/52)**i) #probability of drawing an ace at least once : 1-(48/52)\n\nDrawsDF = pd.DataFrame({\"# of Draws\": nDraws, \"Probability of drawing an ace at least once\": probs})\nDrawsDF",
"_____no_output_____"
],
[
"DrawsDF.plot.scatter(x=\"# of Draws\", y=\"Probability of drawing an ace at least once\")",
"_____no_output_____"
]
],
[
[
"___\n### Example: \n- A) Write a function to find the probability of an event in percentage form based on given outcomes and sample space\n- B) Use the function and compute the probability of rolling a 4 with a D6\n- C) Use the function and compute the probability of drawing a King from a standard deck of cards\n- D) Use the function and compute the probability of drawing the King of Hearts from a standard deck of cards\n- E) Use the function and compute the probability of drawing an ace after drawing a king\n- F) Use the function and compute the probability of drawing an ace after drawing an ace\n- G) Use the function and compute the probability of drawing a heart OR a club\n- F) Use the function and compute the probability of drawing a Royal Flush <br>\n*hint: (in poker) a straight flush including ace, king, queen, jack, and ten all in the same suit, which is the hand of the highest possible value\n\n__This problem is designed based on an example by *Daniel Poston* from DataCamp, accessible @ *https://www.datacamp.com/community/tutorials/statistics-python-tutorial-probability-1*__",
"_____no_output_____"
]
],
[
[
"# A\n# Create function that returns probability percent rounded to one decimal place\ndef Prob(outcome, sampspace):\n probability = (outcome / sampspace) * 100\n return round(probability, 1)",
"_____no_output_____"
],
[
"# B\noutcome = 1 #Rolling a 4 is only one of the possible outcomes\nspace = 6 #Rolling a D6 can have 6 different outcomes\nProb(outcome, space)",
"_____no_output_____"
],
[
"# C\noutcome = 4 #Drawing a king is four of the possible outcomes\nspace = 52 #Drawing from a standard deck of cards can have 52 different outcomes\nProb(outcome, space)",
"_____no_output_____"
],
[
"# D\noutcome = 1 #Drawing the king of hearts is only 1 of the possible outcomes\nspace = 52 #Drawing from a standard deck of cards can have 52 different outcomes\nProb(outcome, space)",
"_____no_output_____"
],
[
"# E\noutcome = 4 #Drawing an ace is 4 of the possible outcomes\nspace = 51 #One card has been drawn\nProb(outcome, space)",
"_____no_output_____"
],
[
"# F\noutcome = 3 #Once Ace is already drawn\nspace = 51 #One card has been drawn\nProb(outcome, space)",
"_____no_output_____"
],
[
"# G\nhearts = 13 #13 cards of hearts in a deck\nspace = 52 #total number of cards in a deck\nclubs = 13 #13 cards of clubs in a deck\nProb_heartsORclubs= Prob(hearts, space) + Prob(clubs, space)\nprint(\"Probability of drawing a heart or a club is\",Prob_heartsORclubs,\"%\")",
"Probability of drawing a heart or a club is 50.0 %\n"
],
[
"# F\ndraw1 = 5 #5 cards are needed\nspace1 = 52 #out of the possible 52 cards\ndraw2 = 4 #4 cards are needed\nspace2 = 51 #out of the possible 51 cards\ndraw3 = 3 #3 cards are needed\nspace3 = 50 #out of the possible 50 cards\ndraw4 = 2 #2 cards are needed\nspace4 = 49 #out of the possible 49 cards\ndraw5 = 1 #1 cards is needed\nspace5 = 48 #out of the possible 48 cards\n\n#Probability of a getting a Royal Flush\nProb_RF= 4*(Prob(draw1, space1)/100) * (Prob(draw2, space2)/100) * (Prob(draw3, space3)/100) * (Prob(draw4, space4)/100) * (Prob(draw5, space5)/100) \nprint(\"Probability of drawing a royal flush is\",Prob_RF,\"%\")",
"Probability of drawing a royal flush is 1.5473203199999998e-06 %\n"
]
],
[
[
"___\n### Example: Two unbiased dice are thrown once and the total score is observed. Define an appropriate function and use a simulation to find the estimated probability that :\n- the total score is greater than 10?\n- the total score is even and greater than 7?\n\n\n__This problem is designed based on an example by *Elliott Saslow*\nfrom Medium.com, accessible @ *https://medium.com/future-vision/simulating-probability-events-in-python-5dd29e34e381*__",
"_____no_output_____"
]
],
[
[
"import numpy as np\ndef DiceRoll1(nSimulation):\n count =0\n dice = np.array([1,2,3,4,5,6]) #create a numpy array with values of a D6\n for i in range(nSimulation):\n die1 = np.random.choice(dice,1) #randomly selecting a value from dice - throw the D6 once\n die2 = np.random.choice(dice,1) #randomly selecting a value from dice - throw the D6 once again!\n score = die1 + die2 #summing them up\n if score > 10: #if it meets our desired condition:\n count +=1 #add one to the \"count\"\n return count/nSimulation #compute the probability of the desired event by dividing count by the total number of trials\n\nnSimulation = 10000\nprint(\"The probability of rolling a number greater than 10 after\",nSimulation,\"rolld is:\",DiceRoll1(nSimulation)*100,\"%\")\n",
"The probability of rolling a number greater than 10 after 10000 rolld is: 8.35 %\n"
],
[
"import numpy as np\ndef DiceRoll2(nSimulation):\n count =0\n dice = np.array([1,2,3,4,5,6]) #create a numpy array with values of a D6\n for i in range(nSimulation):\n die1 = np.random.choice(dice,1) #randomly selecting a value from dice - throw the D6 once\n die2 = np.random.choice(dice,1) #randomly selecting a value from dice - throw the D6 once again!\n score = die1 + die2\n if score %2 ==0 and score > 7: #the total score is even and greater than 7\n count +=1\n return count/nSimulation\n\nnSimulation = 10000\nprint(\"The probability of rolling an even number and greater than 7 after\",nSimulation,\" rolls is:\",DiceRoll2(nSimulation)*100,\"%\")",
"The probability of rolling an even number and greater than 7 after 10000 rolls is: 24.77 %\n"
]
],
[
[
"___\n### Example: An urn contains 10 white balls, 20 reds and 30 greens. We want to draw 5 balls with replacement. Use a simulation (10000 trials) to find the estimated probability that:\n- we draw 3 white and 2 red balls\n- we draw 5 balls of the same color\n\n\n__This problem is designed based on an example by *Elliott Saslow*\nfrom Medium.com, accessible @ *https://medium.com/future-vision/simulating-probability-events-in-python-5dd29e34e381*__",
"_____no_output_____"
]
],
[
[
"# A\nimport numpy as np\nimport random\nd = {} #Create an empty dictionary to associate numbers and colors\nfor i in range(0,60,1): #total of 60 balls\n if i <10: #10 white balls\n d[i]=\"White\"\n elif i>9 and i<30: #20 red balls\n d[i]=\"Red\"\n else: #60-30=30 green balls\n d[i]=\"Green\"\n#\nnSimulation= 10000 #How many trials?\noutcome1= 0 #initial value on the desired outcome counter\n\nfor i in range(nSimulation):\n draw=[] #an empty list for the draws\n for i in range(5): #how many balls we want to draw?\n draw.append(d[random.randint(0,59)]) #randomly choose a number from 0 to 59- simulation of drawing balls\n drawarray = np.array(draw) #convert the list into a numpy array\n white = sum(drawarray== \"White\") #count the white balls\n red = sum(drawarray== \"Red\") #count the red balls\n green = sum(drawarray== \"Green\") #count the green balls\n if white ==3 and red==2: #If the desired condition is met, add one to the counter\n outcome1 +=1\nprint(\"The probability of drawing 3 white and 2 red balls is\",(outcome1/nSimulation)*100,\"%\")",
"The probability of drawing 3 white and 2 red balls is 0.54 %\n"
],
[
"# B\nimport numpy as np\nimport random\nd = {}\nfor i in range(0,60,1):\n if i <10:\n d[i]=\"White\"\n elif i>9 and i<30:\n d[i]=\"Red\"\n else:\n d[i]=\"Green\"\n#\nnSimulation= 10000\noutcome1= 0\noutcome2= 0 #we can consider multiple desired outcomes\n\n\nfor i in range(nSimulation):\n draw=[]\n for i in range(5):\n draw.append(d[random.randint(0,59)])\n drawarray = np.array(draw)\n white = sum(drawarray== \"White\")\n red = sum(drawarray== \"Red\")\n green = sum(drawarray== \"Green\")\n if white ==3 and red==2:\n outcome1 +=1\n if white ==5 or red==5 or green==5:\n outcome2 +=1\n\nprint(\"The probability of drawing 3 white and 2 red balls is\",(outcome1/nSimulation)*100,\"%\")\nprint(\"The probability of drawing 5 balls of the same color is\",(outcome2/nSimulation)*100,\"%\")\n",
"The probability of drawing 3 white and 2 red balls is 0.53 %\nThe probability of drawing 5 balls of the same color is 3.8 %\n"
]
],
[
[
"___\n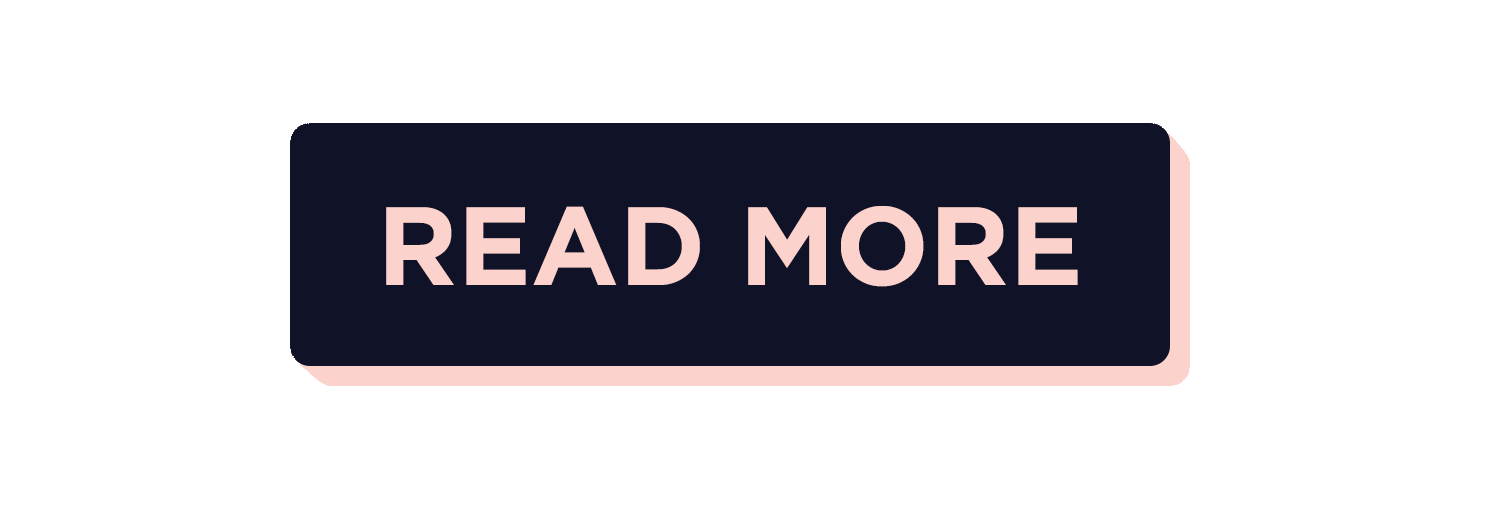 <br>\n\n*Here are some of the resources used for creating this notebook:* \n\n\n- __\"Poker Probability and Statistics with Python\"__ by __Daniel Poston__ available at *https://www.datacamp.com/community/tutorials/statistics-python-tutorial-probability-1*<br>\n- __\"Simulating probability events in Python\"__ by __Elliott Saslow__ available at *https://medium.com/future-vision/simulating-probability-events-in-python-5dd29e34e381*<br>\n\n\n*Here are some great reads on this topic:* \n- __\"Simulate the Monty Hall Problem Using Python\"__ by __randerson112358__ available at *https://medium.com/swlh/simulate-the-monty-hall-problem-using-python-7b76b943640e* <br>\n- __\"The Monty Hall problem\"__ available at *https://scipython.com/book/chapter-4-the-core-python-language-ii/examples/the-monty-hall-problem/*<br>\n- __\"Introduction to Probability Using Python\"__ by __Lisandra Melo__ available at *https://medium.com/future-vision/simulating-probability-events-in-python-5dd29e34e381* <br>\n- __\"Introduction to probability and statistics for Data Scientists and machine learning using python : Part-1\"__ by __Arun Singh__ available at *https://medium.com/@anayan/introduction-to-probability-and-statistics-for-data-scientists-and-machine-learning-using-python-377a9b082487*<br>\n\n*Here are some great videos on these topics:* \n- __\"Monty Hall Problem - Numberphile\"__ by __Numberphile__ available at *https://www.youtube.com/watch?v=4Lb-6rxZxx0* <br>\n- __\"The Monty Hall Problem\"__ by __D!NG__ available at *https://www.youtube.com/watch?v=TVq2ivVpZgQ* <br>\n- __\"21 - Monty Hall - PROPENSITY BASED THEORETICAL MODEL PROBABILITY - MATHEMATICS in the MOVIES\"__ by __Motivating Mathematical Education and STEM__ available at *https://www.youtube.com/watch?v=iBdjqtR2iK4* <br>\n- __\"The Monty Hall Problem\"__ by __niansenx__ available at *https://www.youtube.com/watch?v=mhlc7peGlGg* <br>\n- __\"The Monty Hall Problem - Explained\"__ by __AsapSCIENCE__ available at *https://www.youtube.com/watch?v=9vRUxbzJZ9Y* <br>\n- __\"Introduction to Probability | 365 Data Science Online Course\"__ by __365 Data Science__ available at *https://www.youtube.com/watch?v=soZRfdnkUQg* <br>\n- __\"Probability explained | Independent and dependent events | Probability and Statistics | Khan Academy\"__ by __Khan Academy__ available at *https://www.youtube.com/watch?v=uzkc-qNVoOk* <br>\n- __\"Math Antics - Basic Probability\"__ by __mathantics__ available at *https://www.youtube.com/watch?v=KzfWUEJjG18* <br>",
"_____no_output_____"
],
[
"___\n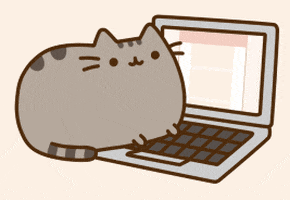 <br>\n",
"_____no_output_____"
],
[
"## Exercise: Risk or Probability <br>\n\n### Are they the same? Are they different? Discuss your opinion. \n\n#### _Make sure to cite any resources that you may use._ ",
"_____no_output_____"
],
[
"",
"_____no_output_____"
]
]
] | [
"markdown",
"code",
"markdown",
"code",
"markdown",
"code",
"markdown",
"code",
"markdown",
"code",
"markdown",
"code",
"markdown",
"code",
"markdown",
"code",
"markdown",
"code",
"markdown",
"code",
"markdown",
"code",
"markdown",
"code",
"markdown",
"code",
"markdown"
] | [
[
"markdown",
"markdown"
],
[
"code"
],
[
"markdown",
"markdown",
"markdown",
"markdown",
"markdown"
],
[
"code",
"code",
"code"
],
[
"markdown",
"markdown"
],
[
"code"
],
[
"markdown"
],
[
"code",
"code",
"code",
"code"
],
[
"markdown",
"markdown",
"markdown",
"markdown",
"markdown",
"markdown",
"markdown"
],
[
"code",
"code",
"code",
"code",
"code",
"code",
"code"
],
[
"markdown",
"markdown",
"markdown",
"markdown",
"markdown"
],
[
"code"
],
[
"markdown"
],
[
"code",
"code",
"code",
"code"
],
[
"markdown"
],
[
"code",
"code"
],
[
"markdown"
],
[
"code",
"code",
"code",
"code",
"code"
],
[
"markdown"
],
[
"code",
"code",
"code",
"code",
"code"
],
[
"markdown"
],
[
"code",
"code",
"code",
"code",
"code",
"code",
"code",
"code"
],
[
"markdown"
],
[
"code",
"code"
],
[
"markdown"
],
[
"code",
"code"
],
[
"markdown",
"markdown",
"markdown",
"markdown"
]
] |
e77f427cf839e580a7c1108e21ede5f5fe8f79a5 | 3,290 | ipynb | Jupyter Notebook | NOMIS API Test.ipynb | FoxyAI/urb-studies-predicting-gentrification | a23cab40d0a72ab44e5f0fa24355b3a3795aa69c | [
"MIT"
] | 1 | 2019-08-19T20:19:19.000Z | 2019-08-19T20:19:19.000Z | NOMIS API Test.ipynb | FoxyAI/urb-studies-predicting-gentrification | a23cab40d0a72ab44e5f0fa24355b3a3795aa69c | [
"MIT"
] | null | null | null | NOMIS API Test.ipynb | FoxyAI/urb-studies-predicting-gentrification | a23cab40d0a72ab44e5f0fa24355b3a3795aa69c | [
"MIT"
] | null | null | null | 43.289474 | 449 | 0.652888 | [
[
[
"# NOMIS API Development\n\nShortly after this paper was accepted, I received a very helpful reply from NOMIS relating to the fact that I couldn't seem to get their API helper to give me the query needed to retrieve each of the data sets used in this research directly (which would make replication much, much faster and easier). I've not had time to refactor the code to incorporate their guidance but here is an overview of the process that will need to be followed:\n\n* Select your dataset\n* Select only the region in your query and not the LSOAs - so in the case of your query, choose the \"London\" region as an individual selection (not using \"areas within\").\n* Continue to select other options to build up your query as normal\n* Select the \"Nomis API\" download format and you should get the page with the API links (ignore the first tab and go to the \"Complete list of API links\" page to get the URL)\n* When you get the URL, modify it as in the example below\n\nExample of modifying the URL is below, the change involves simply telling the API you want all LSOAs in the area you have selected, you do this by adding the text \"TYPE304\" (which is the code for 2001 LSOAs) to the geography code. The code for 2011 LSOAs is TYPE298.\n\nOriginal URL for the query London region:\nhttp://www.nomisweb.co.uk/api/v01/dataset/NM_1673_1.bulk.csv?date=latest&geography=2013265927&dwelling_type=0,3...5,7...9&measures=20100\n\nModified URL for the query selecting all LSOAs in London region:\nhttp://www.nomisweb.co.uk/api/v01/dataset/NM_1673_1.bulk.csv?date=latest&geography=2013265927TYPE304&dwelling_type=0,3...5,7...9&measures=20100\n",
"_____no_output_____"
]
],
[
[
"# 2011 TTW Data (need a different geography TYPE304 for 2001)\nurl = ('http://www.nomisweb.co.uk/api/v01/dataset/',\n 'NM_568_1.bulk.csv?',\n 'date=latest&',\n 'geography=2013265927TYPE298&',\n 'rural_urban=0&',\n 'cell=0...12&',\n 'measures=20100&',\n 'select=date_name,geography_name,geography_code,rural_urban_name,cell_name,measures_name,obs_value,obs_status_name')\n\nprint(\"\".join(url))",
"http://www.nomisweb.co.uk/api/v01/dataset/NM_568_1.bulk.csv?date=latest&geography=2013265927TYPE304&rural_urban=0&cell=0...12&measures=20100&select=date_name,geography_name,geography_code,rural_urban_name,cell_name,measures_name,obs_value,obs_status_name\n"
]
]
] | [
"markdown",
"code"
] | [
[
"markdown"
],
[
"code"
]
] |
e77f4b1a2e84c548a53b1a464291f6afbc2b7df0 | 238,810 | ipynb | Jupyter Notebook | Chapter24/nbs/01_paper-vs-zero_goal.ipynb | bezineb5/Deep-Reinforcement-Learning-Hands-On-Second-Edition | 3ebbd9cab1e936a05a1e8c5b384d552e6819e7a9 | [
"MIT"
] | 621 | 2019-07-27T19:24:56.000Z | 2022-03-31T14:19:52.000Z | Chapter24/nbs/01_paper-vs-zero_goal.ipynb | bezineb5/Deep-Reinforcement-Learning-Hands-On-Second-Edition | 3ebbd9cab1e936a05a1e8c5b384d552e6819e7a9 | [
"MIT"
] | 40 | 2019-09-01T09:45:22.000Z | 2022-03-24T13:13:00.000Z | Chapter24/nbs/01_paper-vs-zero_goal.ipynb | bezineb5/Deep-Reinforcement-Learning-Hands-On-Second-Edition | 3ebbd9cab1e936a05a1e8c5b384d552e6819e7a9 | [
"MIT"
] | 346 | 2019-07-26T15:16:56.000Z | 2022-03-30T15:33:20.000Z | 191.815261 | 36,724 | 0.848964 | [
[
[
"import pandas as pd\nimport matplotlib.pylab as plt\nimport seaborn as sns\nsns.set()\n%pylab inline",
"Populating the interactive namespace from numpy and matplotlib\n"
],
[
"c2_paper_df = pd.read_csv(\"../csvs/c2x2-paper-d200-t1.csv\")\nc2_zg_df = pd.read_csv(\"../csvs/c2x2-zero-goal-d200-t1.csv\")",
"_____no_output_____"
],
[
"c2_paper_df.head()",
"_____no_output_____"
],
[
"sns.lineplot('depth', 'duration', data=c2_paper_df);\nsns.lineplot('depth', 'duration', data=c2_zg_df);",
"_____no_output_____"
],
[
"sns.lineplot('depth', 'is_solved', data=c2_paper_df);\nsns.lineplot('depth', 'is_solved', data=c2_zg_df);",
"_____no_output_____"
],
[
"sns.lineplot('depth', 'solve_steps', data=c2_paper_df);\nsns.lineplot('depth', 'solve_steps', data=c2_zg_df);",
"_____no_output_____"
],
[
"sns.lineplot('depth', 'solve_steps', data=c2_paper_df[c2_paper_df.is_solved == 1]);\nsns.lineplot('depth', 'solve_steps', data=c2_zg_df[c2_zg_df.is_solved == 1]);",
"_____no_output_____"
],
[
"sns.lineplot('depth', 'solve_steps', data=c2_paper_df[c2_paper_df.is_solved == 0]);\nsns.lineplot('depth', 'solve_steps', data=c2_zg_df[c2_zg_df.is_solved == 0]);",
"_____no_output_____"
],
[
"sns.lineplot('depth', 'solve_steps', data=c2_zg_df[c2_zg_df.is_solved == 1]);",
"/home/shmuma/anaconda3/envs/art_01_cube/lib/python3.6/site-packages/scipy/stats/stats.py:1713: FutureWarning: Using a non-tuple sequence for multidimensional indexing is deprecated; use `arr[tuple(seq)]` instead of `arr[seq]`. In the future this will be interpreted as an array index, `arr[np.array(seq)]`, which will result either in an error or a different result.\n return np.add.reduce(sorted[indexer] * weights, axis=axis) / sumval\n"
],
[
"c2_zg_df[c2_zg_df.is_solved == 0]",
"_____no_output_____"
]
],
[
[
"Error in steps limit, rerun tests",
"_____no_output_____"
]
]
] | [
"code",
"markdown"
] | [
[
"code",
"code",
"code",
"code",
"code",
"code",
"code",
"code",
"code",
"code"
],
[
"markdown"
]
] |
e77f64ccdc7b7f318c05b2174ede202f1aaaed81 | 24,865 | ipynb | Jupyter Notebook | solutions by participants/ex1/ex1-InnanNouhaila-79cost.ipynb | fazliberkordek/ibm-quantum-challenge-2021 | 2206a364e354965b749dcda7c5d62631f571d718 | [
"Apache-2.0"
] | 136 | 2021-05-20T14:07:53.000Z | 2022-03-19T17:19:31.000Z | solutions by participants/ex1/ex1-InnanNouhaila-79cost.ipynb | fazliberkordek/ibm-quantum-challenge-2021 | 2206a364e354965b749dcda7c5d62631f571d718 | [
"Apache-2.0"
] | 106 | 2021-05-21T15:41:13.000Z | 2021-11-08T08:29:25.000Z | solutions by participants/ex1/ex1-InnanNouhaila-79cost.ipynb | fazliberkordek/ibm-quantum-challenge-2021 | 2206a364e354965b749dcda7c5d62631f571d718 | [
"Apache-2.0"
] | 190 | 2021-05-20T14:02:09.000Z | 2022-03-27T16:31:20.000Z | 65.60686 | 13,848 | 0.790227 | [
[
[
"# Exercise 1 - Toffoli gate\n",
"_____no_output_____"
]
],
[
[
"from qiskit import QuantumRegister, ClassicalRegister, QuantumCircuit\nfrom qiskit import IBMQ, Aer, execute\nfrom ibm_quantum_widgets import CircuitComposer\neditorEx = CircuitComposer() \neditorEx\nimport math\npi=math.pi",
"_____no_output_____"
],
[
"# You can also build your circuit programmatically using Qiskit code\ncircuit = QuantumCircuit(3)\ntheta = pi/4 # Theta can be anything (pi chosen arbitrarily)\n# WRITE YOUR CODE BETWEEN THESE LINES - START\n\n### Hadamard ###\ncircuit.rz(pi/2, 2)\ncircuit.sx(2)\ncircuit.rz(pi/2, 2)\n\ncircuit.cx(1,2)\n\ncircuit.rz(-theta, 2)\n\ncircuit.cx(0,2)\n\ncircuit.rz(theta, 2)\n\ncircuit.cx(1,2)\n\ncircuit.rz(-theta, 2)\n\ncircuit.cx(0,2)\n\ncircuit.rz(theta, 1)\ncircuit.rz(theta, 2)\n\n### Hadamard ###\ncircuit.rz(pi/2, 2)\ncircuit.sx(2)\ncircuit.rz(pi/2, 2)\n\ncircuit.cnot(0,1)\ncircuit.rz(theta, 0)\ncircuit.rz(-theta, 1)\ncircuit.cnot(0,1)\n\n# WRITE YOUR CODE BETWEEN THESE LINES - END",
"_____no_output_____"
],
[
"# Checking the resulting circuit\nqc = editorEx.circuit \nqc = circuit # Uncomment this line if you want to submit the circuit built using Qiskit code\n\nqc.draw(output='mpl')",
"_____no_output_____"
]
],
[
[
"## By Innan Nouhaila",
"_____no_output_____"
]
]
] | [
"markdown",
"code",
"markdown"
] | [
[
"markdown"
],
[
"code",
"code",
"code"
],
[
"markdown"
]
] |
e77f66982f3e073fbdb3f506d5092d5d997bb8d1 | 11,327 | ipynb | Jupyter Notebook | notebooks/.ipynb_checkpoints/Baseline Experiments-checkpoint.ipynb | stahl085/bracketology | 3311241fa82eb41a5529ac5e126171850f715032 | [
"MIT"
] | 2 | 2020-02-26T07:02:04.000Z | 2020-03-09T19:21:37.000Z | notebooks/.ipynb_checkpoints/Baseline Experiments-checkpoint.ipynb | stahl085/bracketology | 3311241fa82eb41a5529ac5e126171850f715032 | [
"MIT"
] | 1 | 2020-03-09T02:39:25.000Z | 2021-03-16T02:56:58.000Z | notebooks/.ipynb_checkpoints/Baseline Experiments-checkpoint.ipynb | stahl085/bracketology | 3311241fa82eb41a5529ac5e126171850f715032 | [
"MIT"
] | 4 | 2020-02-26T03:35:39.000Z | 2021-04-09T00:46:33.000Z | 28.969309 | 85 | 0.47144 | [
[
[
"from bracketology import Bracket",
"_____no_output_____"
],
[
"import random\ndef upset_prob(p):\n \"\"\"\n Given a probability between 0-1 will return a function that can\n be as an algorithm to fill out an NCAA bracket with `p` as \n the probability of an upset\n \n Parameters\n ----------\n p : (float)\n The probability of an upset\n \n Returns\n -------\n scoring_func : (function)\n function to pick an upset of a Game with probability `p`\n \"\"\"\n assert type(p) == float, \"p must be a float\"\n assert p <= 1.0, \"p must be <= 1.0\"\n assert p >= 0.0, \"p must be >= 0.0\"\n \n def scoring_func(the_game):\n team1 = the_game.top_team\n team2 = the_game.bottom_team\n\n team1_seed = team1.seed\n team2_seed = team2.seed\n \n team1_is_higher_seed = (team1_seed <= team2_seed)\n is_upset = (random.random() < p)\n \n if team1_is_higher_seed:\n if is_upset:\n winner = team2\n else:\n winner = team1\n else:\n if is_upset:\n winner = team1\n else:\n winner = team2\n return winner\n\n return scoring_func\n ",
"_____no_output_____"
],
[
"bracket19 = Bracket(year=2019)",
"_____no_output_____"
],
[
"bracket19.score(upset_prob(0.3))",
"Number of games correct: 29/63\nTotal Score: 59/192\n"
],
[
"bracket19",
"_____no_output_____"
],
[
"import json\nwith open('brackets.json', 'r') as f:\n brackets = json.load(f)",
"_____no_output_____"
],
[
"brackets['2019']",
"_____no_output_____"
]
]
] | [
"code"
] | [
[
"code",
"code",
"code",
"code",
"code",
"code",
"code"
]
] |
e77f69047b1771fb65046838fcfdee4a2d3980bf | 3,705 | ipynb | Jupyter Notebook | Bash Demo.ipynb | Carreau/JGI-demo | 829f0e371633e303f77fc7d2051796c1bd23b2bc | [
"CC0-1.0",
"CC-BY-4.0"
] | null | null | null | Bash Demo.ipynb | Carreau/JGI-demo | 829f0e371633e303f77fc7d2051796c1bd23b2bc | [
"CC0-1.0",
"CC-BY-4.0"
] | null | null | null | Bash Demo.ipynb | Carreau/JGI-demo | 829f0e371633e303f77fc7d2051796c1bd23b2bc | [
"CC0-1.0",
"CC-BY-4.0"
] | null | null | null | 21.171429 | 181 | 0.492308 | [
[
[
"empty"
]
]
] | [
"empty"
] | [
[
"empty"
]
] |
e77f7727f7bbc7a36bf5a2e5794ce939b8c08ee5 | 93,562 | ipynb | Jupyter Notebook | dacon_covid-19/ML/COVID_ML_05_XGBoost-GridSearchCV-final-model.ipynb | sam351/competitions | 617b7c0b76d96847336632fb6b0beff7031328da | [
"MIT"
] | 3 | 2020-03-31T07:48:55.000Z | 2020-07-01T05:38:30.000Z | dacon_covid-19/ML/COVID_ML_05_XGBoost-GridSearchCV-final-model.ipynb | sam351/competitions | 617b7c0b76d96847336632fb6b0beff7031328da | [
"MIT"
] | null | null | null | dacon_covid-19/ML/COVID_ML_05_XGBoost-GridSearchCV-final-model.ipynb | sam351/competitions | 617b7c0b76d96847336632fb6b0beff7031328da | [
"MIT"
] | 1 | 2020-04-02T03:12:16.000Z | 2020-04-02T03:12:16.000Z | 74.909528 | 47,956 | 0.707135 | [
[
[
"import numpy as np\nimport pandas as pd\nimport datetime\nimport time\n\nfrom sklearn.model_selection import train_test_split\nfrom sklearn.preprocessing import LabelEncoder\nfrom sklearn.model_selection import GridSearchCV\nfrom xgboost import XGBClassifier\nfrom xgboost import plot_importance\n\nimport matplotlib.pyplot as plt\nfrom matplotlib import font_manager as fm\nfrom matplotlib import rc\nfont_path = 'C:/Windows/Fonts/malgun.ttf'\nrc('font', family=fm.FontProperties(fname=font_path).get_name())",
"_____no_output_____"
]
],
[
[
"## 1. Load data\n- I'm using the files that were updated at **April 21st**\n- ref : https://github.com/jihoo-kim/Data-Science-for-COVID-19\n<br><br>\n- datasets\n - Region.csv → Region_sido_addPop_addHospital.csv\n - PatientInfo.csv\n - 흡연율-성-연령별.csv",
"_____no_output_____"
]
],
[
[
"# Prepare dataset\nRegion_df = pd.read_csv('../dataset/Region_sido_addPop_addHospital.csv')[['province', 'safe_hospitals_count', 'infection_hospitals_count', 'infection_hospitals_bed_num']]\n\nSmoke_df = pd.read_csv('../dataset/흡연율_성_연령별.csv')\nSmoke_df = Smoke_df.rename(columns={'성별':'sex','연령별':'age','분율':'smoking_rate'})\nSmoke_df.sex.replace('여성','female',inplace=True)\nSmoke_df.sex.replace('남성','male',inplace=True)\nSmoke_df = Smoke_df[['sex','age','smoking_rate']]\n\nPatientInfo_df = pd.read_csv('../dataset/Patient/PatientInfo.csv')\nPatientInfo_df = pd.merge(PatientInfo_df, Smoke_df, how='left', on=['sex','age'])\n\nprint(f'PatientInfo.csv shape : {PatientInfo_df.shape}')\n\nPatientInfo_df = PatientInfo_df[PatientInfo_df.state.isin(['released', 'deceased'])]\nprint(f' → datset shape : {PatientInfo_df.shape}')\n\nPatientInfo_df['symptom_onset_date'] = pd.to_datetime(PatientInfo_df['symptom_onset_date']) # convert data type\nPatientInfo_df['confirmed_date'] = pd.to_datetime(PatientInfo_df['confirmed_date']) # convert data type\nPatientInfo_df['disease'] = PatientInfo_df['disease'].astype(float) # convert data type\n\ndisplay(PatientInfo_df.head(3))\n\n\n# Save patient_id list\npresent_patients = PatientInfo_df.patient_id.astype(str).tolist()\nwith open('patients_id_0421.txt', 'w') as fp:\n fp.write('\\n'.join(present_patients))",
"PatientInfo.csv shape : (3326, 19)\n → datset shape : (1704, 19)\n"
],
[
"# Check Null values\nPatientInfo_df.contact_number.value_counts(dropna=False, normalize=True) * 100\nPatientInfo_df.symptom_onset_date.value_counts(dropna=False, normalize=True) * 100\nPatientInfo_df.infection_order.value_counts(dropna=False, normalize=True) * 100\n# PatientInfo_df.disease.value_counts(dropna=False, normalize=True) * 100\n# PatientInfo_df.country.value_counts(dropna=False, normalize=True) * 100",
"_____no_output_____"
]
],
[
[
"## 2. Preprocess data\n- selected features : **'sex', 'birth_year', 'age', 'province', 'disease (98.9% NaN)', 'infection_case (34.1% NaN)', 'symptom_onset_date (86.2% NaN)', 'confirmed_date', 'contact_number (75.4% NaN)''**\n- dropped features after validation : 'country', 'city', 'infection_order (98.3% NaN)\n- handling nan\n - drop nan from 'sex' & 'age'\n - replace with mean of same age group in birth_year\n - replace with 'non-reported' in infection_case\n - fill -1 in disease\n - fill -1 in contact_number\n - fill -1 in days_btw_symptom_confirm\n- new features : 'years_after_birth', 'days_after_first_date', 'days_btw_symptom_confirm', 'days_after_first_date_province', 'province_safe_hospitals_count', 'province_infection_hospitals_count', 'province_infection_bed_count'\n - (ohter new features were created but deleted after evaluation - 'smoking rate')\n- feature encoding (categorical)\n - age : convert to integer (0~10) - label encoding\n - other categorical columns : one-hot encoding",
"_____no_output_____"
]
],
[
[
"# Select features\nX_features = PatientInfo_df[['sex', 'birth_year', 'age', 'province', 'disease', 'infection_case',\n 'symptom_onset_date', 'confirmed_date', 'contact_number']].copy()\ny_target = PatientInfo_df[['state']].copy()\n\nprint(f'X_features.shape : {X_features.shape}')\nprint(f'y_target.shape : {y_target.shape}')\nX_features.head(3)",
"X_features.shape : (1704, 9)\ny_target.shape : (1704, 1)\n"
],
[
"print('\\n<< no of nan table (before handling) >>')\nprint(X_features.isna().sum())\n\n# Handle nan - sex & age\ny_target = y_target[~X_features.sex.isna() & ~X_features.age.isna()]\nX_features = X_features[~X_features.sex.isna() & ~X_features.age.isna()]\n\n# Handle nan - birth_year\nmean_year_list = dict(X_features.groupby('age')['birth_year'].mean().round().reset_index().values)\nX_features.loc[X_features.birth_year.isna(), 'birth_year'] = X_features.loc[X_features.birth_year.isna(), 'age'].map( lambda x : mean_year_list[ x ] )\n\n# Handle nan - infection_case\nX_features.loc[X_features.infection_case.isna(), 'infection_case'] = 'not-reported'\n\n# Handle nan - disease, contact_number\nX_features.disease = X_features.disease.fillna(-1)\nX_features.contact_number = X_features.contact_number.fillna(-1)\n\n\nprint('\\n<< no of nan table (after handling) >>')\nprint(X_features.isna().sum())\n\n\nprint(f'\\n\\nX_features.shape : {X_features.shape}')\nprint(f'y_target.shape : {y_target.shape}')",
"\n<< no of nan table (before handling) >>\nsex 7\nbirth_year 224\nage 10\nprovince 0\ndisease 1686\ninfection_case 582\nsymptom_onset_date 1469\nconfirmed_date 0\ncontact_number 1285\ndtype: int64\n\n<< no of nan table (after handling) >>\nsex 0\nbirth_year 0\nage 0\nprovince 0\ndisease 0\ninfection_case 0\nsymptom_onset_date 1459\nconfirmed_date 0\ncontact_number 0\ndtype: int64\n\n\nX_features.shape : (1694, 9)\ny_target.shape : (1694, 1)\n"
],
[
"# Create new features\nX_features['years_after_birth'] = datetime.date.today().year - X_features['birth_year']\n\nX_features['days_after_first_date'] = X_features['confirmed_date'] - X_features['confirmed_date'].min()\nX_features['days_after_first_date'] = X_features['days_after_first_date'].dt.days\n\nX_features['days_btw_symptom_confirm'] = (X_features['confirmed_date'] - X_features['symptom_onset_date']).dt.days\nX_features['days_btw_symptom_confirm'] = X_features['days_btw_symptom_confirm'].fillna(-1) # Handle nan\n\n# deleted after evaluation - days_after_first_date_province\nfirst_date_province_dict = dict(PatientInfo_df.groupby('province')['confirmed_date'].min().reset_index().values)\nX_features['first_date_province'] = X_features['province'].map( lambda x : first_date_province_dict[x] )\nX_features['days_after_first_date_province'] = X_features['confirmed_date'] - X_features['first_date_province']\nX_features['days_after_first_date_province'] = X_features['days_after_first_date_province'].dt.days\n\n# deleted after evaluation - province infomations\nprovince_info_dict = { item_list[0]:item_list[1:] for item_list in Region_df.values }\nX_features['province_safe_hospitals_count'] = X_features.province.map(lambda x : province_info_dict[x][0])\nX_features['province_infection_hospitals_count'] = X_features.province.map(lambda x : province_info_dict[x][1])\nX_features['province_infection_bed_count'] = X_features.province.map(lambda x : province_info_dict[x][2])\n\nX_features = X_features.drop(columns=['birth_year', 'symptom_onset_date', 'confirmed_date', 'first_date_province'])\n\nX_features.head(3)",
"_____no_output_____"
],
[
"# feature encoding - X_features\nX_features_processed = X_features.copy()\nX_features_processed = pd.concat([X_features_processed, pd.get_dummies(X_features_processed[['sex', 'province', 'infection_case']])], \n axis=1) # one-hot encoding\nX_features_processed = X_features_processed.drop(columns=['sex', 'province', 'infection_case'])\nX_features_processed['age'] = X_features.age.str.replace('s','').astype(int)//10 # label encoding\n\ndisplay(X_features_processed.head(3))\nprint()\n\n\n# feature encoding - y_target\ny_target_processed = pd.get_dummies(y_target)['state_deceased']\ndisplay(y_target_processed.head(3))",
"_____no_output_____"
]
],
[
[
"## 3. Split data into train, val, test\n- It's important that **labels are highly unbalanced** (only about 3% is deceased)\n- Since the dataset is quite small(1704 records), I will split the date into **6:2:2** for now (test data could be added from the next file update)\n- Since the labels are highly imbalanced, it's better to use **stratified random sampling**.",
"_____no_output_____"
]
],
[
[
"# Get train dataset\nX_train_val, X_test, y_train_val, y_test = train_test_split(X_features_processed, y_target_processed, test_size=0.2, \n random_state=0, stratify=y_target_processed)\n\n# Get val & test dataset\nX_train, X_val, y_train, y_val = train_test_split(X_train_val, y_train_val, test_size=0.33, random_state=0, stratify=y_train_val)\n\n\n# Check the labels of each dataset\nprint('< Percentage of each label (whole dataset) >')\nprint(y_target_processed.value_counts(normalize=True) * 100)\n\nprint('\\n< Percentage of each label (Train dataset) > - size of dataset :', y_train.shape[0])\nprint(y_train.value_counts(normalize=True) * 100)\n\nprint('\\n< Percentage of each label (Validation dataset) > - size of dataset :', y_val.shape[0])\nprint(y_val.value_counts(normalize=True) * 100)\n\nprint('\\n< Percentage of each label (Test dataset) > - size of dataset :', y_test.shape[0])\nprint(y_test.value_counts(normalize=True) * 100)",
"< Percentage of each label (whole dataset) >\n0 96.044864\n1 3.955136\nName: state_deceased, dtype: float64\n\n< Percentage of each label (Train dataset) > - size of dataset : 907\n0 96.030871\n1 3.969129\nName: state_deceased, dtype: float64\n\n< Percentage of each label (Validation dataset) > - size of dataset : 448\n0 95.982143\n1 4.017857\nName: state_deceased, dtype: float64\n"
]
],
[
[
"## 4. Train model - XGBoost with GridSearchCV",
"_____no_output_____"
]
],
[
[
"# Look for best hyper-parameters\nstart = time.time()\n\n# Train model\nxgb = XGBClassifier(random_state=0, n_jobs=-1)\nxgb_param = {\n 'n_estimators': [100, 200, 300, 400],\n 'min_child_weight': [1, 2, 3],\n 'gamma': [1.5, 2, 2.5, 3],\n 'colsample_bytree': [0.7, 0.8, 0.9],\n 'max_depth': [5, 6, 7, 8, 9, 10]\n}\n\ngrid_xgb = GridSearchCV(xgb, param_grid=xgb_param, scoring='f1', cv=5)\ngrid_xgb.fit(X_train_val, y_train_val)\n\nprint('time elapsed :', time.time()-start)\n\nprint('GridSearchCV 최적 파라미터:', grid_xgb.best_params_)\nprint('GridSearchCV 최고 정확도: {0:.4f}'.format(grid_xgb.best_score_))",
"time elapsed : 6163.060415029526\nGridSearchCV 최적 파라미터: {'colsample_bytree': 0.8, 'gamma': 2, 'max_depth': 6, 'min_child_weight': 1, 'n_estimators': 100, 'subsample': 0.6}\nGridSearchCV 최고 정확도: 0.6731\n"
],
[
"scores_df = pd.DataFrame(grid_xgb.cv_results_).sort_values('rank_test_score')\nscores_df[['params', 'mean_test_score', 'rank_test_score',\n 'split0_test_score', 'split2_test_score', 'split4_test_score']]",
"_____no_output_____"
],
[
"# Train final model\nxgb_final = XGBClassifier(random_state=0, n_jobs=-1, n_estimators=100, colsample_bytree=0.8, gamma=2, max_depth=6, min_child_weight=1, subsample=0.6)\nxgb_final.fit(X_train, y_train)",
"_____no_output_____"
],
[
"# Evaluate final model\ndef get_clf_eval(y_test , pred):\n from sklearn.metrics import confusion_matrix, accuracy_score\n from sklearn.metrics import precision_score, recall_score\n from sklearn.metrics import f1_score, roc_auc_score\n\n confusion = confusion_matrix( y_test, pred)\n accuracy = accuracy_score(y_test , pred)\n precision = precision_score(y_test , pred)\n recall = recall_score(y_test , pred)\n f1 = f1_score(y_test,pred)\n roc_auc = roc_auc_score(y_test, pred)\n print('오차 행렬')\n print(confusion)\n print('정확도: {0:.4f}, 정밀도: {1:.4f}, 재현율: {2:.4f},\\\n F1: {3:.4f}, AUC:{4:.4f}'.format(accuracy, precision, recall, f1, roc_auc))\n pass\n\nxgb_preds = xgb_final.predict(X_val)\nget_clf_eval(y_val , xgb_preds)",
"오차 행렬\n[[427 3]\n [ 9 9]]\n정확도: 0.9732, 정밀도: 0.7500, 재현율: 0.5000, F1: 0.6000, AUC:0.7465\n"
],
[
"# Visualize the feature importance\nfig, ax = plt.subplots(figsize=(10, 7))\nplot_importance(xgb_final, ax=ax)\nax.set_title('Feature importance', size=13)\nax.tick_params(axis='y', labelsize=15)\n\nplt.show()",
"_____no_output_____"
]
]
] | [
"code",
"markdown",
"code",
"markdown",
"code",
"markdown",
"code",
"markdown",
"code"
] | [
[
"code"
],
[
"markdown"
],
[
"code",
"code"
],
[
"markdown"
],
[
"code",
"code",
"code",
"code"
],
[
"markdown"
],
[
"code"
],
[
"markdown"
],
[
"code",
"code",
"code",
"code",
"code"
]
] |
e77f9fc328645f93cc4a8161879da08b317e7c8d | 822,759 | ipynb | Jupyter Notebook | NeuralStyleTransfer/StyleTransfer.ipynb | Frostday/Small-Tensorflow-Projects | 63a1fd3fa71a10ad1d35b45334141940c2f234e7 | [
"MIT"
] | 9 | 2021-03-04T03:28:45.000Z | 2022-01-11T23:01:24.000Z | NeuralStyleTransfer/StyleTransfer.ipynb | Frostday/Small-Tensorflow-Projects | 63a1fd3fa71a10ad1d35b45334141940c2f234e7 | [
"MIT"
] | 2 | 2020-11-01T14:29:40.000Z | 2021-03-15T08:08:32.000Z | NeuralStyleTransfer/StyleTransfer.ipynb | Frostday/Small-Tensorflow-Projects | 63a1fd3fa71a10ad1d35b45334141940c2f234e7 | [
"MIT"
] | 7 | 2020-10-30T04:49:58.000Z | 2022-01-17T08:33:16.000Z | 1,082.577632 | 245,634 | 0.935307 | [
[
[
"# Style Transfer\n\nWe are optimising the input image to reduce loss between output of convolutional layers(compared with output from layers when we pass the style/content image) of VGG16 model(we don't optimise weights since they are pretrained). Also we use the optimized input image as final prediction instead of output from convolutional layers.",
"_____no_output_____"
]
],
[
[
"import tensorflow as tf\nif tf.__version__.startswith('2'):\n tf.compat.v1.disable_eager_execution()\n\nfrom tensorflow.keras.layers import Input, Lambda, Dense, Flatten\nfrom tensorflow.keras.layers import AveragePooling2D, MaxPooling2D\nfrom tensorflow.keras.layers import Conv2D\nfrom tensorflow.keras.models import Model, Sequential\nfrom tensorflow.keras.applications.vgg16 import VGG16\nfrom tensorflow.keras.applications.vgg16 import preprocess_input\nfrom tensorflow.keras.preprocessing import image\nimport tensorflow.keras.backend as K\n\nimport numpy as np\nimport matplotlib.pyplot as plt\n\nfrom scipy.optimize import fmin_l_bfgs_b\nfrom datetime import datetime",
"_____no_output_____"
]
],
[
[
"# Transfer Learning Part",
"_____no_output_____"
]
],
[
[
"def VGG16_AvgPool(shape):\n # we want to account for features across the entire image so get rid of the maxpool which throws away information and use average # pooling instead.\n vgg = VGG16(input_shape=shape, weights='imagenet', include_top=False)\n\n i = vgg.input\n x = i\n for layer in vgg.layers:\n if layer.__class__ == MaxPooling2D:\n # replace it with average pooling\n x = AveragePooling2D()(x)\n else:\n x = layer(x)\n\n return Model(i, x)",
"_____no_output_____"
],
[
"def VGG16_AvgPool_CutOff(shape, num_convs):\n # this function creates a partial model because we don't need the full VGG network instead we need to stop at an intermediate \n # convolution. Therefore this function allows us to specify how many convolutions we need\n # there are 13 convolutions in total we can pick any of them as the \"output\" of our content model\n\n if num_convs < 1 or num_convs > 13:\n print(\"num_convs must be in the range [1, 13]\")\n return None\n\n model = VGG16_AvgPool(shape)\n\n n = 0\n output = None\n for layer in model.layers:\n if layer.__class__ == Conv2D:\n n += 1\n if n >= num_convs:\n output = layer.output\n break\n\n return Model(model.input, output)",
"_____no_output_____"
]
],
[
[
"# Processing",
"_____no_output_____"
]
],
[
[
"# load the content image\ndef load_img_and_preprocess(path, shape=None):\n img = image.load_img(path, target_size=shape)\n\n # convert image to array and preprocess for vgg\n x = image.img_to_array(img)\n x = np.expand_dims(x, axis=0)\n x = preprocess_input(x)\n\n return x",
"_____no_output_____"
],
[
"# since VGG accepts BGR this function allows us to convert our values back to RGB so we can plot it using matplotlib\n# so this basically reverses the keras function - preprocess input\ndef unpreprocess(img):\n img[..., 0] += 103.939\n img[..., 1] += 116.779\n img[..., 2] += 126.68\n img = img[..., ::-1]\n return img\n\ndef scale_img(x):\n x = x - x.min()\n x = x / x.max()\n return x",
"_____no_output_____"
]
],
[
[
"# Style loss\n\nConverting output to gram matrix and calculating style loss",
"_____no_output_____"
]
],
[
[
"def gram_matrix(img):\n # input is (H, W, C) (C = # feature maps)\n # we first need to convert it to (C, H*W)\n X = K.batch_flatten(K.permute_dimensions(img, (2, 0, 1)))\n \n # now, calculate the gram matrix\n # gram = XX^T / N\n # the constant is not important since we'll be weighting these\n G = K.dot(X, K.transpose(X)) / img.get_shape().num_elements()\n return G",
"_____no_output_____"
],
[
"def style_loss(y, t):\n return K.mean(K.square(gram_matrix(y) - gram_matrix(t)))",
"_____no_output_____"
]
],
[
[
"# Minimizing loss and optimising image(training)",
"_____no_output_____"
]
],
[
[
"# function to minimise loss by optimising input image\ndef minimize(fn, epochs, batch_shape, content_image):\n t0 = datetime.now()\n losses = []\n # x = np.random.randn(np.prod(batch_shape))\n x = content_image\n for i in range(epochs):\n x, l, _ = fmin_l_bfgs_b(\n func=fn,\n x0=x,\n maxfun=20\n )\n x = np.clip(x, -127, 127)\n print(\"iter=%s, loss=%s\" % (i+1, l))\n losses.append(l)\n\n print(\"duration:\", datetime.now() - t0)\n plt.plot(losses)\n plt.show()\n\n newimg = x.reshape(*batch_shape)\n final_img = unpreprocess(newimg)\n return final_img[0]",
"_____no_output_____"
]
],
[
[
"# Modelling",
"_____no_output_____"
]
],
[
[
"content_path = 'images/content/elephant.jpg'\nstyle_path = 'images/style/lesdemoisellesdavignon.jpg'\n\nx = load_img_and_preprocess(content_path)\nh, w = x.shape[1:3]\n\n# reduce image size while keeping ratio of dimensions same\ni = 2\nh_new = h\nw_new = w\nwhile h_new > 400 or w_new > 400:\n h_new = h/i\n w_new = w/i\n i += 1\n\nh = int(h_new)\nw = int(w_new)\n\nprint(h, w)",
"225 300\n"
],
[
"fig = plt.figure()\n\nimg_content = image.load_img(content_path, target_size=(h, w))\nax1 = fig.add_subplot(1, 2, 1)\nax1.imshow(img_content)\n\nimg_style = image.load_img(style_path, target_size=(h, w))\nax2 = fig.add_subplot(1, 2, 2)\nax2.imshow(img_style)\n\nplt.show()",
"_____no_output_____"
],
[
"# loading and preprocessing input images\ncontent_img = load_img_and_preprocess(content_path, (h, w))\nstyle_img = load_img_and_preprocess(style_path, (h, w))\n\nprint(content_img.shape)\nprint(style_img.shape)",
"(1, 225, 300, 3)\n(1, 225, 300, 3)\n"
],
[
"batch_shape = content_img.shape\nshape = content_img.shape[1:]\n\nprint(batch_shape)\nprint(shape)",
"(1, 225, 300, 3)\n(225, 300, 3)\n"
],
[
"# load the complete model\nvgg = VGG16_AvgPool(shape)",
"WARNING:tensorflow:From D:\\Programs\\anaconda3\\envs\\py37\\lib\\site-packages\\tensorflow\\python\\ops\\resource_variable_ops.py:1666: calling BaseResourceVariable.__init__ (from tensorflow.python.ops.resource_variable_ops) with constraint is deprecated and will be removed in a future version.\nInstructions for updating:\nIf using Keras pass *_constraint arguments to layers.\nWARNING:tensorflow:Model inputs must come from `tf.keras.Input` (thus holding past layer metadata), they cannot be the output of a previous non-Input layer. Here, a tensor specified as input to \"model\" was not an Input tensor, it was generated by layer input_1.\nNote that input tensors are instantiated via `tensor = tf.keras.Input(shape)`.\nThe tensor that caused the issue was: input_1:0\n"
],
[
"# load the content model and we only want one output from this which is from 13th layer\ncontent_model = Model(vgg.input, vgg.layers[13].get_output_at(0))\n\n# target outputs from content image\ncontent_target = K.variable(content_model.predict(content_img))\n\ncontent_model.summary()",
"WARNING:tensorflow:Model inputs must come from `tf.keras.Input` (thus holding past layer metadata), they cannot be the output of a previous non-Input layer. Here, a tensor specified as input to \"model_1\" was not an Input tensor, it was generated by layer input_1.\nNote that input tensors are instantiated via `tensor = tf.keras.Input(shape)`.\nThe tensor that caused the issue was: input_1:0\nModel: \"model_1\"\n_________________________________________________________________\nLayer (type) Output Shape Param # \n=================================================================\ninput_1 (InputLayer) multiple 0 \n_________________________________________________________________\nblock1_conv1 (Conv2D) (None, 225, 300, 64) 1792 \n_________________________________________________________________\nblock1_conv2 (Conv2D) (None, 225, 300, 64) 36928 \n_________________________________________________________________\nblock1_pool (MaxPooling2D) (None, 112, 150, 64) 0 \n_________________________________________________________________\nblock2_conv1 (Conv2D) (None, 112, 150, 128) 73856 \n_________________________________________________________________\nblock2_conv2 (Conv2D) (None, 112, 150, 128) 147584 \n_________________________________________________________________\nblock2_pool (MaxPooling2D) (None, 56, 75, 128) 0 \n_________________________________________________________________\nblock3_conv1 (Conv2D) (None, 56, 75, 256) 295168 \n_________________________________________________________________\nblock3_conv2 (Conv2D) (None, 56, 75, 256) 590080 \n_________________________________________________________________\nblock3_conv3 (Conv2D) (None, 56, 75, 256) 590080 \n_________________________________________________________________\nblock3_pool (MaxPooling2D) (None, 28, 37, 256) 0 \n_________________________________________________________________\nblock4_conv1 (Conv2D) (None, 28, 37, 512) 1180160 \n_________________________________________________________________\nblock4_conv2 (Conv2D) (None, 28, 37, 512) 2359808 \n_________________________________________________________________\nblock4_conv3 (Conv2D) (None, 28, 37, 512) 2359808 \n=================================================================\nTotal params: 7,635,264\nTrainable params: 7,635,264\nNon-trainable params: 0\n_________________________________________________________________\n"
],
[
"# index 0 correspond to the original vgg with maxpool so we do get_output_at(1) which corresponds to vgg with avg pool\nsymbolic_conv_outputs = [\n layer.get_output_at(1) for layer in vgg.layers \\\n if layer.name.endswith('conv1')\n]\n\n# we collect all the convolutional layers in this list because we will need to take output from all of them\nsymbolic_conv_outputs",
"_____no_output_____"
],
[
"# make a big model that outputs multiple layers' outputs(outputs from all layers stored in list symbolic_conv_outputs)\nstyle_model = Model(vgg.input, symbolic_conv_outputs)\n\n# calculate the targets from convolutional outputs at each layer in symbolic_conv_outputs\nstyle_layers_outputs = [K.variable(y) for y in style_model.predict(style_img)]\n\nstyle_model.summary()",
"WARNING:tensorflow:Model inputs must come from `tf.keras.Input` (thus holding past layer metadata), they cannot be the output of a previous non-Input layer. Here, a tensor specified as input to \"model_2\" was not an Input tensor, it was generated by layer input_1.\nNote that input tensors are instantiated via `tensor = tf.keras.Input(shape)`.\nThe tensor that caused the issue was: input_1:0\nModel: \"model_2\"\n__________________________________________________________________________________________________\nLayer (type) Output Shape Param # Connected to \n==================================================================================================\ninput_1 (InputLayer) multiple 0 input_1[0][0] \n__________________________________________________________________________________________________\nblock1_conv1 (Conv2D) (None, 225, 300, 64) 1792 input_1[1][0] \n__________________________________________________________________________________________________\nblock1_conv2 (Conv2D) (None, 225, 300, 64) 36928 block1_conv1[1][0] \n__________________________________________________________________________________________________\naverage_pooling2d (AveragePooli (None, 112, 150, 64) 0 block1_conv2[1][0] \n__________________________________________________________________________________________________\nblock2_conv1 (Conv2D) (None, 112, 150, 128 73856 average_pooling2d[0][0] \n__________________________________________________________________________________________________\nblock2_conv2 (Conv2D) (None, 112, 150, 128 147584 block2_conv1[1][0] \n__________________________________________________________________________________________________\naverage_pooling2d_1 (AveragePoo (None, 56, 75, 128) 0 block2_conv2[1][0] \n__________________________________________________________________________________________________\nblock3_conv1 (Conv2D) (None, 56, 75, 256) 295168 average_pooling2d_1[0][0] \n__________________________________________________________________________________________________\nblock3_conv2 (Conv2D) (None, 56, 75, 256) 590080 block3_conv1[1][0] \n__________________________________________________________________________________________________\nblock3_conv3 (Conv2D) (None, 56, 75, 256) 590080 block3_conv2[1][0] \n__________________________________________________________________________________________________\naverage_pooling2d_2 (AveragePoo (None, 28, 37, 256) 0 block3_conv3[1][0] \n__________________________________________________________________________________________________\nblock4_conv1 (Conv2D) (None, 28, 37, 512) 1180160 average_pooling2d_2[0][0] \n__________________________________________________________________________________________________\nblock4_conv2 (Conv2D) (None, 28, 37, 512) 2359808 block4_conv1[1][0] \n__________________________________________________________________________________________________\nblock4_conv3 (Conv2D) (None, 28, 37, 512) 2359808 block4_conv2[1][0] \n__________________________________________________________________________________________________\naverage_pooling2d_3 (AveragePoo (None, 14, 18, 512) 0 block4_conv3[1][0] \n__________________________________________________________________________________________________\nblock5_conv1 (Conv2D) (None, 14, 18, 512) 2359808 average_pooling2d_3[0][0] \n==================================================================================================\nTotal params: 9,995,072\nTrainable params: 9,995,072\nNon-trainable params: 0\n__________________________________________________________________________________________________\n"
],
[
"# we will assume the weight of the content loss is 1 and only weight the style losses\n# style_weights = [0.2, 0.4, 0.3, 0.5, 0.2]\nstyle_weights = [5, 4, 8, 7, 9]",
"_____no_output_____"
],
[
"# create the total loss which is the sum of content + style loss\n\nloss = K.mean(K.square(content_model.output - content_target))\n\nfor w, symbolic, actual in zip(style_weights, symbolic_conv_outputs, style_layers_outputs):\n # gram_matrix() expects a (H, W, C) as input\n loss += w * style_loss(symbolic[0], actual[0])",
"_____no_output_____"
],
[
"content_model.input",
"_____no_output_____"
],
[
"style_model.input",
"_____no_output_____"
],
[
"content_model.output",
"_____no_output_____"
],
[
"style_model.output",
"_____no_output_____"
],
[
"# NOTE: it doesn't matter which model's input you use they are both pointing to the same keras Input layer in memory\ngrads = K.gradients(loss, vgg.input)",
"_____no_output_____"
],
[
"get_loss_and_grads = K.function(\n inputs=[vgg.input],\n outputs=[loss] + grads\n)\n\ndef get_loss_and_grads_wrapper(x_vec):\n l, g = get_loss_and_grads([x_vec.reshape(*batch_shape)])\n return l.astype(np.float64), g.flatten().astype(np.float64)",
"_____no_output_____"
],
[
"# converting image shape to 1d array\nimg = np.reshape(content_img, (-1))\nimg.shape",
"_____no_output_____"
],
[
"final_img = minimize(get_loss_and_grads_wrapper, 10, batch_shape, img)",
"iter=1, loss=14908.75390625\niter=2, loss=6712.1474609375\niter=3, loss=4878.84375\niter=4, loss=4097.85888671875\niter=5, loss=3610.35009765625\niter=6, loss=3316.75\niter=7, loss=3111.816650390625\niter=8, loss=2973.6328125\niter=9, loss=2869.751708984375\niter=10, loss=2791.61083984375\nduration: 0:00:29.018460\n"
],
[
"plt.imshow(scale_img(final_img))\nplt.show()",
"_____no_output_____"
]
]
] | [
"markdown",
"code",
"markdown",
"code",
"markdown",
"code",
"markdown",
"code",
"markdown",
"code",
"markdown",
"code"
] | [
[
"markdown"
],
[
"code"
],
[
"markdown"
],
[
"code",
"code"
],
[
"markdown"
],
[
"code",
"code"
],
[
"markdown"
],
[
"code",
"code"
],
[
"markdown"
],
[
"code"
],
[
"markdown"
],
[
"code",
"code",
"code",
"code",
"code",
"code",
"code",
"code",
"code",
"code",
"code",
"code",
"code",
"code",
"code",
"code",
"code",
"code",
"code"
]
] |
e77fafdd0ead9dc78187f6c77235bd2c9c58bb0e | 71,122 | ipynb | Jupyter Notebook | Cap05_IntegracionNumerica.ipynb | Youngermaster/Numerical-Analysis | 15e9fe32b21f73f845e02d6214991bdcbcb650b8 | [
"MIT"
] | null | null | null | Cap05_IntegracionNumerica.ipynb | Youngermaster/Numerical-Analysis | 15e9fe32b21f73f845e02d6214991bdcbcb650b8 | [
"MIT"
] | null | null | null | Cap05_IntegracionNumerica.ipynb | Youngermaster/Numerical-Analysis | 15e9fe32b21f73f845e02d6214991bdcbcb650b8 | [
"MIT"
] | null | null | null | 37.650609 | 7,786 | 0.563061 | [
[
[
"<p float=\"center\">\n <img src=\"https://github.com/carlosalvarezh/Analisis_Numerico/blob/master/images/C00_Img00_logo.png?raw=true\" width=\"350\" />\n</p>\n<h1 align=\"center\">ST0256 - Análisis Numérico</h1>\n<h1 align=\"center\">Capítulo 5: Diferenciación e integración numérica</h1>\n<h1 align=\"center\">2021/01</h1>\n<h1 align=\"center\">MEDELLÍN - COLOMBIA </h1>",
"_____no_output_____"
],
[
"<table>\n <tr align=left><td><img align=left src=\"./images/CC-BY.png\">\n <td>Text provided under a Creative Commons Attribution license, CC-BY. All code is made available under the FSF-approved MIT license.(c) Carlos Alberto Alvarez Henao</td>\n</table>",
"_____no_output_____"
],
[
"*** \n\n***Docente:*** Carlos Alberto Álvarez Henao, I.C. D.Sc.\n\n***e-mail:*** [email protected]\n\n***skype:*** carlos.alberto.alvarez.henao\n\n***Herramienta:*** [Jupyter notebook](http://jupyter.org/)\n\n***Kernel:*** Python 3.8\n\n\n***",
"_____no_output_____"
],
[
"<a id='TOC'></a>",
"_____no_output_____"
],
[
"<h1>Tabla de Contenidos<span class=\"tocSkip\"></span></h1>\n<div class=\"toc\"><ul class=\"toc-item\"><li><span><a href=\"#Diferenciación-Numérica\" data-toc-modified-id=\"Diferenciación-Numérica-1\"><span class=\"toc-item-num\">1 </span>Diferenciación Numérica</a></span><ul class=\"toc-item\"><li><span><a href=\"#Introducción\" data-toc-modified-id=\"Introducción-1.1\"><span class=\"toc-item-num\">1.1 </span>Introducción</a></span></li><li><span><a href=\"#Series-de-Taylor\" data-toc-modified-id=\"Series-de-Taylor-1.2\"><span class=\"toc-item-num\">1.2 </span>Series de Taylor</a></span></li><li><span><a href=\"#Esquemas-de-diferencias-finitas-para-la-primera-derivada\" data-toc-modified-id=\"Esquemas-de-diferencias-finitas-para-la-primera-derivada-1.3\"><span class=\"toc-item-num\">1.3 </span>Esquemas de diferencias finitas para la primera derivada</a></span><ul class=\"toc-item\"><li><span><a href=\"#Esquema-de-primer-orden-hacia-adelante-(forward)\" data-toc-modified-id=\"Esquema-de-primer-orden-hacia-adelante-(forward)-1.3.1\"><span class=\"toc-item-num\">1.3.1 </span>Esquema de primer orden hacia adelante (forward)</a></span></li><li><span><a href=\"#Esquema-de-primer-orden-hacia-atrás-(backward)\" data-toc-modified-id=\"Esquema-de-primer-orden-hacia-atrás-(backward)-1.3.2\"><span class=\"toc-item-num\">1.3.2 </span>Esquema de primer orden hacia atrás (backward)</a></span></li><li><span><a href=\"#Esquema-de-segundo-orden-(central)\" data-toc-modified-id=\"Esquema-de-segundo-orden-(central)-1.3.3\"><span class=\"toc-item-num\">1.3.3 </span>Esquema de segundo orden (central)</a></span></li><li><span><a href=\"#Resumen-esquemas-diferencias-finitas-para-la-primera-derivada\" data-toc-modified-id=\"Resumen-esquemas-diferencias-finitas-para-la-primera-derivada-1.3.4\"><span class=\"toc-item-num\">1.3.4 </span>Resumen esquemas diferencias finitas para la primera derivada</a></span></li></ul></li><li><span><a href=\"#Esquemas-de-diferencias-finitas-para-la-segunda-derivada\" data-toc-modified-id=\"Esquemas-de-diferencias-finitas-para-la-segunda-derivada-1.4\"><span class=\"toc-item-num\">1.4 </span>Esquemas de diferencias finitas para la segunda derivada</a></span></li><li><span><a href=\"#Implementación-computacional-de-algunos-esquemas-de-diferencias-finitas\" data-toc-modified-id=\"Implementación-computacional-de-algunos-esquemas-de-diferencias-finitas-1.5\"><span class=\"toc-item-num\">1.5 </span>Implementación computacional de algunos esquemas de diferencias finitas</a></span></li></ul></li><li><span><a href=\"#Integración-Numérica\" data-toc-modified-id=\"Integración-Numérica-2\"><span class=\"toc-item-num\">2 </span>Integración Numérica</a></span><ul class=\"toc-item\"><li><span><a href=\"#Introducción\" data-toc-modified-id=\"Introducción-2.1\"><span class=\"toc-item-num\">2.1 </span>Introducción</a></span></li><li><span><a href=\"#Fórmulas-de-integración-de-Newton---Cotes\" data-toc-modified-id=\"Fórmulas-de-integración-de-Newton---Cotes-2.2\"><span class=\"toc-item-num\">2.2 </span>Fórmulas de integración de <em>Newton - Cotes</em></a></span></li><li><span><a href=\"#Regla-trapezoidal\" data-toc-modified-id=\"Regla-trapezoidal-2.3\"><span class=\"toc-item-num\">2.3 </span>Regla trapezoidal</a></span><ul class=\"toc-item\"><li><span><a href=\"#Regla-trapezoidal-de-aplicación-simple\" data-toc-modified-id=\"Regla-trapezoidal-de-aplicación-simple-2.3.1\"><span class=\"toc-item-num\">2.3.1 </span>Regla trapezoidal de aplicación simple</a></span></li><li><span><a href=\"#Regla-trapezoidal-de-aplicación-múltiple\" data-toc-modified-id=\"Regla-trapezoidal-de-aplicación-múltiple-2.3.2\"><span class=\"toc-item-num\">2.3.2 </span>Regla trapezoidal de aplicación múltiple</a></span></li><li><span><a href=\"#Implementación-computacional\" data-toc-modified-id=\"Implementación-computacional-2.3.3\"><span class=\"toc-item-num\">2.3.3 </span>Implementación computacional</a></span></li><li><span><a href=\"#Error-en-la-aplicación-de-la-regla-trapezoidal\" data-toc-modified-id=\"Error-en-la-aplicación-de-la-regla-trapezoidal-2.3.4\"><span class=\"toc-item-num\">2.3.4 </span>Error en la aplicación de la regla trapezoidal</a></span></li></ul></li><li><span><a href=\"#Reglas-de-Simpson\" data-toc-modified-id=\"Reglas-de-Simpson-2.4\"><span class=\"toc-item-num\">2.4 </span>Reglas de Simpson</a></span><ul class=\"toc-item\"><li><span><a href=\"#Regla-de-Simpson1/3-de-aplicación-simple\" data-toc-modified-id=\"Regla-de-Simpson1/3-de-aplicación-simple-2.4.1\"><span class=\"toc-item-num\">2.4.1 </span>Regla de Simpson1/3 de aplicación simple</a></span></li><li><span><a href=\"#Error-en-la-regla-de-Simpson-1/3-de-aplicación-simple\" data-toc-modified-id=\"Error-en-la-regla-de-Simpson-1/3-de-aplicación-simple-2.4.2\"><span class=\"toc-item-num\">2.4.2 </span>Error en la regla de Simpson 1/3 de aplicación simple</a></span></li><li><span><a href=\"#Regla-de-simpson1/3-de-aplicación-múltiple\" data-toc-modified-id=\"Regla-de-simpson1/3-de-aplicación-múltiple-2.4.3\"><span class=\"toc-item-num\">2.4.3 </span>Regla de simpson1/3 de aplicación múltiple</a></span></li><li><span><a href=\"#Implementación-computacional-regla-de-Simpson1/3-de-aplicación-múltiple\" data-toc-modified-id=\"Implementación-computacional-regla-de-Simpson1/3-de-aplicación-múltiple-2.4.4\"><span class=\"toc-item-num\">2.4.4 </span>Implementación computacional regla de Simpson1/3 de aplicación múltiple</a></span></li><li><span><a href=\"#Regla-de-Simpson-3/8-de-aplicación-simple\" data-toc-modified-id=\"Regla-de-Simpson-3/8-de-aplicación-simple-2.4.5\"><span class=\"toc-item-num\">2.4.5 </span>Regla de Simpson 3/8 de aplicación simple</a></span></li><li><span><a href=\"#Regla-de-Simpson3/8-de-aplicación-múltiple\" data-toc-modified-id=\"Regla-de-Simpson3/8-de-aplicación-múltiple-2.4.6\"><span class=\"toc-item-num\">2.4.6 </span>Regla de Simpson3/8 de aplicación múltiple</a></span></li><li><span><a href=\"#Implementación-computacional-de-la-regla-de-Simpson3/8-de-aplicación-múltiple\" data-toc-modified-id=\"Implementación-computacional-de-la-regla-de-Simpson3/8-de-aplicación-múltiple-2.4.7\"><span class=\"toc-item-num\">2.4.7 </span>Implementación computacional de la regla de Simpson3/8 de aplicación múltiple</a></span></li></ul></li><li><span><a href=\"#Cuadratura-de-Gauss\" data-toc-modified-id=\"Cuadratura-de-Gauss-2.5\"><span class=\"toc-item-num\">2.5 </span>Cuadratura de Gauss</a></span><ul class=\"toc-item\"><li><span><a href=\"#Introducción\" data-toc-modified-id=\"Introducción-2.5.1\"><span class=\"toc-item-num\">2.5.1 </span>Introducción</a></span></li><li><span><a href=\"#Determinación-de-los-coeficientes\" data-toc-modified-id=\"Determinación-de-los-coeficientes-2.5.2\"><span class=\"toc-item-num\">2.5.2 </span>Determinación de los coeficientes</a></span></li><li><span><a href=\"#Cambios-de-los-límites-de-integración\" data-toc-modified-id=\"Cambios-de-los-límites-de-integración-2.5.3\"><span class=\"toc-item-num\">2.5.3 </span>Cambios de los límites de integración</a></span></li><li><span><a href=\"#Fórmulas-de-punto-superior\" data-toc-modified-id=\"Fórmulas-de-punto-superior-2.5.4\"><span class=\"toc-item-num\">2.5.4 </span>Fórmulas de punto superior</a></span></li><li><span><a href=\"#Ejemplo-Cuadratura-de-Gauss\" data-toc-modified-id=\"Ejemplo-Cuadratura-de-Gauss-2.5.5\"><span class=\"toc-item-num\">2.5.5 </span>Ejemplo Cuadratura de Gauss</a></span></li></ul></li></ul></li></ul></div>",
"_____no_output_____"
],
[
"## Diferenciación Numérica",
"_____no_output_____"
],
[
"### Introducción",
"_____no_output_____"
],
[
"La [diferenciación numérica](https://en.wikipedia.org/wiki/Numerical_differentiation) se emplea para determinar (estimar) el valor de la derivada de una función en un punto específico. No confundir con la derivada de una función, pues lo que se obtendrá es un valor puntual y no una función. En este capítulo nos centraremos únicamente en ecuiaciones unidimensionales. ",
"_____no_output_____"
],
[
"[Volver a la Tabla de Contenido](#TOC)",
"_____no_output_____"
],
[
"### Series de Taylor",
"_____no_output_____"
],
[
"De la [serie de Taylor](https://en.wikipedia.org/wiki/Taylor_series) \n\n<a id='Ec5_1'></a>\n\\begin{equation*}\nf(x_{i \\pm 1}) = f(x_i) \\pm f'(x_i)h + \\frac{f''(x_i)h^2}{2!} \\pm \\frac{f'''(x_i)h^3}{3!} + \\ldots\n\\label{eq:Ec5_1} \\tag{5.1}\n\\end{equation*}\n\ncon $h=\\Delta x = x_{i+1}-x_i$ siendo el tamaño de paso.\n\nDada que la serie contiene infinitos términos, partir de la ecuación ($5.1$) se pueden obtener infinitos esquemas numéricos para determinar cada una de las infinitas derivadas de dicho polinomio. En este curso usaremos la técnica de [Diferencias Finitas](https://en.wikipedia.org/wiki/Finite_difference) para desarrollarlas.",
"_____no_output_____"
],
[
"[Volver a la Tabla de Contenido](#TOC)",
"_____no_output_____"
],
[
"### Esquemas de diferencias finitas para la primera derivada",
"_____no_output_____"
],
[
"#### Esquema de primer orden hacia adelante (forward)",
"_____no_output_____"
],
[
"De la ecuación [(5.1)](#Ec5_1) tomando los valores positivos, que involucran únicamente términos hacia adelante, se trunca la serie hasta la primera derivada y se realiza un despeje algebraico para llegar a:\n\n<a id='Ec5_2'></a>\n\\begin{equation*}\nf'(x_i) = \\frac{f(x_{i+1})-f(x_i)}{h} + \\mathcal{O}(h)\n\\label{eq:Ec5_2} \\tag{5.2}\n\\end{equation*}\n\nse puede observar que el término $\\mathcal{O}(h)$ indica que el error es de orden lineal, es decir, si se reduce el tamaño de paso, $h$, a la mitad, el error se reducirá a la mitad. Si se reduc el tamaño de paso a una cuarta parte, el error se reducirá, linealmente, una cuarta parte.",
"_____no_output_____"
],
[
"[Volver a la Tabla de Contenido](#TOC)",
"_____no_output_____"
],
[
"#### Esquema de primer orden hacia atrás (backward)",
"_____no_output_____"
],
[
"De la ecuación [(5.1)](#Ec5_1) tomando los valores negativos, que involucran únicamente términos hacia atrás (backward), se trunca la serie hasta la primera derivada y se realiza un despeje algebraico para llegar a:\n\n<a id='Ec5_1'></a>\n\\begin{equation*}\nf'(x_i) = \\frac{f(x_{i})-f(x_{i-1})}{h} + \\mathcal{O}(h)\n\\label{eq:Ec5_3} \\tag{5.3}\n\\end{equation*}\n\nse observa que se llega a una expresión similar a la de la ecuación [(5.2)](#Ec5_2), pero de esta vez, se tiene en cuenta es el valor anterior al punto $x_i$. También se observa que el error es de orden lineal, por lo que se mantiene un esquema de primer orden.\n\n",
"_____no_output_____"
],
[
"[Volver a la Tabla de Contenido](#TOC)",
"_____no_output_____"
],
[
"#### Esquema de segundo orden (central)",
"_____no_output_____"
],
[
"Una forma de aumentar el orden de estos esquemas, es realizar el truncamiento de la *serie de Taylor* hasta la segunda derivada, hacia adelante y hacia atras, y realizar su resta aritmética.\n\n<a id='Ec5_4'></a>\n\\begin{equation*}\n\\begin{split}\nf(x_{i+1}) & = f(x_i) + f'(x_i)h + \\frac{f''(x_i)h^2}{2!} \\\\\n- \\\\\nf(x_{i-1}) & = f(x_i) - f'(x_i)h + \\frac{f''(x_i)h^2}{2!} \\\\\n\\hline \\\\\nf(x_{i+1}) - f(x_{i-1}) & = 2 f'(x_i)h\n\\end{split}\n\\label{eq:Ec5_4} \\tag{5.4}\n\\end{equation*}\n \nde la anterior ecuación, despejando el término que corresponde a la primera derivada queda:\n\n<a id='Ec5_5'></a>\n\\begin{equation*}\n\\begin{split}\nf'(x_i) = \\frac{f(x_{i+1}) - f(x_{i-1})}{2h} + \\mathcal{O}(h^2)\n\\end{split}\n\\label{eq:Ec5_5} \\tag{5.5}\n\\end{equation*}\n\nse llega al esquema de diferencias finitas central para la primera derivada, que es de orden dos, es decir, si se disminuye el tamaño de paso, $h$, a la mitad, el error se disminuye una cuarta partes. En principio, esta es una mejor aproximación que los dos esquemas anteriores. La selección del esquema dependerá de la disponibilidad de puntos y del fenómeno físico a tratar.",
"_____no_output_____"
],
[
"[Volver a la Tabla de Contenido](#TOC)",
"_____no_output_____"
],
[
"#### Resumen esquemas diferencias finitas para la primera derivada",
"_____no_output_____"
],
[
"Como la serie de Taylor es infinita, se podrían determinar infinitos esquemas de diferentes ordenes para la primera derivada. En la siguiente tabla se presentan algunos esquemas de diferencias finitas para la primera derivada de diferentes órdenes. Se deja al estudiante la consulta de otros esquemas.\n\n|***Esquema***|***Función***|***Error***|\n|:-----:|:-----:|:---:|\n|***Forward***|$$f´(x_0)=\\frac{f(x_0+h)-f(x_0)}{h}$$|$$\\mathcal{O}(h)$$|\n| |$$f´(x_0)=\\frac{-3f(x_0)+4f(x_0+h)-f(x_0+2h)}{2h}$$|$$\\mathcal{O}(h^2)$$|\n|***Central***|$$f´(x_0)=\\frac{f(x_0+h)-f(x_0-h)}{2h}$$|$$\\mathcal{O}(h^2)$$|\n| |$$f´(x_0)=\\frac{f(x_0-2h)-8f(x_0-h)+8f(x_0+h)-f(x_0+2h)}{12h}$$|$$\\mathcal{O}(h^4)$$|\n|***Backward***|$$f´(x_0)=\\frac{f(x_0)-f(x_0-h)}{h}$$|$$\\mathcal{O}(h)$$|\n| |$$f´(x_0)=\\frac{f(x_0-2h)-4f(x_0-h)+3f(x_0)}{2h}$$|$$\\mathcal{O}(h^2)$$|\n",
"_____no_output_____"
],
[
"[Volver a la Tabla de Contenido](#TOC)",
"_____no_output_____"
],
[
"### Esquemas de diferencias finitas para la segunda derivada",
"_____no_output_____"
],
[
"Siguiendo con la misma forma de abordar el problema para la primera derivada, si se amplian los términos en la serie de Taylor hasta la tercera derivada tanto hacia adelante como hacia atrás, y se suman, se llega a:\n\n\\begin{equation*}\n\\begin{split}\nf(x_{i+1}) & = f(x_i) + f'(x_i)h + \\frac{f''(x_i)h^2}{2!} + \\frac{f'''(x_i)h^3}{3!}\\\\\n+ \\\\\nf(x_{i-1}) & = f(x_i) - f'(x_i)h + \\frac{f''(x_i)h^2}{2!} - \\frac{f'''(x_i)h^3}{3!}\\\\\n\\hline \\\\\nf(x_{i+1}) + f(x_{i-1}) & = 2 f(x_i) + 2f''(x_i)\\frac{h^2}{2!} + \\mathcal{O}(h^3)\n\\end{split}\n\\label{eq:Ec5_6} \\tag{5.6}\n\\end{equation*}\n \nDespejando para el término de la segunda derivada, se llega a:\n\n<a id='Ec5_7'></a>\n\\begin{equation*}\n\\begin{split}\nf''(x_i) = \\frac{f(x_{i+1}) - 2f(x_i) + f(x_{i-1})}{h^2} + \\mathcal{O}(h^3)\n\\end{split}\n\\label{eq:Ec5_7} \\tag{5.7}\n\\end{equation*}\n\nQue corresponde a un esquema de diferencias finitas de segundo orden para la segunda derivada. A este esquema también se le llama \"*molécula de tres puntos*\"\n\nIgual que para la primera derivada, se pueden determinar infinitos esquemas de diferentes órdenes para la segunda derivada, y derivadas superiores. A continuación se muestra un cuadro resumen de algunos esquemas de diferencias finitas para la segunda derivada. Se deja al estudiante la revisión de esquemas de mayor orden para la segunda derivada y derivadas superiores.\n\n|***Esquema***|***Función***|***Error***|\n|:-----:|:-----:|:---:|\n|***Forward***|$$f''(x_0)=\\frac{f(x_0)-2f(x_0+h)+f(x_0+2h)}{h^2}$$|$$\\mathcal{O}(h)$$|\n| |$$f''(x_0)=\\frac{2f(x_0)-5f(x_0+h)+4f(x_0+2h)-f(x_0+3h)}{h^2}$$|$$\\mathcal{O}(h^2)$$|\n|***Central***|$$f''(x_0)=\\frac{f(x_0-h)-2f(x_0)+f(x_0+h)}{h^2}$$|$$\\mathcal{O}(h^2)$$|\n| |$$f''(x_0)=\\frac{-f(x_0-2h)+16f(x_0-h)-30f(x_0)+16f(x_0+h)-f(x_0+2h)}{12h^2}$$|$$\\mathcal{O}(h^4)$$|\n|***Backward***|$$f''(x_0)=\\frac{f(x_0-2h)-2f(x_0-h)+f(x_0)}{h}$$|$$\\mathcal{O}(h^2)$$|\n| |$$f''(x_0)=\\frac{-f(x_0-3h)+4f(x_0-2h)-5f(x_0-h)+2f(x_0)}{h^2}$$|$$\\mathcal{O}(h^2)$$|\n",
"_____no_output_____"
],
[
"[Volver a la Tabla de Contenido](#TOC)",
"_____no_output_____"
],
[
"### Implementación computacional de algunos esquemas de diferencias finitas",
"_____no_output_____"
],
[
"A manera de ejemplo, se implementarán algunos esquemas simples de diferencias finitas para la primera derivada. Se deja como actividad a los estudiantes la implementación de otros esquemas para las diferentes derivadas.",
"_____no_output_____"
]
],
[
[
"import numpy as np\nimport matplotlib.pyplot as plt\nimport sympy as sym\n\nsym.init_printing()",
"_____no_output_____"
],
[
"#Esquemas de diferencias finitas para la primera derivada\n\ndef df1df(x0, h):\n # Esquema de diferencias finitas para la primera derivada hacia adelante (forward)\n return (f(x0 + h) - f(x0)) / h\n\ndef df1db(x0, h):\n # Esquema de diferencias finitas para la primera derivada hacia atrás (backward)\n return (f(x0) - f(x0 - h) ) / h\n\ndef df1dc(x0,h):\n # Esquema de diferencias finitas para la primera derivada central (central)\n return (f(x0 + h) - f(x0 - h) ) / (2 * h)\n",
"_____no_output_____"
],
[
"#funcion a determinar el valor de la derivada\ndef f(x):\n return 2*x**3 - 3*x**2 + 5*x+0.8",
"_____no_output_____"
],
[
"#cálculo y evaluación de la primera derivada empleando cálculo simbólico\n\ndef df1de(x0):\n\n x = sym.Symbol('x')\n df = sym.diff(f(x), x)\n #print(df)\n df1 = df.evalf(subs={x:x0})\n return df1",
"_____no_output_____"
],
[
"h = 0.1\nx0 = 0.8\n\nprint(\"1st derivative \\t Value \\t\\t Error(%)\")\nprint('---------------------------------------')\n\npde = df1de(x0)\n\npdf = df1df(x0, h)\nepdf = abs((pde - pdf) / pde * 100) \nprint(\"forward \\t {0:6.4f} \\t {1:6.2f}\".format(pdf,epdf))\n\npdb = df1db(x0, h)\nepdb = abs((pde - pdb) / pde * 100) \nprint(\"backward \\t {0:6.4f} \\t {1:6.2f}\".format(pdb,epdb))\n\npdc = df1dc(x0,h)\nepdc = abs((pde - pdc) / pde * 100) \nprint(\"central \\t {0:6.4f} \\t {1:6.2f}\".format(pdc, epdc))\n\nprint(\"exacta \\t\\t {0:6.4f} \\t {1}\".format(pde, ' -'))",
"_____no_output_____"
]
],
[
[
"[Volver a la Tabla de Contenido](#TOC)",
"_____no_output_____"
],
[
"## Integración Numérica",
"_____no_output_____"
],
[
"### Introducción",
"_____no_output_____"
],
[
"La [integración numérica](https://en.wikipedia.org/wiki/Numerical_integration) aborda una amplia gama de algoritmos para determinar el valor numérico (aproximado) de una integral definida. En este curso nos centraremos principalmente en los métodos de cuadratura, tanto de interpolación como [gaussiana](https://en.wikipedia.org/wiki/Gaussian_quadrature), como dos ejemplos de dichos algoritmos. \n\nEl problema a tratar en este capítulo es la solución aproximada de la función\n\n<a id='Ec5_8'></a>\n\\begin{equation*}\n\\begin{split}\nI = \\int_a^b f(x) dx\n\\end{split}\n\\label{eq:Ec5_8} \\tag{5.8}\n\\end{equation*}\n",
"_____no_output_____"
],
[
"[Volver a la Tabla de Contenido](#TOC)",
"_____no_output_____"
],
[
"### Fórmulas de integración de *Newton - Cotes*",
"_____no_output_____"
],
[
"La idea básica en la integración numérica es cambiar una función difícil de integrar, $f(x)$, dada por la ecuación [(5.8)](#Ec5_8), por una función más simple, $p_n(x)$,\n\n<a id='Ec5_9'></a>\n\\begin{equation*}\n\\begin{split}\n\\widetilde{I} \\approx \\int_{a=x_0}^{b=x_n} p_{n}(x) dx\n\\end{split}\n\\label{eq:Ec5_9} \\tag{5.9}\n\\end{equation*}\n\nCabe resaltar que en integración numérica no se conocerá la función a integrar, solo se dispondrá de una serie de $n+1$ puntos $(x_i, y_i), i = 0, 1, 2, \\ldots, n$, y a partir de ellos se construye un polinomio interpolante de grado $n$, $p_n$, entre los valores de los límites de integración $a = x_0$ y $b=x_n$. $p_n(x)$ es un polinomio de interpolación de la forma\n\n<a id='Ec5_10'></a>\n\\begin{equation*}\n\\begin{split}\np_n(x)=a_0+a_1x+a_2x^2+\\ldots+a_{n-1}x^{n-1}+a_nx^n\n\\end{split}\n\\label{eq:Ec5_10} \\tag{5.10}\n\\end{equation*}\n\nLas fórmulas de integración de [*Newton - Cotes*](https://en.wikipedia.org/wiki/Newton%E2%80%93Cotes_formulas), también llamadas de <a id='Quadrature'></a>[cuadratura](https://en.wikipedia.org/wiki/Quadrature_(mathematics)), son un grupo de fórmulas de integración numérica de tipo interpolación, evaluando la función en puntos equidistantes, para determinar un valor aproximado de la integral. Si no se tienen puntos espaciados, otros métodos deben ser usados, como por ejemplo cuadratura gaussiana, que se verá al final del capítulo.\n\nLa forma general de las fórmulas de Newton - Cotes está dada por la función:\n\n<a id='Ec5_11'></a>\n\\begin{equation*}\n\\begin{split}\np_n(x)=\\sum \\limits_{i=0}^n f(x_i)L_{in}(x)\n\\end{split}\n\\label{eq:Ec5_11} \\tag{5.11}\n\\end{equation*}\n\ndonde\n\n<a id='Ec5_12'></a>\n\\begin{equation*}\n\\begin{split}\nL_{in}(x)=\\frac{(x-x_0)\\ldots(x-x_{i-1})(x-x_{i+1})\\ldots(x-x_n)}{(x_i-x_0)\\ldots(x_i-x_{i-1})(x_i-x_{i+1})\\ldots(x_i-x_n)}\n\\end{split}\n\\label{eq:Ec5_12} \\tag{5.12}\n\\end{equation*}\n\nes el polinomio de Lagrange, de donde se deduce que:\n\n<a id='Ec5_13'></a>\n\\begin{equation*}\n\\begin{split}\n\\int_a^b p(x)dx=(b-a)\\sum \\limits_{i=0}^n f(x_i) \\frac{1}{(b-a)} \\int_a^b L_{in}(x)dx\n\\end{split}\n\\label{eq:Ec5_13} \\tag{5.13}\n\\end{equation*}\n\nentonces,\n\n<a id='Ec5_14'></a>\n\\begin{equation*}\n\\begin{split}\n\\int_a^b f(x)dx \\approx \\int_a^b p(x)dx=(b-a)\\sum \\limits_{i=0}^n w_if(x_i) \n\\end{split}\n\\label{eq:Ec5_14} \\tag{5.14}\n\\end{equation*}\n\ndonde los pesos, $w_i$ de la función son representados por\n\n<a id='Ec5_15'></a>\n\\begin{equation*}\n\\begin{split}\nw_i=\\frac{1}{(b-a)} \\int_a^b L_{in}(x)dx\n\\end{split}\n\\label{eq:Ec5_15} \\tag{5.15}\n\\end{equation*}\n\nA partir de esta idea se obtienen los diferentes esquemas de integración numérica de *Newton - Cotes*",
"_____no_output_____"
],
[
"[Volver a la Tabla de Contenido](#TOC)",
"_____no_output_____"
],
[
"### Regla trapezoidal",
"_____no_output_____"
],
[
"#### Regla trapezoidal de aplicación simple",
"_____no_output_____"
],
[
"La [regla trapezoidal](https://en.wikipedia.org/wiki/Trapezoidal_rule) emplea una aproximación de la función mediante una línea recta\n\n<p float=\"center\">\n <img src=\"https://github.com/carlosalvarezh/Analisis_Numerico/blob/master/images/C05_Img03_TrapezoidalRule.PNG?raw=true\" width=\"250\" />\n</p>\n\n<div style=\"text-align: right\"> Fuente: <a href=\"https://upload.wikimedia.org/wikipedia/commons/4/40/Trapezoidal_rule_illustration.svg\">wikipedia.com</a> </div>\n\ny corresponde al caso en el que el polinomio en la ecuación [(5.11)](#Ec5_11) es de primer orden\n\n\n\\begin{equation*}\n\\begin{split}\nI=\\int_{a}^{b}f(x)dx \\approx \\int_a^b \\left[ f(a) + \\frac{f(b)-f(a)}{b-a}(x-a)\\right]dx\n= (b-a)\\frac{f(a)+f(b)}{2}\n\\end{split}\n\\label{eq:Ec5_16} \\tag{5.16}\n\\end{equation*}\n\nGeométricamente, es equivalente a aproximar el área del trapezoide bajo la línea recta que conecta $f(a)$ y $f(b)$. La integral se representa como:\n\n$$I ≈ \\text{ancho} \\times \\text{altura promedio}$$\n\nEl error en la regla trapezoidal simple se puede determinar como:\n\n\\begin{equation*}\n\\begin{split}\nE_t=-\\frac{1}{12}f''(\\xi)(b-a)^3\n\\end{split}\n\\label{eq:Ec5_17} \\tag{5.17}\n\\end{equation*}\n",
"_____no_output_____"
],
[
"[Volver a la Tabla de Contenido](#TOC)",
"_____no_output_____"
],
[
"#### Regla trapezoidal de aplicación múltiple",
"_____no_output_____"
],
[
"Una manera de mejorar la exactitud de la regla trapezoidal es dividir el intervalo de integración de $a$ a $b$ en un número $n$ de segmentos y aplicar el método a cada uno de ellos. Las ecuaciones resultantes son llamadas fórmulas de integración de múltiple aplicación o compuestas.\n\n<p float=\"center\">\n <img src=\"https://github.com/carlosalvarezh/Analisis_Numerico/blob/master/images/C05_Img04_TrapezoidalRuleMultiple.gif?raw=true\" width=\"350\" />\n</p>\n\n<div style=\"text-align: right\"> Fuente: <a href=\"https://en.wikipedia.org/wiki/Trapezoidal_rule#/media/File:Trapezium2.gif\">wikipedia.com</a> </div>\n\nHay $n+1$ puntos base igualmente espaciados $(x_0, x_1, x_2, \\ldots, x_n)$. En consecuencia hay $n$ segmentos de igual anchura: $h = (b–a) / n$. Si $a$ y $b$ son designados como $x_0$ y $x_n$ respectivamente, la integral total se representará como:\n\n\\begin{equation*}\n\\begin{split}\nI=\\int_{x_0}^{x_1}f(x)dx+\\int_{x_1}^{x_2}f(x)dx+\\int_{x_2}^{x_3}f(x)dx+\\ldots+\\int_{x_{n-2}}^{x_{n-1}}f(x)dx+\\int_{x_{n-1}}^{x_n}f(x)dx\n\\end{split}\n\\label{eq:Ec5_18} \\tag{5.18}\n\\end{equation*}\n\nAl sustituir la regla trapezoidal simple en cada integrando, se tiene\n\n\\begin{equation*}\n\\begin{split}\nI\\approx \\left(f(x_0)+f(x_1)\\right)\\frac{h}{2}+\\left(f(x_1)+f(x_2)\\right)\\frac{h}{2}+\\left(f(x_2)+f(x_3)\\right)\\frac{h}{2}+\\ldots\\left(f(x_{n-2})+f(x_{n-1})\\right)\\frac{h}{2}+\\left(f(x_{n-1})+f(x_n)\\right)\\frac{h}{2}\n\\end{split}\n\\label{eq:Ec5_19} \\tag{5.19}\n\\end{equation*}\n\nahora, agrupando términos\n\n\\begin{equation*}\n\\begin{split}\nI\\approx \\frac{h}{2}\\left[ f(x_0) + 2\\sum_{i=1}^{n-1}f(x_i)+f(x_n) \\right]\n\\end{split}\n\\label{eq:Ec5_20} \\tag{5.20}\n\\end{equation*}\n\ndonde $h=(b-a)/n$",
"_____no_output_____"
],
[
"[Volver a la Tabla de Contenido](#TOC)",
"_____no_output_____"
],
[
"#### Implementación computacional",
"_____no_output_____"
]
],
[
[
"import numpy as np\nimport matplotlib.pyplot as plt",
"_____no_output_____"
],
[
"def trapezoidal(x):\n n = len(x)\n h = (x[-1] - x[0]) / n\n \n suma = 0\n for i in range(1, n-1):\n suma += funcion(x[i])\n \n return h * (funcion(x[0]) + 2 * suma + funcion(x[-1])) / 2",
"_____no_output_____"
],
[
"def funcion(x):\n return 4 / (1 + x**2)",
"_____no_output_____"
],
[
"a = 0\nb = 1\nn = 1000\nx = np.linspace(a, b, n+1)\nI = trapezoidal(x)\nI",
"_____no_output_____"
]
],
[
[
"[Volver a la Tabla de Contenido](#TOC)",
"_____no_output_____"
],
[
"#### Error en la aplicación de la regla trapezoidal",
"_____no_output_____"
],
[
"Recordando que estos esquemas provienen de la serie truncada de Taylor, el error se puede obtener determinando el primer término truncado en el esquema, que para la regla trapezoidal de aplicación simple corresponde a:\n\n\\begin{equation*}\n\\begin{split}\nE_t=-\\frac{1}{12}f''(\\xi)(b-a)^3\n\\end{split}\n\\label{eq:Ec5_21} \\tag{5.21}\n\\end{equation*}\n\ndonde $f''(\\xi)$ es la segunda derivada en el punto $\\xi$ en el intervalo $[a,b]$, y $\\xi$ es un valor que maximiza la evaluación de esta segunda derivada. \n\nGeneralizando este concepto a la aplicación múltiple de la regla trapezoidal, se pueden sumar cada uno de los errores en cada segmento para dar:\n\n\\begin{equation*}\n\\begin{split}\nE_t=-\\frac{(b-a)^3}{12n^3}\\sum\\limits_{i=1}^n f''(\\xi_i)\n\\end{split}\n\\label{eq:Ec5_22} \\tag{5.22}\n\\end{equation*}\n\nel anterior resultado se puede simplificar estimando la media, o valor promedio, de la segunda derivada para todo el intervalo\n\n<a id='Ec5_23'></a>\n\\begin{equation*}\n\\begin{split}\n\\bar{f''} \\approx \\frac{\\sum \\limits_{i=1}^n f''(\\xi_i)}{n}\n\\end{split}\n\\label{eq:Ec5_23} \\tag{5.23}\n\\end{equation*}\n\nde esta ecuación se tiene que $\\sum f''(\\xi_i)\\approx nf''$, y reemplazando en la ecuación [(5.23)](#Ec5_23)\n\n\\begin{equation*}\n\\begin{split}\nE_t \\approx \\frac{(b-a)^3}{12n^2}\\bar{f''}\n\\end{split}\n\\label{eq:Ec5_24} \\tag{5.24}\n\\end{equation*}\n\nDe este resultado se observa que si se duplica el número de segmentos, el error de truncamiento se disminuirá a una cuarta parte.",
"_____no_output_____"
],
[
"[Volver a la Tabla de Contenido](#TOC)",
"_____no_output_____"
],
[
"### Reglas de Simpson",
"_____no_output_____"
],
[
"Las [reglas de Simpson](https://en.wikipedia.org/wiki/Simpson%27s_rule) son esquemas de integración numérica en honor al matemático [*Thomas Simpson*](https://en.wikipedia.org/wiki/Thomas_Simpson), utilizado para obtener la aproximación de la integral empleando interpolación polinomial sustituyendo a $f(x)$. \n",
"_____no_output_____"
],
[
"[Volver a la Tabla de Contenido](#TOC)",
"_____no_output_____"
],
[
"#### Regla de Simpson1/3 de aplicación simple",
"_____no_output_____"
],
[
"La primera regla corresponde a una interpolación polinomial de segundo orden sustituida en la ecuación [(5.8)](#Ec5_8)\n\n<p float=\"center\">\n <img src=\"https://github.com/carlosalvarezh/Analisis_Numerico/blob/master/images/C05_Img05_SimpsonRule13.PNG?raw=true\" width=\"350\" />\n</p>\n\n<div style=\"text-align: right\"> Fuente: <a href=\"https://upload.wikimedia.org/wikipedia/commons/c/ca/Simpsons_method_illustration.svg\">wikipedia.com</a> </div>\n\n\n\\begin{equation*}\n\\begin{split}\nI=\\int_a^b f(x)dx \\approx \\int_a^b p_2(x)dx\n\\end{split}\n\\label{eq:Ec5_25} \\tag{5.25}\n\\end{equation*}\n\ndel esquema de interpolación de Lagrange para un polinomio de segundo grado, visto en el capitulo anterior, y remplazando en la integral arriba, se llega a \n\n\\begin{equation*}\n\\begin{split}\nI\\approx\\int_{x0}^{x2} \\left[\\frac{(x-x_1)(x-x_2)}{(x_0-x_1)(x_0-x_2)}f(x_0)+\\frac{(x-x_0)(x-x_2)}{(x_1-x_0)(x_1-x_2)}f(x_1)+\\frac{(x-x_0)(x-x_1)}{(x_2-x_0)(x_2-x_1)}f(x_2)\\right]dx\n\\end{split}\n\\label{eq:Ec5_26} \\tag{5.26}\n\\end{equation*}\n\nrealizando la integración de forma analítica y un manejo algebraico, resulta\n\n\\begin{equation*}\n\\begin{split}\nI\\approx\\frac{h}{3} \\left[ f(x_0)+4f(x_1)+f(x_2)\\right]\n\\end{split}\n\\label{eq:Ec5_27} \\tag{5.27}\n\\end{equation*}\n\ndonde $h=(b-a)/2$ y los $x_{i+1} = x_i + h$",
"_____no_output_____"
],
[
"A continuación, vamos a comparar graficamente las funciones \"exacta\" (con muchos puntos) y una aproximada empleando alguna técnica de interpolación para $n=3$ puntos (Polinomio interpolante de orden $2$).",
"_____no_output_____"
]
],
[
[
"from scipy.interpolate import barycentric_interpolate\n\n# usaremos uno de los tantos métodos de interpolación dispobibles en las bibliotecas de Python\n\nn = 3 # puntos a interpolar para un polinomio de grado 2\n\nxp = np.linspace(a,b,n) # generación de n puntos igualmente espaciados para la interpolación\nfp = funcion(xp) # evaluación de la función en los n puntos generados\nx = np.linspace(a, b, 100) # generación de 100 puntos igualmente espaciados\ny = barycentric_interpolate(xp, fp, x) # interpolación numérica empleando el método del Baricentro\n\nfig = plt.figure(figsize=(9, 6), dpi= 80, facecolor='w', edgecolor='k')\nax = fig.add_subplot(111)\n\nl, = plt.plot(x, y)\nplt.plot(x, funcion(x), '-', c='red')\nplt.plot(xp, fp, 'o', c=l.get_color())\nplt.annotate('Función \"Real\"', xy=(.63, 1.5), xytext=(0.8, 1.25),arrowprops=dict(facecolor='black', shrink=0.05),)\nplt.annotate('Función interpolada', xy=(.72, 1.75), xytext=(0.4, 2),arrowprops=dict(facecolor='black', shrink=0.05),)\nplt.grid(True) # muestra la malla de fondo\nplt.show() # muestra la gráfica",
"_____no_output_____"
]
],
[
[
"Se observa que hay una gran diferencia entre las áreas que se estarían abarcando en la función llamada \"*real*\" (que se emplearon $100$ puntos para su generación) y la función *interpolada* (con únicamente $3$ puntos para su generación) que será la empleada en la integración numérica (aproximada) mediante la regla de *Simpson $1/3$*.\n\nConscientes de esto, procederemos entonces a realizar el cálculo del área bajo la curva del $p_3(x)$ empleando el método de *Simpson $1/3$*",
"_____no_output_____"
],
[
"Creemos un programa en *Python* para que nos sirva para cualquier función $f(x)$ que queramos integrar en cualquier intervalo $[a,b]$ empleando la regla de integración de *Simpson $1/3$*:",
"_____no_output_____"
]
],
[
[
"# se ingresan los valores del intervalo [a,b]\na = float(input('Ingrese el valor del límite inferior: '))\nb = float(input('Ingrese el valor del límite superior: '))",
"_____no_output_____"
],
[
"# cuerpo del programa por la regla de Simpson 1/3\nh = (b-a)/2 # cálculo del valor de h\n\nx0 = a # valor del primer punto para la fórmula de S1/3 \nx1 = x0 + h # Valor del punto intermedio en la fórmula de S1/3\nx2 = b # valor del tercer punto para la fórmula de S1/3 \n\nfx0 = funcion(x0) # evaluación de la función en el punto x0\nfx1 = funcion(x1) # evaluación de la función en el punto x1\nfx2 = funcion(x2) # evaluación de la función en el punto x2\n\nint_S13 = h / 3 * (fx0 + 4*fx1 + fx2)\n\n#erel = np.abs(exacta - int_S13) / exacta * 100\n\nprint('el valor aproximado de la integral por la regla de Simpson1/3 es: ', int_S13, '\\n')\n#print('el error relativo entre el valor real y el calculado es: ', erel,'%')",
"_____no_output_____"
]
],
[
[
"[Volver a la Tabla de Contenido](#TOC)",
"_____no_output_____"
],
[
"#### Error en la regla de Simpson 1/3 de aplicación simple",
"_____no_output_____"
],
[
"El problema de calcular el error de esta forma es que realmente no conocemos el valor exacto. Para poder calcular el error al usar la regla de *Simpson 1/3*:\n\n\\begin{equation*}\n\\begin{split}\n-\\frac{h^5}{90}f^{(4)}(\\xi)\n\\end{split}\n\\label{eq:Ec5_28} \\tag{5.28}\n\\end{equation*}\n\nserá necesario derivar cuatro veces la función original: $f(x)=e^{x^2}$. Para esto, vamos a usar nuevamente el cálculo simbólico (siempre deben verificar que la respuesta obtenida es la correcta!!!):",
"_____no_output_____"
]
],
[
[
"from sympy import *\nx = symbols('x')",
"_____no_output_____"
]
],
[
[
"Derivamos cuatro veces la función $f(x)$ con respecto a $x$:",
"_____no_output_____"
]
],
[
[
"deriv4 = diff(4 / (1 + x**2),x,4)\nderiv4",
"_____no_output_____"
]
],
[
[
"y evaluamos esta función de la cuarta derivada en un punto $0 \\leq \\xi \\leq 1$. Como la función $f{^{(4)}}(x)$ es creciente en el intervalo $[0,1]$ (compruébelo gráficamente y/o por las técnicas vistas en cálculo diferencial), entonces, el valor que hace máxima la cuarta derivada en el intervalo dado es:",
"_____no_output_____"
]
],
[
[
"x0 = 1.0\nevald4 = deriv4.evalf(subs={x: x0})\nprint('El valor de la cuarta derivada de f en x0={0:6.2f} es {1:6.4f}: '.format(x0, evald4))",
"_____no_output_____"
]
],
[
[
"Calculamos el error en la regla de *Simpson$1/3$*",
"_____no_output_____"
]
],
[
[
"errorS13 = abs(h**5*evald4/90)\nprint('El error al usar la regla de Simpson 1/3 es: {0:6.6f}'.format(errorS13))",
"_____no_output_____"
]
],
[
[
"Entonces, podemos expresar el valor de la integral de la función $f(x)=e^{x^2}$ en el intervalo $[0,1]$ usando la *Regla de Simpson $1/3$* como:\n\n<div class=\"alert alert-block alert-warning\">\n$$\\color{blue}{\\int_0^1 \\frac{4}{1 + x^2}dx} = \\color{green}{3,133333} \\color{red}{+ 0.004167}$$\n</div>",
"_____no_output_____"
],
[
"Si lo fuéramos a hacer \"a mano\" $\\ldots$ aplicando la fórmula directamente, con los siguientes datos:\n\n$h = \\frac{(1.0 - 0.0)}{2.0} = 0.5$\n\n$x_0 = 0.0$\n\n$x_1 = 0.5$\n\n$x_2 = 1.0$\n\n$f(x) = \\frac{4}{1 + x^2}$\n\nsustituyendo estos valores en la fórmula dada:\n\n\n$\\int_0^1\\frac{4}{1 + x^2}dx \\approx \\frac{0.5}{3} \\left[f(0)+4f(0.5)+f(1)\\right]$\n\n$\\int_0^1\\frac{4}{1 + x^2}dx \\approx \\frac{0.5}{3} \\left[ \\frac{4}{1 + 0^2} + 4\\frac{4}{1 + 0.5^2} + \\frac{4}{1 + 1^2} \\right] \\approx 3.133333$",
"_____no_output_____"
],
[
"[Volver a la Tabla de Contenido](#TOC)",
"_____no_output_____"
],
[
"#### Regla de simpson1/3 de aplicación múltiple",
"_____no_output_____"
],
[
"Al igual que en la regla Trapezoidal, las reglas de Simpson también cuentan con un esquema de aplicación múltiple (llamada también compuesta). Supongamos que se divide el intervalo $[a,b]$ se divide en $n$ sub intervalos, con $n$ par, quedando la integral\n\n\\begin{equation*}\n\\begin{split}\nI=\\int_{x_0}^{x_2}f(x)dx+\\int_{x_2}^{x_4}f(x)dx+\\ldots+\\int_{x_{n-2}}^{x_n}f(x)dx\n\\end{split}\n\\label{eq:Ec5_29} \\tag{5.29}\n\\end{equation*}\n\ny sustituyendo en cada una de ellas la regla de Simpson1/3, se llega a\n\n\\begin{equation*}\n\\begin{split}\nI \\approx 2h\\frac{f(x_0)+4f(x_1)+f(x_2)}{6}+2h\\frac{f(x_2)+4f(x_3)+f(x_4)}{6}+\\ldots+2h\\frac{f(x_{n-2})+4f(x_{n-1})+f(x_n)}{6}\n\\end{split}\n\\label{eq:Ec5_30} \\tag{5.30}\n\\end{equation*}\n\n\nentonces la regla de Simpson compuesta (o de aplicación múltiple) se escribe como:\n\n\\begin{equation*}\n\\begin{split}\nI=\\int_a^bf(x)dx\\approx \\frac{h}{3}\\left[f(x_0) + 2 \\sum \\limits_{j=1}^{n/2-1} f(x_{2j}) + 4 \\sum \\limits_{j=1}^{n/2} f(x_{2j-1})+f(x_n)\\right]\n\\end{split}\n\\label{eq:Ec5_31} \\tag{5.31}\n\\end{equation*}\n\ndonde $x_j=a+jh$ para $j=0,1,2, \\ldots, n-1, n$ con $h=(b-a)/n$, $x_0=a$ y $x_n=b$.",
"_____no_output_____"
],
[
"[Volver a la Tabla de Contenido](#TOC)",
"_____no_output_____"
],
[
"#### Implementación computacional regla de Simpson1/3 de aplicación múltiple",
"_____no_output_____"
],
[
"[Volver a la Tabla de Contenido](#TOC)",
"_____no_output_____"
],
[
"#### Regla de Simpson 3/8 de aplicación simple",
"_____no_output_____"
],
[
"Resulta cuando se sustituye la función $f(x)$ por una interpolación de tercer orden:\n\n\\begin{equation*}\n\\begin{split}\nI=\\int_{a}^{b}f(x)dx = \\frac{3h}{8}\\left[ f(x_0)+3f(x_1)+3f(x_2)+f(x_3) \\right]\n\\end{split}\n\\label{eq:Ec5_32} \\tag{5.32}\n\\end{equation*}\n",
"_____no_output_____"
],
[
"Realizando un procedimiento similar al usado para la regla de *Simpson $1/3$*, pero esta vez empleando $n=4$ puntos:",
"_____no_output_____"
]
],
[
[
"# usaremos uno de los tantos métodos de interpolación dispobibles en las bibliotecas de Python\n\nn = 4 # puntos a interpolar para un polinomio de grado 2\n\nxp = np.linspace(0,1,n) # generación de n puntos igualmente espaciados para la interpolación\nfp = funcion(xp) # evaluación de la función en los n puntos generados\nx = np.linspace(0, 1, 100) # generación de 100 puntos igualmente espaciados\ny = barycentric_interpolate(xp, fp, x) # interpolación numérica empleando el método del Baricentro\n\nfig = plt.figure(figsize=(9, 6), dpi= 80, facecolor='w', edgecolor='k')\nax = fig.add_subplot(111)\n\nl, = plt.plot(x, y)\nplt.plot(x, funcion(x), '-', c='red')\nplt.plot(xp, fp, 'o', c=l.get_color())\nplt.annotate('\"Real\"', xy=(.63, 1.5), xytext=(0.8, 1.25),arrowprops=dict(facecolor='black', shrink=0.05),)\nplt.annotate('Interpolación', xy=(.72, 1.75), xytext=(0.4, 2),arrowprops=dict(facecolor='black', shrink=0.05),)\nplt.grid(True) # muestra la malla de fondo\nplt.show() # muestra la gráfica",
"_____no_output_____"
],
[
"# cuerpo del programa por la regla de Simpson 3/8\nh = (b - a) / 3 # cálculo del valor de h\n\nint_S38 = 3 * h / 8 * (funcion(a) + 3*funcion(a + h) + 3*funcion(a + 2*h) + funcion(a + 3*h))\n\nerel = np.abs(np.pi - int_S38) / np.pi * 100\n\nprint('el valor aproximado de la integral utilizando la regla de Simpson 3/8 es: ', int_S38, '\\n')\nprint('el error relativo entre el valor real y el calculado es: ', erel,'%')",
"_____no_output_____"
]
],
[
[
"Para poder calcular el error al usar la regla de *Simpson 3/8*:\n\n<div class=\"alert alert-block alert-warning\">\n$$\\color{red}{-\\frac{3h^5}{80}f^{(4)}(\\xi)}$$\n</div>\n\nserá necesario derivar cuatro veces la función original. Para esto, vamos a usar nuevamente el cálculo simbólico (siempre deben verificar que la respuesta obtenida es la correcta!!!):",
"_____no_output_____"
]
],
[
[
"errorS38 = 3*h**5*evald4/80\nprint('El error al usar la regla de Simpson 3/8 es: ',errorS38)",
"_____no_output_____"
]
],
[
[
"Entonces, podemos expresar el valor de la integral de la función $f(x)=e^{x^2}$ en el intervalo $[0,1]$ usando la *Regla de Simpson $3/8$* como:\n\n<div class=\"alert alert-block alert-warning\">\n$$\\color{blue}{\\int_0^1\\frac{4}{1 + x^2}dx} = \\color{green}{3.138462} \\color{red}{- 0.001852}$$\n</div>",
"_____no_output_____"
],
[
"Aplicando la fórmula directamente, con los siguientes datos:\n\n$h = \\frac{(1.0 - 0.0)}{3.0} = 0.33$\n\n$x_0 = 0.0$, $x_1 = 0.33$, $x_2 = 0.66$, $x_3 = 1.00$\n\n$f(x) = \\frac{4}{1 + x^2}$\n\nsustituyendo estos valores en la fórmula dada:\n\n$\\int_0^1\\frac{4}{1 + x^2}dx \\approx \\frac{3\\times0.3333}{8} \\left[ \\frac{4}{1 + 0^2} + 3\\frac{4}{1 + 0.3333^2} +3\\frac{4}{1 + 0.6666^2} + \\frac{4}{1 + 1^2} \\right] \\approx 3.138462$\n\n\nEsta sería la respuesta si solo nos conformamos con lo que podemos hacer usando word...",
"_____no_output_____"
],
[
"[Volver a la Tabla de Contenido](#TOC)",
"_____no_output_____"
],
[
"#### Regla de Simpson3/8 de aplicación múltiple",
"_____no_output_____"
],
[
"Dividiendo el intervalo $[a,b]$ en $n$ sub intervalos de longitud $h=(b-a)/n$, con $n$ múltiplo de 3, quedando la integral\n\n\\begin{equation*}\n\\begin{split}\nI=\\int_{x_0}^{x_3}f(x)dx+\\int_{x_3}^{x_6}f(x)dx+\\ldots+\\int_{x_{n-3}}^{x_n}f(x)dx\n\\end{split}\n\\label{eq:Ec5_33} \\tag{5.33}\n\\end{equation*}\n\nsustituyendo en cada una de ellas la regla de Simpson3/8, se llega a\n\n\\begin{equation*}\n\\begin{split}\nI=\\int_a^bf(x)dx\\approx \\frac{3h}{8}\\left[f(x_0) + 3 \\sum \\limits_{i=0}^{n/3-1} f(x_{3i+1}) + 3 \\sum \\limits_{i=0}^{n/3-1}f(x_{3i+2})+2 \\sum \\limits_{i=0}^{n/3-2} f(x_{3i+3})+f(x_n)\\right]\n\\end{split}\n\\label{eq:Ec5_34} \\tag{5.34}\n\\end{equation*}\n\ndonde en cada sumatoria se deben tomar los valores de $i$ cumpliendo que $i=i+3$.",
"_____no_output_____"
],
[
"[Volver a la Tabla de Contenido](#TOC)",
"_____no_output_____"
],
[
"#### Implementación computacional de la regla de Simpson3/8 de aplicación múltiple",
"_____no_output_____"
]
],
[
[
"# ",
"_____no_output_____"
]
],
[
[
"[Volver a la Tabla de Contenido](#TOC)",
"_____no_output_____"
],
[
"### Cuadratura de Gauss",
"_____no_output_____"
],
[
"#### Introducción",
"_____no_output_____"
],
[
"Retomando la idea inicial de los esquemas de [cuadratura](#Quadrature), el valor de la integral definida se estima de la siguiente manera:\n\n<a id='Ec5_35'></a>\n\\begin{equation*}\n\\begin{split}\nI=\\int_a^b f(x)dx \\approx \\sum \\limits_{i=0}^n c_if(x_i)\n\\end{split}\n\\label{eq:Ec5_35} \\tag{5.35}\n\\end{equation*}\n\nHasta ahora hemos visto los métodos de la regla trapezoidal y las reglas de Simpson más empleadas. En estos esquemas, la idea central es la distribución uniforme de los puntos que siguen la regla $x_i=x_0+ih$, con $i=0,1,2, \\ldots, n$ y la evaluación de la función en estos puntos.\n\nSupongamos ahora que la restricción de la uniformidad en el espaciamiento de esos puntos fijos no es más considerada y se tiene la libertad de evaluar el área bajo una recta que conecte a dos puntos cualesquiera sobre la curva. Al ubicar estos puntos en forma “inteligente”, se puede definir una línea recta que equilibre los errores negativos y positivos\n\n<p float=\"center\">\n <img src=\"https://github.com/carlosalvarezh/Analisis_Numerico/blob/master/images/C05_Img06_GQ01.PNG?raw=true\" width=\"500\" />\n</p>\n\n<div style=\"text-align: right\"> Fuente: <a href=\"http://artemisa.unicauca.edu.co/~cardila/Chapra.pdf\">Chapra, S., Canale, R. Métodos Numéricos para ingenieros, 5a Ed. Mc. Graw Hill. 2007</a> </div>\n\nDe la figura de la derecha, se disponen de los puntos $x_0$ y $x_1$ para evaluar la función $f(x)$. Expresando la integral bajo la curva de forma aproximada dada en la la ecuación ([5.35](#Ec5_35)), y empleando los límites de integración en el intervalo $[-1,1]$ por simplicidad (después se generalizará el concepto a un intervalo $[a,b]$), se tiene\n\n<a id='Ec5_36'></a>\n\\begin{equation*}\n\\begin{split}\nI=\\int_{-1}^1 f(x)dx \\approx c_0f(x_0)+c_1f(x_1)\n\\end{split}\n\\label{eq:Ec5_36} \\tag{5.36}\n\\end{equation*}\n",
"_____no_output_____"
],
[
"[Volver a la Tabla de Contenido](#TOC)",
"_____no_output_____"
],
[
"#### Determinación de los coeficientes",
"_____no_output_____"
],
[
"se tiene una ecuación con cuatro incógnitas ($c_0, c_1, x_0$ y $x_1$) que se deben determinar. Para ello, supongamos que disponemos de un polinomio de hasta grado 3, $f_3(x)$, de donde podemos construir cuatro ecuaciones con cuatro incógnitas de la siguiente manera:\n\n- $f_3(x)=1$:\n\n<a id='Ec5_37'></a>\n\\begin{equation*}\n\\begin{split}\n\\int_{-1}^1 1dx = c_0 \\times 1 + c_1 \\times 1 = c_0 + c_1 = 2\n\\end{split}\n\\label{eq:Ec5_37} \\tag{5.37}\n\\end{equation*}\n\n- $f_3(x)=x$:\n\n<a id='Ec5_38'></a>\n\\begin{equation*}\n\\begin{split}\n\\int_{-1}^1 xdx = c_0x_0 + c_1x_1 = 0\n\\end{split}\n\\label{eq:Ec5_38} \\tag{5.38}\n\\end{equation*}\n\n- $f_3(x)=x^2$:\n\n<a id='Ec5_39'></a>\n\\begin{equation*}\n\\begin{split}\n\\int_{-1}^1 x^2dx = c_0x^2_0 + c_1x^2_1 = \\frac{2}{3}\n\\end{split}\n\\label{eq:Ec5_39} \\tag{5.39}\n\\end{equation*}\n\ny por último\n\n- $f_3(x)=x^3$:\n\n<a id='Ec5_40'></a>\n\\begin{equation*}\n\\begin{split}\n\\int_{-1}^1 x^3dx = c_0x^3_0 + c_1x^3_1 = 0\n\\end{split}\n\\label{eq:Ec5_40} \\tag{5.40}\n\\end{equation*}\n\nresolviendo simultáneamente las dos primeras ecuaciones para $c_0$ y $c_1$ en térm,inos de $x_0$ y $x_1$, se llega a\n\n<a id='Ec5_41'></a>\n\\begin{equation*}\n\\begin{split}\nc_0=\\frac{2x_1}{x_1-x_0}, \\quad c_1=-\\frac{2x_0}{x_1-x_0}\\end{split}\n\\label{eq:Ec5_41} \\tag{5.41}\n\\end{equation*}\n\nreemplazamos estos dos valores en las siguientes dos ecuaciones\n\n<a id='Ec5_42'></a>\n\\begin{equation*}\n\\begin{split}\n\\frac{2}{3}=\\frac{2x_0^2x_1}{x_1-x_0}-\\frac{2x_0x_1^2}{x_1-x_0}\n\\end{split}\n\\label{eq:Ec5_42} \\tag{5.42}\n\\end{equation*}\n\n<a id='Ec5_43'></a>\n\\begin{equation*}\n\\begin{split}\n0=\\frac{2x_0^3x_1}{x_1-x_0}-\\frac{2x_0x_1^3}{x_1-x_0}\n\\end{split}\n\\label{eq:Ec5_43} \\tag{5.43}\n\\end{equation*}\n\nde la ecuación ([5.43](#Ec5_43)) se tiene\n\n<a id='Ec5_44'></a>\n\\begin{equation*}\n\\begin{split}\nx_0^3x_1&=x_0x_1^3 \\\\\nx_0^2 &= x_1^2\n\\end{split}\n\\label{eq:Ec5_44} \\tag{5.44}\n\\end{equation*}\n\nde aquí se tiene que $|x_0|=|x_1|$ (para considerar las raíces negativas recuerde que $\\sqrt{a^2}= \\pm a = |a|$), y como se asumió que $x_0<x_1$, entonces $x_0<0$ y $x_1>0$ (trabajando en el intervalo $[-1,1]$), llegándose finalmente a que $x_0=-x_1$. Reemplazando este resultado en la ecuación ([5.42](#Ec5_42))\n\n<a id='Ec5_45'></a>\n\\begin{equation*}\n\\begin{split}\n\\frac{2}{3}=2\\frac{x_1^3+x_1^3}{2x_1}\n\\end{split}\n\\label{eq:Ec5_45} \\tag{5.45}\n\\end{equation*}\n\ndespejando, $x_1^2=1/3$, y por último se llega a que\n\n<a id='Ec5_46'></a>\n\\begin{equation*}\n\\begin{split}\nx_0=-\\frac{\\sqrt{3}}{3}, \\quad x_1=\\frac{\\sqrt{3}}{3}\n\\end{split}\n\\label{eq:Ec5_46} \\tag{5.46}\n\\end{equation*}\n\nreemplazando estos resultados en la ecuación ([5.41](#Ec5_41)) y de la ecuación ([5.37](#Ec5_37)), se tiene que $c_0=c_1=1$. Reescribiendo la ecuación ([5.36](#Ec5_36)) con los valores encontrados se llega por último a:\n\n<a id='Ec5_47'></a>\n\\begin{equation*}\n\\begin{split}\nI=\\int_{-1}^1 f(x)dx &\\approx c_0f(x_0)+c_1f(x_1) \\\\\n&= f \\left( \\frac{-\\sqrt{3}}{3}\\right)+f \\left( \\frac{\\sqrt{3}}{3}\\right)\n\\end{split}\n\\label{eq:Ec5_47} \\tag{5.47}\n\\end{equation*}\n\n\nEsta aproximación realizada es \"exacta\" para polinomios de grado menor o igual a tres ($3$). La aproximación trapezoidal es exacta solo para polinomios de grado uno ($1$).\n\n***Ejemplo:*** Calcule la integral de la función $f(x)=x^3+2x^2+1$ en el intervalo $[-1,1]$ empleando tanto las técnicas analíticas como la cuadratura de Gauss vista.\n\n\n- ***Solución analítica (exacta)***\n\n$$\\int_{-1}^1 (x^3+2x^2+1)dx=\\left.\\frac{x^4}{4}+\\frac{2x^3}{3}+x \\right |_{-1}^1=\\frac{10}{3}$$\n\n\n- ***Aproximación numérica por Cuadratura de Gauss***\n\n\\begin{equation*}\n\\begin{split}\n\\int_{-1}^1 (x^3+2x^2+1)dx &\\approx1f\\left(-\\frac{\\sqrt{3}}{3} \\right)+1f\\left(\\frac{\\sqrt{3}}{3} \\right) \\\\\n&=-\\frac{3\\sqrt{3}}{27}+\\frac{2\\times 3}{9}+1+\\frac{3\\sqrt{3}}{27}+\\frac{2\\times 3}{9}+1 \\\\\n&=2+\\frac{4}{3} \\\\\n&= \\frac{10}{3}\n\\end{split}\n\\end{equation*}\n",
"_____no_output_____"
],
[
"[Volver a la Tabla de Contenido](#TOC)",
"_____no_output_____"
],
[
"#### Cambios de los límites de integración",
"_____no_output_____"
],
[
"Obsérvese que los límites de integración de la ecuación ([5.47](#Ec5_47)) son de $-1$ a $1$. Esto se hizo para simplificar las matemáticas y para hacer la formulación tan general como fuera posible. Asumamos ahora que se desea determinar el valor de la integral entre dos límites cualesquiera $a$ y $b$. Supongamos también, que una nueva variable $x_d$ se relaciona con la variable original $x$ de forma lineal,\n\n<a id='Ec5_48'></a>\n\\begin{equation*}\n\\begin{split}\nx=a_0+a_1x_d\n\\end{split}\n\\label{eq:Ec5_48} \\tag{5.48}\n\\end{equation*}\n\nsi el límite inferior, $x=a$, corresponde a $x_d=-1$, estos valores podrán sustituirse en la ecuación ([5.48](#Ec5_48)) para obtener\n\n<a id='Ec5_49'></a>\n\\begin{equation*}\n\\begin{split}\na=a_0+a_1(-1)\n\\end{split}\n\\label{eq:Ec5_49} \\tag{5.49}\n\\end{equation*}\n\nde manera similar, el límite superior, $x=b$, corresponde a $x_d=1$, para dar\n\n<a id='Ec5_50'></a>\n\\begin{equation*}\n\\begin{split}\nb=a_0+a_1(1)\n\\end{split}\n\\label{eq:Ec5_50} \\tag{5.50}\n\\end{equation*}\n\nresolviendo estas ecuaciones simultáneamente,\n\n<a id='Ec5_51'></a>\n\\begin{equation*}\n\\begin{split}\na_0=(b+a)/2, \\quad a_1=(b-a)/2\n\\end{split}\n\\label{eq:Ec5_51} \\tag{5.51}\n\\end{equation*}\n\nsustituyendo en la ecuación ([5.48](#Ec5_48))\n\n<a id='Ec5_52'></a>\n\\begin{equation*}\n\\begin{split}\nx=\\frac{(b+a)+(b-a)x_d}{2}\n\\end{split}\n\\label{eq:Ec5_52} \\tag{5.52}\n\\end{equation*}\n\nderivando la ecuación ([5.52](#Ec5_52)),\n\n<a id='Ec5_53'></a>\n\\begin{equation*}\n\\begin{split}\ndx=\\frac{b-a}{2}dx_d\n\\end{split}\n\\label{eq:Ec5_53} \\tag{5.53}\n\\end{equation*}\n\nLas ecuacio es ([5.51](#Ec5_51)) y ([5.52](#Ec5_52)) se pueden sustituir para $x$ y $dx$, respectivamente, en la evaluación de la integral. Estas sustituciones transforman el intervalo de integración sin cambiar el valor de la integral. En este caso\n\n<a id='Ec5_54'></a>\n\\begin{equation*}\n\\begin{split}\n\\int_a^b f(x)dx = \\frac{b-a}{2} \\int_{-1}^1 f \\left( \\frac{(b+a)+(b-a)x_d}{2}\\right)dx_d\n\\end{split}\n\\label{eq:Ec5_54} \\tag{5.54}\n\\end{equation*}\n\nEsta integral se puede aproximar como,\n\n<a id='Ec5_55'></a>\n\\begin{equation*}\n\\begin{split}\n\\int_a^b f(x)dx \\approx \\frac{b-a}{2} \\left[f\\left( \\frac{(b+a)+(b-a)x_0}{2}\\right)+f\\left( \\frac{(b+a)+(b-a)x_1}{2}\\right) \\right]\n\\end{split}\n\\label{eq:Ec5_55} \\tag{5.55}\n\\end{equation*}",
"_____no_output_____"
],
[
"[Volver a la Tabla de Contenido](#TOC)",
"_____no_output_____"
],
[
"#### Fórmulas de punto superior",
"_____no_output_____"
],
[
"La fórmula anterior para la cuadratura de Gauss era de dos puntos. Se pueden desarrollar versiones de punto superior en la forma general:\n\n<a id='Ec5_56'></a>\n\\begin{equation*}\n\\begin{split}\nI \\approx c_0f(x_0) + c_1f(x_1) + c_2f(x_2) +\\ldots+ c_{n-1}f(x_{n-1})\n\\end{split}\n\\label{eq:Ec5_56} \\tag{5.56}\n\\end{equation*}\n\ncon $n$, el número de puntos.\n\nDebido a que la cuadratura de Gauss requiere evaluaciones de la función en puntos espaciados uniformemente dentro del intervalo de integración, no es apropiada para casos donde se desconoce la función. Si se conoce la función, su ventaja es decisiva.\n\nEn la siguiente tabla se presentan los valores de los parámertros para $1, 2, 3, 4$ y $5$ puntos. \n\n|$$n$$ | $$c_i$$ | $$x_i$$ |\n|:----:|:----------:|:-------------:|\n|$$1$$ |$$2.000000$$| $$0.000000$$ |\n|$$2$$ |$$1.000000$$|$$\\pm0.577350$$|\n|$$3$$ |$$0.555556$$|$$\\pm0.774597$$|\n| |$$0.888889$$| $$0.000000$$ |\n|$$4$$ |$$0.347855$$|$$\\pm0.861136$$|\n| |$$0.652145$$|$$\\pm0.339981$$|\n|$$5$$ |$$0.236927$$|$$\\pm0.906180$$|\n| |$$0.478629$$|$$\\pm0.538469$$|\n| |$$0.568889$$| $$0.000000$$ |\n",
"_____no_output_____"
]
],
[
[
"import numpy as np\nimport pandas as pd\nGaussTable = [[[0], [2]], [[-1/np.sqrt(3), 1/np.sqrt(3)], [1, 1]], [[-np.sqrt(3/5), 0, np.sqrt(3/5)], [5/9, 8/9, 5/9]], [[-0.861136, -0.339981, 0.339981, 0.861136], [0.347855, 0.652145, 0.652145, 0.347855]], [[-0.90618, -0.538469, 0, 0.538469, 0.90618], [0.236927, 0.478629, 0.568889, 0.478629, 0.236927]], [[-0.93247, -0.661209, -0.238619, 0.238619, 0.661209, 0.93247], [0.171324, 0.360762, 0.467914, 0.467914, 0.360762, 0.171324]]]\ndisplay(pd.DataFrame(GaussTable, columns=[\"Integration Points\", \"Corresponding Weights\"]))\ndef IG(f, n):\n n = int(n)\n return sum([GaussTable[n - 1][1][i]*f(GaussTable[n - 1][0][i]) for i in range(n)])\ndef f(x): return x**9 + x**8\nIG(f, 5.0)",
"_____no_output_____"
]
],
[
[
"[Volver a la Tabla de Contenido](#TOC)",
"_____no_output_____"
],
[
"#### Ejemplo Cuadratura de Gauss",
"_____no_output_____"
],
[
"Determine el valor aproximado de:\n\n$$\\int_0^1 \\frac{4}{1+x^2}dx$$\n\nempleando cuadratura gaussiana de dos puntos.\n\nReemplazando los parámetros requeridos en la ecuación ([5.55](#Ec5_55)), donde $a=0$, $b=1$, $x_0=-\\sqrt{3}/3$ y $x_1=\\sqrt{3}/3$\n\n\\begin{equation*}\n\\begin{split}\n\\int_0^1 f(x)dx &\\approx \\frac{1-0}{2} \\left[f\\left( \\frac{(1+0)+(1-0)\\left(-\\frac{\\sqrt{3}}{3}\\right)}{2}\\right)+f\\left( \\frac{(1+0)+(1-0)\\left(\\frac{\\sqrt{3}}{3}\\right)}{2}\\right) \\right]\\\\\n&= \\frac{1}{2} \\left[f\\left( \\frac{1-\\frac{\\sqrt{3}}{3}}{2}\\right)+f\\left( \\frac{1+\\frac{\\sqrt{3}}{3}}{2}\\right) \\right]\\\\\n&= \\frac{1}{2} \\left[ \\frac{4}{1 + \\left( \\frac{1-\\frac{\\sqrt{3}}{3}}{2} \\right)^2}+\\frac{4}{1 + \\left( \\frac{1+\\frac{\\sqrt{3}}{3}}{2} \\right)^2} \\right]\\\\\n&=3.147541\n\\end{split}\n\\end{equation*}\n\nAhora veamos una breve implementación computacional",
"_____no_output_____"
]
],
[
[
"import numpy as np",
"_____no_output_____"
],
[
"def fxG(a, b, x):\n xG = ((b + a) + (b - a) * x) / 2\n return funcion(xG)",
"_____no_output_____"
],
[
"def GQ2(a,b):\n c0 = 1.0\n c1 = 1.0\n x0 = -1.0 / np.sqrt(3)\n x1 = 1.0 / np.sqrt(3)\n \n return (b - a) / 2 * (c0 * fxG(a,b,x0) + c1 * fxG(a,b,x1))\n",
"_____no_output_____"
],
[
"print(GQ2(a,b))",
"_____no_output_____"
]
],
[
[
"[Volver a la Tabla de Contenido](#TOC)",
"_____no_output_____"
]
],
[
[
"from IPython.core.display import HTML\ndef css_styling():\n styles = open('./nb_style.css', 'r').read()\n return HTML(styles)\ncss_styling()",
"_____no_output_____"
]
]
] | [
"markdown",
"code",
"markdown",
"code",
"markdown",
"code",
"markdown",
"code",
"markdown",
"code",
"markdown",
"code",
"markdown",
"code",
"markdown",
"code",
"markdown",
"code",
"markdown",
"code",
"markdown",
"code",
"markdown",
"code",
"markdown",
"code",
"markdown",
"code"
] | [
[
"markdown",
"markdown",
"markdown",
"markdown",
"markdown",
"markdown",
"markdown",
"markdown",
"markdown",
"markdown",
"markdown",
"markdown",
"markdown",
"markdown",
"markdown",
"markdown",
"markdown",
"markdown",
"markdown",
"markdown",
"markdown",
"markdown",
"markdown",
"markdown",
"markdown",
"markdown",
"markdown",
"markdown",
"markdown",
"markdown"
],
[
"code",
"code",
"code",
"code",
"code"
],
[
"markdown",
"markdown",
"markdown",
"markdown",
"markdown",
"markdown",
"markdown",
"markdown",
"markdown",
"markdown",
"markdown",
"markdown",
"markdown",
"markdown",
"markdown",
"markdown"
],
[
"code",
"code",
"code",
"code"
],
[
"markdown",
"markdown",
"markdown",
"markdown",
"markdown",
"markdown",
"markdown",
"markdown",
"markdown",
"markdown"
],
[
"code"
],
[
"markdown",
"markdown"
],
[
"code",
"code"
],
[
"markdown",
"markdown",
"markdown"
],
[
"code"
],
[
"markdown"
],
[
"code"
],
[
"markdown"
],
[
"code"
],
[
"markdown"
],
[
"code"
],
[
"markdown",
"markdown",
"markdown",
"markdown",
"markdown",
"markdown",
"markdown",
"markdown",
"markdown",
"markdown",
"markdown"
],
[
"code",
"code"
],
[
"markdown"
],
[
"code"
],
[
"markdown",
"markdown",
"markdown",
"markdown",
"markdown",
"markdown",
"markdown"
],
[
"code"
],
[
"markdown",
"markdown",
"markdown",
"markdown",
"markdown",
"markdown",
"markdown",
"markdown",
"markdown",
"markdown",
"markdown",
"markdown",
"markdown"
],
[
"code"
],
[
"markdown",
"markdown",
"markdown"
],
[
"code",
"code",
"code",
"code"
],
[
"markdown"
],
[
"code"
]
] |
e77fc19e8c09da1308c74dd5f30991365b53bb2b | 303,998 | ipynb | Jupyter Notebook | 5_word2vec_skip-gram.ipynb | ramborra/Udacity-Deep-Learning | b78d6479f403657a43d3fcd2b4accfe5000ceedf | [
"MIT"
] | null | null | null | 5_word2vec_skip-gram.ipynb | ramborra/Udacity-Deep-Learning | b78d6479f403657a43d3fcd2b4accfe5000ceedf | [
"MIT"
] | null | null | null | 5_word2vec_skip-gram.ipynb | ramborra/Udacity-Deep-Learning | b78d6479f403657a43d3fcd2b4accfe5000ceedf | [
"MIT"
] | null | null | null | 422.219444 | 267,892 | 0.915026 | [
[
[
"# Deep Learning\n## Assignment 5\nThe goal of this assignment is to train a Word2Vec skip-gram model over Text8 data.",
"_____no_output_____"
],
[
"Word2vec is a group of related models that are used to produce word embeddings. These models are shallow, two-layer neural networks that are trained to reconstruct linguistic contexts of words. Word2vec takes as its input a large corpus of text and produces a vector space, typically of several hundred dimensions, with each unique word in the corpus being assigned a corresponding vector in the space. Word vectors are positioned in the vector space such that words that share common contexts in the corpus are located in close proximity to one another in the space\n\nWord2vec was created by a team of researchers led by Tomas Mikolov at Google. \n\nWord2vec can utilize either of two model architectures to produce a distributed representation of words: continuous bag-of-words (CBOW) or continuous skip-gram. In the continuous bag-of-words architecture, the model predicts the current word from a window of surrounding context words. The order of context words does not influence prediction (bag-of-words assumption). In the continuous skip-gram architecture, the model uses the current word to predict the surrounding window of context words. The skip-gram architecture weighs nearby context words more heavily than more distant context words.[1][4] According to the authors' note,[5] CBOW is faster while skip-gram is slower but does a better job for infrequent words.\n\nReferences :\n\n i. Wikipedia\n \nii. http://mccormickml.com/2016/04/27/word2vec-resources/",
"_____no_output_____"
]
],
[
[
"# These are all the modules we'll be using later. Make sure you can import them\n# before proceeding further.\n%matplotlib inline\nfrom __future__ import print_function\nimport collections\nimport math\nimport numpy as np\nimport os\nimport random\nimport tensorflow as tf\nimport zipfile\nfrom matplotlib import pylab\nfrom six.moves import range\nfrom six.moves.urllib.request import urlretrieve\nfrom sklearn.manifold import TSNE",
"_____no_output_____"
]
],
[
[
"Download the data from the source website if necessary.",
"_____no_output_____"
]
],
[
[
"url = 'http://mattmahoney.net/dc/'\n\ndef maybe_download(filename, expected_bytes):\n \"\"\"Download a file if not present, and make sure it's the right size.\"\"\"\n if not os.path.exists(filename):\n filename, _ = urlretrieve(url + filename, filename)\n statinfo = os.stat(filename)\n if statinfo.st_size == expected_bytes:\n print('Found and verified %s' % filename)\n else:\n print(statinfo.st_size)\n raise Exception(\n 'Failed to verify ' + filename + '. Can you get to it with a browser?')\n return filename\n\nfilename = maybe_download('text8.zip', 31344016)",
"Found and verified text8.zip\n"
]
],
[
[
"Read the data into a string.",
"_____no_output_____"
]
],
[
[
"def read_data(filename):\n \"\"\"Extract the first file enclosed in a zip file as a list of words\"\"\"\n with zipfile.ZipFile(filename) as f:\n data = tf.compat.as_str(f.read(f.namelist()[0])).split()\n return data\n \nwords = read_data(filename)\nprint('Data size %d' % len(words))",
"Data size 17005207\n"
]
],
[
[
"Build the dictionary and replace rare words with UNK token. (UNK - Unknown Words)",
"_____no_output_____"
]
],
[
[
"vocabulary_size = 50000\n\ndef build_dataset(words):\n count = [['UNK', -1]]\n count.extend(collections.Counter(words).most_common(vocabulary_size - 1))\n dictionary = dict()\n for word, _ in count:\n dictionary[word] = len(dictionary)\n data = list()\n unk_count = 0\n for word in words:\n if word in dictionary:\n index = dictionary[word]\n else:\n index = 0 # dictionary['UNK']\n unk_count = unk_count + 1\n data.append(index)\n count[0][1] = unk_count\n reverse_dictionary = dict(zip(dictionary.values(), dictionary.keys())) \n return data, count, dictionary, reverse_dictionary\n\ndata, count, dictionary, reverse_dictionary = build_dataset(words)\nprint('Most common words (+UNK)', count[:5])\nprint('Sample data', data[:10])\ndel words # Hint to reduce memory.",
"Most common words (+UNK) [['UNK', 418391], ('the', 1061396), ('of', 593677), ('and', 416629), ('one', 411764)]\nSample data [5239, 3084, 12, 6, 195, 2, 3137, 46, 59, 156]\n"
],
[
"# Printing some sample data\nprint(data[:20])\nprint(count[:20])\nprint(dictionary.items()[:20])\nprint(reverse_dictionary.items()[:20])",
"_____no_output_____"
]
],
[
[
"Function to generate a training batch for the skip-gram model.",
"_____no_output_____"
]
],
[
[
"data_index = 0\n\ndef generate_batch(batch_size, num_skips, skip_window):\n global data_index\n assert batch_size % num_skips == 0\n assert num_skips <= 2 * skip_window\n batch = np.ndarray(shape=(batch_size), dtype=np.int32)\n labels = np.ndarray(shape=(batch_size, 1), dtype=np.int32)\n span = 2 * skip_window + 1 # [ skip_window target skip_window ]\n buffer = collections.deque(maxlen=span)\n for _ in range(span):\n buffer.append(data[data_index])\n data_index = (data_index + 1) % len(data)\n for i in range(batch_size // num_skips):\n target = skip_window # target label at the center of the buffer\n targets_to_avoid = [ skip_window ]\n for j in range(num_skips):\n while target in targets_to_avoid:\n target = random.randint(0, span - 1)\n targets_to_avoid.append(target)\n batch[i * num_skips + j] = buffer[skip_window]\n labels[i * num_skips + j, 0] = buffer[target]\n buffer.append(data[data_index])\n data_index = (data_index + 1) % len(data)\n return batch, labels\n\nprint('data:', [reverse_dictionary[di] for di in data[:8]])\n\nfor num_skips, skip_window in [(2, 1), (4, 2)]:\n data_index = 0\n batch, labels = generate_batch(batch_size=8, num_skips=num_skips, skip_window=skip_window)\n print('\\nwith num_skips = %d and skip_window = %d:' % (num_skips, skip_window))\n print(' batch:', [reverse_dictionary[bi] for bi in batch])\n print(' labels:', [reverse_dictionary[li] for li in labels.reshape(8)])",
"data: ['anarchism', 'originated', 'as', 'a', 'term', 'of', 'abuse', 'first']\n\nwith num_skips = 2 and skip_window = 1:\n batch: ['originated', 'originated', 'as', 'as', 'a', 'a', 'term', 'term']\n labels: ['anarchism', 'as', 'originated', 'a', 'term', 'as', 'a', 'of']\n\nwith num_skips = 4 and skip_window = 2:\n batch: ['as', 'as', 'as', 'as', 'a', 'a', 'a', 'a']\n labels: ['originated', 'term', 'a', 'anarchism', 'as', 'originated', 'term', 'of']\n"
]
],
[
[
"Train a skip-gram model.",
"_____no_output_____"
]
],
[
[
"batch_size = 128\nembedding_size = 128 # Dimension of the embedding vector.\nskip_window = 1 # How many words to consider left and right.\nnum_skips = 2 # How many times to reuse an input to generate a label.\n# We pick a random validation set to sample nearest neighbors. here we limit the\n# validation samples to the words that have a low numeric ID, which by\n# construction are also the most frequent. \nvalid_size = 16 # Random set of words to evaluate similarity on.\nvalid_window = 100 # Only pick dev samples in the head of the distribution.\nvalid_examples = np.array(random.sample(range(valid_window), valid_size))\nnum_sampled = 64 # Number of negative examples to sample.\n\ngraph = tf.Graph()\n\nwith graph.as_default(), tf.device('/cpu:0'):\n\n # Input data.\n train_dataset = tf.placeholder(tf.int32, shape=[batch_size])\n train_labels = tf.placeholder(tf.int32, shape=[batch_size, 1])\n valid_dataset = tf.constant(valid_examples, dtype=tf.int32)\n \n # Variables.\n embeddings = tf.Variable(\n tf.random_uniform([vocabulary_size, embedding_size], -1.0, 1.0))\n softmax_weights = tf.Variable(\n tf.truncated_normal([vocabulary_size, embedding_size],\n stddev=1.0 / math.sqrt(embedding_size)))\n softmax_biases = tf.Variable(tf.zeros([vocabulary_size]))\n \n # Model.\n # Look up embeddings for inputs.\n embed = tf.nn.embedding_lookup(embeddings, train_dataset)\n # Compute the softmax loss, using a sample of the negative labels each time.\n loss = tf.reduce_mean(\n tf.nn.sampled_softmax_loss(weights=softmax_weights, biases=softmax_biases, inputs=embed,\n labels=train_labels, num_sampled=num_sampled, num_classes=vocabulary_size))\n\n # Optimizer.\n # Note: The optimizer will optimize the softmax_weights AND the embeddings.\n # This is because the embeddings are defined as a variable quantity and the\n # optimizer's `minimize` method will by default modify all variable quantities \n # that contribute to the tensor it is passed.\n # See docs on `tf.train.Optimizer.minimize()` for more details.\n optimizer = tf.train.AdagradOptimizer(1.0).minimize(loss)\n \n # Compute the similarity between minibatch examples and all embeddings.\n # We use the cosine distance:\n norm = tf.sqrt(tf.reduce_sum(tf.square(embeddings), 1, keep_dims=True))\n normalized_embeddings = embeddings / norm\n valid_embeddings = tf.nn.embedding_lookup(\n normalized_embeddings, valid_dataset)\n similarity = tf.matmul(valid_embeddings, tf.transpose(normalized_embeddings))",
"_____no_output_____"
],
[
"num_steps = 100001\n\nwith tf.Session(graph=graph) as session:\n tf.global_variables_initializer().run()\n print('Initialized')\n average_loss = 0\n for step in range(num_steps):\n batch_data, batch_labels = generate_batch(\n batch_size, num_skips, skip_window)\n feed_dict = {train_dataset : batch_data, train_labels : batch_labels}\n _, l = session.run([optimizer, loss], feed_dict=feed_dict)\n average_loss += l\n if step % 2000 == 0:\n if step > 0:\n average_loss = average_loss / 2000\n # The average loss is an estimate of the loss over the last 2000 batches.\n print('Average loss at step %d: %f' % (step, average_loss))\n average_loss = 0\n # note that this is expensive (~20% slowdown if computed every 500 steps)\n if step % 10000 == 0:\n sim = similarity.eval()\n for i in range(valid_size):\n valid_word = reverse_dictionary[valid_examples[i]]\n top_k = 8 # number of nearest neighbors\n nearest = (-sim[i, :]).argsort()[1:top_k+1]\n log = 'Nearest to %s:' % valid_word\n for k in range(top_k):\n close_word = reverse_dictionary[nearest[k]]\n log = '%s %s,' % (log, close_word)\n print(log)\n final_embeddings = normalized_embeddings.eval()",
"Initialized\nAverage loss at step 0: 7.724723\nNearest to six: histone, sentenced, bethlehem, sib, frg, racing, troll, greeted,\nNearest to when: sheedy, hendricks, condensate, dragoons, submarines, decalogue, crete, cutting,\nNearest to people: excerpts, leningrad, nieve, vanadium, diametrically, homeopathy, gadamer, bondage,\nNearest to by: piety, calves, deltas, annulled, clr, egon, mistresses, orfeo,\nNearest to is: fps, tolvaj, kunst, arrogance, fieldwork, client, rcito, grenoble,\nNearest to they: effeminate, polidori, variables, holders, commodus, dsm, traitor, villas,\nNearest to d: belisarius, armando, pai, bat, murakami, bolland, sane, fulfilment,\nNearest to these: federico, missy, royals, dewan, kournikova, ili, sheffield, sorghum,\nNearest to world: hamilcar, nagano, grosse, fencers, discrediting, mobster, kharkov, cupid,\nNearest to see: cosmological, juggalo, detailed, stances, cumbersome, germanic, astronaut, teletext,\nNearest to system: ibero, const, he, sheltered, bereshit, fathom, firestorm, roswell,\nNearest to which: baseball, andre, exploitative, alley, ridicule, erowid, wally, hardcore,\nNearest to two: fertilisation, scrap, myoglobin, ripe, raceway, bradford, sounds, sncf,\nNearest to history: nsh, titans, myasthenic, quasar, natal, vulgar, ansgar, schneider,\nNearest to states: fibres, fisc, biochemistry, murmansk, undergo, covenants, sort, formulas,\nNearest to his: posed, anatomist, lettres, experienced, fugues, boasts, krieg, lintel,\nAverage loss at step 2000: 4.366180\nAverage loss at step 4000: 3.866667\nAverage loss at step 6000: 3.790487\nAverage loss at step 8000: 3.689255\nAverage loss at step 10000: 3.614477\nNearest to six: eight, four, five, seven, nine, three, two, zero,\nNearest to when: bathing, geos, macroeconomic, stylistic, integration, spiked, refugees, acura,\nNearest to people: gad, peek, breed, benefits, peer, areas, gazing, gadamer,\nNearest to by: was, drift, on, been, as, in, from, were,\nNearest to is: was, are, has, grotesque, actuated, be, arnaz, horowitz,\nNearest to they: he, there, geologists, genoese, commodus, villas, not, we,\nNearest to d: wye, namek, antimicrobial, murakami, pap, melanogaster, oxygenated, fremantle,\nNearest to these: some, many, irritate, missy, cedes, afghanistan, eisteddfod, metrodome,\nNearest to world: same, hamilcar, casablanca, nagano, cathy, budd, band, kharkov,\nNearest to see: cosmological, edmonds, juggalo, detailed, bangkok, bello, superconductivity, campaigning,\nNearest to system: fathom, heroically, const, drags, ibero, tobin, stylized, references,\nNearest to which: this, who, also, baseball, it, that, caesars, tones,\nNearest to two: five, three, four, six, nine, eight, seven, zero,\nNearest to history: titans, quasar, nsh, limnic, spotted, flutie, schneider, folder,\nNearest to states: formulas, frisians, malpractice, migrants, inhibited, snorri, defeats, yes,\nNearest to his: their, her, s, the, its, plucked, swan, mystical,\nAverage loss at step 12000: 3.604422\nAverage loss at step 14000: 3.571794\nAverage loss at step 16000: 3.413121\nAverage loss at step 18000: 3.452368\nAverage loss at step 20000: 3.542887\nNearest to six: eight, seven, five, nine, four, three, zero, two,\nNearest to when: where, was, keats, cartridge, after, holocaust, gravitons, rik,\nNearest to people: gad, peek, insist, benefits, peer, newman, areas, precautions,\nNearest to by: be, were, lining, into, histones, been, was, from,\nNearest to is: was, are, has, be, tolvaj, grotesque, allowing, but,\nNearest to they: there, he, we, it, who, you, ballast, not,\nNearest to d: b, antimicrobial, murakami, powders, vilna, pap, taino, cellular,\nNearest to these: many, some, several, all, warship, their, such, other,\nNearest to world: hamilcar, cathy, same, kharkov, body, band, rest, usurp,\nNearest to see: cosmological, bangkok, shrinking, timesharing, mma, dont, can, reveal,\nNearest to system: fathom, heroically, dialogues, stylized, shang, function, spinoffs, drags,\nNearest to which: this, that, who, also, it, then, still, but,\nNearest to two: three, four, five, six, seven, one, eight, nine,\nNearest to history: parts, limnic, titans, emedicine, spotted, folder, talked, nsh,\nNearest to states: formulas, montparnasse, nbs, malpractice, yes, frisians, snorri, consonances,\nNearest to his: their, her, its, the, aisha, swan, torts, several,\nAverage loss at step 22000: 3.505388\nAverage loss at step 24000: 3.489771\nAverage loss at step 26000: 3.479666\nAverage loss at step 28000: 3.482867\nAverage loss at step 30000: 3.505132\nNearest to six: seven, five, four, eight, nine, three, zero, two,\nNearest to when: if, where, but, after, though, was, wastes, starfighter,\nNearest to people: peek, newman, gad, areas, insist, troops, peer, micronation,\nNearest to by: from, were, under, in, as, with, was, blower,\nNearest to is: was, are, has, does, be, were, became, had,\nNearest to they: there, we, he, who, you, it, not, these,\nNearest to d: b, murakami, nine, malwa, tragic, ctesiphon, mil, pap,\nNearest to these: some, many, several, their, such, they, both, are,\nNearest to world: kharkov, history, cathy, same, payable, hamilcar, cosets, times,\nNearest to see: cosmological, shrinking, bangkok, timesharing, mistakenly, mma, reveal, parasitic,\nNearest to system: undone, fathom, stylized, shops, ascribed, cola, asset, analysed,\nNearest to which: this, that, also, who, what, then, it, still,\nNearest to two: four, three, one, five, six, seven, eight, nine,\nNearest to history: western, world, emedicine, origin, confirms, heteronyms, atc, modern,\nNearest to states: formulas, migrants, montparnasse, jethro, frisians, multiplications, diglossia, nbs,\nNearest to his: their, her, its, the, aisha, s, hoosiers, almoravids,\nAverage loss at step 32000: 3.502142\nAverage loss at step 34000: 3.494313\nAverage loss at step 36000: 3.452985\nAverage loss at step 38000: 3.305134\nAverage loss at step 40000: 3.431321\nNearest to six: seven, five, four, eight, nine, three, two, one,\nNearest to when: if, where, after, while, cartridge, but, before, though,\nNearest to people: peek, areas, newman, humayun, leaders, today, medici, gad,\nNearest to by: with, cartridge, compressors, plunges, as, histones, be, pick,\nNearest to is: was, has, are, be, if, grotesque, while, szab,\nNearest to they: we, there, he, you, it, who, i, not,\nNearest to d: b, mil, rex, protesters, malwa, tragic, murakami, k,\nNearest to these: some, many, several, such, both, their, antipopes, which,\nNearest to world: hamilcar, cathy, memoriam, rebellious, presidency, electrophilic, continent, pounds,\nNearest to see: bangkok, timesharing, include, can, shrinking, cosmological, parasitic, dont,\nNearest to system: systems, undone, havoc, jarman, shops, asset, spinoffs, interception,\nNearest to which: that, this, also, who, still, but, it, what,\nNearest to two: three, four, five, six, seven, eight, one, nine,\nNearest to history: list, berlin, western, lavoisier, precedent, emedicine, folder, origin,\nNearest to states: kingdom, formulas, actinides, nations, inhibited, diglossia, nami, amicus,\nNearest to his: their, her, its, s, plucked, the, hoosiers, unknowns,\nAverage loss at step 42000: 3.437590\nAverage loss at step 44000: 3.453154\nAverage loss at step 46000: 3.454886\nAverage loss at step 48000: 3.350647\nAverage loss at step 50000: 3.384952\nNearest to six: eight, seven, four, nine, five, three, zero, two,\nNearest to when: if, after, while, where, but, though, before, however,\nNearest to people: troops, benefits, residents, individuals, today, gad, animals, medici,\nNearest to by: histones, powerless, was, princip, during, mithraic, on, with,\nNearest to is: was, are, has, be, grotesque, although, wrecks, became,\nNearest to they: we, he, there, you, it, who, i, she,\nNearest to d: b, m, k, showing, tragic, j, rex, mil,\nNearest to these: some, several, many, such, both, different, all, various,\nNearest to world: presidency, memoriam, hamilcar, kharkov, continent, rebellious, casablanca, kashmir,\nNearest to see: include, bangkok, timesharing, references, cosmological, sgh, mma, parasitic,\nNearest to system: systems, undone, jarman, mantle, shops, fathom, differed, perpendicular,\nNearest to which: this, that, also, what, who, still, but, usually,\nNearest to two: three, four, one, six, five, eight, seven, zero,\nNearest to history: folder, list, busway, origin, chordal, berlin, repelled, precedent,\nNearest to states: kingdom, formulas, nations, montparnasse, diglossia, consonances, thrace, migrants,\nNearest to his: their, her, its, my, our, the, your, hoosiers,\n"
],
[
"# Printing Embeddings (They are all Normalized)\nprint(final_embeddings[0])\nprint(np.sum(np.square(final_embeddings[0])))",
"[-0.01360553 -0.01019099 0.03855132 -0.03409449 -0.12169676 -0.12752521\n -0.10353041 -0.07640228 0.04199962 0.08179612 0.05778846 0.16043897\n -0.06100744 0.15375856 0.10971404 0.10871226 0.06779686 0.02113919\n 0.10539305 -0.01174314 -0.02712017 -0.00601637 0.13712344 0.01578297\n -0.01427499 -0.00438345 0.18935508 -0.03581193 0.04085848 -0.18240088\n 0.05349829 -0.03963359 0.1155377 0.05598118 -0.08191323 0.14696647\n 0.03320661 0.07635265 0.04807431 0.01518252 0.13613747 -0.03814896\n -0.07563203 0.091968 -0.04192531 -0.09706993 0.19997264 0.04855323\n 0.06087693 0.01783208 -0.04071165 -0.02659828 -0.03287474 -0.01833199\n -0.06165279 -0.00613207 -0.11647256 0.06613162 0.096601 0.06109566\n 0.103825 0.03232143 -0.06224754 0.06665117 -0.02050134 -0.02712018\n 0.02533103 0.017258 0.07307839 0.20411792 -0.04445328 -0.02164487\n 0.07405864 -0.03746444 -0.11190646 -0.12785195 0.03590243 0.04973493\n 0.06141543 0.00486605 -0.07174163 -0.07946865 0.04910036 -0.03822383\n -0.12346336 -0.02079795 0.02773008 0.00204804 0.1728252 -0.1250184\n 0.1228409 0.15913655 0.09738228 0.16502139 0.01705573 -0.02964674\n -0.06245537 0.06271623 0.10218396 0.09405631 0.19697966 0.03631858\n 0.08743694 0.07386836 -0.12952395 0.0823264 0.04942108 0.05366875\n 0.03391527 0.03196386 -0.08627483 0.14543229 -0.00330158 0.07375387\n -0.06120221 0.08391016 -0.10126602 0.07451268 -0.03156544 -0.18034153\n -0.08461367 0.04095527 0.02925331 0.18251559 -0.15508997 0.0636633\n -0.07148023 -0.10428033]\n1.0\n"
],
[
"num_points = 400\n\ntsne = TSNE(perplexity=30, n_components=2, init='pca', n_iter=5000, method='exact')\ntwo_d_embeddings = tsne.fit_transform(final_embeddings[1:num_points+1, :])",
"_____no_output_____"
],
[
"def plot(embeddings, labels):\n assert embeddings.shape[0] >= len(labels), 'More labels than embeddings'\n pylab.figure(figsize=(15,15)) # in inches\n for i, label in enumerate(labels):\n x, y = embeddings[i,:]\n pylab.scatter(x, y)\n pylab.annotate(label, xy=(x, y), xytext=(5, 2), textcoords='offset points',\n ha='right', va='bottom')\n pylab.show()\n\nwords = [reverse_dictionary[i] for i in range(1, num_points+1)]\nplot(two_d_embeddings, words)",
"_____no_output_____"
]
],
[
[
"If you observe the scatter plot above, we see that the words that share common contexts in the corpus are located in close proximity to one another in the space.\nEx : one, two, three...\n ",
"_____no_output_____"
]
]
] | [
"markdown",
"code",
"markdown",
"code",
"markdown",
"code",
"markdown",
"code",
"markdown",
"code",
"markdown",
"code",
"markdown"
] | [
[
"markdown",
"markdown"
],
[
"code"
],
[
"markdown"
],
[
"code"
],
[
"markdown"
],
[
"code"
],
[
"markdown"
],
[
"code",
"code"
],
[
"markdown"
],
[
"code"
],
[
"markdown"
],
[
"code",
"code",
"code",
"code",
"code"
],
[
"markdown"
]
] |
e77fd223c698719b2e115866350c1751247bf7b7 | 445,581 | ipynb | Jupyter Notebook | Exercise/stocks-analysis.ipynb | JSJeong-me/Machine_Learning | 8b8c83f58e39b78c3a3bb8d5cb8626d799ec6b17 | [
"MIT"
] | null | null | null | Exercise/stocks-analysis.ipynb | JSJeong-me/Machine_Learning | 8b8c83f58e39b78c3a3bb8d5cb8626d799ec6b17 | [
"MIT"
] | null | null | null | Exercise/stocks-analysis.ipynb | JSJeong-me/Machine_Learning | 8b8c83f58e39b78c3a3bb8d5cb8626d799ec6b17 | [
"MIT"
] | null | null | null | 602.951286 | 102,254 | 0.900884 | [
[
[
"<a href=\"https://colab.research.google.com/github/JSJeong-me/Machine_Learning/blob/main/Exercise/stocks-analysis.ipynb\" target=\"_parent\"><img src=\"https://colab.research.google.com/assets/colab-badge.svg\" alt=\"Open In Colab\"/></a>",
"_____no_output_____"
],
[
"### https://towardsdatascience.com/3-basic-steps-of-stock-market-analysis-in-python-917787012143",
"_____no_output_____"
]
],
[
[
"%matplotlib inline",
"_____no_output_____"
],
[
"!pip install yfinance",
"Requirement already satisfied: yfinance in /usr/local/lib/python3.7/dist-packages (0.1.70)\nRequirement already satisfied: multitasking>=0.0.7 in /usr/local/lib/python3.7/dist-packages (from yfinance) (0.0.10)\nRequirement already satisfied: lxml>=4.5.1 in /usr/local/lib/python3.7/dist-packages (from yfinance) (4.8.0)\nRequirement already satisfied: numpy>=1.15 in /usr/local/lib/python3.7/dist-packages (from yfinance) (1.21.5)\nRequirement already satisfied: pandas>=0.24.0 in /usr/local/lib/python3.7/dist-packages (from yfinance) (1.3.5)\nRequirement already satisfied: requests>=2.26 in /usr/local/lib/python3.7/dist-packages (from yfinance) (2.27.1)\nRequirement already satisfied: pytz>=2017.3 in /usr/local/lib/python3.7/dist-packages (from pandas>=0.24.0->yfinance) (2018.9)\nRequirement already satisfied: python-dateutil>=2.7.3 in /usr/local/lib/python3.7/dist-packages (from pandas>=0.24.0->yfinance) (2.8.2)\nRequirement already satisfied: six>=1.5 in /usr/local/lib/python3.7/dist-packages (from python-dateutil>=2.7.3->pandas>=0.24.0->yfinance) (1.15.0)\nRequirement already satisfied: idna<4,>=2.5 in /usr/local/lib/python3.7/dist-packages (from requests>=2.26->yfinance) (2.10)\nRequirement already satisfied: certifi>=2017.4.17 in /usr/local/lib/python3.7/dist-packages (from requests>=2.26->yfinance) (2021.10.8)\nRequirement already satisfied: urllib3<1.27,>=1.21.1 in /usr/local/lib/python3.7/dist-packages (from requests>=2.26->yfinance) (1.24.3)\nRequirement already satisfied: charset-normalizer~=2.0.0 in /usr/local/lib/python3.7/dist-packages (from requests>=2.26->yfinance) (2.0.12)\n"
],
[
"!wget http://prdownloads.sourceforge.net/ta-lib/ta-lib-0.4.0-src.tar.gz\n!tar -xzvf ta-lib-0.4.0-src.tar.gz\n%cd ta-lib\n!./configure --prefix=/usr\n!make\n!make install\n!pip install Ta-Lib",
"_____no_output_____"
],
[
"# from yahoofinancials import YahooFinancials\nimport matplotlib.pyplot as plt\nimport pandas as pd\nimport talib\nimport yfinance as yf",
"_____no_output_____"
],
[
"plt.rcParams['figure.facecolor'] = 'w'",
"_____no_output_____"
],
[
"df = yf.download(\"TSLA\", start=\"2018-11-01\", end=\"2022-03-03\", interval=\"1d\")\ndf.shape",
"\r[*********************100%***********************] 1 of 1 completed\n"
],
[
"t = yf.Ticker(\"T\")\n\nt.dividends",
"_____no_output_____"
],
[
"t.dividends.plot(figsize=(14, 7))",
"_____no_output_____"
],
[
"df.head()",
"_____no_output_____"
],
[
"df[df.index >= \"2019-11-01\"].Close.plot(figsize=(14, 7))",
"_____no_output_____"
],
[
"df.loc[:, \"rsi\"] = talib.RSI(df.Close, 14)",
"_____no_output_____"
],
[
"df.loc[:, 'ma20'] = df.Close.rolling(20).mean()\ndf.loc[:, 'ma200'] = df.Close.rolling(200).mean()",
"_____no_output_____"
],
[
"df[[\"Close\", \"ma20\", \"ma200\"]].plot(figsize=(14, 7))",
"_____no_output_____"
],
[
"",
"_____no_output_____"
],
[
"fig, ax = plt.subplots(1, 2, figsize=(21, 7))\n\n\nax0 = df[[\"rsi\"]].plot(ax=ax[0])\nax0.axhline(30, color=\"black\")\nax0.axhline(70, color=\"black\")\n\ndf[[\"Close\"]].plot(ax=ax[1])",
"_____no_output_____"
],
[
"df[df.index >= \"2019-11-01\"].Volume.plot(kind=\"bar\", figsize=(14, 4))",
"_____no_output_____"
],
[
"df",
"_____no_output_____"
],
[
"#import plotly.offline as pyo\n\n# Set notebook mode to work in offline\n#pyo.init_notebook_mode()",
"_____no_output_____"
],
[
"import plotly.graph_objects as go\n\nfig = go.Figure(\n data=go.Ohlc(\n x=df.index,\n open=df[\"Open\"],\n high=df[\"High\"],\n low=df[\"Low\"],\n close=df[\"Close\"],\n )\n)\nfig.show()",
"_____no_output_____"
],
[
"",
"_____no_output_____"
]
]
] | [
"markdown",
"code"
] | [
[
"markdown",
"markdown"
],
[
"code",
"code",
"code",
"code",
"code",
"code",
"code",
"code",
"code",
"code",
"code",
"code",
"code",
"code",
"code",
"code",
"code",
"code",
"code",
"code"
]
] |
e77fd7afe240531caa93b8704880028f5ccd4be0 | 264,066 | ipynb | Jupyter Notebook | analysis/tower selection analysis.ipynb | cogtoolslab/projection_block_construction | faae126fbb7da45f2c5f4df05a2ff606a2b86d59 | [
"MIT"
] | null | null | null | analysis/tower selection analysis.ipynb | cogtoolslab/projection_block_construction | faae126fbb7da45f2c5f4df05a2ff606a2b86d59 | [
"MIT"
] | null | null | null | analysis/tower selection analysis.ipynb | cogtoolslab/projection_block_construction | faae126fbb7da45f2c5f4df05a2ff606a2b86d59 | [
"MIT"
] | null | null | null | 84.935992 | 17,698 | 0.65576 | [
[
[
"# Analysis notebook comparing scoping vs no-scoping for tower selection\nPurpose of this notebook is to categorize and analyze generated towers.\n\nRequires:\n* `.pkl` generated by `stimuli/score_towers.py`\n\nSee also:\n* `stimuli/generate_towers.ipynb` for plotting code and a similar analysis in the same place as the tower generation code. This notebook supersedes it.",
"_____no_output_____"
]
],
[
[
"# set up imports\nimport os\nimport sys\n__file__ = os.getcwd()\nproj_dir = os.path.dirname(os.path.realpath(__file__))\nsys.path.append(proj_dir)\nutils_dir = os.path.join(proj_dir, 'utils')\nsys.path.append(utils_dir)\nanalysis_dir = os.path.join(proj_dir, 'analysis')\nanalysis_utils_dir = os.path.join(analysis_dir, 'utils')\nsys.path.append(analysis_utils_dir)\nagent_dir = os.path.join(proj_dir, 'model')\nsys.path.append(agent_dir)\nagent_util_dir = os.path.join(agent_dir, 'utils')\nsys.path.append(agent_util_dir)\nexperiments_dir = os.path.join(proj_dir, 'experiments')\nsys.path.append(experiments_dir)\ndf_dir = os.path.join(proj_dir, 'results/dataframes')\nstim_dir = os.path.join(proj_dir, 'stimuli')\n",
"_____no_output_____"
],
[
"import tqdm\n\nimport pickle\n\nimport math\n\nimport numpy as np\nimport matplotlib.pyplot as plt\nimport seaborn as sns\nimport pandas as pd\n\nimport scipy.stats as stats\nfrom scipy.stats import sem as sem\n\nfrom utils.blockworld_library import *\nfrom utils.blockworld import *\n\nfrom model.BFS_Lookahead_Agent import BFS_Lookahead_Agent\nfrom model.BFS_Agent import BFS_Agent\nfrom model.Astar_Agent import Astar_Agent\n",
"_____no_output_____"
],
[
"# show all columns in dataframe\npd.set_option('display.max_columns', None)\n",
"_____no_output_____"
],
[
"# some helper functions\n\n# look at towers\ndef visualize_towers(towers, text_parameters=None):\n fig, axes = plt.subplots(math.ceil(len(towers)/5),\n 5, figsize=(20, 15*math.ceil(len(towers)/20)))\n for axis, tower in zip(axes.flatten(), towers):\n axis.imshow(tower['bitmap']*1.0)\n if text_parameters is not None:\n if type(text_parameters) is not list:\n text_parameters = [text_parameters]\n for y_offset, text_parameter in enumerate(text_parameters):\n axis.text(0, y_offset*1., str(text_parameter+\": \" +\n str(tower[text_parameter])), color='gray', fontsize=20)\n plt.tight_layout()\n plt.show()\n",
"_____no_output_____"
]
],
[
[
"Load in data (we might have multiple dfs)",
"_____no_output_____"
]
],
[
[
"path_to_dfs = [os.path.join(df_dir, f)\n for f in [\"RLDM_main_experiment.pkl\"]]\ndfs = [pd.read_pickle(path_to_df) for path_to_df in path_to_dfs]\nprint(\"Read {} dataframes: {}\".format(len(dfs), path_to_dfs))\n# merge dfs\ndf = pd.concat(dfs)\nprint(\"Merged dataframes: {}\".format(df.shape))\n",
"Read 1 dataframes: ['/Users/felixbinder/Cloud/Grad School/Fan Lab/Block Construction/tools_block_construction/results/dataframes/RLDM_main_experiment.pkl']\nMerged dataframes: (1508, 51)\n"
],
[
"# do a few things to add helpful columns and such\n# use either solution_cost or states_evaluated as cost\ndf['cost'] = np.maximum(df['solution_cost'].fillna(0),\n df['states_evaluated'].fillna(0))\n# do the same for total cost\ndf['total_cost'] = np.maximum(df['all_sequences_planning_cost'].fillna(\n 0), df['states_evaluated'].fillna(0))\n",
"_____no_output_____"
],
[
"df.columns\n",
"_____no_output_____"
],
[
"# summarize the runs into a run df\ndef summarize_df(df):\n summary_df = df.groupby('run_ID').agg({\n 'agent': 'first',\n 'label': 'first',\n 'world': 'first',\n 'action': 'count',\n 'blockmap': 'last',\n 'states_evaluated': ['sum', 'mean', sem],\n 'partial_solution_cost': ['sum', 'mean', sem],\n 'solution_cost': ['sum', 'mean', sem],\n 'all_sequences_planning_cost': ['sum', 'mean', sem],\n 'perfect': 'last',\n 'cost': ['sum', 'mean', sem],\n 'total_cost': ['sum', 'mean', sem],\n # 'avg_cost_per_step_for_run': ['sum', 'mean', sem],\n })\n return summary_df\n",
"_____no_output_____"
],
[
"sum_df = summarize_df(df)\n",
"/Users/felixbinder/opt/anaconda3/envs/scoping/lib/python3.9/site-packages/numpy/core/_methods.py:262: RuntimeWarning: Degrees of freedom <= 0 for slice\n ret = _var(a, axis=axis, dtype=dtype, out=out, ddof=ddof,\n/Users/felixbinder/opt/anaconda3/envs/scoping/lib/python3.9/site-packages/numpy/core/_methods.py:254: RuntimeWarning: invalid value encountered in double_scalars\n ret = ret.dtype.type(ret / rcount)\n"
]
],
[
[
"Let's explore the data a little bit",
"_____no_output_____"
]
],
[
[
"sum_df\n",
"_____no_output_____"
],
[
"sum_df.groupby([('label', 'first')]).mean()\n",
"_____no_output_____"
],
[
"sum_df.groupby([('label', 'first')]).count()\n",
"_____no_output_____"
]
],
[
[
"What is the rate of success?",
"_____no_output_____"
]
],
[
[
"display(sum_df.groupby([('label', 'first')]).mean()[('perfect', 'last')])\nsum_df.groupby([('label', 'first')]).mean()[('perfect', 'last')].plot(\n kind='bar', title='Rate of perfect solutions')\nplt.show()\n",
"_____no_output_____"
]
],
[
[
"What is the difference in cost between the two conditions?",
"_____no_output_____"
]
],
[
[
"display(sum_df.groupby([('label', 'first')]).mean()[('cost', 'sum')])\nsum_df.groupby([('label', 'first')]).mean()[('cost', 'sum')].plot(\n kind='bar', title='Mean action planning cost (for chosen solution', yerr=sum_df.groupby([('label', 'first')]).mean()[('cost', 'sem')])\nplt.yscale('log')\nplt.show()\n",
"_____no_output_____"
]
],
[
[
"What about the total cost?",
"_____no_output_____"
]
],
[
[
"display(sum_df.groupby([('label', 'first')]).mean()[('total_cost', 'sum')])\nsum_df.groupby([('label', 'first')]).mean()[('total_cost', 'sum')].plot(\n kind='bar', title='Total planning cost', yerr=sum_df.groupby([('label', 'first')]).mean()[('total_cost', 'sem')])\nplt.yscale('log')\nplt.show()\n",
"_____no_output_____"
],
[
"df[df['label'] == 'Full Subgoal Decomposition 2']['_world'].tail(1).item().silhouette",
"_____no_output_____"
]
],
[
[
"Is there a difference between the depth of found solutions?",
"_____no_output_____"
]
],
[
[
"display(sum_df.groupby([('label', 'first')]).mean()[('action', 'count')])\nsum_df.groupby([('label', 'first')]).mean()[('action', 'count')\n ].plot(kind='bar', title='Mean number of actions')\n",
"_____no_output_____"
]
],
[
[
"## Tower analysis\nNow that we have explored the data, let's look at the distribution over towers.",
"_____no_output_____"
],
[
"Let's make a scatterplot over subgoal and no subgoal costs.",
"_____no_output_____"
]
],
[
[
"tower_sum_df = df.groupby(['label', 'world']).agg({\n 'cost': ['sum', 'mean', sem],\n 'total_cost': ['sum', 'mean', sem],\n})\n# flatten the index\ntower_sum_df.reset_index(inplace=True)\n",
"_____no_output_____"
],
[
"tower_sum_df\n",
"_____no_output_____"
],
[
"# for the scatterplots, we can only show two agents at the same time.\nlabel1 = 'Full Subgoal Planning'\nlabel2 = 'Best First Search'\n",
"_____no_output_____"
],
[
"plt.scatter(\n x=tower_sum_df[tower_sum_df['label'] == label1]['cost']['sum'],\n y=tower_sum_df[tower_sum_df['label'] == label2]['cost']['sum'],\n c=tower_sum_df[tower_sum_df['label'] == label1]['world'])\nplt.title(\"Action planning cost of solving a tower with and without subgoals\")\nplt.xlabel(\"Cost of solving without subgoals\")\nplt.ylabel(\"Cost of solving with subgoals\")\n# log log\nplt.xscale('log')\nplt.yscale('log')\nplt.show()\n",
"_____no_output_____"
]
],
[
[
"The same for the total subgoal planning cost",
"_____no_output_____"
]
],
[
[
"plt.scatter(\n x=tower_sum_df[tower_sum_df['label'] == label1]['total_cost']['sum'],\n y=tower_sum_df[tower_sum_df['label'] == label2]['total_cost']['sum'],\n c=tower_sum_df[tower_sum_df['label'] == label1]['world'])\nplt.title(\"Action planning cost of solving a tower with and without subgoals\")\nplt.xlabel(\"Cost of solving without subgoals\")\nplt.ylabel(\"Cost of solving with subgoals\")\n# log log\nplt.xscale('log')\nplt.yscale('log')\nplt.show()\n",
"_____no_output_____"
]
],
[
[
"Can we see a pattern between the relation of the solution and total subgoal planning cost for the subgoal agent?",
"_____no_output_____"
]
],
[
[
"plt.scatter(\n x=tower_sum_df[tower_sum_df['label'] == label1]['cost']['sum'],\n y=tower_sum_df[tower_sum_df['label'] == label2]['total_cost']['sum'],\n c=tower_sum_df[tower_sum_df['label'] == label1]['world'])\nplt.title(\"Cost of the found solution versus costs of all sequences of subgoals\")\nplt.xlabel(\"Cost of the found solution\")\nplt.ylabel(\"Cost of all subgoals\")\n# log log\nplt.xscale('log')\nplt.yscale('log')\nplt.show()\n",
"_____no_output_____"
]
],
[
[
"Looks like there are some **outliers**—let's look at those",
"_____no_output_____"
],
[
"Do we have towers that can't be solved using a subgoal decomposition?",
"_____no_output_____"
]
],
[
[
"failed_df = df[(df['perfect'] == False)]\ndisplay(failed_df)\n",
"_____no_output_____"
],
[
"bad_ID = list(df[df['world_status'] == 'Fail']['run_ID'])[1]\n",
"_____no_output_____"
],
[
"bad_ID\n",
"_____no_output_____"
],
[
"df[df['run_ID'] == bad_ID]\n",
"_____no_output_____"
],
[
"df[df['run_ID'] == bad_ID]['_chosen_subgoal_sequence'].dropna(\n).values[-1].visual_display()\n",
"_____no_output_____"
],
[
"df[df['run_ID'] == bad_ID]['_chosen_subgoal_sequence'].dropna(\n).values[0][0].visual_display()\n",
"_____no_output_____"
],
[
"failed_df['_world'].tail(1).item().silhouette\n",
"_____no_output_____"
],
[
"failed_df['_world'].head(1).item().silhouette\n",
"_____no_output_____"
]
]
] | [
"markdown",
"code",
"markdown",
"code",
"markdown",
"code",
"markdown",
"code",
"markdown",
"code",
"markdown",
"code",
"markdown",
"code",
"markdown",
"code",
"markdown",
"code",
"markdown",
"code",
"markdown",
"code"
] | [
[
"markdown"
],
[
"code",
"code",
"code",
"code"
],
[
"markdown"
],
[
"code",
"code",
"code",
"code",
"code"
],
[
"markdown"
],
[
"code",
"code",
"code"
],
[
"markdown"
],
[
"code"
],
[
"markdown"
],
[
"code"
],
[
"markdown"
],
[
"code",
"code"
],
[
"markdown"
],
[
"code"
],
[
"markdown",
"markdown"
],
[
"code",
"code",
"code",
"code"
],
[
"markdown"
],
[
"code"
],
[
"markdown"
],
[
"code"
],
[
"markdown",
"markdown"
],
[
"code",
"code",
"code",
"code",
"code",
"code",
"code",
"code"
]
] |
e77fddc1c700f2c491651976f46656009f596c23 | 221,876 | ipynb | Jupyter Notebook | Music Generation.ipynb | karandevtyagi/AI-Music-Generator | a02b4b1645ec66be7abccebb0f0475c420b0ba7a | [
"MIT"
] | null | null | null | Music Generation.ipynb | karandevtyagi/AI-Music-Generator | a02b4b1645ec66be7abccebb0f0475c420b0ba7a | [
"MIT"
] | null | null | null | Music Generation.ipynb | karandevtyagi/AI-Music-Generator | a02b4b1645ec66be7abccebb0f0475c420b0ba7a | [
"MIT"
] | null | null | null | 52.169292 | 25,976 | 0.576633 | [
[
[
"### Imports",
"_____no_output_____"
]
],
[
[
"from music21 import converter, instrument, note, chord, stream\nimport glob\nimport pickle\nimport numpy as np\nfrom keras.utils import np_utils",
"Using TensorFlow backend.\n"
]
],
[
[
"## Read a Midi File",
"_____no_output_____"
]
],
[
[
"midi = converter.parse(\"midi_songs/EyesOnMePiano.mid\")",
"_____no_output_____"
],
[
"midi",
"_____no_output_____"
],
[
"midi.show('midi')",
"_____no_output_____"
],
[
"midi.show('text')",
"{0.0} <music21.stream.Part 0x23616a14408>\n {0.0} <music21.instrument.Piano 'Piano'>\n {0.0} <music21.tempo.MetronomeMark Quarter=95.0>\n {0.0} <music21.key.Key of D major>\n {0.0} <music21.meter.TimeSignature 4/4>\n {0.0} <music21.stream.Voice 0x23616a50948>\n {0.0} <music21.note.Note A>\n {0.0} <music21.note.Note A>\n {0.6667} <music21.note.Note G>\n {1.5} <music21.note.Note D>\n {2.0} <music21.note.Note A>\n {2.5} <music21.note.Note G>\n {3.0} <music21.note.Note D>\n {3.5} <music21.note.Note A>\n {4.0} <music21.note.Note A>\n {6.0} <music21.note.Note A>\n {8.0} <music21.note.Note F#>\n {10.0} <music21.chord.Chord F#4 C#5>\n {11.0} <music21.note.Note A>\n {11.6667} <music21.note.Note D>\n {13.3333} <music21.note.Note D>\n {13.6667} <music21.note.Note C#>\n {14.0} <music21.note.Note D>\n {15.0} <music21.note.Note D>\n {16.0} <music21.note.Note G>\n {16.6667} <music21.note.Note F#>\n {17.6667} <music21.note.Note D>\n {19.0} <music21.note.Note F#>\n {19.6667} <music21.note.Note E>\n {22.0} <music21.note.Note C#>\n {24.0} <music21.note.Note A>\n {27.0} <music21.note.Note A>\n {27.0} <music21.note.Note A>\n {31.6667} <music21.note.Note A>\n {33.0} <music21.note.Note E>\n {34.0} <music21.note.Note F#>\n {35.0} <music21.note.Note A>\n {35.6667} <music21.note.Note F#>\n {39.0} <music21.note.Note D>\n {40.0} <music21.note.Note E>\n {40.6667} <music21.note.Note F#>\n {43.0} <music21.note.Note B>\n {43.6667} <music21.note.Note D>\n {45.6667} <music21.chord.Chord A3 C#4>\n {47.0} <music21.chord.Chord G3 B3>\n {48.0} <music21.note.Note D>\n {49.0} <music21.note.Note E>\n {50.0} <music21.note.Note F#>\n {51.0} <music21.note.Note A>\n {51.6667} <music21.note.Note C#>\n {55.0} <music21.note.Note A>\n {56.0} <music21.note.Note C#>\n {56.6667} <music21.note.Note D>\n {58.6667} <music21.note.Note A>\n {59.6667} <music21.note.Note A>\n {63.0} <music21.note.Note A>\n {64.0} <music21.note.Note D>\n {65.6667} <music21.note.Note D>\n {67.0} <music21.note.Note B>\n {67.6667} <music21.note.Note B>\n {69.6667} <music21.note.Note E>\n {71.0} <music21.note.Note C#>\n {72.0} <music21.note.Note B>\n {73.6667} <music21.note.Note B>\n {75.0} <music21.note.Note G>\n {76.0} <music21.note.Note G>\n {76.6667} <music21.note.Note E>\n {79.6667} <music21.note.Note F#>\n {81.0} <music21.note.Note E>\n {81.6667} <music21.note.Note F#>\n {83.0} <music21.note.Note F#>\n {83.6667} <music21.note.Note E>\n {84.6667} <music21.note.Note D>\n {87.0} <music21.note.Note B>\n {87.6667} <music21.note.Note D>\n {91.0} <music21.note.Note F#>\n {91.6667} <music21.note.Note A>\n {92.75} <music21.note.Note A>\n {93.5} <music21.note.Note F#>\n {94.0} <music21.note.Note A>\n {94.3333} <music21.note.Note G>\n {94.6667} <music21.note.Note F#>\n {95.0} <music21.note.Note A>\n {96.0} <music21.note.Note D>\n {97.0} <music21.note.Note E>\n {98.0} <music21.note.Note F#>\n {99.0} <music21.note.Note A>\n {99.6667} <music21.note.Note F#>\n {104.0} <music21.chord.Chord G4 B4>\n {107.0} <music21.note.Note G>\n {108.5} <music21.note.Note D>\n {110.0} <music21.note.Note D>\n {110.6667} <music21.note.Note B>\n {111.6667} <music21.note.Note A>\n {113.0} <music21.note.Note E>\n {114.0} <music21.note.Note F#>\n {115.0} <music21.note.Note A>\n {115.6667} <music21.note.Note C#>\n {120.0} <music21.chord.Chord D5 G5>\n {125.6667} <music21.chord.Chord D4 G4 B4>\n {127.0} <music21.chord.Chord E4 B4 C#5>\n {128.0} <music21.chord.Chord D5 F#5 D6>\n {129.6667} <music21.chord.Chord D5 F#5 D6>\n {131.0} <music21.chord.Chord B4 F#5 B5>\n {131.6667} <music21.chord.Chord B4 F#5 B5>\n {132.6667} <music21.chord.Chord A4 E5 A5>\n {135.0} <music21.chord.Chord F#4 C#5>\n {136.0} <music21.chord.Chord B4 D5>\n {139.0} <music21.chord.Chord F#4 B4>\n {142.0} <music21.note.Note C#>\n {142.6667} <music21.note.Note E>\n {143.6667} <music21.note.Note C#>\n {145.0} <music21.note.Note E>\n {146.0} <music21.note.Note F#>\n {147.0} <music21.note.Note G>\n {147.6667} <music21.note.Note A>\n {150.6667} <music21.note.Note A>\n {151.6667} <music21.note.Note D>\n {153.0} <music21.chord.Chord B4 D5>\n {153.6667} <music21.chord.Chord A4 C#5>\n {155.0} <music21.chord.Chord D4 E4 G4 B4>\n {156.0} <music21.chord.Chord D4 F#4>\n {158.0} <music21.note.Note G>\n {158.6667} <music21.note.Note F#>\n {160.0} <music21.chord.Chord D4 F#4>\n {162.0} <music21.note.Note D>\n {162.75} <music21.note.Note F#>\n {163.5} <music21.note.Note A>\n {165.6667} <music21.note.Note B>\n {170.0} <music21.note.Note B>\n {170.75} <music21.note.Note C#>\n {171.5} <music21.note.Note D>\n {173.6667} <music21.note.Note A>\n {178.0} <music21.note.Note F#>\n {178.75} <music21.note.Note F#>\n {179.5} <music21.note.Note G>\n {181.6667} <music21.note.Note G>\n {182.0} <music21.note.Note G>\n {186.0} <music21.chord.Chord E5 G5>\n {186.75} <music21.chord.Chord F#5 A5>\n {187.5} <music21.chord.Chord G5 B5>\n {189.6667} <music21.chord.Chord A4 A5>\n {194.0} <music21.chord.Chord D4 D5>\n {194.75} <music21.chord.Chord F#4 F#5>\n {195.5} <music21.chord.Chord A4 A5>\n {197.6667} <music21.chord.Chord B4 B5>\n {202.0} <music21.chord.Chord B4 B5>\n {202.75} <music21.chord.Chord C#5 C#6>\n {203.5} <music21.chord.Chord D5 D6>\n {205.6667} <music21.chord.Chord A4 A5>\n {207.0} <music21.chord.Chord C#5 C#6>\n {208.0} <music21.chord.Chord B4 B5>\n {211.6667} <music21.note.Note A>\n {212.6667} <music21.note.Note G>\n {214.0} <music21.note.Note G>\n {215.6667} <music21.note.Note D>\n {216.0} <music21.note.Note F#>\n {217.6667} <music21.note.Note F#>\n {218.6667} <music21.note.Note E>\n {219.6667} <music21.note.Note C#>\n {223.6667} <music21.note.Note D>\n {226.0} <music21.chord.Chord D4 A4>\n {227.0} <music21.chord.Chord D4 G4>\n {228.0} <music21.chord.Chord D4 F#4>\n {234.0} <music21.note.Note E>\n {234.6667} <music21.note.Note D>\n {235.6667} <music21.note.Note A>\n {236.0} <music21.chord.Chord D5 F#5>\n {240.0} <music21.chord.Chord B4 E5>\n {242.0} <music21.chord.Chord D5 B5>\n {242.75} <music21.chord.Chord E5 C#6>\n {243.5} <music21.chord.Chord F5 D6>\n {244.0} <music21.chord.Chord C#5 F#5>\n {248.0} <music21.note.Note C#>\n {250.0} <music21.note.Note B>\n {252.0} <music21.chord.Chord B4 D5 A5>\n {252.6667} <music21.chord.Chord B4 D5 G5>\n {254.0} <music21.note.Note G>\n {255.6667} <music21.note.Note D>\n {256.0} <music21.chord.Chord G4 D5>\n {259.0} <music21.note.Note G>\n {260.0} <music21.note.Note E>\n {263.6667} <music21.note.Note D>\n {266.0} <music21.note.Note E>\n {266.6667} <music21.note.Note D>\n {267.6667} <music21.note.Note D>\n {269.0} <music21.note.Note E>\n {269.25} <music21.note.Note D>\n {269.5} <music21.note.Note C#>\n {269.75} <music21.note.Note G#>\n {270.0} <music21.note.Note A>\n {270.25} <music21.note.Note A>\n {270.5} <music21.note.Note G#>\n {270.75} <music21.note.Note D>\n {271.0} <music21.note.Note D>\n {271.25} <music21.note.Note E>\n {271.5} <music21.note.Note G#>\n {276.0} <music21.note.Note B>\n {0.0} <music21.stream.Voice 0x23616c7a0c8>\n {0.0} <music21.note.Rest rest>\n {1.0} <music21.note.Note F#>\n {1.0} <music21.note.Rest rest>\n {1.75} <music21.note.Note C#>\n {2.75} <music21.note.Note F#>\n {3.75} <music21.note.Note D>\n {5.0} <music21.note.Note E>\n {5.6667} <music21.note.Note F#>\n {7.0} <music21.note.Note C#>\n {7.75} <music21.note.Rest rest>\n {8.0} <music21.note.Note B>\n {9.0} <music21.note.Note C#>\n {9.6667} <music21.note.Note D>\n {12.0} <music21.note.Note B>\n {13.75} <music21.note.Note D>\n {14.0} <music21.note.Note A>\n {14.6667} <music21.note.Note D>\n {15.6667} <music21.note.Note D>\n {17.0} <music21.note.Note G>\n {18.0} <music21.note.Note D>\n {22.0} <music21.note.Note F>\n {22.75} <music21.note.Note C#>\n {23.5} <music21.note.Note B>\n {32.0} <music21.note.Note D>\n {37.6667} <music21.note.Note E>\n {41.0} <music21.note.Note D>\n {44.0} <music21.note.Note E>\n {47.6667} <music21.note.Note A>\n {53.6667} <music21.note.Note E>\n {57.0} <music21.note.Note B>\n {59.0} <music21.note.Note B>\n {66.0} <music21.note.Note C#>\n {68.6667} <music21.note.Note A>\n {71.0} <music21.note.Note F#>\n {71.6667} <music21.note.Note A>\n {74.0} <music21.note.Note A>\n {77.0} <music21.note.Note F#>\n {80.0} <music21.note.Note F#>\n {82.0} <music21.note.Note G>\n {84.0} <music21.note.Note E>\n {89.5} <music21.chord.Chord C#5 F#5>\n {91.0} <music21.chord.Chord D5 B5>\n {92.0} <music21.chord.Chord B-3 E4>\n {94.75} <music21.note.Note D>\n {101.0} <music21.chord.Chord F#4 A4>\n {102.6667} <music21.note.Note F#>\n {104.0} <music21.note.Note E>\n {104.6667} <music21.note.Note F#>\n {107.0} <music21.note.Note B>\n {107.6667} <music21.note.Note D>\n {108.5} <music21.chord.Chord G4 B4>\n {111.0} <music21.note.Note C#>\n {112.0} <music21.note.Note D>\n {117.0} <music21.chord.Chord E4 A4>\n {118.6667} <music21.note.Note D>\n {120.0} <music21.note.Note C#>\n {120.6667} <music21.note.Note D>\n {122.6667} <music21.note.Note A>\n {123.6667} <music21.note.Note A>\n {127.6667} <music21.note.Note A>\n {130.0} <music21.chord.Chord C#5 F#5 C#6>\n {135.0} <music21.note.Note F#>\n {135.6667} <music21.note.Note A>\n {137.6667} <music21.note.Note B>\n {138.6667} <music21.note.Note G>\n {139.6667} <music21.note.Note F#>\n {140.6667} <music21.note.Note E>\n {144.0} <music21.note.Note D>\n {151.0} <music21.note.Note G>\n {152.0} <music21.note.Note F#>\n {155.0} <music21.note.Note E>\n {155.6667} <music21.note.Note D>\n {159.0} <music21.note.Note E>\n {164.0} <music21.note.Note C#>\n {166.0} <music21.note.Note B>\n {172.0} <music21.note.Note B>\n {174.0} <music21.note.Note A>\n {180.0} <music21.note.Note A>\n {181.75} <music21.note.Note F#>\n {188.0} <music21.chord.Chord B4 D5 F#5 B5>\n {190.0} <music21.chord.Chord A4 B4 D5 A5>\n {196.0} <music21.chord.Chord C#5 F#5 C#6>\n {198.0} <music21.chord.Chord B4 D5 F#5 B5>\n {204.0} <music21.chord.Chord C#5 E5 A5 C#6>\n {212.0} <music21.note.Note A>\n {213.0} <music21.chord.Chord D5 G5 D6>\n {215.75} <music21.note.Note E>\n {219.0} <music21.note.Note D>\n {220.0} <music21.note.Note E>\n {224.0} <music21.note.Note D>\n {235.0} <music21.note.Note F#>\n {236.0} <music21.note.Note C#>\n {237.6667} <music21.note.Note B>\n {240.0} <music21.note.Note G#>\n {244.0} <music21.note.Note C#>\n {245.6667} <music21.note.Note A>\n {247.0} <music21.note.Note C#>\n {248.0} <music21.chord.Chord F#5 B5>\n {251.0} <music21.note.Note A>\n {253.0} <music21.chord.Chord D5 F#5 D6>\n {255.75} <music21.note.Note E>\n {256.0} <music21.note.Note F#>\n {257.6667} <music21.note.Note F#>\n {259.0} <music21.note.Note D>\n {259.6667} <music21.note.Note C#>\n {264.0} <music21.chord.Chord F#4 D5>\n {266.0} <music21.note.Note A>\n {267.0} <music21.note.Note F>\n {268.0} <music21.chord.Chord F#4 A4>\n {271.75} <music21.note.Rest rest>\n {0.0} <music21.stream.Voice 0x23617406988>\n {0.0} <music21.note.Rest rest>\n {4.0} <music21.note.Note D>\n {4.0} <music21.note.Rest rest>\n {6.0} <music21.note.Note E>\n {12.0} <music21.note.Note E>\n {15.0} <music21.note.Note G#>\n {70.6667} <music21.note.Note D>\n {89.6667} <music21.note.Note C#>\n {93.0} <music21.note.Note G>\n {93.75} <music21.note.Note D>\n {101.0} <music21.note.Note E>\n {101.3333} <music21.note.Note D>\n {101.6667} <music21.note.Note C#>\n {105.0} <music21.note.Note D>\n {108.6667} <music21.note.Note E>\n {117.0} <music21.note.Note E>\n {117.3333} <music21.note.Note D>\n {117.6667} <music21.note.Note C#>\n {119.0} <music21.note.Note A>\n {121.0} <music21.note.Note B>\n {123.0} <music21.note.Note B>\n {136.0} <music21.note.Note B>\n {138.0} <music21.note.Note A>\n {139.0} <music21.note.Note D>\n {143.0} <music21.note.Note A>\n {154.0} <music21.chord.Chord G4 B4>\n {156.0} <music21.note.Note D>\n {238.0} <music21.note.Note B>\n {258.6667} <music21.note.Note E>\n {267.0} <music21.note.Note C>\n {268.0} <music21.note.Note C#>\n {271.6667} <music21.note.Note E>\n {271.9167} <music21.note.Rest rest>\n {0.0} <music21.stream.Voice 0x23616ba4608>\n {0.0} <music21.note.Rest rest>\n {90.6667} <music21.note.Note E>\n {90.6667} <music21.note.Rest rest>\n {268.0} <music21.note.Note E>\n {270.5} <music21.note.Rest rest>\n {0.0} <music21.stream.Voice 0x23616ba0688>\n {269.6667} <music21.note.Note A>\n {270.0} <music21.note.Note B>\n {1.75} <music21.tempo.MetronomeMark Quarter=96.0>\n {2.0} <music21.tempo.MetronomeMark Quarter=97.0>\n {2.0} <music21.tempo.MetronomeMark Quarter=98.0>\n {2.25} <music21.tempo.MetronomeMark Quarter=99.0>\n {2.25} <music21.tempo.MetronomeMark Quarter=100.0>\n {2.5} <music21.tempo.MetronomeMark Quarter=101.0>\n {2.5} <music21.tempo.MetronomeMark Quarter=102.0>\n {2.6667} <music21.tempo.MetronomeMark Quarter=103.0>\n {2.75} <music21.tempo.MetronomeMark Quarter=104.0>\n {3.0} <music21.tempo.MetronomeMark Quarter=105.0>\n {3.0} <music21.tempo.MetronomeMark allegretto Quarter=106.0>\n {3.25} <music21.tempo.MetronomeMark allegretto Quarter=107.0>\n {3.3333} <music21.tempo.MetronomeMark allegretto Quarter=108.0>\n {3.5} <music21.tempo.MetronomeMark allegretto Quarter=109.0>\n {3.6667} <music21.tempo.MetronomeMark allegretto Quarter=110.0>\n {4.6667} <music21.tempo.MetronomeMark Quarter=98.0>\n {12.25} <music21.tempo.MetronomeMark Quarter=99.0>\n {12.25} <music21.tempo.MetronomeMark Quarter=100.0>\n {12.3333} <music21.tempo.MetronomeMark Quarter=101.0>\n {12.5} <music21.tempo.MetronomeMark Quarter=102.0>\n {12.5} <music21.tempo.MetronomeMark Quarter=103.0>\n {12.6667} <music21.tempo.MetronomeMark Quarter=104.0>\n {12.6667} <music21.tempo.MetronomeMark Quarter=105.0>\n {12.75} <music21.tempo.MetronomeMark allegretto Quarter=106.0>\n {12.75} <music21.tempo.MetronomeMark allegretto Quarter=107.0>\n {13.0} <music21.tempo.MetronomeMark allegretto Quarter=108.0>\n {13.0} <music21.tempo.MetronomeMark allegretto Quarter=109.0>\n {13.0} <music21.tempo.MetronomeMark allegretto Quarter=110.0>\n {13.25} <music21.tempo.MetronomeMark allegretto Quarter=109.0>\n {13.25} <music21.tempo.MetronomeMark allegretto Quarter=108.0>\n {13.3333} <music21.tempo.MetronomeMark allegretto Quarter=107.0>\n {13.5} <music21.tempo.MetronomeMark allegretto Quarter=106.0>\n {13.5} <music21.tempo.MetronomeMark Quarter=105.0>\n {13.6667} <music21.tempo.MetronomeMark Quarter=104.0>\n {13.6667} <music21.tempo.MetronomeMark Quarter=103.0>\n {13.75} <music21.tempo.MetronomeMark Quarter=102.0>\n {13.75} <music21.tempo.MetronomeMark Quarter=101.0>\n {14.0} <music21.tempo.MetronomeMark Quarter=100.0>\n {14.0} <music21.tempo.MetronomeMark Quarter=99.0>\n {14.0} <music21.tempo.MetronomeMark Quarter=98.0>\n {14.25} <music21.tempo.MetronomeMark Quarter=97.0>\n {14.25} <music21.tempo.MetronomeMark Quarter=96.0>\n {14.3333} <music21.tempo.MetronomeMark Quarter=95.0>\n {14.3333} <music21.tempo.MetronomeMark moderate Quarter=94.0>\n {14.5} <music21.tempo.MetronomeMark moderate Quarter=93.0>\n {14.5} <music21.tempo.MetronomeMark moderate Quarter=92.0>\n {14.6667} <music21.tempo.MetronomeMark moderate Quarter=91.0>\n {14.75} <music21.tempo.MetronomeMark maestoso Quarter=90.0>\n {14.75} <music21.tempo.MetronomeMark maestoso Quarter=89.0>\n {15.0} <music21.tempo.MetronomeMark maestoso Quarter=88.0>\n {15.0} <music21.tempo.MetronomeMark maestoso Quarter=87.0>\n {15.0} <music21.tempo.MetronomeMark maestoso Quarter=86.0>\n {15.0} <music21.tempo.MetronomeMark andante moderato Quarter=85.0>\n {15.6667} <music21.tempo.MetronomeMark Quarter=75.0>\n {16.6667} <music21.tempo.MetronomeMark Quarter=95.0>\n {17.0} <music21.tempo.MetronomeMark Quarter=100.0>\n {18.0} <music21.tempo.MetronomeMark Quarter=98.0>\n {20.25} <music21.tempo.MetronomeMark Quarter=97.0>\n {20.25} <music21.tempo.MetronomeMark Quarter=96.0>\n {20.3333} <music21.tempo.MetronomeMark Quarter=95.0>\n {20.3333} <music21.tempo.MetronomeMark moderate Quarter=94.0>\n {20.5} <music21.tempo.MetronomeMark moderate Quarter=93.0>\n {20.5} <music21.tempo.MetronomeMark moderate Quarter=92.0>\n {20.6667} <music21.tempo.MetronomeMark moderate Quarter=91.0>\n {20.6667} <music21.tempo.MetronomeMark maestoso Quarter=90.0>\n {20.75} <music21.tempo.MetronomeMark maestoso Quarter=89.0>\n {20.75} <music21.tempo.MetronomeMark maestoso Quarter=88.0>\n {21.0} <music21.tempo.MetronomeMark maestoso Quarter=87.0>\n {21.0} <music21.tempo.MetronomeMark maestoso Quarter=86.0>\n {21.0} <music21.tempo.MetronomeMark andante moderato Quarter=85.0>\n {21.0} <music21.tempo.MetronomeMark andante moderato Quarter=84.0>\n {21.25} <music21.tempo.MetronomeMark andante moderato Quarter=83.0>\n {21.25} <music21.tempo.MetronomeMark andantino Quarter=82.0>\n {21.3333} <music21.tempo.MetronomeMark andantino Quarter=81.0>\n {21.3333} <music21.tempo.MetronomeMark andantino Quarter=80.0>\n {21.5} <music21.tempo.MetronomeMark andantino Quarter=79.0>\n {21.5} <music21.tempo.MetronomeMark andantino Quarter=78.0>\n {21.6667} <music21.tempo.MetronomeMark Quarter=77.0>\n {21.6667} <music21.tempo.MetronomeMark Quarter=76.0>\n {21.75} <music21.tempo.MetronomeMark Quarter=75.0>\n {21.75} <music21.tempo.MetronomeMark andante Quarter=74.0>\n {22.0} <music21.tempo.MetronomeMark andante Quarter=73.0>\n {22.0} <music21.tempo.MetronomeMark andante Quarter=72.0>\n {22.0} <music21.tempo.MetronomeMark andante Quarter=71.0>\n {22.0} <music21.tempo.MetronomeMark andante Quarter=70.0>\n {23.0} <music21.tempo.MetronomeMark adagietto Quarter=67.0>\n {24.0} <music21.tempo.MetronomeMark Quarter=100.0>\n {25.75} <music21.tempo.MetronomeMark Quarter=99.0>\n {26.3333} <music21.tempo.MetronomeMark Quarter=98.0>\n {27.0} <music21.tempo.MetronomeMark Quarter=97.0>\n {27.5} <music21.tempo.MetronomeMark Quarter=96.0>\n {28.0} <music21.tempo.MetronomeMark Quarter=95.0>\n {44.5} <music21.tempo.MetronomeMark moderate Quarter=94.0>\n {45.0} <music21.tempo.MetronomeMark moderate Quarter=93.0>\n {45.3333} <music21.tempo.MetronomeMark moderate Quarter=92.0>\n {45.75} <music21.tempo.MetronomeMark moderate Quarter=91.0>\n {46.0} <music21.tempo.MetronomeMark maestoso Quarter=90.0>\n {46.5} <music21.tempo.MetronomeMark moderate Quarter=91.0>\n {47.0} <music21.tempo.MetronomeMark moderate Quarter=92.0>\n {47.3333} <music21.tempo.MetronomeMark moderate Quarter=93.0>\n {47.75} <music21.tempo.MetronomeMark moderate Quarter=94.0>\n {48.0} <music21.tempo.MetronomeMark Quarter=95.0>\n {84.6667} <music21.tempo.MetronomeMark moderate Quarter=94.0>\n {85.25} <music21.tempo.MetronomeMark moderate Quarter=93.0>\n {85.75} <music21.tempo.MetronomeMark moderate Quarter=92.0>\n {86.3333} <music21.tempo.MetronomeMark moderate Quarter=91.0>\n {87.0} <music21.tempo.MetronomeMark maestoso Quarter=90.0>\n {87.5} <music21.tempo.MetronomeMark maestoso Quarter=89.0>\n {88.0} <music21.tempo.MetronomeMark maestoso Quarter=88.0>\n {90.3333} <music21.tempo.MetronomeMark maestoso Quarter=87.0>\n {90.6667} <music21.tempo.MetronomeMark maestoso Quarter=86.0>\n {90.75} <music21.tempo.MetronomeMark andante moderato Quarter=85.0>\n {91.0} <music21.tempo.MetronomeMark andante moderato Quarter=84.0>\n {91.3333} <music21.tempo.MetronomeMark andante moderato Quarter=83.0>\n {91.6667} <music21.tempo.MetronomeMark andantino Quarter=82.0>\n {92.0} <music21.tempo.MetronomeMark larghetto Quarter=60.0>\n {92.25} <music21.tempo.MetronomeMark larghetto Quarter=61.0>\n {92.25} <music21.tempo.MetronomeMark larghetto Quarter=62.0>\n {92.25} <music21.tempo.MetronomeMark Quarter=63.0>\n {92.3333} <music21.tempo.MetronomeMark adagietto Quarter=64.0>\n {92.3333} <music21.tempo.MetronomeMark adagietto Quarter=65.0>\n {92.5} <music21.tempo.MetronomeMark adagietto Quarter=66.0>\n {92.5} <music21.tempo.MetronomeMark adagietto Quarter=67.0>\n {92.5} <music21.tempo.MetronomeMark adagietto Quarter=68.0>\n {92.6667} <music21.tempo.MetronomeMark Quarter=69.0>\n {92.6667} <music21.tempo.MetronomeMark andante Quarter=70.0>\n {92.6667} <music21.tempo.MetronomeMark andante Quarter=71.0>\n {92.75} <music21.tempo.MetronomeMark andante Quarter=72.0>\n {92.75} <music21.tempo.MetronomeMark andante Quarter=73.0>\n {92.75} <music21.tempo.MetronomeMark andante Quarter=74.0>\n {93.0} <music21.tempo.MetronomeMark Quarter=75.0>\n {93.0} <music21.tempo.MetronomeMark Quarter=76.0>\n {93.0} <music21.tempo.MetronomeMark Quarter=77.0>\n {93.0} <music21.tempo.MetronomeMark andantino Quarter=78.0>\n {93.0} <music21.tempo.MetronomeMark andantino Quarter=79.0>\n {93.25} <music21.tempo.MetronomeMark andantino Quarter=80.0>\n {93.25} <music21.tempo.MetronomeMark andantino Quarter=81.0>\n {93.25} <music21.tempo.MetronomeMark andantino Quarter=82.0>\n {93.3333} <music21.tempo.MetronomeMark andante moderato Quarter=83.0>\n {93.3333} <music21.tempo.MetronomeMark andante moderato Quarter=84.0>\n {93.5} <music21.tempo.MetronomeMark andante moderato Quarter=85.0>\n {93.5} <music21.tempo.MetronomeMark maestoso Quarter=86.0>\n {93.5} <music21.tempo.MetronomeMark maestoso Quarter=87.0>\n {93.6667} <music21.tempo.MetronomeMark maestoso Quarter=88.0>\n {93.6667} <music21.tempo.MetronomeMark maestoso Quarter=89.0>\n {93.6667} <music21.tempo.MetronomeMark maestoso Quarter=90.0>\n {93.75} <music21.tempo.MetronomeMark moderate Quarter=91.0>\n {93.75} <music21.tempo.MetronomeMark moderate Quarter=92.0>\n {93.75} <music21.tempo.MetronomeMark moderate Quarter=93.0>\n {94.0} <music21.tempo.MetronomeMark moderate Quarter=94.0>\n {94.0} <music21.tempo.MetronomeMark Quarter=95.0>\n {94.0} <music21.tempo.MetronomeMark Quarter=96.0>\n {94.0} <music21.tempo.MetronomeMark Quarter=97.0>\n {94.0} <music21.tempo.MetronomeMark Quarter=98.0>\n {95.0} <music21.tempo.MetronomeMark andantino Quarter=80.0>\n {96.25} <music21.tempo.MetronomeMark andantino Quarter=81.0>\n {96.3333} <music21.tempo.MetronomeMark andantino Quarter=82.0>\n {96.5} <music21.tempo.MetronomeMark andante moderato Quarter=83.0>\n {96.6667} <music21.tempo.MetronomeMark andante moderato Quarter=84.0>\n {96.75} <music21.tempo.MetronomeMark andante moderato Quarter=85.0>\n {97.0} <music21.tempo.MetronomeMark maestoso Quarter=86.0>\n {97.0} <music21.tempo.MetronomeMark maestoso Quarter=87.0>\n {97.25} <music21.tempo.MetronomeMark maestoso Quarter=88.0>\n {97.3333} <music21.tempo.MetronomeMark maestoso Quarter=89.0>\n {97.5} <music21.tempo.MetronomeMark maestoso Quarter=90.0>\n {97.6667} <music21.tempo.MetronomeMark moderate Quarter=91.0>\n {97.75} <music21.tempo.MetronomeMark moderate Quarter=92.0>\n {97.75} <music21.tempo.MetronomeMark moderate Quarter=93.0>\n {98.0} <music21.tempo.MetronomeMark moderate Quarter=94.0>\n {98.0} <music21.tempo.MetronomeMark Quarter=95.0>\n {137.0} <music21.tempo.MetronomeMark moderate Quarter=94.0>\n {137.75} <music21.tempo.MetronomeMark moderate Quarter=93.0>\n {138.5} <music21.tempo.MetronomeMark moderate Quarter=92.0>\n {139.3333} <music21.tempo.MetronomeMark moderate Quarter=91.0>\n {140.0} <music21.tempo.MetronomeMark maestoso Quarter=90.0>\n {140.5} <music21.tempo.MetronomeMark maestoso Quarter=89.0>\n {141.0} <music21.tempo.MetronomeMark maestoso Quarter=88.0>\n {141.3333} <music21.tempo.MetronomeMark maestoso Quarter=87.0>\n {141.75} <music21.tempo.MetronomeMark maestoso Quarter=86.0>\n {142.0} <music21.tempo.MetronomeMark andante moderato Quarter=85.0>\n {142.5} <music21.tempo.MetronomeMark andante moderato Quarter=84.0>\n {143.0} <music21.tempo.MetronomeMark andante moderato Quarter=83.0>\n {143.3333} <music21.tempo.MetronomeMark andantino Quarter=82.0>\n {143.75} <music21.tempo.MetronomeMark andantino Quarter=81.0>\n {144.0} <music21.tempo.MetronomeMark andantino Quarter=80.0>\n {146.25} <music21.tempo.MetronomeMark andantino Quarter=79.0>\n {146.5} <music21.tempo.MetronomeMark andantino Quarter=78.0>\n {146.5} <music21.tempo.MetronomeMark Quarter=77.0>\n {146.75} <music21.tempo.MetronomeMark Quarter=76.0>\n {147.0} <music21.tempo.MetronomeMark Quarter=75.0>\n {147.0} <music21.tempo.MetronomeMark andante Quarter=74.0>\n {147.25} <music21.tempo.MetronomeMark andante Quarter=73.0>\n {147.3333} <music21.tempo.MetronomeMark andante Quarter=72.0>\n {147.5} <music21.tempo.MetronomeMark andante Quarter=71.0>\n {147.6667} <music21.tempo.MetronomeMark andante Quarter=70.0>\n {147.75} <music21.tempo.MetronomeMark Quarter=69.0>\n {148.0} <music21.tempo.MetronomeMark adagietto Quarter=68.0>\n {148.0} <music21.tempo.MetronomeMark adagietto Quarter=67.0>\n {148.0} <music21.tempo.MetronomeMark moderate Quarter=94.0>\n {148.6667} <music21.tempo.MetronomeMark Quarter=105.0>\n {149.0} <music21.tempo.MetronomeMark Quarter=115.0>\n {150.0} <music21.tempo.MetronomeMark Quarter=20.0>\n {150.6667} <music21.tempo.MetronomeMark lento Quarter=50.0>\n {151.0} <music21.tempo.MetronomeMark Quarter=75.0>\n {152.0} <music21.tempo.MetronomeMark adagietto Quarter=65.0>\n {153.0} <music21.tempo.MetronomeMark andantino Quarter=78.0>\n {154.0} <music21.tempo.MetronomeMark larghetto Quarter=60.0>\n {156.25} <music21.tempo.MetronomeMark larghetto Quarter=61.0>\n {156.25} <music21.tempo.MetronomeMark larghetto Quarter=62.0>\n {156.25} <music21.tempo.MetronomeMark Quarter=63.0>\n {156.25} <music21.tempo.MetronomeMark adagietto Quarter=64.0>\n {156.3333} <music21.tempo.MetronomeMark adagietto Quarter=65.0>\n {156.3333} <music21.tempo.MetronomeMark adagietto Quarter=66.0>\n {156.3333} <music21.tempo.MetronomeMark adagietto Quarter=67.0>\n {156.5} <music21.tempo.MetronomeMark adagietto Quarter=68.0>\n {156.5} <music21.tempo.MetronomeMark Quarter=69.0>\n {156.5} <music21.tempo.MetronomeMark andante Quarter=70.0>\n {156.5} <music21.tempo.MetronomeMark andante Quarter=71.0>\n {156.6667} <music21.tempo.MetronomeMark andante Quarter=72.0>\n {156.6667} <music21.tempo.MetronomeMark andante Quarter=73.0>\n {156.6667} <music21.tempo.MetronomeMark andante Quarter=74.0>\n {156.75} <music21.tempo.MetronomeMark Quarter=75.0>\n {156.75} <music21.tempo.MetronomeMark Quarter=76.0>\n {156.75} <music21.tempo.MetronomeMark Quarter=77.0>\n {156.75} <music21.tempo.MetronomeMark andantino Quarter=78.0>\n {157.0} <music21.tempo.MetronomeMark andantino Quarter=79.0>\n {157.0} <music21.tempo.MetronomeMark andantino Quarter=80.0>\n {157.0} <music21.tempo.MetronomeMark andantino Quarter=81.0>\n {157.0} <music21.tempo.MetronomeMark andantino Quarter=82.0>\n {157.0} <music21.tempo.MetronomeMark andante moderato Quarter=83.0>\n {157.0} <music21.tempo.MetronomeMark andante moderato Quarter=84.0>\n {157.0} <music21.tempo.MetronomeMark andante moderato Quarter=85.0>\n {159.0} <music21.tempo.MetronomeMark andantino Quarter=78.0>\n {160.0} <music21.tempo.MetronomeMark andante Quarter=70.0>\n {162.25} <music21.tempo.MetronomeMark andante Quarter=71.0>\n {162.3333} <music21.tempo.MetronomeMark andante Quarter=72.0>\n {162.5} <music21.tempo.MetronomeMark andante Quarter=73.0>\n {162.6667} <music21.tempo.MetronomeMark andante Quarter=74.0>\n {162.75} <music21.tempo.MetronomeMark Quarter=75.0>\n {163.0} <music21.tempo.MetronomeMark Quarter=76.0>\n {163.0} <music21.tempo.MetronomeMark Quarter=77.0>\n {163.25} <music21.tempo.MetronomeMark andantino Quarter=78.0>\n {163.3333} <music21.tempo.MetronomeMark andantino Quarter=79.0>\n {163.5} <music21.tempo.MetronomeMark andantino Quarter=80.0>\n {163.6667} <music21.tempo.MetronomeMark andantino Quarter=81.0>\n {163.75} <music21.tempo.MetronomeMark andantino Quarter=82.0>\n {163.75} <music21.tempo.MetronomeMark andante moderato Quarter=83.0>\n {164.0} <music21.tempo.MetronomeMark andante moderato Quarter=84.0>\n {164.0} <music21.tempo.MetronomeMark andante moderato Quarter=85.0>\n {166.3333} <music21.tempo.MetronomeMark maestoso Quarter=86.0>\n {166.5} <music21.tempo.MetronomeMark maestoso Quarter=87.0>\n {166.75} <music21.tempo.MetronomeMark maestoso Quarter=88.0>\n {167.0} <music21.tempo.MetronomeMark maestoso Quarter=89.0>\n {167.0} <music21.tempo.MetronomeMark maestoso Quarter=90.0>\n {167.3333} <music21.tempo.MetronomeMark moderate Quarter=91.0>\n {167.5} <music21.tempo.MetronomeMark moderate Quarter=92.0>\n {167.75} <music21.tempo.MetronomeMark moderate Quarter=93.0>\n {168.0} <music21.tempo.MetronomeMark moderate Quarter=94.0>\n {168.0} <music21.tempo.MetronomeMark Quarter=95.0>\n {202.0} <music21.tempo.MetronomeMark andante moderato Quarter=85.0>\n {204.0} <music21.tempo.MetronomeMark moderate Quarter=92.0>\n {209.25} <music21.tempo.MetronomeMark moderate Quarter=91.0>\n {209.3333} <music21.tempo.MetronomeMark maestoso Quarter=90.0>\n {209.3333} <music21.tempo.MetronomeMark maestoso Quarter=89.0>\n {209.5} <music21.tempo.MetronomeMark maestoso Quarter=88.0>\n {209.5} <music21.tempo.MetronomeMark maestoso Quarter=87.0>\n {209.6667} <music21.tempo.MetronomeMark maestoso Quarter=86.0>\n {209.75} <music21.tempo.MetronomeMark andante moderato Quarter=85.0>\n {209.75} <music21.tempo.MetronomeMark andante moderato Quarter=84.0>\n {210.0} <music21.tempo.MetronomeMark andante moderato Quarter=83.0>\n {210.0} <music21.tempo.MetronomeMark andantino Quarter=82.0>\n {210.0} <music21.tempo.MetronomeMark andantino Quarter=81.0>\n {210.25} <music21.tempo.MetronomeMark andantino Quarter=80.0>\n {210.3333} <music21.tempo.MetronomeMark andantino Quarter=79.0>\n {210.3333} <music21.tempo.MetronomeMark andantino Quarter=78.0>\n {210.5} <music21.tempo.MetronomeMark Quarter=77.0>\n {210.5} <music21.tempo.MetronomeMark Quarter=76.0>\n {210.6667} <music21.tempo.MetronomeMark Quarter=75.0>\n {210.75} <music21.tempo.MetronomeMark andante Quarter=74.0>\n {210.75} <music21.tempo.MetronomeMark andante Quarter=73.0>\n {211.0} <music21.tempo.MetronomeMark andante Quarter=72.0>\n {211.0} <music21.tempo.MetronomeMark andante Quarter=71.0>\n {211.0} <music21.tempo.MetronomeMark andante Quarter=70.0>\n {211.6667} <music21.tempo.MetronomeMark lento Quarter=50.0>\n {212.0} <music21.tempo.MetronomeMark larghetto Quarter=60.0>\n {214.0} <music21.tempo.MetronomeMark lento Quarter=50.0>\n {215.6667} <music21.tempo.MetronomeMark maestoso Quarter=90.0>\n {216.0} <music21.tempo.MetronomeMark andante moderato Quarter=85.0>\n {218.3333} <music21.tempo.MetronomeMark maestoso Quarter=86.0>\n {218.5} <music21.tempo.MetronomeMark maestoso Quarter=87.0>\n {218.75} <music21.tempo.MetronomeMark maestoso Quarter=88.0>\n {219.0} <music21.tempo.MetronomeMark maestoso Quarter=89.0>\n {219.0} <music21.tempo.MetronomeMark maestoso Quarter=90.0>\n {219.3333} <music21.tempo.MetronomeMark moderate Quarter=91.0>\n {219.5} <music21.tempo.MetronomeMark moderate Quarter=92.0>\n {219.75} <music21.tempo.MetronomeMark moderate Quarter=93.0>\n {220.0} <music21.tempo.MetronomeMark moderate Quarter=94.0>\n {220.0} <music21.tempo.MetronomeMark Quarter=95.0>\n {220.0} <music21.tempo.MetronomeMark andante moderato Quarter=85.0>\n {220.25} <music21.tempo.MetronomeMark andante moderato Quarter=84.0>\n {220.3333} <music21.tempo.MetronomeMark andante moderato Quarter=83.0>\n {220.5} <music21.tempo.MetronomeMark andantino Quarter=82.0>\n {220.5} <music21.tempo.MetronomeMark andantino Quarter=81.0>\n {220.6667} <music21.tempo.MetronomeMark andantino Quarter=80.0>\n {220.75} <music21.tempo.MetronomeMark andantino Quarter=79.0>\n {220.75} <music21.tempo.MetronomeMark andantino Quarter=78.0>\n {221.0} <music21.tempo.MetronomeMark Quarter=77.0>\n {221.0} <music21.tempo.MetronomeMark Quarter=76.0>\n {221.0} <music21.tempo.MetronomeMark Quarter=75.0>\n {221.25} <music21.tempo.MetronomeMark andante Quarter=74.0>\n {221.3333} <music21.tempo.MetronomeMark andante Quarter=73.0>\n {221.5} <music21.tempo.MetronomeMark andante Quarter=72.0>\n {221.5} <music21.tempo.MetronomeMark andante Quarter=71.0>\n {221.6667} <music21.tempo.MetronomeMark andante Quarter=70.0>\n {221.75} <music21.tempo.MetronomeMark Quarter=69.0>\n {221.75} <music21.tempo.MetronomeMark adagietto Quarter=68.0>\n {222.0} <music21.tempo.MetronomeMark adagietto Quarter=67.0>\n {222.0} <music21.tempo.MetronomeMark adagietto Quarter=66.0>\n {222.0} <music21.tempo.MetronomeMark adagietto Quarter=65.0>\n {222.25} <music21.tempo.MetronomeMark adagietto Quarter=64.0>\n {222.3333} <music21.tempo.MetronomeMark Quarter=63.0>\n {222.5} <music21.tempo.MetronomeMark larghetto Quarter=62.0>\n {222.5} <music21.tempo.MetronomeMark larghetto Quarter=61.0>\n {222.6667} <music21.tempo.MetronomeMark larghetto Quarter=60.0>\n {222.75} <music21.tempo.MetronomeMark larghetto Quarter=59.0>\n {222.75} <music21.tempo.MetronomeMark adagio Quarter=58.0>\n {223.0} <music21.tempo.MetronomeMark adagio Quarter=57.0>\n {223.0} <music21.tempo.MetronomeMark adagio Quarter=56.0>\n {223.0} <music21.tempo.MetronomeMark adagio Quarter=55.0>\n {223.25} <music21.tempo.MetronomeMark lento Quarter=54.0>\n {223.3333} <music21.tempo.MetronomeMark lento Quarter=53.0>\n {223.5} <music21.tempo.MetronomeMark lento Quarter=52.0>\n {223.5} <music21.tempo.MetronomeMark lento Quarter=51.0>\n {223.6667} <music21.tempo.MetronomeMark lento Quarter=50.0>\n {223.6667} <music21.tempo.MetronomeMark grave Quarter=40.0>\n {224.0} <music21.tempo.MetronomeMark maestoso Quarter=88.0>\n {228.0} <music21.tempo.MetronomeMark andantino Quarter=80.0>\n {230.3333} <music21.tempo.MetronomeMark andantino Quarter=81.0>\n {230.5} <music21.tempo.MetronomeMark andantino Quarter=82.0>\n {230.75} <music21.tempo.MetronomeMark andante moderato Quarter=83.0>\n {231.0} <music21.tempo.MetronomeMark andante moderato Quarter=84.0>\n {231.0} <music21.tempo.MetronomeMark andante moderato Quarter=85.0>\n {231.3333} <music21.tempo.MetronomeMark maestoso Quarter=86.0>\n {231.5} <music21.tempo.MetronomeMark maestoso Quarter=87.0>\n {231.75} <music21.tempo.MetronomeMark maestoso Quarter=88.0>\n {232.0} <music21.tempo.MetronomeMark maestoso Quarter=89.0>\n {232.0} <music21.tempo.MetronomeMark maestoso Quarter=90.0>\n {232.25} <music21.tempo.MetronomeMark maestoso Quarter=89.0>\n {232.3333} <music21.tempo.MetronomeMark maestoso Quarter=88.0>\n {232.5} <music21.tempo.MetronomeMark maestoso Quarter=87.0>\n {232.6667} <music21.tempo.MetronomeMark maestoso Quarter=86.0>\n {232.6667} <music21.tempo.MetronomeMark andante moderato Quarter=85.0>\n {232.75} <music21.tempo.MetronomeMark andante moderato Quarter=84.0>\n {233.0} <music21.tempo.MetronomeMark andante moderato Quarter=83.0>\n {233.0} <music21.tempo.MetronomeMark andantino Quarter=82.0>\n {233.25} <music21.tempo.MetronomeMark andantino Quarter=81.0>\n {233.25} <music21.tempo.MetronomeMark andantino Quarter=80.0>\n {233.3333} <music21.tempo.MetronomeMark andantino Quarter=79.0>\n {233.5} <music21.tempo.MetronomeMark andantino Quarter=78.0>\n {233.6667} <music21.tempo.MetronomeMark Quarter=77.0>\n {233.75} <music21.tempo.MetronomeMark Quarter=76.0>\n {233.75} <music21.tempo.MetronomeMark Quarter=75.0>\n {234.0} <music21.tempo.MetronomeMark andante Quarter=74.0>\n {234.0} <music21.tempo.MetronomeMark andante Quarter=73.0>\n {234.25} <music21.tempo.MetronomeMark andante Quarter=72.0>\n {234.3333} <music21.tempo.MetronomeMark andante Quarter=71.0>\n {234.5} <music21.tempo.MetronomeMark andante Quarter=70.0>\n {234.5} <music21.tempo.MetronomeMark Quarter=69.0>\n {234.6667} <music21.tempo.MetronomeMark adagietto Quarter=68.0>\n {234.75} <music21.tempo.MetronomeMark adagietto Quarter=67.0>\n {235.0} <music21.tempo.MetronomeMark adagietto Quarter=66.0>\n {235.0} <music21.tempo.MetronomeMark adagietto Quarter=65.0>\n {235.25} <music21.tempo.MetronomeMark adagietto Quarter=64.0>\n {235.25} <music21.tempo.MetronomeMark Quarter=63.0>\n {235.3333} <music21.tempo.MetronomeMark larghetto Quarter=62.0>\n {235.5} <music21.tempo.MetronomeMark larghetto Quarter=61.0>\n {235.6667} <music21.tempo.MetronomeMark larghetto Quarter=60.0>\n {235.6667} <music21.tempo.MetronomeMark lento Quarter=50.0>\n {236.0} <music21.tempo.MetronomeMark andantino Quarter=80.0>\n {252.3333} <music21.tempo.MetronomeMark andantino Quarter=79.0>\n {252.5} <music21.tempo.MetronomeMark andantino Quarter=78.0>\n {252.75} <music21.tempo.MetronomeMark Quarter=77.0>\n {253.0} <music21.tempo.MetronomeMark Quarter=76.0>\n {253.0} <music21.tempo.MetronomeMark Quarter=75.0>\n {253.3333} <music21.tempo.MetronomeMark andante Quarter=74.0>\n {253.5} <music21.tempo.MetronomeMark andante Quarter=73.0>\n {253.75} <music21.tempo.MetronomeMark andante Quarter=72.0>\n {254.0} <music21.tempo.MetronomeMark andante Quarter=71.0>\n {254.0} <music21.tempo.MetronomeMark andante Quarter=70.0>\n {255.0} <music21.tempo.MetronomeMark adagietto Quarter=65.0>\n {256.0} <music21.tempo.MetronomeMark andantino Quarter=80.0>\n {256.25} <music21.tempo.MetronomeMark andantino Quarter=81.0>\n {256.3333} <music21.tempo.MetronomeMark andantino Quarter=82.0>\n {256.5} <music21.tempo.MetronomeMark andante moderato Quarter=83.0>\n {256.5} <music21.tempo.MetronomeMark andante moderato Quarter=84.0>\n {256.6667} <music21.tempo.MetronomeMark andante moderato Quarter=85.0>\n {256.75} <music21.tempo.MetronomeMark maestoso Quarter=86.0>\n {256.75} <music21.tempo.MetronomeMark maestoso Quarter=87.0>\n {257.0} <music21.tempo.MetronomeMark maestoso Quarter=88.0>\n {257.0} <music21.tempo.MetronomeMark maestoso Quarter=89.0>\n {257.0} <music21.tempo.MetronomeMark maestoso Quarter=90.0>\n {258.3333} <music21.tempo.MetronomeMark maestoso Quarter=89.0>\n {258.5} <music21.tempo.MetronomeMark maestoso Quarter=88.0>\n {258.75} <music21.tempo.MetronomeMark maestoso Quarter=87.0>\n {259.0} <music21.tempo.MetronomeMark maestoso Quarter=86.0>\n {259.0} <music21.tempo.MetronomeMark andante moderato Quarter=85.0>\n {259.3333} <music21.tempo.MetronomeMark andante moderato Quarter=84.0>\n {259.5} <music21.tempo.MetronomeMark andante moderato Quarter=83.0>\n {259.75} <music21.tempo.MetronomeMark andantino Quarter=82.0>\n {260.0} <music21.tempo.MetronomeMark andantino Quarter=81.0>\n {260.0} <music21.tempo.MetronomeMark andantino Quarter=80.0>\n {260.5} <music21.tempo.MetronomeMark andantino Quarter=79.0>\n {261.0} <music21.tempo.MetronomeMark andantino Quarter=78.0>\n {261.3333} <music21.tempo.MetronomeMark Quarter=77.0>\n {261.75} <music21.tempo.MetronomeMark Quarter=76.0>\n {262.0} <music21.tempo.MetronomeMark Quarter=75.0>\n {262.5} <music21.tempo.MetronomeMark andante Quarter=74.0>\n {263.0} <music21.tempo.MetronomeMark andante Quarter=73.0>\n {263.3333} <music21.tempo.MetronomeMark andante Quarter=72.0>\n {263.75} <music21.tempo.MetronomeMark andante Quarter=71.0>\n {264.0} <music21.tempo.MetronomeMark andante Quarter=70.0>\n {264.0} <music21.tempo.MetronomeMark andantino Quarter=80.0>\n {264.25} <music21.tempo.MetronomeMark andantino Quarter=79.0>\n {264.3333} <music21.tempo.MetronomeMark andantino Quarter=78.0>\n {264.5} <music21.tempo.MetronomeMark Quarter=77.0>\n {264.6667} <music21.tempo.MetronomeMark Quarter=76.0>\n {264.75} <music21.tempo.MetronomeMark Quarter=75.0>\n {265.0} <music21.tempo.MetronomeMark andante Quarter=74.0>\n {265.0} <music21.tempo.MetronomeMark andante Quarter=73.0>\n {265.25} <music21.tempo.MetronomeMark andante Quarter=72.0>\n {265.3333} <music21.tempo.MetronomeMark andante Quarter=71.0>\n {265.5} <music21.tempo.MetronomeMark andante Quarter=70.0>\n {265.6667} <music21.tempo.MetronomeMark Quarter=69.0>\n {265.75} <music21.tempo.MetronomeMark adagietto Quarter=68.0>\n {265.75} <music21.tempo.MetronomeMark adagietto Quarter=67.0>\n {266.0} <music21.tempo.MetronomeMark adagietto Quarter=66.0>\n {266.0} <music21.tempo.MetronomeMark adagietto Quarter=65.0>\n {266.25} <music21.tempo.MetronomeMark adagietto Quarter=64.0>\n {266.3333} <music21.tempo.MetronomeMark Quarter=63.0>\n {266.5} <music21.tempo.MetronomeMark larghetto Quarter=62.0>\n {266.6667} <music21.tempo.MetronomeMark larghetto Quarter=61.0>\n {266.75} <music21.tempo.MetronomeMark larghetto Quarter=60.0>\n {267.0} <music21.tempo.MetronomeMark larghetto Quarter=59.0>\n {267.0} <music21.tempo.MetronomeMark adagio Quarter=58.0>\n {267.25} <music21.tempo.MetronomeMark adagio Quarter=57.0>\n {267.3333} <music21.tempo.MetronomeMark adagio Quarter=56.0>\n {267.5} <music21.tempo.MetronomeMark adagio Quarter=55.0>\n {267.6667} <music21.tempo.MetronomeMark lento Quarter=54.0>\n {267.75} <music21.tempo.MetronomeMark lento Quarter=53.0>\n {267.75} <music21.tempo.MetronomeMark lento Quarter=52.0>\n {268.0} <music21.tempo.MetronomeMark lento Quarter=51.0>\n {268.0} <music21.tempo.MetronomeMark lento Quarter=50.0>\n {268.0} <music21.tempo.MetronomeMark larghetto Quarter=60.0>\n {269.0} <music21.tempo.MetronomeMark lento Quarter=50.0>\n {269.25} <music21.tempo.MetronomeMark lento Quarter=51.0>\n {269.25} <music21.tempo.MetronomeMark lento Quarter=52.0>\n {269.25} <music21.tempo.MetronomeMark lento Quarter=53.0>\n {269.3333} <music21.tempo.MetronomeMark lento Quarter=54.0>\n {269.3333} <music21.tempo.MetronomeMark adagio Quarter=55.0>\n {269.5} <music21.tempo.MetronomeMark adagio Quarter=56.0>\n {269.5} <music21.tempo.MetronomeMark adagio Quarter=57.0>\n {269.5} <music21.tempo.MetronomeMark adagio Quarter=58.0>\n {269.5} <music21.tempo.MetronomeMark larghetto Quarter=59.0>\n {269.6667} <music21.tempo.MetronomeMark larghetto Quarter=60.0>\n {269.6667} <music21.tempo.MetronomeMark larghetto Quarter=61.0>\n {269.75} <music21.tempo.MetronomeMark larghetto Quarter=62.0>\n {269.75} <music21.tempo.MetronomeMark Quarter=63.0>\n {269.75} <music21.tempo.MetronomeMark adagietto Quarter=64.0>\n {269.75} <music21.tempo.MetronomeMark adagietto Quarter=65.0>\n {270.0} <music21.tempo.MetronomeMark adagietto Quarter=66.0>\n {270.0} <music21.tempo.MetronomeMark adagietto Quarter=67.0>\n {270.0} <music21.tempo.MetronomeMark adagietto Quarter=68.0>\n {270.0} <music21.tempo.MetronomeMark Quarter=69.0>\n {270.0} <music21.tempo.MetronomeMark andante Quarter=70.0>\n {270.25} <music21.tempo.MetronomeMark Quarter=69.0>\n {270.3333} <music21.tempo.MetronomeMark adagietto Quarter=68.0>\n {270.5} <music21.tempo.MetronomeMark adagietto Quarter=67.0>\n {270.5} <music21.tempo.MetronomeMark adagietto Quarter=66.0>\n {270.6667} <music21.tempo.MetronomeMark adagietto Quarter=65.0>\n {270.75} <music21.tempo.MetronomeMark adagietto Quarter=64.0>\n {270.75} <music21.tempo.MetronomeMark Quarter=63.0>\n {271.0} <music21.tempo.MetronomeMark larghetto Quarter=62.0>\n {271.0} <music21.tempo.MetronomeMark larghetto Quarter=61.0>\n {271.0} <music21.tempo.MetronomeMark larghetto Quarter=60.0>\n {271.25} <music21.tempo.MetronomeMark larghetto Quarter=59.0>\n {271.25} <music21.tempo.MetronomeMark adagio Quarter=58.0>\n {271.25} <music21.tempo.MetronomeMark adagio Quarter=57.0>\n {271.3333} <music21.tempo.MetronomeMark adagio Quarter=56.0>\n {271.3333} <music21.tempo.MetronomeMark adagio Quarter=55.0>\n {271.5} <music21.tempo.MetronomeMark lento Quarter=54.0>\n {271.5} <music21.tempo.MetronomeMark lento Quarter=53.0>\n {271.5} <music21.tempo.MetronomeMark lento Quarter=52.0>\n {271.5} <music21.tempo.MetronomeMark lento Quarter=51.0>\n {271.6667} <music21.tempo.MetronomeMark lento Quarter=50.0>\n {271.6667} <music21.tempo.MetronomeMark Quarter=49.0>\n {271.75} <music21.tempo.MetronomeMark largo Quarter=48.0>\n {271.75} <music21.tempo.MetronomeMark largo Quarter=47.0>\n {271.75} <music21.tempo.MetronomeMark largo Quarter=46.0>\n {271.75} <music21.tempo.MetronomeMark largo Quarter=45.0>\n {272.0} <music21.tempo.MetronomeMark largo Quarter=44.0>\n {272.0} <music21.tempo.MetronomeMark Quarter=43.0>\n {272.0} <music21.tempo.MetronomeMark grave Quarter=42.0>\n {272.0} <music21.tempo.MetronomeMark grave Quarter=41.0>\n {272.0} <music21.tempo.MetronomeMark grave Quarter=40.0>\n{0.0} <music21.stream.Part 0x23617b2abc8>\n {0.0} <music21.instrument.Piano 'Piano'>\n {0.0} <music21.key.Key of D major>\n {0.0} <music21.meter.TimeSignature 4/4>\n {0.0} <music21.stream.Voice 0x23617b24448>\n {0.0} <music21.note.Rest rest>\n {4.0} <music21.note.Note B>\n {4.0} <music21.note.Rest rest>\n {5.0} <music21.note.Note C#>\n {5.6667} <music21.note.Note D>\n {7.0} <music21.note.Note A>\n {7.75} <music21.note.Rest rest>\n {8.0} <music21.note.Note G>\n {9.0} <music21.note.Note A>\n {9.6667} <music21.note.Note B>\n {12.0} <music21.note.Note G>\n {16.0} <music21.note.Note E>\n {20.0} <music21.note.Note A>\n {22.0} <music21.chord.Chord A2 G3>\n {24.0} <music21.note.Note D>\n {28.0} <music21.note.Note D>\n {32.0} <music21.note.Note D>\n {36.0} <music21.note.Note B>\n {40.0} <music21.note.Note E>\n {43.0} <music21.note.Note G>\n {44.0} <music21.note.Note A>\n {48.0} <music21.note.Note D>\n {52.0} <music21.note.Note F#>\n {57.0} <music21.note.Note G>\n {60.0} <music21.note.Note A>\n {64.0} <music21.note.Note B>\n {68.0} <music21.note.Note F#>\n {72.0} <music21.note.Note G>\n {76.0} <music21.note.Note D>\n {77.6667} <music21.note.Note A>\n {78.6667} <music21.note.Note A>\n {79.6667} <music21.note.Note D>\n {80.6667} <music21.note.Note G>\n {81.6667} <music21.note.Note D>\n {82.5} <music21.chord.Chord D5 F#5>\n {84.6667} <music21.note.Note C#>\n {85.6667} <music21.note.Note E>\n {86.6667} <music21.note.Note D>\n {88.0} <music21.chord.Chord G#2 F#3>\n {92.0} <music21.chord.Chord G2 D3>\n {96.0} <music21.note.Note D>\n {99.0} <music21.note.Note C#>\n {100.0} <music21.note.Note B>\n {104.0} <music21.note.Note E>\n {108.0} <music21.note.Note A>\n {111.0} <music21.chord.Chord A2 G3>\n {112.0} <music21.note.Note D>\n {116.0} <music21.note.Note F#>\n {120.0} <music21.note.Note G>\n {124.0} <music21.note.Note A>\n {128.0} <music21.note.Note B>\n {132.0} <music21.note.Note F#>\n {136.0} <music21.note.Note G>\n {140.0} <music21.note.Note A>\n {142.0} <music21.note.Note G>\n {144.0} <music21.note.Note F#>\n {148.0} <music21.note.Note E>\n {153.0} <music21.chord.Chord G3 F#4>\n {153.6667} <music21.chord.Chord F#3 E4>\n {155.0} <music21.note.Note A>\n {156.0} <music21.note.Note D>\n {160.0} <music21.chord.Chord D3 A3>\n {164.0} <music21.note.Note G>\n {166.0} <music21.note.Note G>\n {168.0} <music21.note.Note G>\n {170.0} <music21.note.Note G>\n {172.0} <music21.note.Note D>\n {174.0} <music21.note.Note D>\n {176.0} <music21.note.Note D>\n {178.0} <music21.note.Note D>\n {180.0} <music21.note.Note C>\n {182.0} <music21.note.Note C>\n {184.0} <music21.note.Note A>\n {186.0} <music21.note.Note A>\n {188.0} <music21.note.Note D>\n {190.0} <music21.note.Note E>\n {192.0} <music21.note.Note F>\n {195.0} <music21.chord.Chord D2 D3>\n {196.0} <music21.note.Note G>\n {200.0} <music21.note.Note G#>\n {204.0} <music21.note.Note F#>\n {208.0} <music21.note.Note G>\n {208.6667} <music21.note.Note F#>\n {209.6667} <music21.note.Note B>\n {210.6667} <music21.note.Note F#>\n {211.6667} <music21.note.Note B>\n {213.0} <music21.chord.Chord G3 B3 D4 F#4>\n {214.0} <music21.chord.Chord E3 B-3 D4>\n {216.0} <music21.note.Note A>\n {220.6667} <music21.note.Note G>\n {226.0} <music21.chord.Chord F#3 C#4>\n {227.0} <music21.chord.Chord E3 B3>\n {228.0} <music21.chord.Chord D3 A3>\n {230.0} <music21.note.Note D>\n {236.0} <music21.chord.Chord D4 G4>\n {240.0} <music21.chord.Chord B3 F4>\n {244.0} <music21.note.Note A>\n {248.0} <music21.note.Note E>\n {250.0} <music21.note.Note E->\n {252.0} <music21.chord.Chord F#4 G4>\n {252.6667} <music21.chord.Chord E4 G4>\n {254.0} <music21.chord.Chord C4 E4 B-4>\n {256.0} <music21.note.Note B->\n {260.0} <music21.note.Note G>\n {264.0} <music21.note.Note D>\n {268.0} <music21.note.Note D>\n {275.75} <music21.note.Rest rest>\n {0.0} <music21.stream.Voice 0x23617559948>\n {0.0} <music21.note.Rest rest>\n {4.0} <music21.note.Note G>\n {6.0} <music21.note.Note C#>\n {8.0} <music21.note.Note E>\n {10.0} <music21.chord.Chord A3 D4>\n {12.6667} <music21.note.Note D>\n {14.0} <music21.chord.Chord F#3 A3>\n {15.0} <music21.chord.Chord F3 B3>\n {16.6667} <music21.note.Note B>\n {17.6667} <music21.note.Note G>\n {20.6667} <music21.note.Note E>\n {21.6667} <music21.note.Note B>\n {24.6667} <music21.note.Note A>\n {25.6667} <music21.chord.Chord F#3 A3>\n {28.6667} <music21.note.Note A>\n {29.6667} <music21.chord.Chord F#3 A3>\n {32.6667} <music21.note.Note A>\n {33.6667} <music21.chord.Chord F#3 A3>\n {36.6667} <music21.note.Note F#>\n {37.6667} <music21.note.Note C#>\n {38.6667} <music21.note.Note F#>\n {40.6667} <music21.note.Note B>\n {41.6667} <music21.note.Note G>\n {44.6667} <music21.note.Note E>\n {46.6667} <music21.note.Note E>\n {48.6667} <music21.note.Note A>\n {49.6667} <music21.chord.Chord F#3 A3>\n {52.6667} <music21.note.Note E>\n {53.6667} <music21.note.Note C#>\n {56.0} <music21.chord.Chord G3 A3 D4>\n {57.6667} <music21.note.Note D>\n {58.6667} <music21.note.Note A>\n {60.6667} <music21.note.Note E>\n {61.6667} <music21.note.Note D>\n {62.6667} <music21.note.Note E>\n {64.6667} <music21.note.Note F#>\n {65.6667} <music21.chord.Chord D4 F#4>\n {67.0} <music21.note.Note D>\n {68.6667} <music21.note.Note E>\n {69.6667} <music21.note.Note C#>\n {71.0} <music21.note.Note A>\n {72.6667} <music21.note.Note D>\n {73.6667} <music21.note.Note G>\n {74.6667} <music21.note.Note D>\n {75.6667} <music21.note.Note B>\n {78.0} <music21.note.Note C#>\n {79.0} <music21.note.Note B>\n {80.0} <music21.note.Note C>\n {85.0} <music21.note.Note A>\n {86.0} <music21.note.Note F#>\n {87.0} <music21.note.Note A>\n {96.6667} <music21.note.Note A>\n {97.6667} <music21.note.Note A>\n {98.6667} <music21.note.Note A>\n {99.6667} <music21.note.Note A>\n {100.6667} <music21.note.Note F#>\n {101.6667} <music21.note.Note A>\n {102.6667} <music21.note.Note D>\n {103.6667} <music21.note.Note F#>\n {104.6667} <music21.note.Note B>\n {105.6667} <music21.note.Note F#>\n {106.6667} <music21.note.Note F#>\n {107.6667} <music21.note.Note D>\n {108.6667} <music21.note.Note E>\n {109.6667} <music21.note.Note B>\n {112.6667} <music21.note.Note A>\n {113.6667} <music21.note.Note A>\n {114.6667} <music21.note.Note A>\n {115.6667} <music21.note.Note A>\n {116.6667} <music21.note.Note E>\n {117.6667} <music21.note.Note A>\n {119.0} <music21.note.Note F#>\n {119.6667} <music21.note.Note A>\n {120.6667} <music21.note.Note D>\n {121.6667} <music21.note.Note A>\n {122.6667} <music21.note.Note D>\n {124.6667} <music21.note.Note E>\n {126.6667} <music21.note.Note E>\n {128.6667} <music21.note.Note F#>\n {129.6667} <music21.note.Note F#>\n {130.6667} <music21.note.Note F#>\n {131.6667} <music21.note.Note F#>\n {132.6667} <music21.note.Note E>\n {133.6667} <music21.note.Note E>\n {134.6667} <music21.note.Note B>\n {136.6667} <music21.note.Note D>\n {137.6667} <music21.note.Note G>\n {139.0} <music21.note.Note G#>\n {140.6667} <music21.note.Note E>\n {141.6667} <music21.note.Note D>\n {144.6667} <music21.note.Note D>\n {145.6667} <music21.note.Note E>\n {148.5} <music21.note.Note B>\n {149.0} <music21.note.Note F#>\n {149.5} <music21.note.Note G>\n {150.0} <music21.note.Note B>\n {154.0} <music21.chord.Chord E3 D4>\n {156.6667} <music21.note.Note A>\n {157.6667} <music21.note.Note C#>\n {158.6667} <music21.chord.Chord A3 C#4>\n {164.6667} <music21.note.Note B>\n {165.6667} <music21.note.Note A>\n {166.6667} <music21.note.Note B>\n {167.6667} <music21.note.Note G>\n {168.6667} <music21.note.Note B>\n {169.6667} <music21.note.Note F#>\n {170.6667} <music21.note.Note B>\n {171.6667} <music21.note.Note E>\n {172.6667} <music21.note.Note A>\n {173.6667} <music21.note.Note G>\n {174.6667} <music21.note.Note A>\n {175.6667} <music21.note.Note F#>\n {176.6667} <music21.note.Note A>\n {177.6667} <music21.note.Note E>\n {178.6667} <music21.note.Note A>\n {179.6667} <music21.note.Note D>\n {180.6667} <music21.note.Note G>\n {181.6667} <music21.note.Note E>\n {182.6667} <music21.note.Note G>\n {183.6667} <music21.note.Note D>\n {184.6667} <music21.note.Note E>\n {185.6667} <music21.note.Note D>\n {186.6667} <music21.note.Note E>\n {187.6667} <music21.note.Note C#>\n {188.6667} <music21.note.Note A>\n {189.6667} <music21.note.Note F#>\n {190.6667} <music21.note.Note D>\n {191.6667} <music21.note.Note B>\n {192.6667} <music21.note.Note G#>\n {193.6667} <music21.note.Note B>\n {196.6667} <music21.note.Note D>\n {197.6667} <music21.note.Note A>\n {198.6667} <music21.note.Note D>\n {200.6667} <music21.note.Note F#>\n {201.6667} <music21.note.Note D>\n {203.0} <music21.note.Note D>\n {204.6667} <music21.note.Note E>\n {205.6667} <music21.note.Note B>\n {206.6667} <music21.note.Note E>\n {207.6667} <music21.note.Note E>\n {209.0} <music21.note.Note E->\n {210.0} <music21.note.Note A>\n {211.0} <music21.note.Note E->\n {212.0} <music21.note.Note E>\n {216.6667} <music21.note.Note F#>\n {217.6667} <music21.note.Note D>\n {219.0} <music21.note.Note E>\n {221.0} <music21.note.Note D>\n {221.6667} <music21.note.Note G>\n {222.6667} <music21.note.Note G>\n {230.6667} <music21.note.Note A>\n {231.6667} <music21.note.Note D>\n {232.6667} <music21.note.Note E>\n {233.6667} <music21.note.Note A>\n {236.0} <music21.note.Note B>\n {240.0} <music21.note.Note G#>\n {244.0} <music21.note.Note F#>\n {248.0} <music21.chord.Chord F#4 A4>\n {253.0} <music21.chord.Chord D4 G4 B4>\n {254.0} <music21.note.Note D>\n {256.6667} <music21.note.Note G>\n {257.6667} <music21.note.Note B->\n {258.6667} <music21.note.Note A>\n {260.6667} <music21.note.Note D>\n {261.6667} <music21.note.Note A>\n {262.6667} <music21.note.Note D>\n {264.6667} <music21.note.Note A>\n {265.6667} <music21.note.Note A>\n {267.0} <music21.chord.Chord B-2 G#3>\n {268.6667} <music21.note.Note A>\n {0.0} <music21.stream.Voice 0x236175b4d48>\n {0.0} <music21.note.Rest rest>\n {6.0} <music21.note.Note F#>\n {6.75} <music21.note.Rest rest>\n {13.0} <music21.note.Note G>\n {17.0} <music21.note.Note E>\n {18.0} <music21.note.Note B>\n {21.0} <music21.note.Note G>\n {25.0} <music21.note.Note D>\n {29.0} <music21.note.Note D>\n {33.0} <music21.note.Note D>\n {37.0} <music21.note.Note A>\n {39.0} <music21.note.Note B>\n {41.0} <music21.note.Note E>\n {45.0} <music21.note.Note G>\n {49.0} <music21.note.Note D>\n {53.0} <music21.note.Note A>\n {58.0} <music21.note.Note G>\n {59.0} <music21.note.Note D>\n {61.0} <music21.note.Note G>\n {65.0} <music21.note.Note E>\n {66.6667} <music21.note.Note F#>\n {69.0} <music21.note.Note A>\n {70.6667} <music21.note.Note B>\n {73.0} <music21.note.Note G>\n {74.0} <music21.note.Note F#>\n {75.0} <music21.note.Note E>\n {78.0} <music21.note.Note E>\n {79.0} <music21.note.Note D>\n {81.0} <music21.note.Note B->\n {82.6667} <music21.note.Note A>\n {97.0} <music21.note.Note C#>\n {98.0} <music21.note.Note D>\n {101.0} <music21.note.Note C#>\n {102.0} <music21.note.Note E>\n {103.0} <music21.note.Note C#>\n {105.0} <music21.note.Note E>\n {106.0} <music21.note.Note G>\n {107.0} <music21.note.Note E>\n {109.0} <music21.note.Note G>\n {113.0} <music21.note.Note C#>\n {114.0} <music21.note.Note D>\n {115.0} <music21.note.Note F#>\n {117.0} <music21.note.Note C#>\n {118.0} <music21.note.Note E>\n {121.0} <music21.note.Note G>\n {122.0} <music21.note.Note B>\n {123.0} <music21.note.Note G>\n {125.0} <music21.note.Note A>\n {127.0} <music21.note.Note A>\n {129.0} <music21.note.Note C#>\n {130.0} <music21.note.Note E>\n {131.0} <music21.note.Note D>\n {133.0} <music21.note.Note B>\n {134.0} <music21.note.Note C#>\n {135.0} <music21.chord.Chord A3 D4>\n {137.0} <music21.note.Note A>\n {138.0} <music21.note.Note B>\n {141.0} <music21.note.Note A>\n {145.0} <music21.note.Note A>\n {146.0} <music21.note.Note D>\n {148.75} <music21.note.Note E>\n {149.75} <music21.note.Note D>\n {157.0} <music21.note.Note D>\n {158.0} <music21.chord.Chord B3 D4>\n {159.0} <music21.chord.Chord G3 B3>\n {165.0} <music21.note.Note D>\n {167.0} <music21.note.Note D>\n {169.0} <music21.note.Note D>\n {171.0} <music21.note.Note D>\n {173.0} <music21.note.Note D>\n {175.0} <music21.note.Note D>\n {177.0} <music21.note.Note C#>\n {179.0} <music21.note.Note B>\n {181.0} <music21.note.Note B>\n {183.0} <music21.note.Note B>\n {185.0} <music21.note.Note A>\n {187.0} <music21.note.Note A>\n {189.0} <music21.note.Note D>\n {191.0} <music21.note.Note G>\n {193.0} <music21.chord.Chord F3 D4>\n {194.0} <music21.chord.Chord E3 G3 C4>\n {197.0} <music21.note.Note G>\n {198.0} <music21.note.Note B>\n {199.0} <music21.note.Note G>\n {201.0} <music21.note.Note B>\n {202.0} <music21.chord.Chord F3 G#3 B3>\n {205.0} <music21.note.Note A>\n {206.0} <music21.note.Note C#>\n {207.0} <music21.note.Note A>\n {214.0} <music21.chord.Chord G4 D5>\n {217.0} <music21.note.Note A>\n {218.0} <music21.chord.Chord G3 A3>\n {222.0} <music21.note.Note A>\n {223.0} <music21.chord.Chord D4 F#4>\n {231.0} <music21.note.Note E>\n {232.0} <music21.note.Note A>\n {233.0} <music21.note.Note D>\n {257.0} <music21.note.Note A>\n {258.0} <music21.note.Note D>\n {259.0} <music21.chord.Chord A3 E4>\n {261.0} <music21.note.Note G>\n {262.0} <music21.note.Note B>\n {263.0} <music21.note.Note F>\n {265.0} <music21.note.Note D>\n {266.0} <music21.chord.Chord C3 B-3>\n {269.0} <music21.note.Note D>\n {275.75} <music21.note.Rest rest>\n {0.0} <music21.stream.Voice 0x236175fdbc8>\n {62.0} <music21.note.Note C#>\n {202.0} <music21.note.Note E>\n {218.0} <music21.note.Note C#>\n{0.0} <music21.stream.Part 0x23617600608>\n {0.0} <music21.key.Key of D major>\n {0.0} <music21.meter.TimeSignature 4/4>\n {0.0} <music21.stream.Voice 0x2361760ab88>\n {0.0} <music21.note.Note A>\n {0.25} <music21.note.Note A>\n {1.25} <music21.note.Note F#>\n {1.75} <music21.note.Note C#>\n {2.25} <music21.note.Note A>\n {2.75} <music21.note.Note F#>\n {3.25} <music21.note.Note D>\n {3.75} <music21.note.Note D>\n {4.25} <music21.note.Note A>\n {6.25} <music21.note.Note A>\n {8.25} <music21.note.Note F#>\n {10.25} <music21.chord.Chord F#4 C#5>\n {11.25} <music21.note.Note A>\n {12.25} <music21.note.Note B>\n {14.0} <music21.note.Note D>\n {14.25} <music21.note.Note D>\n {15.25} <music21.note.Note D>\n {16.25} <music21.note.Note G>\n {17.25} <music21.note.Note G>\n {18.25} <music21.note.Note D>\n {19.25} <music21.note.Note F#>\n {22.25} <music21.note.Note C#>\n {24.25} <music21.note.Note A>\n {27.0} <music21.note.Note A>\n {27.25} <music21.note.Note A>\n {31.6667} <music21.note.Note A>\n {32.25} <music21.note.Note D>\n {33.25} <music21.note.Note E>\n {34.25} <music21.note.Note F#>\n {35.25} <music21.note.Note A>\n {37.6667} <music21.note.Note E>\n {39.25} <music21.note.Note D>\n {40.25} <music21.note.Note E>\n {41.25} <music21.note.Note D>\n {43.25} <music21.note.Note B>\n {44.25} <music21.note.Note E>\n {47.25} <music21.chord.Chord G3 B3>\n {48.25} <music21.note.Note D>\n {49.25} <music21.note.Note E>\n {50.25} <music21.note.Note F#>\n {51.25} <music21.note.Note A>\n {53.6667} <music21.note.Note E>\n {55.25} <music21.note.Note A>\n {56.25} <music21.note.Note C#>\n {57.25} <music21.note.Note B>\n {59.25} <music21.note.Note B>\n {63.25} <music21.note.Note A>\n {64.25} <music21.note.Note D>\n {66.25} <music21.note.Note C#>\n {67.25} <music21.note.Note B>\n {68.6667} <music21.note.Note A>\n {71.25} <music21.note.Note C#>\n {72.25} <music21.note.Note B>\n {74.25} <music21.note.Note A>\n {75.25} <music21.note.Note G>\n {76.25} <music21.note.Note G>\n {77.25} <music21.note.Note F#>\n {79.6667} <music21.note.Note F#>\n {80.25} <music21.note.Note F#>\n {81.25} <music21.note.Note E>\n {82.25} <music21.note.Note G>\n {83.25} <music21.note.Note F#>\n {84.25} <music21.note.Note E>\n {87.25} <music21.note.Note B>\n {89.5} <music21.chord.Chord C#5 F#5>\n {91.25} <music21.note.Note F#>\n {92.25} <music21.chord.Chord B-3 E4>\n {95.0} <music21.note.Note D>\n {95.25} <music21.note.Note A>\n {96.25} <music21.note.Note D>\n {97.25} <music21.note.Note E>\n {98.25} <music21.note.Note F#>\n {99.25} <music21.note.Note A>\n {101.25} <music21.chord.Chord F#4 A4>\n {102.6667} <music21.note.Note F#>\n {104.25} <music21.chord.Chord G4 B4>\n {107.25} <music21.note.Note G>\n {108.5} <music21.note.Note D>\n {110.25} <music21.note.Note D>\n {111.25} <music21.note.Note C#>\n {112.25} <music21.note.Note D>\n {113.25} <music21.note.Note E>\n {114.25} <music21.note.Note F#>\n {115.25} <music21.note.Note A>\n {117.25} <music21.chord.Chord E4 A4>\n {118.6667} <music21.note.Note D>\n {119.25} <music21.note.Note A>\n {120.25} <music21.chord.Chord D5 G5>\n {125.6667} <music21.chord.Chord D4 G4 B4>\n {127.25} <music21.chord.Chord E4 B4 C#5>\n {128.25} <music21.chord.Chord D5 F#5 D6>\n {130.25} <music21.chord.Chord C#5 F#5 C#6>\n {131.25} <music21.chord.Chord B4 F#5 B5>\n {132.6667} <music21.chord.Chord A4 E5 A5>\n {135.25} <music21.chord.Chord F#4 C#5>\n {136.25} <music21.chord.Chord B4 D5>\n {139.25} <music21.chord.Chord F#4 B4>\n {142.25} <music21.note.Note C#>\n {143.25} <music21.note.Note A>\n {144.25} <music21.note.Note D>\n {145.25} <music21.note.Note E>\n {146.25} <music21.note.Note F#>\n {147.25} <music21.note.Note G>\n {150.6667} <music21.note.Note A>\n {151.25} <music21.note.Note G>\n {152.25} <music21.note.Note F#>\n {155.25} <music21.chord.Chord D4 E4 G4 B4>\n {156.25} <music21.chord.Chord D4 F#4>\n {158.25} <music21.note.Note G>\n {159.25} <music21.note.Note E>\n {160.25} <music21.chord.Chord D4 F#4>\n {162.25} <music21.note.Note D>\n {163.5} <music21.note.Note A>\n {164.25} <music21.note.Note C#>\n {166.25} <music21.note.Note B>\n {170.25} <music21.note.Note B>\n {171.5} <music21.note.Note D>\n {172.25} <music21.note.Note B>\n {174.25} <music21.note.Note A>\n {178.25} <music21.note.Note F#>\n {179.5} <music21.note.Note G>\n {180.25} <music21.note.Note A>\n {182.0} <music21.note.Note F#>\n {182.25} <music21.note.Note G>\n {186.25} <music21.chord.Chord E5 G5>\n {187.5} <music21.chord.Chord G5 B5>\n {188.25} <music21.chord.Chord B4 D5 F#5 B5>\n {189.6667} <music21.chord.Chord A4 A5>\n {190.25} <music21.chord.Chord A4 B4 D5 A5>\n {194.25} <music21.chord.Chord D4 D5>\n {195.5} <music21.chord.Chord A4 A5>\n {196.25} <music21.chord.Chord C#5 F#5 C#6>\n {197.6667} <music21.chord.Chord B4 B5>\n {198.25} <music21.chord.Chord B4 D5 F#5 B5>\n {202.25} <music21.chord.Chord B4 B5>\n {203.5} <music21.chord.Chord D5 D6>\n {204.25} <music21.chord.Chord C#5 E5 A5 C#6>\n {205.6667} <music21.chord.Chord A4 A5>\n {207.25} <music21.chord.Chord C#5 C#6>\n {208.25} <music21.chord.Chord B4 B5>\n {211.6667} <music21.note.Note A>\n {212.25} <music21.note.Note A>\n {213.25} <music21.chord.Chord D5 G5 D6>\n {214.25} <music21.chord.Chord G4 D5 G5>\n {215.6667} <music21.note.Note D>\n {216.0} <music21.note.Note E>\n {216.25} <music21.note.Note F#>\n {217.6667} <music21.note.Note F#>\n {218.6667} <music21.note.Note E>\n {219.25} <music21.note.Note D>\n {220.25} <music21.note.Note E>\n {224.25} <music21.note.Note D>\n {234.25} <music21.note.Note E>\n {235.25} <music21.note.Note F#>\n {236.0} <music21.chord.Chord D5 F#5>\n {240.0} <music21.chord.Chord B4 E5>\n {242.25} <music21.chord.Chord D5 B5>\n {243.5} <music21.chord.Chord F5 D6>\n {244.0} <music21.chord.Chord C#5 F#5>\n {248.0} <music21.note.Note C#>\n {250.25} <music21.note.Note B>\n {252.25} <music21.chord.Chord B4 D5 A5>\n {253.25} <music21.chord.Chord D5 F#5 D6>\n {254.25} <music21.note.Note G>\n {255.6667} <music21.note.Note D>\n {256.0} <music21.note.Note E>\n {256.25} <music21.chord.Chord G4 D5>\n {259.25} <music21.note.Note G>\n {260.25} <music21.note.Note E>\n {264.25} <music21.chord.Chord F#4 D5>\n {266.25} <music21.note.Note E>\n {267.25} <music21.note.Note F>\n {268.25} <music21.chord.Chord F#4 A4>\n {0.0} <music21.stream.Voice 0x236176e3688>\n {0.0} <music21.note.Rest rest>\n {0.6667} <music21.note.Note G>\n {0.6667} <music21.note.Rest rest>\n {1.0} <music21.note.Rest rest>\n {1.5} <music21.note.Note D>\n {2.5} <music21.note.Note G>\n {3.5} <music21.note.Note A>\n {4.25} <music21.note.Note D>\n {4.25} <music21.note.Rest rest>\n {5.25} <music21.note.Note E>\n {6.25} <music21.note.Note E>\n {7.25} <music21.note.Note C#>\n {8.25} <music21.note.Note B>\n {9.25} <music21.note.Note C#>\n {11.6667} <music21.note.Note D>\n {12.25} <music21.note.Note E>\n {13.5} <music21.note.Note D>\n {14.25} <music21.note.Note A>\n {15.25} <music21.note.Note G#>\n {16.6667} <music21.note.Note F#>\n {17.6667} <music21.note.Note D>\n {19.6667} <music21.note.Note E>\n {22.25} <music21.note.Note F>\n {23.5} <music21.note.Note B>\n {35.6667} <music21.note.Note F#>\n {40.6667} <music21.note.Note F#>\n {43.6667} <music21.note.Note D>\n {45.6667} <music21.chord.Chord A3 C#4>\n {47.6667} <music21.note.Note A>\n {51.6667} <music21.note.Note C#>\n {56.6667} <music21.note.Note D>\n {58.6667} <music21.note.Note A>\n {59.6667} <music21.note.Note A>\n {65.6667} <music21.note.Note D>\n {67.6667} <music21.note.Note B>\n {69.6667} <music21.note.Note E>\n {71.25} <music21.note.Note F#>\n {73.6667} <music21.note.Note B>\n {76.6667} <music21.note.Note E>\n {81.6667} <music21.note.Note F#>\n {83.6667} <music21.note.Note E>\n {84.6667} <music21.note.Note D>\n {87.6667} <music21.note.Note D>\n {91.25} <music21.chord.Chord D5 B5>\n {92.75} <music21.note.Note A>\n {93.25} <music21.note.Note G>\n {93.75} <music21.note.Note D>\n {94.25} <music21.note.Note A>\n {94.5} <music21.note.Note G>\n {99.6667} <music21.note.Note F#>\n {104.25} <music21.note.Note E>\n {105.25} <music21.note.Note D>\n {107.25} <music21.note.Note B>\n {108.5} <music21.chord.Chord G4 B4>\n {111.6667} <music21.note.Note A>\n {115.6667} <music21.note.Note C#>\n {120.25} <music21.note.Note C#>\n {121.25} <music21.note.Note B>\n {123.25} <music21.note.Note B>\n {127.6667} <music21.note.Note A>\n {129.6667} <music21.chord.Chord D5 F#5 D6>\n {131.6667} <music21.chord.Chord B4 F#5 B5>\n {135.25} <music21.note.Note F#>\n {136.25} <music21.note.Note B>\n {138.25} <music21.note.Note A>\n {139.25} <music21.note.Note D>\n {140.6667} <music21.note.Note E>\n {147.6667} <music21.note.Note A>\n {151.6667} <music21.note.Note D>\n {153.25} <music21.chord.Chord B4 D5>\n {154.25} <music21.chord.Chord G4 B4>\n {155.25} <music21.note.Note E>\n {156.25} <music21.note.Note D>\n {162.75} <music21.note.Note F#>\n {165.6667} <music21.note.Note B>\n {170.75} <music21.note.Note C#>\n {173.6667} <music21.note.Note A>\n {178.75} <music21.note.Note F#>\n {181.6667} <music21.note.Note G>\n {186.75} <music21.chord.Chord F#5 A5>\n {194.75} <music21.chord.Chord F#4 F#5>\n {202.75} <music21.chord.Chord C#5 C#6>\n {212.6667} <music21.note.Note G>\n {219.6667} <music21.note.Note C#>\n {223.6667} <music21.note.Note D>\n {226.25} <music21.chord.Chord D4 A4>\n {227.25} <music21.chord.Chord D4 G4>\n {228.25} <music21.chord.Chord D4 F#4>\n {234.6667} <music21.note.Note D>\n {235.6667} <music21.note.Note A>\n {236.25} <music21.note.Note C#>\n {237.6667} <music21.note.Note B>\n {238.25} <music21.note.Note B>\n {242.75} <music21.chord.Chord E5 C#6>\n {244.25} <music21.note.Note C#>\n {245.6667} <music21.note.Note A>\n {247.25} <music21.note.Note C#>\n {248.0} <music21.chord.Chord F#5 B5>\n {251.25} <music21.note.Note A>\n {252.6667} <music21.chord.Chord B4 D5 G5>\n {256.25} <music21.note.Note F#>\n {258.6667} <music21.note.Note E>\n {259.25} <music21.note.Note D>\n {263.6667} <music21.note.Note D>\n {266.25} <music21.note.Note A>\n {267.25} <music21.note.Note C>\n {268.25} <music21.note.Note C#>\n {271.25} <music21.note.Note D>\n {271.5} <music21.note.Note G#>\n {271.75} <music21.note.Rest rest>\n {0.0} <music21.stream.Voice 0x23617979a48>\n {0.0} <music21.note.Rest rest>\n {5.6667} <music21.note.Note F#>\n {5.6667} <music21.note.Rest rest>\n {9.6667} <music21.note.Note D>\n {13.6667} <music21.note.Note C#>\n {14.6667} <music21.note.Note D>\n {15.6667} <music21.note.Note D>\n {22.75} <music21.note.Note C#>\n {70.6667} <music21.note.Note D>\n {71.6667} <music21.note.Note A>\n {89.6667} <music21.note.Note C#>\n {91.6667} <music21.note.Note A>\n {93.5} <music21.note.Note F#>\n {94.6667} <music21.note.Note F#>\n {101.25} <music21.note.Note E>\n {101.5} <music21.note.Note D>\n {104.6667} <music21.note.Note F#>\n {107.6667} <music21.note.Note D>\n {108.6667} <music21.note.Note E>\n {117.25} <music21.note.Note E>\n {117.5} <music21.note.Note D>\n {120.6667} <music21.note.Note D>\n {122.6667} <music21.note.Note A>\n {123.6667} <music21.note.Note A>\n {135.6667} <music21.note.Note A>\n {137.6667} <music21.note.Note B>\n {138.6667} <music21.note.Note G>\n {139.6667} <music21.note.Note F#>\n {142.6667} <music21.note.Note E>\n {143.6667} <music21.note.Note C#>\n {153.6667} <music21.chord.Chord A4 C#5>\n {155.6667} <music21.note.Note D>\n {158.6667} <music21.note.Note F#>\n {240.25} <music21.note.Note G#>\n {257.6667} <music21.note.Note F#>\n {259.6667} <music21.note.Note C#>\n {266.6667} <music21.note.Note D>\n {267.6667} <music21.note.Note D>\n {268.25} <music21.note.Note E>\n {270.75} <music21.note.Note D>\n {271.3333} <music21.note.Note E>\n {271.6667} <music21.note.Note E>\n {271.9167} <music21.note.Rest rest>\n {0.0} <music21.stream.Voice 0x236179a3bc8>\n {0.0} <music21.note.Rest rest>\n {90.6667} <music21.note.Note E>\n {90.6667} <music21.note.Rest rest>\n {101.6667} <music21.note.Note C#>\n {110.6667} <music21.note.Note B>\n {117.6667} <music21.note.Note C#>\n {269.25} <music21.note.Note E>\n {269.5} <music21.note.Note C#>\n {269.75} <music21.note.Note G#>\n {270.0} <music21.note.Note A>\n {270.25} <music21.note.Note B>\n {270.5} <music21.note.Note G#>\n {270.75} <music21.note.Rest rest>\n {0.0} <music21.stream.Voice 0x236179b3408>\n {269.3333} <music21.note.Note D>\n {269.6667} <music21.note.Note A>\n {270.3333} <music21.note.Note A>\n{0.0} <music21.stream.Part 0x236179b3f88>\n {0.0} <music21.key.Key of D major>\n {0.0} <music21.meter.TimeSignature 4/4>\n {0.0} <music21.stream.Voice 0x236179c9548>\n {0.0} <music21.note.Rest rest>\n {4.25} <music21.note.Note B>\n {5.25} <music21.note.Note C#>\n {6.25} <music21.note.Note C#>\n {7.25} <music21.note.Note A>\n {8.25} <music21.note.Note G>\n {9.25} <music21.note.Note A>\n {10.25} <music21.chord.Chord A3 D4>\n {12.25} <music21.note.Note G>\n {13.25} <music21.note.Note G>\n {14.25} <music21.chord.Chord F#3 A3>\n {15.25} <music21.chord.Chord F3 B3>\n {16.25} <music21.note.Note E>\n {20.25} <music21.note.Note A>\n {22.25} <music21.chord.Chord A2 G3>\n {24.25} <music21.note.Note D>\n {28.25} <music21.note.Note D>\n {32.25} <music21.note.Note D>\n {36.25} <music21.note.Note B>\n {40.25} <music21.note.Note E>\n {43.25} <music21.note.Note G>\n {44.25} <music21.note.Note A>\n {48.25} <music21.note.Note D>\n {52.25} <music21.note.Note F#>\n {57.25} <music21.note.Note G>\n {60.25} <music21.note.Note A>\n {64.25} <music21.note.Note B>\n {68.25} <music21.note.Note F#>\n {72.25} <music21.note.Note G>\n {76.25} <music21.note.Note D>\n {77.6667} <music21.note.Note A>\n {78.25} <music21.note.Note C#>\n {79.25} <music21.note.Note B>\n {80.25} <music21.note.Note C>\n {84.6667} <music21.note.Note C#>\n {85.25} <music21.note.Note A>\n {86.25} <music21.note.Note F#>\n {87.25} <music21.note.Note A>\n {88.25} <music21.chord.Chord G#2 F#3>\n {92.25} <music21.chord.Chord G2 D3>\n {96.25} <music21.note.Note D>\n {99.25} <music21.note.Note C#>\n {100.25} <music21.note.Note B>\n {104.25} <music21.note.Note E>\n {108.25} <music21.note.Note A>\n {111.25} <music21.chord.Chord A2 G3>\n {112.25} <music21.note.Note D>\n {116.25} <music21.note.Note F#>\n {120.25} <music21.note.Note G>\n {124.25} <music21.note.Note A>\n {128.25} <music21.note.Note B>\n {132.25} <music21.note.Note F#>\n {136.25} <music21.note.Note G>\n {140.25} <music21.note.Note A>\n {142.25} <music21.note.Note G>\n {144.25} <music21.note.Note F#>\n {148.25} <music21.note.Note E>\n {153.25} <music21.chord.Chord G3 F#4>\n {154.25} <music21.chord.Chord E3 D4>\n {155.25} <music21.note.Note A>\n {156.25} <music21.note.Note D>\n {160.25} <music21.chord.Chord D3 A3>\n {164.25} <music21.note.Note G>\n {166.25} <music21.note.Note G>\n {168.25} <music21.note.Note G>\n {170.25} <music21.note.Note G>\n {172.25} <music21.note.Note D>\n {174.25} <music21.note.Note D>\n {176.25} <music21.note.Note D>\n {178.25} <music21.note.Note D>\n {180.25} <music21.note.Note C>\n {182.25} <music21.note.Note C>\n {184.25} <music21.note.Note A>\n {186.25} <music21.note.Note A>\n {188.25} <music21.note.Note D>\n {190.25} <music21.note.Note E>\n {192.25} <music21.note.Note F>\n {195.25} <music21.chord.Chord D2 D3>\n {196.25} <music21.note.Note G>\n {200.25} <music21.note.Note G#>\n {204.25} <music21.note.Note F#>\n {208.25} <music21.note.Note G>\n {209.25} <music21.note.Note E->\n {210.25} <music21.note.Note A>\n {211.25} <music21.note.Note E->\n {212.25} <music21.note.Note E>\n {216.25} <music21.note.Note A>\n {220.6667} <music21.note.Note G>\n {226.25} <music21.chord.Chord F#3 C#4>\n {227.25} <music21.chord.Chord E3 B3>\n {228.25} <music21.chord.Chord D3 A3>\n {230.25} <music21.note.Note D>\n {236.0} <music21.chord.Chord D4 G4>\n {240.0} <music21.chord.Chord B3 F4>\n {244.0} <music21.note.Note A>\n {248.0} <music21.note.Note E>\n {250.25} <music21.note.Note E->\n {252.25} <music21.chord.Chord F#4 G4>\n {253.25} <music21.chord.Chord D4 G4 B4>\n {254.25} <music21.chord.Chord C4 E4 B-4>\n {256.25} <music21.note.Note B->\n {260.25} <music21.note.Note G>\n {264.25} <music21.note.Note D>\n {268.25} <music21.note.Note D>\n {0.0} <music21.stream.Voice 0x23617a4c608>\n {0.0} <music21.note.Rest rest>\n {4.25} <music21.note.Note G>\n {4.25} <music21.note.Rest rest>\n {6.25} <music21.note.Note F#>\n {8.25} <music21.note.Note E>\n {12.6667} <music21.note.Note D>\n {16.6667} <music21.note.Note B>\n {17.25} <music21.note.Note E>\n {18.25} <music21.note.Note B>\n {20.6667} <music21.note.Note E>\n {21.25} <music21.note.Note G>\n {24.6667} <music21.note.Note A>\n {25.25} <music21.note.Note D>\n {28.6667} <music21.note.Note A>\n {29.25} <music21.note.Note D>\n {32.6667} <music21.note.Note A>\n {33.25} <music21.note.Note D>\n {36.6667} <music21.note.Note F#>\n {37.25} <music21.note.Note A>\n {38.6667} <music21.note.Note F#>\n {39.25} <music21.note.Note B>\n {40.6667} <music21.note.Note B>\n {41.25} <music21.note.Note E>\n {44.6667} <music21.note.Note E>\n {45.25} <music21.note.Note G>\n {48.6667} <music21.note.Note A>\n {49.25} <music21.note.Note D>\n {52.6667} <music21.note.Note E>\n {53.25} <music21.note.Note A>\n {56.25} <music21.chord.Chord G3 A3 D4>\n {57.6667} <music21.note.Note D>\n {58.25} <music21.note.Note G>\n {59.25} <music21.note.Note D>\n {60.6667} <music21.note.Note E>\n {61.25} <music21.note.Note G>\n {64.6667} <music21.note.Note F#>\n {65.25} <music21.note.Note E>\n {66.6667} <music21.note.Note F#>\n {67.25} <music21.note.Note D>\n {68.6667} <music21.note.Note E>\n {69.25} <music21.note.Note A>\n {70.6667} <music21.note.Note B>\n {71.25} <music21.note.Note A>\n {72.6667} <music21.note.Note D>\n {73.25} <music21.note.Note G>\n {74.25} <music21.note.Note F#>\n {75.25} <music21.note.Note E>\n {78.25} <music21.note.Note E>\n {79.25} <music21.note.Note D>\n {80.6667} <music21.note.Note G>\n {81.25} <music21.note.Note B->\n {82.5} <music21.chord.Chord D5 F#5>\n {85.6667} <music21.note.Note E>\n {86.6667} <music21.note.Note D>\n {96.6667} <music21.note.Note A>\n {97.25} <music21.note.Note C#>\n {98.25} <music21.note.Note D>\n {99.6667} <music21.note.Note A>\n {100.6667} <music21.note.Note F#>\n {101.25} <music21.note.Note C#>\n {102.25} <music21.note.Note E>\n {103.25} <music21.note.Note C#>\n {104.6667} <music21.note.Note B>\n {105.25} <music21.note.Note E>\n {106.25} <music21.note.Note G>\n {107.25} <music21.note.Note E>\n {108.6667} <music21.note.Note E>\n {109.25} <music21.note.Note G>\n {112.6667} <music21.note.Note A>\n {113.25} <music21.note.Note C#>\n {114.25} <music21.note.Note D>\n {115.25} <music21.note.Note F#>\n {116.6667} <music21.note.Note E>\n {117.25} <music21.note.Note C#>\n {118.25} <music21.note.Note E>\n {119.25} <music21.note.Note F#>\n {120.6667} <music21.note.Note D>\n {121.25} <music21.note.Note G>\n {122.25} <music21.note.Note B>\n {123.25} <music21.note.Note G>\n {124.6667} <music21.note.Note E>\n {125.25} <music21.note.Note A>\n {127.25} <music21.note.Note A>\n {128.6667} <music21.note.Note F#>\n {129.25} <music21.note.Note C#>\n {130.25} <music21.note.Note E>\n {131.25} <music21.note.Note D>\n {132.6667} <music21.note.Note E>\n {133.25} <music21.note.Note B>\n {134.25} <music21.note.Note C#>\n {135.25} <music21.chord.Chord A3 D4>\n {136.6667} <music21.note.Note D>\n {137.25} <music21.note.Note A>\n {138.25} <music21.note.Note B>\n {139.25} <music21.note.Note G#>\n {140.6667} <music21.note.Note E>\n {141.25} <music21.note.Note A>\n {144.6667} <music21.note.Note D>\n {145.25} <music21.note.Note A>\n {146.25} <music21.note.Note D>\n {148.5} <music21.note.Note B>\n {149.25} <music21.note.Note F#>\n {149.75} <music21.note.Note D>\n {150.25} <music21.note.Note B>\n {153.6667} <music21.chord.Chord F#3 E4>\n {156.6667} <music21.note.Note A>\n {157.25} <music21.note.Note D>\n {158.25} <music21.chord.Chord B3 D4>\n {159.25} <music21.chord.Chord G3 B3>\n {164.6667} <music21.note.Note B>\n {165.25} <music21.note.Note D>\n {166.6667} <music21.note.Note B>\n {167.25} <music21.note.Note D>\n {168.6667} <music21.note.Note B>\n {169.25} <music21.note.Note D>\n {170.6667} <music21.note.Note B>\n {171.25} <music21.note.Note D>\n {172.6667} <music21.note.Note A>\n {173.25} <music21.note.Note D>\n {174.6667} <music21.note.Note A>\n {175.25} <music21.note.Note D>\n {176.6667} <music21.note.Note A>\n {177.25} <music21.note.Note C#>\n {178.6667} <music21.note.Note A>\n {179.25} <music21.note.Note B>\n {180.6667} <music21.note.Note G>\n {181.25} <music21.note.Note B>\n {182.6667} <music21.note.Note G>\n {183.25} <music21.note.Note B>\n {184.6667} <music21.note.Note E>\n {185.25} <music21.note.Note A>\n {186.6667} <music21.note.Note E>\n {187.25} <music21.note.Note A>\n {188.6667} <music21.note.Note A>\n {189.25} <music21.note.Note D>\n {190.6667} <music21.note.Note D>\n {191.25} <music21.note.Note G>\n {192.6667} <music21.note.Note G#>\n {193.25} <music21.chord.Chord F3 D4>\n {194.25} <music21.chord.Chord E3 G3 C4>\n {196.6667} <music21.note.Note D>\n {197.25} <music21.note.Note G>\n {198.25} <music21.note.Note B>\n {199.25} <music21.note.Note G>\n {200.6667} <music21.note.Note F#>\n {201.25} <music21.note.Note B>\n {202.25} <music21.chord.Chord F3 G#3 B3>\n {204.6667} <music21.note.Note E>\n {205.25} <music21.note.Note A>\n {206.25} <music21.note.Note C#>\n {207.25} <music21.note.Note A>\n {208.6667} <music21.note.Note F#>\n {209.6667} <music21.note.Note B>\n {210.6667} <music21.note.Note F#>\n {211.6667} <music21.note.Note B>\n {213.25} <music21.chord.Chord G3 B3 D4 F#4>\n {214.25} <music21.chord.Chord E3 B-3 D4>\n {216.6667} <music21.note.Note F#>\n {217.25} <music21.note.Note A>\n {218.25} <music21.chord.Chord G3 A3>\n {221.25} <music21.note.Note D>\n {222.25} <music21.note.Note A>\n {223.25} <music21.chord.Chord D4 F#4>\n {230.6667} <music21.note.Note A>\n {231.25} <music21.note.Note E>\n {232.25} <music21.note.Note A>\n {233.25} <music21.note.Note D>\n {236.25} <music21.note.Note B>\n {240.25} <music21.note.Note G#>\n {244.25} <music21.note.Note F#>\n {248.0} <music21.chord.Chord F#4 A4>\n {252.6667} <music21.chord.Chord E4 G4>\n {254.25} <music21.note.Note D>\n {256.6667} <music21.note.Note G>\n {257.25} <music21.note.Note A>\n {258.25} <music21.note.Note D>\n {259.25} <music21.chord.Chord A3 E4>\n {260.6667} <music21.note.Note D>\n {261.25} <music21.note.Note G>\n {262.25} <music21.note.Note B>\n {263.25} <music21.note.Note F>\n {264.6667} <music21.note.Note A>\n {265.25} <music21.note.Note D>\n {266.25} <music21.chord.Chord C3 B-3>\n {267.25} <music21.chord.Chord B-2 G#3>\n {268.6667} <music21.note.Note A>\n {271.9167} <music21.note.Rest rest>\n {0.0} <music21.stream.Voice 0x23617f23748>\n {0.0} <music21.note.Rest rest>\n {5.6667} <music21.note.Note D>\n {9.6667} <music21.note.Note B>\n {17.6667} <music21.note.Note G>\n {21.6667} <music21.note.Note B>\n {25.6667} <music21.chord.Chord F#3 A3>\n {29.6667} <music21.chord.Chord F#3 A3>\n {33.6667} <music21.chord.Chord F#3 A3>\n {37.6667} <music21.note.Note C#>\n {41.6667} <music21.note.Note G>\n {46.6667} <music21.note.Note E>\n {49.6667} <music21.chord.Chord F#3 A3>\n {53.6667} <music21.note.Note C#>\n {58.6667} <music21.note.Note A>\n {61.6667} <music21.note.Note D>\n {62.25} <music21.note.Note C#>\n {65.6667} <music21.chord.Chord D4 F#4>\n {69.6667} <music21.note.Note C#>\n {73.6667} <music21.note.Note G>\n {74.6667} <music21.note.Note D>\n {75.6667} <music21.note.Note B>\n {78.6667} <music21.note.Note A>\n {79.6667} <music21.note.Note D>\n {81.6667} <music21.note.Note D>\n {82.6667} <music21.note.Note A>\n {97.6667} <music21.note.Note A>\n {98.6667} <music21.note.Note A>\n {101.6667} <music21.note.Note A>\n {102.6667} <music21.note.Note D>\n {103.6667} <music21.note.Note F#>\n {105.6667} <music21.note.Note F#>\n {106.6667} <music21.note.Note F#>\n {107.6667} <music21.note.Note D>\n {109.6667} <music21.note.Note B>\n {113.6667} <music21.note.Note A>\n {114.6667} <music21.note.Note A>\n {115.6667} <music21.note.Note A>\n {117.6667} <music21.note.Note A>\n {119.6667} <music21.note.Note A>\n {121.6667} <music21.note.Note A>\n {122.6667} <music21.note.Note D>\n {126.6667} <music21.note.Note E>\n {129.6667} <music21.note.Note F#>\n {130.6667} <music21.note.Note F#>\n {131.6667} <music21.note.Note F#>\n {133.6667} <music21.note.Note E>\n {134.6667} <music21.note.Note B>\n {137.6667} <music21.note.Note G>\n {141.6667} <music21.note.Note D>\n {145.6667} <music21.note.Note E>\n {148.75} <music21.note.Note E>\n {149.5} <music21.note.Note G>\n {157.6667} <music21.note.Note C#>\n {158.6667} <music21.chord.Chord A3 C#4>\n {165.6667} <music21.note.Note A>\n {167.6667} <music21.note.Note G>\n {169.6667} <music21.note.Note F#>\n {171.6667} <music21.note.Note E>\n {173.6667} <music21.note.Note G>\n {175.6667} <music21.note.Note F#>\n {177.6667} <music21.note.Note E>\n {179.6667} <music21.note.Note D>\n {181.6667} <music21.note.Note E>\n {183.6667} <music21.note.Note D>\n {185.6667} <music21.note.Note D>\n {187.6667} <music21.note.Note C#>\n {189.6667} <music21.note.Note F#>\n {191.6667} <music21.note.Note B>\n {193.6667} <music21.note.Note B>\n {197.6667} <music21.note.Note A>\n {198.6667} <music21.note.Note D>\n {201.6667} <music21.note.Note D>\n {202.25} <music21.note.Note E>\n {203.25} <music21.note.Note D>\n {205.6667} <music21.note.Note B>\n {206.6667} <music21.note.Note E>\n {207.6667} <music21.note.Note E>\n {214.25} <music21.chord.Chord G4 D5>\n {217.6667} <music21.note.Note D>\n {218.25} <music21.note.Note C#>\n {219.25} <music21.note.Note E>\n {221.6667} <music21.note.Note G>\n {222.6667} <music21.note.Note G>\n {231.6667} <music21.note.Note D>\n {232.6667} <music21.note.Note E>\n {233.6667} <music21.note.Note A>\n {257.6667} <music21.note.Note B->\n {258.6667} <music21.note.Note A>\n {261.6667} <music21.note.Note A>\n {262.6667} <music21.note.Note D>\n {265.6667} <music21.note.Note A>\n {269.25} <music21.note.Note D>\n {0.0} <music21.stream.Voice 0x23617f8cc88>\n {62.6667} <music21.note.Note E>\n"
],
[
"# Flat all the elements\nelements_to_parse = midi.flat.notes",
"_____no_output_____"
],
[
"len(elements_to_parse)",
"_____no_output_____"
],
[
"for e in elements_to_parse:\n print(e, e.offset)",
"<music21.note.Note A> 0.0\n<music21.note.Note A> 0.0\n<music21.note.Note A> 0.0\n<music21.note.Note A> 0.25\n<music21.note.Note G> 2/3\n<music21.note.Note G> 2/3\n<music21.note.Note F#> 1.0\n<music21.note.Note F#> 1.25\n<music21.note.Note D> 1.5\n<music21.note.Note D> 1.5\n<music21.note.Note C#> 1.75\n<music21.note.Note C#> 1.75\n<music21.note.Note A> 2.0\n<music21.note.Note A> 2.25\n<music21.note.Note G> 2.5\n<music21.note.Note G> 2.5\n<music21.note.Note F#> 2.75\n<music21.note.Note F#> 2.75\n<music21.note.Note D> 3.0\n<music21.note.Note D> 3.25\n<music21.note.Note A> 3.5\n<music21.note.Note A> 3.5\n<music21.note.Note D> 3.75\n<music21.note.Note D> 3.75\n<music21.note.Note A> 4.0\n<music21.note.Note D> 4.0\n<music21.note.Note B> 4.0\n<music21.note.Note G> 4.0\n<music21.note.Note A> 4.25\n<music21.note.Note D> 4.25\n<music21.note.Note B> 4.25\n<music21.note.Note G> 4.25\n<music21.note.Note E> 5.0\n<music21.note.Note C#> 5.0\n<music21.note.Note E> 5.25\n<music21.note.Note C#> 5.25\n<music21.note.Note F#> 17/3\n<music21.note.Note D> 17/3\n<music21.note.Note F#> 17/3\n<music21.note.Note D> 17/3\n<music21.note.Note A> 6.0\n<music21.note.Note E> 6.0\n<music21.note.Note C#> 6.0\n<music21.note.Note F#> 6.0\n<music21.note.Note A> 6.25\n<music21.note.Note E> 6.25\n<music21.note.Note C#> 6.25\n<music21.note.Note F#> 6.25\n<music21.note.Note C#> 7.0\n<music21.note.Note A> 7.0\n<music21.note.Note C#> 7.25\n<music21.note.Note A> 7.25\n<music21.note.Note F#> 8.0\n<music21.note.Note B> 8.0\n<music21.note.Note G> 8.0\n<music21.note.Note E> 8.0\n<music21.note.Note F#> 8.25\n<music21.note.Note B> 8.25\n<music21.note.Note G> 8.25\n<music21.note.Note E> 8.25\n<music21.note.Note C#> 9.0\n<music21.note.Note A> 9.0\n<music21.note.Note C#> 9.25\n<music21.note.Note A> 9.25\n<music21.note.Note D> 29/3\n<music21.note.Note B> 29/3\n<music21.note.Note D> 29/3\n<music21.note.Note B> 29/3\n<music21.chord.Chord F#4 C#5> 10.0\n<music21.chord.Chord A3 D4> 10.0\n<music21.chord.Chord F#4 C#5> 10.25\n<music21.chord.Chord A3 D4> 10.25\n<music21.note.Note A> 11.0\n<music21.note.Note A> 11.25\n<music21.note.Note D> 35/3\n<music21.note.Note D> 35/3\n<music21.note.Note B> 12.0\n<music21.note.Note E> 12.0\n<music21.note.Note G> 12.0\n<music21.note.Note B> 12.25\n<music21.note.Note E> 12.25\n<music21.note.Note G> 12.25\n<music21.note.Note D> 38/3\n<music21.note.Note D> 38/3\n<music21.note.Note G> 13.0\n<music21.note.Note G> 13.25\n<music21.note.Note D> 40/3\n<music21.note.Note D> 13.5\n<music21.note.Note C#> 41/3\n<music21.note.Note C#> 41/3\n<music21.note.Note D> 13.75\n<music21.note.Note D> 14.0\n<music21.note.Note A> 14.0\n<music21.chord.Chord F#3 A3> 14.0\n<music21.note.Note D> 14.0\n<music21.note.Note D> 14.25\n<music21.note.Note A> 14.25\n<music21.chord.Chord F#3 A3> 14.25\n<music21.note.Note D> 44/3\n<music21.note.Note D> 44/3\n<music21.note.Note D> 15.0\n<music21.note.Note G#> 15.0\n<music21.chord.Chord F3 B3> 15.0\n<music21.note.Note D> 15.25\n<music21.note.Note G#> 15.25\n<music21.chord.Chord F3 B3> 15.25\n<music21.note.Note D> 47/3\n<music21.note.Note D> 47/3\n<music21.note.Note G> 16.0\n<music21.note.Note E> 16.0\n<music21.note.Note G> 16.25\n<music21.note.Note E> 16.25\n<music21.note.Note F#> 50/3\n<music21.note.Note B> 50/3\n<music21.note.Note F#> 50/3\n<music21.note.Note B> 50/3\n<music21.note.Note G> 17.0\n<music21.note.Note E> 17.0\n<music21.note.Note G> 17.25\n<music21.note.Note E> 17.25\n<music21.note.Note D> 53/3\n<music21.note.Note G> 53/3\n<music21.note.Note D> 53/3\n<music21.note.Note G> 53/3\n<music21.note.Note D> 18.0\n<music21.note.Note B> 18.0\n<music21.note.Note D> 18.25\n<music21.note.Note B> 18.25\n<music21.note.Note F#> 19.0\n<music21.note.Note F#> 19.25\n<music21.note.Note E> 59/3\n<music21.note.Note E> 59/3\n<music21.note.Note A> 20.0\n<music21.note.Note A> 20.25\n<music21.note.Note E> 62/3\n<music21.note.Note E> 62/3\n<music21.note.Note G> 21.0\n<music21.note.Note G> 21.25\n<music21.note.Note B> 65/3\n<music21.note.Note B> 65/3\n<music21.note.Note C#> 22.0\n<music21.note.Note F> 22.0\n<music21.chord.Chord A2 G3> 22.0\n<music21.note.Note C#> 22.25\n<music21.note.Note F> 22.25\n<music21.chord.Chord A2 G3> 22.25\n<music21.note.Note C#> 22.75\n<music21.note.Note C#> 22.75\n<music21.note.Note B> 23.5\n<music21.note.Note B> 23.5\n<music21.note.Note A> 24.0\n<music21.note.Note D> 24.0\n<music21.note.Note A> 24.25\n<music21.note.Note D> 24.25\n<music21.note.Note A> 74/3\n<music21.note.Note A> 74/3\n<music21.note.Note D> 25.0\n<music21.note.Note D> 25.25\n<music21.chord.Chord F#3 A3> 77/3\n<music21.chord.Chord F#3 A3> 77/3\n<music21.note.Note A> 27.0\n<music21.note.Note A> 27.0\n<music21.note.Note A> 27.0\n<music21.note.Note A> 27.25\n<music21.note.Note D> 28.0\n<music21.note.Note D> 28.25\n<music21.note.Note A> 86/3\n<music21.note.Note A> 86/3\n<music21.note.Note D> 29.0\n<music21.note.Note D> 29.25\n<music21.chord.Chord F#3 A3> 89/3\n<music21.chord.Chord F#3 A3> 89/3\n<music21.note.Note A> 95/3\n<music21.note.Note A> 95/3\n<music21.note.Note D> 32.0\n<music21.note.Note D> 32.0\n<music21.note.Note D> 32.25\n<music21.note.Note D> 32.25\n<music21.note.Note A> 98/3\n<music21.note.Note A> 98/3\n<music21.note.Note E> 33.0\n<music21.note.Note D> 33.0\n<music21.note.Note E> 33.25\n<music21.note.Note D> 33.25\n<music21.chord.Chord F#3 A3> 101/3\n<music21.chord.Chord F#3 A3> 101/3\n<music21.note.Note F#> 34.0\n<music21.note.Note F#> 34.25\n<music21.note.Note A> 35.0\n<music21.note.Note A> 35.25\n<music21.note.Note F#> 107/3\n<music21.note.Note F#> 107/3\n<music21.note.Note B> 36.0\n<music21.note.Note B> 36.25\n<music21.note.Note F#> 110/3\n<music21.note.Note F#> 110/3\n<music21.note.Note A> 37.0\n<music21.note.Note A> 37.25\n<music21.note.Note E> 113/3\n<music21.note.Note C#> 113/3\n<music21.note.Note E> 113/3\n<music21.note.Note C#> 113/3\n<music21.note.Note F#> 116/3\n<music21.note.Note F#> 116/3\n<music21.note.Note D> 39.0\n<music21.note.Note B> 39.0\n<music21.note.Note D> 39.25\n<music21.note.Note B> 39.25\n<music21.note.Note E> 40.0\n<music21.note.Note E> 40.0\n<music21.note.Note E> 40.25\n<music21.note.Note E> 40.25\n<music21.note.Note F#> 122/3\n<music21.note.Note B> 122/3\n<music21.note.Note F#> 122/3\n<music21.note.Note B> 122/3\n<music21.note.Note D> 41.0\n<music21.note.Note E> 41.0\n<music21.note.Note D> 41.25\n<music21.note.Note E> 41.25\n<music21.note.Note G> 125/3\n<music21.note.Note G> 125/3\n<music21.note.Note B> 43.0\n<music21.note.Note G> 43.0\n<music21.note.Note B> 43.25\n<music21.note.Note G> 43.25\n<music21.note.Note D> 131/3\n<music21.note.Note D> 131/3\n<music21.note.Note E> 44.0\n<music21.note.Note A> 44.0\n<music21.note.Note E> 44.25\n<music21.note.Note A> 44.25\n<music21.note.Note E> 134/3\n<music21.note.Note E> 134/3\n<music21.note.Note G> 45.0\n<music21.note.Note G> 45.25\n<music21.chord.Chord A3 C#4> 137/3\n<music21.chord.Chord A3 C#4> 137/3\n<music21.note.Note E> 140/3\n<music21.note.Note E> 140/3\n<music21.chord.Chord G3 B3> 47.0\n<music21.chord.Chord G3 B3> 47.25\n<music21.note.Note A> 143/3\n<music21.note.Note A> 143/3\n<music21.note.Note D> 48.0\n<music21.note.Note D> 48.0\n<music21.note.Note D> 48.25\n<music21.note.Note D> 48.25\n<music21.note.Note A> 146/3\n<music21.note.Note A> 146/3\n<music21.note.Note E> 49.0\n<music21.note.Note D> 49.0\n<music21.note.Note E> 49.25\n<music21.note.Note D> 49.25\n<music21.chord.Chord F#3 A3> 149/3\n<music21.chord.Chord F#3 A3> 149/3\n<music21.note.Note F#> 50.0\n<music21.note.Note F#> 50.25\n<music21.note.Note A> 51.0\n<music21.note.Note A> 51.25\n<music21.note.Note C#> 155/3\n<music21.note.Note C#> 155/3\n<music21.note.Note F#> 52.0\n<music21.note.Note F#> 52.25\n<music21.note.Note E> 158/3\n<music21.note.Note E> 158/3\n<music21.note.Note A> 53.0\n<music21.note.Note A> 53.25\n<music21.note.Note E> 161/3\n<music21.note.Note C#> 161/3\n<music21.note.Note E> 161/3\n<music21.note.Note C#> 161/3\n<music21.note.Note A> 55.0\n<music21.note.Note A> 55.25\n<music21.note.Note C#> 56.0\n<music21.chord.Chord G3 A3 D4> 56.0\n<music21.note.Note C#> 56.25\n<music21.chord.Chord G3 A3 D4> 56.25\n<music21.note.Note D> 170/3\n<music21.note.Note D> 170/3\n<music21.note.Note B> 57.0\n<music21.note.Note G> 57.0\n<music21.note.Note B> 57.25\n<music21.note.Note G> 57.25\n<music21.note.Note D> 173/3\n<music21.note.Note D> 173/3\n<music21.note.Note G> 58.0\n<music21.note.Note G> 58.25\n<music21.note.Note A> 176/3\n<music21.note.Note A> 176/3\n<music21.note.Note A> 176/3\n<music21.note.Note A> 176/3\n<music21.note.Note B> 59.0\n<music21.note.Note D> 59.0\n<music21.note.Note B> 59.25\n<music21.note.Note D> 59.25\n<music21.note.Note A> 179/3\n<music21.note.Note A> 179/3\n<music21.note.Note A> 60.0\n<music21.note.Note A> 60.25\n<music21.note.Note E> 182/3\n<music21.note.Note E> 182/3\n<music21.note.Note G> 61.0\n<music21.note.Note G> 61.25\n<music21.note.Note D> 185/3\n<music21.note.Note D> 185/3\n<music21.note.Note C#> 62.0\n<music21.note.Note C#> 62.25\n<music21.note.Note E> 188/3\n<music21.note.Note E> 188/3\n<music21.note.Note A> 63.0\n<music21.note.Note A> 63.25\n<music21.note.Note D> 64.0\n<music21.note.Note B> 64.0\n<music21.note.Note D> 64.25\n<music21.note.Note B> 64.25\n<music21.note.Note F#> 194/3\n<music21.note.Note F#> 194/3\n<music21.note.Note E> 65.0\n<music21.note.Note E> 65.25\n<music21.note.Note D> 197/3\n<music21.chord.Chord D4 F#4> 197/3\n<music21.note.Note D> 197/3\n<music21.chord.Chord D4 F#4> 197/3\n<music21.note.Note C#> 66.0\n<music21.note.Note C#> 66.25\n<music21.note.Note F#> 200/3\n<music21.note.Note F#> 200/3\n<music21.note.Note B> 67.0\n<music21.note.Note D> 67.0\n<music21.note.Note B> 67.25\n<music21.note.Note D> 67.25\n<music21.note.Note B> 203/3\n<music21.note.Note B> 203/3\n<music21.note.Note F#> 68.0\n<music21.note.Note F#> 68.25\n<music21.note.Note A> 206/3\n<music21.note.Note E> 206/3\n<music21.note.Note A> 206/3\n<music21.note.Note E> 206/3\n<music21.note.Note A> 69.0\n<music21.note.Note A> 69.25\n<music21.note.Note E> 209/3\n<music21.note.Note C#> 209/3\n<music21.note.Note E> 209/3\n<music21.note.Note C#> 209/3\n<music21.note.Note D> 212/3\n<music21.note.Note B> 212/3\n<music21.note.Note D> 212/3\n<music21.note.Note B> 212/3\n<music21.note.Note C#> 71.0\n<music21.note.Note F#> 71.0\n<music21.note.Note A> 71.0\n<music21.note.Note C#> 71.25\n<music21.note.Note F#> 71.25\n<music21.note.Note A> 71.25\n<music21.note.Note A> 215/3\n<music21.note.Note A> 215/3\n<music21.note.Note B> 72.0\n<music21.note.Note G> 72.0\n<music21.note.Note B> 72.25\n<music21.note.Note G> 72.25\n<music21.note.Note D> 218/3\n<music21.note.Note D> 218/3\n<music21.note.Note G> 73.0\n<music21.note.Note G> 73.25\n<music21.note.Note B> 221/3\n<music21.note.Note G> 221/3\n<music21.note.Note B> 221/3\n<music21.note.Note G> 221/3\n<music21.note.Note A> 74.0\n<music21.note.Note F#> 74.0\n<music21.note.Note A> 74.25\n<music21.note.Note F#> 74.25\n<music21.note.Note D> 224/3\n<music21.note.Note D> 224/3\n<music21.note.Note G> 75.0\n<music21.note.Note E> 75.0\n<music21.note.Note G> 75.25\n<music21.note.Note E> 75.25\n<music21.note.Note B> 227/3\n<music21.note.Note B> 227/3\n<music21.note.Note G> 76.0\n<music21.note.Note D> 76.0\n<music21.note.Note G> 76.25\n<music21.note.Note D> 76.25\n<music21.note.Note E> 230/3\n<music21.note.Note E> 230/3\n<music21.note.Note F#> 77.0\n<music21.note.Note F#> 77.25\n<music21.note.Note A> 233/3\n<music21.note.Note A> 233/3\n<music21.note.Note C#> 78.0\n<music21.note.Note E> 78.0\n<music21.note.Note C#> 78.25\n<music21.note.Note E> 78.25\n<music21.note.Note A> 236/3\n<music21.note.Note A> 236/3\n<music21.note.Note B> 79.0\n<music21.note.Note D> 79.0\n<music21.note.Note B> 79.25\n<music21.note.Note D> 79.25\n<music21.note.Note F#> 239/3\n<music21.note.Note D> 239/3\n<music21.note.Note F#> 239/3\n<music21.note.Note D> 239/3\n<music21.note.Note F#> 80.0\n<music21.note.Note C> 80.0\n<music21.note.Note F#> 80.25\n<music21.note.Note C> 80.25\n<music21.note.Note G> 242/3\n<music21.note.Note G> 242/3\n<music21.note.Note E> 81.0\n<music21.note.Note B-> 81.0\n<music21.note.Note E> 81.25\n<music21.note.Note B-> 81.25\n<music21.note.Note F#> 245/3\n<music21.note.Note D> 245/3\n<music21.note.Note F#> 245/3\n<music21.note.Note D> 245/3\n<music21.note.Note G> 82.0\n<music21.note.Note G> 82.25\n<music21.chord.Chord D5 F#5> 82.5\n<music21.chord.Chord D5 F#5> 82.5\n<music21.note.Note A> 248/3\n<music21.note.Note A> 248/3\n<music21.note.Note F#> 83.0\n<music21.note.Note F#> 83.25\n<music21.note.Note E> 251/3\n<music21.note.Note E> 251/3\n<music21.note.Note E> 84.0\n<music21.note.Note E> 84.25\n<music21.note.Note D> 254/3\n<music21.note.Note C#> 254/3\n<music21.note.Note D> 254/3\n<music21.note.Note C#> 254/3\n<music21.note.Note A> 85.0\n<music21.note.Note A> 85.25\n<music21.note.Note E> 257/3\n<music21.note.Note E> 257/3\n<music21.note.Note F#> 86.0\n<music21.note.Note F#> 86.25\n<music21.note.Note D> 260/3\n<music21.note.Note D> 260/3\n<music21.note.Note B> 87.0\n<music21.note.Note A> 87.0\n<music21.note.Note B> 87.25\n<music21.note.Note A> 87.25\n<music21.note.Note D> 263/3\n<music21.note.Note D> 263/3\n<music21.chord.Chord G#2 F#3> 88.0\n<music21.chord.Chord G#2 F#3> 88.25\n<music21.chord.Chord C#5 F#5> 89.5\n<music21.chord.Chord C#5 F#5> 89.5\n<music21.note.Note C#> 269/3\n<music21.note.Note C#> 269/3\n<music21.note.Note E> 272/3\n<music21.note.Note E> 272/3\n<music21.note.Note F#> 91.0\n<music21.chord.Chord D5 B5> 91.0\n<music21.note.Note F#> 91.25\n<music21.chord.Chord D5 B5> 91.25\n<music21.note.Note A> 275/3\n<music21.note.Note A> 275/3\n<music21.chord.Chord B-3 E4> 92.0\n<music21.chord.Chord G2 D3> 92.0\n<music21.chord.Chord B-3 E4> 92.25\n<music21.chord.Chord G2 D3> 92.25\n<music21.note.Note A> 92.75\n<music21.note.Note A> 92.75\n<music21.note.Note G> 93.0\n<music21.note.Note G> 93.25\n<music21.note.Note F#> 93.5\n<music21.note.Note F#> 93.5\n<music21.note.Note D> 93.75\n<music21.note.Note D> 93.75\n<music21.note.Note A> 94.0\n<music21.note.Note A> 94.25\n<music21.note.Note G> 283/3\n<music21.note.Note G> 94.5\n<music21.note.Note F#> 284/3\n<music21.note.Note F#> 284/3\n<music21.note.Note D> 94.75\n<music21.note.Note A> 95.0\n<music21.note.Note D> 95.0\n<music21.note.Note A> 95.25\n<music21.note.Note D> 96.0\n<music21.note.Note D> 96.0\n<music21.note.Note D> 96.25\n<music21.note.Note D> 96.25\n<music21.note.Note A> 290/3\n<music21.note.Note A> 290/3\n<music21.note.Note E> 97.0\n<music21.note.Note C#> 97.0\n<music21.note.Note E> 97.25\n<music21.note.Note C#> 97.25\n<music21.note.Note A> 293/3\n<music21.note.Note A> 293/3\n<music21.note.Note F#> 98.0\n<music21.note.Note D> 98.0\n<music21.note.Note F#> 98.25\n<music21.note.Note D> 98.25\n<music21.note.Note A> 296/3\n<music21.note.Note A> 296/3\n<music21.note.Note A> 99.0\n<music21.note.Note C#> 99.0\n<music21.note.Note A> 99.25\n<music21.note.Note C#> 99.25\n<music21.note.Note F#> 299/3\n<music21.note.Note A> 299/3\n<music21.note.Note F#> 299/3\n<music21.note.Note A> 299/3\n<music21.note.Note B> 100.0\n<music21.note.Note B> 100.25\n<music21.note.Note F#> 302/3\n<music21.note.Note F#> 302/3\n<music21.chord.Chord F#4 A4> 101.0\n<music21.note.Note E> 101.0\n<music21.note.Note C#> 101.0\n<music21.chord.Chord F#4 A4> 101.25\n<music21.note.Note E> 101.25\n<music21.note.Note C#> 101.25\n<music21.note.Note D> 304/3\n<music21.note.Note D> 101.5\n<music21.note.Note C#> 305/3\n<music21.note.Note A> 305/3\n<music21.note.Note C#> 305/3\n<music21.note.Note A> 305/3\n<music21.note.Note E> 102.0\n<music21.note.Note E> 102.25\n<music21.note.Note F#> 308/3\n<music21.note.Note D> 308/3\n<music21.note.Note F#> 308/3\n<music21.note.Note D> 308/3\n<music21.note.Note C#> 103.0\n<music21.note.Note C#> 103.25\n<music21.note.Note F#> 311/3\n<music21.note.Note F#> 311/3\n<music21.chord.Chord G4 B4> 104.0\n<music21.note.Note E> 104.0\n<music21.note.Note E> 104.0\n<music21.chord.Chord G4 B4> 104.25\n<music21.note.Note E> 104.25\n<music21.note.Note E> 104.25\n<music21.note.Note F#> 314/3\n<music21.note.Note B> 314/3\n<music21.note.Note F#> 314/3\n<music21.note.Note B> 314/3\n<music21.note.Note D> 105.0\n<music21.note.Note E> 105.0\n<music21.note.Note D> 105.25\n<music21.note.Note E> 105.25\n<music21.note.Note F#> 317/3\n<music21.note.Note F#> 317/3\n<music21.note.Note G> 106.0\n<music21.note.Note G> 106.25\n<music21.note.Note F#> 320/3\n<music21.note.Note F#> 320/3\n<music21.note.Note G> 107.0\n<music21.note.Note B> 107.0\n<music21.note.Note E> 107.0\n<music21.note.Note G> 107.25\n<music21.note.Note B> 107.25\n<music21.note.Note E> 107.25\n<music21.note.Note D> 323/3\n<music21.note.Note D> 323/3\n<music21.note.Note D> 323/3\n<music21.note.Note D> 323/3\n<music21.note.Note A> 108.0\n<music21.note.Note A> 108.25\n<music21.note.Note D> 108.5\n<music21.chord.Chord G4 B4> 108.5\n<music21.note.Note D> 108.5\n<music21.chord.Chord G4 B4> 108.5\n<music21.note.Note E> 326/3\n<music21.note.Note E> 326/3\n<music21.note.Note E> 326/3\n<music21.note.Note E> 326/3\n<music21.note.Note G> 109.0\n<music21.note.Note G> 109.25\n<music21.note.Note B> 329/3\n<music21.note.Note B> 329/3\n<music21.note.Note D> 110.0\n<music21.note.Note D> 110.25\n<music21.note.Note B> 332/3\n<music21.note.Note B> 332/3\n<music21.note.Note C#> 111.0\n<music21.chord.Chord A2 G3> 111.0\n<music21.note.Note C#> 111.25\n<music21.chord.Chord A2 G3> 111.25\n<music21.note.Note A> 335/3\n<music21.note.Note A> 335/3\n<music21.note.Note D> 112.0\n<music21.note.Note D> 112.0\n<music21.note.Note D> 112.25\n<music21.note.Note D> 112.25\n<music21.note.Note A> 338/3\n<music21.note.Note A> 338/3\n<music21.note.Note E> 113.0\n<music21.note.Note C#> 113.0\n<music21.note.Note E> 113.25\n<music21.note.Note C#> 113.25\n<music21.note.Note A> 341/3\n<music21.note.Note A> 341/3\n<music21.note.Note F#> 114.0\n<music21.note.Note D> 114.0\n<music21.note.Note F#> 114.25\n<music21.note.Note D> 114.25\n<music21.note.Note A> 344/3\n<music21.note.Note A> 344/3\n<music21.note.Note A> 115.0\n<music21.note.Note F#> 115.0\n<music21.note.Note A> 115.25\n<music21.note.Note F#> 115.25\n<music21.note.Note C#> 347/3\n<music21.note.Note A> 347/3\n<music21.note.Note C#> 347/3\n<music21.note.Note A> 347/3\n<music21.note.Note F#> 116.0\n<music21.note.Note F#> 116.25\n<music21.note.Note E> 350/3\n<music21.note.Note E> 350/3\n<music21.chord.Chord E4 A4> 117.0\n"
],
[
"notes_demo = []\n\nfor ele in elements_to_parse:\n # If the element is a Note, then store it's pitch\n if isinstance(ele, note.Note):\n notes_demo.append(str(ele.pitch))\n \n # If the element is a Chord, split each note of chord and join them with +\n elif isinstance(ele, chord.Chord):\n notes_demo.append(\"+\".join(str(n) for n in ele.normalOrder))",
"_____no_output_____"
],
[
"len(notes_demo)",
"_____no_output_____"
],
[
"isinstance(elements_to_parse[68], chord.Chord)",
"_____no_output_____"
]
],
[
[
"# Preprocessing all Files",
"_____no_output_____"
]
],
[
[
"notes = []\n\nfor file in glob.glob(\"midi_songs/*.mid\"):\n midi = converter.parse(file) # Convert file into stream.Score Object\n \n# print(\"parsing %s\"%file)\n \n elements_to_parse = midi.flat.notes\n \n \n for ele in elements_to_parse:\n # If the element is a Note, then store it's pitch\n if isinstance(ele, note.Note):\n notes.append(str(ele.pitch))\n\n # If the element is a Chord, split each note of chord and join them with +\n elif isinstance(ele, chord.Chord):\n notes.append(\"+\".join(str(n) for n in ele.normalOrder))",
"_____no_output_____"
],
[
"len(notes)",
"_____no_output_____"
],
[
"with open(\"notes\", 'wb') as filepath:\n pickle.dump(notes, filepath)",
"_____no_output_____"
],
[
"with open(\"notes\", 'rb') as f:\n notes= pickle.load(f)",
"_____no_output_____"
],
[
"n_vocab = len(set(notes))",
"_____no_output_____"
],
[
"print(\"Total notes- \", len(notes))\nprint(\"Unique notes- \", n_vocab)",
"Total notes- 60498\nUnique notes- 359\n"
],
[
"print(notes[100:200])",
"['1+5+9', 'G#2', '1+5+9', '1+5+9', 'F3', 'F2', 'F2', 'F2', 'F2', 'F2', '4+9', 'E5', '4+9', 'C5', '4+9', 'A5', '4+9', '5+9', 'F5', '5+9', 'C5', '5+9', 'A5', '5+9', '4+9', 'E5', '4+9', 'C5', '4+9', 'A5', '4+9', 'F5', '5+9', 'C5', '5+9', 'E5', '5+9', 'D5', '5+9', 'E5', '4+9', 'E-5', '4+9', 'B5', '4+9', '4+9', 'A5', '5+9', '5+9', '5+9', '5+9', '4+9', '4+9', '4+9', '4+9', '5+9', '5+9', '5+9', '5+9', 'B4', '4+9', 'A4', '4+9', 'E5', '4+9', '4+9', 'E-5', '5+9', '5+9', '5+9', '5+9', '4+9', '4+9', '4+9', '4+9', '5+9', '5+9', '5+9', '5+9', 'E5', '4', 'E-5', 'C6', 'E5', '5', 'E-5', 'B5', 'E5', '6', 'E-5', 'C6', 'A5', '5', 'A4', '4', 'C5', 'E5', 'F5', 'E5', '5']\n"
]
],
[
[
"# Prepare Sequential Data for LSTM",
"_____no_output_____"
]
],
[
[
"# Hoe many elements LSTM input should consider\nsequence_length = 100",
"_____no_output_____"
],
[
"# All unique classes\npitchnames = sorted(set(notes))",
"_____no_output_____"
],
[
"# Mapping between ele to int value\nele_to_int = dict( (ele, num) for num, ele in enumerate(pitchnames) )",
"_____no_output_____"
],
[
"network_input = []\nnetwork_output = []",
"_____no_output_____"
],
[
"for i in range(len(notes) - sequence_length):\n seq_in = notes[i : i+sequence_length] # contains 100 values\n seq_out = notes[i + sequence_length]\n \n network_input.append([ele_to_int[ch] for ch in seq_in])\n network_output.append(ele_to_int[seq_out])",
"_____no_output_____"
],
[
"# No. of examples\nn_patterns = len(network_input)\nprint(n_patterns)",
"60398\n"
],
[
"# Desired shape for LSTM\nnetwork_input = np.reshape(network_input, (n_patterns, sequence_length, 1))\nprint(network_input.shape)",
"(60398, 100, 1)\n"
],
[
"normalised_network_input = network_input/float(n_vocab)",
"_____no_output_____"
],
[
"# Network output are the classes, encode into one hot vector\nnetwork_output = np_utils.to_categorical(network_output)",
"_____no_output_____"
],
[
"network_output.shape",
"_____no_output_____"
],
[
"print(normalised_network_input.shape)\nprint(network_output.shape)",
"(60398, 100, 1)\n(60398, 359)\n"
]
],
[
[
"# Create Model",
"_____no_output_____"
]
],
[
[
"from keras.models import Sequential, load_model\nfrom keras.layers import *\nfrom keras.callbacks import ModelCheckpoint, EarlyStopping",
"_____no_output_____"
],
[
"model = Sequential()\nmodel.add( LSTM(units=512,\n input_shape = (normalised_network_input.shape[1], normalised_network_input.shape[2]),\n return_sequences = True) )\nmodel.add( Dropout(0.3) )\nmodel.add( LSTM(512, return_sequences=True) )\nmodel.add( Dropout(0.3) )\nmodel.add( LSTM(512) )\nmodel.add( Dense(256) )\nmodel.add( Dropout(0.3) )\nmodel.add( Dense(n_vocab, activation=\"softmax\") )",
"_____no_output_____"
],
[
"model.compile(loss=\"categorical_crossentropy\", optimizer=\"adam\")",
"_____no_output_____"
],
[
"model.summary()",
"Model: \"sequential_1\"\n_________________________________________________________________\nLayer (type) Output Shape Param # \n=================================================================\nlstm_1 (LSTM) (None, 100, 512) 1052672 \n_________________________________________________________________\ndropout_1 (Dropout) (None, 100, 512) 0 \n_________________________________________________________________\nlstm_2 (LSTM) (None, 100, 512) 2099200 \n_________________________________________________________________\ndropout_2 (Dropout) (None, 100, 512) 0 \n_________________________________________________________________\nlstm_3 (LSTM) (None, 512) 2099200 \n_________________________________________________________________\ndense_1 (Dense) (None, 256) 131328 \n_________________________________________________________________\ndropout_3 (Dropout) (None, 256) 0 \n_________________________________________________________________\ndense_2 (Dense) (None, 359) 92263 \n=================================================================\nTotal params: 5,474,663\nTrainable params: 5,474,663\nNon-trainable params: 0\n_________________________________________________________________\n"
],
[
"#Trained on google colab\ncheckpoint = ModelCheckpoint(\"model.hdf5\", monitor='loss', verbose=0, save_best_only=True, mode='min')\n\n\nmodel_his = model.fit(normalised_network_input, network_output, epochs=100, batch_size=64, callbacks=[checkpoint])",
"_____no_output_____"
],
[
"model = load_model(\"new_weights.hdf5\")",
"_____no_output_____"
]
],
[
[
"# Predictions",
"_____no_output_____"
]
],
[
[
"sequence_length = 100\nnetwork_input = []\n\nfor i in range(len(notes) - sequence_length):\n seq_in = notes[i : i+sequence_length] # contains 100 values\n network_input.append([ele_to_int[ch] for ch in seq_in])",
"_____no_output_____"
],
[
"# Any random start index\nstart = np.random.randint(len(network_input) - 1)\n\n# Mapping int_to_ele\nint_to_ele = dict((num, ele) for num, ele in enumerate(pitchnames))\n\n# Initial pattern \npattern = network_input[start]\nprediction_output = []\n\n# generate 200 elements\nfor note_index in range(200):\n prediction_input = np.reshape(pattern, (1, len(pattern), 1)) # convert into numpy desired shape \n prediction_input = prediction_input/float(n_vocab) # normalise\n \n prediction = model.predict(prediction_input, verbose=0)\n \n idx = np.argmax(prediction)\n result = int_to_ele[idx]\n prediction_output.append(result) \n \n # Remove the first value, and append the recent value.. \n # This way input is moving forward step-by-step with time..\n pattern.append(idx)\n pattern = pattern[1:]",
"_____no_output_____"
],
[
"print(prediction_output)",
"['D2', 'D5', 'D3', 'C5', 'B4', 'D2', 'A4', 'D3', 'G#4', 'E5', '4+9', 'C5', 'A4', '0+5', 'C5', 'A4', 'F#5', 'C5', 'A4', '0+5', 'C5', 'A4', 'E5', 'C5', 'B4', 'D5', 'E5', '4+9', 'C5', 'A4', '0+5', '4+9', 'C5', 'A4', 'F#5', 'C5', 'A4', '0+5', 'C5', 'A4', 'E5', 'E3', 'C5', 'B2', 'B4', 'C3', 'D5', 'G#2', 'E5', '4+9', 'C5', 'A4', '0+5', '4+9', 'C5', 'A4', 'F#5', '4+9', 'C5', 'A4', '0+5', '4+9', 'C5', 'A4', 'E5', '4+9', 'C5', 'B4', '7', 'D5', 'E5', '4+9', 'C5', 'A4', '0+5', '4+9', 'C5', 'A4', 'F#5', '4+9', 'C5', 'A4', '0+5', '4+9', 'C5', 'A4', 'E5', '4+9', 'C5', 'B4', '7', 'D5', '9+0', '5', '4+7', '2+5', '7', '0+4', '11+2', '4', '0+4', 'E4', '2+5', '11', '11+2', '5', '7+11', '7', '9+0', '9', '11', '0', '4', 'A4', '5', 'F4', 'E5', 'A4', 'D5', '7', 'C5', 'A4', 'B4', '4', 'G4', 'C5', 'E4', 'D5', '11', 'G4', 'B4', '5', 'E4', 'G4', '7', 'E4', '4+9', '4+9', '4+9', '4+9', '4+9', '4+9', '2+7', '4+9', '4+9', '4+9', '4+9', '4+9', '2+7', 'E4', '4+9', 'A4', 'B4', 'C5', '4+9', 'B4', 'A4', 'E4', '4+9', 'C4', 'B3', '4+9', 'C4', 'A3', '4+9', 'C4', 'B3', '7', 'C4', 'D4', '5', 'E4', 'C4', '5', 'E4', 'D4', '4', 'E4', 'F4', 'B3', '2', 'C4', 'F4', '4+9', '4', 'D4', '4+8', '4', 'D4', 'A4', '4+9', 'E4', 'A4', 'C5', '4+9', 'B4', 'A4', 'E4', '4+9', 'C4']\n"
]
],
[
[
"# Create Midi File",
"_____no_output_____"
]
],
[
[
"offset = 0 # Time\noutput_notes = []\n\nfor pattern in prediction_output:\n \n # if the pattern is a chord\n if ('+' in pattern) or pattern.isdigit():\n notes_in_chord = pattern.split('+')\n temp_notes = []\n for current_note in notes_in_chord:\n new_note = note.Note(int(current_note)) # create Note object for each note in the chord\n new_note.storedInstrument = instrument.Piano()\n temp_notes.append(new_note)\n \n \n new_chord = chord.Chord(temp_notes) # creates the chord() from the list of notes\n new_chord.offset = offset\n output_notes.append(new_chord)\n \n else:\n # if the pattern is a note\n new_note = note.Note(pattern)\n new_note.offset = offset\n new_note.storedInstrument = instrument.Piano()\n output_notes.append(new_note)\n \n offset += 0.5",
"_____no_output_____"
],
[
"# create a stream object from the generated notes\nmidi_stream = stream.Stream(output_notes)\nmidi_stream.write('midi', fp = \"test_output.mid\")",
"_____no_output_____"
],
[
"midi_stream.show('midi')",
"_____no_output_____"
]
]
] | [
"markdown",
"code",
"markdown",
"code",
"markdown",
"code",
"markdown",
"code",
"markdown",
"code",
"markdown",
"code",
"markdown",
"code"
] | [
[
"markdown"
],
[
"code"
],
[
"markdown"
],
[
"code",
"code",
"code",
"code",
"code",
"code",
"code",
"code",
"code",
"code"
],
[
"markdown"
],
[
"code",
"code",
"code",
"code",
"code",
"code",
"code"
],
[
"markdown"
],
[
"code",
"code",
"code",
"code",
"code",
"code",
"code",
"code",
"code",
"code",
"code"
],
[
"markdown"
],
[
"code",
"code",
"code",
"code",
"code",
"code"
],
[
"markdown"
],
[
"code",
"code",
"code"
],
[
"markdown"
],
[
"code",
"code",
"code"
]
] |
e77fe92d687e4f01b94cc26d2ea894b5841b992a | 27,920 | ipynb | Jupyter Notebook | dev_nb/experiments/lm_checks.ipynb | gurvindersingh/fastai_v1 | 18c6170f7fa852f6f24c03badb1bdb03f40c5be9 | [
"Apache-2.0"
] | 1 | 2018-10-23T20:45:41.000Z | 2018-10-23T20:45:41.000Z | dev_nb/experiments/lm_checks.ipynb | lesscomfortable/fastai_v1 | bbc5c37329cf45f59bd2daaa2f56723cb7565643 | [
"Apache-2.0"
] | null | null | null | dev_nb/experiments/lm_checks.ipynb | lesscomfortable/fastai_v1 | bbc5c37329cf45f59bd2daaa2f56723cb7565643 | [
"Apache-2.0"
] | null | null | null | 26.464455 | 125 | 0.528976 | [
[
[
"%load_ext autoreload\n%autoreload 2",
"_____no_output_____"
],
[
"from fastai.text import *",
"_____no_output_____"
],
[
"EOS = '<eos>'\nPATH=Path('../data/wikitext')",
"_____no_output_____"
]
],
[
[
"Small helper function to read the tokens.",
"_____no_output_____"
]
],
[
[
"def read_file(filename):\n tokens = []\n with open(PATH/filename, encoding='utf8') as f:\n for line in f:\n tokens.append(line.split() + [EOS])\n return np.array(tokens)",
"_____no_output_____"
],
[
"trn_tok = read_file('wiki.train.tokens')\nval_tok = read_file('wiki.valid.tokens')\ntst_tok = read_file('wiki.test.tokens')",
"_____no_output_____"
],
[
"len(trn_tok), len(val_tok), len(tst_tok)",
"_____no_output_____"
],
[
"' '.join(trn_tok[4][:20])",
"_____no_output_____"
],
[
"cnt = Counter(word for sent in trn_tok for word in sent)\ncnt.most_common(10)",
"_____no_output_____"
]
],
[
[
"Give an id to each token and add the pad token (just in case we need it).",
"_____no_output_____"
]
],
[
[
"itos = [o for o,c in cnt.most_common()]\nitos.insert(0,'<pad>')",
"_____no_output_____"
],
[
"vocab_size = len(itos); vocab_size",
"_____no_output_____"
]
],
[
[
"Creates the mapping from token to id then numericalizing our datasets.",
"_____no_output_____"
]
],
[
[
"stoi = collections.defaultdict(lambda : 5, {w:i for i,w in enumerate(itos)})",
"_____no_output_____"
],
[
"trn_ids = np.array([([stoi[w] for w in s]) for s in trn_tok])\nval_ids = np.array([([stoi[w] for w in s]) for s in val_tok])\ntst_ids = np.array([([stoi[w] for w in s]) for s in tst_tok])",
"_____no_output_____"
]
],
[
[
"## Testing WeightDropout",
"_____no_output_____"
],
[
"Create a bunch of parameters for deterministic tests.",
"_____no_output_____"
]
],
[
[
"module = nn.LSTM(20, 20)\ntst_input = torch.randn(2,5,20)\ntst_output = torch.randint(0,20,(10,)).long()\nsave_params = {}\nfor n,p in module._parameters.items(): save_params[n] = p.clone()",
"_____no_output_____"
]
],
[
[
"### Old WeightDropout",
"_____no_output_____"
]
],
[
[
"module = nn.LSTM(20, 20)\nfor n,p in save_params.items(): module._parameters[n] = nn.Parameter(p.clone())\ndp_module = WeightDrop(module, 0.5)\nopt = optim.SGD(dp_module.parameters(), 10)\ndp_module.train()",
"_____no_output_____"
],
[
"torch.manual_seed(7)",
"_____no_output_____"
],
[
"x = tst_input.clone()\nx.requires_grad_(requires_grad=True)\nh = (torch.zeros(1,5,20), torch.zeros(1,5,20))\nfor _ in range(5): x,h = dp_module(x,h)",
"_____no_output_____"
],
[
"getattr(dp_module.module, 'weight_hh_l0'),getattr(dp_module.module,'weight_hh_l0_raw')",
"_____no_output_____"
],
[
"target = tst_output.clone()\nloss = F.nll_loss(x.view(-1,20), target)\nloss.backward()\nopt.step()",
"_____no_output_____"
],
[
"w, w_raw = getattr(dp_module.module, 'weight_hh_l0'),getattr(dp_module.module,'weight_hh_l0_raw')\nw.grad, w_raw.grad",
"_____no_output_____"
],
[
"getattr(dp_module.module, 'weight_hh_l0'),getattr(dp_module.module,'weight_hh_l0_raw')",
"_____no_output_____"
]
],
[
[
"### New WeightDropout",
"_____no_output_____"
]
],
[
[
"class WeightDropout(nn.Module):\n \"A module that warps another layer in which some weights will be replaced by 0 during training.\"\n \n def __init__(self, module, dropout, layer_names=['weight_hh_l0']):\n super().__init__()\n self.module,self.dropout,self.layer_names = module,dropout,layer_names\n for layer in self.layer_names:\n #Makes a copy of the weights of the selected layers.\n w = getattr(self.module, layer)\n self.register_parameter(f'{layer}_raw', nn.Parameter(w.data))\n \n def _setweights(self):\n for layer in self.layer_names:\n raw_w = getattr(self, f'{layer}_raw')\n self.module._parameters[layer] = F.dropout(raw_w, p=self.dropout, training=self.training)\n \n def forward(self, *args):\n self._setweights()\n return self.module.forward(*args)\n \n def reset(self):\n if hasattr(self.module, 'reset'): self.module.reset()",
"_____no_output_____"
],
[
"module = nn.LSTM(20, 20)\nfor n,p in save_params.items(): module._parameters[n] = nn.Parameter(p.clone())\ndp_module = WeightDropout(module, 0.5)\nopt = optim.SGD(dp_module.parameters(), 10)\ndp_module.train()",
"_____no_output_____"
],
[
"torch.manual_seed(7)",
"_____no_output_____"
],
[
"x = tst_input.clone()\nx.requires_grad_(requires_grad=True)\nh = (torch.zeros(1,5,20), torch.zeros(1,5,20))\nfor _ in range(5): x,h = dp_module(x,h)",
"_____no_output_____"
],
[
"getattr(dp_module.module, 'weight_hh_l0'),getattr(dp_module,'weight_hh_l0_raw')",
"_____no_output_____"
],
[
"target = tst_output.clone()\nloss = F.nll_loss(x.view(-1,20), target)\nloss.backward()\nopt.step()",
"_____no_output_____"
],
[
"w, w_raw = getattr(dp_module.module, 'weight_hh_l0'),getattr(dp_module,'weight_hh_l0_raw')\nw.grad, w_raw.grad",
"_____no_output_____"
],
[
"getattr(dp_module.module, 'weight_hh_l0'),getattr(dp_module,'weight_hh_l0_raw')",
"_____no_output_____"
]
],
[
[
"## Testing EmbeddingDropout",
"_____no_output_____"
],
[
"Create a bunch of parameters for deterministic tests.",
"_____no_output_____"
]
],
[
[
"enc = nn.Embedding(100,20, padding_idx=0)\ntst_input = torch.randint(0,100,(25,)).long()\nsave_params = enc.weight.clone()",
"_____no_output_____"
]
],
[
[
"### Old EmbeddingDropout",
"_____no_output_____"
]
],
[
[
"enc = nn.Embedding(100,20, padding_idx=0)\nenc.weight = nn.Parameter(save_params.clone())\nenc_dp = EmbeddingDropout(enc)",
"_____no_output_____"
],
[
"torch.manual_seed(7)",
"_____no_output_____"
],
[
"x = tst_input.clone()\nenc_dp(x, dropout=0.5)",
"_____no_output_____"
]
],
[
[
"### New EmbeddingDropout",
"_____no_output_____"
]
],
[
[
"def dropout_mask(x, sz, p):\n \"Returns a dropout mask of the same type as x, size sz, with probability p to cancel an element.\"\n return x.new(*sz).bernoulli_(1-p)/(1-p)",
"_____no_output_____"
],
[
"class EmbeddingDropout1(nn.Module):\n\n \"Applies dropout in the embedding layer by zeroing out some elements of the embedding vector.\"\n def __init__(self, emb, dropout):\n super().__init__()\n self.emb,self.dropout = emb,dropout\n self.pad_idx = self.emb.padding_idx\n if self.pad_idx is None: self.pad_idx = -1\n\n def forward(self, words, dropout=0.1, scale=None):\n if self.training and self.dropout != 0:\n size = (self.emb.weight.size(0),1)\n mask = dropout_mask(self.emb.weight.data, size, self.dropout)\n masked_emb_weight = mask * self.emb.weight\n else: masked_emb_weight = self.emb.weight\n if scale: masked_emb_weight = scale * masked_emb_weight\n return F.embedding(words, masked_emb_weight, self.pad_idx, self.emb.max_norm,\n self.emb.norm_type, self.emb.scale_grad_by_freq, self.emb.sparse)",
"_____no_output_____"
],
[
"enc = nn.Embedding(100,20, padding_idx=0)\nenc.weight = nn.Parameter(save_params.clone())\nenc_dp = EmbeddingDropout1(enc, 0.5)",
"_____no_output_____"
],
[
"torch.manual_seed(7)",
"_____no_output_____"
],
[
"x = tst_input.clone()\nenc_dp(x)",
"_____no_output_____"
]
],
[
[
"## Testing RNN model",
"_____no_output_____"
],
[
"Creating a bunch of parameters for deterministic testing.",
"_____no_output_____"
]
],
[
[
"tst_model = get_language_model(500, 20, 100, 2, 0, bias=True)\nsave_parameters = {}\nfor n,p in tst_model.state_dict().items(): save_parameters[n] = p.clone()\ntst_input = torch.randint(0, 500, (10,5)).long()\ntst_output = torch.randint(0, 500, (50,)).long()",
"_____no_output_____"
]
],
[
[
"### Old RNN model",
"_____no_output_____"
]
],
[
[
"tst_model = get_language_model(500, 20, 100, 2, 0, bias=True, dropout=0.4, dropoute=0.1, dropouth=0.2, \n dropouti=0.6, wdrop=0.5)\nstate_dict = OrderedDict()\nfor n,p in save_parameters.items(): state_dict[n] = p.clone()\ntst_model.load_state_dict(state_dict)\nopt = optim.SGD(tst_model.parameters(), lr=10)",
"_____no_output_____"
],
[
"torch.manual_seed(7)",
"_____no_output_____"
],
[
"x = tst_input.clone()\nz = tst_model(x)\nz",
"_____no_output_____"
],
[
"y = tst_output.clone()\nloss = F.nll_loss(z[0], y)\nloss.backward()\nopt.step()",
"_____no_output_____"
],
[
"tst_model[0].rnns[0].module._parameters['weight_hh_l0_raw']",
"_____no_output_____"
]
],
[
[
"### New RNN model",
"_____no_output_____"
]
],
[
[
"class RNNDropout(nn.Module):\n def __init__(self, p=0.5):\n super().__init__()\n self.p=p\n\n def forward(self, x):\n if not self.training or not self.p: return x\n m = dropout_mask(x.data, (1, x.size(1), x.size(2)), self.p)\n return m * x",
"_____no_output_____"
],
[
"def repackage_var1(h):\n \"Detaches h from its history.\"\n return h.detach() if type(h) == torch.Tensor else tuple(repackage_var(v) for v in h)",
"_____no_output_____"
],
[
"class RNNCore(nn.Module):\n \"AWD-LSTM/QRNN inspired by https://arxiv.org/abs/1708.02182\"\n\n initrange=0.1\n\n def __init__(self, vocab_sz, emb_sz, n_hid, n_layers, pad_token, bidir=False,\n hidden_p=0.2, input_p=0.6, embed_p=0.1, weight_p=0.5, qrnn=False):\n \n super().__init__()\n self.bs,self.qrnn,self.ndir = 1, qrnn,(2 if bidir else 1)\n self.emb_sz,self.n_hid,self.n_layers = emb_sz,n_hid,n_layers\n self.encoder = nn.Embedding(vocab_sz, emb_sz, padding_idx=pad_token)\n self.dp_encoder = EmbeddingDropout1(self.encoder, embed_p)\n if self.qrnn:\n #Using QRNN requires cupy: https://github.com/cupy/cupy\n from .torchqrnn.qrnn import QRNNLayer\n self.rnns = [QRNNLayer(emb_sz if l == 0 else n_hid, (n_hid if l != n_layers - 1 else emb_sz)//self.ndir,\n save_prev_x=True, zoneout=0, window=2 if l == 0 else 1, output_gate=True) for l in range(n_layers)]\n if weight_p != 0.:\n for rnn in self.rnns:\n rnn.linear = WeightDropout(rnn.linear, weight_p, layer_names=['weight'])\n else:\n self.rnns = [nn.LSTM(emb_sz if l == 0 else n_hid, (n_hid if l != n_layers - 1 else emb_sz)//self.ndir,\n 1, bidirectional=bidir) for l in range(n_layers)]\n if weight_p != 0.: self.rnns = [WeightDropout(rnn, weight_p) for rnn in self.rnns]\n self.rnns = torch.nn.ModuleList(self.rnns)\n self.encoder.weight.data.uniform_(-self.initrange, self.initrange)\n self.dropouti = RNNDropout(input_p)\n self.dropouths = nn.ModuleList([RNNDropout(hidden_p) for l in range(n_layers)])\n\n def forward(self, input):\n sl,bs = input.size()\n if bs!=self.bs:\n self.bs=bs\n self.reset()\n raw_output = self.dropouti(self.dp_encoder(input))\n new_hidden,raw_outputs,outputs = [],[],[]\n for l, (rnn,drop) in enumerate(zip(self.rnns, self.dropouths)):\n with warnings.catch_warnings():\n #To avoid the warning that comes because the weights aren't flattened.\n warnings.simplefilter(\"ignore\")\n raw_output, new_h = rnn(raw_output, self.hidden[l])\n new_hidden.append(new_h)\n raw_outputs.append(raw_output)\n if l != self.n_layers - 1: raw_output = drop(raw_output)\n outputs.append(raw_output)\n self.hidden = repackage_var1(new_hidden)\n return raw_outputs, outputs\n\n def one_hidden(self, l):\n nh = (self.n_hid if l != self.n_layers - 1 else self.emb_sz)//self.ndir\n return self.weights.new(self.ndir, self.bs, nh).zero_()\n\n def reset(self):\n [r.reset() for r in self.rnns if hasattr(r, 'reset')]\n self.weights = next(self.parameters()).data\n if self.qrnn: self.hidden = [self.one_hidden(l) for l in range(self.n_layers)]\n else: self.hidden = [(self.one_hidden(l), self.one_hidden(l)) for l in range(self.n_layers)]",
"_____no_output_____"
],
[
"class LinearDecoder1(nn.Module):\n \"To go on top of a RNN_Core module\"\n \n initrange=0.1\n \n def __init__(self, n_out, n_hid, output_p, tie_encoder=None, bias=True):\n super().__init__()\n self.decoder = nn.Linear(n_hid, n_out, bias=bias)\n self.decoder.weight.data.uniform_(-self.initrange, self.initrange)\n self.dropout = RNNDropout(output_p)\n if bias: self.decoder.bias.data.zero_()\n if tie_encoder: self.decoder.weight = tie_encoder.weight\n\n def forward(self, input):\n raw_outputs, outputs = input\n output = self.dropout(outputs[-1])\n decoded = self.decoder(output.view(output.size(0)*output.size(1), output.size(2)))\n return decoded, raw_outputs, outputs",
"_____no_output_____"
],
[
"class SequentialRNN1(nn.Sequential):\n def reset(self):\n for c in self.children():\n if hasattr(c, 'reset'): c.reset()",
"_____no_output_____"
],
[
"def get_language_model1(vocab_sz, emb_sz, n_hid, n_layers, pad_token, tie_weights=True, qrnn=False, bias=True,\n output_p=0.4, hidden_p=0.2, input_p=0.6, embed_p=0.1, weight_p=0.5):\n \"To create a full AWD-LSTM\"\n rnn_enc = RNNCore(vocab_sz, emb_sz, n_hid=n_hid, n_layers=n_layers, pad_token=pad_token, qrnn=qrnn,\n hidden_p=hidden_p, input_p=input_p, embed_p=embed_p, weight_p=weight_p)\n enc = rnn_enc.encoder if tie_weights else None\n return SequentialRNN1(rnn_enc, LinearDecoder1(vocab_sz, emb_sz, output_p, tie_encoder=enc, bias=bias))",
"_____no_output_____"
]
],
[
[
"The new model has weights that are organized a bit differently.",
"_____no_output_____"
]
],
[
[
"save_parameters1 = {}\nfor n,p in save_parameters.items(): \n if 'weight_hh_l0' not in n and n!='0.encoder_with_dropout.embed.weight': save_parameters1[n] = p.clone()\n elif n=='0.encoder_with_dropout.embed.weight': save_parameters1['0.dp_encoder.emb.weight'] = p.clone()\n else: \n save_parameters1[n[:-4]] = p.clone()\n splits = n.split('.')\n splits.remove(splits[-2])\n n1 = '.'.join(splits)\n save_parameters1[n1] = p.clone()",
"_____no_output_____"
],
[
"tst_model = get_language_model1(500, 20, 100, 2, 0)\ntst_model.load_state_dict(save_parameters1)\nopt = optim.SGD(tst_model.parameters(), lr=10)",
"_____no_output_____"
],
[
"torch.manual_seed(7)",
"_____no_output_____"
],
[
"x = tst_input.clone()\nz = tst_model(x)\nz",
"_____no_output_____"
],
[
"y = tst_output.clone()\nloss = F.nll_loss(z[0], y)\nloss.backward()\nopt.step()",
"_____no_output_____"
],
[
"tst_model[0].rnns[0]._parameters['weight_hh_l0_raw']",
"_____no_output_____"
]
],
[
[
"## Regularization",
"_____no_output_____"
],
[
"We'll keep the same param as before.",
"_____no_output_____"
],
[
"### Old reg",
"_____no_output_____"
]
],
[
[
"tst_model = get_language_model(500, 20, 100, 2, 0, bias=True, dropout=0.4, dropoute=0.1, dropouth=0.2, \n dropouti=0.6, wdrop=0.5)\nstate_dict = OrderedDict()\nfor n,p in save_parameters.items(): state_dict[n] = p.clone()\ntst_model.load_state_dict(state_dict)\nopt = optim.SGD(tst_model.parameters(), lr=10, weight_decay=1)",
"_____no_output_____"
],
[
"torch.manual_seed(7)",
"_____no_output_____"
],
[
"x = tst_input.clone()\nz = tst_model(x)\ny = tst_output.clone()\nloss = F.nll_loss(z[0], y)",
"_____no_output_____"
],
[
"loss = seq2seq_reg(z[0], z[1:], loss, 2, 1)\nloss.item()",
"_____no_output_____"
],
[
"loss.backward()\nnn.utils.clip_grad_norm_(tst_model.parameters(), 0.1)\nopt.step()",
"_____no_output_____"
],
[
"tst_model[0].rnns[0].module._parameters['weight_hh_l0_raw']",
"_____no_output_____"
]
],
[
[
"### New reg",
"_____no_output_____"
]
],
[
[
"from dataclasses import dataclass",
"_____no_output_____"
],
[
"@dataclass\nclass RNNTrainer(Callback):\n model:nn.Module\n bptt:int\n clip:float=None\n alpha:float=0.\n beta:float=0.\n \n def on_loss_begin(self, last_output, **kwargs):\n #Save the extra outputs for later and only returns the true output.\n self.raw_out,self.out = last_output[1],last_output[2]\n return last_output[0]\n \n def on_backward_begin(self, last_loss, last_input, last_output, **kwargs):\n #Adjusts the lr to the bptt selected\n #self.learn.opt.lr *= last_input.size(0) / self.bptt\n #AR and TAR\n if self.alpha != 0.: last_loss += (self.alpha * self.out[-1].pow(2).mean()).sum()\n if self.beta != 0.:\n h = self.raw_out[-1]\n if len(h)>1: last_loss += (self.beta * (h[1:] - h[:-1]).pow(2).mean()).sum()\n return last_loss\n \n def on_backward_end(self, **kwargs):\n if self.clip: nn.utils.clip_grad_norm_(self.model.parameters(), self.clip)",
"_____no_output_____"
],
[
"save_parameters1 = {}\nfor n,p in save_parameters.items(): \n if 'weight_hh_l0' not in n and n!='0.encoder_with_dropout.embed.weight': save_parameters1[n] = p.clone()\n elif n=='0.encoder_with_dropout.embed.weight': save_parameters1['0.dp_encoder.embed.weight'] = p.clone()\n else: \n save_parameters1[n[:-4]] = p.clone()\n splits = n.split('.')\n splits.remove(splits[-2])\n n1 = '.'.join(splits)\n save_parameters1[n1] = p.clone()",
"_____no_output_____"
],
[
"tst_model = get_language_model1(500, 20, 100, 2, 0)\ntst_model.load_state_dict(save_parameters1)\nopt = optim.SGD(tst_model.parameters(), lr=10, weight_decay=1)",
"_____no_output_____"
],
[
"torch.manual_seed(7)",
"_____no_output_____"
],
[
"cb = RNNTrainer(tst_model, 10, 0.1, 2, 1)",
"_____no_output_____"
],
[
"x = tst_input.clone()\nz = tst_model(x)\ny = tst_output.clone()\nz = cb.on_loss_begin(z)\nloss = F.nll_loss(z, y)\nloss = cb.on_backward_begin(loss, x, z)\nloss.item()",
"_____no_output_____"
],
[
"loss.backward()\ncb.on_backward_end()\nopt.step()",
"_____no_output_____"
],
[
"tst_model[0].rnns[0]._parameters['weight_hh_l0_raw']",
"_____no_output_____"
]
]
] | [
"code",
"markdown",
"code",
"markdown",
"code",
"markdown",
"code",
"markdown",
"code",
"markdown",
"code",
"markdown",
"code",
"markdown",
"code",
"markdown",
"code",
"markdown",
"code",
"markdown",
"code",
"markdown",
"code",
"markdown",
"code",
"markdown",
"code",
"markdown",
"code",
"markdown",
"code"
] | [
[
"code",
"code",
"code"
],
[
"markdown"
],
[
"code",
"code",
"code",
"code",
"code"
],
[
"markdown"
],
[
"code",
"code"
],
[
"markdown"
],
[
"code",
"code"
],
[
"markdown",
"markdown"
],
[
"code"
],
[
"markdown"
],
[
"code",
"code",
"code",
"code",
"code",
"code",
"code"
],
[
"markdown"
],
[
"code",
"code",
"code",
"code",
"code",
"code",
"code",
"code"
],
[
"markdown",
"markdown"
],
[
"code"
],
[
"markdown"
],
[
"code",
"code",
"code"
],
[
"markdown"
],
[
"code",
"code",
"code",
"code",
"code"
],
[
"markdown",
"markdown"
],
[
"code"
],
[
"markdown"
],
[
"code",
"code",
"code",
"code",
"code"
],
[
"markdown"
],
[
"code",
"code",
"code",
"code",
"code",
"code"
],
[
"markdown"
],
[
"code",
"code",
"code",
"code",
"code",
"code"
],
[
"markdown",
"markdown",
"markdown"
],
[
"code",
"code",
"code",
"code",
"code",
"code"
],
[
"markdown"
],
[
"code",
"code",
"code",
"code",
"code",
"code",
"code",
"code",
"code"
]
] |
e77ff222b7d44bff6b490292a6f296827af5b3ac | 15,222 | ipynb | Jupyter Notebook | sean2.ipynb | Sean8322/mindUPCODE | 204a0dc3d2bf822790a1e284f3ed20950299b034 | [
"BSD-3-Clause",
"MIT"
] | 1 | 2020-04-26T03:01:46.000Z | 2020-04-26T03:01:46.000Z | sean2.ipynb | Sean8322/mindUP | 82dfdc4aeff74fd97659457a10f9cbf8523e983c | [
"BSD-3-Clause",
"MIT"
] | null | null | null | sean2.ipynb | Sean8322/mindUP | 82dfdc4aeff74fd97659457a10f9cbf8523e983c | [
"BSD-3-Clause",
"MIT"
] | null | null | null | 40.592 | 248 | 0.53922 | [
[
[
"import numpy as np\nfrom scipy.stats import ttest_1samp, wilcoxon, ttest_ind, mannwhitneyu\nimport pickle\nimport os",
"_____no_output_____"
],
[
"# mean values\ndaily_intake = np.array([5260,5470,5640,6180,6390,6515,\n 6805,7515,7515,8230,8770])\n\n# one sample t-test\n# null hypothesis: expected value =\nt_statistic, p_value = ttest_1samp(daily_intake, 7725)\n\n# p_value < 0.05 => alternative hypothesis:\n# data deviate significantly from the hypothesis that the mean\n# is ___ at the 5% level of significance\nprint \"one-sample t-test\", p_value\n\n# one sample wilcoxon-test\nz_statistic, p_value = wilcoxon(daily_intake - 7725)\nprint \"one-sample wilcoxon-test\", p_value",
"_____no_output_____"
],
[
"stress = np.array([\n# data\n[9.21, 0],\n[7.53, 1],\n[7.48, 1],\n[8.08, 1],\n[8.09, 1],\n[10.15, 1],\n[8.40, 1],\n[10.88, 1],\n[6.13, 1],\n[7.90, 1],\n[11.51, 0],\n[12.79, 0],\n[7.05, 1],\n[11.85, 0],\n[9.97, 0],\n[7.48, 1],\n[8.79, 0],\n[9.69, 0],\n[9.68, 0],\n[7.58, 1],\n[9.19, 0],\n[8.11, 1]])\n\n# similar to expend ~ stature in R\ngroup1 = stress[:, 1] == 0\ngroup1 = stress[group1][:, 0]\ngroup2 = stress[:, 1] == 1\ngroup2 = stress[group2][:, 0]\n\n# two-sample t-test\n# null hypothesis: the two groups have the same mean\n# this test assumes the two groups have the same variance...\n# (can be checked with tests for equal variance)\n# independent groups: e.g., how boys and girls fare at an exam\n# dependent groups: e.g., how the same class fare at 2 different exams\nt_statistic, p_value = ttest_ind(group1, group2)",
"_____no_output_____"
],
[
"\n# p_value < 0.05 => alternative hypothesis:\n# they don't have the same mean at the 5% significance level\nprint \"two-sample t-test\", p_value\n\n# two-sample wilcoxon test\n# a.k.a Mann Whitney U\nu, p_value = mannwhitneyu(group1, group2)\nprint \"two-sample wilcoxon-test\", p_value\n\n# pre and post-stress\nintake = np.array([\n[5260, 3910],\n[5470, 4220],\n[5640, 3885],\n[6180, 5160],\n[6390, 5645],\n[6515, 4680],\n[6805, 5265],\n[7515, 5975],\n[7515, 6790],\n[8230, 6900],\n[8770, 7335],\n])\n\npre = intake[:, 0]\npost = intake[:, 1]\n\n# paired t-test: doing two measurments on the same experimental unit\n# (before and after a treatment)\nt_statistic, p_value = ttest_1samp(post - pre, 0)\n\n# p < 0.05 => alternative hypothesis:\n# the difference in mean is not equal to 0\nprint \"paired t-test\", p_value\n\n# alternative to paired t-test when data has an ordinary scale or when not\n# normally distributed\nz_statistic, p_value = wilcoxon(post - pre)\n\nprint \"paired wilcoxon-test\", p_value",
"_____no_output_____"
],
[
"baseFolder='./pickled-filt'\nfiles=[f for f in os.listdir(baseFolder) if not f.startswith('.')]\nfiles",
"_____no_output_____"
],
[
"data=pickle.load(open('pickled-filt/'+files[0], 'rb'))\n",
"_____no_output_____"
],
[
"data[0]",
"_____no_output_____"
],
[
"targetTimes=[\n (10, 20),\n (40, 80)\n]\n\n\nmaster=[]\nfor file in files:\n master.append(pickle.load(open('pickled-filt/'+file, 'rb')))\n\nnewData=[]\nfor participant in master:\n for target in targetTimes:\n out=[]\n for i in range(target[0]*125, target[1]*125):\n out.append(participant[i][1])\n out.append(participant[i][19]['bpm'])\n newData.append(out)",
"_____no_output_____"
],
[
"matrix={\n 'one':{'traitAnxiety':69, \n 'stressor1': {'reportedStress':1, 'reportedStress+TraitAnxietyBias':0.420}, \n 'UB1': {'reportedStress':1, 'reportedStress+TraitAnxietyBias':0.420}, \n 'stressor2': {'reportedStress':1, 'reportedStress+TraitAnxietyBias':0.420}, \n 'GB1': {'reportedStress':1, 'reportedStress+TraitAnxietyBias':0.420}, \n 'stressor3': {'reportedStress':1, 'reportedStress+TraitAnxietyBias':0.420}, \n 'UB2': {'reportedStress':1, 'reportedStress+TraitAnxietyBias':0.420}, \n 'stressor4': {'reportedStress':1, 'reportedStress+TraitAnxietyBias':0.420}, \n 'GB2': {'reportedStress':1, 'reportedStress+TraitAnxietyBias':0.420}, \n },\n 'two':{'traitAnxiety':69, \n 'stressor1': {'reportedStress':1, 'reportedStress+TraitAnxietyBias':0.420}, \n 'UB1': {'reportedStress':1, 'reportedStress+TraitAnxietyBias':0.420}, \n 'stressor2': {'reportedStress':1, 'reportedStress+TraitAnxietyBias':0.420}, \n 'GB1': {'reportedStress':1, 'reportedStress+TraitAnxietyBias':0.420}, \n 'stressor3': {'reportedStress':1, 'reportedStress+TraitAnxietyBias':0.420}, \n 'UB2': {'reportedStress':1, 'reportedStress+TraitAnxietyBias':0.420}, \n 'stressor4': {'reportedStress':1, 'reportedStress+TraitAnxietyBias':0.420}, \n 'GB2': {'reportedStress':1, 'reportedStress+TraitAnxietyBias':0.420}, \n },\n 'three':{'traitAnxiety':69, \n 'stressor1': {'reportedStress':1, 'reportedStress+TraitAnxietyBias':0.420}, \n 'UB1': {'reportedStress':1, 'reportedStress+TraitAnxietyBias':0.420}, \n 'stressor2': {'reportedStress':1, 'reportedStress+TraitAnxietyBias':0.420}, \n 'GB1': {'reportedStress':1, 'reportedStress+TraitAnxietyBias':0.420}, \n 'stressor3': {'reportedStress':1, 'reportedStress+TraitAnxietyBias':0.420}, \n 'UB2': {'reportedStress':1, 'reportedStress+TraitAnxietyBias':0.420}, \n 'stressor4': {'reportedStress':1, 'reportedStress+TraitAnxietyBias':0.420}, \n 'GB2': {'reportedStress':1, 'reportedStress+TraitAnxietyBias':0.420}, \n },\n 'four':{'traitAnxiety':69, \n 'stressor1': {'reportedStress':1, 'reportedStress+TraitAnxietyBias':0.420}, \n 'UB1': {'reportedStress':1, 'reportedStress+TraitAnxietyBias':0.420}, \n 'stressor2': {'reportedStress':1, 'reportedStress+TraitAnxietyBias':0.420}, \n 'GB1': {'reportedStress':1, 'reportedStress+TraitAnxietyBias':0.420}, \n 'stressor3': {'reportedStress':1, 'reportedStress+TraitAnxietyBias':0.420}, \n 'UB2': {'reportedStress':1, 'reportedStress+TraitAnxietyBias':0.420}, \n 'stressor4': {'reportedStress':1, 'reportedStress+TraitAnxietyBias':0.420}, \n 'GB2': {'reportedStress':1, 'reportedStress+TraitAnxietyBias':0.420}, \n },\n 'five':{'traitAnxiety':69, \n 'stressor1': {'reportedStress':1, 'reportedStress+TraitAnxietyBias':0.420}, \n 'UB1': {'reportedStress':1, 'reportedStress+TraitAnxietyBias':0.420}, \n 'stressor2': {'reportedStress':1, 'reportedStress+TraitAnxietyBias':0.420}, \n 'GB1': {'reportedStress':1, 'reportedStress+TraitAnxietyBias':0.420}, \n 'stressor3': {'reportedStress':1, 'reportedStress+TraitAnxietyBias':0.420}, \n 'UB2': {'reportedStress':1, 'reportedStress+TraitAnxietyBias':0.420}, \n 'stressor4': {'reportedStress':1, 'reportedStress+TraitAnxietyBias':0.420}, \n 'GB2': {'reportedStress':1, 'reportedStress+TraitAnxietyBias':0.420}, \n },\n 'six':{'traitAnxiety':69, \n 'stressor1': {'reportedStress':1, 'reportedStress+TraitAnxietyBias':0.420}, \n 'UB1': {'reportedStress':1, 'reportedStress+TraitAnxietyBias':0.420}, \n 'stressor2': {'reportedStress':1, 'reportedStress+TraitAnxietyBias':0.420}, \n 'GB1': {'reportedStress':1, 'reportedStress+TraitAnxietyBias':0.420}, \n 'stressor3': {'reportedStress':1, 'reportedStress+TraitAnxietyBias':0.420}, \n 'UB2': {'reportedStress':1, 'reportedStress+TraitAnxietyBias':0.420}, \n 'stressor4': {'reportedStress':1, 'reportedStress+TraitAnxietyBias':0.420}, \n 'GB2': {'reportedStress':1, 'reportedStress+TraitAnxietyBias':0.420}, \n },\n 'seven':{'traitAnxiety':69, \n 'stressor1': {'reportedStress':1, 'reportedStress+TraitAnxietyBias':0.420}, \n 'UB1': {'reportedStress':1, 'reportedStress+TraitAnxietyBias':0.420}, \n 'stressor2': {'reportedStress':1, 'reportedStress+TraitAnxietyBias':0.420}, \n 'GB1': {'reportedStress':1, 'reportedStress+TraitAnxietyBias':0.420}, \n 'stressor3': {'reportedStress':1, 'reportedStress+TraitAnxietyBias':0.420}, \n 'UB2': {'reportedStress':1, 'reportedStress+TraitAnxietyBias':0.420}, \n 'stressor4': {'reportedStress':1, 'reportedStress+TraitAnxietyBias':0.420}, \n 'GB2': {'reportedStress':1, 'reportedStress+TraitAnxietyBias':0.420}, \n },\n 'eight':{'traitAnxiety':69, \n 'stressor1': {'reportedStress':1, 'reportedStress+TraitAnxietyBias':0.420}, \n 'UB1': {'reportedStress':1, 'reportedStress+TraitAnxietyBias':0.420}, \n 'stressor2': {'reportedStress':1, 'reportedStress+TraitAnxietyBias':0.420}, \n 'GB1': {'reportedStress':1, 'reportedStress+TraitAnxietyBias':0.420}, \n 'stressor3': {'reportedStress':1, 'reportedStress+TraitAnxietyBias':0.420}, \n 'UB2': {'reportedStress':1, 'reportedStress+TraitAnxietyBias':0.420}, \n 'stressor4': {'reportedStress':1, 'reportedStress+TraitAnxietyBias':0.420}, \n 'GB2': {'reportedStress':1, 'reportedStress+TraitAnxietyBias':0.420}, \n },\n \n}",
"_____no_output_____"
]
]
] | [
"code"
] | [
[
"code",
"code",
"code",
"code",
"code",
"code",
"code",
"code",
"code"
]
] |
e78012463c34604cc1dbf8e0bb482921c35d8a3b | 11,307 | ipynb | Jupyter Notebook | mission_to_mars.ipynb | Juan-S-Galindo/Web-Scraping-Challenge | 4f351cecf8f81e8ebb785656728adb7e75afae3c | [
"ADSL"
] | null | null | null | mission_to_mars.ipynb | Juan-S-Galindo/Web-Scraping-Challenge | 4f351cecf8f81e8ebb785656728adb7e75afae3c | [
"ADSL"
] | null | null | null | mission_to_mars.ipynb | Juan-S-Galindo/Web-Scraping-Challenge | 4f351cecf8f81e8ebb785656728adb7e75afae3c | [
"ADSL"
] | null | null | null | 41.266423 | 3,239 | 0.587158 | [
[
[
"from bs4 import BeautifulSoup as bs\nimport time\nimport requests\n\nfrom selenium import webdriver #splinter does not work so I had to use selenium in order to get the final html since the nasa website usees psudo elements such as ::before and ::after to load the latest news.as\n\nimport pandas as pd\n\n#Settings for headless mode.\noptions = webdriver.ChromeOptions()\noptions.add_argument('headless')\n\n#path to the driver and load the options.\nbrowser = webdriver.Chrome(\"/Users/Sebastian/Documents/GitHub/Data Visualization Bootcamp/Sebastian Homework/Web-Scraping-Challenge/chromedriver\",chrome_options = options)\n\nmarsInfo_dict = {}",
"_____no_output_____"
],
[
"#Code to get NASA Mars News\n\nurl = \"https://mars.nasa.gov/news/?page=0&per_page=40&order=publish_date+desc%2Ccreated_at+desc&search=&year=2020%3Apublish_date&category=19%2C165%2C184%2C204&blank_scope=Latest\"\n\n#Open url.\nbrowser.get(url)\n\n#Time to let the website load all the elements\ntime.sleep(4)\n\n#save the html source.\nhtml = browser.page_source\n\n#Use bs4 to parse the html response.\nsoup = bs(html, \"html.parser\")\n\n#Collect the latest news title\nnews_title = soup.find_all('li', class_=\"slide\")[0].find(class_=\"content_title\").text\n\nnews_p = soup.find_all('li', class_=\"slide\")[0].text\n\nprint(news_title)\nprint(\"\\n\")\nprint(news_p)\n\nmarsInfo_dict['news_title'] = news_title\nmarsInfo_dict['news_p'] = news_p\n ",
"A New Video Captures the Science of NASA's Perseverance Mars Rover\n\n\nWith a targeted launch date of July 30, the next robotic scientist NASA is sending to the to the Red Planet has big ambitions.A New Video Captures the Science of NASA's Perseverance Mars RoverJuly 27, 2020A New Video Captures the Science of NASA's Perseverance Mars RoverWith a targeted launch date of July 30, the next robotic scientist NASA is sending to the to the Red Planet has big ambitions.\n"
],
[
"\n#Code to get JPL Mars Space Images - Featured Image\n\nurl = \"https://www.jpl.nasa.gov/spaceimages/?search=&category=Mars\"\n\n#Opens the url.\nbrowser.get(url)\n\n#Interact with the FULL IMAGE BUTTON\nbrowser.find_element_by_id(\"full_image\").click()\n\ntime.sleep(4)\n\nhtml = browser.page_source\n\n#Use bs4 to parse the html response.\nsoup = bs(html, \"html.parser\")\n\nfeatured_image_url = \"https://www.jpl.nasa.gov/\" + soup.find_all('img', class_=\"fancybox-image\")[0]['src']\n\nmarsInfo_dict['featured_image_url'] = featured_image_url\n\nprint(featured_image_url)",
"https://www.jpl.nasa.gov//spaceimages/images/mediumsize/PIA19685_ip.jpg\n"
],
[
"#Mars Weather\n\nurl = \"https://twitter.com/marswxreport?lang=en\"\noptions = webdriver.ChromeOptions()\noptions.add_argument('headless')\n\n#Open the url.\nbrowser.get(url)\n\n#Time to let the website load all the elements\ntime.sleep(4)\n\n#save the html source.\nhtml = browser.page_source\n\n#Use bs4 to parse the html response.\nsoup = bs(html, \"html.parser\")\n\nmars_weather = soup.find_all('article', class_=\"css-1dbjc4n r-1loqt21 r-18u37iz r-1ny4l3l r-o7ynqc r-6416eg\")[0].text.strip().replace('Mars Weather@MarsWxReport·19hInSight ','')\n\nmarsInfo_dict['mars_weather'] = mars_weather\n\nprint(mars_weather)",
"Mars Weather@MarsWxReport·Jul 26InSight sol 591 (2020-07-25) low -91.2ºC (-132.2ºF) high -15.5ºC (4.2ºF)\nwinds from the WNW at 7.5 m/s (16.9 mph) gusting to 19.0 m/s (42.5 mph)\npressure at 7.90 hPa1029\n"
],
[
"# Mars Facts\n\nurl = 'http://space-facts.com/mars/'\n\n#Load url to pandas read html.\ntables = pd.read_html(url)\n\n#Tables\nmarsFacts_df = tables[0]\nearthMars_df = tables[1]\n\n#Rename columns\nmarsFacts_df.columns = ['Facts', 'Values']\n\n\n#Outpout\nhtml_outputFacts = marsFacts_df.to_html(index = False)\nhtml_outputFacts = html_outputFacts.replace('\\n', '')\n\nhtml_outputMarsEarth = earthMars_df.to_html(index = False)\nhtml_outputMarsEarth = html_outputMarsEarth.replace('\\n', '')\n\nmarsInfo_dict['html_outputFacts'] = html_outputFacts\nmarsInfo_dict['html_outputMarsEarth'] = html_outputMarsEarth\n",
"_____no_output_____"
],
[
"#hemisphereImages\ntemp_list = []\n\nurl = \"https://astrogeology.usgs.gov/search/results?q=hemisphere+enhanced&k1=target&v1=Mars\"\n\n# start web browser\nbrowser = webdriver.Chrome(\"/Users/Sebastian/Documents/GitHub/Data Visualization Bootcamp/Sebastian Homework/Web-Scraping-Challenge/chromedriver\",chrome_options=options)\n\n#Opens the url.\nbrowser.get(url)\n\ntime.sleep(4)\n\nhtml = browser.page_source\n\n# close web browser\nbrowser.close()\n\n#Use bs4 to parse the html response.\nsoup = bs(html, \"html.parser\")\n\nlinks = soup.find_all('div', class_=\"description\")\n\nfor link in links:\n\n highDef_url = f\"https://astrogeology.usgs.gov{link.find('a')['href']}\"\n\n responseHighDef = requests.get(highDef_url)\n\n soupHighDef = bs(responseHighDef.text, 'html.parser')\n\n highDef_url = soupHighDef.find_all(\"div\", class_=\"downloads\")[0].find('a')['href']\n\n title = link.find('h3').text \n\n temp_list.append({\"title\" : title, \"img_url\" : highDef_url})\n\nmarsInfo_dict['hemisphere_image_urls'] = temp_list",
"_____no_output_____"
],
[
"marsInfo_dict",
"_____no_output_____"
]
]
] | [
"code"
] | [
[
"code",
"code",
"code",
"code",
"code",
"code",
"code"
]
] |
e7803038fbf39519e45050bfd6e8b679f39588ef | 37,982 | ipynb | Jupyter Notebook | 03- General Pandas/06-Merging-Joining-and-Concatenating.ipynb | rikimarutsui/Python-for-Finance-Repo | cd4553da2df56e3552251fdcaeb5c0dcfc378bc5 | [
"Apache-2.0"
] | null | null | null | 03- General Pandas/06-Merging-Joining-and-Concatenating.ipynb | rikimarutsui/Python-for-Finance-Repo | cd4553da2df56e3552251fdcaeb5c0dcfc378bc5 | [
"Apache-2.0"
] | null | null | null | 03- General Pandas/06-Merging-Joining-and-Concatenating.ipynb | rikimarutsui/Python-for-Finance-Repo | cd4553da2df56e3552251fdcaeb5c0dcfc378bc5 | [
"Apache-2.0"
] | null | null | null | 25.997262 | 223 | 0.294034 | [
[
[
"___\n\n<a href='http://www.pieriandata.com'> <img src='../Pierian_Data_Logo.png' /></a>\n___",
"_____no_output_____"
],
[
"# Merging, Joining, and Concatenating\n\nThere are 3 main ways of combining DataFrames together: Merging, Joining and Concatenating. In this lecture we will discuss these 3 methods with examples.\n\n____",
"_____no_output_____"
],
[
"### Example DataFrames",
"_____no_output_____"
]
],
[
[
"import pandas as pd",
"_____no_output_____"
],
[
"df1 = pd.DataFrame({'A': ['A0', 'A1', 'A2', 'A3'],\n 'B': ['B0', 'B1', 'B2', 'B3'],\n 'C': ['C0', 'C1', 'C2', 'C3'],\n 'D': ['D0', 'D1', 'D2', 'D3']},\n index=[0, 1, 2, 3])",
"_____no_output_____"
],
[
"df2 = pd.DataFrame({'A': ['A4', 'A5', 'A6', 'A7'],\n 'B': ['B4', 'B5', 'B6', 'B7'],\n 'C': ['C4', 'C5', 'C6', 'C7'],\n 'D': ['D4', 'D5', 'D6', 'D7']},\n index=[4, 5, 6, 7]) ",
"_____no_output_____"
],
[
"df3 = pd.DataFrame({'A': ['A8', 'A9', 'A10', 'A11'],\n 'B': ['B8', 'B9', 'B10', 'B11'],\n 'C': ['C8', 'C9', 'C10', 'C11'],\n 'D': ['D8', 'D9', 'D10', 'D11']},\n index=[8, 9, 10, 11])",
"_____no_output_____"
],
[
"df1",
"_____no_output_____"
],
[
"df2",
"_____no_output_____"
],
[
"df3",
"_____no_output_____"
]
],
[
[
"## Concatenation\n\nConcatenation basically glues together DataFrames. Keep in mind that dimensions should match along the axis you are concatenating on. You can use **pd.concat** and pass in a list of DataFrames to concatenate together:",
"_____no_output_____"
]
],
[
[
"pd.concat([df1,df2,df3])",
"_____no_output_____"
],
[
"pd.concat([df1,df2,df3],axis=1)",
"_____no_output_____"
]
],
[
[
"_____\n## Example DataFrames",
"_____no_output_____"
]
],
[
[
"left = pd.DataFrame({'key': ['K0', 'K1', 'K2', 'K3'],\n 'A': ['A0', 'A1', 'A2', 'A3'],\n 'B': ['B0', 'B1', 'B2', 'B3']})\n \nright = pd.DataFrame({'key': ['K0', 'K1', 'K2', 'K3'],\n 'C': ['C0', 'C1', 'C2', 'C3'],\n 'D': ['D0', 'D1', 'D2', 'D3']}) ",
"_____no_output_____"
],
[
"left",
"_____no_output_____"
],
[
"right",
"_____no_output_____"
]
],
[
[
"___",
"_____no_output_____"
],
[
"## Merging\n\nThe **merge** function allows you to merge DataFrames together using a similar logic as merging SQL Tables together. For example:",
"_____no_output_____"
]
],
[
[
"pd.merge(left,right,how='inner',on='key')",
"_____no_output_____"
]
],
[
[
"Or to show a more complicated example:",
"_____no_output_____"
]
],
[
[
"left = pd.DataFrame({'key1': ['K0', 'K0', 'K1', 'K2'],\n 'key2': ['K0', 'K1', 'K0', 'K1'],\n 'A': ['A0', 'A1', 'A2', 'A3'],\n 'B': ['B0', 'B1', 'B2', 'B3']})\n \nright = pd.DataFrame({'key1': ['K0', 'K1', 'K1', 'K2'],\n 'key2': ['K0', 'K0', 'K0', 'K0'],\n 'C': ['C0', 'C1', 'C2', 'C3'],\n 'D': ['D0', 'D1', 'D2', 'D3']})",
"_____no_output_____"
],
[
"pd.merge(left, right, on=['key1', 'key2'])",
"_____no_output_____"
],
[
"pd.merge(left, right, how='outer', on=['key1', 'key2'])",
"_____no_output_____"
],
[
"pd.merge(left, right, how='right', on=['key1', 'key2'])",
"_____no_output_____"
],
[
"pd.merge(left, right, how='left', on=['key1', 'key2'])",
"_____no_output_____"
]
],
[
[
"## Joining\nJoining is a convenient method for combining the columns of two potentially differently-indexed DataFrames into a single result DataFrame.",
"_____no_output_____"
]
],
[
[
"left = pd.DataFrame({'A': ['A0', 'A1', 'A2'],\n 'B': ['B0', 'B1', 'B2']},\n index=['K0', 'K1', 'K2']) \n\nright = pd.DataFrame({'C': ['C0', 'C2', 'C3'],\n 'D': ['D0', 'D2', 'D3']},\n index=['K0', 'K2', 'K3'])",
"_____no_output_____"
],
[
"left.join(right)",
"_____no_output_____"
],
[
"left.join(right, how='outer')",
"_____no_output_____"
]
],
[
[
"# Great Job!",
"_____no_output_____"
]
]
] | [
"markdown",
"code",
"markdown",
"code",
"markdown",
"code",
"markdown",
"code",
"markdown",
"code",
"markdown",
"code",
"markdown"
] | [
[
"markdown",
"markdown",
"markdown"
],
[
"code",
"code",
"code",
"code",
"code",
"code",
"code"
],
[
"markdown"
],
[
"code",
"code"
],
[
"markdown"
],
[
"code",
"code",
"code"
],
[
"markdown",
"markdown"
],
[
"code"
],
[
"markdown"
],
[
"code",
"code",
"code",
"code",
"code"
],
[
"markdown"
],
[
"code",
"code",
"code"
],
[
"markdown"
]
] |
e78032ee710c3233ab6a272047c816b63218a0c3 | 491,418 | ipynb | Jupyter Notebook | code/floating Duck Problem.ipynb | duncanmazza/ModSimPy | 07a76c46fb2af172e4c3b279b04762f2c664c7b7 | [
"MIT"
] | null | null | null | code/floating Duck Problem.ipynb | duncanmazza/ModSimPy | 07a76c46fb2af172e4c3b279b04762f2c664c7b7 | [
"MIT"
] | null | null | null | code/floating Duck Problem.ipynb | duncanmazza/ModSimPy | 07a76c46fb2af172e4c3b279b04762f2c664c7b7 | [
"MIT"
] | null | null | null | 45.841231 | 19,876 | 0.425391 | [
[
[
"from modsim import *\nimport numpy as np",
"_____no_output_____"
],
[
"def make_system():\n system = System(pi = np.pi,\n r = 5,\n density_duck = 0.3, # g/cm^3\n density_water = 1.0, # g/cm^3)\n )\n return system\n\ndef error_func(d):\n system = System(pi = np.pi,\n r = 5,\n density_duck = 0.3, # g/cm^3\n density_water = 1.0, # g/cm^3)\n )\n vol_duck_submerged = (system.pi / 3) * (3 * system.r * (d ** 2) - (d ** 3))\n vol_duck = (4/3)*(system.pi * (system.r ** 3))\n mass_duck = vol_duck * system.density_duck\n mass_water_displaced = vol_duck_submerged * system.density_water\n return mass_duck - mass_water_displaced\n\nsolution = fsolve(error_func, 3)\nsolution",
"_____no_output_____"
],
[
"error = []\nindex = []\nfor i in range(-500, 500):\n error.append(error_func(i / 100))\n index.append(i / 100)\nplot(index, error)\ndecorate(title = 'Error Function',\n xlabel = 'd (submerged distance in cm)',\n ylabel = 'Error (g)')",
"_____no_output_____"
],
[
"help(State)",
"Help on class State in module modsim:\n\nclass State(System)\n | Contains state variables and their values.\n | \n | Takes keyword arguments and stores them as rows.\n | \n | Method resolution order:\n | State\n | System\n | ModSimSeries\n | pandas.core.series.Series\n | pandas.core.base.IndexOpsMixin\n | pandas.core.generic.NDFrame\n | pandas.core.base.PandasObject\n | pandas.core.base.StringMixin\n | pandas.core.accessor.DirNamesMixin\n | pandas.core.base.SelectionMixin\n | builtins.object\n | \n | Methods inherited from System:\n | \n | __init__(self, *args, **kwargs)\n | Initialize the series.\n | \n | If there are no positional arguments, use kwargs.\n | \n | If there is one positional argument, copy it and add\n | in the kwargs.\n | \n | More than one positional argument is an error.\n | \n | ----------------------------------------------------------------------\n | Methods inherited from ModSimSeries:\n | \n | __copy__(self, deep=True)\n | \n | copy = __copy__(self, deep=True)\n | \n | set(self, **kwargs)\n | Uses keyword arguments to update the Series in place.\n | \n | Example: series.set(a=1, b=2)\n | \n | ----------------------------------------------------------------------\n | Data descriptors inherited from ModSimSeries:\n | \n | T\n | Intercept the Series accessor object so we can use `T`\n | as a row label and access it using dot notation.\n | \n | https://pandas.pydata.org/pandas-docs/stable/generated/pandas.Series.T.html\n | \n | dt\n | Intercept the Series accessor object so we can use `dt`\n | as a row label and access it using dot notation.\n | \n | https://pandas.pydata.org/pandas-docs/stable/generated/pandas.Series.dt.html\n | \n | ----------------------------------------------------------------------\n | Methods inherited from pandas.core.series.Series:\n | \n | __add__ = wrapper(left, right)\n | \n | __and__ = wrapper(self, other)\n | \n | __array__(self, result=None)\n | the array interface, return my values\n | \n | __array_prepare__(self, result, context=None)\n | Gets called prior to a ufunc\n | \n | __array_wrap__(self, result, context=None)\n | Gets called after a ufunc\n | \n | __div__ = wrapper(left, right)\n | \n | __divmod__ = wrapper(left, right)\n | \n | __eq__ = wrapper(self, other, axis=None)\n | \n | __float__ = wrapper(self)\n | \n | __floordiv__ = wrapper(left, right)\n | \n | __ge__ = wrapper(self, other, axis=None)\n | \n | __getitem__(self, key)\n | \n | __gt__ = wrapper(self, other, axis=None)\n | \n | __iadd__ = f(self, other)\n | \n | __iand__ = f(self, other)\n | \n | __ifloordiv__ = f(self, other)\n | \n | __imod__ = f(self, other)\n | \n | __imul__ = f(self, other)\n | \n | __int__ = wrapper(self)\n | \n | __ior__ = f(self, other)\n | \n | __ipow__ = f(self, other)\n | \n | __isub__ = f(self, other)\n | \n | __itruediv__ = f(self, other)\n | \n | __ixor__ = f(self, other)\n | \n | __le__ = wrapper(self, other, axis=None)\n | \n | __len__(self)\n | return the length of the Series\n | \n | __long__ = wrapper(self)\n | \n | __lt__ = wrapper(self, other, axis=None)\n | \n | __matmul__(self, other)\n | Matrix multiplication using binary `@` operator in Python>=3.5\n | \n | __mod__ = wrapper(left, right)\n | \n | __mul__ = wrapper(left, right)\n | \n | __ne__ = wrapper(self, other, axis=None)\n | \n | __or__ = wrapper(self, other)\n | \n | __pow__ = wrapper(left, right)\n | \n | __radd__ = wrapper(left, right)\n | \n | __rand__ = wrapper(self, other)\n | \n | __rdiv__ = wrapper(left, right)\n | \n | __rfloordiv__ = wrapper(left, right)\n | \n | __rmatmul__(self, other)\n | Matrix multiplication using binary `@` operator in Python>=3.5\n | \n | __rmod__ = wrapper(left, right)\n | \n | __rmul__ = wrapper(left, right)\n | \n | __ror__ = wrapper(self, other)\n | \n | __rpow__ = wrapper(left, right)\n | \n | __rsub__ = wrapper(left, right)\n | \n | __rtruediv__ = wrapper(left, right)\n | \n | __rxor__ = wrapper(self, other)\n | \n | __setitem__(self, key, value)\n | \n | __sub__ = wrapper(left, right)\n | \n | __truediv__ = wrapper(left, right)\n | \n | __unicode__(self)\n | Return a string representation for a particular DataFrame\n | \n | Invoked by unicode(df) in py2 only. Yields a Unicode String in both\n | py2/py3.\n | \n | __xor__ = wrapper(self, other)\n | \n | add(self, other, level=None, fill_value=None, axis=0)\n | Addition of series and other, element-wise (binary operator `add`).\n | \n | Equivalent to ``series + other``, but with support to substitute a fill_value for\n | missing data in one of the inputs.\n | \n | Parameters\n | ----------\n | other : Series or scalar value\n | fill_value : None or float value, default None (NaN)\n | Fill existing missing (NaN) values, and any new element needed for\n | successful Series alignment, with this value before computation.\n | If data in both corresponding Series locations is missing\n | the result will be missing\n | level : int or name\n | Broadcast across a level, matching Index values on the\n | passed MultiIndex level\n | \n | Returns\n | -------\n | result : Series\n | \n | Examples\n | --------\n | >>> a = pd.Series([1, 1, 1, np.nan], index=['a', 'b', 'c', 'd'])\n | >>> a\n | a 1.0\n | b 1.0\n | c 1.0\n | d NaN\n | dtype: float64\n | >>> b = pd.Series([1, np.nan, 1, np.nan], index=['a', 'b', 'd', 'e'])\n | >>> b\n | a 1.0\n | b NaN\n | d 1.0\n | e NaN\n | dtype: float64\n | >>> a.add(b, fill_value=0)\n | a 2.0\n | b 1.0\n | c 1.0\n | d 1.0\n | e NaN\n | dtype: float64\n | \n | See also\n | --------\n | Series.radd\n | \n | agg = aggregate(self, func, axis=0, *args, **kwargs)\n | Aggregate using one or more operations over the specified axis.\n | \n | .. versionadded:: 0.20.0\n | \n | Parameters\n | ----------\n | func : function, string, dictionary, or list of string/functions\n | Function to use for aggregating the data. If a function, must either\n | work when passed a Series or when passed to Series.apply. For\n | a DataFrame, can pass a dict, if the keys are DataFrame column names.\n | \n | Accepted combinations are:\n | \n | - string function name.\n | - function.\n | - list of functions.\n | - dict of column names -> functions (or list of functions).\n | \n | \n | axis : {0 or 'index'}\n | Parameter needed for compatibility with DataFrame.\n | \n | *args\n | Positional arguments to pass to `func`.\n | **kwargs\n | Keyword arguments to pass to `func`.\n | \n | Returns\n | -------\n | aggregated : Series\n | \n | Notes\n | -----\n | `agg` is an alias for `aggregate`. Use the alias.\n | \n | A passed user-defined-function will be passed a Series for evaluation.\n | \n | Examples\n | --------\n | \n | >>> s = Series(np.random.randn(10))\n | \n | >>> s.agg('min')\n | -1.3018049988556679\n | \n | >>> s.agg(['min', 'max'])\n | min -1.301805\n | max 1.127688\n | dtype: float64\n | \n | See also\n | --------\n | pandas.Series.apply\n | pandas.Series.transform\n | \n | aggregate(self, func, axis=0, *args, **kwargs)\n | Aggregate using one or more operations over the specified axis.\n | \n | .. versionadded:: 0.20.0\n | \n | Parameters\n | ----------\n | func : function, string, dictionary, or list of string/functions\n | Function to use for aggregating the data. If a function, must either\n | work when passed a Series or when passed to Series.apply. For\n | a DataFrame, can pass a dict, if the keys are DataFrame column names.\n | \n | Accepted combinations are:\n | \n | - string function name.\n | - function.\n | - list of functions.\n | - dict of column names -> functions (or list of functions).\n | \n | \n | axis : {0 or 'index'}\n | Parameter needed for compatibility with DataFrame.\n | \n | *args\n | Positional arguments to pass to `func`.\n | **kwargs\n | Keyword arguments to pass to `func`.\n | \n | Returns\n | -------\n | aggregated : Series\n | \n | Notes\n | -----\n | `agg` is an alias for `aggregate`. Use the alias.\n | \n | A passed user-defined-function will be passed a Series for evaluation.\n | \n | Examples\n | --------\n | \n | >>> s = Series(np.random.randn(10))\n | \n | >>> s.agg('min')\n | -1.3018049988556679\n | \n | >>> s.agg(['min', 'max'])\n | min -1.301805\n | max 1.127688\n | dtype: float64\n | \n | See also\n | --------\n | pandas.Series.apply\n | pandas.Series.transform\n | \n | align(self, other, join='outer', axis=None, level=None, copy=True, fill_value=None, method=None, limit=None, fill_axis=0, broadcast_axis=None)\n | Align two objects on their axes with the\n | specified join method for each axis Index\n | \n | Parameters\n | ----------\n | other : DataFrame or Series\n | join : {'outer', 'inner', 'left', 'right'}, default 'outer'\n | axis : allowed axis of the other object, default None\n | Align on index (0), columns (1), or both (None)\n | level : int or level name, default None\n | Broadcast across a level, matching Index values on the\n | passed MultiIndex level\n | copy : boolean, default True\n | Always returns new objects. If copy=False and no reindexing is\n | required then original objects are returned.\n | fill_value : scalar, default np.NaN\n | Value to use for missing values. Defaults to NaN, but can be any\n | \"compatible\" value\n | method : str, default None\n | limit : int, default None\n | fill_axis : {0 or 'index'}, default 0\n | Filling axis, method and limit\n | broadcast_axis : {0 or 'index'}, default None\n | Broadcast values along this axis, if aligning two objects of\n | different dimensions\n | \n | Returns\n | -------\n | (left, right) : (Series, type of other)\n | Aligned objects\n | \n | all(self, axis=0, bool_only=None, skipna=True, level=None, **kwargs)\n | Return whether all elements are True, potentially over an axis.\n | \n | Returns True if all elements within a series or along a Dataframe\n | axis are non-zero, not-empty or not-False.\n | \n | Parameters\n | ----------\n | axis : {0 or 'index', 1 or 'columns', None}, default 0\n | Indicate which axis or axes should be reduced.\n | \n | * 0 / 'index' : reduce the index, return a Series whose index is the\n | original column labels.\n | * 1 / 'columns' : reduce the columns, return a Series whose index is the\n | original index.\n | * None : reduce all axes, return a scalar.\n | \n | skipna : boolean, default True\n | Exclude NA/null values. If an entire row/column is NA, the result\n | will be NA.\n | level : int or level name, default None\n | If the axis is a MultiIndex (hierarchical), count along a\n | particular level, collapsing into a scalar.\n | bool_only : boolean, default None\n | Include only boolean columns. If None, will attempt to use everything,\n | then use only boolean data. Not implemented for Series.\n | **kwargs : any, default None\n | Additional keywords have no effect but might be accepted for\n | compatibility with NumPy.\n | \n | Returns\n | -------\n | all : scalar or Series (if level specified)\n | \n | See also\n | --------\n | pandas.Series.all : Return True if all elements are True\n | pandas.DataFrame.any : Return True if one (or more) elements are True\n | \n | Examples\n | --------\n | Series\n | \n | >>> pd.Series([True, True]).all()\n | True\n | >>> pd.Series([True, False]).all()\n | False\n | \n | DataFrames\n | \n | Create a dataframe from a dictionary.\n | \n | >>> df = pd.DataFrame({'col1': [True, True], 'col2': [True, False]})\n | >>> df\n | col1 col2\n | 0 True True\n | 1 True False\n | \n | Default behaviour checks if column-wise values all return True.\n | \n | >>> df.all()\n | col1 True\n | col2 False\n | dtype: bool\n | \n | Specify ``axis='columns'`` to check if row-wise values all return True.\n | \n | >>> df.all(axis='columns')\n | 0 True\n | 1 False\n | dtype: bool\n | \n | Or ``axis=None`` for whether every value is True.\n | \n | >>> df.all(axis=None)\n | False\n | \n | any(self, axis=0, bool_only=None, skipna=True, level=None, **kwargs)\n | Return whether any element is True over requested axis.\n | \n | Unlike :meth:`DataFrame.all`, this performs an *or* operation. If any of the\n | values along the specified axis is True, this will return True.\n | \n | Parameters\n | ----------\n | axis : {0 or 'index', 1 or 'columns', None}, default 0\n | Indicate which axis or axes should be reduced.\n | \n | * 0 / 'index' : reduce the index, return a Series whose index is the\n | original column labels.\n | * 1 / 'columns' : reduce the columns, return a Series whose index is the\n | original index.\n | * None : reduce all axes, return a scalar.\n | \n | skipna : boolean, default True\n | Exclude NA/null values. If an entire row/column is NA, the result\n | will be NA.\n | level : int or level name, default None\n | If the axis is a MultiIndex (hierarchical), count along a\n | particular level, collapsing into a scalar.\n | bool_only : boolean, default None\n | Include only boolean columns. If None, will attempt to use everything,\n | then use only boolean data. Not implemented for Series.\n | **kwargs : any, default None\n | Additional keywords have no effect but might be accepted for\n | compatibility with NumPy.\n | \n | Returns\n | -------\n | any : scalar or Series (if level specified)\n | \n | See Also\n | --------\n | pandas.DataFrame.all : Return whether all elements are True.\n | \n | Examples\n | --------\n | **Series**\n | \n | For Series input, the output is a scalar indicating whether any element\n | is True.\n | \n | >>> pd.Series([True, False]).any()\n | True\n | \n | **DataFrame**\n | \n | Whether each column contains at least one True element (the default).\n | \n | >>> df = pd.DataFrame({\"A\": [1, 2], \"B\": [0, 2], \"C\": [0, 0]})\n | >>> df\n | A B C\n | 0 1 0 0\n | 1 2 2 0\n | \n | >>> df.any()\n | A True\n | B True\n | C False\n | dtype: bool\n | \n | Aggregating over the columns.\n | \n | >>> df = pd.DataFrame({\"A\": [True, False], \"B\": [1, 2]})\n | >>> df\n | A B\n | 0 True 1\n | 1 False 2\n | \n | >>> df.any(axis='columns')\n | 0 True\n | 1 True\n | dtype: bool\n | \n | >>> df = pd.DataFrame({\"A\": [True, False], \"B\": [1, 0]})\n | >>> df\n | A B\n | 0 True 1\n | 1 False 0\n | \n | >>> df.any(axis='columns')\n | 0 True\n | 1 False\n | dtype: bool\n | \n | Aggregating over the entire DataFrame with ``axis=None``.\n | \n | >>> df.any(axis=None)\n | True\n | \n | `any` for an empty DataFrame is an empty Series.\n | \n | >>> pd.DataFrame([]).any()\n | Series([], dtype: bool)\n | \n | append(self, to_append, ignore_index=False, verify_integrity=False)\n | Concatenate two or more Series.\n | \n | Parameters\n | ----------\n | to_append : Series or list/tuple of Series\n | ignore_index : boolean, default False\n | If True, do not use the index labels.\n | \n | .. versionadded:: 0.19.0\n | \n | verify_integrity : boolean, default False\n | If True, raise Exception on creating index with duplicates\n | \n | Notes\n | -----\n | Iteratively appending to a Series can be more computationally intensive\n | than a single concatenate. A better solution is to append values to a\n | list and then concatenate the list with the original Series all at\n | once.\n | \n | See also\n | --------\n | pandas.concat : General function to concatenate DataFrame, Series\n | or Panel objects\n | \n | Returns\n | -------\n | appended : Series\n | \n | Examples\n | --------\n | >>> s1 = pd.Series([1, 2, 3])\n | >>> s2 = pd.Series([4, 5, 6])\n | >>> s3 = pd.Series([4, 5, 6], index=[3,4,5])\n | >>> s1.append(s2)\n | 0 1\n | 1 2\n | 2 3\n | 0 4\n | 1 5\n | 2 6\n | dtype: int64\n | \n | >>> s1.append(s3)\n | 0 1\n | 1 2\n | 2 3\n | 3 4\n | 4 5\n | 5 6\n | dtype: int64\n | \n | With `ignore_index` set to True:\n | \n | >>> s1.append(s2, ignore_index=True)\n | 0 1\n | 1 2\n | 2 3\n | 3 4\n | 4 5\n | 5 6\n | dtype: int64\n | \n | With `verify_integrity` set to True:\n | \n | >>> s1.append(s2, verify_integrity=True)\n | Traceback (most recent call last):\n | ...\n | ValueError: Indexes have overlapping values: [0, 1, 2]\n | \n | apply(self, func, convert_dtype=True, args=(), **kwds)\n | Invoke function on values of Series. Can be ufunc (a NumPy function\n | that applies to the entire Series) or a Python function that only works\n | on single values\n | \n | Parameters\n | ----------\n | func : function\n | convert_dtype : boolean, default True\n | Try to find better dtype for elementwise function results. If\n | False, leave as dtype=object\n | args : tuple\n | Positional arguments to pass to function in addition to the value\n | Additional keyword arguments will be passed as keywords to the function\n | \n | Returns\n | -------\n | y : Series or DataFrame if func returns a Series\n | \n | See also\n | --------\n | Series.map: For element-wise operations\n | Series.agg: only perform aggregating type operations\n | Series.transform: only perform transformating type operations\n | \n | Examples\n | --------\n | \n | Create a series with typical summer temperatures for each city.\n | \n | >>> import pandas as pd\n | >>> import numpy as np\n | >>> series = pd.Series([20, 21, 12], index=['London',\n | ... 'New York','Helsinki'])\n | >>> series\n | London 20\n | New York 21\n | Helsinki 12\n | dtype: int64\n | \n | Square the values by defining a function and passing it as an\n | argument to ``apply()``.\n | \n | >>> def square(x):\n | ... return x**2\n | >>> series.apply(square)\n | London 400\n | New York 441\n | Helsinki 144\n | dtype: int64\n | \n | Square the values by passing an anonymous function as an\n | argument to ``apply()``.\n | \n | >>> series.apply(lambda x: x**2)\n | London 400\n | New York 441\n | Helsinki 144\n | dtype: int64\n | \n | Define a custom function that needs additional positional\n | arguments and pass these additional arguments using the\n | ``args`` keyword.\n | \n | >>> def subtract_custom_value(x, custom_value):\n | ... return x-custom_value\n | \n | >>> series.apply(subtract_custom_value, args=(5,))\n | London 15\n | New York 16\n | Helsinki 7\n | dtype: int64\n | \n | Define a custom function that takes keyword arguments\n | and pass these arguments to ``apply``.\n | \n | >>> def add_custom_values(x, **kwargs):\n | ... for month in kwargs:\n | ... x+=kwargs[month]\n | ... return x\n | \n | >>> series.apply(add_custom_values, june=30, july=20, august=25)\n | London 95\n | New York 96\n | Helsinki 87\n | dtype: int64\n | \n | Use a function from the Numpy library.\n | \n | >>> series.apply(np.log)\n | London 2.995732\n | New York 3.044522\n | Helsinki 2.484907\n | dtype: float64\n | \n | argmax = idxmax(self, axis=0, skipna=True, *args, **kwargs)\n | .. deprecated:: 0.21.0\n | \n | 'argmax' is deprecated, use 'idxmax' instead. The behavior of 'argmax'\n | will be corrected to return the positional maximum in the future. Use\n | 'series.values.argmax' to get the position of the maximum now.\n | \n | \n | Return the row label of the maximum value.\n | \n | If multiple values equal the maximum, the first row label with that\n | value is returned.\n | \n | Parameters\n | ----------\n | skipna : boolean, default True\n | Exclude NA/null values. If the entire Series is NA, the result\n | will be NA.\n | axis : int, default 0\n | For compatibility with DataFrame.idxmax. Redundant for application\n | on Series.\n | *args, **kwargs\n | Additional keywors have no effect but might be accepted\n | for compatibility with NumPy.\n | \n | Returns\n | -------\n | idxmax : Index of maximum of values.\n | \n | Raises\n | ------\n | ValueError\n | If the Series is empty.\n | \n | Notes\n | -----\n | This method is the Series version of ``ndarray.argmax``. This method\n | returns the label of the maximum, while ``ndarray.argmax`` returns\n | the position. To get the position, use ``series.values.argmax()``.\n | \n | See Also\n | --------\n | numpy.argmax : Return indices of the maximum values\n | along the given axis.\n | DataFrame.idxmax : Return index of first occurrence of maximum\n | over requested axis.\n | Series.idxmin : Return index *label* of the first occurrence\n | of minimum of values.\n | \n | Examples\n | --------\n | >>> s = pd.Series(data=[1, None, 4, 3, 4],\n | ... index=['A', 'B', 'C', 'D', 'E'])\n | >>> s\n | A 1.0\n | B NaN\n | C 4.0\n | D 3.0\n | E 4.0\n | dtype: float64\n | \n | >>> s.idxmax()\n | 'C'\n | \n | If `skipna` is False and there is an NA value in the data,\n | the function returns ``nan``.\n | \n | >>> s.idxmax(skipna=False)\n | nan\n | \n | argmin = idxmin(self, axis=None, skipna=True, *args, **kwargs)\n | .. deprecated:: 0.21.0\n | \n | 'argmin' is deprecated, use 'idxmin' instead. The behavior of 'argmin'\n | will be corrected to return the positional minimum in the future. Use\n | 'series.values.argmin' to get the position of the minimum now.\n | \n | \n | Return the row label of the minimum value.\n | \n | If multiple values equal the minimum, the first row label with that\n | value is returned.\n | \n | Parameters\n | ----------\n | skipna : boolean, default True\n | Exclude NA/null values. If the entire Series is NA, the result\n | will be NA.\n | axis : int, default 0\n | For compatibility with DataFrame.idxmin. Redundant for application\n | on Series.\n | *args, **kwargs\n | Additional keywors have no effect but might be accepted\n | for compatibility with NumPy.\n | \n | Returns\n | -------\n | idxmin : Index of minimum of values.\n | \n | Raises\n | ------\n | ValueError\n | If the Series is empty.\n | \n | Notes\n | -----\n | This method is the Series version of ``ndarray.argmin``. This method\n | returns the label of the minimum, while ``ndarray.argmin`` returns\n | the position. To get the position, use ``series.values.argmin()``.\n | \n | See Also\n | --------\n | numpy.argmin : Return indices of the minimum values\n | along the given axis.\n | DataFrame.idxmin : Return index of first occurrence of minimum\n | over requested axis.\n | Series.idxmax : Return index *label* of the first occurrence\n | of maximum of values.\n | \n | Examples\n | --------\n | >>> s = pd.Series(data=[1, None, 4, 1],\n | ... index=['A' ,'B' ,'C' ,'D'])\n | >>> s\n | A 1.0\n | B NaN\n | C 4.0\n | D 1.0\n | dtype: float64\n | \n | >>> s.idxmin()\n | 'A'\n | \n | If `skipna` is False and there is an NA value in the data,\n | the function returns ``nan``.\n | \n | >>> s.idxmin(skipna=False)\n | nan\n | \n | argsort(self, axis=0, kind='quicksort', order=None)\n | Overrides ndarray.argsort. Argsorts the value, omitting NA/null values,\n | and places the result in the same locations as the non-NA values\n | \n | Parameters\n | ----------\n | axis : int (can only be zero)\n | kind : {'mergesort', 'quicksort', 'heapsort'}, default 'quicksort'\n | Choice of sorting algorithm. See np.sort for more\n | information. 'mergesort' is the only stable algorithm\n | order : ignored\n | \n | Returns\n | -------\n | argsorted : Series, with -1 indicated where nan values are present\n | \n | See also\n | --------\n | numpy.ndarray.argsort\n | \n | autocorr(self, lag=1)\n | Lag-N autocorrelation\n | \n | Parameters\n | ----------\n | lag : int, default 1\n | Number of lags to apply before performing autocorrelation.\n | \n | Returns\n | -------\n | autocorr : float\n | \n | between(self, left, right, inclusive=True)\n | Return boolean Series equivalent to left <= series <= right.\n | \n | This function returns a boolean vector containing `True` wherever the\n | corresponding Series element is between the boundary values `left` and\n | `right`. NA values are treated as `False`.\n | \n | Parameters\n | ----------\n | left : scalar\n | Left boundary.\n | right : scalar\n | Right boundary.\n | inclusive : bool, default True\n | Include boundaries.\n | \n | Returns\n | -------\n | Series\n | Each element will be a boolean.\n | \n | Notes\n | -----\n | This function is equivalent to ``(left <= ser) & (ser <= right)``\n | \n | See Also\n | --------\n | pandas.Series.gt : Greater than of series and other\n | pandas.Series.lt : Less than of series and other\n | \n | Examples\n | --------\n | >>> s = pd.Series([2, 0, 4, 8, np.nan])\n | \n | Boundary values are included by default:\n | \n | >>> s.between(1, 4)\n | 0 True\n | 1 False\n | 2 True\n | 3 False\n | 4 False\n | dtype: bool\n | \n | With `inclusive` set to ``False`` boundary values are excluded:\n | \n | >>> s.between(1, 4, inclusive=False)\n | 0 True\n | 1 False\n | 2 False\n | 3 False\n | 4 False\n | dtype: bool\n | \n | `left` and `right` can be any scalar value:\n | \n | >>> s = pd.Series(['Alice', 'Bob', 'Carol', 'Eve'])\n | >>> s.between('Anna', 'Daniel')\n | 0 False\n | 1 True\n | 2 True\n | 3 False\n | dtype: bool\n | \n | combine(self, other, func, fill_value=nan)\n | Perform elementwise binary operation on two Series using given function\n | with optional fill value when an index is missing from one Series or\n | the other\n | \n | Parameters\n | ----------\n | other : Series or scalar value\n | func : function\n | Function that takes two scalars as inputs and return a scalar\n | fill_value : scalar value\n | \n | Returns\n | -------\n | result : Series\n | \n | Examples\n | --------\n | >>> s1 = Series([1, 2])\n | >>> s2 = Series([0, 3])\n | >>> s1.combine(s2, lambda x1, x2: x1 if x1 < x2 else x2)\n | 0 0\n | 1 2\n | dtype: int64\n | \n | See Also\n | --------\n | Series.combine_first : Combine Series values, choosing the calling\n | Series's values first\n | \n | combine_first(self, other)\n | Combine Series values, choosing the calling Series's values\n | first. Result index will be the union of the two indexes\n | \n | Parameters\n | ----------\n | other : Series\n | \n | Returns\n | -------\n | combined : Series\n | \n | Examples\n | --------\n | >>> s1 = pd.Series([1, np.nan])\n | >>> s2 = pd.Series([3, 4])\n | >>> s1.combine_first(s2)\n | 0 1.0\n | 1 4.0\n | dtype: float64\n | \n | See Also\n | --------\n | Series.combine : Perform elementwise operation on two Series\n | using a given function\n | \n | compound(self, axis=None, skipna=None, level=None)\n | Return the compound percentage of the values for the requested axis\n | \n | Parameters\n | ----------\n | axis : {index (0)}\n | skipna : boolean, default True\n | Exclude NA/null values when computing the result.\n | level : int or level name, default None\n | If the axis is a MultiIndex (hierarchical), count along a\n | particular level, collapsing into a scalar\n | numeric_only : boolean, default None\n | Include only float, int, boolean columns. If None, will attempt to use\n | everything, then use only numeric data. Not implemented for Series.\n | \n | Returns\n | -------\n | compounded : scalar or Series (if level specified)\n | \n | compress(self, condition, *args, **kwargs)\n | Return selected slices of an array along given axis as a Series\n | \n | See also\n | --------\n | numpy.ndarray.compress\n | \n | corr(self, other, method='pearson', min_periods=None)\n | Compute correlation with `other` Series, excluding missing values\n | \n | Parameters\n | ----------\n | other : Series\n | method : {'pearson', 'kendall', 'spearman'}\n | * pearson : standard correlation coefficient\n | * kendall : Kendall Tau correlation coefficient\n | * spearman : Spearman rank correlation\n | min_periods : int, optional\n | Minimum number of observations needed to have a valid result\n | \n | \n | Returns\n | -------\n | correlation : float\n | \n | count(self, level=None)\n | Return number of non-NA/null observations in the Series\n | \n | Parameters\n | ----------\n | level : int or level name, default None\n | If the axis is a MultiIndex (hierarchical), count along a\n | particular level, collapsing into a smaller Series\n | \n | Returns\n | -------\n | nobs : int or Series (if level specified)\n | \n | cov(self, other, min_periods=None)\n | Compute covariance with Series, excluding missing values\n | \n | Parameters\n | ----------\n | other : Series\n | min_periods : int, optional\n | Minimum number of observations needed to have a valid result\n | \n | Returns\n | -------\n | covariance : float\n | \n | Normalized by N-1 (unbiased estimator).\n | \n | cummax(self, axis=None, skipna=True, *args, **kwargs)\n | Return cumulative maximum over a DataFrame or Series axis.\n | \n | Returns a DataFrame or Series of the same size containing the cumulative\n | maximum.\n | \n | Parameters\n | ----------\n | axis : {0 or 'index', 1 or 'columns'}, default 0\n | The index or the name of the axis. 0 is equivalent to None or 'index'.\n | skipna : boolean, default True\n | Exclude NA/null values. If an entire row/column is NA, the result\n | will be NA.\n | *args, **kwargs :\n | Additional keywords have no effect but might be accepted for\n | compatibility with NumPy.\n | \n | Returns\n | -------\n | cummax : scalar or Series\n | \n | Examples\n | --------\n | **Series**\n | \n | >>> s = pd.Series([2, np.nan, 5, -1, 0])\n | >>> s\n | 0 2.0\n | 1 NaN\n | 2 5.0\n | 3 -1.0\n | 4 0.0\n | dtype: float64\n | \n | By default, NA values are ignored.\n | \n | >>> s.cummax()\n | 0 2.0\n | 1 NaN\n | 2 5.0\n | 3 5.0\n | 4 5.0\n | dtype: float64\n | \n | To include NA values in the operation, use ``skipna=False``\n | \n | >>> s.cummax(skipna=False)\n | 0 2.0\n | 1 NaN\n | 2 NaN\n | 3 NaN\n | 4 NaN\n | dtype: float64\n | \n | **DataFrame**\n | \n | >>> df = pd.DataFrame([[2.0, 1.0],\n | ... [3.0, np.nan],\n | ... [1.0, 0.0]],\n | ... columns=list('AB'))\n | >>> df\n | A B\n | 0 2.0 1.0\n | 1 3.0 NaN\n | 2 1.0 0.0\n | \n | By default, iterates over rows and finds the maximum\n | in each column. This is equivalent to ``axis=None`` or ``axis='index'``.\n | \n | >>> df.cummax()\n | A B\n | 0 2.0 1.0\n | 1 3.0 NaN\n | 2 3.0 1.0\n | \n | To iterate over columns and find the maximum in each row,\n | use ``axis=1``\n | \n | >>> df.cummax(axis=1)\n | A B\n | 0 2.0 2.0\n | 1 3.0 NaN\n | 2 1.0 1.0\n | \n | See also\n | --------\n | pandas.core.window.Expanding.max : Similar functionality\n | but ignores ``NaN`` values.\n | Series.max : Return the maximum over\n | Series axis.\n | Series.cummax : Return cumulative maximum over Series axis.\n | Series.cummin : Return cumulative minimum over Series axis.\n | Series.cumsum : Return cumulative sum over Series axis.\n | Series.cumprod : Return cumulative product over Series axis.\n | \n | cummin(self, axis=None, skipna=True, *args, **kwargs)\n | Return cumulative minimum over a DataFrame or Series axis.\n | \n | Returns a DataFrame or Series of the same size containing the cumulative\n | minimum.\n | \n | Parameters\n | ----------\n | axis : {0 or 'index', 1 or 'columns'}, default 0\n | The index or the name of the axis. 0 is equivalent to None or 'index'.\n | skipna : boolean, default True\n | Exclude NA/null values. If an entire row/column is NA, the result\n | will be NA.\n | *args, **kwargs :\n | Additional keywords have no effect but might be accepted for\n | compatibility with NumPy.\n | \n | Returns\n | -------\n | cummin : scalar or Series\n | \n | Examples\n | --------\n | **Series**\n | \n | >>> s = pd.Series([2, np.nan, 5, -1, 0])\n | >>> s\n | 0 2.0\n | 1 NaN\n | 2 5.0\n | 3 -1.0\n | 4 0.0\n | dtype: float64\n | \n | By default, NA values are ignored.\n | \n | >>> s.cummin()\n | 0 2.0\n | 1 NaN\n | 2 2.0\n | 3 -1.0\n | 4 -1.0\n | dtype: float64\n | \n | To include NA values in the operation, use ``skipna=False``\n | \n | >>> s.cummin(skipna=False)\n | 0 2.0\n | 1 NaN\n | 2 NaN\n | 3 NaN\n | 4 NaN\n | dtype: float64\n | \n | **DataFrame**\n | \n | >>> df = pd.DataFrame([[2.0, 1.0],\n | ... [3.0, np.nan],\n | ... [1.0, 0.0]],\n | ... columns=list('AB'))\n | >>> df\n | A B\n | 0 2.0 1.0\n | 1 3.0 NaN\n | 2 1.0 0.0\n | \n | By default, iterates over rows and finds the minimum\n | in each column. This is equivalent to ``axis=None`` or ``axis='index'``.\n | \n | >>> df.cummin()\n | A B\n | 0 2.0 1.0\n | 1 2.0 NaN\n | 2 1.0 0.0\n | \n | To iterate over columns and find the minimum in each row,\n | use ``axis=1``\n | \n | >>> df.cummin(axis=1)\n | A B\n | 0 2.0 1.0\n | 1 3.0 NaN\n | 2 1.0 0.0\n | \n | See also\n | --------\n | pandas.core.window.Expanding.min : Similar functionality\n | but ignores ``NaN`` values.\n | Series.min : Return the minimum over\n | Series axis.\n | Series.cummax : Return cumulative maximum over Series axis.\n | Series.cummin : Return cumulative minimum over Series axis.\n | Series.cumsum : Return cumulative sum over Series axis.\n | Series.cumprod : Return cumulative product over Series axis.\n | \n | cumprod(self, axis=None, skipna=True, *args, **kwargs)\n | Return cumulative product over a DataFrame or Series axis.\n | \n | Returns a DataFrame or Series of the same size containing the cumulative\n | product.\n | \n | Parameters\n | ----------\n | axis : {0 or 'index', 1 or 'columns'}, default 0\n | The index or the name of the axis. 0 is equivalent to None or 'index'.\n | skipna : boolean, default True\n | Exclude NA/null values. If an entire row/column is NA, the result\n | will be NA.\n | *args, **kwargs :\n | Additional keywords have no effect but might be accepted for\n | compatibility with NumPy.\n | \n | Returns\n | -------\n | cumprod : scalar or Series\n | \n | Examples\n | --------\n | **Series**\n | \n | >>> s = pd.Series([2, np.nan, 5, -1, 0])\n | >>> s\n | 0 2.0\n | 1 NaN\n | 2 5.0\n | 3 -1.0\n | 4 0.0\n | dtype: float64\n | \n | By default, NA values are ignored.\n | \n | >>> s.cumprod()\n | 0 2.0\n | 1 NaN\n | 2 10.0\n | 3 -10.0\n | 4 -0.0\n | dtype: float64\n | \n | To include NA values in the operation, use ``skipna=False``\n | \n | >>> s.cumprod(skipna=False)\n | 0 2.0\n | 1 NaN\n | 2 NaN\n | 3 NaN\n | 4 NaN\n | dtype: float64\n | \n | **DataFrame**\n | \n | >>> df = pd.DataFrame([[2.0, 1.0],\n | ... [3.0, np.nan],\n | ... [1.0, 0.0]],\n | ... columns=list('AB'))\n | >>> df\n | A B\n | 0 2.0 1.0\n | 1 3.0 NaN\n | 2 1.0 0.0\n | \n | By default, iterates over rows and finds the product\n | in each column. This is equivalent to ``axis=None`` or ``axis='index'``.\n | \n | >>> df.cumprod()\n | A B\n | 0 2.0 1.0\n | 1 6.0 NaN\n | 2 6.0 0.0\n | \n | To iterate over columns and find the product in each row,\n | use ``axis=1``\n | \n | >>> df.cumprod(axis=1)\n | A B\n | 0 2.0 2.0\n | 1 3.0 NaN\n | 2 1.0 0.0\n | \n | See also\n | --------\n | pandas.core.window.Expanding.prod : Similar functionality\n | but ignores ``NaN`` values.\n | Series.prod : Return the product over\n | Series axis.\n | Series.cummax : Return cumulative maximum over Series axis.\n | Series.cummin : Return cumulative minimum over Series axis.\n | Series.cumsum : Return cumulative sum over Series axis.\n | Series.cumprod : Return cumulative product over Series axis.\n | \n | cumsum(self, axis=None, skipna=True, *args, **kwargs)\n | Return cumulative sum over a DataFrame or Series axis.\n | \n | Returns a DataFrame or Series of the same size containing the cumulative\n | sum.\n | \n | Parameters\n | ----------\n | axis : {0 or 'index', 1 or 'columns'}, default 0\n | The index or the name of the axis. 0 is equivalent to None or 'index'.\n | skipna : boolean, default True\n | Exclude NA/null values. If an entire row/column is NA, the result\n | will be NA.\n | *args, **kwargs :\n | Additional keywords have no effect but might be accepted for\n | compatibility with NumPy.\n | \n | Returns\n | -------\n | cumsum : scalar or Series\n | \n | Examples\n | --------\n | **Series**\n | \n | >>> s = pd.Series([2, np.nan, 5, -1, 0])\n | >>> s\n | 0 2.0\n | 1 NaN\n | 2 5.0\n | 3 -1.0\n | 4 0.0\n | dtype: float64\n | \n | By default, NA values are ignored.\n | \n | >>> s.cumsum()\n | 0 2.0\n | 1 NaN\n | 2 7.0\n | 3 6.0\n | 4 6.0\n | dtype: float64\n | \n | To include NA values in the operation, use ``skipna=False``\n | \n | >>> s.cumsum(skipna=False)\n | 0 2.0\n | 1 NaN\n | 2 NaN\n | 3 NaN\n | 4 NaN\n | dtype: float64\n | \n | **DataFrame**\n | \n | >>> df = pd.DataFrame([[2.0, 1.0],\n | ... [3.0, np.nan],\n | ... [1.0, 0.0]],\n | ... columns=list('AB'))\n | >>> df\n | A B\n | 0 2.0 1.0\n | 1 3.0 NaN\n | 2 1.0 0.0\n | \n | By default, iterates over rows and finds the sum\n | in each column. This is equivalent to ``axis=None`` or ``axis='index'``.\n | \n | >>> df.cumsum()\n | A B\n | 0 2.0 1.0\n | 1 5.0 NaN\n | 2 6.0 1.0\n | \n | To iterate over columns and find the sum in each row,\n | use ``axis=1``\n | \n | >>> df.cumsum(axis=1)\n | A B\n | 0 2.0 3.0\n | 1 3.0 NaN\n | 2 1.0 1.0\n | \n | See also\n | --------\n | pandas.core.window.Expanding.sum : Similar functionality\n | but ignores ``NaN`` values.\n | Series.sum : Return the sum over\n | Series axis.\n | Series.cummax : Return cumulative maximum over Series axis.\n | Series.cummin : Return cumulative minimum over Series axis.\n | Series.cumsum : Return cumulative sum over Series axis.\n | Series.cumprod : Return cumulative product over Series axis.\n | \n | diff(self, periods=1)\n | First discrete difference of element.\n | \n | Calculates the difference of a Series element compared with another\n | element in the Series (default is element in previous row).\n | \n | Parameters\n | ----------\n | periods : int, default 1\n | Periods to shift for calculating difference, accepts negative\n | values.\n | \n | Returns\n | -------\n | diffed : Series\n | \n | See Also\n | --------\n | Series.pct_change: Percent change over given number of periods.\n | Series.shift: Shift index by desired number of periods with an\n | optional time freq.\n | DataFrame.diff: First discrete difference of object\n | \n | Examples\n | --------\n | Difference with previous row\n | \n | >>> s = pd.Series([1, 1, 2, 3, 5, 8])\n | >>> s.diff()\n | 0 NaN\n | 1 0.0\n | 2 1.0\n | 3 1.0\n | 4 2.0\n | 5 3.0\n | dtype: float64\n | \n | Difference with 3rd previous row\n | \n | >>> s.diff(periods=3)\n | 0 NaN\n | 1 NaN\n | 2 NaN\n | 3 2.0\n | 4 4.0\n | 5 6.0\n | dtype: float64\n | \n | Difference with following row\n | \n | >>> s.diff(periods=-1)\n | 0 0.0\n | 1 -1.0\n | 2 -1.0\n | 3 -2.0\n | 4 -3.0\n | 5 NaN\n | dtype: float64\n | \n | div = truediv(self, other, level=None, fill_value=None, axis=0)\n | Floating division of series and other, element-wise (binary operator `truediv`).\n | \n | Equivalent to ``series / other``, but with support to substitute a fill_value for\n | missing data in one of the inputs.\n | \n | Parameters\n | ----------\n | other : Series or scalar value\n | fill_value : None or float value, default None (NaN)\n | Fill existing missing (NaN) values, and any new element needed for\n | successful Series alignment, with this value before computation.\n | If data in both corresponding Series locations is missing\n | the result will be missing\n | level : int or name\n | Broadcast across a level, matching Index values on the\n | passed MultiIndex level\n | \n | Returns\n | -------\n | result : Series\n | \n | Examples\n | --------\n | >>> a = pd.Series([1, 1, 1, np.nan], index=['a', 'b', 'c', 'd'])\n | >>> a\n | a 1.0\n | b 1.0\n | c 1.0\n | d NaN\n | dtype: float64\n | >>> b = pd.Series([1, np.nan, 1, np.nan], index=['a', 'b', 'd', 'e'])\n | >>> b\n | a 1.0\n | b NaN\n | d 1.0\n | e NaN\n | dtype: float64\n | >>> a.add(b, fill_value=0)\n | a 2.0\n | b 1.0\n | c 1.0\n | d 1.0\n | e NaN\n | dtype: float64\n | \n | See also\n | --------\n | Series.rtruediv\n | \n | divide = truediv(self, other, level=None, fill_value=None, axis=0)\n | Floating division of series and other, element-wise (binary operator `truediv`).\n | \n | Equivalent to ``series / other``, but with support to substitute a fill_value for\n | missing data in one of the inputs.\n | \n | Parameters\n | ----------\n | other : Series or scalar value\n | fill_value : None or float value, default None (NaN)\n | Fill existing missing (NaN) values, and any new element needed for\n | successful Series alignment, with this value before computation.\n | If data in both corresponding Series locations is missing\n | the result will be missing\n | level : int or name\n | Broadcast across a level, matching Index values on the\n | passed MultiIndex level\n | \n | Returns\n | -------\n | result : Series\n | \n | Examples\n | --------\n | >>> a = pd.Series([1, 1, 1, np.nan], index=['a', 'b', 'c', 'd'])\n | >>> a\n | a 1.0\n | b 1.0\n | c 1.0\n | d NaN\n | dtype: float64\n | >>> b = pd.Series([1, np.nan, 1, np.nan], index=['a', 'b', 'd', 'e'])\n | >>> b\n | a 1.0\n | b NaN\n | d 1.0\n | e NaN\n | dtype: float64\n | >>> a.add(b, fill_value=0)\n | a 2.0\n | b 1.0\n | c 1.0\n | d 1.0\n | e NaN\n | dtype: float64\n | \n | See also\n | --------\n | Series.rtruediv\n | \n | divmod(self, other, level=None, fill_value=None, axis=0)\n | Integer division and modulo of series and other, element-wise (binary operator `divmod`).\n | \n | Equivalent to ``series divmod other``, but with support to substitute a fill_value for\n | missing data in one of the inputs.\n | \n | Parameters\n | ----------\n | other : Series or scalar value\n | fill_value : None or float value, default None (NaN)\n | Fill existing missing (NaN) values, and any new element needed for\n | successful Series alignment, with this value before computation.\n | If data in both corresponding Series locations is missing\n | the result will be missing\n | level : int or name\n | Broadcast across a level, matching Index values on the\n | passed MultiIndex level\n | \n | Returns\n | -------\n | result : Series\n | \n | Examples\n | --------\n | >>> a = pd.Series([1, 1, 1, np.nan], index=['a', 'b', 'c', 'd'])\n | >>> a\n | a 1.0\n | b 1.0\n | c 1.0\n | d NaN\n | dtype: float64\n | >>> b = pd.Series([1, np.nan, 1, np.nan], index=['a', 'b', 'd', 'e'])\n | >>> b\n | a 1.0\n | b NaN\n | d 1.0\n | e NaN\n | dtype: float64\n | >>> a.add(b, fill_value=0)\n | a 2.0\n | b 1.0\n | c 1.0\n | d 1.0\n | e NaN\n | dtype: float64\n | \n | See also\n | --------\n | Series.None\n | \n | dot(self, other)\n | Matrix multiplication with DataFrame or inner-product with Series\n | objects. Can also be called using `self @ other` in Python >= 3.5.\n | \n | Parameters\n | ----------\n | other : Series or DataFrame\n | \n | Returns\n | -------\n | dot_product : scalar or Series\n | \n | drop(self, labels=None, axis=0, index=None, columns=None, level=None, inplace=False, errors='raise')\n | Return Series with specified index labels removed.\n | \n | Remove elements of a Series based on specifying the index labels.\n | When using a multi-index, labels on different levels can be removed\n | by specifying the level.\n | \n | Parameters\n | ----------\n | labels : single label or list-like\n | Index labels to drop.\n | axis : 0, default 0\n | Redundant for application on Series.\n | index, columns : None\n | Redundant for application on Series, but index can be used instead\n | of labels.\n | \n | .. versionadded:: 0.21.0\n | level : int or level name, optional\n | For MultiIndex, level for which the labels will be removed.\n | inplace : bool, default False\n | If True, do operation inplace and return None.\n | errors : {'ignore', 'raise'}, default 'raise'\n | If 'ignore', suppress error and only existing labels are dropped.\n | \n | Returns\n | -------\n | dropped : pandas.Series\n | \n | See Also\n | --------\n | Series.reindex : Return only specified index labels of Series.\n | Series.dropna : Return series without null values.\n | Series.drop_duplicates : Return Series with duplicate values removed.\n | DataFrame.drop : Drop specified labels from rows or columns.\n | \n | Raises\n | ------\n | KeyError\n | If none of the labels are found in the index.\n | \n | Examples\n | --------\n | >>> s = pd.Series(data=np.arange(3), index=['A','B','C'])\n | >>> s\n | A 0\n | B 1\n | C 2\n | dtype: int64\n | \n | Drop labels B en C\n | \n | >>> s.drop(labels=['B','C'])\n | A 0\n | dtype: int64\n | \n | Drop 2nd level label in MultiIndex Series\n | \n | >>> midx = pd.MultiIndex(levels=[['lama', 'cow', 'falcon'],\n | ... ['speed', 'weight', 'length']],\n | ... labels=[[0, 0, 0, 1, 1, 1, 2, 2, 2],\n | ... [0, 1, 2, 0, 1, 2, 0, 1, 2]])\n | >>> s = pd.Series([45, 200, 1.2, 30, 250, 1.5, 320, 1, 0.3],\n | ... index=midx)\n | >>> s\n | lama speed 45.0\n | weight 200.0\n | length 1.2\n | cow speed 30.0\n | weight 250.0\n | length 1.5\n | falcon speed 320.0\n | weight 1.0\n | length 0.3\n | dtype: float64\n | \n | >>> s.drop(labels='weight', level=1)\n | lama speed 45.0\n | length 1.2\n | cow speed 30.0\n | length 1.5\n | falcon speed 320.0\n | length 0.3\n | dtype: float64\n | \n | drop_duplicates(self, keep='first', inplace=False)\n | Return Series with duplicate values removed.\n | \n | Parameters\n | ----------\n | keep : {'first', 'last', ``False``}, default 'first'\n | - 'first' : Drop duplicates except for the first occurrence.\n | - 'last' : Drop duplicates except for the last occurrence.\n | - ``False`` : Drop all duplicates.\n | inplace : boolean, default ``False``\n | If ``True``, performs operation inplace and returns None.\n | \n | Returns\n | -------\n | deduplicated : Series\n | \n | See Also\n | --------\n | Index.drop_duplicates : equivalent method on Index\n | DataFrame.drop_duplicates : equivalent method on DataFrame\n | Series.duplicated : related method on Series, indicating duplicate\n | Series values.\n | \n | Examples\n | --------\n | Generate an Series with duplicated entries.\n | \n | >>> s = pd.Series(['lama', 'cow', 'lama', 'beetle', 'lama', 'hippo'],\n | ... name='animal')\n | >>> s\n | 0 lama\n | 1 cow\n | 2 lama\n | 3 beetle\n | 4 lama\n | 5 hippo\n | Name: animal, dtype: object\n | \n | With the 'keep' parameter, the selection behaviour of duplicated values\n | can be changed. The value 'first' keeps the first occurrence for each\n | set of duplicated entries. The default value of keep is 'first'.\n | \n | >>> s.drop_duplicates()\n | 0 lama\n | 1 cow\n | 3 beetle\n | 5 hippo\n | Name: animal, dtype: object\n | \n | The value 'last' for parameter 'keep' keeps the last occurrence for\n | each set of duplicated entries.\n | \n | >>> s.drop_duplicates(keep='last')\n | 1 cow\n | 3 beetle\n | 4 lama\n | 5 hippo\n | Name: animal, dtype: object\n | \n | The value ``False`` for parameter 'keep' discards all sets of\n | duplicated entries. Setting the value of 'inplace' to ``True`` performs\n | the operation inplace and returns ``None``.\n | \n | >>> s.drop_duplicates(keep=False, inplace=True)\n | >>> s\n | 1 cow\n | 3 beetle\n | 5 hippo\n | Name: animal, dtype: object\n | \n | dropna(self, axis=0, inplace=False, **kwargs)\n | Return a new Series with missing values removed.\n | \n | See the :ref:`User Guide <missing_data>` for more on which values are\n | considered missing, and how to work with missing data.\n | \n | Parameters\n | ----------\n | axis : {0 or 'index'}, default 0\n | There is only one axis to drop values from.\n | inplace : bool, default False\n | If True, do operation inplace and return None.\n | **kwargs\n | Not in use.\n | \n | Returns\n | -------\n | Series\n | Series with NA entries dropped from it.\n | \n | See Also\n | --------\n | Series.isna: Indicate missing values.\n | Series.notna : Indicate existing (non-missing) values.\n | Series.fillna : Replace missing values.\n | DataFrame.dropna : Drop rows or columns which contain NA values.\n | Index.dropna : Drop missing indices.\n | \n | Examples\n | --------\n | >>> ser = pd.Series([1., 2., np.nan])\n | >>> ser\n | 0 1.0\n | 1 2.0\n | 2 NaN\n | dtype: float64\n | \n | Drop NA values from a Series.\n | \n | >>> ser.dropna()\n | 0 1.0\n | 1 2.0\n | dtype: float64\n | \n | Keep the Series with valid entries in the same variable.\n | \n | >>> ser.dropna(inplace=True)\n | >>> ser\n | 0 1.0\n | 1 2.0\n | dtype: float64\n | \n | Empty strings are not considered NA values. ``None`` is considered an\n | NA value.\n | \n | >>> ser = pd.Series([np.NaN, 2, pd.NaT, '', None, 'I stay'])\n | >>> ser\n | 0 NaN\n | 1 2\n | 2 NaT\n | 3\n | 4 None\n | 5 I stay\n | dtype: object\n | >>> ser.dropna()\n | 1 2\n | 3\n | 5 I stay\n | dtype: object\n | \n | duplicated(self, keep='first')\n | Indicate duplicate Series values.\n | \n | Duplicated values are indicated as ``True`` values in the resulting\n | Series. Either all duplicates, all except the first or all except the\n | last occurrence of duplicates can be indicated.\n | \n | Parameters\n | ----------\n | keep : {'first', 'last', False}, default 'first'\n | - 'first' : Mark duplicates as ``True`` except for the first\n | occurrence.\n | - 'last' : Mark duplicates as ``True`` except for the last\n | occurrence.\n | - ``False`` : Mark all duplicates as ``True``.\n | \n | Examples\n | --------\n | By default, for each set of duplicated values, the first occurrence is\n | set on False and all others on True:\n | \n | >>> animals = pd.Series(['lama', 'cow', 'lama', 'beetle', 'lama'])\n | >>> animals.duplicated()\n | 0 False\n | 1 False\n | 2 True\n | 3 False\n | 4 True\n | dtype: bool\n | \n | which is equivalent to\n | \n | >>> animals.duplicated(keep='first')\n | 0 False\n | 1 False\n | 2 True\n | 3 False\n | 4 True\n | dtype: bool\n | \n | By using 'last', the last occurrence of each set of duplicated values\n | is set on False and all others on True:\n | \n | >>> animals.duplicated(keep='last')\n | 0 True\n | 1 False\n | 2 True\n | 3 False\n | 4 False\n | dtype: bool\n | \n | By setting keep on ``False``, all duplicates are True:\n | \n | >>> animals.duplicated(keep=False)\n | 0 True\n | 1 False\n | 2 True\n | 3 False\n | 4 True\n | dtype: bool\n | \n | Returns\n | -------\n | pandas.core.series.Series\n | \n | See Also\n | --------\n | pandas.Index.duplicated : Equivalent method on pandas.Index\n | pandas.DataFrame.duplicated : Equivalent method on pandas.DataFrame\n | pandas.Series.drop_duplicates : Remove duplicate values from Series\n | \n | eq(self, other, level=None, fill_value=None, axis=0)\n | Equal to of series and other, element-wise (binary operator `eq`).\n | \n | Equivalent to ``series == other``, but with support to substitute a fill_value for\n | missing data in one of the inputs.\n | \n | Parameters\n | ----------\n | other : Series or scalar value\n | fill_value : None or float value, default None (NaN)\n | Fill existing missing (NaN) values, and any new element needed for\n | successful Series alignment, with this value before computation.\n | If data in both corresponding Series locations is missing\n | the result will be missing\n | level : int or name\n | Broadcast across a level, matching Index values on the\n | passed MultiIndex level\n | \n | Returns\n | -------\n | result : Series\n | \n | Examples\n | --------\n | >>> a = pd.Series([1, 1, 1, np.nan], index=['a', 'b', 'c', 'd'])\n | >>> a\n | a 1.0\n | b 1.0\n | c 1.0\n | d NaN\n | dtype: float64\n | >>> b = pd.Series([1, np.nan, 1, np.nan], index=['a', 'b', 'd', 'e'])\n | >>> b\n | a 1.0\n | b NaN\n | d 1.0\n | e NaN\n | dtype: float64\n | >>> a.add(b, fill_value=0)\n | a 2.0\n | b 1.0\n | c 1.0\n | d 1.0\n | e NaN\n | dtype: float64\n | \n | See also\n | --------\n | Series.None\n | \n | ewm(self, com=None, span=None, halflife=None, alpha=None, min_periods=0, adjust=True, ignore_na=False, axis=0)\n | Provides exponential weighted functions\n | \n | .. versionadded:: 0.18.0\n | \n | Parameters\n | ----------\n | com : float, optional\n | Specify decay in terms of center of mass,\n | :math:`\\alpha = 1 / (1 + com),\\text{ for } com \\geq 0`\n | span : float, optional\n | Specify decay in terms of span,\n | :math:`\\alpha = 2 / (span + 1),\\text{ for } span \\geq 1`\n | halflife : float, optional\n | Specify decay in terms of half-life,\n | :math:`\\alpha = 1 - exp(log(0.5) / halflife),\\text{ for } halflife > 0`\n | alpha : float, optional\n | Specify smoothing factor :math:`\\alpha` directly,\n | :math:`0 < \\alpha \\leq 1`\n | \n | .. versionadded:: 0.18.0\n | \n | min_periods : int, default 0\n | Minimum number of observations in window required to have a value\n | (otherwise result is NA).\n | adjust : boolean, default True\n | Divide by decaying adjustment factor in beginning periods to account\n | for imbalance in relative weightings (viewing EWMA as a moving average)\n | ignore_na : boolean, default False\n | Ignore missing values when calculating weights;\n | specify True to reproduce pre-0.15.0 behavior\n | \n | Returns\n | -------\n | a Window sub-classed for the particular operation\n | \n | Examples\n | --------\n | \n | >>> df = DataFrame({'B': [0, 1, 2, np.nan, 4]})\n | B\n | 0 0.0\n | 1 1.0\n | 2 2.0\n | 3 NaN\n | 4 4.0\n | \n | >>> df.ewm(com=0.5).mean()\n | B\n | 0 0.000000\n | 1 0.750000\n | 2 1.615385\n | 3 1.615385\n | 4 3.670213\n | \n | Notes\n | -----\n | Exactly one of center of mass, span, half-life, and alpha must be provided.\n | Allowed values and relationship between the parameters are specified in the\n | parameter descriptions above; see the link at the end of this section for\n | a detailed explanation.\n | \n | When adjust is True (default), weighted averages are calculated using\n | weights (1-alpha)**(n-1), (1-alpha)**(n-2), ..., 1-alpha, 1.\n | \n | When adjust is False, weighted averages are calculated recursively as:\n | weighted_average[0] = arg[0];\n | weighted_average[i] = (1-alpha)*weighted_average[i-1] + alpha*arg[i].\n | \n | When ignore_na is False (default), weights are based on absolute positions.\n | For example, the weights of x and y used in calculating the final weighted\n | average of [x, None, y] are (1-alpha)**2 and 1 (if adjust is True), and\n | (1-alpha)**2 and alpha (if adjust is False).\n | \n | When ignore_na is True (reproducing pre-0.15.0 behavior), weights are based\n | on relative positions. For example, the weights of x and y used in\n | calculating the final weighted average of [x, None, y] are 1-alpha and 1\n | (if adjust is True), and 1-alpha and alpha (if adjust is False).\n | \n | More details can be found at\n | http://pandas.pydata.org/pandas-docs/stable/computation.html#exponentially-weighted-windows\n | \n | See Also\n | --------\n | rolling : Provides rolling window calculations\n | expanding : Provides expanding transformations.\n | \n | expanding(self, min_periods=1, center=False, axis=0)\n | Provides expanding transformations.\n | \n | .. versionadded:: 0.18.0\n | \n | Parameters\n | ----------\n | min_periods : int, default 1\n | Minimum number of observations in window required to have a value\n | (otherwise result is NA).\n | center : boolean, default False\n | Set the labels at the center of the window.\n | axis : int or string, default 0\n | \n | Returns\n | -------\n | a Window sub-classed for the particular operation\n | \n | Examples\n | --------\n | \n | >>> df = DataFrame({'B': [0, 1, 2, np.nan, 4]})\n | B\n | 0 0.0\n | 1 1.0\n | 2 2.0\n | 3 NaN\n | 4 4.0\n | \n | >>> df.expanding(2).sum()\n | B\n | 0 NaN\n | 1 1.0\n | 2 3.0\n | 3 3.0\n | 4 7.0\n | \n | Notes\n | -----\n | By default, the result is set to the right edge of the window. This can be\n | changed to the center of the window by setting ``center=True``.\n | \n | See Also\n | --------\n | rolling : Provides rolling window calculations\n | ewm : Provides exponential weighted functions\n | \n | fillna(self, value=None, method=None, axis=None, inplace=False, limit=None, downcast=None, **kwargs)\n | Fill NA/NaN values using the specified method\n | \n | Parameters\n | ----------\n | value : scalar, dict, Series, or DataFrame\n | Value to use to fill holes (e.g. 0), alternately a\n | dict/Series/DataFrame of values specifying which value to use for\n | each index (for a Series) or column (for a DataFrame). (values not\n | in the dict/Series/DataFrame will not be filled). This value cannot\n | be a list.\n | method : {'backfill', 'bfill', 'pad', 'ffill', None}, default None\n | Method to use for filling holes in reindexed Series\n | pad / ffill: propagate last valid observation forward to next valid\n | backfill / bfill: use NEXT valid observation to fill gap\n | axis : {0 or 'index'}\n | inplace : boolean, default False\n | If True, fill in place. Note: this will modify any\n | other views on this object, (e.g. a no-copy slice for a column in a\n | DataFrame).\n | limit : int, default None\n | If method is specified, this is the maximum number of consecutive\n | NaN values to forward/backward fill. In other words, if there is\n | a gap with more than this number of consecutive NaNs, it will only\n | be partially filled. If method is not specified, this is the\n | maximum number of entries along the entire axis where NaNs will be\n | filled. Must be greater than 0 if not None.\n | downcast : dict, default is None\n | a dict of item->dtype of what to downcast if possible,\n | or the string 'infer' which will try to downcast to an appropriate\n | equal type (e.g. float64 to int64 if possible)\n | \n | See Also\n | --------\n | interpolate : Fill NaN values using interpolation.\n | reindex, asfreq\n | \n | Returns\n | -------\n | filled : Series\n | \n | Examples\n | --------\n | >>> df = pd.DataFrame([[np.nan, 2, np.nan, 0],\n | ... [3, 4, np.nan, 1],\n | ... [np.nan, np.nan, np.nan, 5],\n | ... [np.nan, 3, np.nan, 4]],\n | ... columns=list('ABCD'))\n | >>> df\n | A B C D\n | 0 NaN 2.0 NaN 0\n | 1 3.0 4.0 NaN 1\n | 2 NaN NaN NaN 5\n | 3 NaN 3.0 NaN 4\n | \n | Replace all NaN elements with 0s.\n | \n | >>> df.fillna(0)\n | A B C D\n | 0 0.0 2.0 0.0 0\n | 1 3.0 4.0 0.0 1\n | 2 0.0 0.0 0.0 5\n | 3 0.0 3.0 0.0 4\n | \n | We can also propagate non-null values forward or backward.\n | \n | >>> df.fillna(method='ffill')\n | A B C D\n | 0 NaN 2.0 NaN 0\n | 1 3.0 4.0 NaN 1\n | 2 3.0 4.0 NaN 5\n | 3 3.0 3.0 NaN 4\n | \n | Replace all NaN elements in column 'A', 'B', 'C', and 'D', with 0, 1,\n | 2, and 3 respectively.\n | \n | >>> values = {'A': 0, 'B': 1, 'C': 2, 'D': 3}\n | >>> df.fillna(value=values)\n | A B C D\n | 0 0.0 2.0 2.0 0\n | 1 3.0 4.0 2.0 1\n | 2 0.0 1.0 2.0 5\n | 3 0.0 3.0 2.0 4\n | \n | Only replace the first NaN element.\n | \n | >>> df.fillna(value=values, limit=1)\n | A B C D\n | 0 0.0 2.0 2.0 0\n | 1 3.0 4.0 NaN 1\n | 2 NaN 1.0 NaN 5\n | 3 NaN 3.0 NaN 4\n | \n | floordiv(self, other, level=None, fill_value=None, axis=0)\n | Integer division of series and other, element-wise (binary operator `floordiv`).\n | \n | Equivalent to ``series // other``, but with support to substitute a fill_value for\n | missing data in one of the inputs.\n | \n | Parameters\n | ----------\n | other : Series or scalar value\n | fill_value : None or float value, default None (NaN)\n | Fill existing missing (NaN) values, and any new element needed for\n | successful Series alignment, with this value before computation.\n | If data in both corresponding Series locations is missing\n | the result will be missing\n | level : int or name\n | Broadcast across a level, matching Index values on the\n | passed MultiIndex level\n | \n | Returns\n | -------\n | result : Series\n | \n | Examples\n | --------\n | >>> a = pd.Series([1, 1, 1, np.nan], index=['a', 'b', 'c', 'd'])\n | >>> a\n | a 1.0\n | b 1.0\n | c 1.0\n | d NaN\n | dtype: float64\n | >>> b = pd.Series([1, np.nan, 1, np.nan], index=['a', 'b', 'd', 'e'])\n | >>> b\n | a 1.0\n | b NaN\n | d 1.0\n | e NaN\n | dtype: float64\n | >>> a.add(b, fill_value=0)\n | a 2.0\n | b 1.0\n | c 1.0\n | d 1.0\n | e NaN\n | dtype: float64\n | \n | See also\n | --------\n | Series.rfloordiv\n | \n | ge(self, other, level=None, fill_value=None, axis=0)\n | Greater than or equal to of series and other, element-wise (binary operator `ge`).\n | \n | Equivalent to ``series >= other``, but with support to substitute a fill_value for\n | missing data in one of the inputs.\n | \n | Parameters\n | ----------\n | other : Series or scalar value\n | fill_value : None or float value, default None (NaN)\n | Fill existing missing (NaN) values, and any new element needed for\n | successful Series alignment, with this value before computation.\n | If data in both corresponding Series locations is missing\n | the result will be missing\n | level : int or name\n | Broadcast across a level, matching Index values on the\n | passed MultiIndex level\n | \n | Returns\n | -------\n | result : Series\n | \n | Examples\n | --------\n | >>> a = pd.Series([1, 1, 1, np.nan], index=['a', 'b', 'c', 'd'])\n | >>> a\n | a 1.0\n | b 1.0\n | c 1.0\n | d NaN\n | dtype: float64\n | >>> b = pd.Series([1, np.nan, 1, np.nan], index=['a', 'b', 'd', 'e'])\n | >>> b\n | a 1.0\n | b NaN\n | d 1.0\n | e NaN\n | dtype: float64\n | >>> a.add(b, fill_value=0)\n | a 2.0\n | b 1.0\n | c 1.0\n | d 1.0\n | e NaN\n | dtype: float64\n | \n | See also\n | --------\n | Series.None\n | \n | get_value(self, label, takeable=False)\n | Quickly retrieve single value at passed index label\n | \n | .. deprecated:: 0.21.0\n | Please use .at[] or .iat[] accessors.\n | \n | Parameters\n | ----------\n | label : object\n | takeable : interpret the index as indexers, default False\n | \n | Returns\n | -------\n | value : scalar value\n | \n | get_values(self)\n | same as values (but handles sparseness conversions); is a view\n | \n | gt(self, other, level=None, fill_value=None, axis=0)\n | Greater than of series and other, element-wise (binary operator `gt`).\n | \n | Equivalent to ``series > other``, but with support to substitute a fill_value for\n | missing data in one of the inputs.\n | \n | Parameters\n | ----------\n | other : Series or scalar value\n | fill_value : None or float value, default None (NaN)\n | Fill existing missing (NaN) values, and any new element needed for\n | successful Series alignment, with this value before computation.\n | If data in both corresponding Series locations is missing\n | the result will be missing\n | level : int or name\n | Broadcast across a level, matching Index values on the\n | passed MultiIndex level\n | \n | Returns\n | -------\n | result : Series\n | \n | Examples\n | --------\n | >>> a = pd.Series([1, 1, 1, np.nan], index=['a', 'b', 'c', 'd'])\n | >>> a\n | a 1.0\n | b 1.0\n | c 1.0\n | d NaN\n | dtype: float64\n | >>> b = pd.Series([1, np.nan, 1, np.nan], index=['a', 'b', 'd', 'e'])\n | >>> b\n | a 1.0\n | b NaN\n | d 1.0\n | e NaN\n | dtype: float64\n | >>> a.add(b, fill_value=0)\n | a 2.0\n | b 1.0\n | c 1.0\n | d 1.0\n | e NaN\n | dtype: float64\n | \n | See also\n | --------\n | Series.None\n | \n | hist = hist_series(self, by=None, ax=None, grid=True, xlabelsize=None, xrot=None, ylabelsize=None, yrot=None, figsize=None, bins=10, **kwds)\n | Draw histogram of the input series using matplotlib\n | \n | Parameters\n | ----------\n | by : object, optional\n | If passed, then used to form histograms for separate groups\n | ax : matplotlib axis object\n | If not passed, uses gca()\n | grid : boolean, default True\n | Whether to show axis grid lines\n | xlabelsize : int, default None\n | If specified changes the x-axis label size\n | xrot : float, default None\n | rotation of x axis labels\n | ylabelsize : int, default None\n | If specified changes the y-axis label size\n | yrot : float, default None\n | rotation of y axis labels\n | figsize : tuple, default None\n | figure size in inches by default\n | bins : integer or sequence, default 10\n | Number of histogram bins to be used. If an integer is given, bins + 1\n | bin edges are calculated and returned. If bins is a sequence, gives\n | bin edges, including left edge of first bin and right edge of last\n | bin. In this case, bins is returned unmodified.\n | bins: integer, default 10\n | Number of histogram bins to be used\n | `**kwds` : keywords\n | To be passed to the actual plotting function\n | \n | See Also\n | --------\n | matplotlib.axes.Axes.hist : Plot a histogram using matplotlib.\n | \n | idxmax(self, axis=0, skipna=True, *args, **kwargs)\n | Return the row label of the maximum value.\n | \n | If multiple values equal the maximum, the first row label with that\n | value is returned.\n | \n | Parameters\n | ----------\n | skipna : boolean, default True\n | Exclude NA/null values. If the entire Series is NA, the result\n | will be NA.\n | axis : int, default 0\n | For compatibility with DataFrame.idxmax. Redundant for application\n | on Series.\n | *args, **kwargs\n | Additional keywors have no effect but might be accepted\n | for compatibility with NumPy.\n | \n | Returns\n | -------\n | idxmax : Index of maximum of values.\n | \n | Raises\n | ------\n | ValueError\n | If the Series is empty.\n | \n | Notes\n | -----\n | This method is the Series version of ``ndarray.argmax``. This method\n | returns the label of the maximum, while ``ndarray.argmax`` returns\n | the position. To get the position, use ``series.values.argmax()``.\n | \n | See Also\n | --------\n | numpy.argmax : Return indices of the maximum values\n | along the given axis.\n | DataFrame.idxmax : Return index of first occurrence of maximum\n | over requested axis.\n | Series.idxmin : Return index *label* of the first occurrence\n | of minimum of values.\n | \n | Examples\n | --------\n | >>> s = pd.Series(data=[1, None, 4, 3, 4],\n | ... index=['A', 'B', 'C', 'D', 'E'])\n | >>> s\n | A 1.0\n | B NaN\n | C 4.0\n | D 3.0\n | E 4.0\n | dtype: float64\n | \n | >>> s.idxmax()\n | 'C'\n | \n | If `skipna` is False and there is an NA value in the data,\n | the function returns ``nan``.\n | \n | >>> s.idxmax(skipna=False)\n | nan\n | \n | idxmin(self, axis=None, skipna=True, *args, **kwargs)\n | Return the row label of the minimum value.\n | \n | If multiple values equal the minimum, the first row label with that\n | value is returned.\n | \n | Parameters\n | ----------\n | skipna : boolean, default True\n | Exclude NA/null values. If the entire Series is NA, the result\n | will be NA.\n | axis : int, default 0\n | For compatibility with DataFrame.idxmin. Redundant for application\n | on Series.\n | *args, **kwargs\n | Additional keywors have no effect but might be accepted\n | for compatibility with NumPy.\n | \n | Returns\n | -------\n | idxmin : Index of minimum of values.\n | \n | Raises\n | ------\n | ValueError\n | If the Series is empty.\n | \n | Notes\n | -----\n | This method is the Series version of ``ndarray.argmin``. This method\n | returns the label of the minimum, while ``ndarray.argmin`` returns\n | the position. To get the position, use ``series.values.argmin()``.\n | \n | See Also\n | --------\n | numpy.argmin : Return indices of the minimum values\n | along the given axis.\n | DataFrame.idxmin : Return index of first occurrence of minimum\n | over requested axis.\n | Series.idxmax : Return index *label* of the first occurrence\n | of maximum of values.\n | \n | Examples\n | --------\n | >>> s = pd.Series(data=[1, None, 4, 1],\n | ... index=['A' ,'B' ,'C' ,'D'])\n | >>> s\n | A 1.0\n | B NaN\n | C 4.0\n | D 1.0\n | dtype: float64\n | \n | >>> s.idxmin()\n | 'A'\n | \n | If `skipna` is False and there is an NA value in the data,\n | the function returns ``nan``.\n | \n | >>> s.idxmin(skipna=False)\n | nan\n | \n | isin(self, values)\n | Check whether `values` are contained in Series.\n | \n | Return a boolean Series showing whether each element in the Series\n | matches an element in the passed sequence of `values` exactly.\n | \n | Parameters\n | ----------\n | values : set or list-like\n | The sequence of values to test. Passing in a single string will\n | raise a ``TypeError``. Instead, turn a single string into a\n | list of one element.\n | \n | .. versionadded:: 0.18.1\n | \n | Support for values as a set.\n | \n | Returns\n | -------\n | isin : Series (bool dtype)\n | \n | Raises\n | ------\n | TypeError\n | * If `values` is a string\n | \n | See Also\n | --------\n | pandas.DataFrame.isin : equivalent method on DataFrame\n | \n | Examples\n | --------\n | \n | >>> s = pd.Series(['lama', 'cow', 'lama', 'beetle', 'lama',\n | ... 'hippo'], name='animal')\n | >>> s.isin(['cow', 'lama'])\n | 0 True\n | 1 True\n | 2 True\n | 3 False\n | 4 True\n | 5 False\n | Name: animal, dtype: bool\n | \n | Passing a single string as ``s.isin('lama')`` will raise an error. Use\n | a list of one element instead:\n | \n | >>> s.isin(['lama'])\n | 0 True\n | 1 False\n | 2 True\n | 3 False\n | 4 True\n | 5 False\n | Name: animal, dtype: bool\n | \n | isna(self)\n | Detect missing values.\n | \n | Return a boolean same-sized object indicating if the values are NA.\n | NA values, such as None or :attr:`numpy.NaN`, gets mapped to True\n | values.\n | Everything else gets mapped to False values. Characters such as empty\n | strings ``''`` or :attr:`numpy.inf` are not considered NA values\n | (unless you set ``pandas.options.mode.use_inf_as_na = True``).\n | \n | Returns\n | -------\n | Series\n | Mask of bool values for each element in Series that\n | indicates whether an element is not an NA value.\n | \n | See Also\n | --------\n | Series.isnull : alias of isna\n | Series.notna : boolean inverse of isna\n | Series.dropna : omit axes labels with missing values\n | isna : top-level isna\n | \n | Examples\n | --------\n | Show which entries in a DataFrame are NA.\n | \n | >>> df = pd.DataFrame({'age': [5, 6, np.NaN],\n | ... 'born': [pd.NaT, pd.Timestamp('1939-05-27'),\n | ... pd.Timestamp('1940-04-25')],\n | ... 'name': ['Alfred', 'Batman', ''],\n | ... 'toy': [None, 'Batmobile', 'Joker']})\n | >>> df\n | age born name toy\n | 0 5.0 NaT Alfred None\n | 1 6.0 1939-05-27 Batman Batmobile\n | 2 NaN 1940-04-25 Joker\n | \n | >>> df.isna()\n | age born name toy\n | 0 False True False True\n | 1 False False False False\n | 2 True False False False\n | \n | Show which entries in a Series are NA.\n | \n | >>> ser = pd.Series([5, 6, np.NaN])\n | >>> ser\n | 0 5.0\n | 1 6.0\n | 2 NaN\n | dtype: float64\n | \n | >>> ser.isna()\n | 0 False\n | 1 False\n | 2 True\n | dtype: bool\n | \n | isnull(self)\n | Detect missing values.\n | \n | Return a boolean same-sized object indicating if the values are NA.\n | NA values, such as None or :attr:`numpy.NaN`, gets mapped to True\n | values.\n | Everything else gets mapped to False values. Characters such as empty\n | strings ``''`` or :attr:`numpy.inf` are not considered NA values\n | (unless you set ``pandas.options.mode.use_inf_as_na = True``).\n | \n | Returns\n | -------\n | Series\n | Mask of bool values for each element in Series that\n | indicates whether an element is not an NA value.\n | \n | See Also\n | --------\n | Series.isnull : alias of isna\n | Series.notna : boolean inverse of isna\n | Series.dropna : omit axes labels with missing values\n | isna : top-level isna\n | \n | Examples\n | --------\n | Show which entries in a DataFrame are NA.\n | \n | >>> df = pd.DataFrame({'age': [5, 6, np.NaN],\n | ... 'born': [pd.NaT, pd.Timestamp('1939-05-27'),\n | ... pd.Timestamp('1940-04-25')],\n | ... 'name': ['Alfred', 'Batman', ''],\n | ... 'toy': [None, 'Batmobile', 'Joker']})\n | >>> df\n | age born name toy\n | 0 5.0 NaT Alfred None\n | 1 6.0 1939-05-27 Batman Batmobile\n | 2 NaN 1940-04-25 Joker\n | \n | >>> df.isna()\n | age born name toy\n | 0 False True False True\n | 1 False False False False\n | 2 True False False False\n | \n | Show which entries in a Series are NA.\n | \n | >>> ser = pd.Series([5, 6, np.NaN])\n | >>> ser\n | 0 5.0\n | 1 6.0\n | 2 NaN\n | dtype: float64\n | \n | >>> ser.isna()\n | 0 False\n | 1 False\n | 2 True\n | dtype: bool\n | \n | items = iteritems(self)\n | Lazily iterate over (index, value) tuples\n | \n | iteritems(self)\n | Lazily iterate over (index, value) tuples\n | \n | keys(self)\n | Alias for index\n | \n | kurt(self, axis=None, skipna=None, level=None, numeric_only=None, **kwargs)\n | Return unbiased kurtosis over requested axis using Fisher's definition of\n | kurtosis (kurtosis of normal == 0.0). Normalized by N-1\n | \n | \n | Parameters\n | ----------\n | axis : {index (0)}\n | skipna : boolean, default True\n | Exclude NA/null values when computing the result.\n | level : int or level name, default None\n | If the axis is a MultiIndex (hierarchical), count along a\n | particular level, collapsing into a scalar\n | numeric_only : boolean, default None\n | Include only float, int, boolean columns. If None, will attempt to use\n | everything, then use only numeric data. Not implemented for Series.\n | \n | Returns\n | -------\n | kurt : scalar or Series (if level specified)\n | \n | kurtosis = kurt(self, axis=None, skipna=None, level=None, numeric_only=None, **kwargs)\n | Return unbiased kurtosis over requested axis using Fisher's definition of\n | kurtosis (kurtosis of normal == 0.0). Normalized by N-1\n | \n | \n | Parameters\n | ----------\n | axis : {index (0)}\n | skipna : boolean, default True\n | Exclude NA/null values when computing the result.\n | level : int or level name, default None\n | If the axis is a MultiIndex (hierarchical), count along a\n | particular level, collapsing into a scalar\n | numeric_only : boolean, default None\n | Include only float, int, boolean columns. If None, will attempt to use\n | everything, then use only numeric data. Not implemented for Series.\n | \n | Returns\n | -------\n | kurt : scalar or Series (if level specified)\n | \n | le(self, other, level=None, fill_value=None, axis=0)\n | Less than or equal to of series and other, element-wise (binary operator `le`).\n | \n | Equivalent to ``series <= other``, but with support to substitute a fill_value for\n | missing data in one of the inputs.\n | \n | Parameters\n | ----------\n | other : Series or scalar value\n | fill_value : None or float value, default None (NaN)\n | Fill existing missing (NaN) values, and any new element needed for\n | successful Series alignment, with this value before computation.\n | If data in both corresponding Series locations is missing\n | the result will be missing\n | level : int or name\n | Broadcast across a level, matching Index values on the\n | passed MultiIndex level\n | \n | Returns\n | -------\n | result : Series\n | \n | Examples\n | --------\n | >>> a = pd.Series([1, 1, 1, np.nan], index=['a', 'b', 'c', 'd'])\n | >>> a\n | a 1.0\n | b 1.0\n | c 1.0\n | d NaN\n | dtype: float64\n | >>> b = pd.Series([1, np.nan, 1, np.nan], index=['a', 'b', 'd', 'e'])\n | >>> b\n | a 1.0\n | b NaN\n | d 1.0\n | e NaN\n | dtype: float64\n | >>> a.add(b, fill_value=0)\n | a 2.0\n | b 1.0\n | c 1.0\n | d 1.0\n | e NaN\n | dtype: float64\n | \n | See also\n | --------\n | Series.None\n | \n | lt(self, other, level=None, fill_value=None, axis=0)\n | Less than of series and other, element-wise (binary operator `lt`).\n | \n | Equivalent to ``series < other``, but with support to substitute a fill_value for\n | missing data in one of the inputs.\n | \n | Parameters\n | ----------\n | other : Series or scalar value\n | fill_value : None or float value, default None (NaN)\n | Fill existing missing (NaN) values, and any new element needed for\n | successful Series alignment, with this value before computation.\n | If data in both corresponding Series locations is missing\n | the result will be missing\n | level : int or name\n | Broadcast across a level, matching Index values on the\n | passed MultiIndex level\n | \n | Returns\n | -------\n | result : Series\n | \n | Examples\n | --------\n | >>> a = pd.Series([1, 1, 1, np.nan], index=['a', 'b', 'c', 'd'])\n | >>> a\n | a 1.0\n | b 1.0\n | c 1.0\n | d NaN\n | dtype: float64\n | >>> b = pd.Series([1, np.nan, 1, np.nan], index=['a', 'b', 'd', 'e'])\n | >>> b\n | a 1.0\n | b NaN\n | d 1.0\n | e NaN\n | dtype: float64\n | >>> a.add(b, fill_value=0)\n | a 2.0\n | b 1.0\n | c 1.0\n | d 1.0\n | e NaN\n | dtype: float64\n | \n | See also\n | --------\n | Series.None\n | \n | mad(self, axis=None, skipna=None, level=None)\n | Return the mean absolute deviation of the values for the requested axis\n | \n | Parameters\n | ----------\n | axis : {index (0)}\n | skipna : boolean, default True\n | Exclude NA/null values when computing the result.\n | level : int or level name, default None\n | If the axis is a MultiIndex (hierarchical), count along a\n | particular level, collapsing into a scalar\n | numeric_only : boolean, default None\n | Include only float, int, boolean columns. If None, will attempt to use\n | everything, then use only numeric data. Not implemented for Series.\n | \n | Returns\n | -------\n | mad : scalar or Series (if level specified)\n | \n | map(self, arg, na_action=None)\n | Map values of Series using input correspondence (a dict, Series, or\n | function).\n | \n | Parameters\n | ----------\n | arg : function, dict, or Series\n | Mapping correspondence.\n | na_action : {None, 'ignore'}\n | If 'ignore', propagate NA values, without passing them to the\n | mapping correspondence.\n | \n | Returns\n | -------\n | y : Series\n | Same index as caller.\n | \n | Examples\n | --------\n | \n | Map inputs to outputs (both of type `Series`):\n | \n | >>> x = pd.Series([1,2,3], index=['one', 'two', 'three'])\n | >>> x\n | one 1\n | two 2\n | three 3\n | dtype: int64\n | \n | >>> y = pd.Series(['foo', 'bar', 'baz'], index=[1,2,3])\n | >>> y\n | 1 foo\n | 2 bar\n | 3 baz\n | \n | >>> x.map(y)\n | one foo\n | two bar\n | three baz\n | \n | If `arg` is a dictionary, return a new Series with values converted\n | according to the dictionary's mapping:\n | \n | >>> z = {1: 'A', 2: 'B', 3: 'C'}\n | \n | >>> x.map(z)\n | one A\n | two B\n | three C\n | \n | Use na_action to control whether NA values are affected by the mapping\n | function.\n | \n | >>> s = pd.Series([1, 2, 3, np.nan])\n | \n | >>> s2 = s.map('this is a string {}'.format, na_action=None)\n | 0 this is a string 1.0\n | 1 this is a string 2.0\n | 2 this is a string 3.0\n | 3 this is a string nan\n | dtype: object\n | \n | >>> s3 = s.map('this is a string {}'.format, na_action='ignore')\n | 0 this is a string 1.0\n | 1 this is a string 2.0\n | 2 this is a string 3.0\n | 3 NaN\n | dtype: object\n | \n | See Also\n | --------\n | Series.apply : For applying more complex functions on a Series.\n | DataFrame.apply : Apply a function row-/column-wise.\n | DataFrame.applymap : Apply a function elementwise on a whole DataFrame.\n | \n | Notes\n | -----\n | When `arg` is a dictionary, values in Series that are not in the\n | dictionary (as keys) are converted to ``NaN``. However, if the\n | dictionary is a ``dict`` subclass that defines ``__missing__`` (i.e.\n | provides a method for default values), then this default is used\n | rather than ``NaN``:\n | \n | >>> from collections import Counter\n | >>> counter = Counter()\n | >>> counter['bar'] += 1\n | >>> y.map(counter)\n | 1 0\n | 2 1\n | 3 0\n | dtype: int64\n | \n | max(self, axis=None, skipna=None, level=None, numeric_only=None, **kwargs)\n | This method returns the maximum of the values in the object.\n | If you want the *index* of the maximum, use ``idxmax``. This is\n | the equivalent of the ``numpy.ndarray`` method ``argmax``.\n | \n | Parameters\n | ----------\n | axis : {index (0)}\n | skipna : boolean, default True\n | Exclude NA/null values when computing the result.\n | level : int or level name, default None\n | If the axis is a MultiIndex (hierarchical), count along a\n | particular level, collapsing into a scalar\n | numeric_only : boolean, default None\n | Include only float, int, boolean columns. If None, will attempt to use\n | everything, then use only numeric data. Not implemented for Series.\n | \n | Returns\n | -------\n | max : scalar or Series (if level specified)\n | \n | mean(self, axis=None, skipna=None, level=None, numeric_only=None, **kwargs)\n | Return the mean of the values for the requested axis\n | \n | Parameters\n | ----------\n | axis : {index (0)}\n | skipna : boolean, default True\n | Exclude NA/null values when computing the result.\n | level : int or level name, default None\n | If the axis is a MultiIndex (hierarchical), count along a\n | particular level, collapsing into a scalar\n | numeric_only : boolean, default None\n | Include only float, int, boolean columns. If None, will attempt to use\n | everything, then use only numeric data. Not implemented for Series.\n | \n | Returns\n | -------\n | mean : scalar or Series (if level specified)\n | \n | median(self, axis=None, skipna=None, level=None, numeric_only=None, **kwargs)\n | Return the median of the values for the requested axis\n | \n | Parameters\n | ----------\n | axis : {index (0)}\n | skipna : boolean, default True\n | Exclude NA/null values when computing the result.\n | level : int or level name, default None\n | If the axis is a MultiIndex (hierarchical), count along a\n | particular level, collapsing into a scalar\n | numeric_only : boolean, default None\n | Include only float, int, boolean columns. If None, will attempt to use\n | everything, then use only numeric data. Not implemented for Series.\n | \n | Returns\n | -------\n | median : scalar or Series (if level specified)\n | \n | memory_usage(self, index=True, deep=False)\n | Return the memory usage of the Series.\n | \n | The memory usage can optionally include the contribution of\n | the index and of elements of `object` dtype.\n | \n | Parameters\n | ----------\n | index : bool, default True\n | Specifies whether to include the memory usage of the Series index.\n | deep : bool, default False\n | If True, introspect the data deeply by interrogating\n | `object` dtypes for system-level memory consumption, and include\n | it in the returned value.\n | \n | Returns\n | -------\n | int\n | Bytes of memory consumed.\n | \n | See Also\n | --------\n | numpy.ndarray.nbytes : Total bytes consumed by the elements of the\n | array.\n | DataFrame.memory_usage : Bytes consumed by a DataFrame.\n | \n | Examples\n | --------\n | \n | >>> s = pd.Series(range(3))\n | >>> s.memory_usage()\n | 104\n | \n | Not including the index gives the size of the rest of the data, which\n | is necessarily smaller:\n | \n | >>> s.memory_usage(index=False)\n | 24\n | \n | The memory footprint of `object` values is ignored by default:\n | \n | >>> s = pd.Series([\"a\", \"b\"])\n | >>> s.values\n | array(['a', 'b'], dtype=object)\n | >>> s.memory_usage()\n | 96\n | >>> s.memory_usage(deep=True)\n | 212\n | \n | min(self, axis=None, skipna=None, level=None, numeric_only=None, **kwargs)\n | This method returns the minimum of the values in the object.\n | If you want the *index* of the minimum, use ``idxmin``. This is\n | the equivalent of the ``numpy.ndarray`` method ``argmin``.\n | \n | Parameters\n | ----------\n | axis : {index (0)}\n | skipna : boolean, default True\n | Exclude NA/null values when computing the result.\n | level : int or level name, default None\n | If the axis is a MultiIndex (hierarchical), count along a\n | particular level, collapsing into a scalar\n | numeric_only : boolean, default None\n | Include only float, int, boolean columns. If None, will attempt to use\n | everything, then use only numeric data. Not implemented for Series.\n | \n | Returns\n | -------\n | min : scalar or Series (if level specified)\n | \n | mod(self, other, level=None, fill_value=None, axis=0)\n | Modulo of series and other, element-wise (binary operator `mod`).\n | \n | Equivalent to ``series % other``, but with support to substitute a fill_value for\n | missing data in one of the inputs.\n | \n | Parameters\n | ----------\n | other : Series or scalar value\n | fill_value : None or float value, default None (NaN)\n | Fill existing missing (NaN) values, and any new element needed for\n | successful Series alignment, with this value before computation.\n | If data in both corresponding Series locations is missing\n | the result will be missing\n | level : int or name\n | Broadcast across a level, matching Index values on the\n | passed MultiIndex level\n | \n | Returns\n | -------\n | result : Series\n | \n | Examples\n | --------\n | >>> a = pd.Series([1, 1, 1, np.nan], index=['a', 'b', 'c', 'd'])\n | >>> a\n | a 1.0\n | b 1.0\n | c 1.0\n | d NaN\n | dtype: float64\n | >>> b = pd.Series([1, np.nan, 1, np.nan], index=['a', 'b', 'd', 'e'])\n | >>> b\n | a 1.0\n | b NaN\n | d 1.0\n | e NaN\n | dtype: float64\n | >>> a.add(b, fill_value=0)\n | a 2.0\n | b 1.0\n | c 1.0\n | d 1.0\n | e NaN\n | dtype: float64\n | \n | See also\n | --------\n | Series.rmod\n | \n | mode(self)\n | Return the mode(s) of the dataset.\n | \n | Always returns Series even if only one value is returned.\n | \n | Returns\n | -------\n | modes : Series (sorted)\n | \n | mul(self, other, level=None, fill_value=None, axis=0)\n | Multiplication of series and other, element-wise (binary operator `mul`).\n | \n | Equivalent to ``series * other``, but with support to substitute a fill_value for\n | missing data in one of the inputs.\n | \n | Parameters\n | ----------\n | other : Series or scalar value\n | fill_value : None or float value, default None (NaN)\n | Fill existing missing (NaN) values, and any new element needed for\n | successful Series alignment, with this value before computation.\n | If data in both corresponding Series locations is missing\n | the result will be missing\n | level : int or name\n | Broadcast across a level, matching Index values on the\n | passed MultiIndex level\n | \n | Returns\n | -------\n | result : Series\n | \n | Examples\n | --------\n | >>> a = pd.Series([1, 1, 1, np.nan], index=['a', 'b', 'c', 'd'])\n | >>> a\n | a 1.0\n | b 1.0\n | c 1.0\n | d NaN\n | dtype: float64\n | >>> b = pd.Series([1, np.nan, 1, np.nan], index=['a', 'b', 'd', 'e'])\n | >>> b\n | a 1.0\n | b NaN\n | d 1.0\n | e NaN\n | dtype: float64\n | >>> a.add(b, fill_value=0)\n | a 2.0\n | b 1.0\n | c 1.0\n | d 1.0\n | e NaN\n | dtype: float64\n | \n | See also\n | --------\n | Series.rmul\n | \n | multiply = mul(self, other, level=None, fill_value=None, axis=0)\n | Multiplication of series and other, element-wise (binary operator `mul`).\n | \n | Equivalent to ``series * other``, but with support to substitute a fill_value for\n | missing data in one of the inputs.\n | \n | Parameters\n | ----------\n | other : Series or scalar value\n | fill_value : None or float value, default None (NaN)\n | Fill existing missing (NaN) values, and any new element needed for\n | successful Series alignment, with this value before computation.\n | If data in both corresponding Series locations is missing\n | the result will be missing\n | level : int or name\n | Broadcast across a level, matching Index values on the\n | passed MultiIndex level\n | \n | Returns\n | -------\n | result : Series\n | \n | Examples\n | --------\n | >>> a = pd.Series([1, 1, 1, np.nan], index=['a', 'b', 'c', 'd'])\n | >>> a\n | a 1.0\n | b 1.0\n | c 1.0\n | d NaN\n | dtype: float64\n | >>> b = pd.Series([1, np.nan, 1, np.nan], index=['a', 'b', 'd', 'e'])\n | >>> b\n | a 1.0\n | b NaN\n | d 1.0\n | e NaN\n | dtype: float64\n | >>> a.add(b, fill_value=0)\n | a 2.0\n | b 1.0\n | c 1.0\n | d 1.0\n | e NaN\n | dtype: float64\n | \n | See also\n | --------\n | Series.rmul\n | \n | ne(self, other, level=None, fill_value=None, axis=0)\n | Not equal to of series and other, element-wise (binary operator `ne`).\n | \n | Equivalent to ``series != other``, but with support to substitute a fill_value for\n | missing data in one of the inputs.\n | \n | Parameters\n | ----------\n | other : Series or scalar value\n | fill_value : None or float value, default None (NaN)\n | Fill existing missing (NaN) values, and any new element needed for\n | successful Series alignment, with this value before computation.\n | If data in both corresponding Series locations is missing\n | the result will be missing\n | level : int or name\n | Broadcast across a level, matching Index values on the\n | passed MultiIndex level\n | \n | Returns\n | -------\n | result : Series\n | \n | Examples\n | --------\n | >>> a = pd.Series([1, 1, 1, np.nan], index=['a', 'b', 'c', 'd'])\n | >>> a\n | a 1.0\n | b 1.0\n | c 1.0\n | d NaN\n | dtype: float64\n | >>> b = pd.Series([1, np.nan, 1, np.nan], index=['a', 'b', 'd', 'e'])\n | >>> b\n | a 1.0\n | b NaN\n | d 1.0\n | e NaN\n | dtype: float64\n | >>> a.add(b, fill_value=0)\n | a 2.0\n | b 1.0\n | c 1.0\n | d 1.0\n | e NaN\n | dtype: float64\n | \n | See also\n | --------\n | Series.None\n | \n | nlargest(self, n=5, keep='first')\n | Return the largest `n` elements.\n | \n | Parameters\n | ----------\n | n : int\n | Return this many descending sorted values\n | keep : {'first', 'last'}, default 'first'\n | Where there are duplicate values:\n | - ``first`` : take the first occurrence.\n | - ``last`` : take the last occurrence.\n | \n | Returns\n | -------\n | top_n : Series\n | The n largest values in the Series, in sorted order\n | \n | Notes\n | -----\n | Faster than ``.sort_values(ascending=False).head(n)`` for small `n`\n | relative to the size of the ``Series`` object.\n | \n | See Also\n | --------\n | Series.nsmallest\n | \n | Examples\n | --------\n | >>> import pandas as pd\n | >>> import numpy as np\n | >>> s = pd.Series(np.random.randn(10**6))\n | >>> s.nlargest(10) # only sorts up to the N requested\n | 219921 4.644710\n | 82124 4.608745\n | 421689 4.564644\n | 425277 4.447014\n | 718691 4.414137\n | 43154 4.403520\n | 283187 4.313922\n | 595519 4.273635\n | 503969 4.250236\n | 121637 4.240952\n | dtype: float64\n | \n | nonzero(self)\n | Return the *integer* indices of the elements that are non-zero\n | \n | This method is equivalent to calling `numpy.nonzero` on the\n | series data. For compatibility with NumPy, the return value is\n | the same (a tuple with an array of indices for each dimension),\n | but it will always be a one-item tuple because series only have\n | one dimension.\n | \n | Examples\n | --------\n | >>> s = pd.Series([0, 3, 0, 4])\n | >>> s.nonzero()\n | (array([1, 3]),)\n | >>> s.iloc[s.nonzero()[0]]\n | 1 3\n | 3 4\n | dtype: int64\n | \n | >>> s = pd.Series([0, 3, 0, 4], index=['a', 'b', 'c', 'd'])\n | # same return although index of s is different\n | >>> s.nonzero()\n | (array([1, 3]),)\n | >>> s.iloc[s.nonzero()[0]]\n | b 3\n | d 4\n | dtype: int64\n | \n | See Also\n | --------\n | numpy.nonzero\n | \n | notna(self)\n | Detect existing (non-missing) values.\n | \n | Return a boolean same-sized object indicating if the values are not NA.\n | Non-missing values get mapped to True. Characters such as empty\n | strings ``''`` or :attr:`numpy.inf` are not considered NA values\n | (unless you set ``pandas.options.mode.use_inf_as_na = True``).\n | NA values, such as None or :attr:`numpy.NaN`, get mapped to False\n | values.\n | \n | Returns\n | -------\n | Series\n | Mask of bool values for each element in Series that\n | indicates whether an element is not an NA value.\n | \n | See Also\n | --------\n | Series.notnull : alias of notna\n | Series.isna : boolean inverse of notna\n | Series.dropna : omit axes labels with missing values\n | notna : top-level notna\n | \n | Examples\n | --------\n | Show which entries in a DataFrame are not NA.\n | \n | >>> df = pd.DataFrame({'age': [5, 6, np.NaN],\n | ... 'born': [pd.NaT, pd.Timestamp('1939-05-27'),\n | ... pd.Timestamp('1940-04-25')],\n | ... 'name': ['Alfred', 'Batman', ''],\n | ... 'toy': [None, 'Batmobile', 'Joker']})\n | >>> df\n | age born name toy\n | 0 5.0 NaT Alfred None\n | 1 6.0 1939-05-27 Batman Batmobile\n | 2 NaN 1940-04-25 Joker\n | \n | >>> df.notna()\n | age born name toy\n | 0 True False True False\n | 1 True True True True\n | 2 False True True True\n | \n | Show which entries in a Series are not NA.\n | \n | >>> ser = pd.Series([5, 6, np.NaN])\n | >>> ser\n | 0 5.0\n | 1 6.0\n | 2 NaN\n | dtype: float64\n | \n | >>> ser.notna()\n | 0 True\n | 1 True\n | 2 False\n | dtype: bool\n | \n | notnull(self)\n | Detect existing (non-missing) values.\n | \n | Return a boolean same-sized object indicating if the values are not NA.\n | Non-missing values get mapped to True. Characters such as empty\n | strings ``''`` or :attr:`numpy.inf` are not considered NA values\n | (unless you set ``pandas.options.mode.use_inf_as_na = True``).\n | NA values, such as None or :attr:`numpy.NaN`, get mapped to False\n | values.\n | \n | Returns\n | -------\n | Series\n | Mask of bool values for each element in Series that\n | indicates whether an element is not an NA value.\n | \n | See Also\n | --------\n | Series.notnull : alias of notna\n | Series.isna : boolean inverse of notna\n | Series.dropna : omit axes labels with missing values\n | notna : top-level notna\n | \n | Examples\n | --------\n | Show which entries in a DataFrame are not NA.\n | \n | >>> df = pd.DataFrame({'age': [5, 6, np.NaN],\n | ... 'born': [pd.NaT, pd.Timestamp('1939-05-27'),\n | ... pd.Timestamp('1940-04-25')],\n | ... 'name': ['Alfred', 'Batman', ''],\n | ... 'toy': [None, 'Batmobile', 'Joker']})\n | >>> df\n | age born name toy\n | 0 5.0 NaT Alfred None\n | 1 6.0 1939-05-27 Batman Batmobile\n | 2 NaN 1940-04-25 Joker\n | \n | >>> df.notna()\n | age born name toy\n | 0 True False True False\n | 1 True True True True\n | 2 False True True True\n | \n | Show which entries in a Series are not NA.\n | \n | >>> ser = pd.Series([5, 6, np.NaN])\n | >>> ser\n | 0 5.0\n | 1 6.0\n | 2 NaN\n | dtype: float64\n | \n | >>> ser.notna()\n | 0 True\n | 1 True\n | 2 False\n | dtype: bool\n | \n | nsmallest(self, n=5, keep='first')\n | Return the smallest `n` elements.\n | \n | Parameters\n | ----------\n | n : int\n | Return this many ascending sorted values\n | keep : {'first', 'last'}, default 'first'\n | Where there are duplicate values:\n | - ``first`` : take the first occurrence.\n | - ``last`` : take the last occurrence.\n | \n | Returns\n | -------\n | bottom_n : Series\n | The n smallest values in the Series, in sorted order\n | \n | Notes\n | -----\n | Faster than ``.sort_values().head(n)`` for small `n` relative to\n | the size of the ``Series`` object.\n | \n | See Also\n | --------\n | Series.nlargest\n | \n | Examples\n | --------\n | >>> import pandas as pd\n | >>> import numpy as np\n | >>> s = pd.Series(np.random.randn(10**6))\n | >>> s.nsmallest(10) # only sorts up to the N requested\n | 288532 -4.954580\n | 732345 -4.835960\n | 64803 -4.812550\n | 446457 -4.609998\n | 501225 -4.483945\n | 669476 -4.472935\n | 973615 -4.401699\n | 621279 -4.355126\n | 773916 -4.347355\n | 359919 -4.331927\n | dtype: float64\n | \n | pow(self, other, level=None, fill_value=None, axis=0)\n | Exponential power of series and other, element-wise (binary operator `pow`).\n | \n | Equivalent to ``series ** other``, but with support to substitute a fill_value for\n | missing data in one of the inputs.\n | \n | Parameters\n | ----------\n | other : Series or scalar value\n | fill_value : None or float value, default None (NaN)\n | Fill existing missing (NaN) values, and any new element needed for\n | successful Series alignment, with this value before computation.\n | If data in both corresponding Series locations is missing\n | the result will be missing\n | level : int or name\n | Broadcast across a level, matching Index values on the\n | passed MultiIndex level\n | \n | Returns\n | -------\n | result : Series\n | \n | Examples\n | --------\n | >>> a = pd.Series([1, 1, 1, np.nan], index=['a', 'b', 'c', 'd'])\n | >>> a\n | a 1.0\n | b 1.0\n | c 1.0\n | d NaN\n | dtype: float64\n | >>> b = pd.Series([1, np.nan, 1, np.nan], index=['a', 'b', 'd', 'e'])\n | >>> b\n | a 1.0\n | b NaN\n | d 1.0\n | e NaN\n | dtype: float64\n | >>> a.add(b, fill_value=0)\n | a 2.0\n | b 1.0\n | c 1.0\n | d 1.0\n | e NaN\n | dtype: float64\n | \n | See also\n | --------\n | Series.rpow\n | \n | prod(self, axis=None, skipna=None, level=None, numeric_only=None, min_count=0, **kwargs)\n | Return the product of the values for the requested axis\n | \n | Parameters\n | ----------\n | axis : {index (0)}\n | skipna : boolean, default True\n | Exclude NA/null values when computing the result.\n | level : int or level name, default None\n | If the axis is a MultiIndex (hierarchical), count along a\n | particular level, collapsing into a scalar\n | numeric_only : boolean, default None\n | Include only float, int, boolean columns. If None, will attempt to use\n | everything, then use only numeric data. Not implemented for Series.\n | min_count : int, default 0\n | The required number of valid values to perform the operation. If fewer than\n | ``min_count`` non-NA values are present the result will be NA.\n | \n | .. versionadded :: 0.22.0\n | \n | Added with the default being 0. This means the sum of an all-NA\n | or empty Series is 0, and the product of an all-NA or empty\n | Series is 1.\n | \n | Returns\n | -------\n | prod : scalar or Series (if level specified)\n | \n | Examples\n | --------\n | By default, the product of an empty or all-NA Series is ``1``\n | \n | >>> pd.Series([]).prod()\n | 1.0\n | \n | This can be controlled with the ``min_count`` parameter\n | \n | >>> pd.Series([]).prod(min_count=1)\n | nan\n | \n | Thanks to the ``skipna`` parameter, ``min_count`` handles all-NA and\n | empty series identically.\n | \n | >>> pd.Series([np.nan]).prod()\n | 1.0\n | \n | >>> pd.Series([np.nan]).prod(min_count=1)\n | nan\n | \n | product = prod(self, axis=None, skipna=None, level=None, numeric_only=None, min_count=0, **kwargs)\n | Return the product of the values for the requested axis\n | \n | Parameters\n | ----------\n | axis : {index (0)}\n | skipna : boolean, default True\n | Exclude NA/null values when computing the result.\n | level : int or level name, default None\n | If the axis is a MultiIndex (hierarchical), count along a\n | particular level, collapsing into a scalar\n | numeric_only : boolean, default None\n | Include only float, int, boolean columns. If None, will attempt to use\n | everything, then use only numeric data. Not implemented for Series.\n | min_count : int, default 0\n | The required number of valid values to perform the operation. If fewer than\n | ``min_count`` non-NA values are present the result will be NA.\n | \n | .. versionadded :: 0.22.0\n | \n | Added with the default being 0. This means the sum of an all-NA\n | or empty Series is 0, and the product of an all-NA or empty\n | Series is 1.\n | \n | Returns\n | -------\n | prod : scalar or Series (if level specified)\n | \n | Examples\n | --------\n | By default, the product of an empty or all-NA Series is ``1``\n | \n | >>> pd.Series([]).prod()\n | 1.0\n | \n | This can be controlled with the ``min_count`` parameter\n | \n | >>> pd.Series([]).prod(min_count=1)\n | nan\n | \n | Thanks to the ``skipna`` parameter, ``min_count`` handles all-NA and\n | empty series identically.\n | \n | >>> pd.Series([np.nan]).prod()\n | 1.0\n | \n | >>> pd.Series([np.nan]).prod(min_count=1)\n | nan\n | \n | ptp(self, axis=None, skipna=None, level=None, numeric_only=None, **kwargs)\n | Returns the difference between the maximum value and the\n | minimum value in the object. This is the equivalent of the\n | ``numpy.ndarray`` method ``ptp``.\n | \n | Parameters\n | ----------\n | axis : {index (0)}\n | skipna : boolean, default True\n | Exclude NA/null values when computing the result.\n | level : int or level name, default None\n | If the axis is a MultiIndex (hierarchical), count along a\n | particular level, collapsing into a scalar\n | numeric_only : boolean, default None\n | Include only float, int, boolean columns. If None, will attempt to use\n | everything, then use only numeric data. Not implemented for Series.\n | \n | Returns\n | -------\n | ptp : scalar or Series (if level specified)\n | \n | put(self, *args, **kwargs)\n | Applies the `put` method to its `values` attribute\n | if it has one.\n | \n | See also\n | --------\n | numpy.ndarray.put\n | \n | quantile(self, q=0.5, interpolation='linear')\n | Return value at the given quantile, a la numpy.percentile.\n | \n | Parameters\n | ----------\n | q : float or array-like, default 0.5 (50% quantile)\n | 0 <= q <= 1, the quantile(s) to compute\n | interpolation : {'linear', 'lower', 'higher', 'midpoint', 'nearest'}\n | .. versionadded:: 0.18.0\n | \n | This optional parameter specifies the interpolation method to use,\n | when the desired quantile lies between two data points `i` and `j`:\n | \n | * linear: `i + (j - i) * fraction`, where `fraction` is the\n | fractional part of the index surrounded by `i` and `j`.\n | * lower: `i`.\n | * higher: `j`.\n | * nearest: `i` or `j` whichever is nearest.\n | * midpoint: (`i` + `j`) / 2.\n | \n | Returns\n | -------\n | quantile : float or Series\n | if ``q`` is an array, a Series will be returned where the\n | index is ``q`` and the values are the quantiles.\n | \n | Examples\n | --------\n | >>> s = Series([1, 2, 3, 4])\n | >>> s.quantile(.5)\n | 2.5\n | >>> s.quantile([.25, .5, .75])\n | 0.25 1.75\n | 0.50 2.50\n | 0.75 3.25\n | dtype: float64\n | \n | See Also\n | --------\n | pandas.core.window.Rolling.quantile\n | \n | radd(self, other, level=None, fill_value=None, axis=0)\n | Addition of series and other, element-wise (binary operator `radd`).\n | \n | Equivalent to ``other + series``, but with support to substitute a fill_value for\n | missing data in one of the inputs.\n | \n | Parameters\n | ----------\n | other : Series or scalar value\n | fill_value : None or float value, default None (NaN)\n | Fill existing missing (NaN) values, and any new element needed for\n | successful Series alignment, with this value before computation.\n | If data in both corresponding Series locations is missing\n | the result will be missing\n | level : int or name\n | Broadcast across a level, matching Index values on the\n | passed MultiIndex level\n | \n | Returns\n | -------\n | result : Series\n | \n | Examples\n | --------\n | >>> a = pd.Series([1, 1, 1, np.nan], index=['a', 'b', 'c', 'd'])\n | >>> a\n | a 1.0\n | b 1.0\n | c 1.0\n | d NaN\n | dtype: float64\n | >>> b = pd.Series([1, np.nan, 1, np.nan], index=['a', 'b', 'd', 'e'])\n | >>> b\n | a 1.0\n | b NaN\n | d 1.0\n | e NaN\n | dtype: float64\n | >>> a.add(b, fill_value=0)\n | a 2.0\n | b 1.0\n | c 1.0\n | d 1.0\n | e NaN\n | dtype: float64\n | \n | See also\n | --------\n | Series.add\n | \n | ravel(self, order='C')\n | Return the flattened underlying data as an ndarray\n | \n | See also\n | --------\n | numpy.ndarray.ravel\n | \n | rdiv = rtruediv(self, other, level=None, fill_value=None, axis=0)\n | Floating division of series and other, element-wise (binary operator `rtruediv`).\n | \n | Equivalent to ``other / series``, but with support to substitute a fill_value for\n | missing data in one of the inputs.\n | \n | Parameters\n | ----------\n | other : Series or scalar value\n | fill_value : None or float value, default None (NaN)\n | Fill existing missing (NaN) values, and any new element needed for\n | successful Series alignment, with this value before computation.\n | If data in both corresponding Series locations is missing\n | the result will be missing\n | level : int or name\n | Broadcast across a level, matching Index values on the\n | passed MultiIndex level\n | \n | Returns\n | -------\n | result : Series\n | \n | Examples\n | --------\n | >>> a = pd.Series([1, 1, 1, np.nan], index=['a', 'b', 'c', 'd'])\n | >>> a\n | a 1.0\n | b 1.0\n | c 1.0\n | d NaN\n | dtype: float64\n | >>> b = pd.Series([1, np.nan, 1, np.nan], index=['a', 'b', 'd', 'e'])\n | >>> b\n | a 1.0\n | b NaN\n | d 1.0\n | e NaN\n | dtype: float64\n | >>> a.add(b, fill_value=0)\n | a 2.0\n | b 1.0\n | c 1.0\n | d 1.0\n | e NaN\n | dtype: float64\n | \n | See also\n | --------\n | Series.truediv\n | \n | reindex(self, index=None, **kwargs)\n | Conform Series to new index with optional filling logic, placing\n | NA/NaN in locations having no value in the previous index. A new object\n | is produced unless the new index is equivalent to the current one and\n | copy=False\n | \n | Parameters\n | ----------\n | \n | index : array-like, optional (should be specified using keywords)\n | New labels / index to conform to. Preferably an Index object to\n | avoid duplicating data\n | \n | method : {None, 'backfill'/'bfill', 'pad'/'ffill', 'nearest'}, optional\n | method to use for filling holes in reindexed DataFrame.\n | Please note: this is only applicable to DataFrames/Series with a\n | monotonically increasing/decreasing index.\n | \n | * default: don't fill gaps\n | * pad / ffill: propagate last valid observation forward to next\n | valid\n | * backfill / bfill: use next valid observation to fill gap\n | * nearest: use nearest valid observations to fill gap\n | \n | copy : boolean, default True\n | Return a new object, even if the passed indexes are the same\n | level : int or name\n | Broadcast across a level, matching Index values on the\n | passed MultiIndex level\n | fill_value : scalar, default np.NaN\n | Value to use for missing values. Defaults to NaN, but can be any\n | \"compatible\" value\n | limit : int, default None\n | Maximum number of consecutive elements to forward or backward fill\n | tolerance : optional\n | Maximum distance between original and new labels for inexact\n | matches. The values of the index at the matching locations most\n | satisfy the equation ``abs(index[indexer] - target) <= tolerance``.\n | \n | Tolerance may be a scalar value, which applies the same tolerance\n | to all values, or list-like, which applies variable tolerance per\n | element. List-like includes list, tuple, array, Series, and must be\n | the same size as the index and its dtype must exactly match the\n | index's type.\n | \n | .. versionadded:: 0.21.0 (list-like tolerance)\n | \n | Examples\n | --------\n | \n | ``DataFrame.reindex`` supports two calling conventions\n | \n | * ``(index=index_labels, columns=column_labels, ...)``\n | * ``(labels, axis={'index', 'columns'}, ...)``\n | \n | We *highly* recommend using keyword arguments to clarify your\n | intent.\n | \n | Create a dataframe with some fictional data.\n | \n | >>> index = ['Firefox', 'Chrome', 'Safari', 'IE10', 'Konqueror']\n | >>> df = pd.DataFrame({\n | ... 'http_status': [200,200,404,404,301],\n | ... 'response_time': [0.04, 0.02, 0.07, 0.08, 1.0]},\n | ... index=index)\n | >>> df\n | http_status response_time\n | Firefox 200 0.04\n | Chrome 200 0.02\n | Safari 404 0.07\n | IE10 404 0.08\n | Konqueror 301 1.00\n | \n | Create a new index and reindex the dataframe. By default\n | values in the new index that do not have corresponding\n | records in the dataframe are assigned ``NaN``.\n | \n | >>> new_index= ['Safari', 'Iceweasel', 'Comodo Dragon', 'IE10',\n | ... 'Chrome']\n | >>> df.reindex(new_index)\n | http_status response_time\n | Safari 404.0 0.07\n | Iceweasel NaN NaN\n | Comodo Dragon NaN NaN\n | IE10 404.0 0.08\n | Chrome 200.0 0.02\n | \n | We can fill in the missing values by passing a value to\n | the keyword ``fill_value``. Because the index is not monotonically\n | increasing or decreasing, we cannot use arguments to the keyword\n | ``method`` to fill the ``NaN`` values.\n | \n | >>> df.reindex(new_index, fill_value=0)\n | http_status response_time\n | Safari 404 0.07\n | Iceweasel 0 0.00\n | Comodo Dragon 0 0.00\n | IE10 404 0.08\n | Chrome 200 0.02\n | \n | >>> df.reindex(new_index, fill_value='missing')\n | http_status response_time\n | Safari 404 0.07\n | Iceweasel missing missing\n | Comodo Dragon missing missing\n | IE10 404 0.08\n | Chrome 200 0.02\n | \n | We can also reindex the columns.\n | \n | >>> df.reindex(columns=['http_status', 'user_agent'])\n | http_status user_agent\n | Firefox 200 NaN\n | Chrome 200 NaN\n | Safari 404 NaN\n | IE10 404 NaN\n | Konqueror 301 NaN\n | \n | Or we can use \"axis-style\" keyword arguments\n | \n | >>> df.reindex(['http_status', 'user_agent'], axis=\"columns\")\n | http_status user_agent\n | Firefox 200 NaN\n | Chrome 200 NaN\n | Safari 404 NaN\n | IE10 404 NaN\n | Konqueror 301 NaN\n | \n | To further illustrate the filling functionality in\n | ``reindex``, we will create a dataframe with a\n | monotonically increasing index (for example, a sequence\n | of dates).\n | \n | >>> date_index = pd.date_range('1/1/2010', periods=6, freq='D')\n | >>> df2 = pd.DataFrame({\"prices\": [100, 101, np.nan, 100, 89, 88]},\n | ... index=date_index)\n | >>> df2\n | prices\n | 2010-01-01 100\n | 2010-01-02 101\n | 2010-01-03 NaN\n | 2010-01-04 100\n | 2010-01-05 89\n | 2010-01-06 88\n | \n | Suppose we decide to expand the dataframe to cover a wider\n | date range.\n | \n | >>> date_index2 = pd.date_range('12/29/2009', periods=10, freq='D')\n | >>> df2.reindex(date_index2)\n | prices\n | 2009-12-29 NaN\n | 2009-12-30 NaN\n | 2009-12-31 NaN\n | 2010-01-01 100\n | 2010-01-02 101\n | 2010-01-03 NaN\n | 2010-01-04 100\n | 2010-01-05 89\n | 2010-01-06 88\n | 2010-01-07 NaN\n | \n | The index entries that did not have a value in the original data frame\n | (for example, '2009-12-29') are by default filled with ``NaN``.\n | If desired, we can fill in the missing values using one of several\n | options.\n | \n | For example, to backpropagate the last valid value to fill the ``NaN``\n | values, pass ``bfill`` as an argument to the ``method`` keyword.\n | \n | >>> df2.reindex(date_index2, method='bfill')\n | prices\n | 2009-12-29 100\n | 2009-12-30 100\n | 2009-12-31 100\n | 2010-01-01 100\n | 2010-01-02 101\n | 2010-01-03 NaN\n | 2010-01-04 100\n | 2010-01-05 89\n | 2010-01-06 88\n | 2010-01-07 NaN\n | \n | Please note that the ``NaN`` value present in the original dataframe\n | (at index value 2010-01-03) will not be filled by any of the\n | value propagation schemes. This is because filling while reindexing\n | does not look at dataframe values, but only compares the original and\n | desired indexes. If you do want to fill in the ``NaN`` values present\n | in the original dataframe, use the ``fillna()`` method.\n | \n | See the :ref:`user guide <basics.reindexing>` for more.\n | \n | Returns\n | -------\n | reindexed : Series\n | \n | reindex_axis(self, labels, axis=0, **kwargs)\n | Conform Series to new index with optional filling logic.\n | \n | .. deprecated:: 0.21.0\n | Use ``Series.reindex`` instead.\n | \n | rename(self, index=None, **kwargs)\n | Alter Series index labels or name\n | \n | Function / dict values must be unique (1-to-1). Labels not contained in\n | a dict / Series will be left as-is. Extra labels listed don't throw an\n | error.\n | \n | Alternatively, change ``Series.name`` with a scalar value.\n | \n | See the :ref:`user guide <basics.rename>` for more.\n | \n | Parameters\n | ----------\n | index : scalar, hashable sequence, dict-like or function, optional\n | dict-like or functions are transformations to apply to\n | the index.\n | Scalar or hashable sequence-like will alter the ``Series.name``\n | attribute.\n | copy : boolean, default True\n | Also copy underlying data\n | inplace : boolean, default False\n | Whether to return a new Series. If True then value of copy is\n | ignored.\n | level : int or level name, default None\n | In case of a MultiIndex, only rename labels in the specified\n | level.\n | \n | Returns\n | -------\n | renamed : Series (new object)\n | \n | See Also\n | --------\n | pandas.Series.rename_axis\n | \n | Examples\n | --------\n | \n | >>> s = pd.Series([1, 2, 3])\n | >>> s\n | 0 1\n | 1 2\n | 2 3\n | dtype: int64\n | >>> s.rename(\"my_name\") # scalar, changes Series.name\n | 0 1\n | 1 2\n | 2 3\n | Name: my_name, dtype: int64\n | >>> s.rename(lambda x: x ** 2) # function, changes labels\n | 0 1\n | 1 2\n | 4 3\n | dtype: int64\n | >>> s.rename({1: 3, 2: 5}) # mapping, changes labels\n | 0 1\n | 3 2\n | 5 3\n | dtype: int64\n | \n | reorder_levels(self, order)\n | Rearrange index levels using input order. May not drop or duplicate\n | levels\n | \n | Parameters\n | ----------\n | order : list of int representing new level order.\n | (reference level by number or key)\n | axis : where to reorder levels\n | \n | Returns\n | -------\n | type of caller (new object)\n | \n | repeat(self, repeats, *args, **kwargs)\n | Repeat elements of an Series. Refer to `numpy.ndarray.repeat`\n | for more information about the `repeats` argument.\n | \n | See also\n | --------\n | numpy.ndarray.repeat\n | \n | replace(self, to_replace=None, value=None, inplace=False, limit=None, regex=False, method='pad')\n | Replace values given in `to_replace` with `value`.\n | \n | Values of the Series are replaced with other values dynamically.\n | This differs from updating with ``.loc`` or ``.iloc``, which require\n | you to specify a location to update with some value.\n | \n | Parameters\n | ----------\n | to_replace : str, regex, list, dict, Series, int, float, or None\n | How to find the values that will be replaced.\n | \n | * numeric, str or regex:\n | \n | - numeric: numeric values equal to `to_replace` will be\n | replaced with `value`\n | - str: string exactly matching `to_replace` will be replaced\n | with `value`\n | - regex: regexs matching `to_replace` will be replaced with\n | `value`\n | \n | * list of str, regex, or numeric:\n | \n | - First, if `to_replace` and `value` are both lists, they\n | **must** be the same length.\n | - Second, if ``regex=True`` then all of the strings in **both**\n | lists will be interpreted as regexs otherwise they will match\n | directly. This doesn't matter much for `value` since there\n | are only a few possible substitution regexes you can use.\n | - str, regex and numeric rules apply as above.\n | \n | * dict:\n | \n | - Dicts can be used to specify different replacement values\n | for different existing values. For example,\n | ``{'a': 'b', 'y': 'z'}`` replaces the value 'a' with 'b' and\n | 'y' with 'z'. To use a dict in this way the `value`\n | parameter should be `None`.\n | - For a DataFrame a dict can specify that different values\n | should be replaced in different columns. For example,\n | ``{'a': 1, 'b': 'z'}`` looks for the value 1 in column 'a'\n | and the value 'z' in column 'b' and replaces these values\n | with whatever is specified in `value`. The `value` parameter\n | should not be ``None`` in this case. You can treat this as a\n | special case of passing two lists except that you are\n | specifying the column to search in.\n | - For a DataFrame nested dictionaries, e.g.,\n | ``{'a': {'b': np.nan}}``, are read as follows: look in column\n | 'a' for the value 'b' and replace it with NaN. The `value`\n | parameter should be ``None`` to use a nested dict in this\n | way. You can nest regular expressions as well. Note that\n | column names (the top-level dictionary keys in a nested\n | dictionary) **cannot** be regular expressions.\n | \n | * None:\n | \n | - This means that the `regex` argument must be a string,\n | compiled regular expression, or list, dict, ndarray or\n | Series of such elements. If `value` is also ``None`` then\n | this **must** be a nested dictionary or Series.\n | \n | See the examples section for examples of each of these.\n | value : scalar, dict, list, str, regex, default None\n | Value to replace any values matching `to_replace` with.\n | For a DataFrame a dict of values can be used to specify which\n | value to use for each column (columns not in the dict will not be\n | filled). Regular expressions, strings and lists or dicts of such\n | objects are also allowed.\n | inplace : boolean, default False\n | If True, in place. Note: this will modify any\n | other views on this object (e.g. a column from a DataFrame).\n | Returns the caller if this is True.\n | limit : int, default None\n | Maximum size gap to forward or backward fill.\n | regex : bool or same types as `to_replace`, default False\n | Whether to interpret `to_replace` and/or `value` as regular\n | expressions. If this is ``True`` then `to_replace` *must* be a\n | string. Alternatively, this could be a regular expression or a\n | list, dict, or array of regular expressions in which case\n | `to_replace` must be ``None``.\n | method : {'pad', 'ffill', 'bfill', `None`}\n | The method to use when for replacement, when `to_replace` is a\n | scalar, list or tuple and `value` is ``None``.\n | \n | .. versionchanged:: 0.23.0\n | Added to DataFrame.\n | \n | See Also\n | --------\n | Series.fillna : Fill NA values\n | Series.where : Replace values based on boolean condition\n | Series.str.replace : Simple string replacement.\n | \n | Returns\n | -------\n | Series\n | Object after replacement.\n | \n | Raises\n | ------\n | AssertionError\n | * If `regex` is not a ``bool`` and `to_replace` is not\n | ``None``.\n | TypeError\n | * If `to_replace` is a ``dict`` and `value` is not a ``list``,\n | ``dict``, ``ndarray``, or ``Series``\n | * If `to_replace` is ``None`` and `regex` is not compilable\n | into a regular expression or is a list, dict, ndarray, or\n | Series.\n | * When replacing multiple ``bool`` or ``datetime64`` objects and\n | the arguments to `to_replace` does not match the type of the\n | value being replaced\n | ValueError\n | * If a ``list`` or an ``ndarray`` is passed to `to_replace` and\n | `value` but they are not the same length.\n | \n | Notes\n | -----\n | * Regex substitution is performed under the hood with ``re.sub``. The\n | rules for substitution for ``re.sub`` are the same.\n | * Regular expressions will only substitute on strings, meaning you\n | cannot provide, for example, a regular expression matching floating\n | point numbers and expect the columns in your frame that have a\n | numeric dtype to be matched. However, if those floating point\n | numbers *are* strings, then you can do this.\n | * This method has *a lot* of options. You are encouraged to experiment\n | and play with this method to gain intuition about how it works.\n | * When dict is used as the `to_replace` value, it is like\n | key(s) in the dict are the to_replace part and\n | value(s) in the dict are the value parameter.\n | \n | Examples\n | --------\n | \n | **Scalar `to_replace` and `value`**\n | \n | >>> s = pd.Series([0, 1, 2, 3, 4])\n | >>> s.replace(0, 5)\n | 0 5\n | 1 1\n | 2 2\n | 3 3\n | 4 4\n | dtype: int64\n | \n | >>> df = pd.DataFrame({'A': [0, 1, 2, 3, 4],\n | ... 'B': [5, 6, 7, 8, 9],\n | ... 'C': ['a', 'b', 'c', 'd', 'e']})\n | >>> df.replace(0, 5)\n | A B C\n | 0 5 5 a\n | 1 1 6 b\n | 2 2 7 c\n | 3 3 8 d\n | 4 4 9 e\n | \n | **List-like `to_replace`**\n | \n | >>> df.replace([0, 1, 2, 3], 4)\n | A B C\n | 0 4 5 a\n | 1 4 6 b\n | 2 4 7 c\n | 3 4 8 d\n | 4 4 9 e\n | \n | >>> df.replace([0, 1, 2, 3], [4, 3, 2, 1])\n | A B C\n | 0 4 5 a\n | 1 3 6 b\n | 2 2 7 c\n | 3 1 8 d\n | 4 4 9 e\n | \n | >>> s.replace([1, 2], method='bfill')\n | 0 0\n | 1 3\n | 2 3\n | 3 3\n | 4 4\n | dtype: int64\n | \n | **dict-like `to_replace`**\n | \n | >>> df.replace({0: 10, 1: 100})\n | A B C\n | 0 10 5 a\n | 1 100 6 b\n | 2 2 7 c\n | 3 3 8 d\n | 4 4 9 e\n | \n | >>> df.replace({'A': 0, 'B': 5}, 100)\n | A B C\n | 0 100 100 a\n | 1 1 6 b\n | 2 2 7 c\n | 3 3 8 d\n | 4 4 9 e\n | \n | >>> df.replace({'A': {0: 100, 4: 400}})\n | A B C\n | 0 100 5 a\n | 1 1 6 b\n | 2 2 7 c\n | 3 3 8 d\n | 4 400 9 e\n | \n | **Regular expression `to_replace`**\n | \n | >>> df = pd.DataFrame({'A': ['bat', 'foo', 'bait'],\n | ... 'B': ['abc', 'bar', 'xyz']})\n | >>> df.replace(to_replace=r'^ba.$', value='new', regex=True)\n | A B\n | 0 new abc\n | 1 foo new\n | 2 bait xyz\n | \n | >>> df.replace({'A': r'^ba.$'}, {'A': 'new'}, regex=True)\n | A B\n | 0 new abc\n | 1 foo bar\n | 2 bait xyz\n | \n | >>> df.replace(regex=r'^ba.$', value='new')\n | A B\n | 0 new abc\n | 1 foo new\n | 2 bait xyz\n | \n | >>> df.replace(regex={r'^ba.$':'new', 'foo':'xyz'})\n | A B\n | 0 new abc\n | 1 xyz new\n | 2 bait xyz\n | \n | >>> df.replace(regex=[r'^ba.$', 'foo'], value='new')\n | A B\n | 0 new abc\n | 1 new new\n | 2 bait xyz\n | \n | Note that when replacing multiple ``bool`` or ``datetime64`` objects,\n | the data types in the `to_replace` parameter must match the data\n | type of the value being replaced:\n | \n | >>> df = pd.DataFrame({'A': [True, False, True],\n | ... 'B': [False, True, False]})\n | >>> df.replace({'a string': 'new value', True: False}) # raises\n | Traceback (most recent call last):\n | ...\n | TypeError: Cannot compare types 'ndarray(dtype=bool)' and 'str'\n | \n | This raises a ``TypeError`` because one of the ``dict`` keys is not of\n | the correct type for replacement.\n | \n | Compare the behavior of ``s.replace({'a': None})`` and\n | ``s.replace('a', None)`` to understand the pecularities\n | of the `to_replace` parameter:\n | \n | >>> s = pd.Series([10, 'a', 'a', 'b', 'a'])\n | \n | When one uses a dict as the `to_replace` value, it is like the\n | value(s) in the dict are equal to the `value` parameter.\n | ``s.replace({'a': None})`` is equivalent to\n | ``s.replace(to_replace={'a': None}, value=None, method=None)``:\n | \n | >>> s.replace({'a': None})\n | 0 10\n | 1 None\n | 2 None\n | 3 b\n | 4 None\n | dtype: object\n | \n | When ``value=None`` and `to_replace` is a scalar, list or\n | tuple, `replace` uses the method parameter (default 'pad') to do the\n | replacement. So this is why the 'a' values are being replaced by 10\n | in rows 1 and 2 and 'b' in row 4 in this case.\n | The command ``s.replace('a', None)`` is actually equivalent to\n | ``s.replace(to_replace='a', value=None, method='pad')``:\n | \n | >>> s.replace('a', None)\n | 0 10\n | 1 10\n | 2 10\n | 3 b\n | 4 b\n | dtype: object\n | \n | reset_index(self, level=None, drop=False, name=None, inplace=False)\n | Generate a new DataFrame or Series with the index reset.\n | \n | This is useful when the index needs to be treated as a column, or\n | when the index is meaningless and needs to be reset to the default\n | before another operation.\n | \n | Parameters\n | ----------\n | level : int, str, tuple, or list, default optional\n | For a Series with a MultiIndex, only remove the specified levels\n | from the index. Removes all levels by default.\n | drop : bool, default False\n | Just reset the index, without inserting it as a column in\n | the new DataFrame.\n | name : object, optional\n | The name to use for the column containing the original Series\n | values. Uses ``self.name`` by default. This argument is ignored\n | when `drop` is True.\n | inplace : bool, default False\n | Modify the Series in place (do not create a new object).\n | \n | Returns\n | -------\n | Series or DataFrame\n | When `drop` is False (the default), a DataFrame is returned.\n | The newly created columns will come first in the DataFrame,\n | followed by the original Series values.\n | When `drop` is True, a `Series` is returned.\n | In either case, if ``inplace=True``, no value is returned.\n | \n | See Also\n | --------\n | DataFrame.reset_index: Analogous function for DataFrame.\n | \n | Examples\n | --------\n | \n | >>> s = pd.Series([1, 2, 3, 4], name='foo',\n | ... index=pd.Index(['a', 'b', 'c', 'd'], name='idx'))\n | \n | Generate a DataFrame with default index.\n | \n | >>> s.reset_index()\n | idx foo\n | 0 a 1\n | 1 b 2\n | 2 c 3\n | 3 d 4\n | \n | To specify the name of the new column use `name`.\n | \n | >>> s.reset_index(name='values')\n | idx values\n | 0 a 1\n | 1 b 2\n | 2 c 3\n | 3 d 4\n | \n | To generate a new Series with the default set `drop` to True.\n | \n | >>> s.reset_index(drop=True)\n | 0 1\n | 1 2\n | 2 3\n | 3 4\n | Name: foo, dtype: int64\n | \n | To update the Series in place, without generating a new one\n | set `inplace` to True. Note that it also requires ``drop=True``.\n | \n | >>> s.reset_index(inplace=True, drop=True)\n | >>> s\n | 0 1\n | 1 2\n | 2 3\n | 3 4\n | Name: foo, dtype: int64\n | \n | The `level` parameter is interesting for Series with a multi-level\n | index.\n | \n | >>> arrays = [np.array(['bar', 'bar', 'baz', 'baz']),\n | ... np.array(['one', 'two', 'one', 'two'])]\n | >>> s2 = pd.Series(\n | ... range(4), name='foo',\n | ... index=pd.MultiIndex.from_arrays(arrays,\n | ... names=['a', 'b']))\n | \n | To remove a specific level from the Index, use `level`.\n | \n | >>> s2.reset_index(level='a')\n | a foo\n | b\n | one bar 0\n | two bar 1\n | one baz 2\n | two baz 3\n | \n | If `level` is not set, all levels are removed from the Index.\n | \n | >>> s2.reset_index()\n | a b foo\n | 0 bar one 0\n | 1 bar two 1\n | 2 baz one 2\n | 3 baz two 3\n | \n | rfloordiv(self, other, level=None, fill_value=None, axis=0)\n | Integer division of series and other, element-wise (binary operator `rfloordiv`).\n | \n | Equivalent to ``other // series``, but with support to substitute a fill_value for\n | missing data in one of the inputs.\n | \n | Parameters\n | ----------\n | other : Series or scalar value\n | fill_value : None or float value, default None (NaN)\n | Fill existing missing (NaN) values, and any new element needed for\n | successful Series alignment, with this value before computation.\n | If data in both corresponding Series locations is missing\n | the result will be missing\n | level : int or name\n | Broadcast across a level, matching Index values on the\n | passed MultiIndex level\n | \n | Returns\n | -------\n | result : Series\n | \n | Examples\n | --------\n | >>> a = pd.Series([1, 1, 1, np.nan], index=['a', 'b', 'c', 'd'])\n | >>> a\n | a 1.0\n | b 1.0\n | c 1.0\n | d NaN\n | dtype: float64\n | >>> b = pd.Series([1, np.nan, 1, np.nan], index=['a', 'b', 'd', 'e'])\n | >>> b\n | a 1.0\n | b NaN\n | d 1.0\n | e NaN\n | dtype: float64\n | >>> a.add(b, fill_value=0)\n | a 2.0\n | b 1.0\n | c 1.0\n | d 1.0\n | e NaN\n | dtype: float64\n | \n | See also\n | --------\n | Series.floordiv\n | \n | rmod(self, other, level=None, fill_value=None, axis=0)\n | Modulo of series and other, element-wise (binary operator `rmod`).\n | \n | Equivalent to ``other % series``, but with support to substitute a fill_value for\n | missing data in one of the inputs.\n | \n | Parameters\n | ----------\n | other : Series or scalar value\n | fill_value : None or float value, default None (NaN)\n | Fill existing missing (NaN) values, and any new element needed for\n | successful Series alignment, with this value before computation.\n | If data in both corresponding Series locations is missing\n | the result will be missing\n | level : int or name\n | Broadcast across a level, matching Index values on the\n | passed MultiIndex level\n | \n | Returns\n | -------\n | result : Series\n | \n | Examples\n | --------\n | >>> a = pd.Series([1, 1, 1, np.nan], index=['a', 'b', 'c', 'd'])\n | >>> a\n | a 1.0\n | b 1.0\n | c 1.0\n | d NaN\n | dtype: float64\n | >>> b = pd.Series([1, np.nan, 1, np.nan], index=['a', 'b', 'd', 'e'])\n | >>> b\n | a 1.0\n | b NaN\n | d 1.0\n | e NaN\n | dtype: float64\n | >>> a.add(b, fill_value=0)\n | a 2.0\n | b 1.0\n | c 1.0\n | d 1.0\n | e NaN\n | dtype: float64\n | \n | See also\n | --------\n | Series.mod\n | \n | rmul(self, other, level=None, fill_value=None, axis=0)\n | Multiplication of series and other, element-wise (binary operator `rmul`).\n | \n | Equivalent to ``other * series``, but with support to substitute a fill_value for\n | missing data in one of the inputs.\n | \n | Parameters\n | ----------\n | other : Series or scalar value\n | fill_value : None or float value, default None (NaN)\n | Fill existing missing (NaN) values, and any new element needed for\n | successful Series alignment, with this value before computation.\n | If data in both corresponding Series locations is missing\n | the result will be missing\n | level : int or name\n | Broadcast across a level, matching Index values on the\n | passed MultiIndex level\n | \n | Returns\n | -------\n | result : Series\n | \n | Examples\n | --------\n | >>> a = pd.Series([1, 1, 1, np.nan], index=['a', 'b', 'c', 'd'])\n | >>> a\n | a 1.0\n | b 1.0\n | c 1.0\n | d NaN\n | dtype: float64\n | >>> b = pd.Series([1, np.nan, 1, np.nan], index=['a', 'b', 'd', 'e'])\n | >>> b\n | a 1.0\n | b NaN\n | d 1.0\n | e NaN\n | dtype: float64\n | >>> a.add(b, fill_value=0)\n | a 2.0\n | b 1.0\n | c 1.0\n | d 1.0\n | e NaN\n | dtype: float64\n | \n | See also\n | --------\n | Series.mul\n | \n | rolling(self, window, min_periods=None, center=False, win_type=None, on=None, axis=0, closed=None)\n | Provides rolling window calculations.\n | \n | .. versionadded:: 0.18.0\n | \n | Parameters\n | ----------\n | window : int, or offset\n | Size of the moving window. This is the number of observations used for\n | calculating the statistic. Each window will be a fixed size.\n | \n | If its an offset then this will be the time period of each window. Each\n | window will be a variable sized based on the observations included in\n | the time-period. This is only valid for datetimelike indexes. This is\n | new in 0.19.0\n | min_periods : int, default None\n | Minimum number of observations in window required to have a value\n | (otherwise result is NA). For a window that is specified by an offset,\n | this will default to 1.\n | center : boolean, default False\n | Set the labels at the center of the window.\n | win_type : string, default None\n | Provide a window type. If ``None``, all points are evenly weighted.\n | See the notes below for further information.\n | on : string, optional\n | For a DataFrame, column on which to calculate\n | the rolling window, rather than the index\n | closed : string, default None\n | Make the interval closed on the 'right', 'left', 'both' or\n | 'neither' endpoints.\n | For offset-based windows, it defaults to 'right'.\n | For fixed windows, defaults to 'both'. Remaining cases not implemented\n | for fixed windows.\n | \n | .. versionadded:: 0.20.0\n | \n | axis : int or string, default 0\n | \n | Returns\n | -------\n | a Window or Rolling sub-classed for the particular operation\n | \n | Examples\n | --------\n | \n | >>> df = pd.DataFrame({'B': [0, 1, 2, np.nan, 4]})\n | >>> df\n | B\n | 0 0.0\n | 1 1.0\n | 2 2.0\n | 3 NaN\n | 4 4.0\n | \n | Rolling sum with a window length of 2, using the 'triang'\n | window type.\n | \n | >>> df.rolling(2, win_type='triang').sum()\n | B\n | 0 NaN\n | 1 1.0\n | 2 2.5\n | 3 NaN\n | 4 NaN\n | \n | Rolling sum with a window length of 2, min_periods defaults\n | to the window length.\n | \n | >>> df.rolling(2).sum()\n | B\n | 0 NaN\n | 1 1.0\n | 2 3.0\n | 3 NaN\n | 4 NaN\n | \n | Same as above, but explicitly set the min_periods\n | \n | >>> df.rolling(2, min_periods=1).sum()\n | B\n | 0 0.0\n | 1 1.0\n | 2 3.0\n | 3 2.0\n | 4 4.0\n | \n | A ragged (meaning not-a-regular frequency), time-indexed DataFrame\n | \n | >>> df = pd.DataFrame({'B': [0, 1, 2, np.nan, 4]},\n | ... index = [pd.Timestamp('20130101 09:00:00'),\n | ... pd.Timestamp('20130101 09:00:02'),\n | ... pd.Timestamp('20130101 09:00:03'),\n | ... pd.Timestamp('20130101 09:00:05'),\n | ... pd.Timestamp('20130101 09:00:06')])\n | \n | >>> df\n | B\n | 2013-01-01 09:00:00 0.0\n | 2013-01-01 09:00:02 1.0\n | 2013-01-01 09:00:03 2.0\n | 2013-01-01 09:00:05 NaN\n | 2013-01-01 09:00:06 4.0\n | \n | \n | Contrasting to an integer rolling window, this will roll a variable\n | length window corresponding to the time period.\n | The default for min_periods is 1.\n | \n | >>> df.rolling('2s').sum()\n | B\n | 2013-01-01 09:00:00 0.0\n | 2013-01-01 09:00:02 1.0\n | 2013-01-01 09:00:03 3.0\n | 2013-01-01 09:00:05 NaN\n | 2013-01-01 09:00:06 4.0\n | \n | Notes\n | -----\n | By default, the result is set to the right edge of the window. This can be\n | changed to the center of the window by setting ``center=True``.\n | \n | To learn more about the offsets & frequency strings, please see `this link\n | <http://pandas.pydata.org/pandas-docs/stable/timeseries.html#offset-aliases>`__.\n | \n | The recognized win_types are:\n | \n | * ``boxcar``\n | * ``triang``\n | * ``blackman``\n | * ``hamming``\n | * ``bartlett``\n | * ``parzen``\n | * ``bohman``\n | * ``blackmanharris``\n | * ``nuttall``\n | * ``barthann``\n | * ``kaiser`` (needs beta)\n | * ``gaussian`` (needs std)\n | * ``general_gaussian`` (needs power, width)\n | * ``slepian`` (needs width).\n | \n | If ``win_type=None`` all points are evenly weighted. To learn more about\n | different window types see `scipy.signal window functions\n | <https://docs.scipy.org/doc/scipy/reference/signal.html#window-functions>`__.\n | \n | See Also\n | --------\n | expanding : Provides expanding transformations.\n | ewm : Provides exponential weighted functions\n | \n | round(self, decimals=0, *args, **kwargs)\n | Round each value in a Series to the given number of decimals.\n | \n | Parameters\n | ----------\n | decimals : int\n | Number of decimal places to round to (default: 0).\n | If decimals is negative, it specifies the number of\n | positions to the left of the decimal point.\n | \n | Returns\n | -------\n | Series object\n | \n | See Also\n | --------\n | numpy.around\n | DataFrame.round\n | \n | rpow(self, other, level=None, fill_value=None, axis=0)\n | Exponential power of series and other, element-wise (binary operator `rpow`).\n | \n | Equivalent to ``other ** series``, but with support to substitute a fill_value for\n | missing data in one of the inputs.\n | \n | Parameters\n | ----------\n | other : Series or scalar value\n | fill_value : None or float value, default None (NaN)\n | Fill existing missing (NaN) values, and any new element needed for\n | successful Series alignment, with this value before computation.\n | If data in both corresponding Series locations is missing\n | the result will be missing\n | level : int or name\n | Broadcast across a level, matching Index values on the\n | passed MultiIndex level\n | \n | Returns\n | -------\n | result : Series\n | \n | Examples\n | --------\n | >>> a = pd.Series([1, 1, 1, np.nan], index=['a', 'b', 'c', 'd'])\n | >>> a\n | a 1.0\n | b 1.0\n | c 1.0\n | d NaN\n | dtype: float64\n | >>> b = pd.Series([1, np.nan, 1, np.nan], index=['a', 'b', 'd', 'e'])\n | >>> b\n | a 1.0\n | b NaN\n | d 1.0\n | e NaN\n | dtype: float64\n | >>> a.add(b, fill_value=0)\n | a 2.0\n | b 1.0\n | c 1.0\n | d 1.0\n | e NaN\n | dtype: float64\n | \n | See also\n | --------\n | Series.pow\n | \n | rsub(self, other, level=None, fill_value=None, axis=0)\n | Subtraction of series and other, element-wise (binary operator `rsub`).\n | \n | Equivalent to ``other - series``, but with support to substitute a fill_value for\n | missing data in one of the inputs.\n | \n | Parameters\n | ----------\n | other : Series or scalar value\n | fill_value : None or float value, default None (NaN)\n | Fill existing missing (NaN) values, and any new element needed for\n | successful Series alignment, with this value before computation.\n | If data in both corresponding Series locations is missing\n | the result will be missing\n | level : int or name\n | Broadcast across a level, matching Index values on the\n | passed MultiIndex level\n | \n | Returns\n | -------\n | result : Series\n | \n | Examples\n | --------\n | >>> a = pd.Series([1, 1, 1, np.nan], index=['a', 'b', 'c', 'd'])\n | >>> a\n | a 1.0\n | b 1.0\n | c 1.0\n | d NaN\n | dtype: float64\n | >>> b = pd.Series([1, np.nan, 1, np.nan], index=['a', 'b', 'd', 'e'])\n | >>> b\n | a 1.0\n | b NaN\n | d 1.0\n | e NaN\n | dtype: float64\n | >>> a.add(b, fill_value=0)\n | a 2.0\n | b 1.0\n | c 1.0\n | d 1.0\n | e NaN\n | dtype: float64\n | \n | See also\n | --------\n | Series.sub\n | \n | rtruediv(self, other, level=None, fill_value=None, axis=0)\n | Floating division of series and other, element-wise (binary operator `rtruediv`).\n | \n | Equivalent to ``other / series``, but with support to substitute a fill_value for\n | missing data in one of the inputs.\n | \n | Parameters\n | ----------\n | other : Series or scalar value\n | fill_value : None or float value, default None (NaN)\n | Fill existing missing (NaN) values, and any new element needed for\n | successful Series alignment, with this value before computation.\n | If data in both corresponding Series locations is missing\n | the result will be missing\n | level : int or name\n | Broadcast across a level, matching Index values on the\n | passed MultiIndex level\n | \n | Returns\n | -------\n | result : Series\n | \n | Examples\n | --------\n | >>> a = pd.Series([1, 1, 1, np.nan], index=['a', 'b', 'c', 'd'])\n | >>> a\n | a 1.0\n | b 1.0\n | c 1.0\n | d NaN\n | dtype: float64\n | >>> b = pd.Series([1, np.nan, 1, np.nan], index=['a', 'b', 'd', 'e'])\n | >>> b\n | a 1.0\n | b NaN\n | d 1.0\n | e NaN\n | dtype: float64\n | >>> a.add(b, fill_value=0)\n | a 2.0\n | b 1.0\n | c 1.0\n | d 1.0\n | e NaN\n | dtype: float64\n | \n | See also\n | --------\n | Series.truediv\n | \n | searchsorted(self, value, side='left', sorter=None)\n | Find indices where elements should be inserted to maintain order.\n | \n | Find the indices into a sorted Series `self` such that, if the\n | corresponding elements in `value` were inserted before the indices,\n | the order of `self` would be preserved.\n | \n | Parameters\n | ----------\n | value : array_like\n | Values to insert into `self`.\n | side : {'left', 'right'}, optional\n | If 'left', the index of the first suitable location found is given.\n | If 'right', return the last such index. If there is no suitable\n | index, return either 0 or N (where N is the length of `self`).\n | sorter : 1-D array_like, optional\n | Optional array of integer indices that sort `self` into ascending\n | order. They are typically the result of ``np.argsort``.\n | \n | Returns\n | -------\n | indices : array of ints\n | Array of insertion points with the same shape as `value`.\n | \n | See Also\n | --------\n | numpy.searchsorted\n | \n | Notes\n | -----\n | Binary search is used to find the required insertion points.\n | \n | Examples\n | --------\n | \n | >>> x = pd.Series([1, 2, 3])\n | >>> x\n | 0 1\n | 1 2\n | 2 3\n | dtype: int64\n | \n | >>> x.searchsorted(4)\n | array([3])\n | \n | >>> x.searchsorted([0, 4])\n | array([0, 3])\n | \n | >>> x.searchsorted([1, 3], side='left')\n | array([0, 2])\n | \n | >>> x.searchsorted([1, 3], side='right')\n | array([1, 3])\n | \n | >>> x = pd.Categorical(['apple', 'bread', 'bread',\n | 'cheese', 'milk'], ordered=True)\n | [apple, bread, bread, cheese, milk]\n | Categories (4, object): [apple < bread < cheese < milk]\n | \n | >>> x.searchsorted('bread')\n | array([1]) # Note: an array, not a scalar\n | \n | >>> x.searchsorted(['bread'], side='right')\n | array([3])\n | \n | sem(self, axis=None, skipna=None, level=None, ddof=1, numeric_only=None, **kwargs)\n | Return unbiased standard error of the mean over requested axis.\n | \n | Normalized by N-1 by default. This can be changed using the ddof argument\n | \n | Parameters\n | ----------\n | axis : {index (0)}\n | skipna : boolean, default True\n | Exclude NA/null values. If an entire row/column is NA, the result\n | will be NA\n | level : int or level name, default None\n | If the axis is a MultiIndex (hierarchical), count along a\n | particular level, collapsing into a scalar\n | ddof : int, default 1\n | Delta Degrees of Freedom. The divisor used in calculations is N - ddof,\n | where N represents the number of elements.\n | numeric_only : boolean, default None\n | Include only float, int, boolean columns. If None, will attempt to use\n | everything, then use only numeric data. Not implemented for Series.\n | \n | Returns\n | -------\n | sem : scalar or Series (if level specified)\n | \n | set_value(self, label, value, takeable=False)\n | Quickly set single value at passed label. If label is not contained,\n | a new object is created with the label placed at the end of the result\n | index.\n | \n | .. deprecated:: 0.21.0\n | Please use .at[] or .iat[] accessors.\n | \n | Parameters\n | ----------\n | label : object\n | Partial indexing with MultiIndex not allowed\n | value : object\n | Scalar value\n | takeable : interpret the index as indexers, default False\n | \n | Returns\n | -------\n | series : Series\n | If label is contained, will be reference to calling Series,\n | otherwise a new object\n | \n | shift(self, periods=1, freq=None, axis=0)\n | Shift index by desired number of periods with an optional time freq\n | \n | Parameters\n | ----------\n | periods : int\n | Number of periods to move, can be positive or negative\n | freq : DateOffset, timedelta, or time rule string, optional\n | Increment to use from the tseries module or time rule (e.g. 'EOM').\n | See Notes.\n | axis : {0 or 'index'}\n | \n | Notes\n | -----\n | If freq is specified then the index values are shifted but the data\n | is not realigned. That is, use freq if you would like to extend the\n | index when shifting and preserve the original data.\n | \n | Returns\n | -------\n | shifted : Series\n | \n | skew(self, axis=None, skipna=None, level=None, numeric_only=None, **kwargs)\n | Return unbiased skew over requested axis\n | Normalized by N-1\n | \n | Parameters\n | ----------\n | axis : {index (0)}\n | skipna : boolean, default True\n | Exclude NA/null values when computing the result.\n | level : int or level name, default None\n | If the axis is a MultiIndex (hierarchical), count along a\n | particular level, collapsing into a scalar\n | numeric_only : boolean, default None\n | Include only float, int, boolean columns. If None, will attempt to use\n | everything, then use only numeric data. Not implemented for Series.\n | \n | Returns\n | -------\n | skew : scalar or Series (if level specified)\n | \n | sort_index(self, axis=0, level=None, ascending=True, inplace=False, kind='quicksort', na_position='last', sort_remaining=True)\n | Sort Series by index labels.\n | \n | Returns a new Series sorted by label if `inplace` argument is\n | ``False``, otherwise updates the original series and returns None.\n | \n | Parameters\n | ----------\n | axis : int, default 0\n | Axis to direct sorting. This can only be 0 for Series.\n | level : int, optional\n | If not None, sort on values in specified index level(s).\n | ascending : bool, default true\n | Sort ascending vs. descending.\n | inplace : bool, default False\n | If True, perform operation in-place.\n | kind : {'quicksort', 'mergesort', 'heapsort'}, default 'quicksort'\n | Choice of sorting algorithm. See also :func:`numpy.sort` for more\n | information. 'mergesort' is the only stable algorithm. For\n | DataFrames, this option is only applied when sorting on a single\n | column or label.\n | na_position : {'first', 'last'}, default 'last'\n | If 'first' puts NaNs at the beginning, 'last' puts NaNs at the end.\n | Not implemented for MultiIndex.\n | sort_remaining : bool, default True\n | If true and sorting by level and index is multilevel, sort by other\n | levels too (in order) after sorting by specified level.\n | \n | Returns\n | -------\n | pandas.Series\n | The original Series sorted by the labels\n | \n | See Also\n | --------\n | DataFrame.sort_index: Sort DataFrame by the index\n | DataFrame.sort_values: Sort DataFrame by the value\n | Series.sort_values : Sort Series by the value\n | \n | Examples\n | --------\n | >>> s = pd.Series(['a', 'b', 'c', 'd'], index=[3, 2, 1, 4])\n | >>> s.sort_index()\n | 1 c\n | 2 b\n | 3 a\n | 4 d\n | dtype: object\n | \n | Sort Descending\n | \n | >>> s.sort_index(ascending=False)\n | 4 d\n | 3 a\n | 2 b\n | 1 c\n | dtype: object\n | \n | Sort Inplace\n | \n | >>> s.sort_index(inplace=True)\n | >>> s\n | 1 c\n | 2 b\n | 3 a\n | 4 d\n | dtype: object\n | \n | By default NaNs are put at the end, but use `na_position` to place\n | them at the beginning\n | \n | >>> s = pd.Series(['a', 'b', 'c', 'd'], index=[3, 2, 1, np.nan])\n | >>> s.sort_index(na_position='first')\n | NaN d\n | 1.0 c\n | 2.0 b\n | 3.0 a\n | dtype: object\n | \n | Specify index level to sort\n | \n | >>> arrays = [np.array(['qux', 'qux', 'foo', 'foo',\n | ... 'baz', 'baz', 'bar', 'bar']),\n | ... np.array(['two', 'one', 'two', 'one',\n | ... 'two', 'one', 'two', 'one'])]\n | >>> s = pd.Series([1, 2, 3, 4, 5, 6, 7, 8], index=arrays)\n | >>> s.sort_index(level=1)\n | bar one 8\n | baz one 6\n | foo one 4\n | qux one 2\n | bar two 7\n | baz two 5\n | foo two 3\n | qux two 1\n | dtype: int64\n | \n | Does not sort by remaining levels when sorting by levels\n | \n | >>> s.sort_index(level=1, sort_remaining=False)\n | qux one 2\n | foo one 4\n | baz one 6\n | bar one 8\n | qux two 1\n | foo two 3\n | baz two 5\n | bar two 7\n | dtype: int64\n | \n | sort_values(self, axis=0, ascending=True, inplace=False, kind='quicksort', na_position='last')\n | Sort by the values.\n | \n | Sort a Series in ascending or descending order by some\n | criterion.\n | \n | Parameters\n | ----------\n | axis : {0 or 'index'}, default 0\n | Axis to direct sorting. The value 'index' is accepted for\n | compatibility with DataFrame.sort_values.\n | ascending : bool, default True\n | If True, sort values in ascending order, otherwise descending.\n | inplace : bool, default False\n | If True, perform operation in-place.\n | kind : {'quicksort', 'mergesort' or 'heapsort'}, default 'quicksort'\n | Choice of sorting algorithm. See also :func:`numpy.sort` for more\n | information. 'mergesort' is the only stable algorithm.\n | na_position : {'first' or 'last'}, default 'last'\n | Argument 'first' puts NaNs at the beginning, 'last' puts NaNs at\n | the end.\n | \n | Returns\n | -------\n | Series\n | Series ordered by values.\n | \n | See Also\n | --------\n | Series.sort_index : Sort by the Series indices.\n | DataFrame.sort_values : Sort DataFrame by the values along either axis.\n | DataFrame.sort_index : Sort DataFrame by indices.\n | \n | Examples\n | --------\n | >>> s = pd.Series([np.nan, 1, 3, 10, 5])\n | >>> s\n | 0 NaN\n | 1 1.0\n | 2 3.0\n | 3 10.0\n | 4 5.0\n | dtype: float64\n | \n | Sort values ascending order (default behaviour)\n | \n | >>> s.sort_values(ascending=True)\n | 1 1.0\n | 2 3.0\n | 4 5.0\n | 3 10.0\n | 0 NaN\n | dtype: float64\n | \n | Sort values descending order\n | \n | >>> s.sort_values(ascending=False)\n | 3 10.0\n | 4 5.0\n | 2 3.0\n | 1 1.0\n | 0 NaN\n | dtype: float64\n | \n | Sort values inplace\n | \n | >>> s.sort_values(ascending=False, inplace=True)\n | >>> s\n | 3 10.0\n | 4 5.0\n | 2 3.0\n | 1 1.0\n | 0 NaN\n | dtype: float64\n | \n | Sort values putting NAs first\n | \n | >>> s.sort_values(na_position='first')\n | 0 NaN\n | 1 1.0\n | 2 3.0\n | 4 5.0\n | 3 10.0\n | dtype: float64\n | \n | Sort a series of strings\n | \n | >>> s = pd.Series(['z', 'b', 'd', 'a', 'c'])\n | >>> s\n | 0 z\n | 1 b\n | 2 d\n | 3 a\n | 4 c\n | dtype: object\n | \n | >>> s.sort_values()\n | 3 a\n | 1 b\n | 4 c\n | 2 d\n | 0 z\n | dtype: object\n | \n | sortlevel(self, level=0, ascending=True, sort_remaining=True)\n | Sort Series with MultiIndex by chosen level. Data will be\n | lexicographically sorted by the chosen level followed by the other\n | levels (in order),\n | \n | .. deprecated:: 0.20.0\n | Use :meth:`Series.sort_index`\n | \n | Parameters\n | ----------\n | level : int or level name, default None\n | ascending : bool, default True\n | \n | Returns\n | -------\n | sorted : Series\n | \n | See Also\n | --------\n | Series.sort_index(level=...)\n | \n | std(self, axis=None, skipna=None, level=None, ddof=1, numeric_only=None, **kwargs)\n | Return sample standard deviation over requested axis.\n | \n | Normalized by N-1 by default. This can be changed using the ddof argument\n | \n | Parameters\n | ----------\n | axis : {index (0)}\n | skipna : boolean, default True\n | Exclude NA/null values. If an entire row/column is NA, the result\n | will be NA\n | level : int or level name, default None\n | If the axis is a MultiIndex (hierarchical), count along a\n | particular level, collapsing into a scalar\n | ddof : int, default 1\n | Delta Degrees of Freedom. The divisor used in calculations is N - ddof,\n | where N represents the number of elements.\n | numeric_only : boolean, default None\n | Include only float, int, boolean columns. If None, will attempt to use\n | everything, then use only numeric data. Not implemented for Series.\n | \n | Returns\n | -------\n | std : scalar or Series (if level specified)\n | \n | sub(self, other, level=None, fill_value=None, axis=0)\n | Subtraction of series and other, element-wise (binary operator `sub`).\n | \n | Equivalent to ``series - other``, but with support to substitute a fill_value for\n | missing data in one of the inputs.\n | \n | Parameters\n | ----------\n | other : Series or scalar value\n | fill_value : None or float value, default None (NaN)\n | Fill existing missing (NaN) values, and any new element needed for\n | successful Series alignment, with this value before computation.\n | If data in both corresponding Series locations is missing\n | the result will be missing\n | level : int or name\n | Broadcast across a level, matching Index values on the\n | passed MultiIndex level\n | \n | Returns\n | -------\n | result : Series\n | \n | Examples\n | --------\n | >>> a = pd.Series([1, 1, 1, np.nan], index=['a', 'b', 'c', 'd'])\n | >>> a\n | a 1.0\n | b 1.0\n | c 1.0\n | d NaN\n | dtype: float64\n | >>> b = pd.Series([1, np.nan, 1, np.nan], index=['a', 'b', 'd', 'e'])\n | >>> b\n | a 1.0\n | b NaN\n | d 1.0\n | e NaN\n | dtype: float64\n | >>> a.add(b, fill_value=0)\n | a 2.0\n | b 1.0\n | c 1.0\n | d 1.0\n | e NaN\n | dtype: float64\n | \n | See also\n | --------\n | Series.rsub\n | \n | subtract = sub(self, other, level=None, fill_value=None, axis=0)\n | Subtraction of series and other, element-wise (binary operator `sub`).\n | \n | Equivalent to ``series - other``, but with support to substitute a fill_value for\n | missing data in one of the inputs.\n | \n | Parameters\n | ----------\n | other : Series or scalar value\n | fill_value : None or float value, default None (NaN)\n | Fill existing missing (NaN) values, and any new element needed for\n | successful Series alignment, with this value before computation.\n | If data in both corresponding Series locations is missing\n | the result will be missing\n | level : int or name\n | Broadcast across a level, matching Index values on the\n | passed MultiIndex level\n | \n | Returns\n | -------\n | result : Series\n | \n | Examples\n | --------\n | >>> a = pd.Series([1, 1, 1, np.nan], index=['a', 'b', 'c', 'd'])\n | >>> a\n | a 1.0\n | b 1.0\n | c 1.0\n | d NaN\n | dtype: float64\n | >>> b = pd.Series([1, np.nan, 1, np.nan], index=['a', 'b', 'd', 'e'])\n | >>> b\n | a 1.0\n | b NaN\n | d 1.0\n | e NaN\n | dtype: float64\n | >>> a.add(b, fill_value=0)\n | a 2.0\n | b 1.0\n | c 1.0\n | d 1.0\n | e NaN\n | dtype: float64\n | \n | See also\n | --------\n | Series.rsub\n | \n | sum(self, axis=None, skipna=None, level=None, numeric_only=None, min_count=0, **kwargs)\n | Return the sum of the values for the requested axis\n | \n | Parameters\n | ----------\n | axis : {index (0)}\n | skipna : boolean, default True\n | Exclude NA/null values when computing the result.\n | level : int or level name, default None\n | If the axis is a MultiIndex (hierarchical), count along a\n | particular level, collapsing into a scalar\n | numeric_only : boolean, default None\n | Include only float, int, boolean columns. If None, will attempt to use\n | everything, then use only numeric data. Not implemented for Series.\n | min_count : int, default 0\n | The required number of valid values to perform the operation. If fewer than\n | ``min_count`` non-NA values are present the result will be NA.\n | \n | .. versionadded :: 0.22.0\n | \n | Added with the default being 0. This means the sum of an all-NA\n | or empty Series is 0, and the product of an all-NA or empty\n | Series is 1.\n | \n | Returns\n | -------\n | sum : scalar or Series (if level specified)\n | \n | Examples\n | --------\n | By default, the sum of an empty or all-NA Series is ``0``.\n | \n | >>> pd.Series([]).sum() # min_count=0 is the default\n | 0.0\n | \n | This can be controlled with the ``min_count`` parameter. For example, if\n | you'd like the sum of an empty series to be NaN, pass ``min_count=1``.\n | \n | >>> pd.Series([]).sum(min_count=1)\n | nan\n | \n | Thanks to the ``skipna`` parameter, ``min_count`` handles all-NA and\n | empty series identically.\n | \n | >>> pd.Series([np.nan]).sum()\n | 0.0\n | \n | >>> pd.Series([np.nan]).sum(min_count=1)\n | nan\n | \n | swaplevel(self, i=-2, j=-1, copy=True)\n | Swap levels i and j in a MultiIndex\n | \n | Parameters\n | ----------\n | i, j : int, string (can be mixed)\n | Level of index to be swapped. Can pass level name as string.\n | \n | Returns\n | -------\n | swapped : Series\n | \n | .. versionchanged:: 0.18.1\n | \n | The indexes ``i`` and ``j`` are now optional, and default to\n | the two innermost levels of the index.\n | \n | to_csv(self, path=None, index=True, sep=',', na_rep='', float_format=None, header=False, index_label=None, mode='w', encoding=None, compression=None, date_format=None, decimal='.')\n | Write Series to a comma-separated values (csv) file\n | \n | Parameters\n | ----------\n | path : string or file handle, default None\n | File path or object, if None is provided the result is returned as\n | a string.\n | na_rep : string, default ''\n | Missing data representation\n | float_format : string, default None\n | Format string for floating point numbers\n | header : boolean, default False\n | Write out series name\n | index : boolean, default True\n | Write row names (index)\n | index_label : string or sequence, default None\n | Column label for index column(s) if desired. If None is given, and\n | `header` and `index` are True, then the index names are used. A\n | sequence should be given if the DataFrame uses MultiIndex.\n | mode : Python write mode, default 'w'\n | sep : character, default \",\"\n | Field delimiter for the output file.\n | encoding : string, optional\n | a string representing the encoding to use if the contents are\n | non-ascii, for python versions prior to 3\n | compression : string, optional\n | A string representing the compression to use in the output file.\n | Allowed values are 'gzip', 'bz2', 'zip', 'xz'. This input is only\n | used when the first argument is a filename.\n | date_format: string, default None\n | Format string for datetime objects.\n | decimal: string, default '.'\n | Character recognized as decimal separator. E.g. use ',' for\n | European data\n | \n | to_dict(self, into=<class 'dict'>)\n | Convert Series to {label -> value} dict or dict-like object.\n | \n | Parameters\n | ----------\n | into : class, default dict\n | The collections.Mapping subclass to use as the return\n | object. Can be the actual class or an empty\n | instance of the mapping type you want. If you want a\n | collections.defaultdict, you must pass it initialized.\n | \n | .. versionadded:: 0.21.0\n | \n | Returns\n | -------\n | value_dict : collections.Mapping\n | \n | Examples\n | --------\n | >>> s = pd.Series([1, 2, 3, 4])\n | >>> s.to_dict()\n | {0: 1, 1: 2, 2: 3, 3: 4}\n | >>> from collections import OrderedDict, defaultdict\n | >>> s.to_dict(OrderedDict)\n | OrderedDict([(0, 1), (1, 2), (2, 3), (3, 4)])\n | >>> dd = defaultdict(list)\n | >>> s.to_dict(dd)\n | defaultdict(<type 'list'>, {0: 1, 1: 2, 2: 3, 3: 4})\n | \n | to_excel(self, excel_writer, sheet_name='Sheet1', na_rep='', float_format=None, columns=None, header=True, index=True, index_label=None, startrow=0, startcol=0, engine=None, merge_cells=True, encoding=None, inf_rep='inf', verbose=True)\n | Write Series to an excel sheet\n | \n | .. versionadded:: 0.20.0\n | \n | \n | Parameters\n | ----------\n | excel_writer : string or ExcelWriter object\n | File path or existing ExcelWriter\n | sheet_name : string, default 'Sheet1'\n | Name of sheet which will contain DataFrame\n | na_rep : string, default ''\n | Missing data representation\n | float_format : string, default None\n | Format string for floating point numbers\n | columns : sequence, optional\n | Columns to write\n | header : boolean or list of string, default True\n | Write out the column names. If a list of strings is given it is\n | assumed to be aliases for the column names\n | index : boolean, default True\n | Write row names (index)\n | index_label : string or sequence, default None\n | Column label for index column(s) if desired. If None is given, and\n | `header` and `index` are True, then the index names are used. A\n | sequence should be given if the DataFrame uses MultiIndex.\n | startrow :\n | upper left cell row to dump data frame\n | startcol :\n | upper left cell column to dump data frame\n | engine : string, default None\n | write engine to use - you can also set this via the options\n | ``io.excel.xlsx.writer``, ``io.excel.xls.writer``, and\n | ``io.excel.xlsm.writer``.\n | merge_cells : boolean, default True\n | Write MultiIndex and Hierarchical Rows as merged cells.\n | encoding: string, default None\n | encoding of the resulting excel file. Only necessary for xlwt,\n | other writers support unicode natively.\n | inf_rep : string, default 'inf'\n | Representation for infinity (there is no native representation for\n | infinity in Excel)\n | freeze_panes : tuple of integer (length 2), default None\n | Specifies the one-based bottommost row and rightmost column that\n | is to be frozen\n | \n | .. versionadded:: 0.20.0\n | \n | Notes\n | -----\n | If passing an existing ExcelWriter object, then the sheet will be added\n | to the existing workbook. This can be used to save different\n | DataFrames to one workbook:\n | \n | >>> writer = pd.ExcelWriter('output.xlsx')\n | >>> df1.to_excel(writer,'Sheet1')\n | >>> df2.to_excel(writer,'Sheet2')\n | >>> writer.save()\n | \n | For compatibility with to_csv, to_excel serializes lists and dicts to\n | strings before writing.\n | \n | to_frame(self, name=None)\n | Convert Series to DataFrame\n | \n | Parameters\n | ----------\n | name : object, default None\n | The passed name should substitute for the series name (if it has\n | one).\n | \n | Returns\n | -------\n | data_frame : DataFrame\n | \n | to_period(self, freq=None, copy=True)\n | Convert Series from DatetimeIndex to PeriodIndex with desired\n | frequency (inferred from index if not passed)\n | \n | Parameters\n | ----------\n | freq : string, default\n | \n | Returns\n | -------\n | ts : Series with PeriodIndex\n | \n | to_sparse(self, kind='block', fill_value=None)\n | Convert Series to SparseSeries\n | \n | Parameters\n | ----------\n | kind : {'block', 'integer'}\n | fill_value : float, defaults to NaN (missing)\n | \n | Returns\n | -------\n | sp : SparseSeries\n | \n | to_string(self, buf=None, na_rep='NaN', float_format=None, header=True, index=True, length=False, dtype=False, name=False, max_rows=None)\n | Render a string representation of the Series\n | \n | Parameters\n | ----------\n | buf : StringIO-like, optional\n | buffer to write to\n | na_rep : string, optional\n | string representation of NAN to use, default 'NaN'\n | float_format : one-parameter function, optional\n | formatter function to apply to columns' elements if they are floats\n | default None\n | header: boolean, default True\n | Add the Series header (index name)\n | index : bool, optional\n | Add index (row) labels, default True\n | length : boolean, default False\n | Add the Series length\n | dtype : boolean, default False\n | Add the Series dtype\n | name : boolean, default False\n | Add the Series name if not None\n | max_rows : int, optional\n | Maximum number of rows to show before truncating. If None, show\n | all.\n | \n | Returns\n | -------\n | formatted : string (if not buffer passed)\n | \n | to_timestamp(self, freq=None, how='start', copy=True)\n | Cast to datetimeindex of timestamps, at *beginning* of period\n | \n | Parameters\n | ----------\n | freq : string, default frequency of PeriodIndex\n | Desired frequency\n | how : {'s', 'e', 'start', 'end'}\n | Convention for converting period to timestamp; start of period\n | vs. end\n | \n | Returns\n | -------\n | ts : Series with DatetimeIndex\n | \n | transform(self, func, *args, **kwargs)\n | Call function producing a like-indexed NDFrame\n | and return a NDFrame with the transformed values\n | \n | .. versionadded:: 0.20.0\n | \n | Parameters\n | ----------\n | func : callable, string, dictionary, or list of string/callables\n | To apply to column\n | \n | Accepted Combinations are:\n | \n | - string function name\n | - function\n | - list of functions\n | - dict of column names -> functions (or list of functions)\n | \n | Returns\n | -------\n | transformed : NDFrame\n | \n | Examples\n | --------\n | >>> df = pd.DataFrame(np.random.randn(10, 3), columns=['A', 'B', 'C'],\n | ... index=pd.date_range('1/1/2000', periods=10))\n | df.iloc[3:7] = np.nan\n | \n | >>> df.transform(lambda x: (x - x.mean()) / x.std())\n | A B C\n | 2000-01-01 0.579457 1.236184 0.123424\n | 2000-01-02 0.370357 -0.605875 -1.231325\n | 2000-01-03 1.455756 -0.277446 0.288967\n | 2000-01-04 NaN NaN NaN\n | 2000-01-05 NaN NaN NaN\n | 2000-01-06 NaN NaN NaN\n | 2000-01-07 NaN NaN NaN\n | 2000-01-08 -0.498658 1.274522 1.642524\n | 2000-01-09 -0.540524 -1.012676 -0.828968\n | 2000-01-10 -1.366388 -0.614710 0.005378\n | \n | See also\n | --------\n | pandas.NDFrame.aggregate\n | pandas.NDFrame.apply\n | \n | truediv(self, other, level=None, fill_value=None, axis=0)\n | Floating division of series and other, element-wise (binary operator `truediv`).\n | \n | Equivalent to ``series / other``, but with support to substitute a fill_value for\n | missing data in one of the inputs.\n | \n | Parameters\n | ----------\n | other : Series or scalar value\n | fill_value : None or float value, default None (NaN)\n | Fill existing missing (NaN) values, and any new element needed for\n | successful Series alignment, with this value before computation.\n | If data in both corresponding Series locations is missing\n | the result will be missing\n | level : int or name\n | Broadcast across a level, matching Index values on the\n | passed MultiIndex level\n | \n | Returns\n | -------\n | result : Series\n | \n | Examples\n | --------\n | >>> a = pd.Series([1, 1, 1, np.nan], index=['a', 'b', 'c', 'd'])\n | >>> a\n | a 1.0\n | b 1.0\n | c 1.0\n | d NaN\n | dtype: float64\n | >>> b = pd.Series([1, np.nan, 1, np.nan], index=['a', 'b', 'd', 'e'])\n | >>> b\n | a 1.0\n | b NaN\n | d 1.0\n | e NaN\n | dtype: float64\n | >>> a.add(b, fill_value=0)\n | a 2.0\n | b 1.0\n | c 1.0\n | d 1.0\n | e NaN\n | dtype: float64\n | \n | See also\n | --------\n | Series.rtruediv\n | \n | unique(self)\n | Return unique values of Series object.\n | \n | Uniques are returned in order of appearance. Hash table-based unique,\n | therefore does NOT sort.\n | \n | Returns\n | -------\n | ndarray or Categorical\n | The unique values returned as a NumPy array. In case of categorical\n | data type, returned as a Categorical.\n | \n | See Also\n | --------\n | pandas.unique : top-level unique method for any 1-d array-like object.\n | Index.unique : return Index with unique values from an Index object.\n | \n | Examples\n | --------\n | >>> pd.Series([2, 1, 3, 3], name='A').unique()\n | array([2, 1, 3])\n | \n | >>> pd.Series([pd.Timestamp('2016-01-01') for _ in range(3)]).unique()\n | array(['2016-01-01T00:00:00.000000000'], dtype='datetime64[ns]')\n | \n | >>> pd.Series([pd.Timestamp('2016-01-01', tz='US/Eastern')\n | ... for _ in range(3)]).unique()\n | array([Timestamp('2016-01-01 00:00:00-0500', tz='US/Eastern')],\n | dtype=object)\n | \n | An unordered Categorical will return categories in the order of\n | appearance.\n | \n | >>> pd.Series(pd.Categorical(list('baabc'))).unique()\n | [b, a, c]\n | Categories (3, object): [b, a, c]\n | \n | An ordered Categorical preserves the category ordering.\n | \n | >>> pd.Series(pd.Categorical(list('baabc'), categories=list('abc'),\n | ... ordered=True)).unique()\n | [b, a, c]\n | Categories (3, object): [a < b < c]\n | \n | unstack(self, level=-1, fill_value=None)\n | Unstack, a.k.a. pivot, Series with MultiIndex to produce DataFrame.\n | The level involved will automatically get sorted.\n | \n | Parameters\n | ----------\n | level : int, string, or list of these, default last level\n | Level(s) to unstack, can pass level name\n | fill_value : replace NaN with this value if the unstack produces\n | missing values\n | \n | .. versionadded:: 0.18.0\n | \n | Examples\n | --------\n | >>> s = pd.Series([1, 2, 3, 4],\n | ... index=pd.MultiIndex.from_product([['one', 'two'], ['a', 'b']]))\n | >>> s\n | one a 1\n | b 2\n | two a 3\n | b 4\n | dtype: int64\n | \n | >>> s.unstack(level=-1)\n | a b\n | one 1 2\n | two 3 4\n | \n | >>> s.unstack(level=0)\n | one two\n | a 1 3\n | b 2 4\n | \n | Returns\n | -------\n | unstacked : DataFrame\n | \n | update(self, other)\n | Modify Series in place using non-NA values from passed\n | Series. Aligns on index\n | \n | Parameters\n | ----------\n | other : Series\n | \n | Examples\n | --------\n | >>> s = pd.Series([1, 2, 3])\n | >>> s.update(pd.Series([4, 5, 6]))\n | >>> s\n | 0 4\n | 1 5\n | 2 6\n | dtype: int64\n | \n | >>> s = pd.Series(['a', 'b', 'c'])\n | >>> s.update(pd.Series(['d', 'e'], index=[0, 2]))\n | >>> s\n | 0 d\n | 1 b\n | 2 e\n | dtype: object\n | \n | >>> s = pd.Series([1, 2, 3])\n | >>> s.update(pd.Series([4, 5, 6, 7, 8]))\n | >>> s\n | 0 4\n | 1 5\n | 2 6\n | dtype: int64\n | \n | If ``other`` contains NaNs the corresponding values are not updated\n | in the original Series.\n | \n | >>> s = pd.Series([1, 2, 3])\n | >>> s.update(pd.Series([4, np.nan, 6]))\n | >>> s\n | 0 4\n | 1 2\n | 2 6\n | dtype: int64\n | \n | valid(self, inplace=False, **kwargs)\n | Return Series without null values.\n | \n | .. deprecated:: 0.23.0\n | Use :meth:`Series.dropna` instead.\n | \n | var(self, axis=None, skipna=None, level=None, ddof=1, numeric_only=None, **kwargs)\n | Return unbiased variance over requested axis.\n | \n | Normalized by N-1 by default. This can be changed using the ddof argument\n | \n | Parameters\n | ----------\n | axis : {index (0)}\n | skipna : boolean, default True\n | Exclude NA/null values. If an entire row/column is NA, the result\n | will be NA\n | level : int or level name, default None\n | If the axis is a MultiIndex (hierarchical), count along a\n | particular level, collapsing into a scalar\n | ddof : int, default 1\n | Delta Degrees of Freedom. The divisor used in calculations is N - ddof,\n | where N represents the number of elements.\n | numeric_only : boolean, default None\n | Include only float, int, boolean columns. If None, will attempt to use\n | everything, then use only numeric data. Not implemented for Series.\n | \n | Returns\n | -------\n | var : scalar or Series (if level specified)\n | \n | view(self, dtype=None)\n | Create a new view of the Series.\n | \n | This function will return a new Series with a view of the same\n | underlying values in memory, optionally reinterpreted with a new data\n | type. The new data type must preserve the same size in bytes as to not\n | cause index misalignment.\n | \n | Parameters\n | ----------\n | dtype : data type\n | Data type object or one of their string representations.\n | \n | Returns\n | -------\n | Series\n | A new Series object as a view of the same data in memory.\n | \n | See Also\n | --------\n | numpy.ndarray.view : Equivalent numpy function to create a new view of\n | the same data in memory.\n | \n | Notes\n | -----\n | Series are instantiated with ``dtype=float64`` by default. While\n | ``numpy.ndarray.view()`` will return a view with the same data type as\n | the original array, ``Series.view()`` (without specified dtype)\n | will try using ``float64`` and may fail if the original data type size\n | in bytes is not the same.\n | \n | Examples\n | --------\n | >>> s = pd.Series([-2, -1, 0, 1, 2], dtype='int8')\n | >>> s\n | 0 -2\n | 1 -1\n | 2 0\n | 3 1\n | 4 2\n | dtype: int8\n | \n | The 8 bit signed integer representation of `-1` is `0b11111111`, but\n | the same bytes represent 255 if read as an 8 bit unsigned integer:\n | \n | >>> us = s.view('uint8')\n | >>> us\n | 0 254\n | 1 255\n | 2 0\n | 3 1\n | 4 2\n | dtype: uint8\n | \n | The views share the same underlying values:\n | \n | >>> us[0] = 128\n | >>> s\n | 0 -128\n | 1 -1\n | 2 0\n | 3 1\n | 4 2\n | dtype: int8\n | \n | ----------------------------------------------------------------------\n | Class methods inherited from pandas.core.series.Series:\n | \n | from_array(arr, index=None, name=None, dtype=None, copy=False, fastpath=False) from builtins.type\n | Construct Series from array.\n | \n | .. deprecated :: 0.23.0\n | Use pd.Series(..) constructor instead.\n | \n | from_csv(path, sep=',', parse_dates=True, header=None, index_col=0, encoding=None, infer_datetime_format=False) from builtins.type\n | Read CSV file.\n | \n | .. deprecated:: 0.21.0\n | Use :func:`pandas.read_csv` instead.\n | \n | It is preferable to use the more powerful :func:`pandas.read_csv`\n | for most general purposes, but ``from_csv`` makes for an easy\n | roundtrip to and from a file (the exact counterpart of\n | ``to_csv``), especially with a time Series.\n | \n | This method only differs from :func:`pandas.read_csv` in some defaults:\n | \n | - `index_col` is ``0`` instead of ``None`` (take first column as index\n | by default)\n | - `header` is ``None`` instead of ``0`` (the first row is not used as\n | the column names)\n | - `parse_dates` is ``True`` instead of ``False`` (try parsing the index\n | as datetime by default)\n | \n | With :func:`pandas.read_csv`, the option ``squeeze=True`` can be used\n | to return a Series like ``from_csv``.\n | \n | Parameters\n | ----------\n | path : string file path or file handle / StringIO\n | sep : string, default ','\n | Field delimiter\n | parse_dates : boolean, default True\n | Parse dates. Different default from read_table\n | header : int, default None\n | Row to use as header (skip prior rows)\n | index_col : int or sequence, default 0\n | Column to use for index. If a sequence is given, a MultiIndex\n | is used. Different default from read_table\n | encoding : string, optional\n | a string representing the encoding to use if the contents are\n | non-ascii, for python versions prior to 3\n | infer_datetime_format: boolean, default False\n | If True and `parse_dates` is True for a column, try to infer the\n | datetime format based on the first datetime string. If the format\n | can be inferred, there often will be a large parsing speed-up.\n | \n | See also\n | --------\n | pandas.read_csv\n | \n | Returns\n | -------\n | y : Series\n | \n | ----------------------------------------------------------------------\n | Data descriptors inherited from pandas.core.series.Series:\n | \n | asobject\n | Return object Series which contains boxed values.\n | \n | .. deprecated :: 0.23.0\n | \n | Use ``astype(object)`` instead.\n | \n | *this is an internal non-public method*\n | \n | axes\n | Return a list of the row axis labels\n | \n | dtype\n | return the dtype object of the underlying data\n | \n | dtypes\n | return the dtype object of the underlying data\n | \n | ftype\n | return if the data is sparse|dense\n | \n | ftypes\n | return if the data is sparse|dense\n | \n | imag\n | \n | index\n | The index (axis labels) of the Series.\n | \n | name\n | \n | real\n | \n | values\n | Return Series as ndarray or ndarray-like\n | depending on the dtype\n | \n | Returns\n | -------\n | arr : numpy.ndarray or ndarray-like\n | \n | Examples\n | --------\n | >>> pd.Series([1, 2, 3]).values\n | array([1, 2, 3])\n | \n | >>> pd.Series(list('aabc')).values\n | array(['a', 'a', 'b', 'c'], dtype=object)\n | \n | >>> pd.Series(list('aabc')).astype('category').values\n | [a, a, b, c]\n | Categories (3, object): [a, b, c]\n | \n | Timezone aware datetime data is converted to UTC:\n | \n | >>> pd.Series(pd.date_range('20130101', periods=3,\n | ... tz='US/Eastern')).values\n | array(['2013-01-01T05:00:00.000000000',\n | '2013-01-02T05:00:00.000000000',\n | '2013-01-03T05:00:00.000000000'], dtype='datetime64[ns]')\n | \n | ----------------------------------------------------------------------\n | Data and other attributes inherited from pandas.core.series.Series:\n | \n | cat = <class 'pandas.core.arrays.categorical.CategoricalAccessor'>\n | Accessor object for categorical properties of the Series values.\n | \n | Be aware that assigning to `categories` is a inplace operation, while all\n | methods return new categorical data per default (but can be called with\n | `inplace=True`).\n | \n | Parameters\n | ----------\n | data : Series or CategoricalIndex\n | \n | Examples\n | --------\n | >>> s.cat.categories\n | >>> s.cat.categories = list('abc')\n | >>> s.cat.rename_categories(list('cab'))\n | >>> s.cat.reorder_categories(list('cab'))\n | >>> s.cat.add_categories(['d','e'])\n | >>> s.cat.remove_categories(['d'])\n | >>> s.cat.remove_unused_categories()\n | >>> s.cat.set_categories(list('abcde'))\n | >>> s.cat.as_ordered()\n | >>> s.cat.as_unordered()\n | \n | plot = <class 'pandas.plotting._core.SeriesPlotMethods'>\n | Series plotting accessor and method\n | \n | Examples\n | --------\n | >>> s.plot.line()\n | >>> s.plot.bar()\n | >>> s.plot.hist()\n | \n | Plotting methods can also be accessed by calling the accessor as a method\n | with the ``kind`` argument:\n | ``s.plot(kind='line')`` is equivalent to ``s.plot.line()``\n | \n | str = <class 'pandas.core.strings.StringMethods'>\n | Vectorized string functions for Series and Index. NAs stay NA unless\n | handled otherwise by a particular method. Patterned after Python's string\n | methods, with some inspiration from R's stringr package.\n | \n | Examples\n | --------\n | >>> s.str.split('_')\n | >>> s.str.replace('_', '')\n | \n | ----------------------------------------------------------------------\n | Methods inherited from pandas.core.base.IndexOpsMixin:\n | \n | __iter__(self)\n | Return an iterator of the values.\n | \n | These are each a scalar type, which is a Python scalar\n | (for str, int, float) or a pandas scalar\n | (for Timestamp/Timedelta/Interval/Period)\n | \n | factorize(self, sort=False, na_sentinel=-1)\n | Encode the object as an enumerated type or categorical variable.\n | \n | This method is useful for obtaining a numeric representation of an\n | array when all that matters is identifying distinct values. `factorize`\n | is available as both a top-level function :func:`pandas.factorize`,\n | and as a method :meth:`Series.factorize` and :meth:`Index.factorize`.\n | \n | Parameters\n | ----------\n | sort : boolean, default False\n | Sort `uniques` and shuffle `labels` to maintain the\n | relationship.\n | \n | na_sentinel : int, default -1\n | Value to mark \"not found\".\n | \n | Returns\n | -------\n | labels : ndarray\n | An integer ndarray that's an indexer into `uniques`.\n | ``uniques.take(labels)`` will have the same values as `values`.\n | uniques : ndarray, Index, or Categorical\n | The unique valid values. When `values` is Categorical, `uniques`\n | is a Categorical. When `values` is some other pandas object, an\n | `Index` is returned. Otherwise, a 1-D ndarray is returned.\n | \n | .. note ::\n | \n | Even if there's a missing value in `values`, `uniques` will\n | *not* contain an entry for it.\n | \n | See Also\n | --------\n | pandas.cut : Discretize continuous-valued array.\n | pandas.unique : Find the unique valuse in an array.\n | \n | Examples\n | --------\n | These examples all show factorize as a top-level method like\n | ``pd.factorize(values)``. The results are identical for methods like\n | :meth:`Series.factorize`.\n | \n | >>> labels, uniques = pd.factorize(['b', 'b', 'a', 'c', 'b'])\n | >>> labels\n | array([0, 0, 1, 2, 0])\n | >>> uniques\n | array(['b', 'a', 'c'], dtype=object)\n | \n | With ``sort=True``, the `uniques` will be sorted, and `labels` will be\n | shuffled so that the relationship is the maintained.\n | \n | >>> labels, uniques = pd.factorize(['b', 'b', 'a', 'c', 'b'], sort=True)\n | >>> labels\n | array([1, 1, 0, 2, 1])\n | >>> uniques\n | array(['a', 'b', 'c'], dtype=object)\n | \n | Missing values are indicated in `labels` with `na_sentinel`\n | (``-1`` by default). Note that missing values are never\n | included in `uniques`.\n | \n | >>> labels, uniques = pd.factorize(['b', None, 'a', 'c', 'b'])\n | >>> labels\n | array([ 0, -1, 1, 2, 0])\n | >>> uniques\n | array(['b', 'a', 'c'], dtype=object)\n | \n | Thus far, we've only factorized lists (which are internally coerced to\n | NumPy arrays). When factorizing pandas objects, the type of `uniques`\n | will differ. For Categoricals, a `Categorical` is returned.\n | \n | >>> cat = pd.Categorical(['a', 'a', 'c'], categories=['a', 'b', 'c'])\n | >>> labels, uniques = pd.factorize(cat)\n | >>> labels\n | array([0, 0, 1])\n | >>> uniques\n | [a, c]\n | Categories (3, object): [a, b, c]\n | \n | Notice that ``'b'`` is in ``uniques.categories``, desipite not being\n | present in ``cat.values``.\n | \n | For all other pandas objects, an Index of the appropriate type is\n | returned.\n | \n | >>> cat = pd.Series(['a', 'a', 'c'])\n | >>> labels, uniques = pd.factorize(cat)\n | >>> labels\n | array([0, 0, 1])\n | >>> uniques\n | Index(['a', 'c'], dtype='object')\n | \n | item(self)\n | return the first element of the underlying data as a python\n | scalar\n | \n | nunique(self, dropna=True)\n | Return number of unique elements in the object.\n | \n | Excludes NA values by default.\n | \n | Parameters\n | ----------\n | dropna : boolean, default True\n | Don't include NaN in the count.\n | \n | Returns\n | -------\n | nunique : int\n | \n | tolist(self)\n | Return a list of the values.\n | \n | These are each a scalar type, which is a Python scalar\n | (for str, int, float) or a pandas scalar\n | (for Timestamp/Timedelta/Interval/Period)\n | \n | See Also\n | --------\n | numpy.ndarray.tolist\n | \n | transpose(self, *args, **kwargs)\n | return the transpose, which is by definition self\n | \n | value_counts(self, normalize=False, sort=True, ascending=False, bins=None, dropna=True)\n | Returns object containing counts of unique values.\n | \n | The resulting object will be in descending order so that the\n | first element is the most frequently-occurring element.\n | Excludes NA values by default.\n | \n | Parameters\n | ----------\n | normalize : boolean, default False\n | If True then the object returned will contain the relative\n | frequencies of the unique values.\n | sort : boolean, default True\n | Sort by values\n | ascending : boolean, default False\n | Sort in ascending order\n | bins : integer, optional\n | Rather than count values, group them into half-open bins,\n | a convenience for pd.cut, only works with numeric data\n | dropna : boolean, default True\n | Don't include counts of NaN.\n | \n | Returns\n | -------\n | counts : Series\n | \n | ----------------------------------------------------------------------\n | Data descriptors inherited from pandas.core.base.IndexOpsMixin:\n | \n | __dict__\n | dictionary for instance variables (if defined)\n | \n | __weakref__\n | list of weak references to the object (if defined)\n | \n | base\n | return the base object if the memory of the underlying data is\n | shared\n | \n | data\n | return the data pointer of the underlying data\n | \n | empty\n | \n | flags\n | return the ndarray.flags for the underlying data\n | \n | hasnans\n | return if I have any nans; enables various perf speedups\n | \n | is_monotonic\n | Return boolean if values in the object are\n | monotonic_increasing\n | \n | .. versionadded:: 0.19.0\n | \n | Returns\n | -------\n | is_monotonic : boolean\n | \n | is_monotonic_decreasing\n | Return boolean if values in the object are\n | monotonic_decreasing\n | \n | .. versionadded:: 0.19.0\n | \n | Returns\n | -------\n | is_monotonic_decreasing : boolean\n | \n | is_monotonic_increasing\n | Return boolean if values in the object are\n | monotonic_increasing\n | \n | .. versionadded:: 0.19.0\n | \n | Returns\n | -------\n | is_monotonic : boolean\n | \n | is_unique\n | Return boolean if values in the object are unique\n | \n | Returns\n | -------\n | is_unique : boolean\n | \n | itemsize\n | return the size of the dtype of the item of the underlying data\n | \n | nbytes\n | return the number of bytes in the underlying data\n | \n | ndim\n | return the number of dimensions of the underlying data,\n | by definition 1\n | \n | shape\n | return a tuple of the shape of the underlying data\n | \n | size\n | return the number of elements in the underlying data\n | \n | strides\n | return the strides of the underlying data\n | \n | ----------------------------------------------------------------------\n | Data and other attributes inherited from pandas.core.base.IndexOpsMixin:\n | \n | __array_priority__ = 1000\n | \n | ----------------------------------------------------------------------\n | Methods inherited from pandas.core.generic.NDFrame:\n | \n | __abs__(self)\n | \n | __bool__ = __nonzero__(self)\n | \n | __contains__(self, key)\n | True if the key is in the info axis\n | \n | __deepcopy__(self, memo=None)\n | \n | __delitem__(self, key)\n | Delete item\n | \n | __finalize__(self, other, method=None, **kwargs)\n | Propagate metadata from other to self.\n | \n | Parameters\n | ----------\n | other : the object from which to get the attributes that we are going\n | to propagate\n | method : optional, a passed method name ; possibly to take different\n | types of propagation actions based on this\n | \n | __getattr__(self, name)\n | After regular attribute access, try looking up the name\n | This allows simpler access to columns for interactive use.\n | \n | __getstate__(self)\n | \n | __hash__(self)\n | Return hash(self).\n | \n | __invert__(self)\n | \n | __neg__(self)\n | \n | __nonzero__(self)\n | \n | __pos__(self)\n | \n | __round__(self, decimals=0)\n | \n | __setattr__(self, name, value)\n | After regular attribute access, try setting the name\n | This allows simpler access to columns for interactive use.\n | \n | __setstate__(self, state)\n | \n | abs(self)\n | Return a Series/DataFrame with absolute numeric value of each element.\n | \n | This function only applies to elements that are all numeric.\n | \n | Returns\n | -------\n | abs\n | Series/DataFrame containing the absolute value of each element.\n | \n | Notes\n | -----\n | For ``complex`` inputs, ``1.2 + 1j``, the absolute value is\n | :math:`\\sqrt{ a^2 + b^2 }`.\n | \n | Examples\n | --------\n | Absolute numeric values in a Series.\n | \n | >>> s = pd.Series([-1.10, 2, -3.33, 4])\n | >>> s.abs()\n | 0 1.10\n | 1 2.00\n | 2 3.33\n | 3 4.00\n | dtype: float64\n | \n | Absolute numeric values in a Series with complex numbers.\n | \n | >>> s = pd.Series([1.2 + 1j])\n | >>> s.abs()\n | 0 1.56205\n | dtype: float64\n | \n | Absolute numeric values in a Series with a Timedelta element.\n | \n | >>> s = pd.Series([pd.Timedelta('1 days')])\n | >>> s.abs()\n | 0 1 days\n | dtype: timedelta64[ns]\n | \n | Select rows with data closest to certain value using argsort (from\n | `StackOverflow <https://stackoverflow.com/a/17758115>`__).\n | \n | >>> df = pd.DataFrame({\n | ... 'a': [4, 5, 6, 7],\n | ... 'b': [10, 20, 30, 40],\n | ... 'c': [100, 50, -30, -50]\n | ... })\n | >>> df\n | a b c\n | 0 4 10 100\n | 1 5 20 50\n | 2 6 30 -30\n | 3 7 40 -50\n | >>> df.loc[(df.c - 43).abs().argsort()]\n | a b c\n | 1 5 20 50\n | 0 4 10 100\n | 2 6 30 -30\n | 3 7 40 -50\n | \n | See Also\n | --------\n | numpy.absolute : calculate the absolute value element-wise.\n | \n | add_prefix(self, prefix)\n | Prefix labels with string `prefix`.\n | \n | For Series, the row labels are prefixed.\n | For DataFrame, the column labels are prefixed.\n | \n | Parameters\n | ----------\n | prefix : str\n | The string to add before each label.\n | \n | Returns\n | -------\n | Series or DataFrame\n | New Series or DataFrame with updated labels.\n | \n | See Also\n | --------\n | Series.add_suffix: Suffix row labels with string `suffix`.\n | DataFrame.add_suffix: Suffix column labels with string `suffix`.\n | \n | Examples\n | --------\n | >>> s = pd.Series([1, 2, 3, 4])\n | >>> s\n | 0 1\n | 1 2\n | 2 3\n | 3 4\n | dtype: int64\n | \n | >>> s.add_prefix('item_')\n | item_0 1\n | item_1 2\n | item_2 3\n | item_3 4\n | dtype: int64\n | \n | >>> df = pd.DataFrame({'A': [1, 2, 3, 4], 'B': [3, 4, 5, 6]})\n | >>> df\n | A B\n | 0 1 3\n | 1 2 4\n | 2 3 5\n | 3 4 6\n | \n | >>> df.add_prefix('col_')\n | col_A col_B\n | 0 1 3\n | 1 2 4\n | 2 3 5\n | 3 4 6\n | \n | add_suffix(self, suffix)\n | Suffix labels with string `suffix`.\n | \n | For Series, the row labels are suffixed.\n | For DataFrame, the column labels are suffixed.\n | \n | Parameters\n | ----------\n | suffix : str\n | The string to add after each label.\n | \n | Returns\n | -------\n | Series or DataFrame\n | New Series or DataFrame with updated labels.\n | \n | See Also\n | --------\n | Series.add_prefix: Prefix row labels with string `prefix`.\n | DataFrame.add_prefix: Prefix column labels with string `prefix`.\n | \n | Examples\n | --------\n | >>> s = pd.Series([1, 2, 3, 4])\n | >>> s\n | 0 1\n | 1 2\n | 2 3\n | 3 4\n | dtype: int64\n | \n | >>> s.add_suffix('_item')\n | 0_item 1\n | 1_item 2\n | 2_item 3\n | 3_item 4\n | dtype: int64\n | \n | >>> df = pd.DataFrame({'A': [1, 2, 3, 4], 'B': [3, 4, 5, 6]})\n | >>> df\n | A B\n | 0 1 3\n | 1 2 4\n | 2 3 5\n | 3 4 6\n | \n | >>> df.add_suffix('_col')\n | A_col B_col\n | 0 1 3\n | 1 2 4\n | 2 3 5\n | 3 4 6\n | \n | as_blocks(self, copy=True)\n | Convert the frame to a dict of dtype -> Constructor Types that each has\n | a homogeneous dtype.\n | \n | .. deprecated:: 0.21.0\n | \n | NOTE: the dtypes of the blocks WILL BE PRESERVED HERE (unlike in\n | as_matrix)\n | \n | Parameters\n | ----------\n | copy : boolean, default True\n | \n | Returns\n | -------\n | values : a dict of dtype -> Constructor Types\n | \n | as_matrix(self, columns=None)\n | Convert the frame to its Numpy-array representation.\n | \n | .. deprecated:: 0.23.0\n | Use :meth:`DataFrame.values` instead.\n | \n | Parameters\n | ----------\n | columns: list, optional, default:None\n | If None, return all columns, otherwise, returns specified columns.\n | \n | Returns\n | -------\n | values : ndarray\n | If the caller is heterogeneous and contains booleans or objects,\n | the result will be of dtype=object. See Notes.\n | \n | \n | Notes\n | -----\n | Return is NOT a Numpy-matrix, rather, a Numpy-array.\n | \n | The dtype will be a lower-common-denominator dtype (implicit\n | upcasting); that is to say if the dtypes (even of numeric types)\n | are mixed, the one that accommodates all will be chosen. Use this\n | with care if you are not dealing with the blocks.\n | \n | e.g. If the dtypes are float16 and float32, dtype will be upcast to\n | float32. If dtypes are int32 and uint8, dtype will be upcase to\n | int32. By numpy.find_common_type convention, mixing int64 and uint64\n | will result in a flot64 dtype.\n | \n | This method is provided for backwards compatibility. Generally,\n | it is recommended to use '.values'.\n | \n | See Also\n | --------\n | pandas.DataFrame.values\n | \n | asfreq(self, freq, method=None, how=None, normalize=False, fill_value=None)\n | Convert TimeSeries to specified frequency.\n | \n | Optionally provide filling method to pad/backfill missing values.\n | \n | Returns the original data conformed to a new index with the specified\n | frequency. ``resample`` is more appropriate if an operation, such as\n | summarization, is necessary to represent the data at the new frequency.\n | \n | Parameters\n | ----------\n | freq : DateOffset object, or string\n | method : {'backfill'/'bfill', 'pad'/'ffill'}, default None\n | Method to use for filling holes in reindexed Series (note this\n | does not fill NaNs that already were present):\n | \n | * 'pad' / 'ffill': propagate last valid observation forward to next\n | valid\n | * 'backfill' / 'bfill': use NEXT valid observation to fill\n | how : {'start', 'end'}, default end\n | For PeriodIndex only, see PeriodIndex.asfreq\n | normalize : bool, default False\n | Whether to reset output index to midnight\n | fill_value: scalar, optional\n | Value to use for missing values, applied during upsampling (note\n | this does not fill NaNs that already were present).\n | \n | .. versionadded:: 0.20.0\n | \n | Returns\n | -------\n | converted : type of caller\n | \n | Examples\n | --------\n | \n | Start by creating a series with 4 one minute timestamps.\n | \n | >>> index = pd.date_range('1/1/2000', periods=4, freq='T')\n | >>> series = pd.Series([0.0, None, 2.0, 3.0], index=index)\n | >>> df = pd.DataFrame({'s':series})\n | >>> df\n | s\n | 2000-01-01 00:00:00 0.0\n | 2000-01-01 00:01:00 NaN\n | 2000-01-01 00:02:00 2.0\n | 2000-01-01 00:03:00 3.0\n | \n | Upsample the series into 30 second bins.\n | \n | >>> df.asfreq(freq='30S')\n | s\n | 2000-01-01 00:00:00 0.0\n | 2000-01-01 00:00:30 NaN\n | 2000-01-01 00:01:00 NaN\n | 2000-01-01 00:01:30 NaN\n | 2000-01-01 00:02:00 2.0\n | 2000-01-01 00:02:30 NaN\n | 2000-01-01 00:03:00 3.0\n | \n | Upsample again, providing a ``fill value``.\n | \n | >>> df.asfreq(freq='30S', fill_value=9.0)\n | s\n | 2000-01-01 00:00:00 0.0\n | 2000-01-01 00:00:30 9.0\n | 2000-01-01 00:01:00 NaN\n | 2000-01-01 00:01:30 9.0\n | 2000-01-01 00:02:00 2.0\n | 2000-01-01 00:02:30 9.0\n | 2000-01-01 00:03:00 3.0\n | \n | Upsample again, providing a ``method``.\n | \n | >>> df.asfreq(freq='30S', method='bfill')\n | s\n | 2000-01-01 00:00:00 0.0\n | 2000-01-01 00:00:30 NaN\n | 2000-01-01 00:01:00 NaN\n | 2000-01-01 00:01:30 2.0\n | 2000-01-01 00:02:00 2.0\n | 2000-01-01 00:02:30 3.0\n | 2000-01-01 00:03:00 3.0\n | \n | See Also\n | --------\n | reindex\n | \n | Notes\n | -----\n | To learn more about the frequency strings, please see `this link\n | <http://pandas.pydata.org/pandas-docs/stable/timeseries.html#offset-aliases>`__.\n | \n | asof(self, where, subset=None)\n | The last row without any NaN is taken (or the last row without\n | NaN considering only the subset of columns in the case of a DataFrame)\n | \n | .. versionadded:: 0.19.0 For DataFrame\n | \n | If there is no good value, NaN is returned for a Series\n | a Series of NaN values for a DataFrame\n | \n | Parameters\n | ----------\n | where : date or array of dates\n | subset : string or list of strings, default None\n | if not None use these columns for NaN propagation\n | \n | Notes\n | -----\n | Dates are assumed to be sorted\n | Raises if this is not the case\n | \n | Returns\n | -------\n | where is scalar\n | \n | - value or NaN if input is Series\n | - Series if input is DataFrame\n | \n | where is Index: same shape object as input\n | \n | See Also\n | --------\n | merge_asof\n | \n | astype(self, dtype, copy=True, errors='raise', **kwargs)\n | Cast a pandas object to a specified dtype ``dtype``.\n | \n | Parameters\n | ----------\n | dtype : data type, or dict of column name -> data type\n | Use a numpy.dtype or Python type to cast entire pandas object to\n | the same type. Alternatively, use {col: dtype, ...}, where col is a\n | column label and dtype is a numpy.dtype or Python type to cast one\n | or more of the DataFrame's columns to column-specific types.\n | copy : bool, default True.\n | Return a copy when ``copy=True`` (be very careful setting\n | ``copy=False`` as changes to values then may propagate to other\n | pandas objects).\n | errors : {'raise', 'ignore'}, default 'raise'.\n | Control raising of exceptions on invalid data for provided dtype.\n | \n | - ``raise`` : allow exceptions to be raised\n | - ``ignore`` : suppress exceptions. On error return original object\n | \n | .. versionadded:: 0.20.0\n | \n | raise_on_error : raise on invalid input\n | .. deprecated:: 0.20.0\n | Use ``errors`` instead\n | kwargs : keyword arguments to pass on to the constructor\n | \n | Returns\n | -------\n | casted : type of caller\n | \n | Examples\n | --------\n | >>> ser = pd.Series([1, 2], dtype='int32')\n | >>> ser\n | 0 1\n | 1 2\n | dtype: int32\n | >>> ser.astype('int64')\n | 0 1\n | 1 2\n | dtype: int64\n | \n | Convert to categorical type:\n | \n | >>> ser.astype('category')\n | 0 1\n | 1 2\n | dtype: category\n | Categories (2, int64): [1, 2]\n | \n | Convert to ordered categorical type with custom ordering:\n | \n | >>> ser.astype('category', ordered=True, categories=[2, 1])\n | 0 1\n | 1 2\n | dtype: category\n | Categories (2, int64): [2 < 1]\n | \n | Note that using ``copy=False`` and changing data on a new\n | pandas object may propagate changes:\n | \n | >>> s1 = pd.Series([1,2])\n | >>> s2 = s1.astype('int64', copy=False)\n | >>> s2[0] = 10\n | >>> s1 # note that s1[0] has changed too\n | 0 10\n | 1 2\n | dtype: int64\n | \n | See also\n | --------\n | pandas.to_datetime : Convert argument to datetime.\n | pandas.to_timedelta : Convert argument to timedelta.\n | pandas.to_numeric : Convert argument to a numeric type.\n | numpy.ndarray.astype : Cast a numpy array to a specified type.\n | \n | at_time(self, time, asof=False)\n | Select values at particular time of day (e.g. 9:30AM).\n | \n | Raises\n | ------\n | TypeError\n | If the index is not a :class:`DatetimeIndex`\n | \n | Parameters\n | ----------\n | time : datetime.time or string\n | \n | Returns\n | -------\n | values_at_time : type of caller\n | \n | Examples\n | --------\n | >>> i = pd.date_range('2018-04-09', periods=4, freq='12H')\n | >>> ts = pd.DataFrame({'A': [1,2,3,4]}, index=i)\n | >>> ts\n | A\n | 2018-04-09 00:00:00 1\n | 2018-04-09 12:00:00 2\n | 2018-04-10 00:00:00 3\n | 2018-04-10 12:00:00 4\n | \n | >>> ts.at_time('12:00')\n | A\n | 2018-04-09 12:00:00 2\n | 2018-04-10 12:00:00 4\n | \n | See Also\n | --------\n | between_time : Select values between particular times of the day\n | first : Select initial periods of time series based on a date offset\n | last : Select final periods of time series based on a date offset\n | DatetimeIndex.indexer_at_time : Get just the index locations for\n | values at particular time of the day\n | \n | between_time(self, start_time, end_time, include_start=True, include_end=True)\n | Select values between particular times of the day (e.g., 9:00-9:30 AM).\n | \n | By setting ``start_time`` to be later than ``end_time``,\n | you can get the times that are *not* between the two times.\n | \n | Raises\n | ------\n | TypeError\n | If the index is not a :class:`DatetimeIndex`\n | \n | Parameters\n | ----------\n | start_time : datetime.time or string\n | end_time : datetime.time or string\n | include_start : boolean, default True\n | include_end : boolean, default True\n | \n | Returns\n | -------\n | values_between_time : type of caller\n | \n | Examples\n | --------\n | >>> i = pd.date_range('2018-04-09', periods=4, freq='1D20min')\n | >>> ts = pd.DataFrame({'A': [1,2,3,4]}, index=i)\n | >>> ts\n | A\n | 2018-04-09 00:00:00 1\n | 2018-04-10 00:20:00 2\n | 2018-04-11 00:40:00 3\n | 2018-04-12 01:00:00 4\n | \n | >>> ts.between_time('0:15', '0:45')\n | A\n | 2018-04-10 00:20:00 2\n | 2018-04-11 00:40:00 3\n | \n | You get the times that are *not* between two times by setting\n | ``start_time`` later than ``end_time``:\n | \n | >>> ts.between_time('0:45', '0:15')\n | A\n | 2018-04-09 00:00:00 1\n | 2018-04-12 01:00:00 4\n | \n | See Also\n | --------\n | at_time : Select values at a particular time of the day\n | first : Select initial periods of time series based on a date offset\n | last : Select final periods of time series based on a date offset\n | DatetimeIndex.indexer_between_time : Get just the index locations for\n | values between particular times of the day\n | \n | bfill(self, axis=None, inplace=False, limit=None, downcast=None)\n | Synonym for :meth:`DataFrame.fillna(method='bfill') <DataFrame.fillna>`\n | \n | bool(self)\n | Return the bool of a single element PandasObject.\n | \n | This must be a boolean scalar value, either True or False. Raise a\n | ValueError if the PandasObject does not have exactly 1 element, or that\n | element is not boolean\n | \n | clip(self, lower=None, upper=None, axis=None, inplace=False, *args, **kwargs)\n | Trim values at input threshold(s).\n | \n | Assigns values outside boundary to boundary values. Thresholds\n | can be singular values or array like, and in the latter case\n | the clipping is performed element-wise in the specified axis.\n | \n | Parameters\n | ----------\n | lower : float or array_like, default None\n | Minimum threshold value. All values below this\n | threshold will be set to it.\n | upper : float or array_like, default None\n | Maximum threshold value. All values above this\n | threshold will be set to it.\n | axis : int or string axis name, optional\n | Align object with lower and upper along the given axis.\n | inplace : boolean, default False\n | Whether to perform the operation in place on the data.\n | \n | .. versionadded:: 0.21.0\n | *args, **kwargs\n | Additional keywords have no effect but might be accepted\n | for compatibility with numpy.\n | \n | See Also\n | --------\n | clip_lower : Clip values below specified threshold(s).\n | clip_upper : Clip values above specified threshold(s).\n | \n | Returns\n | -------\n | Series or DataFrame\n | Same type as calling object with the values outside the\n | clip boundaries replaced\n | \n | Examples\n | --------\n | >>> data = {'col_0': [9, -3, 0, -1, 5], 'col_1': [-2, -7, 6, 8, -5]}\n | >>> df = pd.DataFrame(data)\n | >>> df\n | col_0 col_1\n | 0 9 -2\n | 1 -3 -7\n | 2 0 6\n | 3 -1 8\n | 4 5 -5\n | \n | Clips per column using lower and upper thresholds:\n | \n | >>> df.clip(-4, 6)\n | col_0 col_1\n | 0 6 -2\n | 1 -3 -4\n | 2 0 6\n | 3 -1 6\n | 4 5 -4\n | \n | Clips using specific lower and upper thresholds per column element:\n | \n | >>> t = pd.Series([2, -4, -1, 6, 3])\n | >>> t\n | 0 2\n | 1 -4\n | 2 -1\n | 3 6\n | 4 3\n | dtype: int64\n | \n | >>> df.clip(t, t + 4, axis=0)\n | col_0 col_1\n | 0 6 2\n | 1 -3 -4\n | 2 0 3\n | 3 6 8\n | 4 5 3\n | \n | clip_lower(self, threshold, axis=None, inplace=False)\n | Return copy of the input with values below a threshold truncated.\n | \n | Parameters\n | ----------\n | threshold : numeric or array-like\n | Minimum value allowed. All values below threshold will be set to\n | this value.\n | \n | * float : every value is compared to `threshold`.\n | * array-like : The shape of `threshold` should match the object\n | it's compared to. When `self` is a Series, `threshold` should be\n | the length. When `self` is a DataFrame, `threshold` should 2-D\n | and the same shape as `self` for ``axis=None``, or 1-D and the\n | same length as the axis being compared.\n | \n | axis : {0 or 'index', 1 or 'columns'}, default 0\n | Align `self` with `threshold` along the given axis.\n | \n | inplace : boolean, default False\n | Whether to perform the operation in place on the data.\n | \n | .. versionadded:: 0.21.0\n | \n | See Also\n | --------\n | Series.clip : Return copy of input with values below and above\n | thresholds truncated.\n | Series.clip_upper : Return copy of input with values above\n | threshold truncated.\n | \n | Returns\n | -------\n | clipped : same type as input\n | \n | Examples\n | --------\n | Series single threshold clipping:\n | \n | >>> s = pd.Series([5, 6, 7, 8, 9])\n | >>> s.clip_lower(8)\n | 0 8\n | 1 8\n | 2 8\n | 3 8\n | 4 9\n | dtype: int64\n | \n | Series clipping element-wise using an array of thresholds. `threshold`\n | should be the same length as the Series.\n | \n | >>> elemwise_thresholds = [4, 8, 7, 2, 5]\n | >>> s.clip_lower(elemwise_thresholds)\n | 0 5\n | 1 8\n | 2 7\n | 3 8\n | 4 9\n | dtype: int64\n | \n | DataFrames can be compared to a scalar.\n | \n | >>> df = pd.DataFrame({\"A\": [1, 3, 5], \"B\": [2, 4, 6]})\n | >>> df\n | A B\n | 0 1 2\n | 1 3 4\n | 2 5 6\n | \n | >>> df.clip_lower(3)\n | A B\n | 0 3 3\n | 1 3 4\n | 2 5 6\n | \n | Or to an array of values. By default, `threshold` should be the same\n | shape as the DataFrame.\n | \n | >>> df.clip_lower(np.array([[3, 4], [2, 2], [6, 2]]))\n | A B\n | 0 3 4\n | 1 3 4\n | 2 6 6\n | \n | Control how `threshold` is broadcast with `axis`. In this case\n | `threshold` should be the same length as the axis specified by\n | `axis`.\n | \n | >>> df.clip_lower(np.array([3, 3, 5]), axis='index')\n | A B\n | 0 3 3\n | 1 3 4\n | 2 5 6\n | \n | >>> df.clip_lower(np.array([4, 5]), axis='columns')\n | A B\n | 0 4 5\n | 1 4 5\n | 2 5 6\n | \n | clip_upper(self, threshold, axis=None, inplace=False)\n | Return copy of input with values above given value(s) truncated.\n | \n | Parameters\n | ----------\n | threshold : float or array_like\n | axis : int or string axis name, optional\n | Align object with threshold along the given axis.\n | inplace : boolean, default False\n | Whether to perform the operation in place on the data\n | \n | .. versionadded:: 0.21.0\n | \n | See Also\n | --------\n | clip\n | \n | Returns\n | -------\n | clipped : same type as input\n | \n | consolidate(self, inplace=False)\n | Compute NDFrame with \"consolidated\" internals (data of each dtype\n | grouped together in a single ndarray).\n | \n | .. deprecated:: 0.20.0\n | Consolidate will be an internal implementation only.\n | \n | convert_objects(self, convert_dates=True, convert_numeric=False, convert_timedeltas=True, copy=True)\n | Attempt to infer better dtype for object columns.\n | \n | .. deprecated:: 0.21.0\n | \n | Parameters\n | ----------\n | convert_dates : boolean, default True\n | If True, convert to date where possible. If 'coerce', force\n | conversion, with unconvertible values becoming NaT.\n | convert_numeric : boolean, default False\n | If True, attempt to coerce to numbers (including strings), with\n | unconvertible values becoming NaN.\n | convert_timedeltas : boolean, default True\n | If True, convert to timedelta where possible. If 'coerce', force\n | conversion, with unconvertible values becoming NaT.\n | copy : boolean, default True\n | If True, return a copy even if no copy is necessary (e.g. no\n | conversion was done). Note: This is meant for internal use, and\n | should not be confused with inplace.\n | \n | See Also\n | --------\n | pandas.to_datetime : Convert argument to datetime.\n | pandas.to_timedelta : Convert argument to timedelta.\n | pandas.to_numeric : Return a fixed frequency timedelta index,\n | with day as the default.\n | \n | Returns\n | -------\n | converted : same as input object\n | \n | describe(self, percentiles=None, include=None, exclude=None)\n | Generates descriptive statistics that summarize the central tendency,\n | dispersion and shape of a dataset's distribution, excluding\n | ``NaN`` values.\n | \n | Analyzes both numeric and object series, as well\n | as ``DataFrame`` column sets of mixed data types. The output\n | will vary depending on what is provided. Refer to the notes\n | below for more detail.\n | \n | Parameters\n | ----------\n | percentiles : list-like of numbers, optional\n | The percentiles to include in the output. All should\n | fall between 0 and 1. The default is\n | ``[.25, .5, .75]``, which returns the 25th, 50th, and\n | 75th percentiles.\n | include : 'all', list-like of dtypes or None (default), optional\n | A white list of data types to include in the result. Ignored\n | for ``Series``. Here are the options:\n | \n | - 'all' : All columns of the input will be included in the output.\n | - A list-like of dtypes : Limits the results to the\n | provided data types.\n | To limit the result to numeric types submit\n | ``numpy.number``. To limit it instead to object columns submit\n | the ``numpy.object`` data type. Strings\n | can also be used in the style of\n | ``select_dtypes`` (e.g. ``df.describe(include=['O'])``). To\n | select pandas categorical columns, use ``'category'``\n | - None (default) : The result will include all numeric columns.\n | exclude : list-like of dtypes or None (default), optional,\n | A black list of data types to omit from the result. Ignored\n | for ``Series``. Here are the options:\n | \n | - A list-like of dtypes : Excludes the provided data types\n | from the result. To exclude numeric types submit\n | ``numpy.number``. To exclude object columns submit the data\n | type ``numpy.object``. Strings can also be used in the style of\n | ``select_dtypes`` (e.g. ``df.describe(include=['O'])``). To\n | exclude pandas categorical columns, use ``'category'``\n | - None (default) : The result will exclude nothing.\n | \n | Returns\n | -------\n | summary: Series/DataFrame of summary statistics\n | \n | Notes\n | -----\n | For numeric data, the result's index will include ``count``,\n | ``mean``, ``std``, ``min``, ``max`` as well as lower, ``50`` and\n | upper percentiles. By default the lower percentile is ``25`` and the\n | upper percentile is ``75``. The ``50`` percentile is the\n | same as the median.\n | \n | For object data (e.g. strings or timestamps), the result's index\n | will include ``count``, ``unique``, ``top``, and ``freq``. The ``top``\n | is the most common value. The ``freq`` is the most common value's\n | frequency. Timestamps also include the ``first`` and ``last`` items.\n | \n | If multiple object values have the highest count, then the\n | ``count`` and ``top`` results will be arbitrarily chosen from\n | among those with the highest count.\n | \n | For mixed data types provided via a ``DataFrame``, the default is to\n | return only an analysis of numeric columns. If the dataframe consists\n | only of object and categorical data without any numeric columns, the\n | default is to return an analysis of both the object and categorical\n | columns. If ``include='all'`` is provided as an option, the result\n | will include a union of attributes of each type.\n | \n | The `include` and `exclude` parameters can be used to limit\n | which columns in a ``DataFrame`` are analyzed for the output.\n | The parameters are ignored when analyzing a ``Series``.\n | \n | Examples\n | --------\n | Describing a numeric ``Series``.\n | \n | >>> s = pd.Series([1, 2, 3])\n | >>> s.describe()\n | count 3.0\n | mean 2.0\n | std 1.0\n | min 1.0\n | 25% 1.5\n | 50% 2.0\n | 75% 2.5\n | max 3.0\n | \n | Describing a categorical ``Series``.\n | \n | >>> s = pd.Series(['a', 'a', 'b', 'c'])\n | >>> s.describe()\n | count 4\n | unique 3\n | top a\n | freq 2\n | dtype: object\n | \n | Describing a timestamp ``Series``.\n | \n | >>> s = pd.Series([\n | ... np.datetime64(\"2000-01-01\"),\n | ... np.datetime64(\"2010-01-01\"),\n | ... np.datetime64(\"2010-01-01\")\n | ... ])\n | >>> s.describe()\n | count 3\n | unique 2\n | top 2010-01-01 00:00:00\n | freq 2\n | first 2000-01-01 00:00:00\n | last 2010-01-01 00:00:00\n | dtype: object\n | \n | Describing a ``DataFrame``. By default only numeric fields\n | are returned.\n | \n | >>> df = pd.DataFrame({ 'object': ['a', 'b', 'c'],\n | ... 'numeric': [1, 2, 3],\n | ... 'categorical': pd.Categorical(['d','e','f'])\n | ... })\n | >>> df.describe()\n | numeric\n | count 3.0\n | mean 2.0\n | std 1.0\n | min 1.0\n | 25% 1.5\n | 50% 2.0\n | 75% 2.5\n | max 3.0\n | \n | Describing all columns of a ``DataFrame`` regardless of data type.\n | \n | >>> df.describe(include='all')\n | categorical numeric object\n | count 3 3.0 3\n | unique 3 NaN 3\n | top f NaN c\n | freq 1 NaN 1\n | mean NaN 2.0 NaN\n | std NaN 1.0 NaN\n | min NaN 1.0 NaN\n | 25% NaN 1.5 NaN\n | 50% NaN 2.0 NaN\n | 75% NaN 2.5 NaN\n | max NaN 3.0 NaN\n | \n | Describing a column from a ``DataFrame`` by accessing it as\n | an attribute.\n | \n | >>> df.numeric.describe()\n | count 3.0\n | mean 2.0\n | std 1.0\n | min 1.0\n | 25% 1.5\n | 50% 2.0\n | 75% 2.5\n | max 3.0\n | Name: numeric, dtype: float64\n | \n | Including only numeric columns in a ``DataFrame`` description.\n | \n | >>> df.describe(include=[np.number])\n | numeric\n | count 3.0\n | mean 2.0\n | std 1.0\n | min 1.0\n | 25% 1.5\n | 50% 2.0\n | 75% 2.5\n | max 3.0\n | \n | Including only string columns in a ``DataFrame`` description.\n | \n | >>> df.describe(include=[np.object])\n | object\n | count 3\n | unique 3\n | top c\n | freq 1\n | \n | Including only categorical columns from a ``DataFrame`` description.\n | \n | >>> df.describe(include=['category'])\n | categorical\n | count 3\n | unique 3\n | top f\n | freq 1\n | \n | Excluding numeric columns from a ``DataFrame`` description.\n | \n | >>> df.describe(exclude=[np.number])\n | categorical object\n | count 3 3\n | unique 3 3\n | top f c\n | freq 1 1\n | \n | Excluding object columns from a ``DataFrame`` description.\n | \n | >>> df.describe(exclude=[np.object])\n | categorical numeric\n | count 3 3.0\n | unique 3 NaN\n | top f NaN\n | freq 1 NaN\n | mean NaN 2.0\n | std NaN 1.0\n | min NaN 1.0\n | 25% NaN 1.5\n | 50% NaN 2.0\n | 75% NaN 2.5\n | max NaN 3.0\n | \n | See Also\n | --------\n | DataFrame.count\n | DataFrame.max\n | DataFrame.min\n | DataFrame.mean\n | DataFrame.std\n | DataFrame.select_dtypes\n | \n | equals(self, other)\n | Determines if two NDFrame objects contain the same elements. NaNs in\n | the same location are considered equal.\n | \n | ffill(self, axis=None, inplace=False, limit=None, downcast=None)\n | Synonym for :meth:`DataFrame.fillna(method='ffill') <DataFrame.fillna>`\n | \n | filter(self, items=None, like=None, regex=None, axis=None)\n | Subset rows or columns of dataframe according to labels in\n | the specified index.\n | \n | Note that this routine does not filter a dataframe on its\n | contents. The filter is applied to the labels of the index.\n | \n | Parameters\n | ----------\n | items : list-like\n | List of info axis to restrict to (must not all be present)\n | like : string\n | Keep info axis where \"arg in col == True\"\n | regex : string (regular expression)\n | Keep info axis with re.search(regex, col) == True\n | axis : int or string axis name\n | The axis to filter on. By default this is the info axis,\n | 'index' for Series, 'columns' for DataFrame\n | \n | Returns\n | -------\n | same type as input object\n | \n | Examples\n | --------\n | >>> df\n | one two three\n | mouse 1 2 3\n | rabbit 4 5 6\n | \n | >>> # select columns by name\n | >>> df.filter(items=['one', 'three'])\n | one three\n | mouse 1 3\n | rabbit 4 6\n | \n | >>> # select columns by regular expression\n | >>> df.filter(regex='e$', axis=1)\n | one three\n | mouse 1 3\n | rabbit 4 6\n | \n | >>> # select rows containing 'bbi'\n | >>> df.filter(like='bbi', axis=0)\n | one two three\n | rabbit 4 5 6\n | \n | See Also\n | --------\n | pandas.DataFrame.loc\n | \n | Notes\n | -----\n | The ``items``, ``like``, and ``regex`` parameters are\n | enforced to be mutually exclusive.\n | \n | ``axis`` defaults to the info axis that is used when indexing\n | with ``[]``.\n | \n | first(self, offset)\n | Convenience method for subsetting initial periods of time series data\n | based on a date offset.\n | \n | Raises\n | ------\n | TypeError\n | If the index is not a :class:`DatetimeIndex`\n | \n | Parameters\n | ----------\n | offset : string, DateOffset, dateutil.relativedelta\n | \n | Examples\n | --------\n | >>> i = pd.date_range('2018-04-09', periods=4, freq='2D')\n | >>> ts = pd.DataFrame({'A': [1,2,3,4]}, index=i)\n | >>> ts\n | A\n | 2018-04-09 1\n | 2018-04-11 2\n | 2018-04-13 3\n | 2018-04-15 4\n | \n | Get the rows for the first 3 days:\n | \n | >>> ts.first('3D')\n | A\n | 2018-04-09 1\n | 2018-04-11 2\n | \n | Notice the data for 3 first calender days were returned, not the first\n | 3 days observed in the dataset, and therefore data for 2018-04-13 was\n | not returned.\n | \n | Returns\n | -------\n | subset : type of caller\n | \n | See Also\n | --------\n | last : Select final periods of time series based on a date offset\n | at_time : Select values at a particular time of the day\n | between_time : Select values between particular times of the day\n | \n | first_valid_index(self)\n | Return index for first non-NA/null value.\n | \n | Notes\n | --------\n | If all elements are non-NA/null, returns None.\n | Also returns None for empty NDFrame.\n | \n | Returns\n | --------\n | scalar : type of index\n | \n | get(self, key, default=None)\n | Get item from object for given key (DataFrame column, Panel slice,\n | etc.). Returns default value if not found.\n | \n | Parameters\n | ----------\n | key : object\n | \n | Returns\n | -------\n | value : type of items contained in object\n | \n | get_dtype_counts(self)\n | Return counts of unique dtypes in this object.\n | \n | Returns\n | -------\n | dtype : Series\n | Series with the count of columns with each dtype.\n | \n | See Also\n | --------\n | dtypes : Return the dtypes in this object.\n | \n | Examples\n | --------\n | >>> a = [['a', 1, 1.0], ['b', 2, 2.0], ['c', 3, 3.0]]\n | >>> df = pd.DataFrame(a, columns=['str', 'int', 'float'])\n | >>> df\n | str int float\n | 0 a 1 1.0\n | 1 b 2 2.0\n | 2 c 3 3.0\n | \n | >>> df.get_dtype_counts()\n | float64 1\n | int64 1\n | object 1\n | dtype: int64\n | \n | get_ftype_counts(self)\n | Return counts of unique ftypes in this object.\n | \n | .. deprecated:: 0.23.0\n | \n | This is useful for SparseDataFrame or for DataFrames containing\n | sparse arrays.\n | \n | Returns\n | -------\n | dtype : Series\n | Series with the count of columns with each type and\n | sparsity (dense/sparse)\n | \n | See Also\n | --------\n | ftypes : Return ftypes (indication of sparse/dense and dtype) in\n | this object.\n | \n | Examples\n | --------\n | >>> a = [['a', 1, 1.0], ['b', 2, 2.0], ['c', 3, 3.0]]\n | >>> df = pd.DataFrame(a, columns=['str', 'int', 'float'])\n | >>> df\n | str int float\n | 0 a 1 1.0\n | 1 b 2 2.0\n | 2 c 3 3.0\n | \n | >>> df.get_ftype_counts()\n | float64:dense 1\n | int64:dense 1\n | object:dense 1\n | dtype: int64\n | \n | groupby(self, by=None, axis=0, level=None, as_index=True, sort=True, group_keys=True, squeeze=False, observed=False, **kwargs)\n | Group series using mapper (dict or key function, apply given function\n | to group, return result as series) or by a series of columns.\n | \n | Parameters\n | ----------\n | by : mapping, function, label, or list of labels\n | Used to determine the groups for the groupby.\n | If ``by`` is a function, it's called on each value of the object's\n | index. If a dict or Series is passed, the Series or dict VALUES\n | will be used to determine the groups (the Series' values are first\n | aligned; see ``.align()`` method). If an ndarray is passed, the\n | values are used as-is determine the groups. A label or list of\n | labels may be passed to group by the columns in ``self``. Notice\n | that a tuple is interpreted a (single) key.\n | axis : int, default 0\n | level : int, level name, or sequence of such, default None\n | If the axis is a MultiIndex (hierarchical), group by a particular\n | level or levels\n | as_index : boolean, default True\n | For aggregated output, return object with group labels as the\n | index. Only relevant for DataFrame input. as_index=False is\n | effectively \"SQL-style\" grouped output\n | sort : boolean, default True\n | Sort group keys. Get better performance by turning this off.\n | Note this does not influence the order of observations within each\n | group. groupby preserves the order of rows within each group.\n | group_keys : boolean, default True\n | When calling apply, add group keys to index to identify pieces\n | squeeze : boolean, default False\n | reduce the dimensionality of the return type if possible,\n | otherwise return a consistent type\n | observed : boolean, default False\n | This only applies if any of the groupers are Categoricals\n | If True: only show observed values for categorical groupers.\n | If False: show all values for categorical groupers.\n | \n | .. versionadded:: 0.23.0\n | \n | Returns\n | -------\n | GroupBy object\n | \n | Examples\n | --------\n | DataFrame results\n | \n | >>> data.groupby(func, axis=0).mean()\n | >>> data.groupby(['col1', 'col2'])['col3'].mean()\n | \n | DataFrame with hierarchical index\n | \n | >>> data.groupby(['col1', 'col2']).mean()\n | \n | Notes\n | -----\n | See the `user guide\n | <http://pandas.pydata.org/pandas-docs/stable/groupby.html>`_ for more.\n | \n | See also\n | --------\n | resample : Convenience method for frequency conversion and resampling\n | of time series.\n | \n | head(self, n=5)\n | Return the first `n` rows.\n | \n | This function returns the first `n` rows for the object based\n | on position. It is useful for quickly testing if your object\n | has the right type of data in it.\n | \n | Parameters\n | ----------\n | n : int, default 5\n | Number of rows to select.\n | \n | Returns\n | -------\n | obj_head : type of caller\n | The first `n` rows of the caller object.\n | \n | See Also\n | --------\n | pandas.DataFrame.tail: Returns the last `n` rows.\n | \n | Examples\n | --------\n | >>> df = pd.DataFrame({'animal':['alligator', 'bee', 'falcon', 'lion',\n | ... 'monkey', 'parrot', 'shark', 'whale', 'zebra']})\n | >>> df\n | animal\n | 0 alligator\n | 1 bee\n | 2 falcon\n | 3 lion\n | 4 monkey\n | 5 parrot\n | 6 shark\n | 7 whale\n | 8 zebra\n | \n | Viewing the first 5 lines\n | \n | >>> df.head()\n | animal\n | 0 alligator\n | 1 bee\n | 2 falcon\n | 3 lion\n | 4 monkey\n | \n | Viewing the first `n` lines (three in this case)\n | \n | >>> df.head(3)\n | animal\n | 0 alligator\n | 1 bee\n | 2 falcon\n | \n | infer_objects(self)\n | Attempt to infer better dtypes for object columns.\n | \n | Attempts soft conversion of object-dtyped\n | columns, leaving non-object and unconvertible\n | columns unchanged. The inference rules are the\n | same as during normal Series/DataFrame construction.\n | \n | .. versionadded:: 0.21.0\n | \n | See Also\n | --------\n | pandas.to_datetime : Convert argument to datetime.\n | pandas.to_timedelta : Convert argument to timedelta.\n | pandas.to_numeric : Convert argument to numeric typeR\n | \n | Returns\n | -------\n | converted : same type as input object\n | \n | Examples\n | --------\n | >>> df = pd.DataFrame({\"A\": [\"a\", 1, 2, 3]})\n | >>> df = df.iloc[1:]\n | >>> df\n | A\n | 1 1\n | 2 2\n | 3 3\n | \n | >>> df.dtypes\n | A object\n | dtype: object\n | \n | >>> df.infer_objects().dtypes\n | A int64\n | dtype: object\n | \n | interpolate(self, method='linear', axis=0, limit=None, inplace=False, limit_direction='forward', limit_area=None, downcast=None, **kwargs)\n | Interpolate values according to different methods.\n | \n | Please note that only ``method='linear'`` is supported for\n | DataFrames/Series with a MultiIndex.\n | \n | Parameters\n | ----------\n | method : {'linear', 'time', 'index', 'values', 'nearest', 'zero',\n | 'slinear', 'quadratic', 'cubic', 'barycentric', 'krogh',\n | 'polynomial', 'spline', 'piecewise_polynomial',\n | 'from_derivatives', 'pchip', 'akima'}\n | \n | * 'linear': ignore the index and treat the values as equally\n | spaced. This is the only method supported on MultiIndexes.\n | default\n | * 'time': interpolation works on daily and higher resolution\n | data to interpolate given length of interval\n | * 'index', 'values': use the actual numerical values of the index\n | * 'nearest', 'zero', 'slinear', 'quadratic', 'cubic',\n | 'barycentric', 'polynomial' is passed to\n | ``scipy.interpolate.interp1d``. Both 'polynomial' and 'spline'\n | require that you also specify an `order` (int),\n | e.g. df.interpolate(method='polynomial', order=4).\n | These use the actual numerical values of the index.\n | * 'krogh', 'piecewise_polynomial', 'spline', 'pchip' and 'akima'\n | are all wrappers around the scipy interpolation methods of\n | similar names. These use the actual numerical values of the\n | index. For more information on their behavior, see the\n | `scipy documentation\n | <http://docs.scipy.org/doc/scipy/reference/interpolate.html#univariate-interpolation>`__\n | and `tutorial documentation\n | <http://docs.scipy.org/doc/scipy/reference/tutorial/interpolate.html>`__\n | * 'from_derivatives' refers to BPoly.from_derivatives which\n | replaces 'piecewise_polynomial' interpolation method in\n | scipy 0.18\n | \n | .. versionadded:: 0.18.1\n | \n | Added support for the 'akima' method\n | Added interpolate method 'from_derivatives' which replaces\n | 'piecewise_polynomial' in scipy 0.18; backwards-compatible with\n | scipy < 0.18\n | \n | axis : {0, 1}, default 0\n | * 0: fill column-by-column\n | * 1: fill row-by-row\n | limit : int, default None.\n | Maximum number of consecutive NaNs to fill. Must be greater than 0.\n | limit_direction : {'forward', 'backward', 'both'}, default 'forward'\n | limit_area : {'inside', 'outside'}, default None\n | * None: (default) no fill restriction\n | * 'inside' Only fill NaNs surrounded by valid values (interpolate).\n | * 'outside' Only fill NaNs outside valid values (extrapolate).\n | \n | If limit is specified, consecutive NaNs will be filled in this\n | direction.\n | \n | .. versionadded:: 0.21.0\n | inplace : bool, default False\n | Update the NDFrame in place if possible.\n | downcast : optional, 'infer' or None, defaults to None\n | Downcast dtypes if possible.\n | kwargs : keyword arguments to pass on to the interpolating function.\n | \n | Returns\n | -------\n | Series or DataFrame of same shape interpolated at the NaNs\n | \n | See Also\n | --------\n | reindex, replace, fillna\n | \n | Examples\n | --------\n | \n | Filling in NaNs\n | \n | >>> s = pd.Series([0, 1, np.nan, 3])\n | >>> s.interpolate()\n | 0 0\n | 1 1\n | 2 2\n | 3 3\n | dtype: float64\n | \n | last(self, offset)\n | Convenience method for subsetting final periods of time series data\n | based on a date offset.\n | \n | Raises\n | ------\n | TypeError\n | If the index is not a :class:`DatetimeIndex`\n | \n | Parameters\n | ----------\n | offset : string, DateOffset, dateutil.relativedelta\n | \n | Examples\n | --------\n | >>> i = pd.date_range('2018-04-09', periods=4, freq='2D')\n | >>> ts = pd.DataFrame({'A': [1,2,3,4]}, index=i)\n | >>> ts\n | A\n | 2018-04-09 1\n | 2018-04-11 2\n | 2018-04-13 3\n | 2018-04-15 4\n | \n | Get the rows for the last 3 days:\n | \n | >>> ts.last('3D')\n | A\n | 2018-04-13 3\n | 2018-04-15 4\n | \n | Notice the data for 3 last calender days were returned, not the last\n | 3 observed days in the dataset, and therefore data for 2018-04-11 was\n | not returned.\n | \n | Returns\n | -------\n | subset : type of caller\n | \n | See Also\n | --------\n | first : Select initial periods of time series based on a date offset\n | at_time : Select values at a particular time of the day\n | between_time : Select values between particular times of the day\n | \n | last_valid_index(self)\n | Return index for last non-NA/null value.\n | \n | Notes\n | --------\n | If all elements are non-NA/null, returns None.\n | Also returns None for empty NDFrame.\n | \n | Returns\n | --------\n | scalar : type of index\n | \n | mask(self, cond, other=nan, inplace=False, axis=None, level=None, errors='raise', try_cast=False, raise_on_error=None)\n | Return an object of same shape as self and whose corresponding\n | entries are from self where `cond` is False and otherwise are from\n | `other`.\n | \n | Parameters\n | ----------\n | cond : boolean NDFrame, array-like, or callable\n | Where `cond` is False, keep the original value. Where\n | True, replace with corresponding value from `other`.\n | If `cond` is callable, it is computed on the NDFrame and\n | should return boolean NDFrame or array. The callable must\n | not change input NDFrame (though pandas doesn't check it).\n | \n | .. versionadded:: 0.18.1\n | A callable can be used as cond.\n | \n | other : scalar, NDFrame, or callable\n | Entries where `cond` is True are replaced with\n | corresponding value from `other`.\n | If other is callable, it is computed on the NDFrame and\n | should return scalar or NDFrame. The callable must not\n | change input NDFrame (though pandas doesn't check it).\n | \n | .. versionadded:: 0.18.1\n | A callable can be used as other.\n | \n | inplace : boolean, default False\n | Whether to perform the operation in place on the data\n | axis : alignment axis if needed, default None\n | level : alignment level if needed, default None\n | errors : str, {'raise', 'ignore'}, default 'raise'\n | - ``raise`` : allow exceptions to be raised\n | - ``ignore`` : suppress exceptions. On error return original object\n | \n | Note that currently this parameter won't affect\n | the results and will always coerce to a suitable dtype.\n | \n | try_cast : boolean, default False\n | try to cast the result back to the input type (if possible),\n | raise_on_error : boolean, default True\n | Whether to raise on invalid data types (e.g. trying to where on\n | strings)\n | \n | .. deprecated:: 0.21.0\n | \n | Returns\n | -------\n | wh : same type as caller\n | \n | Notes\n | -----\n | The mask method is an application of the if-then idiom. For each\n | element in the calling DataFrame, if ``cond`` is ``False`` the\n | element is used; otherwise the corresponding element from the DataFrame\n | ``other`` is used.\n | \n | The signature for :func:`DataFrame.where` differs from\n | :func:`numpy.where`. Roughly ``df1.where(m, df2)`` is equivalent to\n | ``np.where(m, df1, df2)``.\n | \n | For further details and examples see the ``mask`` documentation in\n | :ref:`indexing <indexing.where_mask>`.\n | \n | Examples\n | --------\n | >>> s = pd.Series(range(5))\n | >>> s.where(s > 0)\n | 0 NaN\n | 1 1.0\n | 2 2.0\n | 3 3.0\n | 4 4.0\n | \n | >>> s.mask(s > 0)\n | 0 0.0\n | 1 NaN\n | 2 NaN\n | 3 NaN\n | 4 NaN\n | \n | >>> s.where(s > 1, 10)\n | 0 10.0\n | 1 10.0\n | 2 2.0\n | 3 3.0\n | 4 4.0\n | \n | >>> df = pd.DataFrame(np.arange(10).reshape(-1, 2), columns=['A', 'B'])\n | >>> m = df % 3 == 0\n | >>> df.where(m, -df)\n | A B\n | 0 0 -1\n | 1 -2 3\n | 2 -4 -5\n | 3 6 -7\n | 4 -8 9\n | >>> df.where(m, -df) == np.where(m, df, -df)\n | A B\n | 0 True True\n | 1 True True\n | 2 True True\n | 3 True True\n | 4 True True\n | >>> df.where(m, -df) == df.mask(~m, -df)\n | A B\n | 0 True True\n | 1 True True\n | 2 True True\n | 3 True True\n | 4 True True\n | \n | See Also\n | --------\n | :func:`DataFrame.where`\n | \n | pct_change(self, periods=1, fill_method='pad', limit=None, freq=None, **kwargs)\n | Percentage change between the current and a prior element.\n | \n | Computes the percentage change from the immediately previous row by\n | default. This is useful in comparing the percentage of change in a time\n | series of elements.\n | \n | Parameters\n | ----------\n | periods : int, default 1\n | Periods to shift for forming percent change.\n | fill_method : str, default 'pad'\n | How to handle NAs before computing percent changes.\n | limit : int, default None\n | The number of consecutive NAs to fill before stopping.\n | freq : DateOffset, timedelta, or offset alias string, optional\n | Increment to use from time series API (e.g. 'M' or BDay()).\n | **kwargs\n | Additional keyword arguments are passed into\n | `DataFrame.shift` or `Series.shift`.\n | \n | Returns\n | -------\n | chg : Series or DataFrame\n | The same type as the calling object.\n | \n | See Also\n | --------\n | Series.diff : Compute the difference of two elements in a Series.\n | DataFrame.diff : Compute the difference of two elements in a DataFrame.\n | Series.shift : Shift the index by some number of periods.\n | DataFrame.shift : Shift the index by some number of periods.\n | \n | Examples\n | --------\n | **Series**\n | \n | >>> s = pd.Series([90, 91, 85])\n | >>> s\n | 0 90\n | 1 91\n | 2 85\n | dtype: int64\n | \n | >>> s.pct_change()\n | 0 NaN\n | 1 0.011111\n | 2 -0.065934\n | dtype: float64\n | \n | >>> s.pct_change(periods=2)\n | 0 NaN\n | 1 NaN\n | 2 -0.055556\n | dtype: float64\n | \n | See the percentage change in a Series where filling NAs with last\n | valid observation forward to next valid.\n | \n | >>> s = pd.Series([90, 91, None, 85])\n | >>> s\n | 0 90.0\n | 1 91.0\n | 2 NaN\n | 3 85.0\n | dtype: float64\n | \n | >>> s.pct_change(fill_method='ffill')\n | 0 NaN\n | 1 0.011111\n | 2 0.000000\n | 3 -0.065934\n | dtype: float64\n | \n | **DataFrame**\n | \n | Percentage change in French franc, Deutsche Mark, and Italian lira from\n | 1980-01-01 to 1980-03-01.\n | \n | >>> df = pd.DataFrame({\n | ... 'FR': [4.0405, 4.0963, 4.3149],\n | ... 'GR': [1.7246, 1.7482, 1.8519],\n | ... 'IT': [804.74, 810.01, 860.13]},\n | ... index=['1980-01-01', '1980-02-01', '1980-03-01'])\n | >>> df\n | FR GR IT\n | 1980-01-01 4.0405 1.7246 804.74\n | 1980-02-01 4.0963 1.7482 810.01\n | 1980-03-01 4.3149 1.8519 860.13\n | \n | >>> df.pct_change()\n | FR GR IT\n | 1980-01-01 NaN NaN NaN\n | 1980-02-01 0.013810 0.013684 0.006549\n | 1980-03-01 0.053365 0.059318 0.061876\n | \n | Percentage of change in GOOG and APPL stock volume. Shows computing\n | the percentage change between columns.\n | \n | >>> df = pd.DataFrame({\n | ... '2016': [1769950, 30586265],\n | ... '2015': [1500923, 40912316],\n | ... '2014': [1371819, 41403351]},\n | ... index=['GOOG', 'APPL'])\n | >>> df\n | 2016 2015 2014\n | GOOG 1769950 1500923 1371819\n | APPL 30586265 40912316 41403351\n | \n | >>> df.pct_change(axis='columns')\n | 2016 2015 2014\n | GOOG NaN -0.151997 -0.086016\n | APPL NaN 0.337604 0.012002\n | \n | pipe(self, func, *args, **kwargs)\n | Apply func(self, \\*args, \\*\\*kwargs)\n | \n | Parameters\n | ----------\n | func : function\n | function to apply to the NDFrame.\n | ``args``, and ``kwargs`` are passed into ``func``.\n | Alternatively a ``(callable, data_keyword)`` tuple where\n | ``data_keyword`` is a string indicating the keyword of\n | ``callable`` that expects the NDFrame.\n | args : iterable, optional\n | positional arguments passed into ``func``.\n | kwargs : mapping, optional\n | a dictionary of keyword arguments passed into ``func``.\n | \n | Returns\n | -------\n | object : the return type of ``func``.\n | \n | Notes\n | -----\n | \n | Use ``.pipe`` when chaining together functions that expect\n | Series, DataFrames or GroupBy objects. Instead of writing\n | \n | >>> f(g(h(df), arg1=a), arg2=b, arg3=c)\n | \n | You can write\n | \n | >>> (df.pipe(h)\n | ... .pipe(g, arg1=a)\n | ... .pipe(f, arg2=b, arg3=c)\n | ... )\n | \n | If you have a function that takes the data as (say) the second\n | argument, pass a tuple indicating which keyword expects the\n | data. For example, suppose ``f`` takes its data as ``arg2``:\n | \n | >>> (df.pipe(h)\n | ... .pipe(g, arg1=a)\n | ... .pipe((f, 'arg2'), arg1=a, arg3=c)\n | ... )\n | \n | See Also\n | --------\n | pandas.DataFrame.apply\n | pandas.DataFrame.applymap\n | pandas.Series.map\n | \n | pop(self, item)\n | Return item and drop from frame. Raise KeyError if not found.\n | \n | Parameters\n | ----------\n | item : str\n | Column label to be popped\n | \n | Returns\n | -------\n | popped : Series\n | \n | Examples\n | --------\n | >>> df = pd.DataFrame([('falcon', 'bird', 389.0),\n | ... ('parrot', 'bird', 24.0),\n | ... ('lion', 'mammal', 80.5),\n | ... ('monkey', 'mammal', np.nan)],\n | ... columns=('name', 'class', 'max_speed'))\n | >>> df\n | name class max_speed\n | 0 falcon bird 389.0\n | 1 parrot bird 24.0\n | 2 lion mammal 80.5\n | 3 monkey mammal NaN\n | \n | >>> df.pop('class')\n | 0 bird\n | 1 bird\n | 2 mammal\n | 3 mammal\n | Name: class, dtype: object\n | \n | >>> df\n | name max_speed\n | 0 falcon 389.0\n | 1 parrot 24.0\n | 2 lion 80.5\n | 3 monkey NaN\n | \n | rank(self, axis=0, method='average', numeric_only=None, na_option='keep', ascending=True, pct=False)\n | Compute numerical data ranks (1 through n) along axis. Equal values are\n | assigned a rank that is the average of the ranks of those values\n | \n | Parameters\n | ----------\n | axis : {0 or 'index', 1 or 'columns'}, default 0\n | index to direct ranking\n | method : {'average', 'min', 'max', 'first', 'dense'}\n | * average: average rank of group\n | * min: lowest rank in group\n | * max: highest rank in group\n | * first: ranks assigned in order they appear in the array\n | * dense: like 'min', but rank always increases by 1 between groups\n | numeric_only : boolean, default None\n | Include only float, int, boolean data. Valid only for DataFrame or\n | Panel objects\n | na_option : {'keep', 'top', 'bottom'}\n | * keep: leave NA values where they are\n | * top: smallest rank if ascending\n | * bottom: smallest rank if descending\n | ascending : boolean, default True\n | False for ranks by high (1) to low (N)\n | pct : boolean, default False\n | Computes percentage rank of data\n | \n | Returns\n | -------\n | ranks : same type as caller\n | \n | reindex_like(self, other, method=None, copy=True, limit=None, tolerance=None)\n | Return an object with matching indices to myself.\n | \n | Parameters\n | ----------\n | other : Object\n | method : string or None\n | copy : boolean, default True\n | limit : int, default None\n | Maximum number of consecutive labels to fill for inexact matches.\n | tolerance : optional\n | Maximum distance between labels of the other object and this\n | object for inexact matches. Can be list-like.\n | \n | .. versionadded:: 0.21.0 (list-like tolerance)\n | \n | Notes\n | -----\n | Like calling s.reindex(index=other.index, columns=other.columns,\n | method=...)\n | \n | Returns\n | -------\n | reindexed : same as input\n | \n | rename_axis(self, mapper, axis=0, copy=True, inplace=False)\n | Alter the name of the index or columns.\n | \n | Parameters\n | ----------\n | mapper : scalar, list-like, optional\n | Value to set as the axis name attribute.\n | axis : {0 or 'index', 1 or 'columns'}, default 0\n | The index or the name of the axis.\n | copy : boolean, default True\n | Also copy underlying data.\n | inplace : boolean, default False\n | Modifies the object directly, instead of creating a new Series\n | or DataFrame.\n | \n | Returns\n | -------\n | renamed : Series, DataFrame, or None\n | The same type as the caller or None if `inplace` is True.\n | \n | Notes\n | -----\n | Prior to version 0.21.0, ``rename_axis`` could also be used to change\n | the axis *labels* by passing a mapping or scalar. This behavior is\n | deprecated and will be removed in a future version. Use ``rename``\n | instead.\n | \n | See Also\n | --------\n | pandas.Series.rename : Alter Series index labels or name\n | pandas.DataFrame.rename : Alter DataFrame index labels or name\n | pandas.Index.rename : Set new names on index\n | \n | Examples\n | --------\n | **Series**\n | \n | >>> s = pd.Series([1, 2, 3])\n | >>> s.rename_axis(\"foo\")\n | foo\n | 0 1\n | 1 2\n | 2 3\n | dtype: int64\n | \n | **DataFrame**\n | \n | >>> df = pd.DataFrame({\"A\": [1, 2, 3], \"B\": [4, 5, 6]})\n | >>> df.rename_axis(\"foo\")\n | A B\n | foo\n | 0 1 4\n | 1 2 5\n | 2 3 6\n | \n | >>> df.rename_axis(\"bar\", axis=\"columns\")\n | bar A B\n | 0 1 4\n | 1 2 5\n | 2 3 6\n | \n | resample(self, rule, how=None, axis=0, fill_method=None, closed=None, label=None, convention='start', kind=None, loffset=None, limit=None, base=0, on=None, level=None)\n | Convenience method for frequency conversion and resampling of time\n | series. Object must have a datetime-like index (DatetimeIndex,\n | PeriodIndex, or TimedeltaIndex), or pass datetime-like values\n | to the on or level keyword.\n | \n | Parameters\n | ----------\n | rule : string\n | the offset string or object representing target conversion\n | axis : int, optional, default 0\n | closed : {'right', 'left'}\n | Which side of bin interval is closed. The default is 'left'\n | for all frequency offsets except for 'M', 'A', 'Q', 'BM',\n | 'BA', 'BQ', and 'W' which all have a default of 'right'.\n | label : {'right', 'left'}\n | Which bin edge label to label bucket with. The default is 'left'\n | for all frequency offsets except for 'M', 'A', 'Q', 'BM',\n | 'BA', 'BQ', and 'W' which all have a default of 'right'.\n | convention : {'start', 'end', 's', 'e'}\n | For PeriodIndex only, controls whether to use the start or end of\n | `rule`\n | kind: {'timestamp', 'period'}, optional\n | Pass 'timestamp' to convert the resulting index to a\n | ``DateTimeIndex`` or 'period' to convert it to a ``PeriodIndex``.\n | By default the input representation is retained.\n | loffset : timedelta\n | Adjust the resampled time labels\n | base : int, default 0\n | For frequencies that evenly subdivide 1 day, the \"origin\" of the\n | aggregated intervals. For example, for '5min' frequency, base could\n | range from 0 through 4. Defaults to 0\n | on : string, optional\n | For a DataFrame, column to use instead of index for resampling.\n | Column must be datetime-like.\n | \n | .. versionadded:: 0.19.0\n | \n | level : string or int, optional\n | For a MultiIndex, level (name or number) to use for\n | resampling. Level must be datetime-like.\n | \n | .. versionadded:: 0.19.0\n | \n | Returns\n | -------\n | Resampler object\n | \n | Notes\n | -----\n | See the `user guide\n | <http://pandas.pydata.org/pandas-docs/stable/timeseries.html#resampling>`_\n | for more.\n | \n | To learn more about the offset strings, please see `this link\n | <http://pandas.pydata.org/pandas-docs/stable/timeseries.html#offset-aliases>`__.\n | \n | Examples\n | --------\n | \n | Start by creating a series with 9 one minute timestamps.\n | \n | >>> index = pd.date_range('1/1/2000', periods=9, freq='T')\n | >>> series = pd.Series(range(9), index=index)\n | >>> series\n | 2000-01-01 00:00:00 0\n | 2000-01-01 00:01:00 1\n | 2000-01-01 00:02:00 2\n | 2000-01-01 00:03:00 3\n | 2000-01-01 00:04:00 4\n | 2000-01-01 00:05:00 5\n | 2000-01-01 00:06:00 6\n | 2000-01-01 00:07:00 7\n | 2000-01-01 00:08:00 8\n | Freq: T, dtype: int64\n | \n | Downsample the series into 3 minute bins and sum the values\n | of the timestamps falling into a bin.\n | \n | >>> series.resample('3T').sum()\n | 2000-01-01 00:00:00 3\n | 2000-01-01 00:03:00 12\n | 2000-01-01 00:06:00 21\n | Freq: 3T, dtype: int64\n | \n | Downsample the series into 3 minute bins as above, but label each\n | bin using the right edge instead of the left. Please note that the\n | value in the bucket used as the label is not included in the bucket,\n | which it labels. For example, in the original series the\n | bucket ``2000-01-01 00:03:00`` contains the value 3, but the summed\n | value in the resampled bucket with the label ``2000-01-01 00:03:00``\n | does not include 3 (if it did, the summed value would be 6, not 3).\n | To include this value close the right side of the bin interval as\n | illustrated in the example below this one.\n | \n | >>> series.resample('3T', label='right').sum()\n | 2000-01-01 00:03:00 3\n | 2000-01-01 00:06:00 12\n | 2000-01-01 00:09:00 21\n | Freq: 3T, dtype: int64\n | \n | Downsample the series into 3 minute bins as above, but close the right\n | side of the bin interval.\n | \n | >>> series.resample('3T', label='right', closed='right').sum()\n | 2000-01-01 00:00:00 0\n | 2000-01-01 00:03:00 6\n | 2000-01-01 00:06:00 15\n | 2000-01-01 00:09:00 15\n | Freq: 3T, dtype: int64\n | \n | Upsample the series into 30 second bins.\n | \n | >>> series.resample('30S').asfreq()[0:5] #select first 5 rows\n | 2000-01-01 00:00:00 0.0\n | 2000-01-01 00:00:30 NaN\n | 2000-01-01 00:01:00 1.0\n | 2000-01-01 00:01:30 NaN\n | 2000-01-01 00:02:00 2.0\n | Freq: 30S, dtype: float64\n | \n | Upsample the series into 30 second bins and fill the ``NaN``\n | values using the ``pad`` method.\n | \n | >>> series.resample('30S').pad()[0:5]\n | 2000-01-01 00:00:00 0\n | 2000-01-01 00:00:30 0\n | 2000-01-01 00:01:00 1\n | 2000-01-01 00:01:30 1\n | 2000-01-01 00:02:00 2\n | Freq: 30S, dtype: int64\n | \n | Upsample the series into 30 second bins and fill the\n | ``NaN`` values using the ``bfill`` method.\n | \n | >>> series.resample('30S').bfill()[0:5]\n | 2000-01-01 00:00:00 0\n | 2000-01-01 00:00:30 1\n | 2000-01-01 00:01:00 1\n | 2000-01-01 00:01:30 2\n | 2000-01-01 00:02:00 2\n | Freq: 30S, dtype: int64\n | \n | Pass a custom function via ``apply``\n | \n | >>> def custom_resampler(array_like):\n | ... return np.sum(array_like)+5\n | \n | >>> series.resample('3T').apply(custom_resampler)\n | 2000-01-01 00:00:00 8\n | 2000-01-01 00:03:00 17\n | 2000-01-01 00:06:00 26\n | Freq: 3T, dtype: int64\n | \n | For a Series with a PeriodIndex, the keyword `convention` can be\n | used to control whether to use the start or end of `rule`.\n | \n | >>> s = pd.Series([1, 2], index=pd.period_range('2012-01-01',\n | freq='A',\n | periods=2))\n | >>> s\n | 2012 1\n | 2013 2\n | Freq: A-DEC, dtype: int64\n | \n | Resample by month using 'start' `convention`. Values are assigned to\n | the first month of the period.\n | \n | >>> s.resample('M', convention='start').asfreq().head()\n | 2012-01 1.0\n | 2012-02 NaN\n | 2012-03 NaN\n | 2012-04 NaN\n | 2012-05 NaN\n | Freq: M, dtype: float64\n | \n | Resample by month using 'end' `convention`. Values are assigned to\n | the last month of the period.\n | \n | >>> s.resample('M', convention='end').asfreq()\n | 2012-12 1.0\n | 2013-01 NaN\n | 2013-02 NaN\n | 2013-03 NaN\n | 2013-04 NaN\n | 2013-05 NaN\n | 2013-06 NaN\n | 2013-07 NaN\n | 2013-08 NaN\n | 2013-09 NaN\n | 2013-10 NaN\n | 2013-11 NaN\n | 2013-12 2.0\n | Freq: M, dtype: float64\n | \n | For DataFrame objects, the keyword ``on`` can be used to specify the\n | column instead of the index for resampling.\n | \n | >>> df = pd.DataFrame(data=9*[range(4)], columns=['a', 'b', 'c', 'd'])\n | >>> df['time'] = pd.date_range('1/1/2000', periods=9, freq='T')\n | >>> df.resample('3T', on='time').sum()\n | a b c d\n | time\n | 2000-01-01 00:00:00 0 3 6 9\n | 2000-01-01 00:03:00 0 3 6 9\n | 2000-01-01 00:06:00 0 3 6 9\n | \n | For a DataFrame with MultiIndex, the keyword ``level`` can be used to\n | specify on level the resampling needs to take place.\n | \n | >>> time = pd.date_range('1/1/2000', periods=5, freq='T')\n | >>> df2 = pd.DataFrame(data=10*[range(4)],\n | columns=['a', 'b', 'c', 'd'],\n | index=pd.MultiIndex.from_product([time, [1, 2]])\n | )\n | >>> df2.resample('3T', level=0).sum()\n | a b c d\n | 2000-01-01 00:00:00 0 6 12 18\n | 2000-01-01 00:03:00 0 4 8 12\n | \n | See also\n | --------\n | groupby : Group by mapping, function, label, or list of labels.\n | \n | sample(self, n=None, frac=None, replace=False, weights=None, random_state=None, axis=None)\n | Return a random sample of items from an axis of object.\n | \n | You can use `random_state` for reproducibility.\n | \n | Parameters\n | ----------\n | n : int, optional\n | Number of items from axis to return. Cannot be used with `frac`.\n | Default = 1 if `frac` = None.\n | frac : float, optional\n | Fraction of axis items to return. Cannot be used with `n`.\n | replace : boolean, optional\n | Sample with or without replacement. Default = False.\n | weights : str or ndarray-like, optional\n | Default 'None' results in equal probability weighting.\n | If passed a Series, will align with target object on index. Index\n | values in weights not found in sampled object will be ignored and\n | index values in sampled object not in weights will be assigned\n | weights of zero.\n | If called on a DataFrame, will accept the name of a column\n | when axis = 0.\n | Unless weights are a Series, weights must be same length as axis\n | being sampled.\n | If weights do not sum to 1, they will be normalized to sum to 1.\n | Missing values in the weights column will be treated as zero.\n | inf and -inf values not allowed.\n | random_state : int or numpy.random.RandomState, optional\n | Seed for the random number generator (if int), or numpy RandomState\n | object.\n | axis : int or string, optional\n | Axis to sample. Accepts axis number or name. Default is stat axis\n | for given data type (0 for Series and DataFrames, 1 for Panels).\n | \n | Returns\n | -------\n | A new object of same type as caller.\n | \n | Examples\n | --------\n | Generate an example ``Series`` and ``DataFrame``:\n | \n | >>> s = pd.Series(np.random.randn(50))\n | >>> s.head()\n | 0 -0.038497\n | 1 1.820773\n | 2 -0.972766\n | 3 -1.598270\n | 4 -1.095526\n | dtype: float64\n | >>> df = pd.DataFrame(np.random.randn(50, 4), columns=list('ABCD'))\n | >>> df.head()\n | A B C D\n | 0 0.016443 -2.318952 -0.566372 -1.028078\n | 1 -1.051921 0.438836 0.658280 -0.175797\n | 2 -1.243569 -0.364626 -0.215065 0.057736\n | 3 1.768216 0.404512 -0.385604 -1.457834\n | 4 1.072446 -1.137172 0.314194 -0.046661\n | \n | Next extract a random sample from both of these objects...\n | \n | 3 random elements from the ``Series``:\n | \n | >>> s.sample(n=3)\n | 27 -0.994689\n | 55 -1.049016\n | 67 -0.224565\n | dtype: float64\n | \n | And a random 10% of the ``DataFrame`` with replacement:\n | \n | >>> df.sample(frac=0.1, replace=True)\n | A B C D\n | 35 1.981780 0.142106 1.817165 -0.290805\n | 49 -1.336199 -0.448634 -0.789640 0.217116\n | 40 0.823173 -0.078816 1.009536 1.015108\n | 15 1.421154 -0.055301 -1.922594 -0.019696\n | 6 -0.148339 0.832938 1.787600 -1.383767\n | \n | You can use `random state` for reproducibility:\n | \n | >>> df.sample(random_state=1)\n | A B C D\n | 37 -2.027662 0.103611 0.237496 -0.165867\n | 43 -0.259323 -0.583426 1.516140 -0.479118\n | 12 -1.686325 -0.579510 0.985195 -0.460286\n | 8 1.167946 0.429082 1.215742 -1.636041\n | 9 1.197475 -0.864188 1.554031 -1.505264\n | \n | select(self, crit, axis=0)\n | Return data corresponding to axis labels matching criteria\n | \n | .. deprecated:: 0.21.0\n | Use df.loc[df.index.map(crit)] to select via labels\n | \n | Parameters\n | ----------\n | crit : function\n | To be called on each index (label). Should return True or False\n | axis : int\n | \n | Returns\n | -------\n | selection : type of caller\n | \n | set_axis(self, labels, axis=0, inplace=None)\n | Assign desired index to given axis.\n | \n | Indexes for column or row labels can be changed by assigning\n | a list-like or Index.\n | \n | .. versionchanged:: 0.21.0\n | \n | The signature is now `labels` and `axis`, consistent with\n | the rest of pandas API. Previously, the `axis` and `labels`\n | arguments were respectively the first and second positional\n | arguments.\n | \n | Parameters\n | ----------\n | labels : list-like, Index\n | The values for the new index.\n | \n | axis : {0 or 'index', 1 or 'columns'}, default 0\n | The axis to update. The value 0 identifies the rows, and 1\n | identifies the columns.\n | \n | inplace : boolean, default None\n | Whether to return a new %(klass)s instance.\n | \n | .. warning::\n | \n | ``inplace=None`` currently falls back to to True, but in a\n | future version, will default to False. Use inplace=True\n | explicitly rather than relying on the default.\n | \n | Returns\n | -------\n | renamed : %(klass)s or None\n | An object of same type as caller if inplace=False, None otherwise.\n | \n | See Also\n | --------\n | pandas.DataFrame.rename_axis : Alter the name of the index or columns.\n | \n | Examples\n | --------\n | **Series**\n | \n | >>> s = pd.Series([1, 2, 3])\n | >>> s\n | 0 1\n | 1 2\n | 2 3\n | dtype: int64\n | \n | >>> s.set_axis(['a', 'b', 'c'], axis=0, inplace=False)\n | a 1\n | b 2\n | c 3\n | dtype: int64\n | \n | The original object is not modified.\n | \n | >>> s\n | 0 1\n | 1 2\n | 2 3\n | dtype: int64\n | \n | **DataFrame**\n | \n | >>> df = pd.DataFrame({\"A\": [1, 2, 3], \"B\": [4, 5, 6]})\n | \n | Change the row labels.\n | \n | >>> df.set_axis(['a', 'b', 'c'], axis='index', inplace=False)\n | A B\n | a 1 4\n | b 2 5\n | c 3 6\n | \n | Change the column labels.\n | \n | >>> df.set_axis(['I', 'II'], axis='columns', inplace=False)\n | I II\n | 0 1 4\n | 1 2 5\n | 2 3 6\n | \n | Now, update the labels inplace.\n | \n | >>> df.set_axis(['i', 'ii'], axis='columns', inplace=True)\n | >>> df\n | i ii\n | 0 1 4\n | 1 2 5\n | 2 3 6\n | \n | slice_shift(self, periods=1, axis=0)\n | Equivalent to `shift` without copying data. The shifted data will\n | not include the dropped periods and the shifted axis will be smaller\n | than the original.\n | \n | Parameters\n | ----------\n | periods : int\n | Number of periods to move, can be positive or negative\n | \n | Notes\n | -----\n | While the `slice_shift` is faster than `shift`, you may pay for it\n | later during alignment.\n | \n | Returns\n | -------\n | shifted : same type as caller\n | \n | squeeze(self, axis=None)\n | Squeeze length 1 dimensions.\n | \n | Parameters\n | ----------\n | axis : None, integer or string axis name, optional\n | The axis to squeeze if 1-sized.\n | \n | .. versionadded:: 0.20.0\n | \n | Returns\n | -------\n | scalar if 1-sized, else original object\n | \n | swapaxes(self, axis1, axis2, copy=True)\n | Interchange axes and swap values axes appropriately\n | \n | Returns\n | -------\n | y : same as input\n | \n | tail(self, n=5)\n | Return the last `n` rows.\n | \n | This function returns last `n` rows from the object based on\n | position. It is useful for quickly verifying data, for example,\n | after sorting or appending rows.\n | \n | Parameters\n | ----------\n | n : int, default 5\n | Number of rows to select.\n | \n | Returns\n | -------\n | type of caller\n | The last `n` rows of the caller object.\n | \n | See Also\n | --------\n | pandas.DataFrame.head : The first `n` rows of the caller object.\n | \n | Examples\n | --------\n | >>> df = pd.DataFrame({'animal':['alligator', 'bee', 'falcon', 'lion',\n | ... 'monkey', 'parrot', 'shark', 'whale', 'zebra']})\n | >>> df\n | animal\n | 0 alligator\n | 1 bee\n | 2 falcon\n | 3 lion\n | 4 monkey\n | 5 parrot\n | 6 shark\n | 7 whale\n | 8 zebra\n | \n | Viewing the last 5 lines\n | \n | >>> df.tail()\n | animal\n | 4 monkey\n | 5 parrot\n | 6 shark\n | 7 whale\n | 8 zebra\n | \n | Viewing the last `n` lines (three in this case)\n | \n | >>> df.tail(3)\n | animal\n | 6 shark\n | 7 whale\n | 8 zebra\n | \n | take(self, indices, axis=0, convert=None, is_copy=True, **kwargs)\n | Return the elements in the given *positional* indices along an axis.\n | \n | This means that we are not indexing according to actual values in\n | the index attribute of the object. We are indexing according to the\n | actual position of the element in the object.\n | \n | Parameters\n | ----------\n | indices : array-like\n | An array of ints indicating which positions to take.\n | axis : {0 or 'index', 1 or 'columns', None}, default 0\n | The axis on which to select elements. ``0`` means that we are\n | selecting rows, ``1`` means that we are selecting columns.\n | convert : bool, default True\n | Whether to convert negative indices into positive ones.\n | For example, ``-1`` would map to the ``len(axis) - 1``.\n | The conversions are similar to the behavior of indexing a\n | regular Python list.\n | \n | .. deprecated:: 0.21.0\n | In the future, negative indices will always be converted.\n | \n | is_copy : bool, default True\n | Whether to return a copy of the original object or not.\n | **kwargs\n | For compatibility with :meth:`numpy.take`. Has no effect on the\n | output.\n | \n | Returns\n | -------\n | taken : type of caller\n | An array-like containing the elements taken from the object.\n | \n | See Also\n | --------\n | DataFrame.loc : Select a subset of a DataFrame by labels.\n | DataFrame.iloc : Select a subset of a DataFrame by positions.\n | numpy.take : Take elements from an array along an axis.\n | \n | Examples\n | --------\n | >>> df = pd.DataFrame([('falcon', 'bird', 389.0),\n | ... ('parrot', 'bird', 24.0),\n | ... ('lion', 'mammal', 80.5),\n | ... ('monkey', 'mammal', np.nan)],\n | ... columns=['name', 'class', 'max_speed'],\n | ... index=[0, 2, 3, 1])\n | >>> df\n | name class max_speed\n | 0 falcon bird 389.0\n | 2 parrot bird 24.0\n | 3 lion mammal 80.5\n | 1 monkey mammal NaN\n | \n | Take elements at positions 0 and 3 along the axis 0 (default).\n | \n | Note how the actual indices selected (0 and 1) do not correspond to\n | our selected indices 0 and 3. That's because we are selecting the 0th\n | and 3rd rows, not rows whose indices equal 0 and 3.\n | \n | >>> df.take([0, 3])\n | name class max_speed\n | 0 falcon bird 389.0\n | 1 monkey mammal NaN\n | \n | Take elements at indices 1 and 2 along the axis 1 (column selection).\n | \n | >>> df.take([1, 2], axis=1)\n | class max_speed\n | 0 bird 389.0\n | 2 bird 24.0\n | 3 mammal 80.5\n | 1 mammal NaN\n | \n | We may take elements using negative integers for positive indices,\n | starting from the end of the object, just like with Python lists.\n | \n | >>> df.take([-1, -2])\n | name class max_speed\n | 1 monkey mammal NaN\n | 3 lion mammal 80.5\n | \n | to_clipboard(self, excel=True, sep=None, **kwargs)\n | Copy object to the system clipboard.\n | \n | Write a text representation of object to the system clipboard.\n | This can be pasted into Excel, for example.\n | \n | Parameters\n | ----------\n | excel : bool, default True\n | - True, use the provided separator, writing in a csv format for\n | allowing easy pasting into excel.\n | - False, write a string representation of the object to the\n | clipboard.\n | \n | sep : str, default ``'\\t'``\n | Field delimiter.\n | **kwargs\n | These parameters will be passed to DataFrame.to_csv.\n | \n | See Also\n | --------\n | DataFrame.to_csv : Write a DataFrame to a comma-separated values\n | (csv) file.\n | read_clipboard : Read text from clipboard and pass to read_table.\n | \n | Notes\n | -----\n | Requirements for your platform.\n | \n | - Linux : `xclip`, or `xsel` (with `gtk` or `PyQt4` modules)\n | - Windows : none\n | - OS X : none\n | \n | Examples\n | --------\n | Copy the contents of a DataFrame to the clipboard.\n | \n | >>> df = pd.DataFrame([[1, 2, 3], [4, 5, 6]], columns=['A', 'B', 'C'])\n | >>> df.to_clipboard(sep=',')\n | ... # Wrote the following to the system clipboard:\n | ... # ,A,B,C\n | ... # 0,1,2,3\n | ... # 1,4,5,6\n | \n | We can omit the the index by passing the keyword `index` and setting\n | it to false.\n | \n | >>> df.to_clipboard(sep=',', index=False)\n | ... # Wrote the following to the system clipboard:\n | ... # A,B,C\n | ... # 1,2,3\n | ... # 4,5,6\n | \n | to_dense(self)\n | Return dense representation of NDFrame (as opposed to sparse)\n | \n | to_hdf(self, path_or_buf, key, **kwargs)\n | Write the contained data to an HDF5 file using HDFStore.\n | \n | Hierarchical Data Format (HDF) is self-describing, allowing an\n | application to interpret the structure and contents of a file with\n | no outside information. One HDF file can hold a mix of related objects\n | which can be accessed as a group or as individual objects.\n | \n | In order to add another DataFrame or Series to an existing HDF file\n | please use append mode and a different a key.\n | \n | For more information see the :ref:`user guide <io.hdf5>`.\n | \n | Parameters\n | ----------\n | path_or_buf : str or pandas.HDFStore\n | File path or HDFStore object.\n | key : str\n | Identifier for the group in the store.\n | mode : {'a', 'w', 'r+'}, default 'a'\n | Mode to open file:\n | \n | - 'w': write, a new file is created (an existing file with\n | the same name would be deleted).\n | - 'a': append, an existing file is opened for reading and\n | writing, and if the file does not exist it is created.\n | - 'r+': similar to 'a', but the file must already exist.\n | format : {'fixed', 'table'}, default 'fixed'\n | Possible values:\n | \n | - 'fixed': Fixed format. Fast writing/reading. Not-appendable,\n | nor searchable.\n | - 'table': Table format. Write as a PyTables Table structure\n | which may perform worse but allow more flexible operations\n | like searching / selecting subsets of the data.\n | append : bool, default False\n | For Table formats, append the input data to the existing.\n | data_columns : list of columns or True, optional\n | List of columns to create as indexed data columns for on-disk\n | queries, or True to use all columns. By default only the axes\n | of the object are indexed. See :ref:`io.hdf5-query-data-columns`.\n | Applicable only to format='table'.\n | complevel : {0-9}, optional\n | Specifies a compression level for data.\n | A value of 0 disables compression.\n | complib : {'zlib', 'lzo', 'bzip2', 'blosc'}, default 'zlib'\n | Specifies the compression library to be used.\n | As of v0.20.2 these additional compressors for Blosc are supported\n | (default if no compressor specified: 'blosc:blosclz'):\n | {'blosc:blosclz', 'blosc:lz4', 'blosc:lz4hc', 'blosc:snappy',\n | 'blosc:zlib', 'blosc:zstd'}.\n | Specifying a compression library which is not available issues\n | a ValueError.\n | fletcher32 : bool, default False\n | If applying compression use the fletcher32 checksum.\n | dropna : bool, default False\n | If true, ALL nan rows will not be written to store.\n | errors : str, default 'strict'\n | Specifies how encoding and decoding errors are to be handled.\n | See the errors argument for :func:`open` for a full list\n | of options.\n | \n | See Also\n | --------\n | DataFrame.read_hdf : Read from HDF file.\n | DataFrame.to_parquet : Write a DataFrame to the binary parquet format.\n | DataFrame.to_sql : Write to a sql table.\n | DataFrame.to_feather : Write out feather-format for DataFrames.\n | DataFrame.to_csv : Write out to a csv file.\n | \n | Examples\n | --------\n | >>> df = pd.DataFrame({'A': [1, 2, 3], 'B': [4, 5, 6]},\n | ... index=['a', 'b', 'c'])\n | >>> df.to_hdf('data.h5', key='df', mode='w')\n | \n | We can add another object to the same file:\n | \n | >>> s = pd.Series([1, 2, 3, 4])\n | >>> s.to_hdf('data.h5', key='s')\n | \n | Reading from HDF file:\n | \n | >>> pd.read_hdf('data.h5', 'df')\n | A B\n | a 1 4\n | b 2 5\n | c 3 6\n | >>> pd.read_hdf('data.h5', 's')\n | 0 1\n | 1 2\n | 2 3\n | 3 4\n | dtype: int64\n | \n | Deleting file with data:\n | \n | >>> import os\n | >>> os.remove('data.h5')\n | \n | to_json(self, path_or_buf=None, orient=None, date_format=None, double_precision=10, force_ascii=True, date_unit='ms', default_handler=None, lines=False, compression=None, index=True)\n | Convert the object to a JSON string.\n | \n | Note NaN's and None will be converted to null and datetime objects\n | will be converted to UNIX timestamps.\n | \n | Parameters\n | ----------\n | path_or_buf : string or file handle, optional\n | File path or object. If not specified, the result is returned as\n | a string.\n | orient : string\n | Indication of expected JSON string format.\n | \n | * Series\n | \n | - default is 'index'\n | - allowed values are: {'split','records','index'}\n | \n | * DataFrame\n | \n | - default is 'columns'\n | - allowed values are:\n | {'split','records','index','columns','values'}\n | \n | * The format of the JSON string\n | \n | - 'split' : dict like {'index' -> [index],\n | 'columns' -> [columns], 'data' -> [values]}\n | - 'records' : list like\n | [{column -> value}, ... , {column -> value}]\n | - 'index' : dict like {index -> {column -> value}}\n | - 'columns' : dict like {column -> {index -> value}}\n | - 'values' : just the values array\n | - 'table' : dict like {'schema': {schema}, 'data': {data}}\n | describing the data, and the data component is\n | like ``orient='records'``.\n | \n | .. versionchanged:: 0.20.0\n | \n | date_format : {None, 'epoch', 'iso'}\n | Type of date conversion. 'epoch' = epoch milliseconds,\n | 'iso' = ISO8601. The default depends on the `orient`. For\n | ``orient='table'``, the default is 'iso'. For all other orients,\n | the default is 'epoch'.\n | double_precision : int, default 10\n | The number of decimal places to use when encoding\n | floating point values.\n | force_ascii : boolean, default True\n | Force encoded string to be ASCII.\n | date_unit : string, default 'ms' (milliseconds)\n | The time unit to encode to, governs timestamp and ISO8601\n | precision. One of 's', 'ms', 'us', 'ns' for second, millisecond,\n | microsecond, and nanosecond respectively.\n | default_handler : callable, default None\n | Handler to call if object cannot otherwise be converted to a\n | suitable format for JSON. Should receive a single argument which is\n | the object to convert and return a serialisable object.\n | lines : boolean, default False\n | If 'orient' is 'records' write out line delimited json format. Will\n | throw ValueError if incorrect 'orient' since others are not list\n | like.\n | \n | .. versionadded:: 0.19.0\n | \n | compression : {None, 'gzip', 'bz2', 'zip', 'xz'}\n | A string representing the compression to use in the output file,\n | only used when the first argument is a filename.\n | \n | .. versionadded:: 0.21.0\n | \n | index : boolean, default True\n | Whether to include the index values in the JSON string. Not\n | including the index (``index=False``) is only supported when\n | orient is 'split' or 'table'.\n | \n | .. versionadded:: 0.23.0\n | \n | See Also\n | --------\n | pandas.read_json\n | \n | Examples\n | --------\n | \n | >>> df = pd.DataFrame([['a', 'b'], ['c', 'd']],\n | ... index=['row 1', 'row 2'],\n | ... columns=['col 1', 'col 2'])\n | >>> df.to_json(orient='split')\n | '{\"columns\":[\"col 1\",\"col 2\"],\n | \"index\":[\"row 1\",\"row 2\"],\n | \"data\":[[\"a\",\"b\"],[\"c\",\"d\"]]}'\n | \n | Encoding/decoding a Dataframe using ``'records'`` formatted JSON.\n | Note that index labels are not preserved with this encoding.\n | \n | >>> df.to_json(orient='records')\n | '[{\"col 1\":\"a\",\"col 2\":\"b\"},{\"col 1\":\"c\",\"col 2\":\"d\"}]'\n | \n | Encoding/decoding a Dataframe using ``'index'`` formatted JSON:\n | \n | >>> df.to_json(orient='index')\n | '{\"row 1\":{\"col 1\":\"a\",\"col 2\":\"b\"},\"row 2\":{\"col 1\":\"c\",\"col 2\":\"d\"}}'\n | \n | Encoding/decoding a Dataframe using ``'columns'`` formatted JSON:\n | \n | >>> df.to_json(orient='columns')\n | '{\"col 1\":{\"row 1\":\"a\",\"row 2\":\"c\"},\"col 2\":{\"row 1\":\"b\",\"row 2\":\"d\"}}'\n | \n | Encoding/decoding a Dataframe using ``'values'`` formatted JSON:\n | \n | >>> df.to_json(orient='values')\n | '[[\"a\",\"b\"],[\"c\",\"d\"]]'\n | \n | Encoding with Table Schema\n | \n | >>> df.to_json(orient='table')\n | '{\"schema\": {\"fields\": [{\"name\": \"index\", \"type\": \"string\"},\n | {\"name\": \"col 1\", \"type\": \"string\"},\n | {\"name\": \"col 2\", \"type\": \"string\"}],\n | \"primaryKey\": \"index\",\n | \"pandas_version\": \"0.20.0\"},\n | \"data\": [{\"index\": \"row 1\", \"col 1\": \"a\", \"col 2\": \"b\"},\n | {\"index\": \"row 2\", \"col 1\": \"c\", \"col 2\": \"d\"}]}'\n | \n | to_latex(self, buf=None, columns=None, col_space=None, header=True, index=True, na_rep='NaN', formatters=None, float_format=None, sparsify=None, index_names=True, bold_rows=False, column_format=None, longtable=None, escape=None, encoding=None, decimal='.', multicolumn=None, multicolumn_format=None, multirow=None)\n | Render an object to a tabular environment table. You can splice\n | this into a LaTeX document. Requires \\\\usepackage{booktabs}.\n | \n | .. versionchanged:: 0.20.2\n | Added to Series\n | \n | `to_latex`-specific options:\n | \n | bold_rows : boolean, default False\n | Make the row labels bold in the output\n | column_format : str, default None\n | The columns format as specified in `LaTeX table format\n | <https://en.wikibooks.org/wiki/LaTeX/Tables>`__ e.g 'rcl' for 3\n | columns\n | longtable : boolean, default will be read from the pandas config module\n | Default: False.\n | Use a longtable environment instead of tabular. Requires adding\n | a \\\\usepackage{longtable} to your LaTeX preamble.\n | escape : boolean, default will be read from the pandas config module\n | Default: True.\n | When set to False prevents from escaping latex special\n | characters in column names.\n | encoding : str, default None\n | A string representing the encoding to use in the output file,\n | defaults to 'ascii' on Python 2 and 'utf-8' on Python 3.\n | decimal : string, default '.'\n | Character recognized as decimal separator, e.g. ',' in Europe.\n | \n | .. versionadded:: 0.18.0\n | \n | multicolumn : boolean, default True\n | Use \\multicolumn to enhance MultiIndex columns.\n | The default will be read from the config module.\n | \n | .. versionadded:: 0.20.0\n | \n | multicolumn_format : str, default 'l'\n | The alignment for multicolumns, similar to `column_format`\n | The default will be read from the config module.\n | \n | .. versionadded:: 0.20.0\n | \n | multirow : boolean, default False\n | Use \\multirow to enhance MultiIndex rows.\n | Requires adding a \\\\usepackage{multirow} to your LaTeX preamble.\n | Will print centered labels (instead of top-aligned)\n | across the contained rows, separating groups via clines.\n | The default will be read from the pandas config module.\n | \n | .. versionadded:: 0.20.0\n | \n | to_msgpack(self, path_or_buf=None, encoding='utf-8', **kwargs)\n | msgpack (serialize) object to input file path\n | \n | THIS IS AN EXPERIMENTAL LIBRARY and the storage format\n | may not be stable until a future release.\n | \n | Parameters\n | ----------\n | path : string File path, buffer-like, or None\n | if None, return generated string\n | append : boolean whether to append to an existing msgpack\n | (default is False)\n | compress : type of compressor (zlib or blosc), default to None (no\n | compression)\n | \n | to_pickle(self, path, compression='infer', protocol=4)\n | Pickle (serialize) object to file.\n | \n | Parameters\n | ----------\n | path : str\n | File path where the pickled object will be stored.\n | compression : {'infer', 'gzip', 'bz2', 'zip', 'xz', None}, default 'infer'\n | A string representing the compression to use in the output file. By\n | default, infers from the file extension in specified path.\n | \n | .. versionadded:: 0.20.0\n | protocol : int\n | Int which indicates which protocol should be used by the pickler,\n | default HIGHEST_PROTOCOL (see [1]_ paragraph 12.1.2). The possible\n | values for this parameter depend on the version of Python. For\n | Python 2.x, possible values are 0, 1, 2. For Python>=3.0, 3 is a\n | valid value. For Python >= 3.4, 4 is a valid value. A negative\n | value for the protocol parameter is equivalent to setting its value\n | to HIGHEST_PROTOCOL.\n | \n | .. [1] https://docs.python.org/3/library/pickle.html\n | .. versionadded:: 0.21.0\n | \n | See Also\n | --------\n | read_pickle : Load pickled pandas object (or any object) from file.\n | DataFrame.to_hdf : Write DataFrame to an HDF5 file.\n | DataFrame.to_sql : Write DataFrame to a SQL database.\n | DataFrame.to_parquet : Write a DataFrame to the binary parquet format.\n | \n | Examples\n | --------\n | >>> original_df = pd.DataFrame({\"foo\": range(5), \"bar\": range(5, 10)})\n | >>> original_df\n | foo bar\n | 0 0 5\n | 1 1 6\n | 2 2 7\n | 3 3 8\n | 4 4 9\n | >>> original_df.to_pickle(\"./dummy.pkl\")\n | \n | >>> unpickled_df = pd.read_pickle(\"./dummy.pkl\")\n | >>> unpickled_df\n | foo bar\n | 0 0 5\n | 1 1 6\n | 2 2 7\n | 3 3 8\n | 4 4 9\n | \n | >>> import os\n | >>> os.remove(\"./dummy.pkl\")\n | \n | to_sql(self, name, con, schema=None, if_exists='fail', index=True, index_label=None, chunksize=None, dtype=None)\n | Write records stored in a DataFrame to a SQL database.\n | \n | Databases supported by SQLAlchemy [1]_ are supported. Tables can be\n | newly created, appended to, or overwritten.\n | \n | Parameters\n | ----------\n | name : string\n | Name of SQL table.\n | con : sqlalchemy.engine.Engine or sqlite3.Connection\n | Using SQLAlchemy makes it possible to use any DB supported by that\n | library. Legacy support is provided for sqlite3.Connection objects.\n | schema : string, optional\n | Specify the schema (if database flavor supports this). If None, use\n | default schema.\n | if_exists : {'fail', 'replace', 'append'}, default 'fail'\n | How to behave if the table already exists.\n | \n | * fail: Raise a ValueError.\n | * replace: Drop the table before inserting new values.\n | * append: Insert new values to the existing table.\n | \n | index : boolean, default True\n | Write DataFrame index as a column. Uses `index_label` as the column\n | name in the table.\n | index_label : string or sequence, default None\n | Column label for index column(s). If None is given (default) and\n | `index` is True, then the index names are used.\n | A sequence should be given if the DataFrame uses MultiIndex.\n | chunksize : int, optional\n | Rows will be written in batches of this size at a time. By default,\n | all rows will be written at once.\n | dtype : dict, optional\n | Specifying the datatype for columns. The keys should be the column\n | names and the values should be the SQLAlchemy types or strings for\n | the sqlite3 legacy mode.\n | \n | Raises\n | ------\n | ValueError\n | When the table already exists and `if_exists` is 'fail' (the\n | default).\n | \n | See Also\n | --------\n | pandas.read_sql : read a DataFrame from a table\n | \n | References\n | ----------\n | .. [1] http://docs.sqlalchemy.org\n | .. [2] https://www.python.org/dev/peps/pep-0249/\n | \n | Examples\n | --------\n | \n | Create an in-memory SQLite database.\n | \n | >>> from sqlalchemy import create_engine\n | >>> engine = create_engine('sqlite://', echo=False)\n | \n | Create a table from scratch with 3 rows.\n | \n | >>> df = pd.DataFrame({'name' : ['User 1', 'User 2', 'User 3']})\n | >>> df\n | name\n | 0 User 1\n | 1 User 2\n | 2 User 3\n | \n | >>> df.to_sql('users', con=engine)\n | >>> engine.execute(\"SELECT * FROM users\").fetchall()\n | [(0, 'User 1'), (1, 'User 2'), (2, 'User 3')]\n | \n | >>> df1 = pd.DataFrame({'name' : ['User 4', 'User 5']})\n | >>> df1.to_sql('users', con=engine, if_exists='append')\n | >>> engine.execute(\"SELECT * FROM users\").fetchall()\n | [(0, 'User 1'), (1, 'User 2'), (2, 'User 3'),\n | (0, 'User 4'), (1, 'User 5')]\n | \n | Overwrite the table with just ``df1``.\n | \n | >>> df1.to_sql('users', con=engine, if_exists='replace',\n | ... index_label='id')\n | >>> engine.execute(\"SELECT * FROM users\").fetchall()\n | [(0, 'User 4'), (1, 'User 5')]\n | \n | Specify the dtype (especially useful for integers with missing values).\n | Notice that while pandas is forced to store the data as floating point,\n | the database supports nullable integers. When fetching the data with\n | Python, we get back integer scalars.\n | \n | >>> df = pd.DataFrame({\"A\": [1, None, 2]})\n | >>> df\n | A\n | 0 1.0\n | 1 NaN\n | 2 2.0\n | \n | >>> from sqlalchemy.types import Integer\n | >>> df.to_sql('integers', con=engine, index=False,\n | ... dtype={\"A\": Integer()})\n | \n | >>> engine.execute(\"SELECT * FROM integers\").fetchall()\n | [(1,), (None,), (2,)]\n | \n | to_xarray(self)\n | Return an xarray object from the pandas object.\n | \n | Returns\n | -------\n | a DataArray for a Series\n | a Dataset for a DataFrame\n | a DataArray for higher dims\n | \n | Examples\n | --------\n | >>> df = pd.DataFrame({'A' : [1, 1, 2],\n | 'B' : ['foo', 'bar', 'foo'],\n | 'C' : np.arange(4.,7)})\n | >>> df\n | A B C\n | 0 1 foo 4.0\n | 1 1 bar 5.0\n | 2 2 foo 6.0\n | \n | >>> df.to_xarray()\n | <xarray.Dataset>\n | Dimensions: (index: 3)\n | Coordinates:\n | * index (index) int64 0 1 2\n | Data variables:\n | A (index) int64 1 1 2\n | B (index) object 'foo' 'bar' 'foo'\n | C (index) float64 4.0 5.0 6.0\n | \n | >>> df = pd.DataFrame({'A' : [1, 1, 2],\n | 'B' : ['foo', 'bar', 'foo'],\n | 'C' : np.arange(4.,7)}\n | ).set_index(['B','A'])\n | >>> df\n | C\n | B A\n | foo 1 4.0\n | bar 1 5.0\n | foo 2 6.0\n | \n | >>> df.to_xarray()\n | <xarray.Dataset>\n | Dimensions: (A: 2, B: 2)\n | Coordinates:\n | * B (B) object 'bar' 'foo'\n | * A (A) int64 1 2\n | Data variables:\n | C (B, A) float64 5.0 nan 4.0 6.0\n | \n | >>> p = pd.Panel(np.arange(24).reshape(4,3,2),\n | items=list('ABCD'),\n | major_axis=pd.date_range('20130101', periods=3),\n | minor_axis=['first', 'second'])\n | >>> p\n | <class 'pandas.core.panel.Panel'>\n | Dimensions: 4 (items) x 3 (major_axis) x 2 (minor_axis)\n | Items axis: A to D\n | Major_axis axis: 2013-01-01 00:00:00 to 2013-01-03 00:00:00\n | Minor_axis axis: first to second\n | \n | >>> p.to_xarray()\n | <xarray.DataArray (items: 4, major_axis: 3, minor_axis: 2)>\n | array([[[ 0, 1],\n | [ 2, 3],\n | [ 4, 5]],\n | [[ 6, 7],\n | [ 8, 9],\n | [10, 11]],\n | [[12, 13],\n | [14, 15],\n | [16, 17]],\n | [[18, 19],\n | [20, 21],\n | [22, 23]]])\n | Coordinates:\n | * items (items) object 'A' 'B' 'C' 'D'\n | * major_axis (major_axis) datetime64[ns] 2013-01-01 2013-01-02 2013-01-03 # noqa\n | * minor_axis (minor_axis) object 'first' 'second'\n | \n | Notes\n | -----\n | See the `xarray docs <http://xarray.pydata.org/en/stable/>`__\n | \n | truncate(self, before=None, after=None, axis=None, copy=True)\n | Truncate a Series or DataFrame before and after some index value.\n | \n | This is a useful shorthand for boolean indexing based on index\n | values above or below certain thresholds.\n | \n | Parameters\n | ----------\n | before : date, string, int\n | Truncate all rows before this index value.\n | after : date, string, int\n | Truncate all rows after this index value.\n | axis : {0 or 'index', 1 or 'columns'}, optional\n | Axis to truncate. Truncates the index (rows) by default.\n | copy : boolean, default is True,\n | Return a copy of the truncated section.\n | \n | Returns\n | -------\n | type of caller\n | The truncated Series or DataFrame.\n | \n | See Also\n | --------\n | DataFrame.loc : Select a subset of a DataFrame by label.\n | DataFrame.iloc : Select a subset of a DataFrame by position.\n | \n | Notes\n | -----\n | If the index being truncated contains only datetime values,\n | `before` and `after` may be specified as strings instead of\n | Timestamps.\n | \n | Examples\n | --------\n | >>> df = pd.DataFrame({'A': ['a', 'b', 'c', 'd', 'e'],\n | ... 'B': ['f', 'g', 'h', 'i', 'j'],\n | ... 'C': ['k', 'l', 'm', 'n', 'o']},\n | ... index=[1, 2, 3, 4, 5])\n | >>> df\n | A B C\n | 1 a f k\n | 2 b g l\n | 3 c h m\n | 4 d i n\n | 5 e j o\n | \n | >>> df.truncate(before=2, after=4)\n | A B C\n | 2 b g l\n | 3 c h m\n | 4 d i n\n | \n | The columns of a DataFrame can be truncated.\n | \n | >>> df.truncate(before=\"A\", after=\"B\", axis=\"columns\")\n | A B\n | 1 a f\n | 2 b g\n | 3 c h\n | 4 d i\n | 5 e j\n | \n | For Series, only rows can be truncated.\n | \n | >>> df['A'].truncate(before=2, after=4)\n | 2 b\n | 3 c\n | 4 d\n | Name: A, dtype: object\n | \n | The index values in ``truncate`` can be datetimes or string\n | dates.\n | \n | >>> dates = pd.date_range('2016-01-01', '2016-02-01', freq='s')\n | >>> df = pd.DataFrame(index=dates, data={'A': 1})\n | >>> df.tail()\n | A\n | 2016-01-31 23:59:56 1\n | 2016-01-31 23:59:57 1\n | 2016-01-31 23:59:58 1\n | 2016-01-31 23:59:59 1\n | 2016-02-01 00:00:00 1\n | \n | >>> df.truncate(before=pd.Timestamp('2016-01-05'),\n | ... after=pd.Timestamp('2016-01-10')).tail()\n | A\n | 2016-01-09 23:59:56 1\n | 2016-01-09 23:59:57 1\n | 2016-01-09 23:59:58 1\n | 2016-01-09 23:59:59 1\n | 2016-01-10 00:00:00 1\n | \n | Because the index is a DatetimeIndex containing only dates, we can\n | specify `before` and `after` as strings. They will be coerced to\n | Timestamps before truncation.\n | \n | >>> df.truncate('2016-01-05', '2016-01-10').tail()\n | A\n | 2016-01-09 23:59:56 1\n | 2016-01-09 23:59:57 1\n | 2016-01-09 23:59:58 1\n | 2016-01-09 23:59:59 1\n | 2016-01-10 00:00:00 1\n | \n | Note that ``truncate`` assumes a 0 value for any unspecified time\n | component (midnight). This differs from partial string slicing, which\n | returns any partially matching dates.\n | \n | >>> df.loc['2016-01-05':'2016-01-10', :].tail()\n | A\n | 2016-01-10 23:59:55 1\n | 2016-01-10 23:59:56 1\n | 2016-01-10 23:59:57 1\n | 2016-01-10 23:59:58 1\n | 2016-01-10 23:59:59 1\n | \n | tshift(self, periods=1, freq=None, axis=0)\n | Shift the time index, using the index's frequency if available.\n | \n | Parameters\n | ----------\n | periods : int\n | Number of periods to move, can be positive or negative\n | freq : DateOffset, timedelta, or time rule string, default None\n | Increment to use from the tseries module or time rule (e.g. 'EOM')\n | axis : int or basestring\n | Corresponds to the axis that contains the Index\n | \n | Notes\n | -----\n | If freq is not specified then tries to use the freq or inferred_freq\n | attributes of the index. If neither of those attributes exist, a\n | ValueError is thrown\n | \n | Returns\n | -------\n | shifted : NDFrame\n | \n | tz_convert(self, tz, axis=0, level=None, copy=True)\n | Convert tz-aware axis to target time zone.\n | \n | Parameters\n | ----------\n | tz : string or pytz.timezone object\n | axis : the axis to convert\n | level : int, str, default None\n | If axis ia a MultiIndex, convert a specific level. Otherwise\n | must be None\n | copy : boolean, default True\n | Also make a copy of the underlying data\n | \n | Returns\n | -------\n | \n | Raises\n | ------\n | TypeError\n | If the axis is tz-naive.\n | \n | tz_localize(self, tz, axis=0, level=None, copy=True, ambiguous='raise')\n | Localize tz-naive TimeSeries to target time zone.\n | \n | Parameters\n | ----------\n | tz : string or pytz.timezone object\n | axis : the axis to localize\n | level : int, str, default None\n | If axis ia a MultiIndex, localize a specific level. Otherwise\n | must be None\n | copy : boolean, default True\n | Also make a copy of the underlying data\n | ambiguous : 'infer', bool-ndarray, 'NaT', default 'raise'\n | - 'infer' will attempt to infer fall dst-transition hours based on\n | order\n | - bool-ndarray where True signifies a DST time, False designates\n | a non-DST time (note that this flag is only applicable for\n | ambiguous times)\n | - 'NaT' will return NaT where there are ambiguous times\n | - 'raise' will raise an AmbiguousTimeError if there are ambiguous\n | times\n | \n | Returns\n | -------\n | \n | Raises\n | ------\n | TypeError\n | If the TimeSeries is tz-aware and tz is not None.\n | \n | where(self, cond, other=nan, inplace=False, axis=None, level=None, errors='raise', try_cast=False, raise_on_error=None)\n | Return an object of same shape as self and whose corresponding\n | entries are from self where `cond` is True and otherwise are from\n | `other`.\n | \n | Parameters\n | ----------\n | cond : boolean NDFrame, array-like, or callable\n | Where `cond` is True, keep the original value. Where\n | False, replace with corresponding value from `other`.\n | If `cond` is callable, it is computed on the NDFrame and\n | should return boolean NDFrame or array. The callable must\n | not change input NDFrame (though pandas doesn't check it).\n | \n | .. versionadded:: 0.18.1\n | A callable can be used as cond.\n | \n | other : scalar, NDFrame, or callable\n | Entries where `cond` is False are replaced with\n | corresponding value from `other`.\n | If other is callable, it is computed on the NDFrame and\n | should return scalar or NDFrame. The callable must not\n | change input NDFrame (though pandas doesn't check it).\n | \n | .. versionadded:: 0.18.1\n | A callable can be used as other.\n | \n | inplace : boolean, default False\n | Whether to perform the operation in place on the data\n | axis : alignment axis if needed, default None\n | level : alignment level if needed, default None\n | errors : str, {'raise', 'ignore'}, default 'raise'\n | - ``raise`` : allow exceptions to be raised\n | - ``ignore`` : suppress exceptions. On error return original object\n | \n | Note that currently this parameter won't affect\n | the results and will always coerce to a suitable dtype.\n | \n | try_cast : boolean, default False\n | try to cast the result back to the input type (if possible),\n | raise_on_error : boolean, default True\n | Whether to raise on invalid data types (e.g. trying to where on\n | strings)\n | \n | .. deprecated:: 0.21.0\n | \n | Returns\n | -------\n | wh : same type as caller\n | \n | Notes\n | -----\n | The where method is an application of the if-then idiom. For each\n | element in the calling DataFrame, if ``cond`` is ``True`` the\n | element is used; otherwise the corresponding element from the DataFrame\n | ``other`` is used.\n | \n | The signature for :func:`DataFrame.where` differs from\n | :func:`numpy.where`. Roughly ``df1.where(m, df2)`` is equivalent to\n | ``np.where(m, df1, df2)``.\n | \n | For further details and examples see the ``where`` documentation in\n | :ref:`indexing <indexing.where_mask>`.\n | \n | Examples\n | --------\n | >>> s = pd.Series(range(5))\n | >>> s.where(s > 0)\n | 0 NaN\n | 1 1.0\n | 2 2.0\n | 3 3.0\n | 4 4.0\n | \n | >>> s.mask(s > 0)\n | 0 0.0\n | 1 NaN\n | 2 NaN\n | 3 NaN\n | 4 NaN\n | \n | >>> s.where(s > 1, 10)\n | 0 10.0\n | 1 10.0\n | 2 2.0\n | 3 3.0\n | 4 4.0\n | \n | >>> df = pd.DataFrame(np.arange(10).reshape(-1, 2), columns=['A', 'B'])\n | >>> m = df % 3 == 0\n | >>> df.where(m, -df)\n | A B\n | 0 0 -1\n | 1 -2 3\n | 2 -4 -5\n | 3 6 -7\n | 4 -8 9\n | >>> df.where(m, -df) == np.where(m, df, -df)\n | A B\n | 0 True True\n | 1 True True\n | 2 True True\n | 3 True True\n | 4 True True\n | >>> df.where(m, -df) == df.mask(~m, -df)\n | A B\n | 0 True True\n | 1 True True\n | 2 True True\n | 3 True True\n | 4 True True\n | \n | See Also\n | --------\n | :func:`DataFrame.mask`\n | \n | xs(self, key, axis=0, level=None, drop_level=True)\n | Returns a cross-section (row(s) or column(s)) from the\n | Series/DataFrame. Defaults to cross-section on the rows (axis=0).\n | \n | Parameters\n | ----------\n | key : object\n | Some label contained in the index, or partially in a MultiIndex\n | axis : int, default 0\n | Axis to retrieve cross-section on\n | level : object, defaults to first n levels (n=1 or len(key))\n | In case of a key partially contained in a MultiIndex, indicate\n | which levels are used. Levels can be referred by label or position.\n | drop_level : boolean, default True\n | If False, returns object with same levels as self.\n | \n | Examples\n | --------\n | >>> df\n | A B C\n | a 4 5 2\n | b 4 0 9\n | c 9 7 3\n | >>> df.xs('a')\n | A 4\n | B 5\n | C 2\n | Name: a\n | >>> df.xs('C', axis=1)\n | a 2\n | b 9\n | c 3\n | Name: C\n | \n | >>> df\n | A B C D\n | first second third\n | bar one 1 4 1 8 9\n | two 1 7 5 5 0\n | baz one 1 6 6 8 0\n | three 2 5 3 5 3\n | >>> df.xs(('baz', 'three'))\n | A B C D\n | third\n | 2 5 3 5 3\n | >>> df.xs('one', level=1)\n | A B C D\n | first third\n | bar 1 4 1 8 9\n | baz 1 6 6 8 0\n | >>> df.xs(('baz', 2), level=[0, 'third'])\n | A B C D\n | second\n | three 5 3 5 3\n | \n | Returns\n | -------\n | xs : Series or DataFrame\n | \n | Notes\n | -----\n | xs is only for getting, not setting values.\n | \n | MultiIndex Slicers is a generic way to get/set values on any level or\n | levels. It is a superset of xs functionality, see\n | :ref:`MultiIndex Slicers <advanced.mi_slicers>`\n | \n | ----------------------------------------------------------------------\n | Data descriptors inherited from pandas.core.generic.NDFrame:\n | \n | at\n | Access a single value for a row/column label pair.\n | \n | Similar to ``loc``, in that both provide label-based lookups. Use\n | ``at`` if you only need to get or set a single value in a DataFrame\n | or Series.\n | \n | See Also\n | --------\n | DataFrame.iat : Access a single value for a row/column pair by integer\n | position\n | DataFrame.loc : Access a group of rows and columns by label(s)\n | Series.at : Access a single value using a label\n | \n | Examples\n | --------\n | >>> df = pd.DataFrame([[0, 2, 3], [0, 4, 1], [10, 20, 30]],\n | ... index=[4, 5, 6], columns=['A', 'B', 'C'])\n | >>> df\n | A B C\n | 4 0 2 3\n | 5 0 4 1\n | 6 10 20 30\n | \n | Get value at specified row/column pair\n | \n | >>> df.at[4, 'B']\n | 2\n | \n | Set value at specified row/column pair\n | \n | >>> df.at[4, 'B'] = 10\n | >>> df.at[4, 'B']\n | 10\n | \n | Get value within a Series\n | \n | >>> df.loc[5].at['B']\n | 4\n | \n | Raises\n | ------\n | KeyError\n | When label does not exist in DataFrame\n | \n | blocks\n | Internal property, property synonym for as_blocks()\n | \n | .. deprecated:: 0.21.0\n | \n | iat\n | Access a single value for a row/column pair by integer position.\n | \n | Similar to ``iloc``, in that both provide integer-based lookups. Use\n | ``iat`` if you only need to get or set a single value in a DataFrame\n | or Series.\n | \n | See Also\n | --------\n | DataFrame.at : Access a single value for a row/column label pair\n | DataFrame.loc : Access a group of rows and columns by label(s)\n | DataFrame.iloc : Access a group of rows and columns by integer position(s)\n | \n | Examples\n | --------\n | >>> df = pd.DataFrame([[0, 2, 3], [0, 4, 1], [10, 20, 30]],\n | ... columns=['A', 'B', 'C'])\n | >>> df\n | A B C\n | 0 0 2 3\n | 1 0 4 1\n | 2 10 20 30\n | \n | Get value at specified row/column pair\n | \n | >>> df.iat[1, 2]\n | 1\n | \n | Set value at specified row/column pair\n | \n | >>> df.iat[1, 2] = 10\n | >>> df.iat[1, 2]\n | 10\n | \n | Get value within a series\n | \n | >>> df.loc[0].iat[1]\n | 2\n | \n | Raises\n | ------\n | IndexError\n | When integer position is out of bounds\n | \n | iloc\n | Purely integer-location based indexing for selection by position.\n | \n | ``.iloc[]`` is primarily integer position based (from ``0`` to\n | ``length-1`` of the axis), but may also be used with a boolean\n | array.\n | \n | Allowed inputs are:\n | \n | - An integer, e.g. ``5``.\n | - A list or array of integers, e.g. ``[4, 3, 0]``.\n | - A slice object with ints, e.g. ``1:7``.\n | - A boolean array.\n | - A ``callable`` function with one argument (the calling Series, DataFrame\n | or Panel) and that returns valid output for indexing (one of the above)\n | \n | ``.iloc`` will raise ``IndexError`` if a requested indexer is\n | out-of-bounds, except *slice* indexers which allow out-of-bounds\n | indexing (this conforms with python/numpy *slice* semantics).\n | \n | See more at :ref:`Selection by Position <indexing.integer>`\n | \n | is_copy\n | \n | ix\n | A primarily label-location based indexer, with integer position\n | fallback.\n | \n | Warning: Starting in 0.20.0, the .ix indexer is deprecated, in\n | favor of the more strict .iloc and .loc indexers.\n | \n | ``.ix[]`` supports mixed integer and label based access. It is\n | primarily label based, but will fall back to integer positional\n | access unless the corresponding axis is of integer type.\n | \n | ``.ix`` is the most general indexer and will support any of the\n | inputs in ``.loc`` and ``.iloc``. ``.ix`` also supports floating\n | point label schemes. ``.ix`` is exceptionally useful when dealing\n | with mixed positional and label based hierarchical indexes.\n | \n | However, when an axis is integer based, ONLY label based access\n | and not positional access is supported. Thus, in such cases, it's\n | usually better to be explicit and use ``.iloc`` or ``.loc``.\n | \n | See more at :ref:`Advanced Indexing <advanced>`.\n | \n | loc\n | Access a group of rows and columns by label(s) or a boolean array.\n | \n | ``.loc[]`` is primarily label based, but may also be used with a\n | boolean array.\n | \n | Allowed inputs are:\n | \n | - A single label, e.g. ``5`` or ``'a'``, (note that ``5`` is\n | interpreted as a *label* of the index, and **never** as an\n | integer position along the index).\n | - A list or array of labels, e.g. ``['a', 'b', 'c']``.\n | - A slice object with labels, e.g. ``'a':'f'``.\n | \n | .. warning:: Note that contrary to usual python slices, **both** the\n | start and the stop are included\n | \n | - A boolean array of the same length as the axis being sliced,\n | e.g. ``[True, False, True]``.\n | - A ``callable`` function with one argument (the calling Series, DataFrame\n | or Panel) and that returns valid output for indexing (one of the above)\n | \n | See more at :ref:`Selection by Label <indexing.label>`\n | \n | See Also\n | --------\n | DataFrame.at : Access a single value for a row/column label pair\n | DataFrame.iloc : Access group of rows and columns by integer position(s)\n | DataFrame.xs : Returns a cross-section (row(s) or column(s)) from the\n | Series/DataFrame.\n | Series.loc : Access group of values using labels\n | \n | Examples\n | --------\n | **Getting values**\n | \n | >>> df = pd.DataFrame([[1, 2], [4, 5], [7, 8]],\n | ... index=['cobra', 'viper', 'sidewinder'],\n | ... columns=['max_speed', 'shield'])\n | >>> df\n | max_speed shield\n | cobra 1 2\n | viper 4 5\n | sidewinder 7 8\n | \n | Single label. Note this returns the row as a Series.\n | \n | >>> df.loc['viper']\n | max_speed 4\n | shield 5\n | Name: viper, dtype: int64\n | \n | List of labels. Note using ``[[]]`` returns a DataFrame.\n | \n | >>> df.loc[['viper', 'sidewinder']]\n | max_speed shield\n | viper 4 5\n | sidewinder 7 8\n | \n | Single label for row and column\n | \n | >>> df.loc['cobra', 'shield']\n | 2\n | \n | Slice with labels for row and single label for column. As mentioned\n | above, note that both the start and stop of the slice are included.\n | \n | >>> df.loc['cobra':'viper', 'max_speed']\n | cobra 1\n | viper 4\n | Name: max_speed, dtype: int64\n | \n | Boolean list with the same length as the row axis\n | \n | >>> df.loc[[False, False, True]]\n | max_speed shield\n | sidewinder 7 8\n | \n | Conditional that returns a boolean Series\n | \n | >>> df.loc[df['shield'] > 6]\n | max_speed shield\n | sidewinder 7 8\n | \n | Conditional that returns a boolean Series with column labels specified\n | \n | >>> df.loc[df['shield'] > 6, ['max_speed']]\n | max_speed\n | sidewinder 7\n | \n | Callable that returns a boolean Series\n | \n | >>> df.loc[lambda df: df['shield'] == 8]\n | max_speed shield\n | sidewinder 7 8\n | \n | **Setting values**\n | \n | Set value for all items matching the list of labels\n | \n | >>> df.loc[['viper', 'sidewinder'], ['shield']] = 50\n | >>> df\n | max_speed shield\n | cobra 1 2\n | viper 4 50\n | sidewinder 7 50\n | \n | Set value for an entire row\n | \n | >>> df.loc['cobra'] = 10\n | >>> df\n | max_speed shield\n | cobra 10 10\n | viper 4 50\n | sidewinder 7 50\n | \n | Set value for an entire column\n | \n | >>> df.loc[:, 'max_speed'] = 30\n | >>> df\n | max_speed shield\n | cobra 30 10\n | viper 30 50\n | sidewinder 30 50\n | \n | Set value for rows matching callable condition\n | \n | >>> df.loc[df['shield'] > 35] = 0\n | >>> df\n | max_speed shield\n | cobra 30 10\n | viper 0 0\n | sidewinder 0 0\n | \n | **Getting values on a DataFrame with an index that has integer labels**\n | \n | Another example using integers for the index\n | \n | >>> df = pd.DataFrame([[1, 2], [4, 5], [7, 8]],\n | ... index=[7, 8, 9], columns=['max_speed', 'shield'])\n | >>> df\n | max_speed shield\n | 7 1 2\n | 8 4 5\n | 9 7 8\n | \n | Slice with integer labels for rows. As mentioned above, note that both\n | the start and stop of the slice are included.\n | \n | >>> df.loc[7:9]\n | max_speed shield\n | 7 1 2\n | 8 4 5\n | 9 7 8\n | \n | **Getting values with a MultiIndex**\n | \n | A number of examples using a DataFrame with a MultiIndex\n | \n | >>> tuples = [\n | ... ('cobra', 'mark i'), ('cobra', 'mark ii'),\n | ... ('sidewinder', 'mark i'), ('sidewinder', 'mark ii'),\n | ... ('viper', 'mark ii'), ('viper', 'mark iii')\n | ... ]\n | >>> index = pd.MultiIndex.from_tuples(tuples)\n | >>> values = [[12, 2], [0, 4], [10, 20],\n | ... [1, 4], [7, 1], [16, 36]]\n | >>> df = pd.DataFrame(values, columns=['max_speed', 'shield'], index=index)\n | >>> df\n | max_speed shield\n | cobra mark i 12 2\n | mark ii 0 4\n | sidewinder mark i 10 20\n | mark ii 1 4\n | viper mark ii 7 1\n | mark iii 16 36\n | \n | Single label. Note this returns a DataFrame with a single index.\n | \n | >>> df.loc['cobra']\n | max_speed shield\n | mark i 12 2\n | mark ii 0 4\n | \n | Single index tuple. Note this returns a Series.\n | \n | >>> df.loc[('cobra', 'mark ii')]\n | max_speed 0\n | shield 4\n | Name: (cobra, mark ii), dtype: int64\n | \n | Single label for row and column. Similar to passing in a tuple, this\n | returns a Series.\n | \n | >>> df.loc['cobra', 'mark i']\n | max_speed 12\n | shield 2\n | Name: (cobra, mark i), dtype: int64\n | \n | Single tuple. Note using ``[[]]`` returns a DataFrame.\n | \n | >>> df.loc[[('cobra', 'mark ii')]]\n | max_speed shield\n | cobra mark ii 0 4\n | \n | Single tuple for the index with a single label for the column\n | \n | >>> df.loc[('cobra', 'mark i'), 'shield']\n | 2\n | \n | Slice from index tuple to single label\n | \n | >>> df.loc[('cobra', 'mark i'):'viper']\n | max_speed shield\n | cobra mark i 12 2\n | mark ii 0 4\n | sidewinder mark i 10 20\n | mark ii 1 4\n | viper mark ii 7 1\n | mark iii 16 36\n | \n | Slice from index tuple to index tuple\n | \n | >>> df.loc[('cobra', 'mark i'):('viper', 'mark ii')]\n | max_speed shield\n | cobra mark i 12 2\n | mark ii 0 4\n | sidewinder mark i 10 20\n | mark ii 1 4\n | viper mark ii 7 1\n | \n | Raises\n | ------\n | KeyError:\n | when any items are not found\n | \n | ----------------------------------------------------------------------\n | Methods inherited from pandas.core.base.PandasObject:\n | \n | __sizeof__(self)\n | Generates the total memory usage for an object that returns\n | either a value or Series of values\n | \n | ----------------------------------------------------------------------\n | Methods inherited from pandas.core.base.StringMixin:\n | \n | __bytes__(self)\n | Return a string representation for a particular object.\n | \n | Invoked by bytes(obj) in py3 only.\n | Yields a bytestring in both py2/py3.\n | \n | __repr__(self)\n | Return a string representation for a particular object.\n | \n | Yields Bytestring in Py2, Unicode String in py3.\n | \n | __str__(self)\n | Return a string representation for a particular Object\n | \n | Invoked by str(df) in both py2/py3.\n | Yields Bytestring in Py2, Unicode String in py3.\n | \n | ----------------------------------------------------------------------\n | Methods inherited from pandas.core.accessor.DirNamesMixin:\n | \n | __dir__(self)\n | Provide method name lookup and completion\n | Only provide 'public' methods\n\n"
],
[
"help(linrange)",
"Help on function linrange in module modsim:\n\nlinrange(start=0, stop=None, step=1, **options)\n Returns an array of evenly-spaced values in the interval [start, stop].\n \n This function works best if the space between start and stop\n is divisible by step; otherwise the results might be surprising.\n \n By default, the last value in the array is `stop-step`\n (at least approximately).\n If you provide the keyword argument `endpoint=True`,\n the last value in the array is `stop`.\n \n start: first value\n stop: last value\n step: space between values\n \n returns: array or Quantity\n\n"
]
]
] | [
"code"
] | [
[
"code",
"code",
"code",
"code",
"code"
]
] |
e780490c9e92d9afd6f283c2e6b0988c9eadf6e7 | 200,421 | ipynb | Jupyter Notebook | short_notebook/chapter02_short_ver.ipynb | hedwig100/PRML | 992f2c07e88b2bad331e08303bdba84684f04d40 | [
"MIT"
] | 1 | 2022-02-19T09:44:11.000Z | 2022-02-19T09:44:11.000Z | short_notebook/chapter02_short_ver.ipynb | hedwig100/PRML | 992f2c07e88b2bad331e08303bdba84684f04d40 | [
"MIT"
] | null | null | null | short_notebook/chapter02_short_ver.ipynb | hedwig100/PRML | 992f2c07e88b2bad331e08303bdba84684f04d40 | [
"MIT"
] | 1 | 2022-01-07T11:08:10.000Z | 2022-01-07T11:08:10.000Z | 490.026895 | 25,532 | 0.945899 | [
[
[
"import numpy as np \nimport matplotlib.pyplot as plt \n\nfrom prml.probability_distribution import (\n Binary,\n Multi,\n Gaussian1D,\n plot_student,\n Histgram,\n Parzen,\n KNearestNeighbor,\n KNeighborClassifier\n)",
"_____no_output_____"
]
],
[
[
"# 2.1 Binary Variables",
"_____no_output_____"
],
[
"<h3>\n $$Bern(x|\\mu) = \\mu^x(1-\\mu)^{1-x}$$ <br>\n <br>\n $$Beta(\\mu|a,b) = \\frac{\\Gamma(a+b)}{\\Gamma(a)\\Gamma(b)}\\mu^{a-1}(1-\\mu)^{b-1}$$\n</h3>",
"_____no_output_____"
]
],
[
[
"bern = Binary()\nX = np.array([1,0,0,0,1,1,1,1,1,1,0,1])\nbern.fit(X)\nbern.plot()",
"_____no_output_____"
]
],
[
[
"# 2.2 Multinomial Variables",
"_____no_output_____"
],
[
"<h3>\n $$p(\\boldsymbol{x}|\\boldsymbol{\\mu}) = \\Pi_{k=1}^K \\mu_k^{x_k}$$ <br> \n <br>\n $$Dir(\\boldsymbol{\\mu}|\\boldsymbol{\\alpha}) = \\frac{\\Gamma(\\alpha_0)}{\\Gamma(\\alpha_1) \\cdots \\Gamma(\\alpha_K)}\n \\Pi_{k=1}^K \\mu_k^{\\alpha_k-1}$$\n</h3>",
"_____no_output_____"
],
[
"# 2.3 The Gaussian Distribution",
"_____no_output_____"
],
[
"<h3>\n $$\\mathcal{N}(x|\\mu,\\sigma^2) = \\sqrt{\\frac{1}{2\\pi\\sigma^2}}\\exp{(-\\frac{(x - \\mu)^2}{2\\sigma^2})}$$<br>\n <br>\n $$\\mathcal{N}(\\boldsymbol{x}|\\boldsymbol{\\mu},\\boldsymbol{\\Sigma}) = \\sqrt{\\frac{1}{(2\\pi)^D|\\Sigma|}}\\exp{(-\\frac{1}{2}(\\boldsymbol{x} - \\boldsymbol{\\mu})^T\\Sigma^{-1}(\\boldsymbol{x} - \\boldsymbol{\\mu}))}$$\n</h3>",
"_____no_output_____"
]
],
[
[
"gauss = Gaussian1D()\nX = np.random.randn(100) + 4\ngauss.fit(X)\ngauss.plot()",
"_____no_output_____"
]
],
[
[
"## Student's t-distribution ",
"_____no_output_____"
]
],
[
[
"plot_student(mu = 0,lamda = 2,nu = 3)",
"_____no_output_____"
]
],
[
[
"# 2.5 Nonparametric Methods ",
"_____no_output_____"
]
],
[
[
"hist = Histgram(delta=5e-1)\nX = np.random.randn(100)\nhist.fit(X)\nhist.plot()",
"_____no_output_____"
]
],
[
[
"## Kernel density estimators ",
"_____no_output_____"
]
],
[
[
"parzen_gauss = Parzen()\nX = np.random.randn(100)*2 + 4 \nparzen_gauss.fit(X)\nparzen_gauss.plot()\n\nparzen_hist = Parzen(kernel = \"hist\")\nparzen_hist.fit(X)\nparzen_hist.plot()",
"_____no_output_____"
]
],
[
[
"## Nearest-neighbor methods",
"_____no_output_____"
]
],
[
[
"knn5 = KNearestNeighbor(k=5)\nknn30 = KNearestNeighbor(k=30)\nX = np.random.randn(100)*2.4 + 5.1\nknn5.fit(X)\nknn5.plot()\nknn30.fit(X)\nknn30.plot()",
"_____no_output_____"
],
[
"def load_iris():\n dict = {\n \"Iris-setosa\": 0,\n \"Iris-versicolor\": 1,\n \"Iris-virginica\": 2\n }\n X = []\n y = [] \n with open(\"../data/iris.data\") as f:\n data = f.read()\n \n for line in data.split(\"\\n\"):\n # sepal length | sepal width | petal length | petal width \n if len(line) == 0:\n continue\n sl,sw,pl,pw,cl = line.split(\",\")\n rec = np.array(list(map(float,(sl,sw,pl,pw))))\n cl = dict[cl]\n\n X.append(rec)\n y.append(cl)\n return np.array(X),np.array(y)",
"_____no_output_____"
],
[
"X,y = load_iris()\nX = X[:,:2]\n\nknn10 = KNeighborClassifier()\nknn10.fit(X,y)\nknn10.plot()",
"_____no_output_____"
],
[
"knn30 = KNeighborClassifier(k=30)\nknn30.fit(X,y)\nknn30.plot()",
"_____no_output_____"
]
]
] | [
"code",
"markdown",
"code",
"markdown",
"code",
"markdown",
"code",
"markdown",
"code",
"markdown",
"code",
"markdown",
"code"
] | [
[
"code"
],
[
"markdown",
"markdown"
],
[
"code"
],
[
"markdown",
"markdown",
"markdown",
"markdown"
],
[
"code"
],
[
"markdown"
],
[
"code"
],
[
"markdown"
],
[
"code"
],
[
"markdown"
],
[
"code"
],
[
"markdown"
],
[
"code",
"code",
"code",
"code"
]
] |
e7805593ceea7fa372c85652a6c351309394b319 | 5,246 | ipynb | Jupyter Notebook | ipynb/Germany-Bayern-LK-Weißenburg-Gunzenhausen.ipynb | oscovida/oscovida.github.io | c74d6da79feda1b5ccce107ad3acd48cf0e74c1c | [
"CC-BY-4.0"
] | 2 | 2020-06-19T09:16:14.000Z | 2021-01-24T17:47:56.000Z | ipynb/Germany-Bayern-LK-Weißenburg-Gunzenhausen.ipynb | oscovida/oscovida.github.io | c74d6da79feda1b5ccce107ad3acd48cf0e74c1c | [
"CC-BY-4.0"
] | 8 | 2020-04-20T16:49:49.000Z | 2021-12-25T16:54:19.000Z | ipynb/Germany-Bayern-LK-Weißenburg-Gunzenhausen.ipynb | oscovida/oscovida.github.io | c74d6da79feda1b5ccce107ad3acd48cf0e74c1c | [
"CC-BY-4.0"
] | 4 | 2020-04-20T13:24:45.000Z | 2021-01-29T11:12:12.000Z | 31.413174 | 200 | 0.535265 | [
[
[
"# Germany: LK Weißenburg-Gunzenhausen (Bayern)\n\n* Homepage of project: https://oscovida.github.io\n* Plots are explained at http://oscovida.github.io/plots.html\n* [Execute this Jupyter Notebook using myBinder](https://mybinder.org/v2/gh/oscovida/binder/master?filepath=ipynb/Germany-Bayern-LK-Weißenburg-Gunzenhausen.ipynb)",
"_____no_output_____"
]
],
[
[
"import datetime\nimport time\n\nstart = datetime.datetime.now()\nprint(f\"Notebook executed on: {start.strftime('%d/%m/%Y %H:%M:%S%Z')} {time.tzname[time.daylight]}\")",
"_____no_output_____"
],
[
"%config InlineBackend.figure_formats = ['svg']\nfrom oscovida import *",
"_____no_output_____"
],
[
"overview(country=\"Germany\", subregion=\"LK Weißenburg-Gunzenhausen\", weeks=5);",
"_____no_output_____"
],
[
"overview(country=\"Germany\", subregion=\"LK Weißenburg-Gunzenhausen\");",
"_____no_output_____"
],
[
"compare_plot(country=\"Germany\", subregion=\"LK Weißenburg-Gunzenhausen\", dates=\"2020-03-15:\");\n",
"_____no_output_____"
],
[
"# load the data\ncases, deaths = germany_get_region(landkreis=\"LK Weißenburg-Gunzenhausen\")\n\n# get population of the region for future normalisation:\ninhabitants = population(country=\"Germany\", subregion=\"LK Weißenburg-Gunzenhausen\")\nprint(f'Population of country=\"Germany\", subregion=\"LK Weißenburg-Gunzenhausen\": {inhabitants} people')\n\n# compose into one table\ntable = compose_dataframe_summary(cases, deaths)\n\n# show tables with up to 1000 rows\npd.set_option(\"max_rows\", 1000)\n\n# display the table\ntable",
"_____no_output_____"
]
],
[
[
"# Explore the data in your web browser\n\n- If you want to execute this notebook, [click here to use myBinder](https://mybinder.org/v2/gh/oscovida/binder/master?filepath=ipynb/Germany-Bayern-LK-Weißenburg-Gunzenhausen.ipynb)\n- and wait (~1 to 2 minutes)\n- Then press SHIFT+RETURN to advance code cell to code cell\n- See http://jupyter.org for more details on how to use Jupyter Notebook",
"_____no_output_____"
],
[
"# Acknowledgements:\n\n- Johns Hopkins University provides data for countries\n- Robert Koch Institute provides data for within Germany\n- Atlo Team for gathering and providing data from Hungary (https://atlo.team/koronamonitor/)\n- Open source and scientific computing community for the data tools\n- Github for hosting repository and html files\n- Project Jupyter for the Notebook and binder service\n- The H2020 project Photon and Neutron Open Science Cloud ([PaNOSC](https://www.panosc.eu/))\n\n--------------------",
"_____no_output_____"
]
],
[
[
"print(f\"Download of data from Johns Hopkins university: cases at {fetch_cases_last_execution()} and \"\n f\"deaths at {fetch_deaths_last_execution()}.\")",
"_____no_output_____"
],
[
"# to force a fresh download of data, run \"clear_cache()\"",
"_____no_output_____"
],
[
"print(f\"Notebook execution took: {datetime.datetime.now()-start}\")\n",
"_____no_output_____"
]
]
] | [
"markdown",
"code",
"markdown",
"code"
] | [
[
"markdown"
],
[
"code",
"code",
"code",
"code",
"code",
"code"
],
[
"markdown",
"markdown"
],
[
"code",
"code",
"code"
]
] |
e780564886fcae72ae71dff91d191a7c1acfdd4c | 66,247 | ipynb | Jupyter Notebook | Code/Assignment-10/SubjectSelectionExperiments (rCBF data).ipynb | Upward-Spiral-Science/spect-team | b5876fd76fc1da376b5d1fc6fd9337f620df142c | [
"Apache-2.0"
] | null | null | null | Code/Assignment-10/SubjectSelectionExperiments (rCBF data).ipynb | Upward-Spiral-Science/spect-team | b5876fd76fc1da376b5d1fc6fd9337f620df142c | [
"Apache-2.0"
] | 3 | 2016-02-11T21:18:53.000Z | 2016-04-27T03:50:34.000Z | Code/Assignment-10/SubjectSelectionExperiments (rCBF data).ipynb | Upward-Spiral-Science/spect-team | b5876fd76fc1da376b5d1fc6fd9337f620df142c | [
"Apache-2.0"
] | null | null | null | 129.388672 | 26,616 | 0.869821 | [
[
[
"## Subject Selection Experiments disorder data - Srinivas (handle: thewickedaxe)",
"_____no_output_____"
],
[
"### Initial Data Cleaning",
"_____no_output_____"
]
],
[
[
"# Standard\nimport pandas as pd\nimport numpy as np\n%matplotlib inline\nimport matplotlib.pyplot as plt\n\n# Dimensionality reduction and Clustering\nfrom sklearn.decomposition import PCA\nfrom sklearn.cluster import KMeans\nfrom sklearn.cluster import MeanShift, estimate_bandwidth\nfrom sklearn import manifold, datasets\nfrom itertools import cycle\n\n# Plotting tools and classifiers\nfrom matplotlib.colors import ListedColormap\nfrom sklearn.linear_model import LogisticRegression\nfrom sklearn.cross_validation import train_test_split\nfrom sklearn import preprocessing\nfrom sklearn.datasets import make_moons, make_circles, make_classification\nfrom sklearn.tree import DecisionTreeClassifier\nfrom sklearn.ensemble import RandomForestClassifier\nfrom sklearn.naive_bayes import GaussianNB\nfrom sklearn.discriminant_analysis import LinearDiscriminantAnalysis as LDA\nfrom sklearn.discriminant_analysis import QuadraticDiscriminantAnalysis as QDA\nfrom sklearn import cross_validation\nfrom sklearn.cross_validation import LeaveOneOut\n\n\n# Let's read the data in and clean it\n\ndef get_NaNs(df):\n columns = list(df.columns.get_values()) \n row_metrics = df.isnull().sum(axis=1)\n rows_with_na = []\n for i, x in enumerate(row_metrics):\n if x > 0: rows_with_na.append(i)\n return rows_with_na\n\ndef remove_NaNs(df):\n rows_with_na = get_NaNs(df)\n cleansed_df = df.drop(df.index[rows_with_na], inplace=False) \n return cleansed_df\n\ninitial_data = pd.DataFrame.from_csv('Data_Adults_1_reduced_inv1.csv')\ncleansed_df = remove_NaNs(initial_data)\n\n# Let's also get rid of nominal data\nnumerics = ['int16', 'int32', 'int64', 'float16', 'float32', 'float64']\nX = cleansed_df.select_dtypes(include=numerics)\nprint X.shape",
"(4383, 141)\n"
],
[
"# Let's now clean columns getting rid of certain columns that might not be important to our analysis\n\ncols2drop = ['GROUP_ID', 'doa', 'Baseline_header_id', 'Concentration_header_id', 'Baseline_Reading_id',\n 'Concentration_Reading_id']\nX = X.drop(cols2drop, axis=1, inplace=False)\nprint X.shape\n\n# For our studies children skew the data, it would be cleaner to just analyse adults\nX = X.loc[X['Age'] >= 18]\nY = X.loc[X['race_id'] == 1]\nX = X.loc[X['Gender_id'] == 1]\n\nprint X.shape\nprint Y.shape",
"(4383, 137)\n(2624, 137)\n(2981, 137)\n"
]
],
[
[
"### Extracting the samples we are interested in",
"_____no_output_____"
]
],
[
[
"# Let's extract ADHd and Bipolar patients (mutually exclusive)\n\nADHD_men = X.loc[X['ADHD'] == 1]\nADHD_men = ADHD_men.loc[ADHD_men['Bipolar'] == 0]\n\nBP_men = X.loc[X['Bipolar'] == 1]\nBP_men = BP_men.loc[BP_men['ADHD'] == 0]\n\nADHD_cauc = Y.loc[Y['ADHD'] == 1]\nADHD_cauc = ADHD_cauc.loc[ADHD_cauc['Bipolar'] == 0]\n\nBP_cauc = Y.loc[Y['Bipolar'] == 1]\nBP_cauc = BP_cauc.loc[BP_cauc['ADHD'] == 0]\n\nprint ADHD_men.shape\nprint BP_men.shape\n\nprint ADHD_cauc.shape\nprint BP_cauc.shape\n\n# Keeping a backup of the data frame object because numpy arrays don't play well with certain scikit functions\nADHD_men = pd.DataFrame(ADHD_men.drop(['Patient_ID', 'Gender_id', 'ADHD', 'Bipolar'], axis = 1, inplace = False))\nBP_men = pd.DataFrame(BP_men.drop(['Patient_ID', 'Gender_id', 'ADHD', 'Bipolar'], axis = 1, inplace = False))\n\nADHD_cauc = pd.DataFrame(ADHD_cauc.drop(['Patient_ID', 'race_id', 'ADHD', 'Bipolar'], axis = 1, inplace = False))\nBP_cauc = pd.DataFrame(BP_cauc.drop(['Patient_ID', 'race_id', 'ADHD', 'Bipolar'], axis = 1, inplace = False))",
"(1056, 137)\n(257, 137)\n(1110, 137)\n(323, 137)\n"
]
],
[
[
"### Dimensionality reduction",
"_____no_output_____"
],
[
"#### Manifold Techniques",
"_____no_output_____"
],
[
"##### ISOMAP",
"_____no_output_____"
]
],
[
[
"combined1 = pd.concat([ADHD_men, BP_men])\ncombined2 = pd.concat([ADHD_cauc, BP_cauc])\n\nprint combined1.shape\nprint combined2.shape\n\ncombined1 = preprocessing.scale(combined1)\ncombined2 = preprocessing.scale(combined2)",
"(1313, 133)\n(1433, 133)\n"
],
[
"combined1 = manifold.Isomap(20, 20).fit_transform(combined1)\nADHD_men_iso = combined1[:1056]\nBP_men_iso = combined1[1056:]\n\ncombined2 = manifold.Isomap(20, 20).fit_transform(combined2)\nADHD_cauc_iso = combined2[:1110]\nBP_cauc_iso = combined2[1110:]",
"_____no_output_____"
]
],
[
[
"### Clustering and other grouping experiments",
"_____no_output_____"
],
[
"#### K-Means clustering - iso",
"_____no_output_____"
]
],
[
[
"data1 = pd.concat([pd.DataFrame(ADHD_men_iso), pd.DataFrame(BP_men_iso)])\ndata2 = pd.concat([pd.DataFrame(ADHD_cauc_iso), pd.DataFrame(BP_cauc_iso)])\n\nprint data1.shape\nprint data2.shape",
"(1313, 20)\n(1433, 20)\n"
],
[
"kmeans = KMeans(n_clusters=2)\nkmeans.fit(data1.get_values())\nlabels1 = kmeans.labels_\ncentroids1 = kmeans.cluster_centers_\nprint('Estimated number of clusters: %d' % len(centroids1))\n\nfor label in [0, 1]:\n ds = data1.get_values()[np.where(labels1 == label)] \n plt.plot(ds[:,0], ds[:,1], '.') \n lines = plt.plot(centroids1[label,0], centroids1[label,1], 'o')\n\n",
"Estimated number of clusters: 2\n"
],
[
"kmeans = KMeans(n_clusters=2)\nkmeans.fit(data2.get_values())\nlabels2 = kmeans.labels_\ncentroids2 = kmeans.cluster_centers_\nprint('Estimated number of clusters: %d' % len(centroids2))\n\nfor label in [0, 1]:\n ds2 = data2.get_values()[np.where(labels2 == label)] \n plt.plot(ds2[:,0], ds2[:,1], '.') \n lines = plt.plot(centroids2[label,0], centroids2[label,1], 'o')",
"Estimated number of clusters: 2\n"
]
],
[
[
"As is evident from the above 2 experiments, no clear clustering is apparent.But there is some significant overlap and there 2 clear groups",
"_____no_output_____"
],
[
"### Classification Experiments",
"_____no_output_____"
],
[
"Let's experiment with a bunch of classifiers",
"_____no_output_____"
]
],
[
[
"ADHD_men_iso = pd.DataFrame(ADHD_men_iso)\nBP_men_iso = pd.DataFrame(BP_men_iso)\n\nADHD_cauc_iso = pd.DataFrame(ADHD_cauc_iso)\nBP_cauc_iso = pd.DataFrame(BP_cauc_iso)",
"_____no_output_____"
],
[
"BP_men_iso['ADHD-Bipolar'] = 0\nADHD_men_iso['ADHD-Bipolar'] = 1\n\nBP_cauc_iso['ADHD-Bipolar'] = 0\nADHD_cauc_iso['ADHD-Bipolar'] = 1\n\ndata1 = pd.concat([ADHD_men_iso, BP_men_iso])\ndata2 = pd.concat([ADHD_cauc_iso, BP_cauc_iso])\nclass_labels1 = data1['ADHD-Bipolar']\nclass_labels2 = data2['ADHD-Bipolar']\ndata1 = data1.drop(['ADHD-Bipolar'], axis = 1, inplace = False)\ndata2 = data2.drop(['ADHD-Bipolar'], axis = 1, inplace = False)\ndata1 = data1.get_values()\ndata2 = data2.get_values()",
"_____no_output_____"
],
[
"# Leave one Out cross validation\ndef leave_one_out(classifier, values, labels):\n leave_one_out_validator = LeaveOneOut(len(values))\n classifier_metrics = cross_validation.cross_val_score(classifier, values, labels, cv=leave_one_out_validator)\n accuracy = classifier_metrics.mean()\n deviation = classifier_metrics.std()\n return accuracy, deviation",
"_____no_output_____"
],
[
"rf = RandomForestClassifier(n_estimators = 22) \nqda = QDA()\nlda = LDA()\ngnb = GaussianNB()\nclassifier_accuracy_list = []\nclassifiers = [(rf, \"Random Forest\"), (lda, \"LDA\"), (qda, \"QDA\"), (gnb, \"Gaussian NB\")]\nfor classifier, name in classifiers:\n accuracy, deviation = leave_one_out(classifier, data1, class_labels1)\n print '%s accuracy is %0.4f (+/- %0.3f)' % (name, accuracy, deviation)\n classifier_accuracy_list.append((name, accuracy))",
"Random Forest accuracy is 0.7883 (+/- 0.409)\nLDA accuracy is 0.8043 (+/- 0.397)\nQDA accuracy is 0.7517 (+/- 0.432)\nGaussian NB accuracy is 0.7890 (+/- 0.408)\n"
],
[
"for classifier, name in classifiers:\n accuracy, deviation = leave_one_out(classifier, data2, class_labels2)\n print '%s accuracy is %0.4f (+/- %0.3f)' % (name, accuracy, deviation)\n classifier_accuracy_list.append((name, accuracy))",
"Random Forest accuracy is 0.7565 (+/- 0.429)\nLDA accuracy is 0.7739 (+/- 0.418)\nQDA accuracy is 0.7306 (+/- 0.444)\nGaussian NB accuracy is 0.7558 (+/- 0.430)\n"
]
]
] | [
"markdown",
"code",
"markdown",
"code",
"markdown",
"code",
"markdown",
"code",
"markdown",
"code"
] | [
[
"markdown",
"markdown"
],
[
"code",
"code"
],
[
"markdown"
],
[
"code"
],
[
"markdown",
"markdown",
"markdown"
],
[
"code",
"code"
],
[
"markdown",
"markdown"
],
[
"code",
"code",
"code"
],
[
"markdown",
"markdown",
"markdown"
],
[
"code",
"code",
"code",
"code",
"code"
]
] |
e7807b1ad646edc29a53174335d3a2695a81a70e | 12,651 | ipynb | Jupyter Notebook | Archive/TF_VERSION_MASKRCNN/datasets/ellipse/defectextraction/defectextraction3.ipynb | iphyer/DefectDetection-MaskRCNN | 7d1faf99bb2c4ffdbbaaf4544a996b97b2f3a2f9 | [
"MIT"
] | null | null | null | Archive/TF_VERSION_MASKRCNN/datasets/ellipse/defectextraction/defectextraction3.ipynb | iphyer/DefectDetection-MaskRCNN | 7d1faf99bb2c4ffdbbaaf4544a996b97b2f3a2f9 | [
"MIT"
] | null | null | null | Archive/TF_VERSION_MASKRCNN/datasets/ellipse/defectextraction/defectextraction3.ipynb | iphyer/DefectDetection-MaskRCNN | 7d1faf99bb2c4ffdbbaaf4544a996b97b2f3a2f9 | [
"MIT"
] | null | null | null | 37.651786 | 299 | 0.543831 | [
[
[
"imagefolder = '/u/y/u/yuhanl/Downloads/NextGenMaskRCNN-master/code/datasets/balloon/Data3TypesYminXminYmaxXmax5/images/BF X500K, 05 (3).jpg'\nroot = '/u/y/u/yuhanl/Downloads/NextGenMaskRCNN-master/code/datasets/balloon/Data3TypesYminXminYmaxXmax5'",
"_____no_output_____"
],
[
"import chainer\nfrom chainercv import utils\nfrom chainercv import transforms\nimport os\nimport numpy as np\nfrom skimage import exposure, morphology, measure, draw\nimport json",
"/u/y/u/yuhanl/anaconda3/lib/python3.6/site-packages/h5py/__init__.py:36: FutureWarning: Conversion of the second argument of issubdtype from `float` to `np.floating` is deprecated. In future, it will be treated as `np.float64 == np.dtype(float).type`.\n from ._conv import register_converters as _register_converters\n"
],
[
"img = utils.read_image(imagefolder,color=True)\nimagename = \"BF X500K, 05 (3)\"\nbbs_file = os.path.join(root, 'bounding_boxes', imagename+'.txt')\nbbs = np.stack([line.strip().split() for line in open(bbs_file)]).astype(np.float32)\nlabel = np.stack([0]*bbs.shape[0]).astype(np.int32)\n\n",
"_____no_output_____"
],
[
"\ndef watershed_image(img,flag):\n \"\"\"\n use watershed flooding algorithm to extract the loop contour\n :param img: type(numpy.ndarray) image in CHW format\n :return: type(numpy.ndarray) image in HW format\n \"\"\"\n img_gray = img[1,:,:]\n h, w = img_gray.shape\n img1 = exposure.equalize_hist(img_gray)\n #print(img1.shape)\n # invert the image\n #print(\"====================\")\n #print(np.max(img1).shape)\n img2 = np.max(img1) - img1\n #print(\"====================\")\n #print(img2.shape)\n inner = np.zeros((h, w), np.bool)\n \n\n centroid = [round(a) for a in findCentroid(img2)]\n inner[centroid[0], centroid[1]] = 1\n min_size = round((h + w) / 20)\n kernel = morphology.disk(min_size)\n inner = morphology.dilation(inner, kernel)\n\n out = np.zeros((h,w), np.bool)\n out[0, 0] = 1\n out[h - 1, 0] = 1\n out[0, w - 1] = 1\n out[h - 1, w - 1] = 1\n out = morphology.dilation(out, kernel)\n out[0, :] = 1\n out[h - 1, :] = 1\n out[:, w - 1] = 1\n out[:, 0] = 1\n markers = np.zeros((h, w), np.int)\n \n markers = np.zeros((h, w), np.int)\n markers[inner] = 2\n markers[out] = 1\n\n labels = morphology.watershed(img2, markers)\n \n\n return labels",
"_____no_output_____"
],
[
"def flood_fitting(img,flag):\n \"\"\"\n Use watershed flooding algorithm and regional property analysis\n to output the fitted ellipse parameters\n :param img: (numpy.ndarray) image in CHW format\n :return: region property, where property can be accessed through attributes\n example:\n area, bbox, centroid, major_axis_length, minor_axis_length, orientation\n \"\"\"\n labels = watershed_image(img,flag)\n results = measure.regionprops(labels - 1)\n \n sorted(results, key=lambda k: k['area'],reverse=True)\n # return the one with largest area\n return results[0]",
"_____no_output_____"
],
[
"def cropImage(img, bboxes, expand=True):\n \"\"\"crop images by the given bounding boxes.\n\n Args:\n img (numpy.ndarray): image in CHW format\n bboxes (numpy.ndarray): bounding boxes in the format specified by chainerCV\n expand (bool): whether to expand the bounding boxes or not\n\n Returns:\n a batch of cropped image in CHW format\n The image is in CHW format and its color channel is ordered in\n RGB.\n\n Return type: list\n\n \"\"\"\n\n if expand:\n _, H, W = img.shape\n bboxes = expand_bbox(bboxes, H, W)\n\n subimages = list()\n for bbox in bboxes:\n bbox = bbox.astype(np.int)\n subimages.append(img[:, bbox[1]:bbox[3]+1, bbox[2]:bbox[4]+1])\n return subimages, bboxes\n\ndef expand_bbox(bbox, H, W):\n \"\"\"\n expand the bounding box within the range of height and width of the image\n :param bbox: numpy.ndarray bounding box N by 4\n :param H: int Height of the image\n :param W: int Width of the image\n :return: numpy.ndarray expanded bounding box\n \"\"\"\n b_label = np.zeros(bbox[:, 0].shape)\n b_height = 0.15*(bbox[:, 3] - bbox[:, 1])\n b_width = 0.15*(bbox[:, 4] - bbox[:, 2])\n b_height[b_height < 7] = 7\n b_width[b_width < 7] = 7\n adjust = np.array((b_label, -b_height, -b_width, b_height, b_width)).transpose()\n new_bbox = bbox + adjust\n new_bbox[new_bbox < 0] = 0\n new_bbox[new_bbox[:, 3] >= H, 3] = H - 1\n new_bbox[new_bbox[:, 4] >= W, 4] = W - 1\n\n return new_bbox\n\ndef findCentroid(img):\n \"\"\"\n find the centroid position of a image by weighted method\n :param img: (numpy.ndarray) image in HW format\n :return: (tuple) (y,x) coordinates of the centroid\n \"\"\"\n h, w = img.shape\n # TODO: add weighted method later\n return h/2, w/2",
"_____no_output_____"
],
[
"def load_mask(self, image_id):\n \"\"\"Generate instance masks for an image.\n Returns:\n masks: A bool array of shape [height, width, instance count] with\n one mask per instance.\n class_ids: a 1D array of class IDs of the instance masks.\n \"\"\"\n image_info = self.image_info[image_id]\n if image_info[\"source\"] != \"balloon\":\n return super(self.__class__, self).load_mask(image_id)\n\n # Convert polygons to a bitmap mask of shape\n # [height, width, instance_count]\n info = self.image_info[image_id]\n mask = np.zeros([info[\"height\"], info[\"width\"], len(info[\"polygons\"])],\n dtype=np.uint8)\n for i, p in enumerate(info[\"polygons\"]):\n # Get indexes of pixels inside the polygon and set them to 1\n rr, cc = skimage.draw.polygon(p['all_points_y'], p['all_points_x'])\n mask[rr, cc, i] = 1\n\n # Return mask, and array of class IDs of each instance. Since we have\n # one class ID only, we return an array of 1s\n return mask.astype(np.bool), np.ones([mask.shape[-1]], dtype=np.int32)",
"_____no_output_____"
],
[
"bboxes = bbs\nAll_Image_Defects_List = list()\nsubimages, bboxes = cropImage(img, bboxes)\ncurrent_img_defect_List = list()\ndefects_Dict = dict()\ndefects_X_List = list()\ndefects_Y_List = list()\nfor subim, bbox in zip(subimages, bboxes):\n region1 = flood_fitting(subim,bbox[0])\n if bbox[0] == 0 or bbox[0] == 2:\n continue\n\n result = (int(region1['centroid'][0]+bbox[1]), int(region1['centroid'][1]+bbox[2]),\n int(region1['minor_axis_length'] / 2), int(region1['major_axis_length'] / 2),\n -region1['orientation'])\n rr,cc = draw.ellipse_perimeter(*result)\n\n assert len(rr) == len(cc)\n for i in range(len(cc)):\n defects_X_List.append(cc[i])\n defects_Y_List.append(rr[i])\n defects_Dict['X'] = defects_X_List\n defects_Dict['Y'] = defects_Y_List\n current_img_defect_List.append(defects_Dict)\nAll_Image_Defects_List.append(current_img_defect_List)",
"/u/y/u/yuhanl/anaconda3/lib/python3.6/site-packages/skimage/measure/_regionprops.py:250: UserWarning: regionprops and image moments (including moments, normalized moments, central moments, and inertia tensor) of 2D images will change from xy coordinates to rc coordinates in version 0.16.\nSee http://scikit-image.org/docs/0.14.x/release_notes_and_installation.html#deprecations for details on how to avoid this message.\n warn(XY_TO_RC_DEPRECATION_MESSAGE)\n/u/y/u/yuhanl/anaconda3/lib/python3.6/site-packages/skimage/measure/_regionprops.py:260: UserWarning: regionprops and image moments (including moments, normalized moments, central moments, and inertia tensor) of 2D images will change from xy coordinates to rc coordinates in version 0.16.\nSee http://scikit-image.org/docs/0.14.x/release_notes_and_installation.html#deprecations for details on how to avoid this message.\n warn(XY_TO_RC_DEPRECATION_MESSAGE)\n"
],
[
"with open(\"via_region_data.json\",\"w+\") as jsonfile:\n each_file = dict()\n for i in range(len(All_Image_Defects_List)):\n img_i = All_Image_Defects_List[i]\n file_size = os.path.getsize(imagefolder)\n filename_size = imagename + str(file_size)\n each_file[filename_size] = {'fileref':\"\",'size':file_size,'filename':imagename}\n each_file[filename_size].update({'base64_img_data':\"\",'file_attributes':{},'regions':{}})\n each_bbox = each_file[filename_size]['regions']\n for j in range(len(img_i)):\n each_bbox.update({str(j):{'shape_attributes':{},'region_attributes':{}}})\n each_bbox[str(j)]['shape_attributes'] = {'name':\"polygon\"}\n each_bbox[str(j)]['shape_attributes'].update({'all_points_x':[],'all_points_y':[]})\n each_bbox[str(j)]['shape_attributes']['all_points_x'] = np.asarray(img_i[j]['X']).tolist()#int64Toint32(img_i[j]['X'])\n each_bbox[str(j)]['shape_attributes']['all_points_y'] = np.asarray(img_i[j]['Y']).tolist()#int64Toint32(img_i[j]['Y'])\n\n json.dump(each_file,jsonfile)",
"_____no_output_____"
],
[
"config = balloon.BalloonConfig()\nBALLOON_DIR = os.path.join(ROOT_DIR, \"datasets/balloon\")\n",
"_____no_output_____"
]
]
] | [
"code"
] | [
[
"code",
"code",
"code",
"code",
"code",
"code",
"code",
"code",
"code",
"code"
]
] |
e7807d7904e24579cacb6171458225fd3cb87a27 | 13,667 | ipynb | Jupyter Notebook | examples/interpret_example.ipynb | imoscovitz/ruleset | 14d4e014a0d8159af278740f659a2a3998319cb3 | [
"MIT"
] | 64 | 2019-02-28T08:45:19.000Z | 2022-03-19T21:48:10.000Z | examples/interpret_example.ipynb | imoscovitz/ruleset | 14d4e014a0d8159af278740f659a2a3998319cb3 | [
"MIT"
] | 18 | 2019-03-07T09:12:34.000Z | 2022-01-31T17:05:45.000Z | examples/interpret_example.ipynb | imoscovitz/ruleset | 14d4e014a0d8159af278740f659a2a3998319cb3 | [
"MIT"
] | 17 | 2019-03-15T09:58:43.000Z | 2022-03-16T17:59:42.000Z | 29.328326 | 99 | 0.420063 | [
[
[
"import pandas as pd\nimport numpy as np\n\nfrom sklearn.model_selection import train_test_split\nfrom sklearn.ensemble import RandomForestClassifier\nfrom sklearn.svm import SVC\nfrom sklearn.metrics import precision_score, recall_score, f1_score\nfrom tensorflow.keras import Sequential\nfrom tensorflow.keras.layers import Dense\nimport torch\n\nfrom wittgenstein.irep import IREP\nfrom wittgenstein.ripper import RIPPER\nfrom wittgenstein.interpret import interpret_model, score_fidelity",
"_____no_output_____"
],
[
"# Load data and transform target to binary classification \n\ninpath = ''\ndf = pd.read_csv(inpath + \"wine.csv\")\ndf['Class'] = df['Class'].map(lambda x: True if x==1 else False)\ndf.head()",
"_____no_output_____"
],
[
"# Train-test-split\n\nX, y = df.drop('Class', axis=1), df['Class']\nX_train, X_test, y_train, y_test = train_test_split(\n X, y, random_state=42\n)",
"_____no_output_____"
],
[
"# Create a wittgenstein classifier for (global surrogate) interpretation.\n\ninterpreter = RIPPER(random_state=42)",
"_____no_output_____"
],
[
"# Fit and interpret SVM.\n# interpret_model fits the interpreter to the model.\n\nsvc = SVC(kernel='rbf', random_state=42)\nsvc.fit(X_train, y_train);\n\ninterpret_model(model=svc, X=X_train, interpreter=interpreter).out_pretty()\n",
"[[Proline=>1227.0] V\n[Proline=1048.0-1227.0] V\n[Proline=880.0-1048.0]]\n"
],
[
"# Score how well the interpreter's predictions match the underlying model's\n\nscore_fidelity(\n X_test,\n interpreter,\n model=svc,\n score_function=[precision_score, recall_score, f1_score])",
"_____no_output_____"
],
[
"# Interpret Random Forest\n\nrf = RandomForestClassifier(random_state=42)\nrf.fit(X_train, y_train)\n\ninterpret_model(model=rf, X=X_train, interpreter=interpreter).out_pretty()\n",
"[[Proline=>1227.0] V\n[Proline=1048.0-1227.0] V\n[Proline=880.0-1048.0 ^ Ash=2.0-2.19] V\n[Proline=880.0-1048.0 ^ Malicacid=1.76-1.9] V\n[Colorintensity=5.75-6.78]]\n"
],
[
"score_fidelity(\n X_test,\n interpreter,\n model=rf,\n score_function=[precision_score, recall_score, f1_score])",
"_____no_output_____"
],
[
"# Interpret Tensorflow/Keras model\n\nnp.random.seed(0)\n\nmlp = Sequential()\nmlp.add(Dense(60, input_dim=13, activation='relu'))\nmlp.add(Dense(30, activation='relu'))\nmlp.add(Dense(1, activation='sigmoid'))\nmlp.compile(loss='binary_crossentropy', optimizer='adam', metrics=['accuracy'])\nmlp.fit(\n X_train,\n y_train,\n batch_size=1,\n epochs=10,\n)\n\ninterpret_model(model=mlp, X=X_train, interpreter=interpreter).out_pretty()\n",
"Epoch 1/10\n133/133 [==============================] - 1s 2ms/step - loss: 4.2813 - accuracy: 0.7293\nEpoch 2/10\n133/133 [==============================] - 0s 2ms/step - loss: 1.8663 - accuracy: 0.7744\nEpoch 3/10\n133/133 [==============================] - 0s 1ms/step - loss: 3.7320 - accuracy: 0.7143\nEpoch 4/10\n133/133 [==============================] - 0s 2ms/step - loss: 1.7408 - accuracy: 0.8271\nEpoch 5/10\n133/133 [==============================] - 0s 2ms/step - loss: 1.4441 - accuracy: 0.8271\nEpoch 6/10\n133/133 [==============================] - 0s 2ms/step - loss: 1.7489 - accuracy: 0.8045\nEpoch 7/10\n133/133 [==============================] - 0s 1ms/step - loss: 2.2349 - accuracy: 0.8195\nEpoch 8/10\n133/133 [==============================] - 0s 2ms/step - loss: 1.3922 - accuracy: 0.8421\nEpoch 9/10\n133/133 [==============================] - 0s 3ms/step - loss: 1.1142 - accuracy: 0.8872\nEpoch 10/10\n133/133 [==============================] - 0s 2ms/step - loss: 1.4097 - accuracy: 0.8647\n[[Proline=880.0-1048.0] V\n[Proline=>1227.0] V\n[Proline=1048.0-1227.0] V\n[Alcalinityofash=16.8-17.72]]\n"
],
[
"score_fidelity(\n X_test,\n interpreter,\n model=mlp,\n score_function=[precision_score, recall_score, f1_score])",
"_____no_output_____"
],
[
"# Since interpret_model fits a wittgenstein classifier,\n# we can do with it all the normal things a wittgenstein classifier can do.\n# Here, we'll use it to approximate what in the data the tensorflow model\n# is picking up on when it makes specific positive predictions.\n\npreds = (mlp.predict(X_test.tail()) > .5).flatten()\n_, interpretation = interpreter.predict(X_test.tail(), give_reasons=True)\n\nprint(f'tf preds: {preds}\\n')\nprint('interpretion:')\ninterpretation\n",
"tf preds: [ True False False True False]\n\ninterpretion:\n"
]
]
] | [
"code"
] | [
[
"code",
"code",
"code",
"code",
"code",
"code",
"code",
"code",
"code",
"code",
"code"
]
] |
e780857df16618aac2a418b5a9d3c6303039176f | 417,872 | ipynb | Jupyter Notebook | examples/Tutorial 9.ipynb | xiaolongguo/Riskfolio-Lib | 4e74c4f27a48ced7dcc0ab4a9e96c922cd54f0b4 | [
"BSD-3-Clause"
] | 2 | 2022-02-07T11:16:46.000Z | 2022-02-23T06:57:41.000Z | examples/Tutorial 9.ipynb | xiaolongguo/Riskfolio-Lib | 4e74c4f27a48ced7dcc0ab4a9e96c922cd54f0b4 | [
"BSD-3-Clause"
] | null | null | null | examples/Tutorial 9.ipynb | xiaolongguo/Riskfolio-Lib | 4e74c4f27a48ced7dcc0ab4a9e96c922cd54f0b4 | [
"BSD-3-Clause"
] | 1 | 2022-02-07T11:38:34.000Z | 2022-02-07T11:38:34.000Z | 185.309091 | 80,760 | 0.801047 | [
[
[
"# Riskfolio-Lib Tutorial: \n<br>__[Financionerioncios](https://financioneroncios.wordpress.com)__\n<br>__[Orenji](https://www.orenj-i.net)__\n<br>__[Riskfolio-Lib](https://riskfolio-lib.readthedocs.io/en/latest/)__\n<br>__[Dany Cajas](https://www.linkedin.com/in/dany-cajas/)__\n## Part IX: Portfolio Optimization with Risk Factors and Principal Components Regression (PCR)\n\n## 1. Downloading the data:",
"_____no_output_____"
]
],
[
[
"import numpy as np\nimport pandas as pd\nimport yfinance as yf\nimport warnings\n\nwarnings.filterwarnings(\"ignore\")\n\nyf.pdr_override()\npd.options.display.float_format = '{:.4%}'.format\n\n# Date range\nstart = '2016-01-01'\nend = '2019-12-30'\n\n# Tickers of assets\nassets = ['JCI', 'TGT', 'CMCSA', 'CPB', 'MO', 'NBL', 'APA', 'MMC', 'JPM',\n 'ZION', 'PSA', 'BAX', 'BMY', 'LUV', 'PCAR', 'TXT', 'DHR',\n 'DE', 'MSFT', 'HPQ', 'SEE', 'VZ', 'CNP', 'NI']\nassets.sort()\n\n# Tickers of factors\n\nfactors = ['MTUM', 'QUAL', 'VLUE', 'SIZE', 'USMV']\nfactors.sort()\n\ntickers = assets + factors\ntickers.sort()\n\n# Downloading data\ndata = yf.download(tickers, start = start, end = end)\ndata = data.loc[:,('Adj Close', slice(None))]\ndata.columns = tickers",
"[*********************100%***********************] 29 of 29 completed\n"
],
[
"# Calculating returns\n\nX = data[factors].pct_change().dropna()\nY = data[assets].pct_change().dropna()\n\ndisplay(X.head())",
"_____no_output_____"
]
],
[
[
"## 2. Estimating Mean Variance Portfolios with PCR\n\n### 2.1 Estimating the loadings matrix with PCR.\n\nThis part is just to visualize how Riskfolio-Lib calculates a loadings matrix using PCR.",
"_____no_output_____"
]
],
[
[
"import riskfolio.ParamsEstimation as pe\n\nfeature_selection = 'PCR' # Method to select best model, could be PCR or Stepwise\nn_components = 0.95 # 95% of explained variance. See PCA in scikit learn for more information\n\nloadings = pe.loadings_matrix(X=X, Y=Y, feature_selection=feature_selection,\n n_components=n_components)\n\nloadings.style.format(\"{:.4f}\").background_gradient(cmap='RdYlGn')",
"_____no_output_____"
]
],
[
[
"### 2.2 Calculating the portfolio that maximizes Sharpe ratio.",
"_____no_output_____"
]
],
[
[
"import riskfolio.Portfolio as pf\n\n# Building the portfolio object\nport = pf.Portfolio(returns=Y)\n\n# Calculating optimum portfolio\n\n# Select method and estimate input parameters:\n\nmethod_mu='hist' # Method to estimate expected returns based on historical data.\nmethod_cov='hist' # Method to estimate covariance matrix based on historical data.\n\nport.assets_stats(method_mu=method_mu,\n method_cov=method_cov)\n\nfeature_selection = 'PCR' # Method to select best model, could be PCR or Stepwise\nn_components = 0.95 # 95% of explained variance. See PCA in scikit learn for more information\n\nport.factors = X\nport.factors_stats(method_mu=method_mu,\n method_cov=method_cov,\n feature_selection=feature_selection,\n n_components=n_components\n )\n\n# Estimate optimal portfolio:\n\nmodel='FM' # Factor Model\nrm = 'MV' # Risk measure used, this time will be variance\nobj = 'Sharpe' # Objective function, could be MinRisk, MaxRet, Utility or Sharpe\nhist = False # Use historical scenarios for risk measures that depend on scenarios\nrf = 0 # Risk free rate\nl = 0 # Risk aversion factor, only useful when obj is 'Utility'\n\nw = port.optimization(model=model, rm=rm, obj=obj, rf=rf, l=l, hist=hist)\n\ndisplay(w.T)",
"_____no_output_____"
]
],
[
[
"### 2.3 Plotting portfolio composition",
"_____no_output_____"
]
],
[
[
"import riskfolio.PlotFunctions as plf\n\n# Plotting the composition of the portfolio\n\nax = plf.plot_pie(w=w, title='Sharpe FM Mean Variance', others=0.05, nrow=25, cmap = \"tab20\",\n height=6, width=10, ax=None)",
"_____no_output_____"
]
],
[
[
"### 2.3 Calculate efficient frontier",
"_____no_output_____"
]
],
[
[
"points = 50 # Number of points of the frontier\n\nfrontier = port.efficient_frontier(model=model, rm=rm, points=points, rf=rf, hist=hist)\n\ndisplay(frontier.T.head())",
"_____no_output_____"
],
[
"# Plotting the efficient frontier\n\nlabel = 'Max Risk Adjusted Return Portfolio' # Title of point\nmu = port.mu_fm # Expected returns\ncov = port.cov_fm # Covariance matrix\nreturns = port.returns_fm # Returns of the assets\n\nax = plf.plot_frontier(w_frontier=frontier, mu=mu, cov=cov, returns=returns, rm=rm,\n rf=rf, alpha=0.01, cmap='viridis', w=w, label=label,\n marker='*', s=16, c='r', height=6, width=10, ax=None)",
"_____no_output_____"
],
[
"# Plotting efficient frontier composition\n\nax = plf.plot_frontier_area(w_frontier=frontier, cmap=\"tab20\", height=6, width=10, ax=None)",
"_____no_output_____"
]
],
[
[
"## 3. Estimating Portfolios Using Risk Factors with Other Risk Measures and PCR\n\nIn this part I will calculate optimal portfolios for several risk measures using a __mean estimate based on PCR__. I will find the portfolios that maximize the risk adjusted return for all available risk measures.\n\n### 3.1 Calculate Optimal Portfolios for Several Risk Measures.\n\nI will mantain the constraints on risk factors.",
"_____no_output_____"
]
],
[
[
"# Risk Measures available:\n#\n# 'MV': Standard Deviation.\n# 'MAD': Mean Absolute Deviation.\n# 'MSV': Semi Standard Deviation.\n# 'FLPM': First Lower Partial Moment (Omega Ratio).\n# 'SLPM': Second Lower Partial Moment (Sortino Ratio).\n# 'CVaR': Conditional Value at Risk.\n# 'WR': Worst Realization (Minimax)\n# 'MDD': Maximum Drawdown of uncompounded returns (Calmar Ratio).\n# 'ADD': Average Drawdown of uncompounded returns.\n# 'CDaR': Conditional Drawdown at Risk of uncompounded returns.\n\n# port.reset_linear_constraints() # To reset linear constraints (factor constraints)\n\nrms = ['MV', 'MAD', 'MSV', 'FLPM', 'SLPM',\n 'CVaR', 'WR', 'MDD', 'ADD', 'CDaR']\n\nw_s = pd.DataFrame([])\n\n# When we use hist = True the risk measures all calculated\n# using historical returns, while when hist = False the\n# risk measures are calculated using the expected returns \n# based on risk factor model: R = a + B * F\n\nhist = False\n\nfor i in rms:\n w = port.optimization(model=model, rm=i, obj=obj, rf=rf, l=l, hist=hist)\n w_s = pd.concat([w_s, w], axis=1)\n \nw_s.columns = rms",
"_____no_output_____"
],
[
"w_s.style.format(\"{:.2%}\").background_gradient(cmap='YlGn')",
"_____no_output_____"
],
[
"import matplotlib.pyplot as plt\n\n# Plotting a comparison of assets weights for each portfolio\n\nfig = plt.gcf()\nfig.set_figwidth(14)\nfig.set_figheight(6)\nax = fig.subplots(nrows=1, ncols=1)\n\nw_s.plot.bar(ax=ax)",
"_____no_output_____"
],
[
"w_s = pd.DataFrame([])\n\n# When we use hist = True the risk measures all calculated\n# using historical returns, while when hist = False the\n# risk measures are calculated using the expected returns \n# based on risk factor model: R = a + B * F\n\nhist = True\n\nfor i in rms:\n w = port.optimization(model=model, rm=i, obj=obj, rf=rf, l=l, hist=hist)\n w_s = pd.concat([w_s, w], axis=1)\n \nw_s.columns = rms",
"_____no_output_____"
],
[
"w_s.style.format(\"{:.2%}\").background_gradient(cmap='YlGn')",
"_____no_output_____"
],
[
"import matplotlib.pyplot as plt\n\n# Plotting a comparison of assets weights for each portfolio\n\nfig = plt.gcf()\nfig.set_figwidth(14)\nfig.set_figheight(6)\nax = fig.subplots(nrows=1, ncols=1)\n\nw_s.plot.bar(ax=ax)",
"_____no_output_____"
]
]
] | [
"markdown",
"code",
"markdown",
"code",
"markdown",
"code",
"markdown",
"code",
"markdown",
"code",
"markdown",
"code"
] | [
[
"markdown"
],
[
"code",
"code"
],
[
"markdown"
],
[
"code"
],
[
"markdown"
],
[
"code"
],
[
"markdown"
],
[
"code"
],
[
"markdown"
],
[
"code",
"code",
"code"
],
[
"markdown"
],
[
"code",
"code",
"code",
"code",
"code",
"code"
]
] |
e780bc16734d49dee167960d0d096955281a8357 | 17,108 | ipynb | Jupyter Notebook | Column_Storage.ipynb | arjunrawal4/pandas-memdb | 206b08003c0995549548ad52285aa87529cadc76 | [
"PSF-2.0",
"Apache-2.0",
"BSD-3-Clause-No-Nuclear-License-2014",
"MIT",
"ECL-2.0",
"BSD-3-Clause"
] | null | null | null | Column_Storage.ipynb | arjunrawal4/pandas-memdb | 206b08003c0995549548ad52285aa87529cadc76 | [
"PSF-2.0",
"Apache-2.0",
"BSD-3-Clause-No-Nuclear-License-2014",
"MIT",
"ECL-2.0",
"BSD-3-Clause"
] | null | null | null | Column_Storage.ipynb | arjunrawal4/pandas-memdb | 206b08003c0995549548ad52285aa87529cadc76 | [
"PSF-2.0",
"Apache-2.0",
"BSD-3-Clause-No-Nuclear-License-2014",
"MIT",
"ECL-2.0",
"BSD-3-Clause"
] | null | null | null | 30.333333 | 108 | 0.472352 | [
[
[
"import pandas as pd\nimport resource \nimport pickle as pickle\nimport parquet\nimport pyarrow\nimport feather\nimport json\nimport re\nimport numpy as np\nimport os\n%load_ext memory_profiler\n",
"The memory_profiler extension is already loaded. To reload it, use:\n %reload_ext memory_profiler\n"
],
[
"\nPREC = 2\n\ndef convert_to_categorical(col):\n if (col.nunique() / len(col.index)) < 0.2:\n cat_dtype = pd.api.types.CategoricalDtype(ordered=True)\n col = col.astype(cat_dtype, copy=False)\n return col\n\n\ndef shrink_floats(col):\n if col.dtypes == 'float64':\n col = (col * (10**PREC)).astype('int64', copy=False)\n return col\n\ndef shrink_integers(col):\n if col.dtypes == 'int64':\n min_val = col.min()\n max_val = col.max()\n# print(c, df[c].max(),df[c].min())\n# print(df[c].memory_usage(deep=True)) \n if max_val <= 255 and min_val >= 0:\n col = col.astype('uint8', copy=False)\n elif max_val <= 65535 and min_val >= 0:\n col = col.astype('uint16', copy=False) \n elif max_val <= 4294967295 and min_val >= 0:\n col = col.astype('uint32', copy=False) \n elif max_val <= 127 and min_val >= -128:\n col = col.astype('int8', copy=False) \n elif max_val <= 32767 and min_val >= -32768:\n col = col.astype('int16', copy=False)\n elif max_val <= 2147483647 and min_val >= -2147483648:\n col = col.astype('int32', copy=False)\n else:\n print(\"not worth changing types\")\n return col\n\ndef get_trailing_number(s):\n m = re.search(r'\\d+$', s)\n return int(m.group()) if m else None\n\ndef intersection(lst1, lst2): \n return list(set(lst1) & set(lst2)) \n\ndef union(lst1, lst2): \n return list(set().union(lst1, lst2))\n\ndef read(feather_dir, file, preds, cols, num_chunks):\n indices = []\n for filename in os.listdir(feather_dir):\n for i,col in enumerate(cols):\n if filename.startswith(col) :\n pred = preds[i]\n e = feather.read_dataframe(feather_dir + filename)\n ind = e[pred].index\n ind = np.add(ind, 1 + CHUNK_SIZE * get_trailing_number(filename.split('.')[0]))\n if len(indices) > 0: # only for ands\n indices = union(indices, ind)\n else:\n indices += list(ind)\n df_labels = pd.read_csv(file, nrows=1)\n df = pd.read_csv(file, skiprows=indices, header=0, names=df_labels.columns, index_col=False)\n return df\n\ndef read_cardinality_many(feather_dir, file, preds, cols, num_chunks):\n indices = []\n for i,col in enumerate(cols):\n chunk_inds = []\n for filename in os.listdir(feather_dir):\n if filename.startswith(col) :\n pred = preds[i]\n e = feather.read_dataframe(feather_dir + filename)\n\n ind = e[pred].index\n ind = np.add(ind, CHUNK_SIZE * get_trailing_number(filename.split('.')[0]))\n chunk_inds.extend(ind)\n if len(indices) > 0: # only for ands\n indices = intersection(indices, chunk_inds)\n else:\n indices = chunk_inds\n return len(indices)\n\ndef read_cardinality_one(feather_dir, file, preds, cols, num_chunks):\n indices = []\n total = 0\n for filename in os.listdir(feather_dir):\n for i,col in enumerate(cols):\n if filename.startswith(col) :\n pred = preds[i]\n e = feather.read_dataframe(feather_dir + filename)\n total += len(e[pred])\n return total\n\ndef read_fast(feather_dir, file, preds, cols, num_chunks):\n \n df_all = []\n for i in range(0,num_chunks+1):\n df_part = (feather.read_dataframe(f'{feather_dir}full{i}.f'))\n for pred in preds:\n df_part = df_part[pred]\n df_all.append(df_part)\n df = pd.concat(df_all, ignore_index=True)\n return df\n",
"_____no_output_____"
],
[
"def write_chunk(df, chunk_id):\n for c in df:\n# df[c] = shrink_floats(df[c])\n df[c] = shrink_integers(df[c])\n df[c] = convert_to_categorical(df[c])\n feather.write_dataframe(df[c].to_frame(), f'{FEATHER_DIR}{c}{chunk_id}.f')\n feather.write_dataframe(df, f'{FEATHER_DIR}full{chunk_id}.f')\ndef write_chunks(file, chunk_size):\n num_chunks = 0\n for i, df in enumerate(pd.read_csv(file, iterator=True, chunksize=chunk_size)):\n write_chunk(df, i)\n num_chunks = i\n return num_chunks",
"_____no_output_____"
],
[
"COLS = ['raw_row_number'\n ,'date','county_name','district','subject_race',\n 'subject_sex','department_name','type','violation','arrest_made',\n 'citation_issued','warning_issued','outcome','contraband_found',\n 'frisk_performed','search_conducted','search_person','search_basis',\n 'reason_for_stop','raw_race','raw_search_basis'\n ]\nVALS = [32,'2009-07-01','San Diego County','San Onofre Inspection Facility',\n 'hispanic','male','California Highway Patrol','vehicular','Inspection / Scale Facility',\n 'NA','NA','NA','NA', 'NA','NA',False,False,'NA','Inspection / Scale Facility',\n 'Hispanic','Parole / Probation / Warrant'\n]\n\nPREDS = [lambda x: (x[COLS[0]] != VALS[0]),\n lambda x: (x[COLS[1]] != VALS[1]),\n lambda x: (x[COLS[2]] != VALS[2]),\n lambda x: (x[COLS[3]] != VALS[3]),\n lambda x: (x[COLS[4]] != VALS[4]),\n lambda x: (x[COLS[5]] != VALS[5]),\n lambda x: (x[COLS[6]] != VALS[6]),\n lambda x: (x[COLS[7]] != VALS[7]),\n lambda x: (x[COLS[8]] != VALS[8]),\n lambda x: (~x[COLS[9]].isnull()),\n lambda x: (~x[COLS[10]].isnull()),\n lambda x: (~x[COLS[11]].isnull()),\n lambda x: (~x[COLS[12]].isnull()),\n lambda x: (~x[COLS[13]].isnull()),\n lambda x: (~x[COLS[14]].isnull()),\n lambda x: (x[COLS[15]] != VALS[15]),\n lambda x: (x[COLS[16]] != VALS[16]),\n lambda x: (~x[COLS[17]].isnull()),\n lambda x: (x[COLS[18]] != VALS[18]),\n lambda x: (x[COLS[19]] != VALS[19]),\n lambda x: (x[COLS[20]] != VALS[20]),\n ]\n\nPOS_PREDS = [\n lambda x: (x[COLS[0]] == VALS[0]),\n lambda x: (x[COLS[1]] == VALS[1]),\n lambda x: (x[COLS[2]] == VALS[2]),\n lambda x: (x[COLS[3]] == VALS[3]),\n lambda x: (x[COLS[4]] == VALS[4]),\n lambda x: (x[COLS[5]] == VALS[5]),\n lambda x: (x[COLS[6]] == VALS[6]),\n lambda x: (x[COLS[7]] == VALS[7]),\n lambda x: (x[COLS[8]] == VALS[8]),\n lambda x: (x[COLS[9]].isnull()),\n lambda x: (x[COLS[10]].isnull()),\n lambda x: (x[COLS[11]].isnull()),\n lambda x: (x[COLS[12]].isnull()),\n lambda x: (x[COLS[13]].isnull()),\n lambda x: (x[COLS[14]].isnull()),\n lambda x: (x[COLS[15]] == VALS[15]),\n lambda x: (x[COLS[16]] == VALS[16]),\n lambda x: (x[COLS[17]].isnull()),\n lambda x: (x[COLS[18]] == VALS[18]),\n lambda x: (x[COLS[19]] == VALS[19]),\n lambda x: (x[COLS[20]] == VALS[20]),\n ]\n\n# COLS = ['issue_url','issue_title','body']\n# VALS = ['https://github.com/DungeonKeepers/DnDInventoryManager/issues/2', 'api', 'documentation']\n\n\n# POS_PREDS = [\n# lambda x: (x[COLS[0]].str.contains(VALS[0])),\n# lambda x: (x[COLS[1]].str.contains(VALS[1])),\n# lambda x: (x[COLS[2]].str.contains(VALS[2])),\n# ]\n \n# PREDS = [\n# lambda x: (~x[COLS[0]].str.contains(VALS[0])),\n# lambda x: (~x[COLS[1]].str.contains(VALS[1])),\n# lambda x: (~x[COLS[2]].str.contains(VALS[2])),\n# ]\n\n# COLS = ['LCLid','tstp','energy']\n# VALS = ['MAC000002','2012-10-12 00:30:00.0000000', 0]\n\n\n# POS_PREDS = [\n# lambda x: (x[COLS[0]].str.contains(VALS[0])),\n# lambda x: (x[COLS[1]].str.contains(VALS[1])),\n# lambda x: (x[COLS[2]] == VALS[2]),\n# ]\n \n# PREDS = [\n# lambda x: (~x[COLS[0]].str.contains(VALS[0])),\n# lambda x: (~x[COLS[1]].str.contains(VALS[1])),\n# lambda x: (x[COLS[2]] != VALS[2]),\n# ]\n\n\nCHUNK_SIZE = 5_000_000\nFILE = 'datasets/github_issues.csv'\nFEATHER_DIR = 'datasets/github_issues/'",
"_____no_output_____"
],
[
"%%time\n\nnum_chunks = write_chunks(FILE, CHUNK_SIZE)\n",
"CPU times: user 1min 2s, sys: 55.7 s, total: 1min 58s\nWall time: 2min 52s\n"
],
[
"%%time\n\nprint(read_cardinality_many(FEATHER_DIR, FILE, POS_PREDS[8:13], COLS[8:13], num_chunks))\n# print(read_cardinality_one(FEATHER_DIR, FILE, POS_PREDS[8:9], COLS[8:9], num_chunks))",
"1043872\nCPU times: user 10 s, sys: 1.5 s, total: 11.5 s\nWall time: 12.1 s\n"
],
[
"%%time\n\nnum_chunks = write_chunks(FILE, CHUNK_SIZE)\ndf_read = read_fast(FEATHER_DIR, FILE, PREDS, COLS, num_chunks)\n\nprint(df_read.shape)",
"(528503, 21)\nCPU times: user 6.14 s, sys: 1.05 s, total: 7.19 s\nWall time: 5.3 s\n"
],
[
"%%time\ndf_read = read(FEATHER_DIR, FILE, PREDS[0:3], COLS[0:3], num_chunks)\nprint(df_read.shape)",
"(1, 3)\nCPU times: user 52.5 s, sys: 15.2 s, total: 1min 7s\nWall time: 1min 13s\n"
],
[
"%%time\n\nindices = []\ndf = pd.read_csv(FILE)\n\nfor pred in PREDS[0:3]:\n indices = df[pred].index\n df.drop(indices, inplace=True)\n\nprint(df.shape)",
"(1, 3)\nCPU times: user 23.9 s, sys: 1.71 s, total: 25.6 s\nWall time: 29.6 s\n"
],
[
"%%time\n\ndf_all = []\n\nfor i, df_p in enumerate(pd.read_csv(FILE, iterator=True, chunksize=CHUNK_SIZE)):\n for pred in PREDS[8:9]:\n indices = df_p[pred].index\n df_p.drop(indices, inplace=True)\n df_all.append(df_p)\n\ndf = pd.concat(df_all, ignore_index=True)\nprint(df.shape)",
"(1, 3)\nCPU times: user 23.6 s, sys: 1.4 s, total: 25 s\nWall time: 26.3 s\n"
]
],
[
[
"test across all columns (when is it worth running in this system)\n\nshrinking encodings of different type (approximate queries)\n\nca_police 6.7GB\nfull_files 802MB\ncol_files 802 MB\nparse time 3min 36s\n\n\n\nPREDS[5:6] 22161713\n\nread_fast 8.66 s\nread 1min 24s\nread_chunks 2min 20s\nread_csv 2min 55s\n\nPREDS[4:10] 528503\n\nread_fast 6.58s\nread 2 min 7s\nread_chunks 2min 12s\nread_csv 3min 21s\n\nPREDS[0:20] 1\n\nread_fast 2.66 s\nread 6min 38s\nread_chunks 1min 59s\nread_csv 2min 58s\n\n\ngithub_issues 1min 27s\n\nPREDS[0:3] 1\n\nread_fast 51.9 s\nread 2min 56s\nread_chunks 49.9 s\nread_csv 1min 9s\n\nPREDS[0:1] 102077\n\nread_fast 2min 3s\nread 4min 43s\nread_chunks 1min 23s\nread_csv 2min 26s\n\n\nblock_total 22.6 s\n\nPREDS [2:3] 183368\n\nread_fast 795ms\nread 29.5 s\nread_chunks 21.8 s\nread_csv 17.5 s\n\nPREDS [0:3] 1\n\nread_fast 1.92 s\nread 1min 13s\nread_chunks 26.3 s\nread_csv 29.6 s\n\n\n\nQueries\n\nca_police[8] 82260698\ncardinality_one 234 ms\ncardinality_many 447 ms\nnormal 2-3 min\n\nca_police[8:10]\ncardinality_many 4.1s\nread_fast ~10 s\nnormal ~2 min\n\nca_police[8:11]\ncardinality_many 6.57s\nread_fast ~10 s\nnormal ~2 min\n\nca_police[8:13]\ncardinality_many 12.1s\nread_fast ~10 s\nnormal ~2 min",
"_____no_output_____"
]
],
[
[
"indices = []\n \ndf_all = []\nfor i in range(0,num_chunks+1):\n df_all.append(feather.read_dataframe(f'{FEATHER_DIR}full{i}.f'))\ndf = pd.concat(df_all, ignore_index=True)\nprint('concatted')\nprint(df.index)",
"concatted\nRangeIndex(start=0, stop=4999999, step=1)\n"
]
]
] | [
"code",
"markdown",
"code"
] | [
[
"code",
"code",
"code",
"code",
"code",
"code",
"code",
"code",
"code",
"code"
],
[
"markdown"
],
[
"code"
]
] |
e780c1b8ea12a5fdd13809ac44410430cc7bd857 | 155,503 | ipynb | Jupyter Notebook | notebooks/04-tidy.ipynb | chendaniely-teaching/2019-03-07-python | e56bfa93df4a7061fd640f8fb08ab010daae2893 | [
"MIT"
] | 2 | 2019-10-15T10:19:51.000Z | 2020-09-28T14:03:47.000Z | notebooks/04-tidy.ipynb | chendaniely-teaching/2019-03-07-python | e56bfa93df4a7061fd640f8fb08ab010daae2893 | [
"MIT"
] | null | null | null | notebooks/04-tidy.ipynb | chendaniely-teaching/2019-03-07-python | e56bfa93df4a7061fd640f8fb08ab010daae2893 | [
"MIT"
] | 3 | 2019-07-03T08:40:54.000Z | 2020-09-18T23:51:17.000Z | 32.511604 | 92 | 0.32038 | [
[
[
"import pandas as pd",
"_____no_output_____"
],
[
"pew = pd.read_csv('../data/pew.csv')",
"_____no_output_____"
],
[
"pew",
"_____no_output_____"
],
[
"# pd.melt()\n# pew.melt()",
"_____no_output_____"
],
[
"pew.melt(id_vars='religion', var_name='income', value_name='count')",
"_____no_output_____"
],
[
"billboard = pd.read_csv('../data/billboard.csv')",
"_____no_output_____"
],
[
"billboard.head()",
"_____no_output_____"
],
[
"billboard.columns[:5]",
"_____no_output_____"
],
[
"billboard\\\n .melt(id_vars=['year', 'artist', 'track',\n 'time', 'date.entered']).head()",
"_____no_output_____"
],
[
"artist_avg_rank = (billboard\n .melt(id_vars=['year', 'artist', 'track',\n 'time', 'date.entered'],\n value_name='rank',\n var_name='week')\n .groupby('artist')\n ['rank']\n .mean()\n .sort_values()\n)\nartist_avg_rank",
"_____no_output_____"
],
[
"billboard\\\n .melt(id_vars=['year', 'artist', 'track', 'time', 'date.entered'],\n value_name='rank',\n var_name='week')\\\n .groupby('artist')\\\n ['rank']\\\n .mean()\n",
"_____no_output_____"
],
[
"ebola = pd.read_csv('../data/country_timeseries.csv')",
"_____no_output_____"
],
[
"ebola.head()",
"_____no_output_____"
],
[
"ebola_long = ebola.melt(id_vars=['Date', 'Day'],\n var_name='cd_country',\n value_name='count')",
"_____no_output_____"
],
[
"ebola_long.head()",
"_____no_output_____"
],
[
"var_split = ebola_long['cd_country'].str.split('_')",
"_____no_output_____"
],
[
"# var_split.str[0]",
"_____no_output_____"
],
[
"var_split.str.get(0)",
"_____no_output_____"
],
[
"ebola_long['case_death'] = var_split.str.get(0)\nebola_long['country'] = var_split.str.get(1)",
"_____no_output_____"
],
[
"ebola_long",
"_____no_output_____"
],
[
"var_split_df = ebola_long['cd_country'].str.split('_', expand=True)",
"_____no_output_____"
],
[
"ebola_long[['cd_e', 'c_e']] = var_split_df",
"_____no_output_____"
],
[
"ebola_long.head()",
"_____no_output_____"
],
[
"weather = pd.read_csv('../data/weather.csv')",
"_____no_output_____"
],
[
"weather",
"_____no_output_____"
],
[
"weather_melt = weather.melt(id_vars=['id', 'year', 'month', 'element'],\n var_name='day',\n value_name='temp')",
"_____no_output_____"
],
[
"weather_melt.head()",
"_____no_output_____"
],
[
"weather_tidy = weather_melt.pivot_table(index=['id', 'year', 'month', 'day'],\n columns='element',\n values='temp')",
"_____no_output_____"
],
[
"weather_tidy.reset_index().head()",
"_____no_output_____"
],
[
"100_000_000",
"_____no_output_____"
],
[
"['id',\n #'year',\n #'month',\n #'day'\n]",
"_____no_output_____"
],
[
"billboard_long = billboard\\\n .melt(id_vars=['year', 'artist', 'track',\n 'time', 'date.entered'])",
"_____no_output_____"
],
[
"billboard_long.sort_values(['artist', 'track'])",
"_____no_output_____"
],
[
"billboard_songs = billboard_long[['year', 'artist', 'track', 'time', 'date.entered']]",
"_____no_output_____"
],
[
"billboard_songs = billboard_songs.drop_duplicates()",
"_____no_output_____"
],
[
"billboard_songs.shape",
"_____no_output_____"
],
[
"billboard_songs['id'] = billboard_songs.reset_index().index",
"_____no_output_____"
],
[
"billboard_songs.head(n = 2)",
"_____no_output_____"
],
[
"billboard_long.head(n=2)",
"_____no_output_____"
],
[
"billboard_ratings = billboard_long.merge(billboard_songs,\n on=['year', 'artist', 'track', 'time'])",
"_____no_output_____"
],
[
"billboard_ratings.head(n=2)",
"_____no_output_____"
],
[
"billboard_ratings = billboard_ratings[['id', 'variable', 'value']]",
"_____no_output_____"
],
[
"billboard_ratings.head(n=2)",
"_____no_output_____"
],
[
"billboard_ratings.shape",
"_____no_output_____"
],
[
"billboard_songs.to_csv('billboard_songs.csv', index=False)",
"_____no_output_____"
],
[
"#billboard_ratings.to_excel()",
"_____no_output_____"
],
[
"#billboard_ratings.to_feather()",
"_____no_output_____"
],
[
"#billboard_ratings.to_pickle()",
"_____no_output_____"
],
[
"#billboard_ratings.to_sql()",
"_____no_output_____"
]
]
] | [
"code"
] | [
[
"code",
"code",
"code",
"code",
"code",
"code",
"code",
"code",
"code",
"code",
"code",
"code",
"code",
"code",
"code",
"code",
"code",
"code",
"code",
"code",
"code",
"code",
"code",
"code",
"code",
"code",
"code",
"code",
"code",
"code",
"code",
"code",
"code",
"code",
"code",
"code",
"code",
"code",
"code",
"code",
"code",
"code",
"code",
"code",
"code",
"code",
"code",
"code",
"code"
]
] |
e780d72df5680968f5b4f0d697b7784769515435 | 70,644 | ipynb | Jupyter Notebook | code/5_3d_cgan/2_cgan_analysis/1_cgan3d_analyze-results.ipynb | vmos1/cosmogan_pytorch | 75d3d4f652a92d45d823a051b750b35d802e2317 | [
"BSD-3-Clause-LBNL"
] | 1 | 2020-10-19T18:52:50.000Z | 2020-10-19T18:52:50.000Z | code/5_3d_cgan/2_cgan_analysis/1_cgan3d_analyze-results.ipynb | vmos1/cosmogan_pytorch | 75d3d4f652a92d45d823a051b750b35d802e2317 | [
"BSD-3-Clause-LBNL"
] | 1 | 2020-11-13T22:35:02.000Z | 2020-11-14T02:00:44.000Z | code/5_3d_cgan/2_cgan_analysis/1_cgan3d_analyze-results.ipynb | vmos1/cosmogan_pytorch | 75d3d4f652a92d45d823a051b750b35d802e2317 | [
"BSD-3-Clause-LBNL"
] | null | null | null | 35.481668 | 198 | 0.448248 | [
[
[
"# Analyze results for 3D CGAN\nFeb 22, 2021",
"_____no_output_____"
]
],
[
[
"import numpy as np\nimport matplotlib.pyplot as plt\nimport pandas as pd\n\nimport subprocess as sp\nimport sys\nimport os\nimport glob\nimport pickle \n\nfrom matplotlib.colors import LogNorm, PowerNorm, Normalize\nimport seaborn as sns\nfrom functools import reduce",
"_____no_output_____"
],
[
"from ipywidgets import *",
"_____no_output_____"
],
[
"%matplotlib widget",
"_____no_output_____"
],
[
"sys.path.append('/global/u1/v/vpa/project/jpt_notebooks/Cosmology/Cosmo_GAN/repositories/cosmogan_pytorch/code/modules_image_analysis/')\nfrom modules_img_analysis import *",
"_____no_output_____"
],
[
"sys.path.append('/global/u1/v/vpa/project/jpt_notebooks/Cosmology/Cosmo_GAN/repositories/cosmogan_pytorch/code/5_3d_cgan/1_main_code/')\nimport post_analysis_pandas as post\n",
"_____no_output_____"
],
[
"### Transformation functions for image pixel values\ndef f_transform(x):\n return 2.*x/(x + 4.) - 1.\n\ndef f_invtransform(s):\n return 4.*(1. + s)/(1. - s)\n",
"_____no_output_____"
],
[
"# img_size=64\nimg_size=128",
"_____no_output_____"
],
[
"val_data_dict={'64':'/global/cfs/cdirs/m3363/vayyar/cosmogan_data/raw_data/3d_data/dataset2a_3dcgan_4univs_64cube_simple_splicing',\n '128':'/global/cfs/cdirs/m3363/vayyar/cosmogan_data/raw_data/3d_data/dataset4_smoothing_4univ_cgan_varying_sigma_128cube'}",
"_____no_output_____"
]
],
[
[
"### Read validation data",
"_____no_output_____"
]
],
[
[
"# bins=np.concatenate([np.array([-0.5]),np.arange(0.5,20.5,1),np.arange(20.5,100.5,5),np.arange(100.5,1000.5,50),np.array([2000])]) #bin edges to use\nbins=np.concatenate([np.array([-0.5]),np.arange(0.5,100.5,5),np.arange(100.5,300.5,20),np.arange(300.5,1000.5,50),np.array([2000])]) #bin edges to use\n\nbins=f_transform(bins) ### scale to (-1,1)\n# ### Extract validation data\nsigma_lst=[0.5,0.65,0.8,1.1]\nlabels_lst=range(len(sigma_lst))\nbkgnd_dict={}\nnum_bkgnd=100\n\nfor label in labels_lst:\n fname=val_data_dict[str(img_size)]+'/norm_1_sig_{0}_train_val.npy'.format(sigma_lst[label])\n print(fname)\n samples=np.load(fname,mmap_mode='r')[-num_bkgnd:][:,0,:,:]\n \n dict_val=post.f_compute_hist_spect(samples,bins)\n bkgnd_dict[str(sigma_lst[label])]=dict_val\n del samples",
"/global/cfs/cdirs/m3363/vayyar/cosmogan_data/raw_data/3d_data/dataset4_smoothing_4univ_cgan_varying_sigma_128cube/norm_1_sig_0.5_train_val.npy\n/global/cfs/cdirs/m3363/vayyar/cosmogan_data/raw_data/3d_data/dataset4_smoothing_4univ_cgan_varying_sigma_128cube/norm_1_sig_0.65_train_val.npy\n/global/cfs/cdirs/m3363/vayyar/cosmogan_data/raw_data/3d_data/dataset4_smoothing_4univ_cgan_varying_sigma_128cube/norm_1_sig_0.8_train_val.npy\n/global/cfs/cdirs/m3363/vayyar/cosmogan_data/raw_data/3d_data/dataset4_smoothing_4univ_cgan_varying_sigma_128cube/norm_1_sig_1.1_train_val.npy\n"
]
],
[
[
"## Read data",
"_____no_output_____"
]
],
[
[
"# main_dir='/global/cfs/cdirs/m3363/vayyar/cosmogan_data/results_from_other_code/pytorch/results/128sq/'\n# results_dir=main_dir+'20201002_064327'",
"_____no_output_____"
],
[
"dict1={'64':'/global/cfs/cdirs/m3363/vayyar/cosmogan_data/results_from_other_code/pytorch/results/3d_cGAN/',\n '128':'/global/cfs/cdirs/m3363/vayyar/cosmogan_data/results_from_other_code/pytorch/results/3d_cGAN/'}\n\nu=interactive(lambda x: dict1[x], x=Select(options=dict1.keys()))\n# display(u)\n",
"_____no_output_____"
],
[
"# parent_dir=u.result\nparent_dir=dict1[str(img_size)]\ndir_lst=[i.split('/')[-1] for i in glob.glob(parent_dir+'202107*')]\nn=interactive(lambda x: x, x=Dropdown(options=dir_lst))\ndisplay(n)",
"_____no_output_____"
],
[
"result=n.result\nresult_dir=parent_dir+result\nprint(result_dir)",
"/global/cfs/cdirs/m3363/vayyar/cosmogan_data/results_from_other_code/pytorch/results/3d_cGAN/20210726_173009_cgan_128_nodes1_lr0.000002_finetune\n"
]
],
[
[
"## Plot Losses",
"_____no_output_____"
]
],
[
[
"df_metrics=pd.read_pickle(result_dir+'/df_metrics.pkle').astype(np.float64)\n",
"_____no_output_____"
],
[
"df_metrics.tail(10)",
"_____no_output_____"
],
[
"def f_plot_metrics(df,col_list):\n \n plt.figure()\n for key in col_list:\n plt.plot(df_metrics[key],label=key,marker='*',linestyle='')\n plt.legend()\n \n# col_list=list(col_list)\n# df.plot(kind='line',x='step',y=col_list)\n \n# f_plot_metrics(df_metrics,['spec_chi','hist_chi'])\n\ninteract_manual(f_plot_metrics,df=fixed(df_metrics), col_list=SelectMultiple(options=df_metrics.columns.values))",
"_____no_output_____"
],
[
"\nchi=df_metrics.quantile(q=0.2,axis=0)['hist_chi']\nprint(chi)\ndf_metrics[(df_metrics['hist_chi']<=chi)&(df_metrics.epoch>30)].sort_values(by=['hist_chi']).head(10)",
"-3.003056287765503\n"
],
[
"# display(df_metrics.sort_values(by=['hist_chi']).head(8))\n# display(df_metrics.sort_values(by=['spec_chi']).head(8))",
"_____no_output_____"
]
],
[
[
"<!-- ### Read validation data -->",
"_____no_output_____"
],
[
"## Read stored chi-squares for images",
"_____no_output_____"
]
],
[
[
"## Get sigma list from saved files\nflist=glob.glob(result_dir+'/df_processed*')\nsigma_lst=[i.split('/')[-1].split('df_processed_')[-1].split('.pkle')[0] for i in flist]\nsigma_lst.sort() ### Sorting is important for labels to match !!\n\nlabels_lst=np.arange(len(sigma_lst))",
"_____no_output_____"
],
[
"sigma_lst,labels_lst",
"_____no_output_____"
],
[
"### Create a merged dataframe\n\ndf_list=[]\nfor label in labels_lst:\n df=pd.read_pickle(result_dir+'/df_processed_{0}.pkle'.format(str(sigma_lst[label])))\n df[['epoch','step']]=df[['epoch','step']].astype(int)\n df['label']=df.epoch.astype(str)+'-'+df.step.astype(str) # Add label column for plotting\n df_list.append(df)\n\nfor i,df in enumerate(df_list):\n df1=df.add_suffix('_'+str(i))\n # renaming the columns to be joined on\n keys=['epoch','step','img_type','label']\n rename_cols_dict={key+'_'+str(i):key for key in keys}\n# print(rename_cols_dict)\n df1.rename(columns=rename_cols_dict,inplace=True) \n df_list[i]=df1\n \ndf_merged=reduce(lambda x, y : pd.merge(x, y, on = ['step','epoch','img_type','label']), df_list)\n\n### Get sum of all 4 classes for 3 types of chi-squares\nfor chi_type in ['chi_1','chi_spec1','chi_spec2','chi_1a','chi_1b','chi_1c']:\n keys=[chi_type+'_'+str(label) for label in labels_lst]\n# display(df_merged[keys].sum(axis=1))\n df_merged['sum_'+chi_type]=df_merged[keys].sum(axis=1)\ndel df_list\n",
"_____no_output_____"
],
[
"df",
"_____no_output_____"
]
],
[
[
"### Slice best steps",
"_____no_output_____"
]
],
[
[
"def f_slice_merged_df(df,cutoff=0.2,sort_col='chi_1',col_mode='all',label='all',params_lst=[0,1,2],head=10,epoch_range=[0,None],use_sum=True,display_flag=False):\n ''' View dataframe after slicing\n '''\n\n if epoch_range[1]==None: epoch_range[1]=df.max()['epoch']\n df=df[(df.epoch<=epoch_range[1])&(df.epoch>=epoch_range[0])]\n\n ######### Apply cutoff to keep reasonable chi1 and chispec1\n #### Add chi-square columns to use\n chi_cols=[]\n if use_sum: ## Add sum chi-square columns\n for j in ['chi_1','chi_spec1','chi_1a','chi_1b','chi_1c']: chi_cols.append('sum_'+j)\n \n if label=='all': ### Add chi-squares for all labels\n for j in ['chi_1','chi_spec1','chi_1a','chi_1b','chi_1c']:\n for idx,i in enumerate(params_lst): chi_cols.append(j+'_'+str(idx))\n else: ## Add chi-square for specific label\n assert label in params_lst, \"label %s is not in %s\"%(label,params_lst)\n label_idx=params_lst.index(label)\n print(label_idx)\n for j in ['chi_1','chi_spec1','chi_spec2','chi_1a','chi_1b','chi_1c']: chi_cols.append(j+'_'+str(label_idx))\n# print(chi_cols)\n q_dict=dict(df_merged.quantile(q=cutoff,axis=0)[chi_cols])\n # print(q_dict)\n strg=['%s < %s'%(key,q_dict[key]) for key in chi_cols ]\n query=\" & \".join(strg)\n # print(query)\n df=df.query(query)\n \n # Sort dataframe\n df1=df[df.epoch>0].sort_values(by=sort_col)\n chis=[i for i in df_merged.columns if 'chi' in i]\n col_list=['label']+chis+['epoch','step']\n if (col_mode=='short'): \n col_list=['label']+[i for i in df_merged.columns if i.startswith('sum')]\n col_list=['label']+chi_cols\n df2=df1.head(head)[col_list]\n \n if display_flag: display(df2) # Display df\n \n return df2\n\n# f_slice_merged_df(df_merged,cutoff=0.3,sort_col='sum_chi_1',label=0.65,params_lst=[0.5,0.65,0.8,1.1],use_sum=True,head=2000,display_flag=False,epoch_range=[7,None])",
"_____no_output_____"
],
[
"cols_to_sort=np.unique([i for i in df_merged.columns for j in ['chi_1_','chi_spec1_'] if ((i.startswith(j)) or (i.startswith('sum')))])\n\nw=interactive(f_slice_merged_df,df=fixed(df_merged),\ncutoff=widgets.FloatSlider(value=0.3, min=0, max=1.0, step=0.01), \ncol_mode=['all','short'], display_flag=widgets.Checkbox(value=False),\nuse_sum=widgets.Checkbox(value=True),\nlabel=ToggleButtons(options=['all']+sigma_lst), params_lst=fixed(sigma_lst),\nhead=widgets.IntSlider(value=10,min=1,max=20,step=1),\nepoch_range=widgets.IntRangeSlider(value=[0,np.max(df.epoch.values)],min=0,max=np.max(df.epoch.values),step=1),\nsort_col=cols_to_sort\n)\ndisplay(w)",
"_____no_output_____"
],
[
"df_sliced=w.result\n[int(i.split('-')[1]) for i in df_sliced.label.values]\n\n# df_sliced",
"_____no_output_____"
],
[
"best_step=[]\ndf_test=df_merged.copy()\ndf_test=df_merged[(df_merged.epoch<5000)&(df_merged.epoch>0)]\ncut_off=1.0\n\nbest_step.append(f_slice_merged_df(df_test,cutoff=cut_off,sort_col='sum_chi_1',label='all',use_sum=True,head=4,display_flag=False,epoch_range=[7,None],params_lst=sigma_lst).step.values)\nbest_step.append(f_slice_merged_df(df_test,cutoff=cut_off,sort_col='sum_chi_spec1',label='all',use_sum=True,head=8,display_flag=False,epoch_range=[7,None],params_lst=sigma_lst).step.values)\nbest_step.append(f_slice_merged_df(df_test,cutoff=cut_off,sort_col='sum_chi_spec2',label='all',use_sum=True,head=2,display_flag=False,epoch_range=[7,None],params_lst=sigma_lst).step.values)\nbest_step.append(f_slice_merged_df(df_test,cutoff=cut_off,sort_col='sum_chi_1b',label='all',use_sum=True,head=2,display_flag=False,epoch_range=[7,None],params_lst=sigma_lst).step.values)\n\n# best_step.append([46669,34281])\nbest_step=np.unique([i for j in best_step for i in j])\nprint(best_step)\nbest_step",
"[1640 1650 1740 1950 2330 2580 3010 3020 5590 5780 5890 5940 5950 6090\n 6380 6800]\n"
],
[
"# best_step=[6176]\n# best_step= [23985,24570,25155,25740,26325,26910,27495]\n# best_step=[int(i.split('-')[1]) for i in df_sliced.label.values]\n# best_step=np.arange(40130,40135).astype(int)",
"_____no_output_____"
],
[
"df_best=df_merged[df_merged.step.isin(best_step)]\nprint(df_best.shape)\nprint([(df_best[df_best.step==step].epoch.values[0],df_best[df_best.step==step].step.values[0]) for step in best_step])\n# print([(df_best.loc[idx].epoch,df_best.loc[idx].step) for idx in best_idx])",
"(16, 64)\n[(7, 1640), (7, 1650), (7, 1740), (8, 1950), (9, 2330), (11, 2580), (12, 3010), (12, 3020), (23, 5590), (24, 5780), (25, 5890), (25, 5940), (25, 5950), (26, 6090), (27, 6380), (29, 6800)]\n"
],
[
"col_list=['label']+[i for i in df_merged.columns if i.startswith('sum')]\ndf_best[col_list]",
"_____no_output_____"
]
],
[
[
"### Interactive plot",
"_____no_output_____"
]
],
[
[
"\ndef f_plot_hist_spec(df,param_labels,sigma_lst,steps_list,bkg_dict,plot_type,img_size):\n\n assert plot_type in ['hist','spec','grid','spec_relative'],\"Invalid mode %s\"%(plot_type)\n\n if plot_type in ['hist','spec','spec_relative']: fig=plt.figure(figsize=(6,6))\n for par_label in param_labels:\n df=df[df.step.isin(steps_list)]\n# print(df.shape)\n idx=sigma_lst.index(par_label)\n suffix='_%s'%(idx)\n dict_bkg=bkg_dict[str(par_label)]\n \n for (i,row),marker in zip(df.iterrows(),itertools.cycle('>^*sDHPdpx_')):\n label=row.label+'_'+str(par_label)\n if plot_type=='hist':\n x1=row['hist_bin_centers'+suffix]\n y1=row['hist_val'+suffix]\n yerr1=row['hist_err'+suffix]\n x1=f_invtransform(x1)\n \n plt.errorbar(x1,y1,yerr1,marker=marker,markersize=5,linestyle='',label=label)\n if plot_type=='spec':\n y2=row['spec_val'+suffix]\n yerr2=row['spec_sdev'+suffix]/np.sqrt(row['num_imgs'+suffix])\n x2=np.arange(len(y2))\n y2=x2**2*y2; yerr2=x2**2*yerr2 ## Plot k^2 P(y)\n plt.fill_between(x2, y2 - yerr2, y2 + yerr2, alpha=0.4)\n plt.plot(x2, y2, marker=marker, linestyle=':',label=label)\n\n if plot_type=='spec_relative':\n\n y2=row['spec_val'+suffix]\n yerr2=row['spec_sdev'+suffix]\n x2=np.arange(len(y2))\n\n ### Reference spectrum\n y1,yerr1=dict_bkg['spec_val'],dict_bkg['spec_sdev']\n y=y2/y1\n ## Variance is sum of variance of both variables, since they are uncorrelated\n\n # delta_r= |r| * sqrt(delta_a/a)^2 +(\\delta_b/b)^2) / \\sqrt(N)\n yerr=(np.abs(y))*np.sqrt((yerr1/y1)**2+(yerr2/y2)**2)/np.sqrt(row['num_imgs'+suffix])\n \n plt.fill_between(x2, y - yerr, y + yerr, alpha=0.4)\n plt.plot(x2, y, marker=marker, linestyle=':',label=label)\n\n if plot_type=='grid':\n images=np.load(row['fname'+suffix])[:,0,:,:,0]\n print(images.shape)\n f_plot_grid(images[:8],cols=4,fig_size=(8,4))\n \n ### Plot reference data\n if plot_type=='hist':\n x,y,yerr=dict_bkg['hist_bin_centers'],dict_bkg['hist_val'],dict_bkg['hist_err']\n x=f_invtransform(x)\n plt.errorbar(x, y,yerr,color='k',linestyle='-',label='bkgnd') \n plt.title('Pixel Intensity Histogram')\n plt.xscale('symlog',linthreshx=50)\n \n if plot_type=='spec':\n y,yerr=dict_bkg['spec_val'],dict_bkg['spec_sdev']/np.sqrt(num_bkgnd)\n x=np.arange(len(y))\n y=x**2*y; yerr=x**2*yerr ## Plot k^2 P(y)\n plt.fill_between(x, y - yerr, y + yerr, color='k',alpha=0.8)\n plt.title('Spectrum')\n plt.xlim(0,img_size/2)\n\n if plot_type=='spec_relative':\n plt.axhline(y=1.0,color='k',linestyle='-.')\n plt.title(\"Relative spectrum\")\n plt.xlim(0,img_size/2)\n plt.ylim(0.5,2)\n\n if plot_type in ['hist','spec']: \n plt.yscale('log')\n plt.legend(bbox_to_anchor=(0.3, 0.75),ncol=2, fancybox=True, shadow=True,prop={'size':6})\n\n\n# f_plot_hist_spec(df_merged,[sigma_lst[-1]],sigma_lst,[best_step[0]],bkgnd_dict,'hist')",
"_____no_output_____"
],
[
"interact_manual(f_plot_hist_spec,df=fixed(df_best),\n param_labels=SelectMultiple(options=sigma_lst),sigma_lst=fixed(sigma_lst),\n steps_list=SelectMultiple(options=df_best.step.values),\n img_size=fixed(img_size),\n bkg_dict=fixed(bkgnd_dict),plot_type=ToggleButtons(options=['hist','spec','grid','spec_relative']))",
"_____no_output_____"
],
[
"best_step",
"_____no_output_____"
],
[
"sigma=1.1\nfname=val_data_dict[str(img_size)]+'/norm_1_sig_{0}_train_val.npy'.format(sigma)\nprint(fname)\na1=np.load(fname,mmap_mode='r')[-100:]\na1.shape\n\nimages=a1[:,0,:,:,0]\nf_plot_grid(images[8:16],cols=4,fig_size=(8,4))\n",
"/global/cfs/cdirs/m3363/vayyar/cosmogan_data/raw_data/3d_data/dataset4_smoothing_4univ_cgan_varying_sigma_128cube/norm_1_sig_1.1_train_val.npy\n2 4\n"
]
],
[
[
"### Delete unwanted stored models\n(Since deterministic runs aren't working well )",
"_____no_output_____"
]
],
[
[
"# # fldr='/global/cfs/cdirs/m3363/vayyar/cosmogan_data/results_from_other_code/pytorch/results/128sq/20210119_134802_cgan_predict_0.65_m2/models'\n# fldr=result_dir\n# print(fldr)\n# flist=glob.glob(fldr+'/models/checkpoint_*.tar')\n# len(flist)",
"_____no_output_____"
],
[
"# # Delete unwanted stored images\n# for i in flist:\n# try:\n# step=int(i.split('/')[-1].split('_')[-1].split('.')[0])\n# if step not in best_step:\n# # print(step)\n# os.remove(i)\n# pass\n# else: \n# print(step)\n# # print(i)\n# except Exception as e:\n# # print(e)\n# # print(i)\n# pass",
"_____no_output_____"
],
[
"# best_step",
"_____no_output_____"
],
[
"# ! du -hs /global/cfs/cdirs/m3363/vayyar/cosmogan_data/raw_data/3d_data/dataset2a_3dcgan_4univs_64cube_simple_splicing/norm_1_sig_0.5_train_val.npy",
"_____no_output_____"
],
[
"# fname='/global/cfs/cdirs/m3363/vayyar/cosmogan_data/raw_data/3d_data/dataset4_smoothing_4univ_cgan_varying_sigma_128cube/Om0.3_Sg0.5_H70.0.npy'\n# np.load(fname,mmap_mode='r').shape",
"_____no_output_____"
],
[
"2880/90",
"_____no_output_____"
]
]
] | [
"markdown",
"code",
"markdown",
"code",
"markdown",
"code",
"markdown",
"code",
"markdown",
"code",
"markdown",
"code",
"markdown",
"code",
"markdown",
"code"
] | [
[
"markdown"
],
[
"code",
"code",
"code",
"code",
"code",
"code",
"code",
"code"
],
[
"markdown"
],
[
"code"
],
[
"markdown"
],
[
"code",
"code",
"code",
"code"
],
[
"markdown"
],
[
"code",
"code",
"code",
"code",
"code"
],
[
"markdown",
"markdown"
],
[
"code",
"code",
"code",
"code"
],
[
"markdown"
],
[
"code",
"code",
"code",
"code",
"code",
"code",
"code"
],
[
"markdown"
],
[
"code",
"code",
"code",
"code"
],
[
"markdown"
],
[
"code",
"code",
"code",
"code",
"code",
"code"
]
] |
e780dc8002a7755ed75f4d18d4643dfd2e0645f9 | 19,222 | ipynb | Jupyter Notebook | slides/test_driven_development/tdd_advanced.ipynb | FOSSEE/sees | 0e76356043e3ed28a74ecb5c8f64094bc18f2115 | [
"OLDAP-2.5"
] | 10 | 2015-01-21T13:52:30.000Z | 2021-08-12T08:54:48.000Z | slides/test_driven_development/tdd_advanced.ipynb | FOSSEE/sees | 0e76356043e3ed28a74ecb5c8f64094bc18f2115 | [
"OLDAP-2.5"
] | 3 | 2015-01-22T08:07:08.000Z | 2015-03-12T14:39:57.000Z | slides/test_driven_development/tdd_advanced.ipynb | FOSSEE/sees | 0e76356043e3ed28a74ecb5c8f64094bc18f2115 | [
"OLDAP-2.5"
] | 14 | 2015-01-20T23:03:36.000Z | 2021-08-12T08:54:57.000Z | 22.747929 | 91 | 0.516856 | [
[
[
"# Advanced topics in test driven development",
"_____no_output_____"
],
[
"## Introduction\n\n\n- Already seen the basics\n- Learn some advanced topics",
"_____no_output_____"
],
[
"## The hypothesis package\n\n- http://hypothesis.readthedocs.io\n\n- `pip install hypothesis`\n\n- General idea earlier:\n - Make test data.\n - Perform operations\n - Assert something after operation\n\n- Hypothesis automates this!\n - Describe range of scenarios\n - Computer explores these and tests\n\n- With hypothesis:\n - Generate random data using specification\n - Perform operations\n - assert something about result\n ",
"_____no_output_____"
],
[
"### Example",
"_____no_output_____"
]
],
[
[
"\nfrom hypothesis import given\nfrom hypothesis import strategies as st\n\nfrom gcd import gcd\n\n@given(st.integers(min_value=0), st.integers(min_value=0))\ndef test_gcd(a, b):\n result = gcd(a, b)\n # Now what?\n # assert a%result == 0\n",
"_____no_output_____"
]
],
[
[
"### Example: adding a specific case",
"_____no_output_____"
]
],
[
[
"@given(st.integers(min_value=0), st.integers(min_value=0))\n\n@example(a=44, b=19)\n\ndef test_gcd(a, b):\n result = gcd(a, b)\n # Now what?\n # assert a%result == 0\n",
"_____no_output_____"
]
],
[
[
"### More details\n\n- `given` generates inputs\n- `strategies`: provides a strategy for inputs\n- Different stratiegies\n - `integers`\n - `floats`\n - `text`\n - `booleans`\n - `tuples`\n - `lists`\n - ...\n\n- See: http://hypothesis.readthedocs.io/en/latest/data.html",
"_____no_output_____"
],
[
"### Example exercise\n\n- Write a simple run-length encoding function called `encode`\n- Write another called `decode` to produce the same input from the output of\n `encode`",
"_____no_output_____"
]
],
[
[
"def encode(text):\n return []\n\ndef decode(lst):\n return ''\n\n",
"_____no_output_____"
]
],
[
[
"### The test",
"_____no_output_____"
]
],
[
[
"from hypothesis import given\nfrom hypothesis import strategies as st\n\n@given(st.text())\ndef test_decode_inverts_encode(s):\n assert decode(encode(s)) == s",
"_____no_output_____"
]
],
[
[
"### Summary\n\n- Much easier to test\n- hypothesis does the hard work\n- Can do a lot more!\n- Read the docs for more\n- For some detailed articles:\n http://hypothesis.works/articles/intro/\n- Here in particular is one interesting article:\n http://hypothesis.works/articles/calculating-the-mean/\n\n----\n\n## Unittest module\n\n- Basic idea and style is from JUnit\n- Some consider this old style\n\n\n### How to use unittest\n\n- Subclass `unittest.TestCase`\n- Create test methods\n\n### A simple example\n\n- Let us test gcd.py with unittest",
"_____no_output_____"
]
],
[
[
"# gcd.py\ndef gcd(a, b):\n if b == 0:\n return a\n return gcd(b, a%b)",
"_____no_output_____"
]
],
[
[
"### Writing the test",
"_____no_output_____"
]
],
[
[
"# test_gcd.py\nfrom gcd import gcd\nimport unittest\n\nclass TestGCD(unittest.TestCase):\n def test_gcd_works_for_positive_integers(self):\n self.assertEqual(gcd(48, 64), 16)\n self.assertEqual(gcd(44, 19), 1)\n\nif __name__ == '__main__':\n unittest.main()",
"_____no_output_____"
]
],
[
[
"### Running it\n\n- Just run `python test_gcd.py`\n- Also works with `nosetests` and `pytest`\n\n\n### Notes\n\n- Note the name of the method.\n- Note the use of `self.assertEqual`\n- Also available: `assertNotEqual, assertTrue, assertFalse, assertIs, assertIsNot`\n- `assertIsNone, assertIn, assertIsInstance, assertRaises`\n- `assertAlmostEqual, assertListEqual, assertSequenceEqual ` ...\n\n- https://docs.python.org/2/library/unittest.html\n\n\n### Fixtures\n\n- What if you want to do something common before all tests?\n- Typically called a **fixture**\n\n- Use the `setUp` and `tearDown` methods for method-level fixtures\n\n### Silly fixture example",
"_____no_output_____"
]
],
[
[
"# test_gcd.py\nimport gcd\nimport unittest\n\nclass TestGCD(unittest.TestCase):\n def setUp(self):\n print(\"setUp\")\n def tearDown(self):\n print(\"tearDown\")\n def test_gcd_works_for_positive_integers(self):\n self.assertEqual(gcd(48, 64), 16)\n self.assertEqual(gcd(44, 19), 1)\n\nif __name__ == '__main__':\n unittest.main()\n",
"_____no_output_____"
]
],
[
[
"### Exercise\n\n- Fix bug with negative numbers in gcd.py.\n- Use TDD.\n\n\n### Using hypothesis with unittest",
"_____no_output_____"
]
],
[
[
"# test_gcd.py\nfrom hypothesis import given\nfrom hypothesis import strategies as st\n\nimport gcd\nimport unittest\n\nclass TestGCD(unittest.TestCase):\n @given(a=st.integers(min_value=0), b=st.integers(min_value=0))\n def test_gcd_works_for_positive_integers(self, a, b):\n result = gcd(a, b)\n assert a%result == 0\n assert b%result == 0\n assert result <= a and result <= b\n\nif __name__ == '__main__':\n unittest.main()\n",
"_____no_output_____"
]
],
[
[
"### Some notes on style\n\n- Use descriptive function names\n- Intent matters\n\n- Segregate the test code into the following",
"_____no_output_____"
]
],
[
[
"- Given: what is the context of the test?\n- When: what action is taken to actually test the problem\n- Then: what do we actually ensure.",
"_____no_output_____"
]
],
[
[
"### More on intent driven programming\n\n- \"Programs must be written for people to read, and only incidentally for\n machines to execute.” Harold Abelson\n\n- The code should make the intent clear.\n\nFor example:",
"_____no_output_____"
]
],
[
[
"if self.temperature > 600 and self.pressure > 10e5:\n message = 'hello you have a problem here!'\n message += 'current temp is %s'%(self.temperature)\n print(message)\n self.valve.close()\n self.raise_warning()",
"_____no_output_____"
]
],
[
[
"is totally unclear as to the intent. Instead refactor as follows:",
"_____no_output_____"
]
],
[
[
"if self.reactor_is_critical():\n self.shutdown_with_warning()\n",
"_____no_output_____"
]
],
[
[
"### A more involved testing example\n\n- Motivational problem:\n\n> Find all the git repositories inside a given directory recursively.\n> Make this a command line tool supporting command line use.\n\n- Write tests for the code\n- Some rules:\n\n 0. The test should be runnable by anyone (even by a computer), almost anywhere.\n 1. Don't write anything in the current directory (use a temporary directory).\n 2. Cleanup any files you create while testing.\n 3. Make sure tests do not affect global state too much.\n\n\n### Solution\n\n1. Create some test data.\n2. Test!\n3. Cleanup the test data\n\n\n### Class-level fixtures\n\n- Use `setupClass` and `tearDownClass` classmethods for class level fixtures.\n\n\n### Module-level fixtures\n\n- `setup_module`, `teardown_module`\n- Can be used for a module-level fixture\n\n- http://nose.readthedocs.io/en/latest/writing_tests.html\n\n\n## Coverage\n\n- Assess the amount of code that is covered by testing\n- http://coverage.readthedocs.io/\n- `pip install coverage`\n- Integrates with nosetests/pytest\n\n### Typical coverage usage",
"_____no_output_____"
]
],
[
[
"$ coverage run -m nose.core my_package\n$ coverage report -m\n$ coverage html",
"_____no_output_____"
]
],
[
[
"## mock\n\n- Mocking for advanced testing.\n\n- Example: reading some twitter data\n- Example: function to post an update to facebook or twitter\n- Example: email user when simulation crashes\n\n- Can you test it? How?\n\n### Using mock: the big picture\n\n- Do you really want to post something on facebook?\n- Or do you want to know if the right method was called with the right arguments?\n\n- Idea: \"mock\" the objects that do something and test them\n\n- Quoting from the Python docs:\n\n> It allows you to replace parts of your system under test with mock objects\n> and make assertions about how they have been used.\n\n### Installation\n\n- Built-in on Python >= 3.3",
"_____no_output_____"
]
],
[
[
"- `from unittest import mock`",
"_____no_output_____"
]
],
[
[
"- else `pip install mock`",
"_____no_output_____"
]
],
[
[
"- `import mock`\n",
"_____no_output_____"
]
],
[
[
"### Simple examples\n\nSay we have a class:",
"_____no_output_____"
]
],
[
[
"class ProductionClass(object):\n def method(self, *args):\n # This does something we do not want to actually run in the test\n # ...\n pass",
"_____no_output_____"
]
],
[
[
"To mock the `ProductionClass.method` do this:",
"_____no_output_____"
]
],
[
[
"from unittest.mock import MagicMock\nthing = ProductionClass()\nthing.method = MagicMock(return_value=3)\nthing.method(3, 4, 5, key='value')\nthing.method.assert_called_with(3, 4, 5, key='value')\n",
"_____no_output_____"
]
],
[
[
"### More practical use case\n\n- Mocking a module or system call\n- Mocking an object or method\n- Remember that after testing you want to restore original state\n- Use `mock.patch`\n\n### An example\n\n- Write code to remove generated files from LaTeX compilation, i.e. remove the\n *.aux, *.log, *.pdf etc.\n\nHere is a simple attempt:",
"_____no_output_____"
]
],
[
[
"# clean_tex.py\nimport os\n\ndef cleanup(tex_file_pth):\n base = os.path.splitext(tex_file_pth)[0]\n for ext in ('.aux', '.log'):\n f = base + ext\n if os.path.exists(f):\n os.remove(f)\n",
"_____no_output_____"
]
],
[
[
"### Testing this with mock",
"_____no_output_____"
]
],
[
[
"import mock\n\[email protected]('clean_tex.os.remove')\ndef test_cleanup_removes_extra_files(mock_remove):\n cleanup('foo.tex')\n\n expected = [mock.call('foo.' + x) for x in ('aux', 'log')]\n\n mock_remove.assert_has_calls(expected)\n",
"_____no_output_____"
]
],
[
[
"- Note the mocked argument that is passed.\n- Note that we did not mock `os.remove`\n- Mock where the object is looked up\n\n### Doing more",
"_____no_output_____"
]
],
[
[
"import mock\n\[email protected]('clean_tex.os.path')\[email protected]('clean_tex.os.remove')\ndef test_cleanup_does_not_fail_when_files_dont_exist(mock_remove, mock_path):\n # Setup the mock_path to return False\n mock_path.exists.return_value = False\n\n cleanup('foo.tex')\n\n mock_remove.assert_not_called()",
"_____no_output_____"
]
],
[
[
"- Note the order of the passed arguments\n- Note the name of the method\n\n### Patching instance methods\n\nUse `mock.patch.object` to patch an instance method",
"_____no_output_____"
]
],
[
[
"@mock.patch.object(ProductionClass, 'method')\ndef test_method(mock_method):\n obj = ProductionClass()\n obj.method(1)\n\n mock_method.assert_called_once_with(1)\n",
"_____no_output_____"
]
],
[
[
"Mock works as a context manager:",
"_____no_output_____"
]
],
[
[
"with mock.patch.object(ProductionClass, 'method') as mock_method:\n obj = ProductionClass()\n obj.method(1)\n\nmock_method.assert_called_once_with(1)\n",
"_____no_output_____"
]
],
[
[
"### More articles on mock\n\n- See more here https://docs.python.org/3/library/unittest.mock.html\n- https://www.toptal.com/python/an-introduction-to-mocking-in-python\n\n\n## Pytest\n\nOffers many useful and convenient features that are useful\n\n\n## Odds and ends\n\n### Linters\n\n- `pyflakes`\n- `flake8`\n\n### IPython goodies\n\n- Use `%run`\n- Use `%pdb`\n- `%debug`\n\n### Debugging\n\n- Debug with `%run`\n- pdb.set_trace()\n- IPython set trace:",
"_____no_output_____"
]
],
[
[
"from IPython.core.debugger import Tracer; Tracer()()",
"_____no_output_____"
]
],
[
[
"- See here: http://www.scipy-lectures.org/advanced/debugging/",
"_____no_output_____"
]
]
] | [
"markdown",
"code",
"markdown",
"code",
"markdown",
"code",
"markdown",
"code",
"markdown",
"code",
"markdown",
"code",
"markdown",
"code",
"markdown",
"code",
"markdown",
"code",
"markdown",
"code",
"markdown",
"code",
"markdown",
"code",
"markdown",
"code",
"markdown",
"code",
"markdown",
"code",
"markdown",
"code",
"markdown",
"code",
"markdown",
"code",
"markdown",
"code",
"markdown",
"code",
"markdown",
"code",
"markdown",
"code",
"markdown"
] | [
[
"markdown",
"markdown",
"markdown",
"markdown"
],
[
"code"
],
[
"markdown"
],
[
"code"
],
[
"markdown",
"markdown"
],
[
"code"
],
[
"markdown"
],
[
"code"
],
[
"markdown"
],
[
"code"
],
[
"markdown"
],
[
"code"
],
[
"markdown"
],
[
"code"
],
[
"markdown"
],
[
"code"
],
[
"markdown"
],
[
"code"
],
[
"markdown"
],
[
"code"
],
[
"markdown"
],
[
"code"
],
[
"markdown"
],
[
"code"
],
[
"markdown"
],
[
"code"
],
[
"markdown"
],
[
"code"
],
[
"markdown"
],
[
"code"
],
[
"markdown"
],
[
"code"
],
[
"markdown"
],
[
"code"
],
[
"markdown"
],
[
"code"
],
[
"markdown"
],
[
"code"
],
[
"markdown"
],
[
"code"
],
[
"markdown"
],
[
"code"
],
[
"markdown"
],
[
"code"
],
[
"markdown"
]
] |
e780e5f5ba42a724c07f44af5a5550dc5cb3148c | 153,584 | ipynb | Jupyter Notebook | Regression/Support Vector Machine/SVR_MinMaxScaler.ipynb | PrajwalNimje1997/ds-seed | db23e5d4f99c145ed889b7fc96f7c2239df78eef | [
"Apache-2.0"
] | null | null | null | Regression/Support Vector Machine/SVR_MinMaxScaler.ipynb | PrajwalNimje1997/ds-seed | db23e5d4f99c145ed889b7fc96f7c2239df78eef | [
"Apache-2.0"
] | null | null | null | Regression/Support Vector Machine/SVR_MinMaxScaler.ipynb | PrajwalNimje1997/ds-seed | db23e5d4f99c145ed889b7fc96f7c2239df78eef | [
"Apache-2.0"
] | null | null | null | 223.883382 | 71,236 | 0.896454 | [
[
[
"# Support Vector Regression with MinMaxScaler\n### Required Packages",
"_____no_output_____"
]
],
[
[
"import warnings \nimport numpy as np\nimport pandas as pd \nimport seaborn as se \nimport matplotlib.pyplot as plt \nfrom sklearn.svm import SVR\nfrom sklearn.preprocessing import MinMaxScaler\nfrom sklearn.model_selection import train_test_split \nfrom sklearn.pipeline import make_pipeline\nfrom sklearn.metrics import r2_score, mean_absolute_error, mean_squared_error \nwarnings.filterwarnings('ignore')",
"_____no_output_____"
]
],
[
[
"### Initialization\n\nFilepath of CSV file",
"_____no_output_____"
]
],
[
[
"file_path= \"\"",
"_____no_output_____"
]
],
[
[
"List of features which are required for model training .",
"_____no_output_____"
]
],
[
[
"features = []",
"_____no_output_____"
]
],
[
[
"Target feature for prediction.",
"_____no_output_____"
]
],
[
[
"target=''",
"_____no_output_____"
]
],
[
[
"### Data Fetching\n\nPandas is an open-source, BSD-licensed library providing high-performance, easy-to-use data manipulation and data analysis tools.\n\nWe will use panda's library to read the CSV file using its storage path.And we use the head function to display the initial row or entry.",
"_____no_output_____"
]
],
[
[
"df=pd.read_csv(file_path)\ndf.head()",
"_____no_output_____"
]
],
[
[
"### Feature Selections\n\nIt is the process of reducing the number of input variables when developing a predictive model. Used to reduce the number of input variables to both reduce the computational cost of modelling and, in some cases, to improve the performance of the model.\n\nWe will assign all the required input features to X and target/outcome to Y.",
"_____no_output_____"
]
],
[
[
"X=df[features]\nY=df[target]",
"_____no_output_____"
]
],
[
[
"### Data Preprocessing\n\nSince the majority of the machine learning models in the Sklearn library doesn't handle string category data and Null value, we have to explicitly remove or replace null values. The below snippet have functions, which removes the null value if any exists. And convert the string classes data in the datasets by encoding them to integer classes.\n",
"_____no_output_____"
]
],
[
[
"def NullClearner(df):\n if(isinstance(df, pd.Series) and (df.dtype in [\"float64\",\"int64\"])):\n df.fillna(df.mean(),inplace=True)\n return df\n elif(isinstance(df, pd.Series)):\n df.fillna(df.mode()[0],inplace=True)\n return df\n else:return df\ndef EncodeX(df):\n return pd.get_dummies(df)",
"_____no_output_____"
]
],
[
[
"Calling preprocessing functions on the feature and target set.\n",
"_____no_output_____"
]
],
[
[
"x=X.columns.to_list()\nfor i in x:\n X[i]=NullClearner(X[i])\nX=EncodeX(X)\nY=NullClearner(Y)\nX.head()",
"_____no_output_____"
]
],
[
[
"#### Correlation Map\n\nIn order to check the correlation between the features, we will plot a correlation matrix. It is effective in summarizing a large amount of data where the goal is to see patterns.",
"_____no_output_____"
]
],
[
[
"f,ax = plt.subplots(figsize=(18, 18))\nmatrix = np.triu(X.corr())\nse.heatmap(X.corr(), annot=True, linewidths=.5, fmt= '.1f',ax=ax, mask=matrix)\nplt.show()",
"_____no_output_____"
]
],
[
[
"### Data Splitting\n\nThe train-test split is a procedure for evaluating the performance of an algorithm. The procedure involves taking a dataset and dividing it into two subsets. The first subset is utilized to fit/train the model. The second subset is used for prediction. The main motive is to estimate the performance of the model on new data.",
"_____no_output_____"
]
],
[
[
"x_train,x_test,y_train,y_test=train_test_split(X,Y,test_size=0.2,random_state=123)",
"_____no_output_____"
]
],
[
[
"### Model\nSupport vector machines (SVMs) are a set of supervised learning methods used for classification, regression and outliers detection.\n\nA Support Vector Machine is a discriminative classifier formally defined by a separating hyperplane. In other terms, for a given known/labelled data points, the SVM outputs an appropriate hyperplane that classifies the inputted new cases based on the hyperplane. In 2-Dimensional space, this hyperplane is a line separating a plane into two segments where each class or group occupied on either side.\n\nHere we will use SVR, the svr implementation is based on libsvm. The fit time scales at least quadratically with the number of samples and maybe impractical beyond tens of thousands of samples. \n\n#### Model Tuning Parameters\n\n 1. C : float, default=1.0\n> Regularization parameter. The strength of the regularization is inversely proportional to C. Must be strictly positive. The penalty is a squared l2 penalty.\n\n 2. kernel : {‘linear’, ‘poly’, ‘rbf’, ‘sigmoid’, ‘precomputed’}, default=’rbf’\n> Specifies the kernel type to be used in the algorithm. It must be one of ‘linear’, ‘poly’, ‘rbf’, ‘sigmoid’, ‘precomputed’ or a callable. If none is given, ‘rbf’ will be used. If a callable is given it is used to pre-compute the kernel matrix from data matrices; that matrix should be an array of shape (n_samples, n_samples).\n\n 3. gamma : {‘scale’, ‘auto’} or float, default=’scale’\n> Gamma is a hyperparameter that we have to set before the training model. Gamma decides how much curvature we want in a decision boundary.\n\n 4. degree : int, default=3\n> Degree of the polynomial kernel function (‘poly’). Ignored by all other kernels.Using degree 1 is similar to using a linear kernel. Also, increasing degree parameter leads to higher training times.\n\n\n#### Data Scaling\nMinMaxScaler transforms features by scaling each feature to a given range.\n\nThis estimator scales and translates each feature individually such that it is in the given range on the training set, e.g. between zero and one.\n\nThis transformation is often used as an alternative to zero mean, unit variance scaling.\n\n##### For more information on MinMaxScaler [ click here](https://scikit-learn.org/stable/modules/generated/sklearn.preprocessing.MinMaxScaler.html)",
"_____no_output_____"
]
],
[
[
"model=make_pipeline(MinMaxScaler(),SVR())\nmodel.fit(x_train,y_train)",
"_____no_output_____"
]
],
[
[
"#### Model Accuracy\n\nWe will use the trained model to make a prediction on the test set.Then use the predicted value for measuring the accuracy of our model.\n\n> **score**: The **score** function returns the coefficient of determination <code>R<sup>2</sup></code> of the prediction.",
"_____no_output_____"
]
],
[
[
"print(\"Accuracy score {:.2f} %\\n\".format(model.score(x_test,y_test)*100))",
"Accuracy score 42.32 %\n\n"
]
],
[
[
"> **r2_score**: The **r2_score** function computes the percentage variablility explained by our model, either the fraction or the count of correct predictions. \n\n> **mae**: The **mean abosolute error** function calculates the amount of total error(absolute average distance between the real data and the predicted data) by our model. \n\n> **mse**: The **mean squared error** function squares the error(penalizes the model for large errors) by our model. ",
"_____no_output_____"
]
],
[
[
"y_pred=model.predict(x_test)\nprint(\"R2 Score: {:.2f} %\".format(r2_score(y_test,y_pred)*100))\nprint(\"Mean Absolute Error {:.2f}\".format(mean_absolute_error(y_test,y_pred)))\nprint(\"Mean Squared Error {:.2f}\".format(mean_squared_error(y_test,y_pred)))",
"R2 Score: 42.32 %\nMean Absolute Error 0.48\nMean Squared Error 0.38\n"
]
],
[
[
"#### Prediction Plot\n\nFirst, we make use of a plot to plot the actual observations, with x_train on the x-axis and y_train on the y-axis.\nFor the regression line, we will use x_train on the x-axis and then the predictions of the x_train observations on the y-axis.",
"_____no_output_____"
]
],
[
[
"plt.figure(figsize=(14,10))\nplt.plot(range(20),y_test[0:20], color = \"green\")\nplt.plot(range(20),model.predict(x_test[0:20]), color = \"red\")\nplt.legend([\"Actual\",\"prediction\"]) \nplt.title(\"Predicted vs True Value\")\nplt.xlabel(\"Record number\")\nplt.ylabel(target)\nplt.show()",
"_____no_output_____"
]
],
[
[
"#### Creator: Saharsh Laud , Github: [Profile](https://github.com/SaharshLaud)\n",
"_____no_output_____"
]
]
] | [
"markdown",
"code",
"markdown",
"code",
"markdown",
"code",
"markdown",
"code",
"markdown",
"code",
"markdown",
"code",
"markdown",
"code",
"markdown",
"code",
"markdown",
"code",
"markdown",
"code",
"markdown",
"code",
"markdown",
"code",
"markdown",
"code",
"markdown",
"code",
"markdown"
] | [
[
"markdown"
],
[
"code"
],
[
"markdown"
],
[
"code"
],
[
"markdown"
],
[
"code"
],
[
"markdown"
],
[
"code"
],
[
"markdown"
],
[
"code"
],
[
"markdown"
],
[
"code"
],
[
"markdown"
],
[
"code"
],
[
"markdown"
],
[
"code"
],
[
"markdown"
],
[
"code"
],
[
"markdown"
],
[
"code"
],
[
"markdown"
],
[
"code"
],
[
"markdown"
],
[
"code"
],
[
"markdown"
],
[
"code"
],
[
"markdown"
],
[
"code"
],
[
"markdown"
]
] |
e780e8e94db52d5f25db32cb1c8817e36626ca66 | 103,006 | ipynb | Jupyter Notebook | ai-platform-unified/notebooks/unofficial/gapic/custom/showcase_custom_image_classification_online_ab_testing.ipynb | rastringer/ai-platform-samples | ce9ed78136699d7c9c4ee5d9cc96b56ae011024f | [
"Apache-2.0"
] | 1 | 2021-06-30T17:41:23.000Z | 2021-06-30T17:41:23.000Z | ai-platform-unified/notebooks/unofficial/gapic/custom/showcase_custom_image_classification_online_ab_testing.ipynb | bdoohan-goog/ai-platform-samples | 4022651010de466a3e966c7ca34bbaeb89619460 | [
"Apache-2.0"
] | null | null | null | ai-platform-unified/notebooks/unofficial/gapic/custom/showcase_custom_image_classification_online_ab_testing.ipynb | bdoohan-goog/ai-platform-samples | 4022651010de466a3e966c7ca34bbaeb89619460 | [
"Apache-2.0"
] | null | null | null | 42.11202 | 519 | 0.560628 | [
[
[
"# Copyright 2020 Google LLC\n#\n# Licensed under the Apache License, Version 2.0 (the \"License\");\n# you may not use this file except in compliance with the License.\n# You may obtain a copy of the License at\n#\n# https://www.apache.org/licenses/LICENSE-2.0\n#\n# Unless required by applicable law or agreed to in writing, software\n# distributed under the License is distributed on an \"AS IS\" BASIS,\n# WITHOUT WARRANTIES OR CONDITIONS OF ANY KIND, either express or implied.\n# See the License for the specific language governing permissions and\n# limitations under the License.",
"_____no_output_____"
]
],
[
[
"# Vertex AI client library: Custom training image classification model for online prediction for A/B testing\n\n<table align=\"left\">\n <td>\n <a href=\"https://colab.research.google.com/github/GoogleCloudPlatform/ai-platform-samples/blob/master/ai-platform-unified/notebooks/community/gapic/custom/showcase_custom_image_classification_online_ab_testing.ipynb\">\n <img src=\"https://cloud.google.com/ml-engine/images/colab-logo-32px.png\" alt=\"Colab logo\"> Run in Colab\n </a>\n </td>\n <td>\n <a href=\"https://github.com/GoogleCloudPlatform/ai-platform-samples/blob/master/ai-platform-unified/notebooks/community/gapic/custom/showcase_custom_image_classification_online_ab_testing.ipynb\">\n <img src=\"https://cloud.google.com/ml-engine/images/github-logo-32px.png\" alt=\"GitHub logo\">\n View on GitHub\n </a>\n </td>\n</table>\n<br/><br/><br/>",
"_____no_output_____"
],
[
"## Overview\n\nThis tutorial demonstrates how to use the Vertex AI Python client library to train and deploy a custom image classification model for A/B testing for online prediction.",
"_____no_output_____"
],
[
"### Dataset\n\nThe dataset used for this tutorial is the [CIFAR10 dataset](https://www.tensorflow.org/datasets/catalog/cifar10) from [TensorFlow Datasets](https://www.tensorflow.org/datasets/catalog/overview). The version of the dataset you will use is built into TensorFlow. The trained model predicts which type of class an image is from ten classes: airplane, automobile, bird, cat, deer, dog, frog, horse, ship, truck.",
"_____no_output_____"
],
[
"### Objective\n\nIn this tutorial, you learn how to create multiple instances of a custom model from a Python script in a Docker container using the Vertex AI client library and then deploy for A/B testing\nof online predictions. You can alternatively create custom models from the command line using gcloud or online using Google Cloud Console.\n\nThe steps performed include:\n\n- Create an Vertex AI custom job for training a model.\n- Train two instances (A and B) of the TensorFlow model.\n- Retrieve and load the models artifacts.\n- View the models evaluation.\n- Upload each model instance as a Vertex AI `Model` resource.\n- Deploy the model instances to the same serving `Endpoint` resource.\n- Make a prediction.\n- Review the results from the two model instances.\n- Undeploy the `Model` resources.",
"_____no_output_____"
],
[
"### Costs\n\nThis tutorial uses billable components of Google Cloud (GCP):\n\n* Vertex AI\n* Cloud Storage\n\nLearn about [Vertex AI\npricing](https://cloud.google.com/ai-platform-unified/pricing) and [Cloud Storage\npricing](https://cloud.google.com/storage/pricing), and use the [Pricing\nCalculator](https://cloud.google.com/products/calculator/)\nto generate a cost estimate based on your projected usage.",
"_____no_output_____"
],
[
"## Installation\n\nInstall the latest version of Vertex AI client library.",
"_____no_output_____"
]
],
[
[
"import sys\n\nif \"google.colab\" in sys.modules:\n USER_FLAG = \"\"\nelse:\n USER_FLAG = \"--user\"\n\n! pip3 install -U google-cloud-aiplatform $USER_FLAG",
"_____no_output_____"
]
],
[
[
"Install the latest GA version of *google-cloud-storage* library as well.",
"_____no_output_____"
]
],
[
[
"! pip3 install -U google-cloud-storage $USER_FLAG",
"_____no_output_____"
]
],
[
[
"### Restart the kernel\n\nOnce you've installed the Vertex AI client library and Google *cloud-storage*, you need to restart the notebook kernel so it can find the packages.",
"_____no_output_____"
]
],
[
[
"import os\n\nif not os.getenv(\"IS_TESTING\"):\n # Automatically restart kernel after installs\n import IPython\n\n app = IPython.Application.instance()\n app.kernel.do_shutdown(True)",
"_____no_output_____"
]
],
[
[
"## Before you begin\n\n### GPU runtime\n\n*Make sure you're running this notebook in a GPU runtime if you have that option. In Colab, select* **Runtime > Change Runtime Type > GPU**\n\n### Set up your Google Cloud project\n\n**The following steps are required, regardless of your notebook environment.**\n\n1. [Select or create a Google Cloud project](https://console.cloud.google.com/cloud-resource-manager). When you first create an account, you get a $300 free credit towards your compute/storage costs.\n\n2. [Make sure that billing is enabled for your project.](https://cloud.google.com/billing/docs/how-to/modify-project)\n\n3. [Enable the Vertex AI APIs and Compute Engine APIs.](https://console.cloud.google.com/flows/enableapi?apiid=ml.googleapis.com,compute_component)\n\n4. [The Google Cloud SDK](https://cloud.google.com/sdk) is already installed in Vertex AI Notebooks.\n\n5. Enter your project ID in the cell below. Then run the cell to make sure the\nCloud SDK uses the right project for all the commands in this notebook.\n\n**Note**: Jupyter runs lines prefixed with `!` as shell commands, and it interpolates Python variables prefixed with `$` into these commands.",
"_____no_output_____"
]
],
[
[
"PROJECT_ID = \"[your-project-id]\" # @param {type:\"string\"}",
"_____no_output_____"
],
[
"if PROJECT_ID == \"\" or PROJECT_ID is None or PROJECT_ID == \"[your-project-id]\":\n # Get your GCP project id from gcloud\n shell_output = !gcloud config list --format 'value(core.project)' 2>/dev/null\n PROJECT_ID = shell_output[0]\n print(\"Project ID:\", PROJECT_ID)",
"_____no_output_____"
],
[
"! gcloud config set project $PROJECT_ID",
"_____no_output_____"
]
],
[
[
"#### Region\n\nYou can also change the `REGION` variable, which is used for operations\nthroughout the rest of this notebook. Below are regions supported for Vertex AI. We recommend that you choose the region closest to you.\n\n- Americas: `us-central1`\n- Europe: `europe-west4`\n- Asia Pacific: `asia-east1`\n\nYou may not use a multi-regional bucket for training with Vertex AI. Not all regions provide support for all Vertex AI services. For the latest support per region, see the [Vertex AI locations documentation](https://cloud.google.com/ai-platform-unified/docs/general/locations)",
"_____no_output_____"
]
],
[
[
"REGION = \"us-central1\" # @param {type: \"string\"}",
"_____no_output_____"
]
],
[
[
"#### Timestamp\n\nIf you are in a live tutorial session, you might be using a shared test account or project. To avoid name collisions between users on resources created, you create a timestamp for each instance session, and append onto the name of resources which will be created in this tutorial.",
"_____no_output_____"
]
],
[
[
"from datetime import datetime\n\nTIMESTAMP = datetime.now().strftime(\"%Y%m%d%H%M%S\")",
"_____no_output_____"
]
],
[
[
"### Authenticate your Google Cloud account\n\n**If you are using Vertex AI Notebooks**, your environment is already authenticated. Skip this step.\n\n**If you are using Colab**, run the cell below and follow the instructions when prompted to authenticate your account via oAuth.\n\n**Otherwise**, follow these steps:\n\nIn the Cloud Console, go to the [Create service account key](https://console.cloud.google.com/apis/credentials/serviceaccountkey) page.\n\n**Click Create service account**.\n\nIn the **Service account name** field, enter a name, and click **Create**.\n\nIn the **Grant this service account access to project** section, click the Role drop-down list. Type \"Vertex AI\" into the filter box, and select **Vertex AI Administrator**. Type \"Storage Object Admin\" into the filter box, and select **Storage Object Admin**.\n\nClick Create. A JSON file that contains your key downloads to your local environment.\n\nEnter the path to your service account key as the GOOGLE_APPLICATION_CREDENTIALS variable in the cell below and run the cell.",
"_____no_output_____"
]
],
[
[
"import os\nimport sys\n\n# If you are running this notebook in Colab, run this cell and follow the\n# instructions to authenticate your GCP account. This provides access to your\n# Cloud Storage bucket and lets you submit training jobs and prediction\n# requests.\n\n# If on AI Platform, then don't execute this code\nif not os.path.exists(\"/opt/deeplearning/metadata/env_version\"):\n if \"google.colab\" in sys.modules:\n from google.colab import auth as google_auth\n\n google_auth.authenticate_user()\n\n # If you are running this notebook locally, replace the string below with the\n # path to your service account key and run this cell to authenticate your GCP\n # account.\n elif not os.getenv(\"IS_TESTING\"):\n %env GOOGLE_APPLICATION_CREDENTIALS ''",
"_____no_output_____"
]
],
[
[
"### Create a Cloud Storage bucket\n\n**The following steps are required, regardless of your notebook environment.**\n\nWhen you submit a custom training job using the Vertex AI client library, you upload a Python package\ncontaining your training code to a Cloud Storage bucket. Vertex AI runs\nthe code from this package. In this tutorial, Vertex AI also saves the\ntrained model that results from your job in the same bucket. You can then\ncreate an `Endpoint` resource based on this output in order to serve\nonline predictions.\n\nSet the name of your Cloud Storage bucket below. Bucket names must be globally unique across all Google Cloud projects, including those outside of your organization.",
"_____no_output_____"
]
],
[
[
"BUCKET_NAME = \"gs://[your-bucket-name]\" # @param {type:\"string\"}",
"_____no_output_____"
],
[
"if BUCKET_NAME == \"\" or BUCKET_NAME is None or BUCKET_NAME == \"gs://[your-bucket-name]\":\n BUCKET_NAME = \"gs://\" + PROJECT_ID + \"aip-\" + TIMESTAMP",
"_____no_output_____"
]
],
[
[
"**Only if your bucket doesn't already exist**: Run the following cell to create your Cloud Storage bucket.",
"_____no_output_____"
]
],
[
[
"! gsutil mb -l $REGION $BUCKET_NAME",
"_____no_output_____"
]
],
[
[
"Finally, validate access to your Cloud Storage bucket by examining its contents:",
"_____no_output_____"
]
],
[
[
"! gsutil ls -al $BUCKET_NAME",
"_____no_output_____"
]
],
[
[
"### Set up variables\n\nNext, set up some variables used throughout the tutorial.\n### Import libraries and define constants",
"_____no_output_____"
],
[
"#### Import Vertex AI client library\n\nImport the Vertex AI client library into our Python environment.",
"_____no_output_____"
]
],
[
[
"import os\nimport sys\nimport time\n\nimport google.cloud.aiplatform_v1 as aip\nfrom google.protobuf import json_format\nfrom google.protobuf.json_format import MessageToJson, ParseDict\nfrom google.protobuf.struct_pb2 import Struct, Value",
"_____no_output_____"
]
],
[
[
"#### Vertex AI constants\n\nSetup up the following constants for Vertex AI:\n\n- `API_ENDPOINT`: The Vertex AI API service endpoint for dataset, model, job, pipeline and endpoint services.\n- `PARENT`: The Vertex AI location root path for dataset, model, job, pipeline and endpoint resources.",
"_____no_output_____"
]
],
[
[
"# API service endpoint\nAPI_ENDPOINT = \"{}-aiplatform.googleapis.com\".format(REGION)\n\n# Vertex AI location root path for your dataset, model and endpoint resources\nPARENT = \"projects/\" + PROJECT_ID + \"/locations/\" + REGION",
"_____no_output_____"
]
],
[
[
"#### Hardware Accelerators\n\nSet the hardware accelerators (e.g., GPU), if any, for training and prediction.\n\nSet the variables `TRAIN_GPU/TRAIN_NGPU` and `DEPLOY_GPU/DEPLOY_NGPU` to use a container image supporting a GPU and the number of GPUs allocated to the virtual machine (VM) instance. For example, to use a GPU container image with 4 Nvidia Telsa K80 GPUs allocated to each VM, you would specify:\n\n (aip.AcceleratorType.NVIDIA_TESLA_K80, 4)\n\nFor GPU, available accelerators include:\n - aip.AcceleratorType.NVIDIA_TESLA_K80\n - aip.AcceleratorType.NVIDIA_TESLA_P100\n - aip.AcceleratorType.NVIDIA_TESLA_P4\n - aip.AcceleratorType.NVIDIA_TESLA_T4\n - aip.AcceleratorType.NVIDIA_TESLA_V100\n\n\nOtherwise specify `(None, None)` to use a container image to run on a CPU.\n\n*Note*: TF releases before 2.3 for GPU support will fail to load the custom model in this tutorial. It is a known issue and fixed in TF 2.3 -- which is caused by static graph ops that are generated in the serving function. If you encounter this issue on your own custom models, use a container image for TF 2.3 with GPU support.",
"_____no_output_____"
]
],
[
[
"if os.getenv(\"IS_TESTING_TRAIN_GPU\"):\n TRAIN_GPU, TRAIN_NGPU = (\n aip.AcceleratorType.NVIDIA_TESLA_K80,\n int(os.getenv(\"IS_TESTING_TRAIN_GPU\")),\n )\nelse:\n TRAIN_GPU, TRAIN_NGPU = (aip.AcceleratorType.NVIDIA_TESLA_K80, 1)\n\nif os.getenv(\"IS_TESTING_DEPOLY_GPU\"):\n DEPLOY_GPU, DEPLOY_NGPU = (\n aip.AcceleratorType.NVIDIA_TESLA_K80,\n int(os.getenv(\"IS_TESTING_DEPOLY_GPU\")),\n )\nelse:\n DEPLOY_GPU, DEPLOY_NGPU = (None, None)",
"_____no_output_____"
]
],
[
[
"#### Container (Docker) image\n\nNext, we will set the Docker container images for training and prediction\n\n - TensorFlow 1.15\n - `gcr.io/cloud-aiplatform/training/tf-cpu.1-15:latest`\n - `gcr.io/cloud-aiplatform/training/tf-gpu.1-15:latest`\n - TensorFlow 2.1\n - `gcr.io/cloud-aiplatform/training/tf-cpu.2-1:latest`\n - `gcr.io/cloud-aiplatform/training/tf-gpu.2-1:latest`\n - TensorFlow 2.2\n - `gcr.io/cloud-aiplatform/training/tf-cpu.2-2:latest`\n - `gcr.io/cloud-aiplatform/training/tf-gpu.2-2:latest`\n - TensorFlow 2.3\n - `gcr.io/cloud-aiplatform/training/tf-cpu.2-3:latest`\n - `gcr.io/cloud-aiplatform/training/tf-gpu.2-3:latest`\n - TensorFlow 2.4\n - `gcr.io/cloud-aiplatform/training/tf-cpu.2-4:latest`\n - `gcr.io/cloud-aiplatform/training/tf-gpu.2-4:latest`\n - XGBoost\n - `gcr.io/cloud-aiplatform/training/xgboost-cpu.1-1`\n - Scikit-learn\n - `gcr.io/cloud-aiplatform/training/scikit-learn-cpu.0-23:latest`\n - Pytorch\n - `gcr.io/cloud-aiplatform/training/pytorch-cpu.1-4:latest`\n - `gcr.io/cloud-aiplatform/training/pytorch-cpu.1-5:latest`\n - `gcr.io/cloud-aiplatform/training/pytorch-cpu.1-6:latest`\n - `gcr.io/cloud-aiplatform/training/pytorch-cpu.1-7:latest`\n\nFor the latest list, see [Pre-built containers for training](https://cloud.google.com/ai-platform-unified/docs/training/pre-built-containers).\n\n - TensorFlow 1.15\n - `gcr.io/cloud-aiplatform/prediction/tf-cpu.1-15:latest`\n - `gcr.io/cloud-aiplatform/prediction/tf-gpu.1-15:latest`\n - TensorFlow 2.1\n - `gcr.io/cloud-aiplatform/prediction/tf2-cpu.2-1:latest`\n - `gcr.io/cloud-aiplatform/prediction/tf2-gpu.2-1:latest`\n - TensorFlow 2.2\n - `gcr.io/cloud-aiplatform/prediction/tf2-cpu.2-2:latest`\n - `gcr.io/cloud-aiplatform/prediction/tf2-gpu.2-2:latest`\n - TensorFlow 2.3\n - `gcr.io/cloud-aiplatform/prediction/tf2-cpu.2-3:latest`\n - `gcr.io/cloud-aiplatform/prediction/tf2-gpu.2-3:latest`\n - XGBoost\n - `gcr.io/cloud-aiplatform/prediction/xgboost-cpu.1-2:latest`\n - `gcr.io/cloud-aiplatform/prediction/xgboost-cpu.1-1:latest`\n - `gcr.io/cloud-aiplatform/prediction/xgboost-cpu.0-90:latest`\n - `gcr.io/cloud-aiplatform/prediction/xgboost-cpu.0-82:latest`\n - Scikit-learn\n - `gcr.io/cloud-aiplatform/prediction/sklearn-cpu.0-23:latest`\n - `gcr.io/cloud-aiplatform/prediction/sklearn-cpu.0-22:latest`\n - `gcr.io/cloud-aiplatform/prediction/sklearn-cpu.0-20:latest`\n\nFor the latest list, see [Pre-built containers for prediction](https://cloud.google.com/ai-platform-unified/docs/predictions/pre-built-containers)",
"_____no_output_____"
]
],
[
[
"if os.getenv(\"IS_TESTING_TF\"):\n TF = os.getenv(\"IS_TESTING_TF\")\nelse:\n TF = \"2-1\"\n\nif TF[0] == \"2\":\n if TRAIN_GPU:\n TRAIN_VERSION = \"tf-gpu.{}\".format(TF)\n else:\n TRAIN_VERSION = \"tf-cpu.{}\".format(TF)\n if DEPLOY_GPU:\n DEPLOY_VERSION = \"tf2-gpu.{}\".format(TF)\n else:\n DEPLOY_VERSION = \"tf2-cpu.{}\".format(TF)\nelse:\n if TRAIN_GPU:\n TRAIN_VERSION = \"tf-gpu.{}\".format(TF)\n else:\n TRAIN_VERSION = \"tf-cpu.{}\".format(TF)\n if DEPLOY_GPU:\n DEPLOY_VERSION = \"tf-gpu.{}\".format(TF)\n else:\n DEPLOY_VERSION = \"tf-cpu.{}\".format(TF)\n\nTRAIN_IMAGE = \"gcr.io/cloud-aiplatform/training/{}:latest\".format(TRAIN_VERSION)\nDEPLOY_IMAGE = \"gcr.io/cloud-aiplatform/prediction/{}:latest\".format(DEPLOY_VERSION)\n\nprint(\"Training:\", TRAIN_IMAGE, TRAIN_GPU, TRAIN_NGPU)\nprint(\"Deployment:\", DEPLOY_IMAGE, DEPLOY_GPU, DEPLOY_NGPU)",
"_____no_output_____"
]
],
[
[
"#### Machine Type\n\nNext, set the machine type to use for training and prediction.\n\n- Set the variables `TRAIN_COMPUTE` and `DEPLOY_COMPUTE` to configure the compute resources for the VMs you will use for for training and prediction.\n - `machine type`\n - `n1-standard`: 3.75GB of memory per vCPU.\n - `n1-highmem`: 6.5GB of memory per vCPU\n - `n1-highcpu`: 0.9 GB of memory per vCPU\n - `vCPUs`: number of \\[2, 4, 8, 16, 32, 64, 96 \\]\n\n*Note: The following is not supported for training:*\n\n - `standard`: 2 vCPUs\n - `highcpu`: 2, 4 and 8 vCPUs\n\n*Note: You may also use n2 and e2 machine types for training and deployment, but they do not support GPUs*.",
"_____no_output_____"
]
],
[
[
"if os.getenv(\"IS_TESTING_TRAIN_MACHINE\"):\n MACHINE_TYPE = os.getenv(\"IS_TESTING_TRAIN_MACHINE\")\nelse:\n MACHINE_TYPE = \"n1-standard\"\n\nVCPU = \"4\"\nTRAIN_COMPUTE = MACHINE_TYPE + \"-\" + VCPU\nprint(\"Train machine type\", TRAIN_COMPUTE)\n\nif os.getenv(\"IS_TESTING_DEPLOY_MACHINE\"):\n MACHINE_TYPE = os.getenv(\"IS_TESTING_DEPLOY_MACHINE\")\nelse:\n MACHINE_TYPE = \"n1-standard\"\n\nVCPU = \"4\"\nDEPLOY_COMPUTE = MACHINE_TYPE + \"-\" + VCPU\nprint(\"Deploy machine type\", DEPLOY_COMPUTE)",
"_____no_output_____"
]
],
[
[
"# Tutorial\n\nNow you are ready to start creating your own custom model and training for CIFAR10.",
"_____no_output_____"
],
[
"## Set up clients\n\nThe Vertex AI client library works as a client/server model. On your side (the Python script) you will create a client that sends requests and receives responses from the Vertex AI server.\n\nYou will use different clients in this tutorial for different steps in the workflow. So set them all up upfront.\n\n- Model Service for `Model` resources.\n- Endpoint Service for deployment.\n- Job Service for batch jobs and custom training.\n- Prediction Service for serving.",
"_____no_output_____"
]
],
[
[
"# client options same for all services\nclient_options = {\"api_endpoint\": API_ENDPOINT}\n\n\ndef create_job_client():\n client = aip.JobServiceClient(client_options=client_options)\n return client\n\n\ndef create_model_client():\n client = aip.ModelServiceClient(client_options=client_options)\n return client\n\n\ndef create_endpoint_client():\n client = aip.EndpointServiceClient(client_options=client_options)\n return client\n\n\ndef create_prediction_client():\n client = aip.PredictionServiceClient(client_options=client_options)\n return client\n\n\nclients = {}\nclients[\"job\"] = create_job_client()\nclients[\"model\"] = create_model_client()\nclients[\"endpoint\"] = create_endpoint_client()\nclients[\"prediction\"] = create_prediction_client()\n\nfor client in clients.items():\n print(client)",
"_____no_output_____"
]
],
[
[
"## Train a model\n\nThere are two ways you can train a custom model using a container image:\n\n- **Use a Google Cloud prebuilt container**. If you use a prebuilt container, you will additionally specify a Python package to install into the container image. This Python package contains your code for training a custom model.\n\n- **Use your own custom container image**. If you use your own container, the container needs to contain your code for training a custom model.",
"_____no_output_____"
],
[
"## Prepare your custom job specification\n\nNow that your clients are ready, your first step is to create a Job Specification for your custom training job. The job specification will consist of the following:\n\n- `worker_pool_spec` : The specification of the type of machine(s) you will use for training and how many (single or distributed)\n- `python_package_spec` : The specification of the Python package to be installed with the pre-built container.",
"_____no_output_____"
],
[
"### Prepare your machine specification\n\nNow define the machine specification for your custom training job. This tells Vertex AI what type of machine instance to provision for the training.\n - `machine_type`: The type of GCP instance to provision -- e.g., n1-standard-8.\n - `accelerator_type`: The type, if any, of hardware accelerator. In this tutorial if you previously set the variable `TRAIN_GPU != None`, you are using a GPU; otherwise you will use a CPU.\n - `accelerator_count`: The number of accelerators.",
"_____no_output_____"
]
],
[
[
"if TRAIN_GPU:\n machine_spec = {\n \"machine_type\": TRAIN_COMPUTE,\n \"accelerator_type\": TRAIN_GPU,\n \"accelerator_count\": TRAIN_NGPU,\n }\nelse:\n machine_spec = {\"machine_type\": TRAIN_COMPUTE, \"accelerator_count\": 0}",
"_____no_output_____"
]
],
[
[
"### Prepare your disk specification\n\n(optional) Now define the disk specification for your custom training job. This tells Vertex AI what type and size of disk to provision in each machine instance for the training.\n\n - `boot_disk_type`: Either SSD or Standard. SSD is faster, and Standard is less expensive. Defaults to SSD.\n - `boot_disk_size_gb`: Size of disk in GB.",
"_____no_output_____"
]
],
[
[
"DISK_TYPE = \"pd-ssd\" # [ pd-ssd, pd-standard]\nDISK_SIZE = 200 # GB\n\ndisk_spec = {\"boot_disk_type\": DISK_TYPE, \"boot_disk_size_gb\": DISK_SIZE}",
"_____no_output_____"
]
],
[
[
"### Examine the training package\n\n#### Package layout\n\nBefore you start the training, you will look at how a Python package is assembled for a custom training job. When unarchived, the package contains the following directory/file layout.\n\n- PKG-INFO\n- README.md\n- setup.cfg\n- setup.py\n- trainer\n - \\_\\_init\\_\\_.py\n - task.py\n\nThe files `setup.cfg` and `setup.py` are the instructions for installing the package into the operating environment of the Docker image.\n\nThe file `trainer/task.py` is the Python script for executing the custom training job. *Note*, when we referred to it in the worker pool specification, we replace the directory slash with a dot (`trainer.task`) and dropped the file suffix (`.py`).\n\n#### Package Assembly\n\nIn the following cells, you will assemble the training package.",
"_____no_output_____"
]
],
[
[
"# Make folder for Python training script\n! rm -rf custom\n! mkdir custom\n\n# Add package information\n! touch custom/README.md\n\nsetup_cfg = \"[egg_info]\\n\\ntag_build =\\n\\ntag_date = 0\"\n! echo \"$setup_cfg\" > custom/setup.cfg\n\nsetup_py = \"import setuptools\\n\\nsetuptools.setup(\\n\\n install_requires=[\\n\\n 'tensorflow_datasets==1.3.0',\\n\\n ],\\n\\n packages=setuptools.find_packages())\"\n! echo \"$setup_py\" > custom/setup.py\n\npkg_info = \"Metadata-Version: 1.0\\n\\nName: CIFAR10 image classification\\n\\nVersion: 0.0.0\\n\\nSummary: Demostration training script\\n\\nHome-page: www.google.com\\n\\nAuthor: Google\\n\\nAuthor-email: [email protected]\\n\\nLicense: Public\\n\\nDescription: Demo\\n\\nPlatform: Vertex AI\"\n! echo \"$pkg_info\" > custom/PKG-INFO\n\n# Make the training subfolder\n! mkdir custom/trainer\n! touch custom/trainer/__init__.py",
"_____no_output_____"
]
],
[
[
"#### Task.py contents\n\nIn the next cell, you write the contents of the training script task.py. We won't go into detail, it's just there for you to browse. In summary:\n\n- Get the directory where to save the model artifacts from the command line (`--model_dir`), and if not specified, then from the environment variable `AIP_MODEL_DIR`.\n- Loads CIFAR10 dataset from TF Datasets (tfds).\n- Builds a model using TF.Keras model API.\n- Compiles the model (`compile()`).\n- Sets a training distribution strategy according to the argument `args.distribute`.\n- Trains the model (`fit()`) with epochs and steps according to the arguments `args.epochs` and `args.steps`\n- Saves the trained model (`save(args.model_dir)`) to the specified model directory.",
"_____no_output_____"
]
],
[
[
"%%writefile custom/trainer/task.py\n# Single, Mirror and Multi-Machine Distributed Training for CIFAR-10\n\nimport tensorflow_datasets as tfds\nimport tensorflow as tf\nfrom tensorflow.python.client import device_lib\nimport argparse\nimport os\nimport sys\ntfds.disable_progress_bar()\n\nparser = argparse.ArgumentParser()\nparser.add_argument('--model-dir', dest='model_dir',\n default=os.getenv(\"AIP_MODEL_DIR\"), type=str, help='Model dir.')\nparser.add_argument('--lr', dest='lr',\n default=0.01, type=float,\n help='Learning rate.')\nparser.add_argument('--epochs', dest='epochs',\n default=10, type=int,\n help='Number of epochs.')\nparser.add_argument('--steps', dest='steps',\n default=200, type=int,\n help='Number of steps per epoch.')\nparser.add_argument('--distribute', dest='distribute', type=str, default='single',\n help='distributed training strategy')\nargs = parser.parse_args()\n\nprint('Python Version = {}'.format(sys.version))\nprint('TensorFlow Version = {}'.format(tf.__version__))\nprint('TF_CONFIG = {}'.format(os.environ.get('TF_CONFIG', 'Not found')))\nprint('DEVICES', device_lib.list_local_devices())\n\n# Single Machine, single compute device\nif args.distribute == 'single':\n if tf.test.is_gpu_available():\n strategy = tf.distribute.OneDeviceStrategy(device=\"/gpu:0\")\n else:\n strategy = tf.distribute.OneDeviceStrategy(device=\"/cpu:0\")\n# Single Machine, multiple compute device\nelif args.distribute == 'mirror':\n strategy = tf.distribute.MirroredStrategy()\n# Multiple Machine, multiple compute device\nelif args.distribute == 'multi':\n strategy = tf.distribute.experimental.MultiWorkerMirroredStrategy()\n\n# Multi-worker configuration\nprint('num_replicas_in_sync = {}'.format(strategy.num_replicas_in_sync))\n\n# Preparing dataset\nBUFFER_SIZE = 10000\nBATCH_SIZE = 64\n\ndef make_datasets_unbatched():\n # Scaling CIFAR10 data from (0, 255] to (0., 1.]\n def scale(image, label):\n image = tf.cast(image, tf.float32)\n image /= 255.0\n return image, label\n\n datasets, info = tfds.load(name='cifar10',\n with_info=True,\n as_supervised=True)\n return datasets['train'].map(scale).cache().shuffle(BUFFER_SIZE).repeat()\n\n\n# Build the Keras model\ndef build_and_compile_cnn_model():\n model = tf.keras.Sequential([\n tf.keras.layers.Conv2D(32, 3, activation='relu', input_shape=(32, 32, 3)),\n tf.keras.layers.MaxPooling2D(),\n tf.keras.layers.Conv2D(32, 3, activation='relu'),\n tf.keras.layers.MaxPooling2D(),\n tf.keras.layers.Flatten(),\n tf.keras.layers.Dense(10, activation='softmax')\n ])\n model.compile(\n loss=tf.keras.losses.sparse_categorical_crossentropy,\n optimizer=tf.keras.optimizers.SGD(learning_rate=args.lr),\n metrics=['accuracy'])\n return model\n\n# Train the model\nNUM_WORKERS = strategy.num_replicas_in_sync\n# Here the batch size scales up by number of workers since\n# `tf.data.Dataset.batch` expects the global batch size.\nGLOBAL_BATCH_SIZE = BATCH_SIZE * NUM_WORKERS\ntrain_dataset = make_datasets_unbatched().batch(GLOBAL_BATCH_SIZE)\n\nwith strategy.scope():\n # Creation of dataset, and model building/compiling need to be within\n # `strategy.scope()`.\n model = build_and_compile_cnn_model()\n\nmodel.fit(x=train_dataset, epochs=args.epochs, steps_per_epoch=args.steps)\nmodel.save(args.model_dir)",
"_____no_output_____"
]
],
[
[
"#### Store training script on your Cloud Storage bucket\n\nNext, you package the training folder into a compressed tar ball, and then store it in your Cloud Storage bucket.",
"_____no_output_____"
]
],
[
[
"! rm -f custom.tar custom.tar.gz\n! tar cvf custom.tar custom\n! gzip custom.tar\n! gsutil cp custom.tar.gz $BUCKET_NAME/trainer_cifar10.tar.gz",
"_____no_output_____"
]
],
[
[
"### Define the worker pool specification for Model A\n\nNext, you define the worker pool specification for your custom training job. The worker pool specification will consist of the following:\n\n- `replica_count`: The number of instances to provision of this machine type.\n- `machine_spec`: The hardware specification.\n- `disk_spec` : (optional) The disk storage specification.\n\n- `python_package`: The Python training package to install on the VM instance(s) and which Python module to invoke, along with command line arguments for the Python module.\n\nLet's dive deeper now into the python package specification:\n\n-`executor_image_spec`: This is the docker image which is configured for your custom training job.\n\n-`package_uris`: This is a list of the locations (URIs) of your python training packages to install on the provisioned instance. The locations need to be in a Cloud Storage bucket. These can be either individual python files or a zip (archive) of an entire package. In the later case, the job service will unzip (unarchive) the contents into the docker image.\n\n-`python_module`: The Python module (script) to invoke for running the custom training job. In this example, you will be invoking `trainer.task.py` -- note that it was not neccessary to append the `.py` suffix.\n\n-`args`: The command line arguments to pass to the corresponding Pythom module. In this example, you will be setting:\n - `\"--model-dir=\" + MODEL_DIR` : The Cloud Storage location where to store the model artifacts. There are two ways to tell the training script where to save the model artifacts:\n - direct: You pass the Cloud Storage location as a command line argument to your training script (set variable `DIRECT = True`), or\n - indirect: The service passes the Cloud Storage location as the environment variable `AIP_MODEL_DIR` to your training script (set variable `DIRECT = False`). In this case, you tell the service the model artifact location in the job specification.\n - `\"--epochs=\" + EPOCHS`: The number of epochs for training.\n - `\"--steps=\" + STEPS`: The number of steps (batches) per epoch.\n - `\"--distribute=\" + TRAIN_STRATEGY\"` : The training distribution strategy to use for single or distributed training.\n - `\"single\"`: single device.\n - `\"mirror\"`: all GPU devices on a single compute instance.\n - `\"multi\"`: all GPU devices on all compute instances.",
"_____no_output_____"
]
],
[
[
"JOB_NAME = \"custom_job_A\" + TIMESTAMP\nMODEL_DIR = \"{}/{}\".format(BUCKET_NAME, JOB_NAME)\nMODEL_DIR_A = MODEL_DIR\n\nif not TRAIN_NGPU or TRAIN_NGPU < 2:\n TRAIN_STRATEGY = \"single\"\nelse:\n TRAIN_STRATEGY = \"mirror\"\n\nEPOCHS = 20\nSTEPS = 100\n\nDIRECT = True\nif DIRECT:\n CMDARGS = [\n \"--model-dir=\" + MODEL_DIR,\n \"--epochs=\" + str(EPOCHS),\n \"--steps=\" + str(STEPS),\n \"--distribute=\" + TRAIN_STRATEGY,\n ]\nelse:\n CMDARGS = [\n \"--epochs=\" + str(EPOCHS),\n \"--steps=\" + str(STEPS),\n \"--distribute=\" + TRAIN_STRATEGY,\n ]\n\nworker_pool_spec = [\n {\n \"replica_count\": 1,\n \"machine_spec\": machine_spec,\n \"disk_spec\": disk_spec,\n \"python_package_spec\": {\n \"executor_image_uri\": TRAIN_IMAGE,\n \"package_uris\": [BUCKET_NAME + \"/trainer_cifar10.tar.gz\"],\n \"python_module\": \"trainer.task\",\n \"args\": CMDARGS,\n },\n }\n]",
"_____no_output_____"
]
],
[
[
"### Assemble a job specification\n\nNow assemble the complete description for the custom job specification:\n\n- `display_name`: The human readable name you assign to this custom job.\n- `job_spec`: The specification for the custom job.\n - `worker_pool_specs`: The specification for the machine VM instances.\n - `base_output_directory`: This tells the service the Cloud Storage location where to save the model artifacts (when variable `DIRECT = False`). The service will then pass the location to the training script as the environment variable `AIP_MODEL_DIR`, and the path will be of the form:\n\n <output_uri_prefix>/model",
"_____no_output_____"
]
],
[
[
"if DIRECT:\n job_spec = {\"worker_pool_specs\": worker_pool_spec}\nelse:\n job_spec = {\n \"worker_pool_specs\": worker_pool_spec,\n \"base_output_directory\": {\"output_uri_prefix\": MODEL_DIR},\n }\n\ncustom_job = {\"display_name\": JOB_NAME, \"job_spec\": job_spec}",
"_____no_output_____"
]
],
[
[
"### Train the model\n\n\nNow start the training of your custom training job on Vertex AI. Use this helper function `create_custom_job`, which takes the following parameter:\n\n-`custom_job`: The specification for the custom job.\n\nThe helper function calls job client service's `create_custom_job` method, with the following parameters:\n\n-`parent`: The Vertex AI location path to `Dataset`, `Model` and `Endpoint` resources.\n-`custom_job`: The specification for the custom job.\n\nYou will display a handful of the fields returned in `response` object, with the two that are of most interest are:\n\n`response.name`: The Vertex AI fully qualified identifier assigned to this custom training job. You save this identifier for using in subsequent steps.\n\n`response.state`: The current state of the custom training job.",
"_____no_output_____"
]
],
[
[
"def create_custom_job(custom_job):\n response = clients[\"job\"].create_custom_job(parent=PARENT, custom_job=custom_job)\n print(\"name:\", response.name)\n print(\"display_name:\", response.display_name)\n print(\"state:\", response.state)\n print(\"create_time:\", response.create_time)\n print(\"update_time:\", response.update_time)\n return response\n\n\nresponse = create_custom_job(custom_job)",
"_____no_output_____"
]
],
[
[
"Now get the unique identifier for the custom job you created.",
"_____no_output_____"
]
],
[
[
"# The full unique ID for the custom job\njob_id = response.name\n# The short numeric ID for the custom job\njob_short_id = job_id.split(\"/\")[-1]\n\nprint(job_id)",
"_____no_output_____"
]
],
[
[
"### Get information on a custom job\n\nNext, use this helper function `get_custom_job`, which takes the following parameter:\n\n- `name`: The Vertex AI fully qualified identifier for the custom job.\n\nThe helper function calls the job client service's`get_custom_job` method, with the following parameter:\n\n- `name`: The Vertex AI fully qualified identifier for the custom job.\n\nIf you recall, you got the Vertex AI fully qualified identifier for the custom job in the `response.name` field when you called the `create_custom_job` method, and saved the identifier in the variable `job_id`.",
"_____no_output_____"
]
],
[
[
"def get_custom_job(name, silent=False):\n response = clients[\"job\"].get_custom_job(name=name)\n if silent:\n return response\n\n print(\"name:\", response.name)\n print(\"display_name:\", response.display_name)\n print(\"state:\", response.state)\n print(\"create_time:\", response.create_time)\n print(\"update_time:\", response.update_time)\n return response\n\n\nresponse = get_custom_job(job_id)",
"_____no_output_____"
]
],
[
[
"## Wait for training to complete\n\nTraining the above model may take upwards of 20 minutes time.\n\nOnce your model is done training, you can calculate the actual time it took to train the model by subtracting `end_time` from `start_time`. For your model, we will need to know the location of the saved model, which the Python script saved in your local Cloud Storage bucket at `MODEL_DIR + '/saved_model.pb'`.",
"_____no_output_____"
]
],
[
[
"while True:\n response = get_custom_job(job_id, True)\n if response.state != aip.JobState.JOB_STATE_SUCCEEDED:\n print(\"Training job has not completed:\", response.state)\n model_path_to_deploy_A = None\n if response.state == aip.JobState.JOB_STATE_FAILED:\n break\n else:\n if not DIRECT:\n MODEL_DIR_A = MODEL_DIR_A + \"/model\"\n model_path_to_deploy_A = MODEL_DIR_A\n print(\"Training Time:\", response.update_time - response.create_time)\n break\n time.sleep(60)\n\nprint(\"model_to_deploy:\", model_path_to_deploy_A)",
"_____no_output_____"
]
],
[
[
"### Define the worker pool specification for Model B\n\nNext, you define the worker pool specification for your custom training job. The worker pool specification will consist of the following:\n\n- `replica_count`: The number of instances to provision of this machine type.\n- `machine_spec`: The hardware specification.\n- `disk_spec` : (optional) The disk storage specification.",
"_____no_output_____"
]
],
[
[
"JOB_NAME = \"custom_job_B\" + TIMESTAMP\nMODEL_DIR = \"{}/{}\".format(BUCKET_NAME, JOB_NAME)\nMODEL_DIR_B = MODEL_DIR\n\nif not TRAIN_NGPU or TRAIN_NGPU < 2:\n TRAIN_STRATEGY = \"single\"\nelse:\n TRAIN_STRATEGY = \"mirror\"\n\nEPOCHS = 20\nSTEPS = 100\n\nDIRECT = True\nif DIRECT:\n CMDARGS = [\n \"--model-dir=\" + MODEL_DIR,\n \"--epochs=\" + str(EPOCHS),\n \"--steps=\" + str(STEPS),\n \"--distribute=\" + TRAIN_STRATEGY,\n ]\nelse:\n CMDARGS = [\n \"--epochs=\" + str(EPOCHS),\n \"--steps=\" + str(STEPS),\n \"--distribute=\" + TRAIN_STRATEGY,\n ]\n\nworker_pool_spec = [\n {\n \"replica_count\": 1,\n \"machine_spec\": machine_spec,\n \"disk_spec\": disk_spec,\n \"python_package_spec\": {\n \"executor_image_uri\": TRAIN_IMAGE,\n \"package_uris\": [BUCKET_NAME + \"/trainer_cifar10.tar.gz\"],\n \"python_module\": \"trainer.task\",\n \"args\": CMDARGS,\n },\n }\n]",
"_____no_output_____"
]
],
[
[
"### Assemble a job specification\n\nNow assemble the complete description for the custom job specification:\n\n- `display_name`: The human readable name you assign to this custom job.\n- `job_spec`: The specification for the custom job.\n - `worker_pool_specs`: The specification for the machine VM instances.\n - `base_output_directory`: This tells the service the Cloud Storage location where to save the model artifacts (when variable `DIRECT = False`). The service will then pass the location to the training script as the environment variable `AIP_MODEL_DIR`, and the path will be of the form:\n\n <output_uri_prefix>/model",
"_____no_output_____"
]
],
[
[
"if DIRECT:\n job_spec = {\"worker_pool_specs\": worker_pool_spec}\nelse:\n job_spec = {\n \"worker_pool_specs\": worker_pool_spec,\n \"base_output_directory\": {\"output_uri_prefix\": MODEL_DIR},\n }\n\ncustom_job = {\"display_name\": JOB_NAME, \"job_spec\": job_spec}",
"_____no_output_____"
]
],
[
[
"### Train the model\n\n\nNow start the training of your custom training job on Vertex AI. Use this helper function `create_custom_job`, which takes the following parameter:\n\n-`custom_job`: The specification for the custom job.\n\nThe helper function calls job client service's `create_custom_job` method, with the following parameters:\n\n-`parent`: The Vertex AI location path to `Dataset`, `Model` and `Endpoint` resources.\n-`custom_job`: The specification for the custom job.\n\nYou will display a handful of the fields returned in `response` object, with the two that are of most interest are:\n\n`response.name`: The Vertex AI fully qualified identifier assigned to this custom training job. You save this identifier for using in subsequent steps.\n\n`response.state`: The current state of the custom training job.",
"_____no_output_____"
]
],
[
[
"def create_custom_job(custom_job):\n response = clients[\"job\"].create_custom_job(parent=PARENT, custom_job=custom_job)\n print(\"name:\", response.name)\n print(\"display_name:\", response.display_name)\n print(\"state:\", response.state)\n print(\"create_time:\", response.create_time)\n print(\"update_time:\", response.update_time)\n return response\n\n\nresponse = create_custom_job(custom_job)",
"_____no_output_____"
]
],
[
[
"Now get the unique identifier for the custom job you created.",
"_____no_output_____"
]
],
[
[
"# The full unique ID for the custom job\njob_id = response.name\n# The short numeric ID for the custom job\njob_short_id = job_id.split(\"/\")[-1]\n\nprint(job_id)",
"_____no_output_____"
]
],
[
[
"## Wait for training to complete\n\nTraining the above model may take upwards of 20 minutes time.\n\nOnce your model is done training, you can calculate the actual time it took to train the model by subtracting `end_time` from `start_time`. For your model, we will need to know the location of the saved model, which the Python script saved in your local Cloud Storage bucket at `MODEL_DIR + '/saved_model.pb'`.",
"_____no_output_____"
]
],
[
[
"while True:\n response = get_custom_job(job_id, True)\n if response.state != aip.JobState.JOB_STATE_SUCCEEDED:\n print(\"Training job has not completed:\", response.state)\n model_path_to_deploy_B = None\n if response.state == aip.JobState.JOB_STATE_FAILED:\n break\n else:\n if not DIRECT:\n MODEL_DIR_B = MODEL_DIR_B + \"/model\"\n model_path_to_deploy_B = MODEL_DIR_A\n print(\"Training Time:\", response.update_time - response.create_time)\n break\n time.sleep(60)\n\nprint(\"model_to_deploy:\", model_path_to_deploy_B)",
"_____no_output_____"
]
],
[
[
"## Load the saved model\n\nYour model instances are stored in a TensorFlow SavedModel format in a Cloud Storage bucket. Let's go ahead and load them from the Cloud Storage bucket, and then you can do some things, like evaluate the model, and do a prediction.\n\nTo load, you use the TF.Keras `model.load_model()` method passing it the Cloud Storage path where the model is saved -- specified by `MODEL_DIR_A` and `MODEL_DIR_B`.",
"_____no_output_____"
]
],
[
[
"import tensorflow as tf\n\nmodel_A = tf.keras.models.load_model(MODEL_DIR_A)\nmodel_B = tf.keras.models.load_model(MODEL_DIR_B)",
"_____no_output_____"
]
],
[
[
"## Evaluate the model\n\nNow find out how good the model is.\n\n### Load evaluation data\n\nYou will load the CIFAR10 test (holdout) data from `tf.keras.datasets`, using the method `load_data()`. This will return the dataset as a tuple of two elements. The first element is the training data and the second is the test data. Each element is also a tuple of two elements: the image data, and the corresponding labels.\n\nYou don't need the training data, and hence why we loaded it as `(_, _)`.\n\nBefore you can run the data through evaluation, you need to preprocess it:\n\nx_test:\n1. Normalize (rescaling) the pixel data by dividing each pixel by 255. This will replace each single byte integer pixel with a 32-bit floating point number between 0 and 1.\n\ny_test:<br/>\n2. The labels are currently scalar (sparse). If you look back at the `compile()` step in the `trainer/task.py` script, you will find that it was compiled for sparse labels. So we don't need to do anything more.",
"_____no_output_____"
]
],
[
[
"import numpy as np\nfrom tensorflow.keras.datasets import cifar10\n\n(_, _), (x_test, y_test) = cifar10.load_data()\nx_test = (x_test / 255.0).astype(np.float32)\n\nprint(x_test.shape, y_test.shape)",
"_____no_output_____"
]
],
[
[
"## Adding client instance to model outputs.\n\nFor A/B testing, each model needs to output two items in addition to the prediction:\n\n- The model instance, whether it is A or B.\n- An identifier for the client session where the prediction request originates from.\n\nThe model identifier is already baked into the prediction result returned by the `predict()` method, and you can use the model identifier as the means to determine which model instance A or B made the prediction.\n\nNow, why do you need to know the client session? In A/B testing, your not comparing the model's objective performance -- you've done that already in both model evaluation and post in continuous evaluation. Your comparing a business objective, such as did the customer click through the display ad, did they select a recommendation, was there a transaction conversion, etc. Thus, the business objective is measured on the client session, and you have to associate the model instance with the client session.\n\n\n### Adding client session output for A/B Testing\n\nIn the TF.Keras Functional API, when we build the model using the Model() class, we pass two parameters, the input tensor and the output layer; which I call pulling it all together connecting the inputs to the outputs:\n\n```\nmy_model = Model(inputs, outputs)\n```\n\nWe will use this method to implement passing through client session identification at prediction with your trained model instances. The syntax for specifying both multiple inputs and outputs looks like this:\n\n```\nmy_model = Model( inputs, [outputs1, outputs2])\n```\n\nThis assumes that the application server, which makes the prediction request, will add to the prediction request the client session ID. When the prediction response is received back by the application server, it will record both the model instance and the client session ID. An analysis program will then process these records to measure which model A or B better optimized the business objective.\n\n### Build the Wrapper Model\n\nLet's get started. We can do this in three lines of Keras code!\n\n1. Create a `Lambda()` layer. In this layer, you will take as input the softmax output from the model. You will then output the softmax output along with a numerical identifier representing the client session. Because this is a model, for the identifier, you need to:\n\n- Output as a number.\n- Output the value as graph operator constant using `tf.constant()`.\n- Give it a tensor shape (not-scalar), but specifing the value as a list and then convert to a tensor.\n\n2. Create a wrapper model around the original model, where:\n\n- The input is the original model input.\n- The output is the Lambda layer.\n\nWhen you deploy the model, you will use the wrapper version instead of the original model.",
"_____no_output_____"
]
],
[
[
"import tensorflow as tf\nfrom tensorflow.keras import Input, Model\nfrom tensorflow.keras.layers import Lambda\n\nsoftmax = model_A.outputs[0]\noutputs = Lambda(lambda z: (z, tf.convert_to_tensor([tf.constant(0)])))(softmax)\nwrapper_model_A = Model(model_A.inputs, outputs)\n\nsoftmax = model_B.outputs[0]\noutputs = Lambda(lambda z: (z, tf.convert_to_tensor([tf.constant(1)])))(softmax)\nwrapper_model_B = Model(model_B.inputs, outputs)",
"_____no_output_____"
]
],
[
[
"#### Local Prediction\n\nLet's now do a local prediction with one of your wrapper A/B models. You will pass three instances (images) for prediction, and get back:\n\n- The softmax prediction for each instance request.\n- The model A/B identifier. In this case 0 for A.",
"_____no_output_____"
]
],
[
[
"wrapper_model_A.predict(x_test[0:3])",
"_____no_output_____"
]
],
[
[
"### Serving function for image data\n\nTo pass images to the prediction service, you encode the compressed (e.g., JPEG) image bytes into base 64 -- which makes the content safe from modification while transmitting binary data over the network. Since this deployed model expects input data as raw (uncompressed) bytes, you need to ensure that the base 64 encoded data gets converted back to raw bytes before it is passed as input to the deployed model.\n\nTo resolve this, define a serving function (`serving_fn`) and attach it to the model as a preprocessing step. Add a `@tf.function` decorator so the serving function is fused to the underlying model (instead of upstream on a CPU).\n\nWhen you send a prediction or explanation request, the content of the request is base 64 decoded into a Tensorflow string (`tf.string`), which is passed to the serving function (`serving_fn`). The serving function preprocesses the `tf.string` into raw (uncompressed) numpy bytes (`preprocess_fn`) to match the input requirements of the model:\n- `io.decode_jpeg`- Decompresses the JPG image which is returned as a Tensorflow tensor with three channels (RGB).\n- `image.convert_image_dtype` - Changes integer pixel values to float 32.\n- `image.resize` - Resizes the image to match the input shape for the model.\n- `resized / 255.0` - Rescales (normalization) the pixel data between 0 and 1.\n\nAt this point, the data can be passed to the model (`m_call`).",
"_____no_output_____"
]
],
[
[
"CONCRETE_INPUT = \"numpy_inputs\"\n\n\ndef _preprocess(bytes_input):\n decoded = tf.io.decode_jpeg(bytes_input, channels=3)\n decoded = tf.image.convert_image_dtype(decoded, tf.float32)\n resized = tf.image.resize(decoded, size=(32, 32))\n rescale = tf.cast(resized / 255.0, tf.float32)\n return rescale\n\n\[email protected](input_signature=[tf.TensorSpec([None], tf.string)])\ndef preprocess_fn(bytes_inputs):\n decoded_images = tf.map_fn(\n _preprocess, bytes_inputs, dtype=tf.float32, back_prop=False\n )\n return {\n CONCRETE_INPUT: decoded_images\n } # User needs to make sure the key matches model's input\n\n\[email protected](input_signature=[tf.TensorSpec([None], tf.string)])\ndef serving_fn(bytes_inputs):\n images = preprocess_fn(bytes_inputs)\n prob = m_call(**images)\n return prob\n\n\nm_call = tf.function(wrapper_model_A.call).get_concrete_function(\n [tf.TensorSpec(shape=[None, 32, 32, 3], dtype=tf.float32, name=CONCRETE_INPUT)]\n)\n\ntf.saved_model.save(\n wrapper_model_A, model_path_to_deploy_A, signatures={\"serving_default\": serving_fn}\n)",
"_____no_output_____"
],
[
"CONCRETE_INPUT = \"numpy_inputs\"\n\n\ndef _preprocess(bytes_input):\n decoded = tf.io.decode_jpeg(bytes_input, channels=3)\n decoded = tf.image.convert_image_dtype(decoded, tf.float32)\n resized = tf.image.resize(decoded, size=(32, 32))\n rescale = tf.cast(resized / 255.0, tf.float32)\n return rescale\n\n\[email protected](input_signature=[tf.TensorSpec([None], tf.string)])\ndef preprocess_fn(bytes_inputs):\n decoded_images = tf.map_fn(\n _preprocess, bytes_inputs, dtype=tf.float32, back_prop=False\n )\n return {\n CONCRETE_INPUT: decoded_images\n } # User needs to make sure the key matches model's input\n\n\[email protected](input_signature=[tf.TensorSpec([None], tf.string)])\ndef serving_fn(bytes_inputs):\n images = preprocess_fn(bytes_inputs)\n prob = m_call(**images)\n return prob\n\n\nm_call = tf.function(wrapper_model_B.call).get_concrete_function(\n [tf.TensorSpec(shape=[None, 32, 32, 3], dtype=tf.float32, name=CONCRETE_INPUT)]\n)\n\ntf.saved_model.save(\n wrapper_model_B, model_path_to_deploy_B, signatures={\"serving_default\": serving_fn}\n)",
"_____no_output_____"
],
[
"loaded_A = tf.saved_model.load(model_path_to_deploy_A)\nloaded_B = tf.saved_model.load(model_path_to_deploy_B)\n\nserving_input = list(\n loaded_A.signatures[\"serving_default\"].structured_input_signature[1].keys()\n)[0]\nprint(\"Serving function input:\", serving_input)",
"_____no_output_____"
]
],
[
[
"### Upload the model\n\nUse this helper function `upload_model` to upload your model, stored in SavedModel format, up to the `Model` service, which will instantiate a Vertex AI `Model` resource instance for your model. Once you've done that, you can use the `Model` resource instance in the same way as any other Vertex AI `Model` resource instance, such as deploying to an `Endpoint` resource for serving predictions.\n\nThe helper function takes the following parameters:\n\n- `display_name`: A human readable name for the `Endpoint` service.\n- `image_uri`: The container image for the model deployment.\n- `model_uri`: The Cloud Storage path to our SavedModel artificat. For this tutorial, this is the Cloud Storage location where the `trainer/task.py` saved the model artifacts, which we specified in the variable `MODEL_DIR`.\n\nThe helper function calls the `Model` client service's method `upload_model`, which takes the following parameters:\n\n- `parent`: The Vertex AI location root path for `Dataset`, `Model` and `Endpoint` resources.\n- `model`: The specification for the Vertex AI `Model` resource instance.\n\nLet's now dive deeper into the Vertex AI model specification `model`. This is a dictionary object that consists of the following fields:\n\n- `display_name`: A human readable name for the `Model` resource.\n- `metadata_schema_uri`: Since your model was built without an Vertex AI `Dataset` resource, you will leave this blank (`''`).\n- `artificat_uri`: The Cloud Storage path where the model is stored in SavedModel format.\n- `container_spec`: This is the specification for the Docker container that will be installed on the `Endpoint` resource, from which the `Model` resource will serve predictions. Use the variable you set earlier `DEPLOY_GPU != None` to use a GPU; otherwise only a CPU is allocated.\n\nUploading a model into a Vertex AI Model resource returns a long running operation, since it may take a few moments. You call response.result(), which is a synchronous call and will return when the Vertex AI Model resource is ready.\n\nThe helper function returns the Vertex AI fully qualified identifier for the corresponding Vertex AI Model instance upload_model_response.model. You will save the identifier for subsequent steps in the variable model_to_deploy_id.",
"_____no_output_____"
]
],
[
[
"IMAGE_URI = DEPLOY_IMAGE\n\n\ndef upload_model(display_name, image_uri, model_uri):\n model = {\n \"display_name\": display_name,\n \"metadata_schema_uri\": \"\",\n \"artifact_uri\": model_uri,\n \"container_spec\": {\n \"image_uri\": image_uri,\n \"command\": [],\n \"args\": [],\n \"env\": [{\"name\": \"env_name\", \"value\": \"env_value\"}],\n \"ports\": [{\"container_port\": 8080}],\n \"predict_route\": \"\",\n \"health_route\": \"\",\n },\n }\n response = clients[\"model\"].upload_model(parent=PARENT, model=model)\n print(\"Long running operation:\", response.operation.name)\n upload_model_response = response.result(timeout=180)\n print(\"upload_model_response\")\n print(\" model:\", upload_model_response.model)\n return upload_model_response.model\n\n\nmodel_to_deploy_id_A = upload_model(\n \"cifar10-\" + TIMESTAMP, IMAGE_URI, model_path_to_deploy_A\n)\nmodel_to_deploy_id_B = upload_model(\n \"cifar10-\" + TIMESTAMP, IMAGE_URI, model_path_to_deploy_B\n)",
"_____no_output_____"
]
],
[
[
"## Deploy the `Model` resource\n\nNow deploy the trained Vertex AI custom `Model` resource. This requires two steps:\n\n1. Create an `Endpoint` resource for deploying the `Model` resource to.\n\n2. Deploy the `Model` resource to the `Endpoint` resource.",
"_____no_output_____"
],
[
"### Create an `Endpoint` resource\n\nUse this helper function `create_endpoint` to create an endpoint to deploy the model to for serving predictions, with the following parameter:\n\n- `display_name`: A human readable name for the `Endpoint` resource.\n\nThe helper function uses the endpoint client service's `create_endpoint` method, which takes the following parameter:\n\n- `display_name`: A human readable name for the `Endpoint` resource.\n\nCreating an `Endpoint` resource returns a long running operation, since it may take a few moments to provision the `Endpoint` resource for serving. You call `response.result()`, which is a synchronous call and will return when the Endpoint resource is ready. The helper function returns the Vertex AI fully qualified identifier for the `Endpoint` resource: `response.name`.",
"_____no_output_____"
]
],
[
[
"ENDPOINT_NAME = \"cifar10_endpoint-\" + TIMESTAMP\n\n\ndef create_endpoint(display_name):\n endpoint = {\"display_name\": display_name}\n response = clients[\"endpoint\"].create_endpoint(parent=PARENT, endpoint=endpoint)\n print(\"Long running operation:\", response.operation.name)\n\n result = response.result(timeout=300)\n print(\"result\")\n print(\" name:\", result.name)\n print(\" display_name:\", result.display_name)\n print(\" description:\", result.description)\n print(\" labels:\", result.labels)\n print(\" create_time:\", result.create_time)\n print(\" update_time:\", result.update_time)\n return result\n\n\nresult = create_endpoint(ENDPOINT_NAME)",
"_____no_output_____"
]
],
[
[
"Now get the unique identifier for the `Endpoint` resource you created.",
"_____no_output_____"
]
],
[
[
"# The full unique ID for the endpoint\nendpoint_id = result.name\n# The short numeric ID for the endpoint\nendpoint_short_id = endpoint_id.split(\"/\")[-1]\n\nprint(endpoint_id)",
"_____no_output_____"
]
],
[
[
"### Compute instance scaling\n\nYou have several choices on scaling the compute instances for handling your online prediction requests:\n\n- Single Instance: The online prediction requests are processed on a single compute instance.\n - Set the minimum (`MIN_NODES`) and maximum (`MAX_NODES`) number of compute instances to one.\n\n- Manual Scaling: The online prediction requests are split across a fixed number of compute instances that you manually specified.\n - Set the minimum (`MIN_NODES`) and maximum (`MAX_NODES`) number of compute instances to the same number of nodes. When a model is first deployed to the instance, the fixed number of compute instances are provisioned and online prediction requests are evenly distributed across them.\n\n- Auto Scaling: The online prediction requests are split across a scaleable number of compute instances.\n - Set the minimum (`MIN_NODES`) number of compute instances to provision when a model is first deployed and to de-provision, and set the maximum (`MAX_NODES) number of compute instances to provision, depending on load conditions.\n\nThe minimum number of compute instances corresponds to the field `min_replica_count` and the maximum number of compute instances corresponds to the field `max_replica_count`, in your subsequent deployment request.",
"_____no_output_____"
]
],
[
[
"MIN_NODES = 1\nMAX_NODES = 1",
"_____no_output_____"
]
],
[
[
"### Deploy `Model` resource to the `Endpoint` resource\n\nUse this helper function `deploy_model` to deploy the `Model` resource to the `Endpoint` resource you created for serving predictions, with the following parameters:\n\n- `model`: The Vertex AI fully qualified model identifier of the model to upload (deploy) from the training pipeline.\n- `deploy_model_display_name`: A human readable name for the deployed model.\n- `endpoint`: The Vertex AI fully qualified endpoint identifier to deploy the model to.\n\nThe helper function calls the `Endpoint` client service's method `deploy_model`, which takes the following parameters:\n\n- `endpoint`: The Vertex AI fully qualified `Endpoint` resource identifier to deploy the `Model` resource to.\n- `deployed_model`: The requirements specification for deploying the model.\n- `traffic_split`: Percent of traffic at the endpoint that goes to this model, which is specified as a dictionary of one or more key/value pairs.\n - If only one model, then specify as **{ \"0\": 100 }**, where \"0\" refers to this model being uploaded and 100 means 100% of the traffic.\n - If there are existing models on the endpoint, for which the traffic will be split, then use `model_id` to specify as **{ \"0\": percent, model_id: percent, ... }**, where `model_id` is the model id of an existing model to the deployed endpoint. The percents must add up to 100.\n\nLet's now dive deeper into the `deployed_model` parameter. This parameter is specified as a Python dictionary with the minimum required fields:\n\n- `model`: The Vertex AI fully qualified model identifier of the (upload) model to deploy.\n- `display_name`: A human readable name for the deployed model.\n- `disable_container_logging`: This disables logging of container events, such as execution failures (default is container logging is enabled). Container logging is typically enabled when debugging the deployment and then disabled when deployed for production.\n- `dedicated_resources`: This refers to how many compute instances (replicas) that are scaled for serving prediction requests.\n - `machine_spec`: The compute instance to provision. Use the variable you set earlier `DEPLOY_GPU != None` to use a GPU; otherwise only a CPU is allocated.\n - `min_replica_count`: The number of compute instances to initially provision, which you set earlier as the variable `MIN_NODES`.\n - `max_replica_count`: The maximum number of compute instances to scale to, which you set earlier as the variable `MAX_NODES`.\n\n#### Traffic Split\n\nLet's now dive deeper into the `traffic_split` parameter. This parameter is specified as a Python dictionary. This might at first be a tad bit confusing. Let me explain, you can deploy more than one instance of your model to an endpoint, and then set how much (percent) goes to each instance.\n\nWhy would you do that? Perhaps you already have a previous version deployed in production -- let's call that v1. You got better model evaluation on v2, but you don't know for certain that it is really better until you deploy to production. So in the case of traffic split, you might want to deploy v2 to the same endpoint as v1, but it only get's say 10% of the traffic. That way, you can monitor how well it does without disrupting the majority of users -- until you make a final decision.\n\n#### Response\n\nThe method returns a long running operation `response`. We will wait sychronously for the operation to complete by calling the `response.result()`, which will block until the model is deployed. If this is the first time a model is deployed to the endpoint, it may take a few additional minutes to complete provisioning of resources.",
"_____no_output_____"
]
],
[
[
"DEPLOYED_NAME = \"cifar10_deployed-\" + TIMESTAMP\n\n\ndef deploy_model(\n model, deployed_model_display_name, endpoint, traffic_split={\"0\": 100}\n):\n\n # Accelerators can be used only if the model specifies a GPU image.\n if DEPLOY_GPU:\n machine_spec = {\n \"machine_type\": DEPLOY_COMPUTE,\n \"accelerator_type\": DEPLOY_GPU,\n \"accelerator_count\": DEPLOY_NGPU,\n }\n else:\n machine_spec = {\n \"machine_type\": DEPLOY_COMPUTE,\n \"accelerator_count\": 0,\n }\n\n deployed_model = {\n \"model\": model,\n \"display_name\": deployed_model_display_name,\n \"dedicated_resources\": {\n \"min_replica_count\": MIN_NODES,\n \"max_replica_count\": MAX_NODES,\n \"machine_spec\": machine_spec,\n },\n }\n\n response = clients[\"endpoint\"].deploy_model(\n endpoint=endpoint, deployed_model=deployed_model, traffic_split=traffic_split\n )\n\n print(\"Long running operation:\", response.operation.name)\n result = response.result()\n print(\"result\")\n deployed_model = result.deployed_model\n print(\" deployed_model\")\n print(\" id:\", deployed_model.id)\n print(\" model:\", deployed_model.model)\n print(\" display_name:\", deployed_model.display_name)\n print(\" create_time:\", deployed_model.create_time)\n\n return deployed_model.id\n\n\ndeployed_model_id_A = deploy_model(\n model_to_deploy_id_A, DEPLOYED_NAME + \"-A\", endpoint_id, {\"0\": 100}\n)\ndeployed_model_id_B = deploy_model(\n model_to_deploy_id_B,\n DEPLOYED_NAME + \"-B\",\n endpoint_id,\n {\"0\": 50, deployed_model_id_A: 50},\n)",
"_____no_output_____"
]
],
[
[
"## Make a online prediction request\n\nNow do a online prediction to your deployed model.",
"_____no_output_____"
],
[
"### Get test item\n\nYou will use an example out of the test (holdout) portion of the dataset as a test item.",
"_____no_output_____"
]
],
[
[
"test_image = x_test[0]\ntest_label = y_test[0]\nprint(test_image.shape)",
"_____no_output_____"
]
],
[
[
"### Prepare the request content\nYou are going to send the CIFAR10 image as compressed JPG image, instead of the raw uncompressed bytes:\n\n- `cv2.imwrite`: Use openCV to write the uncompressed image to disk as a compressed JPEG image.\n - Denormalize the image data from \\[0,1) range back to [0,255).\n - Convert the 32-bit floating point values to 8-bit unsigned integers.\n- `tf.io.read_file`: Read the compressed JPG images back into memory as raw bytes.\n- `base64.b64encode`: Encode the raw bytes into a base 64 encoded string.",
"_____no_output_____"
]
],
[
[
"import base64\n\nimport cv2\n\ncv2.imwrite(\"tmp.jpg\", (test_image * 255).astype(np.uint8))\n\nbytes = tf.io.read_file(\"tmp.jpg\")\nb64str = base64.b64encode(bytes.numpy()).decode(\"utf-8\")",
"_____no_output_____"
]
],
[
[
"### Send the prediction request\n\nOk, now you have a test image. Use this helper function `predict_image`, which takes the following parameters:\n\n- `image`: The test image data as a numpy array.\n- `endpoint`: The Vertex AI fully qualified identifier for the `Endpoint` resource where the `Model` resource was deployed to.\n- `parameters_dict`: Additional parameters for serving.\n\nThis function calls the prediction client service `predict` method with the following parameters:\n\n- `endpoint`: The Vertex AI fully qualified identifier for the `Endpoint` resource where the `Model` resource was deployed to.\n- `instances`: A list of instances (encoded images) to predict.\n- `parameters`: Additional parameters for serving.\n\nTo pass the image data to the prediction service, in the previous step you encoded the bytes into base64 -- which makes the content safe from modification when transmitting binary data over the network. You need to tell the serving binary where your model is deployed to, that the content has been base64 encoded, so it will decode it on the other end in the serving binary.\n\nEach instance in the prediction request is a dictionary entry of the form:\n\n {serving_input: {'b64': content}}\n\n- `input_name`: the name of the input layer of the underlying model.\n- `'b64'`: A key that indicates the content is base64 encoded.\n- `content`: The compressed JPG image bytes as a base64 encoded string.\n\nSince the `predict()` service can take multiple images (instances), you will send your single image as a list of one image. As a final step, you package the instances list into Google's protobuf format -- which is what we pass to the `predict()` service.\n\nThe `response` object returns a list, where each element in the list corresponds to the corresponding image in the request. You will see in the output for each prediction:\n\n- `predictions`: Confidence level for the prediction, between 0 and 1, for each of the classes.\n- `output_2`: The client session ID.",
"_____no_output_____"
]
],
[
[
"def predict_image(image, endpoint, parameters_dict):\n # The format of each instance should conform to the deployed model's prediction input schema.\n instances_list = [{serving_input: {\"b64\": image}}]\n instances = [json_format.ParseDict(s, Value()) for s in instances_list]\n\n response = clients[\"prediction\"].predict(\n endpoint=endpoint, instances=instances, parameters=parameters_dict\n )\n print(\"response\")\n print(\" deployed_model_id:\", response.deployed_model_id)\n predictions = response.predictions\n print(\"predictions\")\n for prediction in predictions:\n print(\" prediction:\", dict(prediction))\n\n\npredict_image(b64str, endpoint_id, None)",
"_____no_output_____"
],
[
"def undeploy_model(deployed_model_id, endpoint):\n response = clients[\"endpoint\"].undeploy_model(\n endpoint=endpoint, deployed_model_id=deployed_model_id, traffic_split={}\n )\n print(response)\n\n\nundeploy_model(deployed_model_id_A, endpoint_id)\nundeploy_model(deployed_model_id_B, endpoint_id)",
"_____no_output_____"
]
],
[
[
"# Cleaning up\n\nTo clean up all GCP resources used in this project, you can [delete the GCP\nproject](https://cloud.google.com/resource-manager/docs/creating-managing-projects#shutting_down_projects) you used for the tutorial.\n\nOtherwise, you can delete the individual resources you created in this tutorial:\n\n- Dataset\n- Pipeline\n- Model\n- Endpoint\n- Batch Job\n- Custom Job\n- Hyperparameter Tuning Job\n- Cloud Storage Bucket",
"_____no_output_____"
]
],
[
[
"delete_dataset = True\ndelete_pipeline = True\ndelete_model = True\ndelete_endpoint = True\ndelete_batchjob = True\ndelete_customjob = True\ndelete_hptjob = True\ndelete_bucket = True\n\n# Delete the dataset using the Vertex AI fully qualified identifier for the dataset\ntry:\n if delete_dataset and \"dataset_id\" in globals():\n clients[\"dataset\"].delete_dataset(name=dataset_id)\nexcept Exception as e:\n print(e)\n\n# Delete the training pipeline using the Vertex AI fully qualified identifier for the pipeline\ntry:\n if delete_pipeline and \"pipeline_id\" in globals():\n clients[\"pipeline\"].delete_training_pipeline(name=pipeline_id)\nexcept Exception as e:\n print(e)\n\n# Delete the model using the Vertex AI fully qualified identifier for the model\ntry:\n if delete_model and \"model_to_deploy_id\" in globals():\n clients[\"model\"].delete_model(name=model_to_deploy_id)\nexcept Exception as e:\n print(e)\n\n# Delete the endpoint using the Vertex AI fully qualified identifier for the endpoint\ntry:\n if delete_endpoint and \"endpoint_id\" in globals():\n clients[\"endpoint\"].delete_endpoint(name=endpoint_id)\nexcept Exception as e:\n print(e)\n\n# Delete the batch job using the Vertex AI fully qualified identifier for the batch job\ntry:\n if delete_batchjob and \"batch_job_id\" in globals():\n clients[\"job\"].delete_batch_prediction_job(name=batch_job_id)\nexcept Exception as e:\n print(e)\n\n# Delete the custom job using the Vertex AI fully qualified identifier for the custom job\ntry:\n if delete_customjob and \"job_id\" in globals():\n clients[\"job\"].delete_custom_job(name=job_id)\nexcept Exception as e:\n print(e)\n\n# Delete the hyperparameter tuning job using the Vertex AI fully qualified identifier for the hyperparameter tuning job\ntry:\n if delete_hptjob and \"hpt_job_id\" in globals():\n clients[\"job\"].delete_hyperparameter_tuning_job(name=hpt_job_id)\nexcept Exception as e:\n print(e)\n\nif delete_bucket and \"BUCKET_NAME\" in globals():\n ! gsutil rm -r $BUCKET_NAME",
"_____no_output_____"
]
]
] | [
"code",
"markdown",
"code",
"markdown",
"code",
"markdown",
"code",
"markdown",
"code",
"markdown",
"code",
"markdown",
"code",
"markdown",
"code",
"markdown",
"code",
"markdown",
"code",
"markdown",
"code",
"markdown",
"code",
"markdown",
"code",
"markdown",
"code",
"markdown",
"code",
"markdown",
"code",
"markdown",
"code",
"markdown",
"code",
"markdown",
"code",
"markdown",
"code",
"markdown",
"code",
"markdown",
"code",
"markdown",
"code",
"markdown",
"code",
"markdown",
"code",
"markdown",
"code",
"markdown",
"code",
"markdown",
"code",
"markdown",
"code",
"markdown",
"code",
"markdown",
"code",
"markdown",
"code",
"markdown",
"code",
"markdown",
"code",
"markdown",
"code",
"markdown",
"code",
"markdown",
"code",
"markdown",
"code",
"markdown",
"code",
"markdown",
"code",
"markdown",
"code",
"markdown",
"code",
"markdown",
"code",
"markdown",
"code",
"markdown",
"code",
"markdown",
"code",
"markdown",
"code"
] | [
[
"code"
],
[
"markdown",
"markdown",
"markdown",
"markdown",
"markdown",
"markdown"
],
[
"code"
],
[
"markdown"
],
[
"code"
],
[
"markdown"
],
[
"code"
],
[
"markdown"
],
[
"code",
"code",
"code"
],
[
"markdown"
],
[
"code"
],
[
"markdown"
],
[
"code"
],
[
"markdown"
],
[
"code"
],
[
"markdown"
],
[
"code",
"code"
],
[
"markdown"
],
[
"code"
],
[
"markdown"
],
[
"code"
],
[
"markdown",
"markdown"
],
[
"code"
],
[
"markdown"
],
[
"code"
],
[
"markdown"
],
[
"code"
],
[
"markdown"
],
[
"code"
],
[
"markdown"
],
[
"code"
],
[
"markdown",
"markdown"
],
[
"code"
],
[
"markdown",
"markdown",
"markdown"
],
[
"code"
],
[
"markdown"
],
[
"code"
],
[
"markdown"
],
[
"code"
],
[
"markdown"
],
[
"code"
],
[
"markdown"
],
[
"code"
],
[
"markdown"
],
[
"code"
],
[
"markdown"
],
[
"code"
],
[
"markdown"
],
[
"code"
],
[
"markdown"
],
[
"code"
],
[
"markdown"
],
[
"code"
],
[
"markdown"
],
[
"code"
],
[
"markdown"
],
[
"code"
],
[
"markdown"
],
[
"code"
],
[
"markdown"
],
[
"code"
],
[
"markdown"
],
[
"code"
],
[
"markdown"
],
[
"code"
],
[
"markdown"
],
[
"code"
],
[
"markdown"
],
[
"code"
],
[
"markdown"
],
[
"code"
],
[
"markdown"
],
[
"code"
],
[
"markdown"
],
[
"code",
"code",
"code"
],
[
"markdown"
],
[
"code"
],
[
"markdown",
"markdown"
],
[
"code"
],
[
"markdown"
],
[
"code"
],
[
"markdown"
],
[
"code"
],
[
"markdown"
],
[
"code"
],
[
"markdown",
"markdown"
],
[
"code"
],
[
"markdown"
],
[
"code"
],
[
"markdown"
],
[
"code",
"code"
],
[
"markdown"
],
[
"code"
]
] |
e780f016121f6d74c6b508c3921e9ac62b53bd56 | 522,661 | ipynb | Jupyter Notebook | DeepLearningForVisionSystems_Ch5_InceptionGoogleNet.ipynb | mkkadambi/machine-learning | a43117573040e0be36ef9f3164982e179a293644 | [
"CC0-1.0"
] | 1 | 2021-06-13T13:09:37.000Z | 2021-06-13T13:09:37.000Z | DeepLearningForVisionSystems_Ch5_InceptionGoogleNet.ipynb | mkkadambi/machine-learning | a43117573040e0be36ef9f3164982e179a293644 | [
"CC0-1.0"
] | null | null | null | DeepLearningForVisionSystems_Ch5_InceptionGoogleNet.ipynb | mkkadambi/machine-learning | a43117573040e0be36ef9f3164982e179a293644 | [
"CC0-1.0"
] | null | null | null | 293.960067 | 378,650 | 0.883159 | [
[
[
"This is Tensorflow 2 implementation of GoogleNet model based on the paper in the below link\nhttps://static.googleusercontent.com/media/research.google.com/en//pubs/archive/43022.pdf\n\nFew of the major differences between the paper and this implementation are\n\n\n1. Number of categories for classification here is 10 compared to the paper's 1000\n2. In the paper, conv layers use relu activation layer, but in this implementation elu is used instead\n\n",
"_____no_output_____"
]
],
[
[
"#For any array manipulations\nimport numpy as np\n\n#For plotting graphs\nimport matplotlib.pyplot as plt\n# For loading data from the file system\nimport os\n\n# For randomly selecting data from the dataset\nimport random\n\n# For displaying the confusion matrix in a pretty way\nimport pandas\n\n# loading tensorflow packages\nimport tensorflow as tf\nfrom tensorflow.keras import Model, Input\nfrom tensorflow.keras.layers import Conv2D, MaxPool2D, AveragePooling2D, Concatenate, Dense, BatchNormalization, Dropout, Flatten\nfrom tensorflow.keras.callbacks import ModelCheckpoint\nfrom tensorflow.keras.initializers import RandomNormal\n\nprint(tf.__version__)\n\n",
"2.5.0\n"
]
],
[
[
"# Initializations",
"_____no_output_____"
]
],
[
[
"from tensorflow.python.client import device_lib\n\ndef get_available_gpus():\n local_device_protos = device_lib.list_local_devices()\n return [x.name for x in local_device_protos if x.device_type == 'GPU']\nprint(\"devices =\" , tf.config.list_physical_devices())\n\nprint(get_available_gpus()) ",
"devices = [PhysicalDevice(name='/physical_device:CPU:0', device_type='CPU'), PhysicalDevice(name='/physical_device:GPU:0', device_type='GPU')]\n['/device:GPU:0']\n"
],
[
"# Shape of the input images height=width=224 channels = 3\ninput_shape=(224,224,3) \n\n# batch_size is the number of images in a batch to train and test the model\nbatch_size = 100 \n\n# num_classes is the number of cateegories that input images havee to be classified into\n# This has to be set based on the input dataset\n# For the dataset the paper uses, num_classes = 1000\nnum_classes = 5\n\n\n# Based on computational power availability, size_factor can be varied. \n# This will determine the model complexity and number of trainable parameters\n# This affects the feature map size of all conv layers\n# The model from the paper uses size_factor=64\nsize_factor = 32\n\ncheckpoint_filePath = '/content/drive/MyDrive/MachineLearning/GoogleNet_experiment_1.h5'",
"_____no_output_____"
],
[
"#initializing the random seed so that we get consistent results\ndef set_seed(seed=31415):\n np.random.seed(seed)\n tf.random.set_seed(seed)\n os.environ['PYTHONHASHSEED'] = str(seed)\n os.environ['TF_DETERMINISTIC_OPS'] = '1'\nset_seed()",
"_____no_output_____"
]
],
[
[
"# Data Loading and Preprocessing",
"_____no_output_____"
],
[
"## Procuring the dataset\n",
"_____no_output_____"
]
],
[
[
"path='/content/Linnaeus 5 256X256'\n# Check if the folder with the dataset already exists, if not copy it from the saved location\nif not os.path.isdir(path):\n !cp '/content/drive/MyDrive/MachineLearning/Linnaeus 5 256X256.rar' '/content/' \n get_ipython().system_raw(\"unrar x '/content/Linnaeus 5 256X256.rar'\")\n\n\n\ncategories = os.listdir(os.path.join(path, 'train'))\nprint(len(categories), \" categories found =\", categories)",
"5 categories found = ['dog', 'berry', 'other', 'bird', 'flower']\n"
]
],
[
[
"## Training and Validation Datasets",
"_____no_output_____"
]
],
[
[
"\ntrain_image_dataset = tf.keras.preprocessing.image_dataset_from_directory(\n os.path.join(path, 'train')\n , labels='inferred'\n , label_mode='categorical'\n , class_names=categories\n , batch_size=batch_size\n , image_size=(256, 256)\n , shuffle=True\n , seed=2\n , validation_split=0.1\n , subset= 'training'\n )\n\nvalidation_image_dataset = tf.keras.preprocessing.image_dataset_from_directory(\n os.path.join(path, 'train')\n , labels='inferred'\n , label_mode='categorical'\n , class_names=categories\n , batch_size=batch_size\n , image_size=(256, 256)\n , shuffle=True\n , seed=2\n , validation_split=0.1\n , subset= 'validation'\n )\n\n\nprint(\"Training class names found =\" , train_image_dataset.class_names)\n\ndef crop_images(images, labels):\n '''\n Expecting categories to be names of subfolders and the images belonging to each \n of the subfolders be stored inside them. While reading the images, they are resized to 256x256x3\n and then cropped to 224x224x3 based on the way the paper describes (randomly between 4 corners and center)\n diagnostics: bool (default False), If True it will print a lot of debug information\n\n '''\n # In order to clip the image in either from top-left, top-right, bottom-left, bottom-right or center, \n # we create an array of possible start positions\n corners_list = [0, (256-input_shape[0])//2, 256-input_shape[0]]\n \n # Sampling one number from the list of start positions\n offset_height = offset_width = random.sample(corners_list, 1)[0]\n \n images = tf.image.per_image_standardization(images-127)\n images = images/tf.math.reduce_max(tf.math.abs(images))\n\n # Since there is an auxillary arm of the model, we have to concatenate two labels with each other during training\n return tf.image.crop_to_bounding_box(images, offset_height, offset_width, input_shape[0], input_shape[0]), labels #(labels, labels)\n\n\nvalidation_datasource = validation_image_dataset.map(crop_images)\nvalidation_datasource = validation_datasource.cache().prefetch(buffer_size=tf.data.AUTOTUNE).shuffle(batch_size)\n\ntraining_datasource = train_image_dataset.map(crop_images)\ntraining_datasource = training_datasource.cache().prefetch(buffer_size=tf.data.AUTOTUNE).shuffle(batch_size)\n\n",
"Found 6000 files belonging to 5 classes.\nUsing 5400 files for training.\nFound 6000 files belonging to 5 classes.\nUsing 600 files for validation.\nTraining class names found = ['dog', 'berry', 'other', 'bird', 'flower']\n"
],
[
"for images, labels in training_datasource:\n print(\"images =\", images.shape)\n print(\"labels =\", type(labels))\n break\n\ntraining_datasource = train_image_dataset.map(crop_images)\ntraining_datasource = training_datasource.cache().prefetch(buffer_size=tf.data.AUTOTUNE).shuffle(batch_size)\n",
"images = (100, 224, 224, 3)\nlabels = <class 'tensorflow.python.framework.ops.EagerTensor'>\n"
]
],
[
[
"## Test Data",
"_____no_output_____"
]
],
[
[
"test_image_dataset = tf.keras.preprocessing.image_dataset_from_directory(\n os.path.join(path, 'test')\n , labels='inferred'\n , label_mode='categorical'\n , class_names=categories\n , batch_size=batch_size\n , image_size=(256, 256)\n , seed=2\n )\ndef test_data_crop_images(images, labels):\n '''\n Definiing separate function for test data because labels do not have to be \n concatenated during testing and the map function does not allow multiple function calls\n\n Expecting categories to be names of subfolders and the images belonging to each \n of the subfolders be stored inside them. While reading the images, they are resized to 256x256x3\n and then cropped to 224x224x3 based on the way the paper describes (randomly between 4 corners and center)\n diagnostics: bool (default False), If True it will print a lot of debug information\n\n '''\n # In order to clip the image in either from top-left, top-right, bottom-left, bottom-right or center, \n # we create an array of possible start positions\n corners_list = [0, (256-input_shape[0])//2, 256-input_shape[0]]\n \n # Sampling one number from the list of start positions\n offset_height = offset_width = random.sample(corners_list, 1)[0]\n \n images = tf.image.per_image_standardization(images-127)\n images = images/tf.math.reduce_max(tf.math.abs(images))\n # Since there is an auxillary arm of the model, we have to concatenate two labels with each other during training\n return tf.image.crop_to_bounding_box(images, offset_height, offset_width, input_shape[0], input_shape[0]), labels\n\n\ntest_datasource = test_image_dataset.map(test_data_crop_images)\ntest_datasource = test_datasource.cache().prefetch(buffer_size=tf.data.AUTOTUNE).shuffle(batch_size)",
"Found 2000 files belonging to 5 classes.\n"
]
],
[
[
"# Building the GoogleNet Architecture",
"_____no_output_____"
],
[
"## Define the inception block",
"_____no_output_____"
]
],
[
[
"def inception_block(input, intermediate_filter_size, output_filter_size\n , kernel_initializer, bias_initializer\n , use_bias=True, name_prefix=''):\n '''\n input = input tensor that has to be opeerated on\n intermediate_filter_size = dictionary that keys 3 and 5\n {3: filter size of Conv1x1 in the Conv3x3 pipeline,\n 5: filter size of Conv1x1 in the Conv5x5 pipeline\n } \n output_filter_size = dictionary that have keys 1, 3 and 5\n {1: filter size of the Conv1x1 filter in the Conv1x1 pipeline,\n 3: filter size of the Conv3x3 filter in the Conv3x3 pipeline,\n 5: filter size of the Conv5x5 filter in the Conv5x5 pipeline\n }\n name_prefix = string that will be prefixed with each of the layers' names in the block\n '''\n initializer = RandomNormal(mean=0.5, stddev=0.1, seed = 7)\n\n # Conv 1x1 pipeline taking the input and feeding directly to the output\n conv1 = Conv2D(filters = output_filter_size[1], kernel_size=1, strides = 1\n , activation='elu', padding='same', use_bias=use_bias\n , kernel_initializer = kernel_initializer, bias_initializer=bias_initializer\n , name=name_prefix + 'conv1' ) (input)\n\n # Defining Conv 1x1 -> Conv 3x3 pipeline\n conv1_3 = Conv2D(filters= intermediate_filter_size[3], kernel_size = 1, strides = 1\n , activation='elu', padding='same', use_bias=use_bias\n , kernel_initializer = kernel_initializer, bias_initializer=bias_initializer\n , name=name_prefix + 'conv1_3')(input)\n conv3 = Conv2D(filters = output_filter_size[3], kernel_size = 3, strides = 1\n , activation='elu', padding='same', use_bias=use_bias\n , kernel_initializer = kernel_initializer, bias_initializer=bias_initializer\n , name=name_prefix + 'conv3' )(conv1_3)\n\n # Defining the Conv1x1 -> Conv5x5 pipeline\n conv1_5 = Conv2D(filters= intermediate_filter_size[5], kernel_size = 1, strides = 1\n , activation='elu', padding='same', use_bias=use_bias\n , kernel_initializer = kernel_initializer, bias_initializer=bias_initializer\n , name=name_prefix + 'conv1_5')(input)\n conv5 = Conv2D(filters = output_filter_size[5], kernel_size = 5, strides = 1\n , activation='elu', padding='same', use_bias=use_bias\n , kernel_initializer = kernel_initializer, bias_initializer=bias_initializer\n , name=name_prefix + 'conv5' )(conv1_5)\n\n # Defining the MaxPool pipeline\n max_pool = MaxPool2D(pool_size=3, strides=1, padding='same'\n , name=name_prefix + 'maxpool')(input)\n conv_projection = Conv2D(filters = output_filter_size['proj'], kernel_size=1, strides=1\n , activation='elu', padding='same', use_bias=use_bias\n , kernel_initializer = kernel_initializer, bias_initializer=bias_initializer\n , name=name_prefix + 'proj')(max_pool)\n\n # Concatenating the output of the above pipelines\n output = Concatenate(axis=3\n , name=name_prefix + 'concat')([conv1, conv3, conv5, conv_projection])\n\n return output\n",
"_____no_output_____"
]
],
[
[
"## Defining the Auxillary Branch block",
"_____no_output_____"
]
],
[
[
"def auxillary_branch(input, num_classes, kernel_initializer , bias_initializer\n , filter_size = 128\n \n , use_bias=True, name_prefix=''):\n #initializer = RandomNormal(mean=0.5, stddev=0.1, seed = 7)\n avg_pool = AveragePooling2D(pool_size=5, strides=3, padding='valid'\n , name=name_prefix + 'avg_pool')(input)\n conv = Conv2D(filters= filter_size , kernel_size=1, strides=1\n , padding='same', activation='elu', use_bias=use_bias\n , kernel_initializer = kernel_initializer, bias_initializer=bias_initializer\n , name=name_prefix + 'conv')(avg_pool)\n dense = Dense(units = 1024, activation='elu'\n , kernel_initializer = kernel_initializer, bias_initializer=bias_initializer\n , name=name_prefix + 'fc')(conv)\n dropout = Dropout(0.7, name=name_prefix + 'dropout')(dense)\n flatten = Flatten(name=name_prefix+'flatten')(dropout)\n output = Dense(units = num_classes, activation='softmax'\n , kernel_initializer = kernel_initializer, bias_initializer=bias_initializer\n , name=name_prefix + 'output')(flatten)\n\n return output",
"_____no_output_____"
]
],
[
[
"## Define the actual model",
"_____no_output_____"
]
],
[
[
"def build_googleNet(input_shape, size_factor=64, activation='elu'\n , use_bias=True, num_classes=10):\n '''\n input_shape = tuple of 3 numbers (height, width, channels)\n batch_size = number of images per batch\n\n size_factor = int (default 64). As per the paper, this should be 64. \n Since all the convolutions are sized as multiples of 64, this is made configurable to be able to train a lighter version of the network if needed\n activation = str (default 'elu') Since this is a big network, I have chosen to go with elu activation to give the layers a way our of ending up with dead relus\n '''\n \n kernel_initializer = tf.keras.initializers.GlorotUniform()#(mean=0.5, stddev=0.1, seed = 7)\n bias_initializer = tf.keras.initializers.GlorotUniform() #(0.2)\n input = Input(shape = input_shape, batch_size = batch_size , name=\"main_input\")\n\n # First portion of GoogleNet Architecture is similar to AlexNet/ LeNet \n # i.e. a single pipeline\n # Definining this here\n\n #Conv layer for output 112x112x64\n conv_layer_1 = Conv2D(filters=size_factor, kernel_size = 7, strides = 2, activation='elu'\n , padding= 'same', use_bias=use_bias\n , kernel_initializer = kernel_initializer, bias_initializer=bias_initializer\n , name='conv_layer_1')(input)\n # MaxPool will give out 56x56x64 \n maxpool_1=MaxPool2D(pool_size = 3, strides = 2, padding='same'\n , name='maxpool_1')(conv_layer_1)\n\n # Adding Norm layer as per the textbook\n norm_1 = BatchNormalization(name='norm_1')(maxpool_1)\n\n\n # Paper says the next Conv3x3 layer needs a reduction layer as well\n # Defining the reduction layer of Conv with output 56x56x64\n conv_layer_2a = Conv2D(filters = size_factor, kernel_size = 1, strides = 1, activation ='elu'\n , padding='same', use_bias=use_bias\n , kernel_initializer = kernel_initializer, bias_initializer=bias_initializer\n , name='conv_layer_2') (norm_1)\n\n # Now defining the actual conv3x3 layer output of 28x28x192\n conv_layer_2b = Conv2D(filters = size_factor*3, kernel_size = 3, strides = 1, activation='elu'\n , padding='same', use_bias=use_bias\n , kernel_initializer = kernel_initializer, bias_initializer=bias_initializer\n , name='conv_layer_3')(conv_layer_2a)\n # max_pool should output 28x28x192 \n max_pool_2 = MaxPool2D(pool_size=3, strides = 2, padding='same'\n , name='maxpool_2')(conv_layer_2b)\n\n # Creeating the first inception block 3a with output 28x28x256 \n inception3a = inception_block(max_pool_2, intermediate_filter_size={3:96, 5:16}\n , output_filter_size={1:size_factor\n , 3:size_factor*2\n , 5:size_factor//2\n , 'proj':size_factor//2}\n , use_bias=use_bias\n , kernel_initializer = kernel_initializer\n , bias_initializer=bias_initializer\n , name_prefix='incep_3a_')\n\n # Inception Block 3b with output 28x28x480\n inception3b = inception_block(inception3a, intermediate_filter_size={3:128, 5:32}\n , output_filter_size={1:size_factor*2\n , 3:size_factor*3\n , 5:int(size_factor*1.5)\n , 'proj':size_factor}\n , use_bias=use_bias\n , kernel_initializer = kernel_initializer\n , bias_initializer=bias_initializer\n , name_prefix='incep_3b_')\n\n # Paper specifies a MaxPool layer here output= 14x14x480\n max_pool_3 = MaxPool2D(pool_size=3, strides = 2, padding='same', name='max_pool_3')(inception3b)\n\n #Inception Block 4a, output = 14x14x512\n inception4a = inception_block(max_pool_3, intermediate_filter_size={3:112, 5:24}\n , output_filter_size={1:size_factor*3\n , 3:int(size_factor*3.25) #208\n , 5:int(size_factor * 0.75) #48\n , 'proj':size_factor}\n , use_bias=use_bias\n , kernel_initializer = kernel_initializer\n , bias_initializer=bias_initializer\n , name_prefix='incep_4a_')\n\n # First Auxillary outputs with size 1x1xnum_classes\n #output_aux1 = auxillary_branch(inception4a, num_classes\n # , filter_size=size_factor*2\n # , kernel_initializer = kernel_initializer\n # , bias_initializer=bias_initializer\n # , use_bias=use_bias, name_prefix='aux1_')\n\n #Inception Block 4b with output shape =14x14x512\n inception4b = inception_block(inception4a, intermediate_filter_size={3:96, 5:16}\n , output_filter_size={1:int(size_factor*2.5) #160\n , 3:int(size_factor*3.5) #224\n , 5:size_factor\n , 'proj':size_factor}\n , use_bias=use_bias\n , kernel_initializer = kernel_initializer\n , bias_initializer=bias_initializer\n , name_prefix='incep_4b_')\n\n #Inception Block 4c with output shape = 14x14x512\n inception4c = inception_block(inception4b, intermediate_filter_size={3:144, 5:32}\n , output_filter_size={1:size_factor*2\n , 3:size_factor * 4 #256\n , 5:size_factor\n , 'proj':size_factor}\n , kernel_initializer = kernel_initializer\n , bias_initializer=bias_initializer\n , name_prefix='incep_4c_')\n\n #Inception Block 4d with output shape =14x14x528\n inception4d = inception_block(inception4c, intermediate_filter_size={3:128, 5:24}\n , output_filter_size={1:int(size_factor*1.75) #112\n , 3:int(size_factor*4.5) #288\n , 5:size_factor\n , 'proj':size_factor}\n , use_bias=use_bias\n , kernel_initializer = kernel_initializer\n , bias_initializer=bias_initializer\n , name_prefix='incep_4d_')\n\n # Second Auxillary outputs with output 1x1xnum_classes\n #output_aux2 = auxillary_branch(inception4d, num_classes, filter_size=size_factor*2\n # , kernel_initializer = kernel_initializer, bias_initializer=bias_initializer\n # , use_bias=use_bias, name_prefix='aux2_')\n # The paper later said that just one auxillary branch is sufficient and there is\n # very negligible benefit from the second. Hence removed it here and excluded it from the final output\n\n #Inception Block 4e with output 14x14x832\n inception4e = inception_block(inception4d, intermediate_filter_size={3:160, 5:32}\n , output_filter_size={1:size_factor*4 #256\n , 3:size_factor*5 #320\n , 5:size_factor*2 #128\n , 'proj':size_factor*2 }\n , use_bias=use_bias\n , kernel_initializer = kernel_initializer\n , bias_initializer=bias_initializer\n , name_prefix='incep_4e_')\n\n # Paper specifies a MaxPool layer here with output 7x7x832\n max_pool_4 = MaxPool2D(pool_size=3, strides = 2, padding='same', name='max_pool_4')(inception4e)\n\n #Inception Block 5a with output shape 7x7x832 \n inception5a = inception_block(max_pool_4, intermediate_filter_size={3:160, 5:32}\n , output_filter_size={1:size_factor * 4 #256\n , 3:size_factor * 5 #320\n , 5:size_factor * 2 #128\n , 'proj':size_factor*2}\n , use_bias=use_bias\n , kernel_initializer = kernel_initializer\n , bias_initializer=bias_initializer\n , name_prefix='incep_5a_')\n\n #Inception Block 5b with output shape 7x7x1024\n inception5b = inception_block(inception5a, intermediate_filter_size={3:192, 5:48}\n , output_filter_size={1:size_factor * 6 #384\n , 3:size_factor * 6 #384\n , 5:size_factor * 2 #128\n , 'proj':size_factor * 2}\n , use_bias=use_bias\n , kernel_initializer = kernel_initializer\n , bias_initializer=bias_initializer\n , name_prefix='incep_5b_')\n\n # Avg Pool as specified by the paper, output shape = 1x1x1024\n avg_pool = AveragePooling2D(pool_size=7, strides=1, padding='valid'\n , name='avg_pool')(inception5b)\n\n\n dropout = Dropout(rate=0.4, name='dropout')(avg_pool)\n\n flatten = Flatten(name='flatten')(dropout)\n # Final FC layer with output shape 1x1xnum_classes\n pipeline_output = Dense(units=num_classes, activation='softmax'\n , kernel_initializer = kernel_initializer\n , bias_initializer=bias_initializer\n , name='main_output')(flatten)\n\n # Adding auxillary branches for training purposes based on the paper\n\n # Not using the aux2 branch in the output as the paper suggested\n model = Model (inputs = input, outputs = pipeline_output, name='googleNet')\n\n model.summary()\n return model\n",
"_____no_output_____"
],
[
"model = build_googleNet(input_shape, size_factor=64, activation='elu', num_classes=num_classes, use_bias=True)",
"Model: \"googleNet\"\n__________________________________________________________________________________________________\nLayer (type) Output Shape Param # Connected to \n==================================================================================================\nmain_input (InputLayer) [(100, 224, 224, 3)] 0 \n__________________________________________________________________________________________________\nconv_layer_1 (Conv2D) (100, 112, 112, 64) 9472 main_input[0][0] \n__________________________________________________________________________________________________\nmaxpool_1 (MaxPooling2D) (100, 56, 56, 64) 0 conv_layer_1[0][0] \n__________________________________________________________________________________________________\nnorm_1 (BatchNormalization) (100, 56, 56, 64) 256 maxpool_1[0][0] \n__________________________________________________________________________________________________\nconv_layer_2 (Conv2D) (100, 56, 56, 64) 4160 norm_1[0][0] \n__________________________________________________________________________________________________\nconv_layer_3 (Conv2D) (100, 56, 56, 192) 110784 conv_layer_2[0][0] \n__________________________________________________________________________________________________\nmaxpool_2 (MaxPooling2D) (100, 28, 28, 192) 0 conv_layer_3[0][0] \n__________________________________________________________________________________________________\nincep_3a_conv1_3 (Conv2D) (100, 28, 28, 96) 18528 maxpool_2[0][0] \n__________________________________________________________________________________________________\nincep_3a_conv1_5 (Conv2D) (100, 28, 28, 16) 3088 maxpool_2[0][0] \n__________________________________________________________________________________________________\nincep_3a_maxpool (MaxPooling2D) (100, 28, 28, 192) 0 maxpool_2[0][0] \n__________________________________________________________________________________________________\nincep_3a_conv1 (Conv2D) (100, 28, 28, 64) 12352 maxpool_2[0][0] \n__________________________________________________________________________________________________\nincep_3a_conv3 (Conv2D) (100, 28, 28, 128) 110720 incep_3a_conv1_3[0][0] \n__________________________________________________________________________________________________\nincep_3a_conv5 (Conv2D) (100, 28, 28, 32) 12832 incep_3a_conv1_5[0][0] \n__________________________________________________________________________________________________\nincep_3a_proj (Conv2D) (100, 28, 28, 32) 6176 incep_3a_maxpool[0][0] \n__________________________________________________________________________________________________\nincep_3a_concat (Concatenate) (100, 28, 28, 256) 0 incep_3a_conv1[0][0] \n incep_3a_conv3[0][0] \n incep_3a_conv5[0][0] \n incep_3a_proj[0][0] \n__________________________________________________________________________________________________\nincep_3b_conv1_3 (Conv2D) (100, 28, 28, 128) 32896 incep_3a_concat[0][0] \n__________________________________________________________________________________________________\nincep_3b_conv1_5 (Conv2D) (100, 28, 28, 32) 8224 incep_3a_concat[0][0] \n__________________________________________________________________________________________________\nincep_3b_maxpool (MaxPooling2D) (100, 28, 28, 256) 0 incep_3a_concat[0][0] \n__________________________________________________________________________________________________\nincep_3b_conv1 (Conv2D) (100, 28, 28, 128) 32896 incep_3a_concat[0][0] \n__________________________________________________________________________________________________\nincep_3b_conv3 (Conv2D) (100, 28, 28, 192) 221376 incep_3b_conv1_3[0][0] \n__________________________________________________________________________________________________\nincep_3b_conv5 (Conv2D) (100, 28, 28, 96) 76896 incep_3b_conv1_5[0][0] \n__________________________________________________________________________________________________\nincep_3b_proj (Conv2D) (100, 28, 28, 64) 16448 incep_3b_maxpool[0][0] \n__________________________________________________________________________________________________\nincep_3b_concat (Concatenate) (100, 28, 28, 480) 0 incep_3b_conv1[0][0] \n incep_3b_conv3[0][0] \n incep_3b_conv5[0][0] \n incep_3b_proj[0][0] \n__________________________________________________________________________________________________\nmax_pool_3 (MaxPooling2D) (100, 14, 14, 480) 0 incep_3b_concat[0][0] \n__________________________________________________________________________________________________\nincep_4a_conv1_3 (Conv2D) (100, 14, 14, 112) 53872 max_pool_3[0][0] \n__________________________________________________________________________________________________\nincep_4a_conv1_5 (Conv2D) (100, 14, 14, 24) 11544 max_pool_3[0][0] \n__________________________________________________________________________________________________\nincep_4a_maxpool (MaxPooling2D) (100, 14, 14, 480) 0 max_pool_3[0][0] \n__________________________________________________________________________________________________\nincep_4a_conv1 (Conv2D) (100, 14, 14, 192) 92352 max_pool_3[0][0] \n__________________________________________________________________________________________________\nincep_4a_conv3 (Conv2D) (100, 14, 14, 208) 209872 incep_4a_conv1_3[0][0] \n__________________________________________________________________________________________________\nincep_4a_conv5 (Conv2D) (100, 14, 14, 48) 28848 incep_4a_conv1_5[0][0] \n__________________________________________________________________________________________________\nincep_4a_proj (Conv2D) (100, 14, 14, 64) 30784 incep_4a_maxpool[0][0] \n__________________________________________________________________________________________________\nincep_4a_concat (Concatenate) (100, 14, 14, 512) 0 incep_4a_conv1[0][0] \n incep_4a_conv3[0][0] \n incep_4a_conv5[0][0] \n incep_4a_proj[0][0] \n__________________________________________________________________________________________________\nincep_4b_conv1_3 (Conv2D) (100, 14, 14, 96) 49248 incep_4a_concat[0][0] \n__________________________________________________________________________________________________\nincep_4b_conv1_5 (Conv2D) (100, 14, 14, 16) 8208 incep_4a_concat[0][0] \n__________________________________________________________________________________________________\nincep_4b_maxpool (MaxPooling2D) (100, 14, 14, 512) 0 incep_4a_concat[0][0] \n__________________________________________________________________________________________________\nincep_4b_conv1 (Conv2D) (100, 14, 14, 160) 82080 incep_4a_concat[0][0] \n__________________________________________________________________________________________________\nincep_4b_conv3 (Conv2D) (100, 14, 14, 224) 193760 incep_4b_conv1_3[0][0] \n__________________________________________________________________________________________________\nincep_4b_conv5 (Conv2D) (100, 14, 14, 64) 25664 incep_4b_conv1_5[0][0] \n__________________________________________________________________________________________________\nincep_4b_proj (Conv2D) (100, 14, 14, 64) 32832 incep_4b_maxpool[0][0] \n__________________________________________________________________________________________________\nincep_4b_concat (Concatenate) (100, 14, 14, 512) 0 incep_4b_conv1[0][0] \n incep_4b_conv3[0][0] \n incep_4b_conv5[0][0] \n incep_4b_proj[0][0] \n__________________________________________________________________________________________________\nincep_4c_conv1_3 (Conv2D) (100, 14, 14, 144) 73872 incep_4b_concat[0][0] \n__________________________________________________________________________________________________\nincep_4c_conv1_5 (Conv2D) (100, 14, 14, 32) 16416 incep_4b_concat[0][0] \n__________________________________________________________________________________________________\nincep_4c_maxpool (MaxPooling2D) (100, 14, 14, 512) 0 incep_4b_concat[0][0] \n__________________________________________________________________________________________________\nincep_4c_conv1 (Conv2D) (100, 14, 14, 128) 65664 incep_4b_concat[0][0] \n__________________________________________________________________________________________________\nincep_4c_conv3 (Conv2D) (100, 14, 14, 256) 332032 incep_4c_conv1_3[0][0] \n__________________________________________________________________________________________________\nincep_4c_conv5 (Conv2D) (100, 14, 14, 64) 51264 incep_4c_conv1_5[0][0] \n__________________________________________________________________________________________________\nincep_4c_proj (Conv2D) (100, 14, 14, 64) 32832 incep_4c_maxpool[0][0] \n__________________________________________________________________________________________________\nincep_4c_concat (Concatenate) (100, 14, 14, 512) 0 incep_4c_conv1[0][0] \n incep_4c_conv3[0][0] \n incep_4c_conv5[0][0] \n incep_4c_proj[0][0] \n__________________________________________________________________________________________________\nincep_4d_conv1_3 (Conv2D) (100, 14, 14, 128) 65664 incep_4c_concat[0][0] \n__________________________________________________________________________________________________\nincep_4d_conv1_5 (Conv2D) (100, 14, 14, 24) 12312 incep_4c_concat[0][0] \n__________________________________________________________________________________________________\nincep_4d_maxpool (MaxPooling2D) (100, 14, 14, 512) 0 incep_4c_concat[0][0] \n__________________________________________________________________________________________________\nincep_4d_conv1 (Conv2D) (100, 14, 14, 112) 57456 incep_4c_concat[0][0] \n__________________________________________________________________________________________________\nincep_4d_conv3 (Conv2D) (100, 14, 14, 288) 332064 incep_4d_conv1_3[0][0] \n__________________________________________________________________________________________________\nincep_4d_conv5 (Conv2D) (100, 14, 14, 64) 38464 incep_4d_conv1_5[0][0] \n__________________________________________________________________________________________________\nincep_4d_proj (Conv2D) (100, 14, 14, 64) 32832 incep_4d_maxpool[0][0] \n__________________________________________________________________________________________________\nincep_4d_concat (Concatenate) (100, 14, 14, 528) 0 incep_4d_conv1[0][0] \n incep_4d_conv3[0][0] \n incep_4d_conv5[0][0] \n incep_4d_proj[0][0] \n__________________________________________________________________________________________________\nincep_4e_conv1_3 (Conv2D) (100, 14, 14, 160) 84640 incep_4d_concat[0][0] \n__________________________________________________________________________________________________\nincep_4e_conv1_5 (Conv2D) (100, 14, 14, 32) 16928 incep_4d_concat[0][0] \n__________________________________________________________________________________________________\nincep_4e_maxpool (MaxPooling2D) (100, 14, 14, 528) 0 incep_4d_concat[0][0] \n__________________________________________________________________________________________________\nincep_4e_conv1 (Conv2D) (100, 14, 14, 256) 135424 incep_4d_concat[0][0] \n__________________________________________________________________________________________________\nincep_4e_conv3 (Conv2D) (100, 14, 14, 320) 461120 incep_4e_conv1_3[0][0] \n__________________________________________________________________________________________________\nincep_4e_conv5 (Conv2D) (100, 14, 14, 128) 102528 incep_4e_conv1_5[0][0] \n__________________________________________________________________________________________________\nincep_4e_proj (Conv2D) (100, 14, 14, 128) 67712 incep_4e_maxpool[0][0] \n__________________________________________________________________________________________________\nincep_4e_concat (Concatenate) (100, 14, 14, 832) 0 incep_4e_conv1[0][0] \n incep_4e_conv3[0][0] \n incep_4e_conv5[0][0] \n incep_4e_proj[0][0] \n__________________________________________________________________________________________________\nmax_pool_4 (MaxPooling2D) (100, 7, 7, 832) 0 incep_4e_concat[0][0] \n__________________________________________________________________________________________________\nincep_5a_conv1_3 (Conv2D) (100, 7, 7, 160) 133280 max_pool_4[0][0] \n__________________________________________________________________________________________________\nincep_5a_conv1_5 (Conv2D) (100, 7, 7, 32) 26656 max_pool_4[0][0] \n__________________________________________________________________________________________________\nincep_5a_maxpool (MaxPooling2D) (100, 7, 7, 832) 0 max_pool_4[0][0] \n__________________________________________________________________________________________________\nincep_5a_conv1 (Conv2D) (100, 7, 7, 256) 213248 max_pool_4[0][0] \n__________________________________________________________________________________________________\nincep_5a_conv3 (Conv2D) (100, 7, 7, 320) 461120 incep_5a_conv1_3[0][0] \n__________________________________________________________________________________________________\nincep_5a_conv5 (Conv2D) (100, 7, 7, 128) 102528 incep_5a_conv1_5[0][0] \n__________________________________________________________________________________________________\nincep_5a_proj (Conv2D) (100, 7, 7, 128) 106624 incep_5a_maxpool[0][0] \n__________________________________________________________________________________________________\nincep_5a_concat (Concatenate) (100, 7, 7, 832) 0 incep_5a_conv1[0][0] \n incep_5a_conv3[0][0] \n incep_5a_conv5[0][0] \n incep_5a_proj[0][0] \n__________________________________________________________________________________________________\nincep_5b_conv1_3 (Conv2D) (100, 7, 7, 192) 159936 incep_5a_concat[0][0] \n__________________________________________________________________________________________________\nincep_5b_conv1_5 (Conv2D) (100, 7, 7, 48) 39984 incep_5a_concat[0][0] \n__________________________________________________________________________________________________\nincep_5b_maxpool (MaxPooling2D) (100, 7, 7, 832) 0 incep_5a_concat[0][0] \n__________________________________________________________________________________________________\nincep_5b_conv1 (Conv2D) (100, 7, 7, 384) 319872 incep_5a_concat[0][0] \n__________________________________________________________________________________________________\nincep_5b_conv3 (Conv2D) (100, 7, 7, 384) 663936 incep_5b_conv1_3[0][0] \n__________________________________________________________________________________________________\nincep_5b_conv5 (Conv2D) (100, 7, 7, 128) 153728 incep_5b_conv1_5[0][0] \n__________________________________________________________________________________________________\nincep_5b_proj (Conv2D) (100, 7, 7, 128) 106624 incep_5b_maxpool[0][0] \n__________________________________________________________________________________________________\nincep_5b_concat (Concatenate) (100, 7, 7, 1024) 0 incep_5b_conv1[0][0] \n incep_5b_conv3[0][0] \n incep_5b_conv5[0][0] \n incep_5b_proj[0][0] \n__________________________________________________________________________________________________\navg_pool (AveragePooling2D) (100, 1, 1, 1024) 0 incep_5b_concat[0][0] \n__________________________________________________________________________________________________\ndropout (Dropout) (100, 1, 1, 1024) 0 avg_pool[0][0] \n__________________________________________________________________________________________________\nflatten (Flatten) (100, 1024) 0 dropout[0][0] \n__________________________________________________________________________________________________\nmain_output (Dense) (100, 5) 5125 flatten[0][0] \n==================================================================================================\nTotal params: 5,968,053\nTrainable params: 5,967,925\nNon-trainable params: 128\n__________________________________________________________________________________________________\n"
]
],
[
[
"### Model Figure",
"_____no_output_____"
]
],
[
[
"tf.keras.utils.plot_model(model)",
"_____no_output_____"
]
],
[
[
"# Define Callbacks & Optimizer",
"_____no_output_____"
],
[
"## Learning Rate Modification",
"_____no_output_____"
]
],
[
[
"def lr_schedule(epoch, learning_rate):\n #The paper talks about reducing the learning rate by 4% every 8 epochs\n\n #Checking if 8 epochs are complete\n if epoch > 7 and epoch%8 == 0 :\n # Reducing the learning rate by 4% \n #print(\"lr_schedule: epoch =\",epoch)\n return learning_rate* 0.96\n else:\n return learning_rate\n\nlrScheduler = tf.keras.callbacks.LearningRateScheduler(schedule=lr_schedule, verbose=1)",
"_____no_output_____"
]
],
[
[
"## Checkpoint Definition",
"_____no_output_____"
]
],
[
[
"checkpoint = tf.keras.callbacks.ModelCheckpoint(filepath = checkpoint_filePath\n , monitor='val_loss'\n , verbose = 1\n , save_best_only = True\n , save_weights_only = False\n )\n",
"_____no_output_____"
]
],
[
[
"## EarlyStopping Definition",
"_____no_output_____"
]
],
[
[
"earlyStopper = tf.keras.callbacks.EarlyStopping(monitor='val_loss'\n , min_delta = 0.0001\n , patience = 9\n , verbose=1\n , restore_best_weights=True\n )",
"_____no_output_____"
]
],
[
[
"## Define Callbacks list",
"_____no_output_____"
]
],
[
[
"callbacks = [earlyStopper\n , checkpoint\n , lrScheduler]",
"_____no_output_____"
]
],
[
[
"## Define Optimizer",
"_____no_output_____"
]
],
[
[
"# The paper calls for an SGD with momentum of 0.9\noptimizer = tf.keras.optimizers.SGD(learning_rate=1e-3, momentum=0.9)\n\n#optimizer = tf.keras.optimizers.Adam(learning_rate=1e-5)",
"_____no_output_____"
]
],
[
[
"# Compile the Model",
"_____no_output_____"
]
],
[
[
"#First phase of training will be with aux1 branch as output and ignoring the rest of the model\n\n#aux1_model = Model(inputs=model.get_layer('main_input').input, outputs=model.get_layer('aux1_output').output)\n#aux1_model.summary()",
"_____no_output_____"
],
[
"#aux1_model.reset_states()",
"_____no_output_____"
],
[
"model.compile(optimizer = optimizer \n , loss = 'categorical_crossentropy'\n , metrics = [ 'accuracy']\n )",
"_____no_output_____"
]
],
[
[
"# Train the Model",
"_____no_output_____"
]
],
[
[
"metrics = model.fit(training_datasource\n , batch_size = batch_size\n , epochs= 50\n , callbacks = callbacks\n , validation_data = validation_datasource\n , shuffle=True\n )",
"Epoch 1/50\n\nEpoch 00001: LearningRateScheduler reducing learning rate to 0.0010000000474974513.\n54/54 [==============================] - 59s 1s/step - loss: 1.5524 - accuracy: 0.2978 - val_loss: 1.7891 - val_accuracy: 0.2367\n\nEpoch 00001: val_loss improved from inf to 1.78906, saving model to /content/drive/MyDrive/MachineLearning/GoogleNet_experiment_1.h5\nEpoch 2/50\n\nEpoch 00002: LearningRateScheduler reducing learning rate to 0.0010000000474974513.\n54/54 [==============================] - 56s 1s/step - loss: 1.2865 - accuracy: 0.4633 - val_loss: 2.0464 - val_accuracy: 0.2450\n\nEpoch 00002: val_loss did not improve from 1.78906\nEpoch 3/50\n\nEpoch 00003: LearningRateScheduler reducing learning rate to 0.0010000000474974513.\n54/54 [==============================] - 56s 1s/step - loss: 1.1377 - accuracy: 0.5387 - val_loss: 1.8578 - val_accuracy: 0.2950\n\nEpoch 00003: val_loss did not improve from 1.78906\nEpoch 4/50\n\nEpoch 00004: LearningRateScheduler reducing learning rate to 0.0010000000474974513.\n54/54 [==============================] - 55s 1s/step - loss: 1.0937 - accuracy: 0.5624 - val_loss: 1.8101 - val_accuracy: 0.3300\n\nEpoch 00004: val_loss did not improve from 1.78906\nEpoch 5/50\n\nEpoch 00005: LearningRateScheduler reducing learning rate to 0.0010000000474974513.\n54/54 [==============================] - 55s 1s/step - loss: 1.0085 - accuracy: 0.5993 - val_loss: 1.7051 - val_accuracy: 0.3250\n\nEpoch 00005: val_loss improved from 1.78906 to 1.70514, saving model to /content/drive/MyDrive/MachineLearning/GoogleNet_experiment_1.h5\nEpoch 6/50\n\nEpoch 00006: LearningRateScheduler reducing learning rate to 0.0010000000474974513.\n54/54 [==============================] - 55s 1s/step - loss: 0.9287 - accuracy: 0.6359 - val_loss: 1.7607 - val_accuracy: 0.3233\n\nEpoch 00006: val_loss did not improve from 1.70514\nEpoch 7/50\n\nEpoch 00007: LearningRateScheduler reducing learning rate to 0.0010000000474974513.\n54/54 [==============================] - 55s 1s/step - loss: 0.8747 - accuracy: 0.6622 - val_loss: 1.6034 - val_accuracy: 0.3900\n\nEpoch 00007: val_loss improved from 1.70514 to 1.60342, saving model to /content/drive/MyDrive/MachineLearning/GoogleNet_experiment_1.h5\nEpoch 8/50\n\nEpoch 00008: LearningRateScheduler reducing learning rate to 0.0010000000474974513.\n54/54 [==============================] - 55s 1s/step - loss: 0.8411 - accuracy: 0.6706 - val_loss: 1.2310 - val_accuracy: 0.5550\n\nEpoch 00008: val_loss improved from 1.60342 to 1.23102, saving model to /content/drive/MyDrive/MachineLearning/GoogleNet_experiment_1.h5\nEpoch 9/50\n\nEpoch 00009: LearningRateScheduler reducing learning rate to 0.0009600000455975532.\n54/54 [==============================] - 55s 1s/step - loss: 0.8327 - accuracy: 0.6856 - val_loss: 1.0313 - val_accuracy: 0.5883\n\nEpoch 00009: val_loss improved from 1.23102 to 1.03132, saving model to /content/drive/MyDrive/MachineLearning/GoogleNet_experiment_1.h5\nEpoch 10/50\n\nEpoch 00010: LearningRateScheduler reducing learning rate to 0.0009600000339560211.\n54/54 [==============================] - 55s 1s/step - loss: 0.7771 - accuracy: 0.6974 - val_loss: 1.1018 - val_accuracy: 0.5833\n\nEpoch 00010: val_loss did not improve from 1.03132\nEpoch 11/50\n\nEpoch 00011: LearningRateScheduler reducing learning rate to 0.0009600000339560211.\n54/54 [==============================] - 55s 1s/step - loss: 0.7932 - accuracy: 0.6928 - val_loss: 0.9002 - val_accuracy: 0.6583\n\nEpoch 00011: val_loss improved from 1.03132 to 0.90021, saving model to /content/drive/MyDrive/MachineLearning/GoogleNet_experiment_1.h5\nEpoch 12/50\n\nEpoch 00012: LearningRateScheduler reducing learning rate to 0.0009600000339560211.\n54/54 [==============================] - 55s 1s/step - loss: 0.7454 - accuracy: 0.7152 - val_loss: 0.8373 - val_accuracy: 0.6500\n\nEpoch 00012: val_loss improved from 0.90021 to 0.83734, saving model to /content/drive/MyDrive/MachineLearning/GoogleNet_experiment_1.h5\nEpoch 13/50\n\nEpoch 00013: LearningRateScheduler reducing learning rate to 0.0009600000339560211.\n54/54 [==============================] - 55s 1s/step - loss: 0.7067 - accuracy: 0.7320 - val_loss: 0.8740 - val_accuracy: 0.6683\n\nEpoch 00013: val_loss did not improve from 0.83734\nEpoch 14/50\n\nEpoch 00014: LearningRateScheduler reducing learning rate to 0.0009600000339560211.\n54/54 [==============================] - 55s 1s/step - loss: 0.6830 - accuracy: 0.7398 - val_loss: 0.9047 - val_accuracy: 0.6600\n\nEpoch 00014: val_loss did not improve from 0.83734\nEpoch 15/50\n\nEpoch 00015: LearningRateScheduler reducing learning rate to 0.0009600000339560211.\n54/54 [==============================] - 55s 1s/step - loss: 0.6419 - accuracy: 0.7602 - val_loss: 0.8095 - val_accuracy: 0.6733\n\nEpoch 00015: val_loss improved from 0.83734 to 0.80948, saving model to /content/drive/MyDrive/MachineLearning/GoogleNet_experiment_1.h5\nEpoch 16/50\n\nEpoch 00016: LearningRateScheduler reducing learning rate to 0.0009600000339560211.\n54/54 [==============================] - 55s 1s/step - loss: 0.6372 - accuracy: 0.7576 - val_loss: 0.7508 - val_accuracy: 0.7100\n\nEpoch 00016: val_loss improved from 0.80948 to 0.75078, saving model to /content/drive/MyDrive/MachineLearning/GoogleNet_experiment_1.h5\nEpoch 17/50\n\nEpoch 00017: LearningRateScheduler reducing learning rate to 0.0009216000325977802.\n54/54 [==============================] - 55s 1s/step - loss: 0.6198 - accuracy: 0.7689 - val_loss: 0.8647 - val_accuracy: 0.6667\n\nEpoch 00017: val_loss did not improve from 0.75078\nEpoch 18/50\n\nEpoch 00018: LearningRateScheduler reducing learning rate to 0.0009216000325977802.\n54/54 [==============================] - 55s 1s/step - loss: 0.6092 - accuracy: 0.7615 - val_loss: 0.7914 - val_accuracy: 0.6800\n\nEpoch 00018: val_loss did not improve from 0.75078\nEpoch 19/50\n\nEpoch 00019: LearningRateScheduler reducing learning rate to 0.0009216000325977802.\n54/54 [==============================] - 55s 1s/step - loss: 0.5890 - accuracy: 0.7772 - val_loss: 0.7472 - val_accuracy: 0.7100\n\nEpoch 00019: val_loss improved from 0.75078 to 0.74724, saving model to /content/drive/MyDrive/MachineLearning/GoogleNet_experiment_1.h5\nEpoch 20/50\n\nEpoch 00020: LearningRateScheduler reducing learning rate to 0.0009216000325977802.\n54/54 [==============================] - 55s 1s/step - loss: 0.5666 - accuracy: 0.7867 - val_loss: 0.6939 - val_accuracy: 0.7383\n\nEpoch 00020: val_loss improved from 0.74724 to 0.69395, saving model to /content/drive/MyDrive/MachineLearning/GoogleNet_experiment_1.h5\nEpoch 21/50\n\nEpoch 00021: LearningRateScheduler reducing learning rate to 0.0009216000325977802.\n54/54 [==============================] - 55s 1s/step - loss: 0.5714 - accuracy: 0.7850 - val_loss: 0.7959 - val_accuracy: 0.7050\n\nEpoch 00021: val_loss did not improve from 0.69395\nEpoch 22/50\n\nEpoch 00022: LearningRateScheduler reducing learning rate to 0.0009216000325977802.\n54/54 [==============================] - 55s 1s/step - loss: 0.5251 - accuracy: 0.8037 - val_loss: 0.7059 - val_accuracy: 0.7167\n\nEpoch 00022: val_loss did not improve from 0.69395\nEpoch 23/50\n\nEpoch 00023: LearningRateScheduler reducing learning rate to 0.0009216000325977802.\n54/54 [==============================] - 55s 1s/step - loss: 0.5034 - accuracy: 0.8165 - val_loss: 0.7626 - val_accuracy: 0.7133\n\nEpoch 00023: val_loss did not improve from 0.69395\nEpoch 24/50\n\nEpoch 00024: LearningRateScheduler reducing learning rate to 0.0009216000325977802.\n54/54 [==============================] - 55s 1s/step - loss: 0.5274 - accuracy: 0.7996 - val_loss: 0.8329 - val_accuracy: 0.6783\n\nEpoch 00024: val_loss did not improve from 0.69395\nEpoch 25/50\n\nEpoch 00025: LearningRateScheduler reducing learning rate to 0.000884736031293869.\n54/54 [==============================] - 55s 1s/step - loss: 0.4699 - accuracy: 0.8252 - val_loss: 0.6859 - val_accuracy: 0.7450\n\nEpoch 00025: val_loss improved from 0.69395 to 0.68586, saving model to /content/drive/MyDrive/MachineLearning/GoogleNet_experiment_1.h5\nEpoch 26/50\n\nEpoch 00026: LearningRateScheduler reducing learning rate to 0.000884736014995724.\n54/54 [==============================] - 55s 1s/step - loss: 0.4831 - accuracy: 0.8198 - val_loss: 0.7259 - val_accuracy: 0.7333\n\nEpoch 00026: val_loss did not improve from 0.68586\nEpoch 27/50\n\nEpoch 00027: LearningRateScheduler reducing learning rate to 0.000884736014995724.\n54/54 [==============================] - 55s 1s/step - loss: 0.4490 - accuracy: 0.8376 - val_loss: 0.7365 - val_accuracy: 0.7333\n\nEpoch 00027: val_loss did not improve from 0.68586\nEpoch 28/50\n\nEpoch 00028: LearningRateScheduler reducing learning rate to 0.000884736014995724.\n54/54 [==============================] - 55s 1s/step - loss: 0.4561 - accuracy: 0.8300 - val_loss: 0.7072 - val_accuracy: 0.7300\n\nEpoch 00028: val_loss did not improve from 0.68586\nEpoch 29/50\n\nEpoch 00029: LearningRateScheduler reducing learning rate to 0.000884736014995724.\n54/54 [==============================] - 55s 1s/step - loss: 0.4033 - accuracy: 0.8533 - val_loss: 0.6848 - val_accuracy: 0.7367\n\nEpoch 00029: val_loss improved from 0.68586 to 0.68476, saving model to /content/drive/MyDrive/MachineLearning/GoogleNet_experiment_1.h5\nEpoch 30/50\n\nEpoch 00030: LearningRateScheduler reducing learning rate to 0.000884736014995724.\n54/54 [==============================] - 55s 1s/step - loss: 0.4265 - accuracy: 0.8359 - val_loss: 0.7077 - val_accuracy: 0.7317\n\nEpoch 00030: val_loss did not improve from 0.68476\nEpoch 31/50\n\nEpoch 00031: LearningRateScheduler reducing learning rate to 0.000884736014995724.\n54/54 [==============================] - 56s 1s/step - loss: 0.3834 - accuracy: 0.8644 - val_loss: 0.6988 - val_accuracy: 0.7600\n\nEpoch 00031: val_loss did not improve from 0.68476\nEpoch 32/50\n\nEpoch 00032: LearningRateScheduler reducing learning rate to 0.000884736014995724.\n54/54 [==============================] - 55s 1s/step - loss: 0.3753 - accuracy: 0.8589 - val_loss: 0.7035 - val_accuracy: 0.7417\n\nEpoch 00032: val_loss did not improve from 0.68476\nEpoch 33/50\n\nEpoch 00033: LearningRateScheduler reducing learning rate to 0.000849346574395895.\n54/54 [==============================] - 55s 1s/step - loss: 0.3807 - accuracy: 0.8585 - val_loss: 0.7117 - val_accuracy: 0.7267\n\nEpoch 00033: val_loss did not improve from 0.68476\nEpoch 34/50\n\nEpoch 00034: LearningRateScheduler reducing learning rate to 0.0008493465720675886.\n54/54 [==============================] - 55s 1s/step - loss: 0.3670 - accuracy: 0.8620 - val_loss: 0.7594 - val_accuracy: 0.7300\n\nEpoch 00034: val_loss did not improve from 0.68476\nEpoch 35/50\n\nEpoch 00035: LearningRateScheduler reducing learning rate to 0.0008493465720675886.\n54/54 [==============================] - 55s 1s/step - loss: 0.3761 - accuracy: 0.8570 - val_loss: 0.6519 - val_accuracy: 0.7683\n\nEpoch 00035: val_loss improved from 0.68476 to 0.65189, saving model to /content/drive/MyDrive/MachineLearning/GoogleNet_experiment_1.h5\nEpoch 36/50\n\nEpoch 00036: LearningRateScheduler reducing learning rate to 0.0008493465720675886.\n54/54 [==============================] - 55s 1s/step - loss: 0.3555 - accuracy: 0.8670 - val_loss: 0.6918 - val_accuracy: 0.7650\n\nEpoch 00036: val_loss did not improve from 0.65189\nEpoch 37/50\n\nEpoch 00037: LearningRateScheduler reducing learning rate to 0.0008493465720675886.\n54/54 [==============================] - 55s 1s/step - loss: 0.3167 - accuracy: 0.8891 - val_loss: 0.6478 - val_accuracy: 0.7833\n\nEpoch 00037: val_loss improved from 0.65189 to 0.64783, saving model to /content/drive/MyDrive/MachineLearning/GoogleNet_experiment_1.h5\nEpoch 38/50\n\nEpoch 00038: LearningRateScheduler reducing learning rate to 0.0008493465720675886.\n54/54 [==============================] - 55s 1s/step - loss: 0.3149 - accuracy: 0.8850 - val_loss: 0.5957 - val_accuracy: 0.7900\n\nEpoch 00038: val_loss improved from 0.64783 to 0.59568, saving model to /content/drive/MyDrive/MachineLearning/GoogleNet_experiment_1.h5\nEpoch 39/50\n\nEpoch 00039: LearningRateScheduler reducing learning rate to 0.0008493465720675886.\n54/54 [==============================] - 55s 1s/step - loss: 0.2920 - accuracy: 0.8939 - val_loss: 0.6199 - val_accuracy: 0.7800\n\nEpoch 00039: val_loss did not improve from 0.59568\nEpoch 40/50\n\nEpoch 00040: LearningRateScheduler reducing learning rate to 0.0008493465720675886.\n54/54 [==============================] - 55s 1s/step - loss: 0.2541 - accuracy: 0.9094 - val_loss: 0.6565 - val_accuracy: 0.7650\n\nEpoch 00040: val_loss did not improve from 0.59568\nEpoch 41/50\n\nEpoch 00041: LearningRateScheduler reducing learning rate to 0.0008153727091848849.\n54/54 [==============================] - 55s 1s/step - loss: 0.2474 - accuracy: 0.9124 - val_loss: 0.6770 - val_accuracy: 0.7833\n\nEpoch 00041: val_loss did not improve from 0.59568\nEpoch 42/50\n\nEpoch 00042: LearningRateScheduler reducing learning rate to 0.0008153726812452078.\n54/54 [==============================] - 55s 1s/step - loss: 0.2561 - accuracy: 0.9059 - val_loss: 0.6824 - val_accuracy: 0.7483\n\nEpoch 00042: val_loss did not improve from 0.59568\nEpoch 43/50\n\nEpoch 00043: LearningRateScheduler reducing learning rate to 0.0008153726812452078.\n54/54 [==============================] - 55s 1s/step - loss: 0.2600 - accuracy: 0.9052 - val_loss: 0.6757 - val_accuracy: 0.7500\n\nEpoch 00043: val_loss did not improve from 0.59568\nEpoch 44/50\n\nEpoch 00044: LearningRateScheduler reducing learning rate to 0.0008153726812452078.\n54/54 [==============================] - 55s 1s/step - loss: 0.2710 - accuracy: 0.8970 - val_loss: 0.6145 - val_accuracy: 0.7833\n\nEpoch 00044: val_loss did not improve from 0.59568\nEpoch 45/50\n\nEpoch 00045: LearningRateScheduler reducing learning rate to 0.0008153726812452078.\n54/54 [==============================] - 55s 1s/step - loss: 0.1842 - accuracy: 0.9389 - val_loss: 0.5722 - val_accuracy: 0.8000\n\nEpoch 00045: val_loss improved from 0.59568 to 0.57216, saving model to /content/drive/MyDrive/MachineLearning/GoogleNet_experiment_1.h5\nEpoch 46/50\n\nEpoch 00046: LearningRateScheduler reducing learning rate to 0.0008153726812452078.\n54/54 [==============================] - 55s 1s/step - loss: 0.1756 - accuracy: 0.9417 - val_loss: 0.8662 - val_accuracy: 0.7117\n\nEpoch 00046: val_loss did not improve from 0.57216\nEpoch 47/50\n\nEpoch 00047: LearningRateScheduler reducing learning rate to 0.0008153726812452078.\n54/54 [==============================] - 55s 1s/step - loss: 0.2120 - accuracy: 0.9243 - val_loss: 0.6953 - val_accuracy: 0.7583\n\nEpoch 00047: val_loss did not improve from 0.57216\nEpoch 48/50\n\nEpoch 00048: LearningRateScheduler reducing learning rate to 0.0008153726812452078.\n54/54 [==============================] - 55s 1s/step - loss: 0.2295 - accuracy: 0.9150 - val_loss: 0.7475 - val_accuracy: 0.7767\n\nEpoch 00048: val_loss did not improve from 0.57216\nEpoch 49/50\n\nEpoch 00049: LearningRateScheduler reducing learning rate to 0.0007827577739953995.\n54/54 [==============================] - 55s 1s/step - loss: 0.1823 - accuracy: 0.9372 - val_loss: 0.6702 - val_accuracy: 0.7733\n\nEpoch 00049: val_loss did not improve from 0.57216\nEpoch 50/50\n\nEpoch 00050: LearningRateScheduler reducing learning rate to 0.0007827577646821737.\n54/54 [==============================] - 55s 1s/step - loss: 0.1688 - accuracy: 0.9398 - val_loss: 0.6562 - val_accuracy: 0.7867\n\nEpoch 00050: val_loss did not improve from 0.57216\n"
],
[
"#tf.keras.models.save_model(model, checkpoint_filePath, save_format='h5')",
"_____no_output_____"
]
],
[
[
"## Loss and Accuracy Plots",
"_____no_output_____"
]
],
[
[
"\nacc = metrics.history['accuracy']\nval_acc = metrics.history['val_accuracy']\n\nloss = metrics.history['loss']\nval_loss = metrics.history['val_loss']\n\nepochs_range = range(len(metrics.history['accuracy']))\n\nplt.figure(figsize=(8, 8))\nplt.subplot(1, 2, 1)\nplt.plot(epochs_range, acc, label='Training Accuracy')\nplt.plot(epochs_range, val_acc, label='Validation Accuracy')\nplt.legend(loc='lower right')\nplt.title('Training and Validation Accuracy')\n\nplt.subplot(1, 2, 2)\nplt.plot(epochs_range, loss, label='Training Loss')\nplt.plot(epochs_range, val_loss, label='Validation Loss')\nplt.legend(loc='upper right')\nplt.title('Training and Validation Loss')\nplt.show()",
"_____no_output_____"
]
],
[
[
"# Test the Model",
"_____no_output_____"
]
],
[
[
"predictions = []\nactuals=[]\n\nfor i, (images, labels) in enumerate( test_datasource):\n pred = model(images)\n for j in range(len(labels)):\n actuals.append( labels[j])\n predictions.append(pred[j])\n\n# Printing a few labels and predictions to ensure that there are no dead-Relus\nfor j in range(10):\n print(labels[j].numpy(), \"\\t\", pred[j].numpy())",
"[0. 0. 0. 1. 0.] \t [5.2678269e-01 4.5223912e-04 1.5960732e-01 3.1243265e-01 7.2512066e-04]\n[1. 0. 0. 0. 0.] \t [9.3688345e-01 3.9315761e-05 4.2963952e-02 2.0080591e-02 3.2674830e-05]\n[1. 0. 0. 0. 0.] \t [5.6217247e-01 3.2935925e-05 6.1456640e-03 4.3134734e-01 3.0162817e-04]\n[0. 1. 0. 0. 0.] \t [2.6929042e-08 9.9104989e-01 8.4609836e-03 1.1975218e-05 4.7719607e-04]\n[0. 0. 0. 1. 0.] \t [1.1177908e-04 2.9847620e-09 7.3413408e-05 9.9979931e-01 1.5532069e-05]\n[1. 0. 0. 0. 0.] \t [6.4884788e-01 1.4298371e-07 3.5084713e-01 3.0439568e-04 4.9051403e-07]\n[1. 0. 0. 0. 0.] \t [9.9992180e-01 4.6575969e-06 3.7354968e-07 7.2269759e-05 9.6628423e-07]\n[0. 0. 0. 1. 0.] \t [2.2897586e-01 2.6874379e-03 5.8660603e-01 1.8155751e-01 1.7324543e-04]\n[0. 0. 0. 1. 0.] \t [0.4059488 0.00825622 0.0023726 0.5712473 0.01217511]\n[0. 0. 0. 1. 0.] \t [0.2535905 0.12598905 0.01019515 0.60805327 0.002172 ]\n"
]
],
[
[
"Confusion Matrix",
"_____no_output_____"
]
],
[
[
"import pandas as pd\npd.DataFrame(tf.math.confusion_matrix(\n np.argmax(actuals, axis=1), np.argmax(predictions, axis=1), num_classes=num_classes, dtype=tf.dtypes.int32).numpy()\n , columns = test_image_dataset.class_names\n , index = test_image_dataset.class_names)",
"_____no_output_____"
],
[
"",
"_____no_output_____"
]
]
] | [
"markdown",
"code",
"markdown",
"code",
"markdown",
"code",
"markdown",
"code",
"markdown",
"code",
"markdown",
"code",
"markdown",
"code",
"markdown",
"code",
"markdown",
"code",
"markdown",
"code",
"markdown",
"code",
"markdown",
"code",
"markdown",
"code",
"markdown",
"code",
"markdown",
"code",
"markdown",
"code",
"markdown",
"code",
"markdown",
"code",
"markdown",
"code"
] | [
[
"markdown"
],
[
"code"
],
[
"markdown"
],
[
"code",
"code",
"code"
],
[
"markdown",
"markdown"
],
[
"code"
],
[
"markdown"
],
[
"code",
"code"
],
[
"markdown"
],
[
"code"
],
[
"markdown",
"markdown"
],
[
"code"
],
[
"markdown"
],
[
"code"
],
[
"markdown"
],
[
"code",
"code"
],
[
"markdown"
],
[
"code"
],
[
"markdown",
"markdown"
],
[
"code"
],
[
"markdown"
],
[
"code"
],
[
"markdown"
],
[
"code"
],
[
"markdown"
],
[
"code"
],
[
"markdown"
],
[
"code"
],
[
"markdown"
],
[
"code",
"code",
"code"
],
[
"markdown"
],
[
"code",
"code"
],
[
"markdown"
],
[
"code"
],
[
"markdown"
],
[
"code"
],
[
"markdown"
],
[
"code",
"code"
]
] |
e780f728bc25ee7775ea91141167a1dc7b6d147f | 62,866 | ipynb | Jupyter Notebook | .ipynb_checkpoints/Vereadores RJ 2016 - Votos por Zona-checkpoint.ipynb | vinacius/vereadores_porZona_RJ | b8c9a387273a4c4fe5baea93e1ed2e0133e2989e | [
"MIT"
] | 1 | 2021-09-18T04:10:30.000Z | 2021-09-18T04:10:30.000Z | .ipynb_checkpoints/Vereadores RJ 2016 - Votos por Zona-checkpoint.ipynb | vinacius/vereadores_porZona_RJ | b8c9a387273a4c4fe5baea93e1ed2e0133e2989e | [
"MIT"
] | null | null | null | .ipynb_checkpoints/Vereadores RJ 2016 - Votos por Zona-checkpoint.ipynb | vinacius/vereadores_porZona_RJ | b8c9a387273a4c4fe5baea93e1ed2e0133e2989e | [
"MIT"
] | null | null | null | 124.487129 | 30,968 | 0.833153 | [
[
[
"# Importa as bibliotecas necessárias\n\nimport csv\nimport pandas as pd\nimport os\nimport matplotlib.pyplot as plt\nfrom matplotlib.widgets import Button\nfrom matplotlib.text import Annotation\nimport seaborn as sns\n\n%matplotlib inline",
"_____no_output_____"
],
[
"# Abre o novo arquivo e cria um Dataframe\nver_rj = pd.read_csv(\"output/votacao_filtro_munzona_2016_RJ.csv\", encoding=\"latin-1\")\nvr = pd.DataFrame(ver_rj)",
"_____no_output_____"
],
[
"vr.head()",
"_____no_output_____"
],
[
"# Filtro para separar veradores eleitos e não eleitos.\n\n# Listas que guardam as variáveis de cada tipo de situação eleitoral\nvar_eleitos = ['ELEITO POR MÉDIA', 'ELEITO POR QP']\nvar_nao_eleitos = ['NÃO ELEITO', 'SUPLENTE']\n\n# Resultado booleano gerado analisando se os dados das listas estão\n# ou não nas linhas do Dataframe\nif_eleitos = vr.DS_SIT_TOT_TURNO.isin(var_eleitos)\nif_nao_eleitos = vr.DS_SIT_TOT_TURNO.isin(var_nao_eleitos)\n\n#Variáveis para os filtros no Dataframe\neleitos = vr[if_eleitos]\nnao_eleitos = vr[if_nao_eleitos]\n\neleitos.head(1)",
"_____no_output_____"
],
[
"vr.DS_SIT_TOT_TURNO.isin(var_eleitos)\n\nmq = vr['QT_VOTOS_NOMINAIS']>=7000\n\nvr[mq]",
"_____no_output_____"
],
[
"plt.scatter(eleitos.QT_VOTOS_NOMINAIS, eleitos.NR_ZONA,\n c='#06975e', edgecolors='#FFFFFF')\nplt.xlabel('Votos')\nplt.ylabel('Zonas Eleitorais')\nplt.title('Votos por Zona - Eleitos')",
"_____no_output_____"
],
[
"plt.scatter(nao_eleitos.QT_VOTOS_NOMINAIS, nao_eleitos.NR_ZONA)\nplt.xlabel('Votos')\nplt.ylabel('Zonas Eleitorais')\nplt.title('Votos por Zona - Geral')\n\nvr.count()",
"_____no_output_____"
]
]
] | [
"code"
] | [
[
"code",
"code",
"code",
"code",
"code",
"code",
"code"
]
] |
e781095bb1ecfbc32cd86de872e6c4dedf7357b5 | 59,545 | ipynb | Jupyter Notebook | 4 - Neural Networks/1. Introduction To Neural Nets/1. Gradient Descent/GradientDescent.ipynb | 2series/Artificial-Intelligence | 027951105271886cf29ee9878bac695eadc9742c | [
"MIT"
] | null | null | null | 4 - Neural Networks/1. Introduction To Neural Nets/1. Gradient Descent/GradientDescent.ipynb | 2series/Artificial-Intelligence | 027951105271886cf29ee9878bac695eadc9742c | [
"MIT"
] | null | null | null | 4 - Neural Networks/1. Introduction To Neural Nets/1. Gradient Descent/GradientDescent.ipynb | 2series/Artificial-Intelligence | 027951105271886cf29ee9878bac695eadc9742c | [
"MIT"
] | null | null | null | 139.776995 | 19,684 | 0.874213 | [
[
[
"# Implementing the Gradient Descent Algorithm\n\nIn this lab, we'll implement the basic functions of the Gradient Descent algorithm to find the boundary in a small dataset. First, we'll start with some functions that will help us plot and visualize the data.",
"_____no_output_____"
]
],
[
[
"import matplotlib.pyplot as plt\nimport numpy as np\nimport pandas as pd\n\n#Some helper functions for plotting and drawing lines\n\ndef plot_points(X, y):\n admitted = X[np.argwhere(y==1)]\n rejected = X[np.argwhere(y==0)]\n plt.scatter([s[0][0] for s in rejected], [s[0][1] for s in rejected], s = 25, color = 'blue', edgecolor = 'k')\n plt.scatter([s[0][0] for s in admitted], [s[0][1] for s in admitted], s = 25, color = 'red', edgecolor = 'k')\n\ndef display(m, b, color='g--'):\n plt.xlim(-0.05,1.05)\n plt.ylim(-0.05,1.05)\n x = np.arange(-10, 10, 0.1)\n plt.plot(x, m*x+b, color)",
"_____no_output_____"
]
],
[
[
"## Reading and plotting the data",
"_____no_output_____"
]
],
[
[
"data = pd.read_csv('data.csv', header=None)\nX = np.array(data[[0,1]])\ny = np.array(data[2])\nplot_points(X,y)\nplt.show()",
"_____no_output_____"
]
],
[
[
"## TODO: Implementing the basic functions\nHere is your turn to shine. Implement the following formulas, as explained in the text.\n- Sigmoid activation function\n\n$$\\sigma(x) = \\frac{1}{1+e^{-x}}$$\n\n- Output (prediction) formula\n\n$$\\hat{y} = \\sigma(w_1 x_1 + w_2 x_2 + b)$$\n\n- Error function\n\n$$Error(y, \\hat{y}) = - y \\log(\\hat{y}) - (1-y) \\log(1-\\hat{y})$$\n\n- The function that updates the weights\n\n$$ w_i \\longrightarrow w_i + \\alpha (y - \\hat{y}) x_i$$\n\n$$ b \\longrightarrow b + \\alpha (y - \\hat{y})$$",
"_____no_output_____"
]
],
[
[
"# Implement the following functions\n\n# Activation (sigmoid) function\ndef sigmoid(x):\n return (1 / (1 + np.exp(-x)))\n\n# Output (prediction) formula\ndef output_formula(features, weights, bias):\n return sigmoid(np.matmul(features, weights) + bias)\n \n\n# Error (log-loss) formula\ndef error_formula(y, output):\n return - y * np.log(output) - (1 - y) * np.log(1 - output)\n\n# Gradient descent step\ndef update_weights(x, y, weights, bias, learnrate):\n yhat = output_formula(x, weights, bias)\n weights = weights + learnrate * (y - yhat) * x\n bias = bias + learnrate * (y - yhat)\n return weights, bias",
"_____no_output_____"
]
],
[
[
"## Training function\nThis function will help us iterate the gradient descent algorithm through all the data, for a number of epochs. It will also plot the data, and some of the boundary lines obtained as we run the algorithm.",
"_____no_output_____"
]
],
[
[
"np.random.seed(44)\n\nepochs = 100\nlearnrate = 0.01\n\ndef train(features, targets, epochs, learnrate, graph_lines=False):\n \n errors = []\n n_records, n_features = features.shape\n last_loss = None\n weights = np.random.normal(scale=1 / n_features**.5, size=n_features)\n bias = 0\n for e in range(epochs):\n print(\"starting epoch:{}\".format(e))\n del_w = np.zeros(weights.shape)\n for x, y in zip(features, targets):\n output = output_formula(x, weights, bias)\n error = error_formula(y, output)\n weights, bias = update_weights(x, y, weights, bias, learnrate)\n \n # Printing out the log-loss error on the training set\n out = output_formula(features, weights, bias)\n loss = np.mean(error_formula(targets, out))\n errors.append(loss)\n if e % (epochs / 10) == 0:\n print(\"\\n========== Epoch\", e,\"==========\")\n if last_loss and last_loss < loss:\n print(\"Train loss: \", loss, \" WARNING - Loss Increasing\")\n else:\n print(\"Train loss: \", loss)\n last_loss = loss\n predictions = out > 0.5\n accuracy = np.mean(predictions == targets)\n print(\"Accuracy: \", accuracy)\n \n return weights, bias, errors\n\ndef train_plot(features,targets,weights,bias):\n # Plotting the solution boundary\n plt.title(\"Solution boundary\")\n display(-weights[0]/weights[1], -bias/weights[1], 'black')\n plot_points(features, targets)\n plt.show()\n\ndef train_err(errors):\n plt.title(\"Error Plot\")\n plt.xlabel('Number of epochs')\n plt.ylabel('Error')\n plt.plot(errors)\n plt.show()",
"_____no_output_____"
]
],
[
[
"## Time to train the algorithm!\nWhen we run the function, we'll obtain the following:\n- 10 updates with the current training loss and accuracy\n- A plot of the data and some of the boundary lines obtained. The final one is in black. Notice how the lines get closer and closer to the best fit, as we go through more epochs.\n- A plot of the error function. Notice how it decreases as we go through more epochs.",
"_____no_output_____"
]
],
[
[
"weights, bias, errors = train(X, y, epochs, learnrate, True)\n\n",
"starting epoch:0\n\n========== Epoch 0 ==========\nTrain loss: 0.713584519538\nAccuracy: 0.4\nstarting epoch:1\nstarting epoch:2\nstarting epoch:3\nstarting epoch:4\nstarting epoch:5\nstarting epoch:6\nstarting epoch:7\nstarting epoch:8\nstarting epoch:9\nstarting epoch:10\n\n========== Epoch 10 ==========\nTrain loss: 0.622583521045\nAccuracy: 0.59\nstarting epoch:11\nstarting epoch:12\nstarting epoch:13\nstarting epoch:14\nstarting epoch:15\nstarting epoch:16\nstarting epoch:17\nstarting epoch:18\nstarting epoch:19\nstarting epoch:20\n\n========== Epoch 20 ==========\nTrain loss: 0.554874408367\nAccuracy: 0.74\nstarting epoch:21\nstarting epoch:22\nstarting epoch:23\nstarting epoch:24\nstarting epoch:25\nstarting epoch:26\nstarting epoch:27\nstarting epoch:28\nstarting epoch:29\nstarting epoch:30\n\n========== Epoch 30 ==========\nTrain loss: 0.501606141872\nAccuracy: 0.84\nstarting epoch:31\nstarting epoch:32\nstarting epoch:33\nstarting epoch:34\nstarting epoch:35\nstarting epoch:36\nstarting epoch:37\nstarting epoch:38\nstarting epoch:39\nstarting epoch:40\n\n========== Epoch 40 ==========\nTrain loss: 0.459333464186\nAccuracy: 0.86\nstarting epoch:41\nstarting epoch:42\nstarting epoch:43\nstarting epoch:44\nstarting epoch:45\nstarting epoch:46\nstarting epoch:47\nstarting epoch:48\nstarting epoch:49\nstarting epoch:50\n\n========== Epoch 50 ==========\nTrain loss: 0.425255434335\nAccuracy: 0.93\nstarting epoch:51\nstarting epoch:52\nstarting epoch:53\nstarting epoch:54\nstarting epoch:55\nstarting epoch:56\nstarting epoch:57\nstarting epoch:58\nstarting epoch:59\nstarting epoch:60\n\n========== Epoch 60 ==========\nTrain loss: 0.397346157167\nAccuracy: 0.93\nstarting epoch:61\nstarting epoch:62\nstarting epoch:63\nstarting epoch:64\nstarting epoch:65\nstarting epoch:66\nstarting epoch:67\nstarting epoch:68\nstarting epoch:69\nstarting epoch:70\n\n========== Epoch 70 ==========\nTrain loss: 0.374146976524\nAccuracy: 0.93\nstarting epoch:71\nstarting epoch:72\nstarting epoch:73\nstarting epoch:74\nstarting epoch:75\nstarting epoch:76\nstarting epoch:77\nstarting epoch:78\nstarting epoch:79\nstarting epoch:80\n\n========== Epoch 80 ==========\nTrain loss: 0.354599733682\nAccuracy: 0.94\nstarting epoch:81\nstarting epoch:82\nstarting epoch:83\nstarting epoch:84\nstarting epoch:85\nstarting epoch:86\nstarting epoch:87\nstarting epoch:88\nstarting epoch:89\nstarting epoch:90\n\n========== Epoch 90 ==========\nTrain loss: 0.337927365888\nAccuracy: 0.94\nstarting epoch:91\nstarting epoch:92\nstarting epoch:93\nstarting epoch:94\nstarting epoch:95\nstarting epoch:96\nstarting epoch:97\nstarting epoch:98\nstarting epoch:99\n"
],
[
"train_plot(X, y, weights,bias)",
"_____no_output_____"
],
[
"train_err(errors)",
"_____no_output_____"
]
]
] | [
"markdown",
"code",
"markdown",
"code",
"markdown",
"code",
"markdown",
"code",
"markdown",
"code"
] | [
[
"markdown"
],
[
"code"
],
[
"markdown"
],
[
"code"
],
[
"markdown"
],
[
"code"
],
[
"markdown"
],
[
"code"
],
[
"markdown"
],
[
"code",
"code",
"code"
]
] |
e7810e53142c273e90de169f100508fff8e2d49a | 185,962 | ipynb | Jupyter Notebook | examples/lsc/pcqm4m/.ipynb_checkpoints/triplet-loss-checkpoint.ipynb | edwardelson/ogb | c783060c5ada3641c0f08527acd1d53626f9f9c9 | [
"MIT"
] | 2 | 2021-04-15T10:36:13.000Z | 2021-04-17T05:45:12.000Z | examples/lsc/pcqm4m/.ipynb_checkpoints/triplet-loss-checkpoint.ipynb | edwardelson/ogb | c783060c5ada3641c0f08527acd1d53626f9f9c9 | [
"MIT"
] | null | null | null | examples/lsc/pcqm4m/.ipynb_checkpoints/triplet-loss-checkpoint.ipynb | edwardelson/ogb | c783060c5ada3641c0f08527acd1d53626f9f9c9 | [
"MIT"
] | 1 | 2021-04-10T17:46:25.000Z | 2021-04-10T17:46:25.000Z | 129.952481 | 20,904 | 0.86807 | [
[
[
"import torch\nfrom torch_geometric.data import DataLoader\nimport torch.optim as optim\nimport torch.nn.functional as F\nfrom torch.utils.tensorboard import SummaryWriter\nfrom torch.optim.lr_scheduler import StepLR\n\nfrom gnn import GNN\n\nimport os\nfrom tqdm import tqdm\nimport argparse\nimport time\nimport numpy as np\nimport random\n\ntorch.cuda.is_available()",
"Using backend: pytorch\n"
]
],
[
[
"### hard-coded arguments\n\nexplain GCN model",
"_____no_output_____"
]
],
[
[
"# get args from main_gnn CLI\nclass Argument(object):\n name = \"args\"\n \nargs = Argument()\nargs.batch_size = 256\nargs.num_workers = 0\nargs.num_layers = 5\nargs.emb_dim = 600\nargs.drop_ratio = 0\nargs.graph_pooling = \"sum\"\nargs.checkpoint_dir = \"models/gin-virtual/checkpoint\"\nargs.device = 0",
"_____no_output_____"
],
[
"device = torch.device(\"cuda:\" + str(args.device)) if torch.cuda.is_available() else torch.device(\"cpu\")\n# device = \"cpu\"\ndevice",
"_____no_output_____"
],
[
"shared_params = {\n 'num_layers': args.num_layers,\n 'emb_dim': args.emb_dim,\n 'drop_ratio': args.drop_ratio,\n 'graph_pooling': args.graph_pooling\n}\n",
"_____no_output_____"
]
],
[
[
"### load model",
"_____no_output_____"
]
],
[
[
"from gnn import GNN",
"_____no_output_____"
],
[
"\"\"\"\nLOAD Checkpoint data\n\"\"\"\ncheckpoint = torch.load(os.path.join(args.checkpoint_dir, 'checkpoint.pt'))\ncheckpoint.keys()",
"_____no_output_____"
],
[
"gnn_name = \"gin-virtual\"\ngnn_type = \"gin\"\nvirtual_node = True",
"_____no_output_____"
],
[
"model = GNN(gnn_type = gnn_type, virtual_node = virtual_node, **shared_params).to(device)\nmodel.load_state_dict(checkpoint[\"model_state_dict\"])\nmodel.state_dict()\n\nmodel.eval()\n\ntype(model)",
"_____no_output_____"
],
[
"optimizer = optim.Adam(model.parameters(), lr=0.001)\nscheduler = StepLR(optimizer, step_size=300, gamma=0.25)\nreg_criterion = torch.nn.L1Loss()",
"_____no_output_____"
]
],
[
[
"### load data",
"_____no_output_____"
]
],
[
[
"### importing OGB-LSC\nfrom ogb.lsc import PygPCQM4MDataset, PCQM4MEvaluator\n\ndataset = PygPCQM4MDataset(root = 'dataset/')",
"_____no_output_____"
],
[
"split_idx = dataset.get_idx_split()\nsplit_idx[\"train\"], split_idx[\"test\"], split_idx[\"valid\"]",
"_____no_output_____"
],
[
"valid_loader = DataLoader(dataset[split_idx[\"valid\"]], batch_size=args.batch_size, shuffle=False, num_workers = args.num_workers)\n# valid_loader = DataLoader(dataset[queryID], batch_size=args.batch_size, shuffle=False, num_workers = args.num_workers)\nvalid_loader",
"_____no_output_____"
]
],
[
[
"### triplet loss",
"_____no_output_____"
]
],
[
[
"\"\"\"\nload triplet dataset\n\"\"\"\n\nname = \"valid\"\n\nanchor_loader = DataLoader(dataset[split_idx[name]], batch_size=args.batch_size, shuffle=True, num_workers = args.num_workers)\npositive_loader = DataLoader(dataset[split_idx[name]], batch_size=args.batch_size, shuffle=True, num_workers = args.num_workers)\nnegative_loader = DataLoader(dataset[split_idx[name]], batch_size=args.batch_size, shuffle=True, num_workers = args.num_workers)\n",
"_____no_output_____"
],
[
"\"\"\"\nget embedding\n\"\"\"\nmodel_activation = {}\ndef get_activation(name):\n def hook(model, input, output):\n model_activation[name] = output\n return hook\n\nmodel.gnn_node.register_forward_hook(get_activation('gnn_node'))",
"_____no_output_____"
],
[
"\"\"\"\ndefine triplet loss\n\"\"\"\n\nimport torch\nimport torch.nn as nn\nimport torch.nn.functional as F\nfrom torch import Tensor\nfrom torch_geometric.nn import global_add_pool\n\nclass TripletLossRegression(nn.Module):\n \"\"\"\n anchor, positive, negative are node-level embeddings of a GNN before they are sent to a pooling layer,\n and hence are expected to be matrices.\n anchor_gt, positive_gt, and negative_gt are ground truth tensors that correspond to the ground-truth\n values of the anchor, positive, and negative respectively.\n \"\"\"\n\n def __init__(self, margin: float = 0.0, eps=1e-6):\n super(TripletLossRegression, self).__init__()\n self.margin = margin\n self.eps = eps\n\n def forward(self, anchor_batch, negative_batch, positive_batch,\n anchor: Tensor, negative: Tensor, positive: Tensor,\n anchor_gt: Tensor, negative_gt: Tensor, positive_gt: Tensor) -> Tensor:\n anchor = global_add_pool(anchor, anchor_batch)\n\n positive = global_add_pool(positive, positive_batch)\n\n negative = global_add_pool(negative, negative_batch)\n\n pos_distance = torch.linalg.norm(positive - anchor, dim=1)\n negative_distance = torch.linalg.norm(negative - anchor, dim=1)\n\n coeff = torch.div(torch.abs(negative_gt - anchor_gt) , (torch.abs(positive_gt - anchor_gt) + self.eps))\n loss = F.relu((pos_distance - coeff * negative_distance) + self.margin)\n return torch.mean(loss)\n\n\n",
"_____no_output_____"
],
[
"# def triplet_loss_train(model, device, anchor_loader, negative_loader, positive_loader, optimizer, gnn_name):\nmodel.train()\nloss_accum = 0\ntriplet_loss_criterion = TripletLossRegression()\n\nfor step, (anchor_batch, negative_batch, positive_batch) in \\\n enumerate(zip(tqdm(anchor_loader, desc=\"Iteration\"), negative_loader, positive_loader)):\n anchor_batch = anchor_batch.to(device)\n pred_anchor = model(anchor_batch).view(-1,)\n anchor_embed = model_activation['gnn_node']\n\n negative_batch = negative_batch.to(device)\n pred_neg = model(negative_batch).view(-1,)\n neg_embed = model_activation['gnn_node']\n\n positive_batch = positive_batch.to(device)\n pred_pos= model(positive_batch).view(-1,)\n pos_embed = model_activation['gnn_node']\n\n optimizer.zero_grad()\n mae_loss = reg_criterion(pred_anchor, anchor_batch.y)\n tll_loss = triplet_loss_criterion(anchor_batch.batch, negative_batch.batch, positive_batch.batch,\n anchor_embed, neg_embed, pos_embed,\n anchor_batch.y, negative_batch.y, positive_batch.y)\n loss = mae_loss + tll_loss\n\n if gnn_name == 'gin-virtual-bnn':\n kl_loss = model.get_kl_loss()[0]\n loss += kl_loss\n\n loss.backward()\n optimizer.step()\n\n loss_accum += loss.detach().cpu().item()\n\n# return loss_accum / (step + 1)\nloss_accum / (step + 1)",
"Iteration: 0%| | 0/1487 [00:00<?, ?it/s]\n"
],
[
"raise Exception(\"\")",
"_____no_output_____"
],
[
"\"\"\" \nIMPORTANT: GRAPH QUERY ID\nPick the graph\n\"\"\"\nselectedID = 75088 #0 #131054\nqueryID = split_idx[\"valid\"][selectedID:selectedID + 1]\nqueryID",
"_____no_output_____"
],
[
"list(valid_loader)",
"_____no_output_____"
]
],
[
[
"## predict",
"_____no_output_____"
]
],
[
[
"batch = list(valid_loader)[0]\ndata = batch[0]\ndata",
"_____no_output_____"
],
[
"batch = batch.to(device)\nwith torch.no_grad():\n pred = model(batch).view(-1,)\n \npred",
"_____no_output_____"
],
[
"y_true = data.y.item()\ny_pred = pred.item()\ny_true, y_pred",
"_____no_output_____"
]
],
[
[
"## plot sample",
"_____no_output_____"
]
],
[
[
"import networkx as nx\nimport matplotlib.pyplot as plt",
"_____no_output_____"
],
[
"def plotGraph(data, y_pred, y_true, ax, printnodelabel=False, printedgelabel=False):\n\n edges = data.edge_index.T.tolist()\n edges = np.array(edges)\n edges = [(x[0][0], x[0][1], {\"feat\": str(x[1])}) for x in list(zip(edges.tolist(), data.edge_attr.tolist()))]\n nodes = [(x[0], {\"feat\": str(x[1])}) for x in enumerate(data.x.tolist())]\n\n G = nx.Graph()\n G.add_nodes_from(nodes)\n G.add_edges_from(edges)\n nodelabels = nx.get_node_attributes(G, 'feat') \n edgelabels = nx.get_edge_attributes(G, \"feat\")\n\n pos = nx.spring_layout(G)\n ax.set_title(\"pred={:.2f}, true={:.2f}\".format(y_pred, y_true))\n if printnodelabel:\n nx.draw(G, pos, labels=nodelabels, ax=ax, node_size=40)\n else:\n nx.draw(G, pos, ax=ax, node_size=40)\n \n if printedgelabel:\n nx.draw_networkx_edge_labels(G, pos, ax=ax, edge_labels=edgelabels)\n",
"_____no_output_____"
],
[
"fig, ax = plt.subplots()\nplotGraph(data, y_pred, y_true, ax, False, True)",
"_____no_output_____"
]
],
[
[
"## perturb edge feature",
"_____no_output_____"
],
[
"edge (5, 6, 2) possible dimensions",
"_____no_output_____"
]
],
[
[
"import ogb.utils as utils",
"_____no_output_____"
],
[
"edgeFeatDims = utils.features.get_bond_feature_dims()\nedgeFeatDims",
"_____no_output_____"
],
[
"perturb_data_list = []\n\nfor _ in range(5000):\n # clone original data\n pData = data.clone()\n \n # create random noise\n randomNoise = np.random.randint(low=-4, high=4, size=data.edge_attr.shape)\n randomNoise = torch.tensor(randomNoise)\n\n # add edge_attr noise\n pData.edge_attr += randomNoise\n \n pData.edge_attr[:, 0] = pData.edge_attr[:, 0].clip(0, edgeFeatDims[0]-1)\n pData.edge_attr[:, 1] = pData.edge_attr[:, 1].clip(0, edgeFeatDims[1]-1)\n pData.edge_attr[:, 2] = pData.edge_attr[:, 2].clip(0, edgeFeatDims[2]-1)\n \n perturb_data_list.append(pData)\n \nlen(perturb_data_list)",
"_____no_output_____"
],
[
"valid_loader = DataLoader(perturb_data_list, batch_size=args.batch_size, shuffle=False, num_workers = args.num_workers)\n\n# get data\nbatch = list(valid_loader)[0]\nbatch = batch.to(device)\nwith torch.no_grad():\n pred = model(batch) #.view(-1,)\n \npred.shape",
"_____no_output_____"
],
[
"plt.title(\"Perturb edge features. Label: {:.2f}\".format(y_true))\nplt.hist(pred.view(-1).tolist())\nplt.axvline(y_pred, c=\"r\")\nplt.show()",
"_____no_output_____"
]
],
[
[
"given fixed node features and topology, perturbing edge features don't disturb the output much",
"_____no_output_____"
],
[
"## perturb node features\n",
"_____no_output_____"
]
],
[
[
"nodeDims = utils.features.get_atom_feature_dims()\nnodeDims",
"_____no_output_____"
],
[
"perturb_data_list = []\n\nfor _ in range(1000):\n # clone original data\n pData = data.clone()\n \n # create random noise\n randomNoise = np.random.randint(low=-1, high=1, size=data.x.shape)\n randomNoise = torch.tensor(randomNoise)\n\n # add edge_attr noise\n pData.x += randomNoise\n \n pData.x[:, 0] = pData.x[:, 0].clip(0, nodeDims[0]-1)\n pData.x[:, 1] = pData.x[:, 1].clip(0, nodeDims[1]-1)\n pData.x[:, 2] = pData.x[:, 2].clip(0, nodeDims[2]-1)\n pData.x[:, 3] = pData.x[:, 2].clip(0, nodeDims[3]-1)\n pData.x[:, 4] = pData.x[:, 2].clip(0, nodeDims[4]-1)\n pData.x[:, 5] = pData.x[:, 2].clip(0, nodeDims[5]-1)\n pData.x[:, 6] = pData.x[:, 2].clip(0, nodeDims[6]-1)\n pData.x[:, 7] = pData.x[:, 2].clip(0, nodeDims[7]-1)\n pData.x[:, 8] = pData.x[:, 2].clip(0, nodeDims[8]-1)\n \n perturb_data_list.append(pData)\n \nlen(perturb_data_list)",
"_____no_output_____"
],
[
"# perturb_data_list = [data]\n\n# for i in range(1):\n# pData = data.clone()\n# # pData.x[-1, 0] = torch.tensor(i)\n# pData.x[-1] = torch.tensor([ 5, 0, 4, 5, 3, 0, 2, 0, 0])\n# perturb_data_list.append(pData)\n",
"_____no_output_____"
],
[
"valid_loader = DataLoader(perturb_data_list, batch_size=args.batch_size, shuffle=False, num_workers = args.num_workers)\n\n# get data\nbatch = list(valid_loader)[0]\nbatch = batch.to(device)\nwith torch.no_grad():\n pred = model(batch) #.view(-1,)\n \npred.shape #, pred",
"_____no_output_____"
],
[
"plt.title(\"Perturb node features. Label: {:.2f}\".format(y_true))\nplt.hist(pred.view(-1).tolist())\nplt.axvline(y_pred, c=\"r\")\nplt.show()",
"_____no_output_____"
]
],
[
[
"node features seem very sensitive",
"_____no_output_____"
],
[
"## perturb topology",
"_____no_output_____"
]
],
[
[
"# keep backup\nbackup = data.edge_index.clone()\nbackup",
"_____no_output_____"
],
[
"perturb_data_list = []\n\nfor i in range(1000):\n # clone original data\n pData = data.clone()\n \n # noise parameters\n noEdgeSwap = 3\n\n # create edges\n edges = pData.edge_index.T.tolist()\n edges = np.array(edges)\n edges = [(x[0][0], x[0][1], {\"feat\": str(x[1])}) for x in list(zip(edges.tolist(), pData.edge_attr.tolist()))]\n nodes = [(x[0], {\"feat\": str(x[1])}) for x in enumerate(pData.x.tolist())]\n G = nx.Graph()\n G.add_nodes_from(nodes)\n G.add_edges_from(edges)\n\n # swap edges\n G = nx.double_edge_swap(G, noEdgeSwap)\n # both directions\n newEdges = list(G.edges()) + [(x[1], x[0]) for x in G.edges()]\n newEdges = torch.tensor(newEdges).T\n # set value\n pData.edge_index = newEdges\n\n perturb_data_list.append(pData)\n \n # visualise some graphs\n if i % 50 == 0:\n plt.figure(figsize=(2, 2))\n nx.draw(G)\n plt.show()\n \nlen(perturb_data_list)",
"_____no_output_____"
],
[
"valid_loader = DataLoader(perturb_data_list, batch_size=args.batch_size, shuffle=False, num_workers = args.num_workers)\n\n# get data\nbatch = list(valid_loader)[0]\nbatch = batch.to(device)\nwith torch.no_grad():\n pred = model(batch) #.view(-1,)\n \npred.shape",
"_____no_output_____"
],
[
"plt.title(\"Perturb topology. Label: {:.2f}\".format(y_true))\nplt.hist(pred.view(-1).tolist())\nplt.axvline(y_pred, c=\"r\")\nplt.show()",
"_____no_output_____"
]
],
[
[
"topology doesn't seem to affect the score too",
"_____no_output_____"
]
]
] | [
"code",
"markdown",
"code",
"markdown",
"code",
"markdown",
"code",
"markdown",
"code",
"markdown",
"code",
"markdown",
"code",
"markdown",
"code",
"markdown",
"code",
"markdown",
"code",
"markdown"
] | [
[
"code"
],
[
"markdown"
],
[
"code",
"code",
"code"
],
[
"markdown"
],
[
"code",
"code",
"code",
"code",
"code"
],
[
"markdown"
],
[
"code",
"code",
"code"
],
[
"markdown"
],
[
"code",
"code",
"code",
"code",
"code",
"code",
"code"
],
[
"markdown"
],
[
"code",
"code",
"code"
],
[
"markdown"
],
[
"code",
"code",
"code"
],
[
"markdown",
"markdown"
],
[
"code",
"code",
"code",
"code",
"code"
],
[
"markdown",
"markdown"
],
[
"code",
"code",
"code",
"code",
"code"
],
[
"markdown",
"markdown"
],
[
"code",
"code",
"code",
"code"
],
[
"markdown"
]
] |
e781198f39d7ef26419984f638a67760878df68c | 8,952 | ipynb | Jupyter Notebook | tests/data_preview.ipynb | geek-yang/NEmo | 4f310535c4865f3816155b99b4a2bbb891672cc9 | [
"Apache-2.0"
] | 1 | 2020-05-25T19:06:15.000Z | 2020-05-25T19:06:15.000Z | tests/data_preview.ipynb | geek-yang/IIIDL | 4f310535c4865f3816155b99b4a2bbb891672cc9 | [
"Apache-2.0"
] | null | null | null | tests/data_preview.ipynb | geek-yang/IIIDL | 4f310535c4865f3816155b99b4a2bbb891672cc9 | [
"Apache-2.0"
] | null | null | null | 22.778626 | 364 | 0.367181 | [
[
[
"# Copyright Netherlands eScience Center and Centrum Wiskunde & Informatica <br>\n** Function : Emotion recognition and forecast with BBConvLSTM** <br>\n** Author : Yang Liu** <br>\n** Contributor : Tianyi Zhang (Centrum Wiskunde & Informatica)<br>\n** Last Update : 2021.02.08 ** <br>\n** Last Update : 2021.02.12 ** <br>\n** Library : Pytorth, Numpy, os, DLACs, matplotlib **<br>\nDescription : This notebook serves to test the prediction skill of deep neural networks in emotion recognition and forecast. The Bayesian convolutional Long Short Time Memory neural network with Bernoulli approximate variational inference is used to deal with this spatial-temporal sequence problem. We use Pytorch as the deep learning framework. <br>\n<br>\n** Many to one prediction.** <br>\n\nReturn Values : Time series and figures <br>\n\n**This project is a joint venture between NLeSC and CWI** <br>\n\nThe method comes from the study by Shi et. al. (2015) Convolutional LSTM Network: A Machine Learning Approach for Precipitation Nowcasting. <br>",
"_____no_output_____"
]
],
[
[
"%matplotlib inline\n\nimport sys\nimport numbers\nimport pickle\n\n# for data loading\nimport os\n# for pre-processing and machine learning\nimport numpy as np\nimport csv\n#import sklearn\nfrom scipy.signal import resample\n\n# for visualization\nimport matplotlib\nimport matplotlib.pyplot as plt\nfrom matplotlib.pyplot import cm",
"_____no_output_____"
]
],
[
[
"The testing device is Dell Inspirion 5680 with Intel Core i7-8700 x64 CPU and Nvidia GTX 1060 6GB GPU.<br>\nHere is a benchmark about cpu v.s. gtx 1060 <br>\nhttps://www.analyticsindiamag.com/deep-learning-tensorflow-benchmark-intel-i5-4210u-vs-geforce-nvidia-1060-6gb/",
"_____no_output_____"
]
],
[
[
"################################################################################# \n######### datapath ########\n#################################################################################\n# please specify data path\ndatapath = 'H:\\\\Creator_Zone\\\\Script_craft\\\\NEmo\\\\Data_CASE'\noutput_path = 'H:\\\\Creator_Zone\\\\Script_craft\\\\NEmo\\\\results'\nmodel_path = 'H:\\\\Creator_Zone\\\\Script_craft\\\\NEmo\\\\models'\n# please specify the constants for input data\nwindow_size = 2000 # down-sampling constant\nseq = 20\nv_a = 0 # valance = 0, arousal = 1\n# leave-one-out training and testing\nnum_s = 2",
"_____no_output_____"
],
[
"f = open(os.path.join(datapath, 'data_{}s'.format(int(window_size/100))),'rb')\ndata = pickle.load(f)\nf.close()\n \nsamples = data[\"Samples\"]\nlabels = data[\"label_s\"]\nsubject_id = data[\"Subject_id\"]",
"_____no_output_____"
],
[
"print(subject_id)",
"[[ 1]\n [ 1]\n [ 1]\n [ 1]\n [ 1]\n [ 1]\n [ 1]\n [ 1]\n [ 2]\n [ 2]\n [ 2]\n [ 2]\n [ 2]\n [ 2]\n [ 2]\n [ 2]\n [ 3]\n [ 3]\n [ 3]\n [ 3]\n [ 3]\n [ 3]\n [ 3]\n [ 3]\n [ 4]\n [ 4]\n [ 4]\n [ 4]\n [ 4]\n [ 4]\n [ 4]\n [ 4]\n [ 5]\n [ 5]\n [ 5]\n [ 5]\n [ 5]\n [ 5]\n [ 5]\n [ 5]\n [ 6]\n [ 6]\n [ 6]\n [ 6]\n [ 6]\n [ 6]\n [ 6]\n [ 6]\n [ 7]\n [ 7]\n [ 7]\n [ 7]\n [ 7]\n [ 7]\n [ 7]\n [ 7]\n [ 8]\n [ 8]\n [ 8]\n [ 8]\n [ 8]\n [ 8]\n [ 8]\n [ 8]\n [ 9]\n [ 9]\n [ 9]\n [ 9]\n [ 9]\n [ 9]\n [ 9]\n [ 9]\n [10]\n [10]\n [10]\n [10]\n [10]\n [10]\n [10]\n [10]\n [11]\n [11]\n [11]\n [11]\n [11]\n [11]\n [11]\n [11]\n [12]\n [12]\n [12]\n [12]\n [12]\n [12]\n [12]\n [12]\n [13]\n [13]\n [13]\n [13]\n [13]\n [13]\n [13]\n [13]\n [14]\n [14]\n [14]\n [14]\n [14]\n [14]\n [14]\n [14]\n [15]\n [15]\n [15]\n [15]\n [15]\n [15]\n [15]\n [15]\n [16]\n [16]\n [16]\n [16]\n [16]\n [16]\n [16]\n [16]\n [17]\n [17]\n [17]\n [17]\n [17]\n [17]\n [17]\n [17]\n [18]\n [18]\n [18]\n [18]\n [18]\n [18]\n [18]\n [18]\n [19]\n [19]\n [19]\n [19]\n [19]\n [19]\n [19]\n [19]\n [20]\n [20]\n [20]\n [20]\n [20]\n [20]\n [20]\n [20]\n [21]\n [21]\n [21]\n [21]\n [21]\n [21]\n [21]\n [21]\n [22]\n [22]\n [22]\n [22]\n [22]\n [22]\n [22]\n [22]\n [23]\n [23]\n [23]\n [23]\n [23]\n [23]\n [23]\n [23]\n [24]\n [24]\n [24]\n [24]\n [24]\n [24]\n [24]\n [24]\n [25]\n [25]\n [25]\n [25]\n [25]\n [25]\n [25]\n [25]\n [26]\n [26]\n [26]\n [26]\n [26]\n [26]\n [26]\n [26]\n [27]\n [27]\n [27]\n [27]\n [27]\n [27]\n [27]\n [27]\n [28]\n [28]\n [28]\n [28]\n [28]\n [28]\n [28]\n [28]\n [29]\n [29]\n [29]\n [29]\n [29]\n [29]\n [29]\n [29]\n [30]\n [30]\n [30]\n [30]\n [30]\n [30]\n [30]\n [30]]\n"
],
[
" x_train = samples[np.where(subject_id!=num_s)[0],:,0:4]\n x_test = samples[np.where(subject_id==num_s)[0],:,0:4]\n y_train = np.zeros([0,int(window_size/seq),1])\n y_test = np.zeros([0,int(window_size/seq),1])\n for i in range(len(labels)):\n sig = resample(labels[i][:,v_a],int(window_size/seq)).reshape([1,-1,1])/9\n if subject_id[i] == num_s:\n y_test = np.concatenate([y_test,sig],axis = 0)\n else:\n y_train = np.concatenate([y_train,sig],axis = 0)",
"_____no_output_____"
]
]
] | [
"markdown",
"code",
"markdown",
"code"
] | [
[
"markdown"
],
[
"code"
],
[
"markdown"
],
[
"code",
"code",
"code",
"code"
]
] |
e78125afb773993292142eb2cbe8b85fa4aa3a7f | 432,907 | ipynb | Jupyter Notebook | Experiments/Mars3DOF/Mars_landing_DTM/altimeter_v_mm3-120step.ipynb | CHEN-yongquan/RL-Meta-Learning-ACTA | 57e782af548c15b4067c3ea48fc278cfe63ee43e | [
"MIT"
] | 3 | 2021-06-17T11:02:49.000Z | 2021-12-07T12:10:08.000Z | Experiments/Mars3DOF/Mars_landing_DTM/altimeter_v_mm3-120step.ipynb | Aerospace-AI/RL-Meta-Learning-ACTA | 57e782af548c15b4067c3ea48fc278cfe63ee43e | [
"MIT"
] | null | null | null | Experiments/Mars3DOF/Mars_landing_DTM/altimeter_v_mm3-120step.ipynb | Aerospace-AI/RL-Meta-Learning-ACTA | 57e782af548c15b4067c3ea48fc278cfe63ee43e | [
"MIT"
] | 1 | 2021-11-20T07:54:49.000Z | 2021-11-20T07:54:49.000Z | 84.387329 | 126,075 | 0.710949 | [
[
[
"# Recurrent PPO landing using raw altimeter readings",
"_____no_output_____"
]
],
[
[
"import numpy as np\nimport os,sys\n\n\n\nsys.path.append('../../../RL_lib/Agents/PPO')\nsys.path.append('../../../RL_lib/Utils')\nsys.path.append('../../../Mars3dof_env')\nsys.path.append('../../../Mars_DTM')\n%load_ext autoreload\n%load_ext autoreload\n%autoreload 2\n%matplotlib nbagg\nimport os\nprint(os.getcwd())",
"The autoreload extension is already loaded. To reload it, use:\n %reload_ext autoreload\n/Users/briangaudet/Study/Subjects/MachineLearning/Projects/PCM/PCM_v3/Projects/AAS-19-293/Mars_landing_DTM\n"
],
[
"%%html\n<style>\n.output_wrapper, .output {\n height:auto !important;\n max-height:1000px; /* your desired max-height here */\n}\n.output_scroll {\n box-shadow:none !important;\n webkit-box-shadow:none !important;\n}\n</style>",
"_____no_output_____"
]
],
[
[
"# Optimize Policy",
"_____no_output_____"
]
],
[
[
"from env import Env\nimport env_utils as envu\nfrom dynamics_model import Dynamics_model\nfrom lander_model import Lander_model\nfrom ic_gen2 import Landing_icgen\nimport rl_utils\n\nfrom arch_policy_vf import Arch\n\nfrom model import Model\nfrom policy import Policy\nfrom value_function import Value_function\n\nimport pcm_model_nets as model_nets\nimport policy_nets as policy_nets\nimport valfunc_nets as valfunc_nets\n\nfrom agent import Agent\n\n\nimport torch.nn as nn\n\nfrom flat_constraint import Flat_constraint\nfrom glideslope_constraint import Glideslope_constraint\nfrom reward_terminal_mdr import Reward\n\nfrom dtm_measurement_model3 import DTM_measurement_model\nfrom altimeter_v import Altimeter\ndtm = np.load('../../../Mars_DTM/synth_elevations.npy')\n\nprint(dtm.shape, np.min(dtm), np.max(dtm))\ntarget_position = np.asarray([4000,4000,400])\nmm = DTM_measurement_model(dtm,check_vertical_errors=False)\naltimeter = Altimeter(mm,target_position,theta=np.pi/8)\n\n\narch = Arch()\nlogger = rl_utils.Logger()\ndynamics_model = Dynamics_model()\nlander_model = Lander_model(altimeter=altimeter, apf_tau1=20,apf_tau2=100,apf_vf1=-2,apf_vf2=-1,apf_v0=70,apf_atarg=15.)\nlander_model.get_state_agent = lander_model.get_state_agent_dtm\nobs_dim = 8\nact_dim = 3\nrecurrent_steps = 120\n\n\nreward_object = Reward()\n\nglideslope_constraint = Glideslope_constraint(gs_limit=0.5)\nshape_constraint = Flat_constraint()\nenv = Env(lander_model,dynamics_model,logger,\n reward_object=reward_object,\n glideslope_constraint=glideslope_constraint,\n shape_constraint=shape_constraint,\n tf_limit=100.0,print_every=10,scale_agent_action=True)\n\nenv.ic_gen = Landing_icgen(mass_uncertainty=0.10, \n g_uncertainty=(0.05,0.05),\n adjust_apf_v0=True,\n downrange = (0,2000 , -70, -30), \n crossrange = (-1000,1000 , -30,30), \n altitude = (2300,2400,-90,-70))\n\nenv.ic_gen.show()\n\n\narch = Arch()\n\n\npolicy = Policy(policy_nets.GRU(obs_dim, act_dim, recurrent_steps=recurrent_steps), shuffle=False,\n kl_targ=0.001,epochs=20, beta=0.1, servo_kl=True, max_grad_norm=30,\n init_func=rl_utils.xn_init)\nvalue_function = Value_function(valfunc_nets.GRU(obs_dim, recurrent_steps=recurrent_steps), \n shuffle=False, batch_size=9999999, max_grad_norm=30)\n\n\nagent = Agent(arch, policy, value_function, None, env, logger,\n policy_episodes=30, policy_steps=3000, gamma1=0.95, gamma2=0.995, lam=0.98, \n recurrent_steps=recurrent_steps, monitor=env.rl_stats)\nload_params=True\nfname = \"altimeter_v_mm3-120step\"\nif load_params:\n policy.load_params(fname)\n value_function.load_params(fname)\nelse: \n agent.train(30000)",
"Quaternion_attitude\n(10500, 8000) 0.0 382.8380000000001\nDTM MM: nref fixed: 384 10500 8000\n3-dof dynamics model\nlander model apf\nqueue fixed\nFlat Constraint\n"
],
[
"fname = \"altimeter_v_mm3-120step\"\npolicy.save_params(fname)\nvalue_function.save_params(fname)\nnp.save(fname + \"_history\",env.rl_stats.history)",
"_____no_output_____"
]
],
[
[
"# Test Policy with Realistic Noise\n",
"_____no_output_____"
]
],
[
[
"policy.test_mode=True \nenv.test_policy_batch(agent,1000,print_every=100)",
"DTM Model Miss Ratio: 0.0 0\ni : 100\nCumulative Stats (mean,std,max,argmax)\nthrust |9635.09 |2444.39 |3464.10 |15000.00 | 38\nglideslope | 2.114 | 3.112 | 0.742 |334.760 | 9\nsc_margin |100.000 | 0.000 |100.000 |100.000 | 0\n\nFinal Stats (mean,std,min,max)\nnorm_vf | 27.807 | 2.849 | 24.671 | 41.004\nnorm_rf | 243.9 | 75.3 | 92.7 | 676.8\nposition | -121.0 -200.0 -0.7 | 71.6 73.5 0.4 | -481.5 -475.6 -1.4 | 13.9 -49.4 -0.0\nvelocity | -1.795 -3.098 -24.605 | 8.197 9.527 2.274 | -12.378 -23.837 -31.838 | 28.098 16.953 -19.390\nfuel |233.15 | 18.87 |193.67 |282.39\nglideslope | 3.30 | 8.61 | 0.74 | 88.66\nDTM Model Miss Ratio: 0.0 0\ni : 200\nCumulative Stats (mean,std,max,argmax)\nthrust |9639.64 |2466.74 |3464.10 |15000.00 | 38\nglideslope | 2.190 | 3.291 | 0.597 |339.887 | 142\nsc_margin |100.000 | 0.000 |100.000 |100.000 | 0\n\nFinal Stats (mean,std,min,max)\nnorm_vf | 27.757 | 2.941 | 24.327 | 41.004\nnorm_rf | 247.4 | 116.9 | 92.7 | 1283.3\nposition | -130.4 -199.2 -0.7 | 99.9 90.5 0.4 | -989.3 -817.4 -1.4 | 13.9 -12.7 -0.0\nvelocity | -1.782 -3.001 -24.626 | 8.198 9.404 2.206 | -13.423 -25.991 -31.838 | 28.098 18.916 -19.390\nfuel |232.41 | 18.30 |193.67 |291.53\nglideslope | 3.14 | 6.31 | 0.60 | 88.66\n"
],
[
"len(lander_model.trajectory_list)\ntraj_list = lander_model.trajectory_list[0:100]\nlen(traj_list)\nnp.save(fname + '_100traj',traj_list)",
"_____no_output_____"
],
[
"envu.plot_rf_vf(env.rl_stats.history)",
"_____no_output_____"
]
]
] | [
"markdown",
"code",
"markdown",
"code",
"markdown",
"code"
] | [
[
"markdown"
],
[
"code",
"code"
],
[
"markdown"
],
[
"code",
"code"
],
[
"markdown"
],
[
"code",
"code",
"code"
]
] |
e7813e14e91cc453e99cde11b57dbb5278af9f49 | 22,097 | ipynb | Jupyter Notebook | 1-Flowers/FlowersTransfer-TF-Data-V1.ipynb | bo9zbo9z/MachineLearning | eae74837e1c98c44b9a6b2c1c16c019dd1fba069 | [
"MIT"
] | null | null | null | 1-Flowers/FlowersTransfer-TF-Data-V1.ipynb | bo9zbo9z/MachineLearning | eae74837e1c98c44b9a6b2c1c16c019dd1fba069 | [
"MIT"
] | null | null | null | 1-Flowers/FlowersTransfer-TF-Data-V1.ipynb | bo9zbo9z/MachineLearning | eae74837e1c98c44b9a6b2c1c16c019dd1fba069 | [
"MIT"
] | 1 | 2020-06-25T01:48:18.000Z | 2020-06-25T01:48:18.000Z | 22,097 | 22,097 | 0.656922 | [
[
[
"## Flowers classifier using Transfer Learning and tf.data\n\n\nAccuracy : 0.9090909090909091\n\nClassification Report\n precision recall f1-score support\n\n 0 0.96429 0.90000 0.93103 60\n 1 0.88750 0.98611 0.93421 72\n 2 0.81538 0.89831 0.85484 59\n 3 0.98462 0.86486 0.92086 74\n 4 0.90698 0.89655 0.90173 87\n\n",
"_____no_output_____"
]
],
[
[
"#\"\"\"\n# Google Collab specific stuff....\nfrom google.colab import drive\ndrive.mount('/content/drive')\n\nimport os\n!ls \"/content/drive/My Drive\"\n\nUSING_COLLAB = True\n%tensorflow_version 2.x\n#\"\"\"",
"_____no_output_____"
],
[
"# Setup sys.path to find MachineLearning lib directory\n\ntry: USING_COLLAB\nexcept NameError: USING_COLLAB = False\n\n%load_ext autoreload\n%autoreload 2\n\nimport sys\nif \"MachineLearning\" in sys.path[0]:\n pass\nelse:\n print(sys.path)\n if USING_COLLAB:\n sys.path.insert(0, '/content/drive/My Drive/GitHub/MachineLearning/lib') ###### CHANGE FOR SPECIFIC ENVIRONMENT\n else:\n sys.path.insert(0, '/Users/john/Documents/GitHub/MachineLearning/lib') ###### CHANGE FOR SPECIFIC ENVIRONMENT\n \n print(sys.path)",
"_____no_output_____"
],
[
"from __future__ import absolute_import, division, print_function, unicode_literals\n\nimport os, sys, random, warnings, time, copy, csv, gc\nimport numpy as np \n\nimport IPython.display as display\nfrom PIL import Image\n\nimport matplotlib.pyplot as plt\n%matplotlib inline\n\nimport cv2\nfrom tqdm import tqdm_notebook, tnrange, tqdm\nimport pandas as pd\n\nimport tensorflow as tf\nprint(tf.__version__)\n\nAUTOTUNE = tf.data.experimental.AUTOTUNE\nprint(\"AUTOTUNE: \", AUTOTUNE)\n\nfrom TrainingUtils import *\n\n#warnings.filterwarnings(\"ignore\", category=DeprecationWarning)\n#warnings.filterwarnings(\"ignore\", category=UserWarning)\nwarnings.filterwarnings(\"ignore\", \"(Possibly )?corrupt EXIF data\", UserWarning)",
"_____no_output_____"
]
],
[
[
"## Examine and understand data\n",
"_____no_output_____"
]
],
[
[
"# GLOBALS/CONFIG ITEMS\n\n# Set root directory path to data\nif USING_COLLAB:\n ROOT_PATH = \"/content/drive/My Drive/ImageData/Flowers\" ###### CHANGE FOR SPECIFIC ENVIRONMENT\nelse:\n ROOT_PATH = \"/Users/john/Documents/ImageData/Flowers\" ###### CHANGE FOR SPECIFIC ENVIRONMENT\n \n# Establish global dictionary\nparms = GlobalParms(ROOT_PATH=ROOT_PATH,\n TRAIN_DIR=\"train\", \n SMALL_RUN=False,\n NUM_CLASSES=5,\n IMAGE_ROWS=224,\n IMAGE_COLS=224,\n IMAGE_CHANNELS=3,\n BATCH_SIZE=32,\n EPOCS=10,\n IMAGE_EXT=\".jpg\",\n FINAL_ACTIVATION='sigmoid',\n LOSS='binary_crossentropy',\n METRICS=['accuracy'])\n\nparms.print_contents()",
"_____no_output_____"
],
[
"#\"\"\"\n# If not loaded, uncomment one of these to load the database as needed\n\n# This loads the files into a temporary directory \nload_dir = tf.keras.utils.get_file(origin='https://storage.googleapis.com/download.tensorflow.org/example_images/flower_photos.tgz',\n fname='flower_photos', untar=True)\n\n# This loads the files into a actual directory, WILL TAKE LONGER TO UNZIP AND TRAIN. But stored on Drive\n#load_dir = tf.keras.utils.get_file(origin='https://storage.googleapis.com/download.tensorflow.org/example_images/flower_photos.tgz',\n# fname='flower_photos', untar=True, cache_subdir=parms.TRAIN_PATH)\n\n\n# set new value for TRAIN_PATH\nparms.set_train_path(load_dir) # If we downloaded the images, then overide TRAIN_PATH\nprint(load_dir, parms.TRAIN_PATH)\n\n#\"\"\"",
"_____no_output_____"
],
[
"if parms.SMALL_RUN:\n max_subdir_files = 10\nelse:\n max_subdir_files = 1000000\n \nimages_list, sub_directories = load_file_names_labeled_subdir_Util(parms.TRAIN_PATH, \n parms.IMAGE_EXT, \n max_dir_files=max_subdir_files)\n\nimages_list_len = len(images_list)\nprint(\"Number of images: \", images_list_len)\n\nrandom.shuffle(images_list) # randomize the list\n\n# Set the class names.\nparms.set_class_names(sub_directories)\nprint(\"Classes: \", parms.NUM_CLASSES, \n \" Labels: \", len(parms.CLASS_NAMES), \n \" \", parms.CLASS_NAMES)\n",
"_____no_output_____"
],
[
"# Show a few images\nfor image_path in images_list[:3]:\n print(image_path)\n display.display(Image.open(str(image_path)))",
"_____no_output_____"
],
[
"# Create Dataset from list of images\nfull_dataset = tf.data.Dataset.from_tensor_slices(np.array(images_list))\nfull_dataset = full_dataset.shuffle(images_list_len)\n\n# Verify image paths were loaded and save one path for later in \"some_image\"\nfor f in full_dataset.take(5):\n some_image = f.numpy().decode(\"utf-8\")\n print(f.numpy())\n \nprint(\"Some Image: \", some_image)",
"_____no_output_____"
]
],
[
[
"## Build an input pipeline",
"_____no_output_____"
]
],
[
[
"\ndef get_label(file_path):\n # convert the path to a list of path components\n parts = tf.strings.split(file_path, os.path.sep)\n # The second to last is the class-directory\n return parts[-2] == parms.CLASS_NAMES\n\ndef decode_image(image):\n # convert the compressed string to a 3D uint8 tensor\n image = tf.image.decode_jpeg(image, channels=parms.IMAGE_CHANNELS)\n # Use `convert_image_dtype` to convert to floats in the [0,1] range.\n image = tf.image.convert_image_dtype(image, parms.IMAGE_DTYPE)\n # resize the image to the desired size.\n return tf.image.resize(image, [parms.IMAGE_ROWS, parms.IMAGE_COLS])\n\ndef image_aug(image):\n # do any augmentations\n if tf.random.uniform(()) > 0.25: \n k = tf.random.uniform(shape=[], minval=1, maxval=4, dtype=tf.int32)\n image = tf.image.rot90(image, k) #0-4, 0/270, 90/180/270\n\n image = tf.clip_by_value(image, 0, 1) # always clip back to 0, 1 before returning\n return image\n\ndef process_path_train(file_path):\n label = get_label(file_path)\n # load the raw data from the file as a string\n image = tf.io.read_file(file_path)\n image = decode_image(image)\n # add any augmentations\n image = image_aug(image)\n return image, label\n\ndef process_path_val(file_path):\n label = get_label(file_path)\n # load the raw data from the file as a string\n image = tf.io.read_file(file_path)\n image = decode_image(image)\n return image, label",
"_____no_output_____"
],
[
"def prepare_for_training(ds, cache=True, shuffle_buffer_size=1000):\n # This is a small dataset, only load it once, and keep it in memory.\n # use `.cache(filename)` to cache preprocessing work for datasets that don't\n # fit in memory.\n if cache:\n if isinstance(cache, str):\n ds = ds.cache(cache)\n else:\n ds = ds.cache()\n\n ds = ds.shuffle(buffer_size=shuffle_buffer_size)\n\n # Repeat forever\n ds = ds.repeat()\n ds = ds.batch(parms.BATCH_SIZE)\n\n # `prefetch` lets the dataset fetch batches in the background while the model\n # is training.\n ds = ds.prefetch(buffer_size=AUTOTUNE)\n\n return ds",
"_____no_output_____"
],
[
"# display images.... \ndef show_batch(image_batch, label_batch, number_to_show=25):\n plt.figure(figsize=(10,10))\n show_number = number_to_show\n if parms.BATCH_SIZE < number_to_show:\n show_number = parms.BATCH_SIZE\n \n for n in range(show_number):\n ax = plt.subplot(5,5,n+1)\n plt.imshow(tf.keras.preprocessing.image.array_to_img(image_batch[n]))\n plt.title(parms.CLASS_NAMES[np.argmax(label_batch[n])].title())\n plt.axis('off')",
"_____no_output_____"
],
[
"# split into training and validation sets of images\ntrain_len = int(0.9 * images_list_len)\nval_len = images_list_len - train_len\n\n# Create datasets with new sizes\ntrain_dataset = full_dataset.take(train_len) # Creates dataset with new size\nval_dataset = full_dataset.skip(train_len) # Creates dataset after skipping over the size\n\nprint(\"Total number: \", images_list_len, \" Train number: \", train_len, \" Val number: \", val_len)",
"_____no_output_____"
],
[
"# map training images to processing, includes any augmentation\ntrain_dataset = train_dataset.map(process_path_train, num_parallel_calls=AUTOTUNE)\n\n# Verify the mapping worked\nfor image, label in train_dataset.take(1):\n print(\"Image shape: \", image.numpy().shape)\n print(\"Label: \", label.numpy())\n \n# Ready to be used for training\ntrain_dataset = prepare_for_training(train_dataset)\n",
"_____no_output_____"
],
[
"# map validation images to processing\nval_dataset = val_dataset.map(process_path_val, num_parallel_calls=AUTOTUNE)\n\n# Verify the mapping worked\nfor image, label in val_dataset.take(1):\n print(\"Image shape: \", image.numpy().shape)\n print(\"Label: \", label.numpy())\n \n# Ready to be used for training\nval_dataset = prepare_for_training(val_dataset)\n\n",
"_____no_output_____"
],
[
"# Test Training\n\nimage_batch, label_batch = next(iter(train_dataset))\nshow_batch(image_batch.numpy(), label_batch.numpy())",
"_____no_output_____"
],
[
"# Test Validation\n\nimage_batch, label_batch = next(iter(val_dataset))\nshow_batch(image_batch.numpy(), label_batch.numpy())",
"_____no_output_____"
]
],
[
[
"## Build model\n- add and validate pretrained model as a baseline",
"_____no_output_____"
]
],
[
[
"# Create any call backs for training...These are the most common.\n\nfrom tensorflow.keras.callbacks import EarlyStopping, ModelCheckpoint, ReduceLROnPlateau, CSVLogger\n\nreduce_lr = ReduceLROnPlateau(monitor='loss', patience=2, verbose=1, min_lr=1e-6)\nearlystopper = EarlyStopping(patience=8, verbose=1)\ncheckpointer = ModelCheckpoint(parms.MODEL_PATH, monitor='val_loss', verbose=1, mode=\"auto\", save_best_only=True)\n#csv_logger = CSVLogger(self.cvslogfile, append=True, separator=';')\n\n#from keras.callbacks import TensorBoard\n#tensorboard = TensorBoard(log_dir=\"logs/{}\".format(time()))\n",
"_____no_output_____"
],
[
"# Create model and compile it\n\nfrom tensorflow.keras.models import Sequential, load_model, Model\nfrom tensorflow.keras.layers import Dense, Dropout, Flatten, Input, Conv2D, MaxPooling2D, BatchNormalization, UpSampling2D, Conv2DTranspose, Concatenate, Activation\nfrom tensorflow.keras.losses import binary_crossentropy, categorical_crossentropy\nfrom tensorflow.keras.optimizers import Adadelta, Adam, Nadam, SGD\n########\n#new with transfer learning\nfrom tensorflow.keras.applications import MobileNet, imagenet_utils\nfrom tensorflow.keras.layers import Dense,GlobalAveragePooling2D\n\nactual_MobileNet = tf.keras.applications.mobilenet.MobileNet()\n \ndef set_train_layers(model, train_layers=20): #since 224x224x3, set the first 20 layers of the network to be non-trainable\n if train_layers == 0: #set all non-trainable\n for layer in model.layers:\n layer.trainable=False\n else:\n for layer in model.layers[:train_layers]: \n layer.trainable=False\n for layer in model.layers[train_layers:]:\n layer.trainable=True\n return model\n\ndef predict_image(image): \n image = np.expand_dims(image, axis=0)\n image = tf.keras.applications.mobilenet.preprocess_input(image)\n predictions = actual_MobileNet.predict(image)\n results = imagenet_utils.decode_predictions(predictions)\n return results #list of decoded imagenet results\n\n\ndef build_model(CFG):\n base_model=MobileNet(weights='imagenet',include_top=False, input_shape=parms.IMAGE_DIM) #imports the mobilenet model and discards the last 1000 neuron layer.\n x=base_model.output\n x=GlobalAveragePooling2D()(x)\n x=Dense(1024,activation='relu')(x) #we add dense layers so that the model can learn more complex functions and classify for better results.\n x=Dense(1024,activation='relu')(x) #dense layer 2\n x=Dense(512,activation='relu')(x) #dense layer 3\n preds=Dense(parms.NUM_CLASSES, activation=parms.FINAL_ACTIVATION)(x) #final layer\n model=Model(inputs=base_model.input,outputs=preds)\n return model\n\ndef compile_model(CFG, model):\n model.compile(loss=parms.LOSS,\n #optimizer=SGD(lr=0.001, momentum=0.9),\n optimizer=Adam(),\n metrics=parms.METRICS)\n return model\n",
"_____no_output_____"
],
[
"#test an image just using MobileNet\nfrom tensorflow.keras.preprocessing import image\nimg = image.load_img(some_image, target_size=(224, 224))\nimg_array = image.img_to_array(img)\nresult = predict_image(img_array)\nresult\n\n#str(parms.CLASS_NAMES[0])+'/*'))",
"_____no_output_____"
],
[
"#show the image...\nfrom IPython.display import Image\nImage(filename=some_image)",
"_____no_output_____"
],
[
"# Show the activation layers, can be trained or initial model (BETA)\n#model_raw = build_model(CFG)\n#img_path = os.path.join(parms.TRAIN_PATH, \"Cat/2.jpg\")\n#image_show_seq_model_layers_BETA(img_path, model_raw, parms.IMAGE_DIM, \n# activation_layer_num=0, activation_channel_num=11)\n",
"_____no_output_____"
]
],
[
[
"## Train model",
"_____no_output_____"
]
],
[
[
"# Train model\nsteps_per_epoch = np.ceil(train_len // parms.BATCH_SIZE) # set step sizes based on train & batch\nvalidation_steps = np.ceil(val_len // parms.BATCH_SIZE) # set step sizes based on val & batch\n\nmodel = build_model(parms)\nmodel = compile_model(parms, model)\n\nhistory = model.fit(train_dataset,\n validation_data=val_dataset,\n epochs=parms.EPOCS, \n steps_per_epoch=steps_per_epoch,\n validation_steps=validation_steps,\n callbacks=[reduce_lr, earlystopper, checkpointer] # include any callbacks...\n )\n",
"_____no_output_____"
],
[
"# Plot the training history\nhistory_df = pd.DataFrame(history.history)\nplt.figure()\nhistory_df[['loss', 'val_loss']].plot(title=\"Loss\")\nplt.xlabel('Epocs')\nplt.ylabel('Loss')\nhistory_df[['accuracy', 'val_accuracy']].plot(title=\"Accuracy\")\nplt.xlabel('Epocs')\nplt.ylabel('Accuracy')\nplt.show()",
"_____no_output_____"
]
],
[
[
"## Validate model's predictions\n- Create actual_lables and predict_labels\n- Calculate Confusion Matrix & Accuracy\n- Display results\n",
"_____no_output_____"
]
],
[
[
"#Load saved model\nfrom tensorflow.keras.models import load_model \ndef load_saved_model(model_path):\n model = load_model(model_path)\n print(\"loaded: \", model_path)\n return model\n\nmodel = load_saved_model(parms.MODEL_PATH)",
"_____no_output_____"
],
[
"# Use model to generate predicted labels and probabilities\n#labels, predict_labels, predict_probabilities, bad_results = predictions_using_dataset(model, val_dataset, 1, parms.BATCH_SIZE, create_bad_results_list=False)\nlabels, predict_labels, predict_probabilities, bad_results = predictions_using_dataset(model, val_dataset, validation_steps, parms.BATCH_SIZE, create_bad_results_list=False)\n",
"_____no_output_____"
],
[
"show_confusion_matrix(labels, predict_labels, parms.CLASS_NAMES)",
"_____no_output_____"
],
[
"# Graph the results\n\ndisplay_prediction_results(labels, predict_labels, predict_probabilities, parms.NUM_CLASSES, parms.CLASS_NAMES)\n",
"_____no_output_____"
],
[
"#Create a df from the bad results list, can save as csv or use for further analysis\nbad_results_df = pd.DataFrame(bad_results, columns =['actual', 'predict', 'prob', 'image'])\nbad_results_df.head()",
"_____no_output_____"
],
[
"# default is to not return bad_results, change to include them, create_bad_results_list=True\n\n#bad_act, bad_pred, bad_prob, bad_images = zip(*bad_results)",
"_____no_output_____"
],
[
"# display bad images.... \ndef show_bad_batch(image_batch, bad_act, bad_pred, number_to_show=25):\n plt.figure(figsize=(10,10))\n show_number = number_to_show\n if len(image_batch) < number_to_show:\n show_number = len(image_batch)\n\n for n in range(show_number):\n ax = plt.subplot(5,5,n+1)\n plt.imshow(tf.keras.preprocessing.image.array_to_img(image_batch[n][0]))\n #s = parms.CLASS_NAMES[bad_pred[n][0]]\n s = \"Act: \"+ str(bad_act[n][0]) + \" Pred: \" + str(bad_pred[n][0])\n plt.title(s)\n plt.axis('off')",
"_____no_output_____"
],
[
"print(\" 0)\", parms.CLASS_NAMES[0],\n \" 1)\", parms.CLASS_NAMES[1],\n \" 2)\", parms.CLASS_NAMES[2],\n \" 3)\", parms.CLASS_NAMES[3],\n \" 4)\", parms.CLASS_NAMES[4])\n#show_bad_batch(bad_images, bad_act, bad_pred)",
"_____no_output_____"
]
]
] | [
"markdown",
"code",
"markdown",
"code",
"markdown",
"code",
"markdown",
"code",
"markdown",
"code",
"markdown",
"code"
] | [
[
"markdown"
],
[
"code",
"code",
"code"
],
[
"markdown"
],
[
"code",
"code",
"code",
"code",
"code"
],
[
"markdown"
],
[
"code",
"code",
"code",
"code",
"code",
"code",
"code",
"code"
],
[
"markdown"
],
[
"code",
"code",
"code",
"code",
"code"
],
[
"markdown"
],
[
"code",
"code"
],
[
"markdown"
],
[
"code",
"code",
"code",
"code",
"code",
"code",
"code",
"code"
]
] |
e78145cc1f84c703680b4c94d1472be7a1c71620 | 56,958 | ipynb | Jupyter Notebook | site/en-snapshot/lite/tutorials/pose_classification.ipynb | Icecoffee2500/docs-l10n | a1cb00ac3ade7c7bc6c4dd48e57c7d64ba7a02df | [
"Apache-2.0"
] | 1 | 2021-11-09T10:19:46.000Z | 2021-11-09T10:19:46.000Z | site/en-snapshot/lite/tutorials/pose_classification.ipynb | Icecoffee2500/docs-l10n | a1cb00ac3ade7c7bc6c4dd48e57c7d64ba7a02df | [
"Apache-2.0"
] | null | null | null | site/en-snapshot/lite/tutorials/pose_classification.ipynb | Icecoffee2500/docs-l10n | a1cb00ac3ade7c7bc6c4dd48e57c7d64ba7a02df | [
"Apache-2.0"
] | null | null | null | 42.128698 | 510 | 0.537624 | [
[
[
"##### Copyright 2021 The TensorFlow Authors.",
"_____no_output_____"
]
],
[
[
"#@title Licensed under the Apache License, Version 2.0 (the \"License\");\n# you may not use this file except in compliance with the License.\n# You may obtain a copy of the License at\n#\n# https://www.apache.org/licenses/LICENSE-2.0\n#\n# Unless required by applicable law or agreed to in writing, software\n# distributed under the License is distributed on an \"AS IS\" BASIS,\n# WITHOUT WARRANTIES OR CONDITIONS OF ANY KIND, either express or implied.\n# See the License for the specific language governing permissions and\n# limitations under the License.",
"_____no_output_____"
]
],
[
[
"# Human Pose Classification with MoveNet and TensorFlow Lite\n\nThis notebook teaches you how to train a pose classification model using MoveNet and TensorFlow Lite. The result is a new TensorFlow Lite model that accepts the output from the MoveNet model as its input, and outputs a pose classification, such as the name of a yoga pose.\n\nThe procedure in this notebook consists of 3 parts:\n* Part 1: Preprocess the pose classification training data into a CSV file that specifies the landmarks (body keypoints) detected by the MoveNet model, along with the ground truth pose labels.\n* Part 2: Build and train a pose classification model that takes the landmark coordinates from the CSV file as input, and outputs the predicted labels.\n* Part 3: Convert the pose classification model to TFLite.\n\nBy default, this notebook uses an image dataset with labeled yoga poses, but we've also included a section in Part 1 where you can upload your own image dataset of poses.\n\n<table class=\"tfo-notebook-buttons\" align=\"left\">\n <td>\n <a target=\"_blank\" href=\"https://www.tensorflow.org/lite/tutorials/pose_classification\"><img src=\"https://www.tensorflow.org/images/tf_logo_32px.png\" />View on TensorFlow.org</a>\n </td>\n <td>\n <a target=\"_blank\" href=\"https://colab.research.google.com/github/tensorflow/tensorflow/blob/master/tensorflow/lite/g3doc/tutorials/pose_classification.ipynb\"><img src=\"https://www.tensorflow.org/images/colab_logo_32px.png\" />Run in Google Colab</a>\n </td>\n <td>\n <a target=\"_blank\" href=\"https://github.com/tensorflow/tensorflow/blob/master/tensorflow/lite/g3doc/tutorials/pose_classification.ipynb\"><img src=\"https://www.tensorflow.org/images/GitHub-Mark-32px.png\" />View source on GitHub</a>\n </td>\n <td>\n <a href=\"https://storage.googleapis.com/tensorflow_docs/tensorflow/tensorflow/lite/g3doc/tutorials/pose_classification.ipynb\"><img src=\"https://www.tensorflow.org/images/download_logo_32px.png\" />Download notebook</a>\n </td>\n <td>\n <a href=\"https://tfhub.dev/s?q=movenet\"><img src=\"https://www.tensorflow.org/images/hub_logo_32px.png\" />See TF Hub model</a>\n </td>\n</table>",
"_____no_output_____"
],
[
"## Preparation",
"_____no_output_____"
],
[
"In this section, you'll import the necessary libraries and define several functions to preprocess the training images into a CSV file that contains the landmark coordinates and ground truth labels.\n\nNothing observable happens here, but you can expand the hidden code cells to see the implementation for some of the functions we'll be calling later on.\n\n**If you only want to create the CSV file without knowing all the details, just run this section and proceed to Part 1.**",
"_____no_output_____"
]
],
[
[
"!pip install -q opencv-python",
"_____no_output_____"
],
[
"import csv\nimport cv2\nimport itertools\nimport numpy as np\nimport pandas as pd\nimport os\nimport sys\nimport tempfile\nimport tqdm\n\nfrom matplotlib import pyplot as plt\nfrom matplotlib.collections import LineCollection\n\nimport tensorflow as tf\nimport tensorflow_hub as hub\nfrom tensorflow import keras\n\nfrom sklearn.model_selection import train_test_split\nfrom sklearn.metrics import accuracy_score, classification_report, confusion_matrix",
"_____no_output_____"
]
],
[
[
"### Code to run pose estimation using MoveNet",
"_____no_output_____"
]
],
[
[
"#@title Functions to run pose estimation with MoveNet\n\n#@markdown You'll download the MoveNet Thunder model from [TensorFlow Hub](https://www.google.com/url?sa=D&q=https%3A%2F%2Ftfhub.dev%2Fs%3Fq%3Dmovenet), and reuse some inference and visualization logic from the [MoveNet Raspberry Pi (Python)](https://github.com/tensorflow/examples/tree/master/lite/examples/pose_estimation/raspberry_pi) sample app to detect landmarks (ear, nose, wrist etc.) from the input images.\n\n#@markdown *Note: You should use the most accurate pose estimation model (i.e. MoveNet Thunder) to detect the keypoints and use them to train the pose classification model to achieve the best accuracy. When running inference, you can use a pose estimation model of your choice (e.g. either MoveNet Lightning or Thunder).*\n\n# Download model from TF Hub and check out inference code from GitHub\n!wget -q -O movenet_thunder.tflite https://tfhub.dev/google/lite-model/movenet/singlepose/thunder/tflite/float16/4?lite-format=tflite\n!git clone https://github.com/tensorflow/examples.git\npose_sample_rpi_path = os.path.join(os.getcwd(), 'examples/lite/examples/pose_estimation/raspberry_pi')\nsys.path.append(pose_sample_rpi_path)\n\n# Load MoveNet Thunder model\nimport utils\nfrom data import BodyPart\nfrom ml import Movenet\nmovenet = Movenet('movenet_thunder')\n\n# Define function to run pose estimation using MoveNet Thunder.\n# You'll apply MoveNet's cropping algorithm and run inference multiple times on\n# the input image to improve pose estimation accuracy.\ndef detect(input_tensor, inference_count=3):\n \"\"\"Runs detection on an input image.\n \n Args:\n input_tensor: A [height, width, 3] Tensor of type tf.float32.\n Note that height and width can be anything since the image will be\n immediately resized according to the needs of the model within this\n function.\n inference_count: Number of times the model should run repeatly on the\n same input image to improve detection accuracy.\n \n Returns:\n A Person entity detected by the MoveNet.SinglePose.\n \"\"\"\n image_height, image_width, channel = input_tensor.shape\n \n # Detect pose using the full input image\n movenet.detect(input_tensor.numpy(), reset_crop_region=True)\n \n # Repeatedly using previous detection result to identify the region of\n # interest and only croping that region to improve detection accuracy\n for _ in range(inference_count - 1):\n person = movenet.detect(input_tensor.numpy(), \n reset_crop_region=False)\n\n return person",
"_____no_output_____"
],
[
"#@title Functions to visualize the pose estimation results.\n\ndef draw_prediction_on_image(\n image, person, crop_region=None, close_figure=True,\n keep_input_size=False):\n \"\"\"Draws the keypoint predictions on image.\n \n Args:\n image: An numpy array with shape [height, width, channel] representing the\n pixel values of the input image.\n person: A person entity returned from the MoveNet.SinglePose model.\n close_figure: Whether to close the plt figure after the function returns.\n keep_input_size: Whether to keep the size of the input image.\n \n Returns:\n An numpy array with shape [out_height, out_width, channel] representing the\n image overlaid with keypoint predictions.\n \"\"\"\n # Draw the detection result on top of the image.\n image_np = utils.visualize(image, [person])\n \n # Plot the image with detection results.\n height, width, channel = image.shape\n aspect_ratio = float(width) / height\n fig, ax = plt.subplots(figsize=(12 * aspect_ratio, 12))\n im = ax.imshow(image_np)\n \n if close_figure:\n plt.close(fig)\n \n if not keep_input_size:\n image_np = utils.keep_aspect_ratio_resizer(image_np, (512, 512))\n\n return image_np",
"_____no_output_____"
],
[
"#@title Code to load the images, detect pose landmarks and save them into a CSV file\n\nclass MoveNetPreprocessor(object):\n \"\"\"Helper class to preprocess pose sample images for classification.\"\"\"\n \n def __init__(self,\n images_in_folder,\n images_out_folder,\n csvs_out_path):\n \"\"\"Creates a preprocessor to detection pose from images and save as CSV.\n\n Args:\n images_in_folder: Path to the folder with the input images. It should\n follow this structure:\n yoga_poses\n |__ downdog\n |______ 00000128.jpg\n |______ 00000181.bmp\n |______ ...\n |__ goddess\n |______ 00000243.jpg\n |______ 00000306.jpg\n |______ ...\n ...\n images_out_folder: Path to write the images overlay with detected\n landmarks. These images are useful when you need to debug accuracy\n issues.\n csvs_out_path: Path to write the CSV containing the detected landmark\n coordinates and label of each image that can be used to train a pose\n classification model.\n \"\"\"\n self._images_in_folder = images_in_folder\n self._images_out_folder = images_out_folder\n self._csvs_out_path = csvs_out_path\n self._messages = []\n\n # Create a temp dir to store the pose CSVs per class\n self._csvs_out_folder_per_class = tempfile.mkdtemp()\n \n # Get list of pose classes and print image statistics\n self._pose_class_names = sorted(\n [n for n in os.listdir(self._images_in_folder) if not n.startswith('.')]\n )\n \n def process(self, per_pose_class_limit=None, detection_threshold=0.1):\n \"\"\"Preprocesses images in the given folder.\n Args:\n per_pose_class_limit: Number of images to load. As preprocessing usually\n takes time, this parameter can be specified to make the reduce of the\n dataset for testing.\n detection_threshold: Only keep images with all landmark confidence score\n above this threshold.\n \"\"\"\n # Loop through the classes and preprocess its images\n for pose_class_name in self._pose_class_names:\n print('Preprocessing', pose_class_name, file=sys.stderr)\n\n # Paths for the pose class.\n images_in_folder = os.path.join(self._images_in_folder, pose_class_name)\n images_out_folder = os.path.join(self._images_out_folder, pose_class_name)\n csv_out_path = os.path.join(self._csvs_out_folder_per_class,\n pose_class_name + '.csv')\n if not os.path.exists(images_out_folder):\n os.makedirs(images_out_folder)\n \n # Detect landmarks in each image and write it to a CSV file\n with open(csv_out_path, 'w') as csv_out_file:\n csv_out_writer = csv.writer(csv_out_file, \n delimiter=',', \n quoting=csv.QUOTE_MINIMAL)\n # Get list of images\n image_names = sorted(\n [n for n in os.listdir(images_in_folder) if not n.startswith('.')])\n if per_pose_class_limit is not None:\n image_names = image_names[:per_pose_class_limit]\n\n valid_image_count = 0\n \n # Detect pose landmarks from each image\n for image_name in tqdm.tqdm(image_names):\n image_path = os.path.join(images_in_folder, image_name)\n\n try:\n image = tf.io.read_file(image_path)\n image = tf.io.decode_jpeg(image)\n except:\n self._messages.append('Skipped ' + image_path + '. Invalid image.')\n continue\n else:\n image = tf.io.read_file(image_path)\n image = tf.io.decode_jpeg(image)\n image_height, image_width, channel = image.shape\n \n # Skip images that isn't RGB because Movenet requires RGB images\n if channel != 3:\n self._messages.append('Skipped ' + image_path +\n '. Image isn\\'t in RGB format.')\n continue\n person = detect(image)\n \n # Save landmarks if all landmarks were detected\n min_landmark_score = min(\n [keypoint.score for keypoint in person.keypoints])\n should_keep_image = min_landmark_score >= detection_threshold\n if not should_keep_image:\n self._messages.append('Skipped ' + image_path +\n '. No pose was confidentlly detected.')\n continue\n\n valid_image_count += 1\n\n # Draw the prediction result on top of the image for debugging later\n output_overlay = draw_prediction_on_image(\n image.numpy().astype(np.uint8), person, \n close_figure=True, keep_input_size=True)\n \n # Write detection result into an image file\n output_frame = cv2.cvtColor(output_overlay, cv2.COLOR_RGB2BGR)\n cv2.imwrite(os.path.join(images_out_folder, image_name), output_frame)\n \n # Get landmarks and scale it to the same size as the input image\n pose_landmarks = np.array(\n [[keypoint.coordinate.x, keypoint.coordinate.y, keypoint.score]\n for keypoint in person.keypoints],\n dtype=np.float32)\n\n # Write the landmark coordinates to its per-class CSV file\n coordinates = pose_landmarks.flatten().astype(np.str).tolist()\n csv_out_writer.writerow([image_name] + coordinates)\n\n if not valid_image_count:\n raise RuntimeError(\n 'No valid images found for the \"{}\" class.'\n .format(pose_class_name))\n \n # Print the error message collected during preprocessing.\n print('\\n'.join(self._messages))\n\n # Combine all per-class CSVs into a single output file\n all_landmarks_df = self._all_landmarks_as_dataframe()\n all_landmarks_df.to_csv(self._csvs_out_path, index=False)\n\n def class_names(self):\n \"\"\"List of classes found in the training dataset.\"\"\"\n return self._pose_class_names\n \n def _all_landmarks_as_dataframe(self):\n \"\"\"Merge all per-class CSVs into a single dataframe.\"\"\"\n total_df = None\n for class_index, class_name in enumerate(self._pose_class_names):\n csv_out_path = os.path.join(self._csvs_out_folder_per_class,\n class_name + '.csv')\n per_class_df = pd.read_csv(csv_out_path, header=None)\n \n # Add the labels\n per_class_df['class_no'] = [class_index]*len(per_class_df)\n per_class_df['class_name'] = [class_name]*len(per_class_df)\n\n # Append the folder name to the filename column (first column)\n per_class_df[per_class_df.columns[0]] = (os.path.join(class_name, '') \n + per_class_df[per_class_df.columns[0]].astype(str))\n\n if total_df is None:\n # For the first class, assign its data to the total dataframe\n total_df = per_class_df\n else:\n # Concatenate each class's data into the total dataframe\n total_df = pd.concat([total_df, per_class_df], axis=0)\n \n list_name = [[bodypart.name + '_x', bodypart.name + '_y', \n bodypart.name + '_score'] for bodypart in BodyPart] \n header_name = []\n for columns_name in list_name:\n header_name += columns_name\n header_name = ['file_name'] + header_name\n header_map = {total_df.columns[i]: header_name[i] \n for i in range(len(header_name))}\n \n total_df.rename(header_map, axis=1, inplace=True)\n\n return total_df",
"_____no_output_____"
],
[
"#@title (Optional) Code snippet to try out the Movenet pose estimation logic\n\n#@markdown You can download an image from the internet, run the pose estimation logic on it and plot the detected landmarks on top of the input image. \n\n#@markdown *Note: This code snippet is also useful for debugging when you encounter an image with bad pose classification accuracy. You can run pose estimation on the image and see if the detected landmarks look correct or not before investigating the pose classification logic.*\n\ntest_image_url = \"https://cdn.pixabay.com/photo/2017/03/03/17/30/yoga-2114512_960_720.jpg\" #@param {type:\"string\"}\n!wget -O /tmp/image.jpeg {test_image_url}\n\nif len(test_image_url):\n image = tf.io.read_file('/tmp/image.jpeg')\n image = tf.io.decode_jpeg(image)\n person = detect(image)\n _ = draw_prediction_on_image(image.numpy(), person, crop_region=None, \n close_figure=False, keep_input_size=True)",
"_____no_output_____"
]
],
[
[
"## Part 1: Preprocess the input images\n\nBecause the input for our pose classifier is the *output* landmarks from the MoveNet model, we need to generate our training dataset by running labeled images through MoveNet and then capturing all the landmark data and ground truth labels into a CSV file.\n\nThe dataset we've provided for this tutorial is a CG-generated yoga pose dataset. It contains images of multiple CG-generated models doing 5 different yoga poses. The directory is already split into a `train` dataset and a `test` dataset.\n\nSo in this section, we'll download the yoga dataset and run it through MoveNet so we can capture all the landmarks into a CSV file... **However, it takes about 15 minutes to feed our yoga dataset to MoveNet and generate this CSV file**. So as an alternative, you can download a pre-existing CSV file for the yoga dataset by setting `is_skip_step_1` parameter below to **True**. That way, you'll skip this step and instead download the same CSV file that will be created in this preprocessing step.\n\nOn the other hand, if you want to train the pose classifier with your own image dataset, you need to upload your images and run this preprocessing step (leave `is_skip_step_1` **False**)—follow the instructions below to upload your own pose dataset.",
"_____no_output_____"
]
],
[
[
"is_skip_step_1 = False #@param [\"False\", \"True\"] {type:\"raw\"}",
"_____no_output_____"
]
],
[
[
"### (Optional) Upload your own pose dataset",
"_____no_output_____"
]
],
[
[
"use_custom_dataset = False #@param [\"False\", \"True\"] {type:\"raw\"}\n\ndataset_is_split = False #@param [\"False\", \"True\"] {type:\"raw\"}",
"_____no_output_____"
]
],
[
[
"If you want to train the pose classifier with your own labeled poses (they can be any poses, not just yoga poses), follow these steps:\n\n1. Set the above `use_custom_dataset` option to **True**.\n\n2. Prepare an archive file (ZIP, TAR, or other) that includes a folder with your images dataset. The folder must include sorted images of your poses as follows.\n\n If you've already split your dataset into train and test sets, then set `dataset_is_split` to **True**. That is, your images folder must include \"train\" and \"test\" directories like this:\n\n ```\n yoga_poses/\n |__ train/\n |__ downdog/\n |______ 00000128.jpg\n |______ ...\n |__ test/\n |__ downdog/\n |______ 00000181.jpg\n |______ ...\n ```\n\n Or, if your dataset is NOT split yet, then set\n `dataset_is_split` to **False** and we'll split it up based\n on a specified split fraction. That is, your uploaded images\n folder should look like this:\n\n ```\n yoga_poses/\n |__ downdog/\n |______ 00000128.jpg\n |______ 00000181.jpg\n |______ ...\n |__ goddess/\n |______ 00000243.jpg\n |______ 00000306.jpg\n |______ ...\n ```\n3. Click the **Files** tab on the left (folder icon) and then click **Upload to session storage** (file icon).\n4. Select your archive file and wait until it finishes uploading before you proceed.\n5. Edit the following code block to specify the name of your archive file and images directory. (By default, we expect a ZIP file, so you'll need to also modify that part if your archive is another format.)\n6. Now run the rest of the notebook.",
"_____no_output_____"
]
],
[
[
"#@markdown Be sure you run this cell. It's hiding the `split_into_train_test()` function that's called in the next code block.\n\nimport os\nimport random\nimport shutil\n\ndef split_into_train_test(images_origin, images_dest, test_split):\n \"\"\"Splits a directory of sorted images into training and test sets.\n\n Args:\n images_origin: Path to the directory with your images. This directory\n must include subdirectories for each of your labeled classes. For example:\n yoga_poses/\n |__ downdog/\n |______ 00000128.jpg\n |______ 00000181.jpg\n |______ ...\n |__ goddess/\n |______ 00000243.jpg\n |______ 00000306.jpg\n |______ ...\n ...\n images_dest: Path to a directory where you want the split dataset to be\n saved. The results looks like this:\n split_yoga_poses/\n |__ train/\n |__ downdog/\n |______ 00000128.jpg\n |______ ...\n |__ test/\n |__ downdog/\n |______ 00000181.jpg\n |______ ...\n test_split: Fraction of data to reserve for test (float between 0 and 1).\n \"\"\"\n _, dirs, _ = next(os.walk(images_origin))\n\n TRAIN_DIR = os.path.join(images_dest, 'train')\n TEST_DIR = os.path.join(images_dest, 'test')\n os.makedirs(TRAIN_DIR, exist_ok=True)\n os.makedirs(TEST_DIR, exist_ok=True)\n\n for dir in dirs:\n # Get all filenames for this dir, filtered by filetype\n filenames = os.listdir(os.path.join(images_origin, dir))\n filenames = [os.path.join(images_origin, dir, f) for f in filenames if (\n f.endswith('.png') or f.endswith('.jpg') or f.endswith('.jpeg') or f.endswith('.bmp'))]\n # Shuffle the files, deterministically\n filenames.sort()\n random.seed(42)\n random.shuffle(filenames)\n # Divide them into train/test dirs\n os.makedirs(os.path.join(TEST_DIR, dir), exist_ok=True)\n os.makedirs(os.path.join(TRAIN_DIR, dir), exist_ok=True)\n test_count = int(len(filenames) * test_split)\n for i, file in enumerate(filenames):\n if i < test_count:\n destination = os.path.join(TEST_DIR, dir, os.path.split(file)[1])\n else:\n destination = os.path.join(TRAIN_DIR, dir, os.path.split(file)[1])\n shutil.copyfile(file, destination)\n print(f'Moved {test_count} of {len(filenames)} from class \"{dir}\" into test.')\n print(f'Your split dataset is in \"{images_dest}\"')",
"_____no_output_____"
],
[
"if use_custom_dataset:\n # ATTENTION:\n # You must edit these two lines to match your archive and images folder name:\n # !tar -xf YOUR_DATASET_ARCHIVE_NAME.tar\n !unzip -q YOUR_DATASET_ARCHIVE_NAME.zip\n dataset_in = 'YOUR_DATASET_DIR_NAME'\n\n # You can leave the rest alone:\n if not os.path.isdir(dataset_in):\n raise Exception(\"dataset_in is not a valid directory\")\n if dataset_is_split:\n IMAGES_ROOT = dataset_in\n else:\n dataset_out = 'split_' + dataset_in\n split_into_train_test(dataset_in, dataset_out, test_split=0.2)\n IMAGES_ROOT = dataset_out",
"_____no_output_____"
]
],
[
[
"**Note:** If you're using `split_into_train_test()` to split the dataset, it expects all images to be PNG, JPEG, or BMP—it ignores other file types.",
"_____no_output_____"
],
[
"### Download the yoga dataset",
"_____no_output_____"
]
],
[
[
"if not is_skip_step_1 and not use_custom_dataset:\n !wget -O yoga_poses.zip http://download.tensorflow.org/data/pose_classification/yoga_poses.zip\n !unzip -q yoga_poses.zip -d yoga_cg\n IMAGES_ROOT = \"yoga_cg\"",
"_____no_output_____"
]
],
[
[
"### Preprocess the `TRAIN` dataset",
"_____no_output_____"
]
],
[
[
"if not is_skip_step_1:\n images_in_train_folder = os.path.join(IMAGES_ROOT, 'train')\n images_out_train_folder = 'poses_images_out_train'\n csvs_out_train_path = 'train_data.csv'\n\n preprocessor = MoveNetPreprocessor(\n images_in_folder=images_in_train_folder,\n images_out_folder=images_out_train_folder,\n csvs_out_path=csvs_out_train_path,\n )\n\n preprocessor.process(per_pose_class_limit=None)",
"_____no_output_____"
]
],
[
[
"### Preprocess the `TEST` dataset",
"_____no_output_____"
]
],
[
[
"if not is_skip_step_1:\n images_in_test_folder = os.path.join(IMAGES_ROOT, 'test')\n images_out_test_folder = 'poses_images_out_test'\n csvs_out_test_path = 'test_data.csv'\n\n preprocessor = MoveNetPreprocessor(\n images_in_folder=images_in_test_folder,\n images_out_folder=images_out_test_folder,\n csvs_out_path=csvs_out_test_path,\n )\n\n preprocessor.process(per_pose_class_limit=None)",
"_____no_output_____"
]
],
[
[
"## Part 2: Train a pose classification model that takes the landmark coordinates as input, and output the predicted labels.\n\nYou'll build a TensorFlow model that takes the landmark coordinates and predicts the pose class that the person in the input image performs. The model consists of two submodels:\n\n* Submodel 1 calculates a pose embedding (a.k.a feature vector) from the detected landmark coordinates.\n* Submodel 2 feeds pose embedding through several `Dense` layer to predict the pose class.\n\nYou'll then train the model based on the dataset that were preprocessed in part 1.",
"_____no_output_____"
],
[
"### (Optional) Download the preprocessed dataset if you didn't run part 1",
"_____no_output_____"
]
],
[
[
"# Download the preprocessed CSV files which are the same as the output of step 1\nif is_skip_step_1:\n !wget -O train_data.csv http://download.tensorflow.org/data/pose_classification/yoga_train_data.csv\n !wget -O test_data.csv http://download.tensorflow.org/data/pose_classification/yoga_test_data.csv\n\n csvs_out_train_path = 'train_data.csv'\n csvs_out_test_path = 'test_data.csv'\n is_skipped_step_1 = True",
"_____no_output_____"
]
],
[
[
"### Load the preprocessed CSVs into `TRAIN` and `TEST` datasets.",
"_____no_output_____"
]
],
[
[
"def load_pose_landmarks(csv_path):\n \"\"\"Loads a CSV created by MoveNetPreprocessor.\n \n Returns:\n X: Detected landmark coordinates and scores of shape (N, 17 * 3)\n y: Ground truth labels of shape (N, label_count)\n classes: The list of all class names found in the dataset\n dataframe: The CSV loaded as a Pandas dataframe features (X) and ground\n truth labels (y) to use later to train a pose classification model.\n \"\"\"\n\n # Load the CSV file\n dataframe = pd.read_csv(csv_path)\n df_to_process = dataframe.copy()\n\n # Drop the file_name columns as you don't need it during training.\n df_to_process.drop(columns=['file_name'], inplace=True)\n\n # Extract the list of class names\n classes = df_to_process.pop('class_name').unique()\n\n # Extract the labels\n y = df_to_process.pop('class_no')\n\n # Convert the input features and labels into the correct format for training.\n X = df_to_process.astype('float64')\n y = keras.utils.to_categorical(y)\n\n return X, y, classes, dataframe",
"_____no_output_____"
]
],
[
[
"Load and split the original `TRAIN` dataset into `TRAIN` (85% of the data) and `VALIDATE` (the remaining 15%).",
"_____no_output_____"
]
],
[
[
"# Load the train data\nX, y, class_names, _ = load_pose_landmarks(csvs_out_train_path)\n\n# Split training data (X, y) into (X_train, y_train) and (X_val, y_val)\nX_train, X_val, y_train, y_val = train_test_split(X, y,\n test_size=0.15)",
"_____no_output_____"
],
[
"# Load the test data\nX_test, y_test, _, df_test = load_pose_landmarks(csvs_out_test_path)",
"_____no_output_____"
]
],
[
[
"### Define functions to convert the pose landmarks to a pose embedding (a.k.a. feature vector) for pose classification\n\nNext, convert the landmark coordinates to a feature vector by:\n1. Moving the pose center to the origin.\n2. Scaling the pose so that the pose size becomes 1\n3. Flattening these coordinates into a feature vector\n\nThen use this feature vector to train a neural-network based pose classifier.",
"_____no_output_____"
]
],
[
[
"def get_center_point(landmarks, left_bodypart, right_bodypart):\n \"\"\"Calculates the center point of the two given landmarks.\"\"\"\n\n left = tf.gather(landmarks, left_bodypart.value, axis=1)\n right = tf.gather(landmarks, right_bodypart.value, axis=1)\n center = left * 0.5 + right * 0.5\n return center\n\n\ndef get_pose_size(landmarks, torso_size_multiplier=2.5):\n \"\"\"Calculates pose size.\n\n It is the maximum of two values:\n * Torso size multiplied by `torso_size_multiplier`\n * Maximum distance from pose center to any pose landmark\n \"\"\"\n # Hips center\n hips_center = get_center_point(landmarks, BodyPart.LEFT_HIP, \n BodyPart.RIGHT_HIP)\n\n # Shoulders center\n shoulders_center = get_center_point(landmarks, BodyPart.LEFT_SHOULDER,\n BodyPart.RIGHT_SHOULDER)\n\n # Torso size as the minimum body size\n torso_size = tf.linalg.norm(shoulders_center - hips_center)\n\n # Pose center\n pose_center_new = get_center_point(landmarks, BodyPart.LEFT_HIP, \n BodyPart.RIGHT_HIP)\n pose_center_new = tf.expand_dims(pose_center_new, axis=1)\n # Broadcast the pose center to the same size as the landmark vector to\n # perform substraction\n pose_center_new = tf.broadcast_to(pose_center_new,\n [tf.size(landmarks) // (17*2), 17, 2])\n\n # Dist to pose center\n d = tf.gather(landmarks - pose_center_new, 0, axis=0,\n name=\"dist_to_pose_center\")\n # Max dist to pose center\n max_dist = tf.reduce_max(tf.linalg.norm(d, axis=0))\n\n # Normalize scale\n pose_size = tf.maximum(torso_size * torso_size_multiplier, max_dist)\n\n return pose_size\n\n\ndef normalize_pose_landmarks(landmarks):\n \"\"\"Normalizes the landmarks translation by moving the pose center to (0,0) and\n scaling it to a constant pose size.\n \"\"\"\n # Move landmarks so that the pose center becomes (0,0)\n pose_center = get_center_point(landmarks, BodyPart.LEFT_HIP, \n BodyPart.RIGHT_HIP)\n pose_center = tf.expand_dims(pose_center, axis=1)\n # Broadcast the pose center to the same size as the landmark vector to perform\n # substraction\n pose_center = tf.broadcast_to(pose_center, \n [tf.size(landmarks) // (17*2), 17, 2])\n landmarks = landmarks - pose_center\n\n # Scale the landmarks to a constant pose size\n pose_size = get_pose_size(landmarks)\n landmarks /= pose_size\n\n return landmarks\n\n\ndef landmarks_to_embedding(landmarks_and_scores):\n \"\"\"Converts the input landmarks into a pose embedding.\"\"\"\n # Reshape the flat input into a matrix with shape=(17, 3)\n reshaped_inputs = keras.layers.Reshape((17, 3))(landmarks_and_scores)\n\n # Normalize landmarks 2D\n landmarks = normalize_pose_landmarks(reshaped_inputs[:, :, :2])\n\n # Flatten the normalized landmark coordinates into a vector\n embedding = keras.layers.Flatten()(landmarks)\n\n return embedding",
"_____no_output_____"
]
],
[
[
"### Define a Keras model for pose classification\n\nOur Keras model takes the detected pose landmarks, then calculates the pose embedding and predicts the pose class.",
"_____no_output_____"
]
],
[
[
"# Define the model\ninputs = tf.keras.Input(shape=(51))\nembedding = landmarks_to_embedding(inputs)\n\nlayer = keras.layers.Dense(128, activation=tf.nn.relu6)(embedding)\nlayer = keras.layers.Dropout(0.5)(layer)\nlayer = keras.layers.Dense(64, activation=tf.nn.relu6)(layer)\nlayer = keras.layers.Dropout(0.5)(layer)\noutputs = keras.layers.Dense(5, activation=\"softmax\")(layer)\n\nmodel = keras.Model(inputs, outputs)\nmodel.summary()",
"_____no_output_____"
],
[
"model.compile(\n optimizer='adam',\n loss='categorical_crossentropy',\n metrics=['accuracy']\n)\n\n# Add a checkpoint callback to store the checkpoint that has the highest\n# validation accuracy.\ncheckpoint_path = \"weights.best.hdf5\"\ncheckpoint = keras.callbacks.ModelCheckpoint(checkpoint_path,\n monitor='val_accuracy',\n verbose=1,\n save_best_only=True,\n mode='max')\nearlystopping = keras.callbacks.EarlyStopping(monitor='val_accuracy', \n patience=20)\n\n# Start training\nhistory = model.fit(X_train, y_train,\n epochs=200,\n batch_size=16,\n validation_data=(X_val, y_val),\n callbacks=[checkpoint, earlystopping])",
"_____no_output_____"
],
[
"# Visualize the training history to see whether you're overfitting.\nplt.plot(history.history['accuracy'])\nplt.plot(history.history['val_accuracy'])\nplt.title('Model accuracy')\nplt.ylabel('accuracy')\nplt.xlabel('epoch')\nplt.legend(['TRAIN', 'VAL'], loc='lower right')\nplt.show()",
"_____no_output_____"
],
[
"# Evaluate the model using the TEST dataset\nloss, accuracy = model.evaluate(X_test, y_test)",
"_____no_output_____"
]
],
[
[
"### Draw the confusion matrix to better understand the model performance",
"_____no_output_____"
]
],
[
[
"def plot_confusion_matrix(cm, classes,\n normalize=False,\n title='Confusion matrix',\n cmap=plt.cm.Blues):\n \"\"\"Plots the confusion matrix.\"\"\"\n if normalize:\n cm = cm.astype('float') / cm.sum(axis=1)[:, np.newaxis]\n print(\"Normalized confusion matrix\")\n else:\n print('Confusion matrix, without normalization')\n\n plt.imshow(cm, interpolation='nearest', cmap=cmap)\n plt.title(title)\n plt.colorbar()\n tick_marks = np.arange(len(classes))\n plt.xticks(tick_marks, classes, rotation=55)\n plt.yticks(tick_marks, classes)\n fmt = '.2f' if normalize else 'd'\n thresh = cm.max() / 2.\n for i, j in itertools.product(range(cm.shape[0]), range(cm.shape[1])):\n plt.text(j, i, format(cm[i, j], fmt),\n horizontalalignment=\"center\",\n color=\"white\" if cm[i, j] > thresh else \"black\")\n\n plt.ylabel('True label')\n plt.xlabel('Predicted label')\n plt.tight_layout()\n\n# Classify pose in the TEST dataset using the trained model\ny_pred = model.predict(X_test)\n\n# Convert the prediction result to class name\ny_pred_label = [class_names[i] for i in np.argmax(y_pred, axis=1)]\ny_true_label = [class_names[i] for i in np.argmax(y_test, axis=1)]\n\n# Plot the confusion matrix\ncm = confusion_matrix(np.argmax(y_test, axis=1), np.argmax(y_pred, axis=1))\nplot_confusion_matrix(cm,\n class_names,\n title ='Confusion Matrix of Pose Classification Model')\n\n# Print the classification report\nprint('\\nClassification Report:\\n', classification_report(y_true_label,\n y_pred_label))",
"_____no_output_____"
]
],
[
[
"### (Optional) Investigate incorrect predictions\n\nYou can look at the poses from the `TEST` dataset that were incorrectly predicted to see whether the model accuracy can be improved.\n\nNote: This only works if you have run step 1 because you need the pose image files on your local machine to display them.",
"_____no_output_____"
]
],
[
[
"if is_skip_step_1:\n raise RuntimeError('You must have run step 1 to run this cell.')\n\n# If step 1 was skipped, skip this step.\nIMAGE_PER_ROW = 3\nMAX_NO_OF_IMAGE_TO_PLOT = 30\n\n# Extract the list of incorrectly predicted poses\nfalse_predict = [id_in_df for id_in_df in range(len(y_test)) \\\n if y_pred_label[id_in_df] != y_true_label[id_in_df]]\nif len(false_predict) > MAX_NO_OF_IMAGE_TO_PLOT:\n false_predict = false_predict[:MAX_NO_OF_IMAGE_TO_PLOT]\n\n# Plot the incorrectly predicted images\nrow_count = len(false_predict) // IMAGE_PER_ROW + 1\nfig = plt.figure(figsize=(10 * IMAGE_PER_ROW, 10 * row_count))\nfor i, id_in_df in enumerate(false_predict):\n ax = fig.add_subplot(row_count, IMAGE_PER_ROW, i + 1)\n image_path = os.path.join(images_out_test_folder,\n df_test.iloc[id_in_df]['file_name'])\n\n image = cv2.imread(image_path)\n plt.title(\"Predict: %s; Actual: %s\"\n % (y_pred_label[id_in_df], y_true_label[id_in_df]))\n plt.imshow(cv2.cvtColor(image, cv2.COLOR_BGR2RGB))\nplt.show()",
"_____no_output_____"
]
],
[
[
"## Part 3: Convert the pose classification model to TensorFlow Lite\n\nYou'll convert the Keras pose classification model to the TensorFlow Lite format so that you can deploy it to mobile apps, web browsers and IoT devices. When converting the model, you'll apply [dynamic range quantization](https://www.tensorflow.org/lite/performance/post_training_quant) to reduce the pose classification TensorFlow Lite model size by about 4 times with insignificant accuracy loss.\n\nNote: TensorFlow Lite supports multiple quantization schemes. See the [documentation](https://www.tensorflow.org/lite/performance/model_optimization) if you are interested to learn more.",
"_____no_output_____"
]
],
[
[
"converter = tf.lite.TFLiteConverter.from_keras_model(model)\nconverter.optimizations = [tf.lite.Optimize.DEFAULT]\ntflite_model = converter.convert()\n\nprint('Model size: %dKB' % (len(tflite_model) / 1024))\n\nwith open('pose_classifier.tflite', 'wb') as f:\n f.write(tflite_model)",
"_____no_output_____"
]
],
[
[
"Then you'll write the label file which contains mapping from the class indexes to the human readable class names.",
"_____no_output_____"
]
],
[
[
"with open('pose_labels.txt', 'w') as f:\n f.write('\\n'.join(class_names))",
"_____no_output_____"
]
],
[
[
"As you've applied quantization to reduce the model size, let's evaluate the quantized TFLite model to check whether the accuracy drop is acceptable.",
"_____no_output_____"
]
],
[
[
"def evaluate_model(interpreter, X, y_true):\n \"\"\"Evaluates the given TFLite model and return its accuracy.\"\"\"\n input_index = interpreter.get_input_details()[0][\"index\"]\n output_index = interpreter.get_output_details()[0][\"index\"]\n\n # Run predictions on all given poses.\n y_pred = []\n for i in range(len(y_true)):\n # Pre-processing: add batch dimension and convert to float32 to match with\n # the model's input data format.\n test_image = X[i: i + 1].astype('float32')\n interpreter.set_tensor(input_index, test_image)\n\n # Run inference.\n interpreter.invoke()\n\n # Post-processing: remove batch dimension and find the class with highest\n # probability.\n output = interpreter.tensor(output_index)\n predicted_label = np.argmax(output()[0])\n y_pred.append(predicted_label)\n\n # Compare prediction results with ground truth labels to calculate accuracy.\n y_pred = keras.utils.to_categorical(y_pred)\n return accuracy_score(y_true, y_pred)\n\n# Evaluate the accuracy of the converted TFLite model\nclassifier_interpreter = tf.lite.Interpreter(model_content=tflite_model)\nclassifier_interpreter.allocate_tensors()\nprint('Accuracy of TFLite model: %s' %\n evaluate_model(classifier_interpreter, X_test, y_test))",
"_____no_output_____"
]
],
[
[
"Now you can download the TFLite model (`pose_classifier.tflite`) and the label file (`pose_labels.txt`) to classify custom poses. See the [Android](https://github.com/tensorflow/examples/tree/master/lite/examples/pose_estimation/android) and [Python/Raspberry Pi](https://github.com/tensorflow/examples/tree/master/lite/examples/pose_estimation/raspberry_pi) sample app for an end-to-end example of how to use the TFLite pose classification model.",
"_____no_output_____"
]
],
[
[
"!zip pose_classifier.zip pose_labels.txt pose_classifier.tflite",
"_____no_output_____"
],
[
"# Download the zip archive if running on Colab.\ntry:\n from google.colab import files\n files.download('pose_classifier.zip')\nexcept:\n pass",
"_____no_output_____"
]
]
] | [
"markdown",
"code",
"markdown",
"code",
"markdown",
"code",
"markdown",
"code",
"markdown",
"code",
"markdown",
"code",
"markdown",
"code",
"markdown",
"code",
"markdown",
"code",
"markdown",
"code",
"markdown",
"code",
"markdown",
"code",
"markdown",
"code",
"markdown",
"code",
"markdown",
"code",
"markdown",
"code",
"markdown",
"code",
"markdown",
"code",
"markdown",
"code",
"markdown",
"code"
] | [
[
"markdown"
],
[
"code"
],
[
"markdown",
"markdown",
"markdown"
],
[
"code",
"code"
],
[
"markdown"
],
[
"code",
"code",
"code",
"code"
],
[
"markdown"
],
[
"code"
],
[
"markdown"
],
[
"code"
],
[
"markdown"
],
[
"code",
"code"
],
[
"markdown",
"markdown"
],
[
"code"
],
[
"markdown"
],
[
"code"
],
[
"markdown"
],
[
"code"
],
[
"markdown",
"markdown"
],
[
"code"
],
[
"markdown"
],
[
"code"
],
[
"markdown"
],
[
"code",
"code"
],
[
"markdown"
],
[
"code"
],
[
"markdown"
],
[
"code",
"code",
"code",
"code"
],
[
"markdown"
],
[
"code"
],
[
"markdown"
],
[
"code"
],
[
"markdown"
],
[
"code"
],
[
"markdown"
],
[
"code"
],
[
"markdown"
],
[
"code"
],
[
"markdown"
],
[
"code",
"code"
]
] |
e78151b097a5dacd848bca9cf8d437ff8db05d35 | 154,454 | ipynb | Jupyter Notebook | california_housing.ipynb | crazrycoin/Open-source | 8d4a241b75c429ceb6724b1432f110183c2b3b12 | [
"MIT"
] | null | null | null | california_housing.ipynb | crazrycoin/Open-source | 8d4a241b75c429ceb6724b1432f110183c2b3b12 | [
"MIT"
] | 1 | 2022-03-22T00:52:24.000Z | 2022-03-30T01:20:39.000Z | california_housing.ipynb | crazrycoin/Open-source | 8d4a241b75c429ceb6724b1432f110183c2b3b12 | [
"MIT"
] | null | null | null | 190.683951 | 73,753 | 0.826829 | [
[
[
"<a href=\"https://colab.research.google.com/github/Castlemincode/Open-source/blob/main/california_housing.ipynb\" target=\"_parent\"><img src=\"https://colab.research.google.com/assets/colab-badge.svg\" alt=\"Open In Colab\"/></a>",
"_____no_output_____"
]
],
[
[
"import pandas as pd\nimport numpy as np\nimport matplotlib.pyplot as plt\nimport seaborn as sns",
"_____no_output_____"
],
[
"train = pd.read_csv('/content/sample_data/california_housing_test.csv')\ntest = pd.read_csv('/content/sample_data/california_housing_train.csv')\ntrain.head()",
"_____no_output_____"
],
[
"test.head()",
"_____no_output_____"
],
[
"train.describe()",
"_____no_output_____"
],
[
"train.hist(figsize=(15,13), grid=False, bins=50)\nplt.show()",
"_____no_output_____"
],
[
"correlation = train.corr()",
"_____no_output_____"
],
[
"plt.figure(figsize=(10,10))\nsns.heatmap(correlation , annot=True)\nplt.show()",
"_____no_output_____"
]
]
] | [
"markdown",
"code"
] | [
[
"markdown"
],
[
"code",
"code",
"code",
"code",
"code",
"code",
"code"
]
] |
e7818810e8755e339aeb4d4724d7888bbfc228fc | 528,639 | ipynb | Jupyter Notebook | notebook/06 WRF Python - Boundary layer height plot.ipynb | sonnymetvn/Basic-Python-for-Meteorology | cf98ffe4f92e76b746c1de253c34ef50835bbf26 | [
"MIT"
] | 8 | 2021-09-22T00:39:31.000Z | 2022-03-31T22:49:43.000Z | notebook/06 WRF Python - Boundary layer height plot.ipynb | sonnymetvn/Basic-Python-for-Meteorology | cf98ffe4f92e76b746c1de253c34ef50835bbf26 | [
"MIT"
] | null | null | null | notebook/06 WRF Python - Boundary layer height plot.ipynb | sonnymetvn/Basic-Python-for-Meteorology | cf98ffe4f92e76b746c1de253c34ef50835bbf26 | [
"MIT"
] | 3 | 2021-12-29T11:13:33.000Z | 2022-02-01T11:13:18.000Z | 2,986.661017 | 391,496 | 0.964662 | [
[
[
"from IPython.display import Image\nImage('06overview.png')",
"_____no_output_____"
]
],
[
[
"In this tutorial, we will learn how to plot a variable \"Boundary layer height\" for a particular output of WRF model.\nReferrence: \nhttps://wrf-python.readthedocs.io/en/latest/index.html",
"_____no_output_____"
],
[
"# 1. Import libraries",
"_____no_output_____"
]
],
[
[
"# Loading necessary libraries\nimport numpy as np\nfrom netCDF4 import Dataset\nimport matplotlib.pyplot as plt\nfrom matplotlib.cm import get_cmap\nimport cartopy.crs as crs\nfrom cartopy.feature import NaturalEarthFeature\n\nfrom wrf import (to_np, getvar, smooth2d, get_cartopy, cartopy_xlim,\n cartopy_ylim, latlon_coords, interplevel)",
"_____no_output_____"
]
],
[
[
"# 2. Download data",
"_____no_output_____"
]
],
[
[
"# specify where is the location of the data\npath_in = \"data/\"\npath_out = \"./\"\n# Open the NetCDF file\nncfile = Dataset(path_in + 'wrfout_d01_2016-05-09_00^%00^%00')",
"_____no_output_____"
]
],
[
[
"# 3. Take out the variables",
"_____no_output_____"
]
],
[
[
"# Get the boundary layer height\nPBLH = getvar(ncfile, \"PBLH\")\nprint(PBLH.dims)",
"('south_north', 'west_east')\n"
]
],
[
[
"# 4. Plotting",
"_____no_output_____"
]
],
[
[
"PBLH.plot()",
"_____no_output_____"
]
],
[
[
"## All done !!!\n- Please feel free to let me know if there is any analysis that you would like me to do\n- Please subscribe my youtube channel too\n- Thank you very much",
"_____no_output_____"
]
]
] | [
"code",
"markdown",
"code",
"markdown",
"code",
"markdown",
"code",
"markdown",
"code",
"markdown"
] | [
[
"code"
],
[
"markdown",
"markdown"
],
[
"code"
],
[
"markdown"
],
[
"code"
],
[
"markdown"
],
[
"code"
],
[
"markdown"
],
[
"code"
],
[
"markdown"
]
] |
e78197ea8143acb2a95fd042b1d7fc7009522de7 | 22,305 | ipynb | Jupyter Notebook | first_mask_tissuesegmentation.ipynb | PieterDujardin/AI_pathology | 717e056b4e3d7788d563d24570ab3d683ed44ae4 | [
"MIT"
] | null | null | null | first_mask_tissuesegmentation.ipynb | PieterDujardin/AI_pathology | 717e056b4e3d7788d563d24570ab3d683ed44ae4 | [
"MIT"
] | null | null | null | first_mask_tissuesegmentation.ipynb | PieterDujardin/AI_pathology | 717e056b4e3d7788d563d24570ab3d683ed44ae4 | [
"MIT"
] | null | null | null | 55.074074 | 7,356 | 0.710648 | [
[
[
"import os\nfrom openslide import OpenSlide\nimport matplotlib.pyplot as plt\n\nimport os\nimport sys\nimport glob\nimport random\nimport pickle\nimport numpy as np\nimport pandas as pd\nfrom PIL import Image\nfrom skimage import color\nfrom skimage import filters\nfrom skimage.morphology import disk\nfrom openslide import OpenSlide, OpenSlideUnsupportedFormatError\n\nimport histolab\nfrom histolab.slide import Slide\nfrom histolab.types import CoordinatePair\nfrom histolab.masks import BinaryMask\n\nfrom skimage.color import rgb2hsv\nfrom skimage.filters import threshold_otsu\n\nfrom histolab.tiler import GridTiler",
"_____no_output_____"
],
[
"level = 8 #changes the shape dimensions of the binarymask, makes more sense to do masking on a higher level \nRGB_min = 10 #the smaller the more intensified the yellow map is (bigger mask (more 1s))\n\nclass MaskwithSegmentTissue(BinaryMask):\n def _mask(self, slide): # pragma: no cover\n # This property will be supplied by the inheriting classes individually\n extractedtile = slide.extract_tile(coords = CoordinatePair(0,0,0,0), level = level, tile_size = slide.level_dimensions(level))\n extractedtile= extractedtile.image.convert('RGB')\n\n # note the shape of img_RGB is the transpose of slide.level_dimensions\n# img_RGB = np.transpose(np.array(extractedtile),\n# axes=[1, 0, 2])\n img_RGB = np.array(extractedtile)\n\n\n img_HSV = rgb2hsv(img_RGB)\n\n background_R = img_RGB[:, :, 0] > threshold_otsu(img_RGB[:, :, 0])\n background_G = img_RGB[:, :, 1] > threshold_otsu(img_RGB[:, :, 1])\n background_B = img_RGB[:, :, 2] > threshold_otsu(img_RGB[:, :, 2])\n tissue_RGB = np.logical_not(background_R & background_G & background_B)\n tissue_S = img_HSV[:, :, 1] > threshold_otsu(img_HSV[:, :, 1])\n min_R = img_RGB[:, :, 0] > RGB_min\n min_G = img_RGB[:, :, 1] > RGB_min\n min_B = img_RGB[:, :, 2] > RGB_min\n\n tissue_mask = tissue_S & tissue_RGB & min_R & min_G & min_B\n\n return tissue_mask \n ",
"_____no_output_____"
],
[
"image_path = '/Users/imac/Documents/Documents_Pieter_MacBook_Pro/0MasterAI/THESIS/data/CAMELYON/normal_001.tif'\nprocessed_path = '/Users/imac/Documents/Documents_Pieter_MacBook_Pro/0MasterAI/THESIS/data/CAMELYON/processedimage'\nfirstslide = Slide(image_path,processed_path) #input and output path \n\nbinary_mask = MaskwithSegmentTissue()\nbinary_mask(firstslide)\n# plt.imshow(binary_mask(firstslide))\n\n\n",
"_____no_output_____"
],
[
"firstslide.scaled_image()",
"_____no_output_____"
],
[
"binary_mask = MaskwithSegmentTissue()\nbinary_mask(firstslide).shape #height, width ",
"_____no_output_____"
],
[
"binary_mask = MaskwithSegmentTissue()\nplt.imshow(binary_mask(firstslide))",
"_____no_output_____"
],
[
"firstslide.level_dimensions(6) #Return the slide dimensions (w,h)",
"_____no_output_____"
],
[
"firstslide.locate_mask(binary_mask= MaskwithSegmentTissue())",
"_____no_output_____"
],
[
"# level should vary between 0,1 and 2\ngridtiler = GridTiler(tile_size = (224,224), level=4, tissue_percent=0.75)\ngridtiler.locate_tiles(slide = firstslide, extraction_mask = MaskwithSegmentTissue(), scale_factor=32, alpha = 128 )",
"_____no_output_____"
],
[
"# level should vary between 0,1 and 2\ngridtiler = GridTiler(tile_size = (224,224), level=0, tissue_percent=0.75)\ngridtiler.locate_tiles(slide = firstslide, extraction_mask = MaskwithSegmentTissue(), scale_factor=32, alpha = 128 )",
"_____no_output_____"
],
[
"image_path = '/Users/imac/Documents/Documents_Pieter_MacBook_Pro/0MasterAI/THESIS/data/CAMELYON/normal_001.tif'\nprocessed_path = '/Users/imac/Documents/tryout'\nfirstslide = Slide(image_path,processed_path)\n\n#note: maskwithsegmenttissue has a level, and gritiler has a level\n\ngridtiler = GridTiler(tile_size = (224,224), level = 4, tissue_percent=0.75)\ngridtiler.extract(slide = firstslide, extraction_mask = MaskwithSegmentTissue())\n",
"_____no_output_____"
],
[
"# training set\nfolder = '/Users/imac/Documents/Documents_Pieter_MacBook_Pro/0MasterAI/THESIS/data/CAMELYON'\n\n#first a loop for normal \nindex = 1\nfor idx, image in enumerate(os.listdir(folder)):\n if image[:6] == 'normal':\n print(image[:6])\n image_path = os.path.join(folder, image)\n processed_path = os.path.join('/Users/imac/Documents/tryoutcamelyon/training_data/normal', str(index))\n slide = Slide(image_path, processed_path)\n gridtiler = GridTiler(tile_size = (224,224), level = 4, tissue_percent=0.75)\n gridtiler.extract(slide = slide, extraction_mask = MaskwithSegmentTissue())\n index += 1\n \n\n \n\n#then a loop for tumor \n\n",
"normal\nnormal\nnormal\n"
]
]
] | [
"code"
] | [
[
"code",
"code",
"code",
"code",
"code",
"code",
"code",
"code",
"code",
"code",
"code",
"code"
]
] |
e781a37a0d09e5cffbdde19bc4582baaefddb69a | 18,988 | ipynb | Jupyter Notebook | services/dy-2Dgraph/use-cases/cc/cc-single-cell/notebooks/cc_single_cell.ipynb | GitHK/osparc-services-forked | a8ab08ff7c32de8f1abde015c1515e8cf61426c0 | [
"MIT"
] | 1 | 2019-07-26T02:04:44.000Z | 2019-07-26T02:04:44.000Z | services/dy-2Dgraph/use-cases/cc/cc-single-cell/notebooks/cc_single_cell.ipynb | mguidon/osparc-services | 1cff293fee5e61a6708f1148077ca6a33880c7f4 | [
"MIT"
] | null | null | null | services/dy-2Dgraph/use-cases/cc/cc-single-cell/notebooks/cc_single_cell.ipynb | mguidon/osparc-services | 1cff293fee5e61a6708f1148077ca6a33880c7f4 | [
"MIT"
] | null | null | null | 32.62543 | 152 | 0.494154 | [
[
[
"from IPython.display import HTML\n\nHTML('''<script>\ncode_show=true; \nfunction code_toggle() {\n if (code_show){\n $('div.input').hide();\n } else {\n $('div.input').show();\n }\n code_show = !code_show\n} \n$( document ).ready(code_toggle);\n</script>\n<form action=\"javascript:code_toggle()\"><input type=\"submit\" value=\"Click here to toggle on/off the raw code.\"></form>''')",
"_____no_output_____"
],
[
"%%javascript\n$('#menubar').hide();",
"_____no_output_____"
],
[
"import os",
"_____no_output_____"
],
[
"import plotly.offline as offline\nimport plotly.figure_factory as ff\nimport plotly.graph_objs as go\nfrom plotly import tools\noffline.init_notebook_mode(connected=True)\n\nSLICING = 10\n\ndef create_graphs(data_frames, **kwargs):\n data = [ \n go.Scatter(\n x=data_frames[df_index].iloc[0::SLICING,0],\n y=data_frames[df_index].iloc[0::SLICING,i],\n #opacity=1, \n xaxis=(\"x\" + str(df_index + 1)),\n yaxis=(\"y\" + str(df_index + 1)),\n name=str(data_frames[df_index].columns[i])\n ) for df_index in range(0, len(data_frames)) \n for i in range(1,data_frames[df_index].columns.size)\n ]\n \n layout = go.Layout(**kwargs)\n fig = go.Figure(data=data, layout=layout)\n offline.iplot(fig, config={\"displayModeBar\": False, \"showLink\":False})\n\ndef create_graph(data_frame, title=None, x_axis_title=None, y_axis_title = None):\n data = [ \n go.Scatter(\n x=data_frame.iloc[0::SLICING,0],\n y=data_frame.iloc[0::SLICING,i],\n #opacity=1,\n name=str(data_frame.columns[i])\n ) \n for i in range(1,data_frame.columns.size)\n \n ]\n \n #fig = tools.make_subplots(rows=1, cols=len(data_frames))\n layout = go.Layout(\n title=title, \n showlegend=False,\n xaxis=dict(\n title=x_axis_title\n ),\n yaxis=dict(\n title=y_axis_title\n ) \n )\n fig = go.Figure(data=data, layout=layout)\n offline.iplot(fig, config={\"displayModeBar\": False, \"showLink\":False})",
"_____no_output_____"
],
[
"from simcore_sdk import node_ports\nPORTS = await node_ports.ports()",
"_____no_output_____"
],
[
"# from plotall.m\n\nimport pandas as pd\n\ndata_path_ty = await (await PORTS.inputs)[0].get()\ndata_frame_ty = pd.read_csv(data_path_ty, sep='\\t', header=None)\n\n# scale time\nf = lambda x: x/1000.0\ndata_frame_ty[0] = data_frame_ty[0].apply(f)\nsyids = 9\nyids = [30, 31, 32, 33, 34, 36, 37, 38, 39]\nynid = [0] * 206\nfor id in range(1,syids):\n ynid[yids[id]] = id\n\ndata_path_ar = await (await PORTS.inputs)[1].get()\ndata_frame_ar = pd.read_csv(data_path_ar, sep='\\t', header=None)\n\ntArray = 1\nI_Ca_store = 2\nIto = 3\nItof = 4\nItos = 5\nINa = 6\nIK1 = 7\ns1 = 8\nk1 = 9\nJserca = 10\nIks = 11\nIkr = 12\nJleak = [13,14]\nICFTR = 15\nIncx = 16",
"_____no_output_____"
],
[
"# membrane potential\ntitle=\"Membrane Potential\"\naxis_colums = [0,ynid[39]+1]\nplot_0 = data_frame_ty.filter(items=[data_frame_ty.columns[i] for i in axis_colums])\ncreate_graph(data_frame=plot_0, \n x_axis_title=\"time (sec)\",\n y_axis_title=title)",
"_____no_output_____"
],
[
"# LCC current (ICa)\ntitle=\"I<sub>Ca</sub> (pA/pF)\"\naxis_colums = [0,I_Ca_store-1]\nplot_1 = data_frame_ar.filter(items=[data_frame_ar.columns[i] for i in axis_colums])\ncreate_graph(data_frame=plot_1, \n x_axis_title=\"time (sec)\",\n y_axis_title=title)\n",
"_____no_output_____"
],
[
"# CaSRT & Caj\ndata_frame_casrt = data_frame_ty.filter(items=[data_frame_ty.columns[0], data_frame_ty.columns[ynid[30]+1], data_frame_ty.columns[ynid[31]+1]])\ndata_frame_casrt[3] = data_frame_casrt[1] + data_frame_casrt[2]\nplot_2 = data_frame_casrt.filter(items=[data_frame_casrt.columns[0], data_frame_casrt.columns[3]])\nplot_data = [plot_2]\n\n# \ng = lambda x: x*1000.0\naxis_colums = [0,ynid[36]+1]\ndata_frame_ty[ynid[36]+1] = data_frame_ty[ynid[36]+1].apply(g)\nplot_3 = data_frame_ty.filter(items=[data_frame_ty.columns[i] for i in axis_colums])\nplot_data.append(plot_3)\n\naxis_colums = [0,ynid[37]+1]\nplot_4 = data_frame_ty.filter(items=[data_frame_ty.columns[i] for i in axis_colums])\nplot_data.append(plot_4)\ncreate_graphs(data_frames=plot_data, \n title=None, \n showlegend=False,\n xaxis=dict(\n domain=[0,0.3],\n title=\"time (sec)\"\n ),\n xaxis2=dict(\n domain=[0.4,0.6],\n title=\"time (sec)\"),\n xaxis3=dict(\n domain=[0.7,1.0],\n title=\"time (sec)\"),\n yaxis=dict(\n title=\"[Ca]<sub>SRT</sub> (mM)\"\n ),\n yaxis2=dict(\n title=\"Ca Dyad (\\u00B5M)\", \n anchor=\"x2\"),\n yaxis3=dict(\n title=\"Ca sl (mM)\", \n anchor=\"x3\")\n )",
"_____no_output_____"
],
[
"# Cai\ntitle=\"[Ca]<sub>i</sub> (\\u00B5M)\"\naxis_colums = [0,ynid[38]+1]\nplot_5 = data_frame_ty.filter(items=[data_frame_ty.columns[i] for i in axis_colums])\ncreate_graph(data_frame=plot_5, \n title=None, \n x_axis_title=\"time (sec)\",\n y_axis_title=title)",
"_____no_output_____"
],
[
"# Ito\ntitle=\"I<sub>to</sub> (pA/pF)\"\naxis_colums = [0,Ito-1]\nplot_6 = data_frame_ar.filter(items=[data_frame_ar.columns[i] for i in axis_colums])\ncreate_graph(data_frame=plot_6, \n title=None, \n x_axis_title=\"time (sec)\",\n y_axis_title=title)\n",
"_____no_output_____"
],
[
"# INa\ntitle=\"I<sub>Na</sub> (pA/pF)\"\naxis_colums = [0,INa-1]\nplot_7 = data_frame_ar.filter(items=[data_frame_ar.columns[i] for i in axis_colums])\ncreate_graph(data_frame=plot_7, \n title=None, \n x_axis_title=\"time (sec)\",\n y_axis_title=title)\n",
"_____no_output_____"
],
[
"# IKs and ICFTR\naxis_colums = [0,Iks-1]\nplot_8 = data_frame_ar.filter(items=[data_frame_ar.columns[i] for i in axis_colums])\nplot_data = [plot_8]\n\naxis_colums = [0,ICFTR-1]\nplot_9 = data_frame_ar.filter(items=[data_frame_ar.columns[i] for i in axis_colums])\nplot_data.append(plot_9)\n\ncreate_graphs(data_frames=plot_data, \n title=None, \n showlegend=False,\n #xaxis=dict(title=\"time (sec)\"),\n xaxis2=dict(title=\"time (sec)\", anchor=\"y2\"),\n yaxis=dict(\n domain=[0.6,1.0],\n title=\"I<sub>Ks</sub> (pA/pF)\"\n ),\n yaxis2=dict(\n domain=[0,0.5],\n title=\"I<sub>CFTR</sub>\", \n anchor=\"x2\")\n )",
"_____no_output_____"
],
[
"# IKr and IK1\naxis_colums = [0,Ikr-1]\nplot_10 = data_frame_ar.filter(items=[data_frame_ar.columns[i] for i in axis_colums])\nplot_data = [plot_10]\n\naxis_colums = [0,IK1-1]\nplot_11 = data_frame_ar.filter(items=[data_frame_ar.columns[i] for i in axis_colums])\nplot_data.append(plot_11)\n\ncreate_graphs(data_frames=plot_data, \n title=None, \n showlegend=False,\n #xaxis=dict(title=\"time (sec)\"),\n xaxis2=dict(title=\"time (sec)\", anchor=\"y2\"),\n yaxis=dict(\n domain=[0.6,1.0],\n title=\"I<sub>Kr</sub> (pA/pF)\"\n ),\n yaxis2=dict(\n domain=[0,0.5],\n title=\"I<sub>K1</sub> (pA/pF)\", \n anchor=\"x2\")\n )",
"_____no_output_____"
],
[
"# [Na]\naxis_colums = [0,ynid[32]+1]\nplot_12 = data_frame_ty.filter(items=[data_frame_ty.columns[i] for i in axis_colums])\nplot_data = [plot_12]\n\naxis_colums = [0,ynid[33]+1]\nplot_13 = data_frame_ty.filter(items=[data_frame_ty.columns[i] for i in axis_colums])\nplot_data.append(plot_13)\n\naxis_colums = [0,ynid[34]+1]\nplot_14 = data_frame_ty.filter(items=[data_frame_ty.columns[i] for i in axis_colums])\nplot_data.append(plot_14)\n\ncreate_graphs(data_frames=plot_data, \n title=None, \n showlegend=False,\n xaxis=dict(title=\"time (sec)\", domain=[0,0.3]),\n xaxis2=dict(title=\"time (sec)\", domain=[0.4,0.6]),\n xaxis3=dict(title=\"time (sec)\", domain=[0.7,1.0]),\n yaxis=dict(\n title=\"[Na]<sub>j</sub>\"\n ),\n yaxis2=dict(\n title=\"[Na]<sub>s<sup>l</sup></sub>\", \n anchor=\"x2\"),\n yaxis3=dict(\n title=\"[Na]<sub>i</sub> (mmol/L relevant compartment\", \n anchor=\"x3\")\n )",
"_____no_output_____"
],
[
"# I_NCX\ntitle=\"I<sub>NCX</sub> (pA/pF)\"\naxis_colums = [0,Incx-1]\nplot_15 = data_frame_ar.filter(items=[data_frame_ar.columns[i] for i in axis_colums])\ncreate_graph(data_frame=plot_15, \n title=None, \n x_axis_title=\"time (sec)\",\n y_axis_title=title)\n",
"_____no_output_____"
],
[
"# RyR fluxes\naxis_colums = [0,Jleak[0]-1]\nplot_16 = data_frame_ar.filter(items=[data_frame_ar.columns[i] for i in axis_colums])\nplot_data = [plot_16]\n\naxis_colums = [0,Jleak[1]-1]\nplot_17 = data_frame_ar.filter(items=[data_frame_ar.columns[i] for i in axis_colums])\nplot_data.append(plot_17)\n\nplot_18 = data_frame_ar.filter(items=[data_frame_ar.columns[0]])\nplot_18[1] = data_frame_ar[Jleak[0]-1] - data_frame_ar[Jleak[1]-1]\nplot_data.append(plot_18)\ncreate_graphs(data_frames=plot_data, \n title=None, \n showlegend=False,\n xaxis=dict(title=None),\n xaxis2=dict(title=None),\n xaxis3=dict(title=\"time (sec)\", anchor=\"y3\"),\n yaxis=dict(\n domain=[0.7,1.0],\n title=\"JRyR<sub>tot</sub>\"\n ),\n yaxis2=dict(\n domain=[0.4,0.6],\n title=\"Passive Leak\", \n anchor=\"x2\"),\n yaxis3=dict(\n domain=[0,0.3],\n title=\"SR Ca release\", \n anchor=\"x3\")\n )",
"_____no_output_____"
],
[
"# Export data to CSV\nimport csv_adapter\nawait csv_adapter.pandas_dataframe_to_csv(plot_0, \"Membrane Potential\", [\"time (sec)\", \"Membrane Potential\"], 0)\nawait csv_adapter.pandas_dataframe_to_csv(plot_1, \"I_ca\", [\"time(sec)\", \"I_ca(pA/pF)\"], 1)\nawait csv_adapter.pandas_dataframe_to_csv(plot_2, \"Ca_SRT\", [\"time(sec)\", \"Ca_SRT(mM)\"], 2)\nawait csv_adapter.pandas_dataframe_to_csv(plot_3, \"Ca_Dyad\", [\"time (sec)\", \"Ca_Dyad(uM)\"], 3)\nawait csv_adapter.pandas_dataframe_to_csv(plot_4, \"Ca_sl\", [\"time (sec)\", \"Ca_sl(mM)\"], 4)\nawait csv_adapter.pandas_dataframe_to_csv(plot_5, \"Ca_i\", [\"time (sec)\", \"Ca_i(uM)\"], 5)\nawait csv_adapter.pandas_dataframe_to_csv(plot_6, \"I_to\", [\"time (sec)\", \"I_to(pA/pF)\"], 6)\nawait csv_adapter.pandas_dataframe_to_csv(plot_7, \"I_Na\", [\"time (sec)\", \"I_to(pA/pF)\"], 7)\nawait csv_adapter.pandas_dataframe_to_csv(plot_8, \"I_Ks\", [\"time (sec)\", \"I_Ks(pA/pF)\"], 8)\nawait csv_adapter.pandas_dataframe_to_csv(plot_9, \"I_CFTR\", [\"time (sec)\", \"I_Ks\"], 9)\nawait csv_adapter.pandas_dataframe_to_csv(plot_10, \"I_Kr\", [\"time (sec)\", \"I_Kr(pA/pF)\"], 10)\nawait csv_adapter.pandas_dataframe_to_csv(plot_11, \"I_K1\", [\"time (sec)\", \"I_K1(pA/pF)\"], 11)\nawait csv_adapter.pandas_dataframe_to_csv(plot_12, \"Na_j\", [\"time (sec)\", \"Na_j\"], 12)\nawait csv_adapter.pandas_dataframe_to_csv(plot_13, \"Na_s\", [\"time (sec)\", \"Na_s\"], 13)\nawait csv_adapter.pandas_dataframe_to_csv(plot_14, \"Na_i\", [\"time (sec)\", \"Na_i(mmol/L)\"], 14)\nawait csv_adapter.pandas_dataframe_to_csv(plot_15, \"I_NCX\", [\"time (sec)\", \"I_NCX(pA/pF)\"], 15)\nawait csv_adapter.pandas_dataframe_to_csv(plot_16, \"JRyR_tot\", [\"time (sec)\", \"JRyR_tot\"], 16)\nawait csv_adapter.pandas_dataframe_to_csv(plot_17, \"Passive_Leak\", [\"time (sec)\", \"Passive_Leak\"], 17)\nawait csv_adapter.pandas_dataframe_to_csv(plot_18, \"SR_Ca_realease\", [\"time (sec)\", \"SR_Ca_realease\"], 18)\n",
"_____no_output_____"
]
]
] | [
"code"
] | [
[
"code",
"code",
"code",
"code",
"code",
"code",
"code",
"code",
"code",
"code",
"code",
"code",
"code",
"code",
"code",
"code",
"code",
"code"
]
] |
e781a3a03696a14a602fecd2722bf4aa96240675 | 950,957 | ipynb | Jupyter Notebook | notebooks/ch04-05_Caltech256 - Loading and process Images Data.ipynb | adipolak/ml-with-apache-spark | e6ea10d48531803b4a716a4a041d371b831bab97 | [
"Apache-2.0"
] | 20 | 2021-12-23T05:26:41.000Z | 2022-03-24T23:58:21.000Z | notebooks/ch04-05_Caltech256 - Loading and process Images Data.ipynb | adipolak/ml-with-apache-spark | e6ea10d48531803b4a716a4a041d371b831bab97 | [
"Apache-2.0"
] | null | null | null | notebooks/ch04-05_Caltech256 - Loading and process Images Data.ipynb | adipolak/ml-with-apache-spark | e6ea10d48531803b4a716a4a041d371b831bab97 | [
"Apache-2.0"
] | null | null | null | 558.401057 | 634,396 | 0.936656 | [
[
[
"This notebook shows you how to create and query a table or DataFrame loaded from data stored in Azure Blob storage.",
"_____no_output_____"
]
],
[
[
"from pyspark.sql.functions import lit\nfrom pyspark.sql.types import BinaryType,StringType\nfrom pyspark.sql import SparkSession",
"_____no_output_____"
]
],
[
[
"### Step 1: Set the data location and type\n\nThere are two ways to access Azure Blob storage: account keys and shared access signatures (SAS).\n\nTo get started, we need to set the location and type of the file.",
"_____no_output_____"
]
],
[
[
"file_location = \"256_sampledata/\"",
"_____no_output_____"
]
],
[
[
"### Step 2: Read the data\n\nNow that we have specified our file metadata, we can create a DataFrame. Notice that we use an *option* to specify that we want to infer the schema from the file. We can also explicitly set this to a particular schema if we have one already.\n\nFirst, let's create a DataFrame in Python.",
"_____no_output_____"
]
],
[
[
"! ls -l \"256_sampledata\"\n",
"total 0\r\ndrwxr-xr-x 106 jovyan users 3392 Nov 5 14:35 196.spaghetti\r\ndrwxr-xr-x 138 jovyan users 4416 Nov 5 14:35 212.teapot\r\ndrwxr-xr-x 124 jovyan users 3968 Nov 5 14:35 234.tweezer\r\ndrwxr-xr-x 102 jovyan users 3264 Nov 5 14:35 249.yo-yo\r\n"
],
[
"# start Spark session:\n\nspark = SparkSession \\\n .builder \\\n .appName(\"Marhselling Image data\") \\\n .config(\"spark.memory.offHeap.enabled\",True) \\\n .config(\"spark.memory.offHeap.size\",\"30g\")\\\n .getOrCreate()",
"_____no_output_____"
],
[
"spark.sql(\"set spark.sql.files.ignoreCorruptFiles=true\")\n\ndf = spark.read.format(\"binaryFile\") \\\n .option(\"pathGlobFilter\", \"*.jpg\") \\\n .option(\"recursiveFileLookup\", \"true\") \\\n .load(file_location)\n\n",
"_____no_output_____"
],
[
"df.printSchema()",
"root\n |-- path: string (nullable = true)\n |-- modificationTime: timestamp (nullable = true)\n |-- length: long (nullable = true)\n |-- content: binary (nullable = true)\n\n"
],
[
"# Try image file type to learn about the schema:\n# we are NOT using this DF.\n\nimage_df = spark.read.format(\"image\") \\\n.option(\"pathGlobFilter\", \"*.jpg\") \\\n.option(\"recursiveFileLookup\", \"true\") \\\n.load(file_location)",
"_____no_output_____"
],
[
"image_df.printSchema()",
"root\n |-- image: struct (nullable = true)\n | |-- origin: string (nullable = true)\n | |-- height: integer (nullable = true)\n | |-- width: integer (nullable = true)\n | |-- nChannels: integer (nullable = true)\n | |-- mode: integer (nullable = true)\n | |-- data: binary (nullable = true)\n\n"
],
[
"image_df = None",
"_____no_output_____"
],
[
"df.show(5)",
"+--------------------+--------------------+------+--------------------+\n| path| modificationTime|length| content|\n+--------------------+--------------------+------+--------------------+\n|file:/home/jovyan...|2021-11-05 14:35:...|404028|[FF D8 FF E0 00 1...|\n|file:/home/jovyan...|2021-11-05 14:35:...|291390|[FF D8 FF E0 00 1...|\n|file:/home/jovyan...|2021-11-05 14:35:...|268831|[FF D8 FF E0 00 1...|\n|file:/home/jovyan...|2021-11-05 14:35:...|222657|[FF D8 FF E0 00 1...|\n|file:/home/jovyan...|2021-11-05 14:35:...|221778|[FF D8 FF E0 00 1...|\n+--------------------+--------------------+------+--------------------+\nonly showing top 5 rows\n\n"
],
[
"df.count()",
"_____no_output_____"
]
],
[
[
"## preprocess\n 1. Extract labels\n 2. Extract size\n 3. transform labels to index",
"_____no_output_____"
],
[
"#### Regex expression\nNotice that every path file can be different, you will need to tweak the actual regex experssion to fit your file path. for that, take a look at an example of the file path and experiement with a [regex calculator](https://regexr.com/). ",
"_____no_output_____"
]
],
[
[
"df.select(\"path\").show(5, truncate=False)",
"+---------------------------------------------------------------------+\n|path |\n+---------------------------------------------------------------------+\n|file:/home/jovyan/notebooks/256_sampledata/249.yo-yo/249_0001.jpg |\n|file:/home/jovyan/notebooks/256_sampledata/196.spaghetti/196_0075.jpg|\n|file:/home/jovyan/notebooks/256_sampledata/249.yo-yo/249_0016.jpg |\n|file:/home/jovyan/notebooks/256_sampledata/249.yo-yo/249_0040.jpg |\n|file:/home/jovyan/notebooks/256_sampledata/196.spaghetti/196_0070.jpg|\n+---------------------------------------------------------------------+\nonly showing top 5 rows\n\n"
],
[
"\nimport io\nimport numpy as np\nimport pandas as pd\nimport uuid\nfrom pyspark.sql.functions import col, pandas_udf, regexp_extract\nfrom PIL import Image\n\ndef extract_label(path_col):\n \"\"\"Extract label category number from file path using built-in sql function\"\"\"\n #([^/]+)\n return regexp_extract(path_col,\"256_sampledata/([^/]+)\",1)\n\ndef extract_size(content):\n \"\"\"Extract images size from its raw content\"\"\"\n image = Image.open(io.BytesIO(content))\n return image.size\n\n@pandas_udf(\"width: int, height: int\")\ndef extract_size_udf(content_series):\n sizes = content_series.apply(extract_size)\n return pd.DataFrame(list(sizes))",
"_____no_output_____"
],
[
"images_w_label_size = df.select( \n col(\"path\"),\n extract_label(col(\"path\")).alias(\"label\"),\n extract_size_udf(col(\"content\")).alias(\"size\"),\n col(\"content\"))\n\nimages_w_label_size.show(5)\n",
"+--------------------+-------------+------------+--------------------+\n| path| label| size| content|\n+--------------------+-------------+------------+--------------------+\n|file:/home/jovyan...| 249.yo-yo|{1500, 1500}|[FF D8 FF E0 00 1...|\n|file:/home/jovyan...|196.spaghetti| {630, 537}|[FF D8 FF E0 00 1...|\n|file:/home/jovyan...| 249.yo-yo|{1792, 1200}|[FF D8 FF E0 00 1...|\n|file:/home/jovyan...| 249.yo-yo|{2048, 1536}|[FF D8 FF E0 00 1...|\n|file:/home/jovyan...|196.spaghetti| {696, 806}|[FF D8 FF E0 00 1...|\n+--------------------+-------------+------------+--------------------+\nonly showing top 5 rows\n\n"
]
],
[
[
"#Transform label to index",
"_____no_output_____"
],
[
"### 1st way - the python way",
"_____no_output_____"
]
],
[
[
"labels = images_w_label_size.select(col(\"label\")).distinct().collect()\nlabel_to_idx = {label: index for index,(label,) in enumerate(sorted(labels))}\nnum_classes = len(label_to_idx)\n\n\n@pandas_udf(\"long\")\ndef get_label_idx(labels):\n return labels.map(lambda label: label_to_idx[label])\n\nlabels_idx = images_w_label_size.select( \n col(\"label\"),\n get_label_idx(col(\"label\")).alias(\"label_index\"),\n col(\"content\"),\n col(\"path\"),\n col(\"size\"))\n\nlabels_idx.show(5)",
"+-------------+-----------+--------------------+--------------------+------------+\n| label|label_index| content| path| size|\n+-------------+-----------+--------------------+--------------------+------------+\n| 249.yo-yo| 3|[FF D8 FF E0 00 1...|file:/home/jovyan...|{1500, 1500}|\n|196.spaghetti| 0|[FF D8 FF E0 00 1...|file:/home/jovyan...| {630, 537}|\n| 249.yo-yo| 3|[FF D8 FF E0 00 1...|file:/home/jovyan...|{1792, 1200}|\n| 249.yo-yo| 3|[FF D8 FF E0 00 1...|file:/home/jovyan...|{2048, 1536}|\n|196.spaghetti| 0|[FF D8 FF E0 00 1...|file:/home/jovyan...| {696, 806}|\n+-------------+-----------+--------------------+--------------------+------------+\nonly showing top 5 rows\n\n"
]
],
[
[
"### 2nd way - the mllib way",
"_____no_output_____"
]
],
[
[
"from pyspark.ml.feature import StringIndexer\n\nindexer = StringIndexer(inputCol=\"label\", outputCol=\"label_index\")\nindexed = indexer.fit(images_w_label_size).transform(images_w_label_size)\n\nindexed.show(10)",
"+--------------------+-------------+------------+--------------------+-----------+\n| path| label| size| content|label_index|\n+--------------------+-------------+------------+--------------------+-----------+\n|file:/home/jovyan...| 249.yo-yo|{1500, 1500}|[FF D8 FF E0 00 1...| 3.0|\n|file:/home/jovyan...|196.spaghetti| {630, 537}|[FF D8 FF E0 00 1...| 2.0|\n|file:/home/jovyan...| 249.yo-yo|{1792, 1200}|[FF D8 FF E0 00 1...| 3.0|\n|file:/home/jovyan...| 249.yo-yo|{2048, 1536}|[FF D8 FF E0 00 1...| 3.0|\n|file:/home/jovyan...|196.spaghetti| {696, 806}|[FF D8 FF E0 00 1...| 2.0|\n|file:/home/jovyan...| 249.yo-yo|{1280, 1024}|[FF D8 FF E0 00 1...| 3.0|\n|file:/home/jovyan...|196.spaghetti| {943, 1152}|[FF D8 FF E0 00 1...| 2.0|\n|file:/home/jovyan...| 249.yo-yo| {450, 411}|[FF D8 FF E0 00 1...| 3.0|\n|file:/home/jovyan...|196.spaghetti| {533, 375}|[FF D8 FF E0 00 1...| 2.0|\n|file:/home/jovyan...| 249.yo-yo| {600, 416}|[FF D8 FF E0 00 1...| 3.0|\n+--------------------+-------------+------------+--------------------+-----------+\nonly showing top 10 rows\n\n"
],
[
"indexed.select(\"label_index\").distinct().collect()",
"_____no_output_____"
]
],
[
[
"### 3rd way - from the label itself",
"_____no_output_____"
]
],
[
[
"def extract_index_from_label(label):\n \"\"\"Extract index from label\"\"\"\n return regexp_extract(label,\"^([^.]+)\",1)\n\nlabels_idx = images_w_label_size.select( \n col(\"label\"),\n extract_index_from_label(col(\"label\")).alias(\"label_index\"),\n col(\"content\"),\n col(\"path\"),\n col(\"size\"))\n\nlabels_idx.show(5,truncate=False)",
"IOPub data rate exceeded.\nThe notebook server will temporarily stop sending output\nto the client in order to avoid crashing it.\nTo change this limit, set the config variable\n`--NotebookApp.iopub_data_rate_limit`.\n\nCurrent values:\nNotebookApp.iopub_data_rate_limit=1000000.0 (bytes/sec)\nNotebookApp.rate_limit_window=3.0 (secs)\n\n"
],
[
"images_w_label_size = None",
"_____no_output_____"
],
[
"df = indexed",
"_____no_output_____"
],
[
"labels_idx = None",
"_____no_output_____"
]
],
[
[
"# Step 3: Feature Engineering\nExtracting greyscale images.\nGreyscale is used as an example of feature we might want to extract.",
"_____no_output_____"
]
],
[
[
"df.printSchema()",
"root\n |-- path: string (nullable = true)\n |-- label: string (nullable = true)\n |-- size: struct (nullable = true)\n | |-- width: integer (nullable = true)\n | |-- height: integer (nullable = true)\n |-- content: binary (nullable = true)\n |-- label_index: double (nullable = false)\n\n"
]
],
[
[
"### calculate average image size for each category\n1. flat the column into two columns\n2. calculate average size for category\n3. resize according to average.\n\n",
"_____no_output_____"
]
],
[
[
"# 1st step - flatten the struact \nflattened = df.withColumn('width', col('size')['width'])\nflattened = flattened.withColumn('height', col('size')['height'])\nflattened.select('width','height').show(3, truncate = False)",
"+-----+------+\n|width|height|\n+-----+------+\n|1500 |1500 |\n|630 |537 |\n|1792 |1200 |\n+-----+------+\nonly showing top 3 rows\n\n"
],
[
"# 2 - calculate average size for category",
"_____no_output_____"
],
[
"import pandas as pd\nfrom pyspark.sql.functions import pandas_udf\nfrom pyspark.sql import Window\n\n@pandas_udf(\"int\")\ndef pandas_mean(size: pd.Series) -> (int):\n return size.sum()\n\nflattened.select(pandas_mean(flattened['width'])).show()\nflattened.groupby(\"label\").agg(pandas_mean(flattened['width'])).show()\nflattened.select(pandas_mean(flattened['width']).over(Window.partitionBy('label'))).show()\n\n\nflattened.select(pandas_mean(flattened['height'])).show()\nflattened.groupby(\"label\").agg(pandas_mean(flattened['height'])).show()\nflattened.select(pandas_mean(flattened['height']).over(Window.partitionBy('label'))).show()\n\n",
"+------------------+\n|pandas_mean(width)|\n+------------------+\n| 165992|\n+------------------+\n\n+-------------+------------------+\n| label|pandas_mean(width)|\n+-------------+------------------+\n|196.spaghetti| 39019|\n| 249.yo-yo| 40944|\n| 234.tweezer| 34513|\n| 212.teapot| 51516|\n+-------------+------------------+\n\n+----------------------------------------------------------------+\n|pandas_mean(width) OVER (PARTITION BY label unspecifiedframe$())|\n+----------------------------------------------------------------+\n| 39019|\n| 39019|\n| 39019|\n| 39019|\n| 39019|\n| 39019|\n| 39019|\n| 39019|\n| 39019|\n| 39019|\n| 39019|\n| 39019|\n| 39019|\n| 39019|\n| 39019|\n| 39019|\n| 39019|\n| 39019|\n| 39019|\n| 39019|\n+----------------------------------------------------------------+\nonly showing top 20 rows\n\n+-------------------+\n|pandas_mean(height)|\n+-------------------+\n| 143843|\n+-------------------+\n\n+-------------+-------------------+\n| label|pandas_mean(height)|\n+-------------+-------------------+\n|196.spaghetti| 33160|\n| 249.yo-yo| 37326|\n| 234.tweezer| 27628|\n| 212.teapot| 45729|\n+-------------+-------------------+\n\n+-----------------------------------------------------------------+\n|pandas_mean(height) OVER (PARTITION BY label unspecifiedframe$())|\n+-----------------------------------------------------------------+\n| 33160|\n| 33160|\n| 33160|\n| 33160|\n| 33160|\n| 33160|\n| 33160|\n| 33160|\n| 33160|\n| 33160|\n| 33160|\n| 33160|\n| 33160|\n| 33160|\n| 33160|\n| 33160|\n| 33160|\n| 33160|\n| 33160|\n| 33160|\n+-----------------------------------------------------------------+\nonly showing top 20 rows\n\n"
]
],
[
[
"### Extract greyscale",
"_____no_output_____"
]
],
[
[
"# Sample python native function that can do additional processing - expects pandas df as input and returns pandas df as output.\ndef add_grayscale_img(input_df):\n # Set up return frame. In this case I'll have a row per passed in row. You could be aggregating down to a single image, slicing\n # out columns,or just about anything, here. For this case, I am simply going to return the input_df with some extra columns.\n input_df['grayscale_image'] = input_df.content.apply(lambda image: get_image_bytes(Image.open(io.BytesIO(image)).convert('L'))) \n input_df['grayscale_format'] = \"png\" # Since this is a pandas df, this will assigne png to all rows\n \n return input_df\n\ndef get_image_bytes(image):\n img_bytes = io.BytesIO()\n image.save(img_bytes,format=\"png\")\n return img_bytes.getvalue()",
"_____no_output_____"
],
[
"# Setup the return schema. Add blank columns to match the schema expected after applying the transformation function. Makes the schema definition easy in the function invocation.\nrtn_schema = (df.select('content','label','path')\n .withColumn('grayscale_image', lit(None).cast(BinaryType()))\n .withColumn('grayscale_format', lit(None).cast(StringType()))\n )\n ",
"_____no_output_____"
],
[
"# Reduce df down to data used in the function, the groupBy, and the re-join key respectively. This could include other features as used by your pandas function\nlimited_df = df.select('label','content','path')",
"_____no_output_____"
],
[
"# Returns spark dataframe with transformations applied in parallel for each 'group'\naugmented_df = limited_df.groupBy('label').applyInPandas(add_grayscale_img, schema=rtn_schema.schema)",
"_____no_output_____"
],
[
"# re-join to the full dataset using leftouter in case the image transform needed to skip some rows\noutput_df = df.join(augmented_df.select('path','grayscale_image'),['path'],\"leftouter\") ",
"_____no_output_____"
]
],
[
[
"# Test on small data",
"_____no_output_____"
]
],
[
[
"pd_df = limited_df.limit(5).toPandas()\nprint(pd_df.columns)",
"Index(['label', 'content', 'path'], dtype='object')\n"
],
[
"limited_df = None",
"_____no_output_____"
]
],
[
[
"## Make sure function works correctly",
"_____no_output_____"
]
],
[
[
"\n# Some testing code\ntest_df = pd_df.copy()\nadd_grayscale_img(test_df)\nprint(test_df['grayscale_image'])\n",
"0 b'\\x89PNG\\r\\n\\x1a\\n\\x00\\x00\\x00\\rIHDR\\x00\\x00\\...\n1 b'\\x89PNG\\r\\n\\x1a\\n\\x00\\x00\\x00\\rIHDR\\x00\\x00\\...\n2 b'\\x89PNG\\r\\n\\x1a\\n\\x00\\x00\\x00\\rIHDR\\x00\\x00\\...\n3 b'\\x89PNG\\r\\n\\x1a\\n\\x00\\x00\\x00\\rIHDR\\x00\\x00\\...\n4 b'\\x89PNG\\r\\n\\x1a\\n\\x00\\x00\\x00\\rIHDR\\x00\\x00\\...\nName: grayscale_image, dtype: object\n"
],
[
"\nfrom PIL import ImageFilter\n# Sample python native function that can do additional processing - expects pandas df as input and returns pandas df as output.\ndef add_laplas(input_df):\n # Set up return frame. In this case I'll have a row per passed in row. You could be aggregating down to a single image, slicing\n # out columns,or just about anything, here. For this case, I am simply going to return the input_df with some extra columns.\n input_df['edges_image'] = input_df.grayscale_image.apply(lambda image: get_image_bytes(Image.open(io.BytesIO(image)).filter(ImageFilter.FIND_EDGES)\n)) \n return input_df\n\n",
"_____no_output_____"
],
[
"# Some testing code\nadd_laplas(test_df)\nprint(test_df['edges_image'])",
"0 b'\\x89PNG\\r\\n\\x1a\\n\\x00\\x00\\x00\\rIHDR\\x00\\x00\\...\n1 b'\\x89PNG\\r\\n\\x1a\\n\\x00\\x00\\x00\\rIHDR\\x00\\x00\\...\n2 b'\\x89PNG\\r\\n\\x1a\\n\\x00\\x00\\x00\\rIHDR\\x00\\x00\\...\n3 b'\\x89PNG\\r\\n\\x1a\\n\\x00\\x00\\x00\\rIHDR\\x00\\x00\\...\n4 b'\\x89PNG\\r\\n\\x1a\\n\\x00\\x00\\x00\\rIHDR\\x00\\x00\\...\nName: edges_image, dtype: object\n"
],
[
"print(test_df['path'][4])",
"file:/home/jovyan/notebooks/256_sampledata/196.spaghetti/196_0070.jpg\n"
],
[
"test_df",
"_____no_output_____"
],
[
"print(test_df.columns)",
"Index(['label', 'content', 'path', 'grayscale_image', 'grayscale_format',\n 'edges_image'],\n dtype='object')\n"
],
[
"# display one image\nimport matplotlib.pyplot as plt\nimport matplotlib.image as mpimg\nimport numpy as np\n\ncolor_image = mpimg.imread(io.BytesIO(test_df.loc[1,'content']), format='jpg')\nimage = mpimg.imread(io.BytesIO(test_df.loc[1,'grayscale_image']), format='png')\nedges_image = mpimg.imread(io.BytesIO(test_df.loc[1,'edges_image']), format='png')\nprint('color dimensions = {}'.format(color_image.shape))\nprint('grayscale dimensions = {}'.format(image.shape))\n\nrow_count = test_df.count()[0]\nplt.figure(figsize=(8,20))\nfor label_index,row in test_df.iterrows():\n (_,content,_,grayscale,_,_) = row\n color_image = mpimg.imread(io.BytesIO(content), format='jpg')\n image = mpimg.imread(io.BytesIO(grayscale), format='png')\n\n plt.subplot(row_count,2,label_index*2+1)\n plt.imshow(color_image)\n plt.subplot(row_count,2,label_index*2+2)\n plt.imshow(image,cmap='gray')\n",
"color dimensions = (537, 630, 3)\ngrayscale dimensions = (537, 630)\n"
],
[
"#laplas kernel convolution\nplt.figure(figsize=(8,20))\nfor label_index,row in test_df.iterrows():\n (_,content,_,grayscale,_,edges_image) = row\n edges_image = image = mpimg.imread(io.BytesIO(edges_image), format='png')\n \n plt.subplot(row_count,1,label_index*1+1)\n plt.imshow(edges_image,cmap='gray')",
"_____no_output_____"
]
],
[
[
"# Full Dataset",
"_____no_output_____"
]
],
[
[
"output_df.show(2, truncate=True)",
"+--------------------+-------------+------------+--------------------+-----------+--------------------+\n| path| label| size| content|label_index| grayscale_image|\n+--------------------+-------------+------------+--------------------+-----------+--------------------+\n|file:/home/jovyan...| 249.yo-yo|{1500, 1500}|[FF D8 FF E0 00 1...| 3.0|[89 50 4E 47 0D 0...|\n|file:/home/jovyan...|196.spaghetti| {630, 537}|[FF D8 FF E0 00 1...| 2.0|[89 50 4E 47 0D 0...|\n+--------------------+-------------+------------+--------------------+-----------+--------------------+\nonly showing top 2 rows\n\n"
],
[
"output_df.printSchema()",
"root\n |-- path: string (nullable = true)\n |-- label: string (nullable = true)\n |-- size: struct (nullable = true)\n | |-- width: integer (nullable = true)\n | |-- height: integer (nullable = true)\n |-- content: binary (nullable = true)\n |-- label_index: double (nullable = false)\n |-- grayscale_image: binary (nullable = true)\n\n"
]
],
[
[
"# Step 5: scale the image\n\nFrom the size column, we notice that caltech_256 image size highly varay. To proced with the process, we need to scale the images to have a unannimous size. For tha we will use Spark UDFs with PIL.\n\nThis is a must do part of normalizing and preprocessing image data.",
"_____no_output_____"
]
],
[
[
"from pyspark.sql.types import BinaryType, IntegerType\nfrom pyspark.sql.functions import udf\n\nimg_size = 224\n\ndef scale_image(image_bytes):\n try:\n image = Image.open(io.BytesIO(image_bytes)).resize([img_size, img_size])\n return image.tobytes()\n except:\n return None",
"_____no_output_____"
],
[
"array = output_df.select(\"content\").take(1)",
"_____no_output_____"
],
[
"tmp_scale=scale_image(array[0].content)\nlen(tmp_scale)",
"_____no_output_____"
],
[
"from pyspark.sql.functions import udf\nscale_image_udf = udf(scale_image, BinaryType())",
"_____no_output_____"
],
[
"#image_df = output_df.select(\"label_index\", scale_image_udf(\"content\").alias(\"content\"))\nimage_df = output_df.select(\"label_index\", scale_image_udf(col(\"content\")).alias(\"image\"))",
"_____no_output_____"
],
[
"image_df.printSchema()",
"root\n |-- label_index: double (nullable = false)\n |-- image: binary (nullable = true)\n\n"
],
[
"image_df = image_df.select(\"label_index\",\"image\",col(\"image\").alias(\"content\"))\nimage_df.printSchema()",
"root\n |-- label_index: double (nullable = false)\n |-- image: binary (nullable = true)\n |-- content: binary (nullable = true)\n\n"
],
[
"image_df =image_df.drop(\"image\")\nimage_df.printSchema()",
"root\n |-- label_index: double (nullable = false)\n |-- content: binary (nullable = true)\n\n"
]
],
[
[
"# Step 4: Save and Avoid small files problem\nSave the image data into a file format where you can query and process at scale\n\nSaving the dataset with the greyscale.",
"_____no_output_____"
],
[
"### Repartition and save to **parquet**",
"_____no_output_____"
]
],
[
[
"# incase you are running on a distributed environment, with a large dataset, it's a good idea to partition t\n\n# save the data:\n\nsave_path_augmented = \"images_data/silver/augmented\"\n# Images data is already compressed so we turn off parquet compression\ncompression = spark.conf.get(\"spark.sql.parquet.compression.codec\")\nspark.conf.set(\"spark.sql.parquet.compression.codec\", \"uncompressed\")\n\n",
"_____no_output_____"
],
[
"output_df.write.mode(\"overwrite\").parquet(save_path_augmented)\n",
"_____no_output_____"
],
[
"save_path_filtered = \"images_data/silver/filtered\"\n# parquet.block.size is for Petastorm, later\nimage_df.repartition(2).write.mode(\"overwrite\").option(\"parquet.block.size\", 1024 * 1024).parquet(save_path_filtered)",
"_____no_output_____"
],
[
"spark.conf.set(\"spark.sql.parquet.compression.codec\", compression)",
"_____no_output_____"
]
]
] | [
"markdown",
"code",
"markdown",
"code",
"markdown",
"code",
"markdown",
"code",
"markdown",
"code",
"markdown",
"code",
"markdown",
"code",
"markdown",
"code",
"markdown",
"code",
"markdown",
"code",
"markdown",
"code",
"markdown",
"code",
"markdown",
"code",
"markdown",
"code",
"markdown",
"code"
] | [
[
"markdown"
],
[
"code"
],
[
"markdown"
],
[
"code"
],
[
"markdown"
],
[
"code",
"code",
"code",
"code",
"code",
"code",
"code",
"code",
"code"
],
[
"markdown",
"markdown"
],
[
"code",
"code",
"code"
],
[
"markdown",
"markdown"
],
[
"code"
],
[
"markdown"
],
[
"code",
"code"
],
[
"markdown"
],
[
"code",
"code",
"code",
"code"
],
[
"markdown"
],
[
"code"
],
[
"markdown"
],
[
"code",
"code",
"code"
],
[
"markdown"
],
[
"code",
"code",
"code",
"code",
"code"
],
[
"markdown"
],
[
"code",
"code"
],
[
"markdown"
],
[
"code",
"code",
"code",
"code",
"code",
"code",
"code",
"code"
],
[
"markdown"
],
[
"code",
"code"
],
[
"markdown"
],
[
"code",
"code",
"code",
"code",
"code",
"code",
"code",
"code"
],
[
"markdown",
"markdown"
],
[
"code",
"code",
"code",
"code"
]
] |
e781bdefc868fb9125694bc12aa147a2e4283cb9 | 6,841 | ipynb | Jupyter Notebook | analysis/mf_grc_analysis/share_distribution/distribution_123share_bouton_210519_random_gen_circle_constant_15.ipynb | htem/cb2_project_analysis | a677cbadc7e3bf0074975a94ed1d06b4801899c0 | [
"MIT"
] | null | null | null | analysis/mf_grc_analysis/share_distribution/distribution_123share_bouton_210519_random_gen_circle_constant_15.ipynb | htem/cb2_project_analysis | a677cbadc7e3bf0074975a94ed1d06b4801899c0 | [
"MIT"
] | null | null | null | analysis/mf_grc_analysis/share_distribution/distribution_123share_bouton_210519_random_gen_circle_constant_15.ipynb | htem/cb2_project_analysis | a677cbadc7e3bf0074975a94ed1d06b4801899c0 | [
"MIT"
] | null | null | null | 32.889423 | 125 | 0.500512 | [
[
[
"\nimport os\nimport sys\nimport importlib\nimport copy\nfrom collections import defaultdict\nsys.path.insert(0, '/n/groups/htem/Segmentation/shared-nondev/cb2_segmentation/analysis_mf_grc')\n\nfrom tools_pattern import get_eucledean_dist\n\n# script_n = os.path.basename(__file__).split('.')[0]\nscript_n = 'distribution_123share_bouton_210519_random_gen'\n\nimport my_plot\nimportlib.reload(my_plot)\nfrom my_plot import MyPlotData, my_box_plot\n\ndef to_ng_coord(coord):\n return (\n int(coord[0]/4),\n int(coord[1]/4),\n int(coord[2]/40),\n )\n\nimport compress_pickle\n\n# input_graph = compress_pickle.load('/n/groups/htem/Segmentation/shared-nondev/cb2_segmentation/analysis_mf_grc/'\\\n# 'mf_grc_model/input_graph_201114_restricted_z.gz')\nfname = ('/n/groups/htem/Segmentation/shared-nondev/cb2_segmentation/analysis_mf_grc/' \\\n# 'gen_db/mf_grc/input_graph_210519_all.gz')\n 'gen_db/mf_grc/input_graph_210520_all_100_2.gz')\ninput_graph = compress_pickle.load(fname)\n\n# z_min = 19800\n# z_max = 29800\nz_min = 19800\nz_max = 29800\n# GrCs are fully reconstructed and proofread from 90k to 150k\nx_min = 105*1000*4\nx_max = 135*1000*4\n# radius = 200\n\nn_randoms = 5\nreplication_hist2 = defaultdict(int)\ngrc_ids = set()\nmf_ids = set()\nreplicated_2shares = defaultdict(int)\n\ndef get_prob(in_graph, unique_count=False, count_within_box=True, return_counted=False):\n n_common_pairs = 0\n processed = set()\n total_n_pairs = 0\n hist = defaultdict(int)\n n = 0\n counted_grcs = 0\n for grc_i_id in in_graph.grcs:\n n += 1\n grc_i = in_graph.grcs[grc_i_id]\n x, y, z = grc_i.soma_loc\n if count_within_box:\n if x < x_min or x > x_max:\n continue\n if z < z_min or z > z_max:\n continue\n counted_grcs += 1\n grc_ids.add(grc_i_id)\n rosettes_i = set([mf[1] for mf in grc_i.edges])\n for r in rosettes_i:\n mf_ids.add(r)\n for grc_j_id in in_graph.grcs:\n if grc_i_id == grc_j_id:\n continue\n if unique_count and (grc_i_id, grc_j_id) in processed:\n continue\n processed.add((grc_i_id, grc_j_id))\n processed.add((grc_j_id, grc_i_id))\n grc_j = in_graph.grcs[grc_j_id]\n x, y, z = grc_j.soma_loc\n# if count_within_box:\n# if x < x_min or x > x_max:\n# continue\n# if z < z_min or z > z_max:\n# continue\n common_rosettes = set([mf[1] for mf in grc_j.edges])\n common_rosettes = common_rosettes & rosettes_i\n hist[len(common_rosettes)] += 1\n if len(common_rosettes) == 2:\n replication_hist2[grc_i_id] += 1\n common_rosettes = tuple(sorted(list(common_rosettes)))\n replicated_2shares[common_rosettes] += 1\n for k in hist:\n # fix 0 datapoint plots\n if hist[k] == 0:\n hist[k] = 1\n if return_counted:\n return hist, counted_grcs\n else:\n return hist\n",
"_____no_output_____"
],
[
"n_random = 100\nrounds = []\nfor n in range(n_random):\n print('', end='.')\n input_observed = input_graph\n input_observed.randomize_graph_by_grc2(\n constant_dendrite_length=15000,\n mf_dist_margin=10000,\n seed=n,\n )\n\n replication_hist2 = defaultdict(int)\n hist_data, n_grcs = get_prob(input_observed, count_within_box=True, return_counted=True)\n# print(hist_data)\n\n replication_hist2_list = []\n for grc in grc_ids:\n if grc in replication_hist2:\n replication_hist2_list.append((grc, replication_hist2[grc]))\n else:\n replication_hist2_list.append((grc, 0))\n replication_hist2_list_sorted = sorted(replication_hist2_list, key=lambda x: x[1])\n \n l = []\n for mf_id in replication_hist2_list_sorted:\n mf_id, size = mf_id\n l.append(size)\n rounds.append(l)\n\n# mpd = MyPlotData()\n# mpd_count = MyPlotData()\n# i = 0\n# for grc_id, count in replication_hist2_list_sorted:\n# mpd_count.add_data_point(\n# count=count,\n# grc_id=grc_id,\n# i=i,\n# model='Observed',\n# )\n# i += 1\n",
"...................................................................................................."
],
[
"import compress_pickle\nfname = f'{script_n}_circle_constant15_2_{n_random}.gz'\nprint(fname)\ncompress_pickle.dump(rounds, fname)",
"distribution_123share_bouton_210519_random_gen_circle_constant15_2_100.gz\n"
]
]
] | [
"code"
] | [
[
"code",
"code",
"code"
]
] |
e781c2b1b077c56a7f413795922821f7867cba8a | 161,500 | ipynb | Jupyter Notebook | EmployeeSQL/Working files/SQL BONUS.ipynb | key12pat34/SQL-challenge-hw7 | ef055bf7617bfdaf81383599151ab5c1715e822c | [
"ADSL"
] | null | null | null | EmployeeSQL/Working files/SQL BONUS.ipynb | key12pat34/SQL-challenge-hw7 | ef055bf7617bfdaf81383599151ab5c1715e822c | [
"ADSL"
] | null | null | null | EmployeeSQL/Working files/SQL BONUS.ipynb | key12pat34/SQL-challenge-hw7 | ef055bf7617bfdaf81383599151ab5c1715e822c | [
"ADSL"
] | null | null | null | 66.297209 | 35,036 | 0.652155 | [
[
[
"# pip install psycopg2 sqlalchemy",
"_____no_output_____"
],
[
"# dependencies\nimport pandas as pd\nimport matplotlib.pyplot as plt\nimport numpy as np\nfrom sqlalchemy import create_engine\n%matplotlib notebook\n\n",
"_____no_output_____"
],
[
"# Path to sqlite\n\n\n# Create an engine that can talk to the database\ndbname='SQL_HW_7'\nservername='localhost'\nusername = ''\npassword = ''\nport=5432\nconn_string = f'postgres://{username}:{password}@{servername}:{port}/{dbname}'\nengine = create_engine( conn_string , echo = False)\n\n\n#connection\nconn = engine.connect()",
"_____no_output_____"
],
[
"#salaries table query\nsalary_ranges = pd.read_sql(\"SELECT * FROM salaries\", conn)\nsalary_ranges.head()",
"_____no_output_____"
],
[
"#titles table query\ntitle_names = pd.read_sql(\"select * from titles\", conn)\ntitle_names.head()",
"_____no_output_____"
],
[
"#employees table query\nemployees = pd.read_sql(\"select * from employees\", conn)\nemployees.head()",
"_____no_output_____"
],
[
"#merged employees and salary query\nemp_sal_merged = pd.merge(employees, salary_ranges, on = \"emp_no\", how = \"left\" )\nemp_sal_merged.head()",
"_____no_output_____"
],
[
"#titles merged with emp_sal_merged\nemp_title_merged = pd.merge(emp_sal_merged, title_names, left_on = \"emp_title_id\", right_on = \"title_id\", how = \"left\" )\nemp_title_merged",
"_____no_output_____"
],
[
"#grouping by title and salaries\nsal_title_group = emp_title_merged.groupby(['title']).mean()\nsal_title_group_clean = sal_title_group.drop(columns='emp_no')\nsal_title_group_clean = sal_title_group_clean.reset_index()\nsal_title_group_clean",
"_____no_output_____"
]
],
[
[
"## Histogram\n\n* Create a histogram to visualize the most common salary ranges for employees.",
"_____no_output_____"
]
],
[
[
"# x_axis = sal_title_group_clean['salary']\n# y_axis = \n\nplt.hist(emp_title_merged['salary'], color=\"red\" )\n\nplt.title('Salary Ranges for Employees')\nplt.xlabel('Salary Range ($)')\nplt.ylabel('Employee Count')\n\nplt.grid(alpha=0.5)\nplt.show()\nplt.tight_layout()",
"_____no_output_____"
]
],
[
[
"## Bar Chart\n\n* Create a bar chart of average salary by title. ",
"_____no_output_____"
]
],
[
[
"x_axis = sal_title_group_clean['title']\ny_axis = sal_title_group_clean['salary']\n\nplt.bar(x_axis, y_axis, align = 'center', alpha=0.75, color = ['red','green','blue', 'black', 'orange', 'grey', 'purple'])\nplt.xticks(rotation = 'vertical')\n\nplt.title(\"Average Salary by Title\")\nplt.xlabel(\"Employee Titles\")\nplt.ylabel(\"Salaries ($)\")\n\nplt.grid(alpha=0.25)\nplt.show()\nplt.tight_layout()\n\nplt.savefig()",
"_____no_output_____"
]
]
] | [
"code",
"markdown",
"code",
"markdown",
"code"
] | [
[
"code",
"code",
"code",
"code",
"code",
"code",
"code",
"code",
"code"
],
[
"markdown"
],
[
"code"
],
[
"markdown"
],
[
"code"
]
] |
e781cab19566ab5310f98926753025c3af06846c | 12,932 | ipynb | Jupyter Notebook | Pandas/Dados/extras/extras/Organizando DataFrames (Sort).ipynb | lingsv/alura_ds | a4f0354ef199741726481faa055215d2d1b401c2 | [
"MIT"
] | null | null | null | Pandas/Dados/extras/extras/Organizando DataFrames (Sort).ipynb | lingsv/alura_ds | a4f0354ef199741726481faa055215d2d1b401c2 | [
"MIT"
] | null | null | null | Pandas/Dados/extras/extras/Organizando DataFrames (Sort).ipynb | lingsv/alura_ds | a4f0354ef199741726481faa055215d2d1b401c2 | [
"MIT"
] | null | null | null | 22.068259 | 58 | 0.337071 | [
[
[
"import pandas as pd",
"_____no_output_____"
],
[
"data = [[1,2,3],[4,5,6],[7,8,9]]",
"_____no_output_____"
],
[
"data",
"_____no_output_____"
],
[
"list('321')",
"_____no_output_____"
],
[
"df= pd.DataFrame(data, list('321'), list('ZYX'))",
"_____no_output_____"
],
[
"df",
"_____no_output_____"
],
[
"df.sort_index(inplace=True)",
"_____no_output_____"
],
[
"df.sort_index(axis =1, inplace=True)",
"_____no_output_____"
],
[
"df",
"_____no_output_____"
],
[
"df.sort_values(by=['X', 'Y'], inplace=True)",
"_____no_output_____"
],
[
"df",
"_____no_output_____"
],
[
"df.sort_values(by='3', axis= 1, inplace=True)",
"_____no_output_____"
],
[
"df",
"_____no_output_____"
]
],
[
[
"## Exercícios",
"_____no_output_____"
]
],
[
[
"data = [[1,2,3],[4,5,6],[7,8,9]]\nlist('CBA')\nlist('ZYX')\ndf = pd.DataFrame(data, list('zyx'), list('cba'))\ndf",
"_____no_output_____"
],
[
"df.sort_index()\ndf.sort_index(axis = 1)\ndf",
"_____no_output_____"
]
]
] | [
"code",
"markdown",
"code"
] | [
[
"code",
"code",
"code",
"code",
"code",
"code",
"code",
"code",
"code",
"code",
"code",
"code",
"code"
],
[
"markdown"
],
[
"code",
"code"
]
] |
e781cb361250e1240b84db371e2890dbe3537d04 | 3,313 | ipynb | Jupyter Notebook | pixel_crnn/Untitled1.ipynb | niddal-imam/End-2-End-image-spam-detector-pixel_link | a546b6d55ae611806eef7182c4648be6cce73580 | [
"MIT"
] | null | null | null | pixel_crnn/Untitled1.ipynb | niddal-imam/End-2-End-image-spam-detector-pixel_link | a546b6d55ae611806eef7182c4648be6cce73580 | [
"MIT"
] | null | null | null | pixel_crnn/Untitled1.ipynb | niddal-imam/End-2-End-image-spam-detector-pixel_link | a546b6d55ae611806eef7182c4648be6cce73580 | [
"MIT"
] | null | null | null | 50.19697 | 1,593 | 0.611228 | [
[
[
"#Arabic_datasets\nimport sys\nfile_path = \"/home/niddal/Desktop/PhD_projects/Arabic-text-recognition-master/data/Archive/Annotation_vall.txt\"\noutput_path = \"/home/niddal/Desktop/PhD_projects/Arabic-text-recognition-master/data/Archive/Annotation_val-2.txt\"\nwith open(file_path) as f:\n line = f.readline()\n count= 1\n while line:\n sys.stdout = open(output_path,'a')\n print(line,line.split(\"/\")[10].split(\"_\")[0])\n line = f.readline()\n count += 1\n sys.stdout.close()\n",
"_____no_output_____"
]
]
] | [
"code"
] | [
[
"code"
]
] |
e781e4f701cada6fb049932ab26f7b031862020e | 11,754 | ipynb | Jupyter Notebook | jwst_validation_notebooks/reset/jwst_reset_miri_test/jwst_reset_miri_testing.ipynb | jbhagan/jwst_validation_notebooks | 01062937ba0d5797c5ea08dfca184b3864ff7f1d | [
"BSD-3-Clause"
] | null | null | null | jwst_validation_notebooks/reset/jwst_reset_miri_test/jwst_reset_miri_testing.ipynb | jbhagan/jwst_validation_notebooks | 01062937ba0d5797c5ea08dfca184b3864ff7f1d | [
"BSD-3-Clause"
] | null | null | null | jwst_validation_notebooks/reset/jwst_reset_miri_test/jwst_reset_miri_testing.ipynb | jbhagan/jwst_validation_notebooks | 01062937ba0d5797c5ea08dfca184b3864ff7f1d | [
"BSD-3-Clause"
] | null | null | null | 33.20339 | 659 | 0.598605 | [
[
[
"<a id=\"title_ID\"></a>\n# JWST Pipeline Validation Testing Notebook: Calwebb_detector1, reset step for MIRI\n\n<span style=\"color:red\"> **Instruments Affected**</span>: MIRI\n\n### Table of Contents\n<div style=\"text-align: left\"> \n\n<br> [Imports](#imports_ID) <br> [Introduction](#intro_ID) <br> [Get Documentaion String for Markdown Blocks](#markdown_from_docs) <br> [Loading Data](#data_ID) <br> [Run JWST Pipeline](#pipeline_ID) <br> [Create Figure or Print Output](#residual_ID) <br> [About This Notebook](#about_ID) <br>\n\n</div>",
"_____no_output_____"
],
[
"<a id=\"imports_ID\"></a>\n# Imports\nList the library imports and why they are relevant to this notebook.\n\n* get_bigdata to retrieve data from artifactory\n* jwst.datamodels for building model for JWST Pipeline\n* jwst.module.PipelineStep is the pipeline step being tested\n* matplotlib.pyplot.plt to generate plot\n* numpy\n* inspect to get the docstring of our objects.\n* IPython.display for printing markdown output\n\n\n[Top of Page](#title_ID)",
"_____no_output_____"
]
],
[
[
"from ci_watson.artifactory_helpers import get_bigdata\nimport inspect\nfrom IPython.display import Markdown\nfrom jwst.dq_init import DQInitStep\nfrom jwst.reset import ResetStep\nfrom jwst.datamodels import RampModel\nimport matplotlib.pyplot as plt\nimport numpy as np",
"_____no_output_____"
]
],
[
[
"<a id=\"intro_ID\"></a>\n# Introduction\n\n\nFor this test we are using the reset step in the calwebb_detector1 pipeline. For MIRI exposures, the initial groups in each integration suffer from two effects related to the resetting of the detectors. The first effect is that the first few groups after a reset do not fall on the expected linear accumulation of signal. The most significant deviations ocurr in groups 1 and 2. This behavior is relatively uniform detector-wide. The second effect, on the other hand, is the appearance of significant extra spatial structure in these initial groups, before fading out in later groups. For more information on the pipeline step visit the links below. \n\nStep description: https://jwst-pipeline.readthedocs.io/en/latest/jwst/reset/description.html\n\nPipeline code: https://github.com/spacetelescope/jwst/tree/master/jwst/reset\n\n\n### Calibration WG Requested Algorithm: \n\nA short description and link to the page: https://outerspace.stsci.edu/pages/viewpage.action?spaceKey=JWSTCC&title=Vanilla+MIR+Reset+Anomaly+Correction\n\n\n### Defining Term\nHere is where you will define terms or acronymns that may not be known a general audience (ie a new employee to the institute or an external user). For example\n\nJWST: James Webb Space Telescope\n\nMIRI: Mid Infrared Instrument\n\n\n[Top of Page](#title_ID)",
"_____no_output_____"
],
[
"<a id=\"markdown_from_docs\"></a>\n# Get Documentaion String for Markdown Blocks",
"_____no_output_____"
]
],
[
[
"# Get raw python docstring\nraw = inspect.getdoc(ResetStep)\n\n# To convert to markdown, you need convert line breaks from \\n to <br />\nmarkdown_text = \"<br />\".join(raw.split(\"\\n\"))\n\n# Here you can format markdown as an output using the Markdown method.\nMarkdown(\"\"\"\n# ResetStep\n---\n{}\n\"\"\".format(markdown_text))",
"_____no_output_____"
]
],
[
[
"<a id=\"data_ID\"></a>\n# Loading Data\n\nThe data used to test this step is a dark data file taken as part of pre-launch ground testing. The original file name is MIRV00330001001P0000000002101_1_493_SE_2017-09-07T15h14m25.fits that was renamed to jw02201001001_01101_00001_MIRIMAGE_uncal.fits with a script that updates the file to put it in pipeline ready formatting.\nThis is a dark data file with 40 frames and 4 integrations.\n\n[Top of Page](#title_ID)",
"_____no_output_____"
]
],
[
[
"filename = get_bigdata('jwst_validation_notebooks',\n 'validation_data',\n 'reset',\n 'reset_miri_test', \n 'jw02201001001_01101_00001_MIRIMAGE_uncal.fits')",
"_____no_output_____"
]
],
[
[
"<a id=\"pipeline_ID\"></a>\n# Run JWST Pipeline\n\nTake the initial input file and run it through both dq_init and reset to get the before and after correction versions of the data to run.\n\n[Top of Page](#title_ID)",
"_____no_output_____"
]
],
[
[
"preim = DQInitStep.call(filename)\npostim = ResetStep.call(preim)",
"_____no_output_____"
]
],
[
[
"<a id=\"residual_ID\"></a>\n# Show plots and take statistics before and after correction\n\nFor a specific pixel in the dark data:\n1. Plot the ramps before and after the correction to see if the initial frame values are more in line with the rest of the ramp.\n2. Fit a line to the ramps and calculate the slope and residuals. The slope should be closer to 0 and the residuals should be much smaller after the correction.\n3. Plot the residuals of a single integration before and after the correction to see if they are smaller.\n\n[Top of Page](#title_ID)",
"_____no_output_____"
]
],
[
[
"# set input variables\nprint('Shape of data cube: integrations, groups, ysize, xsize ',preim.shape)\n\nxval = 650\nyval = 550\n\nframenum = 20 # number of frames to plot (reset only corrects first few frames in cube)\nintsnum = 3 # number of integrations to plot (3 should show reset and not crowd)\n \n# put data into proper data models\n# read in images\nwith RampModel(preim) as impre:\n # raises exception if file is not the correct model\n pass\n\n# read in image\nwith RampModel(postim) as impost:\n # raises exception if file is not the correct model\n pass\n ",
"_____no_output_____"
]
],
[
[
"First plot should show that after the correction, the drop at the early part of the ramp has evened out to resemble the data in the rest of the ramp.",
"_____no_output_____"
]
],
[
[
"# Plot frames vs. counts for a dark pixel before and after correction\n\n# loop through integrations\nfor i in range(0, intsnum):\n\n # get locations of flagged pixels within the ramps\n ramp1 = impre.data[i, 0:framenum, yval, xval]\n ramp2 = impost.data[i, 0:framenum, yval, xval]\n\n # plot ramps of selected pixels\n plt.title('Frame values (DN) for a dark pixel')\n plt.xlabel('Frames')\n plt.ylabel('Counts (DN)')\n plt.plot(ramp1+i*10, label='int ' + str(i))\n plt.plot(ramp2+i*10, label='int ' + str(i) + ' after reset')\n\nplt.legend(loc=4)\nplt.show()\n",
"_____no_output_____"
]
],
[
[
"Take a single pixel in the file, before and after the correction, and fit a line to them. After the correction, for a dark, the slope should be closer to zero and the residuals should be much lower.",
"_____no_output_____"
]
],
[
[
"# get array of frame numbers and choose ramps for selected pixel\nframes = np.arange(0, framenum)\n \npreramp = impre.data[0, 0:framenum, yval, xval]\npostramp = impost.data[0, 0:framenum, yval, xval]\n\n# get slopes of selected pixel before and after correction and see if it is more linear\nfit = np.polyfit(frames, preramp, 1, full=True)\n\nslopepre = fit[0][0]\ninterceptpre = fit[0][1]\nresidualspre = fit[1][0]\n\nfitpost = np.polyfit(frames, postramp, 1, full=True)\n\nslopepost = fitpost[0][0]\ninterceptpost = fitpost[0][1]\nresidualspost = fitpost[1][0]\n\n# look at slopes and variances\nprint('The slope of the pixel before correction is: ', slopepre)\nprint('The slope of the pixel after correction is: ', slopepost)\n\nprint('The residuals of the pixel before correction are: ', residualspre)\nprint('The residuals of the pixel after correction are: ', residualspost)\n",
"_____no_output_____"
]
],
[
[
"Plot the residuals for the linear fit before and after correction for the specified pixel to see if the plotted ramp is flatter after the correction.",
"_____no_output_____"
]
],
[
[
"# show line plus residual for 1st int\nyfit = np.polyval(fit[0], frames)\nyfitcorr = np.polyval(fitpost[0], frames)\n\nplt.title('Residuals for ramp (single pixel) before and after reset')\nplt.xlabel('Frames')\nplt.ylabel('Residual: linear fit - data')\nplt.plot(frames, yfit - preramp, label='raw variance')\nplt.plot(frames, yfitcorr - postramp, label='corrected variance')\nplt.legend()\nplt.show()\n",
"_____no_output_____"
]
],
[
[
"<a id=\"about_ID\"></a>\n## About this Notebook\n**Author:** Misty Cracraft, Senior Staff Scientist, MIRI Branch\n<br>**Updated On:** 05/12/2020",
"_____no_output_____"
],
[
"[Top of Page](#title_ID)\n<img style=\"float: right;\" src=\"./stsci_pri_combo_mark_horizonal_white_bkgd.png\" alt=\"stsci_pri_combo_mark_horizonal_white_bkgd\" width=\"200px\"/> ",
"_____no_output_____"
]
]
] | [
"markdown",
"code",
"markdown",
"code",
"markdown",
"code",
"markdown",
"code",
"markdown",
"code",
"markdown",
"code",
"markdown",
"code",
"markdown",
"code",
"markdown"
] | [
[
"markdown",
"markdown"
],
[
"code"
],
[
"markdown",
"markdown"
],
[
"code"
],
[
"markdown"
],
[
"code"
],
[
"markdown"
],
[
"code"
],
[
"markdown"
],
[
"code"
],
[
"markdown"
],
[
"code"
],
[
"markdown"
],
[
"code"
],
[
"markdown"
],
[
"code"
],
[
"markdown",
"markdown"
]
] |
e781ead6266901875140f5f93915c9d904523df0 | 84,546 | ipynb | Jupyter Notebook | Nick's_Copy_of_BW_project_3_27_.ipynb | StephenSpicer/Spotify_Music_Discovery_LS_DS_BW | 2e61e98f45cdfeb064b58e44091ffa16d67d82b2 | [
"MIT"
] | null | null | null | Nick's_Copy_of_BW_project_3_27_.ipynb | StephenSpicer/Spotify_Music_Discovery_LS_DS_BW | 2e61e98f45cdfeb064b58e44091ffa16d67d82b2 | [
"MIT"
] | null | null | null | Nick's_Copy_of_BW_project_3_27_.ipynb | StephenSpicer/Spotify_Music_Discovery_LS_DS_BW | 2e61e98f45cdfeb064b58e44091ffa16d67d82b2 | [
"MIT"
] | 5 | 2021-03-27T21:42:39.000Z | 2021-03-31T15:12:06.000Z | 33.404188 | 2,238 | 0.494039 | [
[
[
"empty"
]
]
] | [
"empty"
] | [
[
"empty"
]
] |
e781fbcfe232ed6761752f16d7548c2746f87119 | 198,605 | ipynb | Jupyter Notebook | python/matplotlib/vector/basic/scipy.ipynb | karng87/nasm_game | a97fdb09459efffc561d2122058c348c93f1dc87 | [
"MIT"
] | null | null | null | python/matplotlib/vector/basic/scipy.ipynb | karng87/nasm_game | a97fdb09459efffc561d2122058c348c93f1dc87 | [
"MIT"
] | null | null | null | python/matplotlib/vector/basic/scipy.ipynb | karng87/nasm_game | a97fdb09459efffc561d2122058c348c93f1dc87 | [
"MIT"
] | null | null | null | 207.963351 | 145,775 | 0.885416 | [
[
[
"import numpy as np\nimport scipy as sp\nimport matplotlib.pyplot as plt\n%matplotlib widget",
"_____no_output_____"
]
],
[
[
"Basic\n Optimization",
"_____no_output_____"
]
],
[
[
"def f(x):\n return (x-3)**2\nsp.optimize.minimize(f,2)",
"_____no_output_____"
],
[
"sp.optimize.minimize(f,2).x",
"_____no_output_____"
],
[
"sp.optimize.minimize(f,2).fun",
"_____no_output_____"
],
[
"sp.optimize.minimize?",
"_____no_output_____"
]
],
[
[
"# $$ f(x,y) = (x-1)^2 + (y-2.5)^2 $$\n$$ x - 2y + 2 \\geq 0 \\\\\n -x - 2y + 6 \\geq 0 \\\\\n -x + 2y + 2 \\geq 0 \\\\\n x \\geq 0 \\\\\n y \\geq 0$$",
"_____no_output_____"
]
],
[
[
"def f(x,y):\n return (x-1)**2 + (y-2.5)**2\ndef g(x,y):\n return x - 2*y + 2\n\ndef h(x,y):\n return -x - 2*y + 6\ndef k(x,y):\n return -x + 2*y +2\n\nx = np.linspace(0,5,100)\nx,y = np.meshgrid(x,x)\nz = f(x,y)\ng = g(x,y)\nh = h(x,y)\nk = k(x,y)\n\nfig = plt.figure()\nax = fig.add_subplot(projection='3d')\nax.plot_surface(x,y,z,cmap='coolwarm',alpha=0.7)\nax.plot_surface(x,y,g,alpha=0.2)\nax.plot_surface(x,y,h,alpha=0.2)\nax.plot_surface(x,y,k,alpha=0.2)\n\n#ax.scatter3D(x,y,z, c=z,cmap='coolwarm')\n\n#############################\n#### optimize.minimize ######\n#############################\n# constraints\n# ineq = inequlity\n# bounds\nl = lambda x: (x[0] - 1)**2 + (x[1] - 2.5)**2\ncons = ({'type':'ineq','fun':lambda x: x[0] - 2*x[1] + 2},\n {'type':'ineq','fun':lambda x: -x[0] - 2*x[1] + 6},\n {'type':'ineq','fun':lambda x: -x[0] + 2*x[1] + 2})\nbnds = ((0,None),(0,None))\nres = sp.optimize.minimize(l,(2,0), bounds=bnds, constraints=cons)\nz3 = f(res.x[0], res.x[1])\n###############################\n\n\nax.scatter3D([res.x[0]],[res.x[1]],[f(res.x[0],res.x[1])])\n",
"_____no_output_____"
]
],
[
[
"# interpolate",
"_____no_output_____"
]
],
[
[
"x = np.linspace(0,10,10)\ny = x**2 * np.sin(x)\n\nfig = plt.figure()\nax = fig.add_subplot()\nplt.scatter(x,y)",
"_____no_output_____"
],
[
"f = sp.interpolate.interp1d(x,y,kind='linear')\nf = sp.interpolate.interp1d(x,y,kind='cubic')\nx_dense = np.linspace(0,10,100)\ny_dense = f(x_dense)\nax.plot(x_dense,y_dense)",
"_____no_output_____"
],
[
"def f(x):\n return x**2 +5\nsp.integrate.quad(f,0,1)",
"_____no_output_____"
],
[
"round(quad(f,0,1)[0], 2)",
"_____no_output_____"
],
[
"quad(lambda x: x**2 + 5, 0,1)",
"_____no_output_____"
],
[
"quad(lambda x:np.exp(-x**2)*np.cos(2*np.pi*x), -np.inf, np.inf)",
"_____no_output_____"
],
[
"n = 1\nquad(lambda x, n: np.exp(-n*x**2),0,np.inf,args=n)",
"_____no_output_____"
],
[
"quad(lambda x, n: np.exp(-n*x**2),0,np.inf,args=n)[0]",
"_____no_output_____"
],
[
"integrals = [quad(lambda x, n: np.exp(-n*x**2),0,np.inf,args=n)[0] for n in range(1,10)]\nintegrals",
"_____no_output_____"
]
]
] | [
"code",
"markdown",
"code",
"markdown",
"code",
"markdown",
"code"
] | [
[
"code"
],
[
"markdown"
],
[
"code",
"code",
"code",
"code"
],
[
"markdown"
],
[
"code"
],
[
"markdown"
],
[
"code",
"code",
"code",
"code",
"code",
"code",
"code",
"code",
"code"
]
] |
e781ff83a85601b33e1aa70f63273afac08fadd0 | 187,238 | ipynb | Jupyter Notebook | monthly_update/generated/book/nteract.ipynb | choldgraf/jupyter-activity-snapshot | 080f8c34e1e3e5081c4b733592b114b01b09b8c0 | [
"BSD-3-Clause"
] | 7 | 2019-08-26T13:19:05.000Z | 2021-11-18T16:34:01.000Z | monthly_update/generated/book/nteract.ipynb | choldgraf/jupyter-activity-snapshot | 080f8c34e1e3e5081c4b733592b114b01b09b8c0 | [
"BSD-3-Clause"
] | 3 | 2019-11-27T19:25:27.000Z | 2021-03-13T01:19:45.000Z | monthly_update/generated/book/nteract.ipynb | choldgraf/jupyter-activity-snapshot | 080f8c34e1e3e5081c4b733592b114b01b09b8c0 | [
"BSD-3-Clause"
] | 4 | 2019-06-20T17:49:53.000Z | 2021-05-21T21:06:18.000Z | 41.32377 | 5,509 | 0.566664 | [
[
[
"# {glue:text}`nteract_github_org`\n\n**Activity from {glue:}`nteract_start` to {glue:}`nteract_stop`**",
"_____no_output_____"
]
],
[
[
"from datetime import date\nfrom dateutil.relativedelta import relativedelta\nfrom myst_nb import glue\nimport seaborn as sns\nimport pandas as pd\nimport numpy as np\nimport altair as alt\nfrom markdown import markdown\nfrom IPython.display import Markdown\nfrom ipywidgets.widgets import HTML, Tab\nfrom ipywidgets import widgets\nfrom datetime import timedelta\nfrom matplotlib import pyplot as plt\nimport os.path as op\n\nfrom warnings import simplefilter\nsimplefilter('ignore')",
"_____no_output_____"
],
[
"# Altair config\ndef author_url(author):\n return f\"https://github.com/{author}\"\n\ndef alt_theme():\n return {\n 'config': {\n 'axisLeft': {\n 'labelFontSize': 15,\n },\n 'axisBottom': {\n 'labelFontSize': 15,\n },\n }\n }\n\nalt.themes.register('my_theme', alt_theme)\nalt.themes.enable(\"my_theme\")\n\n\n# Define colors we'll use for GitHub membership\nauthor_types = ['MEMBER', 'CONTRIBUTOR', 'COLLABORATOR', \"NONE\"]\n\nauthor_palette = np.array(sns.palettes.blend_palette([\"lightgrey\", \"lightgreen\", \"darkgreen\"], 4)) * 256\nauthor_colors = [\"rgb({}, {}, {})\".format(*color) for color in author_palette]\nauthor_color_dict = {key: val for key, val in zip(author_types, author_palette)}",
"_____no_output_____"
],
[
"github_org = \"jupyterhub\"\ntop_n_repos = 15\nn_days = 10",
"_____no_output_____"
],
[
"# Parameters\ngithub_org = \"nteract\"\nn_days = 90\n",
"_____no_output_____"
],
[
"############################################################\n# Variables\nstop = date.today()\nstart = date.today() - relativedelta(days=n_days)\n\n# Strings for use in queries\nstart_date = f\"{start:%Y-%m-%d}\"\nstop_date = f\"{stop:%Y-%m-%d}\"\n\n# Glue variables for use in markdown\nglue(f\"{github_org}_github_org\", github_org, display=False)\nglue(f\"{github_org}_start\", start_date, display=False)\nglue(f\"{github_org}_stop\", stop_date, display=False)",
"_____no_output_____"
]
],
[
[
"## Load data\n\nLoad and clean up the data",
"_____no_output_____"
]
],
[
[
"from pathlib import Path\npath_data = Path(\"../data\")\ncomments = pd.read_csv(path_data.joinpath('comments.csv'), index_col=None).drop_duplicates()\nissues = pd.read_csv(path_data.joinpath('issues.csv'), index_col=None).drop_duplicates()\nprs = pd.read_csv(path_data.joinpath('prs.csv'), index_col=None).drop_duplicates()\n\nfor idata in [comments, issues, prs]:\n idata.query(\"org == @github_org\", inplace=True)",
"_____no_output_____"
],
[
"# What are the top N repos, we will only plot these in the full data plots\ntop_commented_repos = comments.groupby(\"repo\").count().sort_values(\"createdAt\", ascending=False)['createdAt']\nuse_repos = top_commented_repos.head(top_n_repos).index.tolist()",
"_____no_output_____"
]
],
[
[
"## Merged Pull requests\n\nHere's an analysis of **merged pull requests** across each of the repositories in the Jupyter\necosystem.",
"_____no_output_____"
]
],
[
[
"merged = prs.query('state == \"MERGED\" and closedAt > @start_date and closedAt < @stop_date')",
"_____no_output_____"
],
[
"prs_by_repo = merged.groupby(['org', 'repo']).count()['author'].reset_index().sort_values(['org', 'author'], ascending=False)\nalt.Chart(data=prs_by_repo, title=f\"Merged PRs in the last {n_days} days\").mark_bar().encode(\n x=alt.X('repo', sort=prs_by_repo['repo'].values.tolist()),\n y='author',\n color='org'\n)",
"_____no_output_____"
]
],
[
[
"### Authoring and merging stats by repository\n\nLet's see who has been doing most of the PR authoring and merging. The PR author is generally the\nperson that implemented a change in the repository (code, documentation, etc). The PR merger is\nthe person that \"pressed the green button\" and got the change into the main codebase.",
"_____no_output_____"
]
],
[
[
"# Prep our merging DF\nmerged_by_repo = merged.groupby(['repo', 'author'], as_index=False).agg({'id': 'count', 'authorAssociation': 'first'}).rename(columns={'id': \"authored\", 'author': 'username'})\nclosed_by_repo = merged.groupby(['repo', 'mergedBy']).count()['id'].reset_index().rename(columns={'id': \"closed\", \"mergedBy\": \"username\"})",
"_____no_output_____"
],
[
"charts = []\ntitle = f\"PR authors for {github_org} in the last {n_days} days\"\nthis_data = merged_by_repo.replace(np.nan, 0).groupby('username', as_index=False).agg({'authored': 'sum', 'authorAssociation': 'first'})\nthis_data = this_data.sort_values('authored', ascending=False)\nch = alt.Chart(data=this_data, title=title).mark_bar().encode(\n x='username',\n y='authored',\n color=alt.Color('authorAssociation', scale=alt.Scale(domain=author_types, range=author_colors))\n)\nch",
"_____no_output_____"
],
[
"charts = []\ntitle = f\"Merges for {github_org} in the last {n_days} days\"\nch = alt.Chart(data=closed_by_repo.replace(np.nan, 0), title=title).mark_bar().encode(\n x='username',\n y='closed',\n)\nch",
"_____no_output_____"
]
],
[
[
"## Issues\n\nIssues are **conversations** that happen on our GitHub repositories. Here's an\nanalysis of issues across the Jupyter organizations.",
"_____no_output_____"
]
],
[
[
"created = issues.query('state == \"OPEN\" and createdAt > @start_date and createdAt < @stop_date')\nclosed = issues.query('state == \"CLOSED\" and closedAt > @start_date and closedAt < @stop_date')",
"_____no_output_____"
],
[
"created_counts = created.groupby(['org', 'repo']).count()['number'].reset_index()\ncreated_counts['org/repo'] = created_counts.apply(lambda a: a['org'] + '/' + a['repo'], axis=1)\nsorted_vals = created_counts.sort_values(['org', 'number'], ascending=False)['repo'].values\nalt.Chart(data=created_counts, title=f\"Issues created in the last {n_days} days\").mark_bar().encode(\n x=alt.X('repo', sort=alt.Sort(sorted_vals.tolist())),\n y='number',\n)",
"_____no_output_____"
],
[
"closed_counts = closed.groupby(['org', 'repo']).count()['number'].reset_index()\nclosed_counts['org/repo'] = closed_counts.apply(lambda a: a['org'] + '/' + a['repo'], axis=1)\nsorted_vals = closed_counts.sort_values(['number'], ascending=False)['repo'].values\nalt.Chart(data=closed_counts, title=f\"Issues closed in the last {n_days} days\").mark_bar().encode(\n x=alt.X('repo', sort=alt.Sort(sorted_vals.tolist())),\n y='number',\n)",
"_____no_output_____"
],
[
"created_closed = pd.merge(created_counts.rename(columns={'number': 'created'}).drop(columns='org/repo'),\n closed_counts.rename(columns={'number': 'closed'}).drop(columns='org/repo'),\n on=['org', 'repo'], how='outer')\n\ncreated_closed = pd.melt(created_closed, id_vars=['org', 'repo'], var_name=\"kind\", value_name=\"count\").replace(np.nan, 0)",
"_____no_output_____"
],
[
"charts = []\n# Pick the top 10 repositories\ntop_repos = created_closed.groupby(['repo']).sum().sort_values(by='count', ascending=False).head(10).index\nch = alt.Chart(created_closed.query('repo in @top_repos'), width=120).mark_bar().encode(\n x=alt.X(\"kind\", axis=alt.Axis(labelFontSize=15, title=\"\")), \n y=alt.Y('count', axis=alt.Axis(titleFontSize=15, labelFontSize=12)),\n color='kind',\n column=alt.Column(\"repo\", header=alt.Header(title=f\"Issue activity, last {n_days} days for {github_org}\", titleFontSize=15, labelFontSize=12))\n)\nch",
"_____no_output_____"
],
[
"# Set to datetime\nfor kind in ['createdAt', 'closedAt']:\n closed.loc[:, kind] = pd.to_datetime(closed[kind])\n \nclosed.loc[:, 'time_open'] = closed['closedAt'] - closed['createdAt']\nclosed.loc[:, 'time_open'] = closed['time_open'].dt.total_seconds()",
"_____no_output_____"
],
[
"time_open = closed.groupby(['org', 'repo']).agg({'time_open': 'median'}).reset_index()\ntime_open['time_open'] = time_open['time_open'] / (60 * 60 * 24)\ntime_open['org/repo'] = time_open.apply(lambda a: a['org'] + '/' + a['repo'], axis=1)\nsorted_vals = time_open.sort_values(['org', 'time_open'], ascending=False)['repo'].values\nalt.Chart(data=time_open, title=f\"Time to close for issues closed in the last {n_days} days\").mark_bar().encode(\n x=alt.X('repo', sort=alt.Sort(sorted_vals.tolist())),\n y=alt.Y('time_open', title=\"Median Days Open\"),\n)",
"_____no_output_____"
]
],
[
[
"## Most-upvoted issues",
"_____no_output_____"
]
],
[
[
"thumbsup = issues.sort_values(\"thumbsup\", ascending=False).head(25)\nthumbsup = thumbsup[[\"title\", \"url\", \"number\", \"thumbsup\", \"repo\"]]\n\ntext = []\nfor ii, irow in thumbsup.iterrows():\n itext = f\"- ({irow['thumbsup']}) {irow['title']} - {irow['repo']} - [#{irow['number']}]({irow['url']})\"\n text.append(itext)\ntext = '\\n'.join(text)\nHTML(markdown(text))",
"_____no_output_____"
]
],
[
[
"## Commenters across repositories\n\nThese are commenters across all issues and pull requests in the last several days.\nThese are colored by the commenter's association with the organization. For information\nabout what these associations mean, [see this StackOverflow post](https://stackoverflow.com/a/28866914/1927102).",
"_____no_output_____"
]
],
[
[
"commentors = (\n comments\n .query(\"createdAt > @start_date and createdAt < @stop_date\")\n .groupby(['org', 'repo', 'author', 'authorAssociation'])\n .count().rename(columns={'id': 'count'})['count']\n .reset_index()\n .sort_values(['org', 'count'], ascending=False)\n)",
"_____no_output_____"
],
[
"n_plot = 50\ncharts = []\nfor ii, (iorg, idata) in enumerate(commentors.groupby(['org'])):\n title = f\"Top {n_plot} commentors for {iorg} in the last {n_days} days\"\n idata = idata.groupby('author', as_index=False).agg({'count': 'sum', 'authorAssociation': 'first'})\n idata = idata.sort_values('count', ascending=False).head(n_plot)\n ch = alt.Chart(data=idata.head(n_plot), title=title).mark_bar().encode(\n x='author',\n y='count',\n color=alt.Color('authorAssociation', scale=alt.Scale(domain=author_types, range=author_colors))\n )\n charts.append(ch)\nalt.hconcat(*charts)",
"_____no_output_____"
]
],
[
[
"## First responders\n\nFirst responders are the first people to respond to a new issue in one of the repositories.\nThe following plots show first responders for recently-created issues.",
"_____no_output_____"
]
],
[
[
"first_comments = []\nfor (org, repo, issue_id), i_comments in comments.groupby(['org', 'repo', 'id']):\n ix_min = pd.to_datetime(i_comments['createdAt']).idxmin()\n first_comment = i_comments.loc[ix_min]\n if isinstance(first_comment, pd.DataFrame):\n first_comment = first_comment.iloc[0]\n first_comments.append(first_comment)\nfirst_comments = pd.concat(first_comments, axis=1).T\n\n# Make up counts for viz\nfirst_responder_counts = first_comments.groupby(['org', 'author', 'authorAssociation'], as_index=False).\\\n count().rename(columns={'id': 'n_first_responses'}).sort_values(['org', 'n_first_responses'], ascending=False)\n",
"_____no_output_____"
],
[
"n_plot = 50\n\ntitle = f\"Top {n_plot} first responders for {github_org} in the last {n_days} days\"\nidata = first_responder_counts.groupby('author', as_index=False).agg({'n_first_responses': 'sum', 'authorAssociation': 'first'})\nidata = idata.sort_values('n_first_responses', ascending=False).head(n_plot)\nch = alt.Chart(data=idata.head(n_plot), title=title).mark_bar().encode(\n x='author',\n y='n_first_responses',\n color=alt.Color('authorAssociation', scale=alt.Scale(domain=author_types, range=author_colors))\n)\nch",
"_____no_output_____"
]
],
[
[
"## Recent activity\n\n### A list of merged PRs by project\n\nBelow is a tabbed readout of recently-merged PRs. Check out the title to get an idea for what they\nimplemented, and be sure to thank the PR author for their hard work!",
"_____no_output_____"
]
],
[
[
"tabs = widgets.Tab(children=[])\n\nfor ii, ((org, repo), imerged) in enumerate(merged.query(\"repo in @use_repos\").groupby(['org', 'repo'])):\n merged_by = {}\n pr_by = {}\n issue_md = []\n issue_md.append(f\"#### Closed PRs for repo: [{org}/{repo}](https://github.com/{github_org}/{repo})\")\n issue_md.append(\"\")\n issue_md.append(f\"##### \")\n\n for _, ipr in imerged.iterrows():\n user_name = ipr['author']\n user_url = author_url(user_name)\n pr_number = ipr['number']\n pr_html = ipr['url']\n pr_title = ipr['title']\n pr_closedby = ipr['mergedBy']\n pr_closedby_url = f\"https://github.com/{pr_closedby}\"\n if user_name not in pr_by:\n pr_by[user_name] = 1\n else:\n pr_by[user_name] += 1\n\n if pr_closedby not in merged_by:\n merged_by[pr_closedby] = 1\n else:\n merged_by[pr_closedby] += 1\n text = f\"* [(#{pr_number})]({pr_html}): _{pr_title}_ by **[@{user_name}]({user_url})** merged by **[@{pr_closedby}]({pr_closedby_url})**\"\n issue_md.append(text)\n \n issue_md.append('')\n markdown_html = markdown('\\n'.join(issue_md))\n\n children = list(tabs.children)\n children.append(HTML(markdown_html))\n tabs.children = tuple(children)\n tabs.set_title(ii, repo)\ntabs",
"_____no_output_____"
]
],
[
[
"### A list of recent issues\n\nBelow is a list of issues with recent activity in each repository. If they seem of interest\nto you, click on their links and jump in to participate!",
"_____no_output_____"
]
],
[
[
"# Add comment count data to issues and PRs\ncomment_counts = (\n comments\n .query(\"createdAt > @start_date and createdAt < @stop_date\")\n .groupby(['org', 'repo', 'id'])\n .count().iloc[:, 0].to_frame()\n)\ncomment_counts.columns = ['n_comments']\ncomment_counts = comment_counts.reset_index()",
"_____no_output_____"
],
[
"n_plot = 5\ntabs = widgets.Tab(children=[])\n\nfor ii, (repo, i_issues) in enumerate(comment_counts.query(\"repo in @use_repos\").groupby('repo')):\n \n issue_md = []\n issue_md.append(\"\")\n issue_md.append(f\"##### [{github_org}/{repo}](https://github.com/{github_org}/{repo})\")\n\n top_issues = i_issues.sort_values('n_comments', ascending=False).head(n_plot)\n top_issue_list = pd.merge(issues, top_issues, left_on=['org', 'repo', 'id'], right_on=['org', 'repo', 'id'])\n for _, issue in top_issue_list.sort_values('n_comments', ascending=False).head(n_plot).iterrows():\n user_name = issue['author']\n user_url = author_url(user_name)\n issue_number = issue['number']\n issue_html = issue['url']\n issue_title = issue['title']\n\n text = f\"* [(#{issue_number})]({issue_html}): _{issue_title}_ by **[@{user_name}]({user_url})**\"\n issue_md.append(text)\n\n issue_md.append('')\n md_html = HTML(markdown('\\n'.join(issue_md)))\n\n children = list(tabs.children)\n children.append(HTML(markdown('\\n'.join(issue_md))))\n tabs.children = tuple(children)\n tabs.set_title(ii, repo)\n \ndisplay(Markdown(f\"Here are the top {n_plot} active issues in each repository in the last {n_days} days\"))\ndisplay(tabs)",
"_____no_output_____"
]
]
] | [
"markdown",
"code",
"markdown",
"code",
"markdown",
"code",
"markdown",
"code",
"markdown",
"code",
"markdown",
"code",
"markdown",
"code",
"markdown",
"code",
"markdown",
"code",
"markdown",
"code"
] | [
[
"markdown"
],
[
"code",
"code",
"code",
"code",
"code"
],
[
"markdown"
],
[
"code",
"code"
],
[
"markdown"
],
[
"code",
"code"
],
[
"markdown"
],
[
"code",
"code",
"code"
],
[
"markdown"
],
[
"code",
"code",
"code",
"code",
"code",
"code",
"code"
],
[
"markdown"
],
[
"code"
],
[
"markdown"
],
[
"code",
"code"
],
[
"markdown"
],
[
"code",
"code"
],
[
"markdown"
],
[
"code"
],
[
"markdown"
],
[
"code",
"code"
]
] |
e78203d754b103c69315324532125d9329d4aed7 | 1,267 | ipynb | Jupyter Notebook | researchs/No03_dealer_counter/run.ipynb | samacoba/ObjectCounter | 0a4a8f383c2618629f99f5fe81db5db43d4eada0 | [
"MIT"
] | null | null | null | researchs/No03_dealer_counter/run.ipynb | samacoba/ObjectCounter | 0a4a8f383c2618629f99f5fe81db5db43d4eada0 | [
"MIT"
] | null | null | null | researchs/No03_dealer_counter/run.ipynb | samacoba/ObjectCounter | 0a4a8f383c2618629f99f5fe81db5db43d4eada0 | [
"MIT"
] | null | null | null | 18.1 | 73 | 0.502762 | [
[
[
"#メイン関数読み込み\n%run -i run_func.py",
"_____no_output_____"
],
[
"#画像読み込み\nDataA = {'x': data.load_img(fpath = 'img108.png')}\n#Bokeh読み込み\nbokeh_view = data.Bokeh_View(imgs = [DataA['x'][0], DataA['x'][0]])",
"_____no_output_____"
],
[
"#モデルをセット\nrun_set_model()\n#学習開始\nrun_train(nLoop = 200) ",
"_____no_output_____"
]
]
] | [
"code"
] | [
[
"code",
"code",
"code"
]
] |
e78226c1c3c7599e850fa34fd01372d2da1f92c9 | 3,157 | ipynb | Jupyter Notebook | examples/reference/elements/matplotlib/HeatMap.ipynb | stuarteberg/holoviews | 65136173014124b41cee00f5a0fee82acdc78f7f | [
"BSD-3-Clause"
] | null | null | null | examples/reference/elements/matplotlib/HeatMap.ipynb | stuarteberg/holoviews | 65136173014124b41cee00f5a0fee82acdc78f7f | [
"BSD-3-Clause"
] | null | null | null | examples/reference/elements/matplotlib/HeatMap.ipynb | stuarteberg/holoviews | 65136173014124b41cee00f5a0fee82acdc78f7f | [
"BSD-3-Clause"
] | null | null | null | 31.257426 | 240 | 0.591701 | [
[
[
"<div class=\"contentcontainer med left\" style=\"margin-left: -50px;\">\n<dl class=\"dl-horizontal\">\n <dt>Title</dt> <dd> HeatMap Element</dd>\n <dt>Dependencies</dt> <dd>Matplotlib</dd>\n <dt>Backends</dt> <dd><a href='./HeatMap.ipynb'>Matplotlib</a></dd> <dd><a href='../bokeh/HeatMap.ipynb'>Bokeh</a></dd>\n</dl>\n</div>",
"_____no_output_____"
]
],
[
[
"import numpy as np\nimport holoviews as hv\nhv.extension('matplotlib')",
"_____no_output_____"
]
],
[
[
"``HeatMap`` visualises tabular data indexed by two key dimensions as a grid of colored values. This allows spotting correlations in multivariate data and provides a high-level overview of how the two variables are plotted.\n\nThe data for a ``HeatMap`` may be supplied as 2D tabular data with one or more associated value dimensions. The first value dimension will be colormapped, but further value dimensions may be revealed using the hover tool.",
"_____no_output_____"
]
],
[
[
"data = [(chr(65+i), chr(97+j), i*j) for i in range(5) for j in range(5) if i!=j]\nhv.HeatMap(data).sort()",
"_____no_output_____"
]
],
[
[
"It is important to note that the data should be aggregated before plotting as the ``HeatMap`` cannot display multiple values for one coordinate and will simply use the first value it finds for each combination of x- and y-coordinates.",
"_____no_output_____"
]
],
[
[
"heatmap = hv.HeatMap([(0, 0, 1), (0, 0, 10), (1, 0, 2), (1, 1, 3)])\nheatmap + heatmap.aggregate(function=np.max)",
"_____no_output_____"
]
],
[
[
"As the above example shows before aggregating the second value for the (0, 0) is ignored unless we aggregate the data first.\n\nTo reveal the values of a ``HeatMap`` we can enable a ``colorbar`` and if you wish to have interactive hover information, you can use the hover tool in the [Bokeh backend](../bokeh/HeatMap.ipynb):",
"_____no_output_____"
]
],
[
[
"heatmap = hv.HeatMap((np.random.randint(0, 10, 100), np.random.randint(0, 10, 100),\n np.random.randn(100), np.random.randn(100)), vdims=['z', 'z2']).redim.range(z=(-2, 2))\n\nheatmap.opts(colorbar=True, fig_size=250)",
"_____no_output_____"
]
],
[
[
"For full documentation and the available style and plot options, use ``hv.help(hv.HeatMap).``",
"_____no_output_____"
]
]
] | [
"markdown",
"code",
"markdown",
"code",
"markdown",
"code",
"markdown",
"code",
"markdown"
] | [
[
"markdown"
],
[
"code"
],
[
"markdown"
],
[
"code"
],
[
"markdown"
],
[
"code"
],
[
"markdown"
],
[
"code"
],
[
"markdown"
]
] |
e78229a516933660f5c4fcebc4fab96a1155f473 | 575,205 | ipynb | Jupyter Notebook | notebooks/Exploratory/CESAR-School-Database-Exploratory.ipynb | JPMagalhaesCESAR/MLIntro | ea751b340687c4fa7653b513e1138d9d403f0f54 | [
"CC-BY-4.0"
] | null | null | null | notebooks/Exploratory/CESAR-School-Database-Exploratory.ipynb | JPMagalhaesCESAR/MLIntro | ea751b340687c4fa7653b513e1138d9d403f0f54 | [
"CC-BY-4.0"
] | null | null | null | notebooks/Exploratory/CESAR-School-Database-Exploratory.ipynb | JPMagalhaesCESAR/MLIntro | ea751b340687c4fa7653b513e1138d9d403f0f54 | [
"CC-BY-4.0"
] | null | null | null | 236.029955 | 137,964 | 0.883567 | [
[
[
"%matplotlib inline\n\nimport numpy as np\nimport pandas as pd\nimport seaborn as sns\nimport matplotlib.pyplot as plt\n\nfrom sklearn.model_selection import train_test_split\nfrom sklearn.model_selection import GridSearchCV\nfrom sklearn.preprocessing import StandardScaler\n\nfrom sklearn.svm import SVC\nfrom sklearn.neighbors import KNeighborsClassifier\nfrom sklearn.neural_network import MLPClassifier\nfrom sklearn.ensemble import RandomForestClassifier\nfrom sklearn.ensemble import GradientBoostingClassifier\nfrom sklearn.tree import DecisionTreeClassifier",
"_____no_output_____"
],
[
"from pyspark import SparkContext\nfrom pyspark.sql import SQLContext\nfrom pyspark.sql.types import *",
"_____no_output_____"
],
[
"sc = sc = SparkContext.getOrCreate('local')\nlog_txt = sc.textFile(\"BaseCesarSchool.txt\")\nsqlContext = SQLContext(sc)",
"_____no_output_____"
],
[
"header = log_txt.first()\nheader_split = header.split(\"\t\")\nprint(header_split)",
"[u'ID', u'DtRef', u'IND_BOM_1', u'CEP', u'UF', u'IDADE', u'SEXO', u'NIVEL_RELACIONAMENTO_AUTOMOVEL', u'NIVEL_RELACIONAMENTO_SEGUROS01', u'NIVEL_RELACIONAMENTO_CREDITO03', u'NIVEL_RELACIONAMENTO_CREDITO04', u'NIVEL_RELACIONAMENTO_VAREJO', u'NIVEL_RELACIONAMENTO_SEGUROS02', u'NIVEL_RELACIONAMENTO_CREDITO01', u'NIVEL_RELACIONAMENTO_CREDITO02', u'BANCO_REST_IRPF_ULTIMA', u'ATIVIDADE_EMAIL', u'EXPOSICAO_ENDERECO', u'EXPOSICAO_EMAIL', u'EXPOSICAO_TELEFONE', u'ATIVIDADE_ENDERECO', u'ATUALIZACAO_ENDERECO', u'ATUALIZACAO_EMAIL', u'EXPOSICAO_CONSUMIDOR_COBRANCA', u'EXPOSICAO_CONSUMIDOR_EMAILS', u'EXPOSICAO_CONSUMIDOR_TELEFONES', u'ATIVIDADE_TELEFONE', u'VALOR_PARCELA_BOLSA_FAMILIA', u'FLAG_BOLSA_FAMILIA', u'SIGLA_PARTIDO_FILIADO', u'FLAG_FILIADO_PARTIDO_POLITICO', u'REMUNERACAO_SEVIDOR_CIVIL', u'FLAG_SERVIDOR_CIVIL', u'REMUNERACAO_SERVIDOR_MILITAR', u'FLAG_SERVIDOR_MILITAR', u'FLAG_PROUNI', u'RENDA_VIZINHANCA', u'QUANTIDADE_VIZINHANCA', u'COMPARATIVO_RENDA_CEP', u'CLASSE_SOCIAL_CONSUMIDOR', u'ATIVIDADE_CONSUMIDOR_MERCADO_FINANCEIRO', u'ATUALIZACAO_CONSUMIDOR_MERCADO_FINANCEIRO', u'FLAG_PROGRAMAS_SOCIAIS', u'MENOR_DIST_ENDERECO_AEROPORTOS', u'MENOR_DIST_ENDERECO_PARQUES_DIVERSAO', u'MENOR_DIST_ENDERECO_CAIXA_ELETRONICO', u'MENOR_DIST_BANCO', u'MENOR_DIST_ENDERECO_BARES', u'MENOR_DIST_ENDERECO_ESTACAO_ONIBUS', u'MENOR_DIST_CONCESSIONARIA', u'MENOR_DIST_ALUGUEL_CARROS', u'MENOR_DIST_ENDERECO_OFICINAS', u'MENOR_DIST_ENDERECO_LAVA_RAPIDO', u'MENOR_DIST_ENDERECO_CEMITERIO', u'MENOR_DIST_ENDERECO_IGREJA', u'MENOR_DIST_ENDERECO_PREFEITURA', u'MENOR_DIST_ENDERECO_BOMBEIRO', u'MENOR_DIST_ENDERECO_FAVELA', u'MENOR_DIST_ENDERECO_FUNERARIA', u'MENOR_DIST_ENDERECO_POSTO_GASOLINA', u'MENOR_DIST_ENDERECO_SUPERMERCADO', u'MENOR_DIST_ENDERECO_ACADEMIAS', u'MENOR_DIST_ENDERECO_HOSPITAL', u'MENOR_DIST_CORRETOR_SEGUROS', u'MENOR_DIST_ENDERECO_BEBIDAS', u'MENOR_DIST_ENDERECO_HOTEL', u'MENOR_DIST_ENDERECO_CINEMAS', u'MENOR_DIST_ENDERECO_CASA_NOTURNA', u'MENOR_DIST_ENDERECO_PARQUE', u'MENOR_DIST_ESTACIONAMENTOS', u'MENOR_DIST_ENDERECO_POLICIA', u'MENOR_DIST_ENDERECO_CORREIOS', u'MENOR_DIST_ENDERECO_ESCOLAS', u'MENOR_DIST_ENDERECO_SHOPPING', u'MENOR_DIST_ENDERECO_METRO', u'MENOR_DIST_PONTO_TAXI', u'MENOR_DIST_ENDERECO_TREM', u'MENOR_DIST_UNIVERSIDADE', u'MENOR_DIST_ENDERECO_FRONTEIRA_ESTADUAL', u'MENOR_DIST_ENDERECO_FRONTEIRA_MARITIMA', u'MENOR_DIST_ENDERECO_FRONTEIRA_INTERNACIONAL', u'EXPOSICAO_ENDERECO_AEROPORTOS', u'EXPOSICAO_ENDERECO_PARQUES_DIVERSAO', u'EXPOSICAO_ENDERECO_AREA_RISCO', u'EXPOSICAO_ENDERECO_CAIXA_ELETRONICO', u'EXPOSICAO_ENDERECO_BANCOS', u'EXPOSICAO_ENDERECO_BARES', u'EXPOSICAO_ENDERECO_ESTACAO_ONIBUS', u'EXPOSICAO_ENDERECO_CONCESSIONARIA', u'EXPOSICAO_ENDERECO_ALUGUEL_CARROS', u'EXPOSICAO_ENDERECO_OFICINAS', u'EXPOSICAO_ENDERECO_LAVA_RAPIDO', u'EXPOSICAO_ENDERECO_CEMITERIO', u'EXPOSICAO_ENDERECO_IGREJA', u'EXPOSICAO_ENDERECO_PREFEITURA', u'EXPOSICAO_ENDERECO_BOMBEIRO', u'EXPOSICAO_ENDERECO_FAVELAS', u'EXPOSICAO_ENDERECO_FUNERARIA', u'EXPOSICAO_ENDERECO_POSTO_GASOLINA', u'EXPOSICAO_ENDERECO_SUPERMERCADO', u'EXPOSICAO_ENDERECO_ACADEMIAS', u'EXPOSICAO_ENDERECO_HOSPITAL', u'EXPOSICAO_ENDERECO_CORRETOR_SEGUROS', u'EXPOSICAO_ENDERECO_BEBIDAS', u'EXPOSICAO_ENDERECO_HOTEL', u'EXPOSICAO_ENDERECO_CINEMAS', u'EXPOSICAO_ENDERECO_CASA_NOTURNA', u'EXPOSICAO_ENDERECO_PARQUE', u'EXPOSICAO_ENDERECO_ESTACIONAMENTOS', u'EXPOSICAO_ENDERECO_POLICIA', u'EXPOSICAO_ENDERECO_CORREIOS', u'EXPOSICAO_ENDERECO_ESCOLAS', u'EXPOSICAO_ENDERECO_SHOPPING', u'EXPOSICAO_ENDERECO_METRO', u'EXPOSICAO_ENDERECO_PONTO_TAXI', u'EXPOSICAO_ENDERECO_TREM', u'EXPOSICAO_ENDERECO_UNIVERSIDADE', u'FLAG_REDE_SOCIAL', u'FLAG_WEB_ARTES', u'FLAG_WEB_MUSICA', u'FLAG_WEB_TV', u'FLAG_WEB_LIVROS', u'FLAG_WEB_NEGOCIOS', u'FLAG_WEB_NEGOCIOS_SERVICOS', u'FLAG_WEB_NEGOCIOS_MARKETING', u'FLAG_WEB_NEGOCIOS_SERVICOS_UNIVERSIDADES', u'FLAG_WEB_NEGOCIOS_SERVICOS_COMPUTACAO', u'FLAG_WEB_SAUDE', u'FLAG_WEB_NOTICIAS', u'FLAG_WEB_SOCIEDADE', u'FLAG_WEB_SOCIEDADE_GENEALOGIA', u'EXPOSICAO_WEB', u'FLAG_WEB_CIENCIA', u'FLAG_WEB_COMPRAS', u'FLAG_WEB_ESPORTES_FUTEBOL', u'FLAG_WEB', u'CEP1', u'CEP2', u'CEP3', u'CEP4']\n"
],
[
"log_txt = log_txt.filter(lambda line: line != header)\nlog_txt.take(10)[3].split(\"\t\")",
"_____no_output_____"
],
[
"temp_var = log_txt.map(lambda k: k.split(\"\t\"))\nprint \"Number of instances: {}\".format(temp_var.countApprox(1000, 1.0))",
"Number of instances: 518930\n"
],
[
"sample = temp_var.take(5000)",
"_____no_output_____"
],
[
"df = pd.DataFrame(data=sample, columns=header_split)\ndf.head()",
"_____no_output_____"
],
[
"# Vamos ver se pegamos uma amostra interessante com classes balanceadas\nsns.countplot(x='IND_BOM_1', data=df)",
"_____no_output_____"
],
[
"# checking for missing data\nprint('Has missing data: {}'.format(df.isnull().values.any()))",
"Has missing data: False\n"
],
[
"# Empty string is NULL, então vamos dar um replace\ndf.replace(to_replace=' ', value=np.nan, inplace=True)\nprint('Has missing data: {}'.format(df.isnull().values.any()))",
"Has missing data: True\n"
],
[
"df.info()",
"<class 'pandas.core.frame.DataFrame'>\nRangeIndex: 5000 entries, 0 to 4999\nColumns: 140 entries, ID to CEP4\ndtypes: object(140)\nmemory usage: 5.3+ MB\n"
],
[
"df.describe()",
"_____no_output_____"
],
[
"df.head()",
"_____no_output_____"
],
[
"for col in df.columns:\n try:\n df[col] = pd.to_numeric(df[col])\n except:\n pass\n",
"_____no_output_____"
],
[
"plt.figure(figsize=(15, 5))\nsns.countplot(x='UF', data=df)",
"_____no_output_____"
],
[
"plt.figure(figsize=(18,5))\n\n#FAKE PLOTS JUST TO DISPLAY THE LEGEND\nplt.plot([], [], color='yellow', label='MISSING')\nplt.plot([], [], color='purple', label='PRESENT')\n\n# HEATMAP TO DISPLAY THE MISSING DATA\nsns.heatmap(df[sorted(df.columns)].isnull(), yticklabels=False, cbar=False, cmap='viridis')\nplt.legend(bbox_to_anchor=(1.15, 1))\n",
"_____no_output_____"
],
[
"# Target variable does not have missing data\ndf['IND_BOM_1'].isnull().any()",
"_____no_output_____"
],
[
"# vertical elimination\nto_be_eliminated = []\nfor col_name in df.columns: \n # eliminate columns with more than 50% of missing data\n if df[col_name].isnull().any() and sum(df[col_name].isnull()) > int(0.5 * len(df)):\n to_be_eliminated.append(col_name)\n \nprint(to_be_eliminated)",
"[u'NIVEL_RELACIONAMENTO_AUTOMOVEL', u'NIVEL_RELACIONAMENTO_SEGUROS01', u'NIVEL_RELACIONAMENTO_CREDITO04', u'NIVEL_RELACIONAMENTO_VAREJO', u'NIVEL_RELACIONAMENTO_SEGUROS02', u'NIVEL_RELACIONAMENTO_CREDITO02', u'BANCO_REST_IRPF_ULTIMA', u'ATIVIDADE_EMAIL', u'EXPOSICAO_EMAIL', u'ATUALIZACAO_EMAIL', u'EXPOSICAO_CONSUMIDOR_COBRANCA', u'VALOR_PARCELA_BOLSA_FAMILIA', u'SIGLA_PARTIDO_FILIADO', u'REMUNERACAO_SEVIDOR_CIVIL', u'REMUNERACAO_SERVIDOR_MILITAR', u'ATIVIDADE_CONSUMIDOR_MERCADO_FINANCEIRO', u'ATUALIZACAO_CONSUMIDOR_MERCADO_FINANCEIRO', u'FLAG_REDE_SOCIAL']\n"
],
[
"filtered_df = df.drop(labels=to_be_eliminated, axis=1)\n\n# ANALYSING MISSING DATA\nplt.figure(figsize=(18,5))\n\n#FAKE PLOTS JUST TO DISPLAY THE LEGEND\nplt.plot([], [], color='yellow', label='MISSING')\nplt.plot([], [], color='purple', label='PRESENT')\n\n# HEATMAP TO DISPLAY THE MISSING DATA\nsns.heatmap(filtered_df[sorted(filtered_df.columns)].isnull(), yticklabels=False, cbar=False, cmap='viridis')\nplt.legend(bbox_to_anchor=(1.15, 1))",
"_____no_output_____"
],
[
"# horizontal elimination\nfiltered_df.dropna(inplace=True)\n\n# ANALYSING MISSING DATA\nplt.figure(figsize=(18,5))\n\n#FAKE PLOTS JUST TO DISPLAY THE LEGEND\nplt.plot([], [], color='yellow', label='MISSING')\nplt.plot([], [], color='purple', label='PRESENT')\n\n# HEATMAP TO DISPLAY THE MISSING DATA\nsns.heatmap(filtered_df[sorted(filtered_df.columns)].isnull(), yticklabels=False, cbar=False, cmap='viridis')\nplt.legend(bbox_to_anchor=(1.15, 1))",
"_____no_output_____"
],
[
"sns.countplot(x='IND_BOM_1', data=filtered_df)",
"_____no_output_____"
],
[
"filtered_df.info()",
"<class 'pandas.core.frame.DataFrame'>\nInt64Index: 2745 entries, 2 to 4997\nColumns: 122 entries, ID to CEP4\ndtypes: float64(66), int64(52), object(4)\nmemory usage: 2.7+ MB\n"
],
[
"filtered_df.head()",
"_____no_output_____"
],
[
"numerics = ['int16', 'int32', 'int64', 'float16', 'float32', 'float64']\nnewdf = filtered_df.select_dtypes(include=numerics)\n\nprint(newdf.columns)",
"Index([u'ID', u'DtRef', u'IND_BOM_1', u'CEP', u'IDADE',\n u'NIVEL_RELACIONAMENTO_CREDITO03', u'NIVEL_RELACIONAMENTO_CREDITO01',\n u'EXPOSICAO_ENDERECO', u'EXPOSICAO_TELEFONE', u'ATIVIDADE_ENDERECO',\n ...\n u'FLAG_WEB_SOCIEDADE_GENEALOGIA', u'EXPOSICAO_WEB', u'FLAG_WEB_CIENCIA',\n u'FLAG_WEB_COMPRAS', u'FLAG_WEB_ESPORTES_FUTEBOL', u'FLAG_WEB', u'CEP1',\n u'CEP2', u'CEP3', u'CEP4'],\n dtype='object', length=118)\n"
],
[
"newdf.head()",
"_____no_output_____"
],
[
"x = newdf.drop(labels=['IND_BOM_1'], axis=1)\nx.drop(labels=['IDADE', 'ID', 'DtRef', 'CEP', 'CEP1', 'CEP2', 'CEP3', 'CEP4'], axis=1, inplace=True)\n\ny = newdf['IND_BOM_1']",
"_____no_output_____"
],
[
"x.head()",
"_____no_output_____"
],
[
"X_train, X_test, y_train, y_test = train_test_split(x, y, stratify=y, test_size=0.25)",
"_____no_output_____"
],
[
"std = StandardScaler()\n\nX_train_std = std.fit_transform(X_train)\nX_test_std = std.transform(X_test)",
"/usr/local/lib/python2.7/site-packages/sklearn/preprocessing/data.py:625: DataConversionWarning: Data with input dtype int64, float64 were all converted to float64 by StandardScaler.\n return self.partial_fit(X, y)\n/usr/local/lib/python2.7/site-packages/sklearn/base.py:462: DataConversionWarning: Data with input dtype int64, float64 were all converted to float64 by StandardScaler.\n return self.fit(X, **fit_params).transform(X)\n/usr/local/lib/python2.7/site-packages/ipykernel_launcher.py:4: DataConversionWarning: Data with input dtype int64, float64 were all converted to float64 by StandardScaler.\n after removing the cwd from sys.path.\n"
],
[
"knn = KNeighborsClassifier(n_neighbors=3)\nknn.fit(X_train_std, y_train)\n",
"_____no_output_____"
],
[
"knn.score(X_test_std, y_test)",
"_____no_output_____"
],
[
"import numpy as np\nimport operator\n\nfrom sklearn.base import BaseEstimator\nfrom sklearn.base import ClassifierMixin\nfrom sklearn.base import clone\nfrom sklearn.externals import six\nfrom sklearn.pipeline import _name_estimators\nfrom sklearn.preprocessing import LabelEncoder\n\nclass MajorityVoteClassifier(BaseEstimator, ClassifierMixin):\n \n def __init__(self, classifiers, votes='classlabel', weights=None):\n self.classifiers = classifiers\n self.named_classifiers = {key:value for key, value in _name_estimators(classifiers)}\n self.votes = votes\n self.weights = weights\n \n def fit(self, X, y):\n if self.votes not in ('probability', 'classlabel'):\n raise ValueError(\"vote must be 'probability' or 'classlabel'; got (vote=%r)\" % self.vote)\n \n if self.weights and len(self.weights) != len(self.classifiers):\n raise ValueError('Number of classifiers and weights must be equal ; got %d weights, %d classifiers'\n % (len(self.weights), len(self.classifiers)))\n \n self.lablenc_ = LabelEncoder()\n self.lablenc_.fit(y)\n self.classes_ = self.lablenc_.classes_\n self.classifiers_ = []\n for clf in self.classifiers:\n fitted_clf = clone(clf).fit(X, self.lablenc_.transform(y))\n self.classifiers_.append(fitted_clf)\n return self\n \n def predict(self, X):\n if self.votes == 'probability':\n maj_vote = np.argmax(self.predict_proba(X), axis=1)\n else:\n preds = np.asarray([clf.predict(X) for clf in self.classifiers_]).T\n maj_vote = np.apply_along_axis(lambda x : np.argmax(np.bincount(x, weights=self.weights)),\n axis=1, arr=preds)\n maj_vote = self.lablenc_.inverse_transform(maj_vote)\n return maj_vote\n \n def predict_proba(self, X):\n probas = np.asarray([clf.predict_proba(X) for clf in self.classifiers_])\n avg_proba = np.average(probas, axis=0, weights=self.weights)\n return avg_proba\n \n def get_params(self, deep=True):\n if not deep:\n return super(MajorityVoteClassifier, self).get_params(deep=False)\n else:\n out = self.named_classifiers.copy()\n for name, step in six.iteritems(self.named_classifiers):\n for key, value in six.iteritems(step.get_params(deep=True)):\n out['%s__%s' % (name, key)] = value\n return out",
"_____no_output_____"
],
[
"dim = len(x.columns)\n\nclf1 = MLPClassifier(activation='logistic', solver='adam', alpha=1e-5, hidden_layer_sizes=(dim, dim/2, 2*dim),\n max_iter=500)\nclf2 = SVC(kernel='rbf', C=10, gamma=0.001, probability=True)\nclf3 = RandomForestClassifier(n_estimators=100, max_depth=None, min_samples_split=2)\nclfM = MajorityVoteClassifier(classifiers=[clf1, clf2, clf3])\nclf_labels = ['MLP', 'SVM', 'Random Forests', 'Majority Voting']\nclfs = [clf1, clf2, clf3, clfM]",
"_____no_output_____"
],
[
"from sklearn.metrics import auc\nfrom sklearn.metrics import roc_curve\n\ncolors = ['black', 'orange', 'blue', 'green']\nlinestyles = [':', '--', '-.', '-']\n\nplt.figure(figsize=(12,8))\nfor clf, label, clr, ls in zip(clfs, clf_labels, colors, linestyles):\n preds = clf.fit(X_train_std, y_train).predict_proba(X_test_std)[:, 1]\n fpr, tpr, thresholds = roc_curve(y_true=y_test, y_score=preds)\n roc_auc = auc(x=fpr, y=tpr)\n plt.plot(fpr, tpr, color=clr, linestyle=ls, label='%s (auc = %0.2f)' % (label, roc_auc))\nplt.legend(loc='lower right')\nplt.plot([0, 1], [0, 1], linestyle='--', color='gray', linewidth=2)\nplt.xlim([-0.1, 1.1])\nplt.ylim([-0.1, 1.1])\nplt.xlabel('False Positive Rate')\nplt.ylabel('True Positive Rate')",
"_____no_output_____"
],
[
"from sklearn.metrics import confusion_matrix\nfrom sklearn.metrics import classification_report\n\nclf_names = ['MLP', 'SVC', 'Random Forest', 'Majority Vote']\nfor name, clf in zip(clf_names, clfs):\n clf.fit(X_train_std, y_train)\n print 'SCORE: {}'.format(clf.score(X_test_std, y_test))\n preds = clf.predict(X_test_std)\n cfm = confusion_matrix(y_test, preds)\n plt.figure(figsize=(10, 7))\n sns.heatmap(cfm , annot=True, cbar=False, fmt='g', cmap='Blues')\n plt.title('{} CLASSIFIER - MATRIX DE CONFUSAO'.format(name))\n print classification_report(y_test, preds)\n print(\"=\" * 50)\n",
"SCORE: 0.574963609898\n precision recall f1-score support\n\n 0 0.46 0.43 0.44 273\n 1 0.64 0.67 0.66 414\n\n micro avg 0.57 0.57 0.57 687\n macro avg 0.55 0.55 0.55 687\nweighted avg 0.57 0.57 0.57 687\n\n==================================================\nSCORE: 0.604075691412\n precision recall f1-score support\n\n 0 0.51 0.07 0.13 273\n 1 0.61 0.95 0.74 414\n\n micro avg 0.60 0.60 0.60 687\n macro avg 0.56 0.51 0.44 687\nweighted avg 0.57 0.60 0.50 687\n\n==================================================\nSCORE: 0.599708879185\n precision recall f1-score support\n\n 0 0.49 0.23 0.32 273\n 1 0.62 0.84 0.72 414\n\n micro avg 0.60 0.60 0.60 687\n macro avg 0.56 0.54 0.52 687\nweighted avg 0.57 0.60 0.56 687\n\n==================================================\nSCORE: 0.592430858806\n precision recall f1-score support\n\n 0 0.46 0.17 0.25 273\n 1 0.61 0.87 0.72 414\n\n micro avg 0.59 0.59 0.59 687\n macro avg 0.54 0.52 0.48 687\nweighted avg 0.55 0.59 0.53 687\n\n==================================================\n"
],
[
"from sklearn.ensemble import GradientBoostingClassifier\n\ngbc = GradientBoostingClassifier()\ngbc.fit(X_train_std, y_train)\nprint gbc.score(X_test_std, y_test)",
"0.6404657933042213\n"
],
[
"from sklearn.model_selection import GridSearchCV\n\nparam_grid = {\n 'bootstrap': [True],\n 'max_depth': [80, 90, 100, 110],\n 'max_features': [2, 3],\n 'min_samples_leaf': [3, 4, 5],\n 'min_samples_split': [8, 10, 12],\n 'n_estimators': [100, 200, 300, 1000]\n}\n\nrf = RandomForestClassifier()\ngrid_search = GridSearchCV(estimator=rf, param_grid=param_grid, cv=3,\n scoring='roc_auc', n_jobs = -1, verbose = 2)",
"_____no_output_____"
],
[
"grid_search.fit(X_train_std, y_train)",
"Fitting 3 folds for each of 288 candidates, totalling 864 fits\n"
]
]
] | [
"code"
] | [
[
"code",
"code",
"code",
"code",
"code",
"code",
"code",
"code",
"code",
"code",
"code",
"code",
"code",
"code",
"code",
"code",
"code",
"code",
"code",
"code",
"code",
"code",
"code",
"code",
"code",
"code",
"code",
"code",
"code",
"code",
"code",
"code",
"code",
"code",
"code",
"code",
"code",
"code",
"code"
]
] |
e7823baa6fa42598ac780f99023796b54c2030d9 | 171,574 | ipynb | Jupyter Notebook | notebooks/notebooks4ML/DecisionTreeClassifier_RRLyraeExample.ipynb | Astrohackers-TW/IANCUPythonMeetup | 6d7c417c4895b7c8dffc5c4fc7594799e023c4f2 | [
"MIT"
] | null | null | null | notebooks/notebooks4ML/DecisionTreeClassifier_RRLyraeExample.ipynb | Astrohackers-TW/IANCUPythonMeetup | 6d7c417c4895b7c8dffc5c4fc7594799e023c4f2 | [
"MIT"
] | null | null | null | notebooks/notebooks4ML/DecisionTreeClassifier_RRLyraeExample.ipynb | Astrohackers-TW/IANCUPythonMeetup | 6d7c417c4895b7c8dffc5c4fc7594799e023c4f2 | [
"MIT"
] | null | null | null | 187.30786 | 133,207 | 0.855642 | [
[
[
"## 決策樹學習 - 分類樹 (以RR Lyrae變星資料集為例)\n* [程式碼來源](http://www.astroml.org/book_figures/chapter9/fig_rrlyrae_decisiontree.html#book-fig-chapter9-fig-rrlyrae-decisiontree)",
"_____no_output_____"
]
],
[
[
"import numpy as np\nfrom matplotlib import pyplot as plt\nfrom sklearn.tree import DecisionTreeClassifier\nfrom astroML.datasets import fetch_rrlyrae_combined\nfrom astroML.utils import split_samples\nfrom astroML.utils import completeness_contamination\n\n\n#fetch_rrlyrae_combined?\nX, y = fetch_rrlyrae_combined() # 合併RR Lyrae變星和標準星的colors資訊\nprint('Features (u-g, g-r, r-i, i-z colors): ')\nprint(X)\nprint('Labels (標準星-0; RR Lyrae變星-1): ')\nprint(y)",
"Features (u-g, g-r, r-i, i-z colors): \n[[ 1.25099945 0.39400005 0.13700008 0.06199932]\n [ 1.04800034 0.3390007 0.15199852 0.02300072]\n [ 1.00800133 0.34199905 0.12899971 0.20300102]\n ..., \n [ 1.04400063 0.21199989 0.03499985 0.00200081]\n [ 1.06499863 0.17200089 0.04199982 0.00300026]\n [ 1.12599945 0.06500053 -0.0170002 -0.05799866]]\nLabels (標準星-0; RR Lyrae變星-1): \n[ 0. 0. 0. ..., 1. 1. 1.]\n"
],
[
"X = X[:, [1, 0, 2, 3]] # rearrange columns for better 1-color results\n(X_train, X_test), (y_train, y_test) = split_samples(X, y, [0.75, 0.25],\n random_state=0)\nN_tot = len(y)\nN_st = np.sum(y == 0)\nN_rr = N_tot - N_st\nN_train = len(y_train)\nN_test = len(y_test)\nN_plot = 5000 + N_rr",
"_____no_output_____"
],
[
"%matplotlib notebook\n# plot the results\nfig = plt.figure(figsize=(5, 2.5))\nfig.subplots_adjust(bottom=0.15, top=0.95, hspace=0.0,\n left=0.1, right=0.95, wspace=0.2)\n\n# left plot: data and decision boundary\nax = fig.add_subplot(121)\nim = ax.scatter(X[-N_plot:, 1], X[-N_plot:, 0], c=y[-N_plot:],\n s=4, lw=0, cmap=plt.cm.binary, zorder=2)\nim.set_clim(-0.5, 1)\n\n#ax.contour(xx, yy, Z, [0.5], colors='k')\n\n# ax.set_xlim(xlim)\n# ax.set_ylim(ylim)\n\nax.set_xlabel('$u-g$')\nax.set_ylabel('$g-r$')\nplt.show()\n# ax.text(0.02, 0.02, \"depth = %i\" % depths[1],\n# transform=ax.transAxes)",
"_____no_output_____"
]
]
] | [
"markdown",
"code"
] | [
[
"markdown"
],
[
"code",
"code",
"code"
]
] |
e7823d03801b4b969f51c35b3d467be9afa7907a | 48,207 | ipynb | Jupyter Notebook | benchmarks/2_covariates/generate_data_2_covariates.ipynb | bio-datascience/tascCODA_reproducibility | 323c9daab1e08733184431a72bde4e27a4d42208 | [
"BSD-3-Clause"
] | 1 | 2021-12-02T18:40:31.000Z | 2021-12-02T18:40:31.000Z | benchmarks/2_covariates/generate_data_2_covariates.ipynb | mingzehuang/tascCODA_reproducibility | 323c9daab1e08733184431a72bde4e27a4d42208 | [
"BSD-3-Clause"
] | null | null | null | benchmarks/2_covariates/generate_data_2_covariates.ipynb | mingzehuang/tascCODA_reproducibility | 323c9daab1e08733184431a72bde4e27a4d42208 | [
"BSD-3-Clause"
] | 1 | 2021-12-07T20:15:57.000Z | 2021-12-07T20:15:57.000Z | 170.342756 | 26,512 | 0.580227 | [
[
[
"# Generating benchmark data with 2 covariates\n\n## p=30",
"_____no_output_____"
]
],
[
[
"import pandas as pd\nimport toytree as tt\nimport numpy as np\nimport anndata as ad\nimport os\nimport toyplot as tp\nimport toyplot.svg\nimport seaborn as sns\n\nimport benchmarks.scripts.tree_data_generation as tgen",
"_____no_output_____"
],
[
"# tree depth\nd = 5\n\neffect_sizes = [0.3, 0.5, 0.7, 0.9]\n# number of effects\nnum_effects = 3\n# baseline parameter scale\na_abs = 2\n\n# sampling depth\nN = 10000\n# dispersion\ntheta = 499\n# samples per group\nnum_samples = [10]\nreps = 10\n\n\n# counter through all datasets\nid = 0\ndataset_path = os.path.abspath(\"../../../tascCODA_data/benchmarks/2_covariates/datasets/\")\nprint(dataset_path)",
"/Users/johannes.ostner/Documents/PhD/tascCODA/tascCODA_data/benchmarks/2_covariates/datasets\n"
],
[
"# Want everything to be reproducible - set a seed at every block\nnp.random.seed(96)\np = 30\nid = 0\n\nnewick = tgen.generate_tree_levels(p, d)\n\ntree = tt.tree(newick)\ntree.draw(tip_labels_align=True, node_sizes=10, node_labels='idx')",
"_____no_output_____"
],
[
"np.random.seed(76)\neffect_nodes, effect_leaves = tgen.get_effect_nodes(\n newick,\n num_effects=num_effects,\n num_leaves=p\n)\n\nprint(f\"nodes: {effect_nodes}\")\nprint(f\"leaves: {effect_leaves}\")\n\ntlc = [\"red\" if int(i) in effect_leaves else \"blue\" if int(i)==p-1 else \"black\" for i in tree.get_node_values(\"idx\", 1, 1)[-p:]]\ntlc.reverse()\nref_nodes = [p.idx for p in tree.idx_dict[p-1].get_ancestors()][:-1]\nref_nodes.append(p-1)\n\ncanvas = tp.Canvas(width=800, height=1600)\nax0 = canvas.cartesian(bounds=(0, 700, 0, 1600), padding=0)\ntree.draw(\n # tip_labels=False,\n node_sizes=[20 for i in tree.get_node_values(\"name\", 1, 1)],\n node_labels=[x for x in tree.get_node_values(\"idx\", 1, 1)],\n node_colors=[\"lightcoral\" if i in effect_nodes else \"lightblue\" if i in ref_nodes else \"lightgrey\" for i in tree.get_node_values(\"idx\", 1, 1)],\n node_labels_style={\"font-size\": 10},\n width=700,\n height=1600,\n node_style={\"stroke\": \"black\"},\n axes=ax0,\n tip_labels=\"name\",\n tip_labels_colors=tlc,\n)\n# tp.svg.render(canvas, \"./plots/benchmark_tree_30.svg\")",
"effect_nodes: [33, 7, 0]\neffect_leaves: [0, 7, 13, 14, 15, 16]\nnodes: [33, 7, 0]\nleaves: [0, 7, 13, 14, 15, 16]\n"
],
[
"id = 0\n\nx1_nodes = [39]\nx1_leaves = np.arange(13, 24, 1)\nbeta_1 = np.zeros(p)\nbeta_1[x1_leaves] = 3\n\nnp.random.seed(1234)\nfor e in effect_sizes:\n for n in num_samples:\n for r in range(reps):\n\n mu_0, mu_1 = tgen.generate_mu(\n a_abs=a_abs,\n num_leaves=p,\n effect_nodes=effect_nodes,\n effect_leaves=effect_leaves,\n effect_size=e,\n newick=newick\n )\n\n X = pd.DataFrame({\"x_0\": np.repeat([0,1], n), \"x_1\": np.random.uniform(0, 1, 2*n)})\n\n Y = np.zeros((n*2, p))\n for i in range(n):\n #Y[i, :] = np.sum(mu_0) * (mu_0 + beta_1*X.loc[i+n, \"x_1\"])/np.sum(mu_0 + beta_1*X.loc[i+n, \"x_1\"])\n #Y[i+n, :] = np.sum(mu_1) * (mu_1 + beta_1*X.loc[i+n, \"x_1\"])/np.sum(mu_1 + beta_1*X.loc[i+n, \"x_1\"])\n Y[i, :] = np.exp(np.log(mu_0) + beta_1*X.loc[i, \"x_1\"])\n Y[i+n, :] = np.exp(np.log(mu_1) + beta_1*X.loc[i+n, \"x_1\"])\n\n X = X.astype(np.float64)\n Y = Y.astype(np.float64)\n\n test_data = ad.AnnData(\n X=Y,\n obs=X,\n uns={\n \"tree_newick\": newick,\n \"effect_nodes\": effect_nodes,\n \"effect_leaves\": effect_leaves,\n \"effect_size\": e,\n \"num_samples\": n,\n }\n )\n\n # test_data.write_h5ad(dataset_path + f\"/data_{id}\")\n id += 1\n\n",
"/Users/johannes.ostner/opt/anaconda3/envs/scCODA_3/lib/python3.8/site-packages/anndata/_core/anndata.py:120: ImplicitModificationWarning: Transforming to str index.\n warnings.warn(\"Transforming to str index.\", ImplicitModificationWarning)\n"
]
]
] | [
"markdown",
"code"
] | [
[
"markdown"
],
[
"code",
"code",
"code",
"code",
"code"
]
] |
e78259df14f7d373060cfdd13ee1cb4268282184 | 43,178 | ipynb | Jupyter Notebook | docs_src/vision.gan.ipynb | navjotts/fastai | 0eb38bb5654ce2711d64fde1159d11808ea0c9c7 | [
"Apache-2.0"
] | 1 | 2018-12-14T17:35:30.000Z | 2018-12-14T17:35:30.000Z | docs_src/vision.gan.ipynb | navjotts/fastai | 0eb38bb5654ce2711d64fde1159d11808ea0c9c7 | [
"Apache-2.0"
] | 3 | 2021-05-20T19:59:09.000Z | 2022-02-26T09:11:29.000Z | docs_src/vision.gan.ipynb | navjotts/fastai | 0eb38bb5654ce2711d64fde1159d11808ea0c9c7 | [
"Apache-2.0"
] | null | null | null | 32.884996 | 637 | 0.581361 | [
[
[
"# GANs",
"_____no_output_____"
]
],
[
[
"%matplotlib inline\nfrom fastai.gen_doc.nbdoc import *\nfrom fastai import * \nfrom fastai.vision import * \nfrom fastai.vision.gan import *",
"_____no_output_____"
]
],
[
[
"GAN stands for [Generative Adversarial Nets](https://arxiv.org/pdf/1406.2661.pdf) and were invented by Ian Goodfellow. The concept is that we will train two models at the same time: a generator and a critic. The generator will try to make new images similar to the ones in our dataset, and the critic's job will try to classify real images from the fake ones the generator does. The generator returns images, the discriminator a feature map (it can be a single number depending on the input size). Usually the discriminator will be trained to retun 0. everywhere for fake images and 1. everywhere for real ones.\n\nThis module contains all the necessary function to create a GAN.",
"_____no_output_____"
],
[
"We train them against each other in the sense that at each step (more or less), we:\n1. Freeze the generator and train the discriminator for one step by:\n - getting one batch of true images (let's call that `real`)\n - generating one batch of fake images (let's call that `fake`)\n - have the discriminator evaluate each batch and compute a loss function from that; the important part is that it rewards positively the detection of real images and penalizes the fake ones\n - update the weights of the discriminator with the gradients of this loss\n \n \n2. Freeze the discriminator and train the generator for one step by:\n - generating one batch of fake images\n - evaluate the discriminator on it\n - return a loss that rewards posisitivly the discriminator thinking those are real images; the important part is that it rewards positively the detection of real images and penalizes the fake ones\n - update the weights of the generator with the gradients of this loss",
"_____no_output_____"
]
],
[
[
"show_doc(GANLearner)",
"_____no_output_____"
]
],
[
[
"This is the general constructor to create a GAN, you might want to use one of the factory methods that are easier to use. Create a GAN from [`data`](/vision.data.html#vision.data), a `generator` and a `critic`. The [`data`](/vision.data.html#vision.data) should have the inputs the `generator` will expect and the images wanted as targets.\n\n`gen_loss_func` is the loss function that will be applied to the `generator`. It takes three argument `fake_pred`, `target`, `output` and should return a rank 0 tensor. `output` is the result of the `generator` applied to the input (the xs of the batch), `target` is the ys of the batch and `fake_pred` is the result of the `discriminator` being given `output`. `output`and `target` can be used to add a specific loss to the GAN loss (pixel loss, feature loss) and for a good training of the gan, the loss should encourage `fake_pred` to be as close to 1 as possible (the `generator` is trained to fool the `critic`).\n\n`crit_loss_func` is the loss function that will be applied to the `critic`. It takes two arguments `real_pred` and `fake_pred`. `real_pred` is the result of the `critic` on the target images (the ys of the batch) and `fake_pred` is the result of the `critic` applied on a batch of fake, generated byt the `generator` from the xs of the batch.\n\n`switcher` is a [`Callback`](/callback.html#Callback) that should tell the GAN when to switch from critic to generator and vice versa. By default it does 5 iterations of the critic for 1 iteration of the generator. The model begins the training with the `generator` if `gen_first=True`. If `switch_eval=True`, the model that isn't trained is switched on eval mode (left in training mode otherwise, which means some statistics like the running mean in batchnorm layers are updated, or the dropouts are applied).\n\n`clip` should be set to a certain value if one wants to clip the weights (see the [Wassertein GAN](https://arxiv.org/pdf/1701.07875.pdf) for instance).\n\nIf `show_img=True`, one image generated by the GAN is shown at the end of each epoch.",
"_____no_output_____"
],
[
"### Factory methods",
"_____no_output_____"
]
],
[
[
"show_doc(GANLearner.from_learners)",
"_____no_output_____"
]
],
[
[
"Directly creates a [`GANLearner`](/vision.gan.html#GANLearner) from two [`Learner`](/basic_train.html#Learner): one for the `generator` and one for the `critic`. The `switcher` and all `kwargs` will be passed to the initialization of [`GANLearner`](/vision.gan.html#GANLearner) along with the following loss functions:\n\n- `loss_func_crit` is the mean of `learn_crit.loss_func` applied to `real_pred` and a target of ones with `learn_crit.loss_func` applied to `fake_pred` and a target of zeros\n- `loss_func_gen` is the mean of `learn_crit.loss_func` applied to `fake_pred` and a target of ones (to full the discriminator) with `learn_gen.loss_func` applied to `output` and `target`. The weights of each of those contributions can be passed in `weights_gen` (default is 1. and 1.)",
"_____no_output_____"
]
],
[
[
"show_doc(GANLearner.wgan)",
"_____no_output_____"
]
],
[
[
"The Wasserstein GAN is detailed in [this article]. `switcher` and the `kwargs` will be passed to the [`GANLearner`](/vision.gan.html#GANLearner) init, `clip`is the weight clipping.",
"_____no_output_____"
],
[
"## Switchers",
"_____no_output_____"
],
[
"In any GAN training, you will need to tell the [`Learner`](/basic_train.html#Learner) when to switch from generator to critic and vice versa. The two following [`Callback`](/callback.html#Callback) are examples to help you with that.\n\nAs usual, don't call the `on_something` methods directly, the fastai library will do it for you during training.",
"_____no_output_____"
]
],
[
[
"show_doc(FixedGANSwitcher, title_level=3)",
"_____no_output_____"
],
[
"show_doc(FixedGANSwitcher.on_train_begin)",
"_____no_output_____"
],
[
"show_doc(FixedGANSwitcher.on_batch_end)",
"_____no_output_____"
],
[
"show_doc(AdaptiveGANSwitcher, title_level=3)",
"_____no_output_____"
],
[
"show_doc(AdaptiveGANSwitcher.on_batch_end)",
"_____no_output_____"
]
],
[
[
"## Discriminative LR",
"_____no_output_____"
],
[
"If you want to train your critic at a different learning rate than the generator, this will let you do it automatically (even if you have a learning rate schedule).",
"_____no_output_____"
]
],
[
[
"show_doc(GANDiscriminativeLR, title_level=3)",
"_____no_output_____"
],
[
"show_doc(GANDiscriminativeLR.on_batch_begin)",
"_____no_output_____"
],
[
"show_doc(GANDiscriminativeLR.on_step_end)",
"_____no_output_____"
]
],
[
[
"## Specific models",
"_____no_output_____"
]
],
[
[
"show_doc(basic_critic)",
"_____no_output_____"
]
],
[
[
"This model contains a first 4 by 4 convolutional layer of stride 2 from `n_channels` to `n_features` followed by `n_extra_layers` 3 by 3 convolutional layer of stride 1. Then we put as many 4 by 4 convolutional layer of stride 2 with a number of features multiplied by 2 at each stage so that the `in_size` becomes 1. `kwargs` can be used to customize the convolutional layers and are passed to [`conv_layer`](/layers.html#conv_layer).",
"_____no_output_____"
]
],
[
[
"show_doc(basic_generator)",
"_____no_output_____"
]
],
[
[
"This model contains a first 4 by 4 transposed convolutional layer of stride 1 from `noise_size` to the last numbers of features of the corresponding critic. Then we put as many 4 by 4 transposed convolutional layer of stride 2 with a number of features divided by 2 at each stage so that the image ends up being of height and widht `in_size//2`. At the end, we add`n_extra_layers` 3 by 3 convolutional layer of stride 1. The last layer is a transpose convolution of size 4 by 4 and stride 2 followed by `tanh`. `kwargs` can be used to customize the convolutional layers and are passed to [`conv_layer`](/layers.html#conv_layer).",
"_____no_output_____"
]
],
[
[
"show_doc(gan_critic)",
"_____no_output_____"
],
[
"show_doc(GANTrainer)",
"_____no_output_____"
]
],
[
[
"[`LearnerCallback`](/basic_train.html#LearnerCallback) that will be responsible to handle the two different optimizers (one for the generator and one for the critic), and do all the work behind the scenes so that the generator (or the critic) are in training mode with parameters requirement gradients each time we switch.\n\n`switch_eval=True` means that the [`GANTrainer`](/vision.gan.html#GANTrainer) will put the model that isn't training into eval mode (if it's `False` its running statistics like in batchnorm layers will be updated and dropout will be applied). `clip` is the clipping applied to the weights (if not `None`). `beta` is the coefficient for the moving averages as the [`GANTrainer`](/vision.gan.html#GANTrainer)tracks separately the generator loss and the critic loss. `gen_first=True` means the training begins with the generator (with the critic if it's `False`). If `show_img=True` we show a generated image at the end of each epoch.",
"_____no_output_____"
]
],
[
[
"show_doc(GANTrainer.switch)",
"_____no_output_____"
]
],
[
[
"If `gen_mode` is left as `None`, just put the model in the other mode (critic if it was in generator mode and vice versa).",
"_____no_output_____"
]
],
[
[
"show_doc(GANTrainer.on_train_begin)",
"_____no_output_____"
],
[
"show_doc(GANTrainer.on_epoch_begin)",
"_____no_output_____"
],
[
"show_doc(GANTrainer.on_batch_begin)",
"_____no_output_____"
],
[
"show_doc(GANTrainer.on_backward_begin)",
"_____no_output_____"
],
[
"show_doc(GANTrainer.on_epoch_end)",
"_____no_output_____"
],
[
"show_doc(GANTrainer.on_train_end)",
"_____no_output_____"
]
],
[
[
"## Specific modules",
"_____no_output_____"
]
],
[
[
"show_doc(GANModule, title_level=3)",
"_____no_output_____"
]
],
[
[
"If `gen_mode` is left as `None`, just put the model in the other mode (critic if it was in generator mode and vice versa).",
"_____no_output_____"
]
],
[
[
"show_doc(GANModule.switch)",
"_____no_output_____"
],
[
"show_doc(GANLoss, title_level=3)",
"_____no_output_____"
],
[
"show_doc(AdaptiveLoss, title_level=3)",
"_____no_output_____"
],
[
"show_doc(accuracy_thresh_expand)",
"_____no_output_____"
]
],
[
[
"## Data Block API",
"_____no_output_____"
]
],
[
[
"show_doc(NoisyItem, title_level=3)",
"_____no_output_____"
],
[
"show_doc(GANItemList, title_level=3)",
"_____no_output_____"
]
],
[
[
"Inputs will be [`NoisyItem`](/vision.gan.html#NoisyItem) of `noise_sz` while the default class for target is [`ImageItemList`](/vision.data.html#ImageItemList).",
"_____no_output_____"
]
],
[
[
"show_doc(GANItemList.show_xys)",
"_____no_output_____"
],
[
"show_doc(GANItemList.show_xyzs)",
"_____no_output_____"
]
],
[
[
"## Undocumented Methods - Methods moved below this line will intentionally be hidden",
"_____no_output_____"
]
],
[
[
"show_doc(GANLoss.critic)",
"_____no_output_____"
],
[
"show_doc(GANModule.forward)",
"_____no_output_____"
],
[
"show_doc(GANLoss.generator)",
"_____no_output_____"
],
[
"show_doc(NoisyItem.apply_tfms)",
"_____no_output_____"
],
[
"show_doc(AdaptiveLoss.forward)",
"_____no_output_____"
],
[
"show_doc(GANItemList.get)",
"_____no_output_____"
],
[
"show_doc(GANItemList.reconstruct)",
"_____no_output_____"
],
[
"show_doc(AdaptiveLoss.forward)",
"_____no_output_____"
]
],
[
[
"## New Methods - Please document or move to the undocumented section",
"_____no_output_____"
]
]
] | [
"markdown",
"code",
"markdown",
"code",
"markdown",
"code",
"markdown",
"code",
"markdown",
"code",
"markdown",
"code",
"markdown",
"code",
"markdown",
"code",
"markdown",
"code",
"markdown",
"code",
"markdown",
"code",
"markdown",
"code",
"markdown",
"code",
"markdown",
"code",
"markdown",
"code",
"markdown",
"code",
"markdown"
] | [
[
"markdown"
],
[
"code"
],
[
"markdown",
"markdown"
],
[
"code"
],
[
"markdown",
"markdown"
],
[
"code"
],
[
"markdown"
],
[
"code"
],
[
"markdown",
"markdown",
"markdown"
],
[
"code",
"code",
"code",
"code",
"code"
],
[
"markdown",
"markdown"
],
[
"code",
"code",
"code"
],
[
"markdown"
],
[
"code"
],
[
"markdown"
],
[
"code"
],
[
"markdown"
],
[
"code",
"code"
],
[
"markdown"
],
[
"code"
],
[
"markdown"
],
[
"code",
"code",
"code",
"code",
"code",
"code"
],
[
"markdown"
],
[
"code"
],
[
"markdown"
],
[
"code",
"code",
"code",
"code"
],
[
"markdown"
],
[
"code",
"code"
],
[
"markdown"
],
[
"code",
"code"
],
[
"markdown"
],
[
"code",
"code",
"code",
"code",
"code",
"code",
"code",
"code"
],
[
"markdown"
]
] |
e7825c88919c545800ced23c62c362e4b789150d | 49,542 | ipynb | Jupyter Notebook | BeautifulSoup.ipynb | Pytoddler/Web-scraping | c9ce8b84c2dc30917fc9a3ddc84eb8a8d6c583a3 | [
"MIT"
] | null | null | null | BeautifulSoup.ipynb | Pytoddler/Web-scraping | c9ce8b84c2dc30917fc9a3ddc84eb8a8d6c583a3 | [
"MIT"
] | null | null | null | BeautifulSoup.ipynb | Pytoddler/Web-scraping | c9ce8b84c2dc30917fc9a3ddc84eb8a8d6c583a3 | [
"MIT"
] | null | null | null | 31.65623 | 220 | 0.495458 | [
[
[
"# 解析庫",
"_____no_output_____"
]
],
[
[
"BeautifulSoup(markup, \"html.parser\")\nBeautifulSoup(markup, \"lxml\")\nBeautifulSoup(markup, \"xml\")\nBeautifulSoup(markup, \"html5lib\")",
"_____no_output_____"
]
],
[
[
"# 基本使用",
"_____no_output_____"
]
],
[
[
"#引入requests好爬取html檔案給bs4使用\nimport requests\nresponse = requests.get('http://ntumail.cc.ntu.edu.tw')\nresponse.encoding = 'UTF-8' #加入encoding的方法避免中文亂碼\nhtml = response.text\n\nfrom bs4 import BeautifulSoup\nsoup = BeautifulSoup(html,'html.parser')\n\nprint(soup.prettify()) #會把html漂亮輸出\nprint(soup.title.string)",
"\n<!DOCTYPE html PUBLIC \"-//W3C//DTD XHTML 1.0 Transitional//EN\" \"http://www.w3.org/TR/xhtml1/DTD/xhtml1-transitional.dtd\">\n<html xmlns=\"http://www.w3.org/1999/xhtml\">\n <head>\n <meta content=\"text/html; charset=utf-8\" http-equiv=\"Content-Type\"/>\n <title>\n NTU Mail-臺灣大學電子郵件系統\n </title>\n <link href=\"images/style.css\" rel=\"stylesheet\" type=\"text/css\"/>\n </head>\n <body>\n <div id=\"top\">\n |\n <a href=\"http://www.ntu.edu.tw/\">\n 臺大首頁 NTU Home\n </a>\n |\n <a href=\"http://www.cc.ntu.edu.tw/\">\n 計中首頁\n </a>\n |\n </div>\n <div id=\"wrapper\">\n <div id=\"banner\">\n </div>\n <div id=\"mail\">\n <div id=\"imgcss\">\n <img src=\"images/mail20.png\"/>\n </div>\n <div id=\"content\">\n <h1>\n <a href=\"https://mail.ntu.edu.tw/\">\n NTU Mail 2.0\n </a>\n </h1>\n <ul>\n <li>\n <img align=\"absmiddle\" src=\"images/face01-01.gif\"/>\n 服務對象\n <ol>\n <li>\n 教職員帳號 \\ Faculty Account\n </li>\n <li>\n 公務、計畫、及短期帳號 \\ Project and Short Term Account\n </li>\n <li>\n 所有在學學生帳號 \\ Internal Student Account\n </li>\n </ol>\n </li>\n <li>\n <img align=\"absmiddle\" src=\"images/m02-05-2.gif\"/>\n 立即前往 Go to\n <a href=\"https://mail.ntu.edu.tw/\">\n Mail 2.0\n </a>\n </li>\n <li>\n <img align=\"absmiddle\" src=\"images/ic04-04.gif\"/>\n <a href=\"http://www.cc.ntu.edu.tw/mail2.0/\">\n Mail 2.0 FAQ\n </a>\n </li>\n </ul>\n </div>\n <!--content end-->\n </div>\n <!--mail end-->\n <div id=\"webmail\">\n <div id=\"imgcss\">\n <img src=\"images/webmail.png\"/>\n </div>\n <div id=\"content\">\n <h1>\n <a href=\"http://webmail.ntu.edu.tw/\">\n NTU Mail 1.0 (Webmail 1.0)\n </a>\n </h1>\n <ul>\n <li>\n <img align=\"absmiddle\" src=\"images/face01-01.gif\"/>\n 服務對象\n <ol>\n <li>\n 校友帳號 \\ Alumni Account\n </li>\n <li>\n 醫院員工帳號 \\ Hospital Staff Account\n </li>\n </ol>\n </li>\n <li>\n <img align=\"absmiddle\" src=\"images/m02-05-2.gif\"/>\n 立即前往 Go to\n <a href=\"http://webmail.ntu.edu.tw/\">\n Webmail 1.0\n </a>\n </li>\n <li>\n <img align=\"absmiddle\" src=\"images/ic04-04.gif\"/>\n <a href=\"http://jsc.cc.ntu.edu.tw/ntucc/email/\">\n Webmail FAQ\n </a>\n </li>\n </ul>\n </div>\n <!--content end-->\n </div>\n <!--webmail end-->\n </div>\n <!--wrapper end-->\n <div id=\"footer\">\n Copyright 臺灣大學 National Taiwan University\n <br/>\n 諮詢服務電話:(02)3366-5022或3366-5023\n <br/>\n 諮詢服務信箱:[email protected]\n </div>\n </body>\n</html>\n\nNTU Mail-臺灣大學電子郵件系統\n"
]
],
[
[
"# 標籤選擇器",
"_____no_output_____"
],
[
"## 選擇元素",
"_____no_output_____"
]
],
[
[
"#引入requests好爬取html檔案給bs4使用\nimport requests\nresponse = requests.get('http://ntumail.cc.ntu.edu.tw')\nresponse.encoding = 'UTF-8' #加入encoding的方法避免中文亂碼\nhtml = response.text\n\nfrom bs4 import BeautifulSoup\nsoup = BeautifulSoup(html,'lxml')\n\n#印出物包含外框標籤\nprint(soup.title) \nprint(type(soup.title)) #回傳一個tag\nprint(soup.head)\nprint(soup.a) #顯示第一個match的結果",
"<title>NTU Mail-臺灣大學電子郵件系統</title>\n<class 'bs4.element.Tag'>\n<head>\n<meta content=\"text/html; charset=utf-8\" http-equiv=\"Content-Type\"/>\n<title>NTU Mail-臺灣大學電子郵件系統</title>\n<link href=\"images/style.css\" rel=\"stylesheet\" type=\"text/css\"/>\n</head>\n<a href=\"http://www.ntu.edu.tw/\">臺大首頁 NTU Home</a>\n"
]
],
[
[
"## 獲取名稱、內容",
"_____no_output_____"
]
],
[
[
"#引入requests好爬取html檔案給bs4使用\nimport requests\nresponse = requests.get('http://ntumail.cc.ntu.edu.tw')\nresponse.encoding = 'UTF-8' #加入encoding的方法避免中文亂碼\nhtml = response.text\n\nfrom bs4 import BeautifulSoup\nsoup = BeautifulSoup(html,'lxml')\n\nprint(soup.title.name) #列印tag名稱\nprint(soup.title.string) #列印tag裡面的內容",
"title\nNTU Mail-臺灣大學電子郵件系統\n"
]
],
[
[
"## 獲取屬性",
"_____no_output_____"
]
],
[
[
"#引入requests好爬取html檔案給bs4使用\nimport requests\nresponse = requests.get('http://ntumail.cc.ntu.edu.tw')\nresponse.encoding = 'UTF-8' #加入encoding的方法避免中文亂碼\nhtml = response.text\n\nfrom bs4 import BeautifulSoup\nsoup = BeautifulSoup(html,'lxml')\n\n#列印attribute\nprint(soup.img.attrs['src'])\nprint(soup.img['src'])",
"images/mail20.png\nimages/mail20.png\n"
]
],
[
[
"## 嵌套選擇",
"_____no_output_____"
]
],
[
[
"#引入requests好爬取html檔案給bs4使用\nimport requests\nresponse = requests.get('http://ntumail.cc.ntu.edu.tw')\nresponse.encoding = 'UTF-8' #加入encoding的方法避免中文亂碼\nhtml = response.text\n\nfrom bs4 import BeautifulSoup\nsoup = BeautifulSoup(html,'lxml')\n\nprint(soup.head.title.string) #選擇head裡的title的文本",
"NTU Mail-臺灣大學電子郵件系統\n"
]
],
[
[
"## 子節點、子孫節點",
"_____no_output_____"
]
],
[
[
"#引入requests好爬取html檔案給bs4使用\nimport requests\nresponse = requests.get('http://ntumail.cc.ntu.edu.tw')\nresponse.encoding = 'UTF-8' #加入encoding的方法避免中文亂碼\nhtml = response.text\n\nfrom bs4 import BeautifulSoup\nsoup = BeautifulSoup(html,'lxml')\n\n#獲取所有子節點,返回list\nprint(soup.head.contents) #把head裡的文本按照行數讀取出來 ",
"['\\n', <meta content=\"text/html; charset=utf-8\" http-equiv=\"Content-Type\"/>, '\\n', <title>NTU Mail-臺灣大學電子郵件系統</title>, '\\n', <link href=\"images/style.css\" rel=\"stylesheet\" type=\"text/css\"/>, '\\n']\n"
],
[
"#引入requests好爬取html檔案給bs4使用\nimport requests\nresponse = requests.get('http://ntumail.cc.ntu.edu.tw')\nresponse.encoding = 'UTF-8' #加入encoding的方法避免中文亂碼\nhtml = response.text\n\nfrom bs4 import BeautifulSoup\nsoup = BeautifulSoup(html,'lxml')\n\n#獲取所有子節點,返回迭代器,不是list\nprint(soup.head.children)\n\n#i是索引,child是內容\nfor i, child in enumerate(soup.head.children):\n print(i, child) ",
"<list_iterator object at 0x00000290B7304BA8>\n0 \n\n1 <meta content=\"text/html; charset=utf-8\" http-equiv=\"Content-Type\"/>\n2 \n\n3 <title>NTU Mail-臺灣大學電子郵件系統</title>\n4 \n\n5 <link href=\"images/style.css\" rel=\"stylesheet\" type=\"text/css\"/>\n6 \n\n"
],
[
"#引入requests好爬取html檔案給bs4使用\nimport requests\nresponse = requests.get('http://ntumail.cc.ntu.edu.tw')\nresponse.encoding = 'UTF-8' #加入encoding的方法避免中文亂碼\nhtml = response.text\n\nfrom bs4 import BeautifulSoup\nsoup = BeautifulSoup(html,'lxml')\n\n#獲取所有子孫節點,返回迭代器,不是list\nprint(soup.head.descendants)\n\n#i是索引,child是內容\nfor i, child in enumerate(soup.head.descendants):\n print(i, child) ",
"<generator object descendants at 0x00000290B724A0A0>\n0 \n\n1 <meta content=\"text/html; charset=utf-8\" http-equiv=\"Content-Type\"/>\n2 \n\n3 <title>NTU Mail-臺灣大學電子郵件系統</title>\n4 NTU Mail-臺灣大學電子郵件系統\n5 \n\n6 <link href=\"images/style.css\" rel=\"stylesheet\" type=\"text/css\"/>\n7 \n\n"
]
],
[
[
"## 父節點、祖父節點",
"_____no_output_____"
]
],
[
[
"#引入requests好爬取html檔案給bs4使用\nimport requests\nresponse = requests.get('http://ntumail.cc.ntu.edu.tw')\nresponse.encoding = 'UTF-8' #加入encoding的方法避免中文亂碼\nhtml = response.text\n\nfrom bs4 import BeautifulSoup\nsoup = BeautifulSoup(html,'lxml')\n\n#獲取所有父節點\nprint(soup.img.parent)",
"<div id=\"imgcss\"><img src=\"images/mail20.png\"/></div>\n"
],
[
"#引入requests好爬取html檔案給bs4使用\nimport requests\nresponse = requests.get('http://ntumail.cc.ntu.edu.tw')\nresponse.encoding = 'UTF-8' #加入encoding的方法避免中文亂碼\nhtml = response.text\n\nfrom bs4 import BeautifulSoup\nsoup = BeautifulSoup(html,'lxml')\n\n#獲取所有祖先節點,要解析迭代器\nprint(list(enumerate(soup.img.parents)))",
"[(0, <div id=\"imgcss\"><img src=\"images/mail20.png\"/></div>), (1, <div id=\"mail\">\n<div id=\"imgcss\"><img src=\"images/mail20.png\"/></div>\n<div id=\"content\">\n<h1><a href=\"https://mail.ntu.edu.tw/\">NTU Mail 2.0</a></h1>\n<ul>\n<li><img align=\"absmiddle\" src=\"images/face01-01.gif\"/> 服務對象\r\n \t<ol>\n<li>教職員帳號 \\ Faculty Account</li>\n<li>公務、計畫、及短期帳號 \\ Project and Short Term Account</li>\n<li>所有在學學生帳號 \\ Internal Student Account</li>\n</ol>\n</li>\n<li><img align=\"absmiddle\" src=\"images/m02-05-2.gif\"/> 立即前往 Go to <a href=\"https://mail.ntu.edu.tw/\">Mail 2.0</a></li>\n<li><img align=\"absmiddle\" src=\"images/ic04-04.gif\"/> <a href=\"http://www.cc.ntu.edu.tw/mail2.0/\">Mail 2.0 FAQ</a></li>\n</ul>\n</div><!--content end-->\n</div>), (2, <div id=\"wrapper\">\n<div id=\"banner\"></div>\n<div id=\"mail\">\n<div id=\"imgcss\"><img src=\"images/mail20.png\"/></div>\n<div id=\"content\">\n<h1><a href=\"https://mail.ntu.edu.tw/\">NTU Mail 2.0</a></h1>\n<ul>\n<li><img align=\"absmiddle\" src=\"images/face01-01.gif\"/> 服務對象\r\n \t<ol>\n<li>教職員帳號 \\ Faculty Account</li>\n<li>公務、計畫、及短期帳號 \\ Project and Short Term Account</li>\n<li>所有在學學生帳號 \\ Internal Student Account</li>\n</ol>\n</li>\n<li><img align=\"absmiddle\" src=\"images/m02-05-2.gif\"/> 立即前往 Go to <a href=\"https://mail.ntu.edu.tw/\">Mail 2.0</a></li>\n<li><img align=\"absmiddle\" src=\"images/ic04-04.gif\"/> <a href=\"http://www.cc.ntu.edu.tw/mail2.0/\">Mail 2.0 FAQ</a></li>\n</ul>\n</div><!--content end-->\n</div><!--mail end-->\n<div id=\"webmail\">\n<div id=\"imgcss\"><img src=\"images/webmail.png\"/></div>\n<div id=\"content\">\n<h1><a href=\"http://webmail.ntu.edu.tw/\">NTU Mail 1.0 (Webmail 1.0)</a></h1>\n<ul>\n<li><img align=\"absmiddle\" src=\"images/face01-01.gif\"/> 服務對象\r\n \t<ol>\n<li>校友帳號 \\ Alumni Account</li>\n<li>醫院員工帳號 \\ Hospital Staff Account</li>\n</ol>\n</li>\n<li><img align=\"absmiddle\" src=\"images/m02-05-2.gif\"/> 立即前往 Go to <a href=\"http://webmail.ntu.edu.tw/\">Webmail 1.0</a></li>\n<li><img align=\"absmiddle\" src=\"images/ic04-04.gif\"/> <a href=\"http://jsc.cc.ntu.edu.tw/ntucc/email/\">Webmail FAQ</a></li>\n</ul>\n</div><!--content end-->\n</div><!--webmail end-->\n</div>), (3, <body>\n<div id=\"top\">| <a href=\"http://www.ntu.edu.tw/\">臺大首頁 NTU Home</a> | <a href=\"http://www.cc.ntu.edu.tw/\">計中首頁</a> |</div>\n<div id=\"wrapper\">\n<div id=\"banner\"></div>\n<div id=\"mail\">\n<div id=\"imgcss\"><img src=\"images/mail20.png\"/></div>\n<div id=\"content\">\n<h1><a href=\"https://mail.ntu.edu.tw/\">NTU Mail 2.0</a></h1>\n<ul>\n<li><img align=\"absmiddle\" src=\"images/face01-01.gif\"/> 服務對象\r\n \t<ol>\n<li>教職員帳號 \\ Faculty Account</li>\n<li>公務、計畫、及短期帳號 \\ Project and Short Term Account</li>\n<li>所有在學學生帳號 \\ Internal Student Account</li>\n</ol>\n</li>\n<li><img align=\"absmiddle\" src=\"images/m02-05-2.gif\"/> 立即前往 Go to <a href=\"https://mail.ntu.edu.tw/\">Mail 2.0</a></li>\n<li><img align=\"absmiddle\" src=\"images/ic04-04.gif\"/> <a href=\"http://www.cc.ntu.edu.tw/mail2.0/\">Mail 2.0 FAQ</a></li>\n</ul>\n</div><!--content end-->\n</div><!--mail end-->\n<div id=\"webmail\">\n<div id=\"imgcss\"><img src=\"images/webmail.png\"/></div>\n<div id=\"content\">\n<h1><a href=\"http://webmail.ntu.edu.tw/\">NTU Mail 1.0 (Webmail 1.0)</a></h1>\n<ul>\n<li><img align=\"absmiddle\" src=\"images/face01-01.gif\"/> 服務對象\r\n \t<ol>\n<li>校友帳號 \\ Alumni Account</li>\n<li>醫院員工帳號 \\ Hospital Staff Account</li>\n</ol>\n</li>\n<li><img align=\"absmiddle\" src=\"images/m02-05-2.gif\"/> 立即前往 Go to <a href=\"http://webmail.ntu.edu.tw/\">Webmail 1.0</a></li>\n<li><img align=\"absmiddle\" src=\"images/ic04-04.gif\"/> <a href=\"http://jsc.cc.ntu.edu.tw/ntucc/email/\">Webmail FAQ</a></li>\n</ul>\n</div><!--content end-->\n</div><!--webmail end-->\n</div><!--wrapper end-->\n<div id=\"footer\">Copyright 臺灣大學 National Taiwan University<br/>\r\n諮詢服務電話:(02)3366-5022或3366-5023<br/>\r\n諮詢服務信箱:[email protected]</div>\n</body>), (4, <html xmlns=\"http://www.w3.org/1999/xhtml\">\n<head>\n<meta content=\"text/html; charset=utf-8\" http-equiv=\"Content-Type\"/>\n<title>NTU Mail-臺灣大學電子郵件系統</title>\n<link href=\"images/style.css\" rel=\"stylesheet\" type=\"text/css\"/>\n</head>\n<body>\n<div id=\"top\">| <a href=\"http://www.ntu.edu.tw/\">臺大首頁 NTU Home</a> | <a href=\"http://www.cc.ntu.edu.tw/\">計中首頁</a> |</div>\n<div id=\"wrapper\">\n<div id=\"banner\"></div>\n<div id=\"mail\">\n<div id=\"imgcss\"><img src=\"images/mail20.png\"/></div>\n<div id=\"content\">\n<h1><a href=\"https://mail.ntu.edu.tw/\">NTU Mail 2.0</a></h1>\n<ul>\n<li><img align=\"absmiddle\" src=\"images/face01-01.gif\"/> 服務對象\r\n \t<ol>\n<li>教職員帳號 \\ Faculty Account</li>\n<li>公務、計畫、及短期帳號 \\ Project and Short Term Account</li>\n<li>所有在學學生帳號 \\ Internal Student Account</li>\n</ol>\n</li>\n<li><img align=\"absmiddle\" src=\"images/m02-05-2.gif\"/> 立即前往 Go to <a href=\"https://mail.ntu.edu.tw/\">Mail 2.0</a></li>\n<li><img align=\"absmiddle\" src=\"images/ic04-04.gif\"/> <a href=\"http://www.cc.ntu.edu.tw/mail2.0/\">Mail 2.0 FAQ</a></li>\n</ul>\n</div><!--content end-->\n</div><!--mail end-->\n<div id=\"webmail\">\n<div id=\"imgcss\"><img src=\"images/webmail.png\"/></div>\n<div id=\"content\">\n<h1><a href=\"http://webmail.ntu.edu.tw/\">NTU Mail 1.0 (Webmail 1.0)</a></h1>\n<ul>\n<li><img align=\"absmiddle\" src=\"images/face01-01.gif\"/> 服務對象\r\n \t<ol>\n<li>校友帳號 \\ Alumni Account</li>\n<li>醫院員工帳號 \\ Hospital Staff Account</li>\n</ol>\n</li>\n<li><img align=\"absmiddle\" src=\"images/m02-05-2.gif\"/> 立即前往 Go to <a href=\"http://webmail.ntu.edu.tw/\">Webmail 1.0</a></li>\n<li><img align=\"absmiddle\" src=\"images/ic04-04.gif\"/> <a href=\"http://jsc.cc.ntu.edu.tw/ntucc/email/\">Webmail FAQ</a></li>\n</ul>\n</div><!--content end-->\n</div><!--webmail end-->\n</div><!--wrapper end-->\n<div id=\"footer\">Copyright 臺灣大學 National Taiwan University<br/>\r\n諮詢服務電話:(02)3366-5022或3366-5023<br/>\r\n諮詢服務信箱:[email protected]</div>\n</body>\n</html>), (5, <!DOCTYPE html PUBLIC \"-//W3C//DTD XHTML 1.0 Transitional//EN\" \"http://www.w3.org/TR/xhtml1/DTD/xhtml1-transitional.dtd\">\n<html xmlns=\"http://www.w3.org/1999/xhtml\">\n<head>\n<meta content=\"text/html; charset=utf-8\" http-equiv=\"Content-Type\"/>\n<title>NTU Mail-臺灣大學電子郵件系統</title>\n<link href=\"images/style.css\" rel=\"stylesheet\" type=\"text/css\"/>\n</head>\n<body>\n<div id=\"top\">| <a href=\"http://www.ntu.edu.tw/\">臺大首頁 NTU Home</a> | <a href=\"http://www.cc.ntu.edu.tw/\">計中首頁</a> |</div>\n<div id=\"wrapper\">\n<div id=\"banner\"></div>\n<div id=\"mail\">\n<div id=\"imgcss\"><img src=\"images/mail20.png\"/></div>\n<div id=\"content\">\n<h1><a href=\"https://mail.ntu.edu.tw/\">NTU Mail 2.0</a></h1>\n<ul>\n<li><img align=\"absmiddle\" src=\"images/face01-01.gif\"/> 服務對象\r\n \t<ol>\n<li>教職員帳號 \\ Faculty Account</li>\n<li>公務、計畫、及短期帳號 \\ Project and Short Term Account</li>\n<li>所有在學學生帳號 \\ Internal Student Account</li>\n</ol>\n</li>\n<li><img align=\"absmiddle\" src=\"images/m02-05-2.gif\"/> 立即前往 Go to <a href=\"https://mail.ntu.edu.tw/\">Mail 2.0</a></li>\n<li><img align=\"absmiddle\" src=\"images/ic04-04.gif\"/> <a href=\"http://www.cc.ntu.edu.tw/mail2.0/\">Mail 2.0 FAQ</a></li>\n</ul>\n</div><!--content end-->\n</div><!--mail end-->\n<div id=\"webmail\">\n<div id=\"imgcss\"><img src=\"images/webmail.png\"/></div>\n<div id=\"content\">\n<h1><a href=\"http://webmail.ntu.edu.tw/\">NTU Mail 1.0 (Webmail 1.0)</a></h1>\n<ul>\n<li><img align=\"absmiddle\" src=\"images/face01-01.gif\"/> 服務對象\r\n \t<ol>\n<li>校友帳號 \\ Alumni Account</li>\n<li>醫院員工帳號 \\ Hospital Staff Account</li>\n</ol>\n</li>\n<li><img align=\"absmiddle\" src=\"images/m02-05-2.gif\"/> 立即前往 Go to <a href=\"http://webmail.ntu.edu.tw/\">Webmail 1.0</a></li>\n<li><img align=\"absmiddle\" src=\"images/ic04-04.gif\"/> <a href=\"http://jsc.cc.ntu.edu.tw/ntucc/email/\">Webmail FAQ</a></li>\n</ul>\n</div><!--content end-->\n</div><!--webmail end-->\n</div><!--wrapper end-->\n<div id=\"footer\">Copyright 臺灣大學 National Taiwan University<br/>\r\n諮詢服務電話:(02)3366-5022或3366-5023<br/>\r\n諮詢服務信箱:[email protected]</div>\n</body>\n</html>\n)]\n"
]
],
[
[
"## 兄弟節點",
"_____no_output_____"
]
],
[
[
"#引入requests好爬取html檔案給bs4使用\nimport requests\nresponse = requests.get('http://ntumail.cc.ntu.edu.tw')\nresponse.encoding = 'UTF-8' #加入encoding的方法避免中文亂碼\nhtml = response.text\n\nfrom bs4 import BeautifulSoup\nsoup = BeautifulSoup(html,'lxml')\n\n#獲取兄弟節點\nprint(list(enumerate(soup.div.next_siblings)))\nprint(list(enumerate(soup.div.previous_siblings)))",
"[(0, '\\n'), (1, <div id=\"wrapper\">\n<div id=\"banner\"></div>\n<div id=\"mail\">\n<div id=\"imgcss\"><img src=\"images/mail20.png\"/></div>\n<div id=\"content\">\n<h1><a href=\"https://mail.ntu.edu.tw/\">NTU Mail 2.0</a></h1>\n<ul>\n<li><img align=\"absmiddle\" src=\"images/face01-01.gif\"/> 服務對象\r\n \t<ol>\n<li>教職員帳號 \\ Faculty Account</li>\n<li>公務、計畫、及短期帳號 \\ Project and Short Term Account</li>\n<li>所有在學學生帳號 \\ Internal Student Account</li>\n</ol>\n</li>\n<li><img align=\"absmiddle\" src=\"images/m02-05-2.gif\"/> 立即前往 Go to <a href=\"https://mail.ntu.edu.tw/\">Mail 2.0</a></li>\n<li><img align=\"absmiddle\" src=\"images/ic04-04.gif\"/> <a href=\"http://www.cc.ntu.edu.tw/mail2.0/\">Mail 2.0 FAQ</a></li>\n</ul>\n</div><!--content end-->\n</div><!--mail end-->\n<div id=\"webmail\">\n<div id=\"imgcss\"><img src=\"images/webmail.png\"/></div>\n<div id=\"content\">\n<h1><a href=\"http://webmail.ntu.edu.tw/\">NTU Mail 1.0 (Webmail 1.0)</a></h1>\n<ul>\n<li><img align=\"absmiddle\" src=\"images/face01-01.gif\"/> 服務對象\r\n \t<ol>\n<li>校友帳號 \\ Alumni Account</li>\n<li>醫院員工帳號 \\ Hospital Staff Account</li>\n</ol>\n</li>\n<li><img align=\"absmiddle\" src=\"images/m02-05-2.gif\"/> 立即前往 Go to <a href=\"http://webmail.ntu.edu.tw/\">Webmail 1.0</a></li>\n<li><img align=\"absmiddle\" src=\"images/ic04-04.gif\"/> <a href=\"http://jsc.cc.ntu.edu.tw/ntucc/email/\">Webmail FAQ</a></li>\n</ul>\n</div><!--content end-->\n</div><!--webmail end-->\n</div>), (2, 'wrapper end'), (3, '\\n'), (4, <div id=\"footer\">Copyright 臺灣大學 National Taiwan University<br/>\r\n諮詢服務電話:(02)3366-5022或3366-5023<br/>\r\n諮詢服務信箱:[email protected]</div>), (5, '\\n')]\n[(0, '\\n')]\n"
]
],
[
[
"# 標準選擇器",
"_____no_output_____"
],
[
"### find_all(name, attrs, recursive, text, **kwargs),找全部元素",
"_____no_output_____"
],
[
"可以根據標籤名稱,屬性內容查找文檔",
"_____no_output_____"
],
[
"### name",
"_____no_output_____"
]
],
[
[
"import requests\nresponse = requests.get('http://www.pythonscraping.com/pages/page3.html')\nresponse.encoding = 'UTF-8' #加入encoding的方法避免中文亂碼\nhtml = response.text\n\nfrom bs4 import BeautifulSoup\nsoup = BeautifulSoup(html,'lxml')\n\nprint(soup.find_all('td'))\nprint(soup.find_all('td')[0])",
"[<td>\nVegetable Basket\n</td>, <td>\nThis vegetable basket is the perfect gift for your health conscious (or overweight) friends!\n<span class=\"excitingNote\">Now with super-colorful bell peppers!</span>\n</td>, <td>\n$15.00\n</td>, <td>\n<img src=\"../img/gifts/img1.jpg\"/>\n</td>, <td>\nRussian Nesting Dolls\n</td>, <td>\nHand-painted by trained monkeys, these exquisite dolls are priceless! And by \"priceless,\" we mean \"extremely expensive\"! <span class=\"excitingNote\">8 entire dolls per set! Octuple the presents!</span>\n</td>, <td>\n$10,000.52\n</td>, <td>\n<img src=\"../img/gifts/img2.jpg\"/>\n</td>, <td>\nFish Painting\n</td>, <td>\nIf something seems fishy about this painting, it's because it's a fish! <span class=\"excitingNote\">Also hand-painted by trained monkeys!</span>\n</td>, <td>\n$10,005.00\n</td>, <td>\n<img src=\"../img/gifts/img3.jpg\"/>\n</td>, <td>\nDead Parrot\n</td>, <td>\nThis is an ex-parrot! <span class=\"excitingNote\">Or maybe he's only resting?</span>\n</td>, <td>\n$0.50\n</td>, <td>\n<img src=\"../img/gifts/img4.jpg\"/>\n</td>, <td>\nMystery Box\n</td>, <td>\nIf you love suprises, this mystery box is for you! Do not place on light-colored surfaces. May cause oil staining. <span class=\"excitingNote\">Keep your friends guessing!</span>\n</td>, <td>\n$1.50\n</td>, <td>\n<img src=\"../img/gifts/img6.jpg\"/>\n</td>]\n<td>\nVegetable Basket\n</td>\n"
],
[
"import requests\nresponse = requests.get('http://www.pythonscraping.com/pages/page3.html')\nresponse.encoding = 'UTF-8' #加入encoding的方法避免中文亂碼\nhtml = response.text\n\nfrom bs4 import BeautifulSoup\nsoup = BeautifulSoup(html,'lxml')\n\n#從標籤td底下再次提取內容img\nfor td in soup.find_all('td'):\n print(td.find_all('img'))",
"[]\n[]\n[]\n[<img src=\"../img/gifts/img1.jpg\"/>]\n[]\n[]\n[]\n[<img src=\"../img/gifts/img2.jpg\"/>]\n[]\n[]\n[]\n[<img src=\"../img/gifts/img3.jpg\"/>]\n[]\n[]\n[]\n[<img src=\"../img/gifts/img4.jpg\"/>]\n[]\n[]\n[]\n[<img src=\"../img/gifts/img6.jpg\"/>]\n"
]
],
[
[
"### attrs",
"_____no_output_____"
]
],
[
[
"import requests\nresponse = requests.get('http://www.pythonscraping.com/pages/page3.html')\nresponse.encoding = 'UTF-8' #加入encoding的方法避免中文亂碼\nhtml = response.text\n\nfrom bs4 import BeautifulSoup\nsoup = BeautifulSoup(html,'lxml')\n\nprint(soup.find_all(attrs={'id':'gift1'}))\nprint(soup.find_all(attrs={'class':'gift'}))",
"[<tr class=\"gift\" id=\"gift1\"><td>\nVegetable Basket\n</td><td>\nThis vegetable basket is the perfect gift for your health conscious (or overweight) friends!\n<span class=\"excitingNote\">Now with super-colorful bell peppers!</span>\n</td><td>\n$15.00\n</td><td>\n<img src=\"../img/gifts/img1.jpg\"/>\n</td></tr>]\n[<tr class=\"gift\" id=\"gift1\"><td>\nVegetable Basket\n</td><td>\nThis vegetable basket is the perfect gift for your health conscious (or overweight) friends!\n<span class=\"excitingNote\">Now with super-colorful bell peppers!</span>\n</td><td>\n$15.00\n</td><td>\n<img src=\"../img/gifts/img1.jpg\"/>\n</td></tr>, <tr class=\"gift\" id=\"gift2\"><td>\nRussian Nesting Dolls\n</td><td>\nHand-painted by trained monkeys, these exquisite dolls are priceless! And by \"priceless,\" we mean \"extremely expensive\"! <span class=\"excitingNote\">8 entire dolls per set! Octuple the presents!</span>\n</td><td>\n$10,000.52\n</td><td>\n<img src=\"../img/gifts/img2.jpg\"/>\n</td></tr>, <tr class=\"gift\" id=\"gift3\"><td>\nFish Painting\n</td><td>\nIf something seems fishy about this painting, it's because it's a fish! <span class=\"excitingNote\">Also hand-painted by trained monkeys!</span>\n</td><td>\n$10,005.00\n</td><td>\n<img src=\"../img/gifts/img3.jpg\"/>\n</td></tr>, <tr class=\"gift\" id=\"gift4\"><td>\nDead Parrot\n</td><td>\nThis is an ex-parrot! <span class=\"excitingNote\">Or maybe he's only resting?</span>\n</td><td>\n$0.50\n</td><td>\n<img src=\"../img/gifts/img4.jpg\"/>\n</td></tr>, <tr class=\"gift\" id=\"gift5\"><td>\nMystery Box\n</td><td>\nIf you love suprises, this mystery box is for you! Do not place on light-colored surfaces. May cause oil staining. <span class=\"excitingNote\">Keep your friends guessing!</span>\n</td><td>\n$1.50\n</td><td>\n<img src=\"../img/gifts/img6.jpg\"/>\n</td></tr>]\n"
],
[
"import requests\nresponse = requests.get('http://www.pythonscraping.com/pages/page3.html')\nresponse.encoding = 'UTF-8' #加入encoding的方法避免中文亂碼\nhtml = response.text\n\nfrom bs4 import BeautifulSoup\nsoup = BeautifulSoup(html,'lxml')\n\n#特殊屬性可以直接使用\nprint(soup.find_all(id='gift1'))\nprint(soup.find_all(class_='gift'))",
"[<tr class=\"gift\" id=\"gift1\"><td>\nVegetable Basket\n</td><td>\nThis vegetable basket is the perfect gift for your health conscious (or overweight) friends!\n<span class=\"excitingNote\">Now with super-colorful bell peppers!</span>\n</td><td>\n$15.00\n</td><td>\n<img src=\"../img/gifts/img1.jpg\"/>\n</td></tr>]\n[<tr class=\"gift\" id=\"gift1\"><td>\nVegetable Basket\n</td><td>\nThis vegetable basket is the perfect gift for your health conscious (or overweight) friends!\n<span class=\"excitingNote\">Now with super-colorful bell peppers!</span>\n</td><td>\n$15.00\n</td><td>\n<img src=\"../img/gifts/img1.jpg\"/>\n</td></tr>, <tr class=\"gift\" id=\"gift2\"><td>\nRussian Nesting Dolls\n</td><td>\nHand-painted by trained monkeys, these exquisite dolls are priceless! And by \"priceless,\" we mean \"extremely expensive\"! <span class=\"excitingNote\">8 entire dolls per set! Octuple the presents!</span>\n</td><td>\n$10,000.52\n</td><td>\n<img src=\"../img/gifts/img2.jpg\"/>\n</td></tr>, <tr class=\"gift\" id=\"gift3\"><td>\nFish Painting\n</td><td>\nIf something seems fishy about this painting, it's because it's a fish! <span class=\"excitingNote\">Also hand-painted by trained monkeys!</span>\n</td><td>\n$10,005.00\n</td><td>\n<img src=\"../img/gifts/img3.jpg\"/>\n</td></tr>, <tr class=\"gift\" id=\"gift4\"><td>\nDead Parrot\n</td><td>\nThis is an ex-parrot! <span class=\"excitingNote\">Or maybe he's only resting?</span>\n</td><td>\n$0.50\n</td><td>\n<img src=\"../img/gifts/img4.jpg\"/>\n</td></tr>, <tr class=\"gift\" id=\"gift5\"><td>\nMystery Box\n</td><td>\nIf you love suprises, this mystery box is for you! Do not place on light-colored surfaces. May cause oil staining. <span class=\"excitingNote\">Keep your friends guessing!</span>\n</td><td>\n$1.50\n</td><td>\n<img src=\"../img/gifts/img6.jpg\"/>\n</td></tr>]\n"
]
],
[
[
"### text",
"_____no_output_____"
]
],
[
[
"import requests\nresponse = requests.get('http://www.pythonscraping.com/pages/page3.html')\nresponse.encoding = 'UTF-8' #加入encoding的方法避免中文亂碼\nhtml = response.text\n\nfrom bs4 import BeautifulSoup\nsoup = BeautifulSoup(html,'lxml')\n\nprint(soup.find_all(text='trained monkeys'))\n#不知道為什麼找不到",
"[]\n"
]
],
[
[
"### find(name, attrs, recursive, text, **kwargs),返回第一個元素",
"_____no_output_____"
]
],
[
[
"import requests\nresponse = requests.get('http://www.pythonscraping.com/pages/page3.html')\nresponse.encoding = 'UTF-8' #加入encoding的方法避免中文亂碼\nhtml = response.text\n\nfrom bs4 import BeautifulSoup\nsoup = BeautifulSoup(html,'lxml')\n\n#特殊屬性可以直接使用\nprint(soup.find(id='gift1'))\nprint(soup.find(class_='gift'))",
"<tr class=\"gift\" id=\"gift1\"><td>\nVegetable Basket\n</td><td>\nThis vegetable basket is the perfect gift for your health conscious (or overweight) friends!\n<span class=\"excitingNote\">Now with super-colorful bell peppers!</span>\n</td><td>\n$15.00\n</td><td>\n<img src=\"../img/gifts/img1.jpg\"/>\n</td></tr>\n<tr class=\"gift\" id=\"gift1\"><td>\nVegetable Basket\n</td><td>\nThis vegetable basket is the perfect gift for your health conscious (or overweight) friends!\n<span class=\"excitingNote\">Now with super-colorful bell peppers!</span>\n</td><td>\n$15.00\n</td><td>\n<img src=\"../img/gifts/img1.jpg\"/>\n</td></tr>\n"
]
],
[
[
"### find_parents()返回所有祖先節點, find_parent()返回父節點",
"_____no_output_____"
],
[
"### find_next_siblings(), find_next_sibling()",
"_____no_output_____"
],
[
"### find_previous_siblings(), find_previous_sibling()",
"_____no_output_____"
],
[
"### find_all_next(), find_next()",
"_____no_output_____"
],
[
"### find_all_previous(), find_previous()",
"_____no_output_____"
],
[
"# CSS選擇器",
"_____no_output_____"
],
[
"select()可以直接傳入CSS選擇器即可完成",
"_____no_output_____"
]
],
[
[
"import requests\nresponse = requests.get('http://www.pythonscraping.com/pages/page3.html')\nresponse.encoding = 'UTF-8' #加入encoding的方法避免中文亂碼\nhtml = response.text\n\nfrom bs4 import BeautifulSoup\nsoup = BeautifulSoup(html,'lxml')\n\nprint(soup.select('.gift')) #class前面加.\nprint(soup.select('#gift1'))#id前面加#\nprint(soup.select('tr td')) #印出tr中所有td的項目",
"[<tr class=\"gift\" id=\"gift1\"><td>\nVegetable Basket\n</td><td>\nThis vegetable basket is the perfect gift for your health conscious (or overweight) friends!\n<span class=\"excitingNote\">Now with super-colorful bell peppers!</span>\n</td><td>\n$15.00\n</td><td>\n<img src=\"../img/gifts/img1.jpg\"/>\n</td></tr>, <tr class=\"gift\" id=\"gift2\"><td>\nRussian Nesting Dolls\n</td><td>\nHand-painted by trained monkeys, these exquisite dolls are priceless! And by \"priceless,\" we mean \"extremely expensive\"! <span class=\"excitingNote\">8 entire dolls per set! Octuple the presents!</span>\n</td><td>\n$10,000.52\n</td><td>\n<img src=\"../img/gifts/img2.jpg\"/>\n</td></tr>, <tr class=\"gift\" id=\"gift3\"><td>\nFish Painting\n</td><td>\nIf something seems fishy about this painting, it's because it's a fish! <span class=\"excitingNote\">Also hand-painted by trained monkeys!</span>\n</td><td>\n$10,005.00\n</td><td>\n<img src=\"../img/gifts/img3.jpg\"/>\n</td></tr>, <tr class=\"gift\" id=\"gift4\"><td>\nDead Parrot\n</td><td>\nThis is an ex-parrot! <span class=\"excitingNote\">Or maybe he's only resting?</span>\n</td><td>\n$0.50\n</td><td>\n<img src=\"../img/gifts/img4.jpg\"/>\n</td></tr>, <tr class=\"gift\" id=\"gift5\"><td>\nMystery Box\n</td><td>\nIf you love suprises, this mystery box is for you! Do not place on light-colored surfaces. May cause oil staining. <span class=\"excitingNote\">Keep your friends guessing!</span>\n</td><td>\n$1.50\n</td><td>\n<img src=\"../img/gifts/img6.jpg\"/>\n</td></tr>]\n[<tr class=\"gift\" id=\"gift1\"><td>\nVegetable Basket\n</td><td>\nThis vegetable basket is the perfect gift for your health conscious (or overweight) friends!\n<span class=\"excitingNote\">Now with super-colorful bell peppers!</span>\n</td><td>\n$15.00\n</td><td>\n<img src=\"../img/gifts/img1.jpg\"/>\n</td></tr>]\n[<td>\nVegetable Basket\n</td>, <td>\nThis vegetable basket is the perfect gift for your health conscious (or overweight) friends!\n<span class=\"excitingNote\">Now with super-colorful bell peppers!</span>\n</td>, <td>\n$15.00\n</td>, <td>\n<img src=\"../img/gifts/img1.jpg\"/>\n</td>, <td>\nRussian Nesting Dolls\n</td>, <td>\nHand-painted by trained monkeys, these exquisite dolls are priceless! And by \"priceless,\" we mean \"extremely expensive\"! <span class=\"excitingNote\">8 entire dolls per set! Octuple the presents!</span>\n</td>, <td>\n$10,000.52\n</td>, <td>\n<img src=\"../img/gifts/img2.jpg\"/>\n</td>, <td>\nFish Painting\n</td>, <td>\nIf something seems fishy about this painting, it's because it's a fish! <span class=\"excitingNote\">Also hand-painted by trained monkeys!</span>\n</td>, <td>\n$10,005.00\n</td>, <td>\n<img src=\"../img/gifts/img3.jpg\"/>\n</td>, <td>\nDead Parrot\n</td>, <td>\nThis is an ex-parrot! <span class=\"excitingNote\">Or maybe he's only resting?</span>\n</td>, <td>\n$0.50\n</td>, <td>\n<img src=\"../img/gifts/img4.jpg\"/>\n</td>, <td>\nMystery Box\n</td>, <td>\nIf you love suprises, this mystery box is for you! Do not place on light-colored surfaces. May cause oil staining. <span class=\"excitingNote\">Keep your friends guessing!</span>\n</td>, <td>\n$1.50\n</td>, <td>\n<img src=\"../img/gifts/img6.jpg\"/>\n</td>]\n"
],
[
"import requests\nresponse = requests.get('http://www.pythonscraping.com/pages/page3.html')\nresponse.encoding = 'UTF-8' #加入encoding的方法避免中文亂碼\nhtml = response.text\n\nfrom bs4 import BeautifulSoup\nsoup = BeautifulSoup(html,'lxml')\n\nfor tr in soup.select('tr'):\n print(tr.select('td')) #印出每個tr中的td",
"[]\n[<td>\nVegetable Basket\n</td>, <td>\nThis vegetable basket is the perfect gift for your health conscious (or overweight) friends!\n<span class=\"excitingNote\">Now with super-colorful bell peppers!</span>\n</td>, <td>\n$15.00\n</td>, <td>\n<img src=\"../img/gifts/img1.jpg\"/>\n</td>]\n[<td>\nRussian Nesting Dolls\n</td>, <td>\nHand-painted by trained monkeys, these exquisite dolls are priceless! And by \"priceless,\" we mean \"extremely expensive\"! <span class=\"excitingNote\">8 entire dolls per set! Octuple the presents!</span>\n</td>, <td>\n$10,000.52\n</td>, <td>\n<img src=\"../img/gifts/img2.jpg\"/>\n</td>]\n[<td>\nFish Painting\n</td>, <td>\nIf something seems fishy about this painting, it's because it's a fish! <span class=\"excitingNote\">Also hand-painted by trained monkeys!</span>\n</td>, <td>\n$10,005.00\n</td>, <td>\n<img src=\"../img/gifts/img3.jpg\"/>\n</td>]\n[<td>\nDead Parrot\n</td>, <td>\nThis is an ex-parrot! <span class=\"excitingNote\">Or maybe he's only resting?</span>\n</td>, <td>\n$0.50\n</td>, <td>\n<img src=\"../img/gifts/img4.jpg\"/>\n</td>]\n[<td>\nMystery Box\n</td>, <td>\nIf you love suprises, this mystery box is for you! Do not place on light-colored surfaces. May cause oil staining. <span class=\"excitingNote\">Keep your friends guessing!</span>\n</td>, <td>\n$1.50\n</td>, <td>\n<img src=\"../img/gifts/img6.jpg\"/>\n</td>]\n"
]
],
[
[
"### 獲取屬性",
"_____no_output_____"
]
],
[
[
"import requests\nresponse = requests.get('http://ntumail.cc.ntu.edu.tw')\nresponse.encoding = 'UTF-8' #加入encoding的方法避免中文亂碼\nhtml = response.text\n\nfrom bs4 import BeautifulSoup\nsoup = BeautifulSoup(html,'lxml')\nfor div in soup.select('div'):\n print(div['id'])\n print(div.attrs['id'])",
"top\ntop\nwrapper\nwrapper\nbanner\nbanner\nmail\nmail\nimgcss\nimgcss\ncontent\ncontent\nwebmail\nwebmail\nimgcss\nimgcss\ncontent\ncontent\nfooter\nfooter\n"
]
],
[
[
"### 獲取文本內容",
"_____no_output_____"
]
],
[
[
"import requests\nresponse = requests.get('http://ntumail.cc.ntu.edu.tw')\nresponse.encoding = 'UTF-8' #加入encoding的方法避免中文亂碼\nhtml = response.text\n\nfrom bs4 import BeautifulSoup\nsoup = BeautifulSoup(html,'lxml')\n\nfor li in soup.select('li'):\n print(li.get_text())",
" 服務對象\r\n \t\n教職員帳號 \\ Faculty Account\n公務、計畫、及短期帳號 \\ Project and Short Term Account\n所有在學學生帳號 \\ Internal Student Account\n\n\n教職員帳號 \\ Faculty Account\n公務、計畫、及短期帳號 \\ Project and Short Term Account\n所有在學學生帳號 \\ Internal Student Account\n 立即前往 Go to Mail 2.0\n Mail 2.0 FAQ\n 服務對象\r\n \t\n校友帳號 \\ Alumni Account\n醫院員工帳號 \\ Hospital Staff Account\n\n\n校友帳號 \\ Alumni Account\n醫院員工帳號 \\ Hospital Staff Account\n 立即前往 Go to Webmail 1.0\n Webmail FAQ\n"
]
],
[
[
"# 總結",
"_____no_output_____"
]
],
[
[
"推薦使用lxml解析庫,必要時使用html.parser\n標籤選擇篩選功能弱,但是速度快\n建議使用find(), find_all() 查詢匹配單個結果或多個結果\n如果對CSS選擇器熟悉則用select()\n記住常用的獲取attrs和text方法",
"_____no_output_____"
]
]
] | [
"markdown",
"raw",
"markdown",
"code",
"markdown",
"code",
"markdown",
"code",
"markdown",
"code",
"markdown",
"code",
"markdown",
"code",
"markdown",
"code",
"markdown",
"code",
"markdown",
"code",
"markdown",
"code",
"markdown",
"code",
"markdown",
"code",
"markdown",
"code",
"markdown",
"code",
"markdown",
"code",
"markdown",
"raw"
] | [
[
"markdown"
],
[
"raw"
],
[
"markdown"
],
[
"code"
],
[
"markdown",
"markdown"
],
[
"code"
],
[
"markdown"
],
[
"code"
],
[
"markdown"
],
[
"code"
],
[
"markdown"
],
[
"code"
],
[
"markdown"
],
[
"code",
"code",
"code"
],
[
"markdown"
],
[
"code",
"code"
],
[
"markdown"
],
[
"code"
],
[
"markdown",
"markdown",
"markdown",
"markdown"
],
[
"code",
"code"
],
[
"markdown"
],
[
"code",
"code"
],
[
"markdown"
],
[
"code"
],
[
"markdown"
],
[
"code"
],
[
"markdown",
"markdown",
"markdown",
"markdown",
"markdown",
"markdown",
"markdown"
],
[
"code",
"code"
],
[
"markdown"
],
[
"code"
],
[
"markdown"
],
[
"code"
],
[
"markdown"
],
[
"raw"
]
] |
e782601d5774e144da221184638589aea0b56e45 | 34,881 | ipynb | Jupyter Notebook | basicModel/.ipynb_checkpoints/Logistic Regression-checkpoint.ipynb | jamesniro/Prediction-Solar-Wind-with-Machine-Learning | d93ed32cb5c14791fe7979639e7bbddc0c1f372d | [
"MIT"
] | 1 | 2020-09-28T21:33:22.000Z | 2020-09-28T21:33:22.000Z | basicModel/.ipynb_checkpoints/Logistic Regression-checkpoint.ipynb | jamesniro/Prediction-Solar-Wind-with-Machine-Learning | d93ed32cb5c14791fe7979639e7bbddc0c1f372d | [
"MIT"
] | null | null | null | basicModel/.ipynb_checkpoints/Logistic Regression-checkpoint.ipynb | jamesniro/Prediction-Solar-Wind-with-Machine-Learning | d93ed32cb5c14791fe7979639e7bbddc0c1f372d | [
"MIT"
] | null | null | null | 124.131673 | 21,624 | 0.826295 | [
[
[
"#importing packages which we are using for this project\nimport pandas as pd\nimport numpy as np\nfrom sklearn.model_selection import train_test_split\nimport matplotlib.pyplot as plt\nfrom scipy import stats\nfrom sklearn.linear_model import LogisticRegression\nfrom sklearn import preprocessing\nfrom sklearn import utils",
"_____no_output_____"
],
[
"# reading data from CSV\ndata = pd.read_csv(\"../FinalArtemisData.csv\")",
"_____no_output_____"
],
[
"del data['Unnamed: 0']\ndel data['Time_offset_hours']\ndel data['EPOCH_TIME_yyyy-mm-ddThh:mm:ss.sssZ']\ndel data['EPOCH_TIME__yyyy-mm-ddThh:mm:ss.sssZ']\ndel data['new_time']\ndel data['ArtemisIonSpeedKM_S']\ndel data['ArtemisDistanceAU']\ndel data['ArtemisLatDeg']\ndel data['ArtemisLonDeg']",
"_____no_output_____"
],
[
"data.columns = ['Omni latitude', 'Omni longitude', \"Omni speed\", 'Omni Ion Density', 'Artemis Ion Densitity']",
"_____no_output_____"
],
[
"y = np.asarray(data['Artemis Ion Densitity'])\nX = np.asarray(data[['Omni speed', 'Omni Ion Density']])\ny = np.uint32(y)",
"_____no_output_____"
],
[
"X_train,X_test,y_train,y_test=train_test_split(X,y,test_size=0.25,random_state=0)\n",
"_____no_output_____"
],
[
"from sklearn.linear_model import LogisticRegression\n\n# instantiate the model (using the default parameters)\nlogreg = LogisticRegression()\n\nlogreg.fit(X_train,y_train)\n\n#\ny_pred=logreg.predict(X_test)",
"/opt/anaconda3/lib/python3.7/site-packages/sklearn/linear_model/_logistic.py:940: ConvergenceWarning: lbfgs failed to converge (status=1):\nSTOP: TOTAL NO. of ITERATIONS REACHED LIMIT.\n\nIncrease the number of iterations (max_iter) or scale the data as shown in:\n https://scikit-learn.org/stable/modules/preprocessing.html\nPlease also refer to the documentation for alternative solver options:\n https://scikit-learn.org/stable/modules/linear_model.html#logistic-regression\n extra_warning_msg=_LOGISTIC_SOLVER_CONVERGENCE_MSG)\n"
],
[
"from sklearn import metrics\ncnf_matrix = metrics.confusion_matrix(y_test, y_pred)\ncnf_matrix",
"_____no_output_____"
],
[
"import numpy as np\nimport matplotlib.pyplot as plt\nimport seaborn as sns\n%matplotlib inline",
"_____no_output_____"
],
[
"class_names=[0,1] # name of classes\nfig, ax = plt.subplots()\ntick_marks = np.arange(len(class_names))\nplt.xticks(tick_marks, class_names)\nplt.yticks(tick_marks, class_names)\n# create heatmap\nsns.heatmap(pd.DataFrame(cnf_matrix), annot=True, cmap=\"YlGnBu\" ,fmt='g')\nax.xaxis.set_label_position(\"top\")\nplt.tight_layout()\nplt.title('Confusion matrix', y=1.1)\nplt.ylabel('Actual label')\nplt.xlabel('Predicted label')",
"_____no_output_____"
],
[
"print(\"Accuracy:\",metrics.accuracy_score(y_test, y_pred, ))\nprint(\"Precision:\",metrics.precision_score(y_test, y_pred))\nprint(\"Recall:\",metrics.recall_score(y_test, y_pred))",
"_____no_output_____"
]
]
] | [
"code"
] | [
[
"code",
"code",
"code",
"code",
"code",
"code",
"code",
"code",
"code",
"code",
"code"
]
] |
e7826eaaffd9e916d0f343948606878be0567cd7 | 59,830 | ipynb | Jupyter Notebook | toggl/toggl_downloader.ipynb | Zackhardtoname/qs_ledger | 77d15079e90be40429b99be8abaa5a51423585d8 | [
"MIT"
] | 755 | 2018-06-17T08:28:38.000Z | 2022-03-27T05:37:02.000Z | toggl/toggl_downloader.ipynb | Zackhardtoname/qs_ledger | 77d15079e90be40429b99be8abaa5a51423585d8 | [
"MIT"
] | 17 | 2019-03-31T08:26:09.000Z | 2022-03-31T05:33:22.000Z | toggl/toggl_downloader.ipynb | Zackhardtoname/qs_ledger | 77d15079e90be40429b99be8abaa5a51423585d8 | [
"MIT"
] | 195 | 2018-08-30T11:41:28.000Z | 2022-03-31T11:35:20.000Z | 28.572111 | 179 | 0.437523 | [
[
[
"# Toggl Reports Downloader",
"_____no_output_____"
],
[
"Script to Extract from Toggl API and create CSV Export of **Latest and Complete Timelogs** as as well as separate exports of Clients, Projects, Workspace Lists. \n\nUseful for back up purposes or additional data analysis. ",
"_____no_output_____"
],
[
"----",
"_____no_output_____"
],
[
"### Add Dependencies",
"_____no_output_____"
]
],
[
[
"import pandas as pd\nfrom datetime import datetime\nfrom dateutil.parser import parse\nimport time\nimport pytz",
"_____no_output_____"
],
[
"# Toggl Wrapper API \n# https://github.com/matthewdowney/TogglPy\nimport TogglPy",
"_____no_output_____"
]
],
[
[
"----",
"_____no_output_____"
],
[
"## Authentication",
"_____no_output_____"
]
],
[
[
"import json\n\nwith open(\"credentials.json\", \"r\") as file:\n credentials = json.load(file)\n toggl_cr = credentials['toggl']\n APIKEY = toggl_cr['APIKEY']",
"_____no_output_____"
],
[
"toggl = TogglPy.Toggl()\ntoggl.setAPIKey(APIKEY) ",
"_____no_output_____"
]
],
[
[
"-----",
"_____no_output_____"
],
[
"## User Data",
"_____no_output_____"
]
],
[
[
"user = toggl.request(\"https://www.toggl.com/api/v8/me\")",
"_____no_output_____"
],
[
"user_id = user['data']['id']",
"_____no_output_____"
],
[
"user['data']['fullname']",
"_____no_output_____"
],
[
"join_date = parse(user['data']['created_at'])\njoin_date",
"_____no_output_____"
],
[
"# today = datetime.now()\ndef utcnow():\n return datetime.now(tz=pytz.utc)\ntoday = utcnow()\ndates = list(pd.date_range(join_date, today))\nprint(\"Days Since Joining: \" + str(len(dates))) # days since joining",
"Days Since Joining: 2058\n"
]
],
[
[
"-----",
"_____no_output_____"
],
[
"## Clients",
"_____no_output_____"
]
],
[
[
"user_clients = toggl.request(\"https://www.toggl.com/api/v8/clients\")",
"_____no_output_____"
],
[
"clients = pd.DataFrame()\nfor i in list(range(0, len(user_clients))):\n clients_df_temp = pd.DataFrame.from_dict(user_clients)\n clients = pd.concat([clients_df_temp, clients])",
"_____no_output_____"
],
[
"clients.to_csv('data/toggl-clients.csv')",
"_____no_output_____"
]
],
[
[
"-----",
"_____no_output_____"
],
[
"## Workplaces",
"_____no_output_____"
],
[
"API Ref: https://github.com/toggl/toggl_api_docs/blob/master/chapters/workspaces.md#get-workspaces",
"_____no_output_____"
]
],
[
[
"workspaces_list = toggl.request(\"https://www.toggl.com/api/v8/workspaces\")",
"_____no_output_____"
],
[
"len(workspaces_list)",
"_____no_output_____"
],
[
"workspaces = pd.DataFrame.from_dict(workspaces_list)",
"_____no_output_____"
],
[
"workspaces_dict = dict(zip(workspaces.id, workspaces.name))",
"_____no_output_____"
],
[
"workspaces.to_csv('data/toggl-workspaces.csv')",
"_____no_output_____"
]
],
[
[
"----",
"_____no_output_____"
],
[
"## Workplace Projects",
"_____no_output_____"
],
[
"* API Ref: https://github.com/toggl/toggl_api_docs/blob/master/chapters/workspaces.md#get-workspace-projects\n* Endpoint: https://www.toggl.com/api/v8/workspaces/{workspace_id}/projects",
"_____no_output_____"
]
],
[
[
"projects = pd.DataFrame()\nfor i in list(range(0, len(workspaces_list))):\n projects_list = toggl.request(\"https://www.toggl.com/api/v8/workspaces/\" + str(workspaces_list[i]['id']) + \"/projects\")\n projects_df_temp = pd.DataFrame.from_dict(projects_list)\n projects = pd.concat([projects_df_temp, projects])",
"/Users/markkoester/anaconda3/lib/python3.6/site-packages/ipykernel_launcher.py:5: FutureWarning: Sorting because non-concatenation axis is not aligned. A future version\nof pandas will change to not sort by default.\n\nTo accept the future behavior, pass 'sort=False'.\n\nTo retain the current behavior and silence the warning, pass 'sort=True'.\n\n \"\"\"\n"
],
[
"len(projects)",
"_____no_output_____"
],
[
"# map workspace name onto projects\nprojects['workspace_name'] = projects.wid.map(workspaces_dict)",
"_____no_output_____"
],
[
"projects.head(3)",
"_____no_output_____"
],
[
"# total time of active projects\nprojects.actual_hours.sum()",
"_____no_output_____"
],
[
"projects.to_csv('data/toggl-current-projects.csv')",
"_____no_output_____"
]
],
[
[
"----",
"_____no_output_____"
],
[
"# Collect Yearly Export of Detailed Timelogs",
"_____no_output_____"
]
],
[
[
"def get_detailed_reports(wid, since, until): # max 365 days\n uid = user_id\n param = {\n 'workspace_id': wid,\n 'since': since,\n 'until': until,\n 'uid': uid\n }\n #print(str(workspace_id) + \" \" + since)\n toggl.getDetailedReportCSV(param, \"data/detailed/toggl-detailed-report-\" + wid + \"-\" + since + \"-\" + until + \".csv\")",
"_____no_output_____"
],
[
"# years since joinging\nlast_year = today.year + 1\nyears = list(range(join_date.year, last_year))\nyears",
"_____no_output_____"
],
[
"# list of workspace ids\nworkspace_ids = []\nfor i in workspaces_list:\n workspace_ids.append(i['id'])\n# workspace_ids",
"_____no_output_____"
],
[
"workspace_ids",
"_____no_output_____"
],
[
"# Generate Detail CSV Tester\nworkspace_id = \"373504\"\nsince = \"2017-01-01\"\nuntil = \"2017-12-31\"\n\nget_detailed_reports(workspace_id, since, until)",
"_____no_output_____"
],
[
"# generate a yearly report for each workspace\nfor i in workspace_ids:\n wid = str(i)\n for y in years:\n try: \n since = str(y) + \"-01-01\" # \"2013-01-01\"\n until = str(y) + \"-12-31\" # \"2013-12-31\"\n print(\"Generating CSV... \" + \"for Workspace: \" + str(wid) + \" from \" + since + \" until \" + until)\n get_detailed_reports(wid, since, until) \n except:\n print(\"ERROR On: \" + str(uid) + \" \" + str(wid) + \" from \" + since + \" until \" + until)",
"Generating CSV... for Workspace: 341257 from 2013-01-01 until 2013-12-31\nGenerating CSV... for Workspace: 341257 from 2014-01-01 until 2014-12-31\nGenerating CSV... for Workspace: 341257 from 2015-01-01 until 2015-12-31\nGenerating CSV... for Workspace: 341257 from 2016-01-01 until 2016-12-31\nGenerating CSV... for Workspace: 341257 from 2017-01-01 until 2017-12-31\nGenerating CSV... for Workspace: 341257 from 2018-01-01 until 2018-12-31\nGenerating CSV... for Workspace: 373504 from 2013-01-01 until 2013-12-31\nGenerating CSV... for Workspace: 373504 from 2014-01-01 until 2014-12-31\nGenerating CSV... for Workspace: 373504 from 2015-01-01 until 2015-12-31\nGenerating CSV... for Workspace: 373504 from 2016-01-01 until 2016-12-31\nGenerating CSV... for Workspace: 373504 from 2017-01-01 until 2017-12-31\nGenerating CSV... for Workspace: 373504 from 2018-01-01 until 2018-12-31\nGenerating CSV... for Workspace: 1234339 from 2013-01-01 until 2013-12-31\nGenerating CSV... for Workspace: 1234339 from 2014-01-01 until 2014-12-31\nGenerating CSV... for Workspace: 1234339 from 2015-01-01 until 2015-12-31\nGenerating CSV... for Workspace: 1234339 from 2016-01-01 until 2016-12-31\nGenerating CSV... for Workspace: 1234339 from 2017-01-01 until 2017-12-31\nGenerating CSV... for Workspace: 1234339 from 2018-01-01 until 2018-12-31\n"
]
],
[
[
"-----",
"_____no_output_____"
],
[
"## Log of Latest Time Entries for that User ",
"_____no_output_____"
],
[
"* API Ref: https://github.com/toggl/toggl_api_docs/blob/master/chapters/time_entries.md#get-time-entries-started-in-a-specific-time-range\n* Endpoint: https://www.toggl.com/api/v8/time_entries \n* Note: start_date and end_date must be ISO 8601 date and time strings.",
"_____no_output_____"
]
],
[
[
"# latest_time_entries from last 9 days\nlatest_time_entries = toggl.request(\"https://www.toggl.com/api/v8/time_entries\")",
"_____no_output_____"
],
[
"len(latest_time_entries)",
"_____no_output_____"
],
[
"latest_time_entries[-1]",
"_____no_output_____"
],
[
"latest_timelog = pd.DataFrame.from_dict(latest_time_entries)",
"_____no_output_____"
],
[
"latest_timelog.tail()",
"_____no_output_____"
],
[
"latest_timelog.head()",
"_____no_output_____"
],
[
"latest_timelog.to_csv('data/toggl-timelog-latest.csv')",
"_____no_output_____"
]
],
[
[
"-----",
"_____no_output_____"
],
[
"# BONUS: Extract Times Entries for Every Single Day Using Toggl API",
"_____no_output_____"
],
[
"**NOTE:** A bit of a hackish solution. But this is a possible approach to getting individual day logs. ",
"_____no_output_____"
]
],
[
[
"extract_date_start = join_date.strftime(\"%Y-%m-%d\") # join date\nextract_date_end = today.strftime(\"%Y-%m-%d\") # today\n\n# UNCOMMENT TO Overide Full Extract \nextract_date_start = \"2018-05-23\"\n# extract_date_end = \"2018-05-01\".strftime(\"%Y-%m-%d\")\n# extract_date_end = today.strftime(\"%Y-%m-%d\") # today\n\n# Function that turns datetimes back to strings since that's what the API likes\ndef date_only(datetimeVal):\n datePart = datetimeVal.strftime(\"%Y-%m-%d\")\n return datePart\n\n# List of Dates of Dates to Extract Time Entries\ndates_range = list(pd.date_range(extract_date_start, extract_date_end))\ndates_list = [date_only(x) for x in dates_range]",
"_____no_output_____"
],
[
"# Extract Timelogs Between Two Dates and Export to a CSV\ndef toggl_timelog_extractor(input_date1, input_date2):\n date1 = parse(input_date1).isoformat() + '+00:00'\n date2 = parse(input_date2).isoformat() + '+00:00'\n param = {\n 'start_date': date1,\n 'end_date': date2,\n } \n try:\n temp_log = pd.DataFrame.from_dict(toggl.request(\"https://www.toggl.com/api/v8/time_entries\", parameters=param))\n temp_log.to_csv('data/detailed/toggl-time-entries-' + input_date1 + '.csv')\n except: \n # try again if there is an issue the first time\n temp_log = pd.DataFrame.from_dict(toggl.request(\"https://www.toggl.com/api/v8/time_entries\", parameters=param))\n temp_log.to_csv('data/daily-detailed/toggl-time-entries-' + input_date1 + '.csv')",
"_____no_output_____"
],
[
"# UNCOMMENT to Test Between Two Date\n# date1 = '2013-07-23'\n# date2 = '2013-07-24'\n# toggl_timelog_extractor(date1, date2)",
"_____no_output_____"
],
[
"# UNCOMMENT TO RUN\n# Extract All Time Entry Data from Previous Days\n#for count, item in enumerate(dates_list):\n# if item != dates_list[-1]:\n# date1 = item\n# date2 = (dates_list[count + 1])\n# # print(item + \" ~ \"+ date2)\n# time.sleep(1)\n# toggl_timelog_extractor(date1, date2)",
"_____no_output_____"
]
],
[
[
"-----",
"_____no_output_____"
],
[
"# Simple Data Analysis (Using Exported CSV Logs)",
"_____no_output_____"
]
],
[
[
"import glob\nimport os",
"_____no_output_____"
],
[
"# import all days of time entries and create data frame\npath = 'data/detailed/'\nallFiles = glob.glob(path + \"/*.csv\")\ntimelogs = pd.DataFrame()\nlist_ = []\nfor file_ in allFiles:\n df = pd.read_csv(file_,index_col=None, header=0)\n list_.append(df)\ntimelog = pd.concat(list_)",
"/Users/markkoester/anaconda3/lib/python3.6/site-packages/ipykernel_launcher.py:9: FutureWarning: Sorting because non-concatenation axis is not aligned. A future version\nof pandas will change to not sort by default.\n\nTo accept the future behavior, pass 'sort=False'.\n\nTo retain the current behavior and silence the warning, pass 'sort=True'.\n\n if __name__ == '__main__':\n"
],
[
"timelog.head()",
"_____no_output_____"
],
[
"len(timelog)",
"_____no_output_____"
],
[
"# drop unused columns\ntimelog = timelog.drop(['Email', 'User', 'Amount ()', 'Client', 'Billable'], axis=1)",
"_____no_output_____"
],
[
"# helper functions to convert duration string to seconds\ndef get_sec(time_str):\n h, m, s = time_str.split(':')\n return int(h) * 3600 + int(m) * 60 + int(s)\n\n# get_sec(\"01:16:36\")\n\ndef dur2sec(row):\n return get_sec(row['Duration'])\n\n# timelog.apply(dur2sec, axis=1)",
"_____no_output_____"
],
[
"timelog['seconds'] = timelog.apply(dur2sec, axis=1)",
"_____no_output_____"
],
[
"timelog.info()",
"<class 'pandas.core.frame.DataFrame'>\nInt64Index: 17967 entries, 0 to 219\nData columns (total 10 columns):\nDescription 17941 non-null object\nDuration 17967 non-null object\nEnd date 17967 non-null object\nEnd time 17967 non-null object\nProject 17842 non-null object\nStart date 17967 non-null object\nStart time 17967 non-null object\nTags 1148 non-null object\nTask 0 non-null object\nseconds 17967 non-null int64\ndtypes: int64(1), object(9)\nmemory usage: 1.5+ MB\n"
],
[
"timelog.describe()",
"_____no_output_____"
],
[
"timelog.head()",
"_____no_output_____"
],
[
"timelog.tail()",
"_____no_output_____"
],
[
"# Total hours\nround((timelog.seconds.sum() / 60 / 60), 1)",
"_____no_output_____"
],
[
"# total days\nround((timelog.seconds.sum() / 60 / 60 / 24), 1)",
"_____no_output_____"
],
[
"timelog.to_csv(\"data/toggl-detailed-logs-full-export.csv\")",
"_____no_output_____"
]
],
[
[
"-----",
"_____no_output_____"
],
[
"## Combine to a Daily Project Time Number",
"_____no_output_____"
]
],
[
[
"# combine to daily number\ndaily_project_time = timelog.groupby(['Start date'])['seconds'].sum()\nprint('{:,} total project time data'.format(len(daily_project_time)))\ndaily_project_time.to_csv('data/daily_project_time.csv')\ndaily_project_time.tail(5)",
"1,924 total project time data\n"
]
]
] | [
"markdown",
"code",
"markdown",
"code",
"markdown",
"code",
"markdown",
"code",
"markdown",
"code",
"markdown",
"code",
"markdown",
"code",
"markdown",
"code",
"markdown",
"code",
"markdown",
"code",
"markdown",
"code"
] | [
[
"markdown",
"markdown",
"markdown",
"markdown"
],
[
"code",
"code"
],
[
"markdown",
"markdown"
],
[
"code",
"code"
],
[
"markdown",
"markdown"
],
[
"code",
"code",
"code",
"code",
"code"
],
[
"markdown",
"markdown"
],
[
"code",
"code",
"code"
],
[
"markdown",
"markdown",
"markdown"
],
[
"code",
"code",
"code",
"code",
"code"
],
[
"markdown",
"markdown",
"markdown"
],
[
"code",
"code",
"code",
"code",
"code",
"code"
],
[
"markdown",
"markdown"
],
[
"code",
"code",
"code",
"code",
"code",
"code"
],
[
"markdown",
"markdown",
"markdown"
],
[
"code",
"code",
"code",
"code",
"code",
"code",
"code"
],
[
"markdown",
"markdown",
"markdown"
],
[
"code",
"code",
"code",
"code"
],
[
"markdown",
"markdown"
],
[
"code",
"code",
"code",
"code",
"code",
"code",
"code",
"code",
"code",
"code",
"code",
"code",
"code",
"code"
],
[
"markdown",
"markdown"
],
[
"code"
]
] |
e782712a5380ef283638252937505b7b439013bf | 320,965 | ipynb | Jupyter Notebook | notebooks/ImSim - point source sensitivity vs galaxy surface brightness - plots.ipynb | bwgref/duet-astro | 4fe3358bb927c0f03de1b75c01ddf2379b5771b3 | [
"BSD-3-Clause"
] | 1 | 2019-04-15T21:02:57.000Z | 2019-04-15T21:02:57.000Z | notebooks/ImSim - point source sensitivity vs galaxy surface brightness - plots.ipynb | bwgref/duet-astro | 4fe3358bb927c0f03de1b75c01ddf2379b5771b3 | [
"BSD-3-Clause"
] | null | null | null | notebooks/ImSim - point source sensitivity vs galaxy surface brightness - plots.ipynb | bwgref/duet-astro | 4fe3358bb927c0f03de1b75c01ddf2379b5771b3 | [
"BSD-3-Clause"
] | 1 | 2019-04-17T19:46:42.000Z | 2019-04-17T19:46:42.000Z | 1,360.021186 | 141,928 | 0.956117 | [
[
[
"import warnings\nwarnings.simplefilter(action='ignore', category=FutureWarning)\n\n%load_ext autoreload\n%autoreload 2\n\nimport numpy as np\nimport matplotlib.pyplot as plt\nfrom astropy import units as u\nfrom astroduet.bbmag import bb_abmag_fluence\nfrom astroduet.image_utils import construct_image, find, ap_phot, run_daophot\nfrom astroduet.config import Telescope\nfrom astroduet.background import background_pixel_rate\nfrom astroduet.utils import duet_abmag_to_fluence\nfrom astropy.table import Table\nfrom astropy.io import fits",
"The autoreload extension is already loaded. To reload it, use:\n %reload_ext autoreload\n"
],
[
"from astropy.visualization import quantity_support\nimport matplotlib\nfont = {'family' : 'sans',\n 'weight' : 'bold',\n 'size' : 18}\n\nmatplotlib.rc('font', **font)\nplt.rcParams['figure.figsize'] = [15,8]",
"_____no_output_____"
],
[
"# Load fits tables with simulation results\nhdu_src_rates = fits.open('../astroduet/data/src_rate_vs_gal_surfacebrightness.fits')\nhdu_src_lims = fits.open('../astroduet/data/src_det_vs_gal_surfacebrightness.fits')\nsrc_rates = hdu_src_rates[1].data\nsrc_lims = hdu_src_lims[1].data",
"_____no_output_____"
],
[
"src_lims.columns",
"_____no_output_____"
],
[
"# Find detection limits for each galaxy surface brightness:\n# Defined as the source magnitude where 90% of sources are detected\ngalmags = np.arange(15,25)\nlimmags = np.zeros([len(galmags),2])\nfor i, mag in enumerate(galmags):\n limmags[i,0] = src_lims['srcmag'][(src_lims['galmag'] == mag) & (src_lims['src_det_D1'] >= 0.9)][-1]\n limmags[i,1] = src_lims['srcmag'][(src_lims['galmag'] == mag) & (src_lims['src_det_D2'] >= 0.9)][-1]",
"_____no_output_____"
],
[
"plt.plot(galmags,limmags[:,0], linestyle='None', marker='o', markersize=8, label='DUET1')\nplt.plot(galmags,limmags[:,1], linestyle='None', marker='o', markersize=8, label='DUET2')\n\nplt.legend()\nplt.xlabel(r'Surface brightness host galaxy (mag/arcsec$^2$)')\nplt.ylabel('Source magnitude')\nplt.title('Detection limit vs surface brightness, low zodiacal background')\nplt.show()",
"_____no_output_____"
],
[
"for mag in galmags:\n plt.plot(src_lims['srcmag'][src_lims['galmag'] == mag],\n src_lims['av_src_rate_psf_D1_err'][src_lims['galmag'] == mag]/src_lims['av_src_rate_psf_D1'][src_lims['galmag'] == mag],\n label='SB = '+str(mag)+r' mag/arcsec$^2$', linewidth=2.5)\n\nplt.legend()\nplt.ylim(-0.05,1)\nplt.xlim(12,22)\nplt.xlabel(r'Source magnitude')\nplt.ylabel('Photometric precision')\nplt.title('Photometric precision vs source magnitude, DUET1, low zodiacal background')\nplt.show()",
"_____no_output_____"
],
[
"for mag in galmags:\n plt.plot(src_lims['srcmag'][src_lims['galmag'] == mag],\n src_lims['av_src_rate_psf_D2_err'][src_lims['galmag'] == mag]/src_lims['av_src_rate_psf_D2'][src_lims['galmag'] == mag],\n label='SB = '+str(mag)+r' mag/arcsec$^2$', linewidth=2.5)\n\nplt.legend()\nplt.ylim(-0.05,1)\nplt.xlim(12,22)\nplt.xlabel(r'Source magnitude')\nplt.ylabel('Photometric precision')\nplt.title('Photometric precision vs source magnitude, DUET2, low zodiacal background')\nplt.show()",
"_____no_output_____"
]
]
] | [
"code"
] | [
[
"code",
"code",
"code",
"code",
"code",
"code",
"code",
"code"
]
] |
e78278b628f40c32065c2a5b7318fe8a0a470cb1 | 105,345 | ipynb | Jupyter Notebook | Market_Basket_Intro.ipynb | UU-IM-EU/Code_along4 | 1a0a86cbbc177471713caefbdf1907d76adc7167 | [
"Apache-2.0"
] | null | null | null | Market_Basket_Intro.ipynb | UU-IM-EU/Code_along4 | 1a0a86cbbc177471713caefbdf1907d76adc7167 | [
"Apache-2.0"
] | null | null | null | Market_Basket_Intro.ipynb | UU-IM-EU/Code_along4 | 1a0a86cbbc177471713caefbdf1907d76adc7167 | [
"Apache-2.0"
] | 1 | 2021-03-02T12:08:05.000Z | 2021-03-02T12:08:05.000Z | 39.514254 | 566 | 0.365523 | [
[
[
"## Market Basket Analysis Introduction\n\nAttribution Chris Moffitt at http://pbpython.com/",
"_____no_output_____"
],
[
"Assiciationsanalys anses generellt tillhöra de oövervakade inlärningsmetoderna och kan exempelvis användas för att hitta gemensamma mönster bland stora datamängder med transaktionsdata. Ett applikationsområde blir därmed den så kallade *market basket analysis*.\n\nDet finns flera olika algoritmer som kan användas för detta, en av de vanligaste heter apriori. Se mer om market basket analysis exempelvis [här](https://www.youtube.com/watch?v=guVvtZ7ZClw) eller läs en väldigt kort introduktion [här](https://analyticsindiamag.com/hands-on-guide-to-market-basket-analysis-with-python-codes/). Vad det kort handlar om är helt enkelt att vi vill ta reda på hur olika typer av köpmönster, relaterat till produkter, ser ut. Något i stil med att om mina kunder köper hårspray, hur troligt är det då att de också köper schampo? \n\nVad kan vi använda detta till? Ja, i förlängningen kan det vara användbart för att exempelvis ge rekommendationer till kunder, i stil med hur[ Amazon](https://www.amazon.se/Data-Science-John-Kelleher/dp/0262535432/ref=sr_1_1?dchild=1&keywords=data+science&qid=1614593355&sr=8-1) gör.\n\nSe också föreläsningen om oövervakad inlärning, samt [mlxtends dokumentation](http://rasbt.github.io/mlxtend/)",
"_____no_output_____"
]
],
[
[
"import pandas as pd\nfrom mlxtend.frequent_patterns import apriori\nfrom mlxtend.frequent_patterns import association_rules",
"_____no_output_____"
],
[
"df = pd.read_excel('http://archive.ics.uci.edu/ml/machine-learning-databases/00352/Online%20Retail.xlsx')",
"_____no_output_____"
],
[
"df.head()",
"_____no_output_____"
]
],
[
[
"Som vanligt börjar vi med att bekanta oss med det data vi har, vad är det för typ av data?\n\nDärefter behöver vi (som alltid) städa vårt data och se till att dess format passar den typ av analys vi ska genomföra. ",
"_____no_output_____"
]
],
[
[
"# Städa upp mellanslag och ta bort rader som inte har ett giltligt kvitto.\ndf['Description'] = df['Description'].str.strip()\ndf.dropna(axis=0, subset=['InvoiceNo'], inplace=True)",
"_____no_output_____"
],
[
"#Ta bort kvitton från kreditkortstransaktioner\ndf['InvoiceNo'] = df['InvoiceNo'].astype('str')\ndf = df[~df['InvoiceNo'].str.contains('C')]",
"_____no_output_____"
]
],
[
[
"För att kunna köra våra algoritmer behöver vi också se till att ändra om vårt data så att varje rad representerar en transaktion och varje produkt har en egen kolumn. ",
"_____no_output_____"
]
],
[
[
"#Vi startar också med att enbart analysera data från köp gjorda i Frankrike så att det inte blir alltför mycket data.\nbasket = (df[df['Country'] == \"France\"]\n .groupby(['InvoiceNo', 'Description'])['Quantity']\n .sum().unstack().reset_index().fillna(0)\n .set_index('InvoiceNo'))",
"_____no_output_____"
]
],
[
[
"Så här ser vårt dataset ut när vi format om det som vi vill ha det för vår associationsanalys. ",
"_____no_output_____"
]
],
[
[
"basket.head()",
"_____no_output_____"
]
],
[
[
"Hur många produkter säljer företaget i Frankrike?",
"_____no_output_____"
]
],
[
[
"# Titta på några av kolumnerna, vad är det vi ser?\nbasket.iloc[:,[0,1,2,3,4,5,6, 7]].head()",
"_____no_output_____"
]
],
[
[
"Vi behöver också koda om med `one-hot encoding` så att en produkt som inhandlats i en viss transaktion representeras av 1 och frånvaron av en specifik produkt i en transaktion representeras av 0. Det medför att vårt dataset blir väldigt glest, varför? \n\n**OBS!** One hot encoding kan göras på olika sätt! ",
"_____no_output_____"
]
],
[
[
"# Konvertera till 1 för produkt köpt och 0 för produkt inte köpt.\ndef encode_units(x):\n if x <= 0:\n return 0\n if x >= 1:\n return 1 ",
"_____no_output_____"
],
[
"basket_sets = basket.applymap(encode_units)",
"_____no_output_____"
],
[
"# Ta bort onödig data\nbasket_sets.drop('POSTAGE', inplace=True, axis=1)",
"_____no_output_____"
],
[
"basket_sets.head()",
"_____no_output_____"
]
],
[
[
"### Att mäta associeringsregler \n\nFör att ta reda på vilka associationsregler som är värdefulla krävs mycket domänkunskap. Det finns dock också några mätvärden som kan användas för att hjälpa till att avgöra kvaliteten på reglerna och för att veta hur mycket vikt vi bör lägga vid en specifik regel. \n\nDet finns tre huvudsakliga sätt att mäta associeringsregler:\n\n**Support**\n\nSupport är antalet transaktioner som innehåller ett specifierat antal produkter. Ju oftare dessa produkter förekommer gemensamt (alltså idetta fallet köpts gemensamt) desto större blir vikten av supporten.\n\nOm transaktionsdata ser ut enligt följande:\n\n```\nt1: Beef, Carrot, Milk\nt2: Steak, Cheese\nt3: Cheese, Flingor\nt4: Steak, Carrot, Cheese\nt5: Steak, Carrot, Butter, Cheese, Milk\nt6: Carrot, Butter, Milk\nt7: Carrot, Milk, Butter\n```\n\nSkulle supporten för att kombinationen morötter, smör och mjölk köps tillsammans se ut enligt följande:\n\n$$Support(Carrot \\land Butter \\land Milk) = \\frac{3}{7} = 0.43$$\n\ndetta på grund av att en kombination av dessa tre produkter förekommer 3 gånger av 7 möjliga transaktioner. ",
"_____no_output_____"
],
[
"**Confidence**\n\nKonfidens innebär att om vi har en regel som säger följande: $Beef, Chicken \\rightarrow Apple$ med en konfidens på 33%, så innebär det att om det finns biff och kyckling i någons shoppingvagn så är det 33% chans att det också finns äpplen. \n\nKonfidensen beräknas exempelvis såhär: \n\nGivet följande regel: $Butter \\rightarrow Milk, Chicken$\n\n$$Butter \\rightarrow Milk, Chicken = \\frac{Support (Butter \\land Milk \\land Chicken)}{Support (Butter)}$$",
"_____no_output_____"
],
[
"**Lift**\n\nLift ger oss ett mätvärde på hur bra en regel är, baserat enbart på den högra sidan av en regel(alltså $Consequent$). Detta innebär att exempelvis regler som inkluderar vanliga produkter som $Consequent$ så kommer reglen inte säga någoting av värde. Det är alltså inte meningsfullt att ha mjölk, som är en väldigt vanlig produkt, på den högra sidan i en regel. \n\nTumregeln för Lift är följande: \n\nOm Lift är $>1$ så är regeln bättre än att gissa.Om Lift är $\\leq1$ så är regeln ungefär likvärdig med en ren gissning. \n\n\nExempel:\n\n$$Chicken \\rightarrow Milk = \\frac{Support (Chicken \\land Milk)}{Support(Chicken) \\times Support (Milk)} = \\frac{(4 / 7)}{(5 / 7) \\times (4 / 7)} = 1.4$$\n\nDetta implicerar att $Chicken \\rightarrow Milk$ skulle kunna vara en bra regel eftersom $1.4 > 1$. Om vi ändrar support för hur ofta mjölk inhandlas till $6 / 7$ istället så blir resultatet ett annat.\n\n$$Chicken \\rightarrow Milk = \\frac{Support (Chicken \\land Milk)}{Support(Chicken) \\times Support (Milk)} = \\frac{(4 / 7)}{(5 / 7) \\times (6 / 7)} = 0.933$$\n\nNu ser samma regel: $Chicken \\rightarrow Milk$ inte längre ut som en bra regel eftersom $0.933 < 1$. ",
"_____no_output_____"
],
[
"Det finns dessutom ett antal fler mätvärden såsom **Leverage** och **Conviction** som vi kan använda för att avgöra vilka mönster som är intressanta att titta närmare på men dessa kommer vi inte gå igenom i kursen. För den som är intresserad kan ni dock läsa mer [här](https://www.diva-portal.org/smash/get/diva2:956424/FULLTEXT01.pdf), [här](https://michael.hahsler.net/research/recommender/associationrules.html) och [här](https://paginas.fe.up.pt/~ec/files_0506/slides/04_AssociationRules.pdf). \n",
"_____no_output_____"
],
[
"**Enligt mlextendbiblioteket beräknas de olika måtten enligtr följande**\n\n- support(A->C) = support(A+C) [aka 'support'], range: [0, 1]\n\n- confidence(A->C) = support(A+C) / support(A), range: [0, 1]\n\n- lift(A->C) = confidence(A->C) / support(C), range: [0, inf]\n\n- leverage(A->C) = support(A->C) - support(A)*support(C),\nrange: [-1, 1]\n\n- conviction = [1 - support(C)] / [1 - confidence(A->C)],\nrange: [0, inf]",
"_____no_output_____"
],
[
"### Associationsanalys\n\nStarta med att bygga upp vårt `frequent itemset` med hjälp av algoritmen `apriori`. \n\n\nVad anser vi vara gränsen för att vi ska anse att en produkt förekommer ofta?",
"_____no_output_____"
]
],
[
[
"frequent_itemsets = apriori(basket_sets, min_support=0.07, use_colnames=True)",
"_____no_output_____"
],
[
"frequent_itemsets.head()",
"_____no_output_____"
],
[
"# Skapa själva reglerna varvid de olika mätvärdena också beräknas. \nrules = association_rules(frequent_itemsets, metric=\"lift\", min_threshold=1)\nrules",
"_____no_output_____"
],
[
"#Beräkna antal antecendant för varje regel\nrules[\"num_antecedents\"] = rules[\"antecedents\"].apply(lambda x: len(x))\nrules",
"_____no_output_____"
],
[
"grocery_rules_3_items = rules[rules.num_antecedents >= 2]\ngrocery_rules_3_items",
"_____no_output_____"
],
[
"#Strängare regler\nrules[ (rules['lift'] >= 6) &\n (rules['confidence'] >= 0.8) ]",
"_____no_output_____"
],
[
"basket['ALARM CLOCK BAKELIKE GREEN'].sum()",
"_____no_output_____"
],
[
"basket['ALARM CLOCK BAKELIKE RED'].sum()",
"_____no_output_____"
],
[
"Vi tittar på transaktioner från Tyskland också som jämförelse.",
"_____no_output_____"
],
[
"basket2 = (df[df['Country'] ==\"Germany\"]\n .groupby(['InvoiceNo', 'Description'])['Quantity']\n .sum().unstack().reset_index().fillna(0)\n .set_index('InvoiceNo'))",
"_____no_output_____"
],
[
"#Encoding\nbasket_sets2 = basket2.applymap(encode_units)",
"_____no_output_____"
],
[
"basket_sets2.drop('POSTAGE', inplace=True, axis=1)",
"_____no_output_____"
],
[
"#Frekventa artiklar\n\nfrequent_itemsets2 = apriori(basket_sets2, min_support=0.05, use_colnames=True)",
"_____no_output_____"
],
[
"#Regler\nrules2 = association_rules(frequent_itemsets2, metric=\"lift\", min_threshold=1)\nrules2",
"_____no_output_____"
],
[
"rules2[\"num_antecedents\"] = rules2[\"antecedents\"].apply(lambda x: len(x))\nrules2",
"_____no_output_____"
],
[
"grocery_rules_3_items = rules2[rules2.num_antecedents >= 2]\ngrocery_rules_3_items",
"_____no_output_____"
],
[
"#Strängare regler\nrules2[ (rules2['lift'] >= 4) &\n (rules2['confidence'] >= 0.5) ]",
"_____no_output_____"
]
]
] | [
"markdown",
"code",
"markdown",
"code",
"markdown",
"code",
"markdown",
"code",
"markdown",
"code",
"markdown",
"code",
"markdown",
"code"
] | [
[
"markdown",
"markdown"
],
[
"code",
"code",
"code"
],
[
"markdown"
],
[
"code",
"code"
],
[
"markdown"
],
[
"code"
],
[
"markdown"
],
[
"code"
],
[
"markdown"
],
[
"code"
],
[
"markdown"
],
[
"code",
"code",
"code",
"code"
],
[
"markdown",
"markdown",
"markdown",
"markdown",
"markdown",
"markdown"
],
[
"code",
"code",
"code",
"code",
"code",
"code",
"code",
"code",
"code",
"code",
"code",
"code",
"code",
"code",
"code",
"code",
"code"
]
] |
e7827bd54c363d590178e8f905132da643f04ca5 | 1,549 | ipynb | Jupyter Notebook | docs/notebooks/visual_intro.ipynb | clancygeodata/geodatatool | 9b6c805f7ad8e5b3af0d905ebfab3fa1bcc109b6 | [
"MIT"
] | 1 | 2021-03-20T12:19:59.000Z | 2021-03-20T12:19:59.000Z | examples/visual_intro.ipynb | clancygeodata/geodatatool | 9b6c805f7ad8e5b3af0d905ebfab3fa1bcc109b6 | [
"MIT"
] | null | null | null | examples/visual_intro.ipynb | clancygeodata/geodatatool | 9b6c805f7ad8e5b3af0d905ebfab3fa1bcc109b6 | [
"MIT"
] | null | null | null | 18.890244 | 121 | 0.540994 | [
[
[
"## Load an image from a URL",
"_____no_output_____"
]
],
[
[
"from geodatatool import visual",
"_____no_output_____"
],
[
"visual.load_image_from_url(\"https://upload.wikimedia.org/wikipedia/commons/6/61/Remote_Sensing_Illustration.jpg\")",
"_____no_output_____"
]
],
[
[
"## Display a YouTube video",
"_____no_output_____"
]
],
[
[
"from geodatatool import visual",
"_____no_output_____"
],
[
"visual.display_youtube(\"Ezn1ne2Fj6Y\")",
"_____no_output_____"
]
]
] | [
"markdown",
"code",
"markdown",
"code"
] | [
[
"markdown"
],
[
"code",
"code"
],
[
"markdown"
],
[
"code",
"code"
]
] |
e782816aa83e740c90cd533c9323c5918da27207 | 1,385 | ipynb | Jupyter Notebook | notebooks/pr_clips_benchmark.ipynb | pmckenz1/birdclips | 5d2bd0ac35dfc360e4379618be132fbc6c7a0fd0 | [
"MIT"
] | null | null | null | notebooks/pr_clips_benchmark.ipynb | pmckenz1/birdclips | 5d2bd0ac35dfc360e4379618be132fbc6c7a0fd0 | [
"MIT"
] | null | null | null | notebooks/pr_clips_benchmark.ipynb | pmckenz1/birdclips | 5d2bd0ac35dfc360e4379618be132fbc6c7a0fd0 | [
"MIT"
] | null | null | null | 19.785714 | 80 | 0.527076 | [
[
[
"import time\nimport schedule\nimport datetime\nimport subprocess",
"_____no_output_____"
],
[
"def timed_command():\n command = \"bash /pinky/patrick/birdclips/scripts/pr_1hr.sh\"\n process = subprocess.Popen(command.split(), stdout=subprocess.PIPE)\n output, error = process.communicate()",
"_____no_output_____"
],
[
"schedule.every().day.at(\"16:00\").do(timed_command)\n\nwhile True:\n schedule.run_pending()\n time.sleep(1)",
"_____no_output_____"
]
]
] | [
"code"
] | [
[
"code",
"code",
"code"
]
] |
e78288af68d37b6d3cd5729192667cb99229a087 | 159,598 | ipynb | Jupyter Notebook | ImdbTasks.ipynb | cardosorrenan/alura-QuarentenaDados | 29f3e1e36042b99a1a59985329469d327d90c790 | [
"MIT"
] | null | null | null | ImdbTasks.ipynb | cardosorrenan/alura-QuarentenaDados | 29f3e1e36042b99a1a59985329469d327d90c790 | [
"MIT"
] | null | null | null | ImdbTasks.ipynb | cardosorrenan/alura-QuarentenaDados | 29f3e1e36042b99a1a59985329469d327d90c790 | [
"MIT"
] | null | null | null | 172.538378 | 34,238 | 0.851013 | [
[
[
"import pandas as pd\nimport matplotlib.pyplot as plt\nimport seaborn as sns\npd.options.mode.chained_assignment = None ",
"_____no_output_____"
],
[
"imdb = pd.read_csv(\"https://raw.githubusercontent.com/cardosorrenan/alura-QuarentenaDados/master/csv/imdb.csv\")\nimdb.head()",
"_____no_output_____"
]
],
[
[
"#### **Day 3 - Task 1**: Plot the boxplot (column imdb_score) of the colored and bw films",
"_____no_output_____"
]
],
[
[
"imdb_has_attr_color = imdb.dropna(subset=['color'])\nsns.boxplot(data = imdb_has_attr_color, x =\"color\", y=\"imdb_score\")\nplt.gcf().set_size_inches(3, 6)",
"_____no_output_____"
]
],
[
[
"#### **Day 3 - Task 2**: In the graph (budget x gross), we have a point with a high gross value (close to 2.5) and also a high loss, find this movie",
"_____no_output_____"
]
],
[
[
"imdb = imdb.drop_duplicates()\nimdb_usa = imdb.query(\"country == 'USA'\")\nsns.scatterplot(x=\"budget\", y=\"gross\", data = imdb_usa) ",
"_____no_output_____"
],
[
"imdb_usa.query('budget > 250000000 & gross < 100000000')['movie_title']",
"_____no_output_____"
]
],
[
[
"#### **Day 3 - Task 4**: What are the films that came before the 2WW decade and have high gains",
"_____no_output_____"
]
],
[
[
"imdb_usa['earnings'] = imdb_usa['gross'] - imdb_usa['budget']\nsns.scatterplot(x=\"title_year\", y=\"earnings\", data = imdb_usa)\nimdb_usa.query('title_year > 1935 & title_year < 1940 & earnings > 150000000')[['movie_title', 'title_year', 'gross']]",
"_____no_output_____"
]
],
[
[
"#### **Day 3 - Task 5**: In the graph (movies_per_director x gross), we have some strange points between 15 and 20. Confirm Paulo's theory that the director is Woody Allen",
"_____no_output_____"
]
],
[
[
"movies_director = imdb_usa.groupby('director_name')['director_name'].count().rename('movies_director')\ngross_director_movies = imdb_usa[['director_name', 'gross', 'movie_title']].merge(movies_director, on='director_name')\nsns.scatterplot(x=\"movies_director\", y=\"gross\", data = gross_director_movies)\ngross_director_movies.query('movies_director == 18').sort_values('gross').head()\nmovies_director.sort_values()",
"_____no_output_____"
]
],
[
[
"#### **Day 3 - Task 7**: Calculate the correlation of films only after the 2000s",
"_____no_output_____"
]
],
[
[
"imdb_usa_af2000 = imdb_usa.query('title_year > 2000')\nimdb_usa_af2000[[\"gross\", \"budget\", \"earnings\", \"title_year\"]].corr()",
"_____no_output_____"
]
],
[
[
"#### **Day 3 - Task 8**: Try to find a graph that looks like a line",
"_____no_output_____"
]
],
[
[
"sns.lineplot(data = imdb_usa.query('title_year > 2005').groupby('title_year')['gross'].mean())",
"_____no_output_____"
]
],
[
[
"#### **Day 3 - Task 9**: Show the correlation between other variables present in the dataframe. Counting revisions per year can also be a resource.",
"_____no_output_____"
]
],
[
[
"imdb_usa[[\"num_user_for_reviews\", \"num_voted_users\"]].corr()",
"_____no_output_____"
],
[
"imdb_usa[[\"actor_1_facebook_likes\", \"cast_total_facebook_likes\"]].corr()",
"_____no_output_____"
],
[
"sns.lineplot(data = imdb_usa.groupby('title_year')['num_voted_users'].sum())",
"_____no_output_____"
]
]
] | [
"code",
"markdown",
"code",
"markdown",
"code",
"markdown",
"code",
"markdown",
"code",
"markdown",
"code",
"markdown",
"code",
"markdown",
"code"
] | [
[
"code",
"code"
],
[
"markdown"
],
[
"code"
],
[
"markdown"
],
[
"code",
"code"
],
[
"markdown"
],
[
"code"
],
[
"markdown"
],
[
"code"
],
[
"markdown"
],
[
"code"
],
[
"markdown"
],
[
"code"
],
[
"markdown"
],
[
"code",
"code",
"code"
]
] |
e7828918fe4908dc6871defb4a85646feabadce5 | 2,232 | ipynb | Jupyter Notebook | examples/gallery/demos/bokeh/directed_airline_routes.ipynb | ppwadhwa/holoviews | e8e2ec08c669295479f98bb2f46bbd59782786bf | [
"BSD-3-Clause"
] | 864 | 2019-11-13T08:18:27.000Z | 2022-03-31T13:36:13.000Z | examples/gallery/demos/bokeh/directed_airline_routes.ipynb | ppwadhwa/holoviews | e8e2ec08c669295479f98bb2f46bbd59782786bf | [
"BSD-3-Clause"
] | 1,117 | 2019-11-12T16:15:59.000Z | 2022-03-30T22:57:59.000Z | examples/gallery/demos/bokeh/directed_airline_routes.ipynb | ppwadhwa/holoviews | e8e2ec08c669295479f98bb2f46bbd59782786bf | [
"BSD-3-Clause"
] | 180 | 2019-11-19T16:44:44.000Z | 2022-03-28T22:49:18.000Z | 26.891566 | 127 | 0.581541 | [
[
[
"Most examples work across multiple plotting backends, this example is also available for:\n\n* [Matplotlib Directed Airline Routes](../matplotlib/directed_airline_routes.ipynb)",
"_____no_output_____"
]
],
[
[
"import networkx as nx\nimport holoviews as hv\nfrom holoviews import opts\n\nfrom holoviews.element.graphs import layout_nodes\nfrom bokeh.sampledata.airport_routes import routes, airports\n\nhv.extension('bokeh')",
"_____no_output_____"
]
],
[
[
"## Declare data",
"_____no_output_____"
]
],
[
[
"# Create dataset indexed by AirportID and with additional value dimension\nairports = hv.Dataset(airports, ['AirportID'], ['Name', 'IATA', 'City'])\n\nlabel = 'Alaska Airline Routes'\n\n# Select just Alaska Airline routes\nas_graph = hv.Graph((routes[routes.Airline=='AS'], airports), ['SourceID', \"DestinationID\"], 'Airline', label=label)\n\nas_graph = layout_nodes(as_graph, layout=nx.layout.fruchterman_reingold_layout)\nlabels = hv.Labels(as_graph.nodes, ['x', 'y'], ['IATA', 'City'], label=label)",
"_____no_output_____"
]
],
[
[
"## Plot",
"_____no_output_____"
]
],
[
[
"(as_graph * labels).opts(\n opts.Graph(directed=True, node_size=8, bgcolor='gray', xaxis=None, yaxis=None,\n edge_line_color='white', edge_line_width=1, width=800, height=800, arrowhead_length=0.01,\n node_fill_color='white', node_nonselection_fill_color='black'),\n opts.Labels(xoffset=-0.04, yoffset=0.03, text_font_size='10pt'))",
"_____no_output_____"
]
]
] | [
"markdown",
"code",
"markdown",
"code",
"markdown",
"code"
] | [
[
"markdown"
],
[
"code"
],
[
"markdown"
],
[
"code"
],
[
"markdown"
],
[
"code"
]
] |
e7828a95c8cea126f12dbeb2d7e26a9d15e9bc44 | 19,932 | ipynb | Jupyter Notebook | multi_physics/QED/python_bindings/demo_python_bindings.ipynb | RemiLehe/picsar | 8ff12fbf118b9aba7cfe602cb1a5e6da32bf7eef | [
"BSD-3-Clause-LBNL"
] | 20 | 2020-06-22T17:38:17.000Z | 2022-03-11T17:20:30.000Z | multi_physics/QED/python_bindings/demo_python_bindings.ipynb | RemiLehe/picsar | 8ff12fbf118b9aba7cfe602cb1a5e6da32bf7eef | [
"BSD-3-Clause-LBNL"
] | 11 | 2020-11-03T10:55:37.000Z | 2022-02-07T17:00:36.000Z | multi_physics/QED/python_bindings/demo_python_bindings.ipynb | RemiLehe/picsar | 8ff12fbf118b9aba7cfe602cb1a5e6da32bf7eef | [
"BSD-3-Clause-LBNL"
] | 11 | 2020-06-23T13:54:59.000Z | 2022-03-28T21:51:38.000Z | 38.627907 | 121 | 0.610576 | [
[
[
"import numpy as np\nimport scipy as sci\nimport scipy.constants as scicon\nimport pxr_qed\n\nprint(\"pxr_qed is compiled in {:s} precision with {:s} units\".\n format(pxr_qed.PRECISION, pxr_qed.UNITS))\nif(pxr_qed.HAS_OPENMP):\n print(\"pxr_qed has openMP support!\")\nelse:\n print(\"pxr_qed does not have openMP support!\")\n\n#Warning: this notebook is conceived for SI units!!",
"pxr_qed is compiled in double precision with SI units\npxr_qed has openMP support!\n"
],
[
"m_e = scicon.electron_mass\nq_e = scicon.elementary_charge\nhbar = scicon.hbar\nc = scicon.c\num = scicon.micron\nfs = scicon.femto\n\nE_s = m_e**2 * c**3 / (hbar * q_e)\nB_s = E_s/c",
"_____no_output_____"
],
[
"#Gamma functions\n\ngamma_how_many = 10\ngamma_gamma = 10\n\ngamma_px = (np.random.rand(gamma_how_many)-0.5)*2*gamma_gamma*m_e*c;\ngamma_py = (np.random.rand(gamma_how_many)-0.5)*2*gamma_gamma*m_e*c;\ngamma_pz = (np.random.rand(gamma_how_many)-0.5)*2*gamma_gamma*m_e*c;\n\nprint(\"gamma_phot: \", pxr_qed.compute_gamma_photon(\n gamma_px, gamma_py, gamma_pz, 1))\n\nprint(\"gamma_ele_pos: \", pxr_qed.compute_gamma_ele_pos(\n gamma_px, gamma_py, gamma_pz, 1))",
"gamma_phot: [ 4.67604484 11.82685736 8.30917484 14.96331916 9.78242611 11.31114537\n 7.47116378 12.19871847 8.6841809 12.14654068]\ngamma_ele_pos: [ 4.78177743 11.86905872 8.36913296 14.99669698 9.83340534 11.35526352\n 7.53779067 12.23963775 8.74156725 12.18763514]\n"
],
[
"#Chi functions\n\nchi_how_many = 10\nchi_gamma = 10\n\nchi_Ex = (np.random.rand(chi_how_many)-0.5)*2*E_s\nchi_Ey = (np.random.rand(chi_how_many)-0.5)*2*E_s\nchi_Ez = (np.random.rand(chi_how_many)-0.5)*2*E_s\nchi_Bx = (np.random.rand(chi_how_many)-0.5)*2*B_s\nchi_By = (np.random.rand(chi_how_many)-0.5)*2*B_s\nchi_Bz = (np.random.rand(chi_how_many)-0.5)*2*B_s\nchi_px = (np.random.rand(chi_how_many)-0.5)*2*chi_gamma*m_e*c;\nchi_py = (np.random.rand(chi_how_many)-0.5)*2*chi_gamma*m_e*c;\nchi_pz = (np.random.rand(chi_how_many)-0.5)*2*chi_gamma*m_e*c;\n\nprint(\"chi_phot: \", pxr_qed.chi_photon(\n chi_px, chi_py, chi_pz,\n chi_Ex, chi_Ey, chi_Ez,\n chi_Bx, chi_By, chi_Bz, 1))\n\nprint(\"chi_ele_pos: \", pxr_qed.chi_ele_pos(\n chi_px, chi_py, chi_pz, \n chi_Ex, chi_Ey, chi_Ez,\n chi_Bx, chi_By, chi_Bz, 1))",
"chi_phot: [ 7.86550251 0.76186317 5.04807082 11.5553343 6.96356469 17.4901906\n 13.9569598 13.5493148 7.1165209 9.23347205]\nchi_ele_pos: [ 7.91618139 1.26487224 5.09692046 11.60037052 6.99058547 17.54641482\n 13.98964914 13.59008379 7.16717618 9.22869693]\n"
],
[
"#Breit-Wheeler pair production\n\nbw_dndt_params = pxr_qed.bw.dndt_lookup_table_params()\nbw_dndt_params.chi_phot_min = 0.01\nbw_dndt_params.chi_phot_max = 100\nbw_dndt_params.chi_phot_how_many = 128\nprint(bw_dndt_params)\n\nbw_dndt_lookup_table = pxr_qed.bw.dndt_lookup_table(bw_dndt_params)\nprint(bw_dndt_lookup_table)\nbw_dndt_lookup_table.generate()\nprint(bw_dndt_lookup_table)\n\nbw_dndt_lookup_table.save_as(\"bw_dndt.bin\")\nbw_dndt_lookup_table_2 = pxr_qed.bw.dndt_lookup_table()\nprint(bw_dndt_lookup_table_2)\nbw_dndt_lookup_table_2.load_from(\"bw_dndt.bin\");\nprint(bw_dndt_lookup_table_2)\n\nbw_pair_prod_params = pxr_qed.bw.pair_prod_lookup_table_params()\nbw_pair_prod_params.chi_phot_min = 0.01\nbw_pair_prod_params.chi_phot_max = 100\nbw_pair_prod_params.chi_phot_how_many = 64\nbw_pair_prod_params.frac_how_many = 64\nprint(bw_pair_prod_params)\n\nbw_pair_prod_lookup_table = pxr_qed.bw.pair_prod_lookup_table(bw_pair_prod_params)\nprint(bw_pair_prod_lookup_table)\nbw_pair_prod_lookup_table.generate()\nprint(bw_pair_prod_lookup_table)\n\nbw_pair_prod_lookup_table.save_as(\"bw_pairprod.bin\")\nbw_pair_prod_lookup_table_2 = pxr_qed.bw.pair_prod_lookup_table()\nprint(bw_pair_prod_lookup_table_2)\nbw_pair_prod_lookup_table_2.load_from(\"bw_pairprod.bin\");\nprint(bw_pair_prod_lookup_table_2)\n\nbw_how_many = 4\nbw_gamma = 10\nbw_dt = 1e-2*fs\n\nbw_Ex = (np.random.rand(bw_how_many)-0.5)*2*E_s\nbw_Ey = (np.random.rand(bw_how_many)-0.5)*2*E_s\nbw_Ez = (np.random.rand(bw_how_many)-0.5)*2*E_s\nbw_Bx = (np.random.rand(bw_how_many)-0.5)*2*B_s\nbw_By = (np.random.rand(bw_how_many)-0.5)*2*B_s\nbw_Bz = (np.random.rand(bw_how_many)-0.5)*2*B_s\nbw_px = (np.random.rand(bw_how_many)-0.5)*2*bw_gamma*m_e*c;\nbw_py = (np.random.rand(bw_how_many)-0.5)*2*bw_gamma*m_e*c;\nbw_pz = (np.random.rand(bw_how_many)-0.5)*2*bw_gamma*m_e*c\nbw_rand = np.random.rand(bw_how_many)\nbw_ee = np.sqrt(bw_px**2 + bw_py**2 + bw_pz**2)*c;\n\n\nbw_chi = pxr_qed.chi_photon(bw_px, bw_py, bw_pz, bw_Ex, bw_Ey, bw_Ez, bw_Bx, bw_By, bw_Bz)\nprint(\"Chi parameters: \", bw_chi, \"\\n\")\n\nbw_interp_dndt = bw_dndt_lookup_table.interp(bw_chi)\nprint(\"dN/dt lookup table interpolation: \", bw_interp_dndt, \"\\n\")\n\nbw_interp_pair_prod = bw_pair_prod_lookup_table.interp(bw_chi, bw_rand)\nprint(\"Pair production lookup table interpolation: \", bw_interp_pair_prod, \"\\n\")\n\nbw_opt = pxr_qed.bw.get_optical_depth(bw_rand)\nprint(\"Breit-Wheeler optical depth: \", bw_opt, \"\\n\")\n\nbw_dndt = pxr_qed.bw.get_dn_dt(bw_ee, bw_chi, bw_dndt_lookup_table)\nprint(\"Breit-Wheeler dNdt: \", bw_dndt, \"\\n\")\n\nprint(\"Breit-Wheeler opt depth before evolution: \", bw_opt, \"\\n\")\npxr_qed.bw.evolve_optical_depth(bw_ee, bw_chi, bw_dt, bw_opt, bw_dndt_lookup_table)\nprint(\"Breit-Wheeler opt depth after evolution: \", bw_opt, \"\\n\")\n\nbw_ele_px, bw_ele_py, bw_ele_pz, bw_pos_px, bw_pos_py, bw_pos_pz = pxr_qed.bw.generate_breit_wheeler_pairs(\n bw_chi, bw_px, bw_py, bw_pz, bw_rand, bw_pair_prod_lookup_table)\n\nprint(\"Breit-Wheeler electron px: \", bw_ele_px)\nprint(\"Breit-Wheeler electron py: \", bw_ele_py)\nprint(\"Breit-Wheeler electron pz: \", bw_ele_pz)\nprint(\"Breit-Wheeler positron px: \", bw_pos_px)\nprint(\"Breit-Wheeler positron py: \", bw_pos_py)\nprint(\"Breit-Wheeler positron pz: \", bw_pos_pz)",
"bw.dndt_lookup_table_params:\n\tchi_phot_min : 0.01\n\tchi_phot_max : 100\n\tchi_phot_how_many: 128\nbw.dndt_lookup_table:\n\tis initialized? : False\n\nbw.dndt_lookup_table:\n\tis initialized? : True\n\nbw.dndt_lookup_table:\n\tis initialized? : False\n\nbw.dndt_lookup_table:\n\tis initialized? : True\n\nbw.pair_prod_lookup_table_params:\n\tchi_phot_min : 0.01\n\tchi_phot_max : 100\n\tchi_phot_how_many: 64\n\tfrac_how_many : 64\nbw.pair_prod_lookup_table:\n\tis initialized? : False\n\nbw.pair_prod_lookup_table:\n\tis initialized? : True\n\nbw.pair_prod_lookup_table:\n\tis initialized? : False\n\nbw.pair_prod_lookup_table:\n\tis initialized? : True\n\nChi parameters: [ 5.52069331 20.49972262 22.07266127 6.98987992] \n\ndN/dt lookup table interpolation: [0.09874905 0.10486137 0.10386865 0.10432933] \n\nPair production lookup table interpolation: [ 2.64771057 4.62710131 20.55914998 3.6050094 ] \n\nBreit-Wheeler optical depth: [0.65018135 0.3063956 2.62854864 0.72525359] \n\nBreit-Wheeler dNdt: [2.33127565e+17 9.02173278e+17 1.10071850e+18 6.84236681e+17] \n\nBreit-Wheeler opt depth before evolution: [0.65018135 0.3063956 2.62854864 0.72525359] \n\nBreit-Wheeler opt depth after evolution: [-1.6810943 -8.71533718 -8.37863641 -6.11711323] \n\nBreit-Wheeler electron px: [-1.31799211e-22 5.49358908e-22 -2.17854062e-21 -3.71205846e-22]\nBreit-Wheeler electron py: [-1.25710161e-21 -4.94075266e-22 -9.67875049e-23 -6.18616035e-22]\nBreit-Wheeler electron pz: [ 1.17359069e-21 -5.86189907e-22 1.67933159e-21 -3.37058023e-22]\nBreit-Wheeler positron px: [-1.41488322e-22 1.56726089e-21 -2.89643318e-22 -3.54047029e-22]\nBreit-Wheeler positron py: [-1.34951640e-21 -1.40954271e-21 -1.28681805e-23 -5.90020797e-22]\nBreit-Wheeler positron pz: [ 1.25986624e-21 -1.67233571e-21 2.23272024e-22 -3.21477673e-22]\n"
],
[
"# Quantum Synchrotron radiation\n\nqs_dndt_params = pxr_qed.qs.dndt_lookup_table_params()\nqs_dndt_params.chi_part_min = 0.01\nqs_dndt_params.chi_part_max = 100\nqs_dndt_params.chi_part_how_many = 128\nprint(qs_dndt_params)\n\nqs_dndt_lookup_table = pxr_qed.qs.dndt_lookup_table(qs_dndt_params)\nprint(qs_dndt_lookup_table)\nqs_dndt_lookup_table.generate()\nprint(qs_dndt_lookup_table)\n\nqs_dndt_lookup_table.save_as(\"qs_dndt.bin\")\nqs_dndt_lookup_table_2 = pxr_qed.qs.dndt_lookup_table()\nprint(qs_dndt_lookup_table_2)\nqs_dndt_lookup_table_2.load_from(\"qs_dndt.bin\");\nprint(qs_dndt_lookup_table_2)\n\nqs_photem_params = pxr_qed.qs.photon_emission_lookup_table_params()\nqs_photem_params.chi_part_min = 0.01\nqs_photem_params.chi_part_max = 100\nqs_photem_params.frac_min = 1e-12\nqs_photem_params.chi_part_how_many = 64\nqs_photem_params.frac_how_many = 64\nprint(qs_photem_params)\n\nqs_dndt_lookup_table.save_as(\"qs_dndt.bin\")\nqs_dndt_lookup_table_2 = pxr_qed.qs.dndt_lookup_table()\nprint(qs_dndt_lookup_table_2)\nqs_dndt_lookup_table_2.load_from(\"qs_dndt.bin\");\nprint(qs_dndt_lookup_table_2)\n\nqs_photon_emission_lookup_table = pxr_qed.qs.photon_emission_lookup_table(qs_photem_params)\nprint(qs_photon_emission_lookup_table)\nqs_photon_emission_lookup_table.generate()\nprint(qs_photon_emission_lookup_table)\n\nqs_how_many = 4\nqs_gamma = 10\nqs_dt = 1e-2*fs\n\nqs_Ex = (np.random.rand(qs_how_many)-0.5)*2*E_s\nqs_Ey = (np.random.rand(qs_how_many)-0.5)*2*E_s\nqs_Ez = (np.random.rand(qs_how_many)-0.5)*2*E_s\nqs_Bx = (np.random.rand(qs_how_many)-0.5)*2*B_s\nqs_By = (np.random.rand(qs_how_many)-0.5)*2*B_s\nqs_Bz = (np.random.rand(qs_how_many)-0.5)*2*B_s\nqs_px = (np.random.rand(qs_how_many)-0.5)*2*qs_gamma*m_e*c;\nqs_py = (np.random.rand(qs_how_many)-0.5)*2*qs_gamma*m_e*c;\nqs_pz = (np.random.rand(qs_how_many)-0.5)*2*qs_gamma*m_e*c;\nqs_rand = np.random.rand(qs_how_many)\nqs_ee = np.sqrt(1 + (qs_px**2 + qs_py**2 + qs_pz**2)/((m_e*c)**2))*m_e*c**2;\n\n\nqs_chi = pxr_qed.chi_ele_pos(qs_px, qs_py, qs_pz, qs_Ex, qs_Ey, qs_Ez, qs_Bx, qs_By, qs_Bz)\nprint(\"Chi parameters: \", qs_chi, \"\\n\")\n\nqs_interp_dndt = qs_dndt_lookup_table.interp(qs_chi)\nprint(\"dN/dt lookup table interpolation: \", qs_interp_dndt, \"\\n\")\n\nqs_interp_photon_emission = qs_photon_emission_lookup_table.interp(qs_chi, qs_rand)\nprint(\"Pair production lookup table interpolation: \", qs_interp_photon_emission, \"\\n\")\n\nqs_opt = pxr_qed.qs.get_optical_depth(qs_rand)\nprint(\"Quantum synchrotron optical depth: \", qs_opt, \"\\n\")\n\nqs_dndt = pxr_qed.qs.get_dn_dt(qs_ee, qs_chi, qs_dndt_lookup_table)\nprint(\"Quantum synchrotron dNdt: \", qs_dndt, \"\\n\")\n\nprint(\"Quantum synchrotron opt depth before evolution: \", qs_opt, \"\\n\")\npxr_qed.qs.evolve_optical_depth(qs_ee, qs_chi, qs_dt, qs_opt, qs_dndt_lookup_table)\nprint(\"Quantum synchrotron opt depth after evolution: \", qs_opt, \"\\n\")\n\nprint(\"Quantum synchrotron part px (before): \", qs_px)\nprint(\"Quantum synchrotron part py (before): \", qs_py)\nprint(\"Quantum synchrotron part pz (before): \", qs_pz)\n\nqs_phot_px, qs_phot_py, qs_phot_pz = pxr_qed.qs.generate_photon_update_momentum(\n qs_chi, qs_px, qs_py, qs_pz, qs_rand, qs_photon_emission_lookup_table)\n\nprint(\"Quantum synchrotron part px: \", qs_px)\nprint(\"Quantum synchrotron part py: \", qs_py)\nprint(\"Quantum synchrotron part pz: \", qs_pz)\nprint(\"Quantum synchrotron photon px: \", qs_phot_px)\nprint(\"Quantum synchrotron photon py: \", qs_phot_py)\nprint(\"Quantum synchrotron photon pz: \", qs_phot_pz)\n",
"qs.dndt_lookup_table_params:\n\tchi_part_min : 0.01\n\tchi_part_max : 100\n\tchi_part_how_many: 128\nqs.dndt_lookup_table:\n\tis initialized? : False\n\nqs.dndt_lookup_table:\n\tis initialized? : True\n\nqs.dndt_lookup_table:\n\tis initialized? : False\n\nqs.dndt_lookup_table:\n\tis initialized? : True\n\nqs.photon_emission_lookup_table_params:\n\tchi_part_min : 0.01\n\tchi_part_max : 100\n\tfrac_min : 1e-12\n\tchi_part_how_many: 64\n\tfrac_how_many : 64\nqs.dndt_lookup_table:\n\tis initialized? : False\n\nqs.dndt_lookup_table:\n\tis initialized? : True\n\nqs.pair_prod_lookup_table:\n\tis initialized? : False\n\nqs.pair_prod_lookup_table:\n\tis initialized? : True\n\nChi parameters: [5.25052688 6.7088152 9.13282162 6.07430405] \n\ndN/dt lookup table interpolation: [5.70338045 6.84436205 8.58440509 6.35830653] \n\nPair production lookup table interpolation: [1.35492083 5.8118076 0.36467181 1.31991566] \n\nQuantum synchrotron optical depth: [0.31205442 0.02926544 0.91580599 0.36965033] \n\nQuantum synchrotron dNdt: [2.75580636e+18 1.85740858e+18 3.99419588e+18 2.09244207e+18] \n\nQuantum synchrotron opt depth before evolution: [0.31205442 0.02926544 0.91580599 0.36965033] \n\nQuantum synchrotron opt depth after evolution: [-27.24600919 -18.54482035 -39.02615279 -20.55477036] \n\nQuantum synchrotron part px (before): [1.92622282e-21 2.09512463e-21 1.76235868e-21 1.01883823e-21]\nQuantum synchrotron part py (before): [-8.68411094e-22 -2.38967770e-21 -1.09156467e-21 1.81341074e-21]\nQuantum synchrotron part pz (before): [ 1.32565258e-22 -2.06655044e-21 7.36225418e-22 -2.32854237e-21]\nQuantum synchrotron part px: [1.48915370e-21 4.06177946e-22 1.70018369e-21 8.15968516e-22]\nQuantum synchrotron part py: [-6.71364487e-22 -4.63282406e-22 -1.05305490e-21 1.45232681e-21]\nQuantum synchrotron part pz: [ 1.02485571e-22 -4.00638320e-22 7.10251813e-22 -1.86488612e-21]\nQuantum synchrotron photon px: [4.37069117e-22 1.68894668e-21 6.21749909e-23 2.02869712e-22]\nQuantum synchrotron photon py: [-1.97046607e-22 -1.92639529e-21 -3.85097677e-23 3.61083934e-22]\nQuantum synchrotron photon pz: [ 3.00796875e-23 -1.66591212e-21 2.59736052e-23 -4.63656259e-22]\n"
],
[
"#Schwinger pair production\n\nsc_how_many = 10\n\nsc_Ex = (np.random.rand(sc_how_many)-0.5)*2*E_s\nsc_Ey = (np.random.rand(sc_how_many)-0.5)*2*E_s\nsc_Ez = (np.random.rand(sc_how_many)-0.5)*2*E_s\nsc_Bx = (np.random.rand(sc_how_many)-0.5)*2*B_s\nsc_By = (np.random.rand(sc_how_many)-0.5)*2*B_s\nsc_Bz = (np.random.rand(sc_how_many)-0.5)*2*B_s\n\nsc_volume = um**3\nsc_dt = fs\n\npair_production_rate = pxr_qed.sc.pair_production_rate(sc_Ex, sc_Ey, sc_Ez, sc_Bx, sc_By, sc_Bz, 1)\nexp_pair_number = pxr_qed.sc.expected_pair_number(sc_Ex, sc_Ey, sc_Ez, sc_Bx, sc_By, sc_Bz, sc_volume, sc_dt, 1)\n\nprint(\"pair_production_rate:\", pair_production_rate)\n\nprint(\"expected pair number:\", exp_pair_number)",
"pair_production_rate: [3.67909054e+16 1.67669025e+16 3.93542134e+16 2.41222254e+16\n 6.46728241e+15 1.05457518e+17 1.05938614e+16 4.70692444e+16\n 9.10551806e-16 1.01921766e+16]\nexpected pair number: [4.78829683e+21 2.18219436e+21 5.12190862e+21 3.13948174e+21\n 8.41709863e+20 1.37251828e+22 1.37877969e+21 6.12601162e+21\n 1.18507340e-10 1.32650084e+21]\n"
]
]
] | [
"code"
] | [
[
"code",
"code",
"code",
"code",
"code",
"code",
"code"
]
] |
e7829cd3b9b94d120fb927d78adcfa0564593dcb | 395,671 | ipynb | Jupyter Notebook | Capstone/Starbucks_Capstone_notebook.ipynb | mahajan-abhay/Nanodegree | 79ca136b13f355dd426572f6b90e15c5887c9df4 | [
"MIT"
] | null | null | null | Capstone/Starbucks_Capstone_notebook.ipynb | mahajan-abhay/Nanodegree | 79ca136b13f355dd426572f6b90e15c5887c9df4 | [
"MIT"
] | null | null | null | Capstone/Starbucks_Capstone_notebook.ipynb | mahajan-abhay/Nanodegree | 79ca136b13f355dd426572f6b90e15c5887c9df4 | [
"MIT"
] | null | null | null | 54.794488 | 17,340 | 0.588676 | [
[
[
"# Starbucks Capstone Challenge\n\n### Introduction\n\nThis data set contains simulated data that mimics customer behavior on the Starbucks rewards mobile app. Once every few days, Starbucks sends out an offer to users of the mobile app. An offer can be merely an advertisement for a drink or an actual offer such as a discount or BOGO (buy one get one free). Some users might not receive any offer during certain weeks. \n\nNot all users receive the same offer, and that is the challenge to solve with this data set.\n\nYour task is to combine transaction, demographic and offer data to determine which demographic groups respond best to which offer type. This data set is a simplified version of the real Starbucks app because the underlying simulator only has one product whereas Starbucks actually sells dozens of products.\n\nEvery offer has a validity period before the offer expires. As an example, a BOGO offer might be valid for only 5 days. You'll see in the data set that informational offers have a validity period even though these ads are merely providing information about a product; for example, if an informational offer has 7 days of validity, you can assume the customer is feeling the influence of the offer for 7 days after receiving the advertisement.\n\nYou'll be given transactional data showing user purchases made on the app including the timestamp of purchase and the amount of money spent on a purchase. This transactional data also has a record for each offer that a user receives as well as a record for when a user actually views the offer. There are also records for when a user completes an offer. \n\nKeep in mind as well that someone using the app might make a purchase through the app without having received an offer or seen an offer.\n\n### Example\n\nTo give an example, a user could receive a discount offer buy 10 dollars get 2 off on Monday. The offer is valid for 10 days from receipt. If the customer accumulates at least 10 dollars in purchases during the validity period, the customer completes the offer.\n\nHowever, there are a few things to watch out for in this data set. Customers do not opt into the offers that they receive; in other words, a user can receive an offer, never actually view the offer, and still complete the offer. For example, a user might receive the \"buy 10 dollars get 2 dollars off offer\", but the user never opens the offer during the 10 day validity period. The customer spends 15 dollars during those ten days. There will be an offer completion record in the data set; however, the customer was not influenced by the offer because the customer never viewed the offer.\n\n### Cleaning\n\nThis makes data cleaning especially important and tricky.\n\nYou'll also want to take into account that some demographic groups will make purchases even if they don't receive an offer. From a business perspective, if a customer is going to make a 10 dollar purchase without an offer anyway, you wouldn't want to send a buy 10 dollars get 2 dollars off offer. You'll want to try to assess what a certain demographic group will buy when not receiving any offers.\n\n### Final Advice\n\nBecause this is a capstone project, you are free to analyze the data any way you see fit. For example, you could build a machine learning model that predicts how much someone will spend based on demographics and offer type. Or you could build a model that predicts whether or not someone will respond to an offer. Or, you don't need to build a machine learning model at all. You could develop a set of heuristics that determine what offer you should send to each customer (i.e., 75 percent of women customers who were 35 years old responded to offer A vs 40 percent from the same demographic to offer B, so send offer A).",
"_____no_output_____"
],
[
"# Data Sets\n\nThe data is contained in three files:\n\n* portfolio.json - containing offer ids and meta data about each offer (duration, type, etc.)\n* profile.json - demographic data for each customer\n* transcript.json - records for transactions, offers received, offers viewed, and offers completed\n\nHere is the schema and explanation of each variable in the files:\n\n**portfolio.json**\n* id (string) - offer id\n* offer_type (string) - type of offer ie BOGO, discount, informational\n* difficulty (int) - minimum required spend to complete an offer\n* reward (int) - reward given for completing an offer\n* duration (int) - time for offer to be open, in days\n* channels (list of strings)\n\n**profile.json**\n* age (int) - age of the customer \n* became_member_on (int) - date when customer created an app account\n* gender (str) - gender of the customer (note some entries contain 'O' for other rather than M or F)\n* id (str) - customer id\n* income (float) - customer's income\n\n**transcript.json**\n* event (str) - record description (ie transaction, offer received, offer viewed, etc.)\n* person (str) - customer id\n* time (int) - time in hours since start of test. The data begins at time t=0\n* value - (dict of strings) - either an offer id or transaction amount depending on the record\n\n**Note:** If you are using the workspace, you will need to go to the terminal and run the command `conda update pandas` before reading in the files. This is because the version of pandas in the workspace cannot read in the transcript.json file correctly, but the newest version of pandas can. You can access the termnal from the orange icon in the top left of this notebook. \n\nYou can see how to access the terminal and how the install works using the two images below. First you need to access the terminal:\n\n<img src=\"pic1.png\"/>\n\nThen you will want to run the above command:\n\n<img src=\"pic2.png\"/>\n\nFinally, when you enter back into the notebook (use the jupyter icon again), you should be able to run the below cell without any errors.",
"_____no_output_____"
]
],
[
[
"import pandas as pd\nimport numpy as np\nimport math\nimport json\n% matplotlib inline\n\n# read in the json files\nportfolio = pd.read_json('data/portfolio.json', orient='records', lines=True)\nprofile = pd.read_json('data/profile.json', orient='records', lines=True)\ntranscript = pd.read_json('data/transcript.json', orient='records', lines=True)",
"_____no_output_____"
]
],
[
[
"### Reading The Datasets",
"_____no_output_____"
]
],
[
[
"portfolio.head(10)",
"_____no_output_____"
],
[
"portfolio.shape[0]",
"_____no_output_____"
],
[
"portfolio.shape[1]",
"_____no_output_____"
],
[
"print('portfolio: rows = {} ,columns = {}'.format((portfolio.shape[0]),(portfolio.shape[1])))",
"portfolio: rows = 10 ,columns = 6\n"
],
[
"portfolio.describe()",
"_____no_output_____"
],
[
"portfolio.info()",
"<class 'pandas.core.frame.DataFrame'>\nRangeIndex: 10 entries, 0 to 9\nData columns (total 6 columns):\nchannels 10 non-null object\ndifficulty 10 non-null int64\nduration 10 non-null int64\nid 10 non-null object\noffer_type 10 non-null object\nreward 10 non-null int64\ndtypes: int64(3), object(3)\nmemory usage: 560.0+ bytes\n"
],
[
"portfolio.offer_type.value_counts()",
"_____no_output_____"
],
[
"portfolio.reward.value_counts()",
"_____no_output_____"
],
[
"import matplotlib.pyplot as plt\nplt.figure(figsize=[6,6])\nfig, ax = plt.subplots() \ny_counts = portfolio['offer_type'].value_counts()\ny_counts.plot(kind='barh').invert_yaxis()\n \nfor i, v in enumerate(y_counts):\n ax.text(v, i, str(v), fontsize=14)\n plt.title('Different offer types')",
"_____no_output_____"
]
],
[
[
"Discount and bogo are equally given and on maximum times",
"_____no_output_____"
]
],
[
[
"plt.figure(figsize=[6,6])\nfig, ax = plt.subplots() \ny_counts = portfolio['duration'].value_counts()\ny_counts.plot(kind='barh').invert_yaxis()\n \nfor i, v in enumerate(y_counts):\n ax.text(v, i, str(v), color='black', fontsize=14)\n plt.title('Different offer types\\' duartion')",
"_____no_output_____"
]
],
[
[
"Here we can see that most of the offers are for the duration of 7 days",
"_____no_output_____"
],
[
"### Profile",
"_____no_output_____"
]
],
[
[
"profile.head(8)",
"_____no_output_____"
],
[
"print('profile: rows = {} ,columns = {}'.format((profile.shape[0]),(profile.shape[1])))",
"profile: rows = 17000 ,columns = 5\n"
],
[
"profile.describe()",
"_____no_output_____"
],
[
"profile.isnull().sum()\nprofile.shape",
"_____no_output_____"
],
[
"import seaborn as sns",
"_____no_output_____"
],
[
" plt.figure(figsize=[6,6])\n fig, ax = plt.subplots() \n y_counts = profile['gender'].value_counts()\n y_counts.plot(kind='barh').invert_yaxis()\n \n for i, v in enumerate(y_counts):\n ax.text(v, i, str(v), color='black', fontsize=14)\n plt.title('Count of Genders')",
"_____no_output_____"
],
[
"plt.pie(profile['gender'].value_counts() , labels = ['Male' , 'Female' , 'Other'])",
"_____no_output_____"
]
],
[
[
"Mostly male are interested in the offers and they are the major ones",
"_____no_output_____"
],
[
"### Transcript",
"_____no_output_____"
]
],
[
[
"transcript.head(9)",
"_____no_output_____"
],
[
"transcript.describe()",
"_____no_output_____"
],
[
"transcript.info()",
"<class 'pandas.core.frame.DataFrame'>\nRangeIndex: 306534 entries, 0 to 306533\nData columns (total 4 columns):\nevent 306534 non-null object\nperson 306534 non-null object\ntime 306534 non-null int64\nvalue 306534 non-null object\ndtypes: int64(1), object(3)\nmemory usage: 9.4+ MB\n"
],
[
"print('transcript: rows = {} ,columns = {}'.format((profile.shape[0]),(profile.shape[1])))",
"transcript: rows = 17000 ,columns = 5\n"
]
],
[
[
"### Cleaning The Datasets",
"_____no_output_____"
],
[
"#### Portfolio\n\nRenaming 'id' to 'offer_id' ",
"_____no_output_____"
]
],
[
[
"portfolio.columns = ['channels', 'difficulty', 'duration', 'offer_id', 'offer_type', 'reward']",
"_____no_output_____"
],
[
"portfolio.columns",
"_____no_output_____"
],
[
"portfolio.head()",
"_____no_output_____"
]
],
[
[
"# Profile\n\nRenaming 'id' to 'customer_id' , filling the missing values of age and income with mean value , filling the missing values of gender with mode",
"_____no_output_____"
]
],
[
[
"profile.columns",
"_____no_output_____"
],
[
"profile.columns = ['age', 'became_member_on', 'gender', 'customer_id', 'income']",
"_____no_output_____"
],
[
"profile.columns",
"_____no_output_____"
],
[
"profile['age'].fillna(profile['age'].mean()) #filling missing age with average age\nprofile['income'].fillna(profile['income'].mean()) #filling missing income with average income\nprofile['gender'].fillna(profile['gender'].mode()[0]) #filling missing gender with the most occuring gender\nprofile.head()",
"_____no_output_____"
],
[
"profile.isnull().sum()",
"_____no_output_____"
]
],
[
[
"So there is not any missing value remaining in the profile dataframe",
"_____no_output_____"
],
[
"# Transcript\n\nRenaming 'person' to 'customer_id' , splitting the 'value' column based on its keys and\ndropping the unnecessary columns",
"_____no_output_____"
]
],
[
[
"transcript.columns",
"_____no_output_____"
],
[
"transcript.columns = ['event', 'customer_id', 'time', 'value'] #changing the column name",
"_____no_output_____"
],
[
"transcript.head()",
"_____no_output_____"
],
[
"transcript.value.astype('str').value_counts().to_dict() #converting the values in the column 'value' to dictionary",
"_____no_output_____"
],
[
"transcript['offer_id'] = transcript.value.apply(lambda x: x.get('offer_id')) #splitting the 'value' into separate columns.here is 'offer_id'\ntranscript['offer id'] = transcript.value.apply(lambda x: x.get('offer id')) #splitting the 'value' into separate columns.here is 'offer id'\ntranscript['offer_id'] = transcript.apply(lambda x : x['offer id'] if x['offer_id'] == None else x['offer_id'], axis=1) #merging both 'offer id' and 'offer_id' into the same column 'offer_id'\ntranscript.drop('offer id',axis = 1,inplace = True)\n",
"_____no_output_____"
],
[
"transcript.head(10)",
"_____no_output_____"
],
[
"#splitting the reward and amount values in the 'value'\ntranscript['offer_reward'] = transcript['value'].apply(lambda x: x.get('reward'))\ntranscript['amount'] = transcript['value'].apply(lambda x: x.get('amount'))",
"_____no_output_____"
],
[
"transcript.drop('value' ,inplace = True , axis = 1)",
"_____no_output_____"
],
[
"transcript.isnull().sum()",
"_____no_output_____"
],
[
"transcript.fillna(0 , inplace = True) #filling the missing values with 0",
"_____no_output_____"
],
[
"transcript.head(10)",
"_____no_output_____"
]
],
[
[
"### Exploratory Data Analysis\n### Now we will merge the dataframes ",
"_____no_output_____"
]
],
[
[
"merge_df = pd.merge(portfolio, transcript, on='offer_id')#merging portfolio and transcript dataframes on the basis of 'offer_id'\nfinal_df = pd.merge(merge_df, profile, on='customer_id')#merging the merged dataframe of portfolio and transcript with profile dataframe on the basis of 'customer-id'",
"_____no_output_____"
],
[
"#Exploring the final merged dataframe\nfinal_df",
"_____no_output_____"
]
],
[
[
"### Now we will see the different offer types and their counts",
"_____no_output_____"
]
],
[
[
"final_df['offer_type'].value_counts().plot.barh(title = 'Offer types with their counts')",
"_____no_output_____"
]
],
[
[
"So,we can see that discount and bogo are thr most given offer types",
"_____no_output_____"
],
[
"### Now we will see the different events and their counts",
"_____no_output_____"
]
],
[
[
"final_df['event'].value_counts().plot.barh(title = 'Different events and their counts')",
"_____no_output_____"
]
],
[
[
"So,in most of the cases offer is received by the user and it is not completed by him/her,means most of the people just ignore the offers they receive",
"_____no_output_____"
],
[
"### Now we will analyse this data on the basis of the age of the customers ",
"_____no_output_____"
]
],
[
[
"sns.distplot(final_df['age'] , bins = 50 , hist_kws = {'alpha' : 0.4});",
"_____no_output_____"
]
],
[
[
"As we can see that the people after the age of 100 are just acting as outliers,so we will remove them",
"_____no_output_____"
]
],
[
[
"final_df = final_df[final_df['age']<=100] ",
"_____no_output_____"
],
[
"# Now seeing the distortion plot of age\nsns.distplot(final_df['age'] , bins = 50 , hist_kws = {'alpha' : 0.4});",
"_____no_output_____"
]
],
[
[
"We can observe that most of the customers are within the age group of 45-60 are the most frequent customers and more than any other group,this is quite interesting.",
"_____no_output_____"
],
[
"### Now,we will analyse this data on the basis of income of the customers ",
"_____no_output_____"
]
],
[
[
"sns.distplot(final_df['income'] , bins = 50 , hist_kws = {'alpha' : 0.4});",
"_____no_output_____"
],
[
"final_df['income'].mean()",
"_____no_output_____"
]
],
[
[
"Now we can see that most people who are the customers of Starbucks have their income within the range of 55k - 75k with a mean income of 66413.35",
"_____no_output_____"
],
[
"### Now,we will see how our final dataframe is depedent on the 'gender' feature",
"_____no_output_____"
]
],
[
[
"final_df['gender'].value_counts().plot.barh(title = 'Analysing the gender of customers')",
"_____no_output_____"
]
],
[
[
"So,we can see that most of the customers are male",
"_____no_output_____"
],
[
"### We will analyse the dataframe on the basis of 'offer_type' on the basis of gender",
"_____no_output_____"
]
],
[
[
"sns.countplot(x = 'offer_type' , hue = 'gender' , data = final_df)",
"_____no_output_____"
]
],
[
[
"We can see that the count of gender weather it is male or female is approximately equal in the bogo and discount offers",
"_____no_output_____"
],
[
"### Now,we will see the relation between gender and events ",
"_____no_output_____"
]
],
[
[
"sns.countplot(x = 'event' , hue = 'gender' , data = final_df)",
"_____no_output_____"
]
],
[
[
"So,from the exploratory data analysis we can see that most of the customers just receive the offers and they do not view them and the people who complete the offers they receive is quite less and most of the offers made by Starbuks are BOGO and Discount and most of the people that are the customers are within the age group of 45-60 and the most common gender is male and the people who are the customers of Starbucks have their income within the range of 55k - 75k",
"_____no_output_____"
],
[
"# Making a Machine Learning Model",
"_____no_output_____"
],
[
"First analysing our final dataset",
"_____no_output_____"
]
],
[
[
"final_df",
"_____no_output_____"
]
],
[
[
"#### We will now encode the categorical features like 'offer_type' , 'gender' , 'age'\n#### We will encode the offer_id and customer_id",
"_____no_output_____"
]
],
[
[
"final_df = pd.get_dummies(final_df , columns = ['offer_type' , 'gender' , 'age'])\n#processing offer_id \noffer_id = final_df['offer_id'].unique().tolist()\noffer_map = dict( zip(offer_id,range(len(offer_id))) )\nfinal_df.replace({'offer_id': offer_map},inplace=True)\n\n#processing customer_id \ncustomer_id = final_df['customer_id'].unique().tolist()\ncustomer_map = dict( zip(customer_id,range(len(customer_id))) )\nfinal_df.replace({'customer_id': customer_map},inplace=True)",
"_____no_output_____"
],
[
"final_df.head()",
"_____no_output_____"
]
],
[
[
"#### Now we will scale the numerical data including 'income' , 'difficulty' , 'duration' and many more... ",
"_____no_output_____"
]
],
[
[
"from sklearn.preprocessing import MinMaxScaler\nscaler = MinMaxScaler()\nnumerical_columns = ['income' , 'difficulty' , 'duration' , 'offer_reward' , 'time' , 'reward' , 'amount']\nfinal_df[numerical_columns] = scaler.fit_transform(final_df[numerical_columns])",
"_____no_output_____"
],
[
"final_df.head()",
"_____no_output_____"
]
],
[
[
"#### We will encode the values in the 'event' column ",
"_____no_output_____"
]
],
[
[
"final_df['event'] = final_df['event'].map({'offer received':1, 'offer viewed':2, 'offer completed':3})\nfinal_df2 = final_df.drop('event' , axis = 1)",
"_____no_output_____"
]
],
[
[
"#### Now encoding the channels column",
"_____no_output_____"
]
],
[
[
"final_df2['web'] = final_df2['channels'].apply(lambda x : 1 if 'web' in x else 0)\nfinal_df2['mobile'] = final_df2['channels'].apply(lambda x : 1 if 'mobile' in x else 0)\nfinal_df2['social'] = final_df2['channels'].apply(lambda x : 1 if 'social' in x else 0)\nfinal_df2['email'] = final_df2['channels'].apply(lambda x : 1 if 'email' in x else 0)",
"_____no_output_____"
],
[
"#Now dropping the Channels column\nfinal_df2.drop('channels' , axis = 1 , inplace = True)",
"_____no_output_____"
],
[
"final_df2['became_member_on'] = final_df2['became_member_on'].apply(lambda x: pd.to_datetime(str(x), format='%Y%m%d'))\n#adding new columns for month & year\nfinal_df2['month_member'] = final_df2['became_member_on'].apply(lambda x: x.day)\nfinal_df2['year_member'] = final_df2['became_member_on'].apply(lambda x: x.year)\n#dropping the became_member_on column\nfinal_df2.drop('became_member_on',axis=1, inplace=True)",
"_____no_output_____"
],
[
"final_df2.shape",
"_____no_output_____"
]
],
[
[
"# Training Our Dataset ",
"_____no_output_____"
],
[
"### Now splitting our 'final_df' into training and test set ",
"_____no_output_____"
]
],
[
[
"independent_variables = final_df2 #our dataset containing all the independent variables excluding the 'event'\ndependent_variable = final_df['event'] #our final dataset containing the 'event'",
"_____no_output_____"
],
[
"from sklearn.model_selection import train_test_split\n# splitting our dataset into training and test set and the test set being the 30% of the total dataset\nx_train , x_test, y_train , y_test = train_test_split(independent_variables , dependent_variable , test_size = 0.3 , random_state = 1)",
"_____no_output_____"
],
[
"x_train.shape",
"_____no_output_____"
],
[
"x_test.shape",
"_____no_output_____"
]
],
[
[
"# Testing Our Dataset",
"_____no_output_____"
]
],
[
[
"# We will implement a number of classification machine learning methods and will determine which method is best for our model\nfrom sklearn.neighbors import KNeighborsClassifier\nfrom sklearn.linear_model import LogisticRegression\nfrom sklearn.ensemble import RandomForestClassifier\nfrom sklearn.tree import DecisionTreeClassifier",
"_____no_output_____"
],
[
"#We will test the quality of the predicted output on a number of metrics,i.e. accuracy score,f1 score\n#We will use f1 score because it considers the class imbalance pretty well as compared to the accuracy score and is the best metric to evaluate our this model\nfrom sklearn.metrics import confusion_matrix , accuracy_score , fbeta_score\ndef train_test_f1(model):\n \"\"\"\n Returns the F1 score of training and test set of any particular model\n model : model name\n Returns\n f1_score_train : F1 score of training set\n f1_score_test : F1 score of test set\n \"\"\"\n predict_train = (model.fit(x_train , y_train)).predict(x_train)\n predict_test = (model.fit(x_train , y_train)).predict(x_test)\n f1_score_train = fbeta_score(y_train , predict_train , beta = 0.5 , average = 'micro')*100\n f1_score_test = fbeta_score(y_test , predict_test , beta = 0.5 , average = 'micro')*100\n return f1_score_train , f1_score_test",
"_____no_output_____"
]
],
[
[
"### Implementing the KNN Model ",
"_____no_output_____"
]
],
[
[
"knn = KNeighborsClassifier()\nf1_score_train_knn , f1_score_test_knn = train_test_f1(knn)#calculating the F1 scores",
"_____no_output_____"
]
],
[
[
"### Implementing the Logistic Regression",
"_____no_output_____"
]
],
[
[
"logistic = LogisticRegression()\nf1_score_train_logistic , f1_score_test_logistic = train_test_f1(logistic)#calculating the F1 scores",
"_____no_output_____"
]
],
[
[
"### Implementing the Random Forest Classifier\n",
"_____no_output_____"
]
],
[
[
"random_forest = RandomForestClassifier()\nf1_score_train_random , f1_score_test_random = train_test_f1(random_forest)#calculating the F1 scores",
"_____no_output_____"
]
],
[
[
"### Implementing the Decision Tree Classifier ",
"_____no_output_____"
]
],
[
[
"decision_tree = DecisionTreeClassifier()\nf1_score_train_decision , f1_score_test_decision = train_test_f1(decision_tree)#calculating the F1 scores",
"_____no_output_____"
]
],
[
[
"# Concluding from the above models and scores",
"_____no_output_____"
]
],
[
[
"f1_scores_models = {'model_name' : [knn.__class__.__name__ , logistic.__class__.__name__ , random_forest.__class__.__name__ , decision_tree.__class__.__name__] \n , 'Training set F1 Score' : [f1_score_train_knn , f1_score_train_logistic , f1_score_train_random , f1_score_train_decision],\n 'Test set F1 Score' : [f1_score_test_knn , f1_score_test_logistic , f1_score_test_random , f1_score_test_decision]}\nf1_scores_df = pd.DataFrame(f1_scores_models)",
"_____no_output_____"
],
[
"f1_scores_df",
"_____no_output_____"
]
],
[
[
"So,from the above dataframe we can conclude that when we trained our training dataset according to the KNeighborsClassifier our model performed worst,on training the model on RandomForestClassifier ,the training set F1 score is quite good i.e. 93.58 but it performed badly on the test set with a F1 score of 64.266 and when we trained our model on DecisionTreeClassifier our model's performance was best with the training set F1 score of 94.89 and the test set F1 score of 86.02,which means our model was able to classify between the events of offers upto a great extent.As this is a practical case study with real world dataset,we can say that our model has performed successfully.",
"_____no_output_____"
]
]
] | [
"markdown",
"code",
"markdown",
"code",
"markdown",
"code",
"markdown",
"code",
"markdown",
"code",
"markdown",
"code",
"markdown",
"code",
"markdown",
"code",
"markdown",
"code",
"markdown",
"code",
"markdown",
"code",
"markdown",
"code",
"markdown",
"code",
"markdown",
"code",
"markdown",
"code",
"markdown",
"code",
"markdown",
"code",
"markdown",
"code",
"markdown",
"code",
"markdown",
"code",
"markdown",
"code",
"markdown",
"code",
"markdown",
"code",
"markdown",
"code",
"markdown",
"code",
"markdown",
"code",
"markdown",
"code",
"markdown",
"code",
"markdown",
"code",
"markdown"
] | [
[
"markdown",
"markdown"
],
[
"code"
],
[
"markdown"
],
[
"code",
"code",
"code",
"code",
"code",
"code",
"code",
"code",
"code"
],
[
"markdown"
],
[
"code"
],
[
"markdown",
"markdown"
],
[
"code",
"code",
"code",
"code",
"code",
"code",
"code"
],
[
"markdown",
"markdown"
],
[
"code",
"code",
"code",
"code"
],
[
"markdown",
"markdown"
],
[
"code",
"code",
"code"
],
[
"markdown"
],
[
"code",
"code",
"code",
"code",
"code"
],
[
"markdown",
"markdown"
],
[
"code",
"code",
"code",
"code",
"code",
"code",
"code",
"code",
"code",
"code",
"code"
],
[
"markdown"
],
[
"code",
"code"
],
[
"markdown"
],
[
"code"
],
[
"markdown",
"markdown"
],
[
"code"
],
[
"markdown",
"markdown"
],
[
"code"
],
[
"markdown"
],
[
"code",
"code"
],
[
"markdown",
"markdown"
],
[
"code",
"code"
],
[
"markdown",
"markdown"
],
[
"code"
],
[
"markdown",
"markdown"
],
[
"code"
],
[
"markdown",
"markdown"
],
[
"code"
],
[
"markdown",
"markdown",
"markdown"
],
[
"code"
],
[
"markdown"
],
[
"code",
"code"
],
[
"markdown"
],
[
"code",
"code"
],
[
"markdown"
],
[
"code"
],
[
"markdown"
],
[
"code",
"code",
"code",
"code"
],
[
"markdown",
"markdown"
],
[
"code",
"code",
"code",
"code"
],
[
"markdown"
],
[
"code",
"code"
],
[
"markdown"
],
[
"code"
],
[
"markdown"
],
[
"code"
],
[
"markdown"
],
[
"code"
],
[
"markdown"
],
[
"code"
],
[
"markdown"
],
[
"code",
"code"
],
[
"markdown"
]
] |
e782adf19da9ae7faab5a3ad6b363c2a95955616 | 7,437 | ipynb | Jupyter Notebook | .ipynb_checkpoints/my_test-checkpoint.ipynb | jasperhyp/Chemprop4SE | c02b604b63b6766464db829fea0b306c67302e82 | [
"MIT"
] | 1 | 2021-12-15T05:18:07.000Z | 2021-12-15T05:18:07.000Z | .ipynb_checkpoints/my_test-checkpoint.ipynb | jasperhyp/chemprop4SE | c02b604b63b6766464db829fea0b306c67302e82 | [
"MIT"
] | null | null | null | .ipynb_checkpoints/my_test-checkpoint.ipynb | jasperhyp/chemprop4SE | c02b604b63b6766464db829fea0b306c67302e82 | [
"MIT"
] | null | null | null | 29.279528 | 121 | 0.524136 | [
[
[
"from typing import Callable, List, Union\n\nimport numpy as np\n\nfrom rdkit import Chem, DataStructs\nfrom rdkit.Chem import AllChem\n",
"_____no_output_____"
],
[
"Molecule = Union[str, Chem.Mol]\nFeaturesGenerator = Callable[[Molecule], np.ndarray]",
"_____no_output_____"
],
[
"len(a)",
"_____no_output_____"
],
[
"import pandas as pd\na = pd.read_csv(\"./data/mono.csv\")\nb = dict([(a['smiles'][i], a.iloc[i].values[1:]) for i in range(len(a))])\n",
"_____no_output_____"
],
[
"import csv\nfrom tqdm import tqdm\n\nwith open(path:=\"./data/test_random.csv\") as f:\n reader = csv.DictReader(f)\n if target_columns is None:\n target_columns = get_task_names(\n path=path,\n smiles_columns=smiles_columns,\n target_columns=target_columns,\n ignore_columns=ignore_columns,\n )\n\n all_smiles, all_targets, all_rows, all_features, all_phase_features, all_weights = [], [], [], [], [], []\n for i, row in enumerate(tqdm(reader)):\n smiles = [row[c] for c in ['smiles_1', 'smiles_2']]\n\n targets = [float(row[column]) if row[column] not in ['','nan'] else None for column in target_columns]\n\n # Check whether all targets are None and skip if so\n if skip_none_targets and all(x is None for x in targets):\n continue\n\n all_smiles.append(smiles)\n all_targets.append(targets)\n\n if features_data is not None:\n all_features.append(features_data[i])\n\n if phase_features is not None:\n all_phase_features.append(phase_features[i])\n\n if data_weights is not None:\n all_weights.append(data_weights[i])\n\n if store_row:\n all_rows.append(row)\n\n if len(all_smiles) >= max_data_size:\n break\n\n atom_features = None\n atom_descriptors = None\n if args is not None and args.atom_descriptors is not None:\n try:\n descriptors = load_valid_atom_or_bond_features(atom_descriptors_path, [x[0] for x in all_smiles])\n except Exception as e:\n raise ValueError(f'Failed to load or validate custom atomic descriptors or features: {e}')\n\n if args.atom_descriptors == 'feature':\n atom_features = descriptors\n elif args.atom_descriptors == 'descriptor':\n atom_descriptors = descriptors\n\n bond_features = None\n if args is not None and args.bond_features_path is not None:\n try:\n bond_features = load_valid_atom_or_bond_features(bond_features_path, [x[0] for x in all_smiles])\n except Exception as e:\n raise ValueError(f'Failed to load or validate custom bond features: {e}')\n\n data = MoleculeDataset([\n MoleculeDatapoint(\n smiles=smiles,\n targets=targets,\n row=all_rows[i] if store_row else None,\n data_weight=all_weights[i] if data_weights is not None else 1.,\n features_generator=features_generator,\n features=all_features[i] if features_data is not None else None,\n phase_features=all_phase_features[i] if phase_features is not None else None,\n atom_features=atom_features[i] if atom_features is not None else None,\n atom_descriptors=atom_descriptors[i] if atom_descriptors is not None else None,\n bond_features=bond_features[i] if bond_features is not None else None,\n overwrite_default_atom_features=args.overwrite_default_atom_features if args is not None else False,\n overwrite_default_bond_features=args.overwrite_default_bond_features if args is not None else False\n ) for i, (smiles, targets) in tqdm(enumerate(zip(all_smiles, all_targets)),\n total=len(all_smiles))\n ])",
"6347it [00:00, 9920.20it/s]\n"
],
[
"a=\"C[C@@H]1C[C@H]2C3CCC4=CC(=O)C=C[C@@]4([C@]3([C@H](C[C@@]2([C@]1(C(=O)CCl)O)C)O)Cl)C\"\na.split(\">\")[0]",
"_____no_output_____"
],
[
"import torch",
"_____no_output_____"
],
[
"features_batch=[[1,2],[3,4],[5,6]]\ntorch.from_numpy(np.stack(features_batch)).float()",
"_____no_output_____"
]
]
] | [
"code"
] | [
[
"code",
"code",
"code",
"code",
"code",
"code",
"code",
"code"
]
] |
e782b6cfcd16b6cbe5bffd67e5442e03bd1eaffb | 70,486 | ipynb | Jupyter Notebook | _build/html/_sources/notebooks/03/03.06-Unit-Commitment.ipynb | leonlan/MO-book | 9802bac37e6024b5c18fadefb27a16e47e8e75a1 | [
"MIT"
] | null | null | null | _build/html/_sources/notebooks/03/03.06-Unit-Commitment.ipynb | leonlan/MO-book | 9802bac37e6024b5c18fadefb27a16e47e8e75a1 | [
"MIT"
] | null | null | null | _build/html/_sources/notebooks/03/03.06-Unit-Commitment.ipynb | leonlan/MO-book | 9802bac37e6024b5c18fadefb27a16e47e8e75a1 | [
"MIT"
] | null | null | null | 123.012216 | 24,528 | 0.823171 | [
[
[
"# Unit Commitment\n\nKeywords: semi-continuous variables, cbc usage, gdp, disjunctive programming",
"_____no_output_____"
],
[
"## Imports",
"_____no_output_____"
]
],
[
[
"%matplotlib inline\nimport matplotlib.pyplot as plt\nimport numpy as np\nimport pandas as pd\nfrom IPython.display import display, HTML\n\nimport shutil\nimport sys\nimport os.path\n\nif not shutil.which(\"pyomo\"):\n !pip install -q pyomo\n assert(shutil.which(\"pyomo\"))\n\nif not (shutil.which(\"cbc\") or os.path.isfile(\"cbc\")):\n if \"google.colab\" in sys.modules:\n !apt-get install -y -qq coinor-cbc\n else:\n try:\n !conda install -c conda-forge coincbc \n except:\n pass\n\nassert(shutil.which(\"cbc\") or os.path.isfile(\"cbc\"))\nimport pyomo.environ as pyo\nimport pyomo.gdp as gdp",
"_____no_output_____"
]
],
[
[
"## Problem statement\n\nA set of $N$ electrical generating units are available to meet a required demand $d_t$ for time period $t \\in 1, 2, \\ldots, T$. The power generated by unit $n$ for time period $t$ is denoted $x_{n,t}$. Each generating unit is either off, $x_{n,t} = 0$ or else operating in a range $[p_n^{min}, p_n^{max}]$. The incremental cost of operating the generator during period $t$ is $a_n x_{n,t} + b_n$. A binary variable variable $u_{n,t}$ indicates the operational state of a generating unit. \n\nThe unit commmitment problem is then\n\n\\begin{align*}\n\\min \\sum_{n\\in N} \\sum_{t\\in T} a_n x_{n,t} + b_n u_{n,t}\n\\end{align*}\n\nsubject to\n\n\\begin{align*}\n\\sum_{n\\in N} x_{n,t} & = d_t \\qquad \\forall t \\in T \\\\\np_{n}^{min}u_{n,t} & \\leq x_{n,t} \\qquad \\forall n \\in N, \\ \\forall t \\in T \\\\\np_{n}^{max}u_{n,t} & \\geq x_{n,t} \\qquad \\forall n \\in N, \\ \\forall t \\in T \\\\\n\\end{align*}\n\nwhere we use the short-cut notation $T = [1, 2, \\ldots T]$ and $N = [1, 2, \\ldots, N]$.\n\nThis is a minimal model. A realistic model would include additional constraints corresponding to minimum up and down times for generating units, limits on the rate at which power levels can change, maintenance periods, and so forth.\n\n* Sun, Xiaoling, Xiaojin Zheng, and Duan Li. [\"Recent advances in mathematical programming with semi-continuous variables and cardinality constraint.\"](https://link.springer.com/article/10.1007/s40305-013-0004-0) Journal of the Operations Research Society of China 1, no. 1 (2013): 55-77.",
"_____no_output_____"
],
[
"## Model",
"_____no_output_____"
],
[
"### Demand",
"_____no_output_____"
]
],
[
[
"# demand\nT = 20\nT = np.array([t for t in range(0, T)])\nd = np.array([100 + 100*np.random.uniform() for t in T])\n\nfig, ax = plt.subplots(1,1)\nax.bar(T+1, d)\nax.set_xlabel('Time Period')\nax.set_title('Demand')",
"_____no_output_____"
]
],
[
[
"### Generating Units",
"_____no_output_____"
]
],
[
[
"# generating units\nN = 5\npmax = 2*max(d)/N\npmin = 0.6*pmax\n\nN = np.array([n for n in range(0, N)])\na = np.array([0.5 + 0.2*np.random.randn() for n in N])\nb = np.array([10*np.random.uniform() for n in N])\n\np = np.linspace(pmin, pmax)\n\nfig, ax = plt.subplots(1,1)\nfor n in N:\n ax.plot(p, a[n]*p + b[n])\nax.set_xlim(0, pmax)\nax.set_ylim(0, max(a*pmax + b))\nax.set_xlabel('Unit Production')\nax.set_ylabel('Unit Operating Cost')\nax.grid()",
"_____no_output_____"
]
],
[
[
"### Pyomo model 1: Conventional implementation for emi-continuous variables",
"_____no_output_____"
]
],
[
[
"def unit_commitment():\n m = pyo.ConcreteModel()\n\n m.N = pyo.Set(initialize=N)\n m.T = pyo.Set(initialize=T)\n\n m.x = pyo.Var(m.N, m.T, bounds = (0, pmax))\n m.u = pyo.Var(m.N, m.T, domain=pyo.Binary)\n \n # objective\n m.cost = pyo.Objective(expr = sum(m.x[n,t]*a[n] + m.u[n,t]*b[n] for t in m.T for n in m.N), sense=pyo.minimize)\n \n # demand\n m.demand = pyo.Constraint(m.T, rule=lambda m, t: sum(m.x[n,t] for n in N) == d[t])\n \n # semi-continuous\n m.lb = pyo.Constraint(m.N, m.T, rule=lambda m, n, t: pmin*m.u[n,t] <= m.x[n,t])\n m.ub = pyo.Constraint(m.N, m.T, rule=lambda m, n, t: pmax*m.u[n,t] >= m.x[n,t])\n return m\n \nm = unit_commitment()\npyo.SolverFactory('cbc').solve(m).write()\n\nfig, ax = plt.subplots(max(N)+1, 1, figsize=(8, 1.5*max(N)+1))\nfor n in N:\n ax[n].bar(T+1, [m.x[n,t]() for t in T])\n ax[n].set_xlim(0, max(T)+2)\n ax[n].set_ylim(0, 1.1*pmax)\n ax[n].plot(ax[n].get_xlim(), np.array([pmax, pmax]), 'r--')\n ax[n].plot(ax[n].get_xlim(), np.array([pmin, pmin]), 'r--')\n ax[n].set_title('Unit ' + str(n+1))\nfig.tight_layout()",
"# ==========================================================\n# = Solver Results =\n# ==========================================================\n# ----------------------------------------------------------\n# Problem Information\n# ----------------------------------------------------------\nProblem: \n- Name: unknown\n Lower bound: 1018.71533244\n Upper bound: 1018.71533244\n Number of objectives: 1\n Number of constraints: 200\n Number of variables: 180\n Number of binary variables: 100\n Number of integer variables: 100\n Number of nonzeros: 180\n Sense: minimize\n# ----------------------------------------------------------\n# Solver Information\n# ----------------------------------------------------------\nSolver: \n- Status: ok\n User time: -1.0\n System time: 0.06\n Wallclock time: 0.06\n Termination condition: optimal\n Termination message: Model was solved to optimality (subject to tolerances), and an optimal solution is available.\n Statistics: \n Branch and bound: \n Number of bounded subproblems: 0\n Number of created subproblems: 0\n Black box: \n Number of iterations: 0\n Error rc: 0\n Time: 0.07869720458984375\n# ----------------------------------------------------------\n# Solution Information\n# ----------------------------------------------------------\nSolution: \n- number of solutions: 0\n number of solutions displayed: 0\n"
]
],
[
[
"### Pyomo model 2: GDP implementation",
"_____no_output_____"
]
],
[
[
"def unit_commitment_gdp():\n m = pyo.ConcreteModel()\n\n m.N = pyo.Set(initialize=N)\n m.T = pyo.Set(initialize=T)\n\n m.x = pyo.Var(m.N, m.T, bounds = (0, pmax))\n \n # demand\n m.demand = pyo.Constraint(m.T, rule=lambda m, t: sum(m.x[n,t] for n in N) == d[t])\n \n # representing the semicontinous variables as disjuctions\n m.sc1 = gdp.Disjunct(m.N, m.T, rule=lambda d, n, t: d.model().x[n,t] == 0)\n m.sc2 = gdp.Disjunct(m.N, m.T, rule=lambda d, n, t: d.model().x[n,t] >= pmin)\n m.sc = gdp.Disjunction(m.N, m.T, rule=lambda m, n, t: [m.sc1[n,t], m.sc2[n,t]])\n \n # objective. Note use of the disjunct indicator variable\n m.cost = pyo.Objective(expr = sum(m.x[n,t]*a[n] + m.sc2[n,t].indicator_var*b[n] for t in m.T for n in m.N), sense=pyo.minimize)\n\n # alternative formulation. But how to access the indicator variable?\n #m.semicontinuous = gdp.Disjunction(m.N, m.T, rule=lambda m, n, t: [m.x[n,t]==0, m.x[n,t] >= pmin])\n pyo.TransformationFactory('gdp.chull').apply_to(m)\n return m\n \nm_gdp = unit_commitment_gdp()\npyo.SolverFactory('cbc').solve(m_gdp).write()",
"# ==========================================================\n# = Solver Results =\n# ==========================================================\n# ----------------------------------------------------------\n# Problem Information\n# ----------------------------------------------------------\nProblem: \n- Name: unknown\n Lower bound: 863.60019688\n Upper bound: 863.60019688\n Number of objectives: 1\n Number of constraints: 20\n Number of variables: 100\n Number of binary variables: 200\n Number of integer variables: 200\n Number of nonzeros: 100\n Sense: minimize\n# ----------------------------------------------------------\n# Solver Information\n# ----------------------------------------------------------\nSolver: \n- Status: ok\n User time: -1.0\n System time: 0.02\n Wallclock time: 0.03\n Termination condition: optimal\n Termination message: Model was solved to optimality (subject to tolerances), and an optimal solution is available.\n Statistics: \n Branch and bound: \n Number of bounded subproblems: 0\n Number of created subproblems: 0\n Black box: \n Number of iterations: 0\n Error rc: 0\n Time: 0.04323315620422363\n# ----------------------------------------------------------\n# Solution Information\n# ----------------------------------------------------------\nSolution: \n- number of solutions: 0\n number of solutions displayed: 0\n"
]
],
[
[
"### There is a problem here!\n\nWhy are the results different? Somehow it appears values of the indicator variables are being ignored.",
"_____no_output_____"
]
],
[
[
"for n in N:\n for t in T:\n print(\"n = {0:2d} t = {1:2d} {2} {3} {4:5.2f}\".format(n, t, m_gdp.sc1[n,t].indicator_var(), m_gdp.sc2[n,t].indicator_var(), m.x[n,t]()))",
"n = 0 t = 0 1.0 0.0 76.13\nn = 0 t = 1 1.0 0.0 45.86\nn = 0 t = 2 1.0 0.0 45.86\nn = 0 t = 3 1.0 0.0 75.96\nn = 0 t = 4 1.0 0.0 45.86\nn = 0 t = 5 1.0 0.0 45.86\nn = 0 t = 6 1.0 0.0 45.86\nn = 0 t = 7 1.0 0.0 73.80\nn = 0 t = 8 1.0 0.0 68.79\nn = 0 t = 9 1.0 0.0 61.04\nn = 0 t = 10 1.0 0.0 47.89\nn = 0 t = 11 1.0 0.0 56.14\nn = 0 t = 12 1.0 0.0 45.86\nn = 0 t = 13 1.0 0.0 45.86\nn = 0 t = 14 1.0 0.0 45.86\nn = 0 t = 15 1.0 0.0 47.08\nn = 0 t = 16 1.0 0.0 45.86\nn = 0 t = 17 1.0 0.0 53.14\nn = 0 t = 18 1.0 0.0 45.86\nn = 0 t = 19 1.0 0.0 47.56\nn = 1 t = 0 1.0 0.0 0.00\nn = 1 t = 1 1.0 0.0 0.00\nn = 1 t = 2 1.0 0.0 0.00\nn = 1 t = 3 1.0 0.0 0.00\nn = 1 t = 4 1.0 0.0 0.00\nn = 1 t = 5 1.0 0.0 0.00\nn = 1 t = 6 1.0 0.0 0.00\nn = 1 t = 7 1.0 0.0 0.00\nn = 1 t = 8 1.0 0.0 0.00\nn = 1 t = 9 1.0 0.0 0.00\nn = 1 t = 10 1.0 0.0 0.00\nn = 1 t = 11 1.0 0.0 0.00\nn = 1 t = 12 1.0 0.0 0.00\nn = 1 t = 13 1.0 0.0 0.00\nn = 1 t = 14 1.0 0.0 0.00\nn = 1 t = 15 1.0 0.0 0.00\nn = 1 t = 16 1.0 0.0 0.00\nn = 1 t = 17 1.0 0.0 0.00\nn = 1 t = 18 1.0 0.0 0.00\nn = 1 t = 19 1.0 0.0 0.00\nn = 2 t = 0 1.0 0.0 0.00\nn = 2 t = 1 1.0 0.0 0.00\nn = 2 t = 2 1.0 0.0 0.00\nn = 2 t = 3 1.0 0.0 0.00\nn = 2 t = 4 1.0 0.0 0.00\nn = 2 t = 5 1.0 0.0 0.00\nn = 2 t = 6 1.0 0.0 0.00\nn = 2 t = 7 1.0 0.0 0.00\nn = 2 t = 8 1.0 0.0 0.00\nn = 2 t = 9 1.0 0.0 0.00\nn = 2 t = 10 1.0 0.0 0.00\nn = 2 t = 11 1.0 0.0 0.00\nn = 2 t = 12 1.0 0.0 0.00\nn = 2 t = 13 1.0 0.0 0.00\nn = 2 t = 14 1.0 0.0 0.00\nn = 2 t = 15 1.0 0.0 0.00\nn = 2 t = 16 1.0 0.0 0.00\nn = 2 t = 17 1.0 0.0 0.00\nn = 2 t = 18 1.0 0.0 0.00\nn = 2 t = 19 1.0 0.0 0.00\nn = 3 t = 0 1.0 0.0 76.43\nn = 3 t = 1 1.0 0.0 61.93\nn = 3 t = 2 1.0 0.0 64.26\nn = 3 t = 3 1.0 0.0 76.43\nn = 3 t = 4 1.0 0.0 57.86\nn = 3 t = 5 1.0 0.0 67.42\nn = 3 t = 6 1.0 0.0 65.12\nn = 3 t = 7 1.0 0.0 76.43\nn = 3 t = 8 1.0 0.0 76.43\nn = 3 t = 9 1.0 0.0 76.43\nn = 3 t = 10 1.0 0.0 76.43\nn = 3 t = 11 1.0 0.0 76.43\nn = 3 t = 12 1.0 0.0 72.97\nn = 3 t = 13 1.0 0.0 72.25\nn = 3 t = 14 1.0 0.0 72.77\nn = 3 t = 15 1.0 0.0 76.43\nn = 3 t = 16 1.0 0.0 70.91\nn = 3 t = 17 1.0 0.0 76.43\nn = 3 t = 18 1.0 0.0 72.74\nn = 3 t = 19 1.0 0.0 76.43\nn = 4 t = 0 1.0 0.0 0.00\nn = 4 t = 1 1.0 0.0 45.86\nn = 4 t = 2 1.0 0.0 45.86\nn = 4 t = 3 1.0 0.0 0.00\nn = 4 t = 4 1.0 0.0 0.00\nn = 4 t = 5 1.0 0.0 45.86\nn = 4 t = 6 1.0 0.0 45.86\nn = 4 t = 7 1.0 0.0 0.00\nn = 4 t = 8 1.0 0.0 45.86\nn = 4 t = 9 1.0 0.0 0.00\nn = 4 t = 10 1.0 0.0 0.00\nn = 4 t = 11 1.0 0.0 0.00\nn = 4 t = 12 1.0 0.0 45.86\nn = 4 t = 13 1.0 0.0 0.00\nn = 4 t = 14 1.0 0.0 0.00\nn = 4 t = 15 1.0 0.0 45.86\nn = 4 t = 16 1.0 0.0 0.00\nn = 4 t = 17 1.0 0.0 0.00\nn = 4 t = 18 1.0 0.0 45.86\nn = 4 t = 19 1.0 0.0 45.86\n"
]
]
] | [
"markdown",
"code",
"markdown",
"code",
"markdown",
"code",
"markdown",
"code",
"markdown",
"code",
"markdown",
"code"
] | [
[
"markdown",
"markdown"
],
[
"code"
],
[
"markdown",
"markdown",
"markdown"
],
[
"code"
],
[
"markdown"
],
[
"code"
],
[
"markdown"
],
[
"code"
],
[
"markdown"
],
[
"code"
],
[
"markdown"
],
[
"code"
]
] |
e782b92b6a57a221caaedc61400a11707547aa13 | 33,060 | ipynb | Jupyter Notebook | intro-to-pytorch/Part 3 - Training Neural Networks (Solution).ipynb | yangjue-han/deep-learning-v2-pytorch | 8c3b6a4c4fc6457c41a941d2f81b589c1cb25b58 | [
"MIT"
] | null | null | null | intro-to-pytorch/Part 3 - Training Neural Networks (Solution).ipynb | yangjue-han/deep-learning-v2-pytorch | 8c3b6a4c4fc6457c41a941d2f81b589c1cb25b58 | [
"MIT"
] | null | null | null | intro-to-pytorch/Part 3 - Training Neural Networks (Solution).ipynb | yangjue-han/deep-learning-v2-pytorch | 8c3b6a4c4fc6457c41a941d2f81b589c1cb25b58 | [
"MIT"
] | null | null | null | 50.090909 | 7,828 | 0.671809 | [
[
[
"# Training Neural Networks\n\nThe network we built in the previous part isn't so smart, it doesn't know anything about our handwritten digits. Neural networks with non-linear activations work like universal function approximators. There is some function that maps your input to the output. For example, images of handwritten digits to class probabilities. The power of neural networks is that we can train them to approximate this function, and basically any function given enough data and compute time.\n\n<img src=\"assets/function_approx.png\" width=500px>\n\nAt first the network is naive, it doesn't know the function mapping the inputs to the outputs. We train the network by showing it examples of real data, then adjusting the network parameters such that it approximates this function.\n\nTo find these parameters, we need to know how poorly the network is predicting the real outputs. For this we calculate a **loss function** (also called the cost), a measure of our prediction error. For example, the mean squared loss is often used in regression and binary classification problems\n\n$$\n\\large \\ell = \\frac{1}{2n}\\sum_i^n{\\left(y_i - \\hat{y}_i\\right)^2}\n$$\n\nwhere $n$ is the number of training examples, $y_i$ are the true labels, and $\\hat{y}_i$ are the predicted labels.\n\nBy minimizing this loss with respect to the network parameters, we can find configurations where the loss is at a minimum and the network is able to predict the correct labels with high accuracy. We find this minimum using a process called **gradient descent**. The gradient is the slope of the loss function and points in the direction of fastest change. To get to the minimum in the least amount of time, we then want to follow the gradient (downwards). You can think of this like descending a mountain by following the steepest slope to the base.\n\n<img src='assets/gradient_descent.png' width=350px>",
"_____no_output_____"
],
[
"## Backpropagation\n\nFor single layer networks, gradient descent is straightforward to implement. However, it's more complicated for deeper, multilayer neural networks like the one we've built. Complicated enough that it took about 30 years before researchers figured out how to train multilayer networks.\n\nTraining multilayer networks is done through **backpropagation** which is really just an application of the chain rule from calculus. It's easiest to understand if we convert a two layer network into a graph representation.\n\n<img src='assets/backprop_diagram.png' width=550px>\n\nIn the forward pass through the network, our data and operations go from bottom to top here. We pass the input $x$ through a linear transformation $L_1$ with weights $W_1$ and biases $b_1$. The output then goes through the sigmoid operation $S$ and another linear transformation $L_2$. Finally we calculate the loss $\\ell$. We use the loss as a measure of how bad the network's predictions are. The goal then is to adjust the weights and biases to minimize the loss.\n\nTo train the weights with gradient descent, we propagate the gradient of the loss backwards through the network. Each operation has some gradient between the inputs and outputs. As we send the gradients backwards, we multiply the incoming gradient with the gradient for the operation. Mathematically, this is really just calculating the gradient of the loss with respect to the weights using the chain rule.\n\n$$\n\\large \\frac{\\partial \\ell}{\\partial W_1} = \\frac{\\partial L_1}{\\partial W_1} \\frac{\\partial S}{\\partial L_1} \\frac{\\partial L_2}{\\partial S} \\frac{\\partial \\ell}{\\partial L_2}\n$$\n\n**Note:** I'm glossing over a few details here that require some knowledge of vector calculus, but they aren't necessary to understand what's going on.\n\nWe update our weights using this gradient with some learning rate $\\alpha$. \n\n$$\n\\large W^\\prime_1 = W_1 - \\alpha \\frac{\\partial \\ell}{\\partial W_1}\n$$\n\nThe learning rate $\\alpha$ is set such that the weight update steps are small enough that the iterative method settles in a minimum.",
"_____no_output_____"
],
[
"## Losses in PyTorch\n\nLet's start by seeing how we calculate the loss with PyTorch. Through the `nn` module, PyTorch provides losses such as the cross-entropy loss (`nn.CrossEntropyLoss`). You'll usually see the loss assigned to `criterion`. As noted in the last part, with a classification problem such as MNIST, we're using the softmax function to predict class probabilities. With a softmax output, you want to use cross-entropy as the loss. To actually calculate the loss, you first define the criterion then pass in the output of your network and the correct labels.\n\nSomething really important to note here. Looking at [the documentation for `nn.CrossEntropyLoss`](https://pytorch.org/docs/stable/nn.html#torch.nn.CrossEntropyLoss),\n\n> This criterion combines `nn.LogSoftmax()` and `nn.NLLLoss()` in one single class.\n>\n> The input is expected to contain scores for each class.\n\nThis means we need to pass in the raw output of our network into the loss, not the output of the softmax function. This raw output is usually called the *logits* or *scores*. We use the logits because softmax gives you probabilities which will often be very close to zero or one but floating-point numbers can't accurately represent values near zero or one ([read more here](https://docs.python.org/3/tutorial/floatingpoint.html)). It's usually best to avoid doing calculations with probabilities, typically we use log-probabilities.",
"_____no_output_____"
]
],
[
[
"import torch\nfrom torch import nn\nimport torch.nn.functional as F\nfrom torchvision import datasets, transforms\n\n# Define a transform to normalize the data\ntransform = transforms.Compose([transforms.ToTensor(),\n transforms.Normalize((0.5,), (0.5,)),\n ])\n# Download and load the training data\ntrainset = datasets.MNIST('~/.pytorch/MNIST_data/', download=True, train=True, transform=transform)\ntrainloader = torch.utils.data.DataLoader(trainset, batch_size=64, shuffle=True)",
"_____no_output_____"
],
[
"# Build a feed-forward network\nmodel = nn.Sequential(nn.Linear(784, 128),\n nn.ReLU(),\n nn.Linear(128, 64),\n nn.ReLU(),\n nn.Linear(64, 10))\n\n# Define the loss\ncriterion = nn.CrossEntropyLoss()\n\n# Get our data\nimages, labels = next(iter(trainloader))\n# Flatten images\nimages = images.view(images.shape[0], -1)\n\n# Forward pass, get our logits\nlogits = model(images)\n# Calculate the loss with the logits and the labels\nloss = criterion(logits, labels)\n\nprint(loss)",
"tensor(2.3011, grad_fn=<NllLossBackward>)\n"
]
],
[
[
"In my experience it's more convenient to build the model with a log-softmax output using `nn.LogSoftmax` or `F.log_softmax` ([documentation](https://pytorch.org/docs/stable/nn.html#torch.nn.LogSoftmax)). Then you can get the actual probabilites by taking the exponential `torch.exp(output)`. With a log-softmax output, you want to use the negative log likelihood loss, `nn.NLLLoss` ([documentation](https://pytorch.org/docs/stable/nn.html#torch.nn.NLLLoss)).\n\n>**Exercise:** Build a model that returns the log-softmax as the output and calculate the loss using the negative log likelihood loss.",
"_____no_output_____"
]
],
[
[
"## Solution\n\n# Build a feed-forward network\nmodel = nn.Sequential(nn.Linear(784, 128),\n nn.ReLU(),\n nn.Linear(128, 64),\n nn.ReLU(),\n nn.Linear(64, 10),\n nn.LogSoftmax(dim=1))\n\n# Define the loss\ncriterion = nn.NLLLoss()\n\n# Get our data\nimages, labels = next(iter(trainloader))\n# Flatten images\nimages = images.view(images.shape[0], -1)\n\n# Forward pass, get our log-probabilities\nlogps = model(images)\n# Calculate the loss with the logps and the labels\nloss = criterion(logps, labels)\n\nprint(loss)",
"tensor(2.2987, grad_fn=<NllLossBackward>)\n"
]
],
[
[
"## Autograd\n\nNow that we know how to calculate a loss, how do we use it to perform backpropagation? Torch provides a module, `autograd`, for automatically calculating the gradients of tensors. We can use it to calculate the gradients of all our parameters with respect to the loss. Autograd works by keeping track of operations performed on tensors, then going backwards through those operations, calculating gradients along the way. To make sure PyTorch keeps track of operations on a tensor and calculates the gradients, you need to set `requires_grad = True` on a tensor. You can do this at creation with the `requires_grad` keyword, or at any time with `x.requires_grad_(True)`.\n\nYou can turn off gradients for a block of code with the `torch.no_grad()` content:\n```python\nx = torch.zeros(1, requires_grad=True)\n>>> with torch.no_grad():\n... y = x * 2\n>>> y.requires_grad\nFalse\n```\n\nAlso, you can turn on or off gradients altogether with `torch.set_grad_enabled(True|False)`.\n\nThe gradients are computed with respect to some variable `z` with `z.backward()`. This does a backward pass through the operations that created `z`.",
"_____no_output_____"
]
],
[
[
"x = torch.randn(2,2, requires_grad=True)\nprint(x)",
"tensor([[-0.1890, -0.4804],\n [ 1.1457, 1.6178]], requires_grad=True)\n"
],
[
"y = x**2\nprint(y)",
"tensor([[0.0357, 0.2308],\n [1.3125, 2.6173]], grad_fn=<PowBackward0>)\n"
]
],
[
[
"Below we can see the operation that created `y`, a power operation `PowBackward0`.",
"_____no_output_____"
]
],
[
[
"## grad_fn shows the function that generated this variable\nprint(y.grad_fn)",
"<PowBackward0 object at 0x107e2e278>\n"
]
],
[
[
"The autograd module keeps track of these operations and knows how to calculate the gradient for each one. In this way, it's able to calculate the gradients for a chain of operations, with respect to any one tensor. Let's reduce the tensor `y` to a scalar value, the mean.",
"_____no_output_____"
]
],
[
[
"z = y.mean()\nprint(z)",
"tensor(1.0491, grad_fn=<MeanBackward0>)\n"
]
],
[
[
"You can check the gradients for `x` and `y` but they are empty currently.",
"_____no_output_____"
]
],
[
[
"print(x.grad)",
"None\n"
]
],
[
[
"To calculate the gradients, you need to run the `.backward` method on a Variable, `z` for example. This will calculate the gradient for `z` with respect to `x`\n\n$$\n\\frac{\\partial z}{\\partial x} = \\frac{\\partial}{\\partial x}\\left[\\frac{1}{n}\\sum_i^n x_i^2\\right] = \\frac{x}{2}\n$$",
"_____no_output_____"
]
],
[
[
"z.backward()\nprint(x.grad)\nprint(x/2)",
"tensor([[-0.0945, -0.2402],\n [ 0.5728, 0.8089]])\ntensor([[-0.0945, -0.2402],\n [ 0.5728, 0.8089]], grad_fn=<DivBackward0>)\n"
]
],
[
[
"These gradients calculations are particularly useful for neural networks. For training we need the gradients of the weights with respect to the cost. With PyTorch, we run data forward through the network to calculate the loss, then, go backwards to calculate the gradients with respect to the loss. Once we have the gradients we can make a gradient descent step. ",
"_____no_output_____"
],
[
"## Loss and Autograd together\n\nWhen we create a network with PyTorch, all of the parameters are initialized with `requires_grad = True`. This means that when we calculate the loss and call `loss.backward()`, the gradients for the parameters are calculated. These gradients are used to update the weights with gradient descent. Below you can see an example of calculating the gradients using a backwards pass.",
"_____no_output_____"
]
],
[
[
"# Build a feed-forward network\nmodel = nn.Sequential(nn.Linear(784, 128),\n nn.ReLU(),\n nn.Linear(128, 64),\n nn.ReLU(),\n nn.Linear(64, 10),\n nn.LogSoftmax(dim=1))\n\ncriterion = nn.NLLLoss()\nimages, labels = next(iter(trainloader))\nimages = images.view(images.shape[0], -1)\n\nlogps = model(images)\nloss = criterion(logps, labels)",
"_____no_output_____"
],
[
"print('Before backward pass: \\n', model[0].weight.grad)\n\nloss.backward()\n\nprint('After backward pass: \\n', model[0].weight.grad)",
"Before backward pass: \n None\nAfter backward pass: \n tensor([[ 2.9076e-04, 2.9076e-04, 2.9076e-04, ..., 2.9076e-04,\n 2.9076e-04, 2.9076e-04],\n [ 1.8523e-03, 1.8523e-03, 1.8523e-03, ..., 1.8523e-03,\n 1.8523e-03, 1.8523e-03],\n [-1.0316e-03, -1.0316e-03, -1.0316e-03, ..., -1.0316e-03,\n -1.0316e-03, -1.0316e-03],\n ...,\n [-3.6785e-05, -3.6785e-05, -3.6785e-05, ..., -3.6785e-05,\n -3.6785e-05, -3.6785e-05],\n [-1.3995e-03, -1.3995e-03, -1.3995e-03, ..., -1.3995e-03,\n -1.3995e-03, -1.3995e-03],\n [ 0.0000e+00, 0.0000e+00, 0.0000e+00, ..., 0.0000e+00,\n 0.0000e+00, 0.0000e+00]])\n"
]
],
[
[
"## Training the network!\n\nThere's one last piece we need to start training, an optimizer that we'll use to update the weights with the gradients. We get these from PyTorch's [`optim` package](https://pytorch.org/docs/stable/optim.html). For example we can use stochastic gradient descent with `optim.SGD`. You can see how to define an optimizer below.",
"_____no_output_____"
]
],
[
[
"from torch import optim\n\n# Optimizers require the parameters to optimize and a learning rate\noptimizer = optim.SGD(model.parameters(), lr=0.01)",
"_____no_output_____"
]
],
[
[
"Now we know how to use all the individual parts so it's time to see how they work together. Let's consider just one learning step before looping through all the data. The general process with PyTorch:\n\n* Make a forward pass through the network \n* Use the network output to calculate the loss\n* Perform a backward pass through the network with `loss.backward()` to calculate the gradients\n* Take a step with the optimizer to update the weights\n\nBelow I'll go through one training step and print out the weights and gradients so you can see how it changes. Note that I have a line of code `optimizer.zero_grad()`. When you do multiple backwards passes with the same parameters, the gradients are accumulated. This means that you need to zero the gradients on each training pass or you'll retain gradients from previous training batches.",
"_____no_output_____"
]
],
[
[
"print('Initial weights - ', model[0].weight)\n\nimages, labels = next(iter(trainloader))\nimages.resize_(64, 784)\n\n# Clear the gradients, do this because gradients are accumulated\noptimizer.zero_grad()\n\n# Forward pass, then backward pass, then update weights\noutput = model(images)\nloss = criterion(output, labels)\nloss.backward()\nprint('Gradient -', model[0].weight.grad)",
"Initial weights - Parameter containing:\ntensor([[ 0.0134, 0.0305, 0.0163, ..., -0.0268, 0.0101, -0.0027],\n [-0.0333, -0.0089, -0.0294, ..., 0.0047, -0.0106, -0.0214],\n [-0.0068, -0.0275, -0.0132, ..., -0.0203, 0.0075, 0.0117],\n ...,\n [-0.0147, 0.0041, 0.0312, ..., 0.0302, 0.0104, 0.0253],\n [ 0.0122, 0.0233, 0.0090, ..., 0.0184, 0.0041, -0.0196],\n [ 0.0138, 0.0348, 0.0040, ..., -0.0239, -0.0291, 0.0166]],\n requires_grad=True)\nGradient - tensor([[-0.0008, -0.0008, -0.0008, ..., -0.0008, -0.0008, -0.0008],\n [ 0.0029, 0.0029, 0.0029, ..., 0.0029, 0.0029, 0.0029],\n [ 0.0009, 0.0009, 0.0009, ..., 0.0009, 0.0009, 0.0009],\n ...,\n [-0.0008, -0.0008, -0.0008, ..., -0.0008, -0.0008, -0.0008],\n [-0.0002, -0.0002, -0.0002, ..., -0.0002, -0.0002, -0.0002],\n [ 0.0000, 0.0000, 0.0000, ..., 0.0000, 0.0000, 0.0000]])\n"
],
[
"# Take an update step and few the new weights\noptimizer.step()\nprint('Updated weights - ', model[0].weight)",
"Updated weights - Parameter containing:\ntensor([[ 0.0134, 0.0305, 0.0163, ..., -0.0268, 0.0101, -0.0027],\n [-0.0334, -0.0089, -0.0294, ..., 0.0047, -0.0106, -0.0214],\n [-0.0068, -0.0275, -0.0132, ..., -0.0203, 0.0075, 0.0117],\n ...,\n [-0.0147, 0.0041, 0.0312, ..., 0.0302, 0.0105, 0.0253],\n [ 0.0122, 0.0233, 0.0090, ..., 0.0184, 0.0041, -0.0196],\n [ 0.0138, 0.0348, 0.0040, ..., -0.0239, -0.0291, 0.0166]],\n requires_grad=True)\n"
]
],
[
[
"### Training for real\n\nNow we'll put this algorithm into a loop so we can go through all the images. Some nomenclature, one pass through the entire dataset is called an *epoch*. So here we're going to loop through `trainloader` to get our training batches. For each batch, we'll doing a training pass where we calculate the loss, do a backwards pass, and update the weights.\n\n> **Exercise: ** Implement the training pass for our network. If you implemented it correctly, you should see the training loss drop with each epoch.",
"_____no_output_____"
]
],
[
[
"model = nn.Sequential(nn.Linear(784, 128),\n nn.ReLU(),\n nn.Linear(128, 64),\n nn.ReLU(),\n nn.Linear(64, 10),\n nn.LogSoftmax(dim=1))\n\ncriterion = nn.NLLLoss()\noptimizer = optim.SGD(model.parameters(), lr=0.003)\n\nepochs = 5\nfor e in range(epochs):\n running_loss = 0\n for images, labels in trainloader:\n # Flatten MNIST images into a 784 long vector\n images = images.view(images.shape[0], -1)\n \n # TODO: Training pass\n optimizer.zero_grad()\n \n output = model(images)\n loss = criterion(output, labels)\n loss.backward()\n optimizer.step()\n \n running_loss += loss.item()\n else:\n print(f\"Training loss: {running_loss/len(trainloader)}\")",
"_____no_output_____"
]
],
[
[
"With the network trained, we can check out it's predictions.",
"_____no_output_____"
]
],
[
[
"%matplotlib inline\nimport helper\n\nimages, labels = next(iter(trainloader))\n\nimg = images[0].view(1, 784)\n# Turn off gradients to speed up this part\nwith torch.no_grad():\n logps = model(img)\n\n# Output of the network are log-probabilities, need to take exponential for probabilities\nps = torch.exp(logps)\nhelper.view_classify(img.view(1, 28, 28), ps)",
"_____no_output_____"
]
],
[
[
"Now our network is brilliant. It can accurately predict the digits in our images. Next up you'll write the code for training a neural network on a more complex dataset.",
"_____no_output_____"
]
]
] | [
"markdown",
"code",
"markdown",
"code",
"markdown",
"code",
"markdown",
"code",
"markdown",
"code",
"markdown",
"code",
"markdown",
"code",
"markdown",
"code",
"markdown",
"code",
"markdown",
"code",
"markdown",
"code",
"markdown",
"code",
"markdown"
] | [
[
"markdown",
"markdown",
"markdown"
],
[
"code",
"code"
],
[
"markdown"
],
[
"code"
],
[
"markdown"
],
[
"code",
"code"
],
[
"markdown"
],
[
"code"
],
[
"markdown"
],
[
"code"
],
[
"markdown"
],
[
"code"
],
[
"markdown"
],
[
"code"
],
[
"markdown",
"markdown"
],
[
"code",
"code"
],
[
"markdown"
],
[
"code"
],
[
"markdown"
],
[
"code",
"code"
],
[
"markdown"
],
[
"code"
],
[
"markdown"
],
[
"code"
],
[
"markdown"
]
] |
e782bf181a060526824c9a5aef6f57431a761601 | 55,029 | ipynb | Jupyter Notebook | notebooks/richardkim/RK_modeling.ipynb | ConnorHaas03/CDC_capstone | 1a4e3c4290df7d756d4af4f99b191df181307c6e | [
"FTL"
] | null | null | null | notebooks/richardkim/RK_modeling.ipynb | ConnorHaas03/CDC_capstone | 1a4e3c4290df7d756d4af4f99b191df181307c6e | [
"FTL"
] | 3 | 2020-03-13T18:43:39.000Z | 2020-03-13T19:25:10.000Z | notebooks/richardkim/RK_modeling.ipynb | ConnorHaas03/CDC_capstone | 1a4e3c4290df7d756d4af4f99b191df181307c6e | [
"FTL"
] | 3 | 2020-03-09T23:34:01.000Z | 2020-03-11T20:58:16.000Z | 35.640544 | 123 | 0.351124 | [
[
[
"import pandas as pd\nimport numpy as np\n# import random as rn\n# import sklearn\n# from scipy import stats\n# import math\nimport re\n\nfrom sklearn.preprocessing import LabelEncoder #OneHotEncoder\n# from sklearn import ensemble\nfrom sklearn import linear_model\n\n# from sklearn.model_selection import GridSearchCV,cross_val_score\n# from sklearn.metrics import r2_score, mean_squared_error, mean_absolute_error,accuracy_score\n\n# # from sklearn.ensemble import RandomForestClassifier\n# from sklearn import linear_model\n\n# import matplotlib.pyplot as plt\n# import seaborn as sns\n# from sklearn.linear_model import LinearRegression, Ridge, Lasso, ElasticNet, RidgeCV, LassoCV\n\nimport pickle",
"_____no_output_____"
],
[
"totDF = pd.read_csv('../../data/processed/Cleaned_Data_Set.csv')",
"_____no_output_____"
],
[
"totDF.groupby(['birth_year','admit_NICU']).count()",
"_____no_output_____"
]
],
[
[
"## Cleaning / Sampling",
"_____no_output_____"
]
],
[
[
"def cleanDF (df):\n r1 = re.compile('.*reporting')\n r2 = re.compile('.*imputed')\n\n cols_to_drop1 = list(filter((r1.match), df.columns))\n cols_to_drop2 = list(filter((r2.match), df.columns))\n cols_to_drop3 = ['admit_NICU']\n cols_to_drop = cols_to_drop1 + cols_to_drop2 + cols_to_drop3\n\n cols_to_keep = [col for col in df.columns if col not in cols_to_drop]\n\n X_and_target = df[cols_to_keep + ['admit_NICU']].copy()\n\n numerics = ['int16', 'int32', 'int64', 'float16', 'float32', 'float64']\n catDF = X_and_target.select_dtypes(include=object).copy()\n numDF = X_and_target.select_dtypes(include=numerics).copy() #only numeric columns\n\n le = LabelEncoder()\n catDF = catDF.apply(le.fit_transform)\n\n concat_df = pd.concat([numDF,catDF],axis=1)\n return concat_df",
"_____no_output_____"
]
],
[
[
"## Logistic Model Part 1",
"_____no_output_____"
]
],
[
[
"sample_size_list = [100]",
"_____no_output_____"
],
[
"import warnings\nwarnings.filterwarnings('ignore')",
"_____no_output_____"
],
[
"#GLM with Cross Validation\nfor sample_per_year in sample_size_list:\n dwnSmplDF = concat_df.groupby('birth_year',group_keys = False).apply(lambda x: x.sample(sample_per_year))\n \n cl_df = dwnSmplDF[cols_to_keep]\n encoded_target = dwnSmplDF['admit_NICU']\n \n glm_CV = linear_model.LogisticRegressionCV(#Cs = int(1e4),\n cv = 5,\n solver = 'saga',\n n_jobs = -1,\n random_state = 108\n ).fit(cl_df, encoded_target)\n print('sample size : %d\\n' % (sample_per_year*5))\n %time glm_CV.fit(cl_df, encoded_target)\n print('\\nscore : {0}'.format(glm_CV.score(cl_df, encoded_target)))\n print('-'*50)\n",
"_____no_output_____"
],
[
"'''\nsample size : 500\n\nCPU times: user 6min 47s, sys: 1min 26s, total: 8min 14s\nWall time: 1min 54s\n\nscore : 0.932\n--------------------------------------------------\n'''",
"_____no_output_____"
],
[
"#GLM with Lasso Penalty\n\nfor sample_per_year in sample_size_list:\n dwnSmplDF = concat_df.groupby('birth_year',group_keys = False).apply(lambda x: x.sample(sample_per_year))\n \n cl_df = dwnSmplDF[cols_to_keep]\n encoded_target = dwnSmplDF['admit_NICU']\n \n glm_lasso = linear_model.LogisticRegression(penalty = 'l1', \n solver = 'saga', \n multi_class='auto', \n n_jobs = -1,\n C = 1e4)\n print('sample size : %d\\n' % (sample_per_year*5))\n %time glm_lasso.fit(cl_df, encoded_target)\n print('\\nscore : {0}'.format(glm_lasso.score(cl_df, encoded_target)))\n print('-'*50)\n ",
"_____no_output_____"
],
[
"#GLM with Lasso Penalty and Cross Validation\n\nsample_size_list = [100,1000,10000]\n\nfor sample_per_year in sample_size_list:\n dwnSmplDF = concat_df.groupby('birth_year',group_keys = False).apply(lambda x: x.sample(sample_per_year))\n cl_df = dwnSmplDF[cols_to_keep]\n encoded_target = dwnSmplDF['admit_NICU']\n \n glm_lassoCV = linear_model.LogisticRegressionCV(#Cs = int(1e4),\n cv = 5,\n penalty = 'l1',\n solver = 'saga',\n n_jobs = -1,\n random_state = 108\n ).fit(cl_df, encoded_target)\n print('sample size : %d\\n' % (sample_per_year*5))\n %time glm_lassoCV.fit(cl_df, encoded_target)\n print('\\nscore : {0}'.format(glm_lassoCV.score(cl_df, encoded_target)))\n print('-'*50)\n ",
"sample size : 500\n\nCPU times: user 2.87 s, sys: 97.3 ms, total: 2.96 s\nWall time: 571 ms\n\nscore : 0.914\n--------------------------------------------------\nsample size : 5000\n\nCPU times: user 2min, sys: 577 ms, total: 2min 1s\nWall time: 17.4 s\n\nscore : 0.9308\n--------------------------------------------------\nsample size : 50000\n\nCPU times: user 22min 12s, sys: 2.49 s, total: 22min 14s\nWall time: 3min 17s\n\nscore : 0.9245\n--------------------------------------------------\n"
],
[
"#GLM with Lasso Penalty and Cross Validation\n\nsample_size_list = [20000]\n\nfor sample_per_year in sample_size_list:\n dwnSmplDF = concat_df.groupby('birth_year',group_keys = False).apply(lambda x: x.sample(sample_per_year))\n cl_df = dwnSmplDF[cols_to_keep]\n encoded_target = dwnSmplDF['admit_NICU']\n \n glm_lassoCV = linear_model.LogisticRegressionCV(#Cs = int(1e4),\n cv = 5,\n penalty = 'l1',\n solver = 'saga',\n n_jobs = -1,\n random_state = 108\n ).fit(cl_df, encoded_target)\n print('sample size : %d\\n' % (sample_per_year*5))\n %time glm_lassoCV.fit(cl_df, encoded_target)\n print('\\nscore : {0}'.format(glm_lassoCV.score(cl_df, encoded_target)))\n print('-'*50)",
"sample size : 100000\n\nCPU times: user 50min 24s, sys: 20.1 s, total: 50min 45s\nWall time: 8min 27s\n\nscore : 0.92502\n--------------------------------------------------\n"
],
[
"glm_lassoCV",
"_____no_output_____"
],
[
"pickle.dump(glm_lassoCV, open('best_glmlassoCV.sav', 'wb'))\n# glm_lassoCV = pickle.load(open('best_glmlassoCV.sav', 'rb'))",
"_____no_output_____"
],
[
"glm_lassoCV.get_params",
"_____no_output_____"
],
[
"from sklearn.metrics import confusion_matrix\n\nprint(confusion_matrix(encoded_target,y_pred))",
"[[89917 5 355]\n [ 151 1083 6]\n [ 6981 0 1502]]\n"
],
[
"cf = confusion_matrix(encoded_target,y_pred)",
"_____no_output_____"
],
[
"np.set_printoptions(suppress=True)\nprint(cf/1000.)",
"[[89.917 0.005 0.355]\n [ 0.151 1.083 0.006]\n [ 6.981 0. 1.502]]\n"
],
[
"glmLCV_coefs = pd.DataFrame({'col' :list(cl_df.columns), \n 'coef0': glm_lassoCV.coef_[0], \n 'coef1': glm_lassoCV.coef_[1],\n 'coef2': glm_lassoCV.coef_[2]})",
"_____no_output_____"
],
[
"glmLCV_coefs",
"_____no_output_____"
],
[
"glmLCV_coefs['abs_coef0'] = glmLCV_coefs['coef0'].apply(abs)\nglmLCV_coefs['abs_coef1'] = glmLCV_coefs['coef1'].apply(abs)\nglmLCV_coefs['abs_coef2'] = glmLCV_coefs['coef2'].apply(abs)",
"_____no_output_____"
],
[
"top20_coef0 = glmLCV_coefs.nlargest(10,'abs_coef0')['col']\ntop20_coef1 = glmLCV_coefs.nlargest(10,'abs_coef1')['col']\ntop20_coef2 = glmLCV_coefs.nlargest(10,'abs_coef2')['col']",
"_____no_output_____"
],
[
"top20_coef0",
"_____no_output_____"
],
[
"top20_coef1",
"_____no_output_____"
],
[
"top20_coef2",
"_____no_output_____"
],
[
"list(set(top20_coef0).union(set(top20_coef1)).union(set(top20_coef2)))",
"_____no_output_____"
],
[
"list(set(top20_coef0).intersection(set(top20_coef1)).intersection(set(top20_coef2)))",
"_____no_output_____"
],
[
"'''\nsample size : 100000\n\nCPU times: user 54min 1s, sys: 37.3 s, total: 54min 39s\nWall time: 8min 39s\n\nscore : 0.92512\n--------------------------------------------------\n'''",
"_____no_output_____"
],
[
"#GLM with Lasso Penalty and Cross Validation\n\n# sample_size_list = [200000]\n\n# for sample_per_year in sample_size_list:\n# dwnSmplDF = concat_df.groupby('birth_year',group_keys = False).apply(lambda x: x.sample(sample_per_year))\n# cl_df = dwnSmplDF[cols_to_keep]\n# encoded_target = dwnSmplDF['admit_NICU']\n \n# glm_lassoCV = linear_model.LogisticRegressionCV(#Cs = int(1e4),\n# cv = 5,\n# penalty = 'l1',\n# solver = 'saga',\n# n_jobs = -2,\n# random_state = 108\n# ).fit(cl_df, encoded_target)\n# print('sample size : %d\\n' % (sample_per_year*5))\n# %time glm_lassoCV.fit(cl_df, encoded_target)\n# print('\\nscore : {0}'.format(glm_lassoCV.score(cl_df, encoded_target)))\n# print('-'*50)\n ",
"_____no_output_____"
]
],
[
[
"## Logistic Model Part 2",
"_____no_output_____"
],
[
"Sampled in a way that\n1. Unknowns in `admit_NICU` column was thrown away.\n2. There are equal number of `Y`'s and `N`'s in `admit_NICU` column. (balanced sampling)",
"_____no_output_____"
]
],
[
[
"cl_df = cleanDF(totDF)\nnicu_allY = cl_df.loc[cl_df['admit_NICU']==1]\nnicu_allN = cl_df.loc[cl_df['admit_NICU']==0]",
"_____no_output_____"
],
[
"#pure GLM with balanced sample (w/o stratified year)\nsample_size_list = [100]\n\nfor sample_per_class in sample_size_list:\n\n sampN = nicu_allN.sample(sample_per_class)\n sampY = nicu_allY.sample(sample_per_class)\n samp = pd.concat([sampN,sampY],axis=0)\n \n samp_target = samp.admit_NICU\n samp_X = samp.drop('admit_NICU',axis=1)\n \n# bal_dwnSmplY = nicu_allY.groupby('birth_year',group_keys = False).apply(lambda x: x.sample(sample_per_year))\n# bal_dwnSmplN = nicu_allN.groupby('birth_year',group_keys = False).apply(lambda x: x.sample(sample_per_year))\n# bal_dwnSmpl = pd.concat([bal_dwnSmlpY,bal_dwnamlpN],axis=0)\n \n glm = linear_model.LogisticRegression(solver = 'saga', \n multi_class='auto', \n n_jobs = -1,\n C = 1e4)\n print('sample size : %d\\n' % (sample_per_class*2))\n %time glm.fit(samp_X, samp_target)\n print('\\nscore : {0}'.format(glm.score(samp_X, samp_target)))\n print('-'*50)\n ",
"sample size : 200\n\nCPU times: user 19.3 ms, sys: 6.91 ms, total: 26.2 ms\nWall time: 115 ms\n\nscore : 0.925\n--------------------------------------------------\n"
],
[
"#pure GLM with balanced sample (w/ stratified year)\n\nsample_size_class = [100]\n\nfor sample_per_class in sample_size_class:\n\n# sampN = nicu_allN.sample(sample_per_class)\n# sampY = nicu_allY.sample(sample_per_class)\n# samp = pd.concat([sampN,sampY],axis=0)\n \n# samp_target = samp.admit_NICU\n# samp_X = samp.drop('admit_NICU',axis=1)\n \n bal_dwnSmplY = nicu_allY.groupby('birth_year',group_keys = False).apply(lambda x: x.sample(sample_per_class))\n bal_dwnSmplN = nicu_allN.groupby('birth_year',group_keys = False).apply(lambda x: x.sample(sample_per_class))\n bal_dwnSmpl = pd.concat([bal_dwnSmplY,bal_dwnSmplN],axis=0)\n \n bal_target = bal_dwnSmpl.admit_NICU\n bal_X = bal_dwnSmpl.drop('admit_NICU',axis=1)\n \n glm = linear_model.LogisticRegression(solver = 'saga', \n multi_class='auto', \n n_jobs = -1,\n C = 1e4)\n print('sample size : %d\\n' % (sample_per_class*2))\n %time glm.fit(bal_X, bal_target)\n print('\\nscore : {0}'.format(glm.score(bal_X, bal_target)))\n print('-'*50)\n ",
"sample size : 200\n\nCPU times: user 79.9 ms, sys: 1.13 ms, total: 81 ms\nWall time: 108 ms\n\nscore : 0.774\n--------------------------------------------------\n"
]
]
] | [
"code",
"markdown",
"code",
"markdown",
"code",
"markdown",
"code"
] | [
[
"code",
"code",
"code"
],
[
"markdown"
],
[
"code"
],
[
"markdown"
],
[
"code",
"code",
"code",
"code",
"code",
"code",
"code",
"code",
"code",
"code",
"code",
"code",
"code",
"code",
"code",
"code",
"code",
"code",
"code",
"code",
"code",
"code",
"code",
"code"
],
[
"markdown",
"markdown"
],
[
"code",
"code",
"code"
]
] |
e782c519d74182358cbc3a4de3c06f9eed1215c1 | 28,554 | ipynb | Jupyter Notebook | course/chapter7/section3_pt.ipynb | jflam/notebooks | 67aeb5d63d3dfb5a88a33f92cef9cf4d4ea696f7 | [
"Apache-2.0"
] | 1 | 2022-02-05T10:49:14.000Z | 2022-02-05T10:49:14.000Z | course/chapter7/section3_pt.ipynb | cfregly/notebooks | e0dc8cf9770a2d1d4e6108ab5e5c7ea6b27ccc2e | [
"Apache-2.0"
] | null | null | null | course/chapter7/section3_pt.ipynb | cfregly/notebooks | e0dc8cf9770a2d1d4e6108ab5e5c7ea6b27ccc2e | [
"Apache-2.0"
] | null | null | null | 29.930818 | 938 | 0.550011 | [
[
[
"empty"
]
]
] | [
"empty"
] | [
[
"empty"
]
] |
e782dce5a27c58abdfe0ba1436a98859630ddf88 | 9,225 | ipynb | Jupyter Notebook | Session 06 - OOP.ipynb | FOU4D/ITI-Python | 326e7876cfbef1859bd76a3541fe8d18949bdbfe | [
"MIT"
] | null | null | null | Session 06 - OOP.ipynb | FOU4D/ITI-Python | 326e7876cfbef1859bd76a3541fe8d18949bdbfe | [
"MIT"
] | null | null | null | Session 06 - OOP.ipynb | FOU4D/ITI-Python | 326e7876cfbef1859bd76a3541fe8d18949bdbfe | [
"MIT"
] | null | null | null | 21.503497 | 243 | 0.497669 | [
[
[
"<img src=\"https://drive.google.com/uc?id=1v7YY_rNBU2OMaPnbUGmzaBj3PUeddxrw\" alt=\"ITI MCIT EPITA\" style=\"width: 750px;\"/>",
"_____no_output_____"
],
[
"<img src=\"https://drive.google.com/uc?id=1R0-FYpJQW5YFy6Yv-RZ1rpyBslay0251\" alt=\"Python Logo\" style=\"width: 400px;\"/>\n\n-----\n\n## Session 06: OOP",
"_____no_output_____"
],
[
"By: **Mohamed Fouad Fakhruldeen**, [email protected]",
"_____no_output_____"
],
[
"## Class & Object",
"_____no_output_____"
]
],
[
[
"class ClassName:\n attributes\n methods",
"_____no_output_____"
]
],
[
[
"### attributes",
"_____no_output_____"
]
],
[
[
"class My_Class:\n my_attr = \"old Attribute Value Here\"\n \nx = My_Class()\nprint(x)\nprint(x.my_attr)\nx.my_attr = \"New Attribute Value\"\nprint(x.my_attr)",
"<__main__.My_Class object at 0x7f47c80e8130>\nold Attribute Value Here\nNew Attribute Value\n"
]
],
[
[
"### methods",
"_____no_output_____"
]
],
[
[
"class My_Class:\n my_attr = \"New Attribute Value Here\" # class attribute\n def my_method(self):\n print(\"Print my method\")\nx = My_Class()\nprint(x)\nprint(x.my_attr)\nx.my_method()",
"<__main__.My_Class object at 0x7f47c80e8280>\nNew Attribute Value Here\nPrint my method\n"
],
[
"class My_Cars:\n \n def __init__(self, brand, model, year, price): ## instance attributes\n self.brand = brand\n self.model = model\n self.year = year\n self.price = price\n \n \n def description(self):\n return f\"{self.brand} {self.model} made in {self.year} with initial value {self.price}\"\n \n def pricenow(self, condition):\n return f\"{self.description()} has new value {condition*self.price}\"\n \n def __str__(self):\n return \"this only appears while printing\"\n \nfirst_car = My_Cars(\"Toyota\", \"Corolla\", 2016, 5000)\nprint(first_car.brand)\nprint(first_car.description())\nprint(first_car.pricenow(70/100))\nprint(first_car)\nx = str(first_car)\nprint(x)\n",
"Toyota\nToyota Corolla made in 2016 with initial value 5000\nToyota Corolla made in 2016 with initial value 5000 has new value 3500.0\nthis only appears while printing\nthis only appears while printing\n"
]
],
[
[
"### Inheritance",
"_____no_output_____"
],
[
"Child classes can override or extend the attributes and methods of parent classes.",
"_____no_output_____"
],
[
"can also specify attributes and methods that are unique to themselves.",
"_____no_output_____"
]
],
[
[
"class MainClass:\n attr1 = \"this is parent attribute\"\n \nclass ChildClass(MainClass):\n pass\n\nx = ChildClass()\nprint(x.attr1)",
"this is parent attribute\n"
],
[
"class MainClass2:\n attr12 = \"this is parent attribute\"\n \nclass ChildClass2(MainClass2):\n attr12 = \"This one from child\"\n\nx2 = ChildClass2()\nprint(x2.attr12)",
"This one from child\n"
]
],
[
[
"### multiple inheretance",
"_____no_output_____"
]
],
[
[
"class Base1:\n pass\n\nclass Base2:\n pass\n\nclass MultiDerived(Base1, Base2):\n pass",
"_____no_output_____"
],
[
"class Base:\n pass\n\nclass Derived1(Base):\n pass\n\nclass Derived2(Derived1):\n pass",
"_____no_output_____"
]
],
[
[
"check by: ",
"_____no_output_____"
]
],
[
[
"MultiDerived.__mro__\nMultiDerived.mro()",
"_____no_output_____"
]
],
[
[
"### Encapsulation",
"_____no_output_____"
],
[
"we denote private attributes using underscore as the prefix i.e single _ or double __",
"_____no_output_____"
]
],
[
[
"class Cars:\n\n def __init__(self):\n self.__maxprice = 90000\n\n def sell(self):\n print(\"Selling Price: {}\".format(self.__maxprice))\n\n def setMaxPrice(self, price):\n self.__maxprice = price\n\ntoyota = Cars()\ntoyota.sell()\n\n# change the price\ntoyota.__maxprice = 1000\ntoyota.sell()\n\n# using setter function\ntoyota.setMaxPrice(1000)\ntoyota.sell()",
"Selling Price: 90000\nSelling Price: 90000\nSelling Price: 1000\n"
]
],
[
[
"### polymorphism",
"_____no_output_____"
]
],
[
[
"class Parrot:\n\n def fly(self):\n print(\"Parrot can fly\")\n \n def swim(self):\n print(\"Parrot can't swim\")\n\nclass Penguin:\n\n def fly(self):\n print(\"Penguin can't fly\")\n \n def swim(self):\n print(\"Penguin can swim\")\n\n# common interface\ndef flying_test(bird):\n bird.fly()\n\n#instantiate objects\nblu = Parrot()\npeggy = Penguin()\n\n# passing the object\nflying_test(blu)\nflying_test(peggy)",
"Parrot can fly\nPenguin can't fly\n"
]
],
[
[
"In the above program, we defined two classes Parrot and Penguin. Each of them have a common fly() method. However, their functions are different.\n\nTo use polymorphism, we created a common interface i.e flying_test() function that takes any object and calls the object's fly() method. Thus, when we passed the blu and peggy objects in the flying_test() function, it ran effectively.\n\nhttps://www.programiz.com/python-programming/object-oriented-programming",
"_____no_output_____"
]
]
] | [
"markdown",
"raw",
"markdown",
"code",
"markdown",
"code",
"markdown",
"code",
"markdown",
"raw",
"markdown",
"raw",
"markdown",
"code",
"markdown",
"code",
"markdown"
] | [
[
"markdown",
"markdown",
"markdown",
"markdown"
],
[
"raw"
],
[
"markdown"
],
[
"code"
],
[
"markdown"
],
[
"code",
"code"
],
[
"markdown",
"markdown",
"markdown"
],
[
"code",
"code"
],
[
"markdown"
],
[
"raw",
"raw"
],
[
"markdown"
],
[
"raw"
],
[
"markdown",
"markdown"
],
[
"code"
],
[
"markdown"
],
[
"code"
],
[
"markdown"
]
] |
e782e41cd81029850abea6a5557357766458fe67 | 118,795 | ipynb | Jupyter Notebook | demo/Classic/2. Demo - Simulation - Replayer.ipynb | FDR0903/mimicLOB | 0ef79a9a7129fa63a25423e29aba249594ec23b5 | [
"MIT"
] | null | null | null | demo/Classic/2. Demo - Simulation - Replayer.ipynb | FDR0903/mimicLOB | 0ef79a9a7129fa63a25423e29aba249594ec23b5 | [
"MIT"
] | null | null | null | demo/Classic/2. Demo - Simulation - Replayer.ipynb | FDR0903/mimicLOB | 0ef79a9a7129fa63a25423e29aba249594ec23b5 | [
"MIT"
] | null | null | null | 187.966772 | 62,348 | 0.885138 | [
[
[
"# 0. Imports",
"_____no_output_____"
]
],
[
[
"# Imports\nfrom IPython.display import display, HTML\nimport os\nimport pandas as pd, datetime as dt, numpy as np, matplotlib.pyplot as plt\nfrom pandas.tseries.offsets import DateOffset\nimport sys\n\n# Display options\nthisnotebooksys = sys.stdout\npd.set_option('display.width', 1000)\ndisplay(HTML(\"<style>.container { width:100% !important; }</style>\"))\npd.set_option('mode.chained_assignment', None)",
"_____no_output_____"
],
[
"import mimicLOB as mlob",
"_____no_output_____"
]
],
[
[
"## 1. LOB creation",
"_____no_output_____"
]
],
[
[
"# b_tape = True means the LOB \nLOB = mlob.OrderBook(tick_size = 0.5, \n b_tape = True, # tape transactions\n b_tape_LOB = True, # tape lob state at each tick\n verbose = True)",
"_____no_output_____"
]
],
[
[
"## 2. Data\n\n- DTIME : le timestamp de l'ordre\n- ORDER_ID : l'identifiant de l'ordre\n- PRICE \n- QTY\n- ORDER_SIDE\n- ORDER_SIDE\n- ORDER_TYPE : <br>1 pour Market Order; <br>2 pour Limit Order; <br>q pour Quote <br> W pour Market On Open;\n- ACTION_TYPE : <br> I = limit order insertion (passive); <br> C = limit order cacnellations; <br> R = replace order that lose priority; <br> r = replace order that keeps priority; <br> S = replace order that makes the order aggressive (give rise to trade); <br> T = aggressive order (give rise to trade)\n- MATCH_STRATEGY : True/False\n- IS_OPEN_TRADE : True/False",
"_____no_output_____"
]
],
[
[
"df = pd.read_pickle(r'..\\data\\day20160428.pkl')\ndf",
"_____no_output_____"
]
],
[
[
"## 3. Agents Creation",
"_____no_output_____"
]
],
[
[
"auction_config = {'orderbook' : LOB,\n 'id' : 'FDR',\n 'b_record' : False,\n 'historicalOrders' : df[df.DTIME.dt.hour<7]}\n\ncontinuousTrading_config = {'orderbook' : LOB,\n 'id' : 'FDR',\n 'b_record' : False,\n 'historicalOrders' : df[df.DTIME.dt.hour>=7]}\n\nAuctionReplayer = mlob.replayerAgent(**auction_config)\nContReplayer = mlob.replayerAgent(**continuousTrading_config)",
"_____no_output_____"
]
],
[
[
"## 4. Replay orders",
"_____no_output_____"
],
[
"### 4.1. Auction phase\n#### The auction price shall be determined on the basis of the situation of the Central Order Book at the closing of the call phase and shall be the price which produces the highest executable order volume.",
"_____no_output_____"
]
],
[
[
"%%time\n\n# log\nf = open('log_auction.txt','w');\nsys.stdout = f\n\n# Close auction\nLOB.b_auction = True #Open auction\nAuctionReplayer.replayOrders()\n\n# log\nsys.stdout = thisnotebooksys",
"Wall time: 94.1 ms\n"
]
],
[
[
"### 4.2. Auction is over\n\nClosing the auction will result in transactions and a new LOB with unmatched orders will be set.\nThe price is chosen as the one that maximizes volume of transactions.\n\nTrades are executed at the auction price, and according to a time priority. The remaining orders at the auction price are the newest orders.",
"_____no_output_____"
]
],
[
[
"%%time\n\n# log\nf = open('log_auctionClose.txt','w');\nsys.stdout = f\n\nLOB.b_auction = False\n\n# log\nsys.stdout = thisnotebooksys",
"Wall time: 12.4 ms\n"
]
],
[
[
"### 4.3. Lob State\nLOB state before opening the continuous trading",
"_____no_output_____"
]
],
[
[
"LOBstate = AuctionReplayer.getLOBState()\nLOBstate = LOBstate.set_index('Price').sort_index()\nLOBstate.plot.bar(figsize=(20, 7))\nplt.show()",
"_____no_output_____"
]
],
[
[
"### 4.4. Continuous Trading",
"_____no_output_____"
]
],
[
[
"%%time\n\n# log\nf = open('log_continuousTrading.txt','w');\nsys.stdout = f\n\n# Close auction\nContReplayer.replayOrders()\n\n# log\nsys.stdout = thisnotebooksys",
"Wall time: 5min 40s\n"
]
],
[
[
"## 5. Price Tape",
"_____no_output_____"
]
],
[
[
"histoPrices = ContReplayer.getPriceTape().astype(float)\nhistoPrices.plot(figsize=(20,7))\n\n# OHLC\ndisplay(f'open : {histoPrices.iloc[0,0]}')\ndisplay(f'high : {histoPrices.max()[0]}')\ndisplay(f'low : {histoPrices.min()[0]}')\ndisplay(f'close : {histoPrices.iloc[-1, 0]}')\n\nplt.show()",
"_____no_output_____"
]
],
[
[
"## Get Transaction Tape",
"_____no_output_____"
]
],
[
[
"TransactionTape = ContReplayer.getTransactionTape()",
"_____no_output_____"
],
[
"TransactionTape",
"_____no_output_____"
]
],
[
[
"## Get LOB Tape \n\nThe LOB tape is the state of the LOB before each order arrival",
"_____no_output_____"
]
],
[
[
"LOBtape = AuctionReplayer.getLOBTape()",
"_____no_output_____"
],
[
"LOBtape",
"_____no_output_____"
]
]
] | [
"markdown",
"code",
"markdown",
"code",
"markdown",
"code",
"markdown",
"code",
"markdown",
"code",
"markdown",
"code",
"markdown",
"code",
"markdown",
"code",
"markdown",
"code",
"markdown",
"code",
"markdown",
"code"
] | [
[
"markdown"
],
[
"code",
"code"
],
[
"markdown"
],
[
"code"
],
[
"markdown"
],
[
"code"
],
[
"markdown"
],
[
"code"
],
[
"markdown",
"markdown"
],
[
"code"
],
[
"markdown"
],
[
"code"
],
[
"markdown"
],
[
"code"
],
[
"markdown"
],
[
"code"
],
[
"markdown"
],
[
"code"
],
[
"markdown"
],
[
"code",
"code"
],
[
"markdown"
],
[
"code",
"code"
]
] |
e782e9a3832e4c8784007826b4cb6d02cb13942b | 14,911 | ipynb | Jupyter Notebook | modeling/hospital_quickstart.ipynb | Alicegif/covid19-severity-prediction | adb3f483e00444949aaaa46830b0cb5531525cfa | [
"MIT"
] | null | null | null | modeling/hospital_quickstart.ipynb | Alicegif/covid19-severity-prediction | adb3f483e00444949aaaa46830b0cb5531525cfa | [
"MIT"
] | null | null | null | modeling/hospital_quickstart.ipynb | Alicegif/covid19-severity-prediction | adb3f483e00444949aaaa46830b0cb5531525cfa | [
"MIT"
] | null | null | null | 29.468379 | 158 | 0.53142 | [
[
[
"**Note that this notebook uses private hospita-level data, so can't be run publicly**",
"_____no_output_____"
]
],
[
[
"%load_ext autoreload\n%autoreload 2\nimport numpy as np\nimport scipy as sp\nimport pandas as pd\nimport matplotlib.pyplot as plt\nfrom os.path import join as oj\nimport math\nimport pygsheets\nimport pickle as pkl\nimport pandas as pd\nimport seaborn as sns\nimport plotly.express as px\nfrom collections import Counter\nimport plotly\nfrom plotly.subplots import make_subplots\nimport plotly.graph_objects as go\nimport sys\nimport json\nimport os\nimport inspect\n\ncurrentdir = os.path.dirname(os.path.abspath(inspect.getfile(inspect.currentframe())))\nparentdir = os.path.dirname(currentdir)\nsys.path.append(parentdir)\nsys.path.append(parentdir + '/modeling')\n\nimport load_data\nfrom viz import viz_static, viz_interactive, viz_map\nfrom modeling.fit_and_predict import add_preds\nfrom functions import merge_data\nfrom functions import update_severity_index as severity_index\n\nNUM_DAYS_LIST = [1, 2, 3, 4, 5, 6, 7]\ndf_hospital = load_data.load_hospital_level(data_dir=oj(os.path.dirname(parentdir), 'covid-19-private-data'))\ndf_county = load_data.load_county_level(data_dir=oj(parentdir, 'data'))\ndf_county = add_preds(df_county, NUM_DAYS_LIST=NUM_DAYS_LIST, cached_dir=oj(parentdir, 'data')) # adds keys like \"Predicted Deaths 1-day\"\ndf = merge_data.merge_county_and_hosp(df_county, df_hospital)",
"_____no_output_____"
]
],
[
[
"# severity index",
"_____no_output_____"
]
],
[
[
"df = severity_index.add_severity_index(df, NUM_DAYS_LIST)\nd = severity_index.df_to_plot(df, NUM_DAYS_LIST)\nk = 3\ns_hosp = f'Predicted Deaths Hospital {k}-day'\ns_index = f'Severity {k}-day'\nprint('total hospitals', df.shape[0], Counter(df[s_index]))",
"total hospitals 5943 Counter({1: 3412, 2: 1266, 3: 1265})\n"
],
[
"viz_interactive.viz_index_animated(d, [1, 2, 3, 4, 5],\n x_key='Hospital Employees',\n y_key='Predicted (cumulative) deaths at hospital',\n hue='Severity Index',\n out_name=oj(parentdir, 'results', 'hosp_test.html'))",
"_____no_output_____"
],
[
"viz_interactive.viz_index_animated(d, [3],\n by_size=False,\n out_name=oj('results', 'hospital_index_animated_full.html'))",
"_____no_output_____"
],
[
"plt.figure(dpi=500)\nremap = {'High': 'red',\n 'Medium': 'blue',\n 'Low': 'green'}\ndr = d # d[d['Severity Index 1-day']=='Low']\nplt.scatter(dr['Predicted Deaths Hospital 1-day'],\n dr['Surge 1-day'],\n s=(dr['Hospital Employees'] / 500).clip(lower=0.1), alpha=0.9,\n c=[remap[x] for x in dr['Severity Index 1-day']])\n# plt.plot(d['Predicted Deaths Hospital 1-day'], d['Surge 1-day'], '.', )\n# plt.plot(d['Predicted Deaths Hospital 1-day'], d['Surge 1-day'], '.')\n# plt.yscale('log')\n# plt.xscale('log')\nplt.xlim((0, 10))\nplt.ylim((-1, 3))\nplt.xlabel('Predicted Deaths Hospital 1-day')\nplt.ylabel('Surge 1-day')\nplt.show()",
"_____no_output_____"
]
],
[
[
"**start with county-level death predictions**",
"_____no_output_____"
]
],
[
[
"s = f'Predicted Deaths {3}-day' # tot_deaths\n# s = 'tot_deaths'\nnum_days = 1\nnonzero = df[s] > 0\nplt.figure(dpi=300, figsize=(7, 3))\nplt.plot(df_county[s].values, '.', ms=3)\nplt.ylabel(s)\nplt.xlabel('Counties')\nplt.yscale('log')\nplt.tight_layout()\nplt.show()",
"_____no_output_____"
]
],
[
[
"**look at distribution of predicted deaths at hospitals**",
"_____no_output_____"
]
],
[
[
"num_days = 1\nplt.figure(dpi=300, figsize=(7, 3))\n\noffset = 0\nfor i in [5, 4, 3, 2, 1]:\n idxs = (df[s_index] == i)\n plt.plot(np.arange(offset, offset + idxs.sum()), \n np.clip(df[idxs][s_hosp].values, a_min=1, a_max=None), '.-', label=f'{i}: {severity_index.meanings[i]}')\n offset += idxs.sum()\nplt.yscale('log')\nplt.ylabel(s_hosp)\nplt.xlabel('Hospitals')\nplt.legend()\nplt.tight_layout()\nplt.show()",
"_____no_output_____"
],
[
"df.sort_values('Predicted Deaths Hospital 2-day', ascending=False)[['Hospital Name', 'StateName', \n 'Hospital Employees', 'tot_deaths',\n 'Predicted Deaths Hospital 2-day']].head(30)",
"_____no_output_____"
]
],
[
[
"# adjustments",
"_____no_output_____"
],
[
"**different measures of hospital size are pretty consistent**",
"_____no_output_____"
]
],
[
[
"plt.figure(dpi=500, figsize=(7, 3), facecolor='w')\nR, C = 1, 3\nplt.subplot(R, C, 1)\nplt.plot(df['Hospital Employees'], df['Total Average Daily Census'], '.', alpha=0.2, markeredgewidth=0)\nplt.xlabel('Num Hospital Employees')\nplt.ylabel('Total Average Daily Census')\n\nplt.subplot(R, C, 2)\nplt.plot(df['Hospital Employees'], df['Total Beds'], '.', alpha=0.2, markeredgewidth=0)\nplt.xlabel('Num Hospital Employees')\nplt.ylabel('Total Beds')\n\nplt.subplot(R, C, 3)\nplt.plot(df['Hospital Employees'], df['ICU Beds'], '.', alpha=0.2, markeredgewidth=0)\nplt.xlabel('Num Hospital Employees')\nplt.ylabel('ICU Beds')\n\nplt.tight_layout()\nplt.show()",
"_____no_output_____"
]
],
[
[
"**other measures are harder to parse...**",
"_____no_output_____"
]
],
[
[
"ks = ['Predicted Deaths Hospital 2-day', \"Hospital Employees\", 'ICU Beds']\nR, C = 1, len(ks)\nplt.figure(dpi=300, figsize=(C * 3, R * 3))\n\nfor c in range(C):\n plt.subplot(R, C, c + 1)\n if c == 0:\n plt.ylabel('Total Occupancy Rate')\n plt.plot(df[ks[c]], df['Total Occupancy Rate'], '.', alpha=0.5)\n plt.xlabel(ks[c])\n\nplt.tight_layout()\nplt.show()",
"_____no_output_____"
]
],
[
[
"**different hospital types**",
"_____no_output_____"
]
],
[
[
"plt.figure(dpi=500, figsize=(7, 3))\nR, C = 1, 3\na = 0.5\ns = s_hosp\nplt.subplot(R, C, 1)\nidxs = df.IsUrbanHospital == 1\nplt.hist(df[idxs][s], label='Urban', alpha=a)\nplt.hist(df[~idxs][s], label='Rural', alpha=a)\nplt.ylabel('Num Hospitals')\nplt.xlabel(s)\nplt.yscale('log')\nplt.legend()\n\nplt.subplot(R, C, 2)\nidxs = df.IsAcuteCareHospital == 1\nplt.hist(df[idxs][s], label='Acute Care', alpha=a)\nplt.hist(df[~idxs][s], label='Other', alpha=a)\nplt.xlabel(s)\nplt.yscale('log')\nplt.legend()\n\nplt.subplot(R, C, 3)\nidxs = df.IsAcademicHospital == 1\nplt.hist(df[idxs][s], label='Academic', alpha=a)\nplt.hist(df[~idxs][s], label='Other', alpha=a)\nplt.xlabel(s)\nplt.yscale('log')\n\nplt.legend()\nplt.tight_layout()\nplt.show()",
"_____no_output_____"
]
],
[
[
"**rural areas have lower occupancy rates**",
"_____no_output_____"
]
],
[
[
"idxs = df.IsUrbanHospital == 1\nplt.hist(df['Total Occupancy Rate'][idxs], label='urban', alpha=0.5)\nplt.hist(df['Total Occupancy Rate'][~idxs], label='rural', alpha=0.5)\nplt.xlabel('Total Occupancy Rate')\nplt.ylabel('Count')\nplt.legend()\nplt.show()",
"_____no_output_____"
],
[
"ks = ['ICU Beds', 'Total Beds', \n 'Hospital Employees', 'Registered Nurses',\n 'ICU Occupancy Rate', 'Total Occupancy Rate',\n 'Mortality national comparison', 'Total Average Daily Census',\n \n# 'IsAcademicHospital', \n 'IsUrbanHospital', 'IsAcuteCareHospital']\n \n \n\n# ks += [f'Predicted Deaths {n}-day' for n in NUM_DAYS_LIST]\nks += [f'Predicted Deaths Hospital {n}-day' for n in NUM_DAYS_LIST]\n\n# county-level stuff\n# ks += ['unacast_n_grade', Hospital Employees in County', 'tot_deaths', 'tot_cases', 'PopulationDensityperSqMile2010'] \n\n\nviz.corrplot(df[ks], SIZE=6)",
"_____no_output_____"
]
],
[
[
"# look at top counties/hospitals",
"_____no_output_____"
],
[
"**hospitals per county**",
"_____no_output_____"
]
],
[
[
"d = df\n\nR, C = 1, 2\nNUM_COUNTIES = 7\nplt.figure(dpi=300, figsize=(7, 3.5))\n\n\nplt.subplot(R, C, 1)\nc = 'County Name'\ncounty_names = d[c].unique()[:NUM_COUNTIES]\nnum_academic_hospitals = []\n# d = df[outcome_keys + hospital_keys]\n# d = d.sort_values('New Deaths', ascending=False)\nfor county in county_names:\n num_academic_hospitals.append(d[d[c] == county].shape[0])\nplt.barh(county_names[::-1], num_academic_hospitals[::-1]) # reverse to plot top down\nplt.xlabel('Number academic hospitals\\n(for hospitals where we have data)')\n\nplt.subplot(R, C, 2)\nplt.barh(df_county.CountyName[:NUM_COUNTIES].values[::-1], df_county['Hospital Employees in County'][:NUM_COUNTIES][::-1]) # reverse to plot top down\nplt.xlabel('# Hospital Employees')\n\nplt.tight_layout()\nplt.show()",
"_____no_output_____"
],
[
"county_names = d[c].unique()[:NUM_COUNTIES]\nR, C = 4, 1\nplt.figure(figsize=(C * 3, R * 3), dpi=200)\nfor i in range(R * C):\n plt.subplot(R, C, i + 1)\n cn = county_names[i]\n dc = d[d[c] == cn]\n plt.barh(dc['Hospital Name'][::-1], dc['Hospital Employees'][::-1])\n plt.title(cn)\n plt.xlabel('# Hospital Employees')\nplt.tight_layout()\n# plt.subplots_adjust(bottom=1)\nplt.show()",
"_____no_output_____"
]
],
[
[
"## Hospital severity map",
"_____no_output_____"
]
],
[
[
"counties_json = json.load(open(oj(parentdir, \"data\", \"geojson-counties-fips.json\"), \"r\"))\nviz_map.plot_hospital_severity_slider(\n df, df_county=df_county, \n counties_json=counties_json, dark=False,\n filename = oj(parentdir, \"results\", \"severity_map.html\")\n)",
"_____no_output_____"
]
],
[
[
"## hospital contact info gsheet",
"_____no_output_____"
]
],
[
[
"ks_orig = ['countyFIPS', 'CountyName', 'Total Deaths Hospital', 'Hospital Name', 'CMS Certification Number', 'StateName', 'System Affiliation']\nks_contact = ['Phone Number', 'Hospital Employees', 'Website', 'Number to Call (NTC)', 'Donation Phone Number', 'Donation Email', 'Notes']\ndef write_to_gsheets_contact(df, ks_output,\n sheet_name='Contact Info',\n service_file='creds.json'):\n \n d = df[ks_output].fillna('')\n print('writing to gsheets...')\n gc = pygsheets.authorize(service_file=service_file)\n sh = gc.open(sheet_name) # name of the hospital\n wks = sh[0] #select a sheet\n wks.update_value('A1', \"Last updated Apr 14\")\n wks.set_dataframe(d, (3, 1)) #update the first sheet with df, starting at cell B2. \n \nwrite_to_gsheets_contact(df, ks_output=ks_orig + ks_contact)",
"_____no_output_____"
]
]
] | [
"markdown",
"code",
"markdown",
"code",
"markdown",
"code",
"markdown",
"code",
"markdown",
"code",
"markdown",
"code",
"markdown",
"code",
"markdown",
"code",
"markdown",
"code",
"markdown",
"code",
"markdown",
"code"
] | [
[
"markdown"
],
[
"code"
],
[
"markdown"
],
[
"code",
"code",
"code",
"code"
],
[
"markdown"
],
[
"code"
],
[
"markdown"
],
[
"code",
"code"
],
[
"markdown",
"markdown"
],
[
"code"
],
[
"markdown"
],
[
"code"
],
[
"markdown"
],
[
"code"
],
[
"markdown"
],
[
"code",
"code"
],
[
"markdown",
"markdown"
],
[
"code",
"code"
],
[
"markdown"
],
[
"code"
],
[
"markdown"
],
[
"code"
]
] |
e782fa40c8a2d06adc2b7dbdb9e44b2dd38dacb8 | 14,277 | ipynb | Jupyter Notebook | haokai/Speed_Comparison.ipynb | songqsh/Is20f | 42caacb6316feb7f3fde4936a3ce37ba4e249f8b | [
"MIT"
] | null | null | null | haokai/Speed_Comparison.ipynb | songqsh/Is20f | 42caacb6316feb7f3fde4936a3ce37ba4e249f8b | [
"MIT"
] | 7 | 2020-09-05T14:28:47.000Z | 2020-11-18T19:58:10.000Z | haokai/Speed_Comparison.ipynb | songqsh/Is20f | 42caacb6316feb7f3fde4936a3ce37ba4e249f8b | [
"MIT"
] | 3 | 2020-09-09T15:52:34.000Z | 2020-09-16T18:40:05.000Z | 29.018293 | 236 | 0.411361 | [
[
[
"<a href=\"https://colab.research.google.com/github/hhk54250/Is20f/blob/master/haokai/Speed_Comparison.ipynb\" target=\"_parent\"><img src=\"https://colab.research.google.com/assets/colab-badge.svg\" alt=\"Open In Colab\"/></a>",
"_____no_output_____"
]
],
[
[
"import numpy as np\n\ndef BSM_characteristic_function(v, x0, T, r, sigma):\n cf_value = np.exp(((x0 / T + r - 0.5 * sigma ** 2) * 1j * v\n - 0.5 * sigma ** 2 * v ** 2) * T)\n return cf_value\ndef BSM_call_characteristic_function(v,alpha, x0, T, r, sigma):\n res=np.exp(-r*T)/((alpha+1j*v)*(alpha+1j*v+1))\\\n *BSM_characteristic_function((v-(alpha+1)*1j), x0, T, r, sigma)\n return res\n \ndef SimpsonW(N,eta):\n delt = np.zeros(N, dtype=np.float)\n delt[0] = 1\n j = np.arange(1, N + 1, 1)\n SimpsonW = eta*(3 + (-1) ** j - delt) / 3\n return SimpsonW\n \n\ndef Simposon_numerical_integrate(S0, K, T, r, sigma):\n k = np.log(K)\n x0 = np.log(S0)\n N=1024\n B=153.6\n eta=B/N\n W=SimpsonW(N,eta)\n \n alpha=1.5\n sumx=0\n for j in range(N):\n v_j=j*eta\n temp=np.exp(-1j*v_j*k)*\\\n BSM_call_characteristic_function(v_j,alpha, x0, T, r, sigma)*\\\n W[j] \n sumx+=temp.real\n\n \n return sumx*np.exp(-alpha*k)/np.pi",
"_____no_output_____"
],
[
"S0 = 100.0 # index level\nK = 108.52520983216910821762196480844 # option strike\nT = 1.0 # maturity date\nr = 0.0475 # risk-less short rate\nsigma = 0.2 # volatility\n\nprint ('>>>>>>>>>>FT call value is ' + str(Simposon_numerical_integrate(S0, K, T, r, sigma)))",
">>>>>>>>>>FT call value is 6.477779672276538\n"
],
[
"%cd~\n\n!git clone https://github.com/hhk54250/20MA573-HHK.git \npass\n",
"/root\nCloning into '20MA573-HHK'...\nremote: Enumerating objects: 29, done.\u001b[K\nremote: Counting objects: 100% (29/29), done.\u001b[K\nremote: Compressing objects: 100% (26/26), done.\u001b[K\nremote: Total 200 (delta 11), reused 0 (delta 0), pack-reused 171\u001b[K\nReceiving objects: 100% (200/200), 5.79 MiB | 26.82 MiB/s, done.\nResolving deltas: 100% (85/85), done.\n"
],
[
"\n%cd 20MA573-HHK/src/\n%ls",
"/root/20MA573-HHK/src\nbsm.py optiondata.dat prj01.ipynb prj02.ipynb\n"
],
[
"from bsm import *\n\n\n'''===============\nTest bsm_price\n================='''\ngbm1 = Gbm(\n init_state = 100., \n drift_ratio = .0475,\n vol_ratio = .2)\noption1 = VanillaOption(\n otype = 1,\n strike = 108.52520983216910821762196480844, \n maturity = 1.\n) \n\nprint('>>>>>>>>>>BSM call value is ' + str(gbm1.bsm_price(option1)))",
">>>>>>>>>>BSM call value is 6.477779672277251\n"
],
[
"def fft(FFTFunc):\n N=2**10\n eta=0.15\n lambda_ = 2 * np.pi / (N *eta) \n t=np.arange(0, N, 1)\n sumy=np.asarray([np.sum(np.exp(-1j*lambda_*eta*t*m)*FFTFunc) for m in range(N)])\n\n \n return sumy\n\ndef BSM_call_value_FFT(S0, K, T, r, sigma):\n k = np.log(K)\n x0 = np.log(S0)\n N =2**10\n alpha=1.5\n \n eta=0.15\n lambda_ = 2 * np.pi / (N *eta)\n beta=x0-lambda_*N/2\n km=np.asarray([beta+i*lambda_ for i in range(N)])\n W=SimpsonW(N,eta)\n v=np.asarray([i*eta for i in range(N)])\n Psi=np.asarray([BSM_call_characteristic_function(vj,alpha, x0, T, r, sigma) for vj in v])\n FFTFunc=Psi*np.exp(-1j*beta*v)*W\n \n \n y=fft(FFTFunc).real\n \n \n cT=np.exp(-alpha*km)*y/np.pi\n \n return cT",
"_____no_output_____"
],
[
"\nS0 = 100.0 # index level\nK = 110.0 # option strike\nT = 1.0 # maturity date\nr = 0.0475 # risk-less short rate\nsigma = 0.2 # volatility\nprint('>>>>>>>>>>FFT call value is ' + str(BSM_call_value_FFT(S0, K, T, r, sigma)[514]))",
">>>>>>>>>>FFT call value is 6.4777796722766245\n"
],
[
"\"FFT time test\"\nS0 = 100.0 # index level\nK = 110.0 # option strike\nT = 1.0 # maturity date\nr = 0.0475 # risk-less short rate\nsigma = 0.2 # volatility\n%time BSM_call_value_FFT(S0, K, T, r, sigma)",
"CPU times: user 120 ms, sys: 618 µs, total: 120 ms\nWall time: 121 ms\n"
],
[
"\"FT time test\"\nS0 = 100.0 # index level\nT = 1.0 # maturity date\nr = 0.0475 # risk-less short rate\nsigma = 0.2 # volatility\nN =2**10 \neta=0.15\nlambda_ = 2 * np.pi / (N *eta)\nx0 = np.log(S0)\nbeta=x0-lambda_*N/2\nk=np.asarray([np.e**(beta+lambda_*n) for n in range(N)])\n%time np.asarray([Simposon_numerical_integrate(S0, k[n], T, r, sigma) for n in range(N)])\n",
"CPU times: user 13.6 s, sys: 1.28 ms, total: 13.6 s\nWall time: 13.6 s\n"
],
[
"\"BSM time test\"\ngbm1 = Gbm(\n init_state = 100., \n drift_ratio = .0475,\n vol_ratio = .2)\noption1 = VanillaOption(\n otype = 1,\n strike = k, \n maturity = 1.\n) \n\n%time gbm1.bsm_price(option1)",
"CPU times: user 2.04 ms, sys: 0 ns, total: 2.04 ms\nWall time: 1.86 ms\n"
],
[
"def BSM_call_value_NumpyFFT(S0, K, T, r, sigma):\n k = np.log(K)\n x0 = np.log(S0)\n N =2**10\n alpha=1.5\n \n eta=0.15\n lambda_ = 2 * np.pi / (N *eta)\n beta=x0-lambda_*N/2\n km=np.asarray([beta+i*lambda_ for i in range(N)])\n W=SimpsonW(N,eta)\n v=np.asarray([i*eta for i in range(N)])\n Psi=np.asarray([BSM_call_characteristic_function(vj,alpha, x0, T, r, sigma) for vj in v])\n FFTFunc=Psi*np.exp(-1j*beta*v)*W\n \n \n y=np.fft.fft(FFTFunc).real\n \n \n cT=np.exp(-alpha*km)*y/np.pi",
"_____no_output_____"
],
[
"\"FFT time test using Numpy.FFT package\"\nS0 = 100.0 # index level\nK = 110.0 # option strike\nT = 1.0 # maturity date\nr = 0.0475 # risk-less short rate\nsigma = 0.2 # volatility\n%time BSM_call_value_NumpyFFT(S0, K, T, r, sigma)",
"CPU times: user 19.9 ms, sys: 1.02 ms, total: 20.9 ms\nWall time: 22.6 ms\n"
],
[
"",
"_____no_output_____"
]
]
] | [
"markdown",
"code"
] | [
[
"markdown"
],
[
"code",
"code",
"code",
"code",
"code",
"code",
"code",
"code",
"code",
"code",
"code",
"code",
"code"
]
] |
e783073520e1fe4eac2b82b0f04d7443b434bd19 | 88,774 | ipynb | Jupyter Notebook | eda/withdraw_reason.ipynb | Karunya-Manoharan/High-school-drop-out-prediction | 13bf3f10f2344fb066463fe3f0eaaef6894f01c9 | [
"MIT"
] | null | null | null | eda/withdraw_reason.ipynb | Karunya-Manoharan/High-school-drop-out-prediction | 13bf3f10f2344fb066463fe3f0eaaef6894f01c9 | [
"MIT"
] | null | null | null | eda/withdraw_reason.ipynb | Karunya-Manoharan/High-school-drop-out-prediction | 13bf3f10f2344fb066463fe3f0eaaef6894f01c9 | [
"MIT"
] | null | null | null | 82.427112 | 6,592 | 0.377566 | [
[
[
"import sys\nimport psycopg2 as pg2 # Preferred cursor connection\nfrom sqlalchemy import create_engine # preferred for pushing back to DB\nimport yaml\nimport pandas as pd\nimport numpy as np",
"_____no_output_____"
],
[
"# Might need your own path...\nwith open('/data/users/dschnelb/secrets.yaml', 'r') as f:\n # loads contents of secrets.yaml into a python dictionary\n secret_config = yaml.safe_load(f.read())\n\n# Set database connection to `conn`\ndb_params = secret_config['db']\nconn = pg2.connect(host=db_params['host'],\n port=db_params['port'],\n dbname=db_params['dbname'],\n user=db_params['user'],\n password=db_params['password'])\n\n# Connect cursor with psycopg2 database connection\ncur = conn.cursor()",
"_____no_output_____"
]
],
[
[
"# Raw data for all_snapshots, student at grade 10 and above\n\n## use DataFrame `raw`",
"_____no_output_____"
]
],
[
[
"qry = '''\nSELECT * from clean.all_snapshots\nwhere grade >= 10;\n'''\n\ncur.execute(qry)\n\nrows = cur.fetchall()\n\n# Build dataframe from rows\nraw = pd.DataFrame(rows, columns=[name[0] for name in cur.description])\n\n# Make sure student_id is an int\nraw['student_lookup'] = raw['student_lookup'].astype('int')\n\nraw.head()",
"_____no_output_____"
],
[
"raw = raw.replace([None],np.nan)",
"_____no_output_____"
],
[
"all_students = raw[(raw['grade']==12) & (~raw['school_year'].isin([2006, 2007, 2008]))].groupby(['district','school_year']).agg({'student_lookup':'count'})",
"_____no_output_____"
],
[
"withdraw_na = raw[(raw['withdraw_reason'].isna()) & (raw['grade']==12) & (~raw['school_year'].isin([2006, 2007, 2008]))]\nwithdraw_na",
"_____no_output_____"
],
[
"withdraw_na.groupby(['district','school_year']).agg({'student_lookup':'count'})",
"_____no_output_____"
],
[
"all_students.join(withdraw_na.groupby(['district','school_year']).agg({'student_lookup':'count'}), rsuffix='_na')",
"_____no_output_____"
]
],
[
[
"# Certain districts in certain years seem to not use `withdraw_reason` for grade 12 \n\n## Zanesville simply lacks data outside of 2015, but other years where there is effectively total missingness on withdraw reason appear to have `graduation_date` listed for most students, and can likely be used as an imputation.",
"_____no_output_____"
]
],
[
[
"missing_by_district = all_students.join(withdraw_na.groupby(['district','school_year']).agg({'student_lookup':'count'}), rsuffix='_na')\n\nmissing_by_district[missing_by_district['student_lookup_na'].notnull()]",
"_____no_output_____"
]
],
[
[
"# Raw data for withdraw reason for students in 12th grade; also includes any student with a `graduate` withdraw reason since it is possible to graduate early\n\n## use DataFrame `grad_df`",
"_____no_output_____"
]
],
[
[
"qry = '''\nSELECT student_lookup,\n grade,\n school_year,\n withdraw_reason\nfrom clean.all_snapshots\nwhere grade = 12 or withdraw_reason = 'graduate'\norder by student_lookup;\n'''\n\ncur.execute(qry)\n\nrows = cur.fetchall()\n\n# Build dataframe from rows\ngrad_df = pd.DataFrame(rows, columns=[name[0] for name in cur.description])\n\n# Make sure student_id is an int\ngrad_df['student_lookup'] = grad_df['student_lookup'].astype('int')\n\ngrad_df.head()",
"_____no_output_____"
],
[
"len(grad_df)",
"_____no_output_____"
],
[
"grad_df['withdraw_reason'].replace(to_replace=[None], value='Missing', inplace=True)",
"_____no_output_____"
],
[
"cnt_withdraw = grad_df.groupby(['school_year','withdraw_reason']).agg({'student_lookup':'count'})",
"_____no_output_____"
],
[
"# Withdraw reasons for 12th graders by year\ncnt_withdraw.unstack(0).replace(np.nan, 0).astype('int')",
"_____no_output_____"
]
],
[
[
"# All (student, school_year) entering grade 10",
"_____no_output_____"
]
],
[
[
"# Gets all students entering grade 10 at school year\nqry = '''\nSELECT distinct student_lookup,\n grade,\n school_year\nfrom clean.all_snapshots\nwhere grade = 10\norder by student_lookup;\n'''\n\ncur.execute(qry)\n\nrows = cur.fetchall()\n\n# Build dataframe from rows\ndf = pd.DataFrame(rows, columns=[name[0] for name in cur.description])\n\n# Make sure student_id is an int\ndf['student_lookup'] = df['student_lookup'].astype('int')\n\ndf.head()",
"_____no_output_____"
]
],
[
[
"# Links the future \"withdraw reason\" in grade 12 to the student entering 10th grade\n\n## Use DataFrame `grd_10`",
"_____no_output_____"
]
],
[
[
"\n# Left join means it keeps all 10th grade students, even if they didn't appear in the grad_df\ngrd_10 = pd.merge(df, grad_df, how='left', on='student_lookup')\ngrd_10",
"_____no_output_____"
],
[
"grd_10.columns = ['student_lookup',\t'grade_10',\t'yr_grade_10',\t'grade_12',\t'yr_grade_12',\t'grade_12_withdraw']",
"_____no_output_____"
],
[
"grd_10",
"_____no_output_____"
],
[
"grd_10.groupby(['yr_grade_10', 'grade_12_withdraw']).agg({'student_lookup':'count'}).unstack(0).replace(np.nan, 0).astype('int')",
"_____no_output_____"
]
],
[
[
"# Data obtained by the View (sketch.hs_withdraw_info) WITHOUT further deduplication (grade 10)\n\n## Use DataFrame `hs_w`",
"_____no_output_____"
]
],
[
[
"qry = '''\nSELECT * from sketch.hs_withdraw_info\nWHERE grade=10 and entry_year BETWEEN 2007 AND 2013;\n'''\n\ncur.execute(qry)\n\nrows = cur.fetchall()\n\n# Build dataframe from rows\nhs_w = pd.DataFrame(rows, columns=[name[0] for name in cur.description])\n\n# Make sure student_id is an int\nhs_w ['student_lookup'] = hs_w['student_lookup'].astype('int')\n\nhs_w[:10]",
"_____no_output_____"
],
[
"grd_10[grd_10['student_lookup']==47]",
"_____no_output_____"
],
[
"# grd_10 students entering 2007-2013 (our cohorts of interest)\nlen(grd_10[grd_10['yr_grade_10'].isin(list(range(2007,2014)))])",
"_____no_output_____"
]
],
[
[
"# Current data retrieval",
"_____no_output_____"
]
],
[
[
"cur.execute('''\n select *\n from (\n\t\t SELECT *, ROW_NUMBER() OVER\n\t\t (PARTITION BY student_lookup, grade\n ORDER BY student_lookup) AS rnum\n\t\t FROM sketch.hs_withdraw_info hwi) t\n where t.rnum = 1\n and t.grade = 10\n and t.entry_year >= 2007 and t.entry_year <= 2013\n and ((t.grad_year is not null or t.dropout_year is not null)\n \t\tor (t.transfer_out_year is null))\n and ((t.grad_year is not null or t.dropout_year is not null)\n \t\t\tor (t.in_state_transfer_year is null));\n ''')\n\nrows = cur.fetchall()\n\n# Build dataframe from rows\nexisting = pd.DataFrame(rows, columns=[name[0] for name in cur.description])\n\n# Make sure student_id is an int\nexisting['student_lookup'] = existing['student_lookup'].astype('int')\n\nexisting",
"_____no_output_____"
],
[
"list(range(2007,2014))",
"_____no_output_____"
]
]
] | [
"code",
"markdown",
"code",
"markdown",
"code",
"markdown",
"code",
"markdown",
"code",
"markdown",
"code",
"markdown",
"code",
"markdown",
"code"
] | [
[
"code",
"code"
],
[
"markdown"
],
[
"code",
"code",
"code",
"code",
"code",
"code"
],
[
"markdown"
],
[
"code"
],
[
"markdown"
],
[
"code",
"code",
"code",
"code",
"code"
],
[
"markdown"
],
[
"code"
],
[
"markdown"
],
[
"code",
"code",
"code",
"code"
],
[
"markdown"
],
[
"code",
"code",
"code"
],
[
"markdown"
],
[
"code",
"code"
]
] |
e7831d2e3212667e47b81155296b14f5c2ae9f11 | 137,470 | ipynb | Jupyter Notebook | notebooks/Repairer.ipynb | bjrnmath/debuggingbook | 8b6cd36fc75a89464e9252e40e1d4edcb6a70559 | [
"MIT"
] | null | null | null | notebooks/Repairer.ipynb | bjrnmath/debuggingbook | 8b6cd36fc75a89464e9252e40e1d4edcb6a70559 | [
"MIT"
] | null | null | null | notebooks/Repairer.ipynb | bjrnmath/debuggingbook | 8b6cd36fc75a89464e9252e40e1d4edcb6a70559 | [
"MIT"
] | null | null | null | 31.052632 | 556 | 0.553852 | [
[
[
"# Repairing Code Automatically\n\nSo far, we have discussed how to track failures and how to locate defects in code. Let us now discuss how to _repair_ defects – that is, to correct the code such that the failure no longer occurs. We will discuss how to _repair code automatically_ – by systematically searching through possible fixes and evolving the most promising candidates.",
"_____no_output_____"
]
],
[
[
"from bookutils import YouTubeVideo\nYouTubeVideo(\"UJTf7cW0idI\")",
"_____no_output_____"
]
],
[
[
"**Prerequisites**\n\n* Re-read the [introduction to debugging](Intro_Debugging.ipynb), notably on how to properly fix code.\n* We make use of automatic fault localization, as discussed in the [chapter on statistical debugging](StatisticalDebugger.ipynb).\n* We make extensive use of code transformations, as discussed in [the chapter on tracing executions](Tracer.ipynb).\n* We make use of [delta debugging](DeltaDebugger.ipynb).",
"_____no_output_____"
]
],
[
[
"import bookutils",
"_____no_output_____"
]
],
[
[
"## Synopsis\n<!-- Automatically generated. Do not edit. -->\n\nTo [use the code provided in this chapter](Importing.ipynb), write\n\n```python\n>>> from debuggingbook.Repairer import <identifier>\n```\n\nand then make use of the following features.\n\n\nThis chapter provides tools and techniques for automated repair of program code. The `Repairer()` class takes a `RankingDebugger` debugger as input (such as `OchiaiDebugger` from [the chapter on statistical debugging](StatisticalDebugger.ipynb). A typical setup looks like this:\n\n```python\nfrom debuggingbook.StatisticalDebugger import OchiaiDebugger\n\ndebugger = OchiaiDebugger()\nfor inputs in TESTCASES:\n with debugger:\n test_foo(inputs)\n...\n\nrepairer = Repairer(debugger)\n```\nHere, `test_foo()` is a function that raises an exception if the tested function `foo()` fails. If `foo()` passes, `test_foo()` should not raise an exception.\n\nThe `repair()` method of a `Repairer` searches for a repair of the code covered in the debugger (except for methods starting or ending in `test`, such that `foo()`, not `test_foo()` is repaired). `repair()` returns the best fix candidate as a pair `(tree, fitness)` where `tree` is a [Python abstract syntax tree](http://docs.python.org/3/library/ast) (AST) of the fix candidate, and `fitness` is the fitness of the candidate (a value between 0 and 1). A `fitness` of 1.0 means that the candidate passed all tests. A typical usage looks like this:\n\n```python\nimport astor\n\ntree, fitness = repairer.repair()\nprint(astor.to_source(tree), fitness)\n```\n\nHere is a complete example for the `middle()` program. This is the original source code of `middle()`:\n\n```python\ndef middle(x, y, z): # type: ignore\n if y < z:\n if x < y:\n return y\n elif x < z:\n return y\n else:\n if x > y:\n return y\n elif x > z:\n return x\n return z\n```\nWe set up a function `middle_test()` that tests it. The `middle_debugger` collects testcases and outcomes:\n\n```python\n>>> middle_debugger = OchiaiDebugger()\n>>> for x, y, z in MIDDLE_PASSING_TESTCASES + MIDDLE_FAILING_TESTCASES:\n>>> with middle_debugger:\n>>> middle_test(x, y, z)\n```\nThe repairer attempts to repair the invoked function (`middle()`). The returned AST `tree` can be output via `astor.to_source()`:\n\n```python\n>>> middle_repairer = Repairer(middle_debugger)\n>>> tree, fitness = middle_repairer.repair()\n>>> print(astor.to_source(tree), fitness)\ndef middle(x, y, z):\n if y < z:\n if x < z:\n if x < y:\n return y\n else:\n return x\n elif x > y:\n return y\n elif x > z:\n return x\n return z\n 1.0\n\n```\nHere are the classes defined in this chapter. A `Repairer` repairs a program, using a `StatementMutator` and a `CrossoverOperator` to evolve a population of candidates.\n\n\n",
"_____no_output_____"
],
[
"## Automatic Code Repairs\n\nSo far, we have discussed how to locate defects in code, how to track failures back to the defects that caused them, and how to systematically determine failure conditions. Let us now address the last step in debugging – namely, how to _automatically fix code_.\n\nAlready in the [introduction to debugging](Intro_Debugging.ipynb), we have discussed how to fix code manually. Notably, we have established that a _diagnosis_ (which induces a fix) should show _causality_ (i.e, how the defect causes the failure) and _incorrectness_ (how the defect is wrong). Is it possible to obtain such a diagnosis automatically?",
"_____no_output_____"
],
[
"In this chapter, we introduce a technique of _automatic code repair_ – that is, for a given failure, automatically determine a fix that makes the failure go away. To do so, we randomly (but systematically) _mutate_ the program code – that is, insert, change, and delete fragments – until we find a change that actually causes the failing test to pass.",
"_____no_output_____"
],
[
"If this sounds like an audacious idea, that is because it is. But not only is _automated program repair_ one of the hottest topics of software research in the last decade, it is also being increasingly deployed in industry. At Facebook, for instance, every failing test report comes with an automatically generated _repair suggestion_ – a suggestion that already has been validated to work. Programmers can apply the suggestion as is or use it as basis for their own fixes.",
"_____no_output_____"
],
[
"### The middle() Function",
"_____no_output_____"
],
[
"Let us introduce our ongoing example. In the [chapter on statistical debugging](StatisticalDebugger.ipynb), we have introduced the `middle()` function – a function that returns the \"middle\" of three numbers `x`, `y`, and `z`:",
"_____no_output_____"
]
],
[
[
"from StatisticalDebugger import middle",
"_____no_output_____"
],
[
"# ignore\nfrom bookutils import print_content",
"_____no_output_____"
],
[
"# ignore\nimport inspect",
"_____no_output_____"
],
[
"# ignore\n_, first_lineno = inspect.getsourcelines(middle)\nmiddle_source = inspect.getsource(middle)\nprint_content(middle_source, '.py', start_line_number=first_lineno)",
"_____no_output_____"
]
],
[
[
"In most cases, `middle()` just runs fine:",
"_____no_output_____"
]
],
[
[
"middle(4, 5, 6)",
"_____no_output_____"
]
],
[
[
"In some other cases, though, it does not work correctly:",
"_____no_output_____"
]
],
[
[
"middle(2, 1, 3)",
"_____no_output_____"
]
],
[
[
"### Validated Repairs",
"_____no_output_____"
],
[
"Now, if we only want a repair that fixes this one given failure, this would be very easy. All we have to do is to replace the entire body by a single statement:",
"_____no_output_____"
]
],
[
[
"def middle_sort_of_fixed(x, y, z): # type: ignore\n return x",
"_____no_output_____"
]
],
[
[
"You will concur that the failure no longer occurs:",
"_____no_output_____"
]
],
[
[
"middle_sort_of_fixed(2, 1, 3)",
"_____no_output_____"
]
],
[
[
"But this, of course, is not the aim of automatic fixes, nor of fixes in general: We want our fixes not only to make the given failure go away, but we also want the resulting code to be _correct_ (which, of course, is a lot harder).",
"_____no_output_____"
],
[
"Automatic repair techniques therefore assume the existence of a _test suite_ that can check whether an implementation satisfies its requirements. Better yet, one can use the test suite to gradually check _how close_ one is to perfection: A piece of code that satisfies 99% of all tests is better than one that satisfies ~33% of all tests, as `middle_sort_of_fixed()` would do (assuming the test suite evenly checks the input space).",
"_____no_output_____"
],
[
"### Genetic Optimization",
"_____no_output_____"
],
[
"The master plan for automatic repair follows the principle of _genetic optimization_. Roughly spoken, genetic optimization is a _metaheuristic_ inspired by the process of _natural selection_. The idea is to _evolve_ a selection of _candidate solutions_ towards a maximum _fitness_:\n\n1. Have a selection of _candidates_.\n2. Determine the _fitness_ of each candidate.\n3. Retain those candidates with the _highest fitness_.\n4. Create new candidates from the retained candidates, by applying genetic operations:\n * _Mutation_ mutates some aspect of a candidate.\n * _CrossoverOperator_ creates new candidates combining features of two candidates.\n5. Repeat until an optimal solution is found.",
"_____no_output_____"
],
[
"Applied for automated program repair, this means the following steps:\n\n1. Have a _test suite_ with both failing and passing tests that helps asserting correctness of possible solutions.\n2. With the test suite, use [fault localization](StatisticalDebugger.ipynb) to determine potential code locations to be fixed.\n3. Systematically _mutate_ the code (by adding, changing, or deleting code) and _cross_ code to create possible fix candidates.\n4. Identify the _fittest_ fix candidates – that is, those that satisfy the most tests.\n5. _Evolve_ the fittest candidates until a perfect fix is found, or until time resources are depleted.",
"_____no_output_____"
],
[
"Let us illustrate these steps in the following sections.",
"_____no_output_____"
],
[
"## A Test Suite",
"_____no_output_____"
],
[
"In automated repair, the larger and the more thorough the test suite, the higher the quality of the resulting fix (if any). Hence, if we want to repair `middle()` automatically, we need a good test suite – with good inputs, but also with good checks",
"_____no_output_____"
],
[
"For better repair, we will use the test suites introduced in the [chapter on statistical debugging](StatisticalDebugger.ipynb):",
"_____no_output_____"
]
],
[
[
"from StatisticalDebugger import MIDDLE_PASSING_TESTCASES, MIDDLE_FAILING_TESTCASES",
"_____no_output_____"
]
],
[
[
"The `middle_test()` function fails whenever `middle()` returns an incorrect result:",
"_____no_output_____"
]
],
[
[
"def middle_test(x: int, y: int, z: int) -> None:\n m = middle(x, y, z)\n assert m == sorted([x, y, z])[1]",
"_____no_output_____"
],
[
"from ExpectError import ExpectError",
"_____no_output_____"
],
[
"with ExpectError():\n middle_test(2, 1, 3)",
"_____no_output_____"
]
],
[
[
"## Locating the Defect",
"_____no_output_____"
],
[
"Our next step is to find potential defect locations – that is, those locations in the code our mutations should focus upon. Since we already do have two test suites, we can make use of [statistical debugging](StatisticalDebugger.ipynb) to identify likely faulty locations. Our `OchiaiDebugger` ranks individual code lines by how frequently they are executed in failing runs (and not in passing runs).",
"_____no_output_____"
]
],
[
[
"from StatisticalDebugger import OchiaiDebugger, RankingDebugger",
"_____no_output_____"
],
[
"middle_debugger = OchiaiDebugger()\n\nfor x, y, z in MIDDLE_PASSING_TESTCASES + MIDDLE_FAILING_TESTCASES:\n with middle_debugger:\n middle_test(x, y, z)",
"_____no_output_____"
]
],
[
[
"We see that the upper half of the `middle()` code is definitely more suspicious:",
"_____no_output_____"
]
],
[
[
"middle_debugger",
"_____no_output_____"
]
],
[
[
"The most suspicious line is:",
"_____no_output_____"
]
],
[
[
"# ignore\nlocation = middle_debugger.rank()[0]\n(func_name, lineno) = location\nlines, first_lineno = inspect.getsourcelines(middle)\nprint(lineno, end=\"\")\nprint_content(lines[lineno - first_lineno], '.py')",
"_____no_output_____"
]
],
[
[
"with a suspiciousness of:",
"_____no_output_____"
]
],
[
[
"# ignore\nmiddle_debugger.suspiciousness(location)",
"_____no_output_____"
]
],
[
[
"## Random Code Mutations",
"_____no_output_____"
],
[
"Our third step in automatic code repair is to _randomly mutate the code_. Specifically, we want to randomly _delete_, _insert_, and _replace_ statements in the program to be repaired. However, simply synthesizing code _from scratch_ is unlikely to yield anything meaningful – the number of combinations is simply far too high. Already for a three-character identifier name, we have more than 200,000 combinations:",
"_____no_output_____"
]
],
[
[
"import string",
"_____no_output_____"
],
[
"string.ascii_letters",
"_____no_output_____"
],
[
"len(string.ascii_letters + '_') * \\\n len(string.ascii_letters + '_' + string.digits) * \\\n len(string.ascii_letters + '_' + string.digits)",
"_____no_output_____"
]
],
[
[
"Hence, we do _not_ synthesize code from scratch, but instead _reuse_ elements from the program to be fixed, hypothesizing that \"a program that contains an error in one area likely implements the correct behavior elsewhere\" \\cite{LeGoues2012}.",
"_____no_output_____"
],
[
"Furthermore, we do not operate on a _textual_ representation of the program, but rather on a _structural_ representation, which by construction allows us to avoid lexical and syntactical errors in the first place.\n\nThis structural representation is the _abstract syntax tree_ (AST), which we already have seen in various chapters, such as the [chapter on delta debugging](DeltaDebugger.ipynb), the [chapter on tracing](Tracer.ipynb), and excessively in the [chapter on slicing](Slicer.ipynb). The [official Python `ast` reference](http://docs.python.org/3/library/ast) is complete, but a bit brief; the documentation [\"Green Tree Snakes - the missing Python AST docs\"](https://greentreesnakes.readthedocs.io/en/latest/) provides an excellent introduction.\n\nRecapitulating, an AST is a tree representation of the program, showing a hierarchical structure of the program's elements. Here is the AST for our `middle()` function.",
"_____no_output_____"
]
],
[
[
"import ast\nimport astor\nimport inspect",
"_____no_output_____"
],
[
"from bookutils import print_content, show_ast",
"_____no_output_____"
],
[
"def middle_tree() -> ast.AST:\n return ast.parse(inspect.getsource(middle))",
"_____no_output_____"
],
[
"show_ast(middle_tree())",
"_____no_output_____"
]
],
[
[
" You see that it consists of one function definition (`FunctionDef`) with three `arguments` and two statements – one `If` and one `Return`. Each `If` subtree has three branches – one for the condition (`test`), one for the body to be executed if the condition is true (`body`), and one for the `else` case (`orelse`). The `body` and `orelse` branches again are lists of statements.",
"_____no_output_____"
],
[
"An AST can also be shown as text, which is more compact, yet reveals more information. `ast.dump()` gives not only the class names of elements, but also how they are constructed – actually, the whole expression can be used to construct an AST.",
"_____no_output_____"
]
],
[
[
"print(ast.dump(middle_tree()))",
"_____no_output_____"
]
],
[
[
"This is the path to the first `return` statement:",
"_____no_output_____"
]
],
[
[
"ast.dump(middle_tree().body[0].body[0].body[0].body[0]) # type: ignore",
"_____no_output_____"
]
],
[
[
"### Picking Statements",
"_____no_output_____"
],
[
"For our mutation operators, we want to use statements from the program itself. Hence, we need a means to find those very statements. The `StatementVisitor` class iterates through an AST, adding all statements to its `statements` list it finds in function definitions. To do so, it subclasses the Python `ast` `NodeVisitor` class, described in the [official Python `ast` reference](http://docs.python.org/3/library/ast).",
"_____no_output_____"
]
],
[
[
"from ast import NodeVisitor",
"_____no_output_____"
],
[
"# ignore\nfrom typing import Any, Callable, Optional, Type, Tuple\nfrom typing import Dict, Union, Set, List, cast",
"_____no_output_____"
],
[
"class StatementVisitor(NodeVisitor):\n \"\"\"Visit all statements within function defs in an AST\"\"\"\n\n def __init__(self) -> None:\n self.statements: List[Tuple[ast.AST, str]] = []\n self.func_name = \"\"\n self.statements_seen: Set[Tuple[ast.AST, str]] = set()\n super().__init__()\n\n def add_statements(self, node: ast.AST, attr: str) -> None:\n elems: List[ast.AST] = getattr(node, attr, [])\n if not isinstance(elems, list):\n elems = [elems] # type: ignore\n\n for elem in elems:\n stmt = (elem, self.func_name)\n if stmt in self.statements_seen:\n continue\n\n self.statements.append(stmt)\n self.statements_seen.add(stmt)\n\n def visit_node(self, node: ast.AST) -> None:\n # Any node other than the ones listed below\n self.add_statements(node, 'body')\n self.add_statements(node, 'orelse')\n\n def visit_Module(self, node: ast.Module) -> None:\n # Module children are defs, classes and globals - don't add\n super().generic_visit(node)\n\n def visit_ClassDef(self, node: ast.ClassDef) -> None:\n # Class children are defs and globals - don't add\n super().generic_visit(node)\n\n def generic_visit(self, node: ast.AST) -> None:\n self.visit_node(node)\n super().generic_visit(node)\n\n def visit_FunctionDef(self,\n node: Union[ast.FunctionDef, ast.AsyncFunctionDef]) -> None:\n if not self.func_name:\n self.func_name = node.name\n\n self.visit_node(node)\n super().generic_visit(node)\n self.func_name = \"\"\n\n def visit_AsyncFunctionDef(self, node: ast.AsyncFunctionDef) -> None:\n return self.visit_FunctionDef(node)",
"_____no_output_____"
]
],
[
[
"The function `all_statements()` returns all statements in the given AST `tree`. If an `ast` class `tp` is given, it only returns instances of that class.",
"_____no_output_____"
]
],
[
[
"def all_statements_and_functions(tree: ast.AST, \n tp: Optional[Type] = None) -> \\\n List[Tuple[ast.AST, str]]:\n \"\"\"\n Return a list of pairs (`statement`, `function`) for all statements in `tree`.\n If `tp` is given, return only statements of that class.\n \"\"\"\n\n visitor = StatementVisitor()\n visitor.visit(tree)\n statements = visitor.statements\n if tp is not None:\n statements = [s for s in statements if isinstance(s[0], tp)]\n\n return statements",
"_____no_output_____"
],
[
"def all_statements(tree: ast.AST, tp: Optional[Type] = None) -> List[ast.AST]:\n \"\"\"\n Return a list of all statements in `tree`.\n If `tp` is given, return only statements of that class.\n \"\"\"\n\n return [stmt for stmt, func_name in all_statements_and_functions(tree, tp)]",
"_____no_output_____"
]
],
[
[
"Here are all the `return` statements in `middle()`:",
"_____no_output_____"
]
],
[
[
"all_statements(middle_tree(), ast.Return)",
"_____no_output_____"
],
[
"all_statements_and_functions(middle_tree(), ast.If)",
"_____no_output_____"
]
],
[
[
"We can randomly pick an element:",
"_____no_output_____"
]
],
[
[
"import random",
"_____no_output_____"
],
[
"random_node = random.choice(all_statements(middle_tree()))\nastor.to_source(random_node)",
"_____no_output_____"
]
],
[
[
"### Mutating Statements\n\nThe main part in mutation, however, is to actually mutate the code of the program under test. To this end, we introduce a `StatementMutator` class – a subclass of `NodeTransformer`, described in the [official Python `ast` reference](http://docs.python.org/3/library/ast).",
"_____no_output_____"
],
[
"The constructor provides various keyword arguments to configure the mutator.",
"_____no_output_____"
]
],
[
[
"from ast import NodeTransformer",
"_____no_output_____"
],
[
"import copy",
"_____no_output_____"
],
[
"class StatementMutator(NodeTransformer):\n \"\"\"Mutate statements in an AST for automated repair.\"\"\"\n\n def __init__(self, \n suspiciousness_func: \n Optional[Callable[[Tuple[Callable, int]], float]] = None,\n source: Optional[List[ast.AST]] = None, \n log: bool = False) -> None:\n \"\"\"\n Constructor.\n `suspiciousness_func` is a function that takes a location\n (function, line_number) and returns a suspiciousness value\n between 0 and 1.0. If not given, all locations get the same \n suspiciousness of 1.0.\n `source` is a list of statements to choose from.\n \"\"\"\n\n super().__init__()\n self.log = log\n\n if suspiciousness_func is None:\n def suspiciousness_func(location: Tuple[Callable, int]) -> float:\n return 1.0\n assert suspiciousness_func is not None\n\n self.suspiciousness_func: Callable = suspiciousness_func\n\n if source is None:\n source = []\n self.source = source\n\n if self.log > 1:\n for i, node in enumerate(self.source):\n print(f\"Source for repairs #{i}:\")\n print_content(astor.to_source(node), '.py')\n print()\n print()\n\n self.mutations = 0",
"_____no_output_____"
]
],
[
[
"#### Choosing Suspicious Statements to Mutate\n\nWe start with deciding which AST nodes to mutate. The method `node_suspiciousness()` returns the suspiciousness for a given node, by invoking the suspiciousness function `suspiciousness_func` given during initialization.",
"_____no_output_____"
]
],
[
[
"import warnings",
"_____no_output_____"
],
[
"class StatementMutator(StatementMutator):\n def node_suspiciousness(self, stmt: ast.AST, func_name: str) -> float:\n if not hasattr(stmt, 'lineno'):\n warnings.warn(f\"{self.format_node(stmt)}: Expected line number\")\n return 0.0\n\n suspiciousness = self.suspiciousness_func((func_name, stmt.lineno))\n if suspiciousness is None: # not executed\n return 0.0\n\n return suspiciousness\n\n def format_node(self, node: ast.AST) -> str:\n ...",
"_____no_output_____"
]
],
[
[
"The method `node_to_be_mutated()` picks a node (statement) to be mutated. It determines the suspiciousness of all statements, and invokes `random.choices()`, using the suspiciousness as weight. Unsuspicious statements (with zero weight) will not be chosen.",
"_____no_output_____"
]
],
[
[
"class StatementMutator(StatementMutator):\n def node_to_be_mutated(self, tree: ast.AST) -> ast.AST:\n statements = all_statements_and_functions(tree)\n assert len(statements) > 0, \"No statements\"\n\n weights = [self.node_suspiciousness(stmt, func_name) \n for stmt, func_name in statements]\n stmts = [stmt for stmt, func_name in statements]\n\n if self.log > 1:\n print(\"Weights:\")\n for i, stmt in enumerate(statements):\n node, func_name = stmt\n print(f\"{weights[i]:.2} {self.format_node(node)}\")\n\n if sum(weights) == 0.0:\n # No suspicious line\n return random.choice(stmts)\n else:\n return random.choices(stmts, weights=weights)[0]",
"_____no_output_____"
]
],
[
[
"#### Choosing a Mutation Method",
"_____no_output_____"
],
[
"The method `visit()` is invoked on all nodes. For nodes marked with a `mutate_me` attribute, it randomly chooses a mutation method (`choose_op()`) and then invokes it on the node.\n\nAccording to the rules of `NodeTransformer`, the mutation method can return\n\n* a new node or a list of nodes, replacing the current node;\n* `None`, deleting it; or\n* the node itself, keeping things as they are.",
"_____no_output_____"
]
],
[
[
"import re",
"_____no_output_____"
],
[
"RE_SPACE = re.compile(r'[ \\t\\n]+')",
"_____no_output_____"
],
[
"class StatementMutator(StatementMutator):\n def choose_op(self) -> Callable:\n return random.choice([self.insert, self.swap, self.delete])\n\n def visit(self, node: ast.AST) -> ast.AST:\n super().visit(node) # Visits (and transforms?) children\n\n if not node.mutate_me: # type: ignore\n return node\n\n op = self.choose_op()\n new_node = op(node)\n self.mutations += 1\n\n if self.log:\n print(f\"{node.lineno:4}:{op.__name__ + ':':7} \"\n f\"{self.format_node(node)} \"\n f\"becomes {self.format_node(new_node)}\")\n\n return new_node",
"_____no_output_____"
]
],
[
[
"#### Swapping Statements\n\nOur first mutator is `swap()`, which replaces the current node NODE by a random node found in `source` (using a newly defined `choose_statement()`).\n\nAs a rule of thumb, we try to avoid inserting entire subtrees with all attached statements; and try to respect only the first line of a node. If the new node has the form \n\n```python\nif P:\n BODY\n```\n\nwe thus only insert \n\n```python\nif P: \n pass\n```\n\nsince the statements in BODY have a later chance to get inserted. The same holds for all constructs that have a BODY, i.e. `while`, `for`, `try`, `with`, and more.",
"_____no_output_____"
]
],
[
[
"class StatementMutator(StatementMutator):\n def choose_statement(self) -> ast.AST:\n return copy.deepcopy(random.choice(self.source))",
"_____no_output_____"
],
[
"class StatementMutator(StatementMutator):\n def swap(self, node: ast.AST) -> ast.AST:\n \"\"\"Replace `node` with a random node from `source`\"\"\"\n new_node = self.choose_statement()\n\n if isinstance(new_node, ast.stmt):\n # The source `if P: X` is added as `if P: pass`\n if hasattr(new_node, 'body'):\n new_node.body = [ast.Pass()] # type: ignore\n if hasattr(new_node, 'orelse'):\n new_node.orelse = [] # type: ignore\n if hasattr(new_node, 'finalbody'):\n new_node.finalbody = [] # type: ignore\n\n # ast.copy_location(new_node, node)\n return new_node",
"_____no_output_____"
]
],
[
[
"#### Inserting Statements\n\nOur next mutator is `insert()`, which randomly chooses some node from `source` and inserts it after the current node NODE. (If NODE is a `return` statement, then we insert the new node _before_ NODE.)\n\nIf the statement to be inserted has the form\n\n```python\nif P:\n BODY\n```\n\nwe only insert the \"header\" of the `if`, resulting in\n\n```python\nif P: \n NODE\n```\n\nAgain, this applies to all constructs that have a BODY, i.e. `while`, `for`, `try`, `with`, and more.",
"_____no_output_____"
]
],
[
[
"class StatementMutator(StatementMutator):\n def insert(self, node: ast.AST) -> Union[ast.AST, List[ast.AST]]:\n \"\"\"Insert a random node from `source` after `node`\"\"\"\n new_node = self.choose_statement()\n\n if isinstance(new_node, ast.stmt) and hasattr(new_node, 'body'):\n # Inserting `if P: X` as `if P:`\n new_node.body = [node] # type: ignore\n if hasattr(new_node, 'orelse'):\n new_node.orelse = [] # type: ignore\n if hasattr(new_node, 'finalbody'):\n new_node.finalbody = [] # type: ignore\n # ast.copy_location(new_node, node)\n return new_node\n\n # Only insert before `return`, not after it\n if isinstance(node, ast.Return):\n if isinstance(new_node, ast.Return):\n return new_node\n else:\n return [new_node, node]\n\n return [node, new_node]",
"_____no_output_____"
]
],
[
[
"#### Deleting Statements\n\nOur last mutator is `delete()`, which deletes the current node NODE. The standard case is to replace NODE by a `pass` statement.\n\nIf the statement to be deleted has the form\n\n```python\nif P:\n BODY\n```\n\nwe only delete the \"header\" of the `if`, resulting in\n\n```python\nBODY\n```\n\nAgain, this applies to all constructs that have a BODY, i.e. `while`, `for`, `try`, `with`, and more; it also selects a random branch, including `else` branches.",
"_____no_output_____"
]
],
[
[
"class StatementMutator(StatementMutator):\n def delete(self, node: ast.AST) -> None:\n \"\"\"Delete `node`.\"\"\"\n\n branches = [attr for attr in ['body', 'orelse', 'finalbody']\n if hasattr(node, attr) and getattr(node, attr)]\n if branches:\n # Replace `if P: S` by `S`\n branch = random.choice(branches)\n new_node = getattr(node, branch)\n return new_node\n\n if isinstance(node, ast.stmt):\n # Avoid empty bodies; make this a `pass` statement\n new_node = ast.Pass()\n ast.copy_location(new_node, node)\n return new_node\n\n return None # Just delete",
"_____no_output_____"
],
[
"from bookutils import quiz",
"_____no_output_____"
],
[
"quiz(\"Why are statements replaced by `pass` rather than deleted?\",\n [\n \"Because `if P: pass` is valid Python, while `if P:` is not\",\n \"Because in Python, bodies for `if`, `while`, etc. cannot be empty\",\n \"Because a `pass` node makes a target for future mutations\",\n \"Because it causes the tests to pass\"\n ], '[3 ^ n for n in range(3)]')",
"_____no_output_____"
]
],
[
[
"Indeed, Python's `compile()` will fail if any of the bodies is an empty list. Also, it leaves us a statement that can be evolved further.",
"_____no_output_____"
],
[
"#### Helpers\n\nFor logging purposes, we introduce a helper function `format_node()` that returns a short string representation of the node.",
"_____no_output_____"
]
],
[
[
"class StatementMutator(StatementMutator):\n NODE_MAX_LENGTH = 20\n\n def format_node(self, node: ast.AST) -> str:\n \"\"\"Return a string representation for `node`.\"\"\"\n if node is None:\n return \"None\"\n\n if isinstance(node, list):\n return \"; \".join(self.format_node(elem) for elem in node)\n\n s = RE_SPACE.sub(' ', astor.to_source(node)).strip()\n if len(s) > self.NODE_MAX_LENGTH - len(\"...\"):\n s = s[:self.NODE_MAX_LENGTH] + \"...\"\n return repr(s)",
"_____no_output_____"
]
],
[
[
"#### All Together\n\nLet us now create the main entry point, which is `mutate()`. It picks the node to be mutated and marks it with a `mutate_me` attribute. By calling `visit()`, it then sets off the `NodeTransformer` transformation.",
"_____no_output_____"
]
],
[
[
"class StatementMutator(StatementMutator):\n def mutate(self, tree: ast.AST) -> ast.AST:\n \"\"\"Mutate the given AST `tree` in place. Return mutated tree.\"\"\"\n\n assert isinstance(tree, ast.AST)\n\n tree = copy.deepcopy(tree)\n\n if not self.source:\n self.source = all_statements(tree)\n\n for node in ast.walk(tree):\n node.mutate_me = False # type: ignore\n\n node = self.node_to_be_mutated(tree)\n node.mutate_me = True # type: ignore\n\n self.mutations = 0\n\n tree = self.visit(tree)\n\n if self.mutations == 0:\n warnings.warn(\"No mutations found\")\n\n ast.fix_missing_locations(tree)\n return tree",
"_____no_output_____"
]
],
[
[
"Here are a number of transformations applied by `StatementMutator`:",
"_____no_output_____"
]
],
[
[
"mutator = StatementMutator(log=True)\nfor i in range(10):\n new_tree = mutator.mutate(middle_tree())",
"_____no_output_____"
]
],
[
[
"This is the effect of the last mutator applied on `middle`:",
"_____no_output_____"
]
],
[
[
"print_content(astor.to_source(new_tree), '.py')",
"_____no_output_____"
]
],
[
[
"## Fitness\n\nNow that we can apply random mutations to code, let us find out how good these mutations are. Given our test suites for `middle`, we can check for a given code candidate how many of the previously passing test cases it passes, and how many of the failing test cases it passes. The more tests pass, the higher the _fitness_ of the candidate.",
"_____no_output_____"
],
[
"Not all passing tests have the same value, though. We want to prevent _regressions_ – that is, having a fix that breaks a previously passing test. The values of `WEIGHT_PASSING` and `WEIGHT_FAILING` set the relative weight (or importance) of passing vs. failing tests; we see that keeping passing tests passing is far more important then fixing failing tests.",
"_____no_output_____"
]
],
[
[
"WEIGHT_PASSING = 0.99\nWEIGHT_FAILING = 0.01",
"_____no_output_____"
],
[
"def middle_fitness(tree: ast.AST) -> float:\n \"\"\"Compute fitness of a `middle()` candidate given in `tree`\"\"\"\n original_middle = middle\n\n try:\n code = compile(tree, '<fitness>', 'exec')\n except ValueError:\n return 0 # Compilation error\n\n exec(code, globals())\n\n passing_passed = 0\n failing_passed = 0\n\n # Test how many of the passing runs pass\n for x, y, z in MIDDLE_PASSING_TESTCASES:\n try:\n middle_test(x, y, z)\n passing_passed += 1\n except AssertionError:\n pass\n\n passing_ratio = passing_passed / len(MIDDLE_PASSING_TESTCASES)\n\n # Test how many of the failing runs pass\n for x, y, z in MIDDLE_FAILING_TESTCASES:\n try:\n middle_test(x, y, z)\n failing_passed += 1\n except AssertionError:\n pass\n\n failing_ratio = failing_passed / len(MIDDLE_FAILING_TESTCASES)\n\n fitness = (WEIGHT_PASSING * passing_ratio +\n WEIGHT_FAILING * failing_ratio)\n\n globals()['middle'] = original_middle\n return fitness",
"_____no_output_____"
]
],
[
[
"Our faulty `middle()` program has a fitness of `WEIGHT_PASSING` (99%), because it passes all the passing tests (but none of the failing ones).",
"_____no_output_____"
]
],
[
[
"middle_fitness(middle_tree())",
"_____no_output_____"
]
],
[
[
"Our \"sort of fixed\" version of `middle()` gets a much lower fitness:",
"_____no_output_____"
]
],
[
[
"middle_fitness(ast.parse(\"def middle(x, y, z): return x\"))",
"_____no_output_____"
]
],
[
[
"In the [chapter on statistical debugging](StatisticalDebugger), we also defined a fixed version of `middle()`. This gets a fitness of 1.0, passing all tests. (We won't use this fixed version for automated repairs.)",
"_____no_output_____"
]
],
[
[
"from StatisticalDebugger import middle_fixed",
"_____no_output_____"
],
[
"middle_fixed_source = \\\n inspect.getsource(middle_fixed).replace('middle_fixed', 'middle').strip()",
"_____no_output_____"
],
[
"middle_fitness(ast.parse(middle_fixed_source))",
"_____no_output_____"
]
],
[
[
"## Population\n\nWe now set up a _population_ of fix candidates to evolve over time. A higher population size will yield more candidates to check, but also need more time to test; a lower population size will yield fewer candidates, but allow for more evolution steps. We choose a population size of 40 (from \\cite{LeGoues2012}). ",
"_____no_output_____"
]
],
[
[
"POPULATION_SIZE = 40\nmiddle_mutator = StatementMutator()",
"_____no_output_____"
],
[
"MIDDLE_POPULATION = [middle_tree()] + \\\n [middle_mutator.mutate(middle_tree()) for i in range(POPULATION_SIZE - 1)]",
"_____no_output_____"
]
],
[
[
"We sort the fix candidates according to their fitness. This actually runs all tests on all candidates.",
"_____no_output_____"
]
],
[
[
"MIDDLE_POPULATION.sort(key=middle_fitness, reverse=True)",
"_____no_output_____"
]
],
[
[
"The candidate with the highest fitness is still our original (faulty) `middle()` code:",
"_____no_output_____"
]
],
[
[
"print(astor.to_source(MIDDLE_POPULATION[0]),\n middle_fitness(MIDDLE_POPULATION[0]))",
"_____no_output_____"
]
],
[
[
"At the other end of the spectrum, the candidate with the lowest fitness has some vital functionality removed:",
"_____no_output_____"
]
],
[
[
"print(astor.to_source(MIDDLE_POPULATION[-1]),\n middle_fitness(MIDDLE_POPULATION[-1]))",
"_____no_output_____"
]
],
[
[
"## Evolution\n\nTo evolve our population of candidates, we fill up the population with mutations created from the population, using a `StatementMutator` as described above to create these mutations. Then we reduce the population to its original size, keeping the fittest candidates.",
"_____no_output_____"
]
],
[
[
"def evolve_middle() -> None:\n global MIDDLE_POPULATION\n\n source = all_statements(middle_tree())\n mutator = StatementMutator(source=source)\n\n n = len(MIDDLE_POPULATION)\n\n offspring: List[ast.AST] = []\n while len(offspring) < n:\n parent = random.choice(MIDDLE_POPULATION)\n offspring.append(mutator.mutate(parent))\n\n MIDDLE_POPULATION += offspring\n MIDDLE_POPULATION.sort(key=middle_fitness, reverse=True)\n MIDDLE_POPULATION = MIDDLE_POPULATION[:n]",
"_____no_output_____"
]
],
[
[
"This is what happens when evolving our population for the first time; the original source is still our best candidate.",
"_____no_output_____"
]
],
[
[
"evolve_middle()",
"_____no_output_____"
],
[
"tree = MIDDLE_POPULATION[0]\nprint(astor.to_source(tree), middle_fitness(tree))",
"_____no_output_____"
]
],
[
[
"However, nothing keeps us from evolving for a few generations more...",
"_____no_output_____"
]
],
[
[
"for i in range(50):\n evolve_middle()\n best_middle_tree = MIDDLE_POPULATION[0]\n fitness = middle_fitness(best_middle_tree)\n print(f\"\\rIteration {i:2}: fitness = {fitness} \", end=\"\")\n if fitness >= 1.0:\n break",
"_____no_output_____"
]
],
[
[
"Success! We find a candidate that actually passes all tests, including the failing ones. Here is the candidate:",
"_____no_output_____"
]
],
[
[
"print_content(astor.to_source(best_middle_tree), '.py', start_line_number=1)",
"_____no_output_____"
]
],
[
[
"... and yes, it passes all tests:",
"_____no_output_____"
]
],
[
[
"original_middle = middle\ncode = compile(best_middle_tree, '<string>', 'exec')\nexec(code, globals())\n\nfor x, y, z in MIDDLE_PASSING_TESTCASES + MIDDLE_FAILING_TESTCASES:\n middle_test(x, y, z)\n\nmiddle = original_middle",
"_____no_output_____"
]
],
[
[
"As the code is already validated by hundreds of test cases, it is very valuable for the programmer. Even if the programmer decides not to use the code as is, the location gives very strong hints on which code to examine and where to apply a fix.",
"_____no_output_____"
],
[
"However, a closer look at our fix candidate shows that there is some amount of redundancy – that is, superfluous statements.",
"_____no_output_____"
]
],
[
[
"quiz(\"Some of the lines in our fix candidate are redundant. Which are these?\",\n [\n \"Line 3: `if x < y`\",\n \"Line 4: `if x > z`\",\n \"Line 5: `return x`\",\n \"Line 13: `return z`\"\n ], '[eval(chr(100 - x)) for x in [49, 50]]')",
"_____no_output_____"
]
],
[
[
"## Simplifying",
"_____no_output_____"
],
[
"As demonstrated in the chapter on [reducing failure-inducing inputs](DeltaDebugger.ipynb), we can use delta debugging on code to get rid of these superfluous statements.",
"_____no_output_____"
],
[
"The trick for simplification is to have the test function (`test_middle_lines()`) declare a fitness of 1.0 as a \"failure\". Delta debugging will then simplify the input as long as the \"failure\" (and hence the maximum fitness obtained) persists.",
"_____no_output_____"
]
],
[
[
"from DeltaDebugger import DeltaDebugger",
"_____no_output_____"
],
[
"middle_lines = astor.to_source(best_middle_tree).strip().split('\\n')",
"_____no_output_____"
],
[
"def test_middle_lines(lines: List[str]) -> None:\n source = \"\\n\".join(lines)\n tree = ast.parse(source)\n assert middle_fitness(tree) < 1.0 # \"Fail\" only while fitness is 1.0",
"_____no_output_____"
],
[
"with DeltaDebugger() as dd:\n test_middle_lines(middle_lines)",
"_____no_output_____"
],
[
"reduced_lines = dd.min_args()['lines']",
"_____no_output_____"
],
[
"# assert len(reduced_lines) < len(middle_lines)",
"_____no_output_____"
],
[
"reduced_source = \"\\n\".join(reduced_lines)",
"_____no_output_____"
],
[
"repaired_source = astor.to_source(ast.parse(reduced_source)) # normalize\nprint_content(repaired_source, '.py')",
"_____no_output_____"
]
],
[
[
"Success! Delta Debugging has eliminated the superfluous statements. We can present the difference to the original as a patch:",
"_____no_output_____"
]
],
[
[
"original_source = astor.to_source(ast.parse(middle_source)) # normalize",
"_____no_output_____"
],
[
"from ChangeDebugger import diff, print_patch # minor dependency",
"_____no_output_____"
],
[
"for patch in diff(original_source, repaired_source):\n print_patch(patch)",
"_____no_output_____"
]
],
[
[
"We can present this patch to the programmer, who will then immediately know what to fix in the `middle()` code.",
"_____no_output_____"
],
[
"## Crossover\n\nSo far, we have only applied one kind of genetic operators – mutation. There is a second one, though, also inspired by natural selection. \n\nThe *crossover* operation mutates two strands of genes, as illustrated in the following picture. We have two parents (red and blue), each as a sequence of genes. To create \"crossed\" chilren, we pick a _crossover point_ and exchange the strands at this very point:\n\n",
"_____no_output_____"
],
[
"We implement a `CrossoverOperator` class that implements such an operation on two randomly chosen statement lists of two programs. It is used as\n\n```python\ncrossover = CrossoverOperator()\ncrossover.crossover(tree_p1, tree_p2)\n```\n\nwhere `tree_p1` and `tree_p2` are two ASTs that are changed in place.",
"_____no_output_____"
],
[
"### Excursion: Implementing Crossover",
"_____no_output_____"
],
[
"#### Crossing Statement Lists",
"_____no_output_____"
],
[
"Applied on programs, a crossover mutation takes two parents and \"crosses\" a list of statements. As an example, if our \"parents\" `p1()` and `p2()` are defined as follows:",
"_____no_output_____"
]
],
[
[
"def p1(): # type: ignore\n a = 1\n b = 2\n c = 3",
"_____no_output_____"
],
[
"def p2(): # type: ignore\n x = 1\n y = 2\n z = 3",
"_____no_output_____"
]
],
[
[
"Then a crossover operation would produce one child with a body\n\n```python\na = 1\ny = 2\nz = 3\n```\n\nand another child with a body\n\n```python\nx = 1\nb = 2\nc = 3\n```",
"_____no_output_____"
],
[
"We can easily implement this in a `CrossoverOperator` class in a method `cross_bodies()`.",
"_____no_output_____"
]
],
[
[
"class CrossoverOperator:\n \"\"\"A class for performing statement crossover of Python programs\"\"\"\n\n def __init__(self, log: bool = False):\n \"\"\"Constructor. If `log` is set, turn on logging.\"\"\"\n self.log = log\n\n def cross_bodies(self, body_1: List[ast.AST], body_2: List[ast.AST]) -> \\\n Tuple[List[ast.AST], List[ast.AST]]:\n \"\"\"Crossover the statement lists `body_1` x `body_2`. Return new lists.\"\"\"\n\n assert isinstance(body_1, list)\n assert isinstance(body_2, list)\n\n crossover_point_1 = len(body_1) // 2\n crossover_point_2 = len(body_2) // 2\n return (body_1[:crossover_point_1] + body_2[crossover_point_2:],\n body_2[:crossover_point_2] + body_1[crossover_point_1:])",
"_____no_output_____"
]
],
[
[
"Here's the `CrossoverOperatorMutator` applied on `p1` and `p2`:",
"_____no_output_____"
]
],
[
[
"tree_p1: ast.Module = ast.parse(inspect.getsource(p1))\ntree_p2: ast.Module = ast.parse(inspect.getsource(p2))",
"_____no_output_____"
],
[
"body_p1 = tree_p1.body[0].body # type: ignore\nbody_p2 = tree_p2.body[0].body # type: ignore\nbody_p1",
"_____no_output_____"
],
[
"crosser = CrossoverOperator()\ntree_p1.body[0].body, tree_p2.body[0].body = crosser.cross_bodies(body_p1, body_p2) # type: ignore",
"_____no_output_____"
],
[
"print_content(astor.to_source(tree_p1), '.py')",
"_____no_output_____"
],
[
"print_content(astor.to_source(tree_p2), '.py')",
"_____no_output_____"
]
],
[
[
"#### Applying Crossover on Programs\n\nApplying the crossover operation on arbitrary programs is a bit more complex, though. We first have to _find_ lists of statements that we a actually can cross over. The `can_cross()` method returns True if we have a list of statements that we can cross. Python modules and classes are excluded, because changing the ordering of definitions will not have much impact on the program.",
"_____no_output_____"
]
],
[
[
"class CrossoverOperator(CrossoverOperator):\n # In modules and class defs, the ordering of elements does not matter (much)\n SKIP_LIST = {ast.Module, ast.ClassDef}\n\n def can_cross(self, tree: ast.AST, body_attr: str = 'body') -> bool:\n if any(isinstance(tree, cls) for cls in self.SKIP_LIST):\n return False\n\n body = getattr(tree, body_attr, [])\n return body and len(body) >= 2",
"_____no_output_____"
]
],
[
[
"Here comes our method `crossover_attr()` which searches for crossover possibilities. It takes to ASTs `t1` and `t2` and an attribute (typically `'body'`) and retrieves the attribute lists $l_1$ (from `t1.<attr>`) and $l_2$ (from `t2.<attr>`).\n\nIf $l_1$ and $l_2$ can be crossed, it crosses them, and is done. Otherwise\n\n* If there is a pair of elements $e_1 \\in l_1$ and $e_2 \\in l_2$ that has the same name – say, functions of the same name –, it applies itself to $e_1$ and $e_2$.\n* Otherwise, it creates random pairs of elements $e_1 \\in l_1$ and $e_2 \\in l_2$ and applies itself on these very pairs.\n\n`crossover_attr()` changes `t1` and `t2` in place and returns True if a crossover was found; it returns False otherwise.",
"_____no_output_____"
]
],
[
[
"class CrossoverOperator(CrossoverOperator):\n def crossover_attr(self, t1: ast.AST, t2: ast.AST, body_attr: str) -> bool:\n \"\"\"\n Crossover the bodies `body_attr` of two trees `t1` and `t2`.\n Return True if successful.\n \"\"\"\n assert isinstance(t1, ast.AST)\n assert isinstance(t2, ast.AST)\n assert isinstance(body_attr, str)\n\n if not getattr(t1, body_attr, None) or not getattr(t2, body_attr, None):\n return False\n\n if self.crossover_branches(t1, t2):\n return True\n\n if self.log > 1:\n print(f\"Checking {t1}.{body_attr} x {t2}.{body_attr}\")\n\n body_1 = getattr(t1, body_attr)\n body_2 = getattr(t2, body_attr)\n\n # If both trees have the attribute, we can cross their bodies\n if self.can_cross(t1, body_attr) and self.can_cross(t2, body_attr):\n if self.log:\n print(f\"Crossing {t1}.{body_attr} x {t2}.{body_attr}\")\n\n new_body_1, new_body_2 = self.cross_bodies(body_1, body_2)\n setattr(t1, body_attr, new_body_1)\n setattr(t2, body_attr, new_body_2)\n return True\n\n # Strategy 1: Find matches in class/function of same name\n for child_1 in body_1:\n if hasattr(child_1, 'name'):\n for child_2 in body_2:\n if (hasattr(child_2, 'name') and\n child_1.name == child_2.name):\n if self.crossover_attr(child_1, child_2, body_attr):\n return True\n\n # Strategy 2: Find matches anywhere\n for child_1 in random.sample(body_1, len(body_1)):\n for child_2 in random.sample(body_2, len(body_2)):\n if self.crossover_attr(child_1, child_2, body_attr):\n return True\n\n return False",
"_____no_output_____"
]
],
[
[
"We have a special case for `if` nodes, where we can cross their body and `else` branches.",
"_____no_output_____"
]
],
[
[
"class CrossoverOperator(CrossoverOperator):\n def crossover_branches(self, t1: ast.AST, t2: ast.AST) -> bool:\n \"\"\"Special case:\n `t1` = `if P: S1 else: S2` x `t2` = `if P': S1' else: S2'`\n becomes\n `t1` = `if P: S2' else: S1'` and `t2` = `if P': S2 else: S1`\n Returns True if successful.\n \"\"\"\n assert isinstance(t1, ast.AST)\n assert isinstance(t2, ast.AST)\n\n if (hasattr(t1, 'body') and hasattr(t1, 'orelse') and\n hasattr(t2, 'body') and hasattr(t2, 'orelse')):\n\n t1 = cast(ast.If, t1) # keep mypy happy\n t2 = cast(ast.If, t2)\n\n if self.log:\n print(f\"Crossing branches {t1} x {t2}\")\n\n t1.body, t1.orelse, t2.body, t2.orelse = \\\n t2.orelse, t2.body, t1.orelse, t1.body\n return True\n\n return False",
"_____no_output_____"
]
],
[
[
"The method `crossover()` is the main entry point. It checks for the special `if` case as described above; if not, it searches for possible crossover points. It raises `CrossoverError` if not successful.",
"_____no_output_____"
]
],
[
[
"class CrossoverOperator(CrossoverOperator):\n def crossover(self, t1: ast.AST, t2: ast.AST) -> Tuple[ast.AST, ast.AST]:\n \"\"\"Do a crossover of ASTs `t1` and `t2`.\n Raises `CrossoverError` if no crossover is found.\"\"\"\n assert isinstance(t1, ast.AST)\n assert isinstance(t2, ast.AST)\n\n for body_attr in ['body', 'orelse', 'finalbody']:\n if self.crossover_attr(t1, t2, body_attr):\n return t1, t2\n\n raise CrossoverError(\"No crossover found\")",
"_____no_output_____"
],
[
"class CrossoverError(ValueError):\n pass",
"_____no_output_____"
]
],
[
[
"### End of Excursion",
"_____no_output_____"
],
[
"### Crossover in Action",
"_____no_output_____"
],
[
"Let us put our `CrossoverOperator` in action. Here is a test case for crossover, involving more deeply nested structures:",
"_____no_output_____"
]
],
[
[
"def p1(): # type: ignore\n if True:\n print(1)\n print(2)\n print(3)",
"_____no_output_____"
],
[
"def p2(): # type: ignore\n if True:\n print(a)\n print(b)\n else:\n print(c)\n print(d)",
"_____no_output_____"
]
],
[
[
"We invoke the `crossover()` method with two ASTs from `p1` and `p2`:",
"_____no_output_____"
]
],
[
[
"crossover = CrossoverOperator()\ntree_p1 = ast.parse(inspect.getsource(p1))\ntree_p2 = ast.parse(inspect.getsource(p2))\ncrossover.crossover(tree_p1, tree_p2);",
"_____no_output_____"
]
],
[
[
"Here is the crossed offspring, mixing statement lists of `p1` and `p2`:",
"_____no_output_____"
]
],
[
[
"print_content(astor.to_source(tree_p1), '.py')",
"_____no_output_____"
],
[
"print_content(astor.to_source(tree_p2), '.py')",
"_____no_output_____"
]
],
[
[
"Here is our special case for `if` nodes in action, crossing our `middle()` tree with `p2`.",
"_____no_output_____"
]
],
[
[
"middle_t1, middle_t2 = crossover.crossover(middle_tree(),\n ast.parse(inspect.getsource(p2)))",
"_____no_output_____"
]
],
[
[
"We see how the resulting offspring encompasses elements of both sources:",
"_____no_output_____"
]
],
[
[
"print_content(astor.to_source(middle_t1), '.py')",
"_____no_output_____"
],
[
"print_content(astor.to_source(middle_t2), '.py')",
"_____no_output_____"
]
],
[
[
"## A Repairer Class\n\nSo far, we have applied all our techniques on the `middle()` program only. Let us now create a `Repairer` class that applies automatic program repair on arbitrary Python programs. The idea is that you can apply it on some statistical debugger, for which you have gathered passing and failing test cases, and then invoke its `repair()` method to find a \"best\" fix candidate:\n\n```python\ndebugger = OchiaiDebugger()\nwith debugger:\n <passing test>\nwith debugger:\n <failing test>\n...\nrepairer = Repairer(debugger)\nrepairer.repair()\n```",
"_____no_output_____"
],
[
"### Excursion: Implementing Repairer",
"_____no_output_____"
],
[
"The main argument to the `Repairer` constructor is the `debugger` to get information from. On top of that, it also allows to customize the classes used for mutation, crossover, and reduction. Setting `targets` allows to define a set of functions to repair; setting `sources` allows to set a set of sources to take repairs from. The constructor then sets up the environment for running tests and repairing, as described below.",
"_____no_output_____"
]
],
[
[
"from StackInspector import StackInspector # minor dependency",
"_____no_output_____"
],
[
"class Repairer(StackInspector):\n \"\"\"A class for automatic repair of Python programs\"\"\"\n\n def __init__(self, debugger: RankingDebugger, *,\n targets: Optional[List[Any]] = None,\n sources: Optional[List[Any]] = None,\n log: Union[bool, int] = False,\n mutator_class: Type = StatementMutator,\n crossover_class: Type = CrossoverOperator,\n reducer_class: Type = DeltaDebugger,\n globals: Optional[Dict[str, Any]] = None):\n \"\"\"Constructor.\n`debugger`: a `RankingDebugger` to take tests and coverage from.\n`targets`: a list of functions/modules to be repaired.\n (default: the covered functions in `debugger`, except tests)\n`sources`: a list of functions/modules to take repairs from.\n (default: same as `targets`)\n`globals`: if given, a `globals()` dict for executing targets\n (default: `globals()` of caller)\"\"\"\n\n assert isinstance(debugger, RankingDebugger)\n self.debugger = debugger\n self.log = log\n\n if targets is None:\n targets = self.default_functions()\n if not targets:\n raise ValueError(\"No targets to repair\")\n\n if sources is None:\n sources = self.default_functions()\n if not sources:\n raise ValueError(\"No sources to take repairs from\")\n\n if self.debugger.function() is None:\n raise ValueError(\"Multiple entry points observed\")\n\n self.target_tree: ast.AST = self.parse(targets)\n self.source_tree: ast.AST = self.parse(sources)\n\n self.log_tree(\"Target code to be repaired:\", self.target_tree)\n if ast.dump(self.target_tree) != ast.dump(self.source_tree):\n self.log_tree(\"Source code to take repairs from:\", \n self.source_tree)\n\n self.fitness_cache: Dict[str, float] = {}\n\n self.mutator: StatementMutator = \\\n mutator_class(\n source=all_statements(self.source_tree),\n suspiciousness_func=self.debugger.suspiciousness,\n log=(self.log >= 3))\n self.crossover: CrossoverOperator = crossover_class(log=(self.log >= 3))\n self.reducer: DeltaDebugger = reducer_class(log=(self.log >= 3))\n\n if globals is None:\n globals = self.caller_globals() # see below\n\n self.globals = globals",
"_____no_output_____"
]
],
[
[
"When we access or execute functions, we ault_functionso \\todo{What? -- BM} so in the caller's environment, not ours. The `caller_globals()` method from `StackInspector` acts as replacement for `globals()`.",
"_____no_output_____"
],
[
"#### Helper Functions\n\nThe constructor uses a number of helper functions to create its environment.",
"_____no_output_____"
]
],
[
[
"class Repairer(Repairer):\n def getsource(self, item: Union[str, Any]) -> str:\n \"\"\"Get the source for `item`. Can also be a string.\"\"\"\n\n if isinstance(item, str):\n item = self.globals[item]\n return inspect.getsource(item)",
"_____no_output_____"
],
[
"class Repairer(Repairer):\n def default_functions(self) -> List[Callable]:\n \"\"\"Return the set of functions to be repaired.\n Functions whose names start or end in `test` are excluded.\"\"\"\n def is_test(name: str) -> bool:\n return name.startswith('test') or name.endswith('test')\n\n return [func for func in self.debugger.covered_functions()\n if not is_test(func.__name__)]",
"_____no_output_____"
],
[
"class Repairer(Repairer):\n def log_tree(self, description: str, tree: Any) -> None:\n \"\"\"Print out `tree` as source code prefixed by `description`.\"\"\"\n if self.log:\n print(description)\n print_content(astor.to_source(tree), '.py')\n print()\n print()",
"_____no_output_____"
],
[
"class Repairer(Repairer):\n def parse(self, items: List[Any]) -> ast.AST:\n \"\"\"Read in a list of items into a single tree\"\"\"\n tree = ast.parse(\"\")\n for item in items:\n if isinstance(item, str):\n item = self.globals[item]\n\n item_lines, item_first_lineno = inspect.getsourcelines(item)\n\n try:\n item_tree = ast.parse(\"\".join(item_lines))\n except IndentationError:\n # inner function or likewise\n warnings.warn(f\"Can't parse {item.__name__}\")\n continue\n\n ast.increment_lineno(item_tree, item_first_lineno - 1)\n tree.body += item_tree.body\n\n return tree",
"_____no_output_____"
]
],
[
[
"#### Running Tests\n\nNow that we have set the environment for `Repairer`, we can implement one step of automatic repair after the other. The method `run_test_set()` runs the given `test_set` (`DifferenceDebugger.PASS` or `DifferenceDebugger.FAIL`), returning the number of passed tests. If `validate` is set, it checks whether the outcomes are as expected.",
"_____no_output_____"
]
],
[
[
"class Repairer(Repairer):\n def run_test_set(self, test_set: str, validate: bool = False) -> int:\n \"\"\"\n Run given `test_set`\n (`DifferenceDebugger.PASS` or `DifferenceDebugger.FAIL`).\n If `validate` is set, check expectations.\n Return number of passed tests.\n \"\"\"\n passed = 0\n collectors = self.debugger.collectors[test_set]\n function = self.debugger.function()\n assert function is not None\n # FIXME: function may have been redefined\n\n for c in collectors:\n if self.log >= 4:\n print(f\"Testing {c.id()}...\", end=\"\")\n\n try:\n function(**c.args())\n except Exception as err:\n if self.log >= 4:\n print(f\"failed ({err.__class__.__name__})\")\n\n if validate and test_set == self.debugger.PASS:\n raise err.__class__(\n f\"{c.id()} should have passed, but failed\")\n continue\n\n passed += 1\n if self.log >= 4:\n print(\"passed\")\n\n if validate and test_set == self.debugger.FAIL:\n raise FailureNotReproducedError(\n f\"{c.id()} should have failed, but passed\")\n\n return passed",
"_____no_output_____"
],
[
"class FailureNotReproducedError(ValueError):\n pass",
"_____no_output_____"
]
],
[
[
"Here is how we use `run_tests_set()`:",
"_____no_output_____"
]
],
[
[
"repairer = Repairer(middle_debugger)\nassert repairer.run_test_set(middle_debugger.PASS) == \\\n len(MIDDLE_PASSING_TESTCASES)\nassert repairer.run_test_set(middle_debugger.FAIL) == 0",
"_____no_output_____"
]
],
[
[
"The method `run_tests()` runs passing and failing tests, weighing the passed testcases to obtain the overall fitness.",
"_____no_output_____"
]
],
[
[
"class Repairer(Repairer):\n def weight(self, test_set: str) -> float:\n \"\"\"\n Return the weight of `test_set`\n (`DifferenceDebugger.PASS` or `DifferenceDebugger.FAIL`).\n \"\"\"\n return {\n self.debugger.PASS: WEIGHT_PASSING,\n self.debugger.FAIL: WEIGHT_FAILING\n }[test_set]\n\n def run_tests(self, validate: bool = False) -> float:\n \"\"\"Run passing and failing tests, returning weighted fitness.\"\"\"\n fitness = 0.0\n\n for test_set in [self.debugger.PASS, self.debugger.FAIL]:\n passed = self.run_test_set(test_set, validate=validate)\n ratio = passed / len(self.debugger.collectors[test_set])\n fitness += self.weight(test_set) * ratio\n\n return fitness",
"_____no_output_____"
]
],
[
[
"The method `validate()` ensures the observed tests can be adequately reproduced.",
"_____no_output_____"
]
],
[
[
"class Repairer(Repairer):\n def validate(self) -> None:\n fitness = self.run_tests(validate=True)\n assert fitness == self.weight(self.debugger.PASS)",
"_____no_output_____"
],
[
"repairer = Repairer(middle_debugger)\nrepairer.validate()",
"_____no_output_____"
]
],
[
[
"#### (Re)defining Functions\n\nOur `run_tests()` methods above do not yet redefine the function to be repaired. This is done by the `fitness()` function, which compiles and defines the given repair candidate `tree` before testing it. It caches and returns the fitness.",
"_____no_output_____"
]
],
[
[
"class Repairer(Repairer):\n def fitness(self, tree: ast.AST) -> float:\n \"\"\"Test `tree`, returning its fitness\"\"\"\n key = cast(str, ast.dump(tree))\n if key in self.fitness_cache:\n return self.fitness_cache[key]\n\n # Save defs\n original_defs: Dict[str, Any] = {}\n for name in self.toplevel_defs(tree):\n if name in self.globals:\n original_defs[name] = self.globals[name]\n else:\n warnings.warn(f\"Couldn't find definition of {repr(name)}\")\n\n assert original_defs, f\"Couldn't find any definition\"\n\n if self.log >= 3:\n print(\"Repair candidate:\")\n print_content(astor.to_source(tree), '.py')\n print()\n\n # Create new definition\n try:\n code = compile(tree, '<Repairer>', 'exec')\n except ValueError: # Compilation error\n code = None\n\n if code is None:\n if self.log >= 3:\n print(f\"Fitness = 0.0 (compilation error)\")\n\n fitness = 0.0\n return fitness\n\n # Execute new code, defining new functions in `self.globals`\n exec(code, self.globals)\n\n # Set new definitions in the namespace (`__globals__`)\n # of the function we will be calling.\n function = self.debugger.function()\n assert function is not None\n assert hasattr(function, '__globals__')\n\n for name in original_defs:\n function.__globals__[name] = self.globals[name] # type: ignore\n\n fitness = self.run_tests(validate=False)\n\n # Restore definitions\n for name in original_defs:\n function.__globals__[name] = original_defs[name] # type: ignore\n self.globals[name] = original_defs[name]\n\n if self.log >= 3:\n print(f\"Fitness = {fitness}\")\n\n self.fitness_cache[key] = fitness\n return fitness",
"_____no_output_____"
]
],
[
[
"The helper function `toplevel_defs()` helps saving and restoring the environment before and after redefining the function under repair.",
"_____no_output_____"
]
],
[
[
"class Repairer(Repairer):\n def toplevel_defs(self, tree: ast.AST) -> List[str]:\n \"\"\"Return a list of names of defined functions and classes in `tree`\"\"\"\n visitor = DefinitionVisitor()\n visitor.visit(tree)\n assert hasattr(visitor, 'definitions')\n return visitor.definitions",
"_____no_output_____"
],
[
"class DefinitionVisitor(NodeVisitor):\n def __init__(self) -> None:\n self.definitions: List[str] = []\n\n def add_definition(self, node: Union[ast.ClassDef, \n ast.FunctionDef, \n ast.AsyncFunctionDef]) -> None:\n self.definitions.append(node.name)\n\n def visit_FunctionDef(self, node: ast.FunctionDef) -> None:\n self.add_definition(node)\n\n def visit_AsyncFunctionDef(self, node: ast.AsyncFunctionDef) -> None:\n self.add_definition(node)\n\n def visit_ClassDef(self, node: ast.ClassDef) -> None:\n self.add_definition(node)",
"_____no_output_____"
]
],
[
[
"Here's an example for `fitness()`:",
"_____no_output_____"
]
],
[
[
"repairer = Repairer(middle_debugger, log=1)",
"_____no_output_____"
],
[
"good_fitness = repairer.fitness(middle_tree())\ngood_fitness",
"_____no_output_____"
],
[
"# ignore\nassert good_fitness >= 0.99, \"fitness() failed\"",
"_____no_output_____"
],
[
"bad_middle_tree = ast.parse(\"def middle(x, y, z): return x\")\nbad_fitness = repairer.fitness(bad_middle_tree)\nbad_fitness",
"_____no_output_____"
],
[
"# ignore\nassert bad_fitness < 0.5, \"fitness() failed\"",
"_____no_output_____"
]
],
[
[
"#### Repairing\n\nNow for the actual `repair()` method, which creates a `population` and then evolves it until the fitness is 1.0 or the given number of iterations is spent.",
"_____no_output_____"
]
],
[
[
"import traceback",
"_____no_output_____"
],
[
"class Repairer(Repairer):\n def initial_population(self, size: int) -> List[ast.AST]:\n \"\"\"Return an initial population of size `size`\"\"\"\n return [self.target_tree] + \\\n [self.mutator.mutate(copy.deepcopy(self.target_tree))\n for i in range(size - 1)]\n\n def repair(self, population_size: int = POPULATION_SIZE, iterations: int = 100) -> \\\n Tuple[ast.AST, float]:\n \"\"\"\n Repair the function we collected test runs from.\n Use a population size of `population_size` and\n at most `iterations` iterations.\n Returns a pair (`ast`, `fitness`) where \n `ast` is the AST of the repaired function, and\n `fitness` is its fitness (between 0 and 1.0)\n \"\"\"\n self.validate()\n\n population = self.initial_population(population_size)\n\n last_key = ast.dump(self.target_tree)\n\n for iteration in range(iterations):\n population = self.evolve(population)\n\n best_tree = population[0]\n fitness = self.fitness(best_tree)\n\n if self.log:\n print(f\"Evolving population: \"\n f\"iteration{iteration:4}/{iterations} \"\n f\"fitness = {fitness:.5} \\r\", end=\"\")\n\n if self.log >= 2:\n best_key = ast.dump(best_tree)\n if best_key != last_key:\n print()\n print()\n self.log_tree(f\"New best code (fitness = {fitness}):\",\n best_tree)\n last_key = best_key\n\n if fitness >= 1.0:\n break\n\n if self.log:\n print()\n\n if self.log and self.log < 2:\n self.log_tree(f\"Best code (fitness = {fitness}):\", best_tree)\n\n best_tree = self.reduce(best_tree)\n fitness = self.fitness(best_tree)\n\n self.log_tree(f\"Reduced code (fitness = {fitness}):\", best_tree)\n\n return best_tree, fitness",
"_____no_output_____"
]
],
[
[
"#### Evolving\n\nThe evolution of our population takes place in the `evolve()` method. In contrast to the `evolve_middle()` function, above, we use crossover to create the offspring, which we still mutate afterwards.",
"_____no_output_____"
]
],
[
[
"class Repairer(Repairer):\n def evolve(self, population: List[ast.AST]) -> List[ast.AST]:\n \"\"\"Evolve the candidate population by mutating and crossover.\"\"\"\n n = len(population)\n\n # Create offspring as crossover of parents\n offspring: List[ast.AST] = []\n while len(offspring) < n:\n parent_1 = copy.deepcopy(random.choice(population))\n parent_2 = copy.deepcopy(random.choice(population))\n try:\n self.crossover.crossover(parent_1, parent_2)\n except CrossoverError:\n pass # Just keep parents\n offspring += [parent_1, parent_2]\n\n # Mutate offspring\n offspring = [self.mutator.mutate(tree) for tree in offspring]\n\n # Add it to population\n population += offspring\n\n # Keep the fitter part of the population\n population.sort(key=self.fitness_key, reverse=True)\n population = population[:n]\n\n return population",
"_____no_output_____"
]
],
[
[
"A second difference is that we not only sort by fitness, but also by tree size – with equal fitness, a smaller tree thus will be favored. This helps keeping fixes and patches small.",
"_____no_output_____"
]
],
[
[
"class Repairer(Repairer):\n def fitness_key(self, tree: ast.AST) -> Tuple[float, int]:\n \"\"\"Key to be used for sorting the population\"\"\"\n tree_size = len([node for node in ast.walk(tree)])\n return (self.fitness(tree), -tree_size)",
"_____no_output_____"
]
],
[
[
"#### Simplifying\n\nThe last step in repairing is simplifying the code. As demonstrated in the chapter on [reducing failure-inducing inputs](DeltaDebugger.ipynb), we can use delta debugging on code to get rid of superfluous statements. To this end, we convert the tree to lines, run delta debugging on them, and then convert it back to a tree.",
"_____no_output_____"
]
],
[
[
"class Repairer(Repairer):\n def reduce(self, tree: ast.AST) -> ast.AST:\n \"\"\"Simplify `tree` using delta debugging.\"\"\"\n\n original_fitness = self.fitness(tree)\n source_lines = astor.to_source(tree).split('\\n')\n\n with self.reducer:\n self.test_reduce(source_lines, original_fitness)\n\n reduced_lines = self.reducer.min_args()['source_lines']\n reduced_source = \"\\n\".join(reduced_lines)\n\n return ast.parse(reduced_source)",
"_____no_output_____"
]
],
[
[
"As dicussed above, we simplify the code by having the test function (`test_reduce()`) declare reaching the maximum fitness obtained so far as a \"failure\". Delta debugging will then simplify the input as long as the \"failure\" (and hence the maximum fitness obtained) persists.",
"_____no_output_____"
]
],
[
[
"class Repairer(Repairer):\n def test_reduce(self, source_lines: List[str], original_fitness: float) -> None:\n \"\"\"Test function for delta debugging.\"\"\"\n\n try:\n source = \"\\n\".join(source_lines)\n tree = ast.parse(source)\n fitness = self.fitness(tree)\n assert fitness < original_fitness\n\n except AssertionError:\n raise\n except SyntaxError:\n raise\n except IndentationError:\n raise\n except Exception:\n # traceback.print_exc() # Uncomment to see internal errors\n raise",
"_____no_output_____"
]
],
[
[
"### End of Excursion",
"_____no_output_____"
],
[
"### Repairer in Action\n\nLet us go and apply `Repairer` in practice. We initialize it with `middle_debugger`, which has (still) collected the passing and failing runs for `middle_test()`. We also set `log` for some diagnostics along the way.",
"_____no_output_____"
]
],
[
[
"repairer = Repairer(middle_debugger, log=True)",
"_____no_output_____"
]
],
[
[
"We now invoke `repair()` to evolve our population. After a few iterations, we find a best tree with perfect fitness.",
"_____no_output_____"
]
],
[
[
"best_tree, fitness = repairer.repair()",
"_____no_output_____"
],
[
"print_content(astor.to_source(best_tree), '.py')",
"_____no_output_____"
],
[
"fitness",
"_____no_output_____"
]
],
[
[
"Again, we have a perfect solution. Here, we did not even need to simplify the code in the last iteration, as our `fitness_key()` function favors smaller implementations.",
"_____no_output_____"
],
[
"## Removing HTML Markup\n\nLet us apply `Repairer` on our other ongoing example, namely `remove_html_markup()`.",
"_____no_output_____"
]
],
[
[
"def remove_html_markup(s): # type: ignore\n tag = False\n quote = False\n out = \"\"\n\n for c in s:\n if c == '<' and not quote:\n tag = True\n elif c == '>' and not quote:\n tag = False\n elif c == '\"' or c == \"'\" and tag:\n quote = not quote\n elif not tag:\n out = out + c\n\n return out",
"_____no_output_____"
],
[
"def remove_html_markup_tree() -> ast.AST:\n return ast.parse(inspect.getsource(remove_html_markup))",
"_____no_output_____"
]
],
[
[
"To run `Repairer` on `remove_html_markup()`, we need a test and a test suite. `remove_html_markup_test()` raises an exception if applying `remove_html_markup()` on the given `html` string does not yield the `plain` string.",
"_____no_output_____"
]
],
[
[
"def remove_html_markup_test(html: str, plain: str) -> None:\n outcome = remove_html_markup(html)\n assert outcome == plain, \\\n f\"Got {repr(outcome)}, expected {repr(plain)}\"",
"_____no_output_____"
]
],
[
[
"Now for the test suite. We use a simple fuzzing scheme to create dozens of passing and failing test cases in `REMOVE_HTML_PASSING_TESTCASES` and `REMOVE_HTML_FAILING_TESTCASES`, respectively.",
"_____no_output_____"
],
[
"### Excursion: Creating HTML Test Cases",
"_____no_output_____"
]
],
[
[
"def random_string(length: int = 5, start: int = ord(' '), end: int = ord('~')) -> str:\n return \"\".join(chr(random.randrange(start, end + 1)) for i in range(length))",
"_____no_output_____"
],
[
"random_string()",
"_____no_output_____"
],
[
"def random_id(length: int = 2) -> str:\n return random_string(start=ord('a'), end=ord('z'))",
"_____no_output_____"
],
[
"random_id()",
"_____no_output_____"
],
[
"def random_plain() -> str:\n return random_string().replace('<', '').replace('>', '')",
"_____no_output_____"
],
[
"def random_string_noquotes() -> str:\n return random_string().replace('\"', '').replace(\"'\", '')",
"_____no_output_____"
],
[
"def random_html(depth: int = 0) -> Tuple[str, str]:\n prefix = random_plain()\n tag = random_id()\n\n if depth > 0:\n html, plain = random_html(depth - 1)\n else:\n html = plain = random_plain()\n\n attr = random_id()\n value = '\"' + random_string_noquotes() + '\"'\n postfix = random_plain()\n\n return f'{prefix}<{tag} {attr}={value}>{html}</{tag}>{postfix}', \\\n prefix + plain + postfix",
"_____no_output_____"
],
[
"random_html()",
"_____no_output_____"
],
[
"def remove_html_testcase(expected: bool = True) -> Tuple[str, str]:\n while True:\n html, plain = random_html()\n outcome = (remove_html_markup(html) == plain)\n if outcome == expected:\n return html, plain",
"_____no_output_____"
],
[
"REMOVE_HTML_TESTS = 100\nREMOVE_HTML_PASSING_TESTCASES = \\\n [remove_html_testcase(True) for i in range(REMOVE_HTML_TESTS)]\nREMOVE_HTML_FAILING_TESTCASES = \\\n [remove_html_testcase(False) for i in range(REMOVE_HTML_TESTS)]",
"_____no_output_____"
]
],
[
[
"### End of Excursion",
"_____no_output_____"
],
[
"Here is a passing test case:",
"_____no_output_____"
]
],
[
[
"REMOVE_HTML_PASSING_TESTCASES[0]",
"_____no_output_____"
],
[
"html, plain = REMOVE_HTML_PASSING_TESTCASES[0]\nremove_html_markup_test(html, plain)",
"_____no_output_____"
]
],
[
[
"Here is a failing test case (containing a double quote in the plain text)",
"_____no_output_____"
]
],
[
[
"REMOVE_HTML_FAILING_TESTCASES[0]",
"_____no_output_____"
],
[
"with ExpectError():\n html, plain = REMOVE_HTML_FAILING_TESTCASES[0]\n remove_html_markup_test(html, plain)",
"_____no_output_____"
]
],
[
[
"We run our tests, collecting the outcomes in `html_debugger`.",
"_____no_output_____"
]
],
[
[
"html_debugger = OchiaiDebugger()",
"_____no_output_____"
],
[
"for html, plain in (REMOVE_HTML_PASSING_TESTCASES + \n REMOVE_HTML_FAILING_TESTCASES):\n with html_debugger:\n remove_html_markup_test(html, plain)",
"_____no_output_____"
]
],
[
[
"The suspiciousness distribution will not be of much help here – pretty much all lines in `remove_html_markup()` have the same suspiciousness.",
"_____no_output_____"
]
],
[
[
"html_debugger",
"_____no_output_____"
]
],
[
[
"Let us create our repairer and run it.",
"_____no_output_____"
]
],
[
[
"html_repairer = Repairer(html_debugger, log=True)",
"_____no_output_____"
],
[
"best_tree, fitness = html_repairer.repair(iterations=20)",
"_____no_output_____"
]
],
[
[
"We see that the \"best\" code is still our original code, with no changes. And we can set `iterations` to 50, 100, 200... – our `Repairer` won't be able to repair it.",
"_____no_output_____"
]
],
[
[
"quiz(\"Why couldn't `Repairer()` repair `remove_html_markup()`?\",\n [\n \"The population is too small!\",\n \"The suspiciousness is too evenly distributed!\",\n \"We need more test cases!\",\n \"We need more iterations!\",\n \"There is no statement in the source with a correct condition!\",\n \"The population is too big!\",\n ], '5242880 >> 20')",
"_____no_output_____"
]
],
[
[
"You can explore all of the hypotheses above by changing the appropriate parameters, but you won't be able to change the outcome. The problem is that, unlike `middle()`, there is no statement (or combination thereof) in `remove_html_markup()` that could be used to make the failure go away. For this, we need to mutate another aspect of the code, which we will explore in the next section.",
"_____no_output_____"
],
[
"## Mutating Conditions\n\nThe `Repairer` class is very configurable. The individual steps in automated repair can all be replaced by providing own classes in the keyword arguments of its `__init__()` constructor:\n\n* To change fault localization, pass a different `debugger` that is a subclass of `RankingDebugger`.\n* To change the mutation operator, set `mutator_class` to a subclass of `StatementMutator`.\n* To change the crossover operator, set `crossover_class` to a subclass of `CrossoverOperator`.\n* To change the reduction algorithm, set `reducer_class` to a subclass of `Reducer`.\n\nIn this section, we will explore how to extend the mutation operator such that it can mutate _conditions_ for control constructs such as `if`, `while`, or `for`. To this end, we introduce a new class `ConditionMutator` subclassing `StatementMutator`.",
"_____no_output_____"
],
[
"### Collecting Conditions\n\nLet us start with a few simple supporting functions. The function `all_conditions()` retrieves all control conditions from an AST.",
"_____no_output_____"
]
],
[
[
"def all_conditions(trees: Union[ast.AST, List[ast.AST]],\n tp: Optional[Type] = None) -> List[ast.expr]:\n \"\"\"\n Return all conditions from the AST (or AST list) `trees`.\n If `tp` is given, return only elements of that type.\n \"\"\"\n\n if not isinstance(trees, list):\n assert isinstance(trees, ast.AST)\n trees = [trees]\n\n visitor = ConditionVisitor()\n for tree in trees:\n visitor.visit(tree)\n conditions = visitor.conditions\n if tp is not None:\n conditions = [c for c in conditions if isinstance(c, tp)]\n\n return conditions",
"_____no_output_____"
]
],
[
[
"`all_conditions()` uses a `ConditionVisitor` class to walk the tree and collect the conditions:",
"_____no_output_____"
]
],
[
[
"class ConditionVisitor(NodeVisitor):\n def __init__(self) -> None:\n self.conditions: List[ast.expr] = []\n self.conditions_seen: Set[str] = set()\n super().__init__()\n\n def add_conditions(self, node: ast.AST, attr: str) -> None:\n elems = getattr(node, attr, [])\n if not isinstance(elems, list):\n elems = [elems]\n\n elems = cast(List[ast.expr], elems)\n\n for elem in elems:\n elem_str = astor.to_source(elem)\n if elem_str not in self.conditions_seen:\n self.conditions.append(elem)\n self.conditions_seen.add(elem_str)\n\n def visit_BoolOp(self, node: ast.BoolOp) -> ast.AST:\n self.add_conditions(node, 'values')\n return super().generic_visit(node)\n\n def visit_UnaryOp(self, node: ast.UnaryOp) -> ast.AST:\n if isinstance(node.op, ast.Not):\n self.add_conditions(node, 'operand')\n return super().generic_visit(node)\n\n def generic_visit(self, node: ast.AST) -> ast.AST:\n if hasattr(node, 'test'):\n self.add_conditions(node, 'test')\n return super().generic_visit(node)",
"_____no_output_____"
]
],
[
[
"Here are all the conditions in `remove_html_markup()`. This is some material to construct new conditions from.",
"_____no_output_____"
]
],
[
[
"[astor.to_source(cond).strip()\n for cond in all_conditions(remove_html_markup_tree())]",
"_____no_output_____"
]
],
[
[
"### Mutating Conditions\n\nHere comes our `ConditionMutator` class. We subclass from `StatementMutator` and set an attribute `self.conditions` containing all the conditions in the source. The method `choose_condition()` randomly picks a condition.",
"_____no_output_____"
]
],
[
[
"class ConditionMutator(StatementMutator):\n \"\"\"Mutate conditions in an AST\"\"\"\n\n def __init__(self, *args: Any, **kwargs: Any) -> None:\n \"\"\"Constructor. Arguments are as with `StatementMutator` constructor.\"\"\"\n super().__init__(*args, **kwargs)\n self.conditions = all_conditions(self.source)\n if self.log:\n print(\"Found conditions\",\n [astor.to_source(cond).strip() \n for cond in self.conditions])\n\n def choose_condition(self) -> ast.expr:\n \"\"\"Return a random condition from source.\"\"\"\n return copy.deepcopy(random.choice(self.conditions))",
"_____no_output_____"
]
],
[
[
"The actual mutation takes place in the `swap()` method. If the node to be replaced has a `test` attribute (i.e. a controlling predicate), then we pick a random condition `cond` from the source and randomly chose from:\n\n* **set**: We change `test` to `cond`.\n* **not**: We invert `test`.\n* **and**: We replace `test` by `cond and test`.\n* **or**: We replace `test` by `cond or test`.\n\nOver time, this might lead to operators propagating across the population.",
"_____no_output_____"
]
],
[
[
"class ConditionMutator(ConditionMutator):\n def choose_bool_op(self) -> str:\n return random.choice(['set', 'not', 'and', 'or'])\n\n def swap(self, node: ast.AST) -> ast.AST:\n \"\"\"Replace `node` condition by a condition from `source`\"\"\"\n if not hasattr(node, 'test'):\n return super().swap(node)\n\n node = cast(ast.If, node)\n\n cond = self.choose_condition()\n new_test = None\n\n choice = self.choose_bool_op()\n\n if choice == 'set':\n new_test = cond\n elif choice == 'not':\n new_test = ast.UnaryOp(op=ast.Not(), operand=node.test)\n elif choice == 'and':\n new_test = ast.BoolOp(op=ast.And(), values=[cond, node.test])\n elif choice == 'or':\n new_test = ast.BoolOp(op=ast.Or(), values=[cond, node.test])\n else:\n raise ValueError(\"Unknown boolean operand\")\n\n if new_test:\n # ast.copy_location(new_test, node)\n node.test = new_test\n\n return node",
"_____no_output_____"
]
],
[
[
"We can use the mutator just like `StatementMutator`, except that some of the mutations will also include new conditions:",
"_____no_output_____"
]
],
[
[
"mutator = ConditionMutator(source=all_statements(remove_html_markup_tree()),\n log=True)",
"_____no_output_____"
],
[
"for i in range(10):\n new_tree = mutator.mutate(remove_html_markup_tree())",
"_____no_output_____"
]
],
[
[
"Let us put our new mutator to action, again in a `Repairer()`. To activate it, all we need to do is to pass it as `mutator_class` keyword argument.",
"_____no_output_____"
]
],
[
[
"condition_repairer = Repairer(html_debugger,\n mutator_class=ConditionMutator,\n log=2)",
"_____no_output_____"
]
],
[
[
"We might need more iterations for this one. Let us see...",
"_____no_output_____"
]
],
[
[
"best_tree, fitness = condition_repairer.repair(iterations=200)",
"_____no_output_____"
],
[
"repaired_source = astor.to_source(best_tree)",
"_____no_output_____"
],
[
"print_content(repaired_source, '.py')",
"_____no_output_____"
]
],
[
[
"Success again! We have automatically repaired `remove_html_markup()` – the resulting code passes all tests, including those that were previously failing.",
"_____no_output_____"
],
[
"Again, we can present the fix as a patch:",
"_____no_output_____"
]
],
[
[
"original_source = astor.to_source(remove_html_markup_tree())",
"_____no_output_____"
],
[
"for patch in diff(original_source, repaired_source):\n print_patch(patch)",
"_____no_output_____"
]
],
[
[
"However, looking at the patch, one may come up with doubts.",
"_____no_output_____"
]
],
[
[
"quiz(\"Is this actually the best solution?\",\n [\n \"Yes, sure, of course. Why?\",\n \"Err - what happened to single quotes?\"\n ], 1 << 1)",
"_____no_output_____"
]
],
[
[
"Indeed – our solution does not seem to handle single quotes anymore. Why is that so?",
"_____no_output_____"
]
],
[
[
"quiz(\"Why aren't single quotes handled in the solution?\",\n [\n \"Because they're not important. I mean, who uses 'em anyway?\",\n \"Because they are not part of our tests? \"\n \"Let me look up how they are constructed...\"\n ], 1 << 1)",
"_____no_output_____"
]
],
[
[
"Correct! Our test cases do not include single quotes – at least not in the interior of HTML tags – and thus, automatic repair did not care to preserve their handling.",
"_____no_output_____"
],
[
"How can we fix this? An easy way is to include an appropriate test case in our set – a test case that passes with the original `remove_html_markup()`, yet fails with the \"repaired\" `remove_html_markup()` as whosn above.",
"_____no_output_____"
]
],
[
[
"with html_debugger:\n remove_html_markup_test(\"<foo quote='>abc'>me</foo>\", \"me\")",
"_____no_output_____"
]
],
[
[
"Let us repeat the repair with the extended test set:",
"_____no_output_____"
]
],
[
[
"best_tree, fitness = condition_repairer.repair(iterations=200)",
"_____no_output_____"
]
],
[
[
"Here is the final tree:",
"_____no_output_____"
]
],
[
[
"print_content(astor.to_source(best_tree), '.py')",
"_____no_output_____"
]
],
[
[
"And here is its fitness:",
"_____no_output_____"
]
],
[
[
"fitness",
"_____no_output_____"
]
],
[
[
"The revised candidate now passes _all_ tests (including the tricky quote test we added last). Its condition now properly checks for `tag` _and_ both quotes. (The `tag` inside the parentheses is still redundant, but so be it.) From this example, we can learn a few lessons about the possibilities and risks of automated repair:\n\n* First, automatic repair is highly dependent on the quality of the checking tests. The risk is that the repair may overspecialize towards the test.\n* Second, automated repair is highly dependent on the sources that program fragments are chosen from. If there is a hint of a solution somewhere in the code, there is a chance that automated repair will catch it up.\n* Third, automatic repair is a deeply heuristic approach. Its behavior will vary widely with any change to the parameters (and the underlying random number generators)\n* Fourth, automatic repair can take a long time. The examples we have in this chapter take less than a minute to compute, and neither Python nor our implementation is exactly fast. But as the search space grows, automated repair will take much longer.\n\nOn the other hand, even an incomplete automated repair candidate can be much better than nothing at all – it may provide all the essential ingredients (such as the location or the involved variables) for a successful fix. When users of automated repair techniques are aware of its limitations and its assumptions, there is lots of potential in automated repair. Enjoy!",
"_____no_output_____"
],
[
"## Limitations",
"_____no_output_____"
],
[
"The `Repairer` class is hardly tested. Things that do not work include\n\n* Functions with inner functions are not repaired.",
"_____no_output_____"
],
[
"## Synopsis",
"_____no_output_____"
],
[
"This chapter provides tools and techniques for automated repair of program code. The `Repairer()` class takes a `RankingDebugger` debugger as input (such as `OchiaiDebugger` from [the chapter on statistical debugging](StatisticalDebugger.ipynb). A typical setup looks like this:\n\n```python\nfrom debuggingbook.StatisticalDebugger import OchiaiDebugger\n\ndebugger = OchiaiDebugger()\nfor inputs in TESTCASES:\n with debugger:\n test_foo(inputs)\n...\n\nrepairer = Repairer(debugger)\n```\nHere, `test_foo()` is a function that raises an exception if the tested function `foo()` fails. If `foo()` passes, `test_foo()` should not raise an exception.",
"_____no_output_____"
],
[
"The `repair()` method of a `Repairer` searches for a repair of the code covered in the debugger (except for methods starting or ending in `test`, such that `foo()`, not `test_foo()` is repaired). `repair()` returns the best fix candidate as a pair `(tree, fitness)` where `tree` is a [Python abstract syntax tree](http://docs.python.org/3/library/ast) (AST) of the fix candidate, and `fitness` is the fitness of the candidate (a value between 0 and 1). A `fitness` of 1.0 means that the candidate passed all tests. A typical usage looks like this:\n\n```python\nimport astor\n\ntree, fitness = repairer.repair()\nprint(astor.to_source(tree), fitness)\n```",
"_____no_output_____"
],
[
"Here is a complete example for the `middle()` program. This is the original source code of `middle()`:",
"_____no_output_____"
]
],
[
[
"# ignore\nprint_content(middle_source, '.py')",
"_____no_output_____"
]
],
[
[
"We set up a function `middle_test()` that tests it. The `middle_debugger` collects testcases and outcomes:",
"_____no_output_____"
]
],
[
[
"middle_debugger = OchiaiDebugger()",
"_____no_output_____"
],
[
"for x, y, z in MIDDLE_PASSING_TESTCASES + MIDDLE_FAILING_TESTCASES:\n with middle_debugger:\n middle_test(x, y, z)",
"_____no_output_____"
]
],
[
[
"The repairer attempts to repair the invoked function (`middle()`). The returned AST `tree` can be output via `astor.to_source()`:",
"_____no_output_____"
]
],
[
[
"middle_repairer = Repairer(middle_debugger)\ntree, fitness = middle_repairer.repair()\nprint(astor.to_source(tree), fitness)",
"_____no_output_____"
]
],
[
[
"Here are the classes defined in this chapter. A `Repairer` repairs a program, using a `StatementMutator` and a `CrossoverOperator` to evolve a population of candidates.",
"_____no_output_____"
]
],
[
[
"# ignore\nfrom ClassDiagram import display_class_hierarchy",
"_____no_output_____"
],
[
"# ignore\ndisplay_class_hierarchy([Repairer, ConditionMutator, CrossoverOperator],\n abstract_classes=[\n NodeVisitor,\n NodeTransformer\n ],\n public_methods=[\n Repairer.__init__,\n Repairer.repair,\n StatementMutator.__init__,\n StatementMutator.mutate,\n ConditionMutator.__init__,\n CrossoverOperator.__init__,\n CrossoverOperator.crossover,\n ],\n project='debuggingbook')",
"_____no_output_____"
]
],
[
[
"## Lessons Learned\n\n* Automated repair based on genetic optimization uses five ingredients:\n 1. A _test suite_ to determine passing and failing tests\n 2. _Defect localization_ (typically obtained from [statistical debugging](StatisticalDebugger.ipynb) with the test suite) to determine potential locations to be fixed\n 3. _Random code mutations_ and _crossover operations_ to create and evolve a population of inputs\n 4. A _fitness function_ and a _selection strategy_ to determine the part of the population that should be evolved further\n 5. A _reducer_ such as [delta debugging](DeltaDebugger.ipynb) to simplify the final candidate with the highest fitness.\n* The result of automated repair is a _fix candidate_ with the highest fitness for the given tests.\n* A _fix candidate_ is not guaranteed to be correct or optimal, but gives important hints on how to fix the program.\n* All of the above ingredients offer plenty of settings and alternatives to experiment with.",
"_____no_output_____"
],
[
"## Background\n\nThe seminal work in automated repair is [GenProg](https://squareslab.github.io/genprog-code/) \\cite{LeGoues2012}, which heavily inspired our `Repairer` implementation. Major differences between GenProg and `Repairer` include:\n\n* GenProg includes its own defect localization (which is also dynamically updated), whereas `Repairer` builds on earlier statistical debugging.\n* GenProg can apply multiple mutations on programs (or none at all), whereas `Repairer` applies exactly one mutation.\n* The `StatementMutator` used by `Repairer` includes various special cases for program structures (`if`, `for`, `while`...), whereas GenProg operates on statements only.\n* GenProg has been tested on large production programs.\n\nWhile GenProg is _the_ seminal work in the area (and arguably the most important software engineering research contribution of the 2010s), there have been a number of important extensions of automated repair. These include:\n\n* *AutoFix* \\cite{Pei2014} leverages _program contracts_ (pre- and postconditions) to generate tests and assertions automatically. Not only do such [assertions](Assertions.ipynb) help in fault localization, they also allow for much better validation of fix candidates.\n* *SemFix* \\cite{Nguyen2013} presents automated program repair based on _symbolic analysis_ rather than genetic optimization. This allows to leverage program semantics, which GenProg does not consider.\n\nTo learn more about automated program repair, see [program-repair.org](http://program-repair.org), the community page dedicated to research in program repair.",
"_____no_output_____"
],
[
"## Exercises",
"_____no_output_____"
],
[
"### Exercise 1: Automated Repair Parameters\n\nAutomated Repair is influenced by a large number of design choices – the size of the population, the number of iterations, the genetic optimization strategy, and more. How do changes to these design choices affect its effectiveness? \n\n* Consider the constants defined in this chapter (such as `POPULATION_SIZE` or `WEIGHT_PASSING` vs. `WEIGHT_FAILING`). How do changes affect the effectiveness of automated repair?\n* As an effectiveness metric, consider the number of iterations it takes to produce a fix candidate.\n* Since genetic optimization is a random algorithm, you need to determine effectiveness averages over a large number of runs (say, 100).",
"_____no_output_____"
],
[
"### Exercise 2: Elitism\n\n[_Elitism_](https://en.wikipedia.org/wiki/Genetic_algorithm#Elitism) (also known as _elitist selection_) is a variant of genetic selection in which a small fraction of the fittest candidates of the last population are included unchanged in the offspring.\n\n* Implement elitist selection by subclassing the `evolve()` method. Experiment with various fractions (5%, 10%, 25%) of \"elites\" and see how this improves results.",
"_____no_output_____"
],
[
"### Exercise 3: Evolving Values\n\nFollowing the steps of `ConditionMutator`, implement a `ValueMutator` class that replaces one constant value by another one found in the source (say, `0` by `1` or `True` by `False`).\n\nFor validation, consider the following failure in the `square_root()` function from [the chapter on assertions](Assertions.ipynb):",
"_____no_output_____"
]
],
[
[
"from Assertions import square_root # minor dependency",
"_____no_output_____"
],
[
"with ExpectError():\n square_root_of_zero = square_root(0)",
"_____no_output_____"
]
],
[
[
"Can your `ValueMutator` automatically fix this failure?",
"_____no_output_____"
],
[
"**Solution.** Your solution will be effective if it also includes named constants such as `None`.",
"_____no_output_____"
]
],
[
[
"import math",
"_____no_output_____"
],
[
"def square_root_fixed(x): # type: ignore\n assert x >= 0 # precondition\n\n approx = 0 # <-- FIX: Change `None` to 0\n guess = x / 2\n while approx != guess:\n approx = guess\n guess = (approx + x / approx) / 2\n\n assert math.isclose(approx * approx, x)\n return approx",
"_____no_output_____"
],
[
"square_root_fixed(0)",
"_____no_output_____"
]
],
[
[
"### Exercise 4: Evolving Variable Names\n\nFollowing the steps of `ConditionMutator`, implement a `IdentifierMutator` class that replaces one identifier by another one found in the source (say, `y` by `x`). Does it help fixing the `middle()` error?",
"_____no_output_____"
],
[
"### Exercise 5: Parallel Repair\n\nAutomatic Repair is a technique that is embarrassingly parallel – all tests for one candidate can all be run in parallel, and all tests for _all_ candidates can also be run in parallel. Set up an infrastructure for running concurrent tests using Pythons [asyncio](https://docs.python.org/3/library/asyncio.html) library.",
"_____no_output_____"
]
]
] | [
"markdown",
"code",
"markdown",
"code",
"markdown",
"code",
"markdown",
"code",
"markdown",
"code",
"markdown",
"code",
"markdown",
"code",
"markdown",
"code",
"markdown",
"code",
"markdown",
"code",
"markdown",
"code",
"markdown",
"code",
"markdown",
"code",
"markdown",
"code",
"markdown",
"code",
"markdown",
"code",
"markdown",
"code",
"markdown",
"code",
"markdown",
"code",
"markdown",
"code",
"markdown",
"code",
"markdown",
"code",
"markdown",
"code",
"markdown",
"code",
"markdown",
"code",
"markdown",
"code",
"markdown",
"code",
"markdown",
"code",
"markdown",
"code",
"markdown",
"code",
"markdown",
"code",
"markdown",
"code",
"markdown",
"code",
"markdown",
"code",
"markdown",
"code",
"markdown",
"code",
"markdown",
"code",
"markdown",
"code",
"markdown",
"code",
"markdown",
"code",
"markdown",
"code",
"markdown",
"code",
"markdown",
"code",
"markdown",
"code",
"markdown",
"code",
"markdown",
"code",
"markdown",
"code",
"markdown",
"code",
"markdown",
"code",
"markdown",
"code",
"markdown",
"code",
"markdown",
"code",
"markdown",
"code",
"markdown",
"code",
"markdown",
"code",
"markdown",
"code",
"markdown",
"code",
"markdown",
"code",
"markdown",
"code",
"markdown",
"code",
"markdown",
"code",
"markdown",
"code",
"markdown",
"code",
"markdown",
"code",
"markdown",
"code",
"markdown",
"code",
"markdown",
"code",
"markdown",
"code",
"markdown",
"code",
"markdown",
"code",
"markdown",
"code",
"markdown",
"code",
"markdown",
"code",
"markdown",
"code",
"markdown",
"code",
"markdown",
"code",
"markdown",
"code",
"markdown",
"code",
"markdown",
"code",
"markdown",
"code",
"markdown",
"code",
"markdown",
"code",
"markdown",
"code",
"markdown",
"code",
"markdown",
"code",
"markdown",
"code",
"markdown",
"code",
"markdown",
"code",
"markdown",
"code",
"markdown",
"code",
"markdown",
"code",
"markdown",
"code",
"markdown",
"code",
"markdown",
"code",
"markdown",
"code",
"markdown",
"code",
"markdown",
"code",
"markdown",
"code",
"markdown",
"code",
"markdown",
"code",
"markdown",
"code",
"markdown",
"code",
"markdown",
"code",
"markdown",
"code",
"markdown",
"code",
"markdown",
"code",
"markdown"
] | [
[
"markdown"
],
[
"code"
],
[
"markdown"
],
[
"code"
],
[
"markdown",
"markdown",
"markdown",
"markdown",
"markdown",
"markdown"
],
[
"code",
"code",
"code",
"code"
],
[
"markdown"
],
[
"code"
],
[
"markdown"
],
[
"code"
],
[
"markdown",
"markdown"
],
[
"code"
],
[
"markdown"
],
[
"code"
],
[
"markdown",
"markdown",
"markdown",
"markdown",
"markdown",
"markdown",
"markdown",
"markdown",
"markdown"
],
[
"code"
],
[
"markdown"
],
[
"code",
"code",
"code"
],
[
"markdown",
"markdown"
],
[
"code",
"code"
],
[
"markdown"
],
[
"code"
],
[
"markdown"
],
[
"code"
],
[
"markdown"
],
[
"code"
],
[
"markdown",
"markdown"
],
[
"code",
"code",
"code"
],
[
"markdown",
"markdown"
],
[
"code",
"code",
"code",
"code"
],
[
"markdown",
"markdown"
],
[
"code"
],
[
"markdown"
],
[
"code"
],
[
"markdown",
"markdown"
],
[
"code",
"code",
"code"
],
[
"markdown"
],
[
"code",
"code"
],
[
"markdown"
],
[
"code",
"code"
],
[
"markdown"
],
[
"code",
"code"
],
[
"markdown",
"markdown"
],
[
"code",
"code",
"code"
],
[
"markdown"
],
[
"code",
"code"
],
[
"markdown"
],
[
"code"
],
[
"markdown",
"markdown"
],
[
"code",
"code",
"code"
],
[
"markdown"
],
[
"code",
"code"
],
[
"markdown"
],
[
"code"
],
[
"markdown"
],
[
"code",
"code",
"code"
],
[
"markdown",
"markdown"
],
[
"code"
],
[
"markdown"
],
[
"code"
],
[
"markdown"
],
[
"code"
],
[
"markdown"
],
[
"code"
],
[
"markdown",
"markdown"
],
[
"code",
"code"
],
[
"markdown"
],
[
"code"
],
[
"markdown"
],
[
"code"
],
[
"markdown"
],
[
"code",
"code",
"code"
],
[
"markdown"
],
[
"code",
"code"
],
[
"markdown"
],
[
"code"
],
[
"markdown"
],
[
"code"
],
[
"markdown"
],
[
"code"
],
[
"markdown"
],
[
"code"
],
[
"markdown"
],
[
"code",
"code"
],
[
"markdown"
],
[
"code"
],
[
"markdown"
],
[
"code"
],
[
"markdown"
],
[
"code"
],
[
"markdown",
"markdown"
],
[
"code"
],
[
"markdown",
"markdown",
"markdown"
],
[
"code",
"code",
"code",
"code",
"code",
"code",
"code",
"code"
],
[
"markdown"
],
[
"code",
"code",
"code"
],
[
"markdown",
"markdown",
"markdown",
"markdown",
"markdown",
"markdown"
],
[
"code",
"code"
],
[
"markdown",
"markdown"
],
[
"code"
],
[
"markdown"
],
[
"code",
"code",
"code",
"code",
"code"
],
[
"markdown"
],
[
"code"
],
[
"markdown"
],
[
"code"
],
[
"markdown"
],
[
"code"
],
[
"markdown"
],
[
"code",
"code"
],
[
"markdown",
"markdown",
"markdown"
],
[
"code",
"code"
],
[
"markdown"
],
[
"code"
],
[
"markdown"
],
[
"code",
"code"
],
[
"markdown"
],
[
"code"
],
[
"markdown"
],
[
"code",
"code"
],
[
"markdown",
"markdown",
"markdown"
],
[
"code",
"code"
],
[
"markdown",
"markdown"
],
[
"code",
"code",
"code",
"code"
],
[
"markdown"
],
[
"code",
"code"
],
[
"markdown"
],
[
"code"
],
[
"markdown"
],
[
"code"
],
[
"markdown"
],
[
"code",
"code"
],
[
"markdown"
],
[
"code"
],
[
"markdown"
],
[
"code",
"code"
],
[
"markdown"
],
[
"code",
"code",
"code",
"code",
"code"
],
[
"markdown"
],
[
"code",
"code"
],
[
"markdown"
],
[
"code"
],
[
"markdown"
],
[
"code"
],
[
"markdown"
],
[
"code"
],
[
"markdown"
],
[
"code"
],
[
"markdown",
"markdown"
],
[
"code"
],
[
"markdown"
],
[
"code",
"code",
"code"
],
[
"markdown",
"markdown"
],
[
"code",
"code"
],
[
"markdown"
],
[
"code"
],
[
"markdown",
"markdown"
],
[
"code",
"code",
"code",
"code",
"code",
"code",
"code",
"code",
"code",
"code"
],
[
"markdown",
"markdown"
],
[
"code",
"code"
],
[
"markdown"
],
[
"code",
"code"
],
[
"markdown"
],
[
"code",
"code"
],
[
"markdown"
],
[
"code"
],
[
"markdown"
],
[
"code",
"code"
],
[
"markdown"
],
[
"code"
],
[
"markdown",
"markdown",
"markdown"
],
[
"code"
],
[
"markdown"
],
[
"code"
],
[
"markdown"
],
[
"code"
],
[
"markdown"
],
[
"code"
],
[
"markdown"
],
[
"code"
],
[
"markdown"
],
[
"code",
"code"
],
[
"markdown"
],
[
"code"
],
[
"markdown"
],
[
"code",
"code",
"code"
],
[
"markdown",
"markdown"
],
[
"code",
"code"
],
[
"markdown"
],
[
"code"
],
[
"markdown"
],
[
"code"
],
[
"markdown",
"markdown"
],
[
"code"
],
[
"markdown"
],
[
"code"
],
[
"markdown"
],
[
"code"
],
[
"markdown"
],
[
"code"
],
[
"markdown",
"markdown",
"markdown",
"markdown",
"markdown",
"markdown",
"markdown"
],
[
"code"
],
[
"markdown"
],
[
"code",
"code"
],
[
"markdown"
],
[
"code"
],
[
"markdown"
],
[
"code",
"code"
],
[
"markdown",
"markdown",
"markdown",
"markdown",
"markdown",
"markdown"
],
[
"code",
"code"
],
[
"markdown",
"markdown"
],
[
"code",
"code",
"code"
],
[
"markdown",
"markdown"
]
] |
e78335611f1fbe67fdfb19e116bfdb2ba23c993a | 145,388 | ipynb | Jupyter Notebook | apps/networking/ble-localization/onprem/seldon/blerssi-seldon.ipynb | Karthik-Git-Sudo786/cisco-kubeflow-starter-pack | 49013953c0cf0de508bb05f1837809d84e6ea2d2 | [
"Apache-2.0"
] | 60 | 2020-03-20T08:05:32.000Z | 2021-12-17T14:07:53.000Z | apps/networking/ble-localization/onprem/seldon/blerssi-seldon.ipynb | Karthik-Git-Sudo786/cisco-kubeflow-starter-pack | 49013953c0cf0de508bb05f1837809d84e6ea2d2 | [
"Apache-2.0"
] | 84 | 2020-03-18T07:06:20.000Z | 2021-03-02T13:29:20.000Z | apps/networking/ble-localization/onprem/seldon/blerssi-seldon.ipynb | Karthik-Git-Sudo786/cisco-kubeflow-starter-pack | 49013953c0cf0de508bb05f1837809d84e6ea2d2 | [
"Apache-2.0"
] | 90 | 2020-03-17T11:54:05.000Z | 2021-06-03T09:18:58.000Z | 53.451471 | 2,316 | 0.620512 | [
[
[
"## BLERSSI Seldon serving",
"_____no_output_____"
],
[
"## Clone Cisco Kubeflow Starter pack repository",
"_____no_output_____"
]
],
[
[
"BRANCH_NAME=\"master\" #Provide git branch name \"master\" or \"dev\"\n! git clone -b $BRANCH_NAME https://github.com/CiscoAI/cisco-kubeflow-starter-pack.git",
"Cloning into 'cisco-kubeflow-starter-pack'...\nremote: Enumerating objects: 63, done.\u001b[K\nremote: Counting objects: 100% (63/63), done.\u001b[K\nremote: Compressing objects: 100% (44/44), done.\u001b[K\nremote: Total 4630 (delta 16), reused 44 (delta 11), pack-reused 4567\u001b[K\nReceiving objects: 100% (4630/4630), 17.61 MiB | 48.72 MiB/s, done.\nResolving deltas: 100% (1745/1745), done.\n"
]
],
[
[
"## Install the required packages",
"_____no_output_____"
]
],
[
[
"! pip install pandas sklearn seldon_core dill alibi==0.3.2 --user",
"Collecting pandas\n Downloading pandas-1.0.5-cp36-cp36m-manylinux1_x86_64.whl (10.1 MB)\n\u001b[K |████████████████████████████████| 10.1 MB 21.3 MB/s eta 0:00:01\n\u001b[?25hCollecting sklearn\n Downloading sklearn-0.0.tar.gz (1.1 kB)\nCollecting seldon_core\n Downloading seldon_core-1.2.1-py3-none-any.whl (104 kB)\n\u001b[K |████████████████████████████████| 104 kB 134.7 MB/s eta 0:00:01\n\u001b[?25hCollecting dill\n Downloading dill-0.3.2.zip (177 kB)\n\u001b[K |████████████████████████████████| 177 kB 53.4 MB/s eta 0:00:01\n\u001b[?25hCollecting alibi==0.3.2\n Downloading alibi-0.3.2-py3-none-any.whl (81 kB)\n\u001b[K |████████████████████████████████| 81 kB 103 kB/s eta 0:00:01\n\u001b[?25hRequirement already satisfied: numpy>=1.13.3 in /usr/local/lib/python3.6/dist-packages (from pandas) (1.18.1)\nRequirement already satisfied: python-dateutil>=2.6.1 in /usr/local/lib/python3.6/dist-packages (from pandas) (2.8.1)\nRequirement already satisfied: pytz>=2017.2 in /usr/local/lib/python3.6/dist-packages (from pandas) (2019.3)\nCollecting scikit-learn\n Downloading scikit_learn-0.23.1-cp36-cp36m-manylinux1_x86_64.whl (6.8 MB)\n\u001b[K |████████████████████████████████| 6.8 MB 135.5 MB/s eta 0:00:01\n\u001b[?25hCollecting Flask<2.0.0\n Downloading Flask-1.1.2-py2.py3-none-any.whl (94 kB)\n\u001b[K |████████████████████████████████| 94 kB 16.7 MB/s eta 0:00:01\n\u001b[?25hCollecting Flask-OpenTracing<1.2.0,>=1.1.0\n Downloading Flask-OpenTracing-1.1.0.tar.gz (8.2 kB)\nRequirement already satisfied: requests<3.0.0 in /usr/local/lib/python3.6/dist-packages (from seldon_core) (2.22.0)\nCollecting minio<6.0.0,>=4.0.9\n Downloading minio-5.0.10-py2.py3-none-any.whl (75 kB)\n\u001b[K |████████████████████████████████| 75 kB 3.9 MB/s s eta 0:00:01\n\u001b[?25hRequirement already satisfied: jsonschema<4.0.0 in /usr/local/lib/python3.6/dist-packages (from seldon_core) (3.2.0)\nRequirement already satisfied: PyYAML<5.4 in /usr/local/lib/python3.6/dist-packages (from seldon_core) (5.3)\nCollecting grpcio-opentracing<1.2.0,>=1.1.4\n Downloading grpcio_opentracing-1.1.4-py3-none-any.whl (14 kB)\nCollecting azure-storage-blob<3.0.0,>=2.0.1\n Downloading azure_storage_blob-2.1.0-py2.py3-none-any.whl (88 kB)\n\u001b[K |████████████████████████████████| 88 kB 21.2 MB/s eta 0:00:01\n\u001b[?25hCollecting opentracing<2.3.0,>=2.2.0\n Downloading opentracing-2.2.0.tar.gz (47 kB)\n\u001b[K |████████████████████████████████| 47 kB 24.3 MB/s eta 0:00:01\n\u001b[?25hRequirement already satisfied: setuptools>=41.0.0 in /usr/local/lib/python3.6/dist-packages (from seldon_core) (45.1.0)\nCollecting redis<4.0.0\n Downloading redis-3.5.3-py2.py3-none-any.whl (72 kB)\n\u001b[K |████████████████████████████████| 72 kB 2.4 MB/s s eta 0:00:01\n\u001b[?25hCollecting flatbuffers<2.0.0\n Downloading flatbuffers-1.12-py2.py3-none-any.whl (15 kB)\nCollecting jaeger-client<4.2.0,>=4.1.0\n Downloading jaeger-client-4.1.0.tar.gz (80 kB)\n\u001b[K |████████████████████████████████| 80 kB 31.8 MB/s eta 0:00:01\n\u001b[?25hRequirement already satisfied: grpcio<2.0.0 in /usr/local/lib/python3.6/dist-packages (from seldon_core) (1.26.0)\nRequirement already satisfied: protobuf<4.0.0 in /usr/local/lib/python3.6/dist-packages (from seldon_core) (3.11.2)\nRequirement already satisfied: prometheus-client<0.9.0,>=0.7.1 in /usr/local/lib/python3.6/dist-packages (from seldon_core) (0.7.1)\nCollecting Flask-cors<4.0.0\n Downloading Flask_Cors-3.0.8-py2.py3-none-any.whl (14 kB)\nCollecting gunicorn<20.1.0,>=19.9.0\n Downloading gunicorn-20.0.4-py2.py3-none-any.whl (77 kB)\n\u001b[K |████████████████████████████████| 77 kB 27.1 MB/s eta 0:00:01\n\u001b[?25hCollecting beautifulsoup4\n Downloading beautifulsoup4-4.9.1-py3-none-any.whl (115 kB)\n\u001b[K |████████████████████████████████| 115 kB 158.3 MB/s eta 0:00:01\n\u001b[?25hRequirement already satisfied: tensorflow<2.0 in /usr/local/lib/python3.6/dist-packages (from alibi==0.3.2) (1.15.2)\nCollecting scikit-image\n Downloading scikit_image-0.17.2-cp36-cp36m-manylinux1_x86_64.whl (12.4 MB)\n\u001b[K |████████████████████████████████| 12.4 MB 71.0 MB/s eta 0:00:01\n\u001b[?25hCollecting spacy\n Downloading spacy-2.3.2-cp36-cp36m-manylinux1_x86_64.whl (9.9 MB)\n\u001b[K |████████████████████████████████| 9.9 MB 36.7 MB/s eta 0:00:01\n\u001b[?25hCollecting Pillow\n Downloading Pillow-7.2.0-cp36-cp36m-manylinux1_x86_64.whl (2.2 MB)\n\u001b[K |████████████████████████████████| 2.2 MB 68.8 MB/s eta 0:00:01\n\u001b[?25hRequirement already satisfied: six>=1.5 in /usr/lib/python3/dist-packages (from python-dateutil>=2.6.1->pandas) (1.11.0)\nRequirement already satisfied: scipy>=0.19.1 in /usr/local/lib/python3.6/dist-packages (from scikit-learn->sklearn) (1.4.1)\nCollecting joblib>=0.11\n Downloading joblib-0.16.0-py3-none-any.whl (300 kB)\n\u001b[K |████████████████████████████████| 300 kB 150.5 MB/s eta 0:00:01\n\u001b[?25hCollecting threadpoolctl>=2.0.0\n Downloading threadpoolctl-2.1.0-py3-none-any.whl (12 kB)\nCollecting click>=5.1\n Downloading click-7.1.2-py2.py3-none-any.whl (82 kB)\n\u001b[K |████████████████████████████████| 82 kB 5.3 MB/s s eta 0:00:01\n\u001b[?25hRequirement already satisfied: Werkzeug>=0.15 in /usr/local/lib/python3.6/dist-packages (from Flask<2.0.0->seldon_core) (0.16.1)\nCollecting itsdangerous>=0.24\n Downloading itsdangerous-1.1.0-py2.py3-none-any.whl (16 kB)\nRequirement already satisfied: Jinja2>=2.10.1 in /usr/local/lib/python3.6/dist-packages (from Flask<2.0.0->seldon_core) (2.11.0)\nRequirement already satisfied: idna<2.9,>=2.5 in /usr/lib/python3/dist-packages (from requests<3.0.0->seldon_core) (2.6)\nRequirement already satisfied: chardet<3.1.0,>=3.0.2 in /usr/local/lib/python3.6/dist-packages (from requests<3.0.0->seldon_core) (3.0.4)\nRequirement already satisfied: urllib3!=1.25.0,!=1.25.1,<1.26,>=1.21.1 in /usr/local/lib/python3.6/dist-packages (from requests<3.0.0->seldon_core) (1.25.8)\nRequirement already satisfied: certifi>=2017.4.17 in /usr/local/lib/python3.6/dist-packages (from requests<3.0.0->seldon_core) (2019.11.28)\nCollecting configparser\n Downloading configparser-5.0.0-py3-none-any.whl (22 kB)\nRequirement already satisfied: importlib-metadata; python_version < \"3.8\" in /usr/local/lib/python3.6/dist-packages (from jsonschema<4.0.0->seldon_core) (1.4.0)\nRequirement already satisfied: attrs>=17.4.0 in /usr/local/lib/python3.6/dist-packages (from jsonschema<4.0.0->seldon_core) (19.3.0)\nRequirement already satisfied: pyrsistent>=0.14.0 in /usr/local/lib/python3.6/dist-packages (from jsonschema<4.0.0->seldon_core) (0.15.7)\nCollecting azure-common>=1.1.5\n Downloading azure_common-1.1.25-py2.py3-none-any.whl (12 kB)\nCollecting azure-storage-common~=2.1\n Downloading azure_storage_common-2.1.0-py2.py3-none-any.whl (47 kB)\n\u001b[K |████████████████████████████████| 47 kB 11.2 MB/s eta 0:00:01\n\u001b[?25hCollecting threadloop<2,>=1\n Downloading threadloop-1.0.2.tar.gz (4.9 kB)\nCollecting thrift\n Downloading thrift-0.13.0.tar.gz (59 kB)\n\u001b[K |████████████████████████████████| 59 kB 19.0 MB/s eta 0:00:01\n\u001b[?25hRequirement already satisfied: tornado<6,>=4.3 in /usr/local/lib/python3.6/dist-packages (from jaeger-client<4.2.0,>=4.1.0->seldon_core) (5.1.1)\nCollecting soupsieve>1.2\n Downloading soupsieve-2.0.1-py3-none-any.whl (32 kB)\nRequirement already satisfied: absl-py>=0.7.0 in /usr/local/lib/python3.6/dist-packages (from tensorflow<2.0->alibi==0.3.2) (0.9.0)\nRequirement already satisfied: keras-applications>=1.0.8 in /usr/local/lib/python3.6/dist-packages (from tensorflow<2.0->alibi==0.3.2) (1.0.8)\nRequirement already satisfied: google-pasta>=0.1.6 in /usr/local/lib/python3.6/dist-packages (from tensorflow<2.0->alibi==0.3.2) (0.1.8)\nRequirement already satisfied: tensorboard<1.16.0,>=1.15.0 in /usr/local/lib/python3.6/dist-packages (from tensorflow<2.0->alibi==0.3.2) (1.15.0)\nRequirement already satisfied: gast==0.2.2 in /usr/local/lib/python3.6/dist-packages (from tensorflow<2.0->alibi==0.3.2) (0.2.2)\nRequirement already satisfied: tensorflow-estimator==1.15.1 in /usr/local/lib/python3.6/dist-packages (from tensorflow<2.0->alibi==0.3.2) (1.15.1)\nRequirement already satisfied: keras-preprocessing>=1.0.5 in /usr/local/lib/python3.6/dist-packages (from tensorflow<2.0->alibi==0.3.2) (1.1.0)\nRequirement already satisfied: termcolor>=1.1.0 in /usr/local/lib/python3.6/dist-packages (from tensorflow<2.0->alibi==0.3.2) (1.1.0)\nRequirement already satisfied: wrapt>=1.11.1 in /usr/local/lib/python3.6/dist-packages (from tensorflow<2.0->alibi==0.3.2) (1.11.2)\nRequirement already satisfied: astor>=0.6.0 in /usr/local/lib/python3.6/dist-packages (from tensorflow<2.0->alibi==0.3.2) (0.8.1)\nRequirement already satisfied: opt-einsum>=2.3.2 in /usr/local/lib/python3.6/dist-packages (from tensorflow<2.0->alibi==0.3.2) (3.1.0)\nRequirement already satisfied: wheel>=0.26; python_version >= \"3\" in /usr/lib/python3/dist-packages (from tensorflow<2.0->alibi==0.3.2) (0.30.0)\nRequirement already satisfied: matplotlib!=3.0.0,>=2.0.0 in /usr/local/lib/python3.6/dist-packages (from scikit-image->alibi==0.3.2) (3.1.2)\nCollecting PyWavelets>=1.1.1\n Downloading PyWavelets-1.1.1-cp36-cp36m-manylinux1_x86_64.whl (4.4 MB)\n\u001b[K |████████████████████████████████| 4.4 MB 121.5 MB/s eta 0:00:01\n\u001b[?25hCollecting networkx>=2.0\n Downloading networkx-2.4-py3-none-any.whl (1.6 MB)\n\u001b[K |████████████████████████████████| 1.6 MB 49.3 MB/s eta 0:00:01\n\u001b[?25hCollecting imageio>=2.3.0\n Downloading imageio-2.9.0-py3-none-any.whl (3.3 MB)\n\u001b[K |████████████████████████████████| 3.3 MB 94.7 MB/s eta 0:00:01\n\u001b[?25hCollecting tifffile>=2019.7.26\n Downloading tifffile-2020.7.22-py3-none-any.whl (145 kB)\n\u001b[K |████████████████████████████████| 145 kB 132.5 MB/s eta 0:00:01\n\u001b[?25hCollecting catalogue<1.1.0,>=0.0.7\n Downloading catalogue-1.0.0-py2.py3-none-any.whl (7.7 kB)\nCollecting srsly<1.1.0,>=1.0.2\n Downloading srsly-1.0.2-cp36-cp36m-manylinux1_x86_64.whl (185 kB)\n\u001b[K |████████████████████████████████| 185 kB 68.3 MB/s eta 0:00:01\n\u001b[?25hCollecting plac<1.2.0,>=0.9.6\n Downloading plac-1.1.3-py2.py3-none-any.whl (20 kB)\nCollecting thinc==7.4.1\n Downloading thinc-7.4.1-cp36-cp36m-manylinux1_x86_64.whl (2.1 MB)\n\u001b[K |████████████████████████████████| 2.1 MB 55.5 MB/s eta 0:00:01\n\u001b[?25hCollecting preshed<3.1.0,>=3.0.2\n Downloading preshed-3.0.2-cp36-cp36m-manylinux1_x86_64.whl (119 kB)\n\u001b[K |████████████████████████████████| 119 kB 36.9 MB/s eta 0:00:01\n\u001b[?25hCollecting blis<0.5.0,>=0.4.0\n Downloading blis-0.4.1-cp36-cp36m-manylinux1_x86_64.whl (3.7 MB)\n\u001b[K |████████████████████████████████| 3.7 MB 48.7 MB/s eta 0:00:01\n\u001b[?25hCollecting cymem<2.1.0,>=2.0.2\n Downloading cymem-2.0.3-cp36-cp36m-manylinux1_x86_64.whl (32 kB)\nCollecting wasabi<1.1.0,>=0.4.0\n Downloading wasabi-0.7.1.tar.gz (22 kB)\nCollecting tqdm<5.0.0,>=4.38.0\n Downloading tqdm-4.48.0-py2.py3-none-any.whl (67 kB)\n\u001b[K |████████████████████████████████| 67 kB 12.5 MB/s eta 0:00:01\n\u001b[?25hCollecting murmurhash<1.1.0,>=0.28.0\n Downloading murmurhash-1.0.2-cp36-cp36m-manylinux1_x86_64.whl (19 kB)\nRequirement already satisfied: MarkupSafe>=0.23 in /usr/local/lib/python3.6/dist-packages (from Jinja2>=2.10.1->Flask<2.0.0->seldon_core) (1.1.1)\nRequirement already satisfied: zipp>=0.5 in /usr/local/lib/python3.6/dist-packages (from importlib-metadata; python_version < \"3.8\"->jsonschema<4.0.0->seldon_core) (2.1.0)\nRequirement already satisfied: cryptography in /usr/lib/python3/dist-packages (from azure-storage-common~=2.1->azure-storage-blob<3.0.0,>=2.0.1->seldon_core) (2.1.4)\nRequirement already satisfied: h5py in /usr/local/lib/python3.6/dist-packages (from keras-applications>=1.0.8->tensorflow<2.0->alibi==0.3.2) (2.10.0)\nRequirement already satisfied: markdown>=2.6.8 in /usr/local/lib/python3.6/dist-packages (from tensorboard<1.16.0,>=1.15.0->tensorflow<2.0->alibi==0.3.2) (3.1.1)\nRequirement already satisfied: pyparsing!=2.0.4,!=2.1.2,!=2.1.6,>=2.0.1 in /usr/local/lib/python3.6/dist-packages (from matplotlib!=3.0.0,>=2.0.0->scikit-image->alibi==0.3.2) (2.4.6)\nRequirement already satisfied: cycler>=0.10 in /usr/local/lib/python3.6/dist-packages (from matplotlib!=3.0.0,>=2.0.0->scikit-image->alibi==0.3.2) (0.10.0)\nRequirement already satisfied: kiwisolver>=1.0.1 in /usr/local/lib/python3.6/dist-packages (from matplotlib!=3.0.0,>=2.0.0->scikit-image->alibi==0.3.2) (1.1.0)\nRequirement already satisfied: decorator>=4.3.0 in /usr/local/lib/python3.6/dist-packages (from networkx>=2.0->scikit-image->alibi==0.3.2) (4.4.1)\nBuilding wheels for collected packages: sklearn, dill, Flask-OpenTracing, opentracing, jaeger-client, threadloop, thrift, wasabi\n Building wheel for sklearn (setup.py) ... \u001b[?25ldone\n\u001b[?25h Created wheel for sklearn: filename=sklearn-0.0-py2.py3-none-any.whl size=2397 sha256=9c4b3edf39f181793582f584d48c1ca5a85a2e388006ca3ca493a769b6932fbb\n Stored in directory: /home/jovyan/.cache/pip/wheels/23/9d/42/5ec745cbbb17517000a53cecc49d6a865450d1f5cb16dc8a9c\n Building wheel for dill (setup.py) ... \u001b[?25ldone\n\u001b[?25h Created wheel for dill: filename=dill-0.3.2-py3-none-any.whl size=81196 sha256=8cbd79c7ddd7d5fe4056acae1344793eaeb8c261c5621821b8f6003708767e47\n Stored in directory: /home/jovyan/.cache/pip/wheels/02/49/cf/660924cd9bc5fcddc3a0246fe39800c83028d3ccea244de352\n Building wheel for Flask-OpenTracing (setup.py) ... \u001b[?25ldone\n\u001b[?25h Created wheel for Flask-OpenTracing: filename=Flask_OpenTracing-1.1.0-py3-none-any.whl size=11453 sha256=8c805121698d33d2d8a5a3039009a7996375a41955c5fdd7036abebf085443c4\n Stored in directory: /home/jovyan/.cache/pip/wheels/ad/4b/2d/24ff0da0a0b53c7c77ce59b843bcceaf644c88703241e59615\n Building wheel for opentracing (setup.py) ... \u001b[?25ldone\n\u001b[?25h Created wheel for opentracing: filename=opentracing-2.2.0-py3-none-any.whl size=49672 sha256=46329cbf55c5a47d098ee96b8dc65b78c6837928ff1d23417578f5480f0feb0b\n Stored in directory: /home/jovyan/.cache/pip/wheels/39/40/44/8bace79f4514e99786236c31f1df8d1b814ff02c1e08b1d697\n Building wheel for jaeger-client (setup.py) ... \u001b[?25ldone\n\u001b[?25h Created wheel for jaeger-client: filename=jaeger_client-4.1.0-py3-none-any.whl size=65467 sha256=c938ed0e6c88ee63b32d750aa0b28a830a894bc9911d8d695d3ca3370824dff4\n Stored in directory: /home/jovyan/.cache/pip/wheels/e9/9b/8c/503d0cc13b39a551c054515683ba1d15b40324c863dc442e66\n Building wheel for threadloop (setup.py) ... \u001b[?25ldone\n\u001b[?25h Created wheel for threadloop: filename=threadloop-1.0.2-py3-none-any.whl size=4261 sha256=991c49878e61284d0c1fef5b8406b68effb1e676262985d9b91d0aefda8f4122\n Stored in directory: /home/jovyan/.cache/pip/wheels/02/54/65/9f87de48fe8fcaaee30f279973d946ad55f9df56b93b3e78da\n Building wheel for thrift (setup.py) ... \u001b[?25ldone\n\u001b[?25h Created wheel for thrift: filename=thrift-0.13.0-cp36-cp36m-linux_x86_64.whl size=346198 sha256=e48920c920dbf1ef2fdcb6f8a5b769e83b798553e76a6b6933cf722dfc4c282b\n Stored in directory: /home/jovyan/.cache/pip/wheels/e0/38/fc/472fe18756b177b42096961f8bd3ff2dc5c5620ac399fce52d\n Building wheel for wasabi (setup.py) ... \u001b[?25ldone\n\u001b[?25h Created wheel for wasabi: filename=wasabi-0.7.1-py3-none-any.whl size=26108 sha256=4cd99c88f99f06afb0ceac55f7c0df730c9c2621f634da573ebefb084c19fc0b\n Stored in directory: /home/jovyan/.cache/pip/wheels/81/48/90/cf81833b3dfce6eaf7eab4bd5fdc0e75dbca4418b263f444b8\nSuccessfully built sklearn dill Flask-OpenTracing opentracing jaeger-client threadloop thrift wasabi\nInstalling collected packages: pandas, joblib, threadpoolctl, scikit-learn, sklearn, click, itsdangerous, Flask, opentracing, Flask-OpenTracing, configparser, minio, grpcio-opentracing, azure-common, azure-storage-common, azure-storage-blob, redis, flatbuffers, threadloop, thrift, jaeger-client, Flask-cors, gunicorn, seldon-core, dill, soupsieve, beautifulsoup4, Pillow, PyWavelets, networkx, imageio, tifffile, scikit-image, catalogue, srsly, plac, cymem, murmurhash, preshed, blis, wasabi, tqdm, thinc, spacy, alibi\n\u001b[33m WARNING: The script flask is installed in '/home/jovyan/.local/bin' which is not on PATH.\n Consider adding this directory to PATH or, if you prefer to suppress this warning, use --no-warn-script-location.\u001b[0m\n\u001b[33m WARNING: The script gunicorn is installed in '/home/jovyan/.local/bin' which is not on PATH.\n Consider adding this directory to PATH or, if you prefer to suppress this warning, use --no-warn-script-location.\u001b[0m\n\u001b[33m WARNING: The scripts seldon-batch-processor, seldon-core-api-tester, seldon-core-microservice, seldon-core-microservice-tester and seldon-core-tester are installed in '/home/jovyan/.local/bin' which is not on PATH.\n Consider adding this directory to PATH or, if you prefer to suppress this warning, use --no-warn-script-location.\u001b[0m\n\u001b[33m WARNING: The scripts imageio_download_bin and imageio_remove_bin are installed in '/home/jovyan/.local/bin' which is not on PATH.\n Consider adding this directory to PATH or, if you prefer to suppress this warning, use --no-warn-script-location.\u001b[0m\n\u001b[33m WARNING: The scripts lsm2bin and tifffile are installed in '/home/jovyan/.local/bin' which is not on PATH.\n Consider adding this directory to PATH or, if you prefer to suppress this warning, use --no-warn-script-location.\u001b[0m\n\u001b[33m WARNING: The script skivi is installed in '/home/jovyan/.local/bin' which is not on PATH.\n Consider adding this directory to PATH or, if you prefer to suppress this warning, use --no-warn-script-location.\u001b[0m\n\u001b[33m WARNING: The script tqdm is installed in '/home/jovyan/.local/bin' which is not on PATH.\n Consider adding this directory to PATH or, if you prefer to suppress this warning, use --no-warn-script-location.\u001b[0m\nSuccessfully installed Flask-1.1.2 Flask-OpenTracing-1.1.0 Flask-cors-3.0.8 Pillow-7.2.0 PyWavelets-1.1.1 alibi-0.3.2 azure-common-1.1.25 azure-storage-blob-2.1.0 azure-storage-common-2.1.0 beautifulsoup4-4.9.1 blis-0.4.1 catalogue-1.0.0 click-7.1.2 configparser-5.0.0 cymem-2.0.3 dill-0.3.2 flatbuffers-1.12 grpcio-opentracing-1.1.4 gunicorn-20.0.4 imageio-2.9.0 itsdangerous-1.1.0 jaeger-client-4.1.0 joblib-0.16.0 minio-5.0.10 murmurhash-1.0.2 networkx-2.4 opentracing-2.2.0 pandas-1.0.5 plac-1.1.3 preshed-3.0.2 redis-3.5.3 scikit-image-0.17.2 scikit-learn-0.23.1 seldon-core-1.2.1 sklearn-0.0 soupsieve-2.0.1 spacy-2.3.2 srsly-1.0.2 thinc-7.4.1 threadloop-1.0.2 threadpoolctl-2.1.0 thrift-0.13.0 tifffile-2020.7.22 tqdm-4.48.0 wasabi-0.7.1\n\u001b[33mWARNING: You are using pip version 20.0.2; however, version 20.1.1 is available.\nYou should consider upgrading via the '/usr/bin/python3 -m pip install --upgrade pip' command.\u001b[0m\n"
]
],
[
[
"## Restart Notebook kernel",
"_____no_output_____"
]
],
[
[
"from IPython.display import display_html\ndisplay_html(\"<script>Jupyter.notebook.kernel.restart()</script>\",raw=True)",
"_____no_output_____"
]
],
[
[
"## Import Libraries",
"_____no_output_____"
]
],
[
[
"from __future__ import division\nfrom __future__ import print_function\n\nimport tensorflow as tf\nimport pandas as pd\nimport numpy as np\nimport shutil\nimport yaml\nimport random\nimport re\nimport os\nimport dill\nimport logging\nimport requests\nimport json\nfrom time import sleep\nfrom sklearn.preprocessing import OneHotEncoder\nfrom alibi.explainers import AnchorTabular\n\nfrom kubernetes import client as k8s_client\nfrom kubernetes import config as k8s_config\nfrom kubernetes.client.rest import ApiException\n\nk8s_config.load_incluster_config()\napi_client = k8s_client.CoreV1Api()\ncustom_api=k8s_client.CustomObjectsApi()",
"_____no_output_____"
]
],
[
[
"## Get Namespace\nGet current k8s namespace",
"_____no_output_____"
]
],
[
[
"def is_running_in_k8s():\n return os.path.isdir('/var/run/secrets/kubernetes.io/')\n\ndef get_current_k8s_namespace():\n with open('/var/run/secrets/kubernetes.io/serviceaccount/namespace', 'r') as f:\n return f.readline()\n\ndef get_default_target_namespace():\n if not is_running_in_k8s():\n return 'default'\n return get_current_k8s_namespace()\n\nnamespace = get_default_target_namespace()\nprint(namespace)",
"anonymous\n"
]
],
[
[
"## Check GPUs availability",
"_____no_output_____"
]
],
[
[
"gpus = len(tf.config.experimental.list_physical_devices('GPU'))\nif gpus == 0:\n print(\"Model will be trained using CPU\")\nelif gpus >= 0:\n print(\"Num GPUs Available: \", len(tf.config.experimental.list_physical_devices('GPU')))\n tf.config.experimental.list_physical_devices('GPU')\n print(\"Model will be trained using GPU\")",
"Model will be trained using CPU\n"
]
],
[
[
"## Declare Variables",
"_____no_output_____"
]
],
[
[
"path=\"cisco-kubeflow-starter-pack/apps/networking/ble-localization/onprem\"\nBLE_RSSI = pd.read_csv(os.path.join(path, \"data/iBeacon_RSSI_Labeled.csv\")) #Labeled dataset\n\n# Configure model options\nTF_DATA_DIR = os.getenv(\"TF_DATA_DIR\", \"/tmp/data/\")\nTF_MODEL_DIR = os.getenv(\"TF_MODEL_DIR\", \"blerssi/\")\nTF_EXPORT_DIR = os.getenv(\"TF_EXPORT_DIR\", \"blerssi/\")\nTF_MODEL_TYPE = os.getenv(\"TF_MODEL_TYPE\", \"DNN\")\nTF_TRAIN_STEPS = int(os.getenv(\"TF_TRAIN_STEPS\", 5000))\nTF_BATCH_SIZE = int(os.getenv(\"TF_BATCH_SIZE\", 128))\nTF_LEARNING_RATE = float(os.getenv(\"TF_LEARNING_RATE\", 0.001))\n\n\n# Feature columns\nCOLUMNS = list(BLE_RSSI.columns)\nFEATURES = COLUMNS[2:]\ndef make_feature_cols():\n input_columns = [tf.feature_column.numeric_column(k) for k in FEATURES]\n return input_columns",
"_____no_output_____"
]
],
[
[
"## BLERSSI Input Dataset\n### Attribute Information\nlocation: The location of receiving RSSIs from ibeacons b3001 to b3013; \n symbolic values showing the column and row of the location on the map (e.g., A01 stands for column A, row 1).\ndate: Datetime in the format of ‘d-m-yyyy hh:mm:ss’\nb3001 - b3013: RSSI readings corresponding to the iBeacons; numeric, integers only.",
"_____no_output_____"
]
],
[
[
"BLE_RSSI.head(10)",
"_____no_output_____"
]
],
[
[
"## Definition of Serving Input Receiver Function",
"_____no_output_____"
]
],
[
[
"feature_columns = make_feature_cols()\ninputs = {}\nfor feat in feature_columns:\n inputs[feat.name] = tf.placeholder(shape=[None], dtype=feat.dtype)\nserving_input_receiver_fn = tf.estimator.export.build_raw_serving_input_receiver_fn(inputs)",
"_____no_output_____"
]
],
[
[
"## Train and Save BLE RSSI Model",
"_____no_output_____"
]
],
[
[
"# Feature columns\nCOLUMNS = list(BLE_RSSI.columns)\nFEATURES = COLUMNS[2:]\nLABEL = [COLUMNS[0]]\n\nb3001 = tf.feature_column.numeric_column(key='b3001',dtype=tf.float64)\nb3002 = tf.feature_column.numeric_column(key='b3002',dtype=tf.float64)\nb3003 = tf.feature_column.numeric_column(key='b3003',dtype=tf.float64)\nb3004 = tf.feature_column.numeric_column(key='b3004',dtype=tf.float64)\nb3005 = tf.feature_column.numeric_column(key='b3005',dtype=tf.float64)\nb3006 = tf.feature_column.numeric_column(key='b3006',dtype=tf.float64)\nb3007 = tf.feature_column.numeric_column(key='b3007',dtype=tf.float64)\nb3008 = tf.feature_column.numeric_column(key='b3008',dtype=tf.float64)\nb3009 = tf.feature_column.numeric_column(key='b3009',dtype=tf.float64)\nb3010 = tf.feature_column.numeric_column(key='b3010',dtype=tf.float64)\nb3011 = tf.feature_column.numeric_column(key='b3011',dtype=tf.float64)\nb3012 = tf.feature_column.numeric_column(key='b3012',dtype=tf.float64)\nb3013 = tf.feature_column.numeric_column(key='b3013',dtype=tf.float64)\nfeature_columns = [b3001, b3002, b3003, b3004, b3005, b3006, b3007, b3008, b3009, b3010, b3011, b3012, b3013]\n\ndf_full = pd.read_csv(os.path.join(path, \"data/iBeacon_RSSI_Labeled.csv\")) #Labeled dataset\n\n# Input Data Preprocessing \ndf_full = df_full.drop(['date'],axis = 1)\ndf_full[FEATURES] = (df_full[FEATURES])/(-200)\n\n\n#Output Data Preprocessing\ndict = {'O02': 0,'P01': 1,'P02': 2,'R01': 3,'R02': 4,'S01': 5,'S02': 6,'T01': 7,'U02': 8,'U01': 9,'J03': 10,'K03': 11,'L03': 12,'M03': 13,'N03': 14,'O03': 15,'P03': 16,'Q03': 17,'R03': 18,'S03': 19,'T03': 20,'U03': 21,'U04': 22,'T04': 23,'S04': 24,'R04': 25,'Q04': 26,'P04': 27,'O04': 28,'N04': 29,'M04': 30,'L04': 31,'K04': 32,'J04': 33,'I04': 34,'I05': 35,'J05': 36,'K05': 37,'L05': 38,'M05': 39,'N05': 40,'O05': 41,'P05': 42,'Q05': 43,'R05': 44,'S05': 45,'T05': 46,'U05': 47,'S06': 48,'R06': 49,'Q06': 50,'P06': 51,'O06': 52,'N06': 53,'M06': 54,'L06': 55,'K06': 56,'J06': 57,'I06': 58,'F08': 59,'J02': 60,'J07': 61,'I07': 62,'I10': 63,'J10': 64,'D15': 65,'E15': 66,'G15': 67,'J15': 68,'L15': 69,'R15': 70,'T15': 71,'W15': 72,'I08': 73,'I03': 74,'J08': 75,'I01': 76,'I02': 77,'J01': 78,'K01': 79,'K02': 80,'L01': 81,'L02': 82,'M01': 83,'M02': 84,'N01': 85,'N02': 86,'O01': 87,'I09': 88,'D14': 89,'D13': 90,'K07': 91,'K08': 92,'N15': 93,'P15': 94,'I15': 95,'S15': 96,'U15': 97,'V15': 98,'S07': 99,'S08': 100,'L09': 101,'L08': 102,'Q02': 103,'Q01': 104}\ndf_full['location'] = df_full['location'].map(dict)\ndf_train=df_full.sample(frac=0.8,random_state=200)\ndf_valid=df_full.drop(df_train.index)\n\nlocation_counts = BLE_RSSI.location.value_counts()\nx1 = np.asarray(df_train[FEATURES])\ny1 = np.asarray(df_train['location'])\n\nx2 = np.asarray(df_valid[FEATURES])\ny2 = np.asarray(df_valid['location'])\n\ndef formatFeatures(features):\n formattedFeatures = {}\n numColumns = features.shape[1]\n\n for i in range(0, numColumns):\n formattedFeatures[\"b\"+str(3001+i)] = features[:, i]\n\n return formattedFeatures\n\ntrainingFeatures = formatFeatures(x1)\ntrainingCategories = y1\n\ntestFeatures = formatFeatures(x2)\ntestCategories = y2\n\n# Train Input Function\ndef train_input_fn():\n dataset = tf.data.Dataset.from_tensor_slices((trainingFeatures, y1))\n dataset = dataset.repeat(1000).batch(TF_BATCH_SIZE)\n return dataset\n\n# Test Input Function\ndef eval_input_fn():\n dataset = tf.data.Dataset.from_tensor_slices((testFeatures, y2))\n return dataset.repeat(1000).batch(TF_BATCH_SIZE)\n\n# Provide list of GPUs should be used to train the model\n\ndistribution=tf.distribute.experimental.ParameterServerStrategy()\nprint('Number of devices: {}'.format(distribution.num_replicas_in_sync))\n\n# Configuration of training model\n\nconfig = tf.estimator.RunConfig(train_distribute=distribution, model_dir=TF_MODEL_DIR, save_summary_steps=100, save_checkpoints_steps=100)\n\n# Build 3 layer DNN classifier\n\nmodel = tf.estimator.DNNClassifier(hidden_units = [13,65,110],\n feature_columns = feature_columns,\n model_dir = TF_MODEL_DIR,\n n_classes=105, config=config\n )\n\nexport_final = tf.estimator.FinalExporter(TF_EXPORT_DIR, serving_input_receiver_fn=serving_input_receiver_fn)\n\ntrain_spec = tf.estimator.TrainSpec(input_fn=train_input_fn, \n max_steps=TF_TRAIN_STEPS)\n\neval_spec = tf.estimator.EvalSpec(input_fn=eval_input_fn,\n steps=100,\n exporters=export_final,\n throttle_secs=1,\n start_delay_secs=1)\n\n# Train and Evaluate the model\n\ntf.estimator.train_and_evaluate(model, train_spec, eval_spec)",
"INFO:tensorflow:ParameterServerStrategy with compute_devices = ('/device:CPU:0',), variable_device = '/device:CPU:0'\nNumber of devices: 1\nINFO:tensorflow:Initializing RunConfig with distribution strategies.\nINFO:tensorflow:Not using Distribute Coordinator.\nINFO:tensorflow:Using config: {'_model_dir': 'blerssi/', '_tf_random_seed': None, '_save_summary_steps': 100, '_save_checkpoints_steps': 100, '_save_checkpoints_secs': None, '_session_config': allow_soft_placement: true\ngraph_options {\n rewrite_options {\n meta_optimizer_iterations: ONE\n }\n}\n, '_keep_checkpoint_max': 5, '_keep_checkpoint_every_n_hours': 10000, '_log_step_count_steps': 100, '_train_distribute': <tensorflow.python.distribute.parameter_server_strategy.ParameterServerStrategyV1 object at 0x7f93ec2959b0>, '_device_fn': None, '_protocol': None, '_eval_distribute': None, '_experimental_distribute': None, '_experimental_max_worker_delay_secs': None, '_session_creation_timeout_secs': 7200, '_service': None, '_cluster_spec': <tensorflow.python.training.server_lib.ClusterSpec object at 0x7f93ec295c50>, '_task_type': 'worker', '_task_id': 0, '_global_id_in_cluster': 0, '_master': '', '_evaluation_master': '', '_is_chief': True, '_num_ps_replicas': 0, '_num_worker_replicas': 1, '_distribute_coordinator_mode': None}\nINFO:tensorflow:Not using Distribute Coordinator.\nINFO:tensorflow:Running training and evaluation locally (non-distributed).\nINFO:tensorflow:Start train and evaluate loop. The evaluate will happen after every checkpoint. Checkpoint frequency is determined based on RunConfig arguments: save_checkpoints_steps 100 or save_checkpoints_secs None.\nWARNING:tensorflow:From /usr/local/lib/python3.6/dist-packages/tensorflow_core/python/ops/resource_variable_ops.py:1630: calling BaseResourceVariable.__init__ (from tensorflow.python.ops.resource_variable_ops) with constraint is deprecated and will be removed in a future version.\nInstructions for updating:\nIf using Keras pass *_constraint arguments to layers.\nINFO:tensorflow:Calling model_fn.\nWARNING:tensorflow:From /usr/local/lib/python3.6/dist-packages/tensorflow_estimator/python/estimator/canned/head.py:437: to_float (from tensorflow.python.ops.math_ops) is deprecated and will be removed in a future version.\nInstructions for updating:\nUse `tf.cast` instead.\nWARNING:tensorflow:From /usr/local/lib/python3.6/dist-packages/tensorflow_core/python/training/adagrad.py:76: calling Constant.__init__ (from tensorflow.python.ops.init_ops) with dtype is deprecated and will be removed in a future version.\nInstructions for updating:\nCall initializer instance with the dtype argument instead of passing it to the constructor\nINFO:tensorflow:Done calling model_fn.\nWARNING:tensorflow:From /usr/local/lib/python3.6/dist-packages/tensorflow_core/python/ops/array_ops.py:1475: where (from tensorflow.python.ops.array_ops) is deprecated and will be removed in a future version.\nInstructions for updating:\nUse tf.where in 2.0, which has the same broadcast rule as np.where\nINFO:tensorflow:Create CheckpointSaverHook.\nINFO:tensorflow:Graph was finalized.\nINFO:tensorflow:Running local_init_op.\nINFO:tensorflow:Done running local_init_op.\nINFO:tensorflow:Saving checkpoints for 0 into blerssi/model.ckpt.\nINFO:tensorflow:loss = 594.5514, step = 0\nINFO:tensorflow:Saving checkpoints for 100 into blerssi/model.ckpt.\nINFO:tensorflow:Calling model_fn.\nINFO:tensorflow:Done calling model_fn.\nINFO:tensorflow:Starting evaluation at 2020-07-27T12:04:16Z\nINFO:tensorflow:Graph was finalized.\nINFO:tensorflow:Restoring parameters from blerssi/model.ckpt-100\nINFO:tensorflow:Running local_init_op.\nINFO:tensorflow:Done running local_init_op.\nINFO:tensorflow:Evaluation [10/100]\nINFO:tensorflow:Evaluation [20/100]\nINFO:tensorflow:Evaluation [30/100]\nINFO:tensorflow:Evaluation [40/100]\nINFO:tensorflow:Evaluation [50/100]\nINFO:tensorflow:Evaluation [60/100]\nINFO:tensorflow:Evaluation [70/100]\nINFO:tensorflow:Evaluation [80/100]\nINFO:tensorflow:Evaluation [90/100]\nINFO:tensorflow:Evaluation [100/100]\nINFO:tensorflow:Finished evaluation at 2020-07-27-12:04:16\nINFO:tensorflow:Saving dict for global step 100: accuracy = 0.1478125, average_loss = 3.0438955, global_step = 100, loss = 389.61862\nINFO:tensorflow:Saving 'checkpoint_path' summary for global step 100: blerssi/model.ckpt-100\nINFO:tensorflow:global_step/sec: 74.5088\nINFO:tensorflow:loss = 371.56686, step = 100 (1.342 sec)\nINFO:tensorflow:Saving checkpoints for 200 into blerssi/model.ckpt.\nINFO:tensorflow:Calling model_fn.\nINFO:tensorflow:Done calling model_fn.\nINFO:tensorflow:Starting evaluation at 2020-07-27T12:04:17Z\nINFO:tensorflow:Graph was finalized.\nINFO:tensorflow:Restoring parameters from blerssi/model.ckpt-200\nINFO:tensorflow:Running local_init_op.\nINFO:tensorflow:Done running local_init_op.\nINFO:tensorflow:Evaluation [10/100]\nINFO:tensorflow:Evaluation [20/100]\nINFO:tensorflow:Evaluation [30/100]\nINFO:tensorflow:Evaluation [40/100]\nINFO:tensorflow:Evaluation [50/100]\nINFO:tensorflow:Evaluation [60/100]\nINFO:tensorflow:Evaluation [70/100]\nINFO:tensorflow:Evaluation [80/100]\nINFO:tensorflow:Evaluation [90/100]\nINFO:tensorflow:Evaluation [100/100]\nINFO:tensorflow:Finished evaluation at 2020-07-27-12:04:17\nINFO:tensorflow:Saving dict for global step 200: accuracy = 0.16195312, average_loss = 2.8027809, global_step = 200, loss = 358.75595\nINFO:tensorflow:Saving 'checkpoint_path' summary for global step 200: blerssi/model.ckpt-200\nINFO:tensorflow:global_step/sec: 79.6341\nINFO:tensorflow:loss = 342.82297, step = 200 (1.257 sec)\nINFO:tensorflow:Saving checkpoints for 300 into blerssi/model.ckpt.\nINFO:tensorflow:Calling model_fn.\nINFO:tensorflow:Done calling model_fn.\nINFO:tensorflow:Starting evaluation at 2020-07-27T12:04:18Z\nINFO:tensorflow:Graph was finalized.\nINFO:tensorflow:Restoring parameters from blerssi/model.ckpt-300\nINFO:tensorflow:Running local_init_op.\nINFO:tensorflow:Done running local_init_op.\nINFO:tensorflow:Evaluation [10/100]\nINFO:tensorflow:Evaluation [20/100]\nINFO:tensorflow:Evaluation [30/100]\nINFO:tensorflow:Evaluation [40/100]\nINFO:tensorflow:Evaluation [50/100]\nINFO:tensorflow:Evaluation [60/100]\nINFO:tensorflow:Evaluation [70/100]\nINFO:tensorflow:Evaluation [80/100]\nINFO:tensorflow:Evaluation [90/100]\nINFO:tensorflow:Evaluation [100/100]\nINFO:tensorflow:Finished evaluation at 2020-07-27-12:04:19\nINFO:tensorflow:Saving dict for global step 300: accuracy = 0.14773437, average_loss = 2.9204443, global_step = 300, loss = 373.81686\nINFO:tensorflow:Saving 'checkpoint_path' summary for global step 300: blerssi/model.ckpt-300\nINFO:tensorflow:global_step/sec: 82.1163\nINFO:tensorflow:loss = 350.08023, step = 300 (1.217 sec)\nINFO:tensorflow:Saving checkpoints for 400 into blerssi/model.ckpt.\nINFO:tensorflow:Calling model_fn.\nINFO:tensorflow:Done calling model_fn.\nINFO:tensorflow:Starting evaluation at 2020-07-27T12:04:19Z\nINFO:tensorflow:Graph was finalized.\nINFO:tensorflow:Restoring parameters from blerssi/model.ckpt-400\nINFO:tensorflow:Running local_init_op.\nINFO:tensorflow:Done running local_init_op.\nINFO:tensorflow:Evaluation [10/100]\nINFO:tensorflow:Evaluation [20/100]\nINFO:tensorflow:Evaluation [30/100]\nINFO:tensorflow:Evaluation [40/100]\nINFO:tensorflow:Evaluation [50/100]\nINFO:tensorflow:Evaluation [60/100]\nINFO:tensorflow:Evaluation [70/100]\nINFO:tensorflow:Evaluation [80/100]\nINFO:tensorflow:Evaluation [90/100]\nINFO:tensorflow:Evaluation [100/100]\nINFO:tensorflow:Finished evaluation at 2020-07-27-12:04:20\nINFO:tensorflow:Saving dict for global step 400: accuracy = 0.17234375, average_loss = 2.7928438, global_step = 400, loss = 357.484\nINFO:tensorflow:Saving 'checkpoint_path' summary for global step 400: blerssi/model.ckpt-400\nINFO:tensorflow:global_step/sec: 81.5196\nINFO:tensorflow:loss = 330.38446, step = 400 (1.226 sec)\nINFO:tensorflow:Saving checkpoints for 500 into blerssi/model.ckpt.\nWARNING:tensorflow:From /usr/local/lib/python3.6/dist-packages/tensorflow_core/python/training/saver.py:963: remove_checkpoint (from tensorflow.python.training.checkpoint_management) is deprecated and will be removed in a future version.\nInstructions for updating:\nUse standard file APIs to delete files with this prefix.\nINFO:tensorflow:Calling model_fn.\nINFO:tensorflow:Done calling model_fn.\nINFO:tensorflow:Starting evaluation at 2020-07-27T12:04:20Z\nINFO:tensorflow:Graph was finalized.\nINFO:tensorflow:Restoring parameters from blerssi/model.ckpt-500\nINFO:tensorflow:Running local_init_op.\nINFO:tensorflow:Done running local_init_op.\nINFO:tensorflow:Evaluation [10/100]\nINFO:tensorflow:Evaluation [20/100]\nINFO:tensorflow:Evaluation [30/100]\nINFO:tensorflow:Evaluation [40/100]\nINFO:tensorflow:Evaluation [50/100]\nINFO:tensorflow:Evaluation [60/100]\nINFO:tensorflow:Evaluation [70/100]\nINFO:tensorflow:Evaluation [80/100]\nINFO:tensorflow:Evaluation [90/100]\nINFO:tensorflow:Evaluation [100/100]\nINFO:tensorflow:Finished evaluation at 2020-07-27-12:04:21\nINFO:tensorflow:Saving dict for global step 500: accuracy = 0.16554688, average_loss = 2.8326373, global_step = 500, loss = 362.57758\nINFO:tensorflow:Saving 'checkpoint_path' summary for global step 500: blerssi/model.ckpt-500\nINFO:tensorflow:global_step/sec: 72.9833\nINFO:tensorflow:loss = 309.4389, step = 500 (1.370 sec)\nINFO:tensorflow:Saving checkpoints for 600 into blerssi/model.ckpt.\nINFO:tensorflow:Calling model_fn.\nINFO:tensorflow:Done calling model_fn.\nINFO:tensorflow:Starting evaluation at 2020-07-27T12:04:22Z\nINFO:tensorflow:Graph was finalized.\nINFO:tensorflow:Restoring parameters from blerssi/model.ckpt-600\nINFO:tensorflow:Running local_init_op.\nINFO:tensorflow:Done running local_init_op.\nINFO:tensorflow:Evaluation [10/100]\nINFO:tensorflow:Evaluation [20/100]\nINFO:tensorflow:Evaluation [30/100]\nINFO:tensorflow:Evaluation [40/100]\nINFO:tensorflow:Evaluation [50/100]\nINFO:tensorflow:Evaluation [60/100]\nINFO:tensorflow:Evaluation [70/100]\nINFO:tensorflow:Evaluation [80/100]\nINFO:tensorflow:Evaluation [90/100]\nINFO:tensorflow:Evaluation [100/100]\nINFO:tensorflow:Finished evaluation at 2020-07-27-12:04:22\nINFO:tensorflow:Saving dict for global step 600: accuracy = 0.16882813, average_loss = 2.8005483, global_step = 600, loss = 358.47018\nINFO:tensorflow:Saving 'checkpoint_path' summary for global step 600: blerssi/model.ckpt-600\nINFO:tensorflow:global_step/sec: 82.3567\nINFO:tensorflow:loss = 317.98203, step = 600 (1.214 sec)\nINFO:tensorflow:Saving checkpoints for 700 into blerssi/model.ckpt.\nINFO:tensorflow:Calling model_fn.\nINFO:tensorflow:Done calling model_fn.\nINFO:tensorflow:Starting evaluation at 2020-07-27T12:04:23Z\nINFO:tensorflow:Graph was finalized.\nINFO:tensorflow:Restoring parameters from blerssi/model.ckpt-700\nINFO:tensorflow:Running local_init_op.\nINFO:tensorflow:Done running local_init_op.\nINFO:tensorflow:Evaluation [10/100]\nINFO:tensorflow:Evaluation [20/100]\nINFO:tensorflow:Evaluation [30/100]\nINFO:tensorflow:Evaluation [40/100]\nINFO:tensorflow:Evaluation [50/100]\nINFO:tensorflow:Evaluation [60/100]\nINFO:tensorflow:Evaluation [70/100]\nINFO:tensorflow:Evaluation [80/100]\nINFO:tensorflow:Evaluation [90/100]\nINFO:tensorflow:Evaluation [100/100]\nINFO:tensorflow:Finished evaluation at 2020-07-27-12:04:24\nINFO:tensorflow:Saving dict for global step 700: accuracy = 0.18289062, average_loss = 2.8308408, global_step = 700, loss = 362.34763\nINFO:tensorflow:Saving 'checkpoint_path' summary for global step 700: blerssi/model.ckpt-700\nINFO:tensorflow:global_step/sec: 81.834\nINFO:tensorflow:loss = 326.9156, step = 700 (1.222 sec)\nINFO:tensorflow:Saving checkpoints for 800 into blerssi/model.ckpt.\nINFO:tensorflow:Calling model_fn.\nINFO:tensorflow:Done calling model_fn.\nINFO:tensorflow:Starting evaluation at 2020-07-27T12:04:24Z\nINFO:tensorflow:Graph was finalized.\nINFO:tensorflow:Restoring parameters from blerssi/model.ckpt-800\nINFO:tensorflow:Running local_init_op.\nINFO:tensorflow:Done running local_init_op.\nINFO:tensorflow:Evaluation [10/100]\nINFO:tensorflow:Evaluation [20/100]\nINFO:tensorflow:Evaluation [30/100]\nINFO:tensorflow:Evaluation [40/100]\nINFO:tensorflow:Evaluation [50/100]\nINFO:tensorflow:Evaluation [60/100]\nINFO:tensorflow:Evaluation [70/100]\nINFO:tensorflow:Evaluation [80/100]\nINFO:tensorflow:Evaluation [90/100]\nINFO:tensorflow:Evaluation [100/100]\nINFO:tensorflow:Finished evaluation at 2020-07-27-12:04:25\nINFO:tensorflow:Saving dict for global step 800: accuracy = 0.15484375, average_loss = 2.9795644, global_step = 800, loss = 381.38425\nINFO:tensorflow:Saving 'checkpoint_path' summary for global step 800: blerssi/model.ckpt-800\nINFO:tensorflow:global_step/sec: 82.8517\nINFO:tensorflow:loss = 326.4861, step = 800 (1.206 sec)\nINFO:tensorflow:Saving checkpoints for 900 into blerssi/model.ckpt.\nINFO:tensorflow:Calling model_fn.\nINFO:tensorflow:Done calling model_fn.\nINFO:tensorflow:Starting evaluation at 2020-07-27T12:04:25Z\nINFO:tensorflow:Graph was finalized.\nINFO:tensorflow:Restoring parameters from blerssi/model.ckpt-900\nINFO:tensorflow:Running local_init_op.\nINFO:tensorflow:Done running local_init_op.\nINFO:tensorflow:Evaluation [10/100]\nINFO:tensorflow:Evaluation [20/100]\nINFO:tensorflow:Evaluation [30/100]\nINFO:tensorflow:Evaluation [40/100]\nINFO:tensorflow:Evaluation [50/100]\nINFO:tensorflow:Evaluation [60/100]\nINFO:tensorflow:Evaluation [70/100]\nINFO:tensorflow:Evaluation [80/100]\nINFO:tensorflow:Evaluation [90/100]\nINFO:tensorflow:Evaluation [100/100]\nINFO:tensorflow:Finished evaluation at 2020-07-27-12:04:26\nINFO:tensorflow:Saving dict for global step 900: accuracy = 0.19359376, average_loss = 2.8420234, global_step = 900, loss = 363.779\nINFO:tensorflow:Saving 'checkpoint_path' summary for global step 900: blerssi/model.ckpt-900\nINFO:tensorflow:global_step/sec: 81.6693\nINFO:tensorflow:loss = 288.16187, step = 900 (1.227 sec)\nINFO:tensorflow:Saving checkpoints for 1000 into blerssi/model.ckpt.\nINFO:tensorflow:Calling model_fn.\nINFO:tensorflow:Done calling model_fn.\nINFO:tensorflow:Starting evaluation at 2020-07-27T12:04:27Z\nINFO:tensorflow:Graph was finalized.\nINFO:tensorflow:Restoring parameters from blerssi/model.ckpt-1000\nINFO:tensorflow:Running local_init_op.\nINFO:tensorflow:Done running local_init_op.\nINFO:tensorflow:Evaluation [10/100]\nINFO:tensorflow:Evaluation [20/100]\nINFO:tensorflow:Evaluation [30/100]\nINFO:tensorflow:Evaluation [40/100]\nINFO:tensorflow:Evaluation [50/100]\nINFO:tensorflow:Evaluation [60/100]\nINFO:tensorflow:Evaluation [70/100]\nINFO:tensorflow:Evaluation [80/100]\nINFO:tensorflow:Evaluation [90/100]\nINFO:tensorflow:Evaluation [100/100]\nINFO:tensorflow:Finished evaluation at 2020-07-27-12:04:27\nINFO:tensorflow:Saving dict for global step 1000: accuracy = 0.18296875, average_loss = 2.8350174, global_step = 1000, loss = 362.88223\nINFO:tensorflow:Saving 'checkpoint_path' summary for global step 1000: blerssi/model.ckpt-1000\nINFO:tensorflow:global_step/sec: 80.4219\nINFO:tensorflow:loss = 305.5712, step = 1000 (1.243 sec)\nINFO:tensorflow:Saving checkpoints for 1100 into blerssi/model.ckpt.\nINFO:tensorflow:Calling model_fn.\nINFO:tensorflow:Done calling model_fn.\nINFO:tensorflow:Starting evaluation at 2020-07-27T12:04:28Z\nINFO:tensorflow:Graph was finalized.\nINFO:tensorflow:Restoring parameters from blerssi/model.ckpt-1100\nINFO:tensorflow:Running local_init_op.\nINFO:tensorflow:Done running local_init_op.\nINFO:tensorflow:Evaluation [10/100]\nINFO:tensorflow:Evaluation [20/100]\nINFO:tensorflow:Evaluation [30/100]\nINFO:tensorflow:Evaluation [40/100]\nINFO:tensorflow:Evaluation [50/100]\nINFO:tensorflow:Evaluation [60/100]\nINFO:tensorflow:Evaluation [70/100]\nINFO:tensorflow:Evaluation [80/100]\nINFO:tensorflow:Evaluation [90/100]\nINFO:tensorflow:Evaluation [100/100]\nINFO:tensorflow:Finished evaluation at 2020-07-27-12:04:29\nINFO:tensorflow:Saving dict for global step 1100: accuracy = 0.18640625, average_loss = 2.840476, global_step = 1100, loss = 363.58093\nINFO:tensorflow:Saving 'checkpoint_path' summary for global step 1100: blerssi/model.ckpt-1100\nINFO:tensorflow:global_step/sec: 83.3038\nINFO:tensorflow:loss = 309.84857, step = 1100 (1.199 sec)\nINFO:tensorflow:Saving checkpoints for 1200 into blerssi/model.ckpt.\nINFO:tensorflow:Calling model_fn.\nINFO:tensorflow:Done calling model_fn.\nINFO:tensorflow:Starting evaluation at 2020-07-27T12:04:29Z\nINFO:tensorflow:Graph was finalized.\nINFO:tensorflow:Restoring parameters from blerssi/model.ckpt-1200\nINFO:tensorflow:Running local_init_op.\nINFO:tensorflow:Done running local_init_op.\nINFO:tensorflow:Evaluation [10/100]\nINFO:tensorflow:Evaluation [20/100]\nINFO:tensorflow:Evaluation [30/100]\nINFO:tensorflow:Evaluation [40/100]\nINFO:tensorflow:Evaluation [50/100]\nINFO:tensorflow:Evaluation [60/100]\nINFO:tensorflow:Evaluation [70/100]\nINFO:tensorflow:Evaluation [80/100]\nINFO:tensorflow:Evaluation [90/100]\nINFO:tensorflow:Evaluation [100/100]\nINFO:tensorflow:Finished evaluation at 2020-07-27-12:04:30\nINFO:tensorflow:Saving dict for global step 1200: accuracy = 0.20078126, average_loss = 2.8759577, global_step = 1200, loss = 368.1226\nINFO:tensorflow:Saving 'checkpoint_path' summary for global step 1200: blerssi/model.ckpt-1200\nINFO:tensorflow:global_step/sec: 80.6584\nINFO:tensorflow:loss = 304.45615, step = 1200 (1.241 sec)\nINFO:tensorflow:Saving checkpoints for 1300 into blerssi/model.ckpt.\nINFO:tensorflow:Calling model_fn.\nINFO:tensorflow:Done calling model_fn.\nINFO:tensorflow:Starting evaluation at 2020-07-27T12:04:30Z\nINFO:tensorflow:Graph was finalized.\nINFO:tensorflow:Restoring parameters from blerssi/model.ckpt-1300\nINFO:tensorflow:Running local_init_op.\nINFO:tensorflow:Done running local_init_op.\nINFO:tensorflow:Evaluation [10/100]\nINFO:tensorflow:Evaluation [20/100]\nINFO:tensorflow:Evaluation [30/100]\nINFO:tensorflow:Evaluation [40/100]\nINFO:tensorflow:Evaluation [50/100]\nINFO:tensorflow:Evaluation [60/100]\nINFO:tensorflow:Evaluation [70/100]\nINFO:tensorflow:Evaluation [80/100]\nINFO:tensorflow:Evaluation [90/100]\nINFO:tensorflow:Evaluation [100/100]\nINFO:tensorflow:Finished evaluation at 2020-07-27-12:04:31\nINFO:tensorflow:Saving dict for global step 1300: accuracy = 0.19710937, average_loss = 2.8712735, global_step = 1300, loss = 367.523\nINFO:tensorflow:Saving 'checkpoint_path' summary for global step 1300: blerssi/model.ckpt-1300\nINFO:tensorflow:global_step/sec: 68.2263\nINFO:tensorflow:loss = 305.92072, step = 1300 (1.466 sec)\nINFO:tensorflow:Saving checkpoints for 1400 into blerssi/model.ckpt.\nINFO:tensorflow:Calling model_fn.\nINFO:tensorflow:Done calling model_fn.\nINFO:tensorflow:Starting evaluation at 2020-07-27T12:04:32Z\nINFO:tensorflow:Graph was finalized.\nINFO:tensorflow:Restoring parameters from blerssi/model.ckpt-1400\nINFO:tensorflow:Running local_init_op.\nINFO:tensorflow:Done running local_init_op.\nINFO:tensorflow:Evaluation [10/100]\nINFO:tensorflow:Evaluation [20/100]\nINFO:tensorflow:Evaluation [30/100]\nINFO:tensorflow:Evaluation [40/100]\nINFO:tensorflow:Evaluation [50/100]\nINFO:tensorflow:Evaluation [60/100]\nINFO:tensorflow:Evaluation [70/100]\nINFO:tensorflow:Evaluation [80/100]\nINFO:tensorflow:Evaluation [90/100]\nINFO:tensorflow:Evaluation [100/100]\nINFO:tensorflow:Finished evaluation at 2020-07-27-12:04:32\nINFO:tensorflow:Saving dict for global step 1400: accuracy = 0.15476562, average_loss = 2.8461099, global_step = 1400, loss = 364.30206\nINFO:tensorflow:Saving 'checkpoint_path' summary for global step 1400: blerssi/model.ckpt-1400\nINFO:tensorflow:global_step/sec: 83.3139\nINFO:tensorflow:loss = 295.6084, step = 1400 (1.200 sec)\nINFO:tensorflow:Saving checkpoints for 1500 into blerssi/model.ckpt.\nINFO:tensorflow:Calling model_fn.\nINFO:tensorflow:Done calling model_fn.\nINFO:tensorflow:Starting evaluation at 2020-07-27T12:04:33Z\nINFO:tensorflow:Graph was finalized.\nINFO:tensorflow:Restoring parameters from blerssi/model.ckpt-1500\nINFO:tensorflow:Running local_init_op.\nINFO:tensorflow:Done running local_init_op.\nINFO:tensorflow:Evaluation [10/100]\nINFO:tensorflow:Evaluation [20/100]\nINFO:tensorflow:Evaluation [30/100]\nINFO:tensorflow:Evaluation [40/100]\nINFO:tensorflow:Evaluation [50/100]\nINFO:tensorflow:Evaluation [60/100]\nINFO:tensorflow:Evaluation [70/100]\nINFO:tensorflow:Evaluation [80/100]\nINFO:tensorflow:Evaluation [90/100]\nINFO:tensorflow:Evaluation [100/100]\nINFO:tensorflow:Finished evaluation at 2020-07-27-12:04:34\nINFO:tensorflow:Saving dict for global step 1500: accuracy = 0.21453124, average_loss = 2.8982835, global_step = 1500, loss = 370.9803\nINFO:tensorflow:Saving 'checkpoint_path' summary for global step 1500: blerssi/model.ckpt-1500\nINFO:tensorflow:global_step/sec: 81.5076\nINFO:tensorflow:loss = 307.38174, step = 1500 (1.227 sec)\nINFO:tensorflow:Saving checkpoints for 1600 into blerssi/model.ckpt.\nINFO:tensorflow:Calling model_fn.\nINFO:tensorflow:Done calling model_fn.\nINFO:tensorflow:Starting evaluation at 2020-07-27T12:04:34Z\nINFO:tensorflow:Graph was finalized.\nINFO:tensorflow:Restoring parameters from blerssi/model.ckpt-1600\nINFO:tensorflow:Running local_init_op.\nINFO:tensorflow:Done running local_init_op.\nINFO:tensorflow:Evaluation [10/100]\nINFO:tensorflow:Evaluation [20/100]\nINFO:tensorflow:Evaluation [30/100]\nINFO:tensorflow:Evaluation [40/100]\nINFO:tensorflow:Evaluation [50/100]\nINFO:tensorflow:Evaluation [60/100]\nINFO:tensorflow:Evaluation [70/100]\nINFO:tensorflow:Evaluation [80/100]\nINFO:tensorflow:Evaluation [90/100]\nINFO:tensorflow:Evaluation [100/100]\nINFO:tensorflow:Finished evaluation at 2020-07-27-12:04:35\nINFO:tensorflow:Saving dict for global step 1600: accuracy = 0.200625, average_loss = 2.9850085, global_step = 1600, loss = 382.0811\nINFO:tensorflow:Saving 'checkpoint_path' summary for global step 1600: blerssi/model.ckpt-1600\nINFO:tensorflow:global_step/sec: 80.8971\nINFO:tensorflow:loss = 290.9291, step = 1600 (1.236 sec)\nINFO:tensorflow:Saving checkpoints for 1700 into blerssi/model.ckpt.\nINFO:tensorflow:Calling model_fn.\nINFO:tensorflow:Done calling model_fn.\nINFO:tensorflow:Starting evaluation at 2020-07-27T12:04:36Z\nINFO:tensorflow:Graph was finalized.\nINFO:tensorflow:Restoring parameters from blerssi/model.ckpt-1700\nINFO:tensorflow:Running local_init_op.\nINFO:tensorflow:Done running local_init_op.\nINFO:tensorflow:Evaluation [10/100]\nINFO:tensorflow:Evaluation [20/100]\nINFO:tensorflow:Evaluation [30/100]\nINFO:tensorflow:Evaluation [40/100]\nINFO:tensorflow:Evaluation [50/100]\nINFO:tensorflow:Evaluation [60/100]\nINFO:tensorflow:Evaluation [70/100]\nINFO:tensorflow:Evaluation [80/100]\nINFO:tensorflow:Evaluation [90/100]\nINFO:tensorflow:Evaluation [100/100]\nINFO:tensorflow:Finished evaluation at 2020-07-27-12:04:36\nINFO:tensorflow:Saving dict for global step 1700: accuracy = 0.19359376, average_loss = 2.8472593, global_step = 1700, loss = 364.4492\nINFO:tensorflow:Saving 'checkpoint_path' summary for global step 1700: blerssi/model.ckpt-1700\nINFO:tensorflow:global_step/sec: 77.9863\nINFO:tensorflow:loss = 302.74707, step = 1700 (1.282 sec)\nINFO:tensorflow:Saving checkpoints for 1800 into blerssi/model.ckpt.\nINFO:tensorflow:Calling model_fn.\nINFO:tensorflow:Done calling model_fn.\nINFO:tensorflow:Starting evaluation at 2020-07-27T12:04:37Z\nINFO:tensorflow:Graph was finalized.\nINFO:tensorflow:Restoring parameters from blerssi/model.ckpt-1800\nINFO:tensorflow:Running local_init_op.\nINFO:tensorflow:Done running local_init_op.\nINFO:tensorflow:Evaluation [10/100]\nINFO:tensorflow:Evaluation [20/100]\nINFO:tensorflow:Evaluation [30/100]\nINFO:tensorflow:Evaluation [40/100]\nINFO:tensorflow:Evaluation [50/100]\nINFO:tensorflow:Evaluation [60/100]\nINFO:tensorflow:Evaluation [70/100]\nINFO:tensorflow:Evaluation [80/100]\nINFO:tensorflow:Evaluation [90/100]\nINFO:tensorflow:Evaluation [100/100]\nINFO:tensorflow:Finished evaluation at 2020-07-27-12:04:37\nINFO:tensorflow:Saving dict for global step 1800: accuracy = 0.18640625, average_loss = 2.8873806, global_step = 1800, loss = 369.58472\nINFO:tensorflow:Saving 'checkpoint_path' summary for global step 1800: blerssi/model.ckpt-1800\nINFO:tensorflow:global_step/sec: 81.522\nINFO:tensorflow:loss = 311.20178, step = 1800 (1.227 sec)\nINFO:tensorflow:Saving checkpoints for 1900 into blerssi/model.ckpt.\nINFO:tensorflow:Calling model_fn.\nINFO:tensorflow:Done calling model_fn.\nINFO:tensorflow:Starting evaluation at 2020-07-27T12:04:38Z\nINFO:tensorflow:Graph was finalized.\nINFO:tensorflow:Restoring parameters from blerssi/model.ckpt-1900\nINFO:tensorflow:Running local_init_op.\nINFO:tensorflow:Done running local_init_op.\nINFO:tensorflow:Evaluation [10/100]\nINFO:tensorflow:Evaluation [20/100]\nINFO:tensorflow:Evaluation [30/100]\nINFO:tensorflow:Evaluation [40/100]\nINFO:tensorflow:Evaluation [50/100]\nINFO:tensorflow:Evaluation [60/100]\nINFO:tensorflow:Evaluation [70/100]\nINFO:tensorflow:Evaluation [80/100]\nINFO:tensorflow:Evaluation [90/100]\nINFO:tensorflow:Evaluation [100/100]\nINFO:tensorflow:Finished evaluation at 2020-07-27-12:04:39\nINFO:tensorflow:Saving dict for global step 1900: accuracy = 0.1934375, average_loss = 2.8413296, global_step = 1900, loss = 363.6902\nINFO:tensorflow:Saving 'checkpoint_path' summary for global step 1900: blerssi/model.ckpt-1900\nINFO:tensorflow:global_step/sec: 82.8489\nINFO:tensorflow:loss = 294.47797, step = 1900 (1.206 sec)\nINFO:tensorflow:Saving checkpoints for 2000 into blerssi/model.ckpt.\nINFO:tensorflow:Calling model_fn.\nINFO:tensorflow:Done calling model_fn.\nINFO:tensorflow:Starting evaluation at 2020-07-27T12:04:39Z\nINFO:tensorflow:Graph was finalized.\nINFO:tensorflow:Restoring parameters from blerssi/model.ckpt-2000\nINFO:tensorflow:Running local_init_op.\nINFO:tensorflow:Done running local_init_op.\nINFO:tensorflow:Evaluation [10/100]\nINFO:tensorflow:Evaluation [20/100]\nINFO:tensorflow:Evaluation [30/100]\nINFO:tensorflow:Evaluation [40/100]\nINFO:tensorflow:Evaluation [50/100]\nINFO:tensorflow:Evaluation [60/100]\nINFO:tensorflow:Evaluation [70/100]\nINFO:tensorflow:Evaluation [80/100]\nINFO:tensorflow:Evaluation [90/100]\nINFO:tensorflow:Evaluation [100/100]\nINFO:tensorflow:Finished evaluation at 2020-07-27-12:04:40\nINFO:tensorflow:Saving dict for global step 2000: accuracy = 0.21117188, average_loss = 2.896705, global_step = 2000, loss = 370.77823\nINFO:tensorflow:Saving 'checkpoint_path' summary for global step 2000: blerssi/model.ckpt-2000\nINFO:tensorflow:global_step/sec: 81.545\nINFO:tensorflow:loss = 300.31937, step = 2000 (1.226 sec)\nINFO:tensorflow:Saving checkpoints for 2100 into blerssi/model.ckpt.\nINFO:tensorflow:Calling model_fn.\nINFO:tensorflow:Done calling model_fn.\nINFO:tensorflow:Starting evaluation at 2020-07-27T12:04:40Z\nINFO:tensorflow:Graph was finalized.\nINFO:tensorflow:Restoring parameters from blerssi/model.ckpt-2100\nINFO:tensorflow:Running local_init_op.\nINFO:tensorflow:Done running local_init_op.\nINFO:tensorflow:Evaluation [10/100]\nINFO:tensorflow:Evaluation [20/100]\nINFO:tensorflow:Evaluation [30/100]\nINFO:tensorflow:Evaluation [40/100]\nINFO:tensorflow:Evaluation [50/100]\nINFO:tensorflow:Evaluation [60/100]\nINFO:tensorflow:Evaluation [70/100]\nINFO:tensorflow:Evaluation [80/100]\nINFO:tensorflow:Evaluation [90/100]\nINFO:tensorflow:Evaluation [100/100]\nINFO:tensorflow:Finished evaluation at 2020-07-27-12:04:41\nINFO:tensorflow:Saving dict for global step 2100: accuracy = 0.21125, average_loss = 2.9106176, global_step = 2100, loss = 372.55905\nINFO:tensorflow:Saving 'checkpoint_path' summary for global step 2100: blerssi/model.ckpt-2100\nINFO:tensorflow:global_step/sec: 72.1539\nINFO:tensorflow:loss = 285.34515, step = 2100 (1.387 sec)\nINFO:tensorflow:Saving checkpoints for 2200 into blerssi/model.ckpt.\nINFO:tensorflow:Calling model_fn.\nINFO:tensorflow:Done calling model_fn.\nINFO:tensorflow:Starting evaluation at 2020-07-27T12:04:42Z\nINFO:tensorflow:Graph was finalized.\nINFO:tensorflow:Restoring parameters from blerssi/model.ckpt-2200\nINFO:tensorflow:Running local_init_op.\nINFO:tensorflow:Done running local_init_op.\nINFO:tensorflow:Evaluation [10/100]\nINFO:tensorflow:Evaluation [20/100]\nINFO:tensorflow:Evaluation [30/100]\nINFO:tensorflow:Evaluation [40/100]\nINFO:tensorflow:Evaluation [50/100]\nINFO:tensorflow:Evaluation [60/100]\nINFO:tensorflow:Evaluation [70/100]\nINFO:tensorflow:Evaluation [80/100]\nINFO:tensorflow:Evaluation [90/100]\nINFO:tensorflow:Evaluation [100/100]\nINFO:tensorflow:Finished evaluation at 2020-07-27-12:04:42\nINFO:tensorflow:Saving dict for global step 2200: accuracy = 0.17585938, average_loss = 2.9142356, global_step = 2200, loss = 373.02216\nINFO:tensorflow:Saving 'checkpoint_path' summary for global step 2200: blerssi/model.ckpt-2200\nINFO:tensorflow:global_step/sec: 82.1222\nINFO:tensorflow:loss = 301.6997, step = 2200 (1.217 sec)\nINFO:tensorflow:Saving checkpoints for 2300 into blerssi/model.ckpt.\nINFO:tensorflow:Calling model_fn.\nINFO:tensorflow:Done calling model_fn.\nINFO:tensorflow:Starting evaluation at 2020-07-27T12:04:43Z\nINFO:tensorflow:Graph was finalized.\nINFO:tensorflow:Restoring parameters from blerssi/model.ckpt-2300\nINFO:tensorflow:Running local_init_op.\nINFO:tensorflow:Done running local_init_op.\nINFO:tensorflow:Evaluation [10/100]\nINFO:tensorflow:Evaluation [20/100]\nINFO:tensorflow:Evaluation [30/100]\nINFO:tensorflow:Evaluation [40/100]\nINFO:tensorflow:Evaluation [50/100]\nINFO:tensorflow:Evaluation [60/100]\nINFO:tensorflow:Evaluation [70/100]\nINFO:tensorflow:Evaluation [80/100]\nINFO:tensorflow:Evaluation [90/100]\nINFO:tensorflow:Evaluation [100/100]\nINFO:tensorflow:Finished evaluation at 2020-07-27-12:04:44\nINFO:tensorflow:Saving dict for global step 2300: accuracy = 0.218125, average_loss = 2.878163, global_step = 2300, loss = 368.40488\nINFO:tensorflow:Saving 'checkpoint_path' summary for global step 2300: blerssi/model.ckpt-2300\nINFO:tensorflow:global_step/sec: 82.9431\nINFO:tensorflow:loss = 308.0114, step = 2300 (1.205 sec)\nINFO:tensorflow:Saving checkpoints for 2400 into blerssi/model.ckpt.\nINFO:tensorflow:Calling model_fn.\nINFO:tensorflow:Done calling model_fn.\nINFO:tensorflow:Starting evaluation at 2020-07-27T12:04:44Z\nINFO:tensorflow:Graph was finalized.\nINFO:tensorflow:Restoring parameters from blerssi/model.ckpt-2400\nINFO:tensorflow:Running local_init_op.\nINFO:tensorflow:Done running local_init_op.\nINFO:tensorflow:Evaluation [10/100]\nINFO:tensorflow:Evaluation [20/100]\nINFO:tensorflow:Evaluation [30/100]\nINFO:tensorflow:Evaluation [40/100]\nINFO:tensorflow:Evaluation [50/100]\nINFO:tensorflow:Evaluation [60/100]\nINFO:tensorflow:Evaluation [70/100]\nINFO:tensorflow:Evaluation [80/100]\nINFO:tensorflow:Evaluation [90/100]\nINFO:tensorflow:Evaluation [100/100]\nINFO:tensorflow:Finished evaluation at 2020-07-27-12:04:45\nINFO:tensorflow:Saving dict for global step 2400: accuracy = 0.21820313, average_loss = 2.900616, global_step = 2400, loss = 371.27884\nINFO:tensorflow:Saving 'checkpoint_path' summary for global step 2400: blerssi/model.ckpt-2400\nINFO:tensorflow:global_step/sec: 83.301\nINFO:tensorflow:loss = 288.26395, step = 2400 (1.201 sec)\nINFO:tensorflow:Saving checkpoints for 2500 into blerssi/model.ckpt.\nINFO:tensorflow:Calling model_fn.\nINFO:tensorflow:Done calling model_fn.\nINFO:tensorflow:Starting evaluation at 2020-07-27T12:04:45Z\nINFO:tensorflow:Graph was finalized.\nINFO:tensorflow:Restoring parameters from blerssi/model.ckpt-2500\nINFO:tensorflow:Running local_init_op.\nINFO:tensorflow:Done running local_init_op.\nINFO:tensorflow:Evaluation [10/100]\nINFO:tensorflow:Evaluation [20/100]\nINFO:tensorflow:Evaluation [30/100]\nINFO:tensorflow:Evaluation [40/100]\nINFO:tensorflow:Evaluation [50/100]\nINFO:tensorflow:Evaluation [60/100]\nINFO:tensorflow:Evaluation [70/100]\nINFO:tensorflow:Evaluation [80/100]\nINFO:tensorflow:Evaluation [90/100]\nINFO:tensorflow:Evaluation [100/100]\nINFO:tensorflow:Finished evaluation at 2020-07-27-12:04:46\nINFO:tensorflow:Saving dict for global step 2500: accuracy = 0.20414062, average_loss = 3.027789, global_step = 2500, loss = 387.557\nINFO:tensorflow:Saving 'checkpoint_path' summary for global step 2500: blerssi/model.ckpt-2500\nINFO:tensorflow:global_step/sec: 82.5175\nINFO:tensorflow:loss = 289.87027, step = 2500 (1.212 sec)\nINFO:tensorflow:Saving checkpoints for 2600 into blerssi/model.ckpt.\nINFO:tensorflow:Calling model_fn.\nINFO:tensorflow:Done calling model_fn.\nINFO:tensorflow:Starting evaluation at 2020-07-27T12:04:47Z\nINFO:tensorflow:Graph was finalized.\nINFO:tensorflow:Restoring parameters from blerssi/model.ckpt-2600\nINFO:tensorflow:Running local_init_op.\nINFO:tensorflow:Done running local_init_op.\nINFO:tensorflow:Evaluation [10/100]\nINFO:tensorflow:Evaluation [20/100]\nINFO:tensorflow:Evaluation [30/100]\nINFO:tensorflow:Evaluation [40/100]\nINFO:tensorflow:Evaluation [50/100]\nINFO:tensorflow:Evaluation [60/100]\nINFO:tensorflow:Evaluation [70/100]\nINFO:tensorflow:Evaluation [80/100]\nINFO:tensorflow:Evaluation [90/100]\nINFO:tensorflow:Evaluation [100/100]\nINFO:tensorflow:Finished evaluation at 2020-07-27-12:04:47\nINFO:tensorflow:Saving dict for global step 2600: accuracy = 0.19703124, average_loss = 2.8862774, global_step = 2600, loss = 369.4435\nINFO:tensorflow:Saving 'checkpoint_path' summary for global step 2600: blerssi/model.ckpt-2600\nINFO:tensorflow:global_step/sec: 85.1553\nINFO:tensorflow:loss = 304.54187, step = 2600 (1.175 sec)\nINFO:tensorflow:Saving checkpoints for 2700 into blerssi/model.ckpt.\nINFO:tensorflow:Calling model_fn.\nINFO:tensorflow:Done calling model_fn.\nINFO:tensorflow:Starting evaluation at 2020-07-27T12:04:48Z\nINFO:tensorflow:Graph was finalized.\nINFO:tensorflow:Restoring parameters from blerssi/model.ckpt-2700\nINFO:tensorflow:Running local_init_op.\nINFO:tensorflow:Done running local_init_op.\nINFO:tensorflow:Evaluation [10/100]\nINFO:tensorflow:Evaluation [20/100]\nINFO:tensorflow:Evaluation [30/100]\nINFO:tensorflow:Evaluation [40/100]\nINFO:tensorflow:Evaluation [50/100]\nINFO:tensorflow:Evaluation [60/100]\nINFO:tensorflow:Evaluation [70/100]\nINFO:tensorflow:Evaluation [80/100]\nINFO:tensorflow:Evaluation [90/100]\nINFO:tensorflow:Evaluation [100/100]\nINFO:tensorflow:Finished evaluation at 2020-07-27-12:04:48\nINFO:tensorflow:Saving dict for global step 2700: accuracy = 0.22179687, average_loss = 2.8361683, global_step = 2700, loss = 363.02954\nINFO:tensorflow:Saving 'checkpoint_path' summary for global step 2700: blerssi/model.ckpt-2700\nINFO:tensorflow:global_step/sec: 82.4599\nINFO:tensorflow:loss = 286.2304, step = 2700 (1.212 sec)\nINFO:tensorflow:Saving checkpoints for 2800 into blerssi/model.ckpt.\nINFO:tensorflow:Calling model_fn.\nINFO:tensorflow:Done calling model_fn.\nINFO:tensorflow:Starting evaluation at 2020-07-27T12:04:49Z\nINFO:tensorflow:Graph was finalized.\nINFO:tensorflow:Restoring parameters from blerssi/model.ckpt-2800\nINFO:tensorflow:Running local_init_op.\nINFO:tensorflow:Done running local_init_op.\nINFO:tensorflow:Evaluation [10/100]\nINFO:tensorflow:Evaluation [20/100]\nINFO:tensorflow:Evaluation [30/100]\nINFO:tensorflow:Evaluation [40/100]\nINFO:tensorflow:Evaluation [50/100]\nINFO:tensorflow:Evaluation [60/100]\nINFO:tensorflow:Evaluation [70/100]\nINFO:tensorflow:Evaluation [80/100]\nINFO:tensorflow:Evaluation [90/100]\nINFO:tensorflow:Evaluation [100/100]\nINFO:tensorflow:Finished evaluation at 2020-07-27-12:04:50\nINFO:tensorflow:Saving dict for global step 2800: accuracy = 0.22179687, average_loss = 2.822359, global_step = 2800, loss = 361.26196\nINFO:tensorflow:Saving 'checkpoint_path' summary for global step 2800: blerssi/model.ckpt-2800\nINFO:tensorflow:global_step/sec: 82.122\nINFO:tensorflow:loss = 292.93854, step = 2800 (1.218 sec)\nINFO:tensorflow:Saving checkpoints for 2900 into blerssi/model.ckpt.\nINFO:tensorflow:Calling model_fn.\nINFO:tensorflow:Done calling model_fn.\nINFO:tensorflow:Starting evaluation at 2020-07-27T12:04:51Z\nINFO:tensorflow:Graph was finalized.\nINFO:tensorflow:Restoring parameters from blerssi/model.ckpt-2900\nINFO:tensorflow:Running local_init_op.\nINFO:tensorflow:Done running local_init_op.\nINFO:tensorflow:Evaluation [10/100]\nINFO:tensorflow:Evaluation [20/100]\nINFO:tensorflow:Evaluation [30/100]\nINFO:tensorflow:Evaluation [40/100]\nINFO:tensorflow:Evaluation [50/100]\nINFO:tensorflow:Evaluation [60/100]\nINFO:tensorflow:Evaluation [70/100]\nINFO:tensorflow:Evaluation [80/100]\nINFO:tensorflow:Evaluation [90/100]\nINFO:tensorflow:Evaluation [100/100]\nINFO:tensorflow:Finished evaluation at 2020-07-27-12:04:51\nINFO:tensorflow:Saving dict for global step 2900: accuracy = 0.2075, average_loss = 2.9061038, global_step = 2900, loss = 371.9813\nINFO:tensorflow:Saving 'checkpoint_path' summary for global step 2900: blerssi/model.ckpt-2900\nINFO:tensorflow:global_step/sec: 70.3029\nINFO:tensorflow:loss = 291.2099, step = 2900 (1.422 sec)\nINFO:tensorflow:Saving checkpoints for 3000 into blerssi/model.ckpt.\nINFO:tensorflow:Calling model_fn.\nINFO:tensorflow:Done calling model_fn.\nINFO:tensorflow:Starting evaluation at 2020-07-27T12:04:52Z\nINFO:tensorflow:Graph was finalized.\nINFO:tensorflow:Restoring parameters from blerssi/model.ckpt-3000\nINFO:tensorflow:Running local_init_op.\nINFO:tensorflow:Done running local_init_op.\nINFO:tensorflow:Evaluation [10/100]\nINFO:tensorflow:Evaluation [20/100]\nINFO:tensorflow:Evaluation [30/100]\nINFO:tensorflow:Evaluation [40/100]\nINFO:tensorflow:Evaluation [50/100]\nINFO:tensorflow:Evaluation [60/100]\nINFO:tensorflow:Evaluation [70/100]\nINFO:tensorflow:Evaluation [80/100]\nINFO:tensorflow:Evaluation [90/100]\nINFO:tensorflow:Evaluation [100/100]\nINFO:tensorflow:Finished evaluation at 2020-07-27-12:04:52\nINFO:tensorflow:Saving dict for global step 3000: accuracy = 0.20398438, average_loss = 2.9259422, global_step = 3000, loss = 374.5206\nINFO:tensorflow:Saving 'checkpoint_path' summary for global step 3000: blerssi/model.ckpt-3000\nINFO:tensorflow:global_step/sec: 80.3511\nINFO:tensorflow:loss = 291.8711, step = 3000 (1.244 sec)\nINFO:tensorflow:Saving checkpoints for 3100 into blerssi/model.ckpt.\nINFO:tensorflow:Calling model_fn.\nINFO:tensorflow:Done calling model_fn.\nINFO:tensorflow:Starting evaluation at 2020-07-27T12:04:53Z\nINFO:tensorflow:Graph was finalized.\nINFO:tensorflow:Restoring parameters from blerssi/model.ckpt-3100\nINFO:tensorflow:Running local_init_op.\nINFO:tensorflow:Done running local_init_op.\nINFO:tensorflow:Evaluation [10/100]\nINFO:tensorflow:Evaluation [20/100]\nINFO:tensorflow:Evaluation [30/100]\nINFO:tensorflow:Evaluation [40/100]\nINFO:tensorflow:Evaluation [50/100]\nINFO:tensorflow:Evaluation [60/100]\nINFO:tensorflow:Evaluation [70/100]\nINFO:tensorflow:Evaluation [80/100]\nINFO:tensorflow:Evaluation [90/100]\nINFO:tensorflow:Evaluation [100/100]\nINFO:tensorflow:Finished evaluation at 2020-07-27-12:04:54\nINFO:tensorflow:Saving dict for global step 3100: accuracy = 0.22523437, average_loss = 2.8671799, global_step = 3100, loss = 366.99902\nINFO:tensorflow:Saving 'checkpoint_path' summary for global step 3100: blerssi/model.ckpt-3100\nINFO:tensorflow:global_step/sec: 82.5228\nINFO:tensorflow:loss = 270.925, step = 3100 (1.212 sec)\nINFO:tensorflow:Saving checkpoints for 3200 into blerssi/model.ckpt.\nINFO:tensorflow:Calling model_fn.\nINFO:tensorflow:Done calling model_fn.\nINFO:tensorflow:Starting evaluation at 2020-07-27T12:04:54Z\nINFO:tensorflow:Graph was finalized.\nINFO:tensorflow:Restoring parameters from blerssi/model.ckpt-3200\nINFO:tensorflow:Running local_init_op.\nINFO:tensorflow:Done running local_init_op.\nINFO:tensorflow:Evaluation [10/100]\nINFO:tensorflow:Evaluation [20/100]\nINFO:tensorflow:Evaluation [30/100]\nINFO:tensorflow:Evaluation [40/100]\nINFO:tensorflow:Evaluation [50/100]\nINFO:tensorflow:Evaluation [60/100]\nINFO:tensorflow:Evaluation [70/100]\nINFO:tensorflow:Evaluation [80/100]\nINFO:tensorflow:Evaluation [90/100]\nINFO:tensorflow:Evaluation [100/100]\nINFO:tensorflow:Finished evaluation at 2020-07-27-12:04:55\nINFO:tensorflow:Saving dict for global step 3200: accuracy = 0.22523437, average_loss = 2.894812, global_step = 3200, loss = 370.53595\nINFO:tensorflow:Saving 'checkpoint_path' summary for global step 3200: blerssi/model.ckpt-3200\nINFO:tensorflow:global_step/sec: 79.6329\nINFO:tensorflow:loss = 294.95923, step = 3200 (1.256 sec)\nINFO:tensorflow:Saving checkpoints for 3300 into blerssi/model.ckpt.\nINFO:tensorflow:Calling model_fn.\nINFO:tensorflow:Done calling model_fn.\nINFO:tensorflow:Starting evaluation at 2020-07-27T12:04:55Z\nINFO:tensorflow:Graph was finalized.\nINFO:tensorflow:Restoring parameters from blerssi/model.ckpt-3300\nINFO:tensorflow:Running local_init_op.\nINFO:tensorflow:Done running local_init_op.\nINFO:tensorflow:Evaluation [10/100]\nINFO:tensorflow:Evaluation [20/100]\nINFO:tensorflow:Evaluation [30/100]\nINFO:tensorflow:Evaluation [40/100]\nINFO:tensorflow:Evaluation [50/100]\nINFO:tensorflow:Evaluation [60/100]\nINFO:tensorflow:Evaluation [70/100]\nINFO:tensorflow:Evaluation [80/100]\nINFO:tensorflow:Evaluation [90/100]\nINFO:tensorflow:Evaluation [100/100]\nINFO:tensorflow:Finished evaluation at 2020-07-27-12:04:56\nINFO:tensorflow:Saving dict for global step 3300: accuracy = 0.2215625, average_loss = 2.9023647, global_step = 3300, loss = 371.5027\nINFO:tensorflow:Saving 'checkpoint_path' summary for global step 3300: blerssi/model.ckpt-3300\nINFO:tensorflow:global_step/sec: 82.8779\nINFO:tensorflow:loss = 299.6723, step = 3300 (1.207 sec)\nINFO:tensorflow:Saving checkpoints for 3400 into blerssi/model.ckpt.\nINFO:tensorflow:Calling model_fn.\nINFO:tensorflow:Done calling model_fn.\nINFO:tensorflow:Starting evaluation at 2020-07-27T12:04:57Z\nINFO:tensorflow:Graph was finalized.\nINFO:tensorflow:Restoring parameters from blerssi/model.ckpt-3400\nINFO:tensorflow:Running local_init_op.\nINFO:tensorflow:Done running local_init_op.\nINFO:tensorflow:Evaluation [10/100]\nINFO:tensorflow:Evaluation [20/100]\nINFO:tensorflow:Evaluation [30/100]\nINFO:tensorflow:Evaluation [40/100]\nINFO:tensorflow:Evaluation [50/100]\nINFO:tensorflow:Evaluation [60/100]\nINFO:tensorflow:Evaluation [70/100]\nINFO:tensorflow:Evaluation [80/100]\nINFO:tensorflow:Evaluation [90/100]\nINFO:tensorflow:Evaluation [100/100]\nINFO:tensorflow:Finished evaluation at 2020-07-27-12:04:57\nINFO:tensorflow:Saving dict for global step 3400: accuracy = 0.2075, average_loss = 2.8652325, global_step = 3400, loss = 366.74976\nINFO:tensorflow:Saving 'checkpoint_path' summary for global step 3400: blerssi/model.ckpt-3400\nINFO:tensorflow:global_step/sec: 85.4935\nINFO:tensorflow:loss = 278.42737, step = 3400 (1.170 sec)\nINFO:tensorflow:Saving checkpoints for 3500 into blerssi/model.ckpt.\nINFO:tensorflow:Calling model_fn.\nINFO:tensorflow:Done calling model_fn.\nINFO:tensorflow:Starting evaluation at 2020-07-27T12:04:58Z\nINFO:tensorflow:Graph was finalized.\nINFO:tensorflow:Restoring parameters from blerssi/model.ckpt-3500\nINFO:tensorflow:Running local_init_op.\nINFO:tensorflow:Done running local_init_op.\nINFO:tensorflow:Evaluation [10/100]\nINFO:tensorflow:Evaluation [20/100]\nINFO:tensorflow:Evaluation [30/100]\nINFO:tensorflow:Evaluation [40/100]\nINFO:tensorflow:Evaluation [50/100]\nINFO:tensorflow:Evaluation [60/100]\nINFO:tensorflow:Evaluation [70/100]\nINFO:tensorflow:Evaluation [80/100]\nINFO:tensorflow:Evaluation [90/100]\nINFO:tensorflow:Evaluation [100/100]\nINFO:tensorflow:Finished evaluation at 2020-07-27-12:04:58\nINFO:tensorflow:Saving dict for global step 3500: accuracy = 0.23226562, average_loss = 2.896808, global_step = 3500, loss = 370.7914\nINFO:tensorflow:Saving 'checkpoint_path' summary for global step 3500: blerssi/model.ckpt-3500\nINFO:tensorflow:global_step/sec: 83.4493\nINFO:tensorflow:loss = 278.02283, step = 3500 (1.198 sec)\nINFO:tensorflow:Saving checkpoints for 3600 into blerssi/model.ckpt.\nINFO:tensorflow:Calling model_fn.\nINFO:tensorflow:Done calling model_fn.\nINFO:tensorflow:Starting evaluation at 2020-07-27T12:04:59Z\nINFO:tensorflow:Graph was finalized.\nINFO:tensorflow:Restoring parameters from blerssi/model.ckpt-3600\nINFO:tensorflow:Running local_init_op.\nINFO:tensorflow:Done running local_init_op.\nINFO:tensorflow:Evaluation [10/100]\nINFO:tensorflow:Evaluation [20/100]\nINFO:tensorflow:Evaluation [30/100]\nINFO:tensorflow:Evaluation [40/100]\nINFO:tensorflow:Evaluation [50/100]\nINFO:tensorflow:Evaluation [60/100]\nINFO:tensorflow:Evaluation [70/100]\nINFO:tensorflow:Evaluation [80/100]\nINFO:tensorflow:Evaluation [90/100]\nINFO:tensorflow:Evaluation [100/100]\nINFO:tensorflow:Finished evaluation at 2020-07-27-12:05:00\nINFO:tensorflow:Saving dict for global step 3600: accuracy = 0.21460937, average_loss = 2.924043, global_step = 3600, loss = 374.2775\nINFO:tensorflow:Saving 'checkpoint_path' summary for global step 3600: blerssi/model.ckpt-3600\nINFO:tensorflow:global_step/sec: 81.4535\nINFO:tensorflow:loss = 279.70343, step = 3600 (1.227 sec)\nINFO:tensorflow:Saving checkpoints for 3700 into blerssi/model.ckpt.\nINFO:tensorflow:Calling model_fn.\nINFO:tensorflow:Done calling model_fn.\nINFO:tensorflow:Starting evaluation at 2020-07-27T12:05:00Z\nINFO:tensorflow:Graph was finalized.\nINFO:tensorflow:Restoring parameters from blerssi/model.ckpt-3700\nINFO:tensorflow:Running local_init_op.\nINFO:tensorflow:Done running local_init_op.\nINFO:tensorflow:Evaluation [10/100]\nINFO:tensorflow:Evaluation [20/100]\nINFO:tensorflow:Evaluation [30/100]\nINFO:tensorflow:Evaluation [40/100]\nINFO:tensorflow:Evaluation [50/100]\nINFO:tensorflow:Evaluation [60/100]\nINFO:tensorflow:Evaluation [70/100]\nINFO:tensorflow:Evaluation [80/100]\nINFO:tensorflow:Evaluation [90/100]\nINFO:tensorflow:Evaluation [100/100]\nINFO:tensorflow:Finished evaluation at 2020-07-27-12:05:01\nINFO:tensorflow:Saving dict for global step 3700: accuracy = 0.1934375, average_loss = 2.9265563, global_step = 3700, loss = 374.5992\nINFO:tensorflow:Saving 'checkpoint_path' summary for global step 3700: blerssi/model.ckpt-3700\nINFO:tensorflow:global_step/sec: 69.8044\nINFO:tensorflow:loss = 289.2801, step = 3700 (1.432 sec)\nINFO:tensorflow:Saving checkpoints for 3800 into blerssi/model.ckpt.\nINFO:tensorflow:Calling model_fn.\nINFO:tensorflow:Done calling model_fn.\nINFO:tensorflow:Starting evaluation at 2020-07-27T12:05:02Z\nINFO:tensorflow:Graph was finalized.\nINFO:tensorflow:Restoring parameters from blerssi/model.ckpt-3800\nINFO:tensorflow:Running local_init_op.\nINFO:tensorflow:Done running local_init_op.\nINFO:tensorflow:Evaluation [10/100]\nINFO:tensorflow:Evaluation [20/100]\nINFO:tensorflow:Evaluation [30/100]\nINFO:tensorflow:Evaluation [40/100]\nINFO:tensorflow:Evaluation [50/100]\nINFO:tensorflow:Evaluation [60/100]\nINFO:tensorflow:Evaluation [70/100]\nINFO:tensorflow:Evaluation [80/100]\nINFO:tensorflow:Evaluation [90/100]\nINFO:tensorflow:Evaluation [100/100]\nINFO:tensorflow:Finished evaluation at 2020-07-27-12:05:02\nINFO:tensorflow:Saving dict for global step 3800: accuracy = 0.2146875, average_loss = 2.8516412, global_step = 3800, loss = 365.01007\nINFO:tensorflow:Saving 'checkpoint_path' summary for global step 3800: blerssi/model.ckpt-3800\nINFO:tensorflow:global_step/sec: 86.0293\nINFO:tensorflow:loss = 288.4405, step = 3800 (1.162 sec)\nINFO:tensorflow:Saving checkpoints for 3900 into blerssi/model.ckpt.\nINFO:tensorflow:Calling model_fn.\nINFO:tensorflow:Done calling model_fn.\nINFO:tensorflow:Starting evaluation at 2020-07-27T12:05:03Z\nINFO:tensorflow:Graph was finalized.\nINFO:tensorflow:Restoring parameters from blerssi/model.ckpt-3900\nINFO:tensorflow:Running local_init_op.\nINFO:tensorflow:Done running local_init_op.\nINFO:tensorflow:Evaluation [10/100]\nINFO:tensorflow:Evaluation [20/100]\nINFO:tensorflow:Evaluation [30/100]\nINFO:tensorflow:Evaluation [40/100]\nINFO:tensorflow:Evaluation [50/100]\nINFO:tensorflow:Evaluation [60/100]\nINFO:tensorflow:Evaluation [70/100]\nINFO:tensorflow:Evaluation [80/100]\nINFO:tensorflow:Evaluation [90/100]\nINFO:tensorflow:Evaluation [100/100]\nINFO:tensorflow:Finished evaluation at 2020-07-27-12:05:03\nINFO:tensorflow:Saving dict for global step 3900: accuracy = 0.23226562, average_loss = 2.8733413, global_step = 3900, loss = 367.7877\nINFO:tensorflow:Saving 'checkpoint_path' summary for global step 3900: blerssi/model.ckpt-3900\nINFO:tensorflow:global_step/sec: 81.837\nINFO:tensorflow:loss = 274.29977, step = 3900 (1.225 sec)\nINFO:tensorflow:Saving checkpoints for 4000 into blerssi/model.ckpt.\nINFO:tensorflow:Calling model_fn.\nINFO:tensorflow:Done calling model_fn.\nINFO:tensorflow:Starting evaluation at 2020-07-27T12:05:04Z\nINFO:tensorflow:Graph was finalized.\nINFO:tensorflow:Restoring parameters from blerssi/model.ckpt-4000\nINFO:tensorflow:Running local_init_op.\nINFO:tensorflow:Done running local_init_op.\nINFO:tensorflow:Evaluation [10/100]\nINFO:tensorflow:Evaluation [20/100]\nINFO:tensorflow:Evaluation [30/100]\nINFO:tensorflow:Evaluation [40/100]\nINFO:tensorflow:Evaluation [50/100]\nINFO:tensorflow:Evaluation [60/100]\nINFO:tensorflow:Evaluation [70/100]\nINFO:tensorflow:Evaluation [80/100]\nINFO:tensorflow:Evaluation [90/100]\nINFO:tensorflow:Evaluation [100/100]\nINFO:tensorflow:Finished evaluation at 2020-07-27-12:05:05\nINFO:tensorflow:Saving dict for global step 4000: accuracy = 0.23929687, average_loss = 2.8829916, global_step = 4000, loss = 369.02292\nINFO:tensorflow:Saving 'checkpoint_path' summary for global step 4000: blerssi/model.ckpt-4000\nINFO:tensorflow:global_step/sec: 79.7979\nINFO:tensorflow:loss = 280.6007, step = 4000 (1.251 sec)\nINFO:tensorflow:Saving checkpoints for 4100 into blerssi/model.ckpt.\nINFO:tensorflow:Calling model_fn.\nINFO:tensorflow:Done calling model_fn.\nINFO:tensorflow:Starting evaluation at 2020-07-27T12:05:05Z\nINFO:tensorflow:Graph was finalized.\nINFO:tensorflow:Restoring parameters from blerssi/model.ckpt-4100\nINFO:tensorflow:Running local_init_op.\nINFO:tensorflow:Done running local_init_op.\nINFO:tensorflow:Evaluation [10/100]\nINFO:tensorflow:Evaluation [20/100]\nINFO:tensorflow:Evaluation [30/100]\nINFO:tensorflow:Evaluation [40/100]\nINFO:tensorflow:Evaluation [50/100]\nINFO:tensorflow:Evaluation [60/100]\nINFO:tensorflow:Evaluation [70/100]\nINFO:tensorflow:Evaluation [80/100]\nINFO:tensorflow:Evaluation [90/100]\nINFO:tensorflow:Evaluation [100/100]\nINFO:tensorflow:Finished evaluation at 2020-07-27-12:05:06\nINFO:tensorflow:Saving dict for global step 4100: accuracy = 0.20398438, average_loss = 2.924492, global_step = 4100, loss = 374.33496\nINFO:tensorflow:Saving 'checkpoint_path' summary for global step 4100: blerssi/model.ckpt-4100\nINFO:tensorflow:global_step/sec: 82.9037\nINFO:tensorflow:loss = 292.06015, step = 4100 (1.207 sec)\nINFO:tensorflow:Saving checkpoints for 4200 into blerssi/model.ckpt.\nINFO:tensorflow:Calling model_fn.\nINFO:tensorflow:Done calling model_fn.\nINFO:tensorflow:Starting evaluation at 2020-07-27T12:05:07Z\nINFO:tensorflow:Graph was finalized.\nINFO:tensorflow:Restoring parameters from blerssi/model.ckpt-4200\nINFO:tensorflow:Running local_init_op.\nINFO:tensorflow:Done running local_init_op.\nINFO:tensorflow:Evaluation [10/100]\nINFO:tensorflow:Evaluation [20/100]\nINFO:tensorflow:Evaluation [30/100]\nINFO:tensorflow:Evaluation [40/100]\nINFO:tensorflow:Evaluation [50/100]\nINFO:tensorflow:Evaluation [60/100]\nINFO:tensorflow:Evaluation [70/100]\nINFO:tensorflow:Evaluation [80/100]\nINFO:tensorflow:Evaluation [90/100]\nINFO:tensorflow:Evaluation [100/100]\nINFO:tensorflow:Finished evaluation at 2020-07-27-12:05:07\nINFO:tensorflow:Saving dict for global step 4200: accuracy = 0.23578125, average_loss = 2.846016, global_step = 4200, loss = 364.29004\nINFO:tensorflow:Saving 'checkpoint_path' summary for global step 4200: blerssi/model.ckpt-4200\nINFO:tensorflow:global_step/sec: 83.7255\nINFO:tensorflow:loss = 272.29013, step = 4200 (1.194 sec)\nINFO:tensorflow:Saving checkpoints for 4300 into blerssi/model.ckpt.\nINFO:tensorflow:Calling model_fn.\nINFO:tensorflow:Done calling model_fn.\nINFO:tensorflow:Starting evaluation at 2020-07-27T12:05:08Z\nINFO:tensorflow:Graph was finalized.\nINFO:tensorflow:Restoring parameters from blerssi/model.ckpt-4300\nINFO:tensorflow:Running local_init_op.\nINFO:tensorflow:Done running local_init_op.\nINFO:tensorflow:Evaluation [10/100]\nINFO:tensorflow:Evaluation [20/100]\nINFO:tensorflow:Evaluation [30/100]\nINFO:tensorflow:Evaluation [40/100]\nINFO:tensorflow:Evaluation [50/100]\nINFO:tensorflow:Evaluation [60/100]\nINFO:tensorflow:Evaluation [70/100]\nINFO:tensorflow:Evaluation [80/100]\nINFO:tensorflow:Evaluation [90/100]\nINFO:tensorflow:Evaluation [100/100]\nINFO:tensorflow:Finished evaluation at 2020-07-27-12:05:08\nINFO:tensorflow:Saving dict for global step 4300: accuracy = 0.239375, average_loss = 2.8495471, global_step = 4300, loss = 364.74203\nINFO:tensorflow:Saving 'checkpoint_path' summary for global step 4300: blerssi/model.ckpt-4300\nINFO:tensorflow:global_step/sec: 83.2944\nINFO:tensorflow:loss = 300.6521, step = 4300 (1.200 sec)\nINFO:tensorflow:Saving checkpoints for 4400 into blerssi/model.ckpt.\nINFO:tensorflow:Calling model_fn.\nINFO:tensorflow:Done calling model_fn.\nINFO:tensorflow:Starting evaluation at 2020-07-27T12:05:09Z\nINFO:tensorflow:Graph was finalized.\nINFO:tensorflow:Restoring parameters from blerssi/model.ckpt-4400\nINFO:tensorflow:Running local_init_op.\nINFO:tensorflow:Done running local_init_op.\nINFO:tensorflow:Evaluation [10/100]\nINFO:tensorflow:Evaluation [20/100]\nINFO:tensorflow:Evaluation [30/100]\nINFO:tensorflow:Evaluation [40/100]\nINFO:tensorflow:Evaluation [50/100]\nINFO:tensorflow:Evaluation [60/100]\nINFO:tensorflow:Evaluation [70/100]\nINFO:tensorflow:Evaluation [80/100]\nINFO:tensorflow:Evaluation [90/100]\nINFO:tensorflow:Evaluation [100/100]\nINFO:tensorflow:Finished evaluation at 2020-07-27-12:05:10\nINFO:tensorflow:Saving dict for global step 4400: accuracy = 0.22507812, average_loss = 2.8738248, global_step = 4400, loss = 367.84958\nINFO:tensorflow:Saving 'checkpoint_path' summary for global step 4400: blerssi/model.ckpt-4400\nINFO:tensorflow:global_step/sec: 82.7437\nINFO:tensorflow:loss = 297.72165, step = 4400 (1.209 sec)\nINFO:tensorflow:Saving checkpoints for 4500 into blerssi/model.ckpt.\nINFO:tensorflow:Calling model_fn.\nINFO:tensorflow:Done calling model_fn.\nINFO:tensorflow:Starting evaluation at 2020-07-27T12:05:10Z\nINFO:tensorflow:Graph was finalized.\nINFO:tensorflow:Restoring parameters from blerssi/model.ckpt-4500\nINFO:tensorflow:Running local_init_op.\nINFO:tensorflow:Done running local_init_op.\nINFO:tensorflow:Evaluation [10/100]\nINFO:tensorflow:Evaluation [20/100]\nINFO:tensorflow:Evaluation [30/100]\nINFO:tensorflow:Evaluation [40/100]\nINFO:tensorflow:Evaluation [50/100]\nINFO:tensorflow:Evaluation [60/100]\nINFO:tensorflow:Evaluation [70/100]\nINFO:tensorflow:Evaluation [80/100]\nINFO:tensorflow:Evaluation [90/100]\nINFO:tensorflow:Evaluation [100/100]\nINFO:tensorflow:Finished evaluation at 2020-07-27-12:05:11\nINFO:tensorflow:Saving dict for global step 4500: accuracy = 0.21453124, average_loss = 3.0025408, global_step = 4500, loss = 384.32523\nINFO:tensorflow:Saving 'checkpoint_path' summary for global step 4500: blerssi/model.ckpt-4500\nINFO:tensorflow:global_step/sec: 67.978\nINFO:tensorflow:loss = 287.15585, step = 4500 (1.471 sec)\nINFO:tensorflow:Saving checkpoints for 4600 into blerssi/model.ckpt.\nINFO:tensorflow:Calling model_fn.\nINFO:tensorflow:Done calling model_fn.\nINFO:tensorflow:Starting evaluation at 2020-07-27T12:05:12Z\nINFO:tensorflow:Graph was finalized.\nINFO:tensorflow:Restoring parameters from blerssi/model.ckpt-4600\nINFO:tensorflow:Running local_init_op.\nINFO:tensorflow:Done running local_init_op.\nINFO:tensorflow:Evaluation [10/100]\nINFO:tensorflow:Evaluation [20/100]\nINFO:tensorflow:Evaluation [30/100]\nINFO:tensorflow:Evaluation [40/100]\nINFO:tensorflow:Evaluation [50/100]\nINFO:tensorflow:Evaluation [60/100]\nINFO:tensorflow:Evaluation [70/100]\nINFO:tensorflow:Evaluation [80/100]\nINFO:tensorflow:Evaluation [90/100]\nINFO:tensorflow:Evaluation [100/100]\nINFO:tensorflow:Finished evaluation at 2020-07-27-12:05:12\nINFO:tensorflow:Saving dict for global step 4600: accuracy = 0.23585938, average_loss = 2.8682337, global_step = 4600, loss = 367.1339\nINFO:tensorflow:Saving 'checkpoint_path' summary for global step 4600: blerssi/model.ckpt-4600\nINFO:tensorflow:global_step/sec: 84.4059\nINFO:tensorflow:loss = 273.37143, step = 4600 (1.185 sec)\nINFO:tensorflow:Saving checkpoints for 4700 into blerssi/model.ckpt.\nINFO:tensorflow:Calling model_fn.\nINFO:tensorflow:Done calling model_fn.\nINFO:tensorflow:Starting evaluation at 2020-07-27T12:05:13Z\nINFO:tensorflow:Graph was finalized.\nINFO:tensorflow:Restoring parameters from blerssi/model.ckpt-4700\nINFO:tensorflow:Running local_init_op.\nINFO:tensorflow:Done running local_init_op.\nINFO:tensorflow:Evaluation [10/100]\nINFO:tensorflow:Evaluation [20/100]\nINFO:tensorflow:Evaluation [30/100]\nINFO:tensorflow:Evaluation [40/100]\nINFO:tensorflow:Evaluation [50/100]\nINFO:tensorflow:Evaluation [60/100]\nINFO:tensorflow:Evaluation [70/100]\nINFO:tensorflow:Evaluation [80/100]\nINFO:tensorflow:Evaluation [90/100]\nINFO:tensorflow:Evaluation [100/100]\nINFO:tensorflow:Finished evaluation at 2020-07-27-12:05:13\nINFO:tensorflow:Saving dict for global step 4700: accuracy = 0.26742187, average_loss = 2.9070816, global_step = 4700, loss = 372.10645\nINFO:tensorflow:Saving 'checkpoint_path' summary for global step 4700: blerssi/model.ckpt-4700\nINFO:tensorflow:global_step/sec: 82.6464\nINFO:tensorflow:loss = 280.7273, step = 4700 (1.210 sec)\nINFO:tensorflow:Saving checkpoints for 4800 into blerssi/model.ckpt.\nINFO:tensorflow:Calling model_fn.\nINFO:tensorflow:Done calling model_fn.\nINFO:tensorflow:Starting evaluation at 2020-07-27T12:05:14Z\nINFO:tensorflow:Graph was finalized.\nINFO:tensorflow:Restoring parameters from blerssi/model.ckpt-4800\nINFO:tensorflow:Running local_init_op.\nINFO:tensorflow:Done running local_init_op.\nINFO:tensorflow:Evaluation [10/100]\nINFO:tensorflow:Evaluation [20/100]\nINFO:tensorflow:Evaluation [30/100]\nINFO:tensorflow:Evaluation [40/100]\nINFO:tensorflow:Evaluation [50/100]\nINFO:tensorflow:Evaluation [60/100]\nINFO:tensorflow:Evaluation [70/100]\nINFO:tensorflow:Evaluation [80/100]\nINFO:tensorflow:Evaluation [90/100]\nINFO:tensorflow:Evaluation [100/100]\nINFO:tensorflow:Finished evaluation at 2020-07-27-12:05:15\nINFO:tensorflow:Saving dict for global step 4800: accuracy = 0.22867188, average_loss = 2.9323123, global_step = 4800, loss = 375.33597\nINFO:tensorflow:Saving 'checkpoint_path' summary for global step 4800: blerssi/model.ckpt-4800\nINFO:tensorflow:global_step/sec: 84.4072\nINFO:tensorflow:loss = 282.58746, step = 4800 (1.185 sec)\nINFO:tensorflow:Saving checkpoints for 4900 into blerssi/model.ckpt.\nINFO:tensorflow:Calling model_fn.\nINFO:tensorflow:Done calling model_fn.\nINFO:tensorflow:Starting evaluation at 2020-07-27T12:05:15Z\nINFO:tensorflow:Graph was finalized.\nINFO:tensorflow:Restoring parameters from blerssi/model.ckpt-4900\nINFO:tensorflow:Running local_init_op.\nINFO:tensorflow:Done running local_init_op.\nINFO:tensorflow:Evaluation [10/100]\nINFO:tensorflow:Evaluation [20/100]\nINFO:tensorflow:Evaluation [30/100]\nINFO:tensorflow:Evaluation [40/100]\nINFO:tensorflow:Evaluation [50/100]\nINFO:tensorflow:Evaluation [60/100]\nINFO:tensorflow:Evaluation [70/100]\nINFO:tensorflow:Evaluation [80/100]\nINFO:tensorflow:Evaluation [90/100]\nINFO:tensorflow:Evaluation [100/100]\nINFO:tensorflow:Finished evaluation at 2020-07-27-12:05:16\nINFO:tensorflow:Saving dict for global step 4900: accuracy = 0.22859375, average_loss = 2.9506714, global_step = 4900, loss = 377.68594\nINFO:tensorflow:Saving 'checkpoint_path' summary for global step 4900: blerssi/model.ckpt-4900\nINFO:tensorflow:global_step/sec: 83.8655\nINFO:tensorflow:loss = 276.4771, step = 4900 (1.192 sec)\nINFO:tensorflow:Saving checkpoints for 5000 into blerssi/model.ckpt.\nINFO:tensorflow:Calling model_fn.\nINFO:tensorflow:Done calling model_fn.\nINFO:tensorflow:Starting evaluation at 2020-07-27T12:05:16Z\nINFO:tensorflow:Graph was finalized.\nINFO:tensorflow:Restoring parameters from blerssi/model.ckpt-5000\nINFO:tensorflow:Running local_init_op.\nINFO:tensorflow:Done running local_init_op.\nINFO:tensorflow:Evaluation [10/100]\nINFO:tensorflow:Evaluation [20/100]\nINFO:tensorflow:Evaluation [30/100]\nINFO:tensorflow:Evaluation [40/100]\nINFO:tensorflow:Evaluation [50/100]\nINFO:tensorflow:Evaluation [60/100]\nINFO:tensorflow:Evaluation [70/100]\nINFO:tensorflow:Evaluation [80/100]\nINFO:tensorflow:Evaluation [90/100]\nINFO:tensorflow:Evaluation [100/100]\nINFO:tensorflow:Finished evaluation at 2020-07-27-12:05:17\nINFO:tensorflow:Saving dict for global step 5000: accuracy = 0.24632813, average_loss = 2.9123015, global_step = 5000, loss = 372.7746\nINFO:tensorflow:Saving 'checkpoint_path' summary for global step 5000: blerssi/model.ckpt-5000\nINFO:tensorflow:Performing the final export in the end of training.\nINFO:tensorflow:Calling model_fn.\nINFO:tensorflow:Done calling model_fn.\nWARNING:tensorflow:From /usr/local/lib/python3.6/dist-packages/tensorflow_core/python/saved_model/signature_def_utils_impl.py:201: build_tensor_info (from tensorflow.python.saved_model.utils_impl) is deprecated and will be removed in a future version.\nInstructions for updating:\nThis function will only be available through the v1 compatibility library as tf.compat.v1.saved_model.utils.build_tensor_info or tf.compat.v1.saved_model.build_tensor_info.\nINFO:tensorflow:Signatures INCLUDED in export for Classify: None\nINFO:tensorflow:Signatures INCLUDED in export for Regress: None\nINFO:tensorflow:Signatures INCLUDED in export for Predict: ['predict']\nINFO:tensorflow:Signatures INCLUDED in export for Train: None\nINFO:tensorflow:Signatures INCLUDED in export for Eval: None\nINFO:tensorflow:Signatures EXCLUDED from export because they cannot be be served via TensorFlow Serving APIs:\nINFO:tensorflow:'serving_default' : Classification input must be a single string Tensor; got {'b3001': <tf.Tensor 'Placeholder:0' shape=(?,) dtype=float32>, 'b3002': <tf.Tensor 'Placeholder_1:0' shape=(?,) dtype=float32>, 'b3003': <tf.Tensor 'Placeholder_2:0' shape=(?,) dtype=float32>, 'b3004': <tf.Tensor 'Placeholder_3:0' shape=(?,) dtype=float32>, 'b3005': <tf.Tensor 'Placeholder_4:0' shape=(?,) dtype=float32>, 'b3006': <tf.Tensor 'Placeholder_5:0' shape=(?,) dtype=float32>, 'b3007': <tf.Tensor 'Placeholder_6:0' shape=(?,) dtype=float32>, 'b3008': <tf.Tensor 'Placeholder_7:0' shape=(?,) dtype=float32>, 'b3009': <tf.Tensor 'Placeholder_8:0' shape=(?,) dtype=float32>, 'b3010': <tf.Tensor 'Placeholder_9:0' shape=(?,) dtype=float32>, 'b3011': <tf.Tensor 'Placeholder_10:0' shape=(?,) dtype=float32>, 'b3012': <tf.Tensor 'Placeholder_11:0' shape=(?,) dtype=float32>, 'b3013': <tf.Tensor 'Placeholder_12:0' shape=(?,) dtype=float32>}\nINFO:tensorflow:'classification' : Classification input must be a single string Tensor; got {'b3001': <tf.Tensor 'Placeholder:0' shape=(?,) dtype=float32>, 'b3002': <tf.Tensor 'Placeholder_1:0' shape=(?,) dtype=float32>, 'b3003': <tf.Tensor 'Placeholder_2:0' shape=(?,) dtype=float32>, 'b3004': <tf.Tensor 'Placeholder_3:0' shape=(?,) dtype=float32>, 'b3005': <tf.Tensor 'Placeholder_4:0' shape=(?,) dtype=float32>, 'b3006': <tf.Tensor 'Placeholder_5:0' shape=(?,) dtype=float32>, 'b3007': <tf.Tensor 'Placeholder_6:0' shape=(?,) dtype=float32>, 'b3008': <tf.Tensor 'Placeholder_7:0' shape=(?,) dtype=float32>, 'b3009': <tf.Tensor 'Placeholder_8:0' shape=(?,) dtype=float32>, 'b3010': <tf.Tensor 'Placeholder_9:0' shape=(?,) dtype=float32>, 'b3011': <tf.Tensor 'Placeholder_10:0' shape=(?,) dtype=float32>, 'b3012': <tf.Tensor 'Placeholder_11:0' shape=(?,) dtype=float32>, 'b3013': <tf.Tensor 'Placeholder_12:0' shape=(?,) dtype=float32>}\nWARNING:tensorflow:Export includes no default signature!\nINFO:tensorflow:Restoring parameters from blerssi/model.ckpt-5000\nINFO:tensorflow:Assets added to graph.\nINFO:tensorflow:No assets to write.\nINFO:tensorflow:SavedModel written to: blerssi/export/blerssi/temp-b'1595851517'/saved_model.pb\nINFO:tensorflow:Loss for final step: 260.41766.\n"
]
],
[
[
"## Define predict function",
"_____no_output_____"
]
],
[
[
"MODEL_EXPORT_PATH= os.path.join(TF_MODEL_DIR, \"export\", TF_EXPORT_DIR)\n\ndef predict(request):\n \"\"\" \n Define custom predict function to be used by local prediction\n and explainer. Set anchor_tabular predict function so it always returns predicted class\n \"\"\"\n # Get model exporter path\n for dir in os.listdir(MODEL_EXPORT_PATH):\n if re.match('[0-9]',dir):\n exported_path=os.path.join(MODEL_EXPORT_PATH,dir)\n break\n else:\n raise Exception(\"Model path not found\")\n\n # Prepare model input data\n feature_cols=[\"b3001\", \"b3002\",\"b3003\",\"b3004\",\"b3005\",\"b3006\",\"b3007\",\"b3008\",\"b3009\",\"b3010\",\"b3011\",\"b3012\",\"b3013\"]\n input={'b3001': [], 'b3002': [], 'b3003': [], 'b3004': [], 'b3005': [], 'b3006': [], 'b3007': [], 'b3008': [], 'b3009': [], 'b3010': [], 'b3011': [], 'b3012': [], 'b3013': []}\n\n X=request\n if np.ndim(X) != 2:\n for i in range(len(X)):\n input[feature_cols[i]].append(X[i])\n else:\n for i in range(len(X)):\n for j in range(len(X[i])):\n input[feature_cols[j]].append(X[i][j])\n\n # Open a Session to predict\n with tf.Session() as sess:\n tf.saved_model.loader.load(sess, [tf.saved_model.tag_constants.SERVING], exported_path)\n predictor= tf.contrib.predictor.from_saved_model(exported_path,signature_def_key='predict')\n output_dict= predictor(input)\n sess.close()\n output={}\n output[\"predictions\"]={\"probabilities\":output_dict[\"probabilities\"].tolist()}\n return np.asarray(output['predictions'][\"probabilities\"])",
"_____no_output_____"
]
],
[
[
"## Initialize and fit\nTo initialize the explainer, we provide a predict function, a list with the feature names to make the anchors easy to understand.",
"_____no_output_____"
]
],
[
[
"feature_cols=[\"b3001\", \"b3002\", \"b3003\", \"b3004\", \"b3005\", \"b3006\", \"b3007\", \"b3008\", \"b3009\", \"b3010\", \"b3011\", \"b3012\", \"b3013\"]\nexplainer = AnchorTabular(predict, feature_cols)",
"WARNING:tensorflow:From <ipython-input-8-69054218b064>:31: load (from tensorflow.python.saved_model.loader_impl) is deprecated and will be removed in a future version.\nInstructions for updating:\nThis function will only be available through the v1 compatibility library as tf.compat.v1.saved_model.loader.load or tf.compat.v1.saved_model.load. There will be a new function for importing SavedModels in Tensorflow 2.0.\nINFO:tensorflow:Restoring parameters from blerssi/export/blerssi/1595851517/variables/variables\nWARNING:tensorflow:\nThe TensorFlow contrib module will not be included in TensorFlow 2.0.\nFor more information, please see:\n * https://github.com/tensorflow/community/blob/master/rfcs/20180907-contrib-sunset.md\n * https://github.com/tensorflow/addons\n * https://github.com/tensorflow/io (for I/O related ops)\nIf you depend on functionality not listed there, please file an issue.\n\nINFO:tensorflow:Restoring parameters from blerssi/export/blerssi/1595851517/variables/variables\n"
]
],
[
[
"Discretize the ordinal features into quartiles. disc_perc is a list with percentiles used for binning",
"_____no_output_____"
]
],
[
[
"explainer.fit(x1, disc_perc=(25, 50, 75))",
"_____no_output_____"
]
],
[
[
"## Save Explainer file\nSave explainer file with .dill extension. It will be used when creating the InferenceService",
"_____no_output_____"
]
],
[
[
"EXPLAINER_PATH=\"explainer\"\nif not os.path.exists(EXPLAINER_PATH):\n os.mkdir(EXPLAINER_PATH)\nwith open(\"%s/explainer.dill\"%EXPLAINER_PATH, 'wb') as f:\n dill.dump(explainer,f)",
"_____no_output_____"
]
],
[
[
"## Create a gateway\nCreate a gateway called kubeflow-gateway in namespace anonymous.",
"_____no_output_____"
]
],
[
[
"gateway=f\"\"\"apiVersion: networking.istio.io/v1alpha3\nkind: Gateway\nmetadata:\n name: kubeflow-gateway\n namespace: {namespace}\nspec:\n selector:\n istio: ingressgateway\n servers:\n - hosts:\n - '*'\n port:\n name: http\n number: 80\n protocol: HTTP\n\"\"\"\ngateway_spec=yaml.safe_load(gateway)",
"_____no_output_____"
],
[
"custom_api.create_namespaced_custom_object(group=\"networking.istio.io\", version=\"v1alpha3\", namespace=namespace, plural=\"gateways\", body=gateway_spec)",
"_____no_output_____"
]
],
[
[
"## Adding a new inference server \nThe list of available inference servers in Seldon Core is maintained in the **seldon-config** configmap, which lives in the same namespace as your Seldon Core operator. In particular, the **predictor_servers** key holds the JSON config for each inference server.\n\n[Refer to for more information](https://docs.seldon.io/projects/seldon-core/en/v1.1.0/servers/custom.html)",
"_____no_output_____"
]
],
[
[
"api_client.patch_namespaced_config_map(name=\"seldon-config\", namespace=\"kubeflow\",pretty=True, body={\"data\":{\"predictor_servers\":'{\"MLFLOW_SERVER\":{\"grpc\":{\"defaultImageVersion\":\"1.2.1\",\"image\":\"seldonio/mlflowserver_grpc\"},\"rest\":{\"defaultImageVersion\":\"1.2.1\",\"image\":\"seldonio/mlflowserver_rest\"}},\"SKLEARN_SERVER\":{\"grpc\":{\"defaultImageVersion\":\"1.2.1\",\"image\":\"seldonio/sklearnserver_grpc\"},\"rest\":{\"defaultImageVersion\":\"1.2.1\",\"image\":\"seldonio/sklearnserver_rest\"}},\"TENSORFLOW_SERVER\":{\"grpc\":{\"defaultImageVersion\":\"1.2.1\",\"image\":\"seldonio/tfserving-proxy_grpc\"},\"rest\":{\"defaultImageVersion\":\"1.2.1\",\"image\":\"seldonio/tfserving-proxy_rest\"},\"tensorflow\":true,\"tfImage\":\"tensorflow/serving:2.1.0\"},\"XGBOOST_SERVER\":{\"grpc\":{\"defaultImageVersion\":\"1.2.1\",\"image\":\"seldonio/xgboostserver_grpc\"},\"rest\":{\"defaultImageVersion\":\"1.2.1\",\"image\":\"seldonio/xgboostserver_rest\"}}, \"CUSTOM_INFERENCE_SERVER\":{\"rest\":{\"defaultImageVersion\":\"1.0\",\"image\":\"samba07/blerssi-seldon\"}}}'}})",
"_____no_output_____"
]
],
[
[
"## Seldon Serving Deployment\nCreate an **SeldonDeployment** with a blerssi model",
"_____no_output_____"
]
],
[
[
"pvcname = !(echo $HOSTNAME | sed 's/.\\{2\\}$//')\npvc = \"workspace-\"+pvcname[0]\nseldon_deploy=f\"\"\"apiVersion: machinelearning.seldon.io/v1alpha2\nkind: SeldonDeployment\nmetadata:\n name: blerssi\n namespace: {namespace}\nspec:\n name: blerssi\n predictors:\n - graph:\n children: []\n implementation: CUSTOM_INFERENCE_SERVER\n modelUri: pvc://{pvc}/{MODEL_EXPORT_PATH}\n name: blerssi\n explainer:\n containerSpec:\n image: seldonio/alibiexplainer:1.2.2-dev\n name: explainer\n type: AnchorTabular\n modelUri: pvc://{pvc}/{EXPLAINER_PATH}\n name: default\n replicas: 1\n\"\"\"\nseldon_deploy_spec=yaml.safe_load(seldon_deploy)",
"_____no_output_____"
],
[
"custom_api.create_namespaced_custom_object(group=\"machinelearning.seldon.io\", version=\"v1alpha2\", namespace=namespace, plural=\"seldondeployments\", body=seldon_deploy_spec)",
"_____no_output_____"
]
],
[
[
"## Wait for state to become available",
"_____no_output_____"
]
],
[
[
"status=False\nwhile True:\n seldon_status=custom_api.get_namespaced_custom_object_status(group=\"machinelearning.seldon.io\", version=\"v1alpha2\", namespace=namespace, plural=\"seldondeployments\", name=seldon_deploy_spec[\"metadata\"][\"name\"])\n if seldon_status[\"status\"][\"state\"] == \"Available\":\n status=True\n print(\"Status: %s\"%seldon_status[\"status\"][\"state\"])\n if status:\n break\n print(\"Status: %s\"%seldon_status[\"status\"][\"state\"])\n sleep(30)",
"Status: Creating\nStatus: Creating\nStatus: Available\n"
]
],
[
[
"## Run a Prediction",
"_____no_output_____"
]
],
[
[
"CLUSTER='ucs' #where your cluster running 'gcp' or 'ucs'",
"_____no_output_____"
],
[
"%%bash -s \"$CLUSTER\" --out NODE_IP\nif [ $1 = \"ucs\" ]\nthen\n echo \"$(kubectl get node -o=jsonpath='{.items[0].status.addresses[0].address}')\"\nelse\n echo \"$(kubectl get node -o=jsonpath='{.items[0].status.addresses[1].address}')\"\nfi",
"_____no_output_____"
],
[
"%%bash --out INGRESS_PORT\nINGRESS_GATEWAY=\"istio-ingressgateway\"\necho \"$(kubectl -n istio-system get service $INGRESS_GATEWAY -o jsonpath='{.spec.ports[1].nodePort}')\"",
"_____no_output_____"
]
],
[
[
"### Data for prediction",
"_____no_output_____"
]
],
[
[
"df_full = pd.read_csv(os.path.join(path,'data/iBeacon_RSSI_Unlabeled_truncated.csv')) #Labeled dataset\n # Input Data Preprocessing \ndf_full = df_full.drop(['date'],axis = 1)\ndf_full = df_full.drop(['location'],axis = 1)\ndf_full[FEATURES] = (df_full[FEATURES])/(-200)\ninput_data=df_full.to_numpy()[:1]\ninput_data",
"_____no_output_____"
],
[
"headers={\"Content-Type\": \"application/json\"}\ndef inference_predict(X):\n data={\"data\":{\"ndarray\":X.tolist()}}\n url = f\"http://{NODE_IP.strip()}:{INGRESS_PORT.strip()}/seldon/{namespace}/%s/api/v1.0/predictions\"%seldon_deploy_spec[\"metadata\"][\"name\"]\n response=requests.post(url, data=json.dumps(data), headers=headers)\n probabilities=response.json()['data']['ndarray']\n for prob in probabilities:\n cls_id=np.argmax(prob)\n print(\"Probability: %s\"%prob[cls_id])\n print(\"Class-id: %s\"%cls_id)\n\ndef explain(X):\n if np.ndim(X)==2:\n data={\"data\":{\"ndarray\":X.tolist()}}\n else:\n data={\"data\":{\"ndarray\":[X.tolist()]}}\n url = f\"http://{NODE_IP.strip()}:{INGRESS_PORT.strip()}/seldon/{namespace}/%s-explainer/default/api/v1.0/explain\"%seldon_deploy_spec[\"metadata\"][\"name\"]\n response=requests.post(url, data=json.dumps(data), headers=headers)\n print('Anchor: %s' % (' AND '.join(response.json()['names'])))\n print('Coverage: %.2f' % response.json()['coverage'])",
"_____no_output_____"
],
[
"inference_predict(input_data)",
"Probability: 0.6692667603492737\nClass-id: 14\n"
]
],
[
[
"## Prediction of the model and explain",
"_____no_output_____"
]
],
[
[
"explain(input_data)",
"Anchor: b3009 <= 1.00 AND 0.40 < b3004 <= 1.00 AND 0.39 < b3002 <= 1.00 AND b3012 <= 1.00 AND b3011 <= 1.00 AND b3013 <= 1.00 AND b3006 <= 1.00 AND b3003 <= 1.00 AND b3010 <= 1.00 AND b3005 <= 1.00 AND b3001 <= 1.00 AND b3007 <= 1.00 AND b3008 <= 1.00\nCoverage: 0.48\n"
]
],
[
[
"## Clean Up\n### Delete a gateway",
"_____no_output_____"
]
],
[
[
"custom_api.delete_namespaced_custom_object(group=\"networking.istio.io\", version=\"v1alpha3\", namespace=namespace, plural=\"gateways\", name=gateway_spec[\"metadata\"][\"name\"],body=k8s_client.V1DeleteOptions())",
"_____no_output_____"
]
],
[
[
"### Delete Seldon Serving Deployment",
"_____no_output_____"
]
],
[
[
"custom_api.delete_namespaced_custom_object(group=\"machinelearning.seldon.io\", version=\"v1alpha2\", namespace=namespace, plural=\"seldondeployments\", name=seldon_deploy_spec[\"metadata\"][\"name\"], body=k8s_client.V1DeleteOptions())",
"_____no_output_____"
]
],
[
[
"### Delete model and explainer folders from notebook",
"_____no_output_____"
]
],
[
[
"!rm -rf $EXPLAINER_PATH\n!rm -rf $TF_MODEL_DIR",
"_____no_output_____"
]
]
] | [
"markdown",
"code",
"markdown",
"code",
"markdown",
"code",
"markdown",
"code",
"markdown",
"code",
"markdown",
"code",
"markdown",
"code",
"markdown",
"code",
"markdown",
"code",
"markdown",
"code",
"markdown",
"code",
"markdown",
"code",
"markdown",
"code",
"markdown",
"code",
"markdown",
"code",
"markdown",
"code",
"markdown",
"code",
"markdown",
"code",
"markdown",
"code",
"markdown",
"code",
"markdown",
"code",
"markdown",
"code",
"markdown",
"code",
"markdown",
"code"
] | [
[
"markdown",
"markdown"
],
[
"code"
],
[
"markdown"
],
[
"code"
],
[
"markdown"
],
[
"code"
],
[
"markdown"
],
[
"code"
],
[
"markdown"
],
[
"code"
],
[
"markdown"
],
[
"code"
],
[
"markdown"
],
[
"code"
],
[
"markdown"
],
[
"code"
],
[
"markdown"
],
[
"code"
],
[
"markdown"
],
[
"code"
],
[
"markdown"
],
[
"code"
],
[
"markdown"
],
[
"code"
],
[
"markdown"
],
[
"code"
],
[
"markdown"
],
[
"code"
],
[
"markdown"
],
[
"code",
"code"
],
[
"markdown"
],
[
"code"
],
[
"markdown"
],
[
"code",
"code"
],
[
"markdown"
],
[
"code"
],
[
"markdown"
],
[
"code",
"code",
"code"
],
[
"markdown"
],
[
"code",
"code",
"code"
],
[
"markdown"
],
[
"code"
],
[
"markdown"
],
[
"code"
],
[
"markdown"
],
[
"code"
],
[
"markdown"
],
[
"code"
]
] |
e7833cb097e7b4600ffd51137abf197d8a92ec57 | 5,175 | ipynb | Jupyter Notebook | mobilecoind/clients/python/jupyter/wallet.ipynb | MCrank/mobilecoin | 384c96f34748c426ba4c6a29bd01a538a29b9aef | [
"Apache-2.0"
] | 140 | 2020-04-15T17:51:12.000Z | 2020-10-02T19:51:57.000Z | mobilecoind/clients/python/jupyter/wallet.ipynb | MCrank/mobilecoin | 384c96f34748c426ba4c6a29bd01a538a29b9aef | [
"Apache-2.0"
] | 292 | 2020-10-22T00:34:35.000Z | 2022-03-29T09:29:14.000Z | mobilecoind/clients/python/jupyter/wallet.ipynb | MCrank/mobilecoin | 384c96f34748c426ba4c6a29bd01a538a29b9aef | [
"Apache-2.0"
] | 32 | 2020-04-15T18:17:07.000Z | 2020-10-19T23:25:42.000Z | 28.278689 | 239 | 0.623768 | [
[
[
"# MobileCoin Example Wallet\n\nThis is an example python client that interacts with `mobilecoind` to manage a MobileCoin wallet.\n\nYou must start the `mobilecoind` daemon in order to run a wallet. See the mobilecoind README for more information.\n\nTo run this notebook, make sure you have the requirements installed, and that you have compiled the grpc protos.\n\n```\ncd mobilecoind/clients/python/jupyter\n./install.sh\njupyter notebook\n```",
"_____no_output_____"
]
],
[
[
"from mobilecoin import Client",
"_____no_output_____"
]
],
[
[
"#### Start the Mob Client\n\nThe client talks to your local mobilecoind. See the mobilecoind/README.md for information on how to set it up.",
"_____no_output_____"
]
],
[
[
"client = Client(\"localhost:4444\", ssl=False)",
"_____no_output_____"
]
],
[
[
"#### Input Root Entropy for Account\n\nNote: The root entropy is sensitive material. It is used as the seed to create your account keys. Anyone with your root entropy can steal your MobileCoin.",
"_____no_output_____"
]
],
[
[
"entropy = \"4ec2c081e764f4189afba528956c05804a448f55f24cc3d04c9ef7e807a93bcd\"\ncredentials_response = client.get_account_key(bytes.fromhex(entropy))",
"_____no_output_____"
]
],
[
[
"#### Monitor your Account\n\nMonitoring an account means that mobilecoind will persist the transactions that belong to you to a local database. This allows you to retrieve your funds and calculate your balance, as well as to construct and submit transactions.\n\nNote: MobileCoin uses accounts and subaddresses for managing funds. You can optionally specify a range of subaddresses to monitor. See mob_client.py for more information.",
"_____no_output_____"
]
],
[
[
"monitor_id_response = client.add_monitor(credentials_response.account_key)",
"_____no_output_____"
]
],
[
[
"#### Check Balance\n\nYou will need to provide a subaddress index. Most people will only use one subaddress, and can default to 0. Exchanges or users who want to generate lots of new public addresses may use multiple subaddresses.",
"_____no_output_____"
]
],
[
[
"subaddress_index = 0\nclient.get_balance(monitor_id_response.monitor_id, subaddress_index)",
"_____no_output_____"
]
],
[
[
"#### Send a Transaction\n\nMobileCoin uses \"request codes\" to wrap public addresses. See below for how to generate request codes.",
"_____no_output_____"
]
],
[
[
"address_code = \"2nTy8m2VE5UMtfqRf12gEjZmFHKNTDEtNufQZNvE713ytYvdu2kqpbcncHJUSLwmgTCkB56Li9fsGwJF9LRYEQvoQCDzqVQEJETDNQKLzqHCzd\"\ntarget_address_response = client.parse_request_code(address_code)\n\n# Construct the transaction\ntxo_list_response = client.get_unspent_tx_output_list(monitor_id_response.monitor_id, subaddress_index)\noutlays = [{\n 'value': 10, \n 'receiver': target_address_response.receiver\n}]\ntx_proposal_response = client.generate_tx(\n monitor_id_response.monitor_id, \n subaddress_index, \n txo_list_response.output_list, \n outlays\n)\n\n# Send the transaction to consensus validators\nclient.submit_tx(tx_proposal_response.tx_proposal)",
"_____no_output_____"
]
],
[
[
"#### Public Address (Request Code)",
"_____no_output_____"
]
],
[
[
"public_address_response = client.get_public_address(monitor_id_response.monitor_id, subaddress_index)\nrequest_code_response = client.create_request_code(public_address_response.public_address)\nprint(f\"Request code = {request_code_response}\")",
"_____no_output_____"
]
]
] | [
"markdown",
"code",
"markdown",
"code",
"markdown",
"code",
"markdown",
"code",
"markdown",
"code",
"markdown",
"code",
"markdown",
"code"
] | [
[
"markdown"
],
[
"code"
],
[
"markdown"
],
[
"code"
],
[
"markdown"
],
[
"code"
],
[
"markdown"
],
[
"code"
],
[
"markdown"
],
[
"code"
],
[
"markdown"
],
[
"code"
],
[
"markdown"
],
[
"code"
]
] |
Subsets and Splits
No community queries yet
The top public SQL queries from the community will appear here once available.