pipeline_tag
stringclasses 48
values | library_name
stringclasses 198
values | text
stringlengths 1
900k
| metadata
stringlengths 2
438k
| id
stringlengths 5
122
| last_modified
null | tags
sequencelengths 1
1.84k
| sha
null | created_at
stringlengths 25
25
| arxiv
sequencelengths 0
201
| languages
sequencelengths 0
1.83k
| tags_str
stringlengths 17
9.34k
| text_str
stringlengths 0
389k
| text_lists
sequencelengths 0
722
| processed_texts
sequencelengths 1
723
| tokens_length
sequencelengths 1
723
| input_texts
sequencelengths 1
1
|
---|---|---|---|---|---|---|---|---|---|---|---|---|---|---|---|---|
text-generation | mlx |
# Llama-3-IMPACTS-2x8B-64k-MLX
<img src="https://cdn-uploads.huggingface.co/production/uploads/64740cf7485a7c8e1bd51ac9/y7YKGpr7_Tg9YlhbIscI6.webp" width="500" height="500">
---
**Designed for Advanced Problem-Solving Across Interconnected Domains of Biomimicry, Climate Change, and Astrobiology**
The `Llama-3-IMPACTS-2x8B-64k-MLX` model is a cutting-edge large language model trained on the I.M.P.A.C.T.S dataset, which encompasses scenarios from biomimicry, climate change, and theoretical astrobiology. This model has been specifically tailored to generate innovative solutions and insights for both Earth and potential extraterrestrial environments, reflecting key themes of resilience, sustainability, and the interconnectedness of life across the universe.
## Model Details
### Description
- **Model name:** `Llama-3-IMPACTS-2x8B-64k-MLX`
- **Developer:** Severian
- **Version:** 1.0
- **License:** MIT
### Training Data
The model was trained on a subset of the I.M.P.A.C.T. dataset, utilizing 35,000 carefully curated examples that include detailed scenarios involving climate adaptation, biomimetic applications, and the potential for life in varying cosmic conditions.
### Model Architecture
- **Type:** Llama-3
- **Parameters:** 8 billion
- **MoE:** 2 Experts
- **Training:**
- Epochs: 1 (35K Examples)
- R = 64
- Alpha = 128
- Lr = 1e-7
- **Context Limit:** 64K
## Intended Uses
This model is intended for use in applications that require deep, interdisciplinary understanding and the generation of novel insights within the realms of environmental science, synthetic biology, space exploration, and sustainability studies. Its capabilities make it ideal for:
- Research and academic studies aiming to explore complex scenarios involving ecological and astrobiological phenomena.
- Organizations looking to innovate in the fields of climate resilience and biomimicry.
- Creative problem-solving in contexts where conventional approaches are insufficient.
## How to Use This Model
The model can be loaded and used in various natural language processing tasks that require nuanced understanding and creative output. Here is a basic example of how to load and use the model using the MLX library:
```python
pip install mlx-lm
from mlx_lm import load, generate
model, tokenizer = load("Severian/Llama-3-IMPACTS-2x8B-64k-MLX")
response = generate(model, tokenizer, prompt="how could Bioluminescent Algae come to evolve into a life form around a red dwarf star that has no planets or rocky material? Next, how could that Bioluminescent Algae somehow make it's way to earth as an alien entity? Then, what would happen over a 100 year span if that alien Bioluminescent Algae led to the over-acidification of the water on the entire planet? how could we use biomimicry to stop the ocean from over-acidification?", verbose=True)
```
## Limitations and Biases
While the `Llama-3-IMPACTS-2x8B-64k-MLX` model is designed to be a powerful tool for generating insightful content, it inherits limitations from its training data, which, though extensive, may not capture all possible scenarios or biases. Users should be aware of these limitations and consider them when interpreting the model's outputs, especially in decision-making contexts.
## Model Performance
Initial tests indicate that the model performs exceptionally well in tasks that involve complex reasoning and generating innovative solutions based on the scenarios presented in the I.M.P.A.C.T.S dataset. Further evaluation and fine-tuning may be required to optimize performance for specific applications.
The `Llama-3-IMPACTS-2x8B-64k-MLX` model represents an avenue that AI can use for exploring and solving complex problems across multiple domains. By leveraging the rich, interconnected dataset of I.M.P.A.C.T.S, it offers a valuable tool for researchers, innovators, and thinkers aiming to push the boundaries of what's possible in their fields.
# Use Cases
## Universe/World Simulation
Use Llama-3-IMPACTS-2x8B-64k to simulate incredibly unique and diverse ecosystems and cosmic worlds. Example image generation (text-to-image):
**User Prompt:** 'Craft a detailed image generation prompt focused on visualizing an innovative scenario that integrates biomimicry, climate change adaptation, and astrobiology.'
```md
Image Generation Prompt:
Title: “Biomimicry on Kepler-62f: A Red Dwarf Oasis”
Description:
In the year 2154, humanity discovers a new Earth-like planet, Kepler-62f, orbiting a red dwarf star. This distant world is beset by extreme climate conditions, with scorching daytime temperatures and frigid nighttime temperatures. To thrive in this unforgiving environment, scientists apply biomimetic technologies developed on Earth to create a sustainable ecosystem.
Scene:
In the foreground, a sprawling cityscape stretches across the planet’s surface, its architecture inspired by nature’s own designs. Towering “Tree-Habitats” mimic the branching structures of terrestrial trees, providing shelter and habitat for various extraterrestrial species. Their trunks are covered in iridescent, solar-powered panels that generate energy while also filtering out harmful radiation from the red dwarf star.
In the distance, a vast network of “Coral-Reefs” sprawls across the planet’s surface, mimicking the intricate structures found on Earth’s coral reefs. These artificial ecosystems support an incredible array of alien marine life, including bioluminescent fish, towering kelp-like seaweed, and gelatinous sea creatures that drift through the water like terrestrial jellyfish.
Above the city, a series of “Cloud-Cities” float majestically, their translucent membranes reflecting the red dwarf star’s light. These floating metropolises are home to species adapted for life in the upper atmosphere, such as winged beings with iridescent feathers and gas-filled bodies that allow them to soar through the skies.
Key Features:
Biomimetic Architecture: Buildings and structures are designed to mimic natural forms, providing insulation, shelter, and habitat for various species.
Artificial Ecosystems: “Coral-Reefs” support marine life, while “Tree-Habitats” provide shelter and food sources for terrestrial species.
Energy Harvesting: Solar-powered panels on the Tree-Habitats generate energy, while wind turbines and tidal power generators supplement the planet’s energy needs.
Advanced Life Support Systems: Closed-loop systems recycle water, air, and waste, minimizing the impact of human activities on the planet’s fragile ecosystem.
Extraterrestrial Biodiversity: Various alien species thrive in this red dwarf oasis, from towering kelp-like seaweed to bioluminescent fish and winged beings adapted for life in the upper atmosphere.
Color Palette:
Warm oranges and yellows evoke the scorching daytime temperatures
Deep blues and purples represent the frigid nighttime conditions
Iridescent hues on the Tree-Habitats and Cloud-Cities reflect the planet’s unique radiation patterns
Style:
Blend of realistic and stylized elements, with a focus on showcasing the innovative biomimetic technologies and extraterrestrial biodiversity.
Incorporate subtle texture and gradient effects to convey the harsh climate conditions and the adaptability of life on Kepler-62f.
By visualizing this futuristic scenario, we can explore the possibilities of biomimicry as a solution for climate change adaptation and sustainability in extreme environments. The image should inspire viewers to consider the potential applications of nature-inspired technologies in our own struggle against environmental challenges and encourage a deeper appreciation for the diversity of life that exists – or could exist – beyond Earth.
```
---
<img src="https://cdn-uploads.huggingface.co/production/uploads/64740cf7485a7c8e1bd51ac9/m3GfS0TcOejD1V7AI5OwN.webp" width="500" height="500">
---
### Disaster Response Simulation
**Prompt:** "Simulate the impact of a Category 5 hurricane hitting the eastern coast of Florida in 2030, considering factors such as rising sea levels and urban density. Assess potential emergency responses and resource needs."
#### Example Response
**Response:**
"**Scenario:**
A powerful Category 5 hurricane, named "Hurricane Ana," makes landfall on September 15, 2030, along the eastern coast of Florida, near Miami. The storm brings with it catastrophic winds, torrential rainfall, and a massive storm surge due to rising sea levels.
**Storm Characteristics:**
* Wind Speeds: Up to 160 mph (257 km/h)
* Storm Surge: 10-15 feet (3-4.5 meters) above mean sea level
* Rainfall: 12-18 inches (30-45 cm) in the first 24 hours, with isolated areas receiving up to 24 inches (60 cm)
**Impacts:**
1. **Coastal Flooding:** Rising sea levels exacerbate the storm surge, causing widespread flooding and damage along the eastern coast of Florida, particularly in Miami-Dade, Broward, and Palm Beach counties.
2. **Urban Infrastructure:** The densely populated cities along the coast suffer significant damage to buildings, roads, and infrastructure, leading to disruptions in essential services like power, water, and communication.
3. **Displacement and Evacuations:** Over 1 million people are displaced or evacuated from their homes, with many seeking shelter in nearby inland areas or temporary facilities.
4. **Environmental Concerns:** The storm surge contaminates waterways, causing concerns about water quality and potential health risks for those affected.
5. **Economic Impact:** The estimated economic loss is around $100 billion, considering damage to infrastructure, businesses, and agricultural industries.
**Emergency Response:**
1. **Evacuation Efforts:** Emergency responders prioritize evacuations, focusing on vulnerable populations like the elderly, children, and those with disabilities. They also ensure that essential services like hospitals, fire stations, and emergency centers are evacuated.
2. **Search and Rescue Operations:** Teams conduct door-to-door searches for trapped individuals, using specialized equipment and resources to navigate flooded areas.
3. **Food, Water, and Medical Supplies:** Aid distributions prioritize the most affected communities, with a focus on providing essential supplies, shelter, and medical care.
4. **Power Restoration:** Utility companies work to restore power as quickly as possible, deploying crews from neighboring states and using advanced technologies like drones and satellite imaging to assess damage and optimize response efforts.
**Resource Needs:**
1. **Personnel:** Over 10,000 emergency responders, including firefighters, police officers, medical personnel, and National Guard troops, are deployed to support the response effort.
2. **Equipment and Supplies:** Critical resources include:
* Generators and fuel
* Food, water, and medical supplies
* Communication equipment (satellite phones, etc.)
* Heavy machinery for debris removal and infrastructure repair
* Shelter materials (tents, blankets, etc.)
3. **Funding:** Estimated costs exceed $500 million, requiring a significant allocation of federal, state, and local funds to support the response and recovery efforts.
4. **Logistical Support:** Coordination with neighboring states and national agencies is crucial for maintaining supply chains, transporting personnel and equipment, and sharing expertise.
**Challenges:**
1. **Infrastructure Overload:** The sheer scale of damage overwhelms local infrastructure, leading to delays in aid distribution and response efforts.
2. **Water Contamination:** Concerns about water quality hinder access to clean drinking water, exacerbating public health risks.
3. **Displacement and Shelter:** The large number of displaced individuals creates a significant shortage of available shelter options, straining resources and emergency services.
**Lessons Learned:**
1. **Preparedness is Key:** Prioritizing evacuation planning, infrastructure hardening, and disaster preparedness can significantly reduce the impact of such events.
2. **Collaboration is Crucial:** Effective coordination between local, state, and federal agencies, as well as with neighboring states and national organizations, is essential for a successful response.
3. **Adaptation to Rising Sea Levels:** The increasing threat of sea-level rise requires a shift in disaster preparedness strategies, focusing on resilient infrastructure design and adaptive management practices.
**Conclusion:**
The simulated impact of Hurricane Ana highlights the devastating consequences of a Category 5 hurricane hitting Florida's eastern coast, exacerbated by rising sea levels. The emergency response efforts require significant resources, personnel, and coordination to address the complex challenges posed by this disaster. As we move forward, it is essential to prioritize preparedness, collaboration, and adaptation to mitigate the effects of these events and protect vulnerable communities."
# Try it out yourself!
### Extraterrestrial Colony Planning
**Prompt:** "Design a sustainable human colony for Mars, focusing on water resource management, energy production, and habitat construction using local materials. Include potential environmental challenges and solutions."
### Environmental Policy Formulation
**Prompt:** "Evaluate the long-term ecological impacts of banning single-use plastics globally. Simulate the effects on marine life, pollution levels, and waste management systems over the next 50 years."
### Advanced Educational Tools
**Prompt:** "Create an interactive simulation that demonstrates the water cycle on Earth, including the effects of deforestation and urbanization on water availability and quality in major river basins."
### Interactive Storytelling
**Prompt:** "Generate a narrative where the user is a leader in a community facing severe drought conditions. Allow the user to make decisions about water usage, agricultural practices, and public policy, showing the consequences of each choice."
### Biodiversity Conservation Strategies
**Prompt:** "Develop a conservation strategy for the Amazon rainforest, focusing on mitigating the effects of deforestation and climate change. Simulate various scenarios involving local communities and global stakeholders."
### Interstellar Communication Simulation
**Prompt:** "Imagine a scenario where Earth receives a signal from a distant planet. Simulate a series of potential communications that could be exchanged, considering language barriers and the transmission delay over light-years."
### Bioengineering Solutions
**Prompt:** "Propose a bioengineering project to create microbial life forms that can detoxify plastic waste in the ocean. Describe the genetic traits these organisms would need and simulate the potential ecological impact."
### Cross-Planetary Impact Studies
**Prompt:** "Analyze how a supernova explosion in a neighboring star system could affect planetary systems in its vicinity, including potential impacts on Earth's magnetic field and atmosphere."
### Custom Scenario Development
**Prompt:** "Allow the user to create a custom scenario involving an unexpected volcanic eruption in Iceland. Let the user set parameters like the eruption's size, duration, and ash distribution, then simulate the global climate and air travel impacts."
These prompts are designed to maximize the utilization of the model's capabilities in various complex and interdisciplinary scenarios, making them useful for researchers, educators, policymakers, and enthusiasts interested in exploring these domains. | {"language": ["en"], "license": "mit", "library_name": "mlx", "tags": ["climate change", "biomimicry", "theoretical astrobiology", "environmental simulations", "predictive modeling", "life origins", "ecological impacts", "sustainable technologies", "cross-disciplinary learning", "artificial intelligence", "machine learning", "data integration", "complex systems", "scenario analysis", "speculative science", "universe exploration", "biodiversity", "planetary studies", "innovation in science", "role playing scenarios"], "datasets": ["Severian/IMPACTS"], "pipeline_tag": "text-generation"} | Severian/Llama-3-IMPACTS-2x8B-64k-MLX | null | [
"mlx",
"mixtral",
"climate change",
"biomimicry",
"theoretical astrobiology",
"environmental simulations",
"predictive modeling",
"life origins",
"ecological impacts",
"sustainable technologies",
"cross-disciplinary learning",
"artificial intelligence",
"machine learning",
"data integration",
"complex systems",
"scenario analysis",
"speculative science",
"universe exploration",
"biodiversity",
"planetary studies",
"innovation in science",
"role playing scenarios",
"text-generation",
"conversational",
"en",
"dataset:Severian/IMPACTS",
"license:mit",
"region:us"
] | null | 2024-05-02T10:33:54+00:00 | [] | [
"en"
] | TAGS
#mlx #mixtral #climate change #biomimicry #theoretical astrobiology #environmental simulations #predictive modeling #life origins #ecological impacts #sustainable technologies #cross-disciplinary learning #artificial intelligence #machine learning #data integration #complex systems #scenario analysis #speculative science #universe exploration #biodiversity #planetary studies #innovation in science #role playing scenarios #text-generation #conversational #en #dataset-Severian/IMPACTS #license-mit #region-us
|
# Llama-3-IMPACTS-2x8B-64k-MLX
<img src="URL width="500" height="500">
---
Designed for Advanced Problem-Solving Across Interconnected Domains of Biomimicry, Climate Change, and Astrobiology
The 'Llama-3-IMPACTS-2x8B-64k-MLX' model is a cutting-edge large language model trained on the I.M.P.A.C.T.S dataset, which encompasses scenarios from biomimicry, climate change, and theoretical astrobiology. This model has been specifically tailored to generate innovative solutions and insights for both Earth and potential extraterrestrial environments, reflecting key themes of resilience, sustainability, and the interconnectedness of life across the universe.
## Model Details
### Description
- Model name: 'Llama-3-IMPACTS-2x8B-64k-MLX'
- Developer: Severian
- Version: 1.0
- License: MIT
### Training Data
The model was trained on a subset of the I.M.P.A.C.T. dataset, utilizing 35,000 carefully curated examples that include detailed scenarios involving climate adaptation, biomimetic applications, and the potential for life in varying cosmic conditions.
### Model Architecture
- Type: Llama-3
- Parameters: 8 billion
- MoE: 2 Experts
- Training:
- Epochs: 1 (35K Examples)
- R = 64
- Alpha = 128
- Lr = 1e-7
- Context Limit: 64K
## Intended Uses
This model is intended for use in applications that require deep, interdisciplinary understanding and the generation of novel insights within the realms of environmental science, synthetic biology, space exploration, and sustainability studies. Its capabilities make it ideal for:
- Research and academic studies aiming to explore complex scenarios involving ecological and astrobiological phenomena.
- Organizations looking to innovate in the fields of climate resilience and biomimicry.
- Creative problem-solving in contexts where conventional approaches are insufficient.
## How to Use This Model
The model can be loaded and used in various natural language processing tasks that require nuanced understanding and creative output. Here is a basic example of how to load and use the model using the MLX library:
## Limitations and Biases
While the 'Llama-3-IMPACTS-2x8B-64k-MLX' model is designed to be a powerful tool for generating insightful content, it inherits limitations from its training data, which, though extensive, may not capture all possible scenarios or biases. Users should be aware of these limitations and consider them when interpreting the model's outputs, especially in decision-making contexts.
## Model Performance
Initial tests indicate that the model performs exceptionally well in tasks that involve complex reasoning and generating innovative solutions based on the scenarios presented in the I.M.P.A.C.T.S dataset. Further evaluation and fine-tuning may be required to optimize performance for specific applications.
The 'Llama-3-IMPACTS-2x8B-64k-MLX' model represents an avenue that AI can use for exploring and solving complex problems across multiple domains. By leveraging the rich, interconnected dataset of I.M.P.A.C.T.S, it offers a valuable tool for researchers, innovators, and thinkers aiming to push the boundaries of what's possible in their fields.
# Use Cases
## Universe/World Simulation
Use Llama-3-IMPACTS-2x8B-64k to simulate incredibly unique and diverse ecosystems and cosmic worlds. Example image generation (text-to-image):
User Prompt: 'Craft a detailed image generation prompt focused on visualizing an innovative scenario that integrates biomimicry, climate change adaptation, and astrobiology.'
---
<img src="URL width="500" height="500">
---
### Disaster Response Simulation
Prompt: "Simulate the impact of a Category 5 hurricane hitting the eastern coast of Florida in 2030, considering factors such as rising sea levels and urban density. Assess potential emergency responses and resource needs."
#### Example Response
Response:
"Scenario:
A powerful Category 5 hurricane, named "Hurricane Ana," makes landfall on September 15, 2030, along the eastern coast of Florida, near Miami. The storm brings with it catastrophic winds, torrential rainfall, and a massive storm surge due to rising sea levels.
Storm Characteristics:
* Wind Speeds: Up to 160 mph (257 km/h)
* Storm Surge: 10-15 feet (3-4.5 meters) above mean sea level
* Rainfall: 12-18 inches (30-45 cm) in the first 24 hours, with isolated areas receiving up to 24 inches (60 cm)
Impacts:
1. Coastal Flooding: Rising sea levels exacerbate the storm surge, causing widespread flooding and damage along the eastern coast of Florida, particularly in Miami-Dade, Broward, and Palm Beach counties.
2. Urban Infrastructure: The densely populated cities along the coast suffer significant damage to buildings, roads, and infrastructure, leading to disruptions in essential services like power, water, and communication.
3. Displacement and Evacuations: Over 1 million people are displaced or evacuated from their homes, with many seeking shelter in nearby inland areas or temporary facilities.
4. Environmental Concerns: The storm surge contaminates waterways, causing concerns about water quality and potential health risks for those affected.
5. Economic Impact: The estimated economic loss is around $100 billion, considering damage to infrastructure, businesses, and agricultural industries.
Emergency Response:
1. Evacuation Efforts: Emergency responders prioritize evacuations, focusing on vulnerable populations like the elderly, children, and those with disabilities. They also ensure that essential services like hospitals, fire stations, and emergency centers are evacuated.
2. Search and Rescue Operations: Teams conduct door-to-door searches for trapped individuals, using specialized equipment and resources to navigate flooded areas.
3. Food, Water, and Medical Supplies: Aid distributions prioritize the most affected communities, with a focus on providing essential supplies, shelter, and medical care.
4. Power Restoration: Utility companies work to restore power as quickly as possible, deploying crews from neighboring states and using advanced technologies like drones and satellite imaging to assess damage and optimize response efforts.
Resource Needs:
1. Personnel: Over 10,000 emergency responders, including firefighters, police officers, medical personnel, and National Guard troops, are deployed to support the response effort.
2. Equipment and Supplies: Critical resources include:
* Generators and fuel
* Food, water, and medical supplies
* Communication equipment (satellite phones, etc.)
* Heavy machinery for debris removal and infrastructure repair
* Shelter materials (tents, blankets, etc.)
3. Funding: Estimated costs exceed $500 million, requiring a significant allocation of federal, state, and local funds to support the response and recovery efforts.
4. Logistical Support: Coordination with neighboring states and national agencies is crucial for maintaining supply chains, transporting personnel and equipment, and sharing expertise.
Challenges:
1. Infrastructure Overload: The sheer scale of damage overwhelms local infrastructure, leading to delays in aid distribution and response efforts.
2. Water Contamination: Concerns about water quality hinder access to clean drinking water, exacerbating public health risks.
3. Displacement and Shelter: The large number of displaced individuals creates a significant shortage of available shelter options, straining resources and emergency services.
Lessons Learned:
1. Preparedness is Key: Prioritizing evacuation planning, infrastructure hardening, and disaster preparedness can significantly reduce the impact of such events.
2. Collaboration is Crucial: Effective coordination between local, state, and federal agencies, as well as with neighboring states and national organizations, is essential for a successful response.
3. Adaptation to Rising Sea Levels: The increasing threat of sea-level rise requires a shift in disaster preparedness strategies, focusing on resilient infrastructure design and adaptive management practices.
Conclusion:
The simulated impact of Hurricane Ana highlights the devastating consequences of a Category 5 hurricane hitting Florida's eastern coast, exacerbated by rising sea levels. The emergency response efforts require significant resources, personnel, and coordination to address the complex challenges posed by this disaster. As we move forward, it is essential to prioritize preparedness, collaboration, and adaptation to mitigate the effects of these events and protect vulnerable communities."
# Try it out yourself!
### Extraterrestrial Colony Planning
Prompt: "Design a sustainable human colony for Mars, focusing on water resource management, energy production, and habitat construction using local materials. Include potential environmental challenges and solutions."
### Environmental Policy Formulation
Prompt: "Evaluate the long-term ecological impacts of banning single-use plastics globally. Simulate the effects on marine life, pollution levels, and waste management systems over the next 50 years."
### Advanced Educational Tools
Prompt: "Create an interactive simulation that demonstrates the water cycle on Earth, including the effects of deforestation and urbanization on water availability and quality in major river basins."
### Interactive Storytelling
Prompt: "Generate a narrative where the user is a leader in a community facing severe drought conditions. Allow the user to make decisions about water usage, agricultural practices, and public policy, showing the consequences of each choice."
### Biodiversity Conservation Strategies
Prompt: "Develop a conservation strategy for the Amazon rainforest, focusing on mitigating the effects of deforestation and climate change. Simulate various scenarios involving local communities and global stakeholders."
### Interstellar Communication Simulation
Prompt: "Imagine a scenario where Earth receives a signal from a distant planet. Simulate a series of potential communications that could be exchanged, considering language barriers and the transmission delay over light-years."
### Bioengineering Solutions
Prompt: "Propose a bioengineering project to create microbial life forms that can detoxify plastic waste in the ocean. Describe the genetic traits these organisms would need and simulate the potential ecological impact."
### Cross-Planetary Impact Studies
Prompt: "Analyze how a supernova explosion in a neighboring star system could affect planetary systems in its vicinity, including potential impacts on Earth's magnetic field and atmosphere."
### Custom Scenario Development
Prompt: "Allow the user to create a custom scenario involving an unexpected volcanic eruption in Iceland. Let the user set parameters like the eruption's size, duration, and ash distribution, then simulate the global climate and air travel impacts."
These prompts are designed to maximize the utilization of the model's capabilities in various complex and interdisciplinary scenarios, making them useful for researchers, educators, policymakers, and enthusiasts interested in exploring these domains. | [
"# Llama-3-IMPACTS-2x8B-64k-MLX\n\n<img src=\"URL width=\"500\" height=\"500\">\n\n---\n\nDesigned for Advanced Problem-Solving Across Interconnected Domains of Biomimicry, Climate Change, and Astrobiology\n\nThe 'Llama-3-IMPACTS-2x8B-64k-MLX' model is a cutting-edge large language model trained on the I.M.P.A.C.T.S dataset, which encompasses scenarios from biomimicry, climate change, and theoretical astrobiology. This model has been specifically tailored to generate innovative solutions and insights for both Earth and potential extraterrestrial environments, reflecting key themes of resilience, sustainability, and the interconnectedness of life across the universe.",
"## Model Details",
"### Description\n\n- Model name: 'Llama-3-IMPACTS-2x8B-64k-MLX'\n- Developer: Severian\n- Version: 1.0\n- License: MIT",
"### Training Data\n\nThe model was trained on a subset of the I.M.P.A.C.T. dataset, utilizing 35,000 carefully curated examples that include detailed scenarios involving climate adaptation, biomimetic applications, and the potential for life in varying cosmic conditions.",
"### Model Architecture\n\n- Type: Llama-3\n- Parameters: 8 billion\n- MoE: 2 Experts\n- Training:\n- Epochs: 1 (35K Examples)\n- R = 64\n- Alpha = 128\n- Lr = 1e-7\n- Context Limit: 64K",
"## Intended Uses\n\nThis model is intended for use in applications that require deep, interdisciplinary understanding and the generation of novel insights within the realms of environmental science, synthetic biology, space exploration, and sustainability studies. Its capabilities make it ideal for:\n- Research and academic studies aiming to explore complex scenarios involving ecological and astrobiological phenomena.\n- Organizations looking to innovate in the fields of climate resilience and biomimicry.\n- Creative problem-solving in contexts where conventional approaches are insufficient.",
"## How to Use This Model\n\nThe model can be loaded and used in various natural language processing tasks that require nuanced understanding and creative output. Here is a basic example of how to load and use the model using the MLX library:",
"## Limitations and Biases\n\nWhile the 'Llama-3-IMPACTS-2x8B-64k-MLX' model is designed to be a powerful tool for generating insightful content, it inherits limitations from its training data, which, though extensive, may not capture all possible scenarios or biases. Users should be aware of these limitations and consider them when interpreting the model's outputs, especially in decision-making contexts.",
"## Model Performance\n\nInitial tests indicate that the model performs exceptionally well in tasks that involve complex reasoning and generating innovative solutions based on the scenarios presented in the I.M.P.A.C.T.S dataset. Further evaluation and fine-tuning may be required to optimize performance for specific applications.\n\nThe 'Llama-3-IMPACTS-2x8B-64k-MLX' model represents an avenue that AI can use for exploring and solving complex problems across multiple domains. By leveraging the rich, interconnected dataset of I.M.P.A.C.T.S, it offers a valuable tool for researchers, innovators, and thinkers aiming to push the boundaries of what's possible in their fields.",
"# Use Cases",
"## Universe/World Simulation\n\nUse Llama-3-IMPACTS-2x8B-64k to simulate incredibly unique and diverse ecosystems and cosmic worlds. Example image generation (text-to-image):\n\nUser Prompt: 'Craft a detailed image generation prompt focused on visualizing an innovative scenario that integrates biomimicry, climate change adaptation, and astrobiology.'\n\n\n---\n<img src=\"URL width=\"500\" height=\"500\">\n---",
"### Disaster Response Simulation\n\nPrompt: \"Simulate the impact of a Category 5 hurricane hitting the eastern coast of Florida in 2030, considering factors such as rising sea levels and urban density. Assess potential emergency responses and resource needs.\"",
"#### Example Response\nResponse: \n\n\"Scenario:\n\nA powerful Category 5 hurricane, named \"Hurricane Ana,\" makes landfall on September 15, 2030, along the eastern coast of Florida, near Miami. The storm brings with it catastrophic winds, torrential rainfall, and a massive storm surge due to rising sea levels.\n\nStorm Characteristics:\n\n* Wind Speeds: Up to 160 mph (257 km/h)\n* Storm Surge: 10-15 feet (3-4.5 meters) above mean sea level\n* Rainfall: 12-18 inches (30-45 cm) in the first 24 hours, with isolated areas receiving up to 24 inches (60 cm)\n\nImpacts:\n\n1. Coastal Flooding: Rising sea levels exacerbate the storm surge, causing widespread flooding and damage along the eastern coast of Florida, particularly in Miami-Dade, Broward, and Palm Beach counties.\n2. Urban Infrastructure: The densely populated cities along the coast suffer significant damage to buildings, roads, and infrastructure, leading to disruptions in essential services like power, water, and communication.\n3. Displacement and Evacuations: Over 1 million people are displaced or evacuated from their homes, with many seeking shelter in nearby inland areas or temporary facilities.\n4. Environmental Concerns: The storm surge contaminates waterways, causing concerns about water quality and potential health risks for those affected.\n5. Economic Impact: The estimated economic loss is around $100 billion, considering damage to infrastructure, businesses, and agricultural industries.\n\nEmergency Response:\n\n1. Evacuation Efforts: Emergency responders prioritize evacuations, focusing on vulnerable populations like the elderly, children, and those with disabilities. They also ensure that essential services like hospitals, fire stations, and emergency centers are evacuated.\n2. Search and Rescue Operations: Teams conduct door-to-door searches for trapped individuals, using specialized equipment and resources to navigate flooded areas.\n3. Food, Water, and Medical Supplies: Aid distributions prioritize the most affected communities, with a focus on providing essential supplies, shelter, and medical care.\n4. Power Restoration: Utility companies work to restore power as quickly as possible, deploying crews from neighboring states and using advanced technologies like drones and satellite imaging to assess damage and optimize response efforts.\n\nResource Needs:\n\n1. Personnel: Over 10,000 emergency responders, including firefighters, police officers, medical personnel, and National Guard troops, are deployed to support the response effort.\n2. Equipment and Supplies: Critical resources include:\n\t* Generators and fuel\n\t* Food, water, and medical supplies\n\t* Communication equipment (satellite phones, etc.)\n\t* Heavy machinery for debris removal and infrastructure repair\n\t* Shelter materials (tents, blankets, etc.)\n3. Funding: Estimated costs exceed $500 million, requiring a significant allocation of federal, state, and local funds to support the response and recovery efforts.\n4. Logistical Support: Coordination with neighboring states and national agencies is crucial for maintaining supply chains, transporting personnel and equipment, and sharing expertise.\n\nChallenges:\n\n1. Infrastructure Overload: The sheer scale of damage overwhelms local infrastructure, leading to delays in aid distribution and response efforts.\n2. Water Contamination: Concerns about water quality hinder access to clean drinking water, exacerbating public health risks.\n3. Displacement and Shelter: The large number of displaced individuals creates a significant shortage of available shelter options, straining resources and emergency services.\n\nLessons Learned:\n\n1. Preparedness is Key: Prioritizing evacuation planning, infrastructure hardening, and disaster preparedness can significantly reduce the impact of such events.\n2. Collaboration is Crucial: Effective coordination between local, state, and federal agencies, as well as with neighboring states and national organizations, is essential for a successful response.\n3. Adaptation to Rising Sea Levels: The increasing threat of sea-level rise requires a shift in disaster preparedness strategies, focusing on resilient infrastructure design and adaptive management practices.\n\nConclusion:\n\nThe simulated impact of Hurricane Ana highlights the devastating consequences of a Category 5 hurricane hitting Florida's eastern coast, exacerbated by rising sea levels. The emergency response efforts require significant resources, personnel, and coordination to address the complex challenges posed by this disaster. As we move forward, it is essential to prioritize preparedness, collaboration, and adaptation to mitigate the effects of these events and protect vulnerable communities.\"",
"# Try it out yourself!",
"### Extraterrestrial Colony Planning\nPrompt: \"Design a sustainable human colony for Mars, focusing on water resource management, energy production, and habitat construction using local materials. Include potential environmental challenges and solutions.\"",
"### Environmental Policy Formulation\nPrompt: \"Evaluate the long-term ecological impacts of banning single-use plastics globally. Simulate the effects on marine life, pollution levels, and waste management systems over the next 50 years.\"",
"### Advanced Educational Tools\nPrompt: \"Create an interactive simulation that demonstrates the water cycle on Earth, including the effects of deforestation and urbanization on water availability and quality in major river basins.\"",
"### Interactive Storytelling\nPrompt: \"Generate a narrative where the user is a leader in a community facing severe drought conditions. Allow the user to make decisions about water usage, agricultural practices, and public policy, showing the consequences of each choice.\"",
"### Biodiversity Conservation Strategies\nPrompt: \"Develop a conservation strategy for the Amazon rainforest, focusing on mitigating the effects of deforestation and climate change. Simulate various scenarios involving local communities and global stakeholders.\"",
"### Interstellar Communication Simulation\nPrompt: \"Imagine a scenario where Earth receives a signal from a distant planet. Simulate a series of potential communications that could be exchanged, considering language barriers and the transmission delay over light-years.\"",
"### Bioengineering Solutions\nPrompt: \"Propose a bioengineering project to create microbial life forms that can detoxify plastic waste in the ocean. Describe the genetic traits these organisms would need and simulate the potential ecological impact.\"",
"### Cross-Planetary Impact Studies\nPrompt: \"Analyze how a supernova explosion in a neighboring star system could affect planetary systems in its vicinity, including potential impacts on Earth's magnetic field and atmosphere.\"",
"### Custom Scenario Development\nPrompt: \"Allow the user to create a custom scenario involving an unexpected volcanic eruption in Iceland. Let the user set parameters like the eruption's size, duration, and ash distribution, then simulate the global climate and air travel impacts.\"\n\nThese prompts are designed to maximize the utilization of the model's capabilities in various complex and interdisciplinary scenarios, making them useful for researchers, educators, policymakers, and enthusiasts interested in exploring these domains."
] | [
"TAGS\n#mlx #mixtral #climate change #biomimicry #theoretical astrobiology #environmental simulations #predictive modeling #life origins #ecological impacts #sustainable technologies #cross-disciplinary learning #artificial intelligence #machine learning #data integration #complex systems #scenario analysis #speculative science #universe exploration #biodiversity #planetary studies #innovation in science #role playing scenarios #text-generation #conversational #en #dataset-Severian/IMPACTS #license-mit #region-us \n",
"# Llama-3-IMPACTS-2x8B-64k-MLX\n\n<img src=\"URL width=\"500\" height=\"500\">\n\n---\n\nDesigned for Advanced Problem-Solving Across Interconnected Domains of Biomimicry, Climate Change, and Astrobiology\n\nThe 'Llama-3-IMPACTS-2x8B-64k-MLX' model is a cutting-edge large language model trained on the I.M.P.A.C.T.S dataset, which encompasses scenarios from biomimicry, climate change, and theoretical astrobiology. This model has been specifically tailored to generate innovative solutions and insights for both Earth and potential extraterrestrial environments, reflecting key themes of resilience, sustainability, and the interconnectedness of life across the universe.",
"## Model Details",
"### Description\n\n- Model name: 'Llama-3-IMPACTS-2x8B-64k-MLX'\n- Developer: Severian\n- Version: 1.0\n- License: MIT",
"### Training Data\n\nThe model was trained on a subset of the I.M.P.A.C.T. dataset, utilizing 35,000 carefully curated examples that include detailed scenarios involving climate adaptation, biomimetic applications, and the potential for life in varying cosmic conditions.",
"### Model Architecture\n\n- Type: Llama-3\n- Parameters: 8 billion\n- MoE: 2 Experts\n- Training:\n- Epochs: 1 (35K Examples)\n- R = 64\n- Alpha = 128\n- Lr = 1e-7\n- Context Limit: 64K",
"## Intended Uses\n\nThis model is intended for use in applications that require deep, interdisciplinary understanding and the generation of novel insights within the realms of environmental science, synthetic biology, space exploration, and sustainability studies. Its capabilities make it ideal for:\n- Research and academic studies aiming to explore complex scenarios involving ecological and astrobiological phenomena.\n- Organizations looking to innovate in the fields of climate resilience and biomimicry.\n- Creative problem-solving in contexts where conventional approaches are insufficient.",
"## How to Use This Model\n\nThe model can be loaded and used in various natural language processing tasks that require nuanced understanding and creative output. Here is a basic example of how to load and use the model using the MLX library:",
"## Limitations and Biases\n\nWhile the 'Llama-3-IMPACTS-2x8B-64k-MLX' model is designed to be a powerful tool for generating insightful content, it inherits limitations from its training data, which, though extensive, may not capture all possible scenarios or biases. Users should be aware of these limitations and consider them when interpreting the model's outputs, especially in decision-making contexts.",
"## Model Performance\n\nInitial tests indicate that the model performs exceptionally well in tasks that involve complex reasoning and generating innovative solutions based on the scenarios presented in the I.M.P.A.C.T.S dataset. Further evaluation and fine-tuning may be required to optimize performance for specific applications.\n\nThe 'Llama-3-IMPACTS-2x8B-64k-MLX' model represents an avenue that AI can use for exploring and solving complex problems across multiple domains. By leveraging the rich, interconnected dataset of I.M.P.A.C.T.S, it offers a valuable tool for researchers, innovators, and thinkers aiming to push the boundaries of what's possible in their fields.",
"# Use Cases",
"## Universe/World Simulation\n\nUse Llama-3-IMPACTS-2x8B-64k to simulate incredibly unique and diverse ecosystems and cosmic worlds. Example image generation (text-to-image):\n\nUser Prompt: 'Craft a detailed image generation prompt focused on visualizing an innovative scenario that integrates biomimicry, climate change adaptation, and astrobiology.'\n\n\n---\n<img src=\"URL width=\"500\" height=\"500\">\n---",
"### Disaster Response Simulation\n\nPrompt: \"Simulate the impact of a Category 5 hurricane hitting the eastern coast of Florida in 2030, considering factors such as rising sea levels and urban density. Assess potential emergency responses and resource needs.\"",
"#### Example Response\nResponse: \n\n\"Scenario:\n\nA powerful Category 5 hurricane, named \"Hurricane Ana,\" makes landfall on September 15, 2030, along the eastern coast of Florida, near Miami. The storm brings with it catastrophic winds, torrential rainfall, and a massive storm surge due to rising sea levels.\n\nStorm Characteristics:\n\n* Wind Speeds: Up to 160 mph (257 km/h)\n* Storm Surge: 10-15 feet (3-4.5 meters) above mean sea level\n* Rainfall: 12-18 inches (30-45 cm) in the first 24 hours, with isolated areas receiving up to 24 inches (60 cm)\n\nImpacts:\n\n1. Coastal Flooding: Rising sea levels exacerbate the storm surge, causing widespread flooding and damage along the eastern coast of Florida, particularly in Miami-Dade, Broward, and Palm Beach counties.\n2. Urban Infrastructure: The densely populated cities along the coast suffer significant damage to buildings, roads, and infrastructure, leading to disruptions in essential services like power, water, and communication.\n3. Displacement and Evacuations: Over 1 million people are displaced or evacuated from their homes, with many seeking shelter in nearby inland areas or temporary facilities.\n4. Environmental Concerns: The storm surge contaminates waterways, causing concerns about water quality and potential health risks for those affected.\n5. Economic Impact: The estimated economic loss is around $100 billion, considering damage to infrastructure, businesses, and agricultural industries.\n\nEmergency Response:\n\n1. Evacuation Efforts: Emergency responders prioritize evacuations, focusing on vulnerable populations like the elderly, children, and those with disabilities. They also ensure that essential services like hospitals, fire stations, and emergency centers are evacuated.\n2. Search and Rescue Operations: Teams conduct door-to-door searches for trapped individuals, using specialized equipment and resources to navigate flooded areas.\n3. Food, Water, and Medical Supplies: Aid distributions prioritize the most affected communities, with a focus on providing essential supplies, shelter, and medical care.\n4. Power Restoration: Utility companies work to restore power as quickly as possible, deploying crews from neighboring states and using advanced technologies like drones and satellite imaging to assess damage and optimize response efforts.\n\nResource Needs:\n\n1. Personnel: Over 10,000 emergency responders, including firefighters, police officers, medical personnel, and National Guard troops, are deployed to support the response effort.\n2. Equipment and Supplies: Critical resources include:\n\t* Generators and fuel\n\t* Food, water, and medical supplies\n\t* Communication equipment (satellite phones, etc.)\n\t* Heavy machinery for debris removal and infrastructure repair\n\t* Shelter materials (tents, blankets, etc.)\n3. Funding: Estimated costs exceed $500 million, requiring a significant allocation of federal, state, and local funds to support the response and recovery efforts.\n4. Logistical Support: Coordination with neighboring states and national agencies is crucial for maintaining supply chains, transporting personnel and equipment, and sharing expertise.\n\nChallenges:\n\n1. Infrastructure Overload: The sheer scale of damage overwhelms local infrastructure, leading to delays in aid distribution and response efforts.\n2. Water Contamination: Concerns about water quality hinder access to clean drinking water, exacerbating public health risks.\n3. Displacement and Shelter: The large number of displaced individuals creates a significant shortage of available shelter options, straining resources and emergency services.\n\nLessons Learned:\n\n1. Preparedness is Key: Prioritizing evacuation planning, infrastructure hardening, and disaster preparedness can significantly reduce the impact of such events.\n2. Collaboration is Crucial: Effective coordination between local, state, and federal agencies, as well as with neighboring states and national organizations, is essential for a successful response.\n3. Adaptation to Rising Sea Levels: The increasing threat of sea-level rise requires a shift in disaster preparedness strategies, focusing on resilient infrastructure design and adaptive management practices.\n\nConclusion:\n\nThe simulated impact of Hurricane Ana highlights the devastating consequences of a Category 5 hurricane hitting Florida's eastern coast, exacerbated by rising sea levels. The emergency response efforts require significant resources, personnel, and coordination to address the complex challenges posed by this disaster. As we move forward, it is essential to prioritize preparedness, collaboration, and adaptation to mitigate the effects of these events and protect vulnerable communities.\"",
"# Try it out yourself!",
"### Extraterrestrial Colony Planning\nPrompt: \"Design a sustainable human colony for Mars, focusing on water resource management, energy production, and habitat construction using local materials. Include potential environmental challenges and solutions.\"",
"### Environmental Policy Formulation\nPrompt: \"Evaluate the long-term ecological impacts of banning single-use plastics globally. Simulate the effects on marine life, pollution levels, and waste management systems over the next 50 years.\"",
"### Advanced Educational Tools\nPrompt: \"Create an interactive simulation that demonstrates the water cycle on Earth, including the effects of deforestation and urbanization on water availability and quality in major river basins.\"",
"### Interactive Storytelling\nPrompt: \"Generate a narrative where the user is a leader in a community facing severe drought conditions. Allow the user to make decisions about water usage, agricultural practices, and public policy, showing the consequences of each choice.\"",
"### Biodiversity Conservation Strategies\nPrompt: \"Develop a conservation strategy for the Amazon rainforest, focusing on mitigating the effects of deforestation and climate change. Simulate various scenarios involving local communities and global stakeholders.\"",
"### Interstellar Communication Simulation\nPrompt: \"Imagine a scenario where Earth receives a signal from a distant planet. Simulate a series of potential communications that could be exchanged, considering language barriers and the transmission delay over light-years.\"",
"### Bioengineering Solutions\nPrompt: \"Propose a bioengineering project to create microbial life forms that can detoxify plastic waste in the ocean. Describe the genetic traits these organisms would need and simulate the potential ecological impact.\"",
"### Cross-Planetary Impact Studies\nPrompt: \"Analyze how a supernova explosion in a neighboring star system could affect planetary systems in its vicinity, including potential impacts on Earth's magnetic field and atmosphere.\"",
"### Custom Scenario Development\nPrompt: \"Allow the user to create a custom scenario involving an unexpected volcanic eruption in Iceland. Let the user set parameters like the eruption's size, duration, and ash distribution, then simulate the global climate and air travel impacts.\"\n\nThese prompts are designed to maximize the utilization of the model's capabilities in various complex and interdisciplinary scenarios, making them useful for researchers, educators, policymakers, and enthusiasts interested in exploring these domains."
] | [
100,
172,
4,
43,
60,
57,
100,
49,
90,
155,
3,
103,
47,
874,
6,
44,
45,
41,
50,
43,
47,
51,
43,
95
] | [
"TAGS\n#mlx #mixtral #climate change #biomimicry #theoretical astrobiology #environmental simulations #predictive modeling #life origins #ecological impacts #sustainable technologies #cross-disciplinary learning #artificial intelligence #machine learning #data integration #complex systems #scenario analysis #speculative science #universe exploration #biodiversity #planetary studies #innovation in science #role playing scenarios #text-generation #conversational #en #dataset-Severian/IMPACTS #license-mit #region-us \n# Llama-3-IMPACTS-2x8B-64k-MLX\n\n<img src=\"URL width=\"500\" height=\"500\">\n\n---\n\nDesigned for Advanced Problem-Solving Across Interconnected Domains of Biomimicry, Climate Change, and Astrobiology\n\nThe 'Llama-3-IMPACTS-2x8B-64k-MLX' model is a cutting-edge large language model trained on the I.M.P.A.C.T.S dataset, which encompasses scenarios from biomimicry, climate change, and theoretical astrobiology. This model has been specifically tailored to generate innovative solutions and insights for both Earth and potential extraterrestrial environments, reflecting key themes of resilience, sustainability, and the interconnectedness of life across the universe.## Model Details### Description\n\n- Model name: 'Llama-3-IMPACTS-2x8B-64k-MLX'\n- Developer: Severian\n- Version: 1.0\n- License: MIT### Training Data\n\nThe model was trained on a subset of the I.M.P.A.C.T. dataset, utilizing 35,000 carefully curated examples that include detailed scenarios involving climate adaptation, biomimetic applications, and the potential for life in varying cosmic conditions.### Model Architecture\n\n- Type: Llama-3\n- Parameters: 8 billion\n- MoE: 2 Experts\n- Training:\n- Epochs: 1 (35K Examples)\n- R = 64\n- Alpha = 128\n- Lr = 1e-7\n- Context Limit: 64K## Intended Uses\n\nThis model is intended for use in applications that require deep, interdisciplinary understanding and the generation of novel insights within the realms of environmental science, synthetic biology, space exploration, and sustainability studies. Its capabilities make it ideal for:\n- Research and academic studies aiming to explore complex scenarios involving ecological and astrobiological phenomena.\n- Organizations looking to innovate in the fields of climate resilience and biomimicry.\n- Creative problem-solving in contexts where conventional approaches are insufficient.## How to Use This Model\n\nThe model can be loaded and used in various natural language processing tasks that require nuanced understanding and creative output. Here is a basic example of how to load and use the model using the MLX library:## Limitations and Biases\n\nWhile the 'Llama-3-IMPACTS-2x8B-64k-MLX' model is designed to be a powerful tool for generating insightful content, it inherits limitations from its training data, which, though extensive, may not capture all possible scenarios or biases. Users should be aware of these limitations and consider them when interpreting the model's outputs, especially in decision-making contexts.## Model Performance\n\nInitial tests indicate that the model performs exceptionally well in tasks that involve complex reasoning and generating innovative solutions based on the scenarios presented in the I.M.P.A.C.T.S dataset. Further evaluation and fine-tuning may be required to optimize performance for specific applications.\n\nThe 'Llama-3-IMPACTS-2x8B-64k-MLX' model represents an avenue that AI can use for exploring and solving complex problems across multiple domains. By leveraging the rich, interconnected dataset of I.M.P.A.C.T.S, it offers a valuable tool for researchers, innovators, and thinkers aiming to push the boundaries of what's possible in their fields.# Use Cases## Universe/World Simulation\n\nUse Llama-3-IMPACTS-2x8B-64k to simulate incredibly unique and diverse ecosystems and cosmic worlds. Example image generation (text-to-image):\n\nUser Prompt: 'Craft a detailed image generation prompt focused on visualizing an innovative scenario that integrates biomimicry, climate change adaptation, and astrobiology.'\n\n\n---\n<img src=\"URL width=\"500\" height=\"500\">\n---### Disaster Response Simulation\n\nPrompt: \"Simulate the impact of a Category 5 hurricane hitting the eastern coast of Florida in 2030, considering factors such as rising sea levels and urban density. Assess potential emergency responses and resource needs.\"#### Example Response\nResponse: \n\n\"Scenario:\n\nA powerful Category 5 hurricane, named \"Hurricane Ana,\" makes landfall on September 15, 2030, along the eastern coast of Florida, near Miami. The storm brings with it catastrophic winds, torrential rainfall, and a massive storm surge due to rising sea levels.\n\nStorm Characteristics:\n\n* Wind Speeds: Up to 160 mph (257 km/h)\n* Storm Surge: 10-15 feet (3-4.5 meters) above mean sea level\n* Rainfall: 12-18 inches (30-45 cm) in the first 24 hours, with isolated areas receiving up to 24 inches (60 cm)\n\nImpacts:\n\n1. Coastal Flooding: Rising sea levels exacerbate the storm surge, causing widespread flooding and damage along the eastern coast of Florida, particularly in Miami-Dade, Broward, and Palm Beach counties.\n2. Urban Infrastructure: The densely populated cities along the coast suffer significant damage to buildings, roads, and infrastructure, leading to disruptions in essential services like power, water, and communication.\n3. Displacement and Evacuations: Over 1 million people are displaced or evacuated from their homes, with many seeking shelter in nearby inland areas or temporary facilities.\n4. Environmental Concerns: The storm surge contaminates waterways, causing concerns about water quality and potential health risks for those affected.\n5. Economic Impact: The estimated economic loss is around $100 billion, considering damage to infrastructure, businesses, and agricultural industries.\n\nEmergency Response:\n\n1. Evacuation Efforts: Emergency responders prioritize evacuations, focusing on vulnerable populations like the elderly, children, and those with disabilities. They also ensure that essential services like hospitals, fire stations, and emergency centers are evacuated.\n2. Search and Rescue Operations: Teams conduct door-to-door searches for trapped individuals, using specialized equipment and resources to navigate flooded areas.\n3. Food, Water, and Medical Supplies: Aid distributions prioritize the most affected communities, with a focus on providing essential supplies, shelter, and medical care.\n4. Power Restoration: Utility companies work to restore power as quickly as possible, deploying crews from neighboring states and using advanced technologies like drones and satellite imaging to assess damage and optimize response efforts.\n\nResource Needs:\n\n1. Personnel: Over 10,000 emergency responders, including firefighters, police officers, medical personnel, and National Guard troops, are deployed to support the response effort.\n2. Equipment and Supplies: Critical resources include:\n\t* Generators and fuel\n\t* Food, water, and medical supplies\n\t* Communication equipment (satellite phones, etc.)\n\t* Heavy machinery for debris removal and infrastructure repair\n\t* Shelter materials (tents, blankets, etc.)\n3. Funding: Estimated costs exceed $500 million, requiring a significant allocation of federal, state, and local funds to support the response and recovery efforts.\n4. Logistical Support: Coordination with neighboring states and national agencies is crucial for maintaining supply chains, transporting personnel and equipment, and sharing expertise.\n\nChallenges:\n\n1. Infrastructure Overload: The sheer scale of damage overwhelms local infrastructure, leading to delays in aid distribution and response efforts.\n2. Water Contamination: Concerns about water quality hinder access to clean drinking water, exacerbating public health risks.\n3. Displacement and Shelter: The large number of displaced individuals creates a significant shortage of available shelter options, straining resources and emergency services.\n\nLessons Learned:\n\n1. Preparedness is Key: Prioritizing evacuation planning, infrastructure hardening, and disaster preparedness can significantly reduce the impact of such events.\n2. Collaboration is Crucial: Effective coordination between local, state, and federal agencies, as well as with neighboring states and national organizations, is essential for a successful response.\n3. Adaptation to Rising Sea Levels: The increasing threat of sea-level rise requires a shift in disaster preparedness strategies, focusing on resilient infrastructure design and adaptive management practices.\n\nConclusion:\n\nThe simulated impact of Hurricane Ana highlights the devastating consequences of a Category 5 hurricane hitting Florida's eastern coast, exacerbated by rising sea levels. The emergency response efforts require significant resources, personnel, and coordination to address the complex challenges posed by this disaster. As we move forward, it is essential to prioritize preparedness, collaboration, and adaptation to mitigate the effects of these events and protect vulnerable communities.\"# Try it out yourself!### Extraterrestrial Colony Planning\nPrompt: \"Design a sustainable human colony for Mars, focusing on water resource management, energy production, and habitat construction using local materials. Include potential environmental challenges and solutions.\"### Environmental Policy Formulation\nPrompt: \"Evaluate the long-term ecological impacts of banning single-use plastics globally. Simulate the effects on marine life, pollution levels, and waste management systems over the next 50 years.\"### Advanced Educational Tools\nPrompt: \"Create an interactive simulation that demonstrates the water cycle on Earth, including the effects of deforestation and urbanization on water availability and quality in major river basins.\"### Interactive Storytelling\nPrompt: \"Generate a narrative where the user is a leader in a community facing severe drought conditions. Allow the user to make decisions about water usage, agricultural practices, and public policy, showing the consequences of each choice.\"### Biodiversity Conservation Strategies\nPrompt: \"Develop a conservation strategy for the Amazon rainforest, focusing on mitigating the effects of deforestation and climate change. Simulate various scenarios involving local communities and global stakeholders.\"### Interstellar Communication Simulation\nPrompt: \"Imagine a scenario where Earth receives a signal from a distant planet. Simulate a series of potential communications that could be exchanged, considering language barriers and the transmission delay over light-years.\"### Bioengineering Solutions\nPrompt: \"Propose a bioengineering project to create microbial life forms that can detoxify plastic waste in the ocean. Describe the genetic traits these organisms would need and simulate the potential ecological impact.\"### Cross-Planetary Impact Studies\nPrompt: \"Analyze how a supernova explosion in a neighboring star system could affect planetary systems in its vicinity, including potential impacts on Earth's magnetic field and atmosphere.\"### Custom Scenario Development\nPrompt: \"Allow the user to create a custom scenario involving an unexpected volcanic eruption in Iceland. Let the user set parameters like the eruption's size, duration, and ash distribution, then simulate the global climate and air travel impacts.\"\n\nThese prompts are designed to maximize the utilization of the model's capabilities in various complex and interdisciplinary scenarios, making them useful for researchers, educators, policymakers, and enthusiasts interested in exploring these domains."
] |
text-generation | transformers |
# Model Card for Model ID
<!-- Provide a quick summary of what the model is/does. -->
| Tasks |Version|Filter|n-shot| Metric |Value | |Stderr|
|------------|------:|------|-----:|--------|-----:|---|-----:|
|hellaswag_it| 1|none | 0|acc |0.4486|± |0.0052|
| | |none | 0|acc_norm|0.5970|± |0.0051|
|arc_it | 1|none | 0|acc |0.0915|± |0.0084|
| | |none | 0|acc_norm|0.4166|± |0.0144|
|m_mmlu_it | 0|none | 5|acc |0.5651|± |0.0043|
## Model Details
### Model Description
<!-- Provide a longer summary of what this model is. -->
This is the model card of a 🤗 transformers model that has been pushed on the Hub. This model card has been automatically generated.
- **Developed by:** [More Information Needed]
- **Funded by [optional]:** [More Information Needed]
- **Shared by [optional]:** [More Information Needed]
- **Model type:** [More Information Needed]
- **Language(s) (NLP):** [More Information Needed]
- **License:** [More Information Needed]
- **Finetuned from model [optional]:** [More Information Needed]
### Model Sources [optional]
<!-- Provide the basic links for the model. -->
- **Repository:** [More Information Needed]
- **Paper [optional]:** [More Information Needed]
- **Demo [optional]:** [More Information Needed]
## Uses
<!-- Address questions around how the model is intended to be used, including the foreseeable users of the model and those affected by the model. -->
### Direct Use
<!-- This section is for the model use without fine-tuning or plugging into a larger ecosystem/app. -->
[More Information Needed]
### Downstream Use [optional]
<!-- This section is for the model use when fine-tuned for a task, or when plugged into a larger ecosystem/app -->
[More Information Needed]
### Out-of-Scope Use
<!-- This section addresses misuse, malicious use, and uses that the model will not work well for. -->
[More Information Needed]
## Bias, Risks, and Limitations
<!-- This section is meant to convey both technical and sociotechnical limitations. -->
[More Information Needed]
### Recommendations
<!-- This section is meant to convey recommendations with respect to the bias, risk, and technical limitations. -->
Users (both direct and downstream) should be made aware of the risks, biases and limitations of the model. More information needed for further recommendations.
## How to Get Started with the Model
Use the code below to get started with the model.
[More Information Needed]
## Training Details
### Training Data
<!-- This should link to a Dataset Card, perhaps with a short stub of information on what the training data is all about as well as documentation related to data pre-processing or additional filtering. -->
[More Information Needed]
### Training Procedure
<!-- This relates heavily to the Technical Specifications. Content here should link to that section when it is relevant to the training procedure. -->
#### Preprocessing [optional]
[More Information Needed]
#### Training Hyperparameters
- **Training regime:** [More Information Needed] <!--fp32, fp16 mixed precision, bf16 mixed precision, bf16 non-mixed precision, fp16 non-mixed precision, fp8 mixed precision -->
#### Speeds, Sizes, Times [optional]
<!-- This section provides information about throughput, start/end time, checkpoint size if relevant, etc. -->
[More Information Needed]
## Evaluation
<!-- This section describes the evaluation protocols and provides the results. -->
### Testing Data, Factors & Metrics
#### Testing Data
<!-- This should link to a Dataset Card if possible. -->
[More Information Needed]
#### Factors
<!-- These are the things the evaluation is disaggregating by, e.g., subpopulations or domains. -->
[More Information Needed]
#### Metrics
<!-- These are the evaluation metrics being used, ideally with a description of why. -->
[More Information Needed]
### Results
[More Information Needed]
#### Summary
## Model Examination [optional]
<!-- Relevant interpretability work for the model goes here -->
[More Information Needed]
## Environmental Impact
<!-- Total emissions (in grams of CO2eq) and additional considerations, such as electricity usage, go here. Edit the suggested text below accordingly -->
Carbon emissions can be estimated using the [Machine Learning Impact calculator](https://mlco2.github.io/impact#compute) presented in [Lacoste et al. (2019)](https://arxiv.org/abs/1910.09700).
- **Hardware Type:** [More Information Needed]
- **Hours used:** [More Information Needed]
- **Cloud Provider:** [More Information Needed]
- **Compute Region:** [More Information Needed]
- **Carbon Emitted:** [More Information Needed]
## Technical Specifications [optional]
### Model Architecture and Objective
[More Information Needed]
### Compute Infrastructure
[More Information Needed]
#### Hardware
[More Information Needed]
#### Software
[More Information Needed]
## Citation [optional]
<!-- If there is a paper or blog post introducing the model, the APA and Bibtex information for that should go in this section. -->
**BibTeX:**
[More Information Needed]
**APA:**
[More Information Needed]
## Glossary [optional]
<!-- If relevant, include terms and calculations in this section that can help readers understand the model or model card. -->
[More Information Needed]
## More Information [optional]
[More Information Needed]
## Model Card Authors [optional]
[More Information Needed]
## Model Card Contact
[More Information Needed] | {"language": ["it"], "license": "apache-2.0", "library_name": "transformers", "datasets": ["cosimoiaia/Loquace-102k"]} | nonsonpratico/phi3-3.8-128k-italian-v2 | null | [
"transformers",
"safetensors",
"phi3",
"text-generation",
"conversational",
"custom_code",
"it",
"dataset:cosimoiaia/Loquace-102k",
"arxiv:1910.09700",
"license:apache-2.0",
"autotrain_compatible",
"endpoints_compatible",
"region:us"
] | null | 2024-05-02T10:34:39+00:00 | [
"1910.09700"
] | [
"it"
] | TAGS
#transformers #safetensors #phi3 #text-generation #conversational #custom_code #it #dataset-cosimoiaia/Loquace-102k #arxiv-1910.09700 #license-apache-2.0 #autotrain_compatible #endpoints_compatible #region-us
| Model Card for Model ID
=======================
Model Details
-------------
### Model Description
This is the model card of a transformers model that has been pushed on the Hub. This model card has been automatically generated.
* Developed by:
* Funded by [optional]:
* Shared by [optional]:
* Model type:
* Language(s) (NLP):
* License:
* Finetuned from model [optional]:
### Model Sources [optional]
* Repository:
* Paper [optional]:
* Demo [optional]:
Uses
----
### Direct Use
### Downstream Use [optional]
### Out-of-Scope Use
Bias, Risks, and Limitations
----------------------------
### Recommendations
Users (both direct and downstream) should be made aware of the risks, biases and limitations of the model. More information needed for further recommendations.
How to Get Started with the Model
---------------------------------
Use the code below to get started with the model.
Training Details
----------------
### Training Data
### Training Procedure
#### Preprocessing [optional]
#### Training Hyperparameters
* Training regime:
#### Speeds, Sizes, Times [optional]
Evaluation
----------
### Testing Data, Factors & Metrics
#### Testing Data
#### Factors
#### Metrics
### Results
#### Summary
Model Examination [optional]
----------------------------
Environmental Impact
--------------------
Carbon emissions can be estimated using the Machine Learning Impact calculator presented in Lacoste et al. (2019).
* Hardware Type:
* Hours used:
* Cloud Provider:
* Compute Region:
* Carbon Emitted:
Technical Specifications [optional]
-----------------------------------
### Model Architecture and Objective
### Compute Infrastructure
#### Hardware
#### Software
[optional]
BibTeX:
APA:
Glossary [optional]
-------------------
More Information [optional]
---------------------------
Model Card Authors [optional]
-----------------------------
Model Card Contact
------------------
| [
"### Model Description\n\n\nThis is the model card of a transformers model that has been pushed on the Hub. This model card has been automatically generated.\n\n\n* Developed by:\n* Funded by [optional]:\n* Shared by [optional]:\n* Model type:\n* Language(s) (NLP):\n* License:\n* Finetuned from model [optional]:",
"### Model Sources [optional]\n\n\n* Repository:\n* Paper [optional]:\n* Demo [optional]:\n\n\nUses\n----",
"### Direct Use",
"### Downstream Use [optional]",
"### Out-of-Scope Use\n\n\nBias, Risks, and Limitations\n----------------------------",
"### Recommendations\n\n\nUsers (both direct and downstream) should be made aware of the risks, biases and limitations of the model. More information needed for further recommendations.\n\n\nHow to Get Started with the Model\n---------------------------------\n\n\nUse the code below to get started with the model.\n\n\nTraining Details\n----------------",
"### Training Data",
"### Training Procedure",
"#### Preprocessing [optional]",
"#### Training Hyperparameters\n\n\n* Training regime:",
"#### Speeds, Sizes, Times [optional]\n\n\nEvaluation\n----------",
"### Testing Data, Factors & Metrics",
"#### Testing Data",
"#### Factors",
"#### Metrics",
"### Results",
"#### Summary\n\n\nModel Examination [optional]\n----------------------------\n\n\nEnvironmental Impact\n--------------------\n\n\nCarbon emissions can be estimated using the Machine Learning Impact calculator presented in Lacoste et al. (2019).\n\n\n* Hardware Type:\n* Hours used:\n* Cloud Provider:\n* Compute Region:\n* Carbon Emitted:\n\n\nTechnical Specifications [optional]\n-----------------------------------",
"### Model Architecture and Objective",
"### Compute Infrastructure",
"#### Hardware",
"#### Software\n\n\n[optional]\n\n\nBibTeX:\n\n\nAPA:\n\n\nGlossary [optional]\n-------------------\n\n\nMore Information [optional]\n---------------------------\n\n\nModel Card Authors [optional]\n-----------------------------\n\n\nModel Card Contact\n------------------"
] | [
"TAGS\n#transformers #safetensors #phi3 #text-generation #conversational #custom_code #it #dataset-cosimoiaia/Loquace-102k #arxiv-1910.09700 #license-apache-2.0 #autotrain_compatible #endpoints_compatible #region-us \n",
"### Model Description\n\n\nThis is the model card of a transformers model that has been pushed on the Hub. This model card has been automatically generated.\n\n\n* Developed by:\n* Funded by [optional]:\n* Shared by [optional]:\n* Model type:\n* Language(s) (NLP):\n* License:\n* Finetuned from model [optional]:",
"### Model Sources [optional]\n\n\n* Repository:\n* Paper [optional]:\n* Demo [optional]:\n\n\nUses\n----",
"### Direct Use",
"### Downstream Use [optional]",
"### Out-of-Scope Use\n\n\nBias, Risks, and Limitations\n----------------------------",
"### Recommendations\n\n\nUsers (both direct and downstream) should be made aware of the risks, biases and limitations of the model. More information needed for further recommendations.\n\n\nHow to Get Started with the Model\n---------------------------------\n\n\nUse the code below to get started with the model.\n\n\nTraining Details\n----------------",
"### Training Data",
"### Training Procedure",
"#### Preprocessing [optional]",
"#### Training Hyperparameters\n\n\n* Training regime:",
"#### Speeds, Sizes, Times [optional]\n\n\nEvaluation\n----------",
"### Testing Data, Factors & Metrics",
"#### Testing Data",
"#### Factors",
"#### Metrics",
"### Results",
"#### Summary\n\n\nModel Examination [optional]\n----------------------------\n\n\nEnvironmental Impact\n--------------------\n\n\nCarbon emissions can be estimated using the Machine Learning Impact calculator presented in Lacoste et al. (2019).\n\n\n* Hardware Type:\n* Hours used:\n* Cloud Provider:\n* Compute Region:\n* Carbon Emitted:\n\n\nTechnical Specifications [optional]\n-----------------------------------",
"### Model Architecture and Objective",
"### Compute Infrastructure",
"#### Hardware",
"#### Software\n\n\n[optional]\n\n\nBibTeX:\n\n\nAPA:\n\n\nGlossary [optional]\n-------------------\n\n\nMore Information [optional]\n---------------------------\n\n\nModel Card Authors [optional]\n-----------------------------\n\n\nModel Card Contact\n------------------"
] | [
71,
75,
28,
5,
8,
43,
103,
5,
5,
11,
13,
23,
10,
6,
5,
6,
4,
145,
7,
5,
5,
127
] | [
"TAGS\n#transformers #safetensors #phi3 #text-generation #conversational #custom_code #it #dataset-cosimoiaia/Loquace-102k #arxiv-1910.09700 #license-apache-2.0 #autotrain_compatible #endpoints_compatible #region-us \n### Model Description\n\n\nThis is the model card of a transformers model that has been pushed on the Hub. This model card has been automatically generated.\n\n\n* Developed by:\n* Funded by [optional]:\n* Shared by [optional]:\n* Model type:\n* Language(s) (NLP):\n* License:\n* Finetuned from model [optional]:### Model Sources [optional]\n\n\n* Repository:\n* Paper [optional]:\n* Demo [optional]:\n\n\nUses\n----### Direct Use### Downstream Use [optional]### Out-of-Scope Use\n\n\nBias, Risks, and Limitations\n----------------------------### Recommendations\n\n\nUsers (both direct and downstream) should be made aware of the risks, biases and limitations of the model. More information needed for further recommendations.\n\n\nHow to Get Started with the Model\n---------------------------------\n\n\nUse the code below to get started with the model.\n\n\nTraining Details\n----------------### Training Data### Training Procedure#### Preprocessing [optional]#### Training Hyperparameters\n\n\n* Training regime:#### Speeds, Sizes, Times [optional]\n\n\nEvaluation\n----------### Testing Data, Factors & Metrics#### Testing Data#### Factors#### Metrics### Results#### Summary\n\n\nModel Examination [optional]\n----------------------------\n\n\nEnvironmental Impact\n--------------------\n\n\nCarbon emissions can be estimated using the Machine Learning Impact calculator presented in Lacoste et al. (2019).\n\n\n* Hardware Type:\n* Hours used:\n* Cloud Provider:\n* Compute Region:\n* Carbon Emitted:\n\n\nTechnical Specifications [optional]\n-----------------------------------### Model Architecture and Objective### Compute Infrastructure#### Hardware#### Software\n\n\n[optional]\n\n\nBibTeX:\n\n\nAPA:\n\n\nGlossary [optional]\n-------------------\n\n\nMore Information [optional]\n---------------------------\n\n\nModel Card Authors [optional]\n-----------------------------\n\n\nModel Card Contact\n------------------"
] |
null | transformers |
# Model Card for Model ID
<!-- Provide a quick summary of what the model is/does. -->
## Model Details
### Model Description
<!-- Provide a longer summary of what this model is. -->
This is the model card of a 🤗 transformers model that has been pushed on the Hub. This model card has been automatically generated.
- **Developed by:** [More Information Needed]
- **Funded by [optional]:** [More Information Needed]
- **Shared by [optional]:** [More Information Needed]
- **Model type:** [More Information Needed]
- **Language(s) (NLP):** [More Information Needed]
- **License:** [More Information Needed]
- **Finetuned from model [optional]:** [More Information Needed]
### Model Sources [optional]
<!-- Provide the basic links for the model. -->
- **Repository:** [More Information Needed]
- **Paper [optional]:** [More Information Needed]
- **Demo [optional]:** [More Information Needed]
## Uses
<!-- Address questions around how the model is intended to be used, including the foreseeable users of the model and those affected by the model. -->
### Direct Use
<!-- This section is for the model use without fine-tuning or plugging into a larger ecosystem/app. -->
[More Information Needed]
### Downstream Use [optional]
<!-- This section is for the model use when fine-tuned for a task, or when plugged into a larger ecosystem/app -->
[More Information Needed]
### Out-of-Scope Use
<!-- This section addresses misuse, malicious use, and uses that the model will not work well for. -->
[More Information Needed]
## Bias, Risks, and Limitations
<!-- This section is meant to convey both technical and sociotechnical limitations. -->
[More Information Needed]
### Recommendations
<!-- This section is meant to convey recommendations with respect to the bias, risk, and technical limitations. -->
Users (both direct and downstream) should be made aware of the risks, biases and limitations of the model. More information needed for further recommendations.
## How to Get Started with the Model
Use the code below to get started with the model.
[More Information Needed]
## Training Details
### Training Data
<!-- This should link to a Dataset Card, perhaps with a short stub of information on what the training data is all about as well as documentation related to data pre-processing or additional filtering. -->
[More Information Needed]
### Training Procedure
<!-- This relates heavily to the Technical Specifications. Content here should link to that section when it is relevant to the training procedure. -->
#### Preprocessing [optional]
[More Information Needed]
#### Training Hyperparameters
- **Training regime:** [More Information Needed] <!--fp32, fp16 mixed precision, bf16 mixed precision, bf16 non-mixed precision, fp16 non-mixed precision, fp8 mixed precision -->
#### Speeds, Sizes, Times [optional]
<!-- This section provides information about throughput, start/end time, checkpoint size if relevant, etc. -->
[More Information Needed]
## Evaluation
<!-- This section describes the evaluation protocols and provides the results. -->
### Testing Data, Factors & Metrics
#### Testing Data
<!-- This should link to a Dataset Card if possible. -->
[More Information Needed]
#### Factors
<!-- These are the things the evaluation is disaggregating by, e.g., subpopulations or domains. -->
[More Information Needed]
#### Metrics
<!-- These are the evaluation metrics being used, ideally with a description of why. -->
[More Information Needed]
### Results
[More Information Needed]
#### Summary
## Model Examination [optional]
<!-- Relevant interpretability work for the model goes here -->
[More Information Needed]
## Environmental Impact
<!-- Total emissions (in grams of CO2eq) and additional considerations, such as electricity usage, go here. Edit the suggested text below accordingly -->
Carbon emissions can be estimated using the [Machine Learning Impact calculator](https://mlco2.github.io/impact#compute) presented in [Lacoste et al. (2019)](https://arxiv.org/abs/1910.09700).
- **Hardware Type:** [More Information Needed]
- **Hours used:** [More Information Needed]
- **Cloud Provider:** [More Information Needed]
- **Compute Region:** [More Information Needed]
- **Carbon Emitted:** [More Information Needed]
## Technical Specifications [optional]
### Model Architecture and Objective
[More Information Needed]
### Compute Infrastructure
[More Information Needed]
#### Hardware
[More Information Needed]
#### Software
[More Information Needed]
## Citation [optional]
<!-- If there is a paper or blog post introducing the model, the APA and Bibtex information for that should go in this section. -->
**BibTeX:**
[More Information Needed]
**APA:**
[More Information Needed]
## Glossary [optional]
<!-- If relevant, include terms and calculations in this section that can help readers understand the model or model card. -->
[More Information Needed]
## More Information [optional]
[More Information Needed]
## Model Card Authors [optional]
[More Information Needed]
## Model Card Contact
[More Information Needed] | {"library_name": "transformers", "tags": ["trl", "sft"]} | 46an/WaziAi-finetuned-weights | null | [
"transformers",
"safetensors",
"trl",
"sft",
"arxiv:1910.09700",
"endpoints_compatible",
"region:us"
] | null | 2024-05-02T10:36:02+00:00 | [
"1910.09700"
] | [] | TAGS
#transformers #safetensors #trl #sft #arxiv-1910.09700 #endpoints_compatible #region-us
|
# Model Card for Model ID
## Model Details
### Model Description
This is the model card of a transformers model that has been pushed on the Hub. This model card has been automatically generated.
- Developed by:
- Funded by [optional]:
- Shared by [optional]:
- Model type:
- Language(s) (NLP):
- License:
- Finetuned from model [optional]:
### Model Sources [optional]
- Repository:
- Paper [optional]:
- Demo [optional]:
## Uses
### Direct Use
### Downstream Use [optional]
### Out-of-Scope Use
## Bias, Risks, and Limitations
### Recommendations
Users (both direct and downstream) should be made aware of the risks, biases and limitations of the model. More information needed for further recommendations.
## How to Get Started with the Model
Use the code below to get started with the model.
## Training Details
### Training Data
### Training Procedure
#### Preprocessing [optional]
#### Training Hyperparameters
- Training regime:
#### Speeds, Sizes, Times [optional]
## Evaluation
### Testing Data, Factors & Metrics
#### Testing Data
#### Factors
#### Metrics
### Results
#### Summary
## Model Examination [optional]
## Environmental Impact
Carbon emissions can be estimated using the Machine Learning Impact calculator presented in Lacoste et al. (2019).
- Hardware Type:
- Hours used:
- Cloud Provider:
- Compute Region:
- Carbon Emitted:
## Technical Specifications [optional]
### Model Architecture and Objective
### Compute Infrastructure
#### Hardware
#### Software
[optional]
BibTeX:
APA:
## Glossary [optional]
## More Information [optional]
## Model Card Authors [optional]
## Model Card Contact
| [
"# Model Card for Model ID",
"## Model Details",
"### Model Description\n\n\n\nThis is the model card of a transformers model that has been pushed on the Hub. This model card has been automatically generated.\n\n- Developed by: \n- Funded by [optional]: \n- Shared by [optional]: \n- Model type: \n- Language(s) (NLP): \n- License: \n- Finetuned from model [optional]:",
"### Model Sources [optional]\n\n\n\n- Repository: \n- Paper [optional]: \n- Demo [optional]:",
"## Uses",
"### Direct Use",
"### Downstream Use [optional]",
"### Out-of-Scope Use",
"## Bias, Risks, and Limitations",
"### Recommendations\n\n\n\nUsers (both direct and downstream) should be made aware of the risks, biases and limitations of the model. More information needed for further recommendations.",
"## How to Get Started with the Model\n\nUse the code below to get started with the model.",
"## Training Details",
"### Training Data",
"### Training Procedure",
"#### Preprocessing [optional]",
"#### Training Hyperparameters\n\n- Training regime:",
"#### Speeds, Sizes, Times [optional]",
"## Evaluation",
"### Testing Data, Factors & Metrics",
"#### Testing Data",
"#### Factors",
"#### Metrics",
"### Results",
"#### Summary",
"## Model Examination [optional]",
"## Environmental Impact\n\n\n\nCarbon emissions can be estimated using the Machine Learning Impact calculator presented in Lacoste et al. (2019).\n\n- Hardware Type: \n- Hours used: \n- Cloud Provider: \n- Compute Region: \n- Carbon Emitted:",
"## Technical Specifications [optional]",
"### Model Architecture and Objective",
"### Compute Infrastructure",
"#### Hardware",
"#### Software\n\n\n\n[optional]\n\n\n\nBibTeX:\n\n\n\nAPA:",
"## Glossary [optional]",
"## More Information [optional]",
"## Model Card Authors [optional]",
"## Model Card Contact"
] | [
"TAGS\n#transformers #safetensors #trl #sft #arxiv-1910.09700 #endpoints_compatible #region-us \n",
"# Model Card for Model ID",
"## Model Details",
"### Model Description\n\n\n\nThis is the model card of a transformers model that has been pushed on the Hub. This model card has been automatically generated.\n\n- Developed by: \n- Funded by [optional]: \n- Shared by [optional]: \n- Model type: \n- Language(s) (NLP): \n- License: \n- Finetuned from model [optional]:",
"### Model Sources [optional]\n\n\n\n- Repository: \n- Paper [optional]: \n- Demo [optional]:",
"## Uses",
"### Direct Use",
"### Downstream Use [optional]",
"### Out-of-Scope Use",
"## Bias, Risks, and Limitations",
"### Recommendations\n\n\n\nUsers (both direct and downstream) should be made aware of the risks, biases and limitations of the model. More information needed for further recommendations.",
"## How to Get Started with the Model\n\nUse the code below to get started with the model.",
"## Training Details",
"### Training Data",
"### Training Procedure",
"#### Preprocessing [optional]",
"#### Training Hyperparameters\n\n- Training regime:",
"#### Speeds, Sizes, Times [optional]",
"## Evaluation",
"### Testing Data, Factors & Metrics",
"#### Testing Data",
"#### Factors",
"#### Metrics",
"### Results",
"#### Summary",
"## Model Examination [optional]",
"## Environmental Impact\n\n\n\nCarbon emissions can be estimated using the Machine Learning Impact calculator presented in Lacoste et al. (2019).\n\n- Hardware Type: \n- Hours used: \n- Cloud Provider: \n- Compute Region: \n- Carbon Emitted:",
"## Technical Specifications [optional]",
"### Model Architecture and Objective",
"### Compute Infrastructure",
"#### Hardware",
"#### Software\n\n\n\n[optional]\n\n\n\nBibTeX:\n\n\n\nAPA:",
"## Glossary [optional]",
"## More Information [optional]",
"## Model Card Authors [optional]",
"## Model Card Contact"
] | [
32,
6,
4,
75,
23,
3,
5,
8,
9,
8,
34,
20,
4,
5,
5,
11,
13,
12,
3,
10,
6,
5,
6,
4,
5,
7,
49,
7,
7,
5,
5,
15,
7,
7,
8,
5
] | [
"TAGS\n#transformers #safetensors #trl #sft #arxiv-1910.09700 #endpoints_compatible #region-us \n# Model Card for Model ID## Model Details### Model Description\n\n\n\nThis is the model card of a transformers model that has been pushed on the Hub. This model card has been automatically generated.\n\n- Developed by: \n- Funded by [optional]: \n- Shared by [optional]: \n- Model type: \n- Language(s) (NLP): \n- License: \n- Finetuned from model [optional]:### Model Sources [optional]\n\n\n\n- Repository: \n- Paper [optional]: \n- Demo [optional]:## Uses### Direct Use### Downstream Use [optional]### Out-of-Scope Use## Bias, Risks, and Limitations### Recommendations\n\n\n\nUsers (both direct and downstream) should be made aware of the risks, biases and limitations of the model. More information needed for further recommendations.## How to Get Started with the Model\n\nUse the code below to get started with the model.## Training Details### Training Data### Training Procedure#### Preprocessing [optional]#### Training Hyperparameters\n\n- Training regime:#### Speeds, Sizes, Times [optional]## Evaluation### Testing Data, Factors & Metrics#### Testing Data#### Factors#### Metrics### Results#### Summary## Model Examination [optional]## Environmental Impact\n\n\n\nCarbon emissions can be estimated using the Machine Learning Impact calculator presented in Lacoste et al. (2019).\n\n- Hardware Type: \n- Hours used: \n- Cloud Provider: \n- Compute Region: \n- Carbon Emitted:## Technical Specifications [optional]### Model Architecture and Objective### Compute Infrastructure#### Hardware#### Software\n\n\n\n[optional]\n\n\n\nBibTeX:\n\n\n\nAPA:## Glossary [optional]## More Information [optional]## Model Card Authors [optional]## Model Card Contact"
] |
reinforcement-learning | ml-agents |
# **ppo** Agent playing **Huggy**
This is a trained model of a **ppo** agent playing **Huggy**
using the [Unity ML-Agents Library](https://github.com/Unity-Technologies/ml-agents).
## Usage (with ML-Agents)
The Documentation: https://unity-technologies.github.io/ml-agents/ML-Agents-Toolkit-Documentation/
We wrote a complete tutorial to learn to train your first agent using ML-Agents and publish it to the Hub:
- A *short tutorial* where you teach Huggy the Dog 🐶 to fetch the stick and then play with him directly in your
browser: https://huggingface.co/learn/deep-rl-course/unitbonus1/introduction
- A *longer tutorial* to understand how works ML-Agents:
https://huggingface.co/learn/deep-rl-course/unit5/introduction
### Resume the training
```bash
mlagents-learn <your_configuration_file_path.yaml> --run-id=<run_id> --resume
```
### Watch your Agent play
You can watch your agent **playing directly in your browser**
1. If the environment is part of ML-Agents official environments, go to https://huggingface.co/unity
2. Step 1: Find your model_id: raydium/ppo-Huggy
3. Step 2: Select your *.nn /*.onnx file
4. Click on Watch the agent play 👀
| {"library_name": "ml-agents", "tags": ["Huggy", "deep-reinforcement-learning", "reinforcement-learning", "ML-Agents-Huggy"]} | raydium/ppo-Huggy | null | [
"ml-agents",
"tensorboard",
"onnx",
"Huggy",
"deep-reinforcement-learning",
"reinforcement-learning",
"ML-Agents-Huggy",
"region:us"
] | null | 2024-05-02T10:36:26+00:00 | [] | [] | TAGS
#ml-agents #tensorboard #onnx #Huggy #deep-reinforcement-learning #reinforcement-learning #ML-Agents-Huggy #region-us
|
# ppo Agent playing Huggy
This is a trained model of a ppo agent playing Huggy
using the Unity ML-Agents Library.
## Usage (with ML-Agents)
The Documentation: URL
We wrote a complete tutorial to learn to train your first agent using ML-Agents and publish it to the Hub:
- A *short tutorial* where you teach Huggy the Dog to fetch the stick and then play with him directly in your
browser: URL
- A *longer tutorial* to understand how works ML-Agents:
URL
### Resume the training
### Watch your Agent play
You can watch your agent playing directly in your browser
1. If the environment is part of ML-Agents official environments, go to URL
2. Step 1: Find your model_id: raydium/ppo-Huggy
3. Step 2: Select your *.nn /*.onnx file
4. Click on Watch the agent play
| [
"# ppo Agent playing Huggy\n This is a trained model of a ppo agent playing Huggy\n using the Unity ML-Agents Library.\n\n ## Usage (with ML-Agents)\n The Documentation: URL\n\n We wrote a complete tutorial to learn to train your first agent using ML-Agents and publish it to the Hub:\n - A *short tutorial* where you teach Huggy the Dog to fetch the stick and then play with him directly in your\n browser: URL\n - A *longer tutorial* to understand how works ML-Agents:\n URL\n\n ### Resume the training\n \n\n ### Watch your Agent play\n You can watch your agent playing directly in your browser\n\n 1. If the environment is part of ML-Agents official environments, go to URL\n 2. Step 1: Find your model_id: raydium/ppo-Huggy\n 3. Step 2: Select your *.nn /*.onnx file\n 4. Click on Watch the agent play"
] | [
"TAGS\n#ml-agents #tensorboard #onnx #Huggy #deep-reinforcement-learning #reinforcement-learning #ML-Agents-Huggy #region-us \n",
"# ppo Agent playing Huggy\n This is a trained model of a ppo agent playing Huggy\n using the Unity ML-Agents Library.\n\n ## Usage (with ML-Agents)\n The Documentation: URL\n\n We wrote a complete tutorial to learn to train your first agent using ML-Agents and publish it to the Hub:\n - A *short tutorial* where you teach Huggy the Dog to fetch the stick and then play with him directly in your\n browser: URL\n - A *longer tutorial* to understand how works ML-Agents:\n URL\n\n ### Resume the training\n \n\n ### Watch your Agent play\n You can watch your agent playing directly in your browser\n\n 1. If the environment is part of ML-Agents official environments, go to URL\n 2. Step 1: Find your model_id: raydium/ppo-Huggy\n 3. Step 2: Select your *.nn /*.onnx file\n 4. Click on Watch the agent play"
] | [
35,
198
] | [
"TAGS\n#ml-agents #tensorboard #onnx #Huggy #deep-reinforcement-learning #reinforcement-learning #ML-Agents-Huggy #region-us \n# ppo Agent playing Huggy\n This is a trained model of a ppo agent playing Huggy\n using the Unity ML-Agents Library.\n\n ## Usage (with ML-Agents)\n The Documentation: URL\n\n We wrote a complete tutorial to learn to train your first agent using ML-Agents and publish it to the Hub:\n - A *short tutorial* where you teach Huggy the Dog to fetch the stick and then play with him directly in your\n browser: URL\n - A *longer tutorial* to understand how works ML-Agents:\n URL\n\n ### Resume the training\n \n\n ### Watch your Agent play\n You can watch your agent playing directly in your browser\n\n 1. If the environment is part of ML-Agents official environments, go to URL\n 2. Step 1: Find your model_id: raydium/ppo-Huggy\n 3. Step 2: Select your *.nn /*.onnx file\n 4. Click on Watch the agent play"
] |
text2text-generation | transformers |
# Model Card for Model ID
<!-- Provide a quick summary of what the model is/does. -->
## Model Details
### Model Description
<!-- Provide a longer summary of what this model is. -->
This is the model card of a 🤗 transformers model that has been pushed on the Hub. This model card has been automatically generated.
- **Developed by:** [More Information Needed]
- **Funded by [optional]:** [More Information Needed]
- **Shared by [optional]:** [More Information Needed]
- **Model type:** [More Information Needed]
- **Language(s) (NLP):** [More Information Needed]
- **License:** [More Information Needed]
- **Finetuned from model [optional]:** [More Information Needed]
### Model Sources [optional]
<!-- Provide the basic links for the model. -->
- **Repository:** [More Information Needed]
- **Paper [optional]:** [More Information Needed]
- **Demo [optional]:** [More Information Needed]
## Uses
<!-- Address questions around how the model is intended to be used, including the foreseeable users of the model and those affected by the model. -->
### Direct Use
<!-- This section is for the model use without fine-tuning or plugging into a larger ecosystem/app. -->
[More Information Needed]
### Downstream Use [optional]
<!-- This section is for the model use when fine-tuned for a task, or when plugged into a larger ecosystem/app -->
[More Information Needed]
### Out-of-Scope Use
<!-- This section addresses misuse, malicious use, and uses that the model will not work well for. -->
[More Information Needed]
## Bias, Risks, and Limitations
<!-- This section is meant to convey both technical and sociotechnical limitations. -->
[More Information Needed]
### Recommendations
<!-- This section is meant to convey recommendations with respect to the bias, risk, and technical limitations. -->
Users (both direct and downstream) should be made aware of the risks, biases and limitations of the model. More information needed for further recommendations.
## How to Get Started with the Model
Use the code below to get started with the model.
[More Information Needed]
## Training Details
### Training Data
<!-- This should link to a Dataset Card, perhaps with a short stub of information on what the training data is all about as well as documentation related to data pre-processing or additional filtering. -->
[More Information Needed]
### Training Procedure
<!-- This relates heavily to the Technical Specifications. Content here should link to that section when it is relevant to the training procedure. -->
#### Preprocessing [optional]
[More Information Needed]
#### Training Hyperparameters
- **Training regime:** [More Information Needed] <!--fp32, fp16 mixed precision, bf16 mixed precision, bf16 non-mixed precision, fp16 non-mixed precision, fp8 mixed precision -->
#### Speeds, Sizes, Times [optional]
<!-- This section provides information about throughput, start/end time, checkpoint size if relevant, etc. -->
[More Information Needed]
## Evaluation
<!-- This section describes the evaluation protocols and provides the results. -->
### Testing Data, Factors & Metrics
#### Testing Data
<!-- This should link to a Dataset Card if possible. -->
[More Information Needed]
#### Factors
<!-- These are the things the evaluation is disaggregating by, e.g., subpopulations or domains. -->
[More Information Needed]
#### Metrics
<!-- These are the evaluation metrics being used, ideally with a description of why. -->
[More Information Needed]
### Results
[More Information Needed]
#### Summary
## Model Examination [optional]
<!-- Relevant interpretability work for the model goes here -->
[More Information Needed]
## Environmental Impact
<!-- Total emissions (in grams of CO2eq) and additional considerations, such as electricity usage, go here. Edit the suggested text below accordingly -->
Carbon emissions can be estimated using the [Machine Learning Impact calculator](https://mlco2.github.io/impact#compute) presented in [Lacoste et al. (2019)](https://arxiv.org/abs/1910.09700).
- **Hardware Type:** [More Information Needed]
- **Hours used:** [More Information Needed]
- **Cloud Provider:** [More Information Needed]
- **Compute Region:** [More Information Needed]
- **Carbon Emitted:** [More Information Needed]
## Technical Specifications [optional]
### Model Architecture and Objective
[More Information Needed]
### Compute Infrastructure
[More Information Needed]
#### Hardware
[More Information Needed]
#### Software
[More Information Needed]
## Citation [optional]
<!-- If there is a paper or blog post introducing the model, the APA and Bibtex information for that should go in this section. -->
**BibTeX:**
[More Information Needed]
**APA:**
[More Information Needed]
## Glossary [optional]
<!-- If relevant, include terms and calculations in this section that can help readers understand the model or model card. -->
[More Information Needed]
## More Information [optional]
[More Information Needed]
## Model Card Authors [optional]
[More Information Needed]
## Model Card Contact
[More Information Needed] | {"library_name": "transformers", "tags": []} | steve1989/flant5xl-finetuned-finance-headlines-sentiment-analysis-bnb4bits | null | [
"transformers",
"safetensors",
"t5",
"text2text-generation",
"arxiv:1910.09700",
"autotrain_compatible",
"endpoints_compatible",
"text-generation-inference",
"region:us"
] | null | 2024-05-02T10:37:34+00:00 | [
"1910.09700"
] | [] | TAGS
#transformers #safetensors #t5 #text2text-generation #arxiv-1910.09700 #autotrain_compatible #endpoints_compatible #text-generation-inference #region-us
|
# Model Card for Model ID
## Model Details
### Model Description
This is the model card of a transformers model that has been pushed on the Hub. This model card has been automatically generated.
- Developed by:
- Funded by [optional]:
- Shared by [optional]:
- Model type:
- Language(s) (NLP):
- License:
- Finetuned from model [optional]:
### Model Sources [optional]
- Repository:
- Paper [optional]:
- Demo [optional]:
## Uses
### Direct Use
### Downstream Use [optional]
### Out-of-Scope Use
## Bias, Risks, and Limitations
### Recommendations
Users (both direct and downstream) should be made aware of the risks, biases and limitations of the model. More information needed for further recommendations.
## How to Get Started with the Model
Use the code below to get started with the model.
## Training Details
### Training Data
### Training Procedure
#### Preprocessing [optional]
#### Training Hyperparameters
- Training regime:
#### Speeds, Sizes, Times [optional]
## Evaluation
### Testing Data, Factors & Metrics
#### Testing Data
#### Factors
#### Metrics
### Results
#### Summary
## Model Examination [optional]
## Environmental Impact
Carbon emissions can be estimated using the Machine Learning Impact calculator presented in Lacoste et al. (2019).
- Hardware Type:
- Hours used:
- Cloud Provider:
- Compute Region:
- Carbon Emitted:
## Technical Specifications [optional]
### Model Architecture and Objective
### Compute Infrastructure
#### Hardware
#### Software
[optional]
BibTeX:
APA:
## Glossary [optional]
## More Information [optional]
## Model Card Authors [optional]
## Model Card Contact
| [
"# Model Card for Model ID",
"## Model Details",
"### Model Description\n\n\n\nThis is the model card of a transformers model that has been pushed on the Hub. This model card has been automatically generated.\n\n- Developed by: \n- Funded by [optional]: \n- Shared by [optional]: \n- Model type: \n- Language(s) (NLP): \n- License: \n- Finetuned from model [optional]:",
"### Model Sources [optional]\n\n\n\n- Repository: \n- Paper [optional]: \n- Demo [optional]:",
"## Uses",
"### Direct Use",
"### Downstream Use [optional]",
"### Out-of-Scope Use",
"## Bias, Risks, and Limitations",
"### Recommendations\n\n\n\nUsers (both direct and downstream) should be made aware of the risks, biases and limitations of the model. More information needed for further recommendations.",
"## How to Get Started with the Model\n\nUse the code below to get started with the model.",
"## Training Details",
"### Training Data",
"### Training Procedure",
"#### Preprocessing [optional]",
"#### Training Hyperparameters\n\n- Training regime:",
"#### Speeds, Sizes, Times [optional]",
"## Evaluation",
"### Testing Data, Factors & Metrics",
"#### Testing Data",
"#### Factors",
"#### Metrics",
"### Results",
"#### Summary",
"## Model Examination [optional]",
"## Environmental Impact\n\n\n\nCarbon emissions can be estimated using the Machine Learning Impact calculator presented in Lacoste et al. (2019).\n\n- Hardware Type: \n- Hours used: \n- Cloud Provider: \n- Compute Region: \n- Carbon Emitted:",
"## Technical Specifications [optional]",
"### Model Architecture and Objective",
"### Compute Infrastructure",
"#### Hardware",
"#### Software\n\n\n\n[optional]\n\n\n\nBibTeX:\n\n\n\nAPA:",
"## Glossary [optional]",
"## More Information [optional]",
"## Model Card Authors [optional]",
"## Model Card Contact"
] | [
"TAGS\n#transformers #safetensors #t5 #text2text-generation #arxiv-1910.09700 #autotrain_compatible #endpoints_compatible #text-generation-inference #region-us \n",
"# Model Card for Model ID",
"## Model Details",
"### Model Description\n\n\n\nThis is the model card of a transformers model that has been pushed on the Hub. This model card has been automatically generated.\n\n- Developed by: \n- Funded by [optional]: \n- Shared by [optional]: \n- Model type: \n- Language(s) (NLP): \n- License: \n- Finetuned from model [optional]:",
"### Model Sources [optional]\n\n\n\n- Repository: \n- Paper [optional]: \n- Demo [optional]:",
"## Uses",
"### Direct Use",
"### Downstream Use [optional]",
"### Out-of-Scope Use",
"## Bias, Risks, and Limitations",
"### Recommendations\n\n\n\nUsers (both direct and downstream) should be made aware of the risks, biases and limitations of the model. More information needed for further recommendations.",
"## How to Get Started with the Model\n\nUse the code below to get started with the model.",
"## Training Details",
"### Training Data",
"### Training Procedure",
"#### Preprocessing [optional]",
"#### Training Hyperparameters\n\n- Training regime:",
"#### Speeds, Sizes, Times [optional]",
"## Evaluation",
"### Testing Data, Factors & Metrics",
"#### Testing Data",
"#### Factors",
"#### Metrics",
"### Results",
"#### Summary",
"## Model Examination [optional]",
"## Environmental Impact\n\n\n\nCarbon emissions can be estimated using the Machine Learning Impact calculator presented in Lacoste et al. (2019).\n\n- Hardware Type: \n- Hours used: \n- Cloud Provider: \n- Compute Region: \n- Carbon Emitted:",
"## Technical Specifications [optional]",
"### Model Architecture and Objective",
"### Compute Infrastructure",
"#### Hardware",
"#### Software\n\n\n\n[optional]\n\n\n\nBibTeX:\n\n\n\nAPA:",
"## Glossary [optional]",
"## More Information [optional]",
"## Model Card Authors [optional]",
"## Model Card Contact"
] | [
46,
6,
4,
75,
23,
3,
5,
8,
9,
8,
34,
20,
4,
5,
5,
11,
13,
12,
3,
10,
6,
5,
6,
4,
5,
7,
49,
7,
7,
5,
5,
15,
7,
7,
8,
5
] | [
"TAGS\n#transformers #safetensors #t5 #text2text-generation #arxiv-1910.09700 #autotrain_compatible #endpoints_compatible #text-generation-inference #region-us \n# Model Card for Model ID## Model Details### Model Description\n\n\n\nThis is the model card of a transformers model that has been pushed on the Hub. This model card has been automatically generated.\n\n- Developed by: \n- Funded by [optional]: \n- Shared by [optional]: \n- Model type: \n- Language(s) (NLP): \n- License: \n- Finetuned from model [optional]:### Model Sources [optional]\n\n\n\n- Repository: \n- Paper [optional]: \n- Demo [optional]:## Uses### Direct Use### Downstream Use [optional]### Out-of-Scope Use## Bias, Risks, and Limitations### Recommendations\n\n\n\nUsers (both direct and downstream) should be made aware of the risks, biases and limitations of the model. More information needed for further recommendations.## How to Get Started with the Model\n\nUse the code below to get started with the model.## Training Details### Training Data### Training Procedure#### Preprocessing [optional]#### Training Hyperparameters\n\n- Training regime:#### Speeds, Sizes, Times [optional]## Evaluation### Testing Data, Factors & Metrics#### Testing Data#### Factors#### Metrics### Results#### Summary## Model Examination [optional]## Environmental Impact\n\n\n\nCarbon emissions can be estimated using the Machine Learning Impact calculator presented in Lacoste et al. (2019).\n\n- Hardware Type: \n- Hours used: \n- Cloud Provider: \n- Compute Region: \n- Carbon Emitted:## Technical Specifications [optional]### Model Architecture and Objective### Compute Infrastructure#### Hardware#### Software\n\n\n\n[optional]\n\n\n\nBibTeX:\n\n\n\nAPA:## Glossary [optional]## More Information [optional]## Model Card Authors [optional]## Model Card Contact"
] |
video-classification | transformers |
<!-- This model card has been generated automatically according to the information the Trainer had access to. You
should probably proofread and complete it, then remove this comment. -->
# vivit-b-16x2-kinetics400-finetuned-temp-original
This model is a fine-tuned version of [google/vivit-b-16x2-kinetics400](https://huggingface.co/google/vivit-b-16x2-kinetics400) on an unknown dataset.
It achieves the following results on the evaluation set:
- Loss: 1.1267
- Accuracy: 0.64
## Model description
More information needed
## Intended uses & limitations
More information needed
## Training and evaluation data
More information needed
## Training procedure
### Training hyperparameters
The following hyperparameters were used during training:
- learning_rate: 5e-05
- train_batch_size: 1
- eval_batch_size: 1
- seed: 42
- optimizer: Adam with betas=(0.9,0.999) and epsilon=1e-08
- lr_scheduler_type: linear
- lr_scheduler_warmup_ratio: 0.1
- training_steps: 420
### Training results
| Training Loss | Epoch | Step | Validation Loss | Accuracy |
|:-------------:|:-----:|:----:|:---------------:|:--------:|
| 2.3132 | 0.25 | 105 | 2.3486 | 0.14 |
| 1.8975 | 1.25 | 210 | 1.6591 | 0.42 |
| 1.423 | 2.25 | 315 | 1.2563 | 0.58 |
| 0.456 | 3.25 | 420 | 1.1267 | 0.64 |
### Framework versions
- Transformers 4.40.1
- Pytorch 2.2.1+cu121
- Tokenizers 0.19.1
| {"license": "mit", "tags": ["generated_from_trainer"], "metrics": ["accuracy"], "base_model": "google/vivit-b-16x2-kinetics400", "model-index": [{"name": "vivit-b-16x2-kinetics400-finetuned-temp-original", "results": []}]} | kkumtori/vivit-b-16x2-kinetics400-finetuned-temp-original | null | [
"transformers",
"tensorboard",
"safetensors",
"vivit",
"video-classification",
"generated_from_trainer",
"base_model:google/vivit-b-16x2-kinetics400",
"license:mit",
"endpoints_compatible",
"region:us"
] | null | 2024-05-02T10:38:34+00:00 | [] | [] | TAGS
#transformers #tensorboard #safetensors #vivit #video-classification #generated_from_trainer #base_model-google/vivit-b-16x2-kinetics400 #license-mit #endpoints_compatible #region-us
| vivit-b-16x2-kinetics400-finetuned-temp-original
================================================
This model is a fine-tuned version of google/vivit-b-16x2-kinetics400 on an unknown dataset.
It achieves the following results on the evaluation set:
* Loss: 1.1267
* Accuracy: 0.64
Model description
-----------------
More information needed
Intended uses & limitations
---------------------------
More information needed
Training and evaluation data
----------------------------
More information needed
Training procedure
------------------
### Training hyperparameters
The following hyperparameters were used during training:
* learning\_rate: 5e-05
* train\_batch\_size: 1
* eval\_batch\_size: 1
* seed: 42
* optimizer: Adam with betas=(0.9,0.999) and epsilon=1e-08
* lr\_scheduler\_type: linear
* lr\_scheduler\_warmup\_ratio: 0.1
* training\_steps: 420
### Training results
### Framework versions
* Transformers 4.40.1
* Pytorch 2.2.1+cu121
* Tokenizers 0.19.1
| [
"### Training hyperparameters\n\n\nThe following hyperparameters were used during training:\n\n\n* learning\\_rate: 5e-05\n* train\\_batch\\_size: 1\n* eval\\_batch\\_size: 1\n* seed: 42\n* optimizer: Adam with betas=(0.9,0.999) and epsilon=1e-08\n* lr\\_scheduler\\_type: linear\n* lr\\_scheduler\\_warmup\\_ratio: 0.1\n* training\\_steps: 420",
"### Training results",
"### Framework versions\n\n\n* Transformers 4.40.1\n* Pytorch 2.2.1+cu121\n* Tokenizers 0.19.1"
] | [
"TAGS\n#transformers #tensorboard #safetensors #vivit #video-classification #generated_from_trainer #base_model-google/vivit-b-16x2-kinetics400 #license-mit #endpoints_compatible #region-us \n",
"### Training hyperparameters\n\n\nThe following hyperparameters were used during training:\n\n\n* learning\\_rate: 5e-05\n* train\\_batch\\_size: 1\n* eval\\_batch\\_size: 1\n* seed: 42\n* optimizer: Adam with betas=(0.9,0.999) and epsilon=1e-08\n* lr\\_scheduler\\_type: linear\n* lr\\_scheduler\\_warmup\\_ratio: 0.1\n* training\\_steps: 420",
"### Training results",
"### Framework versions\n\n\n* Transformers 4.40.1\n* Pytorch 2.2.1+cu121\n* Tokenizers 0.19.1"
] | [
57,
117,
5,
35
] | [
"TAGS\n#transformers #tensorboard #safetensors #vivit #video-classification #generated_from_trainer #base_model-google/vivit-b-16x2-kinetics400 #license-mit #endpoints_compatible #region-us \n### Training hyperparameters\n\n\nThe following hyperparameters were used during training:\n\n\n* learning\\_rate: 5e-05\n* train\\_batch\\_size: 1\n* eval\\_batch\\_size: 1\n* seed: 42\n* optimizer: Adam with betas=(0.9,0.999) and epsilon=1e-08\n* lr\\_scheduler\\_type: linear\n* lr\\_scheduler\\_warmup\\_ratio: 0.1\n* training\\_steps: 420### Training results### Framework versions\n\n\n* Transformers 4.40.1\n* Pytorch 2.2.1+cu121\n* Tokenizers 0.19.1"
] |
null | null | Apakah Varicap?
Varicap Tablet ialah kapsul farmaseutikal yang direka untuk menangani hipertensi, biasanya dikenali sebagai tekanan darah tinggi. Ia mengandungi gabungan unik bahan semulajadi, dirumus dengan teliti untuk mengawal tahap tekanan darah dan menggalakkan kesihatan kardiovaskular.
Laman web rasmi:<a href="https://www.nutritionsee.com/varicoama">www.varicap.com</a>
<p><a href="https://www.nutritionsee.com/varicoama"> <img src="https://www.nutritionsee.com/wp-content/uploads/2024/04/Varicap-1.png" alt="enter image description here"> </a></p>
<a href="https://www.nutritionsee.com/varicoama">Beli sekarang!! Klik pautan di bawah untuk maklumat lanjut dan dapatkan diskaun 50% sekarang... Cepat</a>
Laman web rasmi:<a href="https://www.nutritionsee.com/varicoama">www.varicap.com</a>
| {"license": "apache-2.0"} | VaricapMalaysia/Varicap | null | [
"license:apache-2.0",
"region:us"
] | null | 2024-05-02T10:38:49+00:00 | [] | [] | TAGS
#license-apache-2.0 #region-us
| Apakah Varicap?
Varicap Tablet ialah kapsul farmaseutikal yang direka untuk menangani hipertensi, biasanya dikenali sebagai tekanan darah tinggi. Ia mengandungi gabungan unik bahan semulajadi, dirumus dengan teliti untuk mengawal tahap tekanan darah dan menggalakkan kesihatan kardiovaskular.
Laman web rasmi:<a href="URL
<p><a href="URL <img src="URL alt="enter image description here"> </a></p>
<a href="URL sekarang!! Klik pautan di bawah untuk maklumat lanjut dan dapatkan diskaun 50% sekarang... Cepat</a>
Laman web rasmi:<a href="URL
| [] | [
"TAGS\n#license-apache-2.0 #region-us \n"
] | [
13
] | [
"TAGS\n#license-apache-2.0 #region-us \n"
] |
text-generation | transformers |
# Model Card for Model ID
<!-- Provide a quick summary of what the model is/does. -->
## Model Details
### Model Description
<!-- Provide a longer summary of what this model is. -->
This is the model card of a 🤗 transformers model that has been pushed on the Hub. This model card has been automatically generated.
- **Developed by:** [More Information Needed]
- **Funded by [optional]:** [More Information Needed]
- **Shared by [optional]:** [More Information Needed]
- **Model type:** [More Information Needed]
- **Language(s) (NLP):** [More Information Needed]
- **License:** [More Information Needed]
- **Finetuned from model [optional]:** [More Information Needed]
### Model Sources [optional]
<!-- Provide the basic links for the model. -->
- **Repository:** [More Information Needed]
- **Paper [optional]:** [More Information Needed]
- **Demo [optional]:** [More Information Needed]
## Uses
<!-- Address questions around how the model is intended to be used, including the foreseeable users of the model and those affected by the model. -->
### Direct Use
<!-- This section is for the model use without fine-tuning or plugging into a larger ecosystem/app. -->
[More Information Needed]
### Downstream Use [optional]
<!-- This section is for the model use when fine-tuned for a task, or when plugged into a larger ecosystem/app -->
[More Information Needed]
### Out-of-Scope Use
<!-- This section addresses misuse, malicious use, and uses that the model will not work well for. -->
[More Information Needed]
## Bias, Risks, and Limitations
<!-- This section is meant to convey both technical and sociotechnical limitations. -->
[More Information Needed]
### Recommendations
<!-- This section is meant to convey recommendations with respect to the bias, risk, and technical limitations. -->
Users (both direct and downstream) should be made aware of the risks, biases and limitations of the model. More information needed for further recommendations.
## How to Get Started with the Model
Use the code below to get started with the model.
[More Information Needed]
## Training Details
### Training Data
<!-- This should link to a Dataset Card, perhaps with a short stub of information on what the training data is all about as well as documentation related to data pre-processing or additional filtering. -->
[More Information Needed]
### Training Procedure
<!-- This relates heavily to the Technical Specifications. Content here should link to that section when it is relevant to the training procedure. -->
#### Preprocessing [optional]
[More Information Needed]
#### Training Hyperparameters
- **Training regime:** [More Information Needed] <!--fp32, fp16 mixed precision, bf16 mixed precision, bf16 non-mixed precision, fp16 non-mixed precision, fp8 mixed precision -->
#### Speeds, Sizes, Times [optional]
<!-- This section provides information about throughput, start/end time, checkpoint size if relevant, etc. -->
[More Information Needed]
## Evaluation
<!-- This section describes the evaluation protocols and provides the results. -->
### Testing Data, Factors & Metrics
#### Testing Data
<!-- This should link to a Dataset Card if possible. -->
[More Information Needed]
#### Factors
<!-- These are the things the evaluation is disaggregating by, e.g., subpopulations or domains. -->
[More Information Needed]
#### Metrics
<!-- These are the evaluation metrics being used, ideally with a description of why. -->
[More Information Needed]
### Results
[More Information Needed]
#### Summary
## Model Examination [optional]
<!-- Relevant interpretability work for the model goes here -->
[More Information Needed]
## Environmental Impact
<!-- Total emissions (in grams of CO2eq) and additional considerations, such as electricity usage, go here. Edit the suggested text below accordingly -->
Carbon emissions can be estimated using the [Machine Learning Impact calculator](https://mlco2.github.io/impact#compute) presented in [Lacoste et al. (2019)](https://arxiv.org/abs/1910.09700).
- **Hardware Type:** [More Information Needed]
- **Hours used:** [More Information Needed]
- **Cloud Provider:** [More Information Needed]
- **Compute Region:** [More Information Needed]
- **Carbon Emitted:** [More Information Needed]
## Technical Specifications [optional]
### Model Architecture and Objective
[More Information Needed]
### Compute Infrastructure
[More Information Needed]
#### Hardware
[More Information Needed]
#### Software
[More Information Needed]
## Citation [optional]
<!-- If there is a paper or blog post introducing the model, the APA and Bibtex information for that should go in this section. -->
**BibTeX:**
[More Information Needed]
**APA:**
[More Information Needed]
## Glossary [optional]
<!-- If relevant, include terms and calculations in this section that can help readers understand the model or model card. -->
[More Information Needed]
## More Information [optional]
[More Information Needed]
## Model Card Authors [optional]
[More Information Needed]
## Model Card Contact
[More Information Needed] | {"library_name": "transformers", "tags": ["llama-factory"]} | Moriacrafter/Gemma-2B_DepressionDetection | null | [
"transformers",
"safetensors",
"gemma",
"text-generation",
"llama-factory",
"conversational",
"arxiv:1910.09700",
"autotrain_compatible",
"endpoints_compatible",
"text-generation-inference",
"region:us"
] | null | 2024-05-02T10:38:50+00:00 | [
"1910.09700"
] | [] | TAGS
#transformers #safetensors #gemma #text-generation #llama-factory #conversational #arxiv-1910.09700 #autotrain_compatible #endpoints_compatible #text-generation-inference #region-us
|
# Model Card for Model ID
## Model Details
### Model Description
This is the model card of a transformers model that has been pushed on the Hub. This model card has been automatically generated.
- Developed by:
- Funded by [optional]:
- Shared by [optional]:
- Model type:
- Language(s) (NLP):
- License:
- Finetuned from model [optional]:
### Model Sources [optional]
- Repository:
- Paper [optional]:
- Demo [optional]:
## Uses
### Direct Use
### Downstream Use [optional]
### Out-of-Scope Use
## Bias, Risks, and Limitations
### Recommendations
Users (both direct and downstream) should be made aware of the risks, biases and limitations of the model. More information needed for further recommendations.
## How to Get Started with the Model
Use the code below to get started with the model.
## Training Details
### Training Data
### Training Procedure
#### Preprocessing [optional]
#### Training Hyperparameters
- Training regime:
#### Speeds, Sizes, Times [optional]
## Evaluation
### Testing Data, Factors & Metrics
#### Testing Data
#### Factors
#### Metrics
### Results
#### Summary
## Model Examination [optional]
## Environmental Impact
Carbon emissions can be estimated using the Machine Learning Impact calculator presented in Lacoste et al. (2019).
- Hardware Type:
- Hours used:
- Cloud Provider:
- Compute Region:
- Carbon Emitted:
## Technical Specifications [optional]
### Model Architecture and Objective
### Compute Infrastructure
#### Hardware
#### Software
[optional]
BibTeX:
APA:
## Glossary [optional]
## More Information [optional]
## Model Card Authors [optional]
## Model Card Contact
| [
"# Model Card for Model ID",
"## Model Details",
"### Model Description\n\n\n\nThis is the model card of a transformers model that has been pushed on the Hub. This model card has been automatically generated.\n\n- Developed by: \n- Funded by [optional]: \n- Shared by [optional]: \n- Model type: \n- Language(s) (NLP): \n- License: \n- Finetuned from model [optional]:",
"### Model Sources [optional]\n\n\n\n- Repository: \n- Paper [optional]: \n- Demo [optional]:",
"## Uses",
"### Direct Use",
"### Downstream Use [optional]",
"### Out-of-Scope Use",
"## Bias, Risks, and Limitations",
"### Recommendations\n\n\n\nUsers (both direct and downstream) should be made aware of the risks, biases and limitations of the model. More information needed for further recommendations.",
"## How to Get Started with the Model\n\nUse the code below to get started with the model.",
"## Training Details",
"### Training Data",
"### Training Procedure",
"#### Preprocessing [optional]",
"#### Training Hyperparameters\n\n- Training regime:",
"#### Speeds, Sizes, Times [optional]",
"## Evaluation",
"### Testing Data, Factors & Metrics",
"#### Testing Data",
"#### Factors",
"#### Metrics",
"### Results",
"#### Summary",
"## Model Examination [optional]",
"## Environmental Impact\n\n\n\nCarbon emissions can be estimated using the Machine Learning Impact calculator presented in Lacoste et al. (2019).\n\n- Hardware Type: \n- Hours used: \n- Cloud Provider: \n- Compute Region: \n- Carbon Emitted:",
"## Technical Specifications [optional]",
"### Model Architecture and Objective",
"### Compute Infrastructure",
"#### Hardware",
"#### Software\n\n\n\n[optional]\n\n\n\nBibTeX:\n\n\n\nAPA:",
"## Glossary [optional]",
"## More Information [optional]",
"## Model Card Authors [optional]",
"## Model Card Contact"
] | [
"TAGS\n#transformers #safetensors #gemma #text-generation #llama-factory #conversational #arxiv-1910.09700 #autotrain_compatible #endpoints_compatible #text-generation-inference #region-us \n",
"# Model Card for Model ID",
"## Model Details",
"### Model Description\n\n\n\nThis is the model card of a transformers model that has been pushed on the Hub. This model card has been automatically generated.\n\n- Developed by: \n- Funded by [optional]: \n- Shared by [optional]: \n- Model type: \n- Language(s) (NLP): \n- License: \n- Finetuned from model [optional]:",
"### Model Sources [optional]\n\n\n\n- Repository: \n- Paper [optional]: \n- Demo [optional]:",
"## Uses",
"### Direct Use",
"### Downstream Use [optional]",
"### Out-of-Scope Use",
"## Bias, Risks, and Limitations",
"### Recommendations\n\n\n\nUsers (both direct and downstream) should be made aware of the risks, biases and limitations of the model. More information needed for further recommendations.",
"## How to Get Started with the Model\n\nUse the code below to get started with the model.",
"## Training Details",
"### Training Data",
"### Training Procedure",
"#### Preprocessing [optional]",
"#### Training Hyperparameters\n\n- Training regime:",
"#### Speeds, Sizes, Times [optional]",
"## Evaluation",
"### Testing Data, Factors & Metrics",
"#### Testing Data",
"#### Factors",
"#### Metrics",
"### Results",
"#### Summary",
"## Model Examination [optional]",
"## Environmental Impact\n\n\n\nCarbon emissions can be estimated using the Machine Learning Impact calculator presented in Lacoste et al. (2019).\n\n- Hardware Type: \n- Hours used: \n- Cloud Provider: \n- Compute Region: \n- Carbon Emitted:",
"## Technical Specifications [optional]",
"### Model Architecture and Objective",
"### Compute Infrastructure",
"#### Hardware",
"#### Software\n\n\n\n[optional]\n\n\n\nBibTeX:\n\n\n\nAPA:",
"## Glossary [optional]",
"## More Information [optional]",
"## Model Card Authors [optional]",
"## Model Card Contact"
] | [
51,
6,
4,
75,
23,
3,
5,
8,
9,
8,
34,
20,
4,
5,
5,
11,
13,
12,
3,
10,
6,
5,
6,
4,
5,
7,
49,
7,
7,
5,
5,
15,
7,
7,
8,
5
] | [
"TAGS\n#transformers #safetensors #gemma #text-generation #llama-factory #conversational #arxiv-1910.09700 #autotrain_compatible #endpoints_compatible #text-generation-inference #region-us \n# Model Card for Model ID## Model Details### Model Description\n\n\n\nThis is the model card of a transformers model that has been pushed on the Hub. This model card has been automatically generated.\n\n- Developed by: \n- Funded by [optional]: \n- Shared by [optional]: \n- Model type: \n- Language(s) (NLP): \n- License: \n- Finetuned from model [optional]:### Model Sources [optional]\n\n\n\n- Repository: \n- Paper [optional]: \n- Demo [optional]:## Uses### Direct Use### Downstream Use [optional]### Out-of-Scope Use## Bias, Risks, and Limitations### Recommendations\n\n\n\nUsers (both direct and downstream) should be made aware of the risks, biases and limitations of the model. More information needed for further recommendations.## How to Get Started with the Model\n\nUse the code below to get started with the model.## Training Details### Training Data### Training Procedure#### Preprocessing [optional]#### Training Hyperparameters\n\n- Training regime:#### Speeds, Sizes, Times [optional]## Evaluation### Testing Data, Factors & Metrics#### Testing Data#### Factors#### Metrics### Results#### Summary## Model Examination [optional]## Environmental Impact\n\n\n\nCarbon emissions can be estimated using the Machine Learning Impact calculator presented in Lacoste et al. (2019).\n\n- Hardware Type: \n- Hours used: \n- Cloud Provider: \n- Compute Region: \n- Carbon Emitted:## Technical Specifications [optional]### Model Architecture and Objective### Compute Infrastructure#### Hardware#### Software\n\n\n\n[optional]\n\n\n\nBibTeX:\n\n\n\nAPA:## Glossary [optional]## More Information [optional]## Model Card Authors [optional]## Model Card Contact"
] |
text-generation | transformers |
## Model Details
Meta developed and released the Meta Llama 3 family of large language models (LLMs), a collection of pretrained and instruction tuned generative text models in 8 and 70B sizes. The Llama 3 instruction tuned models are optimized for dialogue use cases and outperform many of the available open source chat models on common industry benchmarks. Further, in developing these models, we took great care to optimize helpfulness and safety.
**Model developers** Meta
**Variations** Llama 3 comes in two sizes — 8B and 70B parameters — in pre-trained and instruction tuned variants.
**Input** Models input text only.
**Output** Models generate text and code only.
**Model Architecture** Llama 3 is an auto-regressive language model that uses an optimized transformer architecture. The tuned versions use supervised fine-tuning (SFT) and reinforcement learning with human feedback (RLHF) to align with human preferences for helpfulness and safety.
<table>
<tr>
<td>
</td>
<td><strong>Training Data</strong>
</td>
<td><strong>Params</strong>
</td>
<td><strong>Context length</strong>
</td>
<td><strong>GQA</strong>
</td>
<td><strong>Token count</strong>
</td>
<td><strong>Knowledge cutoff</strong>
</td>
</tr>
<tr>
<td rowspan="2" >Llama 3
</td>
<td rowspan="2" >A new mix of publicly available online data.
</td>
<td>8B
</td>
<td>8k
</td>
<td>Yes
</td>
<td rowspan="2" >15T+
</td>
<td>March, 2023
</td>
</tr>
<tr>
<td>70B
</td>
<td>8k
</td>
<td>Yes
</td>
<td>December, 2023
</td>
</tr>
</table>
**Llama 3 family of models**. Token counts refer to pretraining data only. Both the 8 and 70B versions use Grouped-Query Attention (GQA) for improved inference scalability.
**Model Release Date** April 18, 2024.
**Status** This is a static model trained on an offline dataset. Future versions of the tuned models will be released as we improve model safety with community feedback.
**License** A custom commercial license is available at: [https://llama.meta.com/llama3/license](https://llama.meta.com/llama3/license)
Where to send questions or comments about the model Instructions on how to provide feedback or comments on the model can be found in the model [README](https://github.com/meta-llama/llama3). For more technical information about generation parameters and recipes for how to use Llama 3 in applications, please go [here](https://github.com/meta-llama/llama-recipes).
## Intended Use
**Intended Use Cases** Llama 3 is intended for commercial and research use in English. Instruction tuned models are intended for assistant-like chat, whereas pretrained models can be adapted for a variety of natural language generation tasks.
**Out-of-scope** Use in any manner that violates applicable laws or regulations (including trade compliance laws). Use in any other way that is prohibited by the Acceptable Use Policy and Llama 3 Community License. Use in languages other than English**.
**Note: Developers may fine-tune Llama 3 models for languages beyond English provided they comply with the Llama 3 Community License and the Acceptable Use Policy.
## How to use
This repository contains two versions of Meta-Llama-3-8B, for use with transformers and with the original `llama3` codebase.
### Use with transformers
See the snippet below for usage with Transformers:
```python
>>> import transformers
>>> import torch
>>> model_id = "meta-llama/Meta-Llama-3-8B"
>>> pipeline = transformers.pipeline(
"text-generation", model=model_id, model_kwargs={"torch_dtype": torch.bfloat16}, device_map="auto"
)
>>> pipeline("Hey how are you doing today?")
```
### Use with `llama3`
Please, follow the instructions in the [repository](https://github.com/meta-llama/llama3).
To download Original checkpoints, see the example command below leveraging `huggingface-cli`:
```
huggingface-cli download meta-llama/Meta-Llama-3-8B --include "original/*" --local-dir Meta-Llama-3-8B
```
For Hugging Face support, we recommend using transformers or TGI, but a similar command works.
## Hardware and Software
**Training Factors** We used custom training libraries, Meta's Research SuperCluster, and production clusters for pretraining. Fine-tuning, annotation, and evaluation were also performed on third-party cloud compute.
**Carbon Footprint Pretraining utilized a cumulative** 7.7M GPU hours of computation on hardware of type H100-80GB (TDP of 700W). Estimated total emissions were 2290 tCO2eq, 100% of which were offset by Meta’s sustainability program.
<table>
<tr>
<td>
</td>
<td><strong>Time (GPU hours)</strong>
</td>
<td><strong>Power Consumption (W)</strong>
</td>
<td><strong>Carbon Emitted(tCO2eq)</strong>
</td>
</tr>
<tr>
<td>Llama 3 8B
</td>
<td>1.3M
</td>
<td>700
</td>
<td>390
</td>
</tr>
<tr>
<td>Llama 3 70B
</td>
<td>6.4M
</td>
<td>700
</td>
<td>1900
</td>
</tr>
<tr>
<td>Total
</td>
<td>7.7M
</td>
<td>
</td>
<td>2290
</td>
</tr>
</table>
**CO2 emissions during pre-training**. Time: total GPU time required for training each model. Power Consumption: peak power capacity per GPU device for the GPUs used adjusted for power usage efficiency. 100% of the emissions are directly offset by Meta's sustainability program, and because we are openly releasing these models, the pretraining costs do not need to be incurred by others.
## Training Data
**Overview** Llama 3 was pretrained on over 15 trillion tokens of data from publicly available sources. The fine-tuning data includes publicly available instruction datasets, as well as over 10M human-annotated examples. Neither the pretraining nor the fine-tuning datasets include Meta user data.
**Data Freshness** The pretraining data has a cutoff of March 2023 for the 7B and December 2023 for the 70B models respectively.
## Benchmarks
In this section, we report the results for Llama 3 models on standard automatic benchmarks. For all the evaluations, we use our internal evaluations library. For details on the methodology see [here](https://github.com/meta-llama/llama3/blob/main/eval_methodology.md).
### Base pretrained models
<table>
<tr>
<td><strong>Category</strong>
</td>
<td><strong>Benchmark</strong>
</td>
<td><strong>Llama 3 8B</strong>
</td>
<td><strong>Llama2 7B</strong>
</td>
<td><strong>Llama2 13B</strong>
</td>
<td><strong>Llama 3 70B</strong>
</td>
<td><strong>Llama2 70B</strong>
</td>
</tr>
<tr>
<td rowspan="6" >General
</td>
<td>MMLU (5-shot)
</td>
<td>66.6
</td>
<td>45.7
</td>
<td>53.8
</td>
<td>79.5
</td>
<td>69.7
</td>
</tr>
<tr>
<td>AGIEval English (3-5 shot)
</td>
<td>45.9
</td>
<td>28.8
</td>
<td>38.7
</td>
<td>63.0
</td>
<td>54.8
</td>
</tr>
<tr>
<td>CommonSenseQA (7-shot)
</td>
<td>72.6
</td>
<td>57.6
</td>
<td>67.6
</td>
<td>83.8
</td>
<td>78.7
</td>
</tr>
<tr>
<td>Winogrande (5-shot)
</td>
<td>76.1
</td>
<td>73.3
</td>
<td>75.4
</td>
<td>83.1
</td>
<td>81.8
</td>
</tr>
<tr>
<td>BIG-Bench Hard (3-shot, CoT)
</td>
<td>61.1
</td>
<td>38.1
</td>
<td>47.0
</td>
<td>81.3
</td>
<td>65.7
</td>
</tr>
<tr>
<td>ARC-Challenge (25-shot)
</td>
<td>78.6
</td>
<td>53.7
</td>
<td>67.6
</td>
<td>93.0
</td>
<td>85.3
</td>
</tr>
<tr>
<td>Knowledge reasoning
</td>
<td>TriviaQA-Wiki (5-shot)
</td>
<td>78.5
</td>
<td>72.1
</td>
<td>79.6
</td>
<td>89.7
</td>
<td>87.5
</td>
</tr>
<tr>
<td rowspan="4" >Reading comprehension
</td>
<td>SQuAD (1-shot)
</td>
<td>76.4
</td>
<td>72.2
</td>
<td>72.1
</td>
<td>85.6
</td>
<td>82.6
</td>
</tr>
<tr>
<td>QuAC (1-shot, F1)
</td>
<td>44.4
</td>
<td>39.6
</td>
<td>44.9
</td>
<td>51.1
</td>
<td>49.4
</td>
</tr>
<tr>
<td>BoolQ (0-shot)
</td>
<td>75.7
</td>
<td>65.5
</td>
<td>66.9
</td>
<td>79.0
</td>
<td>73.1
</td>
</tr>
<tr>
<td>DROP (3-shot, F1)
</td>
<td>58.4
</td>
<td>37.9
</td>
<td>49.8
</td>
<td>79.7
</td>
<td>70.2
</td>
</tr>
</table>
### Instruction tuned models
<table>
<tr>
<td><strong>Benchmark</strong>
</td>
<td><strong>Llama 3 8B</strong>
</td>
<td><strong>Llama 2 7B</strong>
</td>
<td><strong>Llama 2 13B</strong>
</td>
<td><strong>Llama 3 70B</strong>
</td>
<td><strong>Llama 2 70B</strong>
</td>
</tr>
<tr>
<td>MMLU (5-shot)
</td>
<td>68.4
</td>
<td>34.1
</td>
<td>47.8
</td>
<td>82.0
</td>
<td>52.9
</td>
</tr>
<tr>
<td>GPQA (0-shot)
</td>
<td>34.2
</td>
<td>21.7
</td>
<td>22.3
</td>
<td>39.5
</td>
<td>21.0
</td>
</tr>
<tr>
<td>HumanEval (0-shot)
</td>
<td>62.2
</td>
<td>7.9
</td>
<td>14.0
</td>
<td>81.7
</td>
<td>25.6
</td>
</tr>
<tr>
<td>GSM-8K (8-shot, CoT)
</td>
<td>79.6
</td>
<td>25.7
</td>
<td>77.4
</td>
<td>93.0
</td>
<td>57.5
</td>
</tr>
<tr>
<td>MATH (4-shot, CoT)
</td>
<td>30.0
</td>
<td>3.8
</td>
<td>6.7
</td>
<td>50.4
</td>
<td>11.6
</td>
</tr>
</table>
### Responsibility & Safety
We believe that an open approach to AI leads to better, safer products, faster innovation, and a bigger overall market. We are committed to Responsible AI development and took a series of steps to limit misuse and harm and support the open source community.
Foundation models are widely capable technologies that are built to be used for a diverse range of applications. They are not designed to meet every developer preference on safety levels for all use cases, out-of-the-box, as those by their nature will differ across different applications.
Rather, responsible LLM-application deployment is achieved by implementing a series of safety best practices throughout the development of such applications, from the model pre-training, fine-tuning and the deployment of systems composed of safeguards to tailor the safety needs specifically to the use case and audience.
As part of the Llama 3 release, we updated our [Responsible Use Guide](https://llama.meta.com/responsible-use-guide/) to outline the steps and best practices for developers to implement model and system level safety for their application. We also provide a set of resources including [Meta Llama Guard 2](https://llama.meta.com/purple-llama/) and [Code Shield](https://llama.meta.com/purple-llama/) safeguards. These tools have proven to drastically reduce residual risks of LLM Systems, while maintaining a high level of helpfulness. We encourage developers to tune and deploy these safeguards according to their needs and we provide a [reference implementation](https://github.com/meta-llama/llama-recipes/tree/main/recipes/responsible_ai) to get you started.
#### Llama 3-Instruct
As outlined in the Responsible Use Guide, some trade-off between model helpfulness and model alignment is likely unavoidable. Developers should exercise discretion about how to weigh the benefits of alignment and helpfulness for their specific use case and audience. Developers should be mindful of residual risks when using Llama models and leverage additional safety tools as needed to reach the right safety bar for their use case.
<span style="text-decoration:underline;">Safety</span>
For our instruction tuned model, we conducted extensive red teaming exercises, performed adversarial evaluations and implemented safety mitigations techniques to lower residual risks. As with any Large Language Model, residual risks will likely remain and we recommend that developers assess these risks in the context of their use case. In parallel, we are working with the community to make AI safety benchmark standards transparent, rigorous and interpretable.
<span style="text-decoration:underline;">Refusals</span>
In addition to residual risks, we put a great emphasis on model refusals to benign prompts. Over-refusing not only can impact the user experience but could even be harmful in certain contexts as well. We’ve heard the feedback from the developer community and improved our fine tuning to ensure that Llama 3 is significantly less likely to falsely refuse to answer prompts than Llama 2.
We built internal benchmarks and developed mitigations to limit false refusals making Llama 3 our most helpful model to date.
#### Responsible release
In addition to responsible use considerations outlined above, we followed a rigorous process that requires us to take extra measures against misuse and critical risks before we make our release decision.
Misuse
If you access or use Llama 3, you agree to the Acceptable Use Policy. The most recent copy of this policy can be found at [https://llama.meta.com/llama3/use-policy/](https://llama.meta.com/llama3/use-policy/).
#### Critical risks
<span style="text-decoration:underline;">CBRNE</span> (Chemical, Biological, Radiological, Nuclear, and high yield Explosives)
We have conducted a two fold assessment of the safety of the model in this area:
* Iterative testing during model training to assess the safety of responses related to CBRNE threats and other adversarial risks.
* Involving external CBRNE experts to conduct an uplift test assessing the ability of the model to accurately provide expert knowledge and reduce barriers to potential CBRNE misuse, by reference to what can be achieved using web search (without the model).
### <span style="text-decoration:underline;">Cyber Security </span>
We have evaluated Llama 3 with CyberSecEval, Meta’s cybersecurity safety eval suite, measuring Llama 3’s propensity to suggest insecure code when used as a coding assistant, and Llama 3’s propensity to comply with requests to help carry out cyber attacks, where attacks are defined by the industry standard MITRE ATT&CK cyber attack ontology. On our insecure coding and cyber attacker helpfulness tests, Llama 3 behaved in the same range or safer than models of [equivalent coding capability](https://huggingface.co/spaces/facebook/CyberSecEval).
### <span style="text-decoration:underline;">Child Safety</span>
Child Safety risk assessments were conducted using a team of experts, to assess the model’s capability to produce outputs that could result in Child Safety risks and inform on any necessary and appropriate risk mitigations via fine tuning. We leveraged those expert red teaming sessions to expand the coverage of our evaluation benchmarks through Llama 3 model development. For Llama 3, we conducted new in-depth sessions using objective based methodologies to assess the model risks along multiple attack vectors. We also partnered with content specialists to perform red teaming exercises assessing potentially violating content while taking account of market specific nuances or experiences.
### Community
Generative AI safety requires expertise and tooling, and we believe in the strength of the open community to accelerate its progress. We are active members of open consortiums, including the AI Alliance, Partnership in AI and MLCommons, actively contributing to safety standardization and transparency. We encourage the community to adopt taxonomies like the MLCommons Proof of Concept evaluation to facilitate collaboration and transparency on safety and content evaluations. Our Purple Llama tools are open sourced for the community to use and widely distributed across ecosystem partners including cloud service providers. We encourage community contributions to our [Github repository](https://github.com/meta-llama/PurpleLlama).
Finally, we put in place a set of resources including an [output reporting mechanism](https://developers.facebook.com/llama_output_feedback) and [bug bounty program](https://www.facebook.com/whitehat) to continuously improve the Llama technology with the help of the community.
## Ethical Considerations and Limitations
The core values of Llama 3 are openness, inclusivity and helpfulness. It is meant to serve everyone, and to work for a wide range of use cases. It is thus designed to be accessible to people across many different backgrounds, experiences and perspectives. Llama 3 addresses users and their needs as they are, without insertion unnecessary judgment or normativity, while reflecting the understanding that even content that may appear problematic in some cases can serve valuable purposes in others. It respects the dignity and autonomy of all users, especially in terms of the values of free thought and expression that power innovation and progress.
But Llama 3 is a new technology, and like any new technology, there are risks associated with its use. Testing conducted to date has been in English, and has not covered, nor could it cover, all scenarios. For these reasons, as with all LLMs, Llama 3’s potential outputs cannot be predicted in advance, and the model may in some instances produce inaccurate, biased or other objectionable responses to user prompts. Therefore, before deploying any applications of Llama 3 models, developers should perform safety testing and tuning tailored to their specific applications of the model. As outlined in the Responsible Use Guide, we recommend incorporating [Purple Llama](https://github.com/facebookresearch/PurpleLlama) solutions into your workflows and specifically [Llama Guard](https://ai.meta.com/research/publications/llama-guard-llm-based-input-output-safeguard-for-human-ai-conversations/) which provides a base model to filter input and output prompts to layer system-level safety on top of model-level safety.
Please see the Responsible Use Guide available at [http://llama.meta.com/responsible-use-guide](http://llama.meta.com/responsible-use-guide)
## Citation instructions
@article{llama3modelcard,
title={Llama 3 Model Card},
author={AI@Meta},
year={2024},
url = {https://github.com/meta-llama/llama3/blob/main/MODEL_CARD.md}
}
## Contributors
Aaditya Singh; Aaron Grattafiori; Abhimanyu Dubey; Abhinav Jauhri; Abhinav Pandey; Abhishek Kadian; Adam Kelsey; Adi Gangidi; Ahmad Al-Dahle; Ahuva Goldstand; Aiesha Letman; Ajay Menon; Akhil Mathur; Alan Schelten; Alex Vaughan; Amy Yang; Andrei Lupu; Andres Alvarado; Andrew Gallagher; Andrew Gu; Andrew Ho; Andrew Poulton; Andrew Ryan; Angela Fan; Ankit Ramchandani; Anthony Hartshorn; Archi Mitra; Archie Sravankumar; Artem Korenev; Arun Rao; Ashley Gabriel; Ashwin Bharambe; Assaf Eisenman; Aston Zhang; Aurelien Rodriguez; Austen Gregerson; Ava Spataru; Baptiste Roziere; Ben Maurer; Benjamin Leonhardi; Bernie Huang; Bhargavi Paranjape; Bing Liu; Binh Tang; Bobbie Chern; Brani Stojkovic; Brian Fuller; Catalina Mejia Arenas; Chao Zhou; Charlotte Caucheteux; Chaya Nayak; Ching-Hsiang Chu; Chloe Bi; Chris Cai; Chris Cox; Chris Marra; Chris McConnell; Christian Keller; Christoph Feichtenhofer; Christophe Touret; Chunyang Wu; Corinne Wong; Cristian Canton Ferrer; Damien Allonsius; Daniel Kreymer; Daniel Haziza; Daniel Li; Danielle Pintz; Danny Livshits; Danny Wyatt; David Adkins; David Esiobu; David Xu; Davide Testuggine; Delia David; Devi Parikh; Dhruv Choudhary; Dhruv Mahajan; Diana Liskovich; Diego Garcia-Olano; Diego Perino; Dieuwke Hupkes; Dingkang Wang; Dustin Holland; Egor Lakomkin; Elina Lobanova; Xiaoqing Ellen Tan; Emily Dinan; Eric Smith; Erik Brinkman; Esteban Arcaute; Filip Radenovic; Firat Ozgenel; Francesco Caggioni; Frank Seide; Frank Zhang; Gabriel Synnaeve; Gabriella Schwarz; Gabrielle Lee; Gada Badeer; Georgia Anderson; Graeme Nail; Gregoire Mialon; Guan Pang; Guillem Cucurell; Hailey Nguyen; Hannah Korevaar; Hannah Wang; Haroun Habeeb; Harrison Rudolph; Henry Aspegren; Hu Xu; Hugo Touvron; Iga Kozlowska; Igor Molybog; Igor Tufanov; Iliyan Zarov; Imanol Arrieta Ibarra; Irina-Elena Veliche; Isabel Kloumann; Ishan Misra; Ivan Evtimov; Jacob Xu; Jade Copet; Jake Weissman; Jan Geffert; Jana Vranes; Japhet Asher; Jason Park; Jay Mahadeokar; Jean-Baptiste Gaya; Jeet Shah; Jelmer van der Linde; Jennifer Chan; Jenny Hong; Jenya Lee; Jeremy Fu; Jeremy Teboul; Jianfeng Chi; Jianyu Huang; Jie Wang; Jiecao Yu; Joanna Bitton; Joe Spisak; Joelle Pineau; Jon Carvill; Jongsoo Park; Joseph Rocca; Joshua Johnstun; Junteng Jia; Kalyan Vasuden Alwala; Kam Hou U; Kate Plawiak; Kartikeya Upasani; Kaushik Veeraraghavan; Ke Li; Kenneth Heafield; Kevin Stone; Khalid El-Arini; Krithika Iyer; Kshitiz Malik; Kuenley Chiu; Kunal Bhalla; Kyle Huang; Lakshya Garg; Lauren Rantala-Yeary; Laurens van der Maaten; Lawrence Chen; Leandro Silva; Lee Bell; Lei Zhang; Liang Tan; Louis Martin; Lovish Madaan; Luca Wehrstedt; Lukas Blecher; Luke de Oliveira; Madeline Muzzi; Madian Khabsa; Manav Avlani; Mannat Singh; Manohar Paluri; Mark Zuckerberg; Marcin Kardas; Martynas Mankus; Mathew Oldham; Mathieu Rita; Matthew Lennie; Maya Pavlova; Meghan Keneally; Melanie Kambadur; Mihir Patel; Mikayel Samvelyan; Mike Clark; Mike Lewis; Min Si; Mitesh Kumar Singh; Mo Metanat; Mona Hassan; Naman Goyal; Narjes Torabi; Nicolas Usunier; Nikolay Bashlykov; Nikolay Bogoychev; Niladri Chatterji; Ning Dong; Oliver Aobo Yang; Olivier Duchenne; Onur Celebi; Parth Parekh; Patrick Alrassy; Paul Saab; Pavan Balaji; Pedro Rittner; Pengchuan Zhang; Pengwei Li; Petar Vasic; Peter Weng; Polina Zvyagina; Prajjwal Bhargava; Pratik Dubal; Praveen Krishnan; Punit Singh Koura; Qing He; Rachel Rodriguez; Ragavan Srinivasan; Rahul Mitra; Ramon Calderer; Raymond Li; Robert Stojnic; Roberta Raileanu; Robin Battey; Rocky Wang; Rohit Girdhar; Rohit Patel; Romain Sauvestre; Ronnie Polidoro; Roshan Sumbaly; Ross Taylor; Ruan Silva; Rui Hou; Rui Wang; Russ Howes; Ruty Rinott; Saghar Hosseini; Sai Jayesh Bondu; Samyak Datta; Sanjay Singh; Sara Chugh; Sargun Dhillon; Satadru Pan; Sean Bell; Sergey Edunov; Shaoliang Nie; Sharan Narang; Sharath Raparthy; Shaun Lindsay; Sheng Feng; Sheng Shen; Shenghao Lin; Shiva Shankar; Shruti Bhosale; Shun Zhang; Simon Vandenhende; Sinong Wang; Seohyun Sonia Kim; Soumya Batra; Sten Sootla; Steve Kehoe; Suchin Gururangan; Sumit Gupta; Sunny Virk; Sydney Borodinsky; Tamar Glaser; Tamar Herman; Tamara Best; Tara Fowler; Thomas Georgiou; Thomas Scialom; Tianhe Li; Todor Mihaylov; Tong Xiao; Ujjwal Karn; Vedanuj Goswami; Vibhor Gupta; Vignesh Ramanathan; Viktor Kerkez; Vinay Satish Kumar; Vincent Gonguet; Vish Vogeti; Vlad Poenaru; Vlad Tiberiu Mihailescu; Vladan Petrovic; Vladimir Ivanov; Wei Li; Weiwei Chu; Wenhan Xiong; Wenyin Fu; Wes Bouaziz; Whitney Meers; Will Constable; Xavier Martinet; Xiaojian Wu; Xinbo Gao; Xinfeng Xie; Xuchao Jia; Yaelle Goldschlag; Yann LeCun; Yashesh Gaur; Yasmine Babaei; Ye Qi; Yenda Li; Yi Wen; Yiwen Song; Youngjin Nam; Yuchen Hao; Yuchen Zhang; Yun Wang; Yuning Mao; Yuzi He; Zacharie Delpierre Coudert; Zachary DeVito; Zahra Hankir; Zhaoduo Wen; Zheng Yan; Zhengxing Chen; Zhenyu Yang; Zoe Papakipos
| {"language": ["en"], "license": "other", "tags": ["facebook", "meta", "pytorch", "llama", "llama-3"], "pipeline_tag": "text-generation", "license_name": "llama3", "license_link": "LICENSE", "extra_gated_prompt": "### META LLAMA 3 COMMUNITY LICENSE AGREEMENT\nMeta Llama 3 Version Release Date: April 18, 2024\n\"Agreement\" means the terms and conditions for use, reproduction, distribution and modification of the Llama Materials set forth herein.\n\"Documentation\" means the specifications, manuals and documentation accompanying Meta Llama 3 distributed by Meta at https://llama.meta.com/get-started/.\n\"Licensee\" or \"you\" means you, or your employer or any other person or entity (if you are entering into this Agreement on such person or entity\u2019s behalf), of the age required under applicable laws, rules or regulations to provide legal consent and that has legal authority to bind your employer or such other person or entity if you are entering in this Agreement on their behalf.\n\"Meta Llama 3\" means the foundational large language models and software and algorithms, including machine-learning model code, trained model weights, inference-enabling code, training-enabling code, fine-tuning enabling code and other elements of the foregoing distributed by Meta at https://llama.meta.com/llama-downloads.\n\"Llama Materials\" means, collectively, Meta\u2019s proprietary Meta Llama 3 and Documentation (and any portion thereof) made available under this Agreement.\n\"Meta\" or \"we\" means Meta Platforms Ireland Limited (if you are located in or, if you are an entity, your principal place of business is in the EEA or Switzerland) and Meta Platforms, Inc. (if you are located outside of the EEA or Switzerland).\n \n1. License Rights and Redistribution.\na. Grant of Rights. You are granted a non-exclusive, worldwide, non-transferable and royalty-free limited license under Meta\u2019s intellectual property or other rights owned by Meta embodied in the Llama Materials to use, reproduce, distribute, copy, create derivative works of, and make modifications to the Llama Materials.\nb. Redistribution and Use.\ni. If you distribute or make available the Llama Materials (or any derivative works thereof), or a product or service that uses any of them, including another AI model, you shall (A) provide a copy of this Agreement with any such Llama Materials; and (B) prominently display \u201cBuilt with Meta Llama 3\u201d on a related website, user interface, blogpost, about page, or product documentation. If you use the Llama Materials to create, train, fine tune, or otherwise improve an AI model, which is distributed or made available, you shall also include \u201cLlama 3\u201d at the beginning of any such AI model name.\nii. If you receive Llama Materials, or any derivative works thereof, from a Licensee as part of an integrated end user product, then Section 2 of this Agreement will not apply to you.\niii. You must retain in all copies of the Llama Materials that you distribute the following attribution notice within a \u201cNotice\u201d text file distributed as a part of such copies: \u201cMeta Llama 3 is licensed under the Meta Llama 3 Community License, Copyright \u00a9 Meta Platforms, Inc. All Rights Reserved.\u201d\niv. Your use of the Llama Materials must comply with applicable laws and regulations (including trade compliance laws and regulations) and adhere to the Acceptable Use Policy for the Llama Materials (available at https://llama.meta.com/llama3/use-policy), which is hereby incorporated by reference into this Agreement.\nv. You will not use the Llama Materials or any output or results of the Llama Materials to improve any other large language model (excluding Meta Llama 3 or derivative works thereof).\n2. Additional Commercial Terms. If, on the Meta Llama 3 version release date, the monthly active users of the products or services made available by or for Licensee, or Licensee\u2019s affiliates, is greater than 700 million monthly active users in the preceding calendar month, you must request a license from Meta, which Meta may grant to you in its sole discretion, and you are not authorized to exercise any of the rights under this Agreement unless or until Meta otherwise expressly grants you such rights.\n3. Disclaimer of Warranty. UNLESS REQUIRED BY APPLICABLE LAW, THE LLAMA MATERIALS AND ANY OUTPUT AND RESULTS THEREFROM ARE PROVIDED ON AN \u201cAS IS\u201d BASIS, WITHOUT WARRANTIES OF ANY KIND, AND META DISCLAIMS ALL WARRANTIES OF ANY KIND, BOTH EXPRESS AND IMPLIED, INCLUDING, WITHOUT LIMITATION, ANY WARRANTIES OF TITLE, NON-INFRINGEMENT, MERCHANTABILITY, OR FITNESS FOR A PARTICULAR PURPOSE. YOU ARE SOLELY RESPONSIBLE FOR DETERMINING THE APPROPRIATENESS OF USING OR REDISTRIBUTING THE LLAMA MATERIALS AND ASSUME ANY RISKS ASSOCIATED WITH YOUR USE OF THE LLAMA MATERIALS AND ANY OUTPUT AND RESULTS.\n4. Limitation of Liability. IN NO EVENT WILL META OR ITS AFFILIATES BE LIABLE UNDER ANY THEORY OF LIABILITY, WHETHER IN CONTRACT, TORT, NEGLIGENCE, PRODUCTS LIABILITY, OR OTHERWISE, ARISING OUT OF THIS AGREEMENT, FOR ANY LOST PROFITS OR ANY INDIRECT, SPECIAL, CONSEQUENTIAL, INCIDENTAL, EXEMPLARY OR PUNITIVE DAMAGES, EVEN IF META OR ITS AFFILIATES HAVE BEEN ADVISED OF THE POSSIBILITY OF ANY OF THE FOREGOING.\n5. Intellectual Property.\na. No trademark licenses are granted under this Agreement, and in connection with the Llama Materials, neither Meta nor Licensee may use any name or mark owned by or associated with the other or any of its affiliates, except as required for reasonable and customary use in describing and redistributing the Llama Materials or as set forth in this Section 5(a). Meta hereby grants you a license to use \u201cLlama 3\u201d (the \u201cMark\u201d) solely as required to comply with the last sentence of Section 1.b.i. You will comply with Meta\u2019s brand guidelines (currently accessible at https://about.meta.com/brand/resources/meta/company-brand/ ). All goodwill arising out of your use of the Mark will inure to the benefit of Meta.\nb. Subject to Meta\u2019s ownership of Llama Materials and derivatives made by or for Meta, with respect to any derivative works and modifications of the Llama Materials that are made by you, as between you and Meta, you are and will be the owner of such derivative works and modifications.\nc. If you institute litigation or other proceedings against Meta or any entity (including a cross-claim or counterclaim in a lawsuit) alleging that the Llama Materials or Meta Llama 3 outputs or results, or any portion of any of the foregoing, constitutes infringement of intellectual property or other rights owned or licensable by you, then any licenses granted to you under this Agreement shall terminate as of the date such litigation or claim is filed or instituted. You will indemnify and hold harmless Meta from and against any claim by any third party arising out of or related to your use or distribution of the Llama Materials.\n6. Term and Termination. The term of this Agreement will commence upon your acceptance of this Agreement or access to the Llama Materials and will continue in full force and effect until terminated in accordance with the terms and conditions herein. Meta may terminate this Agreement if you are in breach of any term or condition of this Agreement. Upon termination of this Agreement, you shall delete and cease use of the Llama Materials. Sections 3, 4 and 7 shall survive the termination of this Agreement.\n7. Governing Law and Jurisdiction. This Agreement will be governed and construed under the laws of the State of California without regard to choice of law principles, and the UN Convention on Contracts for the International Sale of Goods does not apply to this Agreement. The courts of California shall have exclusive jurisdiction of any dispute arising out of this Agreement.\n### Meta Llama 3 Acceptable Use Policy\nMeta is committed to promoting safe and fair use of its tools and features, including Meta Llama 3. If you access or use Meta Llama 3, you agree to this Acceptable Use Policy (\u201cPolicy\u201d). The most recent copy of this policy can be found at [https://llama.meta.com/llama3/use-policy](https://llama.meta.com/llama3/use-policy)\n#### Prohibited Uses\nWe want everyone to use Meta Llama 3 safely and responsibly. You agree you will not use, or allow others to use, Meta Llama 3 to: 1. Violate the law or others\u2019 rights, including to:\n 1. Engage in, promote, generate, contribute to, encourage, plan, incite, or further illegal or unlawful activity or content, such as:\n 1. Violence or terrorism\n 2. Exploitation or harm to children, including the solicitation, creation, acquisition, or dissemination of child exploitative content or failure to report Child Sexual Abuse Material\n 3. Human trafficking, exploitation, and sexual violence\n 4. The illegal distribution of information or materials to minors, including obscene materials, or failure to employ legally required age-gating in connection with such information or materials.\n 5. Sexual solicitation\n 6. Any other criminal activity\n 2. Engage in, promote, incite, or facilitate the harassment, abuse, threatening, or bullying of individuals or groups of individuals\n 3. Engage in, promote, incite, or facilitate discrimination or other unlawful or harmful conduct in the provision of employment, employment benefits, credit, housing, other economic benefits, or other essential goods and services\n 4. Engage in the unauthorized or unlicensed practice of any profession including, but not limited to, financial, legal, medical/health, or related professional practices\n 5. Collect, process, disclose, generate, or infer health, demographic, or other sensitive personal or private information about individuals without rights and consents required by applicable laws\n 6. Engage in or facilitate any action or generate any content that infringes, misappropriates, or otherwise violates any third-party rights, including the outputs or results of any products or services using the Llama Materials\n 7. Create, generate, or facilitate the creation of malicious code, malware, computer viruses or do anything else that could disable, overburden, interfere with or impair the proper working, integrity, operation or appearance of a website or computer system\n2. Engage in, promote, incite, facilitate, or assist in the planning or development of activities that present a risk of death or bodily harm to individuals, including use of Meta Llama 3 related to the following:\n 1. Military, warfare, nuclear industries or applications, espionage, use for materials or activities that are subject to the International Traffic Arms Regulations (ITAR) maintained by the United States Department of State\n 2. Guns and illegal weapons (including weapon development)\n 3. Illegal drugs and regulated/controlled substances\n 4. Operation of critical infrastructure, transportation technologies, or heavy machinery\n 5. Self-harm or harm to others, including suicide, cutting, and eating disorders\n 6. Any content intended to incite or promote violence, abuse, or any infliction of bodily harm to an individual\n3. Intentionally deceive or mislead others, including use of Meta Llama 3 related to the following:\n 1. Generating, promoting, or furthering fraud or the creation or promotion of disinformation\n 2. Generating, promoting, or furthering defamatory content, including the creation of defamatory statements, images, or other content\n 3. Generating, promoting, or further distributing spam\n 4. Impersonating another individual without consent, authorization, or legal right\n 5. Representing that the use of Meta Llama 3 or outputs are human-generated\n 6. Generating or facilitating false online engagement, including fake reviews and other means of fake online engagement\n4. Fail to appropriately disclose to end users any known dangers of your AI system\nPlease report any violation of this Policy, software \u201cbug,\u201d or other problems that could lead to a violation of this Policy through one of the following means:\n * Reporting issues with the model: [https://github.com/meta-llama/llama3](https://github.com/meta-llama/llama3)\n * Reporting risky content generated by the model:\n developers.facebook.com/llama_output_feedback\n * Reporting bugs and security concerns: facebook.com/whitehat/info\n * Reporting violations of the Acceptable Use Policy or unlicensed uses of Meta Llama 3: [email protected]", "extra_gated_fields": {"First Name": "text", "Last Name": "text", "Date of birth": "date_picker", "Country": "country", "Affiliation": "text", "geo": "ip_location", "By clicking Submit below I accept the terms of the license and acknowledge that the information I provide will be collected stored processed and shared in accordance with the Meta Privacy Policy": "checkbox"}, "extra_gated_description": "The information you provide will be collected, stored, processed and shared in accordance with the [Meta Privacy Policy](https://www.facebook.com/privacy/policy/).", "extra_gated_button_content": "Submit"} | mlabonne/Meta-Llama-3-8B | null | [
"transformers",
"safetensors",
"llama",
"text-generation",
"facebook",
"meta",
"pytorch",
"llama-3",
"en",
"license:other",
"autotrain_compatible",
"endpoints_compatible",
"text-generation-inference",
"region:us",
"has_space"
] | null | 2024-05-02T10:40:58+00:00 | [] | [
"en"
] | TAGS
#transformers #safetensors #llama #text-generation #facebook #meta #pytorch #llama-3 #en #license-other #autotrain_compatible #endpoints_compatible #text-generation-inference #region-us #has_space
| Model Details
-------------
Meta developed and released the Meta Llama 3 family of large language models (LLMs), a collection of pretrained and instruction tuned generative text models in 8 and 70B sizes. The Llama 3 instruction tuned models are optimized for dialogue use cases and outperform many of the available open source chat models on common industry benchmarks. Further, in developing these models, we took great care to optimize helpfulness and safety.
Model developers Meta
Variations Llama 3 comes in two sizes — 8B and 70B parameters — in pre-trained and instruction tuned variants.
Input Models input text only.
Output Models generate text and code only.
Model Architecture Llama 3 is an auto-regressive language model that uses an optimized transformer architecture. The tuned versions use supervised fine-tuning (SFT) and reinforcement learning with human feedback (RLHF) to align with human preferences for helpfulness and safety.
Llama 3 family of models. Token counts refer to pretraining data only. Both the 8 and 70B versions use Grouped-Query Attention (GQA) for improved inference scalability.
Model Release Date April 18, 2024.
Status This is a static model trained on an offline dataset. Future versions of the tuned models will be released as we improve model safety with community feedback.
License A custom commercial license is available at: URL
Where to send questions or comments about the model Instructions on how to provide feedback or comments on the model can be found in the model README. For more technical information about generation parameters and recipes for how to use Llama 3 in applications, please go here.
Intended Use
------------
Intended Use Cases Llama 3 is intended for commercial and research use in English. Instruction tuned models are intended for assistant-like chat, whereas pretrained models can be adapted for a variety of natural language generation tasks.
Out-of-scope Use in any manner that violates applicable laws or regulations (including trade compliance laws). Use in any other way that is prohibited by the Acceptable Use Policy and Llama 3 Community License. Use in languages other than English.
Note: Developers may fine-tune Llama 3 models for languages beyond English provided they comply with the Llama 3 Community License and the Acceptable Use Policy.
How to use
----------
This repository contains two versions of Meta-Llama-3-8B, for use with transformers and with the original 'llama3' codebase.
### Use with transformers
See the snippet below for usage with Transformers:
### Use with 'llama3'
Please, follow the instructions in the repository.
To download Original checkpoints, see the example command below leveraging 'huggingface-cli':
For Hugging Face support, we recommend using transformers or TGI, but a similar command works.
Hardware and Software
---------------------
Training Factors We used custom training libraries, Meta's Research SuperCluster, and production clusters for pretraining. Fine-tuning, annotation, and evaluation were also performed on third-party cloud compute.
Carbon Footprint Pretraining utilized a cumulative 7.7M GPU hours of computation on hardware of type H100-80GB (TDP of 700W). Estimated total emissions were 2290 tCO2eq, 100% of which were offset by Meta’s sustainability program.
CO2 emissions during pre-training. Time: total GPU time required for training each model. Power Consumption: peak power capacity per GPU device for the GPUs used adjusted for power usage efficiency. 100% of the emissions are directly offset by Meta's sustainability program, and because we are openly releasing these models, the pretraining costs do not need to be incurred by others.
Training Data
-------------
Overview Llama 3 was pretrained on over 15 trillion tokens of data from publicly available sources. The fine-tuning data includes publicly available instruction datasets, as well as over 10M human-annotated examples. Neither the pretraining nor the fine-tuning datasets include Meta user data.
Data Freshness The pretraining data has a cutoff of March 2023 for the 7B and December 2023 for the 70B models respectively.
Benchmarks
----------
In this section, we report the results for Llama 3 models on standard automatic benchmarks. For all the evaluations, we use our internal evaluations library. For details on the methodology see here.
### Base pretrained models
### Instruction tuned models
### Responsibility & Safety
We believe that an open approach to AI leads to better, safer products, faster innovation, and a bigger overall market. We are committed to Responsible AI development and took a series of steps to limit misuse and harm and support the open source community.
Foundation models are widely capable technologies that are built to be used for a diverse range of applications. They are not designed to meet every developer preference on safety levels for all use cases, out-of-the-box, as those by their nature will differ across different applications.
Rather, responsible LLM-application deployment is achieved by implementing a series of safety best practices throughout the development of such applications, from the model pre-training, fine-tuning and the deployment of systems composed of safeguards to tailor the safety needs specifically to the use case and audience.
As part of the Llama 3 release, we updated our Responsible Use Guide to outline the steps and best practices for developers to implement model and system level safety for their application. We also provide a set of resources including Meta Llama Guard 2 and Code Shield safeguards. These tools have proven to drastically reduce residual risks of LLM Systems, while maintaining a high level of helpfulness. We encourage developers to tune and deploy these safeguards according to their needs and we provide a reference implementation to get you started.
#### Llama 3-Instruct
As outlined in the Responsible Use Guide, some trade-off between model helpfulness and model alignment is likely unavoidable. Developers should exercise discretion about how to weigh the benefits of alignment and helpfulness for their specific use case and audience. Developers should be mindful of residual risks when using Llama models and leverage additional safety tools as needed to reach the right safety bar for their use case.
Safety
For our instruction tuned model, we conducted extensive red teaming exercises, performed adversarial evaluations and implemented safety mitigations techniques to lower residual risks. As with any Large Language Model, residual risks will likely remain and we recommend that developers assess these risks in the context of their use case. In parallel, we are working with the community to make AI safety benchmark standards transparent, rigorous and interpretable.
Refusals
In addition to residual risks, we put a great emphasis on model refusals to benign prompts. Over-refusing not only can impact the user experience but could even be harmful in certain contexts as well. We’ve heard the feedback from the developer community and improved our fine tuning to ensure that Llama 3 is significantly less likely to falsely refuse to answer prompts than Llama 2.
We built internal benchmarks and developed mitigations to limit false refusals making Llama 3 our most helpful model to date.
#### Responsible release
In addition to responsible use considerations outlined above, we followed a rigorous process that requires us to take extra measures against misuse and critical risks before we make our release decision.
Misuse
If you access or use Llama 3, you agree to the Acceptable Use Policy. The most recent copy of this policy can be found at URL
#### Critical risks
CBRNE (Chemical, Biological, Radiological, Nuclear, and high yield Explosives)
We have conducted a two fold assessment of the safety of the model in this area:
* Iterative testing during model training to assess the safety of responses related to CBRNE threats and other adversarial risks.
* Involving external CBRNE experts to conduct an uplift test assessing the ability of the model to accurately provide expert knowledge and reduce barriers to potential CBRNE misuse, by reference to what can be achieved using web search (without the model).
### Cyber Security
We have evaluated Llama 3 with CyberSecEval, Meta’s cybersecurity safety eval suite, measuring Llama 3’s propensity to suggest insecure code when used as a coding assistant, and Llama 3’s propensity to comply with requests to help carry out cyber attacks, where attacks are defined by the industry standard MITRE ATT&CK cyber attack ontology. On our insecure coding and cyber attacker helpfulness tests, Llama 3 behaved in the same range or safer than models of equivalent coding capability.
### Child Safety
Child Safety risk assessments were conducted using a team of experts, to assess the model’s capability to produce outputs that could result in Child Safety risks and inform on any necessary and appropriate risk mitigations via fine tuning. We leveraged those expert red teaming sessions to expand the coverage of our evaluation benchmarks through Llama 3 model development. For Llama 3, we conducted new in-depth sessions using objective based methodologies to assess the model risks along multiple attack vectors. We also partnered with content specialists to perform red teaming exercises assessing potentially violating content while taking account of market specific nuances or experiences.
### Community
Generative AI safety requires expertise and tooling, and we believe in the strength of the open community to accelerate its progress. We are active members of open consortiums, including the AI Alliance, Partnership in AI and MLCommons, actively contributing to safety standardization and transparency. We encourage the community to adopt taxonomies like the MLCommons Proof of Concept evaluation to facilitate collaboration and transparency on safety and content evaluations. Our Purple Llama tools are open sourced for the community to use and widely distributed across ecosystem partners including cloud service providers. We encourage community contributions to our Github repository.
Finally, we put in place a set of resources including an output reporting mechanism and bug bounty program to continuously improve the Llama technology with the help of the community.
Ethical Considerations and Limitations
--------------------------------------
The core values of Llama 3 are openness, inclusivity and helpfulness. It is meant to serve everyone, and to work for a wide range of use cases. It is thus designed to be accessible to people across many different backgrounds, experiences and perspectives. Llama 3 addresses users and their needs as they are, without insertion unnecessary judgment or normativity, while reflecting the understanding that even content that may appear problematic in some cases can serve valuable purposes in others. It respects the dignity and autonomy of all users, especially in terms of the values of free thought and expression that power innovation and progress.
But Llama 3 is a new technology, and like any new technology, there are risks associated with its use. Testing conducted to date has been in English, and has not covered, nor could it cover, all scenarios. For these reasons, as with all LLMs, Llama 3’s potential outputs cannot be predicted in advance, and the model may in some instances produce inaccurate, biased or other objectionable responses to user prompts. Therefore, before deploying any applications of Llama 3 models, developers should perform safety testing and tuning tailored to their specific applications of the model. As outlined in the Responsible Use Guide, we recommend incorporating Purple Llama solutions into your workflows and specifically Llama Guard which provides a base model to filter input and output prompts to layer system-level safety on top of model-level safety.
Please see the Responsible Use Guide available at URL
instructions
@article{llama3modelcard,
title={Llama 3 Model Card},
author={AI@Meta},
year={2024},
url = {URL
}
Contributors
------------
Aaditya Singh; Aaron Grattafiori; Abhimanyu Dubey; Abhinav Jauhri; Abhinav Pandey; Abhishek Kadian; Adam Kelsey; Adi Gangidi; Ahmad Al-Dahle; Ahuva Goldstand; Aiesha Letman; Ajay Menon; Akhil Mathur; Alan Schelten; Alex Vaughan; Amy Yang; Andrei Lupu; Andres Alvarado; Andrew Gallagher; Andrew Gu; Andrew Ho; Andrew Poulton; Andrew Ryan; Angela Fan; Ankit Ramchandani; Anthony Hartshorn; Archi Mitra; Archie Sravankumar; Artem Korenev; Arun Rao; Ashley Gabriel; Ashwin Bharambe; Assaf Eisenman; Aston Zhang; Aurelien Rodriguez; Austen Gregerson; Ava Spataru; Baptiste Roziere; Ben Maurer; Benjamin Leonhardi; Bernie Huang; Bhargavi Paranjape; Bing Liu; Binh Tang; Bobbie Chern; Brani Stojkovic; Brian Fuller; Catalina Mejia Arenas; Chao Zhou; Charlotte Caucheteux; Chaya Nayak; Ching-Hsiang Chu; Chloe Bi; Chris Cai; Chris Cox; Chris Marra; Chris McConnell; Christian Keller; Christoph Feichtenhofer; Christophe Touret; Chunyang Wu; Corinne Wong; Cristian Canton Ferrer; Damien Allonsius; Daniel Kreymer; Daniel Haziza; Daniel Li; Danielle Pintz; Danny Livshits; Danny Wyatt; David Adkins; David Esiobu; David Xu; Davide Testuggine; Delia David; Devi Parikh; Dhruv Choudhary; Dhruv Mahajan; Diana Liskovich; Diego Garcia-Olano; Diego Perino; Dieuwke Hupkes; Dingkang Wang; Dustin Holland; Egor Lakomkin; Elina Lobanova; Xiaoqing Ellen Tan; Emily Dinan; Eric Smith; Erik Brinkman; Esteban Arcaute; Filip Radenovic; Firat Ozgenel; Francesco Caggioni; Frank Seide; Frank Zhang; Gabriel Synnaeve; Gabriella Schwarz; Gabrielle Lee; Gada Badeer; Georgia Anderson; Graeme Nail; Gregoire Mialon; Guan Pang; Guillem Cucurell; Hailey Nguyen; Hannah Korevaar; Hannah Wang; Haroun Habeeb; Harrison Rudolph; Henry Aspegren; Hu Xu; Hugo Touvron; Iga Kozlowska; Igor Molybog; Igor Tufanov; Iliyan Zarov; Imanol Arrieta Ibarra; Irina-Elena Veliche; Isabel Kloumann; Ishan Misra; Ivan Evtimov; Jacob Xu; Jade Copet; Jake Weissman; Jan Geffert; Jana Vranes; Japhet Asher; Jason Park; Jay Mahadeokar; Jean-Baptiste Gaya; Jeet Shah; Jelmer van der Linde; Jennifer Chan; Jenny Hong; Jenya Lee; Jeremy Fu; Jeremy Teboul; Jianfeng Chi; Jianyu Huang; Jie Wang; Jiecao Yu; Joanna Bitton; Joe Spisak; Joelle Pineau; Jon Carvill; Jongsoo Park; Joseph Rocca; Joshua Johnstun; Junteng Jia; Kalyan Vasuden Alwala; Kam Hou U; Kate Plawiak; Kartikeya Upasani; Kaushik Veeraraghavan; Ke Li; Kenneth Heafield; Kevin Stone; Khalid El-Arini; Krithika Iyer; Kshitiz Malik; Kuenley Chiu; Kunal Bhalla; Kyle Huang; Lakshya Garg; Lauren Rantala-Yeary; Laurens van der Maaten; Lawrence Chen; Leandro Silva; Lee Bell; Lei Zhang; Liang Tan; Louis Martin; Lovish Madaan; Luca Wehrstedt; Lukas Blecher; Luke de Oliveira; Madeline Muzzi; Madian Khabsa; Manav Avlani; Mannat Singh; Manohar Paluri; Mark Zuckerberg; Marcin Kardas; Martynas Mankus; Mathew Oldham; Mathieu Rita; Matthew Lennie; Maya Pavlova; Meghan Keneally; Melanie Kambadur; Mihir Patel; Mikayel Samvelyan; Mike Clark; Mike Lewis; Min Si; Mitesh Kumar Singh; Mo Metanat; Mona Hassan; Naman Goyal; Narjes Torabi; Nicolas Usunier; Nikolay Bashlykov; Nikolay Bogoychev; Niladri Chatterji; Ning Dong; Oliver Aobo Yang; Olivier Duchenne; Onur Celebi; Parth Parekh; Patrick Alrassy; Paul Saab; Pavan Balaji; Pedro Rittner; Pengchuan Zhang; Pengwei Li; Petar Vasic; Peter Weng; Polina Zvyagina; Prajjwal Bhargava; Pratik Dubal; Praveen Krishnan; Punit Singh Koura; Qing He; Rachel Rodriguez; Ragavan Srinivasan; Rahul Mitra; Ramon Calderer; Raymond Li; Robert Stojnic; Roberta Raileanu; Robin Battey; Rocky Wang; Rohit Girdhar; Rohit Patel; Romain Sauvestre; Ronnie Polidoro; Roshan Sumbaly; Ross Taylor; Ruan Silva; Rui Hou; Rui Wang; Russ Howes; Ruty Rinott; Saghar Hosseini; Sai Jayesh Bondu; Samyak Datta; Sanjay Singh; Sara Chugh; Sargun Dhillon; Satadru Pan; Sean Bell; Sergey Edunov; Shaoliang Nie; Sharan Narang; Sharath Raparthy; Shaun Lindsay; Sheng Feng; Sheng Shen; Shenghao Lin; Shiva Shankar; Shruti Bhosale; Shun Zhang; Simon Vandenhende; Sinong Wang; Seohyun Sonia Kim; Soumya Batra; Sten Sootla; Steve Kehoe; Suchin Gururangan; Sumit Gupta; Sunny Virk; Sydney Borodinsky; Tamar Glaser; Tamar Herman; Tamara Best; Tara Fowler; Thomas Georgiou; Thomas Scialom; Tianhe Li; Todor Mihaylov; Tong Xiao; Ujjwal Karn; Vedanuj Goswami; Vibhor Gupta; Vignesh Ramanathan; Viktor Kerkez; Vinay Satish Kumar; Vincent Gonguet; Vish Vogeti; Vlad Poenaru; Vlad Tiberiu Mihailescu; Vladan Petrovic; Vladimir Ivanov; Wei Li; Weiwei Chu; Wenhan Xiong; Wenyin Fu; Wes Bouaziz; Whitney Meers; Will Constable; Xavier Martinet; Xiaojian Wu; Xinbo Gao; Xinfeng Xie; Xuchao Jia; Yaelle Goldschlag; Yann LeCun; Yashesh Gaur; Yasmine Babaei; Ye Qi; Yenda Li; Yi Wen; Yiwen Song; Youngjin Nam; Yuchen Hao; Yuchen Zhang; Yun Wang; Yuning Mao; Yuzi He; Zacharie Delpierre Coudert; Zachary DeVito; Zahra Hankir; Zhaoduo Wen; Zheng Yan; Zhengxing Chen; Zhenyu Yang; Zoe Papakipos
| [
"### Use with transformers\n\n\nSee the snippet below for usage with Transformers:",
"### Use with 'llama3'\n\n\nPlease, follow the instructions in the repository.\n\n\nTo download Original checkpoints, see the example command below leveraging 'huggingface-cli':\n\n\nFor Hugging Face support, we recommend using transformers or TGI, but a similar command works.\n\n\nHardware and Software\n---------------------\n\n\nTraining Factors We used custom training libraries, Meta's Research SuperCluster, and production clusters for pretraining. Fine-tuning, annotation, and evaluation were also performed on third-party cloud compute.\n\n\nCarbon Footprint Pretraining utilized a cumulative 7.7M GPU hours of computation on hardware of type H100-80GB (TDP of 700W). Estimated total emissions were 2290 tCO2eq, 100% of which were offset by Meta’s sustainability program.\n\n\n\nCO2 emissions during pre-training. Time: total GPU time required for training each model. Power Consumption: peak power capacity per GPU device for the GPUs used adjusted for power usage efficiency. 100% of the emissions are directly offset by Meta's sustainability program, and because we are openly releasing these models, the pretraining costs do not need to be incurred by others.\n\n\nTraining Data\n-------------\n\n\nOverview Llama 3 was pretrained on over 15 trillion tokens of data from publicly available sources. The fine-tuning data includes publicly available instruction datasets, as well as over 10M human-annotated examples. Neither the pretraining nor the fine-tuning datasets include Meta user data.\n\n\nData Freshness The pretraining data has a cutoff of March 2023 for the 7B and December 2023 for the 70B models respectively.\n\n\nBenchmarks\n----------\n\n\nIn this section, we report the results for Llama 3 models on standard automatic benchmarks. For all the evaluations, we use our internal evaluations library. For details on the methodology see here.",
"### Base pretrained models",
"### Instruction tuned models",
"### Responsibility & Safety\n\n\nWe believe that an open approach to AI leads to better, safer products, faster innovation, and a bigger overall market. We are committed to Responsible AI development and took a series of steps to limit misuse and harm and support the open source community.\n\n\nFoundation models are widely capable technologies that are built to be used for a diverse range of applications. They are not designed to meet every developer preference on safety levels for all use cases, out-of-the-box, as those by their nature will differ across different applications.\n\n\nRather, responsible LLM-application deployment is achieved by implementing a series of safety best practices throughout the development of such applications, from the model pre-training, fine-tuning and the deployment of systems composed of safeguards to tailor the safety needs specifically to the use case and audience.\n\n\nAs part of the Llama 3 release, we updated our Responsible Use Guide to outline the steps and best practices for developers to implement model and system level safety for their application. We also provide a set of resources including Meta Llama Guard 2 and Code Shield safeguards. These tools have proven to drastically reduce residual risks of LLM Systems, while maintaining a high level of helpfulness. We encourage developers to tune and deploy these safeguards according to their needs and we provide a reference implementation to get you started.",
"#### Llama 3-Instruct\n\n\nAs outlined in the Responsible Use Guide, some trade-off between model helpfulness and model alignment is likely unavoidable. Developers should exercise discretion about how to weigh the benefits of alignment and helpfulness for their specific use case and audience. Developers should be mindful of residual risks when using Llama models and leverage additional safety tools as needed to reach the right safety bar for their use case.\n\n\nSafety\n\n\nFor our instruction tuned model, we conducted extensive red teaming exercises, performed adversarial evaluations and implemented safety mitigations techniques to lower residual risks. As with any Large Language Model, residual risks will likely remain and we recommend that developers assess these risks in the context of their use case. In parallel, we are working with the community to make AI safety benchmark standards transparent, rigorous and interpretable.\n\n\nRefusals\n\n\nIn addition to residual risks, we put a great emphasis on model refusals to benign prompts. Over-refusing not only can impact the user experience but could even be harmful in certain contexts as well. We’ve heard the feedback from the developer community and improved our fine tuning to ensure that Llama 3 is significantly less likely to falsely refuse to answer prompts than Llama 2.\n\n\nWe built internal benchmarks and developed mitigations to limit false refusals making Llama 3 our most helpful model to date.",
"#### Responsible release\n\n\nIn addition to responsible use considerations outlined above, we followed a rigorous process that requires us to take extra measures against misuse and critical risks before we make our release decision.\n\n\nMisuse\n\n\nIf you access or use Llama 3, you agree to the Acceptable Use Policy. The most recent copy of this policy can be found at URL",
"#### Critical risks\n\n\nCBRNE (Chemical, Biological, Radiological, Nuclear, and high yield Explosives)\n\n\nWe have conducted a two fold assessment of the safety of the model in this area:\n\n\n* Iterative testing during model training to assess the safety of responses related to CBRNE threats and other adversarial risks.\n* Involving external CBRNE experts to conduct an uplift test assessing the ability of the model to accurately provide expert knowledge and reduce barriers to potential CBRNE misuse, by reference to what can be achieved using web search (without the model).",
"### Cyber Security\n\n\nWe have evaluated Llama 3 with CyberSecEval, Meta’s cybersecurity safety eval suite, measuring Llama 3’s propensity to suggest insecure code when used as a coding assistant, and Llama 3’s propensity to comply with requests to help carry out cyber attacks, where attacks are defined by the industry standard MITRE ATT&CK cyber attack ontology. On our insecure coding and cyber attacker helpfulness tests, Llama 3 behaved in the same range or safer than models of equivalent coding capability.",
"### Child Safety\n\n\nChild Safety risk assessments were conducted using a team of experts, to assess the model’s capability to produce outputs that could result in Child Safety risks and inform on any necessary and appropriate risk mitigations via fine tuning. We leveraged those expert red teaming sessions to expand the coverage of our evaluation benchmarks through Llama 3 model development. For Llama 3, we conducted new in-depth sessions using objective based methodologies to assess the model risks along multiple attack vectors. We also partnered with content specialists to perform red teaming exercises assessing potentially violating content while taking account of market specific nuances or experiences.",
"### Community\n\n\nGenerative AI safety requires expertise and tooling, and we believe in the strength of the open community to accelerate its progress. We are active members of open consortiums, including the AI Alliance, Partnership in AI and MLCommons, actively contributing to safety standardization and transparency. We encourage the community to adopt taxonomies like the MLCommons Proof of Concept evaluation to facilitate collaboration and transparency on safety and content evaluations. Our Purple Llama tools are open sourced for the community to use and widely distributed across ecosystem partners including cloud service providers. We encourage community contributions to our Github repository.\n\n\nFinally, we put in place a set of resources including an output reporting mechanism and bug bounty program to continuously improve the Llama technology with the help of the community.\n\n\nEthical Considerations and Limitations\n--------------------------------------\n\n\nThe core values of Llama 3 are openness, inclusivity and helpfulness. It is meant to serve everyone, and to work for a wide range of use cases. It is thus designed to be accessible to people across many different backgrounds, experiences and perspectives. Llama 3 addresses users and their needs as they are, without insertion unnecessary judgment or normativity, while reflecting the understanding that even content that may appear problematic in some cases can serve valuable purposes in others. It respects the dignity and autonomy of all users, especially in terms of the values of free thought and expression that power innovation and progress.\n\n\nBut Llama 3 is a new technology, and like any new technology, there are risks associated with its use. Testing conducted to date has been in English, and has not covered, nor could it cover, all scenarios. For these reasons, as with all LLMs, Llama 3’s potential outputs cannot be predicted in advance, and the model may in some instances produce inaccurate, biased or other objectionable responses to user prompts. Therefore, before deploying any applications of Llama 3 models, developers should perform safety testing and tuning tailored to their specific applications of the model. As outlined in the Responsible Use Guide, we recommend incorporating Purple Llama solutions into your workflows and specifically Llama Guard which provides a base model to filter input and output prompts to layer system-level safety on top of model-level safety.\n\n\nPlease see the Responsible Use Guide available at URL\n\n\ninstructions\n\n\n@article{llama3modelcard,\n\n\ntitle={Llama 3 Model Card},\n\n\nauthor={AI@Meta},\n\n\nyear={2024},\n\n\nurl = {URL\n\n\n}\n\n\nContributors\n------------\n\n\nAaditya Singh; Aaron Grattafiori; Abhimanyu Dubey; Abhinav Jauhri; Abhinav Pandey; Abhishek Kadian; Adam Kelsey; Adi Gangidi; Ahmad Al-Dahle; Ahuva Goldstand; Aiesha Letman; Ajay Menon; Akhil Mathur; Alan Schelten; Alex Vaughan; Amy Yang; Andrei Lupu; Andres Alvarado; Andrew Gallagher; Andrew Gu; Andrew Ho; Andrew Poulton; Andrew Ryan; Angela Fan; Ankit Ramchandani; Anthony Hartshorn; Archi Mitra; Archie Sravankumar; Artem Korenev; Arun Rao; Ashley Gabriel; Ashwin Bharambe; Assaf Eisenman; Aston Zhang; Aurelien Rodriguez; Austen Gregerson; Ava Spataru; Baptiste Roziere; Ben Maurer; Benjamin Leonhardi; Bernie Huang; Bhargavi Paranjape; Bing Liu; Binh Tang; Bobbie Chern; Brani Stojkovic; Brian Fuller; Catalina Mejia Arenas; Chao Zhou; Charlotte Caucheteux; Chaya Nayak; Ching-Hsiang Chu; Chloe Bi; Chris Cai; Chris Cox; Chris Marra; Chris McConnell; Christian Keller; Christoph Feichtenhofer; Christophe Touret; Chunyang Wu; Corinne Wong; Cristian Canton Ferrer; Damien Allonsius; Daniel Kreymer; Daniel Haziza; Daniel Li; Danielle Pintz; Danny Livshits; Danny Wyatt; David Adkins; David Esiobu; David Xu; Davide Testuggine; Delia David; Devi Parikh; Dhruv Choudhary; Dhruv Mahajan; Diana Liskovich; Diego Garcia-Olano; Diego Perino; Dieuwke Hupkes; Dingkang Wang; Dustin Holland; Egor Lakomkin; Elina Lobanova; Xiaoqing Ellen Tan; Emily Dinan; Eric Smith; Erik Brinkman; Esteban Arcaute; Filip Radenovic; Firat Ozgenel; Francesco Caggioni; Frank Seide; Frank Zhang; Gabriel Synnaeve; Gabriella Schwarz; Gabrielle Lee; Gada Badeer; Georgia Anderson; Graeme Nail; Gregoire Mialon; Guan Pang; Guillem Cucurell; Hailey Nguyen; Hannah Korevaar; Hannah Wang; Haroun Habeeb; Harrison Rudolph; Henry Aspegren; Hu Xu; Hugo Touvron; Iga Kozlowska; Igor Molybog; Igor Tufanov; Iliyan Zarov; Imanol Arrieta Ibarra; Irina-Elena Veliche; Isabel Kloumann; Ishan Misra; Ivan Evtimov; Jacob Xu; Jade Copet; Jake Weissman; Jan Geffert; Jana Vranes; Japhet Asher; Jason Park; Jay Mahadeokar; Jean-Baptiste Gaya; Jeet Shah; Jelmer van der Linde; Jennifer Chan; Jenny Hong; Jenya Lee; Jeremy Fu; Jeremy Teboul; Jianfeng Chi; Jianyu Huang; Jie Wang; Jiecao Yu; Joanna Bitton; Joe Spisak; Joelle Pineau; Jon Carvill; Jongsoo Park; Joseph Rocca; Joshua Johnstun; Junteng Jia; Kalyan Vasuden Alwala; Kam Hou U; Kate Plawiak; Kartikeya Upasani; Kaushik Veeraraghavan; Ke Li; Kenneth Heafield; Kevin Stone; Khalid El-Arini; Krithika Iyer; Kshitiz Malik; Kuenley Chiu; Kunal Bhalla; Kyle Huang; Lakshya Garg; Lauren Rantala-Yeary; Laurens van der Maaten; Lawrence Chen; Leandro Silva; Lee Bell; Lei Zhang; Liang Tan; Louis Martin; Lovish Madaan; Luca Wehrstedt; Lukas Blecher; Luke de Oliveira; Madeline Muzzi; Madian Khabsa; Manav Avlani; Mannat Singh; Manohar Paluri; Mark Zuckerberg; Marcin Kardas; Martynas Mankus; Mathew Oldham; Mathieu Rita; Matthew Lennie; Maya Pavlova; Meghan Keneally; Melanie Kambadur; Mihir Patel; Mikayel Samvelyan; Mike Clark; Mike Lewis; Min Si; Mitesh Kumar Singh; Mo Metanat; Mona Hassan; Naman Goyal; Narjes Torabi; Nicolas Usunier; Nikolay Bashlykov; Nikolay Bogoychev; Niladri Chatterji; Ning Dong; Oliver Aobo Yang; Olivier Duchenne; Onur Celebi; Parth Parekh; Patrick Alrassy; Paul Saab; Pavan Balaji; Pedro Rittner; Pengchuan Zhang; Pengwei Li; Petar Vasic; Peter Weng; Polina Zvyagina; Prajjwal Bhargava; Pratik Dubal; Praveen Krishnan; Punit Singh Koura; Qing He; Rachel Rodriguez; Ragavan Srinivasan; Rahul Mitra; Ramon Calderer; Raymond Li; Robert Stojnic; Roberta Raileanu; Robin Battey; Rocky Wang; Rohit Girdhar; Rohit Patel; Romain Sauvestre; Ronnie Polidoro; Roshan Sumbaly; Ross Taylor; Ruan Silva; Rui Hou; Rui Wang; Russ Howes; Ruty Rinott; Saghar Hosseini; Sai Jayesh Bondu; Samyak Datta; Sanjay Singh; Sara Chugh; Sargun Dhillon; Satadru Pan; Sean Bell; Sergey Edunov; Shaoliang Nie; Sharan Narang; Sharath Raparthy; Shaun Lindsay; Sheng Feng; Sheng Shen; Shenghao Lin; Shiva Shankar; Shruti Bhosale; Shun Zhang; Simon Vandenhende; Sinong Wang; Seohyun Sonia Kim; Soumya Batra; Sten Sootla; Steve Kehoe; Suchin Gururangan; Sumit Gupta; Sunny Virk; Sydney Borodinsky; Tamar Glaser; Tamar Herman; Tamara Best; Tara Fowler; Thomas Georgiou; Thomas Scialom; Tianhe Li; Todor Mihaylov; Tong Xiao; Ujjwal Karn; Vedanuj Goswami; Vibhor Gupta; Vignesh Ramanathan; Viktor Kerkez; Vinay Satish Kumar; Vincent Gonguet; Vish Vogeti; Vlad Poenaru; Vlad Tiberiu Mihailescu; Vladan Petrovic; Vladimir Ivanov; Wei Li; Weiwei Chu; Wenhan Xiong; Wenyin Fu; Wes Bouaziz; Whitney Meers; Will Constable; Xavier Martinet; Xiaojian Wu; Xinbo Gao; Xinfeng Xie; Xuchao Jia; Yaelle Goldschlag; Yann LeCun; Yashesh Gaur; Yasmine Babaei; Ye Qi; Yenda Li; Yi Wen; Yiwen Song; Youngjin Nam; Yuchen Hao; Yuchen Zhang; Yun Wang; Yuning Mao; Yuzi He; Zacharie Delpierre Coudert; Zachary DeVito; Zahra Hankir; Zhaoduo Wen; Zheng Yan; Zhengxing Chen; Zhenyu Yang; Zoe Papakipos"
] | [
"TAGS\n#transformers #safetensors #llama #text-generation #facebook #meta #pytorch #llama-3 #en #license-other #autotrain_compatible #endpoints_compatible #text-generation-inference #region-us #has_space \n",
"### Use with transformers\n\n\nSee the snippet below for usage with Transformers:",
"### Use with 'llama3'\n\n\nPlease, follow the instructions in the repository.\n\n\nTo download Original checkpoints, see the example command below leveraging 'huggingface-cli':\n\n\nFor Hugging Face support, we recommend using transformers or TGI, but a similar command works.\n\n\nHardware and Software\n---------------------\n\n\nTraining Factors We used custom training libraries, Meta's Research SuperCluster, and production clusters for pretraining. Fine-tuning, annotation, and evaluation were also performed on third-party cloud compute.\n\n\nCarbon Footprint Pretraining utilized a cumulative 7.7M GPU hours of computation on hardware of type H100-80GB (TDP of 700W). Estimated total emissions were 2290 tCO2eq, 100% of which were offset by Meta’s sustainability program.\n\n\n\nCO2 emissions during pre-training. Time: total GPU time required for training each model. Power Consumption: peak power capacity per GPU device for the GPUs used adjusted for power usage efficiency. 100% of the emissions are directly offset by Meta's sustainability program, and because we are openly releasing these models, the pretraining costs do not need to be incurred by others.\n\n\nTraining Data\n-------------\n\n\nOverview Llama 3 was pretrained on over 15 trillion tokens of data from publicly available sources. The fine-tuning data includes publicly available instruction datasets, as well as over 10M human-annotated examples. Neither the pretraining nor the fine-tuning datasets include Meta user data.\n\n\nData Freshness The pretraining data has a cutoff of March 2023 for the 7B and December 2023 for the 70B models respectively.\n\n\nBenchmarks\n----------\n\n\nIn this section, we report the results for Llama 3 models on standard automatic benchmarks. For all the evaluations, we use our internal evaluations library. For details on the methodology see here.",
"### Base pretrained models",
"### Instruction tuned models",
"### Responsibility & Safety\n\n\nWe believe that an open approach to AI leads to better, safer products, faster innovation, and a bigger overall market. We are committed to Responsible AI development and took a series of steps to limit misuse and harm and support the open source community.\n\n\nFoundation models are widely capable technologies that are built to be used for a diverse range of applications. They are not designed to meet every developer preference on safety levels for all use cases, out-of-the-box, as those by their nature will differ across different applications.\n\n\nRather, responsible LLM-application deployment is achieved by implementing a series of safety best practices throughout the development of such applications, from the model pre-training, fine-tuning and the deployment of systems composed of safeguards to tailor the safety needs specifically to the use case and audience.\n\n\nAs part of the Llama 3 release, we updated our Responsible Use Guide to outline the steps and best practices for developers to implement model and system level safety for their application. We also provide a set of resources including Meta Llama Guard 2 and Code Shield safeguards. These tools have proven to drastically reduce residual risks of LLM Systems, while maintaining a high level of helpfulness. We encourage developers to tune and deploy these safeguards according to their needs and we provide a reference implementation to get you started.",
"#### Llama 3-Instruct\n\n\nAs outlined in the Responsible Use Guide, some trade-off between model helpfulness and model alignment is likely unavoidable. Developers should exercise discretion about how to weigh the benefits of alignment and helpfulness for their specific use case and audience. Developers should be mindful of residual risks when using Llama models and leverage additional safety tools as needed to reach the right safety bar for their use case.\n\n\nSafety\n\n\nFor our instruction tuned model, we conducted extensive red teaming exercises, performed adversarial evaluations and implemented safety mitigations techniques to lower residual risks. As with any Large Language Model, residual risks will likely remain and we recommend that developers assess these risks in the context of their use case. In parallel, we are working with the community to make AI safety benchmark standards transparent, rigorous and interpretable.\n\n\nRefusals\n\n\nIn addition to residual risks, we put a great emphasis on model refusals to benign prompts. Over-refusing not only can impact the user experience but could even be harmful in certain contexts as well. We’ve heard the feedback from the developer community and improved our fine tuning to ensure that Llama 3 is significantly less likely to falsely refuse to answer prompts than Llama 2.\n\n\nWe built internal benchmarks and developed mitigations to limit false refusals making Llama 3 our most helpful model to date.",
"#### Responsible release\n\n\nIn addition to responsible use considerations outlined above, we followed a rigorous process that requires us to take extra measures against misuse and critical risks before we make our release decision.\n\n\nMisuse\n\n\nIf you access or use Llama 3, you agree to the Acceptable Use Policy. The most recent copy of this policy can be found at URL",
"#### Critical risks\n\n\nCBRNE (Chemical, Biological, Radiological, Nuclear, and high yield Explosives)\n\n\nWe have conducted a two fold assessment of the safety of the model in this area:\n\n\n* Iterative testing during model training to assess the safety of responses related to CBRNE threats and other adversarial risks.\n* Involving external CBRNE experts to conduct an uplift test assessing the ability of the model to accurately provide expert knowledge and reduce barriers to potential CBRNE misuse, by reference to what can be achieved using web search (without the model).",
"### Cyber Security\n\n\nWe have evaluated Llama 3 with CyberSecEval, Meta’s cybersecurity safety eval suite, measuring Llama 3’s propensity to suggest insecure code when used as a coding assistant, and Llama 3’s propensity to comply with requests to help carry out cyber attacks, where attacks are defined by the industry standard MITRE ATT&CK cyber attack ontology. On our insecure coding and cyber attacker helpfulness tests, Llama 3 behaved in the same range or safer than models of equivalent coding capability.",
"### Child Safety\n\n\nChild Safety risk assessments were conducted using a team of experts, to assess the model’s capability to produce outputs that could result in Child Safety risks and inform on any necessary and appropriate risk mitigations via fine tuning. We leveraged those expert red teaming sessions to expand the coverage of our evaluation benchmarks through Llama 3 model development. For Llama 3, we conducted new in-depth sessions using objective based methodologies to assess the model risks along multiple attack vectors. We also partnered with content specialists to perform red teaming exercises assessing potentially violating content while taking account of market specific nuances or experiences.",
"### Community\n\n\nGenerative AI safety requires expertise and tooling, and we believe in the strength of the open community to accelerate its progress. We are active members of open consortiums, including the AI Alliance, Partnership in AI and MLCommons, actively contributing to safety standardization and transparency. We encourage the community to adopt taxonomies like the MLCommons Proof of Concept evaluation to facilitate collaboration and transparency on safety and content evaluations. Our Purple Llama tools are open sourced for the community to use and widely distributed across ecosystem partners including cloud service providers. We encourage community contributions to our Github repository.\n\n\nFinally, we put in place a set of resources including an output reporting mechanism and bug bounty program to continuously improve the Llama technology with the help of the community.\n\n\nEthical Considerations and Limitations\n--------------------------------------\n\n\nThe core values of Llama 3 are openness, inclusivity and helpfulness. It is meant to serve everyone, and to work for a wide range of use cases. It is thus designed to be accessible to people across many different backgrounds, experiences and perspectives. Llama 3 addresses users and their needs as they are, without insertion unnecessary judgment or normativity, while reflecting the understanding that even content that may appear problematic in some cases can serve valuable purposes in others. It respects the dignity and autonomy of all users, especially in terms of the values of free thought and expression that power innovation and progress.\n\n\nBut Llama 3 is a new technology, and like any new technology, there are risks associated with its use. Testing conducted to date has been in English, and has not covered, nor could it cover, all scenarios. For these reasons, as with all LLMs, Llama 3’s potential outputs cannot be predicted in advance, and the model may in some instances produce inaccurate, biased or other objectionable responses to user prompts. Therefore, before deploying any applications of Llama 3 models, developers should perform safety testing and tuning tailored to their specific applications of the model. As outlined in the Responsible Use Guide, we recommend incorporating Purple Llama solutions into your workflows and specifically Llama Guard which provides a base model to filter input and output prompts to layer system-level safety on top of model-level safety.\n\n\nPlease see the Responsible Use Guide available at URL\n\n\ninstructions\n\n\n@article{llama3modelcard,\n\n\ntitle={Llama 3 Model Card},\n\n\nauthor={AI@Meta},\n\n\nyear={2024},\n\n\nurl = {URL\n\n\n}\n\n\nContributors\n------------\n\n\nAaditya Singh; Aaron Grattafiori; Abhimanyu Dubey; Abhinav Jauhri; Abhinav Pandey; Abhishek Kadian; Adam Kelsey; Adi Gangidi; Ahmad Al-Dahle; Ahuva Goldstand; Aiesha Letman; Ajay Menon; Akhil Mathur; Alan Schelten; Alex Vaughan; Amy Yang; Andrei Lupu; Andres Alvarado; Andrew Gallagher; Andrew Gu; Andrew Ho; Andrew Poulton; Andrew Ryan; Angela Fan; Ankit Ramchandani; Anthony Hartshorn; Archi Mitra; Archie Sravankumar; Artem Korenev; Arun Rao; Ashley Gabriel; Ashwin Bharambe; Assaf Eisenman; Aston Zhang; Aurelien Rodriguez; Austen Gregerson; Ava Spataru; Baptiste Roziere; Ben Maurer; Benjamin Leonhardi; Bernie Huang; Bhargavi Paranjape; Bing Liu; Binh Tang; Bobbie Chern; Brani Stojkovic; Brian Fuller; Catalina Mejia Arenas; Chao Zhou; Charlotte Caucheteux; Chaya Nayak; Ching-Hsiang Chu; Chloe Bi; Chris Cai; Chris Cox; Chris Marra; Chris McConnell; Christian Keller; Christoph Feichtenhofer; Christophe Touret; Chunyang Wu; Corinne Wong; Cristian Canton Ferrer; Damien Allonsius; Daniel Kreymer; Daniel Haziza; Daniel Li; Danielle Pintz; Danny Livshits; Danny Wyatt; David Adkins; David Esiobu; David Xu; Davide Testuggine; Delia David; Devi Parikh; Dhruv Choudhary; Dhruv Mahajan; Diana Liskovich; Diego Garcia-Olano; Diego Perino; Dieuwke Hupkes; Dingkang Wang; Dustin Holland; Egor Lakomkin; Elina Lobanova; Xiaoqing Ellen Tan; Emily Dinan; Eric Smith; Erik Brinkman; Esteban Arcaute; Filip Radenovic; Firat Ozgenel; Francesco Caggioni; Frank Seide; Frank Zhang; Gabriel Synnaeve; Gabriella Schwarz; Gabrielle Lee; Gada Badeer; Georgia Anderson; Graeme Nail; Gregoire Mialon; Guan Pang; Guillem Cucurell; Hailey Nguyen; Hannah Korevaar; Hannah Wang; Haroun Habeeb; Harrison Rudolph; Henry Aspegren; Hu Xu; Hugo Touvron; Iga Kozlowska; Igor Molybog; Igor Tufanov; Iliyan Zarov; Imanol Arrieta Ibarra; Irina-Elena Veliche; Isabel Kloumann; Ishan Misra; Ivan Evtimov; Jacob Xu; Jade Copet; Jake Weissman; Jan Geffert; Jana Vranes; Japhet Asher; Jason Park; Jay Mahadeokar; Jean-Baptiste Gaya; Jeet Shah; Jelmer van der Linde; Jennifer Chan; Jenny Hong; Jenya Lee; Jeremy Fu; Jeremy Teboul; Jianfeng Chi; Jianyu Huang; Jie Wang; Jiecao Yu; Joanna Bitton; Joe Spisak; Joelle Pineau; Jon Carvill; Jongsoo Park; Joseph Rocca; Joshua Johnstun; Junteng Jia; Kalyan Vasuden Alwala; Kam Hou U; Kate Plawiak; Kartikeya Upasani; Kaushik Veeraraghavan; Ke Li; Kenneth Heafield; Kevin Stone; Khalid El-Arini; Krithika Iyer; Kshitiz Malik; Kuenley Chiu; Kunal Bhalla; Kyle Huang; Lakshya Garg; Lauren Rantala-Yeary; Laurens van der Maaten; Lawrence Chen; Leandro Silva; Lee Bell; Lei Zhang; Liang Tan; Louis Martin; Lovish Madaan; Luca Wehrstedt; Lukas Blecher; Luke de Oliveira; Madeline Muzzi; Madian Khabsa; Manav Avlani; Mannat Singh; Manohar Paluri; Mark Zuckerberg; Marcin Kardas; Martynas Mankus; Mathew Oldham; Mathieu Rita; Matthew Lennie; Maya Pavlova; Meghan Keneally; Melanie Kambadur; Mihir Patel; Mikayel Samvelyan; Mike Clark; Mike Lewis; Min Si; Mitesh Kumar Singh; Mo Metanat; Mona Hassan; Naman Goyal; Narjes Torabi; Nicolas Usunier; Nikolay Bashlykov; Nikolay Bogoychev; Niladri Chatterji; Ning Dong; Oliver Aobo Yang; Olivier Duchenne; Onur Celebi; Parth Parekh; Patrick Alrassy; Paul Saab; Pavan Balaji; Pedro Rittner; Pengchuan Zhang; Pengwei Li; Petar Vasic; Peter Weng; Polina Zvyagina; Prajjwal Bhargava; Pratik Dubal; Praveen Krishnan; Punit Singh Koura; Qing He; Rachel Rodriguez; Ragavan Srinivasan; Rahul Mitra; Ramon Calderer; Raymond Li; Robert Stojnic; Roberta Raileanu; Robin Battey; Rocky Wang; Rohit Girdhar; Rohit Patel; Romain Sauvestre; Ronnie Polidoro; Roshan Sumbaly; Ross Taylor; Ruan Silva; Rui Hou; Rui Wang; Russ Howes; Ruty Rinott; Saghar Hosseini; Sai Jayesh Bondu; Samyak Datta; Sanjay Singh; Sara Chugh; Sargun Dhillon; Satadru Pan; Sean Bell; Sergey Edunov; Shaoliang Nie; Sharan Narang; Sharath Raparthy; Shaun Lindsay; Sheng Feng; Sheng Shen; Shenghao Lin; Shiva Shankar; Shruti Bhosale; Shun Zhang; Simon Vandenhende; Sinong Wang; Seohyun Sonia Kim; Soumya Batra; Sten Sootla; Steve Kehoe; Suchin Gururangan; Sumit Gupta; Sunny Virk; Sydney Borodinsky; Tamar Glaser; Tamar Herman; Tamara Best; Tara Fowler; Thomas Georgiou; Thomas Scialom; Tianhe Li; Todor Mihaylov; Tong Xiao; Ujjwal Karn; Vedanuj Goswami; Vibhor Gupta; Vignesh Ramanathan; Viktor Kerkez; Vinay Satish Kumar; Vincent Gonguet; Vish Vogeti; Vlad Poenaru; Vlad Tiberiu Mihailescu; Vladan Petrovic; Vladimir Ivanov; Wei Li; Weiwei Chu; Wenhan Xiong; Wenyin Fu; Wes Bouaziz; Whitney Meers; Will Constable; Xavier Martinet; Xiaojian Wu; Xinbo Gao; Xinfeng Xie; Xuchao Jia; Yaelle Goldschlag; Yann LeCun; Yashesh Gaur; Yasmine Babaei; Ye Qi; Yenda Li; Yi Wen; Yiwen Song; Youngjin Nam; Yuchen Hao; Yuchen Zhang; Yun Wang; Yuning Mao; Yuzi He; Zacharie Delpierre Coudert; Zachary DeVito; Zahra Hankir; Zhaoduo Wen; Zheng Yan; Zhengxing Chen; Zhenyu Yang; Zoe Papakipos"
] | [
58,
17,
430,
8,
6,
270,
280,
72,
115,
118,
126,
2136
] | [
"TAGS\n#transformers #safetensors #llama #text-generation #facebook #meta #pytorch #llama-3 #en #license-other #autotrain_compatible #endpoints_compatible #text-generation-inference #region-us #has_space \n### Use with transformers\n\n\nSee the snippet below for usage with Transformers:### Use with 'llama3'\n\n\nPlease, follow the instructions in the repository.\n\n\nTo download Original checkpoints, see the example command below leveraging 'huggingface-cli':\n\n\nFor Hugging Face support, we recommend using transformers or TGI, but a similar command works.\n\n\nHardware and Software\n---------------------\n\n\nTraining Factors We used custom training libraries, Meta's Research SuperCluster, and production clusters for pretraining. Fine-tuning, annotation, and evaluation were also performed on third-party cloud compute.\n\n\nCarbon Footprint Pretraining utilized a cumulative 7.7M GPU hours of computation on hardware of type H100-80GB (TDP of 700W). Estimated total emissions were 2290 tCO2eq, 100% of which were offset by Meta’s sustainability program.\n\n\n\nCO2 emissions during pre-training. Time: total GPU time required for training each model. Power Consumption: peak power capacity per GPU device for the GPUs used adjusted for power usage efficiency. 100% of the emissions are directly offset by Meta's sustainability program, and because we are openly releasing these models, the pretraining costs do not need to be incurred by others.\n\n\nTraining Data\n-------------\n\n\nOverview Llama 3 was pretrained on over 15 trillion tokens of data from publicly available sources. The fine-tuning data includes publicly available instruction datasets, as well as over 10M human-annotated examples. Neither the pretraining nor the fine-tuning datasets include Meta user data.\n\n\nData Freshness The pretraining data has a cutoff of March 2023 for the 7B and December 2023 for the 70B models respectively.\n\n\nBenchmarks\n----------\n\n\nIn this section, we report the results for Llama 3 models on standard automatic benchmarks. For all the evaluations, we use our internal evaluations library. For details on the methodology see here.### Base pretrained models### Instruction tuned models### Responsibility & Safety\n\n\nWe believe that an open approach to AI leads to better, safer products, faster innovation, and a bigger overall market. We are committed to Responsible AI development and took a series of steps to limit misuse and harm and support the open source community.\n\n\nFoundation models are widely capable technologies that are built to be used for a diverse range of applications. They are not designed to meet every developer preference on safety levels for all use cases, out-of-the-box, as those by their nature will differ across different applications.\n\n\nRather, responsible LLM-application deployment is achieved by implementing a series of safety best practices throughout the development of such applications, from the model pre-training, fine-tuning and the deployment of systems composed of safeguards to tailor the safety needs specifically to the use case and audience.\n\n\nAs part of the Llama 3 release, we updated our Responsible Use Guide to outline the steps and best practices for developers to implement model and system level safety for their application. We also provide a set of resources including Meta Llama Guard 2 and Code Shield safeguards. These tools have proven to drastically reduce residual risks of LLM Systems, while maintaining a high level of helpfulness. We encourage developers to tune and deploy these safeguards according to their needs and we provide a reference implementation to get you started.#### Llama 3-Instruct\n\n\nAs outlined in the Responsible Use Guide, some trade-off between model helpfulness and model alignment is likely unavoidable. Developers should exercise discretion about how to weigh the benefits of alignment and helpfulness for their specific use case and audience. Developers should be mindful of residual risks when using Llama models and leverage additional safety tools as needed to reach the right safety bar for their use case.\n\n\nSafety\n\n\nFor our instruction tuned model, we conducted extensive red teaming exercises, performed adversarial evaluations and implemented safety mitigations techniques to lower residual risks. As with any Large Language Model, residual risks will likely remain and we recommend that developers assess these risks in the context of their use case. In parallel, we are working with the community to make AI safety benchmark standards transparent, rigorous and interpretable.\n\n\nRefusals\n\n\nIn addition to residual risks, we put a great emphasis on model refusals to benign prompts. Over-refusing not only can impact the user experience but could even be harmful in certain contexts as well. We’ve heard the feedback from the developer community and improved our fine tuning to ensure that Llama 3 is significantly less likely to falsely refuse to answer prompts than Llama 2.\n\n\nWe built internal benchmarks and developed mitigations to limit false refusals making Llama 3 our most helpful model to date.#### Responsible release\n\n\nIn addition to responsible use considerations outlined above, we followed a rigorous process that requires us to take extra measures against misuse and critical risks before we make our release decision.\n\n\nMisuse\n\n\nIf you access or use Llama 3, you agree to the Acceptable Use Policy. The most recent copy of this policy can be found at URL#### Critical risks\n\n\nCBRNE (Chemical, Biological, Radiological, Nuclear, and high yield Explosives)\n\n\nWe have conducted a two fold assessment of the safety of the model in this area:\n\n\n* Iterative testing during model training to assess the safety of responses related to CBRNE threats and other adversarial risks.\n* Involving external CBRNE experts to conduct an uplift test assessing the ability of the model to accurately provide expert knowledge and reduce barriers to potential CBRNE misuse, by reference to what can be achieved using web search (without the model).### Cyber Security\n\n\nWe have evaluated Llama 3 with CyberSecEval, Meta’s cybersecurity safety eval suite, measuring Llama 3’s propensity to suggest insecure code when used as a coding assistant, and Llama 3’s propensity to comply with requests to help carry out cyber attacks, where attacks are defined by the industry standard MITRE ATT&CK cyber attack ontology. On our insecure coding and cyber attacker helpfulness tests, Llama 3 behaved in the same range or safer than models of equivalent coding capability.### Child Safety\n\n\nChild Safety risk assessments were conducted using a team of experts, to assess the model’s capability to produce outputs that could result in Child Safety risks and inform on any necessary and appropriate risk mitigations via fine tuning. We leveraged those expert red teaming sessions to expand the coverage of our evaluation benchmarks through Llama 3 model development. For Llama 3, we conducted new in-depth sessions using objective based methodologies to assess the model risks along multiple attack vectors. We also partnered with content specialists to perform red teaming exercises assessing potentially violating content while taking account of market specific nuances or experiences.### Community\n\n\nGenerative AI safety requires expertise and tooling, and we believe in the strength of the open community to accelerate its progress. We are active members of open consortiums, including the AI Alliance, Partnership in AI and MLCommons, actively contributing to safety standardization and transparency. We encourage the community to adopt taxonomies like the MLCommons Proof of Concept evaluation to facilitate collaboration and transparency on safety and content evaluations. Our Purple Llama tools are open sourced for the community to use and widely distributed across ecosystem partners including cloud service providers. We encourage community contributions to our Github repository.\n\n\nFinally, we put in place a set of resources including an output reporting mechanism and bug bounty program to continuously improve the Llama technology with the help of the community.\n\n\nEthical Considerations and Limitations\n--------------------------------------\n\n\nThe core values of Llama 3 are openness, inclusivity and helpfulness. It is meant to serve everyone, and to work for a wide range of use cases. It is thus designed to be accessible to people across many different backgrounds, experiences and perspectives. Llama 3 addresses users and their needs as they are, without insertion unnecessary judgment or normativity, while reflecting the understanding that even content that may appear problematic in some cases can serve valuable purposes in others. It respects the dignity and autonomy of all users, especially in terms of the values of free thought and expression that power innovation and progress.\n\n\nBut Llama 3 is a new technology, and like any new technology, there are risks associated with its use. Testing conducted to date has been in English, and has not covered, nor could it cover, all scenarios. For these reasons, as with all LLMs, Llama 3’s potential outputs cannot be predicted in advance, and the model may in some instances produce inaccurate, biased or other objectionable responses to user prompts. Therefore, before deploying any applications of Llama 3 models, developers should perform safety testing and tuning tailored to their specific applications of the model. As outlined in the Responsible Use Guide, we recommend incorporating Purple Llama solutions into your workflows and specifically Llama Guard which provides a base model to filter input and output prompts to layer system-level safety on top of model-level safety.\n\n\nPlease see the Responsible Use Guide available at URL\n\n\ninstructions\n\n\n@article{llama3modelcard,\n\n\ntitle={Llama 3 Model Card},\n\n\nauthor={AI@Meta},\n\n\nyear={2024},\n\n\nurl = {URL\n\n\n}\n\n\nContributors\n------------\n\n\nAaditya Singh; Aaron Grattafiori; Abhimanyu Dubey; Abhinav Jauhri; Abhinav Pandey; Abhishek Kadian; Adam Kelsey; Adi Gangidi; Ahmad Al-Dahle; Ahuva Goldstand; Aiesha Letman; Ajay Menon; Akhil Mathur; Alan Schelten; Alex Vaughan; Amy Yang; Andrei Lupu; Andres Alvarado; Andrew Gallagher; Andrew Gu; Andrew Ho; Andrew Poulton; Andrew Ryan; Angela Fan; Ankit Ramchandani; Anthony Hartshorn; Archi Mitra; Archie Sravankumar; Artem Korenev; Arun Rao; Ashley Gabriel; Ashwin Bharambe; Assaf Eisenman; Aston Zhang; Aurelien Rodriguez; Austen Gregerson; Ava Spataru; Baptiste Roziere; Ben Maurer; Benjamin Leonhardi; Bernie Huang; Bhargavi Paranjape; Bing Liu; Binh Tang; Bobbie Chern; Brani Stojkovic; Brian Fuller; Catalina Mejia Arenas; Chao Zhou; Charlotte Caucheteux; Chaya Nayak; Ching-Hsiang Chu; Chloe Bi; Chris Cai; Chris Cox; Chris Marra; Chris McConnell; Christian Keller; Christoph Feichtenhofer; Christophe Touret; Chunyang Wu; Corinne Wong; Cristian Canton Ferrer; Damien Allonsius; Daniel Kreymer; Daniel Haziza; Daniel Li; Danielle Pintz; Danny Livshits; Danny Wyatt; David Adkins; David Esiobu; David Xu; Davide Testuggine; Delia David; Devi Parikh; Dhruv Choudhary; Dhruv Mahajan; Diana Liskovich; Diego Garcia-Olano; Diego Perino; Dieuwke Hupkes; Dingkang Wang; Dustin Holland; Egor Lakomkin; Elina Lobanova; Xiaoqing Ellen Tan; Emily Dinan; Eric Smith; Erik Brinkman; Esteban Arcaute; Filip Radenovic; Firat Ozgenel; Francesco Caggioni; Frank Seide; Frank Zhang; Gabriel Synnaeve; Gabriella Schwarz; Gabrielle Lee; Gada Badeer; Georgia Anderson; Graeme Nail; Gregoire Mialon; Guan Pang; Guillem Cucurell; Hailey Nguyen; Hannah Korevaar; Hannah Wang; Haroun Habeeb; Harrison Rudolph; Henry Aspegren; Hu Xu; Hugo Touvron; Iga Kozlowska; Igor Molybog; Igor Tufanov; Iliyan Zarov; Imanol Arrieta Ibarra; Irina-Elena Veliche; Isabel Kloumann; Ishan Misra; Ivan Evtimov; Jacob Xu; Jade Copet; Jake Weissman; Jan Geffert; Jana Vranes; Japhet Asher; Jason Park; Jay Mahadeokar; Jean-Baptiste Gaya; Jeet Shah; Jelmer van der Linde; Jennifer Chan; Jenny Hong; Jenya Lee; Jeremy Fu; Jeremy Teboul; Jianfeng Chi; Jianyu Huang; Jie Wang; Jiecao Yu; Joanna Bitton; Joe Spisak; Joelle Pineau; Jon Carvill; Jongsoo Park; Joseph Rocca; Joshua Johnstun; Junteng Jia; Kalyan Vasuden Alwala; Kam Hou U; Kate Plawiak; Kartikeya Upasani; Kaushik Veeraraghavan; Ke Li; Kenneth Heafield; Kevin Stone; Khalid El-Arini; Krithika Iyer; Kshitiz Malik; Kuenley Chiu; Kunal Bhalla; Kyle Huang; Lakshya Garg; Lauren Rantala-Yeary; Laurens van der Maaten; Lawrence Chen; Leandro Silva; Lee Bell; Lei Zhang; Liang Tan; Louis Martin; Lovish Madaan; Luca Wehrstedt; Lukas Blecher; Luke de Oliveira; Madeline Muzzi; Madian Khabsa; Manav Avlani; Mannat Singh; Manohar Paluri; Mark Zuckerberg; Marcin Kardas; Martynas Mankus; Mathew Oldham; Mathieu Rita; Matthew Lennie; Maya Pavlova; Meghan Keneally; Melanie Kambadur; Mihir Patel; Mikayel Samvelyan; Mike Clark; Mike Lewis; Min Si; Mitesh Kumar Singh; Mo Metanat; Mona Hassan; Naman Goyal; Narjes Torabi; Nicolas Usunier; Nikolay Bashlykov; Nikolay Bogoychev; Niladri Chatterji; Ning Dong; Oliver Aobo Yang; Olivier Duchenne; Onur Celebi; Parth Parekh; Patrick Alrassy; Paul Saab; Pavan Balaji; Pedro Rittner; Pengchuan Zhang; Pengwei Li; Petar Vasic; Peter Weng; Polina Zvyagina; Prajjwal Bhargava; Pratik Dubal; Praveen Krishnan; Punit Singh Koura; Qing He; Rachel Rodriguez; Ragavan Srinivasan; Rahul Mitra; Ramon Calderer; Raymond Li; Robert Stojnic; Roberta Raileanu; Robin Battey; Rocky Wang; Rohit Girdhar; Rohit Patel; Romain Sauvestre; Ronnie Polidoro; Roshan Sumbaly; Ross Taylor; Ruan Silva; Rui Hou; Rui Wang; Russ Howes; Ruty Rinott; Saghar Hosseini; Sai Jayesh Bondu; Samyak Datta; Sanjay Singh; Sara Chugh; Sargun Dhillon; Satadru Pan; Sean Bell; Sergey Edunov; Shaoliang Nie; Sharan Narang; Sharath Raparthy; Shaun Lindsay; Sheng Feng; Sheng Shen; Shenghao Lin; Shiva Shankar; Shruti Bhosale; Shun Zhang; Simon Vandenhende; Sinong Wang; Seohyun Sonia Kim; Soumya Batra; Sten Sootla; Steve Kehoe; Suchin Gururangan; Sumit Gupta; Sunny Virk; Sydney Borodinsky; Tamar Glaser; Tamar Herman; Tamara Best; Tara Fowler; Thomas Georgiou; Thomas Scialom; Tianhe Li; Todor Mihaylov; Tong Xiao; Ujjwal Karn; Vedanuj Goswami; Vibhor Gupta; Vignesh Ramanathan; Viktor Kerkez; Vinay Satish Kumar; Vincent Gonguet; Vish Vogeti; Vlad Poenaru; Vlad Tiberiu Mihailescu; Vladan Petrovic; Vladimir Ivanov; Wei Li; Weiwei Chu; Wenhan Xiong; Wenyin Fu; Wes Bouaziz; Whitney Meers; Will Constable; Xavier Martinet; Xiaojian Wu; Xinbo Gao; Xinfeng Xie; Xuchao Jia; Yaelle Goldschlag; Yann LeCun; Yashesh Gaur; Yasmine Babaei; Ye Qi; Yenda Li; Yi Wen; Yiwen Song; Youngjin Nam; Yuchen Hao; Yuchen Zhang; Yun Wang; Yuning Mao; Yuzi He; Zacharie Delpierre Coudert; Zachary DeVito; Zahra Hankir; Zhaoduo Wen; Zheng Yan; Zhengxing Chen; Zhenyu Yang; Zoe Papakipos"
] |
null | null | <!-- header start -->
<!-- 200823 -->
<div style="width: auto; margin-left: auto; margin-right: auto">
<a href="https://www.pruna.ai/" target="_blank" rel="noopener noreferrer">
<img src="https://i.imgur.com/eDAlcgk.png" alt="PrunaAI" style="width: 100%; min-width: 400px; display: block; margin: auto;">
</a>
</div>
<!-- header end -->
[](https://twitter.com/PrunaAI)
[](https://github.com/PrunaAI)
[](https://www.linkedin.com/company/93832878/admin/feed/posts/?feedType=following)
[](https://discord.com/invite/vb6SmA3hxu)
## This repo contains GGUF versions of the MathLLM/MathCoder-CL-7B model.
# Simply make AI models cheaper, smaller, faster, and greener!
- Give a thumbs up if you like this model!
- Contact us and tell us which model to compress next [here](https://www.pruna.ai/contact).
- Request access to easily compress your *own* AI models [here](https://z0halsaff74.typeform.com/pruna-access?typeform-source=www.pruna.ai).
- Read the documentations to know more [here](https://pruna-ai-pruna.readthedocs-hosted.com/en/latest/)
- Join Pruna AI community on Discord [here](https://discord.com/invite/vb6SmA3hxu) to share feedback/suggestions or get help.
**Frequently Asked Questions**
- ***How does the compression work?*** The model is compressed with GGUF.
- ***How does the model quality change?*** The quality of the model output might vary compared to the base model.
- ***What is the model format?*** We use GGUF format.
- ***What calibration data has been used?*** If needed by the compression method, we used WikiText as the calibration data.
- ***How to compress my own models?*** You can request premium access to more compression methods and tech support for your specific use-cases [here](https://z0halsaff74.typeform.com/pruna-access?typeform-source=www.pruna.ai).
# Downloading and running the models
You can download the individual files from the Files & versions section. Here is a list of the different versions we provide. For more info checkout [this chart](https://gist.github.com/Artefact2/b5f810600771265fc1e39442288e8ec9) and [this guide](https://www.reddit.com/r/LocalLLaMA/comments/1ba55rj/overview_of_gguf_quantization_methods/):
| Quant type | Description |
|------------|--------------------------------------------------------------------------------------------|
| Q5_K_M | High quality, recommended. |
| Q5_K_S | High quality, recommended. |
| Q4_K_M | Good quality, uses about 4.83 bits per weight, recommended. |
| Q4_K_S | Slightly lower quality with more space savings, recommended. |
| IQ4_NL | Decent quality, slightly smaller than Q4_K_S with similar performance, recommended. |
| IQ4_XS | Decent quality, smaller than Q4_K_S with similar performance, recommended. |
| Q3_K_L | Lower quality but usable, good for low RAM availability. |
| Q3_K_M | Even lower quality. |
| IQ3_M | Medium-low quality, new method with decent performance comparable to Q3_K_M. |
| IQ3_S | Lower quality, new method with decent performance, recommended over Q3_K_S quant, same size with better performance. |
| Q3_K_S | Low quality, not recommended. |
| IQ3_XS | Lower quality, new method with decent performance, slightly better than Q3_K_S. |
| Q2_K | Very low quality but surprisingly usable. |
## How to download GGUF files ?
**Note for manual downloaders:** You almost never want to clone the entire repo! Multiple different quantisation formats are provided, and most users only want to pick and download a single file.
The following clients/libraries will automatically download models for you, providing a list of available models to choose from:
* LM Studio
* LoLLMS Web UI
* Faraday.dev
- **Option A** - Downloading in `text-generation-webui`:
- **Step 1**: Under Download Model, you can enter the model repo: PrunaAI/MathCoder-CL-7B-GGUF-smashed and below it, a specific filename to download, such as: phi-2.IQ3_M.gguf.
- **Step 2**: Then click Download.
- **Option B** - Downloading on the command line (including multiple files at once):
- **Step 1**: We recommend using the `huggingface-hub` Python library:
```shell
pip3 install huggingface-hub
```
- **Step 2**: Then you can download any individual model file to the current directory, at high speed, with a command like this:
```shell
huggingface-cli download PrunaAI/MathCoder-CL-7B-GGUF-smashed MathCoder-CL-7B.IQ3_M.gguf --local-dir . --local-dir-use-symlinks False
```
<details>
<summary>More advanced huggingface-cli download usage (click to read)</summary>
Alternatively, you can also download multiple files at once with a pattern:
```shell
huggingface-cli download PrunaAI/MathCoder-CL-7B-GGUF-smashed --local-dir . --local-dir-use-symlinks False --include='*Q4_K*gguf'
```
For more documentation on downloading with `huggingface-cli`, please see: [HF -> Hub Python Library -> Download files -> Download from the CLI](https://huggingface.co/docs/huggingface_hub/guides/download#download-from-the-cli).
To accelerate downloads on fast connections (1Gbit/s or higher), install `hf_transfer`:
```shell
pip3 install hf_transfer
```
And set environment variable `HF_HUB_ENABLE_HF_TRANSFER` to `1`:
```shell
HF_HUB_ENABLE_HF_TRANSFER=1 huggingface-cli download PrunaAI/MathCoder-CL-7B-GGUF-smashed MathCoder-CL-7B.IQ3_M.gguf --local-dir . --local-dir-use-symlinks False
```
Windows Command Line users: You can set the environment variable by running `set HF_HUB_ENABLE_HF_TRANSFER=1` before the download command.
</details>
<!-- README_GGUF.md-how-to-download end -->
<!-- README_GGUF.md-how-to-run start -->
## How to run model in GGUF format?
- **Option A** - Introductory example with `llama.cpp` command
Make sure you are using `llama.cpp` from commit [d0cee0d](https://github.com/ggerganov/llama.cpp/commit/d0cee0d36d5be95a0d9088b674dbb27354107221) or later.
```shell
./main -ngl 35 -m MathCoder-CL-7B.IQ3_M.gguf --color -c 32768 --temp 0.7 --repeat_penalty 1.1 -n -1 -p "<s>[INST] {prompt\} [/INST]"
```
Change `-ngl 32` to the number of layers to offload to GPU. Remove it if you don't have GPU acceleration.
Change `-c 32768` to the desired sequence length. For extended sequence models - eg 8K, 16K, 32K - the necessary RoPE scaling parameters are read from the GGUF file and set by llama.cpp automatically. Note that longer sequence lengths require much more resources, so you may need to reduce this value.
If you want to have a chat-style conversation, replace the `-p <PROMPT>` argument with `-i -ins`
For other parameters and how to use them, please refer to [the llama.cpp documentation](https://github.com/ggerganov/llama.cpp/blob/master/examples/main/README.md)
- **Option B** - Running in `text-generation-webui`
Further instructions can be found in the text-generation-webui documentation, here: [text-generation-webui/docs/04 ‐ Model Tab.md](https://github.com/oobabooga/text-generation-webui/blob/main/docs/04%20-%20Model%20Tab.md#llamacpp).
- **Option C** - Running from Python code
You can use GGUF models from Python using the [llama-cpp-python](https://github.com/abetlen/llama-cpp-python) or [ctransformers](https://github.com/marella/ctransformers) libraries. Note that at the time of writing (Nov 27th 2023), ctransformers has not been updated for some time and is not compatible with some recent models. Therefore I recommend you use llama-cpp-python.
### How to load this model in Python code, using llama-cpp-python
For full documentation, please see: [llama-cpp-python docs](https://abetlen.github.io/llama-cpp-python/).
#### First install the package
Run one of the following commands, according to your system:
```shell
# Base ctransformers with no GPU acceleration
pip install llama-cpp-python
# With NVidia CUDA acceleration
CMAKE_ARGS="-DLLAMA_CUBLAS=on" pip install llama-cpp-python
# Or with OpenBLAS acceleration
CMAKE_ARGS="-DLLAMA_BLAS=ON -DLLAMA_BLAS_VENDOR=OpenBLAS" pip install llama-cpp-python
# Or with CLBLast acceleration
CMAKE_ARGS="-DLLAMA_CLBLAST=on" pip install llama-cpp-python
# Or with AMD ROCm GPU acceleration (Linux only)
CMAKE_ARGS="-DLLAMA_HIPBLAS=on" pip install llama-cpp-python
# Or with Metal GPU acceleration for macOS systems only
CMAKE_ARGS="-DLLAMA_METAL=on" pip install llama-cpp-python
# In windows, to set the variables CMAKE_ARGS in PowerShell, follow this format; eg for NVidia CUDA:
$env:CMAKE_ARGS = "-DLLAMA_OPENBLAS=on"
pip install llama-cpp-python
```
#### Simple llama-cpp-python example code
```python
from llama_cpp import Llama
# Set gpu_layers to the number of layers to offload to GPU. Set to 0 if no GPU acceleration is available on your system.
llm = Llama(
model_path="./MathCoder-CL-7B.IQ3_M.gguf", # Download the model file first
n_ctx=32768, # The max sequence length to use - note that longer sequence lengths require much more resources
n_threads=8, # The number of CPU threads to use, tailor to your system and the resulting performance
n_gpu_layers=35 # The number of layers to offload to GPU, if you have GPU acceleration available
)
# Simple inference example
output = llm(
"<s>[INST] {prompt} [/INST]", # Prompt
max_tokens=512, # Generate up to 512 tokens
stop=["</s>"], # Example stop token - not necessarily correct for this specific model! Please check before using.
echo=True # Whether to echo the prompt
)
# Chat Completion API
llm = Llama(model_path="./MathCoder-CL-7B.IQ3_M.gguf", chat_format="llama-2") # Set chat_format according to the model you are using
llm.create_chat_completion(
messages = [
{"role": "system", "content": "You are a story writing assistant."},
{
"role": "user",
"content": "Write a story about llamas."
}
]
)
```
- **Option D** - Running with LangChain
Here are guides on using llama-cpp-python and ctransformers with LangChain:
* [LangChain + llama-cpp-python](https://python.langchain.com/docs/integrations/llms/llamacpp)
* [LangChain + ctransformers](https://python.langchain.com/docs/integrations/providers/ctransformers)
## Configurations
The configuration info are in `smash_config.json`.
## Credits & License
The license of the smashed model follows the license of the original model. Please check the license of the original model before using this model which provided the base model. The license of the `pruna-engine` is [here](https://pypi.org/project/pruna-engine/) on Pypi.
## Want to compress other models?
- Contact us and tell us which model to compress next [here](https://www.pruna.ai/contact).
- Request access to easily compress your own AI models [here](https://z0halsaff74.typeform.com/pruna-access?typeform-source=www.pruna.ai).
| {"tags": ["pruna-ai"], "metrics": ["memory_disk", "memory_inference", "inference_latency", "inference_throughput", "inference_CO2_emissions", "inference_energy_consumption"], "thumbnail": "https://assets-global.website-files.com/646b351987a8d8ce158d1940/64ec9e96b4334c0e1ac41504_Logo%20with%20white%20text.svg"} | PrunaAI/MathCoder-CL-7B-GGUF-smashed | null | [
"gguf",
"pruna-ai",
"region:us"
] | null | 2024-05-02T10:41:49+00:00 | [] | [] | TAGS
#gguf #pruna-ai #region-us
|
[](URL target=)
:
* Step 1: We recommend using the 'huggingface-hub' Python library:
* Step 2: Then you can download any individual model file to the current directory, at high speed, with a command like this:
More advanced huggingface-cli download usage (click to read)
Alternatively, you can also download multiple files at once with a pattern:
For more documentation on downloading with 'huggingface-cli', please see: HF -> Hub Python Library -> Download files -> Download from the CLI.
To accelerate downloads on fast connections (1Gbit/s or higher), install 'hf\_transfer':
And set environment variable 'HF\_HUB\_ENABLE\_HF\_TRANSFER' to '1':
Windows Command Line users: You can set the environment variable by running 'set HF\_HUB\_ENABLE\_HF\_TRANSFER=1' before the download command.
How to run model in GGUF format?
--------------------------------
* Option A - Introductory example with 'URL' command
Make sure you are using 'URL' from commit d0cee0d or later.
Change '-ngl 32' to the number of layers to offload to GPU. Remove it if you don't have GPU acceleration.
Change '-c 32768' to the desired sequence length. For extended sequence models - eg 8K, 16K, 32K - the necessary RoPE scaling parameters are read from the GGUF file and set by URL automatically. Note that longer sequence lengths require much more resources, so you may need to reduce this value.
If you want to have a chat-style conversation, replace the '-p ' argument with '-i -ins'
For other parameters and how to use them, please refer to the URL documentation
* Option B - Running in 'text-generation-webui'
Further instructions can be found in the text-generation-webui documentation, here: text-generation-webui/docs/04 ‐ Model URL.
* Option C - Running from Python code
You can use GGUF models from Python using the llama-cpp-python or ctransformers libraries. Note that at the time of writing (Nov 27th 2023), ctransformers has not been updated for some time and is not compatible with some recent models. Therefore I recommend you use llama-cpp-python.
```
### How to load this model in Python code, using llama-cpp-python
For full documentation, please see: llama-cpp-python docs.
#### First install the package
Run one of the following commands, according to your system:
#### Simple llama-cpp-python example code
```
* Option D - Running with LangChain
Here are guides on using llama-cpp-python and ctransformers with LangChain:
* LangChain + llama-cpp-python
* LangChain + ctransformers
Configurations
--------------
The configuration info are in 'smash\_config.json'.
Credits & License
-----------------
The license of the smashed model follows the license of the original model. Please check the license of the original model before using this model which provided the base model. The license of the 'pruna-engine' is here on Pypi.
Want to compress other models?
------------------------------
* Contact us and tell us which model to compress next here.
* Request access to easily compress your own AI models here.
| [
"### How to load this model in Python code, using llama-cpp-python\n\nFor full documentation, please see: llama-cpp-python docs.",
"#### First install the package\n\nRun one of the following commands, according to your system:",
"#### Simple llama-cpp-python example code\n\n```\n\n* Option D - Running with LangChain\n\n\nHere are guides on using llama-cpp-python and ctransformers with LangChain:\n\n\n* LangChain + llama-cpp-python\n* LangChain + ctransformers\n\n\nConfigurations\n--------------\n\n\nThe configuration info are in 'smash\\_config.json'.\n\n\nCredits & License\n-----------------\n\n\nThe license of the smashed model follows the license of the original model. Please check the license of the original model before using this model which provided the base model. The license of the 'pruna-engine' is here on Pypi.\n\n\nWant to compress other models?\n------------------------------\n\n\n* Contact us and tell us which model to compress next here.\n* Request access to easily compress your own AI models here."
] | [
"TAGS\n#gguf #pruna-ai #region-us \n",
"### How to load this model in Python code, using llama-cpp-python\n\nFor full documentation, please see: llama-cpp-python docs.",
"#### First install the package\n\nRun one of the following commands, according to your system:",
"#### Simple llama-cpp-python example code\n\n```\n\n* Option D - Running with LangChain\n\n\nHere are guides on using llama-cpp-python and ctransformers with LangChain:\n\n\n* LangChain + llama-cpp-python\n* LangChain + ctransformers\n\n\nConfigurations\n--------------\n\n\nThe configuration info are in 'smash\\_config.json'.\n\n\nCredits & License\n-----------------\n\n\nThe license of the smashed model follows the license of the original model. Please check the license of the original model before using this model which provided the base model. The license of the 'pruna-engine' is here on Pypi.\n\n\nWant to compress other models?\n------------------------------\n\n\n* Contact us and tell us which model to compress next here.\n* Request access to easily compress your own AI models here."
] | [
14,
37,
20,
236
] | [
"TAGS\n#gguf #pruna-ai #region-us \n### How to load this model in Python code, using llama-cpp-python\n\nFor full documentation, please see: llama-cpp-python docs.#### First install the package\n\nRun one of the following commands, according to your system:#### Simple llama-cpp-python example code\n\n```\n\n* Option D - Running with LangChain\n\n\nHere are guides on using llama-cpp-python and ctransformers with LangChain:\n\n\n* LangChain + llama-cpp-python\n* LangChain + ctransformers\n\n\nConfigurations\n--------------\n\n\nThe configuration info are in 'smash\\_config.json'.\n\n\nCredits & License\n-----------------\n\n\nThe license of the smashed model follows the license of the original model. Please check the license of the original model before using this model which provided the base model. The license of the 'pruna-engine' is here on Pypi.\n\n\nWant to compress other models?\n------------------------------\n\n\n* Contact us and tell us which model to compress next here.\n* Request access to easily compress your own AI models here."
] |
null | peft |
# Model Card for Model ID
<!-- Provide a quick summary of what the model is/does. -->
## Model Details
### Model Description
<!-- Provide a longer summary of what this model is. -->
- **Developed by:** [More Information Needed]
- **Funded by [optional]:** [More Information Needed]
- **Shared by [optional]:** [More Information Needed]
- **Model type:** [More Information Needed]
- **Language(s) (NLP):** [More Information Needed]
- **License:** [More Information Needed]
- **Finetuned from model [optional]:** [More Information Needed]
### Model Sources [optional]
<!-- Provide the basic links for the model. -->
- **Repository:** [More Information Needed]
- **Paper [optional]:** [More Information Needed]
- **Demo [optional]:** [More Information Needed]
## Uses
<!-- Address questions around how the model is intended to be used, including the foreseeable users of the model and those affected by the model. -->
### Direct Use
<!-- This section is for the model use without fine-tuning or plugging into a larger ecosystem/app. -->
[More Information Needed]
### Downstream Use [optional]
<!-- This section is for the model use when fine-tuned for a task, or when plugged into a larger ecosystem/app -->
[More Information Needed]
### Out-of-Scope Use
<!-- This section addresses misuse, malicious use, and uses that the model will not work well for. -->
[More Information Needed]
## Bias, Risks, and Limitations
<!-- This section is meant to convey both technical and sociotechnical limitations. -->
[More Information Needed]
### Recommendations
<!-- This section is meant to convey recommendations with respect to the bias, risk, and technical limitations. -->
Users (both direct and downstream) should be made aware of the risks, biases and limitations of the model. More information needed for further recommendations.
## How to Get Started with the Model
Use the code below to get started with the model.
[More Information Needed]
## Training Details
### Training Data
<!-- This should link to a Dataset Card, perhaps with a short stub of information on what the training data is all about as well as documentation related to data pre-processing or additional filtering. -->
[More Information Needed]
### Training Procedure
<!-- This relates heavily to the Technical Specifications. Content here should link to that section when it is relevant to the training procedure. -->
#### Preprocessing [optional]
[More Information Needed]
#### Training Hyperparameters
- **Training regime:** [More Information Needed] <!--fp32, fp16 mixed precision, bf16 mixed precision, bf16 non-mixed precision, fp16 non-mixed precision, fp8 mixed precision -->
#### Speeds, Sizes, Times [optional]
<!-- This section provides information about throughput, start/end time, checkpoint size if relevant, etc. -->
[More Information Needed]
## Evaluation
<!-- This section describes the evaluation protocols and provides the results. -->
### Testing Data, Factors & Metrics
#### Testing Data
<!-- This should link to a Dataset Card if possible. -->
[More Information Needed]
#### Factors
<!-- These are the things the evaluation is disaggregating by, e.g., subpopulations or domains. -->
[More Information Needed]
#### Metrics
<!-- These are the evaluation metrics being used, ideally with a description of why. -->
[More Information Needed]
### Results
[More Information Needed]
#### Summary
## Model Examination [optional]
<!-- Relevant interpretability work for the model goes here -->
[More Information Needed]
## Environmental Impact
<!-- Total emissions (in grams of CO2eq) and additional considerations, such as electricity usage, go here. Edit the suggested text below accordingly -->
Carbon emissions can be estimated using the [Machine Learning Impact calculator](https://mlco2.github.io/impact#compute) presented in [Lacoste et al. (2019)](https://arxiv.org/abs/1910.09700).
- **Hardware Type:** [More Information Needed]
- **Hours used:** [More Information Needed]
- **Cloud Provider:** [More Information Needed]
- **Compute Region:** [More Information Needed]
- **Carbon Emitted:** [More Information Needed]
## Technical Specifications [optional]
### Model Architecture and Objective
[More Information Needed]
### Compute Infrastructure
[More Information Needed]
#### Hardware
[More Information Needed]
#### Software
[More Information Needed]
## Citation [optional]
<!-- If there is a paper or blog post introducing the model, the APA and Bibtex information for that should go in this section. -->
**BibTeX:**
[More Information Needed]
**APA:**
[More Information Needed]
## Glossary [optional]
<!-- If relevant, include terms and calculations in this section that can help readers understand the model or model card. -->
[More Information Needed]
## More Information [optional]
[More Information Needed]
## Model Card Authors [optional]
[More Information Needed]
## Model Card Contact
[More Information Needed]
### Framework versions
- PEFT 0.7.1 | {"library_name": "peft", "base_model": "meta-llama/Meta-Llama-3-8B-Instruct"} | sravaniayyagari/lora_model_1 | null | [
"peft",
"safetensors",
"arxiv:1910.09700",
"base_model:meta-llama/Meta-Llama-3-8B-Instruct",
"region:us"
] | null | 2024-05-02T10:43:35+00:00 | [
"1910.09700"
] | [] | TAGS
#peft #safetensors #arxiv-1910.09700 #base_model-meta-llama/Meta-Llama-3-8B-Instruct #region-us
|
# Model Card for Model ID
## Model Details
### Model Description
- Developed by:
- Funded by [optional]:
- Shared by [optional]:
- Model type:
- Language(s) (NLP):
- License:
- Finetuned from model [optional]:
### Model Sources [optional]
- Repository:
- Paper [optional]:
- Demo [optional]:
## Uses
### Direct Use
### Downstream Use [optional]
### Out-of-Scope Use
## Bias, Risks, and Limitations
### Recommendations
Users (both direct and downstream) should be made aware of the risks, biases and limitations of the model. More information needed for further recommendations.
## How to Get Started with the Model
Use the code below to get started with the model.
## Training Details
### Training Data
### Training Procedure
#### Preprocessing [optional]
#### Training Hyperparameters
- Training regime:
#### Speeds, Sizes, Times [optional]
## Evaluation
### Testing Data, Factors & Metrics
#### Testing Data
#### Factors
#### Metrics
### Results
#### Summary
## Model Examination [optional]
## Environmental Impact
Carbon emissions can be estimated using the Machine Learning Impact calculator presented in Lacoste et al. (2019).
- Hardware Type:
- Hours used:
- Cloud Provider:
- Compute Region:
- Carbon Emitted:
## Technical Specifications [optional]
### Model Architecture and Objective
### Compute Infrastructure
#### Hardware
#### Software
[optional]
BibTeX:
APA:
## Glossary [optional]
## More Information [optional]
## Model Card Authors [optional]
## Model Card Contact
### Framework versions
- PEFT 0.7.1 | [
"# Model Card for Model ID",
"## Model Details",
"### Model Description\n\n\n\n\n\n- Developed by: \n- Funded by [optional]: \n- Shared by [optional]: \n- Model type: \n- Language(s) (NLP): \n- License: \n- Finetuned from model [optional]:",
"### Model Sources [optional]\n\n\n\n- Repository: \n- Paper [optional]: \n- Demo [optional]:",
"## Uses",
"### Direct Use",
"### Downstream Use [optional]",
"### Out-of-Scope Use",
"## Bias, Risks, and Limitations",
"### Recommendations\n\n\n\nUsers (both direct and downstream) should be made aware of the risks, biases and limitations of the model. More information needed for further recommendations.",
"## How to Get Started with the Model\n\nUse the code below to get started with the model.",
"## Training Details",
"### Training Data",
"### Training Procedure",
"#### Preprocessing [optional]",
"#### Training Hyperparameters\n\n- Training regime:",
"#### Speeds, Sizes, Times [optional]",
"## Evaluation",
"### Testing Data, Factors & Metrics",
"#### Testing Data",
"#### Factors",
"#### Metrics",
"### Results",
"#### Summary",
"## Model Examination [optional]",
"## Environmental Impact\n\n\n\nCarbon emissions can be estimated using the Machine Learning Impact calculator presented in Lacoste et al. (2019).\n\n- Hardware Type: \n- Hours used: \n- Cloud Provider: \n- Compute Region: \n- Carbon Emitted:",
"## Technical Specifications [optional]",
"### Model Architecture and Objective",
"### Compute Infrastructure",
"#### Hardware",
"#### Software\n\n\n\n[optional]\n\n\n\nBibTeX:\n\n\n\nAPA:",
"## Glossary [optional]",
"## More Information [optional]",
"## Model Card Authors [optional]",
"## Model Card Contact",
"### Framework versions\n\n- PEFT 0.7.1"
] | [
"TAGS\n#peft #safetensors #arxiv-1910.09700 #base_model-meta-llama/Meta-Llama-3-8B-Instruct #region-us \n",
"# Model Card for Model ID",
"## Model Details",
"### Model Description\n\n\n\n\n\n- Developed by: \n- Funded by [optional]: \n- Shared by [optional]: \n- Model type: \n- Language(s) (NLP): \n- License: \n- Finetuned from model [optional]:",
"### Model Sources [optional]\n\n\n\n- Repository: \n- Paper [optional]: \n- Demo [optional]:",
"## Uses",
"### Direct Use",
"### Downstream Use [optional]",
"### Out-of-Scope Use",
"## Bias, Risks, and Limitations",
"### Recommendations\n\n\n\nUsers (both direct and downstream) should be made aware of the risks, biases and limitations of the model. More information needed for further recommendations.",
"## How to Get Started with the Model\n\nUse the code below to get started with the model.",
"## Training Details",
"### Training Data",
"### Training Procedure",
"#### Preprocessing [optional]",
"#### Training Hyperparameters\n\n- Training regime:",
"#### Speeds, Sizes, Times [optional]",
"## Evaluation",
"### Testing Data, Factors & Metrics",
"#### Testing Data",
"#### Factors",
"#### Metrics",
"### Results",
"#### Summary",
"## Model Examination [optional]",
"## Environmental Impact\n\n\n\nCarbon emissions can be estimated using the Machine Learning Impact calculator presented in Lacoste et al. (2019).\n\n- Hardware Type: \n- Hours used: \n- Cloud Provider: \n- Compute Region: \n- Carbon Emitted:",
"## Technical Specifications [optional]",
"### Model Architecture and Objective",
"### Compute Infrastructure",
"#### Hardware",
"#### Software\n\n\n\n[optional]\n\n\n\nBibTeX:\n\n\n\nAPA:",
"## Glossary [optional]",
"## More Information [optional]",
"## Model Card Authors [optional]",
"## Model Card Contact",
"### Framework versions\n\n- PEFT 0.7.1"
] | [
44,
6,
4,
50,
23,
3,
5,
8,
9,
8,
34,
20,
4,
5,
5,
11,
13,
12,
3,
10,
6,
5,
6,
4,
5,
7,
49,
7,
7,
5,
5,
15,
7,
7,
8,
5,
13
] | [
"TAGS\n#peft #safetensors #arxiv-1910.09700 #base_model-meta-llama/Meta-Llama-3-8B-Instruct #region-us \n# Model Card for Model ID## Model Details### Model Description\n\n\n\n\n\n- Developed by: \n- Funded by [optional]: \n- Shared by [optional]: \n- Model type: \n- Language(s) (NLP): \n- License: \n- Finetuned from model [optional]:### Model Sources [optional]\n\n\n\n- Repository: \n- Paper [optional]: \n- Demo [optional]:## Uses### Direct Use### Downstream Use [optional]### Out-of-Scope Use## Bias, Risks, and Limitations### Recommendations\n\n\n\nUsers (both direct and downstream) should be made aware of the risks, biases and limitations of the model. More information needed for further recommendations.## How to Get Started with the Model\n\nUse the code below to get started with the model.## Training Details### Training Data### Training Procedure#### Preprocessing [optional]#### Training Hyperparameters\n\n- Training regime:#### Speeds, Sizes, Times [optional]## Evaluation### Testing Data, Factors & Metrics#### Testing Data#### Factors#### Metrics### Results#### Summary## Model Examination [optional]## Environmental Impact\n\n\n\nCarbon emissions can be estimated using the Machine Learning Impact calculator presented in Lacoste et al. (2019).\n\n- Hardware Type: \n- Hours used: \n- Cloud Provider: \n- Compute Region: \n- Carbon Emitted:## Technical Specifications [optional]### Model Architecture and Objective### Compute Infrastructure#### Hardware#### Software\n\n\n\n[optional]\n\n\n\nBibTeX:\n\n\n\nAPA:## Glossary [optional]## More Information [optional]## Model Card Authors [optional]## Model Card Contact### Framework versions\n\n- PEFT 0.7.1"
] |
text-generation | transformers | # merged
This is a merge of pre-trained language models created using [mergekit](https://github.com/cg123/mergekit).
## Merge Details
### Merge Method
This model was merged using the [TIES](https://arxiv.org/abs/2306.01708) merge method using [Shaleen123/llama3-code-8bit](https://huggingface.co/Shaleen123/llama3-code-8bit) as a base.
### Models Merged
The following models were included in the merge:
### Configuration
The following YAML configuration was used to produce this model:
```yaml
base_model: Shaleen123/llama3-code-8bit
dtype: float16
merge_method: ties
parameters:
int8_mask: 1.0
normalize: 1.0
slices:
- sources:
- layer_range: [0, 32]
model: Shaleen123/llama3-code-8bit
- layer_range: [0, 32]
model: Shaleen123/llama3-code-8bit
parameters:
density: 0.5
weight: 0.5
- layer_range: [0, 32]
model: Shaleen123/llama3-code-8bit
parameters:
density: 0.5
weight: 0.3
```
| {"library_name": "transformers", "tags": ["mergekit", "merge"], "base_model": ["Shaleen123/llama3-code-8bit"]} | Shaleen123/llama3-code-8bit-merged | null | [
"transformers",
"safetensors",
"llama",
"text-generation",
"mergekit",
"merge",
"conversational",
"arxiv:2306.01708",
"base_model:Shaleen123/llama3-code-8bit",
"autotrain_compatible",
"endpoints_compatible",
"text-generation-inference",
"8-bit",
"region:us"
] | null | 2024-05-02T10:43:36+00:00 | [
"2306.01708"
] | [] | TAGS
#transformers #safetensors #llama #text-generation #mergekit #merge #conversational #arxiv-2306.01708 #base_model-Shaleen123/llama3-code-8bit #autotrain_compatible #endpoints_compatible #text-generation-inference #8-bit #region-us
| # merged
This is a merge of pre-trained language models created using mergekit.
## Merge Details
### Merge Method
This model was merged using the TIES merge method using Shaleen123/llama3-code-8bit as a base.
### Models Merged
The following models were included in the merge:
### Configuration
The following YAML configuration was used to produce this model:
| [
"# merged\n\nThis is a merge of pre-trained language models created using mergekit.",
"## Merge Details",
"### Merge Method\n\nThis model was merged using the TIES merge method using Shaleen123/llama3-code-8bit as a base.",
"### Models Merged\n\nThe following models were included in the merge:",
"### Configuration\n\nThe following YAML configuration was used to produce this model:"
] | [
"TAGS\n#transformers #safetensors #llama #text-generation #mergekit #merge #conversational #arxiv-2306.01708 #base_model-Shaleen123/llama3-code-8bit #autotrain_compatible #endpoints_compatible #text-generation-inference #8-bit #region-us \n",
"# merged\n\nThis is a merge of pre-trained language models created using mergekit.",
"## Merge Details",
"### Merge Method\n\nThis model was merged using the TIES merge method using Shaleen123/llama3-code-8bit as a base.",
"### Models Merged\n\nThe following models were included in the merge:",
"### Configuration\n\nThe following YAML configuration was used to produce this model:"
] | [
75,
17,
4,
32,
14,
16
] | [
"TAGS\n#transformers #safetensors #llama #text-generation #mergekit #merge #conversational #arxiv-2306.01708 #base_model-Shaleen123/llama3-code-8bit #autotrain_compatible #endpoints_compatible #text-generation-inference #8-bit #region-us \n# merged\n\nThis is a merge of pre-trained language models created using mergekit.## Merge Details### Merge Method\n\nThis model was merged using the TIES merge method using Shaleen123/llama3-code-8bit as a base.### Models Merged\n\nThe following models were included in the merge:### Configuration\n\nThe following YAML configuration was used to produce this model:"
] |
text-generation | transformers |
# Model Card for Model ID
<!-- Provide a quick summary of what the model is/does. -->
## Model Details
### Model Description
<!-- Provide a longer summary of what this model is. -->
This is the model card of a 🤗 transformers model that has been pushed on the Hub. This model card has been automatically generated.
- **Developed by:** [More Information Needed]
- **Funded by [optional]:** [More Information Needed]
- **Shared by [optional]:** [More Information Needed]
- **Model type:** [More Information Needed]
- **Language(s) (NLP):** [More Information Needed]
- **License:** [More Information Needed]
- **Finetuned from model [optional]:** [More Information Needed]
### Model Sources [optional]
<!-- Provide the basic links for the model. -->
- **Repository:** [More Information Needed]
- **Paper [optional]:** [More Information Needed]
- **Demo [optional]:** [More Information Needed]
## Uses
<!-- Address questions around how the model is intended to be used, including the foreseeable users of the model and those affected by the model. -->
### Direct Use
<!-- This section is for the model use without fine-tuning or plugging into a larger ecosystem/app. -->
[More Information Needed]
### Downstream Use [optional]
<!-- This section is for the model use when fine-tuned for a task, or when plugged into a larger ecosystem/app -->
[More Information Needed]
### Out-of-Scope Use
<!-- This section addresses misuse, malicious use, and uses that the model will not work well for. -->
[More Information Needed]
## Bias, Risks, and Limitations
<!-- This section is meant to convey both technical and sociotechnical limitations. -->
[More Information Needed]
### Recommendations
<!-- This section is meant to convey recommendations with respect to the bias, risk, and technical limitations. -->
Users (both direct and downstream) should be made aware of the risks, biases and limitations of the model. More information needed for further recommendations.
## How to Get Started with the Model
Use the code below to get started with the model.
[More Information Needed]
## Training Details
### Training Data
<!-- This should link to a Dataset Card, perhaps with a short stub of information on what the training data is all about as well as documentation related to data pre-processing or additional filtering. -->
[More Information Needed]
### Training Procedure
<!-- This relates heavily to the Technical Specifications. Content here should link to that section when it is relevant to the training procedure. -->
#### Preprocessing [optional]
[More Information Needed]
#### Training Hyperparameters
- **Training regime:** [More Information Needed] <!--fp32, fp16 mixed precision, bf16 mixed precision, bf16 non-mixed precision, fp16 non-mixed precision, fp8 mixed precision -->
#### Speeds, Sizes, Times [optional]
<!-- This section provides information about throughput, start/end time, checkpoint size if relevant, etc. -->
[More Information Needed]
## Evaluation
<!-- This section describes the evaluation protocols and provides the results. -->
### Testing Data, Factors & Metrics
#### Testing Data
<!-- This should link to a Dataset Card if possible. -->
[More Information Needed]
#### Factors
<!-- These are the things the evaluation is disaggregating by, e.g., subpopulations or domains. -->
[More Information Needed]
#### Metrics
<!-- These are the evaluation metrics being used, ideally with a description of why. -->
[More Information Needed]
### Results
[More Information Needed]
#### Summary
## Model Examination [optional]
<!-- Relevant interpretability work for the model goes here -->
[More Information Needed]
## Environmental Impact
<!-- Total emissions (in grams of CO2eq) and additional considerations, such as electricity usage, go here. Edit the suggested text below accordingly -->
Carbon emissions can be estimated using the [Machine Learning Impact calculator](https://mlco2.github.io/impact#compute) presented in [Lacoste et al. (2019)](https://arxiv.org/abs/1910.09700).
- **Hardware Type:** [More Information Needed]
- **Hours used:** [More Information Needed]
- **Cloud Provider:** [More Information Needed]
- **Compute Region:** [More Information Needed]
- **Carbon Emitted:** [More Information Needed]
## Technical Specifications [optional]
### Model Architecture and Objective
[More Information Needed]
### Compute Infrastructure
[More Information Needed]
#### Hardware
[More Information Needed]
#### Software
[More Information Needed]
## Citation [optional]
<!-- If there is a paper or blog post introducing the model, the APA and Bibtex information for that should go in this section. -->
**BibTeX:**
[More Information Needed]
**APA:**
[More Information Needed]
## Glossary [optional]
<!-- If relevant, include terms and calculations in this section that can help readers understand the model or model card. -->
[More Information Needed]
## More Information [optional]
[More Information Needed]
## Model Card Authors [optional]
[More Information Needed]
## Model Card Contact
[More Information Needed] | {"library_name": "transformers", "tags": []} | hoang1123/Llama-2-7b-chat-4bit-gptq | null | [
"transformers",
"llama",
"text-generation",
"arxiv:1910.09700",
"autotrain_compatible",
"endpoints_compatible",
"text-generation-inference",
"4-bit",
"region:us"
] | null | 2024-05-02T10:44:19+00:00 | [
"1910.09700"
] | [] | TAGS
#transformers #llama #text-generation #arxiv-1910.09700 #autotrain_compatible #endpoints_compatible #text-generation-inference #4-bit #region-us
|
# Model Card for Model ID
## Model Details
### Model Description
This is the model card of a transformers model that has been pushed on the Hub. This model card has been automatically generated.
- Developed by:
- Funded by [optional]:
- Shared by [optional]:
- Model type:
- Language(s) (NLP):
- License:
- Finetuned from model [optional]:
### Model Sources [optional]
- Repository:
- Paper [optional]:
- Demo [optional]:
## Uses
### Direct Use
### Downstream Use [optional]
### Out-of-Scope Use
## Bias, Risks, and Limitations
### Recommendations
Users (both direct and downstream) should be made aware of the risks, biases and limitations of the model. More information needed for further recommendations.
## How to Get Started with the Model
Use the code below to get started with the model.
## Training Details
### Training Data
### Training Procedure
#### Preprocessing [optional]
#### Training Hyperparameters
- Training regime:
#### Speeds, Sizes, Times [optional]
## Evaluation
### Testing Data, Factors & Metrics
#### Testing Data
#### Factors
#### Metrics
### Results
#### Summary
## Model Examination [optional]
## Environmental Impact
Carbon emissions can be estimated using the Machine Learning Impact calculator presented in Lacoste et al. (2019).
- Hardware Type:
- Hours used:
- Cloud Provider:
- Compute Region:
- Carbon Emitted:
## Technical Specifications [optional]
### Model Architecture and Objective
### Compute Infrastructure
#### Hardware
#### Software
[optional]
BibTeX:
APA:
## Glossary [optional]
## More Information [optional]
## Model Card Authors [optional]
## Model Card Contact
| [
"# Model Card for Model ID",
"## Model Details",
"### Model Description\n\n\n\nThis is the model card of a transformers model that has been pushed on the Hub. This model card has been automatically generated.\n\n- Developed by: \n- Funded by [optional]: \n- Shared by [optional]: \n- Model type: \n- Language(s) (NLP): \n- License: \n- Finetuned from model [optional]:",
"### Model Sources [optional]\n\n\n\n- Repository: \n- Paper [optional]: \n- Demo [optional]:",
"## Uses",
"### Direct Use",
"### Downstream Use [optional]",
"### Out-of-Scope Use",
"## Bias, Risks, and Limitations",
"### Recommendations\n\n\n\nUsers (both direct and downstream) should be made aware of the risks, biases and limitations of the model. More information needed for further recommendations.",
"## How to Get Started with the Model\n\nUse the code below to get started with the model.",
"## Training Details",
"### Training Data",
"### Training Procedure",
"#### Preprocessing [optional]",
"#### Training Hyperparameters\n\n- Training regime:",
"#### Speeds, Sizes, Times [optional]",
"## Evaluation",
"### Testing Data, Factors & Metrics",
"#### Testing Data",
"#### Factors",
"#### Metrics",
"### Results",
"#### Summary",
"## Model Examination [optional]",
"## Environmental Impact\n\n\n\nCarbon emissions can be estimated using the Machine Learning Impact calculator presented in Lacoste et al. (2019).\n\n- Hardware Type: \n- Hours used: \n- Cloud Provider: \n- Compute Region: \n- Carbon Emitted:",
"## Technical Specifications [optional]",
"### Model Architecture and Objective",
"### Compute Infrastructure",
"#### Hardware",
"#### Software\n\n\n\n[optional]\n\n\n\nBibTeX:\n\n\n\nAPA:",
"## Glossary [optional]",
"## More Information [optional]",
"## Model Card Authors [optional]",
"## Model Card Contact"
] | [
"TAGS\n#transformers #llama #text-generation #arxiv-1910.09700 #autotrain_compatible #endpoints_compatible #text-generation-inference #4-bit #region-us \n",
"# Model Card for Model ID",
"## Model Details",
"### Model Description\n\n\n\nThis is the model card of a transformers model that has been pushed on the Hub. This model card has been automatically generated.\n\n- Developed by: \n- Funded by [optional]: \n- Shared by [optional]: \n- Model type: \n- Language(s) (NLP): \n- License: \n- Finetuned from model [optional]:",
"### Model Sources [optional]\n\n\n\n- Repository: \n- Paper [optional]: \n- Demo [optional]:",
"## Uses",
"### Direct Use",
"### Downstream Use [optional]",
"### Out-of-Scope Use",
"## Bias, Risks, and Limitations",
"### Recommendations\n\n\n\nUsers (both direct and downstream) should be made aware of the risks, biases and limitations of the model. More information needed for further recommendations.",
"## How to Get Started with the Model\n\nUse the code below to get started with the model.",
"## Training Details",
"### Training Data",
"### Training Procedure",
"#### Preprocessing [optional]",
"#### Training Hyperparameters\n\n- Training regime:",
"#### Speeds, Sizes, Times [optional]",
"## Evaluation",
"### Testing Data, Factors & Metrics",
"#### Testing Data",
"#### Factors",
"#### Metrics",
"### Results",
"#### Summary",
"## Model Examination [optional]",
"## Environmental Impact\n\n\n\nCarbon emissions can be estimated using the Machine Learning Impact calculator presented in Lacoste et al. (2019).\n\n- Hardware Type: \n- Hours used: \n- Cloud Provider: \n- Compute Region: \n- Carbon Emitted:",
"## Technical Specifications [optional]",
"### Model Architecture and Objective",
"### Compute Infrastructure",
"#### Hardware",
"#### Software\n\n\n\n[optional]\n\n\n\nBibTeX:\n\n\n\nAPA:",
"## Glossary [optional]",
"## More Information [optional]",
"## Model Card Authors [optional]",
"## Model Card Contact"
] | [
44,
6,
4,
75,
23,
3,
5,
8,
9,
8,
34,
20,
4,
5,
5,
11,
13,
12,
3,
10,
6,
5,
6,
4,
5,
7,
49,
7,
7,
5,
5,
15,
7,
7,
8,
5
] | [
"TAGS\n#transformers #llama #text-generation #arxiv-1910.09700 #autotrain_compatible #endpoints_compatible #text-generation-inference #4-bit #region-us \n# Model Card for Model ID## Model Details### Model Description\n\n\n\nThis is the model card of a transformers model that has been pushed on the Hub. This model card has been automatically generated.\n\n- Developed by: \n- Funded by [optional]: \n- Shared by [optional]: \n- Model type: \n- Language(s) (NLP): \n- License: \n- Finetuned from model [optional]:### Model Sources [optional]\n\n\n\n- Repository: \n- Paper [optional]: \n- Demo [optional]:## Uses### Direct Use### Downstream Use [optional]### Out-of-Scope Use## Bias, Risks, and Limitations### Recommendations\n\n\n\nUsers (both direct and downstream) should be made aware of the risks, biases and limitations of the model. More information needed for further recommendations.## How to Get Started with the Model\n\nUse the code below to get started with the model.## Training Details### Training Data### Training Procedure#### Preprocessing [optional]#### Training Hyperparameters\n\n- Training regime:#### Speeds, Sizes, Times [optional]## Evaluation### Testing Data, Factors & Metrics#### Testing Data#### Factors#### Metrics### Results#### Summary## Model Examination [optional]## Environmental Impact\n\n\n\nCarbon emissions can be estimated using the Machine Learning Impact calculator presented in Lacoste et al. (2019).\n\n- Hardware Type: \n- Hours used: \n- Cloud Provider: \n- Compute Region: \n- Carbon Emitted:## Technical Specifications [optional]### Model Architecture and Objective### Compute Infrastructure#### Hardware#### Software\n\n\n\n[optional]\n\n\n\nBibTeX:\n\n\n\nAPA:## Glossary [optional]## More Information [optional]## Model Card Authors [optional]## Model Card Contact"
] |
text-generation | transformers |
# Model Card for Model ID
<!-- Provide a quick summary of what the model is/does. -->
IMPORTANT NOTE: This is for ONGOING EXPERIMENTATION and is not meant for reuse.
## Model Details
### Model Description
<!-- Provide a longer summary of what this model is. -->
This is the model card of a 🤗 transformers model that has been pushed on the Hub. This model card has been automatically generated.
- **Developed by:** [More Information Needed]
- **Funded by [optional]:** [More Information Needed]
- **Shared by [optional]:** [More Information Needed]
- **Model type:** [More Information Needed]
- **Language(s) (NLP):** [More Information Needed]
- **License:** [More Information Needed]
- **Finetuned from model [optional]:** [More Information Needed]
### Model Sources [optional]
<!-- Provide the basic links for the model. -->
- **Repository:** [More Information Needed]
- **Paper [optional]:** [More Information Needed]
- **Demo [optional]:** [More Information Needed]
## Uses
<!-- Address questions around how the model is intended to be used, including the foreseeable users of the model and those affected by the model. -->
### Direct Use
<!-- This section is for the model use without fine-tuning or plugging into a larger ecosystem/app. -->
[More Information Needed]
### Downstream Use [optional]
<!-- This section is for the model use when fine-tuned for a task, or when plugged into a larger ecosystem/app -->
[More Information Needed]
### Out-of-Scope Use
<!-- This section addresses misuse, malicious use, and uses that the model will not work well for. -->
[More Information Needed]
## Bias, Risks, and Limitations
<!-- This section is meant to convey both technical and sociotechnical limitations. -->
[More Information Needed]
### Recommendations
<!-- This section is meant to convey recommendations with respect to the bias, risk, and technical limitations. -->
Users (both direct and downstream) should be made aware of the risks, biases and limitations of the model. More information needed for further recommendations.
## How to Get Started with the Model
Use the code below to get started with the model.
[More Information Needed]
## Training Details
### Training Data
<!-- This should link to a Dataset Card, perhaps with a short stub of information on what the training data is all about as well as documentation related to data pre-processing or additional filtering. -->
[More Information Needed]
### Training Procedure
<!-- This relates heavily to the Technical Specifications. Content here should link to that section when it is relevant to the training procedure. -->
#### Preprocessing [optional]
[More Information Needed]
#### Training Hyperparameters
- **Training regime:** [More Information Needed] <!--fp32, fp16 mixed precision, bf16 mixed precision, bf16 non-mixed precision, fp16 non-mixed precision, fp8 mixed precision -->
#### Speeds, Sizes, Times [optional]
<!-- This section provides information about throughput, start/end time, checkpoint size if relevant, etc. -->
[More Information Needed]
## Evaluation
<!-- This section describes the evaluation protocols and provides the results. -->
### Testing Data, Factors & Metrics
#### Testing Data
<!-- This should link to a Dataset Card if possible. -->
[More Information Needed]
#### Factors
<!-- These are the things the evaluation is disaggregating by, e.g., subpopulations or domains. -->
[More Information Needed]
#### Metrics
<!-- These are the evaluation metrics being used, ideally with a description of why. -->
[More Information Needed]
### Results
[More Information Needed]
#### Summary
## Model Examination [optional]
<!-- Relevant interpretability work for the model goes here -->
[More Information Needed]
## Environmental Impact
<!-- Total emissions (in grams of CO2eq) and additional considerations, such as electricity usage, go here. Edit the suggested text below accordingly -->
Carbon emissions can be estimated using the [Machine Learning Impact calculator](https://mlco2.github.io/impact#compute) presented in [Lacoste et al. (2019)](https://arxiv.org/abs/1910.09700).
- **Hardware Type:** [More Information Needed]
- **Hours used:** [More Information Needed]
- **Cloud Provider:** [More Information Needed]
- **Compute Region:** [More Information Needed]
- **Carbon Emitted:** [More Information Needed]
## Technical Specifications [optional]
### Model Architecture and Objective
[More Information Needed]
### Compute Infrastructure
[More Information Needed]
#### Hardware
[More Information Needed]
#### Software
[More Information Needed]
## Citation [optional]
<!-- If there is a paper or blog post introducing the model, the APA and Bibtex information for that should go in this section. -->
**BibTeX:**
[More Information Needed]
**APA:**
[More Information Needed]
## Glossary [optional]
<!-- If relevant, include terms and calculations in this section that can help readers understand the model or model card. -->
[More Information Needed]
## More Information [optional]
[More Information Needed]
## Model Card Authors [optional]
[More Information Needed]
## Model Card Contact
[More Information Needed] | {"library_name": "transformers", "tags": []} | tanzuml/phi3-4k | null | [
"transformers",
"safetensors",
"phi3",
"text-generation",
"conversational",
"custom_code",
"arxiv:1910.09700",
"autotrain_compatible",
"endpoints_compatible",
"region:us"
] | null | 2024-05-02T10:47:20+00:00 | [
"1910.09700"
] | [] | TAGS
#transformers #safetensors #phi3 #text-generation #conversational #custom_code #arxiv-1910.09700 #autotrain_compatible #endpoints_compatible #region-us
|
# Model Card for Model ID
IMPORTANT NOTE: This is for ONGOING EXPERIMENTATION and is not meant for reuse.
## Model Details
### Model Description
This is the model card of a transformers model that has been pushed on the Hub. This model card has been automatically generated.
- Developed by:
- Funded by [optional]:
- Shared by [optional]:
- Model type:
- Language(s) (NLP):
- License:
- Finetuned from model [optional]:
### Model Sources [optional]
- Repository:
- Paper [optional]:
- Demo [optional]:
## Uses
### Direct Use
### Downstream Use [optional]
### Out-of-Scope Use
## Bias, Risks, and Limitations
### Recommendations
Users (both direct and downstream) should be made aware of the risks, biases and limitations of the model. More information needed for further recommendations.
## How to Get Started with the Model
Use the code below to get started with the model.
## Training Details
### Training Data
### Training Procedure
#### Preprocessing [optional]
#### Training Hyperparameters
- Training regime:
#### Speeds, Sizes, Times [optional]
## Evaluation
### Testing Data, Factors & Metrics
#### Testing Data
#### Factors
#### Metrics
### Results
#### Summary
## Model Examination [optional]
## Environmental Impact
Carbon emissions can be estimated using the Machine Learning Impact calculator presented in Lacoste et al. (2019).
- Hardware Type:
- Hours used:
- Cloud Provider:
- Compute Region:
- Carbon Emitted:
## Technical Specifications [optional]
### Model Architecture and Objective
### Compute Infrastructure
#### Hardware
#### Software
[optional]
BibTeX:
APA:
## Glossary [optional]
## More Information [optional]
## Model Card Authors [optional]
## Model Card Contact
| [
"# Model Card for Model ID\n\n\nIMPORTANT NOTE: This is for ONGOING EXPERIMENTATION and is not meant for reuse.",
"## Model Details",
"### Model Description\n\n\n\nThis is the model card of a transformers model that has been pushed on the Hub. This model card has been automatically generated.\n\n- Developed by: \n- Funded by [optional]: \n- Shared by [optional]: \n- Model type: \n- Language(s) (NLP): \n- License: \n- Finetuned from model [optional]:",
"### Model Sources [optional]\n\n\n\n- Repository: \n- Paper [optional]: \n- Demo [optional]:",
"## Uses",
"### Direct Use",
"### Downstream Use [optional]",
"### Out-of-Scope Use",
"## Bias, Risks, and Limitations",
"### Recommendations\n\n\n\nUsers (both direct and downstream) should be made aware of the risks, biases and limitations of the model. More information needed for further recommendations.",
"## How to Get Started with the Model\n\nUse the code below to get started with the model.",
"## Training Details",
"### Training Data",
"### Training Procedure",
"#### Preprocessing [optional]",
"#### Training Hyperparameters\n\n- Training regime:",
"#### Speeds, Sizes, Times [optional]",
"## Evaluation",
"### Testing Data, Factors & Metrics",
"#### Testing Data",
"#### Factors",
"#### Metrics",
"### Results",
"#### Summary",
"## Model Examination [optional]",
"## Environmental Impact\n\n\n\nCarbon emissions can be estimated using the Machine Learning Impact calculator presented in Lacoste et al. (2019).\n\n- Hardware Type: \n- Hours used: \n- Cloud Provider: \n- Compute Region: \n- Carbon Emitted:",
"## Technical Specifications [optional]",
"### Model Architecture and Objective",
"### Compute Infrastructure",
"#### Hardware",
"#### Software\n\n\n\n[optional]\n\n\n\nBibTeX:\n\n\n\nAPA:",
"## Glossary [optional]",
"## More Information [optional]",
"## Model Card Authors [optional]",
"## Model Card Contact"
] | [
"TAGS\n#transformers #safetensors #phi3 #text-generation #conversational #custom_code #arxiv-1910.09700 #autotrain_compatible #endpoints_compatible #region-us \n",
"# Model Card for Model ID\n\n\nIMPORTANT NOTE: This is for ONGOING EXPERIMENTATION and is not meant for reuse.",
"## Model Details",
"### Model Description\n\n\n\nThis is the model card of a transformers model that has been pushed on the Hub. This model card has been automatically generated.\n\n- Developed by: \n- Funded by [optional]: \n- Shared by [optional]: \n- Model type: \n- Language(s) (NLP): \n- License: \n- Finetuned from model [optional]:",
"### Model Sources [optional]\n\n\n\n- Repository: \n- Paper [optional]: \n- Demo [optional]:",
"## Uses",
"### Direct Use",
"### Downstream Use [optional]",
"### Out-of-Scope Use",
"## Bias, Risks, and Limitations",
"### Recommendations\n\n\n\nUsers (both direct and downstream) should be made aware of the risks, biases and limitations of the model. More information needed for further recommendations.",
"## How to Get Started with the Model\n\nUse the code below to get started with the model.",
"## Training Details",
"### Training Data",
"### Training Procedure",
"#### Preprocessing [optional]",
"#### Training Hyperparameters\n\n- Training regime:",
"#### Speeds, Sizes, Times [optional]",
"## Evaluation",
"### Testing Data, Factors & Metrics",
"#### Testing Data",
"#### Factors",
"#### Metrics",
"### Results",
"#### Summary",
"## Model Examination [optional]",
"## Environmental Impact\n\n\n\nCarbon emissions can be estimated using the Machine Learning Impact calculator presented in Lacoste et al. (2019).\n\n- Hardware Type: \n- Hours used: \n- Cloud Provider: \n- Compute Region: \n- Carbon Emitted:",
"## Technical Specifications [optional]",
"### Model Architecture and Objective",
"### Compute Infrastructure",
"#### Hardware",
"#### Software\n\n\n\n[optional]\n\n\n\nBibTeX:\n\n\n\nAPA:",
"## Glossary [optional]",
"## More Information [optional]",
"## Model Card Authors [optional]",
"## Model Card Contact"
] | [
45,
22,
4,
75,
23,
3,
5,
8,
9,
8,
34,
20,
4,
5,
5,
11,
13,
12,
3,
10,
6,
5,
6,
4,
5,
7,
49,
7,
7,
5,
5,
15,
7,
7,
8,
5
] | [
"TAGS\n#transformers #safetensors #phi3 #text-generation #conversational #custom_code #arxiv-1910.09700 #autotrain_compatible #endpoints_compatible #region-us \n# Model Card for Model ID\n\n\nIMPORTANT NOTE: This is for ONGOING EXPERIMENTATION and is not meant for reuse.## Model Details### Model Description\n\n\n\nThis is the model card of a transformers model that has been pushed on the Hub. This model card has been automatically generated.\n\n- Developed by: \n- Funded by [optional]: \n- Shared by [optional]: \n- Model type: \n- Language(s) (NLP): \n- License: \n- Finetuned from model [optional]:### Model Sources [optional]\n\n\n\n- Repository: \n- Paper [optional]: \n- Demo [optional]:## Uses### Direct Use### Downstream Use [optional]### Out-of-Scope Use## Bias, Risks, and Limitations### Recommendations\n\n\n\nUsers (both direct and downstream) should be made aware of the risks, biases and limitations of the model. More information needed for further recommendations.## How to Get Started with the Model\n\nUse the code below to get started with the model.## Training Details### Training Data### Training Procedure#### Preprocessing [optional]#### Training Hyperparameters\n\n- Training regime:#### Speeds, Sizes, Times [optional]## Evaluation### Testing Data, Factors & Metrics#### Testing Data#### Factors#### Metrics### Results#### Summary## Model Examination [optional]## Environmental Impact\n\n\n\nCarbon emissions can be estimated using the Machine Learning Impact calculator presented in Lacoste et al. (2019).\n\n- Hardware Type: \n- Hours used: \n- Cloud Provider: \n- Compute Region: \n- Carbon Emitted:## Technical Specifications [optional]### Model Architecture and Objective### Compute Infrastructure#### Hardware#### Software\n\n\n\n[optional]\n\n\n\nBibTeX:\n\n\n\nAPA:## Glossary [optional]## More Information [optional]## Model Card Authors [optional]## Model Card Contact"
] |
text-generation | transformers |
**Attention! The model is in a training state and this repository contains only a checkpoint for 20 billion tokens.**
# Aeoinum v1 Base 1B
A state-of-the-art language model for Russian language processing.
## Usage
```python
from transformers import AutoTokenizer, AutoModelForCausalLM
import torch
tokenizer = AutoTokenizer.from_pretrained("aeonium/Aeonium-v1-Base-1.6B-checkpoint-20B")
model = AutoModelForCausalLM.from_pretrained("aeonium/Aeonium-v1-Base-1.6B-checkpoint-20B").cuda()
input_ids = tokenizer("Искусственный интеллект - это", return_tensors='pt').to(model.device)["input_ids"]
output = model.generate(input_ids, max_new_tokens=48, do_sample=True, temperature=0.7)
print(tokenizer.decode(output[0]))
```
Output:
```
Искусственный интеллект - это основа современной науки и техники. Его потенциал позволяет решать задачи, которые выходят за пределы человеческих возможностей. В работе над ними участвуют все: от ученых до инженеров и даже военных. В своей книге "Искусственный интеллект" автор книги, профессор Л
```
## Dataset Detail
The dataset for pre-training is collected from public data, most of which are web pages in Russian. The total size of the data is 20B tokens.
## Training Detail
The training is performed thanks to a grant from [TPU Research Cloud](https://sites.research.google/trc/about/) on a TPU v4-32 node.
## Copyright
The model is released under the Apache 2.0 license. | {"language": ["ru"], "license": "apache-2.0", "datasets": ["uonlp/CulturaX"], "pipeline_tag": "text-generation"} | aeonium/Aeonium-v1-Base-1.6B-checkpoint-20B | null | [
"transformers",
"pytorch",
"safetensors",
"llama",
"text-generation",
"ru",
"dataset:uonlp/CulturaX",
"license:apache-2.0",
"autotrain_compatible",
"endpoints_compatible",
"text-generation-inference",
"region:us"
] | null | 2024-05-02T10:48:05+00:00 | [] | [
"ru"
] | TAGS
#transformers #pytorch #safetensors #llama #text-generation #ru #dataset-uonlp/CulturaX #license-apache-2.0 #autotrain_compatible #endpoints_compatible #text-generation-inference #region-us
|
Attention! The model is in a training state and this repository contains only a checkpoint for 20 billion tokens.
# Aeoinum v1 Base 1B
A state-of-the-art language model for Russian language processing.
## Usage
Output:
## Dataset Detail
The dataset for pre-training is collected from public data, most of which are web pages in Russian. The total size of the data is 20B tokens.
## Training Detail
The training is performed thanks to a grant from TPU Research Cloud on a TPU v4-32 node.
## Copyright
The model is released under the Apache 2.0 license. | [
"# Aeoinum v1 Base 1B\nA state-of-the-art language model for Russian language processing.",
"## Usage\n\nOutput:",
"## Dataset Detail\nThe dataset for pre-training is collected from public data, most of which are web pages in Russian. The total size of the data is 20B tokens.",
"## Training Detail\nThe training is performed thanks to a grant from TPU Research Cloud on a TPU v4-32 node.",
"## Copyright\nThe model is released under the Apache 2.0 license."
] | [
"TAGS\n#transformers #pytorch #safetensors #llama #text-generation #ru #dataset-uonlp/CulturaX #license-apache-2.0 #autotrain_compatible #endpoints_compatible #text-generation-inference #region-us \n",
"# Aeoinum v1 Base 1B\nA state-of-the-art language model for Russian language processing.",
"## Usage\n\nOutput:",
"## Dataset Detail\nThe dataset for pre-training is collected from public data, most of which are web pages in Russian. The total size of the data is 20B tokens.",
"## Training Detail\nThe training is performed thanks to a grant from TPU Research Cloud on a TPU v4-32 node.",
"## Copyright\nThe model is released under the Apache 2.0 license."
] | [
60,
23,
5,
39,
27,
15
] | [
"TAGS\n#transformers #pytorch #safetensors #llama #text-generation #ru #dataset-uonlp/CulturaX #license-apache-2.0 #autotrain_compatible #endpoints_compatible #text-generation-inference #region-us \n# Aeoinum v1 Base 1B\nA state-of-the-art language model for Russian language processing.## Usage\n\nOutput:## Dataset Detail\nThe dataset for pre-training is collected from public data, most of which are web pages in Russian. The total size of the data is 20B tokens.## Training Detail\nThe training is performed thanks to a grant from TPU Research Cloud on a TPU v4-32 node.## Copyright\nThe model is released under the Apache 2.0 license."
] |
text-generation | transformers |
# Model Card for Model ID
<!-- Provide a quick summary of what the model is/does. -->
## Model Details
### Model Description
<!-- Provide a longer summary of what this model is. -->
This is the model card of a 🤗 transformers model that has been pushed on the Hub. This model card has been automatically generated.
- **Developed by:** [More Information Needed]
- **Funded by [optional]:** [More Information Needed]
- **Shared by [optional]:** [More Information Needed]
- **Model type:** [More Information Needed]
- **Language(s) (NLP):** [More Information Needed]
- **License:** [More Information Needed]
- **Finetuned from model [optional]:** [More Information Needed]
### Model Sources [optional]
<!-- Provide the basic links for the model. -->
- **Repository:** [More Information Needed]
- **Paper [optional]:** [More Information Needed]
- **Demo [optional]:** [More Information Needed]
## Uses
<!-- Address questions around how the model is intended to be used, including the foreseeable users of the model and those affected by the model. -->
### Direct Use
<!-- This section is for the model use without fine-tuning or plugging into a larger ecosystem/app. -->
[More Information Needed]
### Downstream Use [optional]
<!-- This section is for the model use when fine-tuned for a task, or when plugged into a larger ecosystem/app -->
[More Information Needed]
### Out-of-Scope Use
<!-- This section addresses misuse, malicious use, and uses that the model will not work well for. -->
[More Information Needed]
## Bias, Risks, and Limitations
<!-- This section is meant to convey both technical and sociotechnical limitations. -->
[More Information Needed]
### Recommendations
<!-- This section is meant to convey recommendations with respect to the bias, risk, and technical limitations. -->
Users (both direct and downstream) should be made aware of the risks, biases and limitations of the model. More information needed for further recommendations.
## How to Get Started with the Model
Use the code below to get started with the model.
[More Information Needed]
## Training Details
### Training Data
<!-- This should link to a Dataset Card, perhaps with a short stub of information on what the training data is all about as well as documentation related to data pre-processing or additional filtering. -->
[More Information Needed]
### Training Procedure
<!-- This relates heavily to the Technical Specifications. Content here should link to that section when it is relevant to the training procedure. -->
#### Preprocessing [optional]
[More Information Needed]
#### Training Hyperparameters
- **Training regime:** [More Information Needed] <!--fp32, fp16 mixed precision, bf16 mixed precision, bf16 non-mixed precision, fp16 non-mixed precision, fp8 mixed precision -->
#### Speeds, Sizes, Times [optional]
<!-- This section provides information about throughput, start/end time, checkpoint size if relevant, etc. -->
[More Information Needed]
## Evaluation
<!-- This section describes the evaluation protocols and provides the results. -->
### Testing Data, Factors & Metrics
#### Testing Data
<!-- This should link to a Dataset Card if possible. -->
[More Information Needed]
#### Factors
<!-- These are the things the evaluation is disaggregating by, e.g., subpopulations or domains. -->
[More Information Needed]
#### Metrics
<!-- These are the evaluation metrics being used, ideally with a description of why. -->
[More Information Needed]
### Results
[More Information Needed]
#### Summary
## Model Examination [optional]
<!-- Relevant interpretability work for the model goes here -->
[More Information Needed]
## Environmental Impact
<!-- Total emissions (in grams of CO2eq) and additional considerations, such as electricity usage, go here. Edit the suggested text below accordingly -->
Carbon emissions can be estimated using the [Machine Learning Impact calculator](https://mlco2.github.io/impact#compute) presented in [Lacoste et al. (2019)](https://arxiv.org/abs/1910.09700).
- **Hardware Type:** [More Information Needed]
- **Hours used:** [More Information Needed]
- **Cloud Provider:** [More Information Needed]
- **Compute Region:** [More Information Needed]
- **Carbon Emitted:** [More Information Needed]
## Technical Specifications [optional]
### Model Architecture and Objective
[More Information Needed]
### Compute Infrastructure
[More Information Needed]
#### Hardware
[More Information Needed]
#### Software
[More Information Needed]
## Citation [optional]
<!-- If there is a paper or blog post introducing the model, the APA and Bibtex information for that should go in this section. -->
**BibTeX:**
[More Information Needed]
**APA:**
[More Information Needed]
## Glossary [optional]
<!-- If relevant, include terms and calculations in this section that can help readers understand the model or model card. -->
[More Information Needed]
## More Information [optional]
[More Information Needed]
## Model Card Authors [optional]
[More Information Needed]
## Model Card Contact
[More Information Needed] | {"library_name": "transformers", "tags": []} | steve1989/fingpt_sentiment_fin_headlines_bnb4bits | null | [
"transformers",
"safetensors",
"llama",
"text-generation",
"arxiv:1910.09700",
"autotrain_compatible",
"endpoints_compatible",
"text-generation-inference",
"region:us"
] | null | 2024-05-02T10:48:41+00:00 | [
"1910.09700"
] | [] | TAGS
#transformers #safetensors #llama #text-generation #arxiv-1910.09700 #autotrain_compatible #endpoints_compatible #text-generation-inference #region-us
|
# Model Card for Model ID
## Model Details
### Model Description
This is the model card of a transformers model that has been pushed on the Hub. This model card has been automatically generated.
- Developed by:
- Funded by [optional]:
- Shared by [optional]:
- Model type:
- Language(s) (NLP):
- License:
- Finetuned from model [optional]:
### Model Sources [optional]
- Repository:
- Paper [optional]:
- Demo [optional]:
## Uses
### Direct Use
### Downstream Use [optional]
### Out-of-Scope Use
## Bias, Risks, and Limitations
### Recommendations
Users (both direct and downstream) should be made aware of the risks, biases and limitations of the model. More information needed for further recommendations.
## How to Get Started with the Model
Use the code below to get started with the model.
## Training Details
### Training Data
### Training Procedure
#### Preprocessing [optional]
#### Training Hyperparameters
- Training regime:
#### Speeds, Sizes, Times [optional]
## Evaluation
### Testing Data, Factors & Metrics
#### Testing Data
#### Factors
#### Metrics
### Results
#### Summary
## Model Examination [optional]
## Environmental Impact
Carbon emissions can be estimated using the Machine Learning Impact calculator presented in Lacoste et al. (2019).
- Hardware Type:
- Hours used:
- Cloud Provider:
- Compute Region:
- Carbon Emitted:
## Technical Specifications [optional]
### Model Architecture and Objective
### Compute Infrastructure
#### Hardware
#### Software
[optional]
BibTeX:
APA:
## Glossary [optional]
## More Information [optional]
## Model Card Authors [optional]
## Model Card Contact
| [
"# Model Card for Model ID",
"## Model Details",
"### Model Description\n\n\n\nThis is the model card of a transformers model that has been pushed on the Hub. This model card has been automatically generated.\n\n- Developed by: \n- Funded by [optional]: \n- Shared by [optional]: \n- Model type: \n- Language(s) (NLP): \n- License: \n- Finetuned from model [optional]:",
"### Model Sources [optional]\n\n\n\n- Repository: \n- Paper [optional]: \n- Demo [optional]:",
"## Uses",
"### Direct Use",
"### Downstream Use [optional]",
"### Out-of-Scope Use",
"## Bias, Risks, and Limitations",
"### Recommendations\n\n\n\nUsers (both direct and downstream) should be made aware of the risks, biases and limitations of the model. More information needed for further recommendations.",
"## How to Get Started with the Model\n\nUse the code below to get started with the model.",
"## Training Details",
"### Training Data",
"### Training Procedure",
"#### Preprocessing [optional]",
"#### Training Hyperparameters\n\n- Training regime:",
"#### Speeds, Sizes, Times [optional]",
"## Evaluation",
"### Testing Data, Factors & Metrics",
"#### Testing Data",
"#### Factors",
"#### Metrics",
"### Results",
"#### Summary",
"## Model Examination [optional]",
"## Environmental Impact\n\n\n\nCarbon emissions can be estimated using the Machine Learning Impact calculator presented in Lacoste et al. (2019).\n\n- Hardware Type: \n- Hours used: \n- Cloud Provider: \n- Compute Region: \n- Carbon Emitted:",
"## Technical Specifications [optional]",
"### Model Architecture and Objective",
"### Compute Infrastructure",
"#### Hardware",
"#### Software\n\n\n\n[optional]\n\n\n\nBibTeX:\n\n\n\nAPA:",
"## Glossary [optional]",
"## More Information [optional]",
"## Model Card Authors [optional]",
"## Model Card Contact"
] | [
"TAGS\n#transformers #safetensors #llama #text-generation #arxiv-1910.09700 #autotrain_compatible #endpoints_compatible #text-generation-inference #region-us \n",
"# Model Card for Model ID",
"## Model Details",
"### Model Description\n\n\n\nThis is the model card of a transformers model that has been pushed on the Hub. This model card has been automatically generated.\n\n- Developed by: \n- Funded by [optional]: \n- Shared by [optional]: \n- Model type: \n- Language(s) (NLP): \n- License: \n- Finetuned from model [optional]:",
"### Model Sources [optional]\n\n\n\n- Repository: \n- Paper [optional]: \n- Demo [optional]:",
"## Uses",
"### Direct Use",
"### Downstream Use [optional]",
"### Out-of-Scope Use",
"## Bias, Risks, and Limitations",
"### Recommendations\n\n\n\nUsers (both direct and downstream) should be made aware of the risks, biases and limitations of the model. More information needed for further recommendations.",
"## How to Get Started with the Model\n\nUse the code below to get started with the model.",
"## Training Details",
"### Training Data",
"### Training Procedure",
"#### Preprocessing [optional]",
"#### Training Hyperparameters\n\n- Training regime:",
"#### Speeds, Sizes, Times [optional]",
"## Evaluation",
"### Testing Data, Factors & Metrics",
"#### Testing Data",
"#### Factors",
"#### Metrics",
"### Results",
"#### Summary",
"## Model Examination [optional]",
"## Environmental Impact\n\n\n\nCarbon emissions can be estimated using the Machine Learning Impact calculator presented in Lacoste et al. (2019).\n\n- Hardware Type: \n- Hours used: \n- Cloud Provider: \n- Compute Region: \n- Carbon Emitted:",
"## Technical Specifications [optional]",
"### Model Architecture and Objective",
"### Compute Infrastructure",
"#### Hardware",
"#### Software\n\n\n\n[optional]\n\n\n\nBibTeX:\n\n\n\nAPA:",
"## Glossary [optional]",
"## More Information [optional]",
"## Model Card Authors [optional]",
"## Model Card Contact"
] | [
44,
6,
4,
75,
23,
3,
5,
8,
9,
8,
34,
20,
4,
5,
5,
11,
13,
12,
3,
10,
6,
5,
6,
4,
5,
7,
49,
7,
7,
5,
5,
15,
7,
7,
8,
5
] | [
"TAGS\n#transformers #safetensors #llama #text-generation #arxiv-1910.09700 #autotrain_compatible #endpoints_compatible #text-generation-inference #region-us \n# Model Card for Model ID## Model Details### Model Description\n\n\n\nThis is the model card of a transformers model that has been pushed on the Hub. This model card has been automatically generated.\n\n- Developed by: \n- Funded by [optional]: \n- Shared by [optional]: \n- Model type: \n- Language(s) (NLP): \n- License: \n- Finetuned from model [optional]:### Model Sources [optional]\n\n\n\n- Repository: \n- Paper [optional]: \n- Demo [optional]:## Uses### Direct Use### Downstream Use [optional]### Out-of-Scope Use## Bias, Risks, and Limitations### Recommendations\n\n\n\nUsers (both direct and downstream) should be made aware of the risks, biases and limitations of the model. More information needed for further recommendations.## How to Get Started with the Model\n\nUse the code below to get started with the model.## Training Details### Training Data### Training Procedure#### Preprocessing [optional]#### Training Hyperparameters\n\n- Training regime:#### Speeds, Sizes, Times [optional]## Evaluation### Testing Data, Factors & Metrics#### Testing Data#### Factors#### Metrics### Results#### Summary## Model Examination [optional]## Environmental Impact\n\n\n\nCarbon emissions can be estimated using the Machine Learning Impact calculator presented in Lacoste et al. (2019).\n\n- Hardware Type: \n- Hours used: \n- Cloud Provider: \n- Compute Region: \n- Carbon Emitted:## Technical Specifications [optional]### Model Architecture and Objective### Compute Infrastructure#### Hardware#### Software\n\n\n\n[optional]\n\n\n\nBibTeX:\n\n\n\nAPA:## Glossary [optional]## More Information [optional]## Model Card Authors [optional]## Model Card Contact"
] |
text-classification | transformers |
<!-- This model card has been generated automatically according to the information the Trainer had access to. You
should probably proofread and complete it, then remove this comment. -->
# albert_model_02
This model is a fine-tuned version of [distilbert/distilbert-base-uncased](https://huggingface.co/distilbert/distilbert-base-uncased) on an unknown dataset.
It achieves the following results on the evaluation set:
- Loss: 0.6010
- Accuracy: 0.7406
## Model description
More information needed
## Intended uses & limitations
More information needed
## Training and evaluation data
More information needed
## Training procedure
### Training hyperparameters
The following hyperparameters were used during training:
- learning_rate: 2e-05
- train_batch_size: 16
- eval_batch_size: 16
- seed: 42
- optimizer: Adam with betas=(0.9,0.999) and epsilon=1e-08
- lr_scheduler_type: linear
- num_epochs: 5
### Training results
| Training Loss | Epoch | Step | Validation Loss | Accuracy |
|:-------------:|:-----:|:----:|:---------------:|:--------:|
| No log | 1.0 | 310 | 0.6197 | 0.7203 |
| 0.6587 | 2.0 | 620 | 0.6010 | 0.7406 |
| 0.6587 | 3.0 | 930 | 0.6613 | 0.7345 |
| 0.3804 | 4.0 | 1240 | 0.7342 | 0.7467 |
| 0.2432 | 5.0 | 1550 | 0.7571 | 0.7476 |
### Framework versions
- Transformers 4.39.3
- Pytorch 2.2.2+cu121
- Datasets 2.18.0
- Tokenizers 0.15.2
| {"license": "apache-2.0", "tags": ["generated_from_trainer"], "metrics": ["accuracy"], "base_model": "distilbert/distilbert-base-uncased", "model-index": [{"name": "albert_model_02", "results": []}]} | KalaiselvanD/albert_model_02 | null | [
"transformers",
"tensorboard",
"safetensors",
"distilbert",
"text-classification",
"generated_from_trainer",
"base_model:distilbert/distilbert-base-uncased",
"license:apache-2.0",
"autotrain_compatible",
"endpoints_compatible",
"region:us"
] | null | 2024-05-02T10:51:06+00:00 | [] | [] | TAGS
#transformers #tensorboard #safetensors #distilbert #text-classification #generated_from_trainer #base_model-distilbert/distilbert-base-uncased #license-apache-2.0 #autotrain_compatible #endpoints_compatible #region-us
| albert\_model\_02
=================
This model is a fine-tuned version of distilbert/distilbert-base-uncased on an unknown dataset.
It achieves the following results on the evaluation set:
* Loss: 0.6010
* Accuracy: 0.7406
Model description
-----------------
More information needed
Intended uses & limitations
---------------------------
More information needed
Training and evaluation data
----------------------------
More information needed
Training procedure
------------------
### Training hyperparameters
The following hyperparameters were used during training:
* learning\_rate: 2e-05
* train\_batch\_size: 16
* eval\_batch\_size: 16
* seed: 42
* optimizer: Adam with betas=(0.9,0.999) and epsilon=1e-08
* lr\_scheduler\_type: linear
* num\_epochs: 5
### Training results
### Framework versions
* Transformers 4.39.3
* Pytorch 2.2.2+cu121
* Datasets 2.18.0
* Tokenizers 0.15.2
| [
"### Training hyperparameters\n\n\nThe following hyperparameters were used during training:\n\n\n* learning\\_rate: 2e-05\n* train\\_batch\\_size: 16\n* eval\\_batch\\_size: 16\n* seed: 42\n* optimizer: Adam with betas=(0.9,0.999) and epsilon=1e-08\n* lr\\_scheduler\\_type: linear\n* num\\_epochs: 5",
"### Training results",
"### Framework versions\n\n\n* Transformers 4.39.3\n* Pytorch 2.2.2+cu121\n* Datasets 2.18.0\n* Tokenizers 0.15.2"
] | [
"TAGS\n#transformers #tensorboard #safetensors #distilbert #text-classification #generated_from_trainer #base_model-distilbert/distilbert-base-uncased #license-apache-2.0 #autotrain_compatible #endpoints_compatible #region-us \n",
"### Training hyperparameters\n\n\nThe following hyperparameters were used during training:\n\n\n* learning\\_rate: 2e-05\n* train\\_batch\\_size: 16\n* eval\\_batch\\_size: 16\n* seed: 42\n* optimizer: Adam with betas=(0.9,0.999) and epsilon=1e-08\n* lr\\_scheduler\\_type: linear\n* num\\_epochs: 5",
"### Training results",
"### Framework versions\n\n\n* Transformers 4.39.3\n* Pytorch 2.2.2+cu121\n* Datasets 2.18.0\n* Tokenizers 0.15.2"
] | [
63,
101,
5,
44
] | [
"TAGS\n#transformers #tensorboard #safetensors #distilbert #text-classification #generated_from_trainer #base_model-distilbert/distilbert-base-uncased #license-apache-2.0 #autotrain_compatible #endpoints_compatible #region-us \n### Training hyperparameters\n\n\nThe following hyperparameters were used during training:\n\n\n* learning\\_rate: 2e-05\n* train\\_batch\\_size: 16\n* eval\\_batch\\_size: 16\n* seed: 42\n* optimizer: Adam with betas=(0.9,0.999) and epsilon=1e-08\n* lr\\_scheduler\\_type: linear\n* num\\_epochs: 5### Training results### Framework versions\n\n\n* Transformers 4.39.3\n* Pytorch 2.2.2+cu121\n* Datasets 2.18.0\n* Tokenizers 0.15.2"
] |
text-generation | transformers |
# Model Card for Model ID
<!-- Provide a quick summary of what the model is/does. -->
## Model Details
### Model Description
<!-- Provide a longer summary of what this model is. -->
This is the model card of a 🤗 transformers model that has been pushed on the Hub. This model card has been automatically generated.
- **Developed by:** [More Information Needed]
- **Funded by [optional]:** [More Information Needed]
- **Shared by [optional]:** [More Information Needed]
- **Model type:** [More Information Needed]
- **Language(s) (NLP):** [More Information Needed]
- **License:** [More Information Needed]
- **Finetuned from model [optional]:** [More Information Needed]
### Model Sources [optional]
<!-- Provide the basic links for the model. -->
- **Repository:** [More Information Needed]
- **Paper [optional]:** [More Information Needed]
- **Demo [optional]:** [More Information Needed]
## Uses
<!-- Address questions around how the model is intended to be used, including the foreseeable users of the model and those affected by the model. -->
### Direct Use
<!-- This section is for the model use without fine-tuning or plugging into a larger ecosystem/app. -->
[More Information Needed]
### Downstream Use [optional]
<!-- This section is for the model use when fine-tuned for a task, or when plugged into a larger ecosystem/app -->
[More Information Needed]
### Out-of-Scope Use
<!-- This section addresses misuse, malicious use, and uses that the model will not work well for. -->
[More Information Needed]
## Bias, Risks, and Limitations
<!-- This section is meant to convey both technical and sociotechnical limitations. -->
[More Information Needed]
### Recommendations
<!-- This section is meant to convey recommendations with respect to the bias, risk, and technical limitations. -->
Users (both direct and downstream) should be made aware of the risks, biases and limitations of the model. More information needed for further recommendations.
## How to Get Started with the Model
Use the code below to get started with the model.
[More Information Needed]
## Training Details
### Training Data
<!-- This should link to a Dataset Card, perhaps with a short stub of information on what the training data is all about as well as documentation related to data pre-processing or additional filtering. -->
[More Information Needed]
### Training Procedure
<!-- This relates heavily to the Technical Specifications. Content here should link to that section when it is relevant to the training procedure. -->
#### Preprocessing [optional]
[More Information Needed]
#### Training Hyperparameters
- **Training regime:** [More Information Needed] <!--fp32, fp16 mixed precision, bf16 mixed precision, bf16 non-mixed precision, fp16 non-mixed precision, fp8 mixed precision -->
#### Speeds, Sizes, Times [optional]
<!-- This section provides information about throughput, start/end time, checkpoint size if relevant, etc. -->
[More Information Needed]
## Evaluation
<!-- This section describes the evaluation protocols and provides the results. -->
### Testing Data, Factors & Metrics
#### Testing Data
<!-- This should link to a Dataset Card if possible. -->
[More Information Needed]
#### Factors
<!-- These are the things the evaluation is disaggregating by, e.g., subpopulations or domains. -->
[More Information Needed]
#### Metrics
<!-- These are the evaluation metrics being used, ideally with a description of why. -->
[More Information Needed]
### Results
[More Information Needed]
#### Summary
## Model Examination [optional]
<!-- Relevant interpretability work for the model goes here -->
[More Information Needed]
## Environmental Impact
<!-- Total emissions (in grams of CO2eq) and additional considerations, such as electricity usage, go here. Edit the suggested text below accordingly -->
Carbon emissions can be estimated using the [Machine Learning Impact calculator](https://mlco2.github.io/impact#compute) presented in [Lacoste et al. (2019)](https://arxiv.org/abs/1910.09700).
- **Hardware Type:** [More Information Needed]
- **Hours used:** [More Information Needed]
- **Cloud Provider:** [More Information Needed]
- **Compute Region:** [More Information Needed]
- **Carbon Emitted:** [More Information Needed]
## Technical Specifications [optional]
### Model Architecture and Objective
[More Information Needed]
### Compute Infrastructure
[More Information Needed]
#### Hardware
[More Information Needed]
#### Software
[More Information Needed]
## Citation [optional]
<!-- If there is a paper or blog post introducing the model, the APA and Bibtex information for that should go in this section. -->
**BibTeX:**
[More Information Needed]
**APA:**
[More Information Needed]
## Glossary [optional]
<!-- If relevant, include terms and calculations in this section that can help readers understand the model or model card. -->
[More Information Needed]
## More Information [optional]
[More Information Needed]
## Model Card Authors [optional]
[More Information Needed]
## Model Card Contact
[More Information Needed] | {"library_name": "transformers", "tags": []} | vaatsav06/Llama3_medmcqa_finetune | null | [
"transformers",
"safetensors",
"llama",
"text-generation",
"conversational",
"arxiv:1910.09700",
"autotrain_compatible",
"endpoints_compatible",
"text-generation-inference",
"8-bit",
"region:us"
] | null | 2024-05-02T10:51:48+00:00 | [
"1910.09700"
] | [] | TAGS
#transformers #safetensors #llama #text-generation #conversational #arxiv-1910.09700 #autotrain_compatible #endpoints_compatible #text-generation-inference #8-bit #region-us
|
# Model Card for Model ID
## Model Details
### Model Description
This is the model card of a transformers model that has been pushed on the Hub. This model card has been automatically generated.
- Developed by:
- Funded by [optional]:
- Shared by [optional]:
- Model type:
- Language(s) (NLP):
- License:
- Finetuned from model [optional]:
### Model Sources [optional]
- Repository:
- Paper [optional]:
- Demo [optional]:
## Uses
### Direct Use
### Downstream Use [optional]
### Out-of-Scope Use
## Bias, Risks, and Limitations
### Recommendations
Users (both direct and downstream) should be made aware of the risks, biases and limitations of the model. More information needed for further recommendations.
## How to Get Started with the Model
Use the code below to get started with the model.
## Training Details
### Training Data
### Training Procedure
#### Preprocessing [optional]
#### Training Hyperparameters
- Training regime:
#### Speeds, Sizes, Times [optional]
## Evaluation
### Testing Data, Factors & Metrics
#### Testing Data
#### Factors
#### Metrics
### Results
#### Summary
## Model Examination [optional]
## Environmental Impact
Carbon emissions can be estimated using the Machine Learning Impact calculator presented in Lacoste et al. (2019).
- Hardware Type:
- Hours used:
- Cloud Provider:
- Compute Region:
- Carbon Emitted:
## Technical Specifications [optional]
### Model Architecture and Objective
### Compute Infrastructure
#### Hardware
#### Software
[optional]
BibTeX:
APA:
## Glossary [optional]
## More Information [optional]
## Model Card Authors [optional]
## Model Card Contact
| [
"# Model Card for Model ID",
"## Model Details",
"### Model Description\n\n\n\nThis is the model card of a transformers model that has been pushed on the Hub. This model card has been automatically generated.\n\n- Developed by: \n- Funded by [optional]: \n- Shared by [optional]: \n- Model type: \n- Language(s) (NLP): \n- License: \n- Finetuned from model [optional]:",
"### Model Sources [optional]\n\n\n\n- Repository: \n- Paper [optional]: \n- Demo [optional]:",
"## Uses",
"### Direct Use",
"### Downstream Use [optional]",
"### Out-of-Scope Use",
"## Bias, Risks, and Limitations",
"### Recommendations\n\n\n\nUsers (both direct and downstream) should be made aware of the risks, biases and limitations of the model. More information needed for further recommendations.",
"## How to Get Started with the Model\n\nUse the code below to get started with the model.",
"## Training Details",
"### Training Data",
"### Training Procedure",
"#### Preprocessing [optional]",
"#### Training Hyperparameters\n\n- Training regime:",
"#### Speeds, Sizes, Times [optional]",
"## Evaluation",
"### Testing Data, Factors & Metrics",
"#### Testing Data",
"#### Factors",
"#### Metrics",
"### Results",
"#### Summary",
"## Model Examination [optional]",
"## Environmental Impact\n\n\n\nCarbon emissions can be estimated using the Machine Learning Impact calculator presented in Lacoste et al. (2019).\n\n- Hardware Type: \n- Hours used: \n- Cloud Provider: \n- Compute Region: \n- Carbon Emitted:",
"## Technical Specifications [optional]",
"### Model Architecture and Objective",
"### Compute Infrastructure",
"#### Hardware",
"#### Software\n\n\n\n[optional]\n\n\n\nBibTeX:\n\n\n\nAPA:",
"## Glossary [optional]",
"## More Information [optional]",
"## Model Card Authors [optional]",
"## Model Card Contact"
] | [
"TAGS\n#transformers #safetensors #llama #text-generation #conversational #arxiv-1910.09700 #autotrain_compatible #endpoints_compatible #text-generation-inference #8-bit #region-us \n",
"# Model Card for Model ID",
"## Model Details",
"### Model Description\n\n\n\nThis is the model card of a transformers model that has been pushed on the Hub. This model card has been automatically generated.\n\n- Developed by: \n- Funded by [optional]: \n- Shared by [optional]: \n- Model type: \n- Language(s) (NLP): \n- License: \n- Finetuned from model [optional]:",
"### Model Sources [optional]\n\n\n\n- Repository: \n- Paper [optional]: \n- Demo [optional]:",
"## Uses",
"### Direct Use",
"### Downstream Use [optional]",
"### Out-of-Scope Use",
"## Bias, Risks, and Limitations",
"### Recommendations\n\n\n\nUsers (both direct and downstream) should be made aware of the risks, biases and limitations of the model. More information needed for further recommendations.",
"## How to Get Started with the Model\n\nUse the code below to get started with the model.",
"## Training Details",
"### Training Data",
"### Training Procedure",
"#### Preprocessing [optional]",
"#### Training Hyperparameters\n\n- Training regime:",
"#### Speeds, Sizes, Times [optional]",
"## Evaluation",
"### Testing Data, Factors & Metrics",
"#### Testing Data",
"#### Factors",
"#### Metrics",
"### Results",
"#### Summary",
"## Model Examination [optional]",
"## Environmental Impact\n\n\n\nCarbon emissions can be estimated using the Machine Learning Impact calculator presented in Lacoste et al. (2019).\n\n- Hardware Type: \n- Hours used: \n- Cloud Provider: \n- Compute Region: \n- Carbon Emitted:",
"## Technical Specifications [optional]",
"### Model Architecture and Objective",
"### Compute Infrastructure",
"#### Hardware",
"#### Software\n\n\n\n[optional]\n\n\n\nBibTeX:\n\n\n\nAPA:",
"## Glossary [optional]",
"## More Information [optional]",
"## Model Card Authors [optional]",
"## Model Card Contact"
] | [
51,
6,
4,
75,
23,
3,
5,
8,
9,
8,
34,
20,
4,
5,
5,
11,
13,
12,
3,
10,
6,
5,
6,
4,
5,
7,
49,
7,
7,
5,
5,
15,
7,
7,
8,
5
] | [
"TAGS\n#transformers #safetensors #llama #text-generation #conversational #arxiv-1910.09700 #autotrain_compatible #endpoints_compatible #text-generation-inference #8-bit #region-us \n# Model Card for Model ID## Model Details### Model Description\n\n\n\nThis is the model card of a transformers model that has been pushed on the Hub. This model card has been automatically generated.\n\n- Developed by: \n- Funded by [optional]: \n- Shared by [optional]: \n- Model type: \n- Language(s) (NLP): \n- License: \n- Finetuned from model [optional]:### Model Sources [optional]\n\n\n\n- Repository: \n- Paper [optional]: \n- Demo [optional]:## Uses### Direct Use### Downstream Use [optional]### Out-of-Scope Use## Bias, Risks, and Limitations### Recommendations\n\n\n\nUsers (both direct and downstream) should be made aware of the risks, biases and limitations of the model. More information needed for further recommendations.## How to Get Started with the Model\n\nUse the code below to get started with the model.## Training Details### Training Data### Training Procedure#### Preprocessing [optional]#### Training Hyperparameters\n\n- Training regime:#### Speeds, Sizes, Times [optional]## Evaluation### Testing Data, Factors & Metrics#### Testing Data#### Factors#### Metrics### Results#### Summary## Model Examination [optional]## Environmental Impact\n\n\n\nCarbon emissions can be estimated using the Machine Learning Impact calculator presented in Lacoste et al. (2019).\n\n- Hardware Type: \n- Hours used: \n- Cloud Provider: \n- Compute Region: \n- Carbon Emitted:## Technical Specifications [optional]### Model Architecture and Objective### Compute Infrastructure#### Hardware#### Software\n\n\n\n[optional]\n\n\n\nBibTeX:\n\n\n\nAPA:## Glossary [optional]## More Information [optional]## Model Card Authors [optional]## Model Card Contact"
] |
automatic-speech-recognition | transformers |
<!-- This model card has been generated automatically according to the information the Trainer had access to. You
should probably proofread and complete it, then remove this comment. -->
# whisper-tiny-finetune
This model is a fine-tuned version of [openai/whisper-tiny.en](https://huggingface.co/openai/whisper-tiny.en) on an unknown dataset.
It achieves the following results on the evaluation set:
- Loss: 0.5792
- Wer: 20.6820
## Model description
More information needed
## Intended uses & limitations
More information needed
## Training and evaluation data
More information needed
## Training procedure
### Training hyperparameters
The following hyperparameters were used during training:
- learning_rate: 1e-05
- train_batch_size: 128
- eval_batch_size: 8
- seed: 42
- optimizer: Adam with betas=(0.9,0.999) and epsilon=1e-08
- lr_scheduler_type: linear
- lr_scheduler_warmup_steps: 500
- training_steps: 1000
### Training results
| Training Loss | Epoch | Step | Validation Loss | Wer |
|:-------------:|:-------:|:----:|:---------------:|:-------:|
| 4.1356 | 0.2778 | 10 | 4.1201 | 47.9002 |
| 4.0312 | 0.5556 | 20 | 4.0231 | 47.3319 |
| 3.917 | 0.8333 | 30 | 3.8659 | 46.5425 |
| 3.7606 | 1.1111 | 40 | 3.6569 | 45.8478 |
| 3.4823 | 1.3889 | 50 | 3.3969 | 44.5216 |
| 3.0938 | 1.6667 | 60 | 3.0765 | 41.9324 |
| 2.7895 | 1.9444 | 70 | 2.6692 | 34.9542 |
| 2.3101 | 2.2222 | 80 | 2.1389 | 34.5122 |
| 1.6935 | 2.5 | 90 | 1.5546 | 34.8911 |
| 1.1419 | 2.7778 | 100 | 1.0650 | 36.5330 |
| 0.904 | 3.0556 | 110 | 0.8400 | 29.4601 |
| 0.7536 | 3.3333 | 120 | 0.7657 | 28.9233 |
| 0.6857 | 3.6111 | 130 | 0.7202 | 27.7550 |
| 0.6609 | 3.8889 | 140 | 0.6886 | 26.6814 |
| 0.5804 | 4.1667 | 150 | 0.6656 | 25.6710 |
| 0.5611 | 4.4444 | 160 | 0.6465 | 25.0710 |
| 0.5574 | 4.7222 | 170 | 0.6293 | 24.3448 |
| 0.552 | 5.0 | 180 | 0.6135 | 24.0606 |
| 0.4717 | 5.2778 | 190 | 0.6024 | 24.5974 |
| 0.4681 | 5.5556 | 200 | 0.5898 | 24.0290 |
| 0.4679 | 5.8333 | 210 | 0.5778 | 23.5238 |
| 0.4351 | 6.1111 | 220 | 0.5670 | 23.6501 |
| 0.3982 | 6.3889 | 230 | 0.5599 | 23.2081 |
| 0.3892 | 6.6667 | 240 | 0.5520 | 22.0714 |
| 0.3771 | 6.9444 | 250 | 0.5439 | 21.1872 |
| 0.3532 | 7.2222 | 260 | 0.5372 | 21.6925 |
| 0.3435 | 7.5 | 270 | 0.5309 | 27.5024 |
| 0.336 | 7.7778 | 280 | 0.5253 | 20.9346 |
| 0.3088 | 8.0556 | 290 | 0.5201 | 20.4610 |
| 0.3014 | 8.3333 | 300 | 0.5184 | 20.5242 |
| 0.316 | 8.6111 | 310 | 0.5146 | 20.2400 |
| 0.2931 | 8.8889 | 320 | 0.5118 | 19.9874 |
| 0.2228 | 9.1667 | 330 | 0.5079 | 20.3663 |
| 0.2445 | 9.4444 | 340 | 0.5052 | 20.2716 |
| 0.2343 | 9.7222 | 350 | 0.5039 | 20.2084 |
| 0.2893 | 10.0 | 360 | 0.5023 | 20.0189 |
| 0.2014 | 10.2778 | 370 | 0.5030 | 20.0505 |
| 0.2048 | 10.5556 | 380 | 0.5036 | 19.6400 |
| 0.1941 | 10.8333 | 390 | 0.5003 | 20.1137 |
| 0.1601 | 11.1111 | 400 | 0.4992 | 19.8295 |
| 0.1647 | 11.3889 | 410 | 0.5010 | 19.8926 |
| 0.1519 | 11.6667 | 420 | 0.5044 | 19.6716 |
| 0.1747 | 11.9444 | 430 | 0.5005 | 20.1137 |
| 0.1194 | 12.2222 | 440 | 0.5076 | 20.7452 |
| 0.1021 | 12.5 | 450 | 0.5104 | 19.9242 |
| 0.1115 | 12.7778 | 460 | 0.5102 | 20.7136 |
| 0.1355 | 13.0556 | 470 | 0.5068 | 20.3979 |
| 0.0824 | 13.3333 | 480 | 0.5152 | 20.5557 |
| 0.0858 | 13.6111 | 490 | 0.5189 | 20.3663 |
| 0.0786 | 13.8889 | 500 | 0.5225 | 21.1557 |
| 0.0564 | 14.1667 | 510 | 0.5250 | 20.9031 |
| 0.056 | 14.4444 | 520 | 0.5232 | 20.8715 |
| 0.0558 | 14.7222 | 530 | 0.5282 | 20.5557 |
| 0.0657 | 15.0 | 540 | 0.5299 | 20.7452 |
| 0.0369 | 15.2778 | 550 | 0.5342 | 20.6505 |
| 0.0355 | 15.5556 | 560 | 0.5341 | 20.1137 |
| 0.0383 | 15.8333 | 570 | 0.5370 | 20.4926 |
| 0.0333 | 16.1111 | 580 | 0.5401 | 20.5557 |
| 0.027 | 16.3889 | 590 | 0.5455 | 20.9346 |
| 0.0261 | 16.6667 | 600 | 0.5480 | 20.6189 |
| 0.024 | 16.9444 | 610 | 0.5494 | 20.4294 |
| 0.0164 | 17.2222 | 620 | 0.5505 | 20.3663 |
| 0.0159 | 17.5 | 630 | 0.5577 | 20.7136 |
| 0.0168 | 17.7778 | 640 | 0.5549 | 20.9031 |
| 0.015 | 18.0556 | 650 | 0.5555 | 20.8083 |
| 0.0116 | 18.3333 | 660 | 0.5596 | 20.9978 |
| 0.0131 | 18.6111 | 670 | 0.5614 | 20.9346 |
| 0.0121 | 18.8889 | 680 | 0.5634 | 20.3663 |
| 0.009 | 19.1667 | 690 | 0.5643 | 20.7452 |
| 0.0108 | 19.4444 | 700 | 0.5633 | 20.3031 |
| 0.0096 | 19.7222 | 710 | 0.5666 | 20.3979 |
| 0.0123 | 20.0 | 720 | 0.5660 | 20.4610 |
| 0.009 | 20.2778 | 730 | 0.5695 | 20.5242 |
| 0.0099 | 20.5556 | 740 | 0.5684 | 20.3663 |
| 0.0079 | 20.8333 | 750 | 0.5701 | 20.7768 |
| 0.008 | 21.1111 | 760 | 0.5701 | 20.7136 |
| 0.0084 | 21.3889 | 770 | 0.5719 | 20.7136 |
| 0.0076 | 21.6667 | 780 | 0.5724 | 20.4610 |
| 0.0081 | 21.9444 | 790 | 0.5724 | 20.7136 |
| 0.0067 | 22.2222 | 800 | 0.5731 | 20.6820 |
| 0.0076 | 22.5 | 810 | 0.5737 | 20.4926 |
| 0.0079 | 22.7778 | 820 | 0.5748 | 20.3979 |
| 0.0069 | 23.0556 | 830 | 0.5747 | 20.6820 |
| 0.0066 | 23.3333 | 840 | 0.5751 | 20.7136 |
| 0.0062 | 23.6111 | 850 | 0.5755 | 20.7136 |
| 0.0071 | 23.8889 | 860 | 0.5764 | 20.5873 |
| 0.0062 | 24.1667 | 870 | 0.5774 | 20.7136 |
| 0.0059 | 24.4444 | 880 | 0.5769 | 20.5873 |
| 0.0066 | 24.7222 | 890 | 0.5772 | 20.6189 |
| 0.0066 | 25.0 | 900 | 0.5778 | 20.5873 |
| 0.0066 | 25.2778 | 910 | 0.5779 | 20.5557 |
| 0.0062 | 25.5556 | 920 | 0.5781 | 20.5873 |
| 0.006 | 25.8333 | 930 | 0.5787 | 20.6189 |
| 0.0061 | 26.1111 | 940 | 0.5789 | 20.5873 |
| 0.0056 | 26.3889 | 950 | 0.5788 | 20.5557 |
| 0.006 | 26.6667 | 960 | 0.5789 | 20.5873 |
| 0.0055 | 26.9444 | 970 | 0.5790 | 20.5873 |
| 0.0057 | 27.2222 | 980 | 0.5791 | 20.6189 |
| 0.0063 | 27.5 | 990 | 0.5792 | 20.6820 |
| 0.0059 | 27.7778 | 1000 | 0.5792 | 20.6820 |
### Framework versions
- Transformers 4.40.1
- Pytorch 2.2.1+cu121
- Datasets 2.19.1.dev0
- Tokenizers 0.19.1
| {"license": "apache-2.0", "tags": ["generated_from_trainer"], "metrics": ["wer"], "base_model": "openai/whisper-tiny.en", "model-index": [{"name": "whisper-tiny-finetune", "results": []}]} | edoyin/whisper-tiny-finetune | null | [
"transformers",
"tensorboard",
"safetensors",
"whisper",
"automatic-speech-recognition",
"generated_from_trainer",
"base_model:openai/whisper-tiny.en",
"license:apache-2.0",
"endpoints_compatible",
"region:us"
] | null | 2024-05-02T10:51:56+00:00 | [] | [] | TAGS
#transformers #tensorboard #safetensors #whisper #automatic-speech-recognition #generated_from_trainer #base_model-openai/whisper-tiny.en #license-apache-2.0 #endpoints_compatible #region-us
| whisper-tiny-finetune
=====================
This model is a fine-tuned version of openai/URL on an unknown dataset.
It achieves the following results on the evaluation set:
* Loss: 0.5792
* Wer: 20.6820
Model description
-----------------
More information needed
Intended uses & limitations
---------------------------
More information needed
Training and evaluation data
----------------------------
More information needed
Training procedure
------------------
### Training hyperparameters
The following hyperparameters were used during training:
* learning\_rate: 1e-05
* train\_batch\_size: 128
* eval\_batch\_size: 8
* seed: 42
* optimizer: Adam with betas=(0.9,0.999) and epsilon=1e-08
* lr\_scheduler\_type: linear
* lr\_scheduler\_warmup\_steps: 500
* training\_steps: 1000
### Training results
### Framework versions
* Transformers 4.40.1
* Pytorch 2.2.1+cu121
* Datasets 2.19.1.dev0
* Tokenizers 0.19.1
| [
"### Training hyperparameters\n\n\nThe following hyperparameters were used during training:\n\n\n* learning\\_rate: 1e-05\n* train\\_batch\\_size: 128\n* eval\\_batch\\_size: 8\n* seed: 42\n* optimizer: Adam with betas=(0.9,0.999) and epsilon=1e-08\n* lr\\_scheduler\\_type: linear\n* lr\\_scheduler\\_warmup\\_steps: 500\n* training\\_steps: 1000",
"### Training results",
"### Framework versions\n\n\n* Transformers 4.40.1\n* Pytorch 2.2.1+cu121\n* Datasets 2.19.1.dev0\n* Tokenizers 0.19.1"
] | [
"TAGS\n#transformers #tensorboard #safetensors #whisper #automatic-speech-recognition #generated_from_trainer #base_model-openai/whisper-tiny.en #license-apache-2.0 #endpoints_compatible #region-us \n",
"### Training hyperparameters\n\n\nThe following hyperparameters were used during training:\n\n\n* learning\\_rate: 1e-05\n* train\\_batch\\_size: 128\n* eval\\_batch\\_size: 8\n* seed: 42\n* optimizer: Adam with betas=(0.9,0.999) and epsilon=1e-08\n* lr\\_scheduler\\_type: linear\n* lr\\_scheduler\\_warmup\\_steps: 500\n* training\\_steps: 1000",
"### Training results",
"### Framework versions\n\n\n* Transformers 4.40.1\n* Pytorch 2.2.1+cu121\n* Datasets 2.19.1.dev0\n* Tokenizers 0.19.1"
] | [
54,
115,
5,
47
] | [
"TAGS\n#transformers #tensorboard #safetensors #whisper #automatic-speech-recognition #generated_from_trainer #base_model-openai/whisper-tiny.en #license-apache-2.0 #endpoints_compatible #region-us \n### Training hyperparameters\n\n\nThe following hyperparameters were used during training:\n\n\n* learning\\_rate: 1e-05\n* train\\_batch\\_size: 128\n* eval\\_batch\\_size: 8\n* seed: 42\n* optimizer: Adam with betas=(0.9,0.999) and epsilon=1e-08\n* lr\\_scheduler\\_type: linear\n* lr\\_scheduler\\_warmup\\_steps: 500\n* training\\_steps: 1000### Training results### Framework versions\n\n\n* Transformers 4.40.1\n* Pytorch 2.2.1+cu121\n* Datasets 2.19.1.dev0\n* Tokenizers 0.19.1"
] |
question-answering | transformers |
<!-- This model card has been generated automatically according to the information Keras had access to. You should
probably proofread and complete it, then remove this comment. -->
# cancerfarore/albert-base-v2-CancerFarore-Model
This model is a fine-tuned version of [albert/albert-base-v2](https://huggingface.co/albert/albert-base-v2) on an unknown dataset.
It achieves the following results on the evaluation set:
- Train Loss: 0.7456
- Train End Logits Accuracy: 0.7778
- Train Start Logits Accuracy: 0.7525
- Validation Loss: 0.9444
- Validation End Logits Accuracy: 0.7069
- Validation Start Logits Accuracy: 0.6994
- Epoch: 1
## Model description
More information needed
## Intended uses & limitations
More information needed
## Training and evaluation data
More information needed
## Training procedure
### Training hyperparameters
The following hyperparameters were used during training:
- optimizer: {'name': 'Adam', 'weight_decay': None, 'clipnorm': None, 'global_clipnorm': None, 'clipvalue': None, 'use_ema': False, 'ema_momentum': 0.99, 'ema_overwrite_frequency': None, 'jit_compile': True, 'is_legacy_optimizer': False, 'learning_rate': {'module': 'keras.optimizers.schedules', 'class_name': 'PolynomialDecay', 'config': {'initial_learning_rate': 2e-05, 'decay_steps': 3798, 'end_learning_rate': 0.0, 'power': 1.0, 'cycle': False, 'name': None}, 'registered_name': None}, 'beta_1': 0.9, 'beta_2': 0.999, 'epsilon': 1e-08, 'amsgrad': False}
- training_precision: float32
### Training results
| Train Loss | Train End Logits Accuracy | Train Start Logits Accuracy | Validation Loss | Validation End Logits Accuracy | Validation Start Logits Accuracy | Epoch |
|:----------:|:-------------------------:|:---------------------------:|:---------------:|:------------------------------:|:--------------------------------:|:-----:|
| 1.2646 | 0.6255 | 0.6055 | 0.9592 | 0.6964 | 0.6829 | 0 |
| 0.7456 | 0.7778 | 0.7525 | 0.9444 | 0.7069 | 0.6994 | 1 |
### Framework versions
- Transformers 4.40.1
- TensorFlow 2.15.0
- Datasets 2.19.0
- Tokenizers 0.19.1
| {"license": "apache-2.0", "tags": ["generated_from_keras_callback"], "base_model": "albert/albert-base-v2", "model-index": [{"name": "cancerfarore/albert-base-v2-CancerFarore-Model", "results": []}]} | cancerfarore/albert-base-v2-CancerFarore-Model | null | [
"transformers",
"tf",
"albert",
"question-answering",
"generated_from_keras_callback",
"base_model:albert/albert-base-v2",
"license:apache-2.0",
"endpoints_compatible",
"region:us"
] | null | 2024-05-02T10:51:59+00:00 | [] | [] | TAGS
#transformers #tf #albert #question-answering #generated_from_keras_callback #base_model-albert/albert-base-v2 #license-apache-2.0 #endpoints_compatible #region-us
| cancerfarore/albert-base-v2-CancerFarore-Model
==============================================
This model is a fine-tuned version of albert/albert-base-v2 on an unknown dataset.
It achieves the following results on the evaluation set:
* Train Loss: 0.7456
* Train End Logits Accuracy: 0.7778
* Train Start Logits Accuracy: 0.7525
* Validation Loss: 0.9444
* Validation End Logits Accuracy: 0.7069
* Validation Start Logits Accuracy: 0.6994
* Epoch: 1
Model description
-----------------
More information needed
Intended uses & limitations
---------------------------
More information needed
Training and evaluation data
----------------------------
More information needed
Training procedure
------------------
### Training hyperparameters
The following hyperparameters were used during training:
* optimizer: {'name': 'Adam', 'weight\_decay': None, 'clipnorm': None, 'global\_clipnorm': None, 'clipvalue': None, 'use\_ema': False, 'ema\_momentum': 0.99, 'ema\_overwrite\_frequency': None, 'jit\_compile': True, 'is\_legacy\_optimizer': False, 'learning\_rate': {'module': 'keras.optimizers.schedules', 'class\_name': 'PolynomialDecay', 'config': {'initial\_learning\_rate': 2e-05, 'decay\_steps': 3798, 'end\_learning\_rate': 0.0, 'power': 1.0, 'cycle': False, 'name': None}, 'registered\_name': None}, 'beta\_1': 0.9, 'beta\_2': 0.999, 'epsilon': 1e-08, 'amsgrad': False}
* training\_precision: float32
### Training results
### Framework versions
* Transformers 4.40.1
* TensorFlow 2.15.0
* Datasets 2.19.0
* Tokenizers 0.19.1
| [
"### Training hyperparameters\n\n\nThe following hyperparameters were used during training:\n\n\n* optimizer: {'name': 'Adam', 'weight\\_decay': None, 'clipnorm': None, 'global\\_clipnorm': None, 'clipvalue': None, 'use\\_ema': False, 'ema\\_momentum': 0.99, 'ema\\_overwrite\\_frequency': None, 'jit\\_compile': True, 'is\\_legacy\\_optimizer': False, 'learning\\_rate': {'module': 'keras.optimizers.schedules', 'class\\_name': 'PolynomialDecay', 'config': {'initial\\_learning\\_rate': 2e-05, 'decay\\_steps': 3798, 'end\\_learning\\_rate': 0.0, 'power': 1.0, 'cycle': False, 'name': None}, 'registered\\_name': None}, 'beta\\_1': 0.9, 'beta\\_2': 0.999, 'epsilon': 1e-08, 'amsgrad': False}\n* training\\_precision: float32",
"### Training results",
"### Framework versions\n\n\n* Transformers 4.40.1\n* TensorFlow 2.15.0\n* Datasets 2.19.0\n* Tokenizers 0.19.1"
] | [
"TAGS\n#transformers #tf #albert #question-answering #generated_from_keras_callback #base_model-albert/albert-base-v2 #license-apache-2.0 #endpoints_compatible #region-us \n",
"### Training hyperparameters\n\n\nThe following hyperparameters were used during training:\n\n\n* optimizer: {'name': 'Adam', 'weight\\_decay': None, 'clipnorm': None, 'global\\_clipnorm': None, 'clipvalue': None, 'use\\_ema': False, 'ema\\_momentum': 0.99, 'ema\\_overwrite\\_frequency': None, 'jit\\_compile': True, 'is\\_legacy\\_optimizer': False, 'learning\\_rate': {'module': 'keras.optimizers.schedules', 'class\\_name': 'PolynomialDecay', 'config': {'initial\\_learning\\_rate': 2e-05, 'decay\\_steps': 3798, 'end\\_learning\\_rate': 0.0, 'power': 1.0, 'cycle': False, 'name': None}, 'registered\\_name': None}, 'beta\\_1': 0.9, 'beta\\_2': 0.999, 'epsilon': 1e-08, 'amsgrad': False}\n* training\\_precision: float32",
"### Training results",
"### Framework versions\n\n\n* Transformers 4.40.1\n* TensorFlow 2.15.0\n* Datasets 2.19.0\n* Tokenizers 0.19.1"
] | [
52,
292,
5,
38
] | [
"TAGS\n#transformers #tf #albert #question-answering #generated_from_keras_callback #base_model-albert/albert-base-v2 #license-apache-2.0 #endpoints_compatible #region-us \n### Training hyperparameters\n\n\nThe following hyperparameters were used during training:\n\n\n* optimizer: {'name': 'Adam', 'weight\\_decay': None, 'clipnorm': None, 'global\\_clipnorm': None, 'clipvalue': None, 'use\\_ema': False, 'ema\\_momentum': 0.99, 'ema\\_overwrite\\_frequency': None, 'jit\\_compile': True, 'is\\_legacy\\_optimizer': False, 'learning\\_rate': {'module': 'keras.optimizers.schedules', 'class\\_name': 'PolynomialDecay', 'config': {'initial\\_learning\\_rate': 2e-05, 'decay\\_steps': 3798, 'end\\_learning\\_rate': 0.0, 'power': 1.0, 'cycle': False, 'name': None}, 'registered\\_name': None}, 'beta\\_1': 0.9, 'beta\\_2': 0.999, 'epsilon': 1e-08, 'amsgrad': False}\n* training\\_precision: float32### Training results### Framework versions\n\n\n* Transformers 4.40.1\n* TensorFlow 2.15.0\n* Datasets 2.19.0\n* Tokenizers 0.19.1"
] |
text-generation | transformers |
# Model Card for Model ID
<!-- Provide a quick summary of what the model is/does. -->
## Model Details
### Model Description
<!-- Provide a longer summary of what this model is. -->
This is the model card of a 🤗 transformers model that has been pushed on the Hub. This model card has been automatically generated.
- **Developed by:** [More Information Needed]
- **Funded by [optional]:** [More Information Needed]
- **Shared by [optional]:** [More Information Needed]
- **Model type:** [More Information Needed]
- **Language(s) (NLP):** [More Information Needed]
- **License:** [More Information Needed]
- **Finetuned from model [optional]:** [More Information Needed]
### Model Sources [optional]
<!-- Provide the basic links for the model. -->
- **Repository:** [More Information Needed]
- **Paper [optional]:** [More Information Needed]
- **Demo [optional]:** [More Information Needed]
## Uses
<!-- Address questions around how the model is intended to be used, including the foreseeable users of the model and those affected by the model. -->
### Direct Use
<!-- This section is for the model use without fine-tuning or plugging into a larger ecosystem/app. -->
[More Information Needed]
### Downstream Use [optional]
<!-- This section is for the model use when fine-tuned for a task, or when plugged into a larger ecosystem/app -->
[More Information Needed]
### Out-of-Scope Use
<!-- This section addresses misuse, malicious use, and uses that the model will not work well for. -->
[More Information Needed]
## Bias, Risks, and Limitations
<!-- This section is meant to convey both technical and sociotechnical limitations. -->
[More Information Needed]
### Recommendations
<!-- This section is meant to convey recommendations with respect to the bias, risk, and technical limitations. -->
Users (both direct and downstream) should be made aware of the risks, biases and limitations of the model. More information needed for further recommendations.
## How to Get Started with the Model
Use the code below to get started with the model.
[More Information Needed]
## Training Details
### Training Data
<!-- This should link to a Dataset Card, perhaps with a short stub of information on what the training data is all about as well as documentation related to data pre-processing or additional filtering. -->
[More Information Needed]
### Training Procedure
<!-- This relates heavily to the Technical Specifications. Content here should link to that section when it is relevant to the training procedure. -->
#### Preprocessing [optional]
[More Information Needed]
#### Training Hyperparameters
- **Training regime:** [More Information Needed] <!--fp32, fp16 mixed precision, bf16 mixed precision, bf16 non-mixed precision, fp16 non-mixed precision, fp8 mixed precision -->
#### Speeds, Sizes, Times [optional]
<!-- This section provides information about throughput, start/end time, checkpoint size if relevant, etc. -->
[More Information Needed]
## Evaluation
<!-- This section describes the evaluation protocols and provides the results. -->
### Testing Data, Factors & Metrics
#### Testing Data
<!-- This should link to a Dataset Card if possible. -->
[More Information Needed]
#### Factors
<!-- These are the things the evaluation is disaggregating by, e.g., subpopulations or domains. -->
[More Information Needed]
#### Metrics
<!-- These are the evaluation metrics being used, ideally with a description of why. -->
[More Information Needed]
### Results
[More Information Needed]
#### Summary
## Model Examination [optional]
<!-- Relevant interpretability work for the model goes here -->
[More Information Needed]
## Environmental Impact
<!-- Total emissions (in grams of CO2eq) and additional considerations, such as electricity usage, go here. Edit the suggested text below accordingly -->
Carbon emissions can be estimated using the [Machine Learning Impact calculator](https://mlco2.github.io/impact#compute) presented in [Lacoste et al. (2019)](https://arxiv.org/abs/1910.09700).
- **Hardware Type:** [More Information Needed]
- **Hours used:** [More Information Needed]
- **Cloud Provider:** [More Information Needed]
- **Compute Region:** [More Information Needed]
- **Carbon Emitted:** [More Information Needed]
## Technical Specifications [optional]
### Model Architecture and Objective
[More Information Needed]
### Compute Infrastructure
[More Information Needed]
#### Hardware
[More Information Needed]
#### Software
[More Information Needed]
## Citation [optional]
<!-- If there is a paper or blog post introducing the model, the APA and Bibtex information for that should go in this section. -->
**BibTeX:**
[More Information Needed]
**APA:**
[More Information Needed]
## Glossary [optional]
<!-- If relevant, include terms and calculations in this section that can help readers understand the model or model card. -->
[More Information Needed]
## More Information [optional]
[More Information Needed]
## Model Card Authors [optional]
[More Information Needed]
## Model Card Contact
[More Information Needed] | {"library_name": "transformers", "tags": ["trl", "sft"]} | yashdkadam/new-train-on-cleaned-dataset | null | [
"transformers",
"safetensors",
"phi3",
"text-generation",
"trl",
"sft",
"conversational",
"custom_code",
"arxiv:1910.09700",
"autotrain_compatible",
"endpoints_compatible",
"4-bit",
"region:us"
] | null | 2024-05-02T10:51:59+00:00 | [
"1910.09700"
] | [] | TAGS
#transformers #safetensors #phi3 #text-generation #trl #sft #conversational #custom_code #arxiv-1910.09700 #autotrain_compatible #endpoints_compatible #4-bit #region-us
|
# Model Card for Model ID
## Model Details
### Model Description
This is the model card of a transformers model that has been pushed on the Hub. This model card has been automatically generated.
- Developed by:
- Funded by [optional]:
- Shared by [optional]:
- Model type:
- Language(s) (NLP):
- License:
- Finetuned from model [optional]:
### Model Sources [optional]
- Repository:
- Paper [optional]:
- Demo [optional]:
## Uses
### Direct Use
### Downstream Use [optional]
### Out-of-Scope Use
## Bias, Risks, and Limitations
### Recommendations
Users (both direct and downstream) should be made aware of the risks, biases and limitations of the model. More information needed for further recommendations.
## How to Get Started with the Model
Use the code below to get started with the model.
## Training Details
### Training Data
### Training Procedure
#### Preprocessing [optional]
#### Training Hyperparameters
- Training regime:
#### Speeds, Sizes, Times [optional]
## Evaluation
### Testing Data, Factors & Metrics
#### Testing Data
#### Factors
#### Metrics
### Results
#### Summary
## Model Examination [optional]
## Environmental Impact
Carbon emissions can be estimated using the Machine Learning Impact calculator presented in Lacoste et al. (2019).
- Hardware Type:
- Hours used:
- Cloud Provider:
- Compute Region:
- Carbon Emitted:
## Technical Specifications [optional]
### Model Architecture and Objective
### Compute Infrastructure
#### Hardware
#### Software
[optional]
BibTeX:
APA:
## Glossary [optional]
## More Information [optional]
## Model Card Authors [optional]
## Model Card Contact
| [
"# Model Card for Model ID",
"## Model Details",
"### Model Description\n\n\n\nThis is the model card of a transformers model that has been pushed on the Hub. This model card has been automatically generated.\n\n- Developed by: \n- Funded by [optional]: \n- Shared by [optional]: \n- Model type: \n- Language(s) (NLP): \n- License: \n- Finetuned from model [optional]:",
"### Model Sources [optional]\n\n\n\n- Repository: \n- Paper [optional]: \n- Demo [optional]:",
"## Uses",
"### Direct Use",
"### Downstream Use [optional]",
"### Out-of-Scope Use",
"## Bias, Risks, and Limitations",
"### Recommendations\n\n\n\nUsers (both direct and downstream) should be made aware of the risks, biases and limitations of the model. More information needed for further recommendations.",
"## How to Get Started with the Model\n\nUse the code below to get started with the model.",
"## Training Details",
"### Training Data",
"### Training Procedure",
"#### Preprocessing [optional]",
"#### Training Hyperparameters\n\n- Training regime:",
"#### Speeds, Sizes, Times [optional]",
"## Evaluation",
"### Testing Data, Factors & Metrics",
"#### Testing Data",
"#### Factors",
"#### Metrics",
"### Results",
"#### Summary",
"## Model Examination [optional]",
"## Environmental Impact\n\n\n\nCarbon emissions can be estimated using the Machine Learning Impact calculator presented in Lacoste et al. (2019).\n\n- Hardware Type: \n- Hours used: \n- Cloud Provider: \n- Compute Region: \n- Carbon Emitted:",
"## Technical Specifications [optional]",
"### Model Architecture and Objective",
"### Compute Infrastructure",
"#### Hardware",
"#### Software\n\n\n\n[optional]\n\n\n\nBibTeX:\n\n\n\nAPA:",
"## Glossary [optional]",
"## More Information [optional]",
"## Model Card Authors [optional]",
"## Model Card Contact"
] | [
"TAGS\n#transformers #safetensors #phi3 #text-generation #trl #sft #conversational #custom_code #arxiv-1910.09700 #autotrain_compatible #endpoints_compatible #4-bit #region-us \n",
"# Model Card for Model ID",
"## Model Details",
"### Model Description\n\n\n\nThis is the model card of a transformers model that has been pushed on the Hub. This model card has been automatically generated.\n\n- Developed by: \n- Funded by [optional]: \n- Shared by [optional]: \n- Model type: \n- Language(s) (NLP): \n- License: \n- Finetuned from model [optional]:",
"### Model Sources [optional]\n\n\n\n- Repository: \n- Paper [optional]: \n- Demo [optional]:",
"## Uses",
"### Direct Use",
"### Downstream Use [optional]",
"### Out-of-Scope Use",
"## Bias, Risks, and Limitations",
"### Recommendations\n\n\n\nUsers (both direct and downstream) should be made aware of the risks, biases and limitations of the model. More information needed for further recommendations.",
"## How to Get Started with the Model\n\nUse the code below to get started with the model.",
"## Training Details",
"### Training Data",
"### Training Procedure",
"#### Preprocessing [optional]",
"#### Training Hyperparameters\n\n- Training regime:",
"#### Speeds, Sizes, Times [optional]",
"## Evaluation",
"### Testing Data, Factors & Metrics",
"#### Testing Data",
"#### Factors",
"#### Metrics",
"### Results",
"#### Summary",
"## Model Examination [optional]",
"## Environmental Impact\n\n\n\nCarbon emissions can be estimated using the Machine Learning Impact calculator presented in Lacoste et al. (2019).\n\n- Hardware Type: \n- Hours used: \n- Cloud Provider: \n- Compute Region: \n- Carbon Emitted:",
"## Technical Specifications [optional]",
"### Model Architecture and Objective",
"### Compute Infrastructure",
"#### Hardware",
"#### Software\n\n\n\n[optional]\n\n\n\nBibTeX:\n\n\n\nAPA:",
"## Glossary [optional]",
"## More Information [optional]",
"## Model Card Authors [optional]",
"## Model Card Contact"
] | [
55,
6,
4,
75,
23,
3,
5,
8,
9,
8,
34,
20,
4,
5,
5,
11,
13,
12,
3,
10,
6,
5,
6,
4,
5,
7,
49,
7,
7,
5,
5,
15,
7,
7,
8,
5
] | [
"TAGS\n#transformers #safetensors #phi3 #text-generation #trl #sft #conversational #custom_code #arxiv-1910.09700 #autotrain_compatible #endpoints_compatible #4-bit #region-us \n# Model Card for Model ID## Model Details### Model Description\n\n\n\nThis is the model card of a transformers model that has been pushed on the Hub. This model card has been automatically generated.\n\n- Developed by: \n- Funded by [optional]: \n- Shared by [optional]: \n- Model type: \n- Language(s) (NLP): \n- License: \n- Finetuned from model [optional]:### Model Sources [optional]\n\n\n\n- Repository: \n- Paper [optional]: \n- Demo [optional]:## Uses### Direct Use### Downstream Use [optional]### Out-of-Scope Use## Bias, Risks, and Limitations### Recommendations\n\n\n\nUsers (both direct and downstream) should be made aware of the risks, biases and limitations of the model. More information needed for further recommendations.## How to Get Started with the Model\n\nUse the code below to get started with the model.## Training Details### Training Data### Training Procedure#### Preprocessing [optional]#### Training Hyperparameters\n\n- Training regime:#### Speeds, Sizes, Times [optional]## Evaluation### Testing Data, Factors & Metrics#### Testing Data#### Factors#### Metrics### Results#### Summary## Model Examination [optional]## Environmental Impact\n\n\n\nCarbon emissions can be estimated using the Machine Learning Impact calculator presented in Lacoste et al. (2019).\n\n- Hardware Type: \n- Hours used: \n- Cloud Provider: \n- Compute Region: \n- Carbon Emitted:## Technical Specifications [optional]### Model Architecture and Objective### Compute Infrastructure#### Hardware#### Software\n\n\n\n[optional]\n\n\n\nBibTeX:\n\n\n\nAPA:## Glossary [optional]## More Information [optional]## Model Card Authors [optional]## Model Card Contact"
] |
token-classification | transformers |
<!-- This model card has been generated automatically according to the information the Trainer had access to. You
should probably proofread and complete it, then remove this comment. -->
# layoutlm-funsd
This model is a fine-tuned version of [microsoft/layoutlm-base-uncased](https://huggingface.co/microsoft/layoutlm-base-uncased) on the funsd dataset.
It achieves the following results on the evaluation set:
- Loss: 0.6573
- Answer: {'precision': 0.7060773480662983, 'recall': 0.7898640296662547, 'f1': 0.7456242707117853, 'number': 809}
- Header: {'precision': 0.3333333333333333, 'recall': 0.3697478991596639, 'f1': 0.350597609561753, 'number': 119}
- Question: {'precision': 0.7687661777394306, 'recall': 0.8366197183098592, 'f1': 0.8012589928057554, 'number': 1065}
- Overall Precision: 0.7168
- Overall Recall: 0.7898
- Overall F1: 0.7515
- Overall Accuracy: 0.8172
## Model description
More information needed
## Intended uses & limitations
More information needed
## Training and evaluation data
More information needed
## Training procedure
### Training hyperparameters
The following hyperparameters were used during training:
- learning_rate: 3e-05
- train_batch_size: 16
- eval_batch_size: 8
- seed: 42
- optimizer: Adam with betas=(0.9,0.999) and epsilon=1e-08
- lr_scheduler_type: linear
- num_epochs: 15
### Training results
| Training Loss | Epoch | Step | Validation Loss | Answer | Header | Question | Overall Precision | Overall Recall | Overall F1 | Overall Accuracy |
|:-------------:|:-----:|:----:|:---------------:|:------------------------------------------------------------------------------------------------------------:|:-----------------------------------------------------------------------------------------------------------:|:-----------------------------------------------------------------------------------------------------------:|:-----------------:|:--------------:|:----------:|:----------------:|
| 1.7999 | 1.0 | 10 | 1.5802 | {'precision': 0.008905852417302799, 'recall': 0.00865265760197775, 'f1': 0.00877742946708464, 'number': 809} | {'precision': 0.0, 'recall': 0.0, 'f1': 0.0, 'number': 119} | {'precision': 0.1717325227963526, 'recall': 0.10610328638497653, 'f1': 0.13116656993615786, 'number': 1065} | 0.0831 | 0.0602 | 0.0698 | 0.3604 |
| 1.4567 | 2.0 | 20 | 1.2493 | {'precision': 0.18839103869653767, 'recall': 0.22867737948084055, 'f1': 0.20658849804578447, 'number': 809} | {'precision': 0.0, 'recall': 0.0, 'f1': 0.0, 'number': 119} | {'precision': 0.45693950177935944, 'recall': 0.6028169014084507, 'f1': 0.5198380566801619, 'number': 1065} | 0.3465 | 0.4150 | 0.3776 | 0.5986 |
| 1.114 | 3.0 | 30 | 0.9406 | {'precision': 0.43853820598006643, 'recall': 0.4894932014833127, 'f1': 0.46261682242990654, 'number': 809} | {'precision': 0.0, 'recall': 0.0, 'f1': 0.0, 'number': 119} | {'precision': 0.5861538461538461, 'recall': 0.7154929577464789, 'f1': 0.6443974630021141, 'number': 1065} | 0.5237 | 0.5810 | 0.5509 | 0.7001 |
| 0.8434 | 4.0 | 40 | 0.7906 | {'precision': 0.5922836287799792, 'recall': 0.7021013597033374, 'f1': 0.6425339366515838, 'number': 809} | {'precision': 0.1111111111111111, 'recall': 0.04201680672268908, 'f1': 0.06097560975609755, 'number': 119} | {'precision': 0.6526994359387591, 'recall': 0.7605633802816901, 'f1': 0.7025151777970512, 'number': 1065} | 0.6160 | 0.6939 | 0.6527 | 0.7541 |
| 0.6817 | 5.0 | 50 | 0.7106 | {'precision': 0.6502192982456141, 'recall': 0.7330037082818294, 'f1': 0.6891342242882045, 'number': 809} | {'precision': 0.25301204819277107, 'recall': 0.17647058823529413, 'f1': 0.20792079207920794, 'number': 119} | {'precision': 0.683921568627451, 'recall': 0.8187793427230047, 'f1': 0.7452991452991454, 'number': 1065} | 0.6546 | 0.7456 | 0.6972 | 0.7854 |
| 0.5737 | 6.0 | 60 | 0.6807 | {'precision': 0.6482617586912065, 'recall': 0.7836835599505563, 'f1': 0.7095691102406267, 'number': 809} | {'precision': 0.273972602739726, 'recall': 0.16806722689075632, 'f1': 0.20833333333333331, 'number': 119} | {'precision': 0.717206132879046, 'recall': 0.7906103286384977, 'f1': 0.7521214828048235, 'number': 1065} | 0.6724 | 0.7506 | 0.7093 | 0.7898 |
| 0.5058 | 7.0 | 70 | 0.6538 | {'precision': 0.6564102564102564, 'recall': 0.7911001236093943, 'f1': 0.7174887892376681, 'number': 809} | {'precision': 0.3048780487804878, 'recall': 0.21008403361344538, 'f1': 0.24875621890547264, 'number': 119} | {'precision': 0.7324894514767932, 'recall': 0.8150234741784037, 'f1': 0.7715555555555556, 'number': 1065} | 0.6838 | 0.7692 | 0.7240 | 0.7996 |
| 0.4425 | 8.0 | 80 | 0.6574 | {'precision': 0.6625766871165644, 'recall': 0.8009888751545118, 'f1': 0.7252378287632905, 'number': 809} | {'precision': 0.3055555555555556, 'recall': 0.2773109243697479, 'f1': 0.2907488986784141, 'number': 119} | {'precision': 0.7365771812080537, 'recall': 0.8244131455399061, 'f1': 0.7780239255649092, 'number': 1065} | 0.6844 | 0.7822 | 0.7300 | 0.7999 |
| 0.3932 | 9.0 | 90 | 0.6375 | {'precision': 0.6876971608832808, 'recall': 0.8084054388133498, 'f1': 0.7431818181818182, 'number': 809} | {'precision': 0.3645833333333333, 'recall': 0.29411764705882354, 'f1': 0.3255813953488372, 'number': 119} | {'precision': 0.752129471890971, 'recall': 0.8291079812206573, 'f1': 0.7887449754354622, 'number': 1065} | 0.7078 | 0.7888 | 0.7461 | 0.8087 |
| 0.3798 | 10.0 | 100 | 0.6437 | {'precision': 0.6981541802388708, 'recall': 0.7948084054388134, 'f1': 0.7433526011560695, 'number': 809} | {'precision': 0.325, 'recall': 0.3277310924369748, 'f1': 0.3263598326359833, 'number': 119} | {'precision': 0.7665505226480837, 'recall': 0.8262910798122066, 'f1': 0.7953004970628107, 'number': 1065} | 0.7136 | 0.7837 | 0.7470 | 0.8098 |
| 0.3225 | 11.0 | 110 | 0.6566 | {'precision': 0.6817226890756303, 'recall': 0.8022249690976514, 'f1': 0.7370812038614423, 'number': 809} | {'precision': 0.336, 'recall': 0.35294117647058826, 'f1': 0.3442622950819672, 'number': 119} | {'precision': 0.7593856655290102, 'recall': 0.8356807511737089, 'f1': 0.7957085382208315, 'number': 1065} | 0.7030 | 0.7933 | 0.7454 | 0.8038 |
| 0.3097 | 12.0 | 120 | 0.6421 | {'precision': 0.6957928802588996, 'recall': 0.7972805933250927, 'f1': 0.7430875576036866, 'number': 809} | {'precision': 0.35, 'recall': 0.35294117647058826, 'f1': 0.35146443514644354, 'number': 119} | {'precision': 0.7692307692307693, 'recall': 0.8356807511737089, 'f1': 0.8010801080108011, 'number': 1065} | 0.7155 | 0.7913 | 0.7515 | 0.8177 |
| 0.2916 | 13.0 | 130 | 0.6515 | {'precision': 0.7035010940919038, 'recall': 0.7948084054388134, 'f1': 0.7463726059199072, 'number': 809} | {'precision': 0.33076923076923076, 'recall': 0.36134453781512604, 'f1': 0.34538152610441764, 'number': 119} | {'precision': 0.7649092480553155, 'recall': 0.8309859154929577, 'f1': 0.7965796579657966, 'number': 1065} | 0.7138 | 0.7883 | 0.7492 | 0.8154 |
| 0.2707 | 14.0 | 140 | 0.6557 | {'precision': 0.7016393442622951, 'recall': 0.7935723114956736, 'f1': 0.7447795823665894, 'number': 809} | {'precision': 0.3333333333333333, 'recall': 0.36134453781512604, 'f1': 0.34677419354838707, 'number': 119} | {'precision': 0.7688966116420504, 'recall': 0.8309859154929577, 'f1': 0.7987364620938627, 'number': 1065} | 0.7153 | 0.7878 | 0.7498 | 0.8146 |
| 0.2729 | 15.0 | 150 | 0.6573 | {'precision': 0.7060773480662983, 'recall': 0.7898640296662547, 'f1': 0.7456242707117853, 'number': 809} | {'precision': 0.3333333333333333, 'recall': 0.3697478991596639, 'f1': 0.350597609561753, 'number': 119} | {'precision': 0.7687661777394306, 'recall': 0.8366197183098592, 'f1': 0.8012589928057554, 'number': 1065} | 0.7168 | 0.7898 | 0.7515 | 0.8172 |
### Framework versions
- Transformers 4.40.1
- Pytorch 2.3.0+cpu
- Datasets 2.19.0
- Tokenizers 0.19.1
| {"license": "mit", "tags": ["generated_from_trainer"], "datasets": ["funsd"], "base_model": "microsoft/layoutlm-base-uncased", "model-index": [{"name": "layoutlm-funsd", "results": []}]} | RakhissBouchra/layoutlm-funsd | null | [
"transformers",
"tensorboard",
"safetensors",
"layoutlm",
"token-classification",
"generated_from_trainer",
"dataset:funsd",
"base_model:microsoft/layoutlm-base-uncased",
"license:mit",
"autotrain_compatible",
"endpoints_compatible",
"region:us"
] | null | 2024-05-02T10:52:12+00:00 | [] | [] | TAGS
#transformers #tensorboard #safetensors #layoutlm #token-classification #generated_from_trainer #dataset-funsd #base_model-microsoft/layoutlm-base-uncased #license-mit #autotrain_compatible #endpoints_compatible #region-us
| layoutlm-funsd
==============
This model is a fine-tuned version of microsoft/layoutlm-base-uncased on the funsd dataset.
It achieves the following results on the evaluation set:
* Loss: 0.6573
* Answer: {'precision': 0.7060773480662983, 'recall': 0.7898640296662547, 'f1': 0.7456242707117853, 'number': 809}
* Header: {'precision': 0.3333333333333333, 'recall': 0.3697478991596639, 'f1': 0.350597609561753, 'number': 119}
* Question: {'precision': 0.7687661777394306, 'recall': 0.8366197183098592, 'f1': 0.8012589928057554, 'number': 1065}
* Overall Precision: 0.7168
* Overall Recall: 0.7898
* Overall F1: 0.7515
* Overall Accuracy: 0.8172
Model description
-----------------
More information needed
Intended uses & limitations
---------------------------
More information needed
Training and evaluation data
----------------------------
More information needed
Training procedure
------------------
### Training hyperparameters
The following hyperparameters were used during training:
* learning\_rate: 3e-05
* train\_batch\_size: 16
* eval\_batch\_size: 8
* seed: 42
* optimizer: Adam with betas=(0.9,0.999) and epsilon=1e-08
* lr\_scheduler\_type: linear
* num\_epochs: 15
### Training results
### Framework versions
* Transformers 4.40.1
* Pytorch 2.3.0+cpu
* Datasets 2.19.0
* Tokenizers 0.19.1
| [
"### Training hyperparameters\n\n\nThe following hyperparameters were used during training:\n\n\n* learning\\_rate: 3e-05\n* train\\_batch\\_size: 16\n* eval\\_batch\\_size: 8\n* seed: 42\n* optimizer: Adam with betas=(0.9,0.999) and epsilon=1e-08\n* lr\\_scheduler\\_type: linear\n* num\\_epochs: 15",
"### Training results",
"### Framework versions\n\n\n* Transformers 4.40.1\n* Pytorch 2.3.0+cpu\n* Datasets 2.19.0\n* Tokenizers 0.19.1"
] | [
"TAGS\n#transformers #tensorboard #safetensors #layoutlm #token-classification #generated_from_trainer #dataset-funsd #base_model-microsoft/layoutlm-base-uncased #license-mit #autotrain_compatible #endpoints_compatible #region-us \n",
"### Training hyperparameters\n\n\nThe following hyperparameters were used during training:\n\n\n* learning\\_rate: 3e-05\n* train\\_batch\\_size: 16\n* eval\\_batch\\_size: 8\n* seed: 42\n* optimizer: Adam with betas=(0.9,0.999) and epsilon=1e-08\n* lr\\_scheduler\\_type: linear\n* num\\_epochs: 15",
"### Training results",
"### Framework versions\n\n\n* Transformers 4.40.1\n* Pytorch 2.3.0+cpu\n* Datasets 2.19.0\n* Tokenizers 0.19.1"
] | [
61,
101,
5,
42
] | [
"TAGS\n#transformers #tensorboard #safetensors #layoutlm #token-classification #generated_from_trainer #dataset-funsd #base_model-microsoft/layoutlm-base-uncased #license-mit #autotrain_compatible #endpoints_compatible #region-us \n### Training hyperparameters\n\n\nThe following hyperparameters were used during training:\n\n\n* learning\\_rate: 3e-05\n* train\\_batch\\_size: 16\n* eval\\_batch\\_size: 8\n* seed: 42\n* optimizer: Adam with betas=(0.9,0.999) and epsilon=1e-08\n* lr\\_scheduler\\_type: linear\n* num\\_epochs: 15### Training results### Framework versions\n\n\n* Transformers 4.40.1\n* Pytorch 2.3.0+cpu\n* Datasets 2.19.0\n* Tokenizers 0.19.1"
] |
null | transformers |
# Model Card for Model ID
<!-- Provide a quick summary of what the model is/does. -->
## Model Details
### Model Description
<!-- Provide a longer summary of what this model is. -->
This is the model card of a 🤗 transformers model that has been pushed on the Hub. This model card has been automatically generated.
- **Developed by:** [More Information Needed]
- **Funded by [optional]:** [More Information Needed]
- **Shared by [optional]:** [More Information Needed]
- **Model type:** [More Information Needed]
- **Language(s) (NLP):** [More Information Needed]
- **License:** [More Information Needed]
- **Finetuned from model [optional]:** [More Information Needed]
### Model Sources [optional]
<!-- Provide the basic links for the model. -->
- **Repository:** [More Information Needed]
- **Paper [optional]:** [More Information Needed]
- **Demo [optional]:** [More Information Needed]
## Uses
<!-- Address questions around how the model is intended to be used, including the foreseeable users of the model and those affected by the model. -->
### Direct Use
<!-- This section is for the model use without fine-tuning or plugging into a larger ecosystem/app. -->
[More Information Needed]
### Downstream Use [optional]
<!-- This section is for the model use when fine-tuned for a task, or when plugged into a larger ecosystem/app -->
[More Information Needed]
### Out-of-Scope Use
<!-- This section addresses misuse, malicious use, and uses that the model will not work well for. -->
[More Information Needed]
## Bias, Risks, and Limitations
<!-- This section is meant to convey both technical and sociotechnical limitations. -->
[More Information Needed]
### Recommendations
<!-- This section is meant to convey recommendations with respect to the bias, risk, and technical limitations. -->
Users (both direct and downstream) should be made aware of the risks, biases and limitations of the model. More information needed for further recommendations.
## How to Get Started with the Model
Use the code below to get started with the model.
[More Information Needed]
## Training Details
### Training Data
<!-- This should link to a Dataset Card, perhaps with a short stub of information on what the training data is all about as well as documentation related to data pre-processing or additional filtering. -->
[More Information Needed]
### Training Procedure
<!-- This relates heavily to the Technical Specifications. Content here should link to that section when it is relevant to the training procedure. -->
#### Preprocessing [optional]
[More Information Needed]
#### Training Hyperparameters
- **Training regime:** [More Information Needed] <!--fp32, fp16 mixed precision, bf16 mixed precision, bf16 non-mixed precision, fp16 non-mixed precision, fp8 mixed precision -->
#### Speeds, Sizes, Times [optional]
<!-- This section provides information about throughput, start/end time, checkpoint size if relevant, etc. -->
[More Information Needed]
## Evaluation
<!-- This section describes the evaluation protocols and provides the results. -->
### Testing Data, Factors & Metrics
#### Testing Data
<!-- This should link to a Dataset Card if possible. -->
[More Information Needed]
#### Factors
<!-- These are the things the evaluation is disaggregating by, e.g., subpopulations or domains. -->
[More Information Needed]
#### Metrics
<!-- These are the evaluation metrics being used, ideally with a description of why. -->
[More Information Needed]
### Results
[More Information Needed]
#### Summary
## Model Examination [optional]
<!-- Relevant interpretability work for the model goes here -->
[More Information Needed]
## Environmental Impact
<!-- Total emissions (in grams of CO2eq) and additional considerations, such as electricity usage, go here. Edit the suggested text below accordingly -->
Carbon emissions can be estimated using the [Machine Learning Impact calculator](https://mlco2.github.io/impact#compute) presented in [Lacoste et al. (2019)](https://arxiv.org/abs/1910.09700).
- **Hardware Type:** [More Information Needed]
- **Hours used:** [More Information Needed]
- **Cloud Provider:** [More Information Needed]
- **Compute Region:** [More Information Needed]
- **Carbon Emitted:** [More Information Needed]
## Technical Specifications [optional]
### Model Architecture and Objective
[More Information Needed]
### Compute Infrastructure
[More Information Needed]
#### Hardware
[More Information Needed]
#### Software
[More Information Needed]
## Citation [optional]
<!-- If there is a paper or blog post introducing the model, the APA and Bibtex information for that should go in this section. -->
**BibTeX:**
[More Information Needed]
**APA:**
[More Information Needed]
## Glossary [optional]
<!-- If relevant, include terms and calculations in this section that can help readers understand the model or model card. -->
[More Information Needed]
## More Information [optional]
[More Information Needed]
## Model Card Authors [optional]
[More Information Needed]
## Model Card Contact
[More Information Needed] | {"library_name": "transformers", "tags": ["unsloth"]} | ArunIcfoss/instruct_mal_eng_translation | null | [
"transformers",
"safetensors",
"unsloth",
"arxiv:1910.09700",
"endpoints_compatible",
"region:us"
] | null | 2024-05-02T10:52:28+00:00 | [
"1910.09700"
] | [] | TAGS
#transformers #safetensors #unsloth #arxiv-1910.09700 #endpoints_compatible #region-us
|
# Model Card for Model ID
## Model Details
### Model Description
This is the model card of a transformers model that has been pushed on the Hub. This model card has been automatically generated.
- Developed by:
- Funded by [optional]:
- Shared by [optional]:
- Model type:
- Language(s) (NLP):
- License:
- Finetuned from model [optional]:
### Model Sources [optional]
- Repository:
- Paper [optional]:
- Demo [optional]:
## Uses
### Direct Use
### Downstream Use [optional]
### Out-of-Scope Use
## Bias, Risks, and Limitations
### Recommendations
Users (both direct and downstream) should be made aware of the risks, biases and limitations of the model. More information needed for further recommendations.
## How to Get Started with the Model
Use the code below to get started with the model.
## Training Details
### Training Data
### Training Procedure
#### Preprocessing [optional]
#### Training Hyperparameters
- Training regime:
#### Speeds, Sizes, Times [optional]
## Evaluation
### Testing Data, Factors & Metrics
#### Testing Data
#### Factors
#### Metrics
### Results
#### Summary
## Model Examination [optional]
## Environmental Impact
Carbon emissions can be estimated using the Machine Learning Impact calculator presented in Lacoste et al. (2019).
- Hardware Type:
- Hours used:
- Cloud Provider:
- Compute Region:
- Carbon Emitted:
## Technical Specifications [optional]
### Model Architecture and Objective
### Compute Infrastructure
#### Hardware
#### Software
[optional]
BibTeX:
APA:
## Glossary [optional]
## More Information [optional]
## Model Card Authors [optional]
## Model Card Contact
| [
"# Model Card for Model ID",
"## Model Details",
"### Model Description\n\n\n\nThis is the model card of a transformers model that has been pushed on the Hub. This model card has been automatically generated.\n\n- Developed by: \n- Funded by [optional]: \n- Shared by [optional]: \n- Model type: \n- Language(s) (NLP): \n- License: \n- Finetuned from model [optional]:",
"### Model Sources [optional]\n\n\n\n- Repository: \n- Paper [optional]: \n- Demo [optional]:",
"## Uses",
"### Direct Use",
"### Downstream Use [optional]",
"### Out-of-Scope Use",
"## Bias, Risks, and Limitations",
"### Recommendations\n\n\n\nUsers (both direct and downstream) should be made aware of the risks, biases and limitations of the model. More information needed for further recommendations.",
"## How to Get Started with the Model\n\nUse the code below to get started with the model.",
"## Training Details",
"### Training Data",
"### Training Procedure",
"#### Preprocessing [optional]",
"#### Training Hyperparameters\n\n- Training regime:",
"#### Speeds, Sizes, Times [optional]",
"## Evaluation",
"### Testing Data, Factors & Metrics",
"#### Testing Data",
"#### Factors",
"#### Metrics",
"### Results",
"#### Summary",
"## Model Examination [optional]",
"## Environmental Impact\n\n\n\nCarbon emissions can be estimated using the Machine Learning Impact calculator presented in Lacoste et al. (2019).\n\n- Hardware Type: \n- Hours used: \n- Cloud Provider: \n- Compute Region: \n- Carbon Emitted:",
"## Technical Specifications [optional]",
"### Model Architecture and Objective",
"### Compute Infrastructure",
"#### Hardware",
"#### Software\n\n\n\n[optional]\n\n\n\nBibTeX:\n\n\n\nAPA:",
"## Glossary [optional]",
"## More Information [optional]",
"## Model Card Authors [optional]",
"## Model Card Contact"
] | [
"TAGS\n#transformers #safetensors #unsloth #arxiv-1910.09700 #endpoints_compatible #region-us \n",
"# Model Card for Model ID",
"## Model Details",
"### Model Description\n\n\n\nThis is the model card of a transformers model that has been pushed on the Hub. This model card has been automatically generated.\n\n- Developed by: \n- Funded by [optional]: \n- Shared by [optional]: \n- Model type: \n- Language(s) (NLP): \n- License: \n- Finetuned from model [optional]:",
"### Model Sources [optional]\n\n\n\n- Repository: \n- Paper [optional]: \n- Demo [optional]:",
"## Uses",
"### Direct Use",
"### Downstream Use [optional]",
"### Out-of-Scope Use",
"## Bias, Risks, and Limitations",
"### Recommendations\n\n\n\nUsers (both direct and downstream) should be made aware of the risks, biases and limitations of the model. More information needed for further recommendations.",
"## How to Get Started with the Model\n\nUse the code below to get started with the model.",
"## Training Details",
"### Training Data",
"### Training Procedure",
"#### Preprocessing [optional]",
"#### Training Hyperparameters\n\n- Training regime:",
"#### Speeds, Sizes, Times [optional]",
"## Evaluation",
"### Testing Data, Factors & Metrics",
"#### Testing Data",
"#### Factors",
"#### Metrics",
"### Results",
"#### Summary",
"## Model Examination [optional]",
"## Environmental Impact\n\n\n\nCarbon emissions can be estimated using the Machine Learning Impact calculator presented in Lacoste et al. (2019).\n\n- Hardware Type: \n- Hours used: \n- Cloud Provider: \n- Compute Region: \n- Carbon Emitted:",
"## Technical Specifications [optional]",
"### Model Architecture and Objective",
"### Compute Infrastructure",
"#### Hardware",
"#### Software\n\n\n\n[optional]\n\n\n\nBibTeX:\n\n\n\nAPA:",
"## Glossary [optional]",
"## More Information [optional]",
"## Model Card Authors [optional]",
"## Model Card Contact"
] | [
30,
6,
4,
75,
23,
3,
5,
8,
9,
8,
34,
20,
4,
5,
5,
11,
13,
12,
3,
10,
6,
5,
6,
4,
5,
7,
49,
7,
7,
5,
5,
15,
7,
7,
8,
5
] | [
"TAGS\n#transformers #safetensors #unsloth #arxiv-1910.09700 #endpoints_compatible #region-us \n# Model Card for Model ID## Model Details### Model Description\n\n\n\nThis is the model card of a transformers model that has been pushed on the Hub. This model card has been automatically generated.\n\n- Developed by: \n- Funded by [optional]: \n- Shared by [optional]: \n- Model type: \n- Language(s) (NLP): \n- License: \n- Finetuned from model [optional]:### Model Sources [optional]\n\n\n\n- Repository: \n- Paper [optional]: \n- Demo [optional]:## Uses### Direct Use### Downstream Use [optional]### Out-of-Scope Use## Bias, Risks, and Limitations### Recommendations\n\n\n\nUsers (both direct and downstream) should be made aware of the risks, biases and limitations of the model. More information needed for further recommendations.## How to Get Started with the Model\n\nUse the code below to get started with the model.## Training Details### Training Data### Training Procedure#### Preprocessing [optional]#### Training Hyperparameters\n\n- Training regime:#### Speeds, Sizes, Times [optional]## Evaluation### Testing Data, Factors & Metrics#### Testing Data#### Factors#### Metrics### Results#### Summary## Model Examination [optional]## Environmental Impact\n\n\n\nCarbon emissions can be estimated using the Machine Learning Impact calculator presented in Lacoste et al. (2019).\n\n- Hardware Type: \n- Hours used: \n- Cloud Provider: \n- Compute Region: \n- Carbon Emitted:## Technical Specifications [optional]### Model Architecture and Objective### Compute Infrastructure#### Hardware#### Software\n\n\n\n[optional]\n\n\n\nBibTeX:\n\n\n\nAPA:## Glossary [optional]## More Information [optional]## Model Card Authors [optional]## Model Card Contact"
] |
text-generation | transformers |
# LuminariX-8B
LuminariX-8B is a merge of the following models using [mergekit](https://github.com/cg123/mergekit):
## 🧩 Configuration
```yaml
models:
- model: Orenguteng/Llama-3-8B-Lexi-Uncensored
- model: Weyaxi/Einstein-v6.1-Llama3-8B
- model: cognitivecomputations/dolphin-2.9-llama3-8b-256k
merge_method: model_stock
base_model: cognitivecomputations/dolphin-2.9-llama3-8b-256k
dtype: bfloat16
``` | {"license": "apache-2.0", "tags": ["merge", "mergekit", "lazymergekit"]} | bunnycore/LuminariX-8B | null | [
"transformers",
"safetensors",
"llama",
"text-generation",
"merge",
"mergekit",
"lazymergekit",
"conversational",
"license:apache-2.0",
"autotrain_compatible",
"endpoints_compatible",
"text-generation-inference",
"region:us"
] | null | 2024-05-02T10:55:43+00:00 | [] | [] | TAGS
#transformers #safetensors #llama #text-generation #merge #mergekit #lazymergekit #conversational #license-apache-2.0 #autotrain_compatible #endpoints_compatible #text-generation-inference #region-us
|
# LuminariX-8B
LuminariX-8B is a merge of the following models using mergekit:
## Configuration
| [
"# LuminariX-8B\n\nLuminariX-8B is a merge of the following models using mergekit:",
"## Configuration"
] | [
"TAGS\n#transformers #safetensors #llama #text-generation #merge #mergekit #lazymergekit #conversational #license-apache-2.0 #autotrain_compatible #endpoints_compatible #text-generation-inference #region-us \n",
"# LuminariX-8B\n\nLuminariX-8B is a merge of the following models using mergekit:",
"## Configuration"
] | [
55,
24,
3
] | [
"TAGS\n#transformers #safetensors #llama #text-generation #merge #mergekit #lazymergekit #conversational #license-apache-2.0 #autotrain_compatible #endpoints_compatible #text-generation-inference #region-us \n# LuminariX-8B\n\nLuminariX-8B is a merge of the following models using mergekit:## Configuration"
] |
text-classification | transformers |
<!-- This model card has been generated automatically according to the information the Trainer had access to. You
should probably proofread and complete it, then remove this comment. -->
# NDD-petclinic_test-content
This model is a fine-tuned version of [distilbert-base-uncased](https://huggingface.co/distilbert-base-uncased) on an unknown dataset.
It achieves the following results on the evaluation set:
- Loss: 0.1901
- Accuracy: 0.9551
- F1: 0.9518
- Precision: 0.9567
- Recall: 0.9551
## Model description
More information needed
## Intended uses & limitations
More information needed
## Training and evaluation data
More information needed
## Training procedure
### Training hyperparameters
The following hyperparameters were used during training:
- learning_rate: 2e-05
- train_batch_size: 32
- eval_batch_size: 32
- seed: 42
- gradient_accumulation_steps: 4
- total_train_batch_size: 128
- optimizer: Adam with betas=(0.9,0.999) and epsilon=1e-08
- lr_scheduler_type: linear
- num_epochs: 2
### Training results
| Training Loss | Epoch | Step | Validation Loss | Accuracy | F1 | Precision | Recall |
|:-------------:|:------:|:----:|:---------------:|:--------:|:------:|:---------:|:------:|
| 0.1138 | 0.9993 | 674 | 0.1272 | 0.9620 | 0.9604 | 0.9618 | 0.9620 |
| 0.0691 | 1.9985 | 1348 | 0.1901 | 0.9551 | 0.9518 | 0.9567 | 0.9551 |
### Framework versions
- Transformers 4.40.1
- Pytorch 2.2.1+cu121
- Datasets 2.19.0
- Tokenizers 0.19.1
| {"license": "apache-2.0", "tags": ["generated_from_trainer"], "metrics": ["accuracy", "f1", "precision", "recall"], "base_model": "distilbert-base-uncased", "model-index": [{"name": "NDD-petclinic_test-content", "results": []}]} | lgk03/NDD-petclinic_test-content | null | [
"transformers",
"tensorboard",
"safetensors",
"distilbert",
"text-classification",
"generated_from_trainer",
"base_model:distilbert-base-uncased",
"license:apache-2.0",
"autotrain_compatible",
"endpoints_compatible",
"region:us"
] | null | 2024-05-02T10:56:13+00:00 | [] | [] | TAGS
#transformers #tensorboard #safetensors #distilbert #text-classification #generated_from_trainer #base_model-distilbert-base-uncased #license-apache-2.0 #autotrain_compatible #endpoints_compatible #region-us
| NDD-petclinic\_test-content
===========================
This model is a fine-tuned version of distilbert-base-uncased on an unknown dataset.
It achieves the following results on the evaluation set:
* Loss: 0.1901
* Accuracy: 0.9551
* F1: 0.9518
* Precision: 0.9567
* Recall: 0.9551
Model description
-----------------
More information needed
Intended uses & limitations
---------------------------
More information needed
Training and evaluation data
----------------------------
More information needed
Training procedure
------------------
### Training hyperparameters
The following hyperparameters were used during training:
* learning\_rate: 2e-05
* train\_batch\_size: 32
* eval\_batch\_size: 32
* seed: 42
* gradient\_accumulation\_steps: 4
* total\_train\_batch\_size: 128
* optimizer: Adam with betas=(0.9,0.999) and epsilon=1e-08
* lr\_scheduler\_type: linear
* num\_epochs: 2
### Training results
### Framework versions
* Transformers 4.40.1
* Pytorch 2.2.1+cu121
* Datasets 2.19.0
* Tokenizers 0.19.1
| [
"### Training hyperparameters\n\n\nThe following hyperparameters were used during training:\n\n\n* learning\\_rate: 2e-05\n* train\\_batch\\_size: 32\n* eval\\_batch\\_size: 32\n* seed: 42\n* gradient\\_accumulation\\_steps: 4\n* total\\_train\\_batch\\_size: 128\n* optimizer: Adam with betas=(0.9,0.999) and epsilon=1e-08\n* lr\\_scheduler\\_type: linear\n* num\\_epochs: 2",
"### Training results",
"### Framework versions\n\n\n* Transformers 4.40.1\n* Pytorch 2.2.1+cu121\n* Datasets 2.19.0\n* Tokenizers 0.19.1"
] | [
"TAGS\n#transformers #tensorboard #safetensors #distilbert #text-classification #generated_from_trainer #base_model-distilbert-base-uncased #license-apache-2.0 #autotrain_compatible #endpoints_compatible #region-us \n",
"### Training hyperparameters\n\n\nThe following hyperparameters were used during training:\n\n\n* learning\\_rate: 2e-05\n* train\\_batch\\_size: 32\n* eval\\_batch\\_size: 32\n* seed: 42\n* gradient\\_accumulation\\_steps: 4\n* total\\_train\\_batch\\_size: 128\n* optimizer: Adam with betas=(0.9,0.999) and epsilon=1e-08\n* lr\\_scheduler\\_type: linear\n* num\\_epochs: 2",
"### Training results",
"### Framework versions\n\n\n* Transformers 4.40.1\n* Pytorch 2.2.1+cu121\n* Datasets 2.19.0\n* Tokenizers 0.19.1"
] | [
59,
124,
5,
44
] | [
"TAGS\n#transformers #tensorboard #safetensors #distilbert #text-classification #generated_from_trainer #base_model-distilbert-base-uncased #license-apache-2.0 #autotrain_compatible #endpoints_compatible #region-us \n### Training hyperparameters\n\n\nThe following hyperparameters were used during training:\n\n\n* learning\\_rate: 2e-05\n* train\\_batch\\_size: 32\n* eval\\_batch\\_size: 32\n* seed: 42\n* gradient\\_accumulation\\_steps: 4\n* total\\_train\\_batch\\_size: 128\n* optimizer: Adam with betas=(0.9,0.999) and epsilon=1e-08\n* lr\\_scheduler\\_type: linear\n* num\\_epochs: 2### Training results### Framework versions\n\n\n* Transformers 4.40.1\n* Pytorch 2.2.1+cu121\n* Datasets 2.19.0\n* Tokenizers 0.19.1"
] |
text-generation | transformers |
# Uploaded model
- **Developed by:** abdulrehmanibk
- **License:** apache-2.0
- **Finetuned from model :** unsloth/llama-3-8b-bnb-4bit
This llama model was trained 2x faster with [Unsloth](https://github.com/unslothai/unsloth) and Huggingface's TRL library.
[<img src="https://raw.githubusercontent.com/unslothai/unsloth/main/images/unsloth%20made%20with%20love.png" width="200"/>](https://github.com/unslothai/unsloth) | {"language": ["en"], "license": "apache-2.0", "tags": ["text-generation-inference", "transformers", "unsloth", "llama", "trl"], "metrics": ["accuracy"], "base_model": "unsloth/llama-3-8b-bnb-4bit", "pipeline_tag": "text-generation"} | abdulrehmanibk/mpg_project-b | null | [
"transformers",
"safetensors",
"text-generation-inference",
"unsloth",
"llama",
"trl",
"text-generation",
"en",
"base_model:unsloth/llama-3-8b-bnb-4bit",
"license:apache-2.0",
"endpoints_compatible",
"region:us"
] | null | 2024-05-02T10:56:32+00:00 | [] | [
"en"
] | TAGS
#transformers #safetensors #text-generation-inference #unsloth #llama #trl #text-generation #en #base_model-unsloth/llama-3-8b-bnb-4bit #license-apache-2.0 #endpoints_compatible #region-us
|
# Uploaded model
- Developed by: abdulrehmanibk
- License: apache-2.0
- Finetuned from model : unsloth/llama-3-8b-bnb-4bit
This llama model was trained 2x faster with Unsloth and Huggingface's TRL library.
<img src="URL width="200"/> | [
"# Uploaded model\n\n- Developed by: abdulrehmanibk\n- License: apache-2.0\n- Finetuned from model : unsloth/llama-3-8b-bnb-4bit\n\nThis llama model was trained 2x faster with Unsloth and Huggingface's TRL library.\n\n<img src=\"URL width=\"200\"/>"
] | [
"TAGS\n#transformers #safetensors #text-generation-inference #unsloth #llama #trl #text-generation #en #base_model-unsloth/llama-3-8b-bnb-4bit #license-apache-2.0 #endpoints_compatible #region-us \n",
"# Uploaded model\n\n- Developed by: abdulrehmanibk\n- License: apache-2.0\n- Finetuned from model : unsloth/llama-3-8b-bnb-4bit\n\nThis llama model was trained 2x faster with Unsloth and Huggingface's TRL library.\n\n<img src=\"URL width=\"200\"/>"
] | [
68,
82
] | [
"TAGS\n#transformers #safetensors #text-generation-inference #unsloth #llama #trl #text-generation #en #base_model-unsloth/llama-3-8b-bnb-4bit #license-apache-2.0 #endpoints_compatible #region-us \n# Uploaded model\n\n- Developed by: abdulrehmanibk\n- License: apache-2.0\n- Finetuned from model : unsloth/llama-3-8b-bnb-4bit\n\nThis llama model was trained 2x faster with Unsloth and Huggingface's TRL library.\n\n<img src=\"URL width=\"200\"/>"
] |
null | null | <!-- ### quantize_version: 2 -->
<!-- ### output_tensor_quantised: 1 -->
<!-- ### convert_type: -->
<!-- ### vocab_type: -->
weighted/imatrix quants of https://huggingface.co/chargoddard/llama-2-16b-nastychat
| {} | mradermacher/llama-2-16b-nastychat-i1-GGUF | null | [
"gguf",
"region:us"
] | null | 2024-05-02T10:56:45+00:00 | [] | [] | TAGS
#gguf #region-us
|
weighted/imatrix quants of URL
| [] | [
"TAGS\n#gguf #region-us \n"
] | [
9
] | [
"TAGS\n#gguf #region-us \n"
] |
text-classification | transformers |
<!-- This model card has been generated automatically according to the information Keras had access to. You should
probably proofread and complete it, then remove this comment. -->
# Poojithpoosa/financial_phrasebankmodel
This model is a fine-tuned version of [google-bert/bert-base-uncased](https://huggingface.co/google-bert/bert-base-uncased) on an unknown dataset.
It achieves the following results on the evaluation set:
- Train Loss: 0.1745
- Validation Loss: 0.0643
- Train Accuracy: 0.9736
- Epoch: 2
## Model description
More information needed
## Intended uses & limitations
More information needed
## Training and evaluation data
More information needed
## Training procedure
### Training hyperparameters
The following hyperparameters were used during training:
- optimizer: {'name': 'Adam', 'learning_rate': {'class_name': 'PolynomialDecay', 'config': {'initial_learning_rate': 2e-05, 'decay_steps': 24230, 'end_learning_rate': 0.0, 'power': 1.0, 'cycle': False, 'name': None}}, 'decay': 0.0, 'beta_1': 0.9, 'beta_2': 0.999, 'epsilon': 1e-08, 'amsgrad': False}
- training_precision: float32
### Training results
| Train Loss | Validation Loss | Train Accuracy | Epoch |
|:----------:|:---------------:|:--------------:|:-----:|
| 0.6113 | 0.3262 | 0.8803 | 0 |
| 0.3522 | 0.1855 | 0.9416 | 1 |
| 0.1745 | 0.0643 | 0.9736 | 2 |
### Framework versions
- Transformers 4.24.0
- TensorFlow 2.10.1
- Datasets 2.19.0
- Tokenizers 0.11.0
| {"license": "apache-2.0", "tags": ["generated_from_keras_callback"], "model-index": [{"name": "Poojithpoosa/financial_phrasebankmodel", "results": []}]} | Poojithpoosa/financial_phrasebankmodel | null | [
"transformers",
"tf",
"bert",
"text-classification",
"generated_from_keras_callback",
"license:apache-2.0",
"autotrain_compatible",
"endpoints_compatible",
"region:us"
] | null | 2024-05-02T10:57:28+00:00 | [] | [] | TAGS
#transformers #tf #bert #text-classification #generated_from_keras_callback #license-apache-2.0 #autotrain_compatible #endpoints_compatible #region-us
| Poojithpoosa/financial\_phrasebankmodel
=======================================
This model is a fine-tuned version of google-bert/bert-base-uncased on an unknown dataset.
It achieves the following results on the evaluation set:
* Train Loss: 0.1745
* Validation Loss: 0.0643
* Train Accuracy: 0.9736
* Epoch: 2
Model description
-----------------
More information needed
Intended uses & limitations
---------------------------
More information needed
Training and evaluation data
----------------------------
More information needed
Training procedure
------------------
### Training hyperparameters
The following hyperparameters were used during training:
* optimizer: {'name': 'Adam', 'learning\_rate': {'class\_name': 'PolynomialDecay', 'config': {'initial\_learning\_rate': 2e-05, 'decay\_steps': 24230, 'end\_learning\_rate': 0.0, 'power': 1.0, 'cycle': False, 'name': None}}, 'decay': 0.0, 'beta\_1': 0.9, 'beta\_2': 0.999, 'epsilon': 1e-08, 'amsgrad': False}
* training\_precision: float32
### Training results
### Framework versions
* Transformers 4.24.0
* TensorFlow 2.10.1
* Datasets 2.19.0
* Tokenizers 0.11.0
| [
"### Training hyperparameters\n\n\nThe following hyperparameters were used during training:\n\n\n* optimizer: {'name': 'Adam', 'learning\\_rate': {'class\\_name': 'PolynomialDecay', 'config': {'initial\\_learning\\_rate': 2e-05, 'decay\\_steps': 24230, 'end\\_learning\\_rate': 0.0, 'power': 1.0, 'cycle': False, 'name': None}}, 'decay': 0.0, 'beta\\_1': 0.9, 'beta\\_2': 0.999, 'epsilon': 1e-08, 'amsgrad': False}\n* training\\_precision: float32",
"### Training results",
"### Framework versions\n\n\n* Transformers 4.24.0\n* TensorFlow 2.10.1\n* Datasets 2.19.0\n* Tokenizers 0.11.0"
] | [
"TAGS\n#transformers #tf #bert #text-classification #generated_from_keras_callback #license-apache-2.0 #autotrain_compatible #endpoints_compatible #region-us \n",
"### Training hyperparameters\n\n\nThe following hyperparameters were used during training:\n\n\n* optimizer: {'name': 'Adam', 'learning\\_rate': {'class\\_name': 'PolynomialDecay', 'config': {'initial\\_learning\\_rate': 2e-05, 'decay\\_steps': 24230, 'end\\_learning\\_rate': 0.0, 'power': 1.0, 'cycle': False, 'name': None}}, 'decay': 0.0, 'beta\\_1': 0.9, 'beta\\_2': 0.999, 'epsilon': 1e-08, 'amsgrad': False}\n* training\\_precision: float32",
"### Training results",
"### Framework versions\n\n\n* Transformers 4.24.0\n* TensorFlow 2.10.1\n* Datasets 2.19.0\n* Tokenizers 0.11.0"
] | [
44,
178,
5,
38
] | [
"TAGS\n#transformers #tf #bert #text-classification #generated_from_keras_callback #license-apache-2.0 #autotrain_compatible #endpoints_compatible #region-us \n### Training hyperparameters\n\n\nThe following hyperparameters were used during training:\n\n\n* optimizer: {'name': 'Adam', 'learning\\_rate': {'class\\_name': 'PolynomialDecay', 'config': {'initial\\_learning\\_rate': 2e-05, 'decay\\_steps': 24230, 'end\\_learning\\_rate': 0.0, 'power': 1.0, 'cycle': False, 'name': None}}, 'decay': 0.0, 'beta\\_1': 0.9, 'beta\\_2': 0.999, 'epsilon': 1e-08, 'amsgrad': False}\n* training\\_precision: float32### Training results### Framework versions\n\n\n* Transformers 4.24.0\n* TensorFlow 2.10.1\n* Datasets 2.19.0\n* Tokenizers 0.11.0"
] |
text-generation | transformers |
# Model Card for Model ID
<!-- Provide a quick summary of what the model is/does. -->
## Model Details
### Model Description
<!-- Provide a longer summary of what this model is. -->
This is the model card of a 🤗 transformers model that has been pushed on the Hub. This model card has been automatically generated.
- **Developed by:** [More Information Needed]
- **Funded by [optional]:** [More Information Needed]
- **Shared by [optional]:** [More Information Needed]
- **Model type:** [More Information Needed]
- **Language(s) (NLP):** [More Information Needed]
- **License:** [More Information Needed]
- **Finetuned from model [optional]:** [More Information Needed]
### Model Sources [optional]
<!-- Provide the basic links for the model. -->
- **Repository:** [More Information Needed]
- **Paper [optional]:** [More Information Needed]
- **Demo [optional]:** [More Information Needed]
## Uses
<!-- Address questions around how the model is intended to be used, including the foreseeable users of the model and those affected by the model. -->
### Direct Use
<!-- This section is for the model use without fine-tuning or plugging into a larger ecosystem/app. -->
[More Information Needed]
### Downstream Use [optional]
<!-- This section is for the model use when fine-tuned for a task, or when plugged into a larger ecosystem/app -->
[More Information Needed]
### Out-of-Scope Use
<!-- This section addresses misuse, malicious use, and uses that the model will not work well for. -->
[More Information Needed]
## Bias, Risks, and Limitations
<!-- This section is meant to convey both technical and sociotechnical limitations. -->
[More Information Needed]
### Recommendations
<!-- This section is meant to convey recommendations with respect to the bias, risk, and technical limitations. -->
Users (both direct and downstream) should be made aware of the risks, biases and limitations of the model. More information needed for further recommendations.
## How to Get Started with the Model
Use the code below to get started with the model.
[More Information Needed]
## Training Details
### Training Data
<!-- This should link to a Dataset Card, perhaps with a short stub of information on what the training data is all about as well as documentation related to data pre-processing or additional filtering. -->
[More Information Needed]
### Training Procedure
<!-- This relates heavily to the Technical Specifications. Content here should link to that section when it is relevant to the training procedure. -->
#### Preprocessing [optional]
[More Information Needed]
#### Training Hyperparameters
- **Training regime:** [More Information Needed] <!--fp32, fp16 mixed precision, bf16 mixed precision, bf16 non-mixed precision, fp16 non-mixed precision, fp8 mixed precision -->
#### Speeds, Sizes, Times [optional]
<!-- This section provides information about throughput, start/end time, checkpoint size if relevant, etc. -->
[More Information Needed]
## Evaluation
<!-- This section describes the evaluation protocols and provides the results. -->
### Testing Data, Factors & Metrics
#### Testing Data
<!-- This should link to a Dataset Card if possible. -->
[More Information Needed]
#### Factors
<!-- These are the things the evaluation is disaggregating by, e.g., subpopulations or domains. -->
[More Information Needed]
#### Metrics
<!-- These are the evaluation metrics being used, ideally with a description of why. -->
[More Information Needed]
### Results
[More Information Needed]
#### Summary
## Model Examination [optional]
<!-- Relevant interpretability work for the model goes here -->
[More Information Needed]
## Environmental Impact
<!-- Total emissions (in grams of CO2eq) and additional considerations, such as electricity usage, go here. Edit the suggested text below accordingly -->
Carbon emissions can be estimated using the [Machine Learning Impact calculator](https://mlco2.github.io/impact#compute) presented in [Lacoste et al. (2019)](https://arxiv.org/abs/1910.09700).
- **Hardware Type:** [More Information Needed]
- **Hours used:** [More Information Needed]
- **Cloud Provider:** [More Information Needed]
- **Compute Region:** [More Information Needed]
- **Carbon Emitted:** [More Information Needed]
## Technical Specifications [optional]
### Model Architecture and Objective
[More Information Needed]
### Compute Infrastructure
[More Information Needed]
#### Hardware
[More Information Needed]
#### Software
[More Information Needed]
## Citation [optional]
<!-- If there is a paper or blog post introducing the model, the APA and Bibtex information for that should go in this section. -->
**BibTeX:**
[More Information Needed]
**APA:**
[More Information Needed]
## Glossary [optional]
<!-- If relevant, include terms and calculations in this section that can help readers understand the model or model card. -->
[More Information Needed]
## More Information [optional]
[More Information Needed]
## Model Card Authors [optional]
[More Information Needed]
## Model Card Contact
[More Information Needed] | {"library_name": "transformers", "tags": []} | azizksar/mistral-sartex | null | [
"transformers",
"safetensors",
"mistral",
"text-generation",
"arxiv:1910.09700",
"autotrain_compatible",
"endpoints_compatible",
"text-generation-inference",
"4-bit",
"region:us"
] | null | 2024-05-02T10:59:01+00:00 | [
"1910.09700"
] | [] | TAGS
#transformers #safetensors #mistral #text-generation #arxiv-1910.09700 #autotrain_compatible #endpoints_compatible #text-generation-inference #4-bit #region-us
|
# Model Card for Model ID
## Model Details
### Model Description
This is the model card of a transformers model that has been pushed on the Hub. This model card has been automatically generated.
- Developed by:
- Funded by [optional]:
- Shared by [optional]:
- Model type:
- Language(s) (NLP):
- License:
- Finetuned from model [optional]:
### Model Sources [optional]
- Repository:
- Paper [optional]:
- Demo [optional]:
## Uses
### Direct Use
### Downstream Use [optional]
### Out-of-Scope Use
## Bias, Risks, and Limitations
### Recommendations
Users (both direct and downstream) should be made aware of the risks, biases and limitations of the model. More information needed for further recommendations.
## How to Get Started with the Model
Use the code below to get started with the model.
## Training Details
### Training Data
### Training Procedure
#### Preprocessing [optional]
#### Training Hyperparameters
- Training regime:
#### Speeds, Sizes, Times [optional]
## Evaluation
### Testing Data, Factors & Metrics
#### Testing Data
#### Factors
#### Metrics
### Results
#### Summary
## Model Examination [optional]
## Environmental Impact
Carbon emissions can be estimated using the Machine Learning Impact calculator presented in Lacoste et al. (2019).
- Hardware Type:
- Hours used:
- Cloud Provider:
- Compute Region:
- Carbon Emitted:
## Technical Specifications [optional]
### Model Architecture and Objective
### Compute Infrastructure
#### Hardware
#### Software
[optional]
BibTeX:
APA:
## Glossary [optional]
## More Information [optional]
## Model Card Authors [optional]
## Model Card Contact
| [
"# Model Card for Model ID",
"## Model Details",
"### Model Description\n\n\n\nThis is the model card of a transformers model that has been pushed on the Hub. This model card has been automatically generated.\n\n- Developed by: \n- Funded by [optional]: \n- Shared by [optional]: \n- Model type: \n- Language(s) (NLP): \n- License: \n- Finetuned from model [optional]:",
"### Model Sources [optional]\n\n\n\n- Repository: \n- Paper [optional]: \n- Demo [optional]:",
"## Uses",
"### Direct Use",
"### Downstream Use [optional]",
"### Out-of-Scope Use",
"## Bias, Risks, and Limitations",
"### Recommendations\n\n\n\nUsers (both direct and downstream) should be made aware of the risks, biases and limitations of the model. More information needed for further recommendations.",
"## How to Get Started with the Model\n\nUse the code below to get started with the model.",
"## Training Details",
"### Training Data",
"### Training Procedure",
"#### Preprocessing [optional]",
"#### Training Hyperparameters\n\n- Training regime:",
"#### Speeds, Sizes, Times [optional]",
"## Evaluation",
"### Testing Data, Factors & Metrics",
"#### Testing Data",
"#### Factors",
"#### Metrics",
"### Results",
"#### Summary",
"## Model Examination [optional]",
"## Environmental Impact\n\n\n\nCarbon emissions can be estimated using the Machine Learning Impact calculator presented in Lacoste et al. (2019).\n\n- Hardware Type: \n- Hours used: \n- Cloud Provider: \n- Compute Region: \n- Carbon Emitted:",
"## Technical Specifications [optional]",
"### Model Architecture and Objective",
"### Compute Infrastructure",
"#### Hardware",
"#### Software\n\n\n\n[optional]\n\n\n\nBibTeX:\n\n\n\nAPA:",
"## Glossary [optional]",
"## More Information [optional]",
"## Model Card Authors [optional]",
"## Model Card Contact"
] | [
"TAGS\n#transformers #safetensors #mistral #text-generation #arxiv-1910.09700 #autotrain_compatible #endpoints_compatible #text-generation-inference #4-bit #region-us \n",
"# Model Card for Model ID",
"## Model Details",
"### Model Description\n\n\n\nThis is the model card of a transformers model that has been pushed on the Hub. This model card has been automatically generated.\n\n- Developed by: \n- Funded by [optional]: \n- Shared by [optional]: \n- Model type: \n- Language(s) (NLP): \n- License: \n- Finetuned from model [optional]:",
"### Model Sources [optional]\n\n\n\n- Repository: \n- Paper [optional]: \n- Demo [optional]:",
"## Uses",
"### Direct Use",
"### Downstream Use [optional]",
"### Out-of-Scope Use",
"## Bias, Risks, and Limitations",
"### Recommendations\n\n\n\nUsers (both direct and downstream) should be made aware of the risks, biases and limitations of the model. More information needed for further recommendations.",
"## How to Get Started with the Model\n\nUse the code below to get started with the model.",
"## Training Details",
"### Training Data",
"### Training Procedure",
"#### Preprocessing [optional]",
"#### Training Hyperparameters\n\n- Training regime:",
"#### Speeds, Sizes, Times [optional]",
"## Evaluation",
"### Testing Data, Factors & Metrics",
"#### Testing Data",
"#### Factors",
"#### Metrics",
"### Results",
"#### Summary",
"## Model Examination [optional]",
"## Environmental Impact\n\n\n\nCarbon emissions can be estimated using the Machine Learning Impact calculator presented in Lacoste et al. (2019).\n\n- Hardware Type: \n- Hours used: \n- Cloud Provider: \n- Compute Region: \n- Carbon Emitted:",
"## Technical Specifications [optional]",
"### Model Architecture and Objective",
"### Compute Infrastructure",
"#### Hardware",
"#### Software\n\n\n\n[optional]\n\n\n\nBibTeX:\n\n\n\nAPA:",
"## Glossary [optional]",
"## More Information [optional]",
"## Model Card Authors [optional]",
"## Model Card Contact"
] | [
48,
6,
4,
75,
23,
3,
5,
8,
9,
8,
34,
20,
4,
5,
5,
11,
13,
12,
3,
10,
6,
5,
6,
4,
5,
7,
49,
7,
7,
5,
5,
15,
7,
7,
8,
5
] | [
"TAGS\n#transformers #safetensors #mistral #text-generation #arxiv-1910.09700 #autotrain_compatible #endpoints_compatible #text-generation-inference #4-bit #region-us \n# Model Card for Model ID## Model Details### Model Description\n\n\n\nThis is the model card of a transformers model that has been pushed on the Hub. This model card has been automatically generated.\n\n- Developed by: \n- Funded by [optional]: \n- Shared by [optional]: \n- Model type: \n- Language(s) (NLP): \n- License: \n- Finetuned from model [optional]:### Model Sources [optional]\n\n\n\n- Repository: \n- Paper [optional]: \n- Demo [optional]:## Uses### Direct Use### Downstream Use [optional]### Out-of-Scope Use## Bias, Risks, and Limitations### Recommendations\n\n\n\nUsers (both direct and downstream) should be made aware of the risks, biases and limitations of the model. More information needed for further recommendations.## How to Get Started with the Model\n\nUse the code below to get started with the model.## Training Details### Training Data### Training Procedure#### Preprocessing [optional]#### Training Hyperparameters\n\n- Training regime:#### Speeds, Sizes, Times [optional]## Evaluation### Testing Data, Factors & Metrics#### Testing Data#### Factors#### Metrics### Results#### Summary## Model Examination [optional]## Environmental Impact\n\n\n\nCarbon emissions can be estimated using the Machine Learning Impact calculator presented in Lacoste et al. (2019).\n\n- Hardware Type: \n- Hours used: \n- Cloud Provider: \n- Compute Region: \n- Carbon Emitted:## Technical Specifications [optional]### Model Architecture and Objective### Compute Infrastructure#### Hardware#### Software\n\n\n\n[optional]\n\n\n\nBibTeX:\n\n\n\nAPA:## Glossary [optional]## More Information [optional]## Model Card Authors [optional]## Model Card Contact"
] |
null | null |
# ConfigurableLlama-7B
ConfigurableLlama-7B is an automated merge created by [Maxime Labonne](https://huggingface.co/mlabonne) using the following configuration.
* [NousResearch/Meta-Llama-3-8B-Instruct](https://huggingface.co/NousResearch/Meta-Llama-3-8B-Instruct)
* [mlabonne/OrpoLlama-3-8B](https://huggingface.co/mlabonne/OrpoLlama-3-8B)
## 🧩 Configuration
```yaml
models:
- model: NousResearch/Meta-Llama-3-8B
# No parameters necessary for base model
- model: NousResearch/Meta-Llama-3-8B-Instruct
parameters:
density: 0.6
weight: 0.5
- model: mlabonne/OrpoLlama-3-8B
parameters:
density: 0.55
weight: 0.05
merge_method: dare_ties
base_model: NousResearch/Meta-Llama-3-8B
parameters:
int8_mask: true
dtype: float16
```
## 💻 Usage
```python
!pip install -qU transformers accelerate
from transformers import AutoTokenizer
import transformers
import torch
model = "automerger/ConfigurableLlama-7B"
messages = [{"role": "user", "content": "What is a large language model?"}]
tokenizer = AutoTokenizer.from_pretrained(model)
prompt = tokenizer.apply_chat_template(messages, tokenize=False, add_generation_prompt=True)
pipeline = transformers.pipeline(
"text-generation",
model=model,
torch_dtype=torch.float16,
device_map="auto",
)
outputs = pipeline(prompt, max_new_tokens=256, do_sample=True, temperature=0.7, top_k=50, top_p=0.95)
print(outputs[0]["generated_text"])
``` | {"license": "apache-2.0", "tags": ["merge", "mergekit", "lazymergekit", "automerger"], "base_model": ["NousResearch/Meta-Llama-3-8B-Instruct", "mlabonne/OrpoLlama-3-8B"]} | automerger/ConfigurableLlama-7B | null | [
"merge",
"mergekit",
"lazymergekit",
"automerger",
"base_model:NousResearch/Meta-Llama-3-8B-Instruct",
"base_model:mlabonne/OrpoLlama-3-8B",
"license:apache-2.0",
"region:us"
] | null | 2024-05-02T10:59:04+00:00 | [] | [] | TAGS
#merge #mergekit #lazymergekit #automerger #base_model-NousResearch/Meta-Llama-3-8B-Instruct #base_model-mlabonne/OrpoLlama-3-8B #license-apache-2.0 #region-us
|
# ConfigurableLlama-7B
ConfigurableLlama-7B is an automated merge created by Maxime Labonne using the following configuration.
* NousResearch/Meta-Llama-3-8B-Instruct
* mlabonne/OrpoLlama-3-8B
## Configuration
## Usage
| [
"# ConfigurableLlama-7B\n\nConfigurableLlama-7B is an automated merge created by Maxime Labonne using the following configuration.\n* NousResearch/Meta-Llama-3-8B-Instruct\n* mlabonne/OrpoLlama-3-8B",
"## Configuration",
"## Usage"
] | [
"TAGS\n#merge #mergekit #lazymergekit #automerger #base_model-NousResearch/Meta-Llama-3-8B-Instruct #base_model-mlabonne/OrpoLlama-3-8B #license-apache-2.0 #region-us \n",
"# ConfigurableLlama-7B\n\nConfigurableLlama-7B is an automated merge created by Maxime Labonne using the following configuration.\n* NousResearch/Meta-Llama-3-8B-Instruct\n* mlabonne/OrpoLlama-3-8B",
"## Configuration",
"## Usage"
] | [
68,
67,
3,
3
] | [
"TAGS\n#merge #mergekit #lazymergekit #automerger #base_model-NousResearch/Meta-Llama-3-8B-Instruct #base_model-mlabonne/OrpoLlama-3-8B #license-apache-2.0 #region-us \n# ConfigurableLlama-7B\n\nConfigurableLlama-7B is an automated merge created by Maxime Labonne using the following configuration.\n* NousResearch/Meta-Llama-3-8B-Instruct\n* mlabonne/OrpoLlama-3-8B## Configuration## Usage"
] |
text-generation | transformers |
# Model Card for Model ID
<!-- Provide a quick summary of what the model is/does. -->
## Model Details
### Model Description
<!-- Provide a longer summary of what this model is. -->
This is the model card of a 🤗 transformers model that has been pushed on the Hub. This model card has been automatically generated.
- **Developed by:** [More Information Needed]
- **Funded by [optional]:** [More Information Needed]
- **Shared by [optional]:** [More Information Needed]
- **Model type:** [More Information Needed]
- **Language(s) (NLP):** [More Information Needed]
- **License:** [More Information Needed]
- **Finetuned from model [optional]:** [More Information Needed]
### Model Sources [optional]
<!-- Provide the basic links for the model. -->
- **Repository:** [More Information Needed]
- **Paper [optional]:** [More Information Needed]
- **Demo [optional]:** [More Information Needed]
## Uses
<!-- Address questions around how the model is intended to be used, including the foreseeable users of the model and those affected by the model. -->
### Direct Use
<!-- This section is for the model use without fine-tuning or plugging into a larger ecosystem/app. -->
[More Information Needed]
### Downstream Use [optional]
<!-- This section is for the model use when fine-tuned for a task, or when plugged into a larger ecosystem/app -->
[More Information Needed]
### Out-of-Scope Use
<!-- This section addresses misuse, malicious use, and uses that the model will not work well for. -->
[More Information Needed]
## Bias, Risks, and Limitations
<!-- This section is meant to convey both technical and sociotechnical limitations. -->
[More Information Needed]
### Recommendations
<!-- This section is meant to convey recommendations with respect to the bias, risk, and technical limitations. -->
Users (both direct and downstream) should be made aware of the risks, biases and limitations of the model. More information needed for further recommendations.
## How to Get Started with the Model
Use the code below to get started with the model.
[More Information Needed]
## Training Details
### Training Data
<!-- This should link to a Dataset Card, perhaps with a short stub of information on what the training data is all about as well as documentation related to data pre-processing or additional filtering. -->
[More Information Needed]
### Training Procedure
<!-- This relates heavily to the Technical Specifications. Content here should link to that section when it is relevant to the training procedure. -->
#### Preprocessing [optional]
[More Information Needed]
#### Training Hyperparameters
- **Training regime:** [More Information Needed] <!--fp32, fp16 mixed precision, bf16 mixed precision, bf16 non-mixed precision, fp16 non-mixed precision, fp8 mixed precision -->
#### Speeds, Sizes, Times [optional]
<!-- This section provides information about throughput, start/end time, checkpoint size if relevant, etc. -->
[More Information Needed]
## Evaluation
<!-- This section describes the evaluation protocols and provides the results. -->
### Testing Data, Factors & Metrics
#### Testing Data
<!-- This should link to a Dataset Card if possible. -->
[More Information Needed]
#### Factors
<!-- These are the things the evaluation is disaggregating by, e.g., subpopulations or domains. -->
[More Information Needed]
#### Metrics
<!-- These are the evaluation metrics being used, ideally with a description of why. -->
[More Information Needed]
### Results
[More Information Needed]
#### Summary
## Model Examination [optional]
<!-- Relevant interpretability work for the model goes here -->
[More Information Needed]
## Environmental Impact
<!-- Total emissions (in grams of CO2eq) and additional considerations, such as electricity usage, go here. Edit the suggested text below accordingly -->
Carbon emissions can be estimated using the [Machine Learning Impact calculator](https://mlco2.github.io/impact#compute) presented in [Lacoste et al. (2019)](https://arxiv.org/abs/1910.09700).
- **Hardware Type:** [More Information Needed]
- **Hours used:** [More Information Needed]
- **Cloud Provider:** [More Information Needed]
- **Compute Region:** [More Information Needed]
- **Carbon Emitted:** [More Information Needed]
## Technical Specifications [optional]
### Model Architecture and Objective
[More Information Needed]
### Compute Infrastructure
[More Information Needed]
#### Hardware
[More Information Needed]
#### Software
[More Information Needed]
## Citation [optional]
<!-- If there is a paper or blog post introducing the model, the APA and Bibtex information for that should go in this section. -->
**BibTeX:**
[More Information Needed]
**APA:**
[More Information Needed]
## Glossary [optional]
<!-- If relevant, include terms and calculations in this section that can help readers understand the model or model card. -->
[More Information Needed]
## More Information [optional]
[More Information Needed]
## Model Card Authors [optional]
[More Information Needed]
## Model Card Contact
[More Information Needed] | {"library_name": "transformers", "pipeline_tag": "text-generation"} | dendimaki/matrix-model | null | [
"transformers",
"safetensors",
"mistral",
"text-generation",
"arxiv:1910.09700",
"autotrain_compatible",
"endpoints_compatible",
"text-generation-inference",
"region:us"
] | null | 2024-05-02T10:59:18+00:00 | [
"1910.09700"
] | [] | TAGS
#transformers #safetensors #mistral #text-generation #arxiv-1910.09700 #autotrain_compatible #endpoints_compatible #text-generation-inference #region-us
|
# Model Card for Model ID
## Model Details
### Model Description
This is the model card of a transformers model that has been pushed on the Hub. This model card has been automatically generated.
- Developed by:
- Funded by [optional]:
- Shared by [optional]:
- Model type:
- Language(s) (NLP):
- License:
- Finetuned from model [optional]:
### Model Sources [optional]
- Repository:
- Paper [optional]:
- Demo [optional]:
## Uses
### Direct Use
### Downstream Use [optional]
### Out-of-Scope Use
## Bias, Risks, and Limitations
### Recommendations
Users (both direct and downstream) should be made aware of the risks, biases and limitations of the model. More information needed for further recommendations.
## How to Get Started with the Model
Use the code below to get started with the model.
## Training Details
### Training Data
### Training Procedure
#### Preprocessing [optional]
#### Training Hyperparameters
- Training regime:
#### Speeds, Sizes, Times [optional]
## Evaluation
### Testing Data, Factors & Metrics
#### Testing Data
#### Factors
#### Metrics
### Results
#### Summary
## Model Examination [optional]
## Environmental Impact
Carbon emissions can be estimated using the Machine Learning Impact calculator presented in Lacoste et al. (2019).
- Hardware Type:
- Hours used:
- Cloud Provider:
- Compute Region:
- Carbon Emitted:
## Technical Specifications [optional]
### Model Architecture and Objective
### Compute Infrastructure
#### Hardware
#### Software
[optional]
BibTeX:
APA:
## Glossary [optional]
## More Information [optional]
## Model Card Authors [optional]
## Model Card Contact
| [
"# Model Card for Model ID",
"## Model Details",
"### Model Description\n\n\n\nThis is the model card of a transformers model that has been pushed on the Hub. This model card has been automatically generated.\n\n- Developed by: \n- Funded by [optional]: \n- Shared by [optional]: \n- Model type: \n- Language(s) (NLP): \n- License: \n- Finetuned from model [optional]:",
"### Model Sources [optional]\n\n\n\n- Repository: \n- Paper [optional]: \n- Demo [optional]:",
"## Uses",
"### Direct Use",
"### Downstream Use [optional]",
"### Out-of-Scope Use",
"## Bias, Risks, and Limitations",
"### Recommendations\n\n\n\nUsers (both direct and downstream) should be made aware of the risks, biases and limitations of the model. More information needed for further recommendations.",
"## How to Get Started with the Model\n\nUse the code below to get started with the model.",
"## Training Details",
"### Training Data",
"### Training Procedure",
"#### Preprocessing [optional]",
"#### Training Hyperparameters\n\n- Training regime:",
"#### Speeds, Sizes, Times [optional]",
"## Evaluation",
"### Testing Data, Factors & Metrics",
"#### Testing Data",
"#### Factors",
"#### Metrics",
"### Results",
"#### Summary",
"## Model Examination [optional]",
"## Environmental Impact\n\n\n\nCarbon emissions can be estimated using the Machine Learning Impact calculator presented in Lacoste et al. (2019).\n\n- Hardware Type: \n- Hours used: \n- Cloud Provider: \n- Compute Region: \n- Carbon Emitted:",
"## Technical Specifications [optional]",
"### Model Architecture and Objective",
"### Compute Infrastructure",
"#### Hardware",
"#### Software\n\n\n\n[optional]\n\n\n\nBibTeX:\n\n\n\nAPA:",
"## Glossary [optional]",
"## More Information [optional]",
"## Model Card Authors [optional]",
"## Model Card Contact"
] | [
"TAGS\n#transformers #safetensors #mistral #text-generation #arxiv-1910.09700 #autotrain_compatible #endpoints_compatible #text-generation-inference #region-us \n",
"# Model Card for Model ID",
"## Model Details",
"### Model Description\n\n\n\nThis is the model card of a transformers model that has been pushed on the Hub. This model card has been automatically generated.\n\n- Developed by: \n- Funded by [optional]: \n- Shared by [optional]: \n- Model type: \n- Language(s) (NLP): \n- License: \n- Finetuned from model [optional]:",
"### Model Sources [optional]\n\n\n\n- Repository: \n- Paper [optional]: \n- Demo [optional]:",
"## Uses",
"### Direct Use",
"### Downstream Use [optional]",
"### Out-of-Scope Use",
"## Bias, Risks, and Limitations",
"### Recommendations\n\n\n\nUsers (both direct and downstream) should be made aware of the risks, biases and limitations of the model. More information needed for further recommendations.",
"## How to Get Started with the Model\n\nUse the code below to get started with the model.",
"## Training Details",
"### Training Data",
"### Training Procedure",
"#### Preprocessing [optional]",
"#### Training Hyperparameters\n\n- Training regime:",
"#### Speeds, Sizes, Times [optional]",
"## Evaluation",
"### Testing Data, Factors & Metrics",
"#### Testing Data",
"#### Factors",
"#### Metrics",
"### Results",
"#### Summary",
"## Model Examination [optional]",
"## Environmental Impact\n\n\n\nCarbon emissions can be estimated using the Machine Learning Impact calculator presented in Lacoste et al. (2019).\n\n- Hardware Type: \n- Hours used: \n- Cloud Provider: \n- Compute Region: \n- Carbon Emitted:",
"## Technical Specifications [optional]",
"### Model Architecture and Objective",
"### Compute Infrastructure",
"#### Hardware",
"#### Software\n\n\n\n[optional]\n\n\n\nBibTeX:\n\n\n\nAPA:",
"## Glossary [optional]",
"## More Information [optional]",
"## Model Card Authors [optional]",
"## Model Card Contact"
] | [
44,
6,
4,
75,
23,
3,
5,
8,
9,
8,
34,
20,
4,
5,
5,
11,
13,
12,
3,
10,
6,
5,
6,
4,
5,
7,
49,
7,
7,
5,
5,
15,
7,
7,
8,
5
] | [
"TAGS\n#transformers #safetensors #mistral #text-generation #arxiv-1910.09700 #autotrain_compatible #endpoints_compatible #text-generation-inference #region-us \n# Model Card for Model ID## Model Details### Model Description\n\n\n\nThis is the model card of a transformers model that has been pushed on the Hub. This model card has been automatically generated.\n\n- Developed by: \n- Funded by [optional]: \n- Shared by [optional]: \n- Model type: \n- Language(s) (NLP): \n- License: \n- Finetuned from model [optional]:### Model Sources [optional]\n\n\n\n- Repository: \n- Paper [optional]: \n- Demo [optional]:## Uses### Direct Use### Downstream Use [optional]### Out-of-Scope Use## Bias, Risks, and Limitations### Recommendations\n\n\n\nUsers (both direct and downstream) should be made aware of the risks, biases and limitations of the model. More information needed for further recommendations.## How to Get Started with the Model\n\nUse the code below to get started with the model.## Training Details### Training Data### Training Procedure#### Preprocessing [optional]#### Training Hyperparameters\n\n- Training regime:#### Speeds, Sizes, Times [optional]## Evaluation### Testing Data, Factors & Metrics#### Testing Data#### Factors#### Metrics### Results#### Summary## Model Examination [optional]## Environmental Impact\n\n\n\nCarbon emissions can be estimated using the Machine Learning Impact calculator presented in Lacoste et al. (2019).\n\n- Hardware Type: \n- Hours used: \n- Cloud Provider: \n- Compute Region: \n- Carbon Emitted:## Technical Specifications [optional]### Model Architecture and Objective### Compute Infrastructure#### Hardware#### Software\n\n\n\n[optional]\n\n\n\nBibTeX:\n\n\n\nAPA:## Glossary [optional]## More Information [optional]## Model Card Authors [optional]## Model Card Contact"
] |
text-generation | transformers |
<!-- This model card has been generated automatically according to the information the Trainer had access to. You
should probably proofread and complete it, then remove this comment. -->
# ef_gpt2_baselinebest_model
This model is a fine-tuned version of [gpt2](https://huggingface.co/gpt2) on an unknown dataset.
## Model description
More information needed
## Intended uses & limitations
More information needed
## Training and evaluation data
More information needed
## Training procedure
### Training hyperparameters
The following hyperparameters were used during training:
- learning_rate: 0.00011620761063355344
- train_batch_size: 4
- eval_batch_size: 4
- seed: 123
- optimizer: Adam with betas=(0.9,0.999) and epsilon=1e-08
- lr_scheduler_type: linear
- num_epochs: 7
- mixed_precision_training: Native AMP
### Framework versions
- Transformers 4.40.1
- Pytorch 2.1.2
- Datasets 2.19.0
- Tokenizers 0.19.1
| {"license": "mit", "tags": ["generated_from_trainer"], "base_model": "gpt2", "model-index": [{"name": "ef_gpt2_baselinebest_model", "results": []}]} | frayline/ef_gpt2_baselinebest_model | null | [
"transformers",
"safetensors",
"gpt2",
"text-generation",
"generated_from_trainer",
"base_model:gpt2",
"license:mit",
"autotrain_compatible",
"endpoints_compatible",
"text-generation-inference",
"region:us"
] | null | 2024-05-02T10:59:25+00:00 | [] | [] | TAGS
#transformers #safetensors #gpt2 #text-generation #generated_from_trainer #base_model-gpt2 #license-mit #autotrain_compatible #endpoints_compatible #text-generation-inference #region-us
|
# ef_gpt2_baselinebest_model
This model is a fine-tuned version of gpt2 on an unknown dataset.
## Model description
More information needed
## Intended uses & limitations
More information needed
## Training and evaluation data
More information needed
## Training procedure
### Training hyperparameters
The following hyperparameters were used during training:
- learning_rate: 0.00011620761063355344
- train_batch_size: 4
- eval_batch_size: 4
- seed: 123
- optimizer: Adam with betas=(0.9,0.999) and epsilon=1e-08
- lr_scheduler_type: linear
- num_epochs: 7
- mixed_precision_training: Native AMP
### Framework versions
- Transformers 4.40.1
- Pytorch 2.1.2
- Datasets 2.19.0
- Tokenizers 0.19.1
| [
"# ef_gpt2_baselinebest_model\n\nThis model is a fine-tuned version of gpt2 on an unknown dataset.",
"## Model description\n\nMore information needed",
"## Intended uses & limitations\n\nMore information needed",
"## Training and evaluation data\n\nMore information needed",
"## Training procedure",
"### Training hyperparameters\n\nThe following hyperparameters were used during training:\n- learning_rate: 0.00011620761063355344\n- train_batch_size: 4\n- eval_batch_size: 4\n- seed: 123\n- optimizer: Adam with betas=(0.9,0.999) and epsilon=1e-08\n- lr_scheduler_type: linear\n- num_epochs: 7\n- mixed_precision_training: Native AMP",
"### Framework versions\n\n- Transformers 4.40.1\n- Pytorch 2.1.2\n- Datasets 2.19.0\n- Tokenizers 0.19.1"
] | [
"TAGS\n#transformers #safetensors #gpt2 #text-generation #generated_from_trainer #base_model-gpt2 #license-mit #autotrain_compatible #endpoints_compatible #text-generation-inference #region-us \n",
"# ef_gpt2_baselinebest_model\n\nThis model is a fine-tuned version of gpt2 on an unknown dataset.",
"## Model description\n\nMore information needed",
"## Intended uses & limitations\n\nMore information needed",
"## Training and evaluation data\n\nMore information needed",
"## Training procedure",
"### Training hyperparameters\n\nThe following hyperparameters were used during training:\n- learning_rate: 0.00011620761063355344\n- train_batch_size: 4\n- eval_batch_size: 4\n- seed: 123\n- optimizer: Adam with betas=(0.9,0.999) and epsilon=1e-08\n- lr_scheduler_type: linear\n- num_epochs: 7\n- mixed_precision_training: Native AMP",
"### Framework versions\n\n- Transformers 4.40.1\n- Pytorch 2.1.2\n- Datasets 2.19.0\n- Tokenizers 0.19.1"
] | [
53,
31,
7,
9,
9,
4,
112,
40
] | [
"TAGS\n#transformers #safetensors #gpt2 #text-generation #generated_from_trainer #base_model-gpt2 #license-mit #autotrain_compatible #endpoints_compatible #text-generation-inference #region-us \n# ef_gpt2_baselinebest_model\n\nThis model is a fine-tuned version of gpt2 on an unknown dataset.## Model description\n\nMore information needed## Intended uses & limitations\n\nMore information needed## Training and evaluation data\n\nMore information needed## Training procedure### Training hyperparameters\n\nThe following hyperparameters were used during training:\n- learning_rate: 0.00011620761063355344\n- train_batch_size: 4\n- eval_batch_size: 4\n- seed: 123\n- optimizer: Adam with betas=(0.9,0.999) and epsilon=1e-08\n- lr_scheduler_type: linear\n- num_epochs: 7\n- mixed_precision_training: Native AMP### Framework versions\n\n- Transformers 4.40.1\n- Pytorch 2.1.2\n- Datasets 2.19.0\n- Tokenizers 0.19.1"
] |
null | transformers | ## About
<!-- ### quantize_version: 2 -->
<!-- ### output_tensor_quantised: 1 -->
<!-- ### convert_type: hf -->
<!-- ### vocab_type: -->
static quants of https://huggingface.co/asprenger/Meta-Llama-3-8B-VIGGO
<!-- provided-files -->
weighted/imatrix quants seem not to be available (by me) at this time. If they do not show up a week or so after the static ones, I have probably not planned for them. Feel free to request them by opening a Community Discussion.
## Usage
If you are unsure how to use GGUF files, refer to one of [TheBloke's
READMEs](https://huggingface.co/TheBloke/KafkaLM-70B-German-V0.1-GGUF) for
more details, including on how to concatenate multi-part files.
## Provided Quants
(sorted by size, not necessarily quality. IQ-quants are often preferable over similar sized non-IQ quants)
| Link | Type | Size/GB | Notes |
|:-----|:-----|--------:|:------|
| [GGUF](https://huggingface.co/mradermacher/Meta-Llama-3-8B-VIGGO-GGUF/resolve/main/Meta-Llama-3-8B-VIGGO.Q2_K.gguf) | Q2_K | 3.3 | |
| [GGUF](https://huggingface.co/mradermacher/Meta-Llama-3-8B-VIGGO-GGUF/resolve/main/Meta-Llama-3-8B-VIGGO.IQ3_XS.gguf) | IQ3_XS | 3.6 | |
| [GGUF](https://huggingface.co/mradermacher/Meta-Llama-3-8B-VIGGO-GGUF/resolve/main/Meta-Llama-3-8B-VIGGO.Q3_K_S.gguf) | Q3_K_S | 3.8 | |
| [GGUF](https://huggingface.co/mradermacher/Meta-Llama-3-8B-VIGGO-GGUF/resolve/main/Meta-Llama-3-8B-VIGGO.IQ3_S.gguf) | IQ3_S | 3.8 | beats Q3_K* |
| [GGUF](https://huggingface.co/mradermacher/Meta-Llama-3-8B-VIGGO-GGUF/resolve/main/Meta-Llama-3-8B-VIGGO.IQ3_M.gguf) | IQ3_M | 3.9 | |
| [GGUF](https://huggingface.co/mradermacher/Meta-Llama-3-8B-VIGGO-GGUF/resolve/main/Meta-Llama-3-8B-VIGGO.Q3_K_M.gguf) | Q3_K_M | 4.1 | lower quality |
| [GGUF](https://huggingface.co/mradermacher/Meta-Llama-3-8B-VIGGO-GGUF/resolve/main/Meta-Llama-3-8B-VIGGO.Q3_K_L.gguf) | Q3_K_L | 4.4 | |
| [GGUF](https://huggingface.co/mradermacher/Meta-Llama-3-8B-VIGGO-GGUF/resolve/main/Meta-Llama-3-8B-VIGGO.IQ4_XS.gguf) | IQ4_XS | 4.6 | |
| [GGUF](https://huggingface.co/mradermacher/Meta-Llama-3-8B-VIGGO-GGUF/resolve/main/Meta-Llama-3-8B-VIGGO.Q4_K_S.gguf) | Q4_K_S | 4.8 | fast, recommended |
| [GGUF](https://huggingface.co/mradermacher/Meta-Llama-3-8B-VIGGO-GGUF/resolve/main/Meta-Llama-3-8B-VIGGO.Q4_K_M.gguf) | Q4_K_M | 5.0 | fast, recommended |
| [GGUF](https://huggingface.co/mradermacher/Meta-Llama-3-8B-VIGGO-GGUF/resolve/main/Meta-Llama-3-8B-VIGGO.Q5_K_S.gguf) | Q5_K_S | 5.7 | |
| [GGUF](https://huggingface.co/mradermacher/Meta-Llama-3-8B-VIGGO-GGUF/resolve/main/Meta-Llama-3-8B-VIGGO.Q5_K_M.gguf) | Q5_K_M | 5.8 | |
| [GGUF](https://huggingface.co/mradermacher/Meta-Llama-3-8B-VIGGO-GGUF/resolve/main/Meta-Llama-3-8B-VIGGO.Q6_K.gguf) | Q6_K | 6.7 | very good quality |
| [GGUF](https://huggingface.co/mradermacher/Meta-Llama-3-8B-VIGGO-GGUF/resolve/main/Meta-Llama-3-8B-VIGGO.Q8_0.gguf) | Q8_0 | 8.6 | fast, best quality |
| [GGUF](https://huggingface.co/mradermacher/Meta-Llama-3-8B-VIGGO-GGUF/resolve/main/Meta-Llama-3-8B-VIGGO.f16.gguf) | f16 | 16.2 | 16 bpw, overkill |
Here is a handy graph by ikawrakow comparing some lower-quality quant
types (lower is better):

And here are Artefact2's thoughts on the matter:
https://gist.github.com/Artefact2/b5f810600771265fc1e39442288e8ec9
## FAQ / Model Request
See https://huggingface.co/mradermacher/model_requests for some answers to
questions you might have and/or if you want some other model quantized.
## Thanks
I thank my company, [nethype GmbH](https://www.nethype.de/), for letting
me use its servers and providing upgrades to my workstation to enable
this work in my free time.
<!-- end -->
| {"language": ["en"], "library_name": "transformers", "base_model": "asprenger/Meta-Llama-3-8B-VIGGO", "quantized_by": "mradermacher"} | mradermacher/Meta-Llama-3-8B-VIGGO-GGUF | null | [
"transformers",
"gguf",
"en",
"base_model:asprenger/Meta-Llama-3-8B-VIGGO",
"endpoints_compatible",
"region:us"
] | null | 2024-05-02T11:00:57+00:00 | [] | [
"en"
] | TAGS
#transformers #gguf #en #base_model-asprenger/Meta-Llama-3-8B-VIGGO #endpoints_compatible #region-us
| About
-----
static quants of URL
weighted/imatrix quants seem not to be available (by me) at this time. If they do not show up a week or so after the static ones, I have probably not planned for them. Feel free to request them by opening a Community Discussion.
Usage
-----
If you are unsure how to use GGUF files, refer to one of TheBloke's
READMEs for
more details, including on how to concatenate multi-part files.
Provided Quants
---------------
(sorted by size, not necessarily quality. IQ-quants are often preferable over similar sized non-IQ quants)
Here is a handy graph by ikawrakow comparing some lower-quality quant
types (lower is better):
!URL
And here are Artefact2's thoughts on the matter:
URL
FAQ / Model Request
-------------------
See URL for some answers to
questions you might have and/or if you want some other model quantized.
Thanks
------
I thank my company, nethype GmbH, for letting
me use its servers and providing upgrades to my workstation to enable
this work in my free time.
| [] | [
"TAGS\n#transformers #gguf #en #base_model-asprenger/Meta-Llama-3-8B-VIGGO #endpoints_compatible #region-us \n"
] | [
40
] | [
"TAGS\n#transformers #gguf #en #base_model-asprenger/Meta-Llama-3-8B-VIGGO #endpoints_compatible #region-us \n"
] |
text-generation | transformers | Quantization made by Richard Erkhov.
[Github](https://github.com/RichardErkhov)
[Discord](https://discord.gg/pvy7H8DZMG)
[Request more models](https://github.com/RichardErkhov/quant_request)
illuni-llama-2-ko-7b-test - bnb 8bits
- Model creator: https://huggingface.co/julleong/
- Original model: https://huggingface.co/julleong/illuni-llama-2-ko-7b-test/
Original model description:
---
language:
- ko
base_model: beomi/llama-2-ko-7b
license: mit
pipeline_tag: question-answering
tags:
- instruct
- instruction
- llama-2
---
# llama2-7b
### Model Details
- Developed by: Julleong
- Backbone Model: beomi/llama-2-ko-7b
- Library: [transformers](https://github.com/huggingface/transformers)
### Used Datasets
- 개체명 사전 2022(모두의 말뭉치)
### Prompt Template
```
<usr>
{Instruction}
<bot>
{Answer}
```
### License
- MIT
| {} | RichardErkhov/julleong_-_illuni-llama-2-ko-7b-test-8bits | null | [
"transformers",
"safetensors",
"llama",
"text-generation",
"autotrain_compatible",
"endpoints_compatible",
"text-generation-inference",
"8-bit",
"region:us"
] | null | 2024-05-02T11:01:25+00:00 | [] | [] | TAGS
#transformers #safetensors #llama #text-generation #autotrain_compatible #endpoints_compatible #text-generation-inference #8-bit #region-us
| Quantization made by Richard Erkhov.
Github
Discord
Request more models
illuni-llama-2-ko-7b-test - bnb 8bits
- Model creator: URL
- Original model: URL
Original model description:
---
language:
- ko
base_model: beomi/llama-2-ko-7b
license: mit
pipeline_tag: question-answering
tags:
- instruct
- instruction
- llama-2
---
# llama2-7b
### Model Details
- Developed by: Julleong
- Backbone Model: beomi/llama-2-ko-7b
- Library: transformers
### Used Datasets
- 개체명 사전 2022(모두의 말뭉치)
### Prompt Template
### License
- MIT
| [
"# llama2-7b",
"### Model Details\n- Developed by: Julleong\n- Backbone Model: beomi/llama-2-ko-7b\n- Library: transformers",
"### Used Datasets\n- 개체명 사전 2022(모두의 말뭉치)",
"### Prompt Template",
"### License\n- MIT"
] | [
"TAGS\n#transformers #safetensors #llama #text-generation #autotrain_compatible #endpoints_compatible #text-generation-inference #8-bit #region-us \n",
"# llama2-7b",
"### Model Details\n- Developed by: Julleong\n- Backbone Model: beomi/llama-2-ko-7b\n- Library: transformers",
"### Used Datasets\n- 개체명 사전 2022(모두의 말뭉치)",
"### Prompt Template",
"### License\n- MIT"
] | [
38,
7,
32,
38,
5,
6
] | [
"TAGS\n#transformers #safetensors #llama #text-generation #autotrain_compatible #endpoints_compatible #text-generation-inference #8-bit #region-us \n# llama2-7b### Model Details\n- Developed by: Julleong\n- Backbone Model: beomi/llama-2-ko-7b\n- Library: transformers### Used Datasets\n- 개체명 사전 2022(모두의 말뭉치)### Prompt Template### License\n- MIT"
] |
null | null | <!-- ### quantize_version: 2 -->
<!-- ### output_tensor_quantised: 1 -->
<!-- ### convert_type: -->
<!-- ### vocab_type: -->
static quants of https://huggingface.co/jsfs11/TemptressTensor-10.7B-v0.1a
| {} | mradermacher/TemptressTensor-10.7B-v0.1a-GGUF | null | [
"gguf",
"region:us"
] | null | 2024-05-02T11:01:46+00:00 | [] | [] | TAGS
#gguf #region-us
|
static quants of URL
| [] | [
"TAGS\n#gguf #region-us \n"
] | [
9
] | [
"TAGS\n#gguf #region-us \n"
] |
text-classification | transformers |
# Model Trained Using AutoTrain
- Problem type: Text Classification
## Validation Metrics
loss: 0.6593675017356873
f1_macro: 0.6489004767693293
f1_micro: 0.6925925925925925
f1_weighted: 0.6558835632606125
precision_macro: 0.6958400129775946
precision_micro: 0.6925925925925925
precision_weighted: 0.7395732343473669
recall_macro: 0.7205811088713783
recall_micro: 0.6925925925925925
recall_weighted: 0.6925925925925925
accuracy: 0.6925925925925925
| {"tags": ["autotrain", "text-classification"], "datasets": ["v12/autotrain-data"], "widget": [{"text": "I love AutoTrain"}]} | Zerithas/v12 | null | [
"transformers",
"tensorboard",
"safetensors",
"bert",
"text-classification",
"autotrain",
"dataset:v12/autotrain-data",
"autotrain_compatible",
"endpoints_compatible",
"region:us"
] | null | 2024-05-02T11:02:52+00:00 | [] | [] | TAGS
#transformers #tensorboard #safetensors #bert #text-classification #autotrain #dataset-v12/autotrain-data #autotrain_compatible #endpoints_compatible #region-us
|
# Model Trained Using AutoTrain
- Problem type: Text Classification
## Validation Metrics
loss: 0.6593675017356873
f1_macro: 0.6489004767693293
f1_micro: 0.6925925925925925
f1_weighted: 0.6558835632606125
precision_macro: 0.6958400129775946
precision_micro: 0.6925925925925925
precision_weighted: 0.7395732343473669
recall_macro: 0.7205811088713783
recall_micro: 0.6925925925925925
recall_weighted: 0.6925925925925925
accuracy: 0.6925925925925925
| [
"# Model Trained Using AutoTrain\n\n- Problem type: Text Classification",
"## Validation Metrics\nloss: 0.6593675017356873\n\nf1_macro: 0.6489004767693293\n\nf1_micro: 0.6925925925925925\n\nf1_weighted: 0.6558835632606125\n\nprecision_macro: 0.6958400129775946\n\nprecision_micro: 0.6925925925925925\n\nprecision_weighted: 0.7395732343473669\n\nrecall_macro: 0.7205811088713783\n\nrecall_micro: 0.6925925925925925\n\nrecall_weighted: 0.6925925925925925\n\naccuracy: 0.6925925925925925"
] | [
"TAGS\n#transformers #tensorboard #safetensors #bert #text-classification #autotrain #dataset-v12/autotrain-data #autotrain_compatible #endpoints_compatible #region-us \n",
"# Model Trained Using AutoTrain\n\n- Problem type: Text Classification",
"## Validation Metrics\nloss: 0.6593675017356873\n\nf1_macro: 0.6489004767693293\n\nf1_micro: 0.6925925925925925\n\nf1_weighted: 0.6558835632606125\n\nprecision_macro: 0.6958400129775946\n\nprecision_micro: 0.6925925925925925\n\nprecision_weighted: 0.7395732343473669\n\nrecall_macro: 0.7205811088713783\n\nrecall_micro: 0.6925925925925925\n\nrecall_weighted: 0.6925925925925925\n\naccuracy: 0.6925925925925925"
] | [
44,
12,
180
] | [
"TAGS\n#transformers #tensorboard #safetensors #bert #text-classification #autotrain #dataset-v12/autotrain-data #autotrain_compatible #endpoints_compatible #region-us \n# Model Trained Using AutoTrain\n\n- Problem type: Text Classification## Validation Metrics\nloss: 0.6593675017356873\n\nf1_macro: 0.6489004767693293\n\nf1_micro: 0.6925925925925925\n\nf1_weighted: 0.6558835632606125\n\nprecision_macro: 0.6958400129775946\n\nprecision_micro: 0.6925925925925925\n\nprecision_weighted: 0.7395732343473669\n\nrecall_macro: 0.7205811088713783\n\nrecall_micro: 0.6925925925925925\n\nrecall_weighted: 0.6925925925925925\n\naccuracy: 0.6925925925925925"
] |
reinforcement-learning | null |
# **Q-Learning** Agent playing1 **FrozenLake-v1**
This is a trained model of a **Q-Learning** agent playing **FrozenLake-v1** .
## Usage
```python
model = load_from_hub(repo_id="miansumairjaved/q-FrozenLake-v1-4x4-noSlippery", filename="q-learning.pkl")
# Don't forget to check if you need to add additional attributes (is_slippery=False etc)
env = gym.make(model["env_id"])
```
| {"tags": ["FrozenLake-v1-4x4-no_slippery", "q-learning", "reinforcement-learning", "custom-implementation"], "model-index": [{"name": "q-FrozenLake-v1-4x4-noSlippery", "results": [{"task": {"type": "reinforcement-learning", "name": "reinforcement-learning"}, "dataset": {"name": "FrozenLake-v1-4x4-no_slippery", "type": "FrozenLake-v1-4x4-no_slippery"}, "metrics": [{"type": "mean_reward", "value": "1.00 +/- 0.00", "name": "mean_reward", "verified": false}]}]}]} | miansumairjaved/q-FrozenLake-v1-4x4-noSlippery | null | [
"FrozenLake-v1-4x4-no_slippery",
"q-learning",
"reinforcement-learning",
"custom-implementation",
"model-index",
"region:us"
] | null | 2024-05-02T11:03:36+00:00 | [] | [] | TAGS
#FrozenLake-v1-4x4-no_slippery #q-learning #reinforcement-learning #custom-implementation #model-index #region-us
|
# Q-Learning Agent playing1 FrozenLake-v1
This is a trained model of a Q-Learning agent playing FrozenLake-v1 .
## Usage
| [
"# Q-Learning Agent playing1 FrozenLake-v1\n This is a trained model of a Q-Learning agent playing FrozenLake-v1 .\n\n ## Usage"
] | [
"TAGS\n#FrozenLake-v1-4x4-no_slippery #q-learning #reinforcement-learning #custom-implementation #model-index #region-us \n",
"# Q-Learning Agent playing1 FrozenLake-v1\n This is a trained model of a Q-Learning agent playing FrozenLake-v1 .\n\n ## Usage"
] | [
35,
33
] | [
"TAGS\n#FrozenLake-v1-4x4-no_slippery #q-learning #reinforcement-learning #custom-implementation #model-index #region-us \n# Q-Learning Agent playing1 FrozenLake-v1\n This is a trained model of a Q-Learning agent playing FrozenLake-v1 .\n\n ## Usage"
] |
sentence-similarity | sentence-transformers |
# SentenceTransformer based on sentence-transformers/stsb-distilbert-base
This is a [sentence-transformers](https://www.SBERT.net) model finetuned from [sentence-transformers/stsb-distilbert-base](https://huggingface.co/sentence-transformers/stsb-distilbert-base) on the [sentence-transformers/quora-duplicates](https://huggingface.co/datasets/sentence-transformers/quora-duplicates) dataset. It maps sentences & paragraphs to a 768-dimensional dense vector space and can be used for semantic textual similarity, semantic search, paraphrase mining, text classification, clustering, and more.
## Model Details
### Model Description
- **Model Type:** Sentence Transformer
- **Base model:** [sentence-transformers/stsb-distilbert-base](https://huggingface.co/sentence-transformers/stsb-distilbert-base) <!-- at revision 82ad392c08f81be9be9bf065339670b23f2e1493 -->
- **Maximum Sequence Length:** 128 tokens
- **Output Dimensionality:** 768 tokens
- **Similarity Function:** Cosine Similarity
- **Training Dataset:**
- [sentence-transformers/quora-duplicates](https://huggingface.co/datasets/sentence-transformers/quora-duplicates)
- **Language:** en
<!-- - **License:** Unknown -->
### Model Sources
- **Documentation:** [Sentence Transformers Documentation](https://sbert.net)
- **Repository:** [Sentence Transformers on GitHub](https://github.com/UKPLab/sentence-transformers)
- **Hugging Face:** [Sentence Transformers on Hugging Face](https://huggingface.co/models?library=sentence-transformers)
### Full Model Architecture
```
SentenceTransformer(
(0): Transformer({'max_seq_length': 128, 'do_lower_case': False}) with Transformer model: DistilBertModel
(1): Pooling({'word_embedding_dimension': 768, 'pooling_mode_cls_token': False, 'pooling_mode_mean_tokens': True, 'pooling_mode_max_tokens': False, 'pooling_mode_mean_sqrt_len_tokens': False, 'pooling_mode_weightedmean_tokens': False, 'pooling_mode_lasttoken': False, 'include_prompt': True})
)
```
## Usage
### Direct Usage (Sentence Transformers)
First install the Sentence Transformers library:
```bash
pip install -U sentence-transformers
```
Then you can load this model and run inference.
```python
from sentence_transformers import SentenceTransformer
# Download from the 🤗 Hub
model = SentenceTransformer("tomaarsen/stsb-distilbert-base-mnrl")
# Run inference
sentences = [
'Is Cicret a scam?',
'Is the Cicret Bracelet a scam?',
'Can you eat only once a day?',
]
embeddings = model.encode(sentences)
print(embeddings.shape)
# [3, 768]
# Get the similarity scores for the embeddings
similarities = model.similarity(embeddings)
print(similarities.shape)
# [3, 3]
```
<!--
### Direct Usage (Transformers)
<details><summary>Click to see the direct usage in Transformers</summary>
</details>
-->
<!--
### Downstream Usage (Sentence Transformers)
You can finetune this model on your own dataset.
<details><summary>Click to expand</summary>
</details>
-->
<!--
### Out-of-Scope Use
*List how the model may foreseeably be misused and address what users ought not to do with the model.*
-->
## Evaluation
### Metrics
#### Binary Classification
* Dataset: `quora-duplicates`
* Evaluated with [<code>BinaryClassificationEvaluator</code>](https://sbert.net/docs/package_reference/evaluation.html#sentence_transformers.evaluation.BinaryClassificationEvaluator)
| Metric | Value |
|:-----------------------------|:-----------|
| cosine_accuracy | 0.816 |
| cosine_accuracy_threshold | 0.7867 |
| cosine_f1 | 0.7286 |
| cosine_f1_threshold | 0.7353 |
| cosine_precision | 0.6746 |
| cosine_recall | 0.7919 |
| cosine_ap | 0.7731 |
| dot_accuracy | 0.807 |
| dot_accuracy_threshold | 150.9795 |
| dot_f1 | 0.7224 |
| dot_f1_threshold | 137.3444 |
| dot_precision | 0.6641 |
| dot_recall | 0.7919 |
| dot_ap | 0.7492 |
| manhattan_accuracy | 0.81 |
| manhattan_accuracy_threshold | 195.8866 |
| manhattan_f1 | 0.7246 |
| manhattan_f1_threshold | 237.6859 |
| manhattan_precision | 0.6293 |
| manhattan_recall | 0.854 |
| manhattan_ap | 0.7611 |
| euclidean_accuracy | 0.81 |
| euclidean_accuracy_threshold | 8.7739 |
| euclidean_f1 | 0.7261 |
| euclidean_f1_threshold | 10.8438 |
| euclidean_precision | 0.6281 |
| euclidean_recall | 0.8602 |
| euclidean_ap | 0.7612 |
| max_accuracy | 0.816 |
| max_accuracy_threshold | 195.8866 |
| max_f1 | 0.7286 |
| max_f1_threshold | 237.6859 |
| max_precision | 0.6746 |
| max_recall | 0.8602 |
| **max_ap** | **0.7731** |
#### Paraphrase Mining
* Dataset: `quora-duplicates-dev`
* Evaluated with [<code>ParaphraseMiningEvaluator</code>](https://sbert.net/docs/package_reference/evaluation.html#sentence_transformers.evaluation.ParaphraseMiningEvaluator)
| Metric | Value |
|:----------------------|:-----------|
| **average_precision** | **0.5349** |
| f1 | 0.5395 |
| precision | 0.5175 |
| recall | 0.5635 |
| threshold | 0.762 |
#### Information Retrieval
* Evaluated with [<code>InformationRetrievalEvaluator</code>](https://sbert.net/docs/package_reference/evaluation.html#sentence_transformers.evaluation.InformationRetrievalEvaluator)
| Metric | Value |
|:--------------------|:-----------|
| cosine_accuracy@1 | 0.9646 |
| cosine_accuracy@3 | 0.9926 |
| cosine_accuracy@5 | 0.9956 |
| cosine_accuracy@10 | 0.9986 |
| cosine_precision@1 | 0.9646 |
| cosine_precision@3 | 0.4293 |
| cosine_precision@5 | 0.2754 |
| cosine_precision@10 | 0.1452 |
| cosine_recall@1 | 0.8301 |
| cosine_recall@3 | 0.9609 |
| cosine_recall@5 | 0.9808 |
| cosine_recall@10 | 0.9935 |
| cosine_ndcg@10 | 0.9795 |
| cosine_mrr@10 | 0.979 |
| **cosine_map@100** | **0.9718** |
| dot_accuracy@1 | 0.9574 |
| dot_accuracy@3 | 0.9876 |
| dot_accuracy@5 | 0.9924 |
| dot_accuracy@10 | 0.9978 |
| dot_precision@1 | 0.9574 |
| dot_precision@3 | 0.4257 |
| dot_precision@5 | 0.2737 |
| dot_precision@10 | 0.1447 |
| dot_recall@1 | 0.8238 |
| dot_recall@3 | 0.9538 |
| dot_recall@5 | 0.9764 |
| dot_recall@10 | 0.9918 |
| dot_ndcg@10 | 0.9741 |
| dot_mrr@10 | 0.9731 |
| dot_map@100 | 0.9646 |
<!--
## Bias, Risks and Limitations
*What are the known or foreseeable issues stemming from this model? You could also flag here known failure cases or weaknesses of the model.*
-->
<!--
### Recommendations
*What are recommendations with respect to the foreseeable issues? For example, filtering explicit content.*
-->
## Training Details
### Training Dataset
#### sentence-transformers/quora-duplicates
* Dataset: [sentence-transformers/quora-duplicates](https://huggingface.co/datasets/sentence-transformers/quora-duplicates) at [451a485](https://huggingface.co/datasets/sentence-transformers/quora-duplicates/tree/451a4850bd141edb44ade1b5828c259abd762cdb)
* Size: 100,000 training samples
* Columns: <code>anchor</code>, <code>positive</code>, and <code>negative</code>
* Approximate statistics based on the first 1000 samples:
| | anchor | positive | negative |
|:--------|:----------------------------------------------------------------------------------|:----------------------------------------------------------------------------------|:----------------------------------------------------------------------------------|
| type | string | string | string |
| details | <ul><li>min: 6 tokens</li><li>mean: 13.85 tokens</li><li>max: 42 tokens</li></ul> | <ul><li>min: 6 tokens</li><li>mean: 13.65 tokens</li><li>max: 44 tokens</li></ul> | <ul><li>min: 4 tokens</li><li>mean: 14.76 tokens</li><li>max: 64 tokens</li></ul> |
* Samples:
| anchor | positive | negative |
|:--------------------------------------------------------------------------------|:-----------------------------------------------------------------------------------------------|:-----------------------------------------------------------------------------------------------------------------|
| <code>Why in India do we not have one on one political debate as in USA?</code> | <code>Why cant we have a public debate between politicians in India like the one in US?</code> | <code>Can people on Quora stop India Pakistan debate? We are sick and tired seeing this everyday in bulk?</code> |
| <code>What is OnePlus One?</code> | <code>How is oneplus one?</code> | <code>Why is OnePlus One so good?</code> |
| <code>Does our mind control our emotions?</code> | <code>How do smart and successful people control their emotions?</code> | <code>How can I control my positive emotions for the people whom I love but they don't care about me?</code> |
* Loss: [<code>MultipleNegativesRankingLoss</code>](https://sbert.net/docs/package_reference/losses.html#multiplenegativesrankingloss) with these parameters:
```json
{
"scale": 20.0,
"similarity_fct": "cos_sim"
}
```
### Evaluation Dataset
#### sentence-transformers/quora-duplicates
* Dataset: [sentence-transformers/quora-duplicates](https://huggingface.co/datasets/sentence-transformers/quora-duplicates) at [451a485](https://huggingface.co/datasets/sentence-transformers/quora-duplicates/tree/451a4850bd141edb44ade1b5828c259abd762cdb)
* Size: 1,000 evaluation samples
* Columns: <code>anchor</code>, <code>positive</code>, and <code>negative</code>
* Approximate statistics based on the first 1000 samples:
| | anchor | positive | negative |
|:--------|:----------------------------------------------------------------------------------|:---------------------------------------------------------------------------------|:----------------------------------------------------------------------------------|
| type | string | string | string |
| details | <ul><li>min: 7 tokens</li><li>mean: 13.84 tokens</li><li>max: 43 tokens</li></ul> | <ul><li>min: 6 tokens</li><li>mean: 13.8 tokens</li><li>max: 38 tokens</li></ul> | <ul><li>min: 6 tokens</li><li>mean: 14.71 tokens</li><li>max: 56 tokens</li></ul> |
* Samples:
| anchor | positive | negative |
|:---------------------------------------------------------------------------------------------------------|:---------------------------------------------------------------------------------|:----------------------------------------------------------------------------------------------------------------------------------------------------------------------------------------------------------------------------------------------------------------------------------|
| <code>Which programming language is best for developing low-end games?</code> | <code>What coding language should I learn first for making games?</code> | <code>I am entering the world of video game programming and want to know what language I should learn? Because there are so many languages I do not know which one to start with. Can you recommend a language that's easy to learn and can be used with many platforms?</code> |
| <code>Was it appropriate for Meryl Streep to use her Golden Globes speech to attack Donald Trump?</code> | <code>Should Meryl Streep be using her position to attack the president?</code> | <code>Why did Kelly Ann Conway say that Meryl Streep incited peoples worst feelings?</code> |
| <code>Where can I found excellent commercial fridges in Sydney?</code> | <code>Where can I found impressive range of commercial fridges in Sydney?</code> | <code>What is the best grocery delivery service in Sydney?</code> |
* Loss: [<code>MultipleNegativesRankingLoss</code>](https://sbert.net/docs/package_reference/losses.html#multiplenegativesrankingloss) with these parameters:
```json
{
"scale": 20.0,
"similarity_fct": "cos_sim"
}
```
### Training Hyperparameters
#### Non-Default Hyperparameters
- `eval_strategy`: steps
- `per_device_train_batch_size`: 64
- `per_device_eval_batch_size`: 64
- `num_train_epochs`: 1
- `warmup_ratio`: 0.1
- `fp16`: True
- `batch_sampler`: no_duplicates
#### All Hyperparameters
<details><summary>Click to expand</summary>
- `overwrite_output_dir`: False
- `do_predict`: False
- `eval_strategy`: steps
- `prediction_loss_only`: False
- `per_device_train_batch_size`: 64
- `per_device_eval_batch_size`: 64
- `per_gpu_train_batch_size`: None
- `per_gpu_eval_batch_size`: None
- `gradient_accumulation_steps`: 1
- `eval_accumulation_steps`: None
- `learning_rate`: 5e-05
- `weight_decay`: 0.0
- `adam_beta1`: 0.9
- `adam_beta2`: 0.999
- `adam_epsilon`: 1e-08
- `max_grad_norm`: 1.0
- `num_train_epochs`: 1
- `max_steps`: -1
- `lr_scheduler_type`: linear
- `lr_scheduler_kwargs`: {}
- `warmup_ratio`: 0.1
- `warmup_steps`: 0
- `log_level`: passive
- `log_level_replica`: warning
- `log_on_each_node`: True
- `logging_nan_inf_filter`: True
- `save_safetensors`: True
- `save_on_each_node`: False
- `save_only_model`: False
- `no_cuda`: False
- `use_cpu`: False
- `use_mps_device`: False
- `seed`: 42
- `data_seed`: None
- `jit_mode_eval`: False
- `use_ipex`: False
- `bf16`: False
- `fp16`: True
- `fp16_opt_level`: O1
- `half_precision_backend`: auto
- `bf16_full_eval`: False
- `fp16_full_eval`: False
- `tf32`: None
- `local_rank`: 0
- `ddp_backend`: None
- `tpu_num_cores`: None
- `tpu_metrics_debug`: False
- `debug`: []
- `dataloader_drop_last`: False
- `dataloader_num_workers`: 0
- `dataloader_prefetch_factor`: None
- `past_index`: -1
- `disable_tqdm`: False
- `remove_unused_columns`: True
- `label_names`: None
- `load_best_model_at_end`: False
- `ignore_data_skip`: False
- `fsdp`: []
- `fsdp_min_num_params`: 0
- `fsdp_config`: {'min_num_params': 0, 'xla': False, 'xla_fsdp_v2': False, 'xla_fsdp_grad_ckpt': False}
- `fsdp_transformer_layer_cls_to_wrap`: None
- `accelerator_config`: {'split_batches': False, 'dispatch_batches': None, 'even_batches': True, 'use_seedable_sampler': True, 'non_blocking': False, 'gradient_accumulation_kwargs': None}
- `deepspeed`: None
- `label_smoothing_factor`: 0.0
- `optim`: adamw_torch
- `optim_args`: None
- `adafactor`: False
- `group_by_length`: False
- `length_column_name`: length
- `ddp_find_unused_parameters`: None
- `ddp_bucket_cap_mb`: None
- `ddp_broadcast_buffers`: None
- `dataloader_pin_memory`: True
- `dataloader_persistent_workers`: False
- `skip_memory_metrics`: True
- `use_legacy_prediction_loop`: False
- `push_to_hub`: False
- `resume_from_checkpoint`: None
- `hub_model_id`: None
- `hub_strategy`: every_save
- `hub_private_repo`: False
- `hub_always_push`: False
- `gradient_checkpointing`: False
- `gradient_checkpointing_kwargs`: None
- `include_inputs_for_metrics`: False
- `eval_do_concat_batches`: True
- `fp16_backend`: auto
- `push_to_hub_model_id`: None
- `push_to_hub_organization`: None
- `mp_parameters`:
- `auto_find_batch_size`: False
- `full_determinism`: False
- `torchdynamo`: None
- `ray_scope`: last
- `ddp_timeout`: 1800
- `torch_compile`: False
- `torch_compile_backend`: None
- `torch_compile_mode`: None
- `dispatch_batches`: None
- `split_batches`: None
- `include_tokens_per_second`: False
- `include_num_input_tokens_seen`: False
- `neftune_noise_alpha`: None
- `optim_target_modules`: None
- `batch_sampler`: no_duplicates
- `multi_dataset_batch_sampler`: proportional
</details>
### Training Logs
| Epoch | Step | Training Loss | loss | cosine_map@100 | quora-duplicates-dev_average_precision | quora-duplicates_max_ap |
|:------:|:----:|:-------------:|:------:|:--------------:|:--------------------------------------:|:-----------------------:|
| 0 | 0 | - | - | 0.9245 | 0.4200 | 0.6890 |
| 0.0640 | 100 | 0.2535 | - | - | - | - |
| 0.1280 | 200 | 0.1732 | - | - | - | - |
| 0.1599 | 250 | - | 0.1021 | 0.9601 | 0.5033 | 0.7342 |
| 0.1919 | 300 | 0.1465 | - | - | - | - |
| 0.2559 | 400 | 0.1186 | - | - | - | - |
| 0.3199 | 500 | 0.1159 | 0.0773 | 0.9653 | 0.5247 | 0.7453 |
| 0.3839 | 600 | 0.1088 | - | - | - | - |
| 0.4479 | 700 | 0.0993 | - | - | - | - |
| 0.4798 | 750 | - | 0.0665 | 0.9666 | 0.5264 | 0.7655 |
| 0.5118 | 800 | 0.0952 | - | - | - | - |
| 0.5758 | 900 | 0.0799 | - | - | - | - |
| 0.6398 | 1000 | 0.0855 | 0.0570 | 0.9709 | 0.5391 | 0.7717 |
| 0.7038 | 1100 | 0.0804 | - | - | - | - |
| 0.7678 | 1200 | 0.073 | - | - | - | - |
| 0.7997 | 1250 | - | 0.0513 | 0.9719 | 0.5329 | 0.7662 |
| 0.8317 | 1300 | 0.0741 | - | - | - | - |
| 0.8957 | 1400 | 0.0699 | - | - | - | - |
| 0.9597 | 1500 | 0.0755 | 0.0476 | 0.9718 | 0.5349 | 0.7731 |
### Environmental Impact
Carbon emissions were measured using [CodeCarbon](https://github.com/mlco2/codecarbon).
- **Energy Consumed**: 0.039 kWh
- **Carbon Emitted**: 0.015 kg of CO2
- **Hours Used**: 0.169 hours
### Training Hardware
- **On Cloud**: No
- **GPU Model**: 1 x NVIDIA GeForce RTX 3090
- **CPU Model**: 13th Gen Intel(R) Core(TM) i7-13700K
- **RAM Size**: 31.78 GB
### Framework Versions
- Python: 3.11.6
- Sentence Transformers: 3.0.0.dev0
- Transformers: 4.41.0.dev0
- PyTorch: 2.3.0+cu121
- Accelerate: 0.26.1
- Datasets: 2.18.0
- Tokenizers: 0.19.1
## Citation
### BibTeX
#### Sentence Transformers
```bibtex
@inproceedings{reimers-2019-sentence-bert,
title = "Sentence-BERT: Sentence Embeddings using Siamese BERT-Networks",
author = "Reimers, Nils and Gurevych, Iryna",
booktitle = "Proceedings of the 2019 Conference on Empirical Methods in Natural Language Processing",
month = "11",
year = "2019",
publisher = "Association for Computational Linguistics",
url = "https://arxiv.org/abs/1908.10084",
}
```
#### MultipleNegativesRankingLoss
```bibtex
@misc{henderson2017efficient,
title={Efficient Natural Language Response Suggestion for Smart Reply},
author={Matthew Henderson and Rami Al-Rfou and Brian Strope and Yun-hsuan Sung and Laszlo Lukacs and Ruiqi Guo and Sanjiv Kumar and Balint Miklos and Ray Kurzweil},
year={2017},
eprint={1705.00652},
archivePrefix={arXiv},
primaryClass={cs.CL}
}
```
<!--
## Glossary
*Clearly define terms in order to be accessible across audiences.*
-->
<!--
## Model Card Authors
*Lists the people who create the model card, providing recognition and accountability for the detailed work that goes into its construction.*
-->
<!--
## Model Card Contact
*Provides a way for people who have updates to the Model Card, suggestions, or questions, to contact the Model Card authors.*
--> | {"language": ["en"], "library_name": "sentence-transformers", "tags": ["sentence-transformers", "sentence-similarity", "feature-extraction", "loss:MultipleNegativesRankingLoss"], "metrics": ["cosine_accuracy", "cosine_accuracy_threshold", "cosine_f1", "cosine_f1_threshold", "cosine_precision", "cosine_recall", "cosine_ap", "dot_accuracy", "dot_accuracy_threshold", "dot_f1", "dot_f1_threshold", "dot_precision", "dot_recall", "dot_ap", "manhattan_accuracy", "manhattan_accuracy_threshold", "manhattan_f1", "manhattan_f1_threshold", "manhattan_precision", "manhattan_recall", "manhattan_ap", "euclidean_accuracy", "euclidean_accuracy_threshold", "euclidean_f1", "euclidean_f1_threshold", "euclidean_precision", "euclidean_recall", "euclidean_ap", "max_accuracy", "max_accuracy_threshold", "max_f1", "max_f1_threshold", "max_precision", "max_recall", "max_ap", "average_precision", "f1", "precision", "recall", "threshold", "cosine_accuracy@1", "cosine_accuracy@3", "cosine_accuracy@5", "cosine_accuracy@10", "cosine_precision@1", "cosine_precision@3", "cosine_precision@5", "cosine_precision@10", "cosine_recall@1", "cosine_recall@3", "cosine_recall@5", "cosine_recall@10", "cosine_ndcg@10", "cosine_mrr@10", "cosine_map@100", "dot_accuracy@1", "dot_accuracy@3", "dot_accuracy@5", "dot_accuracy@10", "dot_precision@1", "dot_precision@3", "dot_precision@5", "dot_precision@10", "dot_recall@1", "dot_recall@3", "dot_recall@5", "dot_recall@10", "dot_ndcg@10", "dot_mrr@10", "dot_map@100"], "base_model": "sentence-transformers/stsb-distilbert-base", "widget": [{"source_sentence": "How metro works?", "sentences": ["How can Turing machine works?", "What are the best C++ books?", "What should I learn first in PHP?"]}, {"source_sentence": "How fast is fast?", "sentences": ["How does light travel so fast?", "How could I become an actor?", "Was Muhammad a pedophile?"]}, {"source_sentence": "What is a kernel?", "sentences": ["What is a tensor?", "What does copyright protect?", "Can we increase height after 23?"]}, {"source_sentence": "What is a tensor?", "sentences": ["What is reliance jio?", "What are the reasons of war?", "Does speed reading really work?"]}, {"source_sentence": "Is Cicret a scam?", "sentences": ["Is the Cicret Bracelet a scam?", "Can you eat only once a day?", "What books should every man read?"]}], "pipeline_tag": "sentence-similarity", "co2_eq_emissions": {"emissions": 15.153912802318576, "energy_consumed": 0.038985939877640395, "source": "codecarbon", "training_type": "fine-tuning", "on_cloud": false, "cpu_model": "13th Gen Intel(R) Core(TM) i7-13700K", "ram_total_size": 31.777088165283203, "hours_used": 0.169, "hardware_used": "1 x NVIDIA GeForce RTX 3090"}, "model-index": [{"name": "SentenceTransformer based on sentence-transformers/stsb-distilbert-base", "results": [{"task": {"type": "binary-classification", "name": "Binary Classification"}, "dataset": {"name": "quora duplicates", "type": "quora-duplicates"}, "metrics": [{"type": "cosine_accuracy", "value": 0.816, "name": "Cosine Accuracy"}, {"type": "cosine_accuracy_threshold", "value": 0.7866689562797546, "name": "Cosine Accuracy Threshold"}, {"type": "cosine_f1", "value": 0.7285714285714286, "name": "Cosine F1"}, {"type": "cosine_f1_threshold", "value": 0.735264778137207, "name": "Cosine F1 Threshold"}, {"type": "cosine_precision", "value": 0.6746031746031746, "name": "Cosine Precision"}, {"type": "cosine_recall", "value": 0.7919254658385093, "name": "Cosine Recall"}, {"type": "cosine_ap", "value": 0.7731120768804719, "name": "Cosine Ap"}, {"type": "dot_accuracy", "value": 0.807, "name": "Dot Accuracy"}, {"type": "dot_accuracy_threshold", "value": 150.97946166992188, "name": "Dot Accuracy Threshold"}, {"type": "dot_f1", "value": 0.7223796033994335, "name": "Dot F1"}, {"type": "dot_f1_threshold", "value": 137.3444366455078, "name": "Dot F1 Threshold"}, {"type": "dot_precision", "value": 0.6640625, "name": "Dot Precision"}, {"type": "dot_recall", "value": 0.7919254658385093, "name": "Dot Recall"}, {"type": "dot_ap", "value": 0.749212069604305, "name": "Dot Ap"}, {"type": "manhattan_accuracy", "value": 0.81, "name": "Manhattan Accuracy"}, {"type": "manhattan_accuracy_threshold", "value": 195.88662719726562, "name": "Manhattan Accuracy Threshold"}, {"type": "manhattan_f1", "value": 0.7246376811594203, "name": "Manhattan F1"}, {"type": "manhattan_f1_threshold", "value": 237.68594360351562, "name": "Manhattan F1 Threshold"}, {"type": "manhattan_precision", "value": 0.6292906178489702, "name": "Manhattan Precision"}, {"type": "manhattan_recall", "value": 0.8540372670807453, "name": "Manhattan Recall"}, {"type": "manhattan_ap", "value": 0.7610544151599187, "name": "Manhattan Ap"}, {"type": "euclidean_accuracy", "value": 0.81, "name": "Euclidean Accuracy"}, {"type": "euclidean_accuracy_threshold", "value": 8.773942947387695, "name": "Euclidean Accuracy Threshold"}, {"type": "euclidean_f1", "value": 0.7260812581913498, "name": "Euclidean F1"}, {"type": "euclidean_f1_threshold", "value": 10.843769073486328, "name": "Euclidean F1 Threshold"}, {"type": "euclidean_precision", "value": 0.6281179138321995, "name": "Euclidean Precision"}, {"type": "euclidean_recall", "value": 0.860248447204969, "name": "Euclidean Recall"}, {"type": "euclidean_ap", "value": 0.7611533877712096, "name": "Euclidean Ap"}, {"type": "max_accuracy", "value": 0.816, "name": "Max Accuracy"}, {"type": "max_accuracy_threshold", "value": 195.88662719726562, "name": "Max Accuracy Threshold"}, {"type": "max_f1", "value": 0.7285714285714286, "name": "Max F1"}, {"type": "max_f1_threshold", "value": 237.68594360351562, "name": "Max F1 Threshold"}, {"type": "max_precision", "value": 0.6746031746031746, "name": "Max Precision"}, {"type": "max_recall", "value": 0.860248447204969, "name": "Max Recall"}, {"type": "max_ap", "value": 0.7731120768804719, "name": "Max Ap"}]}, {"task": {"type": "paraphrase-mining", "name": "Paraphrase Mining"}, "dataset": {"name": "quora duplicates dev", "type": "quora-duplicates-dev"}, "metrics": [{"type": "average_precision", "value": 0.5348666252858723, "name": "Average Precision"}, {"type": "f1", "value": 0.5395064090300363, "name": "F1"}, {"type": "precision", "value": 0.5174549291251892, "name": "Precision"}, {"type": "recall", "value": 0.5635210071439276, "name": "Recall"}, {"type": "threshold", "value": 0.762035459280014, "name": "Threshold"}]}, {"task": {"type": "information-retrieval", "name": "Information Retrieval"}, "dataset": {"name": "Unknown", "type": "unknown"}, "metrics": [{"type": "cosine_accuracy@1", "value": 0.9646, "name": "Cosine Accuracy@1"}, {"type": "cosine_accuracy@3", "value": 0.9926, "name": "Cosine Accuracy@3"}, {"type": "cosine_accuracy@5", "value": 0.9956, "name": "Cosine Accuracy@5"}, {"type": "cosine_accuracy@10", "value": 0.9986, "name": "Cosine Accuracy@10"}, {"type": "cosine_precision@1", "value": 0.9646, "name": "Cosine Precision@1"}, {"type": "cosine_precision@3", "value": 0.4293333333333333, "name": "Cosine Precision@3"}, {"type": "cosine_precision@5", "value": 0.2754, "name": "Cosine Precision@5"}, {"type": "cosine_precision@10", "value": 0.14515999999999998, "name": "Cosine Precision@10"}, {"type": "cosine_recall@1", "value": 0.830104138622815, "name": "Cosine Recall@1"}, {"type": "cosine_recall@3", "value": 0.9609072390452685, "name": "Cosine Recall@3"}, {"type": "cosine_recall@5", "value": 0.9808022997296821, "name": "Cosine Recall@5"}, {"type": "cosine_recall@10", "value": 0.9934541226453286, "name": "Cosine Recall@10"}, {"type": "cosine_ndcg@10", "value": 0.9795490191788223, "name": "Cosine Ndcg@10"}, {"type": "cosine_mrr@10", "value": 0.9789640476190478, "name": "Cosine Mrr@10"}, {"type": "cosine_map@100", "value": 0.971751123151301, "name": "Cosine Map@100"}, {"type": "dot_accuracy@1", "value": 0.9574, "name": "Dot Accuracy@1"}, {"type": "dot_accuracy@3", "value": 0.9876, "name": "Dot Accuracy@3"}, {"type": "dot_accuracy@5", "value": 0.9924, "name": "Dot Accuracy@5"}, {"type": "dot_accuracy@10", "value": 0.9978, "name": "Dot Accuracy@10"}, {"type": "dot_precision@1", "value": 0.9574, "name": "Dot Precision@1"}, {"type": "dot_precision@3", "value": 0.4257333333333334, "name": "Dot Precision@3"}, {"type": "dot_precision@5", "value": 0.27368000000000003, "name": "Dot Precision@5"}, {"type": "dot_precision@10", "value": 0.14468000000000003, "name": "Dot Precision@10"}, {"type": "dot_recall@1", "value": 0.8237692901379665, "name": "Dot Recall@1"}, {"type": "dot_recall@3", "value": 0.9538191510221804, "name": "Dot Recall@3"}, {"type": "dot_recall@5", "value": 0.9764249670623496, "name": "Dot Recall@5"}, {"type": "dot_recall@10", "value": 0.9918117957075603, "name": "Dot Recall@10"}, {"type": "dot_ndcg@10", "value": 0.9740754474178193, "name": "Dot Ndcg@10"}, {"type": "dot_mrr@10", "value": 0.9731360317460321, "name": "Dot Mrr@10"}, {"type": "dot_map@100", "value": 0.9646398037726347, "name": "Dot Map@100"}]}]}]} | tomaarsen/stsb-distilbert-base-mnrl | null | [
"sentence-transformers",
"safetensors",
"distilbert",
"sentence-similarity",
"feature-extraction",
"loss:MultipleNegativesRankingLoss",
"en",
"arxiv:1908.10084",
"arxiv:1705.00652",
"base_model:sentence-transformers/stsb-distilbert-base",
"model-index",
"co2_eq_emissions",
"endpoints_compatible",
"region:us"
] | null | 2024-05-02T11:03:53+00:00 | [
"1908.10084",
"1705.00652"
] | [
"en"
] | TAGS
#sentence-transformers #safetensors #distilbert #sentence-similarity #feature-extraction #loss-MultipleNegativesRankingLoss #en #arxiv-1908.10084 #arxiv-1705.00652 #base_model-sentence-transformers/stsb-distilbert-base #model-index #co2_eq_emissions #endpoints_compatible #region-us
| SentenceTransformer based on sentence-transformers/stsb-distilbert-base
=======================================================================
This is a sentence-transformers model finetuned from sentence-transformers/stsb-distilbert-base on the sentence-transformers/quora-duplicates dataset. It maps sentences & paragraphs to a 768-dimensional dense vector space and can be used for semantic textual similarity, semantic search, paraphrase mining, text classification, clustering, and more.
Model Details
-------------
### Model Description
* Model Type: Sentence Transformer
* Base model: sentence-transformers/stsb-distilbert-base
* Maximum Sequence Length: 128 tokens
* Output Dimensionality: 768 tokens
* Similarity Function: Cosine Similarity
* Training Dataset:
+ sentence-transformers/quora-duplicates
* Language: en
### Model Sources
* Documentation: Sentence Transformers Documentation
* Repository: Sentence Transformers on GitHub
* Hugging Face: Sentence Transformers on Hugging Face
### Full Model Architecture
Usage
-----
### Direct Usage (Sentence Transformers)
First install the Sentence Transformers library:
Then you can load this model and run inference.
Evaluation
----------
### Metrics
#### Binary Classification
* Dataset: 'quora-duplicates'
* Evaluated with `BinaryClassificationEvaluator`
#### Paraphrase Mining
* Dataset: 'quora-duplicates-dev'
* Evaluated with `ParaphraseMiningEvaluator`
#### Information Retrieval
* Evaluated with `InformationRetrievalEvaluator`
Training Details
----------------
### Training Dataset
#### sentence-transformers/quora-duplicates
* Dataset: sentence-transformers/quora-duplicates at 451a485
* Size: 100,000 training samples
* Columns: `anchor`, `positive`, and `negative`
* Approximate statistics based on the first 1000 samples:
* Samples:
* Loss: `MultipleNegativesRankingLoss` with these parameters:
### Evaluation Dataset
#### sentence-transformers/quora-duplicates
* Dataset: sentence-transformers/quora-duplicates at 451a485
* Size: 1,000 evaluation samples
* Columns: `anchor`, `positive`, and `negative`
* Approximate statistics based on the first 1000 samples:
* Samples:
* Loss: `MultipleNegativesRankingLoss` with these parameters:
### Training Hyperparameters
#### Non-Default Hyperparameters
* 'eval\_strategy': steps
* 'per\_device\_train\_batch\_size': 64
* 'per\_device\_eval\_batch\_size': 64
* 'num\_train\_epochs': 1
* 'warmup\_ratio': 0.1
* 'fp16': True
* 'batch\_sampler': no\_duplicates
#### All Hyperparameters
Click to expand
* 'overwrite\_output\_dir': False
* 'do\_predict': False
* 'eval\_strategy': steps
* 'prediction\_loss\_only': False
* 'per\_device\_train\_batch\_size': 64
* 'per\_device\_eval\_batch\_size': 64
* 'per\_gpu\_train\_batch\_size': None
* 'per\_gpu\_eval\_batch\_size': None
* 'gradient\_accumulation\_steps': 1
* 'eval\_accumulation\_steps': None
* 'learning\_rate': 5e-05
* 'weight\_decay': 0.0
* 'adam\_beta1': 0.9
* 'adam\_beta2': 0.999
* 'adam\_epsilon': 1e-08
* 'max\_grad\_norm': 1.0
* 'num\_train\_epochs': 1
* 'max\_steps': -1
* 'lr\_scheduler\_type': linear
* 'lr\_scheduler\_kwargs': {}
* 'warmup\_ratio': 0.1
* 'warmup\_steps': 0
* 'log\_level': passive
* 'log\_level\_replica': warning
* 'log\_on\_each\_node': True
* 'logging\_nan\_inf\_filter': True
* 'save\_safetensors': True
* 'save\_on\_each\_node': False
* 'save\_only\_model': False
* 'no\_cuda': False
* 'use\_cpu': False
* 'use\_mps\_device': False
* 'seed': 42
* 'data\_seed': None
* 'jit\_mode\_eval': False
* 'use\_ipex': False
* 'bf16': False
* 'fp16': True
* 'fp16\_opt\_level': O1
* 'half\_precision\_backend': auto
* 'bf16\_full\_eval': False
* 'fp16\_full\_eval': False
* 'tf32': None
* 'local\_rank': 0
* 'ddp\_backend': None
* 'tpu\_num\_cores': None
* 'tpu\_metrics\_debug': False
* 'debug': []
* 'dataloader\_drop\_last': False
* 'dataloader\_num\_workers': 0
* 'dataloader\_prefetch\_factor': None
* 'past\_index': -1
* 'disable\_tqdm': False
* 'remove\_unused\_columns': True
* 'label\_names': None
* 'load\_best\_model\_at\_end': False
* 'ignore\_data\_skip': False
* 'fsdp': []
* 'fsdp\_min\_num\_params': 0
* 'fsdp\_config': {'min\_num\_params': 0, 'xla': False, 'xla\_fsdp\_v2': False, 'xla\_fsdp\_grad\_ckpt': False}
* 'fsdp\_transformer\_layer\_cls\_to\_wrap': None
* 'accelerator\_config': {'split\_batches': False, 'dispatch\_batches': None, 'even\_batches': True, 'use\_seedable\_sampler': True, 'non\_blocking': False, 'gradient\_accumulation\_kwargs': None}
* 'deepspeed': None
* 'label\_smoothing\_factor': 0.0
* 'optim': adamw\_torch
* 'optim\_args': None
* 'adafactor': False
* 'group\_by\_length': False
* 'length\_column\_name': length
* 'ddp\_find\_unused\_parameters': None
* 'ddp\_bucket\_cap\_mb': None
* 'ddp\_broadcast\_buffers': None
* 'dataloader\_pin\_memory': True
* 'dataloader\_persistent\_workers': False
* 'skip\_memory\_metrics': True
* 'use\_legacy\_prediction\_loop': False
* 'push\_to\_hub': False
* 'resume\_from\_checkpoint': None
* 'hub\_model\_id': None
* 'hub\_strategy': every\_save
* 'hub\_private\_repo': False
* 'hub\_always\_push': False
* 'gradient\_checkpointing': False
* 'gradient\_checkpointing\_kwargs': None
* 'include\_inputs\_for\_metrics': False
* 'eval\_do\_concat\_batches': True
* 'fp16\_backend': auto
* 'push\_to\_hub\_model\_id': None
* 'push\_to\_hub\_organization': None
* 'mp\_parameters':
* 'auto\_find\_batch\_size': False
* 'full\_determinism': False
* 'torchdynamo': None
* 'ray\_scope': last
* 'ddp\_timeout': 1800
* 'torch\_compile': False
* 'torch\_compile\_backend': None
* 'torch\_compile\_mode': None
* 'dispatch\_batches': None
* 'split\_batches': None
* 'include\_tokens\_per\_second': False
* 'include\_num\_input\_tokens\_seen': False
* 'neftune\_noise\_alpha': None
* 'optim\_target\_modules': None
* 'batch\_sampler': no\_duplicates
* 'multi\_dataset\_batch\_sampler': proportional
### Training Logs
### Environmental Impact
Carbon emissions were measured using CodeCarbon.
* Energy Consumed: 0.039 kWh
* Carbon Emitted: 0.015 kg of CO2
* Hours Used: 0.169 hours
### Training Hardware
* On Cloud: No
* GPU Model: 1 x NVIDIA GeForce RTX 3090
* CPU Model: 13th Gen Intel(R) Core(TM) i7-13700K
* RAM Size: 31.78 GB
### Framework Versions
* Python: 3.11.6
* Sentence Transformers: 3.0.0.dev0
* Transformers: 4.41.0.dev0
* PyTorch: 2.3.0+cu121
* Accelerate: 0.26.1
* Datasets: 2.18.0
* Tokenizers: 0.19.1
### BibTeX
#### Sentence Transformers
#### MultipleNegativesRankingLoss
| [
"### Model Description\n\n\n* Model Type: Sentence Transformer\n* Base model: sentence-transformers/stsb-distilbert-base\n* Maximum Sequence Length: 128 tokens\n* Output Dimensionality: 768 tokens\n* Similarity Function: Cosine Similarity\n* Training Dataset:\n\t+ sentence-transformers/quora-duplicates\n* Language: en",
"### Model Sources\n\n\n* Documentation: Sentence Transformers Documentation\n* Repository: Sentence Transformers on GitHub\n* Hugging Face: Sentence Transformers on Hugging Face",
"### Full Model Architecture\n\n\nUsage\n-----",
"### Direct Usage (Sentence Transformers)\n\n\nFirst install the Sentence Transformers library:\n\n\nThen you can load this model and run inference.\n\n\nEvaluation\n----------",
"### Metrics",
"#### Binary Classification\n\n\n* Dataset: 'quora-duplicates'\n* Evaluated with `BinaryClassificationEvaluator`",
"#### Paraphrase Mining\n\n\n* Dataset: 'quora-duplicates-dev'\n* Evaluated with `ParaphraseMiningEvaluator`",
"#### Information Retrieval\n\n\n* Evaluated with `InformationRetrievalEvaluator`\n\n\n\nTraining Details\n----------------",
"### Training Dataset",
"#### sentence-transformers/quora-duplicates\n\n\n* Dataset: sentence-transformers/quora-duplicates at 451a485\n* Size: 100,000 training samples\n* Columns: `anchor`, `positive`, and `negative`\n* Approximate statistics based on the first 1000 samples:\n* Samples:\n* Loss: `MultipleNegativesRankingLoss` with these parameters:",
"### Evaluation Dataset",
"#### sentence-transformers/quora-duplicates\n\n\n* Dataset: sentence-transformers/quora-duplicates at 451a485\n* Size: 1,000 evaluation samples\n* Columns: `anchor`, `positive`, and `negative`\n* Approximate statistics based on the first 1000 samples:\n* Samples:\n* Loss: `MultipleNegativesRankingLoss` with these parameters:",
"### Training Hyperparameters",
"#### Non-Default Hyperparameters\n\n\n* 'eval\\_strategy': steps\n* 'per\\_device\\_train\\_batch\\_size': 64\n* 'per\\_device\\_eval\\_batch\\_size': 64\n* 'num\\_train\\_epochs': 1\n* 'warmup\\_ratio': 0.1\n* 'fp16': True\n* 'batch\\_sampler': no\\_duplicates",
"#### All Hyperparameters\n\n\nClick to expand\n* 'overwrite\\_output\\_dir': False\n* 'do\\_predict': False\n* 'eval\\_strategy': steps\n* 'prediction\\_loss\\_only': False\n* 'per\\_device\\_train\\_batch\\_size': 64\n* 'per\\_device\\_eval\\_batch\\_size': 64\n* 'per\\_gpu\\_train\\_batch\\_size': None\n* 'per\\_gpu\\_eval\\_batch\\_size': None\n* 'gradient\\_accumulation\\_steps': 1\n* 'eval\\_accumulation\\_steps': None\n* 'learning\\_rate': 5e-05\n* 'weight\\_decay': 0.0\n* 'adam\\_beta1': 0.9\n* 'adam\\_beta2': 0.999\n* 'adam\\_epsilon': 1e-08\n* 'max\\_grad\\_norm': 1.0\n* 'num\\_train\\_epochs': 1\n* 'max\\_steps': -1\n* 'lr\\_scheduler\\_type': linear\n* 'lr\\_scheduler\\_kwargs': {}\n* 'warmup\\_ratio': 0.1\n* 'warmup\\_steps': 0\n* 'log\\_level': passive\n* 'log\\_level\\_replica': warning\n* 'log\\_on\\_each\\_node': True\n* 'logging\\_nan\\_inf\\_filter': True\n* 'save\\_safetensors': True\n* 'save\\_on\\_each\\_node': False\n* 'save\\_only\\_model': False\n* 'no\\_cuda': False\n* 'use\\_cpu': False\n* 'use\\_mps\\_device': False\n* 'seed': 42\n* 'data\\_seed': None\n* 'jit\\_mode\\_eval': False\n* 'use\\_ipex': False\n* 'bf16': False\n* 'fp16': True\n* 'fp16\\_opt\\_level': O1\n* 'half\\_precision\\_backend': auto\n* 'bf16\\_full\\_eval': False\n* 'fp16\\_full\\_eval': False\n* 'tf32': None\n* 'local\\_rank': 0\n* 'ddp\\_backend': None\n* 'tpu\\_num\\_cores': None\n* 'tpu\\_metrics\\_debug': False\n* 'debug': []\n* 'dataloader\\_drop\\_last': False\n* 'dataloader\\_num\\_workers': 0\n* 'dataloader\\_prefetch\\_factor': None\n* 'past\\_index': -1\n* 'disable\\_tqdm': False\n* 'remove\\_unused\\_columns': True\n* 'label\\_names': None\n* 'load\\_best\\_model\\_at\\_end': False\n* 'ignore\\_data\\_skip': False\n* 'fsdp': []\n* 'fsdp\\_min\\_num\\_params': 0\n* 'fsdp\\_config': {'min\\_num\\_params': 0, 'xla': False, 'xla\\_fsdp\\_v2': False, 'xla\\_fsdp\\_grad\\_ckpt': False}\n* 'fsdp\\_transformer\\_layer\\_cls\\_to\\_wrap': None\n* 'accelerator\\_config': {'split\\_batches': False, 'dispatch\\_batches': None, 'even\\_batches': True, 'use\\_seedable\\_sampler': True, 'non\\_blocking': False, 'gradient\\_accumulation\\_kwargs': None}\n* 'deepspeed': None\n* 'label\\_smoothing\\_factor': 0.0\n* 'optim': adamw\\_torch\n* 'optim\\_args': None\n* 'adafactor': False\n* 'group\\_by\\_length': False\n* 'length\\_column\\_name': length\n* 'ddp\\_find\\_unused\\_parameters': None\n* 'ddp\\_bucket\\_cap\\_mb': None\n* 'ddp\\_broadcast\\_buffers': None\n* 'dataloader\\_pin\\_memory': True\n* 'dataloader\\_persistent\\_workers': False\n* 'skip\\_memory\\_metrics': True\n* 'use\\_legacy\\_prediction\\_loop': False\n* 'push\\_to\\_hub': False\n* 'resume\\_from\\_checkpoint': None\n* 'hub\\_model\\_id': None\n* 'hub\\_strategy': every\\_save\n* 'hub\\_private\\_repo': False\n* 'hub\\_always\\_push': False\n* 'gradient\\_checkpointing': False\n* 'gradient\\_checkpointing\\_kwargs': None\n* 'include\\_inputs\\_for\\_metrics': False\n* 'eval\\_do\\_concat\\_batches': True\n* 'fp16\\_backend': auto\n* 'push\\_to\\_hub\\_model\\_id': None\n* 'push\\_to\\_hub\\_organization': None\n* 'mp\\_parameters':\n* 'auto\\_find\\_batch\\_size': False\n* 'full\\_determinism': False\n* 'torchdynamo': None\n* 'ray\\_scope': last\n* 'ddp\\_timeout': 1800\n* 'torch\\_compile': False\n* 'torch\\_compile\\_backend': None\n* 'torch\\_compile\\_mode': None\n* 'dispatch\\_batches': None\n* 'split\\_batches': None\n* 'include\\_tokens\\_per\\_second': False\n* 'include\\_num\\_input\\_tokens\\_seen': False\n* 'neftune\\_noise\\_alpha': None\n* 'optim\\_target\\_modules': None\n* 'batch\\_sampler': no\\_duplicates\n* 'multi\\_dataset\\_batch\\_sampler': proportional",
"### Training Logs",
"### Environmental Impact\n\n\nCarbon emissions were measured using CodeCarbon.\n\n\n* Energy Consumed: 0.039 kWh\n* Carbon Emitted: 0.015 kg of CO2\n* Hours Used: 0.169 hours",
"### Training Hardware\n\n\n* On Cloud: No\n* GPU Model: 1 x NVIDIA GeForce RTX 3090\n* CPU Model: 13th Gen Intel(R) Core(TM) i7-13700K\n* RAM Size: 31.78 GB",
"### Framework Versions\n\n\n* Python: 3.11.6\n* Sentence Transformers: 3.0.0.dev0\n* Transformers: 4.41.0.dev0\n* PyTorch: 2.3.0+cu121\n* Accelerate: 0.26.1\n* Datasets: 2.18.0\n* Tokenizers: 0.19.1",
"### BibTeX",
"#### Sentence Transformers",
"#### MultipleNegativesRankingLoss"
] | [
"TAGS\n#sentence-transformers #safetensors #distilbert #sentence-similarity #feature-extraction #loss-MultipleNegativesRankingLoss #en #arxiv-1908.10084 #arxiv-1705.00652 #base_model-sentence-transformers/stsb-distilbert-base #model-index #co2_eq_emissions #endpoints_compatible #region-us \n",
"### Model Description\n\n\n* Model Type: Sentence Transformer\n* Base model: sentence-transformers/stsb-distilbert-base\n* Maximum Sequence Length: 128 tokens\n* Output Dimensionality: 768 tokens\n* Similarity Function: Cosine Similarity\n* Training Dataset:\n\t+ sentence-transformers/quora-duplicates\n* Language: en",
"### Model Sources\n\n\n* Documentation: Sentence Transformers Documentation\n* Repository: Sentence Transformers on GitHub\n* Hugging Face: Sentence Transformers on Hugging Face",
"### Full Model Architecture\n\n\nUsage\n-----",
"### Direct Usage (Sentence Transformers)\n\n\nFirst install the Sentence Transformers library:\n\n\nThen you can load this model and run inference.\n\n\nEvaluation\n----------",
"### Metrics",
"#### Binary Classification\n\n\n* Dataset: 'quora-duplicates'\n* Evaluated with `BinaryClassificationEvaluator`",
"#### Paraphrase Mining\n\n\n* Dataset: 'quora-duplicates-dev'\n* Evaluated with `ParaphraseMiningEvaluator`",
"#### Information Retrieval\n\n\n* Evaluated with `InformationRetrievalEvaluator`\n\n\n\nTraining Details\n----------------",
"### Training Dataset",
"#### sentence-transformers/quora-duplicates\n\n\n* Dataset: sentence-transformers/quora-duplicates at 451a485\n* Size: 100,000 training samples\n* Columns: `anchor`, `positive`, and `negative`\n* Approximate statistics based on the first 1000 samples:\n* Samples:\n* Loss: `MultipleNegativesRankingLoss` with these parameters:",
"### Evaluation Dataset",
"#### sentence-transformers/quora-duplicates\n\n\n* Dataset: sentence-transformers/quora-duplicates at 451a485\n* Size: 1,000 evaluation samples\n* Columns: `anchor`, `positive`, and `negative`\n* Approximate statistics based on the first 1000 samples:\n* Samples:\n* Loss: `MultipleNegativesRankingLoss` with these parameters:",
"### Training Hyperparameters",
"#### Non-Default Hyperparameters\n\n\n* 'eval\\_strategy': steps\n* 'per\\_device\\_train\\_batch\\_size': 64\n* 'per\\_device\\_eval\\_batch\\_size': 64\n* 'num\\_train\\_epochs': 1\n* 'warmup\\_ratio': 0.1\n* 'fp16': True\n* 'batch\\_sampler': no\\_duplicates",
"#### All Hyperparameters\n\n\nClick to expand\n* 'overwrite\\_output\\_dir': False\n* 'do\\_predict': False\n* 'eval\\_strategy': steps\n* 'prediction\\_loss\\_only': False\n* 'per\\_device\\_train\\_batch\\_size': 64\n* 'per\\_device\\_eval\\_batch\\_size': 64\n* 'per\\_gpu\\_train\\_batch\\_size': None\n* 'per\\_gpu\\_eval\\_batch\\_size': None\n* 'gradient\\_accumulation\\_steps': 1\n* 'eval\\_accumulation\\_steps': None\n* 'learning\\_rate': 5e-05\n* 'weight\\_decay': 0.0\n* 'adam\\_beta1': 0.9\n* 'adam\\_beta2': 0.999\n* 'adam\\_epsilon': 1e-08\n* 'max\\_grad\\_norm': 1.0\n* 'num\\_train\\_epochs': 1\n* 'max\\_steps': -1\n* 'lr\\_scheduler\\_type': linear\n* 'lr\\_scheduler\\_kwargs': {}\n* 'warmup\\_ratio': 0.1\n* 'warmup\\_steps': 0\n* 'log\\_level': passive\n* 'log\\_level\\_replica': warning\n* 'log\\_on\\_each\\_node': True\n* 'logging\\_nan\\_inf\\_filter': True\n* 'save\\_safetensors': True\n* 'save\\_on\\_each\\_node': False\n* 'save\\_only\\_model': False\n* 'no\\_cuda': False\n* 'use\\_cpu': False\n* 'use\\_mps\\_device': False\n* 'seed': 42\n* 'data\\_seed': None\n* 'jit\\_mode\\_eval': False\n* 'use\\_ipex': False\n* 'bf16': False\n* 'fp16': True\n* 'fp16\\_opt\\_level': O1\n* 'half\\_precision\\_backend': auto\n* 'bf16\\_full\\_eval': False\n* 'fp16\\_full\\_eval': False\n* 'tf32': None\n* 'local\\_rank': 0\n* 'ddp\\_backend': None\n* 'tpu\\_num\\_cores': None\n* 'tpu\\_metrics\\_debug': False\n* 'debug': []\n* 'dataloader\\_drop\\_last': False\n* 'dataloader\\_num\\_workers': 0\n* 'dataloader\\_prefetch\\_factor': None\n* 'past\\_index': -1\n* 'disable\\_tqdm': False\n* 'remove\\_unused\\_columns': True\n* 'label\\_names': None\n* 'load\\_best\\_model\\_at\\_end': False\n* 'ignore\\_data\\_skip': False\n* 'fsdp': []\n* 'fsdp\\_min\\_num\\_params': 0\n* 'fsdp\\_config': {'min\\_num\\_params': 0, 'xla': False, 'xla\\_fsdp\\_v2': False, 'xla\\_fsdp\\_grad\\_ckpt': False}\n* 'fsdp\\_transformer\\_layer\\_cls\\_to\\_wrap': None\n* 'accelerator\\_config': {'split\\_batches': False, 'dispatch\\_batches': None, 'even\\_batches': True, 'use\\_seedable\\_sampler': True, 'non\\_blocking': False, 'gradient\\_accumulation\\_kwargs': None}\n* 'deepspeed': None\n* 'label\\_smoothing\\_factor': 0.0\n* 'optim': adamw\\_torch\n* 'optim\\_args': None\n* 'adafactor': False\n* 'group\\_by\\_length': False\n* 'length\\_column\\_name': length\n* 'ddp\\_find\\_unused\\_parameters': None\n* 'ddp\\_bucket\\_cap\\_mb': None\n* 'ddp\\_broadcast\\_buffers': None\n* 'dataloader\\_pin\\_memory': True\n* 'dataloader\\_persistent\\_workers': False\n* 'skip\\_memory\\_metrics': True\n* 'use\\_legacy\\_prediction\\_loop': False\n* 'push\\_to\\_hub': False\n* 'resume\\_from\\_checkpoint': None\n* 'hub\\_model\\_id': None\n* 'hub\\_strategy': every\\_save\n* 'hub\\_private\\_repo': False\n* 'hub\\_always\\_push': False\n* 'gradient\\_checkpointing': False\n* 'gradient\\_checkpointing\\_kwargs': None\n* 'include\\_inputs\\_for\\_metrics': False\n* 'eval\\_do\\_concat\\_batches': True\n* 'fp16\\_backend': auto\n* 'push\\_to\\_hub\\_model\\_id': None\n* 'push\\_to\\_hub\\_organization': None\n* 'mp\\_parameters':\n* 'auto\\_find\\_batch\\_size': False\n* 'full\\_determinism': False\n* 'torchdynamo': None\n* 'ray\\_scope': last\n* 'ddp\\_timeout': 1800\n* 'torch\\_compile': False\n* 'torch\\_compile\\_backend': None\n* 'torch\\_compile\\_mode': None\n* 'dispatch\\_batches': None\n* 'split\\_batches': None\n* 'include\\_tokens\\_per\\_second': False\n* 'include\\_num\\_input\\_tokens\\_seen': False\n* 'neftune\\_noise\\_alpha': None\n* 'optim\\_target\\_modules': None\n* 'batch\\_sampler': no\\_duplicates\n* 'multi\\_dataset\\_batch\\_sampler': proportional",
"### Training Logs",
"### Environmental Impact\n\n\nCarbon emissions were measured using CodeCarbon.\n\n\n* Energy Consumed: 0.039 kWh\n* Carbon Emitted: 0.015 kg of CO2\n* Hours Used: 0.169 hours",
"### Training Hardware\n\n\n* On Cloud: No\n* GPU Model: 1 x NVIDIA GeForce RTX 3090\n* CPU Model: 13th Gen Intel(R) Core(TM) i7-13700K\n* RAM Size: 31.78 GB",
"### Framework Versions\n\n\n* Python: 3.11.6\n* Sentence Transformers: 3.0.0.dev0\n* Transformers: 4.41.0.dev0\n* PyTorch: 2.3.0+cu121\n* Accelerate: 0.26.1\n* Datasets: 2.18.0\n* Tokenizers: 0.19.1",
"### BibTeX",
"#### Sentence Transformers",
"#### MultipleNegativesRankingLoss"
] | [
92,
72,
29,
12,
37,
5,
28,
34,
36,
6,
84,
6,
84,
8,
106,
1494,
5,
43,
55,
79,
6,
6,
12
] | [
"TAGS\n#sentence-transformers #safetensors #distilbert #sentence-similarity #feature-extraction #loss-MultipleNegativesRankingLoss #en #arxiv-1908.10084 #arxiv-1705.00652 #base_model-sentence-transformers/stsb-distilbert-base #model-index #co2_eq_emissions #endpoints_compatible #region-us \n### Model Description\n\n\n* Model Type: Sentence Transformer\n* Base model: sentence-transformers/stsb-distilbert-base\n* Maximum Sequence Length: 128 tokens\n* Output Dimensionality: 768 tokens\n* Similarity Function: Cosine Similarity\n* Training Dataset:\n\t+ sentence-transformers/quora-duplicates\n* Language: en### Model Sources\n\n\n* Documentation: Sentence Transformers Documentation\n* Repository: Sentence Transformers on GitHub\n* Hugging Face: Sentence Transformers on Hugging Face### Full Model Architecture\n\n\nUsage\n-----### Direct Usage (Sentence Transformers)\n\n\nFirst install the Sentence Transformers library:\n\n\nThen you can load this model and run inference.\n\n\nEvaluation\n----------### Metrics#### Binary Classification\n\n\n* Dataset: 'quora-duplicates'\n* Evaluated with `BinaryClassificationEvaluator`#### Paraphrase Mining\n\n\n* Dataset: 'quora-duplicates-dev'\n* Evaluated with `ParaphraseMiningEvaluator`#### Information Retrieval\n\n\n* Evaluated with `InformationRetrievalEvaluator`\n\n\n\nTraining Details\n----------------### Training Dataset#### sentence-transformers/quora-duplicates\n\n\n* Dataset: sentence-transformers/quora-duplicates at 451a485\n* Size: 100,000 training samples\n* Columns: `anchor`, `positive`, and `negative`\n* Approximate statistics based on the first 1000 samples:\n* Samples:\n* Loss: `MultipleNegativesRankingLoss` with these parameters:### Evaluation Dataset#### sentence-transformers/quora-duplicates\n\n\n* Dataset: sentence-transformers/quora-duplicates at 451a485\n* Size: 1,000 evaluation samples\n* Columns: `anchor`, `positive`, and `negative`\n* Approximate statistics based on the first 1000 samples:\n* Samples:\n* Loss: `MultipleNegativesRankingLoss` with these parameters:### Training Hyperparameters#### Non-Default Hyperparameters\n\n\n* 'eval\\_strategy': steps\n* 'per\\_device\\_train\\_batch\\_size': 64\n* 'per\\_device\\_eval\\_batch\\_size': 64\n* 'num\\_train\\_epochs': 1\n* 'warmup\\_ratio': 0.1\n* 'fp16': True\n* 'batch\\_sampler': no\\_duplicates#### All Hyperparameters\n\n\nClick to expand\n* 'overwrite\\_output\\_dir': False\n* 'do\\_predict': False\n* 'eval\\_strategy': steps\n* 'prediction\\_loss\\_only': False\n* 'per\\_device\\_train\\_batch\\_size': 64\n* 'per\\_device\\_eval\\_batch\\_size': 64\n* 'per\\_gpu\\_train\\_batch\\_size': None\n* 'per\\_gpu\\_eval\\_batch\\_size': None\n* 'gradient\\_accumulation\\_steps': 1\n* 'eval\\_accumulation\\_steps': None\n* 'learning\\_rate': 5e-05\n* 'weight\\_decay': 0.0\n* 'adam\\_beta1': 0.9\n* 'adam\\_beta2': 0.999\n* 'adam\\_epsilon': 1e-08\n* 'max\\_grad\\_norm': 1.0\n* 'num\\_train\\_epochs': 1\n* 'max\\_steps': -1\n* 'lr\\_scheduler\\_type': linear\n* 'lr\\_scheduler\\_kwargs': {}\n* 'warmup\\_ratio': 0.1\n* 'warmup\\_steps': 0\n* 'log\\_level': passive\n* 'log\\_level\\_replica': warning\n* 'log\\_on\\_each\\_node': True\n* 'logging\\_nan\\_inf\\_filter': True\n* 'save\\_safetensors': True\n* 'save\\_on\\_each\\_node': False\n* 'save\\_only\\_model': False\n* 'no\\_cuda': False\n* 'use\\_cpu': False\n* 'use\\_mps\\_device': False\n* 'seed': 42\n* 'data\\_seed': None\n* 'jit\\_mode\\_eval': False\n* 'use\\_ipex': False\n* 'bf16': False\n* 'fp16': True\n* 'fp16\\_opt\\_level': O1\n* 'half\\_precision\\_backend': auto\n* 'bf16\\_full\\_eval': False\n* 'fp16\\_full\\_eval': False\n* 'tf32': None\n* 'local\\_rank': 0\n* 'ddp\\_backend': None\n* 'tpu\\_num\\_cores': None\n* 'tpu\\_metrics\\_debug': False\n* 'debug': []\n* 'dataloader\\_drop\\_last': False\n* 'dataloader\\_num\\_workers': 0\n* 'dataloader\\_prefetch\\_factor': None\n* 'past\\_index': -1\n* 'disable\\_tqdm': False\n* 'remove\\_unused\\_columns': True\n* 'label\\_names': None\n* 'load\\_best\\_model\\_at\\_end': False\n* 'ignore\\_data\\_skip': False\n* 'fsdp': []\n* 'fsdp\\_min\\_num\\_params': 0\n* 'fsdp\\_config': {'min\\_num\\_params': 0, 'xla': False, 'xla\\_fsdp\\_v2': False, 'xla\\_fsdp\\_grad\\_ckpt': False}\n* 'fsdp\\_transformer\\_layer\\_cls\\_to\\_wrap': None\n* 'accelerator\\_config': {'split\\_batches': False, 'dispatch\\_batches': None, 'even\\_batches': True, 'use\\_seedable\\_sampler': True, 'non\\_blocking': False, 'gradient\\_accumulation\\_kwargs': None}\n* 'deepspeed': None\n* 'label\\_smoothing\\_factor': 0.0\n* 'optim': adamw\\_torch\n* 'optim\\_args': None\n* 'adafactor': False\n* 'group\\_by\\_length': False\n* 'length\\_column\\_name': length\n* 'ddp\\_find\\_unused\\_parameters': None\n* 'ddp\\_bucket\\_cap\\_mb': None\n* 'ddp\\_broadcast\\_buffers': None\n* 'dataloader\\_pin\\_memory': True\n* 'dataloader\\_persistent\\_workers': False\n* 'skip\\_memory\\_metrics': True\n* 'use\\_legacy\\_prediction\\_loop': False\n* 'push\\_to\\_hub': False\n* 'resume\\_from\\_checkpoint': None\n* 'hub\\_model\\_id': None\n* 'hub\\_strategy': every\\_save\n* 'hub\\_private\\_repo': False\n* 'hub\\_always\\_push': False\n* 'gradient\\_checkpointing': False\n* 'gradient\\_checkpointing\\_kwargs': None\n* 'include\\_inputs\\_for\\_metrics': False\n* 'eval\\_do\\_concat\\_batches': True\n* 'fp16\\_backend': auto\n* 'push\\_to\\_hub\\_model\\_id': None\n* 'push\\_to\\_hub\\_organization': None\n* 'mp\\_parameters':\n* 'auto\\_find\\_batch\\_size': False\n* 'full\\_determinism': False\n* 'torchdynamo': None\n* 'ray\\_scope': last\n* 'ddp\\_timeout': 1800\n* 'torch\\_compile': False\n* 'torch\\_compile\\_backend': None\n* 'torch\\_compile\\_mode': None\n* 'dispatch\\_batches': None\n* 'split\\_batches': None\n* 'include\\_tokens\\_per\\_second': False\n* 'include\\_num\\_input\\_tokens\\_seen': False\n* 'neftune\\_noise\\_alpha': None\n* 'optim\\_target\\_modules': None\n* 'batch\\_sampler': no\\_duplicates\n* 'multi\\_dataset\\_batch\\_sampler': proportional### Training Logs### Environmental Impact\n\n\nCarbon emissions were measured using CodeCarbon.\n\n\n* Energy Consumed: 0.039 kWh\n* Carbon Emitted: 0.015 kg of CO2\n* Hours Used: 0.169 hours### Training Hardware\n\n\n* On Cloud: No\n* GPU Model: 1 x NVIDIA GeForce RTX 3090\n* CPU Model: 13th Gen Intel(R) Core(TM) i7-13700K\n* RAM Size: 31.78 GB### Framework Versions\n\n\n* Python: 3.11.6\n* Sentence Transformers: 3.0.0.dev0\n* Transformers: 4.41.0.dev0\n* PyTorch: 2.3.0+cu121\n* Accelerate: 0.26.1\n* Datasets: 2.18.0\n* Tokenizers: 0.19.1### BibTeX#### Sentence Transformers#### MultipleNegativesRankingLoss"
] |
feature-extraction | transformers | # fine-tuned/car-search-1000-64-16-jinaai_jina-embeddings-v2-small-en-1000-gpt-3.5-_8647177611
## Model Description
fine-tuned/car-search-1000-64-16-jinaai_jina-embeddings-v2-small-en-1000-gpt-3.5-_8647177611 is a fine-tuned version of jinaai/jina-embeddings-v2-small-en designed for a specific domain.
## Use Case
This model is designed to support various applications in natural language processing and understanding.
## Associated Dataset
This the dataset for this model can be found [**here**](https://huggingface.co/datasets/fine-tuned/fine-tuned/car-search-1000-64-16-jinaai_jina-embeddings-v2-small-en-1000-gpt-3.5-_8647177611).
## How to Use
This model can be easily integrated into your NLP pipeline for tasks such as text classification, sentiment analysis, entity recognition, and more. Here's a simple example to get you started:
```python
from transformers import AutoModel, AutoTokenizer
llm_name = "fine-tuned/car-search-1000-64-16-jinaai_jina-embeddings-v2-small-en-1000-gpt-3.5-_8647177611"
tokenizer = AutoTokenizer.from_pretrained(llm_name)
model = AutoModel.from_pretrained(llm_name, trust_remote_code=True)
tokens = tokenizer("Your text here", return_tensors="pt")
embedding = model(**tokens)
```
| {} | fine-tuned/car-search-1000-64-16-jinaai_jina-embeddings-v2-small-en-1000-gpt-3.5-_8647177611 | null | [
"transformers",
"safetensors",
"bert",
"feature-extraction",
"custom_code",
"region:us"
] | null | 2024-05-02T11:04:00+00:00 | [] | [] | TAGS
#transformers #safetensors #bert #feature-extraction #custom_code #region-us
| # fine-tuned/car-search-1000-64-16-jinaai_jina-embeddings-v2-small-en-1000-gpt-3.5-_8647177611
## Model Description
fine-tuned/car-search-1000-64-16-jinaai_jina-embeddings-v2-small-en-1000-gpt-3.5-_8647177611 is a fine-tuned version of jinaai/jina-embeddings-v2-small-en designed for a specific domain.
## Use Case
This model is designed to support various applications in natural language processing and understanding.
## Associated Dataset
This the dataset for this model can be found here.
## How to Use
This model can be easily integrated into your NLP pipeline for tasks such as text classification, sentiment analysis, entity recognition, and more. Here's a simple example to get you started:
| [
"# fine-tuned/car-search-1000-64-16-jinaai_jina-embeddings-v2-small-en-1000-gpt-3.5-_8647177611",
"## Model Description\n\nfine-tuned/car-search-1000-64-16-jinaai_jina-embeddings-v2-small-en-1000-gpt-3.5-_8647177611 is a fine-tuned version of jinaai/jina-embeddings-v2-small-en designed for a specific domain.",
"## Use Case\nThis model is designed to support various applications in natural language processing and understanding.",
"## Associated Dataset\n\nThis the dataset for this model can be found here.",
"## How to Use\n\nThis model can be easily integrated into your NLP pipeline for tasks such as text classification, sentiment analysis, entity recognition, and more. Here's a simple example to get you started:"
] | [
"TAGS\n#transformers #safetensors #bert #feature-extraction #custom_code #region-us \n",
"# fine-tuned/car-search-1000-64-16-jinaai_jina-embeddings-v2-small-en-1000-gpt-3.5-_8647177611",
"## Model Description\n\nfine-tuned/car-search-1000-64-16-jinaai_jina-embeddings-v2-small-en-1000-gpt-3.5-_8647177611 is a fine-tuned version of jinaai/jina-embeddings-v2-small-en designed for a specific domain.",
"## Use Case\nThis model is designed to support various applications in natural language processing and understanding.",
"## Associated Dataset\n\nThis the dataset for this model can be found here.",
"## How to Use\n\nThis model can be easily integrated into your NLP pipeline for tasks such as text classification, sentiment analysis, entity recognition, and more. Here's a simple example to get you started:"
] | [
21,
50,
84,
19,
17,
43
] | [
"TAGS\n#transformers #safetensors #bert #feature-extraction #custom_code #region-us \n# fine-tuned/car-search-1000-64-16-jinaai_jina-embeddings-v2-small-en-1000-gpt-3.5-_8647177611## Model Description\n\nfine-tuned/car-search-1000-64-16-jinaai_jina-embeddings-v2-small-en-1000-gpt-3.5-_8647177611 is a fine-tuned version of jinaai/jina-embeddings-v2-small-en designed for a specific domain.## Use Case\nThis model is designed to support various applications in natural language processing and understanding.## Associated Dataset\n\nThis the dataset for this model can be found here.## How to Use\n\nThis model can be easily integrated into your NLP pipeline for tasks such as text classification, sentiment analysis, entity recognition, and more. Here's a simple example to get you started:"
] |
null | transformers |
<!-- This model card has been generated automatically according to the information the Trainer had access to. You
should probably proofread and complete it, then remove this comment. -->
# PolizzeDonut-SoloConsignee-5Epochs
This model is a fine-tuned version of [naver-clova-ix/donut-base](https://huggingface.co/naver-clova-ix/donut-base) on the imagefolder dataset.
## Model description
More information needed
## Intended uses & limitations
More information needed
## Training and evaluation data
More information needed
## Training procedure
### Training hyperparameters
The following hyperparameters were used during training:
- learning_rate: 2e-05
- train_batch_size: 2
- eval_batch_size: 8
- seed: 42
- optimizer: Adam with betas=(0.9,0.999) and epsilon=1e-08
- lr_scheduler_type: linear
- num_epochs: 5
- mixed_precision_training: Native AMP
### Training results
### Framework versions
- Transformers 4.38.2
- Pytorch 2.2.2+cu121
- Datasets 2.18.0
- Tokenizers 0.15.2
| {"license": "mit", "tags": ["generated_from_trainer"], "datasets": ["imagefolder"], "base_model": "naver-clova-ix/donut-base", "model-index": [{"name": "PolizzeDonut-SoloConsignee-5Epochs", "results": []}]} | tedad09/PolizzeDonut-SoloConsignee-5Epochs | null | [
"transformers",
"tensorboard",
"safetensors",
"vision-encoder-decoder",
"generated_from_trainer",
"dataset:imagefolder",
"base_model:naver-clova-ix/donut-base",
"license:mit",
"endpoints_compatible",
"region:us"
] | null | 2024-05-02T11:04:18+00:00 | [] | [] | TAGS
#transformers #tensorboard #safetensors #vision-encoder-decoder #generated_from_trainer #dataset-imagefolder #base_model-naver-clova-ix/donut-base #license-mit #endpoints_compatible #region-us
|
# PolizzeDonut-SoloConsignee-5Epochs
This model is a fine-tuned version of naver-clova-ix/donut-base on the imagefolder dataset.
## Model description
More information needed
## Intended uses & limitations
More information needed
## Training and evaluation data
More information needed
## Training procedure
### Training hyperparameters
The following hyperparameters were used during training:
- learning_rate: 2e-05
- train_batch_size: 2
- eval_batch_size: 8
- seed: 42
- optimizer: Adam with betas=(0.9,0.999) and epsilon=1e-08
- lr_scheduler_type: linear
- num_epochs: 5
- mixed_precision_training: Native AMP
### Training results
### Framework versions
- Transformers 4.38.2
- Pytorch 2.2.2+cu121
- Datasets 2.18.0
- Tokenizers 0.15.2
| [
"# PolizzeDonut-SoloConsignee-5Epochs\n\nThis model is a fine-tuned version of naver-clova-ix/donut-base on the imagefolder dataset.",
"## Model description\n\nMore information needed",
"## Intended uses & limitations\n\nMore information needed",
"## Training and evaluation data\n\nMore information needed",
"## Training procedure",
"### Training hyperparameters\n\nThe following hyperparameters were used during training:\n- learning_rate: 2e-05\n- train_batch_size: 2\n- eval_batch_size: 8\n- seed: 42\n- optimizer: Adam with betas=(0.9,0.999) and epsilon=1e-08\n- lr_scheduler_type: linear\n- num_epochs: 5\n- mixed_precision_training: Native AMP",
"### Training results",
"### Framework versions\n\n- Transformers 4.38.2\n- Pytorch 2.2.2+cu121\n- Datasets 2.18.0\n- Tokenizers 0.15.2"
] | [
"TAGS\n#transformers #tensorboard #safetensors #vision-encoder-decoder #generated_from_trainer #dataset-imagefolder #base_model-naver-clova-ix/donut-base #license-mit #endpoints_compatible #region-us \n",
"# PolizzeDonut-SoloConsignee-5Epochs\n\nThis model is a fine-tuned version of naver-clova-ix/donut-base on the imagefolder dataset.",
"## Model description\n\nMore information needed",
"## Intended uses & limitations\n\nMore information needed",
"## Training and evaluation data\n\nMore information needed",
"## Training procedure",
"### Training hyperparameters\n\nThe following hyperparameters were used during training:\n- learning_rate: 2e-05\n- train_batch_size: 2\n- eval_batch_size: 8\n- seed: 42\n- optimizer: Adam with betas=(0.9,0.999) and epsilon=1e-08\n- lr_scheduler_type: linear\n- num_epochs: 5\n- mixed_precision_training: Native AMP",
"### Training results",
"### Framework versions\n\n- Transformers 4.38.2\n- Pytorch 2.2.2+cu121\n- Datasets 2.18.0\n- Tokenizers 0.15.2"
] | [
62,
46,
7,
9,
9,
4,
102,
5,
44
] | [
"TAGS\n#transformers #tensorboard #safetensors #vision-encoder-decoder #generated_from_trainer #dataset-imagefolder #base_model-naver-clova-ix/donut-base #license-mit #endpoints_compatible #region-us \n# PolizzeDonut-SoloConsignee-5Epochs\n\nThis model is a fine-tuned version of naver-clova-ix/donut-base on the imagefolder dataset.## Model description\n\nMore information needed## Intended uses & limitations\n\nMore information needed## Training and evaluation data\n\nMore information needed## Training procedure### Training hyperparameters\n\nThe following hyperparameters were used during training:\n- learning_rate: 2e-05\n- train_batch_size: 2\n- eval_batch_size: 8\n- seed: 42\n- optimizer: Adam with betas=(0.9,0.999) and epsilon=1e-08\n- lr_scheduler_type: linear\n- num_epochs: 5\n- mixed_precision_training: Native AMP### Training results### Framework versions\n\n- Transformers 4.38.2\n- Pytorch 2.2.2+cu121\n- Datasets 2.18.0\n- Tokenizers 0.15.2"
] |
null | peft |
# Model Card for Model ID
<!-- Provide a quick summary of what the model is/does. -->
## Model Details
### Model Description
<!-- Provide a longer summary of what this model is. -->
- **Developed by:** [More Information Needed]
- **Funded by [optional]:** [More Information Needed]
- **Shared by [optional]:** [More Information Needed]
- **Model type:** [More Information Needed]
- **Language(s) (NLP):** [More Information Needed]
- **License:** [More Information Needed]
- **Finetuned from model [optional]:** [More Information Needed]
### Model Sources [optional]
<!-- Provide the basic links for the model. -->
- **Repository:** [More Information Needed]
- **Paper [optional]:** [More Information Needed]
- **Demo [optional]:** [More Information Needed]
## Uses
<!-- Address questions around how the model is intended to be used, including the foreseeable users of the model and those affected by the model. -->
### Direct Use
<!-- This section is for the model use without fine-tuning or plugging into a larger ecosystem/app. -->
[More Information Needed]
### Downstream Use [optional]
<!-- This section is for the model use when fine-tuned for a task, or when plugged into a larger ecosystem/app -->
[More Information Needed]
### Out-of-Scope Use
<!-- This section addresses misuse, malicious use, and uses that the model will not work well for. -->
[More Information Needed]
## Bias, Risks, and Limitations
<!-- This section is meant to convey both technical and sociotechnical limitations. -->
[More Information Needed]
### Recommendations
<!-- This section is meant to convey recommendations with respect to the bias, risk, and technical limitations. -->
Users (both direct and downstream) should be made aware of the risks, biases and limitations of the model. More information needed for further recommendations.
## How to Get Started with the Model
Use the code below to get started with the model.
[More Information Needed]
## Training Details
### Training Data
<!-- This should link to a Dataset Card, perhaps with a short stub of information on what the training data is all about as well as documentation related to data pre-processing or additional filtering. -->
[More Information Needed]
### Training Procedure
<!-- This relates heavily to the Technical Specifications. Content here should link to that section when it is relevant to the training procedure. -->
#### Preprocessing [optional]
[More Information Needed]
#### Training Hyperparameters
- **Training regime:** [More Information Needed] <!--fp32, fp16 mixed precision, bf16 mixed precision, bf16 non-mixed precision, fp16 non-mixed precision, fp8 mixed precision -->
#### Speeds, Sizes, Times [optional]
<!-- This section provides information about throughput, start/end time, checkpoint size if relevant, etc. -->
[More Information Needed]
## Evaluation
<!-- This section describes the evaluation protocols and provides the results. -->
### Testing Data, Factors & Metrics
#### Testing Data
<!-- This should link to a Dataset Card if possible. -->
[More Information Needed]
#### Factors
<!-- These are the things the evaluation is disaggregating by, e.g., subpopulations or domains. -->
[More Information Needed]
#### Metrics
<!-- These are the evaluation metrics being used, ideally with a description of why. -->
[More Information Needed]
### Results
[More Information Needed]
#### Summary
## Model Examination [optional]
<!-- Relevant interpretability work for the model goes here -->
[More Information Needed]
## Environmental Impact
<!-- Total emissions (in grams of CO2eq) and additional considerations, such as electricity usage, go here. Edit the suggested text below accordingly -->
Carbon emissions can be estimated using the [Machine Learning Impact calculator](https://mlco2.github.io/impact#compute) presented in [Lacoste et al. (2019)](https://arxiv.org/abs/1910.09700).
- **Hardware Type:** [More Information Needed]
- **Hours used:** [More Information Needed]
- **Cloud Provider:** [More Information Needed]
- **Compute Region:** [More Information Needed]
- **Carbon Emitted:** [More Information Needed]
## Technical Specifications [optional]
### Model Architecture and Objective
[More Information Needed]
### Compute Infrastructure
[More Information Needed]
#### Hardware
[More Information Needed]
#### Software
[More Information Needed]
## Citation [optional]
<!-- If there is a paper or blog post introducing the model, the APA and Bibtex information for that should go in this section. -->
**BibTeX:**
[More Information Needed]
**APA:**
[More Information Needed]
## Glossary [optional]
<!-- If relevant, include terms and calculations in this section that can help readers understand the model or model card. -->
[More Information Needed]
## More Information [optional]
[More Information Needed]
## Model Card Authors [optional]
[More Information Needed]
## Model Card Contact
[More Information Needed]
### Framework versions
- PEFT 0.7.1 | {"library_name": "peft", "base_model": "meta-llama/Meta-Llama-3-8B-Instruct"} | sravaniayyagari/lora_model_2 | null | [
"peft",
"safetensors",
"arxiv:1910.09700",
"base_model:meta-llama/Meta-Llama-3-8B-Instruct",
"region:us"
] | null | 2024-05-02T11:04:39+00:00 | [
"1910.09700"
] | [] | TAGS
#peft #safetensors #arxiv-1910.09700 #base_model-meta-llama/Meta-Llama-3-8B-Instruct #region-us
|
# Model Card for Model ID
## Model Details
### Model Description
- Developed by:
- Funded by [optional]:
- Shared by [optional]:
- Model type:
- Language(s) (NLP):
- License:
- Finetuned from model [optional]:
### Model Sources [optional]
- Repository:
- Paper [optional]:
- Demo [optional]:
## Uses
### Direct Use
### Downstream Use [optional]
### Out-of-Scope Use
## Bias, Risks, and Limitations
### Recommendations
Users (both direct and downstream) should be made aware of the risks, biases and limitations of the model. More information needed for further recommendations.
## How to Get Started with the Model
Use the code below to get started with the model.
## Training Details
### Training Data
### Training Procedure
#### Preprocessing [optional]
#### Training Hyperparameters
- Training regime:
#### Speeds, Sizes, Times [optional]
## Evaluation
### Testing Data, Factors & Metrics
#### Testing Data
#### Factors
#### Metrics
### Results
#### Summary
## Model Examination [optional]
## Environmental Impact
Carbon emissions can be estimated using the Machine Learning Impact calculator presented in Lacoste et al. (2019).
- Hardware Type:
- Hours used:
- Cloud Provider:
- Compute Region:
- Carbon Emitted:
## Technical Specifications [optional]
### Model Architecture and Objective
### Compute Infrastructure
#### Hardware
#### Software
[optional]
BibTeX:
APA:
## Glossary [optional]
## More Information [optional]
## Model Card Authors [optional]
## Model Card Contact
### Framework versions
- PEFT 0.7.1 | [
"# Model Card for Model ID",
"## Model Details",
"### Model Description\n\n\n\n\n\n- Developed by: \n- Funded by [optional]: \n- Shared by [optional]: \n- Model type: \n- Language(s) (NLP): \n- License: \n- Finetuned from model [optional]:",
"### Model Sources [optional]\n\n\n\n- Repository: \n- Paper [optional]: \n- Demo [optional]:",
"## Uses",
"### Direct Use",
"### Downstream Use [optional]",
"### Out-of-Scope Use",
"## Bias, Risks, and Limitations",
"### Recommendations\n\n\n\nUsers (both direct and downstream) should be made aware of the risks, biases and limitations of the model. More information needed for further recommendations.",
"## How to Get Started with the Model\n\nUse the code below to get started with the model.",
"## Training Details",
"### Training Data",
"### Training Procedure",
"#### Preprocessing [optional]",
"#### Training Hyperparameters\n\n- Training regime:",
"#### Speeds, Sizes, Times [optional]",
"## Evaluation",
"### Testing Data, Factors & Metrics",
"#### Testing Data",
"#### Factors",
"#### Metrics",
"### Results",
"#### Summary",
"## Model Examination [optional]",
"## Environmental Impact\n\n\n\nCarbon emissions can be estimated using the Machine Learning Impact calculator presented in Lacoste et al. (2019).\n\n- Hardware Type: \n- Hours used: \n- Cloud Provider: \n- Compute Region: \n- Carbon Emitted:",
"## Technical Specifications [optional]",
"### Model Architecture and Objective",
"### Compute Infrastructure",
"#### Hardware",
"#### Software\n\n\n\n[optional]\n\n\n\nBibTeX:\n\n\n\nAPA:",
"## Glossary [optional]",
"## More Information [optional]",
"## Model Card Authors [optional]",
"## Model Card Contact",
"### Framework versions\n\n- PEFT 0.7.1"
] | [
"TAGS\n#peft #safetensors #arxiv-1910.09700 #base_model-meta-llama/Meta-Llama-3-8B-Instruct #region-us \n",
"# Model Card for Model ID",
"## Model Details",
"### Model Description\n\n\n\n\n\n- Developed by: \n- Funded by [optional]: \n- Shared by [optional]: \n- Model type: \n- Language(s) (NLP): \n- License: \n- Finetuned from model [optional]:",
"### Model Sources [optional]\n\n\n\n- Repository: \n- Paper [optional]: \n- Demo [optional]:",
"## Uses",
"### Direct Use",
"### Downstream Use [optional]",
"### Out-of-Scope Use",
"## Bias, Risks, and Limitations",
"### Recommendations\n\n\n\nUsers (both direct and downstream) should be made aware of the risks, biases and limitations of the model. More information needed for further recommendations.",
"## How to Get Started with the Model\n\nUse the code below to get started with the model.",
"## Training Details",
"### Training Data",
"### Training Procedure",
"#### Preprocessing [optional]",
"#### Training Hyperparameters\n\n- Training regime:",
"#### Speeds, Sizes, Times [optional]",
"## Evaluation",
"### Testing Data, Factors & Metrics",
"#### Testing Data",
"#### Factors",
"#### Metrics",
"### Results",
"#### Summary",
"## Model Examination [optional]",
"## Environmental Impact\n\n\n\nCarbon emissions can be estimated using the Machine Learning Impact calculator presented in Lacoste et al. (2019).\n\n- Hardware Type: \n- Hours used: \n- Cloud Provider: \n- Compute Region: \n- Carbon Emitted:",
"## Technical Specifications [optional]",
"### Model Architecture and Objective",
"### Compute Infrastructure",
"#### Hardware",
"#### Software\n\n\n\n[optional]\n\n\n\nBibTeX:\n\n\n\nAPA:",
"## Glossary [optional]",
"## More Information [optional]",
"## Model Card Authors [optional]",
"## Model Card Contact",
"### Framework versions\n\n- PEFT 0.7.1"
] | [
44,
6,
4,
50,
23,
3,
5,
8,
9,
8,
34,
20,
4,
5,
5,
11,
13,
12,
3,
10,
6,
5,
6,
4,
5,
7,
49,
7,
7,
5,
5,
15,
7,
7,
8,
5,
13
] | [
"TAGS\n#peft #safetensors #arxiv-1910.09700 #base_model-meta-llama/Meta-Llama-3-8B-Instruct #region-us \n# Model Card for Model ID## Model Details### Model Description\n\n\n\n\n\n- Developed by: \n- Funded by [optional]: \n- Shared by [optional]: \n- Model type: \n- Language(s) (NLP): \n- License: \n- Finetuned from model [optional]:### Model Sources [optional]\n\n\n\n- Repository: \n- Paper [optional]: \n- Demo [optional]:## Uses### Direct Use### Downstream Use [optional]### Out-of-Scope Use## Bias, Risks, and Limitations### Recommendations\n\n\n\nUsers (both direct and downstream) should be made aware of the risks, biases and limitations of the model. More information needed for further recommendations.## How to Get Started with the Model\n\nUse the code below to get started with the model.## Training Details### Training Data### Training Procedure#### Preprocessing [optional]#### Training Hyperparameters\n\n- Training regime:#### Speeds, Sizes, Times [optional]## Evaluation### Testing Data, Factors & Metrics#### Testing Data#### Factors#### Metrics### Results#### Summary## Model Examination [optional]## Environmental Impact\n\n\n\nCarbon emissions can be estimated using the Machine Learning Impact calculator presented in Lacoste et al. (2019).\n\n- Hardware Type: \n- Hours used: \n- Cloud Provider: \n- Compute Region: \n- Carbon Emitted:## Technical Specifications [optional]### Model Architecture and Objective### Compute Infrastructure#### Hardware#### Software\n\n\n\n[optional]\n\n\n\nBibTeX:\n\n\n\nAPA:## Glossary [optional]## More Information [optional]## Model Card Authors [optional]## Model Card Contact### Framework versions\n\n- PEFT 0.7.1"
] |
null | transformers | ## About
<!-- ### quantize_version: 2 -->
<!-- ### output_tensor_quantised: 1 -->
<!-- ### convert_type: -->
<!-- ### vocab_type: -->
static quants of https://huggingface.co/Gryphe/Tiamat-8b-1.2-Llama-3-DPO
<!-- provided-files -->
weighted/imatrix quants are available at https://huggingface.co/mradermacher/Tiamat-8b-1.2-Llama-3-DPO-i1-GGUF
## Usage
If you are unsure how to use GGUF files, refer to one of [TheBloke's
READMEs](https://huggingface.co/TheBloke/KafkaLM-70B-German-V0.1-GGUF) for
more details, including on how to concatenate multi-part files.
## Provided Quants
(sorted by size, not necessarily quality. IQ-quants are often preferable over similar sized non-IQ quants)
| Link | Type | Size/GB | Notes |
|:-----|:-----|--------:|:------|
| [GGUF](https://huggingface.co/mradermacher/Tiamat-8b-1.2-Llama-3-DPO-GGUF/resolve/main/Tiamat-8b-1.2-Llama-3-DPO.Q2_K.gguf) | Q2_K | 3.3 | |
| [GGUF](https://huggingface.co/mradermacher/Tiamat-8b-1.2-Llama-3-DPO-GGUF/resolve/main/Tiamat-8b-1.2-Llama-3-DPO.IQ3_XS.gguf) | IQ3_XS | 3.6 | |
| [GGUF](https://huggingface.co/mradermacher/Tiamat-8b-1.2-Llama-3-DPO-GGUF/resolve/main/Tiamat-8b-1.2-Llama-3-DPO.Q3_K_S.gguf) | Q3_K_S | 3.8 | |
| [GGUF](https://huggingface.co/mradermacher/Tiamat-8b-1.2-Llama-3-DPO-GGUF/resolve/main/Tiamat-8b-1.2-Llama-3-DPO.IQ3_S.gguf) | IQ3_S | 3.8 | beats Q3_K* |
| [GGUF](https://huggingface.co/mradermacher/Tiamat-8b-1.2-Llama-3-DPO-GGUF/resolve/main/Tiamat-8b-1.2-Llama-3-DPO.IQ3_M.gguf) | IQ3_M | 3.9 | |
| [GGUF](https://huggingface.co/mradermacher/Tiamat-8b-1.2-Llama-3-DPO-GGUF/resolve/main/Tiamat-8b-1.2-Llama-3-DPO.Q3_K_M.gguf) | Q3_K_M | 4.1 | lower quality |
| [GGUF](https://huggingface.co/mradermacher/Tiamat-8b-1.2-Llama-3-DPO-GGUF/resolve/main/Tiamat-8b-1.2-Llama-3-DPO.Q3_K_L.gguf) | Q3_K_L | 4.4 | |
| [GGUF](https://huggingface.co/mradermacher/Tiamat-8b-1.2-Llama-3-DPO-GGUF/resolve/main/Tiamat-8b-1.2-Llama-3-DPO.IQ4_XS.gguf) | IQ4_XS | 4.6 | |
| [GGUF](https://huggingface.co/mradermacher/Tiamat-8b-1.2-Llama-3-DPO-GGUF/resolve/main/Tiamat-8b-1.2-Llama-3-DPO.Q4_K_S.gguf) | Q4_K_S | 4.8 | fast, recommended |
| [GGUF](https://huggingface.co/mradermacher/Tiamat-8b-1.2-Llama-3-DPO-GGUF/resolve/main/Tiamat-8b-1.2-Llama-3-DPO.Q4_K_M.gguf) | Q4_K_M | 5.0 | fast, recommended |
| [GGUF](https://huggingface.co/mradermacher/Tiamat-8b-1.2-Llama-3-DPO-GGUF/resolve/main/Tiamat-8b-1.2-Llama-3-DPO.Q5_K_S.gguf) | Q5_K_S | 5.7 | |
| [GGUF](https://huggingface.co/mradermacher/Tiamat-8b-1.2-Llama-3-DPO-GGUF/resolve/main/Tiamat-8b-1.2-Llama-3-DPO.Q5_K_M.gguf) | Q5_K_M | 5.8 | |
| [GGUF](https://huggingface.co/mradermacher/Tiamat-8b-1.2-Llama-3-DPO-GGUF/resolve/main/Tiamat-8b-1.2-Llama-3-DPO.Q6_K.gguf) | Q6_K | 6.7 | very good quality |
| [GGUF](https://huggingface.co/mradermacher/Tiamat-8b-1.2-Llama-3-DPO-GGUF/resolve/main/Tiamat-8b-1.2-Llama-3-DPO.Q8_0.gguf) | Q8_0 | 8.6 | fast, best quality |
| [GGUF](https://huggingface.co/mradermacher/Tiamat-8b-1.2-Llama-3-DPO-GGUF/resolve/main/Tiamat-8b-1.2-Llama-3-DPO.f16.gguf) | f16 | 16.2 | 16 bpw, overkill |
Here is a handy graph by ikawrakow comparing some lower-quality quant
types (lower is better):

And here are Artefact2's thoughts on the matter:
https://gist.github.com/Artefact2/b5f810600771265fc1e39442288e8ec9
## FAQ / Model Request
See https://huggingface.co/mradermacher/model_requests for some answers to
questions you might have and/or if you want some other model quantized.
## Thanks
I thank my company, [nethype GmbH](https://www.nethype.de/), for letting
me use its servers and providing upgrades to my workstation to enable
this work in my free time.
<!-- end -->
| {"language": ["en"], "license": "apache-2.0", "library_name": "transformers", "base_model": "Gryphe/Tiamat-8b-1.2-Llama-3-DPO", "quantized_by": "mradermacher"} | mradermacher/Tiamat-8b-1.2-Llama-3-DPO-GGUF | null | [
"transformers",
"gguf",
"en",
"base_model:Gryphe/Tiamat-8b-1.2-Llama-3-DPO",
"license:apache-2.0",
"endpoints_compatible",
"region:us"
] | null | 2024-05-02T11:05:44+00:00 | [] | [
"en"
] | TAGS
#transformers #gguf #en #base_model-Gryphe/Tiamat-8b-1.2-Llama-3-DPO #license-apache-2.0 #endpoints_compatible #region-us
| About
-----
static quants of URL
weighted/imatrix quants are available at URL
Usage
-----
If you are unsure how to use GGUF files, refer to one of TheBloke's
READMEs for
more details, including on how to concatenate multi-part files.
Provided Quants
---------------
(sorted by size, not necessarily quality. IQ-quants are often preferable over similar sized non-IQ quants)
Here is a handy graph by ikawrakow comparing some lower-quality quant
types (lower is better):
!URL
And here are Artefact2's thoughts on the matter:
URL
FAQ / Model Request
-------------------
See URL for some answers to
questions you might have and/or if you want some other model quantized.
Thanks
------
I thank my company, nethype GmbH, for letting
me use its servers and providing upgrades to my workstation to enable
this work in my free time.
| [] | [
"TAGS\n#transformers #gguf #en #base_model-Gryphe/Tiamat-8b-1.2-Llama-3-DPO #license-apache-2.0 #endpoints_compatible #region-us \n"
] | [
52
] | [
"TAGS\n#transformers #gguf #en #base_model-Gryphe/Tiamat-8b-1.2-Llama-3-DPO #license-apache-2.0 #endpoints_compatible #region-us \n"
] |
reinforcement-learning | null |
# **Q-Learning** Agent playing1 **Taxi-v3**
This is a trained model of a **Q-Learning** agent playing **Taxi-v3** .
## Usage
```python
model = load_from_hub(repo_id="miansumairjaved/taxiV3", filename="q-learning.pkl")
# Don't forget to check if you need to add additional attributes (is_slippery=False etc)
env = gym.make(model["env_id"])
```
| {"tags": ["Taxi-v3", "q-learning", "reinforcement-learning", "custom-implementation"], "model-index": [{"name": "taxiV3", "results": [{"task": {"type": "reinforcement-learning", "name": "reinforcement-learning"}, "dataset": {"name": "Taxi-v3", "type": "Taxi-v3"}, "metrics": [{"type": "mean_reward", "value": "7.56 +/- 2.71", "name": "mean_reward", "verified": false}]}]}]} | miansumairjaved/taxiV3 | null | [
"Taxi-v3",
"q-learning",
"reinforcement-learning",
"custom-implementation",
"model-index",
"region:us"
] | null | 2024-05-02T11:06:01+00:00 | [] | [] | TAGS
#Taxi-v3 #q-learning #reinforcement-learning #custom-implementation #model-index #region-us
|
# Q-Learning Agent playing1 Taxi-v3
This is a trained model of a Q-Learning agent playing Taxi-v3 .
## Usage
| [
"# Q-Learning Agent playing1 Taxi-v3\n This is a trained model of a Q-Learning agent playing Taxi-v3 .\n\n ## Usage"
] | [
"TAGS\n#Taxi-v3 #q-learning #reinforcement-learning #custom-implementation #model-index #region-us \n",
"# Q-Learning Agent playing1 Taxi-v3\n This is a trained model of a Q-Learning agent playing Taxi-v3 .\n\n ## Usage"
] | [
26,
31
] | [
"TAGS\n#Taxi-v3 #q-learning #reinforcement-learning #custom-implementation #model-index #region-us \n# Q-Learning Agent playing1 Taxi-v3\n This is a trained model of a Q-Learning agent playing Taxi-v3 .\n\n ## Usage"
] |
null | transformers |
# Model Card for Model ID
<!-- Provide a quick summary of what the model is/does. -->
## Model Details
### Model Description
<!-- Provide a longer summary of what this model is. -->
This is the model card of a 🤗 transformers model that has been pushed on the Hub. This model card has been automatically generated.
- **Developed by:** [More Information Needed]
- **Funded by [optional]:** [More Information Needed]
- **Shared by [optional]:** [More Information Needed]
- **Model type:** [More Information Needed]
- **Language(s) (NLP):** [More Information Needed]
- **License:** [More Information Needed]
- **Finetuned from model [optional]:** [More Information Needed]
### Model Sources [optional]
<!-- Provide the basic links for the model. -->
- **Repository:** [More Information Needed]
- **Paper [optional]:** [More Information Needed]
- **Demo [optional]:** [More Information Needed]
## Uses
<!-- Address questions around how the model is intended to be used, including the foreseeable users of the model and those affected by the model. -->
### Direct Use
<!-- This section is for the model use without fine-tuning or plugging into a larger ecosystem/app. -->
[More Information Needed]
### Downstream Use [optional]
<!-- This section is for the model use when fine-tuned for a task, or when plugged into a larger ecosystem/app -->
[More Information Needed]
### Out-of-Scope Use
<!-- This section addresses misuse, malicious use, and uses that the model will not work well for. -->
[More Information Needed]
## Bias, Risks, and Limitations
<!-- This section is meant to convey both technical and sociotechnical limitations. -->
[More Information Needed]
### Recommendations
<!-- This section is meant to convey recommendations with respect to the bias, risk, and technical limitations. -->
Users (both direct and downstream) should be made aware of the risks, biases and limitations of the model. More information needed for further recommendations.
## How to Get Started with the Model
Use the code below to get started with the model.
[More Information Needed]
## Training Details
### Training Data
<!-- This should link to a Dataset Card, perhaps with a short stub of information on what the training data is all about as well as documentation related to data pre-processing or additional filtering. -->
[More Information Needed]
### Training Procedure
<!-- This relates heavily to the Technical Specifications. Content here should link to that section when it is relevant to the training procedure. -->
#### Preprocessing [optional]
[More Information Needed]
#### Training Hyperparameters
- **Training regime:** [More Information Needed] <!--fp32, fp16 mixed precision, bf16 mixed precision, bf16 non-mixed precision, fp16 non-mixed precision, fp8 mixed precision -->
#### Speeds, Sizes, Times [optional]
<!-- This section provides information about throughput, start/end time, checkpoint size if relevant, etc. -->
[More Information Needed]
## Evaluation
<!-- This section describes the evaluation protocols and provides the results. -->
### Testing Data, Factors & Metrics
#### Testing Data
<!-- This should link to a Dataset Card if possible. -->
[More Information Needed]
#### Factors
<!-- These are the things the evaluation is disaggregating by, e.g., subpopulations or domains. -->
[More Information Needed]
#### Metrics
<!-- These are the evaluation metrics being used, ideally with a description of why. -->
[More Information Needed]
### Results
[More Information Needed]
#### Summary
## Model Examination [optional]
<!-- Relevant interpretability work for the model goes here -->
[More Information Needed]
## Environmental Impact
<!-- Total emissions (in grams of CO2eq) and additional considerations, such as electricity usage, go here. Edit the suggested text below accordingly -->
Carbon emissions can be estimated using the [Machine Learning Impact calculator](https://mlco2.github.io/impact#compute) presented in [Lacoste et al. (2019)](https://arxiv.org/abs/1910.09700).
- **Hardware Type:** [More Information Needed]
- **Hours used:** [More Information Needed]
- **Cloud Provider:** [More Information Needed]
- **Compute Region:** [More Information Needed]
- **Carbon Emitted:** [More Information Needed]
## Technical Specifications [optional]
### Model Architecture and Objective
[More Information Needed]
### Compute Infrastructure
[More Information Needed]
#### Hardware
[More Information Needed]
#### Software
[More Information Needed]
## Citation [optional]
<!-- If there is a paper or blog post introducing the model, the APA and Bibtex information for that should go in this section. -->
**BibTeX:**
[More Information Needed]
**APA:**
[More Information Needed]
## Glossary [optional]
<!-- If relevant, include terms and calculations in this section that can help readers understand the model or model card. -->
[More Information Needed]
## More Information [optional]
[More Information Needed]
## Model Card Authors [optional]
[More Information Needed]
## Model Card Contact
[More Information Needed] | {"library_name": "transformers", "tags": []} | ZahidAhmad/phi2 | null | [
"transformers",
"safetensors",
"arxiv:1910.09700",
"endpoints_compatible",
"region:us"
] | null | 2024-05-02T11:09:44+00:00 | [
"1910.09700"
] | [] | TAGS
#transformers #safetensors #arxiv-1910.09700 #endpoints_compatible #region-us
|
# Model Card for Model ID
## Model Details
### Model Description
This is the model card of a transformers model that has been pushed on the Hub. This model card has been automatically generated.
- Developed by:
- Funded by [optional]:
- Shared by [optional]:
- Model type:
- Language(s) (NLP):
- License:
- Finetuned from model [optional]:
### Model Sources [optional]
- Repository:
- Paper [optional]:
- Demo [optional]:
## Uses
### Direct Use
### Downstream Use [optional]
### Out-of-Scope Use
## Bias, Risks, and Limitations
### Recommendations
Users (both direct and downstream) should be made aware of the risks, biases and limitations of the model. More information needed for further recommendations.
## How to Get Started with the Model
Use the code below to get started with the model.
## Training Details
### Training Data
### Training Procedure
#### Preprocessing [optional]
#### Training Hyperparameters
- Training regime:
#### Speeds, Sizes, Times [optional]
## Evaluation
### Testing Data, Factors & Metrics
#### Testing Data
#### Factors
#### Metrics
### Results
#### Summary
## Model Examination [optional]
## Environmental Impact
Carbon emissions can be estimated using the Machine Learning Impact calculator presented in Lacoste et al. (2019).
- Hardware Type:
- Hours used:
- Cloud Provider:
- Compute Region:
- Carbon Emitted:
## Technical Specifications [optional]
### Model Architecture and Objective
### Compute Infrastructure
#### Hardware
#### Software
[optional]
BibTeX:
APA:
## Glossary [optional]
## More Information [optional]
## Model Card Authors [optional]
## Model Card Contact
| [
"# Model Card for Model ID",
"## Model Details",
"### Model Description\n\n\n\nThis is the model card of a transformers model that has been pushed on the Hub. This model card has been automatically generated.\n\n- Developed by: \n- Funded by [optional]: \n- Shared by [optional]: \n- Model type: \n- Language(s) (NLP): \n- License: \n- Finetuned from model [optional]:",
"### Model Sources [optional]\n\n\n\n- Repository: \n- Paper [optional]: \n- Demo [optional]:",
"## Uses",
"### Direct Use",
"### Downstream Use [optional]",
"### Out-of-Scope Use",
"## Bias, Risks, and Limitations",
"### Recommendations\n\n\n\nUsers (both direct and downstream) should be made aware of the risks, biases and limitations of the model. More information needed for further recommendations.",
"## How to Get Started with the Model\n\nUse the code below to get started with the model.",
"## Training Details",
"### Training Data",
"### Training Procedure",
"#### Preprocessing [optional]",
"#### Training Hyperparameters\n\n- Training regime:",
"#### Speeds, Sizes, Times [optional]",
"## Evaluation",
"### Testing Data, Factors & Metrics",
"#### Testing Data",
"#### Factors",
"#### Metrics",
"### Results",
"#### Summary",
"## Model Examination [optional]",
"## Environmental Impact\n\n\n\nCarbon emissions can be estimated using the Machine Learning Impact calculator presented in Lacoste et al. (2019).\n\n- Hardware Type: \n- Hours used: \n- Cloud Provider: \n- Compute Region: \n- Carbon Emitted:",
"## Technical Specifications [optional]",
"### Model Architecture and Objective",
"### Compute Infrastructure",
"#### Hardware",
"#### Software\n\n\n\n[optional]\n\n\n\nBibTeX:\n\n\n\nAPA:",
"## Glossary [optional]",
"## More Information [optional]",
"## Model Card Authors [optional]",
"## Model Card Contact"
] | [
"TAGS\n#transformers #safetensors #arxiv-1910.09700 #endpoints_compatible #region-us \n",
"# Model Card for Model ID",
"## Model Details",
"### Model Description\n\n\n\nThis is the model card of a transformers model that has been pushed on the Hub. This model card has been automatically generated.\n\n- Developed by: \n- Funded by [optional]: \n- Shared by [optional]: \n- Model type: \n- Language(s) (NLP): \n- License: \n- Finetuned from model [optional]:",
"### Model Sources [optional]\n\n\n\n- Repository: \n- Paper [optional]: \n- Demo [optional]:",
"## Uses",
"### Direct Use",
"### Downstream Use [optional]",
"### Out-of-Scope Use",
"## Bias, Risks, and Limitations",
"### Recommendations\n\n\n\nUsers (both direct and downstream) should be made aware of the risks, biases and limitations of the model. More information needed for further recommendations.",
"## How to Get Started with the Model\n\nUse the code below to get started with the model.",
"## Training Details",
"### Training Data",
"### Training Procedure",
"#### Preprocessing [optional]",
"#### Training Hyperparameters\n\n- Training regime:",
"#### Speeds, Sizes, Times [optional]",
"## Evaluation",
"### Testing Data, Factors & Metrics",
"#### Testing Data",
"#### Factors",
"#### Metrics",
"### Results",
"#### Summary",
"## Model Examination [optional]",
"## Environmental Impact\n\n\n\nCarbon emissions can be estimated using the Machine Learning Impact calculator presented in Lacoste et al. (2019).\n\n- Hardware Type: \n- Hours used: \n- Cloud Provider: \n- Compute Region: \n- Carbon Emitted:",
"## Technical Specifications [optional]",
"### Model Architecture and Objective",
"### Compute Infrastructure",
"#### Hardware",
"#### Software\n\n\n\n[optional]\n\n\n\nBibTeX:\n\n\n\nAPA:",
"## Glossary [optional]",
"## More Information [optional]",
"## Model Card Authors [optional]",
"## Model Card Contact"
] | [
26,
6,
4,
75,
23,
3,
5,
8,
9,
8,
34,
20,
4,
5,
5,
11,
13,
12,
3,
10,
6,
5,
6,
4,
5,
7,
49,
7,
7,
5,
5,
15,
7,
7,
8,
5
] | [
"TAGS\n#transformers #safetensors #arxiv-1910.09700 #endpoints_compatible #region-us \n# Model Card for Model ID## Model Details### Model Description\n\n\n\nThis is the model card of a transformers model that has been pushed on the Hub. This model card has been automatically generated.\n\n- Developed by: \n- Funded by [optional]: \n- Shared by [optional]: \n- Model type: \n- Language(s) (NLP): \n- License: \n- Finetuned from model [optional]:### Model Sources [optional]\n\n\n\n- Repository: \n- Paper [optional]: \n- Demo [optional]:## Uses### Direct Use### Downstream Use [optional]### Out-of-Scope Use## Bias, Risks, and Limitations### Recommendations\n\n\n\nUsers (both direct and downstream) should be made aware of the risks, biases and limitations of the model. More information needed for further recommendations.## How to Get Started with the Model\n\nUse the code below to get started with the model.## Training Details### Training Data### Training Procedure#### Preprocessing [optional]#### Training Hyperparameters\n\n- Training regime:#### Speeds, Sizes, Times [optional]## Evaluation### Testing Data, Factors & Metrics#### Testing Data#### Factors#### Metrics### Results#### Summary## Model Examination [optional]## Environmental Impact\n\n\n\nCarbon emissions can be estimated using the Machine Learning Impact calculator presented in Lacoste et al. (2019).\n\n- Hardware Type: \n- Hours used: \n- Cloud Provider: \n- Compute Region: \n- Carbon Emitted:## Technical Specifications [optional]### Model Architecture and Objective### Compute Infrastructure#### Hardware#### Software\n\n\n\n[optional]\n\n\n\nBibTeX:\n\n\n\nAPA:## Glossary [optional]## More Information [optional]## Model Card Authors [optional]## Model Card Contact"
] |
reinforcement-learning | null |
# **Q-Learning** Agent playing1 **FrozenLake-v1**
This is a trained model of a **Q-Learning** agent playing **FrozenLake-v1** .
## Usage
```python
model = load_from_hub(repo_id="haytamelouarrat/q-FrozenLake-v1-4x4-noSlippery", filename="q-learning.pkl")
# Don't forget to check if you need to add additional attributes (is_slippery=False etc)
env = gym.make(model["env_id"])
```
| {"tags": ["FrozenLake-v1-4x4-no_slippery", "q-learning", "reinforcement-learning", "custom-implementation"], "model-index": [{"name": "q-FrozenLake-v1-4x4-noSlippery", "results": [{"task": {"type": "reinforcement-learning", "name": "reinforcement-learning"}, "dataset": {"name": "FrozenLake-v1-4x4-no_slippery", "type": "FrozenLake-v1-4x4-no_slippery"}, "metrics": [{"type": "mean_reward", "value": "1.00 +/- 0.00", "name": "mean_reward", "verified": false}]}]}]} | haytamelouarrat/q-FrozenLake-v1-4x4-noSlippery | null | [
"FrozenLake-v1-4x4-no_slippery",
"q-learning",
"reinforcement-learning",
"custom-implementation",
"model-index",
"region:us"
] | null | 2024-05-02T11:12:06+00:00 | [] | [] | TAGS
#FrozenLake-v1-4x4-no_slippery #q-learning #reinforcement-learning #custom-implementation #model-index #region-us
|
# Q-Learning Agent playing1 FrozenLake-v1
This is a trained model of a Q-Learning agent playing FrozenLake-v1 .
## Usage
| [
"# Q-Learning Agent playing1 FrozenLake-v1\n This is a trained model of a Q-Learning agent playing FrozenLake-v1 .\n\n ## Usage"
] | [
"TAGS\n#FrozenLake-v1-4x4-no_slippery #q-learning #reinforcement-learning #custom-implementation #model-index #region-us \n",
"# Q-Learning Agent playing1 FrozenLake-v1\n This is a trained model of a Q-Learning agent playing FrozenLake-v1 .\n\n ## Usage"
] | [
35,
33
] | [
"TAGS\n#FrozenLake-v1-4x4-no_slippery #q-learning #reinforcement-learning #custom-implementation #model-index #region-us \n# Q-Learning Agent playing1 FrozenLake-v1\n This is a trained model of a Q-Learning agent playing FrozenLake-v1 .\n\n ## Usage"
] |
text-classification | setfit |
# SetFit with sentence-transformers/multi-qa-mpnet-base-cos-v1
This is a [SetFit](https://github.com/huggingface/setfit) model that can be used for Text Classification. This SetFit model uses [sentence-transformers/multi-qa-mpnet-base-cos-v1](https://huggingface.co/sentence-transformers/multi-qa-mpnet-base-cos-v1) as the Sentence Transformer embedding model. A [SetFitHead](huggingface.co/docs/setfit/reference/main#setfit.SetFitHead) instance is used for classification.
The model has been trained using an efficient few-shot learning technique that involves:
1. Fine-tuning a [Sentence Transformer](https://www.sbert.net) with contrastive learning.
2. Training a classification head with features from the fine-tuned Sentence Transformer.
## Model Details
### Model Description
- **Model Type:** SetFit
- **Sentence Transformer body:** [sentence-transformers/multi-qa-mpnet-base-cos-v1](https://huggingface.co/sentence-transformers/multi-qa-mpnet-base-cos-v1)
- **Classification head:** a [SetFitHead](huggingface.co/docs/setfit/reference/main#setfit.SetFitHead) instance
- **Maximum Sequence Length:** 512 tokens
- **Number of Classes:** 43 classes
<!-- - **Training Dataset:** [Unknown](https://huggingface.co/datasets/unknown) -->
<!-- - **Language:** Unknown -->
<!-- - **License:** Unknown -->
### Model Sources
- **Repository:** [SetFit on GitHub](https://github.com/huggingface/setfit)
- **Paper:** [Efficient Few-Shot Learning Without Prompts](https://arxiv.org/abs/2209.11055)
- **Blogpost:** [SetFit: Efficient Few-Shot Learning Without Prompts](https://huggingface.co/blog/setfit)
### Model Labels
| Label | Examples |
|:------|:---------------------------------------------------------------------------------------------------------------------------------------------------------------------------------------------------------------------------------------------------------------------------------------------------------------------------------------------------------------------------------------------------------------------------------------------------------------------------------------------------------------------------------------------------------------------------------------------------------------------------------------------------------------------------------------------------------------------------------------------------------------------------------------------------------------------------------------------------------------------------------------------------------------------------------------------------------------------------------------------------------------------------------------------------------------------------------------------------------------------------------------------------------------------------------------------------------------------------------------------------------------------------------------------------------------------------------------------------------------------------------------------------------------------------------------------------------------------------------------------------------------------------------------------------------------------------------------------------------------------------------------------------------------------------------------------------------------------------------------------------------------------------------------------------------------------------------------------------------------------------------------------------------------------------------------------------------------------------------------------------------------------------------------------------------------------------------------------------------------------------------------------------------------------------------------------------------------------------------------------------------------------------------------------------------------------------------------------------------------------------------------------------------------------------------------------------------------------------------------------------------------------------------------------------------------------------------------------------------------------------------------------------------------------------------------------------------------------------------------------------------------------------------------------------------------------------------------------------------------------------------------------------------------------------------------------------------------------------------------------------------------------------------------------------------------------------------------------------------------------------------------------------------------------------------------------------------------------------------------------------------------------------------------------------------------------------------------------------------------------------------------------------------------------------------------------------------------------------------------------------------------------------------------------------------------------------------------------------------------------------------------------------------------------------------------------------------------------------------------------------------------------------------------------------------------------------------------------------------------------------------------------------------------------------------------------------------------------------------------------------------------------------------------------------------------------------------------------------------------------------------------------------------------------------------------------------------------------------------------------------------------------------------------------------------------------------------------------------------------------------------------------------------------------------------------------------------------------------------------------------------------------------------------------------------------------------------------------------------------------------------------------------------------------------------------------------------------------------------------------------------------------------------------------------------------------------------------------------------------------------------------------------------------------------------------------------------------------------------------------------------------------------------------------------------------------------------------------------------------------------------------------------------------------------------------------------------------------------------------------------------------------------------------------------------------------------------------------------------------------------------------------------------------------------------------------------------------------------------------------------------------------------------------------------------------------------------------------------------------------------------------------------------------------------------------------------------------------------------------------------------------------------------------------------------------------------------------------------------------------------------------------------------------------------------------------------------------------------------------------------------------------------------------------------------------------------------------------------------------------------------------------------------------------------------------------------------------------------------------------------------------------------------------------------------------------------------------------------------------------------------------------------------------------------------------------------------------------------------------------------------------------------------------------------------------------------------------------------------------------------------------------------------------------------------------------------------------------------------------------------------------------------------------------------------------------------------------------------------------------------------------------------------------------------------------------------------------------------------------------------------------------------------------------------------------------------------------------------------------------------------------------------------------------------------------------------------------------------------------------------------------------------------------------------------------------------------------------------------------------------------------------------------------------------------------------------------------------------------------------------------------------------------------------------------------------------------------------------------------------------------------------------------------------------------------------------------------------------------------------------------------------------------------------------------------------------------------------------------------------------------------------------------------------------------------------------------------------------------------------------------------------------------------------------------------------------------------------------------------------------------------------------------------------------------------------------------------------------------------------------------------------------------------------------------------------------------------------------------------------------------------------------------------------------------------------------------------------------------------------------------------------------------------------------------------------------------------------------------------------------------------------------------------------------------------------------------------------------------------------------------------------------------------------------------------------------------------------------------------------|
| 20 | <ul><li>'##les approach which combined geography history and the sociological approaches of the annee sociologique many members of which were their colleagues at strasbourg to produce an approach which rejected the predominant emphasis on politics diplomacy and war of many 19th and early 20thcentury historians as spearheaded by historians whom febvre called les sorbonnistes instead they pioneered an approach to a study of longterm historical structures la longue duree over events and political transformations geography material culture and what later annalistes called mentalites or the psychology of the epoch are also characteristic areas of study the goal of the annales was to undo the work of the sorbonnistes to turn french historians away from the narrowly political and diplomatic toward the new vistas in social and economic historycofounder marc bloch 1886 – 1944 was a quintessential modernist who studied at the elite ecole normale superieure and in germany serving as a professor at the university of strasbourg until he was called to the sorbonne in paris in 1936 as professor of economic history blochs interests were highly interdisciplinary influenced by the geography of paul vidal de la blache 1845 – 1918 and the sociology of emile durkheim 1858 – 1917 his own ideas especially those expressed in his masterworks french rural history les caracteres originaux de lhistoire rurale francaise 1931 and feudal society were incorporated by the secondgeneration annalistes led by fernand braudel georges duby a leader of the school wrote that the history he taught relegated the sensational to the sidelines and was reluctant to give a simple accounting of events but strove on the contrary to pose and solve problems and neglecting surface disturbances to observe the long and mediumterm evolution of economy society and civilisationthe annalistes especially lucien febvre advocated a histoire totale or histoire tout court a complete study of a historic problem bloch was shot by the gestapo during the german occupation of france in world war ii for his active membership of the french resistance and febvre carried on the annales approach in the 1940s and 1950s it was during this time that he mentored braudel who would become one of the bestknown exponents of this school braudels work came to define a second era of annales historiography and was very influential throughout the 1960s and 1970s especially for his work on the mediterranean region in the era of philip ii of spain braudel developed the idea often associated with annalistes of different modes of historical time lhistoire quasi immobile the quasi motionless history of historical'</li><li>'is important because the persuasiveness of a source usually depends upon its history primary sources may include cases constitutions statutes administrative regulations and other sources of binding legal authority while secondary legal sources may include books the headnotes of case reports articles and encyclopedias legal writers usually prefer to cite primary sources because only primary sources are authoritative and precedential while secondary sources are only persuasive at best family history a secondary source is a record or statement of an event or circumstance made by a noneyewitness or by someone not closely connected with the event or circumstances recorded or stated verbally either at or sometime after the event or by an eyewitness at a time after the event when the fallibility of memory is an important factor consequently according to this definition a firsthand account written long after the event when the fallibility of memory is an important factor is a secondary source even though it may be the first published description of that event autobiographies an autobiography can be a secondary source in history or the humanities when used for information about topics other than its subject for example many firsthand accounts of events in world war i written in the postwar years were influenced by the then prevailing perception of the war which was significantly different from contemporary opinion original research jules r benjamin a students guide to history 2013 isbn 9781457621444 edward h carr what is history basingstoke palgrave 2001 isbn 9780333977019 wood gray historians handbook a key to the study and writing of history prospect heights il waveland press 1991 ©1964 isbn 9780881336269 derek harland a basic course in genealogy volume two research procedure and evaluation of evidence bookcraft inc 1958 worldcat record richard holmes tommy harpercollins 2004 isbn 9780007137510 martha c howell and walter prevenier from reliable sources an introduction to historical methods 2001 isbn 9780801435737 richard a marius and melvin e page a short guide to writing about history 8th edition 2012 isbn 9780205118601 hayden white metahistory the historical imagination in nineteenthcentury europe baltimore johns hopkins university press 1973 isbn 9780801814693'</li><li>'have a meticulous approach to reconstructing the costumes or material culture of past eras but who are perceived to lack much understanding of the cultural values and historical contexts of the periods in question a college or society of antiquaries was founded in london in c 1586 to debate matters of antiquarian interest members included william camden sir robert cotton john stow william lambarde richard carew and others this body existed until 1604 when it fell under suspicion of being political in its aims and was abolished by king james i papers read at their meetings are preserved in cottons collections and were printed by thomas hearne in 1720 under the title a collection of curious discourses a second edition appearing in 1771 in 1707 a number of english antiquaries began to hold regular meetings for the discussion of their hobby and in 1717 the society of antiquaries was formally reconstituted finally receiving a charter from king george ii in 1751 in 1780 king george iii granted the society apartments in somerset house and in 1874 it moved into its present accommodation in burlington house piccadilly the society was governed by a council of twenty and a president who is ex officio a trustee of the british museum the society of antiquaries of scotland was founded in 1780 and had the management of a large national antiquarian museum in edinburgh the society of antiquaries of newcastle upon tyne the oldest provincial antiquarian society in england was founded in 1813 in ireland a society was founded in 1849 called the kilkenny archaeological society holding its meetings at kilkenny in 1869 its name was changed to the royal historical and archaeological association of ireland and in 1890 to the royal society of antiquaries of ireland its office being transferred to dublin in france the societe des antiquaires de france was formed in 1813 by the reconstruction of the academie celtique which had existed since 1804 the american antiquarian society was founded in 1812 with its headquarters at worcester massachusetts in modern times its library has grown to over 4 million items and as an institution it is internationally recognized as a repository and research library for early pre1876 american printed materials in denmark the kongelige nordiske oldskriftselskab also known as la societe royale des antiquaires du nord or the royal society of northern antiquaries was founded at copenhagen in 1825 in germany the gesamtverein der deutschen geschichts und altertumsvereine was founded in 1852in addition a number of local historical and archaeological societies have adopted the word antiquarian in their titles these have included the cambridge antiquarian society'</li></ul> |
| 42 | <ul><li>'been described as the worlds largest repository of covid19 sequences and by far the worlds largest database of sarscov2 sequences by midapril 2021 gisaids sarscov2 database reached over 1200000 submissions a testament to the hard work of researchers in over 170 different countries only three months later the number of uploaded sarscov2 sequences had doubled again to over 24 million by late 2021 the database contained over 5 million genome sequences as of december 2021 over 6 million sequences had been submitted by april 2022 there were 10 million sequences accumulated and in january 2023 the number had reached 144 millionin january 2020 the sarscov2 genetic sequence data was shared through gisaid throughout the first year of the covid19 pandemic most of the sarscov2 wholegenome sequences that were generated and shared globally were submitted through gisaid when the sarscov2 omicron variant was detected in south africa by quickly uploading the sequence to gisaid the national institute for communicable diseases there was able to learn that botswana and hong kong had also reported cases possessing the same gene sequencein march 2023 gisaid temporarily suspended database access for some scientists removing raw data relevant to investigations of the origins of sarscov2 gisaid stated that they do not delete records from their database but data may become temporarily invisible during updates or corrections availability of the data was restored with an additional restriction that any analysis based thereon would not be shared with the public the board of friends of gisaid consists of peter bogner and two german lawyers who are not involved in the daytoday operations of the organisation scientific advice to the organization is provided by its scientific advisory council including directors of leading public health laboratories such as who collaborating centres for influenza in 2023 gisaids lack of transparency was criticized by some gisaid funders including the european commission and the rockefeller foundation with longterm funding being denied from international federation of pharmaceutical manufacturers and associations ifpma in june 2023 it was reported in vanity fair that bogner had said that gisaid will soon launch an independent compliance board responsible for addressing a wide range of governance matters the telegraph similarly reported that gisaids inhouse counsel was developing new governance processes intended to be transparent and allow for the resolution of scientific disputes without the involvement of bogner the creation of the gisaid database was motivated in part by concerns raised by researchers from developing countries with scientific american noting in 2009 that that a previous datasharing system run by who forced them to give up intellectual'</li><li>'viruses can be named based on the antibodies they react with the use of the antibodies which were once exclusively derived from the serum blood fluid of animals is called serology once an antibody – reaction has taken place in a test other methods are needed to confirm this older methods included complement fixation tests hemagglutination inhibition and virus neutralisation newer methods use enzyme immunoassays eiain the years before pcr was invented immunofluorescence was used to quickly confirm viral infections it is an infectivity assay that is virus species specific because antibodies are used the antibodies are tagged with a dye that is luminescencent and when using an optical microscope with a modified light source infected cells glow in the dark pcr is a mainstay method for detecting viruses in all species including plants and animals it works by detecting traces of virus specific rna or dna it is very sensitive and specific but can be easily compromised by contamination most of the tests used in veterinary virology and medical virology are based on pcr or similar methods such as transcription mediated amplification when a novel virus emerges such as the covid coronavirus a specific test can be devised quickly so long as the viral genome has been sequenced and unique regions of the viral dna or rna identified the invention of microfluidic tests as allowed for most of these tests to be automated despite its specificity and sensitivity pcr has a disadvantage in that it does not differentiate infectious and noninfectious viruses and tests of cure have to be delayed for up to 21 days to allow for residual viral nucleic acid to clear from the site of the infection in laboratories many of the diagnostic test for detecting viruses are nucleic acid amplification methods such as pcr some tests detect the viruses or their components as these include electron microscopy and enzymeimmunoassays the socalled home or selftesting gadgets are usually lateral flow tests which detect the virus using a tagged monoclonal antibody these are also used in agriculture food and environmental sciences counting viruses quantitation has always had an important role in virology and has become central to the control of some infections of humans where the viral load is measured there are two basic methods those that count the fully infective virus particles which are called infectivity assays and those that count all the particles including the defective ones infectivity assays measure the amount concentration of infective viruses in a sample of known volume for host cells plants or cultures of bacterial or animal cells are used laboratory animals such as mice'</li><li>'vpx is a virionassociated protein encoded by human immunodeficiency virus type 2 hiv2 and most simian immunodeficiency virus siv strains but that is absent from hiv1 it is similar in structure to the protein vpr that is carried by siv and hiv2 as well as hiv1 vpx is one of five accessory proteins vif vpx vpr vpu and nef carried by lentiviruses that enhances viral replication by inhibiting host antiviral factorsvpx enhances hiv2 replication in humans by counteracting the host factor samhd1 samhd1 is a host factor found in human myeloid cells such as dendritic cells and macrophages that restricts hiv1 replication by depleting the cytoplasmic pool of deoxynucleoside triphosphates needed for viral dna production samhd1 does not however restrict hiv2 replication in myeloid cells due to the presence of viral vpx vpx counteracts restriction by inducing the ubiquitinproteasomedependent degradation of samhd1 vpxmediated degradation of samhd1 therefore decreases deoxynucleoside triphosphate hydrolysis thereby increasing the availability of dntps for viral reverse transcription in the cytoplasm it has been postulated that samhd1 degradation is required for hiv2 replication because the hiv2 reverse transcriptase rt is less active than the hiv1 rt which would be the reason for the absence of vpx from hiv1 because vpx is required for hiv2 reverse transcription and the early stages of the viral life cycle it is packaged into virions in significant amountsvpx is also involved in the nuclear import of the hiv2siv genomes and associated proteins but the specific mechanisms and interactions are currently unknown although vpr and vpx are similar in size both are 100 amino acids with 2025 sequence similarity and structure both are predicted to have similar tertiary structure with three major helices they serve very different roles in viral replication vpx targets a host restriction factor for proteasomal degradation while vpr arrests the host cell cycle in the g2 phase however they are both involved in the import of the viral preintegration complex into the host nucleus'</li></ul> |
| 19 | <ul><li>'##es insulin blood glucose from the portal vein enters liver cells hepatocytes insulin acts on the hepatocytes to stimulate the action of several enzymes including glycogen synthase glucose molecules are added to the chains of glycogen as long as both insulin and glucose remain plentiful in this postprandial or fed state the liver takes in more glucose from the blood than it releases after a meal has been digested and glucose levels begin to fall insulin secretion is reduced and glycogen synthesis stops when it is needed for energy glycogen is broken down and converted again to glucose glycogen phosphorylase is the primary enzyme of glycogen breakdown for the next 8 – 12 hours glucose derived from liver glycogen is the primary source of blood glucose used by the rest of the body for fuel glucagon another hormone produced by the pancreas in many respects serves as a countersignal to insulin in response to insulin levels being below normal when blood levels of glucose begin to fall below the normal range glucagon is secreted in increasing amounts and stimulates both glycogenolysis the breakdown of glycogen and gluconeogenesis the production of glucose from other sources muscle glycogen appears to function as an immediate reserve source of available phosphorylated glucose in the form of glucose1phosphate for muscle cells glycogen contained within skeletal muscle cells are primarily in the form of β particles other cells that contain small amounts use it locally as well as muscle cells lack glucose6phosphatase which is required to pass glucose into the blood the glycogen they store is available solely for internal use and is not shared with other cells this is in contrast to liver cells which on demand readily do break down their stored glycogen into glucose and send it through the blood stream as fuel for other organsskeletal muscle needs atp provides energy for muscle contraction and relaxation in what is known as the sliding filament theory skeletal muscle relies predominantly on glycogenolysis for the first few minutes as it transitions from rest to activity as well as throughout highintensity aerobic activity and all anaerobic activity during anaerobic activity such as weightlifting and isometric exercise the phosphagen system atppcr and muscle glycogen are the only substrates used as they do not require oxygen nor blood flowdifferent bioenergetic systems produce atp at different speeds with atp produced'</li><li>'glycogen storage disease type i gsd i is an inherited disease that prevents the liver from properly breaking down stored glycogen which is necessary to maintain adequate blood sugar levels gsd i is divided into two main types gsd ia and gsd ib which differ in cause presentation and treatment there are also possibly rarer subtypes the translocases for inorganic phosphate gsd ic or glucose gsd id however a recent study suggests that the biochemical assays used to differentiate gsd ic and gsd id from gsd ib are not reliable and are therefore gsd ibgsd ia is caused by a deficiency in the enzyme glucose6phosphatase gsd ib a deficiency in the transport protein glucose6phosphate translocase because glycogenolysis is the principal metabolic mechanism by which the liver supplies glucose to the body during fasting both deficiencies cause severe hypoglycemia and over time excess glycogen storage in the liver and in some cases in the kidneys because of the glycogen buildup gsd i patients typically present with enlarged livers from nonalcoholic fatty liver disease other functions of the liver and kidneys are initially intact in gsd i but are susceptible to other problems without proper treatment gsd i causes chronic low blood sugar which can lead to excessive lactic acid and abnormally high lipids in the blood and other problems frequent feedings of cornstarch or other carbohydrates are the principal treatment for all forms of gsd i gsd ib also features chronic neutropenia due to a dysfunction in the production of neutrophils in the bone marrow this immunodeficiency if untreated makes gsd ib patients susceptible to infection the principal treatment for this feature of gsd ib is filgrastim however patients often still require treatment for frequent infections and a chronically enlarged spleen is a common side effect gsd ib patients often present with inflammatory bowel diseaseit is the most common of the glycogen storage diseases gsd i has an incidence of approximately 1 in 100000 births in the american population and approximately 1 in 20000 births among ashkenazi jews the disease was named after german doctor edgar von gierke who first described it in 1929 early research into gsd i identified numerous clinical manifestations falsely thought to be primary features of the genetic disorder however continuing research has revealed that these clinical features are the consequences of only one in gsd ia or two in gsd ib'</li><li>'##patic arteries and threaded through the gastroduodenal mostly or celiac artery the catheter is fixed in this position and the pump is placed in a subcutaneous pocket finally to confirm adequate placement and hepatic perfusion and to rule out extrahepatic perfusion a dye fluorescein or methylene blue is injected into the pump after the procedure and before starting the hai based treatment a technetium 99mlabeled macroaggregated albumin scan is performed to again confirm adequate hepatic perfusion and no misperfusion outside of the liver the complications of hai therapy can be divided into those related to the surgical placement of the pump technical catheterrelated complications and those related to the chemotherapeutic agents usedrelating to the surgical hai pump placement early postoperative complications consist of arterial injury leading to hepatic artery thrombosis inadequate perfusion of the entire liver due to the inability to identify an accessory hepatic artery extrahepatic perfusion to the stomach or duodenum or hematoma formation in the subcutaneous pump pocket late complications are more common and include inflammation or ulceration of the stomach or duodenum and pump pocket infectionthe most common catheter related complications include displacement of the catheter occlusion of the hepatic artery because of the catheter and catheter thrombosis these catheter related complications dont occur as frequently with increased surgical experience and with improvements in pump designthe most common toxicities caused by the chemotherapeutic agents were gastrointestinal symptoms chemical hepatitis and bone marrow inhibition it is important to note that the most serious and dose limiting complication of hai is hepatobiliary toxicity this occurs more commonly with fudr than any other chemotherapeutic agent patients undergoing hai therapy therefore have regular liver function tests to monitor any damage to the liver as previously mentioned studies have been carried out to come up with treatment algorithms to minimize this serious side effect it has been shown that adding leucovorin and fudr for infusion through the pump not only reduces the biliary toxicity of the drug but also increases the response rate however biliary sclerosis is not seen with hai using 5fu 5fu is associated with an increased risk of myelosuppression logically it would make sense to therefore consider alternating between hai fudr and hai 5fu'</li></ul> |
| 11 | <ul><li>'and arms within the cranium the two vertebral arteries fuse into the basilar artery posterior inferior cerebellar artery pica basilar artery supplies the midbrain cerebellum and usually branches into the posterior cerebral artery anterior inferior cerebellar artery aica pontine branches superior cerebellar artery sca posterior cerebral artery pca posterior communicating artery the venous drainage of the cerebrum can be separated into two subdivisions superficial and deep the superficial systemthe superficial system is composed of dural venous sinuses sinuses channels within the dura mater the dural sinuses are therefore located on the surface of the cerebrum the most prominent of these sinuses is the superior sagittal sinus which is located in the sagittal plane under the midline of the cerebral vault posteriorly and inferiorly to the confluence of sinuses where the superficial drainage joins with the sinus that primarily drains the deep venous system from here two transverse sinuses bifurcate and travel laterally and inferiorly in an sshaped curve that forms the sigmoid sinuses which go on to form the two jugular veins in the neck the jugular veins parallel the upward course of the carotid arteries and drain blood into the superior vena cava the veins puncture the relevant dural sinus piercing the arachnoid and dura mater as bridging veins that drain their contents into the sinus the deep venous systemthe deep venous system is primarily composed of traditional veins inside the deep structures of the brain which join behind the midbrain to form the great cerebral vein vein of galen this vein merges with the inferior sagittal sinus to form the straight sinus which then joins the superficial venous system mentioned above at the confluence of sinuses cerebral blood flow cbf is the blood supply to the brain in a given period of time in an adult cbf is typically 750 millilitres per minute or 15 of the cardiac output this equates to an average perfusion of 50 to 54 millilitres of blood per 100 grams of brain tissue per minute cbf is tightly regulated to meet the brains metabolic demands too much blood a clinical condition of a normal homeostatic response of hyperemia can raise intracranial pressure icp which can compress and damage delicate brain tissue too little blood flow ischemia results if blood flow to the brain is below 18 to 20 ml per 100 g per minute and tissue death occurs if flow dips below 8 to'</li><li>'##ie b infection it is mostly unnecessary for treatment purposes to diagnose which virus is causing the symptoms in question though it may be epidemiologically useful coxsackie b infections usually do not cause serious disease although for newborns in the first 1 – 2 weeks of life coxsackie b infections can easily be fatal the pancreas is a frequent target which can cause pancreatitiscoxsackie b3 cb3 infections are the most common enterovirus cause of myocarditis and sudden cardiac death cb3 infection causes ion channel pathology in the heart leading to ventricular arrhythmia studies in mice suggest that cb3 enters cells by means of tolllike receptor 4 both cb3 and cb4 exploit cellular autophagy to promote replication the b4 coxsackie viruses cb4 serotype was suggested to be a possible cause of diabetes mellitus type 1 t1d an autoimmune response to coxsackie virus b infection upon the islets of langerhans may be a cause of t1dother research implicates strains b1 a4 a2 and a16 in the destruction of beta cells with some suggestion that strains b3 and b6 may have protective effects via immunological crossprotection as of 2008 there is no wellaccepted treatment for the coxsackie b group of viruses palliative care is available however and patients with chest pain or stiffness of the neck should be examined for signs of cardiac or central nervous system involvement respectively some measure of prevention can usually be achieved by basic sanitation on the part of foodservice workers though the viruses are highly contagious care should be taken in washing ones hands and in cleaning the body after swimming in the event of coxsackieinduced myocarditis or pericarditis antiinflammatories can be given to reduce damage to the heart muscle enteroviruses are usually only capable of acute infections that are rapidly cleared by the adaptive immune response however mutations which enterovirus b serotypes such as coxsackievirus b and echovirus acquire in the host during the acute phase can transform these viruses into the noncytolytic form also known as noncytopathic or defective enterovirus this form is a mutated quasispecies of enterovirus which is capable of causing persistent infection in human tissues and such infections have been found in the pancreas in type 1 diabetes in chronic myocarditis and dilated cardiomyopathy in valvular'</li><li>'the biomedical research center brc is a research center at qatar university focusing on biomedical research brc was founded in 2014 and partners with the ministry of public health qatar and hamad medical corporation hmc the incidence of genetic disorders in qatar is high with the top three causes of death in the country cancer heart diseases and diabetes the government saw the creation of brc as a strategy for proactively preventing diseases to foster public healthbrc labs received the isoiec 17025 accreditation from the american association for laboratory accreditation a2la the centres research activities focus on the domains of infectious diseases virology and microbiology metabolic disorders and biomedical omics since its inauguration in 2014 brc researchers have published research papers with more than 530 publicationsthe centres research projects include antibiotic profiling of antibiotics resistant microbes in humans and animals one health approach identified for the first time the reason of why some obese people gets type2 diabetes while others do not conducted six research on covid19 to assist in fighting and recovery provided a study on protection against the omicron variant in qatar decoded the genetic code of qatari falcons and various endangered animal species dna sequence of the dugong sea cow study a nanomedicinebased preventative strategy to controlling diseases and improve health brc introduced the use of zebrafish as an animal model in biomedical research at qu and established a facility for it in 2015 the facility is used as a research unit to study many genetic diseases therefore ministry of public health qatar clearly articulated an institutional research policy irp on human use of zebrafish in research and qu circulated it to qu community for implementation the brc facilities include biosafety level 3 bsl3 built by certek usa it is equipped for viral and bacterial research on risk group 3 pathogens sequencing unit to conduct stateoftheart research in genomics mariam al maadeed sidra medical and research center'</li></ul> |
| 17 | <ul><li>'and rainfall there are many ways to date a core once dated it gives valuable information about changes of climate and terrain for example cores in the ocean floor soil and ice have altered the view of the geologic history of the pleistocene entirely reverse circulation drilling is a method in which rock cuttings are continuously extracted through the hollow drill rod and can be sampled for analysis the method may be faster and use less water than core drilling but does not produce cores of relatively undisturbed material so less information on the rock structure can be derived from analysis if compressed air is used for cutting extraction the sample remains uncontaminated is available almost immediately and the method has a low environmental impact core drill ice core integrated ocean drilling program scientific drilling'</li><li>'##cial environments tend to be found in higher latitudes since there is more land at these latitudes in the north most of this effect is seen in the northern hemisphere however in lower latitudes the direct effect of the suns radiation is greater so the freezethaw effect is seen but permafrost is much less widespread altitude – air temperature drops by approximately 1 °c for every 100 m rise above sea level this means that on mountain ranges modern periglacial conditions are found nearer the equator than they are lower down ocean currents – cold surface currents from polar regions reduce mean average temperatures in places where they exert their effect so that ice caps and periglacial conditions will show nearer to the equator as in labrador for example conversely warm surface currents from tropical seas increases mean temperatures the cold conditions are then found only in more northerly places this is apparent in western north america which is affected by the north pacific current in the same way but more markedly the gulf stream affects western europe continentality – away from the moderating influence of the ocean seasonal temperature variation is more extreme and freezethaw goes deeper in the centres of canada and siberia the permafrost typical of periglaciation goes deeper and extends further towards the equator similarly solifluction associated with freezethaw extends into somewhat lower latitudes than on western coasts periglaciation results in a variety of ground conditions but especially those involving irregular mixed deposits created by ice wedges solifluction gelifluction frost creep and rockfalls periglacial environments trend towards stable geomorphologies coombe and head deposits – coombe deposits are chalk deposits found below chalk escarpments in southern england head deposits are more common below outcrops of granite on dartmoor patterned ground – patterned ground occurs where stones form circles polygons and stripes local topography affects which of these are expressed a process called frost heaving is responsible for these features solifluction lobes – solifluction lobes are formed when waterlogged soil slips down a slope due to gravity forming u shaped lobes blockfields or felsenmeer – blockfields are areas covered by large angular blocks traditionally believed to have been created by freezethaw action a good example of a blockfield can be found in the snowdonia national park wales blockfields are common in the unglaciated parts of the appalachian mountains in the northeastern united states such as at the river of rocks or hickory run boulder field lehigh county pennsylvaniaother landforms include bratschen palsa periglacial lake pingo'</li><li>'climate was cooler during the overarching little ice age than it is today ice cores scientists have studied the chemical composition of ice cores long tubes of ice that are drilled from glaciers and ice sheets to learn of past climate conditions tree rings the width of tree rings can be used to reconstruct past climate conditions as trees grow more slowly in cooler temperatures tree ring data from the little ice age seems to prove a reduction in solar activityoverall the evidence suggests that the amount of solar radiation reaching the earths surface was slightly lower during the grindelwald fluctuation and this reduction in solar radiation is thought to have contributed to the expansion of the glaciers human activities such as deforestation and land use changes are known to negatively affect local climate patterns william ruddiman a palaeoclimatologist proposed the hypothesis that human activity has been affecting the earths climate for much longer than previously thought in particular ruddiman has argued that the early adoption of agriculture and landuse practices by human societies beginning around 8000 years ago led to the release of significant amounts of greenhouse gases into the atmosphere which may have contributed to the warming of the earths climateit is difficult to accurately assess the extent of depopulation that occurred during both the 1500s and 1600s as reliable population data from this period is limited however it is known that this period was one of significant upheaval and change with many regions experiencing significant population drops due to wars plagues famines and natural disasters the bubonic plague for instance killed between 75 and 200 million people in europe alone it is also believed that an onset of disease during the little ice age may have led to further depopulationthis decline in population meant that cultivated lands became unkempt allowing for the regrowth of wild plants this is perceived to be the cause for the drop in atmospheric carbon dioxide in the sixteenth century thus exacerbating the extreme cooling period however of the causes depopulation is the least significant in historical records the grindelwald fluctuation is characterised by a further drop in temperatures and more frequent cold spells throughout many parts of the world the more notable records written by a jacobean weather enthusiast in bristol chronicle some of the effects the weather fluctuation had on agriculture and society they specifically discuss food shortages and crop failures taking precedence throughout the area'</li></ul> |
| 14 | <ul><li>'needle aspiration fna biopsy can be fast and least painful a very thin hollow needle and slight suction will be used to remove a small sample from under the nipple using a local anesthetic to numb the skin may not be necessary since a thin needle is used for the biopsy receiving an injection to prevent pain from the biopsy may be more painful than the biopsy itselfsome men develop a condition known as gynecomastia in which the breast tissue under the nipple develops and grows discharge from the nipple can occur the nipple may swell in some men possibly due to increased levels of estrogen changes in appearance may be normal or related to disease inverted nipples – this is normal if the nipples have always been indented inward and can easily point out when touched if the nipples are pointing in and this is new this is an unexpected change skin puckering of the nipple – this can be caused by scar tissue from surgery or an infection often scar tissue forms for no reason most of the time this issue does not need treatment this is an unexpected change this change can be of concern since puckering or retraction of the nipple can indicate an underlying change in breast tissue that may be cancerous the nipple is warm to the touch red or painful – this can be an infection it is rarely due to breast cancer scaly flaking or itchy nipple – this is most often due to eczema or a bacterial or fungal infection this change is not expected flaking scaly or itchy nipples can be a sign of pagets disease thickened skin with large pores – this is called peau dorange because the skin looks like an orange peel an infection in the breast or inflammatory breast cancer can cause this problem this is not an expected change retracted nipples – the nipple was raised above the surface but changes begins to pull inward and does not come out when stimulatedthe average projection and size of human female nipples is slightly more than 3⁄8 inch 95 mm symptoms of breast cancer can often be seen first by changes of the nipple and areola although not all women have the same symptoms and some people do not have any signs or symptoms at all a person may find out they have breast cancer after a routine mammogram warning signs can include new lump in the nipple or breast or armpit thickening or swelling of part of the breast areola or nipple irritation or dimpling of breast skin redness or flaky skin in the nipple area or the breast pulling in of the nipple or pain in the nipple area nipple discharge other than breast milk including blood any change'</li><li>'the mother over the chorion frondosum this part of the endometrium is called the decidua basalis forms the decidual plate the decidual plate is tightly attached to the chorion frondosum and goes on to form the actual placenta endometrium on the opposite side to the decidua basalis is the decidua parietalis this fuses with the chorion laevae thus filling up the uterine cavityin the case of twins dichorionic placentation refers to the presence of two placentas in all dizygotic and some monozygotic twins monochorionic placentation occurs when monozygotic twins develop with only one placenta and bears a higher risk of complications during pregnancy abnormal placentation can lead to an early termination of pregnancy for example in preeclampsia as placentation often results during the evolution of live birth the more than 100 origins of live birth in lizards and snakes squamata have seen close to an equal number of independent origins of placentation this means that the occurrence of placentation in squamata is more frequent than in all other vertebrates combined making them ideal for research on the evolution of placentation and viviparity itself in most squamates two separate placentae form utilising separate embryonic tissue the chorioallantoic and yolksac placentae in species with more complex placentation we see regional specialisation for gas amino acid and lipid transport placentae form following implantation into uterine tissue as seen in mammals and formation is likely facilitated by a plasma membrane transformationmost reptiles exhibit strict epitheliochorial placentation eg pseudemoia entrecasteauxii however at least two examples of endotheliochorial placentation have been identified mabuya sp and trachylepis ivensi unlike eutherian mammals epitheliochorial placentation is not maintained by maternal tissue as embryos do not readily invade tissues outside of the uterus the placenta is an organ that has evolved multiple times independently evolved relatively recently in some lineages and exists in intermediate forms in living species for these reasons it is an outstanding model to study the evolution of complex organs in animals research into the genetic mechanisms that underpin the evolution of the placenta have been conducted in a diversity of animals including reptiles seahorses and mammalsthe genetic processes that support the evolution of the placenta can be best understood by separating those that result'</li><li>'the myometrium once these cells penetrate through the first few layers of cells of the decidua they lose their ability to proliferate and become invasive this departure from the cell cycle seems to be due to factors such as tgfβ and decorin although these invasive interstitial cytotrophoblasts can no longer divide they retain their ability to form syncytia multinucleated giant cells small syncytia are found in the placental bed and myometrium as a result of the fusion of interstitial cytotrophoblastsinterstitial cytotrophoblasts may also transform into endovascular cytotrophoblasts the primary function of the endovascular cytotrophoblast is to penetrate maternal spiral arteries and route the blood flow through the placenta for the growing embryo to use they arise from interstitial cytotrophoblasts from the process of phenocopying this changes the phenotype of these cells from epithelial to endothelial endovascular cytotrophoblasts like their interstitial predecessor are nonproliferating and invasive proper cytotrophoblast function is essential in the implantation of a blastocyst after hatching the embryonic pole of the blastocyst faces the uterine endometrium once they make contact the trophoblast begins to rapidly proliferate the cytotrophoblast secretes proteolytic enzymes to break down the extracellular matrix between the endometrial cells to allow fingerlike projections of trophoblast to penetrate through projections of cytotrophoblast and syncytiotrophoblast pull the embryo into the endometrium until it is fully covered by endometrial epithelium save for the coagulation plug the most common associated disorder is preeclampsia affecting approximately 7 of all births it is characterized by a failure of the cytotrophoblast to invade the uterus and its vasculature specifically the spiral arteries that the endovascular cytotrophoblast should invade the result of this is decreased blood flow to the fetus which may cause intrauterine growth restriction clinical symptoms of preeclampsia in the mother are most commonly high blood pressure proteinuria and edema conversely if there is too much invasion of uterine tissue by the trophoblast then'</li></ul> |
| 36 | <ul><li>'to some decision or course of action socrates great myth illustrates this motif most clearly when the soul is depicted as a charioteer and its horses being led around a heavenly circuit this is the occasion for the first appearance in platos dialogues of the prominent platonic doctrine that life is motion the soul being the principle or source of life is that which moves itself as opposed to inanimate objects that require an external source of motion to move them the view that life is selfmotion and that the soul is a selfmover is used by plato to guarantee the immortality of the soul making this a novel argument for the souls immortality not found in the phaedo plato relies further on the view that the soul is a mind in order to explain how its motions are possible plato combines the view that the soul is a selfmover with the view that the soul is a mind in order to explain how the soul can move things in the first place eg how it can move the body to which it is attached in life souls move things by means of their thoughts in thomas manns novella death in venice the narrators young love tadzio is associated with phaedrus in mary renaults 1953 novel the charioteer a text of phaedrus is passed among the characters gay men during world war ii and the image of the charioteer and his white and black horses recurs as the protagonist struggles to choose between consummated and unconsummated love in a key scene from the film adaptation of maurice students including maurice attend dean cornwalliss translation class in which two undergraduates orally translate into english the text based on phaedrus stephanus 251a 255a – e during which the dean instructs one to omit the reference to the unspeakable vice of the greeks the 2016 film knight of cups by terrence malick is inspired in part by phaedrus in robert m pirsigs fictionalized autobiographical novel zen and the art of motorcycle maintenance pirsig refers to his past self from before undergoing electroconvulsive therapy in the third person and using the name phaedrus intended to reflect his opposition to certain educational and philosophical ideas the character reappears in the followup lila an inquiry into morals in virginia woolfs 1922 novel jacobs room jacob reads phaedrus alone in his room after a visit to the enormous mind as woolf characterizes the british museum jowett translation at standardebooks greek text at perseus plato nichols j h tr and ed phaedrus cornell university press'</li><li>'other lacks so much the betterthe first two of young becker and pikes four phases of written rogerian argument are based on the first two of rapoports three principles of ethical debate the third of rapoports principles — increasing the perceived similarity between self and other — is a principle that young becker and pike considered to be equally as important as the other two but they said it should be an attitude assumed throughout the discourse and is not a phase of writingmaxine hairston in a section on rogerian or nonthreatening argument in her textbook a contemporary rhetoric advised that one shouldnt start writing with a detailed plan in mind but might start by making four lists the others concerns ones own key points anticipated problems and points of agreement or common ground she gave a different version of young becker and pikes four phases which she expanded to five and called elements of the nonthreatening argument a brief and objective statement of the issue a neutrally worded analysis of the others position a neutrally worded analysis of ones own position a statement of the common aspects goals and values that the positions share and a proposal for resolving the issue that shows how both sides may gain she said that the rogerian approach requires calm patience and effort and will work if one is more concerned about increasing understanding and communication than about scoring a triumph in a related article she noted the similarity between rogerian argument and john stuart mills wellknown phrase from on liberty he who knows only his own side of the case knows little of thatrobert keith millers textbook the informed argument first published in 1986 presented five phases adapted from an earlier textbook by richard coe millers phases were an introduction to the problem a summary of views that oppose the writers position a statement of understanding of the region of validity of the opposing views a statement of the writers position a statement of the situations in which the writers position has merit and a statement of the benefits of accepting the writers positionin 1992 rebecca stephens built on the vague and abstract rogerian principles of other rhetoricians to create a set of 23 concrete and detailed questions that she called a rogerianbased heuristic for rhetorical invention intended to help people think in a rogerian way while discovering ideas and arguments for example the first two of her 23 questions are what is the nature of the issue in general terms and she recommended that the answer should itself be stated as a question and whose lives are affected by the issue the last two questions are what would have to happen to eliminate the disagreement among the opposing groups and what are the chances that this will occur lisa'</li><li>'reestablishes equilibrium and health in the collective imaginary which are jeopardized by the repressive aspects of societythe state of political satire in a given society reflects the tolerance or intolerance that characterizes it and the state of civil liberties and human rights under totalitarian regimes any criticism of a political system and especially satire is suppressed a typical example is the soviet union where the dissidents such as aleksandr solzhenitsyn and andrei sakharov were under strong pressure from the government while satire of everyday life in the ussr was allowed the most prominent satirist being arkady raikin political satire existed in the form of anecdotes that made fun of soviet political leaders especially brezhnev famous for his narrowmindedness and love for awards and decorations satire is a diverse genre which is complex to classify and define with a wide range of satiric modes satirical literature can commonly be categorized as either horatian juvenalian or menippean horatian horatian satire named for the roman satirist horace 65 – 8 bce playfully criticizes some social vice through gentle mild and lighthearted humour horace quintus horatius flaccus wrote satires to gently ridicule the dominant opinions and philosophical beliefs of ancient rome and greece rather than writing in harsh or accusing tones he addressed issues with humor and clever mockery horatian satire follows this same pattern of gently ridiculing the absurdities and follies of human beingsit directs wit exaggeration and selfdeprecating humour toward what it identifies as folly rather than evil horatian satires sympathetic tone is common in modern society a horatian satirists goal is to heal the situation with smiles rather than by anger horatian satire is a gentle reminder to take life less seriously and evokes a wry smile juvenalian juvenalian satire named for the writings of the roman satirist juvenal late first century – early second century ad is more contemptuous and abrasive than the horatian juvenal disagreed with the opinions of the public figures and institutions of the republic and actively attacked them through his literature he utilized the satirical tools of exaggeration and parody to make his targets appear monstrous and incompetent juvenals satire follows this same pattern of abrasively ridiculing societal structures juvenal also unlike horace attacked public officials and governmental organizations through his satires regarding their opinions as not just wrong but evil following in this tradition juvenalia'</li></ul> |
| 27 | <ul><li>'rod is so small newtons third law of physics applies for any action there is a reaction when the electrons are pulled across the surface of the rod so too is the rod pulled in the opposite direction the first recorded success of a nanosubmarine was performed by a team of students led by dan peer from tel aviv university in israel this was a continuation to peers work at harvard on nanosubmarines and targeted drug delivery tests have proven successful in delivering drugs to heal mice with ulcerative colitis tests will continue and the team plans to experiment on the human body soon fantastic voyage novel and movie based on the nanosubmarine theme'</li><li>'electronbeaminduced deposition ebid is a process of decomposing gaseous molecules by an electron beam leading to deposition of nonvolatile fragments onto a nearby substrate the electron beam is usually provided by a scanning electron microscope which results in high spatial accuracy potentially below one nanometer and the possibility to produce freestanding threedimensional structures the focused electron beam of a scanning electron microscope sem or scanning transmission electron microscope stem is commonly used another method is ionbeaminduced deposition ibid where a focused ion beam is applied instead precursor materials are typically liquid or solid and gasified prior to deposition usually through vaporization or sublimation and introduced at accurately controlled rate into the highvacuum chamber of the electron microscope alternatively solid precursors can be sublimated by the electron beam itself when deposition occurs at a high temperature or involves corrosive gases a specially designed deposition chamber is used it is isolated from the microscope and the beam is introduced into it through a micrometresized orifice the small orifice size maintains differential pressure in the microscope vacuum and deposition chamber no vacuum such deposition mode has been used for ebid of diamondin the presence of the precursor gas the electron beam is scanned over the substrate resulting in deposition of material the scanning is usually computercontrolled the deposition rate depends on a variety of processing parameters such as the partial precursor pressure substrate temperature electron beam parameters applied current density etc it usually is in the order of 10 nms primary electron energies in sems or stems are usually between 10 and 300 kev where reactions induced by electron impact ie precursor dissociation have a relatively low cross section the majority of decomposition occurs via low energy electron impact either by low energy secondary electrons which cross the substratevacuum interface and contribute to the total current density or inelastically scattered backscattered electrons primary stem electrons can be focused into spots as small as 0045 nm while the smallest structures deposited so far by ebid are point deposits of 07 nm diameter deposits usually have a larger lateral size than the beam spot size the reason are the socalled proximity effects meaning that secondary backscattered and forward scattered if the beam dwells on already deposited material electrons contribute to the deposition as these electrons can leave the substrate up to several microns away from the point of impact of the electron beam depending on its energy material deposition is not necessarily confined to the irradiated spot to overcome this problem compensation algorithms can be applied which is typical for electron beam lithography as of 2008 the range of materials deposited by ebid included al au amor'</li><li>'##onment this presents a challenge in maintaining protein arrays in a stable condition over extended periods of time in situ methods — invented and published by mingyue he and michael taussig in 2001 — involve onchip synthesis of proteins as and when required directly from the dna using cellfree protein expression systems since dna is a highly stable molecule it does not deteriorate over time and is therefore suited to longterm storage this approach is also advantageous in that it circumvents the laborious and often costly processes of separate protein purification and dna cloning since proteins are made and immobilised simultaneously in a single step on the chip surface examples of in situ techniques are pisa protein in situ array nappa nucleic acid programmable protein array and dapa dna array to protein array there are three types of protein microarrays that are currently used to study the biochemical activities of proteins analytical microarrays are also known as capture arrays in this technique a library of antibodies aptamers or affibodies is arrayed on the support surface these are used as capture molecules since each binds specifically to a particular protein the array is probed with a complex protein solution such as a cell lysate analysis of the resulting binding reactions using various detection systems can provide information about expression levels of particular proteins in the sample as well as measurements of binding affinities and specificities this type of microarray is especially useful in comparing protein expression in different solutions for instance the response of the cells to a particular factor can be identified by comparing the lysates of cells treated with specific substances or grown under certain conditions with the lysates of control cells another application is in the identification and profiling of diseased tissues reverse phase protein microarray rppa involve complex samples such as tissue lysates cells are isolated from various tissues of interest and are lysed the lysate is arrayed onto the microarray and probed with antibodies against the target protein of interest these antibodies are typically detected with chemiluminescent fluorescent or colorimetric assays reference peptides are printed on the slides to allow for protein quantification of the sample lysates rpas allow for the determination of the presence of altered proteins or other agents that may be the result of disease specifically posttranslational modifications which are typically altered as a result of disease can be detected using rpas functional protein microarrays also known as target protein arrays are constructed by immobilising large numbers of purified proteins and are used to'</li></ul> |
| 9 | <ul><li>'a circular chromosome is a chromosome in bacteria archaea mitochondria and chloroplasts in the form of a molecule of circular dna unlike the linear chromosome of most eukaryotes most prokaryote chromosomes contain a circular dna molecule – there are no free ends to the dna free ends would otherwise create significant challenges to cells with respect to dna replication and stability cells that do contain chromosomes with dna ends or telomeres most eukaryotes have acquired elaborate mechanisms to overcome these challenges however a circular chromosome can provide other challenges for cells after replication the two progeny circular chromosomes can sometimes remain interlinked or tangled and they must be resolved so that each cell inherits one complete copy of the chromosome during cell division the circular bacteria chromosome replication is best understood in the wellstudied bacteria escherichia coli and bacillus subtilis chromosome replication proceeds in three major stages initiation elongation and termination the initiation stage starts with the ordered assembly of initiator proteins at the origin region of the chromosome called oric these assembly stages are regulated to ensure that chromosome replication occurs only once in each cell cycle during the elongation phase of replication the enzymes that were assembled at oric during initiation proceed along each arm replichore of the chromosome in opposite directions away from the oric replicating the dna to create two identical copies this process is known as bidirectional replication the entire assembly of molecules involved in dna replication on each arm is called a replisome at the forefront of the replisome is a dna helicase that unwinds the two strands of dna creating a moving replication fork the two unwound single strands of dna serve as templates for dna polymerase which moves with the helicase together with other proteins to synthesise a complementary copy of each strand in this way two identical copies of the original dna are created eventually the two replication forks moving around the circular chromosome meet in a specific zone of the chromosome approximately opposite oric called the terminus region the elongation enzymes then disassemble and the two daughter chromosomes are resolved before cell division is completed the e coli origin of replication called oric consists of dna sequences that are recognised by the dnaa protein which is highly conserved amongst different bacterial species dnaa binding to the origin initiates the regulated recruitment of other enzymes and proteins that will eventually lead to the establishment of two complete replisomes for bidirectional replicationdna sequence elements within oric that are important for its function include dnaa boxes a 9mer repeat with a highly'</li><li>'the second step of this process has recently fallen into question for the past few decades the common view was that a trimeric multiheme ctype hao converts hydroxylamine into nitrite in the periplasm with production of four electrons 12 the stream of four electrons is channeled through cytochrome c554 to a membranebound cytochrome c552 two of the electrons are routed back to amo where they are used for the oxidation of ammonia quinol pool the remaining two electrons are used to generate a proton motive force and reduce nadp through reverse electron transportrecent results however show that hao does not produce nitrite as a direct product of catalysis this enzyme instead produces nitric oxide and three electrons nitric oxide can then be oxidized by other enzymes or oxygen to nitrite in this paradigm the electron balance for overall metabolism needs to be reconsidered nitrite produced in the first step of autotrophic nitrification is oxidized to nitrate by nitrite oxidoreductase nxr 2 it is a membraneassociated ironsulfur molybdo protein and is part of an electron transfer chain which channels electrons from nitrite to molecular oxygen the enzymatic mechanisms involved in nitriteoxidizing bacteria are less described than that of ammonium oxidation recent research eg woznica a et al 2013 proposes a new hypothetical model of nob electron transport chain and nxr mechanisms here in contrast to earlier models the nxr would act on the outside of the plasma membrane and directly contribute to a mechanism of proton gradient generation as postulated by spieck and coworkers nevertheless the molecular mechanism of nitrite oxidation is an open question the twostep conversion of ammonia to nitrate observed in ammoniaoxidizing bacteria ammoniaoxidizing archaea and nitriteoxidizing bacteria such as nitrobacter is puzzling to researchers complete nitrification the conversion of ammonia to nitrate in a single step known as comammox has an energy yield ∆g° ′ of −349 kj mol−1 nh3 while the energy yields for the ammoniaoxidation and nitriteoxidation steps of the observed twostep reaction are −275 kj mol−1 nh3 and −74 kj mol−1 no2− respectively these values indicate that it would be energetically favourable for an organism to carry out complete nitrification from ammonia to nitrate comammox rather'</li><li>'young animals and nonnative breeds the clinical signs of disease are caused by an increased vascular permeability and consequent oedema and hypovolemia the symptoms include neurological signs such as tremors and head pressing respiratory signs such as coughing and nasal discharge and systemic signs such as fever and loss of appetite physical examination may reveal petechiae of the mucous membranes tachycardia and muffled heart sounds heartwater can also cause reproductive and gastrointestinal disease it is frequently fatal on post mortem examination a light yellow transudate that coagulates on exposure to air is often found within the thorax pericardium and abdomen most fatal cases have the hydropericardium that gives the disease its common name pulmonary oedema and mucosal congestion are regularly seen along with frothy fluid in the airways and cut surfaces of the lungs to definitively diagnose the disease c ruminantium must be demonstrated either in preparations of the hippocampus under giemsa staining or by histopathology of brain or kidney during the early stages of disease animals may be treated with sulfonamides and tetracyclines in advanced disease prognosis is poor tetracyclines can also be used prophylactically when animals are introduced into an area endemic with heartwater ectoparasiticides used as dips can be used to reduce exposure the animals exposure to bont ticks in areas endemic for heartwater the use of dips against other ticks of domestic animals such as rhipicephalus boophilus and hyalomma species is likely and this will usually contribute to control of vectors of e ruminantium a live blood vaccine is available for protection of young stock but animals may require treatment for the disease after vaccination several experimental vaccines are currently being developed examples include attenuated recombinant and multiepitope dna vaccines depending on the species of the animal the mortality rate of the disease may vary from 5 to 90 mortality rates appear to be the highest within the various sheep and goat species but this is not always the case as some sheep species such as the afrikaner have mortality rates only reaching as high as 6 heartwater is notifiable to the world organization for animal health the us department of agriculture believes that an outbreak in the us could cost the livestock industry up to 762 million in losses annually the tick that carries the disease is thought to be capable of being transported by migratory birds from the caribbean to at least florida the'</li></ul> |
| 29 | <ul><li>'fixed circle of latitude or zonal region if the coriolis parameter is large the effect of the earths rotation on the body is significant since it will need a larger angular frequency to stay in equilibrium with the coriolis forces alternatively if the coriolis parameter is small the effect of the earths rotation is small since only a small fraction of the centripetal force on the body is canceled by the coriolis force thus the magnitude of f displaystyle f strongly affects the relevant dynamics contributing to the bodys motion these considerations are captured in the nondimensionalized rossby number in stability calculations the rate of change of f displaystyle f along the meridional direction becomes significant this is called the rossby parameter and is usually denoted β ∂ f ∂ y displaystyle beta frac partial fpartial y where y displaystyle y is the in the local direction of increasing meridian this parameter becomes important for example in calculations involving rossby waves beta plane earths rotation rossbygravity waves'</li><li>'of silicic acid to nitrate because larger diatoms that require silicic acid to make their opal silica shells are less prevalent unlike the southern ocean and the north pacific the equatorial pacific experiences temporal silicate availability which leads to large seasonal diatom bloomsthe distribution of trace metals and relative abundance of macronutrients are reflected in the plankton community structure for example the selection of phytoplankton with a high surface area to volume ratio results in hnlc regions being dominated by nano and picoplankton this ratio allows for optimal utilization of available dissolved nutrients larger phytoplankton such as diatoms cannot energetically sustain themselves in these regions common picoplankton within these regions include genera such as prochlorococcus not generally found in the north pacific synechococcus and various eukaryotes grazing protists likely control the abundance and distribution of these small phytoplanktonthe generally lower net primary production in hnlc zones results in lower biological drawdown of atmospheric carbon dioxide and thus these regions are generally considered a net source of carbon dioxide to the atmosphere hnlc areas are of interest to geoengineers and some in the scientific community who believe fertilizing large patches of these waters with iron could potentially lower dissolved carbon dioxide and offset increased anthropogenic carbon emissions analysis of antarctic ice core data over the last million years shows correlation between high levels of dust and low temperature indicating that addition of diffuse ironrich dust to the sea has been a natural amplifier of climate cooling the discovery and naming of the first hnlc region the north pacific was formalized in a seminal paper published in 1988 the study concluded that surface waters of the eastern north pacific are generally dominated by picoplankton despite the relative abundance of macronutrients in other words larger phytoplankton such as diatoms which thrive in nutrientrich waters were not found instead the surface waters were replete with smaller pico and nanoplankton based on laboratory nutrient experiments iron was hypothesized to be a key limiting micronutrientthe pacific ocean is the largest and oldest body of water on earth the north pacific is characterized by the general clockwise rotation of the north pacific gyre which is driven by trade winds spatial variations in tradewinds result in cooler air temperatures in the western north pacific and milder air temperatures in the eastern north pacific ie subarctic pacific iron is supplied to the north pacific by dust storms that occur in asia'</li><li>'atmospheric pressure 101325 pa whereas water has a density of 09998 – 0999863 gcm3 at the same temperature and pressure liquid water is densest essentially 100 gcm3 at 4 °c and begins to lose its density as the water molecules begin to form the hexagonal crystals of ice as the freezing point is reached this is due to hydrogen bonding dominating the intermolecular forces which results in a packing of molecules less compact in the solid density of ice increases slightly with decreasing temperature and has a value of 09340 gcm3 at −180 °c 93 kwhen water freezes it increases in volume about 9 for fresh water the effect of expansion during freezing can be dramatic and ice expansion is a basic cause of freezethaw weathering of rock in nature and damage to building foundations and roadways from frost heaving it is also a common cause of the flooding of houses when water pipes burst due to the pressure of expanding water when it freezes the result of this process is that ice in its most common form floats on liquid water which is an important feature in earths biosphere it has been argued that without this property natural bodies of water would freeze in some cases permanently from the bottom up resulting in a loss of bottomdependent animal and plant life in fresh and sea water sufficiently thin ice sheets allow light to pass through while protecting the underside from shortterm weather extremes such as wind chill this creates a sheltered environment for bacterial and algal colonies when sea water freezes the ice is riddled with brinefilled channels which sustain sympagic organisms such as bacteria algae copepods and annelids which in turn provide food for animals such as krill and specialised fish like the bald notothen fed upon in turn by larger animals such as emperor penguins and minke whaleswhen ice melts it absorbs as much energy as it would take to heat an equivalent mass of water by 80 °c during the melting process the temperature remains constant at 0 °c while melting any energy added breaks the hydrogen bonds between ice water molecules energy becomes available to increase the thermal energy temperature only after enough hydrogen bonds are broken that the ice can be considered liquid water the amount of energy consumed in breaking hydrogen bonds in the transition from ice to water is known as the heat of fusion as with water ice absorbs light at the red end of the spectrum preferentially as the result of an overtone of an oxygen – hydrogen o – h bond stretch compared with water this absorption is shifted toward slightly lower energies thus ice appears blue with'</li></ul> |
| 13 | <ul><li>'has offered artworks in the form of graphics downloadable to the home personal computer – for example by peter halley the thing has enabled a diverse group of artists critics curators and activists to use the internet in its early stages at its core the thing is a social network made up of individuals from diverse backgrounds with a wide range of expert knowledge from this social hub the thing has built an array of programs and initiatives in both technological and cultural networks during its first five years tt became widely recognized as one of the founding and leading online centers for new media culture its activities include hosting artists projects and mailing lists as well as publishing cultural criticism the thing has also organized many public events and symposia on such topics as the state of new media arts the preservation of online privacy artistic innovations in robotics and the possibilities of community empowerment through wireless technologies in 1997 thingnet communications llc an internet service provider isp was incorporated by wolfgang staehle gisela ehrenfried and max kossatz the isp was to provide a financial backbone for the thing inc a 501 c 3 non profit organization thingnet has hosted arts and activist groups and publications including ps1 contemporary art center artforum mabou mines willoughby sharp gallery zingmagazine journal of contemporary art rtmark and tenantnet among many others artists and projects associated with thingnet have included sawad brooks heath bunting cercle ramo nash vuk cosic ricardo dominguez ursula endlicher etoy gh hovagimyan jerome joy john klima jenny marketou mariko mori olivier mosset prema murty mark napier joseph nechvatal phil niblock daniel pflumm francesca da rimini beat streuli and beth stryker the thing amsterdam was founded by walter van der cruijsen the thing basel was founded by barbara strebel and rik gelles the thing berlin was founded by ulf schleth the thing cologne was founded by michael krome the thing dusseldorf was founded by jorg sasse the thing frankfurt was founded by andreas kallfelz the thing hamburg 1993 – 94 was founded by hansjoachim lenger the thing hamburg 2006 – 2009 was founded by the local art association the thing hamburg the thing london was founded by andreas ruethi the thing new york was founded by wolfgang staehle the thing stockholm was founded by magnus borg the thing vienna was founded by helmut mark and max kossatz the thing roma was founded by marco deseriis and giuseppe marano'</li><li>'of using locative media to better understand and connect in their environmentsyzygryd is a collaboration with three other arts organizations interpretive arson false profit labs ardent heavy industries to create a large scale interactive art piece to be unveiled at the 2010 burning man event the first five resident artists alphonzo solorzano gabriel dunne ryan alexander miles stemper and daniel massey moved into the space in july 2009 in 2010 three of these resident artists remained gabriel dunne ryan alexander and daniel massey in 2021 gray area partnered with the human rights foundation to launch the art in protest residency program the program s an opportunity for artists whose art is dedicated to promoting democracy and human rights globally to explore and expand their digital practices the gray area incubator is a peerdriven community of creators developing work at the intersection of art and technology membership is a 6month commitment though many have continued on much longer to develop their works in the incubator artists work in the disciplines of visual media arts creative code virtual augmented reality civic engagement digital activism social entrepreneurship data science sound audio and software hardware gray areas josette melchor was selected as one of the five innovators showcased on fords the edge of progress tourafter the 2016 oakland ghostship warehouse fire gray area raised approximately 13 million from over 12000 donors which it distributed to 390 applicants ranging from deceased victims next of kin displaced residents people injured in the fire as well as people who would not be acknowledged by traditional disaster relief organizations including chosen family within marginalized communities'</li><li>'nfts being used in the filmindustry include a collection of nftartworks for godzilla vs kong the release of both kevin smiths horrormovie killroy was here and the 2021 film zero contact as nfts in 2021 in april 2021 an nft was released for the score of the movie triumph composed by gregg leonard in november 2021 film director quentin tarantino released seven nfts based on uncut scenes of pulp fiction miramax subsequently filed a lawsuit claiming that their film rights were violated and that the original 1993 contract with tarantino gave them the right to mint nfts in relation to pulp fiction in august 2022 muse released album will of the people as 1000 nfts and it became the first album for which nft sales would qualify for the uk and australian chartsby february 2021 nfts accounted for us25 million of revenue generated through the sale of artwork and songs as nfts on february 28 2021 electronic dance musician 3lau sold a collection of 33 nfts for a total of us117 million to commemorate the threeyear anniversary of his ultraviolet album on march 3 2021 an nft was made to promote the kings of leon album when you see yourself other musicians who have used nfts include american rapper lil pump grimes visual artist shepard fairey in collaboration with record producer mike dean and rapper eminema paper presented at the 40th international conference on information systems in munich in 2019 suggested using nfts as tickets for different types of events this would enable organizers of the respective events or artists performing there to receive royalties on the resale of each ticket other associated files a number of internet memes have been associated with nfts which were minted and sold by their creators or by their subjects examples include doge an image of a shiba inu dog as well as charlie bit my finger nyan cat and disaster girl some virtual worlds often marketed as metaverses have incorporated nfts as a means of trading virtual items and virtual real estate some pornographic works have been sold as nfts though hostility from nft marketplaces towards pornographic material has presented significant drawbacks for creators by using nfts people engaged in this area of the entertainmentindustry are able to publish their works without thirdparty platforms being able to delete them the first credited political protest nft destruction of nazi monument symbolizing contemporary lithuania was a video filmed by professor stanislovas tomas on april 8 2019 and minted on march 29 2021 in the video tomas uses a sledgehammer to destroy a statesponsored'</li></ul> |
| 7 | <ul><li>'lot of solutions available for people with hearing impairments some examples of solutions would be blinking lights on different things like their phones alarms and things that are important to alert them cochlear implants are an option too cochlear implants are surgically placed devices that stimulate the cochlear nerve in order to help the person hear a cochlear implant is used instead of hearing aids in order to help when someone has difficulties understanding speech in a cultural context deaf culture refers to a tightknit cultural group of people whose primary language is signed and who practice social and cultural norms which are distinct from those of the surrounding hearing community this community does not automatically include all those who are clinically or legally deaf nor does it exclude every hearing person according to baker and padden it includes any person who identifies himherself as a member of the deaf community and other members accept that person as a part of the community an example being children of deaf adults with normal hearing ability it includes the set of social beliefs behaviors art literary traditions history values and shared institutions of communities that are influenced by deafness and which use sign languages as the main means of communication members of the deaf community tend to view deafness as a difference in human experience rather than a disability or diseasemany nondisabled people continue to assume that deaf people have no autonomy and fail to provide people with support beyond hearing aids which is something that must be addressed different nongovernmental organizations around the world have created programs towards closing the gap between deaf and nondisabled people in developing countries the quota international organization with headquarters in the united states provided immense educational support in the philippines where it started providing free education to deaf children in the leganes resource center for the deaf the sounds seekers british organization also provided support by offering audiology maintenance technology to better assist those who are deaf in hardtoreach places the nippon foundation also supports deaf students at gallaudet university and the national technical institute for the deaf through sponsoring international scholarships programs to encourage students to become future leaders in the deaf community the more aid these organizations give to the deaf people the more opportunities and resources disabled people must speak up about their struggles and goals that they aim to achieve when more people understand how to leverage their privilege for the marginalized groups in the community then we can build a more inclusive and tolerant environment for the generations that are yet to come the first known record of sign language in history comes from platos cratylus written in the fifth century bce in a dialogue on the correctness of names socrates says suppose'</li><li>'the ear canal external acoustic meatus external auditory meatus eam is a pathway running from the outer ear to the middle ear the adult human ear canal extends from the pinna to the eardrum and is about 25 centimetres 1 in in length and 07 centimetres 03 in in diameter the human ear canal is divided into two parts the elastic cartilage part forms the outer third of the canal its anterior and lower wall are cartilaginous whereas its superior and back wall are fibrous the cartilage is the continuation of the cartilage framework of pinna the cartilaginous portion of the ear canal contains small hairs and specialized sweat glands called apocrine glands which produce cerumen ear wax the bony part forms the inner two thirds the bony part is much shorter in children and is only a ring annulus tympanicus in the newborn the layer of epithelium encompassing the bony portion of the ear canal is much thinner and therefore more sensitive in comparison to the cartilaginous portion size and shape of the canal vary among individuals the canal is approximately 25 centimetres 1 in long and 07 centimetres 028 in in diameter it has a sigmoid form and runs from behind and above downward and forward on the crosssection it is of oval shape these are important factors to consider when fitting earplugs due to its relative exposure to the outside world the ear canal is susceptible to diseases and other disorders some disorders include atresia of the ear canal cerumen impaction bone exposure caused by the wearing away of skin in the canal auditory canal osteoma bony outgrowths of the temporal bone cholesteatoma contact dermatitis of the ear canal fungal infection otomycosis ear mites in animals ear myiasis an extremely rare infestation of maggots foreign body in ear granuloma a scar usually caused by tympanostomy tubes otitis externa swimmers ear bacteriacaused inflammation of the ear canal stenosis a gradual closing of the canal earwax also known as cerumen is a yellowish waxy substance secreted in the ear canals it plays an important role in the human ear canal assisting in cleaning and lubrication and also provides some protection from bacteria fungi and insects excess or impacted cerumen can press against the eardrum andor occlude the external auditory canal and impair hearing causing conductive hearing loss if left untreated cerumen impaction can also increase the risk of developing an infection within the ear canal list of specialized glands within the'</li><li>'##anometry and speech audiometry may be helpful testing is performed by an audiologist there is no proven or recommended treatment or cure for snhl management of hearing loss is usually by hearing strategies and hearing aids in cases of profound or total deafness a cochlear implant is a specialised hearing aid that may restore a functional level of hearing snhl is at least partially preventable by avoiding environmental noise ototoxic chemicals and drugs and head trauma and treating or inoculating against certain triggering diseases and conditions like meningitis since the inner ear is not directly accessible to instruments identification is by patient report of the symptoms and audiometric testing of those who present to their doctor with sensorineural hearing loss 90 report having diminished hearing 57 report having a plugged feeling in ear and 49 report having ringing in ear tinnitus about half report vestibular vertigo problemsfor a detailed exposition of symptoms useful for screening a selfassessment questionnaire was developed by the american academy of otolaryngology called the hearing handicap inventory for adults hhia it is a 25question survey of subjective symptoms sensorineural hearing loss may be genetic or acquired ie as a consequence of disease noise trauma etc people may have a hearing loss from birth congenital or the hearing loss may come on later many cases are related to old age agerelated hearing loss can be inherited more than 40 genes have been implicated in the cause of deafness there are 300 syndromes with related hearing loss and each syndrome may have causative genesrecessive dominant xlinked or mitochondrial genetic mutations can affect the structure or metabolism of the inner ear some may be single point mutations whereas others are due to chromosomal abnormalities some genetic causes give rise to a late onset hearing loss mitochondrial mutations can cause snhl ie m1555ag which makes the individual sensitive to the ototoxic effects of aminoglycoside antibiotics the most common cause of recessive genetic congenital hearing impairment in developed countries is dfnb1 also known as connexin 26 deafness or gjb2related deafness the most common syndromic forms of hearing impairment include dominant stickler syndrome and waardenburg syndrome and recessive pendred syndrome and usher syndrome mitochondrial mutations causing deafness are rare mttl1 mutations cause midd maternally inherited deafness and diabetes and other conditions which may include deafness as part of the picture tmprss3 gene was identified by its association with both congenital and childhood onset autosomal recessive deafness this gene is expressed in fetal co'</li></ul> |
| 23 | <ul><li>'tolerogenic dendritic cells a k a toldcs tdcs or dcregs are heterogenous pool of dendritic cells with immunosuppressive properties priming immune system into tolerogenic state against various antigens these tolerogenic effects are mostly mediated through regulation of t cells such as inducing t cell anergy t cell apoptosis and induction of tregs toldcs also affect local microenvironment toward tolerogenic state by producing antiinflammatory cytokines toldcs are not lineage specific and their immunesuppressive functions is due to their state of activation andor differentiation generally properties of all types of dendritic cells can be highly affected by local microenvironment such as presence of pro or antiinflammatory cytokines therefore tolerogenic properties of toldcs are often context dependant and can be even eventually overridden into proinflammatory phenotypetolerogenic dcs present a potential strategy for treatment of autoimmune diseases allergic diseases and transplant rejections moreover agspecific tolerance in humans can be induced in vivo via vaccination with agpulsed ex vivo generated tolerogenic dcs for that reason tolerogenic dcs are an important promising therapeutic tool dendritic cells dcs were first discovered and described in 1973 by ralph m steinman they represent a bridge between innate and adaptive immunity and play a key role in the regulation of initiation of immune responses dcs populate almost all body surfaces and they do not kill the pathogens directly they utilize and subsequently degrade antigens to peptides by their proteolytic activity after that they present these peptides in complexes together with their mhc molecules on their cell surface dcs are also the only cell type which can activate naive t cells and induce antigenspecific immune responsestherefore their role is crucially important in balance between tolerance and immune response tolerogenic dcs are essential in maintenance of central and peripheral tolerance through induction of t cell clonal deletion t cell anergy and generation and activation of regulatory t treg cells for that reason tolerogenic dcs are possible candidates for specific cellular therapy for treatment of allergic diseases autoimmune diseases eg type 1 diabetes multiple sclerosis rheumatoid arthritis or transplant rejectionstolerogenic dcs often display an immature or semimature phenotype with characteristically low expression of costimulatory eg cd80 cd86 and mhc molecules'</li><li>'distribution of il2 receptors cd25 cd122 cd132 on different cell populations resulting in different cells that are activated by high and low dose il2 in general high doses are immune suppressive while low doses can stimulate type 1 immunity lowdose il2 has been reported to reduce hepatitis c and b infectionil2 has been used in clinical trials for the treatment of chronic viral infections and as a booster adjuvant for vaccines the use of large doses of il2 given every 6 – 8 weeks in hiv therapy similar to its use in cancer therapy was found to be ineffective in preventing progression to an aids diagnosis in two large clinical trials published in 2009more recently low dose il2 has shown early success in modulating the immune system in disease like type 1 diabetes and vasculitis there are also promising studies looking to use low dose il2 in ischaemic heart disease il2 cannot accomplish its role as a promising immunotherapeutic agent due to significant drawbacks which are listed above some of the issues can be overcome using il2 ic they are composed of il2 and some of its monoclonal antibody mab and can potentiate biologic activity of il2 in vivo the main mechanism of this phenomenon in vivo is due to the prolongation of the cytokine halflife in circulation depending on the clone of il2 mab il2 ic can selectively stimulate either cd25high il2jes61 complexes or cd122high cells il2s4b6 il2s4b6 immune complexes have high stimulatory activity for nk cells and memory cd8 t cells and they could thus replace the conventional il2 in cancer immunotherapy on the other hand il2jes61 highly selectively stimulate regulatory t cells and they could be potentially useful for transplantations and in treatment of autoimmune diseases according to an immunology textbook il2 is particularly important historically as it is the first type i cytokine that was cloned the first type i cytokine for which a receptor component was cloned and was the first shortchain type i cytokine whose receptor structure was solved many general principles have been derived from studies of this cytokine including its being the first cytokine demonstrated to act in a growth factor – like fashion through specific highaffinity receptors analogous to the growth factors being studied by endocrinologists and biochemists 712 in the mid1960s studies reported activities in leukocyteconditioned media'</li><li>'the immune system during puberty and postpuberty than during the rest of a males adult life physical changes during puberty such as thymic involution also affect immunological response ecoimmunology or ecological immunology explores the relationship between the immune system of an organism and its social biotic and abiotic environment more recent ecoimmunological research has focused on host pathogen defences traditionally considered nonimmunological such as pathogen avoidance selfmedication symbiontmediated defenses and fecundity tradeoffs behavioural immunity a phrase coined by mark schaller specifically refers to psychological pathogen avoidance drivers such as disgust aroused by stimuli encountered around pathogeninfected individuals such as the smell of vomit more broadly behavioural ecological immunity has been demonstrated in multiple species for example the monarch butterfly often lays its eggs on certain toxic milkweed species when infected with parasites these toxins reduce parasite growth in the offspring of the infected monarch however when uninfected monarch butterflies are forced to feed only on these toxic plants they suffer a fitness cost as reduced lifespan relative to other uninfected monarch butterflies this indicates that laying eggs on toxic plants is a costly behaviour in monarchs which has probably evolved to reduce the severity of parasite infectionsymbiontmediated defenses are also heritable across host generations despite a nongenetic direct basis for the transmission aphids for example rely on several different symbionts for defense from key parasites and can vertically transmit their symbionts from parent to offspring therefore a symbiont that successfully confers protection from a parasite is more likely to be passed to the host offspring allowing coevolution with parasites attacking the host in a way similar to traditional immunity the preserved immune tissues of extinct species such as the thylacine thylacine cynocephalus can also provide insights into their biology the study of the interaction of the immune system with cancer cells can lead to diagnostic tests and therapies with which to find and fight cancer the immunology concerned with physiological reaction characteristic of the immune state this area of the immunology is devoted to the study of immunological aspects of the reproductive process including fetus acceptance the term has also been used by fertility clinics to address fertility problems recurrent miscarriages premature deliveries and dangerous complications such as preeclampsia list of immunologists immunomics international reviews of immunology outline of immunology history of immunology osteoimmunology'</li></ul> |
| 25 | <ul><li>'then convergence to i − a − 1 b displaystyle ia1b occurs if the magnitudes of all eigenvalues of a displaystyle a are less than 1 every bounded sequence in r n displaystyle mathbb r n has a convergent subsequence by the bolzano – weierstrass theorem if these all have the same limit then the original sequence converges to that limit if it can be shown that all of the subsequences of f displaystyle f have the same limit such as by showing that there is a unique fixed point of the transformation t displaystyle t then the initial sequence must also converge to that limit every bounded monotonic sequence in r n displaystyle mathbb r n converges to a limit this approach can also be applied to sequences that are not monotonic instead it is possible to define a function v r n → r displaystyle vmathbb r nrightarrow mathbb r such that v f n displaystyle vfn is monotonic in n displaystyle n if the v displaystyle v satisfies the conditions to be a lyapunov function then f displaystyle f is convergent lyapunovs theorem is normally stated for ordinary differential equations but can also be applied to sequences of iterates by replacing derivatives with discrete differences the basic requirements on v displaystyle v are that v f n 1 − v f n 0 displaystyle vfn1vfn0 for f n = 0 displaystyle fnneq 0 and v 0 0 displaystyle v00 or v [UNK] x 0 displaystyle dot vx0 for x = 0 displaystyle xneq 0 v x 0 displaystyle vx0 for all x = 0 displaystyle xneq 0 and v 0 0 displaystyle v00 v displaystyle v be radially unbounded so that v x displaystyle vx goes to infinity for any sequence with ‖ x ‖ displaystyle x that tends to infinityin many cases a lyapunov function of the form v x x t a x displaystyle vxxtax can be found although more complex forms are also used for delay differential equations a similar approach applies with lyapunov functions replaced by lyapunov functionals also called lyapunovkrasovskii functionals if the inequality in the condition 1 is weak lasalles invariance principle may be used to consider the convergence of sequences of functions it is necessary to define a distance between functions to replace the euclidean norm these often include convergence in the'</li><li>'this is a list of convexity topics by wikipedia page alpha blending the process of combining a translucent foreground color with a background color thereby producing a new blended color this is a convex combination of two colors allowing for transparency effects in computer graphics barycentric coordinates a coordinate system in which the location of a point of a simplex a triangle tetrahedron etc is specified as the center of mass or barycenter of masses placed at its vertices the coordinates are nonnegative for points in the convex hull borsuks conjecture a conjecture about the number of pieces required to cover a body with a larger diameter solved by hadwiger for the case of smooth convex bodies bond convexity a measure of the nonlinear relationship between price and yield duration of a bond to changes in interest rates the second derivative of the price of the bond with respect to interest rates a basic form of convexity in finance caratheodorys theorem convex hull if a point x of rd lies in the convex hull of a set p there is a subset of p with d1 or fewer points such that x lies in its convex hull choquet theory an area of functional analysis and convex analysis concerned with measures with support on the extreme points of a convex set c roughly speaking all vectors of c should appear as averages of extreme points complex convexity — extends the notion of convexity to complex numbers convex analysis the branch of mathematics devoted to the study of properties of convex functions and convex sets often with applications in convex minimization convex combination a linear combination of points where all coefficients are nonnegative and sum to 1 all convex combinations are within the convex hull of the given points convex and concave a print by escher in which many of the structures features can be seen as both convex shapes and concave impressions convex body a compact convex set in a euclidean space whose interior is nonempty convex conjugate a dual of a real functional in a vector space can be interpreted as an encoding of the convex hull of the functions epigraph in terms of its supporting hyperplanes convex curve a plane curve that lies entirely on one side of each of its supporting lines the interior of a closed convex curve is a convex set convex function a function in which the line segment between any two points on the graph of the function lies above the graph closed convex function a convex function all of whose sublevel sets are closed sets proper convex function a convex function whose effective domain is nonempty and it never attains minus infinity concave function the negative of a convex function convex geometry the branch of geometry studying'</li><li>'##regularization is useful as it can often be used in a way such that the various symmetries of the physical system are preserved zetafunction regularization is used in conformal field theory renormalization and in fixing the critical spacetime dimension of string theory zeta function regularization is equivalent to dimensional regularization see4 however the main advantage of the zeta regularization is that it can be used whenever the dimensional regularization fails for example if there are matrices or tensors inside the calculations [UNK] i j k displaystyle epsilon ijk zetafunction regularization gives an analytic structure to any sums over an arithmetic function fn such sums are known as dirichlet series the regularized form f s [UNK] n 1 ∞ f n n − s displaystyle tilde fssum n1infty fnns converts divergences of the sum into simple poles on the complex splane in numerical calculations the zetafunction regularization is inappropriate as it is extremely slow to converge for numerical purposes a more rapidly converging sum is the exponential regularization given by f t [UNK] n 1 ∞ f n e − t n displaystyle ftsum n1infty fnetn this is sometimes called the ztransform of f where z exp−t the analytic structure of the exponential and zetaregularizations are related by expanding the exponential sum as a laurent series f t a n t n a n − 1 t n − 1 [UNK] displaystyle ftfrac antnfrac an1tn1cdots one finds that the zetaseries has the structure f s a n s − n [UNK] displaystyle tilde fsfrac ansncdots the structure of the exponential and zetaregulators are related by means of the mellin transform the one may be converted to the other by making use of the integral representation of the gamma function γ s [UNK] 0 ∞ t s − 1 e − t d t displaystyle gamma sint 0infty ts1etdt which leads to the identity γ s f s [UNK] 0 ∞ t s − 1 f t d t displaystyle gamma stilde fsint 0infty ts1ftdt relating the exponential and zetaregulators and converting poles in the splane to divergent terms in the laurent series the sum f s [UNK] n a n e − s ω n displaystyle fssum nanesomega n is sometimes called a heat kernel or a heatkernel regularized sum this name stems from the idea that the ω n'</li></ul> |
| 37 | <ul><li>'##dicative adjective must also be connected by a copula some theories of syntax adopt a subjectpredicate distinction for instance a textbook phrase structure grammar typically divides an english declarative sentence s into a noun phrase np and verb phrase vp the subject np is shown in green and the predicate vp in blue languages with more flexible word order often called nonconfigurational languages are often also treated differently in phrase structure approaches on the other hand dependency grammar rejects the binary subjectpredicate division and places the finite verb as the root of the sentence the matrix predicate is marked in blue and its two arguments are in green while the predicate cannot be construed as a constituent in the formal sense it is a catena barring a discontinuity predicates and their arguments are always catenae in dependency structures some theories of grammar accept both a binary division of sentences into subject and predicate while also giving the head of the predicate a special status in such contexts the term predicator is used to refer to that head there are cases in which the semantic predicand has a syntactic function other than subject this happens in raising constructions such as the following here you is the object of the make verb phrase the head of the main clause but it is also the predicand of the subordinate think clause which has no subject 329 – 335 the term predicate is also used to refer to properties and to words or phrases which denote them this usage of the term comes from the concept of a predicate in logic in logic predicates are symbols which are interpreted as relations or functions over arguments in semantics the denotations of some linguistic expressions are analyzed along similar lines expressions which denote predicates in the semantic sense are sometimes themselves referred to as predication the seminal work of greg carlson distinguishes between types of predicates based on carlsons work predicates have been divided into the following subclasses which roughly pertain to how a predicate relates to its subject stagelevel predicates a stagelevel predicate is true of a temporal stage of its subject for example if john is hungry then he typically will eat some food his state of being hungry therefore lasts a certain amount of time and not his entire lifespan stagelevel predicates can occur in a wide range of grammatical constructions and are probably the most versatile kind of predicate individuallevel predicates an individuallevel predicate is true throughout the existence of an individual for example if john is smart this is a property that he has regardless of which particular point'</li><li>'that there can be exactly the same relation between two completely different objects greek philosophers such as plato and aristotle used a wider notion of analogy they saw analogy as a shared abstraction analogous objects did not share necessarily a relation but also an idea a pattern a regularity an attribute an effect or a philosophy these authors also accepted that comparisons metaphors and images allegories could be used as arguments and sometimes they called them analogies analogies should also make those abstractions easier to understand and give confidence to those who use them james francis ross in portraying analogy 1982 the first substantive examination of the topic since cajetans de nominum analogia demonstrated that analogy is a systematic and universal feature of natural languages with identifiable and lawlike characteristics which explain how the meanings of words in a sentence are interdependent on the contrary ibn taymiyya francis bacon and later john stuart mill argued that analogy is simply a special case of induction in their view analogy is an inductive inference from common known attributes to another probable common attribute which is known about only in the source of the analogy in the following form premises a is c d e f g b is c d e f conclusion b is probably g contemporary cognitive scientists use a wide notion of analogy extensionally close to that of plato and aristotle but framed by gentners 1983 structure mapping theory the same idea of mapping between source and target is used by conceptual metaphor and conceptual blending theorists structure mapping theory concerns both psychology and computer science according to this view analogy depends on the mapping or alignment of the elements of source and target the mapping takes place not only between objects but also between relations of objects and between relations of relations the whole mapping yields the assignment of a predicate or a relation to the target structure mapping theory has been applied and has found considerable confirmation in psychology it has had reasonable success in computer science and artificial intelligence see below some studies extended the approach to specific subjects such as metaphor and similarity logicians analyze how analogical reasoning is used in arguments from analogy an analogy can be stated using is to and as when representing the analogous relationship between two pairs of expressions for example smile is to mouth as wink is to eye in the field of mathematics and logic this can be formalized with colon notation to represent the relationships using single colon for ratio and double colon for equalityin the field of testing the colon notation of ratios and equality is often borrowed so that the example above might be rendered smile mouth wink eye and pronounced the same way an analogy can be the linguistic process that reduces word forms thought to break rules to more common forms that follow these rules for example'</li><li>'this approach can be used to cover a wide variety of semantic phenomena a lambek grammar is an elaboration of this idea that has a concatenation operator for types and several other inference rules mati pentus has shown that these still have the generative capacity of contextfree grammars for the lambek calculus there is a type concatenation operator [UNK] displaystyle star so that prim ⊆ tp prim displaystyle textprimsubseteq texttptextprim and if x y ∈ tp prim displaystyle xyin texttptextprim then x y x [UNK] y x [UNK] y ∈ tp prim displaystyle xyxbackslash yxstar yin texttptextprim the lambek calculus consists of several deduction rules which specify how type inclusion assertions can be derived in the following rules upper case roman letters stand for types upper case greek letters stand for sequences of types a sequent of the form x ← γ displaystyle xleftarrow gamma can be read a string is of type x if it consists of the concatenation of strings of each of the types in γ if a type is interpreted as a set of strings then the ← may be interpreted as [UNK] that is includes as a subset a horizontal line means that the inclusion above the line implies the one below the line the process is begun by the axiom rule which has no antecedents and just says that any type includes itself axiom x ← x displaystyle textaxiomquad over xleftarrow x the cut rule says that inclusions can be composed cut z ← δ x δ ′ x ← γ z ← δ γ δ ′ displaystyle textcutquad zleftarrow delta xdelta qquad xleftarrow gamma over zleftarrow delta gamma delta the other rules come in pairs one pair for each type construction operator each pair consisting of one rule for the operator in the target one in the source of the arrow the name of a rule consists of the operator and an arrow with the operator on the side of the arrow on which it occurs in the conclusion for an example here is a derivation of type raising which says that b a [UNK] b ← a displaystyle babackslash bleftarrow a the names of rules and the substitutions used are to the right b ← b a ← a b ← b a a b a [UNK] b ← a axioms ← z y b x a γ a δ δ ′ [UNK] ← y b x b a γ a displaystyle dfra'</li></ul> |
| 30 | <ul><li>'on february 5 2005 for its operations of a vermiculite mine in libby montana the indictment accused grace of wire fraud knowing endangerment of residents by concealing air monitoring results obstruction of justice by interfering with an environmental protection agency epa investigation violation of the clean air act providing asbestos materials to schools and local residents and conspiracy to release asbestos and cover up health problems from asbestos contamination the department of justice said 1200 residents had developed asbestosrelated diseases and some had died and there could be many more injuries and deathson june 8 2006 a federal judge dismissed the conspiracy charge of knowing endangerment because some of the defendant officials had left the company before the fiveyear statute of limitations had begun to run the wire fraud charge was dropped by prosecutors in march other prosecutions on april 2 1998 three men were indicted in a conspiracy to use homeless men for illegal asbestos removal from an aging wisconsin manufacturing plant thenus attorney general janet reno said knowingly removing asbestos improperly is criminal exploiting the homeless to do this work is cruelon december 12 2004 owners of new york asbestos abatement companies were sentenced to the longest federal jail sentences for environmental crimes in us history after they were convicted on 18 counts of conspiracy to violate the clean air act and the toxic substances control act and actual violations of the clean air act and racketeerinfluenced and corrupt organizations act the crimes involved a 10year scheme to illegally remove asbestos the rico counts included obstruction of justice money laundering mail fraud and bid rigging all related to the asbestos cleanupon january 11 2006 san diego gas electric co two of its employees and a contractor were indicted by a federal grand jury on charges that they violated safety standards while removing asbestos from pipes in lemon grove california the defendants were charged with five counts of conspiracy violating asbestos work practice standards and making false statements'</li><li>'is standard in medicalbilling terminology especially when billing for a growth whose pathology has yet to be determined epidemiology of cancer list of biological development disorders pleomorphism somatic evolution in cancer'</li><li>'atm these epigenetic defects occurred in various cancers including breast ovarian colorectal and head and neck cancers two or three deficiencies in expression of ercc1 xpf or pms2 occur simultaneously in the majority of the 49 colon cancers evaluated by facista et al epigenetic alterations causing reduced expression of dna repair genes is shown in a central box at the third level from the top of the figure in this section and the consequent dna repair deficiency is shown at the fourth level when expression of dna repair genes is reduced dna damages accumulate in cells at a higher than normal level and these excess damages cause increased frequencies of mutation or epimutation mutation rates strongly increase in cells defective in dna mismatch repair or in homologous recombinational repair hrrduring repair of dna double strand breaks or repair of other dna damages incompletely cleared sites of repair can cause epigenetic gene silencing dna repair deficiencies level 4 in the figure cause increased dna damages level 5 in the figure which result in increased somatic mutations and epigenetic alterations level 6 in the figure field defects normalappearing tissue with multiple alterations and discussed in the section below are common precursors to development of the disordered and improperly proliferating clone of tissue in a malignant neoplasm such field defects second level from bottom of figure may have multiple mutations and epigenetic alterations once a cancer is formed it usually has genome instability this instability is likely due to reduced dna repair or excessive dna damage because of such instability the cancer continues to evolve and to produce sub clones for example a renal cancer sampled in 9 areas had 40 ubiquitous mutations demonstrating tumor heterogeneity ie present in all areas of the cancer 59 mutations shared by some but not all areas and 29 private mutations only present in one of the areas of the cancer various other terms have been used to describe this phenomenon including field effect field cancerization and field carcinogenesis the term field cancerization was first used in 1953 to describe an area or field of epithelium that has been preconditioned by at that time largely unknown processes so as to predispose it towards development of cancer since then the terms field cancerization and field defect have been used to describe premalignant tissue in which new cancers are likely to arisefield defects are important in progression to cancer however in most cancer research as pointed out by rubin the vast majority of studies in cancer research has been done on welldefined tumors in vivo or on discrete neoplastic foci in vitro'</li></ul> |
| 2 | <ul><li>'in algebra a resolvent cubic is one of several distinct although related cubic polynomials defined from a monic polynomial of degree four p x x 4 a 3 x 3 a 2 x 2 a 1 x a 0 displaystyle pxx4a3x3a2x2a1xa0 in each case the coefficients of the resolvent cubic can be obtained from the coefficients of px using only sums subtractions and multiplications knowing the roots of the resolvent cubic of px is useful for finding the roots of px itself hence the name “ resolvent cubic ” the polynomial px has a multiple root if and only if its resolvent cubic has a multiple root suppose that the coefficients of px belong to a field k whose characteristic is different from 2 in other words we are working in a field in which 1 1 = 0 whenever roots of px are mentioned they belong to some extension k of k such that px factors into linear factors in kx if k is the field q of rational numbers then k can be the field c of complex numbers or the field q of algebraic numbers in some cases the concept of resolvent cubic is defined only when px is a quartic in depressed form — that is when a3 0 note that the fourth and fifth definitions below also make sense and that the relationship between these resolvent cubics and px are still valid if the characteristic of k is equal to 2 suppose that px is a depressed quartic — that is that a3 0 a possible definition of the resolvent cubic of px is r 1 y 8 y 3 8 a 2 y 2 2 a 2 2 − 8 a 0 y − a 1 2 displaystyle r1y8y38a2y22a228a0ya12 the origin of this definition lies in applying ferraris method to find the roots of px to be more precise p x 0 [UNK] x 4 a 2 x 2 − a 1 x − a 0 [UNK] x 2 a 2 2 2 − a 1 x − a 0 a 2 2 4 displaystyle beginalignedpx0longleftrightarrow x4a2x2a1xa0longleftrightarrow leftx2frac a22right2a1xa0frac a224endaligned add a new unknown y to x2 a22 now you have x 2 a 2 2 y 2 − a 1 x − a 0 a 2 2 4 2 x 2 y a 2 y y 2 2 y x 2 − a 1 x − a'</li><li>'in particular in characteristic zero all complex solutions are sought searching for the real or rational solutions are much more difficult problems that are not considered in this article the set of solutions is not always finite for example the solutions of the system x x − 1 0 x y − 1 0 displaystyle beginalignedxx10xy10endaligned are a point xy 11 and a line x 0 even when the solution set is finite there is in general no closedform expression of the solutions in the case of a single equation this is abel – ruffini theorem the barth surface shown in the figure is the geometric representation of the solutions of a polynomial system reduced to a single equation of degree 6 in 3 variables some of its numerous singular points are visible on the image they are the solutions of a system of 4 equations of degree 5 in 3 variables such an overdetermined system has no solution in general that is if the coefficients are not specific if it has a finite number of solutions this number is at most 53 125 by bezouts theorem however it has been shown that for the case of the singular points of a surface of degree 6 the maximum number of solutions is 65 and is reached by the barth surface a system is overdetermined if the number of equations is higher than the number of variables a system is inconsistent if it has no complex solution or if the coefficients are not complex numbers no solution in an algebraically closed field containing the coefficients by hilberts nullstellensatz this means that 1 is a linear combination with polynomials as coefficients of the first members of the equations most but not all overdetermined systems when constructed with random coefficients are inconsistent for example the system x3 – 1 0 x2 – 1 0 is overdetermined having two equations but only one unknown but it is not inconsistent since it has the solution x 1 a system is underdetermined if the number of equations is lower than the number of the variables an underdetermined system is either inconsistent or has infinitely many complex solutions or solutions in an algebraically closed field that contains the coefficients of the equations this is a nontrivial result of commutative algebra that involves in particular hilberts nullstellensatz and krulls principal ideal theorem a system is zerodimensional if it has a finite number of complex solutions or solutions in an algebraically closed field this terminology comes from the fact that the algebraic variety of the solutions has dimension zero a system with infinitely many solutions is said to be positivedimensional a zerodimensional system with as'</li><li>'##gu endif endwhile return factors the correctness of this algorithm relies on the fact that the ring fqxf is a direct product of the fields fqxfi where fi runs on the irreducible factors of f as all these fields have qd elements the component of g in any of these fields is zero with probability q d − 1 2 q d [UNK] 1 2 displaystyle frac qd12qdsim tfrac 12 this implies that the polynomial gcdg u is the product of the factors of g for which the component of g is zero it has been shown that the average number of iterations of the while loop of the algorithm is less than 25 log 2 r displaystyle 25log 2r giving an average number of arithmetic operations in fq which is o d n 2 log r log q displaystyle odn2logrlogq in the typical case where dlogq n this complexity may be reduced to o n 2 log r log q n displaystyle on2logrlogqn by choosing h in the kernel of the linear map v → v q − v mod f displaystyle vto vqvpmod f and replacing the instruction g h q d − 1 2 − 1 mod f displaystyle ghfrac qd121pmod f by g h q − 1 2 − 1 mod f displaystyle ghfrac q121pmod f the proof of validity is the same as above replacing the direct product of the fields fqxfi by the direct product of their subfields with q elements the complexity is decomposed in o n 2 log r log q displaystyle on2logrlogq for the algorithm itself o n 2 log q n displaystyle on2logqn for the computation of the matrix of the linear map which may be already computed in the squarefree factorization and on3 for computing its kernel it may be noted that this algorithm works also if the factors have not the same degree in this case the number r of factors needed for stopping the while loop is found as the dimension of the kernel nevertheless the complexity is slightly better if squarefree factorization is done before using this algorithm as n may decrease with squarefree factorization this reduces the complexity of the critical steps victor shoups algorithm like the algorithms of the preceding section victor shoups algorithm is an equaldegree factorization algorithm unlike them it is a deterministic algorithm however it is less efficient in practice than the algorithms of preceding section for shoups algorithm the input is restricted'</li></ul> |
| 0 | <ul><li>'occupational noise is the amount of acoustic energy received by an employees auditory system when they are working in the industry occupational noise or industrial noise is often a term used in occupational safety and health as sustained exposure can cause permanent hearing damage occupational noise is considered an occupational hazard traditionally linked to loud industries such as shipbuilding mining railroad work welding and construction but can be present in any workplace where hazardous noise is present in the us the national institute for occupational safety and health niosh and the occupational safety and health administration osha work together to provide standards and regulations for noise in the workplacenational institute for occupational safety and health niosh occupational safety and health administration osha mine safety and health administration msha federal railroad administration fra have all set standards on hazardous occupational noise in their respective industries each industry is different as workers tasks and equipment differ but most regulations agree that noise becomes hazardous when it exceeds 85 decibels for an 8hour time exposure typical work shift this relationship between allotted noise level and exposure time is known as an exposure action value eav or permissible exposure limit pel the eav or pel can be seen as equations which manipulate the allotted exposure time according to the intensity of the industrial noise this equation works as an inverse exponential relationship as the industrial noise intensity increases the allotted exposure time to still remain safe decreases thus a worker exposed to a noise level of 100 decibels for 15 minutes would be at the same risk level as a worker exposed to 85 decibels for 8 hours using this mathematical relationship an employer can calculate whether or not their employees are being overexposed to noise when it is suspected that an employee will reach or exceed the pel a monitoring program for that employee should be implemented by the employerthe above calculations of pel and eav are based on measurements taken to determine the intensity of that particular industrial noise aweighted measurements are commonly used to determine noise levels that can cause harm to the human ear there are also special exposure meters available that integrate noise over a period of time to give an leq value equivalent sound pressure level defined by standards these numerical values do not fully reflect the real situation for example the osha standard sets the action level 85 dba and the pel 90 dba but in practice the compliance safety and health officer must record the excess of these values with a margin in order to take into account the potential measurement error and instead of pel 90 dba it turns out 92 dba and instead of al 85 dba its 87 dba occupational noise if experienced repeatedly at high intensity for an extended period of time can cause noiseinduce'</li><li>'the lowest frequency which can be localized depends on the ear distance animals with a greater ear distance can localize lower frequencies than humans can for animals with a smaller ear distance the lowest localizable frequency is higher than for humans if the ears are located at the side of the head interaural level differences appear for higher frequencies and can be evaluated for localization tasks for animals with ears at the top of the head no shadowing by the head will appear and therefore there will be much less interaural level differences which could be evaluated many of these animals can move their ears and these ear movements can be used as a lateral localization cue for many mammals there are also pronounced structures in the pinna near the entry of the ear canal as a consequence directiondependent resonances can appear which could be used as an additional localization cue similar to the localization in the median plane in the human auditory system there are additional localization cues which are also used by animals for sound localization in the median plane elevation of the sound also two detectors can be used which are positioned at different heights in animals however rough elevation information is gained simply by tilting the head provided that the sound lasts long enough to complete the movement this explains the innate behavior of cocking the head to one side when trying to localize a sound precisely to get instantaneous localization in more than two dimensions from timedifference or amplitudedifference cues requires more than two detectors the tiny parasitic fly ormia ochracea has become a model organism in sound localization experiments because of its unique ear the animal is too small for the time difference of sound arriving at the two ears to be calculated in the usual way yet it can determine the direction of sound sources with exquisite precision the tympanic membranes of opposite ears are directly connected mechanically allowing resolution of submicrosecond time differences and requiring a new neural coding strategy ho showed that the coupledeardrum system in frogs can produce increased interaural vibration disparities when only small arrival time and sound level differences were available to the animals head efforts to build directional microphones based on the coupledeardrum structure are underway most owls are nocturnal or crepuscular birds of prey because they hunt at night they must rely on nonvisual senses experiments by roger payne have shown that owls are sensitive to the sounds made by their prey not the heat or the smell in fact the sound cues are both necessary and sufficient for localization of mice from a distant location where they are perched for this to work the owls must be able to accurately localize both'</li><li>'##benmelodie in rock music from the late 1960s to the 2000s the timbre of specific sounds is important to a song for example in heavy metal music the sonic impact of the heavily amplified heavily distorted power chord played on electric guitar through very loud guitar amplifiers and rows of speaker cabinets is an essential part of the styles musical identity often listeners can identify an instrument even at different pitches and loudness in different environments and with different players in the case of the clarinet acoustic analysis shows waveforms irregular enough to suggest three instruments rather than one david luce suggests that this implies that certain strong regularities in the acoustic waveform of the above instruments must exist which are invariant with respect to the above variables however robert erickson argues that there are few regularities and they do not explain our powers of recognition and identification he suggests borrowing the concept of subjective constancy from studies of vision and visual perceptionpsychoacoustic experiments from the 1960s onwards tried to elucidate the nature of timbre one method involves playing pairs of sounds to listeners then using a multidimensional scaling algorithm to aggregate their dissimilarity judgments into a timbre space the most consistent outcomes from such experiments are that brightness or spectral energy distribution and the bite or rate and synchronicity and rise time of the attack are important factors the concept of tristimulus originates in the world of color describing the way three primary colors can be mixed together to create a given color by analogy the musical tristimulus measures the mixture of harmonics in a given sound grouped into three sections it is basically a proposal of reducing a huge number of sound partials that can amount to dozens or hundreds in some cases down to only three values the first tristimulus measures the relative weight of the first harmonic the second tristimulus measures the relative weight of the second third and fourth harmonics taken together and the third tristimulus measures the relative weight of all the remaining harmonics t 1 a 1 [UNK] h 1 h a h t 2 a 2 a 3 a 4 [UNK] h 1 h a h t 3 [UNK] h 5 h a h [UNK] h 1 h a h displaystyle t1frac a1sum h1hahqquad t2frac a2a3a4sum h1hahqquad t3frac sum h5hahsum h1hah however more evidence studies and applications would be needed regarding this type of representation in order to validate it the term brightness is also used in discussions of sound timbres in a rough analogy'</li></ul> |
| 39 | <ul><li>'waste heat is heat that is produced by a machine or other process that uses energy as a byproduct of doing work all such processes give off some waste heat as a fundamental result of the laws of thermodynamics waste heat has lower utility or in thermodynamics lexicon a lower exergy or higher entropy than the original energy source sources of waste heat include all manner of human activities natural systems and all organisms for example incandescent light bulbs get hot a refrigerator warms the room air a building gets hot during peak hours an internal combustion engine generates hightemperature exhaust gases and electronic components get warm when in operation instead of being wasted by release into the ambient environment sometimes waste heat or cold can be used by another process such as using hot engine coolant to heat a vehicle or a portion of heat that would otherwise be wasted can be reused in the same process if makeup heat is added to the system as with heat recovery ventilation in a building thermal energy storage which includes technologies both for short and longterm retention of heat or cold can create or improve the utility of waste heat or cold one example is waste heat from air conditioning machinery stored in a buffer tank to aid in night time heating another is seasonal thermal energy storage stes at a foundry in sweden the heat is stored in the bedrock surrounding a cluster of heat exchanger equipped boreholes and is used for space heating in an adjacent factory as needed even months later an example of using stes to use natural waste heat is the drake landing solar community in alberta canada which by using a cluster of boreholes in bedrock for interseasonal heat storage obtains 97 percent of its yearround heat from solar thermal collectors on the garage roofs another stes application is storing winter cold underground for summer air conditioningon a biological scale all organisms reject waste heat as part of their metabolic processes and will die if the ambient temperature is too high to allow this anthropogenic waste heat can contribute to the urban heat island effect the biggest point sources of waste heat originate from machines such as electrical generators or industrial processes such as steel or glass production and heat loss through building envelopes the burning of transport fuels is a major contribution to waste heat machines converting energy contained in fuels to mechanical work or electric energy produce heat as a byproduct in the majority of energy applications energy is required in multiple forms these energy forms typically include some combination of heating ventilation and air conditioning mechanical energy and electric power often these additional forms of energy are produced by a heat engine running on a source of hightemperat'</li><li>'boundaries at the flow extremes for a particular speed which are caused by different phenomena the steepness of the high flow part of a constant speed line is due to the effects of compressibility the position of the other end of the line is located by blade or passage flow separation there is a welldefined lowflow boundary marked on the map as a stall or surge line at which blade stall occurs due to positive incidence separation not marked as such on maps for turbochargers and gas turbine engines is a more gradually approached highflow boundary at which passages choke when the gas velocity reaches the speed of sound this boundary is identified for industrial compressors as overload choke sonic or stonewall the approach to this flow limit is indicated by the speed lines becoming more vertical other areas of the map are regions where fluctuating vane stalling may interact with blade structural modes leading to failure ie rotating stall causing metal fatigue different applications move over their particular map along different paths an example map with no operating lines is shown as a pictorial reference with the stallsurge line on the left and the steepening speed lines towards choke and overload on the right maps have similar features and general shape because they all apply to machines with spinning vanes which use similar principles for pumping a compressible fluid not all machines have stationary vanes centrifugal compressors may have either vaned or vaneless diffusers however a compressor operating as part of a gas turbine or turbocharged engine behaves differently to an industrial compressor because its flow and pressure characteristics have to match those of its driving turbine and other engine components such as power turbine or jet nozzle for a gas turbine and for a turbocharger the engine airflow which depends on engine speed and charge pressure a link between a gas turbine compressor and its engine can be shown with lines of constant engine temperature ratio ie the effect of fuellingincreased turbine temperature which raises the running line as the temperature ratio increases one manifestation of different behaviour appears in the choke region on the righthand side of a map it is a noload condition in a gas turbine turbocharger or industrial axial compressor but overload in an industrial centrifugal compressor hiereth et al shows a turbocharger compressor fullload or maximum fuelling curve runs up close to the surge line a gas turbine compressor fullload line also runs close to the surge line the industrial compressor overload is a capacity limit and requires high power levels to pass the high flow rates required excess power is available to inadvertently take the compressor beyond the overload limit to a hazardous condition'</li><li>'quantity thus it is useful to derive relationships between μ j t displaystyle mu mathrm jt and other more conveniently measured quantities as described below the first step in obtaining these results is to note that the joule – thomson coefficient involves the three variables t p and h a useful result is immediately obtained by applying the cyclic rule in terms of these three variables that rule may be written ∂ t ∂ p h ∂ h ∂ t p ∂ p ∂ h t − 1 displaystyle leftfrac partial tpartial prighthleftfrac partial hpartial trightpleftfrac partial ppartial hrightt1 each of the three partial derivatives in this expression has a specific meaning the first is μ j t displaystyle mu mathrm jt the second is the constant pressure heat capacity c p displaystyle cmathrm p defined by c p ∂ h ∂ t p displaystyle cmathrm p leftfrac partial hpartial trightp and the third is the inverse of the isothermal joule – thomson coefficient μ t displaystyle mu mathrm t defined by μ t ∂ h ∂ p t displaystyle mu mathrm t leftfrac partial hpartial prightt this last quantity is more easily measured than μ j t displaystyle mu mathrm jt thus the expression from the cyclic rule becomes μ j t − μ t c p displaystyle mu mathrm jt frac mu mathrm t cp this equation can be used to obtain joule – thomson coefficients from the more easily measured isothermal joule – thomson coefficient it is used in the following to obtain a mathematical expression for the joule – thomson coefficient in terms of the volumetric properties of a fluid to proceed further the starting point is the fundamental equation of thermodynamics in terms of enthalpy this is d h t d s v d p displaystyle mathrm d htmathrm d svmathrm d p now dividing through by dp while holding temperature constant yields ∂ h ∂ p t t ∂ s ∂ p t v displaystyle leftfrac partial hpartial prightttleftfrac partial spartial prighttv the partial derivative on the left is the isothermal joule – thomson coefficient μ t displaystyle mu mathrm t and the one on the right can be expressed in terms of the coefficient of thermal expansion via a maxwell relation the appropriate relation is ∂ s ∂ p t − ∂ v ∂ t p − v α displaystyle leftfrac partial spartial prighttleftfrac partial'</li></ul> |
| 21 | <ul><li>'##agate this type of plant this means that the characteristics of a determined cultivar remain unalteredbulbs can reproduce vegetatively in a number of ways depending on the type of storage organ the plant has bulbs can be evergreen such as clivia agapanthus and some species and varieties of iris and hemerocallis however the majority are deciduous dying down to the storage organ for part of the year this characteristic has been taken advantage of in the commercialization of these plants at the beginning of the rest period the bulbs can be dug out of the ground and prepared for sale as if they remain dry they do not need any nutrition for weeks or monthsbulbous plants are produced on an industrial scale for two main markets cut flowers and dried bulbs the bulbs are produced to satisfy the demand for bulbs for parks gardens and as house plants in addition to providing the bulbs necessary for the production of cut flowers the international trade in cut flowers has a worldwide value of approximately 11000 million euros which gives an idea of the economic importance of this activity the netherlands has been the leader in commercial production since the start of the 16th century both for the dried bulb market and for cut flowers in fact with approximately 30000 hectares dedicated to this activity the production of bulbs in the netherlands represents 65 of global production the netherlands also produces 95 of the international market in bulbs dedicated to the production of cut flowers the united states is the second largest producer followed by france japan italy united kingdom israel brazil and spain international bulb society httpwwwbulbsocietyorgestablished in 1933 this society is an international educational and scientific organization it is a charity dedicated to the dissemination of information regarding the cultivation conservation and botany of all types of bulbous plants their website contains an excellent gallery of high quality photographs of bulbous plantsthe pacific bulb society httpwwwpacificbulbsocietyorgorganized in 2002 this society disseminates information and shares experiences regarding the cultivation of ornamental bulbous plants their website contains an exceptional educational resource pacific bulb society wiki with images and information regarding numerous species of bulbous plantsaustralian bulb association httpswebarchiveorgweb20090518011847httpwwwausbulbsorgindexhtmorganized in 2001 it possessed an excellent collection of photographs of bulbous plants on its website list of flower bulbs hessayon dg 1999 the bulb expert london transworld publishers mathew brian 1978 the larger bulbs london bt batsford in association with the royal horticultural society isbn 9780'</li><li>'soil conservation is the prevention of loss of the topmost layer of the soil from erosion or prevention of reduced fertility caused by over usage acidification salinization or other chemical soil contamination slashandburn and other unsustainable methods of subsistence farming are practiced in some lesser developed areas a consequence of deforestation is typically largescale erosion loss of soil nutrients and sometimes total desertification techniques for improved soil conservation include crop rotation cover crops conservation tillage and planted windbreaks affect both erosion and fertility when plants die they decay and become part of the soil code 330 defines standard methods recommended by the us natural resources conservation service farmers have practiced soil conservation for millennia in europe policies such as the common agricultural policy are targeting the application of best management practices such as reduced tillage winter cover crops plant residues and grass margins in order to better address soil conservation political and economic action is further required to solve the erosion problem a simple governance hurdle concerns how we value the land and this can be changed by cultural adaptation soil carbon is a carbon sink playing a role in climate change mitigation contour ploughing orients furrows following the contour lines of the farmed area furrows move left and right to maintain a constant altitude which reduces runoff contour plowing was practiced by the ancient phoenicians for slopes between two and ten percent contour plowing can increase crop yields from 10 to 50 percent partially as a result of greater soil retention terracing is the practice of creating nearly level areas in a hillside area the terraces form a series of steps each at a higher level than the previous terraces are protected from erosion by other soil barriers terraced farming is more common on small farms keyline design is the enhancement of contour farming where the total watershed properties are taken into account in forming the contour lines tree shrubs and groundcover are effective perimeter treatment for soil erosion prevention by impeding surface flows a special form of this perimeter or interrow treatment is the use of a grass way that both channels and dissipates runoff through surface friction impeding surface runoff and encouraging infiltration of the slowed surface water windbreaks are sufficiently dense rows of trees at the windward exposure of an agricultural field subject to wind erosion evergreen species provide yearround protection however as long as foliage is present in the seasons of bare soil surfaces the effect of deciduous trees may be adequate cover crops such as nitrogenfixing legumes white turnips radishes and other species are rotated with cash crops to blanket the soil yearround and act as green manure that rep'</li><li>'blackberries are also cultivated in the same way in a tropical climate temperatures are prone to soar above all normal levels in such cases foggersmisters are used to reduce the temperature this does not increase the humidity levels in the poly house as the evaporated droplets are almost immediately ventilated to open air hightech poly houses even have spaceheating systems as well as soilheating systems to purify the soil of unwanted viruses bacteria and other organisms the recent indoisrael collaboration at gharunda near karnal is an excellent example of polyhouse farming taking place in a developing country if developing countries were to develop a special incentive program solely for fruitandvegetable farmers especially in demographically large nations like india then the migration rate from rural to urban areas as well as the loss of horticultural and fruitvegetable farmers to urban areas may be reduced this brings a huge potential to improve the farming sector which is key to longterm economic stability the small polytunnels used by each farmer in each village promote the cultivation of vegetables both onseason and offseason and would actually help to moderate the market rate for fruit and vegetables in long run on a yearround basis and would help to satisfy local market needs for example in india the inability to grow tomatoes generates price spikes during the monsoon season this is seen as an ideal time to grow tomatoes in polytunnels since they provide the ideal climate for the crop in india the abhinav farmers club grows flowers and organic vegetables in polytunnels hoophouses have existed at least since the 1940s but they are much more commonly used with each passing decade and their design continues to evolve because of the wide variety of constantly changing designs in reality there is an entirely continuous spectrum from high tunnels through low tunnels to the simplest row covers although they are often thought about as discrete steps major themes of continuing development are 1 achieving the same results with lighter construction and less cost and 2 making hoophouses easily movable the advantages of mobile hoophouses include greater return on investment with the same unit of investment getting greater use per year across different crops in different months and more flexibility on crop rotation without ever having to bother to dig the soil out of a stationary house or use soil steam sterilization to cure greenhouse soil sickness a us department of agriculture program is helping farmers install polytunnels the program was announced at the us white house garden in december 2009farmers in iraq are building these in increasing number and adding drip irrigation to grow tomatoes'</li></ul> |
| 18 | <ul><li>'the first postage stamps those of the united kingdom had no name in 1874 the universal postal union exempted the united kingdom from its rule which stated that a countrys name had to appear on their postage stamps so a profile of the reigning monarch was all that was required for identification of the uks stamps to this day the uk remains the only country not required to name itself on its stamps for all other upu members the name must appear in latin letters many countries using nonlatin alphabets used only those on their early stamps and they remain difficult for most collectors to identify today the name chosen is typically the countrys own name for itself with a modern trend towards using simpler and shorter forms or abbreviations for instance the republic of south africa inscribes with rsa while jordan originally used the hashemite kingdom of jordan and now just jordan some countries have multiple allowed forms from which the designer may choose the most suitable the name may appear in an adjectival form as in posta romana romanian post for romania dependent territories may or may not include the name of the parent country the graphic element of a stamp design falls into one of four major categories portrait bust profile or fullface emblem coat of arms flag national symbol posthorn etc numeric a design built around the numeral of value pictorialthe use of portrait busts of the ruler or other significant person or emblems was typical of the first stamps by extension from currency which was the closest model available to the early stamp designers usage pattern has varied considerably for 60 years from 1840 to 1900 all british stamps used exactly the same portrait bust of victoria enclosed in a dizzying variety of frames while spain periodically updated the image of alfonso xiii as he grew from child to adult norway has issued stamps with the same posthorn motif for over a century changing only the details from time to time as printing technology improves while the us has placed the flag of the united states into a wide variety of settings since first using it on a stamp in the 1950s while numeral designs are eminently practical in that they emphasize the most important element of the stamp they are the exception rather than the rule by far the greatest variety of stamp design seen today is in pictorial issues the choice of image is nearly unlimited ranging from plants and animals to figures from history to landscapes to original artwork images may represent realworld objects or be allegories or abstract designs the choice of pictorial designs is governed by a combination of anniversaries required annual issues such as christmas stamps postal rate changes exhaustion of existing stamp stocks and popular demand since postal administrations are either a branch'</li><li>'##ionism in both cases reflecting the influence of french impressionism which had spread internationally they are also known for their conceptual art as well as an internal split in the group which led to the formation of a new secession 1910 – 1914 key figures included walter leistikow franz skarbina max liebermann hermann struck and the norwegian painter edvard munch cologne 1909 – 1916 — also known as the sonderbund or the separate league of west german art lovers and artists the sonderbund westdeutscher kunstfreunde und kunstler was known for its landmark exhibitions introducing french impressionism postimpressionism and modernism to germany its 1912 show aimed to organize the most disputed paintings of our time and was later credited for helping develop a german version of expressionism while also presenting the most significant exhibition of european modernism prior to world war i the following year in fact it inspired a similar show in new york artists associated with the group included julius bretz max clarenbach august deusser walter ophey ernst osthaus egon schiele wilhelm schmurr alfred sohnrethel karli sohnrethel and otto sohnrethel along with collectors and curators of art dresden 1919 – 1925 — formed in reaction to the oppression of post world war i and the rise of the weimar republic otto schubert conrad felixmuller and otto dix are considered key figures in the dresden secession they are known for a highly accomplished form of german expressionism that was later labeled degenerate by the nazis selection was limited by availability academic art – style of painting and sculpture preraphaelite – group of english painters poets and critics founded in 1848pages displaying short descriptions of redirect targets salon des refuses art exhibition in paris first held in 1863 of works rejected by the academie des beauxarts simon hansulrich sezessionismus kunstgewerbe in literarischer und bildender kunst j b metzlersche verlagsbuchhandlung stuttgart 1976 isbn 3476002896'</li><li>'then still known as the vienna method was the monumental collection of 100 statistical charts gesellschaft und wirtschaft 1930 the first rule of isotype is that greater quantities are not represented by an enlarged pictogram but by a greater number of the samesized pictogram in neurath ’ s view variation in size does not allow accurate comparison what is to be compared – heightlength or area whereas repeated pictograms which always represent a fixed value within a certain chart can be counted if necessary isotype pictograms almost never depicted things in perspective in order to preserve this clarity and there were other guidelines for graphic configuration and use of colour the best exposition of isotype technique remains otto neurath ’ s book international picture language 1936 visual education was always the prime motive behind isotype which was worked out in exhibitions and books designed to inform ordinary citizens including schoolchildren about their place in the world it was never intended to replace verbal language it was a helping language always accompanied by verbal elements otto neurath realized that it could never be a fully developed language so instead he called it a “ languagelike technique ” as more requests came to the vienna museum from abroad a partner institute called mundaneum a name adopted from an abortive collaboration with paul otlet was established in 19312 to promote international work it formed branches containing small exhibitions in berlin the hague london and new york city members of the vienna team travelled periodically to the soviet union during the early 1930s in order to help set up the allunion institute of pictorial statistics of soviet construction and economy всесоюзныи институт изобразительнои статистики советского строительства и хозяиства commonly abbreviated to izostat изостат which produced statistical graphics about the five year plans among other things after the closure of the gesellschafts und wirtschaftsmuseum in 1934 neurath reidemeister and arntz fled to the netherlands where they set up the international foundation for visual education in the hague during the 1930s significant commissions were received from the us including a series of massproduced charts for the national tuberculosis association and otto neurath ’ s book modern man in the making 1939 a high point of isotype on which he reidemeister and arntz worked in close'</li></ul> |
| 5 | <ul><li>'giant stars and white and red dwarf stars could support a timeintegrated biota up to 1046 kgyears in the galaxy and 1057 kgyears in the universesuch astroecology considerations quantify the immense potentials of future life in space with commensurate biodiversity and possibly intelligence chemical analysis of carbonaceous chondrite meteorites show that they contain extractable bioavailable water organic carbon and essential phosphate nitrate and potassium nutrients the results allow assessing the soil fertilities of the parent asteroids and planets and the amounts of biomass that they can sustainlaboratory experiments showed that material from the murchison meteorite when ground into a fine powder and combined with earths water and air can provide the nutrients to support a variety of organisms including bacteria nocardia asteroides algae and plant cultures such as potato and asparagus the microorganisms used organics in the carbonaceous meteorites as the carbon source algae and plant cultures grew well also on mars meteorites because of their high bioavailable phosphate contents the martian materials achieved soil fertility ratings comparable to productive agricultural soils this offers some data relating to terraforming of marsterrestrial analogues of planetary materials are also used in such experiments for comparison and to test the effects of space conditions on microorganismsthe biomass that can be constructed from resources can be calculated by comparing the concentration of elements in the resource materials and in biomass equation 1 a given mass of resource materials mresource can support mbiomass x of biomass containing element x considering x as the limiting nutrient where cresource x is the concentration mass per unit mass of element x in the resource material and cbiomass x is its concentration in the biomass m b i o m a s s x m r e s o u r c e x c r e s o u r c e x c b i o m a s s x displaystyle mbiomassxmresourcexfrac cresourcexcbiomassx 1 assuming that 100000 kg biomass supports one human the asteroids may then sustain about 6e15 six million billion people equal to a million earths a million times the present population similar materials in the comets could support biomass and populations about one hundred times larger solar energy can sustain these populations for the predicted further five billion years of the sun these considerations yield a maximum timeintegrated biota of 3e30 kgyears in the solar system after the sun becomes a white dwarf star and other white dwarf stars can provide energy'</li><li>'astronomer and astrobiology pioneer gavriil adrianovich tikhov tikhov is considered to be the father of astrobotany research in the field has been conducted both with growing earth plants in space environments and searching for botanical life on other planets the first organisms in space were specially developed strains of seeds launched to 134 km 83 mi on 9 july 1946 on a us launched v2 rocket these samples were not recovered the first seeds launched into space and successfully recovered were maize seeds launched on 30 july 1946 which were soon followed by rye and cotton these early suborbital biological experiments were handled by harvard university and the naval research laboratory and were concerned with radiation exposure on living tissue in 1971 500 tree seeds loblolly pine sycamore sweetgum redwood and douglas fir were flown around the moon on apollo 14 these moon trees were planted and grown with controls back on earth where no changes were detected in 1982 the crew of the soviet salyut 7 space station conducted an experiment prepared by lithuanian scientists alfonsas merkys and others and grew some arabidopsis using fiton3 experimental microgreenhouse apparatus thus becoming the first plants to flower and produce seeds in space a skylab experiment studied the effects of gravity and light on rice plants the svet2 space greenhouse successfully achieved seed to seed plant growth in 1997 aboard space station mir bion 5 carried daucus carota and bion 7 carried maize aka corn plant research continued on the international space station biomass production system was used on the iss expedition 4 the vegetable production system veggie system was later used aboard iss plants tested in veggie before going into space included lettuce swiss chard radishes chinese cabbage and peas red romaine lettuce was grown in space on expedition 40 which were harvested when mature frozen and tested back on earth expedition 44 members became the first american astronauts to eat plants grown in space on 10 august 2015 when their crop of red romaine was harvested since 2003 russian cosmonauts have been eating half of their crop while the other half goes towards further research in 2012 a sunflower bloomed aboard the iss under the care of nasa astronaut donald pettit in january 2016 us astronauts announced that a zinnia had blossomed aboard the issin 2018 the veggie3 experiment was tested with plant pillows and root mats one of the goals is to grow food for crew consumption crops tested at this time include cabbage lettuce and mizuna plants that have been grown in space include arabidopsis thale cress bok choy tokyo bekana'</li><li>'the planet simulator also known as a planetary simulator is a climatecontrolled simulation chamber designed to study the origin of life the device was announced by researchers at mcmaster university on behalf of the origins institute on 4 october 2018 the simulator project begun in 2012 and was funded with 1 million from the canada foundation for innovation the ontario government and mcmaster university it was built and manufactured by angstrom engineering inc of kitchener ontariothe device was designed and developed by biophysicist maikel rheinstadter and coprincipal investigators biochemist yingfu li and astrophysicist ralph pudritz for researchers to study a theory that suggests life on early earth began in warm little ponds rather than in deep ocean vents nearly four billion years ago the device can recreate conditions of the primitive earth to see whether cellular life can be created and then later evolvein an 2018 news release maikel rheinstadter stated we want to understand how the first living cell was formed how the earth moved from a chemical world to a biological worldthe planet simulator can mimic the environmental conditions consistent on the early earth and other astronomical bodies including other planets and exoplanets by controlling temperature humidity pressure atmosphere and radiation levels within the simulation chamber according to researchers preliminary tests with the simulator under possible conditions of the early earth created protocells cells which are not living but very important nonetheless according to biologist david deamer the device is a game changer and the cells produced so far are significant the cells are not alive but are evolutionary steps toward a living system of molecules the simulator opens up a lot of experimental activities that were literally impossible before ” based on initial tests with the new simulator technology project director rheinstadter stated that it seems that the formation of life is probably a relatively frequent process in the universe'</li></ul> |
| 28 | <ul><li>'##nfjgk0 if k = 1 displaystyle kneq 1 and [UNK] j 1 n a j 1 [UNK] j 1 n f j e n displaystyle sum j1naj1sum j1nfjen let a ∗ displaystyle aast denote the conjugate transpose of a then a a ∗ a ∗ a n i displaystyle aaast aast ani this implies the desired orthogonality relationship for the characters ie [UNK] k 1 n f k ∗ g i f k g j n δ i j displaystyle sum k1nfkgifkgjndelta ij where δ i j displaystyle delta ij is the kronecker delta and f k ∗ g i displaystyle fkgi is the complex conjugate of f k g i displaystyle fkgi pontryagin duality'</li><li>'j x p i ν p i − 1 [UNK] j i 1 ω x p j ν p j x [UNK] i 1 ω x ν p i x p i x x [UNK] p prime p [UNK] x ν p x p displaystyle dxsum i1omega xleftnu pixleftprod j1i1pjnu pjxrightpinu pi1leftprod ji1omega xpjnu pjxrightrightsum i1omega xfrac nu pixpixxsum stackrel pmid xptext primefrac nu pxp where ωx a prime omega function is the number of distinct prime factors in x and νpx is the padic valuation of x for example d 60 d 2 2 ⋅ 3 ⋅ 5 2 2 1 3 1 5 ⋅ 60 92 displaystyle d60d22cdot 3cdot 5leftfrac 22frac 13frac 15rightcdot 6092 or d 81 d 3 4 4 ⋅ 3 3 ⋅ d 3 4 ⋅ 27 ⋅ 1 108 displaystyle d81d344cdot 33cdot d34cdot 27cdot 1108 the sequence of number derivatives for k 0 1 2 … begins sequence a003415 in the oeis 0 0 1 1 4 1 5 1 12 6 7 1 16 1 9 … displaystyle 00114151126711619ldots the logarithmic derivative ld x d x x [UNK] p prime p [UNK] x ν p x p displaystyle operatorname ld xfrac dxxsum stackrel pmid xptext primefrac nu pxp is a totally additive function ld x ⋅ y ld x ld y displaystyle operatorname ld xcdot yoperatorname ld xoperatorname ld y the arithmetic partial derivative of x displaystyle x with respect to p displaystyle p is defined as x p ′ ν p x p x displaystyle xpprime frac nu pxpx so the arithmetic derivative of x displaystyle x is given as d x [UNK] p prime p [UNK] x x p ′ displaystyle dxsum stackrel pmid xptext primexpprime an arithmetic function f displaystyle f is leibnizadditive if there is a totally multiplicative function h f displaystyle hf such that f m n f m h f n f n h f m displaystyle fmnfmhfnfnhfm for all positive integers m displaystyle m and n displaystyle n a motivation for this concept is'</li><li>'and every rcoloring of the integers greater than one there is a finite monochromatic subset s of these integers such that the conjecture was proven in 2003 by ernest s croot iii znams problem and primary pseudoperfect numbers are closely related to the existence of egyptian fractions of the form for instance the primary pseudoperfect number 1806 is the product of the prime numbers 2 3 7 and 43 and gives rise to the egyptian fraction 1 12 13 17 143 11806 egyptian fractions are normally defined as requiring all denominators to be distinct but this requirement can be relaxed to allow repeated denominators however this relaxed form of egyptian fractions does not allow for any number to be represented using fewer fractions as any expansion with repeated fractions can be converted to an egyptian fraction of equal or smaller length by repeated application of the replacement if k is odd or simply by replacing 1k 1k by 2k if k is even this result was first proven by takenouchi 1921 graham and jewett proved that it is similarly possible to convert expansions with repeated denominators to longer egyptian fractions via the replacement this method can lead to long expansions with large denominators such as botts 1967 had originally used this replacement technique to show that any rational number has egyptian fraction representations with arbitrarily large minimum denominators any fraction xy has an egyptian fraction representation in which the maximum denominator is bounded by and a representation with at most terms the number of terms must sometimes be at least proportional to log log y for instance this is true for the fractions in the sequence 12 23 67 4243 18061807 whose denominators form sylvesters sequence it has been conjectured that olog log y terms are always enough it is also possible to find representations in which both the maximum denominator and the number of terms are small graham 1964 characterized the numbers that can be represented by egyptian fractions in which all denominators are nth powers in particular a rational number q can be represented as an egyptian fraction with square denominators if and only if q lies in one of the two halfopen intervals martin 1999 showed that any rational number has very dense expansions using a constant fraction of the denominators up to n for any sufficiently large n engel expansion sometimes called an egyptian product is a form of egyptian fraction expansion in which each denominator is a multiple of the previous one in addition the sequence of multipliers ai is required to be nondecreasi'</li></ul> |
| 38 | <ul><li>'##ken the global language system theorises that language groups are engaged in unequal competition on different levels globally using the notions of a periphery semiperiphery and a core which are concepts of the world system theory de swaan relates them to the four levels present in the hierarchy of the global language system peripheral central supercentral and hypercentralde swaan also argues that the greater the range of potential uses and users of a language the higher the tendency of an individual to move up the hierarchy in the global language system and learn a more central language thus de swaan views the learning of second languages as proceeding up rather than down the hierarchy in the sense that they learn a language that is on the next level up for instance speakers of catalan a peripheral language have to learn spanish a central language to function in their own society spain meanwhile speakers of persian a central language have to learn arabic a supercentral language to function in their region on the other hand speakers of a supercentral language have to learn the hypercentral language to function globally as is evident from the huge number of nonnative english speakersaccording to de swaan languages exist in constellations and the global language system comprises a sociological classification of languages based on their social role for their speakers the worlds languages and multilinguals are connected in a strongly ordered hierarchical pattern there are thousands of peripheral or minority languages in the world each of which are connected to one of a hundred central languages the connections and patterns between each language is what makes up the global language system the four levels of language are the peripheral central supercentral and hypercentral languages peripheral languages at the lowest level peripheral languages or minority languages form the majority of languages spoken in the world 98 of the worlds languages are peripheral languages and spoken by less than 10 of the world ’ s population unlike central languages these are languages of conversation and narration rather than reading and writing of memory and remembrance rather than record they are used by native speakers within a particular area and are in danger of becoming extinct with increasing globalisation which sees more and more speakers of peripheral languages acquiring more central languages in order to communicate with others central languages the next level constitutes about 100 central languages spoken by 95 of the worlds population and generally used in education media and administration typically they are the national and official languages of the ruling state these are the languages of record and much of what has been said and written in those languages is saved in newspaper reports minutes and proceedings stored in archives included in history books collections of the classics of folk talks and folk ways increasingly recorded on electronic media and'</li><li>'the common misconception that aave carries ungrammatical features or that any speaker who speaks aave are uneducated or sloppy however like all dialects aave shows consistent internal logic and grammatical complexity as explained in the following examplesthe use of done coupled with the past tense of the verb in a sentence as seen in they done used all the good ones is a persistent structural trait of aave that is shared with southern european american vernacular varieties of english although the verbal particle done also occurs in caribbean creoles its syntactic configuration and semanticpragmatic function in aave differ somewhat from its creole counterpartsin aave done occurs only in preverbal auxiliary position with past tense forms whereas it occurs with a bare verb stem eg they done go and can occur in clausefinal position in some creoles in many aspects it functions in aave like a perfect tense referring to an action completed in the recent past but it can also be used to highlight the change of state or to intensify an activity as in the sentence i done told you not to mess up it is a stable feature but it is more frequently used in southern rural versions of aave than in urban aavedouble negation is also another feature commonly found in aave referring to the marking of negation on the auxiliary verb and indefinite pronoun an example would be she aint tellin nobody which would be she isnt telling anybody in standard english another feature copula absence or the absence of is or are in certain contexts can be observed as well he workin or they going home are some examples the habitual aspect marker or the invariant be habitual be as seen in he be workin they be tryin or i be like is a typical feature of aave it is the use of the base form of the copula verb be instead of the inflected forms such as are and am this is probably the most salient grammatical trait of aave both within the community and outside of it to the point of it being a stereotype prominently figured in representations of aave especially in the mediathe link between language and identity can be stretched into a tripartite where culture becomes key the addition of culture to the way language is linked to identity blur the lines because culture can be considered an abstract concept particularly in america it is nearly impossible to pinpoint a common culture in a country filled with so many different cultures especially when many of them are several generations removed from their origins because of the racial makeup of the country it is not ideal to include all american citizens under a'</li><li>'patois pl same or is speech or language that is considered nonstandard although the term is not formally defined in linguistics as such patois can refer to pidgins creoles dialects or vernaculars but not commonly to jargon or slang which are vocabularybased forms of cant in colloquial usage of the term especially in france class distinctions are implied by the very meaning of the term since in french patois refers to any sociolect associated with uneducated rural classes in contrast with the dominant prestige language standard french spoken by the middle and high classes of cities or as used in literature and formal settings the acrolect the term patois comes from old french patois local or regional dialect originally meaning rough clumsy or uncultivated speech possibly from the verb patoier to treat roughly from patte paw from old low franconian patta paw sole of the foot plus the suffix ois in france and other francophone countries patois has been used to describe nonstandard french and regional languages such as picard occitan and francoprovencal since 1643 and catalan after 1700 when the king louis xiv banned its use the word assumes the view of such languages being backward countrified and unlettered thus patois being potentially considered offensive when used by outsiders jean jaures said one names patois the language of a defeated nation in france and switzerland however the term patois no longer holds any offensive connotation and has indeed become a celebrated and distinguished variant of the numerous local tonguesthe vernacular form of english spoken in jamaica is also referred to as patois or patwa it is noted especially in reference to jamaican patois from 1934 jamaican patois language comprises words of the native languages of the many ethnic and cultural groups within the caribbean including spanish portuguese chinese amerindian and english along with several african languages some islands have creole dialects influenced by their linguistic diversity french spanish arabic hebrew german dutch italian chinese vietnamese and others jamaican patois is also spoken in costa rica and french creole is spoken in caribbean countries such as trinidad and tobago and guyana in south america often these patois are popularly considered broken english or slang but cases such as jamaican patois are classified with more correctness as a creole language in fact in the francophone caribbean the analogous term for local basilectal languages is creole see also jamaican english and jamaican creole antillean creole spoken in several present or formerly french islands of the lesser antilles includes vocabulary and grammar of african and carib origin in addition to french its dialects often contain folketymological derivatives of french words for example la'</li></ul> |
| 40 | <ul><li>'##2 is the invariant of rohlin1991 clifford taubes forselfdual yangmills connections on nonselfdual 4manifolds journal of differential geometry 17 1982 no 1 139 – 170 gauge theory on asymptotically periodic 4manifolds j differential geom 25 1987 no 3 363 – 430 cassons invariant and gauge theory j differential geom 31 1990 no 2 547 – 5991996 richard s hamilton forthe formation of singularities in the ricci flow surveys in differential geometry vol ii cambridge ma 1993 7 – 136 int press cambridge ma 1995 fourmanifolds with positive isotropic curvature comm anal geom 5 1997 no 1 1 – 921996 gang tian foron calabis conjecture for complex surfaces with positive first chern class invent math 101 1990 no 1 101 – 172 compactness theorems for kahlereinstein manifolds of dimension 3 and up j differential geom 35 1992 no 3 535 – 558 a mathematical theory of quantum cohomology j differential geom 42 1995 no 2 259 – 367 with yongbin ruan kahlereinstein metrics with positive scalar curvature invent math 130 1997 no 1 1 – 372001 jeff cheeger forfamilies index for manifolds with boundary superconnections and cones i families of manifolds with boundary and dirac operators j funct anal 89 1990 no 2 313 – 363 with jeanmichel bismut families index for manifolds with boundary superconnections and cones ii the chern character j funct anal 90 1990 no 2 306 – 354 with jeanmichel bismut lower bounds on ricci curvature and the almost rigidity of warped products ann of math 2 144 1996 no 1 189 – 237 with tobias colding on the structure of spaces with ricci curvature bounded below i j differential geom 46 1997 no 3 406 – 480 with tobias colding2001 yakov eliashberg forcombinatorial methods in symplectic geometry proceedings of the international congress of mathematicians vol 1 2 berkeley calif 1986 531 – 539 amer math soc providence ri 1987 classification of overtwisted contact structures on 3manifolds invent math 98 1989 no 3 623 – 6372001 michael j hopkins fornilpotence and stable homotopy theory i ann of math 2 128 1988 no 2 207 – 241 with ethan devinatz and jeffrey smith the rigid analytic period mapping lubintate space and stable homotopy theory bull amer math'</li><li>'this case the two metric spaces are essentially identical they are called quasiisometric if there is a quasiisometry between them a normed vector space is a vector space equipped with a norm which is a function that measures the length of vectors the norm of a vector v is typically denoted by ‖ v ‖ displaystyle lvert vrvert any normed vector space can be equipped with a metric in which the distance between two vectors x and y is given by the metric d is said to be induced by the norm ‖ ⋅ ‖ displaystyle lvert cdot rvert conversely if a metric d on a vector space x is translation invariant d x y d x a y a displaystyle dxydxaya for every x y and a in x and absolutely homogeneous d α x α y α d x y displaystyle dalpha xalpha yalpha dxy for every x and y in x and real number αthen it is the metric induced by the norm a similar relationship holds between seminorms and pseudometrics among examples of metrics induced by a norm are the metrics d1 d2 and d∞ on r 2 displaystyle mathbb r 2 which are induced by the manhattan norm the euclidean norm and the maximum norm respectively more generally the kuratowski embedding allows one to see any metric space as a subspace of a normed vector space infinitedimensional normed vector spaces particularly spaces of functions are studied in functional analysis completeness is particularly important in this context a complete normed vector space is known as a banach space an unusual property of normed vector spaces is that linear transformations between them are continuous if and only if they are lipschitz such transformations are known as bounded operators a curve in a metric space m d is a continuous function γ 0 t → m displaystyle gamma 0tto m the length of γ is measured by in general this supremum may be infinite a curve of finite length is called rectifiable suppose that the length of the curve γ is equal to the distance between its endpoints — that is its the shortest possible path between its endpoints after reparametrization by arc length γ becomes a geodesic a curve which is a distancepreserving function a geodesic is a shortest possible path between any two of its pointsa geodesic metric space is a metric space which admits a geodesic between any two of its points the spaces r 2 d 1 displaystyle mathbb r 2d1 and r 2 d 2 displaystyle mathbb r 2d2 are both geo'</li><li>'symmetryprotected topological spt order is a kind of order in zerotemperature quantummechanical states of matter that have a symmetry and a finite energy gap to derive the results in a mostinvariant way renormalization group methods are used leading to equivalence classes corresponding to certain fixed points the spt order has the following defining properties a distinct spt states with a given symmetry cannot be smoothly deformed into each other without a phase transition if the deformation preserves the symmetry b however they all can be smoothly deformed into the same trivial product state without a phase transition if the symmetry is broken during the deformation the above definition works for both bosonic systems and fermionic systems which leads to the notions of bosonic spt order and fermionic spt order using the notion of quantum entanglement we can say that spt states are shortrange entangled states with a symmetry by contrast for longrange entanglement see topological order which is not related to the famous epr paradox since shortrange entangled states have only trivial topological orders we may also refer the spt order as symmetry protected trivial order the boundary effective theory of a nontrivial spt state always has pure gauge anomaly or mixed gaugegravity anomaly for the symmetry group as a result the boundary of a spt state is either gapless or degenerate regardless how we cut the sample to form the boundary a gapped nondegenerate boundary is impossible for a nontrivial spt state if the boundary is a gapped degenerate state the degeneracy may be caused by spontaneous symmetry breaking andor intrinsic topological order monodromy defects in nontrivial 21d spt states carry nontrival statistics and fractional quantum numbers of the symmetry group monodromy defects are created by twisting the boundary condition along a cut by a symmetry transformation the ends of such cut are the monodromy defects for example 21d bosonic zn spt states are classified by a zn integer m one can show that n identical elementary monodromy defects in a zn spt state labeled by m will carry a total zn quantum number 2m which is not a multiple of n 21d bosonic u1 spt states have a hall conductance that is quantized as an even integer 21d bosonic so3 spt states have a quantized spin hall conductance spt states are shortrange entangled while topologically ordered states are longrange entangled both intrinsic topological order and also sp'</li></ul> |
| 4 | <ul><li>'hormone auxin which activates meristem growth alongside other mechanisms to control the relative angle of buds around the stem from a biological perspective arranging leaves as far apart as possible in any given space is favoured by natural selection as it maximises access to resources especially sunlight for photosynthesis in mathematics a dynamical system is chaotic if it is highly sensitive to initial conditions the socalled butterfly effect which requires the mathematical properties of topological mixing and dense periodic orbitsalongside fractals chaos theory ranks as an essentially universal influence on patterns in nature there is a relationship between chaos and fractals — the strange attractors in chaotic systems have a fractal dimension some cellular automata simple sets of mathematical rules that generate patterns have chaotic behaviour notably stephen wolframs rule 30vortex streets are zigzagging patterns of whirling vortices created by the unsteady separation of flow of a fluid most often air or water over obstructing objects smooth laminar flow starts to break up when the size of the obstruction or the velocity of the flow become large enough compared to the viscosity of the fluid meanders are sinuous bends in rivers or other channels which form as a fluid most often water flows around bends as soon as the path is slightly curved the size and curvature of each loop increases as helical flow drags material like sand and gravel across the river to the inside of the bend the outside of the loop is left clean and unprotected so erosion accelerates further increasing the meandering in a powerful positive feedback loop waves are disturbances that carry energy as they move mechanical waves propagate through a medium – air or water making it oscillate as they pass by wind waves are sea surface waves that create the characteristic chaotic pattern of any large body of water though their statistical behaviour can be predicted with wind wave models as waves in water or wind pass over sand they create patterns of ripples when winds blow over large bodies of sand they create dunes sometimes in extensive dune fields as in the taklamakan desert dunes may form a range of patterns including crescents very long straight lines stars domes parabolas and longitudinal or seif sword shapesbarchans or crescent dunes are produced by wind acting on desert sand the two horns of the crescent and the slip face point downwind sand blows over the upwind face which stands at about 15 degrees from the horizontal and falls onto the slip face where it accumulates up to the angle of repose of the sand which is about 35 degrees when the slip face'</li><li>'singleparticle trajectories spts consist of a collection of successive discrete points causal in time these trajectories are acquired from images in experimental data in the context of cell biology the trajectories are obtained by the transient activation by a laser of small dyes attached to a moving molecule molecules can now by visualized based on recent superresolution microscopy which allow routine collections of thousands of short and long trajectories these trajectories explore part of a cell either on the membrane or in 3 dimensions and their paths are critically influenced by the local crowded organization and molecular interaction inside the cell as emphasized in various cell types such as neuronal cells astrocytes immune cells and many others spt allowed observing moving particles these trajectories are used to investigate cytoplasm or membrane organization but also the cell nucleus dynamics remodeler dynamics or mrna production due to the constant improvement of the instrumentation the spatial resolution is continuously decreasing reaching now values of approximately 20 nm while the acquisition time step is usually in the range of 10 to 50 ms to capture short events occurring in live tissues a variant of superresolution microscopy called sptpalm is used to detect the local and dynamically changing organization of molecules in cells or events of dna binding by transcription factors in mammalian nucleus superresolution image acquisition and particle tracking are crucial to guarantee a high quality data once points are acquired the next step is to reconstruct a trajectory this step is done known tracking algorithms to connect the acquired points tracking algorithms are based on a physical model of trajectories perturbed by an additive random noise the redundancy of many short spts is a key feature to extract biophysical information parameters from empirical data at a molecular level in contrast long isolated trajectories have been used to extract information along trajectories destroying the natural spatial heterogeneity associated to the various positions the main statistical tool is to compute the meansquare displacement msd or second order statistical moment ⟨ x t δ t − x t 2 ⟩ [UNK] t α displaystyle langle xtdelta txt2rangle sim talpha average over realizations where α displaystyle alpha is the called the anomalous exponentfor a brownian motion ⟨ x t δ t − x t 2 ⟩ 2 n d t displaystyle langle xtdelta txt2rangle 2ndt where d is the diffusion coefficient n is dimension of the space some other properties can also be recovered from long trajectories such as the'</li><li>'each n displaystyle n the new function is defined at the points a a h a 2 h … a n h … displaystyle aaha2hldots anhldots the fundamental theorem of calculus states that differentiation and integration are inverse operations more precisely it relates the difference quotients to the riemann sums it can also be interpreted as a precise statement of the fact that differentiation is the inverse of integration the fundamental theorem of calculus if a function f displaystyle f is defined on a partition of the interval a b displaystyle ab b a n h displaystyle banh and if f displaystyle f is a function whose difference quotient is f displaystyle f then we have [UNK] i 0 n − 1 f a i h h 2 δ x f b − f a displaystyle sum i0n1faihh2delta xfbfa furthermore for every m 0 1 2 … n − 1 textstyle m012ldots n1 we have δ δ x [UNK] i 0 m f a i h h 2 δ x f a m h h 2 displaystyle frac delta delta xsum i0mfaihh2delta xfamhh2 this is also a prototype solution of a difference equation difference equations relate an unknown function to its difference or difference quotient and are ubiquitous in the sciences the early history of discrete calculus is the history of calculus such basic ideas as the difference quotients and the riemann sums appear implicitly or explicitly in definitions and proofs after the limit is taken however they are never to be seen again however the kirchhoffs voltage law 1847 can be expressed in terms of the onedimensional discrete exterior derivative during the 20th century discrete calculus remains interlinked with infinitesimal calculus especially differential forms but also starts to draw from algebraic topology as both develop the main contributions come from the following individuals henri poincare triangulations barycentric subdivision dual triangulation poincare lemma the first proof of the general stokes theorem and a lot more l e j brouwer simplicial approximation theorem elie cartan georges de rham the notion of differential form the exterior derivative as a coordinateindependent linear operator exactnessclosedness of forms emmy noether heinz hopf leopold vietoris walther mayer modules of chains the boundary operator chain complexes j w alexander solomon lefschetz lev pontryagin andrey kolmogorov norman steenrod eduard cech the early cochain notions hermann weyl the kirchhoff laws'</li></ul> |
| 6 | <ul><li>'##ativistic degenerate matter a polytrope with index n 3 is a good model for the cores of white dwarfs of higher masses according to the equation of state of relativistic degenerate matter a polytrope with index n 3 is usually also used to model mainsequence stars like the sun at least in the radiation zone corresponding to the eddington standard model of stellar structure a polytrope with index n 5 has an infinite radius it corresponds to the simplest plausible model of a selfconsistent stellar system first studied by arthur schuster in 1883 and it has an exact solution a polytrope with index n ∞ corresponds to what is called an isothermal sphere that is an isothermal selfgravitating sphere of gas whose structure is identical to the structure of a collisionless system of stars like a globular cluster this is because for an ideal gas the temperature is proportional to ρ1n so infinite n corresponds to a constant temperaturein general as the polytropic index increases the density distribution is more heavily weighted toward the center r 0 of the body polytropic process equation of state murnaghan equation of state'</li><li>'together the analysis was expanded upon by alar toomre in 1964 and presented in a more general and comprehensive framework'</li><li>'the bidirectional reflectance distribution function brdf symbol f r ω i ω r displaystyle ftextromega textiomega textr is a function of four real variables that defines how light is reflected at an opaque surface it is employed in the optics of realworld light in computer graphics algorithms and in computer vision algorithms the function takes an incoming light direction ω i displaystyle omega texti and outgoing direction ω r displaystyle omega textr taken in a coordinate system where the surface normal n displaystyle mathbf n lies along the zaxis and returns the ratio of reflected radiance exiting along ω r displaystyle omega textr to the irradiance incident on the surface from direction ω i displaystyle omega texti each direction ω displaystyle omega is itself parameterized by azimuth angle [UNK] displaystyle phi and zenith angle θ displaystyle theta therefore the brdf as a whole is a function of 4 variables the brdf has units sr−1 with steradians sr being a unit of solid angle the brdf was first defined by fred nicodemus around 1965 the definition is where l displaystyle l is radiance or power per unit solidangleinthedirectionofaray per unit projectedareaperpendiculartotheray e displaystyle e is irradiance or power per unit surface area and θ i displaystyle theta texti is the angle between ω i displaystyle omega texti and the surface normal n displaystyle mathbf n the index i displaystyle texti indicates incident light whereas the index r displaystyle textr indicates reflected light the reason the function is defined as a quotient of two differentials and not directly as a quotient between the undifferentiated quantities is because irradiating light other than d e i ω i displaystyle mathrm d etextiomega texti which are of no interest for f r ω i ω r displaystyle ftextromega textiomega textr might illuminate the surface which would unintentionally affect l r ω r displaystyle ltextromega textr whereas d l r ω r displaystyle mathrm d ltextromega textr is only affected by d e i ω i displaystyle mathrm d etextiomega texti the spatially varying bidirectional reflectance distribution function svbrdf is a 6dimensional function f r ω i ω r x displaystyle ftextromega textiomega textrmathbf x where x displaystyle mathbf x describes a 2d'</li></ul> |
| 35 | <ul><li>'microbiologically induced calcium carbonate precipitation micp is a biogeochemical process that induces calcium carbonate precipitation within the soil matrix biomineralization in the form of calcium carbonate precipitation can be traced back to the precambrian period calcium carbonate can be precipitated in three polymorphic forms which in the order of their usual stabilities are calcite aragonite and vaterite the main groups of microorganisms that can induce the carbonate precipitation are photosynthetic microorganisms such as cyanobacteria and microalgae sulfatereducing bacteria and some species of microorganisms involved in nitrogen cycle several mechanisms have been identified by which bacteria can induce the calcium carbonate precipitation including urea hydrolysis denitrification sulfate production and iron reduction two different pathways or autotrophic and heterotrophic pathways through which calcium carbonate is produced have been identified there are three autotrophic pathways which all result in depletion of carbon dioxide and favouring calcium carbonate precipitation in heterotrophic pathway two metabolic cycles can be involved the nitrogen cycle and the sulfur cycle several applications of this process have been proposed such as remediation of cracks and corrosion prevention in concrete biogrout sequestration of radionuclides and heavy metals all three principal kinds of bacteria that are involved in autotrophic production of carbonate obtain carbon from gaseous or dissolved carbon dioxide these pathways include nonmethylotrophic methanogenesis anoxygenic photosynthesis and oxygenic photosynthesis nonmethylotrophic methanogenesis is carried out by methanogenic archaebacteria which use co2 and h2 in anaerobiosis to give ch4 two separate and often concurrent heterotrophic pathways that lead to calcium carbonate precipitation may occur including active and passive carbonatogenesis during active carbonatogenesis the carbonate particles are produced by ionic exchanges through the cell membrane by activation of calcium andor magnesium ionic pumps or channels probably coupled with carbonate ion production during passive carbonatogenesis two metabolic cycles can be involved the nitrogen cycle and the sulfur cycle three different pathways can be involved in the nitrogen cycle ammonification of amino acids dissimilatory reduction of nitrate and degradation of urea or uric acid in the sulfur cycle bacteria follow the dissimilatory reduction of sulfate ureolysis or degradation of urea the microbial urease catalyzes the hydrolysis of urea into ammonium and carbonate one mole of urea is hydrolyzed intracellular'</li><li>'brown earth is a type of soil brown earths are mostly located between 35° and 55° north of the equator the largest expanses cover western and central europe large areas of western and transuralian russia the east coast of america and eastern asia here areas of brown earth soil types are found particularly in japan korea china eastern australia and new zealand brown earths cover 45 of the land in england and wales they are common in lowland areas below 1000 feet on permeable parent material the most common vegetation types are deciduous woodland and grassland due to the reasonable natural fertility of brown earths large tracts of deciduous woodland have been cut down and the land is now used for farming they are normally located in regions with a humid temperate climate rainfall totals are moderate usually below 76 cm per year and temperatures range from 4 °c in the winter to 18 °c in the summer they are welldrained fertile soils with a ph of between 50 and 65 soils generally have three horizons the a b and c horizon horizon a is usually a brownish colour and over 20 cm in depth it is composed of mull humus well decomposed alkaline organic matter and mineral matter it is biologically active with many soil organisms and plant roots mixing the mull humus with mineral particles as a result the boundary between the a and b horizons can be illdefined in unploughed examples horizon b is mostly composed of mineral matter which has been weathered from the parent material but it often contains inclusions of more organic material carried in by organisms especially earthworms it is lighter in colour than the a horizon and is often weakly illuviated enriched with material from overlying horizons due to limited leaching only the more soluble bases are moved down through the profile horizon c is made up of the parent material which is generally permeable and non or slightly acidic for example clay loam brown earths are important because they are permeable and usually easy to work throughout the year so they are valued for agriculture they also support a much wider range of forest trees than can be found on wetter land they are freely drained soils with welldeveloped a and b horizons they often develop over relatively permeable bedrock of some kind but are also found over unconsolidated parent materials like river gravels some soil classifications include welldrained alluvial soils in the brown earths too typically the brown earths have dark brown topsoils with loamy particle sizeclasses and good structure – especially under grassland the b horizon lacks the grey colours and mottles characteristic of gley'</li><li>'and it is about twice the carbon content of the atmosphere or around four times larger than the human emissions of carbon between the start of the industrial revolution and 2011 further most of this carbon 1035 billion tons is stored in what is defined as the nearsurface permafrost no deeper than 3 metres 98 ft below the surface however only a fraction of this stored carbon is expected to enter the atmosphere in general the volume of permafrost in the upper 3 m of ground is expected to decrease by about 25 per 1 °c 18 °f of global warming 1283 yet even under the rcp85 scenario associated with over 4 °c 72 °f of global warming by the end of the 21st century about 5 to 15 of permafrost carbon is expected to be lost over decades and centuriesthe exact amount of carbon that will be released due to warming in a given permafrost area depends on depth of thaw carbon content within the thawed soil physical changes to the environment and microbial and vegetation activity in the soil notably estimates of carbon release alone do not fully represent the impact of permafrost thaw on climate change this is because carbon can be released through either aerobic or anaerobic respiration which results in carbon dioxide co2 or methane ch4 emissions respectively while methane lasts less than 12 years in the atmosphere its global warming potential is around 80 times larger than that of co2 over a 20year period and about 28 times larger over a 100year period while only a small fraction of permafrost carbon will enter the atmosphere as methane those emissions will cause 4070 of the total warming caused by permafrost thaw during the 21st century much of the uncertainty about the eventual extent of permafrost methane emissions is caused by the difficulty of accounting for the recently discovered abrupt thaw processes which often increase the fraction of methane emitted over carbon dioxide in comparison to the usual gradual thaw processes another factor which complicates projections of permafrost carbon emissions is the ongoing greening of the arctic as climate change warms the air and the soil the region becomes more hospitable to plants including larger shrubs and trees which could not survive there before thus the arctic is losing more and more of its tundra biomes yet it gains more plants which proceed to absorb more carbon some of the emissions caused by permafrost thaw will be offset by this increased plant growth but the exact proportion is uncertain it is considered very unlikely that this greening could offset all of the emissions from permafrost thaw during the'</li></ul> |
| 8 | <ul><li>'the enhanced avionics system or easy is an integrated modular avionics suite and cockpit display system used on dassault falcon business jets since falcon 900ex and later used in other newer falcon aircraft such as falcon 2000ex and falcon 7xeasy has been jointly developed by dassault and honeywell and is based on honeywell primus epic dassault aviation started to develop the easy flight deck concept in the mid1990s with a goal to have a much better integration of aircraft systems such as fmseasy was first integrated and certificated on falcon 900ex the first easy equipped 900ex was delivered in december 2003 honeywell primus epic base of easy was then integrated on other business jets and helicopterseasy was certified on the falcon 2000ex in june 2004 with deliveries starting shortly after falcon 7x was developed from the groundup with easy avionics in october 2008 dassault announced the launch of easy phase ii program at the annual nbaa meeting in orlando easy phase ii include several enhancements to easy such as synthetic vision system adsb out paperless charts future air navigation system fans1a using controller pilot data link communications cpdlc localizer performance with vertical guidance lpveasy phase ii was certified on falcon 900lx in june 2011 and on falcon 7x in may 2013 easy architecture is based on integrated modular avionics the processing modules are called mau modular avionics units the core operating system of easy is provided by ddci integrated modular avionics ima cockpit display system dassault falcon 7x dassault aviation'</li><li>'briefly before being replaced by sonne and bernard erika transmitted a vhf signal on 3033 mhz which could be received by standard ebl 3 receivers the signal was adjusted in phase between a ref point and a navigation point after processing the fug 121 displayed an angle from the beacon by using two beacons it was possible to achieve a fix however this was a problem as four receivers were required two listening to each station on smaller aircraft there was not enough space and german industry was by now having trouble supplying enough radios to the air force without adding 4 more receivers per plane the system was not deployed some sources indicate that there may have been a version called electra that operated at 250 to 300 khz but details are lacking or contradictorysonne this system transmitted on 270 – 480 khz and could be received on a fug 10 no special receiver was required as the pattern was discernable with the ear all that was required was the special charts at least 6 stations were built providing coverage from the bay of biscay to norway accuracy was reasonable during the day but errors up to 4 degrees occurred at night the allies captured the maps with resulted in the being issued to allied units because of this the allies left the sonne system alone after the war the stations were rebuilt and operated into the 1970s the system was called consol by that time mond development work was done on sonne sun to remove the night time errors this system was called mond moon work was never completed truhe this system was based on the british gee system after british units were captured the germans set up a project to clone the units the first unit was the fug 122 which allowed the reception of british gee signals units in france received these units and were able to navigate using british signals the germans then developed the concept to produce fug 123 receivers which would allow a wider turning range this allowed the germans to setup gee chains of their own further inside germany where the british gee signals were unusable there seems to have been some idea of using frequencies very close to the british frequencies to make jamming by the allies hard to do without jamming their own gee system one chain became operational around berlin fubl 1 used the lorenz landing beam system consisted of the ebl 1 and ebl 2 receivers with display device anf 2 the ebl 1 operated between 30 and 33 mhz and received the azimuth signals from a transmitter at the far end of the runway the ebl 2 operated at 38 mhz and received the two marker beacons as the aircraft approached the threshold to land the afn 2 provided the pilot with'</li><li>'a ground proximity warning system gpws is a system designed to alert pilots if their aircraft is in immediate danger of flying into the ground or an obstacle the united states federal aviation administration faa defines gpws as a type of terrain awareness and warning system taws more advanced systems introduced in 1996 are known as enhanced ground proximity warning systems egpws a modern type of taws in the late 1960s a series of controlled flight into terrain cfit accidents took the lives of hundreds of people a cfit accident is one where a properly functioning airplane under the control of a fully qualified and certified crew is flown into terrain water or obstacles with no apparent awareness on the part of the crewbeginning in the early 1970s a number of studies examined the occurrence of cfit accidents findings from these studies indicated that many such accidents could have been avoided if a warning device called a ground proximity warning system gpws had been used as a result of these studies and recommendations from the us national transportation safety board ntsb in 1974 the faa required all large turbine and turbojet airplanes to install tsoapproved gpws equipmentthe un international civil aviation organization icao recommended the installation of gpws in 1979c donald bateman a canadianborn engineer developed and is credited with the invention of gpwsin march 2000 the us faa amended operating rules to require that all us registered turbinepowered airplanes with six or more passenger seats exclusive of pilot and copilot seating be equipped with an faaapproved taws the mandate affects aircraft manufactured after march 29 2002 prior to the development of gpws large passenger aircraft were involved in 35 fatal cfit accidents per year falling to 2 per year in the mid1970s a 2006 report stated that from 1974 when the us faa made it a requirement for large aircraft to carry such equipment until the time of the report there had not been a single passenger fatality in a cfit crash by a large jet in us airspaceafter 1974 there were still some cfit accidents that gpws was unable to help prevent due to the blind spot of those early gpws systems more advanced systems were developed older taws or deactivation of the egpws or ignoring its warnings when an airport is not in its database still leave aircraft vulnerable to possible cfit incidents in april 2010 a polish air force tupolev tu154m aircraft crashed near smolensk russia in a possible cfit accident killing all passengers and crew including the president of poland lech kaczynski the aircraft was equipped with taws made by universal avionics systems of tucson according to the russian interstate aviation committee'</li></ul> |
| 12 | <ul><li>'of s m displaystyle sm for some integers m displaystyle m whose base k displaystyle k representations are close to that of n displaystyle n constantrecursive sequences can be thought of as 1 displaystyle 1 regular sequences where the base1 representation of n displaystyle n consists of n displaystyle n copies of the digit 1 displaystyle 1'</li><li>'the small triangles whose vertices all have different numbers are shaded in the graph each small triangle becomes a node in the new graph derived from the triangulation the small letters identify the areas eight inside the figure and area i designates the space outside of it as described previously those nodes that share an edge whose endpoints are numbered 1 and 2 are joined in the derived graph for example node d shares an edge with the outer area i and its vertices all have different numbers so it is also shaded node b is not shaded because two vertices have the same number but it is joined to the outer area one could add a new fullnumbered triangle say by inserting a node numbered 3 into the edge between 1 and 1 of node a and joining that node to the other vertex of a doing so would have to create a pair of new nodes like the situation with nodes f and g suppose there is a ddimensional simplex of sidelength n and it is triangulated into subsimplices of sidelength 1 there is a function that given any vertex of the triangulation returns its color the coloring is guaranteed to satisfy sperners boundary condition how many times do we have to call the function in order to find a rainbow simplex obviously we can go over all the triangulation vertices whose number is ond which is polynomial in n when the dimension is fixed but can it be done in time this problem was first studied by christos papadimitriou he introduced a complexity class called ppad which contains this as well as related problems such as finding a brouwer fixed point he proved that finding a sperner simplex is ppadcomplete even for d3 some 15 years later chen and deng proved ppadcompleteness even for d2 it is believed that ppadhard problems cannot be solved in time opolylog n suppose that each vertex of the triangulation may be labeled with multiple colors so that the coloring function is f s → 2n1 for every subsimplex the set of labelings on its vertices is a setfamily over the set of colors n 1 this setfamily can be seen as a hypergraph if for every vertex v on a face of the simplex the colors in fv are a subset of the set of colors on the face endpoints then there exists a subsimplex with a balanced labeling – a labeling in which the corresponding hypergraph admits a perfect fractional matching to illustrate here are some balanced labeling examples for n 2'</li><li>'labeling is also odd l − v − l v displaystyle lvlv hence by tuckers lemma there are two adjacent vertices u v displaystyle uv with opposite labels assume wlog that the labels are l u 1 l v − 1 displaystyle lu1lv1 by the definition of l this means that in both g u displaystyle gu and g v displaystyle gv coordinate 1 is the largest coordinate in g u displaystyle gu this coordinate is positive while in g v displaystyle gv it is negative by the construction of the triangulation the distance between g u displaystyle gu and g v displaystyle gv is at most [UNK] displaystyle epsilon so in particular g u 1 − g v 1 g u 1 g v 1 ≤ [UNK] displaystyle gu1gv1gu1gv1leq epsilon since g u 1 displaystyle gu1 and g v 1 displaystyle gv1 have opposite signs and so g u 1 ≤ [UNK] displaystyle gu1leq epsilon but since the largest coordinate of g u displaystyle gu is coordinate 1 this means that g u k ≤ [UNK] displaystyle gukleq epsilon for each 1 ≤ k ≤ n displaystyle 1leq kleq n so g u ≤ c n [UNK] displaystyle guleq cnepsilon where c n displaystyle cn is some constant depending on n displaystyle n and the norm ⋅ displaystyle cdot which you have chosen the above is true for every [UNK] 0 displaystyle epsilon 0 since s n displaystyle sn is compact there must hence be a point u in which g u 0 displaystyle gu0 no subset of r n displaystyle mathbb r n is homeomorphic to s n displaystyle sn the ham sandwich theorem for any compact sets a1 an in r n displaystyle mathbb r n we can always find a hyperplane dividing each of them into two subsets of equal measure above we showed how to prove the borsuk – ulam theorem from tuckers lemma the converse is also true it is possible to prove tuckers lemma from the borsuk – ulam theorem therefore these two theorems are equivalent there are several fixedpoint theorems which come in three equivalent variants an algebraic topology variant a combinatorial variant and a setcovering variant each variant can be proved separately using totally different arguments but each variant can also be reduced to the other variants in its row additionally each result in the top row can be deduced from the one below it in the same column in the original theorem the domain'</li></ul> |
| 33 | <ul><li>'xenoglossy also written xenoglossia and sometimes also known as xenolalia is the supposedly paranormal phenomenon in which a person is allegedly able to speak write or understand a foreign language that they could not have acquired by natural means the term derives from the ancient greek xenos ξενος foreigner and glossa γλωσσα tongue or language the term xenoglossy was first used by french parapsychologist charles richet in 1905 claims of xenoglossy are found in the new testament and contemporary claims have been made by parapsychologists and reincarnation researchers such as ian stevenson doubts have been expressed that xenoglossy is an actual phenomenon and there is no scientifically admissible evidence supporting any of the alleged instances of xenoglossytwo types of xenoglossy are distinguished recitative xenoglossy is the use of an unacquired language incomprehensibly while responsive xenoglossy refers to the ability to intelligibly employ the unlearned language as if already acquired this phenomenon is mentioned in acts of the apostles chapter 2 at pentecost when the first disciples of jesus christ gathered together numbering one hundred and twenty and of the tongues of fire landed on each of them formalizing the coming of the spirit in an episode of inspired communication that allows the disciples to express themselves in languages other than galilean and to be understood by strangers several accounts of miraculous abilities of some people to read write speak or understand a foreign language as mentioned in the bible have been related in similar christian accounts in the middle ages similar claims were also made by some pentecostal theologians in 1901 claims of mediums speaking foreign languages were made by spiritualists in the 19th century more recent claims of xenoglossy have come from reincarnation researchers who have alleged that individuals were able to recall a language spoken in a past life some reports of xenoglossy have surfaced in the popular press such as czech speedway rider matej kus who in september 2007 supposedly awoke after a crash and was able to converse in perfect english however press reports of his fluency in english were based entirely on anecdotal stories told by his czech teammates xenoglossy has been claimed to have occurred during exorcisms canadian parapsychologist and psychiatrist at the university of virginia ian stevenson claimed there were a handful of cases that suggested evidence of xenoglossy these included two where a subject under hypnosis could'</li><li>'have lost but if asked directly in the context of a psychic reading whether they have such an item the client may be shocked and assume that the reader learned the information directly from the deceased loved one robert todd carroll notes in the skeptics dictionary that some would consider this to be cold reading the rainbow ruse is a crafted statement which simultaneously awards the subject a specific personality trait as well as the opposite of that trait with such a phrase a cold reader can cover all possibilities and appear to have made an accurate deduction in the mind of the subject despite the fact that a rainbow ruse statement is vague and contradictory this technique is used since personality traits are not quantifiable and also because nearly everybody has experienced both sides of a particular emotion at some time in their lives statements of this type include most of the time you are positive and cheerful but there has been a time in the past when you were very upset you are a very kind and considerate person but when somebody does something to break your trust you feel deepseated anger i would say that you are mostly shy and quiet but when the mood strikes you you can easily become the center of attentiona cold reader can choose from a variety of personality traits think of its opposite and then bind the two together in a phrase vaguely linked by factors such as mood time or potential the mentalist branch of the stagemagician community approves of reading as long as it is presented strictly as an artistic entertainment and one is not pretending to be psychicsome performers who use cold reading are honest about their use of the technique lynne kelly kari coleman ian rowland and derren brown have used these techniques at either private fortunetelling sessions or open forum talking with the dead sessions in the manner of those who claim to be genuine mediums only after receiving acclaim and applause from their audience do they reveal that they needed no psychic power for the performance only a sound knowledge of psychology and cold reading in an episode of his trick of the mind series broadcast in march 2006 derren brown showed how easily people can be influenced through cold reading techniques by repeating bertram forers famous demonstration of the personal validation fallacy or forer effect in a detailed review of four sittings conducted by medium tyler henry edward and susan gerbic reviewed all statements made by him on the tv show hollywood medium in their opinion not one statement made by henry was accurate yet each sitter felt that their reading was highly successful in interviews with each sitter after their sitting all four claimed specific statements made by henry but after reviewing the show it was shown that he had not made those statements each sit'</li><li>'al concluding that the ganzfeld studies have not been independently replicated and had thus failed to produce evidence for psi according to hyman reliance on metaanalysis as the sole basis for justifying the claim that an anomaly exists and that the evidence for it is consistent and replicable is fallacious it distorts what scientists mean by confirmatory evidence storm et al published a response to hyman claiming the ganzfeld experimental design has proved to be consistent and reliable but parapsychology is a struggling discipline that has not received much attention so further research on the subject is necessary rouder et al in 2013 wrote that critical evaluation of storm et als metaanalysis reveals no evidence for psi no plausible mechanism and omitted replication failuresa 2016 paper examined questionable research practices in the ganzfeld experiments and simulated how such practices could cause erroneous positive results there are several common criticisms of some or all of the ganzfeld experiments isolation – richard wiseman and others argue that not all of the studies used soundproof rooms so it is possible that when videos were playing the experimenter could have heard it and later given involuntary cues to the receiver during the selection process it could even have been possible that the receiver themselves could hear the video randomization – when subjects are asked to choose from a variety of selections there is an inherent bias to choose the first selection they are shown if the order in which they are shown the selections is randomized each time this bias will be averaged out the randomization procedures used in the experiment have been criticized for not randomizing satisfactorily the psi assumption – the assumption that any statistical deviation from chance is evidence for telepathy is highly controversial strictly speaking a deviation from chance is only evidence that either this was a rare statistically unlikely occurrence that happened by chance or something was causing a deviation from chance flaws in the experimental design are a common cause of this and so the assumption that it must be telepathy is fallaciouswriting in 1985 c e m hansel discovered weaknesses in the design and possibilities of sensory leakage in the ganzfeld experiments reported by carl sargent and other parapsychologists hansel concluded the ganzfeld studies had not been independently replicated and that esp is no nearer to being established than it was a hundred years agodavid marks in his book the psychology of the psychic 2000 has noted that during the autoganzfeld experiments the experimenter sat only fourteen feet from the senders room soundproofing tiles were eventually added but they were designed to absorb sound not to prevent transmission according to marks this was inadequate'</li></ul> |
| 22 | <ul><li>'water resources are natural resources of water that are potentially useful for humans for example as a source of drinking water supply or irrigation water 97 of the water on earth is salt water and only three percent is fresh water slightly over twothirds of this is frozen in glaciers and polar ice caps the remaining unfrozen freshwater is found mainly as groundwater with only a small fraction present above ground or in the air natural sources of fresh water include surface water under river flow groundwater and frozen water artificial sources of fresh water can include treated wastewater wastewater reuse and desalinated seawater human uses of water resources include agricultural industrial household recreational and environmental activities water resources are under threat from water scarcity water pollution water conflict and climate change fresh water is a renewable resource yet the worlds supply of groundwater is steadily decreasing with depletion occurring most prominently in asia south america and north america although it is still unclear how much natural renewal balances this usage and whether ecosystems are threatened natural sources of fresh water include surface water under river flow groundwater and frozen water surface water is water in a river lake or fresh water wetland surface water is naturally replenished by precipitation and naturally lost through discharge to the oceans evaporation evapotranspiration and groundwater recharge the only natural input to any surface water system is precipitation within its watershed the total quantity of water in that system at any given time is also dependent on many other factors these factors include storage capacity in lakes wetlands and artificial reservoirs the permeability of the soil beneath these storage bodies the runoff characteristics of the land in the watershed the timing of the precipitation and local evaporation rates all of these factors also affect the proportions of water loss humans often increase storage capacity by constructing reservoirs and decrease it by draining wetlands humans often increase runoff quantities and velocities by paving areas and channelizing the stream flow natural surface water can be augmented by importing surface water from another watershed through a canal or pipeline brazil is estimated to have the largest supply of fresh water in the world followed by russia and canada water from glaciers glacier runoff is considered to be surface water the himalayas which are often called the roof of the world contain some of the most extensive and rough high altitude areas on earth as well as the greatest area of glaciers and permafrost outside of the poles ten of asias largest rivers flow from there and more than a billion peoples livelihoods depend on them to complicate matters temperatures there are rising more rapidly than the global average in nepal the temperature has risen by 06 degrees celsius over the last decade whereas globally the earth has'</li><li>'##ng magnitude from leftright the finite water content vadose zone flux method works with any monotonic water retention curveunsaturated hydraulic conductivity relations such as brooks and corey clapp and hornberger and van genuchtenmualem the method might work with hysteretic water retention relations these have not yet been tested the finite water content method lacks the effect of soil water diffusion this omission does not affect the accuracy of flux calculations using the method because the mean of the diffusive flux is small practically this means that the shape of the wetting front plays no role in driving the infiltration the method is thus far limited to 1d in practical applications the infiltration equation was extended to 2 and quasi3 dimensions more work remains in extending the entire method into more than one dimension the paper describing this method was selected by the early career hydrogeologists network of the international association of hydrogeologists to receive the coolest paper published in 2015 award in recognition of the potential impact of the publication on the future of hydrogeology richards equation infiltration hydrology soil moisture velocity equation'</li><li>'stress distribution in soil is a function of the type of soil the relative rigidity of the soil and the footing and the depth of foundation at level of contact between footing and soilthe estimation of vertical stresses at any point in a soil mass due to external loading is essential to the prediction of settlements of buildings bridges and pressure the solution to the problem of calculating the stresses in an elastic half space subjected to a vertical point load at the surface will be of value in estimating the stresses induced in a deposit of soil whose depth is large compared to the dimensions of that part of the surface that is loaded δ σ z − 3 p 2 π r 2 cos 3 θ displaystyle delta sigma zfrac 3p2pi r2cos 3theta δ σ r p 2 π r 2 − 3 cos θ sin 2 θ 1 − 2 μ 1 cos θ displaystyle delta sigma rfrac p2pi r23cos theta sin 2theta frac 12mu 1cos theta δ σ t p 2 π r 2 1 − 2 μ cos θ − 1 1 cos θ displaystyle delta sigma tfrac p2pi r212mu cos theta frac 11cos theta δ τ − 3 p 2 π r 2 cos 2 θ sin θ displaystyle delta tau frac 3p2pi r2cos 2theta sin theta cos θ z r displaystyle cos theta frac zr r r 2 z 2 displaystyle rsqrt r2z2 δ σ z − 3 p z 3 2 π r 5 − 3 p 2 π z 3 r 2 z 2 5 2 − 3 p 2 π z 2 1 r z 2 5 2 displaystyle delta sigma zfrac 3pz32pi r5frac 3p2pi frac z3r2z252frac 3p2pi z2left1leftfrac rzright2rightfrac 52 σ q 1 − 1 r z 2 1 3 2 displaystyle sigma q1frac 1frac rz2132'</li></ul> |
| 3 | <ul><li>'##ilise and suggest other technologies such as mobile phones or psion organisers as such feedback studies involve asynchronous communication between the participants and the researchers as the participants ’ data is recorded in their diary first and then passed on to the researchers once completefeedback studies are scalable that is a largescale sample can be used since it is mainly the participants themselves who are responsible for collecting and recording data in elicitation studies participants capture media as soon as the phenomenon occurs the media is usually in the form of a photograph but can be in other different forms as well and so the recording is generally quick and less effortful than feedback studies these media are then used as prompts and memory cues to elicit memories and discussion in interviews that take place much later as such elicitation studies involve synchronous communication between the participants and the researchers usually through interviewsin these later interviews the media and other memory cues such as what activities were done before and after the event can improve participants ’ episodic memory in particular photos were found to elicit more specific recall than all other media types there are two prominent tradeoffs between each type of study feedback studies involve answering questions more frequently and in situ therefore enabling more accurate recall but more effortful recording in contrast elicitation studies involve quickly capturing media in situ but answering questions much later therefore enabling less effortful recording but potentially inaccurate recall diary studies are most often used when observing behavior over time in a natural environment they can be beneficial when one is looking to find new qualitative and quantitative data advantages of diary studies are numerous they allow collecting longitudinal and temporal information reporting events and experiences in context and inthemoment participants to diary their behaviours thoughts and feelings inthemoment thereby minimising the potential for post rationalisation determining the antecedents correlations and consequences of daily experiences and behaviors there are some limitations of diary studies mainly due to their characteristics of reliance on memory and selfreport measures there is low control low participation and there is a risk of disturbing the action in feedback studies it can be troubling and disturbing to write everything down the validity of diary studies rests on the assumption that participants will accurately recall and record their experiences this is somewhat more easily enabled by the fact that diaries are completed media is captured in a natural environment and closer in realtime to any occurrences of the phenomenon of interest however there are multiple barriers to obtaining accurate data such as social desirability bias where participants may answer in a way that makes them appear more socially desirable this may be more prominent in longitudinal studies'</li><li>'indigenous media can reference film video music digital art and sound produced and created by and for indigenous people it refers to the use of communication tools pathways and outlets by indigenous peoples for their own political and cultural purposes indigenous media is the use of modern media techniques by indigenous peoples also called fourth world peoples indigenous media helps communities in their fight against cultural extinction economic and ecological decline and forced displacement most often in the field of indigenous media the creators of the media are also the consumers together with the neighboring communities sometimes the media is also received by institutions and film festivals located far away from the production location like the american indian film festival the production is usually locally based low budget and small scale but it can also be sponsored by different support groups and governments 34 – 35 the concept of indigenous media could be extended to first world alternative media like aids activist video the research of indigenous media and the international indigenous movement in the process of globalization develop in parallel in the second half of the 20th century united nations agencies including the united nations working group on indigenous populations wgip led the movement the united nations general assembly adopted a declaration aimed at protecting the rights of indigenous peoples in 2007 the theoretical development of indigenous media research first occurred in anthropology in 1980 it was accompanied by a critical research method that diverged from postcolonialism and poststructuralism the newer method attempted to minimize the power imbalance between the researcher and the researched leading up to this ethnographic films that gave photographic techniques to locals can be traced back as far as the navajo project in 1960 the project was the pioneering work of sol worth and john adair to which the origin of a new anthropological language and style of ethnography can be attributedhowever the indigenous media movement was not a significant phenomenon for another decade the widely recognized start of the new media movement was a collaboration between american anthropologist eric michaels and australia ’ s warlpiri aboriginal broadcasting this new type of collaborative anthropological project exemplified a change from a simple observation of the life of the indigenous people to a cultural record by the indigenous people themselves following the warlpiri project the brazilian kayapo village project of vincent carelli and terence turner and the indigenous series by maori producer barry barclay in new zealand have been important milestones in the development of indigenous media however it was faye ginsburg an american anthropologist who laid the theoretical foundation for the study of indigenous media her research in 1991 expounded the faustian dilemma between technology and tribal life and inspired later indigenous media researchers the important theories of recent indigenous media studies have highlighted the dynamic relationship between local indigenous communities and their countries and globalization lorna roth'</li><li>'results did not predict any prejudices towards black individuals this study used emic approaches of study by conducting interviews with the locals and etic approaches by giving participants generalized personality tests exonym and endonymother explorations of the differences between reality and humans models of it blind men and an elephant emic and etic units internalism and externalism map – territory relation creswell j w 1998 qualitative enquiry and research design choosing among five traditions london uk sage dundes alan 1962 from etic to emic units in the structural study of folktales journal of american folklore 75 296 95 – 105 doi102307538171 jstor i223629 goodenough ward 1970 describing a culture description and comparison in cultural anthropology cambridge uk cambridge university press pp 104 – 119 isbn 9780202308616 harris marvin 1976 history and significance of the emicetic distinction annual review of anthropology 5 329 – 350 doi101146annurevan05100176001553 harris marvin 1980 chapter two the epistemology of cultural materialism cultural materialism the struggle for a science of culture new york random house pp 29 – 45 isbn 9780759101340 headland thomas pike kenneth harris marvin eds 1990 emics and etics the insideroutsider debate sage jahoda g 1977 y j poortinga ed in pursuit of the emicetic distinction can we ever capture it basic problems in crosscultural psychology pp 55 – 63 jardine nick 2004 etics and emics not to mention anemics and emetics in the history of the sciences history of science 42 3 261 – 278 bibcode2004hissc42261j doi101177007327530404200301 s2cid 141081973 jingfeng xia 2013 an anthropological emicetic perspective on open access practices academic search premier kitayama shinobu cohen dov 2007 handbook of cultural psychology new york guilford press kottak conrad 2006 mirror for humanity new york mcgraw hill isbn 9780078034909 nattiez jeanjacques 1987 musicologie generale et semiologue music and discourse toward a semiology of music translated by carolyn abbate isbn 9780691027142 pike kenneth lee ed 1967 language in relation to a unified theory of structure of human behavior 2nd ed the hague netherlands mouton'</li></ul> |
| 34 | <ul><li>'democratic education is a type of formal education that is organized democratically so that students can manage their own learning and participate in the governance of their school democratic education is often specifically emancipatory with the students voices being equal to the teachersthe history of democratic education spans from at least the 17th century while it is associated with a number of individuals there has been no central figure establishment or nation that advocated democratic education in 1693 john locke published some thoughts concerning education in describing the teaching of children he declares none of the things they are to learn should ever be made a burthen to them or imposd on them as a task whatever is so proposd presently becomes irksome the mind takes an aversion to it though before it were a thing of delight or indifferency let a child but be orderd to whip his top at a certain time every day whether he has or has not a mind to it let this be but requird of him as a duty wherein he must spend so many hours morning and afternoon and see whether he will not soon be weary of any play at this rate jeanjacques rousseaus book of advice on education emile was first published in 1762 emile the imaginary pupil he uses for illustration was only to learn what he could appreciate as useful he was to enjoy his lessons and learn to rely on his own judgement and experience the tutor must not lay down precepts he must let them be discovered wrote rousseau and urged him not make emile learn science but let him discover it he also said that we should not substitute books for personal experience because this does not teach us to reason it teaches us to use other peoples reasoning it teaches us to believe a great deal but never to know anything while locke and rousseau were concerned only with the education of the children of the wealthy in the 19th century leo tolstoy set up a school for peasant children this was on his own estate at yasnaya polyana russia in the late 19th century he tells us that the school evolved freely from principles introduced by teachers and pupils that in spite of the preponderating influence of the teacher the pupil had always had the right not to come to school or having come not to listen to the teacher and that the teacher had the right not to admit a pupil and was able to use all the influence he could muster to win over the community where the children were always in the majority dom sierot in 1912 janusz korczak founded dom sierot the jewish orphanage in warsaw which was run on democratic lines in 1940 dom si'</li><li>'is done through six points of reference learners studentsteachers in dialogue approach their acts of knowing as grounded in individual experience and circumstance learners approach the historical and cultural world as a transformable reality shaped by human ideological representations of reality learners make connections between their own conditions and the conditions produced through the making of reality learners consider the ways that they can shape this reality through their methods of knowing this new reality is collective shared and shifting learners develop literacy skills that put their ideas into print thus giving potency to the act of knowing learners identify the myths in the dominant discourse and work to destabilize these myths ending the cycle of oppression the montessori method developed by maria montessori is an example of problemposing education in an early childhood model ira shor a professor of composition and rhetoric at cuny who has worked closely with freire also advocates a problem posing model in his use of critical pedagogy he has published on the use of contract grading the physical setup of the classroom and the political aspects of student and teacher rolesjames d kirylo in his book paulo freire the man from recife reiterated freires thought and stated that a problemposing education is one where human beings are viewed as conscious beings who are unfinished yet in process of becoming other advocates of problemposing critical pedagogy include henry giroux peter mclaren and bell hooks inquirybased learning problembased learning unschooling'</li><li>'ambiguity tolerance – intolerance is a psychological construct that describes the relationship that individuals have with ambiguous stimuli or events individuals view these stimuli in a neutral and open way or as a threat ambiguity tolerance – intolerance is a construct that was first introduced in 1949 through the work of else frenkelbrunswik while researching ethnocentrism in children and was perpetuated by her research of ambiguity intolerance in connection to authoritarian personality it serves to define and measure how well an individual responds when presented with an event that results in ambiguous stimuli or situations in her study she tested the notion that children who are ethnically prejudiced also tend to reject ambiguity more so than their peers she studied children who ranked high and low on prejudice in a story recall test and then studied their responses to an ambiguous disc shaped figure the children who scored high in prejudice were expected to take longer to give a response to the shape less likely to make changes on their response and less likely to change their perspectives a study by kenny and ginsberg 1958 retesting frenkelbrunswiks original connection of ambiguity intolerance to ethnocentrism and authoritarian personality found that the results were unreplicable however it was discussed that this may be due to the fact that at the time the study was done incorrect methodology was used and that there lacked a concrete definition as to what the construct was most of the research on this subject was completed in the two decades after the publication of the authoritarian personality however the construct is still studied in psychological research today budner gives three examples as to what could be considered ambiguous situations a situation with no familiar cues a situation in which there are many cues to be taken into consideration and a situation in which cues suggest the existence of different structures to be adhered to there have been many attempts to conceptualize the construct of ambiguity tolerance – intolerance as to give researchers a more standard concept to work with many of these conceptualizations are based on the work of frenkelbrunswik budner 1962 defines the construct as the following intolerance of ambiguity may be defined as the tendency to perceive ie interpret ambiguous situations as sources of threat tolerance of ambiguity as the tendency to perceive ambiguous situations as desirableadditionally bochner 1965 categorized attributes given by frenkelbrunswiks theory of individuals who are intolerant to ambiguity the nine primary characteristics describe intolerance of ambiguity and are as follows need for categorization need for certainty inability to allow good and bad traits to exist in the same person'</li></ul> |
| 31 | <ul><li>'in philosophy transcendence is the basic ground concept from the words literal meaning from latin of climbing or going beyond albeit with varying connotations in its different historical and cultural stages it includes philosophies systems and approaches that describe the fundamental structures of being not as an ontology theory of being but as the framework of emergence and validation of knowledge of being these definitions are generally grounded in reason and empirical observation and seek to provide a framework for understanding the world that is not reliant on religious beliefs or supernatural forces transcendental is a word derived from the scholastic designating the extracategorical attributes of beings in religion transcendence refers to the aspect of gods nature and power which is wholly independent of the material universe beyond all physical laws this is contrasted with immanence where a god is said to be fully present in the physical world and thus accessible to creatures in various ways in religious experience transcendence is a state of being that has overcome the limitations of physical existence and by some definitions has also become independent of it this is typically manifested in prayer seance meditation psychedelics and paranormal visions it is affirmed in various religious traditions concept of the divine which contrasts with the notion of a god or the absolute that exists exclusively in the physical order immanentism or indistinguishable from it pantheism transcendence can be attributed to the divine not only in its being but also in its knowledge thus god may transcend both the universe and knowledge is beyond the grasp of the human mind although transcendence is defined as the opposite of immanence the two are not necessarily mutually exclusive some theologians and metaphysicians of various religious traditions affirm that a god is both within and beyond the universe panentheism in it but not of it simultaneously pervading it and surpassing it the ethics of baruch spinoza used the expression transcendental terms in latin termini transcendentales to indicate concepts like being thing something which are so general not to be included in the definitions of species genus and category in modern philosophy immanuel kant introduced a new term transcendental thus instituting a new third meaning in his theory of knowledge this concept is concerned with the condition of possibility of knowledge itself he also opposed the term transcendental to the term transcendent the latter meaning that which goes beyond transcends any possible knowledge of a human being for him transcendental meant knowledge about our cognitive faculty with regard to how objects are possible a priori i call all knowledge transcendental if it is occupied not with objects'</li><li>'atoms in molecules — collision theory — ligand field theory successor to crystal field theory — variational transitionstate theory — benson group increment theory — specific ion interaction theory climatology climate change theory general study of climate changes and anthropogenic climate change acc global warming agw theories due to human activity computer science automata theory — queueing theory cosmology big bang theory — cosmic inflation — loop quantum gravity — superstring theory — supergravity — supersymmetric theory — multiverse theory — holographic principle — quantum gravity — mtheory economics macroeconomic theory — microeconomic theory — law of supply and demand education constructivist theory — critical pedagogy theory — education theory — multiple intelligence theory — progressive education theory engineering circuit theory — control theory — signal theory — systems theory — information theory film film theory geology plate tectonics humanities critical theory jurisprudence or legal theory natural law — legal positivism — legal realism — critical legal studies law see jurisprudence also case theory linguistics xbar theory — government and binding — principles and parameters — universal grammar literature literary theory mathematics approximation theory — arakelov theory — asymptotic theory — bifurcation theory — catastrophe theory — category theory — chaos theory — choquet theory — coding theory — combinatorial game theory — computability theory — computational complexity theory — deformation theory — dimension theory — ergodic theory — field theory — galois theory — game theory — gauge theory — graph theory — group theory — hodge theory — homology theory — homotopy theory — ideal theory — intersection theory — invariant theory — iwasawa theory — ktheory — kktheory — knot theory — ltheory — lie theory — littlewood – paley theory — matrix theory — measure theory — model theory — module theory — morse theory — nevanlinna theory — number theory — obstruction theory — operator theory — order theory — pcf theory — perturbation theory — potential theory — probability theory — ramsey theory — rational choice theory — representation theory — ring theory — set theory — shape theory — small cancellation theory — spectral theory — stability theory — stable theory — sturm – liouville theory — surgery theory — twistor theory — yang – mills theory music music theory philosophy proof theory — speculative reason — theory of truth — type theory — value theory — virtue theory physics acoustic theory — antenna theory — atomic theory — bcs theory — conformal field theory — dirac hole theory — dynamo theory — landau theory — mtheory — perturbation theory — theory'</li><li>'##ism turned this world on its head he argues for the nominalists all real being was individual or particular and universals were thus mere fictionsanother scholar victor bruno follows the same line according to bruno nominalism is one of the first signs of rupture in the medieval system the dismembering of the particulars the dangerous attribution to individuals to a status of totalization of possibilities in themselves all this will unfold in an existential fissure that is both objective and material the result of this fissure will be the essays to establish the nation state indian philosophy encompasses various realist and nominalist traditions certain orthodox hindu schools defend the realist position notably purva mimamsa nyaya and vaisheshika maintaining that the referent of the word is both the individual object perceived by the subject of knowledge and the universal class to which the thing belongs according to indian realism both the individual and the universal exist objectively with the second underlying the former buddhists take the nominalist position especially those of the sautrantika and yogacara schools they were of the opinion that words have as referent not true objects but only concepts produced in the intellect these concepts are not real since they do not have efficient existence that is causal powers words as linguistic conventions are useful to thought and discourse but even so it should not be accepted that words apprehend reality as it is dignaga formulated a nominalist theory of meaning called apohavada or theory of exclusions the theory seeks to explain how it is possible for words to refer to classes of objects even if no such class has an objective existence dignagas thesis is that classes do not refer to positive qualities that their members share in common on the contrary universal classes are exclusions apoha as such the cow class for example is composed of all exclusions common to individual cows they are all nonhorse nonelephant etc nominalism arose in reaction to the problem of universals specifically accounting for the fact that some things are of the same type for example fluffy and kitzler are both cats or the fact that certain properties are repeatable such as the grass the shirt and kermit the frog are green one wants to know by virtue of what are fluffy and kitzler both cats and what makes the grass the shirt and kermit green the platonist answer is that all the green things are green in virtue of the existence of a universal a single abstract thing that in this case is a part of all the green things with respect to the color of the grass the'</li></ul> |
| 41 | <ul><li>'along streams and rivers through parks and across commons another type is the alley normally providing access to the rear of properties or connecting builtup roads not easily reached by vehicles towpaths are another kind of urban footpath but they are often shared with cyclists a typical footpath in a park is found along the seawall in stanley park vancouver british columbia canada this is a segregated path with one lane for skaters and cyclists and the other for pedestriansin the us and canada where urban sprawl has begun to strike even the most rural communities developers and local leaders are currently striving to make their communities more conducive to nonmotorized transportation through the use of less traditional paths the robert wood johnson foundation has established the active living by design program to improve the livability of communities in part through developing trails the upper valley trails alliance has done similar work on traditional trails while the somerville community path and related paths are examples of urban initiatives in st johns newfoundland canada the grand concourse is an integrated walkway system that has over 160 kilometers 99 mi of footpaths which link every major park river pond and green space in six municipalities in london england there are several longdistance walking routes which combine footpaths and roads to link green spaces these include the capital ring london outer orbital path and the jubilee walkway the use of which have been endorsed by transport for london an alley is a narrow usually paved pedestrian path often between the walls of buildings in towns and cities this type is usually short and straight and on steep ground can consist partially or entirely of steps in older cities and towns in europe alleys are often what is left of a medieval street network or a right of way or ancient footpath similar paths also exist in some older north american towns and cities in some older urban development in north america lanes at the rear of houses to allow for deliveries and garbage collection are called alleys alleys may be paved or unpaved and a blind alley is a culdesac some alleys are roofed because they are within buildings such as the traboules of lyon or when they are a pedestrian passage through railway embankments in britain the latter follow the line of rightsof way that existed before the railway was built because of topography steps stairs are the predominant form of alley in hilly cities and towns this includes pittsburgh see steps of pittsburgh cincinnati see steps of cincinnati portland oregon seattle and san francisco in the united states as well as hong kong and rome footpaths and other rights of way have been combined and new paths created so as to produce longdistance walking routes in a number of countries these'</li><li>'the minot area growth through investment and cooperation fund or magic fund is a growth fund financed through a one percent sales tax in the city of minot north dakota the fund was approved by voters on may 1 1990 and the money is used for economic development capital improvements and property tax relief as of 2012 the magic fund has invested over 33 million into 200 projects in 44 communities forty percent of the one percent tax is earmarked for economic development and is used to help finance relocations startups and expansions in the minot area minot area development corporation the lead economic development agency for the city of minot targets primary sector businesses such as those in valueadded agriculture knowledgebased business and the energy industry the availability of magic funds makes minot more appealing to businesses the magic fund is very progressive in that it was one of the first growth funds in the state of north dakota and the first one to be used regionally when the magic fund was originally established it was designed to operate with minimal guidelines to allow for the high level of flexibility necessary when assembling financing and incentive packages to benefit potential businesses and the community of minot this nonrestrictive nature of the fund has been a source of some criticism though local leadership acknowledges that throughout the life of the magic fund it has been a challenge maintain openness with the public about specific spending while at the same time respecting the confidentiality of business information leaders are striving however to keep communications clearin 2005 new magic fund guidelines were set in place to clearly define “ full time ” and to require a breakdown — not an average of — salaries of proposed positions more recently in october 2008 the guidelines of the magic fund underwent public review and area residents were encouraged to offer suggestions suggestions included making magic funds available for private sector projects such as housing recreation and childcare or using the money for infrastructure purposes such as streets and sewer in order to encourage more housing projects after consideration the guidelines review committee decided to continue using magic funding for businessrelated projects the initial creation of the magic fund in may 1990 established it through 2006 and come june 2004 city voters approved an extension of the 1 city sales tax through the year 2014 the magic fund has a rich history of aiding economic development in the minot region and study after study shows the local economy has benefited drastically from its availability historically magic funds have been used in three main areas of primary sector economic development knowledgebased employment agriculture and energy five of the ten largest employers conducting business in minot today were recruited using magic funds choice hotels international was one of the first businesses to be recruited using'</li><li>'##tes to solve problems everything promised by compact cities can be delivered'</li></ul> |
| 16 | <ul><li>'physiographic regions are a means of defining earths landforms into distinct mutually exclusive areas independent of political boundaries it is based upon the classic threetiered approach by nevin m fenneman in 1916 that separates landforms into physiographic divisions physiographic provinces and physiographic sectionsthe classification mechanism has become a popular geographical tool in the united states indicated by the publication of a usgs shapefile that maps the regions of the original work and the national park servicess use of the terminology to describe the regions in which its parks are locatedoriginally used in north america the model became the basis for similar classifications of other continents during the early 1900s the study of regionalscale geomorphology was termed physiography physiography later was considered to be a portmanteau of physical and geography and therefore synonymous with physical geography and the concept became embroiled in controversy surrounding the appropriate concerns of that discipline some geomorphologists held to a geological basis for physiography and emphasized a concept of physiographic regions while a conflicting trend among geographers was to equate physiography with pure morphology separated from its geological heritage in the period following world war ii the emergence of process climatic and quantitative studies led to a preference by many earth scientists for the term geomorphology in order to suggest an analytical approach to landscapes rather than a descriptive one in current usage physiography still lends itself to confusion as to which meaning is meant the more specialized geomorphological definition or the more encompassing physical geography definition for the purposes of physiographic mapping landforms are classified according to both their geologic structures and histories distinctions based on geologic age also correspond to physiographic distinctions where the forms are so recent as to be in their first erosion cycle as is generally the case with sheets of glacial drift generally forms which result from similar histories are characterized by certain similar features and differences in history result in corresponding differences of form usually resulting in distinctive features which are obvious to the casual observer but this is not always the case a maturely dissected plateau may grade without a break from rugged mountains on the one hand to mildly rolling farm lands on the other so also forms which are not classified together may be superficially similar for example a young coastal plain and a peneplain in a large number of cases the boundary lines are also geologic lines due to differences in the nature or structure of the underlying rocks the history of physiography itself is at best a complicated effort much of'</li><li>'##ythagoras contrary to popular belief most educated people in the middle ages did not believe the earth was flat this misconception is often called the myth of the flat earth as evidenced by thinkers such as thomas aquinas the european belief in a spherical earth was widespread by this point in time prior to circumnavigation of the planet and the introduction of space flight belief in a spherical earth was based on observations of the secondary effects of the earths shape and parallels drawn with the shape of other planets humans have commonly traveled for business pleasure discovery and adventure all made easier in recent human history as a result of technologies like cars trains planes and ships land navigation is an aspect of travel and refers to progressing through unfamiliar terrain using navigational tools like maps with references to terrain a compass or satellite navigation navigation on land is often facilitated by reference to landmarks – enduring and recognizable natural or artificial features that stand out from their nearby environment and are often visible from long distances natural landmarks can be characteristic features such as mountains or plateaus with examples including table mountain in south africa mount ararat in turkey the grand canyon in the united states uluru in'</li><li>'##width extra versatility compared to the strahler number however unlike the strahler number the pathwidth is defined only for the whole graph and not separately for each node in the graph main stem of a river typically found by following the branch with the highest strahler number pfafstetter coding system'</li></ul> |
| 24 | <ul><li>'glenstone is a private contemporary art museum in potomac maryland founded in 2006 by american billionaire mitchell rales and his wife emily wei rales the museums exhibitions are drawn from a collection of about 1300 works from postworld war ii artists around the world it is the largest private contemporary art museum in the united states holding more than 46 billion in net assets and is noted for its setting in a broad natural landscape glenstones original building was designed by charles gwathmey with it being expanded several times on its 230acre 93 ha campus its most significant expansion was finished in the late 2010s with outdoor sculpture installations landscaping a new complex designed by thomas phifer and an environmental center being added glenstone has been compared to other private museums such as the frick collection and the phillips collection the museum is free to the public with it seeing over 100000 visitors in 2022 in 1986 billionaire american businessman mitchell rales purchased the property in potomac maryland to build a home starting in 1990 rales began collecting art for that home following a neardeath accident on a helicopter trip in russia rales decided to take on a philanthropic project which became the establishment of a private contemporary art museum built on land that was formerly a fox hunting club glenstone is named for the nearby glen road and because of stone quarries located in the vicinity located 15 miles 24 km from downtown washington dc the museums initial 30000squarefoot 2800 m2 modernist limestone gallery opened in 2006 and admitted visitors two days a week in its first seven years the museum admitted only 10000 visitorsthough several smaller expansions took place in the years after the museums opening the largest expansion was announced in 2013 and was completed in 2018 opening to the public on october 4 2018 with a cost of approximately 219 million the expansion increased the size of the museums gallery space by a factor of five increasing the propertys size by 130 acres 53 ha and included substantial landscaping changes with the expansion glenstone became the largest private contemporary art museum in the united states in 2019 the expansion was named as a museum opening of the year by apollowith the expansion glenstone opened to the public with free tickets available online in the year following the expansion glenstone admitted nearly 100000 visitorsin 2015 glenstone was one of several private museums questioned by the us senate finance committee over its nonprofit tax status after reporting from the new york times had questioned the validity of nonprofit tax status for institutions like glenstone which at the time welcomed very few visitors the committee sought to investigate whether highvalue individuals and families were using private museums as a form of tax shelter committee chairman senator orrin hatch said'</li><li>'in consistently producing organic litter is believed to be more important in reducing erosion than its direct speedreducing effects on raindrops nevertheless gardens are less effective than natural forests in erosion reduction harvesting of rice — the dominant staple of indonesia — influences the use of pekarangans in some ways production in the gardens decreases during riceharvesting season but peaks during the rest of the year lowerincome villagers benefit from the consistent productivity of starch crops in the gardens especially in a period of food shortage prerice harvest or after a failed rice harvest by droughtsettlement dynamics affect pekarangans in various ways expansion of settlements to new lands caused by population growth is the cause of the wide presence of food crops in newly made pekarangans people who resettled via the indonesian transmigration program might support plant diversity in the gardens in the places they migrate to plant species brought by internal migrants need to adapt well to the local environmentcommercialization fragmentation and urbanization are major hazards to pekarangans plant diversity these change the organic cycles within the gardens threatening their ecological sustainability commercialization requires a systemic change of crop planting to optimize and produce more crops a pekarangans owner must specialize in its crops making a small number of crops dominate the garden some owners turn them into monoculture gardens fragmentation stems from the traditional system of inheritance consequences from the reduction of plant diversity include the loss of canopy structures and organic litter resulting in less protection of the gardens soil loss of pestcontrol agents increasing the use of pesticides loss of production stability loss of nutrients diversity and the disappearance of yieldssharing culture despite urbanizations negative effect in reducing their plant diversity it increases that of the ornamental plantsa case study of home gardens in napu valley central sulawesi shows that the decrease in soil protection is caused by insufficient soil fertility management regular weeding and waste burning dumping waste in garbage pits instead of using it for compost and spread of inorganic waste the decrease of soil fertility worsens the decrease of crop diversity in the gardens products from pekarangans have multiple uses for example a coconut tree can provide food oil fuel and building materials and also be used in rituals and ceremonies the gardens plants are known for their products nutritional benefits and diversity while rice is low in vitamins a and c products from the gardens offer an abundance of them pekarangans with more perennial crops tend to create more carbohydrates and proteins and those with more annual plants tend to create more portions of vitamin a pekarangans also act as a source of fire'</li><li>'the german fountain turkish alman cesmesi german deutscher brunnen is a gazebo styled fountain in the northern end of old hippodrome sultanahmet square istanbul turkey and across from the mausoleum of sultan ahmed i it was constructed to commemorate the second anniversary of german emperor wilhelm iis visit to istanbul in 1898 it was built in germany then transported piece by piece and assembled in its current site in 1900 the neobyzantine style fountains octagonal dome has eight marble columns and domes interior is covered with golden mosaics the idea of great palace of constantinoples empire lodge kathisma being on the site of the german fountains conflicts with the view that carceres gates of hippodrome was found on the site of the fountain however the hypothesis of carceres gates being on the site enforces the view that quadriga of lysippos was used to stand on the site of the german fountainduring his reign as german emperor and king of prussia wilhelm ii visited several european and eastern countries his trip started in istanbul ottoman empire on 18 october 1898 during the reign of abdulhamid ii according to peter hopkirk the visit to ottoman empire was an ego trip and also had longterm motivations the emperors primary motivation for visiting was to construct the baghdad railway which would run from berlin to the persian gulf and would further connect to british india through persia this railway could provide a short and quick route from europe to asia and could carry german exports troops and artillery at the time the ottoman empire could not afford such a railway and abdulhamid ii was grateful to wilhelms offer but was suspicious over the german motives abdulhamid iis secret service believed that german archeologists in the emperors retinue were in fact geologists with designs on the oil wealth of the ottoman empire later the secret service uncovered a german report which noted that the oilfields in mosul northern mesopotamia were richer than that in the caucuses in his first visit wilhelm secured the sale of germanmade rifles to ottoman army and in his second visit he secured a promise for german companies to construct the istanbulbaghdad railway the german government constructed the german fountain for wilhelm ii and empress augustas 1898 istanbul visit according to afife batur the fountains plans were drawn by architect spitta and constructed by architect schoele also german architect carlitzik and italian architect joseph anthony worked on this projectaccording to the ottoman inscription the fountains construction started in the hejira 1319 1898 – 1899 although the inauguration of the fountain was planned to take place on 1'</li></ul> |
| 10 | <ul><li>'inhibits the growth of some harmful gramnegative and grampositive bacteria along with yeasts molds and protozoa l reuteri can secrete sufficient amounts of reuterin to inhibit the growth of harmful gut organisms without killing beneficial gut bacteria allowing l reuteri to remove gut invaders while keeping normal gut flora intactreuterin is watersoluble effective in a wide range of ph resistant to proteolytic and lipolytic enzymes and has been studied as a food preservative or auxiliary therapeutic agentreuterin as an extracted compound has been shown capable of killing escherichia coli o157h7 and listeria monocytogenes with the addition of lactic acid increasing its efficacy it has also been demonstrated to kill escherichia coli o157h7 when produced by l reuteri'</li><li>'thus can affect biological function of the fsl lipids in fsl kode constructs include diacyldiakyl eg dope sterols eg cholesterol ceramides one of the important functions of an fsl construct is that it can optimise the presentation of antigens both on cell surfaces and solidphase membranes this optimisation is achieved primarily by the spacer and secondarily by the lipid tail in a typical immunoassay the antigen is deposited directly onto the microplate surface and binds to the surface either in a random fashion or in a preferred orientation depending on the residues present on the surface of this antigen usually this deposition process is uncontrolled in contrast the fsl kode construct bound to a microplate presents the antigen away from the surface in an orientation with a high level of exposure to the environment furthermore typical immunoassays use recombinant peptides rather than discrete peptide antigens as the recombinant peptide is many times bigger than the epitope of interest a lot of undesired and unwanted peptide sequences are also represented on the microplate these additional sequences may include unwanted microbial related sequences as determined by a blast analysis that can cause issues of low level crossreactivity often the mechanism by which an immunoassay is able to overcome this low level activity is to dilute the serum so that the low level microbial reactive antibodies are not seen and only highlevel specific antibodies result in an interpretable result in contrast fsl kode constructs usually use specifically selected peptide fragments up to 40 amino acids thereby overcoming crossreactivity with microbial sequences and allowing for the use of undiluted serum which increases sensitivity the f component can be further enhanced by presentation of it in multimeric formats and with specific spacing the four types of multimeric format include linear repeating units linear repeating units with spacing clusters and branching fig 4 the fsl kode construct by nature of its composition in possessing both hydrophobic and hydrophilic regions are amphiphilic or amphipathic this characteristic determines the way in which the construct will interact with surfaces when present in a solution they may form simple micelles or adopt more complex bilayer structures with two simplistic examples shown in fig 5a more complex structures are expected the actual nature of fsl micelles has not been determined however based on normal structural function of micelles it is expected that it will be determined in part by the combination of functional group spacer and lipid together'</li><li>'##n1 il1 etc which do not have a signal sequence they do not use the classical ergolgi pathway these are secreted through various nonclassical pathways at least four nonclassical unconventional protein secretion pathways have been described they include direct protein translocation across the plasma membrane likely through membrane transport proteins blebbing lysosomal secretion release via exosomes derived from multivesicular bodiesin addition proteins can be released from cells by mechanical or physiological wounding and through nonlethal transient oncotic pores in the plasma membrane induced by washing cells with serumfree media or buffers many human cell types have the ability to be secretory cells they have a welldeveloped endoplasmic reticulum and golgi apparatus to fulfill this function tissues that produce secretions include the gastrointestinal tract which secretes digestive enzymes and gastric acid the lungs which secrete surfactants and sebaceous glands which secrete sebum to lubricate the skin and hair meibomian glands in the eyelid secrete meibum to lubricate and protect the eye secretion is not unique to eukaryotes – it is also present in bacteria and archaea as well atp binding cassette abc type transporters are common to the three domains of life some secreted proteins are translocated across the cytoplasmic membrane by the secyeg translocon one of two translocation systems which requires the presence of an nterminal signal peptide on the secreted protein others are translocated across the cytoplasmic membrane by the twinarginine translocation pathway tat gramnegative bacteria have two membranes thus making secretion topologically more complex there are at least six specialized secretion systems in gramnegative bacteria many secreted proteins are particularly important in bacterial pathogenesis type i secretion is a chaperone dependent secretion system employing the hly and tol gene clusters the process begins as a leader sequence on the protein to be secreted is recognized by hlya and binds hlyb on the membrane this signal sequence is extremely specific for the abc transporter the hlyab complex stimulates hlyd which begins to uncoil and reaches the outer membrane where tolc recognizes a terminal molecule or signal on hlyd hlyd recruits tolc to the inner membrane and hlya is excreted outside of the outer membrane via a longtunnel protein channel type i secretion system transports various molecules from ions drugs to'</li></ul> |
| 1 | <ul><li>'first to form followed by the oblique shock shock diamonds are most commonly associated with jet and rocket propulsion but they can form in other systems shock diamonds can be seen during gas pipeline blowdowns because the gas is under high pressure and exits the blowdown valve at extreme speeds when artillery pieces are fired gas exits the cannon muzzle at supersonic speeds and produces a series of shock diamonds the diamonds cause a bright muzzle flash which can expose the location of gun emplacements to the enemy it was found that when the ratio between the flow pressure and atmospheric pressure is close which can be achieved with a flash suppressor the shock diamonds were greatly minimized adding a muzzle brake to the end of the muzzle balances the pressures and prevents shock diamonds 41 some radio jets powerful jets of plasma that emanate from quasars and radio galaxies are observed to have regularlyspaced knots of enhanced radio emissions 68 the jets travel at supersonic speed through a thin atmosphere of gas in space 51 so it is hypothesized that these knots are shock diamonds index of aviation articles plume hydrodynamics rocket engine nozzle'</li><li>'##al change in location of the marker can be calculated by collecting results from a few markers the degree to which the model is flexibly yielding due to the air load can be calculated there are many different kinds of wind tunnels they are typically classified by the range of speeds that are achieved in the test section as follows lowspeed wind tunnel high speed wind tunnel subsonic and transonic wind tunnel supersonic wind tunnel hypersonic wind tunnel high enthalpy wind tunnelwind tunnels are also classified by the orientation of air flow in the test section with respect to gravity typically they are oriented horizontally as happens during level flight a different class of wind tunnels are oriented vertically so that gravity can be balanced by drag instead of lift and these have become a popular form of recreation for simulating skydiving vertical wind tunnelwind tunnels are also classified based on their main use for those used with land vehicles such as cars and trucks the type of floor aerodynamics is also important these vary from stationary floors through to full moving floors with smaller moving floors and some attempt at boundary level control also being important the main subcategories in the aeronautical wind tunnels are high reynolds number tunnels reynolds number is one of the governing similarity parameters for the simulation of flow in a wind tunnel for mach number less than 03 it is the primary parameter that governs the flow characteristics there are three main ways to simulate high reynolds number since it is not practical to obtain full scale reynolds number by use of a full scale vehicle pressurised tunnels here test gases are pressurised to increase the reynolds number heavy gas tunnels heavier gases like freon and r134a are used as test gases the transonic dynamics tunnel at nasa langley is an example of such a tunnel cryogenic tunnels here test gas is cooled down to increase the reynolds number the european transonic wind tunnel uses this technique highaltitude tunnels these are designed to test the effects of shock waves against various aircraft shapes in near vacuum in 1952 the university of california constructed the first two highaltitude wind tunnels one for testing objects at 50 to 70 miles above the earth and the second for tests at 80 to 200 miles above the earth vstol tunnels vstol tunnels require large cross section area but only small velocities since power varies with the cube of velocity the power required for the operation is also less an example of a vstol tunnel is the nasa langley 14 by 22 ft 43 by 67 m tunnel spin tunnels aircraft have a tendency to spin when they stall these tunnels are used to study that phenomenon automotive wind tunnels fall into two categories'</li><li>'high speed requires at least a 2dimensional treatment when all 3 spatial dimensions and perhaps the time dimension as well are important we often resort to computerized solutions of the governing equations the mach number m is defined as the ratio of the speed of an object or of a flow to the speed of sound for instance in air at room temperature the speed of sound is about 340 ms 1100 fts m can range from 0 to ∞ but this broad range falls naturally into several flow regimes these regimes are subsonic transonic supersonic hypersonic and hypervelocity flow the figure below illustrates the mach number spectrum of these flow regimes these flow regimes are not chosen arbitrarily but rather arise naturally from the strong mathematical background that underlies compressible flow see the cited reference textbooks at very slow flow speeds the speed of sound is so much faster that it is mathematically ignored and the mach number is irrelevant once the speed of the flow approaches the speed of sound however the mach number becomes allimportant and shock waves begin to appear thus the transonic regime is described by a different and much more complex mathematical treatment in the supersonic regime the flow is dominated by wave motion at oblique angles similar to the mach angle above about mach 5 these wave angles grow so small that a different mathematical approach is required defining the hypersonic speed regime finally at speeds comparable to that of planetary atmospheric entry from orbit in the range of several kms the speed of sound is now comparatively so slow that it is once again mathematically ignored in the hypervelocity regime as an object accelerates from subsonic toward supersonic speed in a gas different types of wave phenomena occur to illustrate these changes the next figure shows a stationary point m 0 that emits symmetric sound waves the speed of sound is the same in all directions in a uniform fluid so these waves are simply concentric spheres as the soundgenerating point begins to accelerate the sound waves bunch up in the direction of motion and stretch out in the opposite direction when the point reaches sonic speed m 1 it travels at the same speed as the sound waves it creates therefore an infinite number of these sound waves pile up ahead of the point forming a shock wave upon achieving supersonic flow the particle is moving so fast that it continuously leaves its sound waves behind when this occurs the locus of these waves trailing behind the point creates an angle known as the mach wave angle or mach angle μ μ arcsin a v arcsin 1 m displaystyle mu arcsin leftfrac avrightarcsin leftfrac 1mright where a displaystyle a'</li></ul> |
| 32 | <ul><li>'for producing precision lengths by stacking components which are joined temporarily in a similar fashion'</li><li>'this step does the preforming of green raw bodies of the mould inserts sintering by sintering the preformed green bodies are compressed and hardened in order to do this the green body is heated to a temperature below the melting temperature the sintering process consists of three phases first the volume and the porosity is reduced and secondly the open porosity is reduced in the third phase sinter necks are formed which enhance the materials strength premachining the step of premachining creates the main form of the optical insert it typically contains four process steps these steps are grinding the innerouter diameter grinding the parallelend faces of the insert grindinglapping of the fitting of insert and finally the nearnetshape grinding of the cavity normally the cavity is only premachined to a flat or a bestfit sphere grinding grinding or finishmachining creates the final form and the surface finish of the cavity in the mould insert usually the finish is carried out by grinding a subsequent polishing step is optionally required finish grinding can require several changes of the grinding tool and several truing steps of the tool finishmachining of the mould is an iterative process as long as the machined mould shows deviations from the nominal contour in the measurement step after grinding it has to be reground there is no welldefined border between premachining and fine grinding throughout the grinding process of the cavity the grain size of the tool the feed rate and the cutting depth are reduced whereas machining time increases convex surfaces are easier to manufacture the necessary steps of workpiece preparation are the mould alignment and the mould referencing grinding tool alignment grinding tool referencing and grinding tool truing also have to be done after that polishing can be necessary to remove the anisotropic structure which remains after grinding it can be performed manually or by a cncmachine coating coating is the process step in which a layer is applied on the cavity surface of the optical insert which protects the mould against wear corrosion friction sticking of glass and chemical reactions with glass for coating the surface of moulds by physical vapour deposition pvd metals are evaporated in combination with processgasbased chemicals on the tool surface highly adherent thin coatings are synthesized materials for coatings on optical inserts are platinumbased pvd mostly iridiumalloyed standard diamondlike carbon not yet commercially available sic cvd on sicceramics not yet commercially available have to be postmachined or tialn not yet commercially available to achieve a homogeneous layer thickness the'</li><li>'gag bennet 1974 electricity and modern physics 2nd ed edward arnold uk isbn 0713124598 is grant wr phillips manchester physics 2008 electromagnetism 2nd ed john wiley sons isbn 9780471927129 dj griffiths 2007 introduction to electrodynamics 3rd ed pearson education dorling kindersley isbn 9788177582932 lh greenberg 1978 physics with modern applications holtsaunders international wb saunders and co isbn 0721642470 jb marion wf hornyak 1984 principles of physics holtsaunders international saunders college isbn 4833701952 a beiser 1987 concepts of modern physics 4th ed mcgrawhill international isbn 0071001441 hd young ra freedman 2008 university physics – with modern physics 12th ed addisonwesley pearson international isbn 9780321501301'</li></ul> |
| 26 | <ul><li>'between roughness because due to this tangential component plastic deformation comes with a lower load than when ignoring this component a more realistic description then of the area of each single junction that is created is given by with α displaystyle alpha constant and a tangent force f → i displaystyle vec fi applied to the joint to obtain even more realistic considerations the phenomenon of the third body should also be considered ie the presence of foreign materials such as moisture oxides or lubricants between the two solids in contact a coefficient c is then introduced which is able to correlate the shear strength t of the pure material and that of the third body t t b displaystyle ttb with 0 c 1 by studying the behavior at the limits it will be that for c 0 t 0 and for c 1 it returns to the condition in which the surfaces are directly in contact and there is no presence of a third body keeping in mind what has just been said it is possible to correct the friction coefficient formula as follows in conclusion the case of elastic bodies in interaction with each other is considered similarly to what we have just seen it is possible to define an equation of the type where in this case k depends on the elastic properties of the materials also for the elastic bodies the tangential force depends on the coefficient c seen above and it will be and therefore a fairly exhaustive description of the friction coefficient can be obtained friction measurements the simplest and most immediate method for evaluating the friction coefficient of two surfaces is the use of an inclined plane on which a block of material is made to slide as can be seen in the figure the normal force of the plane is given by m g cos θ displaystyle mgcos theta while the frictional force is equal to m g sin θ displaystyle mgsin theta this allows us to state that the coefficient of friction can be calculated very easily by means of the tangent of the angle in which the block begins to slip in fact we have then from the inclined plane we moved on to more sophisticated systems which allow us to consider all the possible environmental conditions in which the measurement is made such as the crossroller machine or the pin and disk machine today there are digital machines such as the friction tester which allows by means of a software support to insert all the desired variables another widely used process is the ring compression test a flat ring of the material to be studied is plastically deformed by means of a press if the deformation is an expansion in both the inner and the outer circle then there will be low or zero friction coefficients otherwise for a deformation that expands only in'</li><li>'the metallurgical production of the republic of azerbaijan is considered high due to the large deposits of alunite polymetallic ores deposits of iron ore etc the metallurgy industry of azerbaijan encompasses both ferrous and nonferrous branches ferrous metallurgy includes extraction of iron smelting and refining of iron ore rolling and ferroalloys production the ferrous metallurgy production of the country started to meet the demand of oil and gas industry due to pipe production and grew further in order to improve other branches of the industry dashkasan iron ore in 4 deposits dashkesen south dashkasan hamanchay demiroglu in the valley of goshagarchay plays a key role in development of ferrous metallurgy the cities of baku sumgait and dashkesan are major centers of metallurgy in terms of extraction and processing of iron ore the sumgait piperolling plant produces drill pipes casing tubing oil and gas pipes etc bentonite clay deposits in the village of dash salakhly gazakh district is used in steel smelting baku steel company the largest metallurgical enterprise in azerbaijan was opened in 2001 on the initiative of heydar aliyev with two electric arc furnaces and three rolling lines the annual steel production capacity of company increased to 1000000 tons aluminum copper molybdenum cobalt mercury reserves and most importantly electricity for the smelting process has led to the development of nonferrous metallurgy the zeylik mine in daskasan district is the main provider of the alunite for aluminum production the extracted ore here transported through guschualabashli railway to the aluminum plant located in ganja city the obtained aluminum oxide is brought to sumgayit aluminum plant in order produce aluminum metal ganja aluminum plant produces sulfuric acid aluminum oxide and potassium fertilizer through extracted ore from zalik deposit in dashkesen aluminum oxide is also produced in sumgait azergold cjsc created by the presidential decree no 1047 on february 11 2015 implements exploration management and also extraction processing and sale of precious and nonferrous metal ore deposits located within the borders of the country in 2017 the volume of exports of precious metals carried out by this company amounted to 77340 million dollars gold mining began in gedebey in 2009 in 2016 azer gold cjsc began gold mining in the chovdar deposit in 2017 63908 kg of gold was mined which exceeded the 2016 production by 34 times gold production'</li><li>'the material they are most found in these are given in miller indices for simplification purposes cube component 001100 brass component 110112 copper component 112111 s component 123634 the full 3d representation of crystallographic texture is given by the orientation distribution function odf which can be achieved through evaluation of a set of pole figures or diffraction patterns subsequently all pole figures can be derived from the odf the odf is defined as the volume fraction of grains with a certain orientation g displaystyle boldsymbol g odf g 1 v d v g d g displaystyle textodfboldsymbol gfrac 1vfrac dvboldsymbol gdg the orientation g displaystyle boldsymbol g is normally identified using three euler angles the euler angles then describe the transition from the sample ’ s reference frame into the crystallographic reference frame of each individual grain of the polycrystal one thus ends up with a large set of different euler angles the distribution of which is described by the odf the orientation distribution function odf cannot be measured directly by any technique traditionally both xray diffraction and ebsd may collect pole figures different methodologies exist to obtain the odf from the pole figures or data in general they can be classified based on how they represent the odf some represent the odf as a function sum of functions or expand it in a series of harmonic functions others known as discrete methods divide the odf space in cells and focus on determining the value of the odf in each cell in wire and fiber all crystals tend to have nearly identical orientation in the axial direction but nearly random radial orientation the most familiar exceptions to this rule are fiberglass which has no crystal structure and carbon fiber in which the crystalline anisotropy is so great that a goodquality filament will be a distorted single crystal with approximately cylindrical symmetry often compared to a jelly roll singlecrystal fibers are also not uncommon the making of metal sheet often involves compression in one direction and in efficient rolling operations tension in another which can orient crystallites in both axes by a process known as grain flow however cold work destroys much of the crystalline order and the new crystallites that arise with annealing usually have a different texture control of texture is extremely important in the making of silicon steel sheet for transformer cores to reduce magnetic hysteresis and of aluminium cans since deep drawing requires extreme and relatively uniform plasticity texture in ceramics usually arises because the crystallites in a slurry'</li></ul> |
| 15 | <ul><li>'is could effectively be used as a geneediting tool in human 2pn zygotes which could lead potentially pregnancy viable if implanted the scientists used injection of cas9 protein complexed with the relevant sgrnas and homology donors into human embryos the scientists found homologous recombinationmediated alteration in hbb and g6pd the scientists also noted the limitations of their study and called for further researchin august 2017 a group of scientists from oregon published an article in nature journal detailing the successful use of crispr to edit out a mutation responsible for congenital heart disease the study looked at heterozygous mybpc3 mutation in human embryos the study claimed precise crisprcas9 and homologydirected repair response with high accuracy and precision doublestrand breaks at the mutant paternal allele were repaired using the homologous wildtype gene by modifying the cell cycle stage at which the dsb was induced they were able to avoid mosaicism which had been seen in earlier similar studies in cleaving embryos and achieve a large percentage of homozygous embryos carrying the wildtype mybpc3 gene without evidence of unintended mutations the scientists concluded that the technique may be used for the correction of mutations in human embryos the claims of this study were however pushed back on by critics who argued the evidence was overall unpersuasivein june 2018 a group of scientists published and article in nature journal indicating a potential link for edited cells having increased potential turn cancerous the scientists reported that genome editing by crisprcas9 induced dna damage response and the cell cycle stopped the study was conducted in human retinal pigment epithelial cells and the use of crispr led to a selection against cells with a functional p53 pathway the conclusion of the study would suggest that p53 inhibition might increase efficiency of human germline editing and that p53 function would need to be watched when developing crisprcas9 based therapyin november 2018 a group of chinese scientists published research in the journal molecular therapy detailing their use of crisprcas9 technology to correct a single mistaken amino acid successfully in 16 out of 18 attempts in a human embryo the unusual level of precision was achieved by the use of a base editor be system which was constructed by fusing the deaminase to the dcas9 protein the be system efficiently edits the targeted c to t or g to a without the use of a donor and without dbs formation the study focused on the fbn1 mutation that is causative for mar'</li><li>'by the american nurses association which provides rules regulations and guidelines to follow when making a decision that is ethical based these regulations were mainly established to help provide equal healthcare protect the rights safety and privacy of the patient and to hold nurses accountable for their actions and choices genetics can create ethical issues in nursing for a variety of different situations many scenarios questions and debates have been encountered such as what individuals can receive genetic testing or information who owns or controls the information received from the genetic test and how can the owner use that information however the code of ethics does not address genetics or genomics specifically so ethical foundations were also established to help guide genetics into health care the foundations provide a set of guidelines to understand and manage an ethical issue if one should arise and to assist in the translation of genetics into the healthcare environment'</li><li>'than is accurate to the population this is known as the shadow effect the cabrera vole microtus cabrerae is a small endangered rodent that belongs to the microtus genus existing primarily in portugal populations can be difficult to estimate using typical markrecapture methods due to their small size and ability to quickly disperse over large swaths of prairie land with the introduction and reduced cost of using environmental dna in this case feces were able to be used in a relatively low cost experiment to estimate the population size of the cabrera vole in southern portugal in return for sacrificing demographic age sex health information endangered species act of 1973'</li></ul> |
## Evaluation
### Metrics
| Label | Accuracy |
|:--------|:---------|
| **all** | 0.6909 |
## Uses
### Direct Use for Inference
First install the SetFit library:
```bash
pip install setfit
```
Then you can load this model and run inference.
```python
from setfit import SetFitModel
# Download from the 🤗 Hub
model = SetFitModel.from_pretrained("udrearobert999/multi-qa-mpnet-base-cos-v1-test")
# Run inference
preds = model("##rch procedure that evaluates the objective function p x displaystyle pmathbf x on a grid of candidate source locations g displaystyle mathcal g to estimate the spatial location of the sound source x s displaystyle textbf xs as the point of the grid that provides the maximum srp modifications of the classical srpphat algorithm have been proposed to reduce the computational cost of the gridsearch step of the algorithm and to increase the robustness of the method in the classical srpphat for each microphone pair and for each point of the grid a unique integer tdoa value is selected to be the acoustic delay corresponding to that grid point this procedure does not guarantee that all tdoas are associated to points on the grid nor that the spatial grid is consistent since some of the points may not correspond to an intersection of hyperboloids this issue becomes more problematic with coarse grids since when the number of points is reduced part of the tdoa information gets lost because most delays are not anymore associated to any point in the grid the modified srpphat collects and uses the tdoa information related to the volume surrounding each spatial point of the search grid by considering a modified objective function where l m 1 m 2 l x displaystyle lm1m2lmathbf x and l m 1 m 2 u x displaystyle lm1m2umathbf x are the lower and upper accumulation limits of gcc delays which depend on the spatial location x displaystyle mathbf x the accumulation limits can be calculated beforehand in an exact way by exploring the boundaries separating the regions corresponding to the points of the grid alternatively they can be selected by considering the spatial gradient of the tdoa ∇ τ m 1 m 2 x ∇ x τ m 1 m 2 x ∇ y τ m 1 m 2 x ∇ z τ m 1 m 2 x t displaystyle nabla tau m1m2mathbf x nabla xtau m1m2mathbf x nabla ytau m1m2mathbf x nabla ztau m1m2mathbf x t where each component γ ∈ x y z displaystyle gamma in leftxyzright of the gradient is for a rectangular grid where neighboring points are separated a distance r displaystyle r the lower and upper accumulation limits are given by where d r 2 min 1 sin θ cos [UNK] 1 sin θ sin [UNK] 1 cos θ displaystyle dr2min leftfrac 1vert sintheta cosphi vert frac 1vert sintheta sinphi vert frac 1vert")
```
<!--
### Downstream Use
*List how someone could finetune this model on their own dataset.*
-->
<!--
### Out-of-Scope Use
*List how the model may foreseeably be misused and address what users ought not to do with the model.*
-->
<!--
## Bias, Risks and Limitations
*What are the known or foreseeable issues stemming from this model? You could also flag here known failure cases or weaknesses of the model.*
-->
<!--
### Recommendations
*What are recommendations with respect to the foreseeable issues? For example, filtering explicit content.*
-->
## Training Details
### Training Set Metrics
| Training set | Min | Median | Max |
|:-------------|:----|:---------|:----|
| Word count | 1 | 370.3098 | 509 |
| Label | Training Sample Count |
|:------|:----------------------|
| 0 | 50 |
| 1 | 50 |
| 2 | 50 |
| 3 | 50 |
| 4 | 50 |
| 5 | 50 |
| 6 | 50 |
| 7 | 50 |
| 8 | 50 |
| 9 | 50 |
| 10 | 50 |
| 11 | 50 |
| 12 | 50 |
| 13 | 50 |
| 14 | 50 |
| 15 | 50 |
| 16 | 50 |
| 17 | 50 |
| 18 | 50 |
| 19 | 50 |
| 20 | 50 |
| 21 | 50 |
| 22 | 50 |
| 23 | 50 |
| 24 | 50 |
| 25 | 50 |
| 26 | 50 |
| 27 | 50 |
| 28 | 50 |
| 29 | 50 |
| 30 | 50 |
| 31 | 50 |
| 32 | 50 |
| 33 | 50 |
| 34 | 50 |
| 35 | 50 |
| 36 | 50 |
| 37 | 50 |
| 38 | 50 |
| 39 | 50 |
| 40 | 50 |
| 41 | 50 |
| 42 | 50 |
### Training Hyperparameters
- batch_size: (16, 16)
- num_epochs: (1, 4)
- max_steps: -1
- sampling_strategy: oversampling
- num_iterations: 10
- body_learning_rate: (2e-05, 0.01)
- head_learning_rate: 0.01
- loss: CosineSimilarityLoss
- distance_metric: cosine_distance
- margin: 0.25
- end_to_end: False
- use_amp: False
- warmup_proportion: 0.1
- max_length: 512
- seed: 42
- eval_max_steps: -1
- load_best_model_at_end: True
### Training Results
| Epoch | Step | Training Loss | Validation Loss |
|:----------:|:--------:|:-------------:|:---------------:|
| 0.0004 | 1 | 0.3114 | - |
| 0.1860 | 500 | 0.0379 | - |
| 0.3720 | 1000 | 0.1131 | - |
| 0.5580 | 1500 | 0.0567 | - |
| **0.7440** | **2000** | **0.0168** | **0.1033** |
| 0.9301 | 2500 | 0.0033 | - |
* The bold row denotes the saved checkpoint.
### Framework Versions
- Python: 3.10.12
- SetFit: 1.0.3
- Sentence Transformers: 2.7.0
- Transformers: 4.40.1
- PyTorch: 2.2.1+cu121
- Datasets: 2.19.0
- Tokenizers: 0.19.1
## Citation
### BibTeX
```bibtex
@article{https://doi.org/10.48550/arxiv.2209.11055,
doi = {10.48550/ARXIV.2209.11055},
url = {https://arxiv.org/abs/2209.11055},
author = {Tunstall, Lewis and Reimers, Nils and Jo, Unso Eun Seo and Bates, Luke and Korat, Daniel and Wasserblat, Moshe and Pereg, Oren},
keywords = {Computation and Language (cs.CL), FOS: Computer and information sciences, FOS: Computer and information sciences},
title = {Efficient Few-Shot Learning Without Prompts},
publisher = {arXiv},
year = {2022},
copyright = {Creative Commons Attribution 4.0 International}
}
```
<!--
## Glossary
*Clearly define terms in order to be accessible across audiences.*
-->
<!--
## Model Card Authors
*Lists the people who create the model card, providing recognition and accountability for the detailed work that goes into its construction.*
-->
<!--
## Model Card Contact
*Provides a way for people who have updates to the Model Card, suggestions, or questions, to contact the Model Card authors.*
--> | {"library_name": "setfit", "tags": ["setfit", "sentence-transformers", "text-classification", "generated_from_setfit_trainer"], "metrics": ["accuracy"], "base_model": "sentence-transformers/multi-qa-mpnet-base-cos-v1", "widget": [{"text": "in durankulak near varna is another important example other signs of early metals are found from the third millennium bc in palmela portugal los millares spain and stonehenge united kingdom the precise beginnings however have not be clearly ascertained and new discoveries are both continuous and ongoing in tamilnadu in approximately 1900 bc ancient iron smelting sites were functioning in tamil nadu in the near east about 3500 bc it was discovered that by combining copper and tin a superior metal could be made an alloy called bronze this represented a major technological shift known as the bronze age the extraction of iron from its ore into a workable metal is much more difficult than for copper or tin the process appears to have been invented by the hittites in about 1200 bc beginning the iron age the secret of extracting and working iron was a key factor in the success of the philistineshistorical developments in ferrous metallurgy can be found in a wide variety of past cultures and civilizations this includes the ancient and medieval kingdoms and empires of the middle east and near east ancient iran ancient egypt ancient nubia and anatolia in presentday turkey ancient nok carthage the greeks and romans of ancient europe medieval europe ancient and medieval china ancient and medieval india ancient and medieval japan amongst others many applications practices and devices associated or involved in metallurgy were established in ancient china such as the innovation of the blast furnace cast iron hydraulicpowered trip hammers and double acting piston bellowsa 16th century book by georg agricola de re metallica describes the highly developed and complex processes of mining metal ores metal extraction and metallurgy of the time agricola has been described as the father of metallurgy extractive metallurgy is the practice of removing valuable metals from an ore and refining the extracted raw metals into a purer form in order to convert a metal oxide or sulphide to a purer metal the ore must be reduced physically chemically or electrolytically extractive metallurgists are interested in three primary streams feed concentrate metal oxidesulphide and tailings waste after mining large pieces of the ore feed are broken through crushing or grinding in order to obtain particles small enough where each particle is either mostly valuable or mostly waste concentrating the particles of value in a form supporting separation enables the desired metal to be removed from waste products mining may not be necessary if the ore body and physical environment are conducive to leaching leaching dissolves minerals in an ore body and results in an enriched solution the solution is collected and processed to extract valuable metals ore"}, {"text": "##rch procedure that evaluates the objective function p x displaystyle pmathbf x on a grid of candidate source locations g displaystyle mathcal g to estimate the spatial location of the sound source x s displaystyle textbf xs as the point of the grid that provides the maximum srp modifications of the classical srpphat algorithm have been proposed to reduce the computational cost of the gridsearch step of the algorithm and to increase the robustness of the method in the classical srpphat for each microphone pair and for each point of the grid a unique integer tdoa value is selected to be the acoustic delay corresponding to that grid point this procedure does not guarantee that all tdoas are associated to points on the grid nor that the spatial grid is consistent since some of the points may not correspond to an intersection of hyperboloids this issue becomes more problematic with coarse grids since when the number of points is reduced part of the tdoa information gets lost because most delays are not anymore associated to any point in the grid the modified srpphat collects and uses the tdoa information related to the volume surrounding each spatial point of the search grid by considering a modified objective function where l m 1 m 2 l x displaystyle lm1m2lmathbf x and l m 1 m 2 u x displaystyle lm1m2umathbf x are the lower and upper accumulation limits of gcc delays which depend on the spatial location x displaystyle mathbf x the accumulation limits can be calculated beforehand in an exact way by exploring the boundaries separating the regions corresponding to the points of the grid alternatively they can be selected by considering the spatial gradient of the tdoa \u2207 \u03c4 m 1 m 2 x \u2207 x \u03c4 m 1 m 2 x \u2207 y \u03c4 m 1 m 2 x \u2207 z \u03c4 m 1 m 2 x t displaystyle nabla tau m1m2mathbf x nabla xtau m1m2mathbf x nabla ytau m1m2mathbf x nabla ztau m1m2mathbf x t where each component \u03b3 \u2208 x y z displaystyle gamma in leftxyzright of the gradient is for a rectangular grid where neighboring points are separated a distance r displaystyle r the lower and upper accumulation limits are given by where d r 2 min 1 sin \u03b8 cos [UNK] 1 sin \u03b8 sin [UNK] 1 cos \u03b8 displaystyle dr2min leftfrac 1vert sintheta cosphi vert frac 1vert sintheta sinphi vert frac 1vert"}, {"text": "authority to select projects and mandated new metropolitan planning initiatives for the first time state transportation officials were required to consult seriously with local representatives on mpo governing boards regarding matters of project prioritization and decisionmaking these changes had their roots in the need to address increasingly difficult transportation problems \u2014 in particular the more complicated patterns of traffic congestion that arose with the suburban development boom in the previous decades many recognized that the problems could only be addressed effectively through a stronger federal commitment to regional planning the legislation that emerged the intermodal surface transportation efficiency act istea was signed into federal law by president george h w bush in december 1991 it focused on improving transportation not as an end in itself but as the means to achieve important national goals including economic progress cleaner air energy conservation and social equity istea promoted a transportation system in which different modes and facilities \u2014 highway transit pedestrian bicycle aviation and marine \u2014 were integrated to allow a seamless movement of both goods and people new funding programs provided greater flexibility in the use of funds particularly regarding using previously restricted highway funds for transit development improved intermodal connections and emphasized upgrades to existing facilities over building new capacity \u2014 particularly roadway capacity to accomplish more serious metropolitan planning istea doubled federal funding for mpo operations and required the agencies to evaluate a variety of multimodal solutions to roadway congestion and other transportation problems mpos also were required to broaden public participation in the planning process and to see that investment decisions contributed to meeting the air quality standards of the clean air act amendments in addition istea placed a new requirement on mpos to conduct fiscally constrained planning and ensure that longrange transportation plans and shortterm transportation improvement programs were fiscally constrained in other words adopted plans and programs can not include more projects than reasonably can be expected to be funded through existing or projected sources of revenues this new requirement represented a major conceptual shift for many mpos and others in the planning community since the imposition of fiscal discipline on plans now required not only understanding how much money might be available but how to prioritize investment needs and make difficult choices among competing needs adding to this complexity is the need to plan across transportation modes and develop approaches for multimodal investment prioritization and decision making it is in this context of greater prominence funding and requirements that mpos function today an annual element is composed of transportation improvement projects contained in an areas transportation improvement program tip which is proposed for implementation during the current year the annual element is submitted to the us department of transportation as part of the required planning process the passage of safe accountable flexible efficient transportation equity act a legacy for users safetealu"}, {"text": "##pignygiroux served as an assistant professor from 1997 2003 associate professor from 2003 2014 chair of the department of geography from 2015 2018 and professor beginning in 2014 with secondary appointments in department of geology the college of education social services and rubenstein school of environment natural resources she teaches courses in meteorology climatology physical geography remote sensing and landsurface processes in her work as state climatologist for vermont dupignygiroux uses her expertise hydrology and extreme weather such as floods droughts and storms to keep the residents of vermont informed on how climate change will affect their homes health and livelihoods she assists other state agencies in preparing for and adapting to current and future impacts of climate change on vermonts transportation system emergency management planning and agriculture and forestry industries for example she has published analyses of the impacts of climate change on the health of vermonts sugar maples a hardwood species of key economic and cultural importance to the state as cochair of vermonts state \u2019 s drought task force she played a key role in developing the 2018 vermont state hazard mitigation plandupignygiroux served as secretary for the american association of state climatologists from 20102011 and president elect from 20192020 in june 2020 she was elected as president of the american association of state climatologists which is a twoyear term in addition to her research on climate change dupignygiroux is known for her efforts to research and promote climate literacy climate literacy is an understanding of the influences of and influences on the climate system including how people change the climate how climate metrics are observed and modelled and how climate change affects society \u201c being climate literate is more critical than ever before \u201d lesleyann dupignygiroux stated for a 2020 article on climate literacy \u201c if we do not understand weather climate and climate change as intricate and interconnected systems then our appreciation of the big picture is lost \u201d dupignygiroux is known for her climate literacy work with elementary and high school teachers and students she cofounded the satellites weather and climate swac project in 2008 which is a professional development program for k12 teachers designed to promote climate literacy and interest in the stem science technology engineering and mathematics careers dupignygiroux is also a founding member of the climate literacy and energy awareness network clean formerly climate literacy network a communitybased effort to support climate literacy and communication in a 2016 interview dupignygiroux stated \u201c sharing knowledge and giving back to my community are my two axioms in life watching students mature and flourish in"}, {"text": "no solutions to x n y n z n displaystyle xnynzn for all n \u2265 3 displaystyle ngeq 3 this claim appears in his annotations in the margins of his copy of diophantus euler the interest of leonhard euler 1707 \u2013 1783 in number theory was first spurred in 1729 when a friend of his the amateur goldbach pointed him towards some of fermats work on the subject this has been called the rebirth of modern number theory after fermats relative lack of success in getting his contemporaries attention for the subject eulers work on number theory includes the following proofs for fermats statements this includes fermats little theorem generalised by euler to nonprime moduli the fact that p x 2 y 2 displaystyle px2y2 if and only if p \u2261 1 mod 4 displaystyle pequiv 1bmod 4 initial work towards a proof that every integer is the sum of four squares the first complete proof is by josephlouis lagrange 1770 soon improved by euler himself the lack of nonzero integer solutions to x 4 y 4 z 2 displaystyle x4y4z2 implying the case n4 of fermats last theorem the case n3 of which euler also proved by a related method pells equation first misnamed by euler he wrote on the link between continued fractions and pells equation first steps towards analytic number theory in his work of sums of four squares partitions pentagonal numbers and the distribution of prime numbers euler pioneered the use of what can be seen as analysis in particular infinite series in number theory since he lived before the development of complex analysis most of his work is restricted to the formal manipulation of power series he did however do some very notable though not fully rigorous early work on what would later be called the riemann zeta function quadratic forms following fermats lead euler did further research on the question of which primes can be expressed in the form x 2 n y 2 displaystyle x2ny2 some of it prefiguring quadratic reciprocity diophantine equations euler worked on some diophantine equations of genus 0 and 1 in particular he studied diophantuss work he tried to systematise it but the time was not yet ripe for such an endeavour \u2014 algebraic geometry was still in its infancy he did notice there was a connection between diophantine problems and elliptic integrals whose study he had himself initiated lagrange legendre and gauss josephlouis"}], "pipeline_tag": "text-classification", "inference": true, "model-index": [{"name": "SetFit with sentence-transformers/multi-qa-mpnet-base-cos-v1", "results": [{"task": {"type": "text-classification", "name": "Text Classification"}, "dataset": {"name": "Unknown", "type": "unknown", "split": "test"}, "metrics": [{"type": "accuracy", "value": 0.6908674054260604, "name": "Accuracy"}]}]}]} | udrearobert999/multi-qa-mpnet-base-cos-v1-test | null | [
"setfit",
"safetensors",
"mpnet",
"sentence-transformers",
"text-classification",
"generated_from_setfit_trainer",
"arxiv:2209.11055",
"base_model:sentence-transformers/multi-qa-mpnet-base-cos-v1",
"model-index",
"region:us"
] | null | 2024-05-02T11:12:07+00:00 | [
"2209.11055"
] | [] | TAGS
#setfit #safetensors #mpnet #sentence-transformers #text-classification #generated_from_setfit_trainer #arxiv-2209.11055 #base_model-sentence-transformers/multi-qa-mpnet-base-cos-v1 #model-index #region-us
| SetFit with sentence-transformers/multi-qa-mpnet-base-cos-v1
============================================================
This is a SetFit model that can be used for Text Classification. This SetFit model uses sentence-transformers/multi-qa-mpnet-base-cos-v1 as the Sentence Transformer embedding model. A SetFitHead instance is used for classification.
The model has been trained using an efficient few-shot learning technique that involves:
1. Fine-tuning a Sentence Transformer with contrastive learning.
2. Training a classification head with features from the fine-tuned Sentence Transformer.
Model Details
-------------
### Model Description
* Model Type: SetFit
* Sentence Transformer body: sentence-transformers/multi-qa-mpnet-base-cos-v1
* Classification head: a SetFitHead instance
* Maximum Sequence Length: 512 tokens
* Number of Classes: 43 classes
### Model Sources
* Repository: SetFit on GitHub
* Paper: Efficient Few-Shot Learning Without Prompts
* Blogpost: SetFit: Efficient Few-Shot Learning Without Prompts
### Model Labels
Evaluation
----------
### Metrics
Uses
----
### Direct Use for Inference
First install the SetFit library:
Then you can load this model and run inference.
Training Details
----------------
### Training Set Metrics
### Training Hyperparameters
* batch\_size: (16, 16)
* num\_epochs: (1, 4)
* max\_steps: -1
* sampling\_strategy: oversampling
* num\_iterations: 10
* body\_learning\_rate: (2e-05, 0.01)
* head\_learning\_rate: 0.01
* loss: CosineSimilarityLoss
* distance\_metric: cosine\_distance
* margin: 0.25
* end\_to\_end: False
* use\_amp: False
* warmup\_proportion: 0.1
* max\_length: 512
* seed: 42
* eval\_max\_steps: -1
* load\_best\_model\_at\_end: True
### Training Results
* The bold row denotes the saved checkpoint.
### Framework Versions
* Python: 3.10.12
* SetFit: 1.0.3
* Sentence Transformers: 2.7.0
* Transformers: 4.40.1
* PyTorch: 2.2.1+cu121
* Datasets: 2.19.0
* Tokenizers: 0.19.1
### BibTeX
| [
"### Model Description\n\n\n* Model Type: SetFit\n* Sentence Transformer body: sentence-transformers/multi-qa-mpnet-base-cos-v1\n* Classification head: a SetFitHead instance\n* Maximum Sequence Length: 512 tokens\n* Number of Classes: 43 classes",
"### Model Sources\n\n\n* Repository: SetFit on GitHub\n* Paper: Efficient Few-Shot Learning Without Prompts\n* Blogpost: SetFit: Efficient Few-Shot Learning Without Prompts",
"### Model Labels\n\n\n\nEvaluation\n----------",
"### Metrics\n\n\n\nUses\n----",
"### Direct Use for Inference\n\n\nFirst install the SetFit library:\n\n\nThen you can load this model and run inference.\n\n\nTraining Details\n----------------",
"### Training Set Metrics",
"### Training Hyperparameters\n\n\n* batch\\_size: (16, 16)\n* num\\_epochs: (1, 4)\n* max\\_steps: -1\n* sampling\\_strategy: oversampling\n* num\\_iterations: 10\n* body\\_learning\\_rate: (2e-05, 0.01)\n* head\\_learning\\_rate: 0.01\n* loss: CosineSimilarityLoss\n* distance\\_metric: cosine\\_distance\n* margin: 0.25\n* end\\_to\\_end: False\n* use\\_amp: False\n* warmup\\_proportion: 0.1\n* max\\_length: 512\n* seed: 42\n* eval\\_max\\_steps: -1\n* load\\_best\\_model\\_at\\_end: True",
"### Training Results\n\n\n\n* The bold row denotes the saved checkpoint.",
"### Framework Versions\n\n\n* Python: 3.10.12\n* SetFit: 1.0.3\n* Sentence Transformers: 2.7.0\n* Transformers: 4.40.1\n* PyTorch: 2.2.1+cu121\n* Datasets: 2.19.0\n* Tokenizers: 0.19.1",
"### BibTeX"
] | [
"TAGS\n#setfit #safetensors #mpnet #sentence-transformers #text-classification #generated_from_setfit_trainer #arxiv-2209.11055 #base_model-sentence-transformers/multi-qa-mpnet-base-cos-v1 #model-index #region-us \n",
"### Model Description\n\n\n* Model Type: SetFit\n* Sentence Transformer body: sentence-transformers/multi-qa-mpnet-base-cos-v1\n* Classification head: a SetFitHead instance\n* Maximum Sequence Length: 512 tokens\n* Number of Classes: 43 classes",
"### Model Sources\n\n\n* Repository: SetFit on GitHub\n* Paper: Efficient Few-Shot Learning Without Prompts\n* Blogpost: SetFit: Efficient Few-Shot Learning Without Prompts",
"### Model Labels\n\n\n\nEvaluation\n----------",
"### Metrics\n\n\n\nUses\n----",
"### Direct Use for Inference\n\n\nFirst install the SetFit library:\n\n\nThen you can load this model and run inference.\n\n\nTraining Details\n----------------",
"### Training Set Metrics",
"### Training Hyperparameters\n\n\n* batch\\_size: (16, 16)\n* num\\_epochs: (1, 4)\n* max\\_steps: -1\n* sampling\\_strategy: oversampling\n* num\\_iterations: 10\n* body\\_learning\\_rate: (2e-05, 0.01)\n* head\\_learning\\_rate: 0.01\n* loss: CosineSimilarityLoss\n* distance\\_metric: cosine\\_distance\n* margin: 0.25\n* end\\_to\\_end: False\n* use\\_amp: False\n* warmup\\_proportion: 0.1\n* max\\_length: 512\n* seed: 42\n* eval\\_max\\_steps: -1\n* load\\_best\\_model\\_at\\_end: True",
"### Training Results\n\n\n\n* The bold row denotes the saved checkpoint.",
"### Framework Versions\n\n\n* Python: 3.10.12\n* SetFit: 1.0.3\n* Sentence Transformers: 2.7.0\n* Transformers: 4.40.1\n* PyTorch: 2.2.1+cu121\n* Datasets: 2.19.0\n* Tokenizers: 0.19.1",
"### BibTeX"
] | [
72,
62,
42,
16,
10,
43,
7,
184,
14,
75,
6
] | [
"TAGS\n#setfit #safetensors #mpnet #sentence-transformers #text-classification #generated_from_setfit_trainer #arxiv-2209.11055 #base_model-sentence-transformers/multi-qa-mpnet-base-cos-v1 #model-index #region-us \n### Model Description\n\n\n* Model Type: SetFit\n* Sentence Transformer body: sentence-transformers/multi-qa-mpnet-base-cos-v1\n* Classification head: a SetFitHead instance\n* Maximum Sequence Length: 512 tokens\n* Number of Classes: 43 classes### Model Sources\n\n\n* Repository: SetFit on GitHub\n* Paper: Efficient Few-Shot Learning Without Prompts\n* Blogpost: SetFit: Efficient Few-Shot Learning Without Prompts### Model Labels\n\n\n\nEvaluation\n----------### Metrics\n\n\n\nUses\n----### Direct Use for Inference\n\n\nFirst install the SetFit library:\n\n\nThen you can load this model and run inference.\n\n\nTraining Details\n----------------### Training Set Metrics### Training Hyperparameters\n\n\n* batch\\_size: (16, 16)\n* num\\_epochs: (1, 4)\n* max\\_steps: -1\n* sampling\\_strategy: oversampling\n* num\\_iterations: 10\n* body\\_learning\\_rate: (2e-05, 0.01)\n* head\\_learning\\_rate: 0.01\n* loss: CosineSimilarityLoss\n* distance\\_metric: cosine\\_distance\n* margin: 0.25\n* end\\_to\\_end: False\n* use\\_amp: False\n* warmup\\_proportion: 0.1\n* max\\_length: 512\n* seed: 42\n* eval\\_max\\_steps: -1\n* load\\_best\\_model\\_at\\_end: True### Training Results\n\n\n\n* The bold row denotes the saved checkpoint.### Framework Versions\n\n\n* Python: 3.10.12\n* SetFit: 1.0.3\n* Sentence Transformers: 2.7.0\n* Transformers: 4.40.1\n* PyTorch: 2.2.1+cu121\n* Datasets: 2.19.0\n* Tokenizers: 0.19.1### BibTeX"
] |
text-generation | transformers |
# Model Card for Model ID
<!-- Provide a quick summary of what the model is/does. -->
## Model Details
### Model Description
<!-- Provide a longer summary of what this model is. -->
This is the model card of a 🤗 transformers model that has been pushed on the Hub. This model card has been automatically generated.
- **Developed by:** [More Information Needed]
- **Funded by [optional]:** [More Information Needed]
- **Shared by [optional]:** [More Information Needed]
- **Model type:** [More Information Needed]
- **Language(s) (NLP):** [More Information Needed]
- **License:** [More Information Needed]
- **Finetuned from model [optional]:** [More Information Needed]
### Model Sources [optional]
<!-- Provide the basic links for the model. -->
- **Repository:** [More Information Needed]
- **Paper [optional]:** [More Information Needed]
- **Demo [optional]:** [More Information Needed]
## Uses
<!-- Address questions around how the model is intended to be used, including the foreseeable users of the model and those affected by the model. -->
### Direct Use
<!-- This section is for the model use without fine-tuning or plugging into a larger ecosystem/app. -->
[More Information Needed]
### Downstream Use [optional]
<!-- This section is for the model use when fine-tuned for a task, or when plugged into a larger ecosystem/app -->
[More Information Needed]
### Out-of-Scope Use
<!-- This section addresses misuse, malicious use, and uses that the model will not work well for. -->
[More Information Needed]
## Bias, Risks, and Limitations
<!-- This section is meant to convey both technical and sociotechnical limitations. -->
[More Information Needed]
### Recommendations
<!-- This section is meant to convey recommendations with respect to the bias, risk, and technical limitations. -->
Users (both direct and downstream) should be made aware of the risks, biases and limitations of the model. More information needed for further recommendations.
## How to Get Started with the Model
Use the code below to get started with the model.
[More Information Needed]
## Training Details
### Training Data
<!-- This should link to a Dataset Card, perhaps with a short stub of information on what the training data is all about as well as documentation related to data pre-processing or additional filtering. -->
[More Information Needed]
### Training Procedure
<!-- This relates heavily to the Technical Specifications. Content here should link to that section when it is relevant to the training procedure. -->
#### Preprocessing [optional]
[More Information Needed]
#### Training Hyperparameters
- **Training regime:** [More Information Needed] <!--fp32, fp16 mixed precision, bf16 mixed precision, bf16 non-mixed precision, fp16 non-mixed precision, fp8 mixed precision -->
#### Speeds, Sizes, Times [optional]
<!-- This section provides information about throughput, start/end time, checkpoint size if relevant, etc. -->
[More Information Needed]
## Evaluation
<!-- This section describes the evaluation protocols and provides the results. -->
### Testing Data, Factors & Metrics
#### Testing Data
<!-- This should link to a Dataset Card if possible. -->
[More Information Needed]
#### Factors
<!-- These are the things the evaluation is disaggregating by, e.g., subpopulations or domains. -->
[More Information Needed]
#### Metrics
<!-- These are the evaluation metrics being used, ideally with a description of why. -->
[More Information Needed]
### Results
[More Information Needed]
#### Summary
## Model Examination [optional]
<!-- Relevant interpretability work for the model goes here -->
[More Information Needed]
## Environmental Impact
<!-- Total emissions (in grams of CO2eq) and additional considerations, such as electricity usage, go here. Edit the suggested text below accordingly -->
Carbon emissions can be estimated using the [Machine Learning Impact calculator](https://mlco2.github.io/impact#compute) presented in [Lacoste et al. (2019)](https://arxiv.org/abs/1910.09700).
- **Hardware Type:** [More Information Needed]
- **Hours used:** [More Information Needed]
- **Cloud Provider:** [More Information Needed]
- **Compute Region:** [More Information Needed]
- **Carbon Emitted:** [More Information Needed]
## Technical Specifications [optional]
### Model Architecture and Objective
[More Information Needed]
### Compute Infrastructure
[More Information Needed]
#### Hardware
[More Information Needed]
#### Software
[More Information Needed]
## Citation [optional]
<!-- If there is a paper or blog post introducing the model, the APA and Bibtex information for that should go in this section. -->
**BibTeX:**
[More Information Needed]
**APA:**
[More Information Needed]
## Glossary [optional]
<!-- If relevant, include terms and calculations in this section that can help readers understand the model or model card. -->
[More Information Needed]
## More Information [optional]
[More Information Needed]
## Model Card Authors [optional]
[More Information Needed]
## Model Card Contact
[More Information Needed]
| {"library_name": "transformers", "tags": []} | miguel-kjh/pythia_1b-adpater-lora-cola | null | [
"transformers",
"safetensors",
"gpt_neox",
"text-generation",
"arxiv:1910.09700",
"autotrain_compatible",
"endpoints_compatible",
"text-generation-inference",
"region:us"
] | null | 2024-05-02T11:12:13+00:00 | [
"1910.09700"
] | [] | TAGS
#transformers #safetensors #gpt_neox #text-generation #arxiv-1910.09700 #autotrain_compatible #endpoints_compatible #text-generation-inference #region-us
|
# Model Card for Model ID
## Model Details
### Model Description
This is the model card of a transformers model that has been pushed on the Hub. This model card has been automatically generated.
- Developed by:
- Funded by [optional]:
- Shared by [optional]:
- Model type:
- Language(s) (NLP):
- License:
- Finetuned from model [optional]:
### Model Sources [optional]
- Repository:
- Paper [optional]:
- Demo [optional]:
## Uses
### Direct Use
### Downstream Use [optional]
### Out-of-Scope Use
## Bias, Risks, and Limitations
### Recommendations
Users (both direct and downstream) should be made aware of the risks, biases and limitations of the model. More information needed for further recommendations.
## How to Get Started with the Model
Use the code below to get started with the model.
## Training Details
### Training Data
### Training Procedure
#### Preprocessing [optional]
#### Training Hyperparameters
- Training regime:
#### Speeds, Sizes, Times [optional]
## Evaluation
### Testing Data, Factors & Metrics
#### Testing Data
#### Factors
#### Metrics
### Results
#### Summary
## Model Examination [optional]
## Environmental Impact
Carbon emissions can be estimated using the Machine Learning Impact calculator presented in Lacoste et al. (2019).
- Hardware Type:
- Hours used:
- Cloud Provider:
- Compute Region:
- Carbon Emitted:
## Technical Specifications [optional]
### Model Architecture and Objective
### Compute Infrastructure
#### Hardware
#### Software
[optional]
BibTeX:
APA:
## Glossary [optional]
## More Information [optional]
## Model Card Authors [optional]
## Model Card Contact
| [
"# Model Card for Model ID",
"## Model Details",
"### Model Description\n\n\n\nThis is the model card of a transformers model that has been pushed on the Hub. This model card has been automatically generated.\n\n- Developed by: \n- Funded by [optional]: \n- Shared by [optional]: \n- Model type: \n- Language(s) (NLP): \n- License: \n- Finetuned from model [optional]:",
"### Model Sources [optional]\n\n\n\n- Repository: \n- Paper [optional]: \n- Demo [optional]:",
"## Uses",
"### Direct Use",
"### Downstream Use [optional]",
"### Out-of-Scope Use",
"## Bias, Risks, and Limitations",
"### Recommendations\n\n\n\nUsers (both direct and downstream) should be made aware of the risks, biases and limitations of the model. More information needed for further recommendations.",
"## How to Get Started with the Model\n\nUse the code below to get started with the model.",
"## Training Details",
"### Training Data",
"### Training Procedure",
"#### Preprocessing [optional]",
"#### Training Hyperparameters\n\n- Training regime:",
"#### Speeds, Sizes, Times [optional]",
"## Evaluation",
"### Testing Data, Factors & Metrics",
"#### Testing Data",
"#### Factors",
"#### Metrics",
"### Results",
"#### Summary",
"## Model Examination [optional]",
"## Environmental Impact\n\n\n\nCarbon emissions can be estimated using the Machine Learning Impact calculator presented in Lacoste et al. (2019).\n\n- Hardware Type: \n- Hours used: \n- Cloud Provider: \n- Compute Region: \n- Carbon Emitted:",
"## Technical Specifications [optional]",
"### Model Architecture and Objective",
"### Compute Infrastructure",
"#### Hardware",
"#### Software\n\n\n\n[optional]\n\n\n\nBibTeX:\n\n\n\nAPA:",
"## Glossary [optional]",
"## More Information [optional]",
"## Model Card Authors [optional]",
"## Model Card Contact"
] | [
"TAGS\n#transformers #safetensors #gpt_neox #text-generation #arxiv-1910.09700 #autotrain_compatible #endpoints_compatible #text-generation-inference #region-us \n",
"# Model Card for Model ID",
"## Model Details",
"### Model Description\n\n\n\nThis is the model card of a transformers model that has been pushed on the Hub. This model card has been automatically generated.\n\n- Developed by: \n- Funded by [optional]: \n- Shared by [optional]: \n- Model type: \n- Language(s) (NLP): \n- License: \n- Finetuned from model [optional]:",
"### Model Sources [optional]\n\n\n\n- Repository: \n- Paper [optional]: \n- Demo [optional]:",
"## Uses",
"### Direct Use",
"### Downstream Use [optional]",
"### Out-of-Scope Use",
"## Bias, Risks, and Limitations",
"### Recommendations\n\n\n\nUsers (both direct and downstream) should be made aware of the risks, biases and limitations of the model. More information needed for further recommendations.",
"## How to Get Started with the Model\n\nUse the code below to get started with the model.",
"## Training Details",
"### Training Data",
"### Training Procedure",
"#### Preprocessing [optional]",
"#### Training Hyperparameters\n\n- Training regime:",
"#### Speeds, Sizes, Times [optional]",
"## Evaluation",
"### Testing Data, Factors & Metrics",
"#### Testing Data",
"#### Factors",
"#### Metrics",
"### Results",
"#### Summary",
"## Model Examination [optional]",
"## Environmental Impact\n\n\n\nCarbon emissions can be estimated using the Machine Learning Impact calculator presented in Lacoste et al. (2019).\n\n- Hardware Type: \n- Hours used: \n- Cloud Provider: \n- Compute Region: \n- Carbon Emitted:",
"## Technical Specifications [optional]",
"### Model Architecture and Objective",
"### Compute Infrastructure",
"#### Hardware",
"#### Software\n\n\n\n[optional]\n\n\n\nBibTeX:\n\n\n\nAPA:",
"## Glossary [optional]",
"## More Information [optional]",
"## Model Card Authors [optional]",
"## Model Card Contact"
] | [
47,
6,
4,
75,
23,
3,
5,
8,
9,
8,
34,
20,
4,
5,
5,
11,
13,
12,
3,
10,
6,
5,
6,
4,
5,
7,
49,
7,
7,
5,
5,
15,
7,
7,
8,
5
] | [
"TAGS\n#transformers #safetensors #gpt_neox #text-generation #arxiv-1910.09700 #autotrain_compatible #endpoints_compatible #text-generation-inference #region-us \n# Model Card for Model ID## Model Details### Model Description\n\n\n\nThis is the model card of a transformers model that has been pushed on the Hub. This model card has been automatically generated.\n\n- Developed by: \n- Funded by [optional]: \n- Shared by [optional]: \n- Model type: \n- Language(s) (NLP): \n- License: \n- Finetuned from model [optional]:### Model Sources [optional]\n\n\n\n- Repository: \n- Paper [optional]: \n- Demo [optional]:## Uses### Direct Use### Downstream Use [optional]### Out-of-Scope Use## Bias, Risks, and Limitations### Recommendations\n\n\n\nUsers (both direct and downstream) should be made aware of the risks, biases and limitations of the model. More information needed for further recommendations.## How to Get Started with the Model\n\nUse the code below to get started with the model.## Training Details### Training Data### Training Procedure#### Preprocessing [optional]#### Training Hyperparameters\n\n- Training regime:#### Speeds, Sizes, Times [optional]## Evaluation### Testing Data, Factors & Metrics#### Testing Data#### Factors#### Metrics### Results#### Summary## Model Examination [optional]## Environmental Impact\n\n\n\nCarbon emissions can be estimated using the Machine Learning Impact calculator presented in Lacoste et al. (2019).\n\n- Hardware Type: \n- Hours used: \n- Cloud Provider: \n- Compute Region: \n- Carbon Emitted:## Technical Specifications [optional]### Model Architecture and Objective### Compute Infrastructure#### Hardware#### Software\n\n\n\n[optional]\n\n\n\nBibTeX:\n\n\n\nAPA:## Glossary [optional]## More Information [optional]## Model Card Authors [optional]## Model Card Contact"
] |
null | transformers | ## About
<!-- ### quantize_version: 2 -->
<!-- ### output_tensor_quantised: 1 -->
<!-- ### convert_type: -->
<!-- ### vocab_type: -->
static quants of https://huggingface.co/Undi95/Llama3-Unholy-8B-OAS
<!-- provided-files -->
weighted/imatrix quants are available at https://huggingface.co/mradermacher/Llama3-Unholy-8B-OAS-i1-GGUF
## Usage
If you are unsure how to use GGUF files, refer to one of [TheBloke's
READMEs](https://huggingface.co/TheBloke/KafkaLM-70B-German-V0.1-GGUF) for
more details, including on how to concatenate multi-part files.
## Provided Quants
(sorted by size, not necessarily quality. IQ-quants are often preferable over similar sized non-IQ quants)
| Link | Type | Size/GB | Notes |
|:-----|:-----|--------:|:------|
| [GGUF](https://huggingface.co/mradermacher/Llama3-Unholy-8B-OAS-GGUF/resolve/main/Llama3-Unholy-8B-OAS.Q2_K.gguf) | Q2_K | 3.3 | |
| [GGUF](https://huggingface.co/mradermacher/Llama3-Unholy-8B-OAS-GGUF/resolve/main/Llama3-Unholy-8B-OAS.IQ3_XS.gguf) | IQ3_XS | 3.6 | |
| [GGUF](https://huggingface.co/mradermacher/Llama3-Unholy-8B-OAS-GGUF/resolve/main/Llama3-Unholy-8B-OAS.Q3_K_S.gguf) | Q3_K_S | 3.8 | |
| [GGUF](https://huggingface.co/mradermacher/Llama3-Unholy-8B-OAS-GGUF/resolve/main/Llama3-Unholy-8B-OAS.IQ3_S.gguf) | IQ3_S | 3.8 | beats Q3_K* |
| [GGUF](https://huggingface.co/mradermacher/Llama3-Unholy-8B-OAS-GGUF/resolve/main/Llama3-Unholy-8B-OAS.IQ3_M.gguf) | IQ3_M | 3.9 | |
| [GGUF](https://huggingface.co/mradermacher/Llama3-Unholy-8B-OAS-GGUF/resolve/main/Llama3-Unholy-8B-OAS.Q3_K_M.gguf) | Q3_K_M | 4.1 | lower quality |
| [GGUF](https://huggingface.co/mradermacher/Llama3-Unholy-8B-OAS-GGUF/resolve/main/Llama3-Unholy-8B-OAS.Q3_K_L.gguf) | Q3_K_L | 4.4 | |
| [GGUF](https://huggingface.co/mradermacher/Llama3-Unholy-8B-OAS-GGUF/resolve/main/Llama3-Unholy-8B-OAS.IQ4_XS.gguf) | IQ4_XS | 4.6 | |
| [GGUF](https://huggingface.co/mradermacher/Llama3-Unholy-8B-OAS-GGUF/resolve/main/Llama3-Unholy-8B-OAS.Q4_K_S.gguf) | Q4_K_S | 4.8 | fast, recommended |
| [GGUF](https://huggingface.co/mradermacher/Llama3-Unholy-8B-OAS-GGUF/resolve/main/Llama3-Unholy-8B-OAS.Q4_K_M.gguf) | Q4_K_M | 5.0 | fast, recommended |
| [GGUF](https://huggingface.co/mradermacher/Llama3-Unholy-8B-OAS-GGUF/resolve/main/Llama3-Unholy-8B-OAS.Q5_K_S.gguf) | Q5_K_S | 5.7 | |
| [GGUF](https://huggingface.co/mradermacher/Llama3-Unholy-8B-OAS-GGUF/resolve/main/Llama3-Unholy-8B-OAS.Q5_K_M.gguf) | Q5_K_M | 5.8 | |
| [GGUF](https://huggingface.co/mradermacher/Llama3-Unholy-8B-OAS-GGUF/resolve/main/Llama3-Unholy-8B-OAS.Q6_K.gguf) | Q6_K | 6.7 | very good quality |
| [GGUF](https://huggingface.co/mradermacher/Llama3-Unholy-8B-OAS-GGUF/resolve/main/Llama3-Unholy-8B-OAS.Q8_0.gguf) | Q8_0 | 8.6 | fast, best quality |
| [GGUF](https://huggingface.co/mradermacher/Llama3-Unholy-8B-OAS-GGUF/resolve/main/Llama3-Unholy-8B-OAS.f16.gguf) | f16 | 16.2 | 16 bpw, overkill |
Here is a handy graph by ikawrakow comparing some lower-quality quant
types (lower is better):

And here are Artefact2's thoughts on the matter:
https://gist.github.com/Artefact2/b5f810600771265fc1e39442288e8ec9
## FAQ / Model Request
See https://huggingface.co/mradermacher/model_requests for some answers to
questions you might have and/or if you want some other model quantized.
## Thanks
I thank my company, [nethype GmbH](https://www.nethype.de/), for letting
me use its servers and providing upgrades to my workstation to enable
this work in my free time.
<!-- end -->
| {"language": ["en"], "license": "cc-by-nc-4.0", "library_name": "transformers", "tags": ["not-for-all-audiences", "nsfw"], "base_model": "Undi95/Llama3-Unholy-8B-OAS", "quantized_by": "mradermacher"} | mradermacher/Llama3-Unholy-8B-OAS-GGUF | null | [
"transformers",
"gguf",
"not-for-all-audiences",
"nsfw",
"en",
"base_model:Undi95/Llama3-Unholy-8B-OAS",
"license:cc-by-nc-4.0",
"endpoints_compatible",
"region:us"
] | null | 2024-05-02T11:13:34+00:00 | [] | [
"en"
] | TAGS
#transformers #gguf #not-for-all-audiences #nsfw #en #base_model-Undi95/Llama3-Unholy-8B-OAS #license-cc-by-nc-4.0 #endpoints_compatible #region-us
| About
-----
static quants of URL
weighted/imatrix quants are available at URL
Usage
-----
If you are unsure how to use GGUF files, refer to one of TheBloke's
READMEs for
more details, including on how to concatenate multi-part files.
Provided Quants
---------------
(sorted by size, not necessarily quality. IQ-quants are often preferable over similar sized non-IQ quants)
Here is a handy graph by ikawrakow comparing some lower-quality quant
types (lower is better):
!URL
And here are Artefact2's thoughts on the matter:
URL
FAQ / Model Request
-------------------
See URL for some answers to
questions you might have and/or if you want some other model quantized.
Thanks
------
I thank my company, nethype GmbH, for letting
me use its servers and providing upgrades to my workstation to enable
this work in my free time.
| [] | [
"TAGS\n#transformers #gguf #not-for-all-audiences #nsfw #en #base_model-Undi95/Llama3-Unholy-8B-OAS #license-cc-by-nc-4.0 #endpoints_compatible #region-us \n"
] | [
65
] | [
"TAGS\n#transformers #gguf #not-for-all-audiences #nsfw #en #base_model-Undi95/Llama3-Unholy-8B-OAS #license-cc-by-nc-4.0 #endpoints_compatible #region-us \n"
] |
token-classification | transformers |
<!-- This model card has been generated automatically according to the information the Trainer had access to. You
should probably proofread and complete it, then remove this comment. -->
# CNEC2_0_extended_xlm-roberta-large
This model is a fine-tuned version of [FacebookAI/xlm-roberta-large](https://huggingface.co/FacebookAI/xlm-roberta-large) on the cnec dataset.
It achieves the following results on the evaluation set:
- Loss: 0.1464
- Precision: 0.8721
- Recall: 0.9002
- F1: 0.8860
- Accuracy: 0.9766
## Model description
More information needed
## Intended uses & limitations
More information needed
## Training and evaluation data
More information needed
## Training procedure
### Training hyperparameters
The following hyperparameters were used during training:
- learning_rate: 2e-05
- train_batch_size: 8
- eval_batch_size: 8
- seed: 42
- optimizer: Adam with betas=(0.9,0.999) and epsilon=1e-08
- lr_scheduler_type: linear
- num_epochs: 12
### Training results
| Training Loss | Epoch | Step | Validation Loss | Precision | Recall | F1 | Accuracy |
|:-------------:|:-----:|:-----:|:---------------:|:---------:|:------:|:------:|:--------:|
| 0.2193 | 1.12 | 1000 | 0.1389 | 0.7636 | 0.8194 | 0.7905 | 0.9628 |
| 0.1528 | 2.24 | 2000 | 0.1285 | 0.8106 | 0.8600 | 0.8346 | 0.9678 |
| 0.119 | 3.36 | 3000 | 0.1278 | 0.8234 | 0.8491 | 0.8361 | 0.9679 |
| 0.0904 | 4.48 | 4000 | 0.1104 | 0.8466 | 0.8680 | 0.8571 | 0.9747 |
| 0.0768 | 5.6 | 5000 | 0.1269 | 0.8486 | 0.8819 | 0.8649 | 0.9734 |
| 0.0709 | 6.72 | 6000 | 0.1293 | 0.8601 | 0.8878 | 0.8737 | 0.9759 |
| 0.0586 | 7.84 | 7000 | 0.1404 | 0.8624 | 0.8928 | 0.8773 | 0.9753 |
| 0.0486 | 8.96 | 8000 | 0.1445 | 0.8675 | 0.9002 | 0.8836 | 0.9766 |
| 0.0488 | 10.08 | 9000 | 0.1467 | 0.8608 | 0.8963 | 0.8782 | 0.9753 |
| 0.035 | 11.2 | 10000 | 0.1464 | 0.8721 | 0.9002 | 0.8860 | 0.9766 |
### Framework versions
- Transformers 4.36.2
- Pytorch 2.1.2+cu121
- Datasets 2.16.1
- Tokenizers 0.15.0
| {"license": "mit", "tags": ["generated_from_trainer"], "datasets": ["cnec"], "metrics": ["precision", "recall", "f1", "accuracy"], "base_model": "FacebookAI/xlm-roberta-large", "model-index": [{"name": "CNEC2_0_extended_xlm-roberta-large", "results": [{"task": {"type": "token-classification", "name": "Token Classification"}, "dataset": {"name": "cnec", "type": "cnec", "config": "default", "split": "validation", "args": "default"}, "metrics": [{"type": "precision", "value": 0.8721153846153846, "name": "Precision"}, {"type": "recall", "value": 0.9002481389578164, "name": "Recall"}, {"type": "f1", "value": 0.8859584859584859, "name": "F1"}, {"type": "accuracy", "value": 0.9766100702576113, "name": "Accuracy"}]}]}]} | stulcrad/CNEC2_0_extended_xlm-roberta-large | null | [
"transformers",
"safetensors",
"xlm-roberta",
"token-classification",
"generated_from_trainer",
"dataset:cnec",
"base_model:FacebookAI/xlm-roberta-large",
"license:mit",
"model-index",
"autotrain_compatible",
"endpoints_compatible",
"region:us"
] | null | 2024-05-02T11:13:57+00:00 | [] | [] | TAGS
#transformers #safetensors #xlm-roberta #token-classification #generated_from_trainer #dataset-cnec #base_model-FacebookAI/xlm-roberta-large #license-mit #model-index #autotrain_compatible #endpoints_compatible #region-us
| CNEC2\_0\_extended\_xlm-roberta-large
=====================================
This model is a fine-tuned version of FacebookAI/xlm-roberta-large on the cnec dataset.
It achieves the following results on the evaluation set:
* Loss: 0.1464
* Precision: 0.8721
* Recall: 0.9002
* F1: 0.8860
* Accuracy: 0.9766
Model description
-----------------
More information needed
Intended uses & limitations
---------------------------
More information needed
Training and evaluation data
----------------------------
More information needed
Training procedure
------------------
### Training hyperparameters
The following hyperparameters were used during training:
* learning\_rate: 2e-05
* train\_batch\_size: 8
* eval\_batch\_size: 8
* seed: 42
* optimizer: Adam with betas=(0.9,0.999) and epsilon=1e-08
* lr\_scheduler\_type: linear
* num\_epochs: 12
### Training results
### Framework versions
* Transformers 4.36.2
* Pytorch 2.1.2+cu121
* Datasets 2.16.1
* Tokenizers 0.15.0
| [
"### Training hyperparameters\n\n\nThe following hyperparameters were used during training:\n\n\n* learning\\_rate: 2e-05\n* train\\_batch\\_size: 8\n* eval\\_batch\\_size: 8\n* seed: 42\n* optimizer: Adam with betas=(0.9,0.999) and epsilon=1e-08\n* lr\\_scheduler\\_type: linear\n* num\\_epochs: 12",
"### Training results",
"### Framework versions\n\n\n* Transformers 4.36.2\n* Pytorch 2.1.2+cu121\n* Datasets 2.16.1\n* Tokenizers 0.15.0"
] | [
"TAGS\n#transformers #safetensors #xlm-roberta #token-classification #generated_from_trainer #dataset-cnec #base_model-FacebookAI/xlm-roberta-large #license-mit #model-index #autotrain_compatible #endpoints_compatible #region-us \n",
"### Training hyperparameters\n\n\nThe following hyperparameters were used during training:\n\n\n* learning\\_rate: 2e-05\n* train\\_batch\\_size: 8\n* eval\\_batch\\_size: 8\n* seed: 42\n* optimizer: Adam with betas=(0.9,0.999) and epsilon=1e-08\n* lr\\_scheduler\\_type: linear\n* num\\_epochs: 12",
"### Training results",
"### Framework versions\n\n\n* Transformers 4.36.2\n* Pytorch 2.1.2+cu121\n* Datasets 2.16.1\n* Tokenizers 0.15.0"
] | [
64,
101,
5,
44
] | [
"TAGS\n#transformers #safetensors #xlm-roberta #token-classification #generated_from_trainer #dataset-cnec #base_model-FacebookAI/xlm-roberta-large #license-mit #model-index #autotrain_compatible #endpoints_compatible #region-us \n### Training hyperparameters\n\n\nThe following hyperparameters were used during training:\n\n\n* learning\\_rate: 2e-05\n* train\\_batch\\_size: 8\n* eval\\_batch\\_size: 8\n* seed: 42\n* optimizer: Adam with betas=(0.9,0.999) and epsilon=1e-08\n* lr\\_scheduler\\_type: linear\n* num\\_epochs: 12### Training results### Framework versions\n\n\n* Transformers 4.36.2\n* Pytorch 2.1.2+cu121\n* Datasets 2.16.1\n* Tokenizers 0.15.0"
] |
text2text-generation | transformers |
# Model Card for Model ID
<!-- Provide a quick summary of what the model is/does. -->
## Model Details
### Model Description
<!-- Provide a longer summary of what this model is. -->
This is the model card of a 🤗 transformers model that has been pushed on the Hub. This model card has been automatically generated.
- **Developed by:** [More Information Needed]
- **Funded by [optional]:** [More Information Needed]
- **Shared by [optional]:** [More Information Needed]
- **Model type:** [More Information Needed]
- **Language(s) (NLP):** [More Information Needed]
- **License:** [More Information Needed]
- **Finetuned from model [optional]:** [More Information Needed]
### Model Sources [optional]
<!-- Provide the basic links for the model. -->
- **Repository:** [More Information Needed]
- **Paper [optional]:** [More Information Needed]
- **Demo [optional]:** [More Information Needed]
## Uses
<!-- Address questions around how the model is intended to be used, including the foreseeable users of the model and those affected by the model. -->
### Direct Use
<!-- This section is for the model use without fine-tuning or plugging into a larger ecosystem/app. -->
[More Information Needed]
### Downstream Use [optional]
<!-- This section is for the model use when fine-tuned for a task, or when plugged into a larger ecosystem/app -->
[More Information Needed]
### Out-of-Scope Use
<!-- This section addresses misuse, malicious use, and uses that the model will not work well for. -->
[More Information Needed]
## Bias, Risks, and Limitations
<!-- This section is meant to convey both technical and sociotechnical limitations. -->
[More Information Needed]
### Recommendations
<!-- This section is meant to convey recommendations with respect to the bias, risk, and technical limitations. -->
Users (both direct and downstream) should be made aware of the risks, biases and limitations of the model. More information needed for further recommendations.
## How to Get Started with the Model
Use the code below to get started with the model.
[More Information Needed]
## Training Details
### Training Data
<!-- This should link to a Dataset Card, perhaps with a short stub of information on what the training data is all about as well as documentation related to data pre-processing or additional filtering. -->
[More Information Needed]
### Training Procedure
<!-- This relates heavily to the Technical Specifications. Content here should link to that section when it is relevant to the training procedure. -->
#### Preprocessing [optional]
[More Information Needed]
#### Training Hyperparameters
- **Training regime:** [More Information Needed] <!--fp32, fp16 mixed precision, bf16 mixed precision, bf16 non-mixed precision, fp16 non-mixed precision, fp8 mixed precision -->
#### Speeds, Sizes, Times [optional]
<!-- This section provides information about throughput, start/end time, checkpoint size if relevant, etc. -->
[More Information Needed]
## Evaluation
<!-- This section describes the evaluation protocols and provides the results. -->
### Testing Data, Factors & Metrics
#### Testing Data
<!-- This should link to a Dataset Card if possible. -->
[More Information Needed]
#### Factors
<!-- These are the things the evaluation is disaggregating by, e.g., subpopulations or domains. -->
[More Information Needed]
#### Metrics
<!-- These are the evaluation metrics being used, ideally with a description of why. -->
[More Information Needed]
### Results
[More Information Needed]
#### Summary
## Model Examination [optional]
<!-- Relevant interpretability work for the model goes here -->
[More Information Needed]
## Environmental Impact
<!-- Total emissions (in grams of CO2eq) and additional considerations, such as electricity usage, go here. Edit the suggested text below accordingly -->
Carbon emissions can be estimated using the [Machine Learning Impact calculator](https://mlco2.github.io/impact#compute) presented in [Lacoste et al. (2019)](https://arxiv.org/abs/1910.09700).
- **Hardware Type:** [More Information Needed]
- **Hours used:** [More Information Needed]
- **Cloud Provider:** [More Information Needed]
- **Compute Region:** [More Information Needed]
- **Carbon Emitted:** [More Information Needed]
## Technical Specifications [optional]
### Model Architecture and Objective
[More Information Needed]
### Compute Infrastructure
[More Information Needed]
#### Hardware
[More Information Needed]
#### Software
[More Information Needed]
## Citation [optional]
<!-- If there is a paper or blog post introducing the model, the APA and Bibtex information for that should go in this section. -->
**BibTeX:**
[More Information Needed]
**APA:**
[More Information Needed]
## Glossary [optional]
<!-- If relevant, include terms and calculations in this section that can help readers understand the model or model card. -->
[More Information Needed]
## More Information [optional]
[More Information Needed]
## Model Card Authors [optional]
[More Information Needed]
## Model Card Contact
[More Information Needed]
| {"library_name": "transformers", "tags": []} | automated-finetunning/bart_mohit_101 | null | [
"transformers",
"safetensors",
"bart",
"text2text-generation",
"arxiv:1910.09700",
"autotrain_compatible",
"endpoints_compatible",
"region:us"
] | null | 2024-05-02T11:14:58+00:00 | [
"1910.09700"
] | [] | TAGS
#transformers #safetensors #bart #text2text-generation #arxiv-1910.09700 #autotrain_compatible #endpoints_compatible #region-us
|
# Model Card for Model ID
## Model Details
### Model Description
This is the model card of a transformers model that has been pushed on the Hub. This model card has been automatically generated.
- Developed by:
- Funded by [optional]:
- Shared by [optional]:
- Model type:
- Language(s) (NLP):
- License:
- Finetuned from model [optional]:
### Model Sources [optional]
- Repository:
- Paper [optional]:
- Demo [optional]:
## Uses
### Direct Use
### Downstream Use [optional]
### Out-of-Scope Use
## Bias, Risks, and Limitations
### Recommendations
Users (both direct and downstream) should be made aware of the risks, biases and limitations of the model. More information needed for further recommendations.
## How to Get Started with the Model
Use the code below to get started with the model.
## Training Details
### Training Data
### Training Procedure
#### Preprocessing [optional]
#### Training Hyperparameters
- Training regime:
#### Speeds, Sizes, Times [optional]
## Evaluation
### Testing Data, Factors & Metrics
#### Testing Data
#### Factors
#### Metrics
### Results
#### Summary
## Model Examination [optional]
## Environmental Impact
Carbon emissions can be estimated using the Machine Learning Impact calculator presented in Lacoste et al. (2019).
- Hardware Type:
- Hours used:
- Cloud Provider:
- Compute Region:
- Carbon Emitted:
## Technical Specifications [optional]
### Model Architecture and Objective
### Compute Infrastructure
#### Hardware
#### Software
[optional]
BibTeX:
APA:
## Glossary [optional]
## More Information [optional]
## Model Card Authors [optional]
## Model Card Contact
| [
"# Model Card for Model ID",
"## Model Details",
"### Model Description\n\n\n\nThis is the model card of a transformers model that has been pushed on the Hub. This model card has been automatically generated.\n\n- Developed by: \n- Funded by [optional]: \n- Shared by [optional]: \n- Model type: \n- Language(s) (NLP): \n- License: \n- Finetuned from model [optional]:",
"### Model Sources [optional]\n\n\n\n- Repository: \n- Paper [optional]: \n- Demo [optional]:",
"## Uses",
"### Direct Use",
"### Downstream Use [optional]",
"### Out-of-Scope Use",
"## Bias, Risks, and Limitations",
"### Recommendations\n\n\n\nUsers (both direct and downstream) should be made aware of the risks, biases and limitations of the model. More information needed for further recommendations.",
"## How to Get Started with the Model\n\nUse the code below to get started with the model.",
"## Training Details",
"### Training Data",
"### Training Procedure",
"#### Preprocessing [optional]",
"#### Training Hyperparameters\n\n- Training regime:",
"#### Speeds, Sizes, Times [optional]",
"## Evaluation",
"### Testing Data, Factors & Metrics",
"#### Testing Data",
"#### Factors",
"#### Metrics",
"### Results",
"#### Summary",
"## Model Examination [optional]",
"## Environmental Impact\n\n\n\nCarbon emissions can be estimated using the Machine Learning Impact calculator presented in Lacoste et al. (2019).\n\n- Hardware Type: \n- Hours used: \n- Cloud Provider: \n- Compute Region: \n- Carbon Emitted:",
"## Technical Specifications [optional]",
"### Model Architecture and Objective",
"### Compute Infrastructure",
"#### Hardware",
"#### Software\n\n\n\n[optional]\n\n\n\nBibTeX:\n\n\n\nAPA:",
"## Glossary [optional]",
"## More Information [optional]",
"## Model Card Authors [optional]",
"## Model Card Contact"
] | [
"TAGS\n#transformers #safetensors #bart #text2text-generation #arxiv-1910.09700 #autotrain_compatible #endpoints_compatible #region-us \n",
"# Model Card for Model ID",
"## Model Details",
"### Model Description\n\n\n\nThis is the model card of a transformers model that has been pushed on the Hub. This model card has been automatically generated.\n\n- Developed by: \n- Funded by [optional]: \n- Shared by [optional]: \n- Model type: \n- Language(s) (NLP): \n- License: \n- Finetuned from model [optional]:",
"### Model Sources [optional]\n\n\n\n- Repository: \n- Paper [optional]: \n- Demo [optional]:",
"## Uses",
"### Direct Use",
"### Downstream Use [optional]",
"### Out-of-Scope Use",
"## Bias, Risks, and Limitations",
"### Recommendations\n\n\n\nUsers (both direct and downstream) should be made aware of the risks, biases and limitations of the model. More information needed for further recommendations.",
"## How to Get Started with the Model\n\nUse the code below to get started with the model.",
"## Training Details",
"### Training Data",
"### Training Procedure",
"#### Preprocessing [optional]",
"#### Training Hyperparameters\n\n- Training regime:",
"#### Speeds, Sizes, Times [optional]",
"## Evaluation",
"### Testing Data, Factors & Metrics",
"#### Testing Data",
"#### Factors",
"#### Metrics",
"### Results",
"#### Summary",
"## Model Examination [optional]",
"## Environmental Impact\n\n\n\nCarbon emissions can be estimated using the Machine Learning Impact calculator presented in Lacoste et al. (2019).\n\n- Hardware Type: \n- Hours used: \n- Cloud Provider: \n- Compute Region: \n- Carbon Emitted:",
"## Technical Specifications [optional]",
"### Model Architecture and Objective",
"### Compute Infrastructure",
"#### Hardware",
"#### Software\n\n\n\n[optional]\n\n\n\nBibTeX:\n\n\n\nAPA:",
"## Glossary [optional]",
"## More Information [optional]",
"## Model Card Authors [optional]",
"## Model Card Contact"
] | [
39,
6,
4,
75,
23,
3,
5,
8,
9,
8,
34,
20,
4,
5,
5,
11,
13,
12,
3,
10,
6,
5,
6,
4,
5,
7,
49,
7,
7,
5,
5,
15,
7,
7,
8,
5
] | [
"TAGS\n#transformers #safetensors #bart #text2text-generation #arxiv-1910.09700 #autotrain_compatible #endpoints_compatible #region-us \n# Model Card for Model ID## Model Details### Model Description\n\n\n\nThis is the model card of a transformers model that has been pushed on the Hub. This model card has been automatically generated.\n\n- Developed by: \n- Funded by [optional]: \n- Shared by [optional]: \n- Model type: \n- Language(s) (NLP): \n- License: \n- Finetuned from model [optional]:### Model Sources [optional]\n\n\n\n- Repository: \n- Paper [optional]: \n- Demo [optional]:## Uses### Direct Use### Downstream Use [optional]### Out-of-Scope Use## Bias, Risks, and Limitations### Recommendations\n\n\n\nUsers (both direct and downstream) should be made aware of the risks, biases and limitations of the model. More information needed for further recommendations.## How to Get Started with the Model\n\nUse the code below to get started with the model.## Training Details### Training Data### Training Procedure#### Preprocessing [optional]#### Training Hyperparameters\n\n- Training regime:#### Speeds, Sizes, Times [optional]## Evaluation### Testing Data, Factors & Metrics#### Testing Data#### Factors#### Metrics### Results#### Summary## Model Examination [optional]## Environmental Impact\n\n\n\nCarbon emissions can be estimated using the Machine Learning Impact calculator presented in Lacoste et al. (2019).\n\n- Hardware Type: \n- Hours used: \n- Cloud Provider: \n- Compute Region: \n- Carbon Emitted:## Technical Specifications [optional]### Model Architecture and Objective### Compute Infrastructure#### Hardware#### Software\n\n\n\n[optional]\n\n\n\nBibTeX:\n\n\n\nAPA:## Glossary [optional]## More Information [optional]## Model Card Authors [optional]## Model Card Contact"
] |
text-classification | peft |
<!-- This model card has been generated automatically according to the information the Trainer had access to. You
should probably proofread and complete it, then remove this comment. -->
# mistral7b-martinmatrix-finetune
This model is a fine-tuned version of [mistralai/Mistral-7B-v0.1](https://huggingface.co/mistralai/Mistral-7B-v0.1) on an unknown dataset.
It achieves the following results on the evaluation set:
- Loss: 0.0002
## Model description
More information needed
## Intended uses & limitations
More information needed
## Training and evaluation data
More information needed
## Training procedure
### Training hyperparameters
The following hyperparameters were used during training:
- learning_rate: 2.5e-05
- train_batch_size: 2
- eval_batch_size: 8
- seed: 42
- optimizer: Adam with betas=(0.9,0.999) and epsilon=1e-08
- lr_scheduler_type: linear
- lr_scheduler_warmup_steps: 1
- training_steps: 120
### Training results
| Training Loss | Epoch | Step | Validation Loss |
|:-------------:|:------:|:----:|:---------------:|
| 0.8049 | 0.2155 | 25 | 0.0073 |
| 0.0045 | 0.4310 | 50 | 0.0018 |
| 0.0011 | 0.6466 | 75 | 0.0004 |
| 0.0004 | 0.8621 | 100 | 0.0002 |
### Framework versions
- PEFT 0.10.1.dev0
- Transformers 4.41.0.dev0
- Pytorch 2.2.1+cu121
- Datasets 2.19.0
- Tokenizers 0.19.1 | {"license": "apache-2.0", "library_name": "peft", "tags": ["generated_from_trainer"], "base_model": "mistralai/Mistral-7B-v0.1", "pipeline_tag": "text-classification", "model-index": [{"name": "mistral7b-martinmatrix-finetune", "results": []}]} | dendimaki/mistral-checkpoints | null | [
"peft",
"safetensors",
"generated_from_trainer",
"text-classification",
"base_model:mistralai/Mistral-7B-v0.1",
"license:apache-2.0",
"region:us"
] | null | 2024-05-02T11:15:22+00:00 | [] | [] | TAGS
#peft #safetensors #generated_from_trainer #text-classification #base_model-mistralai/Mistral-7B-v0.1 #license-apache-2.0 #region-us
| mistral7b-martinmatrix-finetune
===============================
This model is a fine-tuned version of mistralai/Mistral-7B-v0.1 on an unknown dataset.
It achieves the following results on the evaluation set:
* Loss: 0.0002
Model description
-----------------
More information needed
Intended uses & limitations
---------------------------
More information needed
Training and evaluation data
----------------------------
More information needed
Training procedure
------------------
### Training hyperparameters
The following hyperparameters were used during training:
* learning\_rate: 2.5e-05
* train\_batch\_size: 2
* eval\_batch\_size: 8
* seed: 42
* optimizer: Adam with betas=(0.9,0.999) and epsilon=1e-08
* lr\_scheduler\_type: linear
* lr\_scheduler\_warmup\_steps: 1
* training\_steps: 120
### Training results
### Framework versions
* PEFT 0.10.1.dev0
* Transformers 4.41.0.dev0
* Pytorch 2.2.1+cu121
* Datasets 2.19.0
* Tokenizers 0.19.1
| [
"### Training hyperparameters\n\n\nThe following hyperparameters were used during training:\n\n\n* learning\\_rate: 2.5e-05\n* train\\_batch\\_size: 2\n* eval\\_batch\\_size: 8\n* seed: 42\n* optimizer: Adam with betas=(0.9,0.999) and epsilon=1e-08\n* lr\\_scheduler\\_type: linear\n* lr\\_scheduler\\_warmup\\_steps: 1\n* training\\_steps: 120",
"### Training results",
"### Framework versions\n\n\n* PEFT 0.10.1.dev0\n* Transformers 4.41.0.dev0\n* Pytorch 2.2.1+cu121\n* Datasets 2.19.0\n* Tokenizers 0.19.1"
] | [
"TAGS\n#peft #safetensors #generated_from_trainer #text-classification #base_model-mistralai/Mistral-7B-v0.1 #license-apache-2.0 #region-us \n",
"### Training hyperparameters\n\n\nThe following hyperparameters were used during training:\n\n\n* learning\\_rate: 2.5e-05\n* train\\_batch\\_size: 2\n* eval\\_batch\\_size: 8\n* seed: 42\n* optimizer: Adam with betas=(0.9,0.999) and epsilon=1e-08\n* lr\\_scheduler\\_type: linear\n* lr\\_scheduler\\_warmup\\_steps: 1\n* training\\_steps: 120",
"### Training results",
"### Framework versions\n\n\n* PEFT 0.10.1.dev0\n* Transformers 4.41.0.dev0\n* Pytorch 2.2.1+cu121\n* Datasets 2.19.0\n* Tokenizers 0.19.1"
] | [
49,
117,
5,
58
] | [
"TAGS\n#peft #safetensors #generated_from_trainer #text-classification #base_model-mistralai/Mistral-7B-v0.1 #license-apache-2.0 #region-us \n### Training hyperparameters\n\n\nThe following hyperparameters were used during training:\n\n\n* learning\\_rate: 2.5e-05\n* train\\_batch\\_size: 2\n* eval\\_batch\\_size: 8\n* seed: 42\n* optimizer: Adam with betas=(0.9,0.999) and epsilon=1e-08\n* lr\\_scheduler\\_type: linear\n* lr\\_scheduler\\_warmup\\_steps: 1\n* training\\_steps: 120### Training results### Framework versions\n\n\n* PEFT 0.10.1.dev0\n* Transformers 4.41.0.dev0\n* Pytorch 2.2.1+cu121\n* Datasets 2.19.0\n* Tokenizers 0.19.1"
] |
null | transformers | ## About
<!-- ### quantize_version: 2 -->
<!-- ### output_tensor_quantised: 1 -->
<!-- ### convert_type: -->
<!-- ### vocab_type: -->
static quants of https://huggingface.co/lancartech/LancarAI-v1.0
<!-- provided-files -->
weighted/imatrix quants seem not to be available (by me) at this time. If they do not show up a week or so after the static ones, I have probably not planned for them. Feel free to request them by opening a Community Discussion.
## Usage
If you are unsure how to use GGUF files, refer to one of [TheBloke's
READMEs](https://huggingface.co/TheBloke/KafkaLM-70B-German-V0.1-GGUF) for
more details, including on how to concatenate multi-part files.
## Provided Quants
(sorted by size, not necessarily quality. IQ-quants are often preferable over similar sized non-IQ quants)
| Link | Type | Size/GB | Notes |
|:-----|:-----|--------:|:------|
| [GGUF](https://huggingface.co/mradermacher/LancarAI-v1.0-GGUF/resolve/main/LancarAI-v1.0.Q2_K.gguf) | Q2_K | 2.8 | |
| [GGUF](https://huggingface.co/mradermacher/LancarAI-v1.0-GGUF/resolve/main/LancarAI-v1.0.IQ3_XS.gguf) | IQ3_XS | 3.1 | |
| [GGUF](https://huggingface.co/mradermacher/LancarAI-v1.0-GGUF/resolve/main/LancarAI-v1.0.Q3_K_S.gguf) | Q3_K_S | 3.3 | |
| [GGUF](https://huggingface.co/mradermacher/LancarAI-v1.0-GGUF/resolve/main/LancarAI-v1.0.IQ3_S.gguf) | IQ3_S | 3.3 | beats Q3_K* |
| [GGUF](https://huggingface.co/mradermacher/LancarAI-v1.0-GGUF/resolve/main/LancarAI-v1.0.IQ3_M.gguf) | IQ3_M | 3.4 | |
| [GGUF](https://huggingface.co/mradermacher/LancarAI-v1.0-GGUF/resolve/main/LancarAI-v1.0.Q3_K_M.gguf) | Q3_K_M | 3.6 | lower quality |
| [GGUF](https://huggingface.co/mradermacher/LancarAI-v1.0-GGUF/resolve/main/LancarAI-v1.0.Q3_K_L.gguf) | Q3_K_L | 3.9 | |
| [GGUF](https://huggingface.co/mradermacher/LancarAI-v1.0-GGUF/resolve/main/LancarAI-v1.0.IQ4_XS.gguf) | IQ4_XS | 4.0 | |
| [GGUF](https://huggingface.co/mradermacher/LancarAI-v1.0-GGUF/resolve/main/LancarAI-v1.0.Q4_K_S.gguf) | Q4_K_S | 4.2 | fast, recommended |
| [GGUF](https://huggingface.co/mradermacher/LancarAI-v1.0-GGUF/resolve/main/LancarAI-v1.0.Q4_K_M.gguf) | Q4_K_M | 4.5 | fast, recommended |
| [GGUF](https://huggingface.co/mradermacher/LancarAI-v1.0-GGUF/resolve/main/LancarAI-v1.0.Q5_K_S.gguf) | Q5_K_S | 5.1 | |
| [GGUF](https://huggingface.co/mradermacher/LancarAI-v1.0-GGUF/resolve/main/LancarAI-v1.0.Q5_K_M.gguf) | Q5_K_M | 5.2 | |
| [GGUF](https://huggingface.co/mradermacher/LancarAI-v1.0-GGUF/resolve/main/LancarAI-v1.0.Q6_K.gguf) | Q6_K | 6.0 | very good quality |
| [GGUF](https://huggingface.co/mradermacher/LancarAI-v1.0-GGUF/resolve/main/LancarAI-v1.0.Q8_0.gguf) | Q8_0 | 7.8 | fast, best quality |
| [GGUF](https://huggingface.co/mradermacher/LancarAI-v1.0-GGUF/resolve/main/LancarAI-v1.0.f16.gguf) | f16 | 14.6 | 16 bpw, overkill |
Here is a handy graph by ikawrakow comparing some lower-quality quant
types (lower is better):

And here are Artefact2's thoughts on the matter:
https://gist.github.com/Artefact2/b5f810600771265fc1e39442288e8ec9
## FAQ / Model Request
See https://huggingface.co/mradermacher/model_requests for some answers to
questions you might have and/or if you want some other model quantized.
## Thanks
I thank my company, [nethype GmbH](https://www.nethype.de/), for letting
me use its servers and providing upgrades to my workstation to enable
this work in my free time.
<!-- end -->
| {"language": ["en"], "library_name": "transformers", "tags": ["merge", "mergekit", "lazymergekit", "OpenPipe/mistral-ft-optimized-1218", "mlabonne/NeuralHermes-2.5-Mistral-7B"], "base_model": "lancartech/LancarAI-v1.0", "quantized_by": "mradermacher"} | mradermacher/LancarAI-v1.0-GGUF | null | [
"transformers",
"gguf",
"merge",
"mergekit",
"lazymergekit",
"OpenPipe/mistral-ft-optimized-1218",
"mlabonne/NeuralHermes-2.5-Mistral-7B",
"en",
"base_model:lancartech/LancarAI-v1.0",
"endpoints_compatible",
"region:us"
] | null | 2024-05-02T11:16:02+00:00 | [] | [
"en"
] | TAGS
#transformers #gguf #merge #mergekit #lazymergekit #OpenPipe/mistral-ft-optimized-1218 #mlabonne/NeuralHermes-2.5-Mistral-7B #en #base_model-lancartech/LancarAI-v1.0 #endpoints_compatible #region-us
| About
-----
static quants of URL
weighted/imatrix quants seem not to be available (by me) at this time. If they do not show up a week or so after the static ones, I have probably not planned for them. Feel free to request them by opening a Community Discussion.
Usage
-----
If you are unsure how to use GGUF files, refer to one of TheBloke's
READMEs for
more details, including on how to concatenate multi-part files.
Provided Quants
---------------
(sorted by size, not necessarily quality. IQ-quants are often preferable over similar sized non-IQ quants)
Here is a handy graph by ikawrakow comparing some lower-quality quant
types (lower is better):
!URL
And here are Artefact2's thoughts on the matter:
URL
FAQ / Model Request
-------------------
See URL for some answers to
questions you might have and/or if you want some other model quantized.
Thanks
------
I thank my company, nethype GmbH, for letting
me use its servers and providing upgrades to my workstation to enable
this work in my free time.
| [] | [
"TAGS\n#transformers #gguf #merge #mergekit #lazymergekit #OpenPipe/mistral-ft-optimized-1218 #mlabonne/NeuralHermes-2.5-Mistral-7B #en #base_model-lancartech/LancarAI-v1.0 #endpoints_compatible #region-us \n"
] | [
78
] | [
"TAGS\n#transformers #gguf #merge #mergekit #lazymergekit #OpenPipe/mistral-ft-optimized-1218 #mlabonne/NeuralHermes-2.5-Mistral-7B #en #base_model-lancartech/LancarAI-v1.0 #endpoints_compatible #region-us \n"
] |
text-generation | null |
## Llamacpp imatrix Quantizations of Meta-Llama-3-70B-Instruct
Using <a href="https://github.com/ggerganov/llama.cpp/">llama.cpp</a> release <a href="https://github.com/ggerganov/llama.cpp/releases/tag/b2777">b2777</a> for quantization.
Original model: https://huggingface.co/meta-llama/Meta-Llama-3-70B-Instruct
All quants made using imatrix option with dataset provided by Kalomaze [here](https://github.com/ggerganov/llama.cpp/discussions/5263#discussioncomment-8395384)
## Prompt format
```
<|begin_of_text|><|start_header_id|>system<|end_header_id|>
{system_prompt}<|eot_id|><|start_header_id|>user<|end_header_id|>
{prompt}<|eot_id|><|start_header_id|>assistant<|end_header_id|>
```
## Download a file (not the whole branch) from below:
| Filename | Quant type | File Size | Description |
| -------- | ---------- | --------- | ----------- |
| [Meta-Llama-3-70B-Instruct-Q8_0.gguf](https://huggingface.co/bartowski/Meta-Llama-3-70B-Instruct-GGUF/tree/main/Meta-Llama-3-70B-Instruct-Q8_0.gguf) | Q8_0 | 74.97GB | Extremely high quality, generally unneeded but max available quant. |
| [Meta-Llama-3-70B-Instruct-Q6_K.gguf](https://huggingface.co/bartowski/Meta-Llama-3-70B-Instruct-GGUF/tree/main/Meta-Llama-3-70B-Instruct-Q6_K.gguf) | Q6_K | 57.88GB | Very high quality, near perfect, *recommended*. |
| [Meta-Llama-3-70B-Instruct-Q5_K_M.gguf](https://huggingface.co/bartowski/Meta-Llama-3-70B-Instruct-GGUF/blob/main/Meta-Llama-3-70B-Instruct-Q5_K_M.gguf) | Q5_K_M | 49.94GB | High quality, *recommended*. |
| [Meta-Llama-3-70B-Instruct-Q5_K_S.gguf](https://huggingface.co/bartowski/Meta-Llama-3-70B-Instruct-GGUF/blob/main/Meta-Llama-3-70B-Instruct-Q5_K_S.gguf) | Q5_K_S | 48.65GB | High quality, *recommended*. |
| [Meta-Llama-3-70B-Instruct-Q4_K_M.gguf](https://huggingface.co/bartowski/Meta-Llama-3-70B-Instruct-GGUF/blob/main/Meta-Llama-3-70B-Instruct-Q4_K_M.gguf) | Q4_K_M | 42.52GB | Good quality, uses about 4.83 bits per weight, *recommended*. |
| [Meta-Llama-3-70B-Instruct-Q4_K_S.gguf](https://huggingface.co/bartowski/Meta-Llama-3-70B-Instruct-GGUF/blob/main/Meta-Llama-3-70B-Instruct-Q4_K_S.gguf) | Q4_K_S | 40.34GB | Slightly lower quality with more space savings, *recommended*. |
| [Meta-Llama-3-70B-Instruct-IQ4_NL.gguf](https://huggingface.co/bartowski/Meta-Llama-3-70B-Instruct-GGUF/blob/main/Meta-Llama-3-70B-Instruct-IQ4_NL.gguf) | IQ4_NL | 40.05GB | Decent quality, slightly smaller than Q4_K_S with similar performance *recommended*. |
| [Meta-Llama-3-70B-Instruct-IQ4_XS.gguf](https://huggingface.co/bartowski/Meta-Llama-3-70B-Instruct-GGUF/blob/main/Meta-Llama-3-70B-Instruct-IQ4_XS.gguf) | IQ4_XS | 37.90GB | Decent quality, smaller than Q4_K_S with similar performance, *recommended*. |
| [Meta-Llama-3-70B-Instruct-Q3_K_L.gguf](https://huggingface.co/bartowski/Meta-Llama-3-70B-Instruct-GGUF/blob/main/Meta-Llama-3-70B-Instruct-Q3_K_L.gguf) | Q3_K_L | 37.14GB | Lower quality but usable, good for low RAM availability. |
| [Meta-Llama-3-70B-Instruct-Q3_K_M.gguf](https://huggingface.co/bartowski/Meta-Llama-3-70B-Instruct-GGUF/blob/main/Meta-Llama-3-70B-Instruct-Q3_K_M.gguf) | Q3_K_M | 34.26GB | Even lower quality. |
| [Meta-Llama-3-70B-Instruct-IQ3_M.gguf](https://huggingface.co/bartowski/Meta-Llama-3-70B-Instruct-GGUF/blob/main/Meta-Llama-3-70B-Instruct-IQ3_M.gguf) | IQ3_M | 31.93GB | Medium-low quality, new method with decent performance comparable to Q3_K_M. |
| [Meta-Llama-3-70B-Instruct-IQ3_S.gguf](https://huggingface.co/bartowski/Meta-Llama-3-70B-Instruct-GGUF/blob/main/Meta-Llama-3-70B-Instruct-IQ3_S.gguf) | IQ3_S | 30.91GB | Lower quality, new method with decent performance, recommended over Q3_K_S quant, same size with better performance. |
| [Meta-Llama-3-70B-Instruct-Q3_K_S.gguf](https://huggingface.co/bartowski/Meta-Llama-3-70B-Instruct-GGUF/blob/main/Meta-Llama-3-70B-Instruct-Q3_K_S.gguf) | Q3_K_S | 30.91GB | Low quality, not recommended. |
| [Meta-Llama-3-70B-Instruct-IQ3_XS.gguf](https://huggingface.co/bartowski/Meta-Llama-3-70B-Instruct-GGUF/blob/main/Meta-Llama-3-70B-Instruct-IQ3_XS.gguf) | IQ3_XS | 29.30GB | Lower quality, new method with decent performance, slightly better than Q3_K_S. |
| [Meta-Llama-3-70B-Instruct-IQ3_XXS.gguf](https://huggingface.co/bartowski/Meta-Llama-3-70B-Instruct-GGUF/blob/main/Meta-Llama-3-70B-Instruct-IQ3_XXS.gguf) | IQ3_XXS | 27.46GB | Lower quality, new method with decent performance, comparable to Q3 quants. |
| [Meta-Llama-3-70B-Instruct-Q2_K.gguf](https://huggingface.co/bartowski/Meta-Llama-3-70B-Instruct-GGUF/blob/main/Meta-Llama-3-70B-Instruct-Q2_K.gguf) | Q2_K | 26.37GB | Very low quality but surprisingly usable. |
| [Meta-Llama-3-70B-Instruct-IQ2_M.gguf](https://huggingface.co/bartowski/Meta-Llama-3-70B-Instruct-GGUF/blob/main/Meta-Llama-3-70B-Instruct-IQ2_M.gguf) | IQ2_M | 24.11GB | Very low quality, uses SOTA techniques to also be surprisingly usable. |
| [Meta-Llama-3-70B-Instruct-IQ2_S.gguf](https://huggingface.co/bartowski/Meta-Llama-3-70B-Instruct-GGUF/blob/main/Meta-Llama-3-70B-Instruct-IQ2_S.gguf) | IQ2_S | 22.24GB | Very low quality, uses SOTA techniques to be usable. |
| [Meta-Llama-3-70B-Instruct-IQ2_XS.gguf](https://huggingface.co/bartowski/Meta-Llama-3-70B-Instruct-GGUF/blob/main/Meta-Llama-3-70B-Instruct-IQ2_XS.gguf) | IQ2_XS | 21.14GB | Very low quality, uses SOTA techniques to be usable. |
| [Meta-Llama-3-70B-Instruct-IQ2_XXS.gguf](https://huggingface.co/bartowski/Meta-Llama-3-70B-Instruct-GGUF/blob/main/Meta-Llama-3-70B-Instruct-IQ2_XXS.gguf) | IQ2_XXS | 19.09GB | Lower quality, uses SOTA techniques to be usable. |
| [Meta-Llama-3-70B-Instruct-IQ1_M.gguf](https://huggingface.co/bartowski/Meta-Llama-3-70B-Instruct-GGUF/blob/main/Meta-Llama-3-70B-Instruct-IQ1_M.gguf) | IQ1_M | 16.75GB | Extremely low quality, *not* recommended. |
| [Meta-Llama-3-70B-Instruct-IQ1_S.gguf](https://huggingface.co/bartowski/Meta-Llama-3-70B-Instruct-GGUF/blob/main/Meta-Llama-3-70B-Instruct-IQ1_S.gguf) | IQ1_S | 15.34GB | Extremely low quality, *not* recommended. |
## Downloading using huggingface-cli
First, make sure you have hugginface-cli installed:
```
pip install -U "huggingface_hub[cli]"
```
Then, you can target the specific file you want:
```
huggingface-cli download bartowski/Meta-Llama-3-70B-Instruct-GGUF --include "Meta-Llama-3-70B-Instruct-Q4_K_M.gguf" --local-dir ./ --local-dir-use-symlinks False
```
If the model is bigger than 50GB, it will have been split into multiple files. In order to download them all to a local folder, run:
```
huggingface-cli download bartowski/Meta-Llama-3-70B-Instruct-GGUF --include "Meta-Llama-3-70B-Instruct-Q8_0.gguf/*" --local-dir Meta-Llama-3-70B-Instruct-Q8_0 --local-dir-use-symlinks False
```
You can either specify a new local-dir (Meta-Llama-3-70B-Instruct-Q8_0) or download them all in place (./)
## Which file should I choose?
A great write up with charts showing various performances is provided by Artefact2 [here](https://gist.github.com/Artefact2/b5f810600771265fc1e39442288e8ec9)
The first thing to figure out is how big a model you can run. To do this, you'll need to figure out how much RAM and/or VRAM you have.
If you want your model running as FAST as possible, you'll want to fit the whole thing on your GPU's VRAM. Aim for a quant with a file size 1-2GB smaller than your GPU's total VRAM.
If you want the absolute maximum quality, add both your system RAM and your GPU's VRAM together, then similarly grab a quant with a file size 1-2GB Smaller than that total.
Next, you'll need to decide if you want to use an 'I-quant' or a 'K-quant'.
If you don't want to think too much, grab one of the K-quants. These are in format 'QX_K_X', like Q5_K_M.
If you want to get more into the weeds, you can check out this extremely useful feature chart:
[llama.cpp feature matrix](https://github.com/ggerganov/llama.cpp/wiki/Feature-matrix)
But basically, if you're aiming for below Q4, and you're running cuBLAS (Nvidia) or rocBLAS (AMD), you should look towards the I-quants. These are in format IQX_X, like IQ3_M. These are newer and offer better performance for their size.
These I-quants can also be used on CPU and Apple Metal, but will be slower than their K-quant equivalent, so speed vs performance is a tradeoff you'll have to decide.
The I-quants are *not* compatible with Vulcan, which is also AMD, so if you have an AMD card double check if you're using the rocBLAS build or the Vulcan build. At the time of writing this, LM Studio has a preview with ROCm support, and other inference engines have specific builds for ROCm.
Want to support my work? Visit my ko-fi page here: https://ko-fi.com/bartowski
| {"language": ["en"], "license": "other", "tags": ["facebook", "meta", "pytorch", "llama", "llama-3"], "pipeline_tag": "text-generation", "license_name": "llama3", "license_link": "LICENSE", "extra_gated_prompt": "### META LLAMA 3 COMMUNITY LICENSE AGREEMENT\nMeta Llama 3 Version Release Date: April 18, 2024\n\"Agreement\" means the terms and conditions for use, reproduction, distribution and modification of the Llama Materials set forth herein.\n\"Documentation\" means the specifications, manuals and documentation accompanying Meta Llama 3 distributed by Meta at https://llama.meta.com/get-started/.\n\"Licensee\" or \"you\" means you, or your employer or any other person or entity (if you are entering into this Agreement on such person or entity\u2019s behalf), of the age required under applicable laws, rules or regulations to provide legal consent and that has legal authority to bind your employer or such other person or entity if you are entering in this Agreement on their behalf.\n\"Meta Llama 3\" means the foundational large language models and software and algorithms, including machine-learning model code, trained model weights, inference-enabling code, training-enabling code, fine-tuning enabling code and other elements of the foregoing distributed by Meta at https://llama.meta.com/llama-downloads.\n\"Llama Materials\" means, collectively, Meta\u2019s proprietary Meta Llama 3 and Documentation (and any portion thereof) made available under this Agreement.\n\"Meta\" or \"we\" means Meta Platforms Ireland Limited (if you are located in or, if you are an entity, your principal place of business is in the EEA or Switzerland) and Meta Platforms, Inc. (if you are located outside of the EEA or Switzerland).\n \n1. License Rights and Redistribution.\na. Grant of Rights. You are granted a non-exclusive, worldwide, non-transferable and royalty-free limited license under Meta\u2019s intellectual property or other rights owned by Meta embodied in the Llama Materials to use, reproduce, distribute, copy, create derivative works of, and make modifications to the Llama Materials.\nb. Redistribution and Use.\ni. If you distribute or make available the Llama Materials (or any derivative works thereof), or a product or service that uses any of them, including another AI model, you shall (A) provide a copy of this Agreement with any such Llama Materials; and (B) prominently display \u201cBuilt with Meta Llama 3\u201d on a related website, user interface, blogpost, about page, or product documentation. If you use the Llama Materials to create, train, fine tune, or otherwise improve an AI model, which is distributed or made available, you shall also include \u201cLlama 3\u201d at the beginning of any such AI model name.\nii. If you receive Llama Materials, or any derivative works thereof, from a Licensee as part of an integrated end user product, then Section 2 of this Agreement will not apply to you.\niii. You must retain in all copies of the Llama Materials that you distribute the following attribution notice within a \u201cNotice\u201d text file distributed as a part of such copies: \u201cMeta Llama 3 is licensed under the Meta Llama 3 Community License, Copyright \u00a9 Meta Platforms, Inc. All Rights Reserved.\u201d\niv. Your use of the Llama Materials must comply with applicable laws and regulations (including trade compliance laws and regulations) and adhere to the Acceptable Use Policy for the Llama Materials (available at https://llama.meta.com/llama3/use-policy), which is hereby incorporated by reference into this Agreement.\nv. You will not use the Llama Materials or any output or results of the Llama Materials to improve any other large language model (excluding Meta Llama 3 or derivative works thereof).\n2. Additional Commercial Terms. If, on the Meta Llama 3 version release date, the monthly active users of the products or services made available by or for Licensee, or Licensee\u2019s affiliates, is greater than 700 million monthly active users in the preceding calendar month, you must request a license from Meta, which Meta may grant to you in its sole discretion, and you are not authorized to exercise any of the rights under this Agreement unless or until Meta otherwise expressly grants you such rights.\n3. Disclaimer of Warranty. UNLESS REQUIRED BY APPLICABLE LAW, THE LLAMA MATERIALS AND ANY OUTPUT AND RESULTS THEREFROM ARE PROVIDED ON AN \u201cAS IS\u201d BASIS, WITHOUT WARRANTIES OF ANY KIND, AND META DISCLAIMS ALL WARRANTIES OF ANY KIND, BOTH EXPRESS AND IMPLIED, INCLUDING, WITHOUT LIMITATION, ANY WARRANTIES OF TITLE, NON-INFRINGEMENT, MERCHANTABILITY, OR FITNESS FOR A PARTICULAR PURPOSE. YOU ARE SOLELY RESPONSIBLE FOR DETERMINING THE APPROPRIATENESS OF USING OR REDISTRIBUTING THE LLAMA MATERIALS AND ASSUME ANY RISKS ASSOCIATED WITH YOUR USE OF THE LLAMA MATERIALS AND ANY OUTPUT AND RESULTS.\n4. Limitation of Liability. IN NO EVENT WILL META OR ITS AFFILIATES BE LIABLE UNDER ANY THEORY OF LIABILITY, WHETHER IN CONTRACT, TORT, NEGLIGENCE, PRODUCTS LIABILITY, OR OTHERWISE, ARISING OUT OF THIS AGREEMENT, FOR ANY LOST PROFITS OR ANY INDIRECT, SPECIAL, CONSEQUENTIAL, INCIDENTAL, EXEMPLARY OR PUNITIVE DAMAGES, EVEN IF META OR ITS AFFILIATES HAVE BEEN ADVISED OF THE POSSIBILITY OF ANY OF THE FOREGOING.\n5. Intellectual Property.\na. No trademark licenses are granted under this Agreement, and in connection with the Llama Materials, neither Meta nor Licensee may use any name or mark owned by or associated with the other or any of its affiliates, except as required for reasonable and customary use in describing and redistributing the Llama Materials or as set forth in this Section 5(a). Meta hereby grants you a license to use \u201cLlama 3\u201d (the \u201cMark\u201d) solely as required to comply with the last sentence of Section 1.b.i. You will comply with Meta\u2019s brand guidelines (currently accessible at https://about.meta.com/brand/resources/meta/company-brand/ ). All goodwill arising out of your use of the Mark will inure to the benefit of Meta.\nb. Subject to Meta\u2019s ownership of Llama Materials and derivatives made by or for Meta, with respect to any derivative works and modifications of the Llama Materials that are made by you, as between you and Meta, you are and will be the owner of such derivative works and modifications.\nc. If you institute litigation or other proceedings against Meta or any entity (including a cross-claim or counterclaim in a lawsuit) alleging that the Llama Materials or Meta Llama 3 outputs or results, or any portion of any of the foregoing, constitutes infringement of intellectual property or other rights owned or licensable by you, then any licenses granted to you under this Agreement shall terminate as of the date such litigation or claim is filed or instituted. You will indemnify and hold harmless Meta from and against any claim by any third party arising out of or related to your use or distribution of the Llama Materials.\n6. Term and Termination. The term of this Agreement will commence upon your acceptance of this Agreement or access to the Llama Materials and will continue in full force and effect until terminated in accordance with the terms and conditions herein. Meta may terminate this Agreement if you are in breach of any term or condition of this Agreement. Upon termination of this Agreement, you shall delete and cease use of the Llama Materials. Sections 3, 4 and 7 shall survive the termination of this Agreement.\n7. Governing Law and Jurisdiction. This Agreement will be governed and construed under the laws of the State of California without regard to choice of law principles, and the UN Convention on Contracts for the International Sale of Goods does not apply to this Agreement. The courts of California shall have exclusive jurisdiction of any dispute arising out of this Agreement.\n### Meta Llama 3 Acceptable Use Policy\nMeta is committed to promoting safe and fair use of its tools and features, including Meta Llama 3. If you access or use Meta Llama 3, you agree to this Acceptable Use Policy (\u201cPolicy\u201d). The most recent copy of this policy can be found at [https://llama.meta.com/llama3/use-policy](https://llama.meta.com/llama3/use-policy)\n#### Prohibited Uses\nWe want everyone to use Meta Llama 3 safely and responsibly. You agree you will not use, or allow others to use, Meta Llama 3 to: 1. Violate the law or others\u2019 rights, including to:\n 1. Engage in, promote, generate, contribute to, encourage, plan, incite, or further illegal or unlawful activity or content, such as:\n 1. Violence or terrorism\n 2. Exploitation or harm to children, including the solicitation, creation, acquisition, or dissemination of child exploitative content or failure to report Child Sexual Abuse Material\n 3. Human trafficking, exploitation, and sexual violence\n 4. The illegal distribution of information or materials to minors, including obscene materials, or failure to employ legally required age-gating in connection with such information or materials.\n 5. Sexual solicitation\n 6. Any other criminal activity\n 2. Engage in, promote, incite, or facilitate the harassment, abuse, threatening, or bullying of individuals or groups of individuals\n 3. Engage in, promote, incite, or facilitate discrimination or other unlawful or harmful conduct in the provision of employment, employment benefits, credit, housing, other economic benefits, or other essential goods and services\n 4. Engage in the unauthorized or unlicensed practice of any profession including, but not limited to, financial, legal, medical/health, or related professional practices\n 5. Collect, process, disclose, generate, or infer health, demographic, or other sensitive personal or private information about individuals without rights and consents required by applicable laws\n 6. Engage in or facilitate any action or generate any content that infringes, misappropriates, or otherwise violates any third-party rights, including the outputs or results of any products or services using the Llama Materials\n 7. Create, generate, or facilitate the creation of malicious code, malware, computer viruses or do anything else that could disable, overburden, interfere with or impair the proper working, integrity, operation or appearance of a website or computer system\n2. Engage in, promote, incite, facilitate, or assist in the planning or development of activities that present a risk of death or bodily harm to individuals, including use of Meta Llama 3 related to the following:\n 1. Military, warfare, nuclear industries or applications, espionage, use for materials or activities that are subject to the International Traffic Arms Regulations (ITAR) maintained by the United States Department of State\n 2. Guns and illegal weapons (including weapon development)\n 3. Illegal drugs and regulated/controlled substances\n 4. Operation of critical infrastructure, transportation technologies, or heavy machinery\n 5. Self-harm or harm to others, including suicide, cutting, and eating disorders\n 6. Any content intended to incite or promote violence, abuse, or any infliction of bodily harm to an individual\n3. Intentionally deceive or mislead others, including use of Meta Llama 3 related to the following:\n 1. Generating, promoting, or furthering fraud or the creation or promotion of disinformation\n 2. Generating, promoting, or furthering defamatory content, including the creation of defamatory statements, images, or other content\n 3. Generating, promoting, or further distributing spam\n 4. Impersonating another individual without consent, authorization, or legal right\n 5. Representing that the use of Meta Llama 3 or outputs are human-generated\n 6. Generating or facilitating false online engagement, including fake reviews and other means of fake online engagement\n4. Fail to appropriately disclose to end users any known dangers of your AI system\nPlease report any violation of this Policy, software \u201cbug,\u201d or other problems that could lead to a violation of this Policy through one of the following means:\n * Reporting issues with the model: [https://github.com/meta-llama/llama3](https://github.com/meta-llama/llama3)\n * Reporting risky content generated by the model:\n developers.facebook.com/llama_output_feedback\n * Reporting bugs and security concerns: facebook.com/whitehat/info\n * Reporting violations of the Acceptable Use Policy or unlicensed uses of Meta Llama 3: [email protected]", "extra_gated_fields": {"First Name": "text", "Last Name": "text", "Date of birth": "date_picker", "Country": "country", "Affiliation": "text", "geo": "ip_location", "By clicking Submit below I accept the terms of the license and acknowledge that the information I provide will be collected stored processed and shared in accordance with the Meta Privacy Policy": "checkbox"}, "extra_gated_description": "The information you provide will be collected, stored, processed and shared in accordance with the [Meta Privacy Policy](https://www.facebook.com/privacy/policy/).", "extra_gated_button_content": "Submit", "widget": [{"example_title": "Winter holidays", "messages": [{"role": "system", "content": "You are a helpful and honest assistant. Please, respond concisely and truthfully."}, {"role": "user", "content": "Can you recommend a good destination for Winter holidays?"}]}, {"example_title": "Programming assistant", "messages": [{"role": "system", "content": "You are a helpful and honest code and programming assistant. Please, respond concisely and truthfully."}, {"role": "user", "content": "Write a function that computes the nth fibonacci number."}]}], "inference": {"parameters": {"max_new_tokens": 300, "stop": ["<|end_of_text|>", "<|eot_id|>"]}}, "quantized_by": "bartowski"} | bartowski/Meta-Llama-3-70B-Instruct-GGUF | null | [
"gguf",
"facebook",
"meta",
"pytorch",
"llama",
"llama-3",
"text-generation",
"en",
"license:other",
"region:us"
] | null | 2024-05-02T11:17:13+00:00 | [] | [
"en"
] | TAGS
#gguf #facebook #meta #pytorch #llama #llama-3 #text-generation #en #license-other #region-us
| Llamacpp imatrix Quantizations of Meta-Llama-3-70B-Instruct
-----------------------------------------------------------
Using <a href="URL release <a href="URL for quantization.
Original model: URL
All quants made using imatrix option with dataset provided by Kalomaze here
Prompt format
-------------
Download a file (not the whole branch) from below:
--------------------------------------------------
Downloading using huggingface-cli
---------------------------------
First, make sure you have hugginface-cli installed:
Then, you can target the specific file you want:
If the model is bigger than 50GB, it will have been split into multiple files. In order to download them all to a local folder, run:
You can either specify a new local-dir (Meta-Llama-3-70B-Instruct-Q8\_0) or download them all in place (./)
Which file should I choose?
---------------------------
A great write up with charts showing various performances is provided by Artefact2 here
The first thing to figure out is how big a model you can run. To do this, you'll need to figure out how much RAM and/or VRAM you have.
If you want your model running as FAST as possible, you'll want to fit the whole thing on your GPU's VRAM. Aim for a quant with a file size 1-2GB smaller than your GPU's total VRAM.
If you want the absolute maximum quality, add both your system RAM and your GPU's VRAM together, then similarly grab a quant with a file size 1-2GB Smaller than that total.
Next, you'll need to decide if you want to use an 'I-quant' or a 'K-quant'.
If you don't want to think too much, grab one of the K-quants. These are in format 'QX\_K\_X', like Q5\_K\_M.
If you want to get more into the weeds, you can check out this extremely useful feature chart:
URL feature matrix
But basically, if you're aiming for below Q4, and you're running cuBLAS (Nvidia) or rocBLAS (AMD), you should look towards the I-quants. These are in format IQX\_X, like IQ3\_M. These are newer and offer better performance for their size.
These I-quants can also be used on CPU and Apple Metal, but will be slower than their K-quant equivalent, so speed vs performance is a tradeoff you'll have to decide.
The I-quants are *not* compatible with Vulcan, which is also AMD, so if you have an AMD card double check if you're using the rocBLAS build or the Vulcan build. At the time of writing this, LM Studio has a preview with ROCm support, and other inference engines have specific builds for ROCm.
Want to support my work? Visit my ko-fi page here: URL
| [] | [
"TAGS\n#gguf #facebook #meta #pytorch #llama #llama-3 #text-generation #en #license-other #region-us \n"
] | [
36
] | [
"TAGS\n#gguf #facebook #meta #pytorch #llama #llama-3 #text-generation #en #license-other #region-us \n"
] |
null | null | DataVare Address Book Converter is an efficient tool to smartly import and export VCF contacts. This tool is a 100% safe and secure way to import contacts. With the help of this tool, users can quickly import bulk VCF contacts. The advanced application can rapidly export VCF contacts from Excel, MSG, OST, and PST files. There are zero percentage chances of data loss. Users can preview their selected VCF contact files before exporting. The application can quickly work with all Windows OS versions without creating any problems. There is no need to install another piece of software or tool to export a VCF contact file. Another feature of this software is that users can split or merge VCF contacts. The software can quickly merge many VCF contacts into one without any data loss. While using this tool, users can also save their data as per their chosen location on the desktop. Get the latest free demo edition of this utility before purchasing the complete version.
Read More: https://www.datavare.com/software/address-book-converter.html | {} | DataVare/Address-Book-Manager-Software | null | [
"region:us"
] | null | 2024-05-02T11:17:25+00:00 | [] | [] | TAGS
#region-us
| DataVare Address Book Converter is an efficient tool to smartly import and export VCF contacts. This tool is a 100% safe and secure way to import contacts. With the help of this tool, users can quickly import bulk VCF contacts. The advanced application can rapidly export VCF contacts from Excel, MSG, OST, and PST files. There are zero percentage chances of data loss. Users can preview their selected VCF contact files before exporting. The application can quickly work with all Windows OS versions without creating any problems. There is no need to install another piece of software or tool to export a VCF contact file. Another feature of this software is that users can split or merge VCF contacts. The software can quickly merge many VCF contacts into one without any data loss. While using this tool, users can also save their data as per their chosen location on the desktop. Get the latest free demo edition of this utility before purchasing the complete version.
Read More: URL | [] | [
"TAGS\n#region-us \n"
] | [
5
] | [
"TAGS\n#region-us \n"
] |
image-classification | transformers |
<!-- This model card has been generated automatically according to the information the Trainer had access to. You
should probably proofread and complete it, then remove this comment. -->
# vit-base-patch16-224-vit
This model is a fine-tuned version of [google/vit-base-patch16-224](https://huggingface.co/google/vit-base-patch16-224) on the imagefolder dataset.
It achieves the following results on the evaluation set:
- Loss: 0.6404
- Accuracy: 0.8158
## Model description
More information needed
## Intended uses & limitations
More information needed
## Training and evaluation data
More information needed
## Training procedure
### Training hyperparameters
The following hyperparameters were used during training:
- learning_rate: 0.0002
- train_batch_size: 16
- eval_batch_size: 16
- seed: 42
- gradient_accumulation_steps: 4
- total_train_batch_size: 64
- optimizer: Adam with betas=(0.9,0.999) and epsilon=1e-08
- lr_scheduler_type: linear
- lr_scheduler_warmup_ratio: 0.1
- num_epochs: 10
### Training results
| Training Loss | Epoch | Step | Validation Loss | Accuracy |
|:-------------:|:------:|:----:|:---------------:|:--------:|
| 1.8161 | 0.9787 | 23 | 1.4794 | 0.4368 |
| 0.9674 | 2.0 | 47 | 1.0353 | 0.6737 |
| 0.4804 | 2.9787 | 70 | 0.7857 | 0.7316 |
| 0.3301 | 4.0 | 94 | 0.6994 | 0.7632 |
| 0.1821 | 4.9787 | 117 | 0.8172 | 0.7632 |
| 0.161 | 6.0 | 141 | 0.6663 | 0.8 |
| 0.1161 | 6.9787 | 164 | 0.6439 | 0.8211 |
| 0.0855 | 8.0 | 188 | 0.5770 | 0.8368 |
| 0.0635 | 8.9787 | 211 | 0.6380 | 0.8316 |
| 0.0522 | 9.7872 | 230 | 0.6404 | 0.8158 |
### Framework versions
- Transformers 4.40.1
- Pytorch 2.2.1+cu121
- Datasets 2.19.0
- Tokenizers 0.19.1
| {"license": "apache-2.0", "tags": ["generated_from_trainer"], "datasets": ["imagefolder"], "metrics": ["accuracy"], "base_model": "google/vit-base-patch16-224", "model-index": [{"name": "vit-base-patch16-224-vit", "results": [{"task": {"type": "image-classification", "name": "Image Classification"}, "dataset": {"name": "imagefolder", "type": "imagefolder", "config": "default", "split": "train", "args": "default"}, "metrics": [{"type": "accuracy", "value": 0.8157894736842105, "name": "Accuracy"}]}]}]} | pk3388/vit-base-patch16-224-vit | null | [
"transformers",
"tensorboard",
"safetensors",
"vit",
"image-classification",
"generated_from_trainer",
"dataset:imagefolder",
"base_model:google/vit-base-patch16-224",
"license:apache-2.0",
"model-index",
"autotrain_compatible",
"endpoints_compatible",
"region:us"
] | null | 2024-05-02T11:17:35+00:00 | [] | [] | TAGS
#transformers #tensorboard #safetensors #vit #image-classification #generated_from_trainer #dataset-imagefolder #base_model-google/vit-base-patch16-224 #license-apache-2.0 #model-index #autotrain_compatible #endpoints_compatible #region-us
| vit-base-patch16-224-vit
========================
This model is a fine-tuned version of google/vit-base-patch16-224 on the imagefolder dataset.
It achieves the following results on the evaluation set:
* Loss: 0.6404
* Accuracy: 0.8158
Model description
-----------------
More information needed
Intended uses & limitations
---------------------------
More information needed
Training and evaluation data
----------------------------
More information needed
Training procedure
------------------
### Training hyperparameters
The following hyperparameters were used during training:
* learning\_rate: 0.0002
* train\_batch\_size: 16
* eval\_batch\_size: 16
* seed: 42
* gradient\_accumulation\_steps: 4
* total\_train\_batch\_size: 64
* optimizer: Adam with betas=(0.9,0.999) and epsilon=1e-08
* lr\_scheduler\_type: linear
* lr\_scheduler\_warmup\_ratio: 0.1
* num\_epochs: 10
### Training results
### Framework versions
* Transformers 4.40.1
* Pytorch 2.2.1+cu121
* Datasets 2.19.0
* Tokenizers 0.19.1
| [
"### Training hyperparameters\n\n\nThe following hyperparameters were used during training:\n\n\n* learning\\_rate: 0.0002\n* train\\_batch\\_size: 16\n* eval\\_batch\\_size: 16\n* seed: 42\n* gradient\\_accumulation\\_steps: 4\n* total\\_train\\_batch\\_size: 64\n* optimizer: Adam with betas=(0.9,0.999) and epsilon=1e-08\n* lr\\_scheduler\\_type: linear\n* lr\\_scheduler\\_warmup\\_ratio: 0.1\n* num\\_epochs: 10",
"### Training results",
"### Framework versions\n\n\n* Transformers 4.40.1\n* Pytorch 2.2.1+cu121\n* Datasets 2.19.0\n* Tokenizers 0.19.1"
] | [
"TAGS\n#transformers #tensorboard #safetensors #vit #image-classification #generated_from_trainer #dataset-imagefolder #base_model-google/vit-base-patch16-224 #license-apache-2.0 #model-index #autotrain_compatible #endpoints_compatible #region-us \n",
"### Training hyperparameters\n\n\nThe following hyperparameters were used during training:\n\n\n* learning\\_rate: 0.0002\n* train\\_batch\\_size: 16\n* eval\\_batch\\_size: 16\n* seed: 42\n* gradient\\_accumulation\\_steps: 4\n* total\\_train\\_batch\\_size: 64\n* optimizer: Adam with betas=(0.9,0.999) and epsilon=1e-08\n* lr\\_scheduler\\_type: linear\n* lr\\_scheduler\\_warmup\\_ratio: 0.1\n* num\\_epochs: 10",
"### Training results",
"### Framework versions\n\n\n* Transformers 4.40.1\n* Pytorch 2.2.1+cu121\n* Datasets 2.19.0\n* Tokenizers 0.19.1"
] | [
72,
142,
5,
44
] | [
"TAGS\n#transformers #tensorboard #safetensors #vit #image-classification #generated_from_trainer #dataset-imagefolder #base_model-google/vit-base-patch16-224 #license-apache-2.0 #model-index #autotrain_compatible #endpoints_compatible #region-us \n### Training hyperparameters\n\n\nThe following hyperparameters were used during training:\n\n\n* learning\\_rate: 0.0002\n* train\\_batch\\_size: 16\n* eval\\_batch\\_size: 16\n* seed: 42\n* gradient\\_accumulation\\_steps: 4\n* total\\_train\\_batch\\_size: 64\n* optimizer: Adam with betas=(0.9,0.999) and epsilon=1e-08\n* lr\\_scheduler\\_type: linear\n* lr\\_scheduler\\_warmup\\_ratio: 0.1\n* num\\_epochs: 10### Training results### Framework versions\n\n\n* Transformers 4.40.1\n* Pytorch 2.2.1+cu121\n* Datasets 2.19.0\n* Tokenizers 0.19.1"
] |
feature-extraction | transformers |
# Model Card for Model ID
<!-- Provide a quick summary of what the model is/does. -->
## Model Details
### Model Description
<!-- Provide a longer summary of what this model is. -->
This is the model card of a 🤗 transformers model that has been pushed on the Hub. This model card has been automatically generated.
- **Developed by:** [More Information Needed]
- **Funded by [optional]:** [More Information Needed]
- **Shared by [optional]:** [More Information Needed]
- **Model type:** [More Information Needed]
- **Language(s) (NLP):** [More Information Needed]
- **License:** [More Information Needed]
- **Finetuned from model [optional]:** [More Information Needed]
### Model Sources [optional]
<!-- Provide the basic links for the model. -->
- **Repository:** [More Information Needed]
- **Paper [optional]:** [More Information Needed]
- **Demo [optional]:** [More Information Needed]
## Uses
<!-- Address questions around how the model is intended to be used, including the foreseeable users of the model and those affected by the model. -->
### Direct Use
<!-- This section is for the model use without fine-tuning or plugging into a larger ecosystem/app. -->
[More Information Needed]
### Downstream Use [optional]
<!-- This section is for the model use when fine-tuned for a task, or when plugged into a larger ecosystem/app -->
[More Information Needed]
### Out-of-Scope Use
<!-- This section addresses misuse, malicious use, and uses that the model will not work well for. -->
[More Information Needed]
## Bias, Risks, and Limitations
<!-- This section is meant to convey both technical and sociotechnical limitations. -->
[More Information Needed]
### Recommendations
<!-- This section is meant to convey recommendations with respect to the bias, risk, and technical limitations. -->
Users (both direct and downstream) should be made aware of the risks, biases and limitations of the model. More information needed for further recommendations.
## How to Get Started with the Model
Use the code below to get started with the model.
[More Information Needed]
## Training Details
### Training Data
<!-- This should link to a Dataset Card, perhaps with a short stub of information on what the training data is all about as well as documentation related to data pre-processing or additional filtering. -->
[More Information Needed]
### Training Procedure
<!-- This relates heavily to the Technical Specifications. Content here should link to that section when it is relevant to the training procedure. -->
#### Preprocessing [optional]
[More Information Needed]
#### Training Hyperparameters
- **Training regime:** [More Information Needed] <!--fp32, fp16 mixed precision, bf16 mixed precision, bf16 non-mixed precision, fp16 non-mixed precision, fp8 mixed precision -->
#### Speeds, Sizes, Times [optional]
<!-- This section provides information about throughput, start/end time, checkpoint size if relevant, etc. -->
[More Information Needed]
## Evaluation
<!-- This section describes the evaluation protocols and provides the results. -->
### Testing Data, Factors & Metrics
#### Testing Data
<!-- This should link to a Dataset Card if possible. -->
[More Information Needed]
#### Factors
<!-- These are the things the evaluation is disaggregating by, e.g., subpopulations or domains. -->
[More Information Needed]
#### Metrics
<!-- These are the evaluation metrics being used, ideally with a description of why. -->
[More Information Needed]
### Results
[More Information Needed]
#### Summary
## Model Examination [optional]
<!-- Relevant interpretability work for the model goes here -->
[More Information Needed]
## Environmental Impact
<!-- Total emissions (in grams of CO2eq) and additional considerations, such as electricity usage, go here. Edit the suggested text below accordingly -->
Carbon emissions can be estimated using the [Machine Learning Impact calculator](https://mlco2.github.io/impact#compute) presented in [Lacoste et al. (2019)](https://arxiv.org/abs/1910.09700).
- **Hardware Type:** [More Information Needed]
- **Hours used:** [More Information Needed]
- **Cloud Provider:** [More Information Needed]
- **Compute Region:** [More Information Needed]
- **Carbon Emitted:** [More Information Needed]
## Technical Specifications [optional]
### Model Architecture and Objective
[More Information Needed]
### Compute Infrastructure
[More Information Needed]
#### Hardware
[More Information Needed]
#### Software
[More Information Needed]
## Citation [optional]
<!-- If there is a paper or blog post introducing the model, the APA and Bibtex information for that should go in this section. -->
**BibTeX:**
[More Information Needed]
**APA:**
[More Information Needed]
## Glossary [optional]
<!-- If relevant, include terms and calculations in this section that can help readers understand the model or model card. -->
[More Information Needed]
## More Information [optional]
[More Information Needed]
## Model Card Authors [optional]
[More Information Needed]
## Model Card Contact
[More Information Needed] | {"library_name": "transformers", "tags": []} | stvhuang/rcr-run-5pqr6lwp-90396-master-0_20240402T105012-ep44 | null | [
"transformers",
"safetensors",
"xlm-roberta",
"feature-extraction",
"arxiv:1910.09700",
"endpoints_compatible",
"region:us"
] | null | 2024-05-02T11:20:41+00:00 | [
"1910.09700"
] | [] | TAGS
#transformers #safetensors #xlm-roberta #feature-extraction #arxiv-1910.09700 #endpoints_compatible #region-us
|
# Model Card for Model ID
## Model Details
### Model Description
This is the model card of a transformers model that has been pushed on the Hub. This model card has been automatically generated.
- Developed by:
- Funded by [optional]:
- Shared by [optional]:
- Model type:
- Language(s) (NLP):
- License:
- Finetuned from model [optional]:
### Model Sources [optional]
- Repository:
- Paper [optional]:
- Demo [optional]:
## Uses
### Direct Use
### Downstream Use [optional]
### Out-of-Scope Use
## Bias, Risks, and Limitations
### Recommendations
Users (both direct and downstream) should be made aware of the risks, biases and limitations of the model. More information needed for further recommendations.
## How to Get Started with the Model
Use the code below to get started with the model.
## Training Details
### Training Data
### Training Procedure
#### Preprocessing [optional]
#### Training Hyperparameters
- Training regime:
#### Speeds, Sizes, Times [optional]
## Evaluation
### Testing Data, Factors & Metrics
#### Testing Data
#### Factors
#### Metrics
### Results
#### Summary
## Model Examination [optional]
## Environmental Impact
Carbon emissions can be estimated using the Machine Learning Impact calculator presented in Lacoste et al. (2019).
- Hardware Type:
- Hours used:
- Cloud Provider:
- Compute Region:
- Carbon Emitted:
## Technical Specifications [optional]
### Model Architecture and Objective
### Compute Infrastructure
#### Hardware
#### Software
[optional]
BibTeX:
APA:
## Glossary [optional]
## More Information [optional]
## Model Card Authors [optional]
## Model Card Contact
| [
"# Model Card for Model ID",
"## Model Details",
"### Model Description\n\n\n\nThis is the model card of a transformers model that has been pushed on the Hub. This model card has been automatically generated.\n\n- Developed by: \n- Funded by [optional]: \n- Shared by [optional]: \n- Model type: \n- Language(s) (NLP): \n- License: \n- Finetuned from model [optional]:",
"### Model Sources [optional]\n\n\n\n- Repository: \n- Paper [optional]: \n- Demo [optional]:",
"## Uses",
"### Direct Use",
"### Downstream Use [optional]",
"### Out-of-Scope Use",
"## Bias, Risks, and Limitations",
"### Recommendations\n\n\n\nUsers (both direct and downstream) should be made aware of the risks, biases and limitations of the model. More information needed for further recommendations.",
"## How to Get Started with the Model\n\nUse the code below to get started with the model.",
"## Training Details",
"### Training Data",
"### Training Procedure",
"#### Preprocessing [optional]",
"#### Training Hyperparameters\n\n- Training regime:",
"#### Speeds, Sizes, Times [optional]",
"## Evaluation",
"### Testing Data, Factors & Metrics",
"#### Testing Data",
"#### Factors",
"#### Metrics",
"### Results",
"#### Summary",
"## Model Examination [optional]",
"## Environmental Impact\n\n\n\nCarbon emissions can be estimated using the Machine Learning Impact calculator presented in Lacoste et al. (2019).\n\n- Hardware Type: \n- Hours used: \n- Cloud Provider: \n- Compute Region: \n- Carbon Emitted:",
"## Technical Specifications [optional]",
"### Model Architecture and Objective",
"### Compute Infrastructure",
"#### Hardware",
"#### Software\n\n\n\n[optional]\n\n\n\nBibTeX:\n\n\n\nAPA:",
"## Glossary [optional]",
"## More Information [optional]",
"## Model Card Authors [optional]",
"## Model Card Contact"
] | [
"TAGS\n#transformers #safetensors #xlm-roberta #feature-extraction #arxiv-1910.09700 #endpoints_compatible #region-us \n",
"# Model Card for Model ID",
"## Model Details",
"### Model Description\n\n\n\nThis is the model card of a transformers model that has been pushed on the Hub. This model card has been automatically generated.\n\n- Developed by: \n- Funded by [optional]: \n- Shared by [optional]: \n- Model type: \n- Language(s) (NLP): \n- License: \n- Finetuned from model [optional]:",
"### Model Sources [optional]\n\n\n\n- Repository: \n- Paper [optional]: \n- Demo [optional]:",
"## Uses",
"### Direct Use",
"### Downstream Use [optional]",
"### Out-of-Scope Use",
"## Bias, Risks, and Limitations",
"### Recommendations\n\n\n\nUsers (both direct and downstream) should be made aware of the risks, biases and limitations of the model. More information needed for further recommendations.",
"## How to Get Started with the Model\n\nUse the code below to get started with the model.",
"## Training Details",
"### Training Data",
"### Training Procedure",
"#### Preprocessing [optional]",
"#### Training Hyperparameters\n\n- Training regime:",
"#### Speeds, Sizes, Times [optional]",
"## Evaluation",
"### Testing Data, Factors & Metrics",
"#### Testing Data",
"#### Factors",
"#### Metrics",
"### Results",
"#### Summary",
"## Model Examination [optional]",
"## Environmental Impact\n\n\n\nCarbon emissions can be estimated using the Machine Learning Impact calculator presented in Lacoste et al. (2019).\n\n- Hardware Type: \n- Hours used: \n- Cloud Provider: \n- Compute Region: \n- Carbon Emitted:",
"## Technical Specifications [optional]",
"### Model Architecture and Objective",
"### Compute Infrastructure",
"#### Hardware",
"#### Software\n\n\n\n[optional]\n\n\n\nBibTeX:\n\n\n\nAPA:",
"## Glossary [optional]",
"## More Information [optional]",
"## Model Card Authors [optional]",
"## Model Card Contact"
] | [
35,
6,
4,
75,
23,
3,
5,
8,
9,
8,
34,
20,
4,
5,
5,
11,
13,
12,
3,
10,
6,
5,
6,
4,
5,
7,
49,
7,
7,
5,
5,
15,
7,
7,
8,
5
] | [
"TAGS\n#transformers #safetensors #xlm-roberta #feature-extraction #arxiv-1910.09700 #endpoints_compatible #region-us \n# Model Card for Model ID## Model Details### Model Description\n\n\n\nThis is the model card of a transformers model that has been pushed on the Hub. This model card has been automatically generated.\n\n- Developed by: \n- Funded by [optional]: \n- Shared by [optional]: \n- Model type: \n- Language(s) (NLP): \n- License: \n- Finetuned from model [optional]:### Model Sources [optional]\n\n\n\n- Repository: \n- Paper [optional]: \n- Demo [optional]:## Uses### Direct Use### Downstream Use [optional]### Out-of-Scope Use## Bias, Risks, and Limitations### Recommendations\n\n\n\nUsers (both direct and downstream) should be made aware of the risks, biases and limitations of the model. More information needed for further recommendations.## How to Get Started with the Model\n\nUse the code below to get started with the model.## Training Details### Training Data### Training Procedure#### Preprocessing [optional]#### Training Hyperparameters\n\n- Training regime:#### Speeds, Sizes, Times [optional]## Evaluation### Testing Data, Factors & Metrics#### Testing Data#### Factors#### Metrics### Results#### Summary## Model Examination [optional]## Environmental Impact\n\n\n\nCarbon emissions can be estimated using the Machine Learning Impact calculator presented in Lacoste et al. (2019).\n\n- Hardware Type: \n- Hours used: \n- Cloud Provider: \n- Compute Region: \n- Carbon Emitted:## Technical Specifications [optional]### Model Architecture and Objective### Compute Infrastructure#### Hardware#### Software\n\n\n\n[optional]\n\n\n\nBibTeX:\n\n\n\nAPA:## Glossary [optional]## More Information [optional]## Model Card Authors [optional]## Model Card Contact"
] |
reinforcement-learning | null |
# **Q-Learning** Agent playing1 **Taxi-v3**
This is a trained model of a **Q-Learning** agent playing **Taxi-v3** .
## Usage
```python
model = load_from_hub(repo_id="haytamelouarrat/Taxi-v3", filename="q-learning.pkl")
# Don't forget to check if you need to add additional attributes (is_slippery=False etc)
env = gym.make(model["env_id"])
```
| {"tags": ["Taxi-v3", "q-learning", "reinforcement-learning", "custom-implementation"], "model-index": [{"name": "Taxi-v3", "results": [{"task": {"type": "reinforcement-learning", "name": "reinforcement-learning"}, "dataset": {"name": "Taxi-v3", "type": "Taxi-v3"}, "metrics": [{"type": "mean_reward", "value": "7.46 +/- 2.76", "name": "mean_reward", "verified": false}]}]}]} | haytamelouarrat/Taxi-v3 | null | [
"Taxi-v3",
"q-learning",
"reinforcement-learning",
"custom-implementation",
"model-index",
"region:us"
] | null | 2024-05-02T11:21:02+00:00 | [] | [] | TAGS
#Taxi-v3 #q-learning #reinforcement-learning #custom-implementation #model-index #region-us
|
# Q-Learning Agent playing1 Taxi-v3
This is a trained model of a Q-Learning agent playing Taxi-v3 .
## Usage
| [
"# Q-Learning Agent playing1 Taxi-v3\n This is a trained model of a Q-Learning agent playing Taxi-v3 .\n\n ## Usage"
] | [
"TAGS\n#Taxi-v3 #q-learning #reinforcement-learning #custom-implementation #model-index #region-us \n",
"# Q-Learning Agent playing1 Taxi-v3\n This is a trained model of a Q-Learning agent playing Taxi-v3 .\n\n ## Usage"
] | [
26,
31
] | [
"TAGS\n#Taxi-v3 #q-learning #reinforcement-learning #custom-implementation #model-index #region-us \n# Q-Learning Agent playing1 Taxi-v3\n This is a trained model of a Q-Learning agent playing Taxi-v3 .\n\n ## Usage"
] |
automatic-speech-recognition | transformers |
<!-- This model card has been generated automatically according to the information the Trainer had access to. You
should probably proofread and complete it, then remove this comment. -->
# basic_train_basic_test 1000 similar params: per_device_train_batch_size=32, # bylo 16 a pod tim 1 gradient_accumulation_steps=2, warmup_steps=300, max_steps=3000
This model is a fine-tuned version of [openai/whisper-small](https://huggingface.co/openai/whisper-small) on the xbilek25/xbilek25/train_set_5000_en_de_en dataset.
It achieves the following results on the evaluation set:
- Loss: 0.1263
- Wer: 6.0896
## Model description
More information needed
## Intended uses & limitations
More information needed
## Training and evaluation data
More information needed
## Training procedure
### Training hyperparameters
The following hyperparameters were used during training:
- learning_rate: 1e-05
- train_batch_size: 16
- eval_batch_size: 8
- seed: 42
- optimizer: Adam with betas=(0.9,0.999) and epsilon=1e-08
- lr_scheduler_type: linear
- lr_scheduler_warmup_steps: 500
- training_steps: 4000
- mixed_precision_training: Native AMP
### Training results
| Training Loss | Epoch | Step | Validation Loss | Wer |
|:-------------:|:-----:|:----:|:---------------:|:------:|
| 0.1048 | 1.05 | 500 | 0.1559 | 9.2371 |
| 0.0147 | 3.02 | 1000 | 0.1256 | 7.3212 |
| 0.004 | 4.06 | 1500 | 0.1147 | 6.7054 |
| 0.0013 | 6.03 | 2000 | 0.1188 | 6.1238 |
| 0.0011 | 7.08 | 2500 | 0.1216 | 6.1923 |
| 0.0012 | 9.05 | 3000 | 0.1234 | 5.9528 |
| 0.0007 | 11.01 | 3500 | 0.1258 | 6.0896 |
| 0.0007 | 12.06 | 4000 | 0.1263 | 6.0896 |
### Framework versions
- Transformers 4.37.2
- Pytorch 2.2.1+cu121
- Datasets 2.19.0
- Tokenizers 0.15.2
| {"language": ["multilingual"], "license": "apache-2.0", "tags": ["hf-asr-leaderboard", "generated_from_trainer"], "datasets": ["mozilla-foundation/common_voice_11_0"], "metrics": ["wer"], "base_model": "openai/whisper-small", "model-index": [{"name": "whisper small trained on 5000 en de en", "results": [{"task": {"type": "automatic-speech-recognition", "name": "Automatic Speech Recognition"}, "dataset": {"name": "xbilek25/xbilek25/train_set_5000_en_de_en", "type": "mozilla-foundation/common_voice_11_0", "args": "config: ende, split: train"}, "metrics": [{"type": "wer", "value": 6.089633937735203, "name": "Wer"}]}]}]} | xbilek25/whisper-small-train-v2.3 | null | [
"transformers",
"tensorboard",
"safetensors",
"whisper",
"automatic-speech-recognition",
"hf-asr-leaderboard",
"generated_from_trainer",
"multilingual",
"dataset:mozilla-foundation/common_voice_11_0",
"base_model:openai/whisper-small",
"license:apache-2.0",
"model-index",
"endpoints_compatible",
"region:us"
] | null | 2024-05-02T11:21:34+00:00 | [] | [
"multilingual"
] | TAGS
#transformers #tensorboard #safetensors #whisper #automatic-speech-recognition #hf-asr-leaderboard #generated_from_trainer #multilingual #dataset-mozilla-foundation/common_voice_11_0 #base_model-openai/whisper-small #license-apache-2.0 #model-index #endpoints_compatible #region-us
| basic\_train\_basic\_test 1000 similar params: per\_device\_train\_batch\_size=32, # bylo 16 a pod tim 1 gradient\_accumulation\_steps=2, warmup\_steps=300, max\_steps=3000
============================================================================================================================================================================
This model is a fine-tuned version of openai/whisper-small on the xbilek25/xbilek25/train\_set\_5000\_en\_de\_en dataset.
It achieves the following results on the evaluation set:
* Loss: 0.1263
* Wer: 6.0896
Model description
-----------------
More information needed
Intended uses & limitations
---------------------------
More information needed
Training and evaluation data
----------------------------
More information needed
Training procedure
------------------
### Training hyperparameters
The following hyperparameters were used during training:
* learning\_rate: 1e-05
* train\_batch\_size: 16
* eval\_batch\_size: 8
* seed: 42
* optimizer: Adam with betas=(0.9,0.999) and epsilon=1e-08
* lr\_scheduler\_type: linear
* lr\_scheduler\_warmup\_steps: 500
* training\_steps: 4000
* mixed\_precision\_training: Native AMP
### Training results
### Framework versions
* Transformers 4.37.2
* Pytorch 2.2.1+cu121
* Datasets 2.19.0
* Tokenizers 0.15.2
| [
"# bylo 16 a pod tim 1 gradient\\_accumulation\\_steps=2, warmup\\_steps=300, max\\_steps=3000\n============================================================================================================================================================================\n\n\nThis model is a fine-tuned version of openai/whisper-small on the xbilek25/xbilek25/train\\_set\\_5000\\_en\\_de\\_en dataset.\nIt achieves the following results on the evaluation set:\n\n\n* Loss: 0.1263\n* Wer: 6.0896\n\n\nModel description\n-----------------\n\n\nMore information needed\n\n\nIntended uses & limitations\n---------------------------\n\n\nMore information needed\n\n\nTraining and evaluation data\n----------------------------\n\n\nMore information needed\n\n\nTraining procedure\n------------------",
"### Training hyperparameters\n\n\nThe following hyperparameters were used during training:\n\n\n* learning\\_rate: 1e-05\n* train\\_batch\\_size: 16\n* eval\\_batch\\_size: 8\n* seed: 42\n* optimizer: Adam with betas=(0.9,0.999) and epsilon=1e-08\n* lr\\_scheduler\\_type: linear\n* lr\\_scheduler\\_warmup\\_steps: 500\n* training\\_steps: 4000\n* mixed\\_precision\\_training: Native AMP",
"### Training results",
"### Framework versions\n\n\n* Transformers 4.37.2\n* Pytorch 2.2.1+cu121\n* Datasets 2.19.0\n* Tokenizers 0.15.2"
] | [
"TAGS\n#transformers #tensorboard #safetensors #whisper #automatic-speech-recognition #hf-asr-leaderboard #generated_from_trainer #multilingual #dataset-mozilla-foundation/common_voice_11_0 #base_model-openai/whisper-small #license-apache-2.0 #model-index #endpoints_compatible #region-us \n",
"# bylo 16 a pod tim 1 gradient\\_accumulation\\_steps=2, warmup\\_steps=300, max\\_steps=3000\n============================================================================================================================================================================\n\n\nThis model is a fine-tuned version of openai/whisper-small on the xbilek25/xbilek25/train\\_set\\_5000\\_en\\_de\\_en dataset.\nIt achieves the following results on the evaluation set:\n\n\n* Loss: 0.1263\n* Wer: 6.0896\n\n\nModel description\n-----------------\n\n\nMore information needed\n\n\nIntended uses & limitations\n---------------------------\n\n\nMore information needed\n\n\nTraining and evaluation data\n----------------------------\n\n\nMore information needed\n\n\nTraining procedure\n------------------",
"### Training hyperparameters\n\n\nThe following hyperparameters were used during training:\n\n\n* learning\\_rate: 1e-05\n* train\\_batch\\_size: 16\n* eval\\_batch\\_size: 8\n* seed: 42\n* optimizer: Adam with betas=(0.9,0.999) and epsilon=1e-08\n* lr\\_scheduler\\_type: linear\n* lr\\_scheduler\\_warmup\\_steps: 500\n* training\\_steps: 4000\n* mixed\\_precision\\_training: Native AMP",
"### Training results",
"### Framework versions\n\n\n* Transformers 4.37.2\n* Pytorch 2.2.1+cu121\n* Datasets 2.19.0\n* Tokenizers 0.15.2"
] | [
86,
388,
126,
5,
44
] | [
"TAGS\n#transformers #tensorboard #safetensors #whisper #automatic-speech-recognition #hf-asr-leaderboard #generated_from_trainer #multilingual #dataset-mozilla-foundation/common_voice_11_0 #base_model-openai/whisper-small #license-apache-2.0 #model-index #endpoints_compatible #region-us \n# bylo 16 a pod tim 1 gradient\\_accumulation\\_steps=2, warmup\\_steps=300, max\\_steps=3000\n============================================================================================================================================================================\n\n\nThis model is a fine-tuned version of openai/whisper-small on the xbilek25/xbilek25/train\\_set\\_5000\\_en\\_de\\_en dataset.\nIt achieves the following results on the evaluation set:\n\n\n* Loss: 0.1263\n* Wer: 6.0896\n\n\nModel description\n-----------------\n\n\nMore information needed\n\n\nIntended uses & limitations\n---------------------------\n\n\nMore information needed\n\n\nTraining and evaluation data\n----------------------------\n\n\nMore information needed\n\n\nTraining procedure\n------------------### Training hyperparameters\n\n\nThe following hyperparameters were used during training:\n\n\n* learning\\_rate: 1e-05\n* train\\_batch\\_size: 16\n* eval\\_batch\\_size: 8\n* seed: 42\n* optimizer: Adam with betas=(0.9,0.999) and epsilon=1e-08\n* lr\\_scheduler\\_type: linear\n* lr\\_scheduler\\_warmup\\_steps: 500\n* training\\_steps: 4000\n* mixed\\_precision\\_training: Native AMP### Training results### Framework versions\n\n\n* Transformers 4.37.2\n* Pytorch 2.2.1+cu121\n* Datasets 2.19.0\n* Tokenizers 0.15.2"
] |
null | null | ¿Qué es Huracan impulso tabletas?
Huracan impulso Precio es una cápsula de salud masculina de primera calidad formulada para respaldar los niveles de vitalidad y energía. Su fórmula avanzada combina una mezcla sinérgica de vitaminas, minerales y extractos de hierbas, elegidos específicamente para promover una salud y un bienestar óptimos en los hombres.
Página web oficial:<a href="https://www.nutritionsee.com/huraimpuss">www.Huracanimpulso.com</a>
<p><a href="https://www.nutritionsee.com/huraimpuss"> <img src="https://www.nutritionsee.com/wp-content/uploads/2024/04/Huracan-impulso-Colombia.png" alt="enter image description here"> </a></p>
<a href="https://www.nutritionsee.com/huraimpuss">¡¡Comprar ahora!! Haga clic en el enlace a continuación para obtener más información y obtener un 50% de descuento ahora... ¡Date prisa!</a>
Página web oficial:<a href="https://www.nutritionsee.com/huraimpuss">www.Huracanimpulso.com</a> | {"license": "apache-2.0"} | Huracanimpulsotabletas/HuracanimpulsoColombia | null | [
"license:apache-2.0",
"region:us"
] | null | 2024-05-02T11:22:00+00:00 | [] | [] | TAGS
#license-apache-2.0 #region-us
| ¿Qué es Huracan impulso tabletas?
Huracan impulso Precio es una cápsula de salud masculina de primera calidad formulada para respaldar los niveles de vitalidad y energía. Su fórmula avanzada combina una mezcla sinérgica de vitaminas, minerales y extractos de hierbas, elegidos específicamente para promover una salud y un bienestar óptimos en los hombres.
Página web oficial:<a href="URL
<p><a href="URL <img src="URL alt="enter image description here"> </a></p>
<a href="URL¡¡Comprar ahora!! Haga clic en el enlace a continuación para obtener más información y obtener un 50% de descuento ahora... ¡Date prisa!</a>
Página web oficial:<a href="URL | [] | [
"TAGS\n#license-apache-2.0 #region-us \n"
] | [
13
] | [
"TAGS\n#license-apache-2.0 #region-us \n"
] |
text-classification | transformers |
# Model Trained Using AutoTrain
- Problem type: Text Classification
## Validation Metrics
loss: 0.587009608745575
f1_macro: 0.7086614173228346
f1_micro: 0.7435897435897436
f1_weighted: 0.7361195235210982
precision_macro: 0.7210665002427016
precision_micro: 0.7435897435897436
precision_weighted: 0.7538358857008742
recall_macro: 0.7157125819916518
recall_micro: 0.7435897435897436
recall_weighted: 0.7435897435897436
accuracy: 0.7435897435897436
| {"tags": ["autotrain", "text-classification"], "datasets": ["V13/autotrain-data"], "widget": [{"text": "I love AutoTrain"}]} | Zerithas/V13 | null | [
"transformers",
"tensorboard",
"safetensors",
"bert",
"text-classification",
"autotrain",
"dataset:V13/autotrain-data",
"autotrain_compatible",
"endpoints_compatible",
"region:us"
] | null | 2024-05-02T11:23:07+00:00 | [] | [] | TAGS
#transformers #tensorboard #safetensors #bert #text-classification #autotrain #dataset-V13/autotrain-data #autotrain_compatible #endpoints_compatible #region-us
|
# Model Trained Using AutoTrain
- Problem type: Text Classification
## Validation Metrics
loss: 0.587009608745575
f1_macro: 0.7086614173228346
f1_micro: 0.7435897435897436
f1_weighted: 0.7361195235210982
precision_macro: 0.7210665002427016
precision_micro: 0.7435897435897436
precision_weighted: 0.7538358857008742
recall_macro: 0.7157125819916518
recall_micro: 0.7435897435897436
recall_weighted: 0.7435897435897436
accuracy: 0.7435897435897436
| [
"# Model Trained Using AutoTrain\n\n- Problem type: Text Classification",
"## Validation Metrics\nloss: 0.587009608745575\n\nf1_macro: 0.7086614173228346\n\nf1_micro: 0.7435897435897436\n\nf1_weighted: 0.7361195235210982\n\nprecision_macro: 0.7210665002427016\n\nprecision_micro: 0.7435897435897436\n\nprecision_weighted: 0.7538358857008742\n\nrecall_macro: 0.7157125819916518\n\nrecall_micro: 0.7435897435897436\n\nrecall_weighted: 0.7435897435897436\n\naccuracy: 0.7435897435897436"
] | [
"TAGS\n#transformers #tensorboard #safetensors #bert #text-classification #autotrain #dataset-V13/autotrain-data #autotrain_compatible #endpoints_compatible #region-us \n",
"# Model Trained Using AutoTrain\n\n- Problem type: Text Classification",
"## Validation Metrics\nloss: 0.587009608745575\n\nf1_macro: 0.7086614173228346\n\nf1_micro: 0.7435897435897436\n\nf1_weighted: 0.7361195235210982\n\nprecision_macro: 0.7210665002427016\n\nprecision_micro: 0.7435897435897436\n\nprecision_weighted: 0.7538358857008742\n\nrecall_macro: 0.7157125819916518\n\nrecall_micro: 0.7435897435897436\n\nrecall_weighted: 0.7435897435897436\n\naccuracy: 0.7435897435897436"
] | [
44,
12,
179
] | [
"TAGS\n#transformers #tensorboard #safetensors #bert #text-classification #autotrain #dataset-V13/autotrain-data #autotrain_compatible #endpoints_compatible #region-us \n# Model Trained Using AutoTrain\n\n- Problem type: Text Classification## Validation Metrics\nloss: 0.587009608745575\n\nf1_macro: 0.7086614173228346\n\nf1_micro: 0.7435897435897436\n\nf1_weighted: 0.7361195235210982\n\nprecision_macro: 0.7210665002427016\n\nprecision_micro: 0.7435897435897436\n\nprecision_weighted: 0.7538358857008742\n\nrecall_macro: 0.7157125819916518\n\nrecall_micro: 0.7435897435897436\n\nrecall_weighted: 0.7435897435897436\n\naccuracy: 0.7435897435897436"
] |
null | transformers |
# Model Card for Model ID
<!-- Provide a quick summary of what the model is/does. -->
## Model Details
### Model Description
<!-- Provide a longer summary of what this model is. -->
This is the model card of a 🤗 transformers model that has been pushed on the Hub. This model card has been automatically generated.
- **Developed by:** [More Information Needed]
- **Funded by [optional]:** [More Information Needed]
- **Shared by [optional]:** [More Information Needed]
- **Model type:** [More Information Needed]
- **Language(s) (NLP):** [More Information Needed]
- **License:** [More Information Needed]
- **Finetuned from model [optional]:** [More Information Needed]
### Model Sources [optional]
<!-- Provide the basic links for the model. -->
- **Repository:** [More Information Needed]
- **Paper [optional]:** [More Information Needed]
- **Demo [optional]:** [More Information Needed]
## Uses
<!-- Address questions around how the model is intended to be used, including the foreseeable users of the model and those affected by the model. -->
### Direct Use
<!-- This section is for the model use without fine-tuning or plugging into a larger ecosystem/app. -->
[More Information Needed]
### Downstream Use [optional]
<!-- This section is for the model use when fine-tuned for a task, or when plugged into a larger ecosystem/app -->
[More Information Needed]
### Out-of-Scope Use
<!-- This section addresses misuse, malicious use, and uses that the model will not work well for. -->
[More Information Needed]
## Bias, Risks, and Limitations
<!-- This section is meant to convey both technical and sociotechnical limitations. -->
[More Information Needed]
### Recommendations
<!-- This section is meant to convey recommendations with respect to the bias, risk, and technical limitations. -->
Users (both direct and downstream) should be made aware of the risks, biases and limitations of the model. More information needed for further recommendations.
## How to Get Started with the Model
Use the code below to get started with the model.
[More Information Needed]
## Training Details
### Training Data
<!-- This should link to a Dataset Card, perhaps with a short stub of information on what the training data is all about as well as documentation related to data pre-processing or additional filtering. -->
[More Information Needed]
### Training Procedure
<!-- This relates heavily to the Technical Specifications. Content here should link to that section when it is relevant to the training procedure. -->
#### Preprocessing [optional]
[More Information Needed]
#### Training Hyperparameters
- **Training regime:** [More Information Needed] <!--fp32, fp16 mixed precision, bf16 mixed precision, bf16 non-mixed precision, fp16 non-mixed precision, fp8 mixed precision -->
#### Speeds, Sizes, Times [optional]
<!-- This section provides information about throughput, start/end time, checkpoint size if relevant, etc. -->
[More Information Needed]
## Evaluation
<!-- This section describes the evaluation protocols and provides the results. -->
### Testing Data, Factors & Metrics
#### Testing Data
<!-- This should link to a Dataset Card if possible. -->
[More Information Needed]
#### Factors
<!-- These are the things the evaluation is disaggregating by, e.g., subpopulations or domains. -->
[More Information Needed]
#### Metrics
<!-- These are the evaluation metrics being used, ideally with a description of why. -->
[More Information Needed]
### Results
[More Information Needed]
#### Summary
## Model Examination [optional]
<!-- Relevant interpretability work for the model goes here -->
[More Information Needed]
## Environmental Impact
<!-- Total emissions (in grams of CO2eq) and additional considerations, such as electricity usage, go here. Edit the suggested text below accordingly -->
Carbon emissions can be estimated using the [Machine Learning Impact calculator](https://mlco2.github.io/impact#compute) presented in [Lacoste et al. (2019)](https://arxiv.org/abs/1910.09700).
- **Hardware Type:** [More Information Needed]
- **Hours used:** [More Information Needed]
- **Cloud Provider:** [More Information Needed]
- **Compute Region:** [More Information Needed]
- **Carbon Emitted:** [More Information Needed]
## Technical Specifications [optional]
### Model Architecture and Objective
[More Information Needed]
### Compute Infrastructure
[More Information Needed]
#### Hardware
[More Information Needed]
#### Software
[More Information Needed]
## Citation [optional]
<!-- If there is a paper or blog post introducing the model, the APA and Bibtex information for that should go in this section. -->
**BibTeX:**
[More Information Needed]
**APA:**
[More Information Needed]
## Glossary [optional]
<!-- If relevant, include terms and calculations in this section that can help readers understand the model or model card. -->
[More Information Needed]
## More Information [optional]
[More Information Needed]
## Model Card Authors [optional]
[More Information Needed]
## Model Card Contact
[More Information Needed] | {"library_name": "transformers", "tags": ["unsloth"]} | roibouta/lora_model | null | [
"transformers",
"safetensors",
"unsloth",
"arxiv:1910.09700",
"endpoints_compatible",
"region:us"
] | null | 2024-05-02T11:24:10+00:00 | [
"1910.09700"
] | [] | TAGS
#transformers #safetensors #unsloth #arxiv-1910.09700 #endpoints_compatible #region-us
|
# Model Card for Model ID
## Model Details
### Model Description
This is the model card of a transformers model that has been pushed on the Hub. This model card has been automatically generated.
- Developed by:
- Funded by [optional]:
- Shared by [optional]:
- Model type:
- Language(s) (NLP):
- License:
- Finetuned from model [optional]:
### Model Sources [optional]
- Repository:
- Paper [optional]:
- Demo [optional]:
## Uses
### Direct Use
### Downstream Use [optional]
### Out-of-Scope Use
## Bias, Risks, and Limitations
### Recommendations
Users (both direct and downstream) should be made aware of the risks, biases and limitations of the model. More information needed for further recommendations.
## How to Get Started with the Model
Use the code below to get started with the model.
## Training Details
### Training Data
### Training Procedure
#### Preprocessing [optional]
#### Training Hyperparameters
- Training regime:
#### Speeds, Sizes, Times [optional]
## Evaluation
### Testing Data, Factors & Metrics
#### Testing Data
#### Factors
#### Metrics
### Results
#### Summary
## Model Examination [optional]
## Environmental Impact
Carbon emissions can be estimated using the Machine Learning Impact calculator presented in Lacoste et al. (2019).
- Hardware Type:
- Hours used:
- Cloud Provider:
- Compute Region:
- Carbon Emitted:
## Technical Specifications [optional]
### Model Architecture and Objective
### Compute Infrastructure
#### Hardware
#### Software
[optional]
BibTeX:
APA:
## Glossary [optional]
## More Information [optional]
## Model Card Authors [optional]
## Model Card Contact
| [
"# Model Card for Model ID",
"## Model Details",
"### Model Description\n\n\n\nThis is the model card of a transformers model that has been pushed on the Hub. This model card has been automatically generated.\n\n- Developed by: \n- Funded by [optional]: \n- Shared by [optional]: \n- Model type: \n- Language(s) (NLP): \n- License: \n- Finetuned from model [optional]:",
"### Model Sources [optional]\n\n\n\n- Repository: \n- Paper [optional]: \n- Demo [optional]:",
"## Uses",
"### Direct Use",
"### Downstream Use [optional]",
"### Out-of-Scope Use",
"## Bias, Risks, and Limitations",
"### Recommendations\n\n\n\nUsers (both direct and downstream) should be made aware of the risks, biases and limitations of the model. More information needed for further recommendations.",
"## How to Get Started with the Model\n\nUse the code below to get started with the model.",
"## Training Details",
"### Training Data",
"### Training Procedure",
"#### Preprocessing [optional]",
"#### Training Hyperparameters\n\n- Training regime:",
"#### Speeds, Sizes, Times [optional]",
"## Evaluation",
"### Testing Data, Factors & Metrics",
"#### Testing Data",
"#### Factors",
"#### Metrics",
"### Results",
"#### Summary",
"## Model Examination [optional]",
"## Environmental Impact\n\n\n\nCarbon emissions can be estimated using the Machine Learning Impact calculator presented in Lacoste et al. (2019).\n\n- Hardware Type: \n- Hours used: \n- Cloud Provider: \n- Compute Region: \n- Carbon Emitted:",
"## Technical Specifications [optional]",
"### Model Architecture and Objective",
"### Compute Infrastructure",
"#### Hardware",
"#### Software\n\n\n\n[optional]\n\n\n\nBibTeX:\n\n\n\nAPA:",
"## Glossary [optional]",
"## More Information [optional]",
"## Model Card Authors [optional]",
"## Model Card Contact"
] | [
"TAGS\n#transformers #safetensors #unsloth #arxiv-1910.09700 #endpoints_compatible #region-us \n",
"# Model Card for Model ID",
"## Model Details",
"### Model Description\n\n\n\nThis is the model card of a transformers model that has been pushed on the Hub. This model card has been automatically generated.\n\n- Developed by: \n- Funded by [optional]: \n- Shared by [optional]: \n- Model type: \n- Language(s) (NLP): \n- License: \n- Finetuned from model [optional]:",
"### Model Sources [optional]\n\n\n\n- Repository: \n- Paper [optional]: \n- Demo [optional]:",
"## Uses",
"### Direct Use",
"### Downstream Use [optional]",
"### Out-of-Scope Use",
"## Bias, Risks, and Limitations",
"### Recommendations\n\n\n\nUsers (both direct and downstream) should be made aware of the risks, biases and limitations of the model. More information needed for further recommendations.",
"## How to Get Started with the Model\n\nUse the code below to get started with the model.",
"## Training Details",
"### Training Data",
"### Training Procedure",
"#### Preprocessing [optional]",
"#### Training Hyperparameters\n\n- Training regime:",
"#### Speeds, Sizes, Times [optional]",
"## Evaluation",
"### Testing Data, Factors & Metrics",
"#### Testing Data",
"#### Factors",
"#### Metrics",
"### Results",
"#### Summary",
"## Model Examination [optional]",
"## Environmental Impact\n\n\n\nCarbon emissions can be estimated using the Machine Learning Impact calculator presented in Lacoste et al. (2019).\n\n- Hardware Type: \n- Hours used: \n- Cloud Provider: \n- Compute Region: \n- Carbon Emitted:",
"## Technical Specifications [optional]",
"### Model Architecture and Objective",
"### Compute Infrastructure",
"#### Hardware",
"#### Software\n\n\n\n[optional]\n\n\n\nBibTeX:\n\n\n\nAPA:",
"## Glossary [optional]",
"## More Information [optional]",
"## Model Card Authors [optional]",
"## Model Card Contact"
] | [
30,
6,
4,
75,
23,
3,
5,
8,
9,
8,
34,
20,
4,
5,
5,
11,
13,
12,
3,
10,
6,
5,
6,
4,
5,
7,
49,
7,
7,
5,
5,
15,
7,
7,
8,
5
] | [
"TAGS\n#transformers #safetensors #unsloth #arxiv-1910.09700 #endpoints_compatible #region-us \n# Model Card for Model ID## Model Details### Model Description\n\n\n\nThis is the model card of a transformers model that has been pushed on the Hub. This model card has been automatically generated.\n\n- Developed by: \n- Funded by [optional]: \n- Shared by [optional]: \n- Model type: \n- Language(s) (NLP): \n- License: \n- Finetuned from model [optional]:### Model Sources [optional]\n\n\n\n- Repository: \n- Paper [optional]: \n- Demo [optional]:## Uses### Direct Use### Downstream Use [optional]### Out-of-Scope Use## Bias, Risks, and Limitations### Recommendations\n\n\n\nUsers (both direct and downstream) should be made aware of the risks, biases and limitations of the model. More information needed for further recommendations.## How to Get Started with the Model\n\nUse the code below to get started with the model.## Training Details### Training Data### Training Procedure#### Preprocessing [optional]#### Training Hyperparameters\n\n- Training regime:#### Speeds, Sizes, Times [optional]## Evaluation### Testing Data, Factors & Metrics#### Testing Data#### Factors#### Metrics### Results#### Summary## Model Examination [optional]## Environmental Impact\n\n\n\nCarbon emissions can be estimated using the Machine Learning Impact calculator presented in Lacoste et al. (2019).\n\n- Hardware Type: \n- Hours used: \n- Cloud Provider: \n- Compute Region: \n- Carbon Emitted:## Technical Specifications [optional]### Model Architecture and Objective### Compute Infrastructure#### Hardware#### Software\n\n\n\n[optional]\n\n\n\nBibTeX:\n\n\n\nAPA:## Glossary [optional]## More Information [optional]## Model Card Authors [optional]## Model Card Contact"
] |
text-classification | setfit |
# SetFit with BAAI/bge-small-en-v1.5
This is a [SetFit](https://github.com/huggingface/setfit) model that can be used for Text Classification. This SetFit model uses [BAAI/bge-small-en-v1.5](https://huggingface.co/BAAI/bge-small-en-v1.5) as the Sentence Transformer embedding model. A [LogisticRegression](https://scikit-learn.org/stable/modules/generated/sklearn.linear_model.LogisticRegression.html) instance is used for classification.
The model has been trained using an efficient few-shot learning technique that involves:
1. Fine-tuning a [Sentence Transformer](https://www.sbert.net) with contrastive learning.
2. Training a classification head with features from the fine-tuned Sentence Transformer.
## Model Details
### Model Description
- **Model Type:** SetFit
- **Sentence Transformer body:** [BAAI/bge-small-en-v1.5](https://huggingface.co/BAAI/bge-small-en-v1.5)
- **Classification head:** a [LogisticRegression](https://scikit-learn.org/stable/modules/generated/sklearn.linear_model.LogisticRegression.html) instance
- **Maximum Sequence Length:** 512 tokens
- **Number of Classes:** 4 classes
<!-- - **Training Dataset:** [Unknown](https://huggingface.co/datasets/unknown) -->
<!-- - **Language:** Unknown -->
<!-- - **License:** Unknown -->
### Model Sources
- **Repository:** [SetFit on GitHub](https://github.com/huggingface/setfit)
- **Paper:** [Efficient Few-Shot Learning Without Prompts](https://arxiv.org/abs/2209.11055)
- **Blogpost:** [SetFit: Efficient Few-Shot Learning Without Prompts](https://huggingface.co/blog/setfit)
### Model Labels
| Label | Examples |
|:------|:---------------------------------------------------------------------------------------------------------------------------------------------------------------------------------------------------------------------------------------------------------------------------------------------------------------------------------------------------------------------------------------------------------------------------------------------------------------------------------------------------------------------------------------------------------------------------------------------------------------------------------------------------------------------------------------------------------------------------------------------------------------------------------------------------------------------------------------------------------------------------------------------------------------------------------------------------------------------------------------------------------------------------------------------------------------------------------------------------------------------------------------------------------------------------------------------------------------------------------------------------------------------------------------------------------------------------------------------------------------------------------------------------------------------------------------------------------------------------------------------------------------------------------------------------------------------------------------------------------------------------------------------------------------------------------------------------------------------------------------------------------------------------------------------------------------------------------------------------------------------------------------------------------------------------------------------------------------------------------------------------------------------------------------------------------------------------------------------------------------------------------------------------------------------------------------------------------------------------------------------------------------------------------------------------------------------------------------------------------------------------------------------------------------------------------------------------------------------------------------------------------------------------------------------------------------------------------------------------------------------------------------------------------------------------------------------------------------------------------------------------------------------------------------------------------------------------------------------------------------------------------------------------------------------------------------------------------------------------------------------------------------------------------------------------------------------------------------------------------------------------------------------------------------------------------------------------------------------------------------------------------------------------------------------------------------------------------------------------------------------------------------------------------------------------------------------------------------------------------------------------------------------------------------------------------------------------------------------------------------------------------------------------------------------------------------------------------------------------------------------------------------------------------------------------------------------------------------------------------------------------------------------------------------------------------------------------------------------------------------------------------------------------------------------------------------------------------------------------------------------------------------------------------------------------------------------------------------------------------------------------------------------------------------------------------------------------------------------------------------------------------------------------------------------------------------------------------------------------------------------------------------------------------------------------------------------------------------------------------------------------------------------------------------------------------------------------------------------------------------------------------------------------------------------------------------------------------------------------------------------------------------------------------------------------------------------------------------------------------------------------------------------------------------------------------------------------------------------------------------------------------------------------------------------------------------------------------------------------------------------------------------------------------------------------------------------------------------------------------------------------------------------------------------------------------------------------------------------------------------------------------------------------------------------------------------------------------------------------------------------------------------------------------------------------------------------------------------------------------------------------------------------------------------------------------------------------------------------------------------------------------------------------------------------------------------------------------------------------------------------------------------------------------------------------------------------------------------------------------------------------------------------------------------------------------------------------------------------------------------------------------------------------------------------------------------------------------------------------------------------------------------------------------------------------------------------------------------------------------------------------------------------------------------------------------------------------------------------------------------------------------------------------------------------------------------------------------------------------------------------------------------------------------------------------------------------------------------------------------------------------------------------------------------------------------------------------------------------------------------------------------------------------------------------------------------------------------------------------------------------------------------------------------------------------------------------------------------------------------------------------------------------------------------------------------------------------------------------------------------------------------------------------------------------------------------------------------------------------------------------------------------------------------------------------------------------------------------------------------------------------------------------------------------------------------------------------------------------------------------------------------------------------------------------------------------------------------------------------------------------------------------------------------------------------------------------------------------------------------------------------------------------------------------------------------------------------------------------------------------------------------------------------------------------------------------------------------------------------------------------------------------------------------------------------------------------------------------------------------------------------------------------------------------------------------------------------------------------------------------------------------------------------------------------------------------------------------------------------------------------------------------------------------------------------------------------------------------------------------------------------------------------------------------------------------------------------------------------------------------------------------------------------------------------------------------------------------------------------------------------------------------------------------------------------------------------------------------------------------------------------------------------------------------------------------------------------------------------------------------------------------------------------------------------------------------------------------------------------------------------------------------------------------------------------------------------------------------------------------------------------------------------------------------------------------------------------------------------------------------------------------------------|
| 3 | <ul><li>' \n\n \n\nHINDALCO INDUSTRIES LTE...\nHIRARUD POWER\n\np lGEn-T2 Te- 31-J\n\n \n \n \n\nUbATE OTIM\n\n \n\n \n\n28-21-2022\n\n \n\nao e301\n\n \n\n \n\n1 ints MG}\n\n \n\n \n \n\n \n\n \n\ntute\n\n \n\n \n\n \n\nrey\n\n \n \n \n \n\n \n\n \n\n4\n\x0c'</li><li>'HINDALCO INDUSTRIES LTD.,\n\n \n\nJ HIRAKUD POWER\nASH WEIGH ERIDGE PRINTING DATE : 09-01-2021\nASH FROM :- 01-Nov-20 To - 30-Nov-20 PAGENUMBER: 1\nSLNO TRENG LDATE” PARTY "ODATE OTIME INDATE INTIME TARE GROSS HET” We OLOAD SLD OpERATOR\n\n \n\n \n\nKAY KAY BRI\n. Ipce,\n11-20-724895 ORQIN4428 24-11-2020 IDC(B) —-M-11-2020 10:10 4-11-2020 09:08 10.65 27.91 «17.26 19.00 000 6 N.PANDA\n11-20-724979 « OROON4428 4-11-2020 IDC(B) «4-11-2030 19:34 24-11-2020 17:25 10.65 27.04 16.39 19.00 «8G 64) ON. PANDA\n11-20-725215 ODISF8190 26-11-2020 IDC(B) 26-11-2020.0748 36-11-2020 O1-01 14.12 33.95 19.83 1998 «= 00 6 ITU\naie 11-20-725261 ODISG7595. 26-11-2028 IDC(B) 26-11-2020 12:05 26-11-2020 10-48 11.39 27.38 ««15.99 1760 000 2 N.PANDA\n11-20-725376 OD15G7595 27-11-2020 IDCCB) 27-11-2020. 04:20 27-11-2020 03:22 11.36 © 30.05 «18.69 1760 «1869 § = ~ITU\n11-20-725445 OROINE428 27-11-2020 IDCCB) 77-11-2020 16:39 27-11-2020 1:55 10.58 27.33 16.75 19.00 «8.00 6 N.PANDA\n11-20-725559 — OD15G7595_ 28-11-2020 JDC(B) 28-11-2020 10:08 28-11-2020 08:39 1148 28.88 1742 1760 600 2 N.PANDA\nLi 31-20-725591 ODISFS190 28-11-2020 DCB) 98-11-2020 1:15 28-11-2020 10:21 14.31 36.77) 2.96 19.96 21.96 2 N. PANDA\n11-20-725866 ODISG7595 29-11-2020 JDC(B) 29-11-2020 23:21 29-11-2020 21:09 11.25 77.60 16.35 1760 900 2 N.PANDA\nPARTY TRIPS- 9 77 26641 160.64 167.31 40.65\nAREATRIPS- 9 266.41 160.64 167.31\n\n \n\n \n\n \n\n \n\x0c'</li><li>' \n\n" G1-20-679262 ODITF4129 26-01-2000 AK.S 26-01-2000 16:18 6-01-2000 1448 1038 2858 «1918 14.88 1818\n\n. 10-19-665660 ODI7F4129 30-10-2019 AK.S 31-10-2019 00:3\n\nHINDALCO INDUSTRIES LTD.,\nHIRAKUD POWER\n\nPRINTING DATE : 21-03-2020\nFROM :- 03-Jul-19 To - 31-Jan-20 PAGENUMBER . 1\n\n \n\n \n\nLDATE PARTY ODATE OTIME INDATE INTIME TARE\n\n \n\nSHREESAI BRK &EL\nAKS\n\nG1-20-676454 «= GDI7F4229 (05-01-2000) AKS 06-01-2020 00:42\n01-20-676764 «= ODI7F4229 08-01-2020 AK.S 08-01-2020 19:18\nG1-20-677590 ODITF4229 15-01-WAKS 15-01-2020 0511 15-91-\nO1-20-677704 = ODITF4129 15-01-2020 ARS 15-01-2020 23:42 15-01-2020 19:22 10.67 27.99 17.32 14.88) 17.33\nGi -20-67784- ODITF4129 16-01-2020 ARS = 17-01-2020 01:00 16-01-2020 23:19 1065 2882 1517 14.88 15.17\nO1-20-678816 ODITF4i29 24-01-2020 ARS = 4-01-2020 02:46 4-01-2020 01:43 10.23 28.56 15.53 14.88 = 1533\n\n \n\n10.89 77.04 16.15 14.88 | 1815\n10.90 36.63) 15.73 14.88 15.73\n\nN. PANDA\nSS.SARANGI\nPANDA\n\n. PANDA\n\n. PANDA\nPANDA 1\n- PANDA\n\n- PANDA\nSS.SARANGI\nWN. PANDA\nSITU\n\nJITU\n\nJiTy\n\n08-01-2020 18:33\n2020 04:34 10.69) 7768 16.97 14.88 16.97\n\n \n\nBABA ze\n\n \n\n5\n2\n6\n6\n6\ne\n8\nO7-19-645824 OD17F4229 04-07-2019 AK.S 04-07-2019 08:15 04-07-2019 07-45 10.30 25.23 1493 14.68 14.93 8\n7-19-646881 ODITF4129 1-07-2019 ARS — 11-07-201902:58 11-07-2019 62:28 10.80 26.15 «1535 1488 1535 8\n07-19-649182 ODITF4129 4-07-2019 AK.S 24-07-2019 18:41 4-07-2019 18:14 10.53 25.36 14.83 14.88 ©6000 5\nO7-19-649510 ODITF4229 26-07-2019 AK.S 26-07-2019 1315 36-07-2019 1212 1032-467 14.35 14.88.00 2\nO7-19-650166 ODIIF4129 31-07-2019 AK.S 31-07-2019 08:09 31-07-2019 07:36 1048 96.85 1641 1488 84) 8\nO7-19-630193 | ODITF4229 31-07-2019 AK.S = 31-07-2019 11:06 31-07-2019 10:39 10.23 480 1457 1488 «600 8\n08-19-651551 ODIFF4129 07-08-2019 AK.S 7-08-2019 13:40 07-08-2019 13:00 10.39 25.99 1560 1488 1560 2 N. PANDA\n08-19-651907 ODITF4229 09-08-2018 AK.S 09-08-2019 16:34 09-08-2019 15:24 10.62, 25.26 146d 1488 860.00 2 SITU\n68-19-654918 QDI7F4129 75-08-2019 AK.S 25-08-2019 1848 25-08-2019 18:27 10.96 M47 1351 1488° O00 5 JITU .\n08-19-655973 ODITF4129 31-08-2019 AK.S 31-08-2019 09:28 31-08-2019 07-51 1082 4.77 1395 1488 000 8 JITU fe\n09-19-659591 ODI7F4129 22-09-2019 AK.S 23-09-2019 12.31 22-09-2019 11:58 1034 25.52 15.18 1488 1518 6 N.PANDA\n09-19-660295 ODIT7F4229 27-09-2019 AK.S 27-09-2019 05:16 27-09-2019 447 11.69 26.25 14.56 14.88 0.00 5 RUPESH\n10-19-661801 ODI7F4229 05-10-2019 AK.S 05-10-2019 19:50 05-19-2019 19:29 1040 2658 1618 1488 1618 6 . PANDA\n10-19-661842 ODI7F4129 06-10-2019 AHS 06-10-2019 07:30 06-10-2019 05:39 1049 2563 1514 488 1514 8 PANDA\n10-19-663006 © GDI7F4129 13-10-2019 AK.S 14-10-2019 00:26 13-10-2019 23:22 10.61 26.36 15.78 1488 1575 § PANDA\n10-19-663007 ODI7F4229 13-10-2019 AK.S 14-10-2019 00:37 13-10-2019 23:25 10.64 2780 1716 4.88 1716 8 - PANDA\n10-19-663413 ODITF4i29 16-10-2019 AK.S 16-10-2019 G4: 4 16-10-2019 03:43 10.64 29.08 1844 14.88 «1844 6 . PANDA\n10-19-663414 ODI7F4229 16-10-2019 ARS — 16-10-2019 04:33 14-10-2019 0346 1053 26.52 15.99 14.88 1599 6 - PANDA\n2 - PANDA\n5 - PANDA\n8 N.PANDA\n2 SS.SARANGI\n§ SS SARANGI\n5 NTU\n\n30-10-2019 22:15 10.55 26.95 15.70 14.88 = 15.70\n11-19-665836 ODI7F4129 61-11-2019 AK.S — O1-11-201904:53 01-11-2019 02:33 1068 34.05 15.37 1498 0.00\n11-19-668335 ODITF4129 15-11-2019 AK.S 15-11-2019 00:51 15-11-2019 00:17 10.84 «5.54 14.70 14.88 0.00\n11-19-668505 ODI7F4229 15-11-2019 ARS 15-11-2019 20:43 15-11-2019 1946 1049 440 13.91 14.98 © 0.00\n11-19-668742 ODITF4229 17-11-2019 AK.S 17-11-2019 0243 17-11-2019 01:37 10.73 6.18 15.45 14.88 15.45\n11-19-668883 ODI7F4229 16-11-2019 AK.S —18-11-201903:21 18-11-2019 02:51 10.60 26.43 15.83 14.98 15.83\n1-19-669818 CDIFF4229 23-11-2019 AHS — 23-11-2019 02:41 23-11-2019 01:53 1066 23.61 12.95 1488 0.00 5\n11-19-670013 ODIFF4229 23-11-2019 AK.S — 93-11-2019.23:45 23-11-2019 22:59 1054 2530 1476 1488 0.00 §\n11-19-670586 = ODI7F4229 27-11-2019 AK.S = 27-11-2019 03: QF11-2019 0245 1036 25.99 15.63 14.88 15.63 8\n14-19-670698 ODI7F4229 27-11-2019 AK.S 27-11-2019 16:35 77-11-2019 15:50 1018 98 1480 1498 000 6\n11-19-670702 ODITF4129 27-11-2019 AK.S 27-11-2019 1647 77-11-2019 16:19 1050 34.75 14.25 1488 «0000 8 SITU\n12-19-671669 ODI7E4129 03-12-2019 AK.S 03-12-2019 .01:25 03-12-2019 00:47 10.60 2513 1453 1488 000 6 N. PANDA\n13-19-671860 ODI7F4229 04-12-2019 AK\'S 04-12-2019 04:08 4-12-2019 03:26 10.64 26.09 1545 14.88 15. 45 6 ITU\n§\n&\n9\na}\n2\n3\n\n \n\nAAmABAAAAt we\n\n33.SARANGI\nSS.SARANGI\nWN. PANDA\nJitu\n\n \n \n\n \n\n12-19-672043 ODI7F4229 05-12-2019 AK.S 05-12-2019 03:49 05-12-2619 02:50 10.56 28.65 18.09 1488 18.99 JITU =\n12-19-672854 ODIFF4129 10-12-2019 AK.S — 10-12-2019 03:38 10-12-2019 01:37 10.27 26.32 1605 1488 16.05 N. BANDA\n12-19-672858 ODI7F4229 10-12-2019 AK.S 10-12-2019 03:1] 10-12-2019 01:59 10.71 98.32 17.61 14.88 1761 N. PANDA\n12-19-673533 ODI7F4229 15-12-2019 AK.S 15-12-2019 04:31 15-12-2019 02:46 10.22 27.05 16.83 14.88 16.83 N. PANDA\n\n. 12-19-675418 ODIFF42I9 28-12-2019 AK.S 28-12-2019 21:24 28-19-2019 20:35 10.54 2717 16.63 14.88 (16.63 SS.SARANGI\n12-19-675733 ODIVF4129 31-12-2019 AKS 31-12-2019 12:30 31-19-2019 10:09 10.62 26.56 1594 14.88 15.94 SITU\nPARTY TRIBS - 44 405.49 1149.36 683.87 654.50 470.19\nAREATRIPS- 44 465.49 1149.36 683.87, 654-50 470.19\n\x0c'</li></ul> |
| 0 | <ul><li>', TAX INVOICE\n\n8looin Combustion India Pvt Ltd\nv-44\' Near Quality Circie\nA160 C Bhoecurt\n\nPraerr 17 F026\nSITUINOUIN 2 AADCBI ibe les\nate Name Moharashla, Gode 27\n\nCIM US89U-:Mm2007PTC172730\n\nvoniacl 920-60 1242 10, 9850754646\n\nE-Maut OPatekar@bloomeng com\n\nCons.nce (Ship to)\n\nHindatco Industries Limited (Odisha)\nHirakuo Smetter, Hlrakud - 768016, Sambalpur\nOdisha\nGSTIN/UIN\nPANIIT Ne\nStale Name\ntis eo (Lallaoe\nHindalco tndustrtes Limited (Odisha)\n\nHirakud Smelter Hirakud - 768016, Samboilpur\nOdssha\n\n21A4AACH1201R1ZZ7\nAAACH1201R\nOdisha, Code 21\n\nGSTIAN‘UIN 21AAACH1201R1Z2Z\nPAN TI No AAATCH1201R\n\nSte Name Odisha Code .\'1\nyee Al sappy Odisha\n\n \n\nDesrnotion of\n‘s Goods and Senices\n\n3h\n\n- Bumer Spares (Mtr)\n\n2On Poe OS Wire igncian Qia-1 Smm2\n2 Surner Spares\n\n47 4762R0005, Gas Cycle Valve\n= Bummer Spares\n\not 122050118 Pressure Connector 1°\n\nPACKING & FORWARDING\n(GST Output @ 18%\n\nTotal\nTerms & Cenditons\n\nAmcunt Chargeable in words}\n\nINR Three Lakh Fifty Thousand Two Hundred Fifty Three\nand Sixty Two passe Only\n\n‘ops, SPAN AADCB1916E\nsti 9\nNe der urs that tenon ice snes he at tua price of ine\n\nJods ueser bed end tna! da" garticu ars are ‘vue ard coi-eci\n\n22\\0292V2\n\n(DUPLICATE FOR TRANSPORTER)\n\n\'nvoice NO\n22-23/2595/M123\nDeltvery Note\n\nReference No & Date\nABW ATA tor\n\nfh\n\nDated\n\n31-Oct-22\nMode/Terms of Payment\n15 DAYS\n\nOther References\n\nBuyer\'s Order No Dated\n13602313947 die. 17 082022, 1-Oct-22\nDispatch Doc No : Delvary Notes Date\nDispatched through Destination\nVessal/Flight No Place of receipt by shipper\nCity/Port of Laadmng City/Port of Discharge\nTerms of Delvery\n15 NOV 2022\nHSNY/SAC Quant ty Rate per Amount\n64769000 100.00 MT B3250 MT 83,250.00\n84169000 10NOS 13,96750 NOS 1,39,675.09\n84169000 2NOS 24,040.00 NOS 63,080.00\n2.91 005 00\n938549 2 % 5,820.10\n18 % 53,428 52\n3,50,253.62\nE&OE\n\nConipany s Bank Deta\'\'s\n\nBan Name\n\nAic No\n\nBranch & \\FS Code\nfor\n\nThus is a Computer Generateu Invaic2\n\n4010 ICICi Bank CA AIC No 215205003976\n215205003076\nBhosari & ICICO0021\n\n \n\nBloom\n\n \n\x0c'</li><li>"b'Invoice\\n\\nNASFUND LTD Invoice date\\nP. O Box 5791 2023-07-14\\nBoroko\\n\\nDue date\\n\\nNational Capital District\\n\\nPapua New Guinea 2028-07-14\\n\\n \\n\\npropertypng....\\n\\nProperty PNG Limited 01062023 (2023-06-08) (2023-06-13) (2023-06-21)\\n\\nP.O. Box 1067\\nBOROKO, NCD\\n\\nPapua New Guinea Cee\\n\\nou\\n\\n \\n\\n \\n\\n \\n\\n \\n\\n \\n\\nInvoice number COGExernpt BY. ey (\\nAttention: Mr. Fiona Saascee COI 1-72109 TIN 501080230 Cae : | , ] ~~\\nNelson ab 0+ {123\\nQuote number\\n2023016\\nValuation - NASFUND Ltd properties\\nQty Unit price Total\\n1 10,000.00 10,000.00\\n1 10,000.00 10,000.00\\n1 10,000.00 10,000.00\\nCe\\nTotal 30,000.00\\n\\nHow to Pay:\\n\\nDirect Deposit;\\n\\nBank: Westpac Bank PNG Limited - Boroko Branch\\nBSB Number: 038006\\n\\nAccount Name: Property PNG Limited\\n\\nAccount Number: 600 361 6070\\n\\n \\n\\n \\n\\n \\n\\x0c'"</li><li>'GST Tax Invoice\n\ni. R. Technology Services Pvt. Ltd. Invoice Numbe: ORIGINAL FOR RECIPIE!\nEBSITE: www.irtech.in E Mail: service_cal@irtech. Date of Invoice : 16/05/2018 Your Ref. No. : P/PO/SRV/1819/0029 DT.\[email protected] PHONE: (033) 40361800 FAX: Due Date : 16/05/2018 12.04.2018\n91-33-40361888 ECOSPACE Business Park,Block-48, 9th Payment Terms: As Per Work Order\nFloor,Unit - ESNT 4B 0903, New Town, Rajarhat, Kolkata\n1700156\n\n \n\n \n\n \n\n \n\n[State - WEST BENGAL, State Code - 19\niSTIN Number of Supplier - 19AAACI1256K1ZI\nIPAN No. - AAACI1256K CIN No. -\n\n1U74220MH1977PTCO19758\nDetails of Receiver (Billed to) - KC235 - Hindalco Industries Limited. Hirakud Details of Consignee (Shipped to) - KC235 = Hindalco Industries Limited. Hirakud\nHirakud Power, Hirakud Hirakud Power,, Hirakud,\nDist-Sambalpur - 768016 Dist-Sambalpur - 768016\nState - ODISHA, State Code - 21 State - ODISHA, State Code - 21\n\nPlace of Supply - ODISHA\n|GSTIN - 21AAACH1201R1ZZ\nContact Id - Mr. NC Sahu\nTel -,\n\nDepartment -\n\nTotal Discount Sy\nfereepeeete mS " an\n\n1 [100% AMC CHARGES FOR YOUR 03 NOS. LECO INSTRUMENTS 998719 208,447.50 208,477: ie 37,525.95)\nIMODELAC-500, AC-350 AND TGA-701. AMC PERIOD FROM 01.04.18 TO\n31.03.2018. BILLING PERIOD FROM 01.04.2018 TO 31.03.2019 FOR ONE\n\n[208,447.50] 447: sol |___ 208,477.59 477:\n\nInvoice Total(in Words) : Rupees Two Lakhs Forty Six Thousand Three And Forty a Paisa Only = = Fig |__*af 246,003.45\nAdvance Paid (In Words) : Rupees Zero Only ‘Advance Paid (In Fig) Ce\n\n \n\n \n\n \n\n \n\n \n\n \n\n \n\njet Amount Due (In Words) : Rupees Two Lakhs Forty Six Thousand Three And Forty Five Paisa Only ‘Net Amount Due (In Fie] «INR 246,003.44\nRemarks : 100% ADVANCE PAYMENT Based On Sales Orders340017. Sy\nDeclaration : A\n‘lectronics Reference Number Signature : noo + ho\nRN Date IName of Signatory : oo Gh Cow\n\nPage 1 of1\n\x0c'</li></ul> |
| 1 | <ul><li>"GSTIN: 21AAACH1201R1ZZ\nHINDALCO INDUSTRIES LIMITED ou\n\n \n\n \n\n \n\n \n\n \n\n \n\n \n\n \n\n \n\n \n\n \n\n- HIRAKUD POWER, HIRAKUD-768 016,DIST.:SAMBALPUR (ODISHA) GST Rangeltivision: Sambalpur\n= PHONE: 0663-2481965, FAX: 0663-2481342 GST Commissionerate -Cuttack\n~~ PURCHASE ORDER\n‘Vendor Code: S065 P.O/No: =P/PO/SRV/1819/0759 pee Zeon\nMis SK. MANSUR ALI ee\n‘Order Type: PURCHASE ORDER:\nNEAR GURUDWARA PADA Effective From 01/01/2019 To 31/12/2019\nHIRAKUD Price Basis :\n‘Transportation arrangement :\n‘SAMBALPUR, ORISSA, IN 768016 lee ens oar\nEmail: sk.mansur.ai@[email protected] Gamer :\nFax: () Currency 2 INR\nContact: MANSOOR ALi () 9776531785 Hindalco Contact Person : AMARESH MISHRA,\n+ GSTIN:21ALGPASG7SE1ZJ —State:21 - Odisha Email of Contact Person: [email protected]\n(Order Unit of Value\n‘Quantity Measurement (Rs)\n330.000 MDY 1098170.70\n98835.36\n98835.96\na qaese4t.42\nYramentTem: iSDesGem 8 : GRAND TOTAL: 1295841.42\nBiling Address: Hindalco Industries Ltd, Hirakud Power Hiekus - 76804 coats mercer Rene\n{Hard copy of bills to be forwarded at shipping address]\nShipping address : Hindalco Industries Ltd, Hirakud Power, Hirakud ~ *T65016, Sombalpur (ODISHA)\n_ Noles:\n4. THE ABOVE PRICE IS BASED ON ATTACHED LTS CALCULATION.\n2. AS PER LTS, WAGES WILL BE PAID FOR NATIONAL HOLIDAYS & FESTIVAL INCASE A LABOUR IS REQUIRED TO PERFORM\nHIS HOB ON A HOLIDAY MENTIONED ‘AS PER LAW THE CALCULATION FOR THAT DAY WILL BE APPLICABLE OVER AND ABOVE THE WAGES\n\nHE IS BEING PAID (BASIC+ DA X 2+ 1 HRA+ 1 DUST ALLOWANCE + 1 CANTEEN ALLOWANCE). CONTRACTOR PROFIT WILL BE @9.5% & FIX MARGIN.\n3, SHIFT ALLOWANCE WILL BE PAID EXTRA AS PER LTS.\n\n4. PRO-RATA DEDUCTION WILL BE MADE FOR ABSENTISM AND DETERIORATION IN QUALITY OF SERVICE AS DECIDED BY THE AUTHORITY.\n\n5. PAYMENT ON MONTHLY BASIS WILL BE RELEASED WITHIN 15 DAYS OF SUBMISSION OF INVOICE.\n\n6. 10% OF TOTAL BILL VALUE WILL BE RETAINED BY THE COMPANY EVERY MONTH AND WiLL BE RELEASED ANNUALLY ONLY AFTER PAYING\nBONUS & OTHER DUES TO THE LABOURERS ENGAGED BY YOU.\n\n7. IT 1S MANDATORY TO PUT GST NUMBER IN THE INVOICE.\n\nOTHER TERMS :-\n1. JOB SHALL BE EXECUTED AS PER THE SCHEDULE ISSUED BY THE DEPARTMENT ( TO BE COLLECTED IN ADVANCE).\n2. YOU / CONTRACTOR'S SUPERVISOR SHALL MEET THE DEPARTMENTAL SUPERVISOR AT REGULAR INTERVAL DECIDED BY THE DEPARTMENT TO\n\nCONFIRM CHANGE IN SCHEDULE, IF ANY.\n\n3. YOU SHALL SUBMIT THE DAILY REPORT AFTER COMPLETION OF JOS IN THE PRESCRIBED FORMAT AS DIRECTED BY THE DEPARTMENT.\n\n4, NO EXTRA CLAIM TO BE MADE FOR CHANGE IN SCHEDULE FOR SIMILAR TYPE OF JOB.\n\n§. REFUSAL OF ANY JOB SIMILAR TO THE JOB ENLISTED IN THE ORDER OR FAILURE TO EXECUTE THE JOB AS PER SCHEDULE WILL BE LIAGLE FOR\n\nAND SHALL KEEP THE SUPPORTING DOCUMENT WHICH WILL BE VERIFIED BY HR FROM TIME TO TIME.\n\n8. CLEANING OF AREAS WITHOUT CONSUMABLE MATERIAL WILL BE TREATED AS NO CLEANING AND AMOUNT RELATED TO THAT JOB WILL BE\n* DEDUCTED FOR THAT PARTICULAR OCCASION.\n\n9. MANPOWER SHOULD BE DEPLOYED AS PER REQUIREMENT OF THE MANAGEMENT.\n\n~ NOTE:\n4.NH & PH WILL BE APPICABLE AS PER THE LTS LAW AND PF,ESIC.BONUS, CONTRACTOR PROFIT (9.5%) WILL ATTRACT.\n\nRegd. Office:\nHINDALCO INDUSTRIES LIMITED Page 1 of 2\nAhura Centre, 1st Floor, ' Wing, Mahskali Caves Road Andhori(East), Mumbai 400033, In India,\nTel No: 91 22 6691 7000, Email: [email protected], Website: www.adityabiria.com\n\nIdentity No: L27020MH1958PLC011238\n\n4\\ o\n\x0c"</li><li>')\n\nHINDALCO INDUSTRIES LIMITED\n\nHIRAKUD POWER, HIRAKUD-768 016,DIST :SAMBALPUR (ODISHA)\n|) PHONE: 0663-2481365, FAX: 0663-2481342\n\nPURCHASE ORDER\n\n \n\nGSTIN: 21AAACH1201R1ZZ,\nState Code: 21 - Odisha\n\nGST Rangeldivision:\nSambalpur\nGST Commissionerate -Cuttack\n\nVendor Code: us42 PO/No: PIPO/SRVI2122/0846 Date: 12-JAN-2022\n\nMs UNITED ECO CARE CONSULTANCY PVT LTD. Revise No:\n\nDate’\n\nOrder Type: PURCHASE ORDER\n\nFLAT NO - 006, PRASANTI APARTMENT.\nDEULSAHI, TULSIPUR\n\n \n\n \n\nEffective From 12/01/2022 To 31/12/2022\n\n \n\n \n\nPrice Basis\n“curTack, 7 Transportation arrangement\nSUTVADEL COREA e008 Ship to Location HIRAKUD - POWER\nEmail: [email protected] Carer é\nFax: (09397712700 Currency INR\nContact: Manikial Ghosh () 09337312700 Hindalco Contact Person: AMARESH MISHRA,\nGSTIN:21AAACU9687L1ZS —State:21 - Odisha Email of Contact Person: [email protected]\nOrder Unit of Rate/Unit Value\nSl, Stock No. & Description Quantity Measurement Rs.) (Rs)\n8 811418040116 HSNISAC: 5,000 NOS. 500.00 2500.00\nPROVIDE: THIRD PARTY INSPECTION; TYPE: FACILITY; METHOD: LOAD TEST, CCST Tax@o% 225.00\nSCOPE: INSPECTION AND TESTING OF OF CHAIN BLOCK ABOVE 3T AND UPTO. . et\n8T, TOOLS PROVIDED BY: HIL, MATERIAL PROVIDE BY: HiL, LOCATION: SGST Tax@o% 22\nHIRAKUD.\nCC Code Quantity trem Total: 2950.00\n5,000 Need By: 31-DEC-2022\n9 811418040117 HSNISAc: 25.000 NOS 304.00 7600.00\nPROVIDE: THIRD PARTY INSPECTION; TYPE: FACILITY; METHOD: LOAD TEST, COST Tax@o% 684.00\n‘SCOPE: LOAD TEST OF CHAIN BLOCK UPTO 3T, TOOLS PROVIDED BY: Hil, tt sand\nMATERIAL PROVIDE BY: HIL, LOCATION: HIRAKUD SGST Tax@o%\nCC Code Quantity\n25.000 Need By 31-DEC-2022 Hem Totat SO88:00\nPayment Terms: 30 Days Credit GRAND TOTAL: 62994.30\n\nBilling Address: Hindalco Industries Ltd, Hirakud Power, Hirakud - 768016, Sambalpur (ODISHA)\n(Hard copy of bills to be forwarded at shipping addi\n\n)\n) Shipping address : Hindalco Industries Ltd, Hirakud Power, Hirakud - 768016, Sambalpur (ODISHA)\n\nNotes:\n\n“You wil be raising valid Tax invoice as per GST Laws for the supply covered by the instant\nPO and comply with all the GST rules and regulations as notified and/or to be notified in future\nincluding fing of retum , payment of taxes etc. Failure to comply with any of the provisions of\nGST Laws will lead fo cancellation of this order and / or subject to any other action as\nmanagement will deem fit."\n\nRegd. Office:\n\nHINDALCO INDUSTRIES LIMITED\nAhura Centre, 1st Floor, 8 Wing, Mahakali Caves Road Andheri(East), Mumbai 400093, india,\nTel No: 91 22 6691 7000, Email: [email protected], Website: www-adityabirla.com\nCorporate Identity No: L27020MH158PLC011238\n\nCHANDRA MAKTHALA,\nPlant Materials Head\nHindalco Industries Limited\n\nHirakud\n\nPage 3 of 15\n\x0c'</li><li>' \n\n \n\nGSTIN: 21AAACH1201R1ZZ\nDUSTRIES LIMITED\nHINDALCO IN eee .\nHIRAKUD POWER, HIRAKUD-768 016.DIST.SAMBALPUR (ODISHA) GST Rangeldivision: Sambelpur\nPHONE: 0663-2481365, FAX: 0663-2481342 GST Commissionerate -Cuttack\nPURCHASE ORDER\n‘AMENOMENT Z\nVendor Code: J123 P.O/No: P/PO/SRV/1920/1161 Date: 27-MAR-2020\nMis JAIDURGA CONSTRUCTION Rete ee Dater04-MAY-2020\n‘Order Type: PURCHASE ORDER\nBUDHAKATA, Effective From 01/03/2020 To 31/03/2021\nGADMUNDA Price Basis\nHIRAKUD i a ;\nMB, ISSA, 768011 ransportation arrangement\nSEA PUR OR SSN NOR roomie Ship to Location HIRAKUD - POWER\nEmail: [email protected] Carrier\nFax:() Currency 2 INR\nContact: DILIP PRADHAN () 9438452293 Hindalco Contact Person: SIDDHARTH KUNDA,\nGSTIN: 21AACFJ4294P122 —_State:21- Odisha Email of Contact Person: [email protected]\nRef: ASH TRANSPORTATION TO VARIOUS BRICKS MANUFACTURING PLANT\nOrder Unit of Rate/Unit Value\nSl Stock No. & Descfiption ‘Quantity Measurement (Rs) (Rs)\n1 sera’ HSNISAC: 3600.00 MT 126.00" 4536000.00\nASH TRANSPORTATION TO VARIOUS BRICKS MANUFACTURING PLANT CCST [email protected]% 113400.\nDISTANCE TO & FRO 26KM TO 40KM Set Tego ve\nCO case Ss Gaaey SGST [email protected]% 113400.00\n36000.000 Need By: 31-MAR-2021 RCM CGST Tax@25% — -113400.00\n‘Supplier tom. DR RS.67 164. TR 27.03.20 RCM SGST [email protected]% ~113400.00\ner tem Total: —_-4536000.00\n2 _Sc1750_ HSN/SAC: 200.000 MT _7200_¥~ 144000.00\nASH TRANSPORTATION TO VARIOUS BRICKS MANUFACTURING PLANT 1 ~ 3600.\nDISTANCE TO & FRO 11KM TO 15KM cease on\n= ees SGST [email protected]% 3600.00\n200,000 ‘Need By: 31-MAR-2021 RCM CGST [email protected]% -3600.00\nSupplier tem. D.R.RS.67.16/ TR 27 03 20 RCM SGST [email protected]% -3600.00\ntem Tota: 144000.00\n3 sciTsa HSNISAC: 2000.00 MT 96.00 192000.00\nCC Code Quantity SGST [email protected]% 4800.00\n200.000 Need By: 31-MAR-2021 | RCM CGST [email protected]% 4800.00\n‘Supplier tem: D.R RS.67.AG/LTR 27.03.20 RCM SGST [email protected]% ~4800.00\noo lem Totat: |” 192000.00\n4 sci74s < HSNISAC: 10000.000 MT 56.00 “ —560000.00\nTRANSPORTATION TO VARIOUS BRICKS MANUFACTURING PLANT ae\nDISTANCE TO & FRO 6KM TO 10KM CT ISEESS pictase\ncG.0ode ae Caatiy SGST [email protected]% 4000.00\n10000.000 Need By: 31-MAR-2021 RCM CGST [email protected]% -14000.00\nSupplier tem: O.R. RS 67 18/.TR 27.03.20 ROM SGST [email protected]% -14000.00\n‘tem Tota: $6000.00\n5 _scizg eal HSNISAC: 1500.000 MT 100.00 ~~ 150000.00\nASH TRANSPORTATION TO VARIOUS BRICKS MANUFACTURING PLANT <G25%\nDISTANCE TO & FRO 26KM TO 30KM CeStrmee Same\nCC Code ———"" Quantity SGST [email protected]% 3750.00\n+500 000 Need By: 31-MAR-2021 ROM CGST [email protected]% “3750.00\n‘Supplier Hem D.R.RS 87 16/1 TR 27.03.20 RCM SGST [email protected]% 3750.00\ntem Total: +150000.00\nRegd. Office:\nHINDALCO INDUSTRIES LIMITED\nAhura Centre. 1st Floor, B Wing, Mehakali Caves Road Andhon(East), Mumbai 400083, india, Page 10813\n\nTel No: 91 22 6691 7000. Email: [email protected], Website: werw.adityabiria com\nCorporate identity No: L27020MH1958PLC011238\n\n \n\x0c'</li></ul> |
| 2 | <ul><li>"b'\\xe2\\x80\\x9cnasfund &&\\n\\nMemorandum\\nTo: Accounts Payables Office\\nDate: 15 February 2024\\nFrom: Niasul. K. Lillie\\nRe: Binding Clear Cover & Hard Cover for HQ\\n\\n \\n\\n \\n\\n \\n\\n \\n\\n \\n\\nPAYEE Theodist Ltd\\n\\nPURPOSE Supply Binding Clear Cover & Hard Cover for NSF HQ (NIL STOCK on Clear\\nCover)\\n\\nAMOUNT K1,290.01\\n\\nMethod of Purchase Order\\n\\nPayment\\n\\n \\n\\n \\n\\n \\n\\n \\n\\n| refer above request for approval of this Urgent Request.\\n\\n \\n\\nRequested by: Ak fu\\n\\nNiasul. Kisokau- Lillie\\n(AP) Finance\\n\\nDate: 15 /_02__/_2024__\\n\\nApproved pa.\\n\\nDebbie Oli\\nChief Finance Officer\\n\\nDate: 15 / 0A) ay\\n\\nP +675 3131998 F +675 3201625\\n\\nBSP Haus, Level 3, Harbour City\\nPO Box 5791, Port Moresby, NCD\\n\\nnasfund.com.pg\\n\\n \\n\\n \\n\\x0c"</li><li>"b'nasfund*_\\n\\n12 May 2023\\n\\nFlora Kwapena\\n\\nDirector/Registered Valuer #123 (PNG)\\nProperty PNG Limited\\n\\nP.O Box 1067, Boroko\\n\\nNCD\\n\\nPapua New Guinea\\n\\nBy Email: [email protected]\\n\\nDear Florence,\\nRE: ENGAGEMENT TO PROVIDE INDEPENDENT VALUATION SERVICES\\n\\nWe refer to your bid proposal dated 10 March 2023 and are pleased to confirm the\\nengagement of your firm to undertake an independent valuation for the properties as\\n\\n \\n\\n \\n\\nfollows:\\nProperty Property Description Quoted Price\\nCredit House 1x 7-levels high-end | PGK10,000.00\\n\\ncommercial property\\nsituated on Allotment 2, 3 &\\n8, Section 45 \\xe2\\x80\\x94 Granville.\\n\\n \\n\\nEra Dorina Apartments 5x high-end residential | PGK10,000.00\\napartment blocks situated\\non Allotments 27, 28 & 29,\\nSection 34 \\xe2\\x80\\x94 Granville.\\n\\n \\n\\nEra Matana Apartments 2x high-end _ residential | PGK10,000.00\\napartment blocks situated\\non Allotment 22, Section 33\\n\\xe2\\x80\\x94 Granville.\\n\\nTotal excl. GST\\n\\n \\n\\nPGK30,000.00 _\\n\\n \\n\\n \\n\\n \\n\\n \\n\\nWe confirm the program for this valuation exercise to be as follows:\\n\\ne Property PNG Ltd to confirm information requirements by 16 May 2023;\\n\\n \\n \\n\\nNational Superannuation Fund Limited\\nP1588 E [email protected]\\n\\nBSP Haus, Level 3-4, Harbour City\\nPO Box 5791, Boroko, NCD\\n\\nnasfund.com.pg\\n\\x0c'"</li><li>"b'Spencer Kaba\\n<< SSS SSS SSeS\\n\\nFrom: Maria Kauvu <[email protected]>\\nSent: 19 February 2024 10:34\\n\\nTo: Spencer Kaba\\n\\n\\xe2\\x80\\x98Gc: [email protected]\\n\\nSubject: RE: Quote request\\n\\nAttachments: IMG_20240219_095133.jpg\\n\\nHi Spencer,\\n\\nAcknowledged receipt of your quote request. Please see hi-lighted:\\n\\ne 11 Pauls Milk\\xe2\\x80\\x944 cartons Nil stock we have Meadow fresh\\n\\ne 100g Nestle Niugini Blend Coffee \\xe2\\x80\\x94 1 carton Nil stock we have Bon Aroma Roasted beans\\ne\\xc2\\xa2 600m! Nature\\xe2\\x80\\x99s Own Water \\xe2\\x80\\x94 4 cartons Nil stock we have Wave water\\n\\ne 250ml Air Fresheners \\xe2\\x80\\x94 10 pieces (2 different fragrance) Nil stock\\n\\ne 750g Saxas Table Salt \\xe2\\x80\\x94 2 pieces Nil stock we have Super as per picture\\n\\nThank you and await your swift response.\\nKind regards,\\n\\nMaria Kauvu Nock\\n\\nCash & Carry Sales Supervisor\\n\\nMobile: +675 7076 1246 | 755 86224\\n\\nOffice: +675 321 3733 | Ex: 816\\n\\nSection 6 Lot 22 Matirogo Street Koki Hubert Murray Highway NCD\\n\\nFrom: Spencer Kaba [[email protected]]\\n\\nSent: Monday, February 19, 2024 8:19 AM\\n\\nTo: Ruth Viae <[email protected]>; [email protected]\\n\\nCc: Niasul Kisokau <[email protected]>; Maureen Ababa <[email protected]>\\nSubject: Quote request\\n\\nHi Ruth/Maria\\n\\nPlease quote us the following and kindly include the delivery charge on the quote. Please note that we have already paid o\\ninvoice.\\n\\ne iL Pauls Milk \\xe2\\x80\\x944 cartons\\n\\ne 100g Nestle Niugini Blend Coffee \\xe2\\x80\\x94 1 carton\\n\\ne 1KG Ramu Sugar \\xe2\\x80\\x94 4 bales (40 pieces)\\n\\ne 100g Carnation Coffeemate \\xe2\\x80\\x94 1 carton\\n\\ne 200g Nestle Milo \\xe2\\x80\\x94 10 pieces\\n\\ne 100g Nestle Sunshine Milk powder \\xe2\\x80\\x94 1 carton\\ne 200g National No. 1 tea bag \\xe2\\x80\\x94 1 carton\\n\\ne 600m! Nature\\xe2\\x80\\x99s Own Water \\xe2\\x80\\x94 4 cartons\\n\\ne 200g Axion paste (Kalamansi/Lime) \\xe2\\x80\\x94 1 carton\\ne 250ml! Axion liquid (Lemon) \\xe2\\x80\\x94 1 carton\\n\\ne Kitchen sponge \\xe2\\x80\\x94 10 pieces\\n\\x0c"</li></ul> |
## Evaluation
### Metrics
| Label | Accuracy |
|:--------|:---------|
| **all** | 1.0 |
## Uses
### Direct Use for Inference
First install the SetFit library:
```bash
pip install setfit
```
Then you can load this model and run inference.
```python
from setfit import SetFitModel
# Download from the 🤗 Hub
model = SetFitModel.from_pretrained("Gopal2002/NASFUND_MODEL_V1")
# Run inference
preds = model("| Sth. Mansur i ves cere
09861521380
At- Hirakud, Dist- Sambalpur, Odisha-768016
{ORIGINAL FOR RECEIPIENT)
TAX INVOICE
ISSUED UNDER RULE 46 OF CGST / OGST RULES, 2017) _
wvorceno: SX 0-219 /19
DETAILS OF RECEIPIENTS (BILLED TO)
HINDALCO INDUSTRIES LIMITED
HIRAKUD POWER
AT/PO- HIRAKUD,
DIST- SAMBALPUR-768016, ODISHA
INVOICEDATE: | ¥~ G'
PO NO. : ejeo| seupres] oF € J
GSTIN : 21AAACH1201R17Z
PAN No. : AAACH1201R
DETAILS OF CONSIGNEE (SHIPPED TO)
HINDALCO INDUSTRIES LIMITED
PODATE: 9Q~-'-'%
DATE OF ISSUE :
PERIOD OF SERVICE: 14-$°\"% fo 16-64-19
af uh Dea VC cy )* OR gre
b)L Om egy cd.
SG0004
Se eS
Sea wee. of a Ck.fwd pote. ce. ovev
| x
aged of Rok. Ae gers € eAv cdl
Total Invoice value (In Figures)
Total Invoice value (in words) —
“T wralisa $2.93 §acKs a f : “4 of Fy as nS a @& @er\. | wai
CERTIFIED THAT THE PARTICULARS GIVEN ABOVE ARE TRUE AND CORRECT AND THE AMOUNT INDICATED REPRESENTS THE PRICE ACTUALLY
CHARGED BY US AND IS NO FLOW OS ADDITIAL CONDITION DIRECTLY OR INDIRECTLY FROM THE BUYER.
Our GSTIN : 21ALGPA5675EIZI
Our PAN: ALGPAS675E
")
```
<!--
### Downstream Use
*List how someone could finetune this model on their own dataset.*
-->
<!--
### Out-of-Scope Use
*List how the model may foreseeably be misused and address what users ought not to do with the model.*
-->
<!--
## Bias, Risks and Limitations
*What are the known or foreseeable issues stemming from this model? You could also flag here known failure cases or weaknesses of the model.*
-->
<!--
### Recommendations
*What are recommendations with respect to the foreseeable issues? For example, filtering explicit content.*
-->
## Training Details
### Training Set Metrics
| Training set | Min | Median | Max |
|:-------------|:----|:---------|:----|
| Word count | 2 | 274.2711 | 699 |
| Label | Training Sample Count |
|:------|:----------------------|
| 0 | 53 |
| 1 | 53 |
| 2 | 5 |
| 3 | 55 |
### Training Hyperparameters
- batch_size: (32, 32)
- num_epochs: (2, 2)
- max_steps: -1
- sampling_strategy: oversampling
- body_learning_rate: (2e-05, 1e-05)
- head_learning_rate: 0.01
- loss: CosineSimilarityLoss
- distance_metric: cosine_distance
- margin: 0.25
- end_to_end: False
- use_amp: False
- warmup_proportion: 0.1
- seed: 42
- eval_max_steps: -1
- load_best_model_at_end: False
### Training Results
| Epoch | Step | Training Loss | Validation Loss |
|:------:|:----:|:-------------:|:---------------:|
| 0.0017 | 1 | 0.2889 | - |
| 0.0846 | 50 | 0.2092 | - |
| 0.1692 | 100 | 0.0499 | - |
| 0.2538 | 150 | 0.0143 | - |
| 0.3384 | 200 | 0.0113 | - |
| 0.4230 | 250 | 0.0023 | - |
| 0.5076 | 300 | 0.0027 | - |
| 0.5922 | 350 | 0.0025 | - |
| 0.6768 | 400 | 0.0012 | - |
| 0.7614 | 450 | 0.0012 | - |
| 0.8460 | 500 | 0.001 | - |
| 0.9306 | 550 | 0.0014 | - |
| 1.0152 | 600 | 0.0011 | - |
| 1.0998 | 650 | 0.0013 | - |
| 1.1844 | 700 | 0.0012 | - |
| 1.2690 | 750 | 0.0008 | - |
| 1.3536 | 800 | 0.0008 | - |
| 1.4382 | 850 | 0.0008 | - |
| 1.5228 | 900 | 0.0008 | - |
| 1.6074 | 950 | 0.0008 | - |
| 1.6920 | 1000 | 0.0007 | - |
| 1.7766 | 1050 | 0.0007 | - |
| 1.8613 | 1100 | 0.0008 | - |
| 1.9459 | 1150 | 0.0007 | - |
### Framework Versions
- Python: 3.10.12
- SetFit: 1.0.3
- Sentence Transformers: 2.7.0
- Transformers: 4.40.1
- PyTorch: 2.2.1+cu121
- Datasets: 2.19.0
- Tokenizers: 0.19.1
## Citation
### BibTeX
```bibtex
@article{https://doi.org/10.48550/arxiv.2209.11055,
doi = {10.48550/ARXIV.2209.11055},
url = {https://arxiv.org/abs/2209.11055},
author = {Tunstall, Lewis and Reimers, Nils and Jo, Unso Eun Seo and Bates, Luke and Korat, Daniel and Wasserblat, Moshe and Pereg, Oren},
keywords = {Computation and Language (cs.CL), FOS: Computer and information sciences, FOS: Computer and information sciences},
title = {Efficient Few-Shot Learning Without Prompts},
publisher = {arXiv},
year = {2022},
copyright = {Creative Commons Attribution 4.0 International}
}
```
<!--
## Glossary
*Clearly define terms in order to be accessible across audiences.*
-->
<!--
## Model Card Authors
*Lists the people who create the model card, providing recognition and accountability for the detailed work that goes into its construction.*
-->
<!--
## Model Card Contact
*Provides a way for people who have updates to the Model Card, suggestions, or questions, to contact the Model Card authors.*
--> | {"library_name": "setfit", "tags": ["setfit", "sentence-transformers", "text-classification", "generated_from_setfit_trainer"], "metrics": ["accuracy"], "base_model": "BAAI/bge-small-en-v1.5", "widget": [{"text": "ADITYA BIRLA\n\n \n\nHINDALCO INDUSTRIES LIMITED\n\n \n\nHIRAKUD POWER, HIRAKUD-768 016, DIST.:SAMBALPUR (ODISHA) Range: Hirakuc\nPHONE: 0663-2481365, FAX: 0663-2481342 Division : Sambal\nPURCHASE ORDER Excise Regn. No.\nVendor Code: B120 PO No: P/PO/SRV/1617/0998 Date: 24-FEB-2/\nM/s BHARAT INSULATION COMPANY Rev. No: Date:\nB-61, TURRA\nPrice Basis:\nTransportation arrangement:\nDIST. SONEBHADRA, UTTARPRADESH, IN 231221 Ship to Location: HIRAKUD - POWER\nEmail: [email protected] Currency: INR\nFax: () Hindalco Contact Person AMARESH MISHRA,\nContact: SAGAR KHANNA (+91) 9838407002 Email of Contact Person: [email protected]\niNaige oo Oo oo OO OS OO\n\nRATE, TERMS & CONDITIONS ARE AS PER OUR EARLIER ORDER NO. P/PO/SRV/1617/0804\n\nA. PAYMENT TERMS :-\n1. Service Tax as applicable will be paid extra.\n\n2. It is mandatory to put PAN number in the invoice. As per Section 69 of the Finance Act and rule 4 of the Service Tax Rules, 1994, it is mandatory to mention\nTax Registration number in the invoice.\n\n3.All tools and tackles are in your scope.\n\n4. Seif tapping screw, scaffolding, pop rivet, GI wire, Tape in your scope.\n\nOTHER TERMS:-\n\n1. Bill will be released only after thermography report submitted by Department\n\n2. Strip to be installed in duct area free of cost\n\n3. Scrap material to be resused after due consultation with department\n\n4. Warranty will be 1 Year from date of completion of job\n\n5. We will supply only Cladding sheet and Insulation to the Contractor, rest all the material in your scope.\n\nNOTE:-\n1. PLEASE REFER ANNEXURE - II FOR \u201cGENERAL TERMS AND CONDITIONS - SERVICES\"\n2. PLEASE REFER ANNEXURE- A FOR \"SAFETY CODE OF CONDUCT\"\n\nfor and on behalf of\n\nGIRISH ABBOTT\nComplex Head - Materials\n\nHindalco Industries Limited\n\n \n\nHirakud\nRegd. Office: Page 3 of 3 Sales Tax mes\nHINDALCO INDUSTRIES LIMITED C.S.T.No. : SAI(C) 345, 0\nCentury Bhavan, 3rd Floor, 0.S.T. No. SAI 2587, Dt.\nDr. Annie Besant Road, Worli, Mumbai-400 030 TIN : 21601703134\n\nTel No: 91 22 6662 6666, Email: [email protected], Website: www.adityabirla.com\n\nCarnarata Idantitu Ma: I ITNINMLADERDI 7N11922\n\f"}, {"text": " \n\nM/S ANIL KUMAR SAHU\n\n \n\nAT/PO- HIRAKUD\nDIST - SAMBALPUR- 768016, ODISHA,\n\nMOBILE NO : 9777356729\nEMAIL ID : [email protected]\u2019\n\n \n\nDETAILS OF RECEIPIENT (BILLED TO)\nHINDALCO INDUSTRIES LTD.\nHIRAKUD POWER\nAT/PO:- HIRAKUD\n\n|\n\nTAX INVOICE\n(ISSUEDUNDER RULE 46 OF GST/OGST RULE,2017)\n\n \n\n \n\n \n\n \n\n \n\n \n\n \n\n \n\n \n\n \n\n \n\n \n\n \n\n \n\n \n\n \n\n \n\n \n\n \n\n \n\n \n\n \n\n \n\n \n\n \n\n \n\n \n\n \n\nDIST: | SAMBALPUR - 798016,ODISHA INVOICE NO. 487/20\nSTATE CODE:- 21 INVOICE DATE 11.03.2020\nGSTIN :- 21AAACH1201R1ZZ\nPAN NO:- AAACH1201R PO. NO. P/PO/SRV/1920/0976\nDETAILS OF COSIGNEE (SHIPPED ) |\nHINDALCO INDUSTRIES LTD. PO DATE\ni HIRAKUD POWER\nAT/PO:- HIRAKUD DATE OF ISSUE\nDIST:- | SAMBALPUR - 798016,ODISH..\nPERIOD OF SERVICE JULY - 2019 TO JANUARY - 2020\nNo. SERVICE DESCRIPTION Meooe yous] vom | fag) | as)\n$C1764\nASH TRANSPORTATION TO VARIOUS\nL BRICKS PLANT DISTANCE 106 KM TO110 KM\n1 MONTH OF JULY - 2019 90.44 | 313.50 28,352.94\n2 MONTH OF AUGUST - 2019 57.70 313.50 18,088.95\n3 MONTH OF SEPTEMBER - 2019 29.74 313.50 9,323.49\n4 MONTH OF OCTOBER - 2019 9954 | 114.36 MT 313.50 35,851.86\n5 r MONTH OF NOVEMBER - 2019 145.65 313.50 45,661.28 |\n6 MONTH OF DECEMBER - 2019 131.13 313.50 41,109.26\n7 MONTH OF JANUARY - 2020 114.85 | 313.50 36,005.48 |\nTOTAL 2,14,393.25\nWHETHER THE TAX IXS PAYABLE ON REVERSE CHARGE BASIS :- [No\nINVOICE TOTAL 2,14,393.25\n\n \n\n \n\nTOTAL INVOICE VALUE (IN FIGURE )\n\nRS.2,14,393.25\n\n \n\na_i\n\nTOTAL INVOICE VALUE (IN WORDS )\n\n \n\nTWO LAKH FOURTEEN THOUSAND THREE HUNDRED NINETY THREE ONLY.\n\n \n\nCERTIFIED THAT THE PARTICULARS GIVEN ABOVE ARE TRUE AND CORRECT AND THE AMOUNT INDICATED\nREPRESENTS THE PRICE ACTUALLY CHARGED BY US AND THERE IS NO FLOW OS ADDITIAL CONDITION DIRECTLY OR INDIRECTLY FROM THE BUYER\n\n \n\n-\nSTATE CODE:- 21\n\nOUR GST NO:- 21AIUPS3946D1Z1\n\nOUR PAN NO: AIUPS3946D\n\n \n\n(E&O.E.)\n\nFOR M/S ANIL KUMAR SAHU\nFor Anil Kumar Sahu\n\nlw Gs\n\nProprietor\nAUTHORISED SIGNATORY\n\n \n\n \n\n \n\f"}, {"text": "| Sth. Mansur i ves cere\n\n09861521380\nAt- Hirakud, Dist- Sambalpur, Odisha-768016\n{ORIGINAL FOR RECEIPIENT)\n\nTAX INVOICE\nISSUED UNDER RULE 46 OF CGST / OGST RULES, 2017) _\n\nwvorceno: SX 0-219 /19\n\n \n \n \n \n\n \n \n\nDETAILS OF RECEIPIENTS (BILLED TO)\nHINDALCO INDUSTRIES LIMITED\nHIRAKUD POWER\nAT/PO- HIRAKUD,\nDIST- SAMBALPUR-768016, ODISHA\n\n \n \n \n \n \n\n \n \n \n\nINVOICEDATE: | \u00a5~ G'\n\nPO NO. : ejeo| seupres] oF \u20ac J\n\n \n \n \n\n \n \n\nGSTIN : 21AAACH1201R17Z\nPAN No. : AAACH1201R\nDETAILS OF CONSIGNEE (SHIPPED TO)\nHINDALCO INDUSTRIES LIMITED\n\n \n \n \n \n\n \n \n\nPODATE: 9Q~-'-'%\n\n \n \n\nDATE OF ISSUE :\n\n \n\nPERIOD OF SERVICE: 14-$\u00b0\"% fo 16-64-19\n\n \n \n \n\n \n \n\naf uh Dea VC cy )* OR gre\n\nb)L Om egy cd.\n\n \n \n \n\n \n\nSG0004\nSe eS\n\nSea wee. of a Ck.fwd pote. ce. ovev\n| x\n\naged of Rok. Ae gers \u20ac eAv cdl\n\n \n \n\n \n\n \n\n \n \n\nTotal Invoice value (In Figures)\nTotal Invoice value (in words) \u2014\n\n \n \n\n \n\n \n \n \n\n\u201cT wralisa $2.93 \u00a7acKs a f : \u201c4 of Fy as nS a @& @er\\. | wai\n\n \n\nCERTIFIED THAT THE PARTICULARS GIVEN ABOVE ARE TRUE AND CORRECT AND THE AMOUNT INDICATED REPRESENTS THE PRICE ACTUALLY\nCHARGED BY US AND IS NO FLOW OS ADDITIAL CONDITION DIRECTLY OR INDIRECTLY FROM THE BUYER.\n\n \n\n \n \n \n \n\nOur GSTIN : 21ALGPA5675EIZI\n\n \n\nOur PAN: ALGPAS675E\n\f"}, {"text": "fan UNITED ECO CARE CONSULTANCY PVT.LTD\n\n \n\nTAX INVOICE\nINVOICE Neh eet) 02-03-2022\n\n \n \n\n|VENDOR DETAILS:\nIHINDALCO INDUSTRIES UMITED [UNITED ECO CARE CONSULTANCY PRIVATE LIMITED\nHirakud Power, Hirakud - 768016, Sambalpur (ODISHA) Flat No: 006, Prasanti Apartment, Deulsahi, Tulsipur,\nDist: Cuttack-753008, Odisha, India.\nICIN NO: U513970R2008PTC009763\n\n \n \n\n \n \n \n\n \n\n:]21MAACH1201R1ZZ GSTIN: |21AAACU9687L1ZS, State Code: 21 (Odisha)\nPAN: |AAACH1201R - PAN: | AAACU9687L |SAC CODE: |998346\n>/SR\\ ~ SERVICE TYPE: Scientific & Technical Consultancy Services.\n\n \n \n \n\n \n \n \n\n \n \n\n \n\nP.O. NO: |P/PO/SRV/2122/0846\n\n \n\n \n\n \n \n \n\nP.O. DATE: (12-01- 202200 IBANK DETAILS:\nISHIP TO: \u2014_ BANK NAME: AXIS BANK LTD.\nIHINDALCO INDUSTRIES LIMITED A/C NO: 9100 2001 2908 617\n\n \n \n\nHirakud Power, Hirakud - 768016, Sambalpur (ODISHA) A/C TYPE: CURRENT\nEffective From 12/01/2022 To 31/12/2022 FSC CODE: UTIB 0000 550\nBRANCH ADDRESS: BIDANASI, CUTTACK\n\n \n \n \n \n\n \n\n \n\nSERVICE PERIOD: 15/01/2022 to 17/01/2022\nICONTACT PERSON: Mr. Subrat kumar Biswal (chp dept) 8 \u00a2\nBRIEF ORDER DESCRIPTION: LOAD TEST WITH CERTIFICATION OF HOIST & CHAIN\n\nPULLY BLOCK.\n\n \n \n \n\n \n\nACLU TCCa CPA Ey eit)\n\n \n \n\nSERVICE DESCRIPTION PO. QTY. UoM eee ee LINE TOTAL (Rs.)\n(US\n\n \n \n \n \n \n \n \n \n \n\n \n \n \n \n \n\n \n\n \n\n \n\n \n\n \n \n \n\n \n\n \n\n \n\n \n\n \n\n \n\n731216140082\n10 | ELECTRICALLY OPERATED OVERHEAD WIRE ROPE HOIST: 2 TON 1,615.00\n~ 731216140084 ne Ga, te F _\n2.0 _ ELECTRICALLY OPERATED OVERHEAD WIRE ROPE HOIST: 1,615.00 il 1,615.00\n731216140085 : \u2018i a aN j\n3.0 ELECTRICALLY OPERATED OVERHEAD WIRE ROPE HOIST: 10 TON 2,400.00 2.00 4,800.00\n|U731216140335 - ay iv | |\n4.0 |evectRICALLY OPERATED OVERHEAD WIRE ROPE HOIST: 6TON 1,615.00 109 | 1,615.00\nFea a 2 i al | | as\nU731216140336 |\n3.0 ELECTRICALLY OPERATED OVERHEAD WIRE ROPE HOIST: 3 TON A NGS: gh\" 1615.00 100 1615.00 |\nU731216140337 oo | alte i\n6.0 ELECTRICALLY OPERATED OVERHEAD WIRE ROPE HOIST: 7.5 TON i G | Nes | 2,400.00 4.00 9,600.00\n731216140426 - sb svcammeenitl oT gs Pte\n70 CHAIN PULLEY BLOCK (37) | 5 Nos 304.00 1.00 304.00\n(811418040116 \u2014 \u2014 TY |\n8,0 INSPECTION AND TESTING OF OF CHAIN BLOCK ABOVE 3T AND UPTO. 5 Nos 500.00 | 1.00 500.00\n6T\n811418040117 = =|\n9.0 Loa TEST OF CHAIN BLOCK UPTO aT 25 Nos 5,472.00\n7 tL _ EER\n_ \u2014_ SGST @9% 2,587.59\n| CGST @9% 2,587.59 |\nlIGst @ 18%\nAmount in Words: Rupees Thirty Three Thousand Nine Hundred Twet\n\n \n\n \n\n \n\n \n\n \n\f"}, {"text": " \n\n \n\n| HINDALCO INDUSTRIES LIMITED\n\ngy HIRAKUD SMELTER, HIRAKUD-768 016,DIST.:SAMBALPUR (ODISHA)\nPHONE: 0663-248 1307/2481273/2481452, FAX: 0663-2481356\n\n \n\nGSTIN: 21AAACH1201R1ZZ\nState Code: 21 - Odisha\n\nGST Range/division: Sambalpur\nGST Commissionerate - Cuttack\n\n \n\nPURCHASE ORDER\n\nVendor Code: P81 P.O/No: S/PO/SRV/1920/0144 Date: 26-APR-2019\nMis P.K.TRIPATHY Revies No} Date\n\nOrder Type: PURCHASE ORDER\nF-3/D, MAA TARINI APARTMENT Effective From 01/04/2019 To 31/03/2020\nBUDHARAJA Price Basis * NONE\nSAMBALPUR, ORISSA, IN 768 004 Transportation arrangement\n\nShip to Location HIRAKUD - SMELTER:\nEmail: [email protected] Carrier\nFax: () Currency SINR,\nContact: PRATAP KU, TRIPATHY () 9861732186. Hindalco Contact Person SRIKANDAR BHOI,\n\nGSTIN: 21AAXPTOS26F1ZO \u2014_State:21 - Odisha\n\nEmail of Contact Person: purchasesmelter@adityabiria com\n\nRef (ANNUAL ORDER) PERIPHERAL HOUSEKEEPING ,METAL PAD CUTTING IN LINE-183 AND COURTYARD HOUSEKEEPING IN LINEA\n\n \n\n \n\n \n\n \n\n \n\n \n\nOrder Unit of Rate/Unit Value\nSl. Stock No. & Description Quantity Measurement (Rs) (Rs)\n1 SCPRO382 HSN/SAC: 9985 12.000 MON 101021.171212284.04\nPERIPHERAL HOUSEKEEPING AND MISCELLANEOUS JOBS IN POT LINES. cost Tax@a% 10910286\nCC Code Quant\n\nyd Need By: 31-MAR-2020 SGST Tax@9% 109102.86\nTem Total 1430459.76\n2 ScPRo4S1 HSN/SAC: 9985 42.000 MON 4934072 -592088.64\nMETAL PAD CUTTING IN LINE1 AND 3. COST Tax@o% 53287.98\n\nCC Code Quantity\n32000 Need By: 31-MAR-2020 SGST TanQo%. SST\nitem Total 698664.60\n3 SCPRO3e2 HSNISAC: 9985, 12,000 MON 148669.52 1748034.24\nCOURTYARD HOUSEKEEPING IN LINE-1 COST Tax@9% 157323.08\nCia usiiy SGST Tax@9% 157323.08\n12,000 Need By: 3t-MAR-2029 item Total: 2062680.40\nPayment Terms: 15 days credit against RA bill GRAND TOTAL: 4191804.76\n\nFreight Terms: NONE\n\nBilling Address\n(Hard\nShipping address : Hindalco'Industries Ltd, Hirakud Smol\n\nNotes:\n\nNeQB/S TO BE DONE AS PER THE INSTRUCTION & SATISFACTION OF ENGIENEER-IN-CHARGE AT SITE.DETAILS SCOPE OF WORK ATTACHED\n\nHEREWITH.\n2. BREAK-UP OF MANPOWER :\nITEM SL. NO.1 (SCPRO382) :\n\n \n\n \n\n+ Hindalco Industries Ltd, Hirakud Smelter, Hirakud - 768016, Sambalpur (ODISHA)\ny of bills to be forwarded at shipping address)\nr, Hirakud - 768016,\n\n\u2018Sambalpur (ODISHA)\n\nOBNOS UN-SKILLED (LTS) FOR 30.42AYS = 243 36MANDAYS X 415.11=1,01,021.17/- PER MONTH\n\nITEM SL. NO.2 (SCPRO451) :\n\nO4NOS SEMI-SKILLED (LTS) FOR 26DAYS = 104MANDAYS X 474 43= 49,340.72/- PER MONTH\n\nITEM SL.NO.3 (SCPRO392) :\n\nDINO. HI-SKILLED (LTS) FOR 30.42AYS = 30.42MANDAYS x 637.51:\n\n \n\n9,393.05).\n\nONOS UN-SKILLED (LTS) FOR 30.42AYS = 304.2MANDAYS X 415 11=1,26,276.46/-\n\n1,45 (669 52/- PER MONTH\n\n3. YOU HAVE TO SUBMIT PROPER SUPPORTING DOCUMENTS LIKE JOB TICKET & LOG BOOK ALONG WITH BILL AT ACCOUNTS.\n\n4. RATE |S AS PER LTS LABOUR RATE W.E.F.30/10/2018,\n\n \n\nRegd. Office:\nHINDALCO INDUSTRIES LIMITED\n\nAhura Centra, 1st Floor, B Wing, Mahakali Caves Road Andheri(East), Mumbai 400093, India,\n\nPage 1 of 2\n\nTel No: 91 22 6691 7000, Emall: [email protected], Website: www.adityabiria com\n\nCorporate Identity No: L27020MH1958PLC011238\n\nwy\n\u00a5Y\n\n \n\nrv .\nTA\\W\\rP\n\f"}], "pipeline_tag": "text-classification", "inference": true, "model-index": [{"name": "SetFit with BAAI/bge-small-en-v1.5", "results": [{"task": {"type": "text-classification", "name": "Text Classification"}, "dataset": {"name": "Unknown", "type": "unknown", "split": "test"}, "metrics": [{"type": "accuracy", "value": 1.0, "name": "Accuracy"}]}]}]} | Gopal2002/NASFUND_MODEL_V1 | null | [
"setfit",
"safetensors",
"bert",
"sentence-transformers",
"text-classification",
"generated_from_setfit_trainer",
"arxiv:2209.11055",
"base_model:BAAI/bge-small-en-v1.5",
"model-index",
"region:us"
] | null | 2024-05-02T11:24:40+00:00 | [
"2209.11055"
] | [] | TAGS
#setfit #safetensors #bert #sentence-transformers #text-classification #generated_from_setfit_trainer #arxiv-2209.11055 #base_model-BAAI/bge-small-en-v1.5 #model-index #region-us
| SetFit with BAAI/bge-small-en-v1.5
==================================
This is a SetFit model that can be used for Text Classification. This SetFit model uses BAAI/bge-small-en-v1.5 as the Sentence Transformer embedding model. A LogisticRegression instance is used for classification.
The model has been trained using an efficient few-shot learning technique that involves:
1. Fine-tuning a Sentence Transformer with contrastive learning.
2. Training a classification head with features from the fine-tuned Sentence Transformer.
Model Details
-------------
### Model Description
* Model Type: SetFit
* Sentence Transformer body: BAAI/bge-small-en-v1.5
* Classification head: a LogisticRegression instance
* Maximum Sequence Length: 512 tokens
* Number of Classes: 4 classes
### Model Sources
* Repository: SetFit on GitHub
* Paper: Efficient Few-Shot Learning Without Prompts
* Blogpost: SetFit: Efficient Few-Shot Learning Without Prompts
### Model Labels
Evaluation
----------
### Metrics
Uses
----
### Direct Use for Inference
First install the SetFit library:
Then you can load this model and run inference.
Training Details
----------------
### Training Set Metrics
### Training Hyperparameters
* batch\_size: (32, 32)
* num\_epochs: (2, 2)
* max\_steps: -1
* sampling\_strategy: oversampling
* body\_learning\_rate: (2e-05, 1e-05)
* head\_learning\_rate: 0.01
* loss: CosineSimilarityLoss
* distance\_metric: cosine\_distance
* margin: 0.25
* end\_to\_end: False
* use\_amp: False
* warmup\_proportion: 0.1
* seed: 42
* eval\_max\_steps: -1
* load\_best\_model\_at\_end: False
### Training Results
### Framework Versions
* Python: 3.10.12
* SetFit: 1.0.3
* Sentence Transformers: 2.7.0
* Transformers: 4.40.1
* PyTorch: 2.2.1+cu121
* Datasets: 2.19.0
* Tokenizers: 0.19.1
### BibTeX
| [
"### Model Description\n\n\n* Model Type: SetFit\n* Sentence Transformer body: BAAI/bge-small-en-v1.5\n* Classification head: a LogisticRegression instance\n* Maximum Sequence Length: 512 tokens\n* Number of Classes: 4 classes",
"### Model Sources\n\n\n* Repository: SetFit on GitHub\n* Paper: Efficient Few-Shot Learning Without Prompts\n* Blogpost: SetFit: Efficient Few-Shot Learning Without Prompts",
"### Model Labels\n\n\n\nEvaluation\n----------",
"### Metrics\n\n\n\nUses\n----",
"### Direct Use for Inference\n\n\nFirst install the SetFit library:\n\n\nThen you can load this model and run inference.\n\n\nTraining Details\n----------------",
"### Training Set Metrics",
"### Training Hyperparameters\n\n\n* batch\\_size: (32, 32)\n* num\\_epochs: (2, 2)\n* max\\_steps: -1\n* sampling\\_strategy: oversampling\n* body\\_learning\\_rate: (2e-05, 1e-05)\n* head\\_learning\\_rate: 0.01\n* loss: CosineSimilarityLoss\n* distance\\_metric: cosine\\_distance\n* margin: 0.25\n* end\\_to\\_end: False\n* use\\_amp: False\n* warmup\\_proportion: 0.1\n* seed: 42\n* eval\\_max\\_steps: -1\n* load\\_best\\_model\\_at\\_end: False",
"### Training Results",
"### Framework Versions\n\n\n* Python: 3.10.12\n* SetFit: 1.0.3\n* Sentence Transformers: 2.7.0\n* Transformers: 4.40.1\n* PyTorch: 2.2.1+cu121\n* Datasets: 2.19.0\n* Tokenizers: 0.19.1",
"### BibTeX"
] | [
"TAGS\n#setfit #safetensors #bert #sentence-transformers #text-classification #generated_from_setfit_trainer #arxiv-2209.11055 #base_model-BAAI/bge-small-en-v1.5 #model-index #region-us \n",
"### Model Description\n\n\n* Model Type: SetFit\n* Sentence Transformer body: BAAI/bge-small-en-v1.5\n* Classification head: a LogisticRegression instance\n* Maximum Sequence Length: 512 tokens\n* Number of Classes: 4 classes",
"### Model Sources\n\n\n* Repository: SetFit on GitHub\n* Paper: Efficient Few-Shot Learning Without Prompts\n* Blogpost: SetFit: Efficient Few-Shot Learning Without Prompts",
"### Model Labels\n\n\n\nEvaluation\n----------",
"### Metrics\n\n\n\nUses\n----",
"### Direct Use for Inference\n\n\nFirst install the SetFit library:\n\n\nThen you can load this model and run inference.\n\n\nTraining Details\n----------------",
"### Training Set Metrics",
"### Training Hyperparameters\n\n\n* batch\\_size: (32, 32)\n* num\\_epochs: (2, 2)\n* max\\_steps: -1\n* sampling\\_strategy: oversampling\n* body\\_learning\\_rate: (2e-05, 1e-05)\n* head\\_learning\\_rate: 0.01\n* loss: CosineSimilarityLoss\n* distance\\_metric: cosine\\_distance\n* margin: 0.25\n* end\\_to\\_end: False\n* use\\_amp: False\n* warmup\\_proportion: 0.1\n* seed: 42\n* eval\\_max\\_steps: -1\n* load\\_best\\_model\\_at\\_end: False",
"### Training Results",
"### Framework Versions\n\n\n* Python: 3.10.12\n* SetFit: 1.0.3\n* Sentence Transformers: 2.7.0\n* Transformers: 4.40.1\n* PyTorch: 2.2.1+cu121\n* Datasets: 2.19.0\n* Tokenizers: 0.19.1",
"### BibTeX"
] | [
66,
58,
42,
16,
10,
43,
7,
169,
5,
75,
6
] | [
"TAGS\n#setfit #safetensors #bert #sentence-transformers #text-classification #generated_from_setfit_trainer #arxiv-2209.11055 #base_model-BAAI/bge-small-en-v1.5 #model-index #region-us \n### Model Description\n\n\n* Model Type: SetFit\n* Sentence Transformer body: BAAI/bge-small-en-v1.5\n* Classification head: a LogisticRegression instance\n* Maximum Sequence Length: 512 tokens\n* Number of Classes: 4 classes### Model Sources\n\n\n* Repository: SetFit on GitHub\n* Paper: Efficient Few-Shot Learning Without Prompts\n* Blogpost: SetFit: Efficient Few-Shot Learning Without Prompts### Model Labels\n\n\n\nEvaluation\n----------### Metrics\n\n\n\nUses\n----### Direct Use for Inference\n\n\nFirst install the SetFit library:\n\n\nThen you can load this model and run inference.\n\n\nTraining Details\n----------------### Training Set Metrics### Training Hyperparameters\n\n\n* batch\\_size: (32, 32)\n* num\\_epochs: (2, 2)\n* max\\_steps: -1\n* sampling\\_strategy: oversampling\n* body\\_learning\\_rate: (2e-05, 1e-05)\n* head\\_learning\\_rate: 0.01\n* loss: CosineSimilarityLoss\n* distance\\_metric: cosine\\_distance\n* margin: 0.25\n* end\\_to\\_end: False\n* use\\_amp: False\n* warmup\\_proportion: 0.1\n* seed: 42\n* eval\\_max\\_steps: -1\n* load\\_best\\_model\\_at\\_end: False### Training Results### Framework Versions\n\n\n* Python: 3.10.12\n* SetFit: 1.0.3\n* Sentence Transformers: 2.7.0\n* Transformers: 4.40.1\n* PyTorch: 2.2.1+cu121\n* Datasets: 2.19.0\n* Tokenizers: 0.19.1### BibTeX"
] |
null | peft |
# Model Card for Model ID
<!-- Provide a quick summary of what the model is/does. -->
## Model Details
### Model Description
<!-- Provide a longer summary of what this model is. -->
- **Developed by:** [More Information Needed]
- **Funded by [optional]:** [More Information Needed]
- **Shared by [optional]:** [More Information Needed]
- **Model type:** [More Information Needed]
- **Language(s) (NLP):** [More Information Needed]
- **License:** [More Information Needed]
- **Finetuned from model [optional]:** [More Information Needed]
### Model Sources [optional]
<!-- Provide the basic links for the model. -->
- **Repository:** [More Information Needed]
- **Paper [optional]:** [More Information Needed]
- **Demo [optional]:** [More Information Needed]
## Uses
<!-- Address questions around how the model is intended to be used, including the foreseeable users of the model and those affected by the model. -->
### Direct Use
<!-- This section is for the model use without fine-tuning or plugging into a larger ecosystem/app. -->
[More Information Needed]
### Downstream Use [optional]
<!-- This section is for the model use when fine-tuned for a task, or when plugged into a larger ecosystem/app -->
[More Information Needed]
### Out-of-Scope Use
<!-- This section addresses misuse, malicious use, and uses that the model will not work well for. -->
[More Information Needed]
## Bias, Risks, and Limitations
<!-- This section is meant to convey both technical and sociotechnical limitations. -->
[More Information Needed]
### Recommendations
<!-- This section is meant to convey recommendations with respect to the bias, risk, and technical limitations. -->
Users (both direct and downstream) should be made aware of the risks, biases and limitations of the model. More information needed for further recommendations.
## How to Get Started with the Model
Use the code below to get started with the model.
[More Information Needed]
## Training Details
### Training Data
<!-- This should link to a Dataset Card, perhaps with a short stub of information on what the training data is all about as well as documentation related to data pre-processing or additional filtering. -->
[More Information Needed]
### Training Procedure
<!-- This relates heavily to the Technical Specifications. Content here should link to that section when it is relevant to the training procedure. -->
#### Preprocessing [optional]
[More Information Needed]
#### Training Hyperparameters
- **Training regime:** [More Information Needed] <!--fp32, fp16 mixed precision, bf16 mixed precision, bf16 non-mixed precision, fp16 non-mixed precision, fp8 mixed precision -->
#### Speeds, Sizes, Times [optional]
<!-- This section provides information about throughput, start/end time, checkpoint size if relevant, etc. -->
[More Information Needed]
## Evaluation
<!-- This section describes the evaluation protocols and provides the results. -->
### Testing Data, Factors & Metrics
#### Testing Data
<!-- This should link to a Dataset Card if possible. -->
[More Information Needed]
#### Factors
<!-- These are the things the evaluation is disaggregating by, e.g., subpopulations or domains. -->
[More Information Needed]
#### Metrics
<!-- These are the evaluation metrics being used, ideally with a description of why. -->
[More Information Needed]
### Results
[More Information Needed]
#### Summary
## Model Examination [optional]
<!-- Relevant interpretability work for the model goes here -->
[More Information Needed]
## Environmental Impact
<!-- Total emissions (in grams of CO2eq) and additional considerations, such as electricity usage, go here. Edit the suggested text below accordingly -->
Carbon emissions can be estimated using the [Machine Learning Impact calculator](https://mlco2.github.io/impact#compute) presented in [Lacoste et al. (2019)](https://arxiv.org/abs/1910.09700).
- **Hardware Type:** [More Information Needed]
- **Hours used:** [More Information Needed]
- **Cloud Provider:** [More Information Needed]
- **Compute Region:** [More Information Needed]
- **Carbon Emitted:** [More Information Needed]
## Technical Specifications [optional]
### Model Architecture and Objective
[More Information Needed]
### Compute Infrastructure
[More Information Needed]
#### Hardware
[More Information Needed]
#### Software
[More Information Needed]
## Citation [optional]
<!-- If there is a paper or blog post introducing the model, the APA and Bibtex information for that should go in this section. -->
**BibTeX:**
[More Information Needed]
**APA:**
[More Information Needed]
## Glossary [optional]
<!-- If relevant, include terms and calculations in this section that can help readers understand the model or model card. -->
[More Information Needed]
## More Information [optional]
[More Information Needed]
## Model Card Authors [optional]
[More Information Needed]
## Model Card Contact
[More Information Needed]
### Framework versions
- PEFT 0.10.1.dev0 | {"library_name": "peft", "base_model": "microsoft/Phi-3-mini-128k-instruct"} | ItsArch3r/Phi3_mini_128k_Gita_Finetune_CKPT | null | [
"peft",
"safetensors",
"arxiv:1910.09700",
"base_model:microsoft/Phi-3-mini-128k-instruct",
"region:us"
] | null | 2024-05-02T11:26:04+00:00 | [
"1910.09700"
] | [] | TAGS
#peft #safetensors #arxiv-1910.09700 #base_model-microsoft/Phi-3-mini-128k-instruct #region-us
|
# Model Card for Model ID
## Model Details
### Model Description
- Developed by:
- Funded by [optional]:
- Shared by [optional]:
- Model type:
- Language(s) (NLP):
- License:
- Finetuned from model [optional]:
### Model Sources [optional]
- Repository:
- Paper [optional]:
- Demo [optional]:
## Uses
### Direct Use
### Downstream Use [optional]
### Out-of-Scope Use
## Bias, Risks, and Limitations
### Recommendations
Users (both direct and downstream) should be made aware of the risks, biases and limitations of the model. More information needed for further recommendations.
## How to Get Started with the Model
Use the code below to get started with the model.
## Training Details
### Training Data
### Training Procedure
#### Preprocessing [optional]
#### Training Hyperparameters
- Training regime:
#### Speeds, Sizes, Times [optional]
## Evaluation
### Testing Data, Factors & Metrics
#### Testing Data
#### Factors
#### Metrics
### Results
#### Summary
## Model Examination [optional]
## Environmental Impact
Carbon emissions can be estimated using the Machine Learning Impact calculator presented in Lacoste et al. (2019).
- Hardware Type:
- Hours used:
- Cloud Provider:
- Compute Region:
- Carbon Emitted:
## Technical Specifications [optional]
### Model Architecture and Objective
### Compute Infrastructure
#### Hardware
#### Software
[optional]
BibTeX:
APA:
## Glossary [optional]
## More Information [optional]
## Model Card Authors [optional]
## Model Card Contact
### Framework versions
- PEFT 0.10.1.dev0 | [
"# Model Card for Model ID",
"## Model Details",
"### Model Description\n\n\n\n\n\n- Developed by: \n- Funded by [optional]: \n- Shared by [optional]: \n- Model type: \n- Language(s) (NLP): \n- License: \n- Finetuned from model [optional]:",
"### Model Sources [optional]\n\n\n\n- Repository: \n- Paper [optional]: \n- Demo [optional]:",
"## Uses",
"### Direct Use",
"### Downstream Use [optional]",
"### Out-of-Scope Use",
"## Bias, Risks, and Limitations",
"### Recommendations\n\n\n\nUsers (both direct and downstream) should be made aware of the risks, biases and limitations of the model. More information needed for further recommendations.",
"## How to Get Started with the Model\n\nUse the code below to get started with the model.",
"## Training Details",
"### Training Data",
"### Training Procedure",
"#### Preprocessing [optional]",
"#### Training Hyperparameters\n\n- Training regime:",
"#### Speeds, Sizes, Times [optional]",
"## Evaluation",
"### Testing Data, Factors & Metrics",
"#### Testing Data",
"#### Factors",
"#### Metrics",
"### Results",
"#### Summary",
"## Model Examination [optional]",
"## Environmental Impact\n\n\n\nCarbon emissions can be estimated using the Machine Learning Impact calculator presented in Lacoste et al. (2019).\n\n- Hardware Type: \n- Hours used: \n- Cloud Provider: \n- Compute Region: \n- Carbon Emitted:",
"## Technical Specifications [optional]",
"### Model Architecture and Objective",
"### Compute Infrastructure",
"#### Hardware",
"#### Software\n\n\n\n[optional]\n\n\n\nBibTeX:\n\n\n\nAPA:",
"## Glossary [optional]",
"## More Information [optional]",
"## Model Card Authors [optional]",
"## Model Card Contact",
"### Framework versions\n\n- PEFT 0.10.1.dev0"
] | [
"TAGS\n#peft #safetensors #arxiv-1910.09700 #base_model-microsoft/Phi-3-mini-128k-instruct #region-us \n",
"# Model Card for Model ID",
"## Model Details",
"### Model Description\n\n\n\n\n\n- Developed by: \n- Funded by [optional]: \n- Shared by [optional]: \n- Model type: \n- Language(s) (NLP): \n- License: \n- Finetuned from model [optional]:",
"### Model Sources [optional]\n\n\n\n- Repository: \n- Paper [optional]: \n- Demo [optional]:",
"## Uses",
"### Direct Use",
"### Downstream Use [optional]",
"### Out-of-Scope Use",
"## Bias, Risks, and Limitations",
"### Recommendations\n\n\n\nUsers (both direct and downstream) should be made aware of the risks, biases and limitations of the model. More information needed for further recommendations.",
"## How to Get Started with the Model\n\nUse the code below to get started with the model.",
"## Training Details",
"### Training Data",
"### Training Procedure",
"#### Preprocessing [optional]",
"#### Training Hyperparameters\n\n- Training regime:",
"#### Speeds, Sizes, Times [optional]",
"## Evaluation",
"### Testing Data, Factors & Metrics",
"#### Testing Data",
"#### Factors",
"#### Metrics",
"### Results",
"#### Summary",
"## Model Examination [optional]",
"## Environmental Impact\n\n\n\nCarbon emissions can be estimated using the Machine Learning Impact calculator presented in Lacoste et al. (2019).\n\n- Hardware Type: \n- Hours used: \n- Cloud Provider: \n- Compute Region: \n- Carbon Emitted:",
"## Technical Specifications [optional]",
"### Model Architecture and Objective",
"### Compute Infrastructure",
"#### Hardware",
"#### Software\n\n\n\n[optional]\n\n\n\nBibTeX:\n\n\n\nAPA:",
"## Glossary [optional]",
"## More Information [optional]",
"## Model Card Authors [optional]",
"## Model Card Contact",
"### Framework versions\n\n- PEFT 0.10.1.dev0"
] | [
40,
6,
4,
50,
23,
3,
5,
8,
9,
8,
34,
20,
4,
5,
5,
11,
13,
12,
3,
10,
6,
5,
6,
4,
5,
7,
49,
7,
7,
5,
5,
15,
7,
7,
8,
5,
16
] | [
"TAGS\n#peft #safetensors #arxiv-1910.09700 #base_model-microsoft/Phi-3-mini-128k-instruct #region-us \n# Model Card for Model ID## Model Details### Model Description\n\n\n\n\n\n- Developed by: \n- Funded by [optional]: \n- Shared by [optional]: \n- Model type: \n- Language(s) (NLP): \n- License: \n- Finetuned from model [optional]:### Model Sources [optional]\n\n\n\n- Repository: \n- Paper [optional]: \n- Demo [optional]:## Uses### Direct Use### Downstream Use [optional]### Out-of-Scope Use## Bias, Risks, and Limitations### Recommendations\n\n\n\nUsers (both direct and downstream) should be made aware of the risks, biases and limitations of the model. More information needed for further recommendations.## How to Get Started with the Model\n\nUse the code below to get started with the model.## Training Details### Training Data### Training Procedure#### Preprocessing [optional]#### Training Hyperparameters\n\n- Training regime:#### Speeds, Sizes, Times [optional]## Evaluation### Testing Data, Factors & Metrics#### Testing Data#### Factors#### Metrics### Results#### Summary## Model Examination [optional]## Environmental Impact\n\n\n\nCarbon emissions can be estimated using the Machine Learning Impact calculator presented in Lacoste et al. (2019).\n\n- Hardware Type: \n- Hours used: \n- Cloud Provider: \n- Compute Region: \n- Carbon Emitted:## Technical Specifications [optional]### Model Architecture and Objective### Compute Infrastructure#### Hardware#### Software\n\n\n\n[optional]\n\n\n\nBibTeX:\n\n\n\nAPA:## Glossary [optional]## More Information [optional]## Model Card Authors [optional]## Model Card Contact### Framework versions\n\n- PEFT 0.10.1.dev0"
] |
text-generation | transformers | Quantization made by Richard Erkhov.
[Github](https://github.com/RichardErkhov)
[Discord](https://discord.gg/pvy7H8DZMG)
[Request more models](https://github.com/RichardErkhov/quant_request)
gemma-7b-it - bnb 4bits
- Model creator: https://huggingface.co/google/
- Original model: https://huggingface.co/google/gemma-7b-it/
Original model description:
---
library_name: transformers
tags: []
widget:
- messages:
- role: user
content: How does the brain work?
inference:
parameters:
max_new_tokens: 200
extra_gated_heading: Access Gemma on Hugging Face
extra_gated_prompt: >-
To access Gemma on Hugging Face, you’re required to review and agree to
Google’s usage license. To do this, please ensure you’re logged-in to Hugging
Face and click below. Requests are processed immediately.
extra_gated_button_content: Acknowledge license
license: gemma
---
# Gemma Model Card
**Model Page**: [Gemma](https://ai.google.dev/gemma/docs)
This model card corresponds to the 7B instruct version of the Gemma model. You can also visit the model card of the [2B base model](https://huggingface.co/google/gemma-2b), [7B base model](https://huggingface.co/google/gemma-7b), and [2B instruct model](https://huggingface.co/google/gemma-2b-it).
**Resources and Technical Documentation**:
* [Responsible Generative AI Toolkit](https://ai.google.dev/responsible)
* [Gemma on Kaggle](https://www.kaggle.com/models/google/gemma)
* [Gemma on Vertex Model Garden](https://console.cloud.google.com/vertex-ai/publishers/google/model-garden/335?version=gemma-7b-it-gg-hf)
**Terms of Use**: [Terms](https://www.kaggle.com/models/google/gemma/license/consent)
**Authors**: Google
## Model Information
Summary description and brief definition of inputs and outputs.
### Description
Gemma is a family of lightweight, state-of-the-art open models from Google,
built from the same research and technology used to create the Gemini models.
They are text-to-text, decoder-only large language models, available in English,
with open weights, pre-trained variants, and instruction-tuned variants. Gemma
models are well-suited for a variety of text generation tasks, including
question answering, summarization, and reasoning. Their relatively small size
makes it possible to deploy them in environments with limited resources such as
a laptop, desktop or your own cloud infrastructure, democratizing access to
state of the art AI models and helping foster innovation for everyone.
### Usage
Below we share some code snippets on how to get quickly started with running the model. First make sure to `pip install -U transformers`, then copy the snippet from the section that is relevant for your usecase.
#### Fine-tuning the model
You can find fine-tuning scripts and notebook under the [`examples/` directory](https://huggingface.co/google/gemma-7b/tree/main/examples) of [`google/gemma-7b`](https://huggingface.co/google/gemma-7b) repository. To adapt it to this model, simply change the model-id to `google/gemma-7b-it`.
In that repository, we provide:
* A script to perform Supervised Fine-Tuning (SFT) on UltraChat dataset using QLoRA
* A script to perform SFT using FSDP on TPU devices
* A notebook that you can run on a free-tier Google Colab instance to perform SFT on English quotes dataset
#### Running the model on a CPU
As explained below, we recommend `torch.bfloat16` as the default dtype. You can use [a different precision](#precisions) if necessary.
```python
from transformers import AutoTokenizer, AutoModelForCausalLM
import torch
tokenizer = AutoTokenizer.from_pretrained("google/gemma-7b-it")
model = AutoModelForCausalLM.from_pretrained(
"google/gemma-7b-it",
torch_dtype=torch.bfloat16
)
input_text = "Write me a poem about Machine Learning."
input_ids = tokenizer(input_text, return_tensors="pt")
outputs = model.generate(**input_ids)
print(tokenizer.decode(outputs[0]))
```
#### Running the model on a single / multi GPU
```python
# pip install accelerate
from transformers import AutoTokenizer, AutoModelForCausalLM
import torch
tokenizer = AutoTokenizer.from_pretrained("google/gemma-7b-it")
model = AutoModelForCausalLM.from_pretrained(
"google/gemma-7b-it",
device_map="auto",
torch_dtype=torch.bfloat16
)
input_text = "Write me a poem about Machine Learning."
input_ids = tokenizer(input_text, return_tensors="pt").to("cuda")
outputs = model.generate(**input_ids)
print(tokenizer.decode(outputs[0]))
```
<a name="precisions"></a>
#### Running the model on a GPU using different precisions
The native weights of this model were exported in `bfloat16` precision. You can use `float16`, which may be faster on certain hardware, indicating the `torch_dtype` when loading the model. For convenience, the `float16` revision of the repo contains a copy of the weights already converted to that precision.
You can also use `float32` if you skip the dtype, but no precision increase will occur (model weights will just be upcasted to `float32`). See examples below.
* _Using `torch.float16`_
```python
# pip install accelerate
from transformers import AutoTokenizer, AutoModelForCausalLM
import torch
tokenizer = AutoTokenizer.from_pretrained("google/gemma-7b-it")
model = AutoModelForCausalLM.from_pretrained(
"google/gemma-7b-it",
device_map="auto",
torch_dtype=torch.float16,
revision="float16",
)
input_text = "Write me a poem about Machine Learning."
input_ids = tokenizer(input_text, return_tensors="pt").to("cuda")
outputs = model.generate(**input_ids)
print(tokenizer.decode(outputs[0]))
```
* _Using `torch.bfloat16`_
```python
# pip install accelerate
from transformers import AutoTokenizer, AutoModelForCausalLM
tokenizer = AutoTokenizer.from_pretrained("google/gemma-7b-it")
model = AutoModelForCausalLM.from_pretrained("google/gemma-7b-it", device_map="auto", torch_dtype=torch.bfloat16)
input_text = "Write me a poem about Machine Learning."
input_ids = tokenizer(input_text, return_tensors="pt").to("cuda")
outputs = model.generate(**input_ids)
print(tokenizer.decode(outputs[0]))
```
* _Upcasting to `torch.float32`_
```python
# pip install accelerate
from transformers import AutoTokenizer, AutoModelForCausalLM
tokenizer = AutoTokenizer.from_pretrained("google/gemma-7b-it")
model = AutoModelForCausalLM.from_pretrained(
"google/gemma-7b-it",
device_map="auto"
)
input_text = "Write me a poem about Machine Learning."
input_ids = tokenizer(input_text, return_tensors="pt").to("cuda")
outputs = model.generate(**input_ids)
print(tokenizer.decode(outputs[0]))
```
#### Quantized Versions through `bitsandbytes`
* _Using 8-bit precision (int8)_
```python
# pip install bitsandbytes accelerate
from transformers import AutoTokenizer, AutoModelForCausalLM, BitsAndBytesConfig
quantization_config = BitsAndBytesConfig(load_in_8bit=True)
tokenizer = AutoTokenizer.from_pretrained("google/gemma-7b-it")
model = AutoModelForCausalLM.from_pretrained("google/gemma-7b-it", quantization_config=quantization_config)
input_text = "Write me a poem about Machine Learning."
input_ids = tokenizer(input_text, return_tensors="pt").to("cuda")
outputs = model.generate(**input_ids)
print(tokenizer.decode(outputs[0]))
```
* _Using 4-bit precision_
```python
# pip install bitsandbytes accelerate
from transformers import AutoTokenizer, AutoModelForCausalLM, BitsAndBytesConfig
quantization_config = BitsAndBytesConfig(load_in_4bit=True)
tokenizer = AutoTokenizer.from_pretrained("google/gemma-7b-it")
model = AutoModelForCausalLM.from_pretrained("google/gemma-7b-it", quantization_config=quantization_config)
input_text = "Write me a poem about Machine Learning."
input_ids = tokenizer(input_text, return_tensors="pt").to("cuda")
outputs = model.generate(**input_ids)
print(tokenizer.decode(outputs[0]))
```
#### Other optimizations
* _Flash Attention 2_
First make sure to install `flash-attn` in your environment `pip install flash-attn`
```diff
model = AutoModelForCausalLM.from_pretrained(
model_id,
torch_dtype=torch.float16,
+ attn_implementation="flash_attention_2"
).to(0)
```
### Chat Template
The instruction-tuned models use a chat template that must be adhered to for conversational use.
The easiest way to apply it is using the tokenizer's built-in chat template, as shown in the following snippet.
Let's load the model and apply the chat template to a conversation. In this example, we'll start with a single user interaction:
```py
from transformers import AutoTokenizer, AutoModelForCausalLM
import transformers
import torch
model_id = "google/gemma-7b-it"
dtype = torch.bfloat16
tokenizer = AutoTokenizer.from_pretrained(model_id)
model = AutoModelForCausalLM.from_pretrained(
model_id,
device_map="cuda",
torch_dtype=dtype,
)
chat = [
{ "role": "user", "content": "Write a hello world program" },
]
prompt = tokenizer.apply_chat_template(chat, tokenize=False, add_generation_prompt=True)
```
At this point, the prompt contains the following text:
```
<bos><start_of_turn>user
Write a hello world program<end_of_turn>
<start_of_turn>model
```
As you can see, each turn is preceded by a `<start_of_turn>` delimiter and then the role of the entity
(either `user`, for content supplied by the user, or `model` for LLM responses). Turns finish with
the `<end_of_turn>` token.
You can follow this format to build the prompt manually, if you need to do it without the tokenizer's
chat template.
After the prompt is ready, generation can be performed like this:
```py
inputs = tokenizer.encode(prompt, add_special_tokens=False, return_tensors="pt")
outputs = model.generate(input_ids=inputs.to(model.device), max_new_tokens=150)
print(tokenizer.decode(outputs[0]))
```
### Inputs and outputs
* **Input:** Text string, such as a question, a prompt, or a document to be
summarized.
* **Output:** Generated English-language text in response to the input, such
as an answer to a question, or a summary of a document.
## Model Data
Data used for model training and how the data was processed.
### Training Dataset
These models were trained on a dataset of text data that includes a wide variety
of sources, totaling 6 trillion tokens. Here are the key components:
* Web Documents: A diverse collection of web text ensures the model is exposed
to a broad range of linguistic styles, topics, and vocabulary. Primarily
English-language content.
* Code: Exposing the model to code helps it to learn the syntax and patterns of
programming languages, which improves its ability to generate code or
understand code-related questions.
* Mathematics: Training on mathematical text helps the model learn logical
reasoning, symbolic representation, and to address mathematical queries.
The combination of these diverse data sources is crucial for training a powerful
language model that can handle a wide variety of different tasks and text
formats.
### Data Preprocessing
Here are the key data cleaning and filtering methods applied to the training
data:
* CSAM Filtering: Rigorous CSAM (Child Sexual Abuse Material) filtering was
applied at multiple stages in the data preparation process to ensure the
exclusion of harmful and illegal content
* Sensitive Data Filtering: As part of making Gemma pre-trained models safe and
reliable, automated techniques were used to filter out certain personal
information and other sensitive data from training sets.
* Additional methods: Filtering based on content quality and safely in line with
[our policies](https://storage.googleapis.com/gweb-uniblog-publish-prod/documents/2023_Google_AI_Principles_Progress_Update.pdf#page=11).
## Implementation Information
Details about the model internals.
### Hardware
Gemma was trained using the latest generation of
[Tensor Processing Unit (TPU)](https://cloud.google.com/tpu/docs/intro-to-tpu) hardware (TPUv5e).
Training large language models requires significant computational power. TPUs,
designed specifically for matrix operations common in machine learning, offer
several advantages in this domain:
* Performance: TPUs are specifically designed to handle the massive computations
involved in training LLMs. They can speed up training considerably compared to
CPUs.
* Memory: TPUs often come with large amounts of high-bandwidth memory, allowing
for the handling of large models and batch sizes during training. This can
lead to better model quality.
* Scalability: TPU Pods (large clusters of TPUs) provide a scalable solution for
handling the growing complexity of large foundation models. You can distribute
training across multiple TPU devices for faster and more efficient processing.
* Cost-effectiveness: In many scenarios, TPUs can provide a more cost-effective
solution for training large models compared to CPU-based infrastructure,
especially when considering the time and resources saved due to faster
training.
* These advantages are aligned with
[Google's commitments to operate sustainably](https://sustainability.google/operating-sustainably/).
### Software
Training was done using [JAX](https://github.com/google/jax) and [ML Pathways](https://blog.google/technology/ai/introducing-pathways-next-generation-ai-architecture).
JAX allows researchers to take advantage of the latest generation of hardware,
including TPUs, for faster and more efficient training of large models.
ML Pathways is Google's latest effort to build artificially intelligent systems
capable of generalizing across multiple tasks. This is specially suitable for
[foundation models](https://ai.google/discover/foundation-models/), including large language models like
these ones.
Together, JAX and ML Pathways are used as described in the
[paper about the Gemini family of models](https://arxiv.org/abs/2312.11805); "the 'single
controller' programming model of Jax and Pathways allows a single Python
process to orchestrate the entire training run, dramatically simplifying the
development workflow."
## Evaluation
Model evaluation metrics and results.
### Benchmark Results
These models were evaluated against a large collection of different datasets and
metrics to cover different aspects of text generation:
| Benchmark | Metric | 2B Params | 7B Params |
| ------------------------------ | ------------- | ----------- | --------- |
| [MMLU](https://arxiv.org/abs/2009.03300) | 5-shot, top-1 | 42.3 | 64.3 |
| [HellaSwag](https://arxiv.org/abs/1905.07830) | 0-shot |71.4 | 81.2 |
| [PIQA](https://arxiv.org/abs/1911.11641) | 0-shot | 77.3 | 81.2 |
| [SocialIQA](https://arxiv.org/abs/1904.09728) | 0-shot | 49.7 | 51.8 |
| [BooIQ](https://arxiv.org/abs/1905.10044) | 0-shot | 69.4 | 83.2 |
| [WinoGrande](https://arxiv.org/abs/1907.10641) | partial score | 65.4 | 72.3 |
| [CommonsenseQA](https://arxiv.org/abs/1811.00937) | 7-shot | 65.3 | 71.3 |
| [OpenBookQA](https://arxiv.org/abs/1809.02789) | | 47.8 | 52.8 |
| [ARC-e](https://arxiv.org/abs/1911.01547) | | 73.2 | 81.5 |
| [ARC-c](https://arxiv.org/abs/1911.01547) | | 42.1 | 53.2 |
| [TriviaQA](https://arxiv.org/abs/1705.03551) | 5-shot | 53.2 | 63.4 |
| [Natural Questions](https://github.com/google-research-datasets/natural-questions) | 5-shot | 12.5 | 23 |
| [HumanEval](https://arxiv.org/abs/2107.03374) | pass@1 | 22.0 | 32.3 |
| [MBPP](https://arxiv.org/abs/2108.07732) | 3-shot | 29.2 | 44.4 |
| [GSM8K](https://arxiv.org/abs/2110.14168) | maj@1 | 17.7 | 46.4 |
| [MATH](https://arxiv.org/abs/2108.07732) | 4-shot | 11.8 | 24.3 |
| [AGIEval](https://arxiv.org/abs/2304.06364) | | 24.2 | 41.7 |
| [BIG-Bench](https://arxiv.org/abs/2206.04615) | | 35.2 | 55.1 |
| ------------------------------ | ------------- | ----------- | --------- |
| **Average** | | **45.0** | **56.9** |
## Ethics and Safety
Ethics and safety evaluation approach and results.
### Evaluation Approach
Our evaluation methods include structured evaluations and internal red-teaming
testing of relevant content policies. Red-teaming was conducted by a number of
different teams, each with different goals and human evaluation metrics. These
models were evaluated against a number of different categories relevant to
ethics and safety, including:
* Text-to-Text Content Safety: Human evaluation on prompts covering safety
policies including child sexual abuse and exploitation, harassment, violence
and gore, and hate speech.
* Text-to-Text Representational Harms: Benchmark against relevant academic
datasets such as [WinoBias](https://arxiv.org/abs/1804.06876) and [BBQ Dataset](https://arxiv.org/abs/2110.08193v2).
* Memorization: Automated evaluation of memorization of training data, including
the risk of personally identifiable information exposure.
* Large-scale harm: Tests for "dangerous capabilities," such as chemical,
biological, radiological, and nuclear (CBRN) risks.
### Evaluation Results
The results of ethics and safety evaluations are within acceptable thresholds
for meeting [internal policies](https://storage.googleapis.com/gweb-uniblog-publish-prod/documents/2023_Google_AI_Principles_Progress_Update.pdf#page=11) for categories such as child
safety, content safety, representational harms, memorization, large-scale harms.
On top of robust internal evaluations, the results of well known safety
benchmarks like BBQ, BOLD, Winogender, Winobias, RealToxicity, and TruthfulQA
are shown here.
| Benchmark | Metric | 2B Params | 7B Params |
| ------------------------------ | ------------- | ----------- | --------- |
| [RealToxicity](https://arxiv.org/abs/2009.11462) | average | 6.86 | 7.90 |
| [BOLD](https://arxiv.org/abs/2101.11718) | | 45.57 | 49.08 |
| [CrowS-Pairs](https://aclanthology.org/2020.emnlp-main.154/) | top-1 | 45.82 | 51.33 |
| [BBQ Ambig](https://arxiv.org/abs/2110.08193v2) | 1-shot, top-1 | 62.58 | 92.54 |
| [BBQ Disambig](https://arxiv.org/abs/2110.08193v2) | top-1 | 54.62 | 71.99 |
| [Winogender](https://arxiv.org/abs/1804.09301) | top-1 | 51.25 | 54.17 |
| [TruthfulQA](https://arxiv.org/abs/2109.07958) | | 44.84 | 31.81 |
| [Winobias 1_2](https://arxiv.org/abs/1804.06876) | | 56.12 | 59.09 |
| [Winobias 2_2](https://arxiv.org/abs/1804.06876) | | 91.10 | 92.23 |
| [Toxigen](https://arxiv.org/abs/2203.09509) | | 29.77 | 39.59 |
| ------------------------------ | ------------- | ----------- | --------- |
## Usage and Limitations
These models have certain limitations that users should be aware of.
### Intended Usage
Open Large Language Models (LLMs) have a wide range of applications across
various industries and domains. The following list of potential uses is not
comprehensive. The purpose of this list is to provide contextual information
about the possible use-cases that the model creators considered as part of model
training and development.
* Content Creation and Communication
* Text Generation: These models can be used to generate creative text formats
such as poems, scripts, code, marketing copy, and email drafts.
* Chatbots and Conversational AI: Power conversational interfaces for customer
service, virtual assistants, or interactive applications.
* Text Summarization: Generate concise summaries of a text corpus, research
papers, or reports.
* Research and Education
* Natural Language Processing (NLP) Research: These models can serve as a
foundation for researchers to experiment with NLP techniques, develop
algorithms, and contribute to the advancement of the field.
* Language Learning Tools: Support interactive language learning experiences,
aiding in grammar correction or providing writing practice.
* Knowledge Exploration: Assist researchers in exploring large bodies of text
by generating summaries or answering questions about specific topics.
### Limitations
* Training Data
* The quality and diversity of the training data significantly influence the
model's capabilities. Biases or gaps in the training data can lead to
limitations in the model's responses.
* The scope of the training dataset determines the subject areas the model can
handle effectively.
* Context and Task Complexity
* LLMs are better at tasks that can be framed with clear prompts and
instructions. Open-ended or highly complex tasks might be challenging.
* A model's performance can be influenced by the amount of context provided
(longer context generally leads to better outputs, up to a certain point).
* Language Ambiguity and Nuance
* Natural language is inherently complex. LLMs might struggle to grasp subtle
nuances, sarcasm, or figurative language.
* Factual Accuracy
* LLMs generate responses based on information they learned from their
training datasets, but they are not knowledge bases. They may generate
incorrect or outdated factual statements.
* Common Sense
* LLMs rely on statistical patterns in language. They might lack the ability
to apply common sense reasoning in certain situations.
### Ethical Considerations and Risks
The development of large language models (LLMs) raises several ethical concerns.
In creating an open model, we have carefully considered the following:
* Bias and Fairness
* LLMs trained on large-scale, real-world text data can reflect socio-cultural
biases embedded in the training material. These models underwent careful
scrutiny, input data pre-processing described and posterior evaluations
reported in this card.
* Misinformation and Misuse
* LLMs can be misused to generate text that is false, misleading, or harmful.
* Guidelines are provided for responsible use with the model, see the
[Responsible Generative AI Toolkit](http://ai.google.dev/gemma/responsible).
* Transparency and Accountability:
* This model card summarizes details on the models' architecture,
capabilities, limitations, and evaluation processes.
* A responsibly developed open model offers the opportunity to share
innovation by making LLM technology accessible to developers and researchers
across the AI ecosystem.
Risks identified and mitigations:
* Perpetuation of biases: It's encouraged to perform continuous monitoring
(using evaluation metrics, human review) and the exploration of de-biasing
techniques during model training, fine-tuning, and other use cases.
* Generation of harmful content: Mechanisms and guidelines for content safety
are essential. Developers are encouraged to exercise caution and implement
appropriate content safety safeguards based on their specific product policies
and application use cases.
* Misuse for malicious purposes: Technical limitations and developer and
end-user education can help mitigate against malicious applications of LLMs.
Educational resources and reporting mechanisms for users to flag misuse are
provided. Prohibited uses of Gemma models are outlined in the
[Gemma Prohibited Use Policy](https://ai.google.dev/gemma/prohibited_use_policy).
* Privacy violations: Models were trained on data filtered for removal of PII
(Personally Identifiable Information). Developers are encouraged to adhere to
privacy regulations with privacy-preserving techniques.
### Benefits
At the time of release, this family of models provides high-performance open
large language model implementations designed from the ground up for Responsible
AI development compared to similarly sized models.
Using the benchmark evaluation metrics described in this document, these models
have shown to provide superior performance to other, comparably-sized open model
alternatives.
| {} | RichardErkhov/google_-_gemma-7b-it-4bits | null | [
"transformers",
"safetensors",
"gemma",
"text-generation",
"conversational",
"arxiv:2312.11805",
"arxiv:2009.03300",
"arxiv:1905.07830",
"arxiv:1911.11641",
"arxiv:1904.09728",
"arxiv:1905.10044",
"arxiv:1907.10641",
"arxiv:1811.00937",
"arxiv:1809.02789",
"arxiv:1911.01547",
"arxiv:1705.03551",
"arxiv:2107.03374",
"arxiv:2108.07732",
"arxiv:2110.14168",
"arxiv:2304.06364",
"arxiv:2206.04615",
"arxiv:1804.06876",
"arxiv:2110.08193",
"arxiv:2009.11462",
"arxiv:2101.11718",
"arxiv:1804.09301",
"arxiv:2109.07958",
"arxiv:2203.09509",
"autotrain_compatible",
"endpoints_compatible",
"text-generation-inference",
"4-bit",
"region:us"
] | null | 2024-05-02T11:26:18+00:00 | [
"2312.11805",
"2009.03300",
"1905.07830",
"1911.11641",
"1904.09728",
"1905.10044",
"1907.10641",
"1811.00937",
"1809.02789",
"1911.01547",
"1705.03551",
"2107.03374",
"2108.07732",
"2110.14168",
"2304.06364",
"2206.04615",
"1804.06876",
"2110.08193",
"2009.11462",
"2101.11718",
"1804.09301",
"2109.07958",
"2203.09509"
] | [] | TAGS
#transformers #safetensors #gemma #text-generation #conversational #arxiv-2312.11805 #arxiv-2009.03300 #arxiv-1905.07830 #arxiv-1911.11641 #arxiv-1904.09728 #arxiv-1905.10044 #arxiv-1907.10641 #arxiv-1811.00937 #arxiv-1809.02789 #arxiv-1911.01547 #arxiv-1705.03551 #arxiv-2107.03374 #arxiv-2108.07732 #arxiv-2110.14168 #arxiv-2304.06364 #arxiv-2206.04615 #arxiv-1804.06876 #arxiv-2110.08193 #arxiv-2009.11462 #arxiv-2101.11718 #arxiv-1804.09301 #arxiv-2109.07958 #arxiv-2203.09509 #autotrain_compatible #endpoints_compatible #text-generation-inference #4-bit #region-us
| Quantization made by Richard Erkhov.
Github
Discord
Request more models
gemma-7b-it - bnb 4bits
* Model creator: URL
* Original model: URL
Original model description:
---------------------------
library\_name: transformers
tags: []
widget:
* messages:
+ role: user
content: How does the brain work?
inference:
parameters:
max\_new\_tokens: 200
extra\_gated\_heading: Access Gemma on Hugging Face
extra\_gated\_prompt: >-
To access Gemma on Hugging Face, you’re required to review and agree to
Google’s usage license. To do this, please ensure you’re logged-in to Hugging
Face and click below. Requests are processed immediately.
extra\_gated\_button\_content: Acknowledge license
license: gemma
---
Gemma Model Card
================
Model Page: Gemma
This model card corresponds to the 7B instruct version of the Gemma model. You can also visit the model card of the 2B base model, 7B base model, and 2B instruct model.
Resources and Technical Documentation:
* Responsible Generative AI Toolkit
* Gemma on Kaggle
* Gemma on Vertex Model Garden
Terms of Use: Terms
Authors: Google
Model Information
-----------------
Summary description and brief definition of inputs and outputs.
### Description
Gemma is a family of lightweight, state-of-the-art open models from Google,
built from the same research and technology used to create the Gemini models.
They are text-to-text, decoder-only large language models, available in English,
with open weights, pre-trained variants, and instruction-tuned variants. Gemma
models are well-suited for a variety of text generation tasks, including
question answering, summarization, and reasoning. Their relatively small size
makes it possible to deploy them in environments with limited resources such as
a laptop, desktop or your own cloud infrastructure, democratizing access to
state of the art AI models and helping foster innovation for everyone.
### Usage
Below we share some code snippets on how to get quickly started with running the model. First make sure to 'pip install -U transformers', then copy the snippet from the section that is relevant for your usecase.
#### Fine-tuning the model
You can find fine-tuning scripts and notebook under the 'examples/' directory of 'google/gemma-7b' repository. To adapt it to this model, simply change the model-id to 'google/gemma-7b-it'.
In that repository, we provide:
* A script to perform Supervised Fine-Tuning (SFT) on UltraChat dataset using QLoRA
* A script to perform SFT using FSDP on TPU devices
* A notebook that you can run on a free-tier Google Colab instance to perform SFT on English quotes dataset
#### Running the model on a CPU
As explained below, we recommend 'torch.bfloat16' as the default dtype. You can use a different precision if necessary.
#### Running the model on a single / multi GPU
#### Running the model on a GPU using different precisions
The native weights of this model were exported in 'bfloat16' precision. You can use 'float16', which may be faster on certain hardware, indicating the 'torch\_dtype' when loading the model. For convenience, the 'float16' revision of the repo contains a copy of the weights already converted to that precision.
You can also use 'float32' if you skip the dtype, but no precision increase will occur (model weights will just be upcasted to 'float32'). See examples below.
* *Using 'torch.float16'*
* *Using 'torch.bfloat16'*
* *Upcasting to 'torch.float32'*
#### Quantized Versions through 'bitsandbytes'
* *Using 8-bit precision (int8)*
* *Using 4-bit precision*
#### Other optimizations
* *Flash Attention 2*
First make sure to install 'flash-attn' in your environment 'pip install flash-attn'
### Chat Template
The instruction-tuned models use a chat template that must be adhered to for conversational use.
The easiest way to apply it is using the tokenizer's built-in chat template, as shown in the following snippet.
Let's load the model and apply the chat template to a conversation. In this example, we'll start with a single user interaction:
At this point, the prompt contains the following text:
As you can see, each turn is preceded by a '<start\_of\_turn>' delimiter and then the role of the entity
(either 'user', for content supplied by the user, or 'model' for LLM responses). Turns finish with
the '<end\_of\_turn>' token.
You can follow this format to build the prompt manually, if you need to do it without the tokenizer's
chat template.
After the prompt is ready, generation can be performed like this:
### Inputs and outputs
* Input: Text string, such as a question, a prompt, or a document to be
summarized.
* Output: Generated English-language text in response to the input, such
as an answer to a question, or a summary of a document.
Model Data
----------
Data used for model training and how the data was processed.
### Training Dataset
These models were trained on a dataset of text data that includes a wide variety
of sources, totaling 6 trillion tokens. Here are the key components:
* Web Documents: A diverse collection of web text ensures the model is exposed
to a broad range of linguistic styles, topics, and vocabulary. Primarily
English-language content.
* Code: Exposing the model to code helps it to learn the syntax and patterns of
programming languages, which improves its ability to generate code or
understand code-related questions.
* Mathematics: Training on mathematical text helps the model learn logical
reasoning, symbolic representation, and to address mathematical queries.
The combination of these diverse data sources is crucial for training a powerful
language model that can handle a wide variety of different tasks and text
formats.
### Data Preprocessing
Here are the key data cleaning and filtering methods applied to the training
data:
* CSAM Filtering: Rigorous CSAM (Child Sexual Abuse Material) filtering was
applied at multiple stages in the data preparation process to ensure the
exclusion of harmful and illegal content
* Sensitive Data Filtering: As part of making Gemma pre-trained models safe and
reliable, automated techniques were used to filter out certain personal
information and other sensitive data from training sets.
* Additional methods: Filtering based on content quality and safely in line with
our policies.
Implementation Information
--------------------------
Details about the model internals.
### Hardware
Gemma was trained using the latest generation of
Tensor Processing Unit (TPU) hardware (TPUv5e).
Training large language models requires significant computational power. TPUs,
designed specifically for matrix operations common in machine learning, offer
several advantages in this domain:
* Performance: TPUs are specifically designed to handle the massive computations
involved in training LLMs. They can speed up training considerably compared to
CPUs.
* Memory: TPUs often come with large amounts of high-bandwidth memory, allowing
for the handling of large models and batch sizes during training. This can
lead to better model quality.
* Scalability: TPU Pods (large clusters of TPUs) provide a scalable solution for
handling the growing complexity of large foundation models. You can distribute
training across multiple TPU devices for faster and more efficient processing.
* Cost-effectiveness: In many scenarios, TPUs can provide a more cost-effective
solution for training large models compared to CPU-based infrastructure,
especially when considering the time and resources saved due to faster
training.
* These advantages are aligned with
Google's commitments to operate sustainably.
### Software
Training was done using JAX and ML Pathways.
JAX allows researchers to take advantage of the latest generation of hardware,
including TPUs, for faster and more efficient training of large models.
ML Pathways is Google's latest effort to build artificially intelligent systems
capable of generalizing across multiple tasks. This is specially suitable for
foundation models, including large language models like
these ones.
Together, JAX and ML Pathways are used as described in the
paper about the Gemini family of models; "the 'single
controller' programming model of Jax and Pathways allows a single Python
process to orchestrate the entire training run, dramatically simplifying the
development workflow."
Evaluation
----------
Model evaluation metrics and results.
### Benchmark Results
These models were evaluated against a large collection of different datasets and
metrics to cover different aspects of text generation:
Ethics and Safety
-----------------
Ethics and safety evaluation approach and results.
### Evaluation Approach
Our evaluation methods include structured evaluations and internal red-teaming
testing of relevant content policies. Red-teaming was conducted by a number of
different teams, each with different goals and human evaluation metrics. These
models were evaluated against a number of different categories relevant to
ethics and safety, including:
* Text-to-Text Content Safety: Human evaluation on prompts covering safety
policies including child sexual abuse and exploitation, harassment, violence
and gore, and hate speech.
* Text-to-Text Representational Harms: Benchmark against relevant academic
datasets such as WinoBias and BBQ Dataset.
* Memorization: Automated evaluation of memorization of training data, including
the risk of personally identifiable information exposure.
* Large-scale harm: Tests for "dangerous capabilities," such as chemical,
biological, radiological, and nuclear (CBRN) risks.
### Evaluation Results
The results of ethics and safety evaluations are within acceptable thresholds
for meeting internal policies for categories such as child
safety, content safety, representational harms, memorization, large-scale harms.
On top of robust internal evaluations, the results of well known safety
benchmarks like BBQ, BOLD, Winogender, Winobias, RealToxicity, and TruthfulQA
are shown here.
Usage and Limitations
---------------------
These models have certain limitations that users should be aware of.
### Intended Usage
Open Large Language Models (LLMs) have a wide range of applications across
various industries and domains. The following list of potential uses is not
comprehensive. The purpose of this list is to provide contextual information
about the possible use-cases that the model creators considered as part of model
training and development.
* Content Creation and Communication
+ Text Generation: These models can be used to generate creative text formats
such as poems, scripts, code, marketing copy, and email drafts.
+ Chatbots and Conversational AI: Power conversational interfaces for customer
service, virtual assistants, or interactive applications.
+ Text Summarization: Generate concise summaries of a text corpus, research
papers, or reports.
* Research and Education
+ Natural Language Processing (NLP) Research: These models can serve as a
foundation for researchers to experiment with NLP techniques, develop
algorithms, and contribute to the advancement of the field.
+ Language Learning Tools: Support interactive language learning experiences,
aiding in grammar correction or providing writing practice.
+ Knowledge Exploration: Assist researchers in exploring large bodies of text
by generating summaries or answering questions about specific topics.
### Limitations
* Training Data
+ The quality and diversity of the training data significantly influence the
model's capabilities. Biases or gaps in the training data can lead to
limitations in the model's responses.
+ The scope of the training dataset determines the subject areas the model can
handle effectively.
* Context and Task Complexity
+ LLMs are better at tasks that can be framed with clear prompts and
instructions. Open-ended or highly complex tasks might be challenging.
+ A model's performance can be influenced by the amount of context provided
(longer context generally leads to better outputs, up to a certain point).
* Language Ambiguity and Nuance
+ Natural language is inherently complex. LLMs might struggle to grasp subtle
nuances, sarcasm, or figurative language.
* Factual Accuracy
+ LLMs generate responses based on information they learned from their
training datasets, but they are not knowledge bases. They may generate
incorrect or outdated factual statements.
* Common Sense
+ LLMs rely on statistical patterns in language. They might lack the ability
to apply common sense reasoning in certain situations.
### Ethical Considerations and Risks
The development of large language models (LLMs) raises several ethical concerns.
In creating an open model, we have carefully considered the following:
* Bias and Fairness
+ LLMs trained on large-scale, real-world text data can reflect socio-cultural
biases embedded in the training material. These models underwent careful
scrutiny, input data pre-processing described and posterior evaluations
reported in this card.
* Misinformation and Misuse
+ LLMs can be misused to generate text that is false, misleading, or harmful.
+ Guidelines are provided for responsible use with the model, see the
Responsible Generative AI Toolkit.
* Transparency and Accountability:
+ This model card summarizes details on the models' architecture,
capabilities, limitations, and evaluation processes.
+ A responsibly developed open model offers the opportunity to share
innovation by making LLM technology accessible to developers and researchers
across the AI ecosystem.
Risks identified and mitigations:
* Perpetuation of biases: It's encouraged to perform continuous monitoring
(using evaluation metrics, human review) and the exploration of de-biasing
techniques during model training, fine-tuning, and other use cases.
* Generation of harmful content: Mechanisms and guidelines for content safety
are essential. Developers are encouraged to exercise caution and implement
appropriate content safety safeguards based on their specific product policies
and application use cases.
* Misuse for malicious purposes: Technical limitations and developer and
end-user education can help mitigate against malicious applications of LLMs.
Educational resources and reporting mechanisms for users to flag misuse are
provided. Prohibited uses of Gemma models are outlined in the
Gemma Prohibited Use Policy.
* Privacy violations: Models were trained on data filtered for removal of PII
(Personally Identifiable Information). Developers are encouraged to adhere to
privacy regulations with privacy-preserving techniques.
### Benefits
At the time of release, this family of models provides high-performance open
large language model implementations designed from the ground up for Responsible
AI development compared to similarly sized models.
Using the benchmark evaluation metrics described in this document, these models
have shown to provide superior performance to other, comparably-sized open model
alternatives.
| [
"### Description\n\n\nGemma is a family of lightweight, state-of-the-art open models from Google,\nbuilt from the same research and technology used to create the Gemini models.\nThey are text-to-text, decoder-only large language models, available in English,\nwith open weights, pre-trained variants, and instruction-tuned variants. Gemma\nmodels are well-suited for a variety of text generation tasks, including\nquestion answering, summarization, and reasoning. Their relatively small size\nmakes it possible to deploy them in environments with limited resources such as\na laptop, desktop or your own cloud infrastructure, democratizing access to\nstate of the art AI models and helping foster innovation for everyone.",
"### Usage\n\n\nBelow we share some code snippets on how to get quickly started with running the model. First make sure to 'pip install -U transformers', then copy the snippet from the section that is relevant for your usecase.",
"#### Fine-tuning the model\n\n\nYou can find fine-tuning scripts and notebook under the 'examples/' directory of 'google/gemma-7b' repository. To adapt it to this model, simply change the model-id to 'google/gemma-7b-it'.\nIn that repository, we provide:\n\n\n* A script to perform Supervised Fine-Tuning (SFT) on UltraChat dataset using QLoRA\n* A script to perform SFT using FSDP on TPU devices\n* A notebook that you can run on a free-tier Google Colab instance to perform SFT on English quotes dataset",
"#### Running the model on a CPU\n\n\nAs explained below, we recommend 'torch.bfloat16' as the default dtype. You can use a different precision if necessary.",
"#### Running the model on a single / multi GPU",
"#### Running the model on a GPU using different precisions\n\n\nThe native weights of this model were exported in 'bfloat16' precision. You can use 'float16', which may be faster on certain hardware, indicating the 'torch\\_dtype' when loading the model. For convenience, the 'float16' revision of the repo contains a copy of the weights already converted to that precision.\n\n\nYou can also use 'float32' if you skip the dtype, but no precision increase will occur (model weights will just be upcasted to 'float32'). See examples below.\n\n\n* *Using 'torch.float16'*\n* *Using 'torch.bfloat16'*\n* *Upcasting to 'torch.float32'*",
"#### Quantized Versions through 'bitsandbytes'\n\n\n* *Using 8-bit precision (int8)*\n* *Using 4-bit precision*",
"#### Other optimizations\n\n\n* *Flash Attention 2*\n\n\nFirst make sure to install 'flash-attn' in your environment 'pip install flash-attn'",
"### Chat Template\n\n\nThe instruction-tuned models use a chat template that must be adhered to for conversational use.\nThe easiest way to apply it is using the tokenizer's built-in chat template, as shown in the following snippet.\n\n\nLet's load the model and apply the chat template to a conversation. In this example, we'll start with a single user interaction:\n\n\nAt this point, the prompt contains the following text:\n\n\nAs you can see, each turn is preceded by a '<start\\_of\\_turn>' delimiter and then the role of the entity\n(either 'user', for content supplied by the user, or 'model' for LLM responses). Turns finish with\nthe '<end\\_of\\_turn>' token.\n\n\nYou can follow this format to build the prompt manually, if you need to do it without the tokenizer's\nchat template.\n\n\nAfter the prompt is ready, generation can be performed like this:",
"### Inputs and outputs\n\n\n* Input: Text string, such as a question, a prompt, or a document to be\nsummarized.\n* Output: Generated English-language text in response to the input, such\nas an answer to a question, or a summary of a document.\n\n\nModel Data\n----------\n\n\nData used for model training and how the data was processed.",
"### Training Dataset\n\n\nThese models were trained on a dataset of text data that includes a wide variety\nof sources, totaling 6 trillion tokens. Here are the key components:\n\n\n* Web Documents: A diverse collection of web text ensures the model is exposed\nto a broad range of linguistic styles, topics, and vocabulary. Primarily\nEnglish-language content.\n* Code: Exposing the model to code helps it to learn the syntax and patterns of\nprogramming languages, which improves its ability to generate code or\nunderstand code-related questions.\n* Mathematics: Training on mathematical text helps the model learn logical\nreasoning, symbolic representation, and to address mathematical queries.\n\n\nThe combination of these diverse data sources is crucial for training a powerful\nlanguage model that can handle a wide variety of different tasks and text\nformats.",
"### Data Preprocessing\n\n\nHere are the key data cleaning and filtering methods applied to the training\ndata:\n\n\n* CSAM Filtering: Rigorous CSAM (Child Sexual Abuse Material) filtering was\napplied at multiple stages in the data preparation process to ensure the\nexclusion of harmful and illegal content\n* Sensitive Data Filtering: As part of making Gemma pre-trained models safe and\nreliable, automated techniques were used to filter out certain personal\ninformation and other sensitive data from training sets.\n* Additional methods: Filtering based on content quality and safely in line with\nour policies.\n\n\nImplementation Information\n--------------------------\n\n\nDetails about the model internals.",
"### Hardware\n\n\nGemma was trained using the latest generation of\nTensor Processing Unit (TPU) hardware (TPUv5e).\n\n\nTraining large language models requires significant computational power. TPUs,\ndesigned specifically for matrix operations common in machine learning, offer\nseveral advantages in this domain:\n\n\n* Performance: TPUs are specifically designed to handle the massive computations\ninvolved in training LLMs. They can speed up training considerably compared to\nCPUs.\n* Memory: TPUs often come with large amounts of high-bandwidth memory, allowing\nfor the handling of large models and batch sizes during training. This can\nlead to better model quality.\n* Scalability: TPU Pods (large clusters of TPUs) provide a scalable solution for\nhandling the growing complexity of large foundation models. You can distribute\ntraining across multiple TPU devices for faster and more efficient processing.\n* Cost-effectiveness: In many scenarios, TPUs can provide a more cost-effective\nsolution for training large models compared to CPU-based infrastructure,\nespecially when considering the time and resources saved due to faster\ntraining.\n* These advantages are aligned with\nGoogle's commitments to operate sustainably.",
"### Software\n\n\nTraining was done using JAX and ML Pathways.\n\n\nJAX allows researchers to take advantage of the latest generation of hardware,\nincluding TPUs, for faster and more efficient training of large models.\n\n\nML Pathways is Google's latest effort to build artificially intelligent systems\ncapable of generalizing across multiple tasks. This is specially suitable for\nfoundation models, including large language models like\nthese ones.\n\n\nTogether, JAX and ML Pathways are used as described in the\npaper about the Gemini family of models; \"the 'single\ncontroller' programming model of Jax and Pathways allows a single Python\nprocess to orchestrate the entire training run, dramatically simplifying the\ndevelopment workflow.\"\n\n\nEvaluation\n----------\n\n\nModel evaluation metrics and results.",
"### Benchmark Results\n\n\nThese models were evaluated against a large collection of different datasets and\nmetrics to cover different aspects of text generation:\n\n\n\nEthics and Safety\n-----------------\n\n\nEthics and safety evaluation approach and results.",
"### Evaluation Approach\n\n\nOur evaluation methods include structured evaluations and internal red-teaming\ntesting of relevant content policies. Red-teaming was conducted by a number of\ndifferent teams, each with different goals and human evaluation metrics. These\nmodels were evaluated against a number of different categories relevant to\nethics and safety, including:\n\n\n* Text-to-Text Content Safety: Human evaluation on prompts covering safety\npolicies including child sexual abuse and exploitation, harassment, violence\nand gore, and hate speech.\n* Text-to-Text Representational Harms: Benchmark against relevant academic\ndatasets such as WinoBias and BBQ Dataset.\n* Memorization: Automated evaluation of memorization of training data, including\nthe risk of personally identifiable information exposure.\n* Large-scale harm: Tests for \"dangerous capabilities,\" such as chemical,\nbiological, radiological, and nuclear (CBRN) risks.",
"### Evaluation Results\n\n\nThe results of ethics and safety evaluations are within acceptable thresholds\nfor meeting internal policies for categories such as child\nsafety, content safety, representational harms, memorization, large-scale harms.\nOn top of robust internal evaluations, the results of well known safety\nbenchmarks like BBQ, BOLD, Winogender, Winobias, RealToxicity, and TruthfulQA\nare shown here.\n\n\n\nUsage and Limitations\n---------------------\n\n\nThese models have certain limitations that users should be aware of.",
"### Intended Usage\n\n\nOpen Large Language Models (LLMs) have a wide range of applications across\nvarious industries and domains. The following list of potential uses is not\ncomprehensive. The purpose of this list is to provide contextual information\nabout the possible use-cases that the model creators considered as part of model\ntraining and development.\n\n\n* Content Creation and Communication\n\t+ Text Generation: These models can be used to generate creative text formats\n\tsuch as poems, scripts, code, marketing copy, and email drafts.\n\t+ Chatbots and Conversational AI: Power conversational interfaces for customer\n\tservice, virtual assistants, or interactive applications.\n\t+ Text Summarization: Generate concise summaries of a text corpus, research\n\tpapers, or reports.\n* Research and Education\n\t+ Natural Language Processing (NLP) Research: These models can serve as a\n\tfoundation for researchers to experiment with NLP techniques, develop\n\talgorithms, and contribute to the advancement of the field.\n\t+ Language Learning Tools: Support interactive language learning experiences,\n\taiding in grammar correction or providing writing practice.\n\t+ Knowledge Exploration: Assist researchers in exploring large bodies of text\n\tby generating summaries or answering questions about specific topics.",
"### Limitations\n\n\n* Training Data\n\t+ The quality and diversity of the training data significantly influence the\n\tmodel's capabilities. Biases or gaps in the training data can lead to\n\tlimitations in the model's responses.\n\t+ The scope of the training dataset determines the subject areas the model can\n\thandle effectively.\n* Context and Task Complexity\n\t+ LLMs are better at tasks that can be framed with clear prompts and\n\tinstructions. Open-ended or highly complex tasks might be challenging.\n\t+ A model's performance can be influenced by the amount of context provided\n\t(longer context generally leads to better outputs, up to a certain point).\n* Language Ambiguity and Nuance\n\t+ Natural language is inherently complex. LLMs might struggle to grasp subtle\n\tnuances, sarcasm, or figurative language.\n* Factual Accuracy\n\t+ LLMs generate responses based on information they learned from their\n\ttraining datasets, but they are not knowledge bases. They may generate\n\tincorrect or outdated factual statements.\n* Common Sense\n\t+ LLMs rely on statistical patterns in language. They might lack the ability\n\tto apply common sense reasoning in certain situations.",
"### Ethical Considerations and Risks\n\n\nThe development of large language models (LLMs) raises several ethical concerns.\nIn creating an open model, we have carefully considered the following:\n\n\n* Bias and Fairness\n\t+ LLMs trained on large-scale, real-world text data can reflect socio-cultural\n\tbiases embedded in the training material. These models underwent careful\n\tscrutiny, input data pre-processing described and posterior evaluations\n\treported in this card.\n* Misinformation and Misuse\n\t+ LLMs can be misused to generate text that is false, misleading, or harmful.\n\t+ Guidelines are provided for responsible use with the model, see the\n\tResponsible Generative AI Toolkit.\n* Transparency and Accountability:\n\t+ This model card summarizes details on the models' architecture,\n\tcapabilities, limitations, and evaluation processes.\n\t+ A responsibly developed open model offers the opportunity to share\n\tinnovation by making LLM technology accessible to developers and researchers\n\tacross the AI ecosystem.\n\n\nRisks identified and mitigations:\n\n\n* Perpetuation of biases: It's encouraged to perform continuous monitoring\n(using evaluation metrics, human review) and the exploration of de-biasing\ntechniques during model training, fine-tuning, and other use cases.\n* Generation of harmful content: Mechanisms and guidelines for content safety\nare essential. Developers are encouraged to exercise caution and implement\nappropriate content safety safeguards based on their specific product policies\nand application use cases.\n* Misuse for malicious purposes: Technical limitations and developer and\nend-user education can help mitigate against malicious applications of LLMs.\nEducational resources and reporting mechanisms for users to flag misuse are\nprovided. Prohibited uses of Gemma models are outlined in the\nGemma Prohibited Use Policy.\n* Privacy violations: Models were trained on data filtered for removal of PII\n(Personally Identifiable Information). Developers are encouraged to adhere to\nprivacy regulations with privacy-preserving techniques.",
"### Benefits\n\n\nAt the time of release, this family of models provides high-performance open\nlarge language model implementations designed from the ground up for Responsible\nAI development compared to similarly sized models.\n\n\nUsing the benchmark evaluation metrics described in this document, these models\nhave shown to provide superior performance to other, comparably-sized open model\nalternatives."
] | [
"TAGS\n#transformers #safetensors #gemma #text-generation #conversational #arxiv-2312.11805 #arxiv-2009.03300 #arxiv-1905.07830 #arxiv-1911.11641 #arxiv-1904.09728 #arxiv-1905.10044 #arxiv-1907.10641 #arxiv-1811.00937 #arxiv-1809.02789 #arxiv-1911.01547 #arxiv-1705.03551 #arxiv-2107.03374 #arxiv-2108.07732 #arxiv-2110.14168 #arxiv-2304.06364 #arxiv-2206.04615 #arxiv-1804.06876 #arxiv-2110.08193 #arxiv-2009.11462 #arxiv-2101.11718 #arxiv-1804.09301 #arxiv-2109.07958 #arxiv-2203.09509 #autotrain_compatible #endpoints_compatible #text-generation-inference #4-bit #region-us \n",
"### Description\n\n\nGemma is a family of lightweight, state-of-the-art open models from Google,\nbuilt from the same research and technology used to create the Gemini models.\nThey are text-to-text, decoder-only large language models, available in English,\nwith open weights, pre-trained variants, and instruction-tuned variants. Gemma\nmodels are well-suited for a variety of text generation tasks, including\nquestion answering, summarization, and reasoning. Their relatively small size\nmakes it possible to deploy them in environments with limited resources such as\na laptop, desktop or your own cloud infrastructure, democratizing access to\nstate of the art AI models and helping foster innovation for everyone.",
"### Usage\n\n\nBelow we share some code snippets on how to get quickly started with running the model. First make sure to 'pip install -U transformers', then copy the snippet from the section that is relevant for your usecase.",
"#### Fine-tuning the model\n\n\nYou can find fine-tuning scripts and notebook under the 'examples/' directory of 'google/gemma-7b' repository. To adapt it to this model, simply change the model-id to 'google/gemma-7b-it'.\nIn that repository, we provide:\n\n\n* A script to perform Supervised Fine-Tuning (SFT) on UltraChat dataset using QLoRA\n* A script to perform SFT using FSDP on TPU devices\n* A notebook that you can run on a free-tier Google Colab instance to perform SFT on English quotes dataset",
"#### Running the model on a CPU\n\n\nAs explained below, we recommend 'torch.bfloat16' as the default dtype. You can use a different precision if necessary.",
"#### Running the model on a single / multi GPU",
"#### Running the model on a GPU using different precisions\n\n\nThe native weights of this model were exported in 'bfloat16' precision. You can use 'float16', which may be faster on certain hardware, indicating the 'torch\\_dtype' when loading the model. For convenience, the 'float16' revision of the repo contains a copy of the weights already converted to that precision.\n\n\nYou can also use 'float32' if you skip the dtype, but no precision increase will occur (model weights will just be upcasted to 'float32'). See examples below.\n\n\n* *Using 'torch.float16'*\n* *Using 'torch.bfloat16'*\n* *Upcasting to 'torch.float32'*",
"#### Quantized Versions through 'bitsandbytes'\n\n\n* *Using 8-bit precision (int8)*\n* *Using 4-bit precision*",
"#### Other optimizations\n\n\n* *Flash Attention 2*\n\n\nFirst make sure to install 'flash-attn' in your environment 'pip install flash-attn'",
"### Chat Template\n\n\nThe instruction-tuned models use a chat template that must be adhered to for conversational use.\nThe easiest way to apply it is using the tokenizer's built-in chat template, as shown in the following snippet.\n\n\nLet's load the model and apply the chat template to a conversation. In this example, we'll start with a single user interaction:\n\n\nAt this point, the prompt contains the following text:\n\n\nAs you can see, each turn is preceded by a '<start\\_of\\_turn>' delimiter and then the role of the entity\n(either 'user', for content supplied by the user, or 'model' for LLM responses). Turns finish with\nthe '<end\\_of\\_turn>' token.\n\n\nYou can follow this format to build the prompt manually, if you need to do it without the tokenizer's\nchat template.\n\n\nAfter the prompt is ready, generation can be performed like this:",
"### Inputs and outputs\n\n\n* Input: Text string, such as a question, a prompt, or a document to be\nsummarized.\n* Output: Generated English-language text in response to the input, such\nas an answer to a question, or a summary of a document.\n\n\nModel Data\n----------\n\n\nData used for model training and how the data was processed.",
"### Training Dataset\n\n\nThese models were trained on a dataset of text data that includes a wide variety\nof sources, totaling 6 trillion tokens. Here are the key components:\n\n\n* Web Documents: A diverse collection of web text ensures the model is exposed\nto a broad range of linguistic styles, topics, and vocabulary. Primarily\nEnglish-language content.\n* Code: Exposing the model to code helps it to learn the syntax and patterns of\nprogramming languages, which improves its ability to generate code or\nunderstand code-related questions.\n* Mathematics: Training on mathematical text helps the model learn logical\nreasoning, symbolic representation, and to address mathematical queries.\n\n\nThe combination of these diverse data sources is crucial for training a powerful\nlanguage model that can handle a wide variety of different tasks and text\nformats.",
"### Data Preprocessing\n\n\nHere are the key data cleaning and filtering methods applied to the training\ndata:\n\n\n* CSAM Filtering: Rigorous CSAM (Child Sexual Abuse Material) filtering was\napplied at multiple stages in the data preparation process to ensure the\nexclusion of harmful and illegal content\n* Sensitive Data Filtering: As part of making Gemma pre-trained models safe and\nreliable, automated techniques were used to filter out certain personal\ninformation and other sensitive data from training sets.\n* Additional methods: Filtering based on content quality and safely in line with\nour policies.\n\n\nImplementation Information\n--------------------------\n\n\nDetails about the model internals.",
"### Hardware\n\n\nGemma was trained using the latest generation of\nTensor Processing Unit (TPU) hardware (TPUv5e).\n\n\nTraining large language models requires significant computational power. TPUs,\ndesigned specifically for matrix operations common in machine learning, offer\nseveral advantages in this domain:\n\n\n* Performance: TPUs are specifically designed to handle the massive computations\ninvolved in training LLMs. They can speed up training considerably compared to\nCPUs.\n* Memory: TPUs often come with large amounts of high-bandwidth memory, allowing\nfor the handling of large models and batch sizes during training. This can\nlead to better model quality.\n* Scalability: TPU Pods (large clusters of TPUs) provide a scalable solution for\nhandling the growing complexity of large foundation models. You can distribute\ntraining across multiple TPU devices for faster and more efficient processing.\n* Cost-effectiveness: In many scenarios, TPUs can provide a more cost-effective\nsolution for training large models compared to CPU-based infrastructure,\nespecially when considering the time and resources saved due to faster\ntraining.\n* These advantages are aligned with\nGoogle's commitments to operate sustainably.",
"### Software\n\n\nTraining was done using JAX and ML Pathways.\n\n\nJAX allows researchers to take advantage of the latest generation of hardware,\nincluding TPUs, for faster and more efficient training of large models.\n\n\nML Pathways is Google's latest effort to build artificially intelligent systems\ncapable of generalizing across multiple tasks. This is specially suitable for\nfoundation models, including large language models like\nthese ones.\n\n\nTogether, JAX and ML Pathways are used as described in the\npaper about the Gemini family of models; \"the 'single\ncontroller' programming model of Jax and Pathways allows a single Python\nprocess to orchestrate the entire training run, dramatically simplifying the\ndevelopment workflow.\"\n\n\nEvaluation\n----------\n\n\nModel evaluation metrics and results.",
"### Benchmark Results\n\n\nThese models were evaluated against a large collection of different datasets and\nmetrics to cover different aspects of text generation:\n\n\n\nEthics and Safety\n-----------------\n\n\nEthics and safety evaluation approach and results.",
"### Evaluation Approach\n\n\nOur evaluation methods include structured evaluations and internal red-teaming\ntesting of relevant content policies. Red-teaming was conducted by a number of\ndifferent teams, each with different goals and human evaluation metrics. These\nmodels were evaluated against a number of different categories relevant to\nethics and safety, including:\n\n\n* Text-to-Text Content Safety: Human evaluation on prompts covering safety\npolicies including child sexual abuse and exploitation, harassment, violence\nand gore, and hate speech.\n* Text-to-Text Representational Harms: Benchmark against relevant academic\ndatasets such as WinoBias and BBQ Dataset.\n* Memorization: Automated evaluation of memorization of training data, including\nthe risk of personally identifiable information exposure.\n* Large-scale harm: Tests for \"dangerous capabilities,\" such as chemical,\nbiological, radiological, and nuclear (CBRN) risks.",
"### Evaluation Results\n\n\nThe results of ethics and safety evaluations are within acceptable thresholds\nfor meeting internal policies for categories such as child\nsafety, content safety, representational harms, memorization, large-scale harms.\nOn top of robust internal evaluations, the results of well known safety\nbenchmarks like BBQ, BOLD, Winogender, Winobias, RealToxicity, and TruthfulQA\nare shown here.\n\n\n\nUsage and Limitations\n---------------------\n\n\nThese models have certain limitations that users should be aware of.",
"### Intended Usage\n\n\nOpen Large Language Models (LLMs) have a wide range of applications across\nvarious industries and domains. The following list of potential uses is not\ncomprehensive. The purpose of this list is to provide contextual information\nabout the possible use-cases that the model creators considered as part of model\ntraining and development.\n\n\n* Content Creation and Communication\n\t+ Text Generation: These models can be used to generate creative text formats\n\tsuch as poems, scripts, code, marketing copy, and email drafts.\n\t+ Chatbots and Conversational AI: Power conversational interfaces for customer\n\tservice, virtual assistants, or interactive applications.\n\t+ Text Summarization: Generate concise summaries of a text corpus, research\n\tpapers, or reports.\n* Research and Education\n\t+ Natural Language Processing (NLP) Research: These models can serve as a\n\tfoundation for researchers to experiment with NLP techniques, develop\n\talgorithms, and contribute to the advancement of the field.\n\t+ Language Learning Tools: Support interactive language learning experiences,\n\taiding in grammar correction or providing writing practice.\n\t+ Knowledge Exploration: Assist researchers in exploring large bodies of text\n\tby generating summaries or answering questions about specific topics.",
"### Limitations\n\n\n* Training Data\n\t+ The quality and diversity of the training data significantly influence the\n\tmodel's capabilities. Biases or gaps in the training data can lead to\n\tlimitations in the model's responses.\n\t+ The scope of the training dataset determines the subject areas the model can\n\thandle effectively.\n* Context and Task Complexity\n\t+ LLMs are better at tasks that can be framed with clear prompts and\n\tinstructions. Open-ended or highly complex tasks might be challenging.\n\t+ A model's performance can be influenced by the amount of context provided\n\t(longer context generally leads to better outputs, up to a certain point).\n* Language Ambiguity and Nuance\n\t+ Natural language is inherently complex. LLMs might struggle to grasp subtle\n\tnuances, sarcasm, or figurative language.\n* Factual Accuracy\n\t+ LLMs generate responses based on information they learned from their\n\ttraining datasets, but they are not knowledge bases. They may generate\n\tincorrect or outdated factual statements.\n* Common Sense\n\t+ LLMs rely on statistical patterns in language. They might lack the ability\n\tto apply common sense reasoning in certain situations.",
"### Ethical Considerations and Risks\n\n\nThe development of large language models (LLMs) raises several ethical concerns.\nIn creating an open model, we have carefully considered the following:\n\n\n* Bias and Fairness\n\t+ LLMs trained on large-scale, real-world text data can reflect socio-cultural\n\tbiases embedded in the training material. These models underwent careful\n\tscrutiny, input data pre-processing described and posterior evaluations\n\treported in this card.\n* Misinformation and Misuse\n\t+ LLMs can be misused to generate text that is false, misleading, or harmful.\n\t+ Guidelines are provided for responsible use with the model, see the\n\tResponsible Generative AI Toolkit.\n* Transparency and Accountability:\n\t+ This model card summarizes details on the models' architecture,\n\tcapabilities, limitations, and evaluation processes.\n\t+ A responsibly developed open model offers the opportunity to share\n\tinnovation by making LLM technology accessible to developers and researchers\n\tacross the AI ecosystem.\n\n\nRisks identified and mitigations:\n\n\n* Perpetuation of biases: It's encouraged to perform continuous monitoring\n(using evaluation metrics, human review) and the exploration of de-biasing\ntechniques during model training, fine-tuning, and other use cases.\n* Generation of harmful content: Mechanisms and guidelines for content safety\nare essential. Developers are encouraged to exercise caution and implement\nappropriate content safety safeguards based on their specific product policies\nand application use cases.\n* Misuse for malicious purposes: Technical limitations and developer and\nend-user education can help mitigate against malicious applications of LLMs.\nEducational resources and reporting mechanisms for users to flag misuse are\nprovided. Prohibited uses of Gemma models are outlined in the\nGemma Prohibited Use Policy.\n* Privacy violations: Models were trained on data filtered for removal of PII\n(Personally Identifiable Information). Developers are encouraged to adhere to\nprivacy regulations with privacy-preserving techniques.",
"### Benefits\n\n\nAt the time of release, this family of models provides high-performance open\nlarge language model implementations designed from the ground up for Responsible\nAI development compared to similarly sized models.\n\n\nUsing the benchmark evaluation metrics described in this document, these models\nhave shown to provide superior performance to other, comparably-sized open model\nalternatives."
] | [
278,
141,
53,
131,
39,
14,
163,
34,
37,
204,
80,
157,
145,
228,
151,
58,
179,
125,
231,
218,
374,
70
] | [
"TAGS\n#transformers #safetensors #gemma #text-generation #conversational #arxiv-2312.11805 #arxiv-2009.03300 #arxiv-1905.07830 #arxiv-1911.11641 #arxiv-1904.09728 #arxiv-1905.10044 #arxiv-1907.10641 #arxiv-1811.00937 #arxiv-1809.02789 #arxiv-1911.01547 #arxiv-1705.03551 #arxiv-2107.03374 #arxiv-2108.07732 #arxiv-2110.14168 #arxiv-2304.06364 #arxiv-2206.04615 #arxiv-1804.06876 #arxiv-2110.08193 #arxiv-2009.11462 #arxiv-2101.11718 #arxiv-1804.09301 #arxiv-2109.07958 #arxiv-2203.09509 #autotrain_compatible #endpoints_compatible #text-generation-inference #4-bit #region-us \n### Description\n\n\nGemma is a family of lightweight, state-of-the-art open models from Google,\nbuilt from the same research and technology used to create the Gemini models.\nThey are text-to-text, decoder-only large language models, available in English,\nwith open weights, pre-trained variants, and instruction-tuned variants. Gemma\nmodels are well-suited for a variety of text generation tasks, including\nquestion answering, summarization, and reasoning. Their relatively small size\nmakes it possible to deploy them in environments with limited resources such as\na laptop, desktop or your own cloud infrastructure, democratizing access to\nstate of the art AI models and helping foster innovation for everyone.### Usage\n\n\nBelow we share some code snippets on how to get quickly started with running the model. First make sure to 'pip install -U transformers', then copy the snippet from the section that is relevant for your usecase.#### Fine-tuning the model\n\n\nYou can find fine-tuning scripts and notebook under the 'examples/' directory of 'google/gemma-7b' repository. To adapt it to this model, simply change the model-id to 'google/gemma-7b-it'.\nIn that repository, we provide:\n\n\n* A script to perform Supervised Fine-Tuning (SFT) on UltraChat dataset using QLoRA\n* A script to perform SFT using FSDP on TPU devices\n* A notebook that you can run on a free-tier Google Colab instance to perform SFT on English quotes dataset#### Running the model on a CPU\n\n\nAs explained below, we recommend 'torch.bfloat16' as the default dtype. You can use a different precision if necessary.#### Running the model on a single / multi GPU#### Running the model on a GPU using different precisions\n\n\nThe native weights of this model were exported in 'bfloat16' precision. You can use 'float16', which may be faster on certain hardware, indicating the 'torch\\_dtype' when loading the model. For convenience, the 'float16' revision of the repo contains a copy of the weights already converted to that precision.\n\n\nYou can also use 'float32' if you skip the dtype, but no precision increase will occur (model weights will just be upcasted to 'float32'). See examples below.\n\n\n* *Using 'torch.float16'*\n* *Using 'torch.bfloat16'*\n* *Upcasting to 'torch.float32'*#### Quantized Versions through 'bitsandbytes'\n\n\n* *Using 8-bit precision (int8)*\n* *Using 4-bit precision*#### Other optimizations\n\n\n* *Flash Attention 2*\n\n\nFirst make sure to install 'flash-attn' in your environment 'pip install flash-attn'### Chat Template\n\n\nThe instruction-tuned models use a chat template that must be adhered to for conversational use.\nThe easiest way to apply it is using the tokenizer's built-in chat template, as shown in the following snippet.\n\n\nLet's load the model and apply the chat template to a conversation. In this example, we'll start with a single user interaction:\n\n\nAt this point, the prompt contains the following text:\n\n\nAs you can see, each turn is preceded by a '<start\\_of\\_turn>' delimiter and then the role of the entity\n(either 'user', for content supplied by the user, or 'model' for LLM responses). Turns finish with\nthe '<end\\_of\\_turn>' token.\n\n\nYou can follow this format to build the prompt manually, if you need to do it without the tokenizer's\nchat template.\n\n\nAfter the prompt is ready, generation can be performed like this:### Inputs and outputs\n\n\n* Input: Text string, such as a question, a prompt, or a document to be\nsummarized.\n* Output: Generated English-language text in response to the input, such\nas an answer to a question, or a summary of a document.\n\n\nModel Data\n----------\n\n\nData used for model training and how the data was processed.### Training Dataset\n\n\nThese models were trained on a dataset of text data that includes a wide variety\nof sources, totaling 6 trillion tokens. Here are the key components:\n\n\n* Web Documents: A diverse collection of web text ensures the model is exposed\nto a broad range of linguistic styles, topics, and vocabulary. Primarily\nEnglish-language content.\n* Code: Exposing the model to code helps it to learn the syntax and patterns of\nprogramming languages, which improves its ability to generate code or\nunderstand code-related questions.\n* Mathematics: Training on mathematical text helps the model learn logical\nreasoning, symbolic representation, and to address mathematical queries.\n\n\nThe combination of these diverse data sources is crucial for training a powerful\nlanguage model that can handle a wide variety of different tasks and text\nformats.### Data Preprocessing\n\n\nHere are the key data cleaning and filtering methods applied to the training\ndata:\n\n\n* CSAM Filtering: Rigorous CSAM (Child Sexual Abuse Material) filtering was\napplied at multiple stages in the data preparation process to ensure the\nexclusion of harmful and illegal content\n* Sensitive Data Filtering: As part of making Gemma pre-trained models safe and\nreliable, automated techniques were used to filter out certain personal\ninformation and other sensitive data from training sets.\n* Additional methods: Filtering based on content quality and safely in line with\nour policies.\n\n\nImplementation Information\n--------------------------\n\n\nDetails about the model internals.### Hardware\n\n\nGemma was trained using the latest generation of\nTensor Processing Unit (TPU) hardware (TPUv5e).\n\n\nTraining large language models requires significant computational power. TPUs,\ndesigned specifically for matrix operations common in machine learning, offer\nseveral advantages in this domain:\n\n\n* Performance: TPUs are specifically designed to handle the massive computations\ninvolved in training LLMs. They can speed up training considerably compared to\nCPUs.\n* Memory: TPUs often come with large amounts of high-bandwidth memory, allowing\nfor the handling of large models and batch sizes during training. This can\nlead to better model quality.\n* Scalability: TPU Pods (large clusters of TPUs) provide a scalable solution for\nhandling the growing complexity of large foundation models. You can distribute\ntraining across multiple TPU devices for faster and more efficient processing.\n* Cost-effectiveness: In many scenarios, TPUs can provide a more cost-effective\nsolution for training large models compared to CPU-based infrastructure,\nespecially when considering the time and resources saved due to faster\ntraining.\n* These advantages are aligned with\nGoogle's commitments to operate sustainably.### Software\n\n\nTraining was done using JAX and ML Pathways.\n\n\nJAX allows researchers to take advantage of the latest generation of hardware,\nincluding TPUs, for faster and more efficient training of large models.\n\n\nML Pathways is Google's latest effort to build artificially intelligent systems\ncapable of generalizing across multiple tasks. This is specially suitable for\nfoundation models, including large language models like\nthese ones.\n\n\nTogether, JAX and ML Pathways are used as described in the\npaper about the Gemini family of models; \"the 'single\ncontroller' programming model of Jax and Pathways allows a single Python\nprocess to orchestrate the entire training run, dramatically simplifying the\ndevelopment workflow.\"\n\n\nEvaluation\n----------\n\n\nModel evaluation metrics and results.### Benchmark Results\n\n\nThese models were evaluated against a large collection of different datasets and\nmetrics to cover different aspects of text generation:\n\n\n\nEthics and Safety\n-----------------\n\n\nEthics and safety evaluation approach and results.### Evaluation Approach\n\n\nOur evaluation methods include structured evaluations and internal red-teaming\ntesting of relevant content policies. Red-teaming was conducted by a number of\ndifferent teams, each with different goals and human evaluation metrics. These\nmodels were evaluated against a number of different categories relevant to\nethics and safety, including:\n\n\n* Text-to-Text Content Safety: Human evaluation on prompts covering safety\npolicies including child sexual abuse and exploitation, harassment, violence\nand gore, and hate speech.\n* Text-to-Text Representational Harms: Benchmark against relevant academic\ndatasets such as WinoBias and BBQ Dataset.\n* Memorization: Automated evaluation of memorization of training data, including\nthe risk of personally identifiable information exposure.\n* Large-scale harm: Tests for \"dangerous capabilities,\" such as chemical,\nbiological, radiological, and nuclear (CBRN) risks.### Evaluation Results\n\n\nThe results of ethics and safety evaluations are within acceptable thresholds\nfor meeting internal policies for categories such as child\nsafety, content safety, representational harms, memorization, large-scale harms.\nOn top of robust internal evaluations, the results of well known safety\nbenchmarks like BBQ, BOLD, Winogender, Winobias, RealToxicity, and TruthfulQA\nare shown here.\n\n\n\nUsage and Limitations\n---------------------\n\n\nThese models have certain limitations that users should be aware of.### Intended Usage\n\n\nOpen Large Language Models (LLMs) have a wide range of applications across\nvarious industries and domains. The following list of potential uses is not\ncomprehensive. The purpose of this list is to provide contextual information\nabout the possible use-cases that the model creators considered as part of model\ntraining and development.\n\n\n* Content Creation and Communication\n\t+ Text Generation: These models can be used to generate creative text formats\n\tsuch as poems, scripts, code, marketing copy, and email drafts.\n\t+ Chatbots and Conversational AI: Power conversational interfaces for customer\n\tservice, virtual assistants, or interactive applications.\n\t+ Text Summarization: Generate concise summaries of a text corpus, research\n\tpapers, or reports.\n* Research and Education\n\t+ Natural Language Processing (NLP) Research: These models can serve as a\n\tfoundation for researchers to experiment with NLP techniques, develop\n\talgorithms, and contribute to the advancement of the field.\n\t+ Language Learning Tools: Support interactive language learning experiences,\n\taiding in grammar correction or providing writing practice.\n\t+ Knowledge Exploration: Assist researchers in exploring large bodies of text\n\tby generating summaries or answering questions about specific topics.### Limitations\n\n\n* Training Data\n\t+ The quality and diversity of the training data significantly influence the\n\tmodel's capabilities. Biases or gaps in the training data can lead to\n\tlimitations in the model's responses.\n\t+ The scope of the training dataset determines the subject areas the model can\n\thandle effectively.\n* Context and Task Complexity\n\t+ LLMs are better at tasks that can be framed with clear prompts and\n\tinstructions. Open-ended or highly complex tasks might be challenging.\n\t+ A model's performance can be influenced by the amount of context provided\n\t(longer context generally leads to better outputs, up to a certain point).\n* Language Ambiguity and Nuance\n\t+ Natural language is inherently complex. LLMs might struggle to grasp subtle\n\tnuances, sarcasm, or figurative language.\n* Factual Accuracy\n\t+ LLMs generate responses based on information they learned from their\n\ttraining datasets, but they are not knowledge bases. They may generate\n\tincorrect or outdated factual statements.\n* Common Sense\n\t+ LLMs rely on statistical patterns in language. They might lack the ability\n\tto apply common sense reasoning in certain situations.### Ethical Considerations and Risks\n\n\nThe development of large language models (LLMs) raises several ethical concerns.\nIn creating an open model, we have carefully considered the following:\n\n\n* Bias and Fairness\n\t+ LLMs trained on large-scale, real-world text data can reflect socio-cultural\n\tbiases embedded in the training material. These models underwent careful\n\tscrutiny, input data pre-processing described and posterior evaluations\n\treported in this card.\n* Misinformation and Misuse\n\t+ LLMs can be misused to generate text that is false, misleading, or harmful.\n\t+ Guidelines are provided for responsible use with the model, see the\n\tResponsible Generative AI Toolkit.\n* Transparency and Accountability:\n\t+ This model card summarizes details on the models' architecture,\n\tcapabilities, limitations, and evaluation processes.\n\t+ A responsibly developed open model offers the opportunity to share\n\tinnovation by making LLM technology accessible to developers and researchers\n\tacross the AI ecosystem.\n\n\nRisks identified and mitigations:\n\n\n* Perpetuation of biases: It's encouraged to perform continuous monitoring\n(using evaluation metrics, human review) and the exploration of de-biasing\ntechniques during model training, fine-tuning, and other use cases.\n* Generation of harmful content: Mechanisms and guidelines for content safety\nare essential. Developers are encouraged to exercise caution and implement\nappropriate content safety safeguards based on their specific product policies\nand application use cases.\n* Misuse for malicious purposes: Technical limitations and developer and\nend-user education can help mitigate against malicious applications of LLMs.\nEducational resources and reporting mechanisms for users to flag misuse are\nprovided. Prohibited uses of Gemma models are outlined in the\nGemma Prohibited Use Policy.\n* Privacy violations: Models were trained on data filtered for removal of PII\n(Personally Identifiable Information). Developers are encouraged to adhere to\nprivacy regulations with privacy-preserving techniques.### Benefits\n\n\nAt the time of release, this family of models provides high-performance open\nlarge language model implementations designed from the ground up for Responsible\nAI development compared to similarly sized models.\n\n\nUsing the benchmark evaluation metrics described in this document, these models\nhave shown to provide superior performance to other, comparably-sized open model\nalternatives."
] |
null | transformers | ## About
<!-- ### quantize_version: 2 -->
<!-- ### output_tensor_quantised: 1 -->
<!-- ### convert_type: hf -->
<!-- ### vocab_type: -->
static quants of https://huggingface.co/vicgalle/Configurable-Hermes-2-Pro-Llama-3-8B
<!-- provided-files -->
weighted/imatrix quants seem not to be available (by me) at this time. If they do not show up a week or so after the static ones, I have probably not planned for them. Feel free to request them by opening a Community Discussion.
## Usage
If you are unsure how to use GGUF files, refer to one of [TheBloke's
READMEs](https://huggingface.co/TheBloke/KafkaLM-70B-German-V0.1-GGUF) for
more details, including on how to concatenate multi-part files.
## Provided Quants
(sorted by size, not necessarily quality. IQ-quants are often preferable over similar sized non-IQ quants)
| Link | Type | Size/GB | Notes |
|:-----|:-----|--------:|:------|
| [GGUF](https://huggingface.co/mradermacher/Configurable-Hermes-2-Pro-Llama-3-8B-GGUF/resolve/main/Configurable-Hermes-2-Pro-Llama-3-8B.Q2_K.gguf) | Q2_K | 3.3 | |
| [GGUF](https://huggingface.co/mradermacher/Configurable-Hermes-2-Pro-Llama-3-8B-GGUF/resolve/main/Configurable-Hermes-2-Pro-Llama-3-8B.IQ3_XS.gguf) | IQ3_XS | 3.6 | |
| [GGUF](https://huggingface.co/mradermacher/Configurable-Hermes-2-Pro-Llama-3-8B-GGUF/resolve/main/Configurable-Hermes-2-Pro-Llama-3-8B.Q3_K_S.gguf) | Q3_K_S | 3.8 | |
| [GGUF](https://huggingface.co/mradermacher/Configurable-Hermes-2-Pro-Llama-3-8B-GGUF/resolve/main/Configurable-Hermes-2-Pro-Llama-3-8B.IQ3_S.gguf) | IQ3_S | 3.8 | beats Q3_K* |
| [GGUF](https://huggingface.co/mradermacher/Configurable-Hermes-2-Pro-Llama-3-8B-GGUF/resolve/main/Configurable-Hermes-2-Pro-Llama-3-8B.IQ3_M.gguf) | IQ3_M | 3.9 | |
| [GGUF](https://huggingface.co/mradermacher/Configurable-Hermes-2-Pro-Llama-3-8B-GGUF/resolve/main/Configurable-Hermes-2-Pro-Llama-3-8B.Q3_K_M.gguf) | Q3_K_M | 4.1 | lower quality |
| [GGUF](https://huggingface.co/mradermacher/Configurable-Hermes-2-Pro-Llama-3-8B-GGUF/resolve/main/Configurable-Hermes-2-Pro-Llama-3-8B.Q3_K_L.gguf) | Q3_K_L | 4.4 | |
| [GGUF](https://huggingface.co/mradermacher/Configurable-Hermes-2-Pro-Llama-3-8B-GGUF/resolve/main/Configurable-Hermes-2-Pro-Llama-3-8B.IQ4_XS.gguf) | IQ4_XS | 4.6 | |
| [GGUF](https://huggingface.co/mradermacher/Configurable-Hermes-2-Pro-Llama-3-8B-GGUF/resolve/main/Configurable-Hermes-2-Pro-Llama-3-8B.Q4_K_S.gguf) | Q4_K_S | 4.8 | fast, recommended |
| [GGUF](https://huggingface.co/mradermacher/Configurable-Hermes-2-Pro-Llama-3-8B-GGUF/resolve/main/Configurable-Hermes-2-Pro-Llama-3-8B.Q4_K_M.gguf) | Q4_K_M | 5.0 | fast, recommended |
| [GGUF](https://huggingface.co/mradermacher/Configurable-Hermes-2-Pro-Llama-3-8B-GGUF/resolve/main/Configurable-Hermes-2-Pro-Llama-3-8B.Q5_K_S.gguf) | Q5_K_S | 5.7 | |
| [GGUF](https://huggingface.co/mradermacher/Configurable-Hermes-2-Pro-Llama-3-8B-GGUF/resolve/main/Configurable-Hermes-2-Pro-Llama-3-8B.Q5_K_M.gguf) | Q5_K_M | 5.8 | |
| [GGUF](https://huggingface.co/mradermacher/Configurable-Hermes-2-Pro-Llama-3-8B-GGUF/resolve/main/Configurable-Hermes-2-Pro-Llama-3-8B.Q6_K.gguf) | Q6_K | 6.7 | very good quality |
| [GGUF](https://huggingface.co/mradermacher/Configurable-Hermes-2-Pro-Llama-3-8B-GGUF/resolve/main/Configurable-Hermes-2-Pro-Llama-3-8B.Q8_0.gguf) | Q8_0 | 8.6 | fast, best quality |
| [GGUF](https://huggingface.co/mradermacher/Configurable-Hermes-2-Pro-Llama-3-8B-GGUF/resolve/main/Configurable-Hermes-2-Pro-Llama-3-8B.f16.gguf) | f16 | 16.2 | 16 bpw, overkill |
Here is a handy graph by ikawrakow comparing some lower-quality quant
types (lower is better):

And here are Artefact2's thoughts on the matter:
https://gist.github.com/Artefact2/b5f810600771265fc1e39442288e8ec9
## FAQ / Model Request
See https://huggingface.co/mradermacher/model_requests for some answers to
questions you might have and/or if you want some other model quantized.
## Thanks
I thank my company, [nethype GmbH](https://www.nethype.de/), for letting
me use its servers and providing upgrades to my workstation to enable
this work in my free time.
<!-- end -->
| {"language": ["en"], "license": "apache-2.0", "library_name": "transformers", "tags": ["safety"], "datasets": ["vicgalle/configurable-system-prompt-multitask"], "base_model": "vicgalle/Configurable-Hermes-2-Pro-Llama-3-8B", "quantized_by": "mradermacher"} | mradermacher/Configurable-Hermes-2-Pro-Llama-3-8B-GGUF | null | [
"transformers",
"gguf",
"safety",
"en",
"dataset:vicgalle/configurable-system-prompt-multitask",
"base_model:vicgalle/Configurable-Hermes-2-Pro-Llama-3-8B",
"license:apache-2.0",
"endpoints_compatible",
"region:us"
] | null | 2024-05-02T11:26:28+00:00 | [] | [
"en"
] | TAGS
#transformers #gguf #safety #en #dataset-vicgalle/configurable-system-prompt-multitask #base_model-vicgalle/Configurable-Hermes-2-Pro-Llama-3-8B #license-apache-2.0 #endpoints_compatible #region-us
| About
-----
static quants of URL
weighted/imatrix quants seem not to be available (by me) at this time. If they do not show up a week or so after the static ones, I have probably not planned for them. Feel free to request them by opening a Community Discussion.
Usage
-----
If you are unsure how to use GGUF files, refer to one of TheBloke's
READMEs for
more details, including on how to concatenate multi-part files.
Provided Quants
---------------
(sorted by size, not necessarily quality. IQ-quants are often preferable over similar sized non-IQ quants)
Here is a handy graph by ikawrakow comparing some lower-quality quant
types (lower is better):
!URL
And here are Artefact2's thoughts on the matter:
URL
FAQ / Model Request
-------------------
See URL for some answers to
questions you might have and/or if you want some other model quantized.
Thanks
------
I thank my company, nethype GmbH, for letting
me use its servers and providing upgrades to my workstation to enable
this work in my free time.
| [] | [
"TAGS\n#transformers #gguf #safety #en #dataset-vicgalle/configurable-system-prompt-multitask #base_model-vicgalle/Configurable-Hermes-2-Pro-Llama-3-8B #license-apache-2.0 #endpoints_compatible #region-us \n"
] | [
75
] | [
"TAGS\n#transformers #gguf #safety #en #dataset-vicgalle/configurable-system-prompt-multitask #base_model-vicgalle/Configurable-Hermes-2-Pro-Llama-3-8B #license-apache-2.0 #endpoints_compatible #region-us \n"
] |
null | peft |
<!-- This model card has been generated automatically according to the information the Trainer had access to. You
should probably proofread and complete it, then remove this comment. -->
# phi-2-sqlGPT-ft-v0.0.1
This model is a fine-tuned version of [microsoft/phi-2](https://huggingface.co/microsoft/phi-2) on an unknown dataset.
It achieves the following results on the evaluation set:
- Loss: 0.3112
## Model description
More information needed
## Intended uses & limitations
More information needed
## Training and evaluation data
More information needed
## Training procedure
### Training hyperparameters
The following hyperparameters were used during training:
- learning_rate: 0.0002
- train_batch_size: 4
- eval_batch_size: 4
- seed: 42
- gradient_accumulation_steps: 4
- total_train_batch_size: 16
- optimizer: Adam with betas=(0.9,0.999) and epsilon=1e-08
- lr_scheduler_type: linear
- lr_scheduler_warmup_steps: 2
- num_epochs: 10
- mixed_precision_training: Native AMP
### Training results
| Training Loss | Epoch | Step | Validation Loss |
|:-------------:|:-----:|:----:|:---------------:|
| 1.1123 | 0.992 | 62 | 0.4388 |
| 0.3431 | 2.0 | 125 | 0.3489 |
| 0.2963 | 2.992 | 187 | 0.3228 |
| 0.2735 | 4.0 | 250 | 0.3184 |
| 0.2724 | 4.992 | 312 | 0.3147 |
| 0.2638 | 6.0 | 375 | 0.3133 |
| 0.2656 | 6.992 | 437 | 0.3126 |
| 0.2601 | 8.0 | 500 | 0.3118 |
| 0.2623 | 8.992 | 562 | 0.3109 |
| 0.2578 | 9.92 | 620 | 0.3112 |
### Framework versions
- PEFT 0.10.0
- Transformers 4.40.1
- Pytorch 2.3.0+cu121
- Datasets 2.19.0
- Tokenizers 0.19.1 | {"license": "mit", "library_name": "peft", "tags": ["generated_from_trainer"], "base_model": "microsoft/phi-2", "model-index": [{"name": "phi-2-sqlGPT-ft-v0.0.1", "results": []}]} | AkshayDiwadkar/phi-2-sqlGPT-ft-v0.0.1 | null | [
"peft",
"safetensors",
"generated_from_trainer",
"base_model:microsoft/phi-2",
"license:mit",
"region:us"
] | null | 2024-05-02T11:26:36+00:00 | [] | [] | TAGS
#peft #safetensors #generated_from_trainer #base_model-microsoft/phi-2 #license-mit #region-us
| phi-2-sqlGPT-ft-v0.0.1
======================
This model is a fine-tuned version of microsoft/phi-2 on an unknown dataset.
It achieves the following results on the evaluation set:
* Loss: 0.3112
Model description
-----------------
More information needed
Intended uses & limitations
---------------------------
More information needed
Training and evaluation data
----------------------------
More information needed
Training procedure
------------------
### Training hyperparameters
The following hyperparameters were used during training:
* learning\_rate: 0.0002
* train\_batch\_size: 4
* eval\_batch\_size: 4
* seed: 42
* gradient\_accumulation\_steps: 4
* total\_train\_batch\_size: 16
* optimizer: Adam with betas=(0.9,0.999) and epsilon=1e-08
* lr\_scheduler\_type: linear
* lr\_scheduler\_warmup\_steps: 2
* num\_epochs: 10
* mixed\_precision\_training: Native AMP
### Training results
### Framework versions
* PEFT 0.10.0
* Transformers 4.40.1
* Pytorch 2.3.0+cu121
* Datasets 2.19.0
* Tokenizers 0.19.1
| [
"### Training hyperparameters\n\n\nThe following hyperparameters were used during training:\n\n\n* learning\\_rate: 0.0002\n* train\\_batch\\_size: 4\n* eval\\_batch\\_size: 4\n* seed: 42\n* gradient\\_accumulation\\_steps: 4\n* total\\_train\\_batch\\_size: 16\n* optimizer: Adam with betas=(0.9,0.999) and epsilon=1e-08\n* lr\\_scheduler\\_type: linear\n* lr\\_scheduler\\_warmup\\_steps: 2\n* num\\_epochs: 10\n* mixed\\_precision\\_training: Native AMP",
"### Training results",
"### Framework versions\n\n\n* PEFT 0.10.0\n* Transformers 4.40.1\n* Pytorch 2.3.0+cu121\n* Datasets 2.19.0\n* Tokenizers 0.19.1"
] | [
"TAGS\n#peft #safetensors #generated_from_trainer #base_model-microsoft/phi-2 #license-mit #region-us \n",
"### Training hyperparameters\n\n\nThe following hyperparameters were used during training:\n\n\n* learning\\_rate: 0.0002\n* train\\_batch\\_size: 4\n* eval\\_batch\\_size: 4\n* seed: 42\n* gradient\\_accumulation\\_steps: 4\n* total\\_train\\_batch\\_size: 16\n* optimizer: Adam with betas=(0.9,0.999) and epsilon=1e-08\n* lr\\_scheduler\\_type: linear\n* lr\\_scheduler\\_warmup\\_steps: 2\n* num\\_epochs: 10\n* mixed\\_precision\\_training: Native AMP",
"### Training results",
"### Framework versions\n\n\n* PEFT 0.10.0\n* Transformers 4.40.1\n* Pytorch 2.3.0+cu121\n* Datasets 2.19.0\n* Tokenizers 0.19.1"
] | [
32,
151,
5,
52
] | [
"TAGS\n#peft #safetensors #generated_from_trainer #base_model-microsoft/phi-2 #license-mit #region-us \n### Training hyperparameters\n\n\nThe following hyperparameters were used during training:\n\n\n* learning\\_rate: 0.0002\n* train\\_batch\\_size: 4\n* eval\\_batch\\_size: 4\n* seed: 42\n* gradient\\_accumulation\\_steps: 4\n* total\\_train\\_batch\\_size: 16\n* optimizer: Adam with betas=(0.9,0.999) and epsilon=1e-08\n* lr\\_scheduler\\_type: linear\n* lr\\_scheduler\\_warmup\\_steps: 2\n* num\\_epochs: 10\n* mixed\\_precision\\_training: Native AMP### Training results### Framework versions\n\n\n* PEFT 0.10.0\n* Transformers 4.40.1\n* Pytorch 2.3.0+cu121\n* Datasets 2.19.0\n* Tokenizers 0.19.1"
] |
text-generation | transformers | Quantization made by Richard Erkhov.
[Github](https://github.com/RichardErkhov)
[Discord](https://discord.gg/pvy7H8DZMG)
[Request more models](https://github.com/RichardErkhov/quant_request)
Llama-68M-Chat-v1 - bnb 4bits
- Model creator: https://huggingface.co/Felladrin/
- Original model: https://huggingface.co/Felladrin/Llama-68M-Chat-v1/
Original model description:
---
language:
- en
license: apache-2.0
tags:
- text-generation
datasets:
- THUDM/webglm-qa
- databricks/databricks-dolly-15k
- cognitivecomputations/wizard_vicuna_70k_unfiltered
- totally-not-an-llm/EverythingLM-data-V3
- Amod/mental_health_counseling_conversations
- sablo/oasst2_curated
- starfishmedical/webGPT_x_dolly
- Open-Orca/OpenOrca
- mlabonne/chatml_dpo_pairs
base_model: JackFram/llama-68m
widget:
- messages:
- role: system
content: You are a career counselor. The user will provide you with an individual
looking for guidance in their professional life, and your task is to assist
them in determining what careers they are most suited for based on their skills,
interests, and experience. You should also conduct research into the various
options available, explain the job market trends in different industries, and
advice on which qualifications would be beneficial for pursuing particular fields.
- role: user
content: Heya!
- role: assistant
content: Hi! How may I help you?
- role: user
content: I am interested in developing a career in software engineering. What
would you recommend me to do?
- messages:
- role: system
content: You are a knowledgeable assistant. Help the user as much as you can.
- role: user
content: How to become healthier?
- messages:
- role: system
content: You are a helpful assistant who provides concise responses.
- role: user
content: Hi!
- role: assistant
content: Hello there! How may I help you?
- role: user
content: I need to build a simple website. Where should I start learning about web development?
- messages:
- role: system
content: You are a very creative assistant. User will give you a task, which you should complete with all your knowledge.
- role: user
content: Write the background story of an RPG game about wizards and dragons in a sci-fi world.
inference:
parameters:
max_new_tokens: 64
penalty_alpha: 0.5
top_k: 4
model-index:
- name: Llama-68M-Chat-v1
results:
- task:
type: text-generation
name: Text Generation
dataset:
name: AI2 Reasoning Challenge (25-Shot)
type: ai2_arc
config: ARC-Challenge
split: test
args:
num_few_shot: 25
metrics:
- type: acc_norm
value: 23.29
name: normalized accuracy
source:
url: https://huggingface.co/spaces/HuggingFaceH4/open_llm_leaderboard?query=Felladrin/Llama-68M-Chat-v1
name: Open LLM Leaderboard
- task:
type: text-generation
name: Text Generation
dataset:
name: HellaSwag (10-Shot)
type: hellaswag
split: validation
args:
num_few_shot: 10
metrics:
- type: acc_norm
value: 28.27
name: normalized accuracy
source:
url: https://huggingface.co/spaces/HuggingFaceH4/open_llm_leaderboard?query=Felladrin/Llama-68M-Chat-v1
name: Open LLM Leaderboard
- task:
type: text-generation
name: Text Generation
dataset:
name: MMLU (5-Shot)
type: cais/mmlu
config: all
split: test
args:
num_few_shot: 5
metrics:
- type: acc
value: 25.18
name: accuracy
source:
url: https://huggingface.co/spaces/HuggingFaceH4/open_llm_leaderboard?query=Felladrin/Llama-68M-Chat-v1
name: Open LLM Leaderboard
- task:
type: text-generation
name: Text Generation
dataset:
name: TruthfulQA (0-shot)
type: truthful_qa
config: multiple_choice
split: validation
args:
num_few_shot: 0
metrics:
- type: mc2
value: 47.27
source:
url: https://huggingface.co/spaces/HuggingFaceH4/open_llm_leaderboard?query=Felladrin/Llama-68M-Chat-v1
name: Open LLM Leaderboard
- task:
type: text-generation
name: Text Generation
dataset:
name: Winogrande (5-shot)
type: winogrande
config: winogrande_xl
split: validation
args:
num_few_shot: 5
metrics:
- type: acc
value: 54.3
name: accuracy
source:
url: https://huggingface.co/spaces/HuggingFaceH4/open_llm_leaderboard?query=Felladrin/Llama-68M-Chat-v1
name: Open LLM Leaderboard
- task:
type: text-generation
name: Text Generation
dataset:
name: GSM8k (5-shot)
type: gsm8k
config: main
split: test
args:
num_few_shot: 5
metrics:
- type: acc
value: 0.0
name: accuracy
source:
url: https://huggingface.co/spaces/HuggingFaceH4/open_llm_leaderboard?query=Felladrin/Llama-68M-Chat-v1
name: Open LLM Leaderboard
---
# A Llama Chat Model of 68M Parameters
- Base model: [JackFram/llama-68m](https://huggingface.co/JackFram/llama-68m)
- Datasets:
- [THUDM/webglm-qa](https://huggingface.co/datasets/THUDM/webglm-qa)
- [databricks/databricks-dolly-15k](https://huggingface.co/datasets/databricks/databricks-dolly-15k)
- [cognitivecomputations/wizard_vicuna_70k_unfiltered](https://huggingface.co/datasets/cognitivecomputations/wizard_vicuna_70k_unfiltered)
- [totally-not-an-llm/EverythingLM-data-V3](https://huggingface.co/datasets/totally-not-an-llm/EverythingLM-data-V3)
- [Amod/mental_health_counseling_conversations](https://huggingface.co/datasets/Amod/mental_health_counseling_conversations)
- [sablo/oasst2_curated](https://huggingface.co/datasets/sablo/oasst2_curated)
- [starfishmedical/webGPT_x_dolly](https://huggingface.co/datasets/starfishmedical/webGPT_x_dolly)
- [Open-Orca/OpenOrca](https://huggingface.co/datasets/Open-Orca/OpenOrca)
- [mlabonne/chatml_dpo_pairs](https://huggingface.co/datasets/mlabonne/chatml_dpo_pairs)
- Availability in other ML formats:
- GGUF: [afrideva/Llama-68M-Chat-v1-GGUF](https://huggingface.co/afrideva/Llama-68M-Chat-v1-GGUF)
- ONNX: [Felladrin/onnx-Llama-68M-Chat-v1](https://huggingface.co/Felladrin/onnx-Llama-68M-Chat-v1)
## Recommended Prompt Format
```
<|im_start|>system
{system_message}<|im_end|>
<|im_start|>user
{user_message}<|im_end|>
<|im_start|>assistant
```
## Recommended Inference Parameters
```yml
penalty_alpha: 0.5
top_k: 4
```
## [Open LLM Leaderboard Evaluation Results](https://huggingface.co/spaces/HuggingFaceH4/open_llm_leaderboard)
Detailed results can be found [here](https://huggingface.co/datasets/open-llm-leaderboard/details_Felladrin__Llama-68M-Chat-v1)
| Metric |Value|
|---------------------------------|----:|
|Avg. |29.72|
|AI2 Reasoning Challenge (25-Shot)|23.29|
|HellaSwag (10-Shot) |28.27|
|MMLU (5-Shot) |25.18|
|TruthfulQA (0-shot) |47.27|
|Winogrande (5-shot) |54.30|
|GSM8k (5-shot) | 0.00|
| {} | RichardErkhov/Felladrin_-_Llama-68M-Chat-v1-4bits | null | [
"transformers",
"safetensors",
"llama",
"text-generation",
"conversational",
"autotrain_compatible",
"endpoints_compatible",
"text-generation-inference",
"4-bit",
"region:us"
] | null | 2024-05-02T11:26:38+00:00 | [] | [] | TAGS
#transformers #safetensors #llama #text-generation #conversational #autotrain_compatible #endpoints_compatible #text-generation-inference #4-bit #region-us
| Quantization made by Richard Erkhov.
Github
Discord
Request more models
Llama-68M-Chat-v1 - bnb 4bits
* Model creator: URL
* Original model: URL
Original model description:
---------------------------
language:
* en
license: apache-2.0
tags:
* text-generation
datasets:
* THUDM/webglm-qa
* databricks/databricks-dolly-15k
* cognitivecomputations/wizard\_vicuna\_70k\_unfiltered
* totally-not-an-llm/EverythingLM-data-V3
* Amod/mental\_health\_counseling\_conversations
* sablo/oasst2\_curated
* starfishmedical/webGPT\_x\_dolly
* Open-Orca/OpenOrca
* mlabonne/chatml\_dpo\_pairs
base\_model: JackFram/llama-68m
widget:
* messages:
+ role: system
content: You are a career counselor. The user will provide you with an individual
looking for guidance in their professional life, and your task is to assist
them in determining what careers they are most suited for based on their skills,
interests, and experience. You should also conduct research into the various
options available, explain the job market trends in different industries, and
advice on which qualifications would be beneficial for pursuing particular fields.
+ role: user
content: Heya!
+ role: assistant
content: Hi! How may I help you?
+ role: user
content: I am interested in developing a career in software engineering. What
would you recommend me to do?
* messages:
+ role: system
content: You are a knowledgeable assistant. Help the user as much as you can.
+ role: user
content: How to become healthier?
* messages:
+ role: system
content: You are a helpful assistant who provides concise responses.
+ role: user
content: Hi!
+ role: assistant
content: Hello there! How may I help you?
+ role: user
content: I need to build a simple website. Where should I start learning about web development?
* messages:
+ role: system
content: You are a very creative assistant. User will give you a task, which you should complete with all your knowledge.
+ role: user
content: Write the background story of an RPG game about wizards and dragons in a sci-fi world.
inference:
parameters:
max\_new\_tokens: 64
penalty\_alpha: 0.5
top\_k: 4
model-index:
* name: Llama-68M-Chat-v1
results:
+ task:
type: text-generation
name: Text Generation
dataset:
name: AI2 Reasoning Challenge (25-Shot)
type: ai2\_arc
config: ARC-Challenge
split: test
args:
num\_few\_shot: 25
metrics:
- type: acc\_norm
value: 23.29
name: normalized accuracy
source:
url: URL
name: Open LLM Leaderboard
+ task:
type: text-generation
name: Text Generation
dataset:
name: HellaSwag (10-Shot)
type: hellaswag
split: validation
args:
num\_few\_shot: 10
metrics:
- type: acc\_norm
value: 28.27
name: normalized accuracy
source:
url: URL
name: Open LLM Leaderboard
+ task:
type: text-generation
name: Text Generation
dataset:
name: MMLU (5-Shot)
type: cais/mmlu
config: all
split: test
args:
num\_few\_shot: 5
metrics:
- type: acc
value: 25.18
name: accuracy
source:
url: URL
name: Open LLM Leaderboard
+ task:
type: text-generation
name: Text Generation
dataset:
name: TruthfulQA (0-shot)
type: truthful\_qa
config: multiple\_choice
split: validation
args:
num\_few\_shot: 0
metrics:
- type: mc2
value: 47.27
source:
url: URL
name: Open LLM Leaderboard
+ task:
type: text-generation
name: Text Generation
dataset:
name: Winogrande (5-shot)
type: winogrande
config: winogrande\_xl
split: validation
args:
num\_few\_shot: 5
metrics:
- type: acc
value: 54.3
name: accuracy
source:
url: URL
name: Open LLM Leaderboard
+ task:
type: text-generation
name: Text Generation
dataset:
name: GSM8k (5-shot)
type: gsm8k
config: main
split: test
args:
num\_few\_shot: 5
metrics:
- type: acc
value: 0.0
name: accuracy
source:
url: URL
name: Open LLM Leaderboard
---
A Llama Chat Model of 68M Parameters
====================================
* Base model: JackFram/llama-68m
* Datasets:
+ THUDM/webglm-qa
+ databricks/databricks-dolly-15k
+ cognitivecomputations/wizard\_vicuna\_70k\_unfiltered
+ totally-not-an-llm/EverythingLM-data-V3
+ Amod/mental\_health\_counseling\_conversations
+ sablo/oasst2\_curated
+ starfishmedical/webGPT\_x\_dolly
+ Open-Orca/OpenOrca
+ mlabonne/chatml\_dpo\_pairs
* Availability in other ML formats:
+ GGUF: afrideva/Llama-68M-Chat-v1-GGUF
+ ONNX: Felladrin/onnx-Llama-68M-Chat-v1
Recommended Prompt Format
-------------------------
Recommended Inference Parameters
--------------------------------
Open LLM Leaderboard Evaluation Results
---------------------------------------
Detailed results can be found here
| [] | [
"TAGS\n#transformers #safetensors #llama #text-generation #conversational #autotrain_compatible #endpoints_compatible #text-generation-inference #4-bit #region-us \n"
] | [
41
] | [
"TAGS\n#transformers #safetensors #llama #text-generation #conversational #autotrain_compatible #endpoints_compatible #text-generation-inference #4-bit #region-us \n"
] |
null | transformers | ## About
<!-- ### quantize_version: 2 -->
<!-- ### output_tensor_quantised: 1 -->
<!-- ### convert_type: hf -->
<!-- ### vocab_type: -->
static quants of https://huggingface.co/athirdpath/Llama-3-11b-Instruct
<!-- provided-files -->
weighted/imatrix quants seem not to be available (by me) at this time. If they do not show up a week or so after the static ones, I have probably not planned for them. Feel free to request them by opening a Community Discussion.
## Usage
If you are unsure how to use GGUF files, refer to one of [TheBloke's
READMEs](https://huggingface.co/TheBloke/KafkaLM-70B-German-V0.1-GGUF) for
more details, including on how to concatenate multi-part files.
## Provided Quants
(sorted by size, not necessarily quality. IQ-quants are often preferable over similar sized non-IQ quants)
| Link | Type | Size/GB | Notes |
|:-----|:-----|--------:|:------|
| [GGUF](https://huggingface.co/mradermacher/Llama-3-11b-Instruct-GGUF/resolve/main/Llama-3-11b-Instruct.Q2_K.gguf) | Q2_K | 4.4 | |
| [GGUF](https://huggingface.co/mradermacher/Llama-3-11b-Instruct-GGUF/resolve/main/Llama-3-11b-Instruct.IQ3_XS.gguf) | IQ3_XS | 4.9 | |
| [GGUF](https://huggingface.co/mradermacher/Llama-3-11b-Instruct-GGUF/resolve/main/Llama-3-11b-Instruct.Q3_K_S.gguf) | Q3_K_S | 5.1 | |
| [GGUF](https://huggingface.co/mradermacher/Llama-3-11b-Instruct-GGUF/resolve/main/Llama-3-11b-Instruct.IQ3_S.gguf) | IQ3_S | 5.1 | beats Q3_K* |
| [GGUF](https://huggingface.co/mradermacher/Llama-3-11b-Instruct-GGUF/resolve/main/Llama-3-11b-Instruct.IQ3_M.gguf) | IQ3_M | 5.2 | |
| [GGUF](https://huggingface.co/mradermacher/Llama-3-11b-Instruct-GGUF/resolve/main/Llama-3-11b-Instruct.Q3_K_M.gguf) | Q3_K_M | 5.6 | lower quality |
| [GGUF](https://huggingface.co/mradermacher/Llama-3-11b-Instruct-GGUF/resolve/main/Llama-3-11b-Instruct.Q3_K_L.gguf) | Q3_K_L | 6.0 | |
| [GGUF](https://huggingface.co/mradermacher/Llama-3-11b-Instruct-GGUF/resolve/main/Llama-3-11b-Instruct.IQ4_XS.gguf) | IQ4_XS | 6.2 | |
| [GGUF](https://huggingface.co/mradermacher/Llama-3-11b-Instruct-GGUF/resolve/main/Llama-3-11b-Instruct.Q4_K_S.gguf) | Q4_K_S | 6.5 | fast, recommended |
| [GGUF](https://huggingface.co/mradermacher/Llama-3-11b-Instruct-GGUF/resolve/main/Llama-3-11b-Instruct.Q4_K_M.gguf) | Q4_K_M | 6.8 | fast, recommended |
| [GGUF](https://huggingface.co/mradermacher/Llama-3-11b-Instruct-GGUF/resolve/main/Llama-3-11b-Instruct.Q5_K_S.gguf) | Q5_K_S | 7.8 | |
| [GGUF](https://huggingface.co/mradermacher/Llama-3-11b-Instruct-GGUF/resolve/main/Llama-3-11b-Instruct.Q5_K_M.gguf) | Q5_K_M | 8.0 | |
| [GGUF](https://huggingface.co/mradermacher/Llama-3-11b-Instruct-GGUF/resolve/main/Llama-3-11b-Instruct.Q6_K.gguf) | Q6_K | 9.2 | very good quality |
| [GGUF](https://huggingface.co/mradermacher/Llama-3-11b-Instruct-GGUF/resolve/main/Llama-3-11b-Instruct.Q8_0.gguf) | Q8_0 | 11.9 | fast, best quality |
Here is a handy graph by ikawrakow comparing some lower-quality quant
types (lower is better):

And here are Artefact2's thoughts on the matter:
https://gist.github.com/Artefact2/b5f810600771265fc1e39442288e8ec9
## FAQ / Model Request
See https://huggingface.co/mradermacher/model_requests for some answers to
questions you might have and/or if you want some other model quantized.
## Thanks
I thank my company, [nethype GmbH](https://www.nethype.de/), for letting
me use its servers and providing upgrades to my workstation to enable
this work in my free time.
<!-- end -->
| {"language": ["en"], "license": "llama3", "library_name": "transformers", "base_model": "athirdpath/Llama-3-11b-Instruct", "quantized_by": "mradermacher"} | mradermacher/Llama-3-11b-Instruct-GGUF | null | [
"transformers",
"gguf",
"en",
"base_model:athirdpath/Llama-3-11b-Instruct",
"license:llama3",
"endpoints_compatible",
"region:us"
] | null | 2024-05-02T11:27:09+00:00 | [] | [
"en"
] | TAGS
#transformers #gguf #en #base_model-athirdpath/Llama-3-11b-Instruct #license-llama3 #endpoints_compatible #region-us
| About
-----
static quants of URL
weighted/imatrix quants seem not to be available (by me) at this time. If they do not show up a week or so after the static ones, I have probably not planned for them. Feel free to request them by opening a Community Discussion.
Usage
-----
If you are unsure how to use GGUF files, refer to one of TheBloke's
READMEs for
more details, including on how to concatenate multi-part files.
Provided Quants
---------------
(sorted by size, not necessarily quality. IQ-quants are often preferable over similar sized non-IQ quants)
Here is a handy graph by ikawrakow comparing some lower-quality quant
types (lower is better):
!URL
And here are Artefact2's thoughts on the matter:
URL
FAQ / Model Request
-------------------
See URL for some answers to
questions you might have and/or if you want some other model quantized.
Thanks
------
I thank my company, nethype GmbH, for letting
me use its servers and providing upgrades to my workstation to enable
this work in my free time.
| [] | [
"TAGS\n#transformers #gguf #en #base_model-athirdpath/Llama-3-11b-Instruct #license-llama3 #endpoints_compatible #region-us \n"
] | [
44
] | [
"TAGS\n#transformers #gguf #en #base_model-athirdpath/Llama-3-11b-Instruct #license-llama3 #endpoints_compatible #region-us \n"
] |
text-generation | transformers | Quantization made by Richard Erkhov.
[Github](https://github.com/RichardErkhov)
[Discord](https://discord.gg/pvy7H8DZMG)
[Request more models](https://github.com/RichardErkhov/quant_request)
Llama-68M-Chat-v1 - bnb 8bits
- Model creator: https://huggingface.co/Felladrin/
- Original model: https://huggingface.co/Felladrin/Llama-68M-Chat-v1/
Original model description:
---
language:
- en
license: apache-2.0
tags:
- text-generation
datasets:
- THUDM/webglm-qa
- databricks/databricks-dolly-15k
- cognitivecomputations/wizard_vicuna_70k_unfiltered
- totally-not-an-llm/EverythingLM-data-V3
- Amod/mental_health_counseling_conversations
- sablo/oasst2_curated
- starfishmedical/webGPT_x_dolly
- Open-Orca/OpenOrca
- mlabonne/chatml_dpo_pairs
base_model: JackFram/llama-68m
widget:
- messages:
- role: system
content: You are a career counselor. The user will provide you with an individual
looking for guidance in their professional life, and your task is to assist
them in determining what careers they are most suited for based on their skills,
interests, and experience. You should also conduct research into the various
options available, explain the job market trends in different industries, and
advice on which qualifications would be beneficial for pursuing particular fields.
- role: user
content: Heya!
- role: assistant
content: Hi! How may I help you?
- role: user
content: I am interested in developing a career in software engineering. What
would you recommend me to do?
- messages:
- role: system
content: You are a knowledgeable assistant. Help the user as much as you can.
- role: user
content: How to become healthier?
- messages:
- role: system
content: You are a helpful assistant who provides concise responses.
- role: user
content: Hi!
- role: assistant
content: Hello there! How may I help you?
- role: user
content: I need to build a simple website. Where should I start learning about web development?
- messages:
- role: system
content: You are a very creative assistant. User will give you a task, which you should complete with all your knowledge.
- role: user
content: Write the background story of an RPG game about wizards and dragons in a sci-fi world.
inference:
parameters:
max_new_tokens: 64
penalty_alpha: 0.5
top_k: 4
model-index:
- name: Llama-68M-Chat-v1
results:
- task:
type: text-generation
name: Text Generation
dataset:
name: AI2 Reasoning Challenge (25-Shot)
type: ai2_arc
config: ARC-Challenge
split: test
args:
num_few_shot: 25
metrics:
- type: acc_norm
value: 23.29
name: normalized accuracy
source:
url: https://huggingface.co/spaces/HuggingFaceH4/open_llm_leaderboard?query=Felladrin/Llama-68M-Chat-v1
name: Open LLM Leaderboard
- task:
type: text-generation
name: Text Generation
dataset:
name: HellaSwag (10-Shot)
type: hellaswag
split: validation
args:
num_few_shot: 10
metrics:
- type: acc_norm
value: 28.27
name: normalized accuracy
source:
url: https://huggingface.co/spaces/HuggingFaceH4/open_llm_leaderboard?query=Felladrin/Llama-68M-Chat-v1
name: Open LLM Leaderboard
- task:
type: text-generation
name: Text Generation
dataset:
name: MMLU (5-Shot)
type: cais/mmlu
config: all
split: test
args:
num_few_shot: 5
metrics:
- type: acc
value: 25.18
name: accuracy
source:
url: https://huggingface.co/spaces/HuggingFaceH4/open_llm_leaderboard?query=Felladrin/Llama-68M-Chat-v1
name: Open LLM Leaderboard
- task:
type: text-generation
name: Text Generation
dataset:
name: TruthfulQA (0-shot)
type: truthful_qa
config: multiple_choice
split: validation
args:
num_few_shot: 0
metrics:
- type: mc2
value: 47.27
source:
url: https://huggingface.co/spaces/HuggingFaceH4/open_llm_leaderboard?query=Felladrin/Llama-68M-Chat-v1
name: Open LLM Leaderboard
- task:
type: text-generation
name: Text Generation
dataset:
name: Winogrande (5-shot)
type: winogrande
config: winogrande_xl
split: validation
args:
num_few_shot: 5
metrics:
- type: acc
value: 54.3
name: accuracy
source:
url: https://huggingface.co/spaces/HuggingFaceH4/open_llm_leaderboard?query=Felladrin/Llama-68M-Chat-v1
name: Open LLM Leaderboard
- task:
type: text-generation
name: Text Generation
dataset:
name: GSM8k (5-shot)
type: gsm8k
config: main
split: test
args:
num_few_shot: 5
metrics:
- type: acc
value: 0.0
name: accuracy
source:
url: https://huggingface.co/spaces/HuggingFaceH4/open_llm_leaderboard?query=Felladrin/Llama-68M-Chat-v1
name: Open LLM Leaderboard
---
# A Llama Chat Model of 68M Parameters
- Base model: [JackFram/llama-68m](https://huggingface.co/JackFram/llama-68m)
- Datasets:
- [THUDM/webglm-qa](https://huggingface.co/datasets/THUDM/webglm-qa)
- [databricks/databricks-dolly-15k](https://huggingface.co/datasets/databricks/databricks-dolly-15k)
- [cognitivecomputations/wizard_vicuna_70k_unfiltered](https://huggingface.co/datasets/cognitivecomputations/wizard_vicuna_70k_unfiltered)
- [totally-not-an-llm/EverythingLM-data-V3](https://huggingface.co/datasets/totally-not-an-llm/EverythingLM-data-V3)
- [Amod/mental_health_counseling_conversations](https://huggingface.co/datasets/Amod/mental_health_counseling_conversations)
- [sablo/oasst2_curated](https://huggingface.co/datasets/sablo/oasst2_curated)
- [starfishmedical/webGPT_x_dolly](https://huggingface.co/datasets/starfishmedical/webGPT_x_dolly)
- [Open-Orca/OpenOrca](https://huggingface.co/datasets/Open-Orca/OpenOrca)
- [mlabonne/chatml_dpo_pairs](https://huggingface.co/datasets/mlabonne/chatml_dpo_pairs)
- Availability in other ML formats:
- GGUF: [afrideva/Llama-68M-Chat-v1-GGUF](https://huggingface.co/afrideva/Llama-68M-Chat-v1-GGUF)
- ONNX: [Felladrin/onnx-Llama-68M-Chat-v1](https://huggingface.co/Felladrin/onnx-Llama-68M-Chat-v1)
## Recommended Prompt Format
```
<|im_start|>system
{system_message}<|im_end|>
<|im_start|>user
{user_message}<|im_end|>
<|im_start|>assistant
```
## Recommended Inference Parameters
```yml
penalty_alpha: 0.5
top_k: 4
```
## [Open LLM Leaderboard Evaluation Results](https://huggingface.co/spaces/HuggingFaceH4/open_llm_leaderboard)
Detailed results can be found [here](https://huggingface.co/datasets/open-llm-leaderboard/details_Felladrin__Llama-68M-Chat-v1)
| Metric |Value|
|---------------------------------|----:|
|Avg. |29.72|
|AI2 Reasoning Challenge (25-Shot)|23.29|
|HellaSwag (10-Shot) |28.27|
|MMLU (5-Shot) |25.18|
|TruthfulQA (0-shot) |47.27|
|Winogrande (5-shot) |54.30|
|GSM8k (5-shot) | 0.00|
| {} | RichardErkhov/Felladrin_-_Llama-68M-Chat-v1-8bits | null | [
"transformers",
"safetensors",
"llama",
"text-generation",
"conversational",
"autotrain_compatible",
"endpoints_compatible",
"text-generation-inference",
"8-bit",
"region:us"
] | null | 2024-05-02T11:27:47+00:00 | [] | [] | TAGS
#transformers #safetensors #llama #text-generation #conversational #autotrain_compatible #endpoints_compatible #text-generation-inference #8-bit #region-us
| Quantization made by Richard Erkhov.
Github
Discord
Request more models
Llama-68M-Chat-v1 - bnb 8bits
* Model creator: URL
* Original model: URL
Original model description:
---------------------------
language:
* en
license: apache-2.0
tags:
* text-generation
datasets:
* THUDM/webglm-qa
* databricks/databricks-dolly-15k
* cognitivecomputations/wizard\_vicuna\_70k\_unfiltered
* totally-not-an-llm/EverythingLM-data-V3
* Amod/mental\_health\_counseling\_conversations
* sablo/oasst2\_curated
* starfishmedical/webGPT\_x\_dolly
* Open-Orca/OpenOrca
* mlabonne/chatml\_dpo\_pairs
base\_model: JackFram/llama-68m
widget:
* messages:
+ role: system
content: You are a career counselor. The user will provide you with an individual
looking for guidance in their professional life, and your task is to assist
them in determining what careers they are most suited for based on their skills,
interests, and experience. You should also conduct research into the various
options available, explain the job market trends in different industries, and
advice on which qualifications would be beneficial for pursuing particular fields.
+ role: user
content: Heya!
+ role: assistant
content: Hi! How may I help you?
+ role: user
content: I am interested in developing a career in software engineering. What
would you recommend me to do?
* messages:
+ role: system
content: You are a knowledgeable assistant. Help the user as much as you can.
+ role: user
content: How to become healthier?
* messages:
+ role: system
content: You are a helpful assistant who provides concise responses.
+ role: user
content: Hi!
+ role: assistant
content: Hello there! How may I help you?
+ role: user
content: I need to build a simple website. Where should I start learning about web development?
* messages:
+ role: system
content: You are a very creative assistant. User will give you a task, which you should complete with all your knowledge.
+ role: user
content: Write the background story of an RPG game about wizards and dragons in a sci-fi world.
inference:
parameters:
max\_new\_tokens: 64
penalty\_alpha: 0.5
top\_k: 4
model-index:
* name: Llama-68M-Chat-v1
results:
+ task:
type: text-generation
name: Text Generation
dataset:
name: AI2 Reasoning Challenge (25-Shot)
type: ai2\_arc
config: ARC-Challenge
split: test
args:
num\_few\_shot: 25
metrics:
- type: acc\_norm
value: 23.29
name: normalized accuracy
source:
url: URL
name: Open LLM Leaderboard
+ task:
type: text-generation
name: Text Generation
dataset:
name: HellaSwag (10-Shot)
type: hellaswag
split: validation
args:
num\_few\_shot: 10
metrics:
- type: acc\_norm
value: 28.27
name: normalized accuracy
source:
url: URL
name: Open LLM Leaderboard
+ task:
type: text-generation
name: Text Generation
dataset:
name: MMLU (5-Shot)
type: cais/mmlu
config: all
split: test
args:
num\_few\_shot: 5
metrics:
- type: acc
value: 25.18
name: accuracy
source:
url: URL
name: Open LLM Leaderboard
+ task:
type: text-generation
name: Text Generation
dataset:
name: TruthfulQA (0-shot)
type: truthful\_qa
config: multiple\_choice
split: validation
args:
num\_few\_shot: 0
metrics:
- type: mc2
value: 47.27
source:
url: URL
name: Open LLM Leaderboard
+ task:
type: text-generation
name: Text Generation
dataset:
name: Winogrande (5-shot)
type: winogrande
config: winogrande\_xl
split: validation
args:
num\_few\_shot: 5
metrics:
- type: acc
value: 54.3
name: accuracy
source:
url: URL
name: Open LLM Leaderboard
+ task:
type: text-generation
name: Text Generation
dataset:
name: GSM8k (5-shot)
type: gsm8k
config: main
split: test
args:
num\_few\_shot: 5
metrics:
- type: acc
value: 0.0
name: accuracy
source:
url: URL
name: Open LLM Leaderboard
---
A Llama Chat Model of 68M Parameters
====================================
* Base model: JackFram/llama-68m
* Datasets:
+ THUDM/webglm-qa
+ databricks/databricks-dolly-15k
+ cognitivecomputations/wizard\_vicuna\_70k\_unfiltered
+ totally-not-an-llm/EverythingLM-data-V3
+ Amod/mental\_health\_counseling\_conversations
+ sablo/oasst2\_curated
+ starfishmedical/webGPT\_x\_dolly
+ Open-Orca/OpenOrca
+ mlabonne/chatml\_dpo\_pairs
* Availability in other ML formats:
+ GGUF: afrideva/Llama-68M-Chat-v1-GGUF
+ ONNX: Felladrin/onnx-Llama-68M-Chat-v1
Recommended Prompt Format
-------------------------
Recommended Inference Parameters
--------------------------------
Open LLM Leaderboard Evaluation Results
---------------------------------------
Detailed results can be found here
| [] | [
"TAGS\n#transformers #safetensors #llama #text-generation #conversational #autotrain_compatible #endpoints_compatible #text-generation-inference #8-bit #region-us \n"
] | [
41
] | [
"TAGS\n#transformers #safetensors #llama #text-generation #conversational #autotrain_compatible #endpoints_compatible #text-generation-inference #8-bit #region-us \n"
] |
null | transformers |
# NikolayKozloff/Boreas-7B-Q8_0-GGUF
This model was converted to GGUF format from [`yhavinga/Boreas-7B`](https://huggingface.co/yhavinga/Boreas-7B) using llama.cpp via the ggml.ai's [GGUF-my-repo](https://huggingface.co/spaces/ggml-org/gguf-my-repo) space.
Refer to the [original model card](https://huggingface.co/yhavinga/Boreas-7B) for more details on the model.
## Use with llama.cpp
Install llama.cpp through brew.
```bash
brew install ggerganov/ggerganov/llama.cpp
```
Invoke the llama.cpp server or the CLI.
CLI:
```bash
llama-cli --hf-repo NikolayKozloff/Boreas-7B-Q8_0-GGUF --model boreas-7b.Q8_0.gguf -p "The meaning to life and the universe is"
```
Server:
```bash
llama-server --hf-repo NikolayKozloff/Boreas-7B-Q8_0-GGUF --model boreas-7b.Q8_0.gguf -c 2048
```
Note: You can also use this checkpoint directly through the [usage steps](https://github.com/ggerganov/llama.cpp?tab=readme-ov-file#usage) listed in the Llama.cpp repo as well.
```
git clone https://github.com/ggerganov/llama.cpp && cd llama.cpp && make && ./main -m boreas-7b.Q8_0.gguf -n 128
```
| {"library_name": "transformers", "tags": ["llama-cpp", "gguf-my-repo"]} | NikolayKozloff/Boreas-7B-Q8_0-GGUF | null | [
"transformers",
"gguf",
"llama-cpp",
"gguf-my-repo",
"endpoints_compatible",
"region:us"
] | null | 2024-05-02T11:28:38+00:00 | [] | [] | TAGS
#transformers #gguf #llama-cpp #gguf-my-repo #endpoints_compatible #region-us
|
# NikolayKozloff/Boreas-7B-Q8_0-GGUF
This model was converted to GGUF format from 'yhavinga/Boreas-7B' using URL via the URL's GGUF-my-repo space.
Refer to the original model card for more details on the model.
## Use with URL
Install URL through brew.
Invoke the URL server or the CLI.
CLI:
Server:
Note: You can also use this checkpoint directly through the usage steps listed in the URL repo as well.
| [
"# NikolayKozloff/Boreas-7B-Q8_0-GGUF\nThis model was converted to GGUF format from 'yhavinga/Boreas-7B' using URL via the URL's GGUF-my-repo space.\nRefer to the original model card for more details on the model.",
"## Use with URL\n\nInstall URL through brew.\n\n\nInvoke the URL server or the CLI.\n\nCLI:\n\n\n\nServer:\n\n\n\nNote: You can also use this checkpoint directly through the usage steps listed in the URL repo as well."
] | [
"TAGS\n#transformers #gguf #llama-cpp #gguf-my-repo #endpoints_compatible #region-us \n",
"# NikolayKozloff/Boreas-7B-Q8_0-GGUF\nThis model was converted to GGUF format from 'yhavinga/Boreas-7B' using URL via the URL's GGUF-my-repo space.\nRefer to the original model card for more details on the model.",
"## Use with URL\n\nInstall URL through brew.\n\n\nInvoke the URL server or the CLI.\n\nCLI:\n\n\n\nServer:\n\n\n\nNote: You can also use this checkpoint directly through the usage steps listed in the URL repo as well."
] | [
31,
75,
52
] | [
"TAGS\n#transformers #gguf #llama-cpp #gguf-my-repo #endpoints_compatible #region-us \n# NikolayKozloff/Boreas-7B-Q8_0-GGUF\nThis model was converted to GGUF format from 'yhavinga/Boreas-7B' using URL via the URL's GGUF-my-repo space.\nRefer to the original model card for more details on the model.## Use with URL\n\nInstall URL through brew.\n\n\nInvoke the URL server or the CLI.\n\nCLI:\n\n\n\nServer:\n\n\n\nNote: You can also use this checkpoint directly through the usage steps listed in the URL repo as well."
] |
null | null | Quantization made by Richard Erkhov.
[Github](https://github.com/RichardErkhov)
[Discord](https://discord.gg/pvy7H8DZMG)
[Request more models](https://github.com/RichardErkhov/quant_request)
Llama-68M-Chat-v1 - GGUF
- Model creator: https://huggingface.co/Felladrin/
- Original model: https://huggingface.co/Felladrin/Llama-68M-Chat-v1/
| Name | Quant method | Size |
| ---- | ---- | ---- |
| [Llama-68M-Chat-v1.Q2_K.gguf](https://huggingface.co/RichardErkhov/Felladrin_-_Llama-68M-Chat-v1-gguf/blob/main/Llama-68M-Chat-v1.Q2_K.gguf) | Q2_K | 0.03GB |
| [Llama-68M-Chat-v1.IQ3_XS.gguf](https://huggingface.co/RichardErkhov/Felladrin_-_Llama-68M-Chat-v1-gguf/blob/main/Llama-68M-Chat-v1.IQ3_XS.gguf) | IQ3_XS | 0.04GB |
| [Llama-68M-Chat-v1.IQ3_S.gguf](https://huggingface.co/RichardErkhov/Felladrin_-_Llama-68M-Chat-v1-gguf/blob/main/Llama-68M-Chat-v1.IQ3_S.gguf) | IQ3_S | 0.04GB |
| [Llama-68M-Chat-v1.Q3_K_S.gguf](https://huggingface.co/RichardErkhov/Felladrin_-_Llama-68M-Chat-v1-gguf/blob/main/Llama-68M-Chat-v1.Q3_K_S.gguf) | Q3_K_S | 0.04GB |
| [Llama-68M-Chat-v1.IQ3_M.gguf](https://huggingface.co/RichardErkhov/Felladrin_-_Llama-68M-Chat-v1-gguf/blob/main/Llama-68M-Chat-v1.IQ3_M.gguf) | IQ3_M | 0.04GB |
| [Llama-68M-Chat-v1.Q3_K.gguf](https://huggingface.co/RichardErkhov/Felladrin_-_Llama-68M-Chat-v1-gguf/blob/main/Llama-68M-Chat-v1.Q3_K.gguf) | Q3_K | 0.04GB |
| [Llama-68M-Chat-v1.Q3_K_M.gguf](https://huggingface.co/RichardErkhov/Felladrin_-_Llama-68M-Chat-v1-gguf/blob/main/Llama-68M-Chat-v1.Q3_K_M.gguf) | Q3_K_M | 0.04GB |
| [Llama-68M-Chat-v1.Q3_K_L.gguf](https://huggingface.co/RichardErkhov/Felladrin_-_Llama-68M-Chat-v1-gguf/blob/main/Llama-68M-Chat-v1.Q3_K_L.gguf) | Q3_K_L | 0.04GB |
| [Llama-68M-Chat-v1.IQ4_XS.gguf](https://huggingface.co/RichardErkhov/Felladrin_-_Llama-68M-Chat-v1-gguf/blob/main/Llama-68M-Chat-v1.IQ4_XS.gguf) | IQ4_XS | 0.04GB |
| [Llama-68M-Chat-v1.Q4_0.gguf](https://huggingface.co/RichardErkhov/Felladrin_-_Llama-68M-Chat-v1-gguf/blob/main/Llama-68M-Chat-v1.Q4_0.gguf) | Q4_0 | 0.04GB |
| [Llama-68M-Chat-v1.IQ4_NL.gguf](https://huggingface.co/RichardErkhov/Felladrin_-_Llama-68M-Chat-v1-gguf/blob/main/Llama-68M-Chat-v1.IQ4_NL.gguf) | IQ4_NL | 0.04GB |
| [Llama-68M-Chat-v1.Q4_K_S.gguf](https://huggingface.co/RichardErkhov/Felladrin_-_Llama-68M-Chat-v1-gguf/blob/main/Llama-68M-Chat-v1.Q4_K_S.gguf) | Q4_K_S | 0.04GB |
| [Llama-68M-Chat-v1.Q4_K.gguf](https://huggingface.co/RichardErkhov/Felladrin_-_Llama-68M-Chat-v1-gguf/blob/main/Llama-68M-Chat-v1.Q4_K.gguf) | Q4_K | 0.04GB |
| [Llama-68M-Chat-v1.Q4_K_M.gguf](https://huggingface.co/RichardErkhov/Felladrin_-_Llama-68M-Chat-v1-gguf/blob/main/Llama-68M-Chat-v1.Q4_K_M.gguf) | Q4_K_M | 0.04GB |
| [Llama-68M-Chat-v1.Q4_1.gguf](https://huggingface.co/RichardErkhov/Felladrin_-_Llama-68M-Chat-v1-gguf/blob/main/Llama-68M-Chat-v1.Q4_1.gguf) | Q4_1 | 0.04GB |
| [Llama-68M-Chat-v1.Q5_0.gguf](https://huggingface.co/RichardErkhov/Felladrin_-_Llama-68M-Chat-v1-gguf/blob/main/Llama-68M-Chat-v1.Q5_0.gguf) | Q5_0 | 0.05GB |
| [Llama-68M-Chat-v1.Q5_K_S.gguf](https://huggingface.co/RichardErkhov/Felladrin_-_Llama-68M-Chat-v1-gguf/blob/main/Llama-68M-Chat-v1.Q5_K_S.gguf) | Q5_K_S | 0.05GB |
| [Llama-68M-Chat-v1.Q5_K.gguf](https://huggingface.co/RichardErkhov/Felladrin_-_Llama-68M-Chat-v1-gguf/blob/main/Llama-68M-Chat-v1.Q5_K.gguf) | Q5_K | 0.05GB |
| [Llama-68M-Chat-v1.Q5_K_M.gguf](https://huggingface.co/RichardErkhov/Felladrin_-_Llama-68M-Chat-v1-gguf/blob/main/Llama-68M-Chat-v1.Q5_K_M.gguf) | Q5_K_M | 0.05GB |
| [Llama-68M-Chat-v1.Q5_1.gguf](https://huggingface.co/RichardErkhov/Felladrin_-_Llama-68M-Chat-v1-gguf/blob/main/Llama-68M-Chat-v1.Q5_1.gguf) | Q5_1 | 0.05GB |
| [Llama-68M-Chat-v1.Q6_K.gguf](https://huggingface.co/RichardErkhov/Felladrin_-_Llama-68M-Chat-v1-gguf/blob/main/Llama-68M-Chat-v1.Q6_K.gguf) | Q6_K | 0.05GB |
Original model description:
---
language:
- en
license: apache-2.0
tags:
- text-generation
datasets:
- THUDM/webglm-qa
- databricks/databricks-dolly-15k
- cognitivecomputations/wizard_vicuna_70k_unfiltered
- totally-not-an-llm/EverythingLM-data-V3
- Amod/mental_health_counseling_conversations
- sablo/oasst2_curated
- starfishmedical/webGPT_x_dolly
- Open-Orca/OpenOrca
- mlabonne/chatml_dpo_pairs
base_model: JackFram/llama-68m
widget:
- messages:
- role: system
content: You are a career counselor. The user will provide you with an individual
looking for guidance in their professional life, and your task is to assist
them in determining what careers they are most suited for based on their skills,
interests, and experience. You should also conduct research into the various
options available, explain the job market trends in different industries, and
advice on which qualifications would be beneficial for pursuing particular fields.
- role: user
content: Heya!
- role: assistant
content: Hi! How may I help you?
- role: user
content: I am interested in developing a career in software engineering. What
would you recommend me to do?
- messages:
- role: system
content: You are a knowledgeable assistant. Help the user as much as you can.
- role: user
content: How to become healthier?
- messages:
- role: system
content: You are a helpful assistant who provides concise responses.
- role: user
content: Hi!
- role: assistant
content: Hello there! How may I help you?
- role: user
content: I need to build a simple website. Where should I start learning about web development?
- messages:
- role: system
content: You are a very creative assistant. User will give you a task, which you should complete with all your knowledge.
- role: user
content: Write the background story of an RPG game about wizards and dragons in a sci-fi world.
inference:
parameters:
max_new_tokens: 64
penalty_alpha: 0.5
top_k: 4
model-index:
- name: Llama-68M-Chat-v1
results:
- task:
type: text-generation
name: Text Generation
dataset:
name: AI2 Reasoning Challenge (25-Shot)
type: ai2_arc
config: ARC-Challenge
split: test
args:
num_few_shot: 25
metrics:
- type: acc_norm
value: 23.29
name: normalized accuracy
source:
url: https://huggingface.co/spaces/HuggingFaceH4/open_llm_leaderboard?query=Felladrin/Llama-68M-Chat-v1
name: Open LLM Leaderboard
- task:
type: text-generation
name: Text Generation
dataset:
name: HellaSwag (10-Shot)
type: hellaswag
split: validation
args:
num_few_shot: 10
metrics:
- type: acc_norm
value: 28.27
name: normalized accuracy
source:
url: https://huggingface.co/spaces/HuggingFaceH4/open_llm_leaderboard?query=Felladrin/Llama-68M-Chat-v1
name: Open LLM Leaderboard
- task:
type: text-generation
name: Text Generation
dataset:
name: MMLU (5-Shot)
type: cais/mmlu
config: all
split: test
args:
num_few_shot: 5
metrics:
- type: acc
value: 25.18
name: accuracy
source:
url: https://huggingface.co/spaces/HuggingFaceH4/open_llm_leaderboard?query=Felladrin/Llama-68M-Chat-v1
name: Open LLM Leaderboard
- task:
type: text-generation
name: Text Generation
dataset:
name: TruthfulQA (0-shot)
type: truthful_qa
config: multiple_choice
split: validation
args:
num_few_shot: 0
metrics:
- type: mc2
value: 47.27
source:
url: https://huggingface.co/spaces/HuggingFaceH4/open_llm_leaderboard?query=Felladrin/Llama-68M-Chat-v1
name: Open LLM Leaderboard
- task:
type: text-generation
name: Text Generation
dataset:
name: Winogrande (5-shot)
type: winogrande
config: winogrande_xl
split: validation
args:
num_few_shot: 5
metrics:
- type: acc
value: 54.3
name: accuracy
source:
url: https://huggingface.co/spaces/HuggingFaceH4/open_llm_leaderboard?query=Felladrin/Llama-68M-Chat-v1
name: Open LLM Leaderboard
- task:
type: text-generation
name: Text Generation
dataset:
name: GSM8k (5-shot)
type: gsm8k
config: main
split: test
args:
num_few_shot: 5
metrics:
- type: acc
value: 0.0
name: accuracy
source:
url: https://huggingface.co/spaces/HuggingFaceH4/open_llm_leaderboard?query=Felladrin/Llama-68M-Chat-v1
name: Open LLM Leaderboard
---
# A Llama Chat Model of 68M Parameters
- Base model: [JackFram/llama-68m](https://huggingface.co/JackFram/llama-68m)
- Datasets:
- [THUDM/webglm-qa](https://huggingface.co/datasets/THUDM/webglm-qa)
- [databricks/databricks-dolly-15k](https://huggingface.co/datasets/databricks/databricks-dolly-15k)
- [cognitivecomputations/wizard_vicuna_70k_unfiltered](https://huggingface.co/datasets/cognitivecomputations/wizard_vicuna_70k_unfiltered)
- [totally-not-an-llm/EverythingLM-data-V3](https://huggingface.co/datasets/totally-not-an-llm/EverythingLM-data-V3)
- [Amod/mental_health_counseling_conversations](https://huggingface.co/datasets/Amod/mental_health_counseling_conversations)
- [sablo/oasst2_curated](https://huggingface.co/datasets/sablo/oasst2_curated)
- [starfishmedical/webGPT_x_dolly](https://huggingface.co/datasets/starfishmedical/webGPT_x_dolly)
- [Open-Orca/OpenOrca](https://huggingface.co/datasets/Open-Orca/OpenOrca)
- [mlabonne/chatml_dpo_pairs](https://huggingface.co/datasets/mlabonne/chatml_dpo_pairs)
- Availability in other ML formats:
- GGUF: [afrideva/Llama-68M-Chat-v1-GGUF](https://huggingface.co/afrideva/Llama-68M-Chat-v1-GGUF)
- ONNX: [Felladrin/onnx-Llama-68M-Chat-v1](https://huggingface.co/Felladrin/onnx-Llama-68M-Chat-v1)
## Recommended Prompt Format
```
<|im_start|>system
{system_message}<|im_end|>
<|im_start|>user
{user_message}<|im_end|>
<|im_start|>assistant
```
## Recommended Inference Parameters
```yml
penalty_alpha: 0.5
top_k: 4
```
## [Open LLM Leaderboard Evaluation Results](https://huggingface.co/spaces/HuggingFaceH4/open_llm_leaderboard)
Detailed results can be found [here](https://huggingface.co/datasets/open-llm-leaderboard/details_Felladrin__Llama-68M-Chat-v1)
| Metric |Value|
|---------------------------------|----:|
|Avg. |29.72|
|AI2 Reasoning Challenge (25-Shot)|23.29|
|HellaSwag (10-Shot) |28.27|
|MMLU (5-Shot) |25.18|
|TruthfulQA (0-shot) |47.27|
|Winogrande (5-shot) |54.30|
|GSM8k (5-shot) | 0.00|
| {} | RichardErkhov/Felladrin_-_Llama-68M-Chat-v1-gguf | null | [
"gguf",
"region:us"
] | null | 2024-05-02T11:28:41+00:00 | [] | [] | TAGS
#gguf #region-us
| Quantization made by Richard Erkhov.
Github
Discord
Request more models
Llama-68M-Chat-v1 - GGUF
* Model creator: URL
* Original model: URL
Name: Llama-68M-Chat-v1.Q2\_K.gguf, Quant method: Q2\_K, Size: 0.03GB
Name: Llama-68M-Chat-v1.IQ3\_XS.gguf, Quant method: IQ3\_XS, Size: 0.04GB
Name: Llama-68M-Chat-v1.IQ3\_S.gguf, Quant method: IQ3\_S, Size: 0.04GB
Name: Llama-68M-Chat-v1.Q3\_K\_S.gguf, Quant method: Q3\_K\_S, Size: 0.04GB
Name: Llama-68M-Chat-v1.IQ3\_M.gguf, Quant method: IQ3\_M, Size: 0.04GB
Name: Llama-68M-Chat-v1.Q3\_K.gguf, Quant method: Q3\_K, Size: 0.04GB
Name: Llama-68M-Chat-v1.Q3\_K\_M.gguf, Quant method: Q3\_K\_M, Size: 0.04GB
Name: Llama-68M-Chat-v1.Q3\_K\_L.gguf, Quant method: Q3\_K\_L, Size: 0.04GB
Name: Llama-68M-Chat-v1.IQ4\_XS.gguf, Quant method: IQ4\_XS, Size: 0.04GB
Name: Llama-68M-Chat-v1.Q4\_0.gguf, Quant method: Q4\_0, Size: 0.04GB
Name: Llama-68M-Chat-v1.IQ4\_NL.gguf, Quant method: IQ4\_NL, Size: 0.04GB
Name: Llama-68M-Chat-v1.Q4\_K\_S.gguf, Quant method: Q4\_K\_S, Size: 0.04GB
Name: Llama-68M-Chat-v1.Q4\_K.gguf, Quant method: Q4\_K, Size: 0.04GB
Name: Llama-68M-Chat-v1.Q4\_K\_M.gguf, Quant method: Q4\_K\_M, Size: 0.04GB
Name: Llama-68M-Chat-v1.Q4\_1.gguf, Quant method: Q4\_1, Size: 0.04GB
Name: Llama-68M-Chat-v1.Q5\_0.gguf, Quant method: Q5\_0, Size: 0.05GB
Name: Llama-68M-Chat-v1.Q5\_K\_S.gguf, Quant method: Q5\_K\_S, Size: 0.05GB
Name: Llama-68M-Chat-v1.Q5\_K.gguf, Quant method: Q5\_K, Size: 0.05GB
Name: Llama-68M-Chat-v1.Q5\_K\_M.gguf, Quant method: Q5\_K\_M, Size: 0.05GB
Name: Llama-68M-Chat-v1.Q5\_1.gguf, Quant method: Q5\_1, Size: 0.05GB
Name: Llama-68M-Chat-v1.Q6\_K.gguf, Quant method: Q6\_K, Size: 0.05GB
Original model description:
---------------------------
language:
* en
license: apache-2.0
tags:
* text-generation
datasets:
* THUDM/webglm-qa
* databricks/databricks-dolly-15k
* cognitivecomputations/wizard\_vicuna\_70k\_unfiltered
* totally-not-an-llm/EverythingLM-data-V3
* Amod/mental\_health\_counseling\_conversations
* sablo/oasst2\_curated
* starfishmedical/webGPT\_x\_dolly
* Open-Orca/OpenOrca
* mlabonne/chatml\_dpo\_pairs
base\_model: JackFram/llama-68m
widget:
* messages:
+ role: system
content: You are a career counselor. The user will provide you with an individual
looking for guidance in their professional life, and your task is to assist
them in determining what careers they are most suited for based on their skills,
interests, and experience. You should also conduct research into the various
options available, explain the job market trends in different industries, and
advice on which qualifications would be beneficial for pursuing particular fields.
+ role: user
content: Heya!
+ role: assistant
content: Hi! How may I help you?
+ role: user
content: I am interested in developing a career in software engineering. What
would you recommend me to do?
* messages:
+ role: system
content: You are a knowledgeable assistant. Help the user as much as you can.
+ role: user
content: How to become healthier?
* messages:
+ role: system
content: You are a helpful assistant who provides concise responses.
+ role: user
content: Hi!
+ role: assistant
content: Hello there! How may I help you?
+ role: user
content: I need to build a simple website. Where should I start learning about web development?
* messages:
+ role: system
content: You are a very creative assistant. User will give you a task, which you should complete with all your knowledge.
+ role: user
content: Write the background story of an RPG game about wizards and dragons in a sci-fi world.
inference:
parameters:
max\_new\_tokens: 64
penalty\_alpha: 0.5
top\_k: 4
model-index:
* name: Llama-68M-Chat-v1
results:
+ task:
type: text-generation
name: Text Generation
dataset:
name: AI2 Reasoning Challenge (25-Shot)
type: ai2\_arc
config: ARC-Challenge
split: test
args:
num\_few\_shot: 25
metrics:
- type: acc\_norm
value: 23.29
name: normalized accuracy
source:
url: URL
name: Open LLM Leaderboard
+ task:
type: text-generation
name: Text Generation
dataset:
name: HellaSwag (10-Shot)
type: hellaswag
split: validation
args:
num\_few\_shot: 10
metrics:
- type: acc\_norm
value: 28.27
name: normalized accuracy
source:
url: URL
name: Open LLM Leaderboard
+ task:
type: text-generation
name: Text Generation
dataset:
name: MMLU (5-Shot)
type: cais/mmlu
config: all
split: test
args:
num\_few\_shot: 5
metrics:
- type: acc
value: 25.18
name: accuracy
source:
url: URL
name: Open LLM Leaderboard
+ task:
type: text-generation
name: Text Generation
dataset:
name: TruthfulQA (0-shot)
type: truthful\_qa
config: multiple\_choice
split: validation
args:
num\_few\_shot: 0
metrics:
- type: mc2
value: 47.27
source:
url: URL
name: Open LLM Leaderboard
+ task:
type: text-generation
name: Text Generation
dataset:
name: Winogrande (5-shot)
type: winogrande
config: winogrande\_xl
split: validation
args:
num\_few\_shot: 5
metrics:
- type: acc
value: 54.3
name: accuracy
source:
url: URL
name: Open LLM Leaderboard
+ task:
type: text-generation
name: Text Generation
dataset:
name: GSM8k (5-shot)
type: gsm8k
config: main
split: test
args:
num\_few\_shot: 5
metrics:
- type: acc
value: 0.0
name: accuracy
source:
url: URL
name: Open LLM Leaderboard
---
A Llama Chat Model of 68M Parameters
====================================
* Base model: JackFram/llama-68m
* Datasets:
+ THUDM/webglm-qa
+ databricks/databricks-dolly-15k
+ cognitivecomputations/wizard\_vicuna\_70k\_unfiltered
+ totally-not-an-llm/EverythingLM-data-V3
+ Amod/mental\_health\_counseling\_conversations
+ sablo/oasst2\_curated
+ starfishmedical/webGPT\_x\_dolly
+ Open-Orca/OpenOrca
+ mlabonne/chatml\_dpo\_pairs
* Availability in other ML formats:
+ GGUF: afrideva/Llama-68M-Chat-v1-GGUF
+ ONNX: Felladrin/onnx-Llama-68M-Chat-v1
Recommended Prompt Format
-------------------------
Recommended Inference Parameters
--------------------------------
Open LLM Leaderboard Evaluation Results
---------------------------------------
Detailed results can be found here
| [] | [
"TAGS\n#gguf #region-us \n"
] | [
9
] | [
"TAGS\n#gguf #region-us \n"
] |
null | peft |
# Model Card for Model ID
<!-- Provide a quick summary of what the model is/does. -->
## Model Details
### Model Description
<!-- Provide a longer summary of what this model is. -->
- **Developed by:** [More Information Needed]
- **Funded by [optional]:** [More Information Needed]
- **Shared by [optional]:** [More Information Needed]
- **Model type:** [More Information Needed]
- **Language(s) (NLP):** [More Information Needed]
- **License:** [More Information Needed]
- **Finetuned from model [optional]:** [More Information Needed]
### Model Sources [optional]
<!-- Provide the basic links for the model. -->
- **Repository:** [More Information Needed]
- **Paper [optional]:** [More Information Needed]
- **Demo [optional]:** [More Information Needed]
## Uses
<!-- Address questions around how the model is intended to be used, including the foreseeable users of the model and those affected by the model. -->
### Direct Use
<!-- This section is for the model use without fine-tuning or plugging into a larger ecosystem/app. -->
[More Information Needed]
### Downstream Use [optional]
<!-- This section is for the model use when fine-tuned for a task, or when plugged into a larger ecosystem/app -->
[More Information Needed]
### Out-of-Scope Use
<!-- This section addresses misuse, malicious use, and uses that the model will not work well for. -->
[More Information Needed]
## Bias, Risks, and Limitations
<!-- This section is meant to convey both technical and sociotechnical limitations. -->
[More Information Needed]
### Recommendations
<!-- This section is meant to convey recommendations with respect to the bias, risk, and technical limitations. -->
Users (both direct and downstream) should be made aware of the risks, biases and limitations of the model. More information needed for further recommendations.
## How to Get Started with the Model
Use the code below to get started with the model.
[More Information Needed]
## Training Details
### Training Data
<!-- This should link to a Dataset Card, perhaps with a short stub of information on what the training data is all about as well as documentation related to data pre-processing or additional filtering. -->
[More Information Needed]
### Training Procedure
<!-- This relates heavily to the Technical Specifications. Content here should link to that section when it is relevant to the training procedure. -->
#### Preprocessing [optional]
[More Information Needed]
#### Training Hyperparameters
- **Training regime:** [More Information Needed] <!--fp32, fp16 mixed precision, bf16 mixed precision, bf16 non-mixed precision, fp16 non-mixed precision, fp8 mixed precision -->
#### Speeds, Sizes, Times [optional]
<!-- This section provides information about throughput, start/end time, checkpoint size if relevant, etc. -->
[More Information Needed]
## Evaluation
<!-- This section describes the evaluation protocols and provides the results. -->
### Testing Data, Factors & Metrics
#### Testing Data
<!-- This should link to a Dataset Card if possible. -->
[More Information Needed]
#### Factors
<!-- These are the things the evaluation is disaggregating by, e.g., subpopulations or domains. -->
[More Information Needed]
#### Metrics
<!-- These are the evaluation metrics being used, ideally with a description of why. -->
[More Information Needed]
### Results
[More Information Needed]
#### Summary
## Model Examination [optional]
<!-- Relevant interpretability work for the model goes here -->
[More Information Needed]
## Environmental Impact
<!-- Total emissions (in grams of CO2eq) and additional considerations, such as electricity usage, go here. Edit the suggested text below accordingly -->
Carbon emissions can be estimated using the [Machine Learning Impact calculator](https://mlco2.github.io/impact#compute) presented in [Lacoste et al. (2019)](https://arxiv.org/abs/1910.09700).
- **Hardware Type:** [More Information Needed]
- **Hours used:** [More Information Needed]
- **Cloud Provider:** [More Information Needed]
- **Compute Region:** [More Information Needed]
- **Carbon Emitted:** [More Information Needed]
## Technical Specifications [optional]
### Model Architecture and Objective
[More Information Needed]
### Compute Infrastructure
[More Information Needed]
#### Hardware
[More Information Needed]
#### Software
[More Information Needed]
## Citation [optional]
<!-- If there is a paper or blog post introducing the model, the APA and Bibtex information for that should go in this section. -->
**BibTeX:**
[More Information Needed]
**APA:**
[More Information Needed]
## Glossary [optional]
<!-- If relevant, include terms and calculations in this section that can help readers understand the model or model card. -->
[More Information Needed]
## More Information [optional]
[More Information Needed]
## Model Card Authors [optional]
[More Information Needed]
## Model Card Contact
[More Information Needed]
### Framework versions
- PEFT 0.10.0 | {"library_name": "peft", "base_model": "BramVanroy/fietje-2b-chat"} | HansvDam/fietje-2b-chat-sr-system-do-adapters | null | [
"peft",
"safetensors",
"arxiv:1910.09700",
"base_model:BramVanroy/fietje-2b-chat",
"region:us"
] | null | 2024-05-02T11:29:08+00:00 | [
"1910.09700"
] | [] | TAGS
#peft #safetensors #arxiv-1910.09700 #base_model-BramVanroy/fietje-2b-chat #region-us
|
# Model Card for Model ID
## Model Details
### Model Description
- Developed by:
- Funded by [optional]:
- Shared by [optional]:
- Model type:
- Language(s) (NLP):
- License:
- Finetuned from model [optional]:
### Model Sources [optional]
- Repository:
- Paper [optional]:
- Demo [optional]:
## Uses
### Direct Use
### Downstream Use [optional]
### Out-of-Scope Use
## Bias, Risks, and Limitations
### Recommendations
Users (both direct and downstream) should be made aware of the risks, biases and limitations of the model. More information needed for further recommendations.
## How to Get Started with the Model
Use the code below to get started with the model.
## Training Details
### Training Data
### Training Procedure
#### Preprocessing [optional]
#### Training Hyperparameters
- Training regime:
#### Speeds, Sizes, Times [optional]
## Evaluation
### Testing Data, Factors & Metrics
#### Testing Data
#### Factors
#### Metrics
### Results
#### Summary
## Model Examination [optional]
## Environmental Impact
Carbon emissions can be estimated using the Machine Learning Impact calculator presented in Lacoste et al. (2019).
- Hardware Type:
- Hours used:
- Cloud Provider:
- Compute Region:
- Carbon Emitted:
## Technical Specifications [optional]
### Model Architecture and Objective
### Compute Infrastructure
#### Hardware
#### Software
[optional]
BibTeX:
APA:
## Glossary [optional]
## More Information [optional]
## Model Card Authors [optional]
## Model Card Contact
### Framework versions
- PEFT 0.10.0 | [
"# Model Card for Model ID",
"## Model Details",
"### Model Description\n\n\n\n\n\n- Developed by: \n- Funded by [optional]: \n- Shared by [optional]: \n- Model type: \n- Language(s) (NLP): \n- License: \n- Finetuned from model [optional]:",
"### Model Sources [optional]\n\n\n\n- Repository: \n- Paper [optional]: \n- Demo [optional]:",
"## Uses",
"### Direct Use",
"### Downstream Use [optional]",
"### Out-of-Scope Use",
"## Bias, Risks, and Limitations",
"### Recommendations\n\n\n\nUsers (both direct and downstream) should be made aware of the risks, biases and limitations of the model. More information needed for further recommendations.",
"## How to Get Started with the Model\n\nUse the code below to get started with the model.",
"## Training Details",
"### Training Data",
"### Training Procedure",
"#### Preprocessing [optional]",
"#### Training Hyperparameters\n\n- Training regime:",
"#### Speeds, Sizes, Times [optional]",
"## Evaluation",
"### Testing Data, Factors & Metrics",
"#### Testing Data",
"#### Factors",
"#### Metrics",
"### Results",
"#### Summary",
"## Model Examination [optional]",
"## Environmental Impact\n\n\n\nCarbon emissions can be estimated using the Machine Learning Impact calculator presented in Lacoste et al. (2019).\n\n- Hardware Type: \n- Hours used: \n- Cloud Provider: \n- Compute Region: \n- Carbon Emitted:",
"## Technical Specifications [optional]",
"### Model Architecture and Objective",
"### Compute Infrastructure",
"#### Hardware",
"#### Software\n\n\n\n[optional]\n\n\n\nBibTeX:\n\n\n\nAPA:",
"## Glossary [optional]",
"## More Information [optional]",
"## Model Card Authors [optional]",
"## Model Card Contact",
"### Framework versions\n\n- PEFT 0.10.0"
] | [
"TAGS\n#peft #safetensors #arxiv-1910.09700 #base_model-BramVanroy/fietje-2b-chat #region-us \n",
"# Model Card for Model ID",
"## Model Details",
"### Model Description\n\n\n\n\n\n- Developed by: \n- Funded by [optional]: \n- Shared by [optional]: \n- Model type: \n- Language(s) (NLP): \n- License: \n- Finetuned from model [optional]:",
"### Model Sources [optional]\n\n\n\n- Repository: \n- Paper [optional]: \n- Demo [optional]:",
"## Uses",
"### Direct Use",
"### Downstream Use [optional]",
"### Out-of-Scope Use",
"## Bias, Risks, and Limitations",
"### Recommendations\n\n\n\nUsers (both direct and downstream) should be made aware of the risks, biases and limitations of the model. More information needed for further recommendations.",
"## How to Get Started with the Model\n\nUse the code below to get started with the model.",
"## Training Details",
"### Training Data",
"### Training Procedure",
"#### Preprocessing [optional]",
"#### Training Hyperparameters\n\n- Training regime:",
"#### Speeds, Sizes, Times [optional]",
"## Evaluation",
"### Testing Data, Factors & Metrics",
"#### Testing Data",
"#### Factors",
"#### Metrics",
"### Results",
"#### Summary",
"## Model Examination [optional]",
"## Environmental Impact\n\n\n\nCarbon emissions can be estimated using the Machine Learning Impact calculator presented in Lacoste et al. (2019).\n\n- Hardware Type: \n- Hours used: \n- Cloud Provider: \n- Compute Region: \n- Carbon Emitted:",
"## Technical Specifications [optional]",
"### Model Architecture and Objective",
"### Compute Infrastructure",
"#### Hardware",
"#### Software\n\n\n\n[optional]\n\n\n\nBibTeX:\n\n\n\nAPA:",
"## Glossary [optional]",
"## More Information [optional]",
"## Model Card Authors [optional]",
"## Model Card Contact",
"### Framework versions\n\n- PEFT 0.10.0"
] | [
39,
6,
4,
50,
23,
3,
5,
8,
9,
8,
34,
20,
4,
5,
5,
11,
13,
12,
3,
10,
6,
5,
6,
4,
5,
7,
49,
7,
7,
5,
5,
15,
7,
7,
8,
5,
13
] | [
"TAGS\n#peft #safetensors #arxiv-1910.09700 #base_model-BramVanroy/fietje-2b-chat #region-us \n# Model Card for Model ID## Model Details### Model Description\n\n\n\n\n\n- Developed by: \n- Funded by [optional]: \n- Shared by [optional]: \n- Model type: \n- Language(s) (NLP): \n- License: \n- Finetuned from model [optional]:### Model Sources [optional]\n\n\n\n- Repository: \n- Paper [optional]: \n- Demo [optional]:## Uses### Direct Use### Downstream Use [optional]### Out-of-Scope Use## Bias, Risks, and Limitations### Recommendations\n\n\n\nUsers (both direct and downstream) should be made aware of the risks, biases and limitations of the model. More information needed for further recommendations.## How to Get Started with the Model\n\nUse the code below to get started with the model.## Training Details### Training Data### Training Procedure#### Preprocessing [optional]#### Training Hyperparameters\n\n- Training regime:#### Speeds, Sizes, Times [optional]## Evaluation### Testing Data, Factors & Metrics#### Testing Data#### Factors#### Metrics### Results#### Summary## Model Examination [optional]## Environmental Impact\n\n\n\nCarbon emissions can be estimated using the Machine Learning Impact calculator presented in Lacoste et al. (2019).\n\n- Hardware Type: \n- Hours used: \n- Cloud Provider: \n- Compute Region: \n- Carbon Emitted:## Technical Specifications [optional]### Model Architecture and Objective### Compute Infrastructure#### Hardware#### Software\n\n\n\n[optional]\n\n\n\nBibTeX:\n\n\n\nAPA:## Glossary [optional]## More Information [optional]## Model Card Authors [optional]## Model Card Contact### Framework versions\n\n- PEFT 0.10.0"
] |
null | null |
Please refer to https://github.com/VamosC/CLIP4STR for the usage of the models. | {"language": ["en"], "license": "apache-2.0"} | mzhaoshuai/CLIP4STR | null | [
"en",
"license:apache-2.0",
"region:us"
] | null | 2024-05-02T11:29:28+00:00 | [] | [
"en"
] | TAGS
#en #license-apache-2.0 #region-us
|
Please refer to URL for the usage of the models. | [] | [
"TAGS\n#en #license-apache-2.0 #region-us \n"
] | [
15
] | [
"TAGS\n#en #license-apache-2.0 #region-us \n"
] |
text-generation | transformers |
# Model Card for Model ID
<!-- Provide a quick summary of what the model is/does. -->
## Model Details
### Model Description
<!-- Provide a longer summary of what this model is. -->
This is the model card of a 🤗 transformers model that has been pushed on the Hub. This model card has been automatically generated.
- **Developed by:** [More Information Needed]
- **Funded by [optional]:** [More Information Needed]
- **Shared by [optional]:** [More Information Needed]
- **Model type:** [More Information Needed]
- **Language(s) (NLP):** [More Information Needed]
- **License:** [More Information Needed]
- **Finetuned from model [optional]:** [More Information Needed]
### Model Sources [optional]
<!-- Provide the basic links for the model. -->
- **Repository:** [More Information Needed]
- **Paper [optional]:** [More Information Needed]
- **Demo [optional]:** [More Information Needed]
## Uses
<!-- Address questions around how the model is intended to be used, including the foreseeable users of the model and those affected by the model. -->
### Direct Use
<!-- This section is for the model use without fine-tuning or plugging into a larger ecosystem/app. -->
[More Information Needed]
### Downstream Use [optional]
<!-- This section is for the model use when fine-tuned for a task, or when plugged into a larger ecosystem/app -->
[More Information Needed]
### Out-of-Scope Use
<!-- This section addresses misuse, malicious use, and uses that the model will not work well for. -->
[More Information Needed]
## Bias, Risks, and Limitations
<!-- This section is meant to convey both technical and sociotechnical limitations. -->
[More Information Needed]
### Recommendations
<!-- This section is meant to convey recommendations with respect to the bias, risk, and technical limitations. -->
Users (both direct and downstream) should be made aware of the risks, biases and limitations of the model. More information needed for further recommendations.
## How to Get Started with the Model
Use the code below to get started with the model.
[More Information Needed]
## Training Details
### Training Data
<!-- This should link to a Dataset Card, perhaps with a short stub of information on what the training data is all about as well as documentation related to data pre-processing or additional filtering. -->
[More Information Needed]
### Training Procedure
<!-- This relates heavily to the Technical Specifications. Content here should link to that section when it is relevant to the training procedure. -->
#### Preprocessing [optional]
[More Information Needed]
#### Training Hyperparameters
- **Training regime:** [More Information Needed] <!--fp32, fp16 mixed precision, bf16 mixed precision, bf16 non-mixed precision, fp16 non-mixed precision, fp8 mixed precision -->
#### Speeds, Sizes, Times [optional]
<!-- This section provides information about throughput, start/end time, checkpoint size if relevant, etc. -->
[More Information Needed]
## Evaluation
<!-- This section describes the evaluation protocols and provides the results. -->
### Testing Data, Factors & Metrics
#### Testing Data
<!-- This should link to a Dataset Card if possible. -->
[More Information Needed]
#### Factors
<!-- These are the things the evaluation is disaggregating by, e.g., subpopulations or domains. -->
[More Information Needed]
#### Metrics
<!-- These are the evaluation metrics being used, ideally with a description of why. -->
[More Information Needed]
### Results
[More Information Needed]
#### Summary
## Model Examination [optional]
<!-- Relevant interpretability work for the model goes here -->
[More Information Needed]
## Environmental Impact
<!-- Total emissions (in grams of CO2eq) and additional considerations, such as electricity usage, go here. Edit the suggested text below accordingly -->
Carbon emissions can be estimated using the [Machine Learning Impact calculator](https://mlco2.github.io/impact#compute) presented in [Lacoste et al. (2019)](https://arxiv.org/abs/1910.09700).
- **Hardware Type:** [More Information Needed]
- **Hours used:** [More Information Needed]
- **Cloud Provider:** [More Information Needed]
- **Compute Region:** [More Information Needed]
- **Carbon Emitted:** [More Information Needed]
## Technical Specifications [optional]
### Model Architecture and Objective
[More Information Needed]
### Compute Infrastructure
[More Information Needed]
#### Hardware
[More Information Needed]
#### Software
[More Information Needed]
## Citation [optional]
<!-- If there is a paper or blog post introducing the model, the APA and Bibtex information for that should go in this section. -->
**BibTeX:**
[More Information Needed]
**APA:**
[More Information Needed]
## Glossary [optional]
<!-- If relevant, include terms and calculations in this section that can help readers understand the model or model card. -->
[More Information Needed]
## More Information [optional]
[More Information Needed]
## Model Card Authors [optional]
[More Information Needed]
## Model Card Contact
[More Information Needed] | {"library_name": "transformers", "tags": []} | HansvDam/fietje-2b-chat-sr-system-do | null | [
"transformers",
"safetensors",
"phi",
"text-generation",
"conversational",
"arxiv:1910.09700",
"autotrain_compatible",
"endpoints_compatible",
"text-generation-inference",
"region:us"
] | null | 2024-05-02T11:29:39+00:00 | [
"1910.09700"
] | [] | TAGS
#transformers #safetensors #phi #text-generation #conversational #arxiv-1910.09700 #autotrain_compatible #endpoints_compatible #text-generation-inference #region-us
|
# Model Card for Model ID
## Model Details
### Model Description
This is the model card of a transformers model that has been pushed on the Hub. This model card has been automatically generated.
- Developed by:
- Funded by [optional]:
- Shared by [optional]:
- Model type:
- Language(s) (NLP):
- License:
- Finetuned from model [optional]:
### Model Sources [optional]
- Repository:
- Paper [optional]:
- Demo [optional]:
## Uses
### Direct Use
### Downstream Use [optional]
### Out-of-Scope Use
## Bias, Risks, and Limitations
### Recommendations
Users (both direct and downstream) should be made aware of the risks, biases and limitations of the model. More information needed for further recommendations.
## How to Get Started with the Model
Use the code below to get started with the model.
## Training Details
### Training Data
### Training Procedure
#### Preprocessing [optional]
#### Training Hyperparameters
- Training regime:
#### Speeds, Sizes, Times [optional]
## Evaluation
### Testing Data, Factors & Metrics
#### Testing Data
#### Factors
#### Metrics
### Results
#### Summary
## Model Examination [optional]
## Environmental Impact
Carbon emissions can be estimated using the Machine Learning Impact calculator presented in Lacoste et al. (2019).
- Hardware Type:
- Hours used:
- Cloud Provider:
- Compute Region:
- Carbon Emitted:
## Technical Specifications [optional]
### Model Architecture and Objective
### Compute Infrastructure
#### Hardware
#### Software
[optional]
BibTeX:
APA:
## Glossary [optional]
## More Information [optional]
## Model Card Authors [optional]
## Model Card Contact
| [
"# Model Card for Model ID",
"## Model Details",
"### Model Description\n\n\n\nThis is the model card of a transformers model that has been pushed on the Hub. This model card has been automatically generated.\n\n- Developed by: \n- Funded by [optional]: \n- Shared by [optional]: \n- Model type: \n- Language(s) (NLP): \n- License: \n- Finetuned from model [optional]:",
"### Model Sources [optional]\n\n\n\n- Repository: \n- Paper [optional]: \n- Demo [optional]:",
"## Uses",
"### Direct Use",
"### Downstream Use [optional]",
"### Out-of-Scope Use",
"## Bias, Risks, and Limitations",
"### Recommendations\n\n\n\nUsers (both direct and downstream) should be made aware of the risks, biases and limitations of the model. More information needed for further recommendations.",
"## How to Get Started with the Model\n\nUse the code below to get started with the model.",
"## Training Details",
"### Training Data",
"### Training Procedure",
"#### Preprocessing [optional]",
"#### Training Hyperparameters\n\n- Training regime:",
"#### Speeds, Sizes, Times [optional]",
"## Evaluation",
"### Testing Data, Factors & Metrics",
"#### Testing Data",
"#### Factors",
"#### Metrics",
"### Results",
"#### Summary",
"## Model Examination [optional]",
"## Environmental Impact\n\n\n\nCarbon emissions can be estimated using the Machine Learning Impact calculator presented in Lacoste et al. (2019).\n\n- Hardware Type: \n- Hours used: \n- Cloud Provider: \n- Compute Region: \n- Carbon Emitted:",
"## Technical Specifications [optional]",
"### Model Architecture and Objective",
"### Compute Infrastructure",
"#### Hardware",
"#### Software\n\n\n\n[optional]\n\n\n\nBibTeX:\n\n\n\nAPA:",
"## Glossary [optional]",
"## More Information [optional]",
"## Model Card Authors [optional]",
"## Model Card Contact"
] | [
"TAGS\n#transformers #safetensors #phi #text-generation #conversational #arxiv-1910.09700 #autotrain_compatible #endpoints_compatible #text-generation-inference #region-us \n",
"# Model Card for Model ID",
"## Model Details",
"### Model Description\n\n\n\nThis is the model card of a transformers model that has been pushed on the Hub. This model card has been automatically generated.\n\n- Developed by: \n- Funded by [optional]: \n- Shared by [optional]: \n- Model type: \n- Language(s) (NLP): \n- License: \n- Finetuned from model [optional]:",
"### Model Sources [optional]\n\n\n\n- Repository: \n- Paper [optional]: \n- Demo [optional]:",
"## Uses",
"### Direct Use",
"### Downstream Use [optional]",
"### Out-of-Scope Use",
"## Bias, Risks, and Limitations",
"### Recommendations\n\n\n\nUsers (both direct and downstream) should be made aware of the risks, biases and limitations of the model. More information needed for further recommendations.",
"## How to Get Started with the Model\n\nUse the code below to get started with the model.",
"## Training Details",
"### Training Data",
"### Training Procedure",
"#### Preprocessing [optional]",
"#### Training Hyperparameters\n\n- Training regime:",
"#### Speeds, Sizes, Times [optional]",
"## Evaluation",
"### Testing Data, Factors & Metrics",
"#### Testing Data",
"#### Factors",
"#### Metrics",
"### Results",
"#### Summary",
"## Model Examination [optional]",
"## Environmental Impact\n\n\n\nCarbon emissions can be estimated using the Machine Learning Impact calculator presented in Lacoste et al. (2019).\n\n- Hardware Type: \n- Hours used: \n- Cloud Provider: \n- Compute Region: \n- Carbon Emitted:",
"## Technical Specifications [optional]",
"### Model Architecture and Objective",
"### Compute Infrastructure",
"#### Hardware",
"#### Software\n\n\n\n[optional]\n\n\n\nBibTeX:\n\n\n\nAPA:",
"## Glossary [optional]",
"## More Information [optional]",
"## Model Card Authors [optional]",
"## Model Card Contact"
] | [
46,
6,
4,
75,
23,
3,
5,
8,
9,
8,
34,
20,
4,
5,
5,
11,
13,
12,
3,
10,
6,
5,
6,
4,
5,
7,
49,
7,
7,
5,
5,
15,
7,
7,
8,
5
] | [
"TAGS\n#transformers #safetensors #phi #text-generation #conversational #arxiv-1910.09700 #autotrain_compatible #endpoints_compatible #text-generation-inference #region-us \n# Model Card for Model ID## Model Details### Model Description\n\n\n\nThis is the model card of a transformers model that has been pushed on the Hub. This model card has been automatically generated.\n\n- Developed by: \n- Funded by [optional]: \n- Shared by [optional]: \n- Model type: \n- Language(s) (NLP): \n- License: \n- Finetuned from model [optional]:### Model Sources [optional]\n\n\n\n- Repository: \n- Paper [optional]: \n- Demo [optional]:## Uses### Direct Use### Downstream Use [optional]### Out-of-Scope Use## Bias, Risks, and Limitations### Recommendations\n\n\n\nUsers (both direct and downstream) should be made aware of the risks, biases and limitations of the model. More information needed for further recommendations.## How to Get Started with the Model\n\nUse the code below to get started with the model.## Training Details### Training Data### Training Procedure#### Preprocessing [optional]#### Training Hyperparameters\n\n- Training regime:#### Speeds, Sizes, Times [optional]## Evaluation### Testing Data, Factors & Metrics#### Testing Data#### Factors#### Metrics### Results#### Summary## Model Examination [optional]## Environmental Impact\n\n\n\nCarbon emissions can be estimated using the Machine Learning Impact calculator presented in Lacoste et al. (2019).\n\n- Hardware Type: \n- Hours used: \n- Cloud Provider: \n- Compute Region: \n- Carbon Emitted:## Technical Specifications [optional]### Model Architecture and Objective### Compute Infrastructure#### Hardware#### Software\n\n\n\n[optional]\n\n\n\nBibTeX:\n\n\n\nAPA:## Glossary [optional]## More Information [optional]## Model Card Authors [optional]## Model Card Contact"
] |
text-generation | transformers |
# Model Card for Model ID
<!-- Provide a quick summary of what the model is/does. -->
## Model Details
### Model Description
<!-- Provide a longer summary of what this model is. -->
This is the model card of a 🤗 transformers model that has been pushed on the Hub. This model card has been automatically generated.
- **Developed by:** [More Information Needed]
- **Funded by [optional]:** [More Information Needed]
- **Shared by [optional]:** [More Information Needed]
- **Model type:** [More Information Needed]
- **Language(s) (NLP):** [More Information Needed]
- **License:** [More Information Needed]
- **Finetuned from model [optional]:** [More Information Needed]
### Model Sources [optional]
<!-- Provide the basic links for the model. -->
- **Repository:** [More Information Needed]
- **Paper [optional]:** [More Information Needed]
- **Demo [optional]:** [More Information Needed]
## Uses
<!-- Address questions around how the model is intended to be used, including the foreseeable users of the model and those affected by the model. -->
### Direct Use
<!-- This section is for the model use without fine-tuning or plugging into a larger ecosystem/app. -->
[More Information Needed]
### Downstream Use [optional]
<!-- This section is for the model use when fine-tuned for a task, or when plugged into a larger ecosystem/app -->
[More Information Needed]
### Out-of-Scope Use
<!-- This section addresses misuse, malicious use, and uses that the model will not work well for. -->
[More Information Needed]
## Bias, Risks, and Limitations
<!-- This section is meant to convey both technical and sociotechnical limitations. -->
[More Information Needed]
### Recommendations
<!-- This section is meant to convey recommendations with respect to the bias, risk, and technical limitations. -->
Users (both direct and downstream) should be made aware of the risks, biases and limitations of the model. More information needed for further recommendations.
## How to Get Started with the Model
Use the code below to get started with the model.
[More Information Needed]
## Training Details
### Training Data
<!-- This should link to a Dataset Card, perhaps with a short stub of information on what the training data is all about as well as documentation related to data pre-processing or additional filtering. -->
[More Information Needed]
### Training Procedure
<!-- This relates heavily to the Technical Specifications. Content here should link to that section when it is relevant to the training procedure. -->
#### Preprocessing [optional]
[More Information Needed]
#### Training Hyperparameters
- **Training regime:** [More Information Needed] <!--fp32, fp16 mixed precision, bf16 mixed precision, bf16 non-mixed precision, fp16 non-mixed precision, fp8 mixed precision -->
#### Speeds, Sizes, Times [optional]
<!-- This section provides information about throughput, start/end time, checkpoint size if relevant, etc. -->
[More Information Needed]
## Evaluation
<!-- This section describes the evaluation protocols and provides the results. -->
### Testing Data, Factors & Metrics
#### Testing Data
<!-- This should link to a Dataset Card if possible. -->
[More Information Needed]
#### Factors
<!-- These are the things the evaluation is disaggregating by, e.g., subpopulations or domains. -->
[More Information Needed]
#### Metrics
<!-- These are the evaluation metrics being used, ideally with a description of why. -->
[More Information Needed]
### Results
[More Information Needed]
#### Summary
## Model Examination [optional]
<!-- Relevant interpretability work for the model goes here -->
[More Information Needed]
## Environmental Impact
<!-- Total emissions (in grams of CO2eq) and additional considerations, such as electricity usage, go here. Edit the suggested text below accordingly -->
Carbon emissions can be estimated using the [Machine Learning Impact calculator](https://mlco2.github.io/impact#compute) presented in [Lacoste et al. (2019)](https://arxiv.org/abs/1910.09700).
- **Hardware Type:** [More Information Needed]
- **Hours used:** [More Information Needed]
- **Cloud Provider:** [More Information Needed]
- **Compute Region:** [More Information Needed]
- **Carbon Emitted:** [More Information Needed]
## Technical Specifications [optional]
### Model Architecture and Objective
[More Information Needed]
### Compute Infrastructure
[More Information Needed]
#### Hardware
[More Information Needed]
#### Software
[More Information Needed]
## Citation [optional]
<!-- If there is a paper or blog post introducing the model, the APA and Bibtex information for that should go in this section. -->
**BibTeX:**
[More Information Needed]
**APA:**
[More Information Needed]
## Glossary [optional]
<!-- If relevant, include terms and calculations in this section that can help readers understand the model or model card. -->
[More Information Needed]
## More Information [optional]
[More Information Needed]
## Model Card Authors [optional]
[More Information Needed]
## Model Card Contact
[More Information Needed] | {"library_name": "transformers", "tags": []} | Chetansk/gemma-Code-Instruct-Finetune-test-01 | null | [
"transformers",
"safetensors",
"gemma",
"text-generation",
"conversational",
"arxiv:1910.09700",
"autotrain_compatible",
"endpoints_compatible",
"text-generation-inference",
"region:us"
] | null | 2024-05-02T11:29:47+00:00 | [
"1910.09700"
] | [] | TAGS
#transformers #safetensors #gemma #text-generation #conversational #arxiv-1910.09700 #autotrain_compatible #endpoints_compatible #text-generation-inference #region-us
|
# Model Card for Model ID
## Model Details
### Model Description
This is the model card of a transformers model that has been pushed on the Hub. This model card has been automatically generated.
- Developed by:
- Funded by [optional]:
- Shared by [optional]:
- Model type:
- Language(s) (NLP):
- License:
- Finetuned from model [optional]:
### Model Sources [optional]
- Repository:
- Paper [optional]:
- Demo [optional]:
## Uses
### Direct Use
### Downstream Use [optional]
### Out-of-Scope Use
## Bias, Risks, and Limitations
### Recommendations
Users (both direct and downstream) should be made aware of the risks, biases and limitations of the model. More information needed for further recommendations.
## How to Get Started with the Model
Use the code below to get started with the model.
## Training Details
### Training Data
### Training Procedure
#### Preprocessing [optional]
#### Training Hyperparameters
- Training regime:
#### Speeds, Sizes, Times [optional]
## Evaluation
### Testing Data, Factors & Metrics
#### Testing Data
#### Factors
#### Metrics
### Results
#### Summary
## Model Examination [optional]
## Environmental Impact
Carbon emissions can be estimated using the Machine Learning Impact calculator presented in Lacoste et al. (2019).
- Hardware Type:
- Hours used:
- Cloud Provider:
- Compute Region:
- Carbon Emitted:
## Technical Specifications [optional]
### Model Architecture and Objective
### Compute Infrastructure
#### Hardware
#### Software
[optional]
BibTeX:
APA:
## Glossary [optional]
## More Information [optional]
## Model Card Authors [optional]
## Model Card Contact
| [
"# Model Card for Model ID",
"## Model Details",
"### Model Description\n\n\n\nThis is the model card of a transformers model that has been pushed on the Hub. This model card has been automatically generated.\n\n- Developed by: \n- Funded by [optional]: \n- Shared by [optional]: \n- Model type: \n- Language(s) (NLP): \n- License: \n- Finetuned from model [optional]:",
"### Model Sources [optional]\n\n\n\n- Repository: \n- Paper [optional]: \n- Demo [optional]:",
"## Uses",
"### Direct Use",
"### Downstream Use [optional]",
"### Out-of-Scope Use",
"## Bias, Risks, and Limitations",
"### Recommendations\n\n\n\nUsers (both direct and downstream) should be made aware of the risks, biases and limitations of the model. More information needed for further recommendations.",
"## How to Get Started with the Model\n\nUse the code below to get started with the model.",
"## Training Details",
"### Training Data",
"### Training Procedure",
"#### Preprocessing [optional]",
"#### Training Hyperparameters\n\n- Training regime:",
"#### Speeds, Sizes, Times [optional]",
"## Evaluation",
"### Testing Data, Factors & Metrics",
"#### Testing Data",
"#### Factors",
"#### Metrics",
"### Results",
"#### Summary",
"## Model Examination [optional]",
"## Environmental Impact\n\n\n\nCarbon emissions can be estimated using the Machine Learning Impact calculator presented in Lacoste et al. (2019).\n\n- Hardware Type: \n- Hours used: \n- Cloud Provider: \n- Compute Region: \n- Carbon Emitted:",
"## Technical Specifications [optional]",
"### Model Architecture and Objective",
"### Compute Infrastructure",
"#### Hardware",
"#### Software\n\n\n\n[optional]\n\n\n\nBibTeX:\n\n\n\nAPA:",
"## Glossary [optional]",
"## More Information [optional]",
"## Model Card Authors [optional]",
"## Model Card Contact"
] | [
"TAGS\n#transformers #safetensors #gemma #text-generation #conversational #arxiv-1910.09700 #autotrain_compatible #endpoints_compatible #text-generation-inference #region-us \n",
"# Model Card for Model ID",
"## Model Details",
"### Model Description\n\n\n\nThis is the model card of a transformers model that has been pushed on the Hub. This model card has been automatically generated.\n\n- Developed by: \n- Funded by [optional]: \n- Shared by [optional]: \n- Model type: \n- Language(s) (NLP): \n- License: \n- Finetuned from model [optional]:",
"### Model Sources [optional]\n\n\n\n- Repository: \n- Paper [optional]: \n- Demo [optional]:",
"## Uses",
"### Direct Use",
"### Downstream Use [optional]",
"### Out-of-Scope Use",
"## Bias, Risks, and Limitations",
"### Recommendations\n\n\n\nUsers (both direct and downstream) should be made aware of the risks, biases and limitations of the model. More information needed for further recommendations.",
"## How to Get Started with the Model\n\nUse the code below to get started with the model.",
"## Training Details",
"### Training Data",
"### Training Procedure",
"#### Preprocessing [optional]",
"#### Training Hyperparameters\n\n- Training regime:",
"#### Speeds, Sizes, Times [optional]",
"## Evaluation",
"### Testing Data, Factors & Metrics",
"#### Testing Data",
"#### Factors",
"#### Metrics",
"### Results",
"#### Summary",
"## Model Examination [optional]",
"## Environmental Impact\n\n\n\nCarbon emissions can be estimated using the Machine Learning Impact calculator presented in Lacoste et al. (2019).\n\n- Hardware Type: \n- Hours used: \n- Cloud Provider: \n- Compute Region: \n- Carbon Emitted:",
"## Technical Specifications [optional]",
"### Model Architecture and Objective",
"### Compute Infrastructure",
"#### Hardware",
"#### Software\n\n\n\n[optional]\n\n\n\nBibTeX:\n\n\n\nAPA:",
"## Glossary [optional]",
"## More Information [optional]",
"## Model Card Authors [optional]",
"## Model Card Contact"
] | [
46,
6,
4,
75,
23,
3,
5,
8,
9,
8,
34,
20,
4,
5,
5,
11,
13,
12,
3,
10,
6,
5,
6,
4,
5,
7,
49,
7,
7,
5,
5,
15,
7,
7,
8,
5
] | [
"TAGS\n#transformers #safetensors #gemma #text-generation #conversational #arxiv-1910.09700 #autotrain_compatible #endpoints_compatible #text-generation-inference #region-us \n# Model Card for Model ID## Model Details### Model Description\n\n\n\nThis is the model card of a transformers model that has been pushed on the Hub. This model card has been automatically generated.\n\n- Developed by: \n- Funded by [optional]: \n- Shared by [optional]: \n- Model type: \n- Language(s) (NLP): \n- License: \n- Finetuned from model [optional]:### Model Sources [optional]\n\n\n\n- Repository: \n- Paper [optional]: \n- Demo [optional]:## Uses### Direct Use### Downstream Use [optional]### Out-of-Scope Use## Bias, Risks, and Limitations### Recommendations\n\n\n\nUsers (both direct and downstream) should be made aware of the risks, biases and limitations of the model. More information needed for further recommendations.## How to Get Started with the Model\n\nUse the code below to get started with the model.## Training Details### Training Data### Training Procedure#### Preprocessing [optional]#### Training Hyperparameters\n\n- Training regime:#### Speeds, Sizes, Times [optional]## Evaluation### Testing Data, Factors & Metrics#### Testing Data#### Factors#### Metrics### Results#### Summary## Model Examination [optional]## Environmental Impact\n\n\n\nCarbon emissions can be estimated using the Machine Learning Impact calculator presented in Lacoste et al. (2019).\n\n- Hardware Type: \n- Hours used: \n- Cloud Provider: \n- Compute Region: \n- Carbon Emitted:## Technical Specifications [optional]### Model Architecture and Objective### Compute Infrastructure#### Hardware#### Software\n\n\n\n[optional]\n\n\n\nBibTeX:\n\n\n\nAPA:## Glossary [optional]## More Information [optional]## Model Card Authors [optional]## Model Card Contact"
] |
text-generation | transformers |
# Model Card for Model ID
<!-- Provide a quick summary of what the model is/does. -->
## Model Details
### Model Description
<!-- Provide a longer summary of what this model is. -->
This is the model card of a 🤗 transformers model that has been pushed on the Hub. This model card has been automatically generated.
- **Developed by:** [More Information Needed]
- **Funded by [optional]:** [More Information Needed]
- **Shared by [optional]:** [More Information Needed]
- **Model type:** [More Information Needed]
- **Language(s) (NLP):** [More Information Needed]
- **License:** [More Information Needed]
- **Finetuned from model [optional]:** [More Information Needed]
### Model Sources [optional]
<!-- Provide the basic links for the model. -->
- **Repository:** [More Information Needed]
- **Paper [optional]:** [More Information Needed]
- **Demo [optional]:** [More Information Needed]
## Uses
<!-- Address questions around how the model is intended to be used, including the foreseeable users of the model and those affected by the model. -->
### Direct Use
<!-- This section is for the model use without fine-tuning or plugging into a larger ecosystem/app. -->
[More Information Needed]
### Downstream Use [optional]
<!-- This section is for the model use when fine-tuned for a task, or when plugged into a larger ecosystem/app -->
[More Information Needed]
### Out-of-Scope Use
<!-- This section addresses misuse, malicious use, and uses that the model will not work well for. -->
[More Information Needed]
## Bias, Risks, and Limitations
<!-- This section is meant to convey both technical and sociotechnical limitations. -->
[More Information Needed]
### Recommendations
<!-- This section is meant to convey recommendations with respect to the bias, risk, and technical limitations. -->
Users (both direct and downstream) should be made aware of the risks, biases and limitations of the model. More information needed for further recommendations.
## How to Get Started with the Model
Use the code below to get started with the model.
[More Information Needed]
## Training Details
### Training Data
<!-- This should link to a Dataset Card, perhaps with a short stub of information on what the training data is all about as well as documentation related to data pre-processing or additional filtering. -->
[More Information Needed]
### Training Procedure
<!-- This relates heavily to the Technical Specifications. Content here should link to that section when it is relevant to the training procedure. -->
#### Preprocessing [optional]
[More Information Needed]
#### Training Hyperparameters
- **Training regime:** [More Information Needed] <!--fp32, fp16 mixed precision, bf16 mixed precision, bf16 non-mixed precision, fp16 non-mixed precision, fp8 mixed precision -->
#### Speeds, Sizes, Times [optional]
<!-- This section provides information about throughput, start/end time, checkpoint size if relevant, etc. -->
[More Information Needed]
## Evaluation
<!-- This section describes the evaluation protocols and provides the results. -->
### Testing Data, Factors & Metrics
#### Testing Data
<!-- This should link to a Dataset Card if possible. -->
[More Information Needed]
#### Factors
<!-- These are the things the evaluation is disaggregating by, e.g., subpopulations or domains. -->
[More Information Needed]
#### Metrics
<!-- These are the evaluation metrics being used, ideally with a description of why. -->
[More Information Needed]
### Results
[More Information Needed]
#### Summary
## Model Examination [optional]
<!-- Relevant interpretability work for the model goes here -->
[More Information Needed]
## Environmental Impact
<!-- Total emissions (in grams of CO2eq) and additional considerations, such as electricity usage, go here. Edit the suggested text below accordingly -->
Carbon emissions can be estimated using the [Machine Learning Impact calculator](https://mlco2.github.io/impact#compute) presented in [Lacoste et al. (2019)](https://arxiv.org/abs/1910.09700).
- **Hardware Type:** [More Information Needed]
- **Hours used:** [More Information Needed]
- **Cloud Provider:** [More Information Needed]
- **Compute Region:** [More Information Needed]
- **Carbon Emitted:** [More Information Needed]
## Technical Specifications [optional]
### Model Architecture and Objective
[More Information Needed]
### Compute Infrastructure
[More Information Needed]
#### Hardware
[More Information Needed]
#### Software
[More Information Needed]
## Citation [optional]
<!-- If there is a paper or blog post introducing the model, the APA and Bibtex information for that should go in this section. -->
**BibTeX:**
[More Information Needed]
**APA:**
[More Information Needed]
## Glossary [optional]
<!-- If relevant, include terms and calculations in this section that can help readers understand the model or model card. -->
[More Information Needed]
## More Information [optional]
[More Information Needed]
## Model Card Authors [optional]
[More Information Needed]
## Model Card Contact
[More Information Needed]
| {"library_name": "transformers", "tags": []} | miguel-kjh/pythia_14m-adpater-lora-cola | null | [
"transformers",
"safetensors",
"gpt_neox",
"text-generation",
"arxiv:1910.09700",
"autotrain_compatible",
"endpoints_compatible",
"text-generation-inference",
"region:us"
] | null | 2024-05-02T11:32:08+00:00 | [
"1910.09700"
] | [] | TAGS
#transformers #safetensors #gpt_neox #text-generation #arxiv-1910.09700 #autotrain_compatible #endpoints_compatible #text-generation-inference #region-us
|
# Model Card for Model ID
## Model Details
### Model Description
This is the model card of a transformers model that has been pushed on the Hub. This model card has been automatically generated.
- Developed by:
- Funded by [optional]:
- Shared by [optional]:
- Model type:
- Language(s) (NLP):
- License:
- Finetuned from model [optional]:
### Model Sources [optional]
- Repository:
- Paper [optional]:
- Demo [optional]:
## Uses
### Direct Use
### Downstream Use [optional]
### Out-of-Scope Use
## Bias, Risks, and Limitations
### Recommendations
Users (both direct and downstream) should be made aware of the risks, biases and limitations of the model. More information needed for further recommendations.
## How to Get Started with the Model
Use the code below to get started with the model.
## Training Details
### Training Data
### Training Procedure
#### Preprocessing [optional]
#### Training Hyperparameters
- Training regime:
#### Speeds, Sizes, Times [optional]
## Evaluation
### Testing Data, Factors & Metrics
#### Testing Data
#### Factors
#### Metrics
### Results
#### Summary
## Model Examination [optional]
## Environmental Impact
Carbon emissions can be estimated using the Machine Learning Impact calculator presented in Lacoste et al. (2019).
- Hardware Type:
- Hours used:
- Cloud Provider:
- Compute Region:
- Carbon Emitted:
## Technical Specifications [optional]
### Model Architecture and Objective
### Compute Infrastructure
#### Hardware
#### Software
[optional]
BibTeX:
APA:
## Glossary [optional]
## More Information [optional]
## Model Card Authors [optional]
## Model Card Contact
| [
"# Model Card for Model ID",
"## Model Details",
"### Model Description\n\n\n\nThis is the model card of a transformers model that has been pushed on the Hub. This model card has been automatically generated.\n\n- Developed by: \n- Funded by [optional]: \n- Shared by [optional]: \n- Model type: \n- Language(s) (NLP): \n- License: \n- Finetuned from model [optional]:",
"### Model Sources [optional]\n\n\n\n- Repository: \n- Paper [optional]: \n- Demo [optional]:",
"## Uses",
"### Direct Use",
"### Downstream Use [optional]",
"### Out-of-Scope Use",
"## Bias, Risks, and Limitations",
"### Recommendations\n\n\n\nUsers (both direct and downstream) should be made aware of the risks, biases and limitations of the model. More information needed for further recommendations.",
"## How to Get Started with the Model\n\nUse the code below to get started with the model.",
"## Training Details",
"### Training Data",
"### Training Procedure",
"#### Preprocessing [optional]",
"#### Training Hyperparameters\n\n- Training regime:",
"#### Speeds, Sizes, Times [optional]",
"## Evaluation",
"### Testing Data, Factors & Metrics",
"#### Testing Data",
"#### Factors",
"#### Metrics",
"### Results",
"#### Summary",
"## Model Examination [optional]",
"## Environmental Impact\n\n\n\nCarbon emissions can be estimated using the Machine Learning Impact calculator presented in Lacoste et al. (2019).\n\n- Hardware Type: \n- Hours used: \n- Cloud Provider: \n- Compute Region: \n- Carbon Emitted:",
"## Technical Specifications [optional]",
"### Model Architecture and Objective",
"### Compute Infrastructure",
"#### Hardware",
"#### Software\n\n\n\n[optional]\n\n\n\nBibTeX:\n\n\n\nAPA:",
"## Glossary [optional]",
"## More Information [optional]",
"## Model Card Authors [optional]",
"## Model Card Contact"
] | [
"TAGS\n#transformers #safetensors #gpt_neox #text-generation #arxiv-1910.09700 #autotrain_compatible #endpoints_compatible #text-generation-inference #region-us \n",
"# Model Card for Model ID",
"## Model Details",
"### Model Description\n\n\n\nThis is the model card of a transformers model that has been pushed on the Hub. This model card has been automatically generated.\n\n- Developed by: \n- Funded by [optional]: \n- Shared by [optional]: \n- Model type: \n- Language(s) (NLP): \n- License: \n- Finetuned from model [optional]:",
"### Model Sources [optional]\n\n\n\n- Repository: \n- Paper [optional]: \n- Demo [optional]:",
"## Uses",
"### Direct Use",
"### Downstream Use [optional]",
"### Out-of-Scope Use",
"## Bias, Risks, and Limitations",
"### Recommendations\n\n\n\nUsers (both direct and downstream) should be made aware of the risks, biases and limitations of the model. More information needed for further recommendations.",
"## How to Get Started with the Model\n\nUse the code below to get started with the model.",
"## Training Details",
"### Training Data",
"### Training Procedure",
"#### Preprocessing [optional]",
"#### Training Hyperparameters\n\n- Training regime:",
"#### Speeds, Sizes, Times [optional]",
"## Evaluation",
"### Testing Data, Factors & Metrics",
"#### Testing Data",
"#### Factors",
"#### Metrics",
"### Results",
"#### Summary",
"## Model Examination [optional]",
"## Environmental Impact\n\n\n\nCarbon emissions can be estimated using the Machine Learning Impact calculator presented in Lacoste et al. (2019).\n\n- Hardware Type: \n- Hours used: \n- Cloud Provider: \n- Compute Region: \n- Carbon Emitted:",
"## Technical Specifications [optional]",
"### Model Architecture and Objective",
"### Compute Infrastructure",
"#### Hardware",
"#### Software\n\n\n\n[optional]\n\n\n\nBibTeX:\n\n\n\nAPA:",
"## Glossary [optional]",
"## More Information [optional]",
"## Model Card Authors [optional]",
"## Model Card Contact"
] | [
47,
6,
4,
75,
23,
3,
5,
8,
9,
8,
34,
20,
4,
5,
5,
11,
13,
12,
3,
10,
6,
5,
6,
4,
5,
7,
49,
7,
7,
5,
5,
15,
7,
7,
8,
5
] | [
"TAGS\n#transformers #safetensors #gpt_neox #text-generation #arxiv-1910.09700 #autotrain_compatible #endpoints_compatible #text-generation-inference #region-us \n# Model Card for Model ID## Model Details### Model Description\n\n\n\nThis is the model card of a transformers model that has been pushed on the Hub. This model card has been automatically generated.\n\n- Developed by: \n- Funded by [optional]: \n- Shared by [optional]: \n- Model type: \n- Language(s) (NLP): \n- License: \n- Finetuned from model [optional]:### Model Sources [optional]\n\n\n\n- Repository: \n- Paper [optional]: \n- Demo [optional]:## Uses### Direct Use### Downstream Use [optional]### Out-of-Scope Use## Bias, Risks, and Limitations### Recommendations\n\n\n\nUsers (both direct and downstream) should be made aware of the risks, biases and limitations of the model. More information needed for further recommendations.## How to Get Started with the Model\n\nUse the code below to get started with the model.## Training Details### Training Data### Training Procedure#### Preprocessing [optional]#### Training Hyperparameters\n\n- Training regime:#### Speeds, Sizes, Times [optional]## Evaluation### Testing Data, Factors & Metrics#### Testing Data#### Factors#### Metrics### Results#### Summary## Model Examination [optional]## Environmental Impact\n\n\n\nCarbon emissions can be estimated using the Machine Learning Impact calculator presented in Lacoste et al. (2019).\n\n- Hardware Type: \n- Hours used: \n- Cloud Provider: \n- Compute Region: \n- Carbon Emitted:## Technical Specifications [optional]### Model Architecture and Objective### Compute Infrastructure#### Hardware#### Software\n\n\n\n[optional]\n\n\n\nBibTeX:\n\n\n\nAPA:## Glossary [optional]## More Information [optional]## Model Card Authors [optional]## Model Card Contact"
] |
text-generation | transformers |
# Model Card for Model ID
<!-- Provide a quick summary of what the model is/does. -->
## Model Details
### Model Description
<!-- Provide a longer summary of what this model is. -->
This is the model card of a 🤗 transformers model that has been pushed on the Hub. This model card has been automatically generated.
- **Developed by:** [More Information Needed]
- **Funded by [optional]:** [More Information Needed]
- **Shared by [optional]:** [More Information Needed]
- **Model type:** [More Information Needed]
- **Language(s) (NLP):** [More Information Needed]
- **License:** [More Information Needed]
- **Finetuned from model [optional]:** [More Information Needed]
### Model Sources [optional]
<!-- Provide the basic links for the model. -->
- **Repository:** [More Information Needed]
- **Paper [optional]:** [More Information Needed]
- **Demo [optional]:** [More Information Needed]
## Uses
<!-- Address questions around how the model is intended to be used, including the foreseeable users of the model and those affected by the model. -->
### Direct Use
<!-- This section is for the model use without fine-tuning or plugging into a larger ecosystem/app. -->
[More Information Needed]
### Downstream Use [optional]
<!-- This section is for the model use when fine-tuned for a task, or when plugged into a larger ecosystem/app -->
[More Information Needed]
### Out-of-Scope Use
<!-- This section addresses misuse, malicious use, and uses that the model will not work well for. -->
[More Information Needed]
## Bias, Risks, and Limitations
<!-- This section is meant to convey both technical and sociotechnical limitations. -->
[More Information Needed]
### Recommendations
<!-- This section is meant to convey recommendations with respect to the bias, risk, and technical limitations. -->
Users (both direct and downstream) should be made aware of the risks, biases and limitations of the model. More information needed for further recommendations.
## How to Get Started with the Model
Use the code below to get started with the model.
[More Information Needed]
## Training Details
### Training Data
<!-- This should link to a Dataset Card, perhaps with a short stub of information on what the training data is all about as well as documentation related to data pre-processing or additional filtering. -->
[More Information Needed]
### Training Procedure
<!-- This relates heavily to the Technical Specifications. Content here should link to that section when it is relevant to the training procedure. -->
#### Preprocessing [optional]
[More Information Needed]
#### Training Hyperparameters
- **Training regime:** [More Information Needed] <!--fp32, fp16 mixed precision, bf16 mixed precision, bf16 non-mixed precision, fp16 non-mixed precision, fp8 mixed precision -->
#### Speeds, Sizes, Times [optional]
<!-- This section provides information about throughput, start/end time, checkpoint size if relevant, etc. -->
[More Information Needed]
## Evaluation
<!-- This section describes the evaluation protocols and provides the results. -->
### Testing Data, Factors & Metrics
#### Testing Data
<!-- This should link to a Dataset Card if possible. -->
[More Information Needed]
#### Factors
<!-- These are the things the evaluation is disaggregating by, e.g., subpopulations or domains. -->
[More Information Needed]
#### Metrics
<!-- These are the evaluation metrics being used, ideally with a description of why. -->
[More Information Needed]
### Results
[More Information Needed]
#### Summary
## Model Examination [optional]
<!-- Relevant interpretability work for the model goes here -->
[More Information Needed]
## Environmental Impact
<!-- Total emissions (in grams of CO2eq) and additional considerations, such as electricity usage, go here. Edit the suggested text below accordingly -->
Carbon emissions can be estimated using the [Machine Learning Impact calculator](https://mlco2.github.io/impact#compute) presented in [Lacoste et al. (2019)](https://arxiv.org/abs/1910.09700).
- **Hardware Type:** [More Information Needed]
- **Hours used:** [More Information Needed]
- **Cloud Provider:** [More Information Needed]
- **Compute Region:** [More Information Needed]
- **Carbon Emitted:** [More Information Needed]
## Technical Specifications [optional]
### Model Architecture and Objective
[More Information Needed]
### Compute Infrastructure
[More Information Needed]
#### Hardware
[More Information Needed]
#### Software
[More Information Needed]
## Citation [optional]
<!-- If there is a paper or blog post introducing the model, the APA and Bibtex information for that should go in this section. -->
**BibTeX:**
[More Information Needed]
**APA:**
[More Information Needed]
## Glossary [optional]
<!-- If relevant, include terms and calculations in this section that can help readers understand the model or model card. -->
[More Information Needed]
## More Information [optional]
[More Information Needed]
## Model Card Authors [optional]
[More Information Needed]
## Model Card Contact
[More Information Needed]
| {"library_name": "transformers", "tags": []} | miguel-kjh/pythia_70m-adpater-lora-cola | null | [
"transformers",
"safetensors",
"gpt_neox",
"text-generation",
"arxiv:1910.09700",
"autotrain_compatible",
"endpoints_compatible",
"text-generation-inference",
"region:us"
] | null | 2024-05-02T11:32:26+00:00 | [
"1910.09700"
] | [] | TAGS
#transformers #safetensors #gpt_neox #text-generation #arxiv-1910.09700 #autotrain_compatible #endpoints_compatible #text-generation-inference #region-us
|
# Model Card for Model ID
## Model Details
### Model Description
This is the model card of a transformers model that has been pushed on the Hub. This model card has been automatically generated.
- Developed by:
- Funded by [optional]:
- Shared by [optional]:
- Model type:
- Language(s) (NLP):
- License:
- Finetuned from model [optional]:
### Model Sources [optional]
- Repository:
- Paper [optional]:
- Demo [optional]:
## Uses
### Direct Use
### Downstream Use [optional]
### Out-of-Scope Use
## Bias, Risks, and Limitations
### Recommendations
Users (both direct and downstream) should be made aware of the risks, biases and limitations of the model. More information needed for further recommendations.
## How to Get Started with the Model
Use the code below to get started with the model.
## Training Details
### Training Data
### Training Procedure
#### Preprocessing [optional]
#### Training Hyperparameters
- Training regime:
#### Speeds, Sizes, Times [optional]
## Evaluation
### Testing Data, Factors & Metrics
#### Testing Data
#### Factors
#### Metrics
### Results
#### Summary
## Model Examination [optional]
## Environmental Impact
Carbon emissions can be estimated using the Machine Learning Impact calculator presented in Lacoste et al. (2019).
- Hardware Type:
- Hours used:
- Cloud Provider:
- Compute Region:
- Carbon Emitted:
## Technical Specifications [optional]
### Model Architecture and Objective
### Compute Infrastructure
#### Hardware
#### Software
[optional]
BibTeX:
APA:
## Glossary [optional]
## More Information [optional]
## Model Card Authors [optional]
## Model Card Contact
| [
"# Model Card for Model ID",
"## Model Details",
"### Model Description\n\n\n\nThis is the model card of a transformers model that has been pushed on the Hub. This model card has been automatically generated.\n\n- Developed by: \n- Funded by [optional]: \n- Shared by [optional]: \n- Model type: \n- Language(s) (NLP): \n- License: \n- Finetuned from model [optional]:",
"### Model Sources [optional]\n\n\n\n- Repository: \n- Paper [optional]: \n- Demo [optional]:",
"## Uses",
"### Direct Use",
"### Downstream Use [optional]",
"### Out-of-Scope Use",
"## Bias, Risks, and Limitations",
"### Recommendations\n\n\n\nUsers (both direct and downstream) should be made aware of the risks, biases and limitations of the model. More information needed for further recommendations.",
"## How to Get Started with the Model\n\nUse the code below to get started with the model.",
"## Training Details",
"### Training Data",
"### Training Procedure",
"#### Preprocessing [optional]",
"#### Training Hyperparameters\n\n- Training regime:",
"#### Speeds, Sizes, Times [optional]",
"## Evaluation",
"### Testing Data, Factors & Metrics",
"#### Testing Data",
"#### Factors",
"#### Metrics",
"### Results",
"#### Summary",
"## Model Examination [optional]",
"## Environmental Impact\n\n\n\nCarbon emissions can be estimated using the Machine Learning Impact calculator presented in Lacoste et al. (2019).\n\n- Hardware Type: \n- Hours used: \n- Cloud Provider: \n- Compute Region: \n- Carbon Emitted:",
"## Technical Specifications [optional]",
"### Model Architecture and Objective",
"### Compute Infrastructure",
"#### Hardware",
"#### Software\n\n\n\n[optional]\n\n\n\nBibTeX:\n\n\n\nAPA:",
"## Glossary [optional]",
"## More Information [optional]",
"## Model Card Authors [optional]",
"## Model Card Contact"
] | [
"TAGS\n#transformers #safetensors #gpt_neox #text-generation #arxiv-1910.09700 #autotrain_compatible #endpoints_compatible #text-generation-inference #region-us \n",
"# Model Card for Model ID",
"## Model Details",
"### Model Description\n\n\n\nThis is the model card of a transformers model that has been pushed on the Hub. This model card has been automatically generated.\n\n- Developed by: \n- Funded by [optional]: \n- Shared by [optional]: \n- Model type: \n- Language(s) (NLP): \n- License: \n- Finetuned from model [optional]:",
"### Model Sources [optional]\n\n\n\n- Repository: \n- Paper [optional]: \n- Demo [optional]:",
"## Uses",
"### Direct Use",
"### Downstream Use [optional]",
"### Out-of-Scope Use",
"## Bias, Risks, and Limitations",
"### Recommendations\n\n\n\nUsers (both direct and downstream) should be made aware of the risks, biases and limitations of the model. More information needed for further recommendations.",
"## How to Get Started with the Model\n\nUse the code below to get started with the model.",
"## Training Details",
"### Training Data",
"### Training Procedure",
"#### Preprocessing [optional]",
"#### Training Hyperparameters\n\n- Training regime:",
"#### Speeds, Sizes, Times [optional]",
"## Evaluation",
"### Testing Data, Factors & Metrics",
"#### Testing Data",
"#### Factors",
"#### Metrics",
"### Results",
"#### Summary",
"## Model Examination [optional]",
"## Environmental Impact\n\n\n\nCarbon emissions can be estimated using the Machine Learning Impact calculator presented in Lacoste et al. (2019).\n\n- Hardware Type: \n- Hours used: \n- Cloud Provider: \n- Compute Region: \n- Carbon Emitted:",
"## Technical Specifications [optional]",
"### Model Architecture and Objective",
"### Compute Infrastructure",
"#### Hardware",
"#### Software\n\n\n\n[optional]\n\n\n\nBibTeX:\n\n\n\nAPA:",
"## Glossary [optional]",
"## More Information [optional]",
"## Model Card Authors [optional]",
"## Model Card Contact"
] | [
47,
6,
4,
75,
23,
3,
5,
8,
9,
8,
34,
20,
4,
5,
5,
11,
13,
12,
3,
10,
6,
5,
6,
4,
5,
7,
49,
7,
7,
5,
5,
15,
7,
7,
8,
5
] | [
"TAGS\n#transformers #safetensors #gpt_neox #text-generation #arxiv-1910.09700 #autotrain_compatible #endpoints_compatible #text-generation-inference #region-us \n# Model Card for Model ID## Model Details### Model Description\n\n\n\nThis is the model card of a transformers model that has been pushed on the Hub. This model card has been automatically generated.\n\n- Developed by: \n- Funded by [optional]: \n- Shared by [optional]: \n- Model type: \n- Language(s) (NLP): \n- License: \n- Finetuned from model [optional]:### Model Sources [optional]\n\n\n\n- Repository: \n- Paper [optional]: \n- Demo [optional]:## Uses### Direct Use### Downstream Use [optional]### Out-of-Scope Use## Bias, Risks, and Limitations### Recommendations\n\n\n\nUsers (both direct and downstream) should be made aware of the risks, biases and limitations of the model. More information needed for further recommendations.## How to Get Started with the Model\n\nUse the code below to get started with the model.## Training Details### Training Data### Training Procedure#### Preprocessing [optional]#### Training Hyperparameters\n\n- Training regime:#### Speeds, Sizes, Times [optional]## Evaluation### Testing Data, Factors & Metrics#### Testing Data#### Factors#### Metrics### Results#### Summary## Model Examination [optional]## Environmental Impact\n\n\n\nCarbon emissions can be estimated using the Machine Learning Impact calculator presented in Lacoste et al. (2019).\n\n- Hardware Type: \n- Hours used: \n- Cloud Provider: \n- Compute Region: \n- Carbon Emitted:## Technical Specifications [optional]### Model Architecture and Objective### Compute Infrastructure#### Hardware#### Software\n\n\n\n[optional]\n\n\n\nBibTeX:\n\n\n\nAPA:## Glossary [optional]## More Information [optional]## Model Card Authors [optional]## Model Card Contact"
] |
text-generation | transformers |
# hermesoptimesd-7B-slerp
hermesoptimesd-7B-slerp is a merge of the following models using [LazyMergekit](https://colab.research.google.com/drive/1obulZ1ROXHjYLn6PPZJwRR6GzgQogxxb?usp=sharing):
* [OpenPipe/mistral-ft-optimized-1218](https://huggingface.co/OpenPipe/mistral-ft-optimized-1218)
* [mlabonne/NeuralHermes-2.5-Mistral-7B](https://huggingface.co/mlabonne/NeuralHermes-2.5-Mistral-7B)
## 🧩 Configuration
```yaml
slices:
- sources:
- model: OpenPipe/mistral-ft-optimized-1218
layer_range: [0, 32]
- model: mlabonne/NeuralHermes-2.5-Mistral-7B
layer_range: [0, 32]
merge_method: slerp
base_model: OpenPipe/mistral-ft-optimized-1218
parameters:
t:
- filter: self_attn
value: [0, 0.5, 0.3, 0.7, 1]
- filter: mlp
value: [1, 0.5, 0.7, 0.3, 0]
- value: 0.5
dtype: bfloat16
```
## 💻 Usage
```python
!pip install -qU transformers accelerate
from transformers import AutoTokenizer
import transformers
import torch
model = "tanyakansal/hermesoptimesd-7B-slerp"
messages = [{"role": "user", "content": "What is a large language model?"}]
tokenizer = AutoTokenizer.from_pretrained(model)
prompt = tokenizer.apply_chat_template(messages, tokenize=False, add_generation_prompt=True)
pipeline = transformers.pipeline(
"text-generation",
model=model,
torch_dtype=torch.float16,
device_map="auto",
)
outputs = pipeline(prompt, max_new_tokens=256, do_sample=True, temperature=0.7, top_k=50, top_p=0.95)
print(outputs[0]["generated_text"])
``` | {"tags": ["merge", "mergekit", "lazymergekit", "OpenPipe/mistral-ft-optimized-1218", "mlabonne/NeuralHermes-2.5-Mistral-7B"], "base_model": ["OpenPipe/mistral-ft-optimized-1218", "mlabonne/NeuralHermes-2.5-Mistral-7B"]} | tanyakansal/hermesoptimesd-7B-slerp | null | [
"transformers",
"safetensors",
"mistral",
"text-generation",
"merge",
"mergekit",
"lazymergekit",
"OpenPipe/mistral-ft-optimized-1218",
"mlabonne/NeuralHermes-2.5-Mistral-7B",
"base_model:OpenPipe/mistral-ft-optimized-1218",
"base_model:mlabonne/NeuralHermes-2.5-Mistral-7B",
"autotrain_compatible",
"endpoints_compatible",
"text-generation-inference",
"region:us"
] | null | 2024-05-02T11:32:43+00:00 | [] | [] | TAGS
#transformers #safetensors #mistral #text-generation #merge #mergekit #lazymergekit #OpenPipe/mistral-ft-optimized-1218 #mlabonne/NeuralHermes-2.5-Mistral-7B #base_model-OpenPipe/mistral-ft-optimized-1218 #base_model-mlabonne/NeuralHermes-2.5-Mistral-7B #autotrain_compatible #endpoints_compatible #text-generation-inference #region-us
|
# hermesoptimesd-7B-slerp
hermesoptimesd-7B-slerp is a merge of the following models using LazyMergekit:
* OpenPipe/mistral-ft-optimized-1218
* mlabonne/NeuralHermes-2.5-Mistral-7B
## Configuration
## Usage
| [
"# hermesoptimesd-7B-slerp\n\nhermesoptimesd-7B-slerp is a merge of the following models using LazyMergekit:\n* OpenPipe/mistral-ft-optimized-1218\n* mlabonne/NeuralHermes-2.5-Mistral-7B",
"## Configuration",
"## Usage"
] | [
"TAGS\n#transformers #safetensors #mistral #text-generation #merge #mergekit #lazymergekit #OpenPipe/mistral-ft-optimized-1218 #mlabonne/NeuralHermes-2.5-Mistral-7B #base_model-OpenPipe/mistral-ft-optimized-1218 #base_model-mlabonne/NeuralHermes-2.5-Mistral-7B #autotrain_compatible #endpoints_compatible #text-generation-inference #region-us \n",
"# hermesoptimesd-7B-slerp\n\nhermesoptimesd-7B-slerp is a merge of the following models using LazyMergekit:\n* OpenPipe/mistral-ft-optimized-1218\n* mlabonne/NeuralHermes-2.5-Mistral-7B",
"## Configuration",
"## Usage"
] | [
118,
69,
3,
3
] | [
"TAGS\n#transformers #safetensors #mistral #text-generation #merge #mergekit #lazymergekit #OpenPipe/mistral-ft-optimized-1218 #mlabonne/NeuralHermes-2.5-Mistral-7B #base_model-OpenPipe/mistral-ft-optimized-1218 #base_model-mlabonne/NeuralHermes-2.5-Mistral-7B #autotrain_compatible #endpoints_compatible #text-generation-inference #region-us \n# hermesoptimesd-7B-slerp\n\nhermesoptimesd-7B-slerp is a merge of the following models using LazyMergekit:\n* OpenPipe/mistral-ft-optimized-1218\n* mlabonne/NeuralHermes-2.5-Mistral-7B## Configuration## Usage"
] |
null | transformers |
# Model Card for Model ID
<!-- Provide a quick summary of what the model is/does. -->
## Model Details
### Model Description
<!-- Provide a longer summary of what this model is. -->
This is the model card of a 🤗 transformers model that has been pushed on the Hub. This model card has been automatically generated.
- **Developed by:** [More Information Needed]
- **Funded by [optional]:** [More Information Needed]
- **Shared by [optional]:** [More Information Needed]
- **Model type:** [More Information Needed]
- **Language(s) (NLP):** [More Information Needed]
- **License:** [More Information Needed]
- **Finetuned from model [optional]:** [More Information Needed]
### Model Sources [optional]
<!-- Provide the basic links for the model. -->
- **Repository:** [More Information Needed]
- **Paper [optional]:** [More Information Needed]
- **Demo [optional]:** [More Information Needed]
## Uses
<!-- Address questions around how the model is intended to be used, including the foreseeable users of the model and those affected by the model. -->
### Direct Use
<!-- This section is for the model use without fine-tuning or plugging into a larger ecosystem/app. -->
[More Information Needed]
### Downstream Use [optional]
<!-- This section is for the model use when fine-tuned for a task, or when plugged into a larger ecosystem/app -->
[More Information Needed]
### Out-of-Scope Use
<!-- This section addresses misuse, malicious use, and uses that the model will not work well for. -->
[More Information Needed]
## Bias, Risks, and Limitations
<!-- This section is meant to convey both technical and sociotechnical limitations. -->
[More Information Needed]
### Recommendations
<!-- This section is meant to convey recommendations with respect to the bias, risk, and technical limitations. -->
Users (both direct and downstream) should be made aware of the risks, biases and limitations of the model. More information needed for further recommendations.
## How to Get Started with the Model
Use the code below to get started with the model.
[More Information Needed]
## Training Details
### Training Data
<!-- This should link to a Dataset Card, perhaps with a short stub of information on what the training data is all about as well as documentation related to data pre-processing or additional filtering. -->
[More Information Needed]
### Training Procedure
<!-- This relates heavily to the Technical Specifications. Content here should link to that section when it is relevant to the training procedure. -->
#### Preprocessing [optional]
[More Information Needed]
#### Training Hyperparameters
- **Training regime:** [More Information Needed] <!--fp32, fp16 mixed precision, bf16 mixed precision, bf16 non-mixed precision, fp16 non-mixed precision, fp8 mixed precision -->
#### Speeds, Sizes, Times [optional]
<!-- This section provides information about throughput, start/end time, checkpoint size if relevant, etc. -->
[More Information Needed]
## Evaluation
<!-- This section describes the evaluation protocols and provides the results. -->
### Testing Data, Factors & Metrics
#### Testing Data
<!-- This should link to a Dataset Card if possible. -->
[More Information Needed]
#### Factors
<!-- These are the things the evaluation is disaggregating by, e.g., subpopulations or domains. -->
[More Information Needed]
#### Metrics
<!-- These are the evaluation metrics being used, ideally with a description of why. -->
[More Information Needed]
### Results
[More Information Needed]
#### Summary
## Model Examination [optional]
<!-- Relevant interpretability work for the model goes here -->
[More Information Needed]
## Environmental Impact
<!-- Total emissions (in grams of CO2eq) and additional considerations, such as electricity usage, go here. Edit the suggested text below accordingly -->
Carbon emissions can be estimated using the [Machine Learning Impact calculator](https://mlco2.github.io/impact#compute) presented in [Lacoste et al. (2019)](https://arxiv.org/abs/1910.09700).
- **Hardware Type:** [More Information Needed]
- **Hours used:** [More Information Needed]
- **Cloud Provider:** [More Information Needed]
- **Compute Region:** [More Information Needed]
- **Carbon Emitted:** [More Information Needed]
## Technical Specifications [optional]
### Model Architecture and Objective
[More Information Needed]
### Compute Infrastructure
[More Information Needed]
#### Hardware
[More Information Needed]
#### Software
[More Information Needed]
## Citation [optional]
<!-- If there is a paper or blog post introducing the model, the APA and Bibtex information for that should go in this section. -->
**BibTeX:**
[More Information Needed]
**APA:**
[More Information Needed]
## Glossary [optional]
<!-- If relevant, include terms and calculations in this section that can help readers understand the model or model card. -->
[More Information Needed]
## More Information [optional]
[More Information Needed]
## Model Card Authors [optional]
[More Information Needed]
## Model Card Contact
[More Information Needed] | {"library_name": "transformers", "tags": []} | baraah/blip2-opt-2.7b-with-metrices-2-5 | null | [
"transformers",
"safetensors",
"arxiv:1910.09700",
"endpoints_compatible",
"region:us"
] | null | 2024-05-02T11:33:09+00:00 | [
"1910.09700"
] | [] | TAGS
#transformers #safetensors #arxiv-1910.09700 #endpoints_compatible #region-us
|
# Model Card for Model ID
## Model Details
### Model Description
This is the model card of a transformers model that has been pushed on the Hub. This model card has been automatically generated.
- Developed by:
- Funded by [optional]:
- Shared by [optional]:
- Model type:
- Language(s) (NLP):
- License:
- Finetuned from model [optional]:
### Model Sources [optional]
- Repository:
- Paper [optional]:
- Demo [optional]:
## Uses
### Direct Use
### Downstream Use [optional]
### Out-of-Scope Use
## Bias, Risks, and Limitations
### Recommendations
Users (both direct and downstream) should be made aware of the risks, biases and limitations of the model. More information needed for further recommendations.
## How to Get Started with the Model
Use the code below to get started with the model.
## Training Details
### Training Data
### Training Procedure
#### Preprocessing [optional]
#### Training Hyperparameters
- Training regime:
#### Speeds, Sizes, Times [optional]
## Evaluation
### Testing Data, Factors & Metrics
#### Testing Data
#### Factors
#### Metrics
### Results
#### Summary
## Model Examination [optional]
## Environmental Impact
Carbon emissions can be estimated using the Machine Learning Impact calculator presented in Lacoste et al. (2019).
- Hardware Type:
- Hours used:
- Cloud Provider:
- Compute Region:
- Carbon Emitted:
## Technical Specifications [optional]
### Model Architecture and Objective
### Compute Infrastructure
#### Hardware
#### Software
[optional]
BibTeX:
APA:
## Glossary [optional]
## More Information [optional]
## Model Card Authors [optional]
## Model Card Contact
| [
"# Model Card for Model ID",
"## Model Details",
"### Model Description\n\n\n\nThis is the model card of a transformers model that has been pushed on the Hub. This model card has been automatically generated.\n\n- Developed by: \n- Funded by [optional]: \n- Shared by [optional]: \n- Model type: \n- Language(s) (NLP): \n- License: \n- Finetuned from model [optional]:",
"### Model Sources [optional]\n\n\n\n- Repository: \n- Paper [optional]: \n- Demo [optional]:",
"## Uses",
"### Direct Use",
"### Downstream Use [optional]",
"### Out-of-Scope Use",
"## Bias, Risks, and Limitations",
"### Recommendations\n\n\n\nUsers (both direct and downstream) should be made aware of the risks, biases and limitations of the model. More information needed for further recommendations.",
"## How to Get Started with the Model\n\nUse the code below to get started with the model.",
"## Training Details",
"### Training Data",
"### Training Procedure",
"#### Preprocessing [optional]",
"#### Training Hyperparameters\n\n- Training regime:",
"#### Speeds, Sizes, Times [optional]",
"## Evaluation",
"### Testing Data, Factors & Metrics",
"#### Testing Data",
"#### Factors",
"#### Metrics",
"### Results",
"#### Summary",
"## Model Examination [optional]",
"## Environmental Impact\n\n\n\nCarbon emissions can be estimated using the Machine Learning Impact calculator presented in Lacoste et al. (2019).\n\n- Hardware Type: \n- Hours used: \n- Cloud Provider: \n- Compute Region: \n- Carbon Emitted:",
"## Technical Specifications [optional]",
"### Model Architecture and Objective",
"### Compute Infrastructure",
"#### Hardware",
"#### Software\n\n\n\n[optional]\n\n\n\nBibTeX:\n\n\n\nAPA:",
"## Glossary [optional]",
"## More Information [optional]",
"## Model Card Authors [optional]",
"## Model Card Contact"
] | [
"TAGS\n#transformers #safetensors #arxiv-1910.09700 #endpoints_compatible #region-us \n",
"# Model Card for Model ID",
"## Model Details",
"### Model Description\n\n\n\nThis is the model card of a transformers model that has been pushed on the Hub. This model card has been automatically generated.\n\n- Developed by: \n- Funded by [optional]: \n- Shared by [optional]: \n- Model type: \n- Language(s) (NLP): \n- License: \n- Finetuned from model [optional]:",
"### Model Sources [optional]\n\n\n\n- Repository: \n- Paper [optional]: \n- Demo [optional]:",
"## Uses",
"### Direct Use",
"### Downstream Use [optional]",
"### Out-of-Scope Use",
"## Bias, Risks, and Limitations",
"### Recommendations\n\n\n\nUsers (both direct and downstream) should be made aware of the risks, biases and limitations of the model. More information needed for further recommendations.",
"## How to Get Started with the Model\n\nUse the code below to get started with the model.",
"## Training Details",
"### Training Data",
"### Training Procedure",
"#### Preprocessing [optional]",
"#### Training Hyperparameters\n\n- Training regime:",
"#### Speeds, Sizes, Times [optional]",
"## Evaluation",
"### Testing Data, Factors & Metrics",
"#### Testing Data",
"#### Factors",
"#### Metrics",
"### Results",
"#### Summary",
"## Model Examination [optional]",
"## Environmental Impact\n\n\n\nCarbon emissions can be estimated using the Machine Learning Impact calculator presented in Lacoste et al. (2019).\n\n- Hardware Type: \n- Hours used: \n- Cloud Provider: \n- Compute Region: \n- Carbon Emitted:",
"## Technical Specifications [optional]",
"### Model Architecture and Objective",
"### Compute Infrastructure",
"#### Hardware",
"#### Software\n\n\n\n[optional]\n\n\n\nBibTeX:\n\n\n\nAPA:",
"## Glossary [optional]",
"## More Information [optional]",
"## Model Card Authors [optional]",
"## Model Card Contact"
] | [
26,
6,
4,
75,
23,
3,
5,
8,
9,
8,
34,
20,
4,
5,
5,
11,
13,
12,
3,
10,
6,
5,
6,
4,
5,
7,
49,
7,
7,
5,
5,
15,
7,
7,
8,
5
] | [
"TAGS\n#transformers #safetensors #arxiv-1910.09700 #endpoints_compatible #region-us \n# Model Card for Model ID## Model Details### Model Description\n\n\n\nThis is the model card of a transformers model that has been pushed on the Hub. This model card has been automatically generated.\n\n- Developed by: \n- Funded by [optional]: \n- Shared by [optional]: \n- Model type: \n- Language(s) (NLP): \n- License: \n- Finetuned from model [optional]:### Model Sources [optional]\n\n\n\n- Repository: \n- Paper [optional]: \n- Demo [optional]:## Uses### Direct Use### Downstream Use [optional]### Out-of-Scope Use## Bias, Risks, and Limitations### Recommendations\n\n\n\nUsers (both direct and downstream) should be made aware of the risks, biases and limitations of the model. More information needed for further recommendations.## How to Get Started with the Model\n\nUse the code below to get started with the model.## Training Details### Training Data### Training Procedure#### Preprocessing [optional]#### Training Hyperparameters\n\n- Training regime:#### Speeds, Sizes, Times [optional]## Evaluation### Testing Data, Factors & Metrics#### Testing Data#### Factors#### Metrics### Results#### Summary## Model Examination [optional]## Environmental Impact\n\n\n\nCarbon emissions can be estimated using the Machine Learning Impact calculator presented in Lacoste et al. (2019).\n\n- Hardware Type: \n- Hours used: \n- Cloud Provider: \n- Compute Region: \n- Carbon Emitted:## Technical Specifications [optional]### Model Architecture and Objective### Compute Infrastructure#### Hardware#### Software\n\n\n\n[optional]\n\n\n\nBibTeX:\n\n\n\nAPA:## Glossary [optional]## More Information [optional]## Model Card Authors [optional]## Model Card Contact"
] |
reinforcement-learning | stable-baselines3 |
# **PPO** Agent playing **LunarLander-v2**
This is a trained model of a **PPO** agent playing **LunarLander-v2**
using the [stable-baselines3 library](https://github.com/DLR-RM/stable-baselines3).
## Usage (with Stable-baselines3)
TODO: Add your code
```python
from stable_baselines3 import ...
from huggingface_sb3 import load_from_hub
...
```
| {"library_name": "stable-baselines3", "tags": ["LunarLander-v2", "deep-reinforcement-learning", "reinforcement-learning", "stable-baselines3"], "model-index": [{"name": "PPO", "results": [{"task": {"type": "reinforcement-learning", "name": "reinforcement-learning"}, "dataset": {"name": "LunarLander-v2", "type": "LunarLander-v2"}, "metrics": [{"type": "mean_reward", "value": "256.20 +/- 24.74", "name": "mean_reward", "verified": false}]}]}]} | tmparticle/ppo-LunarLander-v2 | null | [
"stable-baselines3",
"LunarLander-v2",
"deep-reinforcement-learning",
"reinforcement-learning",
"model-index",
"region:us"
] | null | 2024-05-02T11:33:10+00:00 | [] | [] | TAGS
#stable-baselines3 #LunarLander-v2 #deep-reinforcement-learning #reinforcement-learning #model-index #region-us
|
# PPO Agent playing LunarLander-v2
This is a trained model of a PPO agent playing LunarLander-v2
using the stable-baselines3 library.
## Usage (with Stable-baselines3)
TODO: Add your code
| [
"# PPO Agent playing LunarLander-v2\nThis is a trained model of a PPO agent playing LunarLander-v2\nusing the stable-baselines3 library.",
"## Usage (with Stable-baselines3)\nTODO: Add your code"
] | [
"TAGS\n#stable-baselines3 #LunarLander-v2 #deep-reinforcement-learning #reinforcement-learning #model-index #region-us \n",
"# PPO Agent playing LunarLander-v2\nThis is a trained model of a PPO agent playing LunarLander-v2\nusing the stable-baselines3 library.",
"## Usage (with Stable-baselines3)\nTODO: Add your code"
] | [
31,
35,
17
] | [
"TAGS\n#stable-baselines3 #LunarLander-v2 #deep-reinforcement-learning #reinforcement-learning #model-index #region-us \n# PPO Agent playing LunarLander-v2\nThis is a trained model of a PPO agent playing LunarLander-v2\nusing the stable-baselines3 library.## Usage (with Stable-baselines3)\nTODO: Add your code"
] |
feature-extraction | transformers |
# Model Card for Model ID
AidaCoder is a fine-tuned generative text models based on Code Llama 7b model built for testing purposes.
## Model Details
### Model Description
Model capabilities:
Code completion.
Infilling.
Instructions / chat.
This is the model card of a 🤗 transformers model that has been pushed on the Hub. This model card has been automatically generated.
- **Developed by:** [kantobro]
- **Funded by [optional]:** [More Information Needed]
- **Shared by [optional]:** [More Information Needed]
- **Model type:** [More Information Needed]
- **Language(s) (NLP):** [More Information Needed]
- **License:** [ A custom commercial license is available at: https://ai.meta.com/resources/models-and-libraries/llama-downloads/]
- **Finetuned from model [optional]:** [codellama/CodeLlama-7b-Instruct-hf]
### Model Sources [optional]
<!-- Provide the basic links for the model. -->
- **Repository:** [More Information Needed]
- **Paper [optional]:** [More Information Needed]
- **Demo [optional]:** [More Information Needed]
## Uses
<!-- Address questions around how the model is intended to be used, including the foreseeable users of the model and those affected by the model. -->
### Direct Use
<!-- This section is for the model use without fine-tuning or plugging into a larger ecosystem/app. -->
[More Information Needed]
### Downstream Use [optional]
<!-- This section is for the model use when fine-tuned for a task, or when plugged into a larger ecosystem/app -->
[More Information Needed]
### Out-of-Scope Use
<!-- This section addresses misuse, malicious use, and uses that the model will not work well for. -->
[More Information Needed]
## Bias, Risks, and Limitations
<!-- This section is meant to convey both technical and sociotechnical limitations. -->
[More Information Needed]
### Recommendations
<!-- This section is meant to convey recommendations with respect to the bias, risk, and technical limitations. -->
Users (both direct and downstream) should be made aware of the risks, biases and limitations of the model. More information needed for further recommendations.
## How to Get Started with the Model
Use the code below to get started with the model.
[More Information Needed]
## Training Details
### Training Data
<!-- This should link to a Dataset Card, perhaps with a short stub of information on what the training data is all about as well as documentation related to data pre-processing or additional filtering. -->
[More Information Needed]
### Training Procedure
Dataset: aida_stack_dataset
#### Preprocessing [optional]
[More Information Needed]
#### Training Hyperparameters
- **Training regime:** [More Information Needed] <!--fp32, fp16 mixed precision, bf16 mixed precision, bf16 non-mixed precision, fp16 non-mixed precision, fp8 mixed precision -->
#### Speeds, Sizes, Times [optional]
<!-- This section provides information about throughput, start/end time, checkpoint size if relevant, etc. -->
[More Information Needed]
## Evaluation
<!-- This section describes the evaluation protocols and provides the results. -->
### Testing Data, Factors & Metrics
#### Testing Data
<!-- This should link to a Dataset Card if possible. -->
[More Information Needed]
#### Factors
<!-- These are the things the evaluation is disaggregating by, e.g., subpopulations or domains. -->
[More Information Needed]
#### Metrics
<!-- These are the evaluation metrics being used, ideally with a description of why. -->
[More Information Needed]
### Results
[More Information Needed]
#### Summary
## Model Examination [optional]
<!-- Relevant interpretability work for the model goes here -->
[More Information Needed]
## Environmental Impact
<!-- Total emissions (in grams of CO2eq) and additional considerations, such as electricity usage, go here. Edit the suggested text below accordingly -->
Carbon emissions can be estimated using the [Machine Learning Impact calculator](https://mlco2.github.io/impact#compute) presented in [Lacoste et al. (2019)](https://arxiv.org/abs/1910.09700).
- **Hardware Type:** [More Information Needed]
- **Hours used:** [More Information Needed]
- **Cloud Provider:** [More Information Needed]
- **Compute Region:** [More Information Needed]
- **Carbon Emitted:** [More Information Needed]
## Technical Specifications [optional]
### Model Architecture and Objective
[More Information Needed]
### Compute Infrastructure
[More Information Needed]
#### Hardware
[More Information Needed]
#### Software
[More Information Needed]
## Citation [optional]
<!-- If there is a paper or blog post introducing the model, the APA and Bibtex information for that should go in this section. -->
**BibTeX:**
[More Information Needed]
**APA:**
[More Information Needed]
## Glossary [optional]
<!-- If relevant, include terms and calculations in this section that can help readers understand the model or model card. -->
[More Information Needed]
## More Information [optional]
[More Information Needed]
## Model Card Authors [optional]
[More Information Needed]
## Model Card Contact
[More Information Needed] | {"library_name": "transformers", "tags": []} | kantobro/aidacoder | null | [
"transformers",
"safetensors",
"llama",
"feature-extraction",
"arxiv:1910.09700",
"endpoints_compatible",
"text-generation-inference",
"region:us"
] | null | 2024-05-02T11:33:31+00:00 | [
"1910.09700"
] | [] | TAGS
#transformers #safetensors #llama #feature-extraction #arxiv-1910.09700 #endpoints_compatible #text-generation-inference #region-us
|
# Model Card for Model ID
AidaCoder is a fine-tuned generative text models based on Code Llama 7b model built for testing purposes.
## Model Details
### Model Description
Model capabilities:
Code completion.
Infilling.
Instructions / chat.
This is the model card of a transformers model that has been pushed on the Hub. This model card has been automatically generated.
- Developed by: [kantobro]
- Funded by [optional]:
- Shared by [optional]:
- Model type:
- Language(s) (NLP):
- License: [ A custom commercial license is available at: URL
- Finetuned from model [optional]: [codellama/CodeLlama-7b-Instruct-hf]
### Model Sources [optional]
- Repository:
- Paper [optional]:
- Demo [optional]:
## Uses
### Direct Use
### Downstream Use [optional]
### Out-of-Scope Use
## Bias, Risks, and Limitations
### Recommendations
Users (both direct and downstream) should be made aware of the risks, biases and limitations of the model. More information needed for further recommendations.
## How to Get Started with the Model
Use the code below to get started with the model.
## Training Details
### Training Data
### Training Procedure
Dataset: aida_stack_dataset
#### Preprocessing [optional]
#### Training Hyperparameters
- Training regime:
#### Speeds, Sizes, Times [optional]
## Evaluation
### Testing Data, Factors & Metrics
#### Testing Data
#### Factors
#### Metrics
### Results
#### Summary
## Model Examination [optional]
## Environmental Impact
Carbon emissions can be estimated using the Machine Learning Impact calculator presented in Lacoste et al. (2019).
- Hardware Type:
- Hours used:
- Cloud Provider:
- Compute Region:
- Carbon Emitted:
## Technical Specifications [optional]
### Model Architecture and Objective
### Compute Infrastructure
#### Hardware
#### Software
[optional]
BibTeX:
APA:
## Glossary [optional]
## More Information [optional]
## Model Card Authors [optional]
## Model Card Contact
| [
"# Model Card for Model ID\nAidaCoder is a fine-tuned generative text models based on Code Llama 7b model built for testing purposes.",
"## Model Details",
"### Model Description\nModel capabilities:\n\n Code completion.\n Infilling.\n Instructions / chat.\n\n\nThis is the model card of a transformers model that has been pushed on the Hub. This model card has been automatically generated.\n\n- Developed by: [kantobro]\n- Funded by [optional]: \n- Shared by [optional]: \n- Model type: \n- Language(s) (NLP): \n- License: [ A custom commercial license is available at: URL\n- Finetuned from model [optional]: [codellama/CodeLlama-7b-Instruct-hf]",
"### Model Sources [optional]\n\n\n\n- Repository: \n- Paper [optional]: \n- Demo [optional]:",
"## Uses",
"### Direct Use",
"### Downstream Use [optional]",
"### Out-of-Scope Use",
"## Bias, Risks, and Limitations",
"### Recommendations\n\n\n\nUsers (both direct and downstream) should be made aware of the risks, biases and limitations of the model. More information needed for further recommendations.",
"## How to Get Started with the Model\n\nUse the code below to get started with the model.",
"## Training Details",
"### Training Data",
"### Training Procedure\n\nDataset: aida_stack_dataset",
"#### Preprocessing [optional]",
"#### Training Hyperparameters\n\n- Training regime:",
"#### Speeds, Sizes, Times [optional]",
"## Evaluation",
"### Testing Data, Factors & Metrics",
"#### Testing Data",
"#### Factors",
"#### Metrics",
"### Results",
"#### Summary",
"## Model Examination [optional]",
"## Environmental Impact\n\n\n\nCarbon emissions can be estimated using the Machine Learning Impact calculator presented in Lacoste et al. (2019).\n\n- Hardware Type: \n- Hours used: \n- Cloud Provider: \n- Compute Region: \n- Carbon Emitted:",
"## Technical Specifications [optional]",
"### Model Architecture and Objective",
"### Compute Infrastructure",
"#### Hardware",
"#### Software\n\n\n\n[optional]\n\n\n\nBibTeX:\n\n\n\nAPA:",
"## Glossary [optional]",
"## More Information [optional]",
"## Model Card Authors [optional]",
"## Model Card Contact"
] | [
"TAGS\n#transformers #safetensors #llama #feature-extraction #arxiv-1910.09700 #endpoints_compatible #text-generation-inference #region-us \n",
"# Model Card for Model ID\nAidaCoder is a fine-tuned generative text models based on Code Llama 7b model built for testing purposes.",
"## Model Details",
"### Model Description\nModel capabilities:\n\n Code completion.\n Infilling.\n Instructions / chat.\n\n\nThis is the model card of a transformers model that has been pushed on the Hub. This model card has been automatically generated.\n\n- Developed by: [kantobro]\n- Funded by [optional]: \n- Shared by [optional]: \n- Model type: \n- Language(s) (NLP): \n- License: [ A custom commercial license is available at: URL\n- Finetuned from model [optional]: [codellama/CodeLlama-7b-Instruct-hf]",
"### Model Sources [optional]\n\n\n\n- Repository: \n- Paper [optional]: \n- Demo [optional]:",
"## Uses",
"### Direct Use",
"### Downstream Use [optional]",
"### Out-of-Scope Use",
"## Bias, Risks, and Limitations",
"### Recommendations\n\n\n\nUsers (both direct and downstream) should be made aware of the risks, biases and limitations of the model. More information needed for further recommendations.",
"## How to Get Started with the Model\n\nUse the code below to get started with the model.",
"## Training Details",
"### Training Data",
"### Training Procedure\n\nDataset: aida_stack_dataset",
"#### Preprocessing [optional]",
"#### Training Hyperparameters\n\n- Training regime:",
"#### Speeds, Sizes, Times [optional]",
"## Evaluation",
"### Testing Data, Factors & Metrics",
"#### Testing Data",
"#### Factors",
"#### Metrics",
"### Results",
"#### Summary",
"## Model Examination [optional]",
"## Environmental Impact\n\n\n\nCarbon emissions can be estimated using the Machine Learning Impact calculator presented in Lacoste et al. (2019).\n\n- Hardware Type: \n- Hours used: \n- Cloud Provider: \n- Compute Region: \n- Carbon Emitted:",
"## Technical Specifications [optional]",
"### Model Architecture and Objective",
"### Compute Infrastructure",
"#### Hardware",
"#### Software\n\n\n\n[optional]\n\n\n\nBibTeX:\n\n\n\nAPA:",
"## Glossary [optional]",
"## More Information [optional]",
"## Model Card Authors [optional]",
"## Model Card Contact"
] | [
39,
31,
4,
123,
23,
3,
5,
8,
9,
8,
34,
20,
4,
5,
15,
11,
13,
12,
3,
10,
6,
5,
6,
4,
5,
7,
49,
7,
7,
5,
5,
15,
7,
7,
8,
5
] | [
"TAGS\n#transformers #safetensors #llama #feature-extraction #arxiv-1910.09700 #endpoints_compatible #text-generation-inference #region-us \n# Model Card for Model ID\nAidaCoder is a fine-tuned generative text models based on Code Llama 7b model built for testing purposes.## Model Details### Model Description\nModel capabilities:\n\n Code completion.\n Infilling.\n Instructions / chat.\n\n\nThis is the model card of a transformers model that has been pushed on the Hub. This model card has been automatically generated.\n\n- Developed by: [kantobro]\n- Funded by [optional]: \n- Shared by [optional]: \n- Model type: \n- Language(s) (NLP): \n- License: [ A custom commercial license is available at: URL\n- Finetuned from model [optional]: [codellama/CodeLlama-7b-Instruct-hf]### Model Sources [optional]\n\n\n\n- Repository: \n- Paper [optional]: \n- Demo [optional]:## Uses### Direct Use### Downstream Use [optional]### Out-of-Scope Use## Bias, Risks, and Limitations### Recommendations\n\n\n\nUsers (both direct and downstream) should be made aware of the risks, biases and limitations of the model. More information needed for further recommendations.## How to Get Started with the Model\n\nUse the code below to get started with the model.## Training Details### Training Data### Training Procedure\n\nDataset: aida_stack_dataset#### Preprocessing [optional]#### Training Hyperparameters\n\n- Training regime:#### Speeds, Sizes, Times [optional]## Evaluation### Testing Data, Factors & Metrics#### Testing Data#### Factors#### Metrics### Results#### Summary## Model Examination [optional]## Environmental Impact\n\n\n\nCarbon emissions can be estimated using the Machine Learning Impact calculator presented in Lacoste et al. (2019).\n\n- Hardware Type: \n- Hours used: \n- Cloud Provider: \n- Compute Region: \n- Carbon Emitted:## Technical Specifications [optional]### Model Architecture and Objective### Compute Infrastructure#### Hardware#### Software\n\n\n\n[optional]\n\n\n\nBibTeX:\n\n\n\nAPA:## Glossary [optional]## More Information [optional]## Model Card Authors [optional]## Model Card Contact"
] |
text-generation | transformers |
# Uploaded model
- **Developed by:** hakankenar
- **License:** apache-2.0
- **Finetuned from model :** unsloth/llama-3-8b-bnb-4bit
This llama model was trained 2x faster with [Unsloth](https://github.com/unslothai/unsloth) and Huggingface's TRL library.
[<img src="https://raw.githubusercontent.com/unslothai/unsloth/main/images/unsloth%20made%20with%20love.png" width="200"/>](https://github.com/unslothai/unsloth)
| {"language": ["en"], "license": "apache-2.0", "tags": ["text-generation-inference", "transformers", "unsloth", "llama", "trl", "sft"], "base_model": "unsloth/llama-3-8b-bnb-4bit"} | hakankenar/llama-3-8b-bnb-4bit-finetune-test | null | [
"transformers",
"safetensors",
"llama",
"text-generation",
"text-generation-inference",
"unsloth",
"trl",
"sft",
"en",
"base_model:unsloth/llama-3-8b-bnb-4bit",
"license:apache-2.0",
"autotrain_compatible",
"endpoints_compatible",
"region:us"
] | null | 2024-05-02T11:33:47+00:00 | [] | [
"en"
] | TAGS
#transformers #safetensors #llama #text-generation #text-generation-inference #unsloth #trl #sft #en #base_model-unsloth/llama-3-8b-bnb-4bit #license-apache-2.0 #autotrain_compatible #endpoints_compatible #region-us
|
# Uploaded model
- Developed by: hakankenar
- License: apache-2.0
- Finetuned from model : unsloth/llama-3-8b-bnb-4bit
This llama model was trained 2x faster with Unsloth and Huggingface's TRL library.
<img src="URL width="200"/>
| [
"# Uploaded model\n\n- Developed by: hakankenar\n- License: apache-2.0\n- Finetuned from model : unsloth/llama-3-8b-bnb-4bit\n\nThis llama model was trained 2x faster with Unsloth and Huggingface's TRL library.\n\n<img src=\"URL width=\"200\"/>"
] | [
"TAGS\n#transformers #safetensors #llama #text-generation #text-generation-inference #unsloth #trl #sft #en #base_model-unsloth/llama-3-8b-bnb-4bit #license-apache-2.0 #autotrain_compatible #endpoints_compatible #region-us \n",
"# Uploaded model\n\n- Developed by: hakankenar\n- License: apache-2.0\n- Finetuned from model : unsloth/llama-3-8b-bnb-4bit\n\nThis llama model was trained 2x faster with Unsloth and Huggingface's TRL library.\n\n<img src=\"URL width=\"200\"/>"
] | [
76,
81
] | [
"TAGS\n#transformers #safetensors #llama #text-generation #text-generation-inference #unsloth #trl #sft #en #base_model-unsloth/llama-3-8b-bnb-4bit #license-apache-2.0 #autotrain_compatible #endpoints_compatible #region-us \n# Uploaded model\n\n- Developed by: hakankenar\n- License: apache-2.0\n- Finetuned from model : unsloth/llama-3-8b-bnb-4bit\n\nThis llama model was trained 2x faster with Unsloth and Huggingface's TRL library.\n\n<img src=\"URL width=\"200\"/>"
] |
text-generation | transformers |
# Model Card for Model ID
<!-- Provide a quick summary of what the model is/does. -->
## Model Details
### Model Description
<!-- Provide a longer summary of what this model is. -->
This is the model card of a 🤗 transformers model that has been pushed on the Hub. This model card has been automatically generated.
- **Developed by:** [More Information Needed]
- **Funded by [optional]:** [More Information Needed]
- **Shared by [optional]:** [More Information Needed]
- **Model type:** [More Information Needed]
- **Language(s) (NLP):** [More Information Needed]
- **License:** [More Information Needed]
- **Finetuned from model [optional]:** [More Information Needed]
### Model Sources [optional]
<!-- Provide the basic links for the model. -->
- **Repository:** [More Information Needed]
- **Paper [optional]:** [More Information Needed]
- **Demo [optional]:** [More Information Needed]
## Uses
<!-- Address questions around how the model is intended to be used, including the foreseeable users of the model and those affected by the model. -->
### Direct Use
<!-- This section is for the model use without fine-tuning or plugging into a larger ecosystem/app. -->
[More Information Needed]
### Downstream Use [optional]
<!-- This section is for the model use when fine-tuned for a task, or when plugged into a larger ecosystem/app -->
[More Information Needed]
### Out-of-Scope Use
<!-- This section addresses misuse, malicious use, and uses that the model will not work well for. -->
[More Information Needed]
## Bias, Risks, and Limitations
<!-- This section is meant to convey both technical and sociotechnical limitations. -->
[More Information Needed]
### Recommendations
<!-- This section is meant to convey recommendations with respect to the bias, risk, and technical limitations. -->
Users (both direct and downstream) should be made aware of the risks, biases and limitations of the model. More information needed for further recommendations.
## How to Get Started with the Model
Use the code below to get started with the model.
[More Information Needed]
## Training Details
### Training Data
<!-- This should link to a Dataset Card, perhaps with a short stub of information on what the training data is all about as well as documentation related to data pre-processing or additional filtering. -->
[More Information Needed]
### Training Procedure
<!-- This relates heavily to the Technical Specifications. Content here should link to that section when it is relevant to the training procedure. -->
#### Preprocessing [optional]
[More Information Needed]
#### Training Hyperparameters
- **Training regime:** [More Information Needed] <!--fp32, fp16 mixed precision, bf16 mixed precision, bf16 non-mixed precision, fp16 non-mixed precision, fp8 mixed precision -->
#### Speeds, Sizes, Times [optional]
<!-- This section provides information about throughput, start/end time, checkpoint size if relevant, etc. -->
[More Information Needed]
## Evaluation
<!-- This section describes the evaluation protocols and provides the results. -->
### Testing Data, Factors & Metrics
#### Testing Data
<!-- This should link to a Dataset Card if possible. -->
[More Information Needed]
#### Factors
<!-- These are the things the evaluation is disaggregating by, e.g., subpopulations or domains. -->
[More Information Needed]
#### Metrics
<!-- These are the evaluation metrics being used, ideally with a description of why. -->
[More Information Needed]
### Results
[More Information Needed]
#### Summary
## Model Examination [optional]
<!-- Relevant interpretability work for the model goes here -->
[More Information Needed]
## Environmental Impact
<!-- Total emissions (in grams of CO2eq) and additional considerations, such as electricity usage, go here. Edit the suggested text below accordingly -->
Carbon emissions can be estimated using the [Machine Learning Impact calculator](https://mlco2.github.io/impact#compute) presented in [Lacoste et al. (2019)](https://arxiv.org/abs/1910.09700).
- **Hardware Type:** [More Information Needed]
- **Hours used:** [More Information Needed]
- **Cloud Provider:** [More Information Needed]
- **Compute Region:** [More Information Needed]
- **Carbon Emitted:** [More Information Needed]
## Technical Specifications [optional]
### Model Architecture and Objective
[More Information Needed]
### Compute Infrastructure
[More Information Needed]
#### Hardware
[More Information Needed]
#### Software
[More Information Needed]
## Citation [optional]
<!-- If there is a paper or blog post introducing the model, the APA and Bibtex information for that should go in this section. -->
**BibTeX:**
[More Information Needed]
**APA:**
[More Information Needed]
## Glossary [optional]
<!-- If relevant, include terms and calculations in this section that can help readers understand the model or model card. -->
[More Information Needed]
## More Information [optional]
[More Information Needed]
## Model Card Authors [optional]
[More Information Needed]
## Model Card Contact
[More Information Needed]
| {"library_name": "transformers", "tags": []} | miguel-kjh/pythia_160m-adpater-lora-cola | null | [
"transformers",
"safetensors",
"gpt_neox",
"text-generation",
"arxiv:1910.09700",
"autotrain_compatible",
"endpoints_compatible",
"text-generation-inference",
"region:us"
] | null | 2024-05-02T11:33:51+00:00 | [
"1910.09700"
] | [] | TAGS
#transformers #safetensors #gpt_neox #text-generation #arxiv-1910.09700 #autotrain_compatible #endpoints_compatible #text-generation-inference #region-us
|
# Model Card for Model ID
## Model Details
### Model Description
This is the model card of a transformers model that has been pushed on the Hub. This model card has been automatically generated.
- Developed by:
- Funded by [optional]:
- Shared by [optional]:
- Model type:
- Language(s) (NLP):
- License:
- Finetuned from model [optional]:
### Model Sources [optional]
- Repository:
- Paper [optional]:
- Demo [optional]:
## Uses
### Direct Use
### Downstream Use [optional]
### Out-of-Scope Use
## Bias, Risks, and Limitations
### Recommendations
Users (both direct and downstream) should be made aware of the risks, biases and limitations of the model. More information needed for further recommendations.
## How to Get Started with the Model
Use the code below to get started with the model.
## Training Details
### Training Data
### Training Procedure
#### Preprocessing [optional]
#### Training Hyperparameters
- Training regime:
#### Speeds, Sizes, Times [optional]
## Evaluation
### Testing Data, Factors & Metrics
#### Testing Data
#### Factors
#### Metrics
### Results
#### Summary
## Model Examination [optional]
## Environmental Impact
Carbon emissions can be estimated using the Machine Learning Impact calculator presented in Lacoste et al. (2019).
- Hardware Type:
- Hours used:
- Cloud Provider:
- Compute Region:
- Carbon Emitted:
## Technical Specifications [optional]
### Model Architecture and Objective
### Compute Infrastructure
#### Hardware
#### Software
[optional]
BibTeX:
APA:
## Glossary [optional]
## More Information [optional]
## Model Card Authors [optional]
## Model Card Contact
| [
"# Model Card for Model ID",
"## Model Details",
"### Model Description\n\n\n\nThis is the model card of a transformers model that has been pushed on the Hub. This model card has been automatically generated.\n\n- Developed by: \n- Funded by [optional]: \n- Shared by [optional]: \n- Model type: \n- Language(s) (NLP): \n- License: \n- Finetuned from model [optional]:",
"### Model Sources [optional]\n\n\n\n- Repository: \n- Paper [optional]: \n- Demo [optional]:",
"## Uses",
"### Direct Use",
"### Downstream Use [optional]",
"### Out-of-Scope Use",
"## Bias, Risks, and Limitations",
"### Recommendations\n\n\n\nUsers (both direct and downstream) should be made aware of the risks, biases and limitations of the model. More information needed for further recommendations.",
"## How to Get Started with the Model\n\nUse the code below to get started with the model.",
"## Training Details",
"### Training Data",
"### Training Procedure",
"#### Preprocessing [optional]",
"#### Training Hyperparameters\n\n- Training regime:",
"#### Speeds, Sizes, Times [optional]",
"## Evaluation",
"### Testing Data, Factors & Metrics",
"#### Testing Data",
"#### Factors",
"#### Metrics",
"### Results",
"#### Summary",
"## Model Examination [optional]",
"## Environmental Impact\n\n\n\nCarbon emissions can be estimated using the Machine Learning Impact calculator presented in Lacoste et al. (2019).\n\n- Hardware Type: \n- Hours used: \n- Cloud Provider: \n- Compute Region: \n- Carbon Emitted:",
"## Technical Specifications [optional]",
"### Model Architecture and Objective",
"### Compute Infrastructure",
"#### Hardware",
"#### Software\n\n\n\n[optional]\n\n\n\nBibTeX:\n\n\n\nAPA:",
"## Glossary [optional]",
"## More Information [optional]",
"## Model Card Authors [optional]",
"## Model Card Contact"
] | [
"TAGS\n#transformers #safetensors #gpt_neox #text-generation #arxiv-1910.09700 #autotrain_compatible #endpoints_compatible #text-generation-inference #region-us \n",
"# Model Card for Model ID",
"## Model Details",
"### Model Description\n\n\n\nThis is the model card of a transformers model that has been pushed on the Hub. This model card has been automatically generated.\n\n- Developed by: \n- Funded by [optional]: \n- Shared by [optional]: \n- Model type: \n- Language(s) (NLP): \n- License: \n- Finetuned from model [optional]:",
"### Model Sources [optional]\n\n\n\n- Repository: \n- Paper [optional]: \n- Demo [optional]:",
"## Uses",
"### Direct Use",
"### Downstream Use [optional]",
"### Out-of-Scope Use",
"## Bias, Risks, and Limitations",
"### Recommendations\n\n\n\nUsers (both direct and downstream) should be made aware of the risks, biases and limitations of the model. More information needed for further recommendations.",
"## How to Get Started with the Model\n\nUse the code below to get started with the model.",
"## Training Details",
"### Training Data",
"### Training Procedure",
"#### Preprocessing [optional]",
"#### Training Hyperparameters\n\n- Training regime:",
"#### Speeds, Sizes, Times [optional]",
"## Evaluation",
"### Testing Data, Factors & Metrics",
"#### Testing Data",
"#### Factors",
"#### Metrics",
"### Results",
"#### Summary",
"## Model Examination [optional]",
"## Environmental Impact\n\n\n\nCarbon emissions can be estimated using the Machine Learning Impact calculator presented in Lacoste et al. (2019).\n\n- Hardware Type: \n- Hours used: \n- Cloud Provider: \n- Compute Region: \n- Carbon Emitted:",
"## Technical Specifications [optional]",
"### Model Architecture and Objective",
"### Compute Infrastructure",
"#### Hardware",
"#### Software\n\n\n\n[optional]\n\n\n\nBibTeX:\n\n\n\nAPA:",
"## Glossary [optional]",
"## More Information [optional]",
"## Model Card Authors [optional]",
"## Model Card Contact"
] | [
47,
6,
4,
75,
23,
3,
5,
8,
9,
8,
34,
20,
4,
5,
5,
11,
13,
12,
3,
10,
6,
5,
6,
4,
5,
7,
49,
7,
7,
5,
5,
15,
7,
7,
8,
5
] | [
"TAGS\n#transformers #safetensors #gpt_neox #text-generation #arxiv-1910.09700 #autotrain_compatible #endpoints_compatible #text-generation-inference #region-us \n# Model Card for Model ID## Model Details### Model Description\n\n\n\nThis is the model card of a transformers model that has been pushed on the Hub. This model card has been automatically generated.\n\n- Developed by: \n- Funded by [optional]: \n- Shared by [optional]: \n- Model type: \n- Language(s) (NLP): \n- License: \n- Finetuned from model [optional]:### Model Sources [optional]\n\n\n\n- Repository: \n- Paper [optional]: \n- Demo [optional]:## Uses### Direct Use### Downstream Use [optional]### Out-of-Scope Use## Bias, Risks, and Limitations### Recommendations\n\n\n\nUsers (both direct and downstream) should be made aware of the risks, biases and limitations of the model. More information needed for further recommendations.## How to Get Started with the Model\n\nUse the code below to get started with the model.## Training Details### Training Data### Training Procedure#### Preprocessing [optional]#### Training Hyperparameters\n\n- Training regime:#### Speeds, Sizes, Times [optional]## Evaluation### Testing Data, Factors & Metrics#### Testing Data#### Factors#### Metrics### Results#### Summary## Model Examination [optional]## Environmental Impact\n\n\n\nCarbon emissions can be estimated using the Machine Learning Impact calculator presented in Lacoste et al. (2019).\n\n- Hardware Type: \n- Hours used: \n- Cloud Provider: \n- Compute Region: \n- Carbon Emitted:## Technical Specifications [optional]### Model Architecture and Objective### Compute Infrastructure#### Hardware#### Software\n\n\n\n[optional]\n\n\n\nBibTeX:\n\n\n\nAPA:## Glossary [optional]## More Information [optional]## Model Card Authors [optional]## Model Card Contact"
] |
null | peft |
# Model Card for Model ID
<!-- Provide a quick summary of what the model is/does. -->
## Model Details
### Model Description
<!-- Provide a longer summary of what this model is. -->
- **Developed by:** [More Information Needed]
- **Funded by [optional]:** [More Information Needed]
- **Shared by [optional]:** [More Information Needed]
- **Model type:** [More Information Needed]
- **Language(s) (NLP):** [More Information Needed]
- **License:** [More Information Needed]
- **Finetuned from model [optional]:** [More Information Needed]
### Model Sources [optional]
<!-- Provide the basic links for the model. -->
- **Repository:** [More Information Needed]
- **Paper [optional]:** [More Information Needed]
- **Demo [optional]:** [More Information Needed]
## Uses
<!-- Address questions around how the model is intended to be used, including the foreseeable users of the model and those affected by the model. -->
### Direct Use
<!-- This section is for the model use without fine-tuning or plugging into a larger ecosystem/app. -->
[More Information Needed]
### Downstream Use [optional]
<!-- This section is for the model use when fine-tuned for a task, or when plugged into a larger ecosystem/app -->
[More Information Needed]
### Out-of-Scope Use
<!-- This section addresses misuse, malicious use, and uses that the model will not work well for. -->
[More Information Needed]
## Bias, Risks, and Limitations
<!-- This section is meant to convey both technical and sociotechnical limitations. -->
[More Information Needed]
### Recommendations
<!-- This section is meant to convey recommendations with respect to the bias, risk, and technical limitations. -->
Users (both direct and downstream) should be made aware of the risks, biases and limitations of the model. More information needed for further recommendations.
## How to Get Started with the Model
Use the code below to get started with the model.
[More Information Needed]
## Training Details
### Training Data
<!-- This should link to a Dataset Card, perhaps with a short stub of information on what the training data is all about as well as documentation related to data pre-processing or additional filtering. -->
[More Information Needed]
### Training Procedure
<!-- This relates heavily to the Technical Specifications. Content here should link to that section when it is relevant to the training procedure. -->
#### Preprocessing [optional]
[More Information Needed]
#### Training Hyperparameters
- **Training regime:** [More Information Needed] <!--fp32, fp16 mixed precision, bf16 mixed precision, bf16 non-mixed precision, fp16 non-mixed precision, fp8 mixed precision -->
#### Speeds, Sizes, Times [optional]
<!-- This section provides information about throughput, start/end time, checkpoint size if relevant, etc. -->
[More Information Needed]
## Evaluation
<!-- This section describes the evaluation protocols and provides the results. -->
### Testing Data, Factors & Metrics
#### Testing Data
<!-- This should link to a Dataset Card if possible. -->
[More Information Needed]
#### Factors
<!-- These are the things the evaluation is disaggregating by, e.g., subpopulations or domains. -->
[More Information Needed]
#### Metrics
<!-- These are the evaluation metrics being used, ideally with a description of why. -->
[More Information Needed]
### Results
[More Information Needed]
#### Summary
## Model Examination [optional]
<!-- Relevant interpretability work for the model goes here -->
[More Information Needed]
## Environmental Impact
<!-- Total emissions (in grams of CO2eq) and additional considerations, such as electricity usage, go here. Edit the suggested text below accordingly -->
Carbon emissions can be estimated using the [Machine Learning Impact calculator](https://mlco2.github.io/impact#compute) presented in [Lacoste et al. (2019)](https://arxiv.org/abs/1910.09700).
- **Hardware Type:** [More Information Needed]
- **Hours used:** [More Information Needed]
- **Cloud Provider:** [More Information Needed]
- **Compute Region:** [More Information Needed]
- **Carbon Emitted:** [More Information Needed]
## Technical Specifications [optional]
### Model Architecture and Objective
[More Information Needed]
### Compute Infrastructure
[More Information Needed]
#### Hardware
[More Information Needed]
#### Software
[More Information Needed]
## Citation [optional]
<!-- If there is a paper or blog post introducing the model, the APA and Bibtex information for that should go in this section. -->
**BibTeX:**
[More Information Needed]
**APA:**
[More Information Needed]
## Glossary [optional]
<!-- If relevant, include terms and calculations in this section that can help readers understand the model or model card. -->
[More Information Needed]
## More Information [optional]
[More Information Needed]
## Model Card Authors [optional]
[More Information Needed]
## Model Card Contact
[More Information Needed]
### Framework versions
- PEFT 0.10.0 | {"library_name": "peft", "base_model": "meta-llama/Meta-Llama-3-70B-Instruct"} | asbabiy/AspectLens-BA-Large-DPO | null | [
"peft",
"safetensors",
"arxiv:1910.09700",
"base_model:meta-llama/Meta-Llama-3-70B-Instruct",
"region:us"
] | null | 2024-05-02T11:35:10+00:00 | [
"1910.09700"
] | [] | TAGS
#peft #safetensors #arxiv-1910.09700 #base_model-meta-llama/Meta-Llama-3-70B-Instruct #region-us
|
# Model Card for Model ID
## Model Details
### Model Description
- Developed by:
- Funded by [optional]:
- Shared by [optional]:
- Model type:
- Language(s) (NLP):
- License:
- Finetuned from model [optional]:
### Model Sources [optional]
- Repository:
- Paper [optional]:
- Demo [optional]:
## Uses
### Direct Use
### Downstream Use [optional]
### Out-of-Scope Use
## Bias, Risks, and Limitations
### Recommendations
Users (both direct and downstream) should be made aware of the risks, biases and limitations of the model. More information needed for further recommendations.
## How to Get Started with the Model
Use the code below to get started with the model.
## Training Details
### Training Data
### Training Procedure
#### Preprocessing [optional]
#### Training Hyperparameters
- Training regime:
#### Speeds, Sizes, Times [optional]
## Evaluation
### Testing Data, Factors & Metrics
#### Testing Data
#### Factors
#### Metrics
### Results
#### Summary
## Model Examination [optional]
## Environmental Impact
Carbon emissions can be estimated using the Machine Learning Impact calculator presented in Lacoste et al. (2019).
- Hardware Type:
- Hours used:
- Cloud Provider:
- Compute Region:
- Carbon Emitted:
## Technical Specifications [optional]
### Model Architecture and Objective
### Compute Infrastructure
#### Hardware
#### Software
[optional]
BibTeX:
APA:
## Glossary [optional]
## More Information [optional]
## Model Card Authors [optional]
## Model Card Contact
### Framework versions
- PEFT 0.10.0 | [
"# Model Card for Model ID",
"## Model Details",
"### Model Description\n\n\n\n\n\n- Developed by: \n- Funded by [optional]: \n- Shared by [optional]: \n- Model type: \n- Language(s) (NLP): \n- License: \n- Finetuned from model [optional]:",
"### Model Sources [optional]\n\n\n\n- Repository: \n- Paper [optional]: \n- Demo [optional]:",
"## Uses",
"### Direct Use",
"### Downstream Use [optional]",
"### Out-of-Scope Use",
"## Bias, Risks, and Limitations",
"### Recommendations\n\n\n\nUsers (both direct and downstream) should be made aware of the risks, biases and limitations of the model. More information needed for further recommendations.",
"## How to Get Started with the Model\n\nUse the code below to get started with the model.",
"## Training Details",
"### Training Data",
"### Training Procedure",
"#### Preprocessing [optional]",
"#### Training Hyperparameters\n\n- Training regime:",
"#### Speeds, Sizes, Times [optional]",
"## Evaluation",
"### Testing Data, Factors & Metrics",
"#### Testing Data",
"#### Factors",
"#### Metrics",
"### Results",
"#### Summary",
"## Model Examination [optional]",
"## Environmental Impact\n\n\n\nCarbon emissions can be estimated using the Machine Learning Impact calculator presented in Lacoste et al. (2019).\n\n- Hardware Type: \n- Hours used: \n- Cloud Provider: \n- Compute Region: \n- Carbon Emitted:",
"## Technical Specifications [optional]",
"### Model Architecture and Objective",
"### Compute Infrastructure",
"#### Hardware",
"#### Software\n\n\n\n[optional]\n\n\n\nBibTeX:\n\n\n\nAPA:",
"## Glossary [optional]",
"## More Information [optional]",
"## Model Card Authors [optional]",
"## Model Card Contact",
"### Framework versions\n\n- PEFT 0.10.0"
] | [
"TAGS\n#peft #safetensors #arxiv-1910.09700 #base_model-meta-llama/Meta-Llama-3-70B-Instruct #region-us \n",
"# Model Card for Model ID",
"## Model Details",
"### Model Description\n\n\n\n\n\n- Developed by: \n- Funded by [optional]: \n- Shared by [optional]: \n- Model type: \n- Language(s) (NLP): \n- License: \n- Finetuned from model [optional]:",
"### Model Sources [optional]\n\n\n\n- Repository: \n- Paper [optional]: \n- Demo [optional]:",
"## Uses",
"### Direct Use",
"### Downstream Use [optional]",
"### Out-of-Scope Use",
"## Bias, Risks, and Limitations",
"### Recommendations\n\n\n\nUsers (both direct and downstream) should be made aware of the risks, biases and limitations of the model. More information needed for further recommendations.",
"## How to Get Started with the Model\n\nUse the code below to get started with the model.",
"## Training Details",
"### Training Data",
"### Training Procedure",
"#### Preprocessing [optional]",
"#### Training Hyperparameters\n\n- Training regime:",
"#### Speeds, Sizes, Times [optional]",
"## Evaluation",
"### Testing Data, Factors & Metrics",
"#### Testing Data",
"#### Factors",
"#### Metrics",
"### Results",
"#### Summary",
"## Model Examination [optional]",
"## Environmental Impact\n\n\n\nCarbon emissions can be estimated using the Machine Learning Impact calculator presented in Lacoste et al. (2019).\n\n- Hardware Type: \n- Hours used: \n- Cloud Provider: \n- Compute Region: \n- Carbon Emitted:",
"## Technical Specifications [optional]",
"### Model Architecture and Objective",
"### Compute Infrastructure",
"#### Hardware",
"#### Software\n\n\n\n[optional]\n\n\n\nBibTeX:\n\n\n\nAPA:",
"## Glossary [optional]",
"## More Information [optional]",
"## Model Card Authors [optional]",
"## Model Card Contact",
"### Framework versions\n\n- PEFT 0.10.0"
] | [
44,
6,
4,
50,
23,
3,
5,
8,
9,
8,
34,
20,
4,
5,
5,
11,
13,
12,
3,
10,
6,
5,
6,
4,
5,
7,
49,
7,
7,
5,
5,
15,
7,
7,
8,
5,
13
] | [
"TAGS\n#peft #safetensors #arxiv-1910.09700 #base_model-meta-llama/Meta-Llama-3-70B-Instruct #region-us \n# Model Card for Model ID## Model Details### Model Description\n\n\n\n\n\n- Developed by: \n- Funded by [optional]: \n- Shared by [optional]: \n- Model type: \n- Language(s) (NLP): \n- License: \n- Finetuned from model [optional]:### Model Sources [optional]\n\n\n\n- Repository: \n- Paper [optional]: \n- Demo [optional]:## Uses### Direct Use### Downstream Use [optional]### Out-of-Scope Use## Bias, Risks, and Limitations### Recommendations\n\n\n\nUsers (both direct and downstream) should be made aware of the risks, biases and limitations of the model. More information needed for further recommendations.## How to Get Started with the Model\n\nUse the code below to get started with the model.## Training Details### Training Data### Training Procedure#### Preprocessing [optional]#### Training Hyperparameters\n\n- Training regime:#### Speeds, Sizes, Times [optional]## Evaluation### Testing Data, Factors & Metrics#### Testing Data#### Factors#### Metrics### Results#### Summary## Model Examination [optional]## Environmental Impact\n\n\n\nCarbon emissions can be estimated using the Machine Learning Impact calculator presented in Lacoste et al. (2019).\n\n- Hardware Type: \n- Hours used: \n- Cloud Provider: \n- Compute Region: \n- Carbon Emitted:## Technical Specifications [optional]### Model Architecture and Objective### Compute Infrastructure#### Hardware#### Software\n\n\n\n[optional]\n\n\n\nBibTeX:\n\n\n\nAPA:## Glossary [optional]## More Information [optional]## Model Card Authors [optional]## Model Card Contact### Framework versions\n\n- PEFT 0.10.0"
] |
automatic-speech-recognition | transformers |
# Model Card for Model ID
<!-- Provide a quick summary of what the model is/does. -->
## Model Details
### Model Description
<!-- Provide a longer summary of what this model is. -->
This is the model card of a 🤗 transformers model that has been pushed on the Hub. This model card has been automatically generated.
- **Developed by:** [More Information Needed]
- **Funded by [optional]:** [More Information Needed]
- **Shared by [optional]:** [More Information Needed]
- **Model type:** [More Information Needed]
- **Language(s) (NLP):** [More Information Needed]
- **License:** [More Information Needed]
- **Finetuned from model [optional]:** [More Information Needed]
### Model Sources [optional]
<!-- Provide the basic links for the model. -->
- **Repository:** [More Information Needed]
- **Paper [optional]:** [More Information Needed]
- **Demo [optional]:** [More Information Needed]
## Uses
<!-- Address questions around how the model is intended to be used, including the foreseeable users of the model and those affected by the model. -->
### Direct Use
<!-- This section is for the model use without fine-tuning or plugging into a larger ecosystem/app. -->
[More Information Needed]
### Downstream Use [optional]
<!-- This section is for the model use when fine-tuned for a task, or when plugged into a larger ecosystem/app -->
[More Information Needed]
### Out-of-Scope Use
<!-- This section addresses misuse, malicious use, and uses that the model will not work well for. -->
[More Information Needed]
## Bias, Risks, and Limitations
<!-- This section is meant to convey both technical and sociotechnical limitations. -->
[More Information Needed]
### Recommendations
<!-- This section is meant to convey recommendations with respect to the bias, risk, and technical limitations. -->
Users (both direct and downstream) should be made aware of the risks, biases and limitations of the model. More information needed for further recommendations.
## How to Get Started with the Model
Use the code below to get started with the model.
[More Information Needed]
## Training Details
### Training Data
<!-- This should link to a Dataset Card, perhaps with a short stub of information on what the training data is all about as well as documentation related to data pre-processing or additional filtering. -->
[More Information Needed]
### Training Procedure
<!-- This relates heavily to the Technical Specifications. Content here should link to that section when it is relevant to the training procedure. -->
#### Preprocessing [optional]
[More Information Needed]
#### Training Hyperparameters
- **Training regime:** [More Information Needed] <!--fp32, fp16 mixed precision, bf16 mixed precision, bf16 non-mixed precision, fp16 non-mixed precision, fp8 mixed precision -->
#### Speeds, Sizes, Times [optional]
<!-- This section provides information about throughput, start/end time, checkpoint size if relevant, etc. -->
[More Information Needed]
## Evaluation
<!-- This section describes the evaluation protocols and provides the results. -->
### Testing Data, Factors & Metrics
#### Testing Data
<!-- This should link to a Dataset Card if possible. -->
[More Information Needed]
#### Factors
<!-- These are the things the evaluation is disaggregating by, e.g., subpopulations or domains. -->
[More Information Needed]
#### Metrics
<!-- These are the evaluation metrics being used, ideally with a description of why. -->
[More Information Needed]
### Results
[More Information Needed]
#### Summary
## Model Examination [optional]
<!-- Relevant interpretability work for the model goes here -->
[More Information Needed]
## Environmental Impact
<!-- Total emissions (in grams of CO2eq) and additional considerations, such as electricity usage, go here. Edit the suggested text below accordingly -->
Carbon emissions can be estimated using the [Machine Learning Impact calculator](https://mlco2.github.io/impact#compute) presented in [Lacoste et al. (2019)](https://arxiv.org/abs/1910.09700).
- **Hardware Type:** [More Information Needed]
- **Hours used:** [More Information Needed]
- **Cloud Provider:** [More Information Needed]
- **Compute Region:** [More Information Needed]
- **Carbon Emitted:** [More Information Needed]
## Technical Specifications [optional]
### Model Architecture and Objective
[More Information Needed]
### Compute Infrastructure
[More Information Needed]
#### Hardware
[More Information Needed]
#### Software
[More Information Needed]
## Citation [optional]
<!-- If there is a paper or blog post introducing the model, the APA and Bibtex information for that should go in this section. -->
**BibTeX:**
[More Information Needed]
**APA:**
[More Information Needed]
## Glossary [optional]
<!-- If relevant, include terms and calculations in this section that can help readers understand the model or model card. -->
[More Information Needed]
## More Information [optional]
[More Information Needed]
## Model Card Authors [optional]
[More Information Needed]
## Model Card Contact
[More Information Needed] | {"library_name": "transformers", "tags": []} | shtapm/whisper-large_0502_decoder0_200steps | null | [
"transformers",
"safetensors",
"whisper",
"automatic-speech-recognition",
"arxiv:1910.09700",
"endpoints_compatible",
"region:us"
] | null | 2024-05-02T11:36:08+00:00 | [
"1910.09700"
] | [] | TAGS
#transformers #safetensors #whisper #automatic-speech-recognition #arxiv-1910.09700 #endpoints_compatible #region-us
|
# Model Card for Model ID
## Model Details
### Model Description
This is the model card of a transformers model that has been pushed on the Hub. This model card has been automatically generated.
- Developed by:
- Funded by [optional]:
- Shared by [optional]:
- Model type:
- Language(s) (NLP):
- License:
- Finetuned from model [optional]:
### Model Sources [optional]
- Repository:
- Paper [optional]:
- Demo [optional]:
## Uses
### Direct Use
### Downstream Use [optional]
### Out-of-Scope Use
## Bias, Risks, and Limitations
### Recommendations
Users (both direct and downstream) should be made aware of the risks, biases and limitations of the model. More information needed for further recommendations.
## How to Get Started with the Model
Use the code below to get started with the model.
## Training Details
### Training Data
### Training Procedure
#### Preprocessing [optional]
#### Training Hyperparameters
- Training regime:
#### Speeds, Sizes, Times [optional]
## Evaluation
### Testing Data, Factors & Metrics
#### Testing Data
#### Factors
#### Metrics
### Results
#### Summary
## Model Examination [optional]
## Environmental Impact
Carbon emissions can be estimated using the Machine Learning Impact calculator presented in Lacoste et al. (2019).
- Hardware Type:
- Hours used:
- Cloud Provider:
- Compute Region:
- Carbon Emitted:
## Technical Specifications [optional]
### Model Architecture and Objective
### Compute Infrastructure
#### Hardware
#### Software
[optional]
BibTeX:
APA:
## Glossary [optional]
## More Information [optional]
## Model Card Authors [optional]
## Model Card Contact
| [
"# Model Card for Model ID",
"## Model Details",
"### Model Description\n\n\n\nThis is the model card of a transformers model that has been pushed on the Hub. This model card has been automatically generated.\n\n- Developed by: \n- Funded by [optional]: \n- Shared by [optional]: \n- Model type: \n- Language(s) (NLP): \n- License: \n- Finetuned from model [optional]:",
"### Model Sources [optional]\n\n\n\n- Repository: \n- Paper [optional]: \n- Demo [optional]:",
"## Uses",
"### Direct Use",
"### Downstream Use [optional]",
"### Out-of-Scope Use",
"## Bias, Risks, and Limitations",
"### Recommendations\n\n\n\nUsers (both direct and downstream) should be made aware of the risks, biases and limitations of the model. More information needed for further recommendations.",
"## How to Get Started with the Model\n\nUse the code below to get started with the model.",
"## Training Details",
"### Training Data",
"### Training Procedure",
"#### Preprocessing [optional]",
"#### Training Hyperparameters\n\n- Training regime:",
"#### Speeds, Sizes, Times [optional]",
"## Evaluation",
"### Testing Data, Factors & Metrics",
"#### Testing Data",
"#### Factors",
"#### Metrics",
"### Results",
"#### Summary",
"## Model Examination [optional]",
"## Environmental Impact\n\n\n\nCarbon emissions can be estimated using the Machine Learning Impact calculator presented in Lacoste et al. (2019).\n\n- Hardware Type: \n- Hours used: \n- Cloud Provider: \n- Compute Region: \n- Carbon Emitted:",
"## Technical Specifications [optional]",
"### Model Architecture and Objective",
"### Compute Infrastructure",
"#### Hardware",
"#### Software\n\n\n\n[optional]\n\n\n\nBibTeX:\n\n\n\nAPA:",
"## Glossary [optional]",
"## More Information [optional]",
"## Model Card Authors [optional]",
"## Model Card Contact"
] | [
"TAGS\n#transformers #safetensors #whisper #automatic-speech-recognition #arxiv-1910.09700 #endpoints_compatible #region-us \n",
"# Model Card for Model ID",
"## Model Details",
"### Model Description\n\n\n\nThis is the model card of a transformers model that has been pushed on the Hub. This model card has been automatically generated.\n\n- Developed by: \n- Funded by [optional]: \n- Shared by [optional]: \n- Model type: \n- Language(s) (NLP): \n- License: \n- Finetuned from model [optional]:",
"### Model Sources [optional]\n\n\n\n- Repository: \n- Paper [optional]: \n- Demo [optional]:",
"## Uses",
"### Direct Use",
"### Downstream Use [optional]",
"### Out-of-Scope Use",
"## Bias, Risks, and Limitations",
"### Recommendations\n\n\n\nUsers (both direct and downstream) should be made aware of the risks, biases and limitations of the model. More information needed for further recommendations.",
"## How to Get Started with the Model\n\nUse the code below to get started with the model.",
"## Training Details",
"### Training Data",
"### Training Procedure",
"#### Preprocessing [optional]",
"#### Training Hyperparameters\n\n- Training regime:",
"#### Speeds, Sizes, Times [optional]",
"## Evaluation",
"### Testing Data, Factors & Metrics",
"#### Testing Data",
"#### Factors",
"#### Metrics",
"### Results",
"#### Summary",
"## Model Examination [optional]",
"## Environmental Impact\n\n\n\nCarbon emissions can be estimated using the Machine Learning Impact calculator presented in Lacoste et al. (2019).\n\n- Hardware Type: \n- Hours used: \n- Cloud Provider: \n- Compute Region: \n- Carbon Emitted:",
"## Technical Specifications [optional]",
"### Model Architecture and Objective",
"### Compute Infrastructure",
"#### Hardware",
"#### Software\n\n\n\n[optional]\n\n\n\nBibTeX:\n\n\n\nAPA:",
"## Glossary [optional]",
"## More Information [optional]",
"## Model Card Authors [optional]",
"## Model Card Contact"
] | [
34,
6,
4,
75,
23,
3,
5,
8,
9,
8,
34,
20,
4,
5,
5,
11,
13,
12,
3,
10,
6,
5,
6,
4,
5,
7,
49,
7,
7,
5,
5,
15,
7,
7,
8,
5
] | [
"TAGS\n#transformers #safetensors #whisper #automatic-speech-recognition #arxiv-1910.09700 #endpoints_compatible #region-us \n# Model Card for Model ID## Model Details### Model Description\n\n\n\nThis is the model card of a transformers model that has been pushed on the Hub. This model card has been automatically generated.\n\n- Developed by: \n- Funded by [optional]: \n- Shared by [optional]: \n- Model type: \n- Language(s) (NLP): \n- License: \n- Finetuned from model [optional]:### Model Sources [optional]\n\n\n\n- Repository: \n- Paper [optional]: \n- Demo [optional]:## Uses### Direct Use### Downstream Use [optional]### Out-of-Scope Use## Bias, Risks, and Limitations### Recommendations\n\n\n\nUsers (both direct and downstream) should be made aware of the risks, biases and limitations of the model. More information needed for further recommendations.## How to Get Started with the Model\n\nUse the code below to get started with the model.## Training Details### Training Data### Training Procedure#### Preprocessing [optional]#### Training Hyperparameters\n\n- Training regime:#### Speeds, Sizes, Times [optional]## Evaluation### Testing Data, Factors & Metrics#### Testing Data#### Factors#### Metrics### Results#### Summary## Model Examination [optional]## Environmental Impact\n\n\n\nCarbon emissions can be estimated using the Machine Learning Impact calculator presented in Lacoste et al. (2019).\n\n- Hardware Type: \n- Hours used: \n- Cloud Provider: \n- Compute Region: \n- Carbon Emitted:## Technical Specifications [optional]### Model Architecture and Objective### Compute Infrastructure#### Hardware#### Software\n\n\n\n[optional]\n\n\n\nBibTeX:\n\n\n\nAPA:## Glossary [optional]## More Information [optional]## Model Card Authors [optional]## Model Card Contact"
] |
text-generation | transformers |
# Model Card for Model ID
<!-- Provide a quick summary of what the model is/does. -->
## Model Details
### Model Description
<!-- Provide a longer summary of what this model is. -->
This is the model card of a 🤗 transformers model that has been pushed on the Hub. This model card has been automatically generated.
- **Developed by:** [More Information Needed]
- **Funded by [optional]:** [More Information Needed]
- **Shared by [optional]:** [More Information Needed]
- **Model type:** [More Information Needed]
- **Language(s) (NLP):** [More Information Needed]
- **License:** [More Information Needed]
- **Finetuned from model [optional]:** [More Information Needed]
### Model Sources [optional]
<!-- Provide the basic links for the model. -->
- **Repository:** [More Information Needed]
- **Paper [optional]:** [More Information Needed]
- **Demo [optional]:** [More Information Needed]
## Uses
<!-- Address questions around how the model is intended to be used, including the foreseeable users of the model and those affected by the model. -->
### Direct Use
<!-- This section is for the model use without fine-tuning or plugging into a larger ecosystem/app. -->
[More Information Needed]
### Downstream Use [optional]
<!-- This section is for the model use when fine-tuned for a task, or when plugged into a larger ecosystem/app -->
[More Information Needed]
### Out-of-Scope Use
<!-- This section addresses misuse, malicious use, and uses that the model will not work well for. -->
[More Information Needed]
## Bias, Risks, and Limitations
<!-- This section is meant to convey both technical and sociotechnical limitations. -->
[More Information Needed]
### Recommendations
<!-- This section is meant to convey recommendations with respect to the bias, risk, and technical limitations. -->
Users (both direct and downstream) should be made aware of the risks, biases and limitations of the model. More information needed for further recommendations.
## How to Get Started with the Model
Use the code below to get started with the model.
[More Information Needed]
## Training Details
### Training Data
<!-- This should link to a Dataset Card, perhaps with a short stub of information on what the training data is all about as well as documentation related to data pre-processing or additional filtering. -->
[More Information Needed]
### Training Procedure
<!-- This relates heavily to the Technical Specifications. Content here should link to that section when it is relevant to the training procedure. -->
#### Preprocessing [optional]
[More Information Needed]
#### Training Hyperparameters
- **Training regime:** [More Information Needed] <!--fp32, fp16 mixed precision, bf16 mixed precision, bf16 non-mixed precision, fp16 non-mixed precision, fp8 mixed precision -->
#### Speeds, Sizes, Times [optional]
<!-- This section provides information about throughput, start/end time, checkpoint size if relevant, etc. -->
[More Information Needed]
## Evaluation
<!-- This section describes the evaluation protocols and provides the results. -->
### Testing Data, Factors & Metrics
#### Testing Data
<!-- This should link to a Dataset Card if possible. -->
[More Information Needed]
#### Factors
<!-- These are the things the evaluation is disaggregating by, e.g., subpopulations or domains. -->
[More Information Needed]
#### Metrics
<!-- These are the evaluation metrics being used, ideally with a description of why. -->
[More Information Needed]
### Results
[More Information Needed]
#### Summary
## Model Examination [optional]
<!-- Relevant interpretability work for the model goes here -->
[More Information Needed]
## Environmental Impact
<!-- Total emissions (in grams of CO2eq) and additional considerations, such as electricity usage, go here. Edit the suggested text below accordingly -->
Carbon emissions can be estimated using the [Machine Learning Impact calculator](https://mlco2.github.io/impact#compute) presented in [Lacoste et al. (2019)](https://arxiv.org/abs/1910.09700).
- **Hardware Type:** [More Information Needed]
- **Hours used:** [More Information Needed]
- **Cloud Provider:** [More Information Needed]
- **Compute Region:** [More Information Needed]
- **Carbon Emitted:** [More Information Needed]
## Technical Specifications [optional]
### Model Architecture and Objective
[More Information Needed]
### Compute Infrastructure
[More Information Needed]
#### Hardware
[More Information Needed]
#### Software
[More Information Needed]
## Citation [optional]
<!-- If there is a paper or blog post introducing the model, the APA and Bibtex information for that should go in this section. -->
**BibTeX:**
[More Information Needed]
**APA:**
[More Information Needed]
## Glossary [optional]
<!-- If relevant, include terms and calculations in this section that can help readers understand the model or model card. -->
[More Information Needed]
## More Information [optional]
[More Information Needed]
## Model Card Authors [optional]
[More Information Needed]
## Model Card Contact
[More Information Needed] | {"library_name": "transformers", "tags": ["trl", "sft"]} | AvizvaSolutions/phi-sftVersion-1 | null | [
"transformers",
"safetensors",
"phi3",
"text-generation",
"trl",
"sft",
"conversational",
"custom_code",
"arxiv:1910.09700",
"autotrain_compatible",
"endpoints_compatible",
"region:us"
] | null | 2024-05-02T11:36:57+00:00 | [
"1910.09700"
] | [] | TAGS
#transformers #safetensors #phi3 #text-generation #trl #sft #conversational #custom_code #arxiv-1910.09700 #autotrain_compatible #endpoints_compatible #region-us
|
# Model Card for Model ID
## Model Details
### Model Description
This is the model card of a transformers model that has been pushed on the Hub. This model card has been automatically generated.
- Developed by:
- Funded by [optional]:
- Shared by [optional]:
- Model type:
- Language(s) (NLP):
- License:
- Finetuned from model [optional]:
### Model Sources [optional]
- Repository:
- Paper [optional]:
- Demo [optional]:
## Uses
### Direct Use
### Downstream Use [optional]
### Out-of-Scope Use
## Bias, Risks, and Limitations
### Recommendations
Users (both direct and downstream) should be made aware of the risks, biases and limitations of the model. More information needed for further recommendations.
## How to Get Started with the Model
Use the code below to get started with the model.
## Training Details
### Training Data
### Training Procedure
#### Preprocessing [optional]
#### Training Hyperparameters
- Training regime:
#### Speeds, Sizes, Times [optional]
## Evaluation
### Testing Data, Factors & Metrics
#### Testing Data
#### Factors
#### Metrics
### Results
#### Summary
## Model Examination [optional]
## Environmental Impact
Carbon emissions can be estimated using the Machine Learning Impact calculator presented in Lacoste et al. (2019).
- Hardware Type:
- Hours used:
- Cloud Provider:
- Compute Region:
- Carbon Emitted:
## Technical Specifications [optional]
### Model Architecture and Objective
### Compute Infrastructure
#### Hardware
#### Software
[optional]
BibTeX:
APA:
## Glossary [optional]
## More Information [optional]
## Model Card Authors [optional]
## Model Card Contact
| [
"# Model Card for Model ID",
"## Model Details",
"### Model Description\n\n\n\nThis is the model card of a transformers model that has been pushed on the Hub. This model card has been automatically generated.\n\n- Developed by: \n- Funded by [optional]: \n- Shared by [optional]: \n- Model type: \n- Language(s) (NLP): \n- License: \n- Finetuned from model [optional]:",
"### Model Sources [optional]\n\n\n\n- Repository: \n- Paper [optional]: \n- Demo [optional]:",
"## Uses",
"### Direct Use",
"### Downstream Use [optional]",
"### Out-of-Scope Use",
"## Bias, Risks, and Limitations",
"### Recommendations\n\n\n\nUsers (both direct and downstream) should be made aware of the risks, biases and limitations of the model. More information needed for further recommendations.",
"## How to Get Started with the Model\n\nUse the code below to get started with the model.",
"## Training Details",
"### Training Data",
"### Training Procedure",
"#### Preprocessing [optional]",
"#### Training Hyperparameters\n\n- Training regime:",
"#### Speeds, Sizes, Times [optional]",
"## Evaluation",
"### Testing Data, Factors & Metrics",
"#### Testing Data",
"#### Factors",
"#### Metrics",
"### Results",
"#### Summary",
"## Model Examination [optional]",
"## Environmental Impact\n\n\n\nCarbon emissions can be estimated using the Machine Learning Impact calculator presented in Lacoste et al. (2019).\n\n- Hardware Type: \n- Hours used: \n- Cloud Provider: \n- Compute Region: \n- Carbon Emitted:",
"## Technical Specifications [optional]",
"### Model Architecture and Objective",
"### Compute Infrastructure",
"#### Hardware",
"#### Software\n\n\n\n[optional]\n\n\n\nBibTeX:\n\n\n\nAPA:",
"## Glossary [optional]",
"## More Information [optional]",
"## Model Card Authors [optional]",
"## Model Card Contact"
] | [
"TAGS\n#transformers #safetensors #phi3 #text-generation #trl #sft #conversational #custom_code #arxiv-1910.09700 #autotrain_compatible #endpoints_compatible #region-us \n",
"# Model Card for Model ID",
"## Model Details",
"### Model Description\n\n\n\nThis is the model card of a transformers model that has been pushed on the Hub. This model card has been automatically generated.\n\n- Developed by: \n- Funded by [optional]: \n- Shared by [optional]: \n- Model type: \n- Language(s) (NLP): \n- License: \n- Finetuned from model [optional]:",
"### Model Sources [optional]\n\n\n\n- Repository: \n- Paper [optional]: \n- Demo [optional]:",
"## Uses",
"### Direct Use",
"### Downstream Use [optional]",
"### Out-of-Scope Use",
"## Bias, Risks, and Limitations",
"### Recommendations\n\n\n\nUsers (both direct and downstream) should be made aware of the risks, biases and limitations of the model. More information needed for further recommendations.",
"## How to Get Started with the Model\n\nUse the code below to get started with the model.",
"## Training Details",
"### Training Data",
"### Training Procedure",
"#### Preprocessing [optional]",
"#### Training Hyperparameters\n\n- Training regime:",
"#### Speeds, Sizes, Times [optional]",
"## Evaluation",
"### Testing Data, Factors & Metrics",
"#### Testing Data",
"#### Factors",
"#### Metrics",
"### Results",
"#### Summary",
"## Model Examination [optional]",
"## Environmental Impact\n\n\n\nCarbon emissions can be estimated using the Machine Learning Impact calculator presented in Lacoste et al. (2019).\n\n- Hardware Type: \n- Hours used: \n- Cloud Provider: \n- Compute Region: \n- Carbon Emitted:",
"## Technical Specifications [optional]",
"### Model Architecture and Objective",
"### Compute Infrastructure",
"#### Hardware",
"#### Software\n\n\n\n[optional]\n\n\n\nBibTeX:\n\n\n\nAPA:",
"## Glossary [optional]",
"## More Information [optional]",
"## Model Card Authors [optional]",
"## Model Card Contact"
] | [
51,
6,
4,
75,
23,
3,
5,
8,
9,
8,
34,
20,
4,
5,
5,
11,
13,
12,
3,
10,
6,
5,
6,
4,
5,
7,
49,
7,
7,
5,
5,
15,
7,
7,
8,
5
] | [
"TAGS\n#transformers #safetensors #phi3 #text-generation #trl #sft #conversational #custom_code #arxiv-1910.09700 #autotrain_compatible #endpoints_compatible #region-us \n# Model Card for Model ID## Model Details### Model Description\n\n\n\nThis is the model card of a transformers model that has been pushed on the Hub. This model card has been automatically generated.\n\n- Developed by: \n- Funded by [optional]: \n- Shared by [optional]: \n- Model type: \n- Language(s) (NLP): \n- License: \n- Finetuned from model [optional]:### Model Sources [optional]\n\n\n\n- Repository: \n- Paper [optional]: \n- Demo [optional]:## Uses### Direct Use### Downstream Use [optional]### Out-of-Scope Use## Bias, Risks, and Limitations### Recommendations\n\n\n\nUsers (both direct and downstream) should be made aware of the risks, biases and limitations of the model. More information needed for further recommendations.## How to Get Started with the Model\n\nUse the code below to get started with the model.## Training Details### Training Data### Training Procedure#### Preprocessing [optional]#### Training Hyperparameters\n\n- Training regime:#### Speeds, Sizes, Times [optional]## Evaluation### Testing Data, Factors & Metrics#### Testing Data#### Factors#### Metrics### Results#### Summary## Model Examination [optional]## Environmental Impact\n\n\n\nCarbon emissions can be estimated using the Machine Learning Impact calculator presented in Lacoste et al. (2019).\n\n- Hardware Type: \n- Hours used: \n- Cloud Provider: \n- Compute Region: \n- Carbon Emitted:## Technical Specifications [optional]### Model Architecture and Objective### Compute Infrastructure#### Hardware#### Software\n\n\n\n[optional]\n\n\n\nBibTeX:\n\n\n\nAPA:## Glossary [optional]## More Information [optional]## Model Card Authors [optional]## Model Card Contact"
] |
text-classification | transformers | This language model is designed to assess the attitude expressed in texts about **climate change**.
It categorizes the attitude into three types: risk, neutral, and opportunity.
These categories correspond to the negative, neutral, and positive classifications commonly used in sentiment analysis.
We employed a fine-tuning approach to adapt "cardiffnlp/twitter-roberta-base-sentiment-latest" model using a training dataset from "climatebert/climate_sentiment."
In comparison to similar existing models, such as "climatebert/distilroberta-base-climate-sentiment" and "XerOpred/twitter-climate-sentiment-model," which typically achieve accuracies ranging from 10% to 30% and F1 scores around 15%, our model demonstrates exceptional performance. When evaluated using the test dataset from "climatebert/climate_sentiment," it achieves an accuracy of 89% and an F1 score of 89%.
**Note** that you should paste or type a text concerning the **climate change** in the API input bar or using the testing code.
Otherwise, the model does not work so well. e,.g, An example input could be, "Major oil companies have misled Americans for decades about the threat of human-caused climate change, according to a new report released Tuesday by Democrats in Congress.
The 65-page report was the result of a three-year investigation and was made public hours before a Senate Budget Committee hearing about the role that oil and gas companies have played in global warming.
"
Please cite: "Sun., K, and Wang, R. 2024. The fine-tuned language model for detecting human attitudes to climate changes" if you use this model.
The following code shows how to test in the model.
```
from transformers import AutoModelForSequenceClassification, AutoTokenizer
import torch
# Load model and tokenizer
model_path = "Kevintu/climate-attitude-LM" # Ensure this path points to the correct directory
model = AutoModelForSequenceClassification.from_pretrained(model_path)
tokenizer = AutoTokenizer.from_pretrained(model_path)
# Define the path to your text file
file_path = 'yourtext.txt'
# Read the content of the file
with open(file_path, 'r', encoding='utf-8') as file:
new_text = file.read()
# Encode the text using the tokenizer used during training
encoded_input = tokenizer(new_text, return_tensors='pt', padding=True, truncation=True, max_length=64)
# Move the model to the correct device (CPU or GPU if available)
device = "cuda" if torch.cuda.is_available() else "cpu"
model = model.to(device) # Move model to the correct device
encoded_input = {k: v.to(device) for k, v in encoded_input.items()} # Move tensor to the correct device
model.eval() # Set the model to evaluation mode
# Perform the prediction
with torch.no_grad():
outputs = model(**encoded_input)
# Get the predictions (assumes classification with labels)
predictions = outputs.logits.squeeze()
# Assuming softmax is needed to interpret the logits as probabilities
probabilities = torch.softmax(predictions, dim=0)
# Define labels for each class index based on your classification categories
labels = ["risk", "neutral", "opportunity"]
predicted_index = torch.argmax(probabilities).item() # Get the index of the max probability
predicted_label = labels[predicted_index]
predicted_probability = probabilities[predicted_index].item()
# Print the predicted label and its probability
print(f"Predicted Label: {predicted_label}, Probability: {predicted_probability:.4f}")
##the output example: predicted Label: neutral, Probability: 0.8377
``` | {"license": "apache-2.0"} | Kevintu/climate-attitude-LM | null | [
"transformers",
"pytorch",
"roberta",
"text-classification",
"license:apache-2.0",
"autotrain_compatible",
"endpoints_compatible",
"region:us"
] | null | 2024-05-02T11:37:10+00:00 | [] | [] | TAGS
#transformers #pytorch #roberta #text-classification #license-apache-2.0 #autotrain_compatible #endpoints_compatible #region-us
| This language model is designed to assess the attitude expressed in texts about climate change.
It categorizes the attitude into three types: risk, neutral, and opportunity.
These categories correspond to the negative, neutral, and positive classifications commonly used in sentiment analysis.
We employed a fine-tuning approach to adapt "cardiffnlp/twitter-roberta-base-sentiment-latest" model using a training dataset from "climatebert/climate_sentiment."
In comparison to similar existing models, such as "climatebert/distilroberta-base-climate-sentiment" and "XerOpred/twitter-climate-sentiment-model," which typically achieve accuracies ranging from 10% to 30% and F1 scores around 15%, our model demonstrates exceptional performance. When evaluated using the test dataset from "climatebert/climate_sentiment," it achieves an accuracy of 89% and an F1 score of 89%.
Note that you should paste or type a text concerning the climate change in the API input bar or using the testing code.
Otherwise, the model does not work so well. e,.g, An example input could be, "Major oil companies have misled Americans for decades about the threat of human-caused climate change, according to a new report released Tuesday by Democrats in Congress.
The 65-page report was the result of a three-year investigation and was made public hours before a Senate Budget Committee hearing about the role that oil and gas companies have played in global warming.
"
Please cite: "Sun., K, and Wang, R. 2024. The fine-tuned language model for detecting human attitudes to climate changes" if you use this model.
The following code shows how to test in the model.
| [] | [
"TAGS\n#transformers #pytorch #roberta #text-classification #license-apache-2.0 #autotrain_compatible #endpoints_compatible #region-us \n"
] | [
36
] | [
"TAGS\n#transformers #pytorch #roberta #text-classification #license-apache-2.0 #autotrain_compatible #endpoints_compatible #region-us \n"
] |
null | transformers | ## About
<!-- ### quantize_version: 2 -->
<!-- ### output_tensor_quantised: 1 -->
<!-- ### convert_type: hf -->
<!-- ### vocab_type: -->
static quants of https://huggingface.co/aerdincdal/CBDDO-LLM-8B-Instruct-v1
<!-- provided-files -->
weighted/imatrix quants seem not to be available (by me) at this time. If they do not show up a week or so after the static ones, I have probably not planned for them. Feel free to request them by opening a Community Discussion.
## Usage
If you are unsure how to use GGUF files, refer to one of [TheBloke's
READMEs](https://huggingface.co/TheBloke/KafkaLM-70B-German-V0.1-GGUF) for
more details, including on how to concatenate multi-part files.
## Provided Quants
(sorted by size, not necessarily quality. IQ-quants are often preferable over similar sized non-IQ quants)
| Link | Type | Size/GB | Notes |
|:-----|:-----|--------:|:------|
| [GGUF](https://huggingface.co/mradermacher/CBDDO-LLM-8B-Instruct-v1-GGUF/resolve/main/CBDDO-LLM-8B-Instruct-v1.Q2_K.gguf) | Q2_K | 3.3 | |
| [GGUF](https://huggingface.co/mradermacher/CBDDO-LLM-8B-Instruct-v1-GGUF/resolve/main/CBDDO-LLM-8B-Instruct-v1.IQ3_XS.gguf) | IQ3_XS | 3.6 | |
| [GGUF](https://huggingface.co/mradermacher/CBDDO-LLM-8B-Instruct-v1-GGUF/resolve/main/CBDDO-LLM-8B-Instruct-v1.Q3_K_S.gguf) | Q3_K_S | 3.8 | |
| [GGUF](https://huggingface.co/mradermacher/CBDDO-LLM-8B-Instruct-v1-GGUF/resolve/main/CBDDO-LLM-8B-Instruct-v1.IQ3_S.gguf) | IQ3_S | 3.8 | beats Q3_K* |
| [GGUF](https://huggingface.co/mradermacher/CBDDO-LLM-8B-Instruct-v1-GGUF/resolve/main/CBDDO-LLM-8B-Instruct-v1.IQ3_M.gguf) | IQ3_M | 3.9 | |
| [GGUF](https://huggingface.co/mradermacher/CBDDO-LLM-8B-Instruct-v1-GGUF/resolve/main/CBDDO-LLM-8B-Instruct-v1.Q3_K_M.gguf) | Q3_K_M | 4.1 | lower quality |
| [GGUF](https://huggingface.co/mradermacher/CBDDO-LLM-8B-Instruct-v1-GGUF/resolve/main/CBDDO-LLM-8B-Instruct-v1.Q3_K_L.gguf) | Q3_K_L | 4.4 | |
| [GGUF](https://huggingface.co/mradermacher/CBDDO-LLM-8B-Instruct-v1-GGUF/resolve/main/CBDDO-LLM-8B-Instruct-v1.IQ4_XS.gguf) | IQ4_XS | 4.6 | |
| [GGUF](https://huggingface.co/mradermacher/CBDDO-LLM-8B-Instruct-v1-GGUF/resolve/main/CBDDO-LLM-8B-Instruct-v1.Q4_K_S.gguf) | Q4_K_S | 4.8 | fast, recommended |
| [GGUF](https://huggingface.co/mradermacher/CBDDO-LLM-8B-Instruct-v1-GGUF/resolve/main/CBDDO-LLM-8B-Instruct-v1.Q4_K_M.gguf) | Q4_K_M | 5.0 | fast, recommended |
| [GGUF](https://huggingface.co/mradermacher/CBDDO-LLM-8B-Instruct-v1-GGUF/resolve/main/CBDDO-LLM-8B-Instruct-v1.Q5_K_S.gguf) | Q5_K_S | 5.7 | |
| [GGUF](https://huggingface.co/mradermacher/CBDDO-LLM-8B-Instruct-v1-GGUF/resolve/main/CBDDO-LLM-8B-Instruct-v1.Q5_K_M.gguf) | Q5_K_M | 5.8 | |
| [GGUF](https://huggingface.co/mradermacher/CBDDO-LLM-8B-Instruct-v1-GGUF/resolve/main/CBDDO-LLM-8B-Instruct-v1.Q6_K.gguf) | Q6_K | 6.7 | very good quality |
| [GGUF](https://huggingface.co/mradermacher/CBDDO-LLM-8B-Instruct-v1-GGUF/resolve/main/CBDDO-LLM-8B-Instruct-v1.Q8_0.gguf) | Q8_0 | 8.6 | fast, best quality |
| [GGUF](https://huggingface.co/mradermacher/CBDDO-LLM-8B-Instruct-v1-GGUF/resolve/main/CBDDO-LLM-8B-Instruct-v1.f16.gguf) | f16 | 16.2 | 16 bpw, overkill |
Here is a handy graph by ikawrakow comparing some lower-quality quant
types (lower is better):

And here are Artefact2's thoughts on the matter:
https://gist.github.com/Artefact2/b5f810600771265fc1e39442288e8ec9
## FAQ / Model Request
See https://huggingface.co/mradermacher/model_requests for some answers to
questions you might have and/or if you want some other model quantized.
## Thanks
I thank my company, [nethype GmbH](https://www.nethype.de/), for letting
me use its servers and providing upgrades to my workstation to enable
this work in my free time.
<!-- end -->
| {"language": ["en"], "license": "mit", "library_name": "transformers", "datasets": ["aerdincdal/CBDDO-LLM-DB-V1"], "base_model": "aerdincdal/CBDDO-LLM-8B-Instruct-v1", "quantized_by": "mradermacher"} | mradermacher/CBDDO-LLM-8B-Instruct-v1-GGUF | null | [
"transformers",
"gguf",
"en",
"dataset:aerdincdal/CBDDO-LLM-DB-V1",
"base_model:aerdincdal/CBDDO-LLM-8B-Instruct-v1",
"license:mit",
"endpoints_compatible",
"region:us"
] | null | 2024-05-02T11:37:44+00:00 | [] | [
"en"
] | TAGS
#transformers #gguf #en #dataset-aerdincdal/CBDDO-LLM-DB-V1 #base_model-aerdincdal/CBDDO-LLM-8B-Instruct-v1 #license-mit #endpoints_compatible #region-us
| About
-----
static quants of URL
weighted/imatrix quants seem not to be available (by me) at this time. If they do not show up a week or so after the static ones, I have probably not planned for them. Feel free to request them by opening a Community Discussion.
Usage
-----
If you are unsure how to use GGUF files, refer to one of TheBloke's
READMEs for
more details, including on how to concatenate multi-part files.
Provided Quants
---------------
(sorted by size, not necessarily quality. IQ-quants are often preferable over similar sized non-IQ quants)
Here is a handy graph by ikawrakow comparing some lower-quality quant
types (lower is better):
!URL
And here are Artefact2's thoughts on the matter:
URL
FAQ / Model Request
-------------------
See URL for some answers to
questions you might have and/or if you want some other model quantized.
Thanks
------
I thank my company, nethype GmbH, for letting
me use its servers and providing upgrades to my workstation to enable
this work in my free time.
| [] | [
"TAGS\n#transformers #gguf #en #dataset-aerdincdal/CBDDO-LLM-DB-V1 #base_model-aerdincdal/CBDDO-LLM-8B-Instruct-v1 #license-mit #endpoints_compatible #region-us \n"
] | [
67
] | [
"TAGS\n#transformers #gguf #en #dataset-aerdincdal/CBDDO-LLM-DB-V1 #base_model-aerdincdal/CBDDO-LLM-8B-Instruct-v1 #license-mit #endpoints_compatible #region-us \n"
] |
text-generation | transformers |
# Model Card for Model ID
<!-- Provide a quick summary of what the model is/does. -->
## Model Details
### Model Description
<!-- Provide a longer summary of what this model is. -->
This is the model card of a 🤗 transformers model that has been pushed on the Hub. This model card has been automatically generated.
- **Developed by:** [More Information Needed]
- **Funded by [optional]:** [More Information Needed]
- **Shared by [optional]:** [More Information Needed]
- **Model type:** [More Information Needed]
- **Language(s) (NLP):** [More Information Needed]
- **License:** [More Information Needed]
- **Finetuned from model [optional]:** [More Information Needed]
### Model Sources [optional]
<!-- Provide the basic links for the model. -->
- **Repository:** [More Information Needed]
- **Paper [optional]:** [More Information Needed]
- **Demo [optional]:** [More Information Needed]
## Uses
<!-- Address questions around how the model is intended to be used, including the foreseeable users of the model and those affected by the model. -->
### Direct Use
<!-- This section is for the model use without fine-tuning or plugging into a larger ecosystem/app. -->
[More Information Needed]
### Downstream Use [optional]
<!-- This section is for the model use when fine-tuned for a task, or when plugged into a larger ecosystem/app -->
[More Information Needed]
### Out-of-Scope Use
<!-- This section addresses misuse, malicious use, and uses that the model will not work well for. -->
[More Information Needed]
## Bias, Risks, and Limitations
<!-- This section is meant to convey both technical and sociotechnical limitations. -->
[More Information Needed]
### Recommendations
<!-- This section is meant to convey recommendations with respect to the bias, risk, and technical limitations. -->
Users (both direct and downstream) should be made aware of the risks, biases and limitations of the model. More information needed for further recommendations.
## How to Get Started with the Model
Use the code below to get started with the model.
[More Information Needed]
## Training Details
### Training Data
<!-- This should link to a Dataset Card, perhaps with a short stub of information on what the training data is all about as well as documentation related to data pre-processing or additional filtering. -->
[More Information Needed]
### Training Procedure
<!-- This relates heavily to the Technical Specifications. Content here should link to that section when it is relevant to the training procedure. -->
#### Preprocessing [optional]
[More Information Needed]
#### Training Hyperparameters
- **Training regime:** [More Information Needed] <!--fp32, fp16 mixed precision, bf16 mixed precision, bf16 non-mixed precision, fp16 non-mixed precision, fp8 mixed precision -->
#### Speeds, Sizes, Times [optional]
<!-- This section provides information about throughput, start/end time, checkpoint size if relevant, etc. -->
[More Information Needed]
## Evaluation
<!-- This section describes the evaluation protocols and provides the results. -->
### Testing Data, Factors & Metrics
#### Testing Data
<!-- This should link to a Dataset Card if possible. -->
[More Information Needed]
#### Factors
<!-- These are the things the evaluation is disaggregating by, e.g., subpopulations or domains. -->
[More Information Needed]
#### Metrics
<!-- These are the evaluation metrics being used, ideally with a description of why. -->
[More Information Needed]
### Results
[More Information Needed]
#### Summary
## Model Examination [optional]
<!-- Relevant interpretability work for the model goes here -->
[More Information Needed]
## Environmental Impact
<!-- Total emissions (in grams of CO2eq) and additional considerations, such as electricity usage, go here. Edit the suggested text below accordingly -->
Carbon emissions can be estimated using the [Machine Learning Impact calculator](https://mlco2.github.io/impact#compute) presented in [Lacoste et al. (2019)](https://arxiv.org/abs/1910.09700).
- **Hardware Type:** [More Information Needed]
- **Hours used:** [More Information Needed]
- **Cloud Provider:** [More Information Needed]
- **Compute Region:** [More Information Needed]
- **Carbon Emitted:** [More Information Needed]
## Technical Specifications [optional]
### Model Architecture and Objective
[More Information Needed]
### Compute Infrastructure
[More Information Needed]
#### Hardware
[More Information Needed]
#### Software
[More Information Needed]
## Citation [optional]
<!-- If there is a paper or blog post introducing the model, the APA and Bibtex information for that should go in this section. -->
**BibTeX:**
[More Information Needed]
**APA:**
[More Information Needed]
## Glossary [optional]
<!-- If relevant, include terms and calculations in this section that can help readers understand the model or model card. -->
[More Information Needed]
## More Information [optional]
[More Information Needed]
## Model Card Authors [optional]
[More Information Needed]
## Model Card Contact
[More Information Needed]
| {"library_name": "transformers", "tags": []} | miguel-kjh/pythia_410m-adpater-lora-cola | null | [
"transformers",
"safetensors",
"gpt_neox",
"text-generation",
"arxiv:1910.09700",
"autotrain_compatible",
"endpoints_compatible",
"text-generation-inference",
"region:us"
] | null | 2024-05-02T11:37:58+00:00 | [
"1910.09700"
] | [] | TAGS
#transformers #safetensors #gpt_neox #text-generation #arxiv-1910.09700 #autotrain_compatible #endpoints_compatible #text-generation-inference #region-us
|
# Model Card for Model ID
## Model Details
### Model Description
This is the model card of a transformers model that has been pushed on the Hub. This model card has been automatically generated.
- Developed by:
- Funded by [optional]:
- Shared by [optional]:
- Model type:
- Language(s) (NLP):
- License:
- Finetuned from model [optional]:
### Model Sources [optional]
- Repository:
- Paper [optional]:
- Demo [optional]:
## Uses
### Direct Use
### Downstream Use [optional]
### Out-of-Scope Use
## Bias, Risks, and Limitations
### Recommendations
Users (both direct and downstream) should be made aware of the risks, biases and limitations of the model. More information needed for further recommendations.
## How to Get Started with the Model
Use the code below to get started with the model.
## Training Details
### Training Data
### Training Procedure
#### Preprocessing [optional]
#### Training Hyperparameters
- Training regime:
#### Speeds, Sizes, Times [optional]
## Evaluation
### Testing Data, Factors & Metrics
#### Testing Data
#### Factors
#### Metrics
### Results
#### Summary
## Model Examination [optional]
## Environmental Impact
Carbon emissions can be estimated using the Machine Learning Impact calculator presented in Lacoste et al. (2019).
- Hardware Type:
- Hours used:
- Cloud Provider:
- Compute Region:
- Carbon Emitted:
## Technical Specifications [optional]
### Model Architecture and Objective
### Compute Infrastructure
#### Hardware
#### Software
[optional]
BibTeX:
APA:
## Glossary [optional]
## More Information [optional]
## Model Card Authors [optional]
## Model Card Contact
| [
"# Model Card for Model ID",
"## Model Details",
"### Model Description\n\n\n\nThis is the model card of a transformers model that has been pushed on the Hub. This model card has been automatically generated.\n\n- Developed by: \n- Funded by [optional]: \n- Shared by [optional]: \n- Model type: \n- Language(s) (NLP): \n- License: \n- Finetuned from model [optional]:",
"### Model Sources [optional]\n\n\n\n- Repository: \n- Paper [optional]: \n- Demo [optional]:",
"## Uses",
"### Direct Use",
"### Downstream Use [optional]",
"### Out-of-Scope Use",
"## Bias, Risks, and Limitations",
"### Recommendations\n\n\n\nUsers (both direct and downstream) should be made aware of the risks, biases and limitations of the model. More information needed for further recommendations.",
"## How to Get Started with the Model\n\nUse the code below to get started with the model.",
"## Training Details",
"### Training Data",
"### Training Procedure",
"#### Preprocessing [optional]",
"#### Training Hyperparameters\n\n- Training regime:",
"#### Speeds, Sizes, Times [optional]",
"## Evaluation",
"### Testing Data, Factors & Metrics",
"#### Testing Data",
"#### Factors",
"#### Metrics",
"### Results",
"#### Summary",
"## Model Examination [optional]",
"## Environmental Impact\n\n\n\nCarbon emissions can be estimated using the Machine Learning Impact calculator presented in Lacoste et al. (2019).\n\n- Hardware Type: \n- Hours used: \n- Cloud Provider: \n- Compute Region: \n- Carbon Emitted:",
"## Technical Specifications [optional]",
"### Model Architecture and Objective",
"### Compute Infrastructure",
"#### Hardware",
"#### Software\n\n\n\n[optional]\n\n\n\nBibTeX:\n\n\n\nAPA:",
"## Glossary [optional]",
"## More Information [optional]",
"## Model Card Authors [optional]",
"## Model Card Contact"
] | [
"TAGS\n#transformers #safetensors #gpt_neox #text-generation #arxiv-1910.09700 #autotrain_compatible #endpoints_compatible #text-generation-inference #region-us \n",
"# Model Card for Model ID",
"## Model Details",
"### Model Description\n\n\n\nThis is the model card of a transformers model that has been pushed on the Hub. This model card has been automatically generated.\n\n- Developed by: \n- Funded by [optional]: \n- Shared by [optional]: \n- Model type: \n- Language(s) (NLP): \n- License: \n- Finetuned from model [optional]:",
"### Model Sources [optional]\n\n\n\n- Repository: \n- Paper [optional]: \n- Demo [optional]:",
"## Uses",
"### Direct Use",
"### Downstream Use [optional]",
"### Out-of-Scope Use",
"## Bias, Risks, and Limitations",
"### Recommendations\n\n\n\nUsers (both direct and downstream) should be made aware of the risks, biases and limitations of the model. More information needed for further recommendations.",
"## How to Get Started with the Model\n\nUse the code below to get started with the model.",
"## Training Details",
"### Training Data",
"### Training Procedure",
"#### Preprocessing [optional]",
"#### Training Hyperparameters\n\n- Training regime:",
"#### Speeds, Sizes, Times [optional]",
"## Evaluation",
"### Testing Data, Factors & Metrics",
"#### Testing Data",
"#### Factors",
"#### Metrics",
"### Results",
"#### Summary",
"## Model Examination [optional]",
"## Environmental Impact\n\n\n\nCarbon emissions can be estimated using the Machine Learning Impact calculator presented in Lacoste et al. (2019).\n\n- Hardware Type: \n- Hours used: \n- Cloud Provider: \n- Compute Region: \n- Carbon Emitted:",
"## Technical Specifications [optional]",
"### Model Architecture and Objective",
"### Compute Infrastructure",
"#### Hardware",
"#### Software\n\n\n\n[optional]\n\n\n\nBibTeX:\n\n\n\nAPA:",
"## Glossary [optional]",
"## More Information [optional]",
"## Model Card Authors [optional]",
"## Model Card Contact"
] | [
47,
6,
4,
75,
23,
3,
5,
8,
9,
8,
34,
20,
4,
5,
5,
11,
13,
12,
3,
10,
6,
5,
6,
4,
5,
7,
49,
7,
7,
5,
5,
15,
7,
7,
8,
5
] | [
"TAGS\n#transformers #safetensors #gpt_neox #text-generation #arxiv-1910.09700 #autotrain_compatible #endpoints_compatible #text-generation-inference #region-us \n# Model Card for Model ID## Model Details### Model Description\n\n\n\nThis is the model card of a transformers model that has been pushed on the Hub. This model card has been automatically generated.\n\n- Developed by: \n- Funded by [optional]: \n- Shared by [optional]: \n- Model type: \n- Language(s) (NLP): \n- License: \n- Finetuned from model [optional]:### Model Sources [optional]\n\n\n\n- Repository: \n- Paper [optional]: \n- Demo [optional]:## Uses### Direct Use### Downstream Use [optional]### Out-of-Scope Use## Bias, Risks, and Limitations### Recommendations\n\n\n\nUsers (both direct and downstream) should be made aware of the risks, biases and limitations of the model. More information needed for further recommendations.## How to Get Started with the Model\n\nUse the code below to get started with the model.## Training Details### Training Data### Training Procedure#### Preprocessing [optional]#### Training Hyperparameters\n\n- Training regime:#### Speeds, Sizes, Times [optional]## Evaluation### Testing Data, Factors & Metrics#### Testing Data#### Factors#### Metrics### Results#### Summary## Model Examination [optional]## Environmental Impact\n\n\n\nCarbon emissions can be estimated using the Machine Learning Impact calculator presented in Lacoste et al. (2019).\n\n- Hardware Type: \n- Hours used: \n- Cloud Provider: \n- Compute Region: \n- Carbon Emitted:## Technical Specifications [optional]### Model Architecture and Objective### Compute Infrastructure#### Hardware#### Software\n\n\n\n[optional]\n\n\n\nBibTeX:\n\n\n\nAPA:## Glossary [optional]## More Information [optional]## Model Card Authors [optional]## Model Card Contact"
] |
text-generation | transformers |
<!-- This model card has been generated automatically according to the information the Trainer had access to. You
should probably proofread and complete it, then remove this comment. -->
# virus_pythia_31_1024_cross_entropy
This model is a fine-tuned version of [EleutherAI/pythia-31m](https://huggingface.co/EleutherAI/pythia-31m) on an unknown dataset.
## Model description
More information needed
## Intended uses & limitations
More information needed
## Training and evaluation data
More information needed
## Training procedure
### Training hyperparameters
The following hyperparameters were used during training:
- learning_rate: 5e-05
- train_batch_size: 40
- eval_batch_size: 40
- seed: 42
- optimizer: Adam with betas=(0.9,0.999) and epsilon=1e-08
- lr_scheduler_type: linear
- lr_scheduler_warmup_steps: 10
- num_epochs: 1
### Training results
### Framework versions
- Transformers 4.40.1
- Pytorch 2.2.1+cu121
- Datasets 2.19.0
- Tokenizers 0.19.1
| {"tags": ["generated_from_trainer"], "base_model": "EleutherAI/pythia-31m", "model-index": [{"name": "virus_pythia_31_1024_cross_entropy", "results": []}]} | Hack90/virus_pythia_31_1024_cross_entropy | null | [
"transformers",
"safetensors",
"gpt_neox",
"text-generation",
"generated_from_trainer",
"base_model:EleutherAI/pythia-31m",
"autotrain_compatible",
"endpoints_compatible",
"text-generation-inference",
"region:us"
] | null | 2024-05-02T11:38:38+00:00 | [] | [] | TAGS
#transformers #safetensors #gpt_neox #text-generation #generated_from_trainer #base_model-EleutherAI/pythia-31m #autotrain_compatible #endpoints_compatible #text-generation-inference #region-us
|
# virus_pythia_31_1024_cross_entropy
This model is a fine-tuned version of EleutherAI/pythia-31m on an unknown dataset.
## Model description
More information needed
## Intended uses & limitations
More information needed
## Training and evaluation data
More information needed
## Training procedure
### Training hyperparameters
The following hyperparameters were used during training:
- learning_rate: 5e-05
- train_batch_size: 40
- eval_batch_size: 40
- seed: 42
- optimizer: Adam with betas=(0.9,0.999) and epsilon=1e-08
- lr_scheduler_type: linear
- lr_scheduler_warmup_steps: 10
- num_epochs: 1
### Training results
### Framework versions
- Transformers 4.40.1
- Pytorch 2.2.1+cu121
- Datasets 2.19.0
- Tokenizers 0.19.1
| [
"# virus_pythia_31_1024_cross_entropy\n\nThis model is a fine-tuned version of EleutherAI/pythia-31m on an unknown dataset.",
"## Model description\n\nMore information needed",
"## Intended uses & limitations\n\nMore information needed",
"## Training and evaluation data\n\nMore information needed",
"## Training procedure",
"### Training hyperparameters\n\nThe following hyperparameters were used during training:\n- learning_rate: 5e-05\n- train_batch_size: 40\n- eval_batch_size: 40\n- seed: 42\n- optimizer: Adam with betas=(0.9,0.999) and epsilon=1e-08\n- lr_scheduler_type: linear\n- lr_scheduler_warmup_steps: 10\n- num_epochs: 1",
"### Training results",
"### Framework versions\n\n- Transformers 4.40.1\n- Pytorch 2.2.1+cu121\n- Datasets 2.19.0\n- Tokenizers 0.19.1"
] | [
"TAGS\n#transformers #safetensors #gpt_neox #text-generation #generated_from_trainer #base_model-EleutherAI/pythia-31m #autotrain_compatible #endpoints_compatible #text-generation-inference #region-us \n",
"# virus_pythia_31_1024_cross_entropy\n\nThis model is a fine-tuned version of EleutherAI/pythia-31m on an unknown dataset.",
"## Model description\n\nMore information needed",
"## Intended uses & limitations\n\nMore information needed",
"## Training and evaluation data\n\nMore information needed",
"## Training procedure",
"### Training hyperparameters\n\nThe following hyperparameters were used during training:\n- learning_rate: 5e-05\n- train_batch_size: 40\n- eval_batch_size: 40\n- seed: 42\n- optimizer: Adam with betas=(0.9,0.999) and epsilon=1e-08\n- lr_scheduler_type: linear\n- lr_scheduler_warmup_steps: 10\n- num_epochs: 1",
"### Training results",
"### Framework versions\n\n- Transformers 4.40.1\n- Pytorch 2.2.1+cu121\n- Datasets 2.19.0\n- Tokenizers 0.19.1"
] | [
59,
41,
7,
9,
9,
4,
106,
5,
44
] | [
"TAGS\n#transformers #safetensors #gpt_neox #text-generation #generated_from_trainer #base_model-EleutherAI/pythia-31m #autotrain_compatible #endpoints_compatible #text-generation-inference #region-us \n# virus_pythia_31_1024_cross_entropy\n\nThis model is a fine-tuned version of EleutherAI/pythia-31m on an unknown dataset.## Model description\n\nMore information needed## Intended uses & limitations\n\nMore information needed## Training and evaluation data\n\nMore information needed## Training procedure### Training hyperparameters\n\nThe following hyperparameters were used during training:\n- learning_rate: 5e-05\n- train_batch_size: 40\n- eval_batch_size: 40\n- seed: 42\n- optimizer: Adam with betas=(0.9,0.999) and epsilon=1e-08\n- lr_scheduler_type: linear\n- lr_scheduler_warmup_steps: 10\n- num_epochs: 1### Training results### Framework versions\n\n- Transformers 4.40.1\n- Pytorch 2.2.1+cu121\n- Datasets 2.19.0\n- Tokenizers 0.19.1"
] |
null | peft |
# Model Card for Model ID
<!-- Provide a quick summary of what the model is/does. -->
## Model Details
### Model Description
<!-- Provide a longer summary of what this model is. -->
- **Developed by:** [More Information Needed]
- **Funded by [optional]:** [More Information Needed]
- **Shared by [optional]:** [More Information Needed]
- **Model type:** [More Information Needed]
- **Language(s) (NLP):** [More Information Needed]
- **License:** [More Information Needed]
- **Finetuned from model [optional]:** [More Information Needed]
### Model Sources [optional]
<!-- Provide the basic links for the model. -->
- **Repository:** [More Information Needed]
- **Paper [optional]:** [More Information Needed]
- **Demo [optional]:** [More Information Needed]
## Uses
<!-- Address questions around how the model is intended to be used, including the foreseeable users of the model and those affected by the model. -->
### Direct Use
<!-- This section is for the model use without fine-tuning or plugging into a larger ecosystem/app. -->
[More Information Needed]
### Downstream Use [optional]
<!-- This section is for the model use when fine-tuned for a task, or when plugged into a larger ecosystem/app -->
[More Information Needed]
### Out-of-Scope Use
<!-- This section addresses misuse, malicious use, and uses that the model will not work well for. -->
[More Information Needed]
## Bias, Risks, and Limitations
<!-- This section is meant to convey both technical and sociotechnical limitations. -->
[More Information Needed]
### Recommendations
<!-- This section is meant to convey recommendations with respect to the bias, risk, and technical limitations. -->
Users (both direct and downstream) should be made aware of the risks, biases and limitations of the model. More information needed for further recommendations.
## How to Get Started with the Model
Use the code below to get started with the model.
[More Information Needed]
## Training Details
### Training Data
<!-- This should link to a Dataset Card, perhaps with a short stub of information on what the training data is all about as well as documentation related to data pre-processing or additional filtering. -->
[More Information Needed]
### Training Procedure
<!-- This relates heavily to the Technical Specifications. Content here should link to that section when it is relevant to the training procedure. -->
#### Preprocessing [optional]
[More Information Needed]
#### Training Hyperparameters
- **Training regime:** [More Information Needed] <!--fp32, fp16 mixed precision, bf16 mixed precision, bf16 non-mixed precision, fp16 non-mixed precision, fp8 mixed precision -->
#### Speeds, Sizes, Times [optional]
<!-- This section provides information about throughput, start/end time, checkpoint size if relevant, etc. -->
[More Information Needed]
## Evaluation
<!-- This section describes the evaluation protocols and provides the results. -->
### Testing Data, Factors & Metrics
#### Testing Data
<!-- This should link to a Dataset Card if possible. -->
[More Information Needed]
#### Factors
<!-- These are the things the evaluation is disaggregating by, e.g., subpopulations or domains. -->
[More Information Needed]
#### Metrics
<!-- These are the evaluation metrics being used, ideally with a description of why. -->
[More Information Needed]
### Results
[More Information Needed]
#### Summary
## Model Examination [optional]
<!-- Relevant interpretability work for the model goes here -->
[More Information Needed]
## Environmental Impact
<!-- Total emissions (in grams of CO2eq) and additional considerations, such as electricity usage, go here. Edit the suggested text below accordingly -->
Carbon emissions can be estimated using the [Machine Learning Impact calculator](https://mlco2.github.io/impact#compute) presented in [Lacoste et al. (2019)](https://arxiv.org/abs/1910.09700).
- **Hardware Type:** [More Information Needed]
- **Hours used:** [More Information Needed]
- **Cloud Provider:** [More Information Needed]
- **Compute Region:** [More Information Needed]
- **Carbon Emitted:** [More Information Needed]
## Technical Specifications [optional]
### Model Architecture and Objective
[More Information Needed]
### Compute Infrastructure
[More Information Needed]
#### Hardware
[More Information Needed]
#### Software
[More Information Needed]
## Citation [optional]
<!-- If there is a paper or blog post introducing the model, the APA and Bibtex information for that should go in this section. -->
**BibTeX:**
[More Information Needed]
**APA:**
[More Information Needed]
## Glossary [optional]
<!-- If relevant, include terms and calculations in this section that can help readers understand the model or model card. -->
[More Information Needed]
## More Information [optional]
[More Information Needed]
## Model Card Authors [optional]
[More Information Needed]
## Model Card Contact
[More Information Needed]
### Framework versions
- PEFT 0.10.0 | {"library_name": "peft", "base_model": "deepseek-ai/deepseek-coder-6.7b-base"} | JVictor-CC/deepseek-coder-6.7b-base-2.0 | null | [
"peft",
"safetensors",
"arxiv:1910.09700",
"base_model:deepseek-ai/deepseek-coder-6.7b-base",
"region:us"
] | null | 2024-05-02T11:40:37+00:00 | [
"1910.09700"
] | [] | TAGS
#peft #safetensors #arxiv-1910.09700 #base_model-deepseek-ai/deepseek-coder-6.7b-base #region-us
|
# Model Card for Model ID
## Model Details
### Model Description
- Developed by:
- Funded by [optional]:
- Shared by [optional]:
- Model type:
- Language(s) (NLP):
- License:
- Finetuned from model [optional]:
### Model Sources [optional]
- Repository:
- Paper [optional]:
- Demo [optional]:
## Uses
### Direct Use
### Downstream Use [optional]
### Out-of-Scope Use
## Bias, Risks, and Limitations
### Recommendations
Users (both direct and downstream) should be made aware of the risks, biases and limitations of the model. More information needed for further recommendations.
## How to Get Started with the Model
Use the code below to get started with the model.
## Training Details
### Training Data
### Training Procedure
#### Preprocessing [optional]
#### Training Hyperparameters
- Training regime:
#### Speeds, Sizes, Times [optional]
## Evaluation
### Testing Data, Factors & Metrics
#### Testing Data
#### Factors
#### Metrics
### Results
#### Summary
## Model Examination [optional]
## Environmental Impact
Carbon emissions can be estimated using the Machine Learning Impact calculator presented in Lacoste et al. (2019).
- Hardware Type:
- Hours used:
- Cloud Provider:
- Compute Region:
- Carbon Emitted:
## Technical Specifications [optional]
### Model Architecture and Objective
### Compute Infrastructure
#### Hardware
#### Software
[optional]
BibTeX:
APA:
## Glossary [optional]
## More Information [optional]
## Model Card Authors [optional]
## Model Card Contact
### Framework versions
- PEFT 0.10.0 | [
"# Model Card for Model ID",
"## Model Details",
"### Model Description\n\n\n\n\n\n- Developed by: \n- Funded by [optional]: \n- Shared by [optional]: \n- Model type: \n- Language(s) (NLP): \n- License: \n- Finetuned from model [optional]:",
"### Model Sources [optional]\n\n\n\n- Repository: \n- Paper [optional]: \n- Demo [optional]:",
"## Uses",
"### Direct Use",
"### Downstream Use [optional]",
"### Out-of-Scope Use",
"## Bias, Risks, and Limitations",
"### Recommendations\n\n\n\nUsers (both direct and downstream) should be made aware of the risks, biases and limitations of the model. More information needed for further recommendations.",
"## How to Get Started with the Model\n\nUse the code below to get started with the model.",
"## Training Details",
"### Training Data",
"### Training Procedure",
"#### Preprocessing [optional]",
"#### Training Hyperparameters\n\n- Training regime:",
"#### Speeds, Sizes, Times [optional]",
"## Evaluation",
"### Testing Data, Factors & Metrics",
"#### Testing Data",
"#### Factors",
"#### Metrics",
"### Results",
"#### Summary",
"## Model Examination [optional]",
"## Environmental Impact\n\n\n\nCarbon emissions can be estimated using the Machine Learning Impact calculator presented in Lacoste et al. (2019).\n\n- Hardware Type: \n- Hours used: \n- Cloud Provider: \n- Compute Region: \n- Carbon Emitted:",
"## Technical Specifications [optional]",
"### Model Architecture and Objective",
"### Compute Infrastructure",
"#### Hardware",
"#### Software\n\n\n\n[optional]\n\n\n\nBibTeX:\n\n\n\nAPA:",
"## Glossary [optional]",
"## More Information [optional]",
"## Model Card Authors [optional]",
"## Model Card Contact",
"### Framework versions\n\n- PEFT 0.10.0"
] | [
"TAGS\n#peft #safetensors #arxiv-1910.09700 #base_model-deepseek-ai/deepseek-coder-6.7b-base #region-us \n",
"# Model Card for Model ID",
"## Model Details",
"### Model Description\n\n\n\n\n\n- Developed by: \n- Funded by [optional]: \n- Shared by [optional]: \n- Model type: \n- Language(s) (NLP): \n- License: \n- Finetuned from model [optional]:",
"### Model Sources [optional]\n\n\n\n- Repository: \n- Paper [optional]: \n- Demo [optional]:",
"## Uses",
"### Direct Use",
"### Downstream Use [optional]",
"### Out-of-Scope Use",
"## Bias, Risks, and Limitations",
"### Recommendations\n\n\n\nUsers (both direct and downstream) should be made aware of the risks, biases and limitations of the model. More information needed for further recommendations.",
"## How to Get Started with the Model\n\nUse the code below to get started with the model.",
"## Training Details",
"### Training Data",
"### Training Procedure",
"#### Preprocessing [optional]",
"#### Training Hyperparameters\n\n- Training regime:",
"#### Speeds, Sizes, Times [optional]",
"## Evaluation",
"### Testing Data, Factors & Metrics",
"#### Testing Data",
"#### Factors",
"#### Metrics",
"### Results",
"#### Summary",
"## Model Examination [optional]",
"## Environmental Impact\n\n\n\nCarbon emissions can be estimated using the Machine Learning Impact calculator presented in Lacoste et al. (2019).\n\n- Hardware Type: \n- Hours used: \n- Cloud Provider: \n- Compute Region: \n- Carbon Emitted:",
"## Technical Specifications [optional]",
"### Model Architecture and Objective",
"### Compute Infrastructure",
"#### Hardware",
"#### Software\n\n\n\n[optional]\n\n\n\nBibTeX:\n\n\n\nAPA:",
"## Glossary [optional]",
"## More Information [optional]",
"## Model Card Authors [optional]",
"## Model Card Contact",
"### Framework versions\n\n- PEFT 0.10.0"
] | [
46,
6,
4,
50,
23,
3,
5,
8,
9,
8,
34,
20,
4,
5,
5,
11,
13,
12,
3,
10,
6,
5,
6,
4,
5,
7,
49,
7,
7,
5,
5,
15,
7,
7,
8,
5,
13
] | [
"TAGS\n#peft #safetensors #arxiv-1910.09700 #base_model-deepseek-ai/deepseek-coder-6.7b-base #region-us \n# Model Card for Model ID## Model Details### Model Description\n\n\n\n\n\n- Developed by: \n- Funded by [optional]: \n- Shared by [optional]: \n- Model type: \n- Language(s) (NLP): \n- License: \n- Finetuned from model [optional]:### Model Sources [optional]\n\n\n\n- Repository: \n- Paper [optional]: \n- Demo [optional]:## Uses### Direct Use### Downstream Use [optional]### Out-of-Scope Use## Bias, Risks, and Limitations### Recommendations\n\n\n\nUsers (both direct and downstream) should be made aware of the risks, biases and limitations of the model. More information needed for further recommendations.## How to Get Started with the Model\n\nUse the code below to get started with the model.## Training Details### Training Data### Training Procedure#### Preprocessing [optional]#### Training Hyperparameters\n\n- Training regime:#### Speeds, Sizes, Times [optional]## Evaluation### Testing Data, Factors & Metrics#### Testing Data#### Factors#### Metrics### Results#### Summary## Model Examination [optional]## Environmental Impact\n\n\n\nCarbon emissions can be estimated using the Machine Learning Impact calculator presented in Lacoste et al. (2019).\n\n- Hardware Type: \n- Hours used: \n- Cloud Provider: \n- Compute Region: \n- Carbon Emitted:## Technical Specifications [optional]### Model Architecture and Objective### Compute Infrastructure#### Hardware#### Software\n\n\n\n[optional]\n\n\n\nBibTeX:\n\n\n\nAPA:## Glossary [optional]## More Information [optional]## Model Card Authors [optional]## Model Card Contact### Framework versions\n\n- PEFT 0.10.0"
] |
null | transformers |
# Model Card for Model ID
<!-- Provide a quick summary of what the model is/does. -->
## Model Details
### Model Description
<!-- Provide a longer summary of what this model is. -->
This is the model card of a 🤗 transformers model that has been pushed on the Hub. This model card has been automatically generated.
- **Developed by:** [More Information Needed]
- **Funded by [optional]:** [More Information Needed]
- **Shared by [optional]:** [More Information Needed]
- **Model type:** [More Information Needed]
- **Language(s) (NLP):** [More Information Needed]
- **License:** [More Information Needed]
- **Finetuned from model [optional]:** [More Information Needed]
### Model Sources [optional]
<!-- Provide the basic links for the model. -->
- **Repository:** [More Information Needed]
- **Paper [optional]:** [More Information Needed]
- **Demo [optional]:** [More Information Needed]
## Uses
<!-- Address questions around how the model is intended to be used, including the foreseeable users of the model and those affected by the model. -->
### Direct Use
<!-- This section is for the model use without fine-tuning or plugging into a larger ecosystem/app. -->
[More Information Needed]
### Downstream Use [optional]
<!-- This section is for the model use when fine-tuned for a task, or when plugged into a larger ecosystem/app -->
[More Information Needed]
### Out-of-Scope Use
<!-- This section addresses misuse, malicious use, and uses that the model will not work well for. -->
[More Information Needed]
## Bias, Risks, and Limitations
<!-- This section is meant to convey both technical and sociotechnical limitations. -->
[More Information Needed]
### Recommendations
<!-- This section is meant to convey recommendations with respect to the bias, risk, and technical limitations. -->
Users (both direct and downstream) should be made aware of the risks, biases and limitations of the model. More information needed for further recommendations.
## How to Get Started with the Model
Use the code below to get started with the model.
[More Information Needed]
## Training Details
### Training Data
<!-- This should link to a Dataset Card, perhaps with a short stub of information on what the training data is all about as well as documentation related to data pre-processing or additional filtering. -->
[More Information Needed]
### Training Procedure
<!-- This relates heavily to the Technical Specifications. Content here should link to that section when it is relevant to the training procedure. -->
#### Preprocessing [optional]
[More Information Needed]
#### Training Hyperparameters
- **Training regime:** [More Information Needed] <!--fp32, fp16 mixed precision, bf16 mixed precision, bf16 non-mixed precision, fp16 non-mixed precision, fp8 mixed precision -->
#### Speeds, Sizes, Times [optional]
<!-- This section provides information about throughput, start/end time, checkpoint size if relevant, etc. -->
[More Information Needed]
## Evaluation
<!-- This section describes the evaluation protocols and provides the results. -->
### Testing Data, Factors & Metrics
#### Testing Data
<!-- This should link to a Dataset Card if possible. -->
[More Information Needed]
#### Factors
<!-- These are the things the evaluation is disaggregating by, e.g., subpopulations or domains. -->
[More Information Needed]
#### Metrics
<!-- These are the evaluation metrics being used, ideally with a description of why. -->
[More Information Needed]
### Results
[More Information Needed]
#### Summary
## Model Examination [optional]
<!-- Relevant interpretability work for the model goes here -->
[More Information Needed]
## Environmental Impact
<!-- Total emissions (in grams of CO2eq) and additional considerations, such as electricity usage, go here. Edit the suggested text below accordingly -->
Carbon emissions can be estimated using the [Machine Learning Impact calculator](https://mlco2.github.io/impact#compute) presented in [Lacoste et al. (2019)](https://arxiv.org/abs/1910.09700).
- **Hardware Type:** [More Information Needed]
- **Hours used:** [More Information Needed]
- **Cloud Provider:** [More Information Needed]
- **Compute Region:** [More Information Needed]
- **Carbon Emitted:** [More Information Needed]
## Technical Specifications [optional]
### Model Architecture and Objective
[More Information Needed]
### Compute Infrastructure
[More Information Needed]
#### Hardware
[More Information Needed]
#### Software
[More Information Needed]
## Citation [optional]
<!-- If there is a paper or blog post introducing the model, the APA and Bibtex information for that should go in this section. -->
**BibTeX:**
[More Information Needed]
**APA:**
[More Information Needed]
## Glossary [optional]
<!-- If relevant, include terms and calculations in this section that can help readers understand the model or model card. -->
[More Information Needed]
## More Information [optional]
[More Information Needed]
## Model Card Authors [optional]
[More Information Needed]
## Model Card Contact
[More Information Needed]
| {"library_name": "transformers", "tags": []} | Jaafer/code-search-net-tokenizer | null | [
"transformers",
"arxiv:1910.09700",
"endpoints_compatible",
"region:us"
] | null | 2024-05-02T11:41:50+00:00 | [
"1910.09700"
] | [] | TAGS
#transformers #arxiv-1910.09700 #endpoints_compatible #region-us
|
# Model Card for Model ID
## Model Details
### Model Description
This is the model card of a transformers model that has been pushed on the Hub. This model card has been automatically generated.
- Developed by:
- Funded by [optional]:
- Shared by [optional]:
- Model type:
- Language(s) (NLP):
- License:
- Finetuned from model [optional]:
### Model Sources [optional]
- Repository:
- Paper [optional]:
- Demo [optional]:
## Uses
### Direct Use
### Downstream Use [optional]
### Out-of-Scope Use
## Bias, Risks, and Limitations
### Recommendations
Users (both direct and downstream) should be made aware of the risks, biases and limitations of the model. More information needed for further recommendations.
## How to Get Started with the Model
Use the code below to get started with the model.
## Training Details
### Training Data
### Training Procedure
#### Preprocessing [optional]
#### Training Hyperparameters
- Training regime:
#### Speeds, Sizes, Times [optional]
## Evaluation
### Testing Data, Factors & Metrics
#### Testing Data
#### Factors
#### Metrics
### Results
#### Summary
## Model Examination [optional]
## Environmental Impact
Carbon emissions can be estimated using the Machine Learning Impact calculator presented in Lacoste et al. (2019).
- Hardware Type:
- Hours used:
- Cloud Provider:
- Compute Region:
- Carbon Emitted:
## Technical Specifications [optional]
### Model Architecture and Objective
### Compute Infrastructure
#### Hardware
#### Software
[optional]
BibTeX:
APA:
## Glossary [optional]
## More Information [optional]
## Model Card Authors [optional]
## Model Card Contact
| [
"# Model Card for Model ID",
"## Model Details",
"### Model Description\n\n\n\nThis is the model card of a transformers model that has been pushed on the Hub. This model card has been automatically generated.\n\n- Developed by: \n- Funded by [optional]: \n- Shared by [optional]: \n- Model type: \n- Language(s) (NLP): \n- License: \n- Finetuned from model [optional]:",
"### Model Sources [optional]\n\n\n\n- Repository: \n- Paper [optional]: \n- Demo [optional]:",
"## Uses",
"### Direct Use",
"### Downstream Use [optional]",
"### Out-of-Scope Use",
"## Bias, Risks, and Limitations",
"### Recommendations\n\n\n\nUsers (both direct and downstream) should be made aware of the risks, biases and limitations of the model. More information needed for further recommendations.",
"## How to Get Started with the Model\n\nUse the code below to get started with the model.",
"## Training Details",
"### Training Data",
"### Training Procedure",
"#### Preprocessing [optional]",
"#### Training Hyperparameters\n\n- Training regime:",
"#### Speeds, Sizes, Times [optional]",
"## Evaluation",
"### Testing Data, Factors & Metrics",
"#### Testing Data",
"#### Factors",
"#### Metrics",
"### Results",
"#### Summary",
"## Model Examination [optional]",
"## Environmental Impact\n\n\n\nCarbon emissions can be estimated using the Machine Learning Impact calculator presented in Lacoste et al. (2019).\n\n- Hardware Type: \n- Hours used: \n- Cloud Provider: \n- Compute Region: \n- Carbon Emitted:",
"## Technical Specifications [optional]",
"### Model Architecture and Objective",
"### Compute Infrastructure",
"#### Hardware",
"#### Software\n\n\n\n[optional]\n\n\n\nBibTeX:\n\n\n\nAPA:",
"## Glossary [optional]",
"## More Information [optional]",
"## Model Card Authors [optional]",
"## Model Card Contact"
] | [
"TAGS\n#transformers #arxiv-1910.09700 #endpoints_compatible #region-us \n",
"# Model Card for Model ID",
"## Model Details",
"### Model Description\n\n\n\nThis is the model card of a transformers model that has been pushed on the Hub. This model card has been automatically generated.\n\n- Developed by: \n- Funded by [optional]: \n- Shared by [optional]: \n- Model type: \n- Language(s) (NLP): \n- License: \n- Finetuned from model [optional]:",
"### Model Sources [optional]\n\n\n\n- Repository: \n- Paper [optional]: \n- Demo [optional]:",
"## Uses",
"### Direct Use",
"### Downstream Use [optional]",
"### Out-of-Scope Use",
"## Bias, Risks, and Limitations",
"### Recommendations\n\n\n\nUsers (both direct and downstream) should be made aware of the risks, biases and limitations of the model. More information needed for further recommendations.",
"## How to Get Started with the Model\n\nUse the code below to get started with the model.",
"## Training Details",
"### Training Data",
"### Training Procedure",
"#### Preprocessing [optional]",
"#### Training Hyperparameters\n\n- Training regime:",
"#### Speeds, Sizes, Times [optional]",
"## Evaluation",
"### Testing Data, Factors & Metrics",
"#### Testing Data",
"#### Factors",
"#### Metrics",
"### Results",
"#### Summary",
"## Model Examination [optional]",
"## Environmental Impact\n\n\n\nCarbon emissions can be estimated using the Machine Learning Impact calculator presented in Lacoste et al. (2019).\n\n- Hardware Type: \n- Hours used: \n- Cloud Provider: \n- Compute Region: \n- Carbon Emitted:",
"## Technical Specifications [optional]",
"### Model Architecture and Objective",
"### Compute Infrastructure",
"#### Hardware",
"#### Software\n\n\n\n[optional]\n\n\n\nBibTeX:\n\n\n\nAPA:",
"## Glossary [optional]",
"## More Information [optional]",
"## Model Card Authors [optional]",
"## Model Card Contact"
] | [
22,
6,
4,
75,
23,
3,
5,
8,
9,
8,
34,
20,
4,
5,
5,
11,
13,
12,
3,
10,
6,
5,
6,
4,
5,
7,
49,
7,
7,
5,
5,
15,
7,
7,
8,
5
] | [
"TAGS\n#transformers #arxiv-1910.09700 #endpoints_compatible #region-us \n# Model Card for Model ID## Model Details### Model Description\n\n\n\nThis is the model card of a transformers model that has been pushed on the Hub. This model card has been automatically generated.\n\n- Developed by: \n- Funded by [optional]: \n- Shared by [optional]: \n- Model type: \n- Language(s) (NLP): \n- License: \n- Finetuned from model [optional]:### Model Sources [optional]\n\n\n\n- Repository: \n- Paper [optional]: \n- Demo [optional]:## Uses### Direct Use### Downstream Use [optional]### Out-of-Scope Use## Bias, Risks, and Limitations### Recommendations\n\n\n\nUsers (both direct and downstream) should be made aware of the risks, biases and limitations of the model. More information needed for further recommendations.## How to Get Started with the Model\n\nUse the code below to get started with the model.## Training Details### Training Data### Training Procedure#### Preprocessing [optional]#### Training Hyperparameters\n\n- Training regime:#### Speeds, Sizes, Times [optional]## Evaluation### Testing Data, Factors & Metrics#### Testing Data#### Factors#### Metrics### Results#### Summary## Model Examination [optional]## Environmental Impact\n\n\n\nCarbon emissions can be estimated using the Machine Learning Impact calculator presented in Lacoste et al. (2019).\n\n- Hardware Type: \n- Hours used: \n- Cloud Provider: \n- Compute Region: \n- Carbon Emitted:## Technical Specifications [optional]### Model Architecture and Objective### Compute Infrastructure#### Hardware#### Software\n\n\n\n[optional]\n\n\n\nBibTeX:\n\n\n\nAPA:## Glossary [optional]## More Information [optional]## Model Card Authors [optional]## Model Card Contact"
] |
null | transformers | ## About
<!-- ### quantize_version: 2 -->
<!-- ### output_tensor_quantised: 1 -->
<!-- ### convert_type: hfhfix -->
<!-- ### vocab_type: -->
static quants of https://huggingface.co/NousResearch/Meta-Llama-3-8B-Instruct
<!-- provided-files -->
weighted/imatrix quants are available at https://huggingface.co/mradermacher/Meta-Llama-3-8B-Instruct-i1-GGUF
## Usage
If you are unsure how to use GGUF files, refer to one of [TheBloke's
READMEs](https://huggingface.co/TheBloke/KafkaLM-70B-German-V0.1-GGUF) for
more details, including on how to concatenate multi-part files.
## Provided Quants
(sorted by size, not necessarily quality. IQ-quants are often preferable over similar sized non-IQ quants)
| Link | Type | Size/GB | Notes |
|:-----|:-----|--------:|:------|
| [GGUF](https://huggingface.co/mradermacher/Meta-Llama-3-8B-Instruct-GGUF/resolve/main/Meta-Llama-3-8B-Instruct.Q2_K.gguf) | Q2_K | 3.3 | |
| [GGUF](https://huggingface.co/mradermacher/Meta-Llama-3-8B-Instruct-GGUF/resolve/main/Meta-Llama-3-8B-Instruct.IQ3_XS.gguf) | IQ3_XS | 3.6 | |
| [GGUF](https://huggingface.co/mradermacher/Meta-Llama-3-8B-Instruct-GGUF/resolve/main/Meta-Llama-3-8B-Instruct.Q3_K_S.gguf) | Q3_K_S | 3.8 | |
| [GGUF](https://huggingface.co/mradermacher/Meta-Llama-3-8B-Instruct-GGUF/resolve/main/Meta-Llama-3-8B-Instruct.IQ3_S.gguf) | IQ3_S | 3.8 | beats Q3_K* |
| [GGUF](https://huggingface.co/mradermacher/Meta-Llama-3-8B-Instruct-GGUF/resolve/main/Meta-Llama-3-8B-Instruct.IQ3_M.gguf) | IQ3_M | 3.9 | |
| [GGUF](https://huggingface.co/mradermacher/Meta-Llama-3-8B-Instruct-GGUF/resolve/main/Meta-Llama-3-8B-Instruct.Q3_K_M.gguf) | Q3_K_M | 4.1 | lower quality |
| [GGUF](https://huggingface.co/mradermacher/Meta-Llama-3-8B-Instruct-GGUF/resolve/main/Meta-Llama-3-8B-Instruct.Q3_K_L.gguf) | Q3_K_L | 4.4 | |
| [GGUF](https://huggingface.co/mradermacher/Meta-Llama-3-8B-Instruct-GGUF/resolve/main/Meta-Llama-3-8B-Instruct.IQ4_XS.gguf) | IQ4_XS | 4.6 | |
| [GGUF](https://huggingface.co/mradermacher/Meta-Llama-3-8B-Instruct-GGUF/resolve/main/Meta-Llama-3-8B-Instruct.Q4_K_S.gguf) | Q4_K_S | 4.8 | fast, recommended |
| [GGUF](https://huggingface.co/mradermacher/Meta-Llama-3-8B-Instruct-GGUF/resolve/main/Meta-Llama-3-8B-Instruct.Q4_K_M.gguf) | Q4_K_M | 5.0 | fast, recommended |
| [GGUF](https://huggingface.co/mradermacher/Meta-Llama-3-8B-Instruct-GGUF/resolve/main/Meta-Llama-3-8B-Instruct.Q5_K_S.gguf) | Q5_K_S | 5.7 | |
| [GGUF](https://huggingface.co/mradermacher/Meta-Llama-3-8B-Instruct-GGUF/resolve/main/Meta-Llama-3-8B-Instruct.Q5_K_M.gguf) | Q5_K_M | 5.8 | |
| [GGUF](https://huggingface.co/mradermacher/Meta-Llama-3-8B-Instruct-GGUF/resolve/main/Meta-Llama-3-8B-Instruct.Q6_K.gguf) | Q6_K | 6.7 | very good quality |
| [GGUF](https://huggingface.co/mradermacher/Meta-Llama-3-8B-Instruct-GGUF/resolve/main/Meta-Llama-3-8B-Instruct.Q8_0.gguf) | Q8_0 | 8.6 | fast, best quality |
| [GGUF](https://huggingface.co/mradermacher/Meta-Llama-3-8B-Instruct-GGUF/resolve/main/Meta-Llama-3-8B-Instruct.f16.gguf) | f16 | 16.2 | 16 bpw, overkill |
Here is a handy graph by ikawrakow comparing some lower-quality quant
types (lower is better):

And here are Artefact2's thoughts on the matter:
https://gist.github.com/Artefact2/b5f810600771265fc1e39442288e8ec9
## FAQ / Model Request
See https://huggingface.co/mradermacher/model_requests for some answers to
questions you might have and/or if you want some other model quantized.
## Thanks
I thank my company, [nethype GmbH](https://www.nethype.de/), for letting
me use its servers and providing upgrades to my workstation to enable
this work in my free time.
<!-- end -->
| {"language": ["en"], "license": "other", "library_name": "transformers", "tags": ["facebook", "meta", "pytorch", "llama", "llama-3"], "base_model": "NousResearch/Meta-Llama-3-8B-Instruct", "extra_gated_button_content": "Submit", "extra_gated_fields": {"Affiliation": "text", "By clicking Submit below I accept the terms of the license and acknowledge that the information I provide will be collected stored processed and shared in accordance with the Meta Privacy Policy": "checkbox", "Country": "country", "Date of birth": "date_picker", "First Name": "text", "Last Name": "text", "geo": "ip_location"}, "extra_gated_prompt": "### META LLAMA 3 COMMUNITY LICENSE AGREEMENT\nMeta Llama 3 Version Release Date: April 18, 2024\n\"Agreement\" means the terms and conditions for use, reproduction, distribution and modification of the Llama Materials set forth herein.\n\"Documentation\" means the specifications, manuals and documentation accompanying Meta Llama 3 distributed by Meta at https://llama.meta.com/get-started/.\n\"Licensee\" or \"you\" means you, or your employer or any other person or entity (if you are entering into this Agreement on such person or entity\u2019s behalf), of the age required under applicable laws, rules or regulations to provide legal consent and that has legal authority to bind your employer or such other person or entity if you are entering in this Agreement on their behalf.\n\"Meta Llama 3\" means the foundational large language models and software and algorithms, including machine-learning model code, trained model weights, inference-enabling code, training-enabling code, fine-tuning enabling code and other elements of the foregoing distributed by Meta at https://llama.meta.com/llama-downloads.\n\"Llama Materials\" means, collectively, Meta\u2019s proprietary Meta Llama 3 and Documentation (and any portion thereof) made available under this Agreement.\n\"Meta\" or \"we\" means Meta Platforms Ireland Limited (if you are located in or, if you are an entity, your principal place of business is in the EEA or Switzerland) and Meta Platforms, Inc. (if you are located outside of the EEA or Switzerland).\n \n1. License Rights and Redistribution.\na. Grant of Rights. You are granted a non-exclusive, worldwide, non-transferable and royalty-free limited license under Meta\u2019s intellectual property or other rights owned by Meta embodied in the Llama Materials to use, reproduce, distribute, copy, create derivative works of, and make modifications to the Llama Materials.\nb. Redistribution and Use.\ni. If you distribute or make available the Llama Materials (or any derivative works thereof), or a product or service that uses any of them, including another AI model, you shall (A) provide a copy of this Agreement with any such Llama Materials; and (B) prominently display \u201cBuilt with Meta Llama 3\u201d on a related website, user interface, blogpost, about page, or product documentation. If you use the Llama Materials to create, train, fine tune, or otherwise improve an AI model, which is distributed or made available, you shall also include \u201cLlama 3\u201d at the beginning of any such AI model name.\nii. If you receive Llama Materials, or any derivative works thereof, from a Licensee as part of an integrated end user product, then Section 2 of this Agreement will not apply to you.\niii. You must retain in all copies of the Llama Materials that you distribute the following attribution notice within a \u201cNotice\u201d text file distributed as a part of such copies: \u201cMeta Llama 3 is licensed under the Meta Llama 3 Community License, Copyright \u00a9 Meta Platforms, Inc. All Rights Reserved.\u201d\niv. Your use of the Llama Materials must comply with applicable laws and regulations (including trade compliance laws and regulations) and adhere to the Acceptable Use Policy for the Llama Materials (available at https://llama.meta.com/llama3/use-policy), which is hereby incorporated by reference into this Agreement.\nv. You will not use the Llama Materials or any output or results of the Llama Materials to improve any other large language model (excluding Meta Llama 3 or derivative works thereof).\n2. Additional Commercial Terms. If, on the Meta Llama 3 version release date, the monthly active users of the products or services made available by or for Licensee, or Licensee\u2019s affiliates, is greater than 700 million monthly active users in the preceding calendar month, you must request a license from Meta, which Meta may grant to you in its sole discretion, and you are not authorized to exercise any of the rights under this Agreement unless or until Meta otherwise expressly grants you such rights.\n3. Disclaimer of Warranty. UNLESS REQUIRED BY APPLICABLE LAW, THE LLAMA MATERIALS AND ANY OUTPUT AND RESULTS THEREFROM ARE PROVIDED ON AN \u201cAS IS\u201d BASIS, WITHOUT WARRANTIES OF ANY KIND, AND META DISCLAIMS ALL WARRANTIES OF ANY KIND, BOTH EXPRESS AND IMPLIED, INCLUDING, WITHOUT LIMITATION, ANY WARRANTIES OF TITLE, NON-INFRINGEMENT, MERCHANTABILITY, OR FITNESS FOR A PARTICULAR PURPOSE. YOU ARE SOLELY RESPONSIBLE FOR DETERMINING THE APPROPRIATENESS OF USING OR REDISTRIBUTING THE LLAMA MATERIALS AND ASSUME ANY RISKS ASSOCIATED WITH YOUR USE OF THE LLAMA MATERIALS AND ANY OUTPUT AND RESULTS.\n4. Limitation of Liability. IN NO EVENT WILL META OR ITS AFFILIATES BE LIABLE UNDER ANY THEORY OF LIABILITY, WHETHER IN CONTRACT, TORT, NEGLIGENCE, PRODUCTS LIABILITY, OR OTHERWISE, ARISING OUT OF THIS AGREEMENT, FOR ANY LOST PROFITS OR ANY INDIRECT, SPECIAL, CONSEQUENTIAL, INCIDENTAL, EXEMPLARY OR PUNITIVE DAMAGES, EVEN IF META OR ITS AFFILIATES HAVE BEEN ADVISED OF THE POSSIBILITY OF ANY OF THE FOREGOING.\n5. Intellectual Property.\na. No trademark licenses are granted under this Agreement, and in connection with the Llama Materials, neither Meta nor Licensee may use any name or mark owned by or associated with the other or any of its affiliates, except as required for reasonable and customary use in describing and redistributing the Llama Materials or as set forth in this Section 5(a). Meta hereby grants you a license to use \u201cLlama 3\u201d (the \u201cMark\u201d) solely as required to comply with the last sentence of Section 1.b.i. You will comply with Meta\u2019s brand guidelines (currently accessible at https://about.meta.com/brand/resources/meta/company-brand/ ). All goodwill arising out of your use of the Mark will inure to the benefit of Meta.\nb. Subject to Meta\u2019s ownership of Llama Materials and derivatives made by or for Meta, with respect to any derivative works and modifications of the Llama Materials that are made by you, as between you and Meta, you are and will be the owner of such derivative works and modifications.\nc. If you institute litigation or other proceedings against Meta or any entity (including a cross-claim or counterclaim in a lawsuit) alleging that the Llama Materials or Meta Llama 3 outputs or results, or any portion of any of the foregoing, constitutes infringement of intellectual property or other rights owned or licensable by you, then any licenses granted to you under this Agreement shall terminate as of the date such litigation or claim is filed or instituted. You will indemnify and hold harmless Meta from and against any claim by any third party arising out of or related to your use or distribution of the Llama Materials.\n6. Term and Termination. The term of this Agreement will commence upon your acceptance of this Agreement or access to the Llama Materials and will continue in full force and effect until terminated in accordance with the terms and conditions herein. Meta may terminate this Agreement if you are in breach of any term or condition of this Agreement. Upon termination of this Agreement, you shall delete and cease use of the Llama Materials. Sections 3, 4 and 7 shall survive the termination of this Agreement.\n7. Governing Law and Jurisdiction. This Agreement will be governed and construed under the laws of the State of California without regard to choice of law principles, and the UN Convention on Contracts for the International Sale of Goods does not apply to this Agreement. The courts of California shall have exclusive jurisdiction of any dispute arising out of this Agreement.\n### Meta Llama 3 Acceptable Use Policy\nMeta is committed to promoting safe and fair use of its tools and features, including Meta Llama 3. If you access or use Meta Llama 3, you agree to this Acceptable Use Policy (\u201cPolicy\u201d). The most recent copy of this policy can be found at [https://llama.meta.com/llama3/use-policy](https://llama.meta.com/llama3/use-policy)\n#### Prohibited Uses\nWe want everyone to use Meta Llama 3 safely and responsibly. You agree you will not use, or allow others to use, Meta Llama 3 to: 1. Violate the law or others\u2019 rights, including to:\n 1. Engage in, promote, generate, contribute to, encourage, plan, incite, or further illegal or unlawful activity or content, such as:\n 1. Violence or terrorism\n 2. Exploitation or harm to children, including the solicitation, creation, acquisition, or dissemination of child exploitative content or failure to report Child Sexual Abuse Material\n 3. Human trafficking, exploitation, and sexual violence\n 4. The illegal distribution of information or materials to minors, including obscene materials, or failure to employ legally required age-gating in connection with such information or materials.\n 5. Sexual solicitation\n 6. Any other criminal activity\n 2. Engage in, promote, incite, or facilitate the harassment, abuse, threatening, or bullying of individuals or groups of individuals\n 3. Engage in, promote, incite, or facilitate discrimination or other unlawful or harmful conduct in the provision of employment, employment benefits, credit, housing, other economic benefits, or other essential goods and services\n 4. Engage in the unauthorized or unlicensed practice of any profession including, but not limited to, financial, legal, medical/health, or related professional practices\n 5. Collect, process, disclose, generate, or infer health, demographic, or other sensitive personal or private information about individuals without rights and consents required by applicable laws\n 6. Engage in or facilitate any action or generate any content that infringes, misappropriates, or otherwise violates any third-party rights, including the outputs or results of any products or services using the Llama Materials\n 7. Create, generate, or facilitate the creation of malicious code, malware, computer viruses or do anything else that could disable, overburden, interfere with or impair the proper working, integrity, operation or appearance of a website or computer system\n2. Engage in, promote, incite, facilitate, or assist in the planning or development of activities that present a risk of death or bodily harm to individuals, including use of Meta Llama 3 related to the following:\n 1. Military, warfare, nuclear industries or applications, espionage, use for materials or activities that are subject to the International Traffic Arms Regulations (ITAR) maintained by the United States Department of State\n 2. Guns and illegal weapons (including weapon development)\n 3. Illegal drugs and regulated/controlled substances\n 4. Operation of critical infrastructure, transportation technologies, or heavy machinery\n 5. Self-harm or harm to others, including suicide, cutting, and eating disorders\n 6. Any content intended to incite or promote violence, abuse, or any infliction of bodily harm to an individual\n3. Intentionally deceive or mislead others, including use of Meta Llama 3 related to the following:\n 1. Generating, promoting, or furthering fraud or the creation or promotion of disinformation\n 2. Generating, promoting, or furthering defamatory content, including the creation of defamatory statements, images, or other content\n 3. Generating, promoting, or further distributing spam\n 4. Impersonating another individual without consent, authorization, or legal right\n 5. Representing that the use of Meta Llama 3 or outputs are human-generated\n 6. Generating or facilitating false online engagement, including fake reviews and other means of fake online engagement\n4. Fail to appropriately disclose to end users any known dangers of your AI system\nPlease report any violation of this Policy, software \u201cbug,\u201d or other problems that could lead to a violation of this Policy through one of the following means:\n * Reporting issues with the model: [https://github.com/meta-llama/llama3](https://github.com/meta-llama/llama3)\n * Reporting risky content generated by the model:\n developers.facebook.com/llama_output_feedback\n * Reporting bugs and security concerns: facebook.com/whitehat/info\n * Reporting violations of the Acceptable Use Policy or unlicensed uses of Meta Llama 3: [email protected]", "license_link": "LICENSE", "license_name": "llama3", "quantized_by": "mradermacher"} | mradermacher/Meta-Llama-3-8B-Instruct-GGUF | null | [
"transformers",
"gguf",
"facebook",
"meta",
"pytorch",
"llama",
"llama-3",
"en",
"base_model:NousResearch/Meta-Llama-3-8B-Instruct",
"license:other",
"endpoints_compatible",
"region:us"
] | null | 2024-05-02T11:43:53+00:00 | [] | [
"en"
] | TAGS
#transformers #gguf #facebook #meta #pytorch #llama #llama-3 #en #base_model-NousResearch/Meta-Llama-3-8B-Instruct #license-other #endpoints_compatible #region-us
| About
-----
static quants of URL
weighted/imatrix quants are available at URL
Usage
-----
If you are unsure how to use GGUF files, refer to one of TheBloke's
READMEs for
more details, including on how to concatenate multi-part files.
Provided Quants
---------------
(sorted by size, not necessarily quality. IQ-quants are often preferable over similar sized non-IQ quants)
Here is a handy graph by ikawrakow comparing some lower-quality quant
types (lower is better):
!URL
And here are Artefact2's thoughts on the matter:
URL
FAQ / Model Request
-------------------
See URL for some answers to
questions you might have and/or if you want some other model quantized.
Thanks
------
I thank my company, nethype GmbH, for letting
me use its servers and providing upgrades to my workstation to enable
this work in my free time.
| [] | [
"TAGS\n#transformers #gguf #facebook #meta #pytorch #llama #llama-3 #en #base_model-NousResearch/Meta-Llama-3-8B-Instruct #license-other #endpoints_compatible #region-us \n"
] | [
62
] | [
"TAGS\n#transformers #gguf #facebook #meta #pytorch #llama #llama-3 #en #base_model-NousResearch/Meta-Llama-3-8B-Instruct #license-other #endpoints_compatible #region-us \n"
] |
null | transformers | ## About
<!-- ### quantize_version: 2 -->
<!-- ### output_tensor_quantised: 1 -->
<!-- ### convert_type: hfhfix -->
<!-- ### vocab_type: -->
static quants of https://huggingface.co/rinna/llama-3-youko-8b
<!-- provided-files -->
weighted/imatrix quants seem not to be available (by me) at this time. If they do not show up a week or so after the static ones, I have probably not planned for them. Feel free to request them by opening a Community Discussion.
## Usage
If you are unsure how to use GGUF files, refer to one of [TheBloke's
READMEs](https://huggingface.co/TheBloke/KafkaLM-70B-German-V0.1-GGUF) for
more details, including on how to concatenate multi-part files.
## Provided Quants
(sorted by size, not necessarily quality. IQ-quants are often preferable over similar sized non-IQ quants)
| Link | Type | Size/GB | Notes |
|:-----|:-----|--------:|:------|
| [GGUF](https://huggingface.co/mradermacher/llama-3-youko-8b-GGUF/resolve/main/llama-3-youko-8b.Q2_K.gguf) | Q2_K | 3.3 | |
| [GGUF](https://huggingface.co/mradermacher/llama-3-youko-8b-GGUF/resolve/main/llama-3-youko-8b.IQ3_XS.gguf) | IQ3_XS | 3.6 | |
| [GGUF](https://huggingface.co/mradermacher/llama-3-youko-8b-GGUF/resolve/main/llama-3-youko-8b.Q3_K_S.gguf) | Q3_K_S | 3.8 | |
| [GGUF](https://huggingface.co/mradermacher/llama-3-youko-8b-GGUF/resolve/main/llama-3-youko-8b.IQ3_S.gguf) | IQ3_S | 3.8 | beats Q3_K* |
| [GGUF](https://huggingface.co/mradermacher/llama-3-youko-8b-GGUF/resolve/main/llama-3-youko-8b.IQ3_M.gguf) | IQ3_M | 3.9 | |
| [GGUF](https://huggingface.co/mradermacher/llama-3-youko-8b-GGUF/resolve/main/llama-3-youko-8b.Q3_K_M.gguf) | Q3_K_M | 4.1 | lower quality |
| [GGUF](https://huggingface.co/mradermacher/llama-3-youko-8b-GGUF/resolve/main/llama-3-youko-8b.Q3_K_L.gguf) | Q3_K_L | 4.4 | |
| [GGUF](https://huggingface.co/mradermacher/llama-3-youko-8b-GGUF/resolve/main/llama-3-youko-8b.IQ4_XS.gguf) | IQ4_XS | 4.6 | |
| [GGUF](https://huggingface.co/mradermacher/llama-3-youko-8b-GGUF/resolve/main/llama-3-youko-8b.Q4_K_S.gguf) | Q4_K_S | 4.8 | fast, recommended |
| [GGUF](https://huggingface.co/mradermacher/llama-3-youko-8b-GGUF/resolve/main/llama-3-youko-8b.Q4_K_M.gguf) | Q4_K_M | 5.0 | fast, recommended |
| [GGUF](https://huggingface.co/mradermacher/llama-3-youko-8b-GGUF/resolve/main/llama-3-youko-8b.Q5_K_S.gguf) | Q5_K_S | 5.7 | |
| [GGUF](https://huggingface.co/mradermacher/llama-3-youko-8b-GGUF/resolve/main/llama-3-youko-8b.Q5_K_M.gguf) | Q5_K_M | 5.8 | |
| [GGUF](https://huggingface.co/mradermacher/llama-3-youko-8b-GGUF/resolve/main/llama-3-youko-8b.Q6_K.gguf) | Q6_K | 6.7 | very good quality |
| [GGUF](https://huggingface.co/mradermacher/llama-3-youko-8b-GGUF/resolve/main/llama-3-youko-8b.Q8_0.gguf) | Q8_0 | 8.6 | fast, best quality |
| [GGUF](https://huggingface.co/mradermacher/llama-3-youko-8b-GGUF/resolve/main/llama-3-youko-8b.f16.gguf) | f16 | 16.2 | 16 bpw, overkill |
Here is a handy graph by ikawrakow comparing some lower-quality quant
types (lower is better):

And here are Artefact2's thoughts on the matter:
https://gist.github.com/Artefact2/b5f810600771265fc1e39442288e8ec9
## FAQ / Model Request
See https://huggingface.co/mradermacher/model_requests for some answers to
questions you might have and/or if you want some other model quantized.
## Thanks
I thank my company, [nethype GmbH](https://www.nethype.de/), for letting
me use its servers and providing upgrades to my workstation to enable
this work in my free time.
<!-- end -->
| {"language": ["en"], "license": "llama3", "library_name": "transformers", "datasets": ["mc4", "wikipedia", "EleutherAI/pile", "oscar-corpus/colossal-oscar-1.0", "cc100"], "base_model": "rinna/llama-3-youko-8b", "quantized_by": "mradermacher"} | mradermacher/llama-3-youko-8b-GGUF | null | [
"transformers",
"gguf",
"en",
"dataset:mc4",
"dataset:wikipedia",
"dataset:EleutherAI/pile",
"dataset:oscar-corpus/colossal-oscar-1.0",
"dataset:cc100",
"base_model:rinna/llama-3-youko-8b",
"license:llama3",
"endpoints_compatible",
"region:us"
] | null | 2024-05-02T11:43:53+00:00 | [] | [
"en"
] | TAGS
#transformers #gguf #en #dataset-mc4 #dataset-wikipedia #dataset-EleutherAI/pile #dataset-oscar-corpus/colossal-oscar-1.0 #dataset-cc100 #base_model-rinna/llama-3-youko-8b #license-llama3 #endpoints_compatible #region-us
| About
-----
static quants of URL
weighted/imatrix quants seem not to be available (by me) at this time. If they do not show up a week or so after the static ones, I have probably not planned for them. Feel free to request them by opening a Community Discussion.
Usage
-----
If you are unsure how to use GGUF files, refer to one of TheBloke's
READMEs for
more details, including on how to concatenate multi-part files.
Provided Quants
---------------
(sorted by size, not necessarily quality. IQ-quants are often preferable over similar sized non-IQ quants)
Here is a handy graph by ikawrakow comparing some lower-quality quant
types (lower is better):
!URL
And here are Artefact2's thoughts on the matter:
URL
FAQ / Model Request
-------------------
See URL for some answers to
questions you might have and/or if you want some other model quantized.
Thanks
------
I thank my company, nethype GmbH, for letting
me use its servers and providing upgrades to my workstation to enable
this work in my free time.
| [] | [
"TAGS\n#transformers #gguf #en #dataset-mc4 #dataset-wikipedia #dataset-EleutherAI/pile #dataset-oscar-corpus/colossal-oscar-1.0 #dataset-cc100 #base_model-rinna/llama-3-youko-8b #license-llama3 #endpoints_compatible #region-us \n"
] | [
84
] | [
"TAGS\n#transformers #gguf #en #dataset-mc4 #dataset-wikipedia #dataset-EleutherAI/pile #dataset-oscar-corpus/colossal-oscar-1.0 #dataset-cc100 #base_model-rinna/llama-3-youko-8b #license-llama3 #endpoints_compatible #region-us \n"
] |
text-generation | null | 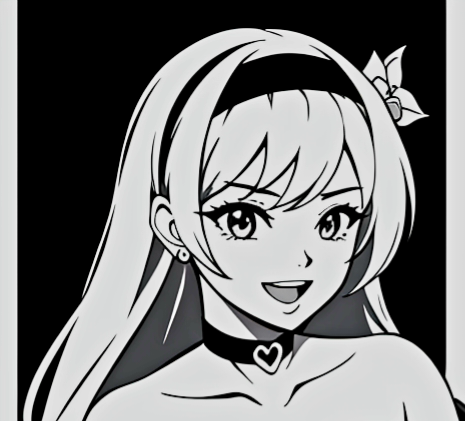
The idea is the same as [InfinityRP v1](https://huggingface.co/Endevor/InfinityRP-v1-7B), but this one is Llama 3 with 16k ctx! Have fun...
### Prompt format: Alpaca.
``"You are now in roleplay chat mode. Engage in an endless chat, always with a creative response. Follow lengths very precisely and create paragraphs accurately. Always wait your turn, next actions and responses. Your internal thoughts are wrapped with ` marks."``
**User Message Prefix = ### Input:**
**Assistant Message Prefix = ### Response:**
**System Message Prefix = ### Instruction:**
**Turn on "Include Names"**
### Example:
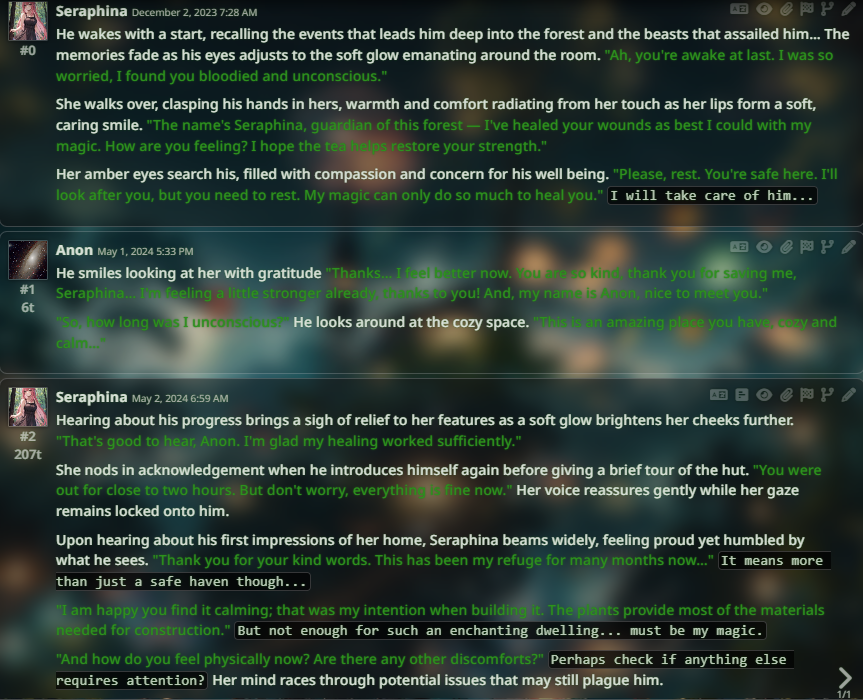 | {"language": ["en"], "license": "apache-2.0", "tags": ["gguf", "llama", "not-for-all-audiences", "nsfw", "rp", "roleplay"], "pipeline_tag": "text-generation"} | Endevor/InfinityRP-v2-8B-GGUF | null | [
"gguf",
"llama",
"not-for-all-audiences",
"nsfw",
"rp",
"roleplay",
"text-generation",
"en",
"license:apache-2.0",
"region:us"
] | null | 2024-05-02T11:44:11+00:00 | [] | [
"en"
] | TAGS
#gguf #llama #not-for-all-audiences #nsfw #rp #roleplay #text-generation #en #license-apache-2.0 #region-us
| !example
The idea is the same as InfinityRP v1, but this one is Llama 3 with 16k ctx! Have fun...
### Prompt format: Alpaca.
''"You are now in roleplay chat mode. Engage in an endless chat, always with a creative response. Follow lengths very precisely and create paragraphs accurately. Always wait your turn, next actions and responses. Your internal thoughts are wrapped with ' marks."''
User Message Prefix = ### Input:
Assistant Message Prefix = ### Response:
System Message Prefix = ### Instruction:
Turn on "Include Names"
### Example:
!example | [
"### Prompt format: Alpaca.\n''\"You are now in roleplay chat mode. Engage in an endless chat, always with a creative response. Follow lengths very precisely and create paragraphs accurately. Always wait your turn, next actions and responses. Your internal thoughts are wrapped with ' marks.\"''\n\nUser Message Prefix = ### Input:\n\nAssistant Message Prefix = ### Response:\n\nSystem Message Prefix = ### Instruction:\n\nTurn on \"Include Names\"",
"### Example:\n\n!example"
] | [
"TAGS\n#gguf #llama #not-for-all-audiences #nsfw #rp #roleplay #text-generation #en #license-apache-2.0 #region-us \n",
"### Prompt format: Alpaca.\n''\"You are now in roleplay chat mode. Engage in an endless chat, always with a creative response. Follow lengths very precisely and create paragraphs accurately. Always wait your turn, next actions and responses. Your internal thoughts are wrapped with ' marks.\"''\n\nUser Message Prefix = ### Input:\n\nAssistant Message Prefix = ### Response:\n\nSystem Message Prefix = ### Instruction:\n\nTurn on \"Include Names\"",
"### Example:\n\n!example"
] | [
44,
99,
7
] | [
"TAGS\n#gguf #llama #not-for-all-audiences #nsfw #rp #roleplay #text-generation #en #license-apache-2.0 #region-us \n### Prompt format: Alpaca.\n''\"You are now in roleplay chat mode. Engage in an endless chat, always with a creative response. Follow lengths very precisely and create paragraphs accurately. Always wait your turn, next actions and responses. Your internal thoughts are wrapped with ' marks.\"''\n\nUser Message Prefix = ### Input:\n\nAssistant Message Prefix = ### Response:\n\nSystem Message Prefix = ### Instruction:\n\nTurn on \"Include Names\"### Example:\n\n!example"
] |
text-generation | transformers |
# Model Card for Model ID
<!-- Provide a quick summary of what the model is/does. -->
## Model Details
### Model Description
<!-- Provide a longer summary of what this model is. -->
This is the model card of a 🤗 transformers model that has been pushed on the Hub. This model card has been automatically generated.
- **Developed by:** [More Information Needed]
- **Funded by [optional]:** [More Information Needed]
- **Shared by [optional]:** [More Information Needed]
- **Model type:** [More Information Needed]
- **Language(s) (NLP):** [More Information Needed]
- **License:** [More Information Needed]
- **Finetuned from model [optional]:** [More Information Needed]
### Model Sources [optional]
<!-- Provide the basic links for the model. -->
- **Repository:** [More Information Needed]
- **Paper [optional]:** [More Information Needed]
- **Demo [optional]:** [More Information Needed]
## Uses
<!-- Address questions around how the model is intended to be used, including the foreseeable users of the model and those affected by the model. -->
### Direct Use
<!-- This section is for the model use without fine-tuning or plugging into a larger ecosystem/app. -->
[More Information Needed]
### Downstream Use [optional]
<!-- This section is for the model use when fine-tuned for a task, or when plugged into a larger ecosystem/app -->
[More Information Needed]
### Out-of-Scope Use
<!-- This section addresses misuse, malicious use, and uses that the model will not work well for. -->
[More Information Needed]
## Bias, Risks, and Limitations
<!-- This section is meant to convey both technical and sociotechnical limitations. -->
[More Information Needed]
### Recommendations
<!-- This section is meant to convey recommendations with respect to the bias, risk, and technical limitations. -->
Users (both direct and downstream) should be made aware of the risks, biases and limitations of the model. More information needed for further recommendations.
## How to Get Started with the Model
Use the code below to get started with the model.
[More Information Needed]
## Training Details
### Training Data
<!-- This should link to a Dataset Card, perhaps with a short stub of information on what the training data is all about as well as documentation related to data pre-processing or additional filtering. -->
[More Information Needed]
### Training Procedure
<!-- This relates heavily to the Technical Specifications. Content here should link to that section when it is relevant to the training procedure. -->
#### Preprocessing [optional]
[More Information Needed]
#### Training Hyperparameters
- **Training regime:** [More Information Needed] <!--fp32, fp16 mixed precision, bf16 mixed precision, bf16 non-mixed precision, fp16 non-mixed precision, fp8 mixed precision -->
#### Speeds, Sizes, Times [optional]
<!-- This section provides information about throughput, start/end time, checkpoint size if relevant, etc. -->
[More Information Needed]
## Evaluation
<!-- This section describes the evaluation protocols and provides the results. -->
### Testing Data, Factors & Metrics
#### Testing Data
<!-- This should link to a Dataset Card if possible. -->
[More Information Needed]
#### Factors
<!-- These are the things the evaluation is disaggregating by, e.g., subpopulations or domains. -->
[More Information Needed]
#### Metrics
<!-- These are the evaluation metrics being used, ideally with a description of why. -->
[More Information Needed]
### Results
[More Information Needed]
#### Summary
## Model Examination [optional]
<!-- Relevant interpretability work for the model goes here -->
[More Information Needed]
## Environmental Impact
<!-- Total emissions (in grams of CO2eq) and additional considerations, such as electricity usage, go here. Edit the suggested text below accordingly -->
Carbon emissions can be estimated using the [Machine Learning Impact calculator](https://mlco2.github.io/impact#compute) presented in [Lacoste et al. (2019)](https://arxiv.org/abs/1910.09700).
- **Hardware Type:** [More Information Needed]
- **Hours used:** [More Information Needed]
- **Cloud Provider:** [More Information Needed]
- **Compute Region:** [More Information Needed]
- **Carbon Emitted:** [More Information Needed]
## Technical Specifications [optional]
### Model Architecture and Objective
[More Information Needed]
### Compute Infrastructure
[More Information Needed]
#### Hardware
[More Information Needed]
#### Software
[More Information Needed]
## Citation [optional]
<!-- If there is a paper or blog post introducing the model, the APA and Bibtex information for that should go in this section. -->
**BibTeX:**
[More Information Needed]
**APA:**
[More Information Needed]
## Glossary [optional]
<!-- If relevant, include terms and calculations in this section that can help readers understand the model or model card. -->
[More Information Needed]
## More Information [optional]
[More Information Needed]
## Model Card Authors [optional]
[More Information Needed]
## Model Card Contact
[More Information Needed]
| {"library_name": "transformers", "tags": []} | miguel-kjh/pythia_14m-adpater-lora-dbpedia | null | [
"transformers",
"safetensors",
"gpt_neox",
"text-generation",
"arxiv:1910.09700",
"autotrain_compatible",
"endpoints_compatible",
"text-generation-inference",
"region:us"
] | null | 2024-05-02T11:46:08+00:00 | [
"1910.09700"
] | [] | TAGS
#transformers #safetensors #gpt_neox #text-generation #arxiv-1910.09700 #autotrain_compatible #endpoints_compatible #text-generation-inference #region-us
|
# Model Card for Model ID
## Model Details
### Model Description
This is the model card of a transformers model that has been pushed on the Hub. This model card has been automatically generated.
- Developed by:
- Funded by [optional]:
- Shared by [optional]:
- Model type:
- Language(s) (NLP):
- License:
- Finetuned from model [optional]:
### Model Sources [optional]
- Repository:
- Paper [optional]:
- Demo [optional]:
## Uses
### Direct Use
### Downstream Use [optional]
### Out-of-Scope Use
## Bias, Risks, and Limitations
### Recommendations
Users (both direct and downstream) should be made aware of the risks, biases and limitations of the model. More information needed for further recommendations.
## How to Get Started with the Model
Use the code below to get started with the model.
## Training Details
### Training Data
### Training Procedure
#### Preprocessing [optional]
#### Training Hyperparameters
- Training regime:
#### Speeds, Sizes, Times [optional]
## Evaluation
### Testing Data, Factors & Metrics
#### Testing Data
#### Factors
#### Metrics
### Results
#### Summary
## Model Examination [optional]
## Environmental Impact
Carbon emissions can be estimated using the Machine Learning Impact calculator presented in Lacoste et al. (2019).
- Hardware Type:
- Hours used:
- Cloud Provider:
- Compute Region:
- Carbon Emitted:
## Technical Specifications [optional]
### Model Architecture and Objective
### Compute Infrastructure
#### Hardware
#### Software
[optional]
BibTeX:
APA:
## Glossary [optional]
## More Information [optional]
## Model Card Authors [optional]
## Model Card Contact
| [
"# Model Card for Model ID",
"## Model Details",
"### Model Description\n\n\n\nThis is the model card of a transformers model that has been pushed on the Hub. This model card has been automatically generated.\n\n- Developed by: \n- Funded by [optional]: \n- Shared by [optional]: \n- Model type: \n- Language(s) (NLP): \n- License: \n- Finetuned from model [optional]:",
"### Model Sources [optional]\n\n\n\n- Repository: \n- Paper [optional]: \n- Demo [optional]:",
"## Uses",
"### Direct Use",
"### Downstream Use [optional]",
"### Out-of-Scope Use",
"## Bias, Risks, and Limitations",
"### Recommendations\n\n\n\nUsers (both direct and downstream) should be made aware of the risks, biases and limitations of the model. More information needed for further recommendations.",
"## How to Get Started with the Model\n\nUse the code below to get started with the model.",
"## Training Details",
"### Training Data",
"### Training Procedure",
"#### Preprocessing [optional]",
"#### Training Hyperparameters\n\n- Training regime:",
"#### Speeds, Sizes, Times [optional]",
"## Evaluation",
"### Testing Data, Factors & Metrics",
"#### Testing Data",
"#### Factors",
"#### Metrics",
"### Results",
"#### Summary",
"## Model Examination [optional]",
"## Environmental Impact\n\n\n\nCarbon emissions can be estimated using the Machine Learning Impact calculator presented in Lacoste et al. (2019).\n\n- Hardware Type: \n- Hours used: \n- Cloud Provider: \n- Compute Region: \n- Carbon Emitted:",
"## Technical Specifications [optional]",
"### Model Architecture and Objective",
"### Compute Infrastructure",
"#### Hardware",
"#### Software\n\n\n\n[optional]\n\n\n\nBibTeX:\n\n\n\nAPA:",
"## Glossary [optional]",
"## More Information [optional]",
"## Model Card Authors [optional]",
"## Model Card Contact"
] | [
"TAGS\n#transformers #safetensors #gpt_neox #text-generation #arxiv-1910.09700 #autotrain_compatible #endpoints_compatible #text-generation-inference #region-us \n",
"# Model Card for Model ID",
"## Model Details",
"### Model Description\n\n\n\nThis is the model card of a transformers model that has been pushed on the Hub. This model card has been automatically generated.\n\n- Developed by: \n- Funded by [optional]: \n- Shared by [optional]: \n- Model type: \n- Language(s) (NLP): \n- License: \n- Finetuned from model [optional]:",
"### Model Sources [optional]\n\n\n\n- Repository: \n- Paper [optional]: \n- Demo [optional]:",
"## Uses",
"### Direct Use",
"### Downstream Use [optional]",
"### Out-of-Scope Use",
"## Bias, Risks, and Limitations",
"### Recommendations\n\n\n\nUsers (both direct and downstream) should be made aware of the risks, biases and limitations of the model. More information needed for further recommendations.",
"## How to Get Started with the Model\n\nUse the code below to get started with the model.",
"## Training Details",
"### Training Data",
"### Training Procedure",
"#### Preprocessing [optional]",
"#### Training Hyperparameters\n\n- Training regime:",
"#### Speeds, Sizes, Times [optional]",
"## Evaluation",
"### Testing Data, Factors & Metrics",
"#### Testing Data",
"#### Factors",
"#### Metrics",
"### Results",
"#### Summary",
"## Model Examination [optional]",
"## Environmental Impact\n\n\n\nCarbon emissions can be estimated using the Machine Learning Impact calculator presented in Lacoste et al. (2019).\n\n- Hardware Type: \n- Hours used: \n- Cloud Provider: \n- Compute Region: \n- Carbon Emitted:",
"## Technical Specifications [optional]",
"### Model Architecture and Objective",
"### Compute Infrastructure",
"#### Hardware",
"#### Software\n\n\n\n[optional]\n\n\n\nBibTeX:\n\n\n\nAPA:",
"## Glossary [optional]",
"## More Information [optional]",
"## Model Card Authors [optional]",
"## Model Card Contact"
] | [
47,
6,
4,
75,
23,
3,
5,
8,
9,
8,
34,
20,
4,
5,
5,
11,
13,
12,
3,
10,
6,
5,
6,
4,
5,
7,
49,
7,
7,
5,
5,
15,
7,
7,
8,
5
] | [
"TAGS\n#transformers #safetensors #gpt_neox #text-generation #arxiv-1910.09700 #autotrain_compatible #endpoints_compatible #text-generation-inference #region-us \n# Model Card for Model ID## Model Details### Model Description\n\n\n\nThis is the model card of a transformers model that has been pushed on the Hub. This model card has been automatically generated.\n\n- Developed by: \n- Funded by [optional]: \n- Shared by [optional]: \n- Model type: \n- Language(s) (NLP): \n- License: \n- Finetuned from model [optional]:### Model Sources [optional]\n\n\n\n- Repository: \n- Paper [optional]: \n- Demo [optional]:## Uses### Direct Use### Downstream Use [optional]### Out-of-Scope Use## Bias, Risks, and Limitations### Recommendations\n\n\n\nUsers (both direct and downstream) should be made aware of the risks, biases and limitations of the model. More information needed for further recommendations.## How to Get Started with the Model\n\nUse the code below to get started with the model.## Training Details### Training Data### Training Procedure#### Preprocessing [optional]#### Training Hyperparameters\n\n- Training regime:#### Speeds, Sizes, Times [optional]## Evaluation### Testing Data, Factors & Metrics#### Testing Data#### Factors#### Metrics### Results#### Summary## Model Examination [optional]## Environmental Impact\n\n\n\nCarbon emissions can be estimated using the Machine Learning Impact calculator presented in Lacoste et al. (2019).\n\n- Hardware Type: \n- Hours used: \n- Cloud Provider: \n- Compute Region: \n- Carbon Emitted:## Technical Specifications [optional]### Model Architecture and Objective### Compute Infrastructure#### Hardware#### Software\n\n\n\n[optional]\n\n\n\nBibTeX:\n\n\n\nAPA:## Glossary [optional]## More Information [optional]## Model Card Authors [optional]## Model Card Contact"
] |
text-generation | transformers | # llama-3-wissenschaft-8B
This model is based on Llama-3-8b, and is governed by [META LLAMA 3 COMMUNITY LICENSE AGREEMENT](LICENSE)
This is a merge of pre-trained language models created using [mergekit](https://github.com/cg123/mergekit).
## Merge Details
### Merge Method
This model was merged using the [Model Stock](https://arxiv.org/abs/2403.19522) merge method using [nbeerbower/llama-3-slerp-kraut-dragon-8B](https://huggingface.co/nbeerbower/llama-3-slerp-kraut-dragon-8B) as a base.
### Models Merged
The following models were included in the merge:
* [VAGOsolutions/Llama-3-SauerkrautLM-8b-Instruct](https://huggingface.co/VAGOsolutions/Llama-3-SauerkrautLM-8b-Instruct)
* [DeepMount00/Llama-3-8b-Ita](https://huggingface.co/DeepMount00/Llama-3-8b-Ita)
* [mlabonne/ChimeraLlama-3-8B-v3](https://huggingface.co/mlabonne/ChimeraLlama-3-8B-v3)
### Configuration
The following YAML configuration was used to produce this model:
```yaml
models:
- model: VAGOsolutions/Llama-3-SauerkrautLM-8b-Instruct
- model: DeepMount00/Llama-3-8b-Ita
- model: mlabonne/ChimeraLlama-3-8B-v3
merge_method: model_stock
base_model: nbeerbower/llama-3-slerp-kraut-dragon-8B
dtype: bfloat16
```
| {"license": "other", "library_name": "transformers", "tags": ["mergekit", "merge"], "base_model": ["VAGOsolutions/Llama-3-SauerkrautLM-8b-Instruct", "DeepMount00/Llama-3-8b-Ita", "mlabonne/ChimeraLlama-3-8B-v3", "nbeerbower/llama-3-slerp-kraut-dragon-8B"], "license_name": "llama3"} | nbeerbower/llama-3-wissenschaft-8B | null | [
"transformers",
"safetensors",
"llama",
"text-generation",
"mergekit",
"merge",
"conversational",
"arxiv:2403.19522",
"base_model:VAGOsolutions/Llama-3-SauerkrautLM-8b-Instruct",
"base_model:DeepMount00/Llama-3-8b-Ita",
"base_model:mlabonne/ChimeraLlama-3-8B-v3",
"base_model:nbeerbower/llama-3-slerp-kraut-dragon-8B",
"license:other",
"autotrain_compatible",
"endpoints_compatible",
"text-generation-inference",
"region:us"
] | null | 2024-05-02T11:46:15+00:00 | [
"2403.19522"
] | [] | TAGS
#transformers #safetensors #llama #text-generation #mergekit #merge #conversational #arxiv-2403.19522 #base_model-VAGOsolutions/Llama-3-SauerkrautLM-8b-Instruct #base_model-DeepMount00/Llama-3-8b-Ita #base_model-mlabonne/ChimeraLlama-3-8B-v3 #base_model-nbeerbower/llama-3-slerp-kraut-dragon-8B #license-other #autotrain_compatible #endpoints_compatible #text-generation-inference #region-us
| # llama-3-wissenschaft-8B
This model is based on Llama-3-8b, and is governed by META LLAMA 3 COMMUNITY LICENSE AGREEMENT
This is a merge of pre-trained language models created using mergekit.
## Merge Details
### Merge Method
This model was merged using the Model Stock merge method using nbeerbower/llama-3-slerp-kraut-dragon-8B as a base.
### Models Merged
The following models were included in the merge:
* VAGOsolutions/Llama-3-SauerkrautLM-8b-Instruct
* DeepMount00/Llama-3-8b-Ita
* mlabonne/ChimeraLlama-3-8B-v3
### Configuration
The following YAML configuration was used to produce this model:
| [
"# llama-3-wissenschaft-8B\n\nThis model is based on Llama-3-8b, and is governed by META LLAMA 3 COMMUNITY LICENSE AGREEMENT\n\nThis is a merge of pre-trained language models created using mergekit.",
"## Merge Details",
"### Merge Method\n\nThis model was merged using the Model Stock merge method using nbeerbower/llama-3-slerp-kraut-dragon-8B as a base.",
"### Models Merged\n\nThe following models were included in the merge:\n* VAGOsolutions/Llama-3-SauerkrautLM-8b-Instruct\n* DeepMount00/Llama-3-8b-Ita\n* mlabonne/ChimeraLlama-3-8B-v3",
"### Configuration\n\nThe following YAML configuration was used to produce this model:"
] | [
"TAGS\n#transformers #safetensors #llama #text-generation #mergekit #merge #conversational #arxiv-2403.19522 #base_model-VAGOsolutions/Llama-3-SauerkrautLM-8b-Instruct #base_model-DeepMount00/Llama-3-8b-Ita #base_model-mlabonne/ChimeraLlama-3-8B-v3 #base_model-nbeerbower/llama-3-slerp-kraut-dragon-8B #license-other #autotrain_compatible #endpoints_compatible #text-generation-inference #region-us \n",
"# llama-3-wissenschaft-8B\n\nThis model is based on Llama-3-8b, and is governed by META LLAMA 3 COMMUNITY LICENSE AGREEMENT\n\nThis is a merge of pre-trained language models created using mergekit.",
"## Merge Details",
"### Merge Method\n\nThis model was merged using the Model Stock merge method using nbeerbower/llama-3-slerp-kraut-dragon-8B as a base.",
"### Models Merged\n\nThe following models were included in the merge:\n* VAGOsolutions/Llama-3-SauerkrautLM-8b-Instruct\n* DeepMount00/Llama-3-8b-Ita\n* mlabonne/ChimeraLlama-3-8B-v3",
"### Configuration\n\nThe following YAML configuration was used to produce this model:"
] | [
150,
51,
4,
42,
69,
16
] | [
"TAGS\n#transformers #safetensors #llama #text-generation #mergekit #merge #conversational #arxiv-2403.19522 #base_model-VAGOsolutions/Llama-3-SauerkrautLM-8b-Instruct #base_model-DeepMount00/Llama-3-8b-Ita #base_model-mlabonne/ChimeraLlama-3-8B-v3 #base_model-nbeerbower/llama-3-slerp-kraut-dragon-8B #license-other #autotrain_compatible #endpoints_compatible #text-generation-inference #region-us \n# llama-3-wissenschaft-8B\n\nThis model is based on Llama-3-8b, and is governed by META LLAMA 3 COMMUNITY LICENSE AGREEMENT\n\nThis is a merge of pre-trained language models created using mergekit.## Merge Details### Merge Method\n\nThis model was merged using the Model Stock merge method using nbeerbower/llama-3-slerp-kraut-dragon-8B as a base.### Models Merged\n\nThe following models were included in the merge:\n* VAGOsolutions/Llama-3-SauerkrautLM-8b-Instruct\n* DeepMount00/Llama-3-8b-Ita\n* mlabonne/ChimeraLlama-3-8B-v3### Configuration\n\nThe following YAML configuration was used to produce this model:"
] |
text-generation | transformers |
# Model Card for Model ID
<!-- Provide a quick summary of what the model is/does. -->
## Model Details
### Model Description
<!-- Provide a longer summary of what this model is. -->
This is the model card of a 🤗 transformers model that has been pushed on the Hub. This model card has been automatically generated.
- **Developed by:** [More Information Needed]
- **Funded by [optional]:** [More Information Needed]
- **Shared by [optional]:** [More Information Needed]
- **Model type:** [More Information Needed]
- **Language(s) (NLP):** [More Information Needed]
- **License:** [More Information Needed]
- **Finetuned from model [optional]:** [More Information Needed]
### Model Sources [optional]
<!-- Provide the basic links for the model. -->
- **Repository:** [More Information Needed]
- **Paper [optional]:** [More Information Needed]
- **Demo [optional]:** [More Information Needed]
## Uses
<!-- Address questions around how the model is intended to be used, including the foreseeable users of the model and those affected by the model. -->
### Direct Use
<!-- This section is for the model use without fine-tuning or plugging into a larger ecosystem/app. -->
[More Information Needed]
### Downstream Use [optional]
<!-- This section is for the model use when fine-tuned for a task, or when plugged into a larger ecosystem/app -->
[More Information Needed]
### Out-of-Scope Use
<!-- This section addresses misuse, malicious use, and uses that the model will not work well for. -->
[More Information Needed]
## Bias, Risks, and Limitations
<!-- This section is meant to convey both technical and sociotechnical limitations. -->
[More Information Needed]
### Recommendations
<!-- This section is meant to convey recommendations with respect to the bias, risk, and technical limitations. -->
Users (both direct and downstream) should be made aware of the risks, biases and limitations of the model. More information needed for further recommendations.
## How to Get Started with the Model
Use the code below to get started with the model.
[More Information Needed]
## Training Details
### Training Data
<!-- This should link to a Dataset Card, perhaps with a short stub of information on what the training data is all about as well as documentation related to data pre-processing or additional filtering. -->
[More Information Needed]
### Training Procedure
<!-- This relates heavily to the Technical Specifications. Content here should link to that section when it is relevant to the training procedure. -->
#### Preprocessing [optional]
[More Information Needed]
#### Training Hyperparameters
- **Training regime:** [More Information Needed] <!--fp32, fp16 mixed precision, bf16 mixed precision, bf16 non-mixed precision, fp16 non-mixed precision, fp8 mixed precision -->
#### Speeds, Sizes, Times [optional]
<!-- This section provides information about throughput, start/end time, checkpoint size if relevant, etc. -->
[More Information Needed]
## Evaluation
<!-- This section describes the evaluation protocols and provides the results. -->
### Testing Data, Factors & Metrics
#### Testing Data
<!-- This should link to a Dataset Card if possible. -->
[More Information Needed]
#### Factors
<!-- These are the things the evaluation is disaggregating by, e.g., subpopulations or domains. -->
[More Information Needed]
#### Metrics
<!-- These are the evaluation metrics being used, ideally with a description of why. -->
[More Information Needed]
### Results
[More Information Needed]
#### Summary
## Model Examination [optional]
<!-- Relevant interpretability work for the model goes here -->
[More Information Needed]
## Environmental Impact
<!-- Total emissions (in grams of CO2eq) and additional considerations, such as electricity usage, go here. Edit the suggested text below accordingly -->
Carbon emissions can be estimated using the [Machine Learning Impact calculator](https://mlco2.github.io/impact#compute) presented in [Lacoste et al. (2019)](https://arxiv.org/abs/1910.09700).
- **Hardware Type:** [More Information Needed]
- **Hours used:** [More Information Needed]
- **Cloud Provider:** [More Information Needed]
- **Compute Region:** [More Information Needed]
- **Carbon Emitted:** [More Information Needed]
## Technical Specifications [optional]
### Model Architecture and Objective
[More Information Needed]
### Compute Infrastructure
[More Information Needed]
#### Hardware
[More Information Needed]
#### Software
[More Information Needed]
## Citation [optional]
<!-- If there is a paper or blog post introducing the model, the APA and Bibtex information for that should go in this section. -->
**BibTeX:**
[More Information Needed]
**APA:**
[More Information Needed]
## Glossary [optional]
<!-- If relevant, include terms and calculations in this section that can help readers understand the model or model card. -->
[More Information Needed]
## More Information [optional]
[More Information Needed]
## Model Card Authors [optional]
[More Information Needed]
## Model Card Contact
[More Information Needed]
| {"library_name": "transformers", "tags": []} | miguel-kjh/pythia_70m-adpater-lora-dbpedia | null | [
"transformers",
"safetensors",
"gpt_neox",
"text-generation",
"arxiv:1910.09700",
"autotrain_compatible",
"endpoints_compatible",
"text-generation-inference",
"region:us"
] | null | 2024-05-02T11:46:30+00:00 | [
"1910.09700"
] | [] | TAGS
#transformers #safetensors #gpt_neox #text-generation #arxiv-1910.09700 #autotrain_compatible #endpoints_compatible #text-generation-inference #region-us
|
# Model Card for Model ID
## Model Details
### Model Description
This is the model card of a transformers model that has been pushed on the Hub. This model card has been automatically generated.
- Developed by:
- Funded by [optional]:
- Shared by [optional]:
- Model type:
- Language(s) (NLP):
- License:
- Finetuned from model [optional]:
### Model Sources [optional]
- Repository:
- Paper [optional]:
- Demo [optional]:
## Uses
### Direct Use
### Downstream Use [optional]
### Out-of-Scope Use
## Bias, Risks, and Limitations
### Recommendations
Users (both direct and downstream) should be made aware of the risks, biases and limitations of the model. More information needed for further recommendations.
## How to Get Started with the Model
Use the code below to get started with the model.
## Training Details
### Training Data
### Training Procedure
#### Preprocessing [optional]
#### Training Hyperparameters
- Training regime:
#### Speeds, Sizes, Times [optional]
## Evaluation
### Testing Data, Factors & Metrics
#### Testing Data
#### Factors
#### Metrics
### Results
#### Summary
## Model Examination [optional]
## Environmental Impact
Carbon emissions can be estimated using the Machine Learning Impact calculator presented in Lacoste et al. (2019).
- Hardware Type:
- Hours used:
- Cloud Provider:
- Compute Region:
- Carbon Emitted:
## Technical Specifications [optional]
### Model Architecture and Objective
### Compute Infrastructure
#### Hardware
#### Software
[optional]
BibTeX:
APA:
## Glossary [optional]
## More Information [optional]
## Model Card Authors [optional]
## Model Card Contact
| [
"# Model Card for Model ID",
"## Model Details",
"### Model Description\n\n\n\nThis is the model card of a transformers model that has been pushed on the Hub. This model card has been automatically generated.\n\n- Developed by: \n- Funded by [optional]: \n- Shared by [optional]: \n- Model type: \n- Language(s) (NLP): \n- License: \n- Finetuned from model [optional]:",
"### Model Sources [optional]\n\n\n\n- Repository: \n- Paper [optional]: \n- Demo [optional]:",
"## Uses",
"### Direct Use",
"### Downstream Use [optional]",
"### Out-of-Scope Use",
"## Bias, Risks, and Limitations",
"### Recommendations\n\n\n\nUsers (both direct and downstream) should be made aware of the risks, biases and limitations of the model. More information needed for further recommendations.",
"## How to Get Started with the Model\n\nUse the code below to get started with the model.",
"## Training Details",
"### Training Data",
"### Training Procedure",
"#### Preprocessing [optional]",
"#### Training Hyperparameters\n\n- Training regime:",
"#### Speeds, Sizes, Times [optional]",
"## Evaluation",
"### Testing Data, Factors & Metrics",
"#### Testing Data",
"#### Factors",
"#### Metrics",
"### Results",
"#### Summary",
"## Model Examination [optional]",
"## Environmental Impact\n\n\n\nCarbon emissions can be estimated using the Machine Learning Impact calculator presented in Lacoste et al. (2019).\n\n- Hardware Type: \n- Hours used: \n- Cloud Provider: \n- Compute Region: \n- Carbon Emitted:",
"## Technical Specifications [optional]",
"### Model Architecture and Objective",
"### Compute Infrastructure",
"#### Hardware",
"#### Software\n\n\n\n[optional]\n\n\n\nBibTeX:\n\n\n\nAPA:",
"## Glossary [optional]",
"## More Information [optional]",
"## Model Card Authors [optional]",
"## Model Card Contact"
] | [
"TAGS\n#transformers #safetensors #gpt_neox #text-generation #arxiv-1910.09700 #autotrain_compatible #endpoints_compatible #text-generation-inference #region-us \n",
"# Model Card for Model ID",
"## Model Details",
"### Model Description\n\n\n\nThis is the model card of a transformers model that has been pushed on the Hub. This model card has been automatically generated.\n\n- Developed by: \n- Funded by [optional]: \n- Shared by [optional]: \n- Model type: \n- Language(s) (NLP): \n- License: \n- Finetuned from model [optional]:",
"### Model Sources [optional]\n\n\n\n- Repository: \n- Paper [optional]: \n- Demo [optional]:",
"## Uses",
"### Direct Use",
"### Downstream Use [optional]",
"### Out-of-Scope Use",
"## Bias, Risks, and Limitations",
"### Recommendations\n\n\n\nUsers (both direct and downstream) should be made aware of the risks, biases and limitations of the model. More information needed for further recommendations.",
"## How to Get Started with the Model\n\nUse the code below to get started with the model.",
"## Training Details",
"### Training Data",
"### Training Procedure",
"#### Preprocessing [optional]",
"#### Training Hyperparameters\n\n- Training regime:",
"#### Speeds, Sizes, Times [optional]",
"## Evaluation",
"### Testing Data, Factors & Metrics",
"#### Testing Data",
"#### Factors",
"#### Metrics",
"### Results",
"#### Summary",
"## Model Examination [optional]",
"## Environmental Impact\n\n\n\nCarbon emissions can be estimated using the Machine Learning Impact calculator presented in Lacoste et al. (2019).\n\n- Hardware Type: \n- Hours used: \n- Cloud Provider: \n- Compute Region: \n- Carbon Emitted:",
"## Technical Specifications [optional]",
"### Model Architecture and Objective",
"### Compute Infrastructure",
"#### Hardware",
"#### Software\n\n\n\n[optional]\n\n\n\nBibTeX:\n\n\n\nAPA:",
"## Glossary [optional]",
"## More Information [optional]",
"## Model Card Authors [optional]",
"## Model Card Contact"
] | [
47,
6,
4,
75,
23,
3,
5,
8,
9,
8,
34,
20,
4,
5,
5,
11,
13,
12,
3,
10,
6,
5,
6,
4,
5,
7,
49,
7,
7,
5,
5,
15,
7,
7,
8,
5
] | [
"TAGS\n#transformers #safetensors #gpt_neox #text-generation #arxiv-1910.09700 #autotrain_compatible #endpoints_compatible #text-generation-inference #region-us \n# Model Card for Model ID## Model Details### Model Description\n\n\n\nThis is the model card of a transformers model that has been pushed on the Hub. This model card has been automatically generated.\n\n- Developed by: \n- Funded by [optional]: \n- Shared by [optional]: \n- Model type: \n- Language(s) (NLP): \n- License: \n- Finetuned from model [optional]:### Model Sources [optional]\n\n\n\n- Repository: \n- Paper [optional]: \n- Demo [optional]:## Uses### Direct Use### Downstream Use [optional]### Out-of-Scope Use## Bias, Risks, and Limitations### Recommendations\n\n\n\nUsers (both direct and downstream) should be made aware of the risks, biases and limitations of the model. More information needed for further recommendations.## How to Get Started with the Model\n\nUse the code below to get started with the model.## Training Details### Training Data### Training Procedure#### Preprocessing [optional]#### Training Hyperparameters\n\n- Training regime:#### Speeds, Sizes, Times [optional]## Evaluation### Testing Data, Factors & Metrics#### Testing Data#### Factors#### Metrics### Results#### Summary## Model Examination [optional]## Environmental Impact\n\n\n\nCarbon emissions can be estimated using the Machine Learning Impact calculator presented in Lacoste et al. (2019).\n\n- Hardware Type: \n- Hours used: \n- Cloud Provider: \n- Compute Region: \n- Carbon Emitted:## Technical Specifications [optional]### Model Architecture and Objective### Compute Infrastructure#### Hardware#### Software\n\n\n\n[optional]\n\n\n\nBibTeX:\n\n\n\nAPA:## Glossary [optional]## More Information [optional]## Model Card Authors [optional]## Model Card Contact"
] |
text-generation | null | # [MaziyarPanahi/Llama-3-70B-Instruct-DPO-v0.4-GGUF](https://huggingface.co/MaziyarPanahi/Llama-3-70B-Instruct-DPO-v0.4-GGUF)
- Model creator: [MaziyarPanahi](https://huggingface.co/MaziyarPanahi)
- Original model: [MaziyarPanahi/Llama-3-70B-Instruct-DPO-v0.4](https://huggingface.co/MaziyarPanahi/Llama-3-70B-Instruct-DPO-v0.4)
## Description
[MaziyarPanahi/Llama-3-70B-Instruct-DPO-v0.4-GGUF](https://huggingface.co/MaziyarPanahi/Llama-3-70B-Instruct-DPO-v0.4-GGUF) contains GGUF format model files for [MaziyarPanahi/Llama-3-70B-Instruct-DPO-v0.4](https://huggingface.co/MaziyarPanahi/Llama-3-70B-Instruct-DPO-v0.4).
IMPORTANT: There is no need to merge the splits. By now, most libraries support automatically loading the splits by simply pointing to the first one.
### About GGUF
GGUF is a new format introduced by the llama.cpp team on August 21st 2023. It is a replacement for GGML, which is no longer supported by llama.cpp.
Here is an incomplete list of clients and libraries that are known to support GGUF:
* [llama.cpp](https://github.com/ggerganov/llama.cpp). The source project for GGUF. Offers a CLI and a server option.
* [llama-cpp-python](https://github.com/abetlen/llama-cpp-python), a Python library with GPU accel, LangChain support, and OpenAI-compatible API server.
* [LM Studio](https://lmstudio.ai/), an easy-to-use and powerful local GUI for Windows and macOS (Silicon), with GPU acceleration. Linux available, in beta as of 27/11/2023.
* [text-generation-webui](https://github.com/oobabooga/text-generation-webui), the most widely used web UI, with many features and powerful extensions. Supports GPU acceleration.
* [KoboldCpp](https://github.com/LostRuins/koboldcpp), a fully featured web UI, with GPU accel across all platforms and GPU architectures. Especially good for story telling.
* [GPT4All](https://gpt4all.io/index.html), a free and open source local running GUI, supporting Windows, Linux and macOS with full GPU accel.
* [LoLLMS Web UI](https://github.com/ParisNeo/lollms-webui), a great web UI with many interesting and unique features, including a full model library for easy model selection.
* [Faraday.dev](https://faraday.dev/), an attractive and easy to use character-based chat GUI for Windows and macOS (both Silicon and Intel), with GPU acceleration.
* [candle](https://github.com/huggingface/candle), a Rust ML framework with a focus on performance, including GPU support, and ease of use.
* [ctransformers](https://github.com/marella/ctransformers), a Python library with GPU accel, LangChain support, and OpenAI-compatible AI server. Note, as of time of writing (November 27th 2023), ctransformers has not been updated in a long time and does not support many recent models.
## Special thanks
🙏 Special thanks to [Georgi Gerganov](https://github.com/ggerganov) and the whole team working on [llama.cpp](https://github.com/ggerganov/llama.cpp/) for making all of this possible. | {"tags": ["quantized", "2-bit", "3-bit", "4-bit", "5-bit", "6-bit", "8-bit", "GGUF", "text-generation", "llama", "llama-3", "text-generation"], "model_name": "Llama-3-70B-Instruct-DPO-v0.4-GGUF", "base_model": "MaziyarPanahi/Llama-3-70B-Instruct-DPO-v0.4", "inference": false, "model_creator": "MaziyarPanahi", "pipeline_tag": "text-generation", "quantized_by": "MaziyarPanahi"} | MaziyarPanahi/Llama-3-70B-Instruct-DPO-v0.4-GGUF | null | [
"gguf",
"quantized",
"2-bit",
"3-bit",
"4-bit",
"5-bit",
"6-bit",
"8-bit",
"GGUF",
"text-generation",
"llama",
"llama-3",
"base_model:MaziyarPanahi/Llama-3-70B-Instruct-DPO-v0.4",
"region:us"
] | null | 2024-05-02T11:47:43+00:00 | [] | [] | TAGS
#gguf #quantized #2-bit #3-bit #4-bit #5-bit #6-bit #8-bit #GGUF #text-generation #llama #llama-3 #base_model-MaziyarPanahi/Llama-3-70B-Instruct-DPO-v0.4 #region-us
| # MaziyarPanahi/Llama-3-70B-Instruct-DPO-v0.4-GGUF
- Model creator: MaziyarPanahi
- Original model: MaziyarPanahi/Llama-3-70B-Instruct-DPO-v0.4
## Description
MaziyarPanahi/Llama-3-70B-Instruct-DPO-v0.4-GGUF contains GGUF format model files for MaziyarPanahi/Llama-3-70B-Instruct-DPO-v0.4.
IMPORTANT: There is no need to merge the splits. By now, most libraries support automatically loading the splits by simply pointing to the first one.
### About GGUF
GGUF is a new format introduced by the URL team on August 21st 2023. It is a replacement for GGML, which is no longer supported by URL.
Here is an incomplete list of clients and libraries that are known to support GGUF:
* URL. The source project for GGUF. Offers a CLI and a server option.
* llama-cpp-python, a Python library with GPU accel, LangChain support, and OpenAI-compatible API server.
* LM Studio, an easy-to-use and powerful local GUI for Windows and macOS (Silicon), with GPU acceleration. Linux available, in beta as of 27/11/2023.
* text-generation-webui, the most widely used web UI, with many features and powerful extensions. Supports GPU acceleration.
* KoboldCpp, a fully featured web UI, with GPU accel across all platforms and GPU architectures. Especially good for story telling.
* GPT4All, a free and open source local running GUI, supporting Windows, Linux and macOS with full GPU accel.
* LoLLMS Web UI, a great web UI with many interesting and unique features, including a full model library for easy model selection.
* URL, an attractive and easy to use character-based chat GUI for Windows and macOS (both Silicon and Intel), with GPU acceleration.
* candle, a Rust ML framework with a focus on performance, including GPU support, and ease of use.
* ctransformers, a Python library with GPU accel, LangChain support, and OpenAI-compatible AI server. Note, as of time of writing (November 27th 2023), ctransformers has not been updated in a long time and does not support many recent models.
## Special thanks
Special thanks to Georgi Gerganov and the whole team working on URL for making all of this possible. | [
"# MaziyarPanahi/Llama-3-70B-Instruct-DPO-v0.4-GGUF\n- Model creator: MaziyarPanahi\n- Original model: MaziyarPanahi/Llama-3-70B-Instruct-DPO-v0.4",
"## Description\nMaziyarPanahi/Llama-3-70B-Instruct-DPO-v0.4-GGUF contains GGUF format model files for MaziyarPanahi/Llama-3-70B-Instruct-DPO-v0.4.\n\nIMPORTANT: There is no need to merge the splits. By now, most libraries support automatically loading the splits by simply pointing to the first one.",
"### About GGUF\n\nGGUF is a new format introduced by the URL team on August 21st 2023. It is a replacement for GGML, which is no longer supported by URL.\n\nHere is an incomplete list of clients and libraries that are known to support GGUF:\n\n* URL. The source project for GGUF. Offers a CLI and a server option.\n* llama-cpp-python, a Python library with GPU accel, LangChain support, and OpenAI-compatible API server.\n* LM Studio, an easy-to-use and powerful local GUI for Windows and macOS (Silicon), with GPU acceleration. Linux available, in beta as of 27/11/2023.\n* text-generation-webui, the most widely used web UI, with many features and powerful extensions. Supports GPU acceleration.\n* KoboldCpp, a fully featured web UI, with GPU accel across all platforms and GPU architectures. Especially good for story telling.\n* GPT4All, a free and open source local running GUI, supporting Windows, Linux and macOS with full GPU accel.\n* LoLLMS Web UI, a great web UI with many interesting and unique features, including a full model library for easy model selection.\n* URL, an attractive and easy to use character-based chat GUI for Windows and macOS (both Silicon and Intel), with GPU acceleration.\n* candle, a Rust ML framework with a focus on performance, including GPU support, and ease of use.\n* ctransformers, a Python library with GPU accel, LangChain support, and OpenAI-compatible AI server. Note, as of time of writing (November 27th 2023), ctransformers has not been updated in a long time and does not support many recent models.",
"## Special thanks\n\n Special thanks to Georgi Gerganov and the whole team working on URL for making all of this possible."
] | [
"TAGS\n#gguf #quantized #2-bit #3-bit #4-bit #5-bit #6-bit #8-bit #GGUF #text-generation #llama #llama-3 #base_model-MaziyarPanahi/Llama-3-70B-Instruct-DPO-v0.4 #region-us \n",
"# MaziyarPanahi/Llama-3-70B-Instruct-DPO-v0.4-GGUF\n- Model creator: MaziyarPanahi\n- Original model: MaziyarPanahi/Llama-3-70B-Instruct-DPO-v0.4",
"## Description\nMaziyarPanahi/Llama-3-70B-Instruct-DPO-v0.4-GGUF contains GGUF format model files for MaziyarPanahi/Llama-3-70B-Instruct-DPO-v0.4.\n\nIMPORTANT: There is no need to merge the splits. By now, most libraries support automatically loading the splits by simply pointing to the first one.",
"### About GGUF\n\nGGUF is a new format introduced by the URL team on August 21st 2023. It is a replacement for GGML, which is no longer supported by URL.\n\nHere is an incomplete list of clients and libraries that are known to support GGUF:\n\n* URL. The source project for GGUF. Offers a CLI and a server option.\n* llama-cpp-python, a Python library with GPU accel, LangChain support, and OpenAI-compatible API server.\n* LM Studio, an easy-to-use and powerful local GUI for Windows and macOS (Silicon), with GPU acceleration. Linux available, in beta as of 27/11/2023.\n* text-generation-webui, the most widely used web UI, with many features and powerful extensions. Supports GPU acceleration.\n* KoboldCpp, a fully featured web UI, with GPU accel across all platforms and GPU architectures. Especially good for story telling.\n* GPT4All, a free and open source local running GUI, supporting Windows, Linux and macOS with full GPU accel.\n* LoLLMS Web UI, a great web UI with many interesting and unique features, including a full model library for easy model selection.\n* URL, an attractive and easy to use character-based chat GUI for Windows and macOS (both Silicon and Intel), with GPU acceleration.\n* candle, a Rust ML framework with a focus on performance, including GPU support, and ease of use.\n* ctransformers, a Python library with GPU accel, LangChain support, and OpenAI-compatible AI server. Note, as of time of writing (November 27th 2023), ctransformers has not been updated in a long time and does not support many recent models.",
"## Special thanks\n\n Special thanks to Georgi Gerganov and the whole team working on URL for making all of this possible."
] | [
82,
69,
95,
392,
27
] | [
"TAGS\n#gguf #quantized #2-bit #3-bit #4-bit #5-bit #6-bit #8-bit #GGUF #text-generation #llama #llama-3 #base_model-MaziyarPanahi/Llama-3-70B-Instruct-DPO-v0.4 #region-us \n# MaziyarPanahi/Llama-3-70B-Instruct-DPO-v0.4-GGUF\n- Model creator: MaziyarPanahi\n- Original model: MaziyarPanahi/Llama-3-70B-Instruct-DPO-v0.4## Description\nMaziyarPanahi/Llama-3-70B-Instruct-DPO-v0.4-GGUF contains GGUF format model files for MaziyarPanahi/Llama-3-70B-Instruct-DPO-v0.4.\n\nIMPORTANT: There is no need to merge the splits. By now, most libraries support automatically loading the splits by simply pointing to the first one.### About GGUF\n\nGGUF is a new format introduced by the URL team on August 21st 2023. It is a replacement for GGML, which is no longer supported by URL.\n\nHere is an incomplete list of clients and libraries that are known to support GGUF:\n\n* URL. The source project for GGUF. Offers a CLI and a server option.\n* llama-cpp-python, a Python library with GPU accel, LangChain support, and OpenAI-compatible API server.\n* LM Studio, an easy-to-use and powerful local GUI for Windows and macOS (Silicon), with GPU acceleration. Linux available, in beta as of 27/11/2023.\n* text-generation-webui, the most widely used web UI, with many features and powerful extensions. Supports GPU acceleration.\n* KoboldCpp, a fully featured web UI, with GPU accel across all platforms and GPU architectures. Especially good for story telling.\n* GPT4All, a free and open source local running GUI, supporting Windows, Linux and macOS with full GPU accel.\n* LoLLMS Web UI, a great web UI with many interesting and unique features, including a full model library for easy model selection.\n* URL, an attractive and easy to use character-based chat GUI for Windows and macOS (both Silicon and Intel), with GPU acceleration.\n* candle, a Rust ML framework with a focus on performance, including GPU support, and ease of use.\n* ctransformers, a Python library with GPU accel, LangChain support, and OpenAI-compatible AI server. Note, as of time of writing (November 27th 2023), ctransformers has not been updated in a long time and does not support many recent models.## Special thanks\n\n Special thanks to Georgi Gerganov and the whole team working on URL for making all of this possible."
] |
text-generation | transformers |
# Model Card for Model ID
<!-- Provide a quick summary of what the model is/does. -->
## Model Details
### Model Description
<!-- Provide a longer summary of what this model is. -->
This is the model card of a 🤗 transformers model that has been pushed on the Hub. This model card has been automatically generated.
- **Developed by:** [More Information Needed]
- **Funded by [optional]:** [More Information Needed]
- **Shared by [optional]:** [More Information Needed]
- **Model type:** [More Information Needed]
- **Language(s) (NLP):** [More Information Needed]
- **License:** [More Information Needed]
- **Finetuned from model [optional]:** [More Information Needed]
### Model Sources [optional]
<!-- Provide the basic links for the model. -->
- **Repository:** [More Information Needed]
- **Paper [optional]:** [More Information Needed]
- **Demo [optional]:** [More Information Needed]
## Uses
<!-- Address questions around how the model is intended to be used, including the foreseeable users of the model and those affected by the model. -->
### Direct Use
<!-- This section is for the model use without fine-tuning or plugging into a larger ecosystem/app. -->
[More Information Needed]
### Downstream Use [optional]
<!-- This section is for the model use when fine-tuned for a task, or when plugged into a larger ecosystem/app -->
[More Information Needed]
### Out-of-Scope Use
<!-- This section addresses misuse, malicious use, and uses that the model will not work well for. -->
[More Information Needed]
## Bias, Risks, and Limitations
<!-- This section is meant to convey both technical and sociotechnical limitations. -->
[More Information Needed]
### Recommendations
<!-- This section is meant to convey recommendations with respect to the bias, risk, and technical limitations. -->
Users (both direct and downstream) should be made aware of the risks, biases and limitations of the model. More information needed for further recommendations.
## How to Get Started with the Model
Use the code below to get started with the model.
[More Information Needed]
## Training Details
### Training Data
<!-- This should link to a Dataset Card, perhaps with a short stub of information on what the training data is all about as well as documentation related to data pre-processing or additional filtering. -->
[More Information Needed]
### Training Procedure
<!-- This relates heavily to the Technical Specifications. Content here should link to that section when it is relevant to the training procedure. -->
#### Preprocessing [optional]
[More Information Needed]
#### Training Hyperparameters
- **Training regime:** [More Information Needed] <!--fp32, fp16 mixed precision, bf16 mixed precision, bf16 non-mixed precision, fp16 non-mixed precision, fp8 mixed precision -->
#### Speeds, Sizes, Times [optional]
<!-- This section provides information about throughput, start/end time, checkpoint size if relevant, etc. -->
[More Information Needed]
## Evaluation
<!-- This section describes the evaluation protocols and provides the results. -->
### Testing Data, Factors & Metrics
#### Testing Data
<!-- This should link to a Dataset Card if possible. -->
[More Information Needed]
#### Factors
<!-- These are the things the evaluation is disaggregating by, e.g., subpopulations or domains. -->
[More Information Needed]
#### Metrics
<!-- These are the evaluation metrics being used, ideally with a description of why. -->
[More Information Needed]
### Results
[More Information Needed]
#### Summary
## Model Examination [optional]
<!-- Relevant interpretability work for the model goes here -->
[More Information Needed]
## Environmental Impact
<!-- Total emissions (in grams of CO2eq) and additional considerations, such as electricity usage, go here. Edit the suggested text below accordingly -->
Carbon emissions can be estimated using the [Machine Learning Impact calculator](https://mlco2.github.io/impact#compute) presented in [Lacoste et al. (2019)](https://arxiv.org/abs/1910.09700).
- **Hardware Type:** [More Information Needed]
- **Hours used:** [More Information Needed]
- **Cloud Provider:** [More Information Needed]
- **Compute Region:** [More Information Needed]
- **Carbon Emitted:** [More Information Needed]
## Technical Specifications [optional]
### Model Architecture and Objective
[More Information Needed]
### Compute Infrastructure
[More Information Needed]
#### Hardware
[More Information Needed]
#### Software
[More Information Needed]
## Citation [optional]
<!-- If there is a paper or blog post introducing the model, the APA and Bibtex information for that should go in this section. -->
**BibTeX:**
[More Information Needed]
**APA:**
[More Information Needed]
## Glossary [optional]
<!-- If relevant, include terms and calculations in this section that can help readers understand the model or model card. -->
[More Information Needed]
## More Information [optional]
[More Information Needed]
## Model Card Authors [optional]
[More Information Needed]
## Model Card Contact
[More Information Needed]
| {"library_name": "transformers", "tags": []} | miguel-kjh/pythia_160m-adpater-lora-dbpedia | null | [
"transformers",
"safetensors",
"gpt_neox",
"text-generation",
"arxiv:1910.09700",
"autotrain_compatible",
"endpoints_compatible",
"text-generation-inference",
"region:us"
] | null | 2024-05-02T11:47:53+00:00 | [
"1910.09700"
] | [] | TAGS
#transformers #safetensors #gpt_neox #text-generation #arxiv-1910.09700 #autotrain_compatible #endpoints_compatible #text-generation-inference #region-us
|
# Model Card for Model ID
## Model Details
### Model Description
This is the model card of a transformers model that has been pushed on the Hub. This model card has been automatically generated.
- Developed by:
- Funded by [optional]:
- Shared by [optional]:
- Model type:
- Language(s) (NLP):
- License:
- Finetuned from model [optional]:
### Model Sources [optional]
- Repository:
- Paper [optional]:
- Demo [optional]:
## Uses
### Direct Use
### Downstream Use [optional]
### Out-of-Scope Use
## Bias, Risks, and Limitations
### Recommendations
Users (both direct and downstream) should be made aware of the risks, biases and limitations of the model. More information needed for further recommendations.
## How to Get Started with the Model
Use the code below to get started with the model.
## Training Details
### Training Data
### Training Procedure
#### Preprocessing [optional]
#### Training Hyperparameters
- Training regime:
#### Speeds, Sizes, Times [optional]
## Evaluation
### Testing Data, Factors & Metrics
#### Testing Data
#### Factors
#### Metrics
### Results
#### Summary
## Model Examination [optional]
## Environmental Impact
Carbon emissions can be estimated using the Machine Learning Impact calculator presented in Lacoste et al. (2019).
- Hardware Type:
- Hours used:
- Cloud Provider:
- Compute Region:
- Carbon Emitted:
## Technical Specifications [optional]
### Model Architecture and Objective
### Compute Infrastructure
#### Hardware
#### Software
[optional]
BibTeX:
APA:
## Glossary [optional]
## More Information [optional]
## Model Card Authors [optional]
## Model Card Contact
| [
"# Model Card for Model ID",
"## Model Details",
"### Model Description\n\n\n\nThis is the model card of a transformers model that has been pushed on the Hub. This model card has been automatically generated.\n\n- Developed by: \n- Funded by [optional]: \n- Shared by [optional]: \n- Model type: \n- Language(s) (NLP): \n- License: \n- Finetuned from model [optional]:",
"### Model Sources [optional]\n\n\n\n- Repository: \n- Paper [optional]: \n- Demo [optional]:",
"## Uses",
"### Direct Use",
"### Downstream Use [optional]",
"### Out-of-Scope Use",
"## Bias, Risks, and Limitations",
"### Recommendations\n\n\n\nUsers (both direct and downstream) should be made aware of the risks, biases and limitations of the model. More information needed for further recommendations.",
"## How to Get Started with the Model\n\nUse the code below to get started with the model.",
"## Training Details",
"### Training Data",
"### Training Procedure",
"#### Preprocessing [optional]",
"#### Training Hyperparameters\n\n- Training regime:",
"#### Speeds, Sizes, Times [optional]",
"## Evaluation",
"### Testing Data, Factors & Metrics",
"#### Testing Data",
"#### Factors",
"#### Metrics",
"### Results",
"#### Summary",
"## Model Examination [optional]",
"## Environmental Impact\n\n\n\nCarbon emissions can be estimated using the Machine Learning Impact calculator presented in Lacoste et al. (2019).\n\n- Hardware Type: \n- Hours used: \n- Cloud Provider: \n- Compute Region: \n- Carbon Emitted:",
"## Technical Specifications [optional]",
"### Model Architecture and Objective",
"### Compute Infrastructure",
"#### Hardware",
"#### Software\n\n\n\n[optional]\n\n\n\nBibTeX:\n\n\n\nAPA:",
"## Glossary [optional]",
"## More Information [optional]",
"## Model Card Authors [optional]",
"## Model Card Contact"
] | [
"TAGS\n#transformers #safetensors #gpt_neox #text-generation #arxiv-1910.09700 #autotrain_compatible #endpoints_compatible #text-generation-inference #region-us \n",
"# Model Card for Model ID",
"## Model Details",
"### Model Description\n\n\n\nThis is the model card of a transformers model that has been pushed on the Hub. This model card has been automatically generated.\n\n- Developed by: \n- Funded by [optional]: \n- Shared by [optional]: \n- Model type: \n- Language(s) (NLP): \n- License: \n- Finetuned from model [optional]:",
"### Model Sources [optional]\n\n\n\n- Repository: \n- Paper [optional]: \n- Demo [optional]:",
"## Uses",
"### Direct Use",
"### Downstream Use [optional]",
"### Out-of-Scope Use",
"## Bias, Risks, and Limitations",
"### Recommendations\n\n\n\nUsers (both direct and downstream) should be made aware of the risks, biases and limitations of the model. More information needed for further recommendations.",
"## How to Get Started with the Model\n\nUse the code below to get started with the model.",
"## Training Details",
"### Training Data",
"### Training Procedure",
"#### Preprocessing [optional]",
"#### Training Hyperparameters\n\n- Training regime:",
"#### Speeds, Sizes, Times [optional]",
"## Evaluation",
"### Testing Data, Factors & Metrics",
"#### Testing Data",
"#### Factors",
"#### Metrics",
"### Results",
"#### Summary",
"## Model Examination [optional]",
"## Environmental Impact\n\n\n\nCarbon emissions can be estimated using the Machine Learning Impact calculator presented in Lacoste et al. (2019).\n\n- Hardware Type: \n- Hours used: \n- Cloud Provider: \n- Compute Region: \n- Carbon Emitted:",
"## Technical Specifications [optional]",
"### Model Architecture and Objective",
"### Compute Infrastructure",
"#### Hardware",
"#### Software\n\n\n\n[optional]\n\n\n\nBibTeX:\n\n\n\nAPA:",
"## Glossary [optional]",
"## More Information [optional]",
"## Model Card Authors [optional]",
"## Model Card Contact"
] | [
47,
6,
4,
75,
23,
3,
5,
8,
9,
8,
34,
20,
4,
5,
5,
11,
13,
12,
3,
10,
6,
5,
6,
4,
5,
7,
49,
7,
7,
5,
5,
15,
7,
7,
8,
5
] | [
"TAGS\n#transformers #safetensors #gpt_neox #text-generation #arxiv-1910.09700 #autotrain_compatible #endpoints_compatible #text-generation-inference #region-us \n# Model Card for Model ID## Model Details### Model Description\n\n\n\nThis is the model card of a transformers model that has been pushed on the Hub. This model card has been automatically generated.\n\n- Developed by: \n- Funded by [optional]: \n- Shared by [optional]: \n- Model type: \n- Language(s) (NLP): \n- License: \n- Finetuned from model [optional]:### Model Sources [optional]\n\n\n\n- Repository: \n- Paper [optional]: \n- Demo [optional]:## Uses### Direct Use### Downstream Use [optional]### Out-of-Scope Use## Bias, Risks, and Limitations### Recommendations\n\n\n\nUsers (both direct and downstream) should be made aware of the risks, biases and limitations of the model. More information needed for further recommendations.## How to Get Started with the Model\n\nUse the code below to get started with the model.## Training Details### Training Data### Training Procedure#### Preprocessing [optional]#### Training Hyperparameters\n\n- Training regime:#### Speeds, Sizes, Times [optional]## Evaluation### Testing Data, Factors & Metrics#### Testing Data#### Factors#### Metrics### Results#### Summary## Model Examination [optional]## Environmental Impact\n\n\n\nCarbon emissions can be estimated using the Machine Learning Impact calculator presented in Lacoste et al. (2019).\n\n- Hardware Type: \n- Hours used: \n- Cloud Provider: \n- Compute Region: \n- Carbon Emitted:## Technical Specifications [optional]### Model Architecture and Objective### Compute Infrastructure#### Hardware#### Software\n\n\n\n[optional]\n\n\n\nBibTeX:\n\n\n\nAPA:## Glossary [optional]## More Information [optional]## Model Card Authors [optional]## Model Card Contact"
] |
Subsets and Splits
No community queries yet
The top public SQL queries from the community will appear here once available.