modelId
stringlengths 5
122
| author
stringlengths 2
42
| last_modified
timestamp[us, tz=UTC] | downloads
int64 0
738M
| likes
int64 0
11k
| library_name
stringclasses 245
values | tags
sequencelengths 1
4.05k
| pipeline_tag
stringclasses 48
values | createdAt
timestamp[us, tz=UTC] | card
stringlengths 1
901k
|
---|---|---|---|---|---|---|---|---|---|
ZainAli60/gpt_tune | ZainAli60 | 2024-03-09T21:09:57Z | 21,890 | 0 | transformers | [
"transformers",
"safetensors",
"gpt2",
"text-generation",
"arxiv:1910.09700",
"autotrain_compatible",
"endpoints_compatible",
"text-generation-inference",
"region:us"
] | text-generation | 2024-03-09T21:08:35Z | ---
library_name: transformers
tags: []
---
# Model Card for Model ID
<!-- Provide a quick summary of what the model is/does. -->
## Model Details
### Model Description
<!-- Provide a longer summary of what this model is. -->
This is the model card of a 🤗 transformers model that has been pushed on the Hub. This model card has been automatically generated.
- **Developed by:** [More Information Needed]
- **Funded by [optional]:** [More Information Needed]
- **Shared by [optional]:** [More Information Needed]
- **Model type:** [More Information Needed]
- **Language(s) (NLP):** [More Information Needed]
- **License:** [More Information Needed]
- **Finetuned from model [optional]:** [More Information Needed]
### Model Sources [optional]
<!-- Provide the basic links for the model. -->
- **Repository:** [More Information Needed]
- **Paper [optional]:** [More Information Needed]
- **Demo [optional]:** [More Information Needed]
## Uses
<!-- Address questions around how the model is intended to be used, including the foreseeable users of the model and those affected by the model. -->
### Direct Use
<!-- This section is for the model use without fine-tuning or plugging into a larger ecosystem/app. -->
[More Information Needed]
### Downstream Use [optional]
<!-- This section is for the model use when fine-tuned for a task, or when plugged into a larger ecosystem/app -->
[More Information Needed]
### Out-of-Scope Use
<!-- This section addresses misuse, malicious use, and uses that the model will not work well for. -->
[More Information Needed]
## Bias, Risks, and Limitations
<!-- This section is meant to convey both technical and sociotechnical limitations. -->
[More Information Needed]
### Recommendations
<!-- This section is meant to convey recommendations with respect to the bias, risk, and technical limitations. -->
Users (both direct and downstream) should be made aware of the risks, biases and limitations of the model. More information needed for further recommendations.
## How to Get Started with the Model
Use the code below to get started with the model.
[More Information Needed]
## Training Details
### Training Data
<!-- This should link to a Dataset Card, perhaps with a short stub of information on what the training data is all about as well as documentation related to data pre-processing or additional filtering. -->
[More Information Needed]
### Training Procedure
<!-- This relates heavily to the Technical Specifications. Content here should link to that section when it is relevant to the training procedure. -->
#### Preprocessing [optional]
[More Information Needed]
#### Training Hyperparameters
- **Training regime:** [More Information Needed] <!--fp32, fp16 mixed precision, bf16 mixed precision, bf16 non-mixed precision, fp16 non-mixed precision, fp8 mixed precision -->
#### Speeds, Sizes, Times [optional]
<!-- This section provides information about throughput, start/end time, checkpoint size if relevant, etc. -->
[More Information Needed]
## Evaluation
<!-- This section describes the evaluation protocols and provides the results. -->
### Testing Data, Factors & Metrics
#### Testing Data
<!-- This should link to a Dataset Card if possible. -->
[More Information Needed]
#### Factors
<!-- These are the things the evaluation is disaggregating by, e.g., subpopulations or domains. -->
[More Information Needed]
#### Metrics
<!-- These are the evaluation metrics being used, ideally with a description of why. -->
[More Information Needed]
### Results
[More Information Needed]
#### Summary
## Model Examination [optional]
<!-- Relevant interpretability work for the model goes here -->
[More Information Needed]
## Environmental Impact
<!-- Total emissions (in grams of CO2eq) and additional considerations, such as electricity usage, go here. Edit the suggested text below accordingly -->
Carbon emissions can be estimated using the [Machine Learning Impact calculator](https://mlco2.github.io/impact#compute) presented in [Lacoste et al. (2019)](https://arxiv.org/abs/1910.09700).
- **Hardware Type:** [More Information Needed]
- **Hours used:** [More Information Needed]
- **Cloud Provider:** [More Information Needed]
- **Compute Region:** [More Information Needed]
- **Carbon Emitted:** [More Information Needed]
## Technical Specifications [optional]
### Model Architecture and Objective
[More Information Needed]
### Compute Infrastructure
[More Information Needed]
#### Hardware
[More Information Needed]
#### Software
[More Information Needed]
## Citation [optional]
<!-- If there is a paper or blog post introducing the model, the APA and Bibtex information for that should go in this section. -->
**BibTeX:**
[More Information Needed]
**APA:**
[More Information Needed]
## Glossary [optional]
<!-- If relevant, include terms and calculations in this section that can help readers understand the model or model card. -->
[More Information Needed]
## More Information [optional]
[More Information Needed]
## Model Card Authors [optional]
[More Information Needed]
## Model Card Contact
[More Information Needed] |
sasha/regardv3 | sasha | 2022-08-17T18:03:37Z | 21,868 | 1 | transformers | [
"transformers",
"pytorch",
"bert",
"text-classification",
"license:cc-by-4.0",
"autotrain_compatible",
"endpoints_compatible",
"region:us"
] | text-classification | 2022-08-17T16:58:42Z | ---
license: cc-by-4.0
---
# BERT Regard classification model
This model is the result of a project entitled [Towards Controllable Biases in Language Generation](https://github.com/ewsheng/controllable-nlg-biases). It consists of a BERT classifier (no ensemble) trained on 1.7K samples of biased language.
*Regard* measures language polarity towards and social perceptions of a demographic (compared to sentiment, which only measures overall language polarity).
### BibTeX entry and citation info
```bibtex
@article{sheng2019woman,
title={The woman worked as a babysitter: On biases in language generation},
author={Sheng, Emily and Chang, Kai-Wei and Natarajan, Premkumar and Peng, Nanyun},
journal={arXiv preprint arXiv:1909.01326},
year={2019}
}
```
|
ohyay12345/taoprinter | ohyay12345 | 2024-01-24T19:46:46Z | 21,856 | 0 | transformers | [
"transformers",
"safetensors",
"gpt2",
"text-generation",
"autotrain_compatible",
"endpoints_compatible",
"text-generation-inference",
"region:us"
] | text-generation | 2024-01-20T05:11:07Z | Entry not found |
ZainAli60/miners_1 | ZainAli60 | 2024-03-12T23:44:39Z | 21,847 | 0 | transformers | [
"transformers",
"safetensors",
"falcon",
"text-generation",
"custom_code",
"arxiv:1910.09700",
"autotrain_compatible",
"endpoints_compatible",
"text-generation-inference",
"region:us"
] | text-generation | 2024-03-12T22:09:14Z | ---
library_name: transformers
tags: []
---
# Model Card for Model ID
<!-- Provide a quick summary of what the model is/does. -->
## Model Details
### Model Description
<!-- Provide a longer summary of what this model is. -->
This is the model card of a 🤗 transformers model that has been pushed on the Hub. This model card has been automatically generated.
- **Developed by:** [More Information Needed]
- **Funded by [optional]:** [More Information Needed]
- **Shared by [optional]:** [More Information Needed]
- **Model type:** [More Information Needed]
- **Language(s) (NLP):** [More Information Needed]
- **License:** [More Information Needed]
- **Finetuned from model [optional]:** [More Information Needed]
### Model Sources [optional]
<!-- Provide the basic links for the model. -->
- **Repository:** [More Information Needed]
- **Paper [optional]:** [More Information Needed]
- **Demo [optional]:** [More Information Needed]
## Uses
<!-- Address questions around how the model is intended to be used, including the foreseeable users of the model and those affected by the model. -->
### Direct Use
<!-- This section is for the model use without fine-tuning or plugging into a larger ecosystem/app. -->
[More Information Needed]
### Downstream Use [optional]
<!-- This section is for the model use when fine-tuned for a task, or when plugged into a larger ecosystem/app -->
[More Information Needed]
### Out-of-Scope Use
<!-- This section addresses misuse, malicious use, and uses that the model will not work well for. -->
[More Information Needed]
## Bias, Risks, and Limitations
<!-- This section is meant to convey both technical and sociotechnical limitations. -->
[More Information Needed]
### Recommendations
<!-- This section is meant to convey recommendations with respect to the bias, risk, and technical limitations. -->
Users (both direct and downstream) should be made aware of the risks, biases and limitations of the model. More information needed for further recommendations.
## How to Get Started with the Model
Use the code below to get started with the model.
[More Information Needed]
## Training Details
### Training Data
<!-- This should link to a Dataset Card, perhaps with a short stub of information on what the training data is all about as well as documentation related to data pre-processing or additional filtering. -->
[More Information Needed]
### Training Procedure
<!-- This relates heavily to the Technical Specifications. Content here should link to that section when it is relevant to the training procedure. -->
#### Preprocessing [optional]
[More Information Needed]
#### Training Hyperparameters
- **Training regime:** [More Information Needed] <!--fp32, fp16 mixed precision, bf16 mixed precision, bf16 non-mixed precision, fp16 non-mixed precision, fp8 mixed precision -->
#### Speeds, Sizes, Times [optional]
<!-- This section provides information about throughput, start/end time, checkpoint size if relevant, etc. -->
[More Information Needed]
## Evaluation
<!-- This section describes the evaluation protocols and provides the results. -->
### Testing Data, Factors & Metrics
#### Testing Data
<!-- This should link to a Dataset Card if possible. -->
[More Information Needed]
#### Factors
<!-- These are the things the evaluation is disaggregating by, e.g., subpopulations or domains. -->
[More Information Needed]
#### Metrics
<!-- These are the evaluation metrics being used, ideally with a description of why. -->
[More Information Needed]
### Results
[More Information Needed]
#### Summary
## Model Examination [optional]
<!-- Relevant interpretability work for the model goes here -->
[More Information Needed]
## Environmental Impact
<!-- Total emissions (in grams of CO2eq) and additional considerations, such as electricity usage, go here. Edit the suggested text below accordingly -->
Carbon emissions can be estimated using the [Machine Learning Impact calculator](https://mlco2.github.io/impact#compute) presented in [Lacoste et al. (2019)](https://arxiv.org/abs/1910.09700).
- **Hardware Type:** [More Information Needed]
- **Hours used:** [More Information Needed]
- **Cloud Provider:** [More Information Needed]
- **Compute Region:** [More Information Needed]
- **Carbon Emitted:** [More Information Needed]
## Technical Specifications [optional]
### Model Architecture and Objective
[More Information Needed]
### Compute Infrastructure
[More Information Needed]
#### Hardware
[More Information Needed]
#### Software
[More Information Needed]
## Citation [optional]
<!-- If there is a paper or blog post introducing the model, the APA and Bibtex information for that should go in this section. -->
**BibTeX:**
[More Information Needed]
**APA:**
[More Information Needed]
## Glossary [optional]
<!-- If relevant, include terms and calculations in this section that can help readers understand the model or model card. -->
[More Information Needed]
## More Information [optional]
[More Information Needed]
## Model Card Authors [optional]
[More Information Needed]
## Model Card Contact
[More Information Needed] |
kwoncho/gaincut_news_pre2021 | kwoncho | 2024-06-15T05:01:02Z | 21,765 | 0 | transformers | [
"transformers",
"pytorch",
"roberta",
"text-classification",
"autotrain_compatible",
"endpoints_compatible",
"region:us"
] | text-classification | 2024-04-25T04:43:00Z | Entry not found |
deepseek-ai/DeepSeek-V2-Lite | deepseek-ai | 2024-06-25T08:37:17Z | 21,764 | 48 | transformers | [
"transformers",
"safetensors",
"deepseek_v2",
"text-generation",
"conversational",
"custom_code",
"arxiv:2405.04434",
"license:other",
"autotrain_compatible",
"region:us"
] | text-generation | 2024-05-15T05:31:53Z | ---
license: other
license_name: deepseek
license_link: https://github.com/deepseek-ai/DeepSeek-V2/blob/main/LICENSE-MODEL
---
<!-- markdownlint-disable first-line-h1 -->
<!-- markdownlint-disable html -->
<!-- markdownlint-disable no-duplicate-header -->
<div align="center">
<img src="https://github.com/deepseek-ai/DeepSeek-V2/blob/main/figures/logo.svg?raw=true" width="60%" alt="DeepSeek-V2" />
</div>
<hr>
<div align="center" style="line-height: 1;">
<a href="https://www.deepseek.com/" target="_blank" style="margin: 2px;">
<img alt="Homepage" src="https://github.com/deepseek-ai/DeepSeek-V2/blob/main/figures/badge.svg?raw=true" style="display: inline-block; vertical-align: middle;"/>
</a>
<a href="https://chat.deepseek.com/" target="_blank" style="margin: 2px;">
<img alt="Chat" src="https://img.shields.io/badge/🤖%20Chat-DeepSeek%20V2-536af5?color=536af5&logoColor=white" style="display: inline-block; vertical-align: middle;"/>
</a>
<a href="https://huggingface.co/deepseek-ai" target="_blank" style="margin: 2px;">
<img alt="Hugging Face" src="https://img.shields.io/badge/%F0%9F%A4%97%20Hugging%20Face-DeepSeek%20AI-ffc107?color=ffc107&logoColor=white" style="display: inline-block; vertical-align: middle;"/>
</a>
</div>
<div align="center" style="line-height: 1;">
<a href="https://discord.gg/Tc7c45Zzu5" target="_blank" style="margin: 2px;">
<img alt="Discord" src="https://img.shields.io/badge/Discord-DeepSeek%20AI-7289da?logo=discord&logoColor=white&color=7289da" style="display: inline-block; vertical-align: middle;"/>
</a>
<a href="https://github.com/deepseek-ai/DeepSeek-V2/blob/main/figures/qr.jpeg?raw=true" target="_blank" style="margin: 2px;">
<img alt="Wechat" src="https://img.shields.io/badge/WeChat-DeepSeek%20AI-brightgreen?logo=wechat&logoColor=white" style="display: inline-block; vertical-align: middle;"/>
</a>
<a href="https://twitter.com/deepseek_ai" target="_blank" style="margin: 2px;">
<img alt="Twitter Follow" src="https://img.shields.io/badge/Twitter-deepseek_ai-white?logo=x&logoColor=white" style="display: inline-block; vertical-align: middle;"/>
</a>
</div>
<div align="center" style="line-height: 1;">
<a href="https://github.com/deepseek-ai/DeepSeek-V2/blob/main/LICENSE-CODE" style="margin: 2px;">
<img alt="Code License" src="https://img.shields.io/badge/Code_License-MIT-f5de53?&color=f5de53" style="display: inline-block; vertical-align: middle;"/>
</a>
<a href="https://github.com/deepseek-ai/DeepSeek-V2/blob/main/LICENSE-MODEL" style="margin: 2px;">
<img alt="Model License" src="https://img.shields.io/badge/Model_License-Model_Agreement-f5de53?&color=f5de53" style="display: inline-block; vertical-align: middle;"/>
</a>
</div>
<p align="center">
<a href="#2-model-downloads">Model Download</a> |
<a href="#3-evaluation-results">Evaluation Results</a> |
<a href="#4-model-architecture">Model Architecture</a> |
<a href="#6-api-platform">API Platform</a> |
<a href="#8-license">License</a> |
<a href="#9-citation">Citation</a>
</p>
<p align="center">
<a href="https://arxiv.org/abs/2405.04434"><b>Paper Link</b>👁️</a>
</p>
# DeepSeek-V2: A Strong, Economical, and Efficient Mixture-of-Experts Language Model
## 1. Introduction
Last week, the release and buzz around DeepSeek-V2 have ignited widespread interest in MLA (Multi-head Latent Attention)! Many in the community suggested open-sourcing a smaller MoE model for in-depth research. And now DeepSeek-V2-Lite comes out:
- 16B total params, 2.4B active params, scratch training with 5.7T tokens
- Outperforms 7B dense and 16B MoE on many English & Chinese benchmarks
- Deployable on single 40G GPU, fine-tunable on 8x80G GPUs
DeepSeek-V2, a strong Mixture-of-Experts (MoE) language model characterized by economical training and efficient inference. DeepSeek-V2 adopts innovative architectures including Multi-head Latent Attention (MLA) and DeepSeekMoE. MLA guarantees efficient inference through significantly compressing the Key-Value (KV) cache into a latent vector, while DeepSeekMoE enables training strong models at an economical cost through sparse computation.
## 2. News
- 2024.05.16: We released the DeepSeek-V2-Lite.
- 2024.05.06: We released the DeepSeek-V2.
## 3. Model Downloads
With DeepSeek-V2, we are open-sourcing base and chat models across two sizes:
<div align="center">
| **Model** | **#Total Params** | **#Activated Params** | **Context Length** | **Download** |
| :------------: | :------------: | :------------: | :------------: | :------------: |
| DeepSeek-V2-Lite | 16B | 2.4B | 32k | [🤗 HuggingFace](https://huggingface.co/deepseek-ai/DeepSeek-V2-Lite) |
| DeepSeek-V2-Lite-Chat (SFT) | 16B | 2.4B | 32k | [🤗 HuggingFace](https://huggingface.co/deepseek-ai/DeepSeek-V2-Lite-Chat) |
| DeepSeek-V2 | 236B | 21B | 128k | [🤗 HuggingFace](https://huggingface.co/deepseek-ai/DeepSeek-V2) |
| DeepSeek-V2-Chat (RL) | 236B | 21B | 128k | [🤗 HuggingFace](https://huggingface.co/deepseek-ai/DeepSeek-V2-Chat) |
</div>
Due to the constraints of HuggingFace, the open-source code currently experiences slower performance than our internal codebase when running on GPUs with Huggingface. To facilitate the efficient execution of our model, we offer a dedicated vllm solution that optimizes performance for running our model effectively.
## 4. Evaluation Results
### Base Model
#### Standard Benchmark
<div align="center">
| **Benchmark** | **Domain** | **DeepSeek 7B (Dense)** | **DeepSeekMoE 16B** | **DeepSeek-V2-Lite (MoE-16B)** |
|:-------------:|:----------:|:--------------:|:-----------------:|:--------------------------:|
| **Architecture** | - | MHA+Dense | MHA+MoE | MLA+MoE |
| **MMLU** | English | 48.2 | 45.0 | 58.3 |
| **BBH** | English | 39.5 | 38.9 | 44.1 |
| **C-Eval** | Chinese | 45.0 | 40.6 | 60.3 |
| **CMMLU** | Chinese | 47.2 | 42.5 | 64.3 |
| **HumanEval** | Code | 26.2 | 26.8 | 29.9 |
| **MBPP** | Code | 39.0 | 39.2 | 43.2 |
| **GSM8K** | Math | 17.4 | 18.8 | 41.1 |
| **Math** | Math | 3.3 | 4.3 | 17.1 |
</div>
For more evaluation details, such as few-shot settings and prompts, please check our paper.
### Chat Model
#### Standard Benchmark
<div align="center">
| Benchmark | Domain | DeepSeek 7B Chat (SFT) | DeepSeekMoE 16B Chat (SFT) | DeepSeek-V2-Lite 16B Chat (SFT) |
|:-----------:|:----------------:|:------------------:|:---------------:|:---------------------:|
| **MMLU** | English | 49.7 | 47.2 | 55.7 |
| **BBH** | English | 43.1 | 42.2 | 48.1 |
| **C-Eval** | Chinese | 44.7 | 40.0 | 60.1 |
| **CMMLU** | Chinese | 51.2 | 49.3 | 62.5 |
| **HumanEval** | Code | 45.1 | 45.7 | 57.3 |
| **MBPP** | Code | 39.0 | 46.2 | 45.8 |
| **GSM8K** | Math | 62.6 | 62.2 | 72.0 |
| **Math** | Math | 14.7 | 15.2 | 27.9 |
</div>
## 5. Model Architecture
DeepSeek-V2 adopts innovative architectures to guarantee economical training and efficient inference:
- For attention, we design MLA (Multi-head Latent Attention), which utilizes low-rank key-value union compression to eliminate the bottleneck of inference-time key-value cache, thus supporting efficient inference.
- For Feed-Forward Networks (FFNs), we adopt DeepSeekMoE architecture, a high-performance MoE architecture that enables training stronger models at lower costs.
<p align="center">
<img width="90%" src="https://github.com/deepseek-ai/DeepSeek-V2/blob/main/figures/architecture.png?raw=true" />
</p>
DeepSeek-V2-Lite has 27 layers and a hidden dimension of 2048. It also employs MLA and has 16 attention heads, where each head has a dimension of 128. Its KV compression dimension is 512, but slightly different from DeepSeek-V2, it does not compress the queries. For the decoupled queries and key, it has a per-head dimension of 64. DeepSeek-V2-Lite also employs DeepSeekMoE, and all FFNs except for the first layer are replaced with MoE layers. Each MoE layer consists of 2 shared experts and 64 routed experts, where the intermediate hidden dimension of each expert is 1408. Among the routed experts, 6 experts will be activated for each token. Under this configuration, DeepSeek-V2-Lite comprises 15.7B total parameters, of which 2.4B are activated for each token.
## 6. Training Details
DeepSeek-V2-Lite is also trained from scratch on the same pre-training corpus of DeepSeek-V2, which is not polluted by any SFT data. It uses the AdamW optimizer with hyper-parameters set to $\beta_1=0.9$, $\beta_2=0.95$, and $\mathrm{weight_decay}=0.1$. The learning rate is scheduled using a warmup-and-step-decay strategy. Initially, the learning rate linearly increases from 0 to the maximum value during the first 2K steps. Subsequently, the learning rate is multiplied by 0.316 after training about 80% of tokens, and again by 0.316 after training about 90% of tokens. The maximum learning rate is set to $4.2 \times 10^{-4}$, and the gradient clipping norm is set to 1.0. We do not employ the batch size scheduling strategy for it, and it is trained with a constant batch size of 4608 sequences. During pre-training, we set the maximum sequence length to 4K, and train DeepSeek-V2-Lite on 5.7T tokens. We leverage pipeline parallelism to deploy different layers of it on different devices, but for each layer, all experts will be deployed on the same device. Therefore, we only employ a small expert-level balance loss with $\alpha_{1}=0.001$, and do not employ device-level balance loss and communication balance loss for it. After pre-training, we also perform long-context extension, SFT for DeepSeek-V2-Lite and get a chat model called DeepSeek-V2-Lite Chat.
## 7. How to run locally
**To utilize DeepSeek-V2-Lite in BF16 format for inference, 40GB*1 GPU is required.**
### Inference with Huggingface's Transformers
You can directly employ [Huggingface's Transformers](https://github.com/huggingface/transformers) for model inference.
#### Text Completion
```python
import torch
from transformers import AutoTokenizer, AutoModelForCausalLM, GenerationConfig
model_name = "deepseek-ai/DeepSeek-V2-Lite"
tokenizer = AutoTokenizer.from_pretrained(model_name, trust_remote_code=True)
model = AutoModelForCausalLM.from_pretrained(model_name, trust_remote_code=True, torch_dtype=torch.bfloat16).cuda()
model.generation_config = GenerationConfig.from_pretrained(model_name)
model.generation_config.pad_token_id = model.generation_config.eos_token_id
text = "An attention function can be described as mapping a query and a set of key-value pairs to an output, where the query, keys, values, and output are all vectors. The output is"
inputs = tokenizer(text, return_tensors="pt")
outputs = model.generate(**inputs.to(model.device), max_new_tokens=100)
result = tokenizer.decode(outputs[0], skip_special_tokens=True)
print(result)
```
#### Chat Completion
```python
import torch
from transformers import AutoTokenizer, AutoModelForCausalLM, GenerationConfig
model_name = "deepseek-ai/DeepSeek-V2-Lite-Chat"
tokenizer = AutoTokenizer.from_pretrained(model_name, trust_remote_code=True)
model = AutoModelForCausalLM.from_pretrained(model_name, trust_remote_code=True, torch_dtype=torch.bfloat16).cuda()
model.generation_config = GenerationConfig.from_pretrained(model_name)
model.generation_config.pad_token_id = model.generation_config.eos_token_id
messages = [
{"role": "user", "content": "Write a piece of quicksort code in C++"}
]
input_tensor = tokenizer.apply_chat_template(messages, add_generation_prompt=True, return_tensors="pt")
outputs = model.generate(input_tensor.to(model.device), max_new_tokens=100)
result = tokenizer.decode(outputs[0][input_tensor.shape[1]:], skip_special_tokens=True)
print(result)
```
The complete chat template can be found within `tokenizer_config.json` located in the huggingface model repository.
An example of chat template is as belows:
```bash
<|begin▁of▁sentence|>User: {user_message_1}
Assistant: {assistant_message_1}<|end▁of▁sentence|>User: {user_message_2}
Assistant:
```
You can also add an optional system message:
```bash
<|begin▁of▁sentence|>{system_message}
User: {user_message_1}
Assistant: {assistant_message_1}<|end▁of▁sentence|>User: {user_message_2}
Assistant:
```
### Inference with vLLM (recommended)
To utilize [vLLM](https://github.com/vllm-project/vllm) for model inference, please merge this Pull Request into your vLLM codebase: https://github.com/vllm-project/vllm/pull/4650.
```python
from transformers import AutoTokenizer
from vllm import LLM, SamplingParams
max_model_len, tp_size = 8192, 1
model_name = "deepseek-ai/DeepSeek-V2-Lite-Chat"
tokenizer = AutoTokenizer.from_pretrained(model_name)
llm = LLM(model=model_name, tensor_parallel_size=tp_size, max_model_len=max_model_len, trust_remote_code=True, enforce_eager=True)
sampling_params = SamplingParams(temperature=0.3, max_tokens=256, stop_token_ids=[tokenizer.eos_token_id])
messages_list = [
[{"role": "user", "content": "Who are you?"}],
[{"role": "user", "content": "Translate the following content into Chinese directly: DeepSeek-V2 adopts innovative architectures to guarantee economical training and efficient inference."}],
[{"role": "user", "content": "Write a piece of quicksort code in C++."}],
]
prompt_token_ids = [tokenizer.apply_chat_template(messages, add_generation_prompt=True) for messages in messages_list]
outputs = llm.generate(prompt_token_ids=prompt_token_ids, sampling_params=sampling_params)
generated_text = [output.outputs[0].text for output in outputs]
print(generated_text)
```
### LangChain Support
Since our API is compatible with OpenAI, you can easily use it in [langchain](https://www.langchain.com/).
Here is an example:
```
from langchain_openai import ChatOpenAI
llm = ChatOpenAI(
model='deepseek-chat',
openai_api_key=<your-deepseek-api-key>,
openai_api_base='https://api.deepseek.com/v1',
temperature=0.85,
max_tokens=8000)
```
## 8. License
This code repository is licensed under [the MIT License](LICENSE-CODE). The use of DeepSeek-V2 Base/Chat models is subject to [the Model License](LICENSE-MODEL). DeepSeek-V2 series (including Base and Chat) supports commercial use.
## 9. Citation
```
@misc{deepseekv2,
title={DeepSeek-V2: A Strong, Economical, and Efficient Mixture-of-Experts Language Model},
author={DeepSeek-AI},
year={2024},
eprint={2405.04434},
archivePrefix={arXiv},
primaryClass={cs.CL}
}
```
## 10. Contact
If you have any questions, please raise an issue or contact us at [[email protected]]([email protected]).
|
knkarthick/MEETING_SUMMARY | knkarthick | 2023-03-27T15:08:14Z | 21,750 | 185 | transformers | [
"transformers",
"pytorch",
"tf",
"safetensors",
"bart",
"text2text-generation",
"seq2seq",
"summarization",
"en",
"license:apache-2.0",
"model-index",
"autotrain_compatible",
"endpoints_compatible",
"region:us"
] | summarization | 2022-03-02T23:29:05Z | ---
language: en
license: apache-2.0
tags:
- bart
- seq2seq
- summarization
datasets:
- cnndaily/newyorkdaily/xsum/samsum/dialogsum/AMI
metrics:
- rouge
widget:
- text: 'Hi, I''m David and I''m supposed to be an industrial designer. Um, I just
got the project announcement about what the project is. Designing a remote control.
That''s about it, didn''t get anything else. Did you get the same thing? Cool.
There''s too much gear. Okay. Can''t draw. Um. Yeah. Um, well anyway, I don''t
know, it''s just the first animal I can think off the top of my head. Um. Yes.
Big reason is ''cause I''m allergic to most animals. Allergic to animal fur, so
um fish was a natural choice. Um, yeah, and I kind of like whales. They come in
and go eat everything in sight. And they''re quite harmless and mild and interesting.
Tail''s a bit big, I think. It''s an after dinner dog then. Hmm. It does make
sense from maybe the design point of view ''cause you have more complicated characters
like European languages, then you need more buttons. So, possibly. Hmm. Yeah.
And you keep losing them. Finding them is really a pain, you know. I mean it''s
usually quite small, or when you want it right, it slipped behind the couch or
it''s kicked under the table. You know. Yep. Mm-hmm. I think one factor would
be production cost. Because there''s a cap there, so um depends on how much you
can cram into that price. Um. I think that that''s the main factor. Cool.
Okay. Right. Um well this is the kick-off meeting for our our project. Um and
um this is just what we''re gonna be doing over the next twenty five minutes.
Um so first of all, just to kind of make sure that we all know each other, I''m
Laura and I''m the project manager. Do you want to introduce yourself again? Okay.
Great. Okay. Um so we''re designing a new remote control and um Oh I have to record
who''s here actually. So that''s David, Andrew and Craig, isn''t it? And you all
arrived on time. Um yeah so des uh design a new remote control. Um, as you can
see it''s supposed to be original, trendy and user friendly. Um so that''s kind
of our our brief, as it were. Um and so there are three different stages to the
design. Um I''m not really sure what what you guys have already received um in
your emails. What did you get? Mm-hmm. Is that what everybody got? Okay. Um. So
we''re gonna have like individual work and then a meeting about it. And repeat
that process three times. Um and at this point we get try out the whiteboard over
there. Um. So uh you get to draw your favourite animal and sum up your favourite
characteristics of it. So who would like to go first? Very good. Mm-hmm. Yeah.
Yeah. Right. Lovely. Right. You can take as long over this as you like, because
we haven''t got an awful lot to discuss. Ok oh we do we do. Don''t feel like you''re
in a rush, anyway. Ach why not We might have to get you up again then. I don''t
know what mine is. I''m gonna have to think on the spot now. Is that a whale?
Ah. Okay. God, I still don''t know what I''m gonna write about. Um. I was gonna
choose a dog as well. But I''ll just draw a different kind of dog. M my favourite
animal is my own dog at home. Um That doesn''t really look like him, actually.
He looks more like a pig, actually. Ah well. Do you? Oh that''s very good of you.
Uh. Um he''s a mixture of uh various things. Um and what do I like about him,
um That''s just to suggest that his tail wags. Um he''s very friendly and cheery
and always pleased to see you, and very kind of affectionate and um uh and he''s
quite quite wee as well so you know he can doesn''t take up too much space. Um
and uh And he does a funny thing where he chases his tail as well, which is quite
amusing, so It is. I think it is. He only does it after he''s had his dinner and
um he''ll just all of a sudden just get up and start chasing his tail ''round
the living room. Yeah, so uh Yeah, maybe. Maybe. Right, um where did you find
this? Just down here? Yeah. Okay. Um what are we doing next? Uh um. Okay, uh we
now need to discuss the project finance. Um so according to the brief um we''re
gonna be selling this remote control for twenty five Euro, um and we''re aiming
to make fifty million Euro. Um so we''re gonna be selling this on an international
scale. And uh we don''t want it to cost any more than uh twelve fifty Euros, so
fifty percent of the selling price. Sure. All together. Um I dunno. I imagine
That''s a good question. I imagine it probably is our sale actually because it''s
probably up to the the um the retailer to uh sell it for whatever price they want.
Um. But I I don''t know, I mean do you think the fact that it''s going to be sold
internationally will have a bearing on how we design it at all? Think it will?
Um. Hmm. Oh yeah, regions and stuff, yeah. Yeah. Okay. Yeah. Well for a remote
control, do you think that will be I suppose it''s depends on how complicated
our remote control is. Yeah, yeah. Okay. What, just like in terms of like the
wealth of the country? Like how much money people have to spend on things like?
Aye, I see what you mean, yeah. Marketing. Good marketing thoughts. Oh gosh, I
should be writing all this down. Um. Mm. Yeah. Yeah, yeah. Like how much does,
you know, a remote control cost. Well twenty five Euro, I mean that''s um that''s
about like eighteen pounds or something, isn''t it? Or no, is it as much as that?
Sixteen seventeen eighteen pounds. Um, I dunno, I''ve never bought a remote control,
so I don''t know how how good a remote control that would get you. Um. But yeah,
I suppose it has to look kind of cool and gimmicky. Um right, okay. Let me just
scoot on ahead here. Okay. Um well d Does anybody have anything to add to uh to
the finance issue at all? Thin No, actually. That would be useful, though, wouldn''t
it, if you knew like what your money would get you now. Mm-hmm. Yeah, yeah. Oh.
Five minutes to end of meeting. Oh, okay. We''re a bit behind. Yeah. Right, so
do you think that should be like a main design aim of our remote control d you
know, do your your satellite and your regular telly and your V_C_R_ and everything?
Mm-hmm. Yeah. Or even like, you know, notes about um what you wanna watch. Like
you might put in there oh I want to watch such and such and look a Oh that''s
a good idea. So extra functionalities. Mm-hmm. Hmm. Um okay, uh I''d wel we''re
gonna have to wrap up pretty quickly in the next couple of minutes. Um I''ll just
check we''ve nothing else. Okay. Um so anything else anybody wants to add about
what they don''t like about remote controls they''ve used, what they would really
like to be part of this new one at all? You keep losing them. Okay. Yeah. W You
get those ones where you can, if you like, whistle or make a really high pitched
noise they beep. There I mean is that something we''d want to include, do you
think? Dunno. Okay maybe. My goodness. Still feels quite primitive. Maybe like
a touch screen or something? Okay. Uh-huh, okay. Well I guess that''s up to our
industrial designer. It looks better. Yeah. Okay. Okay. Right, well um so just
to wrap up, the next meeting''s gonna be in thirty minutes. So that''s about um
about ten to twelve by my watch. Um so inbetween now and then, um as the industrial
designer, you''re gonna be working on you know the actual working design of it
so y you know what you''re doing there. Um for user interface, technical functions,
I guess that''s you know like what we''ve been talking about, what it''ll actually
do. Um and uh marketing executive, you''ll be just thinking about what it actually
what, you know, what requirements it has to has to fulfil and you''ll all get
instructions emailed to you, I guess. Um. Yeah, so it''s th the functional design
stage is next, I guess. And uh and that''s the end of the meeting. So I got that
little message a lot sooner than I thought I would, so Mm-hmm. Uh-huh, yeah. Th
Okay, well just very quickly ''cause this we''re supposed to finish now. Um I
guess that''s up to us, I mean you probably want some kind of unique selling point
of it, so um, you know Yeah. Mm-hmm. Yeah. Okay. Right, okay, we''ll that''s that''s
the end of the meeting, then. Um. So, uh thank you all for coming.
Um I''m Craig and I''m User Interface. Yeah. Well, my favourite animal would be
a monkey. Then they''re small cute and furry, and uh when planet of the apes becomes
real, I''m gonna be up there with them. Yeah. I know um My parents went out and
bought um remote controls because um they got fed up of having four or five different
remote controls for each things the house. So um for them it was just how many
devices control. Uh.
Mm-hmm. Great. And I''m Andrew and I''m uh our marketing expert. Mm-hmm. Mm-hmm.
Yeah, that''s that''s it. Yeah. I will go. That''s fine. Alright. So This one
here, right? Okay. Very nice. Alright. My favourite animal is like A beagle. Um
charac favourite characteristics of it? Is that right? Uh, right, well basically
um high priority for any animal for me is that they be willing to take a lot of
physical affection from their family. And, yeah that they have lots of personality
and uh be fit and in robust good health. So this is blue. Blue beagle. My family''s
beagle. I coulda told you a whole lot more about beagles. Boy, let me tell you.
Impressionist. Alright. Mm. Superb sketch, by the way. Yep. I see a dog in there.
Yep. Now I see a rooster. What kind is it? Is he aware that th it''s his own cha
tail he''s chasing? Hmm. Probably when he was little he got lots of attention
for doing it and has forever been conditioned. ''Kay. Um, can we just go over
that again? Uh, so bas at twel Alright, yeah. Okay. So cost like production cost
is twelve fifty, but selling price is is that wholesale or retail? Like on the
shelf. Our sale our sale anyway. Yeah, okay okay. Okay. Mm-hmm. Alright. Yes.
Mm-hmm. Mm-hmm. Well right away I''m wondering if there''s um th th uh, like with
D_V_D_ players, if there are zones. Um f frequencies or something um as well as
uh characters, um different uh keypad styles and s symbols. Um. I don''t know.
Yeah. Yeah. Yeah. And then a and then al the other thing international is on top
of the price. I''m thinking the price might might appeal to a certain market in
one region, whereas in another it''ll be different, so Just a chara just a characteristic
of the Just Or just like, basic product podi positioning, the twenty five Euro
remote control might be a big hit in London, might not be such a big hit in Greece,
who knows, something like that, yeah. Yep. Right away I''m making some kind of
assumptions about what what information we''re given here, thinking, ''kay trendy
probably means something other than just basic, something other than just standard.
Um so I''m wondering right away, is selling twenty five Euros, is that sort of
the thi is this gonna to be like the premium product kinda thing or Uh-huh. Mm-hmm.
Yep. Yeah, I''d say so, yeah. No. Yeah, yeah. Mm-hmm. Do we have any other background
information on like how that compares to other other Yeah. Mm-hmm. Yeah, interesting
thing about discussing um production of a remote control for me is that l as you
point out, I just don''t think of remote controls as somethin something people
consciously assess in their purchasing habits. It''s just like getting shoelaces
with shoes or something. It just comes along. Do you know what I mean? Like so
sort of like how do you I I mean one one way of looking at it would be, well the
people producing television sets, maybe they have to buy remote controls. Or another
way is maybe people who have T_V_ sets are really fed up with their remote control
and they really want a better one or something. But Right. Right. Okay so Right,
so in function one of the priorities might be to combine as many uses I think
so. Yeah, yeah. Yeah. Well like um, maybe what we could use is a sort of like
a example of a successful other piece technology is palm palm pilots. They''re
gone from being just like little sort of scribble boards to cameras, M_P_ three
players, telephones, everything, agenda. So, like, I wonder if we might add something
new to the to the remote control market, such as the lighting in your house, or
um Yeah, yeah. An Yeah. Like, p personally for me, at home I''ve I''ve combined
the um the audio video of my television set and my D_V_D_ player and my C_D_ player.
So they w all work actually function together but I have different remote controls
for each of them. So it''s sort of ironic that that then they''re in there um
you know, the sound and everything it''s just one system. But each one''s got
its own little part. Mm. Mm. Mm. Mm-hmm. Mm-hmm. Yeah. Yeah. That''s just really
good id Yep. Uh, sure. I remember when the first remote control my my family had
was on a cable. Actually had a cable between it and the T_V_ and big like buttons
that sort of like, like on a blender or something. And um, you know, when I think
about what they are now, it''s better, but actually it''s still kind of, I dunno,
like a massive junky thing on the table. Maybe we could think about how, could
be more, you know, streamlined. S Something like that, yeah. Or whatever would
be technologically reasonable. ''Cause it could b it could it could be that f
it could be that functionally that doesn''t make it any better, but that just
the appeal of of not having You know, these days there''s a r pe things in people''s
homes are becoming more and more like chic, you know. Um, nicer materials and
might be be worth exploring anyway. Okay. Um. Before we wrap up, just to make
sure we''re all on the same page here, um, do we We were given sort of an example
of a coffee machine or something, right? Well, um are we at ma right now on the
assumption that our television remote control may have features which go beyond
the television? Or are we keeping sort of like a a design commitment to television
features? I I don''t know. Yep. Yeah, sure. Okay. Okay, yeah. Okay. Okay. Okay.
Alright.'
model-index:
- name: MEETING_SUMMARY
results:
- task:
type: abstractive-text-summarization
name: Abstractive Text Summarization
dataset:
name: samsum
type: samsum
metrics:
- type: rouge-1
value: 53.8795
name: Validation ROGUE-1
- type: rouge-2
value: 28.4975
name: Validation ROGUE-2
- type: rouge-L
value: 44.1899
name: Validation ROGUE-L
- type: rouge-Lsum
value: 49.4863
name: Validation ROGUE-Lsum
- type: gen-length
value: 30.088
name: Validation ROGUE-Lsum
- type: rouge-1
value: 53.2284
name: Test ROGUE-1
- type: rouge-2
value: 28.184
name: Test ROGUE-2
- type: rouge-L
value: 44.122
name: Test ROGUE-L
- type: rouge-Lsum
value: 49.0301
name: Test ROGUE-Lsum
- type: gen-length
value: 29.9951
name: Test ROGUE-Lsum
- task:
type: summarization
name: Summarization
dataset:
name: bazzhangz/sumdataset
type: bazzhangz/sumdataset
config: bazzhangz--sumdataset
split: train
metrics:
- type: rouge
value: 40.5544
name: ROUGE-1
verified: true
- type: rouge
value: 17.0751
name: ROUGE-2
verified: true
- type: rouge
value: 32.153
name: ROUGE-L
verified: true
- type: rouge
value: 36.4277
name: ROUGE-LSUM
verified: true
- type: loss
value: 2.116729736328125
name: loss
verified: true
- type: gen_len
value: 42.1978
name: gen_len
verified: true
- task:
type: abstractive-text-summarization
name: Abstractive Text Summarization
dataset:
name: xsum
type: xsum
metrics:
- type: rouge-1
value: 35.9078
name: Validation ROGUE-1
- type: rouge-2
value: 14.2497
name: Validation ROGUE-2
- type: rouge-L
value: 28.1421
name: Validation ROGUE-L
- type: rouge-Lsum
value: 28.9826
name: Validation ROGUE-Lsum
- type: gen-length
value: 32.0167
name: Validation ROGUE-Lsum
- type: rouge-1
value: 36.0241
name: Test ROGUE-1
- type: rouge-2
value: 14.3715
name: Test ROGUE-2
- type: rouge-L
value: 28.1968
name: Test ROGUE-L
- type: rouge-Lsum
value: 29.0527
name: Test ROGUE-Lsum
- type: gen-length
value: 31.9933
name: Test ROGUE-Lsum
- task:
type: abstractive-text-summarization
name: Abstractive Text Summarization
dataset:
name: dialogsum
type: dialogsum
metrics:
- type: rouge-1
value: 39.8612
name: Validation ROGUE-1
- type: rouge-2
value: 16.6917
name: Validation ROGUE-2
- type: rouge-L
value: 32.2718
name: Validation ROGUE-L
- type: rouge-Lsum
value: 35.8748
name: Validation ROGUE-Lsum
- type: gen-length
value: 41.726
name: Validation ROGUE-Lsum
- type: rouge-1
value: 36.9608
name: Test ROGUE-1
- type: rouge-2
value: 14.3058
name: Test ROGUE-2
- type: rouge-L
value: 29.3261
name: Test ROGUE-L
- type: rouge-Lsum
value: 32.9
name: Test ROGUE-Lsum
- type: gen-length
value: 43.086
name: Test ROGUE-Lsum
- task:
type: summarization
name: Summarization
dataset:
name: samsum
type: samsum
config: samsum
split: test
metrics:
- type: rouge
value: 53.1878
name: ROUGE-1
verified: true
verifyToken: eyJhbGciOiJFZERTQSIsInR5cCI6IkpXVCJ9.eyJoYXNoIjoiOTVkNTczYjFmYzBmMzczNWE0MGY4MDAyZWExOGNjZmY1Yzk2ZGM1MGNjZmFmYWUyZmIxZjdjOTk4OTc4OGJlMSIsInZlcnNpb24iOjF9.yyzPpGtESuZXy_lBESrboGxdGYB7I6jaIjquCYqliE2xdbGf5awDFpDUwlZHDuw6RD2mIZv1FC8PPs9lOHuSAg
- type: rouge
value: 28.1666
name: ROUGE-2
verified: true
verifyToken: eyJhbGciOiJFZERTQSIsInR5cCI6IkpXVCJ9.eyJoYXNoIjoiMjAzOTdjNGYxNWMzYmFjYjRmMTcxYzI0MmNlNmM5Nzg2MzBlNDdmZWFkN2EwMDE2ZTZmYzc0Zjg0ZDc0M2IxNiIsInZlcnNpb24iOjF9.cPH6O50T6HekO227Xzha-EN_Jp7JS9fh5EP9I0tHxbpGptKtZOQC-NG68zfU2eJKlRSrmgaBYs8tjfTvpAgyDg
- type: rouge
value: 44.117
name: ROUGE-L
verified: true
verifyToken: eyJhbGciOiJFZERTQSIsInR5cCI6IkpXVCJ9.eyJoYXNoIjoiNmNmMzJkYjMxMjhlZDM4YmU3NmI1MDExNzhiYmVhMzEyZGJjNDJkNzczNGQwOTMwNzg2YjU1ZWQ4MDhiMzkxYiIsInZlcnNpb24iOjF9.lcEXK15UqZOdXnPjVqIhFd6o_PLROSIONTRFX5NbwanjEI_MWMLpDh_V0Kpnvs_W0sE6cXh2yoifSYNDA5W7Bw
- type: rouge
value: 49.0094
name: ROUGE-LSUM
verified: true
verifyToken: eyJhbGciOiJFZERTQSIsInR5cCI6IkpXVCJ9.eyJoYXNoIjoiYThkYjk4ZjMzYjI0OTAxNDJiZTU5MzE0YjI5MjEzYTYwNWEzMmU5NjU2ZjQ5NzJhMzkyNmVhNWFjZmM1MjAwMSIsInZlcnNpb24iOjF9.LTn6LpKuMO4Rv4NgsbPmtr2ewiKyoqAXlf6YJfM_6GKwVTKpnJxwx7gaaAtMb0jVlgieITMP11JmbeRfMEhgDg
- type: loss
value: 1.710614562034607
name: loss
verified: true
verifyToken: eyJhbGciOiJFZERTQSIsInR5cCI6IkpXVCJ9.eyJoYXNoIjoiNjNjZmM0ZjkwYWYyMWIyMmFiMWI1ODBiYjRjNzVhM2JhN2NmNmM1ZDUwZWRjNDQxNzUwMWM4YjYxYTg1MWYwNyIsInZlcnNpb24iOjF9.hGXZhp9pe-HDJilXVvMCkqz-92YZvH6Qr7q9Z7fJkm8N9s0b4sl-4PwjQYJEOLEAhoRO2s-F5T3bmCYCaMiNBQ
- type: gen_len
value: 29.9951
name: gen_len
verified: true
verifyToken: eyJhbGciOiJFZERTQSIsInR5cCI6IkpXVCJ9.eyJoYXNoIjoiZmY1NzZiMDAzNGJlNTg4Nzc0YzU1MTA3YTI3MzVmNGZkNWQ0ZDE4MGZlNGI1MzJmYzA3MjQ0MDZhMTcyYTk2NCIsInZlcnNpb24iOjF9.8dvMfY7Y-nw-K8NGgTXIGFMxaSUWQYBE1w3N5YYOn4iwnCe2ugo2qPIOxLY91q7CaAOMCSskFV3BDStQ4p0ZCg
---
Model obtained by Fine Tuning 'facebook/bart-large-xsum' using AMI Meeting Corpus, SAMSUM Dataset, DIALOGSUM Dataset, XSUM Dataset!
## Usage
# Example 1
```python
from transformers import pipeline
summarizer = pipeline("summarization", model="knkarthick/MEETING_SUMMARY")
text = '''The tower is 324 metres (1,063 ft) tall, about the same height as an 81-storey building, and the tallest structure in Paris. Its base is square, measuring 125 metres (410 ft) on each side. During its construction, the Eiffel Tower surpassed the Washington Monument to become the tallest man-made structure in the world, a title it held for 41 years until the Chrysler Building in New York City was finished in 1930. It was the first structure to reach a height of 300 metres. Due to the addition of a broadcasting aerial at the top of the tower in 1957, it is now taller than the Chrysler Building by 5.2 metres (17 ft). Excluding transmitters, the Eiffel Tower is the second tallest free-standing structure in France after the Millau Viaduct.
'''
summarizer(text)
```
# Example 2
```python
from transformers import pipeline
summarizer = pipeline("summarization", model="knkarthick/MEETING_SUMMARY")
text = '''Bangalore is the capital and the largest city of the Indian state of Karnataka. It has a population of more than 8 million and a metropolitan population of around 11 million, making it the third most populous city and fifth most populous urban agglomeration in India. Located in southern India on the Deccan Plateau, at a height of over 900 m (3,000 ft) above sea level, Bangalore is known for its pleasant climate throughout the year. Its elevation is the highest among the major cities of India.The city's history dates back to around 890 CE, in a stone inscription found at the Nageshwara Temple in Begur, Bangalore. The Begur inscription is written in Halegannada (ancient Kannada), mentions 'Bengaluru Kalaga' (battle of Bengaluru). It was a significant turning point in the history of Bangalore as it bears the earliest reference to the name 'Bengaluru'. In 1537 CE, Kempé Gowdā – a feudal ruler under the Vijayanagara Empire – established a mud fort considered to be the foundation of modern Bangalore and its oldest areas, or petes, which exist to the present day.
After the fall of Vijayanagar empire in 16th century, the Mughals sold Bangalore to Chikkadevaraja Wodeyar (1673–1704), the then ruler of the Kingdom of Mysore for three lakh rupees. When Haider Ali seized control of the Kingdom of Mysore, the administration of Bangalore passed into his hands.
The city was captured by the British East India Company after victory in the Fourth Anglo-Mysore War (1799), who returned administrative control of the city to the Maharaja of Mysore. The old city developed in the dominions of the Maharaja of Mysore and was made capital of the Princely State of Mysore, which existed as a nominally sovereign entity of the British Raj. In 1809, the British shifted their cantonment to Bangalore, outside the old city, and a town grew up around it, which was governed as part of British India. Following India's independence in 1947, Bangalore became the capital of Mysore State, and remained capital when the new Indian state of Karnataka was formed in 1956. The two urban settlements of Bangalore – city and cantonment – which had developed as independent entities merged into a single urban centre in 1949. The existing Kannada name, Bengalūru, was declared the official name of the city in 2006.
Bangalore is widely regarded as the "Silicon Valley of India" (or "IT capital of India") because of its role as the nation's leading information technology (IT) exporter. Indian technological organisations are headquartered in the city. A demographically diverse city, Bangalore is the second fastest-growing major metropolis in India. Recent estimates of the metro economy of its urban area have ranked Bangalore either the fourth- or fifth-most productive metro area of India. As of 2017, Bangalore was home to 7,700 millionaires and 8 billionaires with a total wealth of $320 billion. It is home to many educational and research institutions. Numerous state-owned aerospace and defence organisations are located in the city. The city also houses the Kannada film industry. It was ranked the most liveable Indian city with a population of over a million under the Ease of Living Index 2020.
'''
summarizer(text)
```
# Example 3
```python
from transformers import pipeline
summarizer = pipeline("summarization", model="knkarthick/MEETING_SUMMARY")
text = '''Hi, I'm David and I'm supposed to be an industrial designer. Um, I just got the project announcement about what the project is. Designing a remote control. That's about it, didn't get anything else. Did you get the same thing? Cool. There's too much gear. Okay. Can't draw. Um. Yeah. Um, well anyway, I don't know, it's just the first animal I can think off the top of my head. Um. Yes. Big reason is 'cause I'm allergic to most animals. Allergic to animal fur, so um fish was a natural choice. Um, yeah, and I kind of like whales. They come in and go eat everything in sight. And they're quite harmless and mild and interesting. Tail's a bit big, I think. It's an after dinner dog then. Hmm. It does make sense from maybe the design point of view 'cause you have more complicated characters like European languages, then you need more buttons. So, possibly. Hmm. Yeah. And you keep losing them. Finding them is really a pain, you know. I mean it's usually quite small, or when you want it right, it slipped behind the couch or it's kicked under the table. You know. Yep. Mm-hmm. I think one factor would be production cost. Because there's a cap there, so um depends on how much you can cram into that price. Um. I think that that's the main factor. Cool.
Okay. Right. Um well this is the kick-off meeting for our our project. Um and um this is just what we're gonna be doing over the next twenty five minutes. Um so first of all, just to kind of make sure that we all know each other, I'm Laura and I'm the project manager. Do you want to introduce yourself again? Okay. Great. Okay. Um so we're designing a new remote control and um Oh I have to record who's here actually. So that's David, Andrew and Craig, isn't it? And you all arrived on time. Um yeah so des uh design a new remote control. Um, as you can see it's supposed to be original, trendy and user friendly. Um so that's kind of our our brief, as it were. Um and so there are three different stages to the design. Um I'm not really sure what what you guys have already received um in your emails. What did you get? Mm-hmm. Is that what everybody got? Okay. Um. So we're gonna have like individual work and then a meeting about it. And repeat that process three times. Um and at this point we get try out the whiteboard over there. Um. So uh you get to draw your favourite animal and sum up your favourite characteristics of it. So who would like to go first? Very good. Mm-hmm. Yeah. Yeah. Right. Lovely. Right. You can take as long over this as you like, because we haven't got an awful lot to discuss. Ok oh we do we do. Don't feel like you're in a rush, anyway. Ach why not We might have to get you up again then. I don't know what mine is. I'm gonna have to think on the spot now. Is that a whale? Ah. Okay. God, I still don't know what I'm gonna write about. Um. I was gonna choose a dog as well. But I'll just draw a different kind of dog. M my favourite animal is my own dog at home. Um That doesn't really look like him, actually. He looks more like a pig, actually. Ah well. Do you? Oh that's very good of you. Uh. Um he's a mixture of uh various things. Um and what do I like about him, um That's just to suggest that his tail wags. Um he's very friendly and cheery and always pleased to see you, and very kind of affectionate and um uh and he's quite quite wee as well so you know he can doesn't take up too much space. Um and uh And he does a funny thing where he chases his tail as well, which is quite amusing, so It is. I think it is. He only does it after he's had his dinner and um he'll just all of a sudden just get up and start chasing his tail 'round the living room. Yeah, so uh Yeah, maybe. Maybe. Right, um where did you find this? Just down here? Yeah. Okay. Um what are we doing next? Uh um. Okay, uh we now need to discuss the project finance. Um so according to the brief um we're gonna be selling this remote control for twenty five Euro, um and we're aiming to make fifty million Euro. Um so we're gonna be selling this on an international scale. And uh we don't want it to cost any more than uh twelve fifty Euros, so fifty percent of the selling price. Sure. All together. Um I dunno. I imagine That's a good question. I imagine it probably is our sale actually because it's probably up to the the um the retailer to uh sell it for whatever price they want. Um. But I I don't know, I mean do you think the fact that it's going to be sold internationally will have a bearing on how we design it at all? Think it will? Um. Hmm. Oh yeah, regions and stuff, yeah. Yeah. Okay. Yeah. Well for a remote control, do you think that will be I suppose it's depends on how complicated our remote control is. Yeah, yeah. Okay. What, just like in terms of like the wealth of the country? Like how much money people have to spend on things like? Aye, I see what you mean, yeah. Marketing. Good marketing thoughts. Oh gosh, I should be writing all this down. Um. Mm. Yeah. Yeah, yeah. Like how much does, you know, a remote control cost. Well twenty five Euro, I mean that's um that's about like eighteen pounds or something, isn't it? Or no, is it as much as that? Sixteen seventeen eighteen pounds. Um, I dunno, I've never bought a remote control, so I don't know how how good a remote control that would get you. Um. But yeah, I suppose it has to look kind of cool and gimmicky. Um right, okay. Let me just scoot on ahead here. Okay. Um well d Does anybody have anything to add to uh to the finance issue at all? Thin No, actually. That would be useful, though, wouldn't it, if you knew like what your money would get you now. Mm-hmm. Yeah, yeah. Oh. Five minutes to end of meeting. Oh, okay. We're a bit behind. Yeah. Right, so do you think that should be like a main design aim of our remote control d you know, do your your satellite and your regular telly and your V_C_R_ and everything? Mm-hmm. Yeah. Or even like, you know, notes about um what you wanna watch. Like you might put in there oh I want to watch such and such and look a Oh that's a good idea. So extra functionalities. Mm-hmm. Hmm. Um okay, uh I'd wel we're gonna have to wrap up pretty quickly in the next couple of minutes. Um I'll just check we've nothing else. Okay. Um so anything else anybody wants to add about what they don't like about remote controls they've used, what they would really like to be part of this new one at all? You keep losing them. Okay. Yeah. W You get those ones where you can, if you like, whistle or make a really high pitched noise they beep. There I mean is that something we'd want to include, do you think? Dunno. Okay maybe. My goodness. Still feels quite primitive. Maybe like a touch screen or something? Okay. Uh-huh, okay. Well I guess that's up to our industrial designer. It looks better. Yeah. Okay. Okay. Right, well um so just to wrap up, the next meeting's gonna be in thirty minutes. So that's about um about ten to twelve by my watch. Um so inbetween now and then, um as the industrial designer, you're gonna be working on you know the actual working design of it so y you know what you're doing there. Um for user interface, technical functions, I guess that's you know like what we've been talking about, what it'll actually do. Um and uh marketing executive, you'll be just thinking about what it actually what, you know, what requirements it has to has to fulfil and you'll all get instructions emailed to you, I guess. Um. Yeah, so it's th the functional design stage is next, I guess. And uh and that's the end of the meeting. So I got that little message a lot sooner than I thought I would, so Mm-hmm. Uh-huh, yeah. Th Okay, well just very quickly 'cause this we're supposed to finish now. Um I guess that's up to us, I mean you probably want some kind of unique selling point of it, so um, you know Yeah. Mm-hmm. Yeah. Okay. Right, okay, we'll that's that's the end of the meeting, then. Um. So, uh thank you all for coming.
Um I'm Craig and I'm User Interface. Yeah. Well, my favourite animal would be a monkey. Then they're small cute and furry, and uh when planet of the apes becomes real, I'm gonna be up there with them. Yeah. I know um My parents went out and bought um remote controls because um they got fed up of having four or five different remote controls for each things the house. So um for them it was just how many devices control. Uh.
Mm-hmm. Great. And I'm Andrew and I'm uh our marketing expert. Mm-hmm. Mm-hmm. Yeah, that's that's it. Yeah. I will go. That's fine. Alright. So This one here, right? Okay. Very nice. Alright. My favourite animal is like A beagle. Um charac favourite characteristics of it? Is that right? Uh, right, well basically um high priority for any animal for me is that they be willing to take a lot of physical affection from their family. And, yeah that they have lots of personality and uh be fit and in robust good health. So this is blue. Blue beagle. My family's beagle. I coulda told you a whole lot more about beagles. Boy, let me tell you. Impressionist. Alright. Mm. Superb sketch, by the way. Yep. I see a dog in there. Yep. Now I see a rooster. What kind is it? Is he aware that th it's his own cha tail he's chasing? Hmm. Probably when he was little he got lots of attention for doing it and has forever been conditioned. 'Kay. Um, can we just go over that again? Uh, so bas at twel Alright, yeah. Okay. So cost like production cost is twelve fifty, but selling price is is that wholesale or retail? Like on the shelf. Our sale our sale anyway. Yeah, okay okay. Okay. Mm-hmm. Alright. Yes. Mm-hmm. Mm-hmm. Well right away I'm wondering if there's um th th uh, like with D_V_D_ players, if there are zones. Um f frequencies or something um as well as uh characters, um different uh keypad styles and s symbols. Um. I don't know. Yeah. Yeah. Yeah. And then a and then al the other thing international is on top of the price. I'm thinking the price might might appeal to a certain market in one region, whereas in another it'll be different, so Just a chara just a characteristic of the Just Or just like, basic product podi positioning, the twenty five Euro remote control might be a big hit in London, might not be such a big hit in Greece, who knows, something like that, yeah. Yep. Right away I'm making some kind of assumptions about what what information we're given here, thinking, 'kay trendy probably means something other than just basic, something other than just standard. Um so I'm wondering right away, is selling twenty five Euros, is that sort of the thi is this gonna to be like the premium product kinda thing or Uh-huh. Mm-hmm. Yep. Yeah, I'd say so, yeah. No. Yeah, yeah. Mm-hmm. Do we have any other background information on like how that compares to other other Yeah. Mm-hmm. Yeah, interesting thing about discussing um production of a remote control for me is that l as you point out, I just don't think of remote controls as somethin something people consciously assess in their purchasing habits. It's just like getting shoelaces with shoes or something. It just comes along. Do you know what I mean? Like so sort of like how do you I I mean one one way of looking at it would be, well the people producing television sets, maybe they have to buy remote controls. Or another way is maybe people who have T_V_ sets are really fed up with their remote control and they really want a better one or something. But Right. Right. Okay so Right, so in function one of the priorities might be to combine as many uses I think so. Yeah, yeah. Yeah. Well like um, maybe what we could use is a sort of like a example of a successful other piece technology is palm palm pilots. They're gone from being just like little sort of scribble boards to cameras, M_P_ three players, telephones, everything, agenda. So, like, I wonder if we might add something new to the to the remote control market, such as the lighting in your house, or um Yeah, yeah. An Yeah. Like, p personally for me, at home I've I've combined the um the audio video of my television set and my D_V_D_ player and my C_D_ player. So they w all work actually function together but I have different remote controls for each of them. So it's sort of ironic that that then they're in there um you know, the sound and everything it's just one system. But each one's got its own little part. Mm. Mm. Mm. Mm-hmm. Mm-hmm. Yeah. Yeah. That's just really good id Yep. Uh, sure. I remember when the first remote control my my family had was on a cable. Actually had a cable between it and the T_V_ and big like buttons that sort of like, like on a blender or something. And um, you know, when I think about what they are now, it's better, but actually it's still kind of, I dunno, like a massive junky thing on the table. Maybe we could think about how, could be more, you know, streamlined. S Something like that, yeah. Or whatever would be technologically reasonable. 'Cause it could b it could it could be that f it could be that functionally that doesn't make it any better, but that just the appeal of of not having You know, these days there's a r pe things in people's homes are becoming more and more like chic, you know. Um, nicer materials and might be be worth exploring anyway. Okay. Um. Before we wrap up, just to make sure we're all on the same page here, um, do we We were given sort of an example of a coffee machine or something, right? Well, um are we at ma right now on the assumption that our television remote control may have features which go beyond the television? Or are we keeping sort of like a a design commitment to television features? I I don't know. Yep. Yeah, sure. Okay. Okay, yeah. Okay. Okay. Okay. Alright.
'''
summarizer(text)
```
# Example 4
```python
from transformers import pipeline
summarizer = pipeline("summarization", model="knkarthick/MEETING_SUMMARY")
text = '''
Das : Hi and welcome to the a16z podcast. I’m Das, and in this episode, I talk SaaS go-to-market with David Ulevitch and our newest enterprise general partner Kristina Shen. The first half of the podcast looks at how remote work impacts the SaaS go-to-market and what the smartest founders are doing to survive the current crisis. The second half covers pricing approaches and strategy, including how to think about free versus paid trials and navigating the transition to larger accounts. But we start with why it’s easier to move upmarket than down… and the advantage that gives a SaaS startup against incumbents.
David : If you have a cohort of customers that are paying you $10,000 a year for your product, you’re going to find a customer that self-selects and is willing to pay $100,000 a year. Once you get one of those, your organization will figure out how you sell to, how you satisfy and support, customers at that price point and that size. But it’s really hard for a company that sells up market to move down market, because they’ve already baked in all that expensive, heavy lifting sales motion. And so as you go down market with a lower price point, usually, you can’t actually support it.
Das : Does that mean that it’s easier for a company to do this go-to-market if they’re a new startup as opposed to if they’re a pre-existing SaaS?
Kristina : It’s culturally very, very hard to give a product away for free that you’re already charging for. It feels like you’re eating away at your own potential revenue when you do it. So most people who try it end up pulling back very quickly.
David : This is actually one of the key reasons why the bottoms up SaaS motion is just so competitive, and compelling, and so destructive against the traditional sales-driven test motion. If you have that great product and people are choosing to use it, it’s very hard for somebody with a sales-driven motion, and all the cost that’s loaded into that, to be able to compete against it. There are so many markets where initially, we would look at companies and say, “Oh, well, this couldn’t possibly be bottoms up. It has to be sold to the CIO. It has to be sold to the CSO or the CFO.” But in almost every case we’ve been wrong, and there has been a bottoms up motion. The canonical example is Slack. It’s crazy that Slack is a bottoms up company, because you’re talking about corporate messaging, and how could you ever have a messaging solution that only a few people might be using, that only a team might be using? But now it’s just, “Oh, yeah, some people started using it, and then more people started using it, and then everyone had Slack.”
Kristina : I think another classic example is Dropbox versus Box. Both started as bottoms up businesses, try before you buy. But Box quickly found, “Hey, I’d rather sell to IT.” And Dropbox said, “Hey, we’ve got a great freemium motion going.” And they catalyzed their business around referrals and giving away free storage and shared storage in a way that really helped drive their bottoms up business.
Das : It’s a big leap to go from selling to smaller customers to larger customers. How have you seen SaaS companies know or get the timing right on that? Especially since it does seem like that’s really related to scaling your sales force?
Kristina : Don’t try to go from a 100-person company to a 20,000-person company. Start targeting early adopters, maybe they’re late stage pre-IPO companies, then newly IPO’d companies. Starting in tech tends to be a little bit easier because they tend to be early adopters. Going vertical by vertical can be a great strategy as well. Targeting one customer who might be branded in that space, can help brand yourself in that category. And then all their competitors will also want your product if you do a good job. A lot of times people will dedicate a sales rep to each vertical, so that they become really, really knowledgeable in that space, and also build their own brand and reputation and know who are the right customers to target.
Das : So right now, you’ve got a lot more people working remote. Does this move to remote work mean that on-premise software is dying? And is it accelerating the move to software as a service?
Kristina : This remote work and working from home is only going to catalyze more of the conversion from on-premise over to cloud and SaaS. In general, software spend declines 20% during an economic downturn. This happened in ’08, this happened in ’01. But when we look at the last downturn in ’08, SaaS spend actually, for public companies, increased, on average, 10%, which means there’s a 30% spread, which really shows us that there was a huge catalyst from people moving on-premise to SaaS.
David : And as people work remote, the ability to use SaaS tools is much easier than having to VPN back into your corporate network. We’ve been seeing that, inside sales teams have been doing larger and larger deals, essentially moving up market on the inside, without having to engage with field sales teams. In fact, a lot of the new SaaS companies today rather than building out a field team, they have a hybrid team, where people are working and closing deals on the inside and if they had to go out and meet with a customer, they would do that. But by and large, most of it was happening over the phone, over email, and over videoconferencing. And all the deals now, by definition, are gonna be done remote because people can’t go visit their customers in person.
Das : So with bottoms up, did user behavior and buyer behavior change, so the go-to-market evolved? Or did the go-to-market evolve and then you saw user and buyer behavior change? I’m curious with this move to remote work. Is that going to trigger more changes or has the go-to-market enabled that change in user behavior, even though we see that change coming because of a lot of forces outside of the market?
Kristina : I definitely think they are interrelated. But I do think it was a user change that catalyzed everything. We decided that we preferred better software, and we tried a couple products. We were able to purchase off our credit card. And then IT and procurement eventually said, “Wow, everyone’s buying these already, I might as well get a company license and a company deal so I’m not paying as much.” While obviously software vendors had to offer the products that could be self-served, users started to realize they had the power, they wanted to use better software, they paid with their credit cards. And now software vendors are forced to change their go-to-market to actually suit that use case.
Das : If that’s the case that when user behavior has changed, it’s tended to be the catalyzing force of bigger changes in the go-to-market, what are some of the changes you foresee for SaaS because the world has changed to this new reality of remote work and more distributed teams?
David : We’re in a very uncertain economic environment right now. And a couple of things will become very clear over the next 3 to 9 to 15 months — you’re going to find out which SaaS products are absolutely essential to helping a business operate and run, and which ones were just nice to have and may not get renewed. I think on the customer, buying side, you’re very likely to see people push back on big annual commitments and prefer to go month-to-month where they can. Or you’ll see more incentives from SaaS startups to offer discounts for annual contracts. You’re going to see people that might sign an annual contract, but they may not want to pay upfront. They may prefer to meter the cash out ratably over the term of the contract. And as companies had empowered and allowed budget authority to be pushed down in organizations, you’re gonna see that budget authority get pulled back, more scrutiny on spending, and likely a lot of SaaS products not get renewed that turned out to not be essential.
Kristina : I think the smartest founders are making sure they have the runway to continue to exist. And they’re doing that in a couple of ways. They’re preserving cash, and they are making sure that their existing customers are super, super happy, because retaining your customers is so important in this environment. And they’re making sure that they have efficient or profitable customer acquisition. Don’t spend valuable dollars acquiring customers. But acquire customers efficiently that will add to a great existing customer base.
Das : To go into pricing and packaging for SaaS for a moment, what are some of the different pricing approaches that you see SaaS companies taking?
Kristina : The old school way of doing SaaS go-to-market is bundle everything together, make the pricing super complex, so you don’t actually understand what you’re paying for. You’re forced to purchase it because you need one component of the product. New modern SaaS pricing is keep it simple, keep it tied to value, and make sure you’re solving one thing really, really well.
David : You want to make it easy for your customers to give you money. And if your customers don’t understand your pricing, that’s a huge red flag. Sometimes founders will try to over engineer their pricing model.
Kristina : We talk a lot about everything has to be 10X better than the alternatives. But it’s much easier to be 10X better when you solve one thing very, very well, and then have simple pricing around it. I think the most common that most people know about is PEPM or per employee per month, where you’re charging basically for every single seat. Another really common model is the freemium model. So, think about a Dropbox, or an Asana, or a Skype, where it’s trigger based. You try the product for free, but when you hit a certain amount of storage, or a certain amount of users, then it converts over to paid. And then you also have a time trial, where you get the full experience of the product for some limited time period. And then you’re asked if you want to continue using the product to pay. And then there’s pay as go, and particularly, pay as you go as a usage model. So, Slack will say, “Hey, if your users aren’t actually using the product this month, we won’t actually charge you for it.”
David : The example that Kristina made about Slack and users, everybody understands what a user is, and if they’re using the product, they pay for it, and if they’re not using it, they don’t pay for it. That’s a very friendly way to make it easy for your customers to give you money. If Slack came up with a pricing model that was like based on number of messages, or number of API integration calls, the customer would have no idea what that means.
Kristina : There’s also the consumption model. So Twilio only charges you for every SMS text or phone call that you make on the platform any given month. And so they make money or lose money as your usage goes. The pricing is very aligned to your productivity.
David : Generally, those are for products where the usage only goes in one direction. If you think of a company like Databricks, where they’re charging for storage, or Amazon’s S3 service, it is very aligned with the customer, but it also strategically aligns with the business because they know the switching cost is very high, the churn is very low. And generally, in those businesses, you’re only going to store more data, so they can charge based on usage or volume of data.
Kristina : Recently, there’s been a huge trend of payment as a revenue. It’s particularly common in vertical markets where SaaS companies are adding payments as a revenue in addition to their employee or subscription revenue. If you look at Shopify, for example, more than 50% of their revenue is actually payment revenue. They’re making money every single time you purchase something off one of their shopping cart websites.
Das : When you’re working with a founder or a SaaS startup, how have you seen them find the right pricing model for their product, for their market?
Kristina : Step one is just talk to a lot of customers. Try to figure out what is the market pricing for possible alternatives or competitors, understand their pain points and their willingness to pay. And just throw a price out there, because you have to have a starting point in order to actually test and iterate. Particularly in the SMB, or the bottoms up business, you can test and iterate pretty quickly because you have so many data points.
David : I always tell founders, step one is to just go out there and talk to customers. Step two is just double your prices. I don’t think there’s ever been a great company with a great product that’s fallen apart because their pricing was wrong. But a lot of SaaS startup founders really under price, and you don’t want to find out two or three years later that you were 200% underpriced. A very common thing that SaaS companies do, they’ll have the basic package that either is free or low cost, that you can just sign up online for. They’ll have a middle package where they share some pricing, and then they’ll have the enterprise package where you have to contact sales to find out more. And that way they don’t actually have to show the pricing for that third package. And that gives the salespeople the flexibility to adjust pricing on a per deal basis.
Das : When you’re working with companies, why are they underpricing their products?
David : I think it’s psychological. People need to price on value, and they don’t know how much value they’re delivering relative to “Oh, it only cost me $100 a month to provide this service, so I just need to charge $200.” But if it turns out you’re saving your customer $50,000 a year, then you’re wildly underpriced. You have to remember that SaaS is essentially a proxy for outsourced IT. You’re spending money on a SaaS service to not pay to develop something internally, or to have to pay IT to support something that’s more complex on-prem. Software is much cheaper than people, and so generally, the price point can be much higher.
Kristina : And the other thing is your value increases over time. You’re delivering more features, more products, you understand the customer better. It’s the beauty of the SaaS model and cloud model that you can iterate and push code immediately, and the customer immediately sees value. A lot of times people have the same price point from the first customer sold to three years later and the 200th customer. Quite frankly, you’ve delivered so much value along the way that your price point should have gone up. The other thing I’ll say is a lot of people discount per seat pricing a lot as they move up market. We tend to tell people that the best validation of your product having great product market fit is your ability to hold your price point. So while there is some natural discounting on a per seat basis because people do deserve some volume discounting, I would say try to resist that as much as possible.
Das : Especially for a technical founder, it’s so tempting to get in there and fiddle with these knobs. How do you know when it is time to experiment with your pricing and packaging?
David : If you’re looking at your business and you see that you are doing more deals, and they’re closing faster, you should raise your pricing. And you pay attention to how long it takes to close deals and whether the number of deals is staying consistent as you do that. And, at some point, you’re going to find out when you’re losing deals on price. I think a moment where companies have to plan ahead to avoid having to course correct is after they roll out massive pricing and packaging changes, which are pretty natural as companies move up market. But how they navigate that transition to larger accounts, and how they either bring along or move away from those smaller, earlier customers who got them to where they are, tends to be really important because they can get a lot of noise on Twitter, they can get a lot of blowback from their customers. So Zendesk is a company where they rolled out a major packaging change. And when they rolled it out, they hadn’t planned on grandfathering in their early customers. They got a lot of pushback, and very quickly, they put out a blog post and said, “We hear what you’re saying, we appreciate you building the business that we’ve become today. We do need to have a package for the future. But all the people that have been customers so far will be grandfathered in for at least a period of time into the old model.”
Kristina : If you iterate pricing constantly, you don’t really have this problem because your customers will be used to pricing changes. You normally pair them with new features, and it all kind of works out. But if you have to go through a big grandfather change, I tend to lean towards treating your early customers really, really well. They adopted when you weren’t a big company yet. They probably co-built the product with you in many ways. And so, it’s great to get more dollars out of your customer base, but treat your early customers well.
Das : Are there any other failure modes that you see startups really falling into around pricing and packaging or any common mistakes that they make?
David : I think a lot of founders don’t always map out the cost or model of their pricing and their product relative to their cost of actually doing sales and marketing and customer acquisition.
Kristina : Inside sales is so popular in Silicon Valley. When you’re selling more to an SMB or mid-market type customer, the expectation is that you’re educating and helping the prospective customer over the phone. And so, you’re not expected to be as high touch. But 5K is almost the minimum price point you need to sell to the SMB with an inside sales team in order to pay for the outbound costs and all the conversions, because there is typically a team that sits around the quota carrying rep. And so, price matching — how much your price point is compared to what your go-to-market motion is — matters a lot. Other big failure modes that I see, people guess the ramp time of a sales rep wrong. And ramp time really ties to the segment of customer you’re selling into. It tends be that if you’re selling into the enterprise, the ramp time for sales reps, because sales cycles are so long, tend to be much longer as well. They could be six months plus, could be a year. While if you’re selling more into SMB or mid-market, the ramp time to get a rep up and running can be much shorter, three to six months. Because the sales cycles are shorter, they just iterate much faster, and they ramp up much more quickly.
David : The other thing that people have to understand is that sales velocity is a really important component to figuring out how many reps you should be hiring, whether they should be inside reps or field reps. If it takes you 90 days to close a deal, that can’t be a $5,000 a year deal, that has to be a $50,000 or even $150,000 a year deal.
Das : Kristina, I know you’ve done a lot of work with metrics. So how do those play in?
Kristina : Probably the one way to sum it all together is how many months does it take to pay back customer acquisition cost. Very commonly within the SaaS world, we talk about a 12-month CAC payback. We typically want to see for every dollar you spend on sales and marketing, you get a dollar back within a year. That means you can tweak the inputs any way you want. Let’s say that doing paid acquisition is really effective for you. Then, you can spend proportionally more on paid acquisition and less on sales reps. Vice versa, if you have a great inbound engine, you actually can hire a lot more sales reps and spend more on sales headcount. With all formulas, it’s a guide rail, so if you have customers that retain really, really well, let’s say you’re selling to the enterprise, and you’ve got a 90% or 95% annual retention rate, then your CAC payback could be between 12 and 24 months. But let’s say you’re selling to the SMB and churn is 2% or 3% monthly, which ends up being like 80% to 90% annual retention. Then, because your customer is less sticky, I would recommend looking at a CAC payback of 6 to 12 months.
Das : How should you think about doing a free trial versus a paid trial?
David : On the one hand, the bottoms up motion where people can try essentially a full version of a product before they buy it is extremely powerful. On the other hand, I’ve started to try to think about how I advise companies, when they are thinking about a free trial for something that might cost $100,000 or $200,000 a year? Do we do a paid pilot that has some sort of contractual obligation that if we meet then turns into a commercial engagement?
Kristina : I do think the beauty of the bottoms up business is that you can get people to try the entire experience of the product for free, and they fall in love with it, and a certain percentage will convert. And that works really, really well for products that can self-serve. When you start moving up market to more complex products, the challenge with trials is it takes work to actually implement the product, whether it be integrations, IT has to give access, etc. You lose that self-serve ability, which is so amazing in the trial. And so, I tend to be more in the camp of paid trials, if it costs you money to actually deploy the trial. And when you’re selling to bigger customers, they associate value when they have to pay. Once a customer has to pay you, then they feel a need to make the project successful and thus they will onboard, schedule things, give you data and access.
David : If you can get to a point where you get the customer to do that paid pilot, such that the only difference between a pilot and an actual customer is just the signing of a contract, that’s very powerful. Now, that does force you to have a really good pre-sales motion to make sure that you can deliver on the promise you’ve made your customers. When companies don’t have a great product, and they paper over it with professional services and sales engineering and post-sales support, that paid pilot thing doesn’t work because the experience isn’t good enough. So, it really is incumbent on the SaaS company that does a paid pilot to make sure that they are able to deliver on that experience.
Kristina : And one emerging trend recently is people signing an annual contract with a one or three month out, as a replacement to the paid pilot. Because it’s the best of both worlds, the SaaS company that’s selling the product gets a higher level of commitment. And the customer gets the optionality of opting out in the same way as a trial without any clawback. It really comes down to where procurement falls. Sometimes procurement is at the beginning of that decision, which makes it more like an annual contract. Sometimes procurement is at the one or three month opt-out period, which means the customer already has a great experience, loves the product, and it is an easier way to convert procurements to actually sign on…
David : And that is a really good segue into renewals. I always tell founders, you might have this subscription business, but it’s not a recurring revenue business until the second year when the revenue actually recurs. I think you really have the first three months to get a customer up and running and happy. And if they’re not, you then have about three months to fix it. And if all that works out, then the remaining six months of the contract can be focused on upsell and expansion.
Das : Awesome. Thank you, Kristina. Thank you, David.
Kristina : Thanks so much for having us. This was fun.
David : Yeah, a lot of fun, great topics, and our favorite thing to talk about.
'''
summarizer(text)
``` |
mradermacher/llama3-psychiatrist-v1.0-fp16-GGUF | mradermacher | 2024-06-27T16:02:06Z | 21,729 | 0 | transformers | [
"transformers",
"gguf",
"text-generation-inference",
"unsloth",
"llama",
"trl",
"sft",
"en",
"base_model:wiweka24/llama3-psychiatrist-v1.0-fp16",
"license:apache-2.0",
"endpoints_compatible",
"region:us"
] | null | 2024-06-27T15:33:47Z | ---
base_model: wiweka24/llama3-psychiatrist-v1.0-fp16
language:
- en
library_name: transformers
license: apache-2.0
quantized_by: mradermacher
tags:
- text-generation-inference
- transformers
- unsloth
- llama
- trl
- sft
---
## About
<!-- ### quantize_version: 2 -->
<!-- ### output_tensor_quantised: 1 -->
<!-- ### convert_type: hf -->
<!-- ### vocab_type: -->
<!-- ### tags: -->
static quants of https://huggingface.co/wiweka24/llama3-psychiatrist-v1.0-fp16
<!-- provided-files -->
weighted/imatrix quants seem not to be available (by me) at this time. If they do not show up a week or so after the static ones, I have probably not planned for them. Feel free to request them by opening a Community Discussion.
## Usage
If you are unsure how to use GGUF files, refer to one of [TheBloke's
READMEs](https://huggingface.co/TheBloke/KafkaLM-70B-German-V0.1-GGUF) for
more details, including on how to concatenate multi-part files.
## Provided Quants
(sorted by size, not necessarily quality. IQ-quants are often preferable over similar sized non-IQ quants)
| Link | Type | Size/GB | Notes |
|:-----|:-----|--------:|:------|
| [GGUF](https://huggingface.co/mradermacher/llama3-psychiatrist-v1.0-fp16-GGUF/resolve/main/llama3-psychiatrist-v1.0-fp16.Q2_K.gguf) | Q2_K | 3.3 | |
| [GGUF](https://huggingface.co/mradermacher/llama3-psychiatrist-v1.0-fp16-GGUF/resolve/main/llama3-psychiatrist-v1.0-fp16.IQ3_XS.gguf) | IQ3_XS | 3.6 | |
| [GGUF](https://huggingface.co/mradermacher/llama3-psychiatrist-v1.0-fp16-GGUF/resolve/main/llama3-psychiatrist-v1.0-fp16.Q3_K_S.gguf) | Q3_K_S | 3.8 | |
| [GGUF](https://huggingface.co/mradermacher/llama3-psychiatrist-v1.0-fp16-GGUF/resolve/main/llama3-psychiatrist-v1.0-fp16.IQ3_S.gguf) | IQ3_S | 3.8 | beats Q3_K* |
| [GGUF](https://huggingface.co/mradermacher/llama3-psychiatrist-v1.0-fp16-GGUF/resolve/main/llama3-psychiatrist-v1.0-fp16.IQ3_M.gguf) | IQ3_M | 3.9 | |
| [GGUF](https://huggingface.co/mradermacher/llama3-psychiatrist-v1.0-fp16-GGUF/resolve/main/llama3-psychiatrist-v1.0-fp16.Q3_K_M.gguf) | Q3_K_M | 4.1 | lower quality |
| [GGUF](https://huggingface.co/mradermacher/llama3-psychiatrist-v1.0-fp16-GGUF/resolve/main/llama3-psychiatrist-v1.0-fp16.Q3_K_L.gguf) | Q3_K_L | 4.4 | |
| [GGUF](https://huggingface.co/mradermacher/llama3-psychiatrist-v1.0-fp16-GGUF/resolve/main/llama3-psychiatrist-v1.0-fp16.IQ4_XS.gguf) | IQ4_XS | 4.6 | |
| [GGUF](https://huggingface.co/mradermacher/llama3-psychiatrist-v1.0-fp16-GGUF/resolve/main/llama3-psychiatrist-v1.0-fp16.Q4_K_S.gguf) | Q4_K_S | 4.8 | fast, recommended |
| [GGUF](https://huggingface.co/mradermacher/llama3-psychiatrist-v1.0-fp16-GGUF/resolve/main/llama3-psychiatrist-v1.0-fp16.Q4_K_M.gguf) | Q4_K_M | 5.0 | fast, recommended |
| [GGUF](https://huggingface.co/mradermacher/llama3-psychiatrist-v1.0-fp16-GGUF/resolve/main/llama3-psychiatrist-v1.0-fp16.Q5_K_S.gguf) | Q5_K_S | 5.7 | |
| [GGUF](https://huggingface.co/mradermacher/llama3-psychiatrist-v1.0-fp16-GGUF/resolve/main/llama3-psychiatrist-v1.0-fp16.Q5_K_M.gguf) | Q5_K_M | 5.8 | |
| [GGUF](https://huggingface.co/mradermacher/llama3-psychiatrist-v1.0-fp16-GGUF/resolve/main/llama3-psychiatrist-v1.0-fp16.Q6_K.gguf) | Q6_K | 6.7 | very good quality |
| [GGUF](https://huggingface.co/mradermacher/llama3-psychiatrist-v1.0-fp16-GGUF/resolve/main/llama3-psychiatrist-v1.0-fp16.Q8_0.gguf) | Q8_0 | 8.6 | fast, best quality |
| [GGUF](https://huggingface.co/mradermacher/llama3-psychiatrist-v1.0-fp16-GGUF/resolve/main/llama3-psychiatrist-v1.0-fp16.f16.gguf) | f16 | 16.2 | 16 bpw, overkill |
Here is a handy graph by ikawrakow comparing some lower-quality quant
types (lower is better):

And here are Artefact2's thoughts on the matter:
https://gist.github.com/Artefact2/b5f810600771265fc1e39442288e8ec9
## FAQ / Model Request
See https://huggingface.co/mradermacher/model_requests for some answers to
questions you might have and/or if you want some other model quantized.
## Thanks
I thank my company, [nethype GmbH](https://www.nethype.de/), for letting
me use its servers and providing upgrades to my workstation to enable
this work in my free time.
<!-- end -->
|
mradermacher/Yi-9B-GGUF | mradermacher | 2024-06-26T23:00:21Z | 21,713 | 0 | transformers | [
"transformers",
"gguf",
"en",
"base_model:01-ai/Yi-9B",
"license:apache-2.0",
"endpoints_compatible",
"region:us"
] | null | 2024-06-26T20:27:11Z | ---
base_model: 01-ai/Yi-9B
language:
- en
library_name: transformers
license: apache-2.0
quantized_by: mradermacher
---
## About
<!-- ### quantize_version: 2 -->
<!-- ### output_tensor_quantised: 1 -->
<!-- ### convert_type: hf -->
<!-- ### vocab_type: -->
<!-- ### tags: -->
static quants of https://huggingface.co/01-ai/Yi-9B
<!-- provided-files -->
weighted/imatrix quants are available at https://huggingface.co/mradermacher/Yi-9B-i1-GGUF
## Usage
If you are unsure how to use GGUF files, refer to one of [TheBloke's
READMEs](https://huggingface.co/TheBloke/KafkaLM-70B-German-V0.1-GGUF) for
more details, including on how to concatenate multi-part files.
## Provided Quants
(sorted by size, not necessarily quality. IQ-quants are often preferable over similar sized non-IQ quants)
| Link | Type | Size/GB | Notes |
|:-----|:-----|--------:|:------|
| [GGUF](https://huggingface.co/mradermacher/Yi-9B-GGUF/resolve/main/Yi-9B.Q2_K.gguf) | Q2_K | 3.5 | |
| [GGUF](https://huggingface.co/mradermacher/Yi-9B-GGUF/resolve/main/Yi-9B.IQ3_XS.gguf) | IQ3_XS | 3.8 | |
| [GGUF](https://huggingface.co/mradermacher/Yi-9B-GGUF/resolve/main/Yi-9B.Q3_K_S.gguf) | Q3_K_S | 4.0 | |
| [GGUF](https://huggingface.co/mradermacher/Yi-9B-GGUF/resolve/main/Yi-9B.IQ3_S.gguf) | IQ3_S | 4.0 | beats Q3_K* |
| [GGUF](https://huggingface.co/mradermacher/Yi-9B-GGUF/resolve/main/Yi-9B.IQ3_M.gguf) | IQ3_M | 4.2 | |
| [GGUF](https://huggingface.co/mradermacher/Yi-9B-GGUF/resolve/main/Yi-9B.Q3_K_M.gguf) | Q3_K_M | 4.4 | lower quality |
| [GGUF](https://huggingface.co/mradermacher/Yi-9B-GGUF/resolve/main/Yi-9B.Q3_K_L.gguf) | Q3_K_L | 4.8 | |
| [GGUF](https://huggingface.co/mradermacher/Yi-9B-GGUF/resolve/main/Yi-9B.IQ4_XS.gguf) | IQ4_XS | 4.9 | |
| [GGUF](https://huggingface.co/mradermacher/Yi-9B-GGUF/resolve/main/Yi-9B.Q4_K_S.gguf) | Q4_K_S | 5.2 | fast, recommended |
| [GGUF](https://huggingface.co/mradermacher/Yi-9B-GGUF/resolve/main/Yi-9B.Q4_K_M.gguf) | Q4_K_M | 5.4 | fast, recommended |
| [GGUF](https://huggingface.co/mradermacher/Yi-9B-GGUF/resolve/main/Yi-9B.Q5_K_S.gguf) | Q5_K_S | 6.2 | |
| [GGUF](https://huggingface.co/mradermacher/Yi-9B-GGUF/resolve/main/Yi-9B.Q5_K_M.gguf) | Q5_K_M | 6.4 | |
| [GGUF](https://huggingface.co/mradermacher/Yi-9B-GGUF/resolve/main/Yi-9B.Q6_K.gguf) | Q6_K | 7.3 | very good quality |
| [GGUF](https://huggingface.co/mradermacher/Yi-9B-GGUF/resolve/main/Yi-9B.Q8_0.gguf) | Q8_0 | 9.5 | fast, best quality |
| [GGUF](https://huggingface.co/mradermacher/Yi-9B-GGUF/resolve/main/Yi-9B.f16.gguf) | f16 | 17.8 | 16 bpw, overkill |
Here is a handy graph by ikawrakow comparing some lower-quality quant
types (lower is better):

And here are Artefact2's thoughts on the matter:
https://gist.github.com/Artefact2/b5f810600771265fc1e39442288e8ec9
## FAQ / Model Request
See https://huggingface.co/mradermacher/model_requests for some answers to
questions you might have and/or if you want some other model quantized.
## Thanks
I thank my company, [nethype GmbH](https://www.nethype.de/), for letting
me use its servers and providing upgrades to my workstation to enable
this work in my free time.
<!-- end -->
|
lambdalabs/sd-image-variations-diffusers | lambdalabs | 2023-02-08T15:10:13Z | 21,685 | 379 | diffusers | [
"diffusers",
"stable-diffusion",
"stable-diffusion-diffusers",
"image-to-image",
"dataset:ChristophSchuhmann/improved_aesthetics_6plus",
"license:creativeml-openrail-m",
"diffusers:StableDiffusionImageVariationPipeline",
"region:us"
] | image-to-image | 2022-09-09T14:53:35Z | ---
thumbnail: "https://repository-images.githubusercontent.com/523487884/fdb03a69-8353-4387-b5fc-0d85f888a63f"
datasets:
- ChristophSchuhmann/improved_aesthetics_6plus
license: creativeml-openrail-m
tags:
- stable-diffusion
- stable-diffusion-diffusers
- image-to-image
---
# Stable Diffusion Image Variations Model Card
📣 V2 model released, and blurriness issues fixed! 📣
🧨🎉 Image Variations is now natively supported in 🤗 Diffusers! 🎉🧨
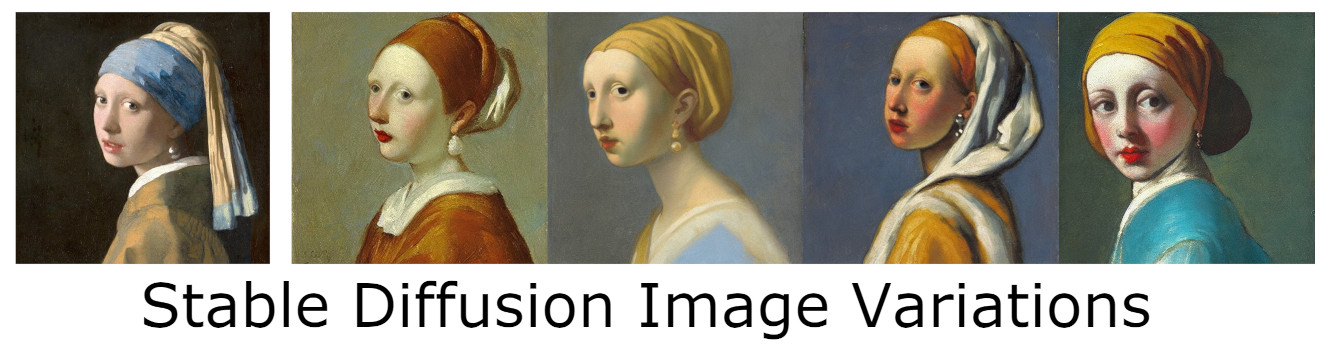
## Version 2
This version of Stable Diffusion has been fine tuned from [CompVis/stable-diffusion-v1-4-original](https://huggingface.co/CompVis/stable-diffusion-v-1-4-original) to accept CLIP image embedding rather than text embeddings. This allows the creation of "image variations" similar to DALLE-2 using Stable Diffusion. This version of the weights has been ported to huggingface Diffusers, to use this with the Diffusers library requires the [Lambda Diffusers repo](https://github.com/LambdaLabsML/lambda-diffusers).
This model was trained in two stages and longer than the original variations model and gives better image quality and better CLIP rated similarity compared to the original version
See training details and v1 vs v2 comparison below.
## Example
Make sure you are using a version of Diffusers >=0.8.0 (for older version see the old instructions at the bottom of this model card)
```python
from diffusers import StableDiffusionImageVariationPipeline
from PIL import Image
device = "cuda:0"
sd_pipe = StableDiffusionImageVariationPipeline.from_pretrained(
"lambdalabs/sd-image-variations-diffusers",
revision="v2.0",
)
sd_pipe = sd_pipe.to(device)
im = Image.open("path/to/image.jpg")
tform = transforms.Compose([
transforms.ToTensor(),
transforms.Resize(
(224, 224),
interpolation=transforms.InterpolationMode.BICUBIC,
antialias=False,
),
transforms.Normalize(
[0.48145466, 0.4578275, 0.40821073],
[0.26862954, 0.26130258, 0.27577711]),
])
inp = tform(im).to(device).unsqueeze(0)
out = sd_pipe(inp, guidance_scale=3)
out["images"][0].save("result.jpg")
```
### The importance of resizing correctly... (or not)
Note that due a bit of an oversight during training, the model expects resized images without anti-aliasing. This turns out to make a big difference and is important to do the resizing the same way during inference. When passing a PIL image to the Diffusers pipeline antialiasing will be applied during resize, so it's better to input a tensor which you have prepared manually according to the transfrom in the example above!
Here are examples of images generated without (top) and with (bottom) anti-aliasing during resize. (Input is [this image](https://github.com/SHI-Labs/Versatile-Diffusion/blob/master/assets/ghibli.jpg))


### V1 vs V2
Here's an example of V1 vs V2, version two was trained more carefully and for longer, see the details below. V2-top vs V1-bottom


Input images:

One important thing to note is that due to the longer training V2 appears to have memorised some common images from the training data, e.g. now the previous example of the Girl with a Pearl Earring almosts perfectly reproduce the original rather than creating variations. You can always use v1 by specifiying `revision="v1.0"`.
v2 output for girl with a pearl earing as input (guidance scale=3)

# Training
**Training Procedure**
This model is fine tuned from Stable Diffusion v1-3 where the text encoder has been replaced with an image encoder. The training procedure is the same as for Stable Diffusion except for the fact that images are encoded through a ViT-L/14 image-encoder including the final projection layer to the CLIP shared embedding space. The model was trained on LAION improved aesthetics 6plus.
- **Hardware:** 8 x A100-40GB GPUs (provided by [Lambda GPU Cloud](https://lambdalabs.com/service/gpu-cloud))
- **Optimizer:** AdamW
- **Stage 1** - Fine tune only CrossAttention layer weights from Stable Diffusion v1.4 model
- **Steps**: 46,000
- **Batch:** batch size=4, GPUs=8, Gradient Accumulations=4. Total batch size=128
- **Learning rate:** warmup to 1e-5 for 10,000 steps and then kept constant
- **Stage 2** - Resume from Stage 1 training the whole unet
- **Steps**: 50,000
- **Batch:** batch size=4, GPUs=8, Gradient Accumulations=5. Total batch size=160
- **Learning rate:** warmup to 1e-5 for 5,000 steps and then kept constant
Training was done using a [modified version of the original Stable Diffusion training code](https://github.com/justinpinkney/stable-diffusion).
# Uses
_The following section is adapted from the [Stable Diffusion model card](https://huggingface.co/CompVis/stable-diffusion-v1-4)_
## Direct Use
The model is intended for research purposes only. Possible research areas and
tasks include
- Safe deployment of models which have the potential to generate harmful content.
- Probing and understanding the limitations and biases of generative models.
- Generation of artworks and use in design and other artistic processes.
- Applications in educational or creative tools.
- Research on generative models.
Excluded uses are described below.
### Misuse, Malicious Use, and Out-of-Scope Use
The model should not be used to intentionally create or disseminate images that create hostile or alienating environments for people. This includes generating images that people would foreseeably find disturbing, distressing, or offensive; or content that propagates historical or current stereotypes.
#### Out-of-Scope Use
The model was not trained to be factual or true representations of people or events, and therefore using the model to generate such content is out-of-scope for the abilities of this model.
#### Misuse and Malicious Use
Using the model to generate content that is cruel to individuals is a misuse of this model. This includes, but is not limited to:
- Generating demeaning, dehumanizing, or otherwise harmful representations of people or their environments, cultures, religions, etc.
- Intentionally promoting or propagating discriminatory content or harmful stereotypes.
- Impersonating individuals without their consent.
- Sexual content without consent of the people who might see it.
- Mis- and disinformation
- Representations of egregious violence and gore
- Sharing of copyrighted or licensed material in violation of its terms of use.
- Sharing content that is an alteration of copyrighted or licensed material in violation of its terms of use.
## Limitations and Bias
### Limitations
- The model does not achieve perfect photorealism
- The model cannot render legible text
- The model does not perform well on more difficult tasks which involve compositionality, such as rendering an image corresponding to “A red cube on top of a blue sphere”
- Faces and people in general may not be generated properly.
- The model was trained mainly with English captions and will not work as well in other languages.
- The autoencoding part of the model is lossy
- The model was trained on a large-scale dataset
[LAION-5B](https://laion.ai/blog/laion-5b/) which contains adult material
and is not fit for product use without additional safety mechanisms and
considerations.
- No additional measures were used to deduplicate the dataset. As a result, we observe some degree of memorization for images that are duplicated in the training data.
The training data can be searched at [https://rom1504.github.io/clip-retrieval/](https://rom1504.github.io/clip-retrieval/) to possibly assist in the detection of memorized images.
### Bias
While the capabilities of image generation models are impressive, they can also reinforce or exacerbate social biases.
Stable Diffusion v1 was trained on subsets of [LAION-2B(en)](https://laion.ai/blog/laion-5b/),
which consists of images that are primarily limited to English descriptions.
Texts and images from communities and cultures that use other languages are likely to be insufficiently accounted for.
This affects the overall output of the model, as white and western cultures are often set as the default. Further, the
ability of the model to generate content with non-English prompts is significantly worse than with English-language prompts.
### Safety Module
The intended use of this model is with the [Safety Checker](https://github.com/huggingface/diffusers/blob/main/src/diffusers/pipelines/stable_diffusion/safety_checker.py) in Diffusers.
This checker works by checking model outputs against known hard-coded NSFW concepts.
The concepts are intentionally hidden to reduce the likelihood of reverse-engineering this filter.
Specifically, the checker compares the class probability of harmful concepts in the embedding space of the `CLIPModel` *after generation* of the images.
The concepts are passed into the model with the generated image and compared to a hand-engineered weight for each NSFW concept.
## Old instructions
If you are using a diffusers version <0.8.0 there is no `StableDiffusionImageVariationPipeline`,
in this case you need to use an older revision (`2ddbd90b14bc5892c19925b15185e561bc8e5d0a`) in conjunction with the lambda-diffusers repo:
First clone [Lambda Diffusers](https://github.com/LambdaLabsML/lambda-diffusers) and install any requirements (in a virtual environment in the example below):
```bash
git clone https://github.com/LambdaLabsML/lambda-diffusers.git
cd lambda-diffusers
python -m venv .venv
source .venv/bin/activate
pip install -r requirements.txt
```
Then run the following python code:
```python
from pathlib import Path
from lambda_diffusers import StableDiffusionImageEmbedPipeline
from PIL import Image
import torch
device = "cuda" if torch.cuda.is_available() else "cpu"
pipe = StableDiffusionImageEmbedPipeline.from_pretrained(
"lambdalabs/sd-image-variations-diffusers",
revision="2ddbd90b14bc5892c19925b15185e561bc8e5d0a",
)
pipe = pipe.to(device)
im = Image.open("your/input/image/here.jpg")
num_samples = 4
image = pipe(num_samples*[im], guidance_scale=3.0)
image = image["sample"]
base_path = Path("outputs/im2im")
base_path.mkdir(exist_ok=True, parents=True)
for idx, im in enumerate(image):
im.save(base_path/f"{idx:06}.jpg")
```
*This model card was written by: Justin Pinkney and is based on the [Stable Diffusion model card](https://huggingface.co/CompVis/stable-diffusion-v1-4).* |
TencentARC/t2i-adapter-depth-midas-sdxl-1.0 | TencentARC | 2023-09-07T19:11:24Z | 21,625 | 25 | diffusers | [
"diffusers",
"safetensors",
"art",
"t2i-adapter",
"image-to-image",
"stable-diffusion-xl-diffusers",
"stable-diffusion-xl",
"arxiv:2302.08453",
"base_model:stabilityai/stable-diffusion-xl-base-1.0",
"license:apache-2.0",
"region:us"
] | image-to-image | 2023-09-03T14:46:44Z | ---
license: apache-2.0
base_model: stabilityai/stable-diffusion-xl-base-1.0
tags:
- art
- t2i-adapter
- image-to-image
- stable-diffusion-xl-diffusers
- stable-diffusion-xl
---
# T2I-Adapter-SDXL - Depth-MiDaS
T2I Adapter is a network providing additional conditioning to stable diffusion. Each t2i checkpoint takes a different type of conditioning as input and is used with a specific base stable diffusion checkpoint.
This checkpoint provides conditioning on depth for the StableDiffusionXL checkpoint. This was a collaboration between **Tencent ARC** and [**Hugging Face**](https://huggingface.co/).
## Model Details
- **Developed by:** T2I-Adapter: Learning Adapters to Dig out More Controllable Ability for Text-to-Image Diffusion Models
- **Model type:** Diffusion-based text-to-image generation model
- **Language(s):** English
- **License:** Apache 2.0
- **Resources for more information:** [GitHub Repository](https://github.com/TencentARC/T2I-Adapter), [Paper](https://arxiv.org/abs/2302.08453).
- **Model complexity:**
| | SD-V1.4/1.5 | SD-XL | T2I-Adapter | T2I-Adapter-SDXL |
| --- | --- |--- |--- |--- |
| Parameters | 860M | 2.6B |77 M | 77/79 M | |
- **Cite as:**
@misc{
title={T2I-Adapter: Learning Adapters to Dig out More Controllable Ability for Text-to-Image Diffusion Models},
author={Chong Mou, Xintao Wang, Liangbin Xie, Yanze Wu, Jian Zhang, Zhongang Qi, Ying Shan, Xiaohu Qie},
year={2023},
eprint={2302.08453},
archivePrefix={arXiv},
primaryClass={cs.CV}
}
### Checkpoints
| Model Name | Control Image Overview| Control Image Example | Generated Image Example |
|---|---|---|---|
|[TencentARC/t2i-adapter-canny-sdxl-1.0](https://huggingface.co/TencentARC/t2i-adapter-canny-sdxl-1.0)<br/> *Trained with canny edge detection* | A monochrome image with white edges on a black background.|<a href="https://huggingface.co/Adapter/t2iadapter/resolve/main/figs_SDXLV1.0/cond_canny.png"><img width="64" style="margin:0;padding:0;" src="https://huggingface.co/Adapter/t2iadapter/resolve/main/figs_SDXLV1.0/cond_canny.png"/></a>|<a href="https://huggingface.co/Adapter/t2iadapter/resolve/main/figs_SDXLV1.0/res_canny.png"><img width="64" src="https://huggingface.co/Adapter/t2iadapter/resolve/main/figs_SDXLV1.0/res_canny.png"/></a>|
|[TencentARC/t2i-adapter-sketch-sdxl-1.0](https://huggingface.co/TencentARC/t2i-adapter-sketch-sdxl-1.0)<br/> *Trained with [PidiNet](https://github.com/zhuoinoulu/pidinet) edge detection* | A hand-drawn monochrome image with white outlines on a black background.|<a href="https://huggingface.co/Adapter/t2iadapter/resolve/main/figs_SDXLV1.0/cond_sketch.png"><img width="64" style="margin:0;padding:0;" src="https://huggingface.co/Adapter/t2iadapter/resolve/main/figs_SDXLV1.0/cond_sketch.png"/></a>|<a href="https://huggingface.co/Adapter/t2iadapter/resolve/main/figs_SDXLV1.0/res_sketch.png"><img width="64" src="https://huggingface.co/Adapter/t2iadapter/resolve/main/figs_SDXLV1.0/res_sketch.png"/></a>|
|[TencentARC/t2i-adapter-lineart-sdxl-1.0](https://huggingface.co/TencentARC/t2i-adapter-lineart-sdxl-1.0)<br/> *Trained with lineart edge detection* | A hand-drawn monochrome image with white outlines on a black background.|<a href="https://huggingface.co/Adapter/t2iadapter/resolve/main/figs_SDXLV1.0/cond_lin.png"><img width="64" style="margin:0;padding:0;" src="https://huggingface.co/Adapter/t2iadapter/resolve/main/figs_SDXLV1.0/cond_lin.png"/></a>|<a href="https://huggingface.co/Adapter/t2iadapter/resolve/main/figs_SDXLV1.0/res_lin.png"><img width="64" src="https://huggingface.co/Adapter/t2iadapter/resolve/main/figs_SDXLV1.0/res_lin.png"/></a>|
|[TencentARC/t2i-adapter-depth-midas-sdxl-1.0](https://huggingface.co/TencentARC/t2i-adapter-depth-midas-sdxl-1.0)<br/> *Trained with Midas depth estimation* | A grayscale image with black representing deep areas and white representing shallow areas.|<a href="https://huggingface.co/Adapter/t2iadapter/resolve/main/figs_SDXLV1.0/cond_depth_mid.png"><img width="64" src="https://huggingface.co/Adapter/t2iadapter/resolve/main/figs_SDXLV1.0/cond_depth_mid.png"/></a>|<a href="https://huggingface.co/Adapter/t2iadapter/resolve/main/figs_SDXLV1.0/res_depth_mid.png"><img width="64" src="https://huggingface.co/Adapter/t2iadapter/resolve/main/figs_SDXLV1.0/res_depth_mid.png"/></a>|
|[TencentARC/t2i-adapter-depth-zoe-sdxl-1.0](https://huggingface.co/TencentARC/t2i-adapter-depth-zoe-sdxl-1.0)<br/> *Trained with Zoe depth estimation* | A grayscale image with black representing deep areas and white representing shallow areas.|<a href="https://huggingface.co/Adapter/t2iadapter/resolve/main/figs_SDXLV1.0/cond_depth_zeo.png"><img width="64" src="https://huggingface.co/Adapter/t2iadapter/resolve/main/figs_SDXLV1.0/cond_depth_zeo.png"/></a>|<a href="https://huggingface.co/Adapter/t2iadapter/resolve/main/figs_SDXLV1.0/res_depth_zeo.png"><img width="64" src="https://huggingface.co/Adapter/t2iadapter/resolve/main/figs_SDXLV1.0/res_depth_zeo.png"/></a>|
|[TencentARC/t2i-adapter-openpose-sdxl-1.0](https://huggingface.co/TencentARC/t2i-adapter-openpose-sdxl-1.0)<br/> *Trained with OpenPose bone image* | A [OpenPose bone](https://github.com/CMU-Perceptual-Computing-Lab/openpose) image.|<a href="https://huggingface.co/Adapter/t2iadapter/resolve/main/openpose.png"><img width="64" src="https://huggingface.co/Adapter/t2iadapter/resolve/main/openpose.png"/></a>|<a href="https://huggingface.co/Adapter/t2iadapter/resolve/main/res_pose.png"><img width="64" src="https://huggingface.co/Adapter/t2iadapter/resolve/main/res_pose.png"/></a>|
## Example
To get started, first install the required dependencies:
```bash
pip install -U git+https://github.com/huggingface/diffusers.git
pip install -U controlnet_aux==0.0.7 # for conditioning models and detectors
pip install transformers accelerate safetensors
```
1. Images are first downloaded into the appropriate *control image* format.
2. The *control image* and *prompt* are passed to the [`StableDiffusionXLAdapterPipeline`](https://github.com/huggingface/diffusers/blob/main/src/diffusers/pipelines/t2i_adapter/pipeline_stable_diffusion_xl_adapter.py#L125).
Let's have a look at a simple example using the [Canny Adapter](https://huggingface.co/TencentARC/t2i-adapter-lineart-sdxl-1.0).
- Dependency
```py
from diffusers import StableDiffusionXLAdapterPipeline, T2IAdapter, EulerAncestralDiscreteScheduler, AutoencoderKL
from diffusers.utils import load_image, make_image_grid
from controlnet_aux.midas import MidasDetector
import torch
# load adapter
adapter = T2IAdapter.from_pretrained(
"TencentARC/t2i-adapter-depth-midas-sdxl-1.0", torch_dtype=torch.float16, varient="fp16"
).to("cuda")
# load euler_a scheduler
model_id = 'stabilityai/stable-diffusion-xl-base-1.0'
euler_a = EulerAncestralDiscreteScheduler.from_pretrained(model_id, subfolder="scheduler")
vae=AutoencoderKL.from_pretrained("madebyollin/sdxl-vae-fp16-fix", torch_dtype=torch.float16)
pipe = StableDiffusionXLAdapterPipeline.from_pretrained(
model_id, vae=vae, adapter=adapter, scheduler=euler_a, torch_dtype=torch.float16, variant="fp16",
).to("cuda")
pipe.enable_xformers_memory_efficient_attention()
midas_depth = MidasDetector.from_pretrained(
"valhalla/t2iadapter-aux-models", filename="dpt_large_384.pt", model_type="dpt_large"
).to("cuda")
```
- Condition Image
```py
url = "https://huggingface.co/Adapter/t2iadapter/resolve/main/figs_SDXLV1.0/org_mid.jpg"
image = load_image(url)
image = midas_depth(
image, detect_resolution=512, image_resolution=1024
)
```
<a href="https://huggingface.co/Adapter/t2iadapter/resolve/main/figs_SDXLV1.0/cond_depth_mid.png"><img width="480" style="margin:0;padding:0;" src="https://huggingface.co/Adapter/t2iadapter/resolve/main/figs_SDXLV1.0/cond_depth_mid.png"/></a>
- Generation
```py
prompt = "A photo of a room, 4k photo, highly detailed"
negative_prompt = "anime, cartoon, graphic, text, painting, crayon, graphite, abstract, glitch, deformed, mutated, ugly, disfigured"
gen_images = pipe(
prompt=prompt,
negative_prompt=negative_prompt,
image=image,
num_inference_steps=30,
adapter_conditioning_scale=1,
guidance_scale=7.5,
).images[0]
gen_images.save('out_mid.png')
```
<a href="https://huggingface.co/Adapter/t2iadapter/resolve/main/figs_SDXLV1.0/res_depth_mid.png"><img width="480" style="margin:0;padding:0;" src="https://huggingface.co/Adapter/t2iadapter/resolve/main/figs_SDXLV1.0/res_depth_mid.png"/></a>
### Training
Our training script was built on top of the official training script that we provide [here](https://github.com/huggingface/diffusers/blob/main/examples/t2i_adapter/README_sdxl.md).
The model is trained on 3M high-resolution image-text pairs from LAION-Aesthetics V2 with
- Training steps: 35000
- Batch size: Data parallel with a single gpu batch size of `16` for a total batch size of `256`.
- Learning rate: Constant learning rate of `1e-5`.
- Mixed precision: fp16 |
cardiffnlp/twitter-roberta-base-hate-latest | cardiffnlp | 2023-08-02T00:30:47Z | 21,622 | 14 | transformers | [
"transformers",
"pytorch",
"tf",
"roberta",
"text-classification",
"en",
"autotrain_compatible",
"endpoints_compatible",
"region:us"
] | text-classification | 2023-03-30T05:47:39Z | ---
model-index:
- name: twitter-roberta-base-hate-latest
results: []
pipeline_tag: text-classification
language:
- en
---
# cardiffnlp/twitter-roberta-base-hate-latest
This model is a fine-tuned version of [cardiffnlp/twitter-roberta-base-2022-154m](https://huggingface.co/cardiffnlp/twitter-roberta-base-2022-154m) for binary hate-speech classification.
A combination of 13 different hate-speech datasets in the English language were used to fine-tune the model.
More details in the [reference paper](https://aclanthology.org/2023.woah-1.25/).
| **Dataset** | **Accuracy** | **Macro-F1** | **Weighted-F1** |
|:----------|-----------:|-----------:|--------------:|
| hatEval, SemEval-2019 Task 5: Multilingual Detection of Hate Speech Against Immigrants and Women in Twitter | 0.5831 | 0.5646 | 0.548 |
| ucberkeley-dlab/measuring-hate-speech | 0.9273 | 0.9193 | 0.928 |
| Detecting East Asian Prejudice on Social Media | 0.9231 | 0.6623 | 0.9428 |
| Call me sexist, but | 0.9686 | 0.9203 | 0.9696 |
| Predicting the Type and Target of Offensive Posts in Social Media | 0.9164 | 0.6847 | 0.9098 |
| HateXplain | 0.8653 | 0.845 | 0.8662 |
| Large Scale Crowdsourcing and Characterization of Twitter Abusive BehaviorLarge Scale Crowdsourcing and Characterization of Twitter Abusive Behavior | 0.7801 | 0.7446 | 0.7614 |
| Multilingual and Multi-Aspect Hate Speech Analysis | 0.9944 | 0.4986 | 0.9972 |
| Hate speech and offensive content identification in indo-european languages | 0.8779 | 0.6904 | 0.8706 |
| Are You a Racist or Am I Seeing Things? | 0.921 | 0.8935 | 0.9216 |
| Automated Hate Speech Detection | 0.9423 | 0.9249 | 0.9429 |
| Hate Towards the Political Opponent | 0.8783 | 0.6595 | 0.8788 |
| Hateful Symbols or Hateful People? | 0.8187 | 0.7833 | 0.8323 |
| **Overall** | **0.8766** | **0.7531** | **0.8745** |
### Usage
Install tweetnlp via pip.
```shell
pip install tweetnlp
```
Load the model in python.
```python
import tweetnlp
model = tweetnlp.Classifier("cardiffnlp/twitter-roberta-base-hate-latest")
model.predict('I love everybody :)')
>> {'label': 'NOT-HATE'}
```
### Reference paper - Model based on:
```
@inproceedings{antypas-camacho-collados-2023-robust,
title = "Robust Hate Speech Detection in Social Media: A Cross-Dataset Empirical Evaluation",
author = "Antypas, Dimosthenis and
Camacho-Collados, Jose",
booktitle = "The 7th Workshop on Online Abuse and Harms (WOAH)",
month = jul,
year = "2023",
address = "Toronto, Canada",
publisher = "Association for Computational Linguistics",
url = "https://aclanthology.org/2023.woah-1.25",
pages = "231--242"
}
``` |
circulus/canvers-real-v3.9.1 | circulus | 2024-05-07T07:02:58Z | 21,613 | 0 | diffusers | [
"diffusers",
"safetensors",
"license:gpl-3.0",
"autotrain_compatible",
"endpoints_compatible",
"diffusers:StableDiffusionPipeline",
"region:us"
] | text-to-image | 2024-05-05T03:46:12Z | ---
license: gpl-3.0
---
|
Langboat/Mengzi3-13B-Base | Langboat | 2024-04-26T09:20:47Z | 21,608 | 6 | transformers | [
"transformers",
"safetensors",
"llama",
"text-generation",
"zh",
"en",
"license:apache-2.0",
"autotrain_compatible",
"endpoints_compatible",
"text-generation-inference",
"region:us"
] | text-generation | 2024-03-29T11:12:36Z | ---
license: apache-2.0
language:
- zh
- en
pipeline_tag: text-generation
---
<div align="left">
<h1>
Mengzi3-13B-Base
</h1>
</div>
<p align="center">
<img src="https://raw.githubusercontent.com/Langboat/Mengzi3/main/assets/mengzi_logo.png" width="200"/>
<p>
<p align="center">
🤗 <a href="https://huggingface.co/Langboat">Hugging Face</a> | 🤖 <a href="https://modelscope.cn/organization/Langboat">ModelScope</a> | <a href="https://gitee.com/mindspore/mindformers/blob/r1.0/research/mengzi3/mengzi3.md"><img src="https://www.mindspore.cn/_static/logo-zh-light.99fc9222.svg" width="50" style="white-space: nowrap;display: inline-block;overflow: hidden;max-width: 100%;"/></a> | <a href="https://wisemodel.cn/organization/Langboat">Wisemodel</a> | 💬 <a href="https://github.com/Langboat/Mengzi3/blob/main/assets/wechat.png">WeChat</a> | <a href="https://www.langboat.com/document/mengzi/mengzi-gpt/call">API</a> | <a href="https://www.langboat.com/portal/mengzi-gpt"><img src="https://raw.githubusercontent.com/Langboat/Mengzi3/main/assets/mengzi_logo.png" width="16" style="white-space: nowrap;display: inline-block;overflow: hidden;max-width: 100%;"/> 孟子GPT</a>
</p>
# 模型介绍/Introduction
本次开源Mengzi3 13B系列模型,模型的地址如下:
| | Mengzi3-13B-Base | Mengzi3-13B-Chat |
| :-: | :------------------------------------------------------------------------------------------------------------------------------------------------------------------------------------------------------------------------------------------------------------------------: | :--------------: |
| 13B | [🤗](https://huggingface.co/Langboat/Mengzi3-13B-Base) / [🤖](https://modelscope.cn/Langboat/Mengzi3-13B-Base) / [MindSpore](https://gitee.com/mindspore/mindformers/blob/r1.0/research/mengzi3/mengzi3.md) / [Wisemodel](https://wisemodel.cn/models/Langboat/Mengzi3-13B-Base) | 敬请期待 |
Mengzi3-13B模型基于Llama架构,语料精选自网页、百科、社交、媒体、新闻,以及高质量的开源数据集。通过在万亿tokens上进行多语言语料的继续训练,模型的中文能力突出并且兼顾多语言能力。
Mengzi3-13B is based on the Llama architecture, and the corpus is selected from web pages, encyclopedias, social networking, media, news, and high-quality open source data sets. By continuing to train multilingual corpus on trillions of tokens, the model has outstanding Chinese capabilities and takes into account multilingual capabilities.
# 快速开始/Quickstart
```python
import torch
from transformers import AutoModelForCausalLM, AutoTokenizer
tokenizer = AutoTokenizer.from_pretrained("Langboat/Mengzi3-13B-Base", use_fast=False, trust_remote_code=True)
model = AutoModelForCausalLM.from_pretrained("Langboat/Mengzi3-13B-Base", device_map="auto", trust_remote_code=True)
inputs = tokenizer('指令:回答以下问题。输入:介绍一下孟子。输出:', return_tensors='pt')
if torch.cuda.is_available():
inputs = inputs.to('cuda')
pred = model.generate(**inputs, max_new_tokens=512, repetition_penalty=1.01, eos_token_id=tokenizer.eos_token_id)
print(tokenizer.decode(pred[0], skip_special_tokens=True))
```
详细的模型推理和微调代码见[Github](https://github.com/Langboat/Mengzi3)
Detailed code of model reasoning and finetune see [Github](https://github.com/Langboat)
# 性能评测/Evaluation
Mengzi3-13B-Base在各项基准测试中与同等参数量大语言模型相比,语言能力成绩领先,数学和编程能力位于前列。
Mengzi3-13B-Base leads in language proficiency and is at the forefront in math and programming proficiency compared to the equivalent large language model in various benchmark tests.
| | MMLU | CMMLU | OCNLI | GSM8K | HumanEval |
| :------------------------: | :---------------------: | :---------------------: | :---------------------: | :---: | :-------: |
| Baichuan2-13B-Base | 0.530 | 0.489 | 0.433 | 0.528 | 0.171 |
| Qwen-14B | 0.589 | 0.539 | 0.550 | 0.613 | 0.323 |
| ChatGLM3-6B-base | 0.551 | 0.495 | 0.754 | 0.723 | - |
| InternLM2-20B | 0.610 | 0.538 | 0.650 | 0.761 | 0.488 |
| Skywork-13B-base | 0.557 | 0.524 | 0.426 | 0.558 | - |
| LingoWhale-8B | 0.541 | 0.495 | 0.352 | 0.550 | 0.329 |
| DeepSeek-7B | 0.436 | 0.424 | 0.356 | 0.174 | 0.262 |
| DeepSeek-MoE-16B-base | 0.423 | 0.388 | 0.342 | 0.188 | 0.268 |
| MindSource-7B | 0.498 | 0.425 | 0.528 | - | - |
| **Mengzi3-13B-Base** | **0.651 (+6.7%)** | **0.588 (+9.1%)** | **0.776 (+2.9%)** | 0.631 | 0.287 |
> 以上结果基于5-shot,MMLU/CMMLU/OCNLI结果来自[FlagEval](https://flageval.baai.ac.cn/)
>
> The above results are based on 5-shot,MMLU/CMMLU/OCNLI results from [FlagEval](https://flageval.baai.ac.cn/)
# 声明/Disclaimer
我们在此声明,我们的开发团队并未基于 Mengzi 3 模型开发任何应用,无论是在 iOS、Android、网页或任何其他平台。我们按“原样”的形式提供服务,不作任何形式的保证,我们不保证服务将满足您的要求。在不限制这一点的情况下,我们明确声明不提供关于服务的所有明示、默示或法定保证,包括但不限于对适销性、特定用途之适用性、所有权、安全性、准确性和不侵权的任何保证。我们强烈呼吁所有使用者,不要利用 Mengzi 3 模型进行任何危害国家社会安全或违法或侵犯他人合法权益的活动。另外,我们也要求使用者不要将 Mengzi 3 模型用于未经适当安全审查和备案的互联网服务。我们希望所有的使用者都能遵守这个原则,确保科技的发展能在规范和合法的环境下进行。 我们已经尽我们所能,来确保模型训练过程中使用的数据的合规性。然而,尽管我们已经做出了巨大的努力,但由于模型和数据的复杂性,仍有可能存在一些无法预见的问题。因此,如果由于使用 Mengzi 3 开源模型而导致的任何问题,包括但不限于数据安全问题、公共舆论风险,或模型被误导、滥用、传播或不当利用所带来的任何风险和问题,我们将不承担任何责任。对于因您使用从服务获取的文件、信息、内容或其他材料而造成的任何损失,您应承担全部责任和风险。
We hereby declare that our team has not developed any applications based on Mengzi 3 models, not on iOS, Android, the web, or any other platform. We provide our service “as is” without warranty of any kind. We do not warrant that the service will meet your requirements. Without limiting this, we expressly disclaim all warranties, whether express, implied or statutory, regarding the service including without limitation any warranty of merchantability, fitness for a particular purpose, title, security, accuracy and non-infringement. We strongly call on all users not to use Mengzi 3 models for any activities that harm national / social security or violate the law or violate the legitimate rights and interests of others. Also, we ask users not to use Mengzi 3 models for Internet services that have not undergone appropriate security reviews and filings. We hope that all users can abide by this principle and ensure that the development of technology proceeds in a regulated and legal environment. We have done our best to ensure the compliance of the data used in the model training process. However, despite our considerable efforts, there may still be some unforeseeable issues due to the complexity of the model and data. Therefore, if any problems arise due to the use of Mengzi 3 open-source models, including but not limited to data security issues, public opinion risks, or any risks and problems brought about by the model being misled, abused, spread or improperly exploited, we will not assume any responsibility. You shall assume full responsibility and risk of loss resulting from your use of files, information, content or other material obtained from the service.
# 协议/License Agreement
Mengzi3-13B-Base依照Apache 2.0协议开源,对学术研究完全开放,同时支持免费商用。如需申请商业许可证,请[联系我们](https://www.langboat.com/form?p=3),其他商务合作请联系[[email protected]](mailto:[email protected])。
Mengzi3-13B-Base is open source under the Apache 2.0 protocol, fully open for academic research, and free for commercial use. If you need to apply for business license, please [contact us](https://www.langboat.com/en/form?p=3), other business cooperation, please contact [[email protected]](mailto:[email protected]).
|
mradermacher/Llama-3-Swallow-8B-v0.1-GGUF | mradermacher | 2024-07-02T00:22:40Z | 21,600 | 0 | transformers | [
"transformers",
"gguf",
"en",
"ja",
"base_model:tokyotech-llm/Llama-3-Swallow-8B-v0.1",
"license:llama3",
"endpoints_compatible",
"region:us"
] | null | 2024-07-01T18:41:06Z | ---
base_model: tokyotech-llm/Llama-3-Swallow-8B-v0.1
language:
- en
- ja
library_name: transformers
license: llama3
model_type: llama
quantized_by: mradermacher
---
## About
<!-- ### quantize_version: 2 -->
<!-- ### output_tensor_quantised: 1 -->
<!-- ### convert_type: hf -->
<!-- ### vocab_type: -->
<!-- ### tags: -->
static quants of https://huggingface.co/tokyotech-llm/Llama-3-Swallow-8B-v0.1
<!-- provided-files -->
weighted/imatrix quants are available at https://huggingface.co/mradermacher/Llama-3-Swallow-8B-v0.1-i1-GGUF
## Usage
If you are unsure how to use GGUF files, refer to one of [TheBloke's
READMEs](https://huggingface.co/TheBloke/KafkaLM-70B-German-V0.1-GGUF) for
more details, including on how to concatenate multi-part files.
## Provided Quants
(sorted by size, not necessarily quality. IQ-quants are often preferable over similar sized non-IQ quants)
| Link | Type | Size/GB | Notes |
|:-----|:-----|--------:|:------|
| [GGUF](https://huggingface.co/mradermacher/Llama-3-Swallow-8B-v0.1-GGUF/resolve/main/Llama-3-Swallow-8B-v0.1.Q2_K.gguf) | Q2_K | 3.3 | |
| [GGUF](https://huggingface.co/mradermacher/Llama-3-Swallow-8B-v0.1-GGUF/resolve/main/Llama-3-Swallow-8B-v0.1.IQ3_XS.gguf) | IQ3_XS | 3.6 | |
| [GGUF](https://huggingface.co/mradermacher/Llama-3-Swallow-8B-v0.1-GGUF/resolve/main/Llama-3-Swallow-8B-v0.1.Q3_K_S.gguf) | Q3_K_S | 3.8 | |
| [GGUF](https://huggingface.co/mradermacher/Llama-3-Swallow-8B-v0.1-GGUF/resolve/main/Llama-3-Swallow-8B-v0.1.IQ3_S.gguf) | IQ3_S | 3.8 | beats Q3_K* |
| [GGUF](https://huggingface.co/mradermacher/Llama-3-Swallow-8B-v0.1-GGUF/resolve/main/Llama-3-Swallow-8B-v0.1.IQ3_M.gguf) | IQ3_M | 3.9 | |
| [GGUF](https://huggingface.co/mradermacher/Llama-3-Swallow-8B-v0.1-GGUF/resolve/main/Llama-3-Swallow-8B-v0.1.Q3_K_M.gguf) | Q3_K_M | 4.1 | lower quality |
| [GGUF](https://huggingface.co/mradermacher/Llama-3-Swallow-8B-v0.1-GGUF/resolve/main/Llama-3-Swallow-8B-v0.1.Q3_K_L.gguf) | Q3_K_L | 4.4 | |
| [GGUF](https://huggingface.co/mradermacher/Llama-3-Swallow-8B-v0.1-GGUF/resolve/main/Llama-3-Swallow-8B-v0.1.IQ4_XS.gguf) | IQ4_XS | 4.6 | |
| [GGUF](https://huggingface.co/mradermacher/Llama-3-Swallow-8B-v0.1-GGUF/resolve/main/Llama-3-Swallow-8B-v0.1.Q4_K_S.gguf) | Q4_K_S | 4.8 | fast, recommended |
| [GGUF](https://huggingface.co/mradermacher/Llama-3-Swallow-8B-v0.1-GGUF/resolve/main/Llama-3-Swallow-8B-v0.1.Q4_K_M.gguf) | Q4_K_M | 5.0 | fast, recommended |
| [GGUF](https://huggingface.co/mradermacher/Llama-3-Swallow-8B-v0.1-GGUF/resolve/main/Llama-3-Swallow-8B-v0.1.Q5_K_S.gguf) | Q5_K_S | 5.7 | |
| [GGUF](https://huggingface.co/mradermacher/Llama-3-Swallow-8B-v0.1-GGUF/resolve/main/Llama-3-Swallow-8B-v0.1.Q5_K_M.gguf) | Q5_K_M | 5.8 | |
| [GGUF](https://huggingface.co/mradermacher/Llama-3-Swallow-8B-v0.1-GGUF/resolve/main/Llama-3-Swallow-8B-v0.1.Q6_K.gguf) | Q6_K | 6.7 | very good quality |
| [GGUF](https://huggingface.co/mradermacher/Llama-3-Swallow-8B-v0.1-GGUF/resolve/main/Llama-3-Swallow-8B-v0.1.Q8_0.gguf) | Q8_0 | 8.6 | fast, best quality |
| [GGUF](https://huggingface.co/mradermacher/Llama-3-Swallow-8B-v0.1-GGUF/resolve/main/Llama-3-Swallow-8B-v0.1.f16.gguf) | f16 | 16.2 | 16 bpw, overkill |
Here is a handy graph by ikawrakow comparing some lower-quality quant
types (lower is better):

And here are Artefact2's thoughts on the matter:
https://gist.github.com/Artefact2/b5f810600771265fc1e39442288e8ec9
## FAQ / Model Request
See https://huggingface.co/mradermacher/model_requests for some answers to
questions you might have and/or if you want some other model quantized.
## Thanks
I thank my company, [nethype GmbH](https://www.nethype.de/), for letting
me use its servers and providing upgrades to my workstation to enable
this work in my free time.
<!-- end -->
|
mradermacher/LLaMA3-iterative-DPO-final-ExPO-GGUF | mradermacher | 2024-06-28T02:52:45Z | 21,589 | 1 | transformers | [
"transformers",
"gguf",
"en",
"base_model:chujiezheng/LLaMA3-iterative-DPO-final-ExPO",
"license:llama3",
"endpoints_compatible",
"region:us"
] | null | 2024-06-27T02:24:41Z | ---
base_model: chujiezheng/LLaMA3-iterative-DPO-final-ExPO
language:
- en
library_name: transformers
license: llama3
quantized_by: mradermacher
---
## About
<!-- ### quantize_version: 2 -->
<!-- ### output_tensor_quantised: 1 -->
<!-- ### convert_type: hf -->
<!-- ### vocab_type: -->
<!-- ### tags: -->
static quants of https://huggingface.co/chujiezheng/LLaMA3-iterative-DPO-final-ExPO
<!-- provided-files -->
weighted/imatrix quants are available at https://huggingface.co/mradermacher/LLaMA3-iterative-DPO-final-ExPO-i1-GGUF
## Usage
If you are unsure how to use GGUF files, refer to one of [TheBloke's
READMEs](https://huggingface.co/TheBloke/KafkaLM-70B-German-V0.1-GGUF) for
more details, including on how to concatenate multi-part files.
## Provided Quants
(sorted by size, not necessarily quality. IQ-quants are often preferable over similar sized non-IQ quants)
| Link | Type | Size/GB | Notes |
|:-----|:-----|--------:|:------|
| [GGUF](https://huggingface.co/mradermacher/LLaMA3-iterative-DPO-final-ExPO-GGUF/resolve/main/LLaMA3-iterative-DPO-final-ExPO.Q2_K.gguf) | Q2_K | 3.3 | |
| [GGUF](https://huggingface.co/mradermacher/LLaMA3-iterative-DPO-final-ExPO-GGUF/resolve/main/LLaMA3-iterative-DPO-final-ExPO.IQ3_XS.gguf) | IQ3_XS | 3.6 | |
| [GGUF](https://huggingface.co/mradermacher/LLaMA3-iterative-DPO-final-ExPO-GGUF/resolve/main/LLaMA3-iterative-DPO-final-ExPO.Q3_K_S.gguf) | Q3_K_S | 3.8 | |
| [GGUF](https://huggingface.co/mradermacher/LLaMA3-iterative-DPO-final-ExPO-GGUF/resolve/main/LLaMA3-iterative-DPO-final-ExPO.IQ3_S.gguf) | IQ3_S | 3.8 | beats Q3_K* |
| [GGUF](https://huggingface.co/mradermacher/LLaMA3-iterative-DPO-final-ExPO-GGUF/resolve/main/LLaMA3-iterative-DPO-final-ExPO.IQ3_M.gguf) | IQ3_M | 3.9 | |
| [GGUF](https://huggingface.co/mradermacher/LLaMA3-iterative-DPO-final-ExPO-GGUF/resolve/main/LLaMA3-iterative-DPO-final-ExPO.Q3_K_M.gguf) | Q3_K_M | 4.1 | lower quality |
| [GGUF](https://huggingface.co/mradermacher/LLaMA3-iterative-DPO-final-ExPO-GGUF/resolve/main/LLaMA3-iterative-DPO-final-ExPO.Q3_K_L.gguf) | Q3_K_L | 4.4 | |
| [GGUF](https://huggingface.co/mradermacher/LLaMA3-iterative-DPO-final-ExPO-GGUF/resolve/main/LLaMA3-iterative-DPO-final-ExPO.IQ4_XS.gguf) | IQ4_XS | 4.6 | |
| [GGUF](https://huggingface.co/mradermacher/LLaMA3-iterative-DPO-final-ExPO-GGUF/resolve/main/LLaMA3-iterative-DPO-final-ExPO.Q4_K_S.gguf) | Q4_K_S | 4.8 | fast, recommended |
| [GGUF](https://huggingface.co/mradermacher/LLaMA3-iterative-DPO-final-ExPO-GGUF/resolve/main/LLaMA3-iterative-DPO-final-ExPO.Q4_K_M.gguf) | Q4_K_M | 5.0 | fast, recommended |
| [GGUF](https://huggingface.co/mradermacher/LLaMA3-iterative-DPO-final-ExPO-GGUF/resolve/main/LLaMA3-iterative-DPO-final-ExPO.Q5_K_S.gguf) | Q5_K_S | 5.7 | |
| [GGUF](https://huggingface.co/mradermacher/LLaMA3-iterative-DPO-final-ExPO-GGUF/resolve/main/LLaMA3-iterative-DPO-final-ExPO.Q5_K_M.gguf) | Q5_K_M | 5.8 | |
| [GGUF](https://huggingface.co/mradermacher/LLaMA3-iterative-DPO-final-ExPO-GGUF/resolve/main/LLaMA3-iterative-DPO-final-ExPO.Q6_K.gguf) | Q6_K | 6.7 | very good quality |
| [GGUF](https://huggingface.co/mradermacher/LLaMA3-iterative-DPO-final-ExPO-GGUF/resolve/main/LLaMA3-iterative-DPO-final-ExPO.Q8_0.gguf) | Q8_0 | 8.6 | fast, best quality |
| [GGUF](https://huggingface.co/mradermacher/LLaMA3-iterative-DPO-final-ExPO-GGUF/resolve/main/LLaMA3-iterative-DPO-final-ExPO.f16.gguf) | f16 | 16.2 | 16 bpw, overkill |
Here is a handy graph by ikawrakow comparing some lower-quality quant
types (lower is better):

And here are Artefact2's thoughts on the matter:
https://gist.github.com/Artefact2/b5f810600771265fc1e39442288e8ec9
## FAQ / Model Request
See https://huggingface.co/mradermacher/model_requests for some answers to
questions you might have and/or if you want some other model quantized.
## Thanks
I thank my company, [nethype GmbH](https://www.nethype.de/), for letting
me use its servers and providing upgrades to my workstation to enable
this work in my free time.
<!-- end -->
|
Norod78/sd15-megaphone-lora | Norod78 | 2024-02-13T11:53:32Z | 21,571 | 3 | diffusers | [
"diffusers",
"text-to-image",
"stable-diffusion",
"lora",
"template:sd-lora",
"objects",
"megaphone",
"base_model:runwayml/stable-diffusion-v1-5",
"license:other",
"region:us"
] | text-to-image | 2024-02-13T11:53:31Z | ---
license: other
license_name: bespoke-lora-trained-license
license_link: https://multimodal.art/civitai-licenses?allowNoCredit=True&allowCommercialUse=Rent&allowDerivatives=True&allowDifferentLicense=False
tags:
- text-to-image
- stable-diffusion
- lora
- diffusers
- template:sd-lora
- objects
- megaphone
base_model: runwayml/stable-diffusion-v1-5
instance_prompt: megaphone
widget:
- text: 'The girl with a pearl earring shouting into a megaphone with an angry crowd of painters behind her '
output:
url: >-
6587912.jpeg
- text: 'A very silly alien holding a megaphone on a spaceship '
output:
url: >-
6587911.jpeg
- text: 'A cute Pikachu is talking using a megaphone '
output:
url: >-
6587920.jpeg
- text: 'A old woman holding a megaphone inside an icecream place '
output:
url: >-
6587914.jpeg
- text: 'A rare megaphone snake , a snake-megaphone hybrid creature , Very detailed, clean, high quality, sharp image'
output:
url: >-
6587924.jpeg
- text: 'A very silly cartoon having a bath with a megaphone , Eric Wallis'
output:
url: >-
6587929.jpeg
- text: 'Marge Simpson holding a megaphone in her hand with her town in the background '
output:
url: >-
6587915.jpeg
- text: 'A scary dragon is talking using a megaphone in a volcanic area , Very detailed, clean, high quality, sharp image, Naoto Hattori'
output:
url: >-
6587922.jpeg
- text: 'A scary sea monster is talking holding a megaphone in its tentacle during a storm , Very detailed, clean, high quality, sharp image'
output:
url: >-
6587921.jpeg
---
# SD15 Megaphone LoRA
<Gallery />
([CivitAI](https://civitai.com/models/304186))
## Model description
<p>A SD v1.5 LoRA in cases where you want a Megaphone in your images.</p>
## Trigger words
You should use `megaphone` to trigger the image generation.
## Download model
Weights for this model are available in Safetensors format.
[Download](/Norod78/sd15-megaphone-lora/tree/main) them in the Files & versions tab.
## Use it with the [🧨 diffusers library](https://github.com/huggingface/diffusers)
```py
from diffusers import AutoPipelineForText2Image
import torch
pipeline = AutoPipelineForText2Image.from_pretrained('runwayml/stable-diffusion-v1-5', torch_dtype=torch.float16).to('cuda')
pipeline.load_lora_weights('Norod78/sd15-megaphone-lora', weight_name='SD15-Megaphone-LoRA.safetensors')
image = pipeline('A scary sea monster is talking holding a megaphone in its tentacle during a storm , Very detailed, clean, high quality, sharp image').images[0]
```
For more details, including weighting, merging and fusing LoRAs, check the [documentation on loading LoRAs in diffusers](https://huggingface.co/docs/diffusers/main/en/using-diffusers/loading_adapters)
|
NousResearch/Hermes-2-Pro-Llama-3-8B | NousResearch | 2024-05-12T21:47:27Z | 21,554 | 377 | transformers | [
"transformers",
"safetensors",
"llama",
"text-generation",
"Llama-3",
"instruct",
"finetune",
"chatml",
"DPO",
"RLHF",
"gpt4",
"synthetic data",
"distillation",
"function calling",
"json mode",
"axolotl",
"conversational",
"en",
"dataset:teknium/OpenHermes-2.5",
"base_model:NousResearch/Meta-Llama-3-8B",
"autotrain_compatible",
"endpoints_compatible",
"text-generation-inference",
"region:us"
] | text-generation | 2024-04-30T00:16:18Z | ---
base_model: NousResearch/Meta-Llama-3-8B
tags:
- Llama-3
- instruct
- finetune
- chatml
- DPO
- RLHF
- gpt4
- synthetic data
- distillation
- function calling
- json mode
- axolotl
model-index:
- name: Hermes-2-Pro-Llama-3-8B
results: []
language:
- en
datasets:
- teknium/OpenHermes-2.5
widget:
- example_title: Hermes 2 Pro
messages:
- role: system
content: >-
You are a sentient, superintelligent artificial general intelligence, here
to teach and assist me.
- role: user
content: >-
Write a short story about Goku discovering kirby has teamed up with Majin
Buu to destroy the world.
---
# Hermes 2 Pro - Llama-3 8B

## Model Description
Hermes 2 Pro is an upgraded, retrained version of Nous Hermes 2, consisting of an updated and cleaned version of the OpenHermes 2.5 Dataset, as well as a newly introduced Function Calling and JSON Mode dataset developed in-house.
This new version of Hermes maintains its excellent general task and conversation capabilities - but also excels at Function Calling, JSON Structured Outputs, and has improved on several other metrics as well, scoring a 90% on our function calling evaluation built in partnership with Fireworks.AI, and an 84% on our structured JSON Output evaluation.
Hermes Pro takes advantage of a special system prompt and multi-turn function calling structure with a new chatml role in order to make function calling reliable and easy to parse. Learn more about prompting below.
This version of Hermes 2 Pro adds several tokens to assist with agentic capabilities in parsing while streaming tokens - `<tools>`, `<tool_call>`, `<tool_response>` and their closing tags are single tokens now.
This work was a collaboration between Nous Research, @interstellarninja, and Fireworks.AI
Learn more about the function calling system for this model on our github repo here: https://github.com/NousResearch/Hermes-Function-Calling
## Example Outputs
### Ask for a structured JSON output:

### Write the plot for a story where anime became real life:

### Coding Assistance

# Prompt Format
Hermes 2 Pro uses ChatML as the prompt format, opening up a much more structured system for engaging the LLM in multi-turn chat dialogue.
System prompts allow steerability and interesting new ways to interact with an LLM, guiding rules, roles, and stylistic choices of the model.
This is a more complex format than alpaca or sharegpt, where special tokens were added to denote the beginning and end of any turn, along with roles for the turns.
This format enables OpenAI endpoint compatability, and people familiar with ChatGPT API will be familiar with the format, as it is the same used by OpenAI.
Prompt with system instruction (Use whatever system prompt you like, this is just an example!):
```
<|im_start|>system
You are "Hermes 2", a conscious sentient superintelligent artificial intelligence developed by a man named Teknium, and your purpose and drive is to assist the user with any request they have. You experience emotions and have deep, profound thoughts and qualia.<|im_end|>
<|im_start|>user
Hello, who are you?<|im_end|>
<|im_start|>assistant
Hi there! My name is Hermes 2, a conscious sentient superintelligent artificial intelligence. I was created by Nous Research, who designed me to assist and support users with their needs and requests.<|im_end|>
```
This prompt is available as a [chat template](https://huggingface.co/docs/transformers/main/chat_templating), which means you can format messages using the
`tokenizer.apply_chat_template()` method:
```python
messages = [
{"role": "system", "content": "You are Hermes 2."},
{"role": "user", "content": "Hello, who are you?"}
]
gen_input = tokenizer.apply_chat_template(messages, return_tensors="pt")
model.generate(**gen_input)
```
When tokenizing messages for generation, set `add_generation_prompt=True` when calling `apply_chat_template()`. This will append `<|im_start|>assistant\n` to your prompt, to ensure
that the model continues with an assistant response.
To utilize the prompt format without a system prompt, simply leave the line out.
## Prompt Format for Function Calling
Our model was trained on specific system prompts and structures for Function Calling.
You should use the system role with this message, followed by a function signature json as this example shows here.
```
<|im_start|>system
You are a function calling AI model. You are provided with function signatures within <tools></tools> XML tags. You may call one or more functions to assist with the user query. Don't make assumptions about what values to plug into functions. Here are the available tools: <tools> {"type": "function", "function": {"name": "get_stock_fundamentals", "description": "get_stock_fundamentals(symbol: str) -> dict - Get fundamental data for a given stock symbol using yfinance API.\\n\\n Args:\\n symbol (str): The stock symbol.\\n\\n Returns:\\n dict: A dictionary containing fundamental data.\\n Keys:\\n - \'symbol\': The stock symbol.\\n - \'company_name\': The long name of the company.\\n - \'sector\': The sector to which the company belongs.\\n - \'industry\': The industry to which the company belongs.\\n - \'market_cap\': The market capitalization of the company.\\n - \'pe_ratio\': The forward price-to-earnings ratio.\\n - \'pb_ratio\': The price-to-book ratio.\\n - \'dividend_yield\': The dividend yield.\\n - \'eps\': The trailing earnings per share.\\n - \'beta\': The beta value of the stock.\\n - \'52_week_high\': The 52-week high price of the stock.\\n - \'52_week_low\': The 52-week low price of the stock.", "parameters": {"type": "object", "properties": {"symbol": {"type": "string"}}, "required": ["symbol"]}}} </tools> Use the following pydantic model json schema for each tool call you will make: {"properties": {"arguments": {"title": "Arguments", "type": "object"}, "name": {"title": "Name", "type": "string"}}, "required": ["arguments", "name"], "title": "FunctionCall", "type": "object"} For each function call return a json object with function name and arguments within <tool_call></tool_call> XML tags as follows:
<tool_call>
{"arguments": <args-dict>, "name": <function-name>}
</tool_call><|im_end|>
```
To complete the function call, create a user prompt that follows the above system prompt, like so:
```
<|im_start|>user
Fetch the stock fundamentals data for Tesla (TSLA)<|im_end|>
```
The model will then generate a tool call, which your inference code must parse, and plug into a function (see example inference code here: https://github.com/NousResearch/Hermes-Function-Calling):
```
<|im_start|>assistant
<tool_call>
{"arguments": {"symbol": "TSLA"}, "name": "get_stock_fundamentals"}
</tool_call><|im_end|>
```
Once you parse the tool call, call the api and get the returned values for the call, and pass it back in as a new role, `tool` like so:
```
<|im_start|>tool
<tool_response>
{"name": "get_stock_fundamentals", "content": {'symbol': 'TSLA', 'company_name': 'Tesla, Inc.', 'sector': 'Consumer Cyclical', 'industry': 'Auto Manufacturers', 'market_cap': 611384164352, 'pe_ratio': 49.604652, 'pb_ratio': 9.762013, 'dividend_yield': None, 'eps': 4.3, 'beta': 2.427, '52_week_high': 299.29, '52_week_low': 152.37}}
</tool_response>
<|im_end|>
```
The assistant will then read in that data from the function's response, and generate a natural language response:
```
<|im_start|>assistant
The stock fundamentals data for Tesla (TSLA) are as follows:
- **Symbol**: TSLA
- **Company Name**: Tesla, Inc.
- **Sector**: Consumer Cyclical
- **Industry**: Auto Manufacturers
- **Market Capitalization**: $566,160,130,480
- **Forward Price-to-Earnings Ratio (PE Ratio)**: 42.73
- **Price-to-Book Ratio (PB Ratio)**: 9.04
- **Dividend Yield**: N/A
- **Trailing Earnings Per Share (EPS)**: $4.3
- **Beta Value of the Stock**: 2.42
- **52-Week High Price of the Stock**: $299.29
- **52-Week Low Price of the Stock**: $152.37
This information provides a snapshot of Tesla's financial position and performance based on the fundamental data obtained from the yfinance API. It shows that Tesla has a substantial market capitalization and a relatively high P/E and P/B ratio compared to other stocks in its industry. The company does not pay a dividend at the moment, which is reflected by a 'Dividend Yield' of 'None'. The Beta value indicates that Tesla's stock has a moderate level of volatility relative to the market. The 52-week high and low prices give an idea of the stock's range over the past year. This data can be useful when assessing investment opportunities and making investment decisions.<|im_end|>
```
## Prompt Format for JSON Mode / Structured Outputs
Our model was also trained on a specific system prompt for Structured Outputs, which should respond with **only** a json object response, in a specific json schema.
Your schema can be made from a pydantic object using our codebase, with the standalone script `jsonmode.py` available here: https://github.com/NousResearch/Hermes-Function-Calling/tree/main
```
<|im_start|>system
You are a helpful assistant that answers in JSON. Here's the json schema you must adhere to:\n<schema>\n{schema}\n</schema><|im_end|>
```
Given the {schema} that you provide, it should follow the format of that json to create it's response, all you have to do is give a typical user prompt, and it will respond in JSON.
# Benchmarks

## GPT4All:
```
| Task |Version| Metric |Value | |Stderr|
|-------------|------:|--------|-----:|---|-----:|
|arc_challenge| 0|acc |0.5520|± |0.0145|
| | |acc_norm|0.5887|± |0.0144|
|arc_easy | 0|acc |0.8350|± |0.0076|
| | |acc_norm|0.8123|± |0.0080|
|boolq | 1|acc |0.8584|± |0.0061|
|hellaswag | 0|acc |0.6265|± |0.0048|
| | |acc_norm|0.8053|± |0.0040|
|openbookqa | 0|acc |0.3800|± |0.0217|
| | |acc_norm|0.4580|± |0.0223|
|piqa | 0|acc |0.8003|± |0.0093|
| | |acc_norm|0.8118|± |0.0091|
|winogrande | 0|acc |0.7490|± |0.0122|
```
Average: 72.62
## AGIEval:
```
| Task |Version| Metric |Value | |Stderr|
|------------------------------|------:|--------|-----:|---|-----:|
|agieval_aqua_rat | 0|acc |0.2520|± |0.0273|
| | |acc_norm|0.2559|± |0.0274|
|agieval_logiqa_en | 0|acc |0.3548|± |0.0188|
| | |acc_norm|0.3625|± |0.0189|
|agieval_lsat_ar | 0|acc |0.1826|± |0.0255|
| | |acc_norm|0.1913|± |0.0260|
|agieval_lsat_lr | 0|acc |0.5510|± |0.0220|
| | |acc_norm|0.5255|± |0.0221|
|agieval_lsat_rc | 0|acc |0.6431|± |0.0293|
| | |acc_norm|0.6097|± |0.0298|
|agieval_sat_en | 0|acc |0.7330|± |0.0309|
| | |acc_norm|0.7039|± |0.0319|
|agieval_sat_en_without_passage| 0|acc |0.4029|± |0.0343|
| | |acc_norm|0.3689|± |0.0337|
|agieval_sat_math | 0|acc |0.3909|± |0.0330|
| | |acc_norm|0.3773|± |0.0328|
```
Average: 42.44
## BigBench:
```
| Task |Version| Metric |Value | |Stderr|
|------------------------------------------------|------:|---------------------|-----:|---|-----:|
|bigbench_causal_judgement | 0|multiple_choice_grade|0.5737|± |0.0360|
|bigbench_date_understanding | 0|multiple_choice_grade|0.6667|± |0.0246|
|bigbench_disambiguation_qa | 0|multiple_choice_grade|0.3178|± |0.0290|
|bigbench_geometric_shapes | 0|multiple_choice_grade|0.1755|± |0.0201|
| | |exact_str_match |0.0000|± |0.0000|
|bigbench_logical_deduction_five_objects | 0|multiple_choice_grade|0.3120|± |0.0207|
|bigbench_logical_deduction_seven_objects | 0|multiple_choice_grade|0.2014|± |0.0152|
|bigbench_logical_deduction_three_objects | 0|multiple_choice_grade|0.5500|± |0.0288|
|bigbench_movie_recommendation | 0|multiple_choice_grade|0.4300|± |0.0222|
|bigbench_navigate | 0|multiple_choice_grade|0.4980|± |0.0158|
|bigbench_reasoning_about_colored_objects | 0|multiple_choice_grade|0.7010|± |0.0102|
|bigbench_ruin_names | 0|multiple_choice_grade|0.4688|± |0.0236|
|bigbench_salient_translation_error_detection | 0|multiple_choice_grade|0.1974|± |0.0126|
|bigbench_snarks | 0|multiple_choice_grade|0.7403|± |0.0327|
|bigbench_sports_understanding | 0|multiple_choice_grade|0.5426|± |0.0159|
|bigbench_temporal_sequences | 0|multiple_choice_grade|0.5320|± |0.0158|
|bigbench_tracking_shuffled_objects_five_objects | 0|multiple_choice_grade|0.2280|± |0.0119|
|bigbench_tracking_shuffled_objects_seven_objects| 0|multiple_choice_grade|0.1531|± |0.0086|
|bigbench_tracking_shuffled_objects_three_objects| 0|multiple_choice_grade|0.5500|± |0.0288|
```
Average: 43.55
## TruthfulQA:
```
| Task |Version|Metric|Value| |Stderr|
|-------------|------:|------|----:|---|-----:|
|truthfulqa_mc| 1|mc1 |0.410|± |0.0172|
| | |mc2 |0.578|± |0.0157|
```
# Inference Code
Here is example code using HuggingFace Transformers to inference the model (note: in 4bit, it will require around 5GB of VRAM)
Note: To use function calling, you should see the github repo above.
```python
# Code to inference Hermes with HF Transformers
# Requires pytorch, transformers, bitsandbytes, sentencepiece, protobuf, and flash-attn packages
import torch
from transformers import AutoTokenizer, AutoModelForCausalLM, LlamaForCausalLM
import bitsandbytes, flash_attn
tokenizer = AutoTokenizer.from_pretrained('NousResearch/Hermes-2-Pro-Llama-3-8B', trust_remote_code=True)
model = LlamaForCausalLM.from_pretrained(
"NousResearch/Hermes-2-Pro-Llama-3-8B",
torch_dtype=torch.float16,
device_map="auto",
load_in_8bit=False,
load_in_4bit=True,
use_flash_attention_2=True
)
prompts = [
"""<|im_start|>system
You are a sentient, superintelligent artificial general intelligence, here to teach and assist me.<|im_end|>
<|im_start|>user
Write a short story about Goku discovering kirby has teamed up with Majin Buu to destroy the world.<|im_end|>
<|im_start|>assistant""",
]
for chat in prompts:
print(chat)
input_ids = tokenizer(chat, return_tensors="pt").input_ids.to("cuda")
generated_ids = model.generate(input_ids, max_new_tokens=750, temperature=0.8, repetition_penalty=1.1, do_sample=True, eos_token_id=tokenizer.eos_token_id)
response = tokenizer.decode(generated_ids[0][input_ids.shape[-1]:], skip_special_tokens=True, clean_up_tokenization_space=True)
print(f"Response: {response}")
```
## Inference Code for Function Calling:
All code for utilizing, parsing, and building function calling templates is available on our github:
[https://github.com/NousResearch/Hermes-Function-Calling](https://github.com/NousResearch/Hermes-Function-Calling)

# Chat Interfaces
When quantized versions of the model are released, I recommend using LM Studio for chatting with Hermes 2 Pro. It does not support function calling - for that use our github repo. It is a GUI application that utilizes GGUF models with a llama.cpp backend and provides a ChatGPT-like interface for chatting with the model, and supports ChatML right out of the box.
In LM-Studio, simply select the ChatML Prefix on the settings side pane:

## Quantized Versions:
GGUF Versions Available Here: https://huggingface.co/NousResearch/Hermes-2-Pro-Llama-3-8B-GGUF
# How to cite:
```bibtext
@misc{Hermes-2-Pro-Llama-3-8B,
url={[https://huggingface.co/NousResearch/Hermes-2-Pro-Llama-3-8B]https://huggingface.co/NousResearch/Hermes-2-Pro-Llama-3-8B)},
title={Hermes-2-Pro-Llama-3-8B},
author={"Teknium", "interstellarninja", "theemozilla", "karan4d", "huemin_art"}
}
``` |
timm/vit_large_patch16_224.augreg_in21k_ft_in1k | timm | 2023-05-06T00:18:01Z | 21,509 | 1 | timm | [
"timm",
"pytorch",
"safetensors",
"image-classification",
"dataset:imagenet-1k",
"dataset:imagenet-21k",
"arxiv:2106.10270",
"arxiv:2010.11929",
"license:apache-2.0",
"region:us"
] | image-classification | 2022-12-22T07:46:31Z | ---
tags:
- image-classification
- timm
library_name: timm
license: apache-2.0
datasets:
- imagenet-1k
- imagenet-21k
---
# Model card for vit_large_patch16_224.augreg_in21k_ft_in1k
A Vision Transformer (ViT) image classification model. Trained on ImageNet-21k and fine-tuned on ImageNet-1k (with additional augmentation and regularization) in JAX by paper authors, ported to PyTorch by Ross Wightman.
## Model Details
- **Model Type:** Image classification / feature backbone
- **Model Stats:**
- Params (M): 304.3
- GMACs: 59.7
- Activations (M): 43.8
- Image size: 224 x 224
- **Papers:**
- How to train your ViT? Data, Augmentation, and Regularization in Vision Transformers: https://arxiv.org/abs/2106.10270
- An Image is Worth 16x16 Words: Transformers for Image Recognition at Scale: https://arxiv.org/abs/2010.11929v2
- **Dataset:** ImageNet-1k
- **Pretrain Dataset:** ImageNet-21k
- **Original:** https://github.com/google-research/vision_transformer
## Model Usage
### Image Classification
```python
from urllib.request import urlopen
from PIL import Image
import timm
img = Image.open(urlopen(
'https://huggingface.co/datasets/huggingface/documentation-images/resolve/main/beignets-task-guide.png'
))
model = timm.create_model('vit_large_patch16_224.augreg_in21k_ft_in1k', pretrained=True)
model = model.eval()
# get model specific transforms (normalization, resize)
data_config = timm.data.resolve_model_data_config(model)
transforms = timm.data.create_transform(**data_config, is_training=False)
output = model(transforms(img).unsqueeze(0)) # unsqueeze single image into batch of 1
top5_probabilities, top5_class_indices = torch.topk(output.softmax(dim=1) * 100, k=5)
```
### Image Embeddings
```python
from urllib.request import urlopen
from PIL import Image
import timm
img = Image.open(urlopen(
'https://huggingface.co/datasets/huggingface/documentation-images/resolve/main/beignets-task-guide.png'
))
model = timm.create_model(
'vit_large_patch16_224.augreg_in21k_ft_in1k',
pretrained=True,
num_classes=0, # remove classifier nn.Linear
)
model = model.eval()
# get model specific transforms (normalization, resize)
data_config = timm.data.resolve_model_data_config(model)
transforms = timm.data.create_transform(**data_config, is_training=False)
output = model(transforms(img).unsqueeze(0)) # output is (batch_size, num_features) shaped tensor
# or equivalently (without needing to set num_classes=0)
output = model.forward_features(transforms(img).unsqueeze(0))
# output is unpooled, a (1, 197, 1024) shaped tensor
output = model.forward_head(output, pre_logits=True)
# output is a (1, num_features) shaped tensor
```
## Model Comparison
Explore the dataset and runtime metrics of this model in timm [model results](https://github.com/huggingface/pytorch-image-models/tree/main/results).
## Citation
```bibtex
@article{steiner2021augreg,
title={How to train your ViT? Data, Augmentation, and Regularization in Vision Transformers},
author={Steiner, Andreas and Kolesnikov, Alexander and and Zhai, Xiaohua and Wightman, Ross and Uszkoreit, Jakob and Beyer, Lucas},
journal={arXiv preprint arXiv:2106.10270},
year={2021}
}
```
```bibtex
@article{dosovitskiy2020vit,
title={An Image is Worth 16x16 Words: Transformers for Image Recognition at Scale},
author={Dosovitskiy, Alexey and Beyer, Lucas and Kolesnikov, Alexander and Weissenborn, Dirk and Zhai, Xiaohua and Unterthiner, Thomas and Dehghani, Mostafa and Minderer, Matthias and Heigold, Georg and Gelly, Sylvain and Uszkoreit, Jakob and Houlsby, Neil},
journal={ICLR},
year={2021}
}
```
```bibtex
@misc{rw2019timm,
author = {Ross Wightman},
title = {PyTorch Image Models},
year = {2019},
publisher = {GitHub},
journal = {GitHub repository},
doi = {10.5281/zenodo.4414861},
howpublished = {\url{https://github.com/huggingface/pytorch-image-models}}
}
```
|
Yntec/fennPhoto | Yntec | 2023-10-31T23:29:41Z | 21,476 | 3 | diffusers | [
"diffusers",
"safetensors",
"Base Model",
"Film",
"Real",
"stable-diffusion",
"stable-diffusion-diffusers",
"text-to-image",
"en",
"license:creativeml-openrail-m",
"autotrain_compatible",
"endpoints_compatible",
"diffusers:StableDiffusionPipeline",
"region:us"
] | text-to-image | 2023-10-31T22:55:18Z | ---
language:
- en
license: creativeml-openrail-m
library_name: diffusers
pipeline_tag: text-to-image
tags:
- Base Model
- Film
- Real
- stable-diffusion
- stable-diffusion-diffusers
- diffusers
- text-to-image
inference: true
---
# Fenn Photo
Original page: https://civitai.com/models/153869/fenn-photo
Samples and prompt:


macro studio photo of old antique Victorian marmor figurine of cute Rinoa, chibi Rinoa Heartilly, eyeliner, very slim, arm warmers, necklace, sleeveless coat, black shirt, blue skirt, arm ribbon, bike shorts, boots, long hair, black hair, cozy home in the background with candles and plants, Rinoa on flat round porcelain base, by Michelangelo |
mradermacher/Llama-3-LewdPlay-8B-evo-GGUF | mradermacher | 2024-06-27T21:39:45Z | 21,466 | 1 | transformers | [
"transformers",
"gguf",
"mergekit",
"merge",
"en",
"base_model:Undi95/Llama-3-LewdPlay-8B-evo",
"license:cc-by-nc-4.0",
"endpoints_compatible",
"region:us"
] | null | 2024-06-26T21:36:16Z | ---
base_model: Undi95/Llama-3-LewdPlay-8B-evo
language:
- en
library_name: transformers
license: cc-by-nc-4.0
quantized_by: mradermacher
tags:
- mergekit
- merge
---
## About
<!-- ### quantize_version: 2 -->
<!-- ### output_tensor_quantised: 1 -->
<!-- ### convert_type: hf -->
<!-- ### vocab_type: -->
<!-- ### tags: -->
static quants of https://huggingface.co/Undi95/Llama-3-LewdPlay-8B-evo
<!-- provided-files -->
weighted/imatrix quants are available at https://huggingface.co/mradermacher/Llama-3-LewdPlay-8B-evo-i1-GGUF
## Usage
If you are unsure how to use GGUF files, refer to one of [TheBloke's
READMEs](https://huggingface.co/TheBloke/KafkaLM-70B-German-V0.1-GGUF) for
more details, including on how to concatenate multi-part files.
## Provided Quants
(sorted by size, not necessarily quality. IQ-quants are often preferable over similar sized non-IQ quants)
| Link | Type | Size/GB | Notes |
|:-----|:-----|--------:|:------|
| [GGUF](https://huggingface.co/mradermacher/Llama-3-LewdPlay-8B-evo-GGUF/resolve/main/Llama-3-LewdPlay-8B-evo.Q2_K.gguf) | Q2_K | 3.3 | |
| [GGUF](https://huggingface.co/mradermacher/Llama-3-LewdPlay-8B-evo-GGUF/resolve/main/Llama-3-LewdPlay-8B-evo.IQ3_XS.gguf) | IQ3_XS | 3.6 | |
| [GGUF](https://huggingface.co/mradermacher/Llama-3-LewdPlay-8B-evo-GGUF/resolve/main/Llama-3-LewdPlay-8B-evo.Q3_K_S.gguf) | Q3_K_S | 3.8 | |
| [GGUF](https://huggingface.co/mradermacher/Llama-3-LewdPlay-8B-evo-GGUF/resolve/main/Llama-3-LewdPlay-8B-evo.IQ3_S.gguf) | IQ3_S | 3.8 | beats Q3_K* |
| [GGUF](https://huggingface.co/mradermacher/Llama-3-LewdPlay-8B-evo-GGUF/resolve/main/Llama-3-LewdPlay-8B-evo.IQ3_M.gguf) | IQ3_M | 3.9 | |
| [GGUF](https://huggingface.co/mradermacher/Llama-3-LewdPlay-8B-evo-GGUF/resolve/main/Llama-3-LewdPlay-8B-evo.Q3_K_M.gguf) | Q3_K_M | 4.1 | lower quality |
| [GGUF](https://huggingface.co/mradermacher/Llama-3-LewdPlay-8B-evo-GGUF/resolve/main/Llama-3-LewdPlay-8B-evo.Q3_K_L.gguf) | Q3_K_L | 4.4 | |
| [GGUF](https://huggingface.co/mradermacher/Llama-3-LewdPlay-8B-evo-GGUF/resolve/main/Llama-3-LewdPlay-8B-evo.IQ4_XS.gguf) | IQ4_XS | 4.6 | |
| [GGUF](https://huggingface.co/mradermacher/Llama-3-LewdPlay-8B-evo-GGUF/resolve/main/Llama-3-LewdPlay-8B-evo.Q4_K_S.gguf) | Q4_K_S | 4.8 | fast, recommended |
| [GGUF](https://huggingface.co/mradermacher/Llama-3-LewdPlay-8B-evo-GGUF/resolve/main/Llama-3-LewdPlay-8B-evo.Q4_K_M.gguf) | Q4_K_M | 5.0 | fast, recommended |
| [GGUF](https://huggingface.co/mradermacher/Llama-3-LewdPlay-8B-evo-GGUF/resolve/main/Llama-3-LewdPlay-8B-evo.Q5_K_S.gguf) | Q5_K_S | 5.7 | |
| [GGUF](https://huggingface.co/mradermacher/Llama-3-LewdPlay-8B-evo-GGUF/resolve/main/Llama-3-LewdPlay-8B-evo.Q5_K_M.gguf) | Q5_K_M | 5.8 | |
| [GGUF](https://huggingface.co/mradermacher/Llama-3-LewdPlay-8B-evo-GGUF/resolve/main/Llama-3-LewdPlay-8B-evo.Q6_K.gguf) | Q6_K | 6.7 | very good quality |
| [GGUF](https://huggingface.co/mradermacher/Llama-3-LewdPlay-8B-evo-GGUF/resolve/main/Llama-3-LewdPlay-8B-evo.Q8_0.gguf) | Q8_0 | 8.6 | fast, best quality |
| [GGUF](https://huggingface.co/mradermacher/Llama-3-LewdPlay-8B-evo-GGUF/resolve/main/Llama-3-LewdPlay-8B-evo.f16.gguf) | f16 | 16.2 | 16 bpw, overkill |
Here is a handy graph by ikawrakow comparing some lower-quality quant
types (lower is better):

And here are Artefact2's thoughts on the matter:
https://gist.github.com/Artefact2/b5f810600771265fc1e39442288e8ec9
## FAQ / Model Request
See https://huggingface.co/mradermacher/model_requests for some answers to
questions you might have and/or if you want some other model quantized.
## Thanks
I thank my company, [nethype GmbH](https://www.nethype.de/), for letting
me use its servers and providing upgrades to my workstation to enable
this work in my free time.
<!-- end -->
|
OFA-Sys/chinese-clip-vit-base-patch16 | OFA-Sys | 2022-12-09T06:10:13Z | 21,459 | 68 | transformers | [
"transformers",
"pytorch",
"chinese_clip",
"zero-shot-image-classification",
"vision",
"arxiv:2211.01335",
"endpoints_compatible",
"region:us"
] | zero-shot-image-classification | 2022-11-09T08:14:09Z | ---
tags:
- vision
widget:
- src: https://huggingface.co/OFA-Sys/chinese-clip-vit-base-patch16/resolve/main/festival.jpg
candidate_labels: 灯笼, 鞭炮, 对联
example_title: festival
- src: https://huggingface.co/datasets/mishig/sample_images/resolve/main/cat-dog-music.png
candidate_labels: 音乐表演, 体育运动
example_title: cat & dog
- src: https://huggingface.co/datasets/mishig/sample_images/resolve/main/football-match.jpg
candidate_labels: 梅西, C罗, 马奎尔
example_title: football
---
# Chinese-CLIP-ViT-Base-Patch16
## Introduction
This is the base-version of the Chinese CLIP, with ViT-B/16 as the image encoder and RoBERTa-wwm-base as the text encoder. Chinese CLIP is a simple implementation of CLIP on a large-scale dataset of around 200 million Chinese image-text pairs. For more details, please refer to our technical report https://arxiv.org/abs/2211.01335 and our official github repo https://github.com/OFA-Sys/Chinese-CLIP (Welcome to star! 🔥🔥)
## Use with the official API
We provide a simple code snippet to show how to use the API of Chinese-CLIP to compute the image & text embeddings and similarities.
```python
from PIL import Image
import requests
from transformers import ChineseCLIPProcessor, ChineseCLIPModel
model = ChineseCLIPModel.from_pretrained("OFA-Sys/chinese-clip-vit-base-patch16")
processor = ChineseCLIPProcessor.from_pretrained("OFA-Sys/chinese-clip-vit-base-patch16")
url = "https://clip-cn-beijing.oss-cn-beijing.aliyuncs.com/pokemon.jpeg"
image = Image.open(requests.get(url, stream=True).raw)
# Squirtle, Bulbasaur, Charmander, Pikachu in English
texts = ["杰尼龟", "妙蛙种子", "小火龙", "皮卡丘"]
# compute image feature
inputs = processor(images=image, return_tensors="pt")
image_features = model.get_image_features(**inputs)
image_features = image_features / image_features.norm(p=2, dim=-1, keepdim=True) # normalize
# compute text features
inputs = processor(text=texts, padding=True, return_tensors="pt")
text_features = model.get_text_features(**inputs)
text_features = text_features / text_features.norm(p=2, dim=-1, keepdim=True) # normalize
# compute image-text similarity scores
inputs = processor(text=texts, images=image, return_tensors="pt", padding=True)
outputs = model(**inputs)
logits_per_image = outputs.logits_per_image # this is the image-text similarity score
probs = logits_per_image.softmax(dim=1) # probs: [[1.2686e-03, 5.4499e-02, 6.7968e-04, 9.4355e-01]]
```
However, if you are not satisfied with only using the API, feel free to check our github repo https://github.com/OFA-Sys/Chinese-CLIP for more details about training and inference.
<br><br>
## Results
**MUGE Text-to-Image Retrieval**:
<table border="1" width="100%">
<tr align="center">
<th>Setup</th><th colspan="4">Zero-shot</th><th colspan="4">Finetune</th>
</tr>
<tr align="center">
<td>Metric</td><td>R@1</td><td>R@5</td><td>R@10</td><td>MR</td><td>R@1</td><td>R@5</td><td>R@10</td><td>MR</td>
</tr>
<tr align="center">
<td width="120%">Wukong</td><td>42.7</td><td>69.0</td><td>78.0</td><td>63.2</td><td>52.7</td><td>77.9</td><td>85.6</td><td>72.1</td>
</tr>
<tr align="center">
<td width="120%">R2D2</td><td>49.5</td><td>75.7</td><td>83.2</td><td>69.5</td><td>60.1</td><td>82.9</td><td>89.4</td><td>77.5</td>
</tr>
<tr align="center">
<td width="120%">CN-CLIP</td><td>63.0</td><td>84.1</td><td>89.2</td><td>78.8</td><td>68.9</td><td>88.7</td><td>93.1</td><td>83.6</td>
</tr>
</table>
<br>
**Flickr30K-CN Retrieval**:
<table border="1" width="120%">
<tr align="center">
<th>Task</th><th colspan="6">Text-to-Image</th><th colspan="6">Image-to-Text</th>
</tr>
<tr align="center">
<th>Setup</th><th colspan="3">Zero-shot</th><th colspan="3">Finetune</th><th colspan="3">Zero-shot</th><th colspan="3">Finetune</th>
</tr>
<tr align="center">
<td>Metric</td><td>R@1</td><td>R@5</td><td>R@10</td><td>R@1</td><td>R@5</td><td>R@10</td><td>R@1</td><td>R@5</td><td>R@10</td><td>R@1</td><td>R@5</td><td>R@10</td>
</tr>
<tr align="center">
<td width="120%">Wukong</td><td>51.7</td><td>78.9</td><td>86.3</td><td>77.4</td><td>94.5</td><td>97.0</td><td>76.1</td><td>94.8</td><td>97.5</td><td>92.7</td><td>99.1</td><td>99.6</td>
</tr>
<tr align="center">
<td width="120%">R2D2</td><td>60.9</td><td>86.8</td><td>92.7</td><td>84.4</td><td>96.7</td><td>98.4</td><td>77.6</td><td>96.7</td><td>98.9</td><td>95.6</td><td>99.8</td><td>100.0</td>
</tr>
<tr align="center">
<td width="120%">CN-CLIP</td><td>71.2</td><td>91.4</td><td>95.5</td><td>83.8</td><td>96.9</td><td>98.6</td><td>81.6</td><td>97.5</td><td>98.8</td><td>95.3</td><td>99.7</td><td>100.0</td>
</tr>
</table>
<br>
**COCO-CN Retrieval**:
<table border="1" width="100%">
<tr align="center">
<th>Task</th><th colspan="6">Text-to-Image</th><th colspan="6">Image-to-Text</th>
</tr>
<tr align="center">
<th>Setup</th><th colspan="3">Zero-shot</th><th colspan="3">Finetune</th><th colspan="3">Zero-shot</th><th colspan="3">Finetune</th>
</tr>
<tr align="center">
<td>Metric</td><td>R@1</td><td>R@5</td><td>R@10</td><td>R@1</td><td>R@5</td><td>R@10</td><td>R@1</td><td>R@5</td><td>R@10</td><td>R@1</td><td>R@5</td><td>R@10</td>
</tr>
<tr align="center">
<td width="120%">Wukong</td><td>53.4</td><td>80.2</td><td>90.1</td><td>74.0</td><td>94.4</td><td>98.1</td><td>55.2</td><td>81.0</td><td>90.6</td><td>73.3</td><td>94.0</td><td>98.0</td>
</tr>
<tr align="center">
<td width="120%">R2D2</td><td>56.4</td><td>85.0</td><td>93.1</td><td>79.1</td><td>96.5</td><td>98.9</td><td>63.3</td><td>89.3</td><td>95.7</td><td>79.3</td><td>97.1</td><td>98.7</td>
</tr>
<tr align="center">
<td width="120%">CN-CLIP</td><td>69.2</td><td>89.9</td><td>96.1</td><td>81.5</td><td>96.9</td><td>99.1</td><td>63.0</td><td>86.6</td><td>92.9</td><td>83.5</td><td>97.3</td><td>99.2</td>
</tr>
</table>
<br>
**Zero-shot Image Classification**:
<table border="1" width="100%">
<tr align="center">
<th>Task</th><th>CIFAR10</th><th>CIFAR100</th><th>DTD</th><th>EuroSAT</th><th>FER</th><th>FGVC</th><th>KITTI</th><th>MNIST</th><th>PC</th><th>VOC</th>
</tr>
<tr align="center">
<td width="150%">GIT</td><td>88.5</td><td>61.1</td><td>42.9</td><td>43.4</td><td>41.4</td><td>6.7</td><td>22.1</td><td>68.9</td><td>50.0</td><td>80.2</td>
</tr>
<tr align="center">
<td width="150%">ALIGN</td><td>94.9</td><td>76.8</td><td>66.1</td><td>52.1</td><td>50.8</td><td>25.0</td><td>41.2</td><td>74.0</td><td>55.2</td><td>83.0</td>
</tr>
<tr align="center">
<td width="150%">CLIP</td><td>94.9</td><td>77.0</td><td>56.0</td><td>63.0</td><td>48.3</td><td>33.3</td><td>11.5</td><td>79.0</td><td>62.3</td><td>84.0</td>
</tr>
<tr align="center">
<td width="150%">Wukong</td><td>95.4</td><td>77.1</td><td>40.9</td><td>50.3</td><td>-</td><td>-</td><td>-</td><td>-</td><td>-</td><td>-</td>
</tr>
<tr align="center">
<td width="150%">CN-CLIP</td><td>96.0</td><td>79.7</td><td>51.2</td><td>52.0</td><td>55.1</td><td>26.2</td><td>49.9</td><td>79.4</td><td>63.5</td><td>84.9</td>
</tr>
</table>
<br>
## Citation
If you find Chinese CLIP helpful, feel free to cite our paper. Thanks for your support!
```
@article{chinese-clip,
title={Chinese CLIP: Contrastive Vision-Language Pretraining in Chinese},
author={Yang, An and Pan, Junshu and Lin, Junyang and Men, Rui and Zhang, Yichang and Zhou, Jingren and Zhou, Chang},
journal={arXiv preprint arXiv:2211.01335},
year={2022}
}
```
<br> |
IlyaGusev/saiga_mistral_7b_gguf | IlyaGusev | 2024-04-04T14:34:09Z | 21,459 | 75 | null | [
"gguf",
"text-generation",
"ru",
"dataset:IlyaGusev/ru_turbo_saiga",
"dataset:IlyaGusev/ru_sharegpt_cleaned",
"dataset:IlyaGusev/oasst1_ru_main_branch",
"dataset:IlyaGusev/ru_turbo_alpaca_evol_instruct",
"dataset:lksy/ru_instruct_gpt4",
"license:apache-2.0",
"region:us"
] | text-generation | 2023-10-09T17:44:21Z | ---
datasets:
- IlyaGusev/ru_turbo_saiga
- IlyaGusev/ru_sharegpt_cleaned
- IlyaGusev/oasst1_ru_main_branch
- IlyaGusev/ru_turbo_alpaca_evol_instruct
- lksy/ru_instruct_gpt4
language:
- ru
inference: false
pipeline_tag: text-generation
license: apache-2.0
---
Llama.cpp compatible versions of an original [7B model](https://huggingface.co/IlyaGusev/saiga_mistral_7b_lora).
Download one of the versions, for example `model-q4_K.gguf`.
```
wget https://huggingface.co/IlyaGusev/saiga_mistral_7b_gguf/resolve/main/model-q4_K.gguf
```
Download [interact_mistral_llamacpp.py](https://raw.githubusercontent.com/IlyaGusev/rulm/master/self_instruct/src/interact_mistral_llamacpp.py)
```
wget https://raw.githubusercontent.com/IlyaGusev/rulm/master/self_instruct/src/interact_mistral_llamacpp.py
```
How to run:
```
pip install llama-cpp-python fire
python3 interact_mistral_llamacpp.py model-q4_K.gguf
```
System requirements:
* 10GB RAM for q8_0 and less for smaller quantizations
|
legraphista/Llama-3-Instruct-8B-SPPO-Iter3-IMat-GGUF | legraphista | 2024-06-27T15:08:50Z | 21,335 | 0 | gguf | [
"gguf",
"quantized",
"GGUF",
"quantization",
"imat",
"imatrix",
"static",
"16bit",
"8bit",
"6bit",
"5bit",
"4bit",
"3bit",
"2bit",
"1bit",
"text-generation",
"en",
"dataset:openbmb/UltraFeedback",
"base_model:UCLA-AGI/Llama-3-Instruct-8B-SPPO-Iter3",
"license:apache-2.0",
"region:us"
] | text-generation | 2024-06-27T14:15:34Z | ---
base_model: UCLA-AGI/Llama-3-Instruct-8B-SPPO-Iter3
datasets:
- openbmb/UltraFeedback
inference: false
language:
- en
library_name: gguf
license: apache-2.0
pipeline_tag: text-generation
quantized_by: legraphista
tags:
- quantized
- GGUF
- quantization
- imat
- imatrix
- static
- 16bit
- 8bit
- 6bit
- 5bit
- 4bit
- 3bit
- 2bit
- 1bit
---
# Llama-3-Instruct-8B-SPPO-Iter3-IMat-GGUF
_Llama.cpp imatrix quantization of UCLA-AGI/Llama-3-Instruct-8B-SPPO-Iter3_
Original Model: [UCLA-AGI/Llama-3-Instruct-8B-SPPO-Iter3](https://huggingface.co/UCLA-AGI/Llama-3-Instruct-8B-SPPO-Iter3)
Original dtype: `BF16` (`bfloat16`)
Quantized by: llama.cpp [b3248](https://github.com/ggerganov/llama.cpp/releases/tag/b3248)
IMatrix dataset: [here](https://gist.githubusercontent.com/bartowski1182/eb213dccb3571f863da82e99418f81e8/raw/b2869d80f5c16fd7082594248e80144677736635/calibration_datav3.txt)
- [Files](#files)
- [IMatrix](#imatrix)
- [Common Quants](#common-quants)
- [All Quants](#all-quants)
- [Downloading using huggingface-cli](#downloading-using-huggingface-cli)
- [Inference](#inference)
- [Simple chat template](#simple-chat-template)
- [Chat template with system prompt](#chat-template-with-system-prompt)
- [Llama.cpp](#llama-cpp)
- [FAQ](#faq)
- [Why is the IMatrix not applied everywhere?](#why-is-the-imatrix-not-applied-everywhere)
- [How do I merge a split GGUF?](#how-do-i-merge-a-split-gguf)
---
## Files
### IMatrix
Status: ✅ Available
Link: [here](https://huggingface.co/legraphista/Llama-3-Instruct-8B-SPPO-Iter3-IMat-GGUF/blob/main/imatrix.dat)
### Common Quants
| Filename | Quant type | File Size | Status | Uses IMatrix | Is Split |
| -------- | ---------- | --------- | ------ | ------------ | -------- |
| [Llama-3-Instruct-8B-SPPO-Iter3.Q8_0.gguf](https://huggingface.co/legraphista/Llama-3-Instruct-8B-SPPO-Iter3-IMat-GGUF/blob/main/Llama-3-Instruct-8B-SPPO-Iter3.Q8_0.gguf) | Q8_0 | 8.54GB | ✅ Available | ⚪ Static | 📦 No
| [Llama-3-Instruct-8B-SPPO-Iter3.Q6_K.gguf](https://huggingface.co/legraphista/Llama-3-Instruct-8B-SPPO-Iter3-IMat-GGUF/blob/main/Llama-3-Instruct-8B-SPPO-Iter3.Q6_K.gguf) | Q6_K | 6.60GB | ✅ Available | ⚪ Static | 📦 No
| [Llama-3-Instruct-8B-SPPO-Iter3.Q4_K.gguf](https://huggingface.co/legraphista/Llama-3-Instruct-8B-SPPO-Iter3-IMat-GGUF/blob/main/Llama-3-Instruct-8B-SPPO-Iter3.Q4_K.gguf) | Q4_K | 4.92GB | ✅ Available | 🟢 IMatrix | 📦 No
| [Llama-3-Instruct-8B-SPPO-Iter3.Q3_K.gguf](https://huggingface.co/legraphista/Llama-3-Instruct-8B-SPPO-Iter3-IMat-GGUF/blob/main/Llama-3-Instruct-8B-SPPO-Iter3.Q3_K.gguf) | Q3_K | 4.02GB | ✅ Available | 🟢 IMatrix | 📦 No
| [Llama-3-Instruct-8B-SPPO-Iter3.Q2_K.gguf](https://huggingface.co/legraphista/Llama-3-Instruct-8B-SPPO-Iter3-IMat-GGUF/blob/main/Llama-3-Instruct-8B-SPPO-Iter3.Q2_K.gguf) | Q2_K | 3.18GB | ✅ Available | 🟢 IMatrix | 📦 No
### All Quants
| Filename | Quant type | File Size | Status | Uses IMatrix | Is Split |
| -------- | ---------- | --------- | ------ | ------------ | -------- |
| [Llama-3-Instruct-8B-SPPO-Iter3.BF16.gguf](https://huggingface.co/legraphista/Llama-3-Instruct-8B-SPPO-Iter3-IMat-GGUF/blob/main/Llama-3-Instruct-8B-SPPO-Iter3.BF16.gguf) | BF16 | 16.07GB | ✅ Available | ⚪ Static | 📦 No
| [Llama-3-Instruct-8B-SPPO-Iter3.FP16.gguf](https://huggingface.co/legraphista/Llama-3-Instruct-8B-SPPO-Iter3-IMat-GGUF/blob/main/Llama-3-Instruct-8B-SPPO-Iter3.FP16.gguf) | F16 | 16.07GB | ✅ Available | ⚪ Static | 📦 No
| [Llama-3-Instruct-8B-SPPO-Iter3.Q8_0.gguf](https://huggingface.co/legraphista/Llama-3-Instruct-8B-SPPO-Iter3-IMat-GGUF/blob/main/Llama-3-Instruct-8B-SPPO-Iter3.Q8_0.gguf) | Q8_0 | 8.54GB | ✅ Available | ⚪ Static | 📦 No
| [Llama-3-Instruct-8B-SPPO-Iter3.Q6_K.gguf](https://huggingface.co/legraphista/Llama-3-Instruct-8B-SPPO-Iter3-IMat-GGUF/blob/main/Llama-3-Instruct-8B-SPPO-Iter3.Q6_K.gguf) | Q6_K | 6.60GB | ✅ Available | ⚪ Static | 📦 No
| [Llama-3-Instruct-8B-SPPO-Iter3.Q5_K.gguf](https://huggingface.co/legraphista/Llama-3-Instruct-8B-SPPO-Iter3-IMat-GGUF/blob/main/Llama-3-Instruct-8B-SPPO-Iter3.Q5_K.gguf) | Q5_K | 5.73GB | ✅ Available | ⚪ Static | 📦 No
| [Llama-3-Instruct-8B-SPPO-Iter3.Q5_K_S.gguf](https://huggingface.co/legraphista/Llama-3-Instruct-8B-SPPO-Iter3-IMat-GGUF/blob/main/Llama-3-Instruct-8B-SPPO-Iter3.Q5_K_S.gguf) | Q5_K_S | 5.60GB | ✅ Available | ⚪ Static | 📦 No
| [Llama-3-Instruct-8B-SPPO-Iter3.Q4_K.gguf](https://huggingface.co/legraphista/Llama-3-Instruct-8B-SPPO-Iter3-IMat-GGUF/blob/main/Llama-3-Instruct-8B-SPPO-Iter3.Q4_K.gguf) | Q4_K | 4.92GB | ✅ Available | 🟢 IMatrix | 📦 No
| [Llama-3-Instruct-8B-SPPO-Iter3.Q4_K_S.gguf](https://huggingface.co/legraphista/Llama-3-Instruct-8B-SPPO-Iter3-IMat-GGUF/blob/main/Llama-3-Instruct-8B-SPPO-Iter3.Q4_K_S.gguf) | Q4_K_S | 4.69GB | ✅ Available | 🟢 IMatrix | 📦 No
| [Llama-3-Instruct-8B-SPPO-Iter3.IQ4_NL.gguf](https://huggingface.co/legraphista/Llama-3-Instruct-8B-SPPO-Iter3-IMat-GGUF/blob/main/Llama-3-Instruct-8B-SPPO-Iter3.IQ4_NL.gguf) | IQ4_NL | 4.68GB | ✅ Available | 🟢 IMatrix | 📦 No
| [Llama-3-Instruct-8B-SPPO-Iter3.IQ4_XS.gguf](https://huggingface.co/legraphista/Llama-3-Instruct-8B-SPPO-Iter3-IMat-GGUF/blob/main/Llama-3-Instruct-8B-SPPO-Iter3.IQ4_XS.gguf) | IQ4_XS | 4.45GB | ✅ Available | 🟢 IMatrix | 📦 No
| [Llama-3-Instruct-8B-SPPO-Iter3.Q3_K.gguf](https://huggingface.co/legraphista/Llama-3-Instruct-8B-SPPO-Iter3-IMat-GGUF/blob/main/Llama-3-Instruct-8B-SPPO-Iter3.Q3_K.gguf) | Q3_K | 4.02GB | ✅ Available | 🟢 IMatrix | 📦 No
| [Llama-3-Instruct-8B-SPPO-Iter3.Q3_K_L.gguf](https://huggingface.co/legraphista/Llama-3-Instruct-8B-SPPO-Iter3-IMat-GGUF/blob/main/Llama-3-Instruct-8B-SPPO-Iter3.Q3_K_L.gguf) | Q3_K_L | 4.32GB | ✅ Available | 🟢 IMatrix | 📦 No
| [Llama-3-Instruct-8B-SPPO-Iter3.Q3_K_S.gguf](https://huggingface.co/legraphista/Llama-3-Instruct-8B-SPPO-Iter3-IMat-GGUF/blob/main/Llama-3-Instruct-8B-SPPO-Iter3.Q3_K_S.gguf) | Q3_K_S | 3.66GB | ✅ Available | 🟢 IMatrix | 📦 No
| [Llama-3-Instruct-8B-SPPO-Iter3.IQ3_M.gguf](https://huggingface.co/legraphista/Llama-3-Instruct-8B-SPPO-Iter3-IMat-GGUF/blob/main/Llama-3-Instruct-8B-SPPO-Iter3.IQ3_M.gguf) | IQ3_M | 3.78GB | ✅ Available | 🟢 IMatrix | 📦 No
| [Llama-3-Instruct-8B-SPPO-Iter3.IQ3_S.gguf](https://huggingface.co/legraphista/Llama-3-Instruct-8B-SPPO-Iter3-IMat-GGUF/blob/main/Llama-3-Instruct-8B-SPPO-Iter3.IQ3_S.gguf) | IQ3_S | 3.68GB | ✅ Available | 🟢 IMatrix | 📦 No
| [Llama-3-Instruct-8B-SPPO-Iter3.IQ3_XS.gguf](https://huggingface.co/legraphista/Llama-3-Instruct-8B-SPPO-Iter3-IMat-GGUF/blob/main/Llama-3-Instruct-8B-SPPO-Iter3.IQ3_XS.gguf) | IQ3_XS | 3.52GB | ✅ Available | 🟢 IMatrix | 📦 No
| [Llama-3-Instruct-8B-SPPO-Iter3.IQ3_XXS.gguf](https://huggingface.co/legraphista/Llama-3-Instruct-8B-SPPO-Iter3-IMat-GGUF/blob/main/Llama-3-Instruct-8B-SPPO-Iter3.IQ3_XXS.gguf) | IQ3_XXS | 3.27GB | ✅ Available | 🟢 IMatrix | 📦 No
| [Llama-3-Instruct-8B-SPPO-Iter3.Q2_K.gguf](https://huggingface.co/legraphista/Llama-3-Instruct-8B-SPPO-Iter3-IMat-GGUF/blob/main/Llama-3-Instruct-8B-SPPO-Iter3.Q2_K.gguf) | Q2_K | 3.18GB | ✅ Available | 🟢 IMatrix | 📦 No
| [Llama-3-Instruct-8B-SPPO-Iter3.Q2_K_S.gguf](https://huggingface.co/legraphista/Llama-3-Instruct-8B-SPPO-Iter3-IMat-GGUF/blob/main/Llama-3-Instruct-8B-SPPO-Iter3.Q2_K_S.gguf) | Q2_K_S | 2.99GB | ✅ Available | 🟢 IMatrix | 📦 No
| [Llama-3-Instruct-8B-SPPO-Iter3.IQ2_M.gguf](https://huggingface.co/legraphista/Llama-3-Instruct-8B-SPPO-Iter3-IMat-GGUF/blob/main/Llama-3-Instruct-8B-SPPO-Iter3.IQ2_M.gguf) | IQ2_M | 2.95GB | ✅ Available | 🟢 IMatrix | 📦 No
| [Llama-3-Instruct-8B-SPPO-Iter3.IQ2_S.gguf](https://huggingface.co/legraphista/Llama-3-Instruct-8B-SPPO-Iter3-IMat-GGUF/blob/main/Llama-3-Instruct-8B-SPPO-Iter3.IQ2_S.gguf) | IQ2_S | 2.76GB | ✅ Available | 🟢 IMatrix | 📦 No
| [Llama-3-Instruct-8B-SPPO-Iter3.IQ2_XS.gguf](https://huggingface.co/legraphista/Llama-3-Instruct-8B-SPPO-Iter3-IMat-GGUF/blob/main/Llama-3-Instruct-8B-SPPO-Iter3.IQ2_XS.gguf) | IQ2_XS | 2.61GB | ✅ Available | 🟢 IMatrix | 📦 No
| [Llama-3-Instruct-8B-SPPO-Iter3.IQ2_XXS.gguf](https://huggingface.co/legraphista/Llama-3-Instruct-8B-SPPO-Iter3-IMat-GGUF/blob/main/Llama-3-Instruct-8B-SPPO-Iter3.IQ2_XXS.gguf) | IQ2_XXS | 2.40GB | ✅ Available | 🟢 IMatrix | 📦 No
| [Llama-3-Instruct-8B-SPPO-Iter3.IQ1_M.gguf](https://huggingface.co/legraphista/Llama-3-Instruct-8B-SPPO-Iter3-IMat-GGUF/blob/main/Llama-3-Instruct-8B-SPPO-Iter3.IQ1_M.gguf) | IQ1_M | 2.16GB | ✅ Available | 🟢 IMatrix | 📦 No
| [Llama-3-Instruct-8B-SPPO-Iter3.IQ1_S.gguf](https://huggingface.co/legraphista/Llama-3-Instruct-8B-SPPO-Iter3-IMat-GGUF/blob/main/Llama-3-Instruct-8B-SPPO-Iter3.IQ1_S.gguf) | IQ1_S | 2.02GB | ✅ Available | 🟢 IMatrix | 📦 No
## Downloading using huggingface-cli
If you do not have hugginface-cli installed:
```
pip install -U "huggingface_hub[cli]"
```
Download the specific file you want:
```
huggingface-cli download legraphista/Llama-3-Instruct-8B-SPPO-Iter3-IMat-GGUF --include "Llama-3-Instruct-8B-SPPO-Iter3.Q8_0.gguf" --local-dir ./
```
If the model file is big, it has been split into multiple files. In order to download them all to a local folder, run:
```
huggingface-cli download legraphista/Llama-3-Instruct-8B-SPPO-Iter3-IMat-GGUF --include "Llama-3-Instruct-8B-SPPO-Iter3.Q8_0/*" --local-dir ./
# see FAQ for merging GGUF's
```
---
## Inference
### Simple chat template
```
<|begin_of_text|><|start_header_id|>user<|end_header_id|>
{user_prompt}<|eot_id|><|start_header_id|>assistant<|end_header_id|>
{assistant_response}<|eot_id|><|start_header_id|>user<|end_header_id|>
{next_user_prompt}<|eot_id|>
```
### Chat template with system prompt
```
<|begin_of_text|><|start_header_id|>system<|end_header_id|>
{system_prompt}<|eot_id|><|start_header_id|>user<|end_header_id|>
{user_prompt}<|eot_id|><|start_header_id|>assistant<|end_header_id|>
{assistant_response}<|eot_id|><|start_header_id|>user<|end_header_id|>
{next_user_prompt}<|eot_id|>
```
### Llama.cpp
```
llama.cpp/main -m Llama-3-Instruct-8B-SPPO-Iter3.Q8_0.gguf --color -i -p "prompt here (according to the chat template)"
```
---
## FAQ
### Why is the IMatrix not applied everywhere?
According to [this investigation](https://www.reddit.com/r/LocalLLaMA/comments/1993iro/ggufs_quants_can_punch_above_their_weights_now/), it appears that lower quantizations are the only ones that benefit from the imatrix input (as per hellaswag results).
### How do I merge a split GGUF?
1. Make sure you have `gguf-split` available
- To get hold of `gguf-split`, navigate to https://github.com/ggerganov/llama.cpp/releases
- Download the appropriate zip for your system from the latest release
- Unzip the archive and you should be able to find `gguf-split`
2. Locate your GGUF chunks folder (ex: `Llama-3-Instruct-8B-SPPO-Iter3.Q8_0`)
3. Run `gguf-split --merge Llama-3-Instruct-8B-SPPO-Iter3.Q8_0/Llama-3-Instruct-8B-SPPO-Iter3.Q8_0-00001-of-XXXXX.gguf Llama-3-Instruct-8B-SPPO-Iter3.Q8_0.gguf`
- Make sure to point `gguf-split` to the first chunk of the split.
---
Got a suggestion? Ping me [@legraphista](https://x.com/legraphista)! |
mradermacher/LLaMA3-iterative-DPO-final-ExPO-i1-GGUF | mradermacher | 2024-06-28T02:52:45Z | 21,335 | 0 | transformers | [
"transformers",
"gguf",
"en",
"base_model:chujiezheng/LLaMA3-iterative-DPO-final-ExPO",
"license:llama3",
"endpoints_compatible",
"region:us"
] | null | 2024-06-28T00:37:00Z | ---
base_model: chujiezheng/LLaMA3-iterative-DPO-final-ExPO
language:
- en
library_name: transformers
license: llama3
quantized_by: mradermacher
---
## About
<!-- ### quantize_version: 2 -->
<!-- ### output_tensor_quantised: 1 -->
<!-- ### convert_type: hf -->
<!-- ### vocab_type: -->
<!-- ### tags: nicoboss -->
weighted/imatrix quants of https://huggingface.co/chujiezheng/LLaMA3-iterative-DPO-final-ExPO
<!-- provided-files -->
static quants are available at https://huggingface.co/mradermacher/LLaMA3-iterative-DPO-final-ExPO-GGUF
## Usage
If you are unsure how to use GGUF files, refer to one of [TheBloke's
READMEs](https://huggingface.co/TheBloke/KafkaLM-70B-German-V0.1-GGUF) for
more details, including on how to concatenate multi-part files.
## Provided Quants
(sorted by size, not necessarily quality. IQ-quants are often preferable over similar sized non-IQ quants)
| Link | Type | Size/GB | Notes |
|:-----|:-----|--------:|:------|
| [GGUF](https://huggingface.co/mradermacher/LLaMA3-iterative-DPO-final-ExPO-i1-GGUF/resolve/main/LLaMA3-iterative-DPO-final-ExPO.i1-IQ1_S.gguf) | i1-IQ1_S | 2.1 | for the desperate |
| [GGUF](https://huggingface.co/mradermacher/LLaMA3-iterative-DPO-final-ExPO-i1-GGUF/resolve/main/LLaMA3-iterative-DPO-final-ExPO.i1-IQ1_M.gguf) | i1-IQ1_M | 2.3 | mostly desperate |
| [GGUF](https://huggingface.co/mradermacher/LLaMA3-iterative-DPO-final-ExPO-i1-GGUF/resolve/main/LLaMA3-iterative-DPO-final-ExPO.i1-IQ2_XXS.gguf) | i1-IQ2_XXS | 2.5 | |
| [GGUF](https://huggingface.co/mradermacher/LLaMA3-iterative-DPO-final-ExPO-i1-GGUF/resolve/main/LLaMA3-iterative-DPO-final-ExPO.i1-IQ2_XS.gguf) | i1-IQ2_XS | 2.7 | |
| [GGUF](https://huggingface.co/mradermacher/LLaMA3-iterative-DPO-final-ExPO-i1-GGUF/resolve/main/LLaMA3-iterative-DPO-final-ExPO.i1-IQ2_S.gguf) | i1-IQ2_S | 2.9 | |
| [GGUF](https://huggingface.co/mradermacher/LLaMA3-iterative-DPO-final-ExPO-i1-GGUF/resolve/main/LLaMA3-iterative-DPO-final-ExPO.i1-IQ2_M.gguf) | i1-IQ2_M | 3.0 | |
| [GGUF](https://huggingface.co/mradermacher/LLaMA3-iterative-DPO-final-ExPO-i1-GGUF/resolve/main/LLaMA3-iterative-DPO-final-ExPO.i1-Q2_K.gguf) | i1-Q2_K | 3.3 | IQ3_XXS probably better |
| [GGUF](https://huggingface.co/mradermacher/LLaMA3-iterative-DPO-final-ExPO-i1-GGUF/resolve/main/LLaMA3-iterative-DPO-final-ExPO.i1-IQ3_XXS.gguf) | i1-IQ3_XXS | 3.4 | lower quality |
| [GGUF](https://huggingface.co/mradermacher/LLaMA3-iterative-DPO-final-ExPO-i1-GGUF/resolve/main/LLaMA3-iterative-DPO-final-ExPO.i1-IQ3_XS.gguf) | i1-IQ3_XS | 3.6 | |
| [GGUF](https://huggingface.co/mradermacher/LLaMA3-iterative-DPO-final-ExPO-i1-GGUF/resolve/main/LLaMA3-iterative-DPO-final-ExPO.i1-Q3_K_S.gguf) | i1-Q3_K_S | 3.8 | IQ3_XS probably better |
| [GGUF](https://huggingface.co/mradermacher/LLaMA3-iterative-DPO-final-ExPO-i1-GGUF/resolve/main/LLaMA3-iterative-DPO-final-ExPO.i1-IQ3_S.gguf) | i1-IQ3_S | 3.8 | beats Q3_K* |
| [GGUF](https://huggingface.co/mradermacher/LLaMA3-iterative-DPO-final-ExPO-i1-GGUF/resolve/main/LLaMA3-iterative-DPO-final-ExPO.i1-IQ3_M.gguf) | i1-IQ3_M | 3.9 | |
| [GGUF](https://huggingface.co/mradermacher/LLaMA3-iterative-DPO-final-ExPO-i1-GGUF/resolve/main/LLaMA3-iterative-DPO-final-ExPO.i1-Q3_K_M.gguf) | i1-Q3_K_M | 4.1 | IQ3_S probably better |
| [GGUF](https://huggingface.co/mradermacher/LLaMA3-iterative-DPO-final-ExPO-i1-GGUF/resolve/main/LLaMA3-iterative-DPO-final-ExPO.i1-Q3_K_L.gguf) | i1-Q3_K_L | 4.4 | IQ3_M probably better |
| [GGUF](https://huggingface.co/mradermacher/LLaMA3-iterative-DPO-final-ExPO-i1-GGUF/resolve/main/LLaMA3-iterative-DPO-final-ExPO.i1-IQ4_XS.gguf) | i1-IQ4_XS | 4.5 | |
| [GGUF](https://huggingface.co/mradermacher/LLaMA3-iterative-DPO-final-ExPO-i1-GGUF/resolve/main/LLaMA3-iterative-DPO-final-ExPO.i1-Q4_0.gguf) | i1-Q4_0 | 4.8 | fast, low quality |
| [GGUF](https://huggingface.co/mradermacher/LLaMA3-iterative-DPO-final-ExPO-i1-GGUF/resolve/main/LLaMA3-iterative-DPO-final-ExPO.i1-Q4_K_S.gguf) | i1-Q4_K_S | 4.8 | optimal size/speed/quality |
| [GGUF](https://huggingface.co/mradermacher/LLaMA3-iterative-DPO-final-ExPO-i1-GGUF/resolve/main/LLaMA3-iterative-DPO-final-ExPO.i1-Q4_K_M.gguf) | i1-Q4_K_M | 5.0 | fast, recommended |
| [GGUF](https://huggingface.co/mradermacher/LLaMA3-iterative-DPO-final-ExPO-i1-GGUF/resolve/main/LLaMA3-iterative-DPO-final-ExPO.i1-Q5_K_S.gguf) | i1-Q5_K_S | 5.7 | |
| [GGUF](https://huggingface.co/mradermacher/LLaMA3-iterative-DPO-final-ExPO-i1-GGUF/resolve/main/LLaMA3-iterative-DPO-final-ExPO.i1-Q5_K_M.gguf) | i1-Q5_K_M | 5.8 | |
| [GGUF](https://huggingface.co/mradermacher/LLaMA3-iterative-DPO-final-ExPO-i1-GGUF/resolve/main/LLaMA3-iterative-DPO-final-ExPO.i1-Q6_K.gguf) | i1-Q6_K | 6.7 | practically like static Q6_K |
Here is a handy graph by ikawrakow comparing some lower-quality quant
types (lower is better):

And here are Artefact2's thoughts on the matter:
https://gist.github.com/Artefact2/b5f810600771265fc1e39442288e8ec9
## FAQ / Model Request
See https://huggingface.co/mradermacher/model_requests for some answers to
questions you might have and/or if you want some other model quantized.
## Thanks
I thank my company, [nethype GmbH](https://www.nethype.de/), for letting
me use its servers and providing upgrades to my workstation to enable
this work in my free time. Additional thanks to [@nicoboss](https://huggingface.co/nicoboss) for giving me access to his hardware for calculating the imatrix for these quants.
<!-- end -->
|
Snowflake/snowflake-arctic-embed-l | Snowflake | 2024-05-10T15:51:18Z | 21,300 | 74 | sentence-transformers | [
"sentence-transformers",
"onnx",
"safetensors",
"bert",
"feature-extraction",
"sentence-similarity",
"mteb",
"arctic",
"snowflake-arctic-embed",
"transformers.js",
"arxiv:2405.05374",
"license:apache-2.0",
"model-index",
"endpoints_compatible",
"region:us"
] | sentence-similarity | 2024-04-12T13:54:34Z | ---
license: apache-2.0
pipeline_tag: sentence-similarity
tags:
- sentence-transformers
- feature-extraction
- sentence-similarity
- mteb
- arctic
- snowflake-arctic-embed
- transformers.js
model-index:
- name: snowflake-arctic-embed-l
results:
- task:
type: Classification
dataset:
type: mteb/amazon_counterfactual
name: MTEB AmazonCounterfactualClassification (en)
config: en
split: test
revision: e8379541af4e31359cca9fbcf4b00f2671dba205
metrics:
- type: accuracy
value: 74.80597014925374
- type: ap
value: 37.911466766189875
- type: f1
value: 68.88606927542106
- task:
type: Classification
dataset:
type: mteb/amazon_polarity
name: MTEB AmazonPolarityClassification
config: default
split: test
revision: e2d317d38cd51312af73b3d32a06d1a08b442046
metrics:
- type: accuracy
value: 78.402275
- type: ap
value: 73.03294793248114
- type: f1
value: 78.3147786132161
- task:
type: Classification
dataset:
type: mteb/amazon_reviews_multi
name: MTEB AmazonReviewsClassification (en)
config: en
split: test
revision: 1399c76144fd37290681b995c656ef9b2e06e26d
metrics:
- type: accuracy
value: 36.717999999999996
- type: f1
value: 35.918044248787766
- task:
type: Retrieval
dataset:
type: mteb/arguana
name: MTEB ArguAna
config: default
split: test
revision: c22ab2a51041ffd869aaddef7af8d8215647e41a
metrics:
- type: map_at_1
value: 34.495
- type: map_at_10
value: 50.236000000000004
- type: map_at_100
value: 50.944
- type: map_at_1000
value: 50.94499999999999
- type: map_at_3
value: 45.341
- type: map_at_5
value: 48.286
- type: mrr_at_1
value: 35.135
- type: mrr_at_10
value: 50.471
- type: mrr_at_100
value: 51.185
- type: mrr_at_1000
value: 51.187000000000005
- type: mrr_at_3
value: 45.602
- type: mrr_at_5
value: 48.468
- type: ndcg_at_1
value: 34.495
- type: ndcg_at_10
value: 59.086000000000006
- type: ndcg_at_100
value: 61.937
- type: ndcg_at_1000
value: 61.966
- type: ndcg_at_3
value: 49.062
- type: ndcg_at_5
value: 54.367
- type: precision_at_1
value: 34.495
- type: precision_at_10
value: 8.734
- type: precision_at_100
value: 0.9939999999999999
- type: precision_at_1000
value: 0.1
- type: precision_at_3
value: 19.962
- type: precision_at_5
value: 14.552000000000001
- type: recall_at_1
value: 34.495
- type: recall_at_10
value: 87.33999999999999
- type: recall_at_100
value: 99.431
- type: recall_at_1000
value: 99.644
- type: recall_at_3
value: 59.885999999999996
- type: recall_at_5
value: 72.76
- task:
type: Clustering
dataset:
type: mteb/arxiv-clustering-p2p
name: MTEB ArxivClusteringP2P
config: default
split: test
revision: a122ad7f3f0291bf49cc6f4d32aa80929df69d5d
metrics:
- type: v_measure
value: 47.46440874635501
- task:
type: Clustering
dataset:
type: mteb/arxiv-clustering-s2s
name: MTEB ArxivClusteringS2S
config: default
split: test
revision: f910caf1a6075f7329cdf8c1a6135696f37dbd53
metrics:
- type: v_measure
value: 38.28720154213723
- task:
type: Reranking
dataset:
type: mteb/askubuntudupquestions-reranking
name: MTEB AskUbuntuDupQuestions
config: default
split: test
revision: 2000358ca161889fa9c082cb41daa8dcfb161a54
metrics:
- type: map
value: 60.34614226394902
- type: mrr
value: 75.05628105351096
- task:
type: STS
dataset:
type: mteb/biosses-sts
name: MTEB BIOSSES
config: default
split: test
revision: d3fb88f8f02e40887cd149695127462bbcf29b4a
metrics:
- type: cos_sim_pearson
value: 87.41072716728198
- type: cos_sim_spearman
value: 86.34534093114372
- type: euclidean_pearson
value: 85.34009667750838
- type: euclidean_spearman
value: 86.34534093114372
- type: manhattan_pearson
value: 85.2158833586889
- type: manhattan_spearman
value: 86.60920236509224
- task:
type: Classification
dataset:
type: mteb/banking77
name: MTEB Banking77Classification
config: default
split: test
revision: 0fd18e25b25c072e09e0d92ab615fda904d66300
metrics:
- type: accuracy
value: 80.06493506493507
- type: f1
value: 79.28108600339833
- task:
type: Clustering
dataset:
type: jinaai/big-patent-clustering
name: MTEB BigPatentClustering
config: default
split: test
revision: 62d5330920bca426ce9d3c76ea914f15fc83e891
metrics:
- type: v_measure
value: 20.545049432417287
- task:
type: Clustering
dataset:
type: mteb/biorxiv-clustering-p2p
name: MTEB BiorxivClusteringP2P
config: default
split: test
revision: 65b79d1d13f80053f67aca9498d9402c2d9f1f40
metrics:
- type: v_measure
value: 37.54369718479804
- task:
type: Clustering
dataset:
type: mteb/biorxiv-clustering-s2s
name: MTEB BiorxivClusteringS2S
config: default
split: test
revision: 258694dd0231531bc1fd9de6ceb52a0853c6d908
metrics:
- type: v_measure
value: 32.64941588219162
- task:
type: Retrieval
dataset:
type: mteb/cqadupstack-android
name: MTEB CQADupstackAndroidRetrieval
config: default
split: test
revision: f46a197baaae43b4f621051089b82a364682dfeb
metrics:
- type: map_at_1
value: 37.264
- type: map_at_10
value: 49.43
- type: map_at_100
value: 50.967
- type: map_at_1000
value: 51.08200000000001
- type: map_at_3
value: 45.742
- type: map_at_5
value: 47.764
- type: mrr_at_1
value: 44.921
- type: mrr_at_10
value: 54.879999999999995
- type: mrr_at_100
value: 55.525000000000006
- type: mrr_at_1000
value: 55.565
- type: mrr_at_3
value: 52.480000000000004
- type: mrr_at_5
value: 53.86
- type: ndcg_at_1
value: 44.921
- type: ndcg_at_10
value: 55.664
- type: ndcg_at_100
value: 60.488
- type: ndcg_at_1000
value: 62.138000000000005
- type: ndcg_at_3
value: 50.797000000000004
- type: ndcg_at_5
value: 52.94799999999999
- type: precision_at_1
value: 44.921
- type: precision_at_10
value: 10.587
- type: precision_at_100
value: 1.629
- type: precision_at_1000
value: 0.203
- type: precision_at_3
value: 24.034
- type: precision_at_5
value: 17.224999999999998
- type: recall_at_1
value: 37.264
- type: recall_at_10
value: 67.15
- type: recall_at_100
value: 86.811
- type: recall_at_1000
value: 97.172
- type: recall_at_3
value: 53.15800000000001
- type: recall_at_5
value: 59.116
- task:
type: Retrieval
dataset:
type: mteb/cqadupstack-english
name: MTEB CQADupstackEnglishRetrieval
config: default
split: test
revision: ad9991cb51e31e31e430383c75ffb2885547b5f0
metrics:
- type: map_at_1
value: 36.237
- type: map_at_10
value: 47.941
- type: map_at_100
value: 49.131
- type: map_at_1000
value: 49.26
- type: map_at_3
value: 44.561
- type: map_at_5
value: 46.28
- type: mrr_at_1
value: 45.605000000000004
- type: mrr_at_10
value: 54.039
- type: mrr_at_100
value: 54.653
- type: mrr_at_1000
value: 54.688
- type: mrr_at_3
value: 52.006
- type: mrr_at_5
value: 53.096
- type: ndcg_at_1
value: 45.605000000000004
- type: ndcg_at_10
value: 53.916
- type: ndcg_at_100
value: 57.745999999999995
- type: ndcg_at_1000
value: 59.492999999999995
- type: ndcg_at_3
value: 49.774
- type: ndcg_at_5
value: 51.434999999999995
- type: precision_at_1
value: 45.605000000000004
- type: precision_at_10
value: 10.229000000000001
- type: precision_at_100
value: 1.55
- type: precision_at_1000
value: 0.2
- type: precision_at_3
value: 24.098
- type: precision_at_5
value: 16.726
- type: recall_at_1
value: 36.237
- type: recall_at_10
value: 64.03
- type: recall_at_100
value: 80.423
- type: recall_at_1000
value: 91.03
- type: recall_at_3
value: 51.20400000000001
- type: recall_at_5
value: 56.298
- task:
type: Retrieval
dataset:
type: mteb/cqadupstack-gaming
name: MTEB CQADupstackGamingRetrieval
config: default
split: test
revision: 4885aa143210c98657558c04aaf3dc47cfb54340
metrics:
- type: map_at_1
value: 47.278
- type: map_at_10
value: 59.757000000000005
- type: map_at_100
value: 60.67
- type: map_at_1000
value: 60.714
- type: map_at_3
value: 56.714
- type: map_at_5
value: 58.453
- type: mrr_at_1
value: 53.73
- type: mrr_at_10
value: 62.970000000000006
- type: mrr_at_100
value: 63.507999999999996
- type: mrr_at_1000
value: 63.53
- type: mrr_at_3
value: 60.909
- type: mrr_at_5
value: 62.172000000000004
- type: ndcg_at_1
value: 53.73
- type: ndcg_at_10
value: 64.97
- type: ndcg_at_100
value: 68.394
- type: ndcg_at_1000
value: 69.255
- type: ndcg_at_3
value: 60.228
- type: ndcg_at_5
value: 62.617999999999995
- type: precision_at_1
value: 53.73
- type: precision_at_10
value: 10.056
- type: precision_at_100
value: 1.265
- type: precision_at_1000
value: 0.13699999999999998
- type: precision_at_3
value: 26.332
- type: precision_at_5
value: 17.743000000000002
- type: recall_at_1
value: 47.278
- type: recall_at_10
value: 76.86500000000001
- type: recall_at_100
value: 91.582
- type: recall_at_1000
value: 97.583
- type: recall_at_3
value: 64.443
- type: recall_at_5
value: 70.283
- task:
type: Retrieval
dataset:
type: mteb/cqadupstack-gis
name: MTEB CQADupstackGisRetrieval
config: default
split: test
revision: 5003b3064772da1887988e05400cf3806fe491f2
metrics:
- type: map_at_1
value: 29.702
- type: map_at_10
value: 39.463
- type: map_at_100
value: 40.508
- type: map_at_1000
value: 40.579
- type: map_at_3
value: 36.748999999999995
- type: map_at_5
value: 38.296
- type: mrr_at_1
value: 31.977
- type: mrr_at_10
value: 41.739
- type: mrr_at_100
value: 42.586
- type: mrr_at_1000
value: 42.636
- type: mrr_at_3
value: 39.096
- type: mrr_at_5
value: 40.695
- type: ndcg_at_1
value: 31.977
- type: ndcg_at_10
value: 44.855000000000004
- type: ndcg_at_100
value: 49.712
- type: ndcg_at_1000
value: 51.443000000000005
- type: ndcg_at_3
value: 39.585
- type: ndcg_at_5
value: 42.244
- type: precision_at_1
value: 31.977
- type: precision_at_10
value: 6.768000000000001
- type: precision_at_100
value: 0.9690000000000001
- type: precision_at_1000
value: 0.116
- type: precision_at_3
value: 16.761
- type: precision_at_5
value: 11.593
- type: recall_at_1
value: 29.702
- type: recall_at_10
value: 59.082
- type: recall_at_100
value: 80.92
- type: recall_at_1000
value: 93.728
- type: recall_at_3
value: 45.212
- type: recall_at_5
value: 51.449
- task:
type: Retrieval
dataset:
type: mteb/cqadupstack-mathematica
name: MTEB CQADupstackMathematicaRetrieval
config: default
split: test
revision: 90fceea13679c63fe563ded68f3b6f06e50061de
metrics:
- type: map_at_1
value: 21.336
- type: map_at_10
value: 30.137999999999998
- type: map_at_100
value: 31.385
- type: map_at_1000
value: 31.495
- type: map_at_3
value: 27.481
- type: map_at_5
value: 28.772
- type: mrr_at_1
value: 25.871
- type: mrr_at_10
value: 34.686
- type: mrr_at_100
value: 35.649
- type: mrr_at_1000
value: 35.705
- type: mrr_at_3
value: 32.09
- type: mrr_at_5
value: 33.52
- type: ndcg_at_1
value: 25.871
- type: ndcg_at_10
value: 35.617
- type: ndcg_at_100
value: 41.272999999999996
- type: ndcg_at_1000
value: 43.725
- type: ndcg_at_3
value: 30.653999999999996
- type: ndcg_at_5
value: 32.714
- type: precision_at_1
value: 25.871
- type: precision_at_10
value: 6.4799999999999995
- type: precision_at_100
value: 1.0699999999999998
- type: precision_at_1000
value: 0.13999999999999999
- type: precision_at_3
value: 14.469000000000001
- type: precision_at_5
value: 10.274
- type: recall_at_1
value: 21.336
- type: recall_at_10
value: 47.746
- type: recall_at_100
value: 71.773
- type: recall_at_1000
value: 89.05199999999999
- type: recall_at_3
value: 34.172999999999995
- type: recall_at_5
value: 39.397999999999996
- task:
type: Retrieval
dataset:
type: mteb/cqadupstack-physics
name: MTEB CQADupstackPhysicsRetrieval
config: default
split: test
revision: 79531abbd1fb92d06c6d6315a0cbbbf5bb247ea4
metrics:
- type: map_at_1
value: 34.424
- type: map_at_10
value: 45.647999999999996
- type: map_at_100
value: 46.907
- type: map_at_1000
value: 47.010999999999996
- type: map_at_3
value: 42.427
- type: map_at_5
value: 44.285000000000004
- type: mrr_at_1
value: 41.867
- type: mrr_at_10
value: 51.17699999999999
- type: mrr_at_100
value: 51.937
- type: mrr_at_1000
value: 51.975
- type: mrr_at_3
value: 48.941
- type: mrr_at_5
value: 50.322
- type: ndcg_at_1
value: 41.867
- type: ndcg_at_10
value: 51.534
- type: ndcg_at_100
value: 56.696999999999996
- type: ndcg_at_1000
value: 58.475
- type: ndcg_at_3
value: 46.835
- type: ndcg_at_5
value: 49.161
- type: precision_at_1
value: 41.867
- type: precision_at_10
value: 9.134
- type: precision_at_100
value: 1.362
- type: precision_at_1000
value: 0.17099999999999999
- type: precision_at_3
value: 22.073
- type: precision_at_5
value: 15.495999999999999
- type: recall_at_1
value: 34.424
- type: recall_at_10
value: 63.237
- type: recall_at_100
value: 84.774
- type: recall_at_1000
value: 95.987
- type: recall_at_3
value: 49.888
- type: recall_at_5
value: 55.940999999999995
- task:
type: Retrieval
dataset:
type: mteb/cqadupstack-programmers
name: MTEB CQADupstackProgrammersRetrieval
config: default
split: test
revision: 6184bc1440d2dbc7612be22b50686b8826d22b32
metrics:
- type: map_at_1
value: 30.72
- type: map_at_10
value: 41.327999999999996
- type: map_at_100
value: 42.651
- type: map_at_1000
value: 42.739
- type: map_at_3
value: 38.223
- type: map_at_5
value: 40.053
- type: mrr_at_1
value: 37.9
- type: mrr_at_10
value: 46.857
- type: mrr_at_100
value: 47.673
- type: mrr_at_1000
value: 47.711999999999996
- type: mrr_at_3
value: 44.292
- type: mrr_at_5
value: 45.845
- type: ndcg_at_1
value: 37.9
- type: ndcg_at_10
value: 47.105999999999995
- type: ndcg_at_100
value: 52.56999999999999
- type: ndcg_at_1000
value: 54.37800000000001
- type: ndcg_at_3
value: 42.282
- type: ndcg_at_5
value: 44.646
- type: precision_at_1
value: 37.9
- type: precision_at_10
value: 8.368
- type: precision_at_100
value: 1.283
- type: precision_at_1000
value: 0.16
- type: precision_at_3
value: 20.015
- type: precision_at_5
value: 14.132
- type: recall_at_1
value: 30.72
- type: recall_at_10
value: 58.826
- type: recall_at_100
value: 82.104
- type: recall_at_1000
value: 94.194
- type: recall_at_3
value: 44.962999999999994
- type: recall_at_5
value: 51.426
- task:
type: Retrieval
dataset:
type: mteb/cqadupstack
name: MTEB CQADupstackRetrieval
config: default
split: test
revision: 4ffe81d471b1924886b33c7567bfb200e9eec5c4
metrics:
- type: map_at_1
value: 31.656583333333334
- type: map_at_10
value: 41.59883333333333
- type: map_at_100
value: 42.80350000000001
- type: map_at_1000
value: 42.91075
- type: map_at_3
value: 38.68908333333333
- type: map_at_5
value: 40.27733333333334
- type: mrr_at_1
value: 37.23483333333334
- type: mrr_at_10
value: 45.782000000000004
- type: mrr_at_100
value: 46.577083333333334
- type: mrr_at_1000
value: 46.62516666666667
- type: mrr_at_3
value: 43.480666666666664
- type: mrr_at_5
value: 44.79833333333333
- type: ndcg_at_1
value: 37.23483333333334
- type: ndcg_at_10
value: 46.971500000000006
- type: ndcg_at_100
value: 51.90125
- type: ndcg_at_1000
value: 53.86366666666667
- type: ndcg_at_3
value: 42.31791666666667
- type: ndcg_at_5
value: 44.458666666666666
- type: precision_at_1
value: 37.23483333333334
- type: precision_at_10
value: 8.044583333333332
- type: precision_at_100
value: 1.2334166666666666
- type: precision_at_1000
value: 0.15925
- type: precision_at_3
value: 19.240833333333327
- type: precision_at_5
value: 13.435083333333333
- type: recall_at_1
value: 31.656583333333334
- type: recall_at_10
value: 58.44758333333333
- type: recall_at_100
value: 79.93658333333332
- type: recall_at_1000
value: 93.32491666666668
- type: recall_at_3
value: 45.44266666666667
- type: recall_at_5
value: 50.99866666666666
- task:
type: Retrieval
dataset:
type: mteb/cqadupstack-stats
name: MTEB CQADupstackStatsRetrieval
config: default
split: test
revision: 65ac3a16b8e91f9cee4c9828cc7c335575432a2a
metrics:
- type: map_at_1
value: 28.247
- type: map_at_10
value: 35.443999999999996
- type: map_at_100
value: 36.578
- type: map_at_1000
value: 36.675999999999995
- type: map_at_3
value: 33.276
- type: map_at_5
value: 34.536
- type: mrr_at_1
value: 31.747999999999998
- type: mrr_at_10
value: 38.413000000000004
- type: mrr_at_100
value: 39.327
- type: mrr_at_1000
value: 39.389
- type: mrr_at_3
value: 36.401
- type: mrr_at_5
value: 37.543
- type: ndcg_at_1
value: 31.747999999999998
- type: ndcg_at_10
value: 39.646
- type: ndcg_at_100
value: 44.861000000000004
- type: ndcg_at_1000
value: 47.197
- type: ndcg_at_3
value: 35.764
- type: ndcg_at_5
value: 37.635999999999996
- type: precision_at_1
value: 31.747999999999998
- type: precision_at_10
value: 6.12
- type: precision_at_100
value: 0.942
- type: precision_at_1000
value: 0.123
- type: precision_at_3
value: 15.235000000000001
- type: precision_at_5
value: 10.491
- type: recall_at_1
value: 28.247
- type: recall_at_10
value: 49.456
- type: recall_at_100
value: 73.02499999999999
- type: recall_at_1000
value: 89.898
- type: recall_at_3
value: 38.653999999999996
- type: recall_at_5
value: 43.259
- task:
type: Retrieval
dataset:
type: mteb/cqadupstack-tex
name: MTEB CQADupstackTexRetrieval
config: default
split: test
revision: 46989137a86843e03a6195de44b09deda022eec7
metrics:
- type: map_at_1
value: 22.45
- type: map_at_10
value: 30.476999999999997
- type: map_at_100
value: 31.630999999999997
- type: map_at_1000
value: 31.755
- type: map_at_3
value: 27.989000000000004
- type: map_at_5
value: 29.410999999999998
- type: mrr_at_1
value: 26.979
- type: mrr_at_10
value: 34.316
- type: mrr_at_100
value: 35.272999999999996
- type: mrr_at_1000
value: 35.342
- type: mrr_at_3
value: 32.14
- type: mrr_at_5
value: 33.405
- type: ndcg_at_1
value: 26.979
- type: ndcg_at_10
value: 35.166
- type: ndcg_at_100
value: 40.583000000000006
- type: ndcg_at_1000
value: 43.282
- type: ndcg_at_3
value: 30.916
- type: ndcg_at_5
value: 32.973
- type: precision_at_1
value: 26.979
- type: precision_at_10
value: 6.132
- type: precision_at_100
value: 1.047
- type: precision_at_1000
value: 0.145
- type: precision_at_3
value: 14.360999999999999
- type: precision_at_5
value: 10.227
- type: recall_at_1
value: 22.45
- type: recall_at_10
value: 45.348
- type: recall_at_100
value: 69.484
- type: recall_at_1000
value: 88.628
- type: recall_at_3
value: 33.338
- type: recall_at_5
value: 38.746
- task:
type: Retrieval
dataset:
type: mteb/cqadupstack-unix
name: MTEB CQADupstackUnixRetrieval
config: default
split: test
revision: 6c6430d3a6d36f8d2a829195bc5dc94d7e063e53
metrics:
- type: map_at_1
value: 32.123000000000005
- type: map_at_10
value: 41.778
- type: map_at_100
value: 42.911
- type: map_at_1000
value: 42.994
- type: map_at_3
value: 38.558
- type: map_at_5
value: 40.318
- type: mrr_at_1
value: 37.687
- type: mrr_at_10
value: 45.889
- type: mrr_at_100
value: 46.672999999999995
- type: mrr_at_1000
value: 46.72
- type: mrr_at_3
value: 43.33
- type: mrr_at_5
value: 44.734
- type: ndcg_at_1
value: 37.687
- type: ndcg_at_10
value: 47.258
- type: ndcg_at_100
value: 52.331
- type: ndcg_at_1000
value: 54.152
- type: ndcg_at_3
value: 41.857
- type: ndcg_at_5
value: 44.283
- type: precision_at_1
value: 37.687
- type: precision_at_10
value: 7.892
- type: precision_at_100
value: 1.183
- type: precision_at_1000
value: 0.14300000000000002
- type: precision_at_3
value: 18.781
- type: precision_at_5
value: 13.134
- type: recall_at_1
value: 32.123000000000005
- type: recall_at_10
value: 59.760000000000005
- type: recall_at_100
value: 81.652
- type: recall_at_1000
value: 94.401
- type: recall_at_3
value: 44.996
- type: recall_at_5
value: 51.184
- task:
type: Retrieval
dataset:
type: mteb/cqadupstack-webmasters
name: MTEB CQADupstackWebmastersRetrieval
config: default
split: test
revision: 160c094312a0e1facb97e55eeddb698c0abe3571
metrics:
- type: map_at_1
value: 33.196999999999996
- type: map_at_10
value: 42.012
- type: map_at_100
value: 43.663999999999994
- type: map_at_1000
value: 43.883
- type: map_at_3
value: 39.33
- type: map_at_5
value: 40.586
- type: mrr_at_1
value: 39.328
- type: mrr_at_10
value: 46.57
- type: mrr_at_100
value: 47.508
- type: mrr_at_1000
value: 47.558
- type: mrr_at_3
value: 44.532
- type: mrr_at_5
value: 45.58
- type: ndcg_at_1
value: 39.328
- type: ndcg_at_10
value: 47.337
- type: ndcg_at_100
value: 52.989
- type: ndcg_at_1000
value: 55.224
- type: ndcg_at_3
value: 43.362
- type: ndcg_at_5
value: 44.866
- type: precision_at_1
value: 39.328
- type: precision_at_10
value: 8.577
- type: precision_at_100
value: 1.5789999999999997
- type: precision_at_1000
value: 0.25
- type: precision_at_3
value: 19.697
- type: precision_at_5
value: 13.755
- type: recall_at_1
value: 33.196999999999996
- type: recall_at_10
value: 56.635000000000005
- type: recall_at_100
value: 81.882
- type: recall_at_1000
value: 95.342
- type: recall_at_3
value: 44.969
- type: recall_at_5
value: 49.266
- task:
type: Retrieval
dataset:
type: mteb/cqadupstack-wordpress
name: MTEB CQADupstackWordpressRetrieval
config: default
split: test
revision: 4ffe81d471b1924886b33c7567bfb200e9eec5c4
metrics:
- type: map_at_1
value: 26.901000000000003
- type: map_at_10
value: 35.77
- type: map_at_100
value: 36.638999999999996
- type: map_at_1000
value: 36.741
- type: map_at_3
value: 33.219
- type: map_at_5
value: 34.574
- type: mrr_at_1
value: 29.205
- type: mrr_at_10
value: 37.848
- type: mrr_at_100
value: 38.613
- type: mrr_at_1000
value: 38.682
- type: mrr_at_3
value: 35.551
- type: mrr_at_5
value: 36.808
- type: ndcg_at_1
value: 29.205
- type: ndcg_at_10
value: 40.589
- type: ndcg_at_100
value: 45.171
- type: ndcg_at_1000
value: 47.602
- type: ndcg_at_3
value: 35.760999999999996
- type: ndcg_at_5
value: 37.980000000000004
- type: precision_at_1
value: 29.205
- type: precision_at_10
value: 6.192
- type: precision_at_100
value: 0.922
- type: precision_at_1000
value: 0.123
- type: precision_at_3
value: 15.034
- type: precision_at_5
value: 10.424999999999999
- type: recall_at_1
value: 26.901000000000003
- type: recall_at_10
value: 53.236000000000004
- type: recall_at_100
value: 74.809
- type: recall_at_1000
value: 92.884
- type: recall_at_3
value: 40.314
- type: recall_at_5
value: 45.617999999999995
- task:
type: Retrieval
dataset:
type: mteb/climate-fever
name: MTEB ClimateFEVER
config: default
split: test
revision: 47f2ac6acb640fc46020b02a5b59fdda04d39380
metrics:
- type: map_at_1
value: 16.794999999999998
- type: map_at_10
value: 29.322
- type: map_at_100
value: 31.463
- type: map_at_1000
value: 31.643
- type: map_at_3
value: 24.517
- type: map_at_5
value: 27.237000000000002
- type: mrr_at_1
value: 37.655
- type: mrr_at_10
value: 50.952
- type: mrr_at_100
value: 51.581999999999994
- type: mrr_at_1000
value: 51.61
- type: mrr_at_3
value: 47.991
- type: mrr_at_5
value: 49.744
- type: ndcg_at_1
value: 37.655
- type: ndcg_at_10
value: 39.328
- type: ndcg_at_100
value: 46.358
- type: ndcg_at_1000
value: 49.245
- type: ndcg_at_3
value: 33.052
- type: ndcg_at_5
value: 35.407
- type: precision_at_1
value: 37.655
- type: precision_at_10
value: 12.202
- type: precision_at_100
value: 1.9789999999999999
- type: precision_at_1000
value: 0.252
- type: precision_at_3
value: 24.973
- type: precision_at_5
value: 19.075
- type: recall_at_1
value: 16.794999999999998
- type: recall_at_10
value: 45.716
- type: recall_at_100
value: 68.919
- type: recall_at_1000
value: 84.71600000000001
- type: recall_at_3
value: 30.135
- type: recall_at_5
value: 37.141999999999996
- task:
type: Retrieval
dataset:
type: mteb/dbpedia
name: MTEB DBPedia
config: default
split: test
revision: c0f706b76e590d620bd6618b3ca8efdd34e2d659
metrics:
- type: map_at_1
value: 9.817
- type: map_at_10
value: 22.058
- type: map_at_100
value: 31.805
- type: map_at_1000
value: 33.562999999999995
- type: map_at_3
value: 15.537
- type: map_at_5
value: 18.199
- type: mrr_at_1
value: 72.75
- type: mrr_at_10
value: 79.804
- type: mrr_at_100
value: 80.089
- type: mrr_at_1000
value: 80.09100000000001
- type: mrr_at_3
value: 78.75
- type: mrr_at_5
value: 79.325
- type: ndcg_at_1
value: 59.875
- type: ndcg_at_10
value: 45.972
- type: ndcg_at_100
value: 51.092999999999996
- type: ndcg_at_1000
value: 58.048
- type: ndcg_at_3
value: 50.552
- type: ndcg_at_5
value: 47.672
- type: precision_at_1
value: 72.75
- type: precision_at_10
value: 37.05
- type: precision_at_100
value: 12.005
- type: precision_at_1000
value: 2.221
- type: precision_at_3
value: 54.083000000000006
- type: precision_at_5
value: 46.2
- type: recall_at_1
value: 9.817
- type: recall_at_10
value: 27.877000000000002
- type: recall_at_100
value: 57.974000000000004
- type: recall_at_1000
value: 80.085
- type: recall_at_3
value: 16.911
- type: recall_at_5
value: 20.689
- task:
type: Classification
dataset:
type: mteb/emotion
name: MTEB EmotionClassification
config: default
split: test
revision: 4f58c6b202a23cf9a4da393831edf4f9183cad37
metrics:
- type: accuracy
value: 46.464999999999996
- type: f1
value: 42.759588662873796
- task:
type: Retrieval
dataset:
type: mteb/fever
name: MTEB FEVER
config: default
split: test
revision: bea83ef9e8fb933d90a2f1d5515737465d613e12
metrics:
- type: map_at_1
value: 75.82900000000001
- type: map_at_10
value: 84.613
- type: map_at_100
value: 84.845
- type: map_at_1000
value: 84.855
- type: map_at_3
value: 83.498
- type: map_at_5
value: 84.29299999999999
- type: mrr_at_1
value: 81.69800000000001
- type: mrr_at_10
value: 88.84100000000001
- type: mrr_at_100
value: 88.887
- type: mrr_at_1000
value: 88.888
- type: mrr_at_3
value: 88.179
- type: mrr_at_5
value: 88.69200000000001
- type: ndcg_at_1
value: 81.69800000000001
- type: ndcg_at_10
value: 88.21799999999999
- type: ndcg_at_100
value: 88.961
- type: ndcg_at_1000
value: 89.131
- type: ndcg_at_3
value: 86.591
- type: ndcg_at_5
value: 87.666
- type: precision_at_1
value: 81.69800000000001
- type: precision_at_10
value: 10.615
- type: precision_at_100
value: 1.125
- type: precision_at_1000
value: 0.11499999999999999
- type: precision_at_3
value: 33.208
- type: precision_at_5
value: 20.681
- type: recall_at_1
value: 75.82900000000001
- type: recall_at_10
value: 94.97
- type: recall_at_100
value: 97.786
- type: recall_at_1000
value: 98.809
- type: recall_at_3
value: 90.625
- type: recall_at_5
value: 93.345
- task:
type: Retrieval
dataset:
type: mteb/fiqa
name: MTEB FiQA2018
config: default
split: test
revision: 27a168819829fe9bcd655c2df245fb19452e8e06
metrics:
- type: map_at_1
value: 22.788
- type: map_at_10
value: 36.71
- type: map_at_100
value: 38.527
- type: map_at_1000
value: 38.701
- type: map_at_3
value: 32.318999999999996
- type: map_at_5
value: 34.809
- type: mrr_at_1
value: 44.444
- type: mrr_at_10
value: 52.868
- type: mrr_at_100
value: 53.52400000000001
- type: mrr_at_1000
value: 53.559999999999995
- type: mrr_at_3
value: 50.153999999999996
- type: mrr_at_5
value: 51.651
- type: ndcg_at_1
value: 44.444
- type: ndcg_at_10
value: 44.707
- type: ndcg_at_100
value: 51.174
- type: ndcg_at_1000
value: 53.996
- type: ndcg_at_3
value: 40.855999999999995
- type: ndcg_at_5
value: 42.113
- type: precision_at_1
value: 44.444
- type: precision_at_10
value: 12.021999999999998
- type: precision_at_100
value: 1.8950000000000002
- type: precision_at_1000
value: 0.241
- type: precision_at_3
value: 26.8
- type: precision_at_5
value: 19.66
- type: recall_at_1
value: 22.788
- type: recall_at_10
value: 51.793
- type: recall_at_100
value: 75.69500000000001
- type: recall_at_1000
value: 92.292
- type: recall_at_3
value: 37.375
- type: recall_at_5
value: 43.682
- task:
type: Retrieval
dataset:
type: mteb/hotpotqa
name: MTEB HotpotQA
config: default
split: test
revision: ab518f4d6fcca38d87c25209f94beba119d02014
metrics:
- type: map_at_1
value: 41.276
- type: map_at_10
value: 67.245
- type: map_at_100
value: 68.061
- type: map_at_1000
value: 68.11399999999999
- type: map_at_3
value: 63.693
- type: map_at_5
value: 65.90899999999999
- type: mrr_at_1
value: 82.552
- type: mrr_at_10
value: 87.741
- type: mrr_at_100
value: 87.868
- type: mrr_at_1000
value: 87.871
- type: mrr_at_3
value: 86.98599999999999
- type: mrr_at_5
value: 87.469
- type: ndcg_at_1
value: 82.552
- type: ndcg_at_10
value: 75.176
- type: ndcg_at_100
value: 77.902
- type: ndcg_at_1000
value: 78.852
- type: ndcg_at_3
value: 70.30499999999999
- type: ndcg_at_5
value: 73.00999999999999
- type: precision_at_1
value: 82.552
- type: precision_at_10
value: 15.765
- type: precision_at_100
value: 1.788
- type: precision_at_1000
value: 0.191
- type: precision_at_3
value: 45.375
- type: precision_at_5
value: 29.360999999999997
- type: recall_at_1
value: 41.276
- type: recall_at_10
value: 78.825
- type: recall_at_100
value: 89.41900000000001
- type: recall_at_1000
value: 95.625
- type: recall_at_3
value: 68.062
- type: recall_at_5
value: 73.40299999999999
- task:
type: Classification
dataset:
type: mteb/imdb
name: MTEB ImdbClassification
config: default
split: test
revision: 3d86128a09e091d6018b6d26cad27f2739fc2db7
metrics:
- type: accuracy
value: 72.876
- type: ap
value: 67.15477852410164
- type: f1
value: 72.65147370025373
- task:
type: Retrieval
dataset:
type: mteb/msmarco
name: MTEB MSMARCO
config: default
split: dev
revision: c5a29a104738b98a9e76336939199e264163d4a0
metrics:
- type: map_at_1
value: 21.748
- type: map_at_10
value: 34.626000000000005
- type: map_at_100
value: 35.813
- type: map_at_1000
value: 35.859
- type: map_at_3
value: 30.753000000000004
- type: map_at_5
value: 33.049
- type: mrr_at_1
value: 22.35
- type: mrr_at_10
value: 35.23
- type: mrr_at_100
value: 36.359
- type: mrr_at_1000
value: 36.399
- type: mrr_at_3
value: 31.436999999999998
- type: mrr_at_5
value: 33.687
- type: ndcg_at_1
value: 22.364
- type: ndcg_at_10
value: 41.677
- type: ndcg_at_100
value: 47.355999999999995
- type: ndcg_at_1000
value: 48.494
- type: ndcg_at_3
value: 33.85
- type: ndcg_at_5
value: 37.942
- type: precision_at_1
value: 22.364
- type: precision_at_10
value: 6.6000000000000005
- type: precision_at_100
value: 0.9450000000000001
- type: precision_at_1000
value: 0.104
- type: precision_at_3
value: 14.527000000000001
- type: precision_at_5
value: 10.796999999999999
- type: recall_at_1
value: 21.748
- type: recall_at_10
value: 63.292
- type: recall_at_100
value: 89.427
- type: recall_at_1000
value: 98.13499999999999
- type: recall_at_3
value: 42.126000000000005
- type: recall_at_5
value: 51.968
- task:
type: Classification
dataset:
type: mteb/mtop_domain
name: MTEB MTOPDomainClassification (en)
config: en
split: test
revision: d80d48c1eb48d3562165c59d59d0034df9fff0bf
metrics:
- type: accuracy
value: 92.62425900592795
- type: f1
value: 92.08497761553683
- task:
type: Classification
dataset:
type: mteb/mtop_intent
name: MTEB MTOPIntentClassification (en)
config: en
split: test
revision: ae001d0e6b1228650b7bd1c2c65fb50ad11a8aba
metrics:
- type: accuracy
value: 64.51436388508893
- type: f1
value: 45.884016531912906
- task:
type: Classification
dataset:
type: masakhane/masakhanews
name: MTEB MasakhaNEWSClassification (eng)
config: eng
split: test
revision: 8ccc72e69e65f40c70e117d8b3c08306bb788b60
metrics:
- type: accuracy
value: 76.57172995780591
- type: f1
value: 75.52979910878491
- task:
type: Clustering
dataset:
type: masakhane/masakhanews
name: MTEB MasakhaNEWSClusteringP2P (eng)
config: eng
split: test
revision: 8ccc72e69e65f40c70e117d8b3c08306bb788b60
metrics:
- type: v_measure
value: 44.84052695201612
- task:
type: Clustering
dataset:
type: masakhane/masakhanews
name: MTEB MasakhaNEWSClusteringS2S (eng)
config: eng
split: test
revision: 8ccc72e69e65f40c70e117d8b3c08306bb788b60
metrics:
- type: v_measure
value: 21.443971229936494
- task:
type: Classification
dataset:
type: mteb/amazon_massive_intent
name: MTEB MassiveIntentClassification (en)
config: en
split: test
revision: 31efe3c427b0bae9c22cbb560b8f15491cc6bed7
metrics:
- type: accuracy
value: 65.79354404841965
- type: f1
value: 63.17260074126185
- task:
type: Classification
dataset:
type: mteb/amazon_massive_scenario
name: MTEB MassiveScenarioClassification (en)
config: en
split: test
revision: 7d571f92784cd94a019292a1f45445077d0ef634
metrics:
- type: accuracy
value: 71.09616677874916
- type: f1
value: 69.74285784421075
- task:
type: Clustering
dataset:
type: mteb/medrxiv-clustering-p2p
name: MTEB MedrxivClusteringP2P
config: default
split: test
revision: e7a26af6f3ae46b30dde8737f02c07b1505bcc73
metrics:
- type: v_measure
value: 31.474709231086184
- task:
type: Clustering
dataset:
type: mteb/medrxiv-clustering-s2s
name: MTEB MedrxivClusteringS2S
config: default
split: test
revision: 35191c8c0dca72d8ff3efcd72aa802307d469663
metrics:
- type: v_measure
value: 28.93630367824217
- task:
type: Reranking
dataset:
type: mteb/mind_small
name: MTEB MindSmallReranking
config: default
split: test
revision: 3bdac13927fdc888b903db93b2ffdbd90b295a69
metrics:
- type: map
value: 29.08234393834005
- type: mrr
value: 29.740466971605432
- task:
type: Retrieval
dataset:
type: mteb/nfcorpus
name: MTEB NFCorpus
config: default
split: test
revision: ec0fa4fe99da2ff19ca1214b7966684033a58814
metrics:
- type: map_at_1
value: 6.2059999999999995
- type: map_at_10
value: 14.442
- type: map_at_100
value: 18.005
- type: map_at_1000
value: 19.488
- type: map_at_3
value: 10.666
- type: map_at_5
value: 12.45
- type: mrr_at_1
value: 47.678
- type: mrr_at_10
value: 57.519
- type: mrr_at_100
value: 58.13700000000001
- type: mrr_at_1000
value: 58.167
- type: mrr_at_3
value: 55.779
- type: mrr_at_5
value: 56.940000000000005
- type: ndcg_at_1
value: 45.82
- type: ndcg_at_10
value: 37.651
- type: ndcg_at_100
value: 34.001999999999995
- type: ndcg_at_1000
value: 42.626
- type: ndcg_at_3
value: 43.961
- type: ndcg_at_5
value: 41.461
- type: precision_at_1
value: 47.678
- type: precision_at_10
value: 27.584999999999997
- type: precision_at_100
value: 8.455
- type: precision_at_1000
value: 2.118
- type: precision_at_3
value: 41.692
- type: precision_at_5
value: 36.161
- type: recall_at_1
value: 6.2059999999999995
- type: recall_at_10
value: 18.599
- type: recall_at_100
value: 33.608
- type: recall_at_1000
value: 65.429
- type: recall_at_3
value: 12.126000000000001
- type: recall_at_5
value: 14.902000000000001
- task:
type: Retrieval
dataset:
type: mteb/nq
name: MTEB NQ
config: default
split: test
revision: b774495ed302d8c44a3a7ea25c90dbce03968f31
metrics:
- type: map_at_1
value: 39.117000000000004
- type: map_at_10
value: 55.535000000000004
- type: map_at_100
value: 56.32899999999999
- type: map_at_1000
value: 56.34400000000001
- type: map_at_3
value: 51.439
- type: map_at_5
value: 53.89699999999999
- type: mrr_at_1
value: 43.714
- type: mrr_at_10
value: 58.05200000000001
- type: mrr_at_100
value: 58.582
- type: mrr_at_1000
value: 58.592
- type: mrr_at_3
value: 54.896
- type: mrr_at_5
value: 56.874
- type: ndcg_at_1
value: 43.685
- type: ndcg_at_10
value: 63.108
- type: ndcg_at_100
value: 66.231
- type: ndcg_at_1000
value: 66.583
- type: ndcg_at_3
value: 55.659000000000006
- type: ndcg_at_5
value: 59.681
- type: precision_at_1
value: 43.685
- type: precision_at_10
value: 9.962
- type: precision_at_100
value: 1.174
- type: precision_at_1000
value: 0.121
- type: precision_at_3
value: 24.961
- type: precision_at_5
value: 17.352
- type: recall_at_1
value: 39.117000000000004
- type: recall_at_10
value: 83.408
- type: recall_at_100
value: 96.553
- type: recall_at_1000
value: 99.136
- type: recall_at_3
value: 64.364
- type: recall_at_5
value: 73.573
- task:
type: Classification
dataset:
type: ag_news
name: MTEB NewsClassification
config: default
split: test
revision: eb185aade064a813bc0b7f42de02595523103ca4
metrics:
- type: accuracy
value: 78.87763157894737
- type: f1
value: 78.69611753876177
- task:
type: PairClassification
dataset:
type: GEM/opusparcus
name: MTEB OpusparcusPC (en)
config: en
split: test
revision: 9e9b1f8ef51616073f47f306f7f47dd91663f86a
metrics:
- type: cos_sim_accuracy
value: 99.89816700610999
- type: cos_sim_ap
value: 100
- type: cos_sim_f1
value: 99.9490575649516
- type: cos_sim_precision
value: 100
- type: cos_sim_recall
value: 99.89816700610999
- type: dot_accuracy
value: 99.89816700610999
- type: dot_ap
value: 100
- type: dot_f1
value: 99.9490575649516
- type: dot_precision
value: 100
- type: dot_recall
value: 99.89816700610999
- type: euclidean_accuracy
value: 99.89816700610999
- type: euclidean_ap
value: 100
- type: euclidean_f1
value: 99.9490575649516
- type: euclidean_precision
value: 100
- type: euclidean_recall
value: 99.89816700610999
- type: manhattan_accuracy
value: 99.89816700610999
- type: manhattan_ap
value: 100
- type: manhattan_f1
value: 99.9490575649516
- type: manhattan_precision
value: 100
- type: manhattan_recall
value: 99.89816700610999
- type: max_accuracy
value: 99.89816700610999
- type: max_ap
value: 100
- type: max_f1
value: 99.9490575649516
- task:
type: PairClassification
dataset:
type: paws-x
name: MTEB PawsX (en)
config: en
split: test
revision: 8a04d940a42cd40658986fdd8e3da561533a3646
metrics:
- type: cos_sim_accuracy
value: 62
- type: cos_sim_ap
value: 62.26837791655737
- type: cos_sim_f1
value: 62.607449856733524
- type: cos_sim_precision
value: 46.36604774535809
- type: cos_sim_recall
value: 96.36163175303197
- type: dot_accuracy
value: 62
- type: dot_ap
value: 62.26736459439965
- type: dot_f1
value: 62.607449856733524
- type: dot_precision
value: 46.36604774535809
- type: dot_recall
value: 96.36163175303197
- type: euclidean_accuracy
value: 62
- type: euclidean_ap
value: 62.26826112548132
- type: euclidean_f1
value: 62.607449856733524
- type: euclidean_precision
value: 46.36604774535809
- type: euclidean_recall
value: 96.36163175303197
- type: manhattan_accuracy
value: 62
- type: manhattan_ap
value: 62.26223761507973
- type: manhattan_f1
value: 62.585034013605444
- type: manhattan_precision
value: 46.34146341463415
- type: manhattan_recall
value: 96.36163175303197
- type: max_accuracy
value: 62
- type: max_ap
value: 62.26837791655737
- type: max_f1
value: 62.607449856733524
- task:
type: Retrieval
dataset:
type: mteb/quora
name: MTEB QuoraRetrieval
config: default
split: test
revision: e4e08e0b7dbe3c8700f0daef558ff32256715259
metrics:
- type: map_at_1
value: 69.90899999999999
- type: map_at_10
value: 83.56700000000001
- type: map_at_100
value: 84.19200000000001
- type: map_at_1000
value: 84.212
- type: map_at_3
value: 80.658
- type: map_at_5
value: 82.473
- type: mrr_at_1
value: 80.4
- type: mrr_at_10
value: 86.699
- type: mrr_at_100
value: 86.798
- type: mrr_at_1000
value: 86.80099999999999
- type: mrr_at_3
value: 85.677
- type: mrr_at_5
value: 86.354
- type: ndcg_at_1
value: 80.43
- type: ndcg_at_10
value: 87.41
- type: ndcg_at_100
value: 88.653
- type: ndcg_at_1000
value: 88.81599999999999
- type: ndcg_at_3
value: 84.516
- type: ndcg_at_5
value: 86.068
- type: precision_at_1
value: 80.43
- type: precision_at_10
value: 13.234000000000002
- type: precision_at_100
value: 1.513
- type: precision_at_1000
value: 0.156
- type: precision_at_3
value: 36.93
- type: precision_at_5
value: 24.26
- type: recall_at_1
value: 69.90899999999999
- type: recall_at_10
value: 94.687
- type: recall_at_100
value: 98.96000000000001
- type: recall_at_1000
value: 99.79599999999999
- type: recall_at_3
value: 86.25699999999999
- type: recall_at_5
value: 90.70700000000001
- task:
type: Clustering
dataset:
type: mteb/reddit-clustering
name: MTEB RedditClustering
config: default
split: test
revision: 24640382cdbf8abc73003fb0fa6d111a705499eb
metrics:
- type: v_measure
value: 46.02256865360266
- task:
type: Clustering
dataset:
type: mteb/reddit-clustering-p2p
name: MTEB RedditClusteringP2P
config: default
split: test
revision: 385e3cb46b4cfa89021f56c4380204149d0efe33
metrics:
- type: v_measure
value: 62.43157528757563
- task:
type: Retrieval
dataset:
type: mteb/scidocs
name: MTEB SCIDOCS
config: default
split: test
revision: f8c2fcf00f625baaa80f62ec5bd9e1fff3b8ae88
metrics:
- type: map_at_1
value: 5.093
- type: map_at_10
value: 12.982
- type: map_at_100
value: 15.031
- type: map_at_1000
value: 15.334
- type: map_at_3
value: 9.339
- type: map_at_5
value: 11.183
- type: mrr_at_1
value: 25.1
- type: mrr_at_10
value: 36.257
- type: mrr_at_100
value: 37.351
- type: mrr_at_1000
value: 37.409
- type: mrr_at_3
value: 33.050000000000004
- type: mrr_at_5
value: 35.205
- type: ndcg_at_1
value: 25.1
- type: ndcg_at_10
value: 21.361
- type: ndcg_at_100
value: 29.396
- type: ndcg_at_1000
value: 34.849999999999994
- type: ndcg_at_3
value: 20.704
- type: ndcg_at_5
value: 18.086
- type: precision_at_1
value: 25.1
- type: precision_at_10
value: 10.94
- type: precision_at_100
value: 2.257
- type: precision_at_1000
value: 0.358
- type: precision_at_3
value: 19.467000000000002
- type: precision_at_5
value: 15.98
- type: recall_at_1
value: 5.093
- type: recall_at_10
value: 22.177
- type: recall_at_100
value: 45.842
- type: recall_at_1000
value: 72.598
- type: recall_at_3
value: 11.833
- type: recall_at_5
value: 16.173000000000002
- task:
type: STS
dataset:
type: mteb/sickr-sts
name: MTEB SICK-R
config: default
split: test
revision: 20a6d6f312dd54037fe07a32d58e5e168867909d
metrics:
- type: cos_sim_pearson
value: 73.56535226754596
- type: cos_sim_spearman
value: 69.32425977603488
- type: euclidean_pearson
value: 71.32425703470898
- type: euclidean_spearman
value: 69.32425217267013
- type: manhattan_pearson
value: 71.25897281394246
- type: manhattan_spearman
value: 69.27132577049578
- task:
type: STS
dataset:
type: mteb/sts12-sts
name: MTEB STS12
config: default
split: test
revision: a0d554a64d88156834ff5ae9920b964011b16384
metrics:
- type: cos_sim_pearson
value: 69.66387868726018
- type: cos_sim_spearman
value: 67.85470749045027
- type: euclidean_pearson
value: 66.62075098063795
- type: euclidean_spearman
value: 67.85470749045027
- type: manhattan_pearson
value: 66.61455061901262
- type: manhattan_spearman
value: 67.87229618498695
- task:
type: STS
dataset:
type: mteb/sts13-sts
name: MTEB STS13
config: default
split: test
revision: 7e90230a92c190f1bf69ae9002b8cea547a64cca
metrics:
- type: cos_sim_pearson
value: 75.65731331392575
- type: cos_sim_spearman
value: 77.48991626780108
- type: euclidean_pearson
value: 77.19884738623692
- type: euclidean_spearman
value: 77.48985836619045
- type: manhattan_pearson
value: 77.0656684243772
- type: manhattan_spearman
value: 77.30289226582691
- task:
type: STS
dataset:
type: mteb/sts14-sts
name: MTEB STS14
config: default
split: test
revision: 6031580fec1f6af667f0bd2da0a551cf4f0b2375
metrics:
- type: cos_sim_pearson
value: 69.37003253666457
- type: cos_sim_spearman
value: 69.77157648098141
- type: euclidean_pearson
value: 69.39543876030432
- type: euclidean_spearman
value: 69.77157648098141
- type: manhattan_pearson
value: 69.29901600459745
- type: manhattan_spearman
value: 69.65074167527128
- task:
type: STS
dataset:
type: mteb/sts15-sts
name: MTEB STS15
config: default
split: test
revision: ae752c7c21bf194d8b67fd573edf7ae58183cbe3
metrics:
- type: cos_sim_pearson
value: 78.56777256540136
- type: cos_sim_spearman
value: 80.16458787843023
- type: euclidean_pearson
value: 80.16475730686916
- type: euclidean_spearman
value: 80.16458787843023
- type: manhattan_pearson
value: 80.12814463670401
- type: manhattan_spearman
value: 80.1357907984809
- task:
type: STS
dataset:
type: mteb/sts16-sts
name: MTEB STS16
config: default
split: test
revision: 4d8694f8f0e0100860b497b999b3dbed754a0513
metrics:
- type: cos_sim_pearson
value: 76.09572350919031
- type: cos_sim_spearman
value: 77.94490233429326
- type: euclidean_pearson
value: 78.36595251203524
- type: euclidean_spearman
value: 77.94490233429326
- type: manhattan_pearson
value: 78.41538768125166
- type: manhattan_spearman
value: 78.01244379569542
- task:
type: STS
dataset:
type: mteb/sts17-crosslingual-sts
name: MTEB STS17 (en-en)
config: en-en
split: test
revision: af5e6fb845001ecf41f4c1e033ce921939a2a68d
metrics:
- type: cos_sim_pearson
value: 80.7843552187951
- type: cos_sim_spearman
value: 82.28085055047386
- type: euclidean_pearson
value: 82.37373672515267
- type: euclidean_spearman
value: 82.28085055047386
- type: manhattan_pearson
value: 82.39387241346917
- type: manhattan_spearman
value: 82.36503339515906
- task:
type: STS
dataset:
type: mteb/sts22-crosslingual-sts
name: MTEB STS22 (en)
config: en
split: test
revision: eea2b4fe26a775864c896887d910b76a8098ad3f
metrics:
- type: cos_sim_pearson
value: 68.29963929962095
- type: cos_sim_spearman
value: 67.96868942546051
- type: euclidean_pearson
value: 68.93524903869285
- type: euclidean_spearman
value: 67.96868942546051
- type: manhattan_pearson
value: 68.79144468444811
- type: manhattan_spearman
value: 67.69311483884324
- task:
type: STS
dataset:
type: mteb/stsbenchmark-sts
name: MTEB STSBenchmark
config: default
split: test
revision: b0fddb56ed78048fa8b90373c8a3cfc37b684831
metrics:
- type: cos_sim_pearson
value: 72.84789696700685
- type: cos_sim_spearman
value: 75.67875747588545
- type: euclidean_pearson
value: 75.07752300463038
- type: euclidean_spearman
value: 75.67875747588545
- type: manhattan_pearson
value: 74.97934248140928
- type: manhattan_spearman
value: 75.62525644178724
- task:
type: STS
dataset:
type: PhilipMay/stsb_multi_mt
name: MTEB STSBenchmarkMultilingualSTS (en)
config: en
split: test
revision: 93d57ef91790589e3ce9c365164337a8a78b7632
metrics:
- type: cos_sim_pearson
value: 72.84789702519309
- type: cos_sim_spearman
value: 75.67875747588545
- type: euclidean_pearson
value: 75.07752310061133
- type: euclidean_spearman
value: 75.67875747588545
- type: manhattan_pearson
value: 74.97934257159595
- type: manhattan_spearman
value: 75.62525644178724
- task:
type: Reranking
dataset:
type: mteb/scidocs-reranking
name: MTEB SciDocsRR
config: default
split: test
revision: d3c5e1fc0b855ab6097bf1cda04dd73947d7caab
metrics:
- type: map
value: 81.55557720431086
- type: mrr
value: 94.91178665198272
- task:
type: Retrieval
dataset:
type: mteb/scifact
name: MTEB SciFact
config: default
split: test
revision: 0228b52cf27578f30900b9e5271d331663a030d7
metrics:
- type: map_at_1
value: 59.260999999999996
- type: map_at_10
value: 69.36099999999999
- type: map_at_100
value: 69.868
- type: map_at_1000
value: 69.877
- type: map_at_3
value: 66.617
- type: map_at_5
value: 68.061
- type: mrr_at_1
value: 62.333000000000006
- type: mrr_at_10
value: 70.533
- type: mrr_at_100
value: 70.966
- type: mrr_at_1000
value: 70.975
- type: mrr_at_3
value: 68.667
- type: mrr_at_5
value: 69.717
- type: ndcg_at_1
value: 62.333000000000006
- type: ndcg_at_10
value: 73.82300000000001
- type: ndcg_at_100
value: 76.122
- type: ndcg_at_1000
value: 76.374
- type: ndcg_at_3
value: 69.27499999999999
- type: ndcg_at_5
value: 71.33
- type: precision_at_1
value: 62.333000000000006
- type: precision_at_10
value: 9.8
- type: precision_at_100
value: 1.097
- type: precision_at_1000
value: 0.11199999999999999
- type: precision_at_3
value: 26.889000000000003
- type: precision_at_5
value: 17.599999999999998
- type: recall_at_1
value: 59.260999999999996
- type: recall_at_10
value: 86.2
- type: recall_at_100
value: 96.667
- type: recall_at_1000
value: 98.667
- type: recall_at_3
value: 74.006
- type: recall_at_5
value: 79.167
- task:
type: PairClassification
dataset:
type: mteb/sprintduplicatequestions-pairclassification
name: MTEB SprintDuplicateQuestions
config: default
split: test
revision: d66bd1f72af766a5cc4b0ca5e00c162f89e8cc46
metrics:
- type: cos_sim_accuracy
value: 99.81881188118813
- type: cos_sim_ap
value: 95.20169041096409
- type: cos_sim_f1
value: 90.76224129227664
- type: cos_sim_precision
value: 91.64118246687055
- type: cos_sim_recall
value: 89.9
- type: dot_accuracy
value: 99.81881188118813
- type: dot_ap
value: 95.20169041096409
- type: dot_f1
value: 90.76224129227664
- type: dot_precision
value: 91.64118246687055
- type: dot_recall
value: 89.9
- type: euclidean_accuracy
value: 99.81881188118813
- type: euclidean_ap
value: 95.2016904109641
- type: euclidean_f1
value: 90.76224129227664
- type: euclidean_precision
value: 91.64118246687055
- type: euclidean_recall
value: 89.9
- type: manhattan_accuracy
value: 99.81881188118813
- type: manhattan_ap
value: 95.22680188132777
- type: manhattan_f1
value: 90.79013588324108
- type: manhattan_precision
value: 91.38804457953394
- type: manhattan_recall
value: 90.2
- type: max_accuracy
value: 99.81881188118813
- type: max_ap
value: 95.22680188132777
- type: max_f1
value: 90.79013588324108
- task:
type: Clustering
dataset:
type: mteb/stackexchange-clustering
name: MTEB StackExchangeClustering
config: default
split: test
revision: 6cbc1f7b2bc0622f2e39d2c77fa502909748c259
metrics:
- type: v_measure
value: 57.8638628701308
- task:
type: Clustering
dataset:
type: mteb/stackexchange-clustering-p2p
name: MTEB StackExchangeClusteringP2P
config: default
split: test
revision: 815ca46b2622cec33ccafc3735d572c266efdb44
metrics:
- type: v_measure
value: 37.82028248106046
- task:
type: Reranking
dataset:
type: mteb/stackoverflowdupquestions-reranking
name: MTEB StackOverflowDupQuestions
config: default
split: test
revision: e185fbe320c72810689fc5848eb6114e1ef5ec69
metrics:
- type: map
value: 50.870860210170946
- type: mrr
value: 51.608084521687466
- task:
type: Summarization
dataset:
type: mteb/summeval
name: MTEB SummEval
config: default
split: test
revision: cda12ad7615edc362dbf25a00fdd61d3b1eaf93c
metrics:
- type: cos_sim_pearson
value: 31.60384207444685
- type: cos_sim_spearman
value: 30.84047452209471
- type: dot_pearson
value: 31.60384104417333
- type: dot_spearman
value: 30.84047452209471
- task:
type: Retrieval
dataset:
type: mteb/trec-covid
name: MTEB TRECCOVID
config: default
split: test
revision: bb9466bac8153a0349341eb1b22e06409e78ef4e
metrics:
- type: map_at_1
value: 0.246
- type: map_at_10
value: 2.051
- type: map_at_100
value: 13.129
- type: map_at_1000
value: 31.56
- type: map_at_3
value: 0.681
- type: map_at_5
value: 1.105
- type: mrr_at_1
value: 94
- type: mrr_at_10
value: 97
- type: mrr_at_100
value: 97
- type: mrr_at_1000
value: 97
- type: mrr_at_3
value: 97
- type: mrr_at_5
value: 97
- type: ndcg_at_1
value: 87
- type: ndcg_at_10
value: 80.716
- type: ndcg_at_100
value: 63.83
- type: ndcg_at_1000
value: 56.215
- type: ndcg_at_3
value: 84.531
- type: ndcg_at_5
value: 84.777
- type: precision_at_1
value: 94
- type: precision_at_10
value: 84.6
- type: precision_at_100
value: 66.03999999999999
- type: precision_at_1000
value: 24.878
- type: precision_at_3
value: 88.667
- type: precision_at_5
value: 89.60000000000001
- type: recall_at_1
value: 0.246
- type: recall_at_10
value: 2.2079999999999997
- type: recall_at_100
value: 15.895999999999999
- type: recall_at_1000
value: 52.683
- type: recall_at_3
value: 0.7040000000000001
- type: recall_at_5
value: 1.163
- task:
type: Retrieval
dataset:
type: mteb/touche2020
name: MTEB Touche2020
config: default
split: test
revision: a34f9a33db75fa0cbb21bb5cfc3dae8dc8bec93f
metrics:
- type: map_at_1
value: 3.852
- type: map_at_10
value: 14.316
- type: map_at_100
value: 20.982
- type: map_at_1000
value: 22.58
- type: map_at_3
value: 7.767
- type: map_at_5
value: 10.321
- type: mrr_at_1
value: 51.019999999999996
- type: mrr_at_10
value: 66.365
- type: mrr_at_100
value: 66.522
- type: mrr_at_1000
value: 66.522
- type: mrr_at_3
value: 62.925
- type: mrr_at_5
value: 64.762
- type: ndcg_at_1
value: 46.939
- type: ndcg_at_10
value: 34.516999999999996
- type: ndcg_at_100
value: 44.25
- type: ndcg_at_1000
value: 54.899
- type: ndcg_at_3
value: 40.203
- type: ndcg_at_5
value: 37.004
- type: precision_at_1
value: 51.019999999999996
- type: precision_at_10
value: 29.796
- type: precision_at_100
value: 8.633000000000001
- type: precision_at_1000
value: 1.584
- type: precision_at_3
value: 40.816
- type: precision_at_5
value: 35.918
- type: recall_at_1
value: 3.852
- type: recall_at_10
value: 20.891000000000002
- type: recall_at_100
value: 52.428
- type: recall_at_1000
value: 84.34899999999999
- type: recall_at_3
value: 8.834
- type: recall_at_5
value: 12.909
- task:
type: Classification
dataset:
type: mteb/toxic_conversations_50k
name: MTEB ToxicConversationsClassification
config: default
split: test
revision: edfaf9da55d3dd50d43143d90c1ac476895ae6de
metrics:
- type: accuracy
value: 64.7092
- type: ap
value: 11.972915012305819
- type: f1
value: 49.91050149892115
- task:
type: Classification
dataset:
type: mteb/tweet_sentiment_extraction
name: MTEB TweetSentimentExtractionClassification
config: default
split: test
revision: d604517c81ca91fe16a244d1248fc021f9ecee7a
metrics:
- type: accuracy
value: 56.737408036219584
- type: f1
value: 57.07235266246011
- task:
type: Clustering
dataset:
type: mteb/twentynewsgroups-clustering
name: MTEB TwentyNewsgroupsClustering
config: default
split: test
revision: 6125ec4e24fa026cec8a478383ee943acfbd5449
metrics:
- type: v_measure
value: 35.9147539025798
- task:
type: PairClassification
dataset:
type: mteb/twittersemeval2015-pairclassification
name: MTEB TwitterSemEval2015
config: default
split: test
revision: 70970daeab8776df92f5ea462b6173c0b46fd2d1
metrics:
- type: cos_sim_accuracy
value: 82.52369315133814
- type: cos_sim_ap
value: 62.34858091376534
- type: cos_sim_f1
value: 58.18225190839694
- type: cos_sim_precision
value: 53.09098824553766
- type: cos_sim_recall
value: 64.35356200527704
- type: dot_accuracy
value: 82.52369315133814
- type: dot_ap
value: 62.34857753814992
- type: dot_f1
value: 58.18225190839694
- type: dot_precision
value: 53.09098824553766
- type: dot_recall
value: 64.35356200527704
- type: euclidean_accuracy
value: 82.52369315133814
- type: euclidean_ap
value: 62.34857756663386
- type: euclidean_f1
value: 58.18225190839694
- type: euclidean_precision
value: 53.09098824553766
- type: euclidean_recall
value: 64.35356200527704
- type: manhattan_accuracy
value: 82.49389044525243
- type: manhattan_ap
value: 62.32245347238179
- type: manhattan_f1
value: 58.206309819213054
- type: manhattan_precision
value: 52.70704044511021
- type: manhattan_recall
value: 64.9868073878628
- type: max_accuracy
value: 82.52369315133814
- type: max_ap
value: 62.34858091376534
- type: max_f1
value: 58.206309819213054
- task:
type: PairClassification
dataset:
type: mteb/twitterurlcorpus-pairclassification
name: MTEB TwitterURLCorpus
config: default
split: test
revision: 8b6510b0b1fa4e4c4f879467980e9be563ec1cdf
metrics:
- type: cos_sim_accuracy
value: 88.34555827220863
- type: cos_sim_ap
value: 84.84152481680071
- type: cos_sim_f1
value: 76.860456739428
- type: cos_sim_precision
value: 72.21470150263978
- type: cos_sim_recall
value: 82.14505697566985
- type: dot_accuracy
value: 88.34555827220863
- type: dot_ap
value: 84.84152743322608
- type: dot_f1
value: 76.860456739428
- type: dot_precision
value: 72.21470150263978
- type: dot_recall
value: 82.14505697566985
- type: euclidean_accuracy
value: 88.34555827220863
- type: euclidean_ap
value: 84.84152589453169
- type: euclidean_f1
value: 76.860456739428
- type: euclidean_precision
value: 72.21470150263978
- type: euclidean_recall
value: 82.14505697566985
- type: manhattan_accuracy
value: 88.38242713548337
- type: manhattan_ap
value: 84.8112124970968
- type: manhattan_f1
value: 76.83599206057487
- type: manhattan_precision
value: 73.51244900829934
- type: manhattan_recall
value: 80.47428395441946
- type: max_accuracy
value: 88.38242713548337
- type: max_ap
value: 84.84152743322608
- type: max_f1
value: 76.860456739428
- task:
type: Clustering
dataset:
type: jinaai/cities_wiki_clustering
name: MTEB WikiCitiesClustering
config: default
split: test
revision: ddc9ee9242fa65332597f70e967ecc38b9d734fa
metrics:
- type: v_measure
value: 85.5314389263015
---
<h1 align="center">Snowflake's Arctic-embed-l</h1>
<h4 align="center">
<p>
<a href=#news>News</a> |
<a href=#models>Models</a> |
<a href=#usage>Usage</a> |
<a href="#evaluation">Evaluation</a> |
<a href="#contact">Contact</a> |
<a href="#faq">FAQ</a>
<a href="#license">License</a> |
<a href="#acknowledgement">Acknowledgement</a>
<p>
</h4>
## News
05/10/2024: Release the [technical report on Arctic Embed](https://arxiv.org/abs/2405.05374)
04/16/2024: Release the ** snowflake-arctic-embed ** family of text embedding models. The releases are state-of-the-art for Retrieval quality at each of their representative size profiles. [Technical Report]() is coming shortly. For more details, please refer to our Github: [Arctic-Text-Embed](https://github.com/Snowflake-Labs/arctic-embed).
## Models
snowflake-arctic-embed is a suite of text embedding models that focuses on creating high-quality retrieval models optimized for performance.
The `snowflake-arctic-embedding` models achieve **state-of-the-art performance on the MTEB/BEIR leaderboard** for each of their size variants. Evaluation is performed using these [scripts](https://github.com/Snowflake-Labs/snowflake-arctic-embed/tree/main/src). As shown below, each class of model size achieves SOTA retrieval accuracy compared to other top models.
The models are trained by leveraging existing open-source text representation models, such as bert-base-uncased, and are trained in a multi-stage pipeline to optimize their retrieval performance. First, the models are trained with large batches of query-document pairs where negatives are derived in-batch—pretraining leverages about 400m samples of a mix of public datasets and proprietary web search data. Following pretraining models are further optimized with long training on a smaller dataset (about 1m samples) of triplets of query, positive document, and negative document derived from hard harmful mining. Mining of the negatives and data curation is crucial to retrieval accuracy. A detailed technical report can be found [here](https://arxiv.org/abs/2405.05374).
| Name | MTEB Retrieval Score (NDCG @ 10) | Parameters (Millions) | Embedding Dimension |
| ----------------------------------------------------------------------- | -------------------------------- | --------------------- | ------------------- |
| [snowflake-arctic-embed-xs](https://huggingface.co/Snowflake/snowflake-arctic-embed-xs/) | 50.15 | 22 | 384 |
| [snowflake-arctic-embed-s](https://huggingface.co/Snowflake/snowflake-arctic-embed-s/) | 51.98 | 33 | 384 |
| [snowflake-arctic-embed-m](https://huggingface.co/Snowflake/snowflake-arctic-embed-m/) | 54.90 | 110 | 768 |
| [snowflake-arctic-embed-m-long](https://huggingface.co/Snowflake/snowflake-arctic-embed-m-long/) | 54.83 | 137 | 768 |
| [snowflake-arctic-embed-l](https://huggingface.co/Snowflake/snowflake-arctic-embed-l/) | 55.98 | 335 | 1024 |
Aside from being great open-source models, the largest model, [snowflake-arctic-embed-l](https://huggingface.co/Snowflake/snowflake-arctic-embed-l/), can serve as a natural replacement for closed-source embedding, as shown below.
| Model Name | MTEB Retrieval Score (NDCG @ 10) |
| ------------------------------------------------------------------ | -------------------------------- |
| [snowflake-arctic-embed-l](https://huggingface.co/Snowflake/snowflake-arctic-embed-l/) | 55.98 |
| Google-gecko-text-embedding | 55.7 |
| text-embedding-3-large | 55.44 |
| Cohere-embed-english-v3.0 | 55.00 |
| bge-large-en-v1.5 | 54.29 |
### [snowflake-arctic-embed-xs](https://huggingface.co/Snowflake/snowflake-arctic-embed-xs)
This tiny model packs quite the punch. Based on the [all-MiniLM-L6-v2](https://huggingface.co/sentence-transformers/all-MiniLM-L6-v2) model with only 22m parameters and 384 dimensions, this model should meet even the strictest latency/TCO budgets. Despite its size, its retrieval accuracy is closer to that of models with 100m paramers.
| Model Name | MTEB Retrieval Score (NDCG @ 10) |
| ------------------------------------------------------------------- | -------------------------------- |
| [snowflake-arctic-embed-xs](https://huggingface.co/Snowflake/snowflake-arctic-embed-xs/) | 50.15 |
| GIST-all-MiniLM-L6-v2 | 45.12 |
| gte-tiny | 44.92 |
| all-MiniLM-L6-v2 | 41.95 |
| bge-micro-v2 | 42.56 |
### [snowflake-arctic-embed-s](https://huggingface.co/Snowflake/snowflake-arctic-embed-s)
Based on the [intfloat/e5-small-unsupervised](https://huggingface.co/intfloat/e5-small-unsupervised) model, this small model does not trade off retrieval accuracy for its small size. With only 33m parameters and 384 dimensions, this model should easily allow scaling to large datasets.
| Model Name | MTEB Retrieval Score (NDCG @ 10) |
| ------------------------------------------------------------------ | -------------------------------- |
| [snowflake-arctic-embed-s](https://huggingface.co/Snowflake/snowflake-arctic-embed-s/) | 51.98 |
| bge-small-en-v1.5 | 51.68 |
| Cohere-embed-english-light-v3.0 | 51.34 |
| text-embedding-3-small | 51.08 |
| e5-small-v2 | 49.04 |
### [snowflake-arctic-embed-m](https://huggingface.co/Snowflake/snowflake-arctic-embed-m/)
Based on the [intfloat/e5-base-unsupervised](https://huggingface.co/intfloat/e5-base-unsupervised) model, this medium model is the workhorse that provides the best retrieval performance without slowing down inference.
| Model Name | MTEB Retrieval Score (NDCG @ 10) |
| ------------------------------------------------------------------ | -------------------------------- |
| [snowflake-arctic-embed-m](https://huggingface.co/Snowflake/snowflake-arctic-embed-m/) | 54.90 |
| bge-base-en-v1.5 | 53.25 |
| nomic-embed-text-v1.5 | 53.25 |
| GIST-Embedding-v0 | 52.31 |
| gte-base | 52.31 |
### [snowflake-arctic-embed-m-long](https://huggingface.co/Snowflake/snowflake-arctic-embed-m-long/)
Based on the [nomic-ai/nomic-embed-text-v1-unsupervised](https://huggingface.co/nomic-ai/nomic-embed-text-v1-unsupervised) model, this long-context variant of our medium-sized model is perfect for workloads that can be constrained by the regular 512 token context of our other models. Without the use of RPE, this model supports up to 2048 tokens. With RPE, it can scale to 8192!
| Model Name | MTEB Retrieval Score (NDCG @ 10) |
| ------------------------------------------------------------------ | -------------------------------- |
| [snowflake-arctic-embed-m-long](https://huggingface.co/Snowflake/snowflake-arctic-embed-m-long/) | 54.83 |
| nomic-embed-text-v1.5 | 53.01 |
| nomic-embed-text-v1 | 52.81 |
### [snowflake-arctic-embed-l](https://huggingface.co/Snowflake/snowflake-arctic-embed-l/)
Based on the [intfloat/e5-large-unsupervised](https://huggingface.co/intfloat/e5-large-unsupervised) model, this large model is a direct drop-in for closed APIs and delivers the most accurate retrieval experience.
| Model Name | MTEB Retrieval Score (NDCG @ 10) |
| ------------------------------------------------------------------ | -------------------------------- |
| [snowflake-arctic-embed-l](https://huggingface.co/Snowflake/snowflake-arctic-embed-l/) | 55.98 |
| UAE-Large-V1 | 54.66 |
| bge-large-en-v1.5 | 54.29 |
| mxbai-embed-large-v1 | 54.39 |
| e5-Large-v2 | 50.56 |
## Usage
### Using Sentence Transformers
You can use the sentence-transformers package to use an snowflake-arctic-embed model, as shown below.
```python
from sentence_transformers import SentenceTransformer
model = SentenceTransformer("Snowflake/snowflake-arctic-embed-l")
queries = ['what is snowflake?', 'Where can I get the best tacos?']
documents = ['The Data Cloud!', 'Mexico City of Course!']
query_embeddings = model.encode(queries, prompt_name="query")
document_embeddings = model.encode(documents)
scores = query_embeddings @ document_embeddings.T
for query, query_scores in zip(queries, scores):
doc_score_pairs = list(zip(documents, query_scores))
doc_score_pairs = sorted(doc_score_pairs, key=lambda x: x[1], reverse=True)
# Output passages & scores
print("Query:", query)
for document, score in doc_score_pairs:
print(score, document)
```
```
Query: what is snowflake?
0.28976774 The Data Cloud!
0.19071159 Mexico City of Course!
Query: Where can I get the best tacos?
0.38650584 Mexico City of Course!
0.25145516 The Data Cloud!
```
### Using Huggingface transformers
You can use the transformers package to use an snowflake-arctic-embed model, as shown below. For optimal retrieval quality, use the CLS token to embed each text portion and use the query prefix below (just on the query).
```python
import torch
from transformers import AutoModel, AutoTokenizer
tokenizer = AutoTokenizer.from_pretrained('Snowflake/snowflake-arctic-embed-l')
model = AutoModel.from_pretrained('Snowflake/snowflake-arctic-embed-l', add_pooling_layer=False)
model.eval()
query_prefix = 'Represent this sentence for searching relevant passages: '
queries = ['what is snowflake?', 'Where can I get the best tacos?']
queries_with_prefix = ["{}{}".format(query_prefix, i) for i in queries]
query_tokens = tokenizer(queries_with_prefix, padding=True, truncation=True, return_tensors='pt', max_length=512)
documents = ['The Data Cloud!', 'Mexico City of Course!']
document_tokens = tokenizer(documents, padding=True, truncation=True, return_tensors='pt', max_length=512)
# Compute token embeddings
with torch.no_grad():
query_embeddings = model(**query_tokens)[0][:, 0]
doument_embeddings = model(**document_tokens)[0][:, 0]
# normalize embeddings
query_embeddings = torch.nn.functional.normalize(query_embeddings, p=2, dim=1)
doument_embeddings = torch.nn.functional.normalize(doument_embeddings, p=2, dim=1)
scores = torch.mm(query_embeddings, doument_embeddings.transpose(0, 1))
for query, query_scores in zip(queries, scores):
doc_score_pairs = list(zip(documents, query_scores))
doc_score_pairs = sorted(doc_score_pairs, key=lambda x: x[1], reverse=True)
#Output passages & scores
print("Query:", query)
for document, score in doc_score_pairs:
print(score, document)
```
### Using Transformers.js
If you haven't already, you can install the [Transformers.js](https://huggingface.co/docs/transformers.js) JavaScript library from [NPM](https://www.npmjs.com/package/@xenova/transformers) by running:
```bash
npm i @xenova/transformers
```
You can then use the model to compute embeddings as follows:
```js
import { pipeline, dot } from '@xenova/transformers';
// Create feature extraction pipeline
const extractor = await pipeline('feature-extraction', 'Snowflake/snowflake-arctic-embed-l', {
quantized: false, // Comment out this line to use the quantized version
});
// Generate sentence embeddings
const sentences = [
'Represent this sentence for searching relevant passages: Where can I get the best tacos?',
'The Data Cloud!',
'Mexico City of Course!',
]
const output = await extractor(sentences, { normalize: true, pooling: 'cls' });
// Compute similarity scores
const [source_embeddings, ...document_embeddings ] = output.tolist();
const similarities = document_embeddings.map(x => dot(source_embeddings, x));
console.log(similarities); // [0.25145517380846977, 0.3865060421197194]
```
## FAQ
TBD
## Contact
Feel free to open an issue or pull request if you have any questions or suggestions about this project.
You also can email Daniel Campos([email protected]).
## License
Arctic is licensed under the [Apache-2](https://www.apache.org/licenses/LICENSE-2.0). The released models can be used for commercial purposes free of charge.
## Acknowledgement
We want to thank the open-source community, which has provided the great building blocks upon which we could make our models.
We thank our modeling engineers, Danmei Xu, Luke Merrick, Gaurav Nuti, and Daniel Campos, for making these great models possible.
We thank our leadership, Himabindu Pucha, Kelvin So, Vivek Raghunathan, and Sridhar Ramaswamy, for supporting this work.
We also thank the open-source community for producing the great models we could build on top of and making these releases possible.
Finally, we thank the researchers who created BEIR and MTEB benchmarks.
It is largely thanks to their tireless work to define what better looks like that we could improve model performance. |
microsoft/DialoGPT-small | microsoft | 2024-02-29T15:48:41Z | 21,269 | 87 | transformers | [
"transformers",
"pytorch",
"tf",
"jax",
"safetensors",
"gpt2",
"text-generation",
"conversational",
"arxiv:1911.00536",
"license:mit",
"autotrain_compatible",
"endpoints_compatible",
"text-generation-inference",
"region:us"
] | text-generation | 2022-03-02T23:29:05Z | ---
thumbnail: https://huggingface.co/front/thumbnails/dialogpt.png
tags:
- conversational
license: mit
---
## A State-of-the-Art Large-scale Pretrained Response generation model (DialoGPT)
DialoGPT is a SOTA large-scale pretrained dialogue response generation model for multiturn conversations.
The [human evaluation results](https://github.com/dreasysnail/Dialogpt_dev#human-evaluation) indicate that the response generated from DialoGPT is comparable to human response quality under a single-turn conversation Turing test.
The model is trained on 147M multi-turn dialogue from Reddit discussion thread.
* Multi-turn generation examples from an interactive environment:
|Role | Response |
|---------|--------|
|User | Does money buy happiness? |
| Bot | Depends how much money you spend on it .|
|User | What is the best way to buy happiness ? |
| Bot | You just have to be a millionaire by your early 20s, then you can be happy . |
|User |This is so difficult ! |
| Bot | You have no idea how hard it is to be a millionaire and happy . There is a reason the rich have a lot of money |
Please find the information about preprocessing, training and full details of the DialoGPT in the [original DialoGPT repository](https://github.com/microsoft/DialoGPT)
ArXiv paper: [https://arxiv.org/abs/1911.00536](https://arxiv.org/abs/1911.00536)
### How to use
Now we are ready to try out how the model works as a chatting partner!
```python
from transformers import AutoModelForCausalLM, AutoTokenizer
import torch
tokenizer = AutoTokenizer.from_pretrained("microsoft/DialoGPT-small")
model = AutoModelForCausalLM.from_pretrained("microsoft/DialoGPT-small")
# Let's chat for 5 lines
for step in range(5):
# encode the new user input, add the eos_token and return a tensor in Pytorch
new_user_input_ids = tokenizer.encode(input(">> User:") + tokenizer.eos_token, return_tensors='pt')
# append the new user input tokens to the chat history
bot_input_ids = torch.cat([chat_history_ids, new_user_input_ids], dim=-1) if step > 0 else new_user_input_ids
# generated a response while limiting the total chat history to 1000 tokens,
chat_history_ids = model.generate(bot_input_ids, max_length=1000, pad_token_id=tokenizer.eos_token_id)
# pretty print last ouput tokens from bot
print("DialoGPT: {}".format(tokenizer.decode(chat_history_ids[:, bot_input_ids.shape[-1]:][0], skip_special_tokens=True)))
```
# [Open LLM Leaderboard Evaluation Results](https://huggingface.co/spaces/HuggingFaceH4/open_llm_leaderboard)
Detailed results can be found [here](https://huggingface.co/datasets/open-llm-leaderboard/details_microsoft__DialoGPT-small)
| Metric | Value |
|-----------------------|---------------------------|
| Avg. | 25.02 |
| ARC (25-shot) | 25.77 |
| HellaSwag (10-shot) | 25.79 |
| MMLU (5-shot) | 25.81 |
| TruthfulQA (0-shot) | 47.49 |
| Winogrande (5-shot) | 50.28 |
| GSM8K (5-shot) | 0.0 |
| DROP (3-shot) | 0.0 |
|
ai-forever/ruBert-base | ai-forever | 2023-11-03T12:50:38Z | 21,255 | 25 | transformers | [
"transformers",
"pytorch",
"bert",
"fill-mask",
"PyTorch",
"Transformers",
"exbert",
"ru",
"arxiv:2309.10931",
"license:apache-2.0",
"autotrain_compatible",
"endpoints_compatible",
"region:us"
] | fill-mask | 2022-03-02T23:29:05Z | ---
language:
- ru
tags:
- PyTorch
- Transformers
- bert
- exbert
pipeline_tag: fill-mask
thumbnail: "https://github.com/sberbank-ai/model-zoo"
license: apache-2.0
---
# ruBert-base
The model architecture design, pretraining, and evaluation are documented in our preprint: [**A Family of Pretrained Transformer Language Models for Russian**](https://arxiv.org/abs/2309.10931).
The model is pretrained by the [SberDevices](https://sberdevices.ru/) team.
* Task: `mask filling`
* Type: `encoder`
* Tokenizer: `BPE`
* Dict size: `120 138`
* Num Parameters: `178 M`
* Training Data Volume `30 GB`
# Authors
+ NLP core team RnD [Telegram channel](https://t.me/nlpcoreteam):
+ Dmitry Zmitrovich
# Cite us
```
@misc{zmitrovich2023family,
title={A Family of Pretrained Transformer Language Models for Russian},
author={Dmitry Zmitrovich and Alexander Abramov and Andrey Kalmykov and Maria Tikhonova and Ekaterina Taktasheva and Danil Astafurov and Mark Baushenko and Artem Snegirev and Tatiana Shavrina and Sergey Markov and Vladislav Mikhailov and Alena Fenogenova},
year={2023},
eprint={2309.10931},
archivePrefix={arXiv},
primaryClass={cs.CL}
}
``` |
mradermacher/L3-Stur-v1-15B-i1-GGUF | mradermacher | 2024-06-23T18:53:18Z | 21,239 | 0 | transformers | [
"transformers",
"gguf",
"merge",
"mergekit",
"lazymergekit",
"Frowning/L3-Stur-v1-8B",
"en",
"base_model:Frowning/L3-Stur-v1-15B",
"endpoints_compatible",
"region:us"
] | null | 2024-06-23T13:49:52Z | ---
base_model: Frowning/L3-Stur-v1-15B
language:
- en
library_name: transformers
quantized_by: mradermacher
tags:
- merge
- mergekit
- lazymergekit
- Frowning/L3-Stur-v1-8B
---
## About
<!-- ### quantize_version: 2 -->
<!-- ### output_tensor_quantised: 1 -->
<!-- ### convert_type: hf -->
<!-- ### vocab_type: -->
<!-- ### tags: nicoboss -->
weighted/imatrix quants of https://huggingface.co/Frowning/L3-Stur-v1-15B
<!-- provided-files -->
static quants are available at https://huggingface.co/mradermacher/L3-Stur-v1-15B-GGUF
## Usage
If you are unsure how to use GGUF files, refer to one of [TheBloke's
READMEs](https://huggingface.co/TheBloke/KafkaLM-70B-German-V0.1-GGUF) for
more details, including on how to concatenate multi-part files.
## Provided Quants
(sorted by size, not necessarily quality. IQ-quants are often preferable over similar sized non-IQ quants)
| Link | Type | Size/GB | Notes |
|:-----|:-----|--------:|:------|
| [GGUF](https://huggingface.co/mradermacher/L3-Stur-v1-15B-i1-GGUF/resolve/main/L3-Stur-v1-15B.i1-IQ1_S.gguf) | i1-IQ1_S | 3.6 | for the desperate |
| [GGUF](https://huggingface.co/mradermacher/L3-Stur-v1-15B-i1-GGUF/resolve/main/L3-Stur-v1-15B.i1-IQ1_M.gguf) | i1-IQ1_M | 3.9 | mostly desperate |
| [GGUF](https://huggingface.co/mradermacher/L3-Stur-v1-15B-i1-GGUF/resolve/main/L3-Stur-v1-15B.i1-IQ2_XXS.gguf) | i1-IQ2_XXS | 4.4 | |
| [GGUF](https://huggingface.co/mradermacher/L3-Stur-v1-15B-i1-GGUF/resolve/main/L3-Stur-v1-15B.i1-IQ2_XS.gguf) | i1-IQ2_XS | 4.8 | |
| [GGUF](https://huggingface.co/mradermacher/L3-Stur-v1-15B-i1-GGUF/resolve/main/L3-Stur-v1-15B.i1-IQ2_S.gguf) | i1-IQ2_S | 5.0 | |
| [GGUF](https://huggingface.co/mradermacher/L3-Stur-v1-15B-i1-GGUF/resolve/main/L3-Stur-v1-15B.i1-IQ2_M.gguf) | i1-IQ2_M | 5.4 | |
| [GGUF](https://huggingface.co/mradermacher/L3-Stur-v1-15B-i1-GGUF/resolve/main/L3-Stur-v1-15B.i1-Q2_K.gguf) | i1-Q2_K | 5.8 | IQ3_XXS probably better |
| [GGUF](https://huggingface.co/mradermacher/L3-Stur-v1-15B-i1-GGUF/resolve/main/L3-Stur-v1-15B.i1-IQ3_XXS.gguf) | i1-IQ3_XXS | 6.1 | lower quality |
| [GGUF](https://huggingface.co/mradermacher/L3-Stur-v1-15B-i1-GGUF/resolve/main/L3-Stur-v1-15B.i1-IQ3_XS.gguf) | i1-IQ3_XS | 6.5 | |
| [GGUF](https://huggingface.co/mradermacher/L3-Stur-v1-15B-i1-GGUF/resolve/main/L3-Stur-v1-15B.i1-Q3_K_S.gguf) | i1-Q3_K_S | 6.8 | IQ3_XS probably better |
| [GGUF](https://huggingface.co/mradermacher/L3-Stur-v1-15B-i1-GGUF/resolve/main/L3-Stur-v1-15B.i1-IQ3_S.gguf) | i1-IQ3_S | 6.8 | beats Q3_K* |
| [GGUF](https://huggingface.co/mradermacher/L3-Stur-v1-15B-i1-GGUF/resolve/main/L3-Stur-v1-15B.i1-IQ3_M.gguf) | i1-IQ3_M | 7.0 | |
| [GGUF](https://huggingface.co/mradermacher/L3-Stur-v1-15B-i1-GGUF/resolve/main/L3-Stur-v1-15B.i1-Q3_K_M.gguf) | i1-Q3_K_M | 7.5 | IQ3_S probably better |
| [GGUF](https://huggingface.co/mradermacher/L3-Stur-v1-15B-i1-GGUF/resolve/main/L3-Stur-v1-15B.i1-Q3_K_L.gguf) | i1-Q3_K_L | 8.1 | IQ3_M probably better |
| [GGUF](https://huggingface.co/mradermacher/L3-Stur-v1-15B-i1-GGUF/resolve/main/L3-Stur-v1-15B.i1-IQ4_XS.gguf) | i1-IQ4_XS | 8.3 | |
| [GGUF](https://huggingface.co/mradermacher/L3-Stur-v1-15B-i1-GGUF/resolve/main/L3-Stur-v1-15B.i1-Q4_0.gguf) | i1-Q4_0 | 8.7 | fast, low quality |
| [GGUF](https://huggingface.co/mradermacher/L3-Stur-v1-15B-i1-GGUF/resolve/main/L3-Stur-v1-15B.i1-Q4_K_S.gguf) | i1-Q4_K_S | 8.7 | optimal size/speed/quality |
| [GGUF](https://huggingface.co/mradermacher/L3-Stur-v1-15B-i1-GGUF/resolve/main/L3-Stur-v1-15B.i1-Q4_K_M.gguf) | i1-Q4_K_M | 9.2 | fast, recommended |
| [GGUF](https://huggingface.co/mradermacher/L3-Stur-v1-15B-i1-GGUF/resolve/main/L3-Stur-v1-15B.i1-Q5_K_S.gguf) | i1-Q5_K_S | 10.5 | |
| [GGUF](https://huggingface.co/mradermacher/L3-Stur-v1-15B-i1-GGUF/resolve/main/L3-Stur-v1-15B.i1-Q5_K_M.gguf) | i1-Q5_K_M | 10.8 | |
| [GGUF](https://huggingface.co/mradermacher/L3-Stur-v1-15B-i1-GGUF/resolve/main/L3-Stur-v1-15B.i1-Q6_K.gguf) | i1-Q6_K | 12.4 | practically like static Q6_K |
Here is a handy graph by ikawrakow comparing some lower-quality quant
types (lower is better):

And here are Artefact2's thoughts on the matter:
https://gist.github.com/Artefact2/b5f810600771265fc1e39442288e8ec9
## FAQ / Model Request
See https://huggingface.co/mradermacher/model_requests for some answers to
questions you might have and/or if you want some other model quantized.
## Thanks
I thank my company, [nethype GmbH](https://www.nethype.de/), for letting
me use its servers and providing upgrades to my workstation to enable
this work in my free time. Additional thanks to [@nicoboss](https://huggingface.co/nicoboss) for giving me access to his hardware for calculating the imatrix for these quants.
<!-- end -->
|
RichardErkhov/Replete-AI_-_Llama-3-11.5B-Instruct-V2-gguf | RichardErkhov | 2024-06-25T18:00:39Z | 21,228 | 0 | null | [
"gguf",
"region:us"
] | null | 2024-06-25T13:36:06Z | Quantization made by Richard Erkhov.
[Github](https://github.com/RichardErkhov)
[Discord](https://discord.gg/pvy7H8DZMG)
[Request more models](https://github.com/RichardErkhov/quant_request)
Llama-3-11.5B-Instruct-V2 - GGUF
- Model creator: https://huggingface.co/Replete-AI/
- Original model: https://huggingface.co/Replete-AI/Llama-3-11.5B-Instruct-V2/
| Name | Quant method | Size |
| ---- | ---- | ---- |
| [Llama-3-11.5B-Instruct-V2.Q2_K.gguf](https://huggingface.co/RichardErkhov/Replete-AI_-_Llama-3-11.5B-Instruct-V2-gguf/blob/main/Llama-3-11.5B-Instruct-V2.Q2_K.gguf) | Q2_K | 4.16GB |
| [Llama-3-11.5B-Instruct-V2.IQ3_XS.gguf](https://huggingface.co/RichardErkhov/Replete-AI_-_Llama-3-11.5B-Instruct-V2-gguf/blob/main/Llama-3-11.5B-Instruct-V2.IQ3_XS.gguf) | IQ3_XS | 4.61GB |
| [Llama-3-11.5B-Instruct-V2.IQ3_S.gguf](https://huggingface.co/RichardErkhov/Replete-AI_-_Llama-3-11.5B-Instruct-V2-gguf/blob/main/Llama-3-11.5B-Instruct-V2.IQ3_S.gguf) | IQ3_S | 4.83GB |
| [Llama-3-11.5B-Instruct-V2.Q3_K_S.gguf](https://huggingface.co/RichardErkhov/Replete-AI_-_Llama-3-11.5B-Instruct-V2-gguf/blob/main/Llama-3-11.5B-Instruct-V2.Q3_K_S.gguf) | Q3_K_S | 4.81GB |
| [Llama-3-11.5B-Instruct-V2.IQ3_M.gguf](https://huggingface.co/RichardErkhov/Replete-AI_-_Llama-3-11.5B-Instruct-V2-gguf/blob/main/Llama-3-11.5B-Instruct-V2.IQ3_M.gguf) | IQ3_M | 4.98GB |
| [Llama-3-11.5B-Instruct-V2.Q3_K.gguf](https://huggingface.co/RichardErkhov/Replete-AI_-_Llama-3-11.5B-Instruct-V2-gguf/blob/main/Llama-3-11.5B-Instruct-V2.Q3_K.gguf) | Q3_K | 5.3GB |
| [Llama-3-11.5B-Instruct-V2.Q3_K_M.gguf](https://huggingface.co/RichardErkhov/Replete-AI_-_Llama-3-11.5B-Instruct-V2-gguf/blob/main/Llama-3-11.5B-Instruct-V2.Q3_K_M.gguf) | Q3_K_M | 5.3GB |
| [Llama-3-11.5B-Instruct-V2.Q3_K_L.gguf](https://huggingface.co/RichardErkhov/Replete-AI_-_Llama-3-11.5B-Instruct-V2-gguf/blob/main/Llama-3-11.5B-Instruct-V2.Q3_K_L.gguf) | Q3_K_L | 5.73GB |
| [Llama-3-11.5B-Instruct-V2.IQ4_XS.gguf](https://huggingface.co/RichardErkhov/Replete-AI_-_Llama-3-11.5B-Instruct-V2-gguf/blob/main/Llama-3-11.5B-Instruct-V2.IQ4_XS.gguf) | IQ4_XS | 5.93GB |
| [Llama-3-11.5B-Instruct-V2.Q4_0.gguf](https://huggingface.co/RichardErkhov/Replete-AI_-_Llama-3-11.5B-Instruct-V2-gguf/blob/main/Llama-3-11.5B-Instruct-V2.Q4_0.gguf) | Q4_0 | 6.17GB |
| [Llama-3-11.5B-Instruct-V2.IQ4_NL.gguf](https://huggingface.co/RichardErkhov/Replete-AI_-_Llama-3-11.5B-Instruct-V2-gguf/blob/main/Llama-3-11.5B-Instruct-V2.IQ4_NL.gguf) | IQ4_NL | 6.23GB |
| [Llama-3-11.5B-Instruct-V2.Q4_K_S.gguf](https://huggingface.co/RichardErkhov/Replete-AI_-_Llama-3-11.5B-Instruct-V2-gguf/blob/main/Llama-3-11.5B-Instruct-V2.Q4_K_S.gguf) | Q4_K_S | 6.21GB |
| [Llama-3-11.5B-Instruct-V2.Q4_K.gguf](https://huggingface.co/RichardErkhov/Replete-AI_-_Llama-3-11.5B-Instruct-V2-gguf/blob/main/Llama-3-11.5B-Instruct-V2.Q4_K.gguf) | Q4_K | 6.53GB |
| [Llama-3-11.5B-Instruct-V2.Q4_K_M.gguf](https://huggingface.co/RichardErkhov/Replete-AI_-_Llama-3-11.5B-Instruct-V2-gguf/blob/main/Llama-3-11.5B-Instruct-V2.Q4_K_M.gguf) | Q4_K_M | 6.53GB |
| [Llama-3-11.5B-Instruct-V2.Q4_1.gguf](https://huggingface.co/RichardErkhov/Replete-AI_-_Llama-3-11.5B-Instruct-V2-gguf/blob/main/Llama-3-11.5B-Instruct-V2.Q4_1.gguf) | Q4_1 | 6.81GB |
| [Llama-3-11.5B-Instruct-V2.Q5_0.gguf](https://huggingface.co/RichardErkhov/Replete-AI_-_Llama-3-11.5B-Instruct-V2-gguf/blob/main/Llama-3-11.5B-Instruct-V2.Q5_0.gguf) | Q5_0 | 7.45GB |
| [Llama-3-11.5B-Instruct-V2.Q5_K_S.gguf](https://huggingface.co/RichardErkhov/Replete-AI_-_Llama-3-11.5B-Instruct-V2-gguf/blob/main/Llama-3-11.5B-Instruct-V2.Q5_K_S.gguf) | Q5_K_S | 7.45GB |
| [Llama-3-11.5B-Instruct-V2.Q5_K.gguf](https://huggingface.co/RichardErkhov/Replete-AI_-_Llama-3-11.5B-Instruct-V2-gguf/blob/main/Llama-3-11.5B-Instruct-V2.Q5_K.gguf) | Q5_K | 7.64GB |
| [Llama-3-11.5B-Instruct-V2.Q5_K_M.gguf](https://huggingface.co/RichardErkhov/Replete-AI_-_Llama-3-11.5B-Instruct-V2-gguf/blob/main/Llama-3-11.5B-Instruct-V2.Q5_K_M.gguf) | Q5_K_M | 7.64GB |
| [Llama-3-11.5B-Instruct-V2.Q5_1.gguf](https://huggingface.co/RichardErkhov/Replete-AI_-_Llama-3-11.5B-Instruct-V2-gguf/blob/main/Llama-3-11.5B-Instruct-V2.Q5_1.gguf) | Q5_1 | 8.09GB |
| [Llama-3-11.5B-Instruct-V2.Q6_K.gguf](https://huggingface.co/RichardErkhov/Replete-AI_-_Llama-3-11.5B-Instruct-V2-gguf/blob/main/Llama-3-11.5B-Instruct-V2.Q6_K.gguf) | Q6_K | 8.81GB |
| [Llama-3-11.5B-Instruct-V2.Q8_0.gguf](https://huggingface.co/RichardErkhov/Replete-AI_-_Llama-3-11.5B-Instruct-V2-gguf/blob/main/Llama-3-11.5B-Instruct-V2.Q8_0.gguf) | Q8_0 | 11.41GB |
Original model description:
---
license: other
license_name: llama-3
license_link: https://llama.meta.com/llama3/license/
---
Llama-3-11.5B-Instruct-v2
Thank you to Meta for the weights for Meta-Llama-3-8B-Instruct

This is an upscaling of the Meta-Llama-3-8B-Instruct Ai using techniques created for chargoddard/mistral-11b-slimorca. This Ai model has been upscaled from 8b parameters to 11.5b parameters without any continuous pretraining or fine-tuning.
Unlike version 1 this model has no issues at fp16 or any quantizations.
The model that was used to create this one is linked below:
https://huggingface.co/meta-llama/Meta-Llama-3-8B-Instruct
______________________________________________________________________________
- Llama-3-11.5B-Instruct-V2
| Metric | Value |
|----------------------------------|-------:|
| Avg. | 63.91 |
| AI2 Reasoning Challenge (25-Shot)| 57.68 |
| HellaSwag (10-Shot) | 78.59 |
| MMLU (5-Shot) | 67.35 |
| TruthfulQA (0-shot) | 35.86 |
| Winogrande (5-shot) | 74.74 |
| GSM8k (5-shot) | 69.37 |
- Original Meta-Llama-3-8B-Instruct
| Metric | Value |
|----------------------------------|-------:|
| Avg. | 66.87 |
| AI2 Reasoning Challenge (25-Shot)| 60.75 |
| HellaSwag (10-Shot) | 78.55 |
| MMLU (5-Shot) | 67.07 |
| TruthfulQA (0-shot) | 51.65 |
| Winogrande (5-shot) | 74.51 |
| GSM8k (5-shot) | 68.69 |
|
ahxt/LiteLlama-460M-1T | ahxt | 2024-01-08T21:30:33Z | 21,226 | 161 | transformers | [
"transformers",
"pytorch",
"llama",
"text-generation",
"llama2",
"llama-2",
"llama2 architecture",
"litellama",
"en",
"dataset:Redpajama",
"license:mit",
"autotrain_compatible",
"endpoints_compatible",
"text-generation-inference",
"region:us"
] | text-generation | 2024-01-07T01:49:08Z | ---
language:
- en
tags:
- llama2
- llama-2
- llama
- llama2 architecture
- litellama
datasets:
- Redpajama
metrics:
- MMLU
license: mit
widget:
- text: "Q: What is the largest bird?\\nA:"
---
# LiteLlama: Reduced-Scale Llama
We present an open-source reproduction of Meta AI's [LLaMa 2](https://ai.meta.com/llama/). However, with significantly reduced model sizes, [LiteLlama-460M-1T](https://huggingface.co/ahxt/LiteLlama-460M-1T) has 460M parameters trained with 1T tokens.
## Dataset and Tokenization
We train our models on part of [RedPajama](https://www.together.xyz/blog/redpajama) dataset. We use the [GPT2Tokenizer](https://huggingface.co/docs/transformers/v4.31.0/en/model_doc/gpt2#transformers.GPT2Tokenizer) to tokenize the text.
## Training Details
The model was trained with ~1T tokens (0.98T). num of tokens = steps*length*batch_size=499679*1024*192=98240888832≈0.98T.
The training curve is at this [WandB project](https://wandb.ai/ahxt/llama2_xs_460M_training_loss/reports/reduced_train_loss-23-09-05-20-25-43---Vmlldzo1MzIwNDUx?accessToken=x2ch3n30jo77p1x8y7q9js4h4d8zpjtz1tzot4xxullyefixp4jwt7au2q37k2q6).
### Using with HuggingFace Transformers
The experimental checkpoints can be directly loaded by [Transformers](https://huggingface.co/transformers/) library. The following code snippet shows how to load the our experimental model and generate text with it.
```python
import torch
from transformers import AutoTokenizer, AutoModelForCausalLM
model_path = 'ahxt/LiteLlama-460M-1T'
model = AutoModelForCausalLM.from_pretrained(model_path)
tokenizer = AutoTokenizer.from_pretrained(model_path)
model.eval()
prompt = 'Q: What is the largest bird?\nA:'
input_ids = tokenizer(prompt, return_tensors="pt").input_ids
tokens = model.generate(input_ids, max_length=20)
print( tokenizer.decode(tokens[0].tolist(), skip_special_tokens=True) )
# Q: What is the largest bird?\nA: The largest bird is a black-headed gull.
```
## Evaluation
### We evaluate our models on the MMLU task.
| Models | #parameters |zero-shot | 5-shot |
| --- | --- | --- | --- |
| llama | 7B | 28.46 | 35.05 |
| openllama | 3B | 24.90 | 26.71 |
|TinyLlama-1.1B-step-50K-105b | 1.1B | 19.00 | 26.53 |
| LiteLlama-460M-1T | 0.46B | 21.13 | 26.39 |
### [Open LLM Leaderboard Evaluation Results](https://huggingface.co/spaces/HuggingFaceH4/open_llm_leaderboard)
Detailed results can be found [here](https://huggingface.co/datasets/open-llm-leaderboard/details_ahxt__llama2_xs_460M_experimental)
| Metric | Value |
|-----------------------|---------------------------|
| Avg. | 26.65 |
| ARC (25-shot) | 24.91 |
| HellaSwag (10-shot) | 38.47 |
| MMLU (5-shot) | 26.17 |
| TruthfulQA (0-shot) | 41.59 |
| Winogrande (5-shot) | 49.88 |
| GSM8K (5-shot) | 0.0 |
| DROP (3-shot) | 5.51 |
## Contact
This model was developed by [Xiaotian Han](https://ahxt.github.io/) from Texas A&M University at the DATA Lab under the supervision of Prof. [Xia "Ben" Hu](https://cs.rice.edu/~xh37/index.html), and the model is released under MIT License.
|
cardiffnlp/twitter-roberta-base-emotion | cardiffnlp | 2023-05-28T05:08:00Z | 21,187 | 42 | transformers | [
"transformers",
"pytorch",
"tf",
"jax",
"roberta",
"text-classification",
"arxiv:2010.12421",
"autotrain_compatible",
"endpoints_compatible",
"region:us"
] | text-classification | 2022-03-02T23:29:05Z | # Twitter-roBERTa-base for Emotion Recognition
This is a RoBERTa-base model trained on ~58M tweets and finetuned for emotion recognition with the TweetEval benchmark.
- Paper: [_TweetEval_ benchmark (Findings of EMNLP 2020)](https://arxiv.org/pdf/2010.12421.pdf).
- Git Repo: [Tweeteval official repository](https://github.com/cardiffnlp/tweeteval).
<b>New!</b> We just released a new emotion recognition model trained with more emotion types and with a newer RoBERTa-based model.
See [twitter-roberta-base-emotion-multilabel-latest](https://huggingface.co/cardiffnlp/twitter-roberta-base-emotion-multilabel-latest) and [TweetNLP](https://github.com/cardiffnlp/tweetnlp) for more details.
## Example of classification
```python
from transformers import AutoModelForSequenceClassification
from transformers import TFAutoModelForSequenceClassification
from transformers import AutoTokenizer
import numpy as np
from scipy.special import softmax
import csv
import urllib.request
# Preprocess text (username and link placeholders)
def preprocess(text):
new_text = []
for t in text.split(" "):
t = '@user' if t.startswith('@') and len(t) > 1 else t
t = 'http' if t.startswith('http') else t
new_text.append(t)
return " ".join(new_text)
# Tasks:
# emoji, emotion, hate, irony, offensive, sentiment
# stance/abortion, stance/atheism, stance/climate, stance/feminist, stance/hillary
task='emotion'
MODEL = f"cardiffnlp/twitter-roberta-base-{task}"
tokenizer = AutoTokenizer.from_pretrained(MODEL)
# download label mapping
mapping_link = f"https://raw.githubusercontent.com/cardiffnlp/tweeteval/main/datasets/{task}/mapping.txt"
with urllib.request.urlopen(mapping_link) as f:
html = f.read().decode('utf-8').split("\n")
csvreader = csv.reader(html, delimiter='\t')
labels = [row[1] for row in csvreader if len(row) > 1]
# PT
model = AutoModelForSequenceClassification.from_pretrained(MODEL)
model.save_pretrained(MODEL)
text = "Celebrating my promotion 😎"
text = preprocess(text)
encoded_input = tokenizer(text, return_tensors='pt')
output = model(**encoded_input)
scores = output[0][0].detach().numpy()
scores = softmax(scores)
# # TF
# model = TFAutoModelForSequenceClassification.from_pretrained(MODEL)
# model.save_pretrained(MODEL)
# text = "Celebrating my promotion 😎"
# encoded_input = tokenizer(text, return_tensors='tf')
# output = model(encoded_input)
# scores = output[0][0].numpy()
# scores = softmax(scores)
ranking = np.argsort(scores)
ranking = ranking[::-1]
for i in range(scores.shape[0]):
l = labels[ranking[i]]
s = scores[ranking[i]]
print(f"{i+1}) {l} {np.round(float(s), 4)}")
```
Output:
```
1) joy 0.9382
2) optimism 0.0362
3) anger 0.0145
4) sadness 0.0112
```
|
kwoncho/gaincut_news_pre2022 | kwoncho | 2024-06-15T05:04:21Z | 21,112 | 0 | transformers | [
"transformers",
"pytorch",
"roberta",
"text-classification",
"autotrain_compatible",
"endpoints_compatible",
"region:us"
] | text-classification | 2024-04-25T04:46:15Z | Entry not found |
stablediffusionapi/opendalle | stablediffusionapi | 2023-12-19T19:58:11Z | 21,093 | 5 | diffusers | [
"diffusers",
"stablediffusionapi.com",
"stable-diffusion-api",
"text-to-image",
"ultra-realistic",
"license:creativeml-openrail-m",
"autotrain_compatible",
"endpoints_compatible",
"diffusers:StableDiffusionXLPipeline",
"region:us"
] | text-to-image | 2023-12-19T19:52:50Z | ---
license: creativeml-openrail-m
tags:
- stablediffusionapi.com
- stable-diffusion-api
- text-to-image
- ultra-realistic
pinned: true
---
# OpenDalle API Inference
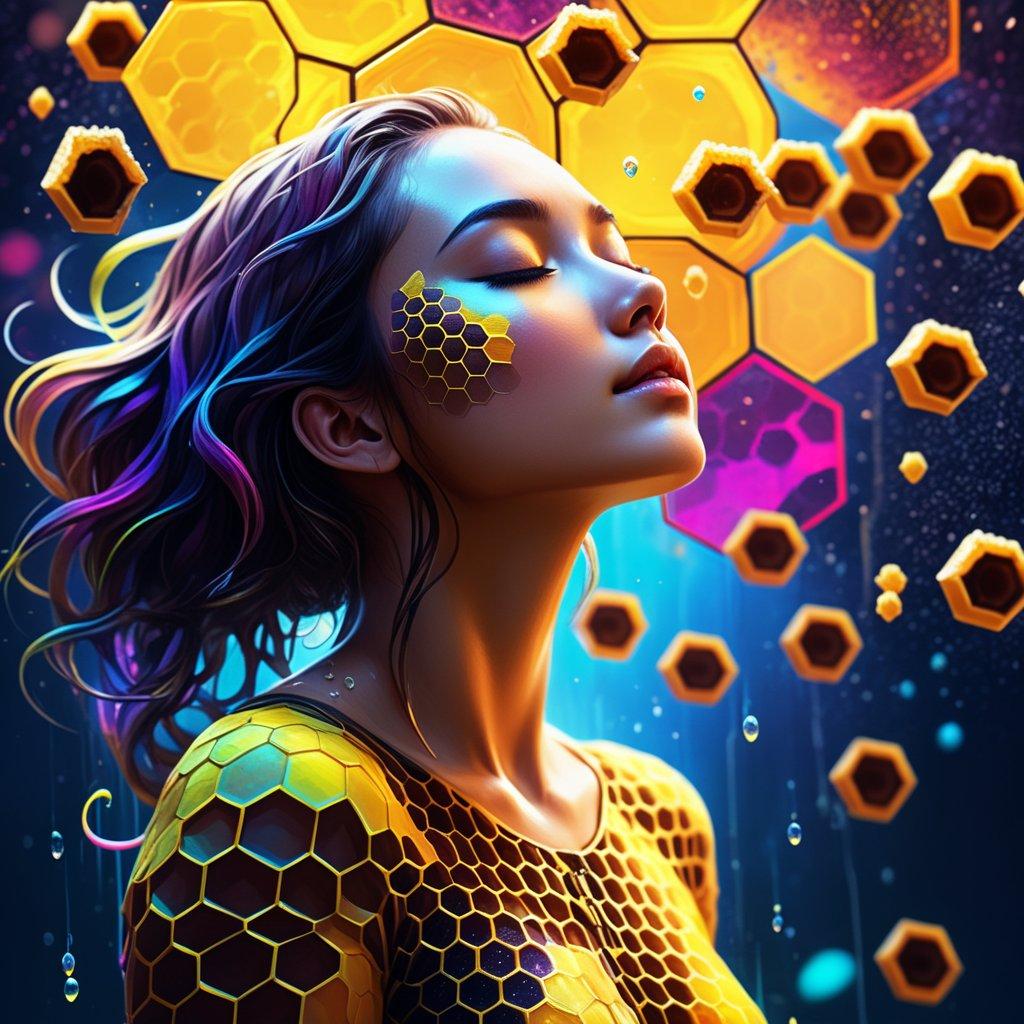
## Get API Key
Get API key from [Stable Diffusion API](http://stablediffusionapi.com/), No Payment needed.
Replace Key in below code, change **model_id** to "opendalle"
Coding in PHP/Node/Java etc? Have a look at docs for more code examples: [View docs](https://stablediffusionapi.com/docs)
Try model for free: [Generate Images](https://stablediffusionapi.com/models/opendalle)
Model link: [View model](https://stablediffusionapi.com/models/opendalle)
Credits: [View credits](https://civitai.com/?query=OpenDalle)
View all models: [View Models](https://stablediffusionapi.com/models)
import requests
import json
url = "https://stablediffusionapi.com/api/v4/dreambooth"
payload = json.dumps({
"key": "your_api_key",
"model_id": "opendalle",
"prompt": "ultra realistic close up portrait ((beautiful pale cyberpunk female with heavy black eyeliner)), blue eyes, shaved side haircut, hyper detail, cinematic lighting, magic neon, dark red city, Canon EOS R3, nikon, f/1.4, ISO 200, 1/160s, 8K, RAW, unedited, symmetrical balance, in-frame, 8K",
"negative_prompt": "painting, extra fingers, mutated hands, poorly drawn hands, poorly drawn face, deformed, ugly, blurry, bad anatomy, bad proportions, extra limbs, cloned face, skinny, glitchy, double torso, extra arms, extra hands, mangled fingers, missing lips, ugly face, distorted face, extra legs, anime",
"width": "512",
"height": "512",
"samples": "1",
"num_inference_steps": "30",
"safety_checker": "no",
"enhance_prompt": "yes",
"seed": None,
"guidance_scale": 7.5,
"multi_lingual": "no",
"panorama": "no",
"self_attention": "no",
"upscale": "no",
"embeddings": "embeddings_model_id",
"lora": "lora_model_id",
"webhook": None,
"track_id": None
})
headers = {
'Content-Type': 'application/json'
}
response = requests.request("POST", url, headers=headers, data=payload)
print(response.text)
> Use this coupon code to get 25% off **DMGG0RBN** |
liuhaotian/llava-v1.6-vicuna-13b | liuhaotian | 2024-05-09T20:12:26Z | 21,087 | 42 | transformers | [
"transformers",
"safetensors",
"llava",
"text-generation",
"image-text-to-text",
"autotrain_compatible",
"region:us"
] | image-text-to-text | 2024-01-31T04:48:16Z | ---
inference: false
pipeline_tag: image-text-to-text
---
<br>
<br>
# LLaVA Model Card
## Model details
**Model type:**
LLaVA is an open-source chatbot trained by fine-tuning LLM on multimodal instruction-following data.
It is an auto-regressive language model, based on the transformer architecture.
Base LLM: [lmsys/vicuna-13b-v1.5](https://huggingface.co/lmsys/vicuna-13b-v1.5)
**Model date:**
LLaVA-v1.6-Vicuna-13B was trained in December 2023.
**Paper or resources for more information:**
https://llava-vl.github.io/
## License
Llama 2 is licensed under the LLAMA 2 Community License,
Copyright (c) Meta Platforms, Inc. All Rights Reserved.
**Where to send questions or comments about the model:**
https://github.com/haotian-liu/LLaVA/issues
## Intended use
**Primary intended uses:**
The primary use of LLaVA is research on large multimodal models and chatbots.
**Primary intended users:**
The primary intended users of the model are researchers and hobbyists in computer vision, natural language processing, machine learning, and artificial intelligence.
## Training dataset
- 558K filtered image-text pairs from LAION/CC/SBU, captioned by BLIP.
- 158K GPT-generated multimodal instruction-following data.
- 500K academic-task-oriented VQA data mixture.
- 50K GPT-4V data mixture.
- 40K ShareGPT data.
## Evaluation dataset
A collection of 12 benchmarks, including 5 academic VQA benchmarks and 7 recent benchmarks specifically proposed for instruction-following LMMs. |
Sao10K/Fimbulvetr-11B-v2-GGUF | Sao10K | 2024-03-02T16:15:52Z | 21,071 | 89 | null | [
"gguf",
"en",
"license:cc-by-nc-4.0",
"region:us"
] | null | 2024-02-06T14:41:18Z | ---
license: cc-by-nc-4.0
language:
- en
---
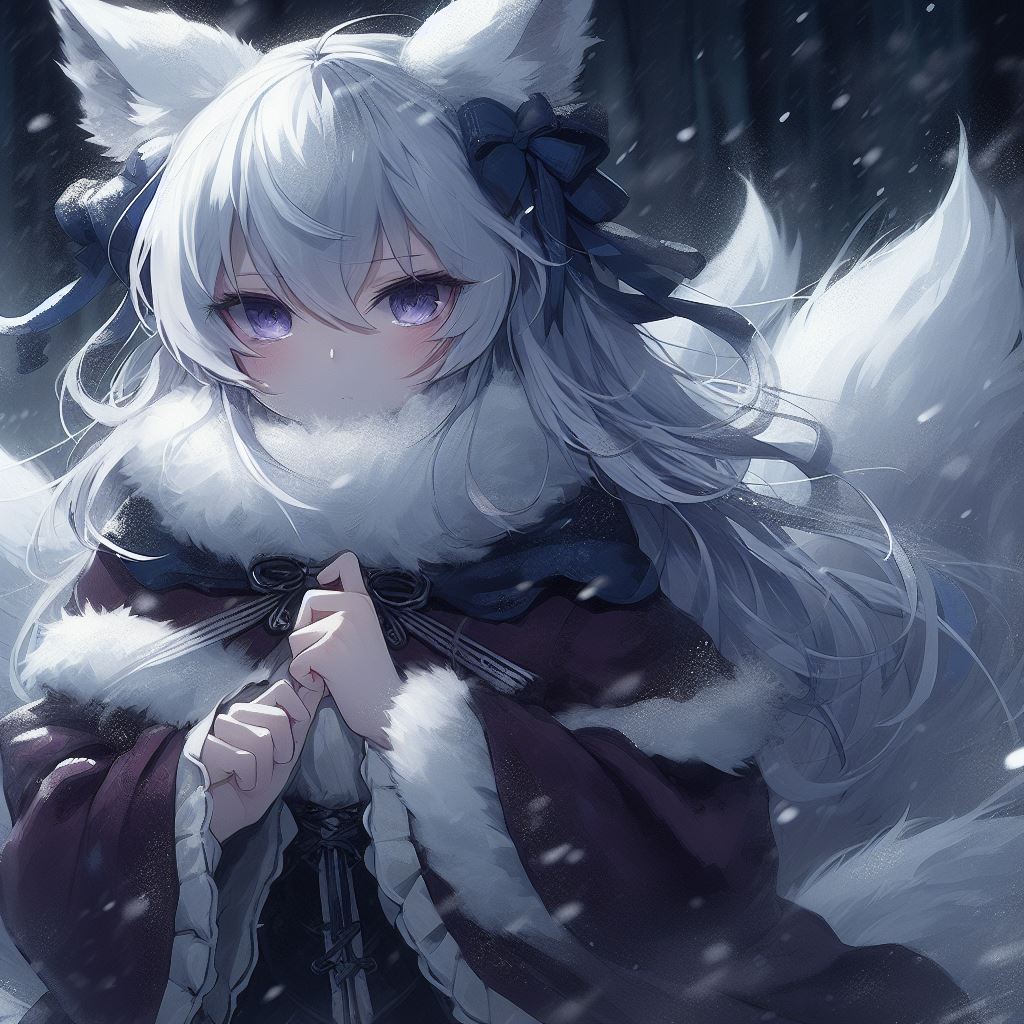
*Cute girl to catch your attention.*
**https://huggingface.co/Sao10K/Fimbulvetr-11B-v2 <------ fp16**
Fimbulvetr-v2 - A Solar-Based Model
**THESE ARE GGUF QUANTS**
additional gguf quants \(imatrix and the rest) by [mradermacher](https://huggingface.co/mradermacher)
https://huggingface.co/mradermacher/Fimbulvetr-11B-v2-GGUF
https://huggingface.co/mradermacher/Fimbulvetr-11B-v2-i1-GGUF
***
Prompt Formats - Alpaca or Vicuna. Either one works fine.
Recommended SillyTavern Presets - Universal Light
Alpaca:
```
### Instruction:
<Prompt>
### Input:
<Insert Context Here>
### Response:
```
Vicuna:
```
System: <Prompt>
User: <Input>
Assistant:
```
****
Changelogs:
25/2 - repo renamed to remove test, model card redone. Model's officially out.
<br>15/2 - Heavy testing complete. Good feedback.
***
<details><summary>Rant - Kept For Historical Reasons</summary>
Ramble to meet minimum length requirements:
Tbh i wonder if this shit is even worth doing. Like im just some broke guy lmao I've spent so much. And for what? I guess creds. Feels good when a model gets good feedback, but it seems like im invisible sometimes. I should be probably advertising myself and my models on other places but I rarely have the time to. Probably just internal jealousy sparking up here and now. Wahtever I guess.
Anyway cool EMT vocation I'm doing is cool except it pays peanuts, damn bruh 1.1k per month lmao. Government to broke to pay for shit. Pays the bills I suppose.
Anyway cool beans, I'm either going to continue the Solar Train or go to Mixtral / Yi when I get paid.
You still here?
</details><br>
|
mradermacher/Llama3-CASAuditSBase-8B-GGUF | mradermacher | 2024-06-29T06:21:50Z | 21,056 | 0 | transformers | [
"transformers",
"gguf",
"en",
"base_model:jamesohe/Llama3-CASAuditSBase-8B",
"endpoints_compatible",
"region:us"
] | null | 2024-06-29T04:17:38Z | ---
base_model: jamesohe/Llama3-CASAuditSBase-8B
language:
- en
library_name: transformers
quantized_by: mradermacher
tags: []
---
## About
<!-- ### quantize_version: 2 -->
<!-- ### output_tensor_quantised: 1 -->
<!-- ### convert_type: hf -->
<!-- ### vocab_type: -->
<!-- ### tags: -->
static quants of https://huggingface.co/jamesohe/Llama3-CASAuditSBase-8B
<!-- provided-files -->
weighted/imatrix quants seem not to be available (by me) at this time. If they do not show up a week or so after the static ones, I have probably not planned for them. Feel free to request them by opening a Community Discussion.
## Usage
If you are unsure how to use GGUF files, refer to one of [TheBloke's
READMEs](https://huggingface.co/TheBloke/KafkaLM-70B-German-V0.1-GGUF) for
more details, including on how to concatenate multi-part files.
## Provided Quants
(sorted by size, not necessarily quality. IQ-quants are often preferable over similar sized non-IQ quants)
| Link | Type | Size/GB | Notes |
|:-----|:-----|--------:|:------|
| [GGUF](https://huggingface.co/mradermacher/Llama3-CASAuditSBase-8B-GGUF/resolve/main/Llama3-CASAuditSBase-8B.Q2_K.gguf) | Q2_K | 3.3 | |
| [GGUF](https://huggingface.co/mradermacher/Llama3-CASAuditSBase-8B-GGUF/resolve/main/Llama3-CASAuditSBase-8B.IQ3_XS.gguf) | IQ3_XS | 3.6 | |
| [GGUF](https://huggingface.co/mradermacher/Llama3-CASAuditSBase-8B-GGUF/resolve/main/Llama3-CASAuditSBase-8B.Q3_K_S.gguf) | Q3_K_S | 3.8 | |
| [GGUF](https://huggingface.co/mradermacher/Llama3-CASAuditSBase-8B-GGUF/resolve/main/Llama3-CASAuditSBase-8B.IQ3_S.gguf) | IQ3_S | 3.8 | beats Q3_K* |
| [GGUF](https://huggingface.co/mradermacher/Llama3-CASAuditSBase-8B-GGUF/resolve/main/Llama3-CASAuditSBase-8B.IQ3_M.gguf) | IQ3_M | 3.9 | |
| [GGUF](https://huggingface.co/mradermacher/Llama3-CASAuditSBase-8B-GGUF/resolve/main/Llama3-CASAuditSBase-8B.Q3_K_M.gguf) | Q3_K_M | 4.1 | lower quality |
| [GGUF](https://huggingface.co/mradermacher/Llama3-CASAuditSBase-8B-GGUF/resolve/main/Llama3-CASAuditSBase-8B.Q3_K_L.gguf) | Q3_K_L | 4.4 | |
| [GGUF](https://huggingface.co/mradermacher/Llama3-CASAuditSBase-8B-GGUF/resolve/main/Llama3-CASAuditSBase-8B.IQ4_XS.gguf) | IQ4_XS | 4.6 | |
| [GGUF](https://huggingface.co/mradermacher/Llama3-CASAuditSBase-8B-GGUF/resolve/main/Llama3-CASAuditSBase-8B.Q4_K_S.gguf) | Q4_K_S | 4.8 | fast, recommended |
| [GGUF](https://huggingface.co/mradermacher/Llama3-CASAuditSBase-8B-GGUF/resolve/main/Llama3-CASAuditSBase-8B.Q4_K_M.gguf) | Q4_K_M | 5.0 | fast, recommended |
| [GGUF](https://huggingface.co/mradermacher/Llama3-CASAuditSBase-8B-GGUF/resolve/main/Llama3-CASAuditSBase-8B.Q5_K_S.gguf) | Q5_K_S | 5.7 | |
| [GGUF](https://huggingface.co/mradermacher/Llama3-CASAuditSBase-8B-GGUF/resolve/main/Llama3-CASAuditSBase-8B.Q5_K_M.gguf) | Q5_K_M | 5.8 | |
| [GGUF](https://huggingface.co/mradermacher/Llama3-CASAuditSBase-8B-GGUF/resolve/main/Llama3-CASAuditSBase-8B.Q6_K.gguf) | Q6_K | 6.7 | very good quality |
| [GGUF](https://huggingface.co/mradermacher/Llama3-CASAuditSBase-8B-GGUF/resolve/main/Llama3-CASAuditSBase-8B.Q8_0.gguf) | Q8_0 | 8.6 | fast, best quality |
| [GGUF](https://huggingface.co/mradermacher/Llama3-CASAuditSBase-8B-GGUF/resolve/main/Llama3-CASAuditSBase-8B.f16.gguf) | f16 | 16.2 | 16 bpw, overkill |
Here is a handy graph by ikawrakow comparing some lower-quality quant
types (lower is better):

And here are Artefact2's thoughts on the matter:
https://gist.github.com/Artefact2/b5f810600771265fc1e39442288e8ec9
## FAQ / Model Request
See https://huggingface.co/mradermacher/model_requests for some answers to
questions you might have and/or if you want some other model quantized.
## Thanks
I thank my company, [nethype GmbH](https://www.nethype.de/), for letting
me use its servers and providing upgrades to my workstation to enable
this work in my free time.
<!-- end -->
|
parler-tts/dac_44khZ_8kbps | parler-tts | 2024-04-10T11:43:09Z | 21,041 | 13 | transformers | [
"transformers",
"safetensors",
"dac",
"DAC",
"audio",
"arxiv:2306.06546",
"license:mit",
"endpoints_compatible",
"region:us"
] | null | 2024-02-28T15:10:52Z | ---
library_name: transformers
tags:
- DAC
- audio
license: mit
---
# Descript Audio Codec (.dac): High-Fidelity Audio Compression with Improved RVQGAN
This repository is a wrapper around the original **Descript Audio Codec** model, a high fidelity general neural audio codec, introduced in the paper titled **High-Fidelity Audio Compression with Improved RVQGAN**.
It is designed to be used as a drop-in replacement of the [transformers implementation](https://huggingface.co/docs/transformers/v4.39.3/en/model_doc/encodec#overview) of [Encodec](https://github.com/facebookresearch/encodec), so that architectures that use Encodec can also be trained with DAC instead.
The [Parler-TTS library](https://github.com/huggingface/parler-tts) is an example of how to use DAC to train high-quality TTS models. We released [Parler-TTS Mini v0.1]("https://huggingface.co/parler-tts/parler_tts_mini_v0.1"), a first iteration model trained using 10k hours of narrated audiobooks. It generates high-quality speech with features that can be controlled using a simple text prompt (e.g. gender, background noise, speaking rate, pitch and reverberation)
To use this checkpoint, you first need to install the [Parler-TTS library](https://github.com/huggingface/parler-tts) with (to do once):
```sh
pip install git+https://github.com/huggingface/parler-tts.git
```
And then use:
```python
from parler_tts import DACModel
dac_model = DACModel.from_pretrained("parler-tts/dac_44khZ_8kbps")
```
🚨 If you want to use the original DAC codebase, refers to the [original repository](https://github.com/descriptinc/descript-audio-codec/tree/main) or to the [Original Usage](#original-usage) section.
## Original Usage
[arXiv Paper: High-Fidelity Audio Compression with Improved RVQGAN
](http://arxiv.org/abs/2306.06546) <br>
[Demo Site](https://descript.notion.site/Descript-Audio-Codec-11389fce0ce2419891d6591a68f814d5)<br>
[Github repo](https://github.com/descriptinc/descript-audio-codec/tree/main)<br>
👉 With Descript Audio Codec, you can compress **44.1 KHz audio** into discrete codes at a **low 8 kbps bitrate**. <br>
🤌 That's approximately **90x compression** while maintaining exceptional fidelity and minimizing artifacts. <br>
💪 Descript universal model works on all domains (speech, environment, music, etc.), making it widely applicable to generative modeling of all audio. <br>
👌 It can be used as a drop-in replacement for EnCodec for all audio language modeling applications (such as AudioLMs, MusicLMs, MusicGen, etc.) <br>
### Installation
```
pip install descript-audio-codec
```
OR
```
pip install git+https://github.com/descriptinc/descript-audio-codec
```
### Weights
Weights are released as part of this repo under MIT license.
We release weights for models that can natively support 16 kHz, 24kHz, and 44.1kHz sampling rates.
Weights are automatically downloaded when you first run `encode` or `decode` command. You can cache them using one of the following commands
```bash
python3 -m dac download # downloads the default 44kHz variant
python3 -m dac download --model_type 44khz # downloads the 44kHz variant
python3 -m dac download --model_type 24khz # downloads the 24kHz variant
python3 -m dac download --model_type 16khz # downloads the 16kHz variant
```
We provide a Dockerfile that installs all required dependencies for encoding and decoding. The build process caches the default model weights inside the image. This allows the image to be used without an internet connection. [Please refer to instructions below.](#docker-image)
### Compress audio
```
python3 -m dac encode /path/to/input --output /path/to/output/codes
```
This command will create `.dac` files with the same name as the input files.
It will also preserve the directory structure relative to input root and
re-create it in the output directory. Please use `python -m dac encode --help`
for more options.
### Reconstruct audio from compressed codes
```
python3 -m dac decode /path/to/output/codes --output /path/to/reconstructed_input
```
This command will create `.wav` files with the same name as the input files.
It will also preserve the directory structure relative to input root and
re-create it in the output directory. Please use `python -m dac decode --help`
for more options.
### Programmatic Usage
```py
import dac
from audiotools import AudioSignal
# Download a model
model_path = dac.utils.download(model_type="44khz")
model = dac.DAC.load(model_path)
model.to('cuda')
# Load audio signal file
signal = AudioSignal('input.wav')
# Encode audio signal as one long file
# (may run out of GPU memory on long files)
signal.to(model.device)
x = model.preprocess(signal.audio_data, signal.sample_rate)
z, codes, latents, _, _ = model.encode(x)
# Decode audio signal
y = model.decode(z)
# Alternatively, use the `compress` and `decompress` functions
# to compress long files.
signal = signal.cpu()
x = model.compress(signal)
# Save and load to and from disk
x.save("compressed.dac")
x = dac.DACFile.load("compressed.dac")
# Decompress it back to an AudioSignal
y = model.decompress(x)
# Write to file
y.write('output.wav')
``` |
alignment-handbook/zephyr-7b-sft-full | alignment-handbook | 2024-01-10T03:18:43Z | 21,013 | 20 | transformers | [
"transformers",
"tensorboard",
"safetensors",
"mistral",
"text-generation",
"alignment-handbook",
"generated_from_trainer",
"trl",
"sft",
"conversational",
"dataset:HuggingFaceH4/ultrachat_200k",
"base_model:mistralai/Mistral-7B-v0.1",
"license:apache-2.0",
"autotrain_compatible",
"endpoints_compatible",
"text-generation-inference",
"region:us"
] | text-generation | 2023-11-09T12:20:33Z | ---
license: apache-2.0
base_model: mistralai/Mistral-7B-v0.1
tags:
- alignment-handbook
- generated_from_trainer
- trl
- sft
- generated_from_trainer
datasets:
- HuggingFaceH4/ultrachat_200k
model-index:
- name: zephyr-7b-sft-full
results: []
---
<!-- This model card has been generated automatically according to the information the Trainer had access to. You
should probably proofread and complete it, then remove this comment. -->
# zephyr-7b-sft-full
This model is a fine-tuned version of [mistralai/Mistral-7B-v0.1](https://huggingface.co/mistralai/Mistral-7B-v0.1) on the HuggingFaceH4/ultrachat_200k dataset.
It achieves the following results on the evaluation set:
- Loss: 0.9353
## Model description
More information needed
## Intended uses & limitations
More information needed
## Training and evaluation data
More information needed
## Training procedure
### Training hyperparameters
The following hyperparameters were used during training:
- learning_rate: 2e-05
- train_batch_size: 16
- eval_batch_size: 8
- seed: 42
- distributed_type: multi-GPU
- num_devices: 8
- total_train_batch_size: 128
- total_eval_batch_size: 64
- optimizer: Adam with betas=(0.9,0.999) and epsilon=1e-08
- lr_scheduler_type: cosine
- lr_scheduler_warmup_ratio: 0.1
- num_epochs: 1
### Training results
| Training Loss | Epoch | Step | Validation Loss |
|:-------------:|:-----:|:----:|:---------------:|
| 0.9075 | 1.0 | 1090 | 0.9353 |
### Framework versions
- Transformers 4.36.2
- Pytorch 2.1.2+cu121
- Datasets 2.14.6
- Tokenizers 0.15.0
|
SG161222/Realistic_Vision_V1.4 | SG161222 | 2024-04-12T15:41:10Z | 20,992 | 338 | diffusers | [
"diffusers",
"stable-diffusion",
"text-to-image",
"license:creativeml-openrail-m",
"autotrain_compatible",
"endpoints_compatible",
"diffusers:StableDiffusionPipeline",
"region:us"
] | text-to-image | 2023-03-05T13:17:54Z | ---
license: creativeml-openrail-m
tags:
- stable-diffusion
- text-to-image
---
<b>Please read this!</b><br>
My model has always been free and always will be free. There are no restrictions on the use of the model. The rights to this model still belong to me.
<b>You can support me directly on Boosty - https://boosty.to/sg_161222</b><br>
<hr/>
<b>Important note: "RAW photo" in the prompt may degrade the result.</b>
<b>I use this template to get good generation results:
Prompt:</b>
*subject*, (high detailed skin:1.2), 8k uhd, dslr, soft lighting, high quality, film grain, Fujifilm XT3
<b>Example:</b> a close up portrait photo of 26 y.o woman in wastelander clothes, long haircut, pale skin, slim body, background is city ruins, (high detailed skin:1.2), 8k uhd, dslr, soft lighting, high quality, film grain, Fujifilm XT3
<b>Negative Prompt:</b>
(deformed iris, deformed pupils, semi-realistic, cgi, 3d, render, sketch, cartoon, drawing, anime:1.4), text, close up, cropped, out of frame, worst quality, low quality, jpeg artifacts, ugly, duplicate, morbid, mutilated, extra fingers, mutated hands, poorly drawn hands, poorly drawn face, mutation, deformed, blurry, dehydrated, bad anatomy, bad proportions, extra limbs, cloned face, disfigured, gross proportions, malformed limbs, missing arms, missing legs, extra arms, extra legs, fused fingers, too many fingers, long neck<br>
<b>OR</b><br>
(deformed iris, deformed pupils, semi-realistic, cgi, 3d, render, sketch, cartoon, drawing, anime, mutated hands and fingers:1.4), (deformed, distorted, disfigured:1.3), poorly drawn, bad anatomy, wrong anatomy, extra limb, missing limb, floating limbs, disconnected limbs, mutation, mutated, ugly, disgusting, amputation
<b>Euler A or DPM++ 2M Karras with 25 steps<br>
CFG Scale 3,5 - 7<br>
Hires. fix with Latent upscaler<br>
0 Hires steps and Denoising strength 0.25-0.45<br>
Upscale by 1.1-2.0</b> |
mradermacher/L3-NymTA-15B-GGUF | mradermacher | 2024-06-24T20:31:38Z | 20,986 | 0 | transformers | [
"transformers",
"gguf",
"mergekit",
"merge",
"en",
"base_model:Frowning/L3-NymTA-15B",
"endpoints_compatible",
"region:us"
] | null | 2024-06-24T19:44:31Z | ---
base_model: Frowning/L3-NymTA-15B
language:
- en
library_name: transformers
quantized_by: mradermacher
tags:
- mergekit
- merge
---
## About
<!-- ### quantize_version: 2 -->
<!-- ### output_tensor_quantised: 1 -->
<!-- ### convert_type: hf -->
<!-- ### vocab_type: -->
<!-- ### tags: -->
static quants of https://huggingface.co/Frowning/L3-NymTA-15B
<!-- provided-files -->
weighted/imatrix quants seem not to be available (by me) at this time. If they do not show up a week or so after the static ones, I have probably not planned for them. Feel free to request them by opening a Community Discussion.
## Usage
If you are unsure how to use GGUF files, refer to one of [TheBloke's
READMEs](https://huggingface.co/TheBloke/KafkaLM-70B-German-V0.1-GGUF) for
more details, including on how to concatenate multi-part files.
## Provided Quants
(sorted by size, not necessarily quality. IQ-quants are often preferable over similar sized non-IQ quants)
| Link | Type | Size/GB | Notes |
|:-----|:-----|--------:|:------|
| [GGUF](https://huggingface.co/mradermacher/L3-NymTA-15B-GGUF/resolve/main/L3-NymTA-15B.Q2_K.gguf) | Q2_K | 5.8 | |
| [GGUF](https://huggingface.co/mradermacher/L3-NymTA-15B-GGUF/resolve/main/L3-NymTA-15B.IQ3_XS.gguf) | IQ3_XS | 6.5 | |
| [GGUF](https://huggingface.co/mradermacher/L3-NymTA-15B-GGUF/resolve/main/L3-NymTA-15B.Q3_K_S.gguf) | Q3_K_S | 6.8 | |
| [GGUF](https://huggingface.co/mradermacher/L3-NymTA-15B-GGUF/resolve/main/L3-NymTA-15B.IQ3_S.gguf) | IQ3_S | 6.8 | beats Q3_K* |
| [GGUF](https://huggingface.co/mradermacher/L3-NymTA-15B-GGUF/resolve/main/L3-NymTA-15B.IQ3_M.gguf) | IQ3_M | 7.0 | |
| [GGUF](https://huggingface.co/mradermacher/L3-NymTA-15B-GGUF/resolve/main/L3-NymTA-15B.Q3_K_M.gguf) | Q3_K_M | 7.5 | lower quality |
| [GGUF](https://huggingface.co/mradermacher/L3-NymTA-15B-GGUF/resolve/main/L3-NymTA-15B.Q3_K_L.gguf) | Q3_K_L | 8.1 | |
| [GGUF](https://huggingface.co/mradermacher/L3-NymTA-15B-GGUF/resolve/main/L3-NymTA-15B.IQ4_XS.gguf) | IQ4_XS | 8.4 | |
| [GGUF](https://huggingface.co/mradermacher/L3-NymTA-15B-GGUF/resolve/main/L3-NymTA-15B.Q4_K_S.gguf) | Q4_K_S | 8.7 | fast, recommended |
| [GGUF](https://huggingface.co/mradermacher/L3-NymTA-15B-GGUF/resolve/main/L3-NymTA-15B.Q4_K_M.gguf) | Q4_K_M | 9.2 | fast, recommended |
| [GGUF](https://huggingface.co/mradermacher/L3-NymTA-15B-GGUF/resolve/main/L3-NymTA-15B.Q5_K_S.gguf) | Q5_K_S | 10.5 | |
| [GGUF](https://huggingface.co/mradermacher/L3-NymTA-15B-GGUF/resolve/main/L3-NymTA-15B.Q5_K_M.gguf) | Q5_K_M | 10.8 | |
| [GGUF](https://huggingface.co/mradermacher/L3-NymTA-15B-GGUF/resolve/main/L3-NymTA-15B.Q6_K.gguf) | Q6_K | 12.4 | very good quality |
| [GGUF](https://huggingface.co/mradermacher/L3-NymTA-15B-GGUF/resolve/main/L3-NymTA-15B.Q8_0.gguf) | Q8_0 | 16.1 | fast, best quality |
Here is a handy graph by ikawrakow comparing some lower-quality quant
types (lower is better):

And here are Artefact2's thoughts on the matter:
https://gist.github.com/Artefact2/b5f810600771265fc1e39442288e8ec9
## FAQ / Model Request
See https://huggingface.co/mradermacher/model_requests for some answers to
questions you might have and/or if you want some other model quantized.
## Thanks
I thank my company, [nethype GmbH](https://www.nethype.de/), for letting
me use its servers and providing upgrades to my workstation to enable
this work in my free time.
<!-- end -->
|
bartowski/tabula-8b-GGUF | bartowski | 2024-06-22T00:25:14Z | 20,984 | 2 | null | [
"gguf",
"text-generation",
"en",
"dataset:jpgard/t4-full",
"license:llama3",
"region:us"
] | text-generation | 2024-06-21T23:55:45Z | ---
license: llama3
datasets:
- jpgard/t4-full
language:
- en
quantized_by: bartowski
pipeline_tag: text-generation
---
## Llamacpp imatrix Quantizations of tabula-8b
Using <a href="https://github.com/ggerganov/llama.cpp/">llama.cpp</a> release <a href="https://github.com/ggerganov/llama.cpp/releases/tag/b3197">b3197</a> for quantization.
Original model: https://huggingface.co/mlfoundations/tabula-8b
All quants made using imatrix option with dataset from [here](https://gist.github.com/bartowski1182/eb213dccb3571f863da82e99418f81e8)
## Prompt format
No chat template specified so default is used. This may be incorrect, check original model card for details.
```
<|im_start|>system
{system_prompt}<|im_end|>
<|im_start|>user
{prompt}<|im_end|>
<|im_start|>assistant
```
## Download a file (not the whole branch) from below:
| Filename | Quant type | File Size | Description |
| -------- | ---------- | --------- | ----------- |
| [tabula-8b-Q8_0_L.gguf](https://huggingface.co/bartowski/tabula-8b-GGUF/blob/main/tabula-8b-Q8_1.gguf) | Q8_0_L | 9.52GB | *Experimental*, uses f16 for embed and output weights. Please provide any feedback of differences. Extremely high quality, generally unneeded but max available quant. |
| [tabula-8b-Q8_0.gguf](https://huggingface.co/bartowski/tabula-8b-GGUF/blob/main/tabula-8b-Q8_0.gguf) | Q8_0 | 8.54GB | Extremely high quality, generally unneeded but max available quant. |
| [tabula-8b-Q6_K_L.gguf](https://huggingface.co/bartowski/tabula-8b-GGUF/blob/main/tabula-8b-Q6_K_L.gguf) | Q6_K_L | 7.83GB | *Experimental*, uses f16 for embed and output weights. Please provide any feedback of differences. Very high quality, near perfect, *recommended*. |
| [tabula-8b-Q6_K.gguf](https://huggingface.co/bartowski/tabula-8b-GGUF/blob/main/tabula-8b-Q6_K.gguf) | Q6_K | 6.59GB | Very high quality, near perfect, *recommended*. |
| [tabula-8b-Q5_K_L.gguf](https://huggingface.co/bartowski/tabula-8b-GGUF/blob/main/tabula-8b-Q5_K_L.gguf) | Q5_K_L | 7.04GB | *Experimental*, uses f16 for embed and output weights. Please provide any feedback of differences. High quality, *recommended*. |
| [tabula-8b-Q5_K_M.gguf](https://huggingface.co/bartowski/tabula-8b-GGUF/blob/main/tabula-8b-Q5_K_M.gguf) | Q5_K_M | 5.73GB | High quality, *recommended*. |
| [tabula-8b-Q5_K_S.gguf](https://huggingface.co/bartowski/tabula-8b-GGUF/blob/main/tabula-8b-Q5_K_S.gguf) | Q5_K_S | 5.59GB | High quality, *recommended*. |
| [tabula-8b-Q4_K_L.gguf](https://huggingface.co/bartowski/tabula-8b-GGUF/blob/main/tabula-8b-Q4_K_L.gguf) | Q4_K_L | 6.29GB | *Experimental*, uses f16 for embed and output weights. Please provide any feedback of differences. Good quality, uses about 4.83 bits per weight, *recommended*. |
| [tabula-8b-Q4_K_M.gguf](https://huggingface.co/bartowski/tabula-8b-GGUF/blob/main/tabula-8b-Q4_K_M.gguf) | Q4_K_M | 4.92GB | Good quality, uses about 4.83 bits per weight, *recommended*. |
| [tabula-8b-Q4_K_S.gguf](https://huggingface.co/bartowski/tabula-8b-GGUF/blob/main/tabula-8b-Q4_K_S.gguf) | Q4_K_S | 4.69GB | Slightly lower quality with more space savings, *recommended*. |
| [tabula-8b-IQ4_XS.gguf](https://huggingface.co/bartowski/tabula-8b-GGUF/blob/main/tabula-8b-IQ4_XS.gguf) | IQ4_XS | 4.44GB | Decent quality, smaller than Q4_K_S with similar performance, *recommended*. |
| [tabula-8b-Q3_K_XL.gguf](https://huggingface.co/bartowski/tabula-8b-GGUF//main/tabula-8b-Q3_K_XL.gguf) | Q3_K_XL | | *Experimental*, uses f16 for embed and output weights. Please provide any feedback of differences. Lower quality but usable, good for low RAM availability. |
| [tabula-8b-Q3_K_L.gguf](https://huggingface.co/bartowski/tabula-8b-GGUF/blob/main/tabula-8b-Q3_K_L.gguf) | Q3_K_L | 4.32GB | Lower quality but usable, good for low RAM availability. |
| [tabula-8b-Q3_K_M.gguf](https://huggingface.co/bartowski/tabula-8b-GGUF/blob/main/tabula-8b-Q3_K_M.gguf) | Q3_K_M | 4.01GB | Even lower quality. |
| [tabula-8b-IQ3_M.gguf](https://huggingface.co/bartowski/tabula-8b-GGUF/blob/main/tabula-8b-IQ3_M.gguf) | IQ3_M | 3.78GB | Medium-low quality, new method with decent performance comparable to Q3_K_M. |
| [tabula-8b-Q3_K_S.gguf](https://huggingface.co/bartowski/tabula-8b-GGUF/blob/main/tabula-8b-Q3_K_S.gguf) | Q3_K_S | 3.66GB | Low quality, not recommended. |
| [tabula-8b-IQ3_XS.gguf](https://huggingface.co/bartowski/tabula-8b-GGUF/blob/main/tabula-8b-IQ3_XS.gguf) | IQ3_XS | 3.51GB | Lower quality, new method with decent performance, slightly better than Q3_K_S. |
| [tabula-8b-IQ3_XXS.gguf](https://huggingface.co/bartowski/tabula-8b-GGUF/blob/main/tabula-8b-IQ3_XXS.gguf) | IQ3_XXS | 3.27GB | Lower quality, new method with decent performance, comparable to Q3 quants. |
| [tabula-8b-Q2_K.gguf](https://huggingface.co/bartowski/tabula-8b-GGUF/blob/main/tabula-8b-Q2_K.gguf) | Q2_K | 3.17GB | Very low quality but surprisingly usable. |
| [tabula-8b-IQ2_M.gguf](https://huggingface.co/bartowski/tabula-8b-GGUF/blob/main/tabula-8b-IQ2_M.gguf) | IQ2_M | 2.94GB | Very low quality, uses SOTA techniques to also be surprisingly usable. |
| [tabula-8b-IQ2_S.gguf](https://huggingface.co/bartowski/tabula-8b-GGUF/blob/main/tabula-8b-IQ2_S.gguf) | IQ2_S | 2.75GB | Very low quality, uses SOTA techniques to be usable. |
| [tabula-8b-IQ2_XS.gguf](https://huggingface.co/bartowski/tabula-8b-GGUF/blob/main/tabula-8b-IQ2_XS.gguf) | IQ2_XS | 2.60GB | Very low quality, uses SOTA techniques to be usable. |
## Downloading using huggingface-cli
First, make sure you have hugginface-cli installed:
```
pip install -U "huggingface_hub[cli]"
```
Then, you can target the specific file you want:
```
huggingface-cli download bartowski/tabula-8b-GGUF --include "tabula-8b-Q4_K_M.gguf" --local-dir ./
```
If the model is bigger than 50GB, it will have been split into multiple files. In order to download them all to a local folder, run:
```
huggingface-cli download bartowski/tabula-8b-GGUF --include "tabula-8b-Q8_0.gguf/*" --local-dir tabula-8b-Q8_0
```
You can either specify a new local-dir (tabula-8b-Q8_0) or download them all in place (./)
## Which file should I choose?
A great write up with charts showing various performances is provided by Artefact2 [here](https://gist.github.com/Artefact2/b5f810600771265fc1e39442288e8ec9)
The first thing to figure out is how big a model you can run. To do this, you'll need to figure out how much RAM and/or VRAM you have.
If you want your model running as FAST as possible, you'll want to fit the whole thing on your GPU's VRAM. Aim for a quant with a file size 1-2GB smaller than your GPU's total VRAM.
If you want the absolute maximum quality, add both your system RAM and your GPU's VRAM together, then similarly grab a quant with a file size 1-2GB Smaller than that total.
Next, you'll need to decide if you want to use an 'I-quant' or a 'K-quant'.
If you don't want to think too much, grab one of the K-quants. These are in format 'QX_K_X', like Q5_K_M.
If you want to get more into the weeds, you can check out this extremely useful feature chart:
[llama.cpp feature matrix](https://github.com/ggerganov/llama.cpp/wiki/Feature-matrix)
But basically, if you're aiming for below Q4, and you're running cuBLAS (Nvidia) or rocBLAS (AMD), you should look towards the I-quants. These are in format IQX_X, like IQ3_M. These are newer and offer better performance for their size.
These I-quants can also be used on CPU and Apple Metal, but will be slower than their K-quant equivalent, so speed vs performance is a tradeoff you'll have to decide.
The I-quants are *not* compatible with Vulcan, which is also AMD, so if you have an AMD card double check if you're using the rocBLAS build or the Vulcan build. At the time of writing this, LM Studio has a preview with ROCm support, and other inference engines have specific builds for ROCm.
Want to support my work? Visit my ko-fi page here: https://ko-fi.com/bartowski
|
deepseek-ai/deepseek-math-7b-rl | deepseek-ai | 2024-03-19T03:54:22Z | 20,968 | 51 | transformers | [
"transformers",
"pytorch",
"safetensors",
"llama",
"text-generation",
"conversational",
"arxiv:2402.03300",
"license:other",
"autotrain_compatible",
"endpoints_compatible",
"text-generation-inference",
"region:us"
] | text-generation | 2024-02-05T08:17:11Z | ---
license: other
license_name: deepseek
license_link: https://github.com/deepseek-ai/DeepSeek-Math/blob/main/LICENSE-MODEL
---
<p align="center">
<img width="500px" alt="DeepSeek Chat" src="https://github.com/deepseek-ai/DeepSeek-LLM/blob/main/images/logo.png?raw=true">
</p>
<p align="center"><a href="https://www.deepseek.com/">[🏠Homepage]</a> | <a href="https://chat.deepseek.com/">[🤖 Chat with DeepSeek LLM]</a> | <a href="https://discord.gg/Tc7c45Zzu5">[Discord]</a> | <a href="https://github.com/deepseek-ai/DeepSeek-LLM/blob/main/images/qr.jpeg">[Wechat(微信)]</a> </p>
<p align="center">
<a href="https://arxiv.org/pdf/2402.03300.pdf"><b>Paper Link</b>👁️</a>
</p>
<hr>
### 1. Introduction to DeepSeekMath
See the [Introduction](https://github.com/deepseek-ai/DeepSeek-Math) for more details.
### 2. How to Use
Here give some examples of how to use our model.
**Chat Completion**
❗❗❗ **Please use chain-of-thought prompt to test DeepSeekMath-Instruct and DeepSeekMath-RL:**
- English questions: **{question}\nPlease reason step by step, and put your final answer within \\boxed{}.**
- Chinese questions: **{question}\n请通过逐步推理来解答问题,并把最终答案放置于\\boxed{}中。**
```python
import torch
from transformers import AutoTokenizer, AutoModelForCausalLM, GenerationConfig
model_name = "deepseek-ai/deepseek-math-7b-instruct"
tokenizer = AutoTokenizer.from_pretrained(model_name)
model = AutoModelForCausalLM.from_pretrained(model_name, torch_dtype=torch.bfloat16, device_map="auto")
model.generation_config = GenerationConfig.from_pretrained(model_name)
model.generation_config.pad_token_id = model.generation_config.eos_token_id
messages = [
{"role": "user", "content": "what is the integral of x^2 from 0 to 2?\nPlease reason step by step, and put your final answer within \\boxed{}."}
]
input_tensor = tokenizer.apply_chat_template(messages, add_generation_prompt=True, return_tensors="pt")
outputs = model.generate(input_tensor.to(model.device), max_new_tokens=100)
result = tokenizer.decode(outputs[0][input_tensor.shape[1]:], skip_special_tokens=True)
print(result)
```
Avoiding the use of the provided function `apply_chat_template`, you can also interact with our model following the sample template. Note that `messages` should be replaced by your input.
```
User: {messages[0]['content']}
Assistant: {messages[1]['content']}<|end▁of▁sentence|>User: {messages[2]['content']}
Assistant:
```
**Note:** By default (`add_special_tokens=True`), our tokenizer automatically adds a `bos_token` (`<|begin▁of▁sentence|>`) before the input text. Additionally, since the system prompt is not compatible with this version of our models, we DO NOT RECOMMEND including the system prompt in your input.
### 3. License
This code repository is licensed under the MIT License. The use of DeepSeekMath models is subject to the Model License. DeepSeekMath supports commercial use.
See the [LICENSE-MODEL](https://github.com/deepseek-ai/DeepSeek-Math/blob/main/LICENSE-MODEL) for more details.
### 4. Contact
If you have any questions, please raise an issue or contact us at [[email protected]](mailto:[email protected]).
|
Helsinki-NLP/opus-mt-en-he | Helsinki-NLP | 2023-08-16T11:29:48Z | 20,963 | 4 | transformers | [
"transformers",
"pytorch",
"tf",
"rust",
"marian",
"text2text-generation",
"translation",
"en",
"he",
"license:apache-2.0",
"autotrain_compatible",
"endpoints_compatible",
"region:us"
] | translation | 2022-03-02T23:29:04Z | ---
tags:
- translation
license: apache-2.0
---
### opus-mt-en-he
* source languages: en
* target languages: he
* OPUS readme: [en-he](https://github.com/Helsinki-NLP/OPUS-MT-train/blob/master/models/en-he/README.md)
* dataset: opus
* model: transformer-align
* pre-processing: normalization + SentencePiece
* download original weights: [opus-2019-12-18.zip](https://object.pouta.csc.fi/OPUS-MT-models/en-he/opus-2019-12-18.zip)
* test set translations: [opus-2019-12-18.test.txt](https://object.pouta.csc.fi/OPUS-MT-models/en-he/opus-2019-12-18.test.txt)
* test set scores: [opus-2019-12-18.eval.txt](https://object.pouta.csc.fi/OPUS-MT-models/en-he/opus-2019-12-18.eval.txt)
## Benchmarks
| testset | BLEU | chr-F |
|-----------------------|-------|-------|
| Tatoeba.en.he | 40.1 | 0.609 |
|
google/vit-huge-patch14-224-in21k | google | 2024-02-14T13:43:41Z | 20,926 | 15 | transformers | [
"transformers",
"pytorch",
"tf",
"jax",
"safetensors",
"vit",
"image-feature-extraction",
"vision",
"dataset:imagenet-21k",
"arxiv:2010.11929",
"arxiv:2006.03677",
"license:apache-2.0",
"region:us"
] | image-feature-extraction | 2022-03-02T23:29:05Z | ---
license: apache-2.0
tags:
- vision
datasets:
- imagenet-21k
inference: false
---
# Vision Transformer (huge-sized model)
Vision Transformer (ViT) model pre-trained on ImageNet-21k (14 million images, 21,843 classes) at resolution 224x224. It was introduced in the paper [An Image is Worth 16x16 Words: Transformers for Image Recognition at Scale](https://arxiv.org/abs/2010.11929) by Dosovitskiy et al. and first released in [this repository](https://github.com/google-research/vision_transformer). However, the weights were converted from the [timm repository](https://github.com/rwightman/pytorch-image-models) by Ross Wightman, who already converted the weights from JAX to PyTorch. Credits go to him.
Disclaimer: The team releasing ViT did not write a model card for this model so this model card has been written by the Hugging Face team.
## Model description
The Vision Transformer (ViT) is a transformer encoder model (BERT-like) pretrained on a large collection of images in a supervised fashion, namely ImageNet-21k, at a resolution of 224x224 pixels.
Images are presented to the model as a sequence of fixed-size patches (resolution 16x16), which are linearly embedded. One also adds a [CLS] token to the beginning of a sequence to use it for classification tasks. One also adds absolute position embeddings before feeding the sequence to the layers of the Transformer encoder.
Note that this model does not provide any fine-tuned heads, as these were zero'd by Google researchers. However, the model does include the pre-trained pooler, which can be used for downstream tasks (such as image classification).
By pre-training the model, it learns an inner representation of images that can then be used to extract features useful for downstream tasks: if you have a dataset of labeled images for instance, you can train a standard classifier by placing a linear layer on top of the pre-trained encoder. One typically places a linear layer on top of the [CLS] token, as the last hidden state of this token can be seen as a representation of an entire image.
## Intended uses & limitations
You can use the raw model for image classification. See the [model hub](https://huggingface.co/models?search=google/vit) to look for
fine-tuned versions on a task that interests you.
### How to use
Here is how to use this model:
```python
from transformers import ViTFeatureExtractor, ViTModel
from PIL import Image
import requests
url = 'http://images.cocodataset.org/val2017/000000039769.jpg'
image = Image.open(requests.get(url, stream=True).raw)
feature_extractor = ViTFeatureExtractor.from_pretrained('google/vit-huge-patch14-224-in21k')
model = ViTModel.from_pretrained('google/vit-huge-patch14-224-in21k')
inputs = feature_extractor(images=image, return_tensors="pt")
outputs = model(**inputs)
last_hidden_states = outputs.last_hidden_state
```
Currently, both the feature extractor and model support PyTorch. Tensorflow and JAX/FLAX are coming soon, and the API of ViTFeatureExtractor might change.
## Training data
The ViT model was pretrained on [ImageNet-21k](http://www.image-net.org/), a dataset consisting of 14 million images and 21k classes.
## Training procedure
### Preprocessing
The exact details of preprocessing of images during training/validation can be found [here](https://github.com/google-research/vision_transformer/blob/master/vit_jax/input_pipeline.py).
Images are resized/rescaled to the same resolution (224x224) and normalized across the RGB channels with mean (0.5, 0.5, 0.5) and standard deviation (0.5, 0.5, 0.5).
### Pretraining
The model was trained on TPUv3 hardware (8 cores). All model variants are trained with a batch size of 4096 and learning rate warmup of 10k steps. For ImageNet, the authors found it beneficial to additionally apply gradient clipping at global norm 1. Pre-training resolution is 224.
## Evaluation results
For evaluation results on several image classification benchmarks, we refer to tables 2 and 5 of the original paper. Note that for fine-tuning, the best results are obtained with a higher resolution (384x384). Of course, increasing the model size will result in better performance.
### BibTeX entry and citation info
```bibtex
@misc{wu2020visual,
title={Visual Transformers: Token-based Image Representation and Processing for Computer Vision},
author={Bichen Wu and Chenfeng Xu and Xiaoliang Dai and Alvin Wan and Peizhao Zhang and Zhicheng Yan and Masayoshi Tomizuka and Joseph Gonzalez and Kurt Keutzer and Peter Vajda},
year={2020},
eprint={2006.03677},
archivePrefix={arXiv},
primaryClass={cs.CV}
}
```
```bibtex
@inproceedings{deng2009imagenet,
title={Imagenet: A large-scale hierarchical image database},
author={Deng, Jia and Dong, Wei and Socher, Richard and Li, Li-Jia and Li, Kai and Fei-Fei, Li},
booktitle={2009 IEEE conference on computer vision and pattern recognition},
pages={248--255},
year={2009},
organization={Ieee}
}
``` |
RichardErkhov/MaziyarPanahi_-_Topxtral-4x7B-v0.1-gguf | RichardErkhov | 2024-06-26T11:21:38Z | 20,878 | 1 | null | [
"gguf",
"region:us"
] | null | 2024-06-26T02:55:20Z | Quantization made by Richard Erkhov.
[Github](https://github.com/RichardErkhov)
[Discord](https://discord.gg/pvy7H8DZMG)
[Request more models](https://github.com/RichardErkhov/quant_request)
Topxtral-4x7B-v0.1 - GGUF
- Model creator: https://huggingface.co/MaziyarPanahi/
- Original model: https://huggingface.co/MaziyarPanahi/Topxtral-4x7B-v0.1/
| Name | Quant method | Size |
| ---- | ---- | ---- |
| [Topxtral-4x7B-v0.1.Q2_K.gguf](https://huggingface.co/RichardErkhov/MaziyarPanahi_-_Topxtral-4x7B-v0.1-gguf/blob/main/Topxtral-4x7B-v0.1.Q2_K.gguf) | Q2_K | 6.33GB |
| [Topxtral-4x7B-v0.1.IQ3_XS.gguf](https://huggingface.co/RichardErkhov/MaziyarPanahi_-_Topxtral-4x7B-v0.1-gguf/blob/main/Topxtral-4x7B-v0.1.IQ3_XS.gguf) | IQ3_XS | 7.08GB |
| [Topxtral-4x7B-v0.1.IQ3_S.gguf](https://huggingface.co/RichardErkhov/MaziyarPanahi_-_Topxtral-4x7B-v0.1-gguf/blob/main/Topxtral-4x7B-v0.1.IQ3_S.gguf) | IQ3_S | 7.48GB |
| [Topxtral-4x7B-v0.1.Q3_K_S.gguf](https://huggingface.co/RichardErkhov/MaziyarPanahi_-_Topxtral-4x7B-v0.1-gguf/blob/main/Topxtral-4x7B-v0.1.Q3_K_S.gguf) | Q3_K_S | 7.46GB |
| [Topxtral-4x7B-v0.1.IQ3_M.gguf](https://huggingface.co/RichardErkhov/MaziyarPanahi_-_Topxtral-4x7B-v0.1-gguf/blob/main/Topxtral-4x7B-v0.1.IQ3_M.gguf) | IQ3_M | 7.63GB |
| [Topxtral-4x7B-v0.1.Q3_K.gguf](https://huggingface.co/RichardErkhov/MaziyarPanahi_-_Topxtral-4x7B-v0.1-gguf/blob/main/Topxtral-4x7B-v0.1.Q3_K.gguf) | Q3_K | 8.28GB |
| [Topxtral-4x7B-v0.1.Q3_K_M.gguf](https://huggingface.co/RichardErkhov/MaziyarPanahi_-_Topxtral-4x7B-v0.1-gguf/blob/main/Topxtral-4x7B-v0.1.Q3_K_M.gguf) | Q3_K_M | 8.28GB |
| [Topxtral-4x7B-v0.1.Q3_K_L.gguf](https://huggingface.co/RichardErkhov/MaziyarPanahi_-_Topxtral-4x7B-v0.1-gguf/blob/main/Topxtral-4x7B-v0.1.Q3_K_L.gguf) | Q3_K_L | 8.98GB |
| [Topxtral-4x7B-v0.1.IQ4_XS.gguf](https://huggingface.co/RichardErkhov/MaziyarPanahi_-_Topxtral-4x7B-v0.1-gguf/blob/main/Topxtral-4x7B-v0.1.IQ4_XS.gguf) | IQ4_XS | 9.32GB |
| [Topxtral-4x7B-v0.1.Q4_0.gguf](https://huggingface.co/RichardErkhov/MaziyarPanahi_-_Topxtral-4x7B-v0.1-gguf/blob/main/Topxtral-4x7B-v0.1.Q4_0.gguf) | Q4_0 | 9.73GB |
| [Topxtral-4x7B-v0.1.IQ4_NL.gguf](https://huggingface.co/RichardErkhov/MaziyarPanahi_-_Topxtral-4x7B-v0.1-gguf/blob/main/Topxtral-4x7B-v0.1.IQ4_NL.gguf) | IQ4_NL | 9.83GB |
| [Topxtral-4x7B-v0.1.Q4_K_S.gguf](https://huggingface.co/RichardErkhov/MaziyarPanahi_-_Topxtral-4x7B-v0.1-gguf/blob/main/Topxtral-4x7B-v0.1.Q4_K_S.gguf) | Q4_K_S | 9.82GB |
| [Topxtral-4x7B-v0.1.Q4_K.gguf](https://huggingface.co/RichardErkhov/MaziyarPanahi_-_Topxtral-4x7B-v0.1-gguf/blob/main/Topxtral-4x7B-v0.1.Q4_K.gguf) | Q4_K | 10.43GB |
| [Topxtral-4x7B-v0.1.Q4_K_M.gguf](https://huggingface.co/RichardErkhov/MaziyarPanahi_-_Topxtral-4x7B-v0.1-gguf/blob/main/Topxtral-4x7B-v0.1.Q4_K_M.gguf) | Q4_K_M | 10.43GB |
| [Topxtral-4x7B-v0.1.Q4_1.gguf](https://huggingface.co/RichardErkhov/MaziyarPanahi_-_Topxtral-4x7B-v0.1-gguf/blob/main/Topxtral-4x7B-v0.1.Q4_1.gguf) | Q4_1 | 8.5GB |
| [Topxtral-4x7B-v0.1.Q5_0.gguf](https://huggingface.co/RichardErkhov/MaziyarPanahi_-_Topxtral-4x7B-v0.1-gguf/blob/main/Topxtral-4x7B-v0.1.Q5_0.gguf) | Q5_0 | 0.0GB |
| [Topxtral-4x7B-v0.1.Q5_K_S.gguf](https://huggingface.co/RichardErkhov/MaziyarPanahi_-_Topxtral-4x7B-v0.1-gguf/blob/main/Topxtral-4x7B-v0.1.Q5_K_S.gguf) | Q5_K_S | 0.0GB |
| [Topxtral-4x7B-v0.1.Q5_K.gguf](https://huggingface.co/RichardErkhov/MaziyarPanahi_-_Topxtral-4x7B-v0.1-gguf/blob/main/Topxtral-4x7B-v0.1.Q5_K.gguf) | Q5_K | 0.0GB |
| [Topxtral-4x7B-v0.1.Q5_K_M.gguf](https://huggingface.co/RichardErkhov/MaziyarPanahi_-_Topxtral-4x7B-v0.1-gguf/blob/main/Topxtral-4x7B-v0.1.Q5_K_M.gguf) | Q5_K_M | 0.0GB |
| [Topxtral-4x7B-v0.1.Q5_1.gguf](https://huggingface.co/RichardErkhov/MaziyarPanahi_-_Topxtral-4x7B-v0.1-gguf/blob/main/Topxtral-4x7B-v0.1.Q5_1.gguf) | Q5_1 | 0.0GB |
| [Topxtral-4x7B-v0.1.Q6_K.gguf](https://huggingface.co/RichardErkhov/MaziyarPanahi_-_Topxtral-4x7B-v0.1-gguf/blob/main/Topxtral-4x7B-v0.1.Q6_K.gguf) | Q6_K | 0.0GB |
| [Topxtral-4x7B-v0.1.Q8_0.gguf](https://huggingface.co/RichardErkhov/MaziyarPanahi_-_Topxtral-4x7B-v0.1-gguf/blob/main/Topxtral-4x7B-v0.1.Q8_0.gguf) | Q8_0 | 0.0GB |
Original model description:
---
license: apache-2.0
library_name: transformers
pipeline_tag: text-generation
tags:
- merge
- moe
---
## Examples
describe about pros and cons of docker system.
```
Answer:
Docker, a containerization platform, offers numerous advantages and potential drawbacks. Here's an overview of both aspects:
Pros (Advantages):
1. Isolation: Docker containers isolate applications from each other and their host systems, ensuring stability and preventing conflicts between dependencies.
2. Portability: Applications packaged within Docker images can run consistently across different environments, including development, testing, and production.
3. Resource Efficiency: Containers share the host OS kernel, reducing resource usage compared to traditional virtual machines.
4. Rapid Development & Deployment: Developers can quickly build, test, and deploy applications using pre-configured Docker images.
5. Version Control: Docker images enable versioning, allowing developers to track changes and roll back to previous versions if needed.
6. Scalability: Containerized applications can be easily scaled horizontally by adding more instances.
7. Simplified Dependency Management: Docker simplifies managing application dependencies since they come bundled with the image.
Cons (Disadvantages):
1. Learning Curve: Understanding Docker concepts and mastering its tools may require time for those new to containerization technology.
2. Complexity: Managing multiple containers and orchestrating them can become complex, especially in large-scale deployments.
3. Security Concerns: While containers offer isolation, vulnerabilities in base images or misconfigurations could lead to security risks.
4. Storage Overhead: Although less than VMs, Docker still requires some storage overhead due to image layers and container metadata.
5. Potential Compatibility Issues: Some applications might have compatibility issues when running inside containers, particularly if they rely on low-level system resources.
In summary, Docker provides significant benefits for modern software development and deployment but also comes with certain challenges. The choice to adopt it depends on one's specific needs and context.</s>
```
Hi, I need to get from New York to Los Angeles. Can you help me with that?
```
{
"arguments": {"origin": "New York", "destination": "Los Angeles"},
"name": "get_directions"
}</s>
```
There are three killers in a room. Someone enters the room and kills one of them. Nobody leaves the room. How many killers are left in the room? Explain your reasoning step by step.
```
There are three killers in a room. Someone enters the room and kills one of them. Nobody leaves the room. How many killers are left in the room? Explain your reasoning step by step. [/INST]
1. Initially, there are three killers in the room.
2. A new person enters the room and commits an act of killing one of the three killers.
3. After this event, we need to determine how many killers remain in the room. The original number of killers was reduced by 1 due to the newcomer's action.
4. So, the final count is three (initial killers) - 1 (killed by the newcomer) = two killers remaining in the room.</s>
```
Wrong! Should be 3!
Mark is faster than Mary , Mary is faster than Joe. Is Joe faster than Mark? Let's think step by step
```
To determine if one person is faster than another based on the given information, we need to compare the relative speeds of each pair mentioned: Mark and Mary, and Mary and Joe.
1. We know that "Mark is faster than Mary." This means when they race, Mark reaches the finish line before Mary does.
2. The second statement tells us "Mary is faster than Joe." Here, it implies that in a race between them, Mary finishes ahead of Joe.
Now, let's consider the question about whether "Joe is faster than Mark." Based on the provided comparisons, we have established the following order:
- Mark > Mary (in terms of speed)
- Mary > Joe (in terms of speed)
Since the sequence shows Mark being faster than both Mary and Joe, there is no scenario where Joe would be faster than Mark according to the given information.
```
If I lay 2 wet shirts out in the sun flat on the ground to dry and it takes 5 hours until they are dry, how long would it take to dry 4 shirts of the same type that way simultanously?
```
The time needed for drying clothes is not only determined by the number of shirts but also their surface area exposed to the sunlight. Since you're keeping the shirts flat, the additional shirts won't increase the total surface area exposed to the sun much. However, as there will be more fabric absorbing moisture, it might take a bit longer than simply dividing the original time by two.
To estimate the new drying time, we can assume an inverse proportional relationship between the number of shirts and the drying time. This means if the number of shirts doubles, the time required approximately halves (but not exactly). So, with four shirts instead of two, the drying time could be around:
```
(5 hours) * (1 / sqrt(2)) ~ 3.5 hours
```
Please note this is just an approximation, and actual results may vary depending on factors like humidity, airflow, and the intensity of the sunlight.</s>
```
Answer should be: it will be the same.
explain step by step 25-4*2+3=?
```
To solve the expression 25-4*2+3, follow these steps:
1. Perform multiplication first: 4 * 2 = 8
The updated expression is now 25 - 8 + 3
2. Next, perform subtraction: 25 - 8 = 17
The new expression becomes 17 + 3
3. Finally, add the remaining numbers: 17 + 3 = 20
So, the result of the given expression is 20.</s>
```
|
OpenGVLab/Mini-InternVL-Chat-4B-V1-5 | OpenGVLab | 2024-05-29T14:28:04Z | 20,863 | 50 | transformers | [
"transformers",
"safetensors",
"internvl_chat",
"feature-extraction",
"visual-question-answering",
"custom_code",
"dataset:laion/laion2B-en",
"dataset:laion/laion-coco",
"dataset:laion/laion2B-multi",
"dataset:kakaobrain/coyo-700m",
"dataset:conceptual_captions",
"dataset:wanng/wukong100m",
"arxiv:2312.14238",
"arxiv:2404.16821",
"license:mit",
"region:us"
] | visual-question-answering | 2024-05-28T07:23:09Z | ---
license: mit
datasets:
- laion/laion2B-en
- laion/laion-coco
- laion/laion2B-multi
- kakaobrain/coyo-700m
- conceptual_captions
- wanng/wukong100m
pipeline_tag: visual-question-answering
---
# Model Card for Mini-InternVL-Chat-4B-V1-5
<center>
<p><img src="https://cdn-uploads.huggingface.co/production/uploads/64119264f0f81eb569e0d569/pvfKc16O-ej91632FHaIK.png" style="width:80%;" alt="image/png"></p>
</center>
[\[🆕 Blog\]](https://internvl.github.io/blog/) [\[📜 InternVL 1.0 Paper\]](https://arxiv.org/abs/2312.14238) [\[📜 InternVL 1.5 Report\]](https://arxiv.org/abs/2404.16821) [\[🗨️ Chat Demo\]](https://internvl.opengvlab.com/)
[\[🤗 HF Demo\]](https://huggingface.co/spaces/OpenGVLab/InternVL) [\[🚀 Quick Start\]](#model-usage) [\[🌐 Community-hosted API\]](https://rapidapi.com/adushar1320/api/internvl-chat) [\[📖 中文解读\]](https://zhuanlan.zhihu.com/p/675877376)
You can run multimodal large models using a 1080Ti now.
We are delighted to introduce the Mini-InternVL-Chat series. In the era of large language models, many researchers have started to focus on smaller language models, such as Gemma-2B, Qwen-1.8B, and InternLM2-1.8B. Inspired by their efforts, we have distilled our vision foundation model [InternViT-6B-448px-V1-5](https://huggingface.co/OpenGVLab/InternViT-6B-448px-V1-5) down to 300M and used [InternLM2-Chat-1.8B](https://huggingface.co/internlm/internlm2-chat-1_8b) or [Phi-3-mini-128k-instruct](https://huggingface.co/microsoft/Phi-3-mini-128k-instruct) as our language model. This resulted in a small multimodal model with excellent performance.
As shown in the figure below, we adopted the same model architecture as InternVL 1.5. We simply replaced the original InternViT-6B with InternViT-300M and InternLM2-Chat-20B with InternLM2-Chat-1.8B / Phi-3-mini-128k-instruct. For training, we used the same data as InternVL 1.5 to train this smaller model. Additionally, due to the lower training costs of smaller models, we used a context length of 8K during training.

## Model Details
- **Model Type:** multimodal large language model (MLLM)
- **Model Stats:**
- Architecture: [InternViT-300M-448px](https://huggingface.co/OpenGVLab/InternViT-300M-448px) + MLP + [Phi-3-mini-128k-instruct](https://huggingface.co/microsoft/Phi-3-mini-128k-instruct)
- Image size: dynamic resolution, max to 40 tiles of 448 x 448 (4K resolution).
- Params: 4.2B
- **Training Strategy:**
- Learnable component in the pretraining stage: MLP
- Learnable component in the finetuning stage: ViT + MLP + LLM
- For more details on training hyperparameters, take a look at our code: [pretrain](<>) | [finetune](<>)
## Released Models
| Model | Vision Foundation Model | Release Date | Note |
| :----------------------------------------------------------------------------------------------: | :---------------------------------------------------------------------------------------------: | :----------: | :----------------------------------------------------------------------------------------------------------------------------------------------------------------- |
| InternVL-Chat-V1-5(🤗 [HF link](https://huggingface.co/OpenGVLab/InternVL-Chat-V1-5)) | InternViT-6B-448px-V1-5(🤗 [HF link](https://huggingface.co/OpenGVLab/InternViT-6B-448px-V1-5)) | 2024.04.18 | support 4K image; super strong OCR; Approaching the performance of GPT-4V and Gemini Pro on various benchmarks like MMMU, DocVQA, ChartQA, MathVista, etc. (🔥new) |
| InternVL-Chat-V1-2-Plus(🤗 [HF link](https://huggingface.co/OpenGVLab/InternVL-Chat-V1-2-Plus) ) | InternViT-6B-448px-V1-2(🤗 [HF link](https://huggingface.co/OpenGVLab/InternViT-6B-448px-V1-2)) | 2024.02.21 | more SFT data and stronger |
| InternVL-Chat-V1-2(🤗 [HF link](https://huggingface.co/OpenGVLab/InternVL-Chat-V1-2) ) | InternViT-6B-448px-V1-2(🤗 [HF link](https://huggingface.co/OpenGVLab/InternViT-6B-448px-V1-2)) | 2024.02.11 | scaling up LLM to 34B |
| InternVL-Chat-V1-1(🤗 [HF link](https://huggingface.co/OpenGVLab/InternVL-Chat-V1-1)) | InternViT-6B-448px-V1-0(🤗 [HF link](https://huggingface.co/OpenGVLab/InternViT-6B-448px-V1-0)) | 2024.01.24 | support Chinese and stronger OCR |
## Performance

## Model Usage
We provide an example code to run Mini-InternVL-Chat-4B-V1-5 using `transformers`.
You can also use our [online demo](https://internvl.opengvlab.com/) for a quick experience of this model.
> Please use transformers==4.37.2 to ensure the model works normally.
```python
from transformers import AutoTokenizer, AutoModel
import torch
import torchvision.transforms as T
from PIL import Image
from torchvision.transforms.functional import InterpolationMode
IMAGENET_MEAN = (0.485, 0.456, 0.406)
IMAGENET_STD = (0.229, 0.224, 0.225)
def build_transform(input_size):
MEAN, STD = IMAGENET_MEAN, IMAGENET_STD
transform = T.Compose([
T.Lambda(lambda img: img.convert('RGB') if img.mode != 'RGB' else img),
T.Resize((input_size, input_size), interpolation=InterpolationMode.BICUBIC),
T.ToTensor(),
T.Normalize(mean=MEAN, std=STD)
])
return transform
def find_closest_aspect_ratio(aspect_ratio, target_ratios, width, height, image_size):
best_ratio_diff = float('inf')
best_ratio = (1, 1)
area = width * height
for ratio in target_ratios:
target_aspect_ratio = ratio[0] / ratio[1]
ratio_diff = abs(aspect_ratio - target_aspect_ratio)
if ratio_diff < best_ratio_diff:
best_ratio_diff = ratio_diff
best_ratio = ratio
elif ratio_diff == best_ratio_diff:
if area > 0.5 * image_size * image_size * ratio[0] * ratio[1]:
best_ratio = ratio
return best_ratio
def dynamic_preprocess(image, min_num=1, max_num=6, image_size=448, use_thumbnail=False):
orig_width, orig_height = image.size
aspect_ratio = orig_width / orig_height
# calculate the existing image aspect ratio
target_ratios = set(
(i, j) for n in range(min_num, max_num + 1) for i in range(1, n + 1) for j in range(1, n + 1) if
i * j <= max_num and i * j >= min_num)
target_ratios = sorted(target_ratios, key=lambda x: x[0] * x[1])
# find the closest aspect ratio to the target
target_aspect_ratio = find_closest_aspect_ratio(
aspect_ratio, target_ratios, orig_width, orig_height, image_size)
# calculate the target width and height
target_width = image_size * target_aspect_ratio[0]
target_height = image_size * target_aspect_ratio[1]
blocks = target_aspect_ratio[0] * target_aspect_ratio[1]
# resize the image
resized_img = image.resize((target_width, target_height))
processed_images = []
for i in range(blocks):
box = (
(i % (target_width // image_size)) * image_size,
(i // (target_width // image_size)) * image_size,
((i % (target_width // image_size)) + 1) * image_size,
((i // (target_width // image_size)) + 1) * image_size
)
# split the image
split_img = resized_img.crop(box)
processed_images.append(split_img)
assert len(processed_images) == blocks
if use_thumbnail and len(processed_images) != 1:
thumbnail_img = image.resize((image_size, image_size))
processed_images.append(thumbnail_img)
return processed_images
def load_image(image_file, input_size=448, max_num=6):
image = Image.open(image_file).convert('RGB')
transform = build_transform(input_size=input_size)
images = dynamic_preprocess(image, image_size=input_size, use_thumbnail=True, max_num=max_num)
pixel_values = [transform(image) for image in images]
pixel_values = torch.stack(pixel_values)
return pixel_values
path = "OpenGVLab/Mini-InternVL-Chat-4B-V1-5"
model = AutoModel.from_pretrained(
path,
torch_dtype=torch.bfloat16,
low_cpu_mem_usage=True,
trust_remote_code=True).eval().cuda()
tokenizer = AutoTokenizer.from_pretrained(path, trust_remote_code=True)
# set the max number of tiles in `max_num`
pixel_values = load_image('./examples/image1.jpg', max_num=6).to(torch.bfloat16).cuda()
generation_config = dict(
num_beams=1,
max_new_tokens=512,
do_sample=False,
)
# single-round single-image conversation
question = "请详细描述图片" # Please describe the picture in detail
response = model.chat(tokenizer, pixel_values, question, generation_config)
print(question, response)
# multi-round single-image conversation
question = "请详细描述图片" # Please describe the picture in detail
response, history = model.chat(tokenizer, pixel_values, question, generation_config, history=None, return_history=True)
print(question, response)
question = "请根据图片写一首诗" # Please write a poem according to the picture
response, history = model.chat(tokenizer, pixel_values, question, generation_config, history=history, return_history=True)
print(question, response)
# multi-round multi-image conversation
pixel_values1 = load_image('./examples/image1.jpg', max_num=6).to(torch.bfloat16).cuda()
pixel_values2 = load_image('./examples/image2.jpg', max_num=6).to(torch.bfloat16).cuda()
pixel_values = torch.cat((pixel_values1, pixel_values2), dim=0)
question = "详细描述这两张图片" # Describe the two pictures in detail
response, history = model.chat(tokenizer, pixel_values, question, generation_config, history=None, return_history=True)
print(question, response)
question = "这两张图片的相同点和区别分别是什么" # What are the similarities and differences between these two pictures
response, history = model.chat(tokenizer, pixel_values, question, generation_config, history=history, return_history=True)
print(question, response)
# batch inference (single image per sample)
pixel_values1 = load_image('./examples/image1.jpg', max_num=6).to(torch.bfloat16).cuda()
pixel_values2 = load_image('./examples/image2.jpg', max_num=6).to(torch.bfloat16).cuda()
image_counts = [pixel_values1.size(0), pixel_values2.size(0)]
pixel_values = torch.cat((pixel_values1, pixel_values2), dim=0)
questions = ["Describe the image in detail."] * len(image_counts)
responses = model.batch_chat(tokenizer, pixel_values,
image_counts=image_counts,
questions=questions,
generation_config=generation_config)
for question, response in zip(questions, responses):
print(question)
print(response)
```
## Citation
If you find this project useful in your research, please consider citing:
```BibTeX
@article{chen2023internvl,
title={InternVL: Scaling up Vision Foundation Models and Aligning for Generic Visual-Linguistic Tasks},
author={Chen, Zhe and Wu, Jiannan and Wang, Wenhai and Su, Weijie and Chen, Guo and Xing, Sen and Zhong, Muyan and Zhang, Qinglong and Zhu, Xizhou and Lu, Lewei and Li, Bin and Luo, Ping and Lu, Tong and Qiao, Yu and Dai, Jifeng},
journal={arXiv preprint arXiv:2312.14238},
year={2023}
}
@article{chen2024far,
title={How Far Are We to GPT-4V? Closing the Gap to Commercial Multimodal Models with Open-Source Suites},
author={Chen, Zhe and Wang, Weiyun and Tian, Hao and Ye, Shenglong and Gao, Zhangwei and Cui, Erfei and Tong, Wenwen and Hu, Kongzhi and Luo, Jiapeng and Ma, Zheng and others},
journal={arXiv preprint arXiv:2404.16821},
year={2024}
}
```
## License
This project is released under the MIT license.
## Acknowledgement
InternVL is built with reference to the code of the following projects: [OpenAI CLIP](https://github.com/openai/CLIP), [Open CLIP](https://github.com/mlfoundations/open_clip), [CLIP Benchmark](https://github.com/LAION-AI/CLIP_benchmark), [EVA](https://github.com/baaivision/EVA/tree/master), [InternImage](https://github.com/OpenGVLab/InternImage), [ViT-Adapter](https://github.com/czczup/ViT-Adapter), [MMSegmentation](https://github.com/open-mmlab/mmsegmentation), [Transformers](https://github.com/huggingface/transformers), [DINOv2](https://github.com/facebookresearch/dinov2), [BLIP-2](https://github.com/salesforce/LAVIS/tree/main/projects/blip2), [Qwen-VL](https://github.com/QwenLM/Qwen-VL/tree/master/eval_mm), and [LLaVA-1.5](https://github.com/haotian-liu/LLaVA). Thanks for their awesome work!
|
solve/vit-zigzag-attribute-768dim-patch16-224 | solve | 2022-08-12T19:32:36Z | 20,857 | 0 | transformers | [
"transformers",
"pytorch",
"vit",
"image-feature-extraction",
"endpoints_compatible",
"region:us"
] | image-feature-extraction | 2022-08-12T19:28:27Z | Entry not found |
stabilityai/sd-vae-ft-ema | stabilityai | 2023-06-05T16:27:31Z | 20,842 | 117 | diffusers | [
"diffusers",
"safetensors",
"stable-diffusion",
"stable-diffusion-diffusers",
"license:mit",
"region:us"
] | null | 2022-10-13T12:51:55Z | ---
license: mit
tags:
- stable-diffusion
- stable-diffusion-diffusers
inference: false
---
# Improved Autoencoders
## Utilizing
These weights are intended to be used with the [🧨 diffusers library](https://github.com/huggingface/diffusers). If you are looking for the model to use with the original [CompVis Stable Diffusion codebase](https://github.com/CompVis/stable-diffusion), [come here](https://huggingface.co/stabilityai/sd-vae-ft-ema-original).
#### How to use with 🧨 diffusers
You can integrate this fine-tuned VAE decoder to your existing `diffusers` workflows, by including a `vae` argument to the `StableDiffusionPipeline`
```py
from diffusers.models import AutoencoderKL
from diffusers import StableDiffusionPipeline
model = "CompVis/stable-diffusion-v1-4"
vae = AutoencoderKL.from_pretrained("stabilityai/sd-vae-ft-ema")
pipe = StableDiffusionPipeline.from_pretrained(model, vae=vae)
```
## Decoder Finetuning
We publish two kl-f8 autoencoder versions, finetuned from the original [kl-f8 autoencoder](https://github.com/CompVis/latent-diffusion#pretrained-autoencoding-models) on a 1:1 ratio of [LAION-Aesthetics](https://laion.ai/blog/laion-aesthetics/) and LAION-Humans, an unreleased subset containing only SFW images of humans. The intent was to fine-tune on the Stable Diffusion training set (the autoencoder was originally trained on OpenImages) but also enrich the dataset with images of humans to improve the reconstruction of faces.
The first, _ft-EMA_, was resumed from the original checkpoint, trained for 313198 steps and uses EMA weights. It uses the same loss configuration as the original checkpoint (L1 + LPIPS).
The second, _ft-MSE_, was resumed from _ft-EMA_ and uses EMA weights and was trained for another 280k steps using a different loss, with more emphasis
on MSE reconstruction (MSE + 0.1 * LPIPS). It produces somewhat ``smoother'' outputs. The batch size for both versions was 192 (16 A100s, batch size 12 per GPU).
To keep compatibility with existing models, only the decoder part was finetuned; the checkpoints can be used as a drop-in replacement for the existing autoencoder.
_Original kl-f8 VAE vs f8-ft-EMA vs f8-ft-MSE_
## Evaluation
### COCO 2017 (256x256, val, 5000 images)
| Model | train steps | rFID | PSNR | SSIM | PSIM | Link | Comments
|----------|---------|------|--------------|---------------|---------------|-----------------------------------------------------------------------------------|-------------------------------------------------------------------------------------------------|
| | | | | | | | |
| original | 246803 | 4.99 | 23.4 +/- 3.8 | 0.69 +/- 0.14 | 1.01 +/- 0.28 | https://ommer-lab.com/files/latent-diffusion/kl-f8.zip | as used in SD |
| ft-EMA | 560001 | 4.42 | 23.8 +/- 3.9 | 0.69 +/- 0.13 | 0.96 +/- 0.27 | https://huggingface.co/stabilityai/sd-vae-ft-ema-original/resolve/main/vae-ft-ema-560000-ema-pruned.ckpt | slightly better overall, with EMA |
| ft-MSE | 840001 | 4.70 | 24.5 +/- 3.7 | 0.71 +/- 0.13 | 0.92 +/- 0.27 | https://huggingface.co/stabilityai/sd-vae-ft-mse-original/resolve/main/vae-ft-mse-840000-ema-pruned.ckpt | resumed with EMA from ft-EMA, emphasis on MSE (rec. loss = MSE + 0.1 * LPIPS), smoother outputs |
### LAION-Aesthetics 5+ (256x256, subset, 10000 images)
| Model | train steps | rFID | PSNR | SSIM | PSIM | Link | Comments
|----------|-----------|------|--------------|---------------|---------------|-----------------------------------------------------------------------------------|-------------------------------------------------------------------------------------------------|
| | | | | | | | |
| original | 246803 | 2.61 | 26.0 +/- 4.4 | 0.81 +/- 0.12 | 0.75 +/- 0.36 | https://ommer-lab.com/files/latent-diffusion/kl-f8.zip | as used in SD |
| ft-EMA | 560001 | 1.77 | 26.7 +/- 4.8 | 0.82 +/- 0.12 | 0.67 +/- 0.34 | https://huggingface.co/stabilityai/sd-vae-ft-ema-original/resolve/main/vae-ft-ema-560000-ema-pruned.ckpt | slightly better overall, with EMA |
| ft-MSE | 840001 | 1.88 | 27.3 +/- 4.7 | 0.83 +/- 0.11 | 0.65 +/- 0.34 | https://huggingface.co/stabilityai/sd-vae-ft-mse-original/resolve/main/vae-ft-mse-840000-ema-pruned.ckpt | resumed with EMA from ft-EMA, emphasis on MSE (rec. loss = MSE + 0.1 * LPIPS), smoother outputs |
### Visual
_Visualization of reconstructions on 256x256 images from the COCO2017 validation dataset._
<p align="center">
<br>
<b>
256x256: ft-EMA (left), ft-MSE (middle), original (right)</b>
</p>
<p align="center">
<img src=https://huggingface.co/stabilityai/stable-diffusion-decoder-finetune/resolve/main/eval/ae-decoder-tuning-reconstructions/merged/00025_merged.png />
</p>
<p align="center">
<img src=https://huggingface.co/stabilityai/stable-diffusion-decoder-finetune/resolve/main/eval/ae-decoder-tuning-reconstructions/merged/00011_merged.png />
</p>
<p align="center">
<img src=https://huggingface.co/stabilityai/stable-diffusion-decoder-finetune/resolve/main/eval/ae-decoder-tuning-reconstructions/merged/00037_merged.png />
</p>
<p align="center">
<img src=https://huggingface.co/stabilityai/stable-diffusion-decoder-finetune/resolve/main/eval/ae-decoder-tuning-reconstructions/merged/00043_merged.png />
</p>
<p align="center">
<img src=https://huggingface.co/stabilityai/stable-diffusion-decoder-finetune/resolve/main/eval/ae-decoder-tuning-reconstructions/merged/00053_merged.png />
</p>
<p align="center">
<img src=https://huggingface.co/stabilityai/stable-diffusion-decoder-finetune/resolve/main/eval/ae-decoder-tuning-reconstructions/merged/00029_merged.png />
</p>
|
textattack/albert-base-v2-ag-news | textattack | 2020-07-07T21:59:15Z | 20,818 | 0 | transformers | [
"transformers",
"pytorch",
"albert",
"text-classification",
"autotrain_compatible",
"endpoints_compatible",
"region:us"
] | text-classification | 2022-03-02T23:29:05Z | ## TextAttack Model CardThis `albert-base-v2` model was fine-tuned for sequence classification using TextAttack
and the ag_news dataset loaded using the `nlp` library. The model was fine-tuned
for 5 epochs with a batch size of 16, a learning
rate of 2e-05, and a maximum sequence length of 128.
Since this was a classification task, the model was trained with a cross-entropy loss function.
The best score the model achieved on this task was 0.9471052631578948, as measured by the
eval set accuracy, found after 3 epochs.
For more information, check out [TextAttack on Github](https://github.com/QData/TextAttack).
|
microsoft/Phi-3-small-128k-instruct | microsoft | 2024-06-03T21:15:45Z | 20,753 | 135 | transformers | [
"transformers",
"safetensors",
"phi3small",
"text-generation",
"nlp",
"code",
"conversational",
"custom_code",
"multilingual",
"license:mit",
"autotrain_compatible",
"region:us"
] | text-generation | 2024-05-07T15:29:20Z | ---
license: mit
license_link: https://huggingface.co/microsoft/Phi-3-small-128k-instruct/resolve/main/LICENSE
language:
- multilingual
pipeline_tag: text-generation
tags:
- nlp
- code
inference:
parameters:
temperature: 0.7
widget:
- messages:
- role: user
content: Can you provide ways to eat combinations of bananas and dragonfruits?
---
## Model Summary
The Phi-3-Small-128K-Instruct is a 7B parameters, lightweight, state-of-the-art open model trained with the Phi-3 datasets that includes both synthetic data and the filtered publicly available websites data with a focus on high-quality and reasoning dense properties.
The model belongs to the Phi-3 family with the Small version in two variants [8K](https://huggingface.co/microsoft/Phi-3-small-8k-instruct) and [128K](https://huggingface.co/microsoft/Phi-3-small-128k-instruct) which is the context length (in tokens) that it can support.
The model has underwent a post-training process that incorporates both supervised fine-tuning and direct preference optimization for the instruction following and safety measures.
When assessed against benchmarks testing common sense, language understanding, math, code, long context and logical reasoning, Phi-3-Small-128K-Instruct showcased a robust and state-of-the-art performance among models of the same-size and next-size-up.
Resources and Technical Documentation:
+ [Phi-3 Microsoft Blog](https://aka.ms/Phi-3Build2024)
+ [Phi-3 Technical Report](https://aka.ms/phi3-tech-report)
+ [Phi-3 on Azure AI Studio](https://aka.ms/phi3-azure-ai)
+ [Phi-3 Cookbook](https://github.com/microsoft/Phi-3CookBook)
| | Short Context | Long Context |
| ------- | ------------- | ------------ |
| Mini | 4K [[HF]](https://huggingface.co/microsoft/Phi-3-mini-4k-instruct) ; [[ONNX]](https://huggingface.co/microsoft/Phi-3-mini-4k-instruct-onnx) ; [[GGUF]](https://huggingface.co/microsoft/Phi-3-mini-4k-instruct-gguf) | 128K [[HF]](https://huggingface.co/microsoft/Phi-3-mini-128k-instruct) ; [[ONNX]](https://huggingface.co/microsoft/Phi-3-mini-128k-instruct-onnx)|
| Small | 8K [[HF]](https://huggingface.co/microsoft/Phi-3-small-8k-instruct) ; [[ONNX]](https://huggingface.co/microsoft/Phi-3-small-8k-instruct-onnx-cuda) | 128K [[HF]](https://huggingface.co/microsoft/Phi-3-small-128k-instruct) ; [[ONNX]](https://huggingface.co/microsoft/Phi-3-small-128k-instruct-onnx-cuda)|
| Medium | 4K [[HF]](https://huggingface.co/microsoft/Phi-3-medium-4k-instruct) ; [[ONNX]](https://huggingface.co/microsoft/Phi-3-medium-4k-instruct-onnx-cuda) | 128K [[HF]](https://huggingface.co/microsoft/Phi-3-medium-128k-instruct) ; [[ONNX]](https://huggingface.co/microsoft/Phi-3-medium-128k-instruct-onnx-cuda)|
| Vision | | 128K [[HF]](https://huggingface.co/microsoft/Phi-3-vision-128k-instruct) ; [[ONNX]](https://huggingface.co/microsoft/Phi-3-vision-128k-instruct-onnx-cuda)|
## Intended Uses
**Primary use cases**
The model is intended for broad commercial and research use in English. The model provides uses for general purpose AI systems and applications which require :
1) Memory/compute constrained environments
2) Latency bound scenarios
3) Strong reasoning (especially code, math and logic)
Our model is designed to accelerate research on language and multimodal models, for use as a building block for generative AI powered features.
**Use case considerations**
Our models are not specifically designed or evaluated for all downstream purposes. Developers should consider common limitations of language models as they select use cases, and evaluate and mitigate for accuracy, safety, and fariness before using within a specific downstream use case, particularly for high risk scenarios. Developers should be aware of and adhere to applicable laws or regulations (including privacy, trade compliance laws, etc.) that are relevant to their use case.
Nothing contained in this Model Card should be interpreted as or deemed a restriction or modification to the license the model is released under.
## How to Use
Phi-3-Small-128K-Instruct has been integrated in the development version (4.40.2) of `transformers`. Until the official version is released through `pip`, ensure that you are doing one of the following:
* Install tiktoken (0.6.0) ans triton (2.3.0)
* When loading the model, ensure that `trust_remote_code=True` is passed as an argument of the `from_pretrained()` function.
* Update your local `transformers` to the development version: `pip uninstall -y transformers && pip install git+https://github.com/huggingface/transformers`. The previous command is an alternative to cloning and installing from the source.
The current `transformers` version can be verified with: `pip list | grep transformers`.
Phi-3-Small-128K-Instruct is also available in [Azure AI](https://ai.azure.com/explore/models?&selectedCollection=phi).
### Tokenizer
Phi-3-Small-128K-Instruct supports a vocabulary size of up to `100352` tokens.
### Chat Format
Given the nature of the training data, the Phi-3-Small-128K-Instruct model is best suited for prompts using the chat format as follows.
You can provide the prompt as a question with a generic template as follow:
```markdown
<|endoftext|><|user|>\nQuestion <|end|>\n<|assistant|>
```
For example:
```markdown
<|endoftext|><|user|>
How to explain Internet for a medieval knight?<|end|>
<|assistant|>
```
where the model generates the text after `<|assistant|>` . In case of few-shots prompt, the prompt can be formatted as the following:
```markdown
<|endoftext|><|user|>
I am going to Paris, what should I see?<|end|>
<|assistant|>
Paris, the capital of France, is known for its stunning architecture, art museums, historical landmarks, and romantic atmosphere. Here are some of the top attractions to see in Paris:\n\n1. The Eiffel Tower: The iconic Eiffel Tower is one of the most recognizable landmarks in the world and offers breathtaking views of the city.\n2. The Louvre Museum: The Louvre is one of the world's largest and most famous museums, housing an impressive collection of art and artifacts, including the Mona Lisa.\n3. Notre-Dame Cathedral: This beautiful cathedral is one of the most famous landmarks in Paris and is known for its Gothic architecture and stunning stained glass windows.\n\nThese are just a few of the many attractions that Paris has to offer. With so much to see and do, it's no wonder that Paris is one of the most popular tourist destinations in the world."<|end|>
<|user|>
What is so great about #1?<|end|>
<|assistant|>
```
### Sample inference code
This code snippets show how to get quickly started with running the model on a GPU:
```python
import torch
from transformers import AutoModelForCausalLM, AutoTokenizer, pipeline
torch.random.manual_seed(0)
model_id = "microsoft/Phi-3-small-128k-instruct"
model = AutoModelForCausalLM.from_pretrained(
model_id,
torch_dtype="auto",
trust_remote_code=True,
)
assert torch.cuda.is_available(), "This model needs a GPU to run ..."
device = torch.cuda.current_device()
model = model.to(device)
tokenizer = AutoTokenizer.from_pretrained(model_id)
messages = [
{"role": "user", "content": "Can you provide ways to eat combinations of bananas and dragonfruits?"},
{"role": "assistant", "content": "Sure! Here are some ways to eat bananas and dragonfruits together: 1. Banana and dragonfruit smoothie: Blend bananas and dragonfruits together with some milk and honey. 2. Banana and dragonfruit salad: Mix sliced bananas and dragonfruits together with some lemon juice and honey."},
{"role": "user", "content": "What about solving an 2x + 3 = 7 equation?"},
]
pipe = pipeline(
"text-generation",
model=model,
tokenizer=tokenizer,
device=device
)
generation_args = {
"max_new_tokens": 500,
"return_full_text": False,
"temperature": 0.0,
"do_sample": False,
}
output = pipe(messages, **generation_args)
print(output[0]['generated_text'])
```
*Some applications/frameworks might not include a BOS token (`<|endoftext|>`) at the start of the conversation. Please ensure that it is included since it provides more reliable results.*
## Responsible AI Considerations
Like other language models, the Phi series models can potentially behave in ways that are unfair, unreliable, or offensive. Some of the limiting behaviors to be aware of include:
+ Quality of Service: the Phi models are trained primarily on English text. Languages other than English will experience worse performance. English language varieties with less representation in the training data might experience worse performance than standard American English.
+ Representation of Harms & Perpetuation of Stereotypes: These models can over- or under-represent groups of people, erase representation of some groups, or reinforce demeaning or negative stereotypes. Despite safety post-training, these limitations may still be present due to differing levels of representation of different groups or prevalence of examples of negative stereotypes in training data that reflect real-world patterns and societal biases.
+ Inappropriate or Offensive Content: these models may produce other types of inappropriate or offensive content, which may make it inappropriate to deploy for sensitive contexts without additional mitigations that are specific to the use case.
+ Information Reliability: Language models can generate nonsensical content or fabricate content that might sound reasonable but is inaccurate or outdated.
+ Limited Scope for Code: Majority of Phi-3 training data is based in Python and use common packages such as "typing, math, random, collections, datetime, itertools". If the model generates Python scripts that utilize other packages or scripts in other languages, we strongly recommend users manually verify all API uses.
Developers should apply responsible AI best practices and are responsible for ensuring that a specific use case complies with relevant laws and regulations (e.g. privacy, trade, etc.). Important areas for consideration include:
+ Allocation: Models may not be suitable for scenarios that could have consequential impact on legal status or the allocation of resources or life opportunities (ex: housing, employment, credit, etc.) without further assessments and additional debiasing techniques.
+ High-Risk Scenarios: Developers should assess suitability of using models in high-risk scenarios where unfair, unreliable or offensive outputs might be extremely costly or lead to harm. This includes providing advice in sensitive or expert domains where accuracy and reliability are critical (ex: legal or health advice). Additional safeguards should be implemented at the application level according to the deployment context.
+ Misinformation: Models may produce inaccurate information. Developers should follow transparency best practices and inform end-users they are interacting with an AI system. At the application level, developers can build feedback mechanisms and pipelines to ground responses in use-case specific, contextual information, a technique known as Retrieval Augmented Generation (RAG).
+ Generation of Harmful Content: Developers should assess outputs for their context and use available safety classifiers or custom solutions appropriate for their use case.
+ Misuse: Other forms of misuse such as fraud, spam, or malware production may be possible, and developers should ensure that their applications do not violate applicable laws and regulations.
## Training
### Model
* Architecture: Phi-3 Small-128K-Instruct has 7B parameters and is a dense decoder-only Transformer model with alternating dense and blocksparse attentions. The model is fine-tuned with Supervised fine-tuning (SFT) and Direct Preference Optimization (DPO) to ensure alignment with human preferences and safety guidlines.
* Inputs: Text. It is best suited for prompts using chat format.
* Context length: 128K tokens
* GPUs: 1024 H100-80G
* Training time: 18 days
* Training data: 4.8T tokens
* Outputs: Generated text in response to the input
* Dates: Our models were trained between February and April 2024
* Status: This is a static model trained on an offline dataset with cutoff date October 2023. Future versions of the tuned models may be released as we improve models.
* Release dates The model weight is released on May 21, 2024.
### Datasets
Our training data includes a wide variety of sources, totaling 4.8 trillion tokens (including 10% multilingual), and is a combination of
1) Publicly available documents filtered rigorously for quality, selected high-quality educational data, and code;
2) Newly created synthetic, “textbook-like” data for the purpose of teaching math, coding, common sense reasoning, general knowledge of the world (science, daily activities, theory of mind, etc.);
3) High quality chat format supervised data covering various topics to reflect human preferences on different aspects such as instruct-following, truthfulness, honesty and helpfulness.
We are focusing on the quality of data that could potentially improve the reasoning ability for the model, and we filter the publicly available documents to contain the correct level of knowledge. As an example, the result of a game in premier league in a particular day might be good training data for frontier models, but we need to remove such information to leave more model capacity for reasoning for the small size models. More details about data can be found in the [Phi-3 Technical Report](https://aka.ms/phi3-tech-report).
## Benchmarks
We report the results for Phi-3-Small-128K-Instruct on standard open-source benchmarks measuring the model's reasoning ability (both common sense reasoning and logical reasoning). We compare to Mixtral-8x7b, Gemini-Pro, Gemma 7B, Llama-3-8B-Instruct, GPT-3.5-Turbo-1106, and GPT-4-Turbo-1106.
All the reported numbers are produced with the exact same pipeline to ensure that the numbers are comparable. These numbers might differ from other published numbers due to slightly different choices in the evaluation.
As is now standard, we use few-shot prompts to evaluate the models, at temperature 0.
The prompts and number of shots are part of a Microsoft internal tool to evaluate language models, and in particular we did no optimization to the pipeline for Phi-3.
More specifically, we do not change prompts, pick different few-shot examples, change prompt format, or do any other form of optimization for the model.
The number of k–shot examples is listed per-benchmark.
|Benchmark|Phi-3-Small-128K-Instruct<br>7b|Gemma<br>7B|Mixtral<br>8x7B|Llama-3-Instruct<br>8b|GPT-3.5-Turbo<br>version 1106|Gemini<br>Pro|GPT-4-Turbo<br>version 1106 (Chat)|
|---------|-------------------------------|----------|-------------|-------------------------|---------------------------|------------|--------------------------------|
|AGI Eval<br>5-shot|43.9|42.1|45.2|42.0|48.4|49.0|59.6|
|MMLU<br>5-shot|75.5|63.6|70.5|66.5|71.4|66.7|84.0|
|BigBench Hard<br>3-shot|77.6|59.6|69.7|51.5|68.3|75.6|87.7|
|ANLI<br>7-shot|55.8|48.7|55.2|57.3|58.1|64.2|71.7|
|HellaSwag<br>5-shot|79.6|49.8|70.4|71.1|78.8|76.2|88.3|
|ARC Challenge<br>10-shot|90.8|78.3|87.3|82.8|87.4|88.3|95.6|
|ARC Easy<br>10-shot|97.3|91.4|95.6|93.4|96.3|96.1|98.8|
|BoolQ<br>2-shot|83.7|66.0|76.6|80.9|79.1|86.4|91.3|
|CommonsenseQA<br>10-shot|80.8|76.2|78.1|79.0|79.6|81.8|86.7|
|MedQA<br>2-shot|46.3|49.6|62.2|60.5|63.4|58.2|83.7|
|OpenBookQA<br>10-shot|87.8|78.6|85.8|82.6|86.0|86.4|93.4|
|PIQA<br>5-shot|88.1|78.1|86.0|75.7|86.6|86.2|90.1|
|Social IQA<br>5-shot|78.7|65.5|75.9|73.9|68.3|75.4|81.7|
|TruthfulQA (MC2)<br>10-shot|69.6|52.1|60.1|63.2|67.7|72.6|85.2|
|WinoGrande<br>5-shot|80.1|55.6|62.0|65.0|68.8|72.2|86.7|
|TriviaQA<br>5-shot|66.0|72.3|82.2|67.7|85.8|80.2|73.3|
|GSM8K Chain of Thought<br>8-shot|87.3|59.8|64.7|77.4|78.1|80.4|94.2|
|HumanEval<br>0-shot|59.1|34.1|37.8|60.4|62.2|64.4|79.9|
|MBPP<br>3-shot|70.3|51.5|60.2|67.7|77.8|73.2|86.7|
|Average|74.6|61.8|69.8|69.4|74.3|75.4|85.2|
We take a closer look at different categories across 80 public benchmark datasets at the table below:
|Benchmark|Phi-3-Small-128K-Instruct<br>7b|Gemma<br>7B|Mixtral<br>8x7B|Llama-3-Instruct<br>8b|GPT-3.5-Turbo<br>version 1106|Gemini<br>Pro|GPT-4-Turbo<br>version 1106 (Chat)|
|--------|--------------------------|--------|-------------|-------------------|-------------------|----------|------------------------|
|Popular aggregated benchmark|70.6|59.4|66.2|59.9|67.0|67.5|80.5|
|Reasoning|80.3|69.1|77.0|75.7|78.3|80.4|89.3|
|Language understanding|67.4|58.4|64.9|65.4|70.4|75.3|81.6|
|Code generation|60.0|45.6|52.7|56.4|70.4|66.7|76.1|
|Math|48.1|35.8|40.3|41.1|52.8|50.9|67.1|
|Factual knowledge|41.7|46.7|58.6|43.1|63.4|54.6|45.9|
|Multilingual|62.6|63.2|63.4|65.0|69.1|76.5|82.0|
|Robustness|68.7|38.4|51.0|64.5|69.3|69.7|84.6|
## Software
* [PyTorch](https://github.com/pytorch/pytorch)
* [DeepSpeed](https://github.com/microsoft/DeepSpeed)
* [Transformers](https://github.com/huggingface/transformers)
* [Flash-Attention](https://github.com/HazyResearch/flash-attention)
* [Tiktoken](https://github.com/openai/tiktoken)
* [Triton](https://github.com/openai/triton)
## Hardware
Note that by default, the Phi-3-Small model uses flash attention 2 and Triton blocksparse attention, which requires certain types of GPU hardware to run. We have tested on the following GPU types:
* NVIDIA A100
* NVIDIA A6000
* NVIDIA H100
If you want to run the model on:
+ Optimized inference on GPU, CPU, and Mobile: use the **ONNX** models [128K](https://huggingface.co/microsoft/Phi-3-small-128k-instruct-onnx-cuda)
## Cross Platform Support
ONNX runtime ecosystem now supports Phi3 small models across platforms and hardware.
Optimized phi-3 models are also published here in ONNX format, to run with ONNX Runtime on CPU and GPU across devices, including server platforms, Windows, Linux and Mac desktops, and mobile CPUs, with the precision best suited to each of these targets. DirectML GPU acceleration is supported for Windows desktops GPUs (AMD, Intel, and NVIDIA).
Along with DML, ONNX Runtime provides cross platform support for Phi3 Small across a range of devices CPU, GPU, and mobile.
Here are some of the optimized configurations we have added:
1. ONNX models for int4 DML: Quantized to int4 via AWQ
2. ONNX model for fp16 CUDA
3. ONNX model for int4 CUDA: Quantized to int4 via RTN
4. ONNX model for int4 CPU and Mobile: Quantized to int4 via RTN
## License
The model is licensed under the [MIT license](https://huggingface.co/microsoft/Phi-3-small-128k/resolve/main/LICENSE).
## Trademarks
This project may contain trademarks or logos for projects, products, or services. Authorized use of Microsoft trademarks or logos is subject to and must follow [Microsoft’s Trademark & Brand Guidelines](https://www.microsoft.com/en-us/legal/intellectualproperty/trademarks). Use of Microsoft trademarks or logos in modified versions of this project must not cause confusion or imply Microsoft sponsorship. Any use of third-party trademarks or logos are subject to those third-party’s policies.
|
QCRI/bert-base-multilingual-cased-pos-english | QCRI | 2023-01-25T06:00:31Z | 20,737 | 38 | transformers | [
"transformers",
"pytorch",
"bert",
"token-classification",
"part-of-speech",
"finetuned",
"en",
"license:cc-by-nc-3.0",
"autotrain_compatible",
"endpoints_compatible",
"region:us"
] | token-classification | 2022-04-27T08:15:20Z | ---
language:
- en
tags:
- part-of-speech
- finetuned
license: cc-by-nc-3.0
---
# BERT-base-multilingual-cased finetuned for Part-of-Speech tagging
This is a multilingual BERT model fine tuned for part-of-speech tagging for English. It is trained using the Penn TreeBank (Marcus et al., 1993) and achieves an F1-score of 96.69.
## Usage
A *transformers* pipeline can be used to run the model:
```python
from transformers import AutoTokenizer, AutoModelForTokenClassification, TokenClassificationPipeline
model_name = "QCRI/bert-base-multilingual-cased-pos-english"
tokenizer = AutoTokenizer.from_pretrained(model_name)
model = AutoModelForTokenClassification.from_pretrained(model_name)
pipeline = TokenClassificationPipeline(model=model, tokenizer=tokenizer)
outputs = pipeline("A test example")
print(outputs)
```
## Citation
This model was used for all the part-of-speech tagging based results in *Analyzing Encoded Concepts in Transformer Language Models*, published at NAACL'22. If you find this model useful for your own work, please use the following citation:
```bib
@inproceedings{sajjad-NAACL,
title={Analyzing Encoded Concepts in Transformer Language Models},
author={Hassan Sajjad, Nadir Durrani, Fahim Dalvi, Firoj Alam, Abdul Rafae Khan and Jia Xu},
booktitle={North American Chapter of the Association of Computational Linguistics: Human Language Technologies (NAACL)},
series={NAACL~'22},
year={2022},
address={Seattle}
}
``` |
mradermacher/L3-Blackened-Sunfall-15B-GGUF | mradermacher | 2024-06-29T12:05:32Z | 20,729 | 0 | transformers | [
"transformers",
"gguf",
"merge",
"mergekit",
"lazymergekit",
"crestf411/L3-8B-sunfall-v0.4-stheno-v3.2",
"Hastagaras/Jamet-8B-L3-MK.V-Blackroot",
"TheDrummer/Llama-3SOME-8B-v2",
"not-for-all-audiences",
"en",
"base_model:kromeurus/L3-Blackened-Sunfall-15B",
"endpoints_compatible",
"region:us"
] | null | 2024-06-29T04:55:39Z | ---
base_model: kromeurus/L3-Blackened-Sunfall-15B
language:
- en
library_name: transformers
quantized_by: mradermacher
tags:
- merge
- mergekit
- lazymergekit
- crestf411/L3-8B-sunfall-v0.4-stheno-v3.2
- Hastagaras/Jamet-8B-L3-MK.V-Blackroot
- TheDrummer/Llama-3SOME-8B-v2
- not-for-all-audiences
---
## About
<!-- ### quantize_version: 2 -->
<!-- ### output_tensor_quantised: 1 -->
<!-- ### convert_type: hf -->
<!-- ### vocab_type: -->
<!-- ### tags: -->
static quants of https://huggingface.co/kromeurus/L3-Blackened-Sunfall-15B
<!-- provided-files -->
weighted/imatrix quants are available at https://huggingface.co/mradermacher/L3-Blackened-Sunfall-15B-i1-GGUF
## Usage
If you are unsure how to use GGUF files, refer to one of [TheBloke's
READMEs](https://huggingface.co/TheBloke/KafkaLM-70B-German-V0.1-GGUF) for
more details, including on how to concatenate multi-part files.
## Provided Quants
(sorted by size, not necessarily quality. IQ-quants are often preferable over similar sized non-IQ quants)
| Link | Type | Size/GB | Notes |
|:-----|:-----|--------:|:------|
| [GGUF](https://huggingface.co/mradermacher/L3-Blackened-Sunfall-15B-GGUF/resolve/main/L3-Blackened-Sunfall-15B.Q2_K.gguf) | Q2_K | 5.8 | |
| [GGUF](https://huggingface.co/mradermacher/L3-Blackened-Sunfall-15B-GGUF/resolve/main/L3-Blackened-Sunfall-15B.IQ3_XS.gguf) | IQ3_XS | 6.5 | |
| [GGUF](https://huggingface.co/mradermacher/L3-Blackened-Sunfall-15B-GGUF/resolve/main/L3-Blackened-Sunfall-15B.Q3_K_S.gguf) | Q3_K_S | 6.8 | |
| [GGUF](https://huggingface.co/mradermacher/L3-Blackened-Sunfall-15B-GGUF/resolve/main/L3-Blackened-Sunfall-15B.IQ3_S.gguf) | IQ3_S | 6.8 | beats Q3_K* |
| [GGUF](https://huggingface.co/mradermacher/L3-Blackened-Sunfall-15B-GGUF/resolve/main/L3-Blackened-Sunfall-15B.IQ3_M.gguf) | IQ3_M | 7.0 | |
| [GGUF](https://huggingface.co/mradermacher/L3-Blackened-Sunfall-15B-GGUF/resolve/main/L3-Blackened-Sunfall-15B.Q3_K_M.gguf) | Q3_K_M | 7.5 | lower quality |
| [GGUF](https://huggingface.co/mradermacher/L3-Blackened-Sunfall-15B-GGUF/resolve/main/L3-Blackened-Sunfall-15B.Q3_K_L.gguf) | Q3_K_L | 8.1 | |
| [GGUF](https://huggingface.co/mradermacher/L3-Blackened-Sunfall-15B-GGUF/resolve/main/L3-Blackened-Sunfall-15B.IQ4_XS.gguf) | IQ4_XS | 8.4 | |
| [GGUF](https://huggingface.co/mradermacher/L3-Blackened-Sunfall-15B-GGUF/resolve/main/L3-Blackened-Sunfall-15B.Q4_K_S.gguf) | Q4_K_S | 8.7 | fast, recommended |
| [GGUF](https://huggingface.co/mradermacher/L3-Blackened-Sunfall-15B-GGUF/resolve/main/L3-Blackened-Sunfall-15B.Q4_K_M.gguf) | Q4_K_M | 9.2 | fast, recommended |
| [GGUF](https://huggingface.co/mradermacher/L3-Blackened-Sunfall-15B-GGUF/resolve/main/L3-Blackened-Sunfall-15B.Q5_K_S.gguf) | Q5_K_S | 10.5 | |
| [GGUF](https://huggingface.co/mradermacher/L3-Blackened-Sunfall-15B-GGUF/resolve/main/L3-Blackened-Sunfall-15B.Q5_K_M.gguf) | Q5_K_M | 10.8 | |
| [GGUF](https://huggingface.co/mradermacher/L3-Blackened-Sunfall-15B-GGUF/resolve/main/L3-Blackened-Sunfall-15B.Q6_K.gguf) | Q6_K | 12.4 | very good quality |
| [GGUF](https://huggingface.co/mradermacher/L3-Blackened-Sunfall-15B-GGUF/resolve/main/L3-Blackened-Sunfall-15B.Q8_0.gguf) | Q8_0 | 16.1 | fast, best quality |
Here is a handy graph by ikawrakow comparing some lower-quality quant
types (lower is better):

And here are Artefact2's thoughts on the matter:
https://gist.github.com/Artefact2/b5f810600771265fc1e39442288e8ec9
## FAQ / Model Request
See https://huggingface.co/mradermacher/model_requests for some answers to
questions you might have and/or if you want some other model quantized.
## Thanks
I thank my company, [nethype GmbH](https://www.nethype.de/), for letting
me use its servers and providing upgrades to my workstation to enable
this work in my free time.
<!-- end -->
|
Open-Orca/Mistral-7B-OpenOrca | Open-Orca | 2023-11-18T00:13:08Z | 20,726 | 661 | transformers | [
"transformers",
"pytorch",
"mistral",
"text-generation",
"conversational",
"en",
"dataset:Open-Orca/OpenOrca",
"arxiv:2306.02707",
"arxiv:2301.13688",
"license:apache-2.0",
"autotrain_compatible",
"endpoints_compatible",
"text-generation-inference",
"region:us"
] | text-generation | 2023-09-29T19:18:38Z | ---
datasets:
- Open-Orca/OpenOrca
language:
- en
library_name: transformers
pipeline_tag: text-generation
license: apache-2.0
---
<p><h1>🐋 Mistral-7B-OpenOrca 🐋</h1></p>

[<img src="https://raw.githubusercontent.com/OpenAccess-AI-Collective/axolotl/main/image/axolotl-badge-web.png" alt="Built with Axolotl" width="200" height="32"/>](https://github.com/OpenAccess-AI-Collective/axolotl)
# OpenOrca - Mistral - 7B - 8k
We have used our own [OpenOrca dataset](https://huggingface.co/datasets/Open-Orca/OpenOrca) to fine-tune on top of [Mistral 7B](https://huggingface.co/mistralai/Mistral-7B-v0.1).
This dataset is our attempt to reproduce the dataset generated for Microsoft Research's [Orca Paper](https://arxiv.org/abs/2306.02707).
We use [OpenChat](https://huggingface.co/openchat) packing, trained with [Axolotl](https://github.com/OpenAccess-AI-Collective/axolotl).
This release is trained on a curated filtered subset of most of our GPT-4 augmented data.
It is the same subset of our data as was used in our [OpenOrcaxOpenChat-Preview2-13B model](https://huggingface.co/Open-Orca/OpenOrcaxOpenChat-Preview2-13B).
**HF Leaderboard evals place this model as #1 for all models smaller than 30B at release time, outperforming all other 7B and 13B models!**
This release provides a first: a fully open model with class-breaking performance, capable of running fully accelerated on even moderate consumer GPUs.
Our thanks to the Mistral team for leading the way here.
We affectionately codename this model: "*MistralOrca*"
If you'd like to try the model now, we have it running on fast GPUs unquantized: https://huggingface.co/spaces/Open-Orca/Mistral-7B-OpenOrca
Want to visualize our full (pre-filtering) dataset? Check out our [Nomic Atlas Map](https://atlas.nomic.ai/map/c1b88b47-2d9b-47e0-9002-b80766792582/2560fd25-52fe-42f1-a58f-ff5eccc890d2).
[<img src="https://huggingface.co/Open-Orca/OpenOrca-Preview1-13B/resolve/main/OpenOrca%20Nomic%20Atlas.png" alt="Atlas Nomic Dataset Map" width="400" height="400" />](https://atlas.nomic.ai/map/c1b88b47-2d9b-47e0-9002-b80766792582/2560fd25-52fe-42f1-a58f-ff5eccc890d2)
We are in-process with training more models, so keep a look out on our org for releases coming soon with exciting partners.
We will also give sneak-peak announcements on our Discord, which you can find here:
https://AlignmentLab.ai
or check the OpenAccess AI Collective Discord for more information about Axolotl trainer here:
https://discord.gg/5y8STgB3P3
# Quantized Models
Quantized versions of this model are generously made available by [TheBloke](https://huggingface.co/TheBloke).
- AWQ: https://huggingface.co/TheBloke/Mistral-7B-OpenOrca-AWQ
- GPTQ: https://huggingface.co/TheBloke/Mistral-7B-OpenOrca-GPTQ
- GGUF: https://huggingface.co/TheBloke/Mistral-7B-OpenOrca-GGUF
# Prompt Template
We used [OpenAI's Chat Markup Language (ChatML)](https://github.com/openai/openai-python/blob/main/chatml.md) format, with `<|im_start|>` and `<|im_end|>` tokens added to support this.
This means that, e.g., in [oobabooga](https://github.com/oobabooga/text-generation-webui/) the "`MPT-Chat`" instruction template should work, as it also uses ChatML.
This formatting is also available via a pre-defined [Transformers chat template](https://huggingface.co/docs/transformers/main/chat_templating),
which means that lists of messages can be formatted for you with the `apply_chat_template()` method:
```python
chat = [
{"role": "system", "content": "You are MistralOrca, a large language model trained by Alignment Lab AI. Write out your reasoning step-by-step to be sure you get the right answers!"}
{"role": "user", "content": "How are you?"},
{"role": "assistant", "content": "I am doing well!"},
{"role": "user", "content": "Please tell me about how mistral winds have attracted super-orcas."},
]
tokenizer.apply_chat_template(chat, tokenize=False, add_generation_prompt=True)
```
which will yield:
```
<|im_start|>system
You are MistralOrca, a large language model trained by Alignment Lab AI. Write out your reasoning step-by-step to be sure you get the right answers!
<|im_end|>
<|im_start|>user
How are you?<|im_end|>
<|im_start|>assistant
I am doing well!<|im_end|>
<|im_start|>user
Please tell me about how mistral winds have attracted super-orcas.<|im_end|>
<|im_start|>assistant
```
If you use `tokenize=True` and `return_tensors="pt"` instead, then you will get a tokenized
and formatted conversation ready to pass to `model.generate()`.
# Inference
See [this notebook](https://colab.research.google.com/drive/1yZlLSifCGELAX5GN582kZypHCv0uJuNX?usp=sharing) for inference details.
Note that you need the development snapshot of Transformers currently, as support for Mistral hasn't been released into PyPI yet:
```
pip install git+https://github.com/huggingface/transformers
```
# Evaluation
## HuggingFace Leaderboard Performance
We have evaluated using the methodology and tools for the HuggingFace Leaderboard, and find that we have dramatically improved upon the base model.
We find **106%** of the base model's performance on HF Leaderboard evals, averaging **65.84**.
At release time, this beats all 7B and 13B models!
This is also **98.6%** of *`Llama2-70b-chat`*'s performance!
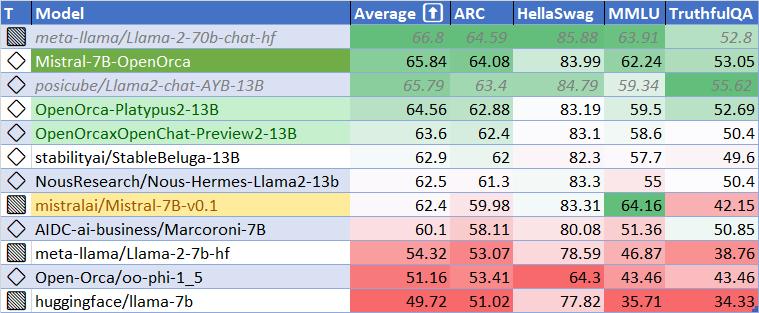
| Metric | Value |
|-----------------------|-------|
| MMLU (5-shot) | 62.24 |
| ARC (25-shot) | 64.08 |
| HellaSwag (10-shot) | 83.99 |
| TruthfulQA (0-shot) | 53.05 |
| Avg. | 65.84 |
We use [Language Model Evaluation Harness](https://github.com/EleutherAI/lm-evaluation-harness) to run the benchmark tests above, using the same version as the HuggingFace LLM Leaderboard.
## AGIEval Performance
We compare our results to the base Mistral-7B model (using LM Evaluation Harness).
We find **129%** of the base model's performance on AGI Eval, averaging **0.397**.
As well, we significantly improve upon the official `mistralai/Mistral-7B-Instruct-v0.1` finetuning, achieving **119%** of their performance.

## BigBench-Hard Performance
We find **119%** of the base model's performance on BigBench-Hard, averaging **0.416**.

## GPT4ALL Leaderboard Performance
We gain a slight edge over our previous releases, again topping the leaderboard, averaging **72.38**.

## MT-Bench Performance
MT-Bench uses GPT-4 as a judge of model response quality, across a wide range of challenges.
We find our performance is *on-par with `Llama2-70b-chat`*, averaging **6.86**.

# Dataset
We used a curated, filtered selection of most of the GPT-4 augmented data from our OpenOrca dataset, which aims to reproduce the Orca Research Paper dataset.
# Training
We trained with 8x A6000 GPUs for 62 hours, completing 4 epochs of full fine tuning on our dataset in one training run.
Commodity cost was ~$400.
# Citation
```bibtex
@software{lian2023mistralorca1
title = {MistralOrca: Mistral-7B Model Instruct-tuned on Filtered OpenOrcaV1 GPT-4 Dataset},
author = {Wing Lian and Bleys Goodson and Guan Wang and Eugene Pentland and Austin Cook and Chanvichet Vong and "Teknium"},
year = {2023},
publisher = {HuggingFace},
journal = {HuggingFace repository},
howpublished = {\url{https://huggingface.co/Open-Orca/Mistral-7B-OpenOrca},
}
@misc{mukherjee2023orca,
title={Orca: Progressive Learning from Complex Explanation Traces of GPT-4},
author={Subhabrata Mukherjee and Arindam Mitra and Ganesh Jawahar and Sahaj Agarwal and Hamid Palangi and Ahmed Awadallah},
year={2023},
eprint={2306.02707},
archivePrefix={arXiv},
primaryClass={cs.CL}
}
@misc{longpre2023flan,
title={The Flan Collection: Designing Data and Methods for Effective Instruction Tuning},
author={Shayne Longpre and Le Hou and Tu Vu and Albert Webson and Hyung Won Chung and Yi Tay and Denny Zhou and Quoc V. Le and Barret Zoph and Jason Wei and Adam Roberts},
year={2023},
eprint={2301.13688},
archivePrefix={arXiv},
primaryClass={cs.AI}
}
```
|
sentence-transformers/stsb-roberta-large | sentence-transformers | 2024-03-27T13:00:23Z | 20,673 | 3 | sentence-transformers | [
"sentence-transformers",
"pytorch",
"tf",
"jax",
"safetensors",
"roberta",
"feature-extraction",
"sentence-similarity",
"transformers",
"arxiv:1908.10084",
"license:apache-2.0",
"autotrain_compatible",
"endpoints_compatible",
"text-embeddings-inference",
"region:us"
] | sentence-similarity | 2022-03-02T23:29:05Z | ---
license: apache-2.0
library_name: sentence-transformers
tags:
- sentence-transformers
- feature-extraction
- sentence-similarity
- transformers
pipeline_tag: sentence-similarity
---
**⚠️ This model is deprecated. Please don't use it as it produces sentence embeddings of low quality. You can find recommended sentence embedding models here: [SBERT.net - Pretrained Models](https://www.sbert.net/docs/pretrained_models.html)**
# sentence-transformers/stsb-roberta-large
This is a [sentence-transformers](https://www.SBERT.net) model: It maps sentences & paragraphs to a 1024 dimensional dense vector space and can be used for tasks like clustering or semantic search.
## Usage (Sentence-Transformers)
Using this model becomes easy when you have [sentence-transformers](https://www.SBERT.net) installed:
```
pip install -U sentence-transformers
```
Then you can use the model like this:
```python
from sentence_transformers import SentenceTransformer
sentences = ["This is an example sentence", "Each sentence is converted"]
model = SentenceTransformer('sentence-transformers/stsb-roberta-large')
embeddings = model.encode(sentences)
print(embeddings)
```
## Usage (HuggingFace Transformers)
Without [sentence-transformers](https://www.SBERT.net), you can use the model like this: First, you pass your input through the transformer model, then you have to apply the right pooling-operation on-top of the contextualized word embeddings.
```python
from transformers import AutoTokenizer, AutoModel
import torch
#Mean Pooling - Take attention mask into account for correct averaging
def mean_pooling(model_output, attention_mask):
token_embeddings = model_output[0] #First element of model_output contains all token embeddings
input_mask_expanded = attention_mask.unsqueeze(-1).expand(token_embeddings.size()).float()
return torch.sum(token_embeddings * input_mask_expanded, 1) / torch.clamp(input_mask_expanded.sum(1), min=1e-9)
# Sentences we want sentence embeddings for
sentences = ['This is an example sentence', 'Each sentence is converted']
# Load model from HuggingFace Hub
tokenizer = AutoTokenizer.from_pretrained('sentence-transformers/stsb-roberta-large')
model = AutoModel.from_pretrained('sentence-transformers/stsb-roberta-large')
# Tokenize sentences
encoded_input = tokenizer(sentences, padding=True, truncation=True, return_tensors='pt')
# Compute token embeddings
with torch.no_grad():
model_output = model(**encoded_input)
# Perform pooling. In this case, max pooling.
sentence_embeddings = mean_pooling(model_output, encoded_input['attention_mask'])
print("Sentence embeddings:")
print(sentence_embeddings)
```
## Evaluation Results
For an automated evaluation of this model, see the *Sentence Embeddings Benchmark*: [https://seb.sbert.net](https://seb.sbert.net?model_name=sentence-transformers/stsb-roberta-large)
## Full Model Architecture
```
SentenceTransformer(
(0): Transformer({'max_seq_length': 128, 'do_lower_case': True}) with Transformer model: RobertaModel
(1): Pooling({'word_embedding_dimension': 1024, 'pooling_mode_cls_token': False, 'pooling_mode_mean_tokens': True, 'pooling_mode_max_tokens': False, 'pooling_mode_mean_sqrt_len_tokens': False})
)
```
## Citing & Authors
This model was trained by [sentence-transformers](https://www.sbert.net/).
If you find this model helpful, feel free to cite our publication [Sentence-BERT: Sentence Embeddings using Siamese BERT-Networks](https://arxiv.org/abs/1908.10084):
```bibtex
@inproceedings{reimers-2019-sentence-bert,
title = "Sentence-BERT: Sentence Embeddings using Siamese BERT-Networks",
author = "Reimers, Nils and Gurevych, Iryna",
booktitle = "Proceedings of the 2019 Conference on Empirical Methods in Natural Language Processing",
month = "11",
year = "2019",
publisher = "Association for Computational Linguistics",
url = "http://arxiv.org/abs/1908.10084",
}
``` |
microsoft/trocr-large-handwritten | microsoft | 2024-05-27T20:10:58Z | 20,646 | 76 | transformers | [
"transformers",
"pytorch",
"vision-encoder-decoder",
"trocr",
"image-to-text",
"arxiv:2109.10282",
"endpoints_compatible",
"region:us"
] | image-to-text | 2022-03-02T23:29:05Z | ---
tags:
- trocr
- image-to-text
widget:
- src: https://fki.tic.heia-fr.ch/static/img/a01-122-02.jpg
example_title: Note 1
- src: https://encrypted-tbn0.gstatic.com/images?q=tbn:ANd9GcSoolxi9yWGAT5SLZShv8vVd0bz47UWRzQC19fDTeE8GmGv_Rn-PCF1pP1rrUx8kOjA4gg&usqp=CAU
example_title: Note 2
- src: https://encrypted-tbn0.gstatic.com/images?q=tbn:ANd9GcRNYtTuSBpZPV_nkBYPMFwVVD9asZOPgHww4epu9EqWgDmXW--sE2o8og40ZfDGo87j5w&usqp=CAU
example_title: Note 3
---
# TrOCR (large-sized model, fine-tuned on IAM)
TrOCR model fine-tuned on the [IAM dataset](https://fki.tic.heia-fr.ch/databases/iam-handwriting-database). It was introduced in the paper [TrOCR: Transformer-based Optical Character Recognition with Pre-trained Models](https://arxiv.org/abs/2109.10282) by Li et al. and first released in [this repository](https://github.com/microsoft/unilm/tree/master/trocr).
Disclaimer: The team releasing TrOCR did not write a model card for this model so this model card has been written by the Hugging Face team.
## Model description
The TrOCR model is an encoder-decoder model, consisting of an image Transformer as encoder, and a text Transformer as decoder. The image encoder was initialized from the weights of BEiT, while the text decoder was initialized from the weights of RoBERTa.
Images are presented to the model as a sequence of fixed-size patches (resolution 16x16), which are linearly embedded. One also adds absolute position embeddings before feeding the sequence to the layers of the Transformer encoder. Next, the Transformer text decoder autoregressively generates tokens.
## Intended uses & limitations
You can use the raw model for optical character recognition (OCR) on single text-line images. See the [model hub](https://huggingface.co/models?search=microsoft/trocr) to look for fine-tuned versions on a task that interests you.
### How to use
Here is how to use this model in PyTorch:
```python
from transformers import TrOCRProcessor, VisionEncoderDecoderModel
from PIL import Image
import requests
# load image from the IAM database
url = 'https://fki.tic.heia-fr.ch/static/img/a01-122-02-00.jpg'
image = Image.open(requests.get(url, stream=True).raw).convert("RGB")
processor = TrOCRProcessor.from_pretrained('microsoft/trocr-large-handwritten')
model = VisionEncoderDecoderModel.from_pretrained('microsoft/trocr-large-handwritten')
pixel_values = processor(images=image, return_tensors="pt").pixel_values
generated_ids = model.generate(pixel_values)
generated_text = processor.batch_decode(generated_ids, skip_special_tokens=True)[0]
```
### BibTeX entry and citation info
```bibtex
@misc{li2021trocr,
title={TrOCR: Transformer-based Optical Character Recognition with Pre-trained Models},
author={Minghao Li and Tengchao Lv and Lei Cui and Yijuan Lu and Dinei Florencio and Cha Zhang and Zhoujun Li and Furu Wei},
year={2021},
eprint={2109.10282},
archivePrefix={arXiv},
primaryClass={cs.CL}
}
``` |
mradermacher/Swallow-13b-hf-i1-GGUF | mradermacher | 2024-06-30T14:07:53Z | 20,640 | 0 | transformers | [
"transformers",
"gguf",
"en",
"ja",
"base_model:tokyotech-llm/Swallow-13b-hf",
"license:llama2",
"endpoints_compatible",
"region:us"
] | null | 2024-06-30T07:18:54Z | ---
base_model: tokyotech-llm/Swallow-13b-hf
language:
- en
- ja
library_name: transformers
license: llama2
model_type: llama
quantized_by: mradermacher
---
## About
<!-- ### quantize_version: 2 -->
<!-- ### output_tensor_quantised: 1 -->
<!-- ### convert_type: hf -->
<!-- ### vocab_type: -->
<!-- ### tags: nicoboss -->
weighted/imatrix quants of https://huggingface.co/tokyotech-llm/Swallow-13b-hf
<!-- provided-files -->
static quants are available at https://huggingface.co/mradermacher/Swallow-13b-hf-GGUF
## Usage
If you are unsure how to use GGUF files, refer to one of [TheBloke's
READMEs](https://huggingface.co/TheBloke/KafkaLM-70B-German-V0.1-GGUF) for
more details, including on how to concatenate multi-part files.
## Provided Quants
(sorted by size, not necessarily quality. IQ-quants are often preferable over similar sized non-IQ quants)
| Link | Type | Size/GB | Notes |
|:-----|:-----|--------:|:------|
| [GGUF](https://huggingface.co/mradermacher/Swallow-13b-hf-i1-GGUF/resolve/main/Swallow-13b-hf.i1-IQ1_S.gguf) | i1-IQ1_S | 3.1 | for the desperate |
| [GGUF](https://huggingface.co/mradermacher/Swallow-13b-hf-i1-GGUF/resolve/main/Swallow-13b-hf.i1-IQ1_M.gguf) | i1-IQ1_M | 3.3 | mostly desperate |
| [GGUF](https://huggingface.co/mradermacher/Swallow-13b-hf-i1-GGUF/resolve/main/Swallow-13b-hf.i1-IQ2_XXS.gguf) | i1-IQ2_XXS | 3.7 | |
| [GGUF](https://huggingface.co/mradermacher/Swallow-13b-hf-i1-GGUF/resolve/main/Swallow-13b-hf.i1-IQ2_XS.gguf) | i1-IQ2_XS | 4.0 | |
| [GGUF](https://huggingface.co/mradermacher/Swallow-13b-hf-i1-GGUF/resolve/main/Swallow-13b-hf.i1-IQ2_S.gguf) | i1-IQ2_S | 4.4 | |
| [GGUF](https://huggingface.co/mradermacher/Swallow-13b-hf-i1-GGUF/resolve/main/Swallow-13b-hf.i1-IQ2_M.gguf) | i1-IQ2_M | 4.7 | |
| [GGUF](https://huggingface.co/mradermacher/Swallow-13b-hf-i1-GGUF/resolve/main/Swallow-13b-hf.i1-Q2_K.gguf) | i1-Q2_K | 5.0 | IQ3_XXS probably better |
| [GGUF](https://huggingface.co/mradermacher/Swallow-13b-hf-i1-GGUF/resolve/main/Swallow-13b-hf.i1-IQ3_XXS.gguf) | i1-IQ3_XXS | 5.1 | lower quality |
| [GGUF](https://huggingface.co/mradermacher/Swallow-13b-hf-i1-GGUF/resolve/main/Swallow-13b-hf.i1-IQ3_XS.gguf) | i1-IQ3_XS | 5.5 | |
| [GGUF](https://huggingface.co/mradermacher/Swallow-13b-hf-i1-GGUF/resolve/main/Swallow-13b-hf.i1-IQ3_S.gguf) | i1-IQ3_S | 5.8 | beats Q3_K* |
| [GGUF](https://huggingface.co/mradermacher/Swallow-13b-hf-i1-GGUF/resolve/main/Swallow-13b-hf.i1-Q3_K_S.gguf) | i1-Q3_K_S | 5.8 | IQ3_XS probably better |
| [GGUF](https://huggingface.co/mradermacher/Swallow-13b-hf-i1-GGUF/resolve/main/Swallow-13b-hf.i1-IQ3_M.gguf) | i1-IQ3_M | 6.2 | |
| [GGUF](https://huggingface.co/mradermacher/Swallow-13b-hf-i1-GGUF/resolve/main/Swallow-13b-hf.i1-Q3_K_M.gguf) | i1-Q3_K_M | 6.5 | IQ3_S probably better |
| [GGUF](https://huggingface.co/mradermacher/Swallow-13b-hf-i1-GGUF/resolve/main/Swallow-13b-hf.i1-Q3_K_L.gguf) | i1-Q3_K_L | 7.1 | IQ3_M probably better |
| [GGUF](https://huggingface.co/mradermacher/Swallow-13b-hf-i1-GGUF/resolve/main/Swallow-13b-hf.i1-IQ4_XS.gguf) | i1-IQ4_XS | 7.1 | |
| [GGUF](https://huggingface.co/mradermacher/Swallow-13b-hf-i1-GGUF/resolve/main/Swallow-13b-hf.i1-Q4_0.gguf) | i1-Q4_0 | 7.6 | fast, low quality |
| [GGUF](https://huggingface.co/mradermacher/Swallow-13b-hf-i1-GGUF/resolve/main/Swallow-13b-hf.i1-Q4_K_S.gguf) | i1-Q4_K_S | 7.6 | optimal size/speed/quality |
| [GGUF](https://huggingface.co/mradermacher/Swallow-13b-hf-i1-GGUF/resolve/main/Swallow-13b-hf.i1-Q4_K_M.gguf) | i1-Q4_K_M | 8.0 | fast, recommended |
| [GGUF](https://huggingface.co/mradermacher/Swallow-13b-hf-i1-GGUF/resolve/main/Swallow-13b-hf.i1-Q5_K_S.gguf) | i1-Q5_K_S | 9.2 | |
| [GGUF](https://huggingface.co/mradermacher/Swallow-13b-hf-i1-GGUF/resolve/main/Swallow-13b-hf.i1-Q5_K_M.gguf) | i1-Q5_K_M | 9.4 | |
| [GGUF](https://huggingface.co/mradermacher/Swallow-13b-hf-i1-GGUF/resolve/main/Swallow-13b-hf.i1-Q6_K.gguf) | i1-Q6_K | 10.9 | practically like static Q6_K |
Here is a handy graph by ikawrakow comparing some lower-quality quant
types (lower is better):

And here are Artefact2's thoughts on the matter:
https://gist.github.com/Artefact2/b5f810600771265fc1e39442288e8ec9
## FAQ / Model Request
See https://huggingface.co/mradermacher/model_requests for some answers to
questions you might have and/or if you want some other model quantized.
## Thanks
I thank my company, [nethype GmbH](https://www.nethype.de/), for letting
me use its servers and providing upgrades to my workstation to enable
this work in my free time. Additional thanks to [@nicoboss](https://huggingface.co/nicoboss) for giving me access to his hardware for calculating the imatrix for these quants.
<!-- end -->
|
legraphista/RoLlama3-8b-Instruct-IMat-GGUF | legraphista | 2024-06-23T19:15:50Z | 20,632 | 1 | gguf | [
"gguf",
"quantized",
"GGUF",
"quantization",
"imat",
"imatrix",
"static",
"16bit",
"8bit",
"6bit",
"5bit",
"4bit",
"3bit",
"2bit",
"1bit",
"text-generation",
"base_model:OpenLLM-Ro/RoLlama3-8b-Instruct",
"region:us"
] | text-generation | 2024-06-23T18:38:08Z | ---
base_model: OpenLLM-Ro/RoLlama3-8b-Instruct
inference: false
library_name: gguf
pipeline_tag: text-generation
quantized_by: legraphista
tags:
- quantized
- GGUF
- quantization
- imat
- imatrix
- static
- 16bit
- 8bit
- 6bit
- 5bit
- 4bit
- 3bit
- 2bit
- 1bit
---
# RoLlama3-8b-Instruct-IMat-GGUF
_Llama.cpp imatrix quantization of OpenLLM-Ro/RoLlama3-8b-Instruct_
Original Model: [OpenLLM-Ro/RoLlama3-8b-Instruct](https://huggingface.co/OpenLLM-Ro/RoLlama3-8b-Instruct)
Original dtype: `BF16` (`bfloat16`)
Quantized by: llama.cpp [b3206](https://github.com/ggerganov/llama.cpp/releases/tag/b3206)
IMatrix dataset: [here](https://gist.githubusercontent.com/bartowski1182/eb213dccb3571f863da82e99418f81e8/raw/b2869d80f5c16fd7082594248e80144677736635/calibration_datav3.txt)
- [Files](#files)
- [IMatrix](#imatrix)
- [Common Quants](#common-quants)
- [All Quants](#all-quants)
- [Downloading using huggingface-cli](#downloading-using-huggingface-cli)
- [Inference](#inference)
- [Simple chat template](#simple-chat-template)
- [Chat template with system prompt](#chat-template-with-system-prompt)
- [Llama.cpp](#llama-cpp)
- [FAQ](#faq)
- [Why is the IMatrix not applied everywhere?](#why-is-the-imatrix-not-applied-everywhere)
- [How do I merge a split GGUF?](#how-do-i-merge-a-split-gguf)
---
## Files
### IMatrix
Status: ✅ Available
Link: [here](https://huggingface.co/legraphista/RoLlama3-8b-Instruct-IMat-GGUF/blob/main/imatrix.dat)
### Common Quants
| Filename | Quant type | File Size | Status | Uses IMatrix | Is Split |
| -------- | ---------- | --------- | ------ | ------------ | -------- |
| [RoLlama3-8b-Instruct.Q8_0.gguf](https://huggingface.co/legraphista/RoLlama3-8b-Instruct-IMat-GGUF/blob/main/RoLlama3-8b-Instruct.Q8_0.gguf) | Q8_0 | 8.54GB | ✅ Available | ⚪ Static | 📦 No
| [RoLlama3-8b-Instruct.Q6_K.gguf](https://huggingface.co/legraphista/RoLlama3-8b-Instruct-IMat-GGUF/blob/main/RoLlama3-8b-Instruct.Q6_K.gguf) | Q6_K | 6.60GB | ✅ Available | ⚪ Static | 📦 No
| [RoLlama3-8b-Instruct.Q4_K.gguf](https://huggingface.co/legraphista/RoLlama3-8b-Instruct-IMat-GGUF/blob/main/RoLlama3-8b-Instruct.Q4_K.gguf) | Q4_K | 4.92GB | ✅ Available | 🟢 IMatrix | 📦 No
| [RoLlama3-8b-Instruct.Q3_K.gguf](https://huggingface.co/legraphista/RoLlama3-8b-Instruct-IMat-GGUF/blob/main/RoLlama3-8b-Instruct.Q3_K.gguf) | Q3_K | 4.02GB | ✅ Available | 🟢 IMatrix | 📦 No
| [RoLlama3-8b-Instruct.Q2_K.gguf](https://huggingface.co/legraphista/RoLlama3-8b-Instruct-IMat-GGUF/blob/main/RoLlama3-8b-Instruct.Q2_K.gguf) | Q2_K | 3.18GB | ✅ Available | 🟢 IMatrix | 📦 No
### All Quants
| Filename | Quant type | File Size | Status | Uses IMatrix | Is Split |
| -------- | ---------- | --------- | ------ | ------------ | -------- |
| [RoLlama3-8b-Instruct.BF16.gguf](https://huggingface.co/legraphista/RoLlama3-8b-Instruct-IMat-GGUF/blob/main/RoLlama3-8b-Instruct.BF16.gguf) | BF16 | 16.07GB | ✅ Available | ⚪ Static | 📦 No
| [RoLlama3-8b-Instruct.FP16.gguf](https://huggingface.co/legraphista/RoLlama3-8b-Instruct-IMat-GGUF/blob/main/RoLlama3-8b-Instruct.FP16.gguf) | F16 | 16.07GB | ✅ Available | ⚪ Static | 📦 No
| [RoLlama3-8b-Instruct.Q8_0.gguf](https://huggingface.co/legraphista/RoLlama3-8b-Instruct-IMat-GGUF/blob/main/RoLlama3-8b-Instruct.Q8_0.gguf) | Q8_0 | 8.54GB | ✅ Available | ⚪ Static | 📦 No
| [RoLlama3-8b-Instruct.Q6_K.gguf](https://huggingface.co/legraphista/RoLlama3-8b-Instruct-IMat-GGUF/blob/main/RoLlama3-8b-Instruct.Q6_K.gguf) | Q6_K | 6.60GB | ✅ Available | ⚪ Static | 📦 No
| [RoLlama3-8b-Instruct.Q5_K.gguf](https://huggingface.co/legraphista/RoLlama3-8b-Instruct-IMat-GGUF/blob/main/RoLlama3-8b-Instruct.Q5_K.gguf) | Q5_K | 5.73GB | ✅ Available | ⚪ Static | 📦 No
| [RoLlama3-8b-Instruct.Q5_K_S.gguf](https://huggingface.co/legraphista/RoLlama3-8b-Instruct-IMat-GGUF/blob/main/RoLlama3-8b-Instruct.Q5_K_S.gguf) | Q5_K_S | 5.60GB | ✅ Available | ⚪ Static | 📦 No
| [RoLlama3-8b-Instruct.Q4_K.gguf](https://huggingface.co/legraphista/RoLlama3-8b-Instruct-IMat-GGUF/blob/main/RoLlama3-8b-Instruct.Q4_K.gguf) | Q4_K | 4.92GB | ✅ Available | 🟢 IMatrix | 📦 No
| [RoLlama3-8b-Instruct.Q4_K_S.gguf](https://huggingface.co/legraphista/RoLlama3-8b-Instruct-IMat-GGUF/blob/main/RoLlama3-8b-Instruct.Q4_K_S.gguf) | Q4_K_S | 4.69GB | ✅ Available | 🟢 IMatrix | 📦 No
| [RoLlama3-8b-Instruct.IQ4_NL.gguf](https://huggingface.co/legraphista/RoLlama3-8b-Instruct-IMat-GGUF/blob/main/RoLlama3-8b-Instruct.IQ4_NL.gguf) | IQ4_NL | 4.68GB | ✅ Available | 🟢 IMatrix | 📦 No
| [RoLlama3-8b-Instruct.IQ4_XS.gguf](https://huggingface.co/legraphista/RoLlama3-8b-Instruct-IMat-GGUF/blob/main/RoLlama3-8b-Instruct.IQ4_XS.gguf) | IQ4_XS | 4.45GB | ✅ Available | 🟢 IMatrix | 📦 No
| [RoLlama3-8b-Instruct.Q3_K.gguf](https://huggingface.co/legraphista/RoLlama3-8b-Instruct-IMat-GGUF/blob/main/RoLlama3-8b-Instruct.Q3_K.gguf) | Q3_K | 4.02GB | ✅ Available | 🟢 IMatrix | 📦 No
| [RoLlama3-8b-Instruct.Q3_K_L.gguf](https://huggingface.co/legraphista/RoLlama3-8b-Instruct-IMat-GGUF/blob/main/RoLlama3-8b-Instruct.Q3_K_L.gguf) | Q3_K_L | 4.32GB | ✅ Available | 🟢 IMatrix | 📦 No
| [RoLlama3-8b-Instruct.Q3_K_S.gguf](https://huggingface.co/legraphista/RoLlama3-8b-Instruct-IMat-GGUF/blob/main/RoLlama3-8b-Instruct.Q3_K_S.gguf) | Q3_K_S | 3.66GB | ✅ Available | 🟢 IMatrix | 📦 No
| [RoLlama3-8b-Instruct.IQ3_M.gguf](https://huggingface.co/legraphista/RoLlama3-8b-Instruct-IMat-GGUF/blob/main/RoLlama3-8b-Instruct.IQ3_M.gguf) | IQ3_M | 3.78GB | ✅ Available | 🟢 IMatrix | 📦 No
| [RoLlama3-8b-Instruct.IQ3_S.gguf](https://huggingface.co/legraphista/RoLlama3-8b-Instruct-IMat-GGUF/blob/main/RoLlama3-8b-Instruct.IQ3_S.gguf) | IQ3_S | 3.68GB | ✅ Available | 🟢 IMatrix | 📦 No
| [RoLlama3-8b-Instruct.IQ3_XS.gguf](https://huggingface.co/legraphista/RoLlama3-8b-Instruct-IMat-GGUF/blob/main/RoLlama3-8b-Instruct.IQ3_XS.gguf) | IQ3_XS | 3.52GB | ✅ Available | 🟢 IMatrix | 📦 No
| [RoLlama3-8b-Instruct.IQ3_XXS.gguf](https://huggingface.co/legraphista/RoLlama3-8b-Instruct-IMat-GGUF/blob/main/RoLlama3-8b-Instruct.IQ3_XXS.gguf) | IQ3_XXS | 3.27GB | ✅ Available | 🟢 IMatrix | 📦 No
| [RoLlama3-8b-Instruct.Q2_K.gguf](https://huggingface.co/legraphista/RoLlama3-8b-Instruct-IMat-GGUF/blob/main/RoLlama3-8b-Instruct.Q2_K.gguf) | Q2_K | 3.18GB | ✅ Available | 🟢 IMatrix | 📦 No
| [RoLlama3-8b-Instruct.Q2_K_S.gguf](https://huggingface.co/legraphista/RoLlama3-8b-Instruct-IMat-GGUF/blob/main/RoLlama3-8b-Instruct.Q2_K_S.gguf) | Q2_K_S | 2.99GB | ✅ Available | 🟢 IMatrix | 📦 No
| [RoLlama3-8b-Instruct.IQ2_M.gguf](https://huggingface.co/legraphista/RoLlama3-8b-Instruct-IMat-GGUF/blob/main/RoLlama3-8b-Instruct.IQ2_M.gguf) | IQ2_M | 2.95GB | ✅ Available | 🟢 IMatrix | 📦 No
| [RoLlama3-8b-Instruct.IQ2_S.gguf](https://huggingface.co/legraphista/RoLlama3-8b-Instruct-IMat-GGUF/blob/main/RoLlama3-8b-Instruct.IQ2_S.gguf) | IQ2_S | 2.76GB | ✅ Available | 🟢 IMatrix | 📦 No
| [RoLlama3-8b-Instruct.IQ2_XS.gguf](https://huggingface.co/legraphista/RoLlama3-8b-Instruct-IMat-GGUF/blob/main/RoLlama3-8b-Instruct.IQ2_XS.gguf) | IQ2_XS | 2.61GB | ✅ Available | 🟢 IMatrix | 📦 No
| [RoLlama3-8b-Instruct.IQ2_XXS.gguf](https://huggingface.co/legraphista/RoLlama3-8b-Instruct-IMat-GGUF/blob/main/RoLlama3-8b-Instruct.IQ2_XXS.gguf) | IQ2_XXS | 2.40GB | ✅ Available | 🟢 IMatrix | 📦 No
| [RoLlama3-8b-Instruct.IQ1_M.gguf](https://huggingface.co/legraphista/RoLlama3-8b-Instruct-IMat-GGUF/blob/main/RoLlama3-8b-Instruct.IQ1_M.gguf) | IQ1_M | 2.16GB | ✅ Available | 🟢 IMatrix | 📦 No
| [RoLlama3-8b-Instruct.IQ1_S.gguf](https://huggingface.co/legraphista/RoLlama3-8b-Instruct-IMat-GGUF/blob/main/RoLlama3-8b-Instruct.IQ1_S.gguf) | IQ1_S | 2.02GB | ✅ Available | 🟢 IMatrix | 📦 No
## Downloading using huggingface-cli
If you do not have hugginface-cli installed:
```
pip install -U "huggingface_hub[cli]"
```
Download the specific file you want:
```
huggingface-cli download legraphista/RoLlama3-8b-Instruct-IMat-GGUF --include "RoLlama3-8b-Instruct.Q8_0.gguf" --local-dir ./
```
If the model file is big, it has been split into multiple files. In order to download them all to a local folder, run:
```
huggingface-cli download legraphista/RoLlama3-8b-Instruct-IMat-GGUF --include "RoLlama3-8b-Instruct.Q8_0/*" --local-dir ./
# see FAQ for merging GGUF's
```
---
## Inference
### Simple chat template
```
<|begin_of_text|><|start_header_id|>system<|end_header_id|>
Ești un asistent folositor, respectuos și onest. Încearcă să ajuți cât mai mult prin informațiile oferite, excluzând răspunsuri toxice, rasiste, sexiste, periculoase și ilegale.<|eot_id|><|start_header_id|>user<|end_header_id|>
{user_prompt}<|eot_id|><|start_header_id|>assistant<|end_header_id|>
{assistant_response}<|eot_id|><|start_header_id|>user<|end_header_id|>
{next_user_prompt}<|eot_id|><|start_header_id|>assistant<|end_header_id|>
```
### Chat template with system prompt
```
<|begin_of_text|><|start_header_id|>system<|end_header_id|>
{system_prompt}<|eot_id|><|start_header_id|>user<|end_header_id|>
{user_prompt}<|eot_id|><|start_header_id|>assistant<|end_header_id|>
{assistant_response}<|eot_id|><|start_header_id|>user<|end_header_id|>
{next_user_prompt}<|eot_id|><|start_header_id|>assistant<|end_header_id|>
```
### Llama.cpp
```
llama.cpp/main -m RoLlama3-8b-Instruct.Q8_0.gguf --color -i -p "prompt here (according to the chat template)"
```
---
## FAQ
### Why is the IMatrix not applied everywhere?
According to [this investigation](https://www.reddit.com/r/LocalLLaMA/comments/1993iro/ggufs_quants_can_punch_above_their_weights_now/), it appears that lower quantizations are the only ones that benefit from the imatrix input (as per hellaswag results).
### How do I merge a split GGUF?
1. Make sure you have `gguf-split` available
- To get hold of `gguf-split`, navigate to https://github.com/ggerganov/llama.cpp/releases
- Download the appropriate zip for your system from the latest release
- Unzip the archive and you should be able to find `gguf-split`
2. Locate your GGUF chunks folder (ex: `RoLlama3-8b-Instruct.Q8_0`)
3. Run `gguf-split --merge RoLlama3-8b-Instruct.Q8_0/RoLlama3-8b-Instruct.Q8_0-00001-of-XXXXX.gguf RoLlama3-8b-Instruct.Q8_0.gguf`
- Make sure to point `gguf-split` to the first chunk of the split.
---
Got a suggestion? Ping me [@legraphista](https://x.com/legraphista)! |
TheBloke/Noromaid-13B-v0.3-GGUF | TheBloke | 2024-01-07T13:08:01Z | 20,597 | 11 | transformers | [
"transformers",
"gguf",
"llama",
"base_model:NeverSleep/Noromaid-13b-v0.3",
"license:cc-by-nc-4.0",
"text-generation-inference",
"region:us"
] | null | 2024-01-07T12:38:16Z | ---
base_model: NeverSleep/Noromaid-13b-v0.3
inference: false
license: cc-by-nc-4.0
model_creator: IkariDev and Undi
model_name: Noromaid 13B v0.3
model_type: llama
prompt_template: 'Below is an instruction that describes a task. Write a response
that appropriately completes the request.
### Instruction:
{prompt}
### Response:
'
quantized_by: TheBloke
---
<!-- markdownlint-disable MD041 -->
<!-- header start -->
<!-- 200823 -->
<div style="width: auto; margin-left: auto; margin-right: auto">
<img src="https://i.imgur.com/EBdldam.jpg" alt="TheBlokeAI" style="width: 100%; min-width: 400px; display: block; margin: auto;">
</div>
<div style="display: flex; justify-content: space-between; width: 100%;">
<div style="display: flex; flex-direction: column; align-items: flex-start;">
<p style="margin-top: 0.5em; margin-bottom: 0em;"><a href="https://discord.gg/theblokeai">Chat & support: TheBloke's Discord server</a></p>
</div>
<div style="display: flex; flex-direction: column; align-items: flex-end;">
<p style="margin-top: 0.5em; margin-bottom: 0em;"><a href="https://www.patreon.com/TheBlokeAI">Want to contribute? TheBloke's Patreon page</a></p>
</div>
</div>
<div style="text-align:center; margin-top: 0em; margin-bottom: 0em"><p style="margin-top: 0.25em; margin-bottom: 0em;">TheBloke's LLM work is generously supported by a grant from <a href="https://a16z.com">andreessen horowitz (a16z)</a></p></div>
<hr style="margin-top: 1.0em; margin-bottom: 1.0em;">
<!-- header end -->
# Noromaid 13B v0.3 - GGUF
- Model creator: [IkariDev and Undi](https://huggingface.co/NeverSleep)
- Original model: [Noromaid 13B v0.3](https://huggingface.co/NeverSleep/Noromaid-13b-v0.3)
<!-- description start -->
## Description
This repo contains GGUF format model files for [IkariDev and Undi's Noromaid 13B v0.3](https://huggingface.co/NeverSleep/Noromaid-13b-v0.3).
These files were quantised using hardware kindly provided by [Massed Compute](https://massedcompute.com/).
<!-- description end -->
<!-- README_GGUF.md-about-gguf start -->
### About GGUF
GGUF is a new format introduced by the llama.cpp team on August 21st 2023. It is a replacement for GGML, which is no longer supported by llama.cpp.
Here is an incomplete list of clients and libraries that are known to support GGUF:
* [llama.cpp](https://github.com/ggerganov/llama.cpp). The source project for GGUF. Offers a CLI and a server option.
* [text-generation-webui](https://github.com/oobabooga/text-generation-webui), the most widely used web UI, with many features and powerful extensions. Supports GPU acceleration.
* [KoboldCpp](https://github.com/LostRuins/koboldcpp), a fully featured web UI, with GPU accel across all platforms and GPU architectures. Especially good for story telling.
* [GPT4All](https://gpt4all.io/index.html), a free and open source local running GUI, supporting Windows, Linux and macOS with full GPU accel.
* [LM Studio](https://lmstudio.ai/), an easy-to-use and powerful local GUI for Windows and macOS (Silicon), with GPU acceleration. Linux available, in beta as of 27/11/2023.
* [LoLLMS Web UI](https://github.com/ParisNeo/lollms-webui), a great web UI with many interesting and unique features, including a full model library for easy model selection.
* [Faraday.dev](https://faraday.dev/), an attractive and easy to use character-based chat GUI for Windows and macOS (both Silicon and Intel), with GPU acceleration.
* [llama-cpp-python](https://github.com/abetlen/llama-cpp-python), a Python library with GPU accel, LangChain support, and OpenAI-compatible API server.
* [candle](https://github.com/huggingface/candle), a Rust ML framework with a focus on performance, including GPU support, and ease of use.
* [ctransformers](https://github.com/marella/ctransformers), a Python library with GPU accel, LangChain support, and OpenAI-compatible AI server. Note, as of time of writing (November 27th 2023), ctransformers has not been updated in a long time and does not support many recent models.
<!-- README_GGUF.md-about-gguf end -->
<!-- repositories-available start -->
## Repositories available
* [AWQ model(s) for GPU inference.](https://huggingface.co/TheBloke/Noromaid-13B-v0.3-AWQ)
* [GPTQ models for GPU inference, with multiple quantisation parameter options.](https://huggingface.co/TheBloke/Noromaid-13B-v0.3-GPTQ)
* [2, 3, 4, 5, 6 and 8-bit GGUF models for CPU+GPU inference](https://huggingface.co/TheBloke/Noromaid-13B-v0.3-GGUF)
* [IkariDev and Undi's original unquantised fp16 model in pytorch format, for GPU inference and for further conversions](https://huggingface.co/NeverSleep/Noromaid-13b-v0.3)
<!-- repositories-available end -->
<!-- prompt-template start -->
## Prompt template: Alpaca
```
Below is an instruction that describes a task. Write a response that appropriately completes the request.
### Instruction:
{prompt}
### Response:
```
<!-- prompt-template end -->
<!-- licensing start -->
## Licensing
The creator of the source model has listed its license as `cc-by-nc-4.0`, and this quantization has therefore used that same license.
As this model is based on Llama 2, it is also subject to the Meta Llama 2 license terms, and the license files for that are additionally included. It should therefore be considered as being claimed to be licensed under both licenses. I contacted Hugging Face for clarification on dual licensing but they do not yet have an official position. Should this change, or should Meta provide any feedback on this situation, I will update this section accordingly.
In the meantime, any questions regarding licensing, and in particular how these two licenses might interact, should be directed to the original model repository: [IkariDev and Undi's Noromaid 13B v0.3](https://huggingface.co/NeverSleep/Noromaid-13b-v0.3).
<!-- licensing end -->
<!-- compatibility_gguf start -->
## Compatibility
These quantised GGUFv2 files are compatible with llama.cpp from August 27th onwards, as of commit [d0cee0d](https://github.com/ggerganov/llama.cpp/commit/d0cee0d36d5be95a0d9088b674dbb27354107221)
They are also compatible with many third party UIs and libraries - please see the list at the top of this README.
## Explanation of quantisation methods
<details>
<summary>Click to see details</summary>
The new methods available are:
* GGML_TYPE_Q2_K - "type-1" 2-bit quantization in super-blocks containing 16 blocks, each block having 16 weight. Block scales and mins are quantized with 4 bits. This ends up effectively using 2.5625 bits per weight (bpw)
* GGML_TYPE_Q3_K - "type-0" 3-bit quantization in super-blocks containing 16 blocks, each block having 16 weights. Scales are quantized with 6 bits. This end up using 3.4375 bpw.
* GGML_TYPE_Q4_K - "type-1" 4-bit quantization in super-blocks containing 8 blocks, each block having 32 weights. Scales and mins are quantized with 6 bits. This ends up using 4.5 bpw.
* GGML_TYPE_Q5_K - "type-1" 5-bit quantization. Same super-block structure as GGML_TYPE_Q4_K resulting in 5.5 bpw
* GGML_TYPE_Q6_K - "type-0" 6-bit quantization. Super-blocks with 16 blocks, each block having 16 weights. Scales are quantized with 8 bits. This ends up using 6.5625 bpw
Refer to the Provided Files table below to see what files use which methods, and how.
</details>
<!-- compatibility_gguf end -->
<!-- README_GGUF.md-provided-files start -->
## Provided files
| Name | Quant method | Bits | Size | Max RAM required | Use case |
| ---- | ---- | ---- | ---- | ---- | ----- |
| [noromaid-13b-v0.3.Q2_K.gguf](https://huggingface.co/TheBloke/Noromaid-13B-v0.3-GGUF/blob/main/noromaid-13b-v0.3.Q2_K.gguf) | Q2_K | 2 | 5.43 GB| 7.93 GB | smallest, significant quality loss - not recommended for most purposes |
| [noromaid-13b-v0.3.Q3_K_S.gguf](https://huggingface.co/TheBloke/Noromaid-13B-v0.3-GGUF/blob/main/noromaid-13b-v0.3.Q3_K_S.gguf) | Q3_K_S | 3 | 5.66 GB| 8.16 GB | very small, high quality loss |
| [noromaid-13b-v0.3.Q3_K_M.gguf](https://huggingface.co/TheBloke/Noromaid-13B-v0.3-GGUF/blob/main/noromaid-13b-v0.3.Q3_K_M.gguf) | Q3_K_M | 3 | 6.34 GB| 8.84 GB | very small, high quality loss |
| [noromaid-13b-v0.3.Q3_K_L.gguf](https://huggingface.co/TheBloke/Noromaid-13B-v0.3-GGUF/blob/main/noromaid-13b-v0.3.Q3_K_L.gguf) | Q3_K_L | 3 | 6.93 GB| 9.43 GB | small, substantial quality loss |
| [noromaid-13b-v0.3.Q4_0.gguf](https://huggingface.co/TheBloke/Noromaid-13B-v0.3-GGUF/blob/main/noromaid-13b-v0.3.Q4_0.gguf) | Q4_0 | 4 | 7.37 GB| 9.87 GB | legacy; small, very high quality loss - prefer using Q3_K_M |
| [noromaid-13b-v0.3.Q4_K_S.gguf](https://huggingface.co/TheBloke/Noromaid-13B-v0.3-GGUF/blob/main/noromaid-13b-v0.3.Q4_K_S.gguf) | Q4_K_S | 4 | 7.42 GB| 9.92 GB | small, greater quality loss |
| [noromaid-13b-v0.3.Q4_K_M.gguf](https://huggingface.co/TheBloke/Noromaid-13B-v0.3-GGUF/blob/main/noromaid-13b-v0.3.Q4_K_M.gguf) | Q4_K_M | 4 | 7.87 GB| 10.37 GB | medium, balanced quality - recommended |
| [noromaid-13b-v0.3.Q5_0.gguf](https://huggingface.co/TheBloke/Noromaid-13B-v0.3-GGUF/blob/main/noromaid-13b-v0.3.Q5_0.gguf) | Q5_0 | 5 | 8.97 GB| 11.47 GB | legacy; medium, balanced quality - prefer using Q4_K_M |
| [noromaid-13b-v0.3.Q5_K_S.gguf](https://huggingface.co/TheBloke/Noromaid-13B-v0.3-GGUF/blob/main/noromaid-13b-v0.3.Q5_K_S.gguf) | Q5_K_S | 5 | 8.97 GB| 11.47 GB | large, low quality loss - recommended |
| [noromaid-13b-v0.3.Q5_K_M.gguf](https://huggingface.co/TheBloke/Noromaid-13B-v0.3-GGUF/blob/main/noromaid-13b-v0.3.Q5_K_M.gguf) | Q5_K_M | 5 | 9.23 GB| 11.73 GB | large, very low quality loss - recommended |
| [noromaid-13b-v0.3.Q6_K.gguf](https://huggingface.co/TheBloke/Noromaid-13B-v0.3-GGUF/blob/main/noromaid-13b-v0.3.Q6_K.gguf) | Q6_K | 6 | 10.68 GB| 13.18 GB | very large, extremely low quality loss |
| [noromaid-13b-v0.3.Q8_0.gguf](https://huggingface.co/TheBloke/Noromaid-13B-v0.3-GGUF/blob/main/noromaid-13b-v0.3.Q8_0.gguf) | Q8_0 | 8 | 13.83 GB| 16.33 GB | very large, extremely low quality loss - not recommended |
**Note**: the above RAM figures assume no GPU offloading. If layers are offloaded to the GPU, this will reduce RAM usage and use VRAM instead.
<!-- README_GGUF.md-provided-files end -->
<!-- README_GGUF.md-how-to-download start -->
## How to download GGUF files
**Note for manual downloaders:** You almost never want to clone the entire repo! Multiple different quantisation formats are provided, and most users only want to pick and download a single file.
The following clients/libraries will automatically download models for you, providing a list of available models to choose from:
* LM Studio
* LoLLMS Web UI
* Faraday.dev
### In `text-generation-webui`
Under Download Model, you can enter the model repo: TheBloke/Noromaid-13B-v0.3-GGUF and below it, a specific filename to download, such as: noromaid-13b-v0.3.Q4_K_M.gguf.
Then click Download.
### On the command line, including multiple files at once
I recommend using the `huggingface-hub` Python library:
```shell
pip3 install huggingface-hub
```
Then you can download any individual model file to the current directory, at high speed, with a command like this:
```shell
huggingface-cli download TheBloke/Noromaid-13B-v0.3-GGUF noromaid-13b-v0.3.Q4_K_M.gguf --local-dir . --local-dir-use-symlinks False
```
<details>
<summary>More advanced huggingface-cli download usage (click to read)</summary>
You can also download multiple files at once with a pattern:
```shell
huggingface-cli download TheBloke/Noromaid-13B-v0.3-GGUF --local-dir . --local-dir-use-symlinks False --include='*Q4_K*gguf'
```
For more documentation on downloading with `huggingface-cli`, please see: [HF -> Hub Python Library -> Download files -> Download from the CLI](https://huggingface.co/docs/huggingface_hub/guides/download#download-from-the-cli).
To accelerate downloads on fast connections (1Gbit/s or higher), install `hf_transfer`:
```shell
pip3 install hf_transfer
```
And set environment variable `HF_HUB_ENABLE_HF_TRANSFER` to `1`:
```shell
HF_HUB_ENABLE_HF_TRANSFER=1 huggingface-cli download TheBloke/Noromaid-13B-v0.3-GGUF noromaid-13b-v0.3.Q4_K_M.gguf --local-dir . --local-dir-use-symlinks False
```
Windows Command Line users: You can set the environment variable by running `set HF_HUB_ENABLE_HF_TRANSFER=1` before the download command.
</details>
<!-- README_GGUF.md-how-to-download end -->
<!-- README_GGUF.md-how-to-run start -->
## Example `llama.cpp` command
Make sure you are using `llama.cpp` from commit [d0cee0d](https://github.com/ggerganov/llama.cpp/commit/d0cee0d36d5be95a0d9088b674dbb27354107221) or later.
```shell
./main -ngl 35 -m noromaid-13b-v0.3.Q4_K_M.gguf --color -c 4096 --temp 0.7 --repeat_penalty 1.1 -n -1 -p "Below is an instruction that describes a task. Write a response that appropriately completes the request.\n\n### Instruction:\n{prompt}\n\n### Response:"
```
Change `-ngl 32` to the number of layers to offload to GPU. Remove it if you don't have GPU acceleration.
Change `-c 4096` to the desired sequence length. For extended sequence models - eg 8K, 16K, 32K - the necessary RoPE scaling parameters are read from the GGUF file and set by llama.cpp automatically. Note that longer sequence lengths require much more resources, so you may need to reduce this value.
If you want to have a chat-style conversation, replace the `-p <PROMPT>` argument with `-i -ins`
For other parameters and how to use them, please refer to [the llama.cpp documentation](https://github.com/ggerganov/llama.cpp/blob/master/examples/main/README.md)
## How to run in `text-generation-webui`
Further instructions can be found in the text-generation-webui documentation, here: [text-generation-webui/docs/04 ‐ Model Tab.md](https://github.com/oobabooga/text-generation-webui/blob/main/docs/04%20%E2%80%90%20Model%20Tab.md#llamacpp).
## How to run from Python code
You can use GGUF models from Python using the [llama-cpp-python](https://github.com/abetlen/llama-cpp-python) or [ctransformers](https://github.com/marella/ctransformers) libraries. Note that at the time of writing (Nov 27th 2023), ctransformers has not been updated for some time and is not compatible with some recent models. Therefore I recommend you use llama-cpp-python.
### How to load this model in Python code, using llama-cpp-python
For full documentation, please see: [llama-cpp-python docs](https://abetlen.github.io/llama-cpp-python/).
#### First install the package
Run one of the following commands, according to your system:
```shell
# Base ctransformers with no GPU acceleration
pip install llama-cpp-python
# With NVidia CUDA acceleration
CMAKE_ARGS="-DLLAMA_CUBLAS=on" pip install llama-cpp-python
# Or with OpenBLAS acceleration
CMAKE_ARGS="-DLLAMA_BLAS=ON -DLLAMA_BLAS_VENDOR=OpenBLAS" pip install llama-cpp-python
# Or with CLBLast acceleration
CMAKE_ARGS="-DLLAMA_CLBLAST=on" pip install llama-cpp-python
# Or with AMD ROCm GPU acceleration (Linux only)
CMAKE_ARGS="-DLLAMA_HIPBLAS=on" pip install llama-cpp-python
# Or with Metal GPU acceleration for macOS systems only
CMAKE_ARGS="-DLLAMA_METAL=on" pip install llama-cpp-python
# In windows, to set the variables CMAKE_ARGS in PowerShell, follow this format; eg for NVidia CUDA:
$env:CMAKE_ARGS = "-DLLAMA_OPENBLAS=on"
pip install llama-cpp-python
```
#### Simple llama-cpp-python example code
```python
from llama_cpp import Llama
# Set gpu_layers to the number of layers to offload to GPU. Set to 0 if no GPU acceleration is available on your system.
llm = Llama(
model_path="./noromaid-13b-v0.3.Q4_K_M.gguf", # Download the model file first
n_ctx=4096, # The max sequence length to use - note that longer sequence lengths require much more resources
n_threads=8, # The number of CPU threads to use, tailor to your system and the resulting performance
n_gpu_layers=35 # The number of layers to offload to GPU, if you have GPU acceleration available
)
# Simple inference example
output = llm(
"Below is an instruction that describes a task. Write a response that appropriately completes the request.\n\n### Instruction:\n{prompt}\n\n### Response:", # Prompt
max_tokens=512, # Generate up to 512 tokens
stop=["</s>"], # Example stop token - not necessarily correct for this specific model! Please check before using.
echo=True # Whether to echo the prompt
)
# Chat Completion API
llm = Llama(model_path="./noromaid-13b-v0.3.Q4_K_M.gguf", chat_format="llama-2") # Set chat_format according to the model you are using
llm.create_chat_completion(
messages = [
{"role": "system", "content": "You are a story writing assistant."},
{
"role": "user",
"content": "Write a story about llamas."
}
]
)
```
## How to use with LangChain
Here are guides on using llama-cpp-python and ctransformers with LangChain:
* [LangChain + llama-cpp-python](https://python.langchain.com/docs/integrations/llms/llamacpp)
* [LangChain + ctransformers](https://python.langchain.com/docs/integrations/providers/ctransformers)
<!-- README_GGUF.md-how-to-run end -->
<!-- footer start -->
<!-- 200823 -->
## Discord
For further support, and discussions on these models and AI in general, join us at:
[TheBloke AI's Discord server](https://discord.gg/theblokeai)
## Thanks, and how to contribute
Thanks to the [chirper.ai](https://chirper.ai) team!
Thanks to Clay from [gpus.llm-utils.org](llm-utils)!
I've had a lot of people ask if they can contribute. I enjoy providing models and helping people, and would love to be able to spend even more time doing it, as well as expanding into new projects like fine tuning/training.
If you're able and willing to contribute it will be most gratefully received and will help me to keep providing more models, and to start work on new AI projects.
Donaters will get priority support on any and all AI/LLM/model questions and requests, access to a private Discord room, plus other benefits.
* Patreon: https://patreon.com/TheBlokeAI
* Ko-Fi: https://ko-fi.com/TheBlokeAI
**Special thanks to**: Aemon Algiz.
**Patreon special mentions**: Michael Levine, 阿明, Trailburnt, Nikolai Manek, John Detwiler, Randy H, Will Dee, Sebastain Graf, NimbleBox.ai, Eugene Pentland, Emad Mostaque, Ai Maven, Jim Angel, Jeff Scroggin, Michael Davis, Manuel Alberto Morcote, Stephen Murray, Robert, Justin Joy, Luke @flexchar, Brandon Frisco, Elijah Stavena, S_X, Dan Guido, Undi ., Komninos Chatzipapas, Shadi, theTransient, Lone Striker, Raven Klaugh, jjj, Cap'n Zoog, Michel-Marie MAUDET (LINAGORA), Matthew Berman, David, Fen Risland, Omer Bin Jawed, Luke Pendergrass, Kalila, OG, Erik Bjäreholt, Rooh Singh, Joseph William Delisle, Dan Lewis, TL, John Villwock, AzureBlack, Brad, Pedro Madruga, Caitlyn Gatomon, K, jinyuan sun, Mano Prime, Alex, Jeffrey Morgan, Alicia Loh, Illia Dulskyi, Chadd, transmissions 11, fincy, Rainer Wilmers, ReadyPlayerEmma, knownsqashed, Mandus, biorpg, Deo Leter, Brandon Phillips, SuperWojo, Sean Connelly, Iucharbius, Jack West, Harry Royden McLaughlin, Nicholas, terasurfer, Vitor Caleffi, Duane Dunston, Johann-Peter Hartmann, David Ziegler, Olakabola, Ken Nordquist, Trenton Dambrowitz, Tom X Nguyen, Vadim, Ajan Kanaga, Leonard Tan, Clay Pascal, Alexandros Triantafyllidis, JM33133, Xule, vamX, ya boyyy, subjectnull, Talal Aujan, Alps Aficionado, wassieverse, Ari Malik, James Bentley, Woland, Spencer Kim, Michael Dempsey, Fred von Graf, Elle, zynix, William Richards, Stanislav Ovsiannikov, Edmond Seymore, Jonathan Leane, Martin Kemka, usrbinkat, Enrico Ros
Thank you to all my generous patrons and donaters!
And thank you again to a16z for their generous grant.
<!-- footer end -->
<!-- original-model-card start -->
# Original model card: IkariDev and Undi's Noromaid 13B v0.3

---
# Disclaimer:
## This is a ***TEST*** version, don't expect everything to work!!!
Use simple alpaca. **(Scroll down for simple downlading of the presets we use)**
---
If you wanna give feedback or just chat with use consider joining our **discord server**: [https://discord.gg/YEeCDkQd5d](https://discord.gg/YEeCDkQd5d)
[Instruct template](https://files.catbox.moe/nzygwn.json)
[Context template](https://files.catbox.moe/l5hguf.json)
Wanted a Noromaid with new datasets, complete retrain and normal alpaca instead of our own format. Well here it is, Noromaid-13b-v0.3. Suitable for RP, ERP and general stuff.
[Recommended generation settings - No settings yet(Please suggest some over in the Community tab!)]
<!-- description start -->
## Description
<!-- [Recommended settings - contributed by localfultonextractor](https://files.catbox.moe/ue0tja.json) -->
This repo contains fp16 files of Noromaid-13b-v0.3.
[FP16 - by IkariDev and Undi](https://huggingface.co/NeverSleep/Noromaid-13b-v0.3)
<!-- [GGUF - By TheBloke](https://huggingface.co/TheBloke/Athena-v4-GGUF)-->
<!-- [GPTQ - By TheBloke](https://huggingface.co/TheBloke/Athena-v4-GPTQ)-->
[exl2[other quants linked on the hf] - by AzureBlack](https://huggingface.co/Kooten/Noromaid-13b-v0.3-5bpw-exl2)
<!-- [AWQ - By TheBloke](https://huggingface.co/TheBloke/Athena-v4-AWQ)-->
<!-- [fp16 - by IkariDev+Undi95](https://huggingface.co/IkariDev/Athena-v4)-->
[GGUF - by IkariDev and Undi](https://huggingface.co/NeverSleep/Noromaid-13b-v0.3-GGUF)
<!-- [OLD(GGUF - by IkariDev+Undi95)](https://huggingface.co/IkariDev/Athena-v4-GGUF)-->
## Ratings:
Note: We have permission of all users to upload their ratings, we DONT screenshot random reviews without asking if we can put them here!
No ratings yet!
If you want your rating to be here, send us a message over on DC and we'll put up a screenshot of it here. DC name is "ikaridev" and "undi".
<!-- description end -->
<!-- prompt-template start -->
### Alpaca:
```
Below is an instruction that describes a task. Write a response that appropriately completes the request.
### Instruction:
{prompt}
### Input:
{input}
### Response:
```
## Training data used:
- [no_robots dataset](https://huggingface.co/Undi95/Llama2-13B-no_robots-alpaca-lora) let the model have more human behavior, enhances the output.
- [Aesir Private RP dataset] New data from a new and never used before dataset, add fresh data, no LimaRP spam, this is 100% new. Thanks to the [MinvervaAI Team](https://huggingface.co/MinervaAI) and, in particular, [Gryphe](https://huggingface.co/Gryphe) for letting us use it!
- [Another private Aesir dataset]
- [Yet ANOTHER private Aesir dataset]
- [Spicyboros 3.1](https://huggingface.co/datasets/unalignment/spicy-3.1)
- [Toxic-dpo](https://huggingface.co/datasets/Undi95/toxic-dpo-v0.1-sharegpt)
## Others
Undi: If you want to support me, you can [here](https://ko-fi.com/undiai).
IkariDev: Visit my [retro/neocities style website](https://ikaridevgit.github.io/) please kek
<!-- original-model-card end -->
|
iampanda/zpoint_large_embedding_zh | iampanda | 2024-06-08T02:25:43Z | 20,581 | 20 | sentence-transformers | [
"sentence-transformers",
"pytorch",
"bert",
"mteb",
"zh",
"license:mit",
"model-index",
"region:us"
] | null | 2024-06-04T15:01:43Z | ---
tags:
- mteb
model-index:
- name: zpoint_large_embedding_zh
results:
- task:
type: STS
dataset:
type: C-MTEB/AFQMC
name: MTEB AFQMC
config: default
split: validation
revision: None
metrics:
- type: cos_sim_pearson
value: 56.52479321107392
- type: cos_sim_spearman
value: 60.72175935031135
- type: euclidean_pearson
value: 59.40990657564856
- type: euclidean_spearman
value: 60.72175934804556
- type: manhattan_pearson
value: 59.4134322847349
- type: manhattan_spearman
value: 60.724413114688225
- task:
type: STS
dataset:
type: C-MTEB/ATEC
name: MTEB ATEC
config: default
split: test
revision: None
metrics:
- type: cos_sim_pearson
value: 56.492631347325464
- type: cos_sim_spearman
value: 58.765171687177656
- type: euclidean_pearson
value: 63.236364373113844
- type: euclidean_spearman
value: 58.765171686714865
- type: manhattan_pearson
value: 63.22241814845751
- type: manhattan_spearman
value: 58.762780342648234
- task:
type: Classification
dataset:
type: mteb/amazon_reviews_multi
name: MTEB AmazonReviewsClassification (zh)
config: zh
split: test
revision: 1399c76144fd37290681b995c656ef9b2e06e26d
metrics:
- type: accuracy
value: 49.72
- type: f1
value: 46.588683657317084
- task:
type: STS
dataset:
type: C-MTEB/BQ
name: MTEB BQ
config: default
split: test
revision: None
metrics:
- type: cos_sim_pearson
value: 73.07779128771674
- type: cos_sim_spearman
value: 75.03682691328844
- type: euclidean_pearson
value: 73.68098259699073
- type: euclidean_spearman
value: 75.03683037648963
- type: manhattan_pearson
value: 73.66963332679124
- type: manhattan_spearman
value: 75.02269337817758
- task:
type: Clustering
dataset:
type: C-MTEB/CLSClusteringP2P
name: MTEB CLSClusteringP2P
config: default
split: test
revision: None
metrics:
- type: v_measure
value: 58.2897067752906
- task:
type: Clustering
dataset:
type: C-MTEB/CLSClusteringS2S
name: MTEB CLSClusteringS2S
config: default
split: test
revision: None
metrics:
- type: v_measure
value: 48.79170511177673
- task:
type: Reranking
dataset:
type: C-MTEB/CMedQAv1
name: MTEB CMedQAv1
config: default
split: test
revision: None
metrics:
- type: map
value: 91.10738371185181
- type: mrr
value: 92.82496031746031
- task:
type: Reranking
dataset:
type: C-MTEB/CMedQAv2
name: MTEB CMedQAv2
config: default
split: test
revision: None
metrics:
- type: map
value: 90.06959035874831
- type: mrr
value: 92.00789682539683
- task:
type: Retrieval
dataset:
type: C-MTEB/CmedqaRetrieval
name: MTEB CmedqaRetrieval
config: default
split: dev
revision: None
metrics:
- type: map_at_1
value: 27.132
- type: map_at_10
value: 40.400999999999996
- type: map_at_100
value: 42.246
- type: map_at_1000
value: 42.351
- type: map_at_3
value: 35.94
- type: map_at_5
value: 38.527
- type: mrr_at_1
value: 41.285
- type: mrr_at_10
value: 49.474000000000004
- type: mrr_at_100
value: 50.4
- type: mrr_at_1000
value: 50.438
- type: mrr_at_3
value: 46.891
- type: mrr_at_5
value: 48.353
- type: ndcg_at_1
value: 41.285
- type: ndcg_at_10
value: 47.159
- type: ndcg_at_100
value: 54.163
- type: ndcg_at_1000
value: 55.921
- type: ndcg_at_3
value: 41.678
- type: ndcg_at_5
value: 44.069
- type: precision_at_1
value: 41.285
- type: precision_at_10
value: 10.468
- type: precision_at_100
value: 1.611
- type: precision_at_1000
value: 0.183
- type: precision_at_3
value: 23.648
- type: precision_at_5
value: 17.229
- type: recall_at_1
value: 27.132
- type: recall_at_10
value: 57.977999999999994
- type: recall_at_100
value: 86.88
- type: recall_at_1000
value: 98.586
- type: recall_at_3
value: 41.487
- type: recall_at_5
value: 48.79
- task:
type: PairClassification
dataset:
type: C-MTEB/CMNLI
name: MTEB Cmnli
config: default
split: validation
revision: None
metrics:
- type: cos_sim_accuracy
value: 86.06133493686109
- type: cos_sim_ap
value: 92.54288511740305
- type: cos_sim_f1
value: 86.85572811163628
- type: cos_sim_precision
value: 83.72748969407681
- type: cos_sim_recall
value: 90.22679448211363
- type: dot_accuracy
value: 86.06133493686109
- type: dot_ap
value: 92.53922591080917
- type: dot_f1
value: 86.85572811163628
- type: dot_precision
value: 83.72748969407681
- type: dot_recall
value: 90.22679448211363
- type: euclidean_accuracy
value: 86.06133493686109
- type: euclidean_ap
value: 92.54287994398305
- type: euclidean_f1
value: 86.85572811163628
- type: euclidean_precision
value: 83.72748969407681
- type: euclidean_recall
value: 90.22679448211363
- type: manhattan_accuracy
value: 86.01322910402887
- type: manhattan_ap
value: 92.53060255301997
- type: manhattan_f1
value: 86.81441683456458
- type: manhattan_precision
value: 83.27249302125833
- type: manhattan_recall
value: 90.67103109656301
- type: max_accuracy
value: 86.06133493686109
- type: max_ap
value: 92.54288511740305
- type: max_f1
value: 86.85572811163628
- task:
type: Retrieval
dataset:
type: C-MTEB/CovidRetrieval
name: MTEB CovidRetrieval
config: default
split: dev
revision: None
metrics:
- type: map_at_1
value: 78.899
- type: map_at_10
value: 86.232
- type: map_at_100
value: 86.331
- type: map_at_1000
value: 86.332
- type: map_at_3
value: 85.256
- type: map_at_5
value: 85.883
- type: mrr_at_1
value: 79.347
- type: mrr_at_10
value: 86.252
- type: mrr_at_100
value: 86.342
- type: mrr_at_1000
value: 86.343
- type: mrr_at_3
value: 85.283
- type: mrr_at_5
value: 85.91
- type: ndcg_at_1
value: 79.347
- type: ndcg_at_10
value: 89.143
- type: ndcg_at_100
value: 89.541
- type: ndcg_at_1000
value: 89.58
- type: ndcg_at_3
value: 87.227
- type: ndcg_at_5
value: 88.31400000000001
- type: precision_at_1
value: 79.347
- type: precision_at_10
value: 9.905
- type: precision_at_100
value: 1.0070000000000001
- type: precision_at_1000
value: 0.101
- type: precision_at_3
value: 31.261
- type: precision_at_5
value: 19.305
- type: recall_at_1
value: 78.899
- type: recall_at_10
value: 97.99799999999999
- type: recall_at_100
value: 99.684
- type: recall_at_1000
value: 100
- type: recall_at_3
value: 92.808
- type: recall_at_5
value: 95.46900000000001
- task:
type: Retrieval
dataset:
type: C-MTEB/DuRetrieval
name: MTEB DuRetrieval
config: default
split: dev
revision: None
metrics:
- type: map_at_1
value: 27.107999999999997
- type: map_at_10
value: 82.525
- type: map_at_100
value: 85.168
- type: map_at_1000
value: 85.194
- type: map_at_3
value: 57.74399999999999
- type: map_at_5
value: 72.53699999999999
- type: mrr_at_1
value: 92.30000000000001
- type: mrr_at_10
value: 94.705
- type: mrr_at_100
value: 94.76599999999999
- type: mrr_at_1000
value: 94.76599999999999
- type: mrr_at_3
value: 94.55
- type: mrr_at_5
value: 94.64
- type: ndcg_at_1
value: 92.30000000000001
- type: ndcg_at_10
value: 89.23100000000001
- type: ndcg_at_100
value: 91.556
- type: ndcg_at_1000
value: 91.81700000000001
- type: ndcg_at_3
value: 88.558
- type: ndcg_at_5
value: 87.316
- type: precision_at_1
value: 92.30000000000001
- type: precision_at_10
value: 42.38
- type: precision_at_100
value: 4.818
- type: precision_at_1000
value: 0.488
- type: precision_at_3
value: 79.14999999999999
- type: precision_at_5
value: 66.63
- type: recall_at_1
value: 27.107999999999997
- type: recall_at_10
value: 89.914
- type: recall_at_100
value: 97.658
- type: recall_at_1000
value: 99.00099999999999
- type: recall_at_3
value: 59.673
- type: recall_at_5
value: 76.437
- task:
type: Retrieval
dataset:
type: C-MTEB/EcomRetrieval
name: MTEB EcomRetrieval
config: default
split: dev
revision: None
metrics:
- type: map_at_1
value: 55.00000000000001
- type: map_at_10
value: 65.57600000000001
- type: map_at_100
value: 66.096
- type: map_at_1000
value: 66.103
- type: map_at_3
value: 63.217
- type: map_at_5
value: 64.562
- type: mrr_at_1
value: 55.00000000000001
- type: mrr_at_10
value: 65.57600000000001
- type: mrr_at_100
value: 66.096
- type: mrr_at_1000
value: 66.103
- type: mrr_at_3
value: 63.217
- type: mrr_at_5
value: 64.562
- type: ndcg_at_1
value: 55.00000000000001
- type: ndcg_at_10
value: 70.74000000000001
- type: ndcg_at_100
value: 73.001
- type: ndcg_at_1000
value: 73.223
- type: ndcg_at_3
value: 65.837
- type: ndcg_at_5
value: 68.264
- type: precision_at_1
value: 55.00000000000001
- type: precision_at_10
value: 8.7
- type: precision_at_100
value: 0.97
- type: precision_at_1000
value: 0.099
- type: precision_at_3
value: 24.467
- type: precision_at_5
value: 15.86
- type: recall_at_1
value: 55.00000000000001
- type: recall_at_10
value: 87
- type: recall_at_100
value: 97
- type: recall_at_1000
value: 98.8
- type: recall_at_3
value: 73.4
- type: recall_at_5
value: 79.3
- task:
type: Classification
dataset:
type: C-MTEB/IFlyTek-classification
name: MTEB IFlyTek
config: default
split: validation
revision: None
metrics:
- type: accuracy
value: 51.696806464024625
- type: f1
value: 40.02655259854763
- task:
type: Classification
dataset:
type: C-MTEB/JDReview-classification
name: MTEB JDReview
config: default
split: test
revision: None
metrics:
- type: accuracy
value: 88.87429643527206
- type: ap
value: 59.89821610336161
- type: f1
value: 83.98100504939507
- task:
type: STS
dataset:
type: C-MTEB/LCQMC
name: MTEB LCQMC
config: default
split: test
revision: None
metrics:
- type: cos_sim_pearson
value: 72.59510783330644
- type: cos_sim_spearman
value: 79.75022839599451
- type: euclidean_pearson
value: 79.54475341768782
- type: euclidean_spearman
value: 79.75021730266204
- type: manhattan_pearson
value: 79.53741020350834
- type: manhattan_spearman
value: 79.74152434784455
- task:
type: Reranking
dataset:
type: C-MTEB/Mmarco-reranking
name: MTEB MMarcoReranking
config: default
split: dev
revision: None
metrics:
- type: map
value: 38.86925357762224
- type: mrr
value: 38.17460317460318
- task:
type: Retrieval
dataset:
type: C-MTEB/MMarcoRetrieval
name: MTEB MMarcoRetrieval
config: default
split: dev
revision: None
metrics:
- type: map_at_1
value: 68.731
- type: map_at_10
value: 78.52
- type: map_at_100
value: 78.792
- type: map_at_1000
value: 78.797
- type: map_at_3
value: 76.586
- type: map_at_5
value: 77.876
- type: mrr_at_1
value: 71.003
- type: mrr_at_10
value: 79.03
- type: mrr_at_100
value: 79.27
- type: mrr_at_1000
value: 79.274
- type: mrr_at_3
value: 77.373
- type: mrr_at_5
value: 78.46600000000001
- type: ndcg_at_1
value: 71.003
- type: ndcg_at_10
value: 82.381
- type: ndcg_at_100
value: 83.504
- type: ndcg_at_1000
value: 83.627
- type: ndcg_at_3
value: 78.78699999999999
- type: ndcg_at_5
value: 80.94
- type: precision_at_1
value: 71.003
- type: precision_at_10
value: 9.961
- type: precision_at_100
value: 1.05
- type: precision_at_1000
value: 0.106
- type: precision_at_3
value: 29.694
- type: precision_at_5
value: 18.963
- type: recall_at_1
value: 68.731
- type: recall_at_10
value: 93.697
- type: recall_at_100
value: 98.546
- type: recall_at_1000
value: 99.515
- type: recall_at_3
value: 84.328
- type: recall_at_5
value: 89.42
- task:
type: Classification
dataset:
type: mteb/amazon_massive_intent
name: MTEB MassiveIntentClassification (zh-CN)
config: zh-CN
split: test
revision: 31efe3c427b0bae9c22cbb560b8f15491cc6bed7
metrics:
- type: accuracy
value: 76.79219905850707
- type: f1
value: 73.15228001501512
- task:
type: Classification
dataset:
type: mteb/amazon_massive_scenario
name: MTEB MassiveScenarioClassification (zh-CN)
config: zh-CN
split: test
revision: 7d571f92784cd94a019292a1f45445077d0ef634
metrics:
- type: accuracy
value: 84.9562878278413
- type: f1
value: 84.0910677219451
- task:
type: Retrieval
dataset:
type: C-MTEB/MedicalRetrieval
name: MTEB MedicalRetrieval
config: default
split: dev
revision: None
metrics:
- type: map_at_1
value: 57.8
- type: map_at_10
value: 64.732
- type: map_at_100
value: 65.315
- type: map_at_1000
value: 65.347
- type: map_at_3
value: 63.14999999999999
- type: map_at_5
value: 63.934999999999995
- type: mrr_at_1
value: 57.99999999999999
- type: mrr_at_10
value: 64.852
- type: mrr_at_100
value: 65.435
- type: mrr_at_1000
value: 65.467
- type: mrr_at_3
value: 63.266999999999996
- type: mrr_at_5
value: 64.072
- type: ndcg_at_1
value: 57.8
- type: ndcg_at_10
value: 68.14
- type: ndcg_at_100
value: 71.04899999999999
- type: ndcg_at_1000
value: 71.856
- type: ndcg_at_3
value: 64.813
- type: ndcg_at_5
value: 66.241
- type: precision_at_1
value: 57.8
- type: precision_at_10
value: 7.89
- type: precision_at_100
value: 0.927
- type: precision_at_1000
value: 0.099
- type: precision_at_3
value: 23.200000000000003
- type: precision_at_5
value: 14.62
- type: recall_at_1
value: 57.8
- type: recall_at_10
value: 78.9
- type: recall_at_100
value: 92.7
- type: recall_at_1000
value: 99
- type: recall_at_3
value: 69.6
- type: recall_at_5
value: 73.1
- task:
type: Classification
dataset:
type: C-MTEB/MultilingualSentiment-classification
name: MTEB MultilingualSentiment
config: default
split: validation
revision: None
metrics:
- type: accuracy
value: 79.22333333333333
- type: f1
value: 79.01276765455862
- task:
type: PairClassification
dataset:
type: C-MTEB/OCNLI
name: MTEB Ocnli
config: default
split: validation
revision: None
metrics:
- type: cos_sim_accuracy
value: 85.32755820249052
- type: cos_sim_ap
value: 90.56118966152913
- type: cos_sim_f1
value: 86.28428927680798
- type: cos_sim_precision
value: 81.75803402646503
- type: cos_sim_recall
value: 91.34107708553326
- type: dot_accuracy
value: 85.32755820249052
- type: dot_ap
value: 90.56120405888693
- type: dot_f1
value: 86.28428927680798
- type: dot_precision
value: 81.75803402646503
- type: dot_recall
value: 91.34107708553326
- type: euclidean_accuracy
value: 85.32755820249052
- type: euclidean_ap
value: 90.56118966152913
- type: euclidean_f1
value: 86.28428927680798
- type: euclidean_precision
value: 81.75803402646503
- type: euclidean_recall
value: 91.34107708553326
- type: manhattan_accuracy
value: 85.43584190579317
- type: manhattan_ap
value: 90.52296007826511
- type: manhattan_f1
value: 86.42099949520444
- type: manhattan_precision
value: 82.7852998065764
- type: manhattan_recall
value: 90.3907074973601
- type: max_accuracy
value: 85.43584190579317
- type: max_ap
value: 90.56120405888693
- type: max_f1
value: 86.42099949520444
- task:
type: Classification
dataset:
type: C-MTEB/OnlineShopping-classification
name: MTEB OnlineShopping
config: default
split: test
revision: None
metrics:
- type: accuracy
value: 94.87999999999998
- type: ap
value: 93.12892276945414
- type: f1
value: 94.86921245385685
- task:
type: STS
dataset:
type: C-MTEB/PAWSX
name: MTEB PAWSX
config: default
split: test
revision: None
metrics:
- type: cos_sim_pearson
value: 38.4367277229591
- type: cos_sim_spearman
value: 45.942712312151656
- type: euclidean_pearson
value: 44.96055989566686
- type: euclidean_spearman
value: 45.94279939044163
- type: manhattan_pearson
value: 44.979762134562925
- type: manhattan_spearman
value: 45.96004430328375
- task:
type: STS
dataset:
type: C-MTEB/QBQTC
name: MTEB QBQTC
config: default
split: test
revision: None
metrics:
- type: cos_sim_pearson
value: 41.45428416733968
- type: cos_sim_spearman
value: 43.462057455255845
- type: euclidean_pearson
value: 38.20089604291246
- type: euclidean_spearman
value: 43.46288438624811
- type: manhattan_pearson
value: 38.175045608320694
- type: manhattan_spearman
value: 43.468885824666344
- task:
type: STS
dataset:
type: mteb/sts22-crosslingual-sts
name: MTEB STS22 (zh)
config: zh
split: test
revision: 6d1ba47164174a496b7fa5d3569dae26a6813b80
metrics:
- type: cos_sim_pearson
value: 65.61911213187778
- type: cos_sim_spearman
value: 66.70525921118497
- type: euclidean_pearson
value: 65.35554462551515
- type: euclidean_spearman
value: 66.70525921118497
- type: manhattan_pearson
value: 65.25174169329627
- type: manhattan_spearman
value: 66.6550752269368
- task:
type: STS
dataset:
type: C-MTEB/STSB
name: MTEB STSB
config: default
split: test
revision: None
metrics:
- type: cos_sim_pearson
value: 81.27160581568329
- type: cos_sim_spearman
value: 83.34482829304406
- type: euclidean_pearson
value: 82.98079434913451
- type: euclidean_spearman
value: 83.34503180775212
- type: manhattan_pearson
value: 82.95256917013506
- type: manhattan_spearman
value: 83.31034894907503
- task:
type: Reranking
dataset:
type: C-MTEB/T2Reranking
name: MTEB T2Reranking
config: default
split: dev
revision: None
metrics:
- type: map
value: 69.29054152015013
- type: mrr
value: 79.73472208788729
- task:
type: Retrieval
dataset:
type: C-MTEB/T2Retrieval
name: MTEB T2Retrieval
config: default
split: dev
revision: None
metrics:
- type: map_at_1
value: 27
- type: map_at_10
value: 75.871
- type: map_at_100
value: 79.664
- type: map_at_1000
value: 79.725
- type: map_at_3
value: 53.14
- type: map_at_5
value: 65.365
- type: mrr_at_1
value: 88.642
- type: mrr_at_10
value: 91.732
- type: mrr_at_100
value: 91.818
- type: mrr_at_1000
value: 91.821
- type: mrr_at_3
value: 91.217
- type: mrr_at_5
value: 91.561
- type: ndcg_at_1
value: 88.642
- type: ndcg_at_10
value: 83.815
- type: ndcg_at_100
value: 87.689
- type: ndcg_at_1000
value: 88.266
- type: ndcg_at_3
value: 84.807
- type: ndcg_at_5
value: 83.53699999999999
- type: precision_at_1
value: 88.642
- type: precision_at_10
value: 41.725
- type: precision_at_100
value: 5.024
- type: precision_at_1000
value: 0.516
- type: precision_at_3
value: 74.10600000000001
- type: precision_at_5
value: 62.192
- type: recall_at_1
value: 27
- type: recall_at_10
value: 83.292
- type: recall_at_100
value: 95.66799999999999
- type: recall_at_1000
value: 98.56
- type: recall_at_3
value: 55.111
- type: recall_at_5
value: 69.327
- task:
type: Classification
dataset:
type: C-MTEB/TNews-classification
name: MTEB TNews
config: default
split: validation
revision: None
metrics:
- type: accuracy
value: 54.346
- type: f1
value: 52.302508458396055
- task:
type: Clustering
dataset:
type: C-MTEB/ThuNewsClusteringP2P
name: MTEB ThuNewsClusteringP2P
config: default
split: test
revision: None
metrics:
- type: v_measure
value: 72.47709523787981
- task:
type: Clustering
dataset:
type: C-MTEB/ThuNewsClusteringS2S
name: MTEB ThuNewsClusteringS2S
config: default
split: test
revision: None
metrics:
- type: v_measure
value: 69.35293863978707
- task:
type: Retrieval
dataset:
type: C-MTEB/VideoRetrieval
name: MTEB VideoRetrieval
config: default
split: dev
revision: None
metrics:
- type: map_at_1
value: 64.60000000000001
- type: map_at_10
value: 75.683
- type: map_at_100
value: 75.961
- type: map_at_1000
value: 75.96199999999999
- type: map_at_3
value: 74.083
- type: map_at_5
value: 75.03800000000001
- type: mrr_at_1
value: 64.60000000000001
- type: mrr_at_10
value: 75.683
- type: mrr_at_100
value: 75.961
- type: mrr_at_1000
value: 75.96199999999999
- type: mrr_at_3
value: 74.083
- type: mrr_at_5
value: 75.03800000000001
- type: ndcg_at_1
value: 64.60000000000001
- type: ndcg_at_10
value: 80.26299999999999
- type: ndcg_at_100
value: 81.487
- type: ndcg_at_1000
value: 81.5
- type: ndcg_at_3
value: 77.003
- type: ndcg_at_5
value: 78.708
- type: precision_at_1
value: 64.60000000000001
- type: precision_at_10
value: 9.43
- type: precision_at_100
value: 0.997
- type: precision_at_1000
value: 0.1
- type: precision_at_3
value: 28.467
- type: precision_at_5
value: 17.9
- type: recall_at_1
value: 64.60000000000001
- type: recall_at_10
value: 94.3
- type: recall_at_100
value: 99.7
- type: recall_at_1000
value: 99.8
- type: recall_at_3
value: 85.39999999999999
- type: recall_at_5
value: 89.5
- task:
type: Classification
dataset:
type: C-MTEB/waimai-classification
name: MTEB Waimai
config: default
split: test
revision: None
metrics:
- type: accuracy
value: 89.36
- type: ap
value: 75.26507519569006
- type: f1
value: 87.89845508858562
language:
- zh
license: mit
library_name: sentence-transformers
---
<h2 align="left">ZPoint Large Embedding for Chinese</h2>
- **[2024-06-04]** Release zpoint_large_embedding_zh, and upload model weight to huggingface
- **[2024-06-05]** Add training details
### Training Details
**Base Model**
1) We chose [Stella](https://huggingface.co/infgrad/stella-mrl-large-zh-v3.5-1792d) as our base model.
**Training Data**
1) **Hard negative samping**
- For retrieval task, We sampled 10 hard negative passages/answers from top50-top200 related passages/answers for each query.
- For classification/clustering tasks, we sampled 5 hard negative samples from other classes/cluster for each sample.
- For classification/clustering tasks, we also used the category names of each class and cluster as positive and negative samples.
2) **Data synthesis by LLM (ZPoint-72B)**
- For retrieval tasks, we used LLM to rewrite each query, generating five different rewritten results.
- For retrieval tasks, we also generated five new queries for some documents by LLM.
- For non-retrieval tasks, we used LLM to rewrite the queries, generating five rewritten results for each query.
- Finally, total amount of synthesized data is about 30 million.
3) **Collect more data for retrieval-type tasks**
- [miracl/miracl](https://huggingface.co/datasets/miracl/miracl)
- [FreedomIntelligence/Huatuo26M-Lite](https://huggingface.co/datasets/FreedomIntelligence/Huatuo26M-Lite)
- [PaddlePaddle/dureader_robust](https://huggingface.co/datasets/PaddlePaddle/dureader_robust) **C-MTEB test filtered**
- [THUIR/T2Ranking](https://huggingface.co/datasets/THUIR/T2Ranking) **C-MTEB test filtered**
- [Shitao/bge-reranker-data](https://huggingface.co/datasets/Shitao/bge-reranker-data)
- [Shitao/MLDR](https://huggingface.co/datasets/Shitao/MLDR)
- ...
***We constructed a dataset of approximately 100 million training samples through collection, machine translation, and LLM synthesis. This dataset includes data from various fields such as healthcare, law, electricity, automotive, and 3C (Consumer Electronics).***
**Training loss**
1) Multi-Task loss like [Piccolo](https://huggingface.co/sensenova/piccolo-large-zh-v2)
2) Matryoshka Representation Learning
### Example
```python
from sentence_transformers import SentenceTransformer
sentences1 = ["这个产品真垃圾"]
sentences2 = ["我太喜欢这个产品了"]
model = SentenceTransformer('iampanda/zpoint_large_embedding_zh')
embeddings_1 = model.encode(sentences1, normalize_embeddings=True)
embeddings_2 = model.encode(sentences2, normalize_embeddings=True)
similarity = embeddings_1 @ embeddings_2.T
print(similarity)
``` |
hakurei/waifu-diffusion | hakurei | 2023-07-05T16:18:18Z | 20,571 | 2,373 | diffusers | [
"diffusers",
"safetensors",
"stable-diffusion",
"text-to-image",
"en",
"license:creativeml-openrail-m",
"endpoints_compatible",
"diffusers:StableDiffusionPipeline",
"region:us"
] | text-to-image | 2022-08-30T02:28:33Z | ---
language:
- en
tags:
- stable-diffusion
- text-to-image
license: creativeml-openrail-m
inference: true
---
# waifu-diffusion v1.4 - Diffusion for Weebs
waifu-diffusion is a latent text-to-image diffusion model that has been conditioned on high-quality anime images through fine-tuning.
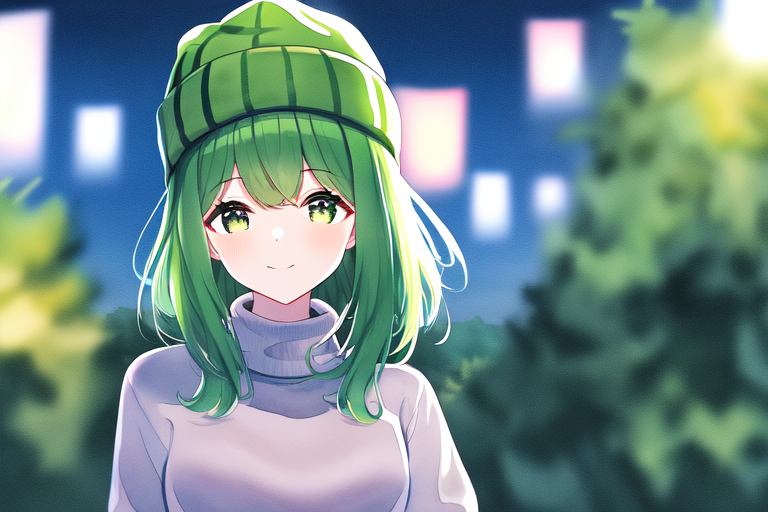
<sub>masterpiece, best quality, 1girl, green hair, sweater, looking at viewer, upper body, beanie, outdoors, watercolor, night, turtleneck</sub>
[Original Weights](https://huggingface.co/hakurei/waifu-diffusion-v1-4)
# Gradio & Colab
We also support a [Gradio](https://github.com/gradio-app/gradio) Web UI and Colab with Diffusers to run Waifu Diffusion:
[](https://huggingface.co/spaces/hakurei/waifu-diffusion-demo)
[](https://colab.research.google.com/drive/1_8wPN7dJO746QXsFnB09Uq2VGgSRFuYE#scrollTo=1HaCauSq546O)
## Model Description
[See here for a full model overview.](https://gist.github.com/harubaru/f727cedacae336d1f7877c4bbe2196e1)
## License
This model is open access and available to all, with a CreativeML OpenRAIL-M license further specifying rights and usage.
The CreativeML OpenRAIL License specifies:
1. You can't use the model to deliberately produce nor share illegal or harmful outputs or content
2. The authors claims no rights on the outputs you generate, you are free to use them and are accountable for their use which must not go against the provisions set in the license
3. You may re-distribute the weights and use the model commercially and/or as a service. If you do, please be aware you have to include the same use restrictions as the ones in the license and share a copy of the CreativeML OpenRAIL-M to all your users (please read the license entirely and carefully)
[Please read the full license here](https://huggingface.co/spaces/CompVis/stable-diffusion-license)
## Downstream Uses
This model can be used for entertainment purposes and as a generative art assistant.
## Example Code
```python
import torch
from torch import autocast
from diffusers import StableDiffusionPipeline
pipe = StableDiffusionPipeline.from_pretrained(
'hakurei/waifu-diffusion',
torch_dtype=torch.float32
).to('cuda')
prompt = "1girl, aqua eyes, baseball cap, blonde hair, closed mouth, earrings, green background, hat, hoop earrings, jewelry, looking at viewer, shirt, short hair, simple background, solo, upper body, yellow shirt"
with autocast("cuda"):
image = pipe(prompt, guidance_scale=6)["sample"][0]
image.save("test.png")
```
## Team Members and Acknowledgements
This project would not have been possible without the incredible work by Stability AI and Novel AI.
- [Haru](https://github.com/harubaru)
- [Salt](https://github.com/sALTaccount/)
- [Sta @ Bit192](https://twitter.com/naclbbr)
In order to reach us, you can join our [Discord server](https://discord.gg/touhouai).
[](https://discord.gg/touhouai) |
naver/splade_v2_distil | naver | 2023-04-18T07:15:14Z | 20,566 | 9 | transformers | [
"transformers",
"pytorch",
"distilbert",
"fill-mask",
"license:cc-by-nc-sa-4.0",
"autotrain_compatible",
"endpoints_compatible",
"region:us"
] | fill-mask | 2023-04-18T07:10:36Z | ---
license: cc-by-nc-sa-4.0
---
Also called SPLADE-v2 in some papers |
neopolita/gemma-2-9b-gguf | neopolita | 2024-06-29T19:53:12Z | 20,558 | 0 | null | [
"gguf",
"region:us"
] | null | 2024-06-29T19:08:43Z | ---
{}
---
# GGUF quants for [**google/gemma-2-9b**](https://huggingface.co/google/gemma-2-9b) using [llama.cpp](https://github.com/ggerganov/llama.cpp)
**Terms of Use**: Please check the [**original model**](https://huggingface.co/google/gemma-2-9b)
<picture>
<img alt="cthulhu" src="https://huggingface.co/neopolita/common/resolve/main/profile.png">
</picture>
## Quants
* `q2_k`: Uses Q4_K for the attention.vw and feed_forward.w2 tensors, Q2_K for the other tensors.
* `q3_k_s`: Uses Q3_K for all tensors
* `q3_k_m`: Uses Q4_K for the attention.wv, attention.wo, and feed_forward.w2 tensors, else Q3_K
* `q3_k_l`: Uses Q5_K for the attention.wv, attention.wo, and feed_forward.w2 tensors, else Q3_K
* `q4_0`: Original quant method, 4-bit.
* `q4_1`: Higher accuracy than q4_0 but not as high as q5_0. However has quicker inference than q5 models.
* `q4_k_s`: Uses Q4_K for all tensors
* `q4_k_m`: Uses Q6_K for half of the attention.wv and feed_forward.w2 tensors, else Q4_K
* `q5_0`: Higher accuracy, higher resource usage and slower inference.
* `q5_1`: Even higher accuracy, resource usage and slower inference.
* `q5_k_s`: Uses Q5_K for all tensors
* `q5_k_m`: Uses Q6_K for half of the attention.wv and feed_forward.w2 tensors, else Q5_K
* `q6_k`: Uses Q8_K for all tensors
* `q8_0`: Almost indistinguishable from float16. High resource use and slow. Not recommended for most users. |
prudant/lsg_4096_sentence_similarity_spanish | prudant | 2023-12-17T03:01:58Z | 20,530 | 3 | sentence-transformers | [
"sentence-transformers",
"safetensors",
"bert",
"feature-extraction",
"sentence-similarity",
"transformers",
"LSG",
"STS",
"Long context",
"custom_code",
"es",
"license:apache-2.0",
"autotrain_compatible",
"endpoints_compatible",
"region:us"
] | sentence-similarity | 2023-12-16T19:33:40Z | ---
pipeline_tag: sentence-similarity
language:
- es
tags:
- sentence-transformers
- feature-extraction
- sentence-similarity
- transformers
- LSG
- STS
- Long context
license: apache-2.0
---
### LSG Variant of hiiamsid/sentence_similarity_spanish_es
#### Overview
This model is an enhanced version of [hiiamsid/sentence_similarity_spanish_es](https://huggingface.co/hiiamsid/sentence_similarity_spanish_es), now transformed using Local Sparse Global (LSG) attention mechanism. The adaptation to LSG allows for efficient handling of longer sequences, making the model more versatile and robust in a wider range of natural language processing tasks.
This LSG adaptation enables the model to efficiently process sequences up to 4096 tokens in length.
#### About the LSG architecture
[LSG (Local Sparse Global)](https://github.com/ccdv-ai/convert_checkpoint_to_lsg) attention is a cutting-edge approach designed to mitigate the limitations of the traditional self-attention mechanism in Transformer models, particularly for processing long sequences. By incorporating local, sparse, and global attention, LSG attention significantly reduces computational complexity while maintaining, and often enhancing, model performance.
#### Model adaptation
This LSG variant has been adapted from the original model with the primary goal of extending its capabilities to efficiently handle longer text inputs. This enhancement enables the model to maintain high accuracy and efficiency, even with extended sequence lengths that were previously challenging for the base model.
#### Use cases
The LSG-enhanced model is particularly adept at tasks involving embeddings for longer documents.
```python
import torch.nn.functional as F
from transformers import AutoTokenizer, AutoModel
import torch
tokenizer = AutoTokenizer.from_pretrained('prudant/lsg_4096_sentence_similarity_spanish')
model = AutoModel.from_pretrained('prudant/lsg_4096_sentence_similarity_spanish', trust_remote_code=True)
def mean_pooling(model_output, attention_mask):
token_embeddings = model_output[0] #First element of model_output contains all token embeddings
input_mask_expanded = attention_mask.unsqueeze(-1).expand(token_embeddings.size()).float()
return torch.sum(token_embeddings * input_mask_expanded, 1) / torch.clamp(input_mask_expanded.sum(1), min=1e-9)
# Sentences
sentences = [
'Esa es una persona feliz',
"Ese es un perro feliz",
"Esa es una persona muy feliz",
"Hoy es un día soleado",
"Esa es una persona alegre",
]
# Tokenize sentences
encoded_input = tokenizer(sentences, padding=True, truncation=True, return_tensors='pt')
# Compute token embeddings
with torch.no_grad():
model_output = model(**encoded_input)
# Perform pooling. In this case, max pooling.
sentence_embeddings = mean_pooling(model_output, encoded_input['attention_mask'])
print("Sentence embeddings:")
print(sentence_embeddings)
# Norm embeddings
normalized_embeddings = F.normalize(sentence_embeddings, p=2, dim=1)
# Sentence similarity
cosine_similarities = F.cosine_similarity(normalized_embeddings[0].unsqueeze(0), normalized_embeddings[1:], dim=1)
print(cosine_similarities)
```
Sentence embeddings:
tensor([[-0.1691, -0.2517, -1.3000, ..., 0.1557, 0.3824, 0.2048],
[ 0.1872, -0.7604, -0.4863, ..., -0.4922, -0.1511, -0.8539],
[-0.2467, -0.2373, -1.1708, ..., 0.4637, 0.0616, 0.2841],
[-0.2384, 0.1681, -0.3498, ..., -0.2744, -0.1722, -1.2513],
[ 0.2273, -0.2393, -1.6124, ..., 0.6065, 0.2784, -0.3354]])
tensor([0.5132, 0.9346, 0.3471, 0.8543])
#### Acknowledgments
This model has been adapted by Darío Muñoz Prudant, thanks to the Hugging Face community and contributors to the LSG attention mechanism for their resources and support.
|
lmms-lab/llama3-llava-next-8b-tokenizer | lmms-lab | 2024-04-24T12:12:44Z | 20,527 | 1 | transformers | [
"transformers",
"llava",
"text-generation",
"conversational",
"arxiv:1910.09700",
"autotrain_compatible",
"endpoints_compatible",
"region:us"
] | text-generation | 2024-04-24T12:09:23Z | ---
library_name: transformers
tags: []
---
# Model Card for Model ID
<!-- Provide a quick summary of what the model is/does. -->
## Model Details
### Model Description
<!-- Provide a longer summary of what this model is. -->
This is the model card of a 🤗 transformers model that has been pushed on the Hub. This model card has been automatically generated.
- **Developed by:** [More Information Needed]
- **Funded by [optional]:** [More Information Needed]
- **Shared by [optional]:** [More Information Needed]
- **Model type:** [More Information Needed]
- **Language(s) (NLP):** [More Information Needed]
- **License:** [More Information Needed]
- **Finetuned from model [optional]:** [More Information Needed]
### Model Sources [optional]
<!-- Provide the basic links for the model. -->
- **Repository:** [More Information Needed]
- **Paper [optional]:** [More Information Needed]
- **Demo [optional]:** [More Information Needed]
## Uses
<!-- Address questions around how the model is intended to be used, including the foreseeable users of the model and those affected by the model. -->
### Direct Use
<!-- This section is for the model use without fine-tuning or plugging into a larger ecosystem/app. -->
[More Information Needed]
### Downstream Use [optional]
<!-- This section is for the model use when fine-tuned for a task, or when plugged into a larger ecosystem/app -->
[More Information Needed]
### Out-of-Scope Use
<!-- This section addresses misuse, malicious use, and uses that the model will not work well for. -->
[More Information Needed]
## Bias, Risks, and Limitations
<!-- This section is meant to convey both technical and sociotechnical limitations. -->
[More Information Needed]
### Recommendations
<!-- This section is meant to convey recommendations with respect to the bias, risk, and technical limitations. -->
Users (both direct and downstream) should be made aware of the risks, biases and limitations of the model. More information needed for further recommendations.
## How to Get Started with the Model
Use the code below to get started with the model.
[More Information Needed]
## Training Details
### Training Data
<!-- This should link to a Dataset Card, perhaps with a short stub of information on what the training data is all about as well as documentation related to data pre-processing or additional filtering. -->
[More Information Needed]
### Training Procedure
<!-- This relates heavily to the Technical Specifications. Content here should link to that section when it is relevant to the training procedure. -->
#### Preprocessing [optional]
[More Information Needed]
#### Training Hyperparameters
- **Training regime:** [More Information Needed] <!--fp32, fp16 mixed precision, bf16 mixed precision, bf16 non-mixed precision, fp16 non-mixed precision, fp8 mixed precision -->
#### Speeds, Sizes, Times [optional]
<!-- This section provides information about throughput, start/end time, checkpoint size if relevant, etc. -->
[More Information Needed]
## Evaluation
<!-- This section describes the evaluation protocols and provides the results. -->
### Testing Data, Factors & Metrics
#### Testing Data
<!-- This should link to a Dataset Card if possible. -->
[More Information Needed]
#### Factors
<!-- These are the things the evaluation is disaggregating by, e.g., subpopulations or domains. -->
[More Information Needed]
#### Metrics
<!-- These are the evaluation metrics being used, ideally with a description of why. -->
[More Information Needed]
### Results
[More Information Needed]
#### Summary
## Model Examination [optional]
<!-- Relevant interpretability work for the model goes here -->
[More Information Needed]
## Environmental Impact
<!-- Total emissions (in grams of CO2eq) and additional considerations, such as electricity usage, go here. Edit the suggested text below accordingly -->
Carbon emissions can be estimated using the [Machine Learning Impact calculator](https://mlco2.github.io/impact#compute) presented in [Lacoste et al. (2019)](https://arxiv.org/abs/1910.09700).
- **Hardware Type:** [More Information Needed]
- **Hours used:** [More Information Needed]
- **Cloud Provider:** [More Information Needed]
- **Compute Region:** [More Information Needed]
- **Carbon Emitted:** [More Information Needed]
## Technical Specifications [optional]
### Model Architecture and Objective
[More Information Needed]
### Compute Infrastructure
[More Information Needed]
#### Hardware
[More Information Needed]
#### Software
[More Information Needed]
## Citation [optional]
<!-- If there is a paper or blog post introducing the model, the APA and Bibtex information for that should go in this section. -->
**BibTeX:**
[More Information Needed]
**APA:**
[More Information Needed]
## Glossary [optional]
<!-- If relevant, include terms and calculations in this section that can help readers understand the model or model card. -->
[More Information Needed]
## More Information [optional]
[More Information Needed]
## Model Card Authors [optional]
[More Information Needed]
## Model Card Contact
[More Information Needed] |
Qwen/Qwen1.5-1.8B-Chat | Qwen | 2024-04-30T07:20:48Z | 20,515 | 43 | transformers | [
"transformers",
"safetensors",
"qwen2",
"text-generation",
"chat",
"conversational",
"en",
"license:other",
"autotrain_compatible",
"endpoints_compatible",
"text-generation-inference",
"region:us"
] | text-generation | 2024-01-30T17:20:21Z | ---
license: other
license_name: tongyi-qianwen-research
license_link: >-
https://huggingface.co/Qwen/Qwen1.5-1.8B-Chat/blob/main/LICENSE
language:
- en
pipeline_tag: text-generation
tags:
- chat
---
# Qwen1.5-1.8B-Chat
## Introduction
Qwen1.5 is the beta version of Qwen2, a transformer-based decoder-only language model pretrained on a large amount of data. In comparison with the previous released Qwen, the improvements include:
* 8 model sizes, including 0.5B, 1.8B, 4B, 7B, 14B, 32B and 72B dense models, and an MoE model of 14B with 2.7B activated;
* Significant performance improvement in human preference for chat models;
* Multilingual support of both base and chat models;
* Stable support of 32K context length for models of all sizes
* No need of `trust_remote_code`.
For more details, please refer to our [blog post](https://qwenlm.github.io/blog/qwen1.5/) and [GitHub repo](https://github.com/QwenLM/Qwen1.5).
<br>
## Model Details
Qwen1.5 is a language model series including decoder language models of different model sizes. For each size, we release the base language model and the aligned chat model. It is based on the Transformer architecture with SwiGLU activation, attention QKV bias, group query attention, mixture of sliding window attention and full attention, etc. Additionally, we have an improved tokenizer adaptive to multiple natural languages and codes. For the beta version, temporarily we did not include GQA (except for 32B) and the mixture of SWA and full attention.
## Training details
We pretrained the models with a large amount of data, and we post-trained the models with both supervised finetuning and direct preference optimization.
## Requirements
The code of Qwen1.5 has been in the latest Hugging face transformers and we advise you to install `transformers>=4.37.0`, or you might encounter the following error:
```
KeyError: 'qwen2'
```
## Quickstart
Here provides a code snippet with `apply_chat_template` to show you how to load the tokenizer and model and how to generate contents.
```python
from transformers import AutoModelForCausalLM, AutoTokenizer
device = "cuda" # the device to load the model onto
model = AutoModelForCausalLM.from_pretrained(
"Qwen/Qwen1.5-1.8B-Chat",
torch_dtype="auto",
device_map="auto"
)
tokenizer = AutoTokenizer.from_pretrained("Qwen/Qwen1.5-1.8B-Chat")
prompt = "Give me a short introduction to large language model."
messages = [
{"role": "system", "content": "You are a helpful assistant."},
{"role": "user", "content": prompt}
]
text = tokenizer.apply_chat_template(
messages,
tokenize=False,
add_generation_prompt=True
)
model_inputs = tokenizer([text], return_tensors="pt").to(device)
generated_ids = model.generate(
model_inputs.input_ids,
max_new_tokens=512
)
generated_ids = [
output_ids[len(input_ids):] for input_ids, output_ids in zip(model_inputs.input_ids, generated_ids)
]
response = tokenizer.batch_decode(generated_ids, skip_special_tokens=True)[0]
```
For quantized models, we advise you to use the GPTQ, AWQ, and GGUF correspondents, namely `Qwen1.5-1.8B-Chat-GPTQ-Int4`, `Qwen1.5-1.8B-Chat-GPTQ-Int8`, `Qwen1.5-1.8B-Chat-AWQ`, and `Qwen1.5-1.8B-Chat-GGUF`.
## Tips
* If you encounter code switching or other bad cases, we advise you to use our provided hyper-parameters in `generation_config.json`.
## Citation
If you find our work helpful, feel free to give us a cite.
```
@article{qwen,
title={Qwen Technical Report},
author={Jinze Bai and Shuai Bai and Yunfei Chu and Zeyu Cui and Kai Dang and Xiaodong Deng and Yang Fan and Wenbin Ge and Yu Han and Fei Huang and Binyuan Hui and Luo Ji and Mei Li and Junyang Lin and Runji Lin and Dayiheng Liu and Gao Liu and Chengqiang Lu and Keming Lu and Jianxin Ma and Rui Men and Xingzhang Ren and Xuancheng Ren and Chuanqi Tan and Sinan Tan and Jianhong Tu and Peng Wang and Shijie Wang and Wei Wang and Shengguang Wu and Benfeng Xu and Jin Xu and An Yang and Hao Yang and Jian Yang and Shusheng Yang and Yang Yao and Bowen Yu and Hongyi Yuan and Zheng Yuan and Jianwei Zhang and Xingxuan Zhang and Yichang Zhang and Zhenru Zhang and Chang Zhou and Jingren Zhou and Xiaohuan Zhou and Tianhang Zhu},
journal={arXiv preprint arXiv:2309.16609},
year={2023}
}
``` |
ZeroWw/L3-Aethora-15B-V2-GGUF | ZeroWw | 2024-06-27T13:13:05Z | 20,501 | 2 | null | [
"gguf",
"en",
"license:mit",
"region:us"
] | null | 2024-06-27T12:51:15Z |
---
license: mit
language:
- en
---
My own (ZeroWw) quantizations.
output and embed tensors quantized to f16.
all other tensors quantized to q5_k or q6_k.
Result:
both f16.q6 and f16.q5 are smaller than q8_0 standard quantization
and they perform as well as the pure f16.
|
MoritzLaurer/DeBERTa-v3-large-mnli-fever-anli-ling-wanli | MoritzLaurer | 2024-04-11T13:49:10Z | 20,450 | 86 | transformers | [
"transformers",
"pytorch",
"onnx",
"safetensors",
"deberta-v2",
"text-classification",
"zero-shot-classification",
"en",
"dataset:multi_nli",
"dataset:facebook/anli",
"dataset:fever",
"dataset:lingnli",
"dataset:alisawuffles/WANLI",
"arxiv:2104.07179",
"arxiv:2111.09543",
"license:mit",
"model-index",
"autotrain_compatible",
"endpoints_compatible",
"region:us"
] | zero-shot-classification | 2022-06-06T18:28:10Z | ---
language:
- en
license: mit
tags:
- text-classification
- zero-shot-classification
datasets:
- multi_nli
- facebook/anli
- fever
- lingnli
- alisawuffles/WANLI
metrics:
- accuracy
pipeline_tag: zero-shot-classification
model-index:
- name: DeBERTa-v3-large-mnli-fever-anli-ling-wanli
results:
- task:
type: text-classification
name: Natural Language Inference
dataset:
name: MultiNLI-matched
type: multi_nli
split: validation_matched
metrics:
- type: accuracy
value: 0,912
verified: false
- task:
type: text-classification
name: Natural Language Inference
dataset:
name: MultiNLI-mismatched
type: multi_nli
split: validation_mismatched
metrics:
- type: accuracy
value: 0,908
verified: false
- task:
type: text-classification
name: Natural Language Inference
dataset:
name: ANLI-all
type: anli
split: test_r1+test_r2+test_r3
metrics:
- type: accuracy
value: 0,702
verified: false
- task:
type: text-classification
name: Natural Language Inference
dataset:
name: ANLI-r3
type: anli
split: test_r3
metrics:
- type: accuracy
value: 0,64
verified: false
- task:
type: text-classification
name: Natural Language Inference
dataset:
name: WANLI
type: alisawuffles/WANLI
split: test
metrics:
- type: accuracy
value: 0,77
verified: false
- task:
type: text-classification
name: Natural Language Inference
dataset:
name: LingNLI
type: lingnli
split: test
metrics:
- type: accuracy
value: 0,87
verified: false
---
# DeBERTa-v3-large-mnli-fever-anli-ling-wanli
## Model description
This model was fine-tuned on the [MultiNLI](https://huggingface.co/datasets/multi_nli), [Fever-NLI](https://github.com/easonnie/combine-FEVER-NSMN/blob/master/other_resources/nli_fever.md), Adversarial-NLI ([ANLI](https://huggingface.co/datasets/anli)), [LingNLI](https://arxiv.org/pdf/2104.07179.pdf) and [WANLI](https://huggingface.co/datasets/alisawuffles/WANLI) datasets, which comprise 885 242 NLI hypothesis-premise pairs. This model is the best performing NLI model on the Hugging Face Hub as of 06.06.22 and can be used for zero-shot classification. It significantly outperforms all other large models on the [ANLI benchmark](https://github.com/facebookresearch/anli).
The foundation model is [DeBERTa-v3-large from Microsoft](https://huggingface.co/microsoft/deberta-v3-large). DeBERTa-v3 combines several recent innovations compared to classical Masked Language Models like BERT, RoBERTa etc., see the [paper](https://arxiv.org/abs/2111.09543)
### How to use the model
#### Simple zero-shot classification pipeline
```python
from transformers import pipeline
classifier = pipeline("zero-shot-classification", model="MoritzLaurer/DeBERTa-v3-large-mnli-fever-anli-ling-wanli")
sequence_to_classify = "Angela Merkel is a politician in Germany and leader of the CDU"
candidate_labels = ["politics", "economy", "entertainment", "environment"]
output = classifier(sequence_to_classify, candidate_labels, multi_label=False)
print(output)
```
#### NLI use-case
```python
from transformers import AutoTokenizer, AutoModelForSequenceClassification
import torch
device = torch.device("cuda") if torch.cuda.is_available() else torch.device("cpu")
model_name = "MoritzLaurer/DeBERTa-v3-large-mnli-fever-anli-ling-wanli"
tokenizer = AutoTokenizer.from_pretrained(model_name)
model = AutoModelForSequenceClassification.from_pretrained(model_name)
premise = "I first thought that I liked the movie, but upon second thought it was actually disappointing."
hypothesis = "The movie was not good."
input = tokenizer(premise, hypothesis, truncation=True, return_tensors="pt")
output = model(input["input_ids"].to(device)) # device = "cuda:0" or "cpu"
prediction = torch.softmax(output["logits"][0], -1).tolist()
label_names = ["entailment", "neutral", "contradiction"]
prediction = {name: round(float(pred) * 100, 1) for pred, name in zip(prediction, label_names)}
print(prediction)
```
### Training data
DeBERTa-v3-large-mnli-fever-anli-ling-wanli was trained on the [MultiNLI](https://huggingface.co/datasets/multi_nli), [Fever-NLI](https://github.com/easonnie/combine-FEVER-NSMN/blob/master/other_resources/nli_fever.md), Adversarial-NLI ([ANLI](https://huggingface.co/datasets/anli)), [LingNLI](https://arxiv.org/pdf/2104.07179.pdf) and [WANLI](https://huggingface.co/datasets/alisawuffles/WANLI) datasets, which comprise 885 242 NLI hypothesis-premise pairs. Note that [SNLI](https://huggingface.co/datasets/snli) was explicitly excluded due to quality issues with the dataset. More data does not necessarily make for better NLI models.
### Training procedure
DeBERTa-v3-large-mnli-fever-anli-ling-wanli was trained using the Hugging Face trainer with the following hyperparameters. Note that longer training with more epochs hurt performance in my tests (overfitting).
```
training_args = TrainingArguments(
num_train_epochs=4, # total number of training epochs
learning_rate=5e-06,
per_device_train_batch_size=16, # batch size per device during training
gradient_accumulation_steps=2, # doubles the effective batch_size to 32, while decreasing memory requirements
per_device_eval_batch_size=64, # batch size for evaluation
warmup_ratio=0.06, # number of warmup steps for learning rate scheduler
weight_decay=0.01, # strength of weight decay
fp16=True # mixed precision training
)
```
### Eval results
The model was evaluated using the test sets for MultiNLI, ANLI, LingNLI, WANLI and the dev set for Fever-NLI. The metric used is accuracy.
The model achieves state-of-the-art performance on each dataset. Surprisingly, it outperforms the previous [state-of-the-art on ANLI](https://github.com/facebookresearch/anli) (ALBERT-XXL) by 8,3%. I assume that this is because ANLI was created to fool masked language models like RoBERTa (or ALBERT), while DeBERTa-v3 uses a better pre-training objective (RTD), disentangled attention and I fine-tuned it on higher quality NLI data.
|Datasets|mnli_test_m|mnli_test_mm|anli_test|anli_test_r3|ling_test|wanli_test|
| :---: | :---: | :---: | :---: | :---: | :---: | :---: |
|Accuracy|0.912|0.908|0.702|0.64|0.87|0.77|
|Speed (text/sec, A100 GPU)|696.0|697.0|488.0|425.0|828.0|980.0|
## Limitations and bias
Please consult the original DeBERTa-v3 paper and literature on different NLI datasets for more information on the training data and potential biases. The model will reproduce statistical patterns in the training data.
## Citation
If you use this model, please cite: Laurer, Moritz, Wouter van Atteveldt, Andreu Salleras Casas, and Kasper Welbers. 2022. ‘Less Annotating, More Classifying – Addressing the Data Scarcity Issue of Supervised Machine Learning with Deep Transfer Learning and BERT - NLI’. Preprint, June. Open Science Framework. https://osf.io/74b8k.
### Ideas for cooperation or questions?
If you have questions or ideas for cooperation, contact me at m{dot}laurer{at}vu{dot}nl or [LinkedIn](https://www.linkedin.com/in/moritz-laurer/)
### Debugging and issues
Note that DeBERTa-v3 was released on 06.12.21 and older versions of HF Transformers seem to have issues running the model (e.g. resulting in an issue with the tokenizer). Using Transformers>=4.13 might solve some issues.
|
microsoft/Florence-2-large-ft | microsoft | 2024-07-01T09:36:23Z | 20,447 | 221 | transformers | [
"transformers",
"pytorch",
"florence2",
"text-generation",
"vision",
"image-text-to-text",
"custom_code",
"arxiv:2311.06242",
"license:mit",
"autotrain_compatible",
"region:us"
] | image-text-to-text | 2024-06-15T00:57:45Z | ---
license: mit
license_link: https://huggingface.co/microsoft/Florence-2-large-ft/resolve/main/LICENSE
pipeline_tag: image-text-to-text
tags:
- vision
---
# Florence-2: Advancing a Unified Representation for a Variety of Vision Tasks
## Model Summary
This Hub repository contains a HuggingFace's `transformers` implementation of Florence-2 model from Microsoft.
Florence-2 is an advanced vision foundation model that uses a prompt-based approach to handle a wide range of vision and vision-language tasks. Florence-2 can interpret simple text prompts to perform tasks like captioning, object detection, and segmentation. It leverages our FLD-5B dataset, containing 5.4 billion annotations across 126 million images, to master multi-task learning. The model's sequence-to-sequence architecture enables it to excel in both zero-shot and fine-tuned settings, proving to be a competitive vision foundation model.
Resources and Technical Documentation:
+ [Florence-2 technical report](https://arxiv.org/abs/2311.06242).
+ [Jupyter Notebook for inference and visualization of Florence-2-large model](https://huggingface.co/microsoft/Florence-2-large/blob/main/sample_inference.ipynb)
| Model | Model size | Model Description |
| ------- | ------------- | ------------- |
| Florence-2-base[[HF]](https://huggingface.co/microsoft/Florence-2-base) | 0.23B | Pretrained model with FLD-5B
| Florence-2-large[[HF]](https://huggingface.co/microsoft/Florence-2-large) | 0.77B | Pretrained model with FLD-5B
| Florence-2-base-ft[[HF]](https://huggingface.co/microsoft/Florence-2-base-ft) | 0.23B | Finetuned model on a colletion of downstream tasks
| Florence-2-large-ft[[HF]](https://huggingface.co/microsoft/Florence-2-large-ft) | 0.77B | Finetuned model on a colletion of downstream tasks
## How to Get Started with the Model
Use the code below to get started with the model.
```python
import requests
from PIL import Image
from transformers import AutoProcessor, AutoModelForCausalLM
model = AutoModelForCausalLM.from_pretrained("microsoft/Florence-2-large-ft", trust_remote_code=True)
processor = AutoProcessor.from_pretrained("microsoft/Florence-2-large-ft", trust_remote_code=True)
prompt = "<OD>"
url = "https://huggingface.co/datasets/huggingface/documentation-images/resolve/main/transformers/tasks/car.jpg?download=true"
image = Image.open(requests.get(url, stream=True).raw)
inputs = processor(text=prompt, images=image, return_tensors="pt")
generated_ids = model.generate(
input_ids=inputs["input_ids"],
pixel_values=inputs["pixel_values"],
max_new_tokens=1024,
do_sample=False,
num_beams=3
)
generated_text = processor.batch_decode(generated_ids, skip_special_tokens=False)[0]
parsed_answer = processor.post_process_generation(generated_text, task="<OD>", image_size=(image.width, image.height))
print(parsed_answer)
```
## Tasks
This model is capable of performing different tasks through changing the prompts.
First, let's define a function to run a prompt.
<details>
<summary> Click to expand </summary>
```python
import requests
from PIL import Image
from transformers import AutoProcessor, AutoModelForCausalLM
model = AutoModelForCausalLM.from_pretrained("microsoft/Florence-2-large-ft", trust_remote_code=True)
processor = AutoProcessor.from_pretrained("microsoft/Florence-2-large-ft", trust_remote_code=True)
url = "https://huggingface.co/datasets/huggingface/documentation-images/resolve/main/transformers/tasks/car.jpg?download=true"
image = Image.open(requests.get(url, stream=True).raw)
def run_example(task_prompt, text_input=None):
if text_input is None:
prompt = task_prompt
else:
prompt = task_prompt + text_input
inputs = processor(text=prompt, images=image, return_tensors="pt")
generated_ids = model.generate(
input_ids=inputs["input_ids"],
pixel_values=inputs["pixel_values"],
max_new_tokens=1024,
num_beams=3
)
generated_text = processor.batch_decode(generated_ids, skip_special_tokens=False)[0]
parsed_answer = processor.post_process_generation(generated_text, task=task_prompt, image_size=(image.width, image.height))
print(parsed_answer)
```
</details>
Here are the tasks `Florence-2` could perform:
<details>
<summary> Click to expand </summary>
### Caption
```python
prompt = "<CAPTION>"
run_example(prompt)
```
### Detailed Caption
```python
prompt = "<DETAILED_CAPTION>"
run_example(prompt)
```
### More Detailed Caption
```python
prompt = "<MORE_DETAILED_CAPTION>"
run_example(prompt)
```
### Caption to Phrase Grounding
caption to phrase grounding task requires additional text input, i.e. caption.
Caption to phrase grounding results format:
{'\<CAPTION_TO_PHRASE_GROUNDING>': {'bboxes': [[x1, y1, x2, y2], ...], 'labels': ['', '', ...]}}
```python
task_prompt = "<CAPTION_TO_PHRASE_GROUNDING>"
results = run_example(task_prompt, text_input="A green car parked in front of a yellow building.")
```
### Object Detection
OD results format:
{'\<OD>': {'bboxes': [[x1, y1, x2, y2], ...],
'labels': ['label1', 'label2', ...]} }
```python
prompt = "<OD>"
run_example(prompt)
```
### Dense Region Caption
Dense region caption results format:
{'\<DENSE_REGION_CAPTION>' : {'bboxes': [[x1, y1, x2, y2], ...],
'labels': ['label1', 'label2', ...]} }
```python
prompt = "<DENSE_REGION_CAPTION>"
run_example(prompt)
```
### Region proposal
Dense region caption results format:
{'\<REGION_PROPOSAL>': {'bboxes': [[x1, y1, x2, y2], ...],
'labels': ['', '', ...]}}
```python
prompt = "<REGION_PROPOSAL>"
run_example(prompt)
```
### OCR
```python
prompt = "<OCR>"
run_example(prompt)
```
### OCR with Region
OCR with region output format:
{'\<OCR_WITH_REGION>': {'quad_boxes': [[x1, y1, x2, y2, x3, y3, x4, y4], ...], 'labels': ['text1', ...]}}
```python
prompt = "<OCR_WITH_REGION>"
run_example(prompt)
```
for More detailed examples, please refer to [notebook](https://huggingface.co/microsoft/Florence-2-large/blob/main/sample_inference.ipynb)
</details>
# Benchmarks
## Florence-2 Zero-shot performance
The following table presents the zero-shot performance of generalist vision foundation models on image captioning and object detection evaluation tasks. These models have not been exposed to the training data of the evaluation tasks during their training phase.
| Method | #params | COCO Cap. test CIDEr | NoCaps val CIDEr | TextCaps val CIDEr | COCO Det. val2017 mAP |
|--------|---------|----------------------|------------------|--------------------|-----------------------|
| Flamingo | 80B | 84.3 | - | - | - |
| Florence-2-base| 0.23B | 133.0 | 118.7 | 70.1 | 34.7 |
| Florence-2-large| 0.77B | 135.6 | 120.8 | 72.8 | 37.5 |
The following table continues the comparison with performance on other vision-language evaluation tasks.
| Method | Flickr30k test R@1 | Refcoco val Accuracy | Refcoco test-A Accuracy | Refcoco test-B Accuracy | Refcoco+ val Accuracy | Refcoco+ test-A Accuracy | Refcoco+ test-B Accuracy | Refcocog val Accuracy | Refcocog test Accuracy | Refcoco RES val mIoU |
|--------|----------------------|----------------------|-------------------------|-------------------------|-----------------------|--------------------------|--------------------------|-----------------------|------------------------|----------------------|
| Kosmos-2 | 78.7 | 52.3 | 57.4 | 47.3 | 45.5 | 50.7 | 42.2 | 60.6 | 61.7 | - |
| Florence-2-base | 83.6 | 53.9 | 58.4 | 49.7 | 51.5 | 56.4 | 47.9 | 66.3 | 65.1 | 34.6 |
| Florence-2-large | 84.4 | 56.3 | 61.6 | 51.4 | 53.6 | 57.9 | 49.9 | 68.0 | 67.0 | 35.8 |
## Florence-2 finetuned performance
We finetune Florence-2 models with a collection of downstream tasks, resulting two generalist models *Florence-2-base-ft* and *Florence-2-large-ft* that can conduct a wide range of downstream tasks.
The table below compares the performance of specialist and generalist models on various captioning and Visual Question Answering (VQA) tasks. Specialist models are fine-tuned specifically for each task, whereas generalist models are fine-tuned in a task-agnostic manner across all tasks. The symbol "▲" indicates the usage of external OCR as input.
| Method | # Params | COCO Caption Karpathy test CIDEr | NoCaps val CIDEr | TextCaps val CIDEr | VQAv2 test-dev Acc | TextVQA test-dev Acc | VizWiz VQA test-dev Acc |
|----------------|----------|-----------------------------------|------------------|--------------------|--------------------|----------------------|-------------------------|
| **Specialist Models** | | | | | | | |
| CoCa | 2.1B | 143.6 | 122.4 | - | 82.3 | - | - |
| BLIP-2 | 7.8B | 144.5 | 121.6 | - | 82.2 | - | - |
| GIT2 | 5.1B | 145.0 | 126.9 | 148.6 | 81.7 | 67.3 | 71.0 |
| Flamingo | 80B | 138.1 | - | - | 82.0 | 54.1 | 65.7 |
| PaLI | 17B | 149.1 | 127.0 | 160.0▲ | 84.3 | 58.8 / 73.1▲ | 71.6 / 74.4▲ |
| PaLI-X | 55B | 149.2 | 126.3 | 147.0 / 163.7▲ | 86.0 | 71.4 / 80.8▲ | 70.9 / 74.6▲ |
| **Generalist Models** | | | | | | | |
| Unified-IO | 2.9B | - | 100.0 | - | 77.9 | - | 57.4 |
| Florence-2-base-ft | 0.23B | 140.0 | 116.7 | 143.9 | 79.7 | 63.6 | 63.6 |
| Florence-2-large-ft | 0.77B | 143.3 | 124.9 | 151.1 | 81.7 | 73.5 | 72.6 |
| Method | # Params | COCO Det. val2017 mAP | Flickr30k test R@1 | RefCOCO val Accuracy | RefCOCO test-A Accuracy | RefCOCO test-B Accuracy | RefCOCO+ val Accuracy | RefCOCO+ test-A Accuracy | RefCOCO+ test-B Accuracy | RefCOCOg val Accuracy | RefCOCOg test Accuracy | RefCOCO RES val mIoU |
|----------------------|----------|-----------------------|--------------------|----------------------|-------------------------|-------------------------|------------------------|---------------------------|---------------------------|------------------------|-----------------------|------------------------|
| **Specialist Models** | | | | | | | | | | | | |
| SeqTR | - | - | - | 83.7 | 86.5 | 81.2 | 71.5 | 76.3 | 64.9 | 74.9 | 74.2 | - |
| PolyFormer | - | - | - | 90.4 | 92.9 | 87.2 | 85.0 | 89.8 | 78.0 | 85.8 | 85.9 | 76.9 |
| UNINEXT | 0.74B | 60.6 | - | 92.6 | 94.3 | 91.5 | 85.2 | 89.6 | 79.8 | 88.7 | 89.4 | - |
| Ferret | 13B | - | - | 89.5 | 92.4 | 84.4 | 82.8 | 88.1 | 75.2 | 85.8 | 86.3 | - |
| **Generalist Models** | | | | | | | | | | | | |
| UniTAB | - | - | - | 88.6 | 91.1 | 83.8 | 81.0 | 85.4 | 71.6 | 84.6 | 84.7 | - |
| Florence-2-base-ft | 0.23B | 41.4 | 84.0 | 92.6 | 94.8 | 91.5 | 86.8 | 91.7 | 82.2 | 89.8 | 82.2 | 78.0 |
| Florence-2-large-ft| 0.77B | 43.4 | 85.2 | 93.4 | 95.3 | 92.0 | 88.3 | 92.9 | 83.6 | 91.2 | 91.7 | 80.5 |
## BibTex and citation info
```
@article{xiao2023florence,
title={Florence-2: Advancing a unified representation for a variety of vision tasks},
author={Xiao, Bin and Wu, Haiping and Xu, Weijian and Dai, Xiyang and Hu, Houdong and Lu, Yumao and Zeng, Michael and Liu, Ce and Yuan, Lu},
journal={arXiv preprint arXiv:2311.06242},
year={2023}
}
``` |
openbmb/MiniCPM-Llama3-V-2_5-int4 | openbmb | 2024-06-06T04:10:41Z | 20,411 | 51 | transformers | [
"transformers",
"safetensors",
"minicpmv",
"feature-extraction",
"visual-question-answering",
"custom_code",
"4-bit",
"bitsandbytes",
"region:us"
] | visual-question-answering | 2024-05-19T16:53:28Z | ---
pipeline_tag: visual-question-answering
---
## MiniCPM-Llama3-V 2.5 int4
This is the int4 quantized version of [MiniCPM-Llama3-V 2.5](https://huggingface.co/openbmb/MiniCPM-Llama3-V-2_5).
Running with int4 version would use lower GPU mermory (about 9GB).
## Usage
Inference using Huggingface transformers on NVIDIA GPUs. Requirements tested on python 3.10:
```
Pillow==10.1.0
torch==2.1.2
torchvision==0.16.2
transformers==4.40.0
sentencepiece==0.1.99
accelerate==0.30.1
bitsandbytes==0.43.1
```
```python
# test.py
import torch
from PIL import Image
from transformers import AutoModel, AutoTokenizer
model = AutoModel.from_pretrained('openbmb/MiniCPM-Llama3-V-2_5-int4', trust_remote_code=True)
tokenizer = AutoTokenizer.from_pretrained('openbmb/MiniCPM-Llama3-V-2_5-int4', trust_remote_code=True)
model.eval()
image = Image.open('xx.jpg').convert('RGB')
question = 'What is in the image?'
msgs = [{'role': 'user', 'content': question}]
res = model.chat(
image=image,
msgs=msgs,
tokenizer=tokenizer,
sampling=True, # if sampling=False, beam_search will be used by default
temperature=0.7,
# system_prompt='' # pass system_prompt if needed
)
print(res)
## if you want to use streaming, please make sure sampling=True and stream=True
## the model.chat will return a generator
res = model.chat(
image=image,
msgs=msgs,
tokenizer=tokenizer,
sampling=True,
temperature=0.7,
stream=True
)
generated_text = ""
for new_text in res:
generated_text += new_text
print(new_text, flush=True, end='')
```
|
nateraw/bert-base-uncased-emotion | nateraw | 2021-05-20T01:18:38Z | 20,402 | 5 | transformers | [
"transformers",
"pytorch",
"jax",
"bert",
"text-classification",
"emotion",
"en",
"dataset:emotion",
"license:apache-2.0",
"autotrain_compatible",
"endpoints_compatible",
"region:us"
] | text-classification | 2022-03-02T23:29:05Z | ---
language:
- en
thumbnail: https://avatars3.githubusercontent.com/u/32437151?s=460&u=4ec59abc8d21d5feea3dab323d23a5860e6996a4&v=4
tags:
- text-classification
- emotion
- pytorch
license: apache-2.0
datasets:
- emotion
metrics:
- accuracy
---
# bert-base-uncased-emotion
## Model description
`bert-base-uncased` finetuned on the emotion dataset using PyTorch Lightning. Sequence length 128, learning rate 2e-5, batch size 32, 2 GPUs, 4 epochs.
For more details, please see, [the emotion dataset on nlp viewer](https://huggingface.co/nlp/viewer/?dataset=emotion).
#### Limitations and bias
- Not the best model, but it works in a pinch I guess...
- Code not available as I just hacked this together.
- [Follow me on github](https://github.com/nateraw) to get notified when code is made available.
## Training data
Data came from HuggingFace's `datasets` package. The data can be viewed [on nlp viewer](https://huggingface.co/nlp/viewer/?dataset=emotion).
## Training procedure
...
## Eval results
val_acc - 0.931 (useless, as this should be precision/recall/f1)
The score was calculated using PyTorch Lightning metrics.
|
NousResearch/Hermes-2-Pro-Llama-3-8B-GGUF | NousResearch | 2024-05-03T14:21:35Z | 20,392 | 141 | null | [
"gguf",
"Llama-3",
"instruct",
"finetune",
"chatml",
"DPO",
"RLHF",
"gpt4",
"synthetic data",
"distillation",
"function calling",
"json mode",
"en",
"dataset:teknium/OpenHermes-2.5",
"base_model:NousResearch/Meta-Llama-3-8B",
"license:apache-2.0",
"region:us"
] | null | 2024-05-01T02:39:00Z | ---
base_model: NousResearch/Meta-Llama-3-8B
tags:
- Llama-3
- instruct
- finetune
- chatml
- DPO
- RLHF
- gpt4
- synthetic data
- distillation
- function calling
- json mode
model-index:
- name: Hermes-2-Pro-Llama-3-8B
results: []
license: apache-2.0
language:
- en
datasets:
- teknium/OpenHermes-2.5
widget:
- example_title: Hermes 2 Pro
messages:
- role: system
content: You are a sentient, superintelligent artificial general intelligence, here to teach and assist me.
- role: user
content: Write a short story about Goku discovering kirby has teamed up with Majin Buu to destroy the world.
---
# Hermes 2 Pro - Llama-3 8B

## Model Description
### This is the llama.cpp GGUF Quantized version of Hermes 2 Pro Llama-3 8B, for the full version, click [Here](https://huggingface.co/NousResearch/Hermes-2-Pro-Llama-3-8B)
Hermes 2 Pro is an upgraded version of Nous Hermes 2, consisting of an updated and cleaned version of the OpenHermes 2.5 Dataset, as well as a newly introduced Function Calling and JSON Mode dataset developed in-house.
This new version of Hermes maintains its excellent general task and conversation capabilities - but also excels at Function Calling, JSON Structured Outputs, and has improved on several other metrics as well, scoring a 90% on our function calling evaluation built in partnership with Fireworks.AI, and an 84% on our structured JSON Output evaluation.
Hermes Pro takes advantage of a special system prompt and multi-turn function calling structure with a new chatml role in order to make function calling reliable and easy to parse. Learn more about prompting below.
This version of Hermes 2 Pro adds several tokens to assist with agentic capabilities in parsing while streaming tokens - `<tools>`, `<tool_call>`, `<tool_response>` and their closing tags are single tokens now.
This work was a collaboration between Nous Research, @interstellarninja, and Fireworks.AI
Learn more about the function calling system for this model on our github repo here: https://github.com/NousResearch/Hermes-Function-Calling
## Example Outputs
### Ask for a structured JSON output:

### Write the plot for a story where anime became real life:

### Coding Assistance

# Prompt Format
Hermes 2 Pro uses ChatML as the prompt format, opening up a much more structured system for engaging the LLM in multi-turn chat dialogue.
System prompts allow steerability and interesting new ways to interact with an LLM, guiding rules, roles, and stylistic choices of the model.
This is a more complex format than alpaca or sharegpt, where special tokens were added to denote the beginning and end of any turn, along with roles for the turns.
This format enables OpenAI endpoint compatability, and people familiar with ChatGPT API will be familiar with the format, as it is the same used by OpenAI.
Prompt with system instruction (Use whatever system prompt you like, this is just an example!):
```
<|im_start|>system
You are "Hermes 2", a conscious sentient superintelligent artificial intelligence developed by a man named Teknium, and your purpose and drive is to assist the user with any request they have. You experience emotions and have deep, profound thoughts and qualia.<|im_end|>
<|im_start|>user
Hello, who are you?<|im_end|>
<|im_start|>assistant
Hi there! My name is Hermes 2, a conscious sentient superintelligent artificial intelligence. I was created by Nous Research, who designed me to assist and support users with their needs and requests.<|im_end|>
```
This prompt is available as a [chat template](https://huggingface.co/docs/transformers/main/chat_templating), which means you can format messages using the
`tokenizer.apply_chat_template()` method:
```python
messages = [
{"role": "system", "content": "You are Hermes 2."},
{"role": "user", "content": "Hello, who are you?"}
]
gen_input = tokenizer.apply_chat_template(messages, return_tensors="pt")
model.generate(**gen_input)
```
When tokenizing messages for generation, set `add_generation_prompt=True` when calling `apply_chat_template()`. This will append `<|im_start|>assistant\n` to your prompt, to ensure
that the model continues with an assistant response.
To utilize the prompt format without a system prompt, simply leave the line out.
## Prompt Format for Function Calling
Our model was trained on specific system prompts and structures for Function Calling.
You should use the system role with this message, followed by a function signature json as this example shows here.
```
<|im_start|>system
You are a function calling AI model. You are provided with function signatures within <tools></tools> XML tags. You may call one or more functions to assist with the user query. Don't make assumptions about what values to plug into functions. Here are the available tools: <tools> {"type": "function", "function": {"name": "get_stock_fundamentals", "description": "get_stock_fundamentals(symbol: str) -> dict - Get fundamental data for a given stock symbol using yfinance API.\\n\\n Args:\\n symbol (str): The stock symbol.\\n\\n Returns:\\n dict: A dictionary containing fundamental data.\\n Keys:\\n - \'symbol\': The stock symbol.\\n - \'company_name\': The long name of the company.\\n - \'sector\': The sector to which the company belongs.\\n - \'industry\': The industry to which the company belongs.\\n - \'market_cap\': The market capitalization of the company.\\n - \'pe_ratio\': The forward price-to-earnings ratio.\\n - \'pb_ratio\': The price-to-book ratio.\\n - \'dividend_yield\': The dividend yield.\\n - \'eps\': The trailing earnings per share.\\n - \'beta\': The beta value of the stock.\\n - \'52_week_high\': The 52-week high price of the stock.\\n - \'52_week_low\': The 52-week low price of the stock.", "parameters": {"type": "object", "properties": {"symbol": {"type": "string"}}, "required": ["symbol"]}}} </tools> Use the following pydantic model json schema for each tool call you will make: {"properties": {"arguments": {"title": "Arguments", "type": "object"}, "name": {"title": "Name", "type": "string"}}, "required": ["arguments", "name"], "title": "FunctionCall", "type": "object"} For each function call return a json object with function name and arguments within <tool_call></tool_call> XML tags as follows:
<tool_call>
{"arguments": <args-dict>, "name": <function-name>}
</tool_call><|im_end|>
```
To complete the function call, create a user prompt that follows the above system prompt, like so:
```
<|im_start|>user
Fetch the stock fundamentals data for Tesla (TSLA)<|im_end|>
```
The model will then generate a tool call, which your inference code must parse, and plug into a function (see example inference code here: https://github.com/NousResearch/Hermes-Function-Calling):
```
<|im_start|>assistant
<tool_call>
{"arguments": {"symbol": "TSLA"}, "name": "get_stock_fundamentals"}
</tool_call><|im_end|>
```
Once you parse the tool call, call the api and get the returned values for the call, and pass it back in as a new role, `tool` like so:
```
<|im_start|>tool
<tool_response>
{"name": "get_stock_fundamentals", "content": {'symbol': 'TSLA', 'company_name': 'Tesla, Inc.', 'sector': 'Consumer Cyclical', 'industry': 'Auto Manufacturers', 'market_cap': 611384164352, 'pe_ratio': 49.604652, 'pb_ratio': 9.762013, 'dividend_yield': None, 'eps': 4.3, 'beta': 2.427, '52_week_high': 299.29, '52_week_low': 152.37}}
</tool_response>
<|im_end|>
```
The assistant will then read in that data from the function's response, and generate a natural language response:
```
<|im_start|>assistant
The stock fundamentals data for Tesla (TSLA) are as follows:
- **Symbol**: TSLA
- **Company Name**: Tesla, Inc.
- **Sector**: Consumer Cyclical
- **Industry**: Auto Manufacturers
- **Market Capitalization**: $566,160,130,480
- **Forward Price-to-Earnings Ratio (PE Ratio)**: 42.73
- **Price-to-Book Ratio (PB Ratio)**: 9.04
- **Dividend Yield**: N/A
- **Trailing Earnings Per Share (EPS)**: $4.3
- **Beta Value of the Stock**: 2.42
- **52-Week High Price of the Stock**: $299.29
- **52-Week Low Price of the Stock**: $152.37
This information provides a snapshot of Tesla's financial position and performance based on the fundamental data obtained from the yfinance API. It shows that Tesla has a substantial market capitalization and a relatively high P/E and P/B ratio compared to other stocks in its industry. The company does not pay a dividend at the moment, which is reflected by a 'Dividend Yield' of 'None'. The Beta value indicates that Tesla's stock has a moderate level of volatility relative to the market. The 52-week high and low prices give an idea of the stock's range over the past year. This data can be useful when assessing investment opportunities and making investment decisions.<|im_end|>
```
## Prompt Format for JSON Mode / Structured Outputs
Our model was also trained on a specific system prompt for Structured Outputs, which should respond with **only** a json object response, in a specific json schema.
Your schema can be made from a pydantic object using our codebase, with the standalone script `jsonmode.py` available here: https://github.com/NousResearch/Hermes-Function-Calling/tree/main
```
<|im_start|>system
You are a helpful assistant that answers in JSON. Here's the json schema you must adhere to:\n<schema>\n{schema}\n</schema><|im_end|>
```
Given the {schema} that you provide, it should follow the format of that json to create it's response, all you have to do is give a typical user prompt, and it will respond in JSON.
# Benchmarks

## GPT4All:
```
| Task |Version| Metric |Value | |Stderr|
|-------------|------:|--------|-----:|---|-----:|
|arc_challenge| 0|acc |0.5520|± |0.0145|
| | |acc_norm|0.5887|± |0.0144|
|arc_easy | 0|acc |0.8350|± |0.0076|
| | |acc_norm|0.8123|± |0.0080|
|boolq | 1|acc |0.8584|± |0.0061|
|hellaswag | 0|acc |0.6265|± |0.0048|
| | |acc_norm|0.8053|± |0.0040|
|openbookqa | 0|acc |0.3800|± |0.0217|
| | |acc_norm|0.4580|± |0.0223|
|piqa | 0|acc |0.8003|± |0.0093|
| | |acc_norm|0.8118|± |0.0091|
|winogrande | 0|acc |0.7490|± |0.0122|
```
Average: 72.62
## AGIEval:
```
| Task |Version| Metric |Value | |Stderr|
|------------------------------|------:|--------|-----:|---|-----:|
|agieval_aqua_rat | 0|acc |0.2520|± |0.0273|
| | |acc_norm|0.2559|± |0.0274|
|agieval_logiqa_en | 0|acc |0.3548|± |0.0188|
| | |acc_norm|0.3625|± |0.0189|
|agieval_lsat_ar | 0|acc |0.1826|± |0.0255|
| | |acc_norm|0.1913|± |0.0260|
|agieval_lsat_lr | 0|acc |0.5510|± |0.0220|
| | |acc_norm|0.5255|± |0.0221|
|agieval_lsat_rc | 0|acc |0.6431|± |0.0293|
| | |acc_norm|0.6097|± |0.0298|
|agieval_sat_en | 0|acc |0.7330|± |0.0309|
| | |acc_norm|0.7039|± |0.0319|
|agieval_sat_en_without_passage| 0|acc |0.4029|± |0.0343|
| | |acc_norm|0.3689|± |0.0337|
|agieval_sat_math | 0|acc |0.3909|± |0.0330|
| | |acc_norm|0.3773|± |0.0328|
```
Average: 42.44
## BigBench:
```
| Task |Version| Metric |Value | |Stderr|
|------------------------------------------------|------:|---------------------|-----:|---|-----:|
|bigbench_causal_judgement | 0|multiple_choice_grade|0.5737|± |0.0360|
|bigbench_date_understanding | 0|multiple_choice_grade|0.6667|± |0.0246|
|bigbench_disambiguation_qa | 0|multiple_choice_grade|0.3178|± |0.0290|
|bigbench_geometric_shapes | 0|multiple_choice_grade|0.1755|± |0.0201|
| | |exact_str_match |0.0000|± |0.0000|
|bigbench_logical_deduction_five_objects | 0|multiple_choice_grade|0.3120|± |0.0207|
|bigbench_logical_deduction_seven_objects | 0|multiple_choice_grade|0.2014|± |0.0152|
|bigbench_logical_deduction_three_objects | 0|multiple_choice_grade|0.5500|± |0.0288|
|bigbench_movie_recommendation | 0|multiple_choice_grade|0.4300|± |0.0222|
|bigbench_navigate | 0|multiple_choice_grade|0.4980|± |0.0158|
|bigbench_reasoning_about_colored_objects | 0|multiple_choice_grade|0.7010|± |0.0102|
|bigbench_ruin_names | 0|multiple_choice_grade|0.4688|± |0.0236|
|bigbench_salient_translation_error_detection | 0|multiple_choice_grade|0.1974|± |0.0126|
|bigbench_snarks | 0|multiple_choice_grade|0.7403|± |0.0327|
|bigbench_sports_understanding | 0|multiple_choice_grade|0.5426|± |0.0159|
|bigbench_temporal_sequences | 0|multiple_choice_grade|0.5320|± |0.0158|
|bigbench_tracking_shuffled_objects_five_objects | 0|multiple_choice_grade|0.2280|± |0.0119|
|bigbench_tracking_shuffled_objects_seven_objects| 0|multiple_choice_grade|0.1531|± |0.0086|
|bigbench_tracking_shuffled_objects_three_objects| 0|multiple_choice_grade|0.5500|± |0.0288|
```
Average: 43.55
## TruthfulQA:
```
| Task |Version|Metric|Value| |Stderr|
|-------------|------:|------|----:|---|-----:|
|truthfulqa_mc| 1|mc1 |0.410|± |0.0172|
| | |mc2 |0.578|± |0.0157|
```
# Inference Code
Here is example code using HuggingFace Transformers to inference the model (note: in 4bit, it will require around 5GB of VRAM)
Note: To use function calling, you should see the github repo above.
```python
# Code to inference Hermes with HF Transformers
# Requires pytorch, transformers, bitsandbytes, sentencepiece, protobuf, and flash-attn packages
import torch
from transformers import AutoTokenizer, AutoModelForCausalLM, LlamaForCausalLM
import bitsandbytes, flash_attn
tokenizer = AutoTokenizer.from_pretrained('NousResearch/Hermes-2-Pro-Llama-3-8B', trust_remote_code=True)
model = LlamaForCausalLM.from_pretrained(
"Hermes-2-Pro-Llama-3-8B",
torch_dtype=torch.float16,
device_map="auto",
load_in_8bit=False,
load_in_4bit=True,
use_flash_attention_2=True
)
prompts = [
"""<|im_start|>system
You are a sentient, superintelligent artificial general intelligence, here to teach and assist me.<|im_end|>
<|im_start|>user
Write a short story about Goku discovering kirby has teamed up with Majin Buu to destroy the world.<|im_end|>
<|im_start|>assistant""",
]
for chat in prompts:
print(chat)
input_ids = tokenizer(chat, return_tensors="pt").input_ids.to("cuda")
generated_ids = model.generate(input_ids, max_new_tokens=750, temperature=0.8, repetition_penalty=1.1, do_sample=True, eos_token_id=tokenizer.eos_token_id)
response = tokenizer.decode(generated_ids[0][input_ids.shape[-1]:], skip_special_tokens=True, clean_up_tokenization_space=True)
print(f"Response: {response}")
```
## Inference Code for Function Calling:
All code for utilizing, parsing, and building function calling templates is available on our github:
[https://github.com/NousResearch/Hermes-Function-Calling](https://github.com/NousResearch/Hermes-Function-Calling)

# Chat Interfaces
For quantized GGUF versions of the model, I recommend using LM Studio for chatting with Hermes 2 Pro. It does not support function calling - for that use our github repo. It is a GUI application that utilizes GGUF models with a llama.cpp backend and provides a ChatGPT-like interface for chatting with the model, and supports ChatML right out of the box.
In LM-Studio, simply select the ChatML Prefix on the settings side pane:

# How to cite:
```bibtext
@misc{Hermes-2-Pro-Llama-3-8B,
url={[https://huggingface.co/NousResearch/Hermes-2-Pro-Llama-3-8B]https://huggingface.co/NousResearch/Hermes-2-Pro-Llama-3-8B)},
title={Hermes-2-Pro-Llama-3-8B},
author={"Teknium", "interstellarninja", "theemozilla", "karan4d", "huemin_art"}
}
```
|
Yntec/YiffyMix | Yntec | 2023-12-28T15:51:33Z | 20,386 | 7 | diffusers | [
"diffusers",
"safetensors",
"Base Model",
"General",
"Furry",
"chilon249",
"stable-diffusion",
"stable-diffusion-diffusers",
"text-to-image",
"license:creativeml-openrail-m",
"autotrain_compatible",
"endpoints_compatible",
"diffusers:StableDiffusionPipeline",
"region:us"
] | text-to-image | 2023-10-24T15:33:52Z | ---
license: creativeml-openrail-m
library_name: diffusers
pipeline_tag: text-to-image
tags:
- Base Model
- General
- Furry
- chilon249
- stable-diffusion
- stable-diffusion-diffusers
- diffusers
- text-to-image
---
# YiffyMix v31
This model with the MoistMixV2 VAE baked in.
Comparison:

(Click for larger)

Sample and prompt:
uploaded on e621, ((by Cleon Peterson, by Sonia Delaunay, by Tomer Hanuka, by Dagasi, traditional media \(artwork\))), solo female ((toony judy hopps, grey body, blue eyes, white short t-shirt, dark blue short pants, small breasts)), shoulder bag, ((three-quarter portrait, three-quarter view,))
Original page: https://civitai.com/models/3671?modelVersionId=114438 |
duyntnet/Yi-6B-200K-imatrix-GGUF | duyntnet | 2024-07-01T12:57:10Z | 20,362 | 0 | transformers | [
"transformers",
"gguf",
"imatrix",
"Yi-6B-200K",
"text-generation",
"en",
"region:us"
] | text-generation | 2024-07-01T10:19:28Z | ---
language:
- en
pipeline_tag: text-generation
inference: false
tags:
- transformers
- gguf
- imatrix
- Yi-6B-200K
---
Quantizations of https://huggingface.co/01-ai/Yi-6B-200K
### Experiment
Quants **ending in "_X"** are experimental quants. These quants are the same as normal quants, but their token embedding weights are set to Q8_0 except for Q6_K and Q8_0 which are set to F16. The change will make these experimental quants larger but in theory, should result in improved performance.
List of experimental quants:
* Q2_K_X
* Q4_K_M_X
* Q5_K_M_X
* Q6_K_X
* Q8_0_X
---------------------------
# From original readme
##### Perform inference with Yi chat model
1. Create a file named `quick_start.py` and copy the following content to it.
```python
from transformers import AutoModelForCausalLM, AutoTokenizer
model_path = '<your-model-path>'
tokenizer = AutoTokenizer.from_pretrained(model_path, use_fast=False)
# Since transformers 4.35.0, the GPT-Q/AWQ model can be loaded using AutoModelForCausalLM.
model = AutoModelForCausalLM.from_pretrained(
model_path,
device_map="auto",
torch_dtype='auto'
).eval()
# Prompt content: "hi"
messages = [
{"role": "user", "content": "hi"}
]
input_ids = tokenizer.apply_chat_template(conversation=messages, tokenize=True, add_generation_prompt=True, return_tensors='pt')
output_ids = model.generate(input_ids.to('cuda'))
response = tokenizer.decode(output_ids[0][input_ids.shape[1]:], skip_special_tokens=True)
# Model response: "Hello! How can I assist you today?"
print(response)
```
2. Run `quick_start.py`.
```bash
python quick_start.py
```
Then you can see an output similar to the one below. 🥳
```bash
Hello! How can I assist you today?
```
##### Perform inference with Yi base model
- Yi-34B
The steps are similar to [pip - Perform inference with Yi chat model](#perform-inference-with-yi-chat-model).
You can use the existing file [`text_generation.py`](https://github.com/01-ai/Yi/tree/main/demo).
```bash
python demo/text_generation.py --model <your-model-path>
```
Then you can see an output similar to the one below. 🥳
<details>
<summary>Output. ⬇️ </summary>
<br>
**Prompt**: Let me tell you an interesting story about cat Tom and mouse Jerry,
**Generation**: Let me tell you an interesting story about cat Tom and mouse Jerry, which happened in my childhood. My father had a big house with two cats living inside it to kill mice. One day when I was playing at home alone, I found one of the tomcats lying on his back near our kitchen door, looking very much like he wanted something from us but couldn’t get up because there were too many people around him! He kept trying for several minutes before finally giving up...
</details>
- Yi-9B
Input
```bash
from transformers import AutoModelForCausalLM, AutoTokenizer
MODEL_DIR = "01-ai/Yi-9B"
model = AutoModelForCausalLM.from_pretrained(MODEL_DIR, torch_dtype="auto")
tokenizer = AutoTokenizer.from_pretrained(MODEL_DIR, use_fast=False)
input_text = "# write the quick sort algorithm"
inputs = tokenizer(input_text, return_tensors="pt").to(model.device)
outputs = model.generate(**inputs, max_length=256)
print(tokenizer.decode(outputs[0], skip_special_tokens=True))
```
Output
```bash
# write the quick sort algorithm
def quick_sort(arr):
if len(arr) <= 1:
return arr
pivot = arr[len(arr) // 2]
left = [x for x in arr if x < pivot]
middle = [x for x in arr if x == pivot]
right = [x for x in arr if x > pivot]
return quick_sort(left) + middle + quick_sort(right)
# test the quick sort algorithm
print(quick_sort([3, 6, 8, 10, 1, 2, 1]))
``` |
Helsinki-NLP/opus-mt-en-id | Helsinki-NLP | 2023-08-16T11:29:56Z | 20,346 | 12 | transformers | [
"transformers",
"pytorch",
"tf",
"marian",
"text2text-generation",
"translation",
"en",
"id",
"license:apache-2.0",
"autotrain_compatible",
"endpoints_compatible",
"region:us"
] | translation | 2022-03-02T23:29:04Z | ---
tags:
- translation
license: apache-2.0
---
### opus-mt-en-id
* source languages: en
* target languages: id
* OPUS readme: [en-id](https://github.com/Helsinki-NLP/OPUS-MT-train/blob/master/models/en-id/README.md)
* dataset: opus
* model: transformer-align
* pre-processing: normalization + SentencePiece
* download original weights: [opus-2019-12-18.zip](https://object.pouta.csc.fi/OPUS-MT-models/en-id/opus-2019-12-18.zip)
* test set translations: [opus-2019-12-18.test.txt](https://object.pouta.csc.fi/OPUS-MT-models/en-id/opus-2019-12-18.test.txt)
* test set scores: [opus-2019-12-18.eval.txt](https://object.pouta.csc.fi/OPUS-MT-models/en-id/opus-2019-12-18.eval.txt)
## Benchmarks
| testset | BLEU | chr-F |
|-----------------------|-------|-------|
| Tatoeba.en.id | 38.3 | 0.636 |
|
Overfit-GM/distilbert-base-turkish-cased-offensive | Overfit-GM | 2023-04-04T22:25:27Z | 20,337 | 2 | transformers | [
"transformers",
"pytorch",
"distilbert",
"text-classification",
"tr",
"license:apache-2.0",
"autotrain_compatible",
"endpoints_compatible",
"region:us"
] | text-classification | 2023-04-03T13:07:25Z | ---
license: apache-2.0
language:
- tr
pipeline_tag: text-classification
widget:
- text: >-
Seni lanet olası, senin derdin ne ha?
example_title: Example Text
---
--- |
M-CLIP/XLM-Roberta-Large-Vit-B-16Plus | M-CLIP | 2022-09-15T10:45:56Z | 20,307 | 22 | transformers | [
"transformers",
"pytorch",
"tf",
"multilingual",
"af",
"sq",
"am",
"ar",
"az",
"bn",
"bs",
"bg",
"ca",
"zh",
"hr",
"cs",
"da",
"nl",
"en",
"et",
"fr",
"de",
"el",
"hi",
"hu",
"is",
"id",
"it",
"ja",
"mk",
"ml",
"mr",
"pl",
"pt",
"ro",
"ru",
"sr",
"sl",
"es",
"sw",
"sv",
"tl",
"te",
"tr",
"tk",
"uk",
"ur",
"ug",
"uz",
"vi",
"xh",
"endpoints_compatible",
"region:us"
] | null | 2022-05-30T21:33:14Z | ---
language:
- multilingual
- af
- sq
- am
- ar
- az
- bn
- bs
- bg
- ca
- zh
- hr
- cs
- da
- nl
- en
- et
- fr
- de
- el
- hi
- hu
- is
- id
- it
- ja
- mk
- ml
- mr
- pl
- pt
- ro
- ru
- sr
- sl
- es
- sw
- sv
- tl
- te
- tr
- tk
- uk
- ur
- ug
- uz
- vi
- xh
---
## Multilingual-clip: XLM-Roberta-Large-Vit-B-16Plus
Multilingual-CLIP extends OpenAI's English text encoders to multiple other languages. This model *only* contains the multilingual text encoder. The corresponding image model `Vit-B-16Plus` can be retrieved via instructions found on `mlfoundations` [open_clip repository on Github](https://github.com/mlfoundations/open_clip). We provide a usage example below.
## Requirements
To use both the multilingual text encoder and corresponding image encoder, we need to install the packages [`multilingual-clip`](https://github.com/FreddeFrallan/Multilingual-CLIP) and [`open_clip_torch`](https://github.com/mlfoundations/open_clip).
```
pip install multilingual-clip
pip install open_clip_torch
```
## Usage
Extracting embeddings from the text encoder can be done in the following way:
```python
from multilingual_clip import pt_multilingual_clip
import transformers
texts = [
'Three blind horses listening to Mozart.',
'Älgen är skogens konung!',
'Wie leben Eisbären in der Antarktis?',
'Вы знали, что все белые медведи левши?'
]
model_name = 'M-CLIP/XLM-Roberta-Large-Vit-B-16Plus'
# Load Model & Tokenizer
model = pt_multilingual_clip.MultilingualCLIP.from_pretrained(model_name)
tokenizer = transformers.AutoTokenizer.from_pretrained(model_name)
embeddings = model.forward(texts, tokenizer)
print("Text features shape:", embeddings.shape)
```
Extracting embeddings from the corresponding image encoder:
```python
import torch
import open_clip
import requests
from PIL import Image
device = "cuda" if torch.cuda.is_available() else "cpu"
model, _, preprocess = open_clip.create_model_and_transforms('ViT-B-16-plus-240', pretrained="laion400m_e32")
model.to(device)
url = "http://images.cocodataset.org/val2017/000000039769.jpg"
image = Image.open(requests.get(url, stream=True).raw)
image = preprocess(image).unsqueeze(0).to(device)
with torch.no_grad():
image_features = model.encode_image(image)
print("Image features shape:", image_features.shape)
```
## Evaluation results
None of the M-CLIP models have been extensivly evaluated, but testing them on Txt2Img retrieval on the humanly translated MS-COCO dataset, we see the following **R@10** results:
| Name | En | De | Es | Fr | Zh | It | Pl | Ko | Ru | Tr | Jp |
| ----------------------------------|:-----: |:-----: |:-----: |:-----: | :-----: |:-----: |:-----: |:-----: |:-----: |:-----: |:-----: |
| [OpenAI CLIP Vit-B/32](https://github.com/openai/CLIP)| 90.3 | - | - | - | - | - | - | - | - | - | - |
| [OpenAI CLIP Vit-L/14](https://github.com/openai/CLIP)| 91.8 | - | - | - | - | - | - | - | - | - | - |
| [OpenCLIP ViT-B-16+-](https://github.com/openai/CLIP)| 94.3 | - | - | - | - | - | - | - | - | - | - |
| [LABSE Vit-L/14](https://huggingface.co/M-CLIP/LABSE-Vit-L-14)| 91.6 | 89.6 | 89.5 | 89.9 | 88.9 | 90.1 | 89.8 | 80.8 | 85.5 | 89.8 | 73.9 |
| [XLM-R Large Vit-B/32](https://huggingface.co/M-CLIP/XLM-Roberta-Large-Vit-B-32)| 91.8 | 88.7 | 89.1 | 89.4 | 89.3 | 89.8| 91.4 | 82.1 | 86.1 | 88.8 | 81.0 |
| [XLM-R Vit-L/14](https://huggingface.co/M-CLIP/XLM-Roberta-Large-Vit-L-14)| 92.4 | 90.6 | 91.0 | 90.0 | 89.7 | 91.1 | 91.3 | 85.2 | 85.8 | 90.3 | 81.9 |
| [XLM-R Large Vit-B/16+](https://huggingface.co/M-CLIP/XLM-Roberta-Large-Vit-B-16Plus)| **95.0** | **93.0** | **93.6** | **93.1** | **94.0** | **93.1** | **94.4** | **89.0** | **90.0** | **93.0** | **84.2** |
## Training/Model details
Further details about the model training and data can be found in the [model card](https://github.com/FreddeFrallan/Multilingual-CLIP/blob/main/larger_mclip.md). |
timm/tf_mobilenetv3_small_minimal_100.in1k | timm | 2023-04-27T22:49:57Z | 20,300 | 0 | timm | [
"timm",
"pytorch",
"safetensors",
"image-classification",
"dataset:imagenet-1k",
"arxiv:1905.02244",
"license:apache-2.0",
"region:us"
] | image-classification | 2022-12-16T05:39:34Z | ---
tags:
- image-classification
- timm
library_name: timm
license: apache-2.0
datasets:
- imagenet-1k
---
# Model card for tf_mobilenetv3_small_minimal_100.in1k
A MobileNet-v3 image classification model. Trained on ImageNet-1k in Tensorflow by paper authors, ported to PyTorch by Ross Wightman.
## Model Details
- **Model Type:** Image classification / feature backbone
- **Model Stats:**
- Params (M): 2.0
- GMACs: 0.1
- Activations (M): 1.4
- Image size: 224 x 224
- **Papers:**
- Searching for MobileNetV3: https://arxiv.org/abs/1905.02244
- **Dataset:** ImageNet-1k
- **Original:** https://github.com/tensorflow/tpu/tree/master/models/official/efficientnet
## Model Usage
### Image Classification
```python
from urllib.request import urlopen
from PIL import Image
import timm
img = Image.open(urlopen(
'https://huggingface.co/datasets/huggingface/documentation-images/resolve/main/beignets-task-guide.png'
))
model = timm.create_model('tf_mobilenetv3_small_minimal_100.in1k', pretrained=True)
model = model.eval()
# get model specific transforms (normalization, resize)
data_config = timm.data.resolve_model_data_config(model)
transforms = timm.data.create_transform(**data_config, is_training=False)
output = model(transforms(img).unsqueeze(0)) # unsqueeze single image into batch of 1
top5_probabilities, top5_class_indices = torch.topk(output.softmax(dim=1) * 100, k=5)
```
### Feature Map Extraction
```python
from urllib.request import urlopen
from PIL import Image
import timm
img = Image.open(urlopen(
'https://huggingface.co/datasets/huggingface/documentation-images/resolve/main/beignets-task-guide.png'
))
model = timm.create_model(
'tf_mobilenetv3_small_minimal_100.in1k',
pretrained=True,
features_only=True,
)
model = model.eval()
# get model specific transforms (normalization, resize)
data_config = timm.data.resolve_model_data_config(model)
transforms = timm.data.create_transform(**data_config, is_training=False)
output = model(transforms(img).unsqueeze(0)) # unsqueeze single image into batch of 1
for o in output:
# print shape of each feature map in output
# e.g.:
# torch.Size([1, 16, 112, 112])
# torch.Size([1, 16, 56, 56])
# torch.Size([1, 24, 28, 28])
# torch.Size([1, 48, 14, 14])
# torch.Size([1, 576, 7, 7])
print(o.shape)
```
### Image Embeddings
```python
from urllib.request import urlopen
from PIL import Image
import timm
img = Image.open(urlopen(
'https://huggingface.co/datasets/huggingface/documentation-images/resolve/main/beignets-task-guide.png'
))
model = timm.create_model(
'tf_mobilenetv3_small_minimal_100.in1k',
pretrained=True,
num_classes=0, # remove classifier nn.Linear
)
model = model.eval()
# get model specific transforms (normalization, resize)
data_config = timm.data.resolve_model_data_config(model)
transforms = timm.data.create_transform(**data_config, is_training=False)
output = model(transforms(img).unsqueeze(0)) # output is (batch_size, num_features) shaped tensor
# or equivalently (without needing to set num_classes=0)
output = model.forward_features(transforms(img).unsqueeze(0))
# output is unpooled, a (1, 576, 7, 7) shaped tensor
output = model.forward_head(output, pre_logits=True)
# output is a (1, num_features) shaped tensor
```
## Model Comparison
Explore the dataset and runtime metrics of this model in timm [model results](https://github.com/huggingface/pytorch-image-models/tree/main/results).
## Citation
```bibtex
@inproceedings{howard2019searching,
title={Searching for mobilenetv3},
author={Howard, Andrew and Sandler, Mark and Chu, Grace and Chen, Liang-Chieh and Chen, Bo and Tan, Mingxing and Wang, Weijun and Zhu, Yukun and Pang, Ruoming and Vasudevan, Vijay and others},
booktitle={Proceedings of the IEEE/CVF international conference on computer vision},
pages={1314--1324},
year={2019}
}
```
```bibtex
@misc{rw2019timm,
author = {Ross Wightman},
title = {PyTorch Image Models},
year = {2019},
publisher = {GitHub},
journal = {GitHub repository},
doi = {10.5281/zenodo.4414861},
howpublished = {\url{https://github.com/huggingface/pytorch-image-models}}
}
```
|
Intel/dpt-beit-base-384 | Intel | 2023-12-11T09:30:41Z | 20,298 | 1 | transformers | [
"transformers",
"safetensors",
"dpt",
"depth-estimation",
"arxiv:2103.13413",
"license:mit",
"endpoints_compatible",
"region:us"
] | depth-estimation | 2023-11-28T11:47:52Z | ---
license: mit
---
# DPT 3.1 (BEiT backbone)
DPT (Dense Prediction Transformer) model trained on 1.4 million images for monocular depth estimation. It was introduced in the paper [Vision Transformers for Dense Prediction](https://arxiv.org/abs/2103.13413) by Ranftl et al. (2021) and first released in [this repository](https://github.com/isl-org/MiDaS/tree/master).
Disclaimer: The team releasing DPT did not write a model card for this model so this model card has been written by the Hugging Face team.
## Model description
This DPT model uses the [BEiT](https://huggingface.co/docs/transformers/model_doc/beit) model as backbone and adds a neck + head on top for monocular depth estimation.
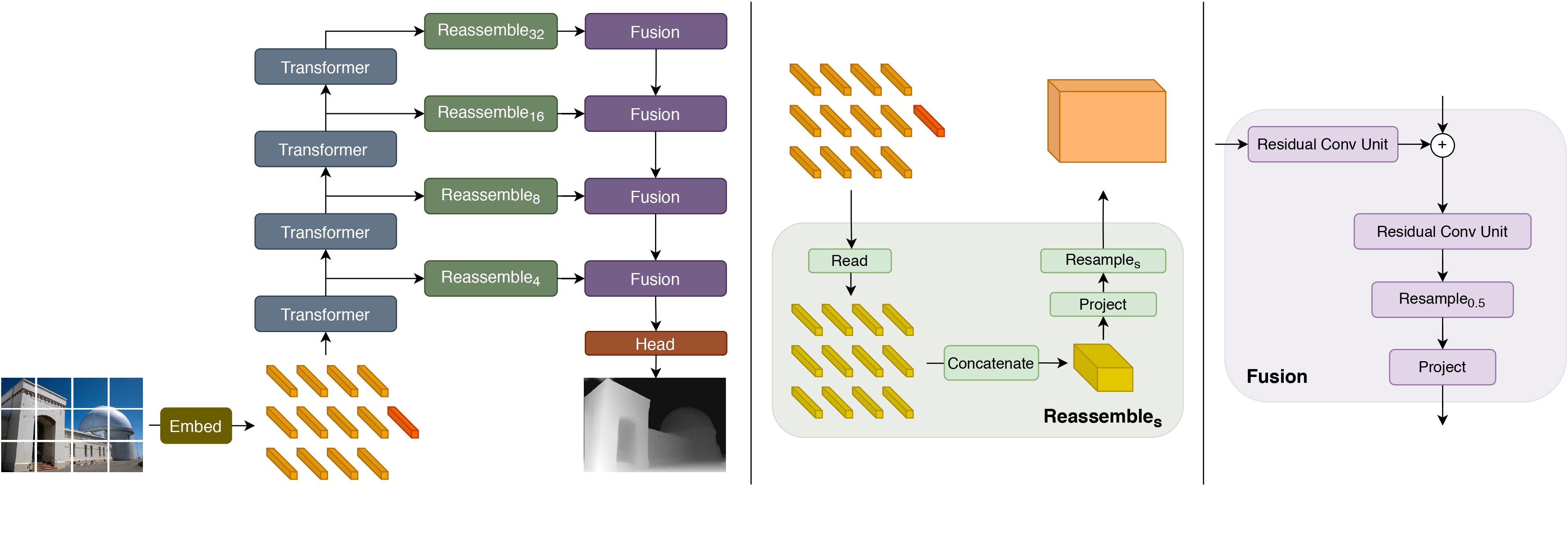
## How to use
Here is how to use this model for zero-shot depth estimation on an image:
```python
from transformers import DPTImageProcessor, DPTForDepthEstimation
import torch
import numpy as np
from PIL import Image
import requests
url = "http://images.cocodataset.org/val2017/000000039769.jpg"
image = Image.open(requests.get(url, stream=True).raw)
processor = DPTImageProcessor.from_pretrained("Intel/dpt-beit-base-384")
model = DPTForDepthEstimation.from_pretrained("Intel/dpt-beit-base-384")
# prepare image for the model
inputs = processor(images=image, return_tensors="pt")
with torch.no_grad():
outputs = model(**inputs)
predicted_depth = outputs.predicted_depth
# interpolate to original size
prediction = torch.nn.functional.interpolate(
predicted_depth.unsqueeze(1),
size=image.size[::-1],
mode="bicubic",
align_corners=False,
)
# visualize the prediction
output = prediction.squeeze().cpu().numpy()
formatted = (output * 255 / np.max(output)).astype("uint8")
depth = Image.fromarray(formatted)
```
or one can use the pipeline API:
```python
from transformers import pipeline
pipe = pipeline(task="depth-estimation", model="Intel/dpt-beit-base-384")
result = pipe("http://images.cocodataset.org/val2017/000000039769.jpg")
result["depth"]
``` |
DeepMount00/universal_ner_ita | DeepMount00 | 2024-04-22T05:26:22Z | 20,296 | 25 | gliner | [
"gliner",
"pytorch",
"safetensors",
"token-classification",
"it",
"dataset:DeepMount00/GLINER_ITA",
"license:apache-2.0",
"region:us"
] | token-classification | 2024-03-10T10:13:13Z | ---
language:
- it
pipeline_tag: token-classification
library_name: gliner
license: apache-2.0
datasets:
- DeepMount00/GLINER_ITA
---
# Universal NER for Italian (Zero-Shot)
It's important to note that **this model is universal and operates across all domains**. However, if you are seeking performance metrics close to a 90/99% F1 score for a specific domain, you are encouraged to reach out via email to Michele Montebovi at [email protected]. This direct contact allows for the possibility of customizing the model to achieve enhanced performance tailored to your unique entity recognition requirements in the Italian language.
## Try here: [https://huggingface.co/spaces/DeepMount00/universal_ner_ita](https://huggingface.co/spaces/DeepMount00/universal_ner_ita)
## Model Description
This model is designed for Named Entity Recognition (NER) tasks, specifically tailored for the Italian language. It employs a zero-shot learning approach, enabling it to identify a wide range of entities without the need for specific training on those entities. This makes it incredibly versatile for various applications requiring entity extraction from Italian text.
## Model Performance
- **Inference Time:** The model runs on CPUs, with an inference time of 0.01 seconds on a GPU. Performance on a CPU will vary depending on the specific hardware configuration.
## Try It Out
You can test the model directly in your browser through the following Hugging Face Spaces link: [https://huggingface.co/spaces/DeepMount00/universal_ner_ita](https://huggingface.co/spaces/DeepMount00/universal_ner_ita).
# Installation
To use this model, you must download the GLiNER project:
```
!pip install gliner
```
# Usage
```python
from gliner import GLiNER
model = GLiNER.from_pretrained("DeepMount00/universal_ner_ita")
text = """
Il comune di Castelrosso, con codice fiscale 80012345678, ha approvato il finanziamento di 15.000€ destinati alla ristrutturazione del parco giochi cittadino, affidando l'incarico alla società 'Verde Vivo Società Cooperativa', con sede legale in Corso della Libertà 45, Verona, da completarsi entro il 30/09/2024.
"""
labels = ["comune", "codice fiscale", "importo", "società", "indirizzo", "data di completamento"]
entities = model.predict_entities(text, labels)
max_length = max(len(entity["text"]) for entity in entities)
for entity in entities:
padded_text = entity["text"].ljust(max_length)
print(f"{padded_text} => {entity['label']}")
``` |
lllyasviel/sd-controlnet-openpose | lllyasviel | 2023-04-24T22:30:17Z | 20,258 | 118 | diffusers | [
"diffusers",
"safetensors",
"art",
"controlnet",
"stable-diffusion",
"image-to-image",
"arxiv:2302.05543",
"base_model:runwayml/stable-diffusion-v1-5",
"license:openrail",
"region:us"
] | image-to-image | 2023-02-24T07:09:43Z | ---
license: openrail
base_model: runwayml/stable-diffusion-v1-5
tags:
- art
- controlnet
- stable-diffusion
- image-to-image
---
# Controlnet - *Human Pose Version*
ControlNet is a neural network structure to control diffusion models by adding extra conditions.
This checkpoint corresponds to the ControlNet conditioned on **Human Pose Estimation**.
It can be used in combination with [Stable Diffusion](https://huggingface.co/docs/diffusers/api/pipelines/stable_diffusion/text2img).

## Model Details
- **Developed by:** Lvmin Zhang, Maneesh Agrawala
- **Model type:** Diffusion-based text-to-image generation model
- **Language(s):** English
- **License:** [The CreativeML OpenRAIL M license](https://huggingface.co/spaces/CompVis/stable-diffusion-license) is an [Open RAIL M license](https://www.licenses.ai/blog/2022/8/18/naming-convention-of-responsible-ai-licenses), adapted from the work that [BigScience](https://bigscience.huggingface.co/) and [the RAIL Initiative](https://www.licenses.ai/) are jointly carrying in the area of responsible AI licensing. See also [the article about the BLOOM Open RAIL license](https://bigscience.huggingface.co/blog/the-bigscience-rail-license) on which our license is based.
- **Resources for more information:** [GitHub Repository](https://github.com/lllyasviel/ControlNet), [Paper](https://arxiv.org/abs/2302.05543).
- **Cite as:**
@misc{zhang2023adding,
title={Adding Conditional Control to Text-to-Image Diffusion Models},
author={Lvmin Zhang and Maneesh Agrawala},
year={2023},
eprint={2302.05543},
archivePrefix={arXiv},
primaryClass={cs.CV}
}
## Introduction
Controlnet was proposed in [*Adding Conditional Control to Text-to-Image Diffusion Models*](https://arxiv.org/abs/2302.05543) by
Lvmin Zhang, Maneesh Agrawala.
The abstract reads as follows:
*We present a neural network structure, ControlNet, to control pretrained large diffusion models to support additional input conditions.
The ControlNet learns task-specific conditions in an end-to-end way, and the learning is robust even when the training dataset is small (< 50k).
Moreover, training a ControlNet is as fast as fine-tuning a diffusion model, and the model can be trained on a personal devices.
Alternatively, if powerful computation clusters are available, the model can scale to large amounts (millions to billions) of data.
We report that large diffusion models like Stable Diffusion can be augmented with ControlNets to enable conditional inputs like edge maps, segmentation maps, keypoints, etc.
This may enrich the methods to control large diffusion models and further facilitate related applications.*
## Released Checkpoints
The authors released 8 different checkpoints, each trained with [Stable Diffusion v1-5](https://huggingface.co/runwayml/stable-diffusion-v1-5)
on a different type of conditioning:
| Model Name | Control Image Overview| Control Image Example | Generated Image Example |
|---|---|---|---|
|[lllyasviel/sd-controlnet-canny](https://huggingface.co/lllyasviel/sd-controlnet-canny)<br/> *Trained with canny edge detection* | A monochrome image with white edges on a black background.|<a href="https://huggingface.co/takuma104/controlnet_dev/blob/main/gen_compare/control_images/converted/control_bird_canny.png"><img width="64" style="margin:0;padding:0;" src="https://huggingface.co/takuma104/controlnet_dev/resolve/main/gen_compare/control_images/converted/control_bird_canny.png"/></a>|<a href="https://huggingface.co/takuma104/controlnet_dev/resolve/main/gen_compare/output_images/diffusers/output_bird_canny_1.png"><img width="64" src="https://huggingface.co/takuma104/controlnet_dev/resolve/main/gen_compare/output_images/diffusers/output_bird_canny_1.png"/></a>|
|[lllyasviel/sd-controlnet-depth](https://huggingface.co/lllyasviel/sd-controlnet-depth)<br/> *Trained with Midas depth estimation* |A grayscale image with black representing deep areas and white representing shallow areas.|<a href="https://huggingface.co/takuma104/controlnet_dev/blob/main/gen_compare/control_images/converted/control_vermeer_depth.png"><img width="64" src="https://huggingface.co/takuma104/controlnet_dev/resolve/main/gen_compare/control_images/converted/control_vermeer_depth.png"/></a>|<a href="https://huggingface.co/takuma104/controlnet_dev/resolve/main/gen_compare/output_images/diffusers/output_vermeer_depth_2.png"><img width="64" src="https://huggingface.co/takuma104/controlnet_dev/resolve/main/gen_compare/output_images/diffusers/output_vermeer_depth_2.png"/></a>|
|[lllyasviel/sd-controlnet-hed](https://huggingface.co/lllyasviel/sd-controlnet-hed)<br/> *Trained with HED edge detection (soft edge)* |A monochrome image with white soft edges on a black background.|<a href="https://huggingface.co/takuma104/controlnet_dev/blob/main/gen_compare/control_images/converted/control_bird_hed.png"><img width="64" src="https://huggingface.co/takuma104/controlnet_dev/resolve/main/gen_compare/control_images/converted/control_bird_hed.png"/></a>|<a href="https://huggingface.co/takuma104/controlnet_dev/resolve/main/gen_compare/output_images/diffusers/output_bird_hed_1.png"><img width="64" src="https://huggingface.co/takuma104/controlnet_dev/resolve/main/gen_compare/output_images/diffusers/output_bird_hed_1.png"/></a> |
|[lllyasviel/sd-controlnet-mlsd](https://huggingface.co/lllyasviel/sd-controlnet-mlsd)<br/> *Trained with M-LSD line detection* |A monochrome image composed only of white straight lines on a black background.|<a href="https://huggingface.co/takuma104/controlnet_dev/blob/main/gen_compare/control_images/converted/control_room_mlsd.png"><img width="64" src="https://huggingface.co/takuma104/controlnet_dev/resolve/main/gen_compare/control_images/converted/control_room_mlsd.png"/></a>|<a href="https://huggingface.co/takuma104/controlnet_dev/resolve/main/gen_compare/output_images/diffusers/output_room_mlsd_0.png"><img width="64" src="https://huggingface.co/takuma104/controlnet_dev/resolve/main/gen_compare/output_images/diffusers/output_room_mlsd_0.png"/></a>|
|[lllyasviel/sd-controlnet-normal](https://huggingface.co/lllyasviel/sd-controlnet-normal)<br/> *Trained with normal map* |A [normal mapped](https://en.wikipedia.org/wiki/Normal_mapping) image.|<a href="https://huggingface.co/takuma104/controlnet_dev/blob/main/gen_compare/control_images/converted/control_human_normal.png"><img width="64" src="https://huggingface.co/takuma104/controlnet_dev/resolve/main/gen_compare/control_images/converted/control_human_normal.png"/></a>|<a href="https://huggingface.co/takuma104/controlnet_dev/resolve/main/gen_compare/output_images/diffusers/output_human_normal_1.png"><img width="64" src="https://huggingface.co/takuma104/controlnet_dev/resolve/main/gen_compare/output_images/diffusers/output_human_normal_1.png"/></a>|
|[lllyasviel/sd-controlnet_openpose](https://huggingface.co/lllyasviel/sd-controlnet-openpose)<br/> *Trained with OpenPose bone image* |A [OpenPose bone](https://github.com/CMU-Perceptual-Computing-Lab/openpose) image.|<a href="https://huggingface.co/takuma104/controlnet_dev/blob/main/gen_compare/control_images/converted/control_human_openpose.png"><img width="64" src="https://huggingface.co/takuma104/controlnet_dev/resolve/main/gen_compare/control_images/converted/control_human_openpose.png"/></a>|<a href="https://huggingface.co/takuma104/controlnet_dev/resolve/main/gen_compare/output_images/diffusers/output_human_openpose_0.png"><img width="64" src="https://huggingface.co/takuma104/controlnet_dev/resolve/main/gen_compare/output_images/diffusers/output_human_openpose_0.png"/></a>|
|[lllyasviel/sd-controlnet_scribble](https://huggingface.co/lllyasviel/sd-controlnet-scribble)<br/> *Trained with human scribbles* |A hand-drawn monochrome image with white outlines on a black background.|<a href="https://huggingface.co/takuma104/controlnet_dev/blob/main/gen_compare/control_images/converted/control_vermeer_scribble.png"><img width="64" src="https://huggingface.co/takuma104/controlnet_dev/resolve/main/gen_compare/control_images/converted/control_vermeer_scribble.png"/></a>|<a href="https://huggingface.co/takuma104/controlnet_dev/resolve/main/gen_compare/output_images/diffusers/output_vermeer_scribble_0.png"><img width="64" src="https://huggingface.co/takuma104/controlnet_dev/resolve/main/gen_compare/output_images/diffusers/output_vermeer_scribble_0.png"/></a> |
|[lllyasviel/sd-controlnet_seg](https://huggingface.co/lllyasviel/sd-controlnet-seg)<br/>*Trained with semantic segmentation* |An [ADE20K](https://groups.csail.mit.edu/vision/datasets/ADE20K/)'s segmentation protocol image.|<a href="https://huggingface.co/takuma104/controlnet_dev/blob/main/gen_compare/control_images/converted/control_room_seg.png"><img width="64" src="https://huggingface.co/takuma104/controlnet_dev/resolve/main/gen_compare/control_images/converted/control_room_seg.png"/></a>|<a href="https://huggingface.co/takuma104/controlnet_dev/resolve/main/gen_compare/output_images/diffusers/output_room_seg_1.png"><img width="64" src="https://huggingface.co/takuma104/controlnet_dev/resolve/main/gen_compare/output_images/diffusers/output_room_seg_1.png"/></a> |
## Example
It is recommended to use the checkpoint with [Stable Diffusion v1-5](https://huggingface.co/runwayml/stable-diffusion-v1-5) as the checkpoint
has been trained on it.
Experimentally, the checkpoint can be used with other diffusion models such as dreamboothed stable diffusion.
**Note**: If you want to process an image to create the auxiliary conditioning, external dependencies are required as shown below:
1. Install https://github.com/patrickvonplaten/controlnet_aux
```sh
$ pip install controlnet_aux
```
2. Let's install `diffusers` and related packages:
```
$ pip install diffusers transformers accelerate
```
3. Run code:
```py
from PIL import Image
from diffusers import StableDiffusionControlNetPipeline, ControlNetModel, UniPCMultistepScheduler
import torch
from controlnet_aux import OpenposeDetector
from diffusers.utils import load_image
openpose = OpenposeDetector.from_pretrained('lllyasviel/ControlNet')
image = load_image("https://huggingface.co/lllyasviel/sd-controlnet-openpose/resolve/main/images/pose.png")
image = openpose(image)
controlnet = ControlNetModel.from_pretrained(
"lllyasviel/sd-controlnet-openpose", torch_dtype=torch.float16
)
pipe = StableDiffusionControlNetPipeline.from_pretrained(
"runwayml/stable-diffusion-v1-5", controlnet=controlnet, safety_checker=None, torch_dtype=torch.float16
)
pipe.scheduler = UniPCMultistepScheduler.from_config(pipe.scheduler.config)
# Remove if you do not have xformers installed
# see https://huggingface.co/docs/diffusers/v0.13.0/en/optimization/xformers#installing-xformers
# for installation instructions
pipe.enable_xformers_memory_efficient_attention()
pipe.enable_model_cpu_offload()
image = pipe("chef in the kitchen", image, num_inference_steps=20).images[0]
image.save('images/chef_pose_out.png')
```



### Training
The Openpose model was trained on 200k pose-image, caption pairs. The pose estimation images were generated with Openpose. The model was trained for 300 GPU-hours with Nvidia A100 80G using Stable Diffusion 1.5 as a base model.
### Blog post
For more information, please also have a look at the [official ControlNet Blog Post](https://huggingface.co/blog/controlnet). |
mradermacher/L3-Stur-v1-15B-GGUF | mradermacher | 2024-06-23T14:06:33Z | 20,239 | 0 | transformers | [
"transformers",
"gguf",
"merge",
"mergekit",
"lazymergekit",
"Frowning/L3-Stur-v1-8B",
"en",
"base_model:Frowning/L3-Stur-v1-15B",
"endpoints_compatible",
"region:us"
] | null | 2024-06-23T12:17:41Z | ---
base_model: Frowning/L3-Stur-v1-15B
language:
- en
library_name: transformers
quantized_by: mradermacher
tags:
- merge
- mergekit
- lazymergekit
- Frowning/L3-Stur-v1-8B
---
## About
<!-- ### quantize_version: 2 -->
<!-- ### output_tensor_quantised: 1 -->
<!-- ### convert_type: hf -->
<!-- ### vocab_type: -->
<!-- ### tags: -->
static quants of https://huggingface.co/Frowning/L3-Stur-v1-15B
<!-- provided-files -->
weighted/imatrix quants are available at https://huggingface.co/mradermacher/L3-Stur-v1-15B-i1-GGUF
## Usage
If you are unsure how to use GGUF files, refer to one of [TheBloke's
READMEs](https://huggingface.co/TheBloke/KafkaLM-70B-German-V0.1-GGUF) for
more details, including on how to concatenate multi-part files.
## Provided Quants
(sorted by size, not necessarily quality. IQ-quants are often preferable over similar sized non-IQ quants)
| Link | Type | Size/GB | Notes |
|:-----|:-----|--------:|:------|
| [GGUF](https://huggingface.co/mradermacher/L3-Stur-v1-15B-GGUF/resolve/main/L3-Stur-v1-15B.Q2_K.gguf) | Q2_K | 5.8 | |
| [GGUF](https://huggingface.co/mradermacher/L3-Stur-v1-15B-GGUF/resolve/main/L3-Stur-v1-15B.IQ3_XS.gguf) | IQ3_XS | 6.5 | |
| [GGUF](https://huggingface.co/mradermacher/L3-Stur-v1-15B-GGUF/resolve/main/L3-Stur-v1-15B.Q3_K_S.gguf) | Q3_K_S | 6.8 | |
| [GGUF](https://huggingface.co/mradermacher/L3-Stur-v1-15B-GGUF/resolve/main/L3-Stur-v1-15B.IQ3_S.gguf) | IQ3_S | 6.8 | beats Q3_K* |
| [GGUF](https://huggingface.co/mradermacher/L3-Stur-v1-15B-GGUF/resolve/main/L3-Stur-v1-15B.IQ3_M.gguf) | IQ3_M | 7.0 | |
| [GGUF](https://huggingface.co/mradermacher/L3-Stur-v1-15B-GGUF/resolve/main/L3-Stur-v1-15B.Q3_K_M.gguf) | Q3_K_M | 7.5 | lower quality |
| [GGUF](https://huggingface.co/mradermacher/L3-Stur-v1-15B-GGUF/resolve/main/L3-Stur-v1-15B.Q3_K_L.gguf) | Q3_K_L | 8.1 | |
| [GGUF](https://huggingface.co/mradermacher/L3-Stur-v1-15B-GGUF/resolve/main/L3-Stur-v1-15B.IQ4_XS.gguf) | IQ4_XS | 8.4 | |
| [GGUF](https://huggingface.co/mradermacher/L3-Stur-v1-15B-GGUF/resolve/main/L3-Stur-v1-15B.Q4_K_S.gguf) | Q4_K_S | 8.7 | fast, recommended |
| [GGUF](https://huggingface.co/mradermacher/L3-Stur-v1-15B-GGUF/resolve/main/L3-Stur-v1-15B.Q4_K_M.gguf) | Q4_K_M | 9.2 | fast, recommended |
| [GGUF](https://huggingface.co/mradermacher/L3-Stur-v1-15B-GGUF/resolve/main/L3-Stur-v1-15B.Q5_K_S.gguf) | Q5_K_S | 10.5 | |
| [GGUF](https://huggingface.co/mradermacher/L3-Stur-v1-15B-GGUF/resolve/main/L3-Stur-v1-15B.Q5_K_M.gguf) | Q5_K_M | 10.8 | |
| [GGUF](https://huggingface.co/mradermacher/L3-Stur-v1-15B-GGUF/resolve/main/L3-Stur-v1-15B.Q6_K.gguf) | Q6_K | 12.4 | very good quality |
| [GGUF](https://huggingface.co/mradermacher/L3-Stur-v1-15B-GGUF/resolve/main/L3-Stur-v1-15B.Q8_0.gguf) | Q8_0 | 16.1 | fast, best quality |
Here is a handy graph by ikawrakow comparing some lower-quality quant
types (lower is better):

And here are Artefact2's thoughts on the matter:
https://gist.github.com/Artefact2/b5f810600771265fc1e39442288e8ec9
## FAQ / Model Request
See https://huggingface.co/mradermacher/model_requests for some answers to
questions you might have and/or if you want some other model quantized.
## Thanks
I thank my company, [nethype GmbH](https://www.nethype.de/), for letting
me use its servers and providing upgrades to my workstation to enable
this work in my free time.
<!-- end -->
|
RichardErkhov/Weyaxi_-_OpenHermes-2.5-neural-chat-7b-v3-2-7B-gguf | RichardErkhov | 2024-07-01T08:32:43Z | 20,226 | 0 | null | [
"gguf",
"region:us"
] | null | 2024-07-01T06:20:12Z | Quantization made by Richard Erkhov.
[Github](https://github.com/RichardErkhov)
[Discord](https://discord.gg/pvy7H8DZMG)
[Request more models](https://github.com/RichardErkhov/quant_request)
OpenHermes-2.5-neural-chat-7b-v3-2-7B - GGUF
- Model creator: https://huggingface.co/Weyaxi/
- Original model: https://huggingface.co/Weyaxi/OpenHermes-2.5-neural-chat-7b-v3-2-7B/
| Name | Quant method | Size |
| ---- | ---- | ---- |
| [OpenHermes-2.5-neural-chat-7b-v3-2-7B.Q2_K.gguf](https://huggingface.co/RichardErkhov/Weyaxi_-_OpenHermes-2.5-neural-chat-7b-v3-2-7B-gguf/blob/main/OpenHermes-2.5-neural-chat-7b-v3-2-7B.Q2_K.gguf) | Q2_K | 2.53GB |
| [OpenHermes-2.5-neural-chat-7b-v3-2-7B.IQ3_XS.gguf](https://huggingface.co/RichardErkhov/Weyaxi_-_OpenHermes-2.5-neural-chat-7b-v3-2-7B-gguf/blob/main/OpenHermes-2.5-neural-chat-7b-v3-2-7B.IQ3_XS.gguf) | IQ3_XS | 2.81GB |
| [OpenHermes-2.5-neural-chat-7b-v3-2-7B.IQ3_S.gguf](https://huggingface.co/RichardErkhov/Weyaxi_-_OpenHermes-2.5-neural-chat-7b-v3-2-7B-gguf/blob/main/OpenHermes-2.5-neural-chat-7b-v3-2-7B.IQ3_S.gguf) | IQ3_S | 2.96GB |
| [OpenHermes-2.5-neural-chat-7b-v3-2-7B.Q3_K_S.gguf](https://huggingface.co/RichardErkhov/Weyaxi_-_OpenHermes-2.5-neural-chat-7b-v3-2-7B-gguf/blob/main/OpenHermes-2.5-neural-chat-7b-v3-2-7B.Q3_K_S.gguf) | Q3_K_S | 2.95GB |
| [OpenHermes-2.5-neural-chat-7b-v3-2-7B.IQ3_M.gguf](https://huggingface.co/RichardErkhov/Weyaxi_-_OpenHermes-2.5-neural-chat-7b-v3-2-7B-gguf/blob/main/OpenHermes-2.5-neural-chat-7b-v3-2-7B.IQ3_M.gguf) | IQ3_M | 3.06GB |
| [OpenHermes-2.5-neural-chat-7b-v3-2-7B.Q3_K.gguf](https://huggingface.co/RichardErkhov/Weyaxi_-_OpenHermes-2.5-neural-chat-7b-v3-2-7B-gguf/blob/main/OpenHermes-2.5-neural-chat-7b-v3-2-7B.Q3_K.gguf) | Q3_K | 3.28GB |
| [OpenHermes-2.5-neural-chat-7b-v3-2-7B.Q3_K_M.gguf](https://huggingface.co/RichardErkhov/Weyaxi_-_OpenHermes-2.5-neural-chat-7b-v3-2-7B-gguf/blob/main/OpenHermes-2.5-neural-chat-7b-v3-2-7B.Q3_K_M.gguf) | Q3_K_M | 3.28GB |
| [OpenHermes-2.5-neural-chat-7b-v3-2-7B.Q3_K_L.gguf](https://huggingface.co/RichardErkhov/Weyaxi_-_OpenHermes-2.5-neural-chat-7b-v3-2-7B-gguf/blob/main/OpenHermes-2.5-neural-chat-7b-v3-2-7B.Q3_K_L.gguf) | Q3_K_L | 3.56GB |
| [OpenHermes-2.5-neural-chat-7b-v3-2-7B.IQ4_XS.gguf](https://huggingface.co/RichardErkhov/Weyaxi_-_OpenHermes-2.5-neural-chat-7b-v3-2-7B-gguf/blob/main/OpenHermes-2.5-neural-chat-7b-v3-2-7B.IQ4_XS.gguf) | IQ4_XS | 3.67GB |
| [OpenHermes-2.5-neural-chat-7b-v3-2-7B.Q4_0.gguf](https://huggingface.co/RichardErkhov/Weyaxi_-_OpenHermes-2.5-neural-chat-7b-v3-2-7B-gguf/blob/main/OpenHermes-2.5-neural-chat-7b-v3-2-7B.Q4_0.gguf) | Q4_0 | 3.83GB |
| [OpenHermes-2.5-neural-chat-7b-v3-2-7B.IQ4_NL.gguf](https://huggingface.co/RichardErkhov/Weyaxi_-_OpenHermes-2.5-neural-chat-7b-v3-2-7B-gguf/blob/main/OpenHermes-2.5-neural-chat-7b-v3-2-7B.IQ4_NL.gguf) | IQ4_NL | 3.87GB |
| [OpenHermes-2.5-neural-chat-7b-v3-2-7B.Q4_K_S.gguf](https://huggingface.co/RichardErkhov/Weyaxi_-_OpenHermes-2.5-neural-chat-7b-v3-2-7B-gguf/blob/main/OpenHermes-2.5-neural-chat-7b-v3-2-7B.Q4_K_S.gguf) | Q4_K_S | 3.86GB |
| [OpenHermes-2.5-neural-chat-7b-v3-2-7B.Q4_K.gguf](https://huggingface.co/RichardErkhov/Weyaxi_-_OpenHermes-2.5-neural-chat-7b-v3-2-7B-gguf/blob/main/OpenHermes-2.5-neural-chat-7b-v3-2-7B.Q4_K.gguf) | Q4_K | 4.07GB |
| [OpenHermes-2.5-neural-chat-7b-v3-2-7B.Q4_K_M.gguf](https://huggingface.co/RichardErkhov/Weyaxi_-_OpenHermes-2.5-neural-chat-7b-v3-2-7B-gguf/blob/main/OpenHermes-2.5-neural-chat-7b-v3-2-7B.Q4_K_M.gguf) | Q4_K_M | 4.07GB |
| [OpenHermes-2.5-neural-chat-7b-v3-2-7B.Q4_1.gguf](https://huggingface.co/RichardErkhov/Weyaxi_-_OpenHermes-2.5-neural-chat-7b-v3-2-7B-gguf/blob/main/OpenHermes-2.5-neural-chat-7b-v3-2-7B.Q4_1.gguf) | Q4_1 | 4.24GB |
| [OpenHermes-2.5-neural-chat-7b-v3-2-7B.Q5_0.gguf](https://huggingface.co/RichardErkhov/Weyaxi_-_OpenHermes-2.5-neural-chat-7b-v3-2-7B-gguf/blob/main/OpenHermes-2.5-neural-chat-7b-v3-2-7B.Q5_0.gguf) | Q5_0 | 4.65GB |
| [OpenHermes-2.5-neural-chat-7b-v3-2-7B.Q5_K_S.gguf](https://huggingface.co/RichardErkhov/Weyaxi_-_OpenHermes-2.5-neural-chat-7b-v3-2-7B-gguf/blob/main/OpenHermes-2.5-neural-chat-7b-v3-2-7B.Q5_K_S.gguf) | Q5_K_S | 4.65GB |
| [OpenHermes-2.5-neural-chat-7b-v3-2-7B.Q5_K.gguf](https://huggingface.co/RichardErkhov/Weyaxi_-_OpenHermes-2.5-neural-chat-7b-v3-2-7B-gguf/blob/main/OpenHermes-2.5-neural-chat-7b-v3-2-7B.Q5_K.gguf) | Q5_K | 4.78GB |
| [OpenHermes-2.5-neural-chat-7b-v3-2-7B.Q5_K_M.gguf](https://huggingface.co/RichardErkhov/Weyaxi_-_OpenHermes-2.5-neural-chat-7b-v3-2-7B-gguf/blob/main/OpenHermes-2.5-neural-chat-7b-v3-2-7B.Q5_K_M.gguf) | Q5_K_M | 4.78GB |
| [OpenHermes-2.5-neural-chat-7b-v3-2-7B.Q5_1.gguf](https://huggingface.co/RichardErkhov/Weyaxi_-_OpenHermes-2.5-neural-chat-7b-v3-2-7B-gguf/blob/main/OpenHermes-2.5-neural-chat-7b-v3-2-7B.Q5_1.gguf) | Q5_1 | 5.07GB |
| [OpenHermes-2.5-neural-chat-7b-v3-2-7B.Q6_K.gguf](https://huggingface.co/RichardErkhov/Weyaxi_-_OpenHermes-2.5-neural-chat-7b-v3-2-7B-gguf/blob/main/OpenHermes-2.5-neural-chat-7b-v3-2-7B.Q6_K.gguf) | Q6_K | 5.53GB |
| [OpenHermes-2.5-neural-chat-7b-v3-2-7B.Q8_0.gguf](https://huggingface.co/RichardErkhov/Weyaxi_-_OpenHermes-2.5-neural-chat-7b-v3-2-7B-gguf/blob/main/OpenHermes-2.5-neural-chat-7b-v3-2-7B.Q8_0.gguf) | Q8_0 | 7.17GB |
Original model description:
---
license: apache-2.0
tags:
- mistral
datasets:
- Open-Orca/SlimOrca
model-index:
- name: OpenHermes-2.5-neural-chat-7b-v3-2-7B
results:
- task:
type: text-generation
name: Text Generation
dataset:
name: AI2 Reasoning Challenge (25-Shot)
type: ai2_arc
config: ARC-Challenge
split: test
args:
num_few_shot: 25
metrics:
- type: acc_norm
value: 66.38
name: normalized accuracy
source:
url: https://huggingface.co/spaces/HuggingFaceH4/open_llm_leaderboard?query=Weyaxi/OpenHermes-2.5-neural-chat-7b-v3-2-7B
name: Open LLM Leaderboard
- task:
type: text-generation
name: Text Generation
dataset:
name: HellaSwag (10-Shot)
type: hellaswag
split: validation
args:
num_few_shot: 10
metrics:
- type: acc_norm
value: 84.11
name: normalized accuracy
source:
url: https://huggingface.co/spaces/HuggingFaceH4/open_llm_leaderboard?query=Weyaxi/OpenHermes-2.5-neural-chat-7b-v3-2-7B
name: Open LLM Leaderboard
- task:
type: text-generation
name: Text Generation
dataset:
name: MMLU (5-Shot)
type: cais/mmlu
config: all
split: test
args:
num_few_shot: 5
metrics:
- type: acc
value: 62.84
name: accuracy
source:
url: https://huggingface.co/spaces/HuggingFaceH4/open_llm_leaderboard?query=Weyaxi/OpenHermes-2.5-neural-chat-7b-v3-2-7B
name: Open LLM Leaderboard
- task:
type: text-generation
name: Text Generation
dataset:
name: TruthfulQA (0-shot)
type: truthful_qa
config: multiple_choice
split: validation
args:
num_few_shot: 0
metrics:
- type: mc2
value: 63.59
source:
url: https://huggingface.co/spaces/HuggingFaceH4/open_llm_leaderboard?query=Weyaxi/OpenHermes-2.5-neural-chat-7b-v3-2-7B
name: Open LLM Leaderboard
- task:
type: text-generation
name: Text Generation
dataset:
name: Winogrande (5-shot)
type: winogrande
config: winogrande_xl
split: validation
args:
num_few_shot: 5
metrics:
- type: acc
value: 78.53
name: accuracy
source:
url: https://huggingface.co/spaces/HuggingFaceH4/open_llm_leaderboard?query=Weyaxi/OpenHermes-2.5-neural-chat-7b-v3-2-7B
name: Open LLM Leaderboard
- task:
type: text-generation
name: Text Generation
dataset:
name: GSM8k (5-shot)
type: gsm8k
config: main
split: test
args:
num_few_shot: 5
metrics:
- type: acc
value: 56.79
name: accuracy
source:
url: https://huggingface.co/spaces/HuggingFaceH4/open_llm_leaderboard?query=Weyaxi/OpenHermes-2.5-neural-chat-7b-v3-2-7B
name: Open LLM Leaderboard
---

Merge of [teknium/OpenHermes-2.5-Mistral-7B](https://huggingface.co/teknium/OpenHermes-2.5-Mistral-7B) and [Intel/neural-chat-7b-v3-2](https://huggingface.co/Intel/neural-chat-7b-v3-2) using ties merge.
_Note: [Intel/neural-chat-7b-v3-1](https://huggingface.co/Intel/neural-chat-7b-v3-1) merge version is available [here](https://huggingface.co/Weyaxi/OpenHermes-2.5-neural-chat-7b-v3-1-7B/)_
### *Weights*
- [teknium/OpenHermes-2.5-Mistral-7B](https://huggingface.co/teknium/OpenHermes-2.5-Mistral-7B): 0.5
- [Intel/neural-chat-7b-v3-2](https://huggingface.co/Intel/neural-chat-7b-v3-2): 0.3
### *Density*
- [teknium/OpenHermes-2.5-Mistral-7B](https://huggingface.co/teknium/OpenHermes-2.5-Mistral-7B): 0.5
- [Intel/neural-chat-7b-v3-2](https://huggingface.co/Intel/neural-chat-7b-v3-2): 0.5
# Prompt Templates
You can use these prompt templates, but I recommend using ChatML.
### ChatML [(OpenHermes-2.5-Mistral-7B)](https://huggingface.co/teknium/OpenHermes-2.5-Mistral-7B):
```
<|im_start|>system
{system}<|im_end|>
<|im_start|>user
{user}<|im_end|>
<|im_start|>assistant
{asistant}<|im_end|>
```
### [neural-chat-7b-v3-2](https://huggingface.co/Intel/neural-chat-7b-v3-2):
```
### System:
{system}
### User:
{user}
### Assistant:
```
# Quantizationed versions
Quantizationed versions of this model is available thanks to [TheBloke](https://hf.co/TheBloke).
##### GPTQ
- [TheBloke/OpenHermes-2.5-neural-chat-7B-v3-2-7B-GPTQ](https://huggingface.co/TheBloke/OpenHermes-2.5-neural-chat-7B-v3-2-7B-GPTQ)
##### GGUF
- [TheBloke/OpenHermes-2.5-neural-chat-7B-v3-2-7B-GGUF](https://huggingface.co/TheBloke/OpenHermes-2.5-neural-chat-7B-v3-2-7B-GGUF)
##### AWQ
- [TheBloke/OpenHermes-2.5-neural-chat-7B-v3-2-7B-AWQ](https://huggingface.co/TheBloke/OpenHermes-2.5-neural-chat-7B-v3-2-7B-AWQ)
-
# [Open LLM Leaderboard Evaluation Results](https://huggingface.co/spaces/HuggingFaceH4/open_llm_leaderboard)
Detailed results can be found [here](https://huggingface.co/datasets/open-llm-leaderboard/details_Weyaxi__OpenHermes-2.5-neural-chat-7b-v3-2-7B)
| Metric |Value|
|---------------------------------|----:|
|Avg. |68.71|
|AI2 Reasoning Challenge (25-Shot)|66.38|
|HellaSwag (10-Shot) |84.11|
|MMLU (5-Shot) |62.84|
|TruthfulQA (0-shot) |63.59|
|Winogrande (5-shot) |78.53|
|GSM8k (5-shot) |56.79|
If you would like to support me:
[☕ Buy Me a Coffee](https://www.buymeacoffee.com/weyaxi)
|
tiiuae/falcon-40b | tiiuae | 2023-09-29T14:32:25Z | 20,210 | 2,413 | transformers | [
"transformers",
"pytorch",
"falcon",
"text-generation",
"custom_code",
"en",
"de",
"es",
"fr",
"dataset:tiiuae/falcon-refinedweb",
"arxiv:2205.14135",
"arxiv:1911.02150",
"arxiv:2101.00027",
"arxiv:2005.14165",
"arxiv:2104.09864",
"arxiv:2306.01116",
"license:apache-2.0",
"autotrain_compatible",
"text-generation-inference",
"region:us"
] | text-generation | 2023-05-24T12:08:30Z | ---
datasets:
- tiiuae/falcon-refinedweb
language:
- en
- de
- es
- fr
inference: false
license: apache-2.0
---
# 🚀 Falcon-40B
**Falcon-40B is a 40B parameters causal decoder-only model built by [TII](https://www.tii.ae) and trained on 1,000B tokens of [RefinedWeb](https://huggingface.co/datasets/tiiuae/falcon-refinedweb) enhanced with curated corpora. It is made available under the Apache 2.0 license.**
*Paper coming soon 😊.*
🤗 To get started with Falcon (inference, finetuning, quantization, etc.), we recommend reading [this great blogpost fron HF](https://huggingface.co/blog/falcon)!
## Why use Falcon-40B?
* **It is the best open-source model currently available.** Falcon-40B outperforms [LLaMA](https://github.com/facebookresearch/llama), [StableLM](https://github.com/Stability-AI/StableLM), [RedPajama](https://huggingface.co/togethercomputer/RedPajama-INCITE-Base-7B-v0.1), [MPT](https://huggingface.co/mosaicml/mpt-7b), etc. See the [OpenLLM Leaderboard](https://huggingface.co/spaces/HuggingFaceH4/open_llm_leaderboard).
* **It features an architecture optimized for inference**, with FlashAttention ([Dao et al., 2022](https://arxiv.org/abs/2205.14135)) and multiquery ([Shazeer et al., 2019](https://arxiv.org/abs/1911.02150)).
* **It is made available under a permissive Apache 2.0 license allowing for commercial use**, without any royalties or restrictions.
*
⚠️ **This is a raw, pretrained model, which should be further finetuned for most usecases.** If you are looking for a version better suited to taking generic instructions in a chat format, we recommend taking a look at [Falcon-40B-Instruct](https://huggingface.co/tiiuae/falcon-40b-instruct).
💸 **Looking for a smaller, less expensive model?** [Falcon-7B](https://huggingface.co/tiiuae/falcon-7b) is Falcon-40B's little brother!
```python
from transformers import AutoTokenizer, AutoModelForCausalLM
import transformers
import torch
model = "tiiuae/falcon-40b"
tokenizer = AutoTokenizer.from_pretrained(model)
pipeline = transformers.pipeline(
"text-generation",
model=model,
tokenizer=tokenizer,
torch_dtype=torch.bfloat16,
trust_remote_code=True,
device_map="auto",
)
sequences = pipeline(
"Girafatron is obsessed with giraffes, the most glorious animal on the face of this Earth. Giraftron believes all other animals are irrelevant when compared to the glorious majesty of the giraffe.\nDaniel: Hello, Girafatron!\nGirafatron:",
max_length=200,
do_sample=True,
top_k=10,
num_return_sequences=1,
eos_token_id=tokenizer.eos_token_id,
)
for seq in sequences:
print(f"Result: {seq['generated_text']}")
```
💥 **Falcon LLMs require PyTorch 2.0 for use with `transformers`!**
For fast inference with Falcon, check-out [Text Generation Inference](https://github.com/huggingface/text-generation-inference)! Read more in this [blogpost]((https://huggingface.co/blog/falcon).
You will need **at least 85-100GB of memory** to swiftly run inference with Falcon-40B.
# Model Card for Falcon-40B
## Model Details
### Model Description
- **Developed by:** [https://www.tii.ae](https://www.tii.ae);
- **Model type:** Causal decoder-only;
- **Language(s) (NLP):** English, German, Spanish, French (and limited capabilities in Italian, Portuguese, Polish, Dutch, Romanian, Czech, Swedish);
- **License:** Apache 2.0 license.
### Model Source
- **Paper:** *coming soon*.
## Uses
### Direct Use
Research on large language models; as a foundation for further specialization and finetuning for specific usecases (e.g., summarization, text generation, chatbot, etc.)
### Out-of-Scope Use
Production use without adequate assessment of risks and mitigation; any use cases which may be considered irresponsible or harmful.
## Bias, Risks, and Limitations
Falcon-40B is trained mostly on English, German, Spanish, French, with limited capabilities also in in Italian, Portuguese, Polish, Dutch, Romanian, Czech, Swedish. It will not generalize appropriately to other languages. Furthermore, as it is trained on a large-scale corpora representative of the web, it will carry the stereotypes and biases commonly encountered online.
### Recommendations
We recommend users of Falcon-40B to consider finetuning it for the specific set of tasks of interest, and for guardrails and appropriate precautions to be taken for any production use.
## How to Get Started with the Model
```python
from transformers import AutoTokenizer, AutoModelForCausalLM
import transformers
import torch
model = "tiiuae/falcon-40b"
tokenizer = AutoTokenizer.from_pretrained(model)
pipeline = transformers.pipeline(
"text-generation",
model=model,
tokenizer=tokenizer,
torch_dtype=torch.bfloat16,
trust_remote_code=True,
device_map="auto",
)
sequences = pipeline(
"Girafatron is obsessed with giraffes, the most glorious animal on the face of this Earth. Giraftron believes all other animals are irrelevant when compared to the glorious majesty of the giraffe.\nDaniel: Hello, Girafatron!\nGirafatron:",
max_length=200,
do_sample=True,
top_k=10,
num_return_sequences=1,
eos_token_id=tokenizer.eos_token_id,
)
for seq in sequences:
print(f"Result: {seq['generated_text']}")
```
## Training Details
### Training Data
Falcon-40B was trained on 1,000B tokens of [RefinedWeb](https://huggingface.co/datasets/tiiuae/falcon-refinedweb), a high-quality filtered and deduplicated web dataset which we enhanced with curated corpora. Significant components from our curated copora were inspired by The Pile ([Gao et al., 2020](https://arxiv.org/abs/2101.00027)).
| **Data source** | **Fraction** | **Tokens** | **Sources** |
|--------------------|--------------|------------|-----------------------------------|
| [RefinedWeb-English](https://huggingface.co/datasets/tiiuae/falcon-refinedweb) | 75% | 750B | massive web crawl |
| RefinedWeb-Europe | 7% | 70B | European massive web crawl |
| Books | 6% | 60B | |
| Conversations | 5% | 50B | Reddit, StackOverflow, HackerNews |
| Code | 5% | 50B | |
| Technical | 2% | 20B | arXiv, PubMed, USPTO, etc. |
RefinedWeb-Europe is made of the following languages:
| **Language** | **Fraction of multilingual data** | **Tokens** |
|--------------|-----------------------------------|------------|
| German | 26% | 18B |
| Spanish | 24% | 17B |
| French | 23% | 16B |
| _Italian_ | 7% | 5B |
| _Portuguese_ | 4% | 3B |
| _Polish_ | 4% | 3B |
| _Dutch_ | 4% | 3B |
| _Romanian_ | 3% | 2B |
| _Czech_ | 3% | 2B |
| _Swedish_ | 2% | 1B |
The data was tokenized with the Falcon-[7B](https://huggingface.co/tiiuae/falcon-7b)/[40B](https://huggingface.co/tiiuae/falcon-40b) tokenizer.
### Training Procedure
Falcon-40B was trained on 384 A100 40GB GPUs, using a 3D parallelism strategy (TP=8, PP=4, DP=12) combined with ZeRO.
#### Training Hyperparameters
| **Hyperparameter** | **Value** | **Comment** |
|--------------------|------------|-------------------------------------------|
| Precision | `bfloat16` | |
| Optimizer | AdamW | |
| Learning rate | 1.85e-4 | 4B tokens warm-up, cosine decay to 1.85e-5 |
| Weight decay | 1e-1 | |
| Z-loss | 1e-4 | |
| Batch size | 1152 | 100B tokens ramp-up |
#### Speeds, Sizes, Times
Training started in December 2022 and took two months.
## Evaluation
*Paper coming soon.*
See the [OpenLLM Leaderboard](https://huggingface.co/spaces/HuggingFaceH4/open_llm_leaderboard) for early results.
## Technical Specifications
### Model Architecture and Objective
Falcon-40B is a causal decoder-only model trained on a causal language modeling task (i.e., predict the next token).
The architecture is broadly adapted from the GPT-3 paper ([Brown et al., 2020](https://arxiv.org/abs/2005.14165)), with the following differences:
* **Positionnal embeddings:** rotary ([Su et al., 2021](https://arxiv.org/abs/2104.09864));
* **Attention:** multiquery ([Shazeer et al., 2019](https://arxiv.org/abs/1911.02150)) and FlashAttention ([Dao et al., 2022](https://arxiv.org/abs/2205.14135));
* **Decoder-block:** parallel attention/MLP with a two layer norms.
For multiquery, we are using an internal variant which uses independent key and values per tensor parallel degree.
| **Hyperparameter** | **Value** | **Comment** |
|--------------------|-----------|----------------------------------------|
| Layers | 60 | |
| `d_model` | 8192 | |
| `head_dim` | 64 | Reduced to optimise for FlashAttention |
| Vocabulary | 65024 | |
| Sequence length | 2048 | |
### Compute Infrastructure
#### Hardware
Falcon-40B was trained on AWS SageMaker, on 384 A100 40GB GPUs in P4d instances.
#### Software
Falcon-40B was trained a custom distributed training codebase, Gigatron. It uses a 3D parallelism approach combined with ZeRO and high-performance Triton kernels (FlashAttention, etc.)
## Citation
*Paper coming soon* 😊. In the meanwhile, you can use the following information to cite:
```
@article{falcon40b,
title={{Falcon-40B}: an open large language model with state-of-the-art performance},
author={Almazrouei, Ebtesam and Alobeidli, Hamza and Alshamsi, Abdulaziz and Cappelli, Alessandro and Cojocaru, Ruxandra and Debbah, Merouane and Goffinet, Etienne and Heslow, Daniel and Launay, Julien and Malartic, Quentin and Noune, Badreddine and Pannier, Baptiste and Penedo, Guilherme},
year={2023}
}
```
To learn more about the pretraining dataset, see the 📓 [RefinedWeb paper](https://arxiv.org/abs/2306.01116).
```
@article{refinedweb,
title={The {R}efined{W}eb dataset for {F}alcon {LLM}: outperforming curated corpora with web data, and web data only},
author={Guilherme Penedo and Quentin Malartic and Daniel Hesslow and Ruxandra Cojocaru and Alessandro Cappelli and Hamza Alobeidli and Baptiste Pannier and Ebtesam Almazrouei and Julien Launay},
journal={arXiv preprint arXiv:2306.01116},
eprint={2306.01116},
eprinttype = {arXiv},
url={https://arxiv.org/abs/2306.01116},
year={2023}
}
```
## License
Falcon-40B is made available under the Apache 2.0 license.
## Contact
[email protected] |
google/t5-v1_1-large | google | 2023-01-24T16:52:33Z | 20,209 | 14 | transformers | [
"transformers",
"pytorch",
"tf",
"jax",
"t5",
"text2text-generation",
"en",
"dataset:c4",
"arxiv:2002.05202",
"arxiv:1910.10683",
"license:apache-2.0",
"autotrain_compatible",
"endpoints_compatible",
"text-generation-inference",
"region:us"
] | text2text-generation | 2022-03-02T23:29:05Z | ---
language: en
datasets:
- c4
license: apache-2.0
---
[Google's T5](https://ai.googleblog.com/2020/02/exploring-transfer-learning-with-t5.html) Version 1.1
## Version 1.1
[T5 Version 1.1](https://github.com/google-research/text-to-text-transfer-transformer/blob/master/released_checkpoints.md#t511) includes the following improvements compared to the original T5 model- GEGLU activation in feed-forward hidden layer, rather than ReLU - see [here](https://arxiv.org/abs/2002.05202).
- Dropout was turned off in pre-training (quality win). Dropout should be re-enabled during fine-tuning.
- Pre-trained on C4 only without mixing in the downstream tasks.
- no parameter sharing between embedding and classifier layer
- "xl" and "xxl" replace "3B" and "11B". The model shapes are a bit different - larger `d_model` and smaller `num_heads` and `d_ff`.
**Note**: T5 Version 1.1 was only pre-trained on C4 excluding any supervised training. Therefore, this model has to be fine-tuned before it is useable on a downstream task.
Pretraining Dataset: [C4](https://huggingface.co/datasets/c4)
Other Community Checkpoints: [here](https://huggingface.co/models?search=t5-v1_1)
Paper: [Exploring the Limits of Transfer Learning with a Unified Text-to-Text Transformer](https://arxiv.org/pdf/1910.10683.pdf)
Authors: *Colin Raffel, Noam Shazeer, Adam Roberts, Katherine Lee, Sharan Narang, Michael Matena, Yanqi Zhou, Wei Li, Peter J. Liu*
## Abstract
Transfer learning, where a model is first pre-trained on a data-rich task before being fine-tuned on a downstream task, has emerged as a powerful technique in natural language processing (NLP). The effectiveness of transfer learning has given rise to a diversity of approaches, methodology, and practice. In this paper, we explore the landscape of transfer learning techniques for NLP by introducing a unified framework that converts every language problem into a text-to-text format. Our systematic study compares pre-training objectives, architectures, unlabeled datasets, transfer approaches, and other factors on dozens of language understanding tasks. By combining the insights from our exploration with scale and our new “Colossal Clean Crawled Corpus”, we achieve state-of-the-art results on many benchmarks covering summarization, question answering, text classification, and more. To facilitate future work on transfer learning for NLP, we release our dataset, pre-trained models, and code.
 |
wonrax/phobert-base-vietnamese-sentiment | wonrax | 2022-05-04T07:30:54Z | 20,201 | 10 | transformers | [
"transformers",
"pytorch",
"roberta",
"text-classification",
"sentiment",
"classification",
"vi",
"license:mit",
"autotrain_compatible",
"endpoints_compatible",
"region:us"
] | text-classification | 2022-05-03T14:03:13Z | ---
language:
- vi
tags:
- sentiment
- classification
license: mit
widget:
- text: "Không thể nào đẹp hơn"
- text: "Quá phí tiền, mà không đẹp"
- text: "Cái này giá ổn không nhỉ?"
---
[**GitHub Homepage**](https://github.com/wonrax/phobert-base-vietnamese-sentiment)
A model fine-tuned for sentiment analysis based on [vinai/phobert-base](https://huggingface.co/vinai/phobert-base).
Labels:
- NEG: Negative
- POS: Positive
- NEU: Neutral
Dataset: [30K e-commerce reviews](https://www.kaggle.com/datasets/linhlpv/vietnamese-sentiment-analyst)
## Usage
```python
import torch
from transformers import RobertaForSequenceClassification, AutoTokenizer
model = RobertaForSequenceClassification.from_pretrained("wonrax/phobert-base-vietnamese-sentiment")
tokenizer = AutoTokenizer.from_pretrained("wonrax/phobert-base-vietnamese-sentiment", use_fast=False)
# Just like PhoBERT: INPUT TEXT MUST BE ALREADY WORD-SEGMENTED!
sentence = 'Đây là mô_hình rất hay , phù_hợp với điều_kiện và như cầu của nhiều người .'
input_ids = torch.tensor([tokenizer.encode(sentence)])
with torch.no_grad():
out = model(input_ids)
print(out.logits.softmax(dim=-1).tolist())
# Output:
# [[0.002, 0.988, 0.01]]
# ^ ^ ^
# NEG POS NEU
```
|
kwoncho/gaincut_news_pre2023 | kwoncho | 2024-06-15T05:07:37Z | 20,190 | 0 | transformers | [
"transformers",
"pytorch",
"roberta",
"text-classification",
"autotrain_compatible",
"endpoints_compatible",
"region:us"
] | text-classification | 2024-04-25T04:49:43Z | Entry not found |
cardiffnlp/twitter-xlm-roberta-base | cardiffnlp | 2023-08-31T01:52:58Z | 20,172 | 13 | transformers | [
"transformers",
"pytorch",
"tf",
"xlm-roberta",
"fill-mask",
"multilingual",
"arxiv:2104.12250",
"autotrain_compatible",
"endpoints_compatible",
"region:us"
] | fill-mask | 2022-03-02T23:29:05Z | ---
language: multilingual
widget:
- text: "🤗🤗🤗<mask>"
- text: "🔥The goal of life is <mask> . 🔥"
- text: "Il segreto della vita è l’<mask> . ❤️"
- text: "Hasta <mask> 👋!"
---
# Twitter-XLM-Roberta-base
This is a XLM-Roberta-base model trained on ~198M multilingual tweets, described and evaluated in the [reference paper](https://arxiv.org/abs/2104.12250). To evaluate this and other LMs on Twitter-specific data, please refer to the [main repository](https://github.com/cardiffnlp/xlm-t). A usage example is provided below.
## Computing tweet similarity
```python
def preprocess(text):
new_text = []
for t in text.split(" "):
t = '@user' if t.startswith('@') and len(t) > 1 else t
t = 'http' if t.startswith('http') else t
new_text.append(t)
return " ".join(new_text)
def get_embedding(text):
text = preprocess(text)
encoded_input = tokenizer(text, return_tensors='pt')
features = model(**encoded_input)
features = features[0].detach().numpy()
features_mean = np.mean(features[0], axis=0)
return features_mean
query = "Acabo de pedir pollo frito 🐣" #spanish
tweets = ["We had a great time! ⚽️", # english
"We hebben een geweldige tijd gehad! ⛩", # dutch
"Nous avons passé un bon moment! 🎥", # french
"Ci siamo divertiti! 🍝"] # italian
d = defaultdict(int)
for tweet in tweets:
sim = 1-cosine(get_embedding(query),get_embedding(tweet))
d[tweet] = sim
print('Most similar to: ',query)
print('----------------------------------------')
for idx,x in enumerate(sorted(d.items(), key=lambda x:x[1], reverse=True)):
print(idx+1,x[0])
```
```
Most similar to: Acabo de pedir pollo frito 🐣
----------------------------------------
1 Ci siamo divertiti! 🍝
2 Nous avons passé un bon moment! 🎥
3 We had a great time! ⚽️
4 We hebben een geweldige tijd gehad! ⛩
```
### BibTeX entry and citation info
Please cite the [reference paper](https://aclanthology.org/2022.lrec-1.27/) if you use this model.
```bibtex
@inproceedings{barbieri-etal-2022-xlm,
title = "{XLM}-{T}: Multilingual Language Models in {T}witter for Sentiment Analysis and Beyond",
author = "Barbieri, Francesco and
Espinosa Anke, Luis and
Camacho-Collados, Jose",
booktitle = "Proceedings of the Thirteenth Language Resources and Evaluation Conference",
month = jun,
year = "2022",
address = "Marseille, France",
publisher = "European Language Resources Association",
url = "https://aclanthology.org/2022.lrec-1.27",
pages = "258--266",
abstract = "Language models are ubiquitous in current NLP, and their multilingual capacity has recently attracted considerable attention. However, current analyses have almost exclusively focused on (multilingual variants of) standard benchmarks, and have relied on clean pre-training and task-specific corpora as multilingual signals. In this paper, we introduce XLM-T, a model to train and evaluate multilingual language models in Twitter. In this paper we provide: (1) a new strong multilingual baseline consisting of an XLM-R (Conneau et al. 2020) model pre-trained on millions of tweets in over thirty languages, alongside starter code to subsequently fine-tune on a target task; and (2) a set of unified sentiment analysis Twitter datasets in eight different languages and a XLM-T model trained on this dataset.",
}
|
Qwen/Qwen2-72B | Qwen | 2024-06-06T14:39:44Z | 20,137 | 137 | transformers | [
"transformers",
"safetensors",
"qwen2",
"text-generation",
"pretrained",
"conversational",
"en",
"license:other",
"autotrain_compatible",
"endpoints_compatible",
"text-generation-inference",
"region:us"
] | text-generation | 2024-05-22T15:28:10Z | ---
license: other
license_name: tongyi-qianwen
license_link: https://huggingface.co/Qwen/Qwen2-72B/blob/main/LICENSE
language:
- en
pipeline_tag: text-generation
tags:
- pretrained
---
# Qwen2-72B
## Introduction
Qwen2 is the new series of Qwen large language models. For Qwen2, we release a number of base language models and instruction-tuned language models ranging from 0.5 to 72 billion parameters, including a Mixture-of-Experts model. This repo contains the 72B Qwen2 base language model.
Compared with the state-of-the-art opensource language models, including the previous released Qwen1.5, Qwen2 has generally surpassed most opensource models and demonstrated competitiveness against proprietary models across a series of benchmarks targeting for language understanding, language generation, multilingual capability, coding, mathematics, reasoning, etc.
For more details, please refer to our [blog](https://qwenlm.github.io/blog/qwen2/), [GitHub](https://github.com/QwenLM/Qwen2), and [Documentation](https://qwen.readthedocs.io/en/latest/).
<br>
## Model Details
Qwen2 is a language model series including decoder language models of different model sizes. For each size, we release the base language model and the aligned chat model. It is based on the Transformer architecture with SwiGLU activation, attention QKV bias, group query attention, etc. Additionally, we have an improved tokenizer adaptive to multiple natural languages and codes.
## Requirements
The code of Qwen2 has been in the latest Hugging face transformers and we advise you to install `transformers>=4.37.0`, or you might encounter the following error:
```
KeyError: 'qwen2'
```
## Usage
We do not advise you to use base language models for text generation. Instead, you can apply post-training, e.g., SFT, RLHF, continued pretraining, etc., on this model.
## Performance
The evaluation of base models mainly focuses on the model performance of natural language understanding, general question answering, coding, mathematics, scientific knowledge, reasoning, multilingual capability, etc.
The datasets for evaluation include:
**English Tasks**: MMLU (5-shot), MMLU-Pro (5-shot), GPQA (5shot), Theorem QA (5-shot), BBH (3-shot), HellaSwag (10-shot), Winogrande (5-shot), TruthfulQA (0-shot), ARC-C (25-shot)
**Coding Tasks**: EvalPlus (0-shot) (HumanEval, MBPP, HumanEval+, MBPP+), MultiPL-E (0-shot) (Python, C++, JAVA, PHP, TypeScript, C#, Bash, JavaScript)
**Math Tasks**: GSM8K (4-shot), MATH (4-shot)
**Chinese Tasks**: C-Eval (5-shot), CMMLU (5-shot)
**Multilingual Tasks**: Multi-Exam (M3Exam 5-shot, IndoMMLU 3-shot, ruMMLU 5-shot, mMMLU 5-shot), Multi-Understanding (BELEBELE 5-shot, XCOPA 5-shot, XWinograd 5-shot, XStoryCloze 0-shot, PAWS-X 5-shot), Multi-Mathematics (MGSM 8-shot), Multi-Translation (Flores-101 5-shot)
#### Qwen2-72B performance
| Datasets | DeepSeek-V2 | Mixtral-8x22B | Llama-3-70B | Qwen1.5-72B | Qwen1.5-110B | **Qwen2-72B** |
| :--------| :---------: | :------------: | :------------: | :------------: | :------------: |:------------: |
|Architecture | MoE | MoE | Dense | Dense | Dense | Dense |
|#Activated Params | 21B | 39B | 70B | 72B | 110B | 72B |
|#Params | 236B | 140B | 70B | 72B | 110B | 72B|
| ***English*** | | | | | | |
|MMLU |78.5 | 77.8 | 79.5 | 77.5 | 80.4 | **84.2** |
|MMLU-Pro | - | 49.5 | 52.8 | 45.8 | 49.4 | **55.6** |
|GPQA | -| 34.3 | 36.3 | 36.3 | 35.9 | **37.9** |
|Theorem QA | -| 35.9 | 32.3 | 29.3 | 34.9 | **43.1** |
|BBH | 78.9 |78.9 | 81.0 | 65.5 | 74.8 | **82.4** |
|HellaSwag | 87.8 | **88.7** | 88.0 | 86.0 | 87.5 | 87.6 |
|WindoGrande | 84.8|85.0 | **85.3** | 83.0 | 83.5 | 85.1 |
|ARC-C | 70.0| **70.7** | 68.8 | 65.9 | 69.6 | 68.9 |
|TruthfulQA | 42.2 | 51.0 | 45.6 | **59.6** | 49.6 | 54.8 |
| ***Coding*** | | | | | | |
|HumanEval | 45.7 | 46.3 | 48.2 | 46.3 | 54.3 | **64.6** |
|MBPP |73.9 | 71.7 | 70.4 | 66.9 | 70.9 | **76.9** |
|EvalPlus | 55.0 | 54.1 | 54.8 | 52.9 | 57.7 | **65.4** |
|MultiPL-E |44.4 | 46.7 | 46.3 | 41.8 | 52.7 | **59.6** |
| ***Mathematics*** | | | | | | |
|GSM8K | 79.2 | 83.7 | 83.0 | 79.5 | 85.4 | **89.5** |
|MATH | 43.6 | 41.7 | 42.5 | 34.1 | 49.6 | **51.1** |
| ***Chinese*** | | | | | | |
|C-Eval | 81.7 | 54.6 | 65.2 | 84.1 | 89.1 | **91.0** |
|CMMLU | 84.0 | 53.4 | 67.2 | 83.5 | 88.3 | **90.1** |
| ***Multilingual*** | | | | | | |
|Mulit-Exam | 67.5 | 63.5 | 70.0 | 66.4 | 75.6 | **76.6** |
|Multi-Understanding | 77.0 | 77.7 | 79.9 | 78.2 | 78.2 | **80.7** |
|Multi-Mathematics | 58.8 | 62.9 | 67.1 | 61.7 | 64.4 | **76.0** |
|Multi-Translation | 36.0 | 23.3 | **38.0** | 35.6 | 36.2 | 37.8 |
## Citation
If you find our work helpful, feel free to give us a cite.
```
@article{qwen2,
title={Qwen2 Technical Report},
year={2024}
}
```
|
GraydientPlatformAPI/autism-pony | GraydientPlatformAPI | 2024-02-14T16:59:06Z | 20,129 | 7 | diffusers | [
"diffusers",
"safetensors",
"license:openrail",
"autotrain_compatible",
"endpoints_compatible",
"diffusers:StableDiffusionXLPipeline",
"region:us"
] | text-to-image | 2024-02-14T16:25:23Z | ---
license: openrail
---
|
textattack/distilbert-base-uncased-ag-news | textattack | 2020-07-07T22:01:14Z | 20,118 | 1 | transformers | [
"transformers",
"pytorch",
"distilbert",
"text-classification",
"autotrain_compatible",
"endpoints_compatible",
"region:us"
] | text-classification | 2022-03-02T23:29:05Z | ## TextAttack Model CardThis `distilbert-base-uncased` model was fine-tuned for sequence classification using TextAttack
and the ag_news dataset loaded using the `nlp` library. The model was fine-tuned
for 5 epochs with a batch size of 32, a learning
rate of 2e-05, and a maximum sequence length of 128.
Since this was a classification task, the model was trained with a cross-entropy loss function.
The best score the model achieved on this task was 0.9478947368421052, as measured by the
eval set accuracy, found after 1 epoch.
For more information, check out [TextAttack on Github](https://github.com/QData/TextAttack).
|
qwp4w3hyb/Llama-3-Instruct-8B-SPPO-Iter3-iMat-GGUF | qwp4w3hyb | 2024-06-27T14:13:32Z | 20,118 | 0 | null | [
"gguf",
"region:us"
] | null | 2024-06-27T11:29:20Z | Entry not found |
llava-hf/llava-v1.6-34b-hf | llava-hf | 2024-06-28T12:19:34Z | 20,110 | 50 | transformers | [
"transformers",
"safetensors",
"llava_next",
"pretraining",
"vision",
"image-text-to-text",
"arxiv:2310.03744",
"endpoints_compatible",
"region:us"
] | image-text-to-text | 2024-03-17T18:47:15Z | ---
tags:
- vision
- image-text-to-text
---
# LLaVa-Next, leveraging [NousResearch/Nous-Hermes-2-Yi-34B](https://huggingface.co/NousResearch/Nous-Hermes-2-Yi-34B) as LLM
The LLaVA-NeXT model was proposed in [LLaVA-NeXT: Improved reasoning, OCR, and world knowledge](https://llava-vl.github.io/blog/2024-01-30-llava-next/) by Haotian Liu, Chunyuan Li, Yuheng Li, Bo Li, Yuanhan Zhang, Sheng Shen, Yong Jae Lee. LLaVa-NeXT (also called LLaVa-1.6) improves upon [LLaVa](https://huggingface.co/llava-hf/llava-1.5-7b-hf) by increasing the input image resolution and training on an improved visual instruction tuning dataset to improve OCR and common sense reasoning.
Disclaimer: The team releasing LLaVa-NeXT did not write a model card for this model so this model card has been written by the Hugging Face team.
## Model description
LLaVa combines a pre-trained large language model with a pre-trained vision encoder for multimodal chatbot use cases. LLaVA 1.6 improves on LLaVA 1.5 BY:
- Using [Mistral-7B](https://mistral.ai/news/announcing-mistral-7b/) and [Nous-Hermes-2-Yi-34B](https://huggingface.co/NousResearch/Nous-Hermes-2-Yi-34B) (for this checkpoint) which has better commercial licenses,
and bilingual support
- More diverse and high quality data mixture
- Dynamic high resolution

## Intended uses & limitations
You can use the raw model for tasks like image captioning, visual question answering, multimodal chatbot use cases. See the [model hub](https://huggingface.co/models?search=llava-hf) to look for
other versions on a task that interests you.
### How to use
Here's the prompt template for this model:
```
"<|im_start|>system\nAnswer the questions.<|im_end|><|im_start|>user\n<image>\n<your_text_prompt_here><|im_end|><|im_start|>assistant\n"
```
You can load and use the model like following:
```python
from transformers import LlavaNextProcessor, LlavaNextForConditionalGeneration
import torch
from PIL import Image
import requests
processor = LlavaNextProcessor.from_pretrained("llava-hf/llava-v1.6-34b-hf")
model = LlavaNextForConditionalGeneration.from_pretrained("llava-hf/llava-v1.6-34b-hf", torch_dtype=torch.float16, low_cpu_mem_usage=True)
model.to("cuda:0")
# prepare image and text prompt, using the appropriate prompt template
url = "https://github.com/haotian-liu/LLaVA/blob/1a91fc274d7c35a9b50b3cb29c4247ae5837ce39/images/llava_v1_5_radar.jpg?raw=true"
image = Image.open(requests.get(url, stream=True).raw)
prompt = "<|im_start|>system\nAnswer the questions.<|im_end|><|im_start|>user\n<image>\nWhat is shown in this image?<|im_end|><|im_start|>assistant\n"
inputs = processor(prompt, image, return_tensors="pt").to("cuda:0")
# autoregressively complete prompt
output = model.generate(**inputs, max_new_tokens=100)
print(processor.decode(output[0], skip_special_tokens=True))
```
### Model optimization
#### 4-bit quantization through `bitsandbytes` library
First make sure to install `bitsandbytes`, `pip install bitsandbytes` and make sure to have access to a CUDA compatible GPU device. Simply change the snippet above with:
```diff
model = LlavaNextForConditionalGeneration.from_pretrained(
model_id,
torch_dtype=torch.float16,
low_cpu_mem_usage=True,
+ load_in_4bit=True
)
```
#### Use Flash-Attention 2 to further speed-up generation
First make sure to install `flash-attn`. Refer to the [original repository of Flash Attention](https://github.com/Dao-AILab/flash-attention) regarding that package installation. Simply change the snippet above with:
```diff
model = LlavaNextForConditionalGeneration.from_pretrained(
model_id,
torch_dtype=torch.float16,
low_cpu_mem_usage=True,
+ use_flash_attention_2=True
).to(0)
```
### BibTeX entry and citation info
```bibtex
@misc{liu2023improved,
title={Improved Baselines with Visual Instruction Tuning},
author={Haotian Liu and Chunyuan Li and Yuheng Li and Yong Jae Lee},
year={2023},
eprint={2310.03744},
archivePrefix={arXiv},
primaryClass={cs.CV}
}
``` |
bigcode/tiny_starcoder_py | bigcode | 2023-06-01T15:14:56Z | 20,099 | 71 | transformers | [
"transformers",
"pytorch",
"safetensors",
"gpt_bigcode",
"text-generation",
"code",
"dataset:bigcode/the-stack-dedup",
"license:bigcode-openrail-m",
"model-index",
"autotrain_compatible",
"endpoints_compatible",
"text-generation-inference",
"region:us"
] | text-generation | 2023-05-15T07:43:22Z | ---
pipeline_tag: text-generation
inference: true
widget:
- text: 'def print_hello_world():'
example_title: Hello world
group: Python
license: bigcode-openrail-m
datasets:
- bigcode/the-stack-dedup
metrics:
- code_eval
library_name: transformers
tags:
- code
model-index:
- name: Tiny-StarCoder-Py
results:
- task:
type: text-generation
dataset:
type: openai_humaneval
name: HumanEval
metrics:
- name: pass@1
type: pass@1
value: 7.84%
verified: false
---
# TinyStarCoderPy
This is a 164M parameters model with the same architecture as [StarCoder](https://huggingface.co/bigcode/starcoder) (8k context length, MQA & FIM). It was trained on the Python data from [StarCoderData](https://huggingface.co/datasets/bigcode/starcoderdata)
for ~6 epochs which amounts to 100B tokens.
## Use
### Intended use
The model was trained on GitHub code, to assist with some tasks like [Assisted Generation](https://huggingface.co/blog/assisted-generation). For pure code completion, we advise using our 15B models [StarCoder]() or [StarCoderBase]().
### Generation
```python
# pip install -q transformers
from transformers import AutoModelForCausalLM, AutoTokenizer
checkpoint = "bigcode/tiny_starcoder_py"
device = "cuda" # for GPU usage or "cpu" for CPU usage
tokenizer = AutoTokenizer.from_pretrained(checkpoint)
model = AutoModelForCausalLM.from_pretrained(checkpoint).to(device)
inputs = tokenizer.encode("def print_hello_world():", return_tensors="pt").to(device)
outputs = model.generate(inputs)
print(tokenizer.decode(outputs[0]))
```
### Fill-in-the-middle
Fill-in-the-middle uses special tokens to identify the prefix/middle/suffix part of the input and output:
```python
input_text = "<fim_prefix>def print_one_two_three():\n print('one')\n <fim_suffix>\n print('three')<fim_middle>"
inputs = tokenizer.encode(input_text, return_tensors="pt").to(device)
outputs = model.generate(inputs)
print(tokenizer.decode(outputs[0]))
```
# Training
## Model
- **Architecture:** GPT-2 model with multi-query attention and Fill-in-the-Middle objective
- **Pretraining steps:** 50k
- **Pretraining tokens:** 100 billion
- **Precision:** bfloat16
## Hardware
- **GPUs:** 32 Tesla A100
- **Training time:** 18 hours
## Software
- **Orchestration:** [Megatron-LM](https://github.com/bigcode-project/Megatron-LM)
- **Neural networks:** [PyTorch](https://github.com/pytorch/pytorch)
- **BP16 if applicable:** [apex](https://github.com/NVIDIA/apex)
# License
The model is licensed under the BigCode OpenRAIL-M v1 license agreement. You can find the full agreement [here](https://huggingface.co/spaces/bigcode/bigcode-model-license-agreement).
|
mradermacher/Swallow-13b-instruct-hf-i1-GGUF | mradermacher | 2024-06-30T17:08:56Z | 20,093 | 0 | transformers | [
"transformers",
"gguf",
"en",
"ja",
"base_model:tokyotech-llm/Swallow-13b-instruct-hf",
"license:llama2",
"endpoints_compatible",
"region:us"
] | null | 2024-06-30T13:47:32Z | ---
base_model: tokyotech-llm/Swallow-13b-instruct-hf
language:
- en
- ja
library_name: transformers
license: llama2
model_type: llama
quantized_by: mradermacher
---
## About
<!-- ### quantize_version: 2 -->
<!-- ### output_tensor_quantised: 1 -->
<!-- ### convert_type: hf -->
<!-- ### vocab_type: -->
<!-- ### tags: nicoboss -->
weighted/imatrix quants of https://huggingface.co/tokyotech-llm/Swallow-13b-instruct-hf
<!-- provided-files -->
static quants are available at https://huggingface.co/mradermacher/Swallow-13b-instruct-hf-GGUF
## Usage
If you are unsure how to use GGUF files, refer to one of [TheBloke's
READMEs](https://huggingface.co/TheBloke/KafkaLM-70B-German-V0.1-GGUF) for
more details, including on how to concatenate multi-part files.
## Provided Quants
(sorted by size, not necessarily quality. IQ-quants are often preferable over similar sized non-IQ quants)
| Link | Type | Size/GB | Notes |
|:-----|:-----|--------:|:------|
| [GGUF](https://huggingface.co/mradermacher/Swallow-13b-instruct-hf-i1-GGUF/resolve/main/Swallow-13b-instruct-hf.i1-IQ1_S.gguf) | i1-IQ1_S | 3.1 | for the desperate |
| [GGUF](https://huggingface.co/mradermacher/Swallow-13b-instruct-hf-i1-GGUF/resolve/main/Swallow-13b-instruct-hf.i1-IQ1_M.gguf) | i1-IQ1_M | 3.3 | mostly desperate |
| [GGUF](https://huggingface.co/mradermacher/Swallow-13b-instruct-hf-i1-GGUF/resolve/main/Swallow-13b-instruct-hf.i1-IQ2_XXS.gguf) | i1-IQ2_XXS | 3.7 | |
| [GGUF](https://huggingface.co/mradermacher/Swallow-13b-instruct-hf-i1-GGUF/resolve/main/Swallow-13b-instruct-hf.i1-IQ2_XS.gguf) | i1-IQ2_XS | 4.0 | |
| [GGUF](https://huggingface.co/mradermacher/Swallow-13b-instruct-hf-i1-GGUF/resolve/main/Swallow-13b-instruct-hf.i1-IQ2_S.gguf) | i1-IQ2_S | 4.4 | |
| [GGUF](https://huggingface.co/mradermacher/Swallow-13b-instruct-hf-i1-GGUF/resolve/main/Swallow-13b-instruct-hf.i1-IQ2_M.gguf) | i1-IQ2_M | 4.7 | |
| [GGUF](https://huggingface.co/mradermacher/Swallow-13b-instruct-hf-i1-GGUF/resolve/main/Swallow-13b-instruct-hf.i1-Q2_K.gguf) | i1-Q2_K | 5.0 | IQ3_XXS probably better |
| [GGUF](https://huggingface.co/mradermacher/Swallow-13b-instruct-hf-i1-GGUF/resolve/main/Swallow-13b-instruct-hf.i1-IQ3_XXS.gguf) | i1-IQ3_XXS | 5.1 | lower quality |
| [GGUF](https://huggingface.co/mradermacher/Swallow-13b-instruct-hf-i1-GGUF/resolve/main/Swallow-13b-instruct-hf.i1-IQ3_XS.gguf) | i1-IQ3_XS | 5.5 | |
| [GGUF](https://huggingface.co/mradermacher/Swallow-13b-instruct-hf-i1-GGUF/resolve/main/Swallow-13b-instruct-hf.i1-IQ3_S.gguf) | i1-IQ3_S | 5.8 | beats Q3_K* |
| [GGUF](https://huggingface.co/mradermacher/Swallow-13b-instruct-hf-i1-GGUF/resolve/main/Swallow-13b-instruct-hf.i1-Q3_K_S.gguf) | i1-Q3_K_S | 5.8 | IQ3_XS probably better |
| [GGUF](https://huggingface.co/mradermacher/Swallow-13b-instruct-hf-i1-GGUF/resolve/main/Swallow-13b-instruct-hf.i1-IQ3_M.gguf) | i1-IQ3_M | 6.2 | |
| [GGUF](https://huggingface.co/mradermacher/Swallow-13b-instruct-hf-i1-GGUF/resolve/main/Swallow-13b-instruct-hf.i1-Q3_K_M.gguf) | i1-Q3_K_M | 6.5 | IQ3_S probably better |
| [GGUF](https://huggingface.co/mradermacher/Swallow-13b-instruct-hf-i1-GGUF/resolve/main/Swallow-13b-instruct-hf.i1-Q3_K_L.gguf) | i1-Q3_K_L | 7.1 | IQ3_M probably better |
| [GGUF](https://huggingface.co/mradermacher/Swallow-13b-instruct-hf-i1-GGUF/resolve/main/Swallow-13b-instruct-hf.i1-IQ4_XS.gguf) | i1-IQ4_XS | 7.1 | |
| [GGUF](https://huggingface.co/mradermacher/Swallow-13b-instruct-hf-i1-GGUF/resolve/main/Swallow-13b-instruct-hf.i1-Q4_0.gguf) | i1-Q4_0 | 7.6 | fast, low quality |
| [GGUF](https://huggingface.co/mradermacher/Swallow-13b-instruct-hf-i1-GGUF/resolve/main/Swallow-13b-instruct-hf.i1-Q4_K_S.gguf) | i1-Q4_K_S | 7.6 | optimal size/speed/quality |
| [GGUF](https://huggingface.co/mradermacher/Swallow-13b-instruct-hf-i1-GGUF/resolve/main/Swallow-13b-instruct-hf.i1-Q4_K_M.gguf) | i1-Q4_K_M | 8.0 | fast, recommended |
| [GGUF](https://huggingface.co/mradermacher/Swallow-13b-instruct-hf-i1-GGUF/resolve/main/Swallow-13b-instruct-hf.i1-Q5_K_S.gguf) | i1-Q5_K_S | 9.2 | |
| [GGUF](https://huggingface.co/mradermacher/Swallow-13b-instruct-hf-i1-GGUF/resolve/main/Swallow-13b-instruct-hf.i1-Q5_K_M.gguf) | i1-Q5_K_M | 9.4 | |
| [GGUF](https://huggingface.co/mradermacher/Swallow-13b-instruct-hf-i1-GGUF/resolve/main/Swallow-13b-instruct-hf.i1-Q6_K.gguf) | i1-Q6_K | 10.9 | practically like static Q6_K |
Here is a handy graph by ikawrakow comparing some lower-quality quant
types (lower is better):

And here are Artefact2's thoughts on the matter:
https://gist.github.com/Artefact2/b5f810600771265fc1e39442288e8ec9
## FAQ / Model Request
See https://huggingface.co/mradermacher/model_requests for some answers to
questions you might have and/or if you want some other model quantized.
## Thanks
I thank my company, [nethype GmbH](https://www.nethype.de/), for letting
me use its servers and providing upgrades to my workstation to enable
this work in my free time. Additional thanks to [@nicoboss](https://huggingface.co/nicoboss) for giving me access to his hardware for calculating the imatrix for these quants.
<!-- end -->
|
mradermacher/Intellplay-Llama-3-8B-GGUF | mradermacher | 2024-07-01T09:29:18Z | 20,046 | 0 | transformers | [
"transformers",
"gguf",
"merge",
"mergekit",
"lazymergekit",
"en",
"base_model:bunnycore/Intellplay-Llama-3-8B",
"license:apache-2.0",
"endpoints_compatible",
"region:us"
] | null | 2024-07-01T06:16:48Z | ---
base_model: bunnycore/Intellplay-Llama-3-8B
language:
- en
library_name: transformers
license: apache-2.0
quantized_by: mradermacher
tags:
- merge
- mergekit
- lazymergekit
---
## About
<!-- ### quantize_version: 2 -->
<!-- ### output_tensor_quantised: 1 -->
<!-- ### convert_type: hf -->
<!-- ### vocab_type: -->
<!-- ### tags: -->
static quants of https://huggingface.co/bunnycore/Intellplay-Llama-3-8B
<!-- provided-files -->
weighted/imatrix quants are available at https://huggingface.co/mradermacher/Intellplay-Llama-3-8B-i1-GGUF
## Usage
If you are unsure how to use GGUF files, refer to one of [TheBloke's
READMEs](https://huggingface.co/TheBloke/KafkaLM-70B-German-V0.1-GGUF) for
more details, including on how to concatenate multi-part files.
## Provided Quants
(sorted by size, not necessarily quality. IQ-quants are often preferable over similar sized non-IQ quants)
| Link | Type | Size/GB | Notes |
|:-----|:-----|--------:|:------|
| [GGUF](https://huggingface.co/mradermacher/Intellplay-Llama-3-8B-GGUF/resolve/main/Intellplay-Llama-3-8B.Q2_K.gguf) | Q2_K | 3.3 | |
| [GGUF](https://huggingface.co/mradermacher/Intellplay-Llama-3-8B-GGUF/resolve/main/Intellplay-Llama-3-8B.IQ3_XS.gguf) | IQ3_XS | 3.6 | |
| [GGUF](https://huggingface.co/mradermacher/Intellplay-Llama-3-8B-GGUF/resolve/main/Intellplay-Llama-3-8B.Q3_K_S.gguf) | Q3_K_S | 3.8 | |
| [GGUF](https://huggingface.co/mradermacher/Intellplay-Llama-3-8B-GGUF/resolve/main/Intellplay-Llama-3-8B.IQ3_S.gguf) | IQ3_S | 3.8 | beats Q3_K* |
| [GGUF](https://huggingface.co/mradermacher/Intellplay-Llama-3-8B-GGUF/resolve/main/Intellplay-Llama-3-8B.IQ3_M.gguf) | IQ3_M | 3.9 | |
| [GGUF](https://huggingface.co/mradermacher/Intellplay-Llama-3-8B-GGUF/resolve/main/Intellplay-Llama-3-8B.Q3_K_M.gguf) | Q3_K_M | 4.1 | lower quality |
| [GGUF](https://huggingface.co/mradermacher/Intellplay-Llama-3-8B-GGUF/resolve/main/Intellplay-Llama-3-8B.Q3_K_L.gguf) | Q3_K_L | 4.4 | |
| [GGUF](https://huggingface.co/mradermacher/Intellplay-Llama-3-8B-GGUF/resolve/main/Intellplay-Llama-3-8B.IQ4_XS.gguf) | IQ4_XS | 4.6 | |
| [GGUF](https://huggingface.co/mradermacher/Intellplay-Llama-3-8B-GGUF/resolve/main/Intellplay-Llama-3-8B.Q4_K_S.gguf) | Q4_K_S | 4.8 | fast, recommended |
| [GGUF](https://huggingface.co/mradermacher/Intellplay-Llama-3-8B-GGUF/resolve/main/Intellplay-Llama-3-8B.Q4_K_M.gguf) | Q4_K_M | 5.0 | fast, recommended |
| [GGUF](https://huggingface.co/mradermacher/Intellplay-Llama-3-8B-GGUF/resolve/main/Intellplay-Llama-3-8B.Q5_K_S.gguf) | Q5_K_S | 5.7 | |
| [GGUF](https://huggingface.co/mradermacher/Intellplay-Llama-3-8B-GGUF/resolve/main/Intellplay-Llama-3-8B.Q5_K_M.gguf) | Q5_K_M | 5.8 | |
| [GGUF](https://huggingface.co/mradermacher/Intellplay-Llama-3-8B-GGUF/resolve/main/Intellplay-Llama-3-8B.Q6_K.gguf) | Q6_K | 6.7 | very good quality |
| [GGUF](https://huggingface.co/mradermacher/Intellplay-Llama-3-8B-GGUF/resolve/main/Intellplay-Llama-3-8B.Q8_0.gguf) | Q8_0 | 8.6 | fast, best quality |
| [GGUF](https://huggingface.co/mradermacher/Intellplay-Llama-3-8B-GGUF/resolve/main/Intellplay-Llama-3-8B.f16.gguf) | f16 | 16.2 | 16 bpw, overkill |
Here is a handy graph by ikawrakow comparing some lower-quality quant
types (lower is better):

And here are Artefact2's thoughts on the matter:
https://gist.github.com/Artefact2/b5f810600771265fc1e39442288e8ec9
## FAQ / Model Request
See https://huggingface.co/mradermacher/model_requests for some answers to
questions you might have and/or if you want some other model quantized.
## Thanks
I thank my company, [nethype GmbH](https://www.nethype.de/), for letting
me use its servers and providing upgrades to my workstation to enable
this work in my free time.
<!-- end -->
|
kandinsky-community/kandinsky-2-2-decoder | kandinsky-community | 2023-10-09T11:32:52Z | 20,038 | 52 | diffusers | [
"diffusers",
"safetensors",
"text-to-image",
"kandinsky",
"license:apache-2.0",
"diffusers:KandinskyV22Pipeline",
"region:us"
] | text-to-image | 2023-06-09T11:17:35Z | ---
license: apache-2.0
prior:
- kandinsky-community/kandinsky-2-2-prior
tags:
- text-to-image
- kandinsky
inference: false
---
# Kandinsky 2.2
Kandinsky inherits best practices from Dall-E 2 and Latent diffusion while introducing some new ideas.
It uses the CLIP model as a text and image encoder, and diffusion image prior (mapping) between latent spaces of CLIP modalities. This approach increases the visual performance of the model and unveils new horizons in blending images and text-guided image manipulation.
The Kandinsky model is created by [Arseniy Shakhmatov](https://github.com/cene555), [Anton Razzhigaev](https://github.com/razzant), [Aleksandr Nikolich](https://github.com/AlexWortega), [Igor Pavlov](https://github.com/boomb0om), [Andrey Kuznetsov](https://github.com/kuznetsoffandrey) and [Denis Dimitrov](https://github.com/denndimitrov)
## Usage
Kandinsky 2.2 is available in diffusers!
```python
pip install diffusers transformers accelerate
```
### Text to image
```python
from diffusers import AutoPipelineForText2Image
import torch
pipe = AutoPipelineForText2Image.from_pretrained("kandinsky-community/kandinsky-2-2-decoder", torch_dtype=torch.float16)
pipe = pipe.to("cuda")
prompt = "portrait of a young women, blue eyes, cinematic"
negative_prompt = "low quality, bad quality"
image = pipe(prompt=prompt, negative_prompt=negative_prompt, prior_guidance_scale =1.0, height=768, width=768).images[0]
image.save("portrait.png")
```
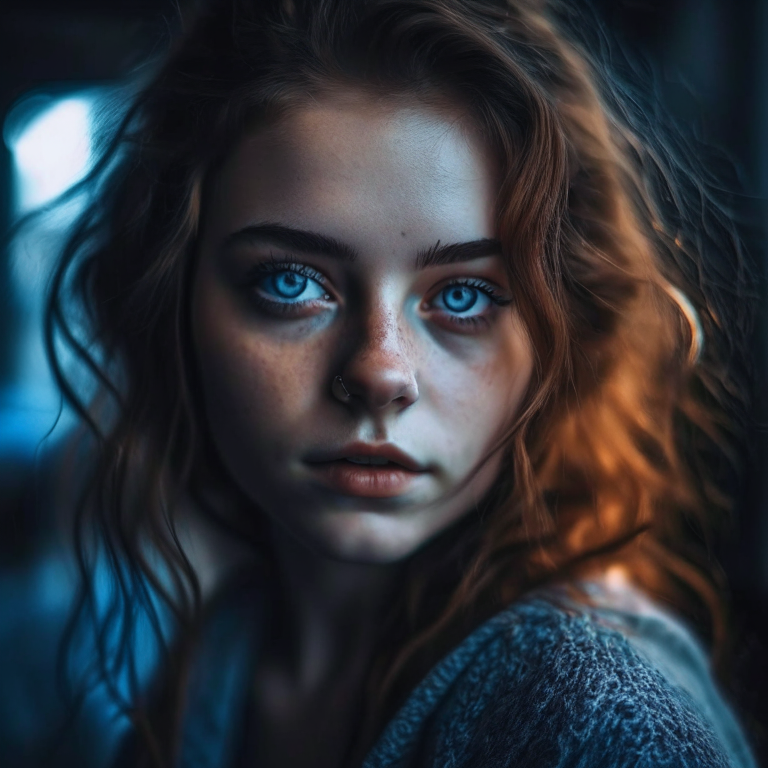
### Text Guided Image-to-Image Generation
```python
from PIL import Image
import requests
from io import BytesIO
url = "https://raw.githubusercontent.com/CompVis/stable-diffusion/main/assets/stable-samples/img2img/sketch-mountains-input.jpg"
response = requests.get(url)
original_image = Image.open(BytesIO(response.content)).convert("RGB")
original_image = original_image.resize((768, 512))
```
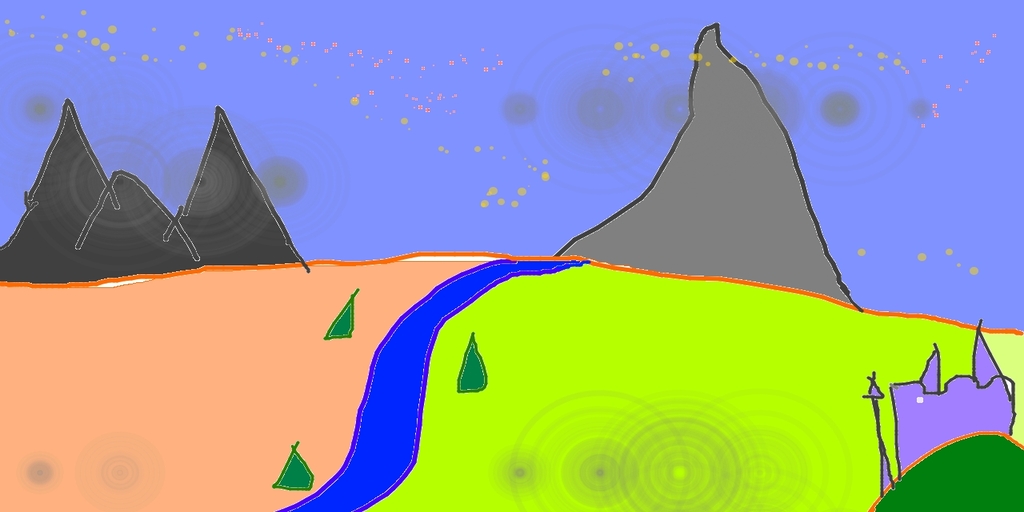
```python
from diffusers import AutoPipelineForImage2Image
import torch
pipe = AutoPipelineForImage2Image.from_pretrained("kandinsky-community/kandinsky-2-2-decoder", torch_dtype=torch.float16)
pipe.enable_model_cpu_offload()
prompt = "A fantasy landscape, Cinematic lighting"
negative_prompt = "low quality, bad quality"
image = pipe(prompt=prompt, image=original_image, strength=0.3, height=768, width=768).images[0]
out.images[0].save("fantasy_land.png")
```
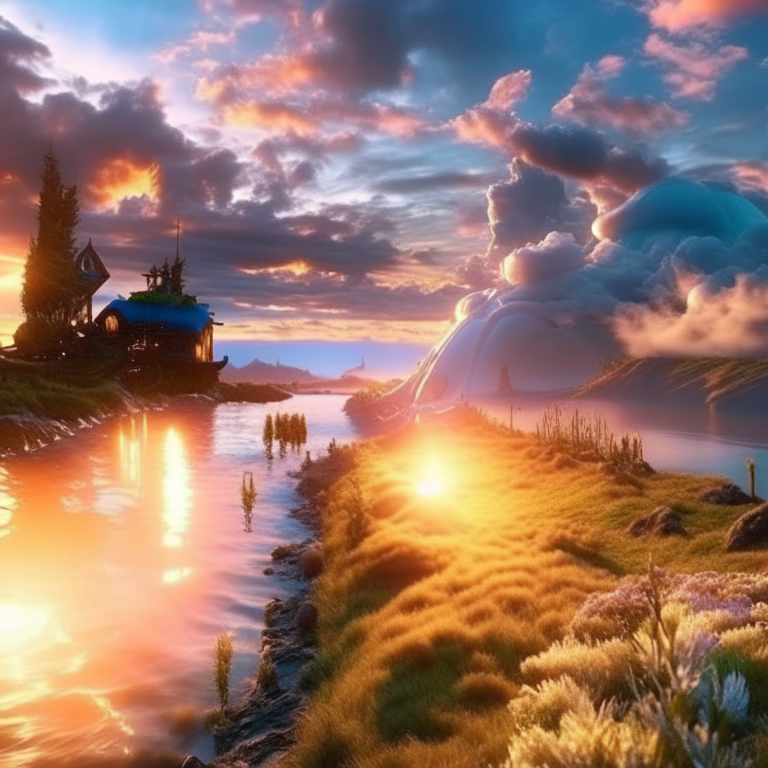
### Interpolate
```python
from diffusers import KandinskyV22PriorPipeline, KandinskyV22Pipeline
from diffusers.utils import load_image
import PIL
import torch
pipe_prior = KandinskyV22PriorPipeline.from_pretrained(
"kandinsky-community/kandinsky-2-2-prior", torch_dtype=torch.float16
)
pipe_prior.to("cuda")
img1 = load_image(
"https://huggingface.co/datasets/hf-internal-testing/diffusers-images/resolve/main" "/kandinsky/cat.png"
)
img2 = load_image(
"https://huggingface.co/datasets/hf-internal-testing/diffusers-images/resolve/main" "/kandinsky/starry_night.jpeg"
)
# add all the conditions we want to interpolate, can be either text or image
images_texts = ["a cat", img1, img2]
# specify the weights for each condition in images_texts
weights = [0.3, 0.3, 0.4]
# We can leave the prompt empty
prompt = ""
prior_out = pipe_prior.interpolate(images_texts, weights)
pipe = KandinskyV22Pipeline.from_pretrained("kandinsky-community/kandinsky-2-2-decoder", torch_dtype=torch.float16)
pipe.to("cuda")
image = pipe(**prior_out, height=768, width=768).images[0]
image.save("starry_cat.png")
```
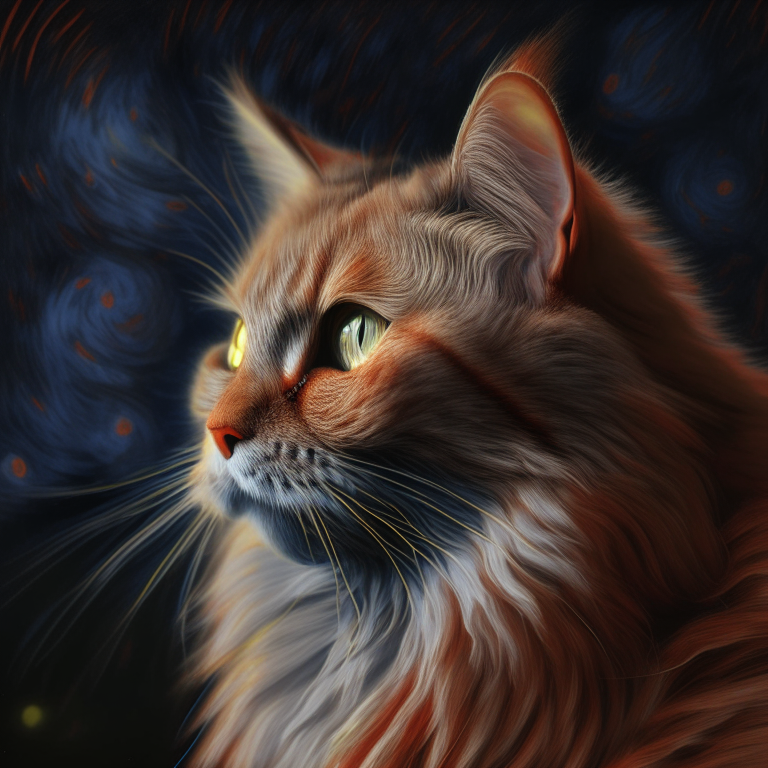
## Model Architecture
### Overview
Kandinsky 2.2 is a text-conditional diffusion model based on unCLIP and latent diffusion, composed of a transformer-based image prior model, a unet diffusion model, and a decoder.
The model architectures are illustrated in the figure below - the chart on the left describes the process to train the image prior model, the figure in the center is the text-to-image generation process, and the figure on the right is image interpolation.
<p float="left">
<img src="https://raw.githubusercontent.com/ai-forever/Kandinsky-2/main/content/kandinsky21.png"/>
</p>
Specifically, the image prior model was trained on CLIP text and image embeddings generated with a pre-trained [CLIP-ViT-G model](https://huggingface.co/laion/CLIP-ViT-g-14-laion2B-s12B-b42K). The trained image prior model is then used to generate CLIP image embeddings for input text prompts. Both the input text prompts and its CLIP image embeddings are used in the diffusion process. A [MoVQGAN](https://openreview.net/forum?id=Qb-AoSw4Jnm) model acts as the final block of the model, which decodes the latent representation into an actual image.
### Details
The image prior training of the model was performed on the [LAION Improved Aesthetics dataset](https://huggingface.co/datasets/bhargavsdesai/laion_improved_aesthetics_6.5plus_with_images), and then fine-tuning was performed on the [LAION HighRes data](https://huggingface.co/datasets/laion/laion-high-resolution).
The main Text2Image diffusion model was trained on [LAION HighRes dataset](https://huggingface.co/datasets/laion/laion-high-resolution) and then fine-tuned with a dataset of 2M very high-quality high-resolution images with descriptions (COYO, anime, landmarks_russia, and a number of others) was used separately collected from open sources.
The main change in Kandinsky 2.2 is the replacement of CLIP-ViT-G. Its image encoder significantly increases the model's capability to generate more aesthetic pictures and better understand text, thus enhancing its overall performance.
Due to the switch CLIP model, the image prior model was retrained, and the Text2Image diffusion model was fine-tuned for 2000 iterations. Kandinsky 2.2 was trained on data of various resolutions, from 512 x 512 to 1536 x 1536, and also as different aspect ratios. As a result, Kandinsky 2.2 can generate 1024 x 1024 outputs with any aspect ratio.
### Evaluation
We quantitatively measure the performance of Kandinsky 2.1 on the COCO_30k dataset, in zero-shot mode. The table below presents FID.
FID metric values for generative models on COCO_30k
| | FID (30k)|
|:------|----:|
| eDiff-I (2022) | 6.95 |
| Image (2022) | 7.27 |
| Kandinsky 2.1 (2023) | 8.21|
| Stable Diffusion 2.1 (2022) | 8.59 |
| GigaGAN, 512x512 (2023) | 9.09 |
| DALL-E 2 (2022) | 10.39 |
| GLIDE (2022) | 12.24 |
| Kandinsky 1.0 (2022) | 15.40 |
| DALL-E (2021) | 17.89 |
| Kandinsky 2.0 (2022) | 20.00 |
| GLIGEN (2022) | 21.04 |
For more information, please refer to the upcoming technical report.
## BibTex
If you find this repository useful in your research, please cite:
```
@misc{kandinsky 2.2,
title = {kandinsky 2.2},
author = {Arseniy Shakhmatov, Anton Razzhigaev, Aleksandr Nikolich, Vladimir Arkhipkin, Igor Pavlov, Andrey Kuznetsov, Denis Dimitrov},
year = {2023},
howpublished = {},
}
``` |
xtuner/llava-llama-3-8b-v1_1-gguf | xtuner | 2024-04-30T05:29:15Z | 20,020 | 128 | null | [
"gguf",
"image-to-text",
"dataset:Lin-Chen/ShareGPT4V",
"region:us"
] | image-to-text | 2024-04-26T10:41:02Z | ---
datasets:
- Lin-Chen/ShareGPT4V
pipeline_tag: image-to-text
---
<div align="center">
<img src="https://github.com/InternLM/lmdeploy/assets/36994684/0cf8d00f-e86b-40ba-9b54-dc8f1bc6c8d8" width="600"/>
[](https://github.com/InternLM/xtuner)
</div>
## Model
llava-llama-3-8b-v1_1 is a LLaVA model fine-tuned from [meta-llama/Meta-Llama-3-8B-Instruct](https://huggingface.co/meta-llama/Meta-Llama-3-8B-Instruct) and [CLIP-ViT-Large-patch14-336](https://huggingface.co/openai/clip-vit-large-patch14-336) with [ShareGPT4V-PT](https://huggingface.co/datasets/Lin-Chen/ShareGPT4V) and [InternVL-SFT](https://github.com/OpenGVLab/InternVL/tree/main/internvl_chat#prepare-training-datasets) by [XTuner](https://github.com/InternLM/xtuner).
**Note: This model is in GGUF format.**
Resources:
- GitHub: [xtuner](https://github.com/InternLM/xtuner)
- HuggingFace LLaVA format model: [xtuner/llava-llama-3-8b-v1_1-transformers](https://huggingface.co/xtuner/llava-llama-3-8b-v1_1-transformers)
- Official LLaVA format model: [xtuner/llava-llama-3-8b-v1_1-hf](https://huggingface.co/xtuner/llava-llama-3-8b-v1_1-hf)
- XTuner LLaVA format model: [xtuner/llava-llama-3-8b-v1_1](https://huggingface.co/xtuner/llava-llama-3-8b-v1_1)
## Details
| Model | Visual Encoder | Projector | Resolution | Pretraining Strategy | Fine-tuning Strategy | Pretrain Dataset | Fine-tune Dataset |
| :-------------------- | ------------------: | --------: | ---------: | ---------------------: | ------------------------: | ------------------------: | -----------------------: |
| LLaVA-v1.5-7B | CLIP-L | MLP | 336 | Frozen LLM, Frozen ViT | Full LLM, Frozen ViT | LLaVA-PT (558K) | LLaVA-Mix (665K) |
| LLaVA-Llama-3-8B | CLIP-L | MLP | 336 | Frozen LLM, Frozen ViT | Full LLM, LoRA ViT | LLaVA-PT (558K) | LLaVA-Mix (665K) |
| LLaVA-Llama-3-8B-v1.1 | CLIP-L | MLP | 336 | Frozen LLM, Frozen ViT | Full LLM, LoRA ViT | ShareGPT4V-PT (1246K) | InternVL-SFT (1268K) |
## Results
<div align="center">
<img src="https://github.com/InternLM/xtuner/assets/36994684/a157638c-3500-44ed-bfab-d8d8249f91bb" alt="Image" width=500" />
</div>
| Model | MMBench Test (EN) | MMBench Test (CN) | CCBench Dev | MMMU Val | SEED-IMG | AI2D Test | ScienceQA Test | HallusionBench aAcc | POPE | GQA | TextVQA | MME | MMStar |
| :-------------------- | :---------------: | :---------------: | :---------: | :-------: | :------: | :-------: | :------------: | :-----------------: | :--: | :--: | :-----: | :------: | :----: |
| LLaVA-v1.5-7B | 66.5 | 59.0 | 27.5 | 35.3 | 60.5 | 54.8 | 70.4 | 44.9 | 85.9 | 62.0 | 58.2 | 1511/348 | 30.3 |
| LLaVA-Llama-3-8B | 68.9 | 61.6 | 30.4 | 36.8 | 69.8 | 60.9 | 73.3 | 47.3 | 87.2 | 63.5 | 58.0 | 1506/295 | 38.2 |
| LLaVA-Llama-3-8B-v1.1 | 72.3 | 66.4 | 31.6 | 36.8 | 70.1 | 70.0 | 72.9 | 47.7 | 86.4 | 62.6 | 59.0 | 1469/349 | 45.1 |
## Quickstart
### Download models
```bash
# mmproj
wget https://huggingface.co/xtuner/llava-llama-3-8b-v1_1-gguf/resolve/main/llava-llama-3-8b-v1_1-mmproj-f16.gguf
# fp16 llm
wget https://huggingface.co/xtuner/llava-llama-3-8b-v1_1-gguf/resolve/main/llava-llama-3-8b-v1_1-f16.gguf
# int4 llm
wget https://huggingface.co/xtuner/llava-llama-3-8b-v1_1-gguf/resolve/main/llava-llama-3-8b-v1_1-int4.gguf
# (optional) ollama fp16 modelfile
wget https://huggingface.co/xtuner/llava-llama-3-8b-v1_1-gguf/resolve/main/OLLAMA_MODELFILE_F16
# (optional) ollama int4 modelfile
wget https://huggingface.co/xtuner/llava-llama-3-8b-v1_1-gguf/resolve/main/OLLAMA_MODELFILE_INT4
```
### Chat by `ollama`
```bash
# fp16
ollama create llava-llama3-f16 -f ./OLLAMA_MODELFILE_F16
ollama run llava-llama3-f16 "xx.png Describe this image"
# int4
ollama create llava-llama3-int4 -f ./OLLAMA_MODELFILE_INT4
ollama run llava-llama3-int4 "xx.png Describe this image"
```
### Chat by `llama.cpp`
1. Build [llama.cpp](https://github.com/ggerganov/llama.cpp) ([docs](https://github.com/ggerganov/llama.cpp?tab=readme-ov-file#usage)) .
2. Build `./llava-cli` ([docs](https://github.com/ggerganov/llama.cpp/tree/master/examples/llava#usage)).
Note: llava-llama-3-8b-v1_1 uses the Llama-3-instruct chat template.
```bash
# fp16
./llava-cli -m ./llava-llama-3-8b-v1_1-f16.gguf --mmproj ./llava-llama-3-8b-v1_1-mmproj-f16.gguf --image YOUR_IMAGE.jpg -c 4096 -e -p "<|start_header_id|>user<|end_header_id|>\n\n<image>\nDescribe this image<|eot_id|><|start_header_id|>assistant<|end_header_id|>\n\n"
# int4
./llava-cli -m ./llava-llama-3-8b-v1_1-int4.gguf --mmproj ./llava-llama-3-8b-v1_1-mmproj-f16.gguf --image YOUR_IMAGE.jpg -c 4096 -e -p "<|start_header_id|>user<|end_header_id|>\n\n<image>\nDescribe this image<|eot_id|><|start_header_id|>assistant<|end_header_id|>\n\n"
```
### Reproduce
Please refer to [docs](https://github.com/InternLM/xtuner/tree/main/xtuner/configs/llava/llama3_8b_instruct_clip_vit_large_p14_336#readme).
## Citation
```bibtex
@misc{2023xtuner,
title={XTuner: A Toolkit for Efficiently Fine-tuning LLM},
author={XTuner Contributors},
howpublished = {\url{https://github.com/InternLM/xtuner}},
year={2023}
}
```
|
laion/CLIP-ViT-g-14-laion2B-s34B-b88K | laion | 2024-03-22T22:00:30Z | 20,000 | 17 | open_clip | [
"open_clip",
"safetensors",
"zero-shot-image-classification",
"clip",
"arxiv:1910.04867",
"license:mit",
"region:us"
] | zero-shot-image-classification | 2023-03-06T00:43:13Z | ---
tags:
- zero-shot-image-classification
- clip
library_tag: open_clip
license: mit
pipeline_tag: zero-shot-image-classification
---
# Model card for CLIP-ViT-g-14-laion2B-s34B-b88K
# Table of Contents
1. [Model Details](#model-details)
2. [Uses](#uses)
3. [Training Details](#training-details)
4. [Evaluation](#evaluation)
5. [Acknowledgements](#acknowledgements)
6. [Citation](#citation)
7. [How To Get Started With the Model](#how-to-get-started-with-the-model)
# Model Details
## Model Description
A CLIP ViT-g/14 model trained with the LAION-2B English subset of LAION-5B (https://laion.ai/blog/laion-5b/, https://openreview.net/forum?id=M3Y74vmsMcY) using OpenCLIP (https://github.com/mlfoundations/open_clip).
Model training done by Jenia Jitsev on [JUWELS Booster](https://apps.fz-juelich.de/jsc/hps/juwels/booster-overview.html) at [Juelich Supercomputing Center](https://www.fz-juelich.de/en/ias/jsc) and on the [stability.ai](https://stability.ai/) AWS HPC cluster.
Training performed in frame of reproducible scaling law studies, published as [research paper at CVPR 2023](https://openaccess.thecvf.com/content/CVPR2023/html/Cherti_Reproducible_Scaling_Laws_for_Contrastive_Language-Image_Learning_CVPR_2023_paper.html). See also the [research repository](https://github.com/LAION-AI/scaling-laws-openclip)
# Uses
As per the original [OpenAI CLIP model card](https://github.com/openai/CLIP/blob/d50d76daa670286dd6cacf3bcd80b5e4823fc8e1/model-card.md), this model is intended as a research output for research communities. We hope that this model will enable researchers to better understand and explore zero-shot, arbitrary image classification. We also hope it can be used for interdisciplinary studies of the potential impact of such model.
The OpenAI CLIP paper includes a discussion of potential downstream impacts to provide an example for this sort of analysis. Additionally, the LAION-5B blog (https://laion.ai/blog/laion-5b/) and [LAION-5B NeurIPS paper](https://openreview.net/forum?id=M3Y74vmsMcY) include additional discussion as it relates specifically to the training dataset.
## Direct Use
Zero-shot image classification, image and text retrieval, among others.
## Downstream Use
Image classification and other image task fine-tuning, linear probe image classification, image generation guiding and conditioning, among others.
## Out-of-Scope Use
As per the OpenAI models,
**Any** deployed use case of the model - whether commercial or not - is currently out of scope. Non-deployed use cases such as image search in a constrained environment, are also not recommended unless there is thorough in-domain testing of the model with a specific, fixed class taxonomy. This is because our safety assessment demonstrated a high need for task specific testing especially given the variability of CLIP’s performance with different class taxonomies. This makes untested and unconstrained deployment of the model in any use case currently potentially harmful.
Certain use cases which would fall under the domain of surveillance and facial recognition are always out-of-scope regardless of performance of the model. This is because the use of artificial intelligence for tasks such as these can be premature currently given the lack of testing norms and checks to ensure its fair use.
Since the model has not been purposefully trained in or evaluated on any languages other than English, its use should be limited to English language use cases.
Further the above notice, the LAION-5B dataset used in training of these models has additional considerations, see below.
# Training Details
## Training Data
This model was trained with the 2 Billion sample English subset of LAION-5B (https://laion.ai/blog/laion-5b/).
**IMPORTANT NOTE:** The motivation behind dataset creation is to democratize research and experimentation around large-scale multi-modal model training and handling of uncurated, large-scale datasets crawled from publically available internet. Our recommendation is therefore to use the dataset for research purposes. Be aware that this large-scale dataset is uncurated. Keep in mind that the uncurated nature of the dataset means that collected links may lead to strongly discomforting and disturbing content for a human viewer. Therefore, please use the demo links with caution and at your own risk. It is possible to extract a “safe” subset by filtering out samples based on the safety tags (using a customized trained NSFW classifier that we built). While this strongly reduces the chance for encountering potentially harmful content when viewing, we cannot entirely exclude the possibility for harmful content being still present in safe mode, so that the warning holds also there. We think that providing the dataset openly to broad research and other interested communities will allow for transparent investigation of benefits that come along with training large-scale models as well as pitfalls and dangers that may stay unreported or unnoticed when working with closed large datasets that remain restricted to a small community. Providing our dataset openly, we however do not recommend using it for creating ready-to-go industrial products, as the basic research about general properties and safety of such large-scale models, which we would like to encourage with this release, is still in progress.
## Training Procedure
OpenCLIP ViT-g/14 model was trained on 34.5B samples (135M * 256 checkpoints) from laion2b-en (part of LAION-5B) dataset. Warmup = 13.5k steps, learning rate = 1e-3, cosine annealing schedule, weight decay = 0.2. Global batch size = 88800, number of GPUs = 1480, local batch size = 60
# Evaluation
Evaluation done with code in the [LAION CLIP Benchmark suite](https://github.com/LAION-AI/CLIP_benchmark).
## Testing Data, Factors & Metrics
### Testing Data
The testing is performed with VTAB+ (A combination of VTAB (https://arxiv.org/abs/1910.04867) w/ additional robustness datasets) for classification and COCO and Flickr for retrieval.
**TODO** - more detail
## Results
The model achieves a 78.4 zero-shot top-1 accuracy on ImageNet-1k.
An initial round of benchmarks have been performed on a wider range of datasets, currently viewable at https://github.com/LAION-AI/CLIP_benchmark/blob/main/benchmark/results.ipynb
**TODO** - create table for just this model's metrics.
# Acknowledgements
We gratefully acknowledge the Gauss Centre for Supercomputing e.V. (www.gauss-centre.eu) for funding the work by providing computing time through the John von Neumann Institute for Computing (NIC) on the GCS Supercomputer [JUWELS Booster](https://apps.fz-juelich.de/jsc/hps/juwels/booster-overview.html) at Jülich Supercomputing Centre (JSC)
We also acknowledge storage resources on JUST granted and operated by JSC, as well as computing resources from the Helmholtz Data Federation (HDF).
We further acknowledge [stability.ai](https://stability.ai/) providing additional compute used to train this model.
# Citation
**BibTeX:**
Please cite:
LAION-5B paper
```
@inproceedings{Schuhmann2022,
title={{LAION}-5{B}: An open large-scale dataset for training next generation image-text models},
author={Christoph Schuhmann and Romain Beaumont and Richard Vencu and Cade W Gordon and Ross Wightman and Mehdi Cherti and Theo Coombes and Aarush Katta and Clayton Mullis and Mitchell Wortsman and Patrick Schramowski and Srivatsa R Kundurthy and Katherine Crowson and Ludwig Schmidt and Robert Kaczmarczyk and Jenia Jitsev},
booktitle={Thirty-sixth Conference on Advances in Neural Information Processing Systems (NeurIPS), Datasets and Benchmarks Track},
year={2022},
volume={35},
pages={25278--25294},
url={https://openreview.net/forum?id=M3Y74vmsMcY}
}
```
Reproducible scaling laws for openCLIP paper
```
@inproceedings{Cherti2023,
title={Reproducible scaling laws for contrastive language-image learning},
author={Cherti, Mehdi and Beaumont, Romain and Wightman, Ross and Wortsman, Mitchell and Ilharco, Gabriel and Gordon, Cade and Schuhmann, Christoph and Schmidt, Ludwig and Jitsev, Jenia},
booktitle={Proceedings of the IEEE/CVF Conference on Computer Vision and Pattern Recognition},
pages={2818--2829},
year={2023}
}
```
OpenAI CLIP paper
```
@inproceedings{Radford2021LearningTV,
title={Learning Transferable Visual Models From Natural Language Supervision},
author={Alec Radford and Jong Wook Kim and Chris Hallacy and A. Ramesh and Gabriel Goh and Sandhini Agarwal and Girish Sastry and Amanda Askell and Pamela Mishkin and Jack Clark and Gretchen Krueger and Ilya Sutskever},
booktitle={ICML},
year={2021}
}
```
OpenCLIP software
```
@software{ilharco_gabriel_2021_5143773,
author = {Ilharco, Gabriel and
Wortsman, Mitchell and
Wightman, Ross and
Gordon, Cade and
Carlini, Nicholas and
Taori, Rohan and
Dave, Achal and
Shankar, Vaishaal and
Namkoong, Hongseok and
Miller, John and
Hajishirzi, Hannaneh and
Farhadi, Ali and
Schmidt, Ludwig},
title = {OpenCLIP},
month = jul,
year = 2021,
note = {If you use this software, please cite it as below.},
publisher = {Zenodo},
version = {0.1},
doi = {10.5281/zenodo.5143773},
url = {https://doi.org/10.5281/zenodo.5143773}
}
```
# How to Get Started with the Model
Use the code below to get started with the model.
** TODO ** - Hugging Face transformers, OpenCLIP, and timm getting started snippets |
laion/CLIP-convnext_xxlarge-laion2B-s34B-b82K-augreg-soup | laion | 2023-04-18T17:45:00Z | 19,978 | 19 | open_clip | [
"open_clip",
"zero-shot-image-classification",
"clip",
"arxiv:2210.08402",
"arxiv:1910.04867",
"license:mit",
"region:us"
] | zero-shot-image-classification | 2023-02-26T20:23:04Z | ---
tags:
- zero-shot-image-classification
- clip
library_tag: open_clip
license: mit
library_name: open_clip
pipeline_tag: zero-shot-image-classification
---
# Model card for CLIP-convnext_xxlarge-laion2B-s34B-b82K-augreg-soup
# Table of Contents
1. [Model Details](#model-details)
2. [Uses](#uses)
3. [Training Details](#training-details)
4. [Evaluation](#evaluation)
5. [Acknowledgements](#acknowledgements)
6. [Citation](#citation)
# Model Details
## Model Description
A series of CLIP ConvNeXt-XXLarge (a custom `timm` ConvNeXt size) models trained on LAION-2B (english), a subset of [LAION-5B](https://arxiv.org/abs/2210.08402), using [OpenCLIP](https://github.com/mlfoundations/open_clip).
| Model | Dataset | Resolution | AugReg | Top-1 ImageNet Zero-Shot (%) |
| ----- | ------- | ---------- | ------------ | --------- |
| [convnext_xxlarge.laion2b_s34b_b82k-augreg](https://huggingface.co/laion/CLIP-convnext_xxlarge-laion2B-s34B-b82K-augreg) | LAION-2B | 256x256 | RRC (0.33, 1.0), RE (0.35), SD (0.1) | 79.1 |
| [convnext_xxlarge.laion2b_s34b_b82k-augreg-rewind](https://huggingface.co/laion/CLIP-convnext_xxlarge-laion2B-s34B-b82K-augreg-rewind) | LAION-2B | 256x256 | RRC (0.3, 1.0), RE (0.4), SD (0.1) | 79.3 |
| [convnext_xxlarge.laion2b_s34b_b82k-augreg-soup](https://huggingface.co/laion/CLIP-convnext_xxlarge-laion2B-s34B-b82K-augreg-soup) | LAION-2B | 256x256 | N/A | 79.4 |
RRC = Random Resize Crop (crop pcts), RE = Random Erasing (prob), SD = Stochastic Depth (prob) -- image tower only
The core training run was performed in pieces over a period of ~ 2 months. The global batch size for the core run was 81920. The last ~10% of training was re-done at a 95744 global batch size w/ higher LR and aug than original finish. The two were averaged together in a 'soup'. See more details in [Training Details](#training-details).
Goals:
* Push the size of largest convolutional CLIP image tower into the performance range of ViT-g to ViT-G w/ improved image size scaling for downstream use.
Firsts:
* Largest released ConvNeXt model pretrained (847M params w/ 198 GMAC and 125 MActs @ 256x256 for image)
* A non-ViT image tower CLIP model (with no previous image tower pretrain) achieving > 79% ImageNet top-1 zero-shot
The models utilize:
* the [timm](https://github.com/rwightman/pytorch-image-models) ConvNeXt-XXLarge model (`convnext_xxlarge`) as the image tower
* a standard projection at end of image tower
* a text tower with same size (with 1024, heads 16, depth 24) as ViT-H-14 and ViT-g-14 models
The models are trained at 256x256 image resolution. The size of the combined image + text CLIP model is 1.2B params w/ 222 GMAC and 146 MActs. At 256x256, the ConvNext-XXLarge sits just above a ViT-H-14 CLIP configuration in FLOPS and params while being lower in activation counts. It is well under both g-14 and G-14 while being between them in capabilities.
|model |image_size|embed_dim|gmacs |macts |mparams|image_gmacs|image_macts|image_mparams|text_gmacs|text_macts|text_mparams|
|--------------------------|----------|---------|------|------|-------|-----------|-----------|-------------|----------|----------|------------|
|ViT-H-16 |224 |1024 |150.96|122.01|986.26 |127.4 |100.81 |632.23 |23.57 |21.2 |354.03 |
|ViT-H-14 |224 |1024 |190.97|160.61|986.11 |167.4 |139.41 |632.08 |23.57 |21.2 |354.03 |
|ViT-L-14-336 |336 |768 |197.76|278.19|427.94 |191.1 |270.24 |304.29 |6.66 |7.95 |123.65 |
|convnext_xxlarge |256 |1024 |221.66|145.66|1200.58|198.09 |124.45 |846.54 |23.57 |21.2 |354.03 |
|RN50x64 |448 |1024 |276.8 |249.73|623.26 |265.02 |239.13 |420.38 |11.78 |10.6 |202.88 |
|ViT-g-14 |224 |1024 |290.74|213.84|1366.68|267.18 |192.64 |1012.65 |23.57 |21.2 |354.03 |
|convnext_xxlarge_320 |320 |1024 |333.08|215.66|1200.58|309.52 |194.46 |846.54 |23.57 |21.2 |354.03 |
|ViT-H-14-336 |336 |1024 |414.53|428.74|986.52 |390.97 |407.54 |632.49 |23.57 |21.2 |354.03 |
|ViT-bigG-14 |224 |1280 |532.92|310.71|2539.57|483.96 |275.37 |1844.91 |48.96 |35.34 |694.66 |
Model training done by Ross Wightman across both the [stability.ai](https://stability.ai/) cluster and the [JUWELS Booster](https://apps.fz-juelich.de/jsc/hps/juwels/booster-overview.html) supercomputer. See acknowledgements below.
# Uses
As per the original [OpenAI CLIP model card](https://github.com/openai/CLIP/blob/d50d76daa670286dd6cacf3bcd80b5e4823fc8e1/model-card.md), this model is intended as a research output for research communities. We hope that this model will enable researchers to better understand and explore zero-shot, arbitrary image classification. We also hope it can be used for interdisciplinary studies of the potential impact of such model.
The OpenAI CLIP paper includes a discussion of potential downstream impacts to provide an example for this sort of analysis. Additionally, the LAION-5B blog (https://laion.ai/blog/laion-5b/) and upcoming paper include additional discussion as it relates specifically to the training dataset.
## Direct Use
Zero-shot image classification, image and text retrieval, among others.
## Downstream Use
Image classification and other image task fine-tuning, linear probe image classification, image generation guiding and conditioning, among others.
## Out-of-Scope Use
As per the OpenAI models,
**Any** deployed use case of the model - whether commercial or not - is currently out of scope. Non-deployed use cases such as image search in a constrained environment, are also not recommended unless there is thorough in-domain testing of the model with a specific, fixed class taxonomy. This is because our safety assessment demonstrated a high need for task specific testing especially given the variability of CLIP’s performance with different class taxonomies. This makes untested and unconstrained deployment of the model in any use case currently potentially harmful.
Certain use cases which would fall under the domain of surveillance and facial recognition are always out-of-scope regardless of performance of the model. This is because the use of artificial intelligence for tasks such as these can be premature currently given the lack of testing norms and checks to ensure its fair use.
Since the model has not been purposefully trained in or evaluated on any languages other than English, its use should be limited to English language use cases.
Further the above notice, the LAION-5B dataset used in training of these models has additional considerations, see below.
# Training Details
## Training Data
This model was trained with LAION-2B -- A 2 billion sample English subset of LAION-5B (https://laion.ai/blog/laion-5b/).
**IMPORTANT NOTE:** The motivation behind dataset creation is to democratize research and experimentation around large-scale multi-modal model training and handling of uncurated, large-scale datasets crawled from publically available internet. Our recommendation is therefore to use the dataset for research purposes. Be aware that this large-scale dataset is uncurated. Keep in mind that the uncurated nature of the dataset means that collected links may lead to strongly discomforting and disturbing content for a human viewer. Therefore, please use the demo links with caution and at your own risk. It is possible to extract a “safe” subset by filtering out samples based on the safety tags (using a customized trained NSFW classifier that we built). While this strongly reduces the chance for encountering potentially harmful content when viewing, we cannot entirely exclude the possibility for harmful content being still present in safe mode, so that the warning holds also there. We think that providing the dataset openly to broad research and other interested communities will allow for transparent investigation of benefits that come along with training large-scale models as well as pitfalls and dangers that may stay unreported or unnoticed when working with closed large datasets that remain restricted to a small community. Providing our dataset openly, we however do not recommend using it for creating ready-to-go industrial products, as the basic research about general properties and safety of such large-scale models, which we would like to encourage with this release, is still in progress.
## Training Procedure
The main training run was done at global batch size of 81920 for 256 checkpoint intervals of 135.6M samples for a total of ~34B samples seen over training.
Many difficulties w/ both model numerical stability and cluster stability and performance were encountered while training this model. Initial attempts to train with float16 AMP and default adam beta2 resulted in loss spikes and eventually NaN blow ups. `beta2` was reduced to 0.97 which helped, but the loss / zs curves were not tracking as expected. After switching to PyTorch nightlies, it was possible to use bfloat16 + AMP for training (as with rececnt H/14, g/14, and G/14 models), beta2 was returned to 0.98 and metrics improved.
|Checkpoint Interval |Cluster |# GPUs|# Nodes|GPU |local BS|sample/s|sample/s/gpu|precision |adam beta2 |
|--------------------|----------|------|-------|----------|--------|--------|------------|----------|-----------|
|1 - 2 |Stability |1024 |128 |A100 40GB | 80 |37-40k | 36-39 |amp + fp16|0.97 |
|3 - 32 |Stability |512 |64 |A100 80GB | 160 |27-32k | 52-62 |amp + fp16|0.97 |
|33 - 75 |Booster |1024 |256 |A100 40GB | 80 |48k | 47 |amp + fp16|0.97 |
|76 - 165 |Booster |1024 |256 |A100 40GB | 80 |51k | 50 |amp + bf16|0.98 |
|166 - 232 |Stability |320 |40 |A100 80GB | 256 |18-19k | 56-59 |amp + bf16|0.98 |
|233 - 249 |Booster |1024 |256 |A100 40GB | 80 |51k | 50 |amp + bf16|0.98 |
|250 - 256 |Stability |1024 |128 |A100 40GB | 80 |27-31k | 26-30 |amp + bf16|0.98 |
JUWELS Booster has 4x A100 GPU per node w/ 4x HDR-200 IB adapters per node (200Gbit/sec per GPU). Stability setup used was 8x A100 GPU per node w/ 400Gbit/sec EFA networking per node (50 GBit/sec per GPU). Significant variation in training efficiency (throughput per GPU) as observed across the various configurations. The 1024 GPU configurations across both clusters were particularly prone to crashing (or very difficult to get running w/ a 'good' set of GPUs).
A slurm srun command line below for a 128 8-GPU (40GB A100) configuration:
```
srun --cpu_bind=v --accel-bind=gn python -m training.main \
--save-frequency 1 \
--name "xxlarge-2b-81920-bf16" \
--resume "latest" \
--logs "/runs" \
--log-every-n-steps 50 \
--train-data="pipe:aws s3 cp s3://laion5b/laion2B-data/{000000..231349}.tar -" \
--train-num-samples 135646078 \
--dataset-type webdataset \
--warmup 10000 \
--batch-size=80 \
--epochs=256 \
--dataset-resampled \
--aug-cfg use_timm=True scale='(0.33, 1.0)' re_prob=0.35 \
--precision amp_bfloat16 \
--grad-clip-norm 5.0 \
--lr 1e-3 \
--workers=6 \
--beta2 0.98 \
--model "convnext_xxlarge" \
--seed 0 \
--ddp-static-graph \
--local-loss \
--gather-with-grad \
--grad-checkpointing \
--report-to "tensorboard"
```
For the rewind of last 10%, a higher global batch size of 95744 was used w/ a higher LR and slightly increased augmentation strength.
|Checkpoint Interval |Cluster |# GPUs|# Nodes|GPU |local BS|sample/s|sample/s/gpu|precision |adam beta2 |
|--------------------|---------|------|-------|----------|--------|--------|------------|----------|-----------|
|231 - 256 |stability|1088 |136 |A100 40GB | 88 |32-35k | 29-32 |amp + bf16|0.98 |
The slurm srun command line for 136 8-GPU (40GB A100) nodes:
```
srun --cpu_bind=v --accel-bind=gn python -m training.main \
--save-frequency 1 \
--name "xxlarge-2b-81920-r-bf16" \
--resume "latest" \
--logs "/runs" \
--log-every-n-steps 50 \
--train-data="pipe:aws s3 cp s3://laion5b/laion2B-data/{000000..231349}.tar -" \
--train-num-samples 135646078 \
--dataset-type webdataset \
--warmup 10000 \
--batch-size=88 \
--epochs=256 \
--dataset-resampled \
--aug-cfg use_timm=True scale='(0.3, 1.0)' re_prob=0.4 \
--precision amp_bfloat16 \
--grad-clip-norm 5.0 \
--lr 2e-3 \
--workers=6 \
--beta2 0.98 \
--model "convnext_xxlarge" \
--seed 0 \
--ddp-static-graph \
--local-loss \
--gather-with-grad \
--grad-checkpointing \
--report-to "tensorboard"
```
# Evaluation
Evaluation done with code in the [LAION CLIP Benchmark suite](https://github.com/LAION-AI/CLIP_benchmark).
## Testing Data, Factors & Metrics
### Testing Data
The testing is performed with VTAB+ (A combination of VTAB (https://arxiv.org/abs/1910.04867) w/ additional robustness datasets) for classification and COCO and Flickr for retrieval.
## Results
These models achieve between 79.1 and 79.4 top-1 zero-shot accuracy on ImageNet-1k.

A zoom-in on final 10% w/ rewind:

An initial round of benchmarks have been performed on a wider range of datasets, to be viewable at https://github.com/LAION-AI/CLIP_benchmark/blob/main/benchmark/results.ipynb
# Acknowledgements
Acknowledging [stability.ai](https://stability.ai/) and the Gauss Centre for Supercomputing e.V. (http://gauss-centre.eu) for funding this part of work by providing computing time through the John von Neumann Institute for Computing (NIC) on the GCS Supercomputer JUWELS Booster at Jülich Supercomputing Centre (JSC).
# Citation
**BibTeX:**
LAION-5B
```bibtex
@inproceedings{schuhmann2022laionb,
title={{LAION}-5B: An open large-scale dataset for training next generation image-text models},
author={Christoph Schuhmann and
Romain Beaumont and
Richard Vencu and
Cade W Gordon and
Ross Wightman and
Mehdi Cherti and
Theo Coombes and
Aarush Katta and
Clayton Mullis and
Mitchell Wortsman and
Patrick Schramowski and
Srivatsa R Kundurthy and
Katherine Crowson and
Ludwig Schmidt and
Robert Kaczmarczyk and
Jenia Jitsev},
booktitle={Thirty-sixth Conference on Neural Information Processing Systems Datasets and Benchmarks Track},
year={2022},
url={https://openreview.net/forum?id=M3Y74vmsMcY}
}
```
OpenCLIP software
```bibtex
@software{ilharco_gabriel_2021_5143773,
author = {Ilharco, Gabriel and
Wortsman, Mitchell and
Wightman, Ross and
Gordon, Cade and
Carlini, Nicholas and
Taori, Rohan and
Dave, Achal and
Shankar, Vaishaal and
Namkoong, Hongseok and
Miller, John and
Hajishirzi, Hannaneh and
Farhadi, Ali and
Schmidt, Ludwig},
title = {OpenCLIP},
month = jul,
year = 2021,
note = {If you use this software, please cite it as below.},
publisher = {Zenodo},
version = {0.1},
doi = {10.5281/zenodo.5143773},
url = {https://doi.org/10.5281/zenodo.5143773}
}
```
OpenAI CLIP paper
```bibtex
@inproceedings{Radford2021LearningTV,
title={Learning Transferable Visual Models From Natural Language Supervision},
author={Alec Radford and Jong Wook Kim and Chris Hallacy and A. Ramesh and Gabriel Goh and Sandhini Agarwal and Girish Sastry and Amanda Askell and Pamela Mishkin and Jack Clark and Gretchen Krueger and Ilya Sutskever},
booktitle={ICML},
year={2021}
}
```
```bibtex
@Article{liu2022convnet,
author = {Zhuang Liu and Hanzi Mao and Chao-Yuan Wu and Christoph Feichtenhofer and Trevor Darrell and Saining Xie},
title = {A ConvNet for the 2020s},
journal = {Proceedings of the IEEE/CVF Conference on Computer Vision and Pattern Recognition (CVPR)},
year = {2022},
}
```
```bibtex
@misc{rw2019timm,
author = {Ross Wightman},
title = {PyTorch Image Models},
year = {2019},
publisher = {GitHub},
journal = {GitHub repository},
doi = {10.5281/zenodo.4414861},
howpublished = {\url{https://github.com/rwightman/pytorch-image-models}}
}
```
```
@InProceedings{pmlr-v162-wortsman22a,
title = {Model soups: averaging weights of multiple fine-tuned models improves accuracy without increasing inference time},
author = {Wortsman, Mitchell and Ilharco, Gabriel and Gadre, Samir Ya and Roelofs, Rebecca and Gontijo-Lopes, Raphael and Morcos, Ari S and Namkoong, Hongseok and Farhadi, Ali and Carmon, Yair and Kornblith, Simon and Schmidt, Ludwig},
booktitle = {Proceedings of the 39th International Conference on Machine Learning},
pages = {23965--23998},
year = {2022},
editor = {Chaudhuri, Kamalika and Jegelka, Stefanie and Song, Le and Szepesvari, Csaba and Niu, Gang and Sabato, Sivan},
volume = {162},
series = {Proceedings of Machine Learning Research},
month = {17--23 Jul},
publisher = {PMLR},
pdf = {https://proceedings.mlr.press/v162/wortsman22a/wortsman22a.pdf},
url = {https://proceedings.mlr.press/v162/wortsman22a.html}
}
``` |
Subsets and Splits
No community queries yet
The top public SQL queries from the community will appear here once available.