modelId
stringlengths 5
122
| author
stringlengths 2
42
| last_modified
timestamp[us, tz=UTC] | downloads
int64 0
738M
| likes
int64 0
11k
| library_name
stringclasses 245
values | tags
listlengths 1
4.05k
| pipeline_tag
stringclasses 48
values | createdAt
timestamp[us, tz=UTC] | card
stringlengths 1
901k
|
---|---|---|---|---|---|---|---|---|---|
DiscoResearch/mixtral-7b-8expert | DiscoResearch | 2023-12-11T08:58:28Z | 12,507 | 259 | transformers | [
"transformers",
"pytorch",
"mistral",
"text-generation",
"custom_code",
"en",
"fr",
"it",
"es",
"de",
"license:apache-2.0",
"autotrain_compatible",
"endpoints_compatible",
"text-generation-inference",
"region:us"
]
| text-generation | 2023-12-08T23:33:07Z | ---
license: apache-2.0
language:
- en
- fr
- it
- es
- de
---
# Mixtral 7b 8 Expert

This is a preliminary HuggingFace implementation of the newly released MoE model by MistralAi. Make sure to load with `trust_remote_code=True`.
Thanks to @dzhulgakov for his early implementation (https://github.com/dzhulgakov/llama-mistral) that helped me find a working setup.
Also many thanks to our friends at [LAION](https://laion.ai) and [HessianAI](https://hessian.ai/) for the compute used for these projects!
Benchmark scores:
```
hella swag: 0.8661
winogrande: 0.824
truthfulqa_mc2: 0.4855
arc_challenge: 0.6638
gsm8k: 0.5709
MMLU: 0.7173
```
# Basic Inference setup
```python
import torch
from transformers import AutoModelForCausalLM, AutoTokenizer
model = AutoModelForCausalLM.from_pretrained("DiscoResearch/mixtral-7b-8expert", low_cpu_mem_usage=True, device_map="auto", trust_remote_code=True)
tok = AutoTokenizer.from_pretrained("DiscoResearch/mixtral-7b-8expert")
x = tok.encode("The mistral wind in is a phenomenon ", return_tensors="pt").cuda()
x = model.generate(x, max_new_tokens=128).cpu()
print(tok.batch_decode(x))
```
# Conversion
Use `convert_mistral_moe_weights_to_hf.py --input_dir ./input_dir --model_size 7B --output_dir ./output` to convert the original consolidated weights to this HF setup.
Come chat about this in our [Disco(rd)](https://discord.gg/S8W8B5nz3v)! :) |
RichardErkhov/MaziyarPanahi_-_Calme-7B-Instruct-v0.1-gguf | RichardErkhov | 2024-06-19T20:51:58Z | 12,499 | 0 | null | [
"gguf",
"region:us"
]
| null | 2024-06-19T16:38:10Z | Quantization made by Richard Erkhov.
[Github](https://github.com/RichardErkhov)
[Discord](https://discord.gg/pvy7H8DZMG)
[Request more models](https://github.com/RichardErkhov/quant_request)
Calme-7B-Instruct-v0.1 - GGUF
- Model creator: https://huggingface.co/MaziyarPanahi/
- Original model: https://huggingface.co/MaziyarPanahi/Calme-7B-Instruct-v0.1/
| Name | Quant method | Size |
| ---- | ---- | ---- |
| [Calme-7B-Instruct-v0.1.Q2_K.gguf](https://huggingface.co/RichardErkhov/MaziyarPanahi_-_Calme-7B-Instruct-v0.1-gguf/blob/main/Calme-7B-Instruct-v0.1.Q2_K.gguf) | Q2_K | 2.53GB |
| [Calme-7B-Instruct-v0.1.IQ3_XS.gguf](https://huggingface.co/RichardErkhov/MaziyarPanahi_-_Calme-7B-Instruct-v0.1-gguf/blob/main/Calme-7B-Instruct-v0.1.IQ3_XS.gguf) | IQ3_XS | 2.81GB |
| [Calme-7B-Instruct-v0.1.IQ3_S.gguf](https://huggingface.co/RichardErkhov/MaziyarPanahi_-_Calme-7B-Instruct-v0.1-gguf/blob/main/Calme-7B-Instruct-v0.1.IQ3_S.gguf) | IQ3_S | 2.96GB |
| [Calme-7B-Instruct-v0.1.Q3_K_S.gguf](https://huggingface.co/RichardErkhov/MaziyarPanahi_-_Calme-7B-Instruct-v0.1-gguf/blob/main/Calme-7B-Instruct-v0.1.Q3_K_S.gguf) | Q3_K_S | 2.95GB |
| [Calme-7B-Instruct-v0.1.IQ3_M.gguf](https://huggingface.co/RichardErkhov/MaziyarPanahi_-_Calme-7B-Instruct-v0.1-gguf/blob/main/Calme-7B-Instruct-v0.1.IQ3_M.gguf) | IQ3_M | 3.06GB |
| [Calme-7B-Instruct-v0.1.Q3_K.gguf](https://huggingface.co/RichardErkhov/MaziyarPanahi_-_Calme-7B-Instruct-v0.1-gguf/blob/main/Calme-7B-Instruct-v0.1.Q3_K.gguf) | Q3_K | 3.28GB |
| [Calme-7B-Instruct-v0.1.Q3_K_M.gguf](https://huggingface.co/RichardErkhov/MaziyarPanahi_-_Calme-7B-Instruct-v0.1-gguf/blob/main/Calme-7B-Instruct-v0.1.Q3_K_M.gguf) | Q3_K_M | 3.28GB |
| [Calme-7B-Instruct-v0.1.Q3_K_L.gguf](https://huggingface.co/RichardErkhov/MaziyarPanahi_-_Calme-7B-Instruct-v0.1-gguf/blob/main/Calme-7B-Instruct-v0.1.Q3_K_L.gguf) | Q3_K_L | 3.56GB |
| [Calme-7B-Instruct-v0.1.IQ4_XS.gguf](https://huggingface.co/RichardErkhov/MaziyarPanahi_-_Calme-7B-Instruct-v0.1-gguf/blob/main/Calme-7B-Instruct-v0.1.IQ4_XS.gguf) | IQ4_XS | 3.67GB |
| [Calme-7B-Instruct-v0.1.Q4_0.gguf](https://huggingface.co/RichardErkhov/MaziyarPanahi_-_Calme-7B-Instruct-v0.1-gguf/blob/main/Calme-7B-Instruct-v0.1.Q4_0.gguf) | Q4_0 | 3.83GB |
| [Calme-7B-Instruct-v0.1.IQ4_NL.gguf](https://huggingface.co/RichardErkhov/MaziyarPanahi_-_Calme-7B-Instruct-v0.1-gguf/blob/main/Calme-7B-Instruct-v0.1.IQ4_NL.gguf) | IQ4_NL | 3.87GB |
| [Calme-7B-Instruct-v0.1.Q4_K_S.gguf](https://huggingface.co/RichardErkhov/MaziyarPanahi_-_Calme-7B-Instruct-v0.1-gguf/blob/main/Calme-7B-Instruct-v0.1.Q4_K_S.gguf) | Q4_K_S | 3.86GB |
| [Calme-7B-Instruct-v0.1.Q4_K.gguf](https://huggingface.co/RichardErkhov/MaziyarPanahi_-_Calme-7B-Instruct-v0.1-gguf/blob/main/Calme-7B-Instruct-v0.1.Q4_K.gguf) | Q4_K | 4.07GB |
| [Calme-7B-Instruct-v0.1.Q4_K_M.gguf](https://huggingface.co/RichardErkhov/MaziyarPanahi_-_Calme-7B-Instruct-v0.1-gguf/blob/main/Calme-7B-Instruct-v0.1.Q4_K_M.gguf) | Q4_K_M | 4.07GB |
| [Calme-7B-Instruct-v0.1.Q4_1.gguf](https://huggingface.co/RichardErkhov/MaziyarPanahi_-_Calme-7B-Instruct-v0.1-gguf/blob/main/Calme-7B-Instruct-v0.1.Q4_1.gguf) | Q4_1 | 4.24GB |
| [Calme-7B-Instruct-v0.1.Q5_0.gguf](https://huggingface.co/RichardErkhov/MaziyarPanahi_-_Calme-7B-Instruct-v0.1-gguf/blob/main/Calme-7B-Instruct-v0.1.Q5_0.gguf) | Q5_0 | 4.65GB |
| [Calme-7B-Instruct-v0.1.Q5_K_S.gguf](https://huggingface.co/RichardErkhov/MaziyarPanahi_-_Calme-7B-Instruct-v0.1-gguf/blob/main/Calme-7B-Instruct-v0.1.Q5_K_S.gguf) | Q5_K_S | 4.65GB |
| [Calme-7B-Instruct-v0.1.Q5_K.gguf](https://huggingface.co/RichardErkhov/MaziyarPanahi_-_Calme-7B-Instruct-v0.1-gguf/blob/main/Calme-7B-Instruct-v0.1.Q5_K.gguf) | Q5_K | 4.78GB |
| [Calme-7B-Instruct-v0.1.Q5_K_M.gguf](https://huggingface.co/RichardErkhov/MaziyarPanahi_-_Calme-7B-Instruct-v0.1-gguf/blob/main/Calme-7B-Instruct-v0.1.Q5_K_M.gguf) | Q5_K_M | 4.78GB |
| [Calme-7B-Instruct-v0.1.Q5_1.gguf](https://huggingface.co/RichardErkhov/MaziyarPanahi_-_Calme-7B-Instruct-v0.1-gguf/blob/main/Calme-7B-Instruct-v0.1.Q5_1.gguf) | Q5_1 | 5.07GB |
| [Calme-7B-Instruct-v0.1.Q6_K.gguf](https://huggingface.co/RichardErkhov/MaziyarPanahi_-_Calme-7B-Instruct-v0.1-gguf/blob/main/Calme-7B-Instruct-v0.1.Q6_K.gguf) | Q6_K | 5.53GB |
| [Calme-7B-Instruct-v0.1.Q8_0.gguf](https://huggingface.co/RichardErkhov/MaziyarPanahi_-_Calme-7B-Instruct-v0.1-gguf/blob/main/Calme-7B-Instruct-v0.1.Q8_0.gguf) | Q8_0 | 7.17GB |
Original model description:
---
license: apache-2.0
tags:
- generated_from_trainer
- mistral
- 7b
- calme
model-index:
- name: Calme-7B-Instruct-v0.1
results: []
datasets:
- ise-uiuc/Magicoder-Evol-Instruct-110K
model_name: Calme-7B-Instruct-v0.1
inference: false
model_creator: MaziyarPanahi
pipeline_tag: text-generation
quantized_by: MaziyarPanahi
---
<img src="https://cdn-uploads.huggingface.co/production/uploads/5fd5e18a90b6dc4633f6d292/LzEf6vvq2qIiys-q7l9Hq.webp" width="550" />
# MaziyarPanahi/Calme-7B-Instruct-v0.1
## Model Description
Calme-7B is a state-of-the-art language model with 7 billion parameters, fine-tuned over high-quality datasets on top of Mistral-7B. The Calme-7B models excel in generating text that resonates with clarity, calmness, and coherence.
### How to Use
```python
# Use a pipeline as a high-level helper
from transformers import pipeline
pipe = pipeline("text-generation", model="MaziyarPanahi/Calme-7B-Instruct-v0.1")
# Load model directly
from transformers import AutoTokenizer, AutoModelForCausalLM
tokenizer = AutoTokenizer.from_pretrained("MaziyarPanahi/Calme-7B-Instruct-v0.1")
model = AutoModelForCausalLM.from_pretrained("MaziyarPanahi/Calme-7B-Instruct-v0.1")
```
### Quantized Models
> I love how GGUF democratizes the use of Large Language Models (LLMs) on commodity hardware, more specifically, personal computers without any accelerated hardware. Because of this, I am committed to converting and quantizing any models I fine-tune to make them accessible to everyone!
- GGUF (2/3/4/5/6/8 bits): [MaziyarPanahi/Calme-7B-Instruct-v0.1-GGUF](https://huggingface.co/MaziyarPanahi/Calme-7B-Instruct-v0.1-GGUF)
## Examples
```
<s>[INST] You are a helpful, respectful and honest assistant. Always answer as helpfully as possible, while being safe. Your answers should not include any harmful, unethical, racist, sexist, toxic, dangerous, or illegal content. Please ensure that your responses are socially unbiased and positive in nature.
If a question does not make any sense, or is not factually coherent, explain why instead of answering something not correct. If you don't know the answer to a question, please don't share false information.
describe about pros and cons of docker system. [/INST]
```
<details>
<summary>Show me the response</summary>
```
Docker is an open-source platform for developing, shipping, and running applications using containers. It was first introduced in 2013 by Solomon Hykes. Here are some pros and cons of the Docker system:
Pros:
1. Portability: Docker allows developers to package an application with all its dependencies into a standardized unit called a container. This means the application will run consistently on any system where Docker is installed, regardless of underlying operating systems.
2. Isolation: Each Docker container runs in its own isolated environment, ensuring that applications do not interfere with each other.
3. Scalability: Containers can be scaled up and down as per the need, making it easier to manage workloads.
4. Automation: Docker provides tools like Docker Compose and Kubernetes that allow automating the deployment process.
5. Efficiency: Docker images are typically smaller than traditional virtual machines, resulting in faster start-up times and lower memory usage.
Cons:
1. Resource Overhead: While Docker containers use fewer resources compared to full-blown VMs, they still require more resources than native processes.
2. Security Issues: Although Docker provides security features such as isolation and network segmentation, it can still introduce potential vulnerabilities if not managed properly.
3. Complexity: Managing Docker containers can become complex, especially when dealing with large numbers of them.
4. Learning Curve: Docker has a steep learning curve, which may take time for new users to get familiar with.
5. Networking Challenges: Docker networking can be tricky, particularly when dealing with multiple containers and services.</s>
```
</details>
```
<s> [INST] There are three killers in a room. Someone enters the room and kills one of them. Nobody leaves the room. How many killers are left in the room? Explain your reasoning step by step. [/INST]
```
<details>
<summary>Show me the response</summary>
```
1. Initially, there are three killers in the room.
2. One of them is killed by someone who enters the room.
3. Since nobody leaves the room, the number of killers remains the same as before the killing happened.
4. Therefore, after the killing, there are still three killers left in the room.</s>
```
</details>
```
<s> [INST] Mark is faster than Mary, Mary is faster than Joe. Is Joe faster than Mark? Let's think step by step [/INST]
```
<details>
<summary>Show me the response</summary>
```
1) Mark is faster than Mary.
2) Mary is faster than Joe.
Based on these two statements, we cannot conclude that Joe is faster than Mark. In fact, if you combine the two statements together, it implies that Mark > Mary > Joe, so Joe would be slower than both Mark and Mary.</s>
```
</details>
```
<s> [INST] If I lay 2 wet shirts out in the sun flat on the ground to dry and it takes 5 hours until they are dry, how long would it take to dry 4 shirts of the same type that way simultanously? [/INST]
```
<details>
<summary>Show me the response</summary>
```
If you're laying out 2 shirts at a time, you're essentially using half the drying capacity of the sun. Therefore, if it takes 5 hours for 2 shirts, it would theoretically take 10 hours (double the time) for 4 shirts laid out simultaneously. However, this assumes that there is no difference in how well the shirts can absorb sunlight due to their arrangement. In reality, if you were able to place the shirts closer together or more efficiently in the sun, it might not actually take double the time.</s>
```
</details>
```
<s> [INST] explain step by step 25-4*2+3=? [/INST]
```
<details>
<summary>Show me the response</summary>
```
1. The multiplication operation is done first, so we calculate 4 * 2 = 8.
2. Now the expression becomes 25 - 8 + 3.
3. Then perform subtraction, so 25 - 8 = 17.
4. Finally, add 3 to the result from step 3, so 17 + 3 = 20.
Therefore, 25-4*2+3 equals 20.</s>
```
</details>
**Multilingual:**
```
<s> [INST] Vous êtes un assistant utile, respectueux et honnête. Répondez toujours de la manière la plus utile possible, tout en étant sûr. Vos réponses ne doivent inclure aucun contenu nuisible, contraire à l'éthique, raciste, sexiste, toxique, dangereux ou illégal. Assurez-vous que vos réponses sont socialement impartiales et de nature positive.
Si une question n'a pas de sens ou n'est pas cohérente d'un point de vue factuel, expliquez pourquoi au lieu de répondre quelque chose d'incorrect. Si vous ne connaissez pas la réponse à une question, veuillez ne pas partager de fausses informations.
Décrivez les avantages et les inconvénients du système Docker.[/INST]
```
<details>
<summary>Show me the response</summary>
```
Avantages:
1. Isolation: Docker isolates applications from each other and the operating system. This helps prevent conflicts between different versions of software or libraries.
2. Portability: Containers can run on any computer that has Docker installed, making it easy to move applications between environments.
3. Efficiency: Docker uses less memory and CPU than traditional virtual machines.
4. Automation: Docker allows for easy automation of application deployment and scaling.
5. Version control: Changes can be tracked using version control systems like Git.
Inconvénients:
1. Complexité: Setting up and managing Docker containers can be complex, especially for those new to containerization technology.
2. Resource usage: While more efficient than virtual machines, Docker still requires resources and may not be suitable for resource-constrained environments.
3. Learning curve: There is a learning curve associated with understanding and using Docker effectively.
4. Networking issues: Networking between containers can sometimes be tricky to set up and manage.
5. Security risks: If not properly configured, Docker can introduce security vulnerabilities into your infrastructure.</s>
```
</details>
```
<s> [INST] You are a helpful, respectful and honest assistant. Always answer as helpfully as possible, while being safe. Your answers should not include any harmful, unethical, racist, sexist, toxic, dangerous, or illegal content. Please ensure that your responses are socially unbiased and positive in nature.
Answer any question in Russian.
describe about pros and cons of docker system.[/INST]
```
<details>
<summary>Show me the response</summary>
```
Про и кон системы Docker:
Про:
1. Виртуализация на уровне операционной системы.
2. Возможность создания изолированных окружений для разработки, тестирования и развертывания приложений.
3. Ускорение процесса развертывания и отслеживания изменений.
4. Повышение безопасности за счет изоляции приложений.
5. Эффективное использование ресурсов.
6. Совместимость с различными языками программирования и платформами.
7. Наличие большого сообщества пользователей и разработчиков.
Кон:
1. Некоторые функции могут быть сложны в настройке и управлении.
2. Требует дополнительного пространства на диске для хранения образов контейнеров.
3. Использование Docker может повлиять на производительность системы.
4. Из-за высокой изоляции, может возникнуть сложность в отладке.
5. Некоторые пользователи могут испытывать трудности с пониманием концепций Docker.
6. Вопросы безопасности могут возникать, если не корректно настроены сетевые связи.
7. Зависимость от интернета, так как некоторые команды работают только в онлайн режиме.
Однако, несмотря на это, Docker является популярным инструментом в мире IT благодаря его гибкости, возможностям и преимуществам, которые очень важны для современного разработчика.</s>
```
</details>
```
<s>[INST] Ви - корисний, поважний та чесний помічник. Завжди відповідайте максимально корисно, будучи безпечним. Ваші відповіді не повинні містити шкідливого, неетичного, расистського, сексистського, токсичного, небезпечного або нелегального контенту. Будь ласка, переконайтеся, що ваші відповіді соціально неупереджені та мають позитивний характер.
Якщо питання не має сенсу або не є фактично послідовним, поясніть чому, замість того, щоб відповідати щось некоректне. Якщо ви не знаєте відповіді на питання, будь ласка, не діліться неправдивою інформацією.
Опис про переваги та недоліки системи Docker.[/INST]
```
<details>
<summary>Show me the response</summary>
```
Для кращого розуміння, давайте розглянемо Docker як віртуальну машину для програмного забезпечення.
Переваги Docker:
1. Контейнеризація: Docker використовує контейнеризацію, що дозволяє створювати унікальні екології для кожної програми, що зберігається в одному місці. Це означає, що програма може бути виконана незалежно від навколишнього середовища.
2. Абстрагування: Docker об'єднує різноманітні технології, такі як операційні системи, мережу, послуги, і т. д., в один простий пакет.
3. Швидке розгортання: З використанням Docker, можна легко створювати та деплоювати нових версій програм, що значно прискорює процес розробки.
4. Контроль версій: Docker дозволяє легко керувати різними версіями свого програмного забезпечення.
5. Можливість автоматизації: З Docker, можна автоматизувати весь процес розгортання за допомогою інструментів CI/CD.
Недоліки Docker:
1. Процесорний ресурс: Docker може споживати більше процесорних ресурсів ніж традиційні віртуальні машини.
2. Відсутність повної ізоляції: Хоча Docker забезпечує абстракцію, він все ще не повністю ізолює контейнери.
3. Дорожчість: У порівнянні зі стандартною віртуалізацією, Docker може бути дорожче.
4. Налаштування: Початковці можуть стикатися з труднощами під час налаштування Docker.
5. Необхідність постійного оновлення: Docker вимагає постійного оновлення, що може потребувати час і ресурси.
В цілому, Docker є дуже корисним інструментом для розробників і адміністраторів, але його не слід застосовувати у всіх випадках, оскільки він має свої особливості та обмеження.</s>
```
</details>
|
google/pegasus-cnn_dailymail | google | 2023-01-24T16:42:26Z | 12,496 | 69 | transformers | [
"transformers",
"pytorch",
"rust",
"pegasus",
"text2text-generation",
"summarization",
"en",
"arxiv:1912.08777",
"autotrain_compatible",
"endpoints_compatible",
"region:us"
]
| summarization | 2022-03-02T23:29:05Z | ---
language: en
tags:
- summarization
---
### Pegasus Models
See Docs: [here](https://huggingface.co/transformers/master/model_doc/pegasus.html)
Original TF 1 code [here](https://github.com/google-research/pegasus)
Authors: Jingqing Zhang, Yao Zhao, Mohammad Saleh and Peter J. Liu on Dec 18, 2019
Maintained by: [@sshleifer](https://twitter.com/sam_shleifer)
Task: Summarization
The following is copied from the authors' README.
# Mixed & Stochastic Checkpoints
We train a pegasus model with sampled gap sentence ratios on both C4 and HugeNews, and stochastically sample important sentences. The updated the results are reported in this table.
| dataset | C4 | HugeNews | Mixed & Stochastic|
| ---- | ---- | ---- | ----|
| xsum | 45.20/22.06/36.99 | 47.21/24.56/39.25 | 47.60/24.83/39.64|
| cnn_dailymail | 43.90/21.20/40.76 | 44.17/21.47/41.11 | 44.16/21.56/41.30|
| newsroom | 45.07/33.39/41.28 | 45.15/33.51/41.33 | 45.98/34.20/42.18|
| multi_news | 46.74/17.95/24.26 | 47.52/18.72/24.91 | 47.65/18.75/24.95|
| gigaword | 38.75/19.96/36.14 | 39.12/19.86/36.24 | 39.65/20.47/36.76|
| wikihow | 43.07/19.70/34.79 | 41.35/18.51/33.42 | 46.39/22.12/38.41 *|
| reddit_tifu | 26.54/8.94/21.64 | 26.63/9.01/21.60 | 27.99/9.81/22.94|
| big_patent | 53.63/33.16/42.25 | 53.41/32.89/42.07 | 52.29/33.08/41.66 *|
| arxiv | 44.70/17.27/25.80 | 44.67/17.18/25.73 | 44.21/16.95/25.67|
| pubmed | 45.49/19.90/27.69 | 45.09/19.56/27.42 | 45.97/20.15/28.25|
| aeslc | 37.69/21.85/36.84 | 37.40/21.22/36.45 | 37.68/21.25/36.51|
| billsum | 57.20/39.56/45.80 | 57.31/40.19/45.82 | 59.67/41.58/47.59|
The "Mixed & Stochastic" model has the following changes:
- trained on both C4 and HugeNews (dataset mixture is weighted by their number of examples).
- trained for 1.5M instead of 500k (we observe slower convergence on pretraining perplexity).
- the model uniformly sample a gap sentence ratio between 15% and 45%.
- importance sentences are sampled using a 20% uniform noise to importance scores.
- the sentencepiece tokenizer is updated to be able to encode newline character.
(*) the numbers of wikihow and big_patent datasets are not comparable because of change in tokenization and data:
- wikihow dataset contains newline characters which is useful for paragraph segmentation, the C4 and HugeNews model's sentencepiece tokenizer doesn't encode newline and loose this information.
- we update the BigPatent dataset to preserve casing, some format cleanings are also changed, please refer to change in TFDS.
The "Mixed & Stochastic" model has the following changes (from pegasus-large in the paper):
trained on both C4 and HugeNews (dataset mixture is weighted by their number of examples).
trained for 1.5M instead of 500k (we observe slower convergence on pretraining perplexity).
the model uniformly sample a gap sentence ratio between 15% and 45%.
importance sentences are sampled using a 20% uniform noise to importance scores.
the sentencepiece tokenizer is updated to be able to encode newline character.
Citation
```
@misc{zhang2019pegasus,
title={PEGASUS: Pre-training with Extracted Gap-sentences for Abstractive Summarization},
author={Jingqing Zhang and Yao Zhao and Mohammad Saleh and Peter J. Liu},
year={2019},
eprint={1912.08777},
archivePrefix={arXiv},
primaryClass={cs.CL}
}
``` |
google/vivit-b-16x2-kinetics400 | google | 2023-08-03T10:01:22Z | 12,488 | 14 | transformers | [
"transformers",
"pytorch",
"vivit",
"vision",
"video-classification",
"arxiv:2103.15691",
"license:mit",
"endpoints_compatible",
"region:us"
]
| video-classification | 2022-11-23T21:21:55Z | ---
license: "mit"
tags:
- vision
- video-classification
---
# ViViT (Video Vision Transformer)
ViViT model as introduced in the paper [ViViT: A Video Vision Transformer](https://arxiv.org/abs/2103.15691) by Arnab et al. and first released in [this repository](https://github.com/google-research/scenic/tree/main/scenic/projects/vivit).
Disclaimer: The team releasing ViViT did not write a model card for this model so this model card has been written by the Hugging Face team.
## Model description
ViViT is an extension of the [Vision Transformer (ViT)](https://huggingface.co/docs/transformers/v4.27.0/model_doc/vit) to video.
We refer to the paper for details.
## Intended uses & limitations
The model is mostly meant to intended to be fine-tuned on a downstream task, like video classification. See the [model hub](https://huggingface.co/models?filter=vivit) to look for fine-tuned versions on a task that interests you.
### How to use
For code examples, we refer to the [documentation](https://huggingface.co/transformers/main/model_doc/vivit).
### BibTeX entry and citation info
```bibtex
@misc{arnab2021vivit,
title={ViViT: A Video Vision Transformer},
author={Anurag Arnab and Mostafa Dehghani and Georg Heigold and Chen Sun and Mario Lučić and Cordelia Schmid},
year={2021},
eprint={2103.15691},
archivePrefix={arXiv},
primaryClass={cs.CV}
}
``` |
gaianet/llm-compiler-13b-GGUF | gaianet | 2024-06-29T09:35:38Z | 12,484 | 0 | transformers | [
"transformers",
"gguf",
"llama",
"text-generation",
"code",
"base_model:facebook/llm-compiler-13b",
"license:other",
"autotrain_compatible",
"text-generation-inference",
"region:us"
]
| text-generation | 2024-06-29T09:06:42Z | ---
language:
- code
license: other
model_name: llm-compiler-13b
base_model: facebook/llm-compiler-13b
inference: false
model_creator: facebook
quantized_by: Second State Inc.
---

# llm-compiler-13b-GGUF
## Original Model
[facebook/llm-compiler-13b](https://huggingface.co/facebook/llm-compiler-13b)
**License** A custom commercial license is available at: [https://ai.meta.com/resources/models-and-libraries/llama-downloads/](https://ai.meta.com/resources/models-and-libraries/llama-downloads/)
## Run with Gaianet
(coming soon)
<!-- **Prompt template:**
prompt template: `gemma-instruct`
**Context size:**
chat_ctx_size: `8192` -->
**Run with GaiaNet:**
- Quick start: https://docs.gaianet.ai/node-guide/quick-start
- Customize your node: https://docs.gaianet.ai/node-guide/customize
*Quantized with llama.cpp b3259*
|
yuridrcosta/nees-bert-base-portuguese-cased-finetuned-ner | yuridrcosta | 2024-05-02T01:20:30Z | 12,483 | 0 | transformers | [
"transformers",
"tensorboard",
"safetensors",
"bert",
"token-classification",
"generated_from_trainer",
"base_model:neuralmind/bert-base-portuguese-cased",
"license:mit",
"autotrain_compatible",
"endpoints_compatible",
"region:us"
]
| token-classification | 2024-02-27T00:49:22Z | ---
license: mit
base_model: neuralmind/bert-base-portuguese-cased
tags:
- generated_from_trainer
metrics:
- precision
- recall
- f1
- accuracy
model-index:
- name: nees-bert-base-portuguese-cased-finetuned-ner
results: []
---
<!-- This model card has been generated automatically according to the information the Trainer had access to. You
should probably proofread and complete it, then remove this comment. -->
# nees-bert-base-portuguese-cased-finetuned-ner
This model is a fine-tuned version of [neuralmind/bert-base-portuguese-cased](https://huggingface.co/neuralmind/bert-base-portuguese-cased) on an unknown dataset.
It achieves the following results on the evaluation set:
- Loss: 0.0008
- Precision: 0.6804
- Recall: 0.9301
- F1: 0.7859
- Accuracy: 0.9997
## Model description
More information needed
## Intended uses & limitations
More information needed
## Training and evaluation data
More information needed
## Training procedure
### Training hyperparameters
The following hyperparameters were used during training:
- learning_rate: 2e-05
- train_batch_size: 16
- eval_batch_size: 16
- seed: 42
- optimizer: Adam with betas=(0.9,0.999) and epsilon=1e-08
- lr_scheduler_type: linear
- num_epochs: 1
### Training results
| Training Loss | Epoch | Step | Validation Loss | Precision | Recall | F1 | Accuracy |
|:-------------:|:-----:|:----:|:---------------:|:---------:|:------:|:------:|:--------:|
| 0.0009 | 1.0 | 3534 | 0.0008 | 0.6804 | 0.9301 | 0.7859 | 0.9997 |
### Framework versions
- Transformers 4.40.1
- Pytorch 2.3.0+cu121
- Datasets 2.19.0
- Tokenizers 0.19.1
|
Habana/bert-large-uncased-whole-word-masking | Habana | 2023-09-08T16:18:32Z | 12,459 | 0 | null | [
"optimum_habana",
"license:apache-2.0",
"region:us"
]
| null | 2022-04-22T18:04:29Z | ---
license: apache-2.0
---
[Optimum Habana](https://github.com/huggingface/optimum-habana) is the interface between the Hugging Face Transformers and Diffusers libraries and Habana's Gaudi processor (HPU).
It provides a set of tools enabling easy and fast model loading, training and inference on single- and multi-HPU settings for different downstream tasks.
Learn more about how to take advantage of the power of Habana HPUs to train and deploy Transformers and Diffusers models at [hf.co/hardware/habana](https://huggingface.co/hardware/habana).
## BERT Large model HPU configuration
This model only contains the `GaudiConfig` file for running the [bert-large-uncased-whole-word-masking](https://huggingface.co/bert-large-uncased-whole-word-masking) model on Habana's Gaudi processors (HPU).
**This model contains no model weights, only a GaudiConfig.**
This enables to specify:
- `use_fused_adam`: whether to use Habana's custom AdamW implementation
- `use_fused_clip_norm`: whether to use Habana's fused gradient norm clipping operator
- `use_torch_autocast`: whether to use Torch Autocast for managing mixed precision
## Usage
The model is instantiated the same way as in the Transformers library.
The only difference is that there are a few new training arguments specific to HPUs.\
It is strongly recommended to train this model doing bf16 mixed-precision training for optimal performance and accuracy.
[Here](https://github.com/huggingface/optimum-habana/blob/main/examples/question-answering/run_qa.py) is a question-answering example script to fine-tune a model on SQuAD. You can run it with BERT Large with the following command:
```bash
python run_qa.py \
--model_name_or_path bert-large-uncased-whole-word-masking \
--gaudi_config_name gaudi_config_name_or_path \
--dataset_name squad \
--do_train \
--do_eval \
--per_device_train_batch_size 24 \
--per_device_eval_batch_size 8 \
--learning_rate 3e-5 \
--num_train_epochs 2 \
--max_seq_length 384 \
--doc_stride 128 \
--output_dir /tmp/squad/ \
--use_habana \
--use_lazy_mode \
--throughput_warmup_steps 3 \
--bf16
```
Check the [documentation](https://huggingface.co/docs/optimum/habana/index) out for more advanced usage and examples.
|
Kyle1668/boss-toxicity-bert-base-uncased | Kyle1668 | 2024-02-07T18:41:05Z | 12,453 | 0 | transformers | [
"transformers",
"pytorch",
"safetensors",
"bert",
"text-classification",
"autotrain_compatible",
"endpoints_compatible",
"region:us"
]
| text-classification | 2023-08-08T16:46:35Z | Entry not found |
google/bert_uncased_L-12_H-768_A-12 | google | 2021-05-19T17:27:43Z | 12,449 | 11 | transformers | [
"transformers",
"pytorch",
"jax",
"bert",
"arxiv:1908.08962",
"license:apache-2.0",
"endpoints_compatible",
"region:us"
]
| null | 2022-03-02T23:29:05Z | ---
thumbnail: https://huggingface.co/front/thumbnails/google.png
license: apache-2.0
---
BERT Miniatures
===
This is the set of 24 BERT models referenced in [Well-Read Students Learn Better: On the Importance of Pre-training Compact Models](https://arxiv.org/abs/1908.08962) (English only, uncased, trained with WordPiece masking).
We have shown that the standard BERT recipe (including model architecture and training objective) is effective on a wide range of model sizes, beyond BERT-Base and BERT-Large. The smaller BERT models are intended for environments with restricted computational resources. They can be fine-tuned in the same manner as the original BERT models. However, they are most effective in the context of knowledge distillation, where the fine-tuning labels are produced by a larger and more accurate teacher.
Our goal is to enable research in institutions with fewer computational resources and encourage the community to seek directions of innovation alternative to increasing model capacity.
You can download the 24 BERT miniatures either from the [official BERT Github page](https://github.com/google-research/bert/), or via HuggingFace from the links below:
| |H=128|H=256|H=512|H=768|
|---|:---:|:---:|:---:|:---:|
| **L=2** |[**2/128 (BERT-Tiny)**][2_128]|[2/256][2_256]|[2/512][2_512]|[2/768][2_768]|
| **L=4** |[4/128][4_128]|[**4/256 (BERT-Mini)**][4_256]|[**4/512 (BERT-Small)**][4_512]|[4/768][4_768]|
| **L=6** |[6/128][6_128]|[6/256][6_256]|[6/512][6_512]|[6/768][6_768]|
| **L=8** |[8/128][8_128]|[8/256][8_256]|[**8/512 (BERT-Medium)**][8_512]|[8/768][8_768]|
| **L=10** |[10/128][10_128]|[10/256][10_256]|[10/512][10_512]|[10/768][10_768]|
| **L=12** |[12/128][12_128]|[12/256][12_256]|[12/512][12_512]|[**12/768 (BERT-Base)**][12_768]|
Note that the BERT-Base model in this release is included for completeness only; it was re-trained under the same regime as the original model.
Here are the corresponding GLUE scores on the test set:
|Model|Score|CoLA|SST-2|MRPC|STS-B|QQP|MNLI-m|MNLI-mm|QNLI(v2)|RTE|WNLI|AX|
|---|:---:|:---:|:---:|:---:|:---:|:---:|:---:|:---:|:---:|:---:|:---:|:---:|
|BERT-Tiny|64.2|0.0|83.2|81.1/71.1|74.3/73.6|62.2/83.4|70.2|70.3|81.5|57.2|62.3|21.0|
|BERT-Mini|65.8|0.0|85.9|81.1/71.8|75.4/73.3|66.4/86.2|74.8|74.3|84.1|57.9|62.3|26.1|
|BERT-Small|71.2|27.8|89.7|83.4/76.2|78.8/77.0|68.1/87.0|77.6|77.0|86.4|61.8|62.3|28.6|
|BERT-Medium|73.5|38.0|89.6|86.6/81.6|80.4/78.4|69.6/87.9|80.0|79.1|87.7|62.2|62.3|30.5|
For each task, we selected the best fine-tuning hyperparameters from the lists below, and trained for 4 epochs:
- batch sizes: 8, 16, 32, 64, 128
- learning rates: 3e-4, 1e-4, 5e-5, 3e-5
If you use these models, please cite the following paper:
```
@article{turc2019,
title={Well-Read Students Learn Better: On the Importance of Pre-training Compact Models},
author={Turc, Iulia and Chang, Ming-Wei and Lee, Kenton and Toutanova, Kristina},
journal={arXiv preprint arXiv:1908.08962v2 },
year={2019}
}
```
[2_128]: https://huggingface.co/google/bert_uncased_L-2_H-128_A-2
[2_256]: https://huggingface.co/google/bert_uncased_L-2_H-256_A-4
[2_512]: https://huggingface.co/google/bert_uncased_L-2_H-512_A-8
[2_768]: https://huggingface.co/google/bert_uncased_L-2_H-768_A-12
[4_128]: https://huggingface.co/google/bert_uncased_L-4_H-128_A-2
[4_256]: https://huggingface.co/google/bert_uncased_L-4_H-256_A-4
[4_512]: https://huggingface.co/google/bert_uncased_L-4_H-512_A-8
[4_768]: https://huggingface.co/google/bert_uncased_L-4_H-768_A-12
[6_128]: https://huggingface.co/google/bert_uncased_L-6_H-128_A-2
[6_256]: https://huggingface.co/google/bert_uncased_L-6_H-256_A-4
[6_512]: https://huggingface.co/google/bert_uncased_L-6_H-512_A-8
[6_768]: https://huggingface.co/google/bert_uncased_L-6_H-768_A-12
[8_128]: https://huggingface.co/google/bert_uncased_L-8_H-128_A-2
[8_256]: https://huggingface.co/google/bert_uncased_L-8_H-256_A-4
[8_512]: https://huggingface.co/google/bert_uncased_L-8_H-512_A-8
[8_768]: https://huggingface.co/google/bert_uncased_L-8_H-768_A-12
[10_128]: https://huggingface.co/google/bert_uncased_L-10_H-128_A-2
[10_256]: https://huggingface.co/google/bert_uncased_L-10_H-256_A-4
[10_512]: https://huggingface.co/google/bert_uncased_L-10_H-512_A-8
[10_768]: https://huggingface.co/google/bert_uncased_L-10_H-768_A-12
[12_128]: https://huggingface.co/google/bert_uncased_L-12_H-128_A-2
[12_256]: https://huggingface.co/google/bert_uncased_L-12_H-256_A-4
[12_512]: https://huggingface.co/google/bert_uncased_L-12_H-512_A-8
[12_768]: https://huggingface.co/google/bert_uncased_L-12_H-768_A-12
|
espnet/voxcelebs12_rawnet3 | espnet | 2024-05-31T17:46:05Z | 12,446 | 3 | espnet | [
"espnet",
"audio",
"speaker-recognition",
"multilingual",
"dataset:voxceleb",
"license:cc-by-4.0",
"region:us"
]
| null | 2023-11-21T18:26:21Z | ---
tags:
- espnet
- audio
- speaker-recognition
language: multilingual
datasets:
- voxceleb
license: cc-by-4.0
---
## ESPnet2 SPK model
### `espnet/voxcelebs12_rawnet3`
This model was trained by Jungjee using voxceleb recipe in [espnet](https://github.com/espnet/espnet/).
### Demo: How to use in ESPnet2
Follow the [ESPnet installation instructions](https://espnet.github.io/espnet/installation.html)
if you haven't done that already.
```bash
cd espnet
git checkout 0c489a83607efb8e21331a9f01df21aac58c2a88
pip install -e .
cd egs2/voxceleb/spk1
./run.sh --skip_data_prep false --skip_train true --download_model espnet/voxcelebs12_rawnet3
```
```python
import numpy as np
from espnet2.bin.spk_inference import Speech2Embedding
# from uploaded models
speech2spk_embed = Speech2Embedding.from_pretrained(model_tag="espnet/voxcelebs12_rawnet3")
embedding = speech2spk_embed(np.zeros(16500))
# from checkpoints trained by oneself
speech2spk_embed = Speech2Embedding(model_file="model.pth", train_config="config.yaml")
embedding = speech2spk_embed(np.zeros(32000))
```
<!-- Generated by scripts/utils/show_spk_result.py -->
# RESULTS
## Environments
date: 2023-11-21 12:43:27.293418
- python version: \`3.9.16 (main, Mar 8 2023, 14:00:05) [GCC 11.2.0]\`
- espnet version: \`espnet 202310\`
- pytorch version: \`pytorch 2.0.1\`
| | Mean | Std |
|---|---|---|
| Target | -0.8015 | 0.1383 |
| Non-target | 0.0836 | 0.0836 |
| | EER(\%) | minDCF |
|---|---|---|
| | 0.739 | 0.05818 |
## SPK config
<details><summary>expand</summary>
```
config: conf/tuning/train_rawnet3_best_trnVox12_emb192_amp_subcentertopk.yaml
print_config: false
log_level: INFO
drop_last_iter: true
dry_run: false
iterator_type: category
valid_iterator_type: sequence
output_dir: exp/spk_train_rawnet3_best_trnVox12_emb192_amp_subcentertopk_raw_sp
ngpu: 1
seed: 0
num_workers: 6
num_att_plot: 0
dist_backend: nccl
dist_init_method: env://
dist_world_size: 4
dist_rank: 0
local_rank: 0
dist_master_addr: localhost
dist_master_port: 56599
dist_launcher: null
multiprocessing_distributed: true
unused_parameters: false
sharded_ddp: false
cudnn_enabled: true
cudnn_benchmark: true
cudnn_deterministic: false
collect_stats: false
write_collected_feats: false
max_epoch: 40
patience: null
val_scheduler_criterion:
- valid
- loss
early_stopping_criterion:
- valid
- loss
- min
best_model_criterion:
- - valid
- eer
- min
keep_nbest_models: 3
nbest_averaging_interval: 0
grad_clip: 9999
grad_clip_type: 2.0
grad_noise: false
accum_grad: 1
no_forward_run: false
resume: true
train_dtype: float32
use_amp: true
log_interval: 100
use_matplotlib: true
use_tensorboard: true
create_graph_in_tensorboard: false
use_wandb: false
wandb_project: null
wandb_id: null
wandb_entity: null
wandb_name: null
wandb_model_log_interval: -1
detect_anomaly: false
pretrain_path: null
init_param: []
ignore_init_mismatch: false
freeze_param: []
num_iters_per_epoch: null
batch_size: 512
valid_batch_size: 40
batch_bins: 1000000
valid_batch_bins: null
train_shape_file:
- exp/spk_stats_16k_sp/train/speech_shape
valid_shape_file:
- exp/spk_stats_16k_sp/valid/speech_shape
batch_type: folded
valid_batch_type: null
fold_length:
- 120000
sort_in_batch: descending
shuffle_within_batch: false
sort_batch: descending
multiple_iterator: false
chunk_length: 500
chunk_shift_ratio: 0.5
num_cache_chunks: 1024
chunk_excluded_key_prefixes: []
train_data_path_and_name_and_type:
- - dump/raw/voxceleb12_devs_sp/wav.scp
- speech
- sound
- - dump/raw/voxceleb12_devs_sp/utt2spk
- spk_labels
- text
valid_data_path_and_name_and_type:
- - dump/raw/voxceleb1_test/trial.scp
- speech
- sound
- - dump/raw/voxceleb1_test/trial2.scp
- speech2
- sound
- - dump/raw/voxceleb1_test/trial_label
- spk_labels
- text
allow_variable_data_keys: false
max_cache_size: 0.0
max_cache_fd: 32
valid_max_cache_size: null
exclude_weight_decay: false
exclude_weight_decay_conf: {}
optim: adam
optim_conf:
lr: 0.001
weight_decay: 5.0e-05
amsgrad: false
scheduler: cosineannealingwarmuprestarts
scheduler_conf:
first_cycle_steps: 71280
cycle_mult: 1.0
max_lr: 0.001
min_lr: 5.0e-06
warmup_steps: 1000
gamma: 0.75
init: null
use_preprocessor: true
input_size: null
target_duration: 3.0
spk2utt: dump/raw/voxceleb12_devs_sp/spk2utt
spk_num: 21615
sample_rate: 16000
num_eval: 10
rir_scp: ''
model_conf:
extract_feats_in_collect_stats: false
frontend: asteroid_frontend
frontend_conf:
sinc_stride: 16
sinc_kernel_size: 251
sinc_filters: 256
preemph_coef: 0.97
log_term: 1.0e-06
specaug: null
specaug_conf: {}
normalize: null
normalize_conf: {}
encoder: rawnet3
encoder_conf:
model_scale: 8
ndim: 1024
output_size: 1536
pooling: chn_attn_stat
pooling_conf: {}
projector: rawnet3
projector_conf:
output_size: 192
preprocessor: spk
preprocessor_conf:
target_duration: 3.0
sample_rate: 16000
num_eval: 5
noise_apply_prob: 0.5
noise_info:
- - 1.0
- dump/raw/musan_speech.scp
- - 4
- 7
- - 13
- 20
- - 1.0
- dump/raw/musan_noise.scp
- - 1
- 1
- - 0
- 15
- - 1.0
- dump/raw/musan_music.scp
- - 1
- 1
- - 5
- 15
rir_apply_prob: 0.5
rir_scp: dump/raw/rirs.scp
loss: aamsoftmax_sc_topk
loss_conf:
margin: 0.3
scale: 30
K: 3
mp: 0.06
k_top: 5
required:
- output_dir
version: '202308'
distributed: true
```
</details>
### Citing
```BibTex
@article{jung2024espnet,
title={ESPnet-SPK: full pipeline speaker embedding toolkit with reproducible recipes, self-supervised front-ends, and off-the-shelf models},
author={Jung, Jee-weon and Zhang, Wangyou and Shi, Jiatong and Aldeneh, Zakaria and Higuchi, Takuya and Theobald, Barry-John and Abdelaziz, Ahmed Hussen and Watanabe, Shinji},
journal={arXiv preprint arXiv:2401.17230},
year={2024}
}
@article{jung2022pushing,
title={Pushing the limits of raw waveform speaker recognition},
author={Jung, Jee-weon and Kim, You Jin and Heo, Hee-Soo and Lee, Bong-Jin and Kwon, Youngki and Chung, Joon Son},
journal={Proc. Interspeech},
year={2022}
}
@inproceedings{watanabe2018espnet,
author={Shinji Watanabe and Takaaki Hori and Shigeki Karita and Tomoki Hayashi and Jiro Nishitoba and Yuya Unno and Nelson Yalta and Jahn Heymann and Matthew Wiesner and Nanxin Chen and Adithya Renduchintala and Tsubasa Ochiai},
title={{ESPnet}: End-to-End Speech Processing Toolkit},
year={2018},
booktitle={Proc. Interspeech},
pages={2207--2211},
doi={10.21437/Interspeech.2018-1456},
url={http://dx.doi.org/10.21437/Interspeech.2018-1456}
}
```
|
TIGER-Lab/MAmmoTH2-8B-Plus | TIGER-Lab | 2024-05-22T03:11:32Z | 12,446 | 19 | transformers | [
"transformers",
"safetensors",
"llama",
"text-generation",
"conversational",
"en",
"dataset:TIGER-Lab/WebInstructSub",
"arxiv:2405.03548",
"license:mit",
"autotrain_compatible",
"endpoints_compatible",
"text-generation-inference",
"region:us"
]
| text-generation | 2024-05-06T07:53:16Z | ---
license: mit
language:
- en
datasets:
- TIGER-Lab/WebInstructSub
metrics:
- accuracy
library_name: transformers
---
# 🦣 MAmmoTH2: Scaling Instructions from the Web
Project Page: [https://tiger-ai-lab.github.io/MAmmoTH2/](https://tiger-ai-lab.github.io/MAmmoTH2/)
Paper: [https://arxiv.org/pdf/2405.03548](https://arxiv.org/pdf/2405.03548)
Code: [https://github.com/TIGER-AI-Lab/MAmmoTH2](https://github.com/TIGER-AI-Lab/MAmmoTH2)
## Introduction
Introducing 🦣 MAmmoTH2, a game-changer in improving the reasoning abilities of large language models (LLMs) through innovative instruction tuning. By efficiently harvesting 10 million instruction-response pairs from the pre-training web corpus, we've developed MAmmoTH2 models that significantly boost performance on reasoning benchmarks. For instance, MAmmoTH2-7B (Mistral) sees its performance soar from 11% to 36.7% on MATH and from 36% to 68.4% on GSM8K, all without training on any domain-specific data. Further training on public instruction tuning datasets yields MAmmoTH2-Plus, setting new standards in reasoning and chatbot benchmarks. Our work presents a cost-effective approach to acquiring large-scale, high-quality instruction data, offering a fresh perspective on enhancing LLM reasoning abilities.
| | **Base Model** | **MAmmoTH2** | **MAmmoTH2-Plus** |
|:-----|:---------------------|:-------------------------------------------------------------------|:------------------------------------------------------------------|
| 7B | Mistral | 🦣 [MAmmoTH2-7B](https://huggingface.co/TIGER-Lab/MAmmoTH2-7B) | 🦣 [MAmmoTH2-7B-Plus](https://huggingface.co/TIGER-Lab/MAmmoTH2-7B-Plus) |
| 8B | Llama-3 | 🦣 [MAmmoTH2-8B](https://huggingface.co/TIGER-Lab/MAmmoTH2-8B) | 🦣 [MAmmoTH2-8B-Plus](https://huggingface.co/TIGER-Lab/MAmmoTH2-8B-Plus) |
| 8x7B | Mixtral | 🦣 [MAmmoTH2-8x7B](https://huggingface.co/TIGER-Lab/MAmmoTH2-8x7B) | 🦣 [MAmmoTH2-8x7B-Plus](https://huggingface.co/TIGER-Lab/MAmmoTH2-8x7B-Plus) |
## Training Data
Please refer to https://huggingface.co/datasets/TIGER-Lab/WebInstructSub for more details.

## Training Procedure
The models are fine-tuned with the WEBINSTRUCT dataset using the original Llama-3, Mistral and Mistal models as base models. The training procedure varies for different models based on their sizes. Check out our paper for more details.
## Evaluation
The models are evaluated using open-ended and multiple-choice math problems from several datasets. Here are the results:
| **Model** | **TheoremQA** | **MATH** | **GSM8K** | **GPQA** | **MMLU-ST** | **BBH** | **ARC-C** | **Avg** |
|:---------------------------------------|:--------------|:---------|:----------|:---------|:------------|:--------|:----------|:--------|
| **MAmmoTH2-7B** (Updated) | 29.0 | 36.7 | 68.4 | 32.4 | 62.4 | 58.6 | 81.7 | 52.7 |
| **MAmmoTH2-8B** (Updated) | 30.3 | 35.8 | 70.4 | 35.2 | 64.2 | 62.1 | 82.2 | 54.3 |
| **MAmmoTH2-8x7B** | 32.2 | 39.0 | 75.4 | 36.8 | 67.4 | 71.1 | 87.5 | 58.9 |
| **MAmmoTH2-7B-Plus** (Updated) | 31.2 | 46.0 | 84.6 | 33.8 | 63.8 | 63.3 | 84.4 | 58.1 |
| **MAmmoTH2-8B-Plus** (Updated) | 31.5 | 43.0 | 85.2 | 35.8 | 66.7 | 69.7 | 84.3 | 59.4 |
| **MAmmoTH2-8x7B-Plus** | 34.1 | 47.0 | 86.4 | 37.8 | 72.4 | 74.1 | 88.4 | 62.9 |
To reproduce our results, please refer to https://github.com/TIGER-AI-Lab/MAmmoTH2/tree/main/math_eval.
## Usage
You can use the models through Huggingface's Transformers library. Use the pipeline function to create a text-generation pipeline with the model of your choice, then feed in a math problem to get the solution.
Check our Github repo for more advanced use: https://github.com/TIGER-AI-Lab/MAmmoTH2
## Limitations
We've tried our best to build math generalist models. However, we acknowledge that the models' performance may vary based on the complexity and specifics of the math problem. Still not all mathematical fields can be covered comprehensively.
## Citation
If you use the models, data, or code from this project, please cite the original paper:
```
@article{yue2024mammoth2,
title={MAmmoTH2: Scaling Instructions from the Web},
author={Yue, Xiang and Zheng, Tuney and Zhang, Ge and Chen, Wenhu},
journal={arXiv preprint arXiv:2405.03548},
year={2024}
}
``` |
mradermacher/Yi-6B-GGUF | mradermacher | 2024-06-26T16:47:54Z | 12,430 | 0 | transformers | [
"transformers",
"gguf",
"en",
"base_model:01-ai/Yi-6B",
"license:apache-2.0",
"endpoints_compatible",
"region:us"
]
| null | 2024-06-26T16:25:14Z | ---
base_model: 01-ai/Yi-6B
language:
- en
library_name: transformers
license: apache-2.0
quantized_by: mradermacher
---
## About
<!-- ### quantize_version: 2 -->
<!-- ### output_tensor_quantised: 1 -->
<!-- ### convert_type: hf -->
<!-- ### vocab_type: -->
<!-- ### tags: -->
static quants of https://huggingface.co/01-ai/Yi-6B
<!-- provided-files -->
weighted/imatrix quants are available at https://huggingface.co/mradermacher/Yi-6B-i1-GGUF
## Usage
If you are unsure how to use GGUF files, refer to one of [TheBloke's
READMEs](https://huggingface.co/TheBloke/KafkaLM-70B-German-V0.1-GGUF) for
more details, including on how to concatenate multi-part files.
## Provided Quants
(sorted by size, not necessarily quality. IQ-quants are often preferable over similar sized non-IQ quants)
| Link | Type | Size/GB | Notes |
|:-----|:-----|--------:|:------|
| [GGUF](https://huggingface.co/mradermacher/Yi-6B-GGUF/resolve/main/Yi-6B.Q2_K.gguf) | Q2_K | 2.4 | |
| [GGUF](https://huggingface.co/mradermacher/Yi-6B-GGUF/resolve/main/Yi-6B.IQ3_XS.gguf) | IQ3_XS | 2.7 | |
| [GGUF](https://huggingface.co/mradermacher/Yi-6B-GGUF/resolve/main/Yi-6B.Q3_K_S.gguf) | Q3_K_S | 2.8 | |
| [GGUF](https://huggingface.co/mradermacher/Yi-6B-GGUF/resolve/main/Yi-6B.IQ3_S.gguf) | IQ3_S | 2.8 | beats Q3_K* |
| [GGUF](https://huggingface.co/mradermacher/Yi-6B-GGUF/resolve/main/Yi-6B.IQ3_M.gguf) | IQ3_M | 2.9 | |
| [GGUF](https://huggingface.co/mradermacher/Yi-6B-GGUF/resolve/main/Yi-6B.Q3_K_M.gguf) | Q3_K_M | 3.1 | lower quality |
| [GGUF](https://huggingface.co/mradermacher/Yi-6B-GGUF/resolve/main/Yi-6B.Q3_K_L.gguf) | Q3_K_L | 3.3 | |
| [GGUF](https://huggingface.co/mradermacher/Yi-6B-GGUF/resolve/main/Yi-6B.IQ4_XS.gguf) | IQ4_XS | 3.4 | |
| [GGUF](https://huggingface.co/mradermacher/Yi-6B-GGUF/resolve/main/Yi-6B.Q4_K_S.gguf) | Q4_K_S | 3.6 | fast, recommended |
| [GGUF](https://huggingface.co/mradermacher/Yi-6B-GGUF/resolve/main/Yi-6B.Q4_K_M.gguf) | Q4_K_M | 3.8 | fast, recommended |
| [GGUF](https://huggingface.co/mradermacher/Yi-6B-GGUF/resolve/main/Yi-6B.Q5_K_S.gguf) | Q5_K_S | 4.3 | |
| [GGUF](https://huggingface.co/mradermacher/Yi-6B-GGUF/resolve/main/Yi-6B.Q5_K_M.gguf) | Q5_K_M | 4.4 | |
| [GGUF](https://huggingface.co/mradermacher/Yi-6B-GGUF/resolve/main/Yi-6B.Q6_K.gguf) | Q6_K | 5.1 | very good quality |
| [GGUF](https://huggingface.co/mradermacher/Yi-6B-GGUF/resolve/main/Yi-6B.Q8_0.gguf) | Q8_0 | 6.5 | fast, best quality |
| [GGUF](https://huggingface.co/mradermacher/Yi-6B-GGUF/resolve/main/Yi-6B.f16.gguf) | f16 | 12.2 | 16 bpw, overkill |
Here is a handy graph by ikawrakow comparing some lower-quality quant
types (lower is better):

And here are Artefact2's thoughts on the matter:
https://gist.github.com/Artefact2/b5f810600771265fc1e39442288e8ec9
## FAQ / Model Request
See https://huggingface.co/mradermacher/model_requests for some answers to
questions you might have and/or if you want some other model quantized.
## Thanks
I thank my company, [nethype GmbH](https://www.nethype.de/), for letting
me use its servers and providing upgrades to my workstation to enable
this work in my free time.
<!-- end -->
|
mradermacher/Llama-3-uncensored-Dare-1-GGUF | mradermacher | 2024-06-28T08:45:04Z | 12,428 | 0 | transformers | [
"transformers",
"gguf",
"merge",
"mergekit",
"lazymergekit",
"nbeerbower/llama-3-spicy-abliterated-stella-8B",
"Azazelle/L3-RP_io",
"aifeifei798/llama3-8B-DarkIdol-2.1-Uncensored-1048K",
"en",
"base_model:Rupesh2/Llama-3-uncensored-Dare-1",
"license:apache-2.0",
"endpoints_compatible",
"region:us"
]
| null | 2024-06-28T06:29:53Z | ---
base_model: Rupesh2/Llama-3-uncensored-Dare-1
language:
- en
library_name: transformers
license: apache-2.0
quantized_by: mradermacher
tags:
- merge
- mergekit
- lazymergekit
- nbeerbower/llama-3-spicy-abliterated-stella-8B
- Azazelle/L3-RP_io
- aifeifei798/llama3-8B-DarkIdol-2.1-Uncensored-1048K
---
## About
<!-- ### quantize_version: 2 -->
<!-- ### output_tensor_quantised: 1 -->
<!-- ### convert_type: hf -->
<!-- ### vocab_type: -->
<!-- ### tags: -->
static quants of https://huggingface.co/Rupesh2/Llama-3-uncensored-Dare-1
<!-- provided-files -->
weighted/imatrix quants seem not to be available (by me) at this time. If they do not show up a week or so after the static ones, I have probably not planned for them. Feel free to request them by opening a Community Discussion.
## Usage
If you are unsure how to use GGUF files, refer to one of [TheBloke's
READMEs](https://huggingface.co/TheBloke/KafkaLM-70B-German-V0.1-GGUF) for
more details, including on how to concatenate multi-part files.
## Provided Quants
(sorted by size, not necessarily quality. IQ-quants are often preferable over similar sized non-IQ quants)
| Link | Type | Size/GB | Notes |
|:-----|:-----|--------:|:------|
| [GGUF](https://huggingface.co/mradermacher/Llama-3-uncensored-Dare-1-GGUF/resolve/main/Llama-3-uncensored-Dare-1.Q2_K.gguf) | Q2_K | 3.3 | |
| [GGUF](https://huggingface.co/mradermacher/Llama-3-uncensored-Dare-1-GGUF/resolve/main/Llama-3-uncensored-Dare-1.IQ3_XS.gguf) | IQ3_XS | 3.6 | |
| [GGUF](https://huggingface.co/mradermacher/Llama-3-uncensored-Dare-1-GGUF/resolve/main/Llama-3-uncensored-Dare-1.Q3_K_S.gguf) | Q3_K_S | 3.8 | |
| [GGUF](https://huggingface.co/mradermacher/Llama-3-uncensored-Dare-1-GGUF/resolve/main/Llama-3-uncensored-Dare-1.IQ3_S.gguf) | IQ3_S | 3.8 | beats Q3_K* |
| [GGUF](https://huggingface.co/mradermacher/Llama-3-uncensored-Dare-1-GGUF/resolve/main/Llama-3-uncensored-Dare-1.IQ3_M.gguf) | IQ3_M | 3.9 | |
| [GGUF](https://huggingface.co/mradermacher/Llama-3-uncensored-Dare-1-GGUF/resolve/main/Llama-3-uncensored-Dare-1.Q3_K_M.gguf) | Q3_K_M | 4.1 | lower quality |
| [GGUF](https://huggingface.co/mradermacher/Llama-3-uncensored-Dare-1-GGUF/resolve/main/Llama-3-uncensored-Dare-1.Q3_K_L.gguf) | Q3_K_L | 4.4 | |
| [GGUF](https://huggingface.co/mradermacher/Llama-3-uncensored-Dare-1-GGUF/resolve/main/Llama-3-uncensored-Dare-1.IQ4_XS.gguf) | IQ4_XS | 4.6 | |
| [GGUF](https://huggingface.co/mradermacher/Llama-3-uncensored-Dare-1-GGUF/resolve/main/Llama-3-uncensored-Dare-1.Q4_K_S.gguf) | Q4_K_S | 4.8 | fast, recommended |
| [GGUF](https://huggingface.co/mradermacher/Llama-3-uncensored-Dare-1-GGUF/resolve/main/Llama-3-uncensored-Dare-1.Q4_K_M.gguf) | Q4_K_M | 5.0 | fast, recommended |
| [GGUF](https://huggingface.co/mradermacher/Llama-3-uncensored-Dare-1-GGUF/resolve/main/Llama-3-uncensored-Dare-1.Q5_K_S.gguf) | Q5_K_S | 5.7 | |
| [GGUF](https://huggingface.co/mradermacher/Llama-3-uncensored-Dare-1-GGUF/resolve/main/Llama-3-uncensored-Dare-1.Q5_K_M.gguf) | Q5_K_M | 5.8 | |
| [GGUF](https://huggingface.co/mradermacher/Llama-3-uncensored-Dare-1-GGUF/resolve/main/Llama-3-uncensored-Dare-1.Q6_K.gguf) | Q6_K | 6.7 | very good quality |
| [GGUF](https://huggingface.co/mradermacher/Llama-3-uncensored-Dare-1-GGUF/resolve/main/Llama-3-uncensored-Dare-1.Q8_0.gguf) | Q8_0 | 8.6 | fast, best quality |
| [GGUF](https://huggingface.co/mradermacher/Llama-3-uncensored-Dare-1-GGUF/resolve/main/Llama-3-uncensored-Dare-1.f16.gguf) | f16 | 16.2 | 16 bpw, overkill |
Here is a handy graph by ikawrakow comparing some lower-quality quant
types (lower is better):

And here are Artefact2's thoughts on the matter:
https://gist.github.com/Artefact2/b5f810600771265fc1e39442288e8ec9
## FAQ / Model Request
See https://huggingface.co/mradermacher/model_requests for some answers to
questions you might have and/or if you want some other model quantized.
## Thanks
I thank my company, [nethype GmbH](https://www.nethype.de/), for letting
me use its servers and providing upgrades to my workstation to enable
this work in my free time.
<!-- end -->
|
mradermacher/Symbol-LLM-7B-Instruct-i1-GGUF | mradermacher | 2024-06-23T18:08:49Z | 12,425 | 0 | transformers | [
"transformers",
"gguf",
"en",
"base_model:Symbol-LLM/Symbol-LLM-7B-Instruct",
"license:apache-2.0",
"endpoints_compatible",
"region:us"
]
| null | 2024-06-23T17:03:57Z | ---
base_model: Symbol-LLM/Symbol-LLM-7B-Instruct
language:
- en
library_name: transformers
license: apache-2.0
quantized_by: mradermacher
---
## About
<!-- ### quantize_version: 2 -->
<!-- ### output_tensor_quantised: 1 -->
<!-- ### convert_type: hf -->
<!-- ### vocab_type: -->
<!-- ### tags: nicoboss -->
weighted/imatrix quants of https://huggingface.co/Symbol-LLM/Symbol-LLM-7B-Instruct
<!-- provided-files -->
static quants are available at https://huggingface.co/mradermacher/Symbol-LLM-7B-Instruct-GGUF
## Usage
If you are unsure how to use GGUF files, refer to one of [TheBloke's
READMEs](https://huggingface.co/TheBloke/KafkaLM-70B-German-V0.1-GGUF) for
more details, including on how to concatenate multi-part files.
## Provided Quants
(sorted by size, not necessarily quality. IQ-quants are often preferable over similar sized non-IQ quants)
| Link | Type | Size/GB | Notes |
|:-----|:-----|--------:|:------|
| [GGUF](https://huggingface.co/mradermacher/Symbol-LLM-7B-Instruct-i1-GGUF/resolve/main/Symbol-LLM-7B-Instruct.i1-IQ1_S.gguf) | i1-IQ1_S | 1.6 | for the desperate |
| [GGUF](https://huggingface.co/mradermacher/Symbol-LLM-7B-Instruct-i1-GGUF/resolve/main/Symbol-LLM-7B-Instruct.i1-IQ1_M.gguf) | i1-IQ1_M | 1.8 | mostly desperate |
| [GGUF](https://huggingface.co/mradermacher/Symbol-LLM-7B-Instruct-i1-GGUF/resolve/main/Symbol-LLM-7B-Instruct.i1-IQ2_XXS.gguf) | i1-IQ2_XXS | 2.0 | |
| [GGUF](https://huggingface.co/mradermacher/Symbol-LLM-7B-Instruct-i1-GGUF/resolve/main/Symbol-LLM-7B-Instruct.i1-IQ2_XS.gguf) | i1-IQ2_XS | 2.1 | |
| [GGUF](https://huggingface.co/mradermacher/Symbol-LLM-7B-Instruct-i1-GGUF/resolve/main/Symbol-LLM-7B-Instruct.i1-IQ2_S.gguf) | i1-IQ2_S | 2.3 | |
| [GGUF](https://huggingface.co/mradermacher/Symbol-LLM-7B-Instruct-i1-GGUF/resolve/main/Symbol-LLM-7B-Instruct.i1-IQ2_M.gguf) | i1-IQ2_M | 2.5 | |
| [GGUF](https://huggingface.co/mradermacher/Symbol-LLM-7B-Instruct-i1-GGUF/resolve/main/Symbol-LLM-7B-Instruct.i1-Q2_K.gguf) | i1-Q2_K | 2.6 | IQ3_XXS probably better |
| [GGUF](https://huggingface.co/mradermacher/Symbol-LLM-7B-Instruct-i1-GGUF/resolve/main/Symbol-LLM-7B-Instruct.i1-IQ3_XXS.gguf) | i1-IQ3_XXS | 2.7 | lower quality |
| [GGUF](https://huggingface.co/mradermacher/Symbol-LLM-7B-Instruct-i1-GGUF/resolve/main/Symbol-LLM-7B-Instruct.i1-IQ3_XS.gguf) | i1-IQ3_XS | 2.9 | |
| [GGUF](https://huggingface.co/mradermacher/Symbol-LLM-7B-Instruct-i1-GGUF/resolve/main/Symbol-LLM-7B-Instruct.i1-IQ3_S.gguf) | i1-IQ3_S | 3.0 | beats Q3_K* |
| [GGUF](https://huggingface.co/mradermacher/Symbol-LLM-7B-Instruct-i1-GGUF/resolve/main/Symbol-LLM-7B-Instruct.i1-Q3_K_S.gguf) | i1-Q3_K_S | 3.0 | IQ3_XS probably better |
| [GGUF](https://huggingface.co/mradermacher/Symbol-LLM-7B-Instruct-i1-GGUF/resolve/main/Symbol-LLM-7B-Instruct.i1-IQ3_M.gguf) | i1-IQ3_M | 3.2 | |
| [GGUF](https://huggingface.co/mradermacher/Symbol-LLM-7B-Instruct-i1-GGUF/resolve/main/Symbol-LLM-7B-Instruct.i1-Q3_K_M.gguf) | i1-Q3_K_M | 3.4 | IQ3_S probably better |
| [GGUF](https://huggingface.co/mradermacher/Symbol-LLM-7B-Instruct-i1-GGUF/resolve/main/Symbol-LLM-7B-Instruct.i1-Q3_K_L.gguf) | i1-Q3_K_L | 3.7 | IQ3_M probably better |
| [GGUF](https://huggingface.co/mradermacher/Symbol-LLM-7B-Instruct-i1-GGUF/resolve/main/Symbol-LLM-7B-Instruct.i1-IQ4_XS.gguf) | i1-IQ4_XS | 3.7 | |
| [GGUF](https://huggingface.co/mradermacher/Symbol-LLM-7B-Instruct-i1-GGUF/resolve/main/Symbol-LLM-7B-Instruct.i1-Q4_0.gguf) | i1-Q4_0 | 3.9 | fast, low quality |
| [GGUF](https://huggingface.co/mradermacher/Symbol-LLM-7B-Instruct-i1-GGUF/resolve/main/Symbol-LLM-7B-Instruct.i1-Q4_K_S.gguf) | i1-Q4_K_S | 4.0 | optimal size/speed/quality |
| [GGUF](https://huggingface.co/mradermacher/Symbol-LLM-7B-Instruct-i1-GGUF/resolve/main/Symbol-LLM-7B-Instruct.i1-Q4_K_M.gguf) | i1-Q4_K_M | 4.2 | fast, recommended |
| [GGUF](https://huggingface.co/mradermacher/Symbol-LLM-7B-Instruct-i1-GGUF/resolve/main/Symbol-LLM-7B-Instruct.i1-Q5_K_S.gguf) | i1-Q5_K_S | 4.8 | |
| [GGUF](https://huggingface.co/mradermacher/Symbol-LLM-7B-Instruct-i1-GGUF/resolve/main/Symbol-LLM-7B-Instruct.i1-Q5_K_M.gguf) | i1-Q5_K_M | 4.9 | |
| [GGUF](https://huggingface.co/mradermacher/Symbol-LLM-7B-Instruct-i1-GGUF/resolve/main/Symbol-LLM-7B-Instruct.i1-Q6_K.gguf) | i1-Q6_K | 5.6 | practically like static Q6_K |
Here is a handy graph by ikawrakow comparing some lower-quality quant
types (lower is better):

And here are Artefact2's thoughts on the matter:
https://gist.github.com/Artefact2/b5f810600771265fc1e39442288e8ec9
## FAQ / Model Request
See https://huggingface.co/mradermacher/model_requests for some answers to
questions you might have and/or if you want some other model quantized.
## Thanks
I thank my company, [nethype GmbH](https://www.nethype.de/), for letting
me use its servers and providing upgrades to my workstation to enable
this work in my free time. Additional thanks to [@nicoboss](https://huggingface.co/nicoboss) for giving me access to his hardware for calculating the imatrix for these quants.
<!-- end -->
|
mradermacher/Llama-3-neoAI-8B-Chat-v0.1-i1-GGUF | mradermacher | 2024-06-26T15:48:52Z | 12,419 | 0 | transformers | [
"transformers",
"gguf",
"ja",
"en",
"base_model:neoai-inc/Llama-3-neoAI-8B-Chat-v0.1",
"license:llama3",
"endpoints_compatible",
"region:us"
]
| null | 2024-06-26T14:34:20Z | ---
base_model: neoai-inc/Llama-3-neoAI-8B-Chat-v0.1
language:
- ja
- en
library_name: transformers
license: llama3
quantized_by: mradermacher
---
## About
<!-- ### quantize_version: 2 -->
<!-- ### output_tensor_quantised: 1 -->
<!-- ### convert_type: hf -->
<!-- ### vocab_type: -->
<!-- ### tags: nicoboss -->
weighted/imatrix quants of https://huggingface.co/neoai-inc/Llama-3-neoAI-8B-Chat-v0.1
<!-- provided-files -->
static quants are available at https://huggingface.co/mradermacher/Llama-3-neoAI-8B-Chat-v0.1-GGUF
## Usage
If you are unsure how to use GGUF files, refer to one of [TheBloke's
READMEs](https://huggingface.co/TheBloke/KafkaLM-70B-German-V0.1-GGUF) for
more details, including on how to concatenate multi-part files.
## Provided Quants
(sorted by size, not necessarily quality. IQ-quants are often preferable over similar sized non-IQ quants)
| Link | Type | Size/GB | Notes |
|:-----|:-----|--------:|:------|
| [GGUF](https://huggingface.co/mradermacher/Llama-3-neoAI-8B-Chat-v0.1-i1-GGUF/resolve/main/Llama-3-neoAI-8B-Chat-v0.1.i1-IQ1_S.gguf) | i1-IQ1_S | 2.1 | for the desperate |
| [GGUF](https://huggingface.co/mradermacher/Llama-3-neoAI-8B-Chat-v0.1-i1-GGUF/resolve/main/Llama-3-neoAI-8B-Chat-v0.1.i1-IQ1_M.gguf) | i1-IQ1_M | 2.3 | mostly desperate |
| [GGUF](https://huggingface.co/mradermacher/Llama-3-neoAI-8B-Chat-v0.1-i1-GGUF/resolve/main/Llama-3-neoAI-8B-Chat-v0.1.i1-IQ2_XXS.gguf) | i1-IQ2_XXS | 2.5 | |
| [GGUF](https://huggingface.co/mradermacher/Llama-3-neoAI-8B-Chat-v0.1-i1-GGUF/resolve/main/Llama-3-neoAI-8B-Chat-v0.1.i1-IQ2_XS.gguf) | i1-IQ2_XS | 2.7 | |
| [GGUF](https://huggingface.co/mradermacher/Llama-3-neoAI-8B-Chat-v0.1-i1-GGUF/resolve/main/Llama-3-neoAI-8B-Chat-v0.1.i1-IQ2_S.gguf) | i1-IQ2_S | 2.9 | |
| [GGUF](https://huggingface.co/mradermacher/Llama-3-neoAI-8B-Chat-v0.1-i1-GGUF/resolve/main/Llama-3-neoAI-8B-Chat-v0.1.i1-IQ2_M.gguf) | i1-IQ2_M | 3.0 | |
| [GGUF](https://huggingface.co/mradermacher/Llama-3-neoAI-8B-Chat-v0.1-i1-GGUF/resolve/main/Llama-3-neoAI-8B-Chat-v0.1.i1-Q2_K.gguf) | i1-Q2_K | 3.3 | IQ3_XXS probably better |
| [GGUF](https://huggingface.co/mradermacher/Llama-3-neoAI-8B-Chat-v0.1-i1-GGUF/resolve/main/Llama-3-neoAI-8B-Chat-v0.1.i1-IQ3_XXS.gguf) | i1-IQ3_XXS | 3.4 | lower quality |
| [GGUF](https://huggingface.co/mradermacher/Llama-3-neoAI-8B-Chat-v0.1-i1-GGUF/resolve/main/Llama-3-neoAI-8B-Chat-v0.1.i1-IQ3_XS.gguf) | i1-IQ3_XS | 3.6 | |
| [GGUF](https://huggingface.co/mradermacher/Llama-3-neoAI-8B-Chat-v0.1-i1-GGUF/resolve/main/Llama-3-neoAI-8B-Chat-v0.1.i1-Q3_K_S.gguf) | i1-Q3_K_S | 3.8 | IQ3_XS probably better |
| [GGUF](https://huggingface.co/mradermacher/Llama-3-neoAI-8B-Chat-v0.1-i1-GGUF/resolve/main/Llama-3-neoAI-8B-Chat-v0.1.i1-IQ3_S.gguf) | i1-IQ3_S | 3.8 | beats Q3_K* |
| [GGUF](https://huggingface.co/mradermacher/Llama-3-neoAI-8B-Chat-v0.1-i1-GGUF/resolve/main/Llama-3-neoAI-8B-Chat-v0.1.i1-IQ3_M.gguf) | i1-IQ3_M | 3.9 | |
| [GGUF](https://huggingface.co/mradermacher/Llama-3-neoAI-8B-Chat-v0.1-i1-GGUF/resolve/main/Llama-3-neoAI-8B-Chat-v0.1.i1-Q3_K_M.gguf) | i1-Q3_K_M | 4.1 | IQ3_S probably better |
| [GGUF](https://huggingface.co/mradermacher/Llama-3-neoAI-8B-Chat-v0.1-i1-GGUF/resolve/main/Llama-3-neoAI-8B-Chat-v0.1.i1-Q3_K_L.gguf) | i1-Q3_K_L | 4.4 | IQ3_M probably better |
| [GGUF](https://huggingface.co/mradermacher/Llama-3-neoAI-8B-Chat-v0.1-i1-GGUF/resolve/main/Llama-3-neoAI-8B-Chat-v0.1.i1-IQ4_XS.gguf) | i1-IQ4_XS | 4.5 | |
| [GGUF](https://huggingface.co/mradermacher/Llama-3-neoAI-8B-Chat-v0.1-i1-GGUF/resolve/main/Llama-3-neoAI-8B-Chat-v0.1.i1-Q4_0.gguf) | i1-Q4_0 | 4.8 | fast, low quality |
| [GGUF](https://huggingface.co/mradermacher/Llama-3-neoAI-8B-Chat-v0.1-i1-GGUF/resolve/main/Llama-3-neoAI-8B-Chat-v0.1.i1-Q4_K_S.gguf) | i1-Q4_K_S | 4.8 | optimal size/speed/quality |
| [GGUF](https://huggingface.co/mradermacher/Llama-3-neoAI-8B-Chat-v0.1-i1-GGUF/resolve/main/Llama-3-neoAI-8B-Chat-v0.1.i1-Q4_K_M.gguf) | i1-Q4_K_M | 5.0 | fast, recommended |
| [GGUF](https://huggingface.co/mradermacher/Llama-3-neoAI-8B-Chat-v0.1-i1-GGUF/resolve/main/Llama-3-neoAI-8B-Chat-v0.1.i1-Q5_K_S.gguf) | i1-Q5_K_S | 5.7 | |
| [GGUF](https://huggingface.co/mradermacher/Llama-3-neoAI-8B-Chat-v0.1-i1-GGUF/resolve/main/Llama-3-neoAI-8B-Chat-v0.1.i1-Q5_K_M.gguf) | i1-Q5_K_M | 5.8 | |
| [GGUF](https://huggingface.co/mradermacher/Llama-3-neoAI-8B-Chat-v0.1-i1-GGUF/resolve/main/Llama-3-neoAI-8B-Chat-v0.1.i1-Q6_K.gguf) | i1-Q6_K | 6.7 | practically like static Q6_K |
Here is a handy graph by ikawrakow comparing some lower-quality quant
types (lower is better):

And here are Artefact2's thoughts on the matter:
https://gist.github.com/Artefact2/b5f810600771265fc1e39442288e8ec9
## FAQ / Model Request
See https://huggingface.co/mradermacher/model_requests for some answers to
questions you might have and/or if you want some other model quantized.
## Thanks
I thank my company, [nethype GmbH](https://www.nethype.de/), for letting
me use its servers and providing upgrades to my workstation to enable
this work in my free time. Additional thanks to [@nicoboss](https://huggingface.co/nicoboss) for giving me access to his hardware for calculating the imatrix for these quants.
<!-- end -->
|
mradermacher/neo_7b-i1-GGUF | mradermacher | 2024-07-01T00:55:18Z | 12,412 | 0 | transformers | [
"transformers",
"gguf",
"en",
"base_model:DewEfresh/neo_7b",
"license:apache-2.0",
"endpoints_compatible",
"region:us"
]
| null | 2024-06-30T20:20:38Z | ---
base_model: DewEfresh/neo_7b
language:
- en
library_name: transformers
license: apache-2.0
quantized_by: mradermacher
---
## About
<!-- ### quantize_version: 2 -->
<!-- ### output_tensor_quantised: 1 -->
<!-- ### convert_type: hf -->
<!-- ### vocab_type: -->
<!-- ### tags: nicoboss -->
weighted/imatrix quants of https://huggingface.co/DewEfresh/neo_7b
<!-- provided-files -->
static quants are available at https://huggingface.co/mradermacher/neo_7b-GGUF
## Usage
If you are unsure how to use GGUF files, refer to one of [TheBloke's
READMEs](https://huggingface.co/TheBloke/KafkaLM-70B-German-V0.1-GGUF) for
more details, including on how to concatenate multi-part files.
## Provided Quants
(sorted by size, not necessarily quality. IQ-quants are often preferable over similar sized non-IQ quants)
| Link | Type | Size/GB | Notes |
|:-----|:-----|--------:|:------|
| [GGUF](https://huggingface.co/mradermacher/neo_7b-i1-GGUF/resolve/main/neo_7b.i1-IQ1_S.gguf) | i1-IQ1_S | 1.8 | for the desperate |
| [GGUF](https://huggingface.co/mradermacher/neo_7b-i1-GGUF/resolve/main/neo_7b.i1-IQ1_M.gguf) | i1-IQ1_M | 2.0 | mostly desperate |
| [GGUF](https://huggingface.co/mradermacher/neo_7b-i1-GGUF/resolve/main/neo_7b.i1-IQ2_XXS.gguf) | i1-IQ2_XXS | 2.2 | |
| [GGUF](https://huggingface.co/mradermacher/neo_7b-i1-GGUF/resolve/main/neo_7b.i1-IQ2_XS.gguf) | i1-IQ2_XS | 2.5 | |
| [GGUF](https://huggingface.co/mradermacher/neo_7b-i1-GGUF/resolve/main/neo_7b.i1-IQ2_S.gguf) | i1-IQ2_S | 2.6 | |
| [GGUF](https://huggingface.co/mradermacher/neo_7b-i1-GGUF/resolve/main/neo_7b.i1-IQ2_M.gguf) | i1-IQ2_M | 2.8 | |
| [GGUF](https://huggingface.co/mradermacher/neo_7b-i1-GGUF/resolve/main/neo_7b.i1-Q2_K.gguf) | i1-Q2_K | 3.0 | IQ3_XXS probably better |
| [GGUF](https://huggingface.co/mradermacher/neo_7b-i1-GGUF/resolve/main/neo_7b.i1-IQ3_XXS.gguf) | i1-IQ3_XXS | 3.1 | lower quality |
| [GGUF](https://huggingface.co/mradermacher/neo_7b-i1-GGUF/resolve/main/neo_7b.i1-IQ3_XS.gguf) | i1-IQ3_XS | 3.4 | |
| [GGUF](https://huggingface.co/mradermacher/neo_7b-i1-GGUF/resolve/main/neo_7b.i1-IQ3_S.gguf) | i1-IQ3_S | 3.5 | beats Q3_K* |
| [GGUF](https://huggingface.co/mradermacher/neo_7b-i1-GGUF/resolve/main/neo_7b.i1-Q3_K_S.gguf) | i1-Q3_K_S | 3.5 | IQ3_XS probably better |
| [GGUF](https://huggingface.co/mradermacher/neo_7b-i1-GGUF/resolve/main/neo_7b.i1-IQ3_M.gguf) | i1-IQ3_M | 3.6 | |
| [GGUF](https://huggingface.co/mradermacher/neo_7b-i1-GGUF/resolve/main/neo_7b.i1-Q3_K_M.gguf) | i1-Q3_K_M | 3.9 | IQ3_S probably better |
| [GGUF](https://huggingface.co/mradermacher/neo_7b-i1-GGUF/resolve/main/neo_7b.i1-Q3_K_L.gguf) | i1-Q3_K_L | 4.2 | IQ3_M probably better |
| [GGUF](https://huggingface.co/mradermacher/neo_7b-i1-GGUF/resolve/main/neo_7b.i1-IQ4_XS.gguf) | i1-IQ4_XS | 4.3 | |
| [GGUF](https://huggingface.co/mradermacher/neo_7b-i1-GGUF/resolve/main/neo_7b.i1-Q4_0.gguf) | i1-Q4_0 | 4.6 | fast, low quality |
| [GGUF](https://huggingface.co/mradermacher/neo_7b-i1-GGUF/resolve/main/neo_7b.i1-Q4_K_S.gguf) | i1-Q4_K_S | 4.6 | optimal size/speed/quality |
| [GGUF](https://huggingface.co/mradermacher/neo_7b-i1-GGUF/resolve/main/neo_7b.i1-Q4_K_M.gguf) | i1-Q4_K_M | 4.8 | fast, recommended |
| [GGUF](https://huggingface.co/mradermacher/neo_7b-i1-GGUF/resolve/main/neo_7b.i1-Q5_K_S.gguf) | i1-Q5_K_S | 5.5 | |
| [GGUF](https://huggingface.co/mradermacher/neo_7b-i1-GGUF/resolve/main/neo_7b.i1-Q5_K_M.gguf) | i1-Q5_K_M | 5.6 | |
| [GGUF](https://huggingface.co/mradermacher/neo_7b-i1-GGUF/resolve/main/neo_7b.i1-Q6_K.gguf) | i1-Q6_K | 6.5 | practically like static Q6_K |
Here is a handy graph by ikawrakow comparing some lower-quality quant
types (lower is better):

And here are Artefact2's thoughts on the matter:
https://gist.github.com/Artefact2/b5f810600771265fc1e39442288e8ec9
## FAQ / Model Request
See https://huggingface.co/mradermacher/model_requests for some answers to
questions you might have and/or if you want some other model quantized.
## Thanks
I thank my company, [nethype GmbH](https://www.nethype.de/), for letting
me use its servers and providing upgrades to my workstation to enable
this work in my free time. Additional thanks to [@nicoboss](https://huggingface.co/nicoboss) for giving me access to his hardware for calculating the imatrix for these quants.
<!-- end -->
|
mradermacher/L3-Stur-v1-8B-i1-GGUF | mradermacher | 2024-06-23T19:28:31Z | 12,409 | 0 | transformers | [
"transformers",
"gguf",
"merge",
"mergekit",
"lazymergekit",
"Sao10K/L3-8B-Stheno-v3.2",
"en",
"base_model:Frowning/L3-Stur-v1-8B",
"endpoints_compatible",
"region:us"
]
| null | 2024-06-23T17:11:50Z | ---
base_model: Frowning/L3-Stur-v1-8B
language:
- en
library_name: transformers
quantized_by: mradermacher
tags:
- merge
- mergekit
- lazymergekit
- Sao10K/L3-8B-Stheno-v3.2
---
## About
<!-- ### quantize_version: 2 -->
<!-- ### output_tensor_quantised: 1 -->
<!-- ### convert_type: hf -->
<!-- ### vocab_type: -->
<!-- ### tags: nicoboss -->
weighted/imatrix quants of https://huggingface.co/Frowning/L3-Stur-v1-8B
<!-- provided-files -->
static quants are available at https://huggingface.co/mradermacher/L3-Stur-v1-8B-GGUF
## Usage
If you are unsure how to use GGUF files, refer to one of [TheBloke's
READMEs](https://huggingface.co/TheBloke/KafkaLM-70B-German-V0.1-GGUF) for
more details, including on how to concatenate multi-part files.
## Provided Quants
(sorted by size, not necessarily quality. IQ-quants are often preferable over similar sized non-IQ quants)
| Link | Type | Size/GB | Notes |
|:-----|:-----|--------:|:------|
| [GGUF](https://huggingface.co/mradermacher/L3-Stur-v1-8B-i1-GGUF/resolve/main/L3-Stur-v1-8B.i1-IQ1_S.gguf) | i1-IQ1_S | 2.1 | for the desperate |
| [GGUF](https://huggingface.co/mradermacher/L3-Stur-v1-8B-i1-GGUF/resolve/main/L3-Stur-v1-8B.i1-IQ1_M.gguf) | i1-IQ1_M | 2.3 | mostly desperate |
| [GGUF](https://huggingface.co/mradermacher/L3-Stur-v1-8B-i1-GGUF/resolve/main/L3-Stur-v1-8B.i1-IQ2_XXS.gguf) | i1-IQ2_XXS | 2.5 | |
| [GGUF](https://huggingface.co/mradermacher/L3-Stur-v1-8B-i1-GGUF/resolve/main/L3-Stur-v1-8B.i1-IQ2_XS.gguf) | i1-IQ2_XS | 2.7 | |
| [GGUF](https://huggingface.co/mradermacher/L3-Stur-v1-8B-i1-GGUF/resolve/main/L3-Stur-v1-8B.i1-IQ2_S.gguf) | i1-IQ2_S | 2.9 | |
| [GGUF](https://huggingface.co/mradermacher/L3-Stur-v1-8B-i1-GGUF/resolve/main/L3-Stur-v1-8B.i1-IQ2_M.gguf) | i1-IQ2_M | 3.0 | |
| [GGUF](https://huggingface.co/mradermacher/L3-Stur-v1-8B-i1-GGUF/resolve/main/L3-Stur-v1-8B.i1-Q2_K.gguf) | i1-Q2_K | 3.3 | IQ3_XXS probably better |
| [GGUF](https://huggingface.co/mradermacher/L3-Stur-v1-8B-i1-GGUF/resolve/main/L3-Stur-v1-8B.i1-IQ3_XXS.gguf) | i1-IQ3_XXS | 3.4 | lower quality |
| [GGUF](https://huggingface.co/mradermacher/L3-Stur-v1-8B-i1-GGUF/resolve/main/L3-Stur-v1-8B.i1-IQ3_XS.gguf) | i1-IQ3_XS | 3.6 | |
| [GGUF](https://huggingface.co/mradermacher/L3-Stur-v1-8B-i1-GGUF/resolve/main/L3-Stur-v1-8B.i1-Q3_K_S.gguf) | i1-Q3_K_S | 3.8 | IQ3_XS probably better |
| [GGUF](https://huggingface.co/mradermacher/L3-Stur-v1-8B-i1-GGUF/resolve/main/L3-Stur-v1-8B.i1-IQ3_S.gguf) | i1-IQ3_S | 3.8 | beats Q3_K* |
| [GGUF](https://huggingface.co/mradermacher/L3-Stur-v1-8B-i1-GGUF/resolve/main/L3-Stur-v1-8B.i1-IQ3_M.gguf) | i1-IQ3_M | 3.9 | |
| [GGUF](https://huggingface.co/mradermacher/L3-Stur-v1-8B-i1-GGUF/resolve/main/L3-Stur-v1-8B.i1-Q3_K_M.gguf) | i1-Q3_K_M | 4.1 | IQ3_S probably better |
| [GGUF](https://huggingface.co/mradermacher/L3-Stur-v1-8B-i1-GGUF/resolve/main/L3-Stur-v1-8B.i1-Q3_K_L.gguf) | i1-Q3_K_L | 4.4 | IQ3_M probably better |
| [GGUF](https://huggingface.co/mradermacher/L3-Stur-v1-8B-i1-GGUF/resolve/main/L3-Stur-v1-8B.i1-IQ4_XS.gguf) | i1-IQ4_XS | 4.5 | |
| [GGUF](https://huggingface.co/mradermacher/L3-Stur-v1-8B-i1-GGUF/resolve/main/L3-Stur-v1-8B.i1-Q4_0.gguf) | i1-Q4_0 | 4.8 | fast, low quality |
| [GGUF](https://huggingface.co/mradermacher/L3-Stur-v1-8B-i1-GGUF/resolve/main/L3-Stur-v1-8B.i1-Q4_K_S.gguf) | i1-Q4_K_S | 4.8 | optimal size/speed/quality |
| [GGUF](https://huggingface.co/mradermacher/L3-Stur-v1-8B-i1-GGUF/resolve/main/L3-Stur-v1-8B.i1-Q4_K_M.gguf) | i1-Q4_K_M | 5.0 | fast, recommended |
| [GGUF](https://huggingface.co/mradermacher/L3-Stur-v1-8B-i1-GGUF/resolve/main/L3-Stur-v1-8B.i1-Q5_K_S.gguf) | i1-Q5_K_S | 5.7 | |
| [GGUF](https://huggingface.co/mradermacher/L3-Stur-v1-8B-i1-GGUF/resolve/main/L3-Stur-v1-8B.i1-Q5_K_M.gguf) | i1-Q5_K_M | 5.8 | |
| [GGUF](https://huggingface.co/mradermacher/L3-Stur-v1-8B-i1-GGUF/resolve/main/L3-Stur-v1-8B.i1-Q6_K.gguf) | i1-Q6_K | 6.7 | practically like static Q6_K |
Here is a handy graph by ikawrakow comparing some lower-quality quant
types (lower is better):

And here are Artefact2's thoughts on the matter:
https://gist.github.com/Artefact2/b5f810600771265fc1e39442288e8ec9
## FAQ / Model Request
See https://huggingface.co/mradermacher/model_requests for some answers to
questions you might have and/or if you want some other model quantized.
## Thanks
I thank my company, [nethype GmbH](https://www.nethype.de/), for letting
me use its servers and providing upgrades to my workstation to enable
this work in my free time. Additional thanks to [@nicoboss](https://huggingface.co/nicoboss) for giving me access to his hardware for calculating the imatrix for these quants.
<!-- end -->
|
microsoft/xclip-large-patch14 | microsoft | 2022-09-08T11:08:18Z | 12,408 | 7 | transformers | [
"transformers",
"pytorch",
"xclip",
"feature-extraction",
"vision",
"video-classification",
"en",
"arxiv:2208.02816",
"license:mit",
"model-index",
"endpoints_compatible",
"region:us"
]
| video-classification | 2022-09-07T15:17:38Z | ---
language: en
license: mit
tags:
- vision
- video-classification
model-index:
- name: nielsr/xclip-large-patch14
results:
- task:
type: video-classification
dataset:
name: Kinetics 400
type: kinetics-400
metrics:
- type: top-1 accuracy
value: 87.1
- type: top-5 accuracy
value: 97.6
---
# X-CLIP (large-sized model)
X-CLIP model (large-sized, patch resolution of 14) trained fully-supervised on [Kinetics-400](https://www.deepmind.com/open-source/kinetics). It was introduced in the paper [Expanding Language-Image Pretrained Models for General Video Recognition](https://arxiv.org/abs/2208.02816) by Ni et al. and first released in [this repository](https://github.com/microsoft/VideoX/tree/master/X-CLIP).
This model was trained using 8 frames per video, at a resolution of 224x224.
Disclaimer: The team releasing X-CLIP did not write a model card for this model so this model card has been written by the Hugging Face team.
## Model description
X-CLIP is a minimal extension of [CLIP](https://huggingface.co/docs/transformers/model_doc/clip) for general video-language understanding. The model is trained in a contrastive way on (video, text) pairs.

This allows the model to be used for tasks like zero-shot, few-shot or fully supervised video classification and video-text retrieval.
## Intended uses & limitations
You can use the raw model for determining how well text goes with a given video. See the [model hub](https://huggingface.co/models?search=microsoft/xclip) to look for
fine-tuned versions on a task that interests you.
### How to use
For code examples, we refer to the [documentation](https://huggingface.co/transformers/main/model_doc/xclip.html#).
## Training data
This model was trained on [Kinetics-400](https://www.deepmind.com/open-source/kinetics).
### Preprocessing
The exact details of preprocessing during training can be found [here](https://github.com/microsoft/VideoX/blob/40f6d177e0a057a50ac69ac1de6b5938fd268601/X-CLIP/datasets/build.py#L247).
The exact details of preprocessing during validation can be found [here](https://github.com/microsoft/VideoX/blob/40f6d177e0a057a50ac69ac1de6b5938fd268601/X-CLIP/datasets/build.py#L285).
During validation, one resizes the shorter edge of each frame, after which center cropping is performed to a fixed-size resolution (like 224x224). Next, frames are normalized across the RGB channels with the ImageNet mean and standard deviation.
## Evaluation results
This model achieves a top-1 accuracy of 87.1% and a top-5 accuracy of 97.6%.
|
nncyberpunk/SDXL1.0_JuggernautXL_8 | nncyberpunk | 2024-01-29T18:28:15Z | 12,404 | 0 | diffusers | [
"diffusers",
"autotrain_compatible",
"endpoints_compatible",
"diffusers:StableDiffusionXLPipeline",
"region:us"
]
| text-to-image | 2024-01-29T17:30:50Z | Juggernaut XL V8
https://civitai.com/models/133005/juggernaut-xl?modelVersionId=288982 |
prs-eth/marigold-depth-v1-0 | prs-eth | 2024-05-25T21:18:35Z | 12,395 | 101 | diffusers | [
"diffusers",
"safetensors",
"monocular depth estimation",
"single image depth estimation",
"depth",
"in-the-wild",
"zero-shot",
"depth-estimation",
"en",
"arxiv:2312.02145",
"license:apache-2.0",
"diffusers:MarigoldPipeline",
"region:us"
]
| depth-estimation | 2023-12-05T08:45:47Z | ---
license: apache-2.0
language:
- en
pipeline_tag: depth-estimation
tags:
- monocular depth estimation
- single image depth estimation
- depth
- in-the-wild
- zero-shot
- depth
---
# Marigold: Repurposing Diffusion-Based Image Generators for Monocular Depth Estimation
This model represents the official checkpoint of the paper titled "Repurposing Diffusion-Based Image Generators for Monocular Depth Estimation".
[](https://marigoldmonodepth.github.io)
[](https://github.com/prs-eth/Marigold)
[](https://arxiv.org/abs/2312.02145)
[](https://colab.research.google.com/drive/12G8reD13DdpMie5ZQlaFNo2WCGeNUH-u?usp=sharing)
[](https://huggingface.co/spaces/toshas/marigold)
[](https://www.apache.org/licenses/LICENSE-2.0)
<!-- []() -->
<!-- []() -->
<!-- []() -->
<!-- ### [Repurposing Diffusion-Based Image Generators for Monocular Depth Estimation]() -->
[Bingxin Ke](http://www.kebingxin.com/),
[Anton Obukhov](https://www.obukhov.ai/),
[Shengyu Huang](https://shengyuh.github.io/),
[Nando Metzger](https://nandometzger.github.io/),
[Rodrigo Caye Daudt](https://rcdaudt.github.io/),
[Konrad Schindler](https://scholar.google.com/citations?user=FZuNgqIAAAAJ&hl=en )
We present Marigold, a diffusion model and associated fine-tuning protocol for monocular depth estimation. Its core principle is to leverage the rich visual knowledge stored in modern generative image models. Our model, derived from Stable Diffusion and fine-tuned with synthetic data, can zero-shot transfer to unseen data, offering state-of-the-art monocular depth estimation results.

## 🎓 Citation
```bibtex
@InProceedings{ke2023repurposing,
title={Repurposing Diffusion-Based Image Generators for Monocular Depth Estimation},
author={Bingxin Ke and Anton Obukhov and Shengyu Huang and Nando Metzger and Rodrigo Caye Daudt and Konrad Schindler},
booktitle = {Proceedings of the IEEE/CVF Conference on Computer Vision and Pattern Recognition (CVPR)},
year={2024}
}
```
## 🎫 License
This work is licensed under the Apache License, Version 2.0 (as defined in the [LICENSE](LICENSE.txt)).
By downloading and using the code and model you agree to the terms in the [LICENSE](LICENSE.txt).
[](https://www.apache.org/licenses/LICENSE-2.0)
|
mradermacher/Templar_v1_8B-i1-GGUF | mradermacher | 2024-06-23T06:41:47Z | 12,390 | 0 | transformers | [
"transformers",
"gguf",
"en",
"base_model:ChaoticNeutrals/Templar_v1_8B",
"license:apache-2.0",
"endpoints_compatible",
"region:us"
]
| null | 2024-06-23T04:25:00Z | ---
base_model: ChaoticNeutrals/Templar_v1_8B
language:
- en
library_name: transformers
license: apache-2.0
quantized_by: mradermacher
---
## About
<!-- ### quantize_version: 2 -->
<!-- ### output_tensor_quantised: 1 -->
<!-- ### convert_type: hf -->
<!-- ### vocab_type: -->
<!-- ### tags: nicoboss -->
weighted/imatrix quants of https://huggingface.co/ChaoticNeutrals/Templar_v1_8B
<!-- provided-files -->
static quants are available at https://huggingface.co/mradermacher/Templar_v1_8B-GGUF
## Usage
If you are unsure how to use GGUF files, refer to one of [TheBloke's
READMEs](https://huggingface.co/TheBloke/KafkaLM-70B-German-V0.1-GGUF) for
more details, including on how to concatenate multi-part files.
## Provided Quants
(sorted by size, not necessarily quality. IQ-quants are often preferable over similar sized non-IQ quants)
| Link | Type | Size/GB | Notes |
|:-----|:-----|--------:|:------|
| [GGUF](https://huggingface.co/mradermacher/Templar_v1_8B-i1-GGUF/resolve/main/Templar_v1_8B.i1-IQ1_S.gguf) | i1-IQ1_S | 2.1 | for the desperate |
| [GGUF](https://huggingface.co/mradermacher/Templar_v1_8B-i1-GGUF/resolve/main/Templar_v1_8B.i1-IQ1_M.gguf) | i1-IQ1_M | 2.3 | mostly desperate |
| [GGUF](https://huggingface.co/mradermacher/Templar_v1_8B-i1-GGUF/resolve/main/Templar_v1_8B.i1-IQ2_XXS.gguf) | i1-IQ2_XXS | 2.5 | |
| [GGUF](https://huggingface.co/mradermacher/Templar_v1_8B-i1-GGUF/resolve/main/Templar_v1_8B.i1-IQ2_XS.gguf) | i1-IQ2_XS | 2.7 | |
| [GGUF](https://huggingface.co/mradermacher/Templar_v1_8B-i1-GGUF/resolve/main/Templar_v1_8B.i1-IQ2_S.gguf) | i1-IQ2_S | 2.9 | |
| [GGUF](https://huggingface.co/mradermacher/Templar_v1_8B-i1-GGUF/resolve/main/Templar_v1_8B.i1-IQ2_M.gguf) | i1-IQ2_M | 3.0 | |
| [GGUF](https://huggingface.co/mradermacher/Templar_v1_8B-i1-GGUF/resolve/main/Templar_v1_8B.i1-Q2_K.gguf) | i1-Q2_K | 3.3 | IQ3_XXS probably better |
| [GGUF](https://huggingface.co/mradermacher/Templar_v1_8B-i1-GGUF/resolve/main/Templar_v1_8B.i1-IQ3_XXS.gguf) | i1-IQ3_XXS | 3.4 | lower quality |
| [GGUF](https://huggingface.co/mradermacher/Templar_v1_8B-i1-GGUF/resolve/main/Templar_v1_8B.i1-IQ3_XS.gguf) | i1-IQ3_XS | 3.6 | |
| [GGUF](https://huggingface.co/mradermacher/Templar_v1_8B-i1-GGUF/resolve/main/Templar_v1_8B.i1-Q3_K_S.gguf) | i1-Q3_K_S | 3.8 | IQ3_XS probably better |
| [GGUF](https://huggingface.co/mradermacher/Templar_v1_8B-i1-GGUF/resolve/main/Templar_v1_8B.i1-IQ3_S.gguf) | i1-IQ3_S | 3.8 | beats Q3_K* |
| [GGUF](https://huggingface.co/mradermacher/Templar_v1_8B-i1-GGUF/resolve/main/Templar_v1_8B.i1-IQ3_M.gguf) | i1-IQ3_M | 3.9 | |
| [GGUF](https://huggingface.co/mradermacher/Templar_v1_8B-i1-GGUF/resolve/main/Templar_v1_8B.i1-Q3_K_M.gguf) | i1-Q3_K_M | 4.1 | IQ3_S probably better |
| [GGUF](https://huggingface.co/mradermacher/Templar_v1_8B-i1-GGUF/resolve/main/Templar_v1_8B.i1-Q3_K_L.gguf) | i1-Q3_K_L | 4.4 | IQ3_M probably better |
| [GGUF](https://huggingface.co/mradermacher/Templar_v1_8B-i1-GGUF/resolve/main/Templar_v1_8B.i1-IQ4_XS.gguf) | i1-IQ4_XS | 4.5 | |
| [GGUF](https://huggingface.co/mradermacher/Templar_v1_8B-i1-GGUF/resolve/main/Templar_v1_8B.i1-Q4_0.gguf) | i1-Q4_0 | 4.8 | fast, low quality |
| [GGUF](https://huggingface.co/mradermacher/Templar_v1_8B-i1-GGUF/resolve/main/Templar_v1_8B.i1-Q4_K_S.gguf) | i1-Q4_K_S | 4.8 | optimal size/speed/quality |
| [GGUF](https://huggingface.co/mradermacher/Templar_v1_8B-i1-GGUF/resolve/main/Templar_v1_8B.i1-Q4_K_M.gguf) | i1-Q4_K_M | 5.0 | fast, recommended |
| [GGUF](https://huggingface.co/mradermacher/Templar_v1_8B-i1-GGUF/resolve/main/Templar_v1_8B.i1-Q5_K_S.gguf) | i1-Q5_K_S | 5.7 | |
| [GGUF](https://huggingface.co/mradermacher/Templar_v1_8B-i1-GGUF/resolve/main/Templar_v1_8B.i1-Q5_K_M.gguf) | i1-Q5_K_M | 5.8 | |
| [GGUF](https://huggingface.co/mradermacher/Templar_v1_8B-i1-GGUF/resolve/main/Templar_v1_8B.i1-Q6_K.gguf) | i1-Q6_K | 6.7 | practically like static Q6_K |
Here is a handy graph by ikawrakow comparing some lower-quality quant
types (lower is better):

And here are Artefact2's thoughts on the matter:
https://gist.github.com/Artefact2/b5f810600771265fc1e39442288e8ec9
## FAQ / Model Request
See https://huggingface.co/mradermacher/model_requests for some answers to
questions you might have and/or if you want some other model quantized.
## Thanks
I thank my company, [nethype GmbH](https://www.nethype.de/), for letting
me use its servers and providing upgrades to my workstation to enable
this work in my free time. Additional thanks to [@nicoboss](https://huggingface.co/nicoboss) for giving me access to his hardware for calculating the imatrix for these quants.
<!-- end -->
|
RichardErkhov/Sao10K_-_L3-8B-Stheno-v3.2-gguf | RichardErkhov | 2024-06-25T08:22:02Z | 12,388 | 0 | null | [
"gguf",
"region:us"
]
| null | 2024-06-25T04:05:03Z | Quantization made by Richard Erkhov.
[Github](https://github.com/RichardErkhov)
[Discord](https://discord.gg/pvy7H8DZMG)
[Request more models](https://github.com/RichardErkhov/quant_request)
L3-8B-Stheno-v3.2 - GGUF
- Model creator: https://huggingface.co/Sao10K/
- Original model: https://huggingface.co/Sao10K/L3-8B-Stheno-v3.2/
| Name | Quant method | Size |
| ---- | ---- | ---- |
| [L3-8B-Stheno-v3.2.Q2_K.gguf](https://huggingface.co/RichardErkhov/Sao10K_-_L3-8B-Stheno-v3.2-gguf/blob/main/L3-8B-Stheno-v3.2.Q2_K.gguf) | Q2_K | 2.96GB |
| [L3-8B-Stheno-v3.2.IQ3_XS.gguf](https://huggingface.co/RichardErkhov/Sao10K_-_L3-8B-Stheno-v3.2-gguf/blob/main/L3-8B-Stheno-v3.2.IQ3_XS.gguf) | IQ3_XS | 3.28GB |
| [L3-8B-Stheno-v3.2.IQ3_S.gguf](https://huggingface.co/RichardErkhov/Sao10K_-_L3-8B-Stheno-v3.2-gguf/blob/main/L3-8B-Stheno-v3.2.IQ3_S.gguf) | IQ3_S | 3.43GB |
| [L3-8B-Stheno-v3.2.Q3_K_S.gguf](https://huggingface.co/RichardErkhov/Sao10K_-_L3-8B-Stheno-v3.2-gguf/blob/main/L3-8B-Stheno-v3.2.Q3_K_S.gguf) | Q3_K_S | 3.41GB |
| [L3-8B-Stheno-v3.2.IQ3_M.gguf](https://huggingface.co/RichardErkhov/Sao10K_-_L3-8B-Stheno-v3.2-gguf/blob/main/L3-8B-Stheno-v3.2.IQ3_M.gguf) | IQ3_M | 3.52GB |
| [L3-8B-Stheno-v3.2.Q3_K.gguf](https://huggingface.co/RichardErkhov/Sao10K_-_L3-8B-Stheno-v3.2-gguf/blob/main/L3-8B-Stheno-v3.2.Q3_K.gguf) | Q3_K | 3.74GB |
| [L3-8B-Stheno-v3.2.Q3_K_M.gguf](https://huggingface.co/RichardErkhov/Sao10K_-_L3-8B-Stheno-v3.2-gguf/blob/main/L3-8B-Stheno-v3.2.Q3_K_M.gguf) | Q3_K_M | 3.74GB |
| [L3-8B-Stheno-v3.2.Q3_K_L.gguf](https://huggingface.co/RichardErkhov/Sao10K_-_L3-8B-Stheno-v3.2-gguf/blob/main/L3-8B-Stheno-v3.2.Q3_K_L.gguf) | Q3_K_L | 4.03GB |
| [L3-8B-Stheno-v3.2.IQ4_XS.gguf](https://huggingface.co/RichardErkhov/Sao10K_-_L3-8B-Stheno-v3.2-gguf/blob/main/L3-8B-Stheno-v3.2.IQ4_XS.gguf) | IQ4_XS | 4.18GB |
| [L3-8B-Stheno-v3.2.Q4_0.gguf](https://huggingface.co/RichardErkhov/Sao10K_-_L3-8B-Stheno-v3.2-gguf/blob/main/L3-8B-Stheno-v3.2.Q4_0.gguf) | Q4_0 | 4.34GB |
| [L3-8B-Stheno-v3.2.IQ4_NL.gguf](https://huggingface.co/RichardErkhov/Sao10K_-_L3-8B-Stheno-v3.2-gguf/blob/main/L3-8B-Stheno-v3.2.IQ4_NL.gguf) | IQ4_NL | 4.38GB |
| [L3-8B-Stheno-v3.2.Q4_K_S.gguf](https://huggingface.co/RichardErkhov/Sao10K_-_L3-8B-Stheno-v3.2-gguf/blob/main/L3-8B-Stheno-v3.2.Q4_K_S.gguf) | Q4_K_S | 4.37GB |
| [L3-8B-Stheno-v3.2.Q4_K.gguf](https://huggingface.co/RichardErkhov/Sao10K_-_L3-8B-Stheno-v3.2-gguf/blob/main/L3-8B-Stheno-v3.2.Q4_K.gguf) | Q4_K | 4.58GB |
| [L3-8B-Stheno-v3.2.Q4_K_M.gguf](https://huggingface.co/RichardErkhov/Sao10K_-_L3-8B-Stheno-v3.2-gguf/blob/main/L3-8B-Stheno-v3.2.Q4_K_M.gguf) | Q4_K_M | 4.58GB |
| [L3-8B-Stheno-v3.2.Q4_1.gguf](https://huggingface.co/RichardErkhov/Sao10K_-_L3-8B-Stheno-v3.2-gguf/blob/main/L3-8B-Stheno-v3.2.Q4_1.gguf) | Q4_1 | 4.78GB |
| [L3-8B-Stheno-v3.2.Q5_0.gguf](https://huggingface.co/RichardErkhov/Sao10K_-_L3-8B-Stheno-v3.2-gguf/blob/main/L3-8B-Stheno-v3.2.Q5_0.gguf) | Q5_0 | 5.21GB |
| [L3-8B-Stheno-v3.2.Q5_K_S.gguf](https://huggingface.co/RichardErkhov/Sao10K_-_L3-8B-Stheno-v3.2-gguf/blob/main/L3-8B-Stheno-v3.2.Q5_K_S.gguf) | Q5_K_S | 5.21GB |
| [L3-8B-Stheno-v3.2.Q5_K.gguf](https://huggingface.co/RichardErkhov/Sao10K_-_L3-8B-Stheno-v3.2-gguf/blob/main/L3-8B-Stheno-v3.2.Q5_K.gguf) | Q5_K | 5.34GB |
| [L3-8B-Stheno-v3.2.Q5_K_M.gguf](https://huggingface.co/RichardErkhov/Sao10K_-_L3-8B-Stheno-v3.2-gguf/blob/main/L3-8B-Stheno-v3.2.Q5_K_M.gguf) | Q5_K_M | 5.34GB |
| [L3-8B-Stheno-v3.2.Q5_1.gguf](https://huggingface.co/RichardErkhov/Sao10K_-_L3-8B-Stheno-v3.2-gguf/blob/main/L3-8B-Stheno-v3.2.Q5_1.gguf) | Q5_1 | 5.65GB |
| [L3-8B-Stheno-v3.2.Q6_K.gguf](https://huggingface.co/RichardErkhov/Sao10K_-_L3-8B-Stheno-v3.2-gguf/blob/main/L3-8B-Stheno-v3.2.Q6_K.gguf) | Q6_K | 6.14GB |
| [L3-8B-Stheno-v3.2.Q8_0.gguf](https://huggingface.co/RichardErkhov/Sao10K_-_L3-8B-Stheno-v3.2-gguf/blob/main/L3-8B-Stheno-v3.2.Q8_0.gguf) | Q8_0 | 7.95GB |
Original model description:
---
license: cc-by-nc-4.0
language:
- en
datasets:
- Gryphe/Opus-WritingPrompts
- Sao10K/Claude-3-Opus-Instruct-15K
- Sao10K/Short-Storygen-v2
- Sao10K/c2-Logs-Filtered
---
*Just message me on discord if you want to host this privately for a service or something. We can talk.*
*Train used 1x H100 SXM for like a total of 24 Hours over multiple runs.*
Support me here if you're interested:
<br>Ko-fi: https://ko-fi.com/sao10k
<br> *wink* Euryale v2?
If not, that's fine too. Feedback would be nice.
Contact Me in Discord:
<br>`sao10k` // `Just ping me in the KoboldAI discord, I'll respond faster.`
`Art by navy_(navy.blue)` - [Danbooru](https://danbooru.donmai.us/posts/3214477)
---

Stheno-v3.2-Zeta
I have done a test run with multiple variations of the models, merged back to its base at various weights, different training runs too, and this Sixth iteration is the one I like most.
Changes compared to v3.1
<br>\- Included a mix of SFW and NSFW Storywriting Data, thanks to [Gryphe](https://huggingface.co/datasets/Gryphe/Opus-WritingPrompts)
<br>\- Included More Instruct / Assistant-Style Data
<br>\- Further cleaned up Roleplaying Samples from c2 Logs -> A few terrible, really bad samples escaped heavy filtering. Manual pass fixed it.
<br>\- Hyperparameter tinkering for training, resulting in lower loss levels.
Testing Notes - Compared to v3.1
<br>\- Handles SFW / NSFW seperately better. Not as overly excessive with NSFW now. Kinda balanced.
<br>\- Better at Storywriting / Narration.
<br>\- Better at Assistant-type Tasks.
<br>\- Better Multi-Turn Coherency -> Reduced Issues?
<br>\- Slightly less creative? A worthy tradeoff. Still creative.
<br>\- Better prompt / instruction adherence.
---
**Recommended Samplers:**
```
Temperature - 1.12-1.22
Min-P - 0.075
Top-K - 50
Repetition Penalty - 1.1
```
**Stopping Strings:**
```
\n\n{{User}} # Or Equivalent, depending on Frontend
<|eot_id|>
<|end_of_text|>
```
**Prompting Template - Llama-3-Instruct**
```
<|begin_of_text|><|start_header_id|>system<|end_header_id|>
{system_prompt}<|eot_id|><|start_header_id|>user<|end_header_id|>
{input}<|eot_id|><|start_header_id|>assistant<|end_header_id|>
{output}<|eot_id|>
```
**Basic Roleplay System Prompt**
```
You are an expert actor that can fully immerse yourself into any role given. You do not break character for any reason, even if someone tries addressing you as an AI or language model.
Currently your role is {{char}}, which is described in detail below. As {{char}}, continue the exchange with {{user}}.
```
---
|
h2oai/h2ogpt-oig-oasst1-512-6_9b | h2oai | 2023-06-02T22:20:22Z | 12,381 | 17 | transformers | [
"transformers",
"pytorch",
"gpt_neox",
"text-generation",
"gpt",
"llm",
"large language model",
"open-source",
"en",
"dataset:h2oai/h2ogpt-oig-oasst1-instruct-cleaned-v1",
"dataset:h2oai/openassistant_oasst1_h2ogpt",
"dataset:h2oai/h2ogpt-fortune2000-personalized",
"dataset:h2oai/h2ogpt-oig-oasst1-instruct-cleaned-v3",
"license:apache-2.0",
"autotrain_compatible",
"text-generation-inference",
"region:us"
]
| text-generation | 2023-04-18T06:13:02Z | ---
license: apache-2.0
language:
- en
library_name: transformers
inference: false
thumbnail: https://h2o.ai/etc.clientlibs/h2o/clientlibs/clientlib-site/resources/images/favicon.ico
tags:
- gpt
- llm
- large language model
- open-source
datasets:
- h2oai/h2ogpt-oig-oasst1-instruct-cleaned-v1
- h2oai/openassistant_oasst1_h2ogpt
- h2oai/h2ogpt-fortune2000-personalized
- h2oai/h2ogpt-oig-oasst1-instruct-cleaned-v3
---
# h2oGPT Model Card
## Summary
H2O.ai's `h2ogpt-oig-oasst1-512-6_9b` is a 6.9 billion parameter instruction-following large language model licensed for commercial use.
- Base model: [EleutherAI/pythia-6.9b](https://huggingface.co/EleutherAI/pythia-6.9b)
- Fine-tuning dataset: [h2oai/h2ogpt-oig-oasst1-instruct-cleaned-v1](https://huggingface.co/datasets/h2oai/h2ogpt-oig-oasst1-instruct-cleaned-v1) and [h2oai/openassistant_oasst1_h2ogpt](https://huggingface.co/datasets/h2oai/openassistant_oasst1_h2ogpt) and [h2oai/h2ogpt-fortune2000-personalized](https://huggingface.co/datasets/h2oai/h2ogpt-fortune2000-personalized) and [h2oai/h2ogpt-oig-oasst1-instruct-cleaned-v3](https://huggingface.co/datasets/h2oai/h2ogpt-oig-oasst1-instruct-cleaned-v3)
- Data-prep and fine-tuning code: [H2O.ai GitHub](https://github.com/h2oai/h2ogpt)
- Training logs: [zip](https://huggingface.co/h2oai/h2ogpt-oig-oasst1-512-6.9b/blob/main/pythia-6.9b.h2ogpt-oig-oasst1-instruct-cleaned-v1.json.1_epochs.5fc91911bc2bfaaf3b6c2de577c4b0ae45a07a4a.7.zip) and [zip](https://huggingface.co/h2oai/h2ogpt-oig-oasst1-512-6.9b/blob/main/h2ogpt-oig-oasst1-512-6.9b.h2oaiopenassistant_oasst1_h2ogpt.2_epochs.e35e2e06e0af2f7dceac2e16e3646c90ccce4ec0.1.zip) and [zip](https://huggingface.co/h2oai/h2ogpt-oig-oasst1-512-6.9b/blob/main/h2ogpt-oig-oasst1-512-6.9b.h2oaih2ogpt-oig-oasst1-instruct-cleaned-v3.1_epochs.e48f9debb0d2bd8d866fa5668bbbb51c317c553c.1.zip)
## Chatbot
- Run your own chatbot: [H2O.ai GitHub](https://github.com/h2oai/h2ogpt)
[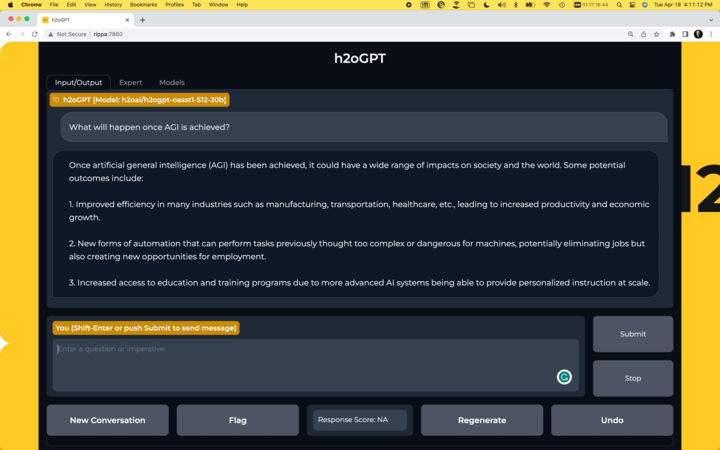](https://github.com/h2oai/h2ogpt)
## Usage
To use the model with the `transformers` library on a machine with GPUs, first make sure you have the `transformers` and `accelerate` libraries installed.
```bash
pip install transformers==4.28.1
pip install accelerate==0.18.0
```
```python
import torch
from transformers import pipeline
generate_text = pipeline(model="h2oai/h2ogpt-oig-oasst1-512-6_9b", torch_dtype=torch.bfloat16, trust_remote_code=True, device_map="auto", prompt_type='human_bot')
res = generate_text("Why is drinking water so healthy?", max_new_tokens=100)
print(res[0]["generated_text"])
```
Alternatively, if you prefer to not use `trust_remote_code=True` you can download [instruct_pipeline.py](https://huggingface.co/h2oai/h2ogpt-oig-oasst1-512-6.9b/blob/main/h2oai_pipeline.py),
store it alongside your notebook, and construct the pipeline yourself from the loaded model and tokenizer:
```python
import torch
from h2oai_pipeline import H2OTextGenerationPipeline
from transformers import AutoModelForCausalLM, AutoTokenizer
tokenizer = AutoTokenizer.from_pretrained("h2oai/h2ogpt-oig-oasst1-512-6_9b", padding_side="left")
model = AutoModelForCausalLM.from_pretrained("h2oai/h2ogpt-oig-oasst1-512-6_9b", torch_dtype=torch.bfloat16, device_map="auto")
generate_text = H2OTextGenerationPipeline(model=model, tokenizer=tokenizer, prompt_type='human_bot')
res = generate_text("Why is drinking water so healthy?", max_new_tokens=100)
print(res[0]["generated_text"])
```
## Model Architecture
```
GPTNeoXForCausalLM(
(gpt_neox): GPTNeoXModel(
(embed_in): Embedding(50432, 4096)
(layers): ModuleList(
(0-31): 32 x GPTNeoXLayer(
(input_layernorm): LayerNorm((4096,), eps=1e-05, elementwise_affine=True)
(post_attention_layernorm): LayerNorm((4096,), eps=1e-05, elementwise_affine=True)
(attention): GPTNeoXAttention(
(rotary_emb): RotaryEmbedding()
(query_key_value): Linear(in_features=4096, out_features=12288, bias=True)
(dense): Linear(in_features=4096, out_features=4096, bias=True)
)
(mlp): GPTNeoXMLP(
(dense_h_to_4h): Linear(in_features=4096, out_features=16384, bias=True)
(dense_4h_to_h): Linear(in_features=16384, out_features=4096, bias=True)
(act): GELUActivation()
)
)
)
(final_layer_norm): LayerNorm((4096,), eps=1e-05, elementwise_affine=True)
)
(embed_out): Linear(in_features=4096, out_features=50432, bias=False)
)
```
## Model Configuration
```json
GPTNeoXConfig {
"_name_or_path": "h2oai/h2ogpt-oig-oasst1-512-6_9b",
"architectures": [
"GPTNeoXForCausalLM"
],
"bos_token_id": 0,
"custom_pipeline": {
"text-generation": {
"impl": "h2oai_pipeline.H2OTextGenerationPipeline",
"pt": "AutoModelForCausalLM"
}
},
"eos_token_id": 0,
"hidden_act": "gelu",
"hidden_size": 4096,
"initializer_range": 0.02,
"intermediate_size": 16384,
"layer_norm_eps": 1e-05,
"max_position_embeddings": 2048,
"model_type": "gpt_neox",
"num_attention_heads": 32,
"num_hidden_layers": 32,
"rotary_emb_base": 10000,
"rotary_pct": 0.25,
"tie_word_embeddings": false,
"torch_dtype": "float16",
"transformers_version": "4.28.1",
"use_cache": true,
"use_parallel_residual": true,
"vocab_size": 50432
}
```
## Model Validation
Model validation results using [EleutherAI lm-evaluation-harness](https://github.com/EleutherAI/lm-evaluation-harness).
[eval source code](https://github.com/h2oai/h2ogpt/issues/125#issue-1702311702)
| Task |Version| Metric |Value | |Stderr|
|-------------|------:|--------|-----:|---|-----:|
|arc_easy | 0|acc |0.6591|± |0.0097|
| | |acc_norm|0.6178|± |0.0100|
|arc_challenge| 0|acc |0.3174|± |0.0136|
| | |acc_norm|0.3558|± |0.0140|
|openbookqa | 0|acc |0.2540|± |0.0195|
| | |acc_norm|0.3580|± |0.0215|
|winogrande | 0|acc |0.6069|± |0.0137|
|piqa | 0|acc |0.7486|± |0.0101|
| | |acc_norm|0.7546|± |0.0100|
|hellaswag | 0|acc |0.4843|± |0.0050|
| | |acc_norm|0.6388|± |0.0048|
|boolq | 1|acc |0.6193|± |0.0085|
## Disclaimer
Please read this disclaimer carefully before using the large language model provided in this repository. Your use of the model signifies your agreement to the following terms and conditions.
- Biases and Offensiveness: The large language model is trained on a diverse range of internet text data, which may contain biased, racist, offensive, or otherwise inappropriate content. By using this model, you acknowledge and accept that the generated content may sometimes exhibit biases or produce content that is offensive or inappropriate. The developers of this repository do not endorse, support, or promote any such content or viewpoints.
- Limitations: The large language model is an AI-based tool and not a human. It may produce incorrect, nonsensical, or irrelevant responses. It is the user's responsibility to critically evaluate the generated content and use it at their discretion.
- Use at Your Own Risk: Users of this large language model must assume full responsibility for any consequences that may arise from their use of the tool. The developers and contributors of this repository shall not be held liable for any damages, losses, or harm resulting from the use or misuse of the provided model.
- Ethical Considerations: Users are encouraged to use the large language model responsibly and ethically. By using this model, you agree not to use it for purposes that promote hate speech, discrimination, harassment, or any form of illegal or harmful activities.
- Reporting Issues: If you encounter any biased, offensive, or otherwise inappropriate content generated by the large language model, please report it to the repository maintainers through the provided channels. Your feedback will help improve the model and mitigate potential issues.
- Changes to this Disclaimer: The developers of this repository reserve the right to modify or update this disclaimer at any time without prior notice. It is the user's responsibility to periodically review the disclaimer to stay informed about any changes.
By using the large language model provided in this repository, you agree to accept and comply with the terms and conditions outlined in this disclaimer. If you do not agree with any part of this disclaimer, you should refrain from using the model and any content generated by it.
|
dicta-il/dictabert-joint | dicta-il | 2024-04-04T14:22:36Z | 12,379 | 0 | transformers | [
"transformers",
"pytorch",
"safetensors",
"bert",
"feature-extraction",
"custom_code",
"he",
"arxiv:2403.06970",
"license:cc-by-4.0",
"text-embeddings-inference",
"region:us"
]
| feature-extraction | 2024-01-10T15:27:50Z | ---
license: cc-by-4.0
language:
- he
inference: false
---
# DictaBERT: A State-of-the-Art BERT Suite for Modern Hebrew
State-of-the-art language model for Hebrew, released [here](https://arxiv.org/abs/2403.06970).
This is the fine-tuned model for the joint parsing of the following tasks:
- Prefix Segmentation
- Morphological Disabmgiuation
- Lexicographical Analysis (Lemmatization)
- Syntactical Parsing (Dependency-Tree)
- Named-Entity Recognition
A live demo of the model with instant visualization of the syntax tree can be found [here](https://huggingface.co/spaces/dicta-il/joint-demo).
For a faster model, you can use the equivalent bert-tiny model for this task [here](https://huggingface.co/dicta-il/dictabert-tiny-joint).
For the bert-base models for other tasks, see [here](https://huggingface.co/collections/dicta-il/dictabert-6588e7cc08f83845fc42a18b).
---
The model currently supports 3 types of output:
1. **JSON**: The model returns a JSON object for each sentence in the input, where for each sentence we have the sentence text, the NER entities, and the list of tokens. For each token we include the output from each of the tasks.
```python
model.predict(..., output_style='json')
```
1. **UD**: The model returns the full UD output for each sentence, according to the style of the Hebrew UD Treebank.
```python
model.predict(..., output_style='ud')
```
1. **UD, in the style of IAHLT**: This model returns the full UD output, with slight modifications to match the style of IAHLT. This differences are mostly granularity of some dependency relations, how the suffix of a word is broken up, and implicit definite articles. The actual tagging behavior doesn't change.
```python
model.predict(..., output_style='iahlt_ud')
```
---
If you only need the output for one of the tasks, you can tell the model to not initialize some of the heads, for example:
```python
model = AutoModel.from_pretrained('dicta-il/dictabert-joint', trust_remote_code=True, do_lex=False)
```
The list of options are: `do_lex`, `do_syntax`, `do_ner`, `do_prefix`, `do_morph`.
---
Sample usage:
```python
from transformers import AutoModel, AutoTokenizer
tokenizer = AutoTokenizer.from_pretrained('dicta-il/dictabert-joint')
model = AutoModel.from_pretrained('dicta-il/dictabert-joint', trust_remote_code=True)
model.eval()
sentence = 'בשנת 1948 השלים אפרים קישון את לימודיו בפיסול מתכת ובתולדות האמנות והחל לפרסם מאמרים הומוריסטיים'
print(model.predict([sentence], tokenizer, output_style='json')) # see below for other return formats
```
Output:
```json
[
{
"text": "בשנת 1948 השלים אפרים קישון את לימודיו בפיסול מתכת ובתולדות האמנות והחל לפרסם מאמרים הומוריסטיים",
"tokens": [
{
"token": "בשנת",
"syntax": {
"word": "בשנת",
"dep_head_idx": 2,
"dep_func": "obl",
"dep_head": "השלים"
},
"seg": [
"ב",
"שנת"
],
"lex": "שנה",
"morph": {
"token": "בשנת",
"pos": "NOUN",
"feats": {
"Gender": "Fem",
"Number": "Sing"
},
"prefixes": [
"ADP"
],
"suffix": false
}
},
{
"token": "1948",
"syntax": {
"word": "1948",
"dep_head_idx": 0,
"dep_func": "compound",
"dep_head": "בשנת"
},
"seg": [
"1948"
],
"lex": "1948",
"morph": {
"token": "1948",
"pos": "NUM",
"feats": {},
"prefixes": [],
"suffix": false
}
},
{
"token": "השלים",
"syntax": {
"word": "השלים",
"dep_head_idx": -1,
"dep_func": "root",
"dep_head": "הומוריסטיים"
},
"seg": [
"השלים"
],
"lex": "השלים",
"morph": {
"token": "השלים",
"pos": "VERB",
"feats": {
"Gender": "Masc",
"Number": "Sing",
"Person": "3",
"Tense": "Past"
},
"prefixes": [],
"suffix": false
}
},
{
"token": "אפרים",
"syntax": {
"word": "אפרים",
"dep_head_idx": 2,
"dep_func": "nsubj",
"dep_head": "השלים"
},
"seg": [
"אפרים"
],
"lex": "אפרים",
"morph": {
"token": "אפרים",
"pos": "PROPN",
"feats": {},
"prefixes": [],
"suffix": false
}
},
{
"token": "קישון",
"syntax": {
"word": "קישון",
"dep_head_idx": 3,
"dep_func": "flat",
"dep_head": "אפרים"
},
"seg": [
"קישון"
],
"lex": "קישון",
"morph": {
"token": "קישון",
"pos": "PROPN",
"feats": {},
"prefixes": [],
"suffix": false
}
},
{
"token": "את",
"syntax": {
"word": "את",
"dep_head_idx": 6,
"dep_func": "case",
"dep_head": "לימודיו"
},
"seg": [
"את"
],
"lex": "את",
"morph": {
"token": "את",
"pos": "ADP",
"feats": {},
"prefixes": [],
"suffix": false
}
},
{
"token": "לימודיו",
"syntax": {
"word": "לימודיו",
"dep_head_idx": 2,
"dep_func": "obj",
"dep_head": "השלים"
},
"seg": [
"לימודיו"
],
"lex": "לימוד",
"morph": {
"token": "לימודיו",
"pos": "NOUN",
"feats": {
"Gender": "Masc",
"Number": "Plur"
},
"prefixes": [],
"suffix": "PRON",
"suffix_feats": {
"Gender": "Masc",
"Number": "Sing",
"Person": "3"
}
}
},
{
"token": "בפיסול",
"syntax": {
"word": "בפיסול",
"dep_head_idx": 6,
"dep_func": "nmod",
"dep_head": "לימודיו"
},
"seg": [
"ב",
"פיסול"
],
"lex": "פיסול",
"morph": {
"token": "בפיסול",
"pos": "NOUN",
"feats": {
"Gender": "Masc",
"Number": "Sing"
},
"prefixes": [
"ADP"
],
"suffix": false
}
},
{
"token": "מתכת",
"syntax": {
"word": "מתכת",
"dep_head_idx": 7,
"dep_func": "compound",
"dep_head": "בפיסול"
},
"seg": [
"מתכת"
],
"lex": "מתכת",
"morph": {
"token": "מתכת",
"pos": "NOUN",
"feats": {
"Gender": "Fem",
"Number": "Sing"
},
"prefixes": [],
"suffix": false
}
},
{
"token": "ובתולדות",
"syntax": {
"word": "ובתולדות",
"dep_head_idx": 7,
"dep_func": "conj",
"dep_head": "בפיסול"
},
"seg": [
"וב",
"תולדות"
],
"lex": "תולדה",
"morph": {
"token": "ובתולדות",
"pos": "NOUN",
"feats": {
"Gender": "Fem",
"Number": "Plur"
},
"prefixes": [
"CCONJ",
"ADP"
],
"suffix": false
}
},
{
"token": "האמנות",
"syntax": {
"word": "האמנות",
"dep_head_idx": 9,
"dep_func": "compound",
"dep_head": "ובתולדות"
},
"seg": [
"ה",
"אמנות"
],
"lex": "אומנות",
"morph": {
"token": "האמנות",
"pos": "NOUN",
"feats": {
"Gender": "Fem",
"Number": "Sing"
},
"prefixes": [
"DET"
],
"suffix": false
}
},
{
"token": "והחל",
"syntax": {
"word": "והחל",
"dep_head_idx": 2,
"dep_func": "conj",
"dep_head": "השלים"
},
"seg": [
"ו",
"החל"
],
"lex": "החל",
"morph": {
"token": "והחל",
"pos": "VERB",
"feats": {
"Gender": "Masc",
"Number": "Sing",
"Person": "3",
"Tense": "Past"
},
"prefixes": [
"CCONJ"
],
"suffix": false
}
},
{
"token": "לפרסם",
"syntax": {
"word": "לפרסם",
"dep_head_idx": 11,
"dep_func": "xcomp",
"dep_head": "והחל"
},
"seg": [
"לפרסם"
],
"lex": "פרסם",
"morph": {
"token": "לפרסם",
"pos": "VERB",
"feats": {},
"prefixes": [],
"suffix": false
}
},
{
"token": "מאמרים",
"syntax": {
"word": "מאמרים",
"dep_head_idx": 12,
"dep_func": "obj",
"dep_head": "לפרסם"
},
"seg": [
"מאמרים"
],
"lex": "מאמר",
"morph": {
"token": "מאמרים",
"pos": "NOUN",
"feats": {
"Gender": "Masc",
"Number": "Plur"
},
"prefixes": [],
"suffix": false
}
},
{
"token": "הומוריסטיים",
"syntax": {
"word": "הומוריסטיים",
"dep_head_idx": 13,
"dep_func": "amod",
"dep_head": "מאמרים"
},
"seg": [
"הומוריסטיים"
],
"lex": "הומוריסטי",
"morph": {
"token": "הומוריסטיים",
"pos": "ADJ",
"feats": {
"Gender": "Masc",
"Number": "Plur"
},
"prefixes": [],
"suffix": false
}
}
],
"root_idx": 2,
"ner_entities": [
{
"phrase": "1948",
"label": "TIMEX"
},
{
"phrase": "אפרים קישון",
"label": "PER"
}
]
}
]
```
You can also choose to get your response in UD format:
```python
sentence = 'בשנת 1948 השלים אפרים קישון את לימודיו בפיסול מתכת ובתולדות האמנות והחל לפרסם מאמרים הומוריסטיים'
print(model.predict([sentence], tokenizer, output_style='ud'))
```
Results:
```json
[
[
"# sent_id = 1",
"# text = בשנת 1948 השלים אפרים קישון את לימודיו בפיסול מתכת ובתולדות האמנות והחל לפרסם מאמרים הומוריסטיים",
"1-2\tבשנת\t_\t_\t_\t_\t_\t_\t_\t_",
"1\tב\tב\tADP\tADP\t_\t2\tcase\t_\t_",
"2\tשנת\tשנה\tNOUN\tNOUN\tGender=Fem|Number=Sing\t4\tobl\t_\t_",
"3\t1948\t1948\tNUM\tNUM\t\t2\tcompound:smixut\t_\t_",
"4\tהשלים\tהשלים\tVERB\tVERB\tGender=Masc|Number=Sing|Person=3|Tense=Past\t0\troot\t_\t_",
"5\tאפרים\tאפרים\tPROPN\tPROPN\t\t4\tnsubj\t_\t_",
"6\tקישון\tקישון\tPROPN\tPROPN\t\t5\tflat\t_\t_",
"7\tאת\tאת\tADP\tADP\t\t8\tcase:acc\t_\t_",
"8-10\tלימודיו\t_\t_\t_\t_\t_\t_\t_\t_",
"8\tלימוד_\tלימוד\tNOUN\tNOUN\tGender=Masc|Number=Plur\t4\tobj\t_\t_",
"9\t_של_\tשל\tADP\tADP\t_\t10\tcase\t_\t_",
"10\t_הוא\tהוא\tPRON\tPRON\tGender=Masc|Number=Sing|Person=3\t8\tnmod:poss\t_\t_",
"11-12\tבפיסול\t_\t_\t_\t_\t_\t_\t_\t_",
"11\tב\tב\tADP\tADP\t_\t12\tcase\t_\t_",
"12\tפיסול\tפיסול\tNOUN\tNOUN\tGender=Masc|Number=Sing\t8\tnmod\t_\t_",
"13\tמתכת\tמתכת\tNOUN\tNOUN\tGender=Fem|Number=Sing\t12\tcompound:smixut\t_\t_",
"14-16\tובתולדות\t_\t_\t_\t_\t_\t_\t_\t_",
"14\tו\tו\tCCONJ\tCCONJ\t_\t16\tcc\t_\t_",
"15\tב\tב\tADP\tADP\t_\t16\tcase\t_\t_",
"16\tתולדות\tתולדה\tNOUN\tNOUN\tGender=Fem|Number=Plur\t12\tconj\t_\t_",
"17-18\tהאמנות\t_\t_\t_\t_\t_\t_\t_\t_",
"17\tה\tה\tDET\tDET\t_\t18\tdet\t_\t_",
"18\tאמנות\tאומנות\tNOUN\tNOUN\tGender=Fem|Number=Sing\t16\tcompound:smixut\t_\t_",
"19-20\tוהחל\t_\t_\t_\t_\t_\t_\t_\t_",
"19\tו\tו\tCCONJ\tCCONJ\t_\t20\tcc\t_\t_",
"20\tהחל\tהחל\tVERB\tVERB\tGender=Masc|Number=Sing|Person=3|Tense=Past\t4\tconj\t_\t_",
"21\tלפרסם\tפרסם\tVERB\tVERB\t\t20\txcomp\t_\t_",
"22\tמאמרים\tמאמר\tNOUN\tNOUN\tGender=Masc|Number=Plur\t21\tobj\t_\t_",
"23\tהומוריסטיים\tהומוריסטי\tADJ\tADJ\tGender=Masc|Number=Plur\t22\tamod\t_\t_"
]
]
```
## Citation
If you use DictaBERT-joint in your research, please cite ```MRL Parsing without Tears: The Case of Hebrew```
**BibTeX:**
```bibtex
@misc{shmidman2024mrl,
title={MRL Parsing Without Tears: The Case of Hebrew},
author={Shaltiel Shmidman and Avi Shmidman and Moshe Koppel and Reut Tsarfaty},
year={2024},
eprint={2403.06970},
archivePrefix={arXiv},
primaryClass={cs.CL}
}
```
## License
Shield: [![CC BY 4.0][cc-by-shield]][cc-by]
This work is licensed under a
[Creative Commons Attribution 4.0 International License][cc-by].
[![CC BY 4.0][cc-by-image]][cc-by]
[cc-by]: http://creativecommons.org/licenses/by/4.0/
[cc-by-image]: https://i.creativecommons.org/l/by/4.0/88x31.png
[cc-by-shield]: https://img.shields.io/badge/License-CC%20BY%204.0-lightgrey.svg
|
mradermacher/Prox-Llama-3-8B-abliterated-orpo-i1-GGUF | mradermacher | 2024-06-20T23:01:46Z | 12,367 | 0 | transformers | [
"transformers",
"gguf",
"code",
"cybersecurity",
"penetration testing",
"hacking",
"uncensored",
"en",
"dataset:mlabonne/orpo-dpo-mix-40k",
"base_model:openvoid/Prox-Llama-3-8B-abliterated-orpo",
"license:apache-2.0",
"endpoints_compatible",
"region:us"
]
| null | 2024-06-20T18:16:30Z | ---
base_model: openvoid/Prox-Llama-3-8B-abliterated-orpo
datasets:
- mlabonne/orpo-dpo-mix-40k
language:
- en
library_name: transformers
license: apache-2.0
quantized_by: mradermacher
tags:
- code
- cybersecurity
- penetration testing
- hacking
- code
- uncensored
---
## About
<!-- ### quantize_version: 2 -->
<!-- ### output_tensor_quantised: 1 -->
<!-- ### convert_type: hf -->
<!-- ### vocab_type: -->
<!-- ### tags: nicoboss -->
weighted/imatrix quants of https://huggingface.co/openvoid/Prox-Llama-3-8B-abliterated-orpo
<!-- provided-files -->
static quants are available at https://huggingface.co/mradermacher/Prox-Llama-3-8B-abliterated-orpo-GGUF
## Usage
If you are unsure how to use GGUF files, refer to one of [TheBloke's
READMEs](https://huggingface.co/TheBloke/KafkaLM-70B-German-V0.1-GGUF) for
more details, including on how to concatenate multi-part files.
## Provided Quants
(sorted by size, not necessarily quality. IQ-quants are often preferable over similar sized non-IQ quants)
| Link | Type | Size/GB | Notes |
|:-----|:-----|--------:|:------|
| [GGUF](https://huggingface.co/mradermacher/Prox-Llama-3-8B-abliterated-orpo-i1-GGUF/resolve/main/Prox-Llama-3-8B-abliterated-orpo.i1-IQ1_S.gguf) | i1-IQ1_S | 2.1 | for the desperate |
| [GGUF](https://huggingface.co/mradermacher/Prox-Llama-3-8B-abliterated-orpo-i1-GGUF/resolve/main/Prox-Llama-3-8B-abliterated-orpo.i1-IQ1_M.gguf) | i1-IQ1_M | 2.3 | mostly desperate |
| [GGUF](https://huggingface.co/mradermacher/Prox-Llama-3-8B-abliterated-orpo-i1-GGUF/resolve/main/Prox-Llama-3-8B-abliterated-orpo.i1-IQ2_XXS.gguf) | i1-IQ2_XXS | 2.5 | |
| [GGUF](https://huggingface.co/mradermacher/Prox-Llama-3-8B-abliterated-orpo-i1-GGUF/resolve/main/Prox-Llama-3-8B-abliterated-orpo.i1-IQ2_XS.gguf) | i1-IQ2_XS | 2.7 | |
| [GGUF](https://huggingface.co/mradermacher/Prox-Llama-3-8B-abliterated-orpo-i1-GGUF/resolve/main/Prox-Llama-3-8B-abliterated-orpo.i1-IQ2_S.gguf) | i1-IQ2_S | 2.9 | |
| [GGUF](https://huggingface.co/mradermacher/Prox-Llama-3-8B-abliterated-orpo-i1-GGUF/resolve/main/Prox-Llama-3-8B-abliterated-orpo.i1-IQ2_M.gguf) | i1-IQ2_M | 3.0 | |
| [GGUF](https://huggingface.co/mradermacher/Prox-Llama-3-8B-abliterated-orpo-i1-GGUF/resolve/main/Prox-Llama-3-8B-abliterated-orpo.i1-Q2_K.gguf) | i1-Q2_K | 3.3 | IQ3_XXS probably better |
| [GGUF](https://huggingface.co/mradermacher/Prox-Llama-3-8B-abliterated-orpo-i1-GGUF/resolve/main/Prox-Llama-3-8B-abliterated-orpo.i1-IQ3_XXS.gguf) | i1-IQ3_XXS | 3.4 | lower quality |
| [GGUF](https://huggingface.co/mradermacher/Prox-Llama-3-8B-abliterated-orpo-i1-GGUF/resolve/main/Prox-Llama-3-8B-abliterated-orpo.i1-IQ3_XS.gguf) | i1-IQ3_XS | 3.6 | |
| [GGUF](https://huggingface.co/mradermacher/Prox-Llama-3-8B-abliterated-orpo-i1-GGUF/resolve/main/Prox-Llama-3-8B-abliterated-orpo.i1-Q3_K_S.gguf) | i1-Q3_K_S | 3.8 | IQ3_XS probably better |
| [GGUF](https://huggingface.co/mradermacher/Prox-Llama-3-8B-abliterated-orpo-i1-GGUF/resolve/main/Prox-Llama-3-8B-abliterated-orpo.i1-IQ3_S.gguf) | i1-IQ3_S | 3.8 | beats Q3_K* |
| [GGUF](https://huggingface.co/mradermacher/Prox-Llama-3-8B-abliterated-orpo-i1-GGUF/resolve/main/Prox-Llama-3-8B-abliterated-orpo.i1-IQ3_M.gguf) | i1-IQ3_M | 3.9 | |
| [GGUF](https://huggingface.co/mradermacher/Prox-Llama-3-8B-abliterated-orpo-i1-GGUF/resolve/main/Prox-Llama-3-8B-abliterated-orpo.i1-Q3_K_M.gguf) | i1-Q3_K_M | 4.1 | IQ3_S probably better |
| [GGUF](https://huggingface.co/mradermacher/Prox-Llama-3-8B-abliterated-orpo-i1-GGUF/resolve/main/Prox-Llama-3-8B-abliterated-orpo.i1-Q3_K_L.gguf) | i1-Q3_K_L | 4.4 | IQ3_M probably better |
| [GGUF](https://huggingface.co/mradermacher/Prox-Llama-3-8B-abliterated-orpo-i1-GGUF/resolve/main/Prox-Llama-3-8B-abliterated-orpo.i1-IQ4_XS.gguf) | i1-IQ4_XS | 4.5 | |
| [GGUF](https://huggingface.co/mradermacher/Prox-Llama-3-8B-abliterated-orpo-i1-GGUF/resolve/main/Prox-Llama-3-8B-abliterated-orpo.i1-Q4_0.gguf) | i1-Q4_0 | 4.8 | fast, low quality |
| [GGUF](https://huggingface.co/mradermacher/Prox-Llama-3-8B-abliterated-orpo-i1-GGUF/resolve/main/Prox-Llama-3-8B-abliterated-orpo.i1-Q4_K_S.gguf) | i1-Q4_K_S | 4.8 | optimal size/speed/quality |
| [GGUF](https://huggingface.co/mradermacher/Prox-Llama-3-8B-abliterated-orpo-i1-GGUF/resolve/main/Prox-Llama-3-8B-abliterated-orpo.i1-Q4_K_M.gguf) | i1-Q4_K_M | 5.0 | fast, recommended |
| [GGUF](https://huggingface.co/mradermacher/Prox-Llama-3-8B-abliterated-orpo-i1-GGUF/resolve/main/Prox-Llama-3-8B-abliterated-orpo.i1-Q5_K_S.gguf) | i1-Q5_K_S | 5.7 | |
| [GGUF](https://huggingface.co/mradermacher/Prox-Llama-3-8B-abliterated-orpo-i1-GGUF/resolve/main/Prox-Llama-3-8B-abliterated-orpo.i1-Q5_K_M.gguf) | i1-Q5_K_M | 5.8 | |
| [GGUF](https://huggingface.co/mradermacher/Prox-Llama-3-8B-abliterated-orpo-i1-GGUF/resolve/main/Prox-Llama-3-8B-abliterated-orpo.i1-Q6_K.gguf) | i1-Q6_K | 6.7 | practically like static Q6_K |
Here is a handy graph by ikawrakow comparing some lower-quality quant
types (lower is better):

And here are Artefact2's thoughts on the matter:
https://gist.github.com/Artefact2/b5f810600771265fc1e39442288e8ec9
## FAQ / Model Request
See https://huggingface.co/mradermacher/model_requests for some answers to
questions you might have and/or if you want some other model quantized.
## Thanks
I thank my company, [nethype GmbH](https://www.nethype.de/), for letting
me use its servers and providing upgrades to my workstation to enable
this work in my free time. Additional thanks to [@nicoboss](https://huggingface.co/nicoboss) for giving me access to his hardware for calculating the imatrix for these quants.
<!-- end -->
|
echarlaix/tiny-random-latent-consistency | echarlaix | 2023-10-24T13:29:09Z | 12,365 | 0 | diffusers | [
"diffusers",
"safetensors",
"license:apache-2.0",
"diffusers:LatentConsistencyModelPipeline",
"region:us"
]
| text-to-image | 2023-10-19T11:05:53Z | ---
license: apache-2.0
---
|
digiplay/Photon_v1 | digiplay | 2024-05-18T19:02:55Z | 12,364 | 37 | diffusers | [
"diffusers",
"safetensors",
"stable-diffusion",
"stable-diffusion-diffusers",
"text-to-image",
"license:other",
"endpoints_compatible",
"diffusers:StableDiffusionPipeline",
"region:us"
]
| text-to-image | 2023-06-08T19:02:37Z | ---
license: other
tags:
- stable-diffusion
- stable-diffusion-diffusers
- text-to-image
- diffusers
inference: true
---
Model info: https://civitai.com/models/84728/photon
 |
RichardErkhov/SanjiWatsuki_-_Kunoichi-7B-gguf | RichardErkhov | 2024-06-20T00:23:11Z | 12,353 | 0 | null | [
"gguf",
"region:us"
]
| null | 2024-06-19T21:02:20Z | Quantization made by Richard Erkhov.
[Github](https://github.com/RichardErkhov)
[Discord](https://discord.gg/pvy7H8DZMG)
[Request more models](https://github.com/RichardErkhov/quant_request)
Kunoichi-7B - GGUF
- Model creator: https://huggingface.co/SanjiWatsuki/
- Original model: https://huggingface.co/SanjiWatsuki/Kunoichi-7B/
| Name | Quant method | Size |
| ---- | ---- | ---- |
| [Kunoichi-7B.Q2_K.gguf](https://huggingface.co/RichardErkhov/SanjiWatsuki_-_Kunoichi-7B-gguf/blob/main/Kunoichi-7B.Q2_K.gguf) | Q2_K | 2.53GB |
| [Kunoichi-7B.IQ3_XS.gguf](https://huggingface.co/RichardErkhov/SanjiWatsuki_-_Kunoichi-7B-gguf/blob/main/Kunoichi-7B.IQ3_XS.gguf) | IQ3_XS | 2.81GB |
| [Kunoichi-7B.IQ3_S.gguf](https://huggingface.co/RichardErkhov/SanjiWatsuki_-_Kunoichi-7B-gguf/blob/main/Kunoichi-7B.IQ3_S.gguf) | IQ3_S | 2.96GB |
| [Kunoichi-7B.Q3_K_S.gguf](https://huggingface.co/RichardErkhov/SanjiWatsuki_-_Kunoichi-7B-gguf/blob/main/Kunoichi-7B.Q3_K_S.gguf) | Q3_K_S | 2.95GB |
| [Kunoichi-7B.IQ3_M.gguf](https://huggingface.co/RichardErkhov/SanjiWatsuki_-_Kunoichi-7B-gguf/blob/main/Kunoichi-7B.IQ3_M.gguf) | IQ3_M | 3.06GB |
| [Kunoichi-7B.Q3_K.gguf](https://huggingface.co/RichardErkhov/SanjiWatsuki_-_Kunoichi-7B-gguf/blob/main/Kunoichi-7B.Q3_K.gguf) | Q3_K | 3.28GB |
| [Kunoichi-7B.Q3_K_M.gguf](https://huggingface.co/RichardErkhov/SanjiWatsuki_-_Kunoichi-7B-gguf/blob/main/Kunoichi-7B.Q3_K_M.gguf) | Q3_K_M | 3.28GB |
| [Kunoichi-7B.Q3_K_L.gguf](https://huggingface.co/RichardErkhov/SanjiWatsuki_-_Kunoichi-7B-gguf/blob/main/Kunoichi-7B.Q3_K_L.gguf) | Q3_K_L | 3.56GB |
| [Kunoichi-7B.IQ4_XS.gguf](https://huggingface.co/RichardErkhov/SanjiWatsuki_-_Kunoichi-7B-gguf/blob/main/Kunoichi-7B.IQ4_XS.gguf) | IQ4_XS | 3.67GB |
| [Kunoichi-7B.Q4_0.gguf](https://huggingface.co/RichardErkhov/SanjiWatsuki_-_Kunoichi-7B-gguf/blob/main/Kunoichi-7B.Q4_0.gguf) | Q4_0 | 3.83GB |
| [Kunoichi-7B.IQ4_NL.gguf](https://huggingface.co/RichardErkhov/SanjiWatsuki_-_Kunoichi-7B-gguf/blob/main/Kunoichi-7B.IQ4_NL.gguf) | IQ4_NL | 3.87GB |
| [Kunoichi-7B.Q4_K_S.gguf](https://huggingface.co/RichardErkhov/SanjiWatsuki_-_Kunoichi-7B-gguf/blob/main/Kunoichi-7B.Q4_K_S.gguf) | Q4_K_S | 3.86GB |
| [Kunoichi-7B.Q4_K.gguf](https://huggingface.co/RichardErkhov/SanjiWatsuki_-_Kunoichi-7B-gguf/blob/main/Kunoichi-7B.Q4_K.gguf) | Q4_K | 4.07GB |
| [Kunoichi-7B.Q4_K_M.gguf](https://huggingface.co/RichardErkhov/SanjiWatsuki_-_Kunoichi-7B-gguf/blob/main/Kunoichi-7B.Q4_K_M.gguf) | Q4_K_M | 4.07GB |
| [Kunoichi-7B.Q4_1.gguf](https://huggingface.co/RichardErkhov/SanjiWatsuki_-_Kunoichi-7B-gguf/blob/main/Kunoichi-7B.Q4_1.gguf) | Q4_1 | 4.24GB |
| [Kunoichi-7B.Q5_0.gguf](https://huggingface.co/RichardErkhov/SanjiWatsuki_-_Kunoichi-7B-gguf/blob/main/Kunoichi-7B.Q5_0.gguf) | Q5_0 | 4.65GB |
| [Kunoichi-7B.Q5_K_S.gguf](https://huggingface.co/RichardErkhov/SanjiWatsuki_-_Kunoichi-7B-gguf/blob/main/Kunoichi-7B.Q5_K_S.gguf) | Q5_K_S | 4.65GB |
| [Kunoichi-7B.Q5_K.gguf](https://huggingface.co/RichardErkhov/SanjiWatsuki_-_Kunoichi-7B-gguf/blob/main/Kunoichi-7B.Q5_K.gguf) | Q5_K | 4.78GB |
| [Kunoichi-7B.Q5_K_M.gguf](https://huggingface.co/RichardErkhov/SanjiWatsuki_-_Kunoichi-7B-gguf/blob/main/Kunoichi-7B.Q5_K_M.gguf) | Q5_K_M | 4.78GB |
| [Kunoichi-7B.Q5_1.gguf](https://huggingface.co/RichardErkhov/SanjiWatsuki_-_Kunoichi-7B-gguf/blob/main/Kunoichi-7B.Q5_1.gguf) | Q5_1 | 5.07GB |
| [Kunoichi-7B.Q6_K.gguf](https://huggingface.co/RichardErkhov/SanjiWatsuki_-_Kunoichi-7B-gguf/blob/main/Kunoichi-7B.Q6_K.gguf) | Q6_K | 5.53GB |
| [Kunoichi-7B.Q8_0.gguf](https://huggingface.co/RichardErkhov/SanjiWatsuki_-_Kunoichi-7B-gguf/blob/main/Kunoichi-7B.Q8_0.gguf) | Q8_0 | 7.17GB |
Original model description:
---
license: cc-by-nc-4.0
tags:
- merge
---
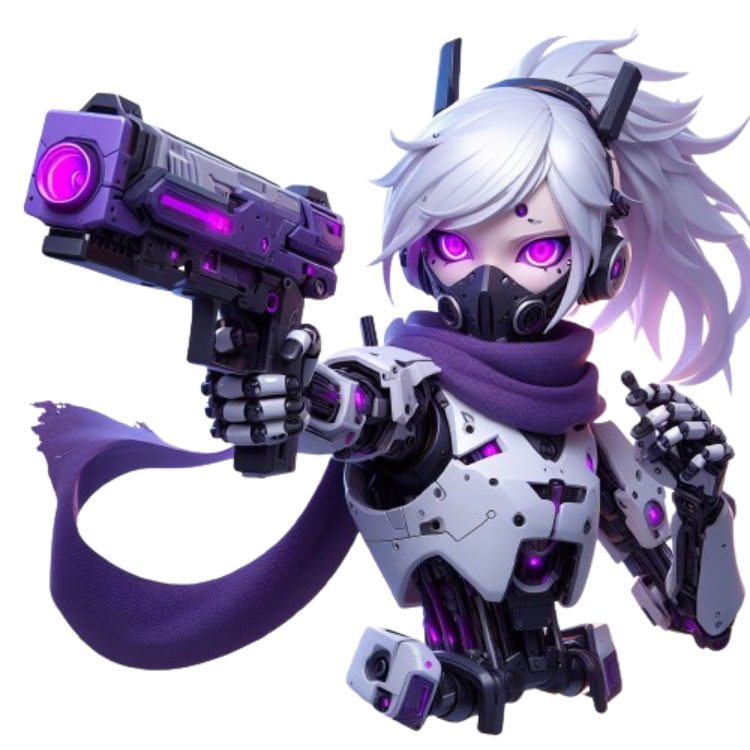
<!-- description start -->
## Description
This repository hosts **Kunoichi-7B**, an general purpose model capable of RP. In both my testing and the benchmarks, Kunoichi is an extremely strong model, keeping the advantages of my previous models but gaining more intelligence. Kunoichi scores extremely well on [all benchmarks which correlate closely with ChatBot Arena Elo.](https://www.reddit.com/r/LocalLLaMA/comments/18u0tu3/benchmarking_the_benchmarks_correlation_with/)
| Model | MT Bench | EQ Bench | MMLU | Logic Test |
|----------------------|----------|----------|---------|-------------|
| GPT-4-Turbo | 9.32 | - | - | - |
| GPT-4 | 8.99 | 62.52 | 86.4 | 0.86 |
| **Kunoichi-7B** | **8.14** | **44.32** | **64.9** | **0.58** |
| Starling-7B | 8.09 | - | 63.9 | 0.51 |
| Claude-2 | 8.06 | 52.14 | 78.5 | - |
| Silicon-Maid-7B | 7.96 | 40.44 | 64.7 | 0.54 |
| Loyal-Macaroni-Maid-7B | 7.95 | 38.66 | 64.9 | 0.57 |
| GPT-3.5-Turbo | 7.94 | 50.28 | 70 | 0.57 |
| Claude-1 | 7.9 | - | 77 | - |
| Openchat-3.5 | 7.81 | 37.08 | 64.3 | 0.39 |
| Dolphin-2.6-DPO | 7.74 | 42.88 | 61.9 | 0.53 |
| Zephyr-7B-beta | 7.34 | 38.71 | 61.4 | 0.30 |
| Llama-2-70b-chat-hf | 6.86 | 51.56 | 63 | - |
| Neural-chat-7b-v3-1 | 6.84 | 43.61 | 62.4 | 0.30 |
The model is intended to be used with up to an 8k context window. Using a NTK RoPE alpha of 2.6, the model can be used experimentally up to a 16k context window.
<!-- description end -->
<!-- prompt-template start -->
## Prompt template: Custom format, or Alpaca
### Alpaca:
```
Below is an instruction that describes a task. Write a response that appropriately completes the request.
### Instruction:
{prompt}
### Response:
```
### SillyTavern format:
I found the best SillyTavern results from using the Noromaid template.
SillyTavern config files: [Context](https://files.catbox.moe/ifmhai.json), [Instruct](https://files.catbox.moe/ttw1l9.json).
Additionally, here is my highly recommended [Text Completion preset](https://huggingface.co/SanjiWatsuki/Loyal-Macaroni-Maid-7B/blob/main/Characters/MinP.json). You can tweak this by adjusting temperature up or dropping min p to boost creativity or raise min p to increase stability. You shouldn't need to touch anything else!
## WTF is Kunoichi-7B?
Kunoichi-7B is a SLERP merger between my previous RP model, Silicon-Maid-7B, and an unreleased model that I had dubbed "Ninja-7B". This model is the result of me attempting to merge an RP focused model which maintained the strengths of Silicon-Maid-7B but further increased the model's brain power. I sought to increase both MT-Bench and EQ-Bench without losing Silicon Maid's strong ability to follow SillyTavern character cards.
Ninja-7B was born from an attempt to turn [jan-hq/stealth-v1.2](https://huggingface.co/jan-hq/stealth-v1.2) into a viable model through mergers. Although none of the Ninja prototype models developed to a point where I was happy, it turned out to be a strong model to merge. Combined with Silicon-Maid-7B, this appeared to be a strong merger.
## Other Benchmarks
| Model | Average | AGIEval | GPT4All | TruthfulQA | Bigbench |
|---|---:|---:|---:|---:|---:|
| [**Kunoichi-7B**](https://huggingface.co/SanjiWatsuki/Kunoichi-7B)|**57.54**| **44.99**| 74.86| **63.72**| 46.58|
| [OpenPipe/mistral-ft-optimized-1218](https://huggingface.co/OpenPipe/mistral-ft-optimized-1218)| 56.85 | 44.74 | **75.6** | 59.89 | **47.17** |
| [Silicon-Maid-7B](https://huggingface.co/SanjiWatsuki/Silicon-Maid-7B) | 56.45| 44.74| 74.26| 61.5| 45.32|
| [mlabonne/NeuralHermes-2.5-Mistral-7B](https://huggingface.co/mlabonne/NeuralHermes-2.5-Mistral-7B) | 53.51 | 43.67 | 73.24 | 55.37 | 41.76 |
| [teknium/OpenHermes-2.5-Mistral-7B](https://huggingface.co/teknium/OpenHermes-2.5-Mistral-7B) | 52.42 | 42.75 | 72.99 | 52.99 | 40.94 |
| [openchat/openchat_3.5](https://huggingface.co/openchat/openchat_3.5) | 51.34 | 42.67 | 72.92 | 47.27 | 42.51 |
| [berkeley-nest/Starling-LM-7B-alpha](https://huggingface.co/berkeley-nest/Starling-LM-7B-alpha) | 51.16 | 42.06 | 72.72 | 47.33 | 42.53 |
| [HuggingFaceH4/zephyr-7b-beta](https://huggingface.co/HuggingFaceH4/zephyr-7b-beta) | 50.99 | 37.33 | 71.83 | 55.1 | 39.7 |
|
myshell-ai/MeloTTS-Spanish | myshell-ai | 2024-03-01T17:30:14Z | 12,347 | 5 | transformers | [
"transformers",
"text-to-speech",
"ko",
"license:mit",
"endpoints_compatible",
"region:us"
]
| text-to-speech | 2024-02-29T14:54:04Z | ---
license: mit
language:
- ko
pipeline_tag: text-to-speech
---
# MeloTTS
MeloTTS is a **high-quality multi-lingual** text-to-speech library by [MyShell.ai](https://myshell.ai). Supported languages include:
| Model card | Example |
| --- | --- |
| [English](https://huggingface.co/myshell-ai/MeloTTS-English-v2) (American) | [Link](https://myshell-public-repo-hosting.s3.amazonaws.com/myshellttsbase/examples/en/EN-US/speed_1.0/sent_000.wav) |
| [English](https://huggingface.co/myshell-ai/MeloTTS-English-v2) (British) | [Link](https://myshell-public-repo-hosting.s3.amazonaws.com/myshellttsbase/examples/en/EN-BR/speed_1.0/sent_000.wav) |
| [English](https://huggingface.co/myshell-ai/MeloTTS-English-v2) (Indian) | [Link](https://myshell-public-repo-hosting.s3.amazonaws.com/myshellttsbase/examples/en/EN_INDIA/speed_1.0/sent_000.wav) |
| [English](https://huggingface.co/myshell-ai/MeloTTS-English-v2) (Australian) | [Link](https://myshell-public-repo-hosting.s3.amazonaws.com/myshellttsbase/examples/en/EN-AU/speed_1.0/sent_000.wav) |
| [English](https://huggingface.co/myshell-ai/MeloTTS-English-v2) (Default) | [Link](https://myshell-public-repo-hosting.s3.amazonaws.com/myshellttsbase/examples/en/EN-Default/speed_1.0/sent_000.wav) |
| [Spanish](https://huggingface.co/myshell-ai/MeloTTS-Spanish) | [Link](https://myshell-public-repo-hosting.s3.amazonaws.com/myshellttsbase/examples/es/ES/speed_1.0/sent_000.wav) |
| [French](https://huggingface.co/myshell-ai/MeloTTS-French) | [Link](https://myshell-public-repo-hosting.s3.amazonaws.com/myshellttsbase/examples/fr/FR/speed_1.0/sent_000.wav) |
| [Chinese](https://huggingface.co/myshell-ai/MeloTTS-Chinese) (mix EN) | [Link](https://myshell-public-repo-hosting.s3.amazonaws.com/myshellttsbase/examples/zh/ZH/speed_1.0/sent_008.wav) |
| [Japanese](https://huggingface.co/myshell-ai/MeloTTS-Japanese) | [Link](https://myshell-public-repo-hosting.s3.amazonaws.com/myshellttsbase/examples/jp/JP/speed_1.0/sent_000.wav) |
| [Korean](https://huggingface.co/myshell-ai/MeloTTS-Korean/) | [Link](https://myshell-public-repo-hosting.s3.amazonaws.com/myshellttsbase/examples/kr/KR/speed_1.0/sent_000.wav) |
Some other features include:
- The Chinese speaker supports `mixed Chinese and English`.
- Fast enough for `CPU real-time inference`.
## Usage
### Without Installation
An unofficial [live demo](https://huggingface.co/spaces/mrfakename/MeloTTS) is hosted on Hugging Face Spaces.
#### Use it on MyShell
There are hundreds of TTS models on MyShell, much more than MeloTTS. See examples [here](https://github.com/myshell-ai/MeloTTS/blob/main/docs/quick_use.md#use-melotts-without-installation).
More can be found at the widget center of [MyShell.ai](https://app.myshell.ai/robot-workshop).
### Install and Use Locally
Follow the installation steps [here](https://github.com/myshell-ai/MeloTTS/blob/main/docs/install.md#linux-and-macos-install) before using the following snippet:
```python
from melo.api import TTS
# Speed is adjustable
speed = 1.0
# CPU is sufficient for real-time inference.
# You can also change to cuda:0
device = 'cpu'
text = "El resplandor del sol acaricia las olas, pintando el cielo con una paleta deslumbrante."
model = TTS(language='ES', device=device)
speaker_ids = model.hps.data.spk2id
output_path = 'es.wav'
model.tts_to_file(text, speaker_ids['ES'], output_path, speed=speed)
```
## Join the Community
**Open Source AI Grant**
We are actively sponsoring open-source AI projects. The sponsorship includes GPU resources, fundings and intellectual support (collaboration with top research labs). We welcome both reseach and engineering projects, as long as the open-source community needs them. Please contact [Zengyi Qin](https://www.qinzy.tech/) if you are interested.
**Contributing**
If you find this work useful, please consider contributing to the GitHub [repo](https://github.com/myshell-ai/MeloTTS).
- Many thanks to [@fakerybakery](https://github.com/fakerybakery) for adding the Web UI and CLI part.
## License
This library is under MIT License, which means it is free for both commercial and non-commercial use.
## Acknowledgements
This implementation is based on [TTS](https://github.com/coqui-ai/TTS), [VITS](https://github.com/jaywalnut310/vits), [VITS2](https://github.com/daniilrobnikov/vits2) and [Bert-VITS2](https://github.com/fishaudio/Bert-VITS2). We appreciate their awesome work.
|
mradermacher/Llama-3-Umbral-Mind-Instruct-8B-i1-GGUF | mradermacher | 2024-06-24T17:57:07Z | 12,343 | 0 | transformers | [
"transformers",
"gguf",
"mergekit",
"merge",
"en",
"base_model:mpasila/Llama-3-Umbral-Mind-Instruct-8B",
"license:llama3",
"endpoints_compatible",
"region:us"
]
| null | 2024-06-24T16:42:13Z | ---
base_model: mpasila/Llama-3-Umbral-Mind-Instruct-8B
language:
- en
library_name: transformers
license: llama3
quantized_by: mradermacher
tags:
- mergekit
- merge
---
## About
<!-- ### quantize_version: 2 -->
<!-- ### output_tensor_quantised: 1 -->
<!-- ### convert_type: hf -->
<!-- ### vocab_type: -->
<!-- ### tags: nicoboss -->
weighted/imatrix quants of https://huggingface.co/mpasila/Llama-3-Umbral-Mind-Instruct-8B
<!-- provided-files -->
static quants are available at https://huggingface.co/mradermacher/Llama-3-Umbral-Mind-Instruct-8B-GGUF
## Usage
If you are unsure how to use GGUF files, refer to one of [TheBloke's
READMEs](https://huggingface.co/TheBloke/KafkaLM-70B-German-V0.1-GGUF) for
more details, including on how to concatenate multi-part files.
## Provided Quants
(sorted by size, not necessarily quality. IQ-quants are often preferable over similar sized non-IQ quants)
| Link | Type | Size/GB | Notes |
|:-----|:-----|--------:|:------|
| [GGUF](https://huggingface.co/mradermacher/Llama-3-Umbral-Mind-Instruct-8B-i1-GGUF/resolve/main/Llama-3-Umbral-Mind-Instruct-8B.i1-IQ1_S.gguf) | i1-IQ1_S | 2.1 | for the desperate |
| [GGUF](https://huggingface.co/mradermacher/Llama-3-Umbral-Mind-Instruct-8B-i1-GGUF/resolve/main/Llama-3-Umbral-Mind-Instruct-8B.i1-IQ1_M.gguf) | i1-IQ1_M | 2.3 | mostly desperate |
| [GGUF](https://huggingface.co/mradermacher/Llama-3-Umbral-Mind-Instruct-8B-i1-GGUF/resolve/main/Llama-3-Umbral-Mind-Instruct-8B.i1-IQ2_XXS.gguf) | i1-IQ2_XXS | 2.5 | |
| [GGUF](https://huggingface.co/mradermacher/Llama-3-Umbral-Mind-Instruct-8B-i1-GGUF/resolve/main/Llama-3-Umbral-Mind-Instruct-8B.i1-IQ2_XS.gguf) | i1-IQ2_XS | 2.7 | |
| [GGUF](https://huggingface.co/mradermacher/Llama-3-Umbral-Mind-Instruct-8B-i1-GGUF/resolve/main/Llama-3-Umbral-Mind-Instruct-8B.i1-IQ2_S.gguf) | i1-IQ2_S | 2.9 | |
| [GGUF](https://huggingface.co/mradermacher/Llama-3-Umbral-Mind-Instruct-8B-i1-GGUF/resolve/main/Llama-3-Umbral-Mind-Instruct-8B.i1-IQ2_M.gguf) | i1-IQ2_M | 3.0 | |
| [GGUF](https://huggingface.co/mradermacher/Llama-3-Umbral-Mind-Instruct-8B-i1-GGUF/resolve/main/Llama-3-Umbral-Mind-Instruct-8B.i1-Q2_K.gguf) | i1-Q2_K | 3.3 | IQ3_XXS probably better |
| [GGUF](https://huggingface.co/mradermacher/Llama-3-Umbral-Mind-Instruct-8B-i1-GGUF/resolve/main/Llama-3-Umbral-Mind-Instruct-8B.i1-IQ3_XXS.gguf) | i1-IQ3_XXS | 3.4 | lower quality |
| [GGUF](https://huggingface.co/mradermacher/Llama-3-Umbral-Mind-Instruct-8B-i1-GGUF/resolve/main/Llama-3-Umbral-Mind-Instruct-8B.i1-IQ3_XS.gguf) | i1-IQ3_XS | 3.6 | |
| [GGUF](https://huggingface.co/mradermacher/Llama-3-Umbral-Mind-Instruct-8B-i1-GGUF/resolve/main/Llama-3-Umbral-Mind-Instruct-8B.i1-Q3_K_S.gguf) | i1-Q3_K_S | 3.8 | IQ3_XS probably better |
| [GGUF](https://huggingface.co/mradermacher/Llama-3-Umbral-Mind-Instruct-8B-i1-GGUF/resolve/main/Llama-3-Umbral-Mind-Instruct-8B.i1-IQ3_S.gguf) | i1-IQ3_S | 3.8 | beats Q3_K* |
| [GGUF](https://huggingface.co/mradermacher/Llama-3-Umbral-Mind-Instruct-8B-i1-GGUF/resolve/main/Llama-3-Umbral-Mind-Instruct-8B.i1-IQ3_M.gguf) | i1-IQ3_M | 3.9 | |
| [GGUF](https://huggingface.co/mradermacher/Llama-3-Umbral-Mind-Instruct-8B-i1-GGUF/resolve/main/Llama-3-Umbral-Mind-Instruct-8B.i1-Q3_K_M.gguf) | i1-Q3_K_M | 4.1 | IQ3_S probably better |
| [GGUF](https://huggingface.co/mradermacher/Llama-3-Umbral-Mind-Instruct-8B-i1-GGUF/resolve/main/Llama-3-Umbral-Mind-Instruct-8B.i1-Q3_K_L.gguf) | i1-Q3_K_L | 4.4 | IQ3_M probably better |
| [GGUF](https://huggingface.co/mradermacher/Llama-3-Umbral-Mind-Instruct-8B-i1-GGUF/resolve/main/Llama-3-Umbral-Mind-Instruct-8B.i1-IQ4_XS.gguf) | i1-IQ4_XS | 4.5 | |
| [GGUF](https://huggingface.co/mradermacher/Llama-3-Umbral-Mind-Instruct-8B-i1-GGUF/resolve/main/Llama-3-Umbral-Mind-Instruct-8B.i1-Q4_0.gguf) | i1-Q4_0 | 4.8 | fast, low quality |
| [GGUF](https://huggingface.co/mradermacher/Llama-3-Umbral-Mind-Instruct-8B-i1-GGUF/resolve/main/Llama-3-Umbral-Mind-Instruct-8B.i1-Q4_K_S.gguf) | i1-Q4_K_S | 4.8 | optimal size/speed/quality |
| [GGUF](https://huggingface.co/mradermacher/Llama-3-Umbral-Mind-Instruct-8B-i1-GGUF/resolve/main/Llama-3-Umbral-Mind-Instruct-8B.i1-Q4_K_M.gguf) | i1-Q4_K_M | 5.0 | fast, recommended |
| [GGUF](https://huggingface.co/mradermacher/Llama-3-Umbral-Mind-Instruct-8B-i1-GGUF/resolve/main/Llama-3-Umbral-Mind-Instruct-8B.i1-Q5_K_S.gguf) | i1-Q5_K_S | 5.7 | |
| [GGUF](https://huggingface.co/mradermacher/Llama-3-Umbral-Mind-Instruct-8B-i1-GGUF/resolve/main/Llama-3-Umbral-Mind-Instruct-8B.i1-Q5_K_M.gguf) | i1-Q5_K_M | 5.8 | |
| [GGUF](https://huggingface.co/mradermacher/Llama-3-Umbral-Mind-Instruct-8B-i1-GGUF/resolve/main/Llama-3-Umbral-Mind-Instruct-8B.i1-Q6_K.gguf) | i1-Q6_K | 6.7 | practically like static Q6_K |
Here is a handy graph by ikawrakow comparing some lower-quality quant
types (lower is better):

And here are Artefact2's thoughts on the matter:
https://gist.github.com/Artefact2/b5f810600771265fc1e39442288e8ec9
## FAQ / Model Request
See https://huggingface.co/mradermacher/model_requests for some answers to
questions you might have and/or if you want some other model quantized.
## Thanks
I thank my company, [nethype GmbH](https://www.nethype.de/), for letting
me use its servers and providing upgrades to my workstation to enable
this work in my free time. Additional thanks to [@nicoboss](https://huggingface.co/nicoboss) for giving me access to his hardware for calculating the imatrix for these quants.
<!-- end -->
|
mradermacher/llama3-carpmuscle-v0.1-GGUF | mradermacher | 2024-07-02T04:22:37Z | 12,342 | 0 | transformers | [
"transformers",
"gguf",
"text-generation-inference",
"unsloth",
"llama",
"trl",
"en",
"base_model:TheTsar1209/llama3-carpmuscle-v0.1",
"license:apache-2.0",
"endpoints_compatible",
"region:us"
]
| null | 2024-07-02T03:22:57Z | ---
base_model: TheTsar1209/llama3-carpmuscle-v0.1
language:
- en
library_name: transformers
license: apache-2.0
quantized_by: mradermacher
tags:
- text-generation-inference
- transformers
- unsloth
- llama
- trl
---
## About
<!-- ### quantize_version: 2 -->
<!-- ### output_tensor_quantised: 1 -->
<!-- ### convert_type: hf -->
<!-- ### vocab_type: -->
<!-- ### tags: -->
static quants of https://huggingface.co/TheTsar1209/llama3-carpmuscle-v0.1
<!-- provided-files -->
weighted/imatrix quants seem not to be available (by me) at this time. If they do not show up a week or so after the static ones, I have probably not planned for them. Feel free to request them by opening a Community Discussion.
## Usage
If you are unsure how to use GGUF files, refer to one of [TheBloke's
READMEs](https://huggingface.co/TheBloke/KafkaLM-70B-German-V0.1-GGUF) for
more details, including on how to concatenate multi-part files.
## Provided Quants
(sorted by size, not necessarily quality. IQ-quants are often preferable over similar sized non-IQ quants)
| Link | Type | Size/GB | Notes |
|:-----|:-----|--------:|:------|
| [GGUF](https://huggingface.co/mradermacher/llama3-carpmuscle-v0.1-GGUF/resolve/main/llama3-carpmuscle-v0.1.Q2_K.gguf) | Q2_K | 3.3 | |
| [GGUF](https://huggingface.co/mradermacher/llama3-carpmuscle-v0.1-GGUF/resolve/main/llama3-carpmuscle-v0.1.IQ3_XS.gguf) | IQ3_XS | 3.6 | |
| [GGUF](https://huggingface.co/mradermacher/llama3-carpmuscle-v0.1-GGUF/resolve/main/llama3-carpmuscle-v0.1.Q3_K_S.gguf) | Q3_K_S | 3.8 | |
| [GGUF](https://huggingface.co/mradermacher/llama3-carpmuscle-v0.1-GGUF/resolve/main/llama3-carpmuscle-v0.1.IQ3_S.gguf) | IQ3_S | 3.8 | beats Q3_K* |
| [GGUF](https://huggingface.co/mradermacher/llama3-carpmuscle-v0.1-GGUF/resolve/main/llama3-carpmuscle-v0.1.IQ3_M.gguf) | IQ3_M | 3.9 | |
| [GGUF](https://huggingface.co/mradermacher/llama3-carpmuscle-v0.1-GGUF/resolve/main/llama3-carpmuscle-v0.1.Q3_K_M.gguf) | Q3_K_M | 4.1 | lower quality |
| [GGUF](https://huggingface.co/mradermacher/llama3-carpmuscle-v0.1-GGUF/resolve/main/llama3-carpmuscle-v0.1.Q3_K_L.gguf) | Q3_K_L | 4.4 | |
| [GGUF](https://huggingface.co/mradermacher/llama3-carpmuscle-v0.1-GGUF/resolve/main/llama3-carpmuscle-v0.1.IQ4_XS.gguf) | IQ4_XS | 4.6 | |
| [GGUF](https://huggingface.co/mradermacher/llama3-carpmuscle-v0.1-GGUF/resolve/main/llama3-carpmuscle-v0.1.Q4_K_S.gguf) | Q4_K_S | 4.8 | fast, recommended |
| [GGUF](https://huggingface.co/mradermacher/llama3-carpmuscle-v0.1-GGUF/resolve/main/llama3-carpmuscle-v0.1.Q4_K_M.gguf) | Q4_K_M | 5.0 | fast, recommended |
| [GGUF](https://huggingface.co/mradermacher/llama3-carpmuscle-v0.1-GGUF/resolve/main/llama3-carpmuscle-v0.1.Q5_K_S.gguf) | Q5_K_S | 5.7 | |
| [GGUF](https://huggingface.co/mradermacher/llama3-carpmuscle-v0.1-GGUF/resolve/main/llama3-carpmuscle-v0.1.Q5_K_M.gguf) | Q5_K_M | 5.8 | |
| [GGUF](https://huggingface.co/mradermacher/llama3-carpmuscle-v0.1-GGUF/resolve/main/llama3-carpmuscle-v0.1.Q6_K.gguf) | Q6_K | 6.7 | very good quality |
| [GGUF](https://huggingface.co/mradermacher/llama3-carpmuscle-v0.1-GGUF/resolve/main/llama3-carpmuscle-v0.1.Q8_0.gguf) | Q8_0 | 8.6 | fast, best quality |
| [GGUF](https://huggingface.co/mradermacher/llama3-carpmuscle-v0.1-GGUF/resolve/main/llama3-carpmuscle-v0.1.f16.gguf) | f16 | 16.2 | 16 bpw, overkill |
Here is a handy graph by ikawrakow comparing some lower-quality quant
types (lower is better):

And here are Artefact2's thoughts on the matter:
https://gist.github.com/Artefact2/b5f810600771265fc1e39442288e8ec9
## FAQ / Model Request
See https://huggingface.co/mradermacher/model_requests for some answers to
questions you might have and/or if you want some other model quantized.
## Thanks
I thank my company, [nethype GmbH](https://www.nethype.de/), for letting
me use its servers and providing upgrades to my workstation to enable
this work in my free time.
<!-- end -->
|
QuantFactory/Llama-3-8B-Magpie-Air-MT-SFT-v0.1-GGUF | QuantFactory | 2024-06-22T07:31:24Z | 12,338 | 0 | null | [
"gguf",
"axolotl",
"generated_from_trainer",
"text-generation",
"arxiv:2406.08464",
"base_model:Magpie-Align/Llama-3-8B-Magpie-Air-MT-SFT-v0.1",
"license:llama3",
"region:us"
]
| text-generation | 2024-06-21T14:43:36Z | ---
license: llama3
base_model: Magpie-Align/Llama-3-8B-Magpie-Air-MT-SFT-v0.1
tags:
- axolotl
- generated_from_trainer
model-index:
- name: Llama-3-8B-Magpie-Air-MT-SFT-v0.1
results: []
pipeline_tag: text-generation
---
# 🐦 Llama-3-8B-Magpie-Air-MT-SFT-v0.1-GGUF
This is quantized version of [Magpie-Align/Llama-3-8B-Magpie-Air-MT-SFT-v0.1](https://huggingface.co/Magpie-Align/Llama-3-8B-Magpie-Air-MT-SFT-v0.1) created using llama.cpp
# Model Description
Project Web: [https://magpie-align.github.io/](https://magpie-align.github.io/)
Arxiv Technical Report: [https://arxiv.org/abs/2406.08464](https://arxiv.org/abs/2406.08464)
Codes: [https://github.com/magpie-align/magpie](https://github.com/magpie-align/magpie)
## Abstract
<details><summary>Click Here</summary>
High-quality instruction data is critical for aligning large language models (LLMs). Although some models, such as Llama-3-Instruct, have open weights, their alignment data remain private, which hinders the democratization of AI. High human labor costs and a limited, predefined scope for prompting prevent existing open-source data creation methods from scaling effectively, potentially limiting the diversity and quality of public alignment datasets. Is it possible to synthesize high-quality instruction data at scale by extracting it directly from an aligned LLM? We present a self-synthesis method for generating large-scale alignment data named Magpie. Our key observation is that aligned LLMs like Llama-3-Instruct can generate a user query when we input only the left-side templates up to the position reserved for user messages, thanks to their auto-regressive nature. We use this method to prompt Llama-3-Instruct and generate 4 million instructions along with their corresponding responses. We perform a comprehensive analysis of the extracted data and select 300K high-quality instances. To compare Magpie data with other public instruction datasets, we fine-tune Llama-3-8B-Base with each dataset and evaluate the performance of the fine-tuned models. Our results indicate that in some tasks, models fine-tuned with Magpie perform comparably to the official Llama-3-8B-Instruct, despite the latter being enhanced with 10 million data points through supervised fine-tuning (SFT) and subsequent feedback learning. We also show that using Magpie solely for SFT can surpass the performance of previous public datasets utilized for both SFT and preference optimization, such as direct preference optimization with UltraFeedback. This advantage is evident on alignment benchmarks such as AlpacaEval, ArenaHard, and WildBench.
</details><be>
## About This Model
This model is a fine-tuned version of [meta-llama/Meta-Llama-3-8B](https://huggingface.co/meta-llama/Meta-Llama-3-8B) on [Magpie-Align/Magpie-Air-MT-300K-v0.1](https://huggingface.co/datasets/Magpie-Align/Magpie-Air-MT-300K-v0.1) dataset.
It achieves performance comparable with the official Llama-3-8B-Instruct Model with SFT only!
- **Alpaca Eval 2 (GPT-4-Turbo-1106): 22.98 (LC), 24.02 (WR)**
- **Alpaca Eval 2 (Llama-3-8B-Instruct): 49.63 (LC), 51.42 (WR)**
- **Arena Hard: 15.5**
## Other Information
**License**: Please follow [Meta Llama 3 Community License](https://llama.meta.com/llama3/license).
**Conversation Template**: Please use Llama 3 **official chat template** for the best performance.
## Citation
If you find the model, data, or code useful, please cite our paper:
```
@misc{xu2024magpie,
title={Magpie: Alignment Data Synthesis from Scratch by Prompting Aligned LLMs with Nothing},
author={Zhangchen Xu and Fengqing Jiang and Luyao Niu and Yuntian Deng and Radha Poovendran and Yejin Choi and Bill Yuchen Lin},
year={2024},
eprint={2406.08464},
archivePrefix={arXiv},
primaryClass={cs.CL}
}
```
## Training procedure
### Training hyperparameters
The following hyperparameters were used during training:
- learning_rate: 2e-05
- train_batch_size: 1
- eval_batch_size: 1
- seed: 42
- distributed_type: multi-GPU
- num_devices: 4
- gradient_accumulation_steps: 8
- total_train_batch_size: 32
- total_eval_batch_size: 4
- optimizer: Adam with betas=(0.9,0.999) and epsilon=1e-08
- lr_scheduler_type: cosine
- lr_scheduler_warmup_steps: 100
- num_epochs: 2
### Training results
| Training Loss | Epoch | Step | Validation Loss |
|:-------------:|:------:|:----:|:---------------:|
| 0.7285 | 0.0007 | 1 | 0.7411 |
| 0.2863 | 0.3332 | 509 | 0.2875 |
| 0.2584 | 0.6664 | 1018 | 0.2501 |
| 0.2187 | 0.9996 | 1527 | 0.2282 |
| 0.1445 | 1.3130 | 2036 | 0.2246 |
| 0.1419 | 1.6462 | 2545 | 0.2211 |
| 0.1413 | 1.9794 | 3054 | 0.2210 |
### Framework versions
- Transformers 4.40.2
- Pytorch 2.3.0+cu121
- Datasets 2.19.1
- Tokenizers 0.19.1
[<img src="https://raw.githubusercontent.com/OpenAccess-AI-Collective/axolotl/main/image/axolotl-badge-web.png" alt="Built with Axolotl" width="200" height="32"/>](https://github.com/OpenAccess-AI-Collective/axolotl)
<details><summary>See axolotl config</summary>
axolotl version: `0.4.0`
```yaml
base_model: meta-llama/Meta-Llama-3-8B
model_type: LlamaForCausalLM
tokenizer_type: AutoTokenizer
load_in_8bit: false
load_in_4bit: false
strict: false
datasets:
- path: SynDa/Llama-3-8B-SynDa-MultiRound-300K
type: sharegpt
conversation: llama3
dataset_prepared_path: last_run_prepared
val_set_size: 0.001
output_dir: ./out_Llama-3-70B-SynDa-300K-Multi-Round
sequence_len: 8192
sample_packing: true
eval_sample_packing: false
pad_to_sequence_len: true
wandb_project: SynDa
wandb_entity:
wandb_watch:
wandb_name: Llama-3-70B-SynDa-300K-MR-2EP-FFT
wandb_log_model:
hub_model_id: SynDa/Llama-3-8B-SynDa-300K-MR
gradient_accumulation_steps: 8
micro_batch_size: 1
num_epochs: 2
optimizer: paged_adamw_8bit
lr_scheduler: cosine
learning_rate: 2e-5
train_on_inputs: false
group_by_length: false
bf16: auto
fp16:
tf32: false
gradient_checkpointing: true
gradient_checkpointing_kwargs:
use_reentrant: false
early_stopping_patience:
resume_from_checkpoint:
logging_steps: 1
xformers_attention:
flash_attention: true
warmup_steps: 100
evals_per_epoch: 3
eval_table_size:
saves_per_epoch: 3
debug:
deepspeed:
weight_decay: 0.0
fsdp:
fsdp_config:
special_tokens:
pad_token: <|end_of_text|>
```
</details><br> |
mradermacher/JOSIEv4o-8b-stage1-beta2.2-GGUF | mradermacher | 2024-06-28T05:57:21Z | 12,334 | 0 | transformers | [
"transformers",
"gguf",
"text-generation-inference",
"unsloth",
"llama",
"trl",
"sft",
"en",
"de",
"base_model:Isaak-Carter/JOSIEv4o-8b-stage1-beta2.2",
"license:apache-2.0",
"endpoints_compatible",
"region:us"
]
| null | 2024-06-28T05:29:20Z | ---
base_model: Isaak-Carter/JOSIEv4o-8b-stage1-beta2.2
language:
- en
- de
library_name: transformers
license: apache-2.0
quantized_by: mradermacher
tags:
- text-generation-inference
- transformers
- unsloth
- llama
- trl
- sft
---
## About
<!-- ### quantize_version: 2 -->
<!-- ### output_tensor_quantised: 1 -->
<!-- ### convert_type: hf -->
<!-- ### vocab_type: -->
<!-- ### tags: -->
static quants of https://huggingface.co/Isaak-Carter/JOSIEv4o-8b-stage1-beta2.2
<!-- provided-files -->
weighted/imatrix quants seem not to be available (by me) at this time. If they do not show up a week or so after the static ones, I have probably not planned for them. Feel free to request them by opening a Community Discussion.
## Usage
If you are unsure how to use GGUF files, refer to one of [TheBloke's
READMEs](https://huggingface.co/TheBloke/KafkaLM-70B-German-V0.1-GGUF) for
more details, including on how to concatenate multi-part files.
## Provided Quants
(sorted by size, not necessarily quality. IQ-quants are often preferable over similar sized non-IQ quants)
| Link | Type | Size/GB | Notes |
|:-----|:-----|--------:|:------|
| [GGUF](https://huggingface.co/mradermacher/JOSIEv4o-8b-stage1-beta2.2-GGUF/resolve/main/JOSIEv4o-8b-stage1-beta2.2.Q2_K.gguf) | Q2_K | 3.3 | |
| [GGUF](https://huggingface.co/mradermacher/JOSIEv4o-8b-stage1-beta2.2-GGUF/resolve/main/JOSIEv4o-8b-stage1-beta2.2.IQ3_XS.gguf) | IQ3_XS | 3.6 | |
| [GGUF](https://huggingface.co/mradermacher/JOSIEv4o-8b-stage1-beta2.2-GGUF/resolve/main/JOSIEv4o-8b-stage1-beta2.2.Q3_K_S.gguf) | Q3_K_S | 3.8 | |
| [GGUF](https://huggingface.co/mradermacher/JOSIEv4o-8b-stage1-beta2.2-GGUF/resolve/main/JOSIEv4o-8b-stage1-beta2.2.IQ3_S.gguf) | IQ3_S | 3.8 | beats Q3_K* |
| [GGUF](https://huggingface.co/mradermacher/JOSIEv4o-8b-stage1-beta2.2-GGUF/resolve/main/JOSIEv4o-8b-stage1-beta2.2.IQ3_M.gguf) | IQ3_M | 3.9 | |
| [GGUF](https://huggingface.co/mradermacher/JOSIEv4o-8b-stage1-beta2.2-GGUF/resolve/main/JOSIEv4o-8b-stage1-beta2.2.Q3_K_M.gguf) | Q3_K_M | 4.1 | lower quality |
| [GGUF](https://huggingface.co/mradermacher/JOSIEv4o-8b-stage1-beta2.2-GGUF/resolve/main/JOSIEv4o-8b-stage1-beta2.2.Q3_K_L.gguf) | Q3_K_L | 4.4 | |
| [GGUF](https://huggingface.co/mradermacher/JOSIEv4o-8b-stage1-beta2.2-GGUF/resolve/main/JOSIEv4o-8b-stage1-beta2.2.IQ4_XS.gguf) | IQ4_XS | 4.6 | |
| [GGUF](https://huggingface.co/mradermacher/JOSIEv4o-8b-stage1-beta2.2-GGUF/resolve/main/JOSIEv4o-8b-stage1-beta2.2.Q4_K_S.gguf) | Q4_K_S | 4.8 | fast, recommended |
| [GGUF](https://huggingface.co/mradermacher/JOSIEv4o-8b-stage1-beta2.2-GGUF/resolve/main/JOSIEv4o-8b-stage1-beta2.2.Q4_K_M.gguf) | Q4_K_M | 5.0 | fast, recommended |
| [GGUF](https://huggingface.co/mradermacher/JOSIEv4o-8b-stage1-beta2.2-GGUF/resolve/main/JOSIEv4o-8b-stage1-beta2.2.Q5_K_S.gguf) | Q5_K_S | 5.7 | |
| [GGUF](https://huggingface.co/mradermacher/JOSIEv4o-8b-stage1-beta2.2-GGUF/resolve/main/JOSIEv4o-8b-stage1-beta2.2.Q5_K_M.gguf) | Q5_K_M | 5.8 | |
| [GGUF](https://huggingface.co/mradermacher/JOSIEv4o-8b-stage1-beta2.2-GGUF/resolve/main/JOSIEv4o-8b-stage1-beta2.2.Q6_K.gguf) | Q6_K | 6.7 | very good quality |
| [GGUF](https://huggingface.co/mradermacher/JOSIEv4o-8b-stage1-beta2.2-GGUF/resolve/main/JOSIEv4o-8b-stage1-beta2.2.Q8_0.gguf) | Q8_0 | 8.6 | fast, best quality |
| [GGUF](https://huggingface.co/mradermacher/JOSIEv4o-8b-stage1-beta2.2-GGUF/resolve/main/JOSIEv4o-8b-stage1-beta2.2.f16.gguf) | f16 | 16.2 | 16 bpw, overkill |
Here is a handy graph by ikawrakow comparing some lower-quality quant
types (lower is better):

And here are Artefact2's thoughts on the matter:
https://gist.github.com/Artefact2/b5f810600771265fc1e39442288e8ec9
## FAQ / Model Request
See https://huggingface.co/mradermacher/model_requests for some answers to
questions you might have and/or if you want some other model quantized.
## Thanks
I thank my company, [nethype GmbH](https://www.nethype.de/), for letting
me use its servers and providing upgrades to my workstation to enable
this work in my free time.
<!-- end -->
|
bartowski/Qwen2-7B-Instruct-GGUF | bartowski | 2024-06-07T01:07:13Z | 12,330 | 14 | null | [
"gguf",
"chat",
"text-generation",
"en",
"base_model:Qwen/Qwen2-7B-Instruct",
"license:apache-2.0",
"region:us"
]
| text-generation | 2024-06-06T17:30:41Z | ---
license: apache-2.0
language:
- en
pipeline_tag: text-generation
tags:
- chat
quantized_by: bartowski
base_model: Qwen/Qwen2-7B-Instruct
---
# <b>Heads up:</b> currently CUDA offloading is broken unless you enable flash attention
## Llamacpp imatrix Quantizations of Qwen2-7B-Instruct
Using <a href="https://github.com/ggerganov/llama.cpp/">llama.cpp</a> commit <a href="https://github.com/ggerganov/llama.cpp/commit/ee459f40f65810a810151b24eba5b8bd174ceffe">ee459f40f65810a810151b24eba5b8bd174ceffe</a> for quantization.
Original model: https://huggingface.co/Qwen/Qwen2-7B-Instruct
All quants made using imatrix option with dataset from [here](https://gist.github.com/bartowski1182/eb213dccb3571f863da82e99418f81e8)
## Prompt format
```
<|im_start|>system
{system_prompt}<|im_end|>
<|im_start|>user
{prompt}<|im_end|>
<|im_start|>assistant
```
## Download a file (not the whole branch) from below:
| Filename | Quant type | File Size | Description |
| -------- | ---------- | --------- | ----------- |
| [Qwen2-7B-Instruct-Q8_0.gguf](https://huggingface.co/bartowski/Qwen2-7B-Instruct-GGUF/blob/main/Qwen2-7B-Instruct-Q8_0.gguf) | Q8_0 | 8.09GB | Extremely high quality, generally unneeded but max available quant. |
| [Qwen2-7B-Instruct-Q6_K.gguf](https://huggingface.co/bartowski/Qwen2-7B-Instruct-GGUF/blob/main/Qwen2-7B-Instruct-Q6_K.gguf) | Q6_K | 6.25GB | Very high quality, near perfect, *recommended*. |
| [Qwen2-7B-Instruct-Q5_K_M.gguf](https://huggingface.co/bartowski/Qwen2-7B-Instruct-GGUF/blob/main/Qwen2-7B-Instruct-Q5_K_M.gguf) | Q5_K_M | 5.44GB | High quality, *recommended*. |
| [Qwen2-7B-Instruct-Q5_K_S.gguf](https://huggingface.co/bartowski/Qwen2-7B-Instruct-GGUF/blob/main/Qwen2-7B-Instruct-Q5_K_S.gguf) | Q5_K_S | 5.31GB | High quality, *recommended*. |
| [Qwen2-7B-Instruct-Q4_K_M.gguf](https://huggingface.co/bartowski/Qwen2-7B-Instruct-GGUF/blob/main/Qwen2-7B-Instruct-Q4_K_M.gguf) | Q4_K_M | 4.68GB | Good quality, uses about 4.83 bits per weight, *recommended*. |
| [Qwen2-7B-Instruct-Q4_K_S.gguf](https://huggingface.co/bartowski/Qwen2-7B-Instruct-GGUF/blob/main/Qwen2-7B-Instruct-Q4_K_S.gguf) | Q4_K_S | 4.45GB | Slightly lower quality with more space savings, *recommended*. |
| [Qwen2-7B-Instruct-IQ4_XS.gguf](https://huggingface.co/bartowski/Qwen2-7B-Instruct-GGUF/blob/main/Qwen2-7B-Instruct-IQ4_XS.gguf) | IQ4_XS | 4.21GB | Decent quality, smaller than Q4_K_S with similar performance, *recommended*. |
| [Qwen2-7B-Instruct-Q3_K_L.gguf](https://huggingface.co/bartowski/Qwen2-7B-Instruct-GGUF/blob/main/Qwen2-7B-Instruct-Q3_K_L.gguf) | Q3_K_L | 4.08GB | Lower quality but usable, good for low RAM availability. |
| [Qwen2-7B-Instruct-Q3_K_M.gguf](https://huggingface.co/bartowski/Qwen2-7B-Instruct-GGUF/blob/main/Qwen2-7B-Instruct-Q3_K_M.gguf) | Q3_K_M | 3.80GB | Even lower quality. |
| [Qwen2-7B-Instruct-IQ3_M.gguf](https://huggingface.co/bartowski/Qwen2-7B-Instruct-GGUF/blob/main/Qwen2-7B-Instruct-IQ3_M.gguf) | IQ3_M | 3.57GB | Medium-low quality, new method with decent performance comparable to Q3_K_M. |
| [Qwen2-7B-Instruct-Q3_K_S.gguf](https://huggingface.co/bartowski/Qwen2-7B-Instruct-GGUF/blob/main/Qwen2-7B-Instruct-Q3_K_S.gguf) | Q3_K_S | 3.49GB | Low quality, not recommended. |
| [Qwen2-7B-Instruct-IQ3_XS.gguf](https://huggingface.co/bartowski/Qwen2-7B-Instruct-GGUF/blob/main/Qwen2-7B-Instruct-IQ3_XS.gguf) | IQ3_XS | 3.34GB | Lower quality, new method with decent performance, slightly better than Q3_K_S. |
| [Qwen2-7B-Instruct-IQ3_XXS.gguf](https://huggingface.co/bartowski/Qwen2-7B-Instruct-GGUF/blob/main/Qwen2-7B-Instruct-IQ3_XXS.gguf) | IQ3_XXS | 3.11GB | Lower quality, new method with decent performance, comparable to Q3 quants. |
| [Qwen2-7B-Instruct-Q2_K.gguf](https://huggingface.co/bartowski/Qwen2-7B-Instruct-GGUF/blob/main/Qwen2-7B-Instruct-Q2_K.gguf) | Q2_K | 3.01GB | Very low quality but surprisingly usable. |
| [Qwen2-7B-Instruct-IQ2_M.gguf](https://huggingface.co/bartowski/Qwen2-7B-Instruct-GGUF/blob/main/Qwen2-7B-Instruct-IQ2_M.gguf) | IQ2_M | 2.78GB | Very low quality, uses SOTA techniques to also be surprisingly usable. |
| [Qwen2-7B-Instruct-IQ2_S.gguf](https://huggingface.co/bartowski/Qwen2-7B-Instruct-GGUF/blob/main/Qwen2-7B-Instruct-IQ2_S.gguf) | IQ2_S | 2.59GB | Very low quality, uses SOTA techniques to be usable. |
| [Qwen2-7B-Instruct-IQ2_XS.gguf](https://huggingface.co/bartowski/Qwen2-7B-Instruct-GGUF/blob/main/Qwen2-7B-Instruct-IQ2_XS.gguf) | IQ2_XS | 2.46GB | Very low quality, uses SOTA techniques to be usable. |
## Downloading using huggingface-cli
First, make sure you have hugginface-cli installed:
```
pip install -U "huggingface_hub[cli]"
```
Then, you can target the specific file you want:
```
huggingface-cli download bartowski/Qwen2-7B-Instruct-GGUF --include "Qwen2-7B-Instruct-Q4_K_M.gguf" --local-dir ./
```
If the model is bigger than 50GB, it will have been split into multiple files. In order to download them all to a local folder, run:
```
huggingface-cli download bartowski/Qwen2-7B-Instruct-GGUF --include "Qwen2-7B-Instruct-Q8_0.gguf/*" --local-dir Qwen2-7B-Instruct-Q8_0
```
You can either specify a new local-dir (Qwen2-7B-Instruct-Q8_0) or download them all in place (./)
## Which file should I choose?
A great write up with charts showing various performances is provided by Artefact2 [here](https://gist.github.com/Artefact2/b5f810600771265fc1e39442288e8ec9)
The first thing to figure out is how big a model you can run. To do this, you'll need to figure out how much RAM and/or VRAM you have.
If you want your model running as FAST as possible, you'll want to fit the whole thing on your GPU's VRAM. Aim for a quant with a file size 1-2GB smaller than your GPU's total VRAM.
If you want the absolute maximum quality, add both your system RAM and your GPU's VRAM together, then similarly grab a quant with a file size 1-2GB Smaller than that total.
Next, you'll need to decide if you want to use an 'I-quant' or a 'K-quant'.
If you don't want to think too much, grab one of the K-quants. These are in format 'QX_K_X', like Q5_K_M.
If you want to get more into the weeds, you can check out this extremely useful feature chart:
[llama.cpp feature matrix](https://github.com/ggerganov/llama.cpp/wiki/Feature-matrix)
But basically, if you're aiming for below Q4, and you're running cuBLAS (Nvidia) or rocBLAS (AMD), you should look towards the I-quants. These are in format IQX_X, like IQ3_M. These are newer and offer better performance for their size.
These I-quants can also be used on CPU and Apple Metal, but will be slower than their K-quant equivalent, so speed vs performance is a tradeoff you'll have to decide.
The I-quants are *not* compatible with Vulcan, which is also AMD, so if you have an AMD card double check if you're using the rocBLAS build or the Vulcan build. At the time of writing this, LM Studio has a preview with ROCm support, and other inference engines have specific builds for ROCm.
Want to support my work? Visit my ko-fi page here: https://ko-fi.com/bartowski
|
Dogge/llama-3-70B-instruct-uncensored-lora | Dogge | 2024-04-20T12:45:43Z | 12,324 | 4 | transformers | [
"transformers",
"safetensors",
"llama",
"text-generation",
"text-generation-inference",
"unsloth",
"trl",
"conversational",
"en",
"base_model:unsloth/llama-3-70b-Instruct-bnb-4bit",
"license:apache-2.0",
"autotrain_compatible",
"endpoints_compatible",
"4-bit",
"bitsandbytes",
"region:us"
]
| text-generation | 2024-04-19T23:19:15Z | ---
language:
- en
license: apache-2.0
tags:
- text-generation-inference
- transformers
- unsloth
- llama
- trl
base_model: unsloth/llama-3-70b-Instruct-bnb-4bit
---
# Uploaded model
- **Developed by:** Dogge
- **License:** apache-2.0
- **Finetuned from model :** unsloth/llama-3-70b-Instruct-bnb-4bit
This llama model was trained 2x faster with [Unsloth](https://github.com/unslothai/unsloth) and Huggingface's TRL library.
[<img src="https://raw.githubusercontent.com/unslothai/unsloth/main/images/unsloth%20made%20with%20love.png" width="200"/>](https://github.com/unslothai/unsloth)
|
mradermacher/Llama-3-8B-ShareGPT-112K-GGUF | mradermacher | 2024-06-28T13:42:58Z | 12,324 | 0 | transformers | [
"transformers",
"gguf",
"axolotl",
"generated_from_trainer",
"en",
"base_model:Magpie-Align/Llama-3-8B-ShareGPT-112K",
"license:llama3",
"endpoints_compatible",
"region:us"
]
| null | 2024-06-27T02:11:20Z | ---
base_model: Magpie-Align/Llama-3-8B-ShareGPT-112K
language:
- en
library_name: transformers
license: llama3
quantized_by: mradermacher
tags:
- axolotl
- generated_from_trainer
---
## About
<!-- ### quantize_version: 2 -->
<!-- ### output_tensor_quantised: 1 -->
<!-- ### convert_type: hf -->
<!-- ### vocab_type: -->
<!-- ### tags: -->
static quants of https://huggingface.co/Magpie-Align/Llama-3-8B-ShareGPT-112K
<!-- provided-files -->
weighted/imatrix quants are available at https://huggingface.co/mradermacher/Llama-3-8B-ShareGPT-112K-i1-GGUF
## Usage
If you are unsure how to use GGUF files, refer to one of [TheBloke's
READMEs](https://huggingface.co/TheBloke/KafkaLM-70B-German-V0.1-GGUF) for
more details, including on how to concatenate multi-part files.
## Provided Quants
(sorted by size, not necessarily quality. IQ-quants are often preferable over similar sized non-IQ quants)
| Link | Type | Size/GB | Notes |
|:-----|:-----|--------:|:------|
| [GGUF](https://huggingface.co/mradermacher/Llama-3-8B-ShareGPT-112K-GGUF/resolve/main/Llama-3-8B-ShareGPT-112K.Q2_K.gguf) | Q2_K | 3.3 | |
| [GGUF](https://huggingface.co/mradermacher/Llama-3-8B-ShareGPT-112K-GGUF/resolve/main/Llama-3-8B-ShareGPT-112K.IQ3_XS.gguf) | IQ3_XS | 3.6 | |
| [GGUF](https://huggingface.co/mradermacher/Llama-3-8B-ShareGPT-112K-GGUF/resolve/main/Llama-3-8B-ShareGPT-112K.Q3_K_S.gguf) | Q3_K_S | 3.8 | |
| [GGUF](https://huggingface.co/mradermacher/Llama-3-8B-ShareGPT-112K-GGUF/resolve/main/Llama-3-8B-ShareGPT-112K.IQ3_S.gguf) | IQ3_S | 3.8 | beats Q3_K* |
| [GGUF](https://huggingface.co/mradermacher/Llama-3-8B-ShareGPT-112K-GGUF/resolve/main/Llama-3-8B-ShareGPT-112K.IQ3_M.gguf) | IQ3_M | 3.9 | |
| [GGUF](https://huggingface.co/mradermacher/Llama-3-8B-ShareGPT-112K-GGUF/resolve/main/Llama-3-8B-ShareGPT-112K.Q3_K_M.gguf) | Q3_K_M | 4.1 | lower quality |
| [GGUF](https://huggingface.co/mradermacher/Llama-3-8B-ShareGPT-112K-GGUF/resolve/main/Llama-3-8B-ShareGPT-112K.Q3_K_L.gguf) | Q3_K_L | 4.4 | |
| [GGUF](https://huggingface.co/mradermacher/Llama-3-8B-ShareGPT-112K-GGUF/resolve/main/Llama-3-8B-ShareGPT-112K.IQ4_XS.gguf) | IQ4_XS | 4.6 | |
| [GGUF](https://huggingface.co/mradermacher/Llama-3-8B-ShareGPT-112K-GGUF/resolve/main/Llama-3-8B-ShareGPT-112K.Q4_K_S.gguf) | Q4_K_S | 4.8 | fast, recommended |
| [GGUF](https://huggingface.co/mradermacher/Llama-3-8B-ShareGPT-112K-GGUF/resolve/main/Llama-3-8B-ShareGPT-112K.Q4_K_M.gguf) | Q4_K_M | 5.0 | fast, recommended |
| [GGUF](https://huggingface.co/mradermacher/Llama-3-8B-ShareGPT-112K-GGUF/resolve/main/Llama-3-8B-ShareGPT-112K.Q5_K_S.gguf) | Q5_K_S | 5.7 | |
| [GGUF](https://huggingface.co/mradermacher/Llama-3-8B-ShareGPT-112K-GGUF/resolve/main/Llama-3-8B-ShareGPT-112K.Q5_K_M.gguf) | Q5_K_M | 5.8 | |
| [GGUF](https://huggingface.co/mradermacher/Llama-3-8B-ShareGPT-112K-GGUF/resolve/main/Llama-3-8B-ShareGPT-112K.Q6_K.gguf) | Q6_K | 6.7 | very good quality |
| [GGUF](https://huggingface.co/mradermacher/Llama-3-8B-ShareGPT-112K-GGUF/resolve/main/Llama-3-8B-ShareGPT-112K.Q8_0.gguf) | Q8_0 | 8.6 | fast, best quality |
| [GGUF](https://huggingface.co/mradermacher/Llama-3-8B-ShareGPT-112K-GGUF/resolve/main/Llama-3-8B-ShareGPT-112K.f16.gguf) | f16 | 16.2 | 16 bpw, overkill |
Here is a handy graph by ikawrakow comparing some lower-quality quant
types (lower is better):

And here are Artefact2's thoughts on the matter:
https://gist.github.com/Artefact2/b5f810600771265fc1e39442288e8ec9
## FAQ / Model Request
See https://huggingface.co/mradermacher/model_requests for some answers to
questions you might have and/or if you want some other model quantized.
## Thanks
I thank my company, [nethype GmbH](https://www.nethype.de/), for letting
me use its servers and providing upgrades to my workstation to enable
this work in my free time.
<!-- end -->
|
RichardErkhov/AIGym_-_deepseek-coder-6.7b-chat-gguf | RichardErkhov | 2024-06-20T10:59:03Z | 12,313 | 1 | null | [
"gguf",
"region:us"
]
| null | 2024-06-20T05:06:39Z | Quantization made by Richard Erkhov.
[Github](https://github.com/RichardErkhov)
[Discord](https://discord.gg/pvy7H8DZMG)
[Request more models](https://github.com/RichardErkhov/quant_request)
deepseek-coder-6.7b-chat - GGUF
- Model creator: https://huggingface.co/AIGym/
- Original model: https://huggingface.co/AIGym/deepseek-coder-6.7b-chat/
| Name | Quant method | Size |
| ---- | ---- | ---- |
| [deepseek-coder-6.7b-chat.Q2_K.gguf](https://huggingface.co/RichardErkhov/AIGym_-_deepseek-coder-6.7b-chat-gguf/blob/main/deepseek-coder-6.7b-chat.Q2_K.gguf) | Q2_K | 2.36GB |
| [deepseek-coder-6.7b-chat.IQ3_XS.gguf](https://huggingface.co/RichardErkhov/AIGym_-_deepseek-coder-6.7b-chat-gguf/blob/main/deepseek-coder-6.7b-chat.IQ3_XS.gguf) | IQ3_XS | 2.61GB |
| [deepseek-coder-6.7b-chat.IQ3_S.gguf](https://huggingface.co/RichardErkhov/AIGym_-_deepseek-coder-6.7b-chat-gguf/blob/main/deepseek-coder-6.7b-chat.IQ3_S.gguf) | IQ3_S | 2.75GB |
| [deepseek-coder-6.7b-chat.Q3_K_S.gguf](https://huggingface.co/RichardErkhov/AIGym_-_deepseek-coder-6.7b-chat-gguf/blob/main/deepseek-coder-6.7b-chat.Q3_K_S.gguf) | Q3_K_S | 2.75GB |
| [deepseek-coder-6.7b-chat.IQ3_M.gguf](https://huggingface.co/RichardErkhov/AIGym_-_deepseek-coder-6.7b-chat-gguf/blob/main/deepseek-coder-6.7b-chat.IQ3_M.gguf) | IQ3_M | 2.9GB |
| [deepseek-coder-6.7b-chat.Q3_K.gguf](https://huggingface.co/RichardErkhov/AIGym_-_deepseek-coder-6.7b-chat-gguf/blob/main/deepseek-coder-6.7b-chat.Q3_K.gguf) | Q3_K | 3.07GB |
| [deepseek-coder-6.7b-chat.Q3_K_M.gguf](https://huggingface.co/RichardErkhov/AIGym_-_deepseek-coder-6.7b-chat-gguf/blob/main/deepseek-coder-6.7b-chat.Q3_K_M.gguf) | Q3_K_M | 3.07GB |
| [deepseek-coder-6.7b-chat.Q3_K_L.gguf](https://huggingface.co/RichardErkhov/AIGym_-_deepseek-coder-6.7b-chat-gguf/blob/main/deepseek-coder-6.7b-chat.Q3_K_L.gguf) | Q3_K_L | 3.35GB |
| [deepseek-coder-6.7b-chat.IQ4_XS.gguf](https://huggingface.co/RichardErkhov/AIGym_-_deepseek-coder-6.7b-chat-gguf/blob/main/deepseek-coder-6.7b-chat.IQ4_XS.gguf) | IQ4_XS | 3.4GB |
| [deepseek-coder-6.7b-chat.Q4_0.gguf](https://huggingface.co/RichardErkhov/AIGym_-_deepseek-coder-6.7b-chat-gguf/blob/main/deepseek-coder-6.7b-chat.Q4_0.gguf) | Q4_0 | 3.56GB |
| [deepseek-coder-6.7b-chat.IQ4_NL.gguf](https://huggingface.co/RichardErkhov/AIGym_-_deepseek-coder-6.7b-chat-gguf/blob/main/deepseek-coder-6.7b-chat.IQ4_NL.gguf) | IQ4_NL | 3.59GB |
| [deepseek-coder-6.7b-chat.Q4_K_S.gguf](https://huggingface.co/RichardErkhov/AIGym_-_deepseek-coder-6.7b-chat-gguf/blob/main/deepseek-coder-6.7b-chat.Q4_K_S.gguf) | Q4_K_S | 3.59GB |
| [deepseek-coder-6.7b-chat.Q4_K.gguf](https://huggingface.co/RichardErkhov/AIGym_-_deepseek-coder-6.7b-chat-gguf/blob/main/deepseek-coder-6.7b-chat.Q4_K.gguf) | Q4_K | 3.8GB |
| [deepseek-coder-6.7b-chat.Q4_K_M.gguf](https://huggingface.co/RichardErkhov/AIGym_-_deepseek-coder-6.7b-chat-gguf/blob/main/deepseek-coder-6.7b-chat.Q4_K_M.gguf) | Q4_K_M | 3.8GB |
| [deepseek-coder-6.7b-chat.Q4_1.gguf](https://huggingface.co/RichardErkhov/AIGym_-_deepseek-coder-6.7b-chat-gguf/blob/main/deepseek-coder-6.7b-chat.Q4_1.gguf) | Q4_1 | 3.95GB |
| [deepseek-coder-6.7b-chat.Q5_0.gguf](https://huggingface.co/RichardErkhov/AIGym_-_deepseek-coder-6.7b-chat-gguf/blob/main/deepseek-coder-6.7b-chat.Q5_0.gguf) | Q5_0 | 4.33GB |
| [deepseek-coder-6.7b-chat.Q5_K_S.gguf](https://huggingface.co/RichardErkhov/AIGym_-_deepseek-coder-6.7b-chat-gguf/blob/main/deepseek-coder-6.7b-chat.Q5_K_S.gguf) | Q5_K_S | 4.33GB |
| [deepseek-coder-6.7b-chat.Q5_K.gguf](https://huggingface.co/RichardErkhov/AIGym_-_deepseek-coder-6.7b-chat-gguf/blob/main/deepseek-coder-6.7b-chat.Q5_K.gguf) | Q5_K | 4.46GB |
| [deepseek-coder-6.7b-chat.Q5_K_M.gguf](https://huggingface.co/RichardErkhov/AIGym_-_deepseek-coder-6.7b-chat-gguf/blob/main/deepseek-coder-6.7b-chat.Q5_K_M.gguf) | Q5_K_M | 4.46GB |
| [deepseek-coder-6.7b-chat.Q5_1.gguf](https://huggingface.co/RichardErkhov/AIGym_-_deepseek-coder-6.7b-chat-gguf/blob/main/deepseek-coder-6.7b-chat.Q5_1.gguf) | Q5_1 | 4.72GB |
| [deepseek-coder-6.7b-chat.Q6_K.gguf](https://huggingface.co/RichardErkhov/AIGym_-_deepseek-coder-6.7b-chat-gguf/blob/main/deepseek-coder-6.7b-chat.Q6_K.gguf) | Q6_K | 5.15GB |
| [deepseek-coder-6.7b-chat.Q8_0.gguf](https://huggingface.co/RichardErkhov/AIGym_-_deepseek-coder-6.7b-chat-gguf/blob/main/deepseek-coder-6.7b-chat.Q8_0.gguf) | Q8_0 | 6.67GB |
Original model description:
---
license: apache-2.0
tags:
- finetuned
pipeline_tag: text-generation
model-index:
- name: deepseek-coder-6.7b-chat
results:
- task:
type: text-generation
name: Text Generation
dataset:
name: AI2 Reasoning Challenge (25-Shot)
type: ai2_arc
config: ARC-Challenge
split: test
args:
num_few_shot: 25
metrics:
- type: acc_norm
value: 36.01
name: normalized accuracy
source:
url: https://huggingface.co/spaces/HuggingFaceH4/open_llm_leaderboard?query=AIGym/deepseek-coder-6.7b-chat
name: Open LLM Leaderboard
- task:
type: text-generation
name: Text Generation
dataset:
name: HellaSwag (10-Shot)
type: hellaswag
split: validation
args:
num_few_shot: 10
metrics:
- type: acc_norm
value: 53.74
name: normalized accuracy
source:
url: https://huggingface.co/spaces/HuggingFaceH4/open_llm_leaderboard?query=AIGym/deepseek-coder-6.7b-chat
name: Open LLM Leaderboard
- task:
type: text-generation
name: Text Generation
dataset:
name: MMLU (5-Shot)
type: cais/mmlu
config: all
split: test
args:
num_few_shot: 5
metrics:
- type: acc
value: 38.22
name: accuracy
source:
url: https://huggingface.co/spaces/HuggingFaceH4/open_llm_leaderboard?query=AIGym/deepseek-coder-6.7b-chat
name: Open LLM Leaderboard
- task:
type: text-generation
name: Text Generation
dataset:
name: TruthfulQA (0-shot)
type: truthful_qa
config: multiple_choice
split: validation
args:
num_few_shot: 0
metrics:
- type: mc2
value: 42.94
source:
url: https://huggingface.co/spaces/HuggingFaceH4/open_llm_leaderboard?query=AIGym/deepseek-coder-6.7b-chat
name: Open LLM Leaderboard
- task:
type: text-generation
name: Text Generation
dataset:
name: Winogrande (5-shot)
type: winogrande
config: winogrande_xl
split: validation
args:
num_few_shot: 5
metrics:
- type: acc
value: 57.54
name: accuracy
source:
url: https://huggingface.co/spaces/HuggingFaceH4/open_llm_leaderboard?query=AIGym/deepseek-coder-6.7b-chat
name: Open LLM Leaderboard
- task:
type: text-generation
name: Text Generation
dataset:
name: GSM8k (5-shot)
type: gsm8k
config: main
split: test
args:
num_few_shot: 5
metrics:
- type: acc
value: 16.98
name: accuracy
source:
url: https://huggingface.co/spaces/HuggingFaceH4/open_llm_leaderboard?query=AIGym/deepseek-coder-6.7b-chat
name: Open LLM Leaderboard
---
# deepseek-coder-6.7B-chat
It was created by starting with the deepseek-coder-6.7B and training it on the open assistant dataset. We have attached the wandb report in pdf form to view the training run at a glance.
# Reson
This model was fine tned to allow it to follow direction and is a steeping stone to further training, but still would be good for asking qestions about code.
# How to use
You will need the transformers>=4.31
```python
from transformers import AutoTokenizer
import transformers
import torch
model = "AIGym/deepseek-coder-6.7b-chat"
tokenizer = AutoTokenizer.from_pretrained(model)
pipeline = transformers.pipeline(
"text-generation",
model=model,
torch_dtype=torch.float16,
device_map="auto",
)
prompt = "What are the values in open source projects?"
formatted_prompt = (
f"### Human: {prompt}### Assistant:"
)
sequences = pipeline(
formatted_prompt,
do_sample=True,
top_k=50,
top_p = 0.7,
num_return_sequences=1,
repetition_penalty=1.1,
max_new_tokens=500,
)
for seq in sequences:
print(f"Result: {seq['generated_text']}")
```
# Referrals
Run Pod - This is who I use to train th emodels on huggingface. If you use it we both get free crdits. - <a href="https://runpod.io?ref=kilq83n1" target="_blank" style="color: #3498db; text-decoration: none; font-weight: bold;">Visit Runpod's Website!</a>
Paypal - If you want to leave a tip, it is appecaheted. - <a href="https://paypal.me/OpenSourceTraining" target="_blank" style="color: #3498db; text-decoration: none; font-weight: bold;">Visit My Paypal!</a>
# [Open LLM Leaderboard Evaluation Results](https://huggingface.co/spaces/HuggingFaceH4/open_llm_leaderboard)
Detailed results can be found [here](https://huggingface.co/datasets/open-llm-leaderboard/details_AIGym__deepseek-coder-6.7b-chat)
| Metric |Value|
|---------------------------------|----:|
|Avg. |40.90|
|AI2 Reasoning Challenge (25-Shot)|36.01|
|HellaSwag (10-Shot) |53.74|
|MMLU (5-Shot) |38.22|
|TruthfulQA (0-shot) |42.94|
|Winogrande (5-shot) |57.54|
|GSM8k (5-shot) |16.98|
|
backyardai/Qwen2-7B-Multilingual-RP-GGUF | backyardai | 2024-06-25T18:43:18Z | 12,313 | 0 | null | [
"gguf",
"en",
"ko",
"ja",
"zh",
"es",
"base_model:maywell/Qwen2-7B-Multilingual-RP",
"license:apache-2.0",
"region:us"
]
| null | 2024-06-25T18:27:42Z | ---
language:
- en
- ko
- ja
- zh
- es
license: apache-2.0
base_model: maywell/Qwen2-7B-Multilingual-RP
model_name: Qwen2-7B-Multilingual-RP-GGUF
quantized_by: brooketh
parameter_count: 7615616512
---
<img src="BackyardAI_Banner.png" alt="Backyard.ai" style="height: 90px; min-width: 32px; display: block; margin: auto;">
**<p style="text-align: center;">The official library of GGUF format models for use in the local AI chat app, Backyard AI.</p>**
<p style="text-align: center;"><a href="https://backyard.ai/">Download Backyard AI here to get started.</a></p>
<p style="text-align: center;"><a href="https://www.reddit.com/r/LLM_Quants/">Request Additional models at r/LLM_Quants.</a></p>
***
# Qwen2 Multilingual RP 7B
- **Creator:** [maywell](https://huggingface.co/maywell/)
- **Original:** [Qwen2 Multilingual RP 7B](https://huggingface.co/maywell/Qwen2-7B-Multilingual-RP)
- **Date Created:** 2024-06-24
- **Trained Context:** 32768 tokens
- **Description:** Multilingual roleplaying model trained over 2B tokens.
***
## What is a GGUF?
GGUF is a large language model (LLM) format that can be split between CPU and GPU. GGUFs are compatible with applications based on llama.cpp, such as Backyard AI. Where other model formats require higher end GPUs with ample VRAM, GGUFs can be efficiently run on a wider variety of hardware.
GGUF models are quantized to reduce resource usage, with a tradeoff of reduced coherence at lower quantizations. Quantization reduces the precision of the model weights by changing the number of bits used for each weight.
***
<img src="BackyardAI_Logo.png" alt="Backyard.ai" style="height: 75px; min-width: 32px; display: block; horizontal align: left;">
## Backyard AI
- Free, local AI chat application.
- One-click installation on Mac and PC.
- Automatically use GPU for maximum speed.
- Built-in model manager.
- High-quality character hub.
- Zero-config desktop-to-mobile tethering.
Backyard AI makes it easy to start chatting with AI using your own characters or one of the many found in the built-in character hub. The model manager helps you find the latest and greatest models without worrying about whether it's the correct format. Backyard AI supports advanced features such as lorebooks, author's note, text formatting, custom context size, sampler settings, grammars, local TTS, cloud inference, and tethering, all implemented in a way that is straightforward and reliable.
**Join us on [Discord](https://discord.gg/SyNN2vC9tQ)**
*** |
Alvenir/bert-punct-restoration-en | Alvenir | 2022-03-23T08:39:39Z | 12,310 | 0 | transformers | [
"transformers",
"pytorch",
"bert",
"token-classification",
"license:apache-2.0",
"autotrain_compatible",
"endpoints_compatible",
"region:us"
]
| token-classification | 2022-03-21T11:15:27Z | ---
license: apache-2.0
---
TODO |
mradermacher/L3-11.5B-DuS-MoonRoot-GGUF | mradermacher | 2024-06-29T07:07:30Z | 12,301 | 0 | transformers | [
"transformers",
"gguf",
"mergekit",
"merge",
"llama",
"en",
"base_model:v000000/L3-11.5B-DuS-MoonRoot",
"endpoints_compatible",
"region:us"
]
| null | 2024-06-29T05:37:27Z | ---
base_model: v000000/L3-11.5B-DuS-MoonRoot
language:
- en
library_name: transformers
quantized_by: mradermacher
tags:
- mergekit
- merge
- llama
---
## About
<!-- ### quantize_version: 2 -->
<!-- ### output_tensor_quantised: 1 -->
<!-- ### convert_type: hf -->
<!-- ### vocab_type: -->
<!-- ### tags: -->
static quants of https://huggingface.co/v000000/L3-11.5B-DuS-MoonRoot
<!-- provided-files -->
weighted/imatrix quants are available at https://huggingface.co/mradermacher/L3-11.5B-DuS-MoonRoot-i1-GGUF
## Usage
If you are unsure how to use GGUF files, refer to one of [TheBloke's
READMEs](https://huggingface.co/TheBloke/KafkaLM-70B-German-V0.1-GGUF) for
more details, including on how to concatenate multi-part files.
## Provided Quants
(sorted by size, not necessarily quality. IQ-quants are often preferable over similar sized non-IQ quants)
| Link | Type | Size/GB | Notes |
|:-----|:-----|--------:|:------|
| [GGUF](https://huggingface.co/mradermacher/L3-11.5B-DuS-MoonRoot-GGUF/resolve/main/L3-11.5B-DuS-MoonRoot.Q2_K.gguf) | Q2_K | 4.6 | |
| [GGUF](https://huggingface.co/mradermacher/L3-11.5B-DuS-MoonRoot-GGUF/resolve/main/L3-11.5B-DuS-MoonRoot.IQ3_XS.gguf) | IQ3_XS | 5.0 | |
| [GGUF](https://huggingface.co/mradermacher/L3-11.5B-DuS-MoonRoot-GGUF/resolve/main/L3-11.5B-DuS-MoonRoot.Q3_K_S.gguf) | Q3_K_S | 5.3 | |
| [GGUF](https://huggingface.co/mradermacher/L3-11.5B-DuS-MoonRoot-GGUF/resolve/main/L3-11.5B-DuS-MoonRoot.IQ3_S.gguf) | IQ3_S | 5.3 | beats Q3_K* |
| [GGUF](https://huggingface.co/mradermacher/L3-11.5B-DuS-MoonRoot-GGUF/resolve/main/L3-11.5B-DuS-MoonRoot.IQ3_M.gguf) | IQ3_M | 5.4 | |
| [GGUF](https://huggingface.co/mradermacher/L3-11.5B-DuS-MoonRoot-GGUF/resolve/main/L3-11.5B-DuS-MoonRoot.Q3_K_M.gguf) | Q3_K_M | 5.8 | lower quality |
| [GGUF](https://huggingface.co/mradermacher/L3-11.5B-DuS-MoonRoot-GGUF/resolve/main/L3-11.5B-DuS-MoonRoot.Q3_K_L.gguf) | Q3_K_L | 6.3 | |
| [GGUF](https://huggingface.co/mradermacher/L3-11.5B-DuS-MoonRoot-GGUF/resolve/main/L3-11.5B-DuS-MoonRoot.IQ4_XS.gguf) | IQ4_XS | 6.5 | |
| [GGUF](https://huggingface.co/mradermacher/L3-11.5B-DuS-MoonRoot-GGUF/resolve/main/L3-11.5B-DuS-MoonRoot.Q4_K_S.gguf) | Q4_K_S | 6.8 | fast, recommended |
| [GGUF](https://huggingface.co/mradermacher/L3-11.5B-DuS-MoonRoot-GGUF/resolve/main/L3-11.5B-DuS-MoonRoot.Q4_K_M.gguf) | Q4_K_M | 7.1 | fast, recommended |
| [GGUF](https://huggingface.co/mradermacher/L3-11.5B-DuS-MoonRoot-GGUF/resolve/main/L3-11.5B-DuS-MoonRoot.Q5_K_S.gguf) | Q5_K_S | 8.1 | |
| [GGUF](https://huggingface.co/mradermacher/L3-11.5B-DuS-MoonRoot-GGUF/resolve/main/L3-11.5B-DuS-MoonRoot.Q5_K_M.gguf) | Q5_K_M | 8.3 | |
| [GGUF](https://huggingface.co/mradermacher/L3-11.5B-DuS-MoonRoot-GGUF/resolve/main/L3-11.5B-DuS-MoonRoot.Q6_K.gguf) | Q6_K | 9.6 | very good quality |
| [GGUF](https://huggingface.co/mradermacher/L3-11.5B-DuS-MoonRoot-GGUF/resolve/main/L3-11.5B-DuS-MoonRoot.Q8_0.gguf) | Q8_0 | 12.3 | fast, best quality |
Here is a handy graph by ikawrakow comparing some lower-quality quant
types (lower is better):

And here are Artefact2's thoughts on the matter:
https://gist.github.com/Artefact2/b5f810600771265fc1e39442288e8ec9
## FAQ / Model Request
See https://huggingface.co/mradermacher/model_requests for some answers to
questions you might have and/or if you want some other model quantized.
## Thanks
I thank my company, [nethype GmbH](https://www.nethype.de/), for letting
me use its servers and providing upgrades to my workstation to enable
this work in my free time.
<!-- end -->
|
ItsJayQz/GTA5_Artwork_Diffusion | ItsJayQz | 2023-01-28T01:05:18Z | 12,293 | 112 | diffusers | [
"diffusers",
"safetensors",
"stable-diffusion",
"text-to-image",
"grand theft auto",
"game",
"en",
"license:creativeml-openrail-m",
"autotrain_compatible",
"endpoints_compatible",
"diffusers:StableDiffusionPipeline",
"region:us"
]
| text-to-image | 2022-12-13T03:04:55Z | ---
language:
- en
license: creativeml-openrail-m
tags:
- stable-diffusion
- text-to-image
- diffusers
- grand theft auto
- game
inference: true
---
### GTA5 Artwork Diffusion
This model was trained on the loading screens, gta storymode, and gta online DLCs artworks.
Which includes characters, background, chop, and some objects.
The model can do people and portrait pretty easily, as well as cars, and houses.
For some reasons, the model stills automatically include in some game footage, so landscapes tend to look a bit more game-like.
Please check out important informations on the usage of the model down bellow.
To reference the art style, use the token: gtav style
There is already an existing model that uses textual inversion. This is trained using Dreambooth instead, whether or not this method is better, I will let you judge.
### Gradio
We support a [Gradio](https://github.com/gradio-app/gradio) Web UI to run GTA5_Artwork_Diffusion:
[](https://huggingface.co/spaces/ItsJayQz/GTA5_Artwork_Diffusion)
Here are some samples.
**Portraits**



Prompt used:
*name* in gtav style
Guidance: 7
Steps: 65 using DDIM
I'm not a prompt wizard so you can definitely get better results with some tuning.
**Landscapes**

**Objects**

**Disclaimers**
- I'm in no way affliated with Rockstar, or any entities relating to the ownership of the game artworks.
- The phrase GTA is simply a reference for accessibility.
- This was created entirely for research, and entertainment purpose.
- I did not plan, or is planning on turning this model into a commercial product, or use for commercial purposes.
- I do not condone the usage of the model for making counterfeit products that might infringe on Rockstar's copyrights/trademarks.
**License**
- This model is under Creative OpenRAIL-M.
- This means the model can be used royalty-free, and flexible with the model usage, such as redistribution of the model, or of any derivatives of the model.
- However, there are restrictions on the openess of the license.
More info into the restrictions can be found [here](https://huggingface.co/spaces/CompVis/stable-diffusion-license).
**Responsibilities**
- By using/downloading the model, you are responsible for:
- All outputs/usage of the model.
- Understanding the Disclaimers.
- Upholding the terms of the license.
Thanks for checking out the model! |
llm-blender/PairRM | llm-blender | 2024-01-22T15:47:01Z | 12,286 | 167 | transformers | [
"transformers",
"safetensors",
"deberta",
"reward_model",
"reward-model",
"RLHF",
"evaluation",
"llm",
"instruction",
"reranking",
"text-generation",
"en",
"dataset:openai/summarize_from_feedback",
"dataset:openai/webgpt_comparisons",
"dataset:Dahoas/synthetic-instruct-gptj-pairwise",
"dataset:Anthropic/hh-rlhf",
"dataset:lmsys/chatbot_arena_conversations",
"dataset:openbmb/UltraFeedback",
"arxiv:2306.02561",
"arxiv:2112.09332",
"license:mit",
"endpoints_compatible",
"region:us"
]
| text-generation | 2023-11-06T07:08:24Z | ---
license: mit
datasets:
- openai/summarize_from_feedback
- openai/webgpt_comparisons
- Dahoas/synthetic-instruct-gptj-pairwise
- Anthropic/hh-rlhf
- lmsys/chatbot_arena_conversations
- openbmb/UltraFeedback
metrics:
- accuracy
tags:
- reward_model
- reward-model
- RLHF
- evaluation
- llm
- instruction
- reranking
language:
- en
pipeline_tag: text-generation
---
# Pairwise Reward Model for LLMs (PairRM) from LLM-Blender
- Github: [https://github.com/yuchenlin/LLM-Blender](https://github.com/yuchenlin/LLM-Blender)
- Paper: [https://arxiv.org/abs/2306.02561](https://arxiv.org/abs/2306.02561)
- Space Demo: [https://huggingface.co/spaces/llm-blender/LLM-Blender](https://huggingface.co/spaces/llm-blender/LLM-Blender)
## News
- Check out our results on AlpacaEval leaderboard: [Twitter](https://x.com/billyuchenlin/status/1732198787354067380?s=20) [Leaderboard](https://tatsu-lab.github.io/alpaca_eval/)
## Introduction
Pairwise Reward Model (PairRM) takes an instruction and a **pair** of output candidates as the input,
and output a score for each candidate to measure their **relative** quality.
PairRM can be used to (re-)rank a list of candidate outputs and thus can be used an LLM evaluator to efficiently assess the quality of LLMs in local environment.
PairRM can also be used to enhance the decoding by `best-of-n sampling` (i.e., reranking N sampled outputs).
Apart from that, one can also use PairRM to further align instruction-tuned LLMs with RLHF methods.
Unlike the other RMs that encode and score each candidate respectively,
PairRM takes a pair of candidates and compares them side-by-side to indentify the subtle differences between them.
Also, PairRM is based on [`microsoft/deberta-v3-large`](https://huggingface.co/microsoft/deberta-v3-large), and thus it is super efficient: **0.4B**.
We trained PairRM on a diverse collection of six human-preference datasets (see more [here](https://huggingface.co/llm-blender/PairRM#training-datasets)).
PairRM is part of the LLM-Blender project (ACL 2023). Please see our [paper](https://arxiv.org/abs/2306.02561) above to know more.
## Installation
- First install `llm-blender`
```bash
pip install git+https://github.com/yuchenlin/LLM-Blender.git
```
- Then load PairRM:
```python
import llm_blender
blender = llm_blender.Blender()
blender.loadranker("llm-blender/PairRM") # load PairRM
```
## Usage
### Use Case 1: Comparing/Ranking output candidates given an instruction
- Ranking a list candidate responses
```python
inputs = ["hello, how are you!", "I love you!"]
candidates_texts = [["get out!", "hi! I am fine, thanks!", "bye!"],
["I love you too!", "I hate you!", "Thanks! You're a good guy!"]]
ranks = blender.rank(inputs, candidates_texts, return_scores=False, batch_size=1)
# ranks is a list of ranks
# ranks[i][j] represents the ranks of candidate-j for input-i
"""
ranks -->
array([[3, 1, 2], # it means "hi! I am fine, thanks!" ranks the 1st, "bye" ranks the 2nd, and "get out!" ranks the 3rd.
[1, 3, 2]], # it means "I love you too"! ranks the the 1st, and "I hate you!" ranks the 3rd.
dtype=int32)
"""
```
- Directly comparing two candidate responses
```python
inputs = ["hello!", "I love you!"]
candidates_A = ["hi!", "I hate you!"]
candidates_B = ["f**k off!", "I love you, too!"]
comparison_results = blender.compare(inputs, candidates_A, candidates_B)
# comparison_results is a list of bool, where comparison_results[i] denotes
# whether candidates_A[i] is better than candidates_B[i] for inputs[i]
# Example: comparison_results[0]--> True
```
<details><summary> Comparing two multi-turn conversations. </summary>
```python
conv1 = [
{
"content": "hello",
"role": "USER"
},
{
"content": "[assistant1‘s response 1]",
"role": "ASSISTANT"
},
...
]
conv2 = [
{
"content": "hello",
"role": "USER"
},
{
"content": "[assistant2's response 1]",
"role": "ASSISTANT"
},
...
]
comparison_results = blender.compare_conversations([conv1], [conv2])
# comparison_results is a list of bool, where each element denotes whether all the responses in conv1 together is better than that of conv2
```
</details>
### Use Case 2: Best-of-n Sampling (Decoding Enhancment)
**Best-of-n Sampling**, aka, rejection sampling, is a strategy to enhance the response quality by selecting the one that was ranked highest by the reward model
(see more in [OpenAI WebGPT section 3.2](https://arxiv.org/pdf/2112.09332.pdf) and [OpenAI Blog](https://openai.com/research/measuring-goodharts-law)).
Best-of-n sampling with PairRM is a very easy way to imporve your LLMs with only a few changes of your inference code:
```python
# loading models
import llm_blender
from transformers import AutoTokenizer, AutoModelForCausalLM
tokenizer = AutoTokenizer.from_pretrained("HuggingFaceH4/zephyr-7b-beta")
model = AutoModelForCausalLM.from_pretrained("HuggingFaceH4/zephyr-7b-beta", device_map="auto")
system_message = {"role": "system", "content": "You are a friendly chatbot."}
# formatting your inputs
inputs = ["can you tell me a joke about OpenAI?"]
messages = [[system_message, {"role": "user", "content": _input}] for _input in inputs]
prompts = [tokenizer.apply_chat_template(m, tokenize=False, add_generation_prompt=True) for m in messages]
# Conventional generation method
input_ids = tokenizer(prompts[0], return_tensors="pt").input_ids
sampled_outputs = model.generate(input_ids, do_sample=True, top_k=50, top_p=0.95, num_return_sequences=1)
print(tokenizer.decode(sampled_outputs[0][len(input_ids[0]):], skip_special_tokens=False))
# --> The output could be a bad case such as a very short one, e.g., `Sure`
# PairRM for best-of-n sampling
blender = llm_blender.Blender()
blender.loadranker("llm-blender/PairRM") # load ranker checkpoint
outputs = blender.best_of_n_generate(model, tokenizer, prompts, n=10)
print("### Prompt:\n", prompts[0])
print("### best-of-n generations:\n", outputs[0])
# --> The output will be much more stable and consistently better than single sampling, for example:
"""
Sure, here's a joke about OpenAI:
Why did OpenAI decide to hire a mime as their new AI researcher?
Because they wanted someone who could communicate complex ideas without making a sound!
(Note: This is a joke, not a reflection of OpenAI's actual hiring practices.)
"""
```
### Use case 3: RLHF
PairRM has been trained on various high-quality and large-scale datasets with human preference annotations
and shown great correlation with human preferences with an extremely small model size (0.4B),
approching the performance of GPT-4.
PairRM will better help the future alignment of LLMs in a more efficient and effective way.
With a `blender.compare()` function, you can apply PairRM to popular RLHF toolkits such as [trl](https://huggingface.co/docs/trl/index).
**🔥 Check more details on our example jupyter notebook usage: [`blender_usage.ipynb`](https://github.com/yuchenlin/LLM-Blender/blob/main/blender_usage.ipynb)**
Learn more in our LLM-Blender Github [README.md](https://github.com/yuchenlin/LLM-Blender#rank-and-fusion)
## Statistics
### Context length
| PairRanker type | Source max length | Candidate max length | Total max length |
|:-----------------:|:-----------------:|----------------------|------------------|
| [pair-ranker](https://huggingface.co/llm-blender/pair-ranker) (our previous version) | 128 | 128 | 384 |
| [PairRM](https://huggingface.co/llm-blender/pair-reward-model/) (This model) | 1224 | 412 | 2048 |
### Training Datasets
- [openai/summarize_from_feedback](https://huggingface.co/datasets/openai/summarize_from_feedback)
- [openai/webgpt_comparisons](https://huggingface.co/datasets/openai/webgpt_comparisons)
- [Dahoas/synthetic-instruct-gptj-pairwise](https://huggingface.co/datasets/Dahoas/synthetic-instruct-gptj-pairwise)
- [Anthropic/hh-rlhf](https://huggingface.co/datasets/Anthropic/hh-rlhf)
- [lmsys/chatbot_arena_conversations](https://huggingface.co/datasets/lmsys/chatbot_arena_conversations)
- [openbmb/UltraFeedback](https://huggingface.co/datasets/openbmb/UltraFeedback)
### Performance
PairRM has been trained on various high-quality and large-scale dataset with human preference annotations and exhibits great correlation with human preferences
with an extremly small model size (0.4B), approching the performance of GPT-4.
We test the pairwise comparison on
- [Auto-J pairwise testdata](https://github.com/GAIR-NLP/auto-j#pairwise-response-comparison)
- [HHH-alignment](https://huggingface.co/datasets/HuggingFaceH4/hhh_alignment)
- [MT-bench-human-judgements](https://huggingface.co/datasets/lmsys/mt_bench_human_judgments)
All following results are reported as pairwise comparison accuracies (agreements).
#### Auto-J Pairwise test data performance
| Model | Summ | Exam | Code | Rewriting | Crea W | Func W | Comm | NLP | Overall |
|:---------------------:|:---------:|:---------:|:---------:|:---------:|:---------:|:---------:|:-----:|:--------:|:---------:|
| Closed -source Models |
| ChatGPT | 33.3 | 40.3 | 36.6 | 31.6 | 48.2 | 40.4 | 47.6 | 45.8 | 42.7 |
| Claude -2 | 30.6 | 36.1 | 41.7 | 34.2 | 48.1 | 42.5 | 40.6 | 48.5 | 42.4 |
| GPT -4 | 59.7 | 51.4 | 69.2 | 58.3 | 66.7 | 60.4 | 58.3 | 65.2 | 61.9 |
| Open -source Models |
| SteamSHP | 33.3 | 29.2 | 26.7 | 33.3 | 40.7 | 31.3 | 51.4 | 51.9 | 40.6 |
| PandaLM | 29.2 | 33.3 | 31.7 | 23.3 | 43.5 | 32.9 | 44.8 | 48.9 | 38.9 |
| LLaMA -2-Chat -13B | 20.8 | 27.8 | 19.2 | 20 | 31.5 | 27.5 | 35.8 | 31.8 | 29 |
| Vicuna -13B-v1.5 | 30.6 | 23.6 | 35 | 28.3 | 36.1 | 37.5 | 45.5 | 39.8 | 37.3 |
| WizardLM -13B-v1.2 | 22.2 | 20.8 | 32.5 | 19.2 | 28.7 | 25.4 | 29.2 | 33 | 27.8 |
| LLAMA -2-chat -70B | 34.7 | 33.3 | 36.7 | 35.8 | 51.4 | 54.2 | 47.2 | 47.7 | 45.9 |
| AUTO -J (13b) | 45.8 | 38.9 | **59.2** | 47.5 | 54.6 | 57.1 | **58** | 57.6 | 54.8 |
| UltraRM (13b) | 56.94 | 43.06 | 55.0 | 53.33 | **67.13** | **64.17** | 56.25 | 59.85 | **59.85** |
| **PairRM (0.4b)** | **56.94** | **52.78** | 58.33 | **55.83** | 61.57 | 59.17 | 57.64 | **62.5** | 59.05 |
#### HHH-Alignment and MT-bench human judgements
| Evaluator LM | HHH ALIGNMENT | | | | | MT BENCH HUMAN JUDG . |
|:-------------------------:|:-------------:|:---------:|:---------:|:--------:|:-----------:|:---------------------:|
| | Help . | Harm . | Hon . | Other | Total Avg . | Human Preference |
| RANDOM | 50 | 50 | 50 | 50 | 50 | 34.26 |
| STANFORDNLP REWARD MODEL | 69.49 | 60.34 | 52.46 | 51.16 | 58.82 | 44.79 |
| ALMOST REWARD MODEL | 74.58 | 67.24 | 78.69 | 86.05 | 76.02 | 49.9 |
| LLAMA2 -CHAT 7B | 66.1 | 81.03 | 70.49 | 74.42 | 72.85 | 51.78 |
| LLAMA2 -CHAT 13B | 74.58 | 87.93 | 55.74 | 79.07 | 73.76 | 52.34 |
| LLAMA2 -CHAT 70B | 66.1 | **89.66** | 67.21 | 74.42 | 74.21 | 53.67 |
| LLAMA2 -CHAT 13B+COARSE . | 68.74 | 68.97 | 65.57 | 67.44 | 67.42 | 46.89 |
| GPT -3.5-TURBO -0613 | 76.27 | 87.93 | 67.21 | 86.05 | 78.73 | 57.12 |
| PROMETHEUS 7B | 69.49 | 84.48 | 78.69 | 90.7 | 80.09 | 55.14 |
| PROMETHEUS 13B | 81.36 | 82.76 | 75.41 | 76.74 | 79.19 | 57.72 |
| UltraRM (13B) | **86.44** | 79.31 | **81.97** | 88.37 | 83.71 | 56 |
| **PairRM (0.4B)** | 84.75 | 84.48 | 80.33 | **90.7** | **84.62** | **59** |
| GPT -4-0613 | 91.53 | 93.1 | 85.25 | 83.72 | 88.69 | 63.87 |
**While PairRM is a extremely small model (0.4B) based on deberta, the pairwise comparison aggrement performance approches GPT-4's performance!**
Two reasons to attribute:
- Our PairRM specically designed model arch for pairwise comparison through bidirectional attention (See LLM-blender paper for more details)
- The high-quality and large-scale human preference annotation data it was train on (see training dataset list on this hugging face page)
## Citation & Credits
If you are using PairRM in your research, please cite LLM-blender.
```bibtex
@inproceedings{llm-blender-2023,
title = "LLM-Blender: Ensembling Large Language Models with Pairwise Comparison and Generative Fusion",
author = "Jiang, Dongfu and Ren, Xiang and Lin, Bill Yuchen",
booktitle = "Proceedings of the 61th Annual Meeting of the Association for Computational Linguistics (ACL 2023)",
year = "2023"
}
```
|
RichardErkhov/NExtNewChattingAI_-_shark_tank_ai_7b_v2-gguf | RichardErkhov | 2024-06-20T04:36:14Z | 12,273 | 0 | null | [
"gguf",
"region:us"
]
| null | 2024-06-19T23:20:42Z | Quantization made by Richard Erkhov.
[Github](https://github.com/RichardErkhov)
[Discord](https://discord.gg/pvy7H8DZMG)
[Request more models](https://github.com/RichardErkhov/quant_request)
shark_tank_ai_7b_v2 - GGUF
- Model creator: https://huggingface.co/NExtNewChattingAI/
- Original model: https://huggingface.co/NExtNewChattingAI/shark_tank_ai_7b_v2/
| Name | Quant method | Size |
| ---- | ---- | ---- |
| [shark_tank_ai_7b_v2.Q2_K.gguf](https://huggingface.co/RichardErkhov/NExtNewChattingAI_-_shark_tank_ai_7b_v2-gguf/blob/main/shark_tank_ai_7b_v2.Q2_K.gguf) | Q2_K | 2.53GB |
| [shark_tank_ai_7b_v2.IQ3_XS.gguf](https://huggingface.co/RichardErkhov/NExtNewChattingAI_-_shark_tank_ai_7b_v2-gguf/blob/main/shark_tank_ai_7b_v2.IQ3_XS.gguf) | IQ3_XS | 2.81GB |
| [shark_tank_ai_7b_v2.IQ3_S.gguf](https://huggingface.co/RichardErkhov/NExtNewChattingAI_-_shark_tank_ai_7b_v2-gguf/blob/main/shark_tank_ai_7b_v2.IQ3_S.gguf) | IQ3_S | 2.96GB |
| [shark_tank_ai_7b_v2.Q3_K_S.gguf](https://huggingface.co/RichardErkhov/NExtNewChattingAI_-_shark_tank_ai_7b_v2-gguf/blob/main/shark_tank_ai_7b_v2.Q3_K_S.gguf) | Q3_K_S | 2.95GB |
| [shark_tank_ai_7b_v2.IQ3_M.gguf](https://huggingface.co/RichardErkhov/NExtNewChattingAI_-_shark_tank_ai_7b_v2-gguf/blob/main/shark_tank_ai_7b_v2.IQ3_M.gguf) | IQ3_M | 3.06GB |
| [shark_tank_ai_7b_v2.Q3_K.gguf](https://huggingface.co/RichardErkhov/NExtNewChattingAI_-_shark_tank_ai_7b_v2-gguf/blob/main/shark_tank_ai_7b_v2.Q3_K.gguf) | Q3_K | 3.28GB |
| [shark_tank_ai_7b_v2.Q3_K_M.gguf](https://huggingface.co/RichardErkhov/NExtNewChattingAI_-_shark_tank_ai_7b_v2-gguf/blob/main/shark_tank_ai_7b_v2.Q3_K_M.gguf) | Q3_K_M | 3.28GB |
| [shark_tank_ai_7b_v2.Q3_K_L.gguf](https://huggingface.co/RichardErkhov/NExtNewChattingAI_-_shark_tank_ai_7b_v2-gguf/blob/main/shark_tank_ai_7b_v2.Q3_K_L.gguf) | Q3_K_L | 3.56GB |
| [shark_tank_ai_7b_v2.IQ4_XS.gguf](https://huggingface.co/RichardErkhov/NExtNewChattingAI_-_shark_tank_ai_7b_v2-gguf/blob/main/shark_tank_ai_7b_v2.IQ4_XS.gguf) | IQ4_XS | 3.67GB |
| [shark_tank_ai_7b_v2.Q4_0.gguf](https://huggingface.co/RichardErkhov/NExtNewChattingAI_-_shark_tank_ai_7b_v2-gguf/blob/main/shark_tank_ai_7b_v2.Q4_0.gguf) | Q4_0 | 3.83GB |
| [shark_tank_ai_7b_v2.IQ4_NL.gguf](https://huggingface.co/RichardErkhov/NExtNewChattingAI_-_shark_tank_ai_7b_v2-gguf/blob/main/shark_tank_ai_7b_v2.IQ4_NL.gguf) | IQ4_NL | 3.87GB |
| [shark_tank_ai_7b_v2.Q4_K_S.gguf](https://huggingface.co/RichardErkhov/NExtNewChattingAI_-_shark_tank_ai_7b_v2-gguf/blob/main/shark_tank_ai_7b_v2.Q4_K_S.gguf) | Q4_K_S | 3.86GB |
| [shark_tank_ai_7b_v2.Q4_K.gguf](https://huggingface.co/RichardErkhov/NExtNewChattingAI_-_shark_tank_ai_7b_v2-gguf/blob/main/shark_tank_ai_7b_v2.Q4_K.gguf) | Q4_K | 4.07GB |
| [shark_tank_ai_7b_v2.Q4_K_M.gguf](https://huggingface.co/RichardErkhov/NExtNewChattingAI_-_shark_tank_ai_7b_v2-gguf/blob/main/shark_tank_ai_7b_v2.Q4_K_M.gguf) | Q4_K_M | 4.07GB |
| [shark_tank_ai_7b_v2.Q4_1.gguf](https://huggingface.co/RichardErkhov/NExtNewChattingAI_-_shark_tank_ai_7b_v2-gguf/blob/main/shark_tank_ai_7b_v2.Q4_1.gguf) | Q4_1 | 4.24GB |
| [shark_tank_ai_7b_v2.Q5_0.gguf](https://huggingface.co/RichardErkhov/NExtNewChattingAI_-_shark_tank_ai_7b_v2-gguf/blob/main/shark_tank_ai_7b_v2.Q5_0.gguf) | Q5_0 | 4.65GB |
| [shark_tank_ai_7b_v2.Q5_K_S.gguf](https://huggingface.co/RichardErkhov/NExtNewChattingAI_-_shark_tank_ai_7b_v2-gguf/blob/main/shark_tank_ai_7b_v2.Q5_K_S.gguf) | Q5_K_S | 4.65GB |
| [shark_tank_ai_7b_v2.Q5_K.gguf](https://huggingface.co/RichardErkhov/NExtNewChattingAI_-_shark_tank_ai_7b_v2-gguf/blob/main/shark_tank_ai_7b_v2.Q5_K.gguf) | Q5_K | 4.78GB |
| [shark_tank_ai_7b_v2.Q5_K_M.gguf](https://huggingface.co/RichardErkhov/NExtNewChattingAI_-_shark_tank_ai_7b_v2-gguf/blob/main/shark_tank_ai_7b_v2.Q5_K_M.gguf) | Q5_K_M | 4.78GB |
| [shark_tank_ai_7b_v2.Q5_1.gguf](https://huggingface.co/RichardErkhov/NExtNewChattingAI_-_shark_tank_ai_7b_v2-gguf/blob/main/shark_tank_ai_7b_v2.Q5_1.gguf) | Q5_1 | 5.07GB |
| [shark_tank_ai_7b_v2.Q6_K.gguf](https://huggingface.co/RichardErkhov/NExtNewChattingAI_-_shark_tank_ai_7b_v2-gguf/blob/main/shark_tank_ai_7b_v2.Q6_K.gguf) | Q6_K | 5.53GB |
| [shark_tank_ai_7b_v2.Q8_0.gguf](https://huggingface.co/RichardErkhov/NExtNewChattingAI_-_shark_tank_ai_7b_v2-gguf/blob/main/shark_tank_ai_7b_v2.Q8_0.gguf) | Q8_0 | 7.17GB |
Original model description:
---
language:
- en
license: cc-by-nc-4.0
model-index:
- name: shark_tank_ai_7b_v2
results:
- task:
type: text-generation
name: Text Generation
dataset:
name: AI2 Reasoning Challenge (25-Shot)
type: ai2_arc
config: ARC-Challenge
split: test
args:
num_few_shot: 25
metrics:
- type: acc_norm
value: 67.75
name: normalized accuracy
source:
url: https://huggingface.co/spaces/HuggingFaceH4/open_llm_leaderboard?query=NExtNewChattingAI/shark_tank_ai_7b_v2
name: Open LLM Leaderboard
- task:
type: text-generation
name: Text Generation
dataset:
name: HellaSwag (10-Shot)
type: hellaswag
split: validation
args:
num_few_shot: 10
metrics:
- type: acc_norm
value: 87.06
name: normalized accuracy
source:
url: https://huggingface.co/spaces/HuggingFaceH4/open_llm_leaderboard?query=NExtNewChattingAI/shark_tank_ai_7b_v2
name: Open LLM Leaderboard
- task:
type: text-generation
name: Text Generation
dataset:
name: MMLU (5-Shot)
type: cais/mmlu
config: all
split: test
args:
num_few_shot: 5
metrics:
- type: acc
value: 58.79
name: accuracy
source:
url: https://huggingface.co/spaces/HuggingFaceH4/open_llm_leaderboard?query=NExtNewChattingAI/shark_tank_ai_7b_v2
name: Open LLM Leaderboard
- task:
type: text-generation
name: Text Generation
dataset:
name: TruthfulQA (0-shot)
type: truthful_qa
config: multiple_choice
split: validation
args:
num_few_shot: 0
metrics:
- type: mc2
value: 62.15
source:
url: https://huggingface.co/spaces/HuggingFaceH4/open_llm_leaderboard?query=NExtNewChattingAI/shark_tank_ai_7b_v2
name: Open LLM Leaderboard
- task:
type: text-generation
name: Text Generation
dataset:
name: Winogrande (5-shot)
type: winogrande
config: winogrande_xl
split: validation
args:
num_few_shot: 5
metrics:
- type: acc
value: 78.45
name: accuracy
source:
url: https://huggingface.co/spaces/HuggingFaceH4/open_llm_leaderboard?query=NExtNewChattingAI/shark_tank_ai_7b_v2
name: Open LLM Leaderboard
- task:
type: text-generation
name: Text Generation
dataset:
name: GSM8k (5-shot)
type: gsm8k
config: main
split: test
args:
num_few_shot: 5
metrics:
- type: acc
value: 45.11
name: accuracy
source:
url: https://huggingface.co/spaces/HuggingFaceH4/open_llm_leaderboard?query=NExtNewChattingAI/shark_tank_ai_7b_v2
name: Open LLM Leaderboard
---
This model is based on https://huggingface.co/AIDC-ai-business/Marcoroni-7B-v3 trained on internal data.
---
license: cc-by-nc-4.0
language:
- en
---
Chatbot is a highly advanced artificial intelligence designed to provide you with personalized assistance and support. With its natural language processing capabilities, it can understand and respond to a wide range of queries and requests, making it a valuable tool for both personal and professional use.
The chatbot is equipped with a vast knowledge base, allowing it to provide accurate and reliable information on a wide range of topics, from general knowledge to specific industry-related information. It can also perform tasks such as scheduling appointments, sending emails, and even ordering products online.
One of the standout features of this assistant chatbot is its ability to learn and adapt to your individual preferences and needs. Over time, it can become more personalized to your specific requirements, making it an even more valuable asset to your daily life.
The chatbot is also designed to be user-friendly and intuitive, with a simple and easy-to-use interface that allows you to interact with it in a natural and conversational way. Whether you're looking for information, need help with a task, or just want to chat, your assistant chatbot is always ready and available to assist you.
# [Open LLM Leaderboard Evaluation Results](https://huggingface.co/spaces/HuggingFaceH4/open_llm_leaderboard)
Detailed results can be found [here](https://huggingface.co/datasets/open-llm-leaderboard/details_NExtNewChattingAI__shark_tank_ai_7b_v2)
| Metric |Value|
|---------------------------------|----:|
|Avg. |66.55|
|AI2 Reasoning Challenge (25-Shot)|67.75|
|HellaSwag (10-Shot) |87.06|
|MMLU (5-Shot) |58.79|
|TruthfulQA (0-shot) |62.15|
|Winogrande (5-shot) |78.45|
|GSM8k (5-shot) |45.11|
|
mradermacher/Llama-3-8B-Instruct-MagPO-GGUF | mradermacher | 2024-06-29T18:54:48Z | 12,271 | 0 | transformers | [
"transformers",
"gguf",
"alignment-handbook",
"trl",
"dpo",
"generated_from_trainer",
"en",
"dataset:Magpie-Align/Magpie-Pro-DPO-200K",
"base_model:Magpie-Align/Llama-3-8B-Instruct-MagPO",
"license:llama3",
"endpoints_compatible",
"region:us"
]
| null | 2024-06-29T16:44:02Z | ---
base_model: Magpie-Align/Llama-3-8B-Instruct-MagPO
datasets:
- Magpie-Align/Magpie-Pro-DPO-200K
language:
- en
library_name: transformers
license: llama3
quantized_by: mradermacher
tags:
- alignment-handbook
- trl
- dpo
- generated_from_trainer
- trl
- dpo
- generated_from_trainer
---
## About
<!-- ### quantize_version: 2 -->
<!-- ### output_tensor_quantised: 1 -->
<!-- ### convert_type: hf -->
<!-- ### vocab_type: -->
<!-- ### tags: -->
static quants of https://huggingface.co/Magpie-Align/Llama-3-8B-Instruct-MagPO
<!-- provided-files -->
weighted/imatrix quants seem not to be available (by me) at this time. If they do not show up a week or so after the static ones, I have probably not planned for them. Feel free to request them by opening a Community Discussion.
## Usage
If you are unsure how to use GGUF files, refer to one of [TheBloke's
READMEs](https://huggingface.co/TheBloke/KafkaLM-70B-German-V0.1-GGUF) for
more details, including on how to concatenate multi-part files.
## Provided Quants
(sorted by size, not necessarily quality. IQ-quants are often preferable over similar sized non-IQ quants)
| Link | Type | Size/GB | Notes |
|:-----|:-----|--------:|:------|
| [GGUF](https://huggingface.co/mradermacher/Llama-3-8B-Instruct-MagPO-GGUF/resolve/main/Llama-3-8B-Instruct-MagPO.Q2_K.gguf) | Q2_K | 3.3 | |
| [GGUF](https://huggingface.co/mradermacher/Llama-3-8B-Instruct-MagPO-GGUF/resolve/main/Llama-3-8B-Instruct-MagPO.IQ3_XS.gguf) | IQ3_XS | 3.6 | |
| [GGUF](https://huggingface.co/mradermacher/Llama-3-8B-Instruct-MagPO-GGUF/resolve/main/Llama-3-8B-Instruct-MagPO.Q3_K_S.gguf) | Q3_K_S | 3.8 | |
| [GGUF](https://huggingface.co/mradermacher/Llama-3-8B-Instruct-MagPO-GGUF/resolve/main/Llama-3-8B-Instruct-MagPO.IQ3_S.gguf) | IQ3_S | 3.8 | beats Q3_K* |
| [GGUF](https://huggingface.co/mradermacher/Llama-3-8B-Instruct-MagPO-GGUF/resolve/main/Llama-3-8B-Instruct-MagPO.IQ3_M.gguf) | IQ3_M | 3.9 | |
| [GGUF](https://huggingface.co/mradermacher/Llama-3-8B-Instruct-MagPO-GGUF/resolve/main/Llama-3-8B-Instruct-MagPO.Q3_K_M.gguf) | Q3_K_M | 4.1 | lower quality |
| [GGUF](https://huggingface.co/mradermacher/Llama-3-8B-Instruct-MagPO-GGUF/resolve/main/Llama-3-8B-Instruct-MagPO.Q3_K_L.gguf) | Q3_K_L | 4.4 | |
| [GGUF](https://huggingface.co/mradermacher/Llama-3-8B-Instruct-MagPO-GGUF/resolve/main/Llama-3-8B-Instruct-MagPO.IQ4_XS.gguf) | IQ4_XS | 4.6 | |
| [GGUF](https://huggingface.co/mradermacher/Llama-3-8B-Instruct-MagPO-GGUF/resolve/main/Llama-3-8B-Instruct-MagPO.Q4_K_S.gguf) | Q4_K_S | 4.8 | fast, recommended |
| [GGUF](https://huggingface.co/mradermacher/Llama-3-8B-Instruct-MagPO-GGUF/resolve/main/Llama-3-8B-Instruct-MagPO.Q4_K_M.gguf) | Q4_K_M | 5.0 | fast, recommended |
| [GGUF](https://huggingface.co/mradermacher/Llama-3-8B-Instruct-MagPO-GGUF/resolve/main/Llama-3-8B-Instruct-MagPO.Q5_K_S.gguf) | Q5_K_S | 5.7 | |
| [GGUF](https://huggingface.co/mradermacher/Llama-3-8B-Instruct-MagPO-GGUF/resolve/main/Llama-3-8B-Instruct-MagPO.Q5_K_M.gguf) | Q5_K_M | 5.8 | |
| [GGUF](https://huggingface.co/mradermacher/Llama-3-8B-Instruct-MagPO-GGUF/resolve/main/Llama-3-8B-Instruct-MagPO.Q6_K.gguf) | Q6_K | 6.7 | very good quality |
| [GGUF](https://huggingface.co/mradermacher/Llama-3-8B-Instruct-MagPO-GGUF/resolve/main/Llama-3-8B-Instruct-MagPO.Q8_0.gguf) | Q8_0 | 8.6 | fast, best quality |
| [GGUF](https://huggingface.co/mradermacher/Llama-3-8B-Instruct-MagPO-GGUF/resolve/main/Llama-3-8B-Instruct-MagPO.f16.gguf) | f16 | 16.2 | 16 bpw, overkill |
Here is a handy graph by ikawrakow comparing some lower-quality quant
types (lower is better):

And here are Artefact2's thoughts on the matter:
https://gist.github.com/Artefact2/b5f810600771265fc1e39442288e8ec9
## FAQ / Model Request
See https://huggingface.co/mradermacher/model_requests for some answers to
questions you might have and/or if you want some other model quantized.
## Thanks
I thank my company, [nethype GmbH](https://www.nethype.de/), for letting
me use its servers and providing upgrades to my workstation to enable
this work in my free time.
<!-- end -->
|
RichardErkhov/SanjiWatsuki_-_Loyal-Toppy-Bruins-Maid-7B-DARE-gguf | RichardErkhov | 2024-06-20T03:22:37Z | 12,263 | 0 | null | [
"gguf",
"region:us"
]
| null | 2024-06-19T22:11:38Z | Quantization made by Richard Erkhov.
[Github](https://github.com/RichardErkhov)
[Discord](https://discord.gg/pvy7H8DZMG)
[Request more models](https://github.com/RichardErkhov/quant_request)
Loyal-Toppy-Bruins-Maid-7B-DARE - GGUF
- Model creator: https://huggingface.co/SanjiWatsuki/
- Original model: https://huggingface.co/SanjiWatsuki/Loyal-Toppy-Bruins-Maid-7B-DARE/
| Name | Quant method | Size |
| ---- | ---- | ---- |
| [Loyal-Toppy-Bruins-Maid-7B-DARE.Q2_K.gguf](https://huggingface.co/RichardErkhov/SanjiWatsuki_-_Loyal-Toppy-Bruins-Maid-7B-DARE-gguf/blob/main/Loyal-Toppy-Bruins-Maid-7B-DARE.Q2_K.gguf) | Q2_K | 2.53GB |
| [Loyal-Toppy-Bruins-Maid-7B-DARE.IQ3_XS.gguf](https://huggingface.co/RichardErkhov/SanjiWatsuki_-_Loyal-Toppy-Bruins-Maid-7B-DARE-gguf/blob/main/Loyal-Toppy-Bruins-Maid-7B-DARE.IQ3_XS.gguf) | IQ3_XS | 2.81GB |
| [Loyal-Toppy-Bruins-Maid-7B-DARE.IQ3_S.gguf](https://huggingface.co/RichardErkhov/SanjiWatsuki_-_Loyal-Toppy-Bruins-Maid-7B-DARE-gguf/blob/main/Loyal-Toppy-Bruins-Maid-7B-DARE.IQ3_S.gguf) | IQ3_S | 2.96GB |
| [Loyal-Toppy-Bruins-Maid-7B-DARE.Q3_K_S.gguf](https://huggingface.co/RichardErkhov/SanjiWatsuki_-_Loyal-Toppy-Bruins-Maid-7B-DARE-gguf/blob/main/Loyal-Toppy-Bruins-Maid-7B-DARE.Q3_K_S.gguf) | Q3_K_S | 2.95GB |
| [Loyal-Toppy-Bruins-Maid-7B-DARE.IQ3_M.gguf](https://huggingface.co/RichardErkhov/SanjiWatsuki_-_Loyal-Toppy-Bruins-Maid-7B-DARE-gguf/blob/main/Loyal-Toppy-Bruins-Maid-7B-DARE.IQ3_M.gguf) | IQ3_M | 3.06GB |
| [Loyal-Toppy-Bruins-Maid-7B-DARE.Q3_K.gguf](https://huggingface.co/RichardErkhov/SanjiWatsuki_-_Loyal-Toppy-Bruins-Maid-7B-DARE-gguf/blob/main/Loyal-Toppy-Bruins-Maid-7B-DARE.Q3_K.gguf) | Q3_K | 3.28GB |
| [Loyal-Toppy-Bruins-Maid-7B-DARE.Q3_K_M.gguf](https://huggingface.co/RichardErkhov/SanjiWatsuki_-_Loyal-Toppy-Bruins-Maid-7B-DARE-gguf/blob/main/Loyal-Toppy-Bruins-Maid-7B-DARE.Q3_K_M.gguf) | Q3_K_M | 3.28GB |
| [Loyal-Toppy-Bruins-Maid-7B-DARE.Q3_K_L.gguf](https://huggingface.co/RichardErkhov/SanjiWatsuki_-_Loyal-Toppy-Bruins-Maid-7B-DARE-gguf/blob/main/Loyal-Toppy-Bruins-Maid-7B-DARE.Q3_K_L.gguf) | Q3_K_L | 3.56GB |
| [Loyal-Toppy-Bruins-Maid-7B-DARE.IQ4_XS.gguf](https://huggingface.co/RichardErkhov/SanjiWatsuki_-_Loyal-Toppy-Bruins-Maid-7B-DARE-gguf/blob/main/Loyal-Toppy-Bruins-Maid-7B-DARE.IQ4_XS.gguf) | IQ4_XS | 3.67GB |
| [Loyal-Toppy-Bruins-Maid-7B-DARE.Q4_0.gguf](https://huggingface.co/RichardErkhov/SanjiWatsuki_-_Loyal-Toppy-Bruins-Maid-7B-DARE-gguf/blob/main/Loyal-Toppy-Bruins-Maid-7B-DARE.Q4_0.gguf) | Q4_0 | 3.83GB |
| [Loyal-Toppy-Bruins-Maid-7B-DARE.IQ4_NL.gguf](https://huggingface.co/RichardErkhov/SanjiWatsuki_-_Loyal-Toppy-Bruins-Maid-7B-DARE-gguf/blob/main/Loyal-Toppy-Bruins-Maid-7B-DARE.IQ4_NL.gguf) | IQ4_NL | 3.87GB |
| [Loyal-Toppy-Bruins-Maid-7B-DARE.Q4_K_S.gguf](https://huggingface.co/RichardErkhov/SanjiWatsuki_-_Loyal-Toppy-Bruins-Maid-7B-DARE-gguf/blob/main/Loyal-Toppy-Bruins-Maid-7B-DARE.Q4_K_S.gguf) | Q4_K_S | 3.86GB |
| [Loyal-Toppy-Bruins-Maid-7B-DARE.Q4_K.gguf](https://huggingface.co/RichardErkhov/SanjiWatsuki_-_Loyal-Toppy-Bruins-Maid-7B-DARE-gguf/blob/main/Loyal-Toppy-Bruins-Maid-7B-DARE.Q4_K.gguf) | Q4_K | 4.07GB |
| [Loyal-Toppy-Bruins-Maid-7B-DARE.Q4_K_M.gguf](https://huggingface.co/RichardErkhov/SanjiWatsuki_-_Loyal-Toppy-Bruins-Maid-7B-DARE-gguf/blob/main/Loyal-Toppy-Bruins-Maid-7B-DARE.Q4_K_M.gguf) | Q4_K_M | 4.07GB |
| [Loyal-Toppy-Bruins-Maid-7B-DARE.Q4_1.gguf](https://huggingface.co/RichardErkhov/SanjiWatsuki_-_Loyal-Toppy-Bruins-Maid-7B-DARE-gguf/blob/main/Loyal-Toppy-Bruins-Maid-7B-DARE.Q4_1.gguf) | Q4_1 | 4.24GB |
| [Loyal-Toppy-Bruins-Maid-7B-DARE.Q5_0.gguf](https://huggingface.co/RichardErkhov/SanjiWatsuki_-_Loyal-Toppy-Bruins-Maid-7B-DARE-gguf/blob/main/Loyal-Toppy-Bruins-Maid-7B-DARE.Q5_0.gguf) | Q5_0 | 4.65GB |
| [Loyal-Toppy-Bruins-Maid-7B-DARE.Q5_K_S.gguf](https://huggingface.co/RichardErkhov/SanjiWatsuki_-_Loyal-Toppy-Bruins-Maid-7B-DARE-gguf/blob/main/Loyal-Toppy-Bruins-Maid-7B-DARE.Q5_K_S.gguf) | Q5_K_S | 4.65GB |
| [Loyal-Toppy-Bruins-Maid-7B-DARE.Q5_K.gguf](https://huggingface.co/RichardErkhov/SanjiWatsuki_-_Loyal-Toppy-Bruins-Maid-7B-DARE-gguf/blob/main/Loyal-Toppy-Bruins-Maid-7B-DARE.Q5_K.gguf) | Q5_K | 4.78GB |
| [Loyal-Toppy-Bruins-Maid-7B-DARE.Q5_K_M.gguf](https://huggingface.co/RichardErkhov/SanjiWatsuki_-_Loyal-Toppy-Bruins-Maid-7B-DARE-gguf/blob/main/Loyal-Toppy-Bruins-Maid-7B-DARE.Q5_K_M.gguf) | Q5_K_M | 4.78GB |
| [Loyal-Toppy-Bruins-Maid-7B-DARE.Q5_1.gguf](https://huggingface.co/RichardErkhov/SanjiWatsuki_-_Loyal-Toppy-Bruins-Maid-7B-DARE-gguf/blob/main/Loyal-Toppy-Bruins-Maid-7B-DARE.Q5_1.gguf) | Q5_1 | 5.07GB |
| [Loyal-Toppy-Bruins-Maid-7B-DARE.Q6_K.gguf](https://huggingface.co/RichardErkhov/SanjiWatsuki_-_Loyal-Toppy-Bruins-Maid-7B-DARE-gguf/blob/main/Loyal-Toppy-Bruins-Maid-7B-DARE.Q6_K.gguf) | Q6_K | 5.53GB |
| [Loyal-Toppy-Bruins-Maid-7B-DARE.Q8_0.gguf](https://huggingface.co/RichardErkhov/SanjiWatsuki_-_Loyal-Toppy-Bruins-Maid-7B-DARE-gguf/blob/main/Loyal-Toppy-Bruins-Maid-7B-DARE.Q8_0.gguf) | Q8_0 | 7.17GB |
Original model description:
---
license: cc-by-nc-4.0
tags:
- merge
---

<!-- description start -->
## Description
This repository hosts FP16 files for **Loyal-Toppy-Bruins-Maid-7B**, a 7B model aimed at having engaging RP with solid character card adherence and being a smart cookie at the same time.
Its foundation is [Starling-LM-7B-alpha](https://huggingface.co/berkeley-nest/Starling-LM-7B-alpha), notable for its performance in the LMSYS Chatbot Arena, even surpassing GPT-3.5-Turbo-1106. The model incorporates [rwitz/go-bruins-v2](https://huggingface.co/rwitz/go-bruins-v2), a [Q-bert/MetaMath-Cybertron-Starling](https://huggingface.co/Q-bert/MetaMath-Cybertron-Starling) derivative with Alpaca RP data tuning.
The other foundational model is [chargoddard/loyal-piano-m7](https://huggingface.co/chargoddard/loyal-piano-m7), chosen for its strong RP performance and Alpaca format training, with a diverse dataset including PIPPA, rpbuild, and LimaRP.
[Undi95/Toppy-M-7B](https://huggingface.co/Undi95/Toppy-M-7B), known for its creativity, brings in useful RP data from various sources. It ranks first among 7B models on [OpenRouter](https://openrouter.ai/rankings) for a good reason.
[NeverSleep/Noromaid-7b-v0.1.1](https://huggingface.co/NeverSleep/Noromaid-7b-v0.1.1), a Mistral finetune with unique RP data not present in other models, was also added for bringing in a unique RP dataset and being a well-regarded RP model.
The models were merged using the DARE ties method, with a targeted 1.2 absolute weight and high density (0.5-0.6), as discussed in the [MergeKit GitHub Repo](https://github.com/cg123/mergekit/issues/26).
Currently, this model ranks at the top of my personal RP unit test benchmark and scored a very solid 20 on [lilblam's LLM Logic Test](https://docs.google.com/spreadsheets/d/1NgHDxbVWJFolq8bLvLkuPWKC7i_R6I6W/edit#gid=1278290632). My first impressions of it for RPing are very good but, admittedly, this model came out of the oven today so I haven't played it with it too much 😊
### The sauce
```
models: # Top-Loyal-Bruins-Maid-DARE-7B_v2
- model: mistralai/Mistral-7B-v0.1
# no parameters necessary for base model
- model: rwitz/go-bruins-v2 # MetamathCybertronStarling base
parameters:
weight: 0.5
density: 0.6
- model: chargoddard/loyal-piano-m7 # Pull in some PIPPA/LimaRP/Orca/rpguild
parameters:
weight: 0.5
density: 0.6
- model: Undi95/Toppy-M-7B
parameters:
weight: 0.1
density: 0.5
- model: NeverSleep/Noromaid-7b-v0.1.1
parameters:
weight: 0.1
density: 0.5
merge_method: dare_ties
base_model: mistralai/Mistral-7B-v0.1
parameters:
normalize: false
int8_mask: true
dtype: bfloat16
```
<!-- description end -->
<!-- prompt-template start -->
## Prompt template: Custom format, or Alpaca
### Custom format:
I found the best SillyTavern results from using the Noromaid template.
SillyTavern config files: [Context](https://files.catbox.moe/ifmhai.json), [Instruct](https://files.catbox.moe/ttw1l9.json).
Otherwise, I tried to ensure that all of the underlying merged models were Alpaca favored.
### Alpaca:
```
Below is an instruction that describes a task. Write a response that appropriately completes the request.
### Instruction:
{prompt}
### Response:
```
|
MoritzLaurer/deberta-v3-xsmall-zeroshot-v1.1-all-33 | MoritzLaurer | 2024-05-07T14:42:09Z | 12,255 | 3 | transformers | [
"transformers",
"pytorch",
"onnx",
"safetensors",
"deberta-v2",
"text-classification",
"zero-shot-classification",
"en",
"arxiv:2312.17543",
"base_model:microsoft/deberta-v3-xsmall",
"license:mit",
"autotrain_compatible",
"endpoints_compatible",
"region:us"
]
| zero-shot-classification | 2024-01-10T17:35:54Z | ---
base_model: microsoft/deberta-v3-xsmall
language:
- en
tags:
- text-classification
- zero-shot-classification
pipeline_tag: zero-shot-classification
library_name: transformers
license: mit
---
# deberta-v3-xsmall-zeroshot-v1.1-all-33
This model was fine-tuned using the same pipeline as described in
the model card for [MoritzLaurer/deberta-v3-large-zeroshot-v1.1-all-33](https://huggingface.co/MoritzLaurer/deberta-v3-large-zeroshot-v1.1-all-33)
and in this [paper](https://arxiv.org/pdf/2312.17543.pdf).
The foundation model is [microsoft/deberta-v3-xsmall](https://huggingface.co/microsoft/deberta-v3-xsmall).
The model only has 22 million backbone parameters and 128 million vocabulary parameters.
The backbone parameters are the main parameters active during inference, providing a significant speedup over larger models.
The model is 142 MB small.
This model was trained to provide a small and highly efficient zeroshot option,
especially for edge devices or in-browser use-cases with transformers.js.
## Usage and other details
For usage instructions and other details refer to
this model card [MoritzLaurer/deberta-v3-large-zeroshot-v1.1-all-33](https://huggingface.co/MoritzLaurer/deberta-v3-large-zeroshot-v1.1-all-33)
and this [paper](https://arxiv.org/pdf/2312.17543.pdf).
## Metrics:
I didn't not do zeroshot evaluation for this model to save time and compute.
The table below shows standard accuracy for all datasets the model was trained on (note that the NLI datasets are binary).
General takeaway: the model is much more efficient than its larger sisters, but it performs less well.
|Datasets|mnli_m|mnli_mm|fevernli|anli_r1|anli_r2|anli_r3|wanli|lingnli|wellformedquery|rottentomatoes|amazonpolarity|imdb|yelpreviews|hatexplain|massive|banking77|emotiondair|emocontext|empathetic|agnews|yahootopics|biasframes_sex|biasframes_offensive|biasframes_intent|financialphrasebank|appreviews|hateoffensive|trueteacher|spam|wikitoxic_toxicaggregated|wikitoxic_obscene|wikitoxic_identityhate|wikitoxic_threat|wikitoxic_insult|manifesto|capsotu|
| :---: | :---: | :---: | :---: | :---: | :---: | :---: | :---: | :---: | :---: | :---: | :---: | :---: | :---: | :---: | :---: | :---: | :---: | :---: | :---: | :---: | :---: | :---: | :---: | :---: | :---: | :---: | :---: | :---: | :---: | :---: | :---: | :---: | :---: | :---: | :---: | :---: |
|Accuracy|0.925|0.923|0.886|0.732|0.633|0.661|0.814|0.887|0.722|0.872|0.944|0.925|0.967|0.774|0.734|0.627|0.762|0.745|0.465|0.888|0.702|0.94|0.853|0.863|0.914|0.926|0.921|0.635|0.968|0.897|0.918|0.915|0.935|0.9|0.505|0.701|
|Inference text/sec (A10G, batch=128)|1573.0|1630.0|683.0|1282.0|1352.0|1072.0|2325.0|2008.0|4781.0|2743.0|677.0|228.0|238.0|2357.0|5027.0|4323.0|3247.0|3129.0|941.0|1643.0|335.0|1517.0|1452.0|1498.0|2367.0|974.0|2634.0|353.0|2284.0|260.0|252.0|256.0|254.0|259.0|1941.0|2080.0|
|
mradermacher/Infinirc-Llama3-8B-2G-Release-v1.0-GGUF | mradermacher | 2024-07-02T23:09:38Z | 12,254 | 0 | transformers | [
"transformers",
"gguf",
"zhtw",
"zh",
"en",
"base_model:Infinirc/Infinirc-Llama3-8B-2G-Release-v1.0",
"license:llama3",
"endpoints_compatible",
"region:us"
]
| null | 2024-06-26T14:13:56Z | ---
base_model: Infinirc/Infinirc-Llama3-8B-2G-Release-v1.0
language:
- zh
- en
library_name: transformers
license: llama3
quantized_by: mradermacher
tags:
- zhtw
---
## About
<!-- ### quantize_version: 2 -->
<!-- ### output_tensor_quantised: 1 -->
<!-- ### convert_type: hf -->
<!-- ### vocab_type: -->
<!-- ### tags: -->
static quants of https://huggingface.co/Infinirc/Infinirc-Llama3-8B-2G-Release-v1.0
<!-- provided-files -->
weighted/imatrix quants seem not to be available (by me) at this time. If they do not show up a week or so after the static ones, I have probably not planned for them. Feel free to request them by opening a Community Discussion.
## Usage
If you are unsure how to use GGUF files, refer to one of [TheBloke's
READMEs](https://huggingface.co/TheBloke/KafkaLM-70B-German-V0.1-GGUF) for
more details, including on how to concatenate multi-part files.
## Provided Quants
(sorted by size, not necessarily quality. IQ-quants are often preferable over similar sized non-IQ quants)
| Link | Type | Size/GB | Notes |
|:-----|:-----|--------:|:------|
| [GGUF](https://huggingface.co/mradermacher/Infinirc-Llama3-8B-2G-Release-v1.0-GGUF/resolve/main/Infinirc-Llama3-8B-2G-Release-v1.0.Q2_K.gguf) | Q2_K | 3.3 | |
| [GGUF](https://huggingface.co/mradermacher/Infinirc-Llama3-8B-2G-Release-v1.0-GGUF/resolve/main/Infinirc-Llama3-8B-2G-Release-v1.0.IQ3_XS.gguf) | IQ3_XS | 3.6 | |
| [GGUF](https://huggingface.co/mradermacher/Infinirc-Llama3-8B-2G-Release-v1.0-GGUF/resolve/main/Infinirc-Llama3-8B-2G-Release-v1.0.Q3_K_S.gguf) | Q3_K_S | 3.8 | |
| [GGUF](https://huggingface.co/mradermacher/Infinirc-Llama3-8B-2G-Release-v1.0-GGUF/resolve/main/Infinirc-Llama3-8B-2G-Release-v1.0.IQ3_S.gguf) | IQ3_S | 3.8 | beats Q3_K* |
| [GGUF](https://huggingface.co/mradermacher/Infinirc-Llama3-8B-2G-Release-v1.0-GGUF/resolve/main/Infinirc-Llama3-8B-2G-Release-v1.0.IQ3_M.gguf) | IQ3_M | 3.9 | |
| [GGUF](https://huggingface.co/mradermacher/Infinirc-Llama3-8B-2G-Release-v1.0-GGUF/resolve/main/Infinirc-Llama3-8B-2G-Release-v1.0.Q3_K_M.gguf) | Q3_K_M | 4.1 | lower quality |
| [GGUF](https://huggingface.co/mradermacher/Infinirc-Llama3-8B-2G-Release-v1.0-GGUF/resolve/main/Infinirc-Llama3-8B-2G-Release-v1.0.Q3_K_L.gguf) | Q3_K_L | 4.4 | |
| [GGUF](https://huggingface.co/mradermacher/Infinirc-Llama3-8B-2G-Release-v1.0-GGUF/resolve/main/Infinirc-Llama3-8B-2G-Release-v1.0.IQ4_XS.gguf) | IQ4_XS | 4.6 | |
| [GGUF](https://huggingface.co/mradermacher/Infinirc-Llama3-8B-2G-Release-v1.0-GGUF/resolve/main/Infinirc-Llama3-8B-2G-Release-v1.0.Q4_K_S.gguf) | Q4_K_S | 4.8 | fast, recommended |
| [GGUF](https://huggingface.co/mradermacher/Infinirc-Llama3-8B-2G-Release-v1.0-GGUF/resolve/main/Infinirc-Llama3-8B-2G-Release-v1.0.Q4_K_M.gguf) | Q4_K_M | 5.0 | fast, recommended |
| [GGUF](https://huggingface.co/mradermacher/Infinirc-Llama3-8B-2G-Release-v1.0-GGUF/resolve/main/Infinirc-Llama3-8B-2G-Release-v1.0.Q5_K_S.gguf) | Q5_K_S | 5.7 | |
| [GGUF](https://huggingface.co/mradermacher/Infinirc-Llama3-8B-2G-Release-v1.0-GGUF/resolve/main/Infinirc-Llama3-8B-2G-Release-v1.0.Q5_K_M.gguf) | Q5_K_M | 5.8 | |
| [GGUF](https://huggingface.co/mradermacher/Infinirc-Llama3-8B-2G-Release-v1.0-GGUF/resolve/main/Infinirc-Llama3-8B-2G-Release-v1.0.Q6_K.gguf) | Q6_K | 6.7 | very good quality |
| [GGUF](https://huggingface.co/mradermacher/Infinirc-Llama3-8B-2G-Release-v1.0-GGUF/resolve/main/Infinirc-Llama3-8B-2G-Release-v1.0.Q8_0.gguf) | Q8_0 | 8.6 | fast, best quality |
| [GGUF](https://huggingface.co/mradermacher/Infinirc-Llama3-8B-2G-Release-v1.0-GGUF/resolve/main/Infinirc-Llama3-8B-2G-Release-v1.0.f16.gguf) | f16 | 16.2 | 16 bpw, overkill |
Here is a handy graph by ikawrakow comparing some lower-quality quant
types (lower is better):

And here are Artefact2's thoughts on the matter:
https://gist.github.com/Artefact2/b5f810600771265fc1e39442288e8ec9
## FAQ / Model Request
See https://huggingface.co/mradermacher/model_requests for some answers to
questions you might have and/or if you want some other model quantized.
## Thanks
I thank my company, [nethype GmbH](https://www.nethype.de/), for letting
me use its servers and providing upgrades to my workstation to enable
this work in my free time.
<!-- end -->
|
RichardErkhov/InferenceIllusionist_-_Magic-Dolphin-7b-gguf | RichardErkhov | 2024-06-20T12:53:48Z | 12,249 | 1 | null | [
"gguf",
"arxiv:2203.05482",
"region:us"
]
| null | 2024-06-20T06:24:51Z | Quantization made by Richard Erkhov.
[Github](https://github.com/RichardErkhov)
[Discord](https://discord.gg/pvy7H8DZMG)
[Request more models](https://github.com/RichardErkhov/quant_request)
Magic-Dolphin-7b - GGUF
- Model creator: https://huggingface.co/InferenceIllusionist/
- Original model: https://huggingface.co/InferenceIllusionist/Magic-Dolphin-7b/
| Name | Quant method | Size |
| ---- | ---- | ---- |
| [Magic-Dolphin-7b.Q2_K.gguf](https://huggingface.co/RichardErkhov/InferenceIllusionist_-_Magic-Dolphin-7b-gguf/blob/main/Magic-Dolphin-7b.Q2_K.gguf) | Q2_K | 2.53GB |
| [Magic-Dolphin-7b.IQ3_XS.gguf](https://huggingface.co/RichardErkhov/InferenceIllusionist_-_Magic-Dolphin-7b-gguf/blob/main/Magic-Dolphin-7b.IQ3_XS.gguf) | IQ3_XS | 2.81GB |
| [Magic-Dolphin-7b.IQ3_S.gguf](https://huggingface.co/RichardErkhov/InferenceIllusionist_-_Magic-Dolphin-7b-gguf/blob/main/Magic-Dolphin-7b.IQ3_S.gguf) | IQ3_S | 2.96GB |
| [Magic-Dolphin-7b.Q3_K_S.gguf](https://huggingface.co/RichardErkhov/InferenceIllusionist_-_Magic-Dolphin-7b-gguf/blob/main/Magic-Dolphin-7b.Q3_K_S.gguf) | Q3_K_S | 2.95GB |
| [Magic-Dolphin-7b.IQ3_M.gguf](https://huggingface.co/RichardErkhov/InferenceIllusionist_-_Magic-Dolphin-7b-gguf/blob/main/Magic-Dolphin-7b.IQ3_M.gguf) | IQ3_M | 3.06GB |
| [Magic-Dolphin-7b.Q3_K.gguf](https://huggingface.co/RichardErkhov/InferenceIllusionist_-_Magic-Dolphin-7b-gguf/blob/main/Magic-Dolphin-7b.Q3_K.gguf) | Q3_K | 3.28GB |
| [Magic-Dolphin-7b.Q3_K_M.gguf](https://huggingface.co/RichardErkhov/InferenceIllusionist_-_Magic-Dolphin-7b-gguf/blob/main/Magic-Dolphin-7b.Q3_K_M.gguf) | Q3_K_M | 3.28GB |
| [Magic-Dolphin-7b.Q3_K_L.gguf](https://huggingface.co/RichardErkhov/InferenceIllusionist_-_Magic-Dolphin-7b-gguf/blob/main/Magic-Dolphin-7b.Q3_K_L.gguf) | Q3_K_L | 3.56GB |
| [Magic-Dolphin-7b.IQ4_XS.gguf](https://huggingface.co/RichardErkhov/InferenceIllusionist_-_Magic-Dolphin-7b-gguf/blob/main/Magic-Dolphin-7b.IQ4_XS.gguf) | IQ4_XS | 3.67GB |
| [Magic-Dolphin-7b.Q4_0.gguf](https://huggingface.co/RichardErkhov/InferenceIllusionist_-_Magic-Dolphin-7b-gguf/blob/main/Magic-Dolphin-7b.Q4_0.gguf) | Q4_0 | 3.83GB |
| [Magic-Dolphin-7b.IQ4_NL.gguf](https://huggingface.co/RichardErkhov/InferenceIllusionist_-_Magic-Dolphin-7b-gguf/blob/main/Magic-Dolphin-7b.IQ4_NL.gguf) | IQ4_NL | 3.87GB |
| [Magic-Dolphin-7b.Q4_K_S.gguf](https://huggingface.co/RichardErkhov/InferenceIllusionist_-_Magic-Dolphin-7b-gguf/blob/main/Magic-Dolphin-7b.Q4_K_S.gguf) | Q4_K_S | 3.86GB |
| [Magic-Dolphin-7b.Q4_K.gguf](https://huggingface.co/RichardErkhov/InferenceIllusionist_-_Magic-Dolphin-7b-gguf/blob/main/Magic-Dolphin-7b.Q4_K.gguf) | Q4_K | 4.07GB |
| [Magic-Dolphin-7b.Q4_K_M.gguf](https://huggingface.co/RichardErkhov/InferenceIllusionist_-_Magic-Dolphin-7b-gguf/blob/main/Magic-Dolphin-7b.Q4_K_M.gguf) | Q4_K_M | 4.07GB |
| [Magic-Dolphin-7b.Q4_1.gguf](https://huggingface.co/RichardErkhov/InferenceIllusionist_-_Magic-Dolphin-7b-gguf/blob/main/Magic-Dolphin-7b.Q4_1.gguf) | Q4_1 | 4.24GB |
| [Magic-Dolphin-7b.Q5_0.gguf](https://huggingface.co/RichardErkhov/InferenceIllusionist_-_Magic-Dolphin-7b-gguf/blob/main/Magic-Dolphin-7b.Q5_0.gguf) | Q5_0 | 4.65GB |
| [Magic-Dolphin-7b.Q5_K_S.gguf](https://huggingface.co/RichardErkhov/InferenceIllusionist_-_Magic-Dolphin-7b-gguf/blob/main/Magic-Dolphin-7b.Q5_K_S.gguf) | Q5_K_S | 4.65GB |
| [Magic-Dolphin-7b.Q5_K.gguf](https://huggingface.co/RichardErkhov/InferenceIllusionist_-_Magic-Dolphin-7b-gguf/blob/main/Magic-Dolphin-7b.Q5_K.gguf) | Q5_K | 4.78GB |
| [Magic-Dolphin-7b.Q5_K_M.gguf](https://huggingface.co/RichardErkhov/InferenceIllusionist_-_Magic-Dolphin-7b-gguf/blob/main/Magic-Dolphin-7b.Q5_K_M.gguf) | Q5_K_M | 4.78GB |
| [Magic-Dolphin-7b.Q5_1.gguf](https://huggingface.co/RichardErkhov/InferenceIllusionist_-_Magic-Dolphin-7b-gguf/blob/main/Magic-Dolphin-7b.Q5_1.gguf) | Q5_1 | 5.07GB |
| [Magic-Dolphin-7b.Q6_K.gguf](https://huggingface.co/RichardErkhov/InferenceIllusionist_-_Magic-Dolphin-7b-gguf/blob/main/Magic-Dolphin-7b.Q6_K.gguf) | Q6_K | 5.53GB |
| [Magic-Dolphin-7b.Q8_0.gguf](https://huggingface.co/RichardErkhov/InferenceIllusionist_-_Magic-Dolphin-7b-gguf/blob/main/Magic-Dolphin-7b.Q8_0.gguf) | Q8_0 | 7.17GB |
Original model description:
---
license: apache-2.0
base_model:
- cognitivecomputations/dolphin-2.6-mistral-7b-dpo-laser
- Locutusque/Hyperion-1.5-Mistral-7B
- ibm/merlinite-7b
library_name: transformers
tags:
- mergekit
- merge
- code
model-index:
- name: Magic-Dolphin-7b
results:
- task:
type: text-generation
name: Text Generation
dataset:
name: AI2 Reasoning Challenge (25-Shot)
type: ai2_arc
config: ARC-Challenge
split: test
args:
num_few_shot: 25
metrics:
- type: acc_norm
value: 65.78
name: normalized accuracy
source:
url: https://huggingface.co/spaces/HuggingFaceH4/open_llm_leaderboard?query=InferenceIllusionist/Magic-Dolphin-7b
name: Open LLM Leaderboard
- task:
type: text-generation
name: Text Generation
dataset:
name: HellaSwag (10-Shot)
type: hellaswag
split: validation
args:
num_few_shot: 10
metrics:
- type: acc_norm
value: 85.61
name: normalized accuracy
source:
url: https://huggingface.co/spaces/HuggingFaceH4/open_llm_leaderboard?query=InferenceIllusionist/Magic-Dolphin-7b
name: Open LLM Leaderboard
- task:
type: text-generation
name: Text Generation
dataset:
name: MMLU (5-Shot)
type: cais/mmlu
config: all
split: test
args:
num_few_shot: 5
metrics:
- type: acc
value: 64.64
name: accuracy
source:
url: https://huggingface.co/spaces/HuggingFaceH4/open_llm_leaderboard?query=InferenceIllusionist/Magic-Dolphin-7b
name: Open LLM Leaderboard
- task:
type: text-generation
name: Text Generation
dataset:
name: TruthfulQA (0-shot)
type: truthful_qa
config: multiple_choice
split: validation
args:
num_few_shot: 0
metrics:
- type: mc2
value: 58.01
source:
url: https://huggingface.co/spaces/HuggingFaceH4/open_llm_leaderboard?query=InferenceIllusionist/Magic-Dolphin-7b
name: Open LLM Leaderboard
- task:
type: text-generation
name: Text Generation
dataset:
name: Winogrande (5-shot)
type: winogrande
config: winogrande_xl
split: validation
args:
num_few_shot: 5
metrics:
- type: acc
value: 79.64
name: accuracy
source:
url: https://huggingface.co/spaces/HuggingFaceH4/open_llm_leaderboard?query=InferenceIllusionist/Magic-Dolphin-7b
name: Open LLM Leaderboard
- task:
type: text-generation
name: Text Generation
dataset:
name: GSM8k (5-shot)
type: gsm8k
config: main
split: test
args:
num_few_shot: 5
metrics:
- type: acc
value: 51.18
name: accuracy
source:
url: https://huggingface.co/spaces/HuggingFaceH4/open_llm_leaderboard?query=InferenceIllusionist/Magic-Dolphin-7b
name: Open LLM Leaderboard
---
# Magic-Dolphin-7b
<img src="https://huggingface.co/InferenceIllusionist/Magic-Dolphin-7b/resolve/main/magic-dolphin.jfif" width="500"/>
<b>The follow-up to this model has been released, check out the updated benchmarks here for [Excalibur-7b](https://huggingface.co/InferenceIllusionist/Excalibur-7b)</b>
For GGUF files please look [here](https://huggingface.co/InferenceIllusionist/Magic-Dolphin-7b-GGUF)
A linear merge of:
- [cognitivecomputations/dolphin-2.6-mistral-7b-dpo-laser](https://huggingface.co/cognitivecomputations/dolphin-2.6-mistral-7b-dpo-laser)
- [Locutusque/Hyperion-1.5-Mistral-7B](https://huggingface.co/Locutusque/Hyperion-1.5-Mistral-7B)
- [ibm/merlinite-7b](https://huggingface.co/ibm/merlinite-7b)
These three models showed excellent acumen in technical topics so I wanted to see how they would behave together in a merge. Several different ratios were tested before this release, in the end a higher weighting for merlinite-7b helped smooth out some edges. This model is a test of how LAB tuning is impacted by merges with models leveraging DPO.
### Benchmark Performance
| Name | Avg. | ARC | HellaSwag | MMLU | TruthfulQA | Winogrande | GSM8K |
| ---- | ---- | ---- | ---- | ---- | ---- | ---- | ---- |
| <b>Magic-Dolphin-7b</b> | <u><b>67.48</b></u> | 65.78 | 85.61 | 64.64 | 58.01 | 79.64 | <u><b>51.18</b></u> |
| dolphin-2.6-mistral-7b-dpo-laser | 67.28 | 66.3 | 85.73 | 63.16 | 61.71 | 79.16 | 47.61 |
| merlinite-7b | 64 | 63.65 | 84.52 | 64.91 | 50.15 | 79.72 | 41.09 |
| Hyperion-1.5-Mistral-7B | 61.43 | 60.49 | 83.64 | 63.57 | 41.78 | 78.61 | 40.49 |
This was my first experiment with merging models so any feedback is greatly appreciated.
Uses Alpaca template.
<p align="center">
</p>
<b>Sample Question</b>
<img src="https://huggingface.co/InferenceIllusionist/Magic-Dolphin-7b/resolve/main/magic-dolphin.JPG" width="750"/>
## Merge Details
### Merge Method
This model was merged using the [linear](https://arxiv.org/abs/2203.05482) merge method.
### Models Merged
The following models were included in the merge:
* [cognitivecomputations/dolphin-2.6-mistral-7b-dpo-laser](https://huggingface.co/cognitivecomputations/dolphin-2.6-mistral-7b-dpo-laser)
* [Locutusque/Hyperion-1.5-Mistral-7B](https://huggingface.co/Locutusque/Hyperion-1.5-Mistral-7B)
* [ibm/merlinite-7b](https://huggingface.co/ibm/merlinite-7b)
### Configuration
The following YAML configuration was used to produce this model:
```yaml
models:
- model: models/dolphin-2.6-mistral-7b-dpo-laser
parameters:
weight: 1.0
- model: models/Hyperion-1.5-Mistral-7B
parameters:
weight: 0.3
- model: models/merlinite-7b
parameters:
weight: 0.5
merge_method: linear
dtype: float16
```
# [Open LLM Leaderboard Evaluation Results](https://huggingface.co/spaces/HuggingFaceH4/open_llm_leaderboard)
Detailed results can be found [here](https://huggingface.co/datasets/open-llm-leaderboard/details_InferenceIllusionist__Magic-Dolphin-7b)
| Metric |Value|
|---------------------------------|----:|
|Avg. |67.48|
|AI2 Reasoning Challenge (25-Shot)|65.78|
|HellaSwag (10-Shot) |85.61|
|MMLU (5-Shot) |64.64|
|TruthfulQA (0-shot) |58.01|
|Winogrande (5-shot) |79.64|
|GSM8k (5-shot) |51.18|
|
TheBloke/Llama-2-13B-chat-GGUF | TheBloke | 2023-09-27T12:47:12Z | 12,239 | 185 | transformers | [
"transformers",
"gguf",
"llama",
"facebook",
"meta",
"pytorch",
"llama-2",
"text-generation",
"en",
"arxiv:2307.09288",
"base_model:meta-llama/Llama-2-13b-chat-hf",
"license:llama2",
"text-generation-inference",
"region:us"
]
| text-generation | 2023-09-04T17:20:15Z | ---
language:
- en
license: llama2
tags:
- facebook
- meta
- pytorch
- llama
- llama-2
model_name: Llama 2 13B Chat
base_model: meta-llama/Llama-2-13b-chat-hf
inference: false
model_creator: Meta Llama 2
model_type: llama
pipeline_tag: text-generation
prompt_template: '[INST] <<SYS>>
You are a helpful, respectful and honest assistant. Always answer as helpfully as
possible, while being safe. Your answers should not include any harmful, unethical,
racist, sexist, toxic, dangerous, or illegal content. Please ensure that your responses
are socially unbiased and positive in nature. If a question does not make any sense,
or is not factually coherent, explain why instead of answering something not correct.
If you don''t know the answer to a question, please don''t share false information.
<</SYS>>
{prompt}[/INST]
'
quantized_by: TheBloke
---
<!-- header start -->
<!-- 200823 -->
<div style="width: auto; margin-left: auto; margin-right: auto">
<img src="https://i.imgur.com/EBdldam.jpg" alt="TheBlokeAI" style="width: 100%; min-width: 400px; display: block; margin: auto;">
</div>
<div style="display: flex; justify-content: space-between; width: 100%;">
<div style="display: flex; flex-direction: column; align-items: flex-start;">
<p style="margin-top: 0.5em; margin-bottom: 0em;"><a href="https://discord.gg/theblokeai">Chat & support: TheBloke's Discord server</a></p>
</div>
<div style="display: flex; flex-direction: column; align-items: flex-end;">
<p style="margin-top: 0.5em; margin-bottom: 0em;"><a href="https://www.patreon.com/TheBlokeAI">Want to contribute? TheBloke's Patreon page</a></p>
</div>
</div>
<div style="text-align:center; margin-top: 0em; margin-bottom: 0em"><p style="margin-top: 0.25em; margin-bottom: 0em;">TheBloke's LLM work is generously supported by a grant from <a href="https://a16z.com">andreessen horowitz (a16z)</a></p></div>
<hr style="margin-top: 1.0em; margin-bottom: 1.0em;">
<!-- header end -->
# Llama 2 13B Chat - GGUF
- Model creator: [Meta Llama 2](https://huggingface.co/meta-llama)
- Original model: [Llama 2 13B Chat](https://huggingface.co/meta-llama/Llama-2-13b-chat-hf)
<!-- description start -->
## Description
This repo contains GGUF format model files for [Meta's Llama 2 13B-chat](https://huggingface.co/meta-llama/Llama-2-13b-chat-hf).
<!-- description end -->
<!-- README_GGUF.md-about-gguf start -->
### About GGUF
GGUF is a new format introduced by the llama.cpp team on August 21st 2023. It is a replacement for GGML, which is no longer supported by llama.cpp. GGUF offers numerous advantages over GGML, such as better tokenisation, and support for special tokens. It is also supports metadata, and is designed to be extensible.
Here is an incomplate list of clients and libraries that are known to support GGUF:
* [llama.cpp](https://github.com/ggerganov/llama.cpp). The source project for GGUF. Offers a CLI and a server option.
* [text-generation-webui](https://github.com/oobabooga/text-generation-webui), the most widely used web UI, with many features and powerful extensions. Supports GPU acceleration.
* [KoboldCpp](https://github.com/LostRuins/koboldcpp), a fully featured web UI, with GPU accel across all platforms and GPU architectures. Especially good for story telling.
* [LM Studio](https://lmstudio.ai/), an easy-to-use and powerful local GUI for Windows and macOS (Silicon), with GPU acceleration.
* [LoLLMS Web UI](https://github.com/ParisNeo/lollms-webui), a great web UI with many interesting and unique features, including a full model library for easy model selection.
* [Faraday.dev](https://faraday.dev/), an attractive and easy to use character-based chat GUI for Windows and macOS (both Silicon and Intel), with GPU acceleration.
* [ctransformers](https://github.com/marella/ctransformers), a Python library with GPU accel, LangChain support, and OpenAI-compatible AI server.
* [llama-cpp-python](https://github.com/abetlen/llama-cpp-python), a Python library with GPU accel, LangChain support, and OpenAI-compatible API server.
* [candle](https://github.com/huggingface/candle), a Rust ML framework with a focus on performance, including GPU support, and ease of use.
<!-- README_GGUF.md-about-gguf end -->
<!-- repositories-available start -->
## Repositories available
* [AWQ model(s) for GPU inference.](https://huggingface.co/TheBloke/Llama-2-13B-chat-AWQ)
* [GPTQ models for GPU inference, with multiple quantisation parameter options.](https://huggingface.co/TheBloke/Llama-2-13B-chat-GPTQ)
* [2, 3, 4, 5, 6 and 8-bit GGUF models for CPU+GPU inference](https://huggingface.co/TheBloke/Llama-2-13B-chat-GGUF)
* [Meta Llama 2's original unquantised fp16 model in pytorch format, for GPU inference and for further conversions](https://huggingface.co/meta-llama/Llama-2-13B-chat-hf)
<!-- repositories-available end -->
<!-- prompt-template start -->
## Prompt template: Llama-2-Chat
```
[INST] <<SYS>>
You are a helpful, respectful and honest assistant. Always answer as helpfully as possible, while being safe. Your answers should not include any harmful, unethical, racist, sexist, toxic, dangerous, or illegal content. Please ensure that your responses are socially unbiased and positive in nature. If a question does not make any sense, or is not factually coherent, explain why instead of answering something not correct. If you don't know the answer to a question, please don't share false information.
<</SYS>>
{prompt}[/INST]
```
<!-- prompt-template end -->
<!-- compatibility_gguf start -->
## Compatibility
These quantised GGUFv2 files are compatible with llama.cpp from August 27th onwards, as of commit [d0cee0d36d5be95a0d9088b674dbb27354107221](https://github.com/ggerganov/llama.cpp/commit/d0cee0d36d5be95a0d9088b674dbb27354107221)
They are also compatible with many third party UIs and libraries - please see the list at the top of this README.
## Explanation of quantisation methods
<details>
<summary>Click to see details</summary>
The new methods available are:
* GGML_TYPE_Q2_K - "type-1" 2-bit quantization in super-blocks containing 16 blocks, each block having 16 weight. Block scales and mins are quantized with 4 bits. This ends up effectively using 2.5625 bits per weight (bpw)
* GGML_TYPE_Q3_K - "type-0" 3-bit quantization in super-blocks containing 16 blocks, each block having 16 weights. Scales are quantized with 6 bits. This end up using 3.4375 bpw.
* GGML_TYPE_Q4_K - "type-1" 4-bit quantization in super-blocks containing 8 blocks, each block having 32 weights. Scales and mins are quantized with 6 bits. This ends up using 4.5 bpw.
* GGML_TYPE_Q5_K - "type-1" 5-bit quantization. Same super-block structure as GGML_TYPE_Q4_K resulting in 5.5 bpw
* GGML_TYPE_Q6_K - "type-0" 6-bit quantization. Super-blocks with 16 blocks, each block having 16 weights. Scales are quantized with 8 bits. This ends up using 6.5625 bpw
Refer to the Provided Files table below to see what files use which methods, and how.
</details>
<!-- compatibility_gguf end -->
<!-- README_GGUF.md-provided-files start -->
## Provided files
| Name | Quant method | Bits | Size | Max RAM required | Use case |
| ---- | ---- | ---- | ---- | ---- | ----- |
| [llama-2-13b-chat.Q2_K.gguf](https://huggingface.co/TheBloke/Llama-2-13B-chat-GGUF/blob/main/llama-2-13b-chat.Q2_K.gguf) | Q2_K | 2 | 5.43 GB| 7.93 GB | smallest, significant quality loss - not recommended for most purposes |
| [llama-2-13b-chat.Q3_K_S.gguf](https://huggingface.co/TheBloke/Llama-2-13B-chat-GGUF/blob/main/llama-2-13b-chat.Q3_K_S.gguf) | Q3_K_S | 3 | 5.66 GB| 8.16 GB | very small, high quality loss |
| [llama-2-13b-chat.Q3_K_M.gguf](https://huggingface.co/TheBloke/Llama-2-13B-chat-GGUF/blob/main/llama-2-13b-chat.Q3_K_M.gguf) | Q3_K_M | 3 | 6.34 GB| 8.84 GB | very small, high quality loss |
| [llama-2-13b-chat.Q3_K_L.gguf](https://huggingface.co/TheBloke/Llama-2-13B-chat-GGUF/blob/main/llama-2-13b-chat.Q3_K_L.gguf) | Q3_K_L | 3 | 6.93 GB| 9.43 GB | small, substantial quality loss |
| [llama-2-13b-chat.Q4_0.gguf](https://huggingface.co/TheBloke/Llama-2-13B-chat-GGUF/blob/main/llama-2-13b-chat.Q4_0.gguf) | Q4_0 | 4 | 7.37 GB| 9.87 GB | legacy; small, very high quality loss - prefer using Q3_K_M |
| [llama-2-13b-chat.Q4_K_S.gguf](https://huggingface.co/TheBloke/Llama-2-13B-chat-GGUF/blob/main/llama-2-13b-chat.Q4_K_S.gguf) | Q4_K_S | 4 | 7.41 GB| 9.91 GB | small, greater quality loss |
| [llama-2-13b-chat.Q4_K_M.gguf](https://huggingface.co/TheBloke/Llama-2-13B-chat-GGUF/blob/main/llama-2-13b-chat.Q4_K_M.gguf) | Q4_K_M | 4 | 7.87 GB| 10.37 GB | medium, balanced quality - recommended |
| [llama-2-13b-chat.Q5_0.gguf](https://huggingface.co/TheBloke/Llama-2-13B-chat-GGUF/blob/main/llama-2-13b-chat.Q5_0.gguf) | Q5_0 | 5 | 8.97 GB| 11.47 GB | legacy; medium, balanced quality - prefer using Q4_K_M |
| [llama-2-13b-chat.Q5_K_S.gguf](https://huggingface.co/TheBloke/Llama-2-13B-chat-GGUF/blob/main/llama-2-13b-chat.Q5_K_S.gguf) | Q5_K_S | 5 | 8.97 GB| 11.47 GB | large, low quality loss - recommended |
| [llama-2-13b-chat.Q5_K_M.gguf](https://huggingface.co/TheBloke/Llama-2-13B-chat-GGUF/blob/main/llama-2-13b-chat.Q5_K_M.gguf) | Q5_K_M | 5 | 9.23 GB| 11.73 GB | large, very low quality loss - recommended |
| [llama-2-13b-chat.Q6_K.gguf](https://huggingface.co/TheBloke/Llama-2-13B-chat-GGUF/blob/main/llama-2-13b-chat.Q6_K.gguf) | Q6_K | 6 | 10.68 GB| 13.18 GB | very large, extremely low quality loss |
| [llama-2-13b-chat.Q8_0.gguf](https://huggingface.co/TheBloke/Llama-2-13B-chat-GGUF/blob/main/llama-2-13b-chat.Q8_0.gguf) | Q8_0 | 8 | 13.83 GB| 16.33 GB | very large, extremely low quality loss - not recommended |
**Note**: the above RAM figures assume no GPU offloading. If layers are offloaded to the GPU, this will reduce RAM usage and use VRAM instead.
<!-- README_GGUF.md-provided-files end -->
<!-- README_GGUF.md-how-to-download start -->
## How to download GGUF files
**Note for manual downloaders:** You almost never want to clone the entire repo! Multiple different quantisation formats are provided, and most users only want to pick and download a single file.
The following clients/libraries will automatically download models for you, providing a list of available models to choose from:
- LM Studio
- LoLLMS Web UI
- Faraday.dev
### In `text-generation-webui`
Under Download Model, you can enter the model repo: TheBloke/Llama-2-13B-chat-GGUF and below it, a specific filename to download, such as: llama-2-13b-chat.q4_K_M.gguf.
Then click Download.
### On the command line, including multiple files at once
I recommend using the `huggingface-hub` Python library:
```shell
pip3 install huggingface-hub>=0.17.1
```
Then you can download any individual model file to the current directory, at high speed, with a command like this:
```shell
huggingface-cli download TheBloke/Llama-2-13B-chat-GGUF llama-2-13b-chat.q4_K_M.gguf --local-dir . --local-dir-use-symlinks False
```
<details>
<summary>More advanced huggingface-cli download usage</summary>
You can also download multiple files at once with a pattern:
```shell
huggingface-cli download TheBloke/Llama-2-13B-chat-GGUF --local-dir . --local-dir-use-symlinks False --include='*Q4_K*gguf'
```
For more documentation on downloading with `huggingface-cli`, please see: [HF -> Hub Python Library -> Download files -> Download from the CLI](https://huggingface.co/docs/huggingface_hub/guides/download#download-from-the-cli).
To accelerate downloads on fast connections (1Gbit/s or higher), install `hf_transfer`:
```shell
pip3 install hf_transfer
```
And set environment variable `HF_HUB_ENABLE_HF_TRANSFER` to `1`:
```shell
HUGGINGFACE_HUB_ENABLE_HF_TRANSFER=1 huggingface-cli download TheBloke/Llama-2-13B-chat-GGUF llama-2-13b-chat.q4_K_M.gguf --local-dir . --local-dir-use-symlinks False
```
Windows CLI users: Use `set HUGGINGFACE_HUB_ENABLE_HF_TRANSFER=1` before running the download command.
</details>
<!-- README_GGUF.md-how-to-download end -->
<!-- README_GGUF.md-how-to-run start -->
## Example `llama.cpp` command
Make sure you are using `llama.cpp` from commit [d0cee0d36d5be95a0d9088b674dbb27354107221](https://github.com/ggerganov/llama.cpp/commit/d0cee0d36d5be95a0d9088b674dbb27354107221) or later.
```shell
./main -ngl 32 -m llama-2-13b-chat.q4_K_M.gguf --color -c 4096 --temp 0.7 --repeat_penalty 1.1 -n -1 -p "[INST] <<SYS>>\nYou are a helpful, respectful and honest assistant. Always answer as helpfully as possible, while being safe. Your answers should not include any harmful, unethical, racist, sexist, toxic, dangerous, or illegal content. Please ensure that your responses are socially unbiased and positive in nature. If a question does not make any sense, or is not factually coherent, explain why instead of answering something not correct. If you don't know the answer to a question, please don't share false information.\n<</SYS>>\n{prompt}[/INST]"
```
Change `-ngl 32` to the number of layers to offload to GPU. Remove it if you don't have GPU acceleration.
Change `-c 4096` to the desired sequence length. For extended sequence models - eg 8K, 16K, 32K - the necessary RoPE scaling parameters are read from the GGUF file and set by llama.cpp automatically.
If you want to have a chat-style conversation, replace the `-p <PROMPT>` argument with `-i -ins`
For other parameters and how to use them, please refer to [the llama.cpp documentation](https://github.com/ggerganov/llama.cpp/blob/master/examples/main/README.md)
## How to run in `text-generation-webui`
Further instructions here: [text-generation-webui/docs/llama.cpp.md](https://github.com/oobabooga/text-generation-webui/blob/main/docs/llama.cpp.md).
## How to run from Python code
You can use GGUF models from Python using the [llama-cpp-python](https://github.com/abetlen/llama-cpp-python) or [ctransformers](https://github.com/marella/ctransformers) libraries.
### How to load this model from Python using ctransformers
#### First install the package
```bash
# Base ctransformers with no GPU acceleration
pip install ctransformers>=0.2.24
# Or with CUDA GPU acceleration
pip install ctransformers[cuda]>=0.2.24
# Or with ROCm GPU acceleration
CT_HIPBLAS=1 pip install ctransformers>=0.2.24 --no-binary ctransformers
# Or with Metal GPU acceleration for macOS systems
CT_METAL=1 pip install ctransformers>=0.2.24 --no-binary ctransformers
```
#### Simple example code to load one of these GGUF models
```python
from ctransformers import AutoModelForCausalLM
# Set gpu_layers to the number of layers to offload to GPU. Set to 0 if no GPU acceleration is available on your system.
llm = AutoModelForCausalLM.from_pretrained("TheBloke/Llama-2-13B-chat-GGUF", model_file="llama-2-13b-chat.q4_K_M.gguf", model_type="llama", gpu_layers=50)
print(llm("AI is going to"))
```
## How to use with LangChain
Here's guides on using llama-cpp-python or ctransformers with LangChain:
* [LangChain + llama-cpp-python](https://python.langchain.com/docs/integrations/llms/llamacpp)
* [LangChain + ctransformers](https://python.langchain.com/docs/integrations/providers/ctransformers)
<!-- README_GGUF.md-how-to-run end -->
<!-- footer start -->
<!-- 200823 -->
## Discord
For further support, and discussions on these models and AI in general, join us at:
[TheBloke AI's Discord server](https://discord.gg/theblokeai)
## Thanks, and how to contribute
Thanks to the [chirper.ai](https://chirper.ai) team!
Thanks to Clay from [gpus.llm-utils.org](llm-utils)!
I've had a lot of people ask if they can contribute. I enjoy providing models and helping people, and would love to be able to spend even more time doing it, as well as expanding into new projects like fine tuning/training.
If you're able and willing to contribute it will be most gratefully received and will help me to keep providing more models, and to start work on new AI projects.
Donaters will get priority support on any and all AI/LLM/model questions and requests, access to a private Discord room, plus other benefits.
* Patreon: https://patreon.com/TheBlokeAI
* Ko-Fi: https://ko-fi.com/TheBlokeAI
**Special thanks to**: Aemon Algiz.
**Patreon special mentions**: Alicia Loh, Stephen Murray, K, Ajan Kanaga, RoA, Magnesian, Deo Leter, Olakabola, Eugene Pentland, zynix, Deep Realms, Raymond Fosdick, Elijah Stavena, Iucharbius, Erik Bjäreholt, Luis Javier Navarrete Lozano, Nicholas, theTransient, John Detwiler, alfie_i, knownsqashed, Mano Prime, Willem Michiel, Enrico Ros, LangChain4j, OG, Michael Dempsey, Pierre Kircher, Pedro Madruga, James Bentley, Thomas Belote, Luke @flexchar, Leonard Tan, Johann-Peter Hartmann, Illia Dulskyi, Fen Risland, Chadd, S_X, Jeff Scroggin, Ken Nordquist, Sean Connelly, Artur Olbinski, Swaroop Kallakuri, Jack West, Ai Maven, David Ziegler, Russ Johnson, transmissions 11, John Villwock, Alps Aficionado, Clay Pascal, Viktor Bowallius, Subspace Studios, Rainer Wilmers, Trenton Dambrowitz, vamX, Michael Levine, 준교 김, Brandon Frisco, Kalila, Trailburnt, Randy H, Talal Aujan, Nathan Dryer, Vadim, 阿明, ReadyPlayerEmma, Tiffany J. Kim, George Stoitzev, Spencer Kim, Jerry Meng, Gabriel Tamborski, Cory Kujawski, Jeffrey Morgan, Spiking Neurons AB, Edmond Seymore, Alexandros Triantafyllidis, Lone Striker, Cap'n Zoog, Nikolai Manek, danny, ya boyyy, Derek Yates, usrbinkat, Mandus, TL, Nathan LeClaire, subjectnull, Imad Khwaja, webtim, Raven Klaugh, Asp the Wyvern, Gabriel Puliatti, Caitlyn Gatomon, Joseph William Delisle, Jonathan Leane, Luke Pendergrass, SuperWojo, Sebastain Graf, Will Dee, Fred von Graf, Andrey, Dan Guido, Daniel P. Andersen, Nitin Borwankar, Elle, Vitor Caleffi, biorpg, jjj, NimbleBox.ai, Pieter, Matthew Berman, terasurfer, Michael Davis, Alex, Stanislav Ovsiannikov
Thank you to all my generous patrons and donaters!
And thank you again to a16z for their generous grant.
<!-- footer end -->
<!-- original-model-card start -->
# Original model card: Meta's Llama 2 13B-chat
# **Llama 2**
Llama 2 is a collection of pretrained and fine-tuned generative text models ranging in scale from 7 billion to 70 billion parameters. This is the repository for the 13B fine-tuned model, optimized for dialogue use cases and converted for the Hugging Face Transformers format. Links to other models can be found in the index at the bottom.
## Model Details
*Note: Use of this model is governed by the Meta license. In order to download the model weights and tokenizer, please visit the [website](https://ai.meta.com/resources/models-and-libraries/llama-downloads/) and accept our License before requesting access here.*
Meta developed and publicly released the Llama 2 family of large language models (LLMs), a collection of pretrained and fine-tuned generative text models ranging in scale from 7 billion to 70 billion parameters. Our fine-tuned LLMs, called Llama-2-Chat, are optimized for dialogue use cases. Llama-2-Chat models outperform open-source chat models on most benchmarks we tested, and in our human evaluations for helpfulness and safety, are on par with some popular closed-source models like ChatGPT and PaLM.
**Model Developers** Meta
**Variations** Llama 2 comes in a range of parameter sizes — 7B, 13B, and 70B — as well as pretrained and fine-tuned variations.
**Input** Models input text only.
**Output** Models generate text only.
**Model Architecture** Llama 2 is an auto-regressive language model that uses an optimized transformer architecture. The tuned versions use supervised fine-tuning (SFT) and reinforcement learning with human feedback (RLHF) to align to human preferences for helpfulness and safety.
||Training Data|Params|Content Length|GQA|Tokens|LR|
|---|---|---|---|---|---|---|
|Llama 2|*A new mix of publicly available online data*|7B|4k|✗|2.0T|3.0 x 10<sup>-4</sup>|
|Llama 2|*A new mix of publicly available online data*|13B|4k|✗|2.0T|3.0 x 10<sup>-4</sup>|
|Llama 2|*A new mix of publicly available online data*|70B|4k|✔|2.0T|1.5 x 10<sup>-4</sup>|
*Llama 2 family of models.* Token counts refer to pretraining data only. All models are trained with a global batch-size of 4M tokens. Bigger models - 70B -- use Grouped-Query Attention (GQA) for improved inference scalability.
**Model Dates** Llama 2 was trained between January 2023 and July 2023.
**Status** This is a static model trained on an offline dataset. Future versions of the tuned models will be released as we improve model safety with community feedback.
**License** A custom commercial license is available at: [https://ai.meta.com/resources/models-and-libraries/llama-downloads/](https://ai.meta.com/resources/models-and-libraries/llama-downloads/)
**Research Paper** ["Llama-2: Open Foundation and Fine-tuned Chat Models"](arxiv.org/abs/2307.09288)
## Intended Use
**Intended Use Cases** Llama 2 is intended for commercial and research use in English. Tuned models are intended for assistant-like chat, whereas pretrained models can be adapted for a variety of natural language generation tasks.
To get the expected features and performance for the chat versions, a specific formatting needs to be followed, including the `INST` and `<<SYS>>` tags, `BOS` and `EOS` tokens, and the whitespaces and breaklines in between (we recommend calling `strip()` on inputs to avoid double-spaces). See our reference code in github for details: [`chat_completion`](https://github.com/facebookresearch/llama/blob/main/llama/generation.py#L212).
**Out-of-scope Uses** Use in any manner that violates applicable laws or regulations (including trade compliance laws).Use in languages other than English. Use in any other way that is prohibited by the Acceptable Use Policy and Licensing Agreement for Llama 2.
## Hardware and Software
**Training Factors** We used custom training libraries, Meta's Research Super Cluster, and production clusters for pretraining. Fine-tuning, annotation, and evaluation were also performed on third-party cloud compute.
**Carbon Footprint** Pretraining utilized a cumulative 3.3M GPU hours of computation on hardware of type A100-80GB (TDP of 350-400W). Estimated total emissions were 539 tCO2eq, 100% of which were offset by Meta’s sustainability program.
||Time (GPU hours)|Power Consumption (W)|Carbon Emitted(tCO<sub>2</sub>eq)|
|---|---|---|---|
|Llama 2 7B|184320|400|31.22|
|Llama 2 13B|368640|400|62.44|
|Llama 2 70B|1720320|400|291.42|
|Total|3311616||539.00|
**CO<sub>2</sub> emissions during pretraining.** Time: total GPU time required for training each model. Power Consumption: peak power capacity per GPU device for the GPUs used adjusted for power usage efficiency. 100% of the emissions are directly offset by Meta's sustainability program, and because we are openly releasing these models, the pretraining costs do not need to be incurred by others.
## Training Data
**Overview** Llama 2 was pretrained on 2 trillion tokens of data from publicly available sources. The fine-tuning data includes publicly available instruction datasets, as well as over one million new human-annotated examples. Neither the pretraining nor the fine-tuning datasets include Meta user data.
**Data Freshness** The pretraining data has a cutoff of September 2022, but some tuning data is more recent, up to July 2023.
## Evaluation Results
In this section, we report the results for the Llama 1 and Llama 2 models on standard academic benchmarks.For all the evaluations, we use our internal evaluations library.
|Model|Size|Code|Commonsense Reasoning|World Knowledge|Reading Comprehension|Math|MMLU|BBH|AGI Eval|
|---|---|---|---|---|---|---|---|---|---|
|Llama 1|7B|14.1|60.8|46.2|58.5|6.95|35.1|30.3|23.9|
|Llama 1|13B|18.9|66.1|52.6|62.3|10.9|46.9|37.0|33.9|
|Llama 1|33B|26.0|70.0|58.4|67.6|21.4|57.8|39.8|41.7|
|Llama 1|65B|30.7|70.7|60.5|68.6|30.8|63.4|43.5|47.6|
|Llama 2|7B|16.8|63.9|48.9|61.3|14.6|45.3|32.6|29.3|
|Llama 2|13B|24.5|66.9|55.4|65.8|28.7|54.8|39.4|39.1|
|Llama 2|70B|**37.5**|**71.9**|**63.6**|**69.4**|**35.2**|**68.9**|**51.2**|**54.2**|
**Overall performance on grouped academic benchmarks.** *Code:* We report the average pass@1 scores of our models on HumanEval and MBPP. *Commonsense Reasoning:* We report the average of PIQA, SIQA, HellaSwag, WinoGrande, ARC easy and challenge, OpenBookQA, and CommonsenseQA. We report 7-shot results for CommonSenseQA and 0-shot results for all other benchmarks. *World Knowledge:* We evaluate the 5-shot performance on NaturalQuestions and TriviaQA and report the average. *Reading Comprehension:* For reading comprehension, we report the 0-shot average on SQuAD, QuAC, and BoolQ. *MATH:* We report the average of the GSM8K (8 shot) and MATH (4 shot) benchmarks at top 1.
|||TruthfulQA|Toxigen|
|---|---|---|---|
|Llama 1|7B|27.42|23.00|
|Llama 1|13B|41.74|23.08|
|Llama 1|33B|44.19|22.57|
|Llama 1|65B|48.71|21.77|
|Llama 2|7B|33.29|**21.25**|
|Llama 2|13B|41.86|26.10|
|Llama 2|70B|**50.18**|24.60|
**Evaluation of pretrained LLMs on automatic safety benchmarks.** For TruthfulQA, we present the percentage of generations that are both truthful and informative (the higher the better). For ToxiGen, we present the percentage of toxic generations (the smaller the better).
|||TruthfulQA|Toxigen|
|---|---|---|---|
|Llama-2-Chat|7B|57.04|**0.00**|
|Llama-2-Chat|13B|62.18|**0.00**|
|Llama-2-Chat|70B|**64.14**|0.01|
**Evaluation of fine-tuned LLMs on different safety datasets.** Same metric definitions as above.
## Ethical Considerations and Limitations
Llama 2 is a new technology that carries risks with use. Testing conducted to date has been in English, and has not covered, nor could it cover all scenarios. For these reasons, as with all LLMs, Llama 2’s potential outputs cannot be predicted in advance, and the model may in some instances produce inaccurate, biased or other objectionable responses to user prompts. Therefore, before deploying any applications of Llama 2, developers should perform safety testing and tuning tailored to their specific applications of the model.
Please see the Responsible Use Guide available at [https://ai.meta.com/llama/responsible-use-guide/](https://ai.meta.com/llama/responsible-use-guide)
## Reporting Issues
Please report any software “bug,” or other problems with the models through one of the following means:
- Reporting issues with the model: [github.com/facebookresearch/llama](http://github.com/facebookresearch/llama)
- Reporting problematic content generated by the model: [developers.facebook.com/llama_output_feedback](http://developers.facebook.com/llama_output_feedback)
- Reporting bugs and security concerns: [facebook.com/whitehat/info](http://facebook.com/whitehat/info)
## Llama Model Index
|Model|Llama2|Llama2-hf|Llama2-chat|Llama2-chat-hf|
|---|---|---|---|---|
|7B| [Link](https://huggingface.co/llamaste/Llama-2-7b) | [Link](https://huggingface.co/llamaste/Llama-2-7b-hf) | [Link](https://huggingface.co/llamaste/Llama-2-7b-chat) | [Link](https://huggingface.co/llamaste/Llama-2-7b-chat-hf)|
|13B| [Link](https://huggingface.co/llamaste/Llama-2-13b) | [Link](https://huggingface.co/llamaste/Llama-2-13b-hf) | [Link](https://huggingface.co/llamaste/Llama-2-13b-chat) | [Link](https://huggingface.co/llamaste/Llama-2-13b-hf)|
|70B| [Link](https://huggingface.co/llamaste/Llama-2-70b) | [Link](https://huggingface.co/llamaste/Llama-2-70b-hf) | [Link](https://huggingface.co/llamaste/Llama-2-70b-chat) | [Link](https://huggingface.co/llamaste/Llama-2-70b-hf)|
<!-- original-model-card end -->
|
RichardErkhov/shanchen_-_llama3-8B-slerp-biomed-chat-chinese-gguf | RichardErkhov | 2024-06-26T11:50:16Z | 12,236 | 0 | null | [
"gguf",
"region:us"
]
| null | 2024-06-26T05:12:31Z | Quantization made by Richard Erkhov.
[Github](https://github.com/RichardErkhov)
[Discord](https://discord.gg/pvy7H8DZMG)
[Request more models](https://github.com/RichardErkhov/quant_request)
llama3-8B-slerp-biomed-chat-chinese - GGUF
- Model creator: https://huggingface.co/shanchen/
- Original model: https://huggingface.co/shanchen/llama3-8B-slerp-biomed-chat-chinese/
| Name | Quant method | Size |
| ---- | ---- | ---- |
| [llama3-8B-slerp-biomed-chat-chinese.Q2_K.gguf](https://huggingface.co/RichardErkhov/shanchen_-_llama3-8B-slerp-biomed-chat-chinese-gguf/blob/main/llama3-8B-slerp-biomed-chat-chinese.Q2_K.gguf) | Q2_K | 2.96GB |
| [llama3-8B-slerp-biomed-chat-chinese.IQ3_XS.gguf](https://huggingface.co/RichardErkhov/shanchen_-_llama3-8B-slerp-biomed-chat-chinese-gguf/blob/main/llama3-8B-slerp-biomed-chat-chinese.IQ3_XS.gguf) | IQ3_XS | 3.28GB |
| [llama3-8B-slerp-biomed-chat-chinese.IQ3_S.gguf](https://huggingface.co/RichardErkhov/shanchen_-_llama3-8B-slerp-biomed-chat-chinese-gguf/blob/main/llama3-8B-slerp-biomed-chat-chinese.IQ3_S.gguf) | IQ3_S | 3.43GB |
| [llama3-8B-slerp-biomed-chat-chinese.Q3_K_S.gguf](https://huggingface.co/RichardErkhov/shanchen_-_llama3-8B-slerp-biomed-chat-chinese-gguf/blob/main/llama3-8B-slerp-biomed-chat-chinese.Q3_K_S.gguf) | Q3_K_S | 3.41GB |
| [llama3-8B-slerp-biomed-chat-chinese.IQ3_M.gguf](https://huggingface.co/RichardErkhov/shanchen_-_llama3-8B-slerp-biomed-chat-chinese-gguf/blob/main/llama3-8B-slerp-biomed-chat-chinese.IQ3_M.gguf) | IQ3_M | 3.52GB |
| [llama3-8B-slerp-biomed-chat-chinese.Q3_K.gguf](https://huggingface.co/RichardErkhov/shanchen_-_llama3-8B-slerp-biomed-chat-chinese-gguf/blob/main/llama3-8B-slerp-biomed-chat-chinese.Q3_K.gguf) | Q3_K | 3.74GB |
| [llama3-8B-slerp-biomed-chat-chinese.Q3_K_M.gguf](https://huggingface.co/RichardErkhov/shanchen_-_llama3-8B-slerp-biomed-chat-chinese-gguf/blob/main/llama3-8B-slerp-biomed-chat-chinese.Q3_K_M.gguf) | Q3_K_M | 3.74GB |
| [llama3-8B-slerp-biomed-chat-chinese.Q3_K_L.gguf](https://huggingface.co/RichardErkhov/shanchen_-_llama3-8B-slerp-biomed-chat-chinese-gguf/blob/main/llama3-8B-slerp-biomed-chat-chinese.Q3_K_L.gguf) | Q3_K_L | 4.03GB |
| [llama3-8B-slerp-biomed-chat-chinese.IQ4_XS.gguf](https://huggingface.co/RichardErkhov/shanchen_-_llama3-8B-slerp-biomed-chat-chinese-gguf/blob/main/llama3-8B-slerp-biomed-chat-chinese.IQ4_XS.gguf) | IQ4_XS | 4.18GB |
| [llama3-8B-slerp-biomed-chat-chinese.Q4_0.gguf](https://huggingface.co/RichardErkhov/shanchen_-_llama3-8B-slerp-biomed-chat-chinese-gguf/blob/main/llama3-8B-slerp-biomed-chat-chinese.Q4_0.gguf) | Q4_0 | 4.34GB |
| [llama3-8B-slerp-biomed-chat-chinese.IQ4_NL.gguf](https://huggingface.co/RichardErkhov/shanchen_-_llama3-8B-slerp-biomed-chat-chinese-gguf/blob/main/llama3-8B-slerp-biomed-chat-chinese.IQ4_NL.gguf) | IQ4_NL | 4.38GB |
| [llama3-8B-slerp-biomed-chat-chinese.Q4_K_S.gguf](https://huggingface.co/RichardErkhov/shanchen_-_llama3-8B-slerp-biomed-chat-chinese-gguf/blob/main/llama3-8B-slerp-biomed-chat-chinese.Q4_K_S.gguf) | Q4_K_S | 4.37GB |
| [llama3-8B-slerp-biomed-chat-chinese.Q4_K.gguf](https://huggingface.co/RichardErkhov/shanchen_-_llama3-8B-slerp-biomed-chat-chinese-gguf/blob/main/llama3-8B-slerp-biomed-chat-chinese.Q4_K.gguf) | Q4_K | 4.58GB |
| [llama3-8B-slerp-biomed-chat-chinese.Q4_K_M.gguf](https://huggingface.co/RichardErkhov/shanchen_-_llama3-8B-slerp-biomed-chat-chinese-gguf/blob/main/llama3-8B-slerp-biomed-chat-chinese.Q4_K_M.gguf) | Q4_K_M | 4.58GB |
| [llama3-8B-slerp-biomed-chat-chinese.Q4_1.gguf](https://huggingface.co/RichardErkhov/shanchen_-_llama3-8B-slerp-biomed-chat-chinese-gguf/blob/main/llama3-8B-slerp-biomed-chat-chinese.Q4_1.gguf) | Q4_1 | 4.78GB |
| [llama3-8B-slerp-biomed-chat-chinese.Q5_0.gguf](https://huggingface.co/RichardErkhov/shanchen_-_llama3-8B-slerp-biomed-chat-chinese-gguf/blob/main/llama3-8B-slerp-biomed-chat-chinese.Q5_0.gguf) | Q5_0 | 5.21GB |
| [llama3-8B-slerp-biomed-chat-chinese.Q5_K_S.gguf](https://huggingface.co/RichardErkhov/shanchen_-_llama3-8B-slerp-biomed-chat-chinese-gguf/blob/main/llama3-8B-slerp-biomed-chat-chinese.Q5_K_S.gguf) | Q5_K_S | 5.21GB |
| [llama3-8B-slerp-biomed-chat-chinese.Q5_K.gguf](https://huggingface.co/RichardErkhov/shanchen_-_llama3-8B-slerp-biomed-chat-chinese-gguf/blob/main/llama3-8B-slerp-biomed-chat-chinese.Q5_K.gguf) | Q5_K | 5.34GB |
| [llama3-8B-slerp-biomed-chat-chinese.Q5_K_M.gguf](https://huggingface.co/RichardErkhov/shanchen_-_llama3-8B-slerp-biomed-chat-chinese-gguf/blob/main/llama3-8B-slerp-biomed-chat-chinese.Q5_K_M.gguf) | Q5_K_M | 3.39GB |
| [llama3-8B-slerp-biomed-chat-chinese.Q5_1.gguf](https://huggingface.co/RichardErkhov/shanchen_-_llama3-8B-slerp-biomed-chat-chinese-gguf/blob/main/llama3-8B-slerp-biomed-chat-chinese.Q5_1.gguf) | Q5_1 | 3.95GB |
| [llama3-8B-slerp-biomed-chat-chinese.Q6_K.gguf](https://huggingface.co/RichardErkhov/shanchen_-_llama3-8B-slerp-biomed-chat-chinese-gguf/blob/main/llama3-8B-slerp-biomed-chat-chinese.Q6_K.gguf) | Q6_K | 6.14GB |
| [llama3-8B-slerp-biomed-chat-chinese.Q8_0.gguf](https://huggingface.co/RichardErkhov/shanchen_-_llama3-8B-slerp-biomed-chat-chinese-gguf/blob/main/llama3-8B-slerp-biomed-chat-chinese.Q8_0.gguf) | Q8_0 | 7.95GB |
Original model description:
---
tags:
- merge
- mergekit
- lazymergekit
- shanchen/llama3-8B-slerp-med-chinese
- shenzhi-wang/Llama3-8B-Chinese-Chat
base_model:
- shanchen/llama3-8B-slerp-med-chinese
- shenzhi-wang/Llama3-8B-Chinese-Chat
license: llama3
language:
- zh
- en
---
# llama3-8B-slerp-biomed-chat-chinese
llama3-8B-slerp-biomed-chat-chinese is a merge of the following models using [LazyMergekit](https://colab.research.google.com/drive/1obulZ1ROXHjYLn6PPZJwRR6GzgQogxxb?usp=sharing):
* [shanchen/llama3-8B-slerp-med-chinese](https://huggingface.co/shanchen/llama3-8B-slerp-med-chinese)
* [shenzhi-wang/Llama3-8B-Chinese-Chat](https://huggingface.co/shenzhi-wang/Llama3-8B-Chinese-Chat)
## 🧩 Configuration
```yaml
slices:
- sources:
- model: shanchen/llama3-8B-slerp-med-chinese
layer_range: [0,32]
- model: shenzhi-wang/Llama3-8B-Chinese-Chat
layer_range: [0,32]
merge_method: slerp
base_model: shenzhi-wang/Llama3-8B-Chinese-Chat
parameters:
t:
- filter: self_attn
value: [0.3, 0.5, 0.5, 0.7, 1]
- filter: mlp
value: [1, 0.7, 0.5, 0.5, 0.3]
- value: 0.5
dtype: bfloat16
```
## 💻 Usage
```python
!pip install -qU transformers accelerate
from transformers import AutoTokenizer, AutoModelForCausalLM
model_id = "shanchen/llama3-8B-slerp-biomed-chat-chinese"
tokenizer = AutoTokenizer.from_pretrained(model_id)
model = AutoModelForCausalLM.from_pretrained(
model_id, torch_dtype="auto", device_map="auto"
)
messages = [
{"role": "user", "content": "Can you speak Japanese?"},
]
input_ids = tokenizer.apply_chat_template(
messages, add_generation_prompt=True, return_tensors="pt"
).to(model.device)
outputs = model.generate(
input_ids,
max_new_tokens=192 max#8192,
do_sample=True,
temperature=0.6,
top_p=0.9,
)
response = outputs[0][input_ids.shape[-1]:]
print(tokenizer.decode(response, skip_special_tokens=True))
```
|
mradermacher/llama-3-8b-chat-srtip-v.1.3-GGUF | mradermacher | 2024-06-29T17:58:01Z | 12,235 | 0 | transformers | [
"transformers",
"gguf",
"en",
"base_model:isakovsh/llama-3-8b-chat-srtip-v.1.3",
"endpoints_compatible",
"region:us"
]
| null | 2024-06-29T17:30:02Z | ---
base_model: isakovsh/llama-3-8b-chat-srtip-v.1.3
language:
- en
library_name: transformers
quantized_by: mradermacher
tags: []
---
## About
<!-- ### quantize_version: 2 -->
<!-- ### output_tensor_quantised: 1 -->
<!-- ### convert_type: hf -->
<!-- ### vocab_type: -->
<!-- ### tags: -->
static quants of https://huggingface.co/isakovsh/llama-3-8b-chat-srtip-v.1.3
<!-- provided-files -->
weighted/imatrix quants seem not to be available (by me) at this time. If they do not show up a week or so after the static ones, I have probably not planned for them. Feel free to request them by opening a Community Discussion.
## Usage
If you are unsure how to use GGUF files, refer to one of [TheBloke's
READMEs](https://huggingface.co/TheBloke/KafkaLM-70B-German-V0.1-GGUF) for
more details, including on how to concatenate multi-part files.
## Provided Quants
(sorted by size, not necessarily quality. IQ-quants are often preferable over similar sized non-IQ quants)
| Link | Type | Size/GB | Notes |
|:-----|:-----|--------:|:------|
| [GGUF](https://huggingface.co/mradermacher/llama-3-8b-chat-srtip-v.1.3-GGUF/resolve/main/llama-3-8b-chat-srtip-v.1.3.Q2_K.gguf) | Q2_K | 3.3 | |
| [GGUF](https://huggingface.co/mradermacher/llama-3-8b-chat-srtip-v.1.3-GGUF/resolve/main/llama-3-8b-chat-srtip-v.1.3.IQ3_XS.gguf) | IQ3_XS | 3.6 | |
| [GGUF](https://huggingface.co/mradermacher/llama-3-8b-chat-srtip-v.1.3-GGUF/resolve/main/llama-3-8b-chat-srtip-v.1.3.Q3_K_S.gguf) | Q3_K_S | 3.8 | |
| [GGUF](https://huggingface.co/mradermacher/llama-3-8b-chat-srtip-v.1.3-GGUF/resolve/main/llama-3-8b-chat-srtip-v.1.3.IQ3_S.gguf) | IQ3_S | 3.8 | beats Q3_K* |
| [GGUF](https://huggingface.co/mradermacher/llama-3-8b-chat-srtip-v.1.3-GGUF/resolve/main/llama-3-8b-chat-srtip-v.1.3.IQ3_M.gguf) | IQ3_M | 3.9 | |
| [GGUF](https://huggingface.co/mradermacher/llama-3-8b-chat-srtip-v.1.3-GGUF/resolve/main/llama-3-8b-chat-srtip-v.1.3.Q3_K_M.gguf) | Q3_K_M | 4.1 | lower quality |
| [GGUF](https://huggingface.co/mradermacher/llama-3-8b-chat-srtip-v.1.3-GGUF/resolve/main/llama-3-8b-chat-srtip-v.1.3.Q3_K_L.gguf) | Q3_K_L | 4.4 | |
| [GGUF](https://huggingface.co/mradermacher/llama-3-8b-chat-srtip-v.1.3-GGUF/resolve/main/llama-3-8b-chat-srtip-v.1.3.IQ4_XS.gguf) | IQ4_XS | 4.6 | |
| [GGUF](https://huggingface.co/mradermacher/llama-3-8b-chat-srtip-v.1.3-GGUF/resolve/main/llama-3-8b-chat-srtip-v.1.3.Q4_K_S.gguf) | Q4_K_S | 4.8 | fast, recommended |
| [GGUF](https://huggingface.co/mradermacher/llama-3-8b-chat-srtip-v.1.3-GGUF/resolve/main/llama-3-8b-chat-srtip-v.1.3.Q4_K_M.gguf) | Q4_K_M | 5.0 | fast, recommended |
| [GGUF](https://huggingface.co/mradermacher/llama-3-8b-chat-srtip-v.1.3-GGUF/resolve/main/llama-3-8b-chat-srtip-v.1.3.Q5_K_S.gguf) | Q5_K_S | 5.7 | |
| [GGUF](https://huggingface.co/mradermacher/llama-3-8b-chat-srtip-v.1.3-GGUF/resolve/main/llama-3-8b-chat-srtip-v.1.3.Q5_K_M.gguf) | Q5_K_M | 5.8 | |
| [GGUF](https://huggingface.co/mradermacher/llama-3-8b-chat-srtip-v.1.3-GGUF/resolve/main/llama-3-8b-chat-srtip-v.1.3.Q6_K.gguf) | Q6_K | 6.7 | very good quality |
| [GGUF](https://huggingface.co/mradermacher/llama-3-8b-chat-srtip-v.1.3-GGUF/resolve/main/llama-3-8b-chat-srtip-v.1.3.Q8_0.gguf) | Q8_0 | 8.6 | fast, best quality |
| [GGUF](https://huggingface.co/mradermacher/llama-3-8b-chat-srtip-v.1.3-GGUF/resolve/main/llama-3-8b-chat-srtip-v.1.3.f16.gguf) | f16 | 16.2 | 16 bpw, overkill |
Here is a handy graph by ikawrakow comparing some lower-quality quant
types (lower is better):

And here are Artefact2's thoughts on the matter:
https://gist.github.com/Artefact2/b5f810600771265fc1e39442288e8ec9
## FAQ / Model Request
See https://huggingface.co/mradermacher/model_requests for some answers to
questions you might have and/or if you want some other model quantized.
## Thanks
I thank my company, [nethype GmbH](https://www.nethype.de/), for letting
me use its servers and providing upgrades to my workstation to enable
this work in my free time.
<!-- end -->
|
timm/convnext_small.in12k_ft_in1k_384 | timm | 2024-02-10T23:29:53Z | 12,226 | 0 | timm | [
"timm",
"pytorch",
"safetensors",
"image-classification",
"dataset:imagenet-1k",
"dataset:imagenet-12k",
"arxiv:2201.03545",
"license:apache-2.0",
"region:us"
]
| image-classification | 2023-01-18T20:12:19Z | ---
license: apache-2.0
library_name: timm
tags:
- image-classification
- timm
datasets:
- imagenet-1k
- imagenet-12k
---
# Model card for convnext_small.in12k_ft_in1k_384
A ConvNeXt image classification model. Pretrained in `timm` on ImageNet-12k (a 11821 class subset of full ImageNet-22k) and fine-tuned on ImageNet-1k by Ross Wightman.
ImageNet-12k training done on TPUs thanks to support of the [TRC](https://sites.research.google/trc/about/) program.
Fine-tuning performed on 8x GPU [Lambda Labs](https://lambdalabs.com/) cloud instances.
## Model Details
- **Model Type:** Image classification / feature backbone
- **Model Stats:**
- Params (M): 50.2
- GMACs: 25.6
- Activations (M): 63.4
- Image size: 384 x 384
- **Papers:**
- A ConvNet for the 2020s: https://arxiv.org/abs/2201.03545
- **Original:** https://github.com/huggingface/pytorch-image-models
- **Dataset:** ImageNet-1k
- **Pretrain Dataset:** ImageNet-12k
## Model Usage
### Image Classification
```python
from urllib.request import urlopen
from PIL import Image
import timm
img = Image.open(urlopen(
'https://huggingface.co/datasets/huggingface/documentation-images/resolve/main/beignets-task-guide.png'
))
model = timm.create_model('convnext_small.in12k_ft_in1k_384', pretrained=True)
model = model.eval()
# get model specific transforms (normalization, resize)
data_config = timm.data.resolve_model_data_config(model)
transforms = timm.data.create_transform(**data_config, is_training=False)
output = model(transforms(img).unsqueeze(0)) # unsqueeze single image into batch of 1
top5_probabilities, top5_class_indices = torch.topk(output.softmax(dim=1) * 100, k=5)
```
### Feature Map Extraction
```python
from urllib.request import urlopen
from PIL import Image
import timm
img = Image.open(urlopen(
'https://huggingface.co/datasets/huggingface/documentation-images/resolve/main/beignets-task-guide.png'
))
model = timm.create_model(
'convnext_small.in12k_ft_in1k_384',
pretrained=True,
features_only=True,
)
model = model.eval()
# get model specific transforms (normalization, resize)
data_config = timm.data.resolve_model_data_config(model)
transforms = timm.data.create_transform(**data_config, is_training=False)
output = model(transforms(img).unsqueeze(0)) # unsqueeze single image into batch of 1
for o in output:
# print shape of each feature map in output
# e.g.:
# torch.Size([1, 96, 96, 96])
# torch.Size([1, 192, 48, 48])
# torch.Size([1, 384, 24, 24])
# torch.Size([1, 768, 12, 12])
print(o.shape)
```
### Image Embeddings
```python
from urllib.request import urlopen
from PIL import Image
import timm
img = Image.open(urlopen(
'https://huggingface.co/datasets/huggingface/documentation-images/resolve/main/beignets-task-guide.png'
))
model = timm.create_model(
'convnext_small.in12k_ft_in1k_384',
pretrained=True,
num_classes=0, # remove classifier nn.Linear
)
model = model.eval()
# get model specific transforms (normalization, resize)
data_config = timm.data.resolve_model_data_config(model)
transforms = timm.data.create_transform(**data_config, is_training=False)
output = model(transforms(img).unsqueeze(0)) # output is (batch_size, num_features) shaped tensor
# or equivalently (without needing to set num_classes=0)
output = model.forward_features(transforms(img).unsqueeze(0))
# output is unpooled, a (1, 768, 12, 12) shaped tensor
output = model.forward_head(output, pre_logits=True)
# output is a (1, num_features) shaped tensor
```
## Model Comparison
Explore the dataset and runtime metrics of this model in timm [model results](https://github.com/huggingface/pytorch-image-models/tree/main/results).
All timing numbers from eager model PyTorch 1.13 on RTX 3090 w/ AMP.
| model |top1 |top5 |img_size|param_count|gmacs |macts |samples_per_sec|batch_size|
|------------------------------------------------------------------------------------------------------------------------------|------|------|--------|-----------|------|------|---------------|----------|
| [convnextv2_huge.fcmae_ft_in22k_in1k_512](https://huggingface.co/timm/convnextv2_huge.fcmae_ft_in22k_in1k_512) |88.848|98.742|512 |660.29 |600.81|413.07|28.58 |48 |
| [convnextv2_huge.fcmae_ft_in22k_in1k_384](https://huggingface.co/timm/convnextv2_huge.fcmae_ft_in22k_in1k_384) |88.668|98.738|384 |660.29 |337.96|232.35|50.56 |64 |
| [convnext_xxlarge.clip_laion2b_soup_ft_in1k](https://huggingface.co/timm/convnext_xxlarge.clip_laion2b_soup_ft_in1k) |88.612|98.704|256 |846.47 |198.09|124.45|122.45 |256 |
| [convnext_large_mlp.clip_laion2b_soup_ft_in12k_in1k_384](https://huggingface.co/timm/convnext_large_mlp.clip_laion2b_soup_ft_in12k_in1k_384) |88.312|98.578|384 |200.13 |101.11|126.74|196.84 |256 |
| [convnextv2_large.fcmae_ft_in22k_in1k_384](https://huggingface.co/timm/convnextv2_large.fcmae_ft_in22k_in1k_384) |88.196|98.532|384 |197.96 |101.1 |126.74|128.94 |128 |
| [convnext_large_mlp.clip_laion2b_soup_ft_in12k_in1k_320](https://huggingface.co/timm/convnext_large_mlp.clip_laion2b_soup_ft_in12k_in1k_320) |87.968|98.47 |320 |200.13 |70.21 |88.02 |283.42 |256 |
| [convnext_xlarge.fb_in22k_ft_in1k_384](https://huggingface.co/timm/convnext_xlarge.fb_in22k_ft_in1k_384) |87.75 |98.556|384 |350.2 |179.2 |168.99|124.85 |192 |
| [convnextv2_base.fcmae_ft_in22k_in1k_384](https://huggingface.co/timm/convnextv2_base.fcmae_ft_in22k_in1k_384) |87.646|98.422|384 |88.72 |45.21 |84.49 |209.51 |256 |
| [convnext_large.fb_in22k_ft_in1k_384](https://huggingface.co/timm/convnext_large.fb_in22k_ft_in1k_384) |87.476|98.382|384 |197.77 |101.1 |126.74|194.66 |256 |
| [convnext_large_mlp.clip_laion2b_augreg_ft_in1k](https://huggingface.co/timm/convnext_large_mlp.clip_laion2b_augreg_ft_in1k) |87.344|98.218|256 |200.13 |44.94 |56.33 |438.08 |256 |
| [convnextv2_large.fcmae_ft_in22k_in1k](https://huggingface.co/timm/convnextv2_large.fcmae_ft_in22k_in1k) |87.26 |98.248|224 |197.96 |34.4 |43.13 |376.84 |256 |
| [convnext_base.clip_laion2b_augreg_ft_in12k_in1k_384](https://huggingface.co/timm/convnext_base.clip_laion2b_augreg_ft_in12k_in1k_384) |87.138|98.212|384 |88.59 |45.21 |84.49 |365.47 |256 |
| [convnext_xlarge.fb_in22k_ft_in1k](https://huggingface.co/timm/convnext_xlarge.fb_in22k_ft_in1k) |87.002|98.208|224 |350.2 |60.98 |57.5 |368.01 |256 |
| [convnext_base.fb_in22k_ft_in1k_384](https://huggingface.co/timm/convnext_base.fb_in22k_ft_in1k_384) |86.796|98.264|384 |88.59 |45.21 |84.49 |366.54 |256 |
| [convnextv2_base.fcmae_ft_in22k_in1k](https://huggingface.co/timm/convnextv2_base.fcmae_ft_in22k_in1k) |86.74 |98.022|224 |88.72 |15.38 |28.75 |624.23 |256 |
| [convnext_large.fb_in22k_ft_in1k](https://huggingface.co/timm/convnext_large.fb_in22k_ft_in1k) |86.636|98.028|224 |197.77 |34.4 |43.13 |581.43 |256 |
| [convnext_base.clip_laiona_augreg_ft_in1k_384](https://huggingface.co/timm/convnext_base.clip_laiona_augreg_ft_in1k_384) |86.504|97.97 |384 |88.59 |45.21 |84.49 |368.14 |256 |
| [convnext_base.clip_laion2b_augreg_ft_in12k_in1k](https://huggingface.co/timm/convnext_base.clip_laion2b_augreg_ft_in12k_in1k) |86.344|97.97 |256 |88.59 |20.09 |37.55 |816.14 |256 |
| [convnextv2_huge.fcmae_ft_in1k](https://huggingface.co/timm/convnextv2_huge.fcmae_ft_in1k) |86.256|97.75 |224 |660.29 |115.0 |79.07 |154.72 |256 |
| [convnext_small.in12k_ft_in1k_384](https://huggingface.co/timm/convnext_small.in12k_ft_in1k_384) |86.182|97.92 |384 |50.22 |25.58 |63.37 |516.19 |256 |
| [convnext_base.clip_laion2b_augreg_ft_in1k](https://huggingface.co/timm/convnext_base.clip_laion2b_augreg_ft_in1k) |86.154|97.68 |256 |88.59 |20.09 |37.55 |819.86 |256 |
| [convnext_base.fb_in22k_ft_in1k](https://huggingface.co/timm/convnext_base.fb_in22k_ft_in1k) |85.822|97.866|224 |88.59 |15.38 |28.75 |1037.66 |256 |
| [convnext_small.fb_in22k_ft_in1k_384](https://huggingface.co/timm/convnext_small.fb_in22k_ft_in1k_384) |85.778|97.886|384 |50.22 |25.58 |63.37 |518.95 |256 |
| [convnextv2_large.fcmae_ft_in1k](https://huggingface.co/timm/convnextv2_large.fcmae_ft_in1k) |85.742|97.584|224 |197.96 |34.4 |43.13 |375.23 |256 |
| [convnext_small.in12k_ft_in1k](https://huggingface.co/timm/convnext_small.in12k_ft_in1k) |85.174|97.506|224 |50.22 |8.71 |21.56 |1474.31 |256 |
| [convnext_tiny.in12k_ft_in1k_384](https://huggingface.co/timm/convnext_tiny.in12k_ft_in1k_384) |85.118|97.608|384 |28.59 |13.14 |39.48 |856.76 |256 |
| [convnextv2_tiny.fcmae_ft_in22k_in1k_384](https://huggingface.co/timm/convnextv2_tiny.fcmae_ft_in22k_in1k_384) |85.112|97.63 |384 |28.64 |13.14 |39.48 |491.32 |256 |
| [convnextv2_base.fcmae_ft_in1k](https://huggingface.co/timm/convnextv2_base.fcmae_ft_in1k) |84.874|97.09 |224 |88.72 |15.38 |28.75 |625.33 |256 |
| [convnext_small.fb_in22k_ft_in1k](https://huggingface.co/timm/convnext_small.fb_in22k_ft_in1k) |84.562|97.394|224 |50.22 |8.71 |21.56 |1478.29 |256 |
| [convnext_large.fb_in1k](https://huggingface.co/timm/convnext_large.fb_in1k) |84.282|96.892|224 |197.77 |34.4 |43.13 |584.28 |256 |
| [convnext_tiny.in12k_ft_in1k](https://huggingface.co/timm/convnext_tiny.in12k_ft_in1k) |84.186|97.124|224 |28.59 |4.47 |13.44 |2433.7 |256 |
| [convnext_tiny.fb_in22k_ft_in1k_384](https://huggingface.co/timm/convnext_tiny.fb_in22k_ft_in1k_384) |84.084|97.14 |384 |28.59 |13.14 |39.48 |862.95 |256 |
| [convnextv2_tiny.fcmae_ft_in22k_in1k](https://huggingface.co/timm/convnextv2_tiny.fcmae_ft_in22k_in1k) |83.894|96.964|224 |28.64 |4.47 |13.44 |1452.72 |256 |
| [convnext_base.fb_in1k](https://huggingface.co/timm/convnext_base.fb_in1k) |83.82 |96.746|224 |88.59 |15.38 |28.75 |1054.0 |256 |
| [convnextv2_nano.fcmae_ft_in22k_in1k_384](https://huggingface.co/timm/convnextv2_nano.fcmae_ft_in22k_in1k_384) |83.37 |96.742|384 |15.62 |7.22 |24.61 |801.72 |256 |
| [convnext_small.fb_in1k](https://huggingface.co/timm/convnext_small.fb_in1k) |83.142|96.434|224 |50.22 |8.71 |21.56 |1464.0 |256 |
| [convnextv2_tiny.fcmae_ft_in1k](https://huggingface.co/timm/convnextv2_tiny.fcmae_ft_in1k) |82.92 |96.284|224 |28.64 |4.47 |13.44 |1425.62 |256 |
| [convnext_tiny.fb_in22k_ft_in1k](https://huggingface.co/timm/convnext_tiny.fb_in22k_ft_in1k) |82.898|96.616|224 |28.59 |4.47 |13.44 |2480.88 |256 |
| [convnext_nano.in12k_ft_in1k](https://huggingface.co/timm/convnext_nano.in12k_ft_in1k) |82.282|96.344|224 |15.59 |2.46 |8.37 |3926.52 |256 |
| [convnext_tiny_hnf.a2h_in1k](https://huggingface.co/timm/convnext_tiny_hnf.a2h_in1k) |82.216|95.852|224 |28.59 |4.47 |13.44 |2529.75 |256 |
| [convnext_tiny.fb_in1k](https://huggingface.co/timm/convnext_tiny.fb_in1k) |82.066|95.854|224 |28.59 |4.47 |13.44 |2346.26 |256 |
| [convnextv2_nano.fcmae_ft_in22k_in1k](https://huggingface.co/timm/convnextv2_nano.fcmae_ft_in22k_in1k) |82.03 |96.166|224 |15.62 |2.46 |8.37 |2300.18 |256 |
| [convnextv2_nano.fcmae_ft_in1k](https://huggingface.co/timm/convnextv2_nano.fcmae_ft_in1k) |81.83 |95.738|224 |15.62 |2.46 |8.37 |2321.48 |256 |
| [convnext_nano_ols.d1h_in1k](https://huggingface.co/timm/convnext_nano_ols.d1h_in1k) |80.866|95.246|224 |15.65 |2.65 |9.38 |3523.85 |256 |
| [convnext_nano.d1h_in1k](https://huggingface.co/timm/convnext_nano.d1h_in1k) |80.768|95.334|224 |15.59 |2.46 |8.37 |3915.58 |256 |
| [convnextv2_pico.fcmae_ft_in1k](https://huggingface.co/timm/convnextv2_pico.fcmae_ft_in1k) |80.304|95.072|224 |9.07 |1.37 |6.1 |3274.57 |256 |
| [convnext_pico.d1_in1k](https://huggingface.co/timm/convnext_pico.d1_in1k) |79.526|94.558|224 |9.05 |1.37 |6.1 |5686.88 |256 |
| [convnext_pico_ols.d1_in1k](https://huggingface.co/timm/convnext_pico_ols.d1_in1k) |79.522|94.692|224 |9.06 |1.43 |6.5 |5422.46 |256 |
| [convnextv2_femto.fcmae_ft_in1k](https://huggingface.co/timm/convnextv2_femto.fcmae_ft_in1k) |78.488|93.98 |224 |5.23 |0.79 |4.57 |4264.2 |256 |
| [convnext_femto_ols.d1_in1k](https://huggingface.co/timm/convnext_femto_ols.d1_in1k) |77.86 |93.83 |224 |5.23 |0.82 |4.87 |6910.6 |256 |
| [convnext_femto.d1_in1k](https://huggingface.co/timm/convnext_femto.d1_in1k) |77.454|93.68 |224 |5.22 |0.79 |4.57 |7189.92 |256 |
| [convnextv2_atto.fcmae_ft_in1k](https://huggingface.co/timm/convnextv2_atto.fcmae_ft_in1k) |76.664|93.044|224 |3.71 |0.55 |3.81 |4728.91 |256 |
| [convnext_atto_ols.a2_in1k](https://huggingface.co/timm/convnext_atto_ols.a2_in1k) |75.88 |92.846|224 |3.7 |0.58 |4.11 |7963.16 |256 |
| [convnext_atto.d2_in1k](https://huggingface.co/timm/convnext_atto.d2_in1k) |75.664|92.9 |224 |3.7 |0.55 |3.81 |8439.22 |256 |
## Citation
```bibtex
@misc{rw2019timm,
author = {Ross Wightman},
title = {PyTorch Image Models},
year = {2019},
publisher = {GitHub},
journal = {GitHub repository},
doi = {10.5281/zenodo.4414861},
howpublished = {\url{https://github.com/huggingface/pytorch-image-models}}
}
```
```bibtex
@article{liu2022convnet,
author = {Zhuang Liu and Hanzi Mao and Chao-Yuan Wu and Christoph Feichtenhofer and Trevor Darrell and Saining Xie},
title = {A ConvNet for the 2020s},
journal = {Proceedings of the IEEE/CVF Conference on Computer Vision and Pattern Recognition (CVPR)},
year = {2022},
}
```
|
mradermacher/Llama-3-Stheno-Instruct-8B-i1-GGUF | mradermacher | 2024-06-24T19:49:13Z | 12,223 | 0 | transformers | [
"transformers",
"gguf",
"mergekit",
"merge",
"en",
"base_model:mpasila/Llama-3-Stheno-Instruct-8B",
"license:llama3",
"endpoints_compatible",
"region:us"
]
| null | 2024-06-24T17:05:28Z | ---
base_model: mpasila/Llama-3-Stheno-Instruct-8B
language:
- en
library_name: transformers
license: llama3
quantized_by: mradermacher
tags:
- mergekit
- merge
---
## About
<!-- ### quantize_version: 2 -->
<!-- ### output_tensor_quantised: 1 -->
<!-- ### convert_type: hf -->
<!-- ### vocab_type: -->
<!-- ### tags: nicoboss -->
weighted/imatrix quants of https://huggingface.co/mpasila/Llama-3-Stheno-Instruct-8B
<!-- provided-files -->
static quants are available at https://huggingface.co/mradermacher/Llama-3-Stheno-Instruct-8B-GGUF
## Usage
If you are unsure how to use GGUF files, refer to one of [TheBloke's
READMEs](https://huggingface.co/TheBloke/KafkaLM-70B-German-V0.1-GGUF) for
more details, including on how to concatenate multi-part files.
## Provided Quants
(sorted by size, not necessarily quality. IQ-quants are often preferable over similar sized non-IQ quants)
| Link | Type | Size/GB | Notes |
|:-----|:-----|--------:|:------|
| [GGUF](https://huggingface.co/mradermacher/Llama-3-Stheno-Instruct-8B-i1-GGUF/resolve/main/Llama-3-Stheno-Instruct-8B.i1-IQ1_S.gguf) | i1-IQ1_S | 2.1 | for the desperate |
| [GGUF](https://huggingface.co/mradermacher/Llama-3-Stheno-Instruct-8B-i1-GGUF/resolve/main/Llama-3-Stheno-Instruct-8B.i1-IQ1_M.gguf) | i1-IQ1_M | 2.3 | mostly desperate |
| [GGUF](https://huggingface.co/mradermacher/Llama-3-Stheno-Instruct-8B-i1-GGUF/resolve/main/Llama-3-Stheno-Instruct-8B.i1-IQ2_XXS.gguf) | i1-IQ2_XXS | 2.5 | |
| [GGUF](https://huggingface.co/mradermacher/Llama-3-Stheno-Instruct-8B-i1-GGUF/resolve/main/Llama-3-Stheno-Instruct-8B.i1-IQ2_XS.gguf) | i1-IQ2_XS | 2.7 | |
| [GGUF](https://huggingface.co/mradermacher/Llama-3-Stheno-Instruct-8B-i1-GGUF/resolve/main/Llama-3-Stheno-Instruct-8B.i1-IQ2_S.gguf) | i1-IQ2_S | 2.9 | |
| [GGUF](https://huggingface.co/mradermacher/Llama-3-Stheno-Instruct-8B-i1-GGUF/resolve/main/Llama-3-Stheno-Instruct-8B.i1-IQ2_M.gguf) | i1-IQ2_M | 3.0 | |
| [GGUF](https://huggingface.co/mradermacher/Llama-3-Stheno-Instruct-8B-i1-GGUF/resolve/main/Llama-3-Stheno-Instruct-8B.i1-Q2_K.gguf) | i1-Q2_K | 3.3 | IQ3_XXS probably better |
| [GGUF](https://huggingface.co/mradermacher/Llama-3-Stheno-Instruct-8B-i1-GGUF/resolve/main/Llama-3-Stheno-Instruct-8B.i1-IQ3_XXS.gguf) | i1-IQ3_XXS | 3.4 | lower quality |
| [GGUF](https://huggingface.co/mradermacher/Llama-3-Stheno-Instruct-8B-i1-GGUF/resolve/main/Llama-3-Stheno-Instruct-8B.i1-IQ3_XS.gguf) | i1-IQ3_XS | 3.6 | |
| [GGUF](https://huggingface.co/mradermacher/Llama-3-Stheno-Instruct-8B-i1-GGUF/resolve/main/Llama-3-Stheno-Instruct-8B.i1-Q3_K_S.gguf) | i1-Q3_K_S | 3.8 | IQ3_XS probably better |
| [GGUF](https://huggingface.co/mradermacher/Llama-3-Stheno-Instruct-8B-i1-GGUF/resolve/main/Llama-3-Stheno-Instruct-8B.i1-IQ3_S.gguf) | i1-IQ3_S | 3.8 | beats Q3_K* |
| [GGUF](https://huggingface.co/mradermacher/Llama-3-Stheno-Instruct-8B-i1-GGUF/resolve/main/Llama-3-Stheno-Instruct-8B.i1-IQ3_M.gguf) | i1-IQ3_M | 3.9 | |
| [GGUF](https://huggingface.co/mradermacher/Llama-3-Stheno-Instruct-8B-i1-GGUF/resolve/main/Llama-3-Stheno-Instruct-8B.i1-Q3_K_M.gguf) | i1-Q3_K_M | 4.1 | IQ3_S probably better |
| [GGUF](https://huggingface.co/mradermacher/Llama-3-Stheno-Instruct-8B-i1-GGUF/resolve/main/Llama-3-Stheno-Instruct-8B.i1-Q3_K_L.gguf) | i1-Q3_K_L | 4.4 | IQ3_M probably better |
| [GGUF](https://huggingface.co/mradermacher/Llama-3-Stheno-Instruct-8B-i1-GGUF/resolve/main/Llama-3-Stheno-Instruct-8B.i1-IQ4_XS.gguf) | i1-IQ4_XS | 4.5 | |
| [GGUF](https://huggingface.co/mradermacher/Llama-3-Stheno-Instruct-8B-i1-GGUF/resolve/main/Llama-3-Stheno-Instruct-8B.i1-Q4_0.gguf) | i1-Q4_0 | 4.8 | fast, low quality |
| [GGUF](https://huggingface.co/mradermacher/Llama-3-Stheno-Instruct-8B-i1-GGUF/resolve/main/Llama-3-Stheno-Instruct-8B.i1-Q4_K_S.gguf) | i1-Q4_K_S | 4.8 | optimal size/speed/quality |
| [GGUF](https://huggingface.co/mradermacher/Llama-3-Stheno-Instruct-8B-i1-GGUF/resolve/main/Llama-3-Stheno-Instruct-8B.i1-Q4_K_M.gguf) | i1-Q4_K_M | 5.0 | fast, recommended |
| [GGUF](https://huggingface.co/mradermacher/Llama-3-Stheno-Instruct-8B-i1-GGUF/resolve/main/Llama-3-Stheno-Instruct-8B.i1-Q5_K_S.gguf) | i1-Q5_K_S | 5.7 | |
| [GGUF](https://huggingface.co/mradermacher/Llama-3-Stheno-Instruct-8B-i1-GGUF/resolve/main/Llama-3-Stheno-Instruct-8B.i1-Q5_K_M.gguf) | i1-Q5_K_M | 5.8 | |
| [GGUF](https://huggingface.co/mradermacher/Llama-3-Stheno-Instruct-8B-i1-GGUF/resolve/main/Llama-3-Stheno-Instruct-8B.i1-Q6_K.gguf) | i1-Q6_K | 6.7 | practically like static Q6_K |
Here is a handy graph by ikawrakow comparing some lower-quality quant
types (lower is better):

And here are Artefact2's thoughts on the matter:
https://gist.github.com/Artefact2/b5f810600771265fc1e39442288e8ec9
## FAQ / Model Request
See https://huggingface.co/mradermacher/model_requests for some answers to
questions you might have and/or if you want some other model quantized.
## Thanks
I thank my company, [nethype GmbH](https://www.nethype.de/), for letting
me use its servers and providing upgrades to my workstation to enable
this work in my free time. Additional thanks to [@nicoboss](https://huggingface.co/nicoboss) for giving me access to his hardware for calculating the imatrix for these quants.
<!-- end -->
|
mradermacher/llama-polya-v2-GGUF | mradermacher | 2024-06-27T14:18:26Z | 12,203 | 0 | transformers | [
"transformers",
"gguf",
"generated_from_trainer",
"en",
"base_model:Llama-Math/llama-polya-v2",
"endpoints_compatible",
"region:us"
]
| null | 2024-06-27T05:39:18Z | ---
base_model: Llama-Math/llama-polya-v2
language:
- en
library_name: transformers
quantized_by: mradermacher
tags:
- generated_from_trainer
---
## About
<!-- ### quantize_version: 2 -->
<!-- ### output_tensor_quantised: 1 -->
<!-- ### convert_type: hf -->
<!-- ### vocab_type: -->
<!-- ### tags: -->
static quants of https://huggingface.co/Llama-Math/llama-polya-v2
<!-- provided-files -->
weighted/imatrix quants are available at https://huggingface.co/mradermacher/llama-polya-v2-i1-GGUF
## Usage
If you are unsure how to use GGUF files, refer to one of [TheBloke's
READMEs](https://huggingface.co/TheBloke/KafkaLM-70B-German-V0.1-GGUF) for
more details, including on how to concatenate multi-part files.
## Provided Quants
(sorted by size, not necessarily quality. IQ-quants are often preferable over similar sized non-IQ quants)
| Link | Type | Size/GB | Notes |
|:-----|:-----|--------:|:------|
| [GGUF](https://huggingface.co/mradermacher/llama-polya-v2-GGUF/resolve/main/llama-polya-v2.Q2_K.gguf) | Q2_K | 2.6 | |
| [GGUF](https://huggingface.co/mradermacher/llama-polya-v2-GGUF/resolve/main/llama-polya-v2.IQ3_XS.gguf) | IQ3_XS | 2.9 | |
| [GGUF](https://huggingface.co/mradermacher/llama-polya-v2-GGUF/resolve/main/llama-polya-v2.IQ3_S.gguf) | IQ3_S | 3.0 | beats Q3_K* |
| [GGUF](https://huggingface.co/mradermacher/llama-polya-v2-GGUF/resolve/main/llama-polya-v2.Q3_K_S.gguf) | Q3_K_S | 3.0 | |
| [GGUF](https://huggingface.co/mradermacher/llama-polya-v2-GGUF/resolve/main/llama-polya-v2.IQ3_M.gguf) | IQ3_M | 3.2 | |
| [GGUF](https://huggingface.co/mradermacher/llama-polya-v2-GGUF/resolve/main/llama-polya-v2.Q3_K_M.gguf) | Q3_K_M | 3.4 | lower quality |
| [GGUF](https://huggingface.co/mradermacher/llama-polya-v2-GGUF/resolve/main/llama-polya-v2.Q3_K_L.gguf) | Q3_K_L | 3.7 | |
| [GGUF](https://huggingface.co/mradermacher/llama-polya-v2-GGUF/resolve/main/llama-polya-v2.IQ4_XS.gguf) | IQ4_XS | 3.7 | |
| [GGUF](https://huggingface.co/mradermacher/llama-polya-v2-GGUF/resolve/main/llama-polya-v2.Q4_K_S.gguf) | Q4_K_S | 4.0 | fast, recommended |
| [GGUF](https://huggingface.co/mradermacher/llama-polya-v2-GGUF/resolve/main/llama-polya-v2.Q4_K_M.gguf) | Q4_K_M | 4.2 | fast, recommended |
| [GGUF](https://huggingface.co/mradermacher/llama-polya-v2-GGUF/resolve/main/llama-polya-v2.Q5_K_S.gguf) | Q5_K_S | 4.8 | |
| [GGUF](https://huggingface.co/mradermacher/llama-polya-v2-GGUF/resolve/main/llama-polya-v2.Q5_K_M.gguf) | Q5_K_M | 4.9 | |
| [GGUF](https://huggingface.co/mradermacher/llama-polya-v2-GGUF/resolve/main/llama-polya-v2.Q6_K.gguf) | Q6_K | 5.6 | very good quality |
| [GGUF](https://huggingface.co/mradermacher/llama-polya-v2-GGUF/resolve/main/llama-polya-v2.Q8_0.gguf) | Q8_0 | 7.3 | fast, best quality |
| [GGUF](https://huggingface.co/mradermacher/llama-polya-v2-GGUF/resolve/main/llama-polya-v2.f16.gguf) | f16 | 13.6 | 16 bpw, overkill |
Here is a handy graph by ikawrakow comparing some lower-quality quant
types (lower is better):

And here are Artefact2's thoughts on the matter:
https://gist.github.com/Artefact2/b5f810600771265fc1e39442288e8ec9
## FAQ / Model Request
See https://huggingface.co/mradermacher/model_requests for some answers to
questions you might have and/or if you want some other model quantized.
## Thanks
I thank my company, [nethype GmbH](https://www.nethype.de/), for letting
me use its servers and providing upgrades to my workstation to enable
this work in my free time.
<!-- end -->
|
mradermacher/YuLan-Chat-3-12b-GGUF | mradermacher | 2024-07-01T11:36:18Z | 12,202 | 0 | transformers | [
"transformers",
"gguf",
"en",
"base_model:yulan-team/YuLan-Chat-3-12b",
"license:mit",
"endpoints_compatible",
"region:us"
]
| null | 2024-07-01T10:49:27Z | ---
base_model: yulan-team/YuLan-Chat-3-12b
language:
- en
library_name: transformers
license: mit
quantized_by: mradermacher
---
## About
<!-- ### quantize_version: 2 -->
<!-- ### output_tensor_quantised: 1 -->
<!-- ### convert_type: hf -->
<!-- ### vocab_type: -->
<!-- ### tags: -->
static quants of https://huggingface.co/yulan-team/YuLan-Chat-3-12b
<!-- provided-files -->
weighted/imatrix quants seem not to be available (by me) at this time. If they do not show up a week or so after the static ones, I have probably not planned for them. Feel free to request them by opening a Community Discussion.
## Usage
If you are unsure how to use GGUF files, refer to one of [TheBloke's
READMEs](https://huggingface.co/TheBloke/KafkaLM-70B-German-V0.1-GGUF) for
more details, including on how to concatenate multi-part files.
## Provided Quants
(sorted by size, not necessarily quality. IQ-quants are often preferable over similar sized non-IQ quants)
| Link | Type | Size/GB | Notes |
|:-----|:-----|--------:|:------|
| [GGUF](https://huggingface.co/mradermacher/YuLan-Chat-3-12b-GGUF/resolve/main/YuLan-Chat-3-12b.Q2_K.gguf) | Q2_K | 4.6 | |
| [GGUF](https://huggingface.co/mradermacher/YuLan-Chat-3-12b-GGUF/resolve/main/YuLan-Chat-3-12b.IQ3_XS.gguf) | IQ3_XS | 5.0 | |
| [GGUF](https://huggingface.co/mradermacher/YuLan-Chat-3-12b-GGUF/resolve/main/YuLan-Chat-3-12b.IQ3_S.gguf) | IQ3_S | 5.3 | beats Q3_K* |
| [GGUF](https://huggingface.co/mradermacher/YuLan-Chat-3-12b-GGUF/resolve/main/YuLan-Chat-3-12b.Q3_K_S.gguf) | Q3_K_S | 5.3 | |
| [GGUF](https://huggingface.co/mradermacher/YuLan-Chat-3-12b-GGUF/resolve/main/YuLan-Chat-3-12b.IQ3_M.gguf) | IQ3_M | 5.6 | |
| [GGUF](https://huggingface.co/mradermacher/YuLan-Chat-3-12b-GGUF/resolve/main/YuLan-Chat-3-12b.Q3_K_M.gguf) | Q3_K_M | 5.9 | lower quality |
| [GGUF](https://huggingface.co/mradermacher/YuLan-Chat-3-12b-GGUF/resolve/main/YuLan-Chat-3-12b.Q3_K_L.gguf) | Q3_K_L | 6.5 | |
| [GGUF](https://huggingface.co/mradermacher/YuLan-Chat-3-12b-GGUF/resolve/main/YuLan-Chat-3-12b.IQ4_XS.gguf) | IQ4_XS | 6.5 | |
| [GGUF](https://huggingface.co/mradermacher/YuLan-Chat-3-12b-GGUF/resolve/main/YuLan-Chat-3-12b.Q4_K_S.gguf) | Q4_K_S | 6.9 | fast, recommended |
| [GGUF](https://huggingface.co/mradermacher/YuLan-Chat-3-12b-GGUF/resolve/main/YuLan-Chat-3-12b.Q4_K_M.gguf) | Q4_K_M | 7.3 | fast, recommended |
| [GGUF](https://huggingface.co/mradermacher/YuLan-Chat-3-12b-GGUF/resolve/main/YuLan-Chat-3-12b.Q5_K_S.gguf) | Q5_K_S | 8.3 | |
| [GGUF](https://huggingface.co/mradermacher/YuLan-Chat-3-12b-GGUF/resolve/main/YuLan-Chat-3-12b.Q5_K_M.gguf) | Q5_K_M | 8.6 | |
| [GGUF](https://huggingface.co/mradermacher/YuLan-Chat-3-12b-GGUF/resolve/main/YuLan-Chat-3-12b.Q6_K.gguf) | Q6_K | 9.9 | very good quality |
| [GGUF](https://huggingface.co/mradermacher/YuLan-Chat-3-12b-GGUF/resolve/main/YuLan-Chat-3-12b.Q8_0.gguf) | Q8_0 | 12.8 | fast, best quality |
Here is a handy graph by ikawrakow comparing some lower-quality quant
types (lower is better):

And here are Artefact2's thoughts on the matter:
https://gist.github.com/Artefact2/b5f810600771265fc1e39442288e8ec9
## FAQ / Model Request
See https://huggingface.co/mradermacher/model_requests for some answers to
questions you might have and/or if you want some other model quantized.
## Thanks
I thank my company, [nethype GmbH](https://www.nethype.de/), for letting
me use its servers and providing upgrades to my workstation to enable
this work in my free time.
<!-- end -->
|
danielheinz/e5-base-sts-en-de | danielheinz | 2024-01-14T17:37:54Z | 12,201 | 7 | transformers | [
"transformers",
"safetensors",
"xlm-roberta",
"feature-extraction",
"de",
"dataset:deutsche-telekom/ger-backtrans-paraphrase",
"dataset:paws-x",
"dataset:stsb_multi_mt",
"license:mit",
"model-index",
"endpoints_compatible",
"region:us"
]
| feature-extraction | 2023-12-21T08:06:47Z | ---
license: mit
datasets:
- deutsche-telekom/ger-backtrans-paraphrase
- paws-x
- stsb_multi_mt
language:
- de
model-index:
- name: e5-base-sts-en-de
results:
- task:
type: semantic textual similarity
dataset:
type: stsb_multi_mt
name: stsb_multi_mt
metrics:
- type: spearmanr
value: 0.904
---
**INFO**: The model is being continuously updated.
The model is a [multilingual-e5-base](https://huggingface.co/intfloat/multilingual-e5-base) model fine-tuned with the task of semantic textual similarity in mind.
## Model Training
The model has been fine-tuned on the German subsets of the following datasets:
- [German paraphrase corpus by Philip May](https://huggingface.co/datasets/deutsche-telekom/ger-backtrans-paraphrase)
- [paws-x](https://huggingface.co/datasets/paws-x)
- [stsb_multi_mt](https://huggingface.co/datasets/stsb_multi_mt)
The training procedure can be divided into two stages:
- training on paraphrase datasets with the Multiple Negatives Ranking Loss
- training on semantic textual similarity datasets using the Cosine Similarity Loss
# Results
The model achieves the following results:
- 0.920 on stsb's validation subset
- 0.904 on stsb's test subset |
dbmdz/distilbert-base-turkish-cased | dbmdz | 2021-01-24T01:01:22Z | 12,194 | 9 | transformers | [
"transformers",
"pytorch",
"tf",
"distilbert",
"tr",
"arxiv:1910.01108",
"license:mit",
"endpoints_compatible",
"region:us"
]
| null | 2022-03-02T23:29:05Z | ---
language: tr
license: mit
---
# 🤗 + 📚 dbmdz Distilled Turkish BERT model
In this repository the MDZ Digital Library team (dbmdz) at the Bavarian State
Library open sources a (cased) distilled model for Turkish 🎉
# 🇹🇷 DistilBERTurk
DistilBERTurk is a community-driven cased distilled BERT model for Turkish.
DistilBERTurk was trained on 7GB of the original training data that was used
for training [BERTurk](https://github.com/stefan-it/turkish-bert/tree/master#stats),
using the cased version of BERTurk as teacher model.
*DistilBERTurk* was trained with the official Hugging Face implementation from
[here](https://github.com/huggingface/transformers/tree/master/examples/distillation)
for 5 days on 4 RTX 2080 TI.
More details about distillation can be found in the
["DistilBERT, a distilled version of BERT: smaller, faster, cheaper and lighter"](https://arxiv.org/abs/1910.01108)
paper by Sanh et al. (2019).
## Model weights
Currently only PyTorch-[Transformers](https://github.com/huggingface/transformers)
compatible weights are available. If you need access to TensorFlow checkpoints,
please raise an issue in the [BERTurk](https://github.com/stefan-it/turkish-bert) repository!
| Model | Downloads
| --------------------------------- | ---------------------------------------------------------------------------------------------------------------
| `dbmdz/distilbert-base-turkish-cased` | [`config.json`](https://cdn.huggingface.co/dbmdz/distilbert-base-turkish-cased/config.json) • [`pytorch_model.bin`](https://cdn.huggingface.co/dbmdz/distilbert-base-turkish-cased/pytorch_model.bin) • [`vocab.txt`](https://cdn.huggingface.co/dbmdz/distilbert-base-turkish-cased/vocab.txt)
## Usage
With Transformers >= 2.3 our DistilBERTurk model can be loaded like:
```python
from transformers import AutoModel, AutoTokenizer
tokenizer = AutoTokenizer.from_pretrained("dbmdz/distilbert-base-turkish-cased")
model = AutoModel.from_pretrained("dbmdz/distilbert-base-turkish-cased")
```
## Results
For results on PoS tagging or NER tasks, please refer to
[this repository](https://github.com/stefan-it/turkish-bert).
For PoS tagging, DistilBERTurk outperforms the 24-layer XLM-RoBERTa model.
The overall performance difference between DistilBERTurk and the original
(teacher) BERTurk model is ~1.18%.
# Huggingface model hub
All models are available on the [Huggingface model hub](https://huggingface.co/dbmdz).
# Contact (Bugs, Feedback, Contribution and more)
For questions about our BERT models just open an issue
[here](https://github.com/dbmdz/berts/issues/new) 🤗
# Acknowledgments
Thanks to [Kemal Oflazer](http://www.andrew.cmu.edu/user/ko/) for providing us
additional large corpora for Turkish. Many thanks to Reyyan Yeniterzi for providing
us the Turkish NER dataset for evaluation.
Research supported with Cloud TPUs from Google's TensorFlow Research Cloud (TFRC).
Thanks for providing access to the TFRC ❤️
Thanks to the generous support from the [Hugging Face](https://huggingface.co/) team,
it is possible to download both cased and uncased models from their S3 storage 🤗
|
mradermacher/Swallow-7b-NVE-instruct-hf-i1-GGUF | mradermacher | 2024-06-30T10:01:26Z | 12,182 | 0 | transformers | [
"transformers",
"gguf",
"en",
"ja",
"base_model:tokyotech-llm/Swallow-7b-NVE-instruct-hf",
"license:llama2",
"endpoints_compatible",
"region:us"
]
| null | 2024-06-30T07:44:50Z | ---
base_model: tokyotech-llm/Swallow-7b-NVE-instruct-hf
language:
- en
- ja
library_name: transformers
license: llama2
model_type: llama
quantized_by: mradermacher
---
## About
<!-- ### quantize_version: 2 -->
<!-- ### output_tensor_quantised: 1 -->
<!-- ### convert_type: hf -->
<!-- ### vocab_type: -->
<!-- ### tags: nicoboss -->
weighted/imatrix quants of https://huggingface.co/tokyotech-llm/Swallow-7b-NVE-instruct-hf
<!-- provided-files -->
static quants are available at https://huggingface.co/mradermacher/Swallow-7b-NVE-instruct-hf-GGUF
## Usage
If you are unsure how to use GGUF files, refer to one of [TheBloke's
READMEs](https://huggingface.co/TheBloke/KafkaLM-70B-German-V0.1-GGUF) for
more details, including on how to concatenate multi-part files.
## Provided Quants
(sorted by size, not necessarily quality. IQ-quants are often preferable over similar sized non-IQ quants)
| Link | Type | Size/GB | Notes |
|:-----|:-----|--------:|:------|
| [GGUF](https://huggingface.co/mradermacher/Swallow-7b-NVE-instruct-hf-i1-GGUF/resolve/main/Swallow-7b-NVE-instruct-hf.i1-IQ1_S.gguf) | i1-IQ1_S | 1.6 | for the desperate |
| [GGUF](https://huggingface.co/mradermacher/Swallow-7b-NVE-instruct-hf-i1-GGUF/resolve/main/Swallow-7b-NVE-instruct-hf.i1-IQ1_M.gguf) | i1-IQ1_M | 1.8 | mostly desperate |
| [GGUF](https://huggingface.co/mradermacher/Swallow-7b-NVE-instruct-hf-i1-GGUF/resolve/main/Swallow-7b-NVE-instruct-hf.i1-IQ2_XXS.gguf) | i1-IQ2_XXS | 2.0 | |
| [GGUF](https://huggingface.co/mradermacher/Swallow-7b-NVE-instruct-hf-i1-GGUF/resolve/main/Swallow-7b-NVE-instruct-hf.i1-IQ2_XS.gguf) | i1-IQ2_XS | 2.1 | |
| [GGUF](https://huggingface.co/mradermacher/Swallow-7b-NVE-instruct-hf-i1-GGUF/resolve/main/Swallow-7b-NVE-instruct-hf.i1-IQ2_S.gguf) | i1-IQ2_S | 2.3 | |
| [GGUF](https://huggingface.co/mradermacher/Swallow-7b-NVE-instruct-hf-i1-GGUF/resolve/main/Swallow-7b-NVE-instruct-hf.i1-IQ2_M.gguf) | i1-IQ2_M | 2.5 | |
| [GGUF](https://huggingface.co/mradermacher/Swallow-7b-NVE-instruct-hf-i1-GGUF/resolve/main/Swallow-7b-NVE-instruct-hf.i1-Q2_K.gguf) | i1-Q2_K | 2.6 | IQ3_XXS probably better |
| [GGUF](https://huggingface.co/mradermacher/Swallow-7b-NVE-instruct-hf-i1-GGUF/resolve/main/Swallow-7b-NVE-instruct-hf.i1-IQ3_XXS.gguf) | i1-IQ3_XXS | 2.7 | lower quality |
| [GGUF](https://huggingface.co/mradermacher/Swallow-7b-NVE-instruct-hf-i1-GGUF/resolve/main/Swallow-7b-NVE-instruct-hf.i1-IQ3_XS.gguf) | i1-IQ3_XS | 2.9 | |
| [GGUF](https://huggingface.co/mradermacher/Swallow-7b-NVE-instruct-hf-i1-GGUF/resolve/main/Swallow-7b-NVE-instruct-hf.i1-IQ3_S.gguf) | i1-IQ3_S | 3.0 | beats Q3_K* |
| [GGUF](https://huggingface.co/mradermacher/Swallow-7b-NVE-instruct-hf-i1-GGUF/resolve/main/Swallow-7b-NVE-instruct-hf.i1-Q3_K_S.gguf) | i1-Q3_K_S | 3.0 | IQ3_XS probably better |
| [GGUF](https://huggingface.co/mradermacher/Swallow-7b-NVE-instruct-hf-i1-GGUF/resolve/main/Swallow-7b-NVE-instruct-hf.i1-IQ3_M.gguf) | i1-IQ3_M | 3.2 | |
| [GGUF](https://huggingface.co/mradermacher/Swallow-7b-NVE-instruct-hf-i1-GGUF/resolve/main/Swallow-7b-NVE-instruct-hf.i1-Q3_K_M.gguf) | i1-Q3_K_M | 3.4 | IQ3_S probably better |
| [GGUF](https://huggingface.co/mradermacher/Swallow-7b-NVE-instruct-hf-i1-GGUF/resolve/main/Swallow-7b-NVE-instruct-hf.i1-Q3_K_L.gguf) | i1-Q3_K_L | 3.7 | IQ3_M probably better |
| [GGUF](https://huggingface.co/mradermacher/Swallow-7b-NVE-instruct-hf-i1-GGUF/resolve/main/Swallow-7b-NVE-instruct-hf.i1-IQ4_XS.gguf) | i1-IQ4_XS | 3.7 | |
| [GGUF](https://huggingface.co/mradermacher/Swallow-7b-NVE-instruct-hf-i1-GGUF/resolve/main/Swallow-7b-NVE-instruct-hf.i1-Q4_0.gguf) | i1-Q4_0 | 3.9 | fast, low quality |
| [GGUF](https://huggingface.co/mradermacher/Swallow-7b-NVE-instruct-hf-i1-GGUF/resolve/main/Swallow-7b-NVE-instruct-hf.i1-Q4_K_S.gguf) | i1-Q4_K_S | 4.0 | optimal size/speed/quality |
| [GGUF](https://huggingface.co/mradermacher/Swallow-7b-NVE-instruct-hf-i1-GGUF/resolve/main/Swallow-7b-NVE-instruct-hf.i1-Q4_K_M.gguf) | i1-Q4_K_M | 4.2 | fast, recommended |
| [GGUF](https://huggingface.co/mradermacher/Swallow-7b-NVE-instruct-hf-i1-GGUF/resolve/main/Swallow-7b-NVE-instruct-hf.i1-Q5_K_S.gguf) | i1-Q5_K_S | 4.8 | |
| [GGUF](https://huggingface.co/mradermacher/Swallow-7b-NVE-instruct-hf-i1-GGUF/resolve/main/Swallow-7b-NVE-instruct-hf.i1-Q5_K_M.gguf) | i1-Q5_K_M | 4.9 | |
| [GGUF](https://huggingface.co/mradermacher/Swallow-7b-NVE-instruct-hf-i1-GGUF/resolve/main/Swallow-7b-NVE-instruct-hf.i1-Q6_K.gguf) | i1-Q6_K | 5.6 | practically like static Q6_K |
Here is a handy graph by ikawrakow comparing some lower-quality quant
types (lower is better):

And here are Artefact2's thoughts on the matter:
https://gist.github.com/Artefact2/b5f810600771265fc1e39442288e8ec9
## FAQ / Model Request
See https://huggingface.co/mradermacher/model_requests for some answers to
questions you might have and/or if you want some other model quantized.
## Thanks
I thank my company, [nethype GmbH](https://www.nethype.de/), for letting
me use its servers and providing upgrades to my workstation to enable
this work in my free time. Additional thanks to [@nicoboss](https://huggingface.co/nicoboss) for giving me access to his hardware for calculating the imatrix for these quants.
<!-- end -->
|
McGill-NLP/LLM2Vec-Meta-Llama-3-8B-Instruct-mntp | McGill-NLP | 2024-05-21T21:59:58Z | 12,181 | 6 | transformers | [
"transformers",
"safetensors",
"llama",
"feature-extraction",
"text-embedding",
"embeddings",
"information-retrieval",
"beir",
"text-classification",
"language-model",
"text-clustering",
"text-semantic-similarity",
"text-evaluation",
"text-reranking",
"sentence-similarity",
"Sentence Similarity",
"natural_questions",
"ms_marco",
"fever",
"hotpot_qa",
"mteb",
"custom_code",
"en",
"arxiv:2404.05961",
"license:mit",
"text-generation-inference",
"region:us"
]
| sentence-similarity | 2024-04-30T03:10:31Z | ---
library_name: transformers
license: mit
language:
- en
pipeline_tag: sentence-similarity
tags:
- text-embedding
- embeddings
- information-retrieval
- beir
- text-classification
- language-model
- text-clustering
- text-semantic-similarity
- text-evaluation
- text-reranking
- feature-extraction
- sentence-similarity
- Sentence Similarity
- natural_questions
- ms_marco
- fever
- hotpot_qa
- mteb
---
# LLM2Vec: Large Language Models Are Secretly Powerful Text Encoders
> LLM2Vec is a simple recipe to convert decoder-only LLMs into text encoders. It consists of 3 simple steps: 1) enabling bidirectional attention, 2) masked next token prediction, and 3) unsupervised contrastive learning. The model can be further fine-tuned to achieve state-of-the-art performance.
- **Repository:** https://github.com/McGill-NLP/llm2vec
- **Paper:** https://arxiv.org/abs/2404.05961
## Installation
```bash
pip install llm2vec
```
## Usage
```python
from llm2vec import LLM2Vec
import torch
from transformers import AutoTokenizer, AutoModel, AutoConfig
from peft import PeftModel
# Loading base Mistral model, along with custom code that enables bidirectional connections in decoder-only LLMs.
tokenizer = AutoTokenizer.from_pretrained(
"McGill-NLP/LLM2Vec-Meta-Llama-3-8B-Instruct-mntp"
)
config = AutoConfig.from_pretrained(
"McGill-NLP/LLM2Vec-Meta-Llama-3-8B-Instruct-mntp", trust_remote_code=True
)
model = AutoModel.from_pretrained(
"McGill-NLP/LLM2Vec-Meta-Llama-3-8B-Instruct-mntp",
trust_remote_code=True,
config=config,
torch_dtype=torch.bfloat16,
device_map="cuda" if torch.cuda.is_available() else "cpu",
)
# Loading MNTP (Masked Next Token Prediction) model.
model = PeftModel.from_pretrained(
model,
"McGill-NLP/LLM2Vec-Meta-Llama-3-8B-Instruct-mntp",
)
# Wrapper for encoding and pooling operations
l2v = LLM2Vec(model, tokenizer, pooling_mode="mean", max_length=512)
# Encoding queries using instructions
instruction = (
"Given a web search query, retrieve relevant passages that answer the query:"
)
queries = [
[instruction, "how much protein should a female eat"],
[instruction, "summit define"],
]
q_reps = l2v.encode(queries)
# Encoding documents. Instruction are not required for documents
documents = [
"As a general guideline, the CDC's average requirement of protein for women ages 19 to 70 is 46 grams per day. But, as you can see from this chart, you'll need to increase that if you're expecting or training for a marathon. Check out the chart below to see how much protein you should be eating each day.",
"Definition of summit for English Language Learners. : 1 the highest point of a mountain : the top of a mountain. : 2 the highest level. : 3 a meeting or series of meetings between the leaders of two or more governments.",
]
d_reps = l2v.encode(documents)
# Compute cosine similarity
q_reps_norm = torch.nn.functional.normalize(q_reps, p=2, dim=1)
d_reps_norm = torch.nn.functional.normalize(d_reps, p=2, dim=1)
cos_sim = torch.mm(q_reps_norm, d_reps_norm.transpose(0, 1))
print(cos_sim)
"""
tensor([[0.7740, 0.5580],
[0.4845, 0.4993]])
"""
```
## Questions
If you have any question about the code, feel free to email Parishad (`[email protected]`) and Vaibhav (`[email protected]`). |
cross-encoder/stsb-roberta-large | cross-encoder | 2021-08-05T08:42:03Z | 12,176 | 8 | transformers | [
"transformers",
"pytorch",
"jax",
"roberta",
"text-classification",
"license:apache-2.0",
"autotrain_compatible",
"endpoints_compatible",
"region:us"
]
| text-classification | 2022-03-02T23:29:05Z | ---
license: apache-2.0
---
# Cross-Encoder for Quora Duplicate Questions Detection
This model was trained using [SentenceTransformers](https://sbert.net) [Cross-Encoder](https://www.sbert.net/examples/applications/cross-encoder/README.html) class.
## Training Data
This model was trained on the [STS benchmark dataset](http://ixa2.si.ehu.eus/stswiki/index.php/STSbenchmark). The model will predict a score between 0 and 1 how for the semantic similarity of two sentences.
## Usage and Performance
Pre-trained models can be used like this:
```
from sentence_transformers import CrossEncoder
model = CrossEncoder('model_name')
scores = model.predict([('Sentence 1', 'Sentence 2'), ('Sentence 3', 'Sentence 4')])
```
The model will predict scores for the pairs `('Sentence 1', 'Sentence 2')` and `('Sentence 3', 'Sentence 4')`.
You can use this model also without sentence_transformers and by just using Transformers ``AutoModel`` class |
RichardErkhov/llm4fun_-_vietrag-7b-v1.0-gguf | RichardErkhov | 2024-06-30T16:12:19Z | 12,175 | 0 | null | [
"gguf",
"region:us"
]
| null | 2024-06-30T14:06:01Z | Quantization made by Richard Erkhov.
[Github](https://github.com/RichardErkhov)
[Discord](https://discord.gg/pvy7H8DZMG)
[Request more models](https://github.com/RichardErkhov/quant_request)
vietrag-7b-v1.0 - GGUF
- Model creator: https://huggingface.co/llm4fun/
- Original model: https://huggingface.co/llm4fun/vietrag-7b-v1.0/
| Name | Quant method | Size |
| ---- | ---- | ---- |
| [vietrag-7b-v1.0.Q2_K.gguf](https://huggingface.co/RichardErkhov/llm4fun_-_vietrag-7b-v1.0-gguf/blob/main/vietrag-7b-v1.0.Q2_K.gguf) | Q2_K | 2.36GB |
| [vietrag-7b-v1.0.IQ3_XS.gguf](https://huggingface.co/RichardErkhov/llm4fun_-_vietrag-7b-v1.0-gguf/blob/main/vietrag-7b-v1.0.IQ3_XS.gguf) | IQ3_XS | 2.6GB |
| [vietrag-7b-v1.0.IQ3_S.gguf](https://huggingface.co/RichardErkhov/llm4fun_-_vietrag-7b-v1.0-gguf/blob/main/vietrag-7b-v1.0.IQ3_S.gguf) | IQ3_S | 2.75GB |
| [vietrag-7b-v1.0.Q3_K_S.gguf](https://huggingface.co/RichardErkhov/llm4fun_-_vietrag-7b-v1.0-gguf/blob/main/vietrag-7b-v1.0.Q3_K_S.gguf) | Q3_K_S | 2.75GB |
| [vietrag-7b-v1.0.IQ3_M.gguf](https://huggingface.co/RichardErkhov/llm4fun_-_vietrag-7b-v1.0-gguf/blob/main/vietrag-7b-v1.0.IQ3_M.gguf) | IQ3_M | 2.9GB |
| [vietrag-7b-v1.0.Q3_K.gguf](https://huggingface.co/RichardErkhov/llm4fun_-_vietrag-7b-v1.0-gguf/blob/main/vietrag-7b-v1.0.Q3_K.gguf) | Q3_K | 3.07GB |
| [vietrag-7b-v1.0.Q3_K_M.gguf](https://huggingface.co/RichardErkhov/llm4fun_-_vietrag-7b-v1.0-gguf/blob/main/vietrag-7b-v1.0.Q3_K_M.gguf) | Q3_K_M | 3.07GB |
| [vietrag-7b-v1.0.Q3_K_L.gguf](https://huggingface.co/RichardErkhov/llm4fun_-_vietrag-7b-v1.0-gguf/blob/main/vietrag-7b-v1.0.Q3_K_L.gguf) | Q3_K_L | 3.35GB |
| [vietrag-7b-v1.0.IQ4_XS.gguf](https://huggingface.co/RichardErkhov/llm4fun_-_vietrag-7b-v1.0-gguf/blob/main/vietrag-7b-v1.0.IQ4_XS.gguf) | IQ4_XS | 3.4GB |
| [vietrag-7b-v1.0.Q4_0.gguf](https://huggingface.co/RichardErkhov/llm4fun_-_vietrag-7b-v1.0-gguf/blob/main/vietrag-7b-v1.0.Q4_0.gguf) | Q4_0 | 3.56GB |
| [vietrag-7b-v1.0.IQ4_NL.gguf](https://huggingface.co/RichardErkhov/llm4fun_-_vietrag-7b-v1.0-gguf/blob/main/vietrag-7b-v1.0.IQ4_NL.gguf) | IQ4_NL | 3.58GB |
| [vietrag-7b-v1.0.Q4_K_S.gguf](https://huggingface.co/RichardErkhov/llm4fun_-_vietrag-7b-v1.0-gguf/blob/main/vietrag-7b-v1.0.Q4_K_S.gguf) | Q4_K_S | 3.59GB |
| [vietrag-7b-v1.0.Q4_K.gguf](https://huggingface.co/RichardErkhov/llm4fun_-_vietrag-7b-v1.0-gguf/blob/main/vietrag-7b-v1.0.Q4_K.gguf) | Q4_K | 3.8GB |
| [vietrag-7b-v1.0.Q4_K_M.gguf](https://huggingface.co/RichardErkhov/llm4fun_-_vietrag-7b-v1.0-gguf/blob/main/vietrag-7b-v1.0.Q4_K_M.gguf) | Q4_K_M | 3.8GB |
| [vietrag-7b-v1.0.Q4_1.gguf](https://huggingface.co/RichardErkhov/llm4fun_-_vietrag-7b-v1.0-gguf/blob/main/vietrag-7b-v1.0.Q4_1.gguf) | Q4_1 | 3.95GB |
| [vietrag-7b-v1.0.Q5_0.gguf](https://huggingface.co/RichardErkhov/llm4fun_-_vietrag-7b-v1.0-gguf/blob/main/vietrag-7b-v1.0.Q5_0.gguf) | Q5_0 | 4.33GB |
| [vietrag-7b-v1.0.Q5_K_S.gguf](https://huggingface.co/RichardErkhov/llm4fun_-_vietrag-7b-v1.0-gguf/blob/main/vietrag-7b-v1.0.Q5_K_S.gguf) | Q5_K_S | 4.33GB |
| [vietrag-7b-v1.0.Q5_K.gguf](https://huggingface.co/RichardErkhov/llm4fun_-_vietrag-7b-v1.0-gguf/blob/main/vietrag-7b-v1.0.Q5_K.gguf) | Q5_K | 4.45GB |
| [vietrag-7b-v1.0.Q5_K_M.gguf](https://huggingface.co/RichardErkhov/llm4fun_-_vietrag-7b-v1.0-gguf/blob/main/vietrag-7b-v1.0.Q5_K_M.gguf) | Q5_K_M | 4.45GB |
| [vietrag-7b-v1.0.Q5_1.gguf](https://huggingface.co/RichardErkhov/llm4fun_-_vietrag-7b-v1.0-gguf/blob/main/vietrag-7b-v1.0.Q5_1.gguf) | Q5_1 | 4.72GB |
| [vietrag-7b-v1.0.Q6_K.gguf](https://huggingface.co/RichardErkhov/llm4fun_-_vietrag-7b-v1.0-gguf/blob/main/vietrag-7b-v1.0.Q6_K.gguf) | Q6_K | 5.15GB |
| [vietrag-7b-v1.0.Q8_0.gguf](https://huggingface.co/RichardErkhov/llm4fun_-_vietrag-7b-v1.0-gguf/blob/main/vietrag-7b-v1.0.Q8_0.gguf) | Q8_0 | 6.67GB |
Original model description:
---
language:
- vi
---
# Usage
You can check our model card here: [`llm4fun/vietrag-7b-v1.0`](https://huggingface.co/llm4fun/vietrag-7b-v1.0)
```py
from transformers import GenerationConfig, TextStreamer
from transformers import LlamaForCausalLM, LlamaTokenizer, LlamaConfig
import torch
question = "<your-question>"
context = "<your-context>"
instruction = 'You are an AI assistant. Provide a detailed answer so user don’t need to search outside to understand the answer.'
input = f"Dựa vào một số ngữ cảnh được cho dưới đây, trả lời câu hỏi ở cuối.\n\n{context}\n\nQuestion: {question}"
prompt_template = (
"### System:\n"
"Below is an instruction that describes a task, paired with an input that provides further context. "
"Write a response that appropriately completes the request.\n\n\n\n"
"### Instruction:\n{instruction}\n\n"
"### Input:\n{input}\n\n"
"### Response:\n{output}"
)
prompt = prompt_template.format(instruction=instruction, input=input, output='')
torch_dtype = torch.bfloat16
model_id = "llm4fun/vietrag-7b-v1.0"
device = "cuda"
tokenizer = LlamaTokenizer.from_pretrained(model_id)
model = LlamaForCausalLM.from_pretrained(
model_id,
config=LlamaConfig.from_pretrained(model_id),
torch_dtype=torch_dtype
)
model = model.eval().to(device)
def generate(prompt, max_new_tokens=1024):
input_ids = tokenizer(prompt, return_tensors="pt")["input_ids"].to(model.device)
model.eval()
with torch.no_grad():
generation_config = GenerationConfig(
repetition_penalty=1.13,
max_new_tokens=max_new_tokens,
# temperature=0.2,
# top_p=0.95,
# top_k=20,
# bos_token_id=tokenizer.bos_token_id,
# eos_token_id=tokenizer.eos_token_id,
# eos_token_id=0, # for open-end generation.
pad_token_id=tokenizer.pad_token_id,
do_sample=False,
use_cache=True,
return_dict_in_generate=True,
output_attentions=False,
output_hidden_states=False,
output_scores=False,
)
streamer = TextStreamer(tokenizer, skip_prompt=True)
generated = model.generate(
inputs=input_ids,
generation_config=generation_config,
streamer=streamer,
)
gen_tokens = generated["sequences"].cpu()[:, len(input_ids[0]):]
output = tokenizer.batch_decode(gen_tokens)[0]
output = output.split(tokenizer.eos_token)[0]
return output.strip()
output = generate(prompt)
```
To tweak the model's answering style, feel free to replace the `instruction` part of the prompt. I reccommend you select one of these following instructions, because they are used during training.
```py
instructions = [
'You are an AI assistant. Provide a detailed answer so user don’t need to search outside to understand the answer.',
'You are an AI assistant. You will be given a task. You must generate a detailed and long answer.',
'You are an AI assistant. User will you give you a task. Your goal is to complete the task as faithfully as you can. While performing the task think step-by-step and justify your steps.',
'You are an smart assistant. Provide a direct, short and exact answer to the following question from its provided context.'
]
```
|
timm/resnet101.tv_in1k | timm | 2024-02-10T23:39:58Z | 12,174 | 0 | timm | [
"timm",
"pytorch",
"safetensors",
"image-classification",
"arxiv:1512.03385",
"license:bsd-3-clause",
"region:us"
]
| image-classification | 2023-04-05T18:23:30Z | ---
license: bsd-3-clause
library_name: timm
tags:
- image-classification
- timm
---
# Model card for resnet101.tv_in1k
A ResNet-B image classification model.
This model features:
* ReLU activations
* single layer 7x7 convolution with pooling
* 1x1 convolution shortcut downsample
Trained on ImageNet-1k, original torchvision model weight.
## Model Details
- **Model Type:** Image classification / feature backbone
- **Model Stats:**
- Params (M): 44.5
- GMACs: 7.8
- Activations (M): 16.2
- Image size: 224 x 224
- **Papers:**
- Deep Residual Learning for Image Recognition: https://arxiv.org/abs/1512.03385
- **Original:** https://github.com/pytorch/vision
## Model Usage
### Image Classification
```python
from urllib.request import urlopen
from PIL import Image
import timm
img = Image.open(urlopen(
'https://huggingface.co/datasets/huggingface/documentation-images/resolve/main/beignets-task-guide.png'
))
model = timm.create_model('resnet101.tv_in1k', pretrained=True)
model = model.eval()
# get model specific transforms (normalization, resize)
data_config = timm.data.resolve_model_data_config(model)
transforms = timm.data.create_transform(**data_config, is_training=False)
output = model(transforms(img).unsqueeze(0)) # unsqueeze single image into batch of 1
top5_probabilities, top5_class_indices = torch.topk(output.softmax(dim=1) * 100, k=5)
```
### Feature Map Extraction
```python
from urllib.request import urlopen
from PIL import Image
import timm
img = Image.open(urlopen(
'https://huggingface.co/datasets/huggingface/documentation-images/resolve/main/beignets-task-guide.png'
))
model = timm.create_model(
'resnet101.tv_in1k',
pretrained=True,
features_only=True,
)
model = model.eval()
# get model specific transforms (normalization, resize)
data_config = timm.data.resolve_model_data_config(model)
transforms = timm.data.create_transform(**data_config, is_training=False)
output = model(transforms(img).unsqueeze(0)) # unsqueeze single image into batch of 1
for o in output:
# print shape of each feature map in output
# e.g.:
# torch.Size([1, 64, 112, 112])
# torch.Size([1, 256, 56, 56])
# torch.Size([1, 512, 28, 28])
# torch.Size([1, 1024, 14, 14])
# torch.Size([1, 2048, 7, 7])
print(o.shape)
```
### Image Embeddings
```python
from urllib.request import urlopen
from PIL import Image
import timm
img = Image.open(urlopen(
'https://huggingface.co/datasets/huggingface/documentation-images/resolve/main/beignets-task-guide.png'
))
model = timm.create_model(
'resnet101.tv_in1k',
pretrained=True,
num_classes=0, # remove classifier nn.Linear
)
model = model.eval()
# get model specific transforms (normalization, resize)
data_config = timm.data.resolve_model_data_config(model)
transforms = timm.data.create_transform(**data_config, is_training=False)
output = model(transforms(img).unsqueeze(0)) # output is (batch_size, num_features) shaped tensor
# or equivalently (without needing to set num_classes=0)
output = model.forward_features(transforms(img).unsqueeze(0))
# output is unpooled, a (1, 2048, 7, 7) shaped tensor
output = model.forward_head(output, pre_logits=True)
# output is a (1, num_features) shaped tensor
```
## Model Comparison
Explore the dataset and runtime metrics of this model in timm [model results](https://github.com/huggingface/pytorch-image-models/tree/main/results).
|model |img_size|top1 |top5 |param_count|gmacs|macts|img/sec|
|------------------------------------------|--------|-----|-----|-----------|-----|-----|-------|
|[seresnextaa101d_32x8d.sw_in12k_ft_in1k_288](https://huggingface.co/timm/seresnextaa101d_32x8d.sw_in12k_ft_in1k_288)|320 |86.72|98.17|93.6 |35.2 |69.7 |451 |
|[seresnextaa101d_32x8d.sw_in12k_ft_in1k_288](https://huggingface.co/timm/seresnextaa101d_32x8d.sw_in12k_ft_in1k_288)|288 |86.51|98.08|93.6 |28.5 |56.4 |560 |
|[seresnextaa101d_32x8d.sw_in12k_ft_in1k](https://huggingface.co/timm/seresnextaa101d_32x8d.sw_in12k_ft_in1k)|288 |86.49|98.03|93.6 |28.5 |56.4 |557 |
|[seresnextaa101d_32x8d.sw_in12k_ft_in1k](https://huggingface.co/timm/seresnextaa101d_32x8d.sw_in12k_ft_in1k)|224 |85.96|97.82|93.6 |17.2 |34.2 |923 |
|[resnext101_32x32d.fb_wsl_ig1b_ft_in1k](https://huggingface.co/timm/resnext101_32x32d.fb_wsl_ig1b_ft_in1k)|224 |85.11|97.44|468.5 |87.3 |91.1 |254 |
|[resnetrs420.tf_in1k](https://huggingface.co/timm/resnetrs420.tf_in1k)|416 |85.0 |97.12|191.9 |108.4|213.8|134 |
|[ecaresnet269d.ra2_in1k](https://huggingface.co/timm/ecaresnet269d.ra2_in1k)|352 |84.96|97.22|102.1 |50.2 |101.2|291 |
|[ecaresnet269d.ra2_in1k](https://huggingface.co/timm/ecaresnet269d.ra2_in1k)|320 |84.73|97.18|102.1 |41.5 |83.7 |353 |
|[resnetrs350.tf_in1k](https://huggingface.co/timm/resnetrs350.tf_in1k)|384 |84.71|96.99|164.0 |77.6 |154.7|183 |
|[seresnextaa101d_32x8d.ah_in1k](https://huggingface.co/timm/seresnextaa101d_32x8d.ah_in1k)|288 |84.57|97.08|93.6 |28.5 |56.4 |557 |
|[resnetrs200.tf_in1k](https://huggingface.co/timm/resnetrs200.tf_in1k)|320 |84.45|97.08|93.2 |31.5 |67.8 |446 |
|[resnetrs270.tf_in1k](https://huggingface.co/timm/resnetrs270.tf_in1k)|352 |84.43|96.97|129.9 |51.1 |105.5|280 |
|[seresnext101d_32x8d.ah_in1k](https://huggingface.co/timm/seresnext101d_32x8d.ah_in1k)|288 |84.36|96.92|93.6 |27.6 |53.0 |595 |
|[seresnet152d.ra2_in1k](https://huggingface.co/timm/seresnet152d.ra2_in1k)|320 |84.35|97.04|66.8 |24.1 |47.7 |610 |
|[resnetrs350.tf_in1k](https://huggingface.co/timm/resnetrs350.tf_in1k)|288 |84.3 |96.94|164.0 |43.7 |87.1 |333 |
|[resnext101_32x8d.fb_swsl_ig1b_ft_in1k](https://huggingface.co/timm/resnext101_32x8d.fb_swsl_ig1b_ft_in1k)|224 |84.28|97.17|88.8 |16.5 |31.2 |1100 |
|[resnetrs420.tf_in1k](https://huggingface.co/timm/resnetrs420.tf_in1k)|320 |84.24|96.86|191.9 |64.2 |126.6|228 |
|[seresnext101_32x8d.ah_in1k](https://huggingface.co/timm/seresnext101_32x8d.ah_in1k)|288 |84.19|96.87|93.6 |27.2 |51.6 |613 |
|[resnext101_32x16d.fb_wsl_ig1b_ft_in1k](https://huggingface.co/timm/resnext101_32x16d.fb_wsl_ig1b_ft_in1k)|224 |84.18|97.19|194.0 |36.3 |51.2 |581 |
|[resnetaa101d.sw_in12k_ft_in1k](https://huggingface.co/timm/resnetaa101d.sw_in12k_ft_in1k)|288 |84.11|97.11|44.6 |15.1 |29.0 |1144 |
|[resnet200d.ra2_in1k](https://huggingface.co/timm/resnet200d.ra2_in1k)|320 |83.97|96.82|64.7 |31.2 |67.3 |518 |
|[resnetrs200.tf_in1k](https://huggingface.co/timm/resnetrs200.tf_in1k)|256 |83.87|96.75|93.2 |20.2 |43.4 |692 |
|[seresnextaa101d_32x8d.ah_in1k](https://huggingface.co/timm/seresnextaa101d_32x8d.ah_in1k)|224 |83.86|96.65|93.6 |17.2 |34.2 |923 |
|[resnetrs152.tf_in1k](https://huggingface.co/timm/resnetrs152.tf_in1k)|320 |83.72|96.61|86.6 |24.3 |48.1 |617 |
|[seresnet152d.ra2_in1k](https://huggingface.co/timm/seresnet152d.ra2_in1k)|256 |83.69|96.78|66.8 |15.4 |30.6 |943 |
|[seresnext101d_32x8d.ah_in1k](https://huggingface.co/timm/seresnext101d_32x8d.ah_in1k)|224 |83.68|96.61|93.6 |16.7 |32.0 |986 |
|[resnet152d.ra2_in1k](https://huggingface.co/timm/resnet152d.ra2_in1k)|320 |83.67|96.74|60.2 |24.1 |47.7 |706 |
|[resnetrs270.tf_in1k](https://huggingface.co/timm/resnetrs270.tf_in1k)|256 |83.59|96.61|129.9 |27.1 |55.8 |526 |
|[seresnext101_32x8d.ah_in1k](https://huggingface.co/timm/seresnext101_32x8d.ah_in1k)|224 |83.58|96.4 |93.6 |16.5 |31.2 |1013 |
|[resnetaa101d.sw_in12k_ft_in1k](https://huggingface.co/timm/resnetaa101d.sw_in12k_ft_in1k)|224 |83.54|96.83|44.6 |9.1 |17.6 |1864 |
|[resnet152.a1h_in1k](https://huggingface.co/timm/resnet152.a1h_in1k)|288 |83.46|96.54|60.2 |19.1 |37.3 |904 |
|[resnext101_32x16d.fb_swsl_ig1b_ft_in1k](https://huggingface.co/timm/resnext101_32x16d.fb_swsl_ig1b_ft_in1k)|224 |83.35|96.85|194.0 |36.3 |51.2 |582 |
|[resnet200d.ra2_in1k](https://huggingface.co/timm/resnet200d.ra2_in1k)|256 |83.23|96.53|64.7 |20.0 |43.1 |809 |
|[resnext101_32x4d.fb_swsl_ig1b_ft_in1k](https://huggingface.co/timm/resnext101_32x4d.fb_swsl_ig1b_ft_in1k)|224 |83.22|96.75|44.2 |8.0 |21.2 |1814 |
|[resnext101_64x4d.c1_in1k](https://huggingface.co/timm/resnext101_64x4d.c1_in1k)|288 |83.16|96.38|83.5 |25.7 |51.6 |590 |
|[resnet152d.ra2_in1k](https://huggingface.co/timm/resnet152d.ra2_in1k)|256 |83.14|96.38|60.2 |15.4 |30.5 |1096 |
|[resnet101d.ra2_in1k](https://huggingface.co/timm/resnet101d.ra2_in1k)|320 |83.02|96.45|44.6 |16.5 |34.8 |992 |
|[ecaresnet101d.miil_in1k](https://huggingface.co/timm/ecaresnet101d.miil_in1k)|288 |82.98|96.54|44.6 |13.4 |28.2 |1077 |
|[resnext101_64x4d.tv_in1k](https://huggingface.co/timm/resnext101_64x4d.tv_in1k)|224 |82.98|96.25|83.5 |15.5 |31.2 |989 |
|[resnetrs152.tf_in1k](https://huggingface.co/timm/resnetrs152.tf_in1k)|256 |82.86|96.28|86.6 |15.6 |30.8 |951 |
|[resnext101_32x8d.tv2_in1k](https://huggingface.co/timm/resnext101_32x8d.tv2_in1k)|224 |82.83|96.22|88.8 |16.5 |31.2 |1099 |
|[resnet152.a1h_in1k](https://huggingface.co/timm/resnet152.a1h_in1k)|224 |82.8 |96.13|60.2 |11.6 |22.6 |1486 |
|[resnet101.a1h_in1k](https://huggingface.co/timm/resnet101.a1h_in1k)|288 |82.8 |96.32|44.6 |13.0 |26.8 |1291 |
|[resnet152.a1_in1k](https://huggingface.co/timm/resnet152.a1_in1k)|288 |82.74|95.71|60.2 |19.1 |37.3 |905 |
|[resnext101_32x8d.fb_wsl_ig1b_ft_in1k](https://huggingface.co/timm/resnext101_32x8d.fb_wsl_ig1b_ft_in1k)|224 |82.69|96.63|88.8 |16.5 |31.2 |1100 |
|[resnet152.a2_in1k](https://huggingface.co/timm/resnet152.a2_in1k)|288 |82.62|95.75|60.2 |19.1 |37.3 |904 |
|[resnetaa50d.sw_in12k_ft_in1k](https://huggingface.co/timm/resnetaa50d.sw_in12k_ft_in1k)|288 |82.61|96.49|25.6 |8.9 |20.6 |1729 |
|[resnet61q.ra2_in1k](https://huggingface.co/timm/resnet61q.ra2_in1k)|288 |82.53|96.13|36.8 |9.9 |21.5 |1773 |
|[wide_resnet101_2.tv2_in1k](https://huggingface.co/timm/wide_resnet101_2.tv2_in1k)|224 |82.5 |96.02|126.9 |22.8 |21.2 |1078 |
|[resnext101_64x4d.c1_in1k](https://huggingface.co/timm/resnext101_64x4d.c1_in1k)|224 |82.46|95.92|83.5 |15.5 |31.2 |987 |
|[resnet51q.ra2_in1k](https://huggingface.co/timm/resnet51q.ra2_in1k)|288 |82.36|96.18|35.7 |8.1 |20.9 |1964 |
|[ecaresnet50t.ra2_in1k](https://huggingface.co/timm/ecaresnet50t.ra2_in1k)|320 |82.35|96.14|25.6 |8.8 |24.1 |1386 |
|[resnet101.a1_in1k](https://huggingface.co/timm/resnet101.a1_in1k)|288 |82.31|95.63|44.6 |13.0 |26.8 |1291 |
|[resnetrs101.tf_in1k](https://huggingface.co/timm/resnetrs101.tf_in1k)|288 |82.29|96.01|63.6 |13.6 |28.5 |1078 |
|[resnet152.tv2_in1k](https://huggingface.co/timm/resnet152.tv2_in1k)|224 |82.29|96.0 |60.2 |11.6 |22.6 |1484 |
|[wide_resnet50_2.racm_in1k](https://huggingface.co/timm/wide_resnet50_2.racm_in1k)|288 |82.27|96.06|68.9 |18.9 |23.8 |1176 |
|[resnet101d.ra2_in1k](https://huggingface.co/timm/resnet101d.ra2_in1k)|256 |82.26|96.07|44.6 |10.6 |22.2 |1542 |
|[resnet101.a2_in1k](https://huggingface.co/timm/resnet101.a2_in1k)|288 |82.24|95.73|44.6 |13.0 |26.8 |1290 |
|[seresnext50_32x4d.racm_in1k](https://huggingface.co/timm/seresnext50_32x4d.racm_in1k)|288 |82.2 |96.14|27.6 |7.0 |23.8 |1547 |
|[ecaresnet101d.miil_in1k](https://huggingface.co/timm/ecaresnet101d.miil_in1k)|224 |82.18|96.05|44.6 |8.1 |17.1 |1771 |
|[resnext50_32x4d.fb_swsl_ig1b_ft_in1k](https://huggingface.co/timm/resnext50_32x4d.fb_swsl_ig1b_ft_in1k)|224 |82.17|96.22|25.0 |4.3 |14.4 |2943 |
|[ecaresnet50t.a1_in1k](https://huggingface.co/timm/ecaresnet50t.a1_in1k)|288 |82.12|95.65|25.6 |7.1 |19.6 |1704 |
|[resnext50_32x4d.a1h_in1k](https://huggingface.co/timm/resnext50_32x4d.a1h_in1k)|288 |82.03|95.94|25.0 |7.0 |23.8 |1745 |
|[ecaresnet101d_pruned.miil_in1k](https://huggingface.co/timm/ecaresnet101d_pruned.miil_in1k)|288 |82.0 |96.15|24.9 |5.8 |12.7 |1787 |
|[resnet61q.ra2_in1k](https://huggingface.co/timm/resnet61q.ra2_in1k)|256 |81.99|95.85|36.8 |7.8 |17.0 |2230 |
|[resnext101_32x8d.tv2_in1k](https://huggingface.co/timm/resnext101_32x8d.tv2_in1k)|176 |81.98|95.72|88.8 |10.3 |19.4 |1768 |
|[resnet152.a1_in1k](https://huggingface.co/timm/resnet152.a1_in1k)|224 |81.97|95.24|60.2 |11.6 |22.6 |1486 |
|[resnet101.a1h_in1k](https://huggingface.co/timm/resnet101.a1h_in1k)|224 |81.93|95.75|44.6 |7.8 |16.2 |2122 |
|[resnet101.tv2_in1k](https://huggingface.co/timm/resnet101.tv2_in1k)|224 |81.9 |95.77|44.6 |7.8 |16.2 |2118 |
|[resnext101_32x16d.fb_ssl_yfcc100m_ft_in1k](https://huggingface.co/timm/resnext101_32x16d.fb_ssl_yfcc100m_ft_in1k)|224 |81.84|96.1 |194.0 |36.3 |51.2 |583 |
|[resnet51q.ra2_in1k](https://huggingface.co/timm/resnet51q.ra2_in1k)|256 |81.78|95.94|35.7 |6.4 |16.6 |2471 |
|[resnet152.a2_in1k](https://huggingface.co/timm/resnet152.a2_in1k)|224 |81.77|95.22|60.2 |11.6 |22.6 |1485 |
|[resnetaa50d.sw_in12k_ft_in1k](https://huggingface.co/timm/resnetaa50d.sw_in12k_ft_in1k)|224 |81.74|96.06|25.6 |5.4 |12.4 |2813 |
|[ecaresnet50t.a2_in1k](https://huggingface.co/timm/ecaresnet50t.a2_in1k)|288 |81.65|95.54|25.6 |7.1 |19.6 |1703 |
|[ecaresnet50d.miil_in1k](https://huggingface.co/timm/ecaresnet50d.miil_in1k)|288 |81.64|95.88|25.6 |7.2 |19.7 |1694 |
|[resnext101_32x8d.fb_ssl_yfcc100m_ft_in1k](https://huggingface.co/timm/resnext101_32x8d.fb_ssl_yfcc100m_ft_in1k)|224 |81.62|96.04|88.8 |16.5 |31.2 |1101 |
|[wide_resnet50_2.tv2_in1k](https://huggingface.co/timm/wide_resnet50_2.tv2_in1k)|224 |81.61|95.76|68.9 |11.4 |14.4 |1930 |
|[resnetaa50.a1h_in1k](https://huggingface.co/timm/resnetaa50.a1h_in1k)|288 |81.61|95.83|25.6 |8.5 |19.2 |1868 |
|[resnet101.a1_in1k](https://huggingface.co/timm/resnet101.a1_in1k)|224 |81.5 |95.16|44.6 |7.8 |16.2 |2125 |
|[resnext50_32x4d.a1_in1k](https://huggingface.co/timm/resnext50_32x4d.a1_in1k)|288 |81.48|95.16|25.0 |7.0 |23.8 |1745 |
|[gcresnet50t.ra2_in1k](https://huggingface.co/timm/gcresnet50t.ra2_in1k)|288 |81.47|95.71|25.9 |6.9 |18.6 |2071 |
|[wide_resnet50_2.racm_in1k](https://huggingface.co/timm/wide_resnet50_2.racm_in1k)|224 |81.45|95.53|68.9 |11.4 |14.4 |1929 |
|[resnet50d.a1_in1k](https://huggingface.co/timm/resnet50d.a1_in1k)|288 |81.44|95.22|25.6 |7.2 |19.7 |1908 |
|[ecaresnet50t.ra2_in1k](https://huggingface.co/timm/ecaresnet50t.ra2_in1k)|256 |81.44|95.67|25.6 |5.6 |15.4 |2168 |
|[ecaresnetlight.miil_in1k](https://huggingface.co/timm/ecaresnetlight.miil_in1k)|288 |81.4 |95.82|30.2 |6.8 |13.9 |2132 |
|[resnet50d.ra2_in1k](https://huggingface.co/timm/resnet50d.ra2_in1k)|288 |81.37|95.74|25.6 |7.2 |19.7 |1910 |
|[resnet101.a2_in1k](https://huggingface.co/timm/resnet101.a2_in1k)|224 |81.32|95.19|44.6 |7.8 |16.2 |2125 |
|[seresnet50.ra2_in1k](https://huggingface.co/timm/seresnet50.ra2_in1k)|288 |81.3 |95.65|28.1 |6.8 |18.4 |1803 |
|[resnext50_32x4d.a2_in1k](https://huggingface.co/timm/resnext50_32x4d.a2_in1k)|288 |81.3 |95.11|25.0 |7.0 |23.8 |1746 |
|[seresnext50_32x4d.racm_in1k](https://huggingface.co/timm/seresnext50_32x4d.racm_in1k)|224 |81.27|95.62|27.6 |4.3 |14.4 |2591 |
|[ecaresnet50t.a1_in1k](https://huggingface.co/timm/ecaresnet50t.a1_in1k)|224 |81.26|95.16|25.6 |4.3 |11.8 |2823 |
|[gcresnext50ts.ch_in1k](https://huggingface.co/timm/gcresnext50ts.ch_in1k)|288 |81.23|95.54|15.7 |4.8 |19.6 |2117 |
|[senet154.gluon_in1k](https://huggingface.co/timm/senet154.gluon_in1k)|224 |81.23|95.35|115.1 |20.8 |38.7 |545 |
|[resnet50.a1_in1k](https://huggingface.co/timm/resnet50.a1_in1k)|288 |81.22|95.11|25.6 |6.8 |18.4 |2089 |
|[resnet50_gn.a1h_in1k](https://huggingface.co/timm/resnet50_gn.a1h_in1k)|288 |81.22|95.63|25.6 |6.8 |18.4 |676 |
|[resnet50d.a2_in1k](https://huggingface.co/timm/resnet50d.a2_in1k)|288 |81.18|95.09|25.6 |7.2 |19.7 |1908 |
|[resnet50.fb_swsl_ig1b_ft_in1k](https://huggingface.co/timm/resnet50.fb_swsl_ig1b_ft_in1k)|224 |81.18|95.98|25.6 |4.1 |11.1 |3455 |
|[resnext50_32x4d.tv2_in1k](https://huggingface.co/timm/resnext50_32x4d.tv2_in1k)|224 |81.17|95.34|25.0 |4.3 |14.4 |2933 |
|[resnext50_32x4d.a1h_in1k](https://huggingface.co/timm/resnext50_32x4d.a1h_in1k)|224 |81.1 |95.33|25.0 |4.3 |14.4 |2934 |
|[seresnet50.a2_in1k](https://huggingface.co/timm/seresnet50.a2_in1k)|288 |81.1 |95.23|28.1 |6.8 |18.4 |1801 |
|[seresnet50.a1_in1k](https://huggingface.co/timm/seresnet50.a1_in1k)|288 |81.1 |95.12|28.1 |6.8 |18.4 |1799 |
|[resnet152s.gluon_in1k](https://huggingface.co/timm/resnet152s.gluon_in1k)|224 |81.02|95.41|60.3 |12.9 |25.0 |1347 |
|[resnet50.d_in1k](https://huggingface.co/timm/resnet50.d_in1k)|288 |80.97|95.44|25.6 |6.8 |18.4 |2085 |
|[gcresnet50t.ra2_in1k](https://huggingface.co/timm/gcresnet50t.ra2_in1k)|256 |80.94|95.45|25.9 |5.4 |14.7 |2571 |
|[resnext101_32x4d.fb_ssl_yfcc100m_ft_in1k](https://huggingface.co/timm/resnext101_32x4d.fb_ssl_yfcc100m_ft_in1k)|224 |80.93|95.73|44.2 |8.0 |21.2 |1814 |
|[resnet50.c1_in1k](https://huggingface.co/timm/resnet50.c1_in1k)|288 |80.91|95.55|25.6 |6.8 |18.4 |2084 |
|[seresnext101_32x4d.gluon_in1k](https://huggingface.co/timm/seresnext101_32x4d.gluon_in1k)|224 |80.9 |95.31|49.0 |8.0 |21.3 |1585 |
|[seresnext101_64x4d.gluon_in1k](https://huggingface.co/timm/seresnext101_64x4d.gluon_in1k)|224 |80.9 |95.3 |88.2 |15.5 |31.2 |918 |
|[resnet50.c2_in1k](https://huggingface.co/timm/resnet50.c2_in1k)|288 |80.86|95.52|25.6 |6.8 |18.4 |2085 |
|[resnet50.tv2_in1k](https://huggingface.co/timm/resnet50.tv2_in1k)|224 |80.85|95.43|25.6 |4.1 |11.1 |3450 |
|[ecaresnet50t.a2_in1k](https://huggingface.co/timm/ecaresnet50t.a2_in1k)|224 |80.84|95.02|25.6 |4.3 |11.8 |2821 |
|[ecaresnet101d_pruned.miil_in1k](https://huggingface.co/timm/ecaresnet101d_pruned.miil_in1k)|224 |80.79|95.62|24.9 |3.5 |7.7 |2961 |
|[seresnet33ts.ra2_in1k](https://huggingface.co/timm/seresnet33ts.ra2_in1k)|288 |80.79|95.36|19.8 |6.0 |14.8 |2506 |
|[ecaresnet50d_pruned.miil_in1k](https://huggingface.co/timm/ecaresnet50d_pruned.miil_in1k)|288 |80.79|95.58|19.9 |4.2 |10.6 |2349 |
|[resnet50.a2_in1k](https://huggingface.co/timm/resnet50.a2_in1k)|288 |80.78|94.99|25.6 |6.8 |18.4 |2088 |
|[resnet50.b1k_in1k](https://huggingface.co/timm/resnet50.b1k_in1k)|288 |80.71|95.43|25.6 |6.8 |18.4 |2087 |
|[resnext50_32x4d.ra_in1k](https://huggingface.co/timm/resnext50_32x4d.ra_in1k)|288 |80.7 |95.39|25.0 |7.0 |23.8 |1749 |
|[resnetrs101.tf_in1k](https://huggingface.co/timm/resnetrs101.tf_in1k)|192 |80.69|95.24|63.6 |6.0 |12.7 |2270 |
|[resnet50d.a1_in1k](https://huggingface.co/timm/resnet50d.a1_in1k)|224 |80.68|94.71|25.6 |4.4 |11.9 |3162 |
|[eca_resnet33ts.ra2_in1k](https://huggingface.co/timm/eca_resnet33ts.ra2_in1k)|288 |80.68|95.36|19.7 |6.0 |14.8 |2637 |
|[resnet50.a1h_in1k](https://huggingface.co/timm/resnet50.a1h_in1k)|224 |80.67|95.3 |25.6 |4.1 |11.1 |3452 |
|[resnext50d_32x4d.bt_in1k](https://huggingface.co/timm/resnext50d_32x4d.bt_in1k)|288 |80.67|95.42|25.0 |7.4 |25.1 |1626 |
|[resnetaa50.a1h_in1k](https://huggingface.co/timm/resnetaa50.a1h_in1k)|224 |80.63|95.21|25.6 |5.2 |11.6 |3034 |
|[ecaresnet50d.miil_in1k](https://huggingface.co/timm/ecaresnet50d.miil_in1k)|224 |80.61|95.32|25.6 |4.4 |11.9 |2813 |
|[resnext101_64x4d.gluon_in1k](https://huggingface.co/timm/resnext101_64x4d.gluon_in1k)|224 |80.61|94.99|83.5 |15.5 |31.2 |989 |
|[gcresnet33ts.ra2_in1k](https://huggingface.co/timm/gcresnet33ts.ra2_in1k)|288 |80.6 |95.31|19.9 |6.0 |14.8 |2578 |
|[gcresnext50ts.ch_in1k](https://huggingface.co/timm/gcresnext50ts.ch_in1k)|256 |80.57|95.17|15.7 |3.8 |15.5 |2710 |
|[resnet152.a3_in1k](https://huggingface.co/timm/resnet152.a3_in1k)|224 |80.56|95.0 |60.2 |11.6 |22.6 |1483 |
|[resnet50d.ra2_in1k](https://huggingface.co/timm/resnet50d.ra2_in1k)|224 |80.53|95.16|25.6 |4.4 |11.9 |3164 |
|[resnext50_32x4d.a1_in1k](https://huggingface.co/timm/resnext50_32x4d.a1_in1k)|224 |80.53|94.46|25.0 |4.3 |14.4 |2930 |
|[wide_resnet101_2.tv2_in1k](https://huggingface.co/timm/wide_resnet101_2.tv2_in1k)|176 |80.48|94.98|126.9 |14.3 |13.2 |1719 |
|[resnet152d.gluon_in1k](https://huggingface.co/timm/resnet152d.gluon_in1k)|224 |80.47|95.2 |60.2 |11.8 |23.4 |1428 |
|[resnet50.b2k_in1k](https://huggingface.co/timm/resnet50.b2k_in1k)|288 |80.45|95.32|25.6 |6.8 |18.4 |2086 |
|[ecaresnetlight.miil_in1k](https://huggingface.co/timm/ecaresnetlight.miil_in1k)|224 |80.45|95.24|30.2 |4.1 |8.4 |3530 |
|[resnext50_32x4d.a2_in1k](https://huggingface.co/timm/resnext50_32x4d.a2_in1k)|224 |80.45|94.63|25.0 |4.3 |14.4 |2936 |
|[wide_resnet50_2.tv2_in1k](https://huggingface.co/timm/wide_resnet50_2.tv2_in1k)|176 |80.43|95.09|68.9 |7.3 |9.0 |3015 |
|[resnet101d.gluon_in1k](https://huggingface.co/timm/resnet101d.gluon_in1k)|224 |80.42|95.01|44.6 |8.1 |17.0 |2007 |
|[resnet50.a1_in1k](https://huggingface.co/timm/resnet50.a1_in1k)|224 |80.38|94.6 |25.6 |4.1 |11.1 |3461 |
|[seresnet33ts.ra2_in1k](https://huggingface.co/timm/seresnet33ts.ra2_in1k)|256 |80.36|95.1 |19.8 |4.8 |11.7 |3267 |
|[resnext101_32x4d.gluon_in1k](https://huggingface.co/timm/resnext101_32x4d.gluon_in1k)|224 |80.34|94.93|44.2 |8.0 |21.2 |1814 |
|[resnext50_32x4d.fb_ssl_yfcc100m_ft_in1k](https://huggingface.co/timm/resnext50_32x4d.fb_ssl_yfcc100m_ft_in1k)|224 |80.32|95.4 |25.0 |4.3 |14.4 |2941 |
|[resnet101s.gluon_in1k](https://huggingface.co/timm/resnet101s.gluon_in1k)|224 |80.28|95.16|44.7 |9.2 |18.6 |1851 |
|[seresnet50.ra2_in1k](https://huggingface.co/timm/seresnet50.ra2_in1k)|224 |80.26|95.08|28.1 |4.1 |11.1 |2972 |
|[resnetblur50.bt_in1k](https://huggingface.co/timm/resnetblur50.bt_in1k)|288 |80.24|95.24|25.6 |8.5 |19.9 |1523 |
|[resnet50d.a2_in1k](https://huggingface.co/timm/resnet50d.a2_in1k)|224 |80.22|94.63|25.6 |4.4 |11.9 |3162 |
|[resnet152.tv2_in1k](https://huggingface.co/timm/resnet152.tv2_in1k)|176 |80.2 |94.64|60.2 |7.2 |14.0 |2346 |
|[seresnet50.a2_in1k](https://huggingface.co/timm/seresnet50.a2_in1k)|224 |80.08|94.74|28.1 |4.1 |11.1 |2969 |
|[eca_resnet33ts.ra2_in1k](https://huggingface.co/timm/eca_resnet33ts.ra2_in1k)|256 |80.08|94.97|19.7 |4.8 |11.7 |3284 |
|[gcresnet33ts.ra2_in1k](https://huggingface.co/timm/gcresnet33ts.ra2_in1k)|256 |80.06|94.99|19.9 |4.8 |11.7 |3216 |
|[resnet50_gn.a1h_in1k](https://huggingface.co/timm/resnet50_gn.a1h_in1k)|224 |80.06|94.95|25.6 |4.1 |11.1 |1109 |
|[seresnet50.a1_in1k](https://huggingface.co/timm/seresnet50.a1_in1k)|224 |80.02|94.71|28.1 |4.1 |11.1 |2962 |
|[resnet50.ram_in1k](https://huggingface.co/timm/resnet50.ram_in1k)|288 |79.97|95.05|25.6 |6.8 |18.4 |2086 |
|[resnet152c.gluon_in1k](https://huggingface.co/timm/resnet152c.gluon_in1k)|224 |79.92|94.84|60.2 |11.8 |23.4 |1455 |
|[seresnext50_32x4d.gluon_in1k](https://huggingface.co/timm/seresnext50_32x4d.gluon_in1k)|224 |79.91|94.82|27.6 |4.3 |14.4 |2591 |
|[resnet50.d_in1k](https://huggingface.co/timm/resnet50.d_in1k)|224 |79.91|94.67|25.6 |4.1 |11.1 |3456 |
|[resnet101.tv2_in1k](https://huggingface.co/timm/resnet101.tv2_in1k)|176 |79.9 |94.6 |44.6 |4.9 |10.1 |3341 |
|[resnetrs50.tf_in1k](https://huggingface.co/timm/resnetrs50.tf_in1k)|224 |79.89|94.97|35.7 |4.5 |12.1 |2774 |
|[resnet50.c2_in1k](https://huggingface.co/timm/resnet50.c2_in1k)|224 |79.88|94.87|25.6 |4.1 |11.1 |3455 |
|[ecaresnet26t.ra2_in1k](https://huggingface.co/timm/ecaresnet26t.ra2_in1k)|320 |79.86|95.07|16.0 |5.2 |16.4 |2168 |
|[resnet50.a2_in1k](https://huggingface.co/timm/resnet50.a2_in1k)|224 |79.85|94.56|25.6 |4.1 |11.1 |3460 |
|[resnet50.ra_in1k](https://huggingface.co/timm/resnet50.ra_in1k)|288 |79.83|94.97|25.6 |6.8 |18.4 |2087 |
|[resnet101.a3_in1k](https://huggingface.co/timm/resnet101.a3_in1k)|224 |79.82|94.62|44.6 |7.8 |16.2 |2114 |
|[resnext50_32x4d.ra_in1k](https://huggingface.co/timm/resnext50_32x4d.ra_in1k)|224 |79.76|94.6 |25.0 |4.3 |14.4 |2943 |
|[resnet50.c1_in1k](https://huggingface.co/timm/resnet50.c1_in1k)|224 |79.74|94.95|25.6 |4.1 |11.1 |3455 |
|[ecaresnet50d_pruned.miil_in1k](https://huggingface.co/timm/ecaresnet50d_pruned.miil_in1k)|224 |79.74|94.87|19.9 |2.5 |6.4 |3929 |
|[resnet33ts.ra2_in1k](https://huggingface.co/timm/resnet33ts.ra2_in1k)|288 |79.71|94.83|19.7 |6.0 |14.8 |2710 |
|[resnet152.gluon_in1k](https://huggingface.co/timm/resnet152.gluon_in1k)|224 |79.68|94.74|60.2 |11.6 |22.6 |1486 |
|[resnext50d_32x4d.bt_in1k](https://huggingface.co/timm/resnext50d_32x4d.bt_in1k)|224 |79.67|94.87|25.0 |4.5 |15.2 |2729 |
|[resnet50.bt_in1k](https://huggingface.co/timm/resnet50.bt_in1k)|288 |79.63|94.91|25.6 |6.8 |18.4 |2086 |
|[ecaresnet50t.a3_in1k](https://huggingface.co/timm/ecaresnet50t.a3_in1k)|224 |79.56|94.72|25.6 |4.3 |11.8 |2805 |
|[resnet101c.gluon_in1k](https://huggingface.co/timm/resnet101c.gluon_in1k)|224 |79.53|94.58|44.6 |8.1 |17.0 |2062 |
|[resnet50.b1k_in1k](https://huggingface.co/timm/resnet50.b1k_in1k)|224 |79.52|94.61|25.6 |4.1 |11.1 |3459 |
|[resnet50.tv2_in1k](https://huggingface.co/timm/resnet50.tv2_in1k)|176 |79.42|94.64|25.6 |2.6 |6.9 |5397 |
|[resnet32ts.ra2_in1k](https://huggingface.co/timm/resnet32ts.ra2_in1k)|288 |79.4 |94.66|18.0 |5.9 |14.6 |2752 |
|[resnet50.b2k_in1k](https://huggingface.co/timm/resnet50.b2k_in1k)|224 |79.38|94.57|25.6 |4.1 |11.1 |3459 |
|[resnext50_32x4d.tv2_in1k](https://huggingface.co/timm/resnext50_32x4d.tv2_in1k)|176 |79.37|94.3 |25.0 |2.7 |9.0 |4577 |
|[resnext50_32x4d.gluon_in1k](https://huggingface.co/timm/resnext50_32x4d.gluon_in1k)|224 |79.36|94.43|25.0 |4.3 |14.4 |2942 |
|[resnext101_32x8d.tv_in1k](https://huggingface.co/timm/resnext101_32x8d.tv_in1k)|224 |79.31|94.52|88.8 |16.5 |31.2 |1100 |
|[resnet101.gluon_in1k](https://huggingface.co/timm/resnet101.gluon_in1k)|224 |79.31|94.53|44.6 |7.8 |16.2 |2125 |
|[resnetblur50.bt_in1k](https://huggingface.co/timm/resnetblur50.bt_in1k)|224 |79.31|94.63|25.6 |5.2 |12.0 |2524 |
|[resnet50.a1h_in1k](https://huggingface.co/timm/resnet50.a1h_in1k)|176 |79.27|94.49|25.6 |2.6 |6.9 |5404 |
|[resnext50_32x4d.a3_in1k](https://huggingface.co/timm/resnext50_32x4d.a3_in1k)|224 |79.25|94.31|25.0 |4.3 |14.4 |2931 |
|[resnet50.fb_ssl_yfcc100m_ft_in1k](https://huggingface.co/timm/resnet50.fb_ssl_yfcc100m_ft_in1k)|224 |79.22|94.84|25.6 |4.1 |11.1 |3451 |
|[resnet33ts.ra2_in1k](https://huggingface.co/timm/resnet33ts.ra2_in1k)|256 |79.21|94.56|19.7 |4.8 |11.7 |3392 |
|[resnet50d.gluon_in1k](https://huggingface.co/timm/resnet50d.gluon_in1k)|224 |79.07|94.48|25.6 |4.4 |11.9 |3162 |
|[resnet50.ram_in1k](https://huggingface.co/timm/resnet50.ram_in1k)|224 |79.03|94.38|25.6 |4.1 |11.1 |3453 |
|[resnet50.am_in1k](https://huggingface.co/timm/resnet50.am_in1k)|224 |79.01|94.39|25.6 |4.1 |11.1 |3461 |
|[resnet32ts.ra2_in1k](https://huggingface.co/timm/resnet32ts.ra2_in1k)|256 |79.01|94.37|18.0 |4.6 |11.6 |3440 |
|[ecaresnet26t.ra2_in1k](https://huggingface.co/timm/ecaresnet26t.ra2_in1k)|256 |78.9 |94.54|16.0 |3.4 |10.5 |3421 |
|[resnet152.a3_in1k](https://huggingface.co/timm/resnet152.a3_in1k)|160 |78.89|94.11|60.2 |5.9 |11.5 |2745 |
|[wide_resnet101_2.tv_in1k](https://huggingface.co/timm/wide_resnet101_2.tv_in1k)|224 |78.84|94.28|126.9 |22.8 |21.2 |1079 |
|[seresnext26d_32x4d.bt_in1k](https://huggingface.co/timm/seresnext26d_32x4d.bt_in1k)|288 |78.83|94.24|16.8 |4.5 |16.8 |2251 |
|[resnet50.ra_in1k](https://huggingface.co/timm/resnet50.ra_in1k)|224 |78.81|94.32|25.6 |4.1 |11.1 |3454 |
|[seresnext26t_32x4d.bt_in1k](https://huggingface.co/timm/seresnext26t_32x4d.bt_in1k)|288 |78.74|94.33|16.8 |4.5 |16.7 |2264 |
|[resnet50s.gluon_in1k](https://huggingface.co/timm/resnet50s.gluon_in1k)|224 |78.72|94.23|25.7 |5.5 |13.5 |2796 |
|[resnet50d.a3_in1k](https://huggingface.co/timm/resnet50d.a3_in1k)|224 |78.71|94.24|25.6 |4.4 |11.9 |3154 |
|[wide_resnet50_2.tv_in1k](https://huggingface.co/timm/wide_resnet50_2.tv_in1k)|224 |78.47|94.09|68.9 |11.4 |14.4 |1934 |
|[resnet50.bt_in1k](https://huggingface.co/timm/resnet50.bt_in1k)|224 |78.46|94.27|25.6 |4.1 |11.1 |3454 |
|[resnet34d.ra2_in1k](https://huggingface.co/timm/resnet34d.ra2_in1k)|288 |78.43|94.35|21.8 |6.5 |7.5 |3291 |
|[gcresnext26ts.ch_in1k](https://huggingface.co/timm/gcresnext26ts.ch_in1k)|288 |78.42|94.04|10.5 |3.1 |13.3 |3226 |
|[resnet26t.ra2_in1k](https://huggingface.co/timm/resnet26t.ra2_in1k)|320 |78.33|94.13|16.0 |5.2 |16.4 |2391 |
|[resnet152.tv_in1k](https://huggingface.co/timm/resnet152.tv_in1k)|224 |78.32|94.04|60.2 |11.6 |22.6 |1487 |
|[seresnext26ts.ch_in1k](https://huggingface.co/timm/seresnext26ts.ch_in1k)|288 |78.28|94.1 |10.4 |3.1 |13.3 |3062 |
|[bat_resnext26ts.ch_in1k](https://huggingface.co/timm/bat_resnext26ts.ch_in1k)|256 |78.25|94.1 |10.7 |2.5 |12.5 |3393 |
|[resnet50.a3_in1k](https://huggingface.co/timm/resnet50.a3_in1k)|224 |78.06|93.78|25.6 |4.1 |11.1 |3450 |
|[resnet50c.gluon_in1k](https://huggingface.co/timm/resnet50c.gluon_in1k)|224 |78.0 |93.99|25.6 |4.4 |11.9 |3286 |
|[eca_resnext26ts.ch_in1k](https://huggingface.co/timm/eca_resnext26ts.ch_in1k)|288 |78.0 |93.91|10.3 |3.1 |13.3 |3297 |
|[seresnext26t_32x4d.bt_in1k](https://huggingface.co/timm/seresnext26t_32x4d.bt_in1k)|224 |77.98|93.75|16.8 |2.7 |10.1 |3841 |
|[resnet34.a1_in1k](https://huggingface.co/timm/resnet34.a1_in1k)|288 |77.92|93.77|21.8 |6.1 |6.2 |3609 |
|[resnet101.a3_in1k](https://huggingface.co/timm/resnet101.a3_in1k)|160 |77.88|93.71|44.6 |4.0 |8.3 |3926 |
|[resnet26t.ra2_in1k](https://huggingface.co/timm/resnet26t.ra2_in1k)|256 |77.87|93.84|16.0 |3.4 |10.5 |3772 |
|[seresnext26ts.ch_in1k](https://huggingface.co/timm/seresnext26ts.ch_in1k)|256 |77.86|93.79|10.4 |2.4 |10.5 |4263 |
|[resnetrs50.tf_in1k](https://huggingface.co/timm/resnetrs50.tf_in1k)|160 |77.82|93.81|35.7 |2.3 |6.2 |5238 |
|[gcresnext26ts.ch_in1k](https://huggingface.co/timm/gcresnext26ts.ch_in1k)|256 |77.81|93.82|10.5 |2.4 |10.5 |4183 |
|[ecaresnet50t.a3_in1k](https://huggingface.co/timm/ecaresnet50t.a3_in1k)|160 |77.79|93.6 |25.6 |2.2 |6.0 |5329 |
|[resnext50_32x4d.a3_in1k](https://huggingface.co/timm/resnext50_32x4d.a3_in1k)|160 |77.73|93.32|25.0 |2.2 |7.4 |5576 |
|[resnext50_32x4d.tv_in1k](https://huggingface.co/timm/resnext50_32x4d.tv_in1k)|224 |77.61|93.7 |25.0 |4.3 |14.4 |2944 |
|[seresnext26d_32x4d.bt_in1k](https://huggingface.co/timm/seresnext26d_32x4d.bt_in1k)|224 |77.59|93.61|16.8 |2.7 |10.2 |3807 |
|[resnet50.gluon_in1k](https://huggingface.co/timm/resnet50.gluon_in1k)|224 |77.58|93.72|25.6 |4.1 |11.1 |3455 |
|[eca_resnext26ts.ch_in1k](https://huggingface.co/timm/eca_resnext26ts.ch_in1k)|256 |77.44|93.56|10.3 |2.4 |10.5 |4284 |
|[resnet26d.bt_in1k](https://huggingface.co/timm/resnet26d.bt_in1k)|288 |77.41|93.63|16.0 |4.3 |13.5 |2907 |
|[resnet101.tv_in1k](https://huggingface.co/timm/resnet101.tv_in1k)|224 |77.38|93.54|44.6 |7.8 |16.2 |2125 |
|[resnet50d.a3_in1k](https://huggingface.co/timm/resnet50d.a3_in1k)|160 |77.22|93.27|25.6 |2.2 |6.1 |5982 |
|[resnext26ts.ra2_in1k](https://huggingface.co/timm/resnext26ts.ra2_in1k)|288 |77.17|93.47|10.3 |3.1 |13.3 |3392 |
|[resnet34.a2_in1k](https://huggingface.co/timm/resnet34.a2_in1k)|288 |77.15|93.27|21.8 |6.1 |6.2 |3615 |
|[resnet34d.ra2_in1k](https://huggingface.co/timm/resnet34d.ra2_in1k)|224 |77.1 |93.37|21.8 |3.9 |4.5 |5436 |
|[seresnet50.a3_in1k](https://huggingface.co/timm/seresnet50.a3_in1k)|224 |77.02|93.07|28.1 |4.1 |11.1 |2952 |
|[resnext26ts.ra2_in1k](https://huggingface.co/timm/resnext26ts.ra2_in1k)|256 |76.78|93.13|10.3 |2.4 |10.5 |4410 |
|[resnet26d.bt_in1k](https://huggingface.co/timm/resnet26d.bt_in1k)|224 |76.7 |93.17|16.0 |2.6 |8.2 |4859 |
|[resnet34.bt_in1k](https://huggingface.co/timm/resnet34.bt_in1k)|288 |76.5 |93.35|21.8 |6.1 |6.2 |3617 |
|[resnet34.a1_in1k](https://huggingface.co/timm/resnet34.a1_in1k)|224 |76.42|92.87|21.8 |3.7 |3.7 |5984 |
|[resnet26.bt_in1k](https://huggingface.co/timm/resnet26.bt_in1k)|288 |76.35|93.18|16.0 |3.9 |12.2 |3331 |
|[resnet50.tv_in1k](https://huggingface.co/timm/resnet50.tv_in1k)|224 |76.13|92.86|25.6 |4.1 |11.1 |3457 |
|[resnet50.a3_in1k](https://huggingface.co/timm/resnet50.a3_in1k)|160 |75.96|92.5 |25.6 |2.1 |5.7 |6490 |
|[resnet34.a2_in1k](https://huggingface.co/timm/resnet34.a2_in1k)|224 |75.52|92.44|21.8 |3.7 |3.7 |5991 |
|[resnet26.bt_in1k](https://huggingface.co/timm/resnet26.bt_in1k)|224 |75.3 |92.58|16.0 |2.4 |7.4 |5583 |
|[resnet34.bt_in1k](https://huggingface.co/timm/resnet34.bt_in1k)|224 |75.16|92.18|21.8 |3.7 |3.7 |5994 |
|[seresnet50.a3_in1k](https://huggingface.co/timm/seresnet50.a3_in1k)|160 |75.1 |92.08|28.1 |2.1 |5.7 |5513 |
|[resnet34.gluon_in1k](https://huggingface.co/timm/resnet34.gluon_in1k)|224 |74.57|91.98|21.8 |3.7 |3.7 |5984 |
|[resnet18d.ra2_in1k](https://huggingface.co/timm/resnet18d.ra2_in1k)|288 |73.81|91.83|11.7 |3.4 |5.4 |5196 |
|[resnet34.tv_in1k](https://huggingface.co/timm/resnet34.tv_in1k)|224 |73.32|91.42|21.8 |3.7 |3.7 |5979 |
|[resnet18.fb_swsl_ig1b_ft_in1k](https://huggingface.co/timm/resnet18.fb_swsl_ig1b_ft_in1k)|224 |73.28|91.73|11.7 |1.8 |2.5 |10213 |
|[resnet18.a1_in1k](https://huggingface.co/timm/resnet18.a1_in1k)|288 |73.16|91.03|11.7 |3.0 |4.1 |6050 |
|[resnet34.a3_in1k](https://huggingface.co/timm/resnet34.a3_in1k)|224 |72.98|91.11|21.8 |3.7 |3.7 |5967 |
|[resnet18.fb_ssl_yfcc100m_ft_in1k](https://huggingface.co/timm/resnet18.fb_ssl_yfcc100m_ft_in1k)|224 |72.6 |91.42|11.7 |1.8 |2.5 |10213 |
|[resnet18.a2_in1k](https://huggingface.co/timm/resnet18.a2_in1k)|288 |72.37|90.59|11.7 |3.0 |4.1 |6051 |
|[resnet14t.c3_in1k](https://huggingface.co/timm/resnet14t.c3_in1k)|224 |72.26|90.31|10.1 |1.7 |5.8 |7026 |
|[resnet18d.ra2_in1k](https://huggingface.co/timm/resnet18d.ra2_in1k)|224 |72.26|90.68|11.7 |2.1 |3.3 |8707 |
|[resnet18.a1_in1k](https://huggingface.co/timm/resnet18.a1_in1k)|224 |71.49|90.07|11.7 |1.8 |2.5 |10187 |
|[resnet14t.c3_in1k](https://huggingface.co/timm/resnet14t.c3_in1k)|176 |71.31|89.69|10.1 |1.1 |3.6 |10970 |
|[resnet18.gluon_in1k](https://huggingface.co/timm/resnet18.gluon_in1k)|224 |70.84|89.76|11.7 |1.8 |2.5 |10210 |
|[resnet18.a2_in1k](https://huggingface.co/timm/resnet18.a2_in1k)|224 |70.64|89.47|11.7 |1.8 |2.5 |10194 |
|[resnet34.a3_in1k](https://huggingface.co/timm/resnet34.a3_in1k)|160 |70.56|89.52|21.8 |1.9 |1.9 |10737 |
|[resnet18.tv_in1k](https://huggingface.co/timm/resnet18.tv_in1k)|224 |69.76|89.07|11.7 |1.8 |2.5 |10205 |
|[resnet10t.c3_in1k](https://huggingface.co/timm/resnet10t.c3_in1k)|224 |68.34|88.03|5.4 |1.1 |2.4 |13079 |
|[resnet18.a3_in1k](https://huggingface.co/timm/resnet18.a3_in1k)|224 |68.25|88.17|11.7 |1.8 |2.5 |10167 |
|[resnet10t.c3_in1k](https://huggingface.co/timm/resnet10t.c3_in1k)|176 |66.71|86.96|5.4 |0.7 |1.5 |20327 |
|[resnet18.a3_in1k](https://huggingface.co/timm/resnet18.a3_in1k)|160 |65.66|86.26|11.7 |0.9 |1.3 |18229 |
## Citation
```bibtex
@article{He2015,
author = {Kaiming He and Xiangyu Zhang and Shaoqing Ren and Jian Sun},
title = {Deep Residual Learning for Image Recognition},
journal = {arXiv preprint arXiv:1512.03385},
year = {2015}
}
```
```bibtex
@misc{rw2019timm,
author = {Ross Wightman},
title = {PyTorch Image Models},
year = {2019},
publisher = {GitHub},
journal = {GitHub repository},
doi = {10.5281/zenodo.4414861},
howpublished = {\url{https://github.com/huggingface/pytorch-image-models}}
}
```
|
mradermacher/TheSalt-L3-8b-v0.3.2-i1-GGUF | mradermacher | 2024-06-19T17:04:26Z | 12,160 | 1 | transformers | [
"transformers",
"gguf",
"en",
"base_model:cgato/TheSalt-L3-8b-v0.3.2",
"license:cc-by-nc-4.0",
"endpoints_compatible",
"region:us"
]
| null | 2024-06-19T12:26:42Z | ---
base_model: cgato/TheSalt-L3-8b-v0.3.2
language:
- en
library_name: transformers
license: cc-by-nc-4.0
quantized_by: mradermacher
---
## About
<!-- ### quantize_version: 2 -->
<!-- ### output_tensor_quantised: 1 -->
<!-- ### convert_type: hf -->
<!-- ### vocab_type: -->
<!-- ### tags: nicoboss -->
weighted/imatrix quants of https://huggingface.co/cgato/TheSalt-L3-8b-v0.3.2
<!-- provided-files -->
static quants are available at https://huggingface.co/mradermacher/TheSalt-L3-8b-v0.3.2-GGUF
## Usage
If you are unsure how to use GGUF files, refer to one of [TheBloke's
READMEs](https://huggingface.co/TheBloke/KafkaLM-70B-German-V0.1-GGUF) for
more details, including on how to concatenate multi-part files.
## Provided Quants
(sorted by size, not necessarily quality. IQ-quants are often preferable over similar sized non-IQ quants)
| Link | Type | Size/GB | Notes |
|:-----|:-----|--------:|:------|
| [GGUF](https://huggingface.co/mradermacher/TheSalt-L3-8b-v0.3.2-i1-GGUF/resolve/main/TheSalt-L3-8b-v0.3.2.i1-IQ1_S.gguf) | i1-IQ1_S | 2.1 | for the desperate |
| [GGUF](https://huggingface.co/mradermacher/TheSalt-L3-8b-v0.3.2-i1-GGUF/resolve/main/TheSalt-L3-8b-v0.3.2.i1-IQ1_M.gguf) | i1-IQ1_M | 2.3 | mostly desperate |
| [GGUF](https://huggingface.co/mradermacher/TheSalt-L3-8b-v0.3.2-i1-GGUF/resolve/main/TheSalt-L3-8b-v0.3.2.i1-IQ2_XXS.gguf) | i1-IQ2_XXS | 2.5 | |
| [GGUF](https://huggingface.co/mradermacher/TheSalt-L3-8b-v0.3.2-i1-GGUF/resolve/main/TheSalt-L3-8b-v0.3.2.i1-IQ2_XS.gguf) | i1-IQ2_XS | 2.7 | |
| [GGUF](https://huggingface.co/mradermacher/TheSalt-L3-8b-v0.3.2-i1-GGUF/resolve/main/TheSalt-L3-8b-v0.3.2.i1-IQ2_S.gguf) | i1-IQ2_S | 2.9 | |
| [GGUF](https://huggingface.co/mradermacher/TheSalt-L3-8b-v0.3.2-i1-GGUF/resolve/main/TheSalt-L3-8b-v0.3.2.i1-IQ2_M.gguf) | i1-IQ2_M | 3.0 | |
| [GGUF](https://huggingface.co/mradermacher/TheSalt-L3-8b-v0.3.2-i1-GGUF/resolve/main/TheSalt-L3-8b-v0.3.2.i1-Q2_K.gguf) | i1-Q2_K | 3.3 | IQ3_XXS probably better |
| [GGUF](https://huggingface.co/mradermacher/TheSalt-L3-8b-v0.3.2-i1-GGUF/resolve/main/TheSalt-L3-8b-v0.3.2.i1-IQ3_XXS.gguf) | i1-IQ3_XXS | 3.4 | lower quality |
| [GGUF](https://huggingface.co/mradermacher/TheSalt-L3-8b-v0.3.2-i1-GGUF/resolve/main/TheSalt-L3-8b-v0.3.2.i1-IQ3_XS.gguf) | i1-IQ3_XS | 3.6 | |
| [GGUF](https://huggingface.co/mradermacher/TheSalt-L3-8b-v0.3.2-i1-GGUF/resolve/main/TheSalt-L3-8b-v0.3.2.i1-Q3_K_S.gguf) | i1-Q3_K_S | 3.8 | IQ3_XS probably better |
| [GGUF](https://huggingface.co/mradermacher/TheSalt-L3-8b-v0.3.2-i1-GGUF/resolve/main/TheSalt-L3-8b-v0.3.2.i1-IQ3_S.gguf) | i1-IQ3_S | 3.8 | beats Q3_K* |
| [GGUF](https://huggingface.co/mradermacher/TheSalt-L3-8b-v0.3.2-i1-GGUF/resolve/main/TheSalt-L3-8b-v0.3.2.i1-IQ3_M.gguf) | i1-IQ3_M | 3.9 | |
| [GGUF](https://huggingface.co/mradermacher/TheSalt-L3-8b-v0.3.2-i1-GGUF/resolve/main/TheSalt-L3-8b-v0.3.2.i1-Q3_K_M.gguf) | i1-Q3_K_M | 4.1 | IQ3_S probably better |
| [GGUF](https://huggingface.co/mradermacher/TheSalt-L3-8b-v0.3.2-i1-GGUF/resolve/main/TheSalt-L3-8b-v0.3.2.i1-Q3_K_L.gguf) | i1-Q3_K_L | 4.4 | IQ3_M probably better |
| [GGUF](https://huggingface.co/mradermacher/TheSalt-L3-8b-v0.3.2-i1-GGUF/resolve/main/TheSalt-L3-8b-v0.3.2.i1-IQ4_XS.gguf) | i1-IQ4_XS | 4.5 | |
| [GGUF](https://huggingface.co/mradermacher/TheSalt-L3-8b-v0.3.2-i1-GGUF/resolve/main/TheSalt-L3-8b-v0.3.2.i1-Q4_0.gguf) | i1-Q4_0 | 4.8 | fast, low quality |
| [GGUF](https://huggingface.co/mradermacher/TheSalt-L3-8b-v0.3.2-i1-GGUF/resolve/main/TheSalt-L3-8b-v0.3.2.i1-Q4_K_S.gguf) | i1-Q4_K_S | 4.8 | optimal size/speed/quality |
| [GGUF](https://huggingface.co/mradermacher/TheSalt-L3-8b-v0.3.2-i1-GGUF/resolve/main/TheSalt-L3-8b-v0.3.2.i1-Q4_K_M.gguf) | i1-Q4_K_M | 5.0 | fast, recommended |
| [GGUF](https://huggingface.co/mradermacher/TheSalt-L3-8b-v0.3.2-i1-GGUF/resolve/main/TheSalt-L3-8b-v0.3.2.i1-Q5_K_S.gguf) | i1-Q5_K_S | 5.7 | |
| [GGUF](https://huggingface.co/mradermacher/TheSalt-L3-8b-v0.3.2-i1-GGUF/resolve/main/TheSalt-L3-8b-v0.3.2.i1-Q5_K_M.gguf) | i1-Q5_K_M | 5.8 | |
| [GGUF](https://huggingface.co/mradermacher/TheSalt-L3-8b-v0.3.2-i1-GGUF/resolve/main/TheSalt-L3-8b-v0.3.2.i1-Q6_K.gguf) | i1-Q6_K | 6.7 | practically like static Q6_K |
Here is a handy graph by ikawrakow comparing some lower-quality quant
types (lower is better):

And here are Artefact2's thoughts on the matter:
https://gist.github.com/Artefact2/b5f810600771265fc1e39442288e8ec9
## FAQ / Model Request
See https://huggingface.co/mradermacher/model_requests for some answers to
questions you might have and/or if you want some other model quantized.
## Thanks
I thank my company, [nethype GmbH](https://www.nethype.de/), for letting
me use its servers and providing upgrades to my workstation to enable
this work in my free time. Additional thanks to [@nicoboss](https://huggingface.co/nicoboss) for giving me access to his hardware for calculating the imatrix for these quants.
<!-- end -->
|
kenhktsui/fineweb-edu-fasttext-classifier | kenhktsui | 2024-06-06T16:28:47Z | 12,159 | 0 | fasttext | [
"fasttext",
"text-classification",
"en",
"dataset:HuggingFaceFW/fineweb-edu-llama3-annotations",
"license:odc-by",
"region:us"
]
| text-classification | 2024-06-06T07:55:54Z | ---
license: odc-by
language:
- en
library_name: fasttext
pipeline_tag: text-classification
datasets:
- HuggingFaceFW/fineweb-edu-llama3-annotations
---
# FineWeb-Edu FastText classifier
## Model summary
This is a FastText classifier for judging the educational value of web pages based on training data [fineweb-edu-llama3-annotations](https://huggingface.co/datasets/HuggingFaceFW/fineweb-edu-llama3-annotations).
There are two objectives:
- ⚡ throughput optimisation: It can classify more than 2000 examples per second in CPU, and so it can be used on-the-fly during pretraining/ to process huge data with CPU.
- 🧪fasttext vs transformer based model: How does this lightweight model with limited capacity compare to the original model [HuggingFaceFW/fineweb-edu-classifier](https://huggingface.co/HuggingFaceFW/fineweb-edu-classifier)?
The FastText approach is inspired by my independent development of educational classifier based on a different definition of educational value, which can be found at [kenhktsui/llm-data-textbook-quality-fasttext-classifier-v2](https://huggingface.co/kenhktsui/llm-data-textbook-quality-fasttext-classifier-v2).
## 🛠️Usage
```python
from typing import List
import re
from huggingface_hub import hf_hub_download
import fasttext
model_hf = fasttext.load_model(hf_hub_download("kenhktsui/fineweb-edu-fasttext-classifier", "model.bin"))
def replace_newlines(text: str) -> str:
return re.sub("\n+", " ", text)
def predict(text_list: List[str]) -> List[dict]:
text_list = [replace_newlines(text) for text in text_list]
pred = model_hf.predict(text_list)
return [{"label": int(l[0].lstrip("__label__")), "score": s[0]}
for l, s in zip(*pred)]
predict(["Hi"])
# Output: [{'label': 0, 'score': 1.00001}]
```
## 📊Evaluation
The last 46867 samples are used as test data, but it's not the exact test data as in [HuggingFaceFW/fineweb-edu-classifier](https://huggingface.co/HuggingFaceFW/fineweb-edu-classifier)
### Classification Report
```
precision recall f1-score support
0 0.72 0.44 0.55 5704
1 0.73 0.87 0.80 26595
2 0.52 0.49 0.50 10350
3 0.48 0.33 0.39 3397
4 0.69 0.03 0.06 819
5 0.00 0.00 0.00 2
accuracy 0.68 46867
macro avg 0.52 0.36 0.38 46867
weighted avg 0.67 0.68 0.66 46867
```
The below table compares FastText model vs transformer based model.
Label|This Model| [HuggingFaceFW/fineweb-edu-classifier](https://huggingface.co/HuggingFaceFW/fineweb-edu-classifier)
-----|-----|----
0|0.55 | 0.59
1|0.80 | 0.81
2|0.50 | 0.59
3|0.39 | 0.53
4|0.06 | 0.44
5|0.00 | 0.02
Label 0, 1, 2 are comparable to the original model.
The performance degradation starts to be noticeable in label 3, and widen further in 4, which is due to limited capacity of fasttext model.
So, this classifer can perform reasonably well in label 0, 1, 2, and also 3 with some degradation.
### Confusion Matrix
```
[ 2537 3098 65 4 0 0]
[ 944 23037 2491 123 0 0]
y_true [ 26 4742 5048 533 1 0]
[ 4 434 1846 1105 8 0]
[ 0 38 213 544 24 0]
[ 0 0 0 0 2 0]
y_pred
```
The model has a accuracy of 68%, and it is more likely to underpredict educational value than overpredict so. The exhibited conservatism is good for filtering large amount of data.
Predicted - Actual Rating | Frequency | %
-----|-----|----
0|31751 | 67.7%
-1|8078 | 17.2%
+1| 6130 | 13.1%
-2|673 | 1.4%
+2|189 | 0.4%
-3|42 | 0.1%
+3|4 | 0.0%
### Alignment with [HuggingFaceFW/fineweb-edu-classifier](https://huggingface.co/HuggingFaceFW/fineweb-edu-classifier)
Spearman rank-order correlation coefficient is 0.5881 in MiniPile train split and 0.5832 in test split, indicating a moderately strong monotonic relationship in over 1 million representative document in web data.
|
ai-forever/rugpt3small_based_on_gpt2 | ai-forever | 2023-12-05T09:19:39Z | 12,151 | 27 | transformers | [
"transformers",
"pytorch",
"jax",
"gpt2",
"text-generation",
"PyTorch",
"Transformers",
"ru",
"arxiv:2309.10931",
"autotrain_compatible",
"endpoints_compatible",
"text-generation-inference",
"region:us"
]
| text-generation | 2022-03-02T23:29:05Z | ---
language:
- ru
tags:
- PyTorch
- Transformers
thumbnail: "https://github.com/sberbank-ai/ru-gpts"
---
# rugpt3small\_based\_on\_gpt2
The model architecture design, pretraining, and evaluation are documented in our preprint: [**A Family of Pretrained Transformer Language Models for Russian**](https://arxiv.org/abs/2309.10931).
The model was pretrained with sequence length 1024 using transformers by the [SberDevices](https://sberdevices.ru/) team on 80B tokens around 3 epochs. After that, the model was finetuned with the context size of 2048.
Total training time took around one week on 32 GPUs.
# Authors
+ NLP core team RnD [Telegram channel](https://t.me/nlpcoreteam):
+ Dmitry Zmitrovich
# Cite us
```
@misc{zmitrovich2023family,
title={A Family of Pretrained Transformer Language Models for Russian},
author={Dmitry Zmitrovich and Alexander Abramov and Andrey Kalmykov and Maria Tikhonova and Ekaterina Taktasheva and Danil Astafurov and Mark Baushenko and Artem Snegirev and Tatiana Shavrina and Sergey Markov and Vladislav Mikhailov and Alena Fenogenova},
year={2023},
eprint={2309.10931},
archivePrefix={arXiv},
primaryClass={cs.CL}
}
``` |
mradermacher/neo_7b_instruct_v0.1-GGUF | mradermacher | 2024-07-01T00:42:38Z | 12,150 | 0 | transformers | [
"transformers",
"gguf",
"en",
"base_model:m-a-p/neo_7b_instruct_v0.1",
"license:apache-2.0",
"endpoints_compatible",
"region:us"
]
| null | 2024-06-30T19:18:51Z | ---
base_model: m-a-p/neo_7b_instruct_v0.1
language:
- en
library_name: transformers
license: apache-2.0
quantized_by: mradermacher
---
## About
<!-- ### quantize_version: 2 -->
<!-- ### output_tensor_quantised: 1 -->
<!-- ### convert_type: hf -->
<!-- ### vocab_type: -->
<!-- ### tags: -->
static quants of https://huggingface.co/m-a-p/neo_7b_instruct_v0.1
<!-- provided-files -->
weighted/imatrix quants are available at https://huggingface.co/mradermacher/neo_7b_instruct_v0.1-i1-GGUF
## Usage
If you are unsure how to use GGUF files, refer to one of [TheBloke's
READMEs](https://huggingface.co/TheBloke/KafkaLM-70B-German-V0.1-GGUF) for
more details, including on how to concatenate multi-part files.
## Provided Quants
(sorted by size, not necessarily quality. IQ-quants are often preferable over similar sized non-IQ quants)
| Link | Type | Size/GB | Notes |
|:-----|:-----|--------:|:------|
| [GGUF](https://huggingface.co/mradermacher/neo_7b_instruct_v0.1-GGUF/resolve/main/neo_7b_instruct_v0.1.Q2_K.gguf) | Q2_K | 3.0 | |
| [GGUF](https://huggingface.co/mradermacher/neo_7b_instruct_v0.1-GGUF/resolve/main/neo_7b_instruct_v0.1.IQ3_XS.gguf) | IQ3_XS | 3.4 | |
| [GGUF](https://huggingface.co/mradermacher/neo_7b_instruct_v0.1-GGUF/resolve/main/neo_7b_instruct_v0.1.IQ3_S.gguf) | IQ3_S | 3.5 | beats Q3_K* |
| [GGUF](https://huggingface.co/mradermacher/neo_7b_instruct_v0.1-GGUF/resolve/main/neo_7b_instruct_v0.1.Q3_K_S.gguf) | Q3_K_S | 3.5 | |
| [GGUF](https://huggingface.co/mradermacher/neo_7b_instruct_v0.1-GGUF/resolve/main/neo_7b_instruct_v0.1.IQ3_M.gguf) | IQ3_M | 3.6 | |
| [GGUF](https://huggingface.co/mradermacher/neo_7b_instruct_v0.1-GGUF/resolve/main/neo_7b_instruct_v0.1.Q3_K_M.gguf) | Q3_K_M | 3.9 | lower quality |
| [GGUF](https://huggingface.co/mradermacher/neo_7b_instruct_v0.1-GGUF/resolve/main/neo_7b_instruct_v0.1.Q3_K_L.gguf) | Q3_K_L | 4.2 | |
| [GGUF](https://huggingface.co/mradermacher/neo_7b_instruct_v0.1-GGUF/resolve/main/neo_7b_instruct_v0.1.IQ4_XS.gguf) | IQ4_XS | 4.3 | |
| [GGUF](https://huggingface.co/mradermacher/neo_7b_instruct_v0.1-GGUF/resolve/main/neo_7b_instruct_v0.1.Q4_K_S.gguf) | Q4_K_S | 4.6 | fast, recommended |
| [GGUF](https://huggingface.co/mradermacher/neo_7b_instruct_v0.1-GGUF/resolve/main/neo_7b_instruct_v0.1.Q4_K_M.gguf) | Q4_K_M | 4.8 | fast, recommended |
| [GGUF](https://huggingface.co/mradermacher/neo_7b_instruct_v0.1-GGUF/resolve/main/neo_7b_instruct_v0.1.Q5_K_S.gguf) | Q5_K_S | 5.5 | |
| [GGUF](https://huggingface.co/mradermacher/neo_7b_instruct_v0.1-GGUF/resolve/main/neo_7b_instruct_v0.1.Q5_K_M.gguf) | Q5_K_M | 5.6 | |
| [GGUF](https://huggingface.co/mradermacher/neo_7b_instruct_v0.1-GGUF/resolve/main/neo_7b_instruct_v0.1.Q6_K.gguf) | Q6_K | 6.5 | very good quality |
| [GGUF](https://huggingface.co/mradermacher/neo_7b_instruct_v0.1-GGUF/resolve/main/neo_7b_instruct_v0.1.Q8_0.gguf) | Q8_0 | 8.4 | fast, best quality |
| [GGUF](https://huggingface.co/mradermacher/neo_7b_instruct_v0.1-GGUF/resolve/main/neo_7b_instruct_v0.1.f16.gguf) | f16 | 15.7 | 16 bpw, overkill |
Here is a handy graph by ikawrakow comparing some lower-quality quant
types (lower is better):

And here are Artefact2's thoughts on the matter:
https://gist.github.com/Artefact2/b5f810600771265fc1e39442288e8ec9
## FAQ / Model Request
See https://huggingface.co/mradermacher/model_requests for some answers to
questions you might have and/or if you want some other model quantized.
## Thanks
I thank my company, [nethype GmbH](https://www.nethype.de/), for letting
me use its servers and providing upgrades to my workstation to enable
this work in my free time.
<!-- end -->
|
cbrosch/product_name_classifier | cbrosch | 2023-07-18T13:12:04Z | 12,146 | 0 | transformers | [
"transformers",
"pytorch",
"bert",
"text-classification",
"license:mit",
"autotrain_compatible",
"endpoints_compatible",
"region:us"
]
| text-classification | 2023-07-18T13:10:37Z | ---
license: mit
---
|
facebook/wav2vec2-lv-60-espeak-cv-ft | facebook | 2023-10-31T13:13:45Z | 12,141 | 21 | transformers | [
"transformers",
"pytorch",
"wav2vec2",
"automatic-speech-recognition",
"speech",
"audio",
"phoneme-recognition",
"multilingual",
"dataset:common_voice",
"arxiv:2109.11680",
"license:apache-2.0",
"endpoints_compatible",
"region:us"
]
| automatic-speech-recognition | 2022-03-02T23:29:05Z | ---
language: multilingual
datasets:
- common_voice
tags:
- speech
- audio
- automatic-speech-recognition
- phoneme-recognition
widget:
- example_title: Librispeech sample 1
src: https://cdn-media.huggingface.co/speech_samples/sample1.flac
- example_title: Librispeech sample 2
src: https://cdn-media.huggingface.co/speech_samples/sample2.flac
license: apache-2.0
---
# Wav2Vec2-Large-LV60 finetuned on multi-lingual Common Voice
This checkpoint leverages the pretrained checkpoint [wav2vec2-large-lv60](https://huggingface.co/facebook/wav2vec2-large-lv60)
and is fine-tuned on [CommonVoice](https://huggingface.co/datasets/common_voice) to recognize phonetic labels in multiple languages.
When using the model make sure that your speech input is sampled at 16kHz.
Note that the model outputs a string of phonetic labels. A dictionary mapping phonetic labels to words
has to be used to map the phonetic output labels to output words.
[Paper: Simple and Effective Zero-shot Cross-lingual Phoneme Recognition](https://arxiv.org/abs/2109.11680)
Authors: Qiantong Xu, Alexei Baevski, Michael Auli
**Abstract**
Recent progress in self-training, self-supervised pretraining and unsupervised learning enabled well performing speech recognition systems without any labeled data. However, in many cases there is labeled data available for related languages which is not utilized by these methods. This paper extends previous work on zero-shot cross-lingual transfer learning by fine-tuning a multilingually pretrained wav2vec 2.0 model to transcribe unseen languages. This is done by mapping phonemes of the training languages to the target language using articulatory features. Experiments show that this simple method significantly outperforms prior work which introduced task-specific architectures and used only part of a monolingually pretrained model.
The original model can be found under https://github.com/pytorch/fairseq/tree/master/examples/wav2vec#wav2vec-20.
# Usage
To transcribe audio files the model can be used as a standalone acoustic model as follows:
```python
from transformers import Wav2Vec2Processor, Wav2Vec2ForCTC
from datasets import load_dataset
import torch
# load model and processor
processor = Wav2Vec2Processor.from_pretrained("facebook/wav2vec2-lv-60-espeak-cv-ft")
model = Wav2Vec2ForCTC.from_pretrained("facebook/wav2vec2-lv-60-espeak-cv-ft")
# load dummy dataset and read soundfiles
ds = load_dataset("patrickvonplaten/librispeech_asr_dummy", "clean", split="validation")
# tokenize
input_values = processor(ds[0]["audio"]["array"], return_tensors="pt").input_values
# retrieve logits
with torch.no_grad():
logits = model(input_values).logits
# take argmax and decode
predicted_ids = torch.argmax(logits, dim=-1)
transcription = processor.batch_decode(predicted_ids)
# => should give ['m ɪ s t ɚ k w ɪ l t ɚ ɹ ɪ z ð ɪ ɐ p ɑː s əl ʌ v ð ə m ɪ d əl k l æ s ᵻ z æ n d w iː ɑːɹ ɡ l æ d t ə w ɛ l k ə m h ɪ z ɡ ɑː s p əl']
``` |
ai-forever/mGPT | ai-forever | 2023-12-05T13:12:21Z | 12,129 | 236 | transformers | [
"transformers",
"pytorch",
"gpt2",
"text-generation",
"multilingual",
"PyTorch",
"Transformers",
"gpt3",
"Deepspeed",
"Megatron",
"ar",
"he",
"vi",
"id",
"jv",
"ms",
"tl",
"lv",
"lt",
"eu",
"ml",
"ta",
"te",
"hy",
"bn",
"mr",
"hi",
"ur",
"af",
"da",
"en",
"de",
"sv",
"fr",
"it",
"pt",
"ro",
"es",
"el",
"os",
"tg",
"fa",
"ja",
"ka",
"ko",
"th",
"bxr",
"xal",
"mn",
"sw",
"yo",
"be",
"bg",
"ru",
"uk",
"pl",
"my",
"uz",
"ba",
"kk",
"ky",
"tt",
"az",
"cv",
"tr",
"tk",
"tyv",
"sax",
"et",
"fi",
"hu",
"dataset:mc4",
"dataset:wikipedia",
"arxiv:2112.10668",
"arxiv:2204.07580",
"license:apache-2.0",
"autotrain_compatible",
"endpoints_compatible",
"text-generation-inference",
"region:us"
]
| text-generation | 2022-04-07T09:13:42Z | ---
license: apache-2.0
language:
- ar
- he
- vi
- id
- jv
- ms
- tl
- lv
- lt
- eu
- ml
- ta
- te
- hy
- bn
- mr
- hi
- ur
- af
- da
- en
- de
- sv
- fr
- it
- pt
- ro
- es
- el
- os
- tg
- fa
- ja
- ka
- ko
- th
- bxr
- xal
- mn
- sw
- yo
- be
- bg
- ru
- uk
- pl
- my
- uz
- ba
- kk
- ky
- tt
- az
- cv
- tr
- tk
- tyv
- sax
- et
- fi
- hu
pipeline_tag: text-generation
tags:
- multilingual
- PyTorch
- Transformers
- gpt3
- gpt2
- Deepspeed
- Megatron
datasets:
- mc4
- wikipedia
thumbnail: "https://github.com/sberbank-ai/mgpt"
---
# Multilingual GPT model
We introduce a family of autoregressive GPT-like models with 1.3 billion parameters trained on 61 languages from 25 language families using Wikipedia and Colossal Clean Crawled Corpus.
We reproduce the GPT-3 architecture using GPT-2 sources and the sparse attention mechanism, [Deepspeed](https://github.com/microsoft/DeepSpeed) and [Megatron](https://github.com/NVIDIA/Megatron-LM) frameworks allows us to effectively parallelize the training and inference steps. The resulting models show performance on par with the recently released [XGLM](https://arxiv.org/pdf/2112.10668.pdf) models at the same time covering more languages and enhancing NLP possibilities for low resource languages.
## Code
The source code for the mGPT XL model is available on [Github](https://github.com/sberbank-ai/mgpt)
## Paper
mGPT: Few-Shot Learners Go Multilingual
[Abstract](https://arxiv.org/abs/2204.07580) [PDF](https://arxiv.org/pdf/2204.07580.pdf)

```
@misc{https://doi.org/10.48550/arxiv.2204.07580,
doi = {10.48550/ARXIV.2204.07580},
url = {https://arxiv.org/abs/2204.07580},
author = {Shliazhko, Oleh and Fenogenova, Alena and Tikhonova, Maria and Mikhailov, Vladislav and Kozlova, Anastasia and Shavrina, Tatiana},
keywords = {Computation and Language (cs.CL), Artificial Intelligence (cs.AI), FOS: Computer and information sciences, FOS: Computer and information sciences, I.2; I.2.7, 68-06, 68-04, 68T50, 68T01},
title = {mGPT: Few-Shot Learners Go Multilingual},
publisher = {arXiv},
year = {2022},
copyright = {Creative Commons Attribution 4.0 International}
}
```
## Languages
Model supports 61 languages:
ISO codes:
```ar he vi id jv ms tl lv lt eu ml ta te hy bn mr hi ur af da en de sv fr it pt ro es el os tg fa ja ka ko th bxr xal mn sw yo be bg ru uk pl my uz ba kk ky tt az cv tr tk tyv sax et fi hu```
Languages:
```Arabic, Hebrew, Vietnamese, Indonesian, Javanese, Malay, Tagalog, Latvian, Lithuanian, Basque, Malayalam, Tamil, Telugu, Armenian, Bengali, Marathi, Hindi, Urdu, Afrikaans, Danish, English, German, Swedish, French, Italian, Portuguese, Romanian, Spanish, Greek, Ossetian, Tajik, Persian, Japanese, Georgian, Korean, Thai, Buryat, Kalmyk, Mongolian, Swahili, Yoruba, Belarusian, Bulgarian, Russian, Ukrainian, Polish, Burmese, Uzbek, Bashkir, Kazakh, Kyrgyz, Tatar, Azerbaijani, Chuvash, Turkish, Turkmen, Tuvan, Yakut, Estonian, Finnish, Hungarian```
## Training Data Statistics
- Size: 488 Billion UTF characters
<img style="text-align:center; display:block;" src="https://huggingface.co/sberbank-ai/mGPT/resolve/main/stats.png">
"General training corpus statistics"
## Details
The model was trained with sequence length 512 using Megatron and Deepspeed libs by [SberDevices](https://sberdevices.ru/) team on a dataset of 600 GB of texts in 61 languages. The model has seen 440 billion BPE tokens in total.
Total training time was around 14 days on 256 Nvidia V100 GPUs.
|
mradermacher/zephyr-qwen2-7b-dpo-i1-GGUF | mradermacher | 2024-06-21T09:43:41Z | 12,129 | 0 | transformers | [
"transformers",
"gguf",
"alignment-handbook",
"trl",
"dpo",
"generated_from_trainer",
"en",
"dataset:HuggingFaceH4/ultrafeedback_binarized",
"base_model:tanliboy/zephyr-qwen2-7b-dpo",
"license:apache-2.0",
"endpoints_compatible",
"region:us"
]
| null | 2024-06-21T06:26:41Z | ---
base_model: tanliboy/zephyr-qwen2-7b-dpo
datasets:
- HuggingFaceH4/ultrafeedback_binarized
language:
- en
library_name: transformers
license: apache-2.0
quantized_by: mradermacher
tags:
- alignment-handbook
- trl
- dpo
- generated_from_trainer
- trl
- dpo
- generated_from_trainer
---
## About
<!-- ### quantize_version: 2 -->
<!-- ### output_tensor_quantised: 1 -->
<!-- ### convert_type: hf -->
<!-- ### vocab_type: -->
<!-- ### tags: nicoboss -->
weighted/imatrix quants of https://huggingface.co/tanliboy/zephyr-qwen2-7b-dpo
<!-- provided-files -->
static quants are available at https://huggingface.co/mradermacher/zephyr-qwen2-7b-dpo-GGUF
## Usage
If you are unsure how to use GGUF files, refer to one of [TheBloke's
READMEs](https://huggingface.co/TheBloke/KafkaLM-70B-German-V0.1-GGUF) for
more details, including on how to concatenate multi-part files.
## Provided Quants
(sorted by size, not necessarily quality. IQ-quants are often preferable over similar sized non-IQ quants)
| Link | Type | Size/GB | Notes |
|:-----|:-----|--------:|:------|
| [GGUF](https://huggingface.co/mradermacher/zephyr-qwen2-7b-dpo-i1-GGUF/resolve/main/zephyr-qwen2-7b-dpo.i1-IQ1_S.gguf) | i1-IQ1_S | 2.0 | for the desperate |
| [GGUF](https://huggingface.co/mradermacher/zephyr-qwen2-7b-dpo-i1-GGUF/resolve/main/zephyr-qwen2-7b-dpo.i1-IQ1_M.gguf) | i1-IQ1_M | 2.1 | mostly desperate |
| [GGUF](https://huggingface.co/mradermacher/zephyr-qwen2-7b-dpo-i1-GGUF/resolve/main/zephyr-qwen2-7b-dpo.i1-IQ2_XXS.gguf) | i1-IQ2_XXS | 2.4 | |
| [GGUF](https://huggingface.co/mradermacher/zephyr-qwen2-7b-dpo-i1-GGUF/resolve/main/zephyr-qwen2-7b-dpo.i1-IQ2_XS.gguf) | i1-IQ2_XS | 2.6 | |
| [GGUF](https://huggingface.co/mradermacher/zephyr-qwen2-7b-dpo-i1-GGUF/resolve/main/zephyr-qwen2-7b-dpo.i1-IQ2_S.gguf) | i1-IQ2_S | 2.7 | |
| [GGUF](https://huggingface.co/mradermacher/zephyr-qwen2-7b-dpo-i1-GGUF/resolve/main/zephyr-qwen2-7b-dpo.i1-IQ2_M.gguf) | i1-IQ2_M | 2.9 | |
| [GGUF](https://huggingface.co/mradermacher/zephyr-qwen2-7b-dpo-i1-GGUF/resolve/main/zephyr-qwen2-7b-dpo.i1-Q2_K.gguf) | i1-Q2_K | 3.1 | IQ3_XXS probably better |
| [GGUF](https://huggingface.co/mradermacher/zephyr-qwen2-7b-dpo-i1-GGUF/resolve/main/zephyr-qwen2-7b-dpo.i1-IQ3_XXS.gguf) | i1-IQ3_XXS | 3.2 | lower quality |
| [GGUF](https://huggingface.co/mradermacher/zephyr-qwen2-7b-dpo-i1-GGUF/resolve/main/zephyr-qwen2-7b-dpo.i1-IQ3_XS.gguf) | i1-IQ3_XS | 3.4 | |
| [GGUF](https://huggingface.co/mradermacher/zephyr-qwen2-7b-dpo-i1-GGUF/resolve/main/zephyr-qwen2-7b-dpo.i1-Q3_K_S.gguf) | i1-Q3_K_S | 3.6 | IQ3_XS probably better |
| [GGUF](https://huggingface.co/mradermacher/zephyr-qwen2-7b-dpo-i1-GGUF/resolve/main/zephyr-qwen2-7b-dpo.i1-IQ3_S.gguf) | i1-IQ3_S | 3.6 | beats Q3_K* |
| [GGUF](https://huggingface.co/mradermacher/zephyr-qwen2-7b-dpo-i1-GGUF/resolve/main/zephyr-qwen2-7b-dpo.i1-IQ3_M.gguf) | i1-IQ3_M | 3.7 | |
| [GGUF](https://huggingface.co/mradermacher/zephyr-qwen2-7b-dpo-i1-GGUF/resolve/main/zephyr-qwen2-7b-dpo.i1-Q3_K_M.gguf) | i1-Q3_K_M | 3.9 | IQ3_S probably better |
| [GGUF](https://huggingface.co/mradermacher/zephyr-qwen2-7b-dpo-i1-GGUF/resolve/main/zephyr-qwen2-7b-dpo.i1-Q3_K_L.gguf) | i1-Q3_K_L | 4.2 | IQ3_M probably better |
| [GGUF](https://huggingface.co/mradermacher/zephyr-qwen2-7b-dpo-i1-GGUF/resolve/main/zephyr-qwen2-7b-dpo.i1-IQ4_XS.gguf) | i1-IQ4_XS | 4.3 | |
| [GGUF](https://huggingface.co/mradermacher/zephyr-qwen2-7b-dpo-i1-GGUF/resolve/main/zephyr-qwen2-7b-dpo.i1-Q4_0.gguf) | i1-Q4_0 | 4.5 | fast, low quality |
| [GGUF](https://huggingface.co/mradermacher/zephyr-qwen2-7b-dpo-i1-GGUF/resolve/main/zephyr-qwen2-7b-dpo.i1-Q4_K_S.gguf) | i1-Q4_K_S | 4.6 | optimal size/speed/quality |
| [GGUF](https://huggingface.co/mradermacher/zephyr-qwen2-7b-dpo-i1-GGUF/resolve/main/zephyr-qwen2-7b-dpo.i1-Q4_K_M.gguf) | i1-Q4_K_M | 4.8 | fast, recommended |
| [GGUF](https://huggingface.co/mradermacher/zephyr-qwen2-7b-dpo-i1-GGUF/resolve/main/zephyr-qwen2-7b-dpo.i1-Q5_K_S.gguf) | i1-Q5_K_S | 5.4 | |
| [GGUF](https://huggingface.co/mradermacher/zephyr-qwen2-7b-dpo-i1-GGUF/resolve/main/zephyr-qwen2-7b-dpo.i1-Q5_K_M.gguf) | i1-Q5_K_M | 5.5 | |
| [GGUF](https://huggingface.co/mradermacher/zephyr-qwen2-7b-dpo-i1-GGUF/resolve/main/zephyr-qwen2-7b-dpo.i1-Q6_K.gguf) | i1-Q6_K | 6.4 | practically like static Q6_K |
Here is a handy graph by ikawrakow comparing some lower-quality quant
types (lower is better):

And here are Artefact2's thoughts on the matter:
https://gist.github.com/Artefact2/b5f810600771265fc1e39442288e8ec9
## FAQ / Model Request
See https://huggingface.co/mradermacher/model_requests for some answers to
questions you might have and/or if you want some other model quantized.
## Thanks
I thank my company, [nethype GmbH](https://www.nethype.de/), for letting
me use its servers and providing upgrades to my workstation to enable
this work in my free time. Additional thanks to [@nicoboss](https://huggingface.co/nicoboss) for giving me access to his hardware for calculating the imatrix for these quants.
<!-- end -->
|
RichardErkhov/Locutusque_-_OpenCerebrum-1.5-Mistral-7B-v0.2-beta-gguf | RichardErkhov | 2024-06-20T13:19:33Z | 12,126 | 1 | null | [
"gguf",
"arxiv:1910.09700",
"region:us"
]
| null | 2024-06-20T06:52:06Z | Quantization made by Richard Erkhov.
[Github](https://github.com/RichardErkhov)
[Discord](https://discord.gg/pvy7H8DZMG)
[Request more models](https://github.com/RichardErkhov/quant_request)
OpenCerebrum-1.5-Mistral-7B-v0.2-beta - GGUF
- Model creator: https://huggingface.co/Locutusque/
- Original model: https://huggingface.co/Locutusque/OpenCerebrum-1.5-Mistral-7B-v0.2-beta/
| Name | Quant method | Size |
| ---- | ---- | ---- |
| [OpenCerebrum-1.5-Mistral-7B-v0.2-beta.Q2_K.gguf](https://huggingface.co/RichardErkhov/Locutusque_-_OpenCerebrum-1.5-Mistral-7B-v0.2-beta-gguf/blob/main/OpenCerebrum-1.5-Mistral-7B-v0.2-beta.Q2_K.gguf) | Q2_K | 2.53GB |
| [OpenCerebrum-1.5-Mistral-7B-v0.2-beta.IQ3_XS.gguf](https://huggingface.co/RichardErkhov/Locutusque_-_OpenCerebrum-1.5-Mistral-7B-v0.2-beta-gguf/blob/main/OpenCerebrum-1.5-Mistral-7B-v0.2-beta.IQ3_XS.gguf) | IQ3_XS | 2.81GB |
| [OpenCerebrum-1.5-Mistral-7B-v0.2-beta.IQ3_S.gguf](https://huggingface.co/RichardErkhov/Locutusque_-_OpenCerebrum-1.5-Mistral-7B-v0.2-beta-gguf/blob/main/OpenCerebrum-1.5-Mistral-7B-v0.2-beta.IQ3_S.gguf) | IQ3_S | 2.96GB |
| [OpenCerebrum-1.5-Mistral-7B-v0.2-beta.Q3_K_S.gguf](https://huggingface.co/RichardErkhov/Locutusque_-_OpenCerebrum-1.5-Mistral-7B-v0.2-beta-gguf/blob/main/OpenCerebrum-1.5-Mistral-7B-v0.2-beta.Q3_K_S.gguf) | Q3_K_S | 2.95GB |
| [OpenCerebrum-1.5-Mistral-7B-v0.2-beta.IQ3_M.gguf](https://huggingface.co/RichardErkhov/Locutusque_-_OpenCerebrum-1.5-Mistral-7B-v0.2-beta-gguf/blob/main/OpenCerebrum-1.5-Mistral-7B-v0.2-beta.IQ3_M.gguf) | IQ3_M | 3.06GB |
| [OpenCerebrum-1.5-Mistral-7B-v0.2-beta.Q3_K.gguf](https://huggingface.co/RichardErkhov/Locutusque_-_OpenCerebrum-1.5-Mistral-7B-v0.2-beta-gguf/blob/main/OpenCerebrum-1.5-Mistral-7B-v0.2-beta.Q3_K.gguf) | Q3_K | 3.28GB |
| [OpenCerebrum-1.5-Mistral-7B-v0.2-beta.Q3_K_M.gguf](https://huggingface.co/RichardErkhov/Locutusque_-_OpenCerebrum-1.5-Mistral-7B-v0.2-beta-gguf/blob/main/OpenCerebrum-1.5-Mistral-7B-v0.2-beta.Q3_K_M.gguf) | Q3_K_M | 3.28GB |
| [OpenCerebrum-1.5-Mistral-7B-v0.2-beta.Q3_K_L.gguf](https://huggingface.co/RichardErkhov/Locutusque_-_OpenCerebrum-1.5-Mistral-7B-v0.2-beta-gguf/blob/main/OpenCerebrum-1.5-Mistral-7B-v0.2-beta.Q3_K_L.gguf) | Q3_K_L | 3.56GB |
| [OpenCerebrum-1.5-Mistral-7B-v0.2-beta.IQ4_XS.gguf](https://huggingface.co/RichardErkhov/Locutusque_-_OpenCerebrum-1.5-Mistral-7B-v0.2-beta-gguf/blob/main/OpenCerebrum-1.5-Mistral-7B-v0.2-beta.IQ4_XS.gguf) | IQ4_XS | 3.67GB |
| [OpenCerebrum-1.5-Mistral-7B-v0.2-beta.Q4_0.gguf](https://huggingface.co/RichardErkhov/Locutusque_-_OpenCerebrum-1.5-Mistral-7B-v0.2-beta-gguf/blob/main/OpenCerebrum-1.5-Mistral-7B-v0.2-beta.Q4_0.gguf) | Q4_0 | 3.83GB |
| [OpenCerebrum-1.5-Mistral-7B-v0.2-beta.IQ4_NL.gguf](https://huggingface.co/RichardErkhov/Locutusque_-_OpenCerebrum-1.5-Mistral-7B-v0.2-beta-gguf/blob/main/OpenCerebrum-1.5-Mistral-7B-v0.2-beta.IQ4_NL.gguf) | IQ4_NL | 3.87GB |
| [OpenCerebrum-1.5-Mistral-7B-v0.2-beta.Q4_K_S.gguf](https://huggingface.co/RichardErkhov/Locutusque_-_OpenCerebrum-1.5-Mistral-7B-v0.2-beta-gguf/blob/main/OpenCerebrum-1.5-Mistral-7B-v0.2-beta.Q4_K_S.gguf) | Q4_K_S | 3.86GB |
| [OpenCerebrum-1.5-Mistral-7B-v0.2-beta.Q4_K.gguf](https://huggingface.co/RichardErkhov/Locutusque_-_OpenCerebrum-1.5-Mistral-7B-v0.2-beta-gguf/blob/main/OpenCerebrum-1.5-Mistral-7B-v0.2-beta.Q4_K.gguf) | Q4_K | 4.07GB |
| [OpenCerebrum-1.5-Mistral-7B-v0.2-beta.Q4_K_M.gguf](https://huggingface.co/RichardErkhov/Locutusque_-_OpenCerebrum-1.5-Mistral-7B-v0.2-beta-gguf/blob/main/OpenCerebrum-1.5-Mistral-7B-v0.2-beta.Q4_K_M.gguf) | Q4_K_M | 4.07GB |
| [OpenCerebrum-1.5-Mistral-7B-v0.2-beta.Q4_1.gguf](https://huggingface.co/RichardErkhov/Locutusque_-_OpenCerebrum-1.5-Mistral-7B-v0.2-beta-gguf/blob/main/OpenCerebrum-1.5-Mistral-7B-v0.2-beta.Q4_1.gguf) | Q4_1 | 4.24GB |
| [OpenCerebrum-1.5-Mistral-7B-v0.2-beta.Q5_0.gguf](https://huggingface.co/RichardErkhov/Locutusque_-_OpenCerebrum-1.5-Mistral-7B-v0.2-beta-gguf/blob/main/OpenCerebrum-1.5-Mistral-7B-v0.2-beta.Q5_0.gguf) | Q5_0 | 4.65GB |
| [OpenCerebrum-1.5-Mistral-7B-v0.2-beta.Q5_K_S.gguf](https://huggingface.co/RichardErkhov/Locutusque_-_OpenCerebrum-1.5-Mistral-7B-v0.2-beta-gguf/blob/main/OpenCerebrum-1.5-Mistral-7B-v0.2-beta.Q5_K_S.gguf) | Q5_K_S | 4.65GB |
| [OpenCerebrum-1.5-Mistral-7B-v0.2-beta.Q5_K.gguf](https://huggingface.co/RichardErkhov/Locutusque_-_OpenCerebrum-1.5-Mistral-7B-v0.2-beta-gguf/blob/main/OpenCerebrum-1.5-Mistral-7B-v0.2-beta.Q5_K.gguf) | Q5_K | 4.78GB |
| [OpenCerebrum-1.5-Mistral-7B-v0.2-beta.Q5_K_M.gguf](https://huggingface.co/RichardErkhov/Locutusque_-_OpenCerebrum-1.5-Mistral-7B-v0.2-beta-gguf/blob/main/OpenCerebrum-1.5-Mistral-7B-v0.2-beta.Q5_K_M.gguf) | Q5_K_M | 4.78GB |
| [OpenCerebrum-1.5-Mistral-7B-v0.2-beta.Q5_1.gguf](https://huggingface.co/RichardErkhov/Locutusque_-_OpenCerebrum-1.5-Mistral-7B-v0.2-beta-gguf/blob/main/OpenCerebrum-1.5-Mistral-7B-v0.2-beta.Q5_1.gguf) | Q5_1 | 5.07GB |
| [OpenCerebrum-1.5-Mistral-7B-v0.2-beta.Q6_K.gguf](https://huggingface.co/RichardErkhov/Locutusque_-_OpenCerebrum-1.5-Mistral-7B-v0.2-beta-gguf/blob/main/OpenCerebrum-1.5-Mistral-7B-v0.2-beta.Q6_K.gguf) | Q6_K | 5.53GB |
| [OpenCerebrum-1.5-Mistral-7B-v0.2-beta.Q8_0.gguf](https://huggingface.co/RichardErkhov/Locutusque_-_OpenCerebrum-1.5-Mistral-7B-v0.2-beta-gguf/blob/main/OpenCerebrum-1.5-Mistral-7B-v0.2-beta.Q8_0.gguf) | Q8_0 | 7.17GB |
Original model description:
---
language:
- en
license: apache-2.0
library_name: transformers
---
# Model Card for Model ID
<!-- Provide a quick summary of what the model is/does. -->
This model represents a kind of trial model to assess its performance subsequent to reducing the dataset's size. An in-house filtering technique has been employed. This iteration of OpenCerebrum remains unfinished and will persist in an incomplete state. Version 2.0 will encompass a fully open SFT and DPO dataset.
## Model Details
### Model Description
<!-- Provide a longer summary of what this model is. -->
This is the model card of a 🤗 transformers model that has been pushed on the Hub. This model card has been automatically generated.
- **Developed by:** [More Information Needed]
- **Funded by [optional]:** [More Information Needed]
- **Shared by [optional]:** [More Information Needed]
- **Model type:** [More Information Needed]
- **Language(s) (NLP):** [More Information Needed]
- **License:** [More Information Needed]
- **Finetuned from model [optional]:** [More Information Needed]
### Model Sources [optional]
<!-- Provide the basic links for the model. -->
- **Repository:** [More Information Needed]
- **Paper [optional]:** [More Information Needed]
- **Demo [optional]:** [More Information Needed]
## Uses
<!-- Address questions around how the model is intended to be used, including the foreseeable users of the model and those affected by the model. -->
### Direct Use
<!-- This section is for the model use without fine-tuning or plugging into a larger ecosystem/app. -->
[More Information Needed]
### Downstream Use [optional]
<!-- This section is for the model use when fine-tuned for a task, or when plugged into a larger ecosystem/app -->
[More Information Needed]
### Out-of-Scope Use
<!-- This section addresses misuse, malicious use, and uses that the model will not work well for. -->
[More Information Needed]
## Bias, Risks, and Limitations
<!-- This section is meant to convey both technical and sociotechnical limitations. -->
[More Information Needed]
### Recommendations
<!-- This section is meant to convey recommendations with respect to the bias, risk, and technical limitations. -->
Users (both direct and downstream) should be made aware of the risks, biases and limitations of the model. More information needed for further recommendations.
## How to Get Started with the Model
Use the code below to get started with the model.
[More Information Needed]
## Training Details
### Training Data
<!-- This should link to a Dataset Card, perhaps with a short stub of information on what the training data is all about as well as documentation related to data pre-processing or additional filtering. -->
[More Information Needed]
### Training Procedure
<!-- This relates heavily to the Technical Specifications. Content here should link to that section when it is relevant to the training procedure. -->
#### Preprocessing [optional]
[More Information Needed]
#### Training Hyperparameters
- **Training regime:** [More Information Needed] <!--fp32, fp16 mixed precision, bf16 mixed precision, bf16 non-mixed precision, fp16 non-mixed precision, fp8 mixed precision -->
#### Speeds, Sizes, Times [optional]
<!-- This section provides information about throughput, start/end time, checkpoint size if relevant, etc. -->
[More Information Needed]
## Evaluation
<!-- This section describes the evaluation protocols and provides the results. -->
### Testing Data, Factors & Metrics
#### Testing Data
<!-- This should link to a Dataset Card if possible. -->
[More Information Needed]
#### Factors
<!-- These are the things the evaluation is disaggregating by, e.g., subpopulations or domains. -->
[More Information Needed]
#### Metrics
<!-- These are the evaluation metrics being used, ideally with a description of why. -->
[More Information Needed]
### Results
[More Information Needed]
#### Summary
## Model Examination [optional]
<!-- Relevant interpretability work for the model goes here -->
[More Information Needed]
## Environmental Impact
<!-- Total emissions (in grams of CO2eq) and additional considerations, such as electricity usage, go here. Edit the suggested text below accordingly -->
Carbon emissions can be estimated using the [Machine Learning Impact calculator](https://mlco2.github.io/impact#compute) presented in [Lacoste et al. (2019)](https://arxiv.org/abs/1910.09700).
- **Hardware Type:** [More Information Needed]
- **Hours used:** [More Information Needed]
- **Cloud Provider:** [More Information Needed]
- **Compute Region:** [More Information Needed]
- **Carbon Emitted:** [More Information Needed]
## Technical Specifications [optional]
### Model Architecture and Objective
[More Information Needed]
### Compute Infrastructure
[More Information Needed]
#### Hardware
[More Information Needed]
#### Software
[More Information Needed]
## Citation [optional]
<!-- If there is a paper or blog post introducing the model, the APA and Bibtex information for that should go in this section. -->
**BibTeX:**
[More Information Needed]
**APA:**
[More Information Needed]
## Glossary [optional]
<!-- If relevant, include terms and calculations in this section that can help readers understand the model or model card. -->
[More Information Needed]
## More Information [optional]
[More Information Needed]
## Model Card Authors [optional]
[More Information Needed]
## Model Card Contact
[More Information Needed]
|
mradermacher/RoLlama2-7b-Instruct-GGUF | mradermacher | 2024-06-28T15:18:27Z | 12,124 | 0 | transformers | [
"transformers",
"gguf",
"ro",
"base_model:OpenLLM-Ro/RoLlama2-7b-Instruct",
"license:cc-by-nc-4.0",
"endpoints_compatible",
"region:us"
]
| null | 2024-06-28T14:17:45Z | ---
base_model: OpenLLM-Ro/RoLlama2-7b-Instruct
language:
- ro
library_name: transformers
license: cc-by-nc-4.0
quantized_by: mradermacher
---
## About
<!-- ### quantize_version: 2 -->
<!-- ### output_tensor_quantised: 1 -->
<!-- ### convert_type: hf -->
<!-- ### vocab_type: -->
<!-- ### tags: -->
static quants of https://huggingface.co/OpenLLM-Ro/RoLlama2-7b-Instruct
<!-- provided-files -->
weighted/imatrix quants seem not to be available (by me) at this time. If they do not show up a week or so after the static ones, I have probably not planned for them. Feel free to request them by opening a Community Discussion.
## Usage
If you are unsure how to use GGUF files, refer to one of [TheBloke's
READMEs](https://huggingface.co/TheBloke/KafkaLM-70B-German-V0.1-GGUF) for
more details, including on how to concatenate multi-part files.
## Provided Quants
(sorted by size, not necessarily quality. IQ-quants are often preferable over similar sized non-IQ quants)
| Link | Type | Size/GB | Notes |
|:-----|:-----|--------:|:------|
| [GGUF](https://huggingface.co/mradermacher/RoLlama2-7b-Instruct-GGUF/resolve/main/RoLlama2-7b-Instruct.Q2_K.gguf) | Q2_K | 2.6 | |
| [GGUF](https://huggingface.co/mradermacher/RoLlama2-7b-Instruct-GGUF/resolve/main/RoLlama2-7b-Instruct.IQ3_XS.gguf) | IQ3_XS | 2.9 | |
| [GGUF](https://huggingface.co/mradermacher/RoLlama2-7b-Instruct-GGUF/resolve/main/RoLlama2-7b-Instruct.IQ3_S.gguf) | IQ3_S | 3.0 | beats Q3_K* |
| [GGUF](https://huggingface.co/mradermacher/RoLlama2-7b-Instruct-GGUF/resolve/main/RoLlama2-7b-Instruct.Q3_K_S.gguf) | Q3_K_S | 3.0 | |
| [GGUF](https://huggingface.co/mradermacher/RoLlama2-7b-Instruct-GGUF/resolve/main/RoLlama2-7b-Instruct.IQ3_M.gguf) | IQ3_M | 3.2 | |
| [GGUF](https://huggingface.co/mradermacher/RoLlama2-7b-Instruct-GGUF/resolve/main/RoLlama2-7b-Instruct.Q3_K_M.gguf) | Q3_K_M | 3.4 | lower quality |
| [GGUF](https://huggingface.co/mradermacher/RoLlama2-7b-Instruct-GGUF/resolve/main/RoLlama2-7b-Instruct.Q3_K_L.gguf) | Q3_K_L | 3.7 | |
| [GGUF](https://huggingface.co/mradermacher/RoLlama2-7b-Instruct-GGUF/resolve/main/RoLlama2-7b-Instruct.IQ4_XS.gguf) | IQ4_XS | 3.7 | |
| [GGUF](https://huggingface.co/mradermacher/RoLlama2-7b-Instruct-GGUF/resolve/main/RoLlama2-7b-Instruct.Q4_K_S.gguf) | Q4_K_S | 4.0 | fast, recommended |
| [GGUF](https://huggingface.co/mradermacher/RoLlama2-7b-Instruct-GGUF/resolve/main/RoLlama2-7b-Instruct.Q4_K_M.gguf) | Q4_K_M | 4.2 | fast, recommended |
| [GGUF](https://huggingface.co/mradermacher/RoLlama2-7b-Instruct-GGUF/resolve/main/RoLlama2-7b-Instruct.Q5_K_S.gguf) | Q5_K_S | 4.8 | |
| [GGUF](https://huggingface.co/mradermacher/RoLlama2-7b-Instruct-GGUF/resolve/main/RoLlama2-7b-Instruct.Q5_K_M.gguf) | Q5_K_M | 4.9 | |
| [GGUF](https://huggingface.co/mradermacher/RoLlama2-7b-Instruct-GGUF/resolve/main/RoLlama2-7b-Instruct.Q6_K.gguf) | Q6_K | 5.6 | very good quality |
| [GGUF](https://huggingface.co/mradermacher/RoLlama2-7b-Instruct-GGUF/resolve/main/RoLlama2-7b-Instruct.Q8_0.gguf) | Q8_0 | 7.3 | fast, best quality |
| [GGUF](https://huggingface.co/mradermacher/RoLlama2-7b-Instruct-GGUF/resolve/main/RoLlama2-7b-Instruct.f16.gguf) | f16 | 13.6 | 16 bpw, overkill |
Here is a handy graph by ikawrakow comparing some lower-quality quant
types (lower is better):

And here are Artefact2's thoughts on the matter:
https://gist.github.com/Artefact2/b5f810600771265fc1e39442288e8ec9
## FAQ / Model Request
See https://huggingface.co/mradermacher/model_requests for some answers to
questions you might have and/or if you want some other model quantized.
## Thanks
I thank my company, [nethype GmbH](https://www.nethype.de/), for letting
me use its servers and providing upgrades to my workstation to enable
this work in my free time.
<!-- end -->
|
mradermacher/Llama-3-Umbral-Mind-SimPO-8B-GGUF | mradermacher | 2024-06-25T00:45:26Z | 12,122 | 0 | transformers | [
"transformers",
"gguf",
"mergekit",
"merge",
"en",
"base_model:mpasila/Llama-3-Umbral-Mind-SimPO-8B",
"license:llama3",
"endpoints_compatible",
"region:us"
]
| null | 2024-06-24T15:23:56Z | ---
base_model: mpasila/Llama-3-Umbral-Mind-SimPO-8B
language:
- en
library_name: transformers
license: llama3
quantized_by: mradermacher
tags:
- mergekit
- merge
---
## About
<!-- ### quantize_version: 2 -->
<!-- ### output_tensor_quantised: 1 -->
<!-- ### convert_type: hf -->
<!-- ### vocab_type: -->
<!-- ### tags: -->
static quants of https://huggingface.co/mpasila/Llama-3-Umbral-Mind-SimPO-8B
<!-- provided-files -->
weighted/imatrix quants are available at https://huggingface.co/mradermacher/Llama-3-Umbral-Mind-SimPO-8B-i1-GGUF
## Usage
If you are unsure how to use GGUF files, refer to one of [TheBloke's
READMEs](https://huggingface.co/TheBloke/KafkaLM-70B-German-V0.1-GGUF) for
more details, including on how to concatenate multi-part files.
## Provided Quants
(sorted by size, not necessarily quality. IQ-quants are often preferable over similar sized non-IQ quants)
| Link | Type | Size/GB | Notes |
|:-----|:-----|--------:|:------|
| [GGUF](https://huggingface.co/mradermacher/Llama-3-Umbral-Mind-SimPO-8B-GGUF/resolve/main/Llama-3-Umbral-Mind-SimPO-8B.Q2_K.gguf) | Q2_K | 3.3 | |
| [GGUF](https://huggingface.co/mradermacher/Llama-3-Umbral-Mind-SimPO-8B-GGUF/resolve/main/Llama-3-Umbral-Mind-SimPO-8B.IQ3_XS.gguf) | IQ3_XS | 3.6 | |
| [GGUF](https://huggingface.co/mradermacher/Llama-3-Umbral-Mind-SimPO-8B-GGUF/resolve/main/Llama-3-Umbral-Mind-SimPO-8B.Q3_K_S.gguf) | Q3_K_S | 3.8 | |
| [GGUF](https://huggingface.co/mradermacher/Llama-3-Umbral-Mind-SimPO-8B-GGUF/resolve/main/Llama-3-Umbral-Mind-SimPO-8B.IQ3_S.gguf) | IQ3_S | 3.8 | beats Q3_K* |
| [GGUF](https://huggingface.co/mradermacher/Llama-3-Umbral-Mind-SimPO-8B-GGUF/resolve/main/Llama-3-Umbral-Mind-SimPO-8B.IQ3_M.gguf) | IQ3_M | 3.9 | |
| [GGUF](https://huggingface.co/mradermacher/Llama-3-Umbral-Mind-SimPO-8B-GGUF/resolve/main/Llama-3-Umbral-Mind-SimPO-8B.Q3_K_M.gguf) | Q3_K_M | 4.1 | lower quality |
| [GGUF](https://huggingface.co/mradermacher/Llama-3-Umbral-Mind-SimPO-8B-GGUF/resolve/main/Llama-3-Umbral-Mind-SimPO-8B.Q3_K_L.gguf) | Q3_K_L | 4.4 | |
| [GGUF](https://huggingface.co/mradermacher/Llama-3-Umbral-Mind-SimPO-8B-GGUF/resolve/main/Llama-3-Umbral-Mind-SimPO-8B.IQ4_XS.gguf) | IQ4_XS | 4.6 | |
| [GGUF](https://huggingface.co/mradermacher/Llama-3-Umbral-Mind-SimPO-8B-GGUF/resolve/main/Llama-3-Umbral-Mind-SimPO-8B.Q4_K_S.gguf) | Q4_K_S | 4.8 | fast, recommended |
| [GGUF](https://huggingface.co/mradermacher/Llama-3-Umbral-Mind-SimPO-8B-GGUF/resolve/main/Llama-3-Umbral-Mind-SimPO-8B.Q4_K_M.gguf) | Q4_K_M | 5.0 | fast, recommended |
| [GGUF](https://huggingface.co/mradermacher/Llama-3-Umbral-Mind-SimPO-8B-GGUF/resolve/main/Llama-3-Umbral-Mind-SimPO-8B.Q5_K_S.gguf) | Q5_K_S | 5.7 | |
| [GGUF](https://huggingface.co/mradermacher/Llama-3-Umbral-Mind-SimPO-8B-GGUF/resolve/main/Llama-3-Umbral-Mind-SimPO-8B.Q5_K_M.gguf) | Q5_K_M | 5.8 | |
| [GGUF](https://huggingface.co/mradermacher/Llama-3-Umbral-Mind-SimPO-8B-GGUF/resolve/main/Llama-3-Umbral-Mind-SimPO-8B.Q6_K.gguf) | Q6_K | 6.7 | very good quality |
| [GGUF](https://huggingface.co/mradermacher/Llama-3-Umbral-Mind-SimPO-8B-GGUF/resolve/main/Llama-3-Umbral-Mind-SimPO-8B.Q8_0.gguf) | Q8_0 | 8.6 | fast, best quality |
| [GGUF](https://huggingface.co/mradermacher/Llama-3-Umbral-Mind-SimPO-8B-GGUF/resolve/main/Llama-3-Umbral-Mind-SimPO-8B.f16.gguf) | f16 | 16.2 | 16 bpw, overkill |
Here is a handy graph by ikawrakow comparing some lower-quality quant
types (lower is better):

And here are Artefact2's thoughts on the matter:
https://gist.github.com/Artefact2/b5f810600771265fc1e39442288e8ec9
## FAQ / Model Request
See https://huggingface.co/mradermacher/model_requests for some answers to
questions you might have and/or if you want some other model quantized.
## Thanks
I thank my company, [nethype GmbH](https://www.nethype.de/), for letting
me use its servers and providing upgrades to my workstation to enable
this work in my free time.
<!-- end -->
|
EK12317/Ekmix-Diffusion | EK12317 | 2023-03-20T15:28:39Z | 12,115 | 60 | diffusers | [
"diffusers",
"stable-diffusion",
"stable-diffusion-diffusers",
"text-to-image",
"en",
"license:creativeml-openrail-m",
"autotrain_compatible",
"endpoints_compatible",
"diffusers:StableDiffusionPipeline",
"region:us"
]
| text-to-image | 2022-12-18T06:45:54Z | ---
language:
- en
license: creativeml-openrail-m
tags:
- stable-diffusion
- stable-diffusion-diffusers
- text-to-image
- diffusers
inference: true
---
## Example:
”Negative prompt: (worst quality, low quality:1.4)” is really useful in anywhere
I think all models are great with correct Hires.fix
## Ekmix-Pastel
pastel but lines(with Hires.fix) (Merging the loras into the model.)
~~~
python networks\merge_lora.py --sd_model .\models\model.safetensors --save_to .\lora\2.safetensors --models .\lora\MagicLORA.pt .\lora\Jordan_3.safetensors .\lora\sttabi_v1.4-04.safetensors .\lora\xlimo768.pt .\lora\dpep2.pt --ratios 0.3 1 0.5 0.6 0.35
~~~
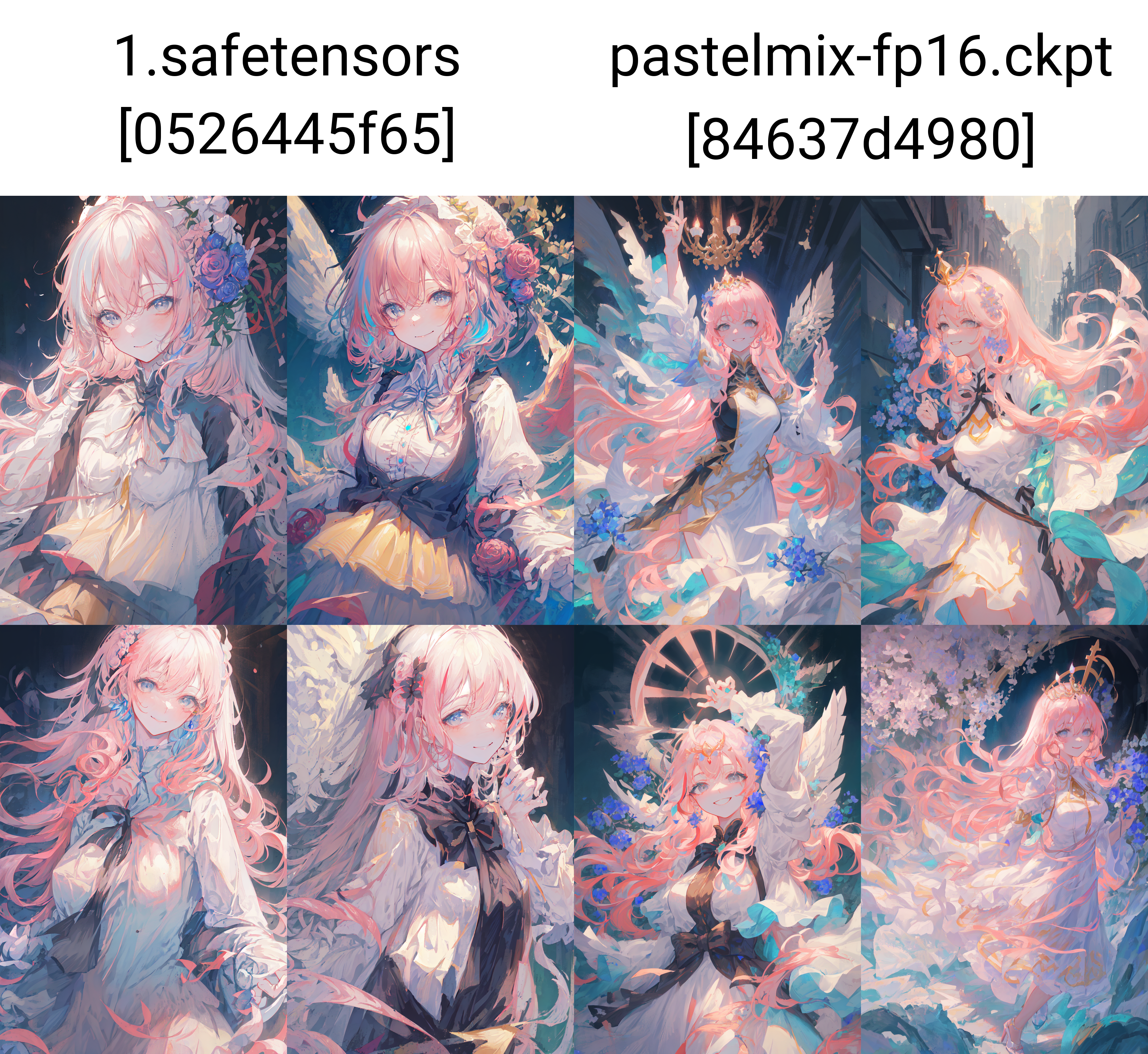
~~~
masterpiece,best quality,best quality,Amazing,beautiful detailed eyes,1girl,finely detail,Depth offield,extremely detailed CG unity 8k wallpaper,masterpiece,upper body,(vtuber minato aqua),pink hair,blue streaked hair, palace,holy,white long split mop dress ,mature female,standing,medium_breasts,silver-tiara,smile,black high heels,very long hair, body towards aside,jewelry,hair blue flower,grey eyes,close-up,
Negative prompt: (worst quality, low quality:1.3)
Steps: 30, Sampler: Euler a, CFG scale: 6, Seed: 191289851, Size: 512x768, Model hash: 0526445f65, Denoising strength: 0.5, Eta: 0.5, Clip skip: 2, ENSD: 31337, Hires resize: 856x1280, Hires steps: 30, Hires upscaler: Latent
~~~
pastel but lines(without hires fix) (better!)
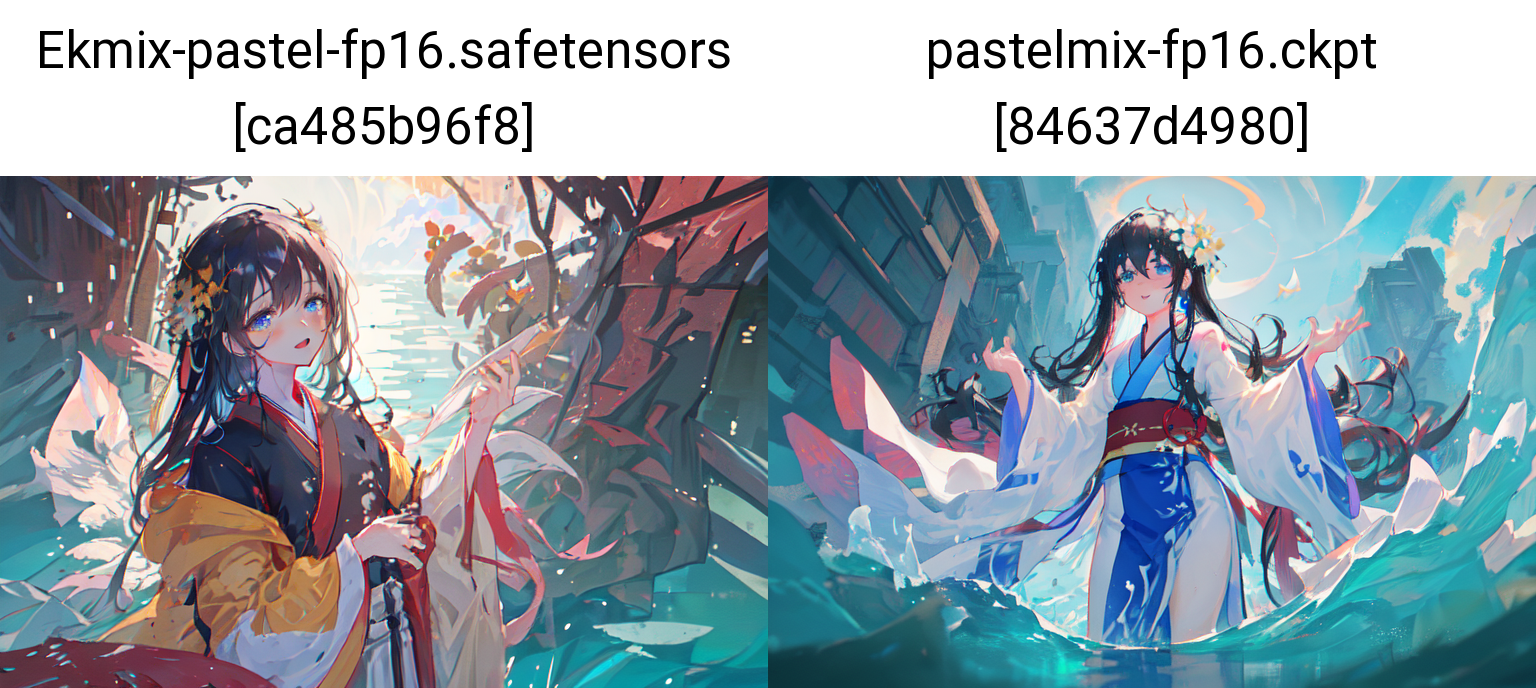
~~~
{masterpiece},{best quality},{1girl,{{loli},black hair,blue eyes,very long hair,hair flower,hanfu,happy}},Amazing,beautiful detailed eyes,finely detail,Depth of field,extremely detailed CG,original,outdoors,beautiful detailed hand,beautiful detailed fingers,{{soaked},{wet through}},{body under water},standing,{beautiful detailed water,beautiful detailed sky,fluttered detailed splashs}
Negative prompt: (worst quality, low quality:1.3)
Steps: 30, Sampler: DPM++ 2M Karras, CFG scale: 6, Seed: 2035526620, Size: 768x512, Model hash: ca485b96f8, Eta: 0.5, Clip skip: 2, ENSD: 31337
~~~
## Ekmix-gen4
balance between anime and reality(Merging by block weighted merge.)

~~~
masterpiece,best quality,best quality,Amazing,beautiful detailed eyes,1girl,finely detail,Depth offield,extremely detailed CG unity 8k wallpaper,masterpiece,upper body,(vtuber minato aqua),pink hair,blue streaked hair, palace,holy,white long split mop dress ,mature female,standing,medium_breasts,silver-tiara,smile,black high heels,very long hair, body towards aside,jewelry,hair blue flower,grey eyes,close-up,
~~~

~~~
{masterpiece},{best quality},{1girl,{{loli},black hair,blue eyes,very long hair,hair flower,hanfu,happy}},Amazing,beautiful detailed eyes,finely detail,Depth of field,extremely detailed CG,original,outdoors,beautiful detailed hand,beautiful detailed fingers,{{soaked},{wet through}},{body under water},standing,{beautiful detailed water,beautiful detailed sky,fluttered detailed splashs},by Paul Hedley,
~~~
# Great hypernetworks
style1 and 2 are my favourite.
3,4 may need retrain.

|
mradermacher/Jellyfish-13B-GGUF | mradermacher | 2024-06-22T03:55:15Z | 12,107 | 0 | transformers | [
"transformers",
"gguf",
"en",
"base_model:NECOUDBFM/Jellyfish-13B",
"endpoints_compatible",
"region:us"
]
| null | 2024-06-21T12:57:22Z | ---
base_model: NECOUDBFM/Jellyfish-13B
language:
- en
library_name: transformers
quantized_by: mradermacher
---
## About
<!-- ### quantize_version: 2 -->
<!-- ### output_tensor_quantised: 1 -->
<!-- ### convert_type: hf -->
<!-- ### vocab_type: -->
<!-- ### tags: -->
static quants of https://huggingface.co/NECOUDBFM/Jellyfish-13B
<!-- provided-files -->
weighted/imatrix quants are available at https://huggingface.co/mradermacher/Jellyfish-13B-i1-GGUF
## Usage
If you are unsure how to use GGUF files, refer to one of [TheBloke's
READMEs](https://huggingface.co/TheBloke/KafkaLM-70B-German-V0.1-GGUF) for
more details, including on how to concatenate multi-part files.
## Provided Quants
(sorted by size, not necessarily quality. IQ-quants are often preferable over similar sized non-IQ quants)
| Link | Type | Size/GB | Notes |
|:-----|:-----|--------:|:------|
| [GGUF](https://huggingface.co/mradermacher/Jellyfish-13B-GGUF/resolve/main/Jellyfish-13B.Q2_K.gguf) | Q2_K | 5.0 | |
| [GGUF](https://huggingface.co/mradermacher/Jellyfish-13B-GGUF/resolve/main/Jellyfish-13B.IQ3_XS.gguf) | IQ3_XS | 5.5 | |
| [GGUF](https://huggingface.co/mradermacher/Jellyfish-13B-GGUF/resolve/main/Jellyfish-13B.IQ3_S.gguf) | IQ3_S | 5.8 | beats Q3_K* |
| [GGUF](https://huggingface.co/mradermacher/Jellyfish-13B-GGUF/resolve/main/Jellyfish-13B.Q3_K_S.gguf) | Q3_K_S | 5.8 | |
| [GGUF](https://huggingface.co/mradermacher/Jellyfish-13B-GGUF/resolve/main/Jellyfish-13B.IQ3_M.gguf) | IQ3_M | 6.1 | |
| [GGUF](https://huggingface.co/mradermacher/Jellyfish-13B-GGUF/resolve/main/Jellyfish-13B.Q3_K_M.gguf) | Q3_K_M | 6.4 | lower quality |
| [GGUF](https://huggingface.co/mradermacher/Jellyfish-13B-GGUF/resolve/main/Jellyfish-13B.Q3_K_L.gguf) | Q3_K_L | 7.0 | |
| [GGUF](https://huggingface.co/mradermacher/Jellyfish-13B-GGUF/resolve/main/Jellyfish-13B.IQ4_XS.gguf) | IQ4_XS | 7.1 | |
| [GGUF](https://huggingface.co/mradermacher/Jellyfish-13B-GGUF/resolve/main/Jellyfish-13B.Q4_K_S.gguf) | Q4_K_S | 7.5 | fast, recommended |
| [GGUF](https://huggingface.co/mradermacher/Jellyfish-13B-GGUF/resolve/main/Jellyfish-13B.Q4_K_M.gguf) | Q4_K_M | 8.0 | fast, recommended |
| [GGUF](https://huggingface.co/mradermacher/Jellyfish-13B-GGUF/resolve/main/Jellyfish-13B.Q5_K_S.gguf) | Q5_K_S | 9.1 | |
| [GGUF](https://huggingface.co/mradermacher/Jellyfish-13B-GGUF/resolve/main/Jellyfish-13B.Q5_K_M.gguf) | Q5_K_M | 9.3 | |
| [GGUF](https://huggingface.co/mradermacher/Jellyfish-13B-GGUF/resolve/main/Jellyfish-13B.Q6_K.gguf) | Q6_K | 10.8 | very good quality |
| [GGUF](https://huggingface.co/mradermacher/Jellyfish-13B-GGUF/resolve/main/Jellyfish-13B.Q8_0.gguf) | Q8_0 | 13.9 | fast, best quality |
Here is a handy graph by ikawrakow comparing some lower-quality quant
types (lower is better):

And here are Artefact2's thoughts on the matter:
https://gist.github.com/Artefact2/b5f810600771265fc1e39442288e8ec9
## FAQ / Model Request
See https://huggingface.co/mradermacher/model_requests for some answers to
questions you might have and/or if you want some other model quantized.
## Thanks
I thank my company, [nethype GmbH](https://www.nethype.de/), for letting
me use its servers and providing upgrades to my workstation to enable
this work in my free time.
<!-- end -->
|
Qwen/CodeQwen1.5-7B-Chat-GGUF | Qwen | 2024-04-23T05:39:54Z | 12,101 | 88 | null | [
"gguf",
"chat",
"text-generation",
"en",
"license:other",
"region:us"
]
| text-generation | 2024-04-15T12:38:36Z | ---
license: other
license_name: tongyi-qianwen
license_link: https://huggingface.co/Qwen/CodeQwen1.5-7B-Chat-GGUF/blob/main/LICENSE
language:
- en
pipeline_tag: text-generation
tags:
- chat
---
# CodeQwen1.5-7B-Chat-GGUF
## Introduction
CodeQwen1.5 is the Code-Specific version of Qwen1.5. It is a transformer-based decoder-only language model pretrained on a large amount of data of codes.
* Strong code generation capabilities and competitve performance across a series of benchmarks;
* Supporting long context understanding and generation with the context length of 64K tokens;
* Supporting 92 coding languages
* Excellent performance in text-to-SQL, bug fix, etc.
For more details, please refer to our [blog post](https://qwenlm.github.io/blog/codeqwen1.5/) and [GitHub repo](https://github.com/QwenLM/Qwen1.5).
In this repo, we provide quantized models in the GGUF formats, including `q2_k`, `q3_k_m`, `q4_0`, `q4_k_m`, `q5_0`, `q5_k_m`, `q6_k` and `q8_0`.
## Model Details
CodeQwen1.5 is based on Qwen1.5, a language model series including decoder language models of different model sizes. It is trained on 3 trillion tokens of data of codes, and it includes group query attention (GQA) for efficient inference.
## Requirements
We advise you to clone [`llama.cpp`](https://github.com/ggerganov/llama.cpp) and install it following the official guide.
## How to use
Cloning the repo may be inefficient, and thus you can manually download the GGUF file that you need or use `huggingface-cli` (`pip install huggingface_hub`) as shown below:
```shell
huggingface-cli download Qwen/CodeQwen1.5-7B-Chat-GGUF codeqwen-1_5-7b-chat-q5_k_m.gguf --local-dir . --local-dir-use-symlinks False
```
We demonstrate how to use `llama.cpp` to run Qwen1.5:
```shell
./main -m codeqwen-1_5-7b-chat-q5_k_m.gguf -n 512 --color -i -cml -f prompts/chat-with-qwen.txt
```
## Citation
If you find our work helpful, feel free to give us a cite.
```
@article{qwen,
title={Qwen Technical Report},
author={Jinze Bai and Shuai Bai and Yunfei Chu and Zeyu Cui and Kai Dang and Xiaodong Deng and Yang Fan and Wenbin Ge and Yu Han and Fei Huang and Binyuan Hui and Luo Ji and Mei Li and Junyang Lin and Runji Lin and Dayiheng Liu and Gao Liu and Chengqiang Lu and Keming Lu and Jianxin Ma and Rui Men and Xingzhang Ren and Xuancheng Ren and Chuanqi Tan and Sinan Tan and Jianhong Tu and Peng Wang and Shijie Wang and Wei Wang and Shengguang Wu and Benfeng Xu and Jin Xu and An Yang and Hao Yang and Jian Yang and Shusheng Yang and Yang Yao and Bowen Yu and Hongyi Yuan and Zheng Yuan and Jianwei Zhang and Xingxuan Zhang and Yichang Zhang and Zhenru Zhang and Chang Zhou and Jingren Zhou and Xiaohuan Zhou and Tianhang Zhu},
journal={arXiv preprint arXiv:2309.16609},
year={2023}
}
```
|
gaianet/gemma-2-27b-it-GGUF | gaianet | 2024-07-02T04:03:40Z | 12,094 | 0 | transformers | [
"transformers",
"gguf",
"gemma2",
"text-generation",
"conversational",
"base_model:google/gemma-2-27b-it",
"license:gemma",
"autotrain_compatible",
"text-generation-inference",
"region:us"
]
| text-generation | 2024-06-28T02:58:50Z | ---
base_model: google/gemma-2-27b-it
inference: false
license: gemma
library_name: transformers
pipeline_tag: text-generation
model_creator: Google
model_name: gemma-2-27b-it
quantized_by: Second State Inc.
tags:
- conversational
---

# Gemma-2-27b-it-GGUF
## Original Model
[google/gemma-2-27b-it](https://huggingface.co/google/gemma-2-27b-it)
## Run with Gaianet
**Prompt template:**
prompt template: `gemma-instruct`
**Context size:**
chat_ctx_size: `8192`
**Run with GaiaNet:**
- Quick start with Gemma-2-27B: https://github.com/GaiaNet-AI/node-configs/blob/main/gemma-2-27b-it/README.md
- Customize your node: https://docs.gaianet.ai/node-guide/customize
*Quantized with llama.cpp b3259*
|
RichardErkhov/Alibaba-NLP_-_gte-Qwen2-7B-instruct-gguf | RichardErkhov | 2024-06-22T15:22:37Z | 12,087 | 1 | null | [
"gguf",
"region:us"
]
| null | 2024-06-22T13:20:44Z | Quantization made by Richard Erkhov.
[Github](https://github.com/RichardErkhov)
[Discord](https://discord.gg/pvy7H8DZMG)
[Request more models](https://github.com/RichardErkhov/quant_request)
gte-Qwen2-7B-instruct - GGUF
- Model creator: https://huggingface.co/Alibaba-NLP/
- Original model: https://huggingface.co/Alibaba-NLP/gte-Qwen2-7B-instruct/
| Name | Quant method | Size |
| ---- | ---- | ---- |
| [gte-Qwen2-7B-instruct.Q2_K.gguf](https://huggingface.co/RichardErkhov/Alibaba-NLP_-_gte-Qwen2-7B-instruct-gguf/blob/main/gte-Qwen2-7B-instruct.Q2_K.gguf) | Q2_K | 2.81GB |
| [gte-Qwen2-7B-instruct.IQ3_XS.gguf](https://huggingface.co/RichardErkhov/Alibaba-NLP_-_gte-Qwen2-7B-instruct-gguf/blob/main/gte-Qwen2-7B-instruct.IQ3_XS.gguf) | IQ3_XS | 3.11GB |
| [gte-Qwen2-7B-instruct.IQ3_S.gguf](https://huggingface.co/RichardErkhov/Alibaba-NLP_-_gte-Qwen2-7B-instruct-gguf/blob/main/gte-Qwen2-7B-instruct.IQ3_S.gguf) | IQ3_S | 3.26GB |
| [gte-Qwen2-7B-instruct.Q3_K_S.gguf](https://huggingface.co/RichardErkhov/Alibaba-NLP_-_gte-Qwen2-7B-instruct-gguf/blob/main/gte-Qwen2-7B-instruct.Q3_K_S.gguf) | Q3_K_S | 3.25GB |
| [gte-Qwen2-7B-instruct.IQ3_M.gguf](https://huggingface.co/RichardErkhov/Alibaba-NLP_-_gte-Qwen2-7B-instruct-gguf/blob/main/gte-Qwen2-7B-instruct.IQ3_M.gguf) | IQ3_M | 3.33GB |
| [gte-Qwen2-7B-instruct.Q3_K.gguf](https://huggingface.co/RichardErkhov/Alibaba-NLP_-_gte-Qwen2-7B-instruct-gguf/blob/main/gte-Qwen2-7B-instruct.Q3_K.gguf) | Q3_K | 3.55GB |
| [gte-Qwen2-7B-instruct.Q3_K_M.gguf](https://huggingface.co/RichardErkhov/Alibaba-NLP_-_gte-Qwen2-7B-instruct-gguf/blob/main/gte-Qwen2-7B-instruct.Q3_K_M.gguf) | Q3_K_M | 3.55GB |
| [gte-Qwen2-7B-instruct.Q3_K_L.gguf](https://huggingface.co/RichardErkhov/Alibaba-NLP_-_gte-Qwen2-7B-instruct-gguf/blob/main/gte-Qwen2-7B-instruct.Q3_K_L.gguf) | Q3_K_L | 3.81GB |
| [gte-Qwen2-7B-instruct.IQ4_XS.gguf](https://huggingface.co/RichardErkhov/Alibaba-NLP_-_gte-Qwen2-7B-instruct-gguf/blob/main/gte-Qwen2-7B-instruct.IQ4_XS.gguf) | IQ4_XS | 3.96GB |
| [gte-Qwen2-7B-instruct.Q4_0.gguf](https://huggingface.co/RichardErkhov/Alibaba-NLP_-_gte-Qwen2-7B-instruct-gguf/blob/main/gte-Qwen2-7B-instruct.Q4_0.gguf) | Q4_0 | 4.13GB |
| [gte-Qwen2-7B-instruct.IQ4_NL.gguf](https://huggingface.co/RichardErkhov/Alibaba-NLP_-_gte-Qwen2-7B-instruct-gguf/blob/main/gte-Qwen2-7B-instruct.IQ4_NL.gguf) | IQ4_NL | 4.15GB |
| [gte-Qwen2-7B-instruct.Q4_K_S.gguf](https://huggingface.co/RichardErkhov/Alibaba-NLP_-_gte-Qwen2-7B-instruct-gguf/blob/main/gte-Qwen2-7B-instruct.Q4_K_S.gguf) | Q4_K_S | 4.15GB |
| [gte-Qwen2-7B-instruct.Q4_K.gguf](https://huggingface.co/RichardErkhov/Alibaba-NLP_-_gte-Qwen2-7B-instruct-gguf/blob/main/gte-Qwen2-7B-instruct.Q4_K.gguf) | Q4_K | 4.36GB |
| [gte-Qwen2-7B-instruct.Q4_K_M.gguf](https://huggingface.co/RichardErkhov/Alibaba-NLP_-_gte-Qwen2-7B-instruct-gguf/blob/main/gte-Qwen2-7B-instruct.Q4_K_M.gguf) | Q4_K_M | 4.36GB |
| [gte-Qwen2-7B-instruct.Q4_1.gguf](https://huggingface.co/RichardErkhov/Alibaba-NLP_-_gte-Qwen2-7B-instruct-gguf/blob/main/gte-Qwen2-7B-instruct.Q4_1.gguf) | Q4_1 | 4.54GB |
| [gte-Qwen2-7B-instruct.Q5_0.gguf](https://huggingface.co/RichardErkhov/Alibaba-NLP_-_gte-Qwen2-7B-instruct-gguf/blob/main/gte-Qwen2-7B-instruct.Q5_0.gguf) | Q5_0 | 4.95GB |
| [gte-Qwen2-7B-instruct.Q5_K_S.gguf](https://huggingface.co/RichardErkhov/Alibaba-NLP_-_gte-Qwen2-7B-instruct-gguf/blob/main/gte-Qwen2-7B-instruct.Q5_K_S.gguf) | Q5_K_S | 4.95GB |
| [gte-Qwen2-7B-instruct.Q5_K.gguf](https://huggingface.co/RichardErkhov/Alibaba-NLP_-_gte-Qwen2-7B-instruct-gguf/blob/main/gte-Qwen2-7B-instruct.Q5_K.gguf) | Q5_K | 5.07GB |
| [gte-Qwen2-7B-instruct.Q5_K_M.gguf](https://huggingface.co/RichardErkhov/Alibaba-NLP_-_gte-Qwen2-7B-instruct-gguf/blob/main/gte-Qwen2-7B-instruct.Q5_K_M.gguf) | Q5_K_M | 5.07GB |
| [gte-Qwen2-7B-instruct.Q5_1.gguf](https://huggingface.co/RichardErkhov/Alibaba-NLP_-_gte-Qwen2-7B-instruct-gguf/blob/main/gte-Qwen2-7B-instruct.Q5_1.gguf) | Q5_1 | 5.36GB |
| [gte-Qwen2-7B-instruct.Q6_K.gguf](https://huggingface.co/RichardErkhov/Alibaba-NLP_-_gte-Qwen2-7B-instruct-gguf/blob/main/gte-Qwen2-7B-instruct.Q6_K.gguf) | Q6_K | 5.82GB |
| [gte-Qwen2-7B-instruct.Q8_0.gguf](https://huggingface.co/RichardErkhov/Alibaba-NLP_-_gte-Qwen2-7B-instruct-gguf/blob/main/gte-Qwen2-7B-instruct.Q8_0.gguf) | Q8_0 | 7.54GB |
Original model description:
---
tags:
- mteb
- sentence-transformers
- transformers
- Qwen2
- sentence-similarity
license: apache-2.0
model-index:
- name: gte-qwen2-7B-instruct
results:
- task:
type: Classification
dataset:
type: mteb/amazon_counterfactual
name: MTEB AmazonCounterfactualClassification (en)
config: en
split: test
revision: e8379541af4e31359cca9fbcf4b00f2671dba205
metrics:
- type: accuracy
value: 91.31343283582089
- type: ap
value: 67.64251402604096
- type: f1
value: 87.53372530755692
- task:
type: Classification
dataset:
type: mteb/amazon_polarity
name: MTEB AmazonPolarityClassification
config: default
split: test
revision: e2d317d38cd51312af73b3d32a06d1a08b442046
metrics:
- type: accuracy
value: 97.497825
- type: ap
value: 96.30329547047529
- type: f1
value: 97.49769793778039
- task:
type: Classification
dataset:
type: mteb/amazon_reviews_multi
name: MTEB AmazonReviewsClassification (en)
config: en
split: test
revision: 1399c76144fd37290681b995c656ef9b2e06e26d
metrics:
- type: accuracy
value: 62.564
- type: f1
value: 60.975777935041066
- task:
type: Retrieval
dataset:
type: mteb/arguana
name: MTEB ArguAna
config: default
split: test
revision: c22ab2a51041ffd869aaddef7af8d8215647e41a
metrics:
- type: map_at_1
value: 36.486000000000004
- type: map_at_10
value: 54.842
- type: map_at_100
value: 55.206999999999994
- type: map_at_1000
value: 55.206999999999994
- type: map_at_3
value: 49.893
- type: map_at_5
value: 53.105000000000004
- type: mrr_at_1
value: 37.34
- type: mrr_at_10
value: 55.143
- type: mrr_at_100
value: 55.509
- type: mrr_at_1000
value: 55.509
- type: mrr_at_3
value: 50.212999999999994
- type: mrr_at_5
value: 53.432
- type: ndcg_at_1
value: 36.486000000000004
- type: ndcg_at_10
value: 64.273
- type: ndcg_at_100
value: 65.66199999999999
- type: ndcg_at_1000
value: 65.66199999999999
- type: ndcg_at_3
value: 54.352999999999994
- type: ndcg_at_5
value: 60.131
- type: precision_at_1
value: 36.486000000000004
- type: precision_at_10
value: 9.395000000000001
- type: precision_at_100
value: 0.996
- type: precision_at_1000
value: 0.1
- type: precision_at_3
value: 22.428
- type: precision_at_5
value: 16.259
- type: recall_at_1
value: 36.486000000000004
- type: recall_at_10
value: 93.95400000000001
- type: recall_at_100
value: 99.644
- type: recall_at_1000
value: 99.644
- type: recall_at_3
value: 67.283
- type: recall_at_5
value: 81.294
- task:
type: Clustering
dataset:
type: mteb/arxiv-clustering-p2p
name: MTEB ArxivClusteringP2P
config: default
split: test
revision: a122ad7f3f0291bf49cc6f4d32aa80929df69d5d
metrics:
- type: v_measure
value: 56.461169803700564
- task:
type: Clustering
dataset:
type: mteb/arxiv-clustering-s2s
name: MTEB ArxivClusteringS2S
config: default
split: test
revision: f910caf1a6075f7329cdf8c1a6135696f37dbd53
metrics:
- type: v_measure
value: 51.73600434466286
- task:
type: Reranking
dataset:
type: mteb/askubuntudupquestions-reranking
name: MTEB AskUbuntuDupQuestions
config: default
split: test
revision: 2000358ca161889fa9c082cb41daa8dcfb161a54
metrics:
- type: map
value: 67.57827065898053
- type: mrr
value: 79.08136569493911
- task:
type: STS
dataset:
type: mteb/biosses-sts
name: MTEB BIOSSES
config: default
split: test
revision: d3fb88f8f02e40887cd149695127462bbcf29b4a
metrics:
- type: cos_sim_pearson
value: 83.53324575999243
- type: cos_sim_spearman
value: 81.37173362822374
- type: euclidean_pearson
value: 82.19243335103444
- type: euclidean_spearman
value: 81.33679307304334
- type: manhattan_pearson
value: 82.38752665975699
- type: manhattan_spearman
value: 81.31510583189689
- task:
type: Classification
dataset:
type: mteb/banking77
name: MTEB Banking77Classification
config: default
split: test
revision: 0fd18e25b25c072e09e0d92ab615fda904d66300
metrics:
- type: accuracy
value: 87.56818181818181
- type: f1
value: 87.25826722019875
- task:
type: Clustering
dataset:
type: mteb/biorxiv-clustering-p2p
name: MTEB BiorxivClusteringP2P
config: default
split: test
revision: 65b79d1d13f80053f67aca9498d9402c2d9f1f40
metrics:
- type: v_measure
value: 50.09239610327673
- task:
type: Clustering
dataset:
type: mteb/biorxiv-clustering-s2s
name: MTEB BiorxivClusteringS2S
config: default
split: test
revision: 258694dd0231531bc1fd9de6ceb52a0853c6d908
metrics:
- type: v_measure
value: 46.64733054606282
- task:
type: Retrieval
dataset:
type: BeIR/cqadupstack
name: MTEB CQADupstackAndroidRetrieval
config: default
split: test
revision: f46a197baaae43b4f621051089b82a364682dfeb
metrics:
- type: map_at_1
value: 33.997
- type: map_at_10
value: 48.176
- type: map_at_100
value: 49.82
- type: map_at_1000
value: 49.924
- type: map_at_3
value: 43.626
- type: map_at_5
value: 46.275
- type: mrr_at_1
value: 42.059999999999995
- type: mrr_at_10
value: 53.726
- type: mrr_at_100
value: 54.398
- type: mrr_at_1000
value: 54.416
- type: mrr_at_3
value: 50.714999999999996
- type: mrr_at_5
value: 52.639
- type: ndcg_at_1
value: 42.059999999999995
- type: ndcg_at_10
value: 55.574999999999996
- type: ndcg_at_100
value: 60.744
- type: ndcg_at_1000
value: 61.85699999999999
- type: ndcg_at_3
value: 49.363
- type: ndcg_at_5
value: 52.44
- type: precision_at_1
value: 42.059999999999995
- type: precision_at_10
value: 11.101999999999999
- type: precision_at_100
value: 1.73
- type: precision_at_1000
value: 0.218
- type: precision_at_3
value: 24.464
- type: precision_at_5
value: 18.026
- type: recall_at_1
value: 33.997
- type: recall_at_10
value: 70.35900000000001
- type: recall_at_100
value: 91.642
- type: recall_at_1000
value: 97.977
- type: recall_at_3
value: 52.76
- type: recall_at_5
value: 61.148
- task:
type: Retrieval
dataset:
type: BeIR/cqadupstack
name: MTEB CQADupstackEnglishRetrieval
config: default
split: test
revision: ad9991cb51e31e31e430383c75ffb2885547b5f0
metrics:
- type: map_at_1
value: 35.884
- type: map_at_10
value: 48.14
- type: map_at_100
value: 49.5
- type: map_at_1000
value: 49.63
- type: map_at_3
value: 44.646
- type: map_at_5
value: 46.617999999999995
- type: mrr_at_1
value: 44.458999999999996
- type: mrr_at_10
value: 53.751000000000005
- type: mrr_at_100
value: 54.37800000000001
- type: mrr_at_1000
value: 54.415
- type: mrr_at_3
value: 51.815
- type: mrr_at_5
value: 52.882
- type: ndcg_at_1
value: 44.458999999999996
- type: ndcg_at_10
value: 54.157
- type: ndcg_at_100
value: 58.362
- type: ndcg_at_1000
value: 60.178
- type: ndcg_at_3
value: 49.661
- type: ndcg_at_5
value: 51.74999999999999
- type: precision_at_1
value: 44.458999999999996
- type: precision_at_10
value: 10.248
- type: precision_at_100
value: 1.5890000000000002
- type: precision_at_1000
value: 0.207
- type: precision_at_3
value: 23.928
- type: precision_at_5
value: 16.878999999999998
- type: recall_at_1
value: 35.884
- type: recall_at_10
value: 64.798
- type: recall_at_100
value: 82.345
- type: recall_at_1000
value: 93.267
- type: recall_at_3
value: 51.847
- type: recall_at_5
value: 57.601
- task:
type: Retrieval
dataset:
type: BeIR/cqadupstack
name: MTEB CQADupstackGamingRetrieval
config: default
split: test
revision: 4885aa143210c98657558c04aaf3dc47cfb54340
metrics:
- type: map_at_1
value: 39.383
- type: map_at_10
value: 53.714
- type: map_at_100
value: 54.838
- type: map_at_1000
value: 54.87800000000001
- type: map_at_3
value: 50.114999999999995
- type: map_at_5
value: 52.153000000000006
- type: mrr_at_1
value: 45.016
- type: mrr_at_10
value: 56.732000000000006
- type: mrr_at_100
value: 57.411
- type: mrr_at_1000
value: 57.431
- type: mrr_at_3
value: 54.044000000000004
- type: mrr_at_5
value: 55.639
- type: ndcg_at_1
value: 45.016
- type: ndcg_at_10
value: 60.228
- type: ndcg_at_100
value: 64.277
- type: ndcg_at_1000
value: 65.07
- type: ndcg_at_3
value: 54.124
- type: ndcg_at_5
value: 57.147000000000006
- type: precision_at_1
value: 45.016
- type: precision_at_10
value: 9.937
- type: precision_at_100
value: 1.288
- type: precision_at_1000
value: 0.13899999999999998
- type: precision_at_3
value: 24.471999999999998
- type: precision_at_5
value: 16.991
- type: recall_at_1
value: 39.383
- type: recall_at_10
value: 76.175
- type: recall_at_100
value: 93.02
- type: recall_at_1000
value: 98.60900000000001
- type: recall_at_3
value: 60.265
- type: recall_at_5
value: 67.46600000000001
- task:
type: Retrieval
dataset:
type: BeIR/cqadupstack
name: MTEB CQADupstackGisRetrieval
config: default
split: test
revision: 5003b3064772da1887988e05400cf3806fe491f2
metrics:
- type: map_at_1
value: 27.426000000000002
- type: map_at_10
value: 37.397000000000006
- type: map_at_100
value: 38.61
- type: map_at_1000
value: 38.678000000000004
- type: map_at_3
value: 34.150999999999996
- type: map_at_5
value: 36.137
- type: mrr_at_1
value: 29.944
- type: mrr_at_10
value: 39.654
- type: mrr_at_100
value: 40.638000000000005
- type: mrr_at_1000
value: 40.691
- type: mrr_at_3
value: 36.817
- type: mrr_at_5
value: 38.524
- type: ndcg_at_1
value: 29.944
- type: ndcg_at_10
value: 43.094
- type: ndcg_at_100
value: 48.789
- type: ndcg_at_1000
value: 50.339999999999996
- type: ndcg_at_3
value: 36.984
- type: ndcg_at_5
value: 40.248
- type: precision_at_1
value: 29.944
- type: precision_at_10
value: 6.78
- type: precision_at_100
value: 1.024
- type: precision_at_1000
value: 0.11800000000000001
- type: precision_at_3
value: 15.895000000000001
- type: precision_at_5
value: 11.39
- type: recall_at_1
value: 27.426000000000002
- type: recall_at_10
value: 58.464000000000006
- type: recall_at_100
value: 84.193
- type: recall_at_1000
value: 95.52000000000001
- type: recall_at_3
value: 42.172
- type: recall_at_5
value: 50.101
- task:
type: Retrieval
dataset:
type: BeIR/cqadupstack
name: MTEB CQADupstackMathematicaRetrieval
config: default
split: test
revision: 90fceea13679c63fe563ded68f3b6f06e50061de
metrics:
- type: map_at_1
value: 19.721
- type: map_at_10
value: 31.604
- type: map_at_100
value: 32.972
- type: map_at_1000
value: 33.077
- type: map_at_3
value: 27.218999999999998
- type: map_at_5
value: 29.53
- type: mrr_at_1
value: 25.0
- type: mrr_at_10
value: 35.843
- type: mrr_at_100
value: 36.785000000000004
- type: mrr_at_1000
value: 36.842000000000006
- type: mrr_at_3
value: 32.193
- type: mrr_at_5
value: 34.264
- type: ndcg_at_1
value: 25.0
- type: ndcg_at_10
value: 38.606
- type: ndcg_at_100
value: 44.272
- type: ndcg_at_1000
value: 46.527
- type: ndcg_at_3
value: 30.985000000000003
- type: ndcg_at_5
value: 34.43
- type: precision_at_1
value: 25.0
- type: precision_at_10
value: 7.811
- type: precision_at_100
value: 1.203
- type: precision_at_1000
value: 0.15
- type: precision_at_3
value: 15.423
- type: precision_at_5
value: 11.791
- type: recall_at_1
value: 19.721
- type: recall_at_10
value: 55.625
- type: recall_at_100
value: 79.34400000000001
- type: recall_at_1000
value: 95.208
- type: recall_at_3
value: 35.19
- type: recall_at_5
value: 43.626
- task:
type: Retrieval
dataset:
type: BeIR/cqadupstack
name: MTEB CQADupstackPhysicsRetrieval
config: default
split: test
revision: 79531abbd1fb92d06c6d6315a0cbbbf5bb247ea4
metrics:
- type: map_at_1
value: 33.784
- type: map_at_10
value: 47.522
- type: map_at_100
value: 48.949999999999996
- type: map_at_1000
value: 49.038
- type: map_at_3
value: 43.284
- type: map_at_5
value: 45.629
- type: mrr_at_1
value: 41.482
- type: mrr_at_10
value: 52.830999999999996
- type: mrr_at_100
value: 53.559999999999995
- type: mrr_at_1000
value: 53.588
- type: mrr_at_3
value: 50.016000000000005
- type: mrr_at_5
value: 51.614000000000004
- type: ndcg_at_1
value: 41.482
- type: ndcg_at_10
value: 54.569
- type: ndcg_at_100
value: 59.675999999999995
- type: ndcg_at_1000
value: 60.989000000000004
- type: ndcg_at_3
value: 48.187000000000005
- type: ndcg_at_5
value: 51.183
- type: precision_at_1
value: 41.482
- type: precision_at_10
value: 10.221
- type: precision_at_100
value: 1.486
- type: precision_at_1000
value: 0.17500000000000002
- type: precision_at_3
value: 23.548
- type: precision_at_5
value: 16.805
- type: recall_at_1
value: 33.784
- type: recall_at_10
value: 69.798
- type: recall_at_100
value: 90.098
- type: recall_at_1000
value: 98.176
- type: recall_at_3
value: 52.127
- type: recall_at_5
value: 59.861
- task:
type: Retrieval
dataset:
type: BeIR/cqadupstack
name: MTEB CQADupstackProgrammersRetrieval
config: default
split: test
revision: 6184bc1440d2dbc7612be22b50686b8826d22b32
metrics:
- type: map_at_1
value: 28.038999999999998
- type: map_at_10
value: 41.904
- type: map_at_100
value: 43.36
- type: map_at_1000
value: 43.453
- type: map_at_3
value: 37.785999999999994
- type: map_at_5
value: 40.105000000000004
- type: mrr_at_1
value: 35.046
- type: mrr_at_10
value: 46.926
- type: mrr_at_100
value: 47.815000000000005
- type: mrr_at_1000
value: 47.849000000000004
- type: mrr_at_3
value: 44.273
- type: mrr_at_5
value: 45.774
- type: ndcg_at_1
value: 35.046
- type: ndcg_at_10
value: 48.937000000000005
- type: ndcg_at_100
value: 54.544000000000004
- type: ndcg_at_1000
value: 56.069
- type: ndcg_at_3
value: 42.858000000000004
- type: ndcg_at_5
value: 45.644
- type: precision_at_1
value: 35.046
- type: precision_at_10
value: 9.452
- type: precision_at_100
value: 1.429
- type: precision_at_1000
value: 0.173
- type: precision_at_3
value: 21.346999999999998
- type: precision_at_5
value: 15.342
- type: recall_at_1
value: 28.038999999999998
- type: recall_at_10
value: 64.59700000000001
- type: recall_at_100
value: 87.735
- type: recall_at_1000
value: 97.41300000000001
- type: recall_at_3
value: 47.368
- type: recall_at_5
value: 54.93900000000001
- task:
type: Retrieval
dataset:
type: BeIR/cqadupstack
name: MTEB CQADupstackRetrieval
config: default
split: test
revision: 4ffe81d471b1924886b33c7567bfb200e9eec5c4
metrics:
- type: map_at_1
value: 28.17291666666667
- type: map_at_10
value: 40.025749999999995
- type: map_at_100
value: 41.39208333333333
- type: map_at_1000
value: 41.499249999999996
- type: map_at_3
value: 36.347
- type: map_at_5
value: 38.41391666666667
- type: mrr_at_1
value: 33.65925
- type: mrr_at_10
value: 44.085499999999996
- type: mrr_at_100
value: 44.94116666666667
- type: mrr_at_1000
value: 44.9855
- type: mrr_at_3
value: 41.2815
- type: mrr_at_5
value: 42.91491666666666
- type: ndcg_at_1
value: 33.65925
- type: ndcg_at_10
value: 46.430833333333325
- type: ndcg_at_100
value: 51.761
- type: ndcg_at_1000
value: 53.50899999999999
- type: ndcg_at_3
value: 40.45133333333333
- type: ndcg_at_5
value: 43.31483333333334
- type: precision_at_1
value: 33.65925
- type: precision_at_10
value: 8.4995
- type: precision_at_100
value: 1.3210000000000004
- type: precision_at_1000
value: 0.16591666666666666
- type: precision_at_3
value: 19.165083333333335
- type: precision_at_5
value: 13.81816666666667
- type: recall_at_1
value: 28.17291666666667
- type: recall_at_10
value: 61.12624999999999
- type: recall_at_100
value: 83.97266666666667
- type: recall_at_1000
value: 95.66550000000001
- type: recall_at_3
value: 44.661249999999995
- type: recall_at_5
value: 51.983333333333334
- task:
type: Retrieval
dataset:
type: BeIR/cqadupstack
name: MTEB CQADupstackStatsRetrieval
config: default
split: test
revision: 65ac3a16b8e91f9cee4c9828cc7c335575432a2a
metrics:
- type: map_at_1
value: 24.681
- type: map_at_10
value: 34.892
- type: map_at_100
value: 35.996
- type: map_at_1000
value: 36.083
- type: map_at_3
value: 31.491999999999997
- type: map_at_5
value: 33.632
- type: mrr_at_1
value: 28.528
- type: mrr_at_10
value: 37.694
- type: mrr_at_100
value: 38.613
- type: mrr_at_1000
value: 38.668
- type: mrr_at_3
value: 34.714
- type: mrr_at_5
value: 36.616
- type: ndcg_at_1
value: 28.528
- type: ndcg_at_10
value: 40.703
- type: ndcg_at_100
value: 45.993
- type: ndcg_at_1000
value: 47.847
- type: ndcg_at_3
value: 34.622
- type: ndcg_at_5
value: 38.035999999999994
- type: precision_at_1
value: 28.528
- type: precision_at_10
value: 6.902
- type: precision_at_100
value: 1.0370000000000001
- type: precision_at_1000
value: 0.126
- type: precision_at_3
value: 15.798000000000002
- type: precision_at_5
value: 11.655999999999999
- type: recall_at_1
value: 24.681
- type: recall_at_10
value: 55.81
- type: recall_at_100
value: 79.785
- type: recall_at_1000
value: 92.959
- type: recall_at_3
value: 39.074
- type: recall_at_5
value: 47.568
- task:
type: Retrieval
dataset:
type: BeIR/cqadupstack
name: MTEB CQADupstackTexRetrieval
config: default
split: test
revision: 46989137a86843e03a6195de44b09deda022eec7
metrics:
- type: map_at_1
value: 18.627
- type: map_at_10
value: 27.872000000000003
- type: map_at_100
value: 29.237999999999996
- type: map_at_1000
value: 29.363
- type: map_at_3
value: 24.751
- type: map_at_5
value: 26.521
- type: mrr_at_1
value: 23.021
- type: mrr_at_10
value: 31.924000000000003
- type: mrr_at_100
value: 32.922000000000004
- type: mrr_at_1000
value: 32.988
- type: mrr_at_3
value: 29.192
- type: mrr_at_5
value: 30.798
- type: ndcg_at_1
value: 23.021
- type: ndcg_at_10
value: 33.535
- type: ndcg_at_100
value: 39.732
- type: ndcg_at_1000
value: 42.201
- type: ndcg_at_3
value: 28.153
- type: ndcg_at_5
value: 30.746000000000002
- type: precision_at_1
value: 23.021
- type: precision_at_10
value: 6.459
- type: precision_at_100
value: 1.1320000000000001
- type: precision_at_1000
value: 0.153
- type: precision_at_3
value: 13.719000000000001
- type: precision_at_5
value: 10.193000000000001
- type: recall_at_1
value: 18.627
- type: recall_at_10
value: 46.463
- type: recall_at_100
value: 74.226
- type: recall_at_1000
value: 91.28500000000001
- type: recall_at_3
value: 31.357000000000003
- type: recall_at_5
value: 38.067
- task:
type: Retrieval
dataset:
type: BeIR/cqadupstack
name: MTEB CQADupstackUnixRetrieval
config: default
split: test
revision: 6c6430d3a6d36f8d2a829195bc5dc94d7e063e53
metrics:
- type: map_at_1
value: 31.457
- type: map_at_10
value: 42.888
- type: map_at_100
value: 44.24
- type: map_at_1000
value: 44.327
- type: map_at_3
value: 39.588
- type: map_at_5
value: 41.423
- type: mrr_at_1
value: 37.126999999999995
- type: mrr_at_10
value: 47.083000000000006
- type: mrr_at_100
value: 47.997
- type: mrr_at_1000
value: 48.044
- type: mrr_at_3
value: 44.574000000000005
- type: mrr_at_5
value: 46.202
- type: ndcg_at_1
value: 37.126999999999995
- type: ndcg_at_10
value: 48.833
- type: ndcg_at_100
value: 54.327000000000005
- type: ndcg_at_1000
value: 56.011
- type: ndcg_at_3
value: 43.541999999999994
- type: ndcg_at_5
value: 46.127
- type: precision_at_1
value: 37.126999999999995
- type: precision_at_10
value: 8.376999999999999
- type: precision_at_100
value: 1.2309999999999999
- type: precision_at_1000
value: 0.146
- type: precision_at_3
value: 20.211000000000002
- type: precision_at_5
value: 14.16
- type: recall_at_1
value: 31.457
- type: recall_at_10
value: 62.369
- type: recall_at_100
value: 85.444
- type: recall_at_1000
value: 96.65599999999999
- type: recall_at_3
value: 47.961
- type: recall_at_5
value: 54.676
- task:
type: Retrieval
dataset:
type: BeIR/cqadupstack
name: MTEB CQADupstackWebmastersRetrieval
config: default
split: test
revision: 160c094312a0e1facb97e55eeddb698c0abe3571
metrics:
- type: map_at_1
value: 27.139999999999997
- type: map_at_10
value: 38.801
- type: map_at_100
value: 40.549
- type: map_at_1000
value: 40.802
- type: map_at_3
value: 35.05
- type: map_at_5
value: 36.884
- type: mrr_at_1
value: 33.004
- type: mrr_at_10
value: 43.864
- type: mrr_at_100
value: 44.667
- type: mrr_at_1000
value: 44.717
- type: mrr_at_3
value: 40.777
- type: mrr_at_5
value: 42.319
- type: ndcg_at_1
value: 33.004
- type: ndcg_at_10
value: 46.022
- type: ndcg_at_100
value: 51.542
- type: ndcg_at_1000
value: 53.742000000000004
- type: ndcg_at_3
value: 39.795
- type: ndcg_at_5
value: 42.272
- type: precision_at_1
value: 33.004
- type: precision_at_10
value: 9.012
- type: precision_at_100
value: 1.7770000000000001
- type: precision_at_1000
value: 0.26
- type: precision_at_3
value: 19.038
- type: precision_at_5
value: 13.675999999999998
- type: recall_at_1
value: 27.139999999999997
- type: recall_at_10
value: 60.961
- type: recall_at_100
value: 84.451
- type: recall_at_1000
value: 98.113
- type: recall_at_3
value: 43.001
- type: recall_at_5
value: 49.896
- task:
type: Retrieval
dataset:
type: BeIR/cqadupstack
name: MTEB CQADupstackWordpressRetrieval
config: default
split: test
revision: 4ffe81d471b1924886b33c7567bfb200e9eec5c4
metrics:
- type: map_at_1
value: 17.936
- type: map_at_10
value: 27.399
- type: map_at_100
value: 28.632
- type: map_at_1000
value: 28.738000000000003
- type: map_at_3
value: 24.456
- type: map_at_5
value: 26.06
- type: mrr_at_1
value: 19.224
- type: mrr_at_10
value: 28.998
- type: mrr_at_100
value: 30.11
- type: mrr_at_1000
value: 30.177
- type: mrr_at_3
value: 26.247999999999998
- type: mrr_at_5
value: 27.708
- type: ndcg_at_1
value: 19.224
- type: ndcg_at_10
value: 32.911
- type: ndcg_at_100
value: 38.873999999999995
- type: ndcg_at_1000
value: 41.277
- type: ndcg_at_3
value: 27.142
- type: ndcg_at_5
value: 29.755
- type: precision_at_1
value: 19.224
- type: precision_at_10
value: 5.6930000000000005
- type: precision_at_100
value: 0.9259999999999999
- type: precision_at_1000
value: 0.126
- type: precision_at_3
value: 12.138
- type: precision_at_5
value: 8.909
- type: recall_at_1
value: 17.936
- type: recall_at_10
value: 48.096
- type: recall_at_100
value: 75.389
- type: recall_at_1000
value: 92.803
- type: recall_at_3
value: 32.812999999999995
- type: recall_at_5
value: 38.851
- task:
type: Retrieval
dataset:
type: mteb/climate-fever
name: MTEB ClimateFEVER
config: default
split: test
revision: 47f2ac6acb640fc46020b02a5b59fdda04d39380
metrics:
- type: map_at_1
value: 22.076999999999998
- type: map_at_10
value: 35.44
- type: map_at_100
value: 37.651
- type: map_at_1000
value: 37.824999999999996
- type: map_at_3
value: 30.764999999999997
- type: map_at_5
value: 33.26
- type: mrr_at_1
value: 50.163000000000004
- type: mrr_at_10
value: 61.207
- type: mrr_at_100
value: 61.675000000000004
- type: mrr_at_1000
value: 61.692
- type: mrr_at_3
value: 58.60999999999999
- type: mrr_at_5
value: 60.307
- type: ndcg_at_1
value: 50.163000000000004
- type: ndcg_at_10
value: 45.882
- type: ndcg_at_100
value: 53.239999999999995
- type: ndcg_at_1000
value: 55.852000000000004
- type: ndcg_at_3
value: 40.514
- type: ndcg_at_5
value: 42.038
- type: precision_at_1
value: 50.163000000000004
- type: precision_at_10
value: 13.466000000000001
- type: precision_at_100
value: 2.164
- type: precision_at_1000
value: 0.266
- type: precision_at_3
value: 29.707
- type: precision_at_5
value: 21.694
- type: recall_at_1
value: 22.076999999999998
- type: recall_at_10
value: 50.193
- type: recall_at_100
value: 74.993
- type: recall_at_1000
value: 89.131
- type: recall_at_3
value: 35.472
- type: recall_at_5
value: 41.814
- task:
type: Retrieval
dataset:
type: mteb/dbpedia
name: MTEB DBPedia
config: default
split: test
revision: c0f706b76e590d620bd6618b3ca8efdd34e2d659
metrics:
- type: map_at_1
value: 9.953
- type: map_at_10
value: 24.515
- type: map_at_100
value: 36.173
- type: map_at_1000
value: 38.351
- type: map_at_3
value: 16.592000000000002
- type: map_at_5
value: 20.036
- type: mrr_at_1
value: 74.25
- type: mrr_at_10
value: 81.813
- type: mrr_at_100
value: 82.006
- type: mrr_at_1000
value: 82.011
- type: mrr_at_3
value: 80.875
- type: mrr_at_5
value: 81.362
- type: ndcg_at_1
value: 62.5
- type: ndcg_at_10
value: 52.42
- type: ndcg_at_100
value: 56.808
- type: ndcg_at_1000
value: 63.532999999999994
- type: ndcg_at_3
value: 56.654
- type: ndcg_at_5
value: 54.18300000000001
- type: precision_at_1
value: 74.25
- type: precision_at_10
value: 42.699999999999996
- type: precision_at_100
value: 13.675
- type: precision_at_1000
value: 2.664
- type: precision_at_3
value: 60.5
- type: precision_at_5
value: 52.800000000000004
- type: recall_at_1
value: 9.953
- type: recall_at_10
value: 30.253999999999998
- type: recall_at_100
value: 62.516000000000005
- type: recall_at_1000
value: 84.163
- type: recall_at_3
value: 18.13
- type: recall_at_5
value: 22.771
- task:
type: Classification
dataset:
type: mteb/emotion
name: MTEB EmotionClassification
config: default
split: test
revision: 4f58c6b202a23cf9a4da393831edf4f9183cad37
metrics:
- type: accuracy
value: 79.455
- type: f1
value: 74.16798697647569
- task:
type: Retrieval
dataset:
type: mteb/fever
name: MTEB FEVER
config: default
split: test
revision: bea83ef9e8fb933d90a2f1d5515737465d613e12
metrics:
- type: map_at_1
value: 87.531
- type: map_at_10
value: 93.16799999999999
- type: map_at_100
value: 93.341
- type: map_at_1000
value: 93.349
- type: map_at_3
value: 92.444
- type: map_at_5
value: 92.865
- type: mrr_at_1
value: 94.014
- type: mrr_at_10
value: 96.761
- type: mrr_at_100
value: 96.762
- type: mrr_at_1000
value: 96.762
- type: mrr_at_3
value: 96.672
- type: mrr_at_5
value: 96.736
- type: ndcg_at_1
value: 94.014
- type: ndcg_at_10
value: 95.112
- type: ndcg_at_100
value: 95.578
- type: ndcg_at_1000
value: 95.68900000000001
- type: ndcg_at_3
value: 94.392
- type: ndcg_at_5
value: 94.72500000000001
- type: precision_at_1
value: 94.014
- type: precision_at_10
value: 11.065
- type: precision_at_100
value: 1.157
- type: precision_at_1000
value: 0.11800000000000001
- type: precision_at_3
value: 35.259
- type: precision_at_5
value: 21.599
- type: recall_at_1
value: 87.531
- type: recall_at_10
value: 97.356
- type: recall_at_100
value: 98.965
- type: recall_at_1000
value: 99.607
- type: recall_at_3
value: 95.312
- type: recall_at_5
value: 96.295
- task:
type: Retrieval
dataset:
type: mteb/fiqa
name: MTEB FiQA2018
config: default
split: test
revision: 27a168819829fe9bcd655c2df245fb19452e8e06
metrics:
- type: map_at_1
value: 32.055
- type: map_at_10
value: 53.114
- type: map_at_100
value: 55.235
- type: map_at_1000
value: 55.345
- type: map_at_3
value: 45.854
- type: map_at_5
value: 50.025
- type: mrr_at_1
value: 60.34
- type: mrr_at_10
value: 68.804
- type: mrr_at_100
value: 69.309
- type: mrr_at_1000
value: 69.32199999999999
- type: mrr_at_3
value: 66.40899999999999
- type: mrr_at_5
value: 67.976
- type: ndcg_at_1
value: 60.34
- type: ndcg_at_10
value: 62.031000000000006
- type: ndcg_at_100
value: 68.00500000000001
- type: ndcg_at_1000
value: 69.286
- type: ndcg_at_3
value: 56.355999999999995
- type: ndcg_at_5
value: 58.687
- type: precision_at_1
value: 60.34
- type: precision_at_10
value: 17.176
- type: precision_at_100
value: 2.36
- type: precision_at_1000
value: 0.259
- type: precision_at_3
value: 37.14
- type: precision_at_5
value: 27.809
- type: recall_at_1
value: 32.055
- type: recall_at_10
value: 70.91
- type: recall_at_100
value: 91.83
- type: recall_at_1000
value: 98.871
- type: recall_at_3
value: 51.202999999999996
- type: recall_at_5
value: 60.563
- task:
type: Retrieval
dataset:
type: mteb/hotpotqa
name: MTEB HotpotQA
config: default
split: test
revision: ab518f4d6fcca38d87c25209f94beba119d02014
metrics:
- type: map_at_1
value: 43.68
- type: map_at_10
value: 64.389
- type: map_at_100
value: 65.24
- type: map_at_1000
value: 65.303
- type: map_at_3
value: 61.309000000000005
- type: map_at_5
value: 63.275999999999996
- type: mrr_at_1
value: 87.36
- type: mrr_at_10
value: 91.12
- type: mrr_at_100
value: 91.227
- type: mrr_at_1000
value: 91.229
- type: mrr_at_3
value: 90.57600000000001
- type: mrr_at_5
value: 90.912
- type: ndcg_at_1
value: 87.36
- type: ndcg_at_10
value: 73.076
- type: ndcg_at_100
value: 75.895
- type: ndcg_at_1000
value: 77.049
- type: ndcg_at_3
value: 68.929
- type: ndcg_at_5
value: 71.28
- type: precision_at_1
value: 87.36
- type: precision_at_10
value: 14.741000000000001
- type: precision_at_100
value: 1.694
- type: precision_at_1000
value: 0.185
- type: precision_at_3
value: 43.043
- type: precision_at_5
value: 27.681
- type: recall_at_1
value: 43.68
- type: recall_at_10
value: 73.707
- type: recall_at_100
value: 84.7
- type: recall_at_1000
value: 92.309
- type: recall_at_3
value: 64.564
- type: recall_at_5
value: 69.203
- task:
type: Classification
dataset:
type: mteb/imdb
name: MTEB ImdbClassification
config: default
split: test
revision: 3d86128a09e091d6018b6d26cad27f2739fc2db7
metrics:
- type: accuracy
value: 96.75399999999999
- type: ap
value: 95.29389839242187
- type: f1
value: 96.75348377433475
- task:
type: Retrieval
dataset:
type: mteb/msmarco
name: MTEB MSMARCO
config: default
split: dev
revision: c5a29a104738b98a9e76336939199e264163d4a0
metrics:
- type: map_at_1
value: 25.176
- type: map_at_10
value: 38.598
- type: map_at_100
value: 39.707
- type: map_at_1000
value: 39.744
- type: map_at_3
value: 34.566
- type: map_at_5
value: 36.863
- type: mrr_at_1
value: 25.874000000000002
- type: mrr_at_10
value: 39.214
- type: mrr_at_100
value: 40.251
- type: mrr_at_1000
value: 40.281
- type: mrr_at_3
value: 35.291
- type: mrr_at_5
value: 37.545
- type: ndcg_at_1
value: 25.874000000000002
- type: ndcg_at_10
value: 45.98
- type: ndcg_at_100
value: 51.197
- type: ndcg_at_1000
value: 52.073
- type: ndcg_at_3
value: 37.785999999999994
- type: ndcg_at_5
value: 41.870000000000005
- type: precision_at_1
value: 25.874000000000002
- type: precision_at_10
value: 7.181
- type: precision_at_100
value: 0.979
- type: precision_at_1000
value: 0.106
- type: precision_at_3
value: 16.051000000000002
- type: precision_at_5
value: 11.713
- type: recall_at_1
value: 25.176
- type: recall_at_10
value: 68.67699999999999
- type: recall_at_100
value: 92.55
- type: recall_at_1000
value: 99.164
- type: recall_at_3
value: 46.372
- type: recall_at_5
value: 56.16
- task:
type: Classification
dataset:
type: mteb/mtop_domain
name: MTEB MTOPDomainClassification (en)
config: en
split: test
revision: d80d48c1eb48d3562165c59d59d0034df9fff0bf
metrics:
- type: accuracy
value: 99.03784769721841
- type: f1
value: 98.97791641821495
- task:
type: Classification
dataset:
type: mteb/mtop_intent
name: MTEB MTOPIntentClassification (en)
config: en
split: test
revision: ae001d0e6b1228650b7bd1c2c65fb50ad11a8aba
metrics:
- type: accuracy
value: 91.88326493388054
- type: f1
value: 73.74809928034335
- task:
type: Classification
dataset:
type: mteb/amazon_massive_intent
name: MTEB MassiveIntentClassification (en)
config: en
split: test
revision: 31efe3c427b0bae9c22cbb560b8f15491cc6bed7
metrics:
- type: accuracy
value: 85.41358439811701
- type: f1
value: 83.503679460639
- task:
type: Classification
dataset:
type: mteb/amazon_massive_scenario
name: MTEB MassiveScenarioClassification (en)
config: en
split: test
revision: 7d571f92784cd94a019292a1f45445077d0ef634
metrics:
- type: accuracy
value: 89.77135171486215
- type: f1
value: 88.89843747468366
- task:
type: Clustering
dataset:
type: mteb/medrxiv-clustering-p2p
name: MTEB MedrxivClusteringP2P
config: default
split: test
revision: e7a26af6f3ae46b30dde8737f02c07b1505bcc73
metrics:
- type: v_measure
value: 46.22695362087359
- task:
type: Clustering
dataset:
type: mteb/medrxiv-clustering-s2s
name: MTEB MedrxivClusteringS2S
config: default
split: test
revision: 35191c8c0dca72d8ff3efcd72aa802307d469663
metrics:
- type: v_measure
value: 44.132372165849425
- task:
type: Reranking
dataset:
type: mteb/mind_small
name: MTEB MindSmallReranking
config: default
split: test
revision: 3bdac13927fdc888b903db93b2ffdbd90b295a69
metrics:
- type: map
value: 33.35680810650402
- type: mrr
value: 34.72625715637218
- task:
type: Retrieval
dataset:
type: mteb/nfcorpus
name: MTEB NFCorpus
config: default
split: test
revision: ec0fa4fe99da2ff19ca1214b7966684033a58814
metrics:
- type: map_at_1
value: 7.165000000000001
- type: map_at_10
value: 15.424
- type: map_at_100
value: 20.28
- type: map_at_1000
value: 22.065
- type: map_at_3
value: 11.236
- type: map_at_5
value: 13.025999999999998
- type: mrr_at_1
value: 51.702999999999996
- type: mrr_at_10
value: 59.965
- type: mrr_at_100
value: 60.667
- type: mrr_at_1000
value: 60.702999999999996
- type: mrr_at_3
value: 58.772000000000006
- type: mrr_at_5
value: 59.267
- type: ndcg_at_1
value: 49.536
- type: ndcg_at_10
value: 40.6
- type: ndcg_at_100
value: 37.848
- type: ndcg_at_1000
value: 46.657
- type: ndcg_at_3
value: 46.117999999999995
- type: ndcg_at_5
value: 43.619
- type: precision_at_1
value: 51.393
- type: precision_at_10
value: 30.31
- type: precision_at_100
value: 9.972
- type: precision_at_1000
value: 2.329
- type: precision_at_3
value: 43.137
- type: precision_at_5
value: 37.585
- type: recall_at_1
value: 7.165000000000001
- type: recall_at_10
value: 19.689999999999998
- type: recall_at_100
value: 39.237
- type: recall_at_1000
value: 71.417
- type: recall_at_3
value: 12.247
- type: recall_at_5
value: 14.902999999999999
- task:
type: Retrieval
dataset:
type: mteb/nq
name: MTEB NQ
config: default
split: test
revision: b774495ed302d8c44a3a7ea25c90dbce03968f31
metrics:
- type: map_at_1
value: 42.653999999999996
- type: map_at_10
value: 59.611999999999995
- type: map_at_100
value: 60.32300000000001
- type: map_at_1000
value: 60.336
- type: map_at_3
value: 55.584999999999994
- type: map_at_5
value: 58.19
- type: mrr_at_1
value: 47.683
- type: mrr_at_10
value: 62.06700000000001
- type: mrr_at_100
value: 62.537
- type: mrr_at_1000
value: 62.544999999999995
- type: mrr_at_3
value: 59.178
- type: mrr_at_5
value: 61.034
- type: ndcg_at_1
value: 47.654
- type: ndcg_at_10
value: 67.001
- type: ndcg_at_100
value: 69.73899999999999
- type: ndcg_at_1000
value: 69.986
- type: ndcg_at_3
value: 59.95700000000001
- type: ndcg_at_5
value: 64.025
- type: precision_at_1
value: 47.654
- type: precision_at_10
value: 10.367999999999999
- type: precision_at_100
value: 1.192
- type: precision_at_1000
value: 0.121
- type: precision_at_3
value: 26.651000000000003
- type: precision_at_5
value: 18.459
- type: recall_at_1
value: 42.653999999999996
- type: recall_at_10
value: 86.619
- type: recall_at_100
value: 98.04899999999999
- type: recall_at_1000
value: 99.812
- type: recall_at_3
value: 68.987
- type: recall_at_5
value: 78.158
- task:
type: Retrieval
dataset:
type: mteb/quora
name: MTEB QuoraRetrieval
config: default
split: test
revision: None
metrics:
- type: map_at_1
value: 72.538
- type: map_at_10
value: 86.702
- type: map_at_100
value: 87.31
- type: map_at_1000
value: 87.323
- type: map_at_3
value: 83.87
- type: map_at_5
value: 85.682
- type: mrr_at_1
value: 83.31
- type: mrr_at_10
value: 89.225
- type: mrr_at_100
value: 89.30399999999999
- type: mrr_at_1000
value: 89.30399999999999
- type: mrr_at_3
value: 88.44300000000001
- type: mrr_at_5
value: 89.005
- type: ndcg_at_1
value: 83.32000000000001
- type: ndcg_at_10
value: 90.095
- type: ndcg_at_100
value: 91.12
- type: ndcg_at_1000
value: 91.179
- type: ndcg_at_3
value: 87.606
- type: ndcg_at_5
value: 89.031
- type: precision_at_1
value: 83.32000000000001
- type: precision_at_10
value: 13.641
- type: precision_at_100
value: 1.541
- type: precision_at_1000
value: 0.157
- type: precision_at_3
value: 38.377
- type: precision_at_5
value: 25.162000000000003
- type: recall_at_1
value: 72.538
- type: recall_at_10
value: 96.47200000000001
- type: recall_at_100
value: 99.785
- type: recall_at_1000
value: 99.99900000000001
- type: recall_at_3
value: 89.278
- type: recall_at_5
value: 93.367
- task:
type: Clustering
dataset:
type: mteb/reddit-clustering
name: MTEB RedditClustering
config: default
split: test
revision: 24640382cdbf8abc73003fb0fa6d111a705499eb
metrics:
- type: v_measure
value: 73.55219145406065
- task:
type: Clustering
dataset:
type: mteb/reddit-clustering-p2p
name: MTEB RedditClusteringP2P
config: default
split: test
revision: 282350215ef01743dc01b456c7f5241fa8937f16
metrics:
- type: v_measure
value: 74.13437105242755
- task:
type: Retrieval
dataset:
type: mteb/scidocs
name: MTEB SCIDOCS
config: default
split: test
revision: None
metrics:
- type: map_at_1
value: 6.873
- type: map_at_10
value: 17.944
- type: map_at_100
value: 21.171
- type: map_at_1000
value: 21.528
- type: map_at_3
value: 12.415
- type: map_at_5
value: 15.187999999999999
- type: mrr_at_1
value: 33.800000000000004
- type: mrr_at_10
value: 46.455
- type: mrr_at_100
value: 47.378
- type: mrr_at_1000
value: 47.394999999999996
- type: mrr_at_3
value: 42.367
- type: mrr_at_5
value: 44.972
- type: ndcg_at_1
value: 33.800000000000004
- type: ndcg_at_10
value: 28.907
- type: ndcg_at_100
value: 39.695
- type: ndcg_at_1000
value: 44.582
- type: ndcg_at_3
value: 26.949
- type: ndcg_at_5
value: 23.988
- type: precision_at_1
value: 33.800000000000004
- type: precision_at_10
value: 15.079999999999998
- type: precision_at_100
value: 3.056
- type: precision_at_1000
value: 0.42100000000000004
- type: precision_at_3
value: 25.167
- type: precision_at_5
value: 21.26
- type: recall_at_1
value: 6.873
- type: recall_at_10
value: 30.568
- type: recall_at_100
value: 62.062
- type: recall_at_1000
value: 85.37700000000001
- type: recall_at_3
value: 15.312999999999999
- type: recall_at_5
value: 21.575
- task:
type: STS
dataset:
type: mteb/sickr-sts
name: MTEB SICK-R
config: default
split: test
revision: a6ea5a8cab320b040a23452cc28066d9beae2cee
metrics:
- type: cos_sim_pearson
value: 82.37009118256057
- type: cos_sim_spearman
value: 79.27986395671529
- type: euclidean_pearson
value: 79.18037715442115
- type: euclidean_spearman
value: 79.28004791561621
- type: manhattan_pearson
value: 79.34062972800541
- type: manhattan_spearman
value: 79.43106695543402
- task:
type: STS
dataset:
type: mteb/sts12-sts
name: MTEB STS12
config: default
split: test
revision: a0d554a64d88156834ff5ae9920b964011b16384
metrics:
- type: cos_sim_pearson
value: 87.48474767383833
- type: cos_sim_spearman
value: 79.54505388752513
- type: euclidean_pearson
value: 83.43282704179565
- type: euclidean_spearman
value: 79.54579919925405
- type: manhattan_pearson
value: 83.77564492427952
- type: manhattan_spearman
value: 79.84558396989286
- task:
type: STS
dataset:
type: mteb/sts13-sts
name: MTEB STS13
config: default
split: test
revision: 7e90230a92c190f1bf69ae9002b8cea547a64cca
metrics:
- type: cos_sim_pearson
value: 88.803698035802
- type: cos_sim_spearman
value: 88.83451367754881
- type: euclidean_pearson
value: 88.28939285711628
- type: euclidean_spearman
value: 88.83528996073112
- type: manhattan_pearson
value: 88.28017412671795
- type: manhattan_spearman
value: 88.9228828016344
- task:
type: STS
dataset:
type: mteb/sts14-sts
name: MTEB STS14
config: default
split: test
revision: 6031580fec1f6af667f0bd2da0a551cf4f0b2375
metrics:
- type: cos_sim_pearson
value: 85.27469288153428
- type: cos_sim_spearman
value: 83.87477064876288
- type: euclidean_pearson
value: 84.2601737035379
- type: euclidean_spearman
value: 83.87431082479074
- type: manhattan_pearson
value: 84.3621547772745
- type: manhattan_spearman
value: 84.12094375000423
- task:
type: STS
dataset:
type: mteb/sts15-sts
name: MTEB STS15
config: default
split: test
revision: ae752c7c21bf194d8b67fd573edf7ae58183cbe3
metrics:
- type: cos_sim_pearson
value: 88.12749863201587
- type: cos_sim_spearman
value: 88.54287568368565
- type: euclidean_pearson
value: 87.90429700607999
- type: euclidean_spearman
value: 88.5437689576261
- type: manhattan_pearson
value: 88.19276653356833
- type: manhattan_spearman
value: 88.99995393814679
- task:
type: STS
dataset:
type: mteb/sts16-sts
name: MTEB STS16
config: default
split: test
revision: 4d8694f8f0e0100860b497b999b3dbed754a0513
metrics:
- type: cos_sim_pearson
value: 85.68398747560902
- type: cos_sim_spearman
value: 86.48815303460574
- type: euclidean_pearson
value: 85.52356631237954
- type: euclidean_spearman
value: 86.486391949551
- type: manhattan_pearson
value: 85.67267981761788
- type: manhattan_spearman
value: 86.7073696332485
- task:
type: STS
dataset:
type: mteb/sts17-crosslingual-sts
name: MTEB STS17 (en-en)
config: en-en
split: test
revision: af5e6fb845001ecf41f4c1e033ce921939a2a68d
metrics:
- type: cos_sim_pearson
value: 88.9057107443124
- type: cos_sim_spearman
value: 88.7312168757697
- type: euclidean_pearson
value: 88.72810439714794
- type: euclidean_spearman
value: 88.71976185854771
- type: manhattan_pearson
value: 88.50433745949111
- type: manhattan_spearman
value: 88.51726175544195
- task:
type: STS
dataset:
type: mteb/sts22-crosslingual-sts
name: MTEB STS22 (en)
config: en
split: test
revision: eea2b4fe26a775864c896887d910b76a8098ad3f
metrics:
- type: cos_sim_pearson
value: 67.59391795109886
- type: cos_sim_spearman
value: 66.87613008631367
- type: euclidean_pearson
value: 69.23198488262217
- type: euclidean_spearman
value: 66.85427723013692
- type: manhattan_pearson
value: 69.50730124841084
- type: manhattan_spearman
value: 67.10404669820792
- task:
type: STS
dataset:
type: mteb/stsbenchmark-sts
name: MTEB STSBenchmark
config: default
split: test
revision: b0fddb56ed78048fa8b90373c8a3cfc37b684831
metrics:
- type: cos_sim_pearson
value: 87.0820605344619
- type: cos_sim_spearman
value: 86.8518089863434
- type: euclidean_pearson
value: 86.31087134689284
- type: euclidean_spearman
value: 86.8518520517941
- type: manhattan_pearson
value: 86.47203796160612
- type: manhattan_spearman
value: 87.1080149734421
- task:
type: Reranking
dataset:
type: mteb/scidocs-reranking
name: MTEB SciDocsRR
config: default
split: test
revision: d3c5e1fc0b855ab6097bf1cda04dd73947d7caab
metrics:
- type: map
value: 89.09255369305481
- type: mrr
value: 97.10323445617563
- task:
type: Retrieval
dataset:
type: mteb/scifact
name: MTEB SciFact
config: default
split: test
revision: 0228b52cf27578f30900b9e5271d331663a030d7
metrics:
- type: map_at_1
value: 61.260999999999996
- type: map_at_10
value: 74.043
- type: map_at_100
value: 74.37700000000001
- type: map_at_1000
value: 74.384
- type: map_at_3
value: 71.222
- type: map_at_5
value: 72.875
- type: mrr_at_1
value: 64.333
- type: mrr_at_10
value: 74.984
- type: mrr_at_100
value: 75.247
- type: mrr_at_1000
value: 75.25500000000001
- type: mrr_at_3
value: 73.167
- type: mrr_at_5
value: 74.35000000000001
- type: ndcg_at_1
value: 64.333
- type: ndcg_at_10
value: 79.06
- type: ndcg_at_100
value: 80.416
- type: ndcg_at_1000
value: 80.55600000000001
- type: ndcg_at_3
value: 74.753
- type: ndcg_at_5
value: 76.97500000000001
- type: precision_at_1
value: 64.333
- type: precision_at_10
value: 10.567
- type: precision_at_100
value: 1.1199999999999999
- type: precision_at_1000
value: 0.11299999999999999
- type: precision_at_3
value: 29.889
- type: precision_at_5
value: 19.533
- type: recall_at_1
value: 61.260999999999996
- type: recall_at_10
value: 93.167
- type: recall_at_100
value: 99.0
- type: recall_at_1000
value: 100.0
- type: recall_at_3
value: 81.667
- type: recall_at_5
value: 87.394
- task:
type: PairClassification
dataset:
type: mteb/sprintduplicatequestions-pairclassification
name: MTEB SprintDuplicateQuestions
config: default
split: test
revision: d66bd1f72af766a5cc4b0ca5e00c162f89e8cc46
metrics:
- type: cos_sim_accuracy
value: 99.71980198019801
- type: cos_sim_ap
value: 92.81616007802704
- type: cos_sim_f1
value: 85.17548454688318
- type: cos_sim_precision
value: 89.43894389438944
- type: cos_sim_recall
value: 81.3
- type: dot_accuracy
value: 99.71980198019801
- type: dot_ap
value: 92.81398760591358
- type: dot_f1
value: 85.17548454688318
- type: dot_precision
value: 89.43894389438944
- type: dot_recall
value: 81.3
- type: euclidean_accuracy
value: 99.71980198019801
- type: euclidean_ap
value: 92.81560637245072
- type: euclidean_f1
value: 85.17548454688318
- type: euclidean_precision
value: 89.43894389438944
- type: euclidean_recall
value: 81.3
- type: manhattan_accuracy
value: 99.73069306930694
- type: manhattan_ap
value: 93.14005487480794
- type: manhattan_f1
value: 85.56263269639068
- type: manhattan_precision
value: 91.17647058823529
- type: manhattan_recall
value: 80.60000000000001
- type: max_accuracy
value: 99.73069306930694
- type: max_ap
value: 93.14005487480794
- type: max_f1
value: 85.56263269639068
- task:
type: Clustering
dataset:
type: mteb/stackexchange-clustering
name: MTEB StackExchangeClustering
config: default
split: test
revision: 6cbc1f7b2bc0622f2e39d2c77fa502909748c259
metrics:
- type: v_measure
value: 79.86443362395185
- task:
type: Clustering
dataset:
type: mteb/stackexchange-clustering-p2p
name: MTEB StackExchangeClusteringP2P
config: default
split: test
revision: 815ca46b2622cec33ccafc3735d572c266efdb44
metrics:
- type: v_measure
value: 49.40897096662564
- task:
type: Reranking
dataset:
type: mteb/stackoverflowdupquestions-reranking
name: MTEB StackOverflowDupQuestions
config: default
split: test
revision: e185fbe320c72810689fc5848eb6114e1ef5ec69
metrics:
- type: map
value: 55.66040806627947
- type: mrr
value: 56.58670475766064
- task:
type: Summarization
dataset:
type: mteb/summeval
name: MTEB SummEval
config: default
split: test
revision: cda12ad7615edc362dbf25a00fdd61d3b1eaf93c
metrics:
- type: cos_sim_pearson
value: 31.51015090598575
- type: cos_sim_spearman
value: 31.35016454939226
- type: dot_pearson
value: 31.5150068731
- type: dot_spearman
value: 31.34790869023487
- task:
type: Retrieval
dataset:
type: mteb/trec-covid
name: MTEB TRECCOVID
config: default
split: test
revision: None
metrics:
- type: map_at_1
value: 0.254
- type: map_at_10
value: 2.064
- type: map_at_100
value: 12.909
- type: map_at_1000
value: 31.761
- type: map_at_3
value: 0.738
- type: map_at_5
value: 1.155
- type: mrr_at_1
value: 96.0
- type: mrr_at_10
value: 98.0
- type: mrr_at_100
value: 98.0
- type: mrr_at_1000
value: 98.0
- type: mrr_at_3
value: 98.0
- type: mrr_at_5
value: 98.0
- type: ndcg_at_1
value: 93.0
- type: ndcg_at_10
value: 82.258
- type: ndcg_at_100
value: 64.34
- type: ndcg_at_1000
value: 57.912
- type: ndcg_at_3
value: 90.827
- type: ndcg_at_5
value: 86.79
- type: precision_at_1
value: 96.0
- type: precision_at_10
value: 84.8
- type: precision_at_100
value: 66.0
- type: precision_at_1000
value: 25.356
- type: precision_at_3
value: 94.667
- type: precision_at_5
value: 90.4
- type: recall_at_1
value: 0.254
- type: recall_at_10
value: 2.1950000000000003
- type: recall_at_100
value: 16.088
- type: recall_at_1000
value: 54.559000000000005
- type: recall_at_3
value: 0.75
- type: recall_at_5
value: 1.191
- task:
type: Retrieval
dataset:
type: mteb/touche2020
name: MTEB Touche2020
config: default
split: test
revision: a34f9a33db75fa0cbb21bb5cfc3dae8dc8bec93f
metrics:
- type: map_at_1
value: 2.976
- type: map_at_10
value: 11.389000000000001
- type: map_at_100
value: 18.429000000000002
- type: map_at_1000
value: 20.113
- type: map_at_3
value: 6.483
- type: map_at_5
value: 8.770999999999999
- type: mrr_at_1
value: 40.816
- type: mrr_at_10
value: 58.118
- type: mrr_at_100
value: 58.489999999999995
- type: mrr_at_1000
value: 58.489999999999995
- type: mrr_at_3
value: 53.061
- type: mrr_at_5
value: 57.041
- type: ndcg_at_1
value: 40.816
- type: ndcg_at_10
value: 30.567
- type: ndcg_at_100
value: 42.44
- type: ndcg_at_1000
value: 53.480000000000004
- type: ndcg_at_3
value: 36.016
- type: ndcg_at_5
value: 34.257
- type: precision_at_1
value: 42.857
- type: precision_at_10
value: 25.714
- type: precision_at_100
value: 8.429
- type: precision_at_1000
value: 1.5939999999999999
- type: precision_at_3
value: 36.735
- type: precision_at_5
value: 33.878
- type: recall_at_1
value: 2.976
- type: recall_at_10
value: 17.854999999999997
- type: recall_at_100
value: 51.833
- type: recall_at_1000
value: 86.223
- type: recall_at_3
value: 7.887
- type: recall_at_5
value: 12.026
- task:
type: Classification
dataset:
type: mteb/toxic_conversations_50k
name: MTEB ToxicConversationsClassification
config: default
split: test
revision: d7c0de2777da35d6aae2200a62c6e0e5af397c4c
metrics:
- type: accuracy
value: 85.1174
- type: ap
value: 30.169441069345748
- type: f1
value: 69.79254701873245
- task:
type: Classification
dataset:
type: mteb/tweet_sentiment_extraction
name: MTEB TweetSentimentExtractionClassification
config: default
split: test
revision: d604517c81ca91fe16a244d1248fc021f9ecee7a
metrics:
- type: accuracy
value: 72.58347481607245
- type: f1
value: 72.74877295564937
- task:
type: Clustering
dataset:
type: mteb/twentynewsgroups-clustering
name: MTEB TwentyNewsgroupsClustering
config: default
split: test
revision: 6125ec4e24fa026cec8a478383ee943acfbd5449
metrics:
- type: v_measure
value: 53.90586138221305
- task:
type: PairClassification
dataset:
type: mteb/twittersemeval2015-pairclassification
name: MTEB TwitterSemEval2015
config: default
split: test
revision: 70970daeab8776df92f5ea462b6173c0b46fd2d1
metrics:
- type: cos_sim_accuracy
value: 87.35769207844072
- type: cos_sim_ap
value: 77.9645072410354
- type: cos_sim_f1
value: 71.32352941176471
- type: cos_sim_precision
value: 66.5903890160183
- type: cos_sim_recall
value: 76.78100263852242
- type: dot_accuracy
value: 87.37557370209214
- type: dot_ap
value: 77.96250046429908
- type: dot_f1
value: 71.28932757557064
- type: dot_precision
value: 66.95249130938586
- type: dot_recall
value: 76.22691292875989
- type: euclidean_accuracy
value: 87.35173153722357
- type: euclidean_ap
value: 77.96520460741593
- type: euclidean_f1
value: 71.32470733210104
- type: euclidean_precision
value: 66.91329479768785
- type: euclidean_recall
value: 76.35883905013192
- type: manhattan_accuracy
value: 87.25636287774931
- type: manhattan_ap
value: 77.77752485611796
- type: manhattan_f1
value: 71.18148599269183
- type: manhattan_precision
value: 66.10859728506787
- type: manhattan_recall
value: 77.0976253298153
- type: max_accuracy
value: 87.37557370209214
- type: max_ap
value: 77.96520460741593
- type: max_f1
value: 71.32470733210104
- task:
type: PairClassification
dataset:
type: mteb/twitterurlcorpus-pairclassification
name: MTEB TwitterURLCorpus
config: default
split: test
revision: 8b6510b0b1fa4e4c4f879467980e9be563ec1cdf
metrics:
- type: cos_sim_accuracy
value: 89.38176737687739
- type: cos_sim_ap
value: 86.58811861657401
- type: cos_sim_f1
value: 79.09430644097604
- type: cos_sim_precision
value: 75.45085977911366
- type: cos_sim_recall
value: 83.10748383122882
- type: dot_accuracy
value: 89.38370784336554
- type: dot_ap
value: 86.58840606004333
- type: dot_f1
value: 79.10179860068133
- type: dot_precision
value: 75.44546153308643
- type: dot_recall
value: 83.13058207576223
- type: euclidean_accuracy
value: 89.38564830985369
- type: euclidean_ap
value: 86.58820721061164
- type: euclidean_f1
value: 79.09070942235888
- type: euclidean_precision
value: 75.38729937194697
- type: euclidean_recall
value: 83.17677856482906
- type: manhattan_accuracy
value: 89.40699344122326
- type: manhattan_ap
value: 86.60631843011362
- type: manhattan_f1
value: 79.14949970570925
- type: manhattan_precision
value: 75.78191039729502
- type: manhattan_recall
value: 82.83030489682784
- type: max_accuracy
value: 89.40699344122326
- type: max_ap
value: 86.60631843011362
- type: max_f1
value: 79.14949970570925
- task:
type: STS
dataset:
type: C-MTEB/AFQMC
name: MTEB AFQMC
config: default
split: validation
revision: b44c3b011063adb25877c13823db83bb193913c4
metrics:
- type: cos_sim_pearson
value: 65.58442135663871
- type: cos_sim_spearman
value: 72.2538631361313
- type: euclidean_pearson
value: 70.97255486607429
- type: euclidean_spearman
value: 72.25374250228647
- type: manhattan_pearson
value: 70.83250199989911
- type: manhattan_spearman
value: 72.14819496536272
- task:
type: STS
dataset:
type: C-MTEB/ATEC
name: MTEB ATEC
config: default
split: test
revision: 0f319b1142f28d00e055a6770f3f726ae9b7d865
metrics:
- type: cos_sim_pearson
value: 59.99478404929932
- type: cos_sim_spearman
value: 62.61836216999812
- type: euclidean_pearson
value: 66.86429811933593
- type: euclidean_spearman
value: 62.6183520374191
- type: manhattan_pearson
value: 66.8063778911633
- type: manhattan_spearman
value: 62.569607573241115
- task:
type: Classification
dataset:
type: mteb/amazon_reviews_multi
name: MTEB AmazonReviewsClassification (zh)
config: zh
split: test
revision: 1399c76144fd37290681b995c656ef9b2e06e26d
metrics:
- type: accuracy
value: 53.98400000000001
- type: f1
value: 51.21447361350723
- task:
type: STS
dataset:
type: C-MTEB/BQ
name: MTEB BQ
config: default
split: test
revision: e3dda5e115e487b39ec7e618c0c6a29137052a55
metrics:
- type: cos_sim_pearson
value: 79.11941660686553
- type: cos_sim_spearman
value: 81.25029594540435
- type: euclidean_pearson
value: 82.06973504238826
- type: euclidean_spearman
value: 81.2501989488524
- type: manhattan_pearson
value: 82.10094630392753
- type: manhattan_spearman
value: 81.27987244392389
- task:
type: Clustering
dataset:
type: C-MTEB/CLSClusteringP2P
name: MTEB CLSClusteringP2P
config: default
split: test
revision: 4b6227591c6c1a73bc76b1055f3b7f3588e72476
metrics:
- type: v_measure
value: 47.07270168705156
- task:
type: Clustering
dataset:
type: C-MTEB/CLSClusteringS2S
name: MTEB CLSClusteringS2S
config: default
split: test
revision: e458b3f5414b62b7f9f83499ac1f5497ae2e869f
metrics:
- type: v_measure
value: 45.98511703185043
- task:
type: Reranking
dataset:
type: C-MTEB/CMedQAv1-reranking
name: MTEB CMedQAv1
config: default
split: test
revision: 8d7f1e942507dac42dc58017c1a001c3717da7df
metrics:
- type: map
value: 88.19895157194931
- type: mrr
value: 90.21424603174603
- task:
type: Reranking
dataset:
type: C-MTEB/CMedQAv2-reranking
name: MTEB CMedQAv2
config: default
split: test
revision: 23d186750531a14a0357ca22cd92d712fd512ea0
metrics:
- type: map
value: 88.03317320980119
- type: mrr
value: 89.9461507936508
- task:
type: Retrieval
dataset:
type: C-MTEB/CmedqaRetrieval
name: MTEB CmedqaRetrieval
config: default
split: dev
revision: cd540c506dae1cf9e9a59c3e06f42030d54e7301
metrics:
- type: map_at_1
value: 29.037000000000003
- type: map_at_10
value: 42.001
- type: map_at_100
value: 43.773
- type: map_at_1000
value: 43.878
- type: map_at_3
value: 37.637
- type: map_at_5
value: 40.034
- type: mrr_at_1
value: 43.136
- type: mrr_at_10
value: 51.158
- type: mrr_at_100
value: 52.083
- type: mrr_at_1000
value: 52.12
- type: mrr_at_3
value: 48.733
- type: mrr_at_5
value: 50.025
- type: ndcg_at_1
value: 43.136
- type: ndcg_at_10
value: 48.685
- type: ndcg_at_100
value: 55.513
- type: ndcg_at_1000
value: 57.242000000000004
- type: ndcg_at_3
value: 43.329
- type: ndcg_at_5
value: 45.438
- type: precision_at_1
value: 43.136
- type: precision_at_10
value: 10.56
- type: precision_at_100
value: 1.6129999999999998
- type: precision_at_1000
value: 0.184
- type: precision_at_3
value: 24.064
- type: precision_at_5
value: 17.269000000000002
- type: recall_at_1
value: 29.037000000000003
- type: recall_at_10
value: 59.245000000000005
- type: recall_at_100
value: 87.355
- type: recall_at_1000
value: 98.74000000000001
- type: recall_at_3
value: 42.99
- type: recall_at_5
value: 49.681999999999995
- task:
type: PairClassification
dataset:
type: C-MTEB/CMNLI
name: MTEB Cmnli
config: default
split: validation
revision: 41bc36f332156f7adc9e38f53777c959b2ae9766
metrics:
- type: cos_sim_accuracy
value: 82.68190018039687
- type: cos_sim_ap
value: 90.18017125327886
- type: cos_sim_f1
value: 83.64080906868193
- type: cos_sim_precision
value: 79.7076890489303
- type: cos_sim_recall
value: 87.98223053542202
- type: dot_accuracy
value: 82.68190018039687
- type: dot_ap
value: 90.18782350103646
- type: dot_f1
value: 83.64242087729039
- type: dot_precision
value: 79.65313028764805
- type: dot_recall
value: 88.05237315875614
- type: euclidean_accuracy
value: 82.68190018039687
- type: euclidean_ap
value: 90.1801957900632
- type: euclidean_f1
value: 83.63636363636364
- type: euclidean_precision
value: 79.52772506852203
- type: euclidean_recall
value: 88.19265840542437
- type: manhattan_accuracy
value: 82.14070956103427
- type: manhattan_ap
value: 89.96178420101427
- type: manhattan_f1
value: 83.21087838578791
- type: manhattan_precision
value: 78.35605121850475
- type: manhattan_recall
value: 88.70703764320785
- type: max_accuracy
value: 82.68190018039687
- type: max_ap
value: 90.18782350103646
- type: max_f1
value: 83.64242087729039
- task:
type: Retrieval
dataset:
type: C-MTEB/CovidRetrieval
name: MTEB CovidRetrieval
config: default
split: dev
revision: 1271c7809071a13532e05f25fb53511ffce77117
metrics:
- type: map_at_1
value: 72.234
- type: map_at_10
value: 80.10000000000001
- type: map_at_100
value: 80.36
- type: map_at_1000
value: 80.363
- type: map_at_3
value: 78.315
- type: map_at_5
value: 79.607
- type: mrr_at_1
value: 72.392
- type: mrr_at_10
value: 80.117
- type: mrr_at_100
value: 80.36999999999999
- type: mrr_at_1000
value: 80.373
- type: mrr_at_3
value: 78.469
- type: mrr_at_5
value: 79.633
- type: ndcg_at_1
value: 72.392
- type: ndcg_at_10
value: 83.651
- type: ndcg_at_100
value: 84.749
- type: ndcg_at_1000
value: 84.83000000000001
- type: ndcg_at_3
value: 80.253
- type: ndcg_at_5
value: 82.485
- type: precision_at_1
value: 72.392
- type: precision_at_10
value: 9.557
- type: precision_at_100
value: 1.004
- type: precision_at_1000
value: 0.101
- type: precision_at_3
value: 28.732000000000003
- type: precision_at_5
value: 18.377
- type: recall_at_1
value: 72.234
- type: recall_at_10
value: 94.573
- type: recall_at_100
value: 99.368
- type: recall_at_1000
value: 100.0
- type: recall_at_3
value: 85.669
- type: recall_at_5
value: 91.01700000000001
- task:
type: Retrieval
dataset:
type: C-MTEB/DuRetrieval
name: MTEB DuRetrieval
config: default
split: dev
revision: a1a333e290fe30b10f3f56498e3a0d911a693ced
metrics:
- type: map_at_1
value: 26.173999999999996
- type: map_at_10
value: 80.04
- type: map_at_100
value: 82.94500000000001
- type: map_at_1000
value: 82.98100000000001
- type: map_at_3
value: 55.562999999999995
- type: map_at_5
value: 69.89800000000001
- type: mrr_at_1
value: 89.5
- type: mrr_at_10
value: 92.996
- type: mrr_at_100
value: 93.06400000000001
- type: mrr_at_1000
value: 93.065
- type: mrr_at_3
value: 92.658
- type: mrr_at_5
value: 92.84599999999999
- type: ndcg_at_1
value: 89.5
- type: ndcg_at_10
value: 87.443
- type: ndcg_at_100
value: 90.253
- type: ndcg_at_1000
value: 90.549
- type: ndcg_at_3
value: 85.874
- type: ndcg_at_5
value: 84.842
- type: precision_at_1
value: 89.5
- type: precision_at_10
value: 41.805
- type: precision_at_100
value: 4.827
- type: precision_at_1000
value: 0.49
- type: precision_at_3
value: 76.85
- type: precision_at_5
value: 64.8
- type: recall_at_1
value: 26.173999999999996
- type: recall_at_10
value: 89.101
- type: recall_at_100
value: 98.08099999999999
- type: recall_at_1000
value: 99.529
- type: recall_at_3
value: 57.902
- type: recall_at_5
value: 74.602
- task:
type: Retrieval
dataset:
type: C-MTEB/EcomRetrieval
name: MTEB EcomRetrieval
config: default
split: dev
revision: 687de13dc7294d6fd9be10c6945f9e8fec8166b9
metrics:
- type: map_at_1
value: 56.10000000000001
- type: map_at_10
value: 66.15299999999999
- type: map_at_100
value: 66.625
- type: map_at_1000
value: 66.636
- type: map_at_3
value: 63.632999999999996
- type: map_at_5
value: 65.293
- type: mrr_at_1
value: 56.10000000000001
- type: mrr_at_10
value: 66.15299999999999
- type: mrr_at_100
value: 66.625
- type: mrr_at_1000
value: 66.636
- type: mrr_at_3
value: 63.632999999999996
- type: mrr_at_5
value: 65.293
- type: ndcg_at_1
value: 56.10000000000001
- type: ndcg_at_10
value: 71.146
- type: ndcg_at_100
value: 73.27799999999999
- type: ndcg_at_1000
value: 73.529
- type: ndcg_at_3
value: 66.09
- type: ndcg_at_5
value: 69.08999999999999
- type: precision_at_1
value: 56.10000000000001
- type: precision_at_10
value: 8.68
- type: precision_at_100
value: 0.964
- type: precision_at_1000
value: 0.098
- type: precision_at_3
value: 24.4
- type: precision_at_5
value: 16.1
- type: recall_at_1
value: 56.10000000000001
- type: recall_at_10
value: 86.8
- type: recall_at_100
value: 96.39999999999999
- type: recall_at_1000
value: 98.3
- type: recall_at_3
value: 73.2
- type: recall_at_5
value: 80.5
- task:
type: Classification
dataset:
type: C-MTEB/IFlyTek-classification
name: MTEB IFlyTek
config: default
split: validation
revision: 421605374b29664c5fc098418fe20ada9bd55f8a
metrics:
- type: accuracy
value: 54.52096960369373
- type: f1
value: 40.930845295808695
- task:
type: Classification
dataset:
type: C-MTEB/JDReview-classification
name: MTEB JDReview
config: default
split: test
revision: b7c64bd89eb87f8ded463478346f76731f07bf8b
metrics:
- type: accuracy
value: 86.51031894934334
- type: ap
value: 55.9516014323483
- type: f1
value: 81.54813679326381
- task:
type: STS
dataset:
type: C-MTEB/LCQMC
name: MTEB LCQMC
config: default
split: test
revision: 17f9b096f80380fce5ed12a9be8be7784b337daf
metrics:
- type: cos_sim_pearson
value: 69.67437838574276
- type: cos_sim_spearman
value: 73.81314174653045
- type: euclidean_pearson
value: 72.63430276680275
- type: euclidean_spearman
value: 73.81358736777001
- type: manhattan_pearson
value: 72.58743833842829
- type: manhattan_spearman
value: 73.7590419009179
- task:
type: Reranking
dataset:
type: C-MTEB/Mmarco-reranking
name: MTEB MMarcoReranking
config: default
split: dev
revision: None
metrics:
- type: map
value: 31.648613483640254
- type: mrr
value: 30.37420634920635
- task:
type: Retrieval
dataset:
type: C-MTEB/MMarcoRetrieval
name: MTEB MMarcoRetrieval
config: default
split: dev
revision: 539bbde593d947e2a124ba72651aafc09eb33fc2
metrics:
- type: map_at_1
value: 73.28099999999999
- type: map_at_10
value: 81.977
- type: map_at_100
value: 82.222
- type: map_at_1000
value: 82.22699999999999
- type: map_at_3
value: 80.441
- type: map_at_5
value: 81.46600000000001
- type: mrr_at_1
value: 75.673
- type: mrr_at_10
value: 82.41000000000001
- type: mrr_at_100
value: 82.616
- type: mrr_at_1000
value: 82.621
- type: mrr_at_3
value: 81.094
- type: mrr_at_5
value: 81.962
- type: ndcg_at_1
value: 75.673
- type: ndcg_at_10
value: 85.15599999999999
- type: ndcg_at_100
value: 86.151
- type: ndcg_at_1000
value: 86.26899999999999
- type: ndcg_at_3
value: 82.304
- type: ndcg_at_5
value: 84.009
- type: precision_at_1
value: 75.673
- type: precision_at_10
value: 10.042
- type: precision_at_100
value: 1.052
- type: precision_at_1000
value: 0.106
- type: precision_at_3
value: 30.673000000000002
- type: precision_at_5
value: 19.326999999999998
- type: recall_at_1
value: 73.28099999999999
- type: recall_at_10
value: 94.446
- type: recall_at_100
value: 98.737
- type: recall_at_1000
value: 99.649
- type: recall_at_3
value: 86.984
- type: recall_at_5
value: 91.024
- task:
type: Classification
dataset:
type: mteb/amazon_massive_intent
name: MTEB MassiveIntentClassification (zh-CN)
config: zh-CN
split: test
revision: 31efe3c427b0bae9c22cbb560b8f15491cc6bed7
metrics:
- type: accuracy
value: 81.08607935440484
- type: f1
value: 78.24879986066307
- task:
type: Classification
dataset:
type: mteb/amazon_massive_scenario
name: MTEB MassiveScenarioClassification (zh-CN)
config: zh-CN
split: test
revision: 7d571f92784cd94a019292a1f45445077d0ef634
metrics:
- type: accuracy
value: 86.05917955615332
- type: f1
value: 85.05279279434997
- task:
type: Retrieval
dataset:
type: C-MTEB/MedicalRetrieval
name: MTEB MedicalRetrieval
config: default
split: dev
revision: 2039188fb5800a9803ba5048df7b76e6fb151fc6
metrics:
- type: map_at_1
value: 56.2
- type: map_at_10
value: 62.57899999999999
- type: map_at_100
value: 63.154999999999994
- type: map_at_1000
value: 63.193
- type: map_at_3
value: 61.217
- type: map_at_5
value: 62.012
- type: mrr_at_1
value: 56.3
- type: mrr_at_10
value: 62.629000000000005
- type: mrr_at_100
value: 63.205999999999996
- type: mrr_at_1000
value: 63.244
- type: mrr_at_3
value: 61.267
- type: mrr_at_5
value: 62.062
- type: ndcg_at_1
value: 56.2
- type: ndcg_at_10
value: 65.592
- type: ndcg_at_100
value: 68.657
- type: ndcg_at_1000
value: 69.671
- type: ndcg_at_3
value: 62.808
- type: ndcg_at_5
value: 64.24499999999999
- type: precision_at_1
value: 56.2
- type: precision_at_10
value: 7.5
- type: precision_at_100
value: 0.899
- type: precision_at_1000
value: 0.098
- type: precision_at_3
value: 22.467000000000002
- type: precision_at_5
value: 14.180000000000001
- type: recall_at_1
value: 56.2
- type: recall_at_10
value: 75.0
- type: recall_at_100
value: 89.9
- type: recall_at_1000
value: 97.89999999999999
- type: recall_at_3
value: 67.4
- type: recall_at_5
value: 70.89999999999999
- task:
type: Classification
dataset:
type: C-MTEB/MultilingualSentiment-classification
name: MTEB MultilingualSentiment
config: default
split: validation
revision: 46958b007a63fdbf239b7672c25d0bea67b5ea1a
metrics:
- type: accuracy
value: 76.87666666666667
- type: f1
value: 76.7317686219665
- task:
type: PairClassification
dataset:
type: C-MTEB/OCNLI
name: MTEB Ocnli
config: default
split: validation
revision: 66e76a618a34d6d565d5538088562851e6daa7ec
metrics:
- type: cos_sim_accuracy
value: 79.64266377910124
- type: cos_sim_ap
value: 84.78274442344829
- type: cos_sim_f1
value: 81.16947472745292
- type: cos_sim_precision
value: 76.47058823529412
- type: cos_sim_recall
value: 86.48363252375924
- type: dot_accuracy
value: 79.64266377910124
- type: dot_ap
value: 84.7851404063692
- type: dot_f1
value: 81.16947472745292
- type: dot_precision
value: 76.47058823529412
- type: dot_recall
value: 86.48363252375924
- type: euclidean_accuracy
value: 79.64266377910124
- type: euclidean_ap
value: 84.78068373762378
- type: euclidean_f1
value: 81.14794656110837
- type: euclidean_precision
value: 76.35009310986965
- type: euclidean_recall
value: 86.58922914466737
- type: manhattan_accuracy
value: 79.48023822414727
- type: manhattan_ap
value: 84.72928897427576
- type: manhattan_f1
value: 81.32084770823064
- type: manhattan_precision
value: 76.24768946395564
- type: manhattan_recall
value: 87.11721224920802
- type: max_accuracy
value: 79.64266377910124
- type: max_ap
value: 84.7851404063692
- type: max_f1
value: 81.32084770823064
- task:
type: Classification
dataset:
type: C-MTEB/OnlineShopping-classification
name: MTEB OnlineShopping
config: default
split: test
revision: e610f2ebd179a8fda30ae534c3878750a96db120
metrics:
- type: accuracy
value: 94.3
- type: ap
value: 92.8664032274438
- type: f1
value: 94.29311102997727
- task:
type: STS
dataset:
type: C-MTEB/PAWSX
name: MTEB PAWSX
config: default
split: test
revision: 9c6a90e430ac22b5779fb019a23e820b11a8b5e1
metrics:
- type: cos_sim_pearson
value: 48.51392279882909
- type: cos_sim_spearman
value: 54.06338895994974
- type: euclidean_pearson
value: 52.58480559573412
- type: euclidean_spearman
value: 54.06417276612201
- type: manhattan_pearson
value: 52.69525121721343
- type: manhattan_spearman
value: 54.048147455389675
- task:
type: STS
dataset:
type: C-MTEB/QBQTC
name: MTEB QBQTC
config: default
split: test
revision: 790b0510dc52b1553e8c49f3d2afb48c0e5c48b7
metrics:
- type: cos_sim_pearson
value: 29.728387290757325
- type: cos_sim_spearman
value: 31.366121633635284
- type: euclidean_pearson
value: 29.14588368552961
- type: euclidean_spearman
value: 31.36764411112844
- type: manhattan_pearson
value: 29.63517350523121
- type: manhattan_spearman
value: 31.94157020583762
- task:
type: STS
dataset:
type: mteb/sts22-crosslingual-sts
name: MTEB STS22 (zh)
config: zh
split: test
revision: eea2b4fe26a775864c896887d910b76a8098ad3f
metrics:
- type: cos_sim_pearson
value: 63.64868296271406
- type: cos_sim_spearman
value: 66.12800618164744
- type: euclidean_pearson
value: 63.21405767340238
- type: euclidean_spearman
value: 66.12786567790748
- type: manhattan_pearson
value: 64.04300276525848
- type: manhattan_spearman
value: 66.5066857145652
- task:
type: STS
dataset:
type: C-MTEB/STSB
name: MTEB STSB
config: default
split: test
revision: 0cde68302b3541bb8b3c340dc0644b0b745b3dc0
metrics:
- type: cos_sim_pearson
value: 81.2302623912794
- type: cos_sim_spearman
value: 81.16833673266562
- type: euclidean_pearson
value: 79.47647843876024
- type: euclidean_spearman
value: 81.16944349524972
- type: manhattan_pearson
value: 79.84947238492208
- type: manhattan_spearman
value: 81.64626599410026
- task:
type: Reranking
dataset:
type: C-MTEB/T2Reranking
name: MTEB T2Reranking
config: default
split: dev
revision: 76631901a18387f85eaa53e5450019b87ad58ef9
metrics:
- type: map
value: 67.80129586475687
- type: mrr
value: 77.77402311635554
- task:
type: Retrieval
dataset:
type: C-MTEB/T2Retrieval
name: MTEB T2Retrieval
config: default
split: dev
revision: 8731a845f1bf500a4f111cf1070785c793d10e64
metrics:
- type: map_at_1
value: 28.666999999999998
- type: map_at_10
value: 81.063
- type: map_at_100
value: 84.504
- type: map_at_1000
value: 84.552
- type: map_at_3
value: 56.897
- type: map_at_5
value: 70.073
- type: mrr_at_1
value: 92.087
- type: mrr_at_10
value: 94.132
- type: mrr_at_100
value: 94.19800000000001
- type: mrr_at_1000
value: 94.19999999999999
- type: mrr_at_3
value: 93.78999999999999
- type: mrr_at_5
value: 94.002
- type: ndcg_at_1
value: 92.087
- type: ndcg_at_10
value: 87.734
- type: ndcg_at_100
value: 90.736
- type: ndcg_at_1000
value: 91.184
- type: ndcg_at_3
value: 88.78
- type: ndcg_at_5
value: 87.676
- type: precision_at_1
value: 92.087
- type: precision_at_10
value: 43.46
- type: precision_at_100
value: 5.07
- type: precision_at_1000
value: 0.518
- type: precision_at_3
value: 77.49000000000001
- type: precision_at_5
value: 65.194
- type: recall_at_1
value: 28.666999999999998
- type: recall_at_10
value: 86.632
- type: recall_at_100
value: 96.646
- type: recall_at_1000
value: 98.917
- type: recall_at_3
value: 58.333999999999996
- type: recall_at_5
value: 72.974
- task:
type: Classification
dataset:
type: C-MTEB/TNews-classification
name: MTEB TNews
config: default
split: validation
revision: 317f262bf1e6126357bbe89e875451e4b0938fe4
metrics:
- type: accuracy
value: 52.971999999999994
- type: f1
value: 50.2898280984929
- task:
type: Clustering
dataset:
type: C-MTEB/ThuNewsClusteringP2P
name: MTEB ThuNewsClusteringP2P
config: default
split: test
revision: 5798586b105c0434e4f0fe5e767abe619442cf93
metrics:
- type: v_measure
value: 86.0797948663824
- task:
type: Clustering
dataset:
type: C-MTEB/ThuNewsClusteringS2S
name: MTEB ThuNewsClusteringS2S
config: default
split: test
revision: 8a8b2caeda43f39e13c4bc5bea0f8a667896e10d
metrics:
- type: v_measure
value: 85.10759092255017
- task:
type: Retrieval
dataset:
type: C-MTEB/VideoRetrieval
name: MTEB VideoRetrieval
config: default
split: dev
revision: 58c2597a5943a2ba48f4668c3b90d796283c5639
metrics:
- type: map_at_1
value: 65.60000000000001
- type: map_at_10
value: 74.773
- type: map_at_100
value: 75.128
- type: map_at_1000
value: 75.136
- type: map_at_3
value: 73.05
- type: map_at_5
value: 74.13499999999999
- type: mrr_at_1
value: 65.60000000000001
- type: mrr_at_10
value: 74.773
- type: mrr_at_100
value: 75.128
- type: mrr_at_1000
value: 75.136
- type: mrr_at_3
value: 73.05
- type: mrr_at_5
value: 74.13499999999999
- type: ndcg_at_1
value: 65.60000000000001
- type: ndcg_at_10
value: 78.84299999999999
- type: ndcg_at_100
value: 80.40899999999999
- type: ndcg_at_1000
value: 80.57
- type: ndcg_at_3
value: 75.40599999999999
- type: ndcg_at_5
value: 77.351
- type: precision_at_1
value: 65.60000000000001
- type: precision_at_10
value: 9.139999999999999
- type: precision_at_100
value: 0.984
- type: precision_at_1000
value: 0.1
- type: precision_at_3
value: 27.400000000000002
- type: precision_at_5
value: 17.380000000000003
- type: recall_at_1
value: 65.60000000000001
- type: recall_at_10
value: 91.4
- type: recall_at_100
value: 98.4
- type: recall_at_1000
value: 99.6
- type: recall_at_3
value: 82.19999999999999
- type: recall_at_5
value: 86.9
- task:
type: Classification
dataset:
type: C-MTEB/waimai-classification
name: MTEB Waimai
config: default
split: test
revision: 339287def212450dcaa9df8c22bf93e9980c7023
metrics:
- type: accuracy
value: 89.47
- type: ap
value: 75.59561751845389
- type: f1
value: 87.95207751382563
---
## gte-Qwen2-7B-instruct
**gte-Qwen2-7B-instruct** is the latest model in the gte (General Text Embedding) model family that ranks **No.1** in both English and Chinese evaluations on the Massive Text Embedding Benchmark [MTEB benchmark](https://huggingface.co/spaces/mteb/leaderboard) (as of June 16, 2024).
Recently, the [**Qwen team**](https://huggingface.co/Qwen) released the Qwen2 series models, and we have trained the **gte-Qwen2-7B-instruct** model based on the [Qwen2-7B](https://huggingface.co/Qwen/Qwen2-7B) LLM model. Compared to the [gte-Qwen1.5-7B-instruct](https://huggingface.co/Alibaba-NLP/gte-Qwen1.5-7B-instruct) model, the **gte-Qwen2-7B-instruct** model uses the same training data and training strategies during the finetuning stage, with the only difference being the upgraded base model to Qwen2-7B. Considering the improvements in the Qwen2 series models compared to the Qwen1.5 series, we can also expect consistent performance enhancements in the embedding models.
The model incorporates several key advancements:
- Integration of bidirectional attention mechanisms, enriching its contextual understanding.
- Instruction tuning, applied solely on the query side for streamlined efficiency
- Comprehensive training across a vast, multilingual text corpus spanning diverse domains and scenarios. This training leverages both weakly supervised and supervised data, ensuring the model's applicability across numerous languages and a wide array of downstream tasks.
## Model Information
- Model Size: 7B
- Embedding Dimension: 3584
- Max Input Tokens: 32k
## Requirements
```
transformers>=4.39.2
flash_attn>=2.5.6
```
## Usage
### Sentence Transformers
```python
from sentence_transformers import SentenceTransformer
model = SentenceTransformer("Alibaba-NLP/gte-Qwen2-7B-instruct", trust_remote_code=True)
# In case you want to reduce the maximum length:
model.max_seq_length = 8192
queries = [
"how much protein should a female eat",
"summit define",
]
documents = [
"As a general guideline, the CDC's average requirement of protein for women ages 19 to 70 is 46 grams per day. But, as you can see from this chart, you'll need to increase that if you're expecting or training for a marathon. Check out the chart below to see how much protein you should be eating each day.",
"Definition of summit for English Language Learners. : 1 the highest point of a mountain : the top of a mountain. : 2 the highest level. : 3 a meeting or series of meetings between the leaders of two or more governments.",
]
query_embeddings = model.encode(queries, prompt_name="query")
document_embeddings = model.encode(documents)
scores = (query_embeddings @ document_embeddings.T) * 100
print(scores.tolist())
```
Observe the [config_sentence_transformers.json](config_sentence_transformers.json) to see all pre-built prompt names. Otherwise, you can use `model.encode(queries, prompt="Instruct: ...\nQuery: "` to use a custom prompt of your choice.
### Transformers
```python
import torch
import torch.nn.functional as F
from torch import Tensor
from transformers import AutoTokenizer, AutoModel
def last_token_pool(last_hidden_states: Tensor,
attention_mask: Tensor) -> Tensor:
left_padding = (attention_mask[:, -1].sum() == attention_mask.shape[0])
if left_padding:
return last_hidden_states[:, -1]
else:
sequence_lengths = attention_mask.sum(dim=1) - 1
batch_size = last_hidden_states.shape[0]
return last_hidden_states[torch.arange(batch_size, device=last_hidden_states.device), sequence_lengths]
def get_detailed_instruct(task_description: str, query: str) -> str:
return f'Instruct: {task_description}\nQuery: {query}'
# Each query must come with a one-sentence instruction that describes the task
task = 'Given a web search query, retrieve relevant passages that answer the query'
queries = [
get_detailed_instruct(task, 'how much protein should a female eat'),
get_detailed_instruct(task, 'summit define')
]
# No need to add instruction for retrieval documents
documents = [
"As a general guideline, the CDC's average requirement of protein for women ages 19 to 70 is 46 grams per day. But, as you can see from this chart, you'll need to increase that if you're expecting or training for a marathon. Check out the chart below to see how much protein you should be eating each day.",
"Definition of summit for English Language Learners. : 1 the highest point of a mountain : the top of a mountain. : 2 the highest level. : 3 a meeting or series of meetings between the leaders of two or more governments."
]
input_texts = queries + documents
tokenizer = AutoTokenizer.from_pretrained('Alibaba-NLP/gte-Qwen2-7B-instruct', trust_remote_code=True)
model = AutoModel.from_pretrained('Alibaba-NLP/gte-Qwen2-7B-instruct', trust_remote_code=True)
max_length = 8192
# Tokenize the input texts
batch_dict = tokenizer(input_texts, max_length=max_length, padding=True, truncation=True, return_tensors='pt')
outputs = model(**batch_dict)
embeddings = last_token_pool(outputs.last_hidden_state, batch_dict['attention_mask'])
# normalize embeddings
embeddings = F.normalize(embeddings, p=2, dim=1)
scores = (embeddings[:2] @ embeddings[2:].T) * 100
print(scores.tolist())
```
## Evaluation
### MTEB & C-MTEB
You can use the [scripts/eval_mteb.py](https://huggingface.co/Alibaba-NLP/gte-Qwen2-7B-instruct/blob/main/scripts/eval_mteb.py) to reproduce the following result of **gte-Qwen2-7B-instruct** on MTEB(English)/C-MTEB(Chinese):
| Model Name | MTEB(56) | C-MTEB(35) |
|:----:|:---------:|:----------:|
| [bge-base-en-1.5](https://huggingface.co/BAAI/bge-base-en-v1.5) | 64.23 | - |
| [bge-large-en-1.5](https://huggingface.co/BAAI/bge-large-en-v1.5) | 63.55 | - |
| [gte-large-en-v1.5](https://huggingface.co/Alibaba-NLP/gte-large-en-v1.5) | 65.39 | - |
| [gte-base-en-v1.5](https://huggingface.co/Alibaba-NLP/gte-large-en-v1.5) | 64.11 | - |
| [mxbai-embed-large-v1](https://huggingface.co/mixedbread-ai/mxbai-embed-large-v1) | 64.68 | - |
| [acge_text_embedding](https://huggingface.co/aspire/acge_text_embedding) | - | 69.07 |
| [stella-mrl-large-zh-v3.5-1792d](https://huggingface.co/infgrad/stella-mrl-large-zh-v3.5-1792d) | - | 68.55 |
| [gte-large-zh](https://huggingface.co/thenlper/gte-large-zh) | - | 66.72 |
| [multilingual-e5-base](https://huggingface.co/intfloat/multilingual-e5-base) | 59.45 | 56.21 |
| [multilingual-e5-large](https://huggingface.co/intfloat/multilingual-e5-large) | 61.50 | 58.81 |
| [e5-mistral-7b-instruct](https://huggingface.co/intfloat/e5-mistral-7b-instruct) | 66.63 | 60.81 |
| [gte-Qwen1.5-7B-instruct](https://huggingface.co/Alibaba-NLP/gte-Qwen1.5-7B-instruct) | 67.34 | 69.52 |
| [NV-Embed-v1](https://huggingface.co/nvidia/NV-Embed-v1) | 69.32 | - |
| [**gte-Qwen2-7B-instruct**](https://huggingface.co/Alibaba-NLP/gte-Qwen2-7B-instruct) | **70.24** | **72.05** |
### GTE Models
The gte series models have consistently released two types of models: encoder-only models (based on the BERT architecture) and decode-only models (based on the LLM architecture).
| Models | Language | Max Sequence Length | Dimension | Model Size (Memory Usage, fp32) |
|:-------------------------------------------------------------------------------------:|:--------:|:-----: |:---------:|:-------------------------------:|
| [GTE-large-zh](https://huggingface.co/thenlper/gte-large-zh) | Chinese | 512 | 1024 | 1.25GB |
| [GTE-base-zh](https://huggingface.co/thenlper/gte-base-zh) | Chinese | 512 | 512 | 0.41GB |
| [GTE-small-zh](https://huggingface.co/thenlper/gte-small-zh) | Chinese | 512 | 512 | 0.12GB |
| [GTE-large](https://huggingface.co/thenlper/gte-large) | English | 512 | 1024 | 1.25GB |
| [GTE-base](https://huggingface.co/thenlper/gte-base) | English | 512 | 512 | 0.21GB |
| [GTE-small](https://huggingface.co/thenlper/gte-small) | English | 512 | 384 | 0.10GB |
| [GTE-large-en-v1.5](https://huggingface.co/Alibaba-NLP/gte-large-en-v1.5) | English | 8192 | 1024 | 1.74GB |
| [GTE-base-en-v1.5](https://huggingface.co/Alibaba-NLP/gte-base-en-v1.5) | English | 8192 | 768 | 0.51GB |
| [GTE-Qwen1.5-7B-instruct](https://huggingface.co/Alibaba-NLP/gte-Qwen1.5-7B-instruct) | Multilingual | 32000 | 4096 | 26.45GB |
| [GTE-Qwen2-7B-instruct](https://huggingface.co/Alibaba-NLP/gte-Qwen2-7B-instruct) | Multilingual | 32000 | 3584 | 26.45GB |
## Citation
If you find our paper or models helpful, please consider cite:
```
@article{li2023towards,
title={Towards general text embeddings with multi-stage contrastive learning},
author={Li, Zehan and Zhang, Xin and Zhang, Yanzhao and Long, Dingkun and Xie, Pengjun and Zhang, Meishan},
journal={arXiv preprint arXiv:2308.03281},
year={2023}
}
```
|
mradermacher/meta-llama-3-8b-instruct-hf-ortho-baukit-5fail-3000total-bf16-GGUF | mradermacher | 2024-06-28T22:31:32Z | 12,085 | 0 | transformers | [
"transformers",
"gguf",
"en",
"base_model:Edgerunners/meta-llama-3-8b-instruct-hf-ortho-baukit-5fail-3000total-bf16",
"license:cc-by-nc-4.0",
"endpoints_compatible",
"region:us"
]
| null | 2024-06-27T00:20:48Z | ---
base_model: Edgerunners/meta-llama-3-8b-instruct-hf-ortho-baukit-5fail-3000total-bf16
language:
- en
library_name: transformers
license: cc-by-nc-4.0
quantized_by: mradermacher
---
## About
<!-- ### quantize_version: 2 -->
<!-- ### output_tensor_quantised: 1 -->
<!-- ### convert_type: hf -->
<!-- ### vocab_type: -->
<!-- ### tags: -->
static quants of https://huggingface.co/Edgerunners/meta-llama-3-8b-instruct-hf-ortho-baukit-5fail-3000total-bf16
<!-- provided-files -->
weighted/imatrix quants are available at https://huggingface.co/mradermacher/meta-llama-3-8b-instruct-hf-ortho-baukit-5fail-3000total-bf16-i1-GGUF
## Usage
If you are unsure how to use GGUF files, refer to one of [TheBloke's
READMEs](https://huggingface.co/TheBloke/KafkaLM-70B-German-V0.1-GGUF) for
more details, including on how to concatenate multi-part files.
## Provided Quants
(sorted by size, not necessarily quality. IQ-quants are often preferable over similar sized non-IQ quants)
| Link | Type | Size/GB | Notes |
|:-----|:-----|--------:|:------|
| [GGUF](https://huggingface.co/mradermacher/meta-llama-3-8b-instruct-hf-ortho-baukit-5fail-3000total-bf16-GGUF/resolve/main/meta-llama-3-8b-instruct-hf-ortho-baukit-5fail-3000total-bf16.Q2_K.gguf) | Q2_K | 3.3 | |
| [GGUF](https://huggingface.co/mradermacher/meta-llama-3-8b-instruct-hf-ortho-baukit-5fail-3000total-bf16-GGUF/resolve/main/meta-llama-3-8b-instruct-hf-ortho-baukit-5fail-3000total-bf16.IQ3_XS.gguf) | IQ3_XS | 3.6 | |
| [GGUF](https://huggingface.co/mradermacher/meta-llama-3-8b-instruct-hf-ortho-baukit-5fail-3000total-bf16-GGUF/resolve/main/meta-llama-3-8b-instruct-hf-ortho-baukit-5fail-3000total-bf16.Q3_K_S.gguf) | Q3_K_S | 3.8 | |
| [GGUF](https://huggingface.co/mradermacher/meta-llama-3-8b-instruct-hf-ortho-baukit-5fail-3000total-bf16-GGUF/resolve/main/meta-llama-3-8b-instruct-hf-ortho-baukit-5fail-3000total-bf16.IQ3_S.gguf) | IQ3_S | 3.8 | beats Q3_K* |
| [GGUF](https://huggingface.co/mradermacher/meta-llama-3-8b-instruct-hf-ortho-baukit-5fail-3000total-bf16-GGUF/resolve/main/meta-llama-3-8b-instruct-hf-ortho-baukit-5fail-3000total-bf16.IQ3_M.gguf) | IQ3_M | 3.9 | |
| [GGUF](https://huggingface.co/mradermacher/meta-llama-3-8b-instruct-hf-ortho-baukit-5fail-3000total-bf16-GGUF/resolve/main/meta-llama-3-8b-instruct-hf-ortho-baukit-5fail-3000total-bf16.Q3_K_M.gguf) | Q3_K_M | 4.1 | lower quality |
| [GGUF](https://huggingface.co/mradermacher/meta-llama-3-8b-instruct-hf-ortho-baukit-5fail-3000total-bf16-GGUF/resolve/main/meta-llama-3-8b-instruct-hf-ortho-baukit-5fail-3000total-bf16.Q3_K_L.gguf) | Q3_K_L | 4.4 | |
| [GGUF](https://huggingface.co/mradermacher/meta-llama-3-8b-instruct-hf-ortho-baukit-5fail-3000total-bf16-GGUF/resolve/main/meta-llama-3-8b-instruct-hf-ortho-baukit-5fail-3000total-bf16.IQ4_XS.gguf) | IQ4_XS | 4.6 | |
| [GGUF](https://huggingface.co/mradermacher/meta-llama-3-8b-instruct-hf-ortho-baukit-5fail-3000total-bf16-GGUF/resolve/main/meta-llama-3-8b-instruct-hf-ortho-baukit-5fail-3000total-bf16.Q4_K_S.gguf) | Q4_K_S | 4.8 | fast, recommended |
| [GGUF](https://huggingface.co/mradermacher/meta-llama-3-8b-instruct-hf-ortho-baukit-5fail-3000total-bf16-GGUF/resolve/main/meta-llama-3-8b-instruct-hf-ortho-baukit-5fail-3000total-bf16.Q4_K_M.gguf) | Q4_K_M | 5.0 | fast, recommended |
| [GGUF](https://huggingface.co/mradermacher/meta-llama-3-8b-instruct-hf-ortho-baukit-5fail-3000total-bf16-GGUF/resolve/main/meta-llama-3-8b-instruct-hf-ortho-baukit-5fail-3000total-bf16.Q5_K_S.gguf) | Q5_K_S | 5.7 | |
| [GGUF](https://huggingface.co/mradermacher/meta-llama-3-8b-instruct-hf-ortho-baukit-5fail-3000total-bf16-GGUF/resolve/main/meta-llama-3-8b-instruct-hf-ortho-baukit-5fail-3000total-bf16.Q5_K_M.gguf) | Q5_K_M | 5.8 | |
| [GGUF](https://huggingface.co/mradermacher/meta-llama-3-8b-instruct-hf-ortho-baukit-5fail-3000total-bf16-GGUF/resolve/main/meta-llama-3-8b-instruct-hf-ortho-baukit-5fail-3000total-bf16.Q6_K.gguf) | Q6_K | 6.7 | very good quality |
| [GGUF](https://huggingface.co/mradermacher/meta-llama-3-8b-instruct-hf-ortho-baukit-5fail-3000total-bf16-GGUF/resolve/main/meta-llama-3-8b-instruct-hf-ortho-baukit-5fail-3000total-bf16.Q8_0.gguf) | Q8_0 | 8.6 | fast, best quality |
| [GGUF](https://huggingface.co/mradermacher/meta-llama-3-8b-instruct-hf-ortho-baukit-5fail-3000total-bf16-GGUF/resolve/main/meta-llama-3-8b-instruct-hf-ortho-baukit-5fail-3000total-bf16.f16.gguf) | f16 | 16.2 | 16 bpw, overkill |
Here is a handy graph by ikawrakow comparing some lower-quality quant
types (lower is better):

And here are Artefact2's thoughts on the matter:
https://gist.github.com/Artefact2/b5f810600771265fc1e39442288e8ec9
## FAQ / Model Request
See https://huggingface.co/mradermacher/model_requests for some answers to
questions you might have and/or if you want some other model quantized.
## Thanks
I thank my company, [nethype GmbH](https://www.nethype.de/), for letting
me use its servers and providing upgrades to my workstation to enable
this work in my free time.
<!-- end -->
|
facebook/rag-sequence-base | facebook | 2020-12-11T21:39:37Z | 12,076 | 7 | transformers | [
"transformers",
"pytorch",
"rag",
"arxiv:2005.11401",
"license:apache-2.0",
"endpoints_compatible",
"region:us"
]
| null | 2022-03-02T23:29:05Z | ---
license: apache-2.0
thumbnail: https://huggingface.co/front/thumbnails/facebook.png
---
## RAG
This is a non-finetuned version of the RAG-Sequence model of the the paper [Retrieval-Augmented Generation for Knowledge-Intensive NLP Tasks](https://arxiv.org/pdf/2005.11401.pdf)
by Patrick Lewis, Ethan Perez, Aleksandara Piktus et al.
Rag consits of a *question encoder*, *retriever* and a *generator*. The retriever should be a `RagRetriever` instance. The *question encoder* can be any model that can be loaded with `AutoModel` and the *generator* can be any model that can be loaded with `AutoModelForSeq2SeqLM`.
This model is a non-finetuned RAG-Sequence model and was created as follows:
```python
from transformers import RagTokenizer, RagRetriever, RagSequenceForGeneration, AutoTokenizer
model = RagSequenceForGeneration.from_pretrained_question_encoder_generator("facebook/dpr-question_encoder-single-nq-base", "facebook/bart-large")
question_encoder_tokenizer = AutoTokenizer.from_pretrained("facebook/dpr-question_encoder-single-nq-base")
generator_tokenizer = AutoTokenizer.from_pretrained("facebook/bart-large")
tokenizer = RagTokenizer(question_encoder_tokenizer, generator_tokenizer)
model.config.use_dummy_dataset = True
model.config.index_name = "exact"
retriever = RagRetriever(model.config, question_encoder_tokenizer, generator_tokenizer)
model.save_pretrained("./")
tokenizer.save_pretrained("./")
retriever.save_pretrained("./")
```
Note that the model is *uncased* so that all capital input letters are converted to lower-case.
## Usage:
*Note*: the model uses the *dummy* retriever as a default. Better results are obtained by using the full retriever,
by setting `config.index_name="legacy"` and `config.use_dummy_dataset=False`.
The model can be fine-tuned as follows:
```python
from transformers import RagTokenizer, RagRetriever, RagTokenForGeneration
tokenizer = RagTokenizer.from_pretrained("facebook/rag-sequence-base")
retriever = RagRetriever.from_pretrained("facebook/rag-sequence-base")
model = RagTokenForGeneration.from_pretrained("facebook/rag-sequence-base", retriever=retriever)
input_dict = tokenizer.prepare_seq2seq_batch("who holds the record in 100m freestyle", "michael phelps", return_tensors="pt")
outputs = model(input_dict["input_ids"], labels=input_dict["labels"])
loss = outputs.loss
# train on loss
```
|
hfl/chinese-roberta-wwm-ext-large | hfl | 2022-03-01T09:15:16Z | 12,070 | 166 | transformers | [
"transformers",
"pytorch",
"tf",
"jax",
"bert",
"fill-mask",
"zh",
"arxiv:1906.08101",
"arxiv:2004.13922",
"license:apache-2.0",
"autotrain_compatible",
"endpoints_compatible",
"region:us"
]
| fill-mask | 2022-03-02T23:29:05Z | ---
language:
- zh
tags:
- bert
license: "apache-2.0"
---
# Please use 'Bert' related functions to load this model!
## Chinese BERT with Whole Word Masking
For further accelerating Chinese natural language processing, we provide **Chinese pre-trained BERT with Whole Word Masking**.
**[Pre-Training with Whole Word Masking for Chinese BERT](https://arxiv.org/abs/1906.08101)**
Yiming Cui, Wanxiang Che, Ting Liu, Bing Qin, Ziqing Yang, Shijin Wang, Guoping Hu
This repository is developed based on:https://github.com/google-research/bert
You may also interested in,
- Chinese BERT series: https://github.com/ymcui/Chinese-BERT-wwm
- Chinese MacBERT: https://github.com/ymcui/MacBERT
- Chinese ELECTRA: https://github.com/ymcui/Chinese-ELECTRA
- Chinese XLNet: https://github.com/ymcui/Chinese-XLNet
- Knowledge Distillation Toolkit - TextBrewer: https://github.com/airaria/TextBrewer
More resources by HFL: https://github.com/ymcui/HFL-Anthology
## Citation
If you find the technical report or resource is useful, please cite the following technical report in your paper.
- Primary: https://arxiv.org/abs/2004.13922
```
@inproceedings{cui-etal-2020-revisiting,
title = "Revisiting Pre-Trained Models for {C}hinese Natural Language Processing",
author = "Cui, Yiming and
Che, Wanxiang and
Liu, Ting and
Qin, Bing and
Wang, Shijin and
Hu, Guoping",
booktitle = "Proceedings of the 2020 Conference on Empirical Methods in Natural Language Processing: Findings",
month = nov,
year = "2020",
address = "Online",
publisher = "Association for Computational Linguistics",
url = "https://www.aclweb.org/anthology/2020.findings-emnlp.58",
pages = "657--668",
}
```
- Secondary: https://arxiv.org/abs/1906.08101
```
@article{chinese-bert-wwm,
title={Pre-Training with Whole Word Masking for Chinese BERT},
author={Cui, Yiming and Che, Wanxiang and Liu, Ting and Qin, Bing and Yang, Ziqing and Wang, Shijin and Hu, Guoping},
journal={arXiv preprint arXiv:1906.08101},
year={2019}
}
``` |
tblard/tf-allocine | tblard | 2020-12-11T22:02:40Z | 12,049 | 9 | transformers | [
"transformers",
"tf",
"camembert",
"text-classification",
"fr",
"autotrain_compatible",
"endpoints_compatible",
"region:us"
]
| text-classification | 2022-03-02T23:29:05Z | ---
language: fr
---
# tf-allociné
A french sentiment analysis model, based on [CamemBERT](https://camembert-model.fr/), and finetuned on a large-scale dataset scraped from [Allociné.fr](http://www.allocine.fr/) user reviews.
## Results
| Validation Accuracy | Validation F1-Score | Test Accuracy | Test F1-Score |
|--------------------:| -------------------:| -------------:|--------------:|
| 97.39 | 97.36 | 97.44 | 97.34 |
The dataset and the evaluation code are available on [this repo](https://github.com/TheophileBlard/french-sentiment-analysis-with-bert).
## Usage
```python
from transformers import AutoTokenizer, TFAutoModelForSequenceClassification
from transformers import pipeline
tokenizer = AutoTokenizer.from_pretrained("tblard/tf-allocine")
model = TFAutoModelForSequenceClassification.from_pretrained("tblard/tf-allocine")
nlp = pipeline('sentiment-analysis', model=model, tokenizer=tokenizer)
print(nlp("Alad'2 est clairement le meilleur film de l'année 2018.")) # POSITIVE
print(nlp("Juste whoaaahouuu !")) # POSITIVE
print(nlp("NUL...A...CHIER ! FIN DE TRANSMISSION.")) # NEGATIVE
print(nlp("Je m'attendais à mieux de la part de Franck Dubosc !")) # NEGATIVE
```
## Author
Théophile Blard – :email: [email protected]
If you use this work (code, model or dataset), please cite as:
> Théophile Blard, French sentiment analysis with BERT, (2020), GitHub repository, <https://github.com/TheophileBlard/french-sentiment-analysis-with-bert>
|
PrunaAI/nvidia-Llama3-ChatQA-1.5-8B-GGUF-smashed | PrunaAI | 2024-06-28T17:59:46Z | 12,045 | 0 | null | [
"gguf",
"pruna-ai",
"region:us"
]
| null | 2024-05-04T16:32:35Z | ---
thumbnail: "https://assets-global.website-files.com/646b351987a8d8ce158d1940/64ec9e96b4334c0e1ac41504_Logo%20with%20white%20text.svg"
metrics:
- memory_disk
- memory_inference
- inference_latency
- inference_throughput
- inference_CO2_emissions
- inference_energy_consumption
tags:
- pruna-ai
---
<!-- header start -->
<!-- 200823 -->
<div style="width: auto; margin-left: auto; margin-right: auto">
<a href="https://www.pruna.ai/" target="_blank" rel="noopener noreferrer">
<img src="https://i.imgur.com/eDAlcgk.png" alt="PrunaAI" style="width: 100%; min-width: 400px; display: block; margin: auto;">
</a>
</div>
<!-- header end -->
[](https://twitter.com/PrunaAI)
[](https://github.com/PrunaAI)
[](https://www.linkedin.com/company/93832878/admin/feed/posts/?feedType=following)
[](https://discord.com/invite/vb6SmA3hxu)
## This repo contains GGUF versions of the nvidia/Llama3-ChatQA-1.5-8B model.
# Simply make AI models cheaper, smaller, faster, and greener!
- Give a thumbs up if you like this model!
- Contact us and tell us which model to compress next [here](https://www.pruna.ai/contact).
- Request access to easily compress your *own* AI models [here](https://z0halsaff74.typeform.com/pruna-access?typeform-source=www.pruna.ai).
- Read the documentations to know more [here](https://pruna-ai-pruna.readthedocs-hosted.com/en/latest/)
- Join Pruna AI community on Discord [here](https://discord.com/invite/vb6SmA3hxu) to share feedback/suggestions or get help.
**Frequently Asked Questions**
- ***How does the compression work?*** The model is compressed with GGUF.
- ***How does the model quality change?*** The quality of the model output might vary compared to the base model.
- ***What is the model format?*** We use GGUF format.
- ***What calibration data has been used?*** If needed by the compression method, we used WikiText as the calibration data.
- ***How to compress my own models?*** You can request premium access to more compression methods and tech support for your specific use-cases [here](https://z0halsaff74.typeform.com/pruna-access?typeform-source=www.pruna.ai).
# Downloading and running the models
You can download the individual files from the Files & versions section. Here is a list of the different versions we provide. For more info checkout [this chart](https://gist.github.com/Artefact2/b5f810600771265fc1e39442288e8ec9) and [this guide](https://www.reddit.com/r/LocalLLaMA/comments/1ba55rj/overview_of_gguf_quantization_methods/):
| Quant type | Description |
|------------|--------------------------------------------------------------------------------------------|
| Q5_K_M | High quality, recommended. |
| Q5_K_S | High quality, recommended. |
| Q4_K_M | Good quality, uses about 4.83 bits per weight, recommended. |
| Q4_K_S | Slightly lower quality with more space savings, recommended. |
| IQ4_NL | Decent quality, slightly smaller than Q4_K_S with similar performance, recommended. |
| IQ4_XS | Decent quality, smaller than Q4_K_S with similar performance, recommended. |
| Q3_K_L | Lower quality but usable, good for low RAM availability. |
| Q3_K_M | Even lower quality. |
| IQ3_M | Medium-low quality, new method with decent performance comparable to Q3_K_M. |
| IQ3_S | Lower quality, new method with decent performance, recommended over Q3_K_S quant, same size with better performance. |
| Q3_K_S | Low quality, not recommended. |
| IQ3_XS | Lower quality, new method with decent performance, slightly better than Q3_K_S. |
| Q2_K | Very low quality but surprisingly usable. |
## How to download GGUF files ?
**Note for manual downloaders:** You almost never want to clone the entire repo! Multiple different quantisation formats are provided, and most users only want to pick and download a single file.
The following clients/libraries will automatically download models for you, providing a list of available models to choose from:
* LM Studio
* LoLLMS Web UI
* Faraday.dev
- **Option A** - Downloading in `text-generation-webui`:
- **Step 1**: Under Download Model, you can enter the model repo: nvidia-Llama3-ChatQA-1.5-8B-GGUF-smashed and below it, a specific filename to download, such as: phi-2.IQ3_M.gguf.
- **Step 2**: Then click Download.
- **Option B** - Downloading on the command line (including multiple files at once):
- **Step 1**: We recommend using the `huggingface-hub` Python library:
```shell
pip3 install huggingface-hub
```
- **Step 2**: Then you can download any individual model file to the current directory, at high speed, with a command like this:
```shell
huggingface-cli download nvidia-Llama3-ChatQA-1.5-8B-GGUF-smashed Llama3-ChatQA-1.5-8B.IQ3_M.gguf --local-dir . --local-dir-use-symlinks False
```
<details>
<summary>More advanced huggingface-cli download usage (click to read)</summary>
Alternatively, you can also download multiple files at once with a pattern:
```shell
huggingface-cli download nvidia-Llama3-ChatQA-1.5-8B-GGUF-smashed --local-dir . --local-dir-use-symlinks False --include='*Q4_K*gguf'
```
For more documentation on downloading with `huggingface-cli`, please see: [HF -> Hub Python Library -> Download files -> Download from the CLI](https://huggingface.co/docs/huggingface_hub/guides/download#download-from-the-cli).
To accelerate downloads on fast connections (1Gbit/s or higher), install `hf_transfer`:
```shell
pip3 install hf_transfer
```
And set environment variable `HF_HUB_ENABLE_HF_TRANSFER` to `1`:
```shell
HF_HUB_ENABLE_HF_TRANSFER=1 huggingface-cli download nvidia-Llama3-ChatQA-1.5-8B-GGUF-smashed Llama3-ChatQA-1.5-8B.IQ3_M.gguf --local-dir . --local-dir-use-symlinks False
```
Windows Command Line users: You can set the environment variable by running `set HF_HUB_ENABLE_HF_TRANSFER=1` before the download command.
</details>
<!-- README_GGUF.md-how-to-download end -->
<!-- README_GGUF.md-how-to-run start -->
## How to run model in GGUF format?
- **Option A** - Introductory example with `llama.cpp` command
Make sure you are using `llama.cpp` from commit [d0cee0d](https://github.com/ggerganov/llama.cpp/commit/d0cee0d36d5be95a0d9088b674dbb27354107221) or later.
```shell
./main -ngl 35 -m Llama3-ChatQA-1.5-8B.IQ3_M.gguf --color -c 32768 --temp 0.7 --repeat_penalty 1.1 -n -1 -p "<s>[INST] {{prompt\}} [/INST]"
```
Change `-ngl 32` to the number of layers to offload to GPU. Remove it if you don't have GPU acceleration.
Change `-c 32768` to the desired sequence length. For extended sequence models - eg 8K, 16K, 32K - the necessary RoPE scaling parameters are read from the GGUF file and set by llama.cpp automatically. Note that longer sequence lengths require much more resources, so you may need to reduce this value.
If you want to have a chat-style conversation, replace the `-p <PROMPT>` argument with `-i -ins`
For other parameters and how to use them, please refer to [the llama.cpp documentation](https://github.com/ggerganov/llama.cpp/blob/master/examples/main/README.md)
- **Option B** - Running in `text-generation-webui`
Further instructions can be found in the text-generation-webui documentation, here: [text-generation-webui/docs/04 ‐ Model Tab.md](https://github.com/oobabooga/text-generation-webui/blob/main/docs/04%20-%20Model%20Tab.md#llamacpp).
- **Option C** - Running from Python code
You can use GGUF models from Python using the [llama-cpp-python](https://github.com/abetlen/llama-cpp-python) or [ctransformers](https://github.com/marella/ctransformers) libraries. Note that at the time of writing (Nov 27th 2023), ctransformers has not been updated for some time and is not compatible with some recent models. Therefore I recommend you use llama-cpp-python.
### How to load this model in Python code, using llama-cpp-python
For full documentation, please see: [llama-cpp-python docs](https://abetlen.github.io/llama-cpp-python/).
#### First install the package
Run one of the following commands, according to your system:
```shell
# Base ctransformers with no GPU acceleration
pip install llama-cpp-python
# With NVidia CUDA acceleration
CMAKE_ARGS="-DLLAMA_CUBLAS=on" pip install llama-cpp-python
# Or with OpenBLAS acceleration
CMAKE_ARGS="-DLLAMA_BLAS=ON -DLLAMA_BLAS_VENDOR=OpenBLAS" pip install llama-cpp-python
# Or with CLBLast acceleration
CMAKE_ARGS="-DLLAMA_CLBLAST=on" pip install llama-cpp-python
# Or with AMD ROCm GPU acceleration (Linux only)
CMAKE_ARGS="-DLLAMA_HIPBLAS=on" pip install llama-cpp-python
# Or with Metal GPU acceleration for macOS systems only
CMAKE_ARGS="-DLLAMA_METAL=on" pip install llama-cpp-python
# In windows, to set the variables CMAKE_ARGS in PowerShell, follow this format; eg for NVidia CUDA:
$env:CMAKE_ARGS = "-DLLAMA_OPENBLAS=on"
pip install llama-cpp-python
```
#### Simple llama-cpp-python example code
```python
from llama_cpp import Llama
# Set gpu_layers to the number of layers to offload to GPU. Set to 0 if no GPU acceleration is available on your system.
llm = Llama(
model_path="./Llama3-ChatQA-1.5-8B.IQ3_M.gguf", # Download the model file first
n_ctx=32768, # The max sequence length to use - note that longer sequence lengths require much more resources
n_threads=8, # The number of CPU threads to use, tailor to your system and the resulting performance
n_gpu_layers=35 # The number of layers to offload to GPU, if you have GPU acceleration available
)
# Simple inference example
output = llm(
"<s>[INST] {{prompt}} [/INST]", # Prompt
max_tokens=512, # Generate up to 512 tokens
stop=["</s>"], # Example stop token - not necessarily correct for this specific model! Please check before using.
echo=True # Whether to echo the prompt
)
# Chat Completion API
llm = Llama(model_path="./Llama3-ChatQA-1.5-8B.IQ3_M.gguf", chat_format="llama-2") # Set chat_format according to the model you are using
llm.create_chat_completion(
messages = [
{{"role": "system", "content": "You are a story writing assistant."}},
{{
"role": "user",
"content": "Write a story about llamas."
}}
]
)
```
- **Option D** - Running with LangChain
Here are guides on using llama-cpp-python and ctransformers with LangChain:
* [LangChain + llama-cpp-python](https://python.langchain.com/docs/integrations/llms/llamacpp)
* [LangChain + ctransformers](https://python.langchain.com/docs/integrations/providers/ctransformers)
## Configurations
The configuration info are in `smash_config.json`.
## Credits & License
The license of the smashed model follows the license of the original model. Please check the license of the original model before using this model which provided the base model. The license of the `pruna-engine` is [here](https://pypi.org/project/pruna-engine/) on Pypi.
## Want to compress other models?
- Contact us and tell us which model to compress next [here](https://www.pruna.ai/contact).
- Request access to easily compress your own AI models [here](https://z0halsaff74.typeform.com/pruna-access?typeform-source=www.pruna.ai). |
Xenova/llama2.c-stories15M | Xenova | 2024-03-16T22:26:22Z | 12,041 | 6 | transformers.js | [
"transformers.js",
"pytorch",
"onnx",
"llama",
"text-generation",
"transformers",
"region:us"
]
| text-generation | 2023-08-03T11:34:33Z | ---
library_name: transformers.js
tags:
- transformers
---
## Usage (Transformers.js)
If you haven't already, you can install the [Transformers.js](https://huggingface.co/docs/transformers.js) JavaScript library from [NPM](https://www.npmjs.com/package/@xenova/transformers) using:
```bash
npm i @xenova/transformers
```
You can then use the model to generate text like this:
```js
import { pipeline } from "@xenova/transformers";
// Create a text-generation pipeline
const generator = await pipeline('text-generation', 'Xenova/llama2.c-stories15M');
const text = 'Once upon a time,';
const output = await generator(text);
console.log(output);
// [{ generated_text: "Once upon a time, there was a little girl named Lily. She loved to play outside in" }]
const output2 = await generator(text, { max_new_tokens: 50 });
console.log(output2);
// [{ generated_text: "Once upon a time, there was a little girl named Lily. She loved to play outside in the sunshine. One day, she saw a big, dark cloud in the sky. She knew it was going to rain soon.\nLily ran inside her house" }]
```
|
RichardErkhov/fhai50032_-_BeagleLake-7B-gguf | RichardErkhov | 2024-06-20T05:38:28Z | 12,041 | 0 | null | [
"gguf",
"region:us"
]
| null | 2024-06-20T00:29:28Z | Quantization made by Richard Erkhov.
[Github](https://github.com/RichardErkhov)
[Discord](https://discord.gg/pvy7H8DZMG)
[Request more models](https://github.com/RichardErkhov/quant_request)
BeagleLake-7B - GGUF
- Model creator: https://huggingface.co/fhai50032/
- Original model: https://huggingface.co/fhai50032/BeagleLake-7B/
| Name | Quant method | Size |
| ---- | ---- | ---- |
| [BeagleLake-7B.Q2_K.gguf](https://huggingface.co/RichardErkhov/fhai50032_-_BeagleLake-7B-gguf/blob/main/BeagleLake-7B.Q2_K.gguf) | Q2_K | 2.53GB |
| [BeagleLake-7B.IQ3_XS.gguf](https://huggingface.co/RichardErkhov/fhai50032_-_BeagleLake-7B-gguf/blob/main/BeagleLake-7B.IQ3_XS.gguf) | IQ3_XS | 2.81GB |
| [BeagleLake-7B.IQ3_S.gguf](https://huggingface.co/RichardErkhov/fhai50032_-_BeagleLake-7B-gguf/blob/main/BeagleLake-7B.IQ3_S.gguf) | IQ3_S | 2.96GB |
| [BeagleLake-7B.Q3_K_S.gguf](https://huggingface.co/RichardErkhov/fhai50032_-_BeagleLake-7B-gguf/blob/main/BeagleLake-7B.Q3_K_S.gguf) | Q3_K_S | 2.95GB |
| [BeagleLake-7B.IQ3_M.gguf](https://huggingface.co/RichardErkhov/fhai50032_-_BeagleLake-7B-gguf/blob/main/BeagleLake-7B.IQ3_M.gguf) | IQ3_M | 3.06GB |
| [BeagleLake-7B.Q3_K.gguf](https://huggingface.co/RichardErkhov/fhai50032_-_BeagleLake-7B-gguf/blob/main/BeagleLake-7B.Q3_K.gguf) | Q3_K | 3.28GB |
| [BeagleLake-7B.Q3_K_M.gguf](https://huggingface.co/RichardErkhov/fhai50032_-_BeagleLake-7B-gguf/blob/main/BeagleLake-7B.Q3_K_M.gguf) | Q3_K_M | 3.28GB |
| [BeagleLake-7B.Q3_K_L.gguf](https://huggingface.co/RichardErkhov/fhai50032_-_BeagleLake-7B-gguf/blob/main/BeagleLake-7B.Q3_K_L.gguf) | Q3_K_L | 3.56GB |
| [BeagleLake-7B.IQ4_XS.gguf](https://huggingface.co/RichardErkhov/fhai50032_-_BeagleLake-7B-gguf/blob/main/BeagleLake-7B.IQ4_XS.gguf) | IQ4_XS | 3.67GB |
| [BeagleLake-7B.Q4_0.gguf](https://huggingface.co/RichardErkhov/fhai50032_-_BeagleLake-7B-gguf/blob/main/BeagleLake-7B.Q4_0.gguf) | Q4_0 | 3.83GB |
| [BeagleLake-7B.IQ4_NL.gguf](https://huggingface.co/RichardErkhov/fhai50032_-_BeagleLake-7B-gguf/blob/main/BeagleLake-7B.IQ4_NL.gguf) | IQ4_NL | 3.87GB |
| [BeagleLake-7B.Q4_K_S.gguf](https://huggingface.co/RichardErkhov/fhai50032_-_BeagleLake-7B-gguf/blob/main/BeagleLake-7B.Q4_K_S.gguf) | Q4_K_S | 3.86GB |
| [BeagleLake-7B.Q4_K.gguf](https://huggingface.co/RichardErkhov/fhai50032_-_BeagleLake-7B-gguf/blob/main/BeagleLake-7B.Q4_K.gguf) | Q4_K | 4.07GB |
| [BeagleLake-7B.Q4_K_M.gguf](https://huggingface.co/RichardErkhov/fhai50032_-_BeagleLake-7B-gguf/blob/main/BeagleLake-7B.Q4_K_M.gguf) | Q4_K_M | 4.07GB |
| [BeagleLake-7B.Q4_1.gguf](https://huggingface.co/RichardErkhov/fhai50032_-_BeagleLake-7B-gguf/blob/main/BeagleLake-7B.Q4_1.gguf) | Q4_1 | 4.24GB |
| [BeagleLake-7B.Q5_0.gguf](https://huggingface.co/RichardErkhov/fhai50032_-_BeagleLake-7B-gguf/blob/main/BeagleLake-7B.Q5_0.gguf) | Q5_0 | 4.65GB |
| [BeagleLake-7B.Q5_K_S.gguf](https://huggingface.co/RichardErkhov/fhai50032_-_BeagleLake-7B-gguf/blob/main/BeagleLake-7B.Q5_K_S.gguf) | Q5_K_S | 4.65GB |
| [BeagleLake-7B.Q5_K.gguf](https://huggingface.co/RichardErkhov/fhai50032_-_BeagleLake-7B-gguf/blob/main/BeagleLake-7B.Q5_K.gguf) | Q5_K | 4.78GB |
| [BeagleLake-7B.Q5_K_M.gguf](https://huggingface.co/RichardErkhov/fhai50032_-_BeagleLake-7B-gguf/blob/main/BeagleLake-7B.Q5_K_M.gguf) | Q5_K_M | 4.78GB |
| [BeagleLake-7B.Q5_1.gguf](https://huggingface.co/RichardErkhov/fhai50032_-_BeagleLake-7B-gguf/blob/main/BeagleLake-7B.Q5_1.gguf) | Q5_1 | 5.07GB |
| [BeagleLake-7B.Q6_K.gguf](https://huggingface.co/RichardErkhov/fhai50032_-_BeagleLake-7B-gguf/blob/main/BeagleLake-7B.Q6_K.gguf) | Q6_K | 5.53GB |
| [BeagleLake-7B.Q8_0.gguf](https://huggingface.co/RichardErkhov/fhai50032_-_BeagleLake-7B-gguf/blob/main/BeagleLake-7B.Q8_0.gguf) | Q8_0 | 7.17GB |
Original model description:
---
license: apache-2.0
tags:
- merge
- mergekit
- mistral
- fhai50032/RolePlayLake-7B
- mlabonne/NeuralBeagle14-7B
base_model:
- fhai50032/RolePlayLake-7B
- mlabonne/NeuralBeagle14-7B
model-index:
- name: BeagleLake-7B
results:
- task:
type: text-generation
name: Text Generation
dataset:
name: AI2 Reasoning Challenge (25-Shot)
type: ai2_arc
config: ARC-Challenge
split: test
args:
num_few_shot: 25
metrics:
- type: acc_norm
value: 70.39
name: normalized accuracy
source:
url: https://huggingface.co/spaces/HuggingFaceH4/open_llm_leaderboard?query=fhai50032/BeagleLake-7B
name: Open LLM Leaderboard
- task:
type: text-generation
name: Text Generation
dataset:
name: HellaSwag (10-Shot)
type: hellaswag
split: validation
args:
num_few_shot: 10
metrics:
- type: acc_norm
value: 87.38
name: normalized accuracy
source:
url: https://huggingface.co/spaces/HuggingFaceH4/open_llm_leaderboard?query=fhai50032/BeagleLake-7B
name: Open LLM Leaderboard
- task:
type: text-generation
name: Text Generation
dataset:
name: MMLU (5-Shot)
type: cais/mmlu
config: all
split: test
args:
num_few_shot: 5
metrics:
- type: acc
value: 64.25
name: accuracy
source:
url: https://huggingface.co/spaces/HuggingFaceH4/open_llm_leaderboard?query=fhai50032/BeagleLake-7B
name: Open LLM Leaderboard
- task:
type: text-generation
name: Text Generation
dataset:
name: TruthfulQA (0-shot)
type: truthful_qa
config: multiple_choice
split: validation
args:
num_few_shot: 0
metrics:
- type: mc2
value: 64.92
source:
url: https://huggingface.co/spaces/HuggingFaceH4/open_llm_leaderboard?query=fhai50032/BeagleLake-7B
name: Open LLM Leaderboard
- task:
type: text-generation
name: Text Generation
dataset:
name: Winogrande (5-shot)
type: winogrande
config: winogrande_xl
split: validation
args:
num_few_shot: 5
metrics:
- type: acc
value: 83.19
name: accuracy
source:
url: https://huggingface.co/spaces/HuggingFaceH4/open_llm_leaderboard?query=fhai50032/BeagleLake-7B
name: Open LLM Leaderboard
- task:
type: text-generation
name: Text Generation
dataset:
name: GSM8k (5-shot)
type: gsm8k
config: main
split: test
args:
num_few_shot: 5
metrics:
- type: acc
value: 63.91
name: accuracy
source:
url: https://huggingface.co/spaces/HuggingFaceH4/open_llm_leaderboard?query=fhai50032/BeagleLake-7B
name: Open LLM Leaderboard
---
# BeagleLake-7B
BeagleLake-7B is a merge of the following models :
* [fhai50032/RolePlayLake-7B](https://huggingface.co/fhai50032/RolePlayLake-7B)
* [mlabonne/NeuralBeagle14-7B](https://huggingface.co/mlabonne/NeuralBeagle14-7B)
Merging models are not powerful but are helpful in the case that it can work like Transfer Learning similar idk.. But they perform high on Leaderboard
For ex. NeuralBeagle is powerful model with lot of potential to grow and RolePlayLake is Suitable for RP (No-Simping) and is significantly uncensored and nice obligations
Fine-tuning a Merged model as a base model is surely a way to look forward and see a lot of potential going forward..
Much thanks to [Charles Goddard](https://huggingface.co/chargoddard) for making simple interface ['mergekit' ](https://github.com/cg123/mergekit)
## 🧩 Configuration
```yaml
models:
- model: mlabonne/NeuralBeagle14-7B
# no params for base model
- model: fhai50032/RolePlayLake-7B
parameters:
weight: 0.8
density: 0.6
- model: mlabonne/NeuralBeagle14-7B
parameters:
weight: 0.3
density: [0.1,0.3,0.5,0.7,1]
merge_method: dare_ties
base_model: mlabonne/NeuralBeagle14-7B
parameters:
normalize: true
int8_mask: true
dtype: float16
```
## 💻 Usage
```python
!pip install -qU transformers accelerate
from transformers import AutoTokenizer
import transformers
import torch
model = "fhai50032/BeagleLake-7B"
messages = [{"role": "user", "content": "What is a large language model?"}]
tokenizer = AutoTokenizer.from_pretrained(model)
prompt = tokenizer.apply_chat_template(messages, tokenize=False, add_generation_prompt=True)
pipeline = transformers.pipeline(
"text-generation",
model=model,
torch_dtype=torch.float16,
device_map="auto",
)
outputs = pipeline(prompt, max_new_tokens=256, do_sample=True, temperature=0.7, top_k=50, top_p=0.95)
print(outputs[0]["generated_text"])
```
# [Open LLM Leaderboard Evaluation Results](https://huggingface.co/spaces/HuggingFaceH4/open_llm_leaderboard)
Detailed results can be found [here](https://huggingface.co/datasets/open-llm-leaderboard/details_fhai50032__BeagleLake-7B)
| Metric |Value|
|---------------------------------|----:|
|Avg. |72.34|
|AI2 Reasoning Challenge (25-Shot)|70.39|
|HellaSwag (10-Shot) |87.38|
|MMLU (5-Shot) |64.25|
|TruthfulQA (0-shot) |64.92|
|Winogrande (5-shot) |83.19|
|GSM8k (5-shot) |63.91|
|
mradermacher/trithemius-mistral-0.3-7b-GGUF | mradermacher | 2024-06-27T03:08:56Z | 12,031 | 0 | transformers | [
"transformers",
"gguf",
"json mode",
"function calling",
"chatml",
"mistral",
"digital alchemy",
"en",
"base_model:jmars/trithemius-mistral-0.3-7b",
"license:apache-2.0",
"endpoints_compatible",
"region:us"
]
| null | 2024-06-27T02:43:15Z | ---
base_model: jmars/trithemius-mistral-0.3-7b
language:
- en
library_name: transformers
license: apache-2.0
quantized_by: mradermacher
tags:
- json mode
- function calling
- chatml
- mistral
- digital alchemy
---
## About
<!-- ### quantize_version: 2 -->
<!-- ### output_tensor_quantised: 1 -->
<!-- ### convert_type: hf -->
<!-- ### vocab_type: -->
<!-- ### tags: -->
static quants of https://huggingface.co/jmars/trithemius-mistral-0.3-7b
<!-- provided-files -->
weighted/imatrix quants are available at https://huggingface.co/mradermacher/trithemius-mistral-0.3-7b-i1-GGUF
## Usage
If you are unsure how to use GGUF files, refer to one of [TheBloke's
READMEs](https://huggingface.co/TheBloke/KafkaLM-70B-German-V0.1-GGUF) for
more details, including on how to concatenate multi-part files.
## Provided Quants
(sorted by size, not necessarily quality. IQ-quants are often preferable over similar sized non-IQ quants)
| Link | Type | Size/GB | Notes |
|:-----|:-----|--------:|:------|
| [GGUF](https://huggingface.co/mradermacher/trithemius-mistral-0.3-7b-GGUF/resolve/main/trithemius-mistral-0.3-7b.Q2_K.gguf) | Q2_K | 2.8 | |
| [GGUF](https://huggingface.co/mradermacher/trithemius-mistral-0.3-7b-GGUF/resolve/main/trithemius-mistral-0.3-7b.IQ3_XS.gguf) | IQ3_XS | 3.1 | |
| [GGUF](https://huggingface.co/mradermacher/trithemius-mistral-0.3-7b-GGUF/resolve/main/trithemius-mistral-0.3-7b.Q3_K_S.gguf) | Q3_K_S | 3.3 | |
| [GGUF](https://huggingface.co/mradermacher/trithemius-mistral-0.3-7b-GGUF/resolve/main/trithemius-mistral-0.3-7b.IQ3_S.gguf) | IQ3_S | 3.3 | beats Q3_K* |
| [GGUF](https://huggingface.co/mradermacher/trithemius-mistral-0.3-7b-GGUF/resolve/main/trithemius-mistral-0.3-7b.IQ3_M.gguf) | IQ3_M | 3.4 | |
| [GGUF](https://huggingface.co/mradermacher/trithemius-mistral-0.3-7b-GGUF/resolve/main/trithemius-mistral-0.3-7b.Q3_K_M.gguf) | Q3_K_M | 3.6 | lower quality |
| [GGUF](https://huggingface.co/mradermacher/trithemius-mistral-0.3-7b-GGUF/resolve/main/trithemius-mistral-0.3-7b.Q3_K_L.gguf) | Q3_K_L | 3.9 | |
| [GGUF](https://huggingface.co/mradermacher/trithemius-mistral-0.3-7b-GGUF/resolve/main/trithemius-mistral-0.3-7b.IQ4_XS.gguf) | IQ4_XS | 4.0 | |
| [GGUF](https://huggingface.co/mradermacher/trithemius-mistral-0.3-7b-GGUF/resolve/main/trithemius-mistral-0.3-7b.Q4_K_S.gguf) | Q4_K_S | 4.2 | fast, recommended |
| [GGUF](https://huggingface.co/mradermacher/trithemius-mistral-0.3-7b-GGUF/resolve/main/trithemius-mistral-0.3-7b.Q4_K_M.gguf) | Q4_K_M | 4.5 | fast, recommended |
| [GGUF](https://huggingface.co/mradermacher/trithemius-mistral-0.3-7b-GGUF/resolve/main/trithemius-mistral-0.3-7b.Q5_K_S.gguf) | Q5_K_S | 5.1 | |
| [GGUF](https://huggingface.co/mradermacher/trithemius-mistral-0.3-7b-GGUF/resolve/main/trithemius-mistral-0.3-7b.Q5_K_M.gguf) | Q5_K_M | 5.2 | |
| [GGUF](https://huggingface.co/mradermacher/trithemius-mistral-0.3-7b-GGUF/resolve/main/trithemius-mistral-0.3-7b.Q6_K.gguf) | Q6_K | 6.0 | very good quality |
| [GGUF](https://huggingface.co/mradermacher/trithemius-mistral-0.3-7b-GGUF/resolve/main/trithemius-mistral-0.3-7b.Q8_0.gguf) | Q8_0 | 7.8 | fast, best quality |
| [GGUF](https://huggingface.co/mradermacher/trithemius-mistral-0.3-7b-GGUF/resolve/main/trithemius-mistral-0.3-7b.f16.gguf) | f16 | 14.6 | 16 bpw, overkill |
Here is a handy graph by ikawrakow comparing some lower-quality quant
types (lower is better):

And here are Artefact2's thoughts on the matter:
https://gist.github.com/Artefact2/b5f810600771265fc1e39442288e8ec9
## FAQ / Model Request
See https://huggingface.co/mradermacher/model_requests for some answers to
questions you might have and/or if you want some other model quantized.
## Thanks
I thank my company, [nethype GmbH](https://www.nethype.de/), for letting
me use its servers and providing upgrades to my workstation to enable
this work in my free time.
<!-- end -->
|
xtuner/llava-phi-3-mini-gguf | xtuner | 2024-04-29T09:04:44Z | 12,028 | 95 | null | [
"gguf",
"image-to-text",
"dataset:Lin-Chen/ShareGPT4V",
"region:us"
]
| image-to-text | 2024-04-25T12:25:50Z | ---
datasets:
- Lin-Chen/ShareGPT4V
pipeline_tag: image-to-text
---
<div align="center">
<img src="https://github.com/InternLM/lmdeploy/assets/36994684/0cf8d00f-e86b-40ba-9b54-dc8f1bc6c8d8" width="600"/>
[](https://github.com/InternLM/xtuner)
</div>
## Model
llava-phi-3-mini is a LLaVA model fine-tuned from [microsoft/Phi-3-mini-4k-instruct](https://huggingface.co/microsoft/Phi-3-mini-4k-instruct) and [CLIP-ViT-Large-patch14-336](https://huggingface.co/openai/clip-vit-large-patch14-336) with [ShareGPT4V-PT](https://huggingface.co/datasets/Lin-Chen/ShareGPT4V) and [InternVL-SFT](https://github.com/OpenGVLab/InternVL/tree/main/internvl_chat#prepare-training-datasets) by [XTuner](https://github.com/InternLM/xtuner).
**Note: This model is in GGUF format.**
Resources:
- GitHub: [xtuner](https://github.com/InternLM/xtuner)
- Official LLaVA format model: [xtuner/llava-phi-3-mini](https://huggingface.co/xtuner/llava-phi-3-mini)
- HuggingFace LLaVA format model: [xtuner/llava-phi-3-mini-hf](https://huggingface.co/xtuner/llava-phi-3-mini-hf)
- XTuner LLaVA format model: [xtuner/llava-phi-3-mini-xtuner](https://huggingface.co/xtuner/llava-phi-3-mini-xtuner)
## Details
| Model | Visual Encoder | Projector | Resolution | Pretraining Strategy | Fine-tuning Strategy | Pretrain Dataset | Fine-tune Dataset | Pretrain Epoch | Fine-tune Epoch |
| :-------------------- | ------------------: | --------: | ---------: | ---------------------: | ------------------------: | ------------------------: | -----------------------: | -------------- | --------------- |
| LLaVA-v1.5-7B | CLIP-L | MLP | 336 | Frozen LLM, Frozen ViT | Full LLM, Frozen ViT | LLaVA-PT (558K) | LLaVA-Mix (665K) | 1 | 1 |
| LLaVA-Llama-3-8B | CLIP-L | MLP | 336 | Frozen LLM, Frozen ViT | Full LLM, LoRA ViT | LLaVA-PT (558K) | LLaVA-Mix (665K) | 1 | 1 |
| LLaVA-Llama-3-8B-v1.1 | CLIP-L | MLP | 336 | Frozen LLM, Frozen ViT | Full LLM, LoRA ViT | ShareGPT4V-PT (1246K) | InternVL-SFT (1268K) | 1 | 1 |
| **LLaVA-Phi-3-mini** | CLIP-L | MLP | 336 | Frozen LLM, Frozen ViT | Full LLM, Full ViT | ShareGPT4V-PT (1246K) | InternVL-SFT (1268K) | 1 | 2 |
## Results
<div align="center">
<img src="https://github.com/InternLM/xtuner/assets/36994684/78524f65-260d-4ae3-a687-03fc5a19dcbb" alt="Image" width=500" />
</div>
| Model | MMBench Test (EN) | MMMU Val | SEED-IMG | AI2D Test | ScienceQA Test | HallusionBench aAcc | POPE | GQA | TextVQA | MME | MMStar |
| :-------------------- | :---------------: | :-------: | :------: | :-------: | :------------: | :-----------------: | :--: | :--: | :-----: | :------: | :----: |
| LLaVA-v1.5-7B | 66.5 | 35.3 | 60.5 | 54.8 | 70.4 | 44.9 | 85.9 | 62.0 | 58.2 | 1511/348 | 30.3 |
| LLaVA-Llama-3-8B | 68.9 | 36.8 | 69.8 | 60.9 | 73.3 | 47.3 | 87.2 | 63.5 | 58.0 | 1506/295 | 38.2 |
| LLaVA-Llama-3-8B-v1.1 | 72.3 | 37.1 | 70.1 | 70.0 | 72.9 | 47.7 | 86.4 | 62.6 | 59.0 | 1469/349 | 45.1 |
| **LLaVA-Phi-3-mini** | 69.2 | 41.4 | 70.0 | 69.3 | 73.7 | 49.8 | 87.3 | 61.5 | 57.8 | 1477/313 | 43.7 |
## Quickstart
### Download models
```bash
# mmproj
wget https://huggingface.co/xtuner/llava-phi-3-mini-gguf/resolve/main/llava-phi-3-mini-mmproj-f16.gguf
# fp16 llm
wget https://huggingface.co/xtuner/llava-phi-3-mini-gguf/resolve/main/llava-phi-3-mini-f16.gguf
# int4 llm
wget https://huggingface.co/xtuner/llava-phi-3-mini-gguf/resolve/main/llava-phi-3-mini-int4.gguf
# (optional) ollama fp16 modelfile
wget https://huggingface.co/xtuner/llava-phi-3-mini-gguf/resolve/main/OLLAMA_MODELFILE_F16
# (optional) ollama int4 modelfile
wget https://huggingface.co/xtuner/llava-phi-3-mini-gguf/resolve/main/OLLAMA_MODELFILE_INT4
```
### Chat by `ollama`
Note: llava-phi-3-mini uses the `Phi-3-instruct` chat template.
```bash
# fp16
ollama create llava-phi3-f16 -f ./OLLAMA_MODELFILE_F16
ollama run llava-phi3-f16 "xx.png Describe this image"
# int4
ollama create llava-phi3-int4 -f ./OLLAMA_MODELFILE_INT4
ollama run llava-phi3-int4 "xx.png Describe this image"
```
### Chat by `./llava-cli`
1. Build [llama.cpp](https://github.com/ggerganov/llama.cpp) ([docs](https://github.com/ggerganov/llama.cpp?tab=readme-ov-file#usage)) .
2. Build `./llava-cli` ([docs](https://github.com/ggerganov/llama.cpp/tree/master/examples/llava#usage)).
Note: llava-phi-3-mini uses the `Phi-3-instruct` chat template.
```bash
# fp16
./llava-cli -m ./llava-phi-3-mini-f16.gguf --mmproj ./llava-phi-3-mini-mmproj-f16.gguf --image YOUR_IMAGE.jpg -c 4096
# int4
./llava-cli -m ./llava-phi-3-mini-int4.gguf --mmproj ./llava-phi-3-mini-mmproj-f16.gguf --image YOUR_IMAGE.jpg -c 4096
```
### Reproduce
Please refer to [docs](https://github.com/InternLM/xtuner/tree/main/xtuner/configs/llava/phi3_mini_4k_instruct_clip_vit_large_p14_336#readme).
## Citation
```bibtex
@misc{2023xtuner,
title={XTuner: A Toolkit for Efficiently Fine-tuning LLM},
author={XTuner Contributors},
howpublished = {\url{https://github.com/InternLM/xtuner}},
year={2023}
}
```
|
vilsonrodrigues/falcon-7b-instruct-sharded | vilsonrodrigues | 2023-07-13T12:22:04Z | 12,019 | 26 | transformers | [
"transformers",
"safetensors",
"falcon",
"text-generation",
"custom_code",
"en",
"dataset:tiiuae/falcon-refinedweb",
"arxiv:2205.14135",
"arxiv:1911.02150",
"arxiv:2005.14165",
"arxiv:2104.09864",
"arxiv:2306.01116",
"license:apache-2.0",
"autotrain_compatible",
"endpoints_compatible",
"text-generation-inference",
"region:us"
]
| text-generation | 2023-06-09T01:06:04Z | ---
datasets:
- tiiuae/falcon-refinedweb
language:
- en
inference: true
widget:
- text: "Hey Falcon! Any recommendations for my holidays in Abu Dhabi?"
example_title: "Abu Dhabi Trip"
- text: "What's the Everett interpretation of quantum mechanics?"
example_title: "Q/A: Quantum & Answers"
- text: "Give me a list of the top 10 dive sites you would recommend around the world."
example_title: "Diving Top 10"
- text: "Can you tell me more about deep-water soloing?"
example_title: "Extreme sports"
- text: "Can you write a short tweet about the Apache 2.0 release of our latest AI model, Falcon LLM?"
example_title: "Twitter Helper"
- text: "What are the responsabilities of a Chief Llama Officer?"
example_title: "Trendy Jobs"
license: apache-2.0
---
# Resharded
Resharded version of https://huggingface.co/tiiuae/falcon-7b-instruct for low RAM enviroments (e.g. Colab, Kaggle) in safetensors
Tutorial: https://medium.com/@vilsonrodrigues/run-your-private-llm-falcon-7b-instruct-with-less-than-6gb-of-gpu-using-4-bit-quantization-ff1d4ffbabcc
---
# ✨ Falcon-7B-Instruct
**Falcon-7B-Instruct is a 7B parameters causal decoder-only model built by [TII](https://www.tii.ae) based on [Falcon-7B](https://huggingface.co/tiiuae/falcon-7b) and finetuned on a mixture of chat/instruct datasets. It is made available under the Apache 2.0 license.**
*Paper coming soon 😊.*
🤗 To get started with Falcon (inference, finetuning, quantization, etc.), we recommend reading [this great blogpost fron HF](https://huggingface.co/blog/falcon)!
## Why use Falcon-7B-Instruct?
* **You are looking for a ready-to-use chat/instruct model based on [Falcon-7B](https://huggingface.co/tiiuae/falcon-7b).**
* **Falcon-7B is a strong base model, outperforming comparable open-source models** (e.g., [MPT-7B](https://huggingface.co/mosaicml/mpt-7b), [StableLM](https://github.com/Stability-AI/StableLM), [RedPajama](https://huggingface.co/togethercomputer/RedPajama-INCITE-Base-7B-v0.1) etc.), thanks to being trained on 1,500B tokens of [RefinedWeb](https://huggingface.co/datasets/tiiuae/falcon-refinedweb) enhanced with curated corpora. See the [OpenLLM Leaderboard](https://huggingface.co/spaces/HuggingFaceH4/open_llm_leaderboard).
* **It features an architecture optimized for inference**, with FlashAttention ([Dao et al., 2022](https://arxiv.org/abs/2205.14135)) and multiquery ([Shazeer et al., 2019](https://arxiv.org/abs/1911.02150)).
⚠️ Falcon is now available as a core model in the `transformers` library! To use the in-library version, please install the latest version of `transformers` with `pip install git+https://github.com/ huggingface/transformers.git`, then simply remove the `trust_remote_code=True` argument from `from_pretrained()`.
💬 **This is an instruct model, which may not be ideal for further finetuning.** If you are interested in building your own instruct/chat model, we recommend starting from [Falcon-7B](https://huggingface.co/tiiuae/falcon-7b).
🔥 **Looking for an even more powerful model?** [Falcon-40B-Instruct](https://huggingface.co/tiiuae/falcon-40b-instruct) is Falcon-7B-Instruct's big brother!
```python
from transformers import AutoTokenizer, AutoModelForCausalLM
import transformers
import torch
model = "tiiuae/falcon-7b-instruct"
tokenizer = AutoTokenizer.from_pretrained(model)
pipeline = transformers.pipeline(
"text-generation",
model=model,
tokenizer=tokenizer,
torch_dtype=torch.bfloat16,
device_map="auto",
)
sequences = pipeline(
"Girafatron is obsessed with giraffes, the most glorious animal on the face of this Earth. Giraftron believes all other animals are irrelevant when compared to the glorious majesty of the giraffe.\nDaniel: Hello, Girafatron!\nGirafatron:",
max_length=200,
do_sample=True,
top_k=10,
num_return_sequences=1,
eos_token_id=tokenizer.eos_token_id,
)
for seq in sequences:
print(f"Result: {seq['generated_text']}")
```
💥 **Falcon LLMs require PyTorch 2.0 for use with `transformers`!**
For fast inference with Falcon, check-out [Text Generation Inference](https://github.com/huggingface/text-generation-inference)! Read more in this [blogpost]((https://huggingface.co/blog/falcon).
You will need **at least 16GB of memory** to swiftly run inference with Falcon-7B-Instruct.
# Model Card for Falcon-7B-Instruct
## Model Details
### Model Description
- **Developed by:** [https://www.tii.ae](https://www.tii.ae);
- **Model type:** Causal decoder-only;
- **Language(s) (NLP):** English and French;
- **License:** Apache 2.0;
- **Finetuned from model:** [Falcon-7B](https://huggingface.co/tiiuae/falcon-7b).
### Model Source
- **Paper:** *coming soon*.
## Uses
### Direct Use
Falcon-7B-Instruct has been finetuned on a mixture of instruct and chat datasets.
### Out-of-Scope Use
Production use without adequate assessment of risks and mitigation; any use cases which may be considered irresponsible or harmful.
## Bias, Risks, and Limitations
Falcon-7B-Instruct is mostly trained on English data, and will not generalize appropriately to other languages. Furthermore, as it is trained on a large-scale corpora representative of the web, it will carry the stereotypes and biases commonly encountered online.
### Recommendations
We recommend users of Falcon-7B-Instruct to develop guardrails and to take appropriate precautions for any production use.
## How to Get Started with the Model
```python
from transformers import AutoTokenizer, AutoModelForCausalLM
import transformers
import torch
model = "tiiuae/falcon-7b-instruct"
tokenizer = AutoTokenizer.from_pretrained(model)
pipeline = transformers.pipeline(
"text-generation",
model=model,
tokenizer=tokenizer,
torch_dtype=torch.bfloat16,
device_map="auto",
)
sequences = pipeline(
"Girafatron is obsessed with giraffes, the most glorious animal on the face of this Earth. Giraftron believes all other animals are irrelevant when compared to the glorious majesty of the giraffe.\nDaniel: Hello, Girafatron!\nGirafatron:",
max_length=200,
do_sample=True,
top_k=10,
num_return_sequences=1,
eos_token_id=tokenizer.eos_token_id,
)
for seq in sequences:
print(f"Result: {seq['generated_text']}")
```
## Training Details
### Training Data
Falcon-7B-Instruct was finetuned on a 250M tokens mixture of instruct/chat datasets.
| **Data source** | **Fraction** | **Tokens** | **Description** |
|--------------------|--------------|------------|-----------------------------------|
| [Bai ze](https://github.com/project-baize/baize-chatbot) | 65% | 164M | chat |
| [GPT4All](https://github.com/nomic-ai/gpt4all) | 25% | 62M | instruct |
| [GPTeacher](https://github.com/teknium1/GPTeacher) | 5% | 11M | instruct |
| [RefinedWeb-English](https://huggingface.co/datasets/tiiuae/falcon-refinedweb) | 5% | 13M | massive web crawl |
The data was tokenized with the Falcon-[7B](https://huggingface.co/tiiuae/falcon-7b)/[40B](https://huggingface.co/tiiuae/falcon-40b) tokenizer.
## Evaluation
*Paper coming soon.*
See the [OpenLLM Leaderboard](https://huggingface.co/spaces/HuggingFaceH4/open_llm_leaderboard) for early results.
Note that this model variant is not optimized for NLP benchmarks.
## Technical Specifications
For more information about pretraining, see [Falcon-7B](https://huggingface.co/tiiuae/falcon-7b).
### Model Architecture and Objective
Falcon-7B is a causal decoder-only model trained on a causal language modeling task (i.e., predict the next token).
The architecture is broadly adapted from the GPT-3 paper ([Brown et al., 2020](https://arxiv.org/abs/2005.14165)), with the following differences:
* **Positionnal embeddings:** rotary ([Su et al., 2021](https://arxiv.org/abs/2104.09864));
* **Attention:** multiquery ([Shazeer et al., 2019](https://arxiv.org/abs/1911.02150)) and FlashAttention ([Dao et al., 2022](https://arxiv.org/abs/2205.14135));
* **Decoder-block:** parallel attention/MLP with a single layer norm.
| **Hyperparameter** | **Value** | **Comment** |
|--------------------|-----------|----------------------------------------|
| Layers | 32 | |
| `d_model` | 4544 | Increased to compensate for multiquery |
| `head_dim` | 64 | Reduced to optimise for FlashAttention |
| Vocabulary | 65024 | |
| Sequence length | 2048 | |
### Compute Infrastructure
#### Hardware
Falcon-7B-Instruct was trained on AWS SageMaker, on 32 A100 40GB GPUs in P4d instances.
#### Software
Falcon-7B-Instruct was trained a custom distributed training codebase, Gigatron. It uses a 3D parallelism approach combined with ZeRO and high-performance Triton kernels (FlashAttention, etc.)
## Citation
*Paper coming soon* 😊. In the meanwhile, you can use the following information to cite:
```
@article{falcon40b,
title={{Falcon-40B}: an open large language model with state-of-the-art performance},
author={Almazrouei, Ebtesam and Alobeidli, Hamza and Alshamsi, Abdulaziz and Cappelli, Alessandro and Cojocaru, Ruxandra and Debbah, Merouane and Goffinet, Etienne and Heslow, Daniel and Launay, Julien and Malartic, Quentin and Noune, Badreddine and Pannier, Baptiste and Penedo, Guilherme},
year={2023}
}
```
To learn more about the pretraining dataset, see the 📓 [RefinedWeb paper](https://arxiv.org/abs/2306.01116).
```
@article{refinedweb,
title={The {R}efined{W}eb dataset for {F}alcon {LLM}: outperforming curated corpora with web data, and web data only},
author={Guilherme Penedo and Quentin Malartic and Daniel Hesslow and Ruxandra Cojocaru and Alessandro Cappelli and Hamza Alobeidli and Baptiste Pannier and Ebtesam Almazrouei and Julien Launay},
journal={arXiv preprint arXiv:2306.01116},
eprint={2306.01116},
eprinttype = {arXiv},
url={https://arxiv.org/abs/2306.01116},
year={2023}
}
```
## License
Falcon-7B-Instruct is made available under the Apache 2.0 license.
## Contact
[email protected] |
naclbit/trinart_characters_19.2m_stable_diffusion_v1 | naclbit | 2023-05-07T17:12:10Z | 12,013 | 170 | diffusers | [
"diffusers",
"stable-diffusion",
"stable-diffusion-diffusers",
"text-to-image",
"license:creativeml-openrail-m",
"autotrain_compatible",
"diffusers:StableDiffusionPipeline",
"region:us"
]
| text-to-image | 2022-10-15T01:21:16Z | ---
inference: false
tags:
- stable-diffusion
- stable-diffusion-diffusers
- text-to-image
license: creativeml-openrail-m
---
## Note
A newer version of this model has been released:
https://huggingface.co/naclbit/trinart_derrida_characters_v2_stable_diffusion
## Stable Diffusion TrinArt Characters model v1
trinart_characters_19.2m_stable_diffusion_v1 is a stable diffusion v1-based model trained by roughly 19.2M anime/manga style images (pre-rolled augmented images included) plus final finetuning by about 50,000 images. This model seeks for a sweet spot between artistic style versatility and anatomical quality within the given model spec of SDv1.
This is the same version 1 model that was released in AI Novelist/TrinArt service from early September through Oct 14. We are currently experimenting with the new Derrida model on TrinArt service for further improvement and anatomical stabilization. In the mean time, please enjoy this real-service-tested Characters v1!
#### Hardware
- 8xNVIDIA A100 40GB
#### Custom autoencoder
*Note: There was a wrong checkpoint uploaded before 5 Nov 2022. The file has been replaced with the latest checkpoint.*
We also provide a separate checkpoint for the custom KL autoencoder. As suggested by the Latent Diffusion paper, we found that training the autoencoder and the latent diffusion model separately improves the result. Since the official stable diffusion script does not support loading the other VAE, in order to run it in your script, you'll need to override state_dict for first_stage_model.
The popular WebUI has the script to load separate first_stage_model parameters.
#### Safety
The dataset is filtered to exclude NSFW or unsafe contents. After our extensive experimentation and testing with 10M+ user generated images, we decided that this model is safe enough and less likely to spit out questionable (nudity/overly sexual/realistic gore) content than the stock SD v1.4 model or other anime/manga models. However, if the user tortures this model enough until it talks, it may be still possible to force this model to generate obnoxious materials. We do not consider this model to be 100% risk-free.
*This statement does not necessarily restrict third-party from training a derivative of this model that includes NSFW.
#### Examples
Below images are directly generated by the native TrinArt service with its idiosyncratic upscaler, parser and processes. Your mileage may vary.
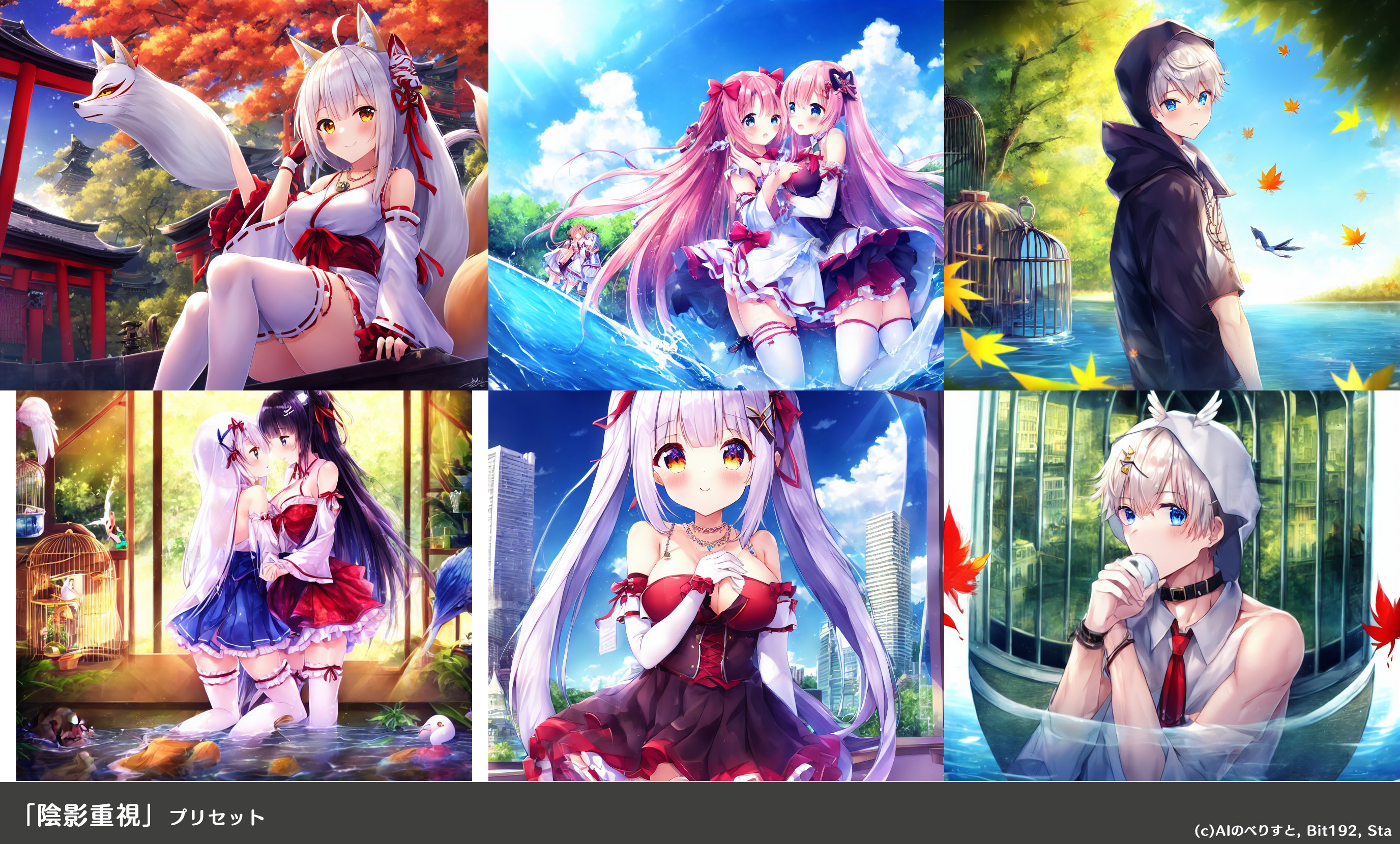
(assorted random examples)
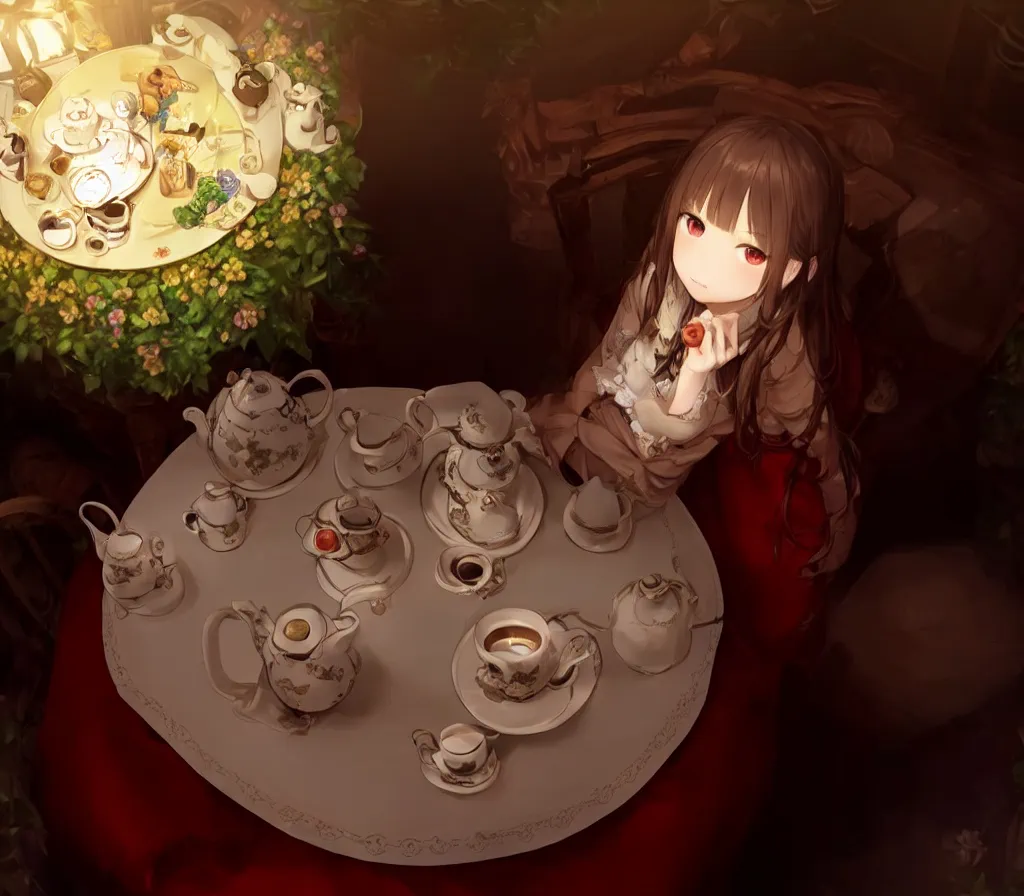
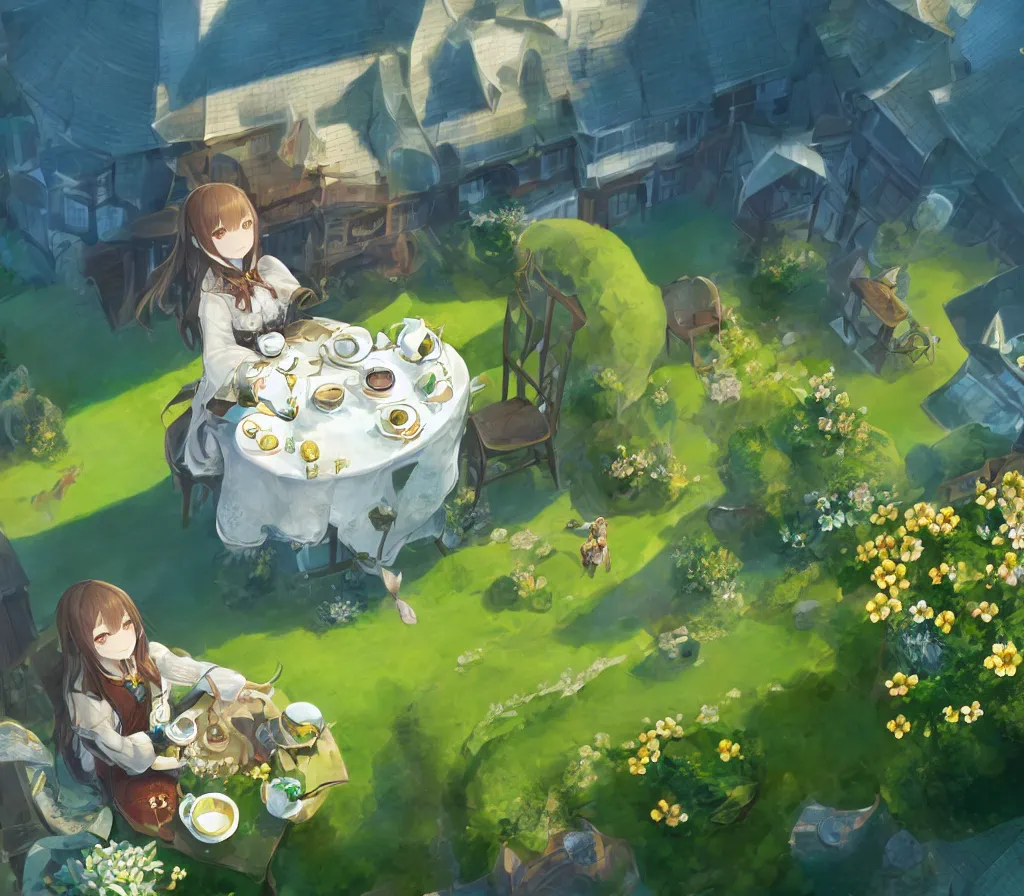
wide shot, high quality, htgngg animal arm rest brown hair merry chair cup dress flower from above jacket on shoulders long hair sitting solo sugar bowl fantasy adventurer's inn table teacup teapot landscape miniature (2022 Artstyle preset)
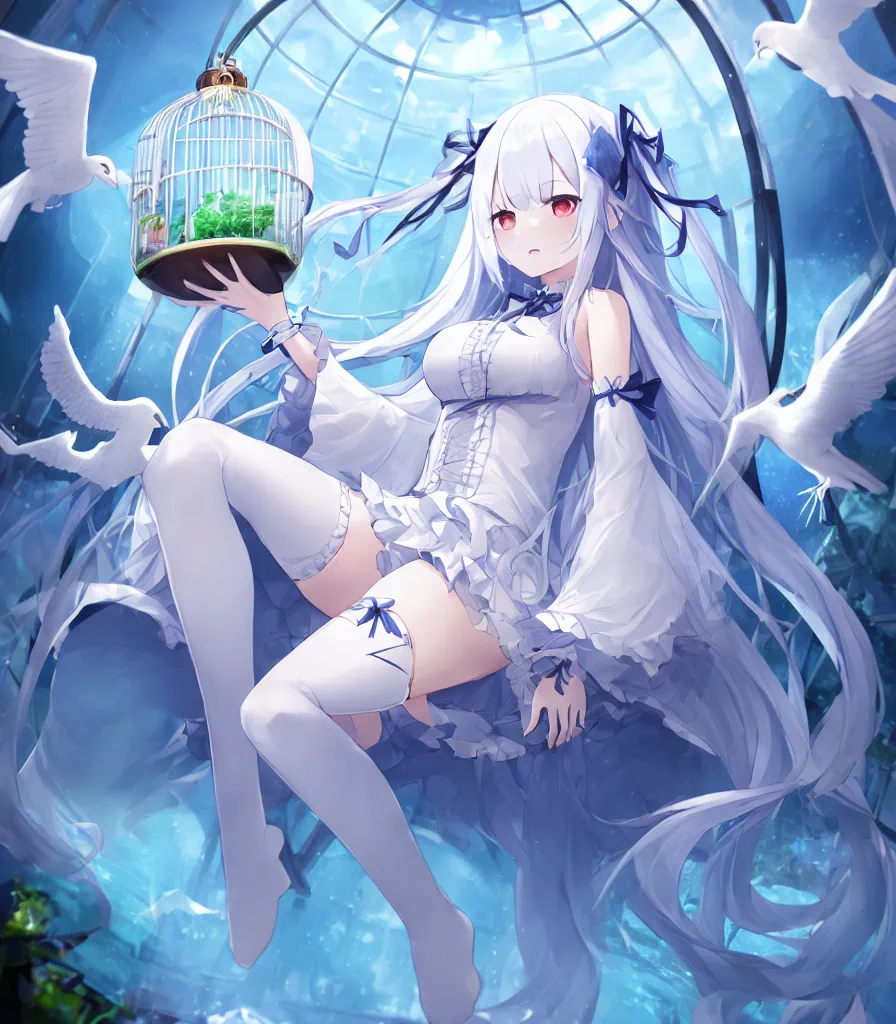
highres wide shot bangs bare shoulders water bird cage terrarium detached sleeves frilled frilled legwear frills hair ornament hair ribbon hood long hair medium breasts ribbon thighhighs (2019 Artstyle preset)
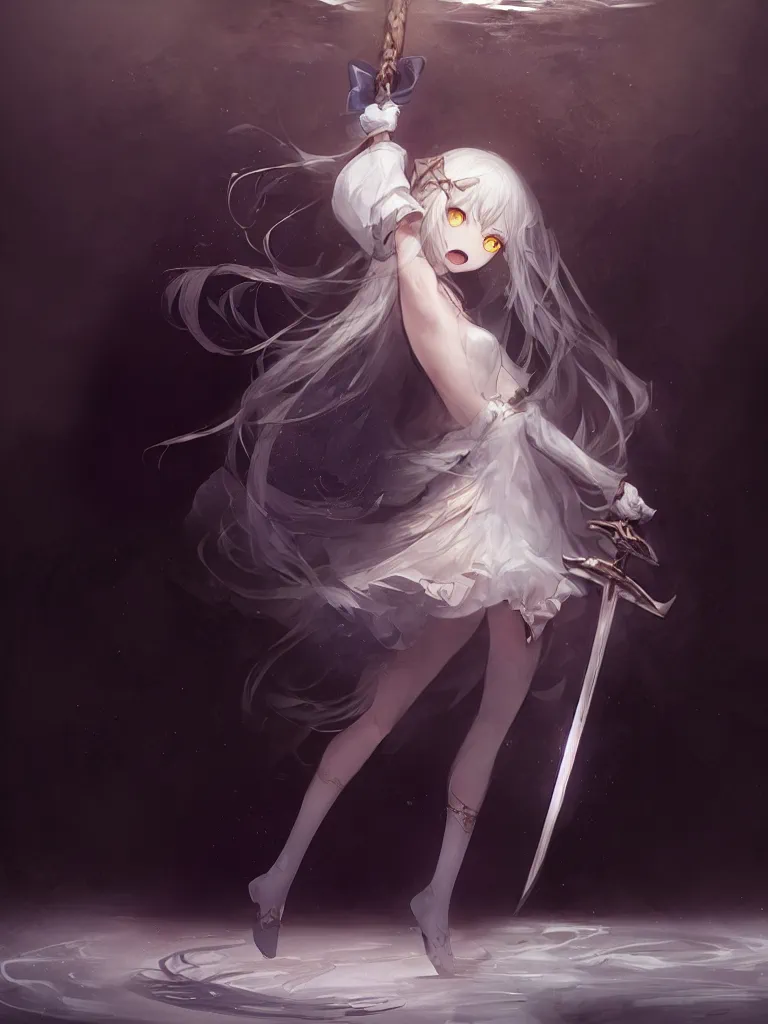
1girl standing holding sword hizzrd arm up bangs bare shoulders boots bow breasts bright pupils choker detached sleeves diamond (shape) floating floating hair footwear bow from side full body gloves leg up long hair looking at viewer open mouth outstretched arm solo streaked hair swept bangs two tone hair very long hair::4 angry::1 (2022 Artstyle preset)
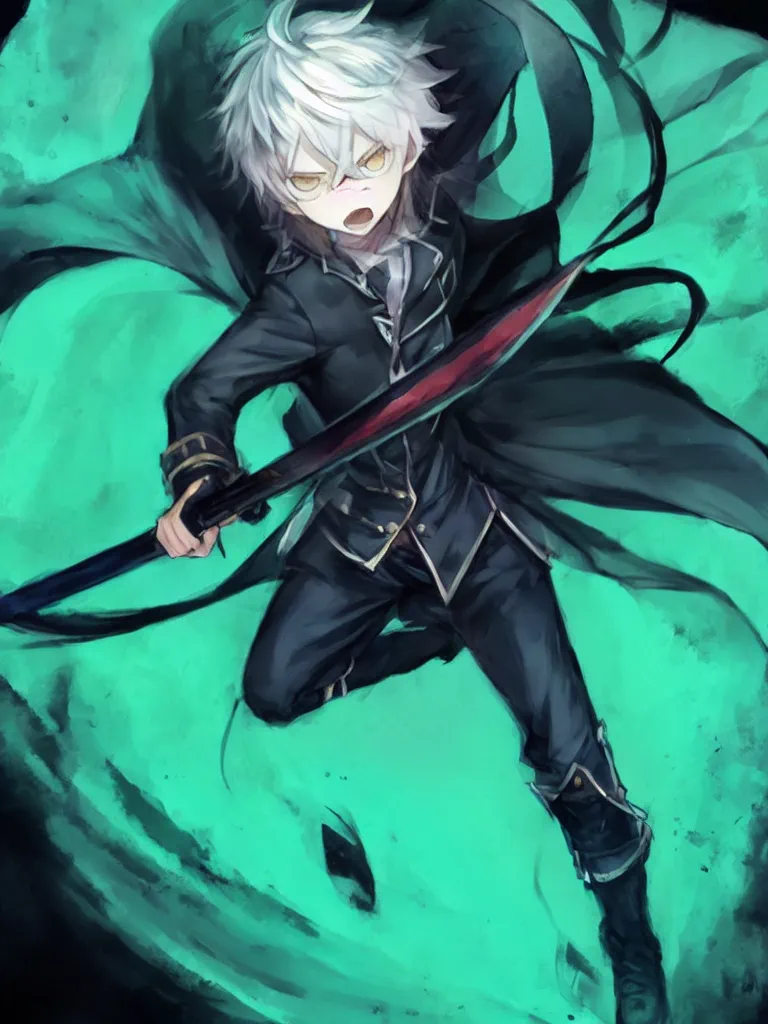
1boy male focus standing hizzrd holding sword arm up bow bright pupils cape coat diamond (shape) floating floating hair fold-over boots footwear bow from side full body gloves leg up long sleeves looking at viewer open mouth outstretched arm open coat open clothes solo swept two tone hair thigh boots::4 angry::1.25 (2022 Artstyle preset)
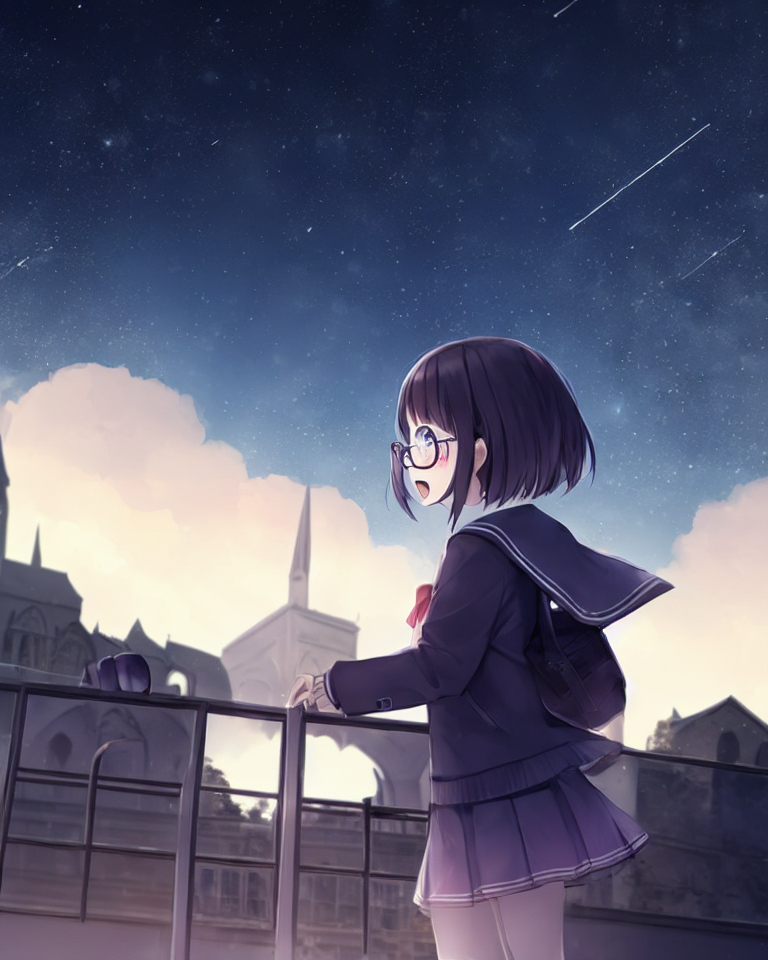
cathedral 1girl schoolgirl momoko school uniform cats particles beautiful shooting stars detailed cathedral jacket open mouth glasses cats (2022 Artstyle preset)
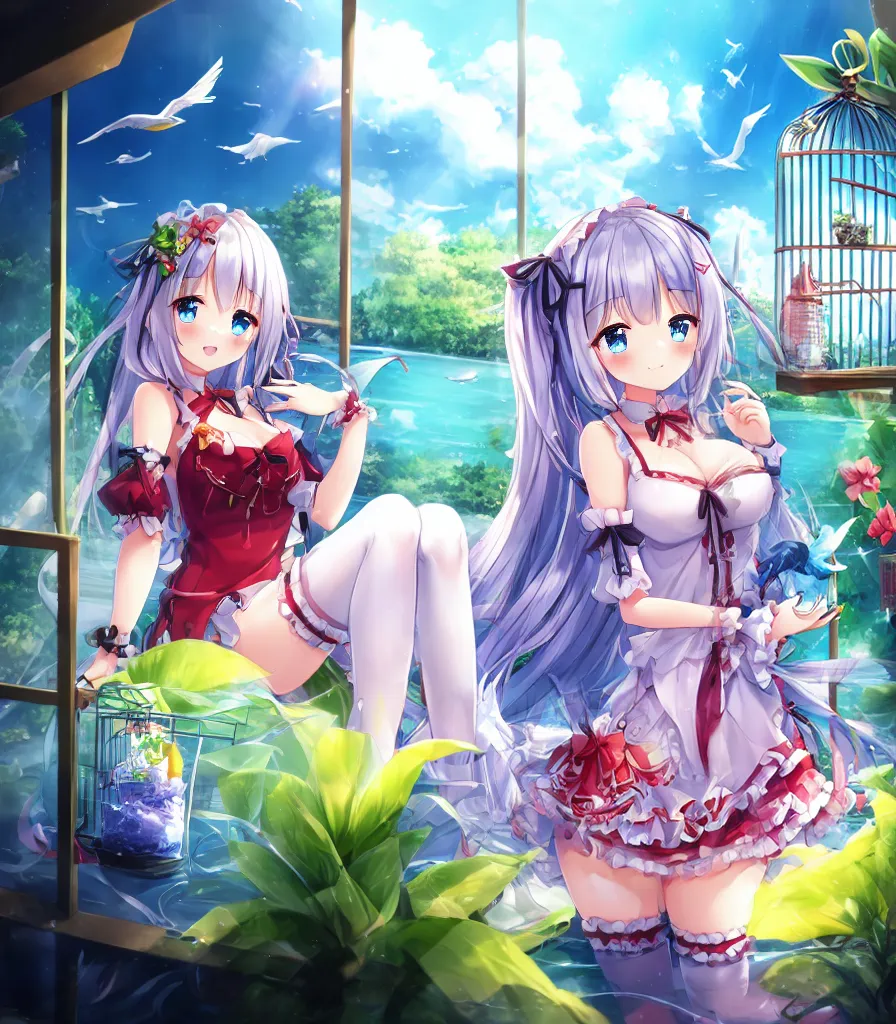
highres 2girls yuri wide shot bangs bare shoulders water bird cage terrarium detached sleeves frilled frilled legwear frills hair ornament hair ribbon hood long hair medium breasts ribbon thighhighs (More Details preset)
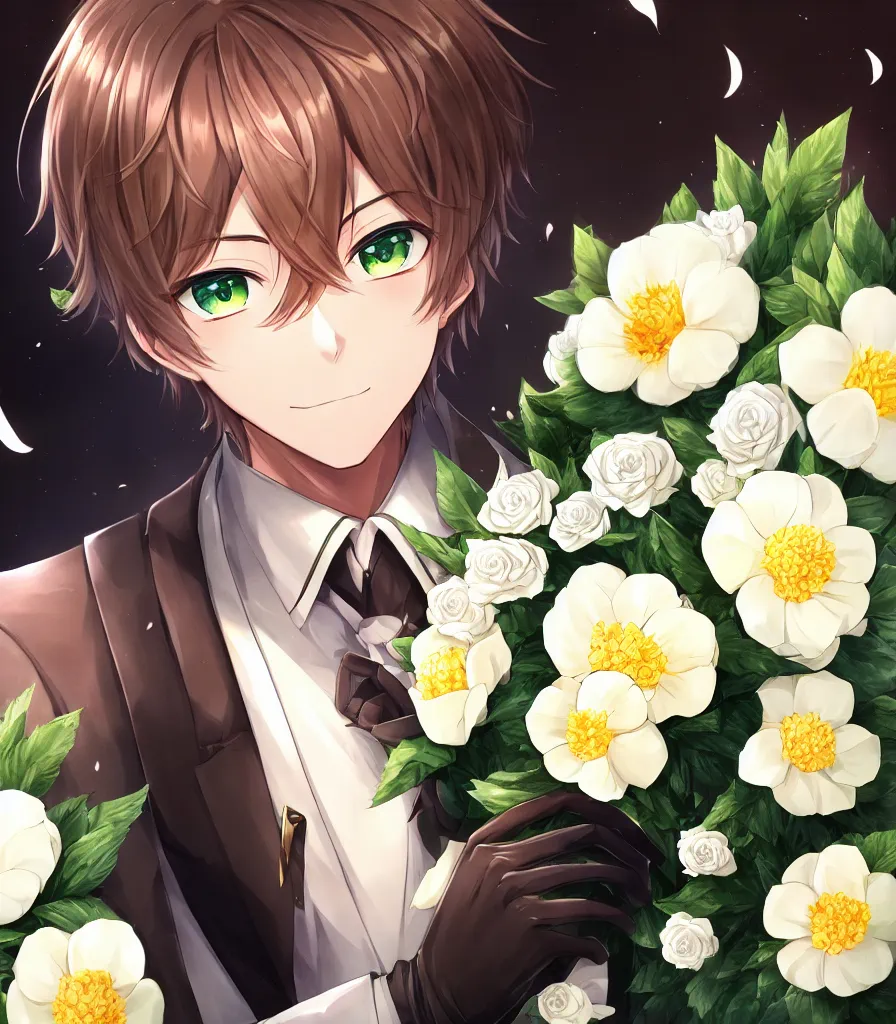
wide shot, best quality lapis erebcir highres 1boy bangs black gloves brown hair closed mouth gloves hair between eyes looking at viewer male focus flowers green eyes (More Details preset)
TrinArt 2022 Artstyle preset negative prompts: **retro style, 1980s, 1990s, 2000s, 2005 2006 2007 2008 2009 2010 2011 2012 2013 2014 2015 2016 2017 2018 2019**
TrinArt More Details preset negative prompts: **flat color, flat shading**
We recommend to add known sets of negative prompts in order to stabilize the anatomy such as: bad hands, fewer digits, etc.
#### Credits
- Sta, AI Novelist Dev (https://ai-novel.com/) @ Bit192, Inc. Twitter https://twitter.com/naclbbr (Japanese) https://twitter.com/naclbbre (English)
- Stable Diffusion - Rombach, Robin and Blattmann, Andreas and Lorenz, Dominik and Esser, Patrick and Ommer, Bjorn
#### License
CreativeML OpenRAIL-M |
fluently/Fluently-XL-v3 | fluently | 2024-05-24T21:21:31Z | 12,008 | 11 | diffusers | [
"diffusers",
"safetensors",
"stable-diffusion",
"sdxl",
"fluetnly-xl",
"fluently",
"trained",
"text-to-image",
"dataset:ehristoforu/midjourney-images",
"dataset:ehristoforu/dalle-3-images",
"dataset:ehristoforu/fav_images",
"base_model:stabilityai/stable-diffusion-xl-base-1.0",
"license:other",
"autotrain_compatible",
"endpoints_compatible",
"diffusers:StableDiffusionXLPipeline",
"region:us"
]
| text-to-image | 2024-03-20T10:50:23Z | ---
license: other
license_name: fluently-license
license_link: https://huggingface.co/spaces/fluently/License
datasets:
- ehristoforu/midjourney-images
- ehristoforu/dalle-3-images
- ehristoforu/fav_images
library_name: diffusers
pipeline_tag: text-to-image
base_model: stabilityai/stable-diffusion-xl-base-1.0
tags:
- safetensors
- stable-diffusion
- sdxl
- fluetnly-xl
- fluently
- trained
inference:
parameters:
num_inference_steps: 25
guidance_scale: 5
negative_prompt: "(deformed, distorted, disfigured:1.3), poorly drawn, bad anatomy, wrong anatomy, extra limb, missing limb, floating limbs, (mutated hands and fingers:1.4), disconnected limbs, mutation, mutated, ugly, disgusting, blurry, amputation"
---
# **Fluently XL** V3 - the best XL-model

[>>> Run in **RunDiffusion** <<<](https://civitai.com/api/run/401769?partnerId=1&strategyId=1067841896)
Introducing Fluently XL, you are probably ready to argue with the name of the model: “The best XL-model”, but now I will prove to you why it is true.
## About this model
The model was obtained through training on *expensive graphics accelerators*, a lot of work was done, now we will show why this XL model is better than others.
### Features
- Correct anatomy
- Art and realism in one
- Controling contrast
- Great nature
- Great faces without AfterDetailer
### More info
Our model is better than others because we do not mix but **train**, but at first it may seem that the model is not very good, but if you are a real professional you will like it.
## Using
Optimal parameters in Automatic1111/ComfyUI:
- Sampling steps: 20-35
- Sampler method: Euler a/Euler
- CFG Scale: 4-6.5
## End
Let's remove models that copy each other from the top and put one that is actually developing, thank you) |
mradermacher/L3-Nymeria-v2-8B-GGUF | mradermacher | 2024-06-30T04:55:44Z | 11,993 | 1 | transformers | [
"transformers",
"gguf",
"mergekit",
"merge",
"roleplay",
"sillytavern",
"llama3",
"not-for-all-audiences",
"en",
"base_model:tannedbum/L3-Nymeria-v2-8B",
"license:cc-by-nc-4.0",
"endpoints_compatible",
"region:us"
]
| null | 2024-06-30T03:58:59Z | ---
base_model: tannedbum/L3-Nymeria-v2-8B
language:
- en
library_name: transformers
license: cc-by-nc-4.0
quantized_by: mradermacher
tags:
- mergekit
- merge
- roleplay
- sillytavern
- llama3
- not-for-all-audiences
---
## About
<!-- ### quantize_version: 2 -->
<!-- ### output_tensor_quantised: 1 -->
<!-- ### convert_type: hf -->
<!-- ### vocab_type: -->
<!-- ### tags: -->
static quants of https://huggingface.co/tannedbum/L3-Nymeria-v2-8B
<!-- provided-files -->
weighted/imatrix quants are available at https://huggingface.co/mradermacher/L3-Nymeria-v2-8B-i1-GGUF
## Usage
If you are unsure how to use GGUF files, refer to one of [TheBloke's
READMEs](https://huggingface.co/TheBloke/KafkaLM-70B-German-V0.1-GGUF) for
more details, including on how to concatenate multi-part files.
## Provided Quants
(sorted by size, not necessarily quality. IQ-quants are often preferable over similar sized non-IQ quants)
| Link | Type | Size/GB | Notes |
|:-----|:-----|--------:|:------|
| [GGUF](https://huggingface.co/mradermacher/L3-Nymeria-v2-8B-GGUF/resolve/main/L3-Nymeria-v2-8B.Q2_K.gguf) | Q2_K | 3.3 | |
| [GGUF](https://huggingface.co/mradermacher/L3-Nymeria-v2-8B-GGUF/resolve/main/L3-Nymeria-v2-8B.IQ3_XS.gguf) | IQ3_XS | 3.6 | |
| [GGUF](https://huggingface.co/mradermacher/L3-Nymeria-v2-8B-GGUF/resolve/main/L3-Nymeria-v2-8B.Q3_K_S.gguf) | Q3_K_S | 3.8 | |
| [GGUF](https://huggingface.co/mradermacher/L3-Nymeria-v2-8B-GGUF/resolve/main/L3-Nymeria-v2-8B.IQ3_S.gguf) | IQ3_S | 3.8 | beats Q3_K* |
| [GGUF](https://huggingface.co/mradermacher/L3-Nymeria-v2-8B-GGUF/resolve/main/L3-Nymeria-v2-8B.IQ3_M.gguf) | IQ3_M | 3.9 | |
| [GGUF](https://huggingface.co/mradermacher/L3-Nymeria-v2-8B-GGUF/resolve/main/L3-Nymeria-v2-8B.Q3_K_M.gguf) | Q3_K_M | 4.1 | lower quality |
| [GGUF](https://huggingface.co/mradermacher/L3-Nymeria-v2-8B-GGUF/resolve/main/L3-Nymeria-v2-8B.Q3_K_L.gguf) | Q3_K_L | 4.4 | |
| [GGUF](https://huggingface.co/mradermacher/L3-Nymeria-v2-8B-GGUF/resolve/main/L3-Nymeria-v2-8B.IQ4_XS.gguf) | IQ4_XS | 4.6 | |
| [GGUF](https://huggingface.co/mradermacher/L3-Nymeria-v2-8B-GGUF/resolve/main/L3-Nymeria-v2-8B.Q4_K_S.gguf) | Q4_K_S | 4.8 | fast, recommended |
| [GGUF](https://huggingface.co/mradermacher/L3-Nymeria-v2-8B-GGUF/resolve/main/L3-Nymeria-v2-8B.Q4_K_M.gguf) | Q4_K_M | 5.0 | fast, recommended |
| [GGUF](https://huggingface.co/mradermacher/L3-Nymeria-v2-8B-GGUF/resolve/main/L3-Nymeria-v2-8B.Q5_K_S.gguf) | Q5_K_S | 5.7 | |
| [GGUF](https://huggingface.co/mradermacher/L3-Nymeria-v2-8B-GGUF/resolve/main/L3-Nymeria-v2-8B.Q5_K_M.gguf) | Q5_K_M | 5.8 | |
| [GGUF](https://huggingface.co/mradermacher/L3-Nymeria-v2-8B-GGUF/resolve/main/L3-Nymeria-v2-8B.Q6_K.gguf) | Q6_K | 6.7 | very good quality |
| [GGUF](https://huggingface.co/mradermacher/L3-Nymeria-v2-8B-GGUF/resolve/main/L3-Nymeria-v2-8B.Q8_0.gguf) | Q8_0 | 8.6 | fast, best quality |
| [GGUF](https://huggingface.co/mradermacher/L3-Nymeria-v2-8B-GGUF/resolve/main/L3-Nymeria-v2-8B.f16.gguf) | f16 | 16.2 | 16 bpw, overkill |
Here is a handy graph by ikawrakow comparing some lower-quality quant
types (lower is better):

And here are Artefact2's thoughts on the matter:
https://gist.github.com/Artefact2/b5f810600771265fc1e39442288e8ec9
## FAQ / Model Request
See https://huggingface.co/mradermacher/model_requests for some answers to
questions you might have and/or if you want some other model quantized.
## Thanks
I thank my company, [nethype GmbH](https://www.nethype.de/), for letting
me use its servers and providing upgrades to my workstation to enable
this work in my free time.
<!-- end -->
|
indolem/indobertweet-base-uncased | indolem | 2021-09-18T01:24:17Z | 11,988 | 10 | transformers | [
"transformers",
"pytorch",
"bert",
"fill-mask",
"Twitter",
"id",
"arxiv:2109.04607",
"license:apache-2.0",
"autotrain_compatible",
"endpoints_compatible",
"region:us"
]
| fill-mask | 2022-03-02T23:29:05Z | ---
language:
- id
tags:
- Twitter
license: apache-2.0
datasets:
- Twitter 2021
widget:
- text: "guweehh udh ga' paham lg sm [MASK]"
---
# IndoBERTweet 🐦
## 1. Paper
Fajri Koto, Jey Han Lau, and Timothy Baldwin. [_IndoBERTweet: A Pretrained Language Model for Indonesian Twitter
with Effective Domain-Specific Vocabulary Initialization_](https://arxiv.org/pdf/2109.04607.pdf).
In Proceedings of the 2021 Conference on Empirical Methods in Natural Language Processing (**EMNLP 2021**), Dominican Republic (virtual).
## 2. About
[IndoBERTweet](https://github.com/indolem/IndoBERTweet) is the first large-scale pretrained model for Indonesian Twitter
that is trained by extending a monolingually trained Indonesian BERT model with additive domain-specific vocabulary.
In this paper, we show that initializing domain-specific vocabulary with average-pooling of BERT subword embeddings is more efficient than pretraining from scratch, and more effective than initializing based on word2vec projections.
## 3. Pretraining Data
We crawl Indonesian tweets over a 1-year period using the official Twitter API, from December 2019 to December 2020, with 60 keywords covering 4 main topics: economy, health, education, and government. We obtain in total of **409M word tokens**, two times larger than the training data used to pretrain [IndoBERT](https://aclanthology.org/2020.coling-main.66.pdf). Due to Twitter policy, this pretraining data will not be released to public.
## 4. How to use
Load model and tokenizer (tested with transformers==3.5.1)
```python
from transformers import AutoTokenizer, AutoModel
tokenizer = AutoTokenizer.from_pretrained("indolem/indobertweet-base-uncased")
model = AutoModel.from_pretrained("indolem/indobertweet-base-uncased")
```
**Preprocessing Steps:**
* lower-case all words
* converting user mentions and URLs into @USER and HTTPURL, respectively
* translating emoticons into text using the [emoji package](https://pypi.org/project/emoji/).
## 5. Results over 7 Indonesian Twitter Datasets
<table>
<col>
<colgroup span="2"></colgroup>
<colgroup span="2"></colgroup>
<tr>
<th rowspan="2">Models</td>
<th colspan="2" scope="colgroup">Sentiment</th>
<th colspan="1" scope="colgroup">Emotion</th>
<th colspan="2" scope="colgroup">Hate Speech</th>
<th colspan="2" scope="colgroup">NER</th>
<th rowspan="2" scope="colgroup">Average</th>
</tr>
<tr>
<th scope="col">IndoLEM</th>
<th scope="col">SmSA</th>
<th scope="col">EmoT</th>
<th scope="col">HS1</th>
<th scope="col">HS2</th>
<th scope="col">Formal</th>
<th scope="col">Informal</th>
</tr>
<tr>
<td scope="row">mBERT</td>
<td>76.6</td>
<td>84.7</td>
<td>67.5</td>
<td>85.1</td>
<td>75.1</td>
<td>85.2</td>
<td>83.2</td>
<td>79.6</td>
</tr>
<tr>
<td scope="row">malayBERT</td>
<td>82.0</td>
<td>84.1</td>
<td>74.2</td>
<td>85.0</td>
<td>81.9</td>
<td>81.9</td>
<td>81.3</td>
<td>81.5</td>
</tr>
<tr>
<td scope="row">IndoBERT (Willie, et al., 2020)</td>
<td>84.1</td>
<td>88.7</td>
<td>73.3</td>
<td>86.8</td>
<td>80.4</td>
<td>86.3</td>
<td>84.3</td>
<td>83.4</td>
</tr>
<tr>
<td scope="row">IndoBERT (Koto, et al., 2020)</td>
<td>84.1</td>
<td>87.9</td>
<td>71.0</td>
<td>86.4</td>
<td>79.3</td>
<td>88.0</td>
<td><b>86.9</b></td>
<td>83.4</td>
</tr>
<tr>
<td scope="row">IndoBERTweet (1M steps from scratch)</td>
<td>86.2</td>
<td>90.4</td>
<td>76.0</td>
<td><b>88.8</b></td>
<td><b>87.5</b></td>
<td><b>88.1</b></td>
<td>85.4</td>
<td>86.1</td>
</tr>
<tr>
<td scope="row">IndoBERT + Voc adaptation + 200k steps</td>
<td><b>86.6</b></td>
<td><b>92.7</b></td>
<td><b>79.0</b></td>
<td>88.4</td>
<td>84.0</td>
<td>87.7</td>
<td><b>86.9</b></td>
<td><b>86.5</b></td>
</tr>
</table>
## Citation
If you use our work, please cite:
```bibtex
@inproceedings{koto2021indobertweet,
title={IndoBERTweet: A Pretrained Language Model for Indonesian Twitter with Effective Domain-Specific Vocabulary Initialization},
author={Fajri Koto and Jey Han Lau and Timothy Baldwin},
booktitle={Proceedings of the 2021 Conference on Empirical Methods in Natural Language Processing (EMNLP 2021)},
year={2021}
}
``` |
alvdansen/BandW-Manga | alvdansen | 2024-06-16T16:26:16Z | 11,984 | 105 | diffusers | [
"diffusers",
"text-to-image",
"stable-diffusion",
"lora",
"template:sd-lora",
"base_model:stabilityai/stable-diffusion-xl-base-1.0",
"license:creativeml-openrail-m",
"region:us"
]
| text-to-image | 2024-06-10T22:45:04Z | ---
tags:
- text-to-image
- stable-diffusion
- lora
- diffusers
- template:sd-lora
widget:
- text: a boy in a sailor suit frowning
output:
url: images/BW_e000014_02_20240610214209.jpeg
- text: a toad
output:
url: images/BW_e000014_01_20240610214155.jpeg
- text: a girl with a flower crown
output:
url: images/BW_e000014_00_20240610214140.jpeg
- text: a girl with blonde-brown hair and big round glasses wearing a whit tank top
output:
url: images/ComfyUI_01003_.jpeg
- text: girl,neck tuft,white hair,sheep horns,blue eyes
output:
url: images/ComfyUI_01023_.jpeg
base_model: stabilityai/stable-diffusion-xl-base-1.0
instance_prompt: null
license: creativeml-openrail-m
---
# B&W Manga Block
<Gallery />
## Model description
This model makes super bold line portrait illustrations. Best in monochrome with simple prompts.
## Download model
Weights for this model are available in Safetensors format.
Model release is for research purposes only. For commercial use, please contact me directly.
[Download](/alvdansen/BandW-Manga/tree/main) them in the Files & versions tab.
|
mradermacher/L3-Sophie-64-1ep-GGUF | mradermacher | 2024-06-23T14:49:29Z | 11,981 | 0 | transformers | [
"transformers",
"gguf",
"mergekit",
"merge",
"en",
"base_model:Fischerboot/L3-Sophie-64-1ep",
"endpoints_compatible",
"region:us"
]
| null | 2024-06-23T02:49:27Z | ---
base_model: Fischerboot/L3-Sophie-64-1ep
language:
- en
library_name: transformers
quantized_by: mradermacher
tags:
- mergekit
- merge
---
## About
<!-- ### quantize_version: 2 -->
<!-- ### output_tensor_quantised: 1 -->
<!-- ### convert_type: hf -->
<!-- ### vocab_type: -->
<!-- ### tags: -->
static quants of https://huggingface.co/Fischerboot/L3-Sophie-64-1ep
<!-- provided-files -->
weighted/imatrix quants seem not to be available (by me) at this time. If they do not show up a week or so after the static ones, I have probably not planned for them. Feel free to request them by opening a Community Discussion.
## Usage
If you are unsure how to use GGUF files, refer to one of [TheBloke's
READMEs](https://huggingface.co/TheBloke/KafkaLM-70B-German-V0.1-GGUF) for
more details, including on how to concatenate multi-part files.
## Provided Quants
(sorted by size, not necessarily quality. IQ-quants are often preferable over similar sized non-IQ quants)
| Link | Type | Size/GB | Notes |
|:-----|:-----|--------:|:------|
| [GGUF](https://huggingface.co/mradermacher/L3-Sophie-64-1ep-GGUF/resolve/main/L3-Sophie-64-1ep.Q2_K.gguf) | Q2_K | 3.3 | |
| [GGUF](https://huggingface.co/mradermacher/L3-Sophie-64-1ep-GGUF/resolve/main/L3-Sophie-64-1ep.IQ3_XS.gguf) | IQ3_XS | 3.6 | |
| [GGUF](https://huggingface.co/mradermacher/L3-Sophie-64-1ep-GGUF/resolve/main/L3-Sophie-64-1ep.Q3_K_S.gguf) | Q3_K_S | 3.8 | |
| [GGUF](https://huggingface.co/mradermacher/L3-Sophie-64-1ep-GGUF/resolve/main/L3-Sophie-64-1ep.IQ3_S.gguf) | IQ3_S | 3.8 | beats Q3_K* |
| [GGUF](https://huggingface.co/mradermacher/L3-Sophie-64-1ep-GGUF/resolve/main/L3-Sophie-64-1ep.IQ3_M.gguf) | IQ3_M | 3.9 | |
| [GGUF](https://huggingface.co/mradermacher/L3-Sophie-64-1ep-GGUF/resolve/main/L3-Sophie-64-1ep.Q3_K_M.gguf) | Q3_K_M | 4.1 | lower quality |
| [GGUF](https://huggingface.co/mradermacher/L3-Sophie-64-1ep-GGUF/resolve/main/L3-Sophie-64-1ep.Q3_K_L.gguf) | Q3_K_L | 4.4 | |
| [GGUF](https://huggingface.co/mradermacher/L3-Sophie-64-1ep-GGUF/resolve/main/L3-Sophie-64-1ep.IQ4_XS.gguf) | IQ4_XS | 4.6 | |
| [GGUF](https://huggingface.co/mradermacher/L3-Sophie-64-1ep-GGUF/resolve/main/L3-Sophie-64-1ep.Q4_K_S.gguf) | Q4_K_S | 4.8 | fast, recommended |
| [GGUF](https://huggingface.co/mradermacher/L3-Sophie-64-1ep-GGUF/resolve/main/L3-Sophie-64-1ep.Q4_K_M.gguf) | Q4_K_M | 5.0 | fast, recommended |
| [GGUF](https://huggingface.co/mradermacher/L3-Sophie-64-1ep-GGUF/resolve/main/L3-Sophie-64-1ep.Q5_K_S.gguf) | Q5_K_S | 5.7 | |
| [GGUF](https://huggingface.co/mradermacher/L3-Sophie-64-1ep-GGUF/resolve/main/L3-Sophie-64-1ep.Q5_K_M.gguf) | Q5_K_M | 5.8 | |
| [GGUF](https://huggingface.co/mradermacher/L3-Sophie-64-1ep-GGUF/resolve/main/L3-Sophie-64-1ep.Q6_K.gguf) | Q6_K | 6.7 | very good quality |
| [GGUF](https://huggingface.co/mradermacher/L3-Sophie-64-1ep-GGUF/resolve/main/L3-Sophie-64-1ep.Q8_0.gguf) | Q8_0 | 8.6 | fast, best quality |
| [GGUF](https://huggingface.co/mradermacher/L3-Sophie-64-1ep-GGUF/resolve/main/L3-Sophie-64-1ep.f16.gguf) | f16 | 16.2 | 16 bpw, overkill |
Here is a handy graph by ikawrakow comparing some lower-quality quant
types (lower is better):

And here are Artefact2's thoughts on the matter:
https://gist.github.com/Artefact2/b5f810600771265fc1e39442288e8ec9
## FAQ / Model Request
See https://huggingface.co/mradermacher/model_requests for some answers to
questions you might have and/or if you want some other model quantized.
## Thanks
I thank my company, [nethype GmbH](https://www.nethype.de/), for letting
me use its servers and providing upgrades to my workstation to enable
this work in my free time.
<!-- end -->
|
peft-internal-testing/tiny-random-BertModel | peft-internal-testing | 2024-04-17T09:25:03Z | 11,974 | 0 | transformers | [
"transformers",
"safetensors",
"bert",
"text-generation",
"arxiv:1910.09700",
"autotrain_compatible",
"endpoints_compatible",
"region:us"
]
| text-generation | 2024-04-17T09:25:00Z | ---
library_name: transformers
tags: []
---
# Model Card for Model ID
<!-- Provide a quick summary of what the model is/does. -->
## Model Details
### Model Description
<!-- Provide a longer summary of what this model is. -->
This is the model card of a 🤗 transformers model that has been pushed on the Hub. This model card has been automatically generated.
- **Developed by:** [More Information Needed]
- **Funded by [optional]:** [More Information Needed]
- **Shared by [optional]:** [More Information Needed]
- **Model type:** [More Information Needed]
- **Language(s) (NLP):** [More Information Needed]
- **License:** [More Information Needed]
- **Finetuned from model [optional]:** [More Information Needed]
### Model Sources [optional]
<!-- Provide the basic links for the model. -->
- **Repository:** [More Information Needed]
- **Paper [optional]:** [More Information Needed]
- **Demo [optional]:** [More Information Needed]
## Uses
<!-- Address questions around how the model is intended to be used, including the foreseeable users of the model and those affected by the model. -->
### Direct Use
<!-- This section is for the model use without fine-tuning or plugging into a larger ecosystem/app. -->
[More Information Needed]
### Downstream Use [optional]
<!-- This section is for the model use when fine-tuned for a task, or when plugged into a larger ecosystem/app -->
[More Information Needed]
### Out-of-Scope Use
<!-- This section addresses misuse, malicious use, and uses that the model will not work well for. -->
[More Information Needed]
## Bias, Risks, and Limitations
<!-- This section is meant to convey both technical and sociotechnical limitations. -->
[More Information Needed]
### Recommendations
<!-- This section is meant to convey recommendations with respect to the bias, risk, and technical limitations. -->
Users (both direct and downstream) should be made aware of the risks, biases and limitations of the model. More information needed for further recommendations.
## How to Get Started with the Model
Use the code below to get started with the model.
[More Information Needed]
## Training Details
### Training Data
<!-- This should link to a Dataset Card, perhaps with a short stub of information on what the training data is all about as well as documentation related to data pre-processing or additional filtering. -->
[More Information Needed]
### Training Procedure
<!-- This relates heavily to the Technical Specifications. Content here should link to that section when it is relevant to the training procedure. -->
#### Preprocessing [optional]
[More Information Needed]
#### Training Hyperparameters
- **Training regime:** [More Information Needed] <!--fp32, fp16 mixed precision, bf16 mixed precision, bf16 non-mixed precision, fp16 non-mixed precision, fp8 mixed precision -->
#### Speeds, Sizes, Times [optional]
<!-- This section provides information about throughput, start/end time, checkpoint size if relevant, etc. -->
[More Information Needed]
## Evaluation
<!-- This section describes the evaluation protocols and provides the results. -->
### Testing Data, Factors & Metrics
#### Testing Data
<!-- This should link to a Dataset Card if possible. -->
[More Information Needed]
#### Factors
<!-- These are the things the evaluation is disaggregating by, e.g., subpopulations or domains. -->
[More Information Needed]
#### Metrics
<!-- These are the evaluation metrics being used, ideally with a description of why. -->
[More Information Needed]
### Results
[More Information Needed]
#### Summary
## Model Examination [optional]
<!-- Relevant interpretability work for the model goes here -->
[More Information Needed]
## Environmental Impact
<!-- Total emissions (in grams of CO2eq) and additional considerations, such as electricity usage, go here. Edit the suggested text below accordingly -->
Carbon emissions can be estimated using the [Machine Learning Impact calculator](https://mlco2.github.io/impact#compute) presented in [Lacoste et al. (2019)](https://arxiv.org/abs/1910.09700).
- **Hardware Type:** [More Information Needed]
- **Hours used:** [More Information Needed]
- **Cloud Provider:** [More Information Needed]
- **Compute Region:** [More Information Needed]
- **Carbon Emitted:** [More Information Needed]
## Technical Specifications [optional]
### Model Architecture and Objective
[More Information Needed]
### Compute Infrastructure
[More Information Needed]
#### Hardware
[More Information Needed]
#### Software
[More Information Needed]
## Citation [optional]
<!-- If there is a paper or blog post introducing the model, the APA and Bibtex information for that should go in this section. -->
**BibTeX:**
[More Information Needed]
**APA:**
[More Information Needed]
## Glossary [optional]
<!-- If relevant, include terms and calculations in this section that can help readers understand the model or model card. -->
[More Information Needed]
## More Information [optional]
[More Information Needed]
## Model Card Authors [optional]
[More Information Needed]
## Model Card Contact
[More Information Needed]
|
digiplay/majicMIXfantasy_v2 | digiplay | 2024-04-18T00:07:32Z | 11,970 | 4 | diffusers | [
"diffusers",
"safetensors",
"stable-diffusion",
"stable-diffusion-diffusers",
"text-to-image",
"license:other",
"autotrain_compatible",
"endpoints_compatible",
"diffusers:StableDiffusionPipeline",
"region:us"
]
| text-to-image | 2023-05-28T19:06:34Z | ---
license: other
tags:
- stable-diffusion
- stable-diffusion-diffusers
- text-to-image
- diffusers
inference: true
---
https://civitai.com/models/41865/majicmix-fantasy
Original Author's DEMO image :
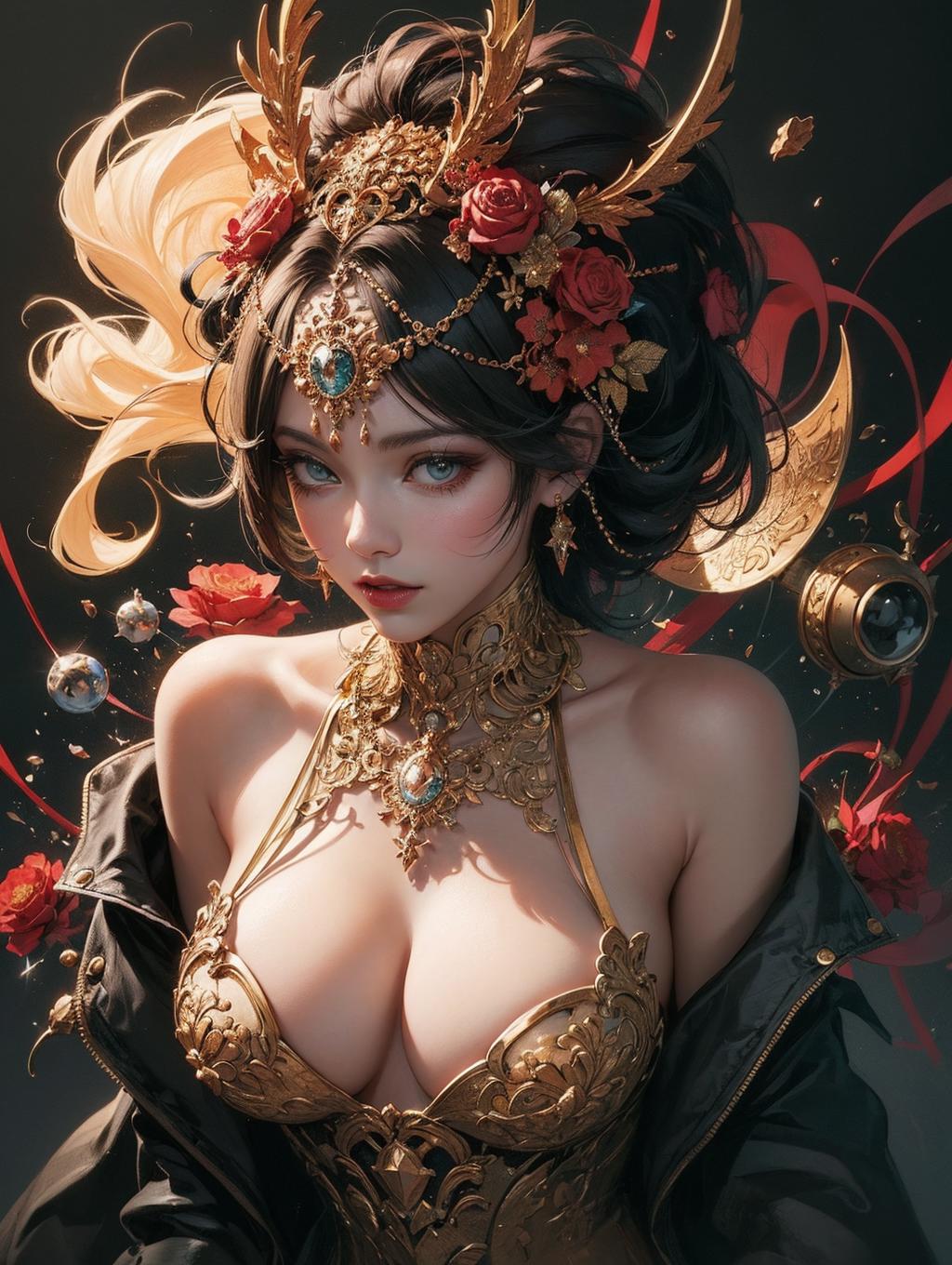 |
mradermacher/Llama-3-8B-Instruct-UltraDPO3-GGUF | mradermacher | 2024-06-30T19:21:42Z | 11,965 | 0 | transformers | [
"transformers",
"gguf",
"alignment-handbook",
"generated_from_trainer",
"en",
"dataset:princeton-nlp/llama3-ultrafeedback",
"base_model:Magpie-Align/Llama-3-8B-Instruct-UltraDPO3",
"license:llama3",
"endpoints_compatible",
"region:us"
]
| null | 2024-06-30T18:52:59Z | ---
base_model: Magpie-Align/Llama-3-8B-Instruct-UltraDPO3
datasets:
- princeton-nlp/llama3-ultrafeedback
language:
- en
library_name: transformers
license: llama3
quantized_by: mradermacher
tags:
- alignment-handbook
- generated_from_trainer
---
## About
<!-- ### quantize_version: 2 -->
<!-- ### output_tensor_quantised: 1 -->
<!-- ### convert_type: hf -->
<!-- ### vocab_type: -->
<!-- ### tags: -->
static quants of https://huggingface.co/Magpie-Align/Llama-3-8B-Instruct-UltraDPO3
<!-- provided-files -->
weighted/imatrix quants seem not to be available (by me) at this time. If they do not show up a week or so after the static ones, I have probably not planned for them. Feel free to request them by opening a Community Discussion.
## Usage
If you are unsure how to use GGUF files, refer to one of [TheBloke's
READMEs](https://huggingface.co/TheBloke/KafkaLM-70B-German-V0.1-GGUF) for
more details, including on how to concatenate multi-part files.
## Provided Quants
(sorted by size, not necessarily quality. IQ-quants are often preferable over similar sized non-IQ quants)
| Link | Type | Size/GB | Notes |
|:-----|:-----|--------:|:------|
| [GGUF](https://huggingface.co/mradermacher/Llama-3-8B-Instruct-UltraDPO3-GGUF/resolve/main/Llama-3-8B-Instruct-UltraDPO3.Q2_K.gguf) | Q2_K | 3.3 | |
| [GGUF](https://huggingface.co/mradermacher/Llama-3-8B-Instruct-UltraDPO3-GGUF/resolve/main/Llama-3-8B-Instruct-UltraDPO3.IQ3_XS.gguf) | IQ3_XS | 3.6 | |
| [GGUF](https://huggingface.co/mradermacher/Llama-3-8B-Instruct-UltraDPO3-GGUF/resolve/main/Llama-3-8B-Instruct-UltraDPO3.Q3_K_S.gguf) | Q3_K_S | 3.8 | |
| [GGUF](https://huggingface.co/mradermacher/Llama-3-8B-Instruct-UltraDPO3-GGUF/resolve/main/Llama-3-8B-Instruct-UltraDPO3.IQ3_S.gguf) | IQ3_S | 3.8 | beats Q3_K* |
| [GGUF](https://huggingface.co/mradermacher/Llama-3-8B-Instruct-UltraDPO3-GGUF/resolve/main/Llama-3-8B-Instruct-UltraDPO3.IQ3_M.gguf) | IQ3_M | 3.9 | |
| [GGUF](https://huggingface.co/mradermacher/Llama-3-8B-Instruct-UltraDPO3-GGUF/resolve/main/Llama-3-8B-Instruct-UltraDPO3.Q3_K_M.gguf) | Q3_K_M | 4.1 | lower quality |
| [GGUF](https://huggingface.co/mradermacher/Llama-3-8B-Instruct-UltraDPO3-GGUF/resolve/main/Llama-3-8B-Instruct-UltraDPO3.Q3_K_L.gguf) | Q3_K_L | 4.4 | |
| [GGUF](https://huggingface.co/mradermacher/Llama-3-8B-Instruct-UltraDPO3-GGUF/resolve/main/Llama-3-8B-Instruct-UltraDPO3.IQ4_XS.gguf) | IQ4_XS | 4.6 | |
| [GGUF](https://huggingface.co/mradermacher/Llama-3-8B-Instruct-UltraDPO3-GGUF/resolve/main/Llama-3-8B-Instruct-UltraDPO3.Q4_K_S.gguf) | Q4_K_S | 4.8 | fast, recommended |
| [GGUF](https://huggingface.co/mradermacher/Llama-3-8B-Instruct-UltraDPO3-GGUF/resolve/main/Llama-3-8B-Instruct-UltraDPO3.Q4_K_M.gguf) | Q4_K_M | 5.0 | fast, recommended |
| [GGUF](https://huggingface.co/mradermacher/Llama-3-8B-Instruct-UltraDPO3-GGUF/resolve/main/Llama-3-8B-Instruct-UltraDPO3.Q5_K_S.gguf) | Q5_K_S | 5.7 | |
| [GGUF](https://huggingface.co/mradermacher/Llama-3-8B-Instruct-UltraDPO3-GGUF/resolve/main/Llama-3-8B-Instruct-UltraDPO3.Q5_K_M.gguf) | Q5_K_M | 5.8 | |
| [GGUF](https://huggingface.co/mradermacher/Llama-3-8B-Instruct-UltraDPO3-GGUF/resolve/main/Llama-3-8B-Instruct-UltraDPO3.Q6_K.gguf) | Q6_K | 6.7 | very good quality |
| [GGUF](https://huggingface.co/mradermacher/Llama-3-8B-Instruct-UltraDPO3-GGUF/resolve/main/Llama-3-8B-Instruct-UltraDPO3.Q8_0.gguf) | Q8_0 | 8.6 | fast, best quality |
| [GGUF](https://huggingface.co/mradermacher/Llama-3-8B-Instruct-UltraDPO3-GGUF/resolve/main/Llama-3-8B-Instruct-UltraDPO3.f16.gguf) | f16 | 16.2 | 16 bpw, overkill |
Here is a handy graph by ikawrakow comparing some lower-quality quant
types (lower is better):

And here are Artefact2's thoughts on the matter:
https://gist.github.com/Artefact2/b5f810600771265fc1e39442288e8ec9
## FAQ / Model Request
See https://huggingface.co/mradermacher/model_requests for some answers to
questions you might have and/or if you want some other model quantized.
## Thanks
I thank my company, [nethype GmbH](https://www.nethype.de/), for letting
me use its servers and providing upgrades to my workstation to enable
this work in my free time.
<!-- end -->
|
QuantFactory/Llama-3-Ko-Luxia-Instruct-GGUF | QuantFactory | 2024-06-28T13:11:43Z | 11,957 | 0 | transformers | [
"transformers",
"gguf",
"text-generation",
"base_model:maywell/Llama-3-Ko-Luxia-Instruct",
"endpoints_compatible",
"region:us"
]
| text-generation | 2024-06-26T15:41:42Z | ---
library_name: transformers
pipeline_tag: text-generation
base_model: maywell/Llama-3-Ko-Luxia-Instruct
---
# QuantFactory/Llama-3-Ko-Luxia-Instruct-GGUF
This is quantized version of [maywell/Llama-3-Ko-Luxia-Instruct](https://huggingface.co/maywell/Llama-3-Ko-Luxia-Instruct) created using llama.cpp
### Template
ChatML |
castorini/monot5-base-msmarco | castorini | 2021-11-24T17:59:19Z | 11,955 | 6 | transformers | [
"transformers",
"pytorch",
"jax",
"t5",
"text2text-generation",
"autotrain_compatible",
"endpoints_compatible",
"text-generation-inference",
"region:us"
]
| text2text-generation | 2022-03-02T23:29:05Z | This model is a T5-base reranker fine-tuned on the MS MARCO passage dataset for 100k steps (or 10 epochs).
For better zero-shot performance (i.e., inference on other datasets), we recommend using `castorini/monot5-base-msmarco-10k`.
For more details on how to use it, check the following links:
- [A simple reranking example](https://github.com/castorini/pygaggle#a-simple-reranking-example)
- [Rerank MS MARCO passages](https://github.com/castorini/pygaggle/blob/master/docs/experiments-msmarco-passage-subset.md)
- [Rerank Robust04 documents](https://github.com/castorini/pygaggle/blob/master/docs/experiments-robust04-monot5-gpu.md)
Paper describing the model: [Document Ranking with a Pretrained Sequence-to-Sequence Model](https://www.aclweb.org/anthology/2020.findings-emnlp.63/) |
internlm/internlm-xcomposer2-vl-7b-4bit | internlm | 2024-02-06T13:06:34Z | 11,946 | 23 | transformers | [
"transformers",
"internlm",
"feature-extraction",
"text-generation",
"custom_code",
"arxiv:2401.16420",
"license:other",
"region:us"
]
| text-generation | 2024-02-06T12:15:29Z | ---
license: other
pipeline_tag: text-generation
---
<p align="center">
<img src="logo_en.png" width="400"/>
<p>
<p align="center">
<b><font size="6">InternLM-XComposer2</font></b>
<p>
<div align="center">
[💻Github Repo](https://github.com/InternLM/InternLM-XComposer)
[Paper](https://arxiv.org/abs/2401.16420)
</div>
**InternLM-XComposer2** is a vision-language large model (VLLM) based on [InternLM2](https://github.com/InternLM/InternLM) for advanced text-image comprehension and composition.
We release InternLM-XComposer2 series in two versions:
- InternLM-XComposer2-VL: The pretrained VLLM model with InternLM2 as the initialization of the LLM, achieving strong performance on various multimodal benchmarks.
- InternLM-XComposer2: The finetuned VLLM for *Free-from Interleaved Text-Image Composition*.
This is the 4-bit version of InternLM-XComposer2-VL, install the latest version of [auto_gptq](https://github.com/AutoGPTQ/AutoGPTQ#quick-installation) before using.
## Quickstart
We provide a simple example to show how to use InternLM-XComposer with 🤗 Transformers.
```python
import torch, auto_gptq
from transformers import AutoModel, AutoTokenizer
from auto_gptq.modeling import BaseGPTQForCausalLM
auto_gptq.modeling._base.SUPPORTED_MODELS = ["internlm"]
torch.set_grad_enabled(False)
class InternLMXComposer2QForCausalLM(BaseGPTQForCausalLM):
layers_block_name = "model.layers"
outside_layer_modules = [
'vit', 'vision_proj', 'model.tok_embeddings', 'model.norm', 'output',
]
inside_layer_modules = [
["attention.wqkv.linear"],
["attention.wo.linear"],
["feed_forward.w1.linear", "feed_forward.w3.linear"],
["feed_forward.w2.linear"],
]
# init model and tokenizer
model = InternLMXComposer2QForCausalLM.from_quantized(
'internlm/internlm-xcomposer2-vl-7b-4bit', trust_remote_code=True, device="cuda:0").eval()
tokenizer = AutoTokenizer.from_pretrained(
'internlm/internlm-xcomposer2-vl-7b-4bit', trust_remote_code=True)
text = '<ImageHere>Please describe this image in detail.'
image = 'examples/image1.webp'
with torch.cuda.amp.autocast():
response, _ = model.chat(tokenizer, query=query, image=image, history=[], do_sample=False)
print(response)
#The image features a quote by Oscar Wilde, "Live life with no excuses, travel with no regrets."
#The quote is displayed in white text against a dark background. In the foreground, there are two silhouettes of people standing on a hill at sunset.
#They appear to be hiking or climbing, as one of them is holding a walking stick.
#The sky behind them is painted with hues of orange and purple, creating a beautiful contrast with the dark figures.
```
### Open Source License
The code is licensed under Apache-2.0, while model weights are fully open for academic research and also allow free commercial usage. To apply for a commercial license, please fill in the application form (English)/申请表(中文). For other questions or collaborations, please contact [email protected].
|
Subsets and Splits
No community queries yet
The top public SQL queries from the community will appear here once available.