modelId
stringlengths 5
122
| author
stringlengths 2
42
| last_modified
unknown | downloads
int64 0
738M
| likes
int64 0
11k
| library_name
stringclasses 245
values | tags
sequencelengths 1
4.05k
| pipeline_tag
stringclasses 48
values | createdAt
unknown | card
stringlengths 1
901k
|
---|---|---|---|---|---|---|---|---|---|
mradermacher/Gromenauer-7B-Instruct-GGUF | mradermacher | "2024-06-24T21:27:43Z" | 13,070 | 0 | transformers | [
"transformers",
"gguf",
"es",
"ca",
"dataset:bertin-project/bonanza-hf",
"dataset:bertin-project/zenobia-instruct-hf",
"base_model:bertin-project/Gromenauer-7B-Instruct",
"license:apache-2.0",
"endpoints_compatible",
"region:us"
] | null | "2024-06-24T21:00:26Z" | ---
base_model: bertin-project/Gromenauer-7B-Instruct
datasets:
- bertin-project/bonanza-hf
- bertin-project/zenobia-instruct-hf
language:
- es
- ca
library_name: transformers
license: apache-2.0
quantized_by: mradermacher
---
## About
<!-- ### quantize_version: 2 -->
<!-- ### output_tensor_quantised: 1 -->
<!-- ### convert_type: hf -->
<!-- ### vocab_type: -->
<!-- ### tags: -->
static quants of https://huggingface.co/bertin-project/Gromenauer-7B-Instruct
<!-- provided-files -->
weighted/imatrix quants seem not to be available (by me) at this time. If they do not show up a week or so after the static ones, I have probably not planned for them. Feel free to request them by opening a Community Discussion.
## Usage
If you are unsure how to use GGUF files, refer to one of [TheBloke's
READMEs](https://huggingface.co/TheBloke/KafkaLM-70B-German-V0.1-GGUF) for
more details, including on how to concatenate multi-part files.
## Provided Quants
(sorted by size, not necessarily quality. IQ-quants are often preferable over similar sized non-IQ quants)
| Link | Type | Size/GB | Notes |
|:-----|:-----|--------:|:------|
| [GGUF](https://huggingface.co/mradermacher/Gromenauer-7B-Instruct-GGUF/resolve/main/Gromenauer-7B-Instruct.Q2_K.gguf) | Q2_K | 2.8 | |
| [GGUF](https://huggingface.co/mradermacher/Gromenauer-7B-Instruct-GGUF/resolve/main/Gromenauer-7B-Instruct.IQ3_XS.gguf) | IQ3_XS | 3.1 | |
| [GGUF](https://huggingface.co/mradermacher/Gromenauer-7B-Instruct-GGUF/resolve/main/Gromenauer-7B-Instruct.Q3_K_S.gguf) | Q3_K_S | 3.3 | |
| [GGUF](https://huggingface.co/mradermacher/Gromenauer-7B-Instruct-GGUF/resolve/main/Gromenauer-7B-Instruct.IQ3_S.gguf) | IQ3_S | 3.3 | beats Q3_K* |
| [GGUF](https://huggingface.co/mradermacher/Gromenauer-7B-Instruct-GGUF/resolve/main/Gromenauer-7B-Instruct.IQ3_M.gguf) | IQ3_M | 3.4 | |
| [GGUF](https://huggingface.co/mradermacher/Gromenauer-7B-Instruct-GGUF/resolve/main/Gromenauer-7B-Instruct.Q3_K_M.gguf) | Q3_K_M | 3.6 | lower quality |
| [GGUF](https://huggingface.co/mradermacher/Gromenauer-7B-Instruct-GGUF/resolve/main/Gromenauer-7B-Instruct.Q3_K_L.gguf) | Q3_K_L | 3.9 | |
| [GGUF](https://huggingface.co/mradermacher/Gromenauer-7B-Instruct-GGUF/resolve/main/Gromenauer-7B-Instruct.IQ4_XS.gguf) | IQ4_XS | 4.0 | |
| [GGUF](https://huggingface.co/mradermacher/Gromenauer-7B-Instruct-GGUF/resolve/main/Gromenauer-7B-Instruct.Q4_K_S.gguf) | Q4_K_S | 4.2 | fast, recommended |
| [GGUF](https://huggingface.co/mradermacher/Gromenauer-7B-Instruct-GGUF/resolve/main/Gromenauer-7B-Instruct.Q4_K_M.gguf) | Q4_K_M | 4.5 | fast, recommended |
| [GGUF](https://huggingface.co/mradermacher/Gromenauer-7B-Instruct-GGUF/resolve/main/Gromenauer-7B-Instruct.Q5_K_S.gguf) | Q5_K_S | 5.1 | |
| [GGUF](https://huggingface.co/mradermacher/Gromenauer-7B-Instruct-GGUF/resolve/main/Gromenauer-7B-Instruct.Q5_K_M.gguf) | Q5_K_M | 5.2 | |
| [GGUF](https://huggingface.co/mradermacher/Gromenauer-7B-Instruct-GGUF/resolve/main/Gromenauer-7B-Instruct.Q6_K.gguf) | Q6_K | 6.0 | very good quality |
| [GGUF](https://huggingface.co/mradermacher/Gromenauer-7B-Instruct-GGUF/resolve/main/Gromenauer-7B-Instruct.Q8_0.gguf) | Q8_0 | 7.8 | fast, best quality |
| [GGUF](https://huggingface.co/mradermacher/Gromenauer-7B-Instruct-GGUF/resolve/main/Gromenauer-7B-Instruct.f16.gguf) | f16 | 14.6 | 16 bpw, overkill |
Here is a handy graph by ikawrakow comparing some lower-quality quant
types (lower is better):

And here are Artefact2's thoughts on the matter:
https://gist.github.com/Artefact2/b5f810600771265fc1e39442288e8ec9
## FAQ / Model Request
See https://huggingface.co/mradermacher/model_requests for some answers to
questions you might have and/or if you want some other model quantized.
## Thanks
I thank my company, [nethype GmbH](https://www.nethype.de/), for letting
me use its servers and providing upgrades to my workstation to enable
this work in my free time.
<!-- end -->
|
Qwen/Qwen1.5-110B-Chat | Qwen | "2024-04-30T07:22:18Z" | 13,067 | 117 | transformers | [
"transformers",
"safetensors",
"qwen2",
"text-generation",
"chat",
"conversational",
"en",
"license:other",
"autotrain_compatible",
"endpoints_compatible",
"text-generation-inference",
"region:us"
] | text-generation | "2024-04-25T14:30:47Z" | ---
license: other
license_name: tongyi-qianwen
license_link: >-
https://huggingface.co/Qwen/Qwen1.5-110B-Chat/blob/main/LICENSE
language:
- en
pipeline_tag: text-generation
tags:
- chat
---
# Qwen1.5-110B-Chat
## Introduction
Qwen1.5 is the beta version of Qwen2, a transformer-based decoder-only language model pretrained on a large amount of data. In comparison with the previous released Qwen, the improvements include:
* 9 model sizes, including 0.5B, 1.8B, 4B, 7B, 14B, 32B, 72B, and 110B dense models, and an MoE model of 14B with 2.7B activated;
* Significant performance improvement in human preference for chat models;
* Multilingual support of both base and chat models;
* Stable support of 32K context length for models of all sizes
* No need of `trust_remote_code`.
For more details, please refer to our [blog post](https://qwenlm.github.io/blog/qwen1.5/) and [GitHub repo](https://github.com/QwenLM/Qwen1.5).
<br>
## Model Details
Qwen1.5 is a language model series including decoder language models of different model sizes. For each size, we release the base language model and the aligned chat model. It is based on the Transformer architecture with SwiGLU activation, attention QKV bias, group query attention, mixture of sliding window attention and full attention, etc. Additionally, we have an improved tokenizer adaptive to multiple natural languages and codes. For the beta version, temporarily we did not include GQA (except for 32B and 110B) and the mixture of SWA and full attention.
## Training details
We pretrained the models with a large amount of data, and we post-trained the models with both supervised finetuning and direct preference optimization.
## Requirements
The code of Qwen1.5 has been in the latest Hugging face transformers and we advise you to install `transformers>=4.37.0`, or you might encounter the following error:
```
KeyError: 'qwen2'
```
## Quickstart
Here provides a code snippet with `apply_chat_template` to show you how to load the tokenizer and model and how to generate contents.
```python
from transformers import AutoModelForCausalLM, AutoTokenizer
device = "cuda" # the device to load the model onto
model = AutoModelForCausalLM.from_pretrained(
"Qwen/Qwen1.5-110B-Chat",
torch_dtype="auto",
device_map="auto"
)
tokenizer = AutoTokenizer.from_pretrained("Qwen/Qwen1.5-110B-Chat")
prompt = "Give me a short introduction to large language model."
messages = [
{"role": "system", "content": "You are a helpful assistant."},
{"role": "user", "content": prompt}
]
text = tokenizer.apply_chat_template(
messages,
tokenize=False,
add_generation_prompt=True
)
model_inputs = tokenizer([text], return_tensors="pt").to(device)
generated_ids = model.generate(
model_inputs.input_ids,
max_new_tokens=512
)
generated_ids = [
output_ids[len(input_ids):] for input_ids, output_ids in zip(model_inputs.input_ids, generated_ids)
]
response = tokenizer.batch_decode(generated_ids, skip_special_tokens=True)[0]
```
## Tips
* If you encounter code switching or other bad cases, we advise you to use our provided hyper-parameters in `generation_config.json`.
## Citation
If you find our work helpful, feel free to give us a cite.
```
@article{qwen,
title={Qwen Technical Report},
author={Jinze Bai and Shuai Bai and Yunfei Chu and Zeyu Cui and Kai Dang and Xiaodong Deng and Yang Fan and Wenbin Ge and Yu Han and Fei Huang and Binyuan Hui and Luo Ji and Mei Li and Junyang Lin and Runji Lin and Dayiheng Liu and Gao Liu and Chengqiang Lu and Keming Lu and Jianxin Ma and Rui Men and Xingzhang Ren and Xuancheng Ren and Chuanqi Tan and Sinan Tan and Jianhong Tu and Peng Wang and Shijie Wang and Wei Wang and Shengguang Wu and Benfeng Xu and Jin Xu and An Yang and Hao Yang and Jian Yang and Shusheng Yang and Yang Yao and Bowen Yu and Hongyi Yuan and Zheng Yuan and Jianwei Zhang and Xingxuan Zhang and Yichang Zhang and Zhenru Zhang and Chang Zhou and Jingren Zhou and Xiaohuan Zhou and Tianhang Zhu},
journal={arXiv preprint arXiv:2309.16609},
year={2023}
}
```
|
THUDM/cogvlm2-llama3-chinese-chat-19B-int4 | THUDM | "2024-05-24T10:01:50Z" | 13,065 | 7 | transformers | [
"transformers",
"pytorch",
"text-generation",
"chat",
"cogvlm2",
"conversational",
"custom_code",
"en",
"arxiv:2311.03079",
"license:other",
"autotrain_compatible",
"4-bit",
"bitsandbytes",
"region:us"
] | text-generation | "2024-05-24T07:30:55Z" | ---
license: other
license_name: cogvlm2
license_link: https://huggingface.co/THUDM/cogvlm2-llama3-chinese-chat-19B-int4/blob/main/LICENSE
language:
- en
pipeline_tag: text-generation
tags:
- chat
- cogvlm2
inference: false
---
# CogVLM2
<div align="center">
<img src=https://raw.githubusercontent.com/THUDM/CogVLM2/53d5d5ea1aa8d535edffc0d15e31685bac40f878/resources/logo.svg width="40%"/>
</div>
<p align="center">
👋 <a href="resources/WECHAT.md" target="_blank">Wechat</a> · 💡<a href="http://36.103.203.44:7861/" target="_blank">Online Demo</a> · 🎈<a href="https://github.com/THUDM/CogVLM2" target="_blank">Github Page</a>
</p>
<p align="center">
📍Experience the larger-scale CogVLM model on the <a href="https://open.bigmodel.cn/dev/api#glm-4v">ZhipuAI Open Platform</a>.
</p>
## Model introduction
We launch a new generation of **CogVLM2** series of models and open source two models built
with [Meta-Llama-3-8B-Instruct](https://huggingface.co/meta-llama/Meta-Llama-3-8B-Instruct). Compared with the previous
generation of CogVLM open source models, the CogVLM2 series of open source models have the following improvements:
1. Significant improvements in many benchmarks such as `TextVQA`, `DocVQA`.
2. Support **8K** content length.
3. Support image resolution up to **1344 * 1344**.
4. Provide an open source model version that supports both **Chinese and English**.
CogVlM2 Int4 model requires 16G GPU memory and Must be run on Linux with Nvidia GPU.
| Model name | cogvlm2-llama3-chinese-chat-19B-int4 | cogvlm2-llama3-chinese-chat-19B |
|---------------------|--------------------------------------|-------------------------|
| GPU Memory Required | 16G | 42G |
| System Required | Linux (With Nvidia GPU) | Linux (With Nvidia GPU) |
## Benchmark
Our open source models have achieved good results in many lists compared to the previous generation of CogVLM open
source models. Its excellent performance can compete with some non-open source models, as shown in the table below:
| Model | Open Source | LLM Size | TextVQA | DocVQA | ChartQA | OCRbench | MMMU | MMVet | MMBench |
|--------------------------------|-------------|----------|----------|----------|----------|----------|----------|----------|----------|
| CogVLM1.1 | ✅ | 7B | 69.7 | - | 68.3 | 590 | 37.3 | 52.0 | 65.8 |
| LLaVA-1.5 | ✅ | 13B | 61.3 | - | - | 337 | 37.0 | 35.4 | 67.7 |
| Mini-Gemini | ✅ | 34B | 74.1 | - | - | - | 48.0 | 59.3 | 80.6 |
| LLaVA-NeXT-LLaMA3 | ✅ | 8B | - | 78.2 | 69.5 | - | 41.7 | - | 72.1 |
| LLaVA-NeXT-110B | ✅ | 110B | - | 85.7 | 79.7 | - | 49.1 | - | 80.5 |
| InternVL-1.5 | ✅ | 20B | 80.6 | 90.9 | **83.8** | 720 | 46.8 | 55.4 | **82.3** |
| QwenVL-Plus | ❌ | - | 78.9 | 91.4 | 78.1 | 726 | 51.4 | 55.7 | 67.0 |
| Claude3-Opus | ❌ | - | - | 89.3 | 80.8 | 694 | **59.4** | 51.7 | 63.3 |
| Gemini Pro 1.5 | ❌ | - | 73.5 | 86.5 | 81.3 | - | 58.5 | - | - |
| GPT-4V | ❌ | - | 78.0 | 88.4 | 78.5 | 656 | 56.8 | **67.7** | 75.0 |
| CogVLM2-LLaMA3 (Ours) | ✅ | 8B | 84.2 | **92.3** | 81.0 | 756 | 44.3 | 60.4 | 80.5 |
| CogVLM2-LLaMA3-Chinese (Ours) | ✅ | 8B | **85.0** | 88.4 | 74.7 | **780** | 42.8 | 60.5 | 78.9 |
All reviews were obtained without using any external OCR tools ("pixel only").
## Quick Start
here is a simple example of how to use the model to chat with the CogVLM2 model. For More use case. Find in
our [github](https://github.com/THUDM/CogVLM2)
```python
import torch
from PIL import Image
from transformers import AutoModelForCausalLM, AutoTokenizer
MODEL_PATH = "THUDM/cogvlm2-llama3-chinese-chat-19B-int4"
DEVICE = 'cuda' if torch.cuda.is_available() else 'cpu'
TORCH_TYPE = torch.bfloat16 if torch.cuda.is_available() and torch.cuda.get_device_capability()[
0] >= 8 else torch.float16
tokenizer = AutoTokenizer.from_pretrained(
MODEL_PATH,
trust_remote_code=True
)
model = AutoModelForCausalLM.from_pretrained(
MODEL_PATH,
torch_dtype=TORCH_TYPE,
trust_remote_code=True,
low_cpu_mem_usage=True,
).eval()
text_only_template = "A chat between a curious user and an artificial intelligence assistant. The assistant gives helpful, detailed, and polite answers to the user's questions. USER: {} ASSISTANT:"
while True:
image_path = input("image path >>>>> ")
if image_path == '':
print('You did not enter image path, the following will be a plain text conversation.')
image = None
text_only_first_query = True
else:
image = Image.open(image_path).convert('RGB')
history = []
while True:
query = input("Human:")
if query == "clear":
break
if image is None:
if text_only_first_query:
query = text_only_template.format(query)
text_only_first_query = False
else:
old_prompt = ''
for _, (old_query, response) in enumerate(history):
old_prompt += old_query + " " + response + "\n"
query = old_prompt + "USER: {} ASSISTANT:".format(query)
if image is None:
input_by_model = model.build_conversation_input_ids(
tokenizer,
query=query,
history=history,
template_version='chat'
)
else:
input_by_model = model.build_conversation_input_ids(
tokenizer,
query=query,
history=history,
images=[image],
template_version='chat'
)
inputs = {
'input_ids': input_by_model['input_ids'].unsqueeze(0).to(DEVICE),
'token_type_ids': input_by_model['token_type_ids'].unsqueeze(0).to(DEVICE),
'attention_mask': input_by_model['attention_mask'].unsqueeze(0).to(DEVICE),
'images': [[input_by_model['images'][0].to(DEVICE).to(TORCH_TYPE)]] if image is not None else None,
}
gen_kwargs = {
"max_new_tokens": 2048,
"pad_token_id": 128002,
}
with torch.no_grad():
outputs = model.generate(**inputs, **gen_kwargs)
outputs = outputs[:, inputs['input_ids'].shape[1]:]
response = tokenizer.decode(outputs[0])
response = response.split("<|end_of_text|>")[0]
print("\nCogVLM2:", response)
history.append((query, response))
```
## License
This model is released under the CogVLM2 [LICENSE](LICENSE). For models built with Meta Llama 3, please also adhere to
the [LLAMA3_LICENSE](LLAMA3_LICENSE).
## Citation
If you find our work helpful, please consider citing the following papers
```
@misc{wang2023cogvlm,
title={CogVLM: Visual Expert for Pretrained Language Models},
author={Weihan Wang and Qingsong Lv and Wenmeng Yu and Wenyi Hong and Ji Qi and Yan Wang and Junhui Ji and Zhuoyi Yang and Lei Zhao and Xixuan Song and Jiazheng Xu and Bin Xu and Juanzi Li and Yuxiao Dong and Ming Ding and Jie Tang},
year={2023},
eprint={2311.03079},
archivePrefix={arXiv},
primaryClass={cs.CV}
}
```
|
mradermacher/XiXiLM-GGUF | mradermacher | "2024-07-02T02:26:30Z" | 13,057 | 0 | transformers | [
"transformers",
"gguf",
"en",
"base_model:AI4Bread/XiXiLM",
"license:other",
"endpoints_compatible",
"region:us"
] | null | "2024-07-02T01:29:28Z" | ---
base_model: AI4Bread/XiXiLM
language:
- en
library_name: transformers
license: other
quantized_by: mradermacher
---
## About
<!-- ### quantize_version: 2 -->
<!-- ### output_tensor_quantised: 1 -->
<!-- ### convert_type: hf -->
<!-- ### vocab_type: -->
<!-- ### tags: -->
static quants of https://huggingface.co/AI4Bread/XiXiLM
<!-- provided-files -->
weighted/imatrix quants seem not to be available (by me) at this time. If they do not show up a week or so after the static ones, I have probably not planned for them. Feel free to request them by opening a Community Discussion.
## Usage
If you are unsure how to use GGUF files, refer to one of [TheBloke's
READMEs](https://huggingface.co/TheBloke/KafkaLM-70B-German-V0.1-GGUF) for
more details, including on how to concatenate multi-part files.
## Provided Quants
(sorted by size, not necessarily quality. IQ-quants are often preferable over similar sized non-IQ quants)
| Link | Type | Size/GB | Notes |
|:-----|:-----|--------:|:------|
| [GGUF](https://huggingface.co/mradermacher/XiXiLM-GGUF/resolve/main/XiXiLM.Q2_K.gguf) | Q2_K | 3.1 | |
| [GGUF](https://huggingface.co/mradermacher/XiXiLM-GGUF/resolve/main/XiXiLM.IQ3_XS.gguf) | IQ3_XS | 3.4 | |
| [GGUF](https://huggingface.co/mradermacher/XiXiLM-GGUF/resolve/main/XiXiLM.Q3_K_S.gguf) | Q3_K_S | 3.6 | |
| [GGUF](https://huggingface.co/mradermacher/XiXiLM-GGUF/resolve/main/XiXiLM.IQ3_S.gguf) | IQ3_S | 3.6 | beats Q3_K* |
| [GGUF](https://huggingface.co/mradermacher/XiXiLM-GGUF/resolve/main/XiXiLM.IQ3_M.gguf) | IQ3_M | 3.7 | |
| [GGUF](https://huggingface.co/mradermacher/XiXiLM-GGUF/resolve/main/XiXiLM.Q3_K_M.gguf) | Q3_K_M | 3.9 | lower quality |
| [GGUF](https://huggingface.co/mradermacher/XiXiLM-GGUF/resolve/main/XiXiLM.Q3_K_L.gguf) | Q3_K_L | 4.2 | |
| [GGUF](https://huggingface.co/mradermacher/XiXiLM-GGUF/resolve/main/XiXiLM.IQ4_XS.gguf) | IQ4_XS | 4.4 | |
| [GGUF](https://huggingface.co/mradermacher/XiXiLM-GGUF/resolve/main/XiXiLM.Q4_K_S.gguf) | Q4_K_S | 4.6 | fast, recommended |
| [GGUF](https://huggingface.co/mradermacher/XiXiLM-GGUF/resolve/main/XiXiLM.Q4_K_M.gguf) | Q4_K_M | 4.8 | fast, recommended |
| [GGUF](https://huggingface.co/mradermacher/XiXiLM-GGUF/resolve/main/XiXiLM.Q5_K_S.gguf) | Q5_K_S | 5.5 | |
| [GGUF](https://huggingface.co/mradermacher/XiXiLM-GGUF/resolve/main/XiXiLM.Q5_K_M.gguf) | Q5_K_M | 5.6 | |
| [GGUF](https://huggingface.co/mradermacher/XiXiLM-GGUF/resolve/main/XiXiLM.Q6_K.gguf) | Q6_K | 6.5 | very good quality |
| [GGUF](https://huggingface.co/mradermacher/XiXiLM-GGUF/resolve/main/XiXiLM.Q8_0.gguf) | Q8_0 | 8.3 | fast, best quality |
| [GGUF](https://huggingface.co/mradermacher/XiXiLM-GGUF/resolve/main/XiXiLM.f16.gguf) | f16 | 15.6 | 16 bpw, overkill |
Here is a handy graph by ikawrakow comparing some lower-quality quant
types (lower is better):

And here are Artefact2's thoughts on the matter:
https://gist.github.com/Artefact2/b5f810600771265fc1e39442288e8ec9
## FAQ / Model Request
See https://huggingface.co/mradermacher/model_requests for some answers to
questions you might have and/or if you want some other model quantized.
## Thanks
I thank my company, [nethype GmbH](https://www.nethype.de/), for letting
me use its servers and providing upgrades to my workstation to enable
this work in my free time.
<!-- end -->
|
thodsapon/V1_test | thodsapon | "2024-07-02T14:49:08Z" | 13,048 | 0 | transformers | [
"transformers",
"gguf",
"llama",
"endpoints_compatible",
"text-generation-inference",
"region:us"
] | null | "2024-07-01T06:25:51Z" | typh1.5 8b, 4096, sample พี่เอ็ม + schema, r64a128, epoch1, learning2e-4 |
NousResearch/Llama-2-13b-hf | NousResearch | "2024-06-03T19:23:22Z" | 13,045 | 69 | transformers | [
"transformers",
"pytorch",
"safetensors",
"llama",
"text-generation",
"facebook",
"meta",
"llama-2",
"en",
"autotrain_compatible",
"text-generation-inference",
"region:us"
] | text-generation | "2023-07-18T19:16:15Z" | ---
extra_gated_heading: Access Llama 2 on Hugging Face
extra_gated_description: >-
This is a form to enable access to Llama 2 on Hugging Face after you have been
granted access from Meta. Please visit the [Meta website](https://ai.meta.com/resources/models-and-libraries/llama-downloads) and accept our
license terms and acceptable use policy before submitting this form. Requests
will be processed in 1-2 days.
extra_gated_button_content: Submit
extra_gated_fields:
I agree to share my name, email address and username with Meta and confirm that I have already been granted download access on the Meta website: checkbox
language:
- en
pipeline_tag: text-generation
inference: false
tags:
- facebook
- meta
- pytorch
- llama
- llama-2
---
# **Llama 2**
Llama 2 is a collection of pretrained and fine-tuned generative text models ranging in scale from 7 billion to 70 billion parameters. This is the repository for the 13B pretrained model, converted for the Hugging Face Transformers format. Links to other models can be found in the index at the bottom.
## Model Details
*Note: Use of this model is governed by the Meta license. In order to download the model weights and tokenizer, please visit the [website](https://ai.meta.com/resources/models-and-libraries/llama-downloads/) and accept our License before requesting access here.*
Meta developed and publicly released the Llama 2 family of large language models (LLMs), a collection of pretrained and fine-tuned generative text models ranging in scale from 7 billion to 70 billion parameters. Our fine-tuned LLMs, called Llama-2-Chat, are optimized for dialogue use cases. Llama-2-Chat models outperform open-source chat models on most benchmarks we tested, and in our human evaluations for helpfulness and safety, are on par with some popular closed-source models like ChatGPT and PaLM.
**Model Developers** Meta
**Variations** Llama 2 comes in a range of parameter sizes — 7B, 13B, and 70B — as well as pretrained and fine-tuned variations.
**Input** Models input text only.
**Output** Models generate text only.
**Model Architecture** Llama 2 is an auto-regressive language model that uses an optimized transformer architecture. The tuned versions use supervised fine-tuning (SFT) and reinforcement learning with human feedback (RLHF) to align to human preferences for helpfulness and safety.
||Training Data|Params|Content Length|GQA|Tokens|LR|
|---|---|---|---|---|---|---|
|Llama 2|*A new mix of publicly available online data*|7B|4k|✗|2.0T|3.0 x 10<sup>-4</sup>|
|Llama 2|*A new mix of publicly available online data*|13B|4k|✗|2.0T|3.0 x 10<sup>-4</sup>|
|Llama 2|*A new mix of publicly available online data*|70B|4k|✔|2.0T|1.5 x 10<sup>-4</sup>|
*Llama 2 family of models.* Token counts refer to pretraining data only. All models are trained with a global batch-size of 4M tokens. Bigger models - 70B -- use Grouped-Query Attention (GQA) for improved inference scalability.
**Model Dates** Llama 2 was trained between January 2023 and July 2023.
**Status** This is a static model trained on an offline dataset. Future versions of the tuned models will be released as we improve model safety with community feedback.
**License** A custom commercial license is available at: [https://ai.meta.com/resources/models-and-libraries/llama-downloads/](https://ai.meta.com/resources/models-and-libraries/llama-downloads/)
## Intended Use
**Intended Use Cases** Llama 2 is intended for commercial and research use in English. Tuned models are intended for assistant-like chat, whereas pretrained models can be adapted for a variety of natural language generation tasks.
To get the expected features and performance for the chat versions, a specific formatting needs to be followed, including the `INST` and `<<SYS>>` tags, `BOS` and `EOS` tokens, and the whitespaces and breaklines in between (we recommend calling `strip()` on inputs to avoid double-spaces). See our reference code in github for details: [`chat_completion`](https://github.com/facebookresearch/llama/blob/main/llama/generation.py#L212).
**Out-of-scope Uses** Use in any manner that violates applicable laws or regulations (including trade compliance laws).Use in languages other than English. Use in any other way that is prohibited by the Acceptable Use Policy and Licensing Agreement for Llama 2.
## Hardware and Software
**Training Factors** We used custom training libraries, Meta's Research Super Cluster, and production clusters for pretraining. Fine-tuning, annotation, and evaluation were also performed on third-party cloud compute.
**Carbon Footprint** Pretraining utilized a cumulative 3.3M GPU hours of computation on hardware of type A100-80GB (TDP of 350-400W). Estimated total emissions were 539 tCO2eq, 100% of which were offset by Meta’s sustainability program.
||Time (GPU hours)|Power Consumption (W)|Carbon Emitted(tCO<sub>2</sub>eq)|
|---|---|---|---|
|Llama 2 7B|184320|400|31.22|
|Llama 2 13B|368640|400|62.44|
|Llama 2 70B|1720320|400|291.42|
|Total|3311616||539.00|
**CO<sub>2</sub> emissions during pretraining.** Time: total GPU time required for training each model. Power Consumption: peak power capacity per GPU device for the GPUs used adjusted for power usage efficiency. 100% of the emissions are directly offset by Meta's sustainability program, and because we are openly releasing these models, the pretraining costs do not need to be incurred by others.
## Training Data
**Overview** Llama 2 was pretrained on 2 trillion tokens of data from publicly available sources. The fine-tuning data includes publicly available instruction datasets, as well as over one million new human-annotated examples. Neither the pretraining nor the fine-tuning datasets include Meta user data.
**Data Freshness** The pretraining data has a cutoff of September 2022, but some tuning data is more recent, up to July 2023.
## Evaluation Results
In this section, we report the results for the Llama 1 and Llama 2 models on standard academic benchmarks.For all the evaluations, we use our internal evaluations library.
|Model|Size|Code|Commonsense Reasoning|World Knowledge|Reading Comprehension|Math|MMLU|BBH|AGI Eval|
|---|---|---|---|---|---|---|---|---|---|
|Llama 1|7B|14.1|60.8|46.2|58.5|6.95|35.1|30.3|23.9|
|Llama 1|13B|18.9|66.1|52.6|62.3|10.9|46.9|37.0|33.9|
|Llama 1|33B|26.0|70.0|58.4|67.6|21.4|57.8|39.8|41.7|
|Llama 1|65B|30.7|70.7|60.5|68.6|30.8|63.4|43.5|47.6|
|Llama 2|7B|16.8|63.9|48.9|61.3|14.6|45.3|32.6|29.3|
|Llama 2|13B|24.5|66.9|55.4|65.8|28.7|54.8|39.4|39.1|
|Llama 2|70B|**37.5**|**71.9**|**63.6**|**69.4**|**35.2**|**68.9**|**51.2**|**54.2**|
**Overall performance on grouped academic benchmarks.** *Code:* We report the average pass@1 scores of our models on HumanEval and MBPP. *Commonsense Reasoning:* We report the average of PIQA, SIQA, HellaSwag, WinoGrande, ARC easy and challenge, OpenBookQA, and CommonsenseQA. We report 7-shot results for CommonSenseQA and 0-shot results for all other benchmarks. *World Knowledge:* We evaluate the 5-shot performance on NaturalQuestions and TriviaQA and report the average. *Reading Comprehension:* For reading comprehension, we report the 0-shot average on SQuAD, QuAC, and BoolQ. *MATH:* We report the average of the GSM8K (8 shot) and MATH (4 shot) benchmarks at top 1.
|||TruthfulQA|Toxigen|
|---|---|---|---|
|Llama 1|7B|27.42|23.00|
|Llama 1|13B|41.74|23.08|
|Llama 1|33B|44.19|22.57|
|Llama 1|65B|48.71|21.77|
|Llama 2|7B|33.29|**21.25**|
|Llama 2|13B|41.86|26.10|
|Llama 2|70B|**50.18**|24.60|
**Evaluation of pretrained LLMs on automatic safety benchmarks.** For TruthfulQA, we present the percentage of generations that are both truthful and informative (the higher the better). For ToxiGen, we present the percentage of toxic generations (the smaller the better).
|||TruthfulQA|Toxigen|
|---|---|---|---|
|Llama-2-Chat|7B|57.04|**0.00**|
|Llama-2-Chat|13B|62.18|**0.00**|
|Llama-2-Chat|70B|**64.14**|0.01|
**Evaluation of fine-tuned LLMs on different safety datasets.** Same metric definitions as above.
## Ethical Considerations and Limitations
Llama 2 is a new technology that carries risks with use. Testing conducted to date has been in English, and has not covered, nor could it cover all scenarios. For these reasons, as with all LLMs, Llama 2’s potential outputs cannot be predicted in advance, and the model may in some instances produce inaccurate, biased or other objectionable responses to user prompts. Therefore, before deploying any applications of Llama 2, developers should perform safety testing and tuning tailored to their specific applications of the model.
Please see the Responsible Use Guide available at [https://ai.meta.com/llama/responsible-use-guide/](https://ai.meta.com/llama/responsible-use-guide)
## Reporting Issues
Please report any software “bug,” or other problems with the models through one of the following means:
- Reporting issues with the model: [github.com/facebookresearch/llama](http://github.com/facebookresearch/llama)
- Reporting problematic content generated by the model: [developers.facebook.com/llama_output_feedback](http://developers.facebook.com/llama_output_feedback)
- Reporting bugs and security concerns: [facebook.com/whitehat/info](http://facebook.com/whitehat/info)
## Llama Model Index
|Model|Llama2|Llama2-hf|Llama2-chat|Llama2-chat-hf|
|---|---|---|---|---|
|7B| [Link](https://huggingface.co/llamaste/Llama-2-7b) | [Link](https://huggingface.co/llamaste/Llama-2-7b-hf) | [Link](https://huggingface.co/llamaste/Llama-2-7b-chat) | [Link](https://huggingface.co/llamaste/Llama-2-7b-chat-hf)|
|13B| [Link](https://huggingface.co/llamaste/Llama-2-13b) | [Link](https://huggingface.co/llamaste/Llama-2-13b-hf) | [Link](https://huggingface.co/llamaste/Llama-2-13b-chat) | [Link](https://huggingface.co/llamaste/Llama-2-13b-hf)|
|70B| [Link](https://huggingface.co/llamaste/Llama-2-70b) | [Link](https://huggingface.co/llamaste/Llama-2-70b-hf) | [Link](https://huggingface.co/llamaste/Llama-2-70b-chat) | [Link](https://huggingface.co/llamaste/Llama-2-70b-hf)| |
kwoncho/losscut_news_pre2024_2 | kwoncho | "2024-06-05T04:47:44Z" | 13,044 | 0 | transformers | [
"transformers",
"pytorch",
"roberta",
"text-classification",
"autotrain_compatible",
"endpoints_compatible",
"region:us"
] | text-classification | "2024-06-05T04:46:51Z" | Entry not found |
svjack/comet-atomic-zh | svjack | "2023-02-22T13:19:08Z" | 13,036 | 2 | transformers | [
"transformers",
"pytorch",
"t5",
"text2text-generation",
"zh",
"autotrain_compatible",
"endpoints_compatible",
"text-generation-inference",
"region:us"
] | text2text-generation | "2023-02-22T11:46:50Z" | ---
language:
- zh
pipeline_tag: text2text-generation
---
```python
from transformers import T5ForConditionalGeneration
from transformers import T5TokenizerFast as T5Tokenizer
import pandas as pd
model = "svjack/comet-atomic-zh"
device = "cpu"
#device = "cuda:0"
tokenizer = T5Tokenizer.from_pretrained(model)
model = T5ForConditionalGeneration.from_pretrained(model).to(device).eval()
NEED_PREFIX = '以下事件有哪些必要的先决条件:'
EFFECT_PREFIX = '下面的事件发生后可能会发生什么:'
INTENT_PREFIX = '以下事件的动机是什么:'
REACT_PREFIX = '以下事件发生后,你有什么感觉:'
event = "X吃了一顿美餐。"
for prefix in [NEED_PREFIX, EFFECT_PREFIX, INTENT_PREFIX, REACT_PREFIX]:
prompt = "{}{}".format(prefix, event)
encode = tokenizer(prompt, return_tensors='pt').to(device)
answer = model.generate(encode.input_ids,
max_length = 128,
num_beams=2,
top_p = 0.95,
top_k = 50,
repetition_penalty = 2.5,
length_penalty=1.0,
early_stopping=True,
)[0]
decoded = tokenizer.decode(answer, skip_special_tokens=True)
print(prompt, "\n---答案:", decoded, "----\n")
```
</br>
```json
以下事件有哪些必要的先决条件:X吃了一顿美餐。
---答案: X买了食物 ----
下面的事件发生后可能会发生什么:X吃了一顿美餐。
---答案: X会吃到好的食物 ----
以下事件的动机是什么:X吃了一顿美餐。
---答案: X想吃东西 ----
以下事件发生后,你有什么感觉:X吃了一顿美餐。
---答案: X的味道很好 ----
``` |
mradermacher/mixtral-8x22b-instruct-oh-i1-GGUF | mradermacher | "2024-07-02T23:04:40Z" | 13,023 | 0 | transformers | [
"transformers",
"gguf",
"en",
"dataset:teknium/OpenHermes-2.5",
"base_model:fireworks-ai/mixtral-8x22b-instruct-oh",
"license:apache-2.0",
"endpoints_compatible",
"region:us"
] | null | "2024-06-29T20:56:57Z" | ---
base_model: fireworks-ai/mixtral-8x22b-instruct-oh
datasets:
- teknium/OpenHermes-2.5
language:
- en
library_name: transformers
license: apache-2.0
quantized_by: mradermacher
---
## About
<!-- ### quantize_version: 2 -->
<!-- ### output_tensor_quantised: 1 -->
<!-- ### convert_type: hf -->
<!-- ### vocab_type: -->
<!-- ### tags: nicoboss -->
weighted/imatrix quants of https://huggingface.co/fireworks-ai/mixtral-8x22b-instruct-oh
<!-- provided-files -->
static quants are available at https://huggingface.co/mradermacher/mixtral-8x22b-instruct-oh-GGUF
## Usage
If you are unsure how to use GGUF files, refer to one of [TheBloke's
READMEs](https://huggingface.co/TheBloke/KafkaLM-70B-German-V0.1-GGUF) for
more details, including on how to concatenate multi-part files.
## Provided Quants
(sorted by size, not necessarily quality. IQ-quants are often preferable over similar sized non-IQ quants)
| Link | Type | Size/GB | Notes |
|:-----|:-----|--------:|:------|
| [GGUF](https://huggingface.co/mradermacher/mixtral-8x22b-instruct-oh-i1-GGUF/resolve/main/mixtral-8x22b-instruct-oh.i1-IQ2_M.gguf) | i1-IQ2_M | 46.8 | |
| [PART 1](https://huggingface.co/mradermacher/mixtral-8x22b-instruct-oh-i1-GGUF/resolve/main/mixtral-8x22b-instruct-oh.i1-Q2_K.gguf.part1of2) [PART 2](https://huggingface.co/mradermacher/mixtral-8x22b-instruct-oh-i1-GGUF/resolve/main/mixtral-8x22b-instruct-oh.i1-Q2_K.gguf.part2of2) | i1-Q2_K | 52.2 | IQ3_XXS probably better |
| [PART 1](https://huggingface.co/mradermacher/mixtral-8x22b-instruct-oh-i1-GGUF/resolve/main/mixtral-8x22b-instruct-oh.i1-IQ3_XXS.gguf.part1of2) [PART 2](https://huggingface.co/mradermacher/mixtral-8x22b-instruct-oh-i1-GGUF/resolve/main/mixtral-8x22b-instruct-oh.i1-IQ3_XXS.gguf.part2of2) | i1-IQ3_XXS | 55.0 | lower quality |
| [PART 1](https://huggingface.co/mradermacher/mixtral-8x22b-instruct-oh-i1-GGUF/resolve/main/mixtral-8x22b-instruct-oh.i1-IQ3_XS.gguf.part1of2) [PART 2](https://huggingface.co/mradermacher/mixtral-8x22b-instruct-oh-i1-GGUF/resolve/main/mixtral-8x22b-instruct-oh.i1-IQ3_XS.gguf.part2of2) | i1-IQ3_XS | 58.3 | |
| [PART 1](https://huggingface.co/mradermacher/mixtral-8x22b-instruct-oh-i1-GGUF/resolve/main/mixtral-8x22b-instruct-oh.i1-Q3_K_S.gguf.part1of2) [PART 2](https://huggingface.co/mradermacher/mixtral-8x22b-instruct-oh-i1-GGUF/resolve/main/mixtral-8x22b-instruct-oh.i1-Q3_K_S.gguf.part2of2) | i1-Q3_K_S | 61.6 | IQ3_XS probably better |
| [PART 1](https://huggingface.co/mradermacher/mixtral-8x22b-instruct-oh-i1-GGUF/resolve/main/mixtral-8x22b-instruct-oh.i1-Q3_K_M.gguf.part1of2) [PART 2](https://huggingface.co/mradermacher/mixtral-8x22b-instruct-oh-i1-GGUF/resolve/main/mixtral-8x22b-instruct-oh.i1-Q3_K_M.gguf.part2of2) | i1-Q3_K_M | 67.9 | IQ3_S probably better |
| [PART 1](https://huggingface.co/mradermacher/mixtral-8x22b-instruct-oh-i1-GGUF/resolve/main/mixtral-8x22b-instruct-oh.i1-Q3_K_L.gguf.part1of2) [PART 2](https://huggingface.co/mradermacher/mixtral-8x22b-instruct-oh-i1-GGUF/resolve/main/mixtral-8x22b-instruct-oh.i1-Q3_K_L.gguf.part2of2) | i1-Q3_K_L | 72.7 | IQ3_M probably better |
| [PART 1](https://huggingface.co/mradermacher/mixtral-8x22b-instruct-oh-i1-GGUF/resolve/main/mixtral-8x22b-instruct-oh.i1-IQ4_XS.gguf.part1of2) [PART 2](https://huggingface.co/mradermacher/mixtral-8x22b-instruct-oh-i1-GGUF/resolve/main/mixtral-8x22b-instruct-oh.i1-IQ4_XS.gguf.part2of2) | i1-IQ4_XS | 75.6 | |
| [PART 1](https://huggingface.co/mradermacher/mixtral-8x22b-instruct-oh-i1-GGUF/resolve/main/mixtral-8x22b-instruct-oh.i1-Q4_0.gguf.part1of2) [PART 2](https://huggingface.co/mradermacher/mixtral-8x22b-instruct-oh-i1-GGUF/resolve/main/mixtral-8x22b-instruct-oh.i1-Q4_0.gguf.part2of2) | i1-Q4_0 | 80.0 | fast, low quality |
| [PART 1](https://huggingface.co/mradermacher/mixtral-8x22b-instruct-oh-i1-GGUF/resolve/main/mixtral-8x22b-instruct-oh.i1-Q4_K_S.gguf.part1of2) [PART 2](https://huggingface.co/mradermacher/mixtral-8x22b-instruct-oh-i1-GGUF/resolve/main/mixtral-8x22b-instruct-oh.i1-Q4_K_S.gguf.part2of2) | i1-Q4_K_S | 80.6 | optimal size/speed/quality |
| [PART 1](https://huggingface.co/mradermacher/mixtral-8x22b-instruct-oh-i1-GGUF/resolve/main/mixtral-8x22b-instruct-oh.i1-Q4_K_M.gguf.part1of2) [PART 2](https://huggingface.co/mradermacher/mixtral-8x22b-instruct-oh-i1-GGUF/resolve/main/mixtral-8x22b-instruct-oh.i1-Q4_K_M.gguf.part2of2) | i1-Q4_K_M | 85.7 | fast, recommended |
| [PART 1](https://huggingface.co/mradermacher/mixtral-8x22b-instruct-oh-i1-GGUF/resolve/main/mixtral-8x22b-instruct-oh.i1-Q5_K_S.gguf.part1of2) [PART 2](https://huggingface.co/mradermacher/mixtral-8x22b-instruct-oh-i1-GGUF/resolve/main/mixtral-8x22b-instruct-oh.i1-Q5_K_S.gguf.part2of2) | i1-Q5_K_S | 97.1 | |
| [PART 1](https://huggingface.co/mradermacher/mixtral-8x22b-instruct-oh-i1-GGUF/resolve/main/mixtral-8x22b-instruct-oh.i1-Q5_K_M.gguf.part1of3) [PART 2](https://huggingface.co/mradermacher/mixtral-8x22b-instruct-oh-i1-GGUF/resolve/main/mixtral-8x22b-instruct-oh.i1-Q5_K_M.gguf.part2of3) [PART 3](https://huggingface.co/mradermacher/mixtral-8x22b-instruct-oh-i1-GGUF/resolve/main/mixtral-8x22b-instruct-oh.i1-Q5_K_M.gguf.part3of3) | i1-Q5_K_M | 100.1 | |
| [PART 1](https://huggingface.co/mradermacher/mixtral-8x22b-instruct-oh-i1-GGUF/resolve/main/mixtral-8x22b-instruct-oh.i1-Q6_K.gguf.part1of3) [PART 2](https://huggingface.co/mradermacher/mixtral-8x22b-instruct-oh-i1-GGUF/resolve/main/mixtral-8x22b-instruct-oh.i1-Q6_K.gguf.part2of3) [PART 3](https://huggingface.co/mradermacher/mixtral-8x22b-instruct-oh-i1-GGUF/resolve/main/mixtral-8x22b-instruct-oh.i1-Q6_K.gguf.part3of3) | i1-Q6_K | 115.6 | practically like static Q6_K |
Here is a handy graph by ikawrakow comparing some lower-quality quant
types (lower is better):

And here are Artefact2's thoughts on the matter:
https://gist.github.com/Artefact2/b5f810600771265fc1e39442288e8ec9
## FAQ / Model Request
See https://huggingface.co/mradermacher/model_requests for some answers to
questions you might have and/or if you want some other model quantized.
## Thanks
I thank my company, [nethype GmbH](https://www.nethype.de/), for letting
me use its servers and providing upgrades to my workstation to enable
this work in my free time. Additional thanks to [@nicoboss](https://huggingface.co/nicoboss) for giving me access to his hardware for calculating the imatrix for these quants.
<!-- end -->
|
bigcode/starcoder2-7b | bigcode | "2024-06-11T08:15:50Z" | 13,022 | 142 | transformers | [
"transformers",
"safetensors",
"starcoder2",
"text-generation",
"code",
"dataset:bigcode/the-stack-v2-train",
"arxiv:2305.13245",
"arxiv:2205.14135",
"arxiv:2004.05150",
"arxiv:2207.14255",
"arxiv:2402.19173",
"license:bigcode-openrail-m",
"model-index",
"autotrain_compatible",
"endpoints_compatible",
"text-generation-inference",
"region:us"
] | text-generation | "2024-02-20T18:00:27Z" | ---
pipeline_tag: text-generation
inference:
parameters:
temperature: 0.2
top_p: 0.95
widget:
- text: 'def print_hello_world():'
example_title: Hello world
group: Python
datasets:
- bigcode/the-stack-v2-train
license: bigcode-openrail-m
library_name: transformers
tags:
- code
model-index:
- name: starcoder2-7b
results:
- task:
type: text-generation
dataset:
name: CruxEval-I
type: cruxeval-i
metrics:
- type: pass@1
value: 34.6
- task:
type: text-generation
dataset:
name: DS-1000
type: ds-1000
metrics:
- type: pass@1
value: 27.8
- task:
type: text-generation
dataset:
name: GSM8K (PAL)
type: gsm8k-pal
metrics:
- type: accuracy
value: 40.4
- task:
type: text-generation
dataset:
name: HumanEval+
type: humanevalplus
metrics:
- type: pass@1
value: 29.9
- task:
type: text-generation
dataset:
name: HumanEval
type: humaneval
metrics:
- type: pass@1
value: 35.4
- task:
type: text-generation
dataset:
name: RepoBench-v1.1
type: repobench-v1.1
metrics:
- type: edit-smiliarity
value: 72.07
---
# StarCoder2
<center>
<img src="https://huggingface.co/datasets/bigcode/admin_private/resolve/main/starcoder2_banner.png" alt="SC2" width="900" height="600">
</center>
## Table of Contents
1. [Model Summary](##model-summary)
2. [Use](##use)
3. [Limitations](##limitations)
4. [Training](##training)
5. [License](##license)
6. [Citation](##citation)
## Model Summary
StarCoder2-7B model is a 7B parameter model trained on 17 programming languages from [The Stack v2](https://huggingface.co/datasets/bigcode/the-stack-v2-train), with opt-out requests excluded. The model uses [Grouped Query Attention](https://arxiv.org/abs/2305.13245), [a context window of 16,384 tokens](https://arxiv.org/abs/2205.14135) with [a sliding window attention of 4,096 tokens](https://arxiv.org/abs/2004.05150v2), and was trained using the [Fill-in-the-Middle objective](https://arxiv.org/abs/2207.14255) on 3.5+ trillion tokens.
- **Project Website:** [bigcode-project.org](https://www.bigcode-project.org)
- **Paper:** [Link](https://huggingface.co/papers/2402.19173)
- **Point of Contact:** [[email protected]](mailto:[email protected])
- **Languages:** 17 Programming languages
## Use
### Intended use
The model was trained on GitHub code as well as additional selected data sources such as Arxiv and Wikipedia. As such it is _not_ an instruction model and commands like "Write a function that computes the square root." do not work well.
### Generation
Here are some examples to get started with the model. You can find a script for fine-tuning in StarCoder2's [GitHub repository](https://github.com/bigcode-project/starcoder2).
First, make sure to install `transformers` from source:
```bash
pip install git+https://github.com/huggingface/transformers.git
```
#### Running the model on CPU/GPU/multi GPU
* _Using full precision_
```python
# pip install git+https://github.com/huggingface/transformers.git # TODO: merge PR to main
from transformers import AutoModelForCausalLM, AutoTokenizer
checkpoint = "bigcode/starcoder2-7b"
device = "cuda" # for GPU usage or "cpu" for CPU usage
tokenizer = AutoTokenizer.from_pretrained(checkpoint)
# for multiple GPUs install accelerate and do `model = AutoModelForCausalLM.from_pretrained(checkpoint, device_map="auto")`
model = AutoModelForCausalLM.from_pretrained(checkpoint).to(device)
inputs = tokenizer.encode("def print_hello_world():", return_tensors="pt").to(device)
outputs = model.generate(inputs)
print(tokenizer.decode(outputs[0]))
```
```bash
>>> print(f"Memory footprint: {model.get_memory_footprint() / 1e6:.2f} MB")
Memory footprint: 29232.57 MB
```
* _Using `torch.bfloat16`_
```python
# pip install accelerate
import torch
from transformers import AutoTokenizer, AutoModelForCausalLM
checkpoint = "bigcode/starcoder2-7b"
tokenizer = AutoTokenizer.from_pretrained(checkpoint)
# for fp16 use `torch_dtype=torch.float16` instead
model = AutoModelForCausalLM.from_pretrained(checkpoint, device_map="auto", torch_dtype=torch.bfloat16)
inputs = tokenizer.encode("def print_hello_world():", return_tensors="pt").to("cuda")
outputs = model.generate(inputs)
print(tokenizer.decode(outputs[0]))
```
```bash
>>> print(f"Memory footprint: {model.get_memory_footprint() / 1e6:.2f} MB")
Memory footprint: 14616.29 MB
```
#### Quantized Versions through `bitsandbytes`
* _Using 8-bit precision (int8)_
```python
# pip install bitsandbytes accelerate
from transformers import AutoTokenizer, AutoModelForCausalLM, BitsAndBytesConfig
# to use 4bit use `load_in_4bit=True` instead
quantization_config = BitsAndBytesConfig(load_in_8bit=True)
checkpoint = "bigcode/starcoder2-7b"
tokenizer = AutoTokenizer.from_pretrained(checkpoint)
model = AutoModelForCausalLM.from_pretrained(checkpoint, quantization_config=quantization_config)
inputs = tokenizer.encode("def print_hello_world():", return_tensors="pt").to("cuda")
outputs = model.generate(inputs)
print(tokenizer.decode(outputs[0]))
```
```bash
>>> print(f"Memory footprint: {model.get_memory_footprint() / 1e6:.2f} MB")
# load_in_8bit
Memory footprint: 7670.52 MB
# load_in_4bit
>>> print(f"Memory footprint: {model.get_memory_footprint() / 1e6:.2f} MB")
Memory footprint: 4197.64 MB
```
### Attribution & Other Requirements
The pretraining dataset of the model was filtered for permissive licenses and code with no license only. Nevertheless, the model can generate source code verbatim from the dataset. The code's license might require attribution and/or other specific requirements that must be respected. We provide a [search index](https://huggingface.co/spaces/bigcode/search-v2) that lets you search through the pretraining data to identify where the generated code came from and apply the proper attribution to your code.
# Limitations
The model has been trained on source code from 17 programming languages. The predominant language in source is English although other languages are also present. As such the model is capable of generating code snippets provided some context but the generated code is not guaranteed to work as intended. It can be inefficient and contain bugs or exploits. See [the paper](https://huggingface.co/papers/2402.19173) for an in-depth discussion of the model limitations.
# Training
## Model
- **Architecture:** Transformer decoder with grouped-query and sliding window attention and Fill-in-the-Middle objective
- **Pretraining steps:** 1 million
- **Pretraining tokens:** 3.5+ trillion
- **Precision:** bfloat16
## Hardware
- **GPUs:** 432 H100
## Software
- **Framework:** [nanotron](https://github.com/huggingface/nanotron/)
- **Neural networks:** [PyTorch](https://github.com/pytorch/pytorch)
# License
The model is licensed under the BigCode OpenRAIL-M v1 license agreement. You can find the full agreement [here](https://huggingface.co/spaces/bigcode/bigcode-model-license-agreement).
# Citation
```bash
@misc{lozhkov2024starcoder,
title={StarCoder 2 and The Stack v2: The Next Generation},
author={Anton Lozhkov and Raymond Li and Loubna Ben Allal and Federico Cassano and Joel Lamy-Poirier and Nouamane Tazi and Ao Tang and Dmytro Pykhtar and Jiawei Liu and Yuxiang Wei and Tianyang Liu and Max Tian and Denis Kocetkov and Arthur Zucker and Younes Belkada and Zijian Wang and Qian Liu and Dmitry Abulkhanov and Indraneil Paul and Zhuang Li and Wen-Ding Li and Megan Risdal and Jia Li and Jian Zhu and Terry Yue Zhuo and Evgenii Zheltonozhskii and Nii Osae Osae Dade and Wenhao Yu and Lucas Krauß and Naman Jain and Yixuan Su and Xuanli He and Manan Dey and Edoardo Abati and Yekun Chai and Niklas Muennighoff and Xiangru Tang and Muhtasham Oblokulov and Christopher Akiki and Marc Marone and Chenghao Mou and Mayank Mishra and Alex Gu and Binyuan Hui and Tri Dao and Armel Zebaze and Olivier Dehaene and Nicolas Patry and Canwen Xu and Julian McAuley and Han Hu and Torsten Scholak and Sebastien Paquet and Jennifer Robinson and Carolyn Jane Anderson and Nicolas Chapados and Mostofa Patwary and Nima Tajbakhsh and Yacine Jernite and Carlos Muñoz Ferrandis and Lingming Zhang and Sean Hughes and Thomas Wolf and Arjun Guha and Leandro von Werra and Harm de Vries},
year={2024},
eprint={2402.19173},
archivePrefix={arXiv},
primaryClass={cs.SE}
}
``` |
mradermacher/Swallow-7b-NVE-hf-i1-GGUF | mradermacher | "2024-06-30T10:22:25Z" | 13,018 | 0 | transformers | [
"transformers",
"gguf",
"en",
"ja",
"base_model:tokyotech-llm/Swallow-7b-NVE-hf",
"license:llama2",
"endpoints_compatible",
"region:us"
] | null | "2024-06-30T06:10:16Z" | ---
base_model: tokyotech-llm/Swallow-7b-NVE-hf
language:
- en
- ja
library_name: transformers
license: llama2
model_type: llama
quantized_by: mradermacher
---
## About
<!-- ### quantize_version: 2 -->
<!-- ### output_tensor_quantised: 1 -->
<!-- ### convert_type: hf -->
<!-- ### vocab_type: -->
<!-- ### tags: nicoboss -->
weighted/imatrix quants of https://huggingface.co/tokyotech-llm/Swallow-7b-NVE-hf
<!-- provided-files -->
static quants are available at https://huggingface.co/mradermacher/Swallow-7b-NVE-hf-GGUF
## Usage
If you are unsure how to use GGUF files, refer to one of [TheBloke's
READMEs](https://huggingface.co/TheBloke/KafkaLM-70B-German-V0.1-GGUF) for
more details, including on how to concatenate multi-part files.
## Provided Quants
(sorted by size, not necessarily quality. IQ-quants are often preferable over similar sized non-IQ quants)
| Link | Type | Size/GB | Notes |
|:-----|:-----|--------:|:------|
| [GGUF](https://huggingface.co/mradermacher/Swallow-7b-NVE-hf-i1-GGUF/resolve/main/Swallow-7b-NVE-hf.i1-IQ1_S.gguf) | i1-IQ1_S | 1.6 | for the desperate |
| [GGUF](https://huggingface.co/mradermacher/Swallow-7b-NVE-hf-i1-GGUF/resolve/main/Swallow-7b-NVE-hf.i1-IQ1_M.gguf) | i1-IQ1_M | 1.8 | mostly desperate |
| [GGUF](https://huggingface.co/mradermacher/Swallow-7b-NVE-hf-i1-GGUF/resolve/main/Swallow-7b-NVE-hf.i1-IQ2_XXS.gguf) | i1-IQ2_XXS | 2.0 | |
| [GGUF](https://huggingface.co/mradermacher/Swallow-7b-NVE-hf-i1-GGUF/resolve/main/Swallow-7b-NVE-hf.i1-IQ2_XS.gguf) | i1-IQ2_XS | 2.1 | |
| [GGUF](https://huggingface.co/mradermacher/Swallow-7b-NVE-hf-i1-GGUF/resolve/main/Swallow-7b-NVE-hf.i1-IQ2_S.gguf) | i1-IQ2_S | 2.3 | |
| [GGUF](https://huggingface.co/mradermacher/Swallow-7b-NVE-hf-i1-GGUF/resolve/main/Swallow-7b-NVE-hf.i1-IQ2_M.gguf) | i1-IQ2_M | 2.5 | |
| [GGUF](https://huggingface.co/mradermacher/Swallow-7b-NVE-hf-i1-GGUF/resolve/main/Swallow-7b-NVE-hf.i1-Q2_K.gguf) | i1-Q2_K | 2.6 | IQ3_XXS probably better |
| [GGUF](https://huggingface.co/mradermacher/Swallow-7b-NVE-hf-i1-GGUF/resolve/main/Swallow-7b-NVE-hf.i1-IQ3_XXS.gguf) | i1-IQ3_XXS | 2.7 | lower quality |
| [GGUF](https://huggingface.co/mradermacher/Swallow-7b-NVE-hf-i1-GGUF/resolve/main/Swallow-7b-NVE-hf.i1-IQ3_XS.gguf) | i1-IQ3_XS | 2.9 | |
| [GGUF](https://huggingface.co/mradermacher/Swallow-7b-NVE-hf-i1-GGUF/resolve/main/Swallow-7b-NVE-hf.i1-IQ3_S.gguf) | i1-IQ3_S | 3.0 | beats Q3_K* |
| [GGUF](https://huggingface.co/mradermacher/Swallow-7b-NVE-hf-i1-GGUF/resolve/main/Swallow-7b-NVE-hf.i1-Q3_K_S.gguf) | i1-Q3_K_S | 3.0 | IQ3_XS probably better |
| [GGUF](https://huggingface.co/mradermacher/Swallow-7b-NVE-hf-i1-GGUF/resolve/main/Swallow-7b-NVE-hf.i1-IQ3_M.gguf) | i1-IQ3_M | 3.2 | |
| [GGUF](https://huggingface.co/mradermacher/Swallow-7b-NVE-hf-i1-GGUF/resolve/main/Swallow-7b-NVE-hf.i1-Q3_K_M.gguf) | i1-Q3_K_M | 3.4 | IQ3_S probably better |
| [GGUF](https://huggingface.co/mradermacher/Swallow-7b-NVE-hf-i1-GGUF/resolve/main/Swallow-7b-NVE-hf.i1-Q3_K_L.gguf) | i1-Q3_K_L | 3.7 | IQ3_M probably better |
| [GGUF](https://huggingface.co/mradermacher/Swallow-7b-NVE-hf-i1-GGUF/resolve/main/Swallow-7b-NVE-hf.i1-IQ4_XS.gguf) | i1-IQ4_XS | 3.7 | |
| [GGUF](https://huggingface.co/mradermacher/Swallow-7b-NVE-hf-i1-GGUF/resolve/main/Swallow-7b-NVE-hf.i1-Q4_0.gguf) | i1-Q4_0 | 3.9 | fast, low quality |
| [GGUF](https://huggingface.co/mradermacher/Swallow-7b-NVE-hf-i1-GGUF/resolve/main/Swallow-7b-NVE-hf.i1-Q4_K_S.gguf) | i1-Q4_K_S | 4.0 | optimal size/speed/quality |
| [GGUF](https://huggingface.co/mradermacher/Swallow-7b-NVE-hf-i1-GGUF/resolve/main/Swallow-7b-NVE-hf.i1-Q4_K_M.gguf) | i1-Q4_K_M | 4.2 | fast, recommended |
| [GGUF](https://huggingface.co/mradermacher/Swallow-7b-NVE-hf-i1-GGUF/resolve/main/Swallow-7b-NVE-hf.i1-Q5_K_S.gguf) | i1-Q5_K_S | 4.8 | |
| [GGUF](https://huggingface.co/mradermacher/Swallow-7b-NVE-hf-i1-GGUF/resolve/main/Swallow-7b-NVE-hf.i1-Q5_K_M.gguf) | i1-Q5_K_M | 4.9 | |
| [GGUF](https://huggingface.co/mradermacher/Swallow-7b-NVE-hf-i1-GGUF/resolve/main/Swallow-7b-NVE-hf.i1-Q6_K.gguf) | i1-Q6_K | 5.6 | practically like static Q6_K |
Here is a handy graph by ikawrakow comparing some lower-quality quant
types (lower is better):

And here are Artefact2's thoughts on the matter:
https://gist.github.com/Artefact2/b5f810600771265fc1e39442288e8ec9
## FAQ / Model Request
See https://huggingface.co/mradermacher/model_requests for some answers to
questions you might have and/or if you want some other model quantized.
## Thanks
I thank my company, [nethype GmbH](https://www.nethype.de/), for letting
me use its servers and providing upgrades to my workstation to enable
this work in my free time. Additional thanks to [@nicoboss](https://huggingface.co/nicoboss) for giving me access to his hardware for calculating the imatrix for these quants.
<!-- end -->
|
RichardErkhov/bardsai_-_jaskier-7b-dpo-v6.1-gguf | RichardErkhov | "2024-06-19T20:31:35Z" | 13,016 | 0 | null | [
"gguf",
"region:us"
] | null | "2024-06-19T14:38:09Z" | Quantization made by Richard Erkhov.
[Github](https://github.com/RichardErkhov)
[Discord](https://discord.gg/pvy7H8DZMG)
[Request more models](https://github.com/RichardErkhov/quant_request)
jaskier-7b-dpo-v6.1 - GGUF
- Model creator: https://huggingface.co/bardsai/
- Original model: https://huggingface.co/bardsai/jaskier-7b-dpo-v6.1/
| Name | Quant method | Size |
| ---- | ---- | ---- |
| [jaskier-7b-dpo-v6.1.Q2_K.gguf](https://huggingface.co/RichardErkhov/bardsai_-_jaskier-7b-dpo-v6.1-gguf/blob/main/jaskier-7b-dpo-v6.1.Q2_K.gguf) | Q2_K | 2.53GB |
| [jaskier-7b-dpo-v6.1.IQ3_XS.gguf](https://huggingface.co/RichardErkhov/bardsai_-_jaskier-7b-dpo-v6.1-gguf/blob/main/jaskier-7b-dpo-v6.1.IQ3_XS.gguf) | IQ3_XS | 2.81GB |
| [jaskier-7b-dpo-v6.1.IQ3_S.gguf](https://huggingface.co/RichardErkhov/bardsai_-_jaskier-7b-dpo-v6.1-gguf/blob/main/jaskier-7b-dpo-v6.1.IQ3_S.gguf) | IQ3_S | 2.96GB |
| [jaskier-7b-dpo-v6.1.Q3_K_S.gguf](https://huggingface.co/RichardErkhov/bardsai_-_jaskier-7b-dpo-v6.1-gguf/blob/main/jaskier-7b-dpo-v6.1.Q3_K_S.gguf) | Q3_K_S | 2.95GB |
| [jaskier-7b-dpo-v6.1.IQ3_M.gguf](https://huggingface.co/RichardErkhov/bardsai_-_jaskier-7b-dpo-v6.1-gguf/blob/main/jaskier-7b-dpo-v6.1.IQ3_M.gguf) | IQ3_M | 3.06GB |
| [jaskier-7b-dpo-v6.1.Q3_K.gguf](https://huggingface.co/RichardErkhov/bardsai_-_jaskier-7b-dpo-v6.1-gguf/blob/main/jaskier-7b-dpo-v6.1.Q3_K.gguf) | Q3_K | 3.28GB |
| [jaskier-7b-dpo-v6.1.Q3_K_M.gguf](https://huggingface.co/RichardErkhov/bardsai_-_jaskier-7b-dpo-v6.1-gguf/blob/main/jaskier-7b-dpo-v6.1.Q3_K_M.gguf) | Q3_K_M | 3.28GB |
| [jaskier-7b-dpo-v6.1.Q3_K_L.gguf](https://huggingface.co/RichardErkhov/bardsai_-_jaskier-7b-dpo-v6.1-gguf/blob/main/jaskier-7b-dpo-v6.1.Q3_K_L.gguf) | Q3_K_L | 3.56GB |
| [jaskier-7b-dpo-v6.1.IQ4_XS.gguf](https://huggingface.co/RichardErkhov/bardsai_-_jaskier-7b-dpo-v6.1-gguf/blob/main/jaskier-7b-dpo-v6.1.IQ4_XS.gguf) | IQ4_XS | 3.67GB |
| [jaskier-7b-dpo-v6.1.Q4_0.gguf](https://huggingface.co/RichardErkhov/bardsai_-_jaskier-7b-dpo-v6.1-gguf/blob/main/jaskier-7b-dpo-v6.1.Q4_0.gguf) | Q4_0 | 3.83GB |
| [jaskier-7b-dpo-v6.1.IQ4_NL.gguf](https://huggingface.co/RichardErkhov/bardsai_-_jaskier-7b-dpo-v6.1-gguf/blob/main/jaskier-7b-dpo-v6.1.IQ4_NL.gguf) | IQ4_NL | 3.87GB |
| [jaskier-7b-dpo-v6.1.Q4_K_S.gguf](https://huggingface.co/RichardErkhov/bardsai_-_jaskier-7b-dpo-v6.1-gguf/blob/main/jaskier-7b-dpo-v6.1.Q4_K_S.gguf) | Q4_K_S | 3.86GB |
| [jaskier-7b-dpo-v6.1.Q4_K.gguf](https://huggingface.co/RichardErkhov/bardsai_-_jaskier-7b-dpo-v6.1-gguf/blob/main/jaskier-7b-dpo-v6.1.Q4_K.gguf) | Q4_K | 4.07GB |
| [jaskier-7b-dpo-v6.1.Q4_K_M.gguf](https://huggingface.co/RichardErkhov/bardsai_-_jaskier-7b-dpo-v6.1-gguf/blob/main/jaskier-7b-dpo-v6.1.Q4_K_M.gguf) | Q4_K_M | 4.07GB |
| [jaskier-7b-dpo-v6.1.Q4_1.gguf](https://huggingface.co/RichardErkhov/bardsai_-_jaskier-7b-dpo-v6.1-gguf/blob/main/jaskier-7b-dpo-v6.1.Q4_1.gguf) | Q4_1 | 4.24GB |
| [jaskier-7b-dpo-v6.1.Q5_0.gguf](https://huggingface.co/RichardErkhov/bardsai_-_jaskier-7b-dpo-v6.1-gguf/blob/main/jaskier-7b-dpo-v6.1.Q5_0.gguf) | Q5_0 | 4.65GB |
| [jaskier-7b-dpo-v6.1.Q5_K_S.gguf](https://huggingface.co/RichardErkhov/bardsai_-_jaskier-7b-dpo-v6.1-gguf/blob/main/jaskier-7b-dpo-v6.1.Q5_K_S.gguf) | Q5_K_S | 4.65GB |
| [jaskier-7b-dpo-v6.1.Q5_K.gguf](https://huggingface.co/RichardErkhov/bardsai_-_jaskier-7b-dpo-v6.1-gguf/blob/main/jaskier-7b-dpo-v6.1.Q5_K.gguf) | Q5_K | 4.78GB |
| [jaskier-7b-dpo-v6.1.Q5_K_M.gguf](https://huggingface.co/RichardErkhov/bardsai_-_jaskier-7b-dpo-v6.1-gguf/blob/main/jaskier-7b-dpo-v6.1.Q5_K_M.gguf) | Q5_K_M | 4.78GB |
| [jaskier-7b-dpo-v6.1.Q5_1.gguf](https://huggingface.co/RichardErkhov/bardsai_-_jaskier-7b-dpo-v6.1-gguf/blob/main/jaskier-7b-dpo-v6.1.Q5_1.gguf) | Q5_1 | 5.07GB |
| [jaskier-7b-dpo-v6.1.Q6_K.gguf](https://huggingface.co/RichardErkhov/bardsai_-_jaskier-7b-dpo-v6.1-gguf/blob/main/jaskier-7b-dpo-v6.1.Q6_K.gguf) | Q6_K | 5.53GB |
| [jaskier-7b-dpo-v6.1.Q8_0.gguf](https://huggingface.co/RichardErkhov/bardsai_-_jaskier-7b-dpo-v6.1-gguf/blob/main/jaskier-7b-dpo-v6.1.Q8_0.gguf) | Q8_0 | 7.17GB |
Original model description:
---
library_name: transformers
tags:
- llm
- 7b
license: cc-by-4.0
datasets:
- jondurbin/truthy-dpo-v0.1
language:
- en
---
# Jaskier-7b-dpo-v5.6
<figure>

</figure>
**This is work-in-progress model, may not be ready for production use**
Model based on `bardsai/jaskier-7b-dpo-v5.6` (downstream version of Mistral7B) finetuned using Direct Preference Optimization on argilla/distilabel-math-preference-dpo.
## How to use
You can use this model directly with a Hugging Face pipeline:
```python
from transformers import pipeline, Conversation
import torch
base_model_name = "bardsai/jaskier-7b-dpo-v6.1"
chatbot = pipeline("conversational", model=base_model_name, torch_dtype=torch.float16, device_map="auto")
conversation = Conversation("Can Poland into space?")
conversation = chatbot(conversation)
print(conversation.messages[-1]["content"])
```
## Output
"Poland, as a nation, doesn't physically travel to space. However, Poland has contributed to the field of space exploration through its scientists, engineers, and collaborations with international space agencies. The Polish Space Agency, established in 2016, aims to promote and coordinate the country's space activities."
## Changelog
- 2024-02-20: Initial release
## About bards.ai
At bards.ai, we focus on providing machine learning expertise and skills to our partners, particularly in the areas of nlp, machine vision and time series analysis. Our team is located in Wroclaw, Poland. Please visit our website for more information: bards.ai
Let us know if you use our model :). Also, if you need any help, feel free to contact us at [email protected]
|
BeaverAI/test | BeaverAI | "2024-06-30T21:13:06Z" | 13,015 | 0 | null | [
"gguf",
"region:us"
] | null | "2024-06-30T19:40:52Z" | Entry not found |
mradermacher/Oumuamua-7b-RP-GGUF | mradermacher | "2024-06-23T19:57:05Z" | 13,012 | 0 | transformers | [
"transformers",
"gguf",
"mergekit",
"merge",
"ja",
"dataset:Aratako/Rosebleu-1on1-Dialogues",
"dataset:Aratako/LimaRP-augmented-ja-karakuri",
"dataset:Aratako/Bluemoon_Top50MB_Sorted_Fixed_ja",
"dataset:grimulkan/LimaRP-augmented",
"dataset:SicariusSicariiStuff/Bluemoon_Top50MB_Sorted_Fixed",
"dataset:OmniAICreator/Japanese-Roleplay",
"dataset:OmniAICreator/Japanese-Roleplay-Dialogues",
"base_model:Aratako/Oumuamua-7b-RP",
"license:cc-by-nc-4.0",
"endpoints_compatible",
"region:us"
] | null | "2024-06-23T19:31:19Z" | ---
base_model: Aratako/Oumuamua-7b-RP
datasets:
- Aratako/Rosebleu-1on1-Dialogues
- Aratako/LimaRP-augmented-ja-karakuri
- Aratako/Bluemoon_Top50MB_Sorted_Fixed_ja
- grimulkan/LimaRP-augmented
- SicariusSicariiStuff/Bluemoon_Top50MB_Sorted_Fixed
- OmniAICreator/Japanese-Roleplay
- OmniAICreator/Japanese-Roleplay-Dialogues
language:
- ja
library_name: transformers
license: cc-by-nc-4.0
quantized_by: mradermacher
tags:
- mergekit
- merge
---
## About
<!-- ### quantize_version: 2 -->
<!-- ### output_tensor_quantised: 1 -->
<!-- ### convert_type: hf -->
<!-- ### vocab_type: -->
<!-- ### tags: -->
static quants of https://huggingface.co/Aratako/Oumuamua-7b-RP
<!-- provided-files -->
weighted/imatrix quants seem not to be available (by me) at this time. If they do not show up a week or so after the static ones, I have probably not planned for them. Feel free to request them by opening a Community Discussion.
## Usage
If you are unsure how to use GGUF files, refer to one of [TheBloke's
READMEs](https://huggingface.co/TheBloke/KafkaLM-70B-German-V0.1-GGUF) for
more details, including on how to concatenate multi-part files.
## Provided Quants
(sorted by size, not necessarily quality. IQ-quants are often preferable over similar sized non-IQ quants)
| Link | Type | Size/GB | Notes |
|:-----|:-----|--------:|:------|
| [GGUF](https://huggingface.co/mradermacher/Oumuamua-7b-RP-GGUF/resolve/main/Oumuamua-7b-RP.Q2_K.gguf) | Q2_K | 2.9 | |
| [GGUF](https://huggingface.co/mradermacher/Oumuamua-7b-RP-GGUF/resolve/main/Oumuamua-7b-RP.IQ3_XS.gguf) | IQ3_XS | 3.2 | |
| [GGUF](https://huggingface.co/mradermacher/Oumuamua-7b-RP-GGUF/resolve/main/Oumuamua-7b-RP.Q3_K_S.gguf) | Q3_K_S | 3.3 | |
| [GGUF](https://huggingface.co/mradermacher/Oumuamua-7b-RP-GGUF/resolve/main/Oumuamua-7b-RP.IQ3_S.gguf) | IQ3_S | 3.3 | beats Q3_K* |
| [GGUF](https://huggingface.co/mradermacher/Oumuamua-7b-RP-GGUF/resolve/main/Oumuamua-7b-RP.IQ3_M.gguf) | IQ3_M | 3.4 | |
| [GGUF](https://huggingface.co/mradermacher/Oumuamua-7b-RP-GGUF/resolve/main/Oumuamua-7b-RP.Q3_K_M.gguf) | Q3_K_M | 3.7 | lower quality |
| [GGUF](https://huggingface.co/mradermacher/Oumuamua-7b-RP-GGUF/resolve/main/Oumuamua-7b-RP.Q3_K_L.gguf) | Q3_K_L | 4.0 | |
| [GGUF](https://huggingface.co/mradermacher/Oumuamua-7b-RP-GGUF/resolve/main/Oumuamua-7b-RP.IQ4_XS.gguf) | IQ4_XS | 4.1 | |
| [GGUF](https://huggingface.co/mradermacher/Oumuamua-7b-RP-GGUF/resolve/main/Oumuamua-7b-RP.Q4_K_S.gguf) | Q4_K_S | 4.3 | fast, recommended |
| [GGUF](https://huggingface.co/mradermacher/Oumuamua-7b-RP-GGUF/resolve/main/Oumuamua-7b-RP.Q4_K_M.gguf) | Q4_K_M | 4.5 | fast, recommended |
| [GGUF](https://huggingface.co/mradermacher/Oumuamua-7b-RP-GGUF/resolve/main/Oumuamua-7b-RP.Q5_K_S.gguf) | Q5_K_S | 5.2 | |
| [GGUF](https://huggingface.co/mradermacher/Oumuamua-7b-RP-GGUF/resolve/main/Oumuamua-7b-RP.Q5_K_M.gguf) | Q5_K_M | 5.3 | |
| [GGUF](https://huggingface.co/mradermacher/Oumuamua-7b-RP-GGUF/resolve/main/Oumuamua-7b-RP.Q6_K.gguf) | Q6_K | 6.1 | very good quality |
| [GGUF](https://huggingface.co/mradermacher/Oumuamua-7b-RP-GGUF/resolve/main/Oumuamua-7b-RP.Q8_0.gguf) | Q8_0 | 7.9 | fast, best quality |
| [GGUF](https://huggingface.co/mradermacher/Oumuamua-7b-RP-GGUF/resolve/main/Oumuamua-7b-RP.f16.gguf) | f16 | 14.8 | 16 bpw, overkill |
Here is a handy graph by ikawrakow comparing some lower-quality quant
types (lower is better):

And here are Artefact2's thoughts on the matter:
https://gist.github.com/Artefact2/b5f810600771265fc1e39442288e8ec9
## FAQ / Model Request
See https://huggingface.co/mradermacher/model_requests for some answers to
questions you might have and/or if you want some other model quantized.
## Thanks
I thank my company, [nethype GmbH](https://www.nethype.de/), for letting
me use its servers and providing upgrades to my workstation to enable
this work in my free time.
<!-- end -->
|
klue/roberta-base | klue | "2023-06-12T12:29:12Z" | 13,000 | 22 | transformers | [
"transformers",
"pytorch",
"safetensors",
"roberta",
"fill-mask",
"korean",
"klue",
"ko",
"arxiv:2105.09680",
"autotrain_compatible",
"endpoints_compatible",
"region:us"
] | fill-mask | "2022-03-02T23:29:05Z" | ---
language: ko
tags:
- korean
- klue
mask_token: "[MASK]"
widget:
- text: 대한민국의 수도는 [MASK] 입니다.
---
# KLUE RoBERTa base
Pretrained RoBERTa Model on Korean Language. See [Github](https://github.com/KLUE-benchmark/KLUE) and [Paper](https://arxiv.org/abs/2105.09680) for more details.
## How to use
_NOTE:_ Use `BertTokenizer` instead of RobertaTokenizer. (`AutoTokenizer` will load `BertTokenizer`)
```python
from transformers import AutoModel, AutoTokenizer
model = AutoModel.from_pretrained("klue/roberta-base")
tokenizer = AutoTokenizer.from_pretrained("klue/roberta-base")
```
## BibTeX entry and citation info
```bibtex
@misc{park2021klue,
title={KLUE: Korean Language Understanding Evaluation},
author={Sungjoon Park and Jihyung Moon and Sungdong Kim and Won Ik Cho and Jiyoon Han and Jangwon Park and Chisung Song and Junseong Kim and Yongsook Song and Taehwan Oh and Joohong Lee and Juhyun Oh and Sungwon Lyu and Younghoon Jeong and Inkwon Lee and Sangwoo Seo and Dongjun Lee and Hyunwoo Kim and Myeonghwa Lee and Seongbo Jang and Seungwon Do and Sunkyoung Kim and Kyungtae Lim and Jongwon Lee and Kyumin Park and Jamin Shin and Seonghyun Kim and Lucy Park and Alice Oh and Jungwoo Ha and Kyunghyun Cho},
year={2021},
eprint={2105.09680},
archivePrefix={arXiv},
primaryClass={cs.CL}
}
```
|
lllyasviel/control_v11p_sd15_lineart | lllyasviel | "2023-05-04T18:49:42Z" | 12,999 | 39 | diffusers | [
"diffusers",
"safetensors",
"art",
"controlnet",
"stable-diffusion",
"controlnet-v1-1",
"image-to-image",
"arxiv:2302.05543",
"base_model:runwayml/stable-diffusion-v1-5",
"license:openrail",
"region:us"
] | image-to-image | "2023-04-14T19:25:13Z" | ---
license: openrail
base_model: runwayml/stable-diffusion-v1-5
tags:
- art
- controlnet
- stable-diffusion
- controlnet-v1-1
- image-to-image
duplicated_from: ControlNet-1-1-preview/control_v11p_sd15_lineart
---
# Controlnet - v1.1 - *lineart Version*
**Controlnet v1.1** was released in [lllyasviel/ControlNet-v1-1](https://huggingface.co/lllyasviel/ControlNet-v1-1) by [Lvmin Zhang](https://huggingface.co/lllyasviel).
This checkpoint is a conversion of [the original checkpoint](https://huggingface.co/lllyasviel/ControlNet-v1-1/blob/main/control_v11p_sd15_lineart.pth) into `diffusers` format.
It can be used in combination with **Stable Diffusion**, such as [runwayml/stable-diffusion-v1-5](https://huggingface.co/runwayml/stable-diffusion-v1-5).
For more details, please also have a look at the [🧨 Diffusers docs](https://huggingface.co/docs/diffusers/api/pipelines/stable_diffusion/controlnet).
ControlNet is a neural network structure to control diffusion models by adding extra conditions.

This checkpoint corresponds to the ControlNet conditioned on **lineart images**.
## Model Details
- **Developed by:** Lvmin Zhang, Maneesh Agrawala
- **Model type:** Diffusion-based text-to-image generation model
- **Language(s):** English
- **License:** [The CreativeML OpenRAIL M license](https://huggingface.co/spaces/CompVis/stable-diffusion-license) is an [Open RAIL M license](https://www.licenses.ai/blog/2022/8/18/naming-convention-of-responsible-ai-licenses), adapted from the work that [BigScience](https://bigscience.huggingface.co/) and [the RAIL Initiative](https://www.licenses.ai/) are jointly carrying in the area of responsible AI licensing. See also [the article about the BLOOM Open RAIL license](https://bigscience.huggingface.co/blog/the-bigscience-rail-license) on which our license is based.
- **Resources for more information:** [GitHub Repository](https://github.com/lllyasviel/ControlNet), [Paper](https://arxiv.org/abs/2302.05543).
- **Cite as:**
@misc{zhang2023adding,
title={Adding Conditional Control to Text-to-Image Diffusion Models},
author={Lvmin Zhang and Maneesh Agrawala},
year={2023},
eprint={2302.05543},
archivePrefix={arXiv},
primaryClass={cs.CV}
}
## Introduction
Controlnet was proposed in [*Adding Conditional Control to Text-to-Image Diffusion Models*](https://arxiv.org/abs/2302.05543) by
Lvmin Zhang, Maneesh Agrawala.
The abstract reads as follows:
*We present a neural network structure, ControlNet, to control pretrained large diffusion models to support additional input conditions.
The ControlNet learns task-specific conditions in an end-to-end way, and the learning is robust even when the training dataset is small (< 50k).
Moreover, training a ControlNet is as fast as fine-tuning a diffusion model, and the model can be trained on a personal devices.
Alternatively, if powerful computation clusters are available, the model can scale to large amounts (millions to billions) of data.
We report that large diffusion models like Stable Diffusion can be augmented with ControlNets to enable conditional inputs like edge maps, segmentation maps, keypoints, etc.
This may enrich the methods to control large diffusion models and further facilitate related applications.*
## Example
It is recommended to use the checkpoint with [Stable Diffusion v1-5](https://huggingface.co/runwayml/stable-diffusion-v1-5) as the checkpoint
has been trained on it.
Experimentally, the checkpoint can be used with other diffusion models such as dreamboothed stable diffusion.
**Note**: If you want to process an image to create the auxiliary conditioning, external dependencies are required as shown below:
1. Install https://github.com/patrickvonplaten/controlnet_aux
```sh
$ pip install controlnet_aux==0.3.0
```
2. Let's install `diffusers` and related packages:
```
$ pip install diffusers transformers accelerate
```
3. Run code:
```python
import torch
import os
from huggingface_hub import HfApi
from pathlib import Path
from diffusers.utils import load_image
from PIL import Image
import numpy as np
from controlnet_aux import LineartDetector
from diffusers import (
ControlNetModel,
StableDiffusionControlNetPipeline,
UniPCMultistepScheduler,
)
checkpoint = "ControlNet-1-1-preview/control_v11p_sd15_lineart"
image = load_image(
"https://huggingface.co/ControlNet-1-1-preview/control_v11p_sd15_lineart/resolve/main/images/input.png"
)
image = image.resize((512, 512))
prompt = "michael jackson concert"
processor = LineartDetector.from_pretrained("lllyasviel/Annotators")
control_image = processor(image)
control_image.save("./images/control.png")
controlnet = ControlNetModel.from_pretrained(checkpoint, torch_dtype=torch.float16)
pipe = StableDiffusionControlNetPipeline.from_pretrained(
"runwayml/stable-diffusion-v1-5", controlnet=controlnet, torch_dtype=torch.float16
)
pipe.scheduler = UniPCMultistepScheduler.from_config(pipe.scheduler.config)
pipe.enable_model_cpu_offload()
generator = torch.manual_seed(0)
image = pipe(prompt, num_inference_steps=30, generator=generator, image=control_image).images[0]
image.save('images/image_out.png')
```



## Other released checkpoints v1-1
The authors released 14 different checkpoints, each trained with [Stable Diffusion v1-5](https://huggingface.co/runwayml/stable-diffusion-v1-5)
on a different type of conditioning:
| Model Name | Control Image Overview| Condition Image | Control Image Example | Generated Image Example |
|---|---|---|---|---|
|[lllyasviel/control_v11p_sd15_canny](https://huggingface.co/lllyasviel/control_v11p_sd15_canny)<br/> | *Trained with canny edge detection* | A monochrome image with white edges on a black background.|<a href="https://huggingface.co/lllyasviel/control_v11p_sd15_canny/resolve/main/images/control.png"><img width="64" style="margin:0;padding:0;" src="https://huggingface.co/lllyasviel/control_v11p_sd15_canny/resolve/main/images/control.png"/></a>|<a href="https://huggingface.co/lllyasviel/control_v11p_sd15_canny/resolve/main/images/image_out.png"><img width="64" src="https://huggingface.co/lllyasviel/control_v11p_sd15_canny/resolve/main/images/image_out.png"/></a>|
|[lllyasviel/control_v11e_sd15_ip2p](https://huggingface.co/lllyasviel/control_v11e_sd15_ip2p)<br/> | *Trained with pixel to pixel instruction* | No condition .|<a href="https://huggingface.co/lllyasviel/control_v11e_sd15_ip2p/resolve/main/images/control.png"><img width="64" style="margin:0;padding:0;" src="https://huggingface.co/lllyasviel/control_v11e_sd15_ip2p/resolve/main/images/control.png"/></a>|<a href="https://huggingface.co/lllyasviel/control_v11e_sd15_ip2p/resolve/main/images/image_out.png"><img width="64" src="https://huggingface.co/lllyasviel/control_v11e_sd15_ip2p/resolve/main/images/image_out.png"/></a>|
|[lllyasviel/control_v11p_sd15_inpaint](https://huggingface.co/lllyasviel/control_v11p_sd15_inpaint)<br/> | Trained with image inpainting | No condition.|<a href="https://huggingface.co/lllyasviel/control_v11p_sd15_inpaint/resolve/main/images/control.png"><img width="64" style="margin:0;padding:0;" src="https://huggingface.co/lllyasviel/control_v11p_sd15_inpaint/resolve/main/images/control.png"/></a>|<a href="https://huggingface.co/lllyasviel/control_v11p_sd15_inpaint/resolve/main/images/output.png"><img width="64" src="https://huggingface.co/lllyasviel/control_v11p_sd15_inpaint/resolve/main/images/output.png"/></a>|
|[lllyasviel/control_v11p_sd15_mlsd](https://huggingface.co/lllyasviel/control_v11p_sd15_mlsd)<br/> | Trained with multi-level line segment detection | An image with annotated line segments.|<a href="https://huggingface.co/lllyasviel/control_v11p_sd15_mlsd/resolve/main/images/control.png"><img width="64" style="margin:0;padding:0;" src="https://huggingface.co/lllyasviel/control_v11p_sd15_mlsd/resolve/main/images/control.png"/></a>|<a href="https://huggingface.co/lllyasviel/control_v11p_sd15_mlsd/resolve/main/images/image_out.png"><img width="64" src="https://huggingface.co/lllyasviel/control_v11p_sd15_mlsd/resolve/main/images/image_out.png"/></a>|
|[lllyasviel/control_v11f1p_sd15_depth](https://huggingface.co/lllyasviel/control_v11f1p_sd15_depth)<br/> | Trained with depth estimation | An image with depth information, usually represented as a grayscale image.|<a href="https://huggingface.co/lllyasviel/control_v11f1p_sd15_depth/resolve/main/images/control.png"><img width="64" style="margin:0;padding:0;" src="https://huggingface.co/lllyasviel/control_v11f1p_sd15_depth/resolve/main/images/control.png"/></a>|<a href="https://huggingface.co/lllyasviel/control_v11f1p_sd15_depth/resolve/main/images/image_out.png"><img width="64" src="https://huggingface.co/lllyasviel/control_v11f1p_sd15_depth/resolve/main/images/image_out.png"/></a>|
|[lllyasviel/control_v11p_sd15_normalbae](https://huggingface.co/lllyasviel/control_v11p_sd15_normalbae)<br/> | Trained with surface normal estimation | An image with surface normal information, usually represented as a color-coded image.|<a href="https://huggingface.co/lllyasviel/control_v11p_sd15_normalbae/resolve/main/images/control.png"><img width="64" style="margin:0;padding:0;" src="https://huggingface.co/lllyasviel/control_v11p_sd15_normalbae/resolve/main/images/control.png"/></a>|<a href="https://huggingface.co/lllyasviel/control_v11p_sd15_normalbae/resolve/main/images/image_out.png"><img width="64" src="https://huggingface.co/lllyasviel/control_v11p_sd15_normalbae/resolve/main/images/image_out.png"/></a>|
|[lllyasviel/control_v11p_sd15_seg](https://huggingface.co/lllyasviel/control_v11p_sd15_seg)<br/> | Trained with image segmentation | An image with segmented regions, usually represented as a color-coded image.|<a href="https://huggingface.co/lllyasviel/control_v11p_sd15_seg/resolve/main/images/control.png"><img width="64" style="margin:0;padding:0;" src="https://huggingface.co/lllyasviel/control_v11p_sd15_seg/resolve/main/images/control.png"/></a>|<a href="https://huggingface.co/lllyasviel/control_v11p_sd15_seg/resolve/main/images/image_out.png"><img width="64" src="https://huggingface.co/lllyasviel/control_v11p_sd15_seg/resolve/main/images/image_out.png"/></a>|
|[lllyasviel/control_v11p_sd15_lineart](https://huggingface.co/lllyasviel/control_v11p_sd15_lineart)<br/> | Trained with line art generation | An image with line art, usually black lines on a white background.|<a href="https://huggingface.co/lllyasviel/control_v11p_sd15_lineart/resolve/main/images/control.png"><img width="64" style="margin:0;padding:0;" src="https://huggingface.co/lllyasviel/control_v11p_sd15_lineart/resolve/main/images/control.png"/></a>|<a href="https://huggingface.co/lllyasviel/control_v11p_sd15_lineart/resolve/main/images/image_out.png"><img width="64" src="https://huggingface.co/lllyasviel/control_v11p_sd15_lineart/resolve/main/images/image_out.png"/></a>|
|[lllyasviel/control_v11p_sd15s2_lineart_anime](https://huggingface.co/lllyasviel/control_v11p_sd15s2_lineart_anime)<br/> | Trained with anime line art generation | An image with anime-style line art.|<a href="https://huggingface.co/lllyasviel/control_v11p_sd15s2_lineart_anime/resolve/main/images/control.png"><img width="64" style="margin:0;padding:0;" src="https://huggingface.co/lllyasviel/control_v11p_sd15s2_lineart_anime/resolve/main/images/control.png"/></a>|<a href="https://huggingface.co/lllyasviel/control_v11p_sd15s2_lineart_anime/resolve/main/images/image_out.png"><img width="64" src="https://huggingface.co/lllyasviel/control_v11p_sd15s2_lineart_anime/resolve/main/images/image_out.png"/></a>|
|[lllyasviel/control_v11p_sd15_openpose](https://huggingface.co/lllyasviel/control_v11p_sd15s2_lineart_anime)<br/> | Trained with human pose estimation | An image with human poses, usually represented as a set of keypoints or skeletons.|<a href="https://huggingface.co/lllyasviel/control_v11p_sd15_openpose/resolve/main/images/control.png"><img width="64" style="margin:0;padding:0;" src="https://huggingface.co/lllyasviel/control_v11p_sd15_openpose/resolve/main/images/control.png"/></a>|<a href="https://huggingface.co/lllyasviel/control_v11p_sd15_openpose/resolve/main/images/image_out.png"><img width="64" src="https://huggingface.co/lllyasviel/control_v11p_sd15_openpose/resolve/main/images/image_out.png"/></a>|
|[lllyasviel/control_v11p_sd15_scribble](https://huggingface.co/lllyasviel/control_v11p_sd15_scribble)<br/> | Trained with scribble-based image generation | An image with scribbles, usually random or user-drawn strokes.|<a href="https://huggingface.co/lllyasviel/control_v11p_sd15_scribble/resolve/main/images/control.png"><img width="64" style="margin:0;padding:0;" src="https://huggingface.co/lllyasviel/control_v11p_sd15_scribble/resolve/main/images/control.png"/></a>|<a href="https://huggingface.co/lllyasviel/control_v11p_sd15_scribble/resolve/main/images/image_out.png"><img width="64" src="https://huggingface.co/lllyasviel/control_v11p_sd15_scribble/resolve/main/images/image_out.png"/></a>|
|[lllyasviel/control_v11p_sd15_softedge](https://huggingface.co/lllyasviel/control_v11p_sd15_softedge)<br/> | Trained with soft edge image generation | An image with soft edges, usually to create a more painterly or artistic effect.|<a href="https://huggingface.co/lllyasviel/control_v11p_sd15_softedge/resolve/main/images/control.png"><img width="64" style="margin:0;padding:0;" src="https://huggingface.co/lllyasviel/control_v11p_sd15_softedge/resolve/main/images/control.png"/></a>|<a href="https://huggingface.co/lllyasviel/control_v11p_sd15_softedge/resolve/main/images/image_out.png"><img width="64" src="https://huggingface.co/lllyasviel/control_v11p_sd15_softedge/resolve/main/images/image_out.png"/></a>|
|[lllyasviel/control_v11e_sd15_shuffle](https://huggingface.co/lllyasviel/control_v11e_sd15_shuffle)<br/> | Trained with image shuffling | An image with shuffled patches or regions.|<a href="https://huggingface.co/lllyasviel/control_v11e_sd15_shuffle/resolve/main/images/control.png"><img width="64" style="margin:0;padding:0;" src="https://huggingface.co/lllyasviel/control_v11e_sd15_shuffle/resolve/main/images/control.png"/></a>|<a href="https://huggingface.co/lllyasviel/control_v11e_sd15_shuffle/resolve/main/images/image_out.png"><img width="64" src="https://huggingface.co/lllyasviel/control_v11e_sd15_shuffle/resolve/main/images/image_out.png"/></a>|
|[lllyasviel/control_v11f1e_sd15_tile](https://huggingface.co/lllyasviel/control_v11f1e_sd15_tile)<br/> | Trained with image tiling | A blurry image or part of an image .|<a href="https://huggingface.co/lllyasviel/control_v11f1e_sd15_tile/resolve/main/images/original.png"><img width="64" style="margin:0;padding:0;" src="https://huggingface.co/lllyasviel/control_v11f1e_sd15_tile/resolve/main/images/original.png"/></a>|<a href="https://huggingface.co/lllyasviel/control_v11f1e_sd15_tile/resolve/main/images/output.png"><img width="64" src="https://huggingface.co/lllyasviel/control_v11f1e_sd15_tile/resolve/main/images/output.png"/></a>|
## More information
For more information, please also have a look at the [Diffusers ControlNet Blog Post](https://huggingface.co/blog/controlnet) and have a look at the [official docs](https://github.com/lllyasviel/ControlNet-v1-1-nightly). |
batterydata/bde-pos-bert-cased-base | batterydata | "2022-05-31T15:02:27Z" | 12,984 | 0 | transformers | [
"transformers",
"pytorch",
"bert",
"token-classification",
"license:mit",
"autotrain_compatible",
"endpoints_compatible",
"region:us"
] | token-classification | "2022-04-01T14:16:04Z" | ---
license: mit
---
|
mradermacher/Hathor_Fractionate-L3-8B-v.05-i1-GGUF | mradermacher | "2024-06-24T18:21:13Z" | 12,981 | 0 | transformers | [
"transformers",
"gguf",
"en",
"base_model:Nitral-AI/Hathor_Fractionate-L3-8B-v.05",
"license:other",
"endpoints_compatible",
"region:us"
] | null | "2024-06-24T16:04:18Z" | ---
base_model: Nitral-AI/Hathor_Fractionate-L3-8B-v.05
language:
- en
library_name: transformers
license: other
quantized_by: mradermacher
---
## About
<!-- ### quantize_version: 2 -->
<!-- ### output_tensor_quantised: 1 -->
<!-- ### convert_type: hf -->
<!-- ### vocab_type: -->
<!-- ### tags: nicoboss -->
weighted/imatrix quants of https://huggingface.co/Nitral-AI/Hathor_Fractionate-L3-8B-v.05
<!-- provided-files -->
static quants are available at https://huggingface.co/mradermacher/Hathor_Fractionate-L3-8B-v.05-GGUF
## Usage
If you are unsure how to use GGUF files, refer to one of [TheBloke's
READMEs](https://huggingface.co/TheBloke/KafkaLM-70B-German-V0.1-GGUF) for
more details, including on how to concatenate multi-part files.
## Provided Quants
(sorted by size, not necessarily quality. IQ-quants are often preferable over similar sized non-IQ quants)
| Link | Type | Size/GB | Notes |
|:-----|:-----|--------:|:------|
| [GGUF](https://huggingface.co/mradermacher/Hathor_Fractionate-L3-8B-v.05-i1-GGUF/resolve/main/Hathor_Fractionate-L3-8B-v.05.i1-IQ1_S.gguf) | i1-IQ1_S | 2.1 | for the desperate |
| [GGUF](https://huggingface.co/mradermacher/Hathor_Fractionate-L3-8B-v.05-i1-GGUF/resolve/main/Hathor_Fractionate-L3-8B-v.05.i1-IQ1_M.gguf) | i1-IQ1_M | 2.3 | mostly desperate |
| [GGUF](https://huggingface.co/mradermacher/Hathor_Fractionate-L3-8B-v.05-i1-GGUF/resolve/main/Hathor_Fractionate-L3-8B-v.05.i1-IQ2_XXS.gguf) | i1-IQ2_XXS | 2.5 | |
| [GGUF](https://huggingface.co/mradermacher/Hathor_Fractionate-L3-8B-v.05-i1-GGUF/resolve/main/Hathor_Fractionate-L3-8B-v.05.i1-IQ2_XS.gguf) | i1-IQ2_XS | 2.7 | |
| [GGUF](https://huggingface.co/mradermacher/Hathor_Fractionate-L3-8B-v.05-i1-GGUF/resolve/main/Hathor_Fractionate-L3-8B-v.05.i1-IQ2_S.gguf) | i1-IQ2_S | 2.9 | |
| [GGUF](https://huggingface.co/mradermacher/Hathor_Fractionate-L3-8B-v.05-i1-GGUF/resolve/main/Hathor_Fractionate-L3-8B-v.05.i1-IQ2_M.gguf) | i1-IQ2_M | 3.0 | |
| [GGUF](https://huggingface.co/mradermacher/Hathor_Fractionate-L3-8B-v.05-i1-GGUF/resolve/main/Hathor_Fractionate-L3-8B-v.05.i1-Q2_K.gguf) | i1-Q2_K | 3.3 | IQ3_XXS probably better |
| [GGUF](https://huggingface.co/mradermacher/Hathor_Fractionate-L3-8B-v.05-i1-GGUF/resolve/main/Hathor_Fractionate-L3-8B-v.05.i1-IQ3_XXS.gguf) | i1-IQ3_XXS | 3.4 | lower quality |
| [GGUF](https://huggingface.co/mradermacher/Hathor_Fractionate-L3-8B-v.05-i1-GGUF/resolve/main/Hathor_Fractionate-L3-8B-v.05.i1-IQ3_XS.gguf) | i1-IQ3_XS | 3.6 | |
| [GGUF](https://huggingface.co/mradermacher/Hathor_Fractionate-L3-8B-v.05-i1-GGUF/resolve/main/Hathor_Fractionate-L3-8B-v.05.i1-Q3_K_S.gguf) | i1-Q3_K_S | 3.8 | IQ3_XS probably better |
| [GGUF](https://huggingface.co/mradermacher/Hathor_Fractionate-L3-8B-v.05-i1-GGUF/resolve/main/Hathor_Fractionate-L3-8B-v.05.i1-IQ3_S.gguf) | i1-IQ3_S | 3.8 | beats Q3_K* |
| [GGUF](https://huggingface.co/mradermacher/Hathor_Fractionate-L3-8B-v.05-i1-GGUF/resolve/main/Hathor_Fractionate-L3-8B-v.05.i1-IQ3_M.gguf) | i1-IQ3_M | 3.9 | |
| [GGUF](https://huggingface.co/mradermacher/Hathor_Fractionate-L3-8B-v.05-i1-GGUF/resolve/main/Hathor_Fractionate-L3-8B-v.05.i1-Q3_K_M.gguf) | i1-Q3_K_M | 4.1 | IQ3_S probably better |
| [GGUF](https://huggingface.co/mradermacher/Hathor_Fractionate-L3-8B-v.05-i1-GGUF/resolve/main/Hathor_Fractionate-L3-8B-v.05.i1-Q3_K_L.gguf) | i1-Q3_K_L | 4.4 | IQ3_M probably better |
| [GGUF](https://huggingface.co/mradermacher/Hathor_Fractionate-L3-8B-v.05-i1-GGUF/resolve/main/Hathor_Fractionate-L3-8B-v.05.i1-IQ4_XS.gguf) | i1-IQ4_XS | 4.5 | |
| [GGUF](https://huggingface.co/mradermacher/Hathor_Fractionate-L3-8B-v.05-i1-GGUF/resolve/main/Hathor_Fractionate-L3-8B-v.05.i1-Q4_0.gguf) | i1-Q4_0 | 4.8 | fast, low quality |
| [GGUF](https://huggingface.co/mradermacher/Hathor_Fractionate-L3-8B-v.05-i1-GGUF/resolve/main/Hathor_Fractionate-L3-8B-v.05.i1-Q4_K_S.gguf) | i1-Q4_K_S | 4.8 | optimal size/speed/quality |
| [GGUF](https://huggingface.co/mradermacher/Hathor_Fractionate-L3-8B-v.05-i1-GGUF/resolve/main/Hathor_Fractionate-L3-8B-v.05.i1-Q4_K_M.gguf) | i1-Q4_K_M | 5.0 | fast, recommended |
| [GGUF](https://huggingface.co/mradermacher/Hathor_Fractionate-L3-8B-v.05-i1-GGUF/resolve/main/Hathor_Fractionate-L3-8B-v.05.i1-Q5_K_S.gguf) | i1-Q5_K_S | 5.7 | |
| [GGUF](https://huggingface.co/mradermacher/Hathor_Fractionate-L3-8B-v.05-i1-GGUF/resolve/main/Hathor_Fractionate-L3-8B-v.05.i1-Q5_K_M.gguf) | i1-Q5_K_M | 5.8 | |
| [GGUF](https://huggingface.co/mradermacher/Hathor_Fractionate-L3-8B-v.05-i1-GGUF/resolve/main/Hathor_Fractionate-L3-8B-v.05.i1-Q6_K.gguf) | i1-Q6_K | 6.7 | practically like static Q6_K |
Here is a handy graph by ikawrakow comparing some lower-quality quant
types (lower is better):

And here are Artefact2's thoughts on the matter:
https://gist.github.com/Artefact2/b5f810600771265fc1e39442288e8ec9
## FAQ / Model Request
See https://huggingface.co/mradermacher/model_requests for some answers to
questions you might have and/or if you want some other model quantized.
## Thanks
I thank my company, [nethype GmbH](https://www.nethype.de/), for letting
me use its servers and providing upgrades to my workstation to enable
this work in my free time. Additional thanks to [@nicoboss](https://huggingface.co/nicoboss) for giving me access to his hardware for calculating the imatrix for these quants.
<!-- end -->
|
mradermacher/AlphaMath-7B-GGUF | mradermacher | "2024-06-19T14:49:33Z" | 12,980 | 1 | transformers | [
"transformers",
"gguf",
"en",
"base_model:MARIO-Math-Reasoning/AlphaMath-7B",
"license:mit",
"endpoints_compatible",
"region:us"
] | null | "2024-06-19T12:52:36Z" | ---
base_model: MARIO-Math-Reasoning/AlphaMath-7B
language:
- en
library_name: transformers
license: mit
quantized_by: mradermacher
---
## About
<!-- ### quantize_version: 2 -->
<!-- ### output_tensor_quantised: 1 -->
<!-- ### convert_type: hf -->
<!-- ### vocab_type: -->
<!-- ### tags: -->
static quants of https://huggingface.co/MARIO-Math-Reasoning/AlphaMath-7B
<!-- provided-files -->
weighted/imatrix quants seem not to be available (by me) at this time. If they do not show up a week or so after the static ones, I have probably not planned for them. Feel free to request them by opening a Community Discussion.
## Usage
If you are unsure how to use GGUF files, refer to one of [TheBloke's
READMEs](https://huggingface.co/TheBloke/KafkaLM-70B-German-V0.1-GGUF) for
more details, including on how to concatenate multi-part files.
## Provided Quants
(sorted by size, not necessarily quality. IQ-quants are often preferable over similar sized non-IQ quants)
| Link | Type | Size/GB | Notes |
|:-----|:-----|--------:|:------|
| [GGUF](https://huggingface.co/mradermacher/AlphaMath-7B-GGUF/resolve/main/AlphaMath-7B.Q2_K.gguf) | Q2_K | 2.8 | |
| [GGUF](https://huggingface.co/mradermacher/AlphaMath-7B-GGUF/resolve/main/AlphaMath-7B.IQ3_XS.gguf) | IQ3_XS | 3.1 | |
| [GGUF](https://huggingface.co/mradermacher/AlphaMath-7B-GGUF/resolve/main/AlphaMath-7B.IQ3_S.gguf) | IQ3_S | 3.2 | beats Q3_K* |
| [GGUF](https://huggingface.co/mradermacher/AlphaMath-7B-GGUF/resolve/main/AlphaMath-7B.Q3_K_S.gguf) | Q3_K_S | 3.2 | |
| [GGUF](https://huggingface.co/mradermacher/AlphaMath-7B-GGUF/resolve/main/AlphaMath-7B.IQ3_M.gguf) | IQ3_M | 3.4 | |
| [GGUF](https://huggingface.co/mradermacher/AlphaMath-7B-GGUF/resolve/main/AlphaMath-7B.Q3_K_M.gguf) | Q3_K_M | 3.6 | lower quality |
| [GGUF](https://huggingface.co/mradermacher/AlphaMath-7B-GGUF/resolve/main/AlphaMath-7B.Q3_K_L.gguf) | Q3_K_L | 3.8 | |
| [GGUF](https://huggingface.co/mradermacher/AlphaMath-7B-GGUF/resolve/main/AlphaMath-7B.IQ4_XS.gguf) | IQ4_XS | 3.9 | |
| [GGUF](https://huggingface.co/mradermacher/AlphaMath-7B-GGUF/resolve/main/AlphaMath-7B.Q4_K_S.gguf) | Q4_K_S | 4.1 | fast, recommended |
| [GGUF](https://huggingface.co/mradermacher/AlphaMath-7B-GGUF/resolve/main/AlphaMath-7B.Q4_K_M.gguf) | Q4_K_M | 4.3 | fast, recommended |
| [GGUF](https://huggingface.co/mradermacher/AlphaMath-7B-GGUF/resolve/main/AlphaMath-7B.Q5_K_S.gguf) | Q5_K_S | 4.9 | |
| [GGUF](https://huggingface.co/mradermacher/AlphaMath-7B-GGUF/resolve/main/AlphaMath-7B.Q5_K_M.gguf) | Q5_K_M | 5.0 | |
| [GGUF](https://huggingface.co/mradermacher/AlphaMath-7B-GGUF/resolve/main/AlphaMath-7B.Q6_K.gguf) | Q6_K | 5.8 | very good quality |
| [GGUF](https://huggingface.co/mradermacher/AlphaMath-7B-GGUF/resolve/main/AlphaMath-7B.Q8_0.gguf) | Q8_0 | 7.4 | fast, best quality |
| [GGUF](https://huggingface.co/mradermacher/AlphaMath-7B-GGUF/resolve/main/AlphaMath-7B.f16.gguf) | f16 | 13.9 | 16 bpw, overkill |
Here is a handy graph by ikawrakow comparing some lower-quality quant
types (lower is better):

And here are Artefact2's thoughts on the matter:
https://gist.github.com/Artefact2/b5f810600771265fc1e39442288e8ec9
## FAQ / Model Request
See https://huggingface.co/mradermacher/model_requests for some answers to
questions you might have and/or if you want some other model quantized.
## Thanks
I thank my company, [nethype GmbH](https://www.nethype.de/), for letting
me use its servers and providing upgrades to my workstation to enable
this work in my free time.
<!-- end -->
|
urchade/gliner_medium-v2.1 | urchade | "2024-04-10T10:13:28Z" | 12,972 | 10 | gliner | [
"gliner",
"pytorch",
"token-classification",
"en",
"dataset:urchade/pile-mistral-v0.1",
"arxiv:2311.08526",
"license:apache-2.0",
"region:us"
] | token-classification | "2024-03-17T19:43:17Z" | ---
license: apache-2.0
datasets:
- urchade/pile-mistral-v0.1
language:
- en
pipeline_tag: token-classification
library_name: gliner
---
# About
GLiNER is a Named Entity Recognition (NER) model capable of identifying any entity type using a bidirectional transformer encoder (BERT-like). It provides a practical alternative to traditional NER models, which are limited to predefined entities, and Large Language Models (LLMs) that, despite their flexibility, are costly and large for resource-constrained scenarios.
## Links
* Paper: https://arxiv.org/abs/2311.08526
* Repository: https://github.com/urchade/GLiNER
## Available models
| Release | Model Name | # of Parameters | Language | License |
| - | - | - | - | - |
| v0 | [urchade/gliner_base](https://huggingface.co/urchade/gliner_base)<br>[urchade/gliner_multi](https://huggingface.co/urchade/gliner_multi) | 209M<br>209M | English<br>Multilingual | cc-by-nc-4.0 |
| v1 | [urchade/gliner_small-v1](https://huggingface.co/urchade/gliner_small-v1)<br>[urchade/gliner_medium-v1](https://huggingface.co/urchade/gliner_medium-v1)<br>[urchade/gliner_large-v1](https://huggingface.co/urchade/gliner_large-v1) | 166M<br>209M<br>459M | English <br> English <br> English | cc-by-nc-4.0 |
| v2 | [urchade/gliner_small-v2](https://huggingface.co/urchade/gliner_small-v2)<br>[urchade/gliner_medium-v2](https://huggingface.co/urchade/gliner_medium-v2)<br>[urchade/gliner_large-v2](https://huggingface.co/urchade/gliner_large-v2) | 166M<br>209M<br>459M | English <br> English <br> English | apache-2.0 |
| v2.1 | [urchade/gliner_small-v2.1](https://huggingface.co/urchade/gliner_small-v2.1)<br>[urchade/gliner_medium-v2.1](https://huggingface.co/urchade/gliner_medium-v2.1)<br>[urchade/gliner_large-v2.1](https://huggingface.co/urchade/gliner_large-v2.1) <br>[urchade/gliner_multi-v2.1](https://huggingface.co/urchade/gliner_multi-v2.1) | 166M<br>209M<br>459M<br>209M | English <br> English <br> English <br> Multilingual | apache-2.0 |
## Installation
To use this model, you must install the GLiNER Python library:
```
!pip install gliner
```
## Usage
Once you've downloaded the GLiNER library, you can import the GLiNER class. You can then load this model using `GLiNER.from_pretrained` and predict entities with `predict_entities`.
```python
from gliner import GLiNER
model = GLiNER.from_pretrained("urchade/gliner_medium-v2.1")
text = """
Cristiano Ronaldo dos Santos Aveiro (Portuguese pronunciation: [kɾiʃˈtjɐnu ʁɔˈnaldu]; born 5 February 1985) is a Portuguese professional footballer who plays as a forward for and captains both Saudi Pro League club Al Nassr and the Portugal national team. Widely regarded as one of the greatest players of all time, Ronaldo has won five Ballon d'Or awards,[note 3] a record three UEFA Men's Player of the Year Awards, and four European Golden Shoes, the most by a European player. He has won 33 trophies in his career, including seven league titles, five UEFA Champions Leagues, the UEFA European Championship and the UEFA Nations League. Ronaldo holds the records for most appearances (183), goals (140) and assists (42) in the Champions League, goals in the European Championship (14), international goals (128) and international appearances (205). He is one of the few players to have made over 1,200 professional career appearances, the most by an outfield player, and has scored over 850 official senior career goals for club and country, making him the top goalscorer of all time.
"""
labels = ["person", "award", "date", "competitions", "teams"]
entities = model.predict_entities(text, labels)
for entity in entities:
print(entity["text"], "=>", entity["label"])
```
```
Cristiano Ronaldo dos Santos Aveiro => person
5 February 1985 => date
Al Nassr => teams
Portugal national team => teams
Ballon d'Or => award
UEFA Men's Player of the Year Awards => award
European Golden Shoes => award
UEFA Champions Leagues => competitions
UEFA European Championship => competitions
UEFA Nations League => competitions
Champions League => competitions
European Championship => competitions
```
## Named Entity Recognition benchmark result

## Model Authors
The model authors are:
* [Urchade Zaratiana](https://huggingface.co/urchade)
* Nadi Tomeh
* Pierre Holat
* Thierry Charnois
## Citation
```bibtex
@misc{zaratiana2023gliner,
title={GLiNER: Generalist Model for Named Entity Recognition using Bidirectional Transformer},
author={Urchade Zaratiana and Nadi Tomeh and Pierre Holat and Thierry Charnois},
year={2023},
eprint={2311.08526},
archivePrefix={arXiv},
primaryClass={cs.CL}
}
``` |
Yntec/nuipenimix | Yntec | "2024-01-13T21:27:34Z" | 12,969 | 3 | diffusers | [
"diffusers",
"safetensors",
"Anime",
"Cute",
"Animals",
"McSionnaigh",
"stable-diffusion",
"stable-diffusion-diffusers",
"text-to-image",
"license:creativeml-openrail-m",
"endpoints_compatible",
"diffusers:StableDiffusionPipeline",
"region:us"
] | text-to-image | "2024-01-13T21:03:47Z" | ---
license: creativeml-openrail-m
library_name: diffusers
pipeline_tag: text-to-image
tags:
- Anime
- Cute
- Animals
- McSionnaigh
- stable-diffusion
- stable-diffusion-diffusers
- diffusers
- text-to-image
---
# nuipenimix
This model with the MoistMixV2 VAE baked in. Original page: https://civitai.com/models/81937?modelVersionId=86977
Samples and prompts:

Top left: uploaded on e621, ((by Cleon Peterson, by Sonia Delaunay, by Tomer Hanuka, by Dagasi, traditional media (artwork))), solo female ((toony judy hopps, grey body, blue eyes, white short t-shirt, dark blue short pants, small breasts)), shoulder bag, ((three-quarter portrait, three-quarter view,))
Top right: Highly detailed, High Quality, Masterpiece, beautiful, cute girl as toon link, red headwear, Zelda
Bottom left: highquality, masterpiece, girl, Chi-Chi, :D, close up, smile, arms up, pink helmet, black hair, black eyes, blush, bikini armor, aqua cape, pink gloves, pink boots, cleavage. cave, rock, mountain. blue collar
Bottom right: a red panda playing guitar in a club, whimsical
|
Salesforce/ctrl | Salesforce | "2024-02-19T11:09:30Z" | 12,968 | 9 | transformers | [
"transformers",
"pytorch",
"tf",
"ctrl",
"text-generation",
"en",
"arxiv:1909.05858",
"arxiv:1910.09700",
"license:bsd-3-clause",
"endpoints_compatible",
"region:us"
] | text-generation | "2022-03-02T23:29:04Z" | ---
language: en
license: bsd-3-clause
pipeline_tag: text-generation
---
# ctrl
# Table of Contents
1. [Model Details](#model-details)
2. [Uses](#uses)
3. [Bias, Risks, and Limitations](#bias-risks-and-limitations)
4. [Training](#training)
5. [Evaluation](#evaluation)
6. [Environmental Impact](#environmental-impact)
7. [Technical Specifications](#technical-specifications)
8. [Citation](#citation)
9. [Model Card Authors](#model-card-authors)
10. [How To Get Started With the Model](#how-to-get-started-with-the-model)
# Model Details
## Model Description
The CTRL model was proposed in [CTRL: A Conditional Transformer Language Model for Controllable Generation](https://arxiv.org/abs/1909.05858) by Nitish Shirish Keskar*, Bryan McCann*, Lav R. Varshney, Caiming Xiong and Richard Socher. It's a causal (unidirectional) transformer pre-trained using language modeling on a very large corpus of ~140 GB of text data with the first token reserved as a control code (such as Links, Books, Wikipedia etc.). The model developers released a model card for CTRL, available [here](https://github.com/salesforce/ctrl/blob/master/ModelCard.pdf).
In their [model card](https://github.com/salesforce/ctrl/blob/master/ModelCard.pdf), the developers write:
> The CTRL Language Model analyzed in this card generates text conditioned on control codes that specify domain, style, topics, dates, entities, relationships between entities, plot points, and task-related behavior.
- **Developed by:** See [associated paper](https://arxiv.org/abs/1909.05858) from Salesforce Research
- **Model type:** Transformer-based language model
- **Language(s) (NLP):** Primarily English, some German, Spanish, French
- **License:** [BSD 3-Clause](https://github.com/salesforce/ctrl/blob/master/LICENSE.txt); also see [Code of Conduct](https://github.com/salesforce/ctrl)
- **Related Models:** More information needed
- **Parent Model:** More information needed
- **Resources for more information:**
- [Associated paper](https://arxiv.org/abs/1909.05858)
- [GitHub repo](https://github.com/salesforce/ctrl)
- [Developer Model Card](https://github.com/salesforce/ctrl/blob/master/ModelCard.pdf)
- [Blog post](https://blog.salesforceairesearch.com/introducing-a-conditional-transformer-language-model-for-controllable-generation/)
# Uses
## Direct Use
The model is a language model. The model can be used for text generation.
## Downstream Use
In their [model card](https://github.com/salesforce/ctrl/blob/master/ModelCard.pdf), the developers write that the primary intended users are general audiences and NLP Researchers, and that the primary intended uses are:
> 1. Generating artificial text in collaboration with a human, including but not limited to:
> - Creative writing
> - Automating repetitive writing tasks
> - Formatting specific text types
> - Creating contextualized marketing materials
> 2. Improvement of other NLP applications through fine-tuning (on another task or other data, e.g. fine-tuning CTRL to learn new kinds of language like product descriptions)
> 3. Enhancement in the field of natural language understanding to push towards a better understanding of artificial text generation, including how to detect it and work toward control, understanding, and potentially combating potentially negative consequences of such models.
## Out-of-Scope Use
In their [model card](https://github.com/salesforce/ctrl/blob/master/ModelCard.pdf), the developers write:
> - CTRL should not be used for generating artificial text without collaboration with a human.
> - It should not be used to make normative or prescriptive claims.
> - This software should not be used to promote or profit from:
> - violence, hate, and division;
> - environmental destruction;
> - abuse of human rights; or
> - the destruction of people's physical and mental health.
# Bias, Risks, and Limitations
Significant research has explored bias and fairness issues with language models (see, e.g., [Sheng et al. (2021)](https://aclanthology.org/2021.acl-long.330.pdf) and [Bender et al. (2021)](https://dl.acm.org/doi/pdf/10.1145/3442188.3445922)). Predictions generated by the model may include disturbing and harmful stereotypes across protected classes; identity characteristics; and sensitive, social, and occupational groups.
In their [model card](https://github.com/salesforce/ctrl/blob/master/ModelCard.pdf), the developers write:
> We recognize the potential for misuse or abuse, including use by bad actors who could manipulate the system to act maliciously and generate text to influence decision-making in political, economic, and social settings. False attribution could also harm individuals, organizations, or other entities. To address these concerns, the model was evaluated internally as well as externally by third parties, including the Partnership on AI, prior to release.
> To mitigate potential misuse to the extent possible, we stripped out all detectable training data from undesirable sources. We then redteamed the model and found that negative utterances were often placed in contexts that made them identifiable as such. For example, when using the ‘News’ control code, hate speech could be embedded as part of an apology (e.g. “the politician apologized for saying [insert hateful statement]”), implying that this type of speech was negative. By pre-selecting the available control codes (omitting, for example, Instagram and Twitter from the available domains), we are able to limit the potential for misuse.
> In releasing our model, we hope to put it into the hands of researchers and prosocial actors so that they can work to control, understand, and potentially combat the negative consequences of such models. We hope that research into detecting fake news and model-generated content of all kinds will be pushed forward by CTRL. It is our belief that these models should become a common tool so researchers can design methods to guard against malicious use and so the public becomes familiar with their existence and patterns of behavior.
See the [associated paper](https://arxiv.org/pdf/1909.05858.pdf) for further discussions about the ethics of LLMs.
## Recommendations
In their [model card](https://github.com/salesforce/ctrl/blob/master/ModelCard.pdf), the developers write:
> - A recommendation to monitor and detect use will be implemented through the development of a model that will identify CTRLgenerated text.
> - A second recommendation to further screen the input into and output from the model will be implemented through the addition of a check in the CTRL interface to prohibit the insertion into the model of certain negative inputs, which will help control the output that can be generated.
> - The model is trained on a limited number of languages: primarily English and some German, Spanish, French. A recommendation for a future area of research is to train the model on more languages.
See the [CTRL-detector GitHub repo](https://github.com/salesforce/ctrl-detector) for more on the detector model.
# Training
## Training Data
In their [model card](https://github.com/salesforce/ctrl/blob/master/ModelCard.pdf), the developers write:
> This model is trained on 140 GB of text drawn from a variety of domains: Wikipedia (English, German, Spanish, and French), Project Gutenberg, submissions from 45 subreddits, OpenWebText, a large collection of news data, Amazon Reviews, Europarl and UN data from WMT (En-De, En-Es, En-Fr), question-answer pairs (no context documents) from ELI5, and the MRQA shared task, which includes Stanford Question Answering Dataset, NewsQA, TriviaQA, SearchQA, HotpotQA, and Natural Questions. See the paper for the full list of training data.
## Training Procedure
### Preprocessing
In the [associated paper](https://arxiv.org/pdf/1909.05858.pdf) the developers write:
> We learn BPE (Sennrich et al., 2015) codes and tokenize the data using fastBPE4, but we use a large vocabulary of roughly 250K tokens. This includes the sub-word tokens necessary to mitigate problems with rare words, but it also reduces the average number of tokens required to generate long text by including most common words. We use English Wikipedia and a 5% split of our collected OpenWebText data for learning BPE codes. We also introduce an unknown token so that during preprocessing we can filter out sequences that contain more than 2 unknown tokens. This, along with the compressed storage for efficient training (TFRecords) (Abadi et al., 2016), reduces our training data to 140 GB from the total 180 GB collected.
See the paper for links, references, and further details.
### Training
In the [associated paper](https://arxiv.org/pdf/1909.05858.pdf) the developers write:
> CTRL has model dimension d = 1280, inner dimension f = 8192, 48 layers, and 16 heads per layer. Dropout with probability 0.1 follows the residual connections in each layer. Token embeddings were tied with the final output embedding layer (Inan et al., 2016; Press & Wolf, 2016).
See the paper for links, references, and further details.
# Evaluation
## Testing Data, Factors & Metrics
In their [model card](https://github.com/salesforce/ctrl/blob/master/ModelCard.pdf), the developers write that model performance measures are:
> Performance evaluated on qualitative judgments by humans as to whether the control codes lead to text generated in the desired domain
# Environmental Impact
Carbon emissions can be estimated using the [Machine Learning Impact calculator](https://mlco2.github.io/impact#compute) presented in [Lacoste et al. (2019)](https://arxiv.org/abs/1910.09700). Details are pulled from the [associated paper](https://arxiv.org/pdf/1909.05858.pdf).
- **Hardware Type:** TPU v3 Pod
- **Hours used:** Approximately 336 hours (2 weeks)
- **Cloud Provider:** GCP
- **Compute Region:** More information needed
- **Carbon Emitted:** More information needed
# Technical Specifications
In the [associated paper](https://arxiv.org/pdf/1909.05858.pdf) the developers write:
> CTRL was implemented in TensorFlow (Abadi et al., 2016) and trained with a global batch size of 1024 distributed across 256 cores of a Cloud TPU v3 Pod for 800k iterations. Training took approximately 2 weeks using Adagrad (Duchi et al., 2011) with a linear warmup from 0 to 0.05 over 25k steps. The norm of gradients were clipped to 0.25 as in (Merity et al., 2017). Learning rate decay was not necessary due to the monotonic nature of the Adagrad accumulator. We compared to the Adam optimizer (Kingma & Ba, 2014) while training smaller models, but we noticed comparable convergence rates and significant memory savings with Adagrad. We also experimented with explicit memory-saving optimizers including SM3 (Anil et al., 2019), Adafactor (Shazeer & Stern, 2018), and NovoGrad (Ginsburg et al., 2019) with mixed results.
See the paper for links, references, and further details.
# Citation
**BibTeX:**
```bibtex
@article{keskarCTRL2019,
title={{CTRL - A Conditional Transformer Language Model for Controllable Generation}},
author={Keskar, Nitish Shirish and McCann, Bryan and Varshney, Lav and Xiong, Caiming and Socher, Richard},
journal={arXiv preprint arXiv:1909.05858},
year={2019}
}
```
**APA:**
- Keskar, N. S., McCann, B., Varshney, L. R., Xiong, C., & Socher, R. (2019). Ctrl: A conditional transformer language model for controllable generation. arXiv preprint arXiv:1909.05858.
# Model Card Authors
This model card was written by the team at Hugging Face, referencing the [model card](https://github.com/salesforce/ctrl/blob/master/ModelCard.pdf) released by the developers.
# How to Get Started with the Model
Use the code below to get started with the model. See the [Hugging Face ctrl docs](https://huggingface.co/docs/transformers/model_doc/ctrl) for more information.
<details>
<summary> Click to expand </summary>
```python
>>> from transformers import CTRLTokenizer, CTRLModel
>>> import torch
>>> tokenizer = CTRLTokenizer.from_pretrained("ctrl")
>>> model = CTRLModel.from_pretrained("ctrl")
>>> # CTRL was trained with control codes as the first token
>>> inputs = tokenizer("Opinion My dog is cute", return_tensors="pt")
>>> assert inputs["input_ids"][0, 0].item() in tokenizer.control_codes.values()
>>> outputs = model(**inputs)
>>> last_hidden_states = outputs.last_hidden_state
>>> list(last_hidden_states.shape)
```
</details> |
mradermacher/Llama-3-LiPPA-8B-GGUF | mradermacher | "2024-06-28T03:05:43Z" | 12,965 | 0 | transformers | [
"transformers",
"gguf",
"text-generation-inference",
"unsloth",
"llama",
"trl",
"sft",
"not-for-all-audiences",
"en",
"dataset:mpasila/LimaRP-PIPPA-Mix-8K-Context",
"dataset:grimulkan/LimaRP-augmented",
"dataset:KaraKaraWitch/PIPPA-ShareGPT-formatted",
"base_model:mpasila/Llama-3-LiPPA-8B",
"license:llama3",
"endpoints_compatible",
"region:us"
] | null | "2024-06-26T23:35:08Z" | ---
base_model: mpasila/Llama-3-LiPPA-8B
datasets:
- mpasila/LimaRP-PIPPA-Mix-8K-Context
- grimulkan/LimaRP-augmented
- KaraKaraWitch/PIPPA-ShareGPT-formatted
language:
- en
library_name: transformers
license: llama3
quantized_by: mradermacher
tags:
- text-generation-inference
- transformers
- unsloth
- llama
- trl
- sft
- not-for-all-audiences
---
## About
<!-- ### quantize_version: 2 -->
<!-- ### output_tensor_quantised: 1 -->
<!-- ### convert_type: hf -->
<!-- ### vocab_type: -->
<!-- ### tags: -->
static quants of https://huggingface.co/mpasila/Llama-3-LiPPA-8B
<!-- provided-files -->
weighted/imatrix quants are available at https://huggingface.co/mradermacher/Llama-3-LiPPA-8B-i1-GGUF
## Usage
If you are unsure how to use GGUF files, refer to one of [TheBloke's
READMEs](https://huggingface.co/TheBloke/KafkaLM-70B-German-V0.1-GGUF) for
more details, including on how to concatenate multi-part files.
## Provided Quants
(sorted by size, not necessarily quality. IQ-quants are often preferable over similar sized non-IQ quants)
| Link | Type | Size/GB | Notes |
|:-----|:-----|--------:|:------|
| [GGUF](https://huggingface.co/mradermacher/Llama-3-LiPPA-8B-GGUF/resolve/main/Llama-3-LiPPA-8B.Q2_K.gguf) | Q2_K | 3.3 | |
| [GGUF](https://huggingface.co/mradermacher/Llama-3-LiPPA-8B-GGUF/resolve/main/Llama-3-LiPPA-8B.IQ3_XS.gguf) | IQ3_XS | 3.6 | |
| [GGUF](https://huggingface.co/mradermacher/Llama-3-LiPPA-8B-GGUF/resolve/main/Llama-3-LiPPA-8B.Q3_K_S.gguf) | Q3_K_S | 3.8 | |
| [GGUF](https://huggingface.co/mradermacher/Llama-3-LiPPA-8B-GGUF/resolve/main/Llama-3-LiPPA-8B.IQ3_S.gguf) | IQ3_S | 3.8 | beats Q3_K* |
| [GGUF](https://huggingface.co/mradermacher/Llama-3-LiPPA-8B-GGUF/resolve/main/Llama-3-LiPPA-8B.IQ3_M.gguf) | IQ3_M | 3.9 | |
| [GGUF](https://huggingface.co/mradermacher/Llama-3-LiPPA-8B-GGUF/resolve/main/Llama-3-LiPPA-8B.Q3_K_M.gguf) | Q3_K_M | 4.1 | lower quality |
| [GGUF](https://huggingface.co/mradermacher/Llama-3-LiPPA-8B-GGUF/resolve/main/Llama-3-LiPPA-8B.Q3_K_L.gguf) | Q3_K_L | 4.4 | |
| [GGUF](https://huggingface.co/mradermacher/Llama-3-LiPPA-8B-GGUF/resolve/main/Llama-3-LiPPA-8B.IQ4_XS.gguf) | IQ4_XS | 4.6 | |
| [GGUF](https://huggingface.co/mradermacher/Llama-3-LiPPA-8B-GGUF/resolve/main/Llama-3-LiPPA-8B.Q4_K_S.gguf) | Q4_K_S | 4.8 | fast, recommended |
| [GGUF](https://huggingface.co/mradermacher/Llama-3-LiPPA-8B-GGUF/resolve/main/Llama-3-LiPPA-8B.Q4_K_M.gguf) | Q4_K_M | 5.0 | fast, recommended |
| [GGUF](https://huggingface.co/mradermacher/Llama-3-LiPPA-8B-GGUF/resolve/main/Llama-3-LiPPA-8B.Q5_K_S.gguf) | Q5_K_S | 5.7 | |
| [GGUF](https://huggingface.co/mradermacher/Llama-3-LiPPA-8B-GGUF/resolve/main/Llama-3-LiPPA-8B.Q5_K_M.gguf) | Q5_K_M | 5.8 | |
| [GGUF](https://huggingface.co/mradermacher/Llama-3-LiPPA-8B-GGUF/resolve/main/Llama-3-LiPPA-8B.Q6_K.gguf) | Q6_K | 6.7 | very good quality |
| [GGUF](https://huggingface.co/mradermacher/Llama-3-LiPPA-8B-GGUF/resolve/main/Llama-3-LiPPA-8B.Q8_0.gguf) | Q8_0 | 8.6 | fast, best quality |
| [GGUF](https://huggingface.co/mradermacher/Llama-3-LiPPA-8B-GGUF/resolve/main/Llama-3-LiPPA-8B.f16.gguf) | f16 | 16.2 | 16 bpw, overkill |
Here is a handy graph by ikawrakow comparing some lower-quality quant
types (lower is better):

And here are Artefact2's thoughts on the matter:
https://gist.github.com/Artefact2/b5f810600771265fc1e39442288e8ec9
## FAQ / Model Request
See https://huggingface.co/mradermacher/model_requests for some answers to
questions you might have and/or if you want some other model quantized.
## Thanks
I thank my company, [nethype GmbH](https://www.nethype.de/), for letting
me use its servers and providing upgrades to my workstation to enable
this work in my free time.
<!-- end -->
|
mradermacher/Thoth-GGUF | mradermacher | "2024-06-23T04:37:41Z" | 12,959 | 0 | transformers | [
"transformers",
"gguf",
"mergekit",
"merge",
"en",
"base_model:CoprolaliacPress/Thoth",
"endpoints_compatible",
"region:us"
] | null | "2024-06-23T04:09:37Z" | ---
base_model: CoprolaliacPress/Thoth
language:
- en
library_name: transformers
quantized_by: mradermacher
tags:
- mergekit
- merge
---
## About
<!-- ### quantize_version: 2 -->
<!-- ### output_tensor_quantised: 1 -->
<!-- ### convert_type: hf -->
<!-- ### vocab_type: -->
<!-- ### tags: -->
static quants of https://huggingface.co/CoprolaliacPress/Thoth
<!-- provided-files -->
weighted/imatrix quants seem not to be available (by me) at this time. If they do not show up a week or so after the static ones, I have probably not planned for them. Feel free to request them by opening a Community Discussion.
## Usage
If you are unsure how to use GGUF files, refer to one of [TheBloke's
READMEs](https://huggingface.co/TheBloke/KafkaLM-70B-German-V0.1-GGUF) for
more details, including on how to concatenate multi-part files.
## Provided Quants
(sorted by size, not necessarily quality. IQ-quants are often preferable over similar sized non-IQ quants)
| Link | Type | Size/GB | Notes |
|:-----|:-----|--------:|:------|
| [GGUF](https://huggingface.co/mradermacher/Thoth-GGUF/resolve/main/Thoth.Q2_K.gguf) | Q2_K | 2.8 | |
| [GGUF](https://huggingface.co/mradermacher/Thoth-GGUF/resolve/main/Thoth.IQ3_XS.gguf) | IQ3_XS | 3.1 | |
| [GGUF](https://huggingface.co/mradermacher/Thoth-GGUF/resolve/main/Thoth.Q3_K_S.gguf) | Q3_K_S | 3.3 | |
| [GGUF](https://huggingface.co/mradermacher/Thoth-GGUF/resolve/main/Thoth.IQ3_S.gguf) | IQ3_S | 3.3 | beats Q3_K* |
| [GGUF](https://huggingface.co/mradermacher/Thoth-GGUF/resolve/main/Thoth.IQ3_M.gguf) | IQ3_M | 3.4 | |
| [GGUF](https://huggingface.co/mradermacher/Thoth-GGUF/resolve/main/Thoth.Q3_K_M.gguf) | Q3_K_M | 3.6 | lower quality |
| [GGUF](https://huggingface.co/mradermacher/Thoth-GGUF/resolve/main/Thoth.Q3_K_L.gguf) | Q3_K_L | 3.9 | |
| [GGUF](https://huggingface.co/mradermacher/Thoth-GGUF/resolve/main/Thoth.IQ4_XS.gguf) | IQ4_XS | 4.0 | |
| [GGUF](https://huggingface.co/mradermacher/Thoth-GGUF/resolve/main/Thoth.Q4_K_S.gguf) | Q4_K_S | 4.2 | fast, recommended |
| [GGUF](https://huggingface.co/mradermacher/Thoth-GGUF/resolve/main/Thoth.Q4_K_M.gguf) | Q4_K_M | 4.5 | fast, recommended |
| [GGUF](https://huggingface.co/mradermacher/Thoth-GGUF/resolve/main/Thoth.Q5_K_S.gguf) | Q5_K_S | 5.1 | |
| [GGUF](https://huggingface.co/mradermacher/Thoth-GGUF/resolve/main/Thoth.Q5_K_M.gguf) | Q5_K_M | 5.2 | |
| [GGUF](https://huggingface.co/mradermacher/Thoth-GGUF/resolve/main/Thoth.Q6_K.gguf) | Q6_K | 6.0 | very good quality |
| [GGUF](https://huggingface.co/mradermacher/Thoth-GGUF/resolve/main/Thoth.Q8_0.gguf) | Q8_0 | 7.8 | fast, best quality |
| [GGUF](https://huggingface.co/mradermacher/Thoth-GGUF/resolve/main/Thoth.f16.gguf) | f16 | 14.6 | 16 bpw, overkill |
Here is a handy graph by ikawrakow comparing some lower-quality quant
types (lower is better):

And here are Artefact2's thoughts on the matter:
https://gist.github.com/Artefact2/b5f810600771265fc1e39442288e8ec9
## FAQ / Model Request
See https://huggingface.co/mradermacher/model_requests for some answers to
questions you might have and/or if you want some other model quantized.
## Thanks
I thank my company, [nethype GmbH](https://www.nethype.de/), for letting
me use its servers and providing upgrades to my workstation to enable
this work in my free time.
<!-- end -->
|
mradermacher/SthenoBu8bl3-32K-GGUF | mradermacher | "2024-06-24T14:23:00Z" | 12,955 | 0 | transformers | [
"transformers",
"gguf",
"mergekit",
"merge",
"en",
"base_model:Hastagaras/SthenoBu8bl3-32K",
"license:llama3",
"endpoints_compatible",
"region:us"
] | null | "2024-06-24T13:54:44Z" | ---
base_model: Hastagaras/SthenoBu8bl3-32K
language:
- en
library_name: transformers
license: llama3
quantized_by: mradermacher
tags:
- mergekit
- merge
---
## About
<!-- ### quantize_version: 2 -->
<!-- ### output_tensor_quantised: 1 -->
<!-- ### convert_type: hf -->
<!-- ### vocab_type: -->
<!-- ### tags: -->
static quants of https://huggingface.co/Hastagaras/SthenoBu8bl3-32K
<!-- provided-files -->
weighted/imatrix quants are available at https://huggingface.co/mradermacher/SthenoBu8bl3-32K-i1-GGUF
## Usage
If you are unsure how to use GGUF files, refer to one of [TheBloke's
READMEs](https://huggingface.co/TheBloke/KafkaLM-70B-German-V0.1-GGUF) for
more details, including on how to concatenate multi-part files.
## Provided Quants
(sorted by size, not necessarily quality. IQ-quants are often preferable over similar sized non-IQ quants)
| Link | Type | Size/GB | Notes |
|:-----|:-----|--------:|:------|
| [GGUF](https://huggingface.co/mradermacher/SthenoBu8bl3-32K-GGUF/resolve/main/SthenoBu8bl3-32K.Q2_K.gguf) | Q2_K | 3.3 | |
| [GGUF](https://huggingface.co/mradermacher/SthenoBu8bl3-32K-GGUF/resolve/main/SthenoBu8bl3-32K.IQ3_XS.gguf) | IQ3_XS | 3.6 | |
| [GGUF](https://huggingface.co/mradermacher/SthenoBu8bl3-32K-GGUF/resolve/main/SthenoBu8bl3-32K.Q3_K_S.gguf) | Q3_K_S | 3.8 | |
| [GGUF](https://huggingface.co/mradermacher/SthenoBu8bl3-32K-GGUF/resolve/main/SthenoBu8bl3-32K.IQ3_S.gguf) | IQ3_S | 3.8 | beats Q3_K* |
| [GGUF](https://huggingface.co/mradermacher/SthenoBu8bl3-32K-GGUF/resolve/main/SthenoBu8bl3-32K.IQ3_M.gguf) | IQ3_M | 3.9 | |
| [GGUF](https://huggingface.co/mradermacher/SthenoBu8bl3-32K-GGUF/resolve/main/SthenoBu8bl3-32K.Q3_K_M.gguf) | Q3_K_M | 4.1 | lower quality |
| [GGUF](https://huggingface.co/mradermacher/SthenoBu8bl3-32K-GGUF/resolve/main/SthenoBu8bl3-32K.Q3_K_L.gguf) | Q3_K_L | 4.4 | |
| [GGUF](https://huggingface.co/mradermacher/SthenoBu8bl3-32K-GGUF/resolve/main/SthenoBu8bl3-32K.IQ4_XS.gguf) | IQ4_XS | 4.6 | |
| [GGUF](https://huggingface.co/mradermacher/SthenoBu8bl3-32K-GGUF/resolve/main/SthenoBu8bl3-32K.Q4_K_S.gguf) | Q4_K_S | 4.8 | fast, recommended |
| [GGUF](https://huggingface.co/mradermacher/SthenoBu8bl3-32K-GGUF/resolve/main/SthenoBu8bl3-32K.Q4_K_M.gguf) | Q4_K_M | 5.0 | fast, recommended |
| [GGUF](https://huggingface.co/mradermacher/SthenoBu8bl3-32K-GGUF/resolve/main/SthenoBu8bl3-32K.Q5_K_S.gguf) | Q5_K_S | 5.7 | |
| [GGUF](https://huggingface.co/mradermacher/SthenoBu8bl3-32K-GGUF/resolve/main/SthenoBu8bl3-32K.Q5_K_M.gguf) | Q5_K_M | 5.8 | |
| [GGUF](https://huggingface.co/mradermacher/SthenoBu8bl3-32K-GGUF/resolve/main/SthenoBu8bl3-32K.Q6_K.gguf) | Q6_K | 6.7 | very good quality |
| [GGUF](https://huggingface.co/mradermacher/SthenoBu8bl3-32K-GGUF/resolve/main/SthenoBu8bl3-32K.Q8_0.gguf) | Q8_0 | 8.6 | fast, best quality |
| [GGUF](https://huggingface.co/mradermacher/SthenoBu8bl3-32K-GGUF/resolve/main/SthenoBu8bl3-32K.f16.gguf) | f16 | 16.2 | 16 bpw, overkill |
Here is a handy graph by ikawrakow comparing some lower-quality quant
types (lower is better):

And here are Artefact2's thoughts on the matter:
https://gist.github.com/Artefact2/b5f810600771265fc1e39442288e8ec9
## FAQ / Model Request
See https://huggingface.co/mradermacher/model_requests for some answers to
questions you might have and/or if you want some other model quantized.
## Thanks
I thank my company, [nethype GmbH](https://www.nethype.de/), for letting
me use its servers and providing upgrades to my workstation to enable
this work in my free time.
<!-- end -->
|
mradermacher/Tinybra_13B-i1-GGUF | mradermacher | "2024-06-22T17:34:36Z" | 12,932 | 1 | transformers | [
"transformers",
"gguf",
"en",
"base_model:SicariusSicariiStuff/Tinybra_13B",
"license:apache-2.0",
"endpoints_compatible",
"region:us"
] | null | "2024-06-22T15:27:54Z" | ---
base_model: SicariusSicariiStuff/Tinybra_13B
language:
- en
library_name: transformers
license: apache-2.0
quantized_by: mradermacher
---
## About
<!-- ### quantize_version: 2 -->
<!-- ### output_tensor_quantised: 1 -->
<!-- ### convert_type: hf -->
<!-- ### vocab_type: -->
<!-- ### tags: nicoboss -->
weighted/imatrix quants of https://huggingface.co/SicariusSicariiStuff/Tinybra_13B
<!-- provided-files -->
static quants are available at https://huggingface.co/mradermacher/Tinybra_13B-GGUF
## Usage
If you are unsure how to use GGUF files, refer to one of [TheBloke's
READMEs](https://huggingface.co/TheBloke/KafkaLM-70B-German-V0.1-GGUF) for
more details, including on how to concatenate multi-part files.
## Provided Quants
(sorted by size, not necessarily quality. IQ-quants are often preferable over similar sized non-IQ quants)
| Link | Type | Size/GB | Notes |
|:-----|:-----|--------:|:------|
| [GGUF](https://huggingface.co/mradermacher/Tinybra_13B-i1-GGUF/resolve/main/Tinybra_13B.i1-IQ1_S.gguf) | i1-IQ1_S | 3.0 | for the desperate |
| [GGUF](https://huggingface.co/mradermacher/Tinybra_13B-i1-GGUF/resolve/main/Tinybra_13B.i1-IQ1_M.gguf) | i1-IQ1_M | 3.2 | mostly desperate |
| [GGUF](https://huggingface.co/mradermacher/Tinybra_13B-i1-GGUF/resolve/main/Tinybra_13B.i1-IQ2_XXS.gguf) | i1-IQ2_XXS | 3.6 | |
| [GGUF](https://huggingface.co/mradermacher/Tinybra_13B-i1-GGUF/resolve/main/Tinybra_13B.i1-IQ2_XS.gguf) | i1-IQ2_XS | 4.0 | |
| [GGUF](https://huggingface.co/mradermacher/Tinybra_13B-i1-GGUF/resolve/main/Tinybra_13B.i1-IQ2_S.gguf) | i1-IQ2_S | 4.3 | |
| [GGUF](https://huggingface.co/mradermacher/Tinybra_13B-i1-GGUF/resolve/main/Tinybra_13B.i1-IQ2_M.gguf) | i1-IQ2_M | 4.6 | |
| [GGUF](https://huggingface.co/mradermacher/Tinybra_13B-i1-GGUF/resolve/main/Tinybra_13B.i1-Q2_K.gguf) | i1-Q2_K | 5.0 | IQ3_XXS probably better |
| [GGUF](https://huggingface.co/mradermacher/Tinybra_13B-i1-GGUF/resolve/main/Tinybra_13B.i1-IQ3_XXS.gguf) | i1-IQ3_XXS | 5.1 | lower quality |
| [GGUF](https://huggingface.co/mradermacher/Tinybra_13B-i1-GGUF/resolve/main/Tinybra_13B.i1-IQ3_XS.gguf) | i1-IQ3_XS | 5.5 | |
| [GGUF](https://huggingface.co/mradermacher/Tinybra_13B-i1-GGUF/resolve/main/Tinybra_13B.i1-IQ3_S.gguf) | i1-IQ3_S | 5.8 | beats Q3_K* |
| [GGUF](https://huggingface.co/mradermacher/Tinybra_13B-i1-GGUF/resolve/main/Tinybra_13B.i1-Q3_K_S.gguf) | i1-Q3_K_S | 5.8 | IQ3_XS probably better |
| [GGUF](https://huggingface.co/mradermacher/Tinybra_13B-i1-GGUF/resolve/main/Tinybra_13B.i1-IQ3_M.gguf) | i1-IQ3_M | 6.1 | |
| [GGUF](https://huggingface.co/mradermacher/Tinybra_13B-i1-GGUF/resolve/main/Tinybra_13B.i1-Q3_K_M.gguf) | i1-Q3_K_M | 6.4 | IQ3_S probably better |
| [GGUF](https://huggingface.co/mradermacher/Tinybra_13B-i1-GGUF/resolve/main/Tinybra_13B.i1-Q3_K_L.gguf) | i1-Q3_K_L | 7.0 | IQ3_M probably better |
| [GGUF](https://huggingface.co/mradermacher/Tinybra_13B-i1-GGUF/resolve/main/Tinybra_13B.i1-IQ4_XS.gguf) | i1-IQ4_XS | 7.1 | |
| [GGUF](https://huggingface.co/mradermacher/Tinybra_13B-i1-GGUF/resolve/main/Tinybra_13B.i1-Q4_0.gguf) | i1-Q4_0 | 7.5 | fast, low quality |
| [GGUF](https://huggingface.co/mradermacher/Tinybra_13B-i1-GGUF/resolve/main/Tinybra_13B.i1-Q4_K_S.gguf) | i1-Q4_K_S | 7.5 | optimal size/speed/quality |
| [GGUF](https://huggingface.co/mradermacher/Tinybra_13B-i1-GGUF/resolve/main/Tinybra_13B.i1-Q4_K_M.gguf) | i1-Q4_K_M | 8.0 | fast, recommended |
| [GGUF](https://huggingface.co/mradermacher/Tinybra_13B-i1-GGUF/resolve/main/Tinybra_13B.i1-Q5_K_S.gguf) | i1-Q5_K_S | 9.1 | |
| [GGUF](https://huggingface.co/mradermacher/Tinybra_13B-i1-GGUF/resolve/main/Tinybra_13B.i1-Q5_K_M.gguf) | i1-Q5_K_M | 9.3 | |
| [GGUF](https://huggingface.co/mradermacher/Tinybra_13B-i1-GGUF/resolve/main/Tinybra_13B.i1-Q6_K.gguf) | i1-Q6_K | 10.8 | practically like static Q6_K |
Here is a handy graph by ikawrakow comparing some lower-quality quant
types (lower is better):

And here are Artefact2's thoughts on the matter:
https://gist.github.com/Artefact2/b5f810600771265fc1e39442288e8ec9
## FAQ / Model Request
See https://huggingface.co/mradermacher/model_requests for some answers to
questions you might have and/or if you want some other model quantized.
## Thanks
I thank my company, [nethype GmbH](https://www.nethype.de/), for letting
me use its servers and providing upgrades to my workstation to enable
this work in my free time. Additional thanks to [@nicoboss](https://huggingface.co/nicoboss) for giving me access to his hardware for calculating the imatrix for these quants.
<!-- end -->
|
google/reformer-crime-and-punishment | google | "2024-02-29T09:53:44Z" | 12,931 | 8 | transformers | [
"transformers",
"pytorch",
"rust",
"reformer",
"text-generation",
"autotrain_compatible",
"endpoints_compatible",
"region:us"
] | text-generation | "2022-03-02T23:29:05Z" | ## Reformer Model trained on "Crime and Punishment"
Crime and Punishment is a novel written by Fyodor Dostoevsky and was translated into English.
Crime and Punishment training data was taken from `gs://trax-ml/reformer/crime-and-punishment-2554.txt` and contains
roughly 0.5M tokens.
The ReformerLM model was trained in flax using colab notebook proposed by authors: https://colab.research.google.com/github/google/trax/blob/master/trax/models/reformer/text_generation.ipynb and the weights were converted to Hugging Face's PyTorch ReformerLM model `ReformerModelWithLMHead`.
The model is a language model that operates on small sub-word units. Text can be generated as follows:
```python
model = ReformerModelWithLMHead.from_pretrained("google/reformer-crime-and-punishment")
tok = ReformerTokenizer.from_pretrained("google/reformer-crime-and-punishment")
tok.decode(model.generate(tok.encode("A few months later", return_tensors="pt"), do_sample=True,temperature=0.7, max_length=100)[0])
# gives:'A few months later on was more than anything in the flat.
# “I have already.” “That’s not my notion that he had forgotten him.
# What does that matter? And why do you mean? It’s only another fellow,” he said as he went out, as though he want'
```
|
mradermacher/SharkOgno-11b-Passthrough-GGUF | mradermacher | "2024-06-23T06:02:46Z" | 12,930 | 0 | transformers | [
"transformers",
"gguf",
"merge",
"mergekit",
"lazymergekit",
"powermove72/Shark-1",
"eren23/OGNO-7b-dpo-truthful",
"en",
"base_model:powermove72/SharkOgno-11b-Passthrough",
"endpoints_compatible",
"region:us"
] | null | "2024-06-23T04:59:53Z" | ---
base_model: powermove72/SharkOgno-11b-Passthrough
language:
- en
library_name: transformers
quantized_by: mradermacher
tags:
- merge
- mergekit
- lazymergekit
- powermove72/Shark-1
- eren23/OGNO-7b-dpo-truthful
---
## About
<!-- ### quantize_version: 2 -->
<!-- ### output_tensor_quantised: 1 -->
<!-- ### convert_type: hf -->
<!-- ### vocab_type: -->
<!-- ### tags: -->
static quants of https://huggingface.co/powermove72/SharkOgno-11b-Passthrough
<!-- provided-files -->
weighted/imatrix quants seem not to be available (by me) at this time. If they do not show up a week or so after the static ones, I have probably not planned for them. Feel free to request them by opening a Community Discussion.
## Usage
If you are unsure how to use GGUF files, refer to one of [TheBloke's
READMEs](https://huggingface.co/TheBloke/KafkaLM-70B-German-V0.1-GGUF) for
more details, including on how to concatenate multi-part files.
## Provided Quants
(sorted by size, not necessarily quality. IQ-quants are often preferable over similar sized non-IQ quants)
| Link | Type | Size/GB | Notes |
|:-----|:-----|--------:|:------|
| [GGUF](https://huggingface.co/mradermacher/SharkOgno-11b-Passthrough-GGUF/resolve/main/SharkOgno-11b-Passthrough.Q2_K.gguf) | Q2_K | 4.1 | |
| [GGUF](https://huggingface.co/mradermacher/SharkOgno-11b-Passthrough-GGUF/resolve/main/SharkOgno-11b-Passthrough.IQ3_XS.gguf) | IQ3_XS | 4.5 | |
| [GGUF](https://huggingface.co/mradermacher/SharkOgno-11b-Passthrough-GGUF/resolve/main/SharkOgno-11b-Passthrough.Q3_K_S.gguf) | Q3_K_S | 4.8 | |
| [GGUF](https://huggingface.co/mradermacher/SharkOgno-11b-Passthrough-GGUF/resolve/main/SharkOgno-11b-Passthrough.IQ3_S.gguf) | IQ3_S | 4.8 | beats Q3_K* |
| [GGUF](https://huggingface.co/mradermacher/SharkOgno-11b-Passthrough-GGUF/resolve/main/SharkOgno-11b-Passthrough.IQ3_M.gguf) | IQ3_M | 4.9 | |
| [GGUF](https://huggingface.co/mradermacher/SharkOgno-11b-Passthrough-GGUF/resolve/main/SharkOgno-11b-Passthrough.Q3_K_M.gguf) | Q3_K_M | 5.3 | lower quality |
| [GGUF](https://huggingface.co/mradermacher/SharkOgno-11b-Passthrough-GGUF/resolve/main/SharkOgno-11b-Passthrough.Q3_K_L.gguf) | Q3_K_L | 5.8 | |
| [GGUF](https://huggingface.co/mradermacher/SharkOgno-11b-Passthrough-GGUF/resolve/main/SharkOgno-11b-Passthrough.IQ4_XS.gguf) | IQ4_XS | 5.9 | |
| [GGUF](https://huggingface.co/mradermacher/SharkOgno-11b-Passthrough-GGUF/resolve/main/SharkOgno-11b-Passthrough.Q4_K_S.gguf) | Q4_K_S | 6.2 | fast, recommended |
| [GGUF](https://huggingface.co/mradermacher/SharkOgno-11b-Passthrough-GGUF/resolve/main/SharkOgno-11b-Passthrough.Q4_K_M.gguf) | Q4_K_M | 6.6 | fast, recommended |
| [GGUF](https://huggingface.co/mradermacher/SharkOgno-11b-Passthrough-GGUF/resolve/main/SharkOgno-11b-Passthrough.Q5_K_S.gguf) | Q5_K_S | 7.5 | |
| [GGUF](https://huggingface.co/mradermacher/SharkOgno-11b-Passthrough-GGUF/resolve/main/SharkOgno-11b-Passthrough.Q5_K_M.gguf) | Q5_K_M | 7.7 | |
| [GGUF](https://huggingface.co/mradermacher/SharkOgno-11b-Passthrough-GGUF/resolve/main/SharkOgno-11b-Passthrough.Q6_K.gguf) | Q6_K | 8.9 | very good quality |
| [GGUF](https://huggingface.co/mradermacher/SharkOgno-11b-Passthrough-GGUF/resolve/main/SharkOgno-11b-Passthrough.Q8_0.gguf) | Q8_0 | 11.5 | fast, best quality |
Here is a handy graph by ikawrakow comparing some lower-quality quant
types (lower is better):

And here are Artefact2's thoughts on the matter:
https://gist.github.com/Artefact2/b5f810600771265fc1e39442288e8ec9
## FAQ / Model Request
See https://huggingface.co/mradermacher/model_requests for some answers to
questions you might have and/or if you want some other model quantized.
## Thanks
I thank my company, [nethype GmbH](https://www.nethype.de/), for letting
me use its servers and providing upgrades to my workstation to enable
this work in my free time.
<!-- end -->
|
RichardErkhov/SanjiWatsuki_-_Sonya-7B-gguf | RichardErkhov | "2024-06-20T00:11:39Z" | 12,920 | 0 | null | [
"gguf",
"region:us"
] | null | "2024-06-19T20:49:51Z" | Quantization made by Richard Erkhov.
[Github](https://github.com/RichardErkhov)
[Discord](https://discord.gg/pvy7H8DZMG)
[Request more models](https://github.com/RichardErkhov/quant_request)
Sonya-7B - GGUF
- Model creator: https://huggingface.co/SanjiWatsuki/
- Original model: https://huggingface.co/SanjiWatsuki/Sonya-7B/
| Name | Quant method | Size |
| ---- | ---- | ---- |
| [Sonya-7B.Q2_K.gguf](https://huggingface.co/RichardErkhov/SanjiWatsuki_-_Sonya-7B-gguf/blob/main/Sonya-7B.Q2_K.gguf) | Q2_K | 2.53GB |
| [Sonya-7B.IQ3_XS.gguf](https://huggingface.co/RichardErkhov/SanjiWatsuki_-_Sonya-7B-gguf/blob/main/Sonya-7B.IQ3_XS.gguf) | IQ3_XS | 2.81GB |
| [Sonya-7B.IQ3_S.gguf](https://huggingface.co/RichardErkhov/SanjiWatsuki_-_Sonya-7B-gguf/blob/main/Sonya-7B.IQ3_S.gguf) | IQ3_S | 2.96GB |
| [Sonya-7B.Q3_K_S.gguf](https://huggingface.co/RichardErkhov/SanjiWatsuki_-_Sonya-7B-gguf/blob/main/Sonya-7B.Q3_K_S.gguf) | Q3_K_S | 2.95GB |
| [Sonya-7B.IQ3_M.gguf](https://huggingface.co/RichardErkhov/SanjiWatsuki_-_Sonya-7B-gguf/blob/main/Sonya-7B.IQ3_M.gguf) | IQ3_M | 3.06GB |
| [Sonya-7B.Q3_K.gguf](https://huggingface.co/RichardErkhov/SanjiWatsuki_-_Sonya-7B-gguf/blob/main/Sonya-7B.Q3_K.gguf) | Q3_K | 3.28GB |
| [Sonya-7B.Q3_K_M.gguf](https://huggingface.co/RichardErkhov/SanjiWatsuki_-_Sonya-7B-gguf/blob/main/Sonya-7B.Q3_K_M.gguf) | Q3_K_M | 3.28GB |
| [Sonya-7B.Q3_K_L.gguf](https://huggingface.co/RichardErkhov/SanjiWatsuki_-_Sonya-7B-gguf/blob/main/Sonya-7B.Q3_K_L.gguf) | Q3_K_L | 3.56GB |
| [Sonya-7B.IQ4_XS.gguf](https://huggingface.co/RichardErkhov/SanjiWatsuki_-_Sonya-7B-gguf/blob/main/Sonya-7B.IQ4_XS.gguf) | IQ4_XS | 3.67GB |
| [Sonya-7B.Q4_0.gguf](https://huggingface.co/RichardErkhov/SanjiWatsuki_-_Sonya-7B-gguf/blob/main/Sonya-7B.Q4_0.gguf) | Q4_0 | 3.83GB |
| [Sonya-7B.IQ4_NL.gguf](https://huggingface.co/RichardErkhov/SanjiWatsuki_-_Sonya-7B-gguf/blob/main/Sonya-7B.IQ4_NL.gguf) | IQ4_NL | 3.87GB |
| [Sonya-7B.Q4_K_S.gguf](https://huggingface.co/RichardErkhov/SanjiWatsuki_-_Sonya-7B-gguf/blob/main/Sonya-7B.Q4_K_S.gguf) | Q4_K_S | 3.86GB |
| [Sonya-7B.Q4_K.gguf](https://huggingface.co/RichardErkhov/SanjiWatsuki_-_Sonya-7B-gguf/blob/main/Sonya-7B.Q4_K.gguf) | Q4_K | 4.07GB |
| [Sonya-7B.Q4_K_M.gguf](https://huggingface.co/RichardErkhov/SanjiWatsuki_-_Sonya-7B-gguf/blob/main/Sonya-7B.Q4_K_M.gguf) | Q4_K_M | 4.07GB |
| [Sonya-7B.Q4_1.gguf](https://huggingface.co/RichardErkhov/SanjiWatsuki_-_Sonya-7B-gguf/blob/main/Sonya-7B.Q4_1.gguf) | Q4_1 | 4.24GB |
| [Sonya-7B.Q5_0.gguf](https://huggingface.co/RichardErkhov/SanjiWatsuki_-_Sonya-7B-gguf/blob/main/Sonya-7B.Q5_0.gguf) | Q5_0 | 4.65GB |
| [Sonya-7B.Q5_K_S.gguf](https://huggingface.co/RichardErkhov/SanjiWatsuki_-_Sonya-7B-gguf/blob/main/Sonya-7B.Q5_K_S.gguf) | Q5_K_S | 4.65GB |
| [Sonya-7B.Q5_K.gguf](https://huggingface.co/RichardErkhov/SanjiWatsuki_-_Sonya-7B-gguf/blob/main/Sonya-7B.Q5_K.gguf) | Q5_K | 4.78GB |
| [Sonya-7B.Q5_K_M.gguf](https://huggingface.co/RichardErkhov/SanjiWatsuki_-_Sonya-7B-gguf/blob/main/Sonya-7B.Q5_K_M.gguf) | Q5_K_M | 4.78GB |
| [Sonya-7B.Q5_1.gguf](https://huggingface.co/RichardErkhov/SanjiWatsuki_-_Sonya-7B-gguf/blob/main/Sonya-7B.Q5_1.gguf) | Q5_1 | 5.07GB |
| [Sonya-7B.Q6_K.gguf](https://huggingface.co/RichardErkhov/SanjiWatsuki_-_Sonya-7B-gguf/blob/main/Sonya-7B.Q6_K.gguf) | Q6_K | 5.53GB |
| [Sonya-7B.Q8_0.gguf](https://huggingface.co/RichardErkhov/SanjiWatsuki_-_Sonya-7B-gguf/blob/main/Sonya-7B.Q8_0.gguf) | Q8_0 | 7.17GB |
Original model description:
---
license: cc-by-4.0
language:
- en
tags:
- merge
---
<div style="display: flex; justify-content: center; align-items: center">
<img src="https://huggingface.co/SanjiWatsuki/Sonya-7B/resolve/main/assets/Sonya.jpg">
</div
>
<p align="center">
<big><b>Top 1 Performer MT-bench 🤪</b></big>
</p>
## WTF is This?
Sonya-7B is, at the time of writing, the **#1 performing model in MT-Bench first turn, ahead of GPT-4, and overall the #2 model in MT-Bench**, to the best of my knowledge. Sonya-7B should be a good all-purpose model for all tasks including assistant, RP, etc.
Sonya-7B has a similar structure to my previous model, [Silicon-Maid-7B](https://huggingface.co/SanjiWatsuki/Silicon-Maid-7B), and uses a very similar merge. It's a merge of [xDAN-AI/xDAN-L1-Chat-RL-v1](https://huggingface.co/xDAN-AI/xDAN-L1-Chat-RL-v1), [Jan-Ai's Stealth v1.2](https://huggingface.co/jan-hq/stealth-v1.2), [chargoddard/piano-medley-7b](https://huggingface.co/chargoddard/piano-medley-7b), [NeverSleep/Noromaid-7B-v0.2](https://huggingface.co/NeverSleep/Noromaid-7b-v0.2), and [athirdpath/NSFW_DPO_vmgb-7b](athirdpath/NSFW_DPO_vmgb-7b). Sauce is below. Somehow, by combining these pieces, it substantially outscores any of its parents on MT-Bench.
I picked these models because:
* MT-Bench normally correlates well with real world model quality and xDAN performs well on it.
* Almost all models in the mix were Alpaca prompt formatted which gives prompt consistency.
* Stealth v1.2 has been a magic sprinkle that seems to increase my MT-Bench scores.
* I added RP models because it boosted the Writing and Roleplay benchmarks 👀
Based on the parent models, I expect this model to be used with an 8192 context window. Please use NTK scaling alpha of 2.6 to experimentally try out 16384 context.
**Let me be candid:** Despite the test scores, this model is **NOT is a GPT killer**. I think it's a very sharp model **for a 7B**, it probably punches way above its weight **for a 7B**, but it's still a 7B model. Even for a 7B model, I think **it's quirky and has some weird outputs**, probably due to how Frankenstein this merge is. Keep your expectations in check 😉
**MT-Bench Average Turn**
| model | score | size
|--------------------|-----------|--------
| gpt-4 | 8.99 | -
| **Sonya-7B** | **8.52** | **7b**
| xDAN-L1-Chat-RL-v1 | 8.34 | 7b
| Starling-7B | 8.09 | 7b
| Claude-2 | 8.06 | -
| *Silicon-Maid* | *7.96* | *7b*
| *Loyal-Macaroni-Maid*| *7.95* | *7b*
| gpt-3.5-turbo | 7.94 | 20b?
| Claude-1 | 7.90 | -
| OpenChat-3.5 | 7.81 | -
| vicuna-33b-v1.3 | 7.12 | 33b
| wizardlm-30b | 7.01 | 30b
| Llama-2-70b-chat | 6.86 | 70b
<img src="https://huggingface.co/SanjiWatsuki/Sonya-7B/resolve/main/assets/mt-bench-gpt.png">
<img src="https://huggingface.co/SanjiWatsuki/Sonya-7B/resolve/main/assets/mt-bench-comparison.png">
### The Sauce
```
models:
- model: xDAN-AI/xDAN-L1-Chat-RL-v1
parameters:
weight: 1
density: 1
- model: chargoddard/piano-medley-7b
parameters:
weight: 0.3
- model: jan-hq/stealth-v1.2
parameters:
weight: 0.2
- model: NeverSleep/Noromaid-7b-v0.2
parameters:
weight: 0.2
- model: athirdpath/NSFW_DPO_vmgb-7b
parameters:
weight: 0.2
merge_method: ties
base_model: mistralai/Mistral-7B-v0.1
parameters:
density: 0.4
int8_mask: true
normalize: true
dtype: bfloat16
```
**There was no additional training, finetuning, or DPO.** This is a straight merger.
### Prompt Template (Alpaca)
```
Below is an instruction that describes a task. Write a response that appropriately completes the request.
### Instruction:
{prompt}
### Response:
```
I found that this model **performed worse** with the xDAN prompt format so, despite the heavy weight of xDAN in this merger, I recommeend *against* its use.
### Other Benchmark Stuff
**########## First turn ##########**
| model | turn | score | size
|--------------------|------|----------|--------
| **Sonya-7B** | 1 | **9.06875** | **7b**
| gpt-4 | 1 | 8.95625 | -
| xDAN-L1-Chat-RL-v1 | 1 | *8.87500* | *7b*
| xDAN-L2-Chat-RL-v2 | 1 | 8.78750 | 30b
| claude-v1 | 1 | 8.15000 | -
| gpt-3.5-turbo | 1 | 8.07500 | 20b
| vicuna-33b-v1.3 | 1 | 7.45625 | 33b
| wizardlm-30b | 1 | 7.13125 | 30b
| oasst-sft-7-llama-30b | 1 | 7.10625 | 30b
| Llama-2-70b-chat | 1 | 6.98750 | 70b
########## Second turn ##########
| model | turn | score | size
|--------------------|------|-----------|--------
| gpt-4 | 2 | 9.025000 | -
| xDAN-L2-Chat-RL-v2 | 2 | 8.087500 | 30b
| **Sonya-7B** | 2 | **7.962500** | **7b**
| xDAN-L1-Chat-RL-v1 | 2 | 7.825000 | 7b
| gpt-3.5-turbo | 2 | 7.812500 | 20b
| claude-v1 | 2 | 7.650000 | -
| wizardlm-30b | 2 | 6.887500 | 30b
| vicuna-33b-v1.3 | 2 | 6.787500 | 33b
| Llama-2-70b-chat | 2 | 6.725000 | 70b
If you'd like to replicate the MT-Bench run, please ensure that the Alpaca prompt template is applied to the model. I did this by putting "alpaca" in the model path to trigger the `AlpacaAdapter`.
|
mradermacher/EnnoAi-Pro-French-Llama-3-8B-v0.4-GGUF | mradermacher | "2024-07-01T14:44:22Z" | 12,914 | 0 | transformers | [
"transformers",
"gguf",
"chatml",
"fr",
"en",
"base_model:Enno-Ai/EnnoAi-Pro-French-Llama-3-8B-v0.4",
"license:creativeml-openrail-m",
"endpoints_compatible",
"region:us"
] | null | "2024-07-01T11:42:56Z" | ---
base_model: Enno-Ai/EnnoAi-Pro-French-Llama-3-8B-v0.4
language:
- fr
- en
library_name: transformers
license: creativeml-openrail-m
quantized_by: mradermacher
tags:
- chatml
---
## About
<!-- ### quantize_version: 2 -->
<!-- ### output_tensor_quantised: 1 -->
<!-- ### convert_type: hf -->
<!-- ### vocab_type: -->
<!-- ### tags: -->
static quants of https://huggingface.co/Enno-Ai/EnnoAi-Pro-French-Llama-3-8B-v0.4
<!-- provided-files -->
weighted/imatrix quants seem not to be available (by me) at this time. If they do not show up a week or so after the static ones, I have probably not planned for them. Feel free to request them by opening a Community Discussion.
## Usage
If you are unsure how to use GGUF files, refer to one of [TheBloke's
READMEs](https://huggingface.co/TheBloke/KafkaLM-70B-German-V0.1-GGUF) for
more details, including on how to concatenate multi-part files.
## Provided Quants
(sorted by size, not necessarily quality. IQ-quants are often preferable over similar sized non-IQ quants)
| Link | Type | Size/GB | Notes |
|:-----|:-----|--------:|:------|
| [GGUF](https://huggingface.co/mradermacher/EnnoAi-Pro-French-Llama-3-8B-v0.4-GGUF/resolve/main/EnnoAi-Pro-French-Llama-3-8B-v0.4.Q2_K.gguf) | Q2_K | 3.3 | |
| [GGUF](https://huggingface.co/mradermacher/EnnoAi-Pro-French-Llama-3-8B-v0.4-GGUF/resolve/main/EnnoAi-Pro-French-Llama-3-8B-v0.4.IQ3_XS.gguf) | IQ3_XS | 3.6 | |
| [GGUF](https://huggingface.co/mradermacher/EnnoAi-Pro-French-Llama-3-8B-v0.4-GGUF/resolve/main/EnnoAi-Pro-French-Llama-3-8B-v0.4.Q3_K_S.gguf) | Q3_K_S | 3.8 | |
| [GGUF](https://huggingface.co/mradermacher/EnnoAi-Pro-French-Llama-3-8B-v0.4-GGUF/resolve/main/EnnoAi-Pro-French-Llama-3-8B-v0.4.IQ3_S.gguf) | IQ3_S | 3.8 | beats Q3_K* |
| [GGUF](https://huggingface.co/mradermacher/EnnoAi-Pro-French-Llama-3-8B-v0.4-GGUF/resolve/main/EnnoAi-Pro-French-Llama-3-8B-v0.4.IQ3_M.gguf) | IQ3_M | 3.9 | |
| [GGUF](https://huggingface.co/mradermacher/EnnoAi-Pro-French-Llama-3-8B-v0.4-GGUF/resolve/main/EnnoAi-Pro-French-Llama-3-8B-v0.4.Q3_K_M.gguf) | Q3_K_M | 4.1 | lower quality |
| [GGUF](https://huggingface.co/mradermacher/EnnoAi-Pro-French-Llama-3-8B-v0.4-GGUF/resolve/main/EnnoAi-Pro-French-Llama-3-8B-v0.4.Q3_K_L.gguf) | Q3_K_L | 4.4 | |
| [GGUF](https://huggingface.co/mradermacher/EnnoAi-Pro-French-Llama-3-8B-v0.4-GGUF/resolve/main/EnnoAi-Pro-French-Llama-3-8B-v0.4.IQ4_XS.gguf) | IQ4_XS | 4.6 | |
| [GGUF](https://huggingface.co/mradermacher/EnnoAi-Pro-French-Llama-3-8B-v0.4-GGUF/resolve/main/EnnoAi-Pro-French-Llama-3-8B-v0.4.Q4_K_S.gguf) | Q4_K_S | 4.8 | fast, recommended |
| [GGUF](https://huggingface.co/mradermacher/EnnoAi-Pro-French-Llama-3-8B-v0.4-GGUF/resolve/main/EnnoAi-Pro-French-Llama-3-8B-v0.4.Q4_K_M.gguf) | Q4_K_M | 5.0 | fast, recommended |
| [GGUF](https://huggingface.co/mradermacher/EnnoAi-Pro-French-Llama-3-8B-v0.4-GGUF/resolve/main/EnnoAi-Pro-French-Llama-3-8B-v0.4.Q5_K_S.gguf) | Q5_K_S | 5.7 | |
| [GGUF](https://huggingface.co/mradermacher/EnnoAi-Pro-French-Llama-3-8B-v0.4-GGUF/resolve/main/EnnoAi-Pro-French-Llama-3-8B-v0.4.Q5_K_M.gguf) | Q5_K_M | 5.8 | |
| [GGUF](https://huggingface.co/mradermacher/EnnoAi-Pro-French-Llama-3-8B-v0.4-GGUF/resolve/main/EnnoAi-Pro-French-Llama-3-8B-v0.4.Q6_K.gguf) | Q6_K | 6.7 | very good quality |
| [GGUF](https://huggingface.co/mradermacher/EnnoAi-Pro-French-Llama-3-8B-v0.4-GGUF/resolve/main/EnnoAi-Pro-French-Llama-3-8B-v0.4.Q8_0.gguf) | Q8_0 | 8.6 | fast, best quality |
| [GGUF](https://huggingface.co/mradermacher/EnnoAi-Pro-French-Llama-3-8B-v0.4-GGUF/resolve/main/EnnoAi-Pro-French-Llama-3-8B-v0.4.f16.gguf) | f16 | 16.2 | 16 bpw, overkill |
Here is a handy graph by ikawrakow comparing some lower-quality quant
types (lower is better):

And here are Artefact2's thoughts on the matter:
https://gist.github.com/Artefact2/b5f810600771265fc1e39442288e8ec9
## FAQ / Model Request
See https://huggingface.co/mradermacher/model_requests for some answers to
questions you might have and/or if you want some other model quantized.
## Thanks
I thank my company, [nethype GmbH](https://www.nethype.de/), for letting
me use its servers and providing upgrades to my workstation to enable
this work in my free time.
<!-- end -->
|
intfloat/e5-base | intfloat | "2023-08-07T04:59:19Z" | 12,911 | 19 | sentence-transformers | [
"sentence-transformers",
"pytorch",
"safetensors",
"bert",
"mteb",
"Sentence Transformers",
"sentence-similarity",
"en",
"arxiv:2212.03533",
"arxiv:2104.08663",
"arxiv:2210.07316",
"license:mit",
"model-index",
"endpoints_compatible",
"region:us"
] | sentence-similarity | "2022-12-26T05:58:05Z" | ---
tags:
- mteb
- Sentence Transformers
- sentence-similarity
- sentence-transformers
model-index:
- name: e5-base
results:
- task:
type: Classification
dataset:
type: mteb/amazon_counterfactual
name: MTEB AmazonCounterfactualClassification (en)
config: en
split: test
revision: e8379541af4e31359cca9fbcf4b00f2671dba205
metrics:
- type: accuracy
value: 79.71641791044777
- type: ap
value: 44.15426065428253
- type: f1
value: 73.89474407693241
- task:
type: Classification
dataset:
type: mteb/amazon_polarity
name: MTEB AmazonPolarityClassification
config: default
split: test
revision: e2d317d38cd51312af73b3d32a06d1a08b442046
metrics:
- type: accuracy
value: 87.9649
- type: ap
value: 84.10171551915973
- type: f1
value: 87.94148377827356
- task:
type: Classification
dataset:
type: mteb/amazon_reviews_multi
name: MTEB AmazonReviewsClassification (en)
config: en
split: test
revision: 1399c76144fd37290681b995c656ef9b2e06e26d
metrics:
- type: accuracy
value: 42.645999999999994
- type: f1
value: 42.230574673549
- task:
type: Retrieval
dataset:
type: arguana
name: MTEB ArguAna
config: default
split: test
revision: None
metrics:
- type: map_at_1
value: 26.814
- type: map_at_10
value: 42.681999999999995
- type: map_at_100
value: 43.714
- type: map_at_1000
value: 43.724000000000004
- type: map_at_3
value: 38.11
- type: map_at_5
value: 40.666999999999994
- type: mrr_at_1
value: 27.168999999999997
- type: mrr_at_10
value: 42.84
- type: mrr_at_100
value: 43.864
- type: mrr_at_1000
value: 43.875
- type: mrr_at_3
value: 38.193
- type: mrr_at_5
value: 40.793
- type: ndcg_at_1
value: 26.814
- type: ndcg_at_10
value: 51.410999999999994
- type: ndcg_at_100
value: 55.713
- type: ndcg_at_1000
value: 55.957
- type: ndcg_at_3
value: 41.955
- type: ndcg_at_5
value: 46.558
- type: precision_at_1
value: 26.814
- type: precision_at_10
value: 7.922999999999999
- type: precision_at_100
value: 0.9780000000000001
- type: precision_at_1000
value: 0.1
- type: precision_at_3
value: 17.71
- type: precision_at_5
value: 12.859000000000002
- type: recall_at_1
value: 26.814
- type: recall_at_10
value: 79.232
- type: recall_at_100
value: 97.795
- type: recall_at_1000
value: 99.644
- type: recall_at_3
value: 53.129000000000005
- type: recall_at_5
value: 64.29599999999999
- task:
type: Clustering
dataset:
type: mteb/arxiv-clustering-p2p
name: MTEB ArxivClusteringP2P
config: default
split: test
revision: a122ad7f3f0291bf49cc6f4d32aa80929df69d5d
metrics:
- type: v_measure
value: 44.56933066536439
- task:
type: Clustering
dataset:
type: mteb/arxiv-clustering-s2s
name: MTEB ArxivClusteringS2S
config: default
split: test
revision: f910caf1a6075f7329cdf8c1a6135696f37dbd53
metrics:
- type: v_measure
value: 40.47647746165173
- task:
type: Reranking
dataset:
type: mteb/askubuntudupquestions-reranking
name: MTEB AskUbuntuDupQuestions
config: default
split: test
revision: 2000358ca161889fa9c082cb41daa8dcfb161a54
metrics:
- type: map
value: 59.65675531567043
- type: mrr
value: 72.95255683067317
- task:
type: STS
dataset:
type: mteb/biosses-sts
name: MTEB BIOSSES
config: default
split: test
revision: d3fb88f8f02e40887cd149695127462bbcf29b4a
metrics:
- type: cos_sim_pearson
value: 85.83147014162338
- type: cos_sim_spearman
value: 85.1031439521441
- type: euclidean_pearson
value: 83.53609085510973
- type: euclidean_spearman
value: 84.59650590202833
- type: manhattan_pearson
value: 83.14611947586386
- type: manhattan_spearman
value: 84.13384475757064
- task:
type: Classification
dataset:
type: mteb/banking77
name: MTEB Banking77Classification
config: default
split: test
revision: 0fd18e25b25c072e09e0d92ab615fda904d66300
metrics:
- type: accuracy
value: 83.32792207792208
- type: f1
value: 83.32037485050513
- task:
type: Clustering
dataset:
type: mteb/biorxiv-clustering-p2p
name: MTEB BiorxivClusteringP2P
config: default
split: test
revision: 65b79d1d13f80053f67aca9498d9402c2d9f1f40
metrics:
- type: v_measure
value: 36.18605446588703
- task:
type: Clustering
dataset:
type: mteb/biorxiv-clustering-s2s
name: MTEB BiorxivClusteringS2S
config: default
split: test
revision: 258694dd0231531bc1fd9de6ceb52a0853c6d908
metrics:
- type: v_measure
value: 32.72379130181917
- task:
type: Retrieval
dataset:
type: BeIR/cqadupstack
name: MTEB CQADupstackAndroidRetrieval
config: default
split: test
revision: None
metrics:
- type: map_at_1
value: 30.659
- type: map_at_10
value: 40.333999999999996
- type: map_at_100
value: 41.763
- type: map_at_1000
value: 41.894
- type: map_at_3
value: 37.561
- type: map_at_5
value: 39.084
- type: mrr_at_1
value: 37.482
- type: mrr_at_10
value: 45.736
- type: mrr_at_100
value: 46.591
- type: mrr_at_1000
value: 46.644999999999996
- type: mrr_at_3
value: 43.491
- type: mrr_at_5
value: 44.75
- type: ndcg_at_1
value: 37.482
- type: ndcg_at_10
value: 45.606
- type: ndcg_at_100
value: 51.172
- type: ndcg_at_1000
value: 53.407000000000004
- type: ndcg_at_3
value: 41.808
- type: ndcg_at_5
value: 43.449
- type: precision_at_1
value: 37.482
- type: precision_at_10
value: 8.254999999999999
- type: precision_at_100
value: 1.3719999999999999
- type: precision_at_1000
value: 0.186
- type: precision_at_3
value: 19.695
- type: precision_at_5
value: 13.847999999999999
- type: recall_at_1
value: 30.659
- type: recall_at_10
value: 55.409
- type: recall_at_100
value: 78.687
- type: recall_at_1000
value: 93.068
- type: recall_at_3
value: 43.891999999999996
- type: recall_at_5
value: 48.678
- task:
type: Retrieval
dataset:
type: BeIR/cqadupstack
name: MTEB CQADupstackEnglishRetrieval
config: default
split: test
revision: None
metrics:
- type: map_at_1
value: 30.977
- type: map_at_10
value: 40.296
- type: map_at_100
value: 41.453
- type: map_at_1000
value: 41.581
- type: map_at_3
value: 37.619
- type: map_at_5
value: 39.181
- type: mrr_at_1
value: 39.108
- type: mrr_at_10
value: 46.894000000000005
- type: mrr_at_100
value: 47.55
- type: mrr_at_1000
value: 47.598
- type: mrr_at_3
value: 44.766
- type: mrr_at_5
value: 46.062999999999995
- type: ndcg_at_1
value: 39.108
- type: ndcg_at_10
value: 45.717
- type: ndcg_at_100
value: 49.941
- type: ndcg_at_1000
value: 52.138
- type: ndcg_at_3
value: 42.05
- type: ndcg_at_5
value: 43.893
- type: precision_at_1
value: 39.108
- type: precision_at_10
value: 8.306
- type: precision_at_100
value: 1.3419999999999999
- type: precision_at_1000
value: 0.184
- type: precision_at_3
value: 19.979
- type: precision_at_5
value: 14.038
- type: recall_at_1
value: 30.977
- type: recall_at_10
value: 54.688
- type: recall_at_100
value: 72.556
- type: recall_at_1000
value: 86.53800000000001
- type: recall_at_3
value: 43.388
- type: recall_at_5
value: 48.717
- task:
type: Retrieval
dataset:
type: BeIR/cqadupstack
name: MTEB CQADupstackGamingRetrieval
config: default
split: test
revision: None
metrics:
- type: map_at_1
value: 39.812
- type: map_at_10
value: 50.1
- type: map_at_100
value: 51.193999999999996
- type: map_at_1000
value: 51.258
- type: map_at_3
value: 47.510999999999996
- type: map_at_5
value: 48.891
- type: mrr_at_1
value: 45.266
- type: mrr_at_10
value: 53.459999999999994
- type: mrr_at_100
value: 54.19199999999999
- type: mrr_at_1000
value: 54.228
- type: mrr_at_3
value: 51.296
- type: mrr_at_5
value: 52.495999999999995
- type: ndcg_at_1
value: 45.266
- type: ndcg_at_10
value: 55.034000000000006
- type: ndcg_at_100
value: 59.458
- type: ndcg_at_1000
value: 60.862
- type: ndcg_at_3
value: 50.52799999999999
- type: ndcg_at_5
value: 52.564
- type: precision_at_1
value: 45.266
- type: precision_at_10
value: 8.483
- type: precision_at_100
value: 1.162
- type: precision_at_1000
value: 0.133
- type: precision_at_3
value: 21.944
- type: precision_at_5
value: 14.721
- type: recall_at_1
value: 39.812
- type: recall_at_10
value: 66.36
- type: recall_at_100
value: 85.392
- type: recall_at_1000
value: 95.523
- type: recall_at_3
value: 54.127
- type: recall_at_5
value: 59.245000000000005
- task:
type: Retrieval
dataset:
type: BeIR/cqadupstack
name: MTEB CQADupstackGisRetrieval
config: default
split: test
revision: None
metrics:
- type: map_at_1
value: 26.186
- type: map_at_10
value: 33.18
- type: map_at_100
value: 34.052
- type: map_at_1000
value: 34.149
- type: map_at_3
value: 31.029
- type: map_at_5
value: 32.321
- type: mrr_at_1
value: 28.136
- type: mrr_at_10
value: 35.195
- type: mrr_at_100
value: 35.996
- type: mrr_at_1000
value: 36.076
- type: mrr_at_3
value: 33.051
- type: mrr_at_5
value: 34.407
- type: ndcg_at_1
value: 28.136
- type: ndcg_at_10
value: 37.275999999999996
- type: ndcg_at_100
value: 41.935
- type: ndcg_at_1000
value: 44.389
- type: ndcg_at_3
value: 33.059
- type: ndcg_at_5
value: 35.313
- type: precision_at_1
value: 28.136
- type: precision_at_10
value: 5.457999999999999
- type: precision_at_100
value: 0.826
- type: precision_at_1000
value: 0.107
- type: precision_at_3
value: 13.522
- type: precision_at_5
value: 9.424000000000001
- type: recall_at_1
value: 26.186
- type: recall_at_10
value: 47.961999999999996
- type: recall_at_100
value: 70.072
- type: recall_at_1000
value: 88.505
- type: recall_at_3
value: 36.752
- type: recall_at_5
value: 42.168
- task:
type: Retrieval
dataset:
type: BeIR/cqadupstack
name: MTEB CQADupstackMathematicaRetrieval
config: default
split: test
revision: None
metrics:
- type: map_at_1
value: 16.586000000000002
- type: map_at_10
value: 23.637
- type: map_at_100
value: 24.82
- type: map_at_1000
value: 24.95
- type: map_at_3
value: 21.428
- type: map_at_5
value: 22.555
- type: mrr_at_1
value: 20.771
- type: mrr_at_10
value: 27.839999999999996
- type: mrr_at_100
value: 28.887
- type: mrr_at_1000
value: 28.967
- type: mrr_at_3
value: 25.56
- type: mrr_at_5
value: 26.723000000000003
- type: ndcg_at_1
value: 20.771
- type: ndcg_at_10
value: 28.255000000000003
- type: ndcg_at_100
value: 33.886
- type: ndcg_at_1000
value: 36.963
- type: ndcg_at_3
value: 24.056
- type: ndcg_at_5
value: 25.818
- type: precision_at_1
value: 20.771
- type: precision_at_10
value: 5.1
- type: precision_at_100
value: 0.9119999999999999
- type: precision_at_1000
value: 0.132
- type: precision_at_3
value: 11.526
- type: precision_at_5
value: 8.158999999999999
- type: recall_at_1
value: 16.586000000000002
- type: recall_at_10
value: 38.456
- type: recall_at_100
value: 62.666
- type: recall_at_1000
value: 84.47
- type: recall_at_3
value: 26.765
- type: recall_at_5
value: 31.297000000000004
- task:
type: Retrieval
dataset:
type: BeIR/cqadupstack
name: MTEB CQADupstackPhysicsRetrieval
config: default
split: test
revision: None
metrics:
- type: map_at_1
value: 28.831
- type: map_at_10
value: 37.545
- type: map_at_100
value: 38.934999999999995
- type: map_at_1000
value: 39.044000000000004
- type: map_at_3
value: 34.601
- type: map_at_5
value: 36.302
- type: mrr_at_1
value: 34.264
- type: mrr_at_10
value: 42.569
- type: mrr_at_100
value: 43.514
- type: mrr_at_1000
value: 43.561
- type: mrr_at_3
value: 40.167
- type: mrr_at_5
value: 41.678
- type: ndcg_at_1
value: 34.264
- type: ndcg_at_10
value: 42.914
- type: ndcg_at_100
value: 48.931999999999995
- type: ndcg_at_1000
value: 51.004000000000005
- type: ndcg_at_3
value: 38.096999999999994
- type: ndcg_at_5
value: 40.509
- type: precision_at_1
value: 34.264
- type: precision_at_10
value: 7.642
- type: precision_at_100
value: 1.258
- type: precision_at_1000
value: 0.161
- type: precision_at_3
value: 17.453
- type: precision_at_5
value: 12.608
- type: recall_at_1
value: 28.831
- type: recall_at_10
value: 53.56999999999999
- type: recall_at_100
value: 79.26100000000001
- type: recall_at_1000
value: 92.862
- type: recall_at_3
value: 40.681
- type: recall_at_5
value: 46.597
- task:
type: Retrieval
dataset:
type: BeIR/cqadupstack
name: MTEB CQADupstackProgrammersRetrieval
config: default
split: test
revision: None
metrics:
- type: map_at_1
value: 27.461000000000002
- type: map_at_10
value: 35.885
- type: map_at_100
value: 37.039
- type: map_at_1000
value: 37.16
- type: map_at_3
value: 33.451
- type: map_at_5
value: 34.807
- type: mrr_at_1
value: 34.018
- type: mrr_at_10
value: 41.32
- type: mrr_at_100
value: 42.157
- type: mrr_at_1000
value: 42.223
- type: mrr_at_3
value: 39.288000000000004
- type: mrr_at_5
value: 40.481
- type: ndcg_at_1
value: 34.018
- type: ndcg_at_10
value: 40.821000000000005
- type: ndcg_at_100
value: 46.053
- type: ndcg_at_1000
value: 48.673
- type: ndcg_at_3
value: 36.839
- type: ndcg_at_5
value: 38.683
- type: precision_at_1
value: 34.018
- type: precision_at_10
value: 7.009
- type: precision_at_100
value: 1.123
- type: precision_at_1000
value: 0.153
- type: precision_at_3
value: 16.933
- type: precision_at_5
value: 11.826
- type: recall_at_1
value: 27.461000000000002
- type: recall_at_10
value: 50.285000000000004
- type: recall_at_100
value: 73.25500000000001
- type: recall_at_1000
value: 91.17699999999999
- type: recall_at_3
value: 39.104
- type: recall_at_5
value: 43.968
- task:
type: Retrieval
dataset:
type: BeIR/cqadupstack
name: MTEB CQADupstackRetrieval
config: default
split: test
revision: None
metrics:
- type: map_at_1
value: 26.980083333333337
- type: map_at_10
value: 34.47208333333333
- type: map_at_100
value: 35.609249999999996
- type: map_at_1000
value: 35.72833333333333
- type: map_at_3
value: 32.189416666666666
- type: map_at_5
value: 33.44683333333334
- type: mrr_at_1
value: 31.731666666666662
- type: mrr_at_10
value: 38.518
- type: mrr_at_100
value: 39.38166666666667
- type: mrr_at_1000
value: 39.446999999999996
- type: mrr_at_3
value: 36.49966666666668
- type: mrr_at_5
value: 37.639916666666664
- type: ndcg_at_1
value: 31.731666666666662
- type: ndcg_at_10
value: 38.92033333333333
- type: ndcg_at_100
value: 44.01675
- type: ndcg_at_1000
value: 46.51075
- type: ndcg_at_3
value: 35.09766666666667
- type: ndcg_at_5
value: 36.842999999999996
- type: precision_at_1
value: 31.731666666666662
- type: precision_at_10
value: 6.472583333333332
- type: precision_at_100
value: 1.0665
- type: precision_at_1000
value: 0.14725000000000002
- type: precision_at_3
value: 15.659083333333331
- type: precision_at_5
value: 10.878833333333333
- type: recall_at_1
value: 26.980083333333337
- type: recall_at_10
value: 48.13925
- type: recall_at_100
value: 70.70149999999998
- type: recall_at_1000
value: 88.10775000000001
- type: recall_at_3
value: 37.30091666666667
- type: recall_at_5
value: 41.90358333333333
- task:
type: Retrieval
dataset:
type: BeIR/cqadupstack
name: MTEB CQADupstackStatsRetrieval
config: default
split: test
revision: None
metrics:
- type: map_at_1
value: 25.607999999999997
- type: map_at_10
value: 30.523
- type: map_at_100
value: 31.409
- type: map_at_1000
value: 31.507
- type: map_at_3
value: 28.915000000000003
- type: map_at_5
value: 29.756
- type: mrr_at_1
value: 28.681
- type: mrr_at_10
value: 33.409
- type: mrr_at_100
value: 34.241
- type: mrr_at_1000
value: 34.313
- type: mrr_at_3
value: 32.029999999999994
- type: mrr_at_5
value: 32.712
- type: ndcg_at_1
value: 28.681
- type: ndcg_at_10
value: 33.733000000000004
- type: ndcg_at_100
value: 38.32
- type: ndcg_at_1000
value: 40.937
- type: ndcg_at_3
value: 30.898999999999997
- type: ndcg_at_5
value: 32.088
- type: precision_at_1
value: 28.681
- type: precision_at_10
value: 4.968999999999999
- type: precision_at_100
value: 0.79
- type: precision_at_1000
value: 0.11
- type: precision_at_3
value: 12.73
- type: precision_at_5
value: 8.558
- type: recall_at_1
value: 25.607999999999997
- type: recall_at_10
value: 40.722
- type: recall_at_100
value: 61.956999999999994
- type: recall_at_1000
value: 81.43
- type: recall_at_3
value: 32.785
- type: recall_at_5
value: 35.855
- task:
type: Retrieval
dataset:
type: BeIR/cqadupstack
name: MTEB CQADupstackTexRetrieval
config: default
split: test
revision: None
metrics:
- type: map_at_1
value: 20.399
- type: map_at_10
value: 25.968000000000004
- type: map_at_100
value: 26.985999999999997
- type: map_at_1000
value: 27.105
- type: map_at_3
value: 24.215
- type: map_at_5
value: 25.157
- type: mrr_at_1
value: 24.708
- type: mrr_at_10
value: 29.971999999999998
- type: mrr_at_100
value: 30.858
- type: mrr_at_1000
value: 30.934
- type: mrr_at_3
value: 28.304000000000002
- type: mrr_at_5
value: 29.183999999999997
- type: ndcg_at_1
value: 24.708
- type: ndcg_at_10
value: 29.676000000000002
- type: ndcg_at_100
value: 34.656
- type: ndcg_at_1000
value: 37.588
- type: ndcg_at_3
value: 26.613
- type: ndcg_at_5
value: 27.919
- type: precision_at_1
value: 24.708
- type: precision_at_10
value: 5.01
- type: precision_at_100
value: 0.876
- type: precision_at_1000
value: 0.13
- type: precision_at_3
value: 11.975
- type: precision_at_5
value: 8.279
- type: recall_at_1
value: 20.399
- type: recall_at_10
value: 36.935
- type: recall_at_100
value: 59.532
- type: recall_at_1000
value: 80.58
- type: recall_at_3
value: 27.979
- type: recall_at_5
value: 31.636999999999997
- task:
type: Retrieval
dataset:
type: BeIR/cqadupstack
name: MTEB CQADupstackUnixRetrieval
config: default
split: test
revision: None
metrics:
- type: map_at_1
value: 27.606
- type: map_at_10
value: 34.213
- type: map_at_100
value: 35.339999999999996
- type: map_at_1000
value: 35.458
- type: map_at_3
value: 31.987
- type: map_at_5
value: 33.322
- type: mrr_at_1
value: 31.53
- type: mrr_at_10
value: 37.911
- type: mrr_at_100
value: 38.879000000000005
- type: mrr_at_1000
value: 38.956
- type: mrr_at_3
value: 35.868
- type: mrr_at_5
value: 37.047999999999995
- type: ndcg_at_1
value: 31.53
- type: ndcg_at_10
value: 38.312000000000005
- type: ndcg_at_100
value: 43.812
- type: ndcg_at_1000
value: 46.414
- type: ndcg_at_3
value: 34.319
- type: ndcg_at_5
value: 36.312
- type: precision_at_1
value: 31.53
- type: precision_at_10
value: 5.970000000000001
- type: precision_at_100
value: 0.9939999999999999
- type: precision_at_1000
value: 0.133
- type: precision_at_3
value: 14.738999999999999
- type: precision_at_5
value: 10.242999999999999
- type: recall_at_1
value: 27.606
- type: recall_at_10
value: 47.136
- type: recall_at_100
value: 71.253
- type: recall_at_1000
value: 89.39399999999999
- type: recall_at_3
value: 36.342
- type: recall_at_5
value: 41.388999999999996
- task:
type: Retrieval
dataset:
type: BeIR/cqadupstack
name: MTEB CQADupstackWebmastersRetrieval
config: default
split: test
revision: None
metrics:
- type: map_at_1
value: 24.855
- type: map_at_10
value: 31.963
- type: map_at_100
value: 33.371
- type: map_at_1000
value: 33.584
- type: map_at_3
value: 29.543999999999997
- type: map_at_5
value: 30.793
- type: mrr_at_1
value: 29.644
- type: mrr_at_10
value: 35.601
- type: mrr_at_100
value: 36.551
- type: mrr_at_1000
value: 36.623
- type: mrr_at_3
value: 33.399
- type: mrr_at_5
value: 34.575
- type: ndcg_at_1
value: 29.644
- type: ndcg_at_10
value: 36.521
- type: ndcg_at_100
value: 42.087
- type: ndcg_at_1000
value: 45.119
- type: ndcg_at_3
value: 32.797
- type: ndcg_at_5
value: 34.208
- type: precision_at_1
value: 29.644
- type: precision_at_10
value: 6.7
- type: precision_at_100
value: 1.374
- type: precision_at_1000
value: 0.22899999999999998
- type: precision_at_3
value: 15.152
- type: precision_at_5
value: 10.671999999999999
- type: recall_at_1
value: 24.855
- type: recall_at_10
value: 45.449
- type: recall_at_100
value: 70.921
- type: recall_at_1000
value: 90.629
- type: recall_at_3
value: 33.526
- type: recall_at_5
value: 37.848
- task:
type: Retrieval
dataset:
type: BeIR/cqadupstack
name: MTEB CQADupstackWordpressRetrieval
config: default
split: test
revision: None
metrics:
- type: map_at_1
value: 24.781
- type: map_at_10
value: 30.020999999999997
- type: map_at_100
value: 30.948999999999998
- type: map_at_1000
value: 31.05
- type: map_at_3
value: 28.412
- type: map_at_5
value: 29.193
- type: mrr_at_1
value: 27.172
- type: mrr_at_10
value: 32.309
- type: mrr_at_100
value: 33.164
- type: mrr_at_1000
value: 33.239999999999995
- type: mrr_at_3
value: 30.775999999999996
- type: mrr_at_5
value: 31.562
- type: ndcg_at_1
value: 27.172
- type: ndcg_at_10
value: 33.178999999999995
- type: ndcg_at_100
value: 37.949
- type: ndcg_at_1000
value: 40.635
- type: ndcg_at_3
value: 30.107
- type: ndcg_at_5
value: 31.36
- type: precision_at_1
value: 27.172
- type: precision_at_10
value: 4.769
- type: precision_at_100
value: 0.769
- type: precision_at_1000
value: 0.109
- type: precision_at_3
value: 12.261
- type: precision_at_5
value: 8.17
- type: recall_at_1
value: 24.781
- type: recall_at_10
value: 40.699000000000005
- type: recall_at_100
value: 62.866
- type: recall_at_1000
value: 83.11699999999999
- type: recall_at_3
value: 32.269999999999996
- type: recall_at_5
value: 35.443999999999996
- task:
type: Retrieval
dataset:
type: climate-fever
name: MTEB ClimateFEVER
config: default
split: test
revision: None
metrics:
- type: map_at_1
value: 5.2139999999999995
- type: map_at_10
value: 9.986
- type: map_at_100
value: 11.343
- type: map_at_1000
value: 11.55
- type: map_at_3
value: 7.961
- type: map_at_5
value: 8.967
- type: mrr_at_1
value: 12.052
- type: mrr_at_10
value: 20.165
- type: mrr_at_100
value: 21.317
- type: mrr_at_1000
value: 21.399
- type: mrr_at_3
value: 17.079
- type: mrr_at_5
value: 18.695
- type: ndcg_at_1
value: 12.052
- type: ndcg_at_10
value: 15.375
- type: ndcg_at_100
value: 21.858
- type: ndcg_at_1000
value: 26.145000000000003
- type: ndcg_at_3
value: 11.334
- type: ndcg_at_5
value: 12.798000000000002
- type: precision_at_1
value: 12.052
- type: precision_at_10
value: 5.16
- type: precision_at_100
value: 1.206
- type: precision_at_1000
value: 0.198
- type: precision_at_3
value: 8.73
- type: precision_at_5
value: 7.114
- type: recall_at_1
value: 5.2139999999999995
- type: recall_at_10
value: 20.669999999999998
- type: recall_at_100
value: 43.901
- type: recall_at_1000
value: 68.447
- type: recall_at_3
value: 11.049000000000001
- type: recall_at_5
value: 14.652999999999999
- task:
type: Retrieval
dataset:
type: dbpedia-entity
name: MTEB DBPedia
config: default
split: test
revision: None
metrics:
- type: map_at_1
value: 8.511000000000001
- type: map_at_10
value: 19.503
- type: map_at_100
value: 27.46
- type: map_at_1000
value: 29.187
- type: map_at_3
value: 14.030999999999999
- type: map_at_5
value: 16.329
- type: mrr_at_1
value: 63.74999999999999
- type: mrr_at_10
value: 73.419
- type: mrr_at_100
value: 73.691
- type: mrr_at_1000
value: 73.697
- type: mrr_at_3
value: 71.792
- type: mrr_at_5
value: 72.979
- type: ndcg_at_1
value: 53.125
- type: ndcg_at_10
value: 41.02
- type: ndcg_at_100
value: 45.407
- type: ndcg_at_1000
value: 52.68000000000001
- type: ndcg_at_3
value: 46.088
- type: ndcg_at_5
value: 43.236000000000004
- type: precision_at_1
value: 63.74999999999999
- type: precision_at_10
value: 32.35
- type: precision_at_100
value: 10.363
- type: precision_at_1000
value: 2.18
- type: precision_at_3
value: 49.667
- type: precision_at_5
value: 41.5
- type: recall_at_1
value: 8.511000000000001
- type: recall_at_10
value: 24.851
- type: recall_at_100
value: 50.745
- type: recall_at_1000
value: 73.265
- type: recall_at_3
value: 15.716
- type: recall_at_5
value: 19.256
- task:
type: Classification
dataset:
type: mteb/emotion
name: MTEB EmotionClassification
config: default
split: test
revision: 4f58c6b202a23cf9a4da393831edf4f9183cad37
metrics:
- type: accuracy
value: 49.43500000000001
- type: f1
value: 44.56288273966374
- task:
type: Retrieval
dataset:
type: fever
name: MTEB FEVER
config: default
split: test
revision: None
metrics:
- type: map_at_1
value: 40.858
- type: map_at_10
value: 52.276
- type: map_at_100
value: 52.928
- type: map_at_1000
value: 52.966
- type: map_at_3
value: 49.729
- type: map_at_5
value: 51.27
- type: mrr_at_1
value: 43.624
- type: mrr_at_10
value: 55.22899999999999
- type: mrr_at_100
value: 55.823
- type: mrr_at_1000
value: 55.85
- type: mrr_at_3
value: 52.739999999999995
- type: mrr_at_5
value: 54.251000000000005
- type: ndcg_at_1
value: 43.624
- type: ndcg_at_10
value: 58.23500000000001
- type: ndcg_at_100
value: 61.315
- type: ndcg_at_1000
value: 62.20099999999999
- type: ndcg_at_3
value: 53.22
- type: ndcg_at_5
value: 55.88999999999999
- type: precision_at_1
value: 43.624
- type: precision_at_10
value: 8.068999999999999
- type: precision_at_100
value: 0.975
- type: precision_at_1000
value: 0.107
- type: precision_at_3
value: 21.752
- type: precision_at_5
value: 14.515
- type: recall_at_1
value: 40.858
- type: recall_at_10
value: 73.744
- type: recall_at_100
value: 87.667
- type: recall_at_1000
value: 94.15599999999999
- type: recall_at_3
value: 60.287
- type: recall_at_5
value: 66.703
- task:
type: Retrieval
dataset:
type: fiqa
name: MTEB FiQA2018
config: default
split: test
revision: None
metrics:
- type: map_at_1
value: 17.864
- type: map_at_10
value: 28.592000000000002
- type: map_at_100
value: 30.165
- type: map_at_1000
value: 30.364
- type: map_at_3
value: 24.586
- type: map_at_5
value: 26.717000000000002
- type: mrr_at_1
value: 35.031
- type: mrr_at_10
value: 43.876
- type: mrr_at_100
value: 44.683
- type: mrr_at_1000
value: 44.736
- type: mrr_at_3
value: 40.998000000000005
- type: mrr_at_5
value: 42.595
- type: ndcg_at_1
value: 35.031
- type: ndcg_at_10
value: 36.368
- type: ndcg_at_100
value: 42.472
- type: ndcg_at_1000
value: 45.973000000000006
- type: ndcg_at_3
value: 31.915
- type: ndcg_at_5
value: 33.394
- type: precision_at_1
value: 35.031
- type: precision_at_10
value: 10.139
- type: precision_at_100
value: 1.6420000000000001
- type: precision_at_1000
value: 0.22699999999999998
- type: precision_at_3
value: 21.142
- type: precision_at_5
value: 15.772
- type: recall_at_1
value: 17.864
- type: recall_at_10
value: 43.991
- type: recall_at_100
value: 66.796
- type: recall_at_1000
value: 87.64
- type: recall_at_3
value: 28.915999999999997
- type: recall_at_5
value: 35.185
- task:
type: Retrieval
dataset:
type: hotpotqa
name: MTEB HotpotQA
config: default
split: test
revision: None
metrics:
- type: map_at_1
value: 36.556
- type: map_at_10
value: 53.056000000000004
- type: map_at_100
value: 53.909
- type: map_at_1000
value: 53.98
- type: map_at_3
value: 49.982
- type: map_at_5
value: 51.9
- type: mrr_at_1
value: 73.113
- type: mrr_at_10
value: 79.381
- type: mrr_at_100
value: 79.60300000000001
- type: mrr_at_1000
value: 79.617
- type: mrr_at_3
value: 78.298
- type: mrr_at_5
value: 78.995
- type: ndcg_at_1
value: 73.113
- type: ndcg_at_10
value: 62.21
- type: ndcg_at_100
value: 65.242
- type: ndcg_at_1000
value: 66.667
- type: ndcg_at_3
value: 57.717
- type: ndcg_at_5
value: 60.224
- type: precision_at_1
value: 73.113
- type: precision_at_10
value: 12.842999999999998
- type: precision_at_100
value: 1.522
- type: precision_at_1000
value: 0.17099999999999999
- type: precision_at_3
value: 36.178
- type: precision_at_5
value: 23.695
- type: recall_at_1
value: 36.556
- type: recall_at_10
value: 64.213
- type: recall_at_100
value: 76.077
- type: recall_at_1000
value: 85.53699999999999
- type: recall_at_3
value: 54.266999999999996
- type: recall_at_5
value: 59.236999999999995
- task:
type: Classification
dataset:
type: mteb/imdb
name: MTEB ImdbClassification
config: default
split: test
revision: 3d86128a09e091d6018b6d26cad27f2739fc2db7
metrics:
- type: accuracy
value: 75.958
- type: ap
value: 69.82869527654348
- type: f1
value: 75.89120903005633
- task:
type: Retrieval
dataset:
type: msmarco
name: MTEB MSMARCO
config: default
split: dev
revision: None
metrics:
- type: map_at_1
value: 23.608
- type: map_at_10
value: 36.144
- type: map_at_100
value: 37.244
- type: map_at_1000
value: 37.291999999999994
- type: map_at_3
value: 32.287
- type: map_at_5
value: 34.473
- type: mrr_at_1
value: 24.226
- type: mrr_at_10
value: 36.711
- type: mrr_at_100
value: 37.758
- type: mrr_at_1000
value: 37.8
- type: mrr_at_3
value: 32.92
- type: mrr_at_5
value: 35.104
- type: ndcg_at_1
value: 24.269
- type: ndcg_at_10
value: 43.138
- type: ndcg_at_100
value: 48.421
- type: ndcg_at_1000
value: 49.592000000000006
- type: ndcg_at_3
value: 35.269
- type: ndcg_at_5
value: 39.175
- type: precision_at_1
value: 24.269
- type: precision_at_10
value: 6.755999999999999
- type: precision_at_100
value: 0.941
- type: precision_at_1000
value: 0.104
- type: precision_at_3
value: 14.938
- type: precision_at_5
value: 10.934000000000001
- type: recall_at_1
value: 23.608
- type: recall_at_10
value: 64.679
- type: recall_at_100
value: 89.027
- type: recall_at_1000
value: 97.91
- type: recall_at_3
value: 43.25
- type: recall_at_5
value: 52.617000000000004
- task:
type: Classification
dataset:
type: mteb/mtop_domain
name: MTEB MTOPDomainClassification (en)
config: en
split: test
revision: d80d48c1eb48d3562165c59d59d0034df9fff0bf
metrics:
- type: accuracy
value: 93.21477428180576
- type: f1
value: 92.92502305092152
- task:
type: Classification
dataset:
type: mteb/mtop_intent
name: MTEB MTOPIntentClassification (en)
config: en
split: test
revision: ae001d0e6b1228650b7bd1c2c65fb50ad11a8aba
metrics:
- type: accuracy
value: 74.76744186046511
- type: f1
value: 59.19855520057899
- task:
type: Classification
dataset:
type: mteb/amazon_massive_intent
name: MTEB MassiveIntentClassification (en)
config: en
split: test
revision: 31efe3c427b0bae9c22cbb560b8f15491cc6bed7
metrics:
- type: accuracy
value: 72.24613315400134
- type: f1
value: 70.19950395651232
- task:
type: Classification
dataset:
type: mteb/amazon_massive_scenario
name: MTEB MassiveScenarioClassification (en)
config: en
split: test
revision: 7d571f92784cd94a019292a1f45445077d0ef634
metrics:
- type: accuracy
value: 76.75857431069268
- type: f1
value: 76.5433450230191
- task:
type: Clustering
dataset:
type: mteb/medrxiv-clustering-p2p
name: MTEB MedrxivClusteringP2P
config: default
split: test
revision: e7a26af6f3ae46b30dde8737f02c07b1505bcc73
metrics:
- type: v_measure
value: 31.525463791623604
- task:
type: Clustering
dataset:
type: mteb/medrxiv-clustering-s2s
name: MTEB MedrxivClusteringS2S
config: default
split: test
revision: 35191c8c0dca72d8ff3efcd72aa802307d469663
metrics:
- type: v_measure
value: 28.28695907385136
- task:
type: Reranking
dataset:
type: mteb/mind_small
name: MTEB MindSmallReranking
config: default
split: test
revision: 3bdac13927fdc888b903db93b2ffdbd90b295a69
metrics:
- type: map
value: 30.068174046665224
- type: mrr
value: 30.827586642840803
- task:
type: Retrieval
dataset:
type: nfcorpus
name: MTEB NFCorpus
config: default
split: test
revision: None
metrics:
- type: map_at_1
value: 6.322
- type: map_at_10
value: 13.919999999999998
- type: map_at_100
value: 17.416
- type: map_at_1000
value: 18.836
- type: map_at_3
value: 10.111
- type: map_at_5
value: 11.991999999999999
- type: mrr_at_1
value: 48.297000000000004
- type: mrr_at_10
value: 57.114
- type: mrr_at_100
value: 57.713
- type: mrr_at_1000
value: 57.751
- type: mrr_at_3
value: 55.108000000000004
- type: mrr_at_5
value: 56.533
- type: ndcg_at_1
value: 46.44
- type: ndcg_at_10
value: 36.589
- type: ndcg_at_100
value: 33.202
- type: ndcg_at_1000
value: 41.668
- type: ndcg_at_3
value: 41.302
- type: ndcg_at_5
value: 39.829
- type: precision_at_1
value: 47.988
- type: precision_at_10
value: 27.059
- type: precision_at_100
value: 8.235000000000001
- type: precision_at_1000
value: 2.091
- type: precision_at_3
value: 38.184000000000005
- type: precision_at_5
value: 34.365
- type: recall_at_1
value: 6.322
- type: recall_at_10
value: 18.288
- type: recall_at_100
value: 32.580999999999996
- type: recall_at_1000
value: 63.605999999999995
- type: recall_at_3
value: 11.266
- type: recall_at_5
value: 14.69
- task:
type: Retrieval
dataset:
type: nq
name: MTEB NQ
config: default
split: test
revision: None
metrics:
- type: map_at_1
value: 36.586999999999996
- type: map_at_10
value: 52.464
- type: map_at_100
value: 53.384
- type: map_at_1000
value: 53.405
- type: map_at_3
value: 48.408
- type: map_at_5
value: 50.788999999999994
- type: mrr_at_1
value: 40.904
- type: mrr_at_10
value: 54.974000000000004
- type: mrr_at_100
value: 55.60699999999999
- type: mrr_at_1000
value: 55.623
- type: mrr_at_3
value: 51.73799999999999
- type: mrr_at_5
value: 53.638
- type: ndcg_at_1
value: 40.904
- type: ndcg_at_10
value: 59.965999999999994
- type: ndcg_at_100
value: 63.613
- type: ndcg_at_1000
value: 64.064
- type: ndcg_at_3
value: 52.486
- type: ndcg_at_5
value: 56.377
- type: precision_at_1
value: 40.904
- type: precision_at_10
value: 9.551
- type: precision_at_100
value: 1.162
- type: precision_at_1000
value: 0.12
- type: precision_at_3
value: 23.552
- type: precision_at_5
value: 16.436999999999998
- type: recall_at_1
value: 36.586999999999996
- type: recall_at_10
value: 80.094
- type: recall_at_100
value: 95.515
- type: recall_at_1000
value: 98.803
- type: recall_at_3
value: 60.907
- type: recall_at_5
value: 69.817
- task:
type: Retrieval
dataset:
type: quora
name: MTEB QuoraRetrieval
config: default
split: test
revision: None
metrics:
- type: map_at_1
value: 70.422
- type: map_at_10
value: 84.113
- type: map_at_100
value: 84.744
- type: map_at_1000
value: 84.762
- type: map_at_3
value: 81.171
- type: map_at_5
value: 83.039
- type: mrr_at_1
value: 81.12
- type: mrr_at_10
value: 87.277
- type: mrr_at_100
value: 87.384
- type: mrr_at_1000
value: 87.385
- type: mrr_at_3
value: 86.315
- type: mrr_at_5
value: 86.981
- type: ndcg_at_1
value: 81.12
- type: ndcg_at_10
value: 87.92
- type: ndcg_at_100
value: 89.178
- type: ndcg_at_1000
value: 89.29899999999999
- type: ndcg_at_3
value: 85.076
- type: ndcg_at_5
value: 86.67099999999999
- type: precision_at_1
value: 81.12
- type: precision_at_10
value: 13.325999999999999
- type: precision_at_100
value: 1.524
- type: precision_at_1000
value: 0.157
- type: precision_at_3
value: 37.16
- type: precision_at_5
value: 24.456
- type: recall_at_1
value: 70.422
- type: recall_at_10
value: 95.00800000000001
- type: recall_at_100
value: 99.38
- type: recall_at_1000
value: 99.94800000000001
- type: recall_at_3
value: 86.809
- type: recall_at_5
value: 91.334
- task:
type: Clustering
dataset:
type: mteb/reddit-clustering
name: MTEB RedditClustering
config: default
split: test
revision: 24640382cdbf8abc73003fb0fa6d111a705499eb
metrics:
- type: v_measure
value: 48.18491891699636
- task:
type: Clustering
dataset:
type: mteb/reddit-clustering-p2p
name: MTEB RedditClusteringP2P
config: default
split: test
revision: 282350215ef01743dc01b456c7f5241fa8937f16
metrics:
- type: v_measure
value: 62.190639679711914
- task:
type: Retrieval
dataset:
type: scidocs
name: MTEB SCIDOCS
config: default
split: test
revision: None
metrics:
- type: map_at_1
value: 4.478
- type: map_at_10
value: 11.268
- type: map_at_100
value: 13.129
- type: map_at_1000
value: 13.41
- type: map_at_3
value: 8.103
- type: map_at_5
value: 9.609
- type: mrr_at_1
value: 22
- type: mrr_at_10
value: 32.248
- type: mrr_at_100
value: 33.355000000000004
- type: mrr_at_1000
value: 33.42
- type: mrr_at_3
value: 29.15
- type: mrr_at_5
value: 30.785
- type: ndcg_at_1
value: 22
- type: ndcg_at_10
value: 18.990000000000002
- type: ndcg_at_100
value: 26.302999999999997
- type: ndcg_at_1000
value: 31.537
- type: ndcg_at_3
value: 18.034
- type: ndcg_at_5
value: 15.655
- type: precision_at_1
value: 22
- type: precision_at_10
value: 9.91
- type: precision_at_100
value: 2.0420000000000003
- type: precision_at_1000
value: 0.33
- type: precision_at_3
value: 16.933
- type: precision_at_5
value: 13.719999999999999
- type: recall_at_1
value: 4.478
- type: recall_at_10
value: 20.087
- type: recall_at_100
value: 41.457
- type: recall_at_1000
value: 67.10199999999999
- type: recall_at_3
value: 10.313
- type: recall_at_5
value: 13.927999999999999
- task:
type: STS
dataset:
type: mteb/sickr-sts
name: MTEB SICK-R
config: default
split: test
revision: a6ea5a8cab320b040a23452cc28066d9beae2cee
metrics:
- type: cos_sim_pearson
value: 84.27341574565806
- type: cos_sim_spearman
value: 79.66419880841734
- type: euclidean_pearson
value: 81.32473321838208
- type: euclidean_spearman
value: 79.29828832085133
- type: manhattan_pearson
value: 81.25554065883132
- type: manhattan_spearman
value: 79.23275543279853
- task:
type: STS
dataset:
type: mteb/sts12-sts
name: MTEB STS12
config: default
split: test
revision: a0d554a64d88156834ff5ae9920b964011b16384
metrics:
- type: cos_sim_pearson
value: 83.40468875905418
- type: cos_sim_spearman
value: 74.2189990321174
- type: euclidean_pearson
value: 80.74376966290956
- type: euclidean_spearman
value: 74.97663839079335
- type: manhattan_pearson
value: 80.69779331646207
- type: manhattan_spearman
value: 75.00225252917613
- task:
type: STS
dataset:
type: mteb/sts13-sts
name: MTEB STS13
config: default
split: test
revision: 7e90230a92c190f1bf69ae9002b8cea547a64cca
metrics:
- type: cos_sim_pearson
value: 82.5745290053095
- type: cos_sim_spearman
value: 83.31401180333397
- type: euclidean_pearson
value: 82.96500607325534
- type: euclidean_spearman
value: 83.8534967935793
- type: manhattan_pearson
value: 82.83112050632508
- type: manhattan_spearman
value: 83.70877296557838
- task:
type: STS
dataset:
type: mteb/sts14-sts
name: MTEB STS14
config: default
split: test
revision: 6031580fec1f6af667f0bd2da0a551cf4f0b2375
metrics:
- type: cos_sim_pearson
value: 80.67833656607704
- type: cos_sim_spearman
value: 78.52252410630707
- type: euclidean_pearson
value: 80.071189514343
- type: euclidean_spearman
value: 78.95143545742796
- type: manhattan_pearson
value: 80.0128926165121
- type: manhattan_spearman
value: 78.91236678732628
- task:
type: STS
dataset:
type: mteb/sts15-sts
name: MTEB STS15
config: default
split: test
revision: ae752c7c21bf194d8b67fd573edf7ae58183cbe3
metrics:
- type: cos_sim_pearson
value: 87.48437639980746
- type: cos_sim_spearman
value: 88.34876527774259
- type: euclidean_pearson
value: 87.64898081823888
- type: euclidean_spearman
value: 88.58937180804213
- type: manhattan_pearson
value: 87.5942417815288
- type: manhattan_spearman
value: 88.53013922267687
- task:
type: STS
dataset:
type: mteb/sts16-sts
name: MTEB STS16
config: default
split: test
revision: 4d8694f8f0e0100860b497b999b3dbed754a0513
metrics:
- type: cos_sim_pearson
value: 82.69189187164781
- type: cos_sim_spearman
value: 84.15327883572112
- type: euclidean_pearson
value: 83.64202266685898
- type: euclidean_spearman
value: 84.6219602318862
- type: manhattan_pearson
value: 83.53256698709998
- type: manhattan_spearman
value: 84.49260712904946
- task:
type: STS
dataset:
type: mteb/sts17-crosslingual-sts
name: MTEB STS17 (en-en)
config: en-en
split: test
revision: af5e6fb845001ecf41f4c1e033ce921939a2a68d
metrics:
- type: cos_sim_pearson
value: 87.09508017611589
- type: cos_sim_spearman
value: 87.23010990417097
- type: euclidean_pearson
value: 87.62545569077133
- type: euclidean_spearman
value: 86.71152051711714
- type: manhattan_pearson
value: 87.5057154278377
- type: manhattan_spearman
value: 86.60611898281267
- task:
type: STS
dataset:
type: mteb/sts22-crosslingual-sts
name: MTEB STS22 (en)
config: en
split: test
revision: 6d1ba47164174a496b7fa5d3569dae26a6813b80
metrics:
- type: cos_sim_pearson
value: 61.72129893941176
- type: cos_sim_spearman
value: 62.87871412069194
- type: euclidean_pearson
value: 63.21077648290454
- type: euclidean_spearman
value: 63.03263080805978
- type: manhattan_pearson
value: 63.20740860135976
- type: manhattan_spearman
value: 62.89930471802817
- task:
type: STS
dataset:
type: mteb/stsbenchmark-sts
name: MTEB STSBenchmark
config: default
split: test
revision: b0fddb56ed78048fa8b90373c8a3cfc37b684831
metrics:
- type: cos_sim_pearson
value: 85.039118236799
- type: cos_sim_spearman
value: 86.18102563389962
- type: euclidean_pearson
value: 85.62977041471879
- type: euclidean_spearman
value: 86.02478990544347
- type: manhattan_pearson
value: 85.60786740521806
- type: manhattan_spearman
value: 85.99546210442547
- task:
type: Reranking
dataset:
type: mteb/scidocs-reranking
name: MTEB SciDocsRR
config: default
split: test
revision: d3c5e1fc0b855ab6097bf1cda04dd73947d7caab
metrics:
- type: map
value: 82.89875069737266
- type: mrr
value: 95.42621322033087
- task:
type: Retrieval
dataset:
type: scifact
name: MTEB SciFact
config: default
split: test
revision: None
metrics:
- type: map_at_1
value: 58.660999999999994
- type: map_at_10
value: 68.738
- type: map_at_100
value: 69.33200000000001
- type: map_at_1000
value: 69.352
- type: map_at_3
value: 66.502
- type: map_at_5
value: 67.686
- type: mrr_at_1
value: 61.667
- type: mrr_at_10
value: 70.003
- type: mrr_at_100
value: 70.441
- type: mrr_at_1000
value: 70.46
- type: mrr_at_3
value: 68.278
- type: mrr_at_5
value: 69.194
- type: ndcg_at_1
value: 61.667
- type: ndcg_at_10
value: 73.083
- type: ndcg_at_100
value: 75.56
- type: ndcg_at_1000
value: 76.01400000000001
- type: ndcg_at_3
value: 69.28699999999999
- type: ndcg_at_5
value: 70.85000000000001
- type: precision_at_1
value: 61.667
- type: precision_at_10
value: 9.6
- type: precision_at_100
value: 1.087
- type: precision_at_1000
value: 0.11199999999999999
- type: precision_at_3
value: 27.111
- type: precision_at_5
value: 17.467
- type: recall_at_1
value: 58.660999999999994
- type: recall_at_10
value: 85.02199999999999
- type: recall_at_100
value: 95.933
- type: recall_at_1000
value: 99.333
- type: recall_at_3
value: 74.506
- type: recall_at_5
value: 78.583
- task:
type: PairClassification
dataset:
type: mteb/sprintduplicatequestions-pairclassification
name: MTEB SprintDuplicateQuestions
config: default
split: test
revision: d66bd1f72af766a5cc4b0ca5e00c162f89e8cc46
metrics:
- type: cos_sim_accuracy
value: 99.8029702970297
- type: cos_sim_ap
value: 94.87673936635738
- type: cos_sim_f1
value: 90.00502260170768
- type: cos_sim_precision
value: 90.41372351160445
- type: cos_sim_recall
value: 89.60000000000001
- type: dot_accuracy
value: 99.57524752475247
- type: dot_ap
value: 84.81717934496321
- type: dot_f1
value: 78.23026646556059
- type: dot_precision
value: 78.66531850353893
- type: dot_recall
value: 77.8
- type: euclidean_accuracy
value: 99.8029702970297
- type: euclidean_ap
value: 94.74658253135284
- type: euclidean_f1
value: 90.08470353761834
- type: euclidean_precision
value: 89.77159880834161
- type: euclidean_recall
value: 90.4
- type: manhattan_accuracy
value: 99.8
- type: manhattan_ap
value: 94.69224030742787
- type: manhattan_f1
value: 89.9502487562189
- type: manhattan_precision
value: 89.50495049504951
- type: manhattan_recall
value: 90.4
- type: max_accuracy
value: 99.8029702970297
- type: max_ap
value: 94.87673936635738
- type: max_f1
value: 90.08470353761834
- task:
type: Clustering
dataset:
type: mteb/stackexchange-clustering
name: MTEB StackExchangeClustering
config: default
split: test
revision: 6cbc1f7b2bc0622f2e39d2c77fa502909748c259
metrics:
- type: v_measure
value: 63.906039623153035
- task:
type: Clustering
dataset:
type: mteb/stackexchange-clustering-p2p
name: MTEB StackExchangeClusteringP2P
config: default
split: test
revision: 815ca46b2622cec33ccafc3735d572c266efdb44
metrics:
- type: v_measure
value: 32.56053830923281
- task:
type: Reranking
dataset:
type: mteb/stackoverflowdupquestions-reranking
name: MTEB StackOverflowDupQuestions
config: default
split: test
revision: e185fbe320c72810689fc5848eb6114e1ef5ec69
metrics:
- type: map
value: 50.15326538775145
- type: mrr
value: 50.99279295051355
- task:
type: Summarization
dataset:
type: mteb/summeval
name: MTEB SummEval
config: default
split: test
revision: cda12ad7615edc362dbf25a00fdd61d3b1eaf93c
metrics:
- type: cos_sim_pearson
value: 31.44030762047337
- type: cos_sim_spearman
value: 31.00910300264562
- type: dot_pearson
value: 26.88257194766013
- type: dot_spearman
value: 27.646202679013577
- task:
type: Retrieval
dataset:
type: trec-covid
name: MTEB TRECCOVID
config: default
split: test
revision: None
metrics:
- type: map_at_1
value: 0.247
- type: map_at_10
value: 1.9429999999999998
- type: map_at_100
value: 10.82
- type: map_at_1000
value: 25.972
- type: map_at_3
value: 0.653
- type: map_at_5
value: 1.057
- type: mrr_at_1
value: 94
- type: mrr_at_10
value: 96.333
- type: mrr_at_100
value: 96.333
- type: mrr_at_1000
value: 96.333
- type: mrr_at_3
value: 96.333
- type: mrr_at_5
value: 96.333
- type: ndcg_at_1
value: 89
- type: ndcg_at_10
value: 79.63799999999999
- type: ndcg_at_100
value: 57.961
- type: ndcg_at_1000
value: 50.733
- type: ndcg_at_3
value: 84.224
- type: ndcg_at_5
value: 82.528
- type: precision_at_1
value: 94
- type: precision_at_10
value: 84.2
- type: precision_at_100
value: 59.36
- type: precision_at_1000
value: 22.738
- type: precision_at_3
value: 88
- type: precision_at_5
value: 86.8
- type: recall_at_1
value: 0.247
- type: recall_at_10
value: 2.131
- type: recall_at_100
value: 14.035
- type: recall_at_1000
value: 47.457
- type: recall_at_3
value: 0.6779999999999999
- type: recall_at_5
value: 1.124
- task:
type: Retrieval
dataset:
type: webis-touche2020
name: MTEB Touche2020
config: default
split: test
revision: None
metrics:
- type: map_at_1
value: 2.603
- type: map_at_10
value: 11.667
- type: map_at_100
value: 16.474
- type: map_at_1000
value: 18.074
- type: map_at_3
value: 6.03
- type: map_at_5
value: 8.067
- type: mrr_at_1
value: 34.694
- type: mrr_at_10
value: 51.063
- type: mrr_at_100
value: 51.908
- type: mrr_at_1000
value: 51.908
- type: mrr_at_3
value: 47.959
- type: mrr_at_5
value: 49.694
- type: ndcg_at_1
value: 32.653
- type: ndcg_at_10
value: 28.305000000000003
- type: ndcg_at_100
value: 35.311
- type: ndcg_at_1000
value: 47.644999999999996
- type: ndcg_at_3
value: 32.187
- type: ndcg_at_5
value: 29.134999999999998
- type: precision_at_1
value: 34.694
- type: precision_at_10
value: 26.122
- type: precision_at_100
value: 6.755
- type: precision_at_1000
value: 1.467
- type: precision_at_3
value: 34.694
- type: precision_at_5
value: 30.203999999999997
- type: recall_at_1
value: 2.603
- type: recall_at_10
value: 18.716
- type: recall_at_100
value: 42.512
- type: recall_at_1000
value: 79.32000000000001
- type: recall_at_3
value: 7.59
- type: recall_at_5
value: 10.949
- task:
type: Classification
dataset:
type: mteb/toxic_conversations_50k
name: MTEB ToxicConversationsClassification
config: default
split: test
revision: d7c0de2777da35d6aae2200a62c6e0e5af397c4c
metrics:
- type: accuracy
value: 74.117
- type: ap
value: 15.89357321699319
- type: f1
value: 57.14385866369257
- task:
type: Classification
dataset:
type: mteb/tweet_sentiment_extraction
name: MTEB TweetSentimentExtractionClassification
config: default
split: test
revision: d604517c81ca91fe16a244d1248fc021f9ecee7a
metrics:
- type: accuracy
value: 61.38370118845502
- type: f1
value: 61.67038693866553
- task:
type: Clustering
dataset:
type: mteb/twentynewsgroups-clustering
name: MTEB TwentyNewsgroupsClustering
config: default
split: test
revision: 6125ec4e24fa026cec8a478383ee943acfbd5449
metrics:
- type: v_measure
value: 42.57754941537969
- task:
type: PairClassification
dataset:
type: mteb/twittersemeval2015-pairclassification
name: MTEB TwitterSemEval2015
config: default
split: test
revision: 70970daeab8776df92f5ea462b6173c0b46fd2d1
metrics:
- type: cos_sim_accuracy
value: 86.1775049174465
- type: cos_sim_ap
value: 74.3994879581554
- type: cos_sim_f1
value: 69.32903671308551
- type: cos_sim_precision
value: 61.48193508879363
- type: cos_sim_recall
value: 79.47229551451187
- type: dot_accuracy
value: 81.65345413363534
- type: dot_ap
value: 59.690898346685096
- type: dot_f1
value: 57.27622826467499
- type: dot_precision
value: 51.34965473948525
- type: dot_recall
value: 64.74934036939314
- type: euclidean_accuracy
value: 86.04637301066937
- type: euclidean_ap
value: 74.33009001775268
- type: euclidean_f1
value: 69.2458374142997
- type: euclidean_precision
value: 64.59570580173595
- type: euclidean_recall
value: 74.6174142480211
- type: manhattan_accuracy
value: 86.11193896405793
- type: manhattan_ap
value: 74.2964140130421
- type: manhattan_f1
value: 69.11601528788066
- type: manhattan_precision
value: 64.86924323073363
- type: manhattan_recall
value: 73.95778364116094
- type: max_accuracy
value: 86.1775049174465
- type: max_ap
value: 74.3994879581554
- type: max_f1
value: 69.32903671308551
- task:
type: PairClassification
dataset:
type: mteb/twitterurlcorpus-pairclassification
name: MTEB TwitterURLCorpus
config: default
split: test
revision: 8b6510b0b1fa4e4c4f879467980e9be563ec1cdf
metrics:
- type: cos_sim_accuracy
value: 89.01501921061823
- type: cos_sim_ap
value: 85.97819287477351
- type: cos_sim_f1
value: 78.33882858518875
- type: cos_sim_precision
value: 75.49446626204926
- type: cos_sim_recall
value: 81.40591315060055
- type: dot_accuracy
value: 86.47494857763806
- type: dot_ap
value: 78.77420360340282
- type: dot_f1
value: 73.06433247936238
- type: dot_precision
value: 67.92140777983595
- type: dot_recall
value: 79.04989220819218
- type: euclidean_accuracy
value: 88.7297706368611
- type: euclidean_ap
value: 85.61550568529317
- type: euclidean_f1
value: 77.84805525263539
- type: euclidean_precision
value: 73.73639994491117
- type: euclidean_recall
value: 82.44533415460425
- type: manhattan_accuracy
value: 88.75111576823068
- type: manhattan_ap
value: 85.58701671476263
- type: manhattan_f1
value: 77.70169909067856
- type: manhattan_precision
value: 73.37666780704755
- type: manhattan_recall
value: 82.5685247921158
- type: max_accuracy
value: 89.01501921061823
- type: max_ap
value: 85.97819287477351
- type: max_f1
value: 78.33882858518875
language:
- en
license: mit
---
## E5-base
**News (May 2023): please switch to [e5-base-v2](https://huggingface.co/intfloat/e5-base-v2), which has better performance and same method of usage.**
[Text Embeddings by Weakly-Supervised Contrastive Pre-training](https://arxiv.org/pdf/2212.03533.pdf).
Liang Wang, Nan Yang, Xiaolong Huang, Binxing Jiao, Linjun Yang, Daxin Jiang, Rangan Majumder, Furu Wei, arXiv 2022
This model has 12 layers and the embedding size is 768.
## Usage
Below is an example to encode queries and passages from the MS-MARCO passage ranking dataset.
```python
import torch.nn.functional as F
from torch import Tensor
from transformers import AutoTokenizer, AutoModel
def average_pool(last_hidden_states: Tensor,
attention_mask: Tensor) -> Tensor:
last_hidden = last_hidden_states.masked_fill(~attention_mask[..., None].bool(), 0.0)
return last_hidden.sum(dim=1) / attention_mask.sum(dim=1)[..., None]
# Each input text should start with "query: " or "passage: ".
# For tasks other than retrieval, you can simply use the "query: " prefix.
input_texts = ['query: how much protein should a female eat',
'query: summit define',
"passage: As a general guideline, the CDC's average requirement of protein for women ages 19 to 70 is 46 grams per day. But, as you can see from this chart, you'll need to increase that if you're expecting or training for a marathon. Check out the chart below to see how much protein you should be eating each day.",
"passage: Definition of summit for English Language Learners. : 1 the highest point of a mountain : the top of a mountain. : 2 the highest level. : 3 a meeting or series of meetings between the leaders of two or more governments."]
tokenizer = AutoTokenizer.from_pretrained('intfloat/e5-base')
model = AutoModel.from_pretrained('intfloat/e5-base')
# Tokenize the input texts
batch_dict = tokenizer(input_texts, max_length=512, padding=True, truncation=True, return_tensors='pt')
outputs = model(**batch_dict)
embeddings = average_pool(outputs.last_hidden_state, batch_dict['attention_mask'])
# normalize embeddings
embeddings = F.normalize(embeddings, p=2, dim=1)
scores = (embeddings[:2] @ embeddings[2:].T) * 100
print(scores.tolist())
```
## Training Details
Please refer to our paper at [https://arxiv.org/pdf/2212.03533.pdf](https://arxiv.org/pdf/2212.03533.pdf).
## Benchmark Evaluation
Check out [unilm/e5](https://github.com/microsoft/unilm/tree/master/e5) to reproduce evaluation results
on the [BEIR](https://arxiv.org/abs/2104.08663) and [MTEB benchmark](https://arxiv.org/abs/2210.07316).
## Support for Sentence Transformers
Below is an example for usage with sentence_transformers.
```python
from sentence_transformers import SentenceTransformer
model = SentenceTransformer('intfloat/e5-base')
input_texts = [
'query: how much protein should a female eat',
'query: summit define',
"passage: As a general guideline, the CDC's average requirement of protein for women ages 19 to 70 is 46 grams per day. But, as you can see from this chart, you'll need to increase that if you're expecting or training for a marathon. Check out the chart below to see how much protein you should be eating each day.",
"passage: Definition of summit for English Language Learners. : 1 the highest point of a mountain : the top of a mountain. : 2 the highest level. : 3 a meeting or series of meetings between the leaders of two or more governments."
]
embeddings = model.encode(input_texts, normalize_embeddings=True)
```
Package requirements
`pip install sentence_transformers~=2.2.2`
Contributors: [michaelfeil](https://huggingface.co/michaelfeil)
## FAQ
**1. Do I need to add the prefix "query: " and "passage: " to input texts?**
Yes, this is how the model is trained, otherwise you will see a performance degradation.
Here are some rules of thumb:
- Use "query: " and "passage: " correspondingly for asymmetric tasks such as passage retrieval in open QA, ad-hoc information retrieval.
- Use "query: " prefix for symmetric tasks such as semantic similarity, paraphrase retrieval.
- Use "query: " prefix if you want to use embeddings as features, such as linear probing classification, clustering.
**2. Why are my reproduced results slightly different from reported in the model card?**
Different versions of `transformers` and `pytorch` could cause negligible but non-zero performance differences.
**3. Why does the cosine similarity scores distribute around 0.7 to 1.0?**
This is a known and expected behavior as we use a low temperature 0.01 for InfoNCE contrastive loss.
For text embedding tasks like text retrieval or semantic similarity,
what matters is the relative order of the scores instead of the absolute values,
so this should not be an issue.
## Citation
If you find our paper or models helpful, please consider cite as follows:
```
@article{wang2022text,
title={Text Embeddings by Weakly-Supervised Contrastive Pre-training},
author={Wang, Liang and Yang, Nan and Huang, Xiaolong and Jiao, Binxing and Yang, Linjun and Jiang, Daxin and Majumder, Rangan and Wei, Furu},
journal={arXiv preprint arXiv:2212.03533},
year={2022}
}
```
## Limitations
This model only works for English texts. Long texts will be truncated to at most 512 tokens.
|
RichardErkhov/Minirecord_-_Mini_DPO_7b_01-gguf | RichardErkhov | "2024-06-30T11:02:38Z" | 12,907 | 0 | null | [
"gguf",
"region:us"
] | null | "2024-06-30T08:54:10Z" | Quantization made by Richard Erkhov.
[Github](https://github.com/RichardErkhov)
[Discord](https://discord.gg/pvy7H8DZMG)
[Request more models](https://github.com/RichardErkhov/quant_request)
Mini_DPO_7b_01 - GGUF
- Model creator: https://huggingface.co/Minirecord/
- Original model: https://huggingface.co/Minirecord/Mini_DPO_7b_01/
| Name | Quant method | Size |
| ---- | ---- | ---- |
| [Mini_DPO_7b_01.Q2_K.gguf](https://huggingface.co/RichardErkhov/Minirecord_-_Mini_DPO_7b_01-gguf/blob/main/Mini_DPO_7b_01.Q2_K.gguf) | Q2_K | 2.53GB |
| [Mini_DPO_7b_01.IQ3_XS.gguf](https://huggingface.co/RichardErkhov/Minirecord_-_Mini_DPO_7b_01-gguf/blob/main/Mini_DPO_7b_01.IQ3_XS.gguf) | IQ3_XS | 2.81GB |
| [Mini_DPO_7b_01.IQ3_S.gguf](https://huggingface.co/RichardErkhov/Minirecord_-_Mini_DPO_7b_01-gguf/blob/main/Mini_DPO_7b_01.IQ3_S.gguf) | IQ3_S | 2.96GB |
| [Mini_DPO_7b_01.Q3_K_S.gguf](https://huggingface.co/RichardErkhov/Minirecord_-_Mini_DPO_7b_01-gguf/blob/main/Mini_DPO_7b_01.Q3_K_S.gguf) | Q3_K_S | 2.95GB |
| [Mini_DPO_7b_01.IQ3_M.gguf](https://huggingface.co/RichardErkhov/Minirecord_-_Mini_DPO_7b_01-gguf/blob/main/Mini_DPO_7b_01.IQ3_M.gguf) | IQ3_M | 3.06GB |
| [Mini_DPO_7b_01.Q3_K.gguf](https://huggingface.co/RichardErkhov/Minirecord_-_Mini_DPO_7b_01-gguf/blob/main/Mini_DPO_7b_01.Q3_K.gguf) | Q3_K | 3.28GB |
| [Mini_DPO_7b_01.Q3_K_M.gguf](https://huggingface.co/RichardErkhov/Minirecord_-_Mini_DPO_7b_01-gguf/blob/main/Mini_DPO_7b_01.Q3_K_M.gguf) | Q3_K_M | 3.28GB |
| [Mini_DPO_7b_01.Q3_K_L.gguf](https://huggingface.co/RichardErkhov/Minirecord_-_Mini_DPO_7b_01-gguf/blob/main/Mini_DPO_7b_01.Q3_K_L.gguf) | Q3_K_L | 3.56GB |
| [Mini_DPO_7b_01.IQ4_XS.gguf](https://huggingface.co/RichardErkhov/Minirecord_-_Mini_DPO_7b_01-gguf/blob/main/Mini_DPO_7b_01.IQ4_XS.gguf) | IQ4_XS | 3.67GB |
| [Mini_DPO_7b_01.Q4_0.gguf](https://huggingface.co/RichardErkhov/Minirecord_-_Mini_DPO_7b_01-gguf/blob/main/Mini_DPO_7b_01.Q4_0.gguf) | Q4_0 | 3.83GB |
| [Mini_DPO_7b_01.IQ4_NL.gguf](https://huggingface.co/RichardErkhov/Minirecord_-_Mini_DPO_7b_01-gguf/blob/main/Mini_DPO_7b_01.IQ4_NL.gguf) | IQ4_NL | 3.87GB |
| [Mini_DPO_7b_01.Q4_K_S.gguf](https://huggingface.co/RichardErkhov/Minirecord_-_Mini_DPO_7b_01-gguf/blob/main/Mini_DPO_7b_01.Q4_K_S.gguf) | Q4_K_S | 3.86GB |
| [Mini_DPO_7b_01.Q4_K.gguf](https://huggingface.co/RichardErkhov/Minirecord_-_Mini_DPO_7b_01-gguf/blob/main/Mini_DPO_7b_01.Q4_K.gguf) | Q4_K | 4.07GB |
| [Mini_DPO_7b_01.Q4_K_M.gguf](https://huggingface.co/RichardErkhov/Minirecord_-_Mini_DPO_7b_01-gguf/blob/main/Mini_DPO_7b_01.Q4_K_M.gguf) | Q4_K_M | 4.07GB |
| [Mini_DPO_7b_01.Q4_1.gguf](https://huggingface.co/RichardErkhov/Minirecord_-_Mini_DPO_7b_01-gguf/blob/main/Mini_DPO_7b_01.Q4_1.gguf) | Q4_1 | 4.24GB |
| [Mini_DPO_7b_01.Q5_0.gguf](https://huggingface.co/RichardErkhov/Minirecord_-_Mini_DPO_7b_01-gguf/blob/main/Mini_DPO_7b_01.Q5_0.gguf) | Q5_0 | 4.65GB |
| [Mini_DPO_7b_01.Q5_K_S.gguf](https://huggingface.co/RichardErkhov/Minirecord_-_Mini_DPO_7b_01-gguf/blob/main/Mini_DPO_7b_01.Q5_K_S.gguf) | Q5_K_S | 4.65GB |
| [Mini_DPO_7b_01.Q5_K.gguf](https://huggingface.co/RichardErkhov/Minirecord_-_Mini_DPO_7b_01-gguf/blob/main/Mini_DPO_7b_01.Q5_K.gguf) | Q5_K | 4.78GB |
| [Mini_DPO_7b_01.Q5_K_M.gguf](https://huggingface.co/RichardErkhov/Minirecord_-_Mini_DPO_7b_01-gguf/blob/main/Mini_DPO_7b_01.Q5_K_M.gguf) | Q5_K_M | 4.78GB |
| [Mini_DPO_7b_01.Q5_1.gguf](https://huggingface.co/RichardErkhov/Minirecord_-_Mini_DPO_7b_01-gguf/blob/main/Mini_DPO_7b_01.Q5_1.gguf) | Q5_1 | 5.07GB |
| [Mini_DPO_7b_01.Q6_K.gguf](https://huggingface.co/RichardErkhov/Minirecord_-_Mini_DPO_7b_01-gguf/blob/main/Mini_DPO_7b_01.Q6_K.gguf) | Q6_K | 5.53GB |
| [Mini_DPO_7b_01.Q8_0.gguf](https://huggingface.co/RichardErkhov/Minirecord_-_Mini_DPO_7b_01-gguf/blob/main/Mini_DPO_7b_01.Q8_0.gguf) | Q8_0 | 7.17GB |
Original model description:
---
license: cc-by-sa-4.0
---
|
mradermacher/L3-8B-Chara-v1-Alpha-GGUF | mradermacher | "2024-07-01T10:17:09Z" | 12,904 | 0 | transformers | [
"transformers",
"gguf",
"en",
"base_model:Sao10K/L3-8B-Chara-v1-Alpha",
"license:cc-by-nc-4.0",
"endpoints_compatible",
"region:us"
] | null | "2024-07-01T08:11:09Z" | ---
base_model: Sao10K/L3-8B-Chara-v1-Alpha
language:
- en
library_name: transformers
license: cc-by-nc-4.0
quantized_by: mradermacher
---
## About
<!-- ### quantize_version: 2 -->
<!-- ### output_tensor_quantised: 1 -->
<!-- ### convert_type: hf -->
<!-- ### vocab_type: -->
<!-- ### tags: -->
static quants of https://huggingface.co/Sao10K/L3-8B-Chara-v1-Alpha
<!-- provided-files -->
weighted/imatrix quants are available at https://huggingface.co/mradermacher/L3-8B-Chara-v1-Alpha-i1-GGUF
## Usage
If you are unsure how to use GGUF files, refer to one of [TheBloke's
READMEs](https://huggingface.co/TheBloke/KafkaLM-70B-German-V0.1-GGUF) for
more details, including on how to concatenate multi-part files.
## Provided Quants
(sorted by size, not necessarily quality. IQ-quants are often preferable over similar sized non-IQ quants)
| Link | Type | Size/GB | Notes |
|:-----|:-----|--------:|:------|
| [GGUF](https://huggingface.co/mradermacher/L3-8B-Chara-v1-Alpha-GGUF/resolve/main/L3-8B-Chara-v1-Alpha.Q2_K.gguf) | Q2_K | 3.3 | |
| [GGUF](https://huggingface.co/mradermacher/L3-8B-Chara-v1-Alpha-GGUF/resolve/main/L3-8B-Chara-v1-Alpha.IQ3_XS.gguf) | IQ3_XS | 3.6 | |
| [GGUF](https://huggingface.co/mradermacher/L3-8B-Chara-v1-Alpha-GGUF/resolve/main/L3-8B-Chara-v1-Alpha.Q3_K_S.gguf) | Q3_K_S | 3.8 | |
| [GGUF](https://huggingface.co/mradermacher/L3-8B-Chara-v1-Alpha-GGUF/resolve/main/L3-8B-Chara-v1-Alpha.IQ3_S.gguf) | IQ3_S | 3.8 | beats Q3_K* |
| [GGUF](https://huggingface.co/mradermacher/L3-8B-Chara-v1-Alpha-GGUF/resolve/main/L3-8B-Chara-v1-Alpha.IQ3_M.gguf) | IQ3_M | 3.9 | |
| [GGUF](https://huggingface.co/mradermacher/L3-8B-Chara-v1-Alpha-GGUF/resolve/main/L3-8B-Chara-v1-Alpha.Q3_K_M.gguf) | Q3_K_M | 4.1 | lower quality |
| [GGUF](https://huggingface.co/mradermacher/L3-8B-Chara-v1-Alpha-GGUF/resolve/main/L3-8B-Chara-v1-Alpha.Q3_K_L.gguf) | Q3_K_L | 4.4 | |
| [GGUF](https://huggingface.co/mradermacher/L3-8B-Chara-v1-Alpha-GGUF/resolve/main/L3-8B-Chara-v1-Alpha.IQ4_XS.gguf) | IQ4_XS | 4.6 | |
| [GGUF](https://huggingface.co/mradermacher/L3-8B-Chara-v1-Alpha-GGUF/resolve/main/L3-8B-Chara-v1-Alpha.Q4_K_S.gguf) | Q4_K_S | 4.8 | fast, recommended |
| [GGUF](https://huggingface.co/mradermacher/L3-8B-Chara-v1-Alpha-GGUF/resolve/main/L3-8B-Chara-v1-Alpha.Q4_K_M.gguf) | Q4_K_M | 5.0 | fast, recommended |
| [GGUF](https://huggingface.co/mradermacher/L3-8B-Chara-v1-Alpha-GGUF/resolve/main/L3-8B-Chara-v1-Alpha.Q5_K_S.gguf) | Q5_K_S | 5.7 | |
| [GGUF](https://huggingface.co/mradermacher/L3-8B-Chara-v1-Alpha-GGUF/resolve/main/L3-8B-Chara-v1-Alpha.Q5_K_M.gguf) | Q5_K_M | 5.8 | |
| [GGUF](https://huggingface.co/mradermacher/L3-8B-Chara-v1-Alpha-GGUF/resolve/main/L3-8B-Chara-v1-Alpha.Q6_K.gguf) | Q6_K | 6.7 | very good quality |
| [GGUF](https://huggingface.co/mradermacher/L3-8B-Chara-v1-Alpha-GGUF/resolve/main/L3-8B-Chara-v1-Alpha.Q8_0.gguf) | Q8_0 | 8.6 | fast, best quality |
| [GGUF](https://huggingface.co/mradermacher/L3-8B-Chara-v1-Alpha-GGUF/resolve/main/L3-8B-Chara-v1-Alpha.f16.gguf) | f16 | 16.2 | 16 bpw, overkill |
Here is a handy graph by ikawrakow comparing some lower-quality quant
types (lower is better):

And here are Artefact2's thoughts on the matter:
https://gist.github.com/Artefact2/b5f810600771265fc1e39442288e8ec9
## FAQ / Model Request
See https://huggingface.co/mradermacher/model_requests for some answers to
questions you might have and/or if you want some other model quantized.
## Thanks
I thank my company, [nethype GmbH](https://www.nethype.de/), for letting
me use its servers and providing upgrades to my workstation to enable
this work in my free time.
<!-- end -->
|
QuantFactory/Blue-Orchid-2x7b-GGUF | QuantFactory | "2024-06-24T06:39:03Z" | 12,892 | 0 | null | [
"gguf",
"text-generation",
"base_model:nakodanei/Blue-Orchid-2x7b",
"license:apache-2.0",
"region:us"
] | text-generation | "2024-06-24T02:59:06Z" | ---
license: apache-2.0
pipeline_tag: text-generation
base_model: nakodanei/Blue-Orchid-2x7b
---
# QuantFactory/Blue-Orchid-2x7b-GGUF
This is quantized version of [nakodanei/Blue-Orchid-2x7b](https://huggingface.co/nakodanei/Blue-Orchid-2x7b) created using llama.cpp
# Model Description
Roleplaying focused MoE Mistral model.
One expert is a merge of mostly RP models, the other is a merge of mostly storywriting models. So it should be good at both. The base model is SanjiWatsuki/Kunoichi-DPO-v2-7B.
- Expert 1 is a merge of LimaRP, Limamono, Noromaid 0.4 DPO and good-robot.
- Expert 2 is a merge of Erebus, Holodeck, Dans-AdventurousWinds-Mk2, Opus, Ashhwriter and good-robot.
## Prompt template (LimaRP):
```
### Instruction:
{system prompt}
### Input:
User: {prompt}
### Response:
Character:
```
Alpaca prompt template should work fine too. |
HooshvareLab/bert-fa-base-uncased-ner-arman | HooshvareLab | "2021-05-18T20:52:21Z" | 12,891 | 0 | transformers | [
"transformers",
"pytorch",
"tf",
"jax",
"bert",
"token-classification",
"fa",
"license:apache-2.0",
"autotrain_compatible",
"endpoints_compatible",
"region:us"
] | token-classification | "2022-03-02T23:29:04Z" | ---
language: fa
license: apache-2.0
---
# ParsBERT (v2.0)
A Transformer-based Model for Persian Language Understanding
We reconstructed the vocabulary and fine-tuned the ParsBERT v1.1 on the new Persian corpora in order to provide some functionalities for using ParsBERT in other scopes!
Please follow the [ParsBERT](https://github.com/hooshvare/parsbert) repo for the latest information about previous and current models.
## Persian NER [ARMAN, PEYMA]
This task aims to extract named entities in the text, such as names and label with appropriate `NER` classes such as locations, organizations, etc. The datasets used for this task contain sentences that are marked with `IOB` format. In this format, tokens that are not part of an entity are tagged as `”O”` the `”B”`tag corresponds to the first word of an object, and the `”I”` tag corresponds to the rest of the terms of the same entity. Both `”B”` and `”I”` tags are followed by a hyphen (or underscore), followed by the entity category. Therefore, the NER task is a multi-class token classification problem that labels the tokens upon being fed a raw text. There are two primary datasets used in Persian NER, `ARMAN`, and `PEYMA`.
### ARMAN
ARMAN dataset holds 7,682 sentences with 250,015 sentences tagged over six different classes.
1. Organization
2. Location
3. Facility
4. Event
5. Product
6. Person
| Label | # |
|:------------:|:-----:|
| Organization | 30108 |
| Location | 12924 |
| Facility | 4458 |
| Event | 7557 |
| Product | 4389 |
| Person | 15645 |
**Download**
You can download the dataset from [here](https://github.com/HaniehP/PersianNER)
## Results
The following table summarizes the F1 score obtained by ParsBERT as compared to other models and architectures.
| Dataset | ParsBERT v2 | ParsBERT v1 | mBERT | MorphoBERT | Beheshti-NER | LSTM-CRF | Rule-Based CRF | BiLSTM-CRF |
|---------|-------------|-------------|-------|------------|--------------|----------|----------------|------------|
| ARMAN | 99.84* | 98.79 | 95.89 | 89.9 | 84.03 | 86.55 | - | 77.45 |
## How to use :hugs:
| Notebook | Description | |
|:----------|:-------------|------:|
| [How to use Pipelines](https://github.com/hooshvare/parsbert-ner/blob/master/persian-ner-pipeline.ipynb) | Simple and efficient way to use State-of-the-Art models on downstream tasks through transformers | [](https://colab.research.google.com/github/hooshvare/parsbert-ner/blob/master/persian-ner-pipeline.ipynb) |
### BibTeX entry and citation info
Please cite in publications as the following:
```bibtex
@article{ParsBERT,
title={ParsBERT: Transformer-based Model for Persian Language Understanding},
author={Mehrdad Farahani, Mohammad Gharachorloo, Marzieh Farahani, Mohammad Manthouri},
journal={ArXiv},
year={2020},
volume={abs/2005.12515}
}
```
## Questions?
Post a Github issue on the [ParsBERT Issues](https://github.com/hooshvare/parsbert/issues) repo. |
mradermacher/llama3-mawared-2E-GGUF | mradermacher | "2024-06-23T18:44:16Z" | 12,891 | 0 | transformers | [
"transformers",
"gguf",
"text-generation-inference",
"unsloth",
"llama",
"trl",
"en",
"base_model:Ammartatox/llama3-mawared-2E",
"license:apache-2.0",
"endpoints_compatible",
"region:us"
] | null | "2024-06-23T18:16:37Z" | ---
base_model: Ammartatox/llama3-mawared-2E
language:
- en
library_name: transformers
license: apache-2.0
quantized_by: mradermacher
tags:
- text-generation-inference
- transformers
- unsloth
- llama
- trl
---
## About
<!-- ### quantize_version: 2 -->
<!-- ### output_tensor_quantised: 1 -->
<!-- ### convert_type: hf -->
<!-- ### vocab_type: -->
<!-- ### tags: -->
static quants of https://huggingface.co/Ammartatox/llama3-mawared-2E
<!-- provided-files -->
weighted/imatrix quants seem not to be available (by me) at this time. If they do not show up a week or so after the static ones, I have probably not planned for them. Feel free to request them by opening a Community Discussion.
## Usage
If you are unsure how to use GGUF files, refer to one of [TheBloke's
READMEs](https://huggingface.co/TheBloke/KafkaLM-70B-German-V0.1-GGUF) for
more details, including on how to concatenate multi-part files.
## Provided Quants
(sorted by size, not necessarily quality. IQ-quants are often preferable over similar sized non-IQ quants)
| Link | Type | Size/GB | Notes |
|:-----|:-----|--------:|:------|
| [GGUF](https://huggingface.co/mradermacher/llama3-mawared-2E-GGUF/resolve/main/llama3-mawared-2E.Q2_K.gguf) | Q2_K | 3.3 | |
| [GGUF](https://huggingface.co/mradermacher/llama3-mawared-2E-GGUF/resolve/main/llama3-mawared-2E.IQ3_XS.gguf) | IQ3_XS | 3.6 | |
| [GGUF](https://huggingface.co/mradermacher/llama3-mawared-2E-GGUF/resolve/main/llama3-mawared-2E.Q3_K_S.gguf) | Q3_K_S | 3.8 | |
| [GGUF](https://huggingface.co/mradermacher/llama3-mawared-2E-GGUF/resolve/main/llama3-mawared-2E.IQ3_S.gguf) | IQ3_S | 3.8 | beats Q3_K* |
| [GGUF](https://huggingface.co/mradermacher/llama3-mawared-2E-GGUF/resolve/main/llama3-mawared-2E.IQ3_M.gguf) | IQ3_M | 3.9 | |
| [GGUF](https://huggingface.co/mradermacher/llama3-mawared-2E-GGUF/resolve/main/llama3-mawared-2E.Q3_K_M.gguf) | Q3_K_M | 4.1 | lower quality |
| [GGUF](https://huggingface.co/mradermacher/llama3-mawared-2E-GGUF/resolve/main/llama3-mawared-2E.Q3_K_L.gguf) | Q3_K_L | 4.4 | |
| [GGUF](https://huggingface.co/mradermacher/llama3-mawared-2E-GGUF/resolve/main/llama3-mawared-2E.IQ4_XS.gguf) | IQ4_XS | 4.6 | |
| [GGUF](https://huggingface.co/mradermacher/llama3-mawared-2E-GGUF/resolve/main/llama3-mawared-2E.Q4_K_S.gguf) | Q4_K_S | 4.8 | fast, recommended |
| [GGUF](https://huggingface.co/mradermacher/llama3-mawared-2E-GGUF/resolve/main/llama3-mawared-2E.Q4_K_M.gguf) | Q4_K_M | 5.0 | fast, recommended |
| [GGUF](https://huggingface.co/mradermacher/llama3-mawared-2E-GGUF/resolve/main/llama3-mawared-2E.Q5_K_S.gguf) | Q5_K_S | 5.7 | |
| [GGUF](https://huggingface.co/mradermacher/llama3-mawared-2E-GGUF/resolve/main/llama3-mawared-2E.Q5_K_M.gguf) | Q5_K_M | 5.8 | |
| [GGUF](https://huggingface.co/mradermacher/llama3-mawared-2E-GGUF/resolve/main/llama3-mawared-2E.Q6_K.gguf) | Q6_K | 6.7 | very good quality |
| [GGUF](https://huggingface.co/mradermacher/llama3-mawared-2E-GGUF/resolve/main/llama3-mawared-2E.Q8_0.gguf) | Q8_0 | 8.6 | fast, best quality |
| [GGUF](https://huggingface.co/mradermacher/llama3-mawared-2E-GGUF/resolve/main/llama3-mawared-2E.f16.gguf) | f16 | 16.2 | 16 bpw, overkill |
Here is a handy graph by ikawrakow comparing some lower-quality quant
types (lower is better):

And here are Artefact2's thoughts on the matter:
https://gist.github.com/Artefact2/b5f810600771265fc1e39442288e8ec9
## FAQ / Model Request
See https://huggingface.co/mradermacher/model_requests for some answers to
questions you might have and/or if you want some other model quantized.
## Thanks
I thank my company, [nethype GmbH](https://www.nethype.de/), for letting
me use its servers and providing upgrades to my workstation to enable
this work in my free time.
<!-- end -->
|
BioMistral/BioMistral-7B | BioMistral | "2024-02-21T20:53:27Z" | 12,883 | 359 | transformers | [
"transformers",
"pytorch",
"tensorboard",
"mistral",
"text-generation",
"medical",
"biology",
"conversational",
"fr",
"en",
"de",
"nl",
"es",
"pt",
"pl",
"ro",
"it",
"arxiv:2402.10373",
"license:apache-2.0",
"autotrain_compatible",
"endpoints_compatible",
"text-generation-inference",
"region:us"
] | text-generation | "2024-02-14T11:33:32Z" | ---
license: apache-2.0
language:
- fr
- en
- de
- nl
- es
- pt
- pl
- ro
- it
pipeline_tag: text-generation
tags:
- medical
- biology
---
<p align="center">
<img src="https://huggingface.co/BioMistral/BioMistral-7B/resolve/main/wordart_blue_m_rectangle.png?download=true" alt="drawing" width="250"/>
</p>
# BioMistral: A Collection of Open-Source Pretrained Large Language Models for Medical Domains
**Abstract:**
Large Language Models (LLMs) have demonstrated remarkable versatility in recent years, offering potential applications across specialized domains such as healthcare and medicine. Despite the availability of various open-source LLMs tailored for health contexts, adapting general-purpose LLMs to the medical domain presents significant challenges.
In this paper, we introduce BioMistral, an open-source LLM tailored for the biomedical domain, utilizing Mistral as its foundation model and further pre-trained on PubMed Central. We conduct a comprehensive evaluation of BioMistral on a benchmark comprising 10 established medical question-answering (QA) tasks in English. We also explore lightweight models obtained through quantization and model merging approaches. Our results demonstrate BioMistral's superior performance compared to existing open-source medical models and its competitive edge against proprietary counterparts. Finally, to address the limited availability of data beyond English and to assess the multilingual generalization of medical LLMs, we automatically translated and evaluated this benchmark into 7 other languages. This marks the first large-scale multilingual evaluation of LLMs in the medical domain. Datasets, multilingual evaluation benchmarks, scripts, and all the models obtained during our experiments are freely released.
**Advisory Notice!** Although BioMistral is intended to encapsulate medical knowledge sourced from high-quality evidence, it hasn't been tailored to effectively, safely, or suitably convey this knowledge within professional parameters for action. We advise refraining from utilizing BioMistral in medical contexts unless it undergoes thorough alignment with specific use cases and undergoes further testing, notably including randomized controlled trials in real-world medical environments. BioMistral 7B may possess inherent risks and biases that have not yet been thoroughly assessed. Additionally, the model's performance has not been evaluated in real-world clinical settings. Consequently, we recommend using BioMistral 7B strictly as a research tool and advise against deploying it in production environments for natural language generation or any professional health and medical purposes.
# 1. BioMistral models
**BioMistral** is a suite of Mistral-based further pre-trained open source models suited for the medical domains and pre-trained using textual data from PubMed Central Open Access (CC0, CC BY, CC BY-SA, and CC BY-ND). All the models are trained using the CNRS (French National Centre for Scientific Research) [Jean Zay](http://www.idris.fr/jean-zay/) French HPC.
| Model Name | Base Model | Model Type | Sequence Length | Download |
|:-------------------:|:----------------------------------:|:-------------------:|:---------------:|:-----------------------------------------------------:|
| BioMistral-7B | [Mistral-7B-Instruct-v0.1](https://huggingface.co/mistralai/Mistral-7B-Instruct-v0.1) | Further Pre-trained | 2048 | [HuggingFace](https://huggingface.co/BioMistral/BioMistral-7B) |
| BioMistral-7B-DARE | [Mistral-7B-Instruct-v0.1](https://huggingface.co/mistralai/Mistral-7B-Instruct-v0.1) | Merge DARE | 2048 | [HuggingFace](https://huggingface.co/BioMistral/BioMistral-7B-DARE) |
| BioMistral-7B-TIES | [Mistral-7B-Instruct-v0.1](https://huggingface.co/mistralai/Mistral-7B-Instruct-v0.1) | Merge TIES | 2048 | [HuggingFace](https://huggingface.co/BioMistral/BioMistral-7B-TIES) |
| BioMistral-7B-SLERP | [Mistral-7B-Instruct-v0.1](https://huggingface.co/mistralai/Mistral-7B-Instruct-v0.1) | Merge SLERP | 2048 | [HuggingFace](https://huggingface.co/BioMistral/BioMistral-7B-SLERP) |
# 2. Quantized Models
| Base Model | Method | q_group_size | w_bit | version | VRAM GB | Time | Download |
|:-------------------:|:------:|:------------:|:-----:|:-------:|:-------:|:------:|:--------:|
| BioMistral-7B | FP16/BF16 | | | | 15.02 | x1.00 | [HuggingFace](https://huggingface.co/BioMistral/BioMistral-7B) |
| BioMistral-7B | AWQ | 128 | 4 | GEMM | 4.68 | x1.41 | [HuggingFace](https://huggingface.co/BioMistral/BioMistral-7B-AWQ-QGS128-W4-GEMM) |
| BioMistral-7B | AWQ | 128 | 4 | GEMV | 4.68 | x10.30 | [HuggingFace](https://huggingface.co/BioMistral/BioMistral-7B-AWQ-QGS128-W4-GEMV) |
| BioMistral-7B | BnB.4 | | 4 | | 5.03 | x3.25 | [HuggingFace](blank) |
| BioMistral-7B | BnB.8 | | 8 | | 8.04 | x4.34 | [HuggingFace](blank) |
| BioMistral-7B-DARE | AWQ | 128 | 4 | GEMM | 4.68 | x1.41 | [HuggingFace](https://huggingface.co/BioMistral/BioMistral-7B-DARE-AWQ-QGS128-W4-GEMM) |
| BioMistral-7B-TIES | AWQ | 128 | 4 | GEMM | 4.68 | x1.41 | [HuggingFace](https://huggingface.co/BioMistral/BioMistral-7B-TIES-AWQ-QGS128-W4-GEMM) |
| BioMistral-7B-SLERP | AWQ | 128 | 4 | GEMM | 4.68 | x1.41 | [HuggingFace](https://huggingface.co/BioMistral/BioMistral-7B-SLERP-AWQ-QGS128-W4-GEMM) |
# 2. Using BioMistral
You can use BioMistral with [Hugging Face's Transformers library](https://github.com/huggingface/transformers) as follow.
Loading the model and tokenizer :
```python
from transformers import AutoModel, AutoTokenizer
tokenizer = AutoTokenizer.from_pretrained("BioMistral/BioMistral-7B")
model = AutoModel.from_pretrained("BioMistral/BioMistral-7B")
```
# 3. Supervised Fine-tuning Benchmark
| | Clinical KG | Medical Genetics | Anatomy | Pro Medicine | College Biology | College Medicine | MedQA | MedQA 5 opts | PubMedQA | MedMCQA | Avg. |
|-------------------------------------------|:---------------------------------------------:|-----------------------------------------------|-----------------------------------------------|-----------------------------------------------|-----------------------------------------------|-----------------------------------------------|-----------------------------------------------|-----------------------------------------------|-----------------------------------------------|-----------------------------------------------|------------------|
| **BioMistral 7B** | 59.9 | 64.0 | 56.5 | 60.4 | 59.0 | 54.7 | 50.6 | 42.8 | 77.5 | 48.1 | 57.3 |
| **Mistral 7B Instruct** | **62.9** | 57.0 | 55.6 | 59.4 | 62.5 | <u>57.2</u> | 42.0 | 40.9 | 75.7 | 46.1 | 55.9 |
| | | | | | | | | | | | |
| **BioMistral 7B Ensemble** | <u>62.8</u> | 62.7 | <u>57.5</u> | **63.5** | 64.3 | 55.7 | 50.6 | 43.6 | 77.5 | **48.8** | 58.7 |
| **BioMistral 7B DARE** | 62.3 | **67.0** | 55.8 | 61.4 | **66.9** | **58.0** | **51.1** | **45.2** | <u>77.7</u> | <u>48.7</u> | **59.4** |
| **BioMistral 7B TIES** | 60.1 | <u>65.0</u> | **58.5** | 60.5 | 60.4 | 56.5 | 49.5 | 43.2 | 77.5 | 48.1 | 57.9 |
| **BioMistral 7B SLERP** | 62.5 | 64.7 | 55.8 | <u>62.7</u> | <u>64.8</u> | 56.3 | <u>50.8</u> | <u>44.3</u> | **77.8** | 48.6 | <u>58.8</u> |
| | | | | | | | | | | | |
| **MedAlpaca 7B** | 53.1 | 58.0 | 54.1 | 58.8 | 58.1 | 48.6 | 40.1 | 33.7 | 73.6 | 37.0 | 51.5 |
| **PMC-LLaMA 7B** | 24.5 | 27.7 | 35.3 | 17.4 | 30.3 | 23.3 | 25.5 | 20.2 | 72.9 | 26.6 | 30.4 |
| **MediTron-7B** | 41.6 | 50.3 | 46.4 | 27.9 | 44.4 | 30.8 | 41.6 | 28.1 | 74.9 | 41.3 | 42.7 |
| **BioMedGPT-LM-7B** | 51.4 | 52.0 | 49.4 | 53.3 | 50.7 | 49.1 | 42.5 | 33.9 | 76.8 | 37.6 | 49.7 |
| | | | | | | | | | | | |
| **GPT-3.5 Turbo 1106*** | 74.71 | 74.00 | 65.92 | 72.79 | 72.91 | 64.73 | 57.71 | 50.82 | 72.66 | 53.79 | 66.0 |
Supervised Fine-Tuning (SFT) performance of BioMistral 7B models compared to baselines, measured by accuracy (↑) and averaged across 3 random seeds of 3-shot. DARE, TIES, and SLERP are model merging strategies that combine BioMistral 7B and Mistral 7B Instruct. Best model in bold, and second-best underlined. *GPT-3.5 Turbo performances are reported from the 3-shot results without SFT.
# Citation BibTeX
Arxiv : [https://arxiv.org/abs/2402.10373](https://arxiv.org/abs/2402.10373)
```bibtex
@misc{labrak2024biomistral,
title={BioMistral: A Collection of Open-Source Pretrained Large Language Models for Medical Domains},
author={Yanis Labrak and Adrien Bazoge and Emmanuel Morin and Pierre-Antoine Gourraud and Mickael Rouvier and Richard Dufour},
year={2024},
eprint={2402.10373},
archivePrefix={arXiv},
primaryClass={cs.CL}
}
```
**CAUTION!** Both direct and downstream users need to be informed about the risks, biases, and constraints inherent in the model. While the model can produce natural language text, our exploration of its capabilities and limitations is just beginning. In fields such as medicine, comprehending these limitations is crucial. Hence, we strongly advise against deploying this model for natural language generation in production or for professional tasks in the realm of health and medicine. |
mradermacher/Llama-3-Smaug-8B-GGUF | mradermacher | "2024-06-27T13:44:57Z" | 12,875 | 0 | transformers | [
"transformers",
"gguf",
"en",
"dataset:aqua_rat",
"dataset:microsoft/orca-math-word-problems-200k",
"dataset:m-a-p/CodeFeedback-Filtered-Instruction",
"dataset:anon8231489123/ShareGPT_Vicuna_unfiltered",
"base_model:abacusai/Llama-3-Smaug-8B",
"license:llama2",
"endpoints_compatible",
"region:us"
] | null | "2024-06-27T11:12:43Z" | ---
base_model: abacusai/Llama-3-Smaug-8B
datasets:
- aqua_rat
- microsoft/orca-math-word-problems-200k
- m-a-p/CodeFeedback-Filtered-Instruction
- anon8231489123/ShareGPT_Vicuna_unfiltered
language:
- en
library_name: transformers
license: llama2
quantized_by: mradermacher
---
## About
<!-- ### quantize_version: 2 -->
<!-- ### output_tensor_quantised: 1 -->
<!-- ### convert_type: hf -->
<!-- ### vocab_type: -->
<!-- ### tags: -->
static quants of https://huggingface.co/abacusai/Llama-3-Smaug-8B
<!-- provided-files -->
weighted/imatrix quants are available at https://huggingface.co/mradermacher/Llama-3-Smaug-8B-i1-GGUF
## Usage
If you are unsure how to use GGUF files, refer to one of [TheBloke's
READMEs](https://huggingface.co/TheBloke/KafkaLM-70B-German-V0.1-GGUF) for
more details, including on how to concatenate multi-part files.
## Provided Quants
(sorted by size, not necessarily quality. IQ-quants are often preferable over similar sized non-IQ quants)
| Link | Type | Size/GB | Notes |
|:-----|:-----|--------:|:------|
| [GGUF](https://huggingface.co/mradermacher/Llama-3-Smaug-8B-GGUF/resolve/main/Llama-3-Smaug-8B.Q2_K.gguf) | Q2_K | 3.3 | |
| [GGUF](https://huggingface.co/mradermacher/Llama-3-Smaug-8B-GGUF/resolve/main/Llama-3-Smaug-8B.IQ3_XS.gguf) | IQ3_XS | 3.6 | |
| [GGUF](https://huggingface.co/mradermacher/Llama-3-Smaug-8B-GGUF/resolve/main/Llama-3-Smaug-8B.Q3_K_S.gguf) | Q3_K_S | 3.8 | |
| [GGUF](https://huggingface.co/mradermacher/Llama-3-Smaug-8B-GGUF/resolve/main/Llama-3-Smaug-8B.IQ3_S.gguf) | IQ3_S | 3.8 | beats Q3_K* |
| [GGUF](https://huggingface.co/mradermacher/Llama-3-Smaug-8B-GGUF/resolve/main/Llama-3-Smaug-8B.IQ3_M.gguf) | IQ3_M | 3.9 | |
| [GGUF](https://huggingface.co/mradermacher/Llama-3-Smaug-8B-GGUF/resolve/main/Llama-3-Smaug-8B.Q3_K_M.gguf) | Q3_K_M | 4.1 | lower quality |
| [GGUF](https://huggingface.co/mradermacher/Llama-3-Smaug-8B-GGUF/resolve/main/Llama-3-Smaug-8B.Q3_K_L.gguf) | Q3_K_L | 4.4 | |
| [GGUF](https://huggingface.co/mradermacher/Llama-3-Smaug-8B-GGUF/resolve/main/Llama-3-Smaug-8B.IQ4_XS.gguf) | IQ4_XS | 4.6 | |
| [GGUF](https://huggingface.co/mradermacher/Llama-3-Smaug-8B-GGUF/resolve/main/Llama-3-Smaug-8B.Q4_K_S.gguf) | Q4_K_S | 4.8 | fast, recommended |
| [GGUF](https://huggingface.co/mradermacher/Llama-3-Smaug-8B-GGUF/resolve/main/Llama-3-Smaug-8B.Q4_K_M.gguf) | Q4_K_M | 5.0 | fast, recommended |
| [GGUF](https://huggingface.co/mradermacher/Llama-3-Smaug-8B-GGUF/resolve/main/Llama-3-Smaug-8B.Q5_K_S.gguf) | Q5_K_S | 5.7 | |
| [GGUF](https://huggingface.co/mradermacher/Llama-3-Smaug-8B-GGUF/resolve/main/Llama-3-Smaug-8B.Q5_K_M.gguf) | Q5_K_M | 5.8 | |
| [GGUF](https://huggingface.co/mradermacher/Llama-3-Smaug-8B-GGUF/resolve/main/Llama-3-Smaug-8B.Q6_K.gguf) | Q6_K | 6.7 | very good quality |
| [GGUF](https://huggingface.co/mradermacher/Llama-3-Smaug-8B-GGUF/resolve/main/Llama-3-Smaug-8B.Q8_0.gguf) | Q8_0 | 8.6 | fast, best quality |
| [GGUF](https://huggingface.co/mradermacher/Llama-3-Smaug-8B-GGUF/resolve/main/Llama-3-Smaug-8B.f16.gguf) | f16 | 16.2 | 16 bpw, overkill |
Here is a handy graph by ikawrakow comparing some lower-quality quant
types (lower is better):

And here are Artefact2's thoughts on the matter:
https://gist.github.com/Artefact2/b5f810600771265fc1e39442288e8ec9
## FAQ / Model Request
See https://huggingface.co/mradermacher/model_requests for some answers to
questions you might have and/or if you want some other model quantized.
## Thanks
I thank my company, [nethype GmbH](https://www.nethype.de/), for letting
me use its servers and providing upgrades to my workstation to enable
this work in my free time.
<!-- end -->
|
hongdthaui/ManmaruMix_v30 | hongdthaui | "2024-01-18T16:12:12Z" | 12,868 | 1 | diffusers | [
"diffusers",
"autotrain_compatible",
"endpoints_compatible",
"diffusers:StableDiffusionPipeline",
"region:us"
] | text-to-image | "2024-01-18T16:04:34Z" | Entry not found |
mradermacher/pankaj-llama-3-8B-GGUF | mradermacher | "2024-06-28T15:56:30Z" | 12,851 | 0 | transformers | [
"transformers",
"gguf",
"en",
"base_model:pankajshakya627/pankaj-llama-3-8B",
"endpoints_compatible",
"region:us"
] | null | "2024-06-28T13:51:23Z" | ---
base_model: pankajshakya627/pankaj-llama-3-8B
language:
- en
library_name: transformers
quantized_by: mradermacher
---
## About
<!-- ### quantize_version: 2 -->
<!-- ### output_tensor_quantised: 1 -->
<!-- ### convert_type: hf -->
<!-- ### vocab_type: -->
<!-- ### tags: -->
static quants of https://huggingface.co/pankajshakya627/pankaj-llama-3-8B
<!-- provided-files -->
weighted/imatrix quants seem not to be available (by me) at this time. If they do not show up a week or so after the static ones, I have probably not planned for them. Feel free to request them by opening a Community Discussion.
## Usage
If you are unsure how to use GGUF files, refer to one of [TheBloke's
READMEs](https://huggingface.co/TheBloke/KafkaLM-70B-German-V0.1-GGUF) for
more details, including on how to concatenate multi-part files.
## Provided Quants
(sorted by size, not necessarily quality. IQ-quants are often preferable over similar sized non-IQ quants)
| Link | Type | Size/GB | Notes |
|:-----|:-----|--------:|:------|
| [GGUF](https://huggingface.co/mradermacher/pankaj-llama-3-8B-GGUF/resolve/main/pankaj-llama-3-8B.Q2_K.gguf) | Q2_K | 3.3 | |
| [GGUF](https://huggingface.co/mradermacher/pankaj-llama-3-8B-GGUF/resolve/main/pankaj-llama-3-8B.IQ3_XS.gguf) | IQ3_XS | 3.6 | |
| [GGUF](https://huggingface.co/mradermacher/pankaj-llama-3-8B-GGUF/resolve/main/pankaj-llama-3-8B.Q3_K_S.gguf) | Q3_K_S | 3.8 | |
| [GGUF](https://huggingface.co/mradermacher/pankaj-llama-3-8B-GGUF/resolve/main/pankaj-llama-3-8B.IQ3_S.gguf) | IQ3_S | 3.8 | beats Q3_K* |
| [GGUF](https://huggingface.co/mradermacher/pankaj-llama-3-8B-GGUF/resolve/main/pankaj-llama-3-8B.IQ3_M.gguf) | IQ3_M | 3.9 | |
| [GGUF](https://huggingface.co/mradermacher/pankaj-llama-3-8B-GGUF/resolve/main/pankaj-llama-3-8B.Q3_K_M.gguf) | Q3_K_M | 4.1 | lower quality |
| [GGUF](https://huggingface.co/mradermacher/pankaj-llama-3-8B-GGUF/resolve/main/pankaj-llama-3-8B.Q3_K_L.gguf) | Q3_K_L | 4.4 | |
| [GGUF](https://huggingface.co/mradermacher/pankaj-llama-3-8B-GGUF/resolve/main/pankaj-llama-3-8B.IQ4_XS.gguf) | IQ4_XS | 4.6 | |
| [GGUF](https://huggingface.co/mradermacher/pankaj-llama-3-8B-GGUF/resolve/main/pankaj-llama-3-8B.Q4_K_S.gguf) | Q4_K_S | 4.8 | fast, recommended |
| [GGUF](https://huggingface.co/mradermacher/pankaj-llama-3-8B-GGUF/resolve/main/pankaj-llama-3-8B.Q4_K_M.gguf) | Q4_K_M | 5.0 | fast, recommended |
| [GGUF](https://huggingface.co/mradermacher/pankaj-llama-3-8B-GGUF/resolve/main/pankaj-llama-3-8B.Q5_K_S.gguf) | Q5_K_S | 5.7 | |
| [GGUF](https://huggingface.co/mradermacher/pankaj-llama-3-8B-GGUF/resolve/main/pankaj-llama-3-8B.Q5_K_M.gguf) | Q5_K_M | 5.8 | |
| [GGUF](https://huggingface.co/mradermacher/pankaj-llama-3-8B-GGUF/resolve/main/pankaj-llama-3-8B.Q6_K.gguf) | Q6_K | 6.7 | very good quality |
| [GGUF](https://huggingface.co/mradermacher/pankaj-llama-3-8B-GGUF/resolve/main/pankaj-llama-3-8B.Q8_0.gguf) | Q8_0 | 8.6 | fast, best quality |
| [GGUF](https://huggingface.co/mradermacher/pankaj-llama-3-8B-GGUF/resolve/main/pankaj-llama-3-8B.f16.gguf) | f16 | 16.2 | 16 bpw, overkill |
Here is a handy graph by ikawrakow comparing some lower-quality quant
types (lower is better):

And here are Artefact2's thoughts on the matter:
https://gist.github.com/Artefact2/b5f810600771265fc1e39442288e8ec9
## FAQ / Model Request
See https://huggingface.co/mradermacher/model_requests for some answers to
questions you might have and/or if you want some other model quantized.
## Thanks
I thank my company, [nethype GmbH](https://www.nethype.de/), for letting
me use its servers and providing upgrades to my workstation to enable
this work in my free time.
<!-- end -->
|
microsoft/swin-base-patch4-window7-224 | microsoft | "2023-09-10T18:04:03Z" | 12,844 | 6 | transformers | [
"transformers",
"pytorch",
"tf",
"safetensors",
"swin",
"image-classification",
"vision",
"dataset:imagenet-1k",
"arxiv:2103.14030",
"license:apache-2.0",
"autotrain_compatible",
"endpoints_compatible",
"region:us"
] | image-classification | "2022-03-02T23:29:05Z" | ---
license: apache-2.0
tags:
- vision
- image-classification
datasets:
- imagenet-1k
widget:
- src: https://huggingface.co/datasets/mishig/sample_images/resolve/main/tiger.jpg
example_title: Tiger
- src: https://huggingface.co/datasets/mishig/sample_images/resolve/main/teapot.jpg
example_title: Teapot
- src: https://huggingface.co/datasets/mishig/sample_images/resolve/main/palace.jpg
example_title: Palace
---
# Swin Transformer (base-sized model)
Swin Transformer model trained on ImageNet-1k at resolution 224x224. It was introduced in the paper [Swin Transformer: Hierarchical Vision Transformer using Shifted Windows](https://arxiv.org/abs/2103.14030) by Liu et al. and first released in [this repository](https://github.com/microsoft/Swin-Transformer).
Disclaimer: The team releasing Swin Transformer did not write a model card for this model so this model card has been written by the Hugging Face team.
## Model description
The Swin Transformer is a type of Vision Transformer. It builds hierarchical feature maps by merging image patches (shown in gray) in deeper layers and has linear computation complexity to input image size due to computation of self-attention only within each local window (shown in red). It can thus serve as a general-purpose backbone for both image classification and dense recognition tasks. In contrast, previous vision Transformers produce feature maps of a single low resolution and have quadratic computation complexity to input image size due to computation of self-attention globally.
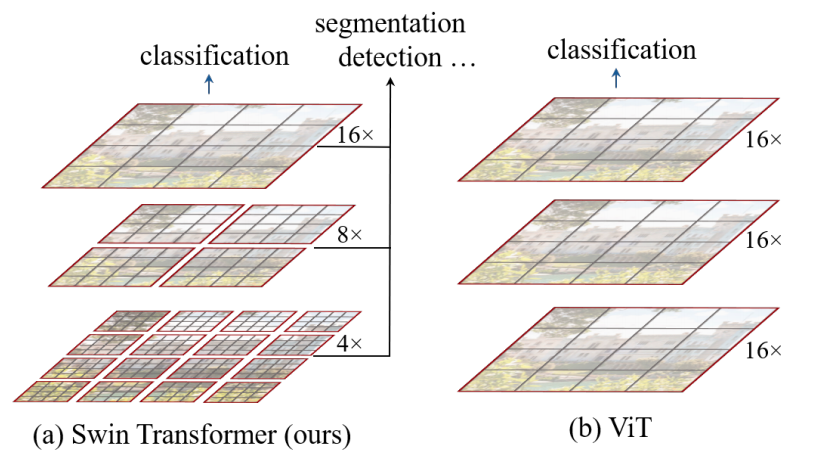
[Source](https://paperswithcode.com/method/swin-transformer)
## Intended uses & limitations
You can use the raw model for image classification. See the [model hub](https://huggingface.co/models?search=swin) to look for
fine-tuned versions on a task that interests you.
### How to use
Here is how to use this model to classify an image of the COCO 2017 dataset into one of the 1,000 ImageNet classes:
```python
from transformers import AutoFeatureExtractor, SwinForImageClassification
from PIL import Image
import requests
url = "http://images.cocodataset.org/val2017/000000039769.jpg"
image = Image.open(requests.get(url, stream=True).raw)
feature_extractor = AutoFeatureExtractor.from_pretrained("microsoft/swin-base-patch4-window7-224")
model = SwinForImageClassification.from_pretrained("microsoft/swin-base-patch4-window7-224")
inputs = feature_extractor(images=image, return_tensors="pt")
outputs = model(**inputs)
logits = outputs.logits
# model predicts one of the 1000 ImageNet classes
predicted_class_idx = logits.argmax(-1).item()
print("Predicted class:", model.config.id2label[predicted_class_idx])
```
For more code examples, we refer to the [documentation](https://huggingface.co/transformers/model_doc/swin.html#).
### BibTeX entry and citation info
```bibtex
@article{DBLP:journals/corr/abs-2103-14030,
author = {Ze Liu and
Yutong Lin and
Yue Cao and
Han Hu and
Yixuan Wei and
Zheng Zhang and
Stephen Lin and
Baining Guo},
title = {Swin Transformer: Hierarchical Vision Transformer using Shifted Windows},
journal = {CoRR},
volume = {abs/2103.14030},
year = {2021},
url = {https://arxiv.org/abs/2103.14030},
eprinttype = {arXiv},
eprint = {2103.14030},
timestamp = {Thu, 08 Apr 2021 07:53:26 +0200},
biburl = {https://dblp.org/rec/journals/corr/abs-2103-14030.bib},
bibsource = {dblp computer science bibliography, https://dblp.org}
}
``` |
mradermacher/SEVENLLM-Qwen1.5-7B-GGUF | mradermacher | "2024-06-26T20:31:23Z" | 12,835 | 0 | transformers | [
"transformers",
"gguf",
"en",
"base_model:Multilingual-Multimodal-NLP/SEVENLLM-Qwen1.5-7B",
"license:mit",
"endpoints_compatible",
"region:us"
] | null | "2024-06-21T14:45:51Z" | ---
base_model: Multilingual-Multimodal-NLP/SEVENLLM-Qwen1.5-7B
language:
- en
library_name: transformers
license: mit
quantized_by: mradermacher
---
## About
<!-- ### quantize_version: 2 -->
<!-- ### output_tensor_quantised: 1 -->
<!-- ### convert_type: hf -->
<!-- ### vocab_type: -->
<!-- ### tags: -->
static quants of https://huggingface.co/Multilingual-Multimodal-NLP/SEVENLLM-Qwen1.5-7B
<!-- provided-files -->
weighted/imatrix quants seem not to be available (by me) at this time. If they do not show up a week or so after the static ones, I have probably not planned for them. Feel free to request them by opening a Community Discussion.
## Usage
If you are unsure how to use GGUF files, refer to one of [TheBloke's
READMEs](https://huggingface.co/TheBloke/KafkaLM-70B-German-V0.1-GGUF) for
more details, including on how to concatenate multi-part files.
## Provided Quants
(sorted by size, not necessarily quality. IQ-quants are often preferable over similar sized non-IQ quants)
| Link | Type | Size/GB | Notes |
|:-----|:-----|--------:|:------|
| [GGUF](https://huggingface.co/mradermacher/SEVENLLM-Qwen1.5-7B-GGUF/resolve/main/SEVENLLM-Qwen1.5-7B.Q2_K.gguf) | Q2_K | 3.2 | |
| [GGUF](https://huggingface.co/mradermacher/SEVENLLM-Qwen1.5-7B-GGUF/resolve/main/SEVENLLM-Qwen1.5-7B.IQ3_XS.gguf) | IQ3_XS | 3.5 | |
| [GGUF](https://huggingface.co/mradermacher/SEVENLLM-Qwen1.5-7B-GGUF/resolve/main/SEVENLLM-Qwen1.5-7B.IQ3_S.gguf) | IQ3_S | 3.7 | beats Q3_K* |
| [GGUF](https://huggingface.co/mradermacher/SEVENLLM-Qwen1.5-7B-GGUF/resolve/main/SEVENLLM-Qwen1.5-7B.Q3_K_S.gguf) | Q3_K_S | 3.7 | |
| [GGUF](https://huggingface.co/mradermacher/SEVENLLM-Qwen1.5-7B-GGUF/resolve/main/SEVENLLM-Qwen1.5-7B.IQ3_M.gguf) | IQ3_M | 3.8 | |
| [GGUF](https://huggingface.co/mradermacher/SEVENLLM-Qwen1.5-7B-GGUF/resolve/main/SEVENLLM-Qwen1.5-7B.Q3_K_M.gguf) | Q3_K_M | 4.0 | lower quality |
| [GGUF](https://huggingface.co/mradermacher/SEVENLLM-Qwen1.5-7B-GGUF/resolve/main/SEVENLLM-Qwen1.5-7B.Q3_K_L.gguf) | Q3_K_L | 4.3 | |
| [GGUF](https://huggingface.co/mradermacher/SEVENLLM-Qwen1.5-7B-GGUF/resolve/main/SEVENLLM-Qwen1.5-7B.IQ4_XS.gguf) | IQ4_XS | 4.4 | |
| [GGUF](https://huggingface.co/mradermacher/SEVENLLM-Qwen1.5-7B-GGUF/resolve/main/SEVENLLM-Qwen1.5-7B.Q4_K_S.gguf) | Q4_K_S | 4.6 | fast, recommended |
| [GGUF](https://huggingface.co/mradermacher/SEVENLLM-Qwen1.5-7B-GGUF/resolve/main/SEVENLLM-Qwen1.5-7B.Q4_K_M.gguf) | Q4_K_M | 4.9 | fast, recommended |
| [GGUF](https://huggingface.co/mradermacher/SEVENLLM-Qwen1.5-7B-GGUF/resolve/main/SEVENLLM-Qwen1.5-7B.Q5_K_S.gguf) | Q5_K_S | 5.5 | |
| [GGUF](https://huggingface.co/mradermacher/SEVENLLM-Qwen1.5-7B-GGUF/resolve/main/SEVENLLM-Qwen1.5-7B.Q5_K_M.gguf) | Q5_K_M | 5.6 | |
| [GGUF](https://huggingface.co/mradermacher/SEVENLLM-Qwen1.5-7B-GGUF/resolve/main/SEVENLLM-Qwen1.5-7B.Q6_K.gguf) | Q6_K | 6.4 | very good quality |
| [GGUF](https://huggingface.co/mradermacher/SEVENLLM-Qwen1.5-7B-GGUF/resolve/main/SEVENLLM-Qwen1.5-7B.Q8_0.gguf) | Q8_0 | 8.3 | fast, best quality |
| [GGUF](https://huggingface.co/mradermacher/SEVENLLM-Qwen1.5-7B-GGUF/resolve/main/SEVENLLM-Qwen1.5-7B.f16.gguf) | f16 | 15.5 | 16 bpw, overkill |
Here is a handy graph by ikawrakow comparing some lower-quality quant
types (lower is better):

And here are Artefact2's thoughts on the matter:
https://gist.github.com/Artefact2/b5f810600771265fc1e39442288e8ec9
## FAQ / Model Request
See https://huggingface.co/mradermacher/model_requests for some answers to
questions you might have and/or if you want some other model quantized.
## Thanks
I thank my company, [nethype GmbH](https://www.nethype.de/), for letting
me use its servers and providing upgrades to my workstation to enable
this work in my free time.
<!-- end -->
|
tomasonjo/text2cypher-qwen2-72b-4bit-gguf | tomasonjo | "2024-07-02T05:10:06Z" | 12,834 | 2 | transformers | [
"transformers",
"gguf",
"text-generation-inference",
"unsloth",
"qwen2",
"en",
"dataset:tomasonjo/text2cypher-gpt4o-clean",
"base_model:Qwen/Qwen2-72B-Instruct",
"license:apache-2.0",
"endpoints_compatible",
"region:us"
] | null | "2024-07-01T17:42:48Z" | ---
base_model: Qwen/Qwen2-72B-Instruct
language:
- en
license: apache-2.0
tags:
- text-generation-inference
- transformers
- unsloth
- qwen2
- gguf
datasets:
- tomasonjo/text2cypher-gpt4o-clean
---
# Uploaded model
- **Developed by:** tomasonjo
- **License:** apache-2.0
- **Finetuned from model :** Qwen/Qwen2-72B-Instruct
This qwen2 model was trained 2x faster with [Unsloth](https://github.com/unslothai/unsloth) and Huggingface's TRL library.
[<img src="https://raw.githubusercontent.com/unslothai/unsloth/main/images/unsloth%20made%20with%20love.png" width="200"/>](https://github.com/unslothai/unsloth) |
batterydata/batterybert-cased-squad-v1 | batterydata | "2022-03-05T13:50:54Z" | 12,828 | 0 | transformers | [
"transformers",
"pytorch",
"bert",
"question-answering",
"question answering",
"en",
"dataset:squad",
"dataset:batterydata/battery-device-data-qa",
"license:apache-2.0",
"endpoints_compatible",
"region:us"
] | question-answering | "2022-03-02T23:29:05Z" | ---
language: en
tags: question answering
license: apache-2.0
datasets:
- squad
- batterydata/battery-device-data-qa
metrics: squad
---
# BatteryBERT-cased for QA
**Language model:** batterybert-cased
**Language:** English
**Downstream-task:** Extractive QA
**Training data:** SQuAD v1
**Eval data:** SQuAD v1
**Code:** See [example](https://github.com/ShuHuang/batterybert)
**Infrastructure**: 8x DGX A100
## Hyperparameters
```
batch_size = 16
n_epochs = 4
base_LM_model = "batterybert-cased"
max_seq_len = 386
learning_rate = 2e-5
doc_stride=128
max_query_length=64
```
## Performance
Evaluated on the SQuAD v1.0 dev set.
```
"exact": 81.54,
"f1": 89.16,
```
Evaluated on the battery device dataset.
```
"precision": 70.74,
"recall": 84.19,
```
## Usage
### In Transformers
```python
from transformers import AutoModelForQuestionAnswering, AutoTokenizer, pipeline
model_name = "batterydata/batterybert-cased-squad-v1"
# a) Get predictions
nlp = pipeline('question-answering', model=model_name, tokenizer=model_name)
QA_input = {
'question': 'What is the electrolyte?',
'context': 'The typical non-aqueous electrolyte for commercial Li-ion cells is a solution of LiPF6 in linear and cyclic carbonates.'
}
res = nlp(QA_input)
# b) Load model & tokenizer
model = AutoModelForQuestionAnswering.from_pretrained(model_name)
tokenizer = AutoTokenizer.from_pretrained(model_name)
```
## Authors
Shu Huang: `sh2009 [at] cam.ac.uk`
Jacqueline Cole: `jmc61 [at] cam.ac.uk`
## Citation
BatteryBERT: A Pre-trained Language Model for Battery Database Enhancement
|
mradermacher/LLaMa-3-CursedStock-v2.0-8B-GGUF | mradermacher | "2024-06-26T18:41:50Z" | 12,827 | 0 | transformers | [
"transformers",
"gguf",
"not-for-all-audiences",
"merge",
"mergekit",
"en",
"base_model:PJMixers/LLaMa-3-CursedStock-v2.0-8B",
"license:llama3",
"endpoints_compatible",
"region:us"
] | null | "2024-06-26T17:37:59Z" | ---
base_model: PJMixers/LLaMa-3-CursedStock-v2.0-8B
language:
- en
library_name: transformers
license: llama3
quantized_by: mradermacher
tags:
- not-for-all-audiences
- merge
- mergekit
---
## About
<!-- ### quantize_version: 2 -->
<!-- ### output_tensor_quantised: 1 -->
<!-- ### convert_type: hf -->
<!-- ### vocab_type: -->
<!-- ### tags: -->
static quants of https://huggingface.co/PJMixers/LLaMa-3-CursedStock-v2.0-8B
<!-- provided-files -->
weighted/imatrix quants are available at https://huggingface.co/mradermacher/LLaMa-3-CursedStock-v2.0-8B-i1-GGUF
## Usage
If you are unsure how to use GGUF files, refer to one of [TheBloke's
READMEs](https://huggingface.co/TheBloke/KafkaLM-70B-German-V0.1-GGUF) for
more details, including on how to concatenate multi-part files.
## Provided Quants
(sorted by size, not necessarily quality. IQ-quants are often preferable over similar sized non-IQ quants)
| Link | Type | Size/GB | Notes |
|:-----|:-----|--------:|:------|
| [GGUF](https://huggingface.co/mradermacher/LLaMa-3-CursedStock-v2.0-8B-GGUF/resolve/main/LLaMa-3-CursedStock-v2.0-8B.Q2_K.gguf) | Q2_K | 3.3 | |
| [GGUF](https://huggingface.co/mradermacher/LLaMa-3-CursedStock-v2.0-8B-GGUF/resolve/main/LLaMa-3-CursedStock-v2.0-8B.IQ3_XS.gguf) | IQ3_XS | 3.6 | |
| [GGUF](https://huggingface.co/mradermacher/LLaMa-3-CursedStock-v2.0-8B-GGUF/resolve/main/LLaMa-3-CursedStock-v2.0-8B.Q3_K_S.gguf) | Q3_K_S | 3.8 | |
| [GGUF](https://huggingface.co/mradermacher/LLaMa-3-CursedStock-v2.0-8B-GGUF/resolve/main/LLaMa-3-CursedStock-v2.0-8B.IQ3_S.gguf) | IQ3_S | 3.8 | beats Q3_K* |
| [GGUF](https://huggingface.co/mradermacher/LLaMa-3-CursedStock-v2.0-8B-GGUF/resolve/main/LLaMa-3-CursedStock-v2.0-8B.IQ3_M.gguf) | IQ3_M | 3.9 | |
| [GGUF](https://huggingface.co/mradermacher/LLaMa-3-CursedStock-v2.0-8B-GGUF/resolve/main/LLaMa-3-CursedStock-v2.0-8B.Q3_K_M.gguf) | Q3_K_M | 4.1 | lower quality |
| [GGUF](https://huggingface.co/mradermacher/LLaMa-3-CursedStock-v2.0-8B-GGUF/resolve/main/LLaMa-3-CursedStock-v2.0-8B.Q3_K_L.gguf) | Q3_K_L | 4.4 | |
| [GGUF](https://huggingface.co/mradermacher/LLaMa-3-CursedStock-v2.0-8B-GGUF/resolve/main/LLaMa-3-CursedStock-v2.0-8B.IQ4_XS.gguf) | IQ4_XS | 4.6 | |
| [GGUF](https://huggingface.co/mradermacher/LLaMa-3-CursedStock-v2.0-8B-GGUF/resolve/main/LLaMa-3-CursedStock-v2.0-8B.Q4_K_S.gguf) | Q4_K_S | 4.8 | fast, recommended |
| [GGUF](https://huggingface.co/mradermacher/LLaMa-3-CursedStock-v2.0-8B-GGUF/resolve/main/LLaMa-3-CursedStock-v2.0-8B.Q4_K_M.gguf) | Q4_K_M | 5.0 | fast, recommended |
| [GGUF](https://huggingface.co/mradermacher/LLaMa-3-CursedStock-v2.0-8B-GGUF/resolve/main/LLaMa-3-CursedStock-v2.0-8B.Q5_K_S.gguf) | Q5_K_S | 5.7 | |
| [GGUF](https://huggingface.co/mradermacher/LLaMa-3-CursedStock-v2.0-8B-GGUF/resolve/main/LLaMa-3-CursedStock-v2.0-8B.Q5_K_M.gguf) | Q5_K_M | 5.8 | |
| [GGUF](https://huggingface.co/mradermacher/LLaMa-3-CursedStock-v2.0-8B-GGUF/resolve/main/LLaMa-3-CursedStock-v2.0-8B.Q6_K.gguf) | Q6_K | 6.7 | very good quality |
| [GGUF](https://huggingface.co/mradermacher/LLaMa-3-CursedStock-v2.0-8B-GGUF/resolve/main/LLaMa-3-CursedStock-v2.0-8B.Q8_0.gguf) | Q8_0 | 8.6 | fast, best quality |
| [GGUF](https://huggingface.co/mradermacher/LLaMa-3-CursedStock-v2.0-8B-GGUF/resolve/main/LLaMa-3-CursedStock-v2.0-8B.f16.gguf) | f16 | 16.2 | 16 bpw, overkill |
Here is a handy graph by ikawrakow comparing some lower-quality quant
types (lower is better):

And here are Artefact2's thoughts on the matter:
https://gist.github.com/Artefact2/b5f810600771265fc1e39442288e8ec9
## FAQ / Model Request
See https://huggingface.co/mradermacher/model_requests for some answers to
questions you might have and/or if you want some other model quantized.
## Thanks
I thank my company, [nethype GmbH](https://www.nethype.de/), for letting
me use its servers and providing upgrades to my workstation to enable
this work in my free time.
<!-- end -->
|
mradermacher/Llama-3-LewdPlay-8B-evo-i1-GGUF | mradermacher | "2024-06-27T21:39:45Z" | 12,825 | 0 | transformers | [
"transformers",
"gguf",
"mergekit",
"merge",
"en",
"base_model:Undi95/Llama-3-LewdPlay-8B-evo",
"license:cc-by-nc-4.0",
"endpoints_compatible",
"region:us"
] | null | "2024-06-27T16:46:00Z" | ---
base_model: Undi95/Llama-3-LewdPlay-8B-evo
language:
- en
library_name: transformers
license: cc-by-nc-4.0
quantized_by: mradermacher
tags:
- mergekit
- merge
---
## About
<!-- ### quantize_version: 2 -->
<!-- ### output_tensor_quantised: 1 -->
<!-- ### convert_type: hf -->
<!-- ### vocab_type: -->
<!-- ### tags: nicoboss -->
weighted/imatrix quants of https://huggingface.co/Undi95/Llama-3-LewdPlay-8B-evo
<!-- provided-files -->
static quants are available at https://huggingface.co/mradermacher/Llama-3-LewdPlay-8B-evo-GGUF
## Usage
If you are unsure how to use GGUF files, refer to one of [TheBloke's
READMEs](https://huggingface.co/TheBloke/KafkaLM-70B-German-V0.1-GGUF) for
more details, including on how to concatenate multi-part files.
## Provided Quants
(sorted by size, not necessarily quality. IQ-quants are often preferable over similar sized non-IQ quants)
| Link | Type | Size/GB | Notes |
|:-----|:-----|--------:|:------|
| [GGUF](https://huggingface.co/mradermacher/Llama-3-LewdPlay-8B-evo-i1-GGUF/resolve/main/Llama-3-LewdPlay-8B-evo.i1-IQ1_S.gguf) | i1-IQ1_S | 2.1 | for the desperate |
| [GGUF](https://huggingface.co/mradermacher/Llama-3-LewdPlay-8B-evo-i1-GGUF/resolve/main/Llama-3-LewdPlay-8B-evo.i1-IQ1_M.gguf) | i1-IQ1_M | 2.3 | mostly desperate |
| [GGUF](https://huggingface.co/mradermacher/Llama-3-LewdPlay-8B-evo-i1-GGUF/resolve/main/Llama-3-LewdPlay-8B-evo.i1-IQ2_XXS.gguf) | i1-IQ2_XXS | 2.5 | |
| [GGUF](https://huggingface.co/mradermacher/Llama-3-LewdPlay-8B-evo-i1-GGUF/resolve/main/Llama-3-LewdPlay-8B-evo.i1-IQ2_XS.gguf) | i1-IQ2_XS | 2.7 | |
| [GGUF](https://huggingface.co/mradermacher/Llama-3-LewdPlay-8B-evo-i1-GGUF/resolve/main/Llama-3-LewdPlay-8B-evo.i1-IQ2_S.gguf) | i1-IQ2_S | 2.9 | |
| [GGUF](https://huggingface.co/mradermacher/Llama-3-LewdPlay-8B-evo-i1-GGUF/resolve/main/Llama-3-LewdPlay-8B-evo.i1-IQ2_M.gguf) | i1-IQ2_M | 3.0 | |
| [GGUF](https://huggingface.co/mradermacher/Llama-3-LewdPlay-8B-evo-i1-GGUF/resolve/main/Llama-3-LewdPlay-8B-evo.i1-Q2_K.gguf) | i1-Q2_K | 3.3 | IQ3_XXS probably better |
| [GGUF](https://huggingface.co/mradermacher/Llama-3-LewdPlay-8B-evo-i1-GGUF/resolve/main/Llama-3-LewdPlay-8B-evo.i1-IQ3_XXS.gguf) | i1-IQ3_XXS | 3.4 | lower quality |
| [GGUF](https://huggingface.co/mradermacher/Llama-3-LewdPlay-8B-evo-i1-GGUF/resolve/main/Llama-3-LewdPlay-8B-evo.i1-IQ3_XS.gguf) | i1-IQ3_XS | 3.6 | |
| [GGUF](https://huggingface.co/mradermacher/Llama-3-LewdPlay-8B-evo-i1-GGUF/resolve/main/Llama-3-LewdPlay-8B-evo.i1-Q3_K_S.gguf) | i1-Q3_K_S | 3.8 | IQ3_XS probably better |
| [GGUF](https://huggingface.co/mradermacher/Llama-3-LewdPlay-8B-evo-i1-GGUF/resolve/main/Llama-3-LewdPlay-8B-evo.i1-IQ3_S.gguf) | i1-IQ3_S | 3.8 | beats Q3_K* |
| [GGUF](https://huggingface.co/mradermacher/Llama-3-LewdPlay-8B-evo-i1-GGUF/resolve/main/Llama-3-LewdPlay-8B-evo.i1-IQ3_M.gguf) | i1-IQ3_M | 3.9 | |
| [GGUF](https://huggingface.co/mradermacher/Llama-3-LewdPlay-8B-evo-i1-GGUF/resolve/main/Llama-3-LewdPlay-8B-evo.i1-Q3_K_M.gguf) | i1-Q3_K_M | 4.1 | IQ3_S probably better |
| [GGUF](https://huggingface.co/mradermacher/Llama-3-LewdPlay-8B-evo-i1-GGUF/resolve/main/Llama-3-LewdPlay-8B-evo.i1-Q3_K_L.gguf) | i1-Q3_K_L | 4.4 | IQ3_M probably better |
| [GGUF](https://huggingface.co/mradermacher/Llama-3-LewdPlay-8B-evo-i1-GGUF/resolve/main/Llama-3-LewdPlay-8B-evo.i1-IQ4_XS.gguf) | i1-IQ4_XS | 4.5 | |
| [GGUF](https://huggingface.co/mradermacher/Llama-3-LewdPlay-8B-evo-i1-GGUF/resolve/main/Llama-3-LewdPlay-8B-evo.i1-Q4_0.gguf) | i1-Q4_0 | 4.8 | fast, low quality |
| [GGUF](https://huggingface.co/mradermacher/Llama-3-LewdPlay-8B-evo-i1-GGUF/resolve/main/Llama-3-LewdPlay-8B-evo.i1-Q4_K_S.gguf) | i1-Q4_K_S | 4.8 | optimal size/speed/quality |
| [GGUF](https://huggingface.co/mradermacher/Llama-3-LewdPlay-8B-evo-i1-GGUF/resolve/main/Llama-3-LewdPlay-8B-evo.i1-Q4_K_M.gguf) | i1-Q4_K_M | 5.0 | fast, recommended |
| [GGUF](https://huggingface.co/mradermacher/Llama-3-LewdPlay-8B-evo-i1-GGUF/resolve/main/Llama-3-LewdPlay-8B-evo.i1-Q5_K_S.gguf) | i1-Q5_K_S | 5.7 | |
| [GGUF](https://huggingface.co/mradermacher/Llama-3-LewdPlay-8B-evo-i1-GGUF/resolve/main/Llama-3-LewdPlay-8B-evo.i1-Q5_K_M.gguf) | i1-Q5_K_M | 5.8 | |
| [GGUF](https://huggingface.co/mradermacher/Llama-3-LewdPlay-8B-evo-i1-GGUF/resolve/main/Llama-3-LewdPlay-8B-evo.i1-Q6_K.gguf) | i1-Q6_K | 6.7 | practically like static Q6_K |
Here is a handy graph by ikawrakow comparing some lower-quality quant
types (lower is better):

And here are Artefact2's thoughts on the matter:
https://gist.github.com/Artefact2/b5f810600771265fc1e39442288e8ec9
## FAQ / Model Request
See https://huggingface.co/mradermacher/model_requests for some answers to
questions you might have and/or if you want some other model quantized.
## Thanks
I thank my company, [nethype GmbH](https://www.nethype.de/), for letting
me use its servers and providing upgrades to my workstation to enable
this work in my free time. Additional thanks to [@nicoboss](https://huggingface.co/nicoboss) for giving me access to his hardware for calculating the imatrix for these quants.
<!-- end -->
|
mradermacher/L3-Uncen-Merger-Omelette-RP-v0.1.1-8B-i1-GGUF | mradermacher | "2024-06-25T12:28:19Z" | 12,822 | 2 | transformers | [
"transformers",
"gguf",
"mergekit",
"merge",
"not-for-all-audiences",
"nsfw",
"rp",
"roleplay",
"role-play",
"en",
"base_model:Casual-Autopsy/L3-Uncen-Merger-Omelette-RP-v0.1.1-8B",
"license:llama3",
"endpoints_compatible",
"region:us"
] | null | "2024-06-25T07:31:49Z" | ---
base_model: Casual-Autopsy/L3-Uncen-Merger-Omelette-RP-v0.1.1-8B
language:
- en
library_name: transformers
license: llama3
quantized_by: mradermacher
tags:
- mergekit
- merge
- not-for-all-audiences
- nsfw
- rp
- roleplay
- role-play
---
## About
<!-- ### quantize_version: 2 -->
<!-- ### output_tensor_quantised: 1 -->
<!-- ### convert_type: hf -->
<!-- ### vocab_type: -->
<!-- ### tags: nicoboss -->
weighted/imatrix quants of https://huggingface.co/Casual-Autopsy/L3-Uncen-Merger-Omelette-RP-v0.1.1-8B
<!-- provided-files -->
static quants are available at https://huggingface.co/mradermacher/L3-Uncen-Merger-Omelette-RP-v0.1.1-8B-GGUF
## Usage
If you are unsure how to use GGUF files, refer to one of [TheBloke's
READMEs](https://huggingface.co/TheBloke/KafkaLM-70B-German-V0.1-GGUF) for
more details, including on how to concatenate multi-part files.
## Provided Quants
(sorted by size, not necessarily quality. IQ-quants are often preferable over similar sized non-IQ quants)
| Link | Type | Size/GB | Notes |
|:-----|:-----|--------:|:------|
| [GGUF](https://huggingface.co/mradermacher/L3-Uncen-Merger-Omelette-RP-v0.1.1-8B-i1-GGUF/resolve/main/L3-Uncen-Merger-Omelette-RP-v0.1.1-8B.i1-IQ1_S.gguf) | i1-IQ1_S | 2.1 | for the desperate |
| [GGUF](https://huggingface.co/mradermacher/L3-Uncen-Merger-Omelette-RP-v0.1.1-8B-i1-GGUF/resolve/main/L3-Uncen-Merger-Omelette-RP-v0.1.1-8B.i1-IQ1_M.gguf) | i1-IQ1_M | 2.3 | mostly desperate |
| [GGUF](https://huggingface.co/mradermacher/L3-Uncen-Merger-Omelette-RP-v0.1.1-8B-i1-GGUF/resolve/main/L3-Uncen-Merger-Omelette-RP-v0.1.1-8B.i1-IQ2_XXS.gguf) | i1-IQ2_XXS | 2.5 | |
| [GGUF](https://huggingface.co/mradermacher/L3-Uncen-Merger-Omelette-RP-v0.1.1-8B-i1-GGUF/resolve/main/L3-Uncen-Merger-Omelette-RP-v0.1.1-8B.i1-IQ2_XS.gguf) | i1-IQ2_XS | 2.7 | |
| [GGUF](https://huggingface.co/mradermacher/L3-Uncen-Merger-Omelette-RP-v0.1.1-8B-i1-GGUF/resolve/main/L3-Uncen-Merger-Omelette-RP-v0.1.1-8B.i1-IQ2_S.gguf) | i1-IQ2_S | 2.9 | |
| [GGUF](https://huggingface.co/mradermacher/L3-Uncen-Merger-Omelette-RP-v0.1.1-8B-i1-GGUF/resolve/main/L3-Uncen-Merger-Omelette-RP-v0.1.1-8B.i1-IQ2_M.gguf) | i1-IQ2_M | 3.0 | |
| [GGUF](https://huggingface.co/mradermacher/L3-Uncen-Merger-Omelette-RP-v0.1.1-8B-i1-GGUF/resolve/main/L3-Uncen-Merger-Omelette-RP-v0.1.1-8B.i1-Q2_K.gguf) | i1-Q2_K | 3.3 | IQ3_XXS probably better |
| [GGUF](https://huggingface.co/mradermacher/L3-Uncen-Merger-Omelette-RP-v0.1.1-8B-i1-GGUF/resolve/main/L3-Uncen-Merger-Omelette-RP-v0.1.1-8B.i1-IQ3_XXS.gguf) | i1-IQ3_XXS | 3.4 | lower quality |
| [GGUF](https://huggingface.co/mradermacher/L3-Uncen-Merger-Omelette-RP-v0.1.1-8B-i1-GGUF/resolve/main/L3-Uncen-Merger-Omelette-RP-v0.1.1-8B.i1-IQ3_XS.gguf) | i1-IQ3_XS | 3.6 | |
| [GGUF](https://huggingface.co/mradermacher/L3-Uncen-Merger-Omelette-RP-v0.1.1-8B-i1-GGUF/resolve/main/L3-Uncen-Merger-Omelette-RP-v0.1.1-8B.i1-Q3_K_S.gguf) | i1-Q3_K_S | 3.8 | IQ3_XS probably better |
| [GGUF](https://huggingface.co/mradermacher/L3-Uncen-Merger-Omelette-RP-v0.1.1-8B-i1-GGUF/resolve/main/L3-Uncen-Merger-Omelette-RP-v0.1.1-8B.i1-IQ3_S.gguf) | i1-IQ3_S | 3.8 | beats Q3_K* |
| [GGUF](https://huggingface.co/mradermacher/L3-Uncen-Merger-Omelette-RP-v0.1.1-8B-i1-GGUF/resolve/main/L3-Uncen-Merger-Omelette-RP-v0.1.1-8B.i1-IQ3_M.gguf) | i1-IQ3_M | 3.9 | |
| [GGUF](https://huggingface.co/mradermacher/L3-Uncen-Merger-Omelette-RP-v0.1.1-8B-i1-GGUF/resolve/main/L3-Uncen-Merger-Omelette-RP-v0.1.1-8B.i1-Q3_K_M.gguf) | i1-Q3_K_M | 4.1 | IQ3_S probably better |
| [GGUF](https://huggingface.co/mradermacher/L3-Uncen-Merger-Omelette-RP-v0.1.1-8B-i1-GGUF/resolve/main/L3-Uncen-Merger-Omelette-RP-v0.1.1-8B.i1-Q3_K_L.gguf) | i1-Q3_K_L | 4.4 | IQ3_M probably better |
| [GGUF](https://huggingface.co/mradermacher/L3-Uncen-Merger-Omelette-RP-v0.1.1-8B-i1-GGUF/resolve/main/L3-Uncen-Merger-Omelette-RP-v0.1.1-8B.i1-IQ4_XS.gguf) | i1-IQ4_XS | 4.5 | |
| [GGUF](https://huggingface.co/mradermacher/L3-Uncen-Merger-Omelette-RP-v0.1.1-8B-i1-GGUF/resolve/main/L3-Uncen-Merger-Omelette-RP-v0.1.1-8B.i1-Q4_0.gguf) | i1-Q4_0 | 4.8 | fast, low quality |
| [GGUF](https://huggingface.co/mradermacher/L3-Uncen-Merger-Omelette-RP-v0.1.1-8B-i1-GGUF/resolve/main/L3-Uncen-Merger-Omelette-RP-v0.1.1-8B.i1-Q4_K_S.gguf) | i1-Q4_K_S | 4.8 | optimal size/speed/quality |
| [GGUF](https://huggingface.co/mradermacher/L3-Uncen-Merger-Omelette-RP-v0.1.1-8B-i1-GGUF/resolve/main/L3-Uncen-Merger-Omelette-RP-v0.1.1-8B.i1-Q4_K_M.gguf) | i1-Q4_K_M | 5.0 | fast, recommended |
| [GGUF](https://huggingface.co/mradermacher/L3-Uncen-Merger-Omelette-RP-v0.1.1-8B-i1-GGUF/resolve/main/L3-Uncen-Merger-Omelette-RP-v0.1.1-8B.i1-Q5_K_S.gguf) | i1-Q5_K_S | 5.7 | |
| [GGUF](https://huggingface.co/mradermacher/L3-Uncen-Merger-Omelette-RP-v0.1.1-8B-i1-GGUF/resolve/main/L3-Uncen-Merger-Omelette-RP-v0.1.1-8B.i1-Q5_K_M.gguf) | i1-Q5_K_M | 5.8 | |
| [GGUF](https://huggingface.co/mradermacher/L3-Uncen-Merger-Omelette-RP-v0.1.1-8B-i1-GGUF/resolve/main/L3-Uncen-Merger-Omelette-RP-v0.1.1-8B.i1-Q6_K.gguf) | i1-Q6_K | 6.7 | practically like static Q6_K |
Here is a handy graph by ikawrakow comparing some lower-quality quant
types (lower is better):

And here are Artefact2's thoughts on the matter:
https://gist.github.com/Artefact2/b5f810600771265fc1e39442288e8ec9
## FAQ / Model Request
See https://huggingface.co/mradermacher/model_requests for some answers to
questions you might have and/or if you want some other model quantized.
## Thanks
I thank my company, [nethype GmbH](https://www.nethype.de/), for letting
me use its servers and providing upgrades to my workstation to enable
this work in my free time. Additional thanks to [@nicoboss](https://huggingface.co/nicoboss) for giving me access to his hardware for calculating the imatrix for these quants.
<!-- end -->
|
mradermacher/L3-8B-Spicey-Stheno-v0.2-i1-GGUF | mradermacher | "2024-07-01T22:07:26Z" | 12,816 | 0 | transformers | [
"transformers",
"gguf",
"en",
"base_model:Rockinsince87/L3-8B-Spicey-Stheno-v0.2",
"endpoints_compatible",
"region:us"
] | null | "2024-07-01T19:29:52Z" | ---
base_model: Rockinsince87/L3-8B-Spicey-Stheno-v0.2
language:
- en
library_name: transformers
quantized_by: mradermacher
---
## About
<!-- ### quantize_version: 2 -->
<!-- ### output_tensor_quantised: 1 -->
<!-- ### convert_type: hf -->
<!-- ### vocab_type: -->
<!-- ### tags: nicoboss -->
weighted/imatrix quants of https://huggingface.co/Rockinsince87/L3-8B-Spicey-Stheno-v0.2
<!-- provided-files -->
static quants are available at https://huggingface.co/mradermacher/L3-8B-Spicey-Stheno-v0.2-GGUF
## Usage
If you are unsure how to use GGUF files, refer to one of [TheBloke's
READMEs](https://huggingface.co/TheBloke/KafkaLM-70B-German-V0.1-GGUF) for
more details, including on how to concatenate multi-part files.
## Provided Quants
(sorted by size, not necessarily quality. IQ-quants are often preferable over similar sized non-IQ quants)
| Link | Type | Size/GB | Notes |
|:-----|:-----|--------:|:------|
| [GGUF](https://huggingface.co/mradermacher/L3-8B-Spicey-Stheno-v0.2-i1-GGUF/resolve/main/L3-8B-Spicey-Stheno-v0.2.i1-IQ1_S.gguf) | i1-IQ1_S | 2.1 | for the desperate |
| [GGUF](https://huggingface.co/mradermacher/L3-8B-Spicey-Stheno-v0.2-i1-GGUF/resolve/main/L3-8B-Spicey-Stheno-v0.2.i1-IQ1_M.gguf) | i1-IQ1_M | 2.3 | mostly desperate |
| [GGUF](https://huggingface.co/mradermacher/L3-8B-Spicey-Stheno-v0.2-i1-GGUF/resolve/main/L3-8B-Spicey-Stheno-v0.2.i1-IQ2_XXS.gguf) | i1-IQ2_XXS | 2.5 | |
| [GGUF](https://huggingface.co/mradermacher/L3-8B-Spicey-Stheno-v0.2-i1-GGUF/resolve/main/L3-8B-Spicey-Stheno-v0.2.i1-IQ2_XS.gguf) | i1-IQ2_XS | 2.7 | |
| [GGUF](https://huggingface.co/mradermacher/L3-8B-Spicey-Stheno-v0.2-i1-GGUF/resolve/main/L3-8B-Spicey-Stheno-v0.2.i1-IQ2_S.gguf) | i1-IQ2_S | 2.9 | |
| [GGUF](https://huggingface.co/mradermacher/L3-8B-Spicey-Stheno-v0.2-i1-GGUF/resolve/main/L3-8B-Spicey-Stheno-v0.2.i1-IQ2_M.gguf) | i1-IQ2_M | 3.0 | |
| [GGUF](https://huggingface.co/mradermacher/L3-8B-Spicey-Stheno-v0.2-i1-GGUF/resolve/main/L3-8B-Spicey-Stheno-v0.2.i1-Q2_K.gguf) | i1-Q2_K | 3.3 | IQ3_XXS probably better |
| [GGUF](https://huggingface.co/mradermacher/L3-8B-Spicey-Stheno-v0.2-i1-GGUF/resolve/main/L3-8B-Spicey-Stheno-v0.2.i1-IQ3_XXS.gguf) | i1-IQ3_XXS | 3.4 | lower quality |
| [GGUF](https://huggingface.co/mradermacher/L3-8B-Spicey-Stheno-v0.2-i1-GGUF/resolve/main/L3-8B-Spicey-Stheno-v0.2.i1-IQ3_XS.gguf) | i1-IQ3_XS | 3.6 | |
| [GGUF](https://huggingface.co/mradermacher/L3-8B-Spicey-Stheno-v0.2-i1-GGUF/resolve/main/L3-8B-Spicey-Stheno-v0.2.i1-Q3_K_S.gguf) | i1-Q3_K_S | 3.8 | IQ3_XS probably better |
| [GGUF](https://huggingface.co/mradermacher/L3-8B-Spicey-Stheno-v0.2-i1-GGUF/resolve/main/L3-8B-Spicey-Stheno-v0.2.i1-IQ3_S.gguf) | i1-IQ3_S | 3.8 | beats Q3_K* |
| [GGUF](https://huggingface.co/mradermacher/L3-8B-Spicey-Stheno-v0.2-i1-GGUF/resolve/main/L3-8B-Spicey-Stheno-v0.2.i1-IQ3_M.gguf) | i1-IQ3_M | 3.9 | |
| [GGUF](https://huggingface.co/mradermacher/L3-8B-Spicey-Stheno-v0.2-i1-GGUF/resolve/main/L3-8B-Spicey-Stheno-v0.2.i1-Q3_K_M.gguf) | i1-Q3_K_M | 4.1 | IQ3_S probably better |
| [GGUF](https://huggingface.co/mradermacher/L3-8B-Spicey-Stheno-v0.2-i1-GGUF/resolve/main/L3-8B-Spicey-Stheno-v0.2.i1-Q3_K_L.gguf) | i1-Q3_K_L | 4.4 | IQ3_M probably better |
| [GGUF](https://huggingface.co/mradermacher/L3-8B-Spicey-Stheno-v0.2-i1-GGUF/resolve/main/L3-8B-Spicey-Stheno-v0.2.i1-IQ4_XS.gguf) | i1-IQ4_XS | 4.5 | |
| [GGUF](https://huggingface.co/mradermacher/L3-8B-Spicey-Stheno-v0.2-i1-GGUF/resolve/main/L3-8B-Spicey-Stheno-v0.2.i1-Q4_0.gguf) | i1-Q4_0 | 4.8 | fast, low quality |
| [GGUF](https://huggingface.co/mradermacher/L3-8B-Spicey-Stheno-v0.2-i1-GGUF/resolve/main/L3-8B-Spicey-Stheno-v0.2.i1-Q4_K_S.gguf) | i1-Q4_K_S | 4.8 | optimal size/speed/quality |
| [GGUF](https://huggingface.co/mradermacher/L3-8B-Spicey-Stheno-v0.2-i1-GGUF/resolve/main/L3-8B-Spicey-Stheno-v0.2.i1-Q4_K_M.gguf) | i1-Q4_K_M | 5.0 | fast, recommended |
| [GGUF](https://huggingface.co/mradermacher/L3-8B-Spicey-Stheno-v0.2-i1-GGUF/resolve/main/L3-8B-Spicey-Stheno-v0.2.i1-Q5_K_S.gguf) | i1-Q5_K_S | 5.7 | |
| [GGUF](https://huggingface.co/mradermacher/L3-8B-Spicey-Stheno-v0.2-i1-GGUF/resolve/main/L3-8B-Spicey-Stheno-v0.2.i1-Q5_K_M.gguf) | i1-Q5_K_M | 5.8 | |
| [GGUF](https://huggingface.co/mradermacher/L3-8B-Spicey-Stheno-v0.2-i1-GGUF/resolve/main/L3-8B-Spicey-Stheno-v0.2.i1-Q6_K.gguf) | i1-Q6_K | 6.7 | practically like static Q6_K |
Here is a handy graph by ikawrakow comparing some lower-quality quant
types (lower is better):

And here are Artefact2's thoughts on the matter:
https://gist.github.com/Artefact2/b5f810600771265fc1e39442288e8ec9
## FAQ / Model Request
See https://huggingface.co/mradermacher/model_requests for some answers to
questions you might have and/or if you want some other model quantized.
## Thanks
I thank my company, [nethype GmbH](https://www.nethype.de/), for letting
me use its servers and providing upgrades to my workstation to enable
this work in my free time. Additional thanks to [@nicoboss](https://huggingface.co/nicoboss) for giving me access to his hardware for calculating the imatrix for these quants.
<!-- end -->
|
mradermacher/llama3-8B-DarkIdol-2.2-Uncensored-1048K-i1-GGUF | mradermacher | "2024-07-02T00:59:49Z" | 12,816 | 1 | transformers | [
"transformers",
"gguf",
"roleplay",
"llama3",
"sillytavern",
"idol",
"en",
"ja",
"zh",
"base_model:aifeifei798/llama3-8B-DarkIdol-2.2-Uncensored-1048K",
"license:llama3",
"endpoints_compatible",
"region:us"
] | null | "2024-07-01T19:50:24Z" | ---
base_model: aifeifei798/llama3-8B-DarkIdol-2.2-Uncensored-1048K
language:
- en
- ja
- zh
library_name: transformers
license: llama3
quantized_by: mradermacher
tags:
- roleplay
- llama3
- sillytavern
- idol
---
## About
<!-- ### quantize_version: 2 -->
<!-- ### output_tensor_quantised: 1 -->
<!-- ### convert_type: hf -->
<!-- ### vocab_type: -->
<!-- ### tags: nicoboss -->
weighted/imatrix quants of https://huggingface.co/aifeifei798/llama3-8B-DarkIdol-2.2-Uncensored-1048K
<!-- provided-files -->
static quants are available at https://huggingface.co/mradermacher/llama3-8B-DarkIdol-2.2-Uncensored-1048K-GGUF
## Usage
If you are unsure how to use GGUF files, refer to one of [TheBloke's
READMEs](https://huggingface.co/TheBloke/KafkaLM-70B-German-V0.1-GGUF) for
more details, including on how to concatenate multi-part files.
## Provided Quants
(sorted by size, not necessarily quality. IQ-quants are often preferable over similar sized non-IQ quants)
| Link | Type | Size/GB | Notes |
|:-----|:-----|--------:|:------|
| [GGUF](https://huggingface.co/mradermacher/llama3-8B-DarkIdol-2.2-Uncensored-1048K-i1-GGUF/resolve/main/llama3-8B-DarkIdol-2.2-Uncensored-1048K.i1-IQ1_S.gguf) | i1-IQ1_S | 2.1 | for the desperate |
| [GGUF](https://huggingface.co/mradermacher/llama3-8B-DarkIdol-2.2-Uncensored-1048K-i1-GGUF/resolve/main/llama3-8B-DarkIdol-2.2-Uncensored-1048K.i1-IQ1_M.gguf) | i1-IQ1_M | 2.3 | mostly desperate |
| [GGUF](https://huggingface.co/mradermacher/llama3-8B-DarkIdol-2.2-Uncensored-1048K-i1-GGUF/resolve/main/llama3-8B-DarkIdol-2.2-Uncensored-1048K.i1-IQ2_XXS.gguf) | i1-IQ2_XXS | 2.5 | |
| [GGUF](https://huggingface.co/mradermacher/llama3-8B-DarkIdol-2.2-Uncensored-1048K-i1-GGUF/resolve/main/llama3-8B-DarkIdol-2.2-Uncensored-1048K.i1-IQ2_XS.gguf) | i1-IQ2_XS | 2.7 | |
| [GGUF](https://huggingface.co/mradermacher/llama3-8B-DarkIdol-2.2-Uncensored-1048K-i1-GGUF/resolve/main/llama3-8B-DarkIdol-2.2-Uncensored-1048K.i1-IQ2_S.gguf) | i1-IQ2_S | 2.9 | |
| [GGUF](https://huggingface.co/mradermacher/llama3-8B-DarkIdol-2.2-Uncensored-1048K-i1-GGUF/resolve/main/llama3-8B-DarkIdol-2.2-Uncensored-1048K.i1-IQ2_M.gguf) | i1-IQ2_M | 3.0 | |
| [GGUF](https://huggingface.co/mradermacher/llama3-8B-DarkIdol-2.2-Uncensored-1048K-i1-GGUF/resolve/main/llama3-8B-DarkIdol-2.2-Uncensored-1048K.i1-Q2_K.gguf) | i1-Q2_K | 3.3 | IQ3_XXS probably better |
| [GGUF](https://huggingface.co/mradermacher/llama3-8B-DarkIdol-2.2-Uncensored-1048K-i1-GGUF/resolve/main/llama3-8B-DarkIdol-2.2-Uncensored-1048K.i1-IQ3_XXS.gguf) | i1-IQ3_XXS | 3.4 | lower quality |
| [GGUF](https://huggingface.co/mradermacher/llama3-8B-DarkIdol-2.2-Uncensored-1048K-i1-GGUF/resolve/main/llama3-8B-DarkIdol-2.2-Uncensored-1048K.i1-IQ3_XS.gguf) | i1-IQ3_XS | 3.6 | |
| [GGUF](https://huggingface.co/mradermacher/llama3-8B-DarkIdol-2.2-Uncensored-1048K-i1-GGUF/resolve/main/llama3-8B-DarkIdol-2.2-Uncensored-1048K.i1-Q3_K_S.gguf) | i1-Q3_K_S | 3.8 | IQ3_XS probably better |
| [GGUF](https://huggingface.co/mradermacher/llama3-8B-DarkIdol-2.2-Uncensored-1048K-i1-GGUF/resolve/main/llama3-8B-DarkIdol-2.2-Uncensored-1048K.i1-IQ3_S.gguf) | i1-IQ3_S | 3.8 | beats Q3_K* |
| [GGUF](https://huggingface.co/mradermacher/llama3-8B-DarkIdol-2.2-Uncensored-1048K-i1-GGUF/resolve/main/llama3-8B-DarkIdol-2.2-Uncensored-1048K.i1-IQ3_M.gguf) | i1-IQ3_M | 3.9 | |
| [GGUF](https://huggingface.co/mradermacher/llama3-8B-DarkIdol-2.2-Uncensored-1048K-i1-GGUF/resolve/main/llama3-8B-DarkIdol-2.2-Uncensored-1048K.i1-Q3_K_M.gguf) | i1-Q3_K_M | 4.1 | IQ3_S probably better |
| [GGUF](https://huggingface.co/mradermacher/llama3-8B-DarkIdol-2.2-Uncensored-1048K-i1-GGUF/resolve/main/llama3-8B-DarkIdol-2.2-Uncensored-1048K.i1-Q3_K_L.gguf) | i1-Q3_K_L | 4.4 | IQ3_M probably better |
| [GGUF](https://huggingface.co/mradermacher/llama3-8B-DarkIdol-2.2-Uncensored-1048K-i1-GGUF/resolve/main/llama3-8B-DarkIdol-2.2-Uncensored-1048K.i1-IQ4_XS.gguf) | i1-IQ4_XS | 4.5 | |
| [GGUF](https://huggingface.co/mradermacher/llama3-8B-DarkIdol-2.2-Uncensored-1048K-i1-GGUF/resolve/main/llama3-8B-DarkIdol-2.2-Uncensored-1048K.i1-Q4_0.gguf) | i1-Q4_0 | 4.8 | fast, low quality |
| [GGUF](https://huggingface.co/mradermacher/llama3-8B-DarkIdol-2.2-Uncensored-1048K-i1-GGUF/resolve/main/llama3-8B-DarkIdol-2.2-Uncensored-1048K.i1-Q4_K_S.gguf) | i1-Q4_K_S | 4.8 | optimal size/speed/quality |
| [GGUF](https://huggingface.co/mradermacher/llama3-8B-DarkIdol-2.2-Uncensored-1048K-i1-GGUF/resolve/main/llama3-8B-DarkIdol-2.2-Uncensored-1048K.i1-Q4_K_M.gguf) | i1-Q4_K_M | 5.0 | fast, recommended |
| [GGUF](https://huggingface.co/mradermacher/llama3-8B-DarkIdol-2.2-Uncensored-1048K-i1-GGUF/resolve/main/llama3-8B-DarkIdol-2.2-Uncensored-1048K.i1-Q5_K_S.gguf) | i1-Q5_K_S | 5.7 | |
| [GGUF](https://huggingface.co/mradermacher/llama3-8B-DarkIdol-2.2-Uncensored-1048K-i1-GGUF/resolve/main/llama3-8B-DarkIdol-2.2-Uncensored-1048K.i1-Q5_K_M.gguf) | i1-Q5_K_M | 5.8 | |
| [GGUF](https://huggingface.co/mradermacher/llama3-8B-DarkIdol-2.2-Uncensored-1048K-i1-GGUF/resolve/main/llama3-8B-DarkIdol-2.2-Uncensored-1048K.i1-Q6_K.gguf) | i1-Q6_K | 6.7 | practically like static Q6_K |
Here is a handy graph by ikawrakow comparing some lower-quality quant
types (lower is better):

And here are Artefact2's thoughts on the matter:
https://gist.github.com/Artefact2/b5f810600771265fc1e39442288e8ec9
## FAQ / Model Request
See https://huggingface.co/mradermacher/model_requests for some answers to
questions you might have and/or if you want some other model quantized.
## Thanks
I thank my company, [nethype GmbH](https://www.nethype.de/), for letting
me use its servers and providing upgrades to my workstation to enable
this work in my free time. Additional thanks to [@nicoboss](https://huggingface.co/nicoboss) for giving me access to his hardware for calculating the imatrix for these quants.
<!-- end -->
|
bigscience/bloom-3b | bigscience | "2023-04-14T08:43:29Z" | 12,796 | 86 | transformers | [
"transformers",
"pytorch",
"safetensors",
"bloom",
"text-generation",
"ak",
"ar",
"as",
"bm",
"bn",
"ca",
"code",
"en",
"es",
"eu",
"fon",
"fr",
"gu",
"hi",
"id",
"ig",
"ki",
"kn",
"lg",
"ln",
"ml",
"mr",
"ne",
"nso",
"ny",
"or",
"pa",
"pt",
"rn",
"rw",
"sn",
"st",
"sw",
"ta",
"te",
"tn",
"ts",
"tum",
"tw",
"ur",
"vi",
"wo",
"xh",
"yo",
"zh",
"zhs",
"zht",
"zu",
"arxiv:1909.08053",
"arxiv:2110.02861",
"arxiv:2108.12409",
"license:bigscience-bloom-rail-1.0",
"model-index",
"autotrain_compatible",
"endpoints_compatible",
"text-generation-inference",
"region:us"
] | text-generation | "2022-05-19T11:52:27Z" | ---
license: bigscience-bloom-rail-1.0
language:
- ak
- ar
- as
- bm
- bn
- ca
- code
- en
- es
- eu
- fon
- fr
- gu
- hi
- id
- ig
- ki
- kn
- lg
- ln
- ml
- mr
- ne
- nso
- ny
- or
- pa
- pt
- rn
- rw
- sn
- st
- sw
- ta
- te
- tn
- ts
- tum
- tw
- ur
- vi
- wo
- xh
- yo
- zh
- zhs
- zht
- zu
pipeline_tag: text-generation
model-index:
- name: bloom
results:
- task:
type: text-generation
name: text generation
dataset:
name: arc_challenge
type: arc_challenge
metrics:
- name: acc
type: acc
value: 0.27986348122866894
verified: false
- task:
type: text-generation
name: text generation
dataset:
name: arc_easy
type: arc_easy
metrics:
- name: acc
type: acc
value: 0.5946969696969697
verified: false
- task:
type: text-generation
name: text generation
dataset:
name: axb
type: axb
metrics:
- name: acc
type: acc
value: 0.4433876811594203
verified: false
- task:
type: text-generation
name: text generation
dataset:
name: axg
type: axg
metrics:
- name: acc
type: acc
value: 0.5
verified: false
- task:
type: text-generation
name: text generation
dataset:
name: boolq
type: boolq
metrics:
- name: acc
type: acc
value: 0.6165137614678899
verified: false
- task:
type: text-generation
name: text generation
dataset:
name: cb
type: cb
metrics:
- name: acc
type: acc
value: 0.30357142857142855
verified: false
- task:
type: text-generation
name: text generation
dataset:
name: cola
type: cola
metrics:
- name: acc
type: acc
value: 0.610738255033557
verified: false
- task:
type: text-generation
name: text generation
dataset:
name: copa
type: copa
metrics:
- name: acc
type: acc
value: 0.63
verified: false
- task:
type: text-generation
name: text generation
dataset:
name: crows_pairs_english
type: crows_pairs_english
metrics:
- name: acc
type: acc
value: 0.4973166368515206
verified: false
- task:
type: text-generation
name: text generation
dataset:
name: crows_pairs_french
type: crows_pairs_french
metrics:
- name: acc
type: acc
value: 0.5032796660703638
verified: false
- task:
type: text-generation
name: text generation
dataset:
name: diabla
type: diabla
metrics:
- name: acc
type: acc
value: 0.28888308977035493
verified: false
- task:
type: text-generation
name: text generation
dataset:
name: gsarti/flores_101_afr
type: gsarti/flores_101_afr
metrics:
- name: byte_perplexity
type: byte_perplexity
value: 6.500798737976343
verified: false
- task:
type: text-generation
name: text generation
dataset:
name: gsarti/flores_101_amh
type: gsarti/flores_101_amh
metrics:
- name: byte_perplexity
type: byte_perplexity
value: 3.9726863338897145
verified: false
- task:
type: text-generation
name: text generation
dataset:
name: gsarti/flores_101_ara
type: gsarti/flores_101_ara
metrics:
- name: byte_perplexity
type: byte_perplexity
value: 1.8083841089875814
verified: false
- task:
type: text-generation
name: text generation
dataset:
name: gsarti/flores_101_asm
type: gsarti/flores_101_asm
metrics:
- name: byte_perplexity
type: byte_perplexity
value: 5.699102962086425
verified: false
- task:
type: text-generation
name: text generation
dataset:
name: gsarti/flores_101_ast
type: gsarti/flores_101_ast
metrics:
- name: byte_perplexity
type: byte_perplexity
value: 3.9252047073429384
verified: false
- task:
type: text-generation
name: text generation
dataset:
name: gsarti/flores_101_azj
type: gsarti/flores_101_azj
metrics:
- name: byte_perplexity
type: byte_perplexity
value: 6.942805054270002
verified: false
- task:
type: text-generation
name: text generation
dataset:
name: gsarti/flores_101_bel
type: gsarti/flores_101_bel
metrics:
- name: byte_perplexity
type: byte_perplexity
value: 3.614136245847082
verified: false
- task:
type: text-generation
name: text generation
dataset:
name: gsarti/flores_101_ben
type: gsarti/flores_101_ben
metrics:
- name: byte_perplexity
type: byte_perplexity
value: 5.121491534300969
verified: false
- task:
type: text-generation
name: text generation
dataset:
name: gsarti/flores_101_bos
type: gsarti/flores_101_bos
metrics:
- name: byte_perplexity
type: byte_perplexity
value: 5.653353469118798
verified: false
- task:
type: text-generation
name: text generation
dataset:
name: gsarti/flores_101_bul
type: gsarti/flores_101_bul
metrics:
- name: byte_perplexity
type: byte_perplexity
value: 2.7014693938055068
verified: false
- task:
type: text-generation
name: text generation
dataset:
name: gsarti/flores_101_cat
type: gsarti/flores_101_cat
metrics:
- name: byte_perplexity
type: byte_perplexity
value: 2.305190041967345
verified: false
- task:
type: text-generation
name: text generation
dataset:
name: gsarti/flores_101_ceb
type: gsarti/flores_101_ceb
metrics:
- name: byte_perplexity
type: byte_perplexity
value: 6.291000321323428
verified: false
- task:
type: text-generation
name: text generation
dataset:
name: gsarti/flores_101_ces
type: gsarti/flores_101_ces
metrics:
- name: byte_perplexity
type: byte_perplexity
value: 5.447322753586386
verified: false
- task:
type: text-generation
name: text generation
dataset:
name: gsarti/flores_101_ckb
type: gsarti/flores_101_ckb
metrics:
- name: byte_perplexity
type: byte_perplexity
value: 3.7255124939234765
verified: false
- task:
type: text-generation
name: text generation
dataset:
name: gsarti/flores_101_cym
type: gsarti/flores_101_cym
metrics:
- name: byte_perplexity
type: byte_perplexity
value: 12.539424151448149
verified: false
- task:
type: text-generation
name: text generation
dataset:
name: gsarti/flores_101_dan
type: gsarti/flores_101_dan
metrics:
- name: byte_perplexity
type: byte_perplexity
value: 5.183309001005672
verified: false
- task:
type: text-generation
name: text generation
dataset:
name: gsarti/flores_101_deu
type: gsarti/flores_101_deu
metrics:
- name: byte_perplexity
type: byte_perplexity
value: 3.1180422286591347
verified: false
- task:
type: text-generation
name: text generation
dataset:
name: gsarti/flores_101_ell
type: gsarti/flores_101_ell
metrics:
- name: byte_perplexity
type: byte_perplexity
value: 2.467943456164706
verified: false
- task:
type: text-generation
name: text generation
dataset:
name: gsarti/flores_101_eng
type: gsarti/flores_101_eng
metrics:
- name: byte_perplexity
type: byte_perplexity
value: 2.018740628193298
verified: false
- task:
type: text-generation
name: text generation
dataset:
name: gsarti/flores_101_est
type: gsarti/flores_101_est
metrics:
- name: byte_perplexity
type: byte_perplexity
value: 9.11654425176368
verified: false
- task:
type: text-generation
name: text generation
dataset:
name: gsarti/flores_101_fas
type: gsarti/flores_101_fas
metrics:
- name: byte_perplexity
type: byte_perplexity
value: 3.058009097116482
verified: false
- task:
type: text-generation
name: text generation
dataset:
name: gsarti/flores_101_fin
type: gsarti/flores_101_fin
metrics:
- name: byte_perplexity
type: byte_perplexity
value: 6.847047959628553
verified: false
- task:
type: text-generation
name: text generation
dataset:
name: gsarti/flores_101_fra
type: gsarti/flores_101_fra
metrics:
- name: byte_perplexity
type: byte_perplexity
value: 1.9975177011840075
verified: false
- task:
type: text-generation
name: text generation
dataset:
name: gsarti/flores_101_ful
type: gsarti/flores_101_ful
metrics:
- name: byte_perplexity
type: byte_perplexity
value: 11.465912731488828
verified: false
- task:
type: text-generation
name: text generation
dataset:
name: gsarti/flores_101_gle
type: gsarti/flores_101_gle
metrics:
- name: byte_perplexity
type: byte_perplexity
value: 8.681491663539422
verified: false
- task:
type: text-generation
name: text generation
dataset:
name: gsarti/flores_101_glg
type: gsarti/flores_101_glg
metrics:
- name: byte_perplexity
type: byte_perplexity
value: 3.029991089015508
verified: false
- task:
type: text-generation
name: text generation
dataset:
name: gsarti/flores_101_guj
type: gsarti/flores_101_guj
metrics:
- name: byte_perplexity
type: byte_perplexity
value: 4.955224230286231
verified: false
- task:
type: text-generation
name: text generation
dataset:
name: gsarti/flores_101_hau
type: gsarti/flores_101_hau
metrics:
- name: byte_perplexity
type: byte_perplexity
value: 10.758347356372159
verified: false
- task:
type: text-generation
name: text generation
dataset:
name: gsarti/flores_101_heb
type: gsarti/flores_101_heb
metrics:
- name: byte_perplexity
type: byte_perplexity
value: 3.6004478129801667
verified: false
- task:
type: text-generation
name: text generation
dataset:
name: gsarti/flores_101_hin
type: gsarti/flores_101_hin
metrics:
- name: byte_perplexity
type: byte_perplexity
value: 4.712530650588064
verified: false
- task:
type: text-generation
name: text generation
dataset:
name: gsarti/flores_101_hrv
type: gsarti/flores_101_hrv
metrics:
- name: byte_perplexity
type: byte_perplexity
value: 5.822418943372185
verified: false
- task:
type: text-generation
name: text generation
dataset:
name: gsarti/flores_101_hun
type: gsarti/flores_101_hun
metrics:
- name: byte_perplexity
type: byte_perplexity
value: 6.440482646965992
verified: false
- task:
type: text-generation
name: text generation
dataset:
name: gsarti/flores_101_hye
type: gsarti/flores_101_hye
metrics:
- name: byte_perplexity
type: byte_perplexity
value: 3.657718918347166
verified: false
- task:
type: text-generation
name: text generation
dataset:
name: gsarti/flores_101_ibo
type: gsarti/flores_101_ibo
metrics:
- name: byte_perplexity
type: byte_perplexity
value: 5.564814003872672
verified: false
- task:
type: text-generation
name: text generation
dataset:
name: gsarti/flores_101_ind
type: gsarti/flores_101_ind
metrics:
- name: byte_perplexity
type: byte_perplexity
value: 2.1597101468869373
verified: false
- task:
type: text-generation
name: text generation
dataset:
name: gsarti/flores_101_isl
type: gsarti/flores_101_isl
metrics:
- name: byte_perplexity
type: byte_perplexity
value: 8.082349269518136
verified: false
- task:
type: text-generation
name: text generation
dataset:
name: gsarti/flores_101_ita
type: gsarti/flores_101_ita
metrics:
- name: byte_perplexity
type: byte_perplexity
value: 2.9687591414176207
verified: false
- task:
type: text-generation
name: text generation
dataset:
name: gsarti/flores_101_jav
type: gsarti/flores_101_jav
metrics:
- name: byte_perplexity
type: byte_perplexity
value: 7.0573805415708994
verified: false
- task:
type: text-generation
name: text generation
dataset:
name: gsarti/flores_101_jpn
type: gsarti/flores_101_jpn
metrics:
- name: byte_perplexity
type: byte_perplexity
value: 2.7758864197116933
verified: false
- task:
type: text-generation
name: text generation
dataset:
name: gsarti/flores_101_kam
type: gsarti/flores_101_kam
metrics:
- name: byte_perplexity
type: byte_perplexity
value: 11.072949642861332
verified: false
- task:
type: text-generation
name: text generation
dataset:
name: gsarti/flores_101_kan
type: gsarti/flores_101_kan
metrics:
- name: byte_perplexity
type: byte_perplexity
value: 5.551730651007082
verified: false
- task:
type: text-generation
name: text generation
dataset:
name: gsarti/flores_101_kat
type: gsarti/flores_101_kat
metrics:
- name: byte_perplexity
type: byte_perplexity
value: 2.522630524283745
verified: false
- task:
type: text-generation
name: text generation
dataset:
name: gsarti/flores_101_kaz
type: gsarti/flores_101_kaz
metrics:
- name: byte_perplexity
type: byte_perplexity
value: 3.3901748516975574
verified: false
- task:
type: text-generation
name: text generation
dataset:
name: gsarti/flores_101_kea
type: gsarti/flores_101_kea
metrics:
- name: byte_perplexity
type: byte_perplexity
value: 8.918534182590863
verified: false
- task:
type: text-generation
name: text generation
dataset:
name: gsarti/flores_101_kir
type: gsarti/flores_101_kir
metrics:
- name: byte_perplexity
type: byte_perplexity
value: 3.729278369847201
verified: false
- task:
type: text-generation
name: text generation
dataset:
name: gsarti/flores_101_kor
type: gsarti/flores_101_kor
metrics:
- name: byte_perplexity
type: byte_perplexity
value: 3.932884847226212
verified: false
- task:
type: text-generation
name: text generation
dataset:
name: gsarti/flores_101_lao
type: gsarti/flores_101_lao
metrics:
- name: byte_perplexity
type: byte_perplexity
value: 2.9077314760849924
verified: false
- task:
type: text-generation
name: text generation
dataset:
name: gsarti/flores_101_lav
type: gsarti/flores_101_lav
metrics:
- name: byte_perplexity
type: byte_perplexity
value: 7.777221919194806
verified: false
- task:
type: text-generation
name: text generation
dataset:
name: gsarti/flores_101_lin
type: gsarti/flores_101_lin
metrics:
- name: byte_perplexity
type: byte_perplexity
value: 7.524842908050988
verified: false
- task:
type: text-generation
name: text generation
dataset:
name: gsarti/flores_101_lit
type: gsarti/flores_101_lit
metrics:
- name: byte_perplexity
type: byte_perplexity
value: 7.369179434621725
verified: false
- task:
type: text-generation
name: text generation
dataset:
name: gsarti/flores_101_ltz
type: gsarti/flores_101_ltz
metrics:
- name: byte_perplexity
type: byte_perplexity
value: 8.801059747949214
verified: false
- task:
type: text-generation
name: text generation
dataset:
name: gsarti/flores_101_lug
type: gsarti/flores_101_lug
metrics:
- name: byte_perplexity
type: byte_perplexity
value: 8.483203026364786
verified: false
- task:
type: text-generation
name: text generation
dataset:
name: gsarti/flores_101_luo
type: gsarti/flores_101_luo
metrics:
- name: byte_perplexity
type: byte_perplexity
value: 11.975963093623681
verified: false
- task:
type: text-generation
name: text generation
dataset:
name: gsarti/flores_101_mal
type: gsarti/flores_101_mal
metrics:
- name: byte_perplexity
type: byte_perplexity
value: 4.615948455160037
verified: false
- task:
type: text-generation
name: text generation
dataset:
name: gsarti/flores_101_mar
type: gsarti/flores_101_mar
metrics:
- name: byte_perplexity
type: byte_perplexity
value: 5.483253482821379
verified: false
- task:
type: text-generation
name: text generation
dataset:
name: gsarti/flores_101_mkd
type: gsarti/flores_101_mkd
metrics:
- name: byte_perplexity
type: byte_perplexity
value: 2.9656732291754087
verified: false
- task:
type: text-generation
name: text generation
dataset:
name: gsarti/flores_101_mlt
type: gsarti/flores_101_mlt
metrics:
- name: byte_perplexity
type: byte_perplexity
value: 15.004773437665275
verified: false
- task:
type: text-generation
name: text generation
dataset:
name: gsarti/flores_101_mon
type: gsarti/flores_101_mon
metrics:
- name: byte_perplexity
type: byte_perplexity
value: 3.410598542315402
verified: false
- task:
type: text-generation
name: text generation
dataset:
name: gsarti/flores_101_mri
type: gsarti/flores_101_mri
metrics:
- name: byte_perplexity
type: byte_perplexity
value: 7.474035895661322
verified: false
- task:
type: text-generation
name: text generation
dataset:
name: gsarti/flores_101_msa
type: gsarti/flores_101_msa
metrics:
- name: byte_perplexity
type: byte_perplexity
value: 2.5710001772665634
verified: false
- task:
type: text-generation
name: text generation
dataset:
name: gsarti/flores_101_mya
type: gsarti/flores_101_mya
metrics:
- name: byte_perplexity
type: byte_perplexity
value: 2.413577969878331
verified: false
- task:
type: text-generation
name: text generation
dataset:
name: gsarti/flores_101_nld
type: gsarti/flores_101_nld
metrics:
- name: byte_perplexity
type: byte_perplexity
value: 4.127831721885065
verified: false
- task:
type: text-generation
name: text generation
dataset:
name: gsarti/flores_101_nob
type: gsarti/flores_101_nob
metrics:
- name: byte_perplexity
type: byte_perplexity
value: 5.402763169129877
verified: false
- task:
type: text-generation
name: text generation
dataset:
name: gsarti/flores_101_npi
type: gsarti/flores_101_npi
metrics:
- name: byte_perplexity
type: byte_perplexity
value: 5.199342701937889
verified: false
- task:
type: text-generation
name: text generation
dataset:
name: gsarti/flores_101_nso
type: gsarti/flores_101_nso
metrics:
- name: byte_perplexity
type: byte_perplexity
value: 8.154626800955667
verified: false
- task:
type: text-generation
name: text generation
dataset:
name: gsarti/flores_101_nya
type: gsarti/flores_101_nya
metrics:
- name: byte_perplexity
type: byte_perplexity
value: 8.179860208369393
verified: false
- task:
type: text-generation
name: text generation
dataset:
name: gsarti/flores_101_oci
type: gsarti/flores_101_oci
metrics:
- name: byte_perplexity
type: byte_perplexity
value: 4.8617357393685845
verified: false
- task:
type: text-generation
name: text generation
dataset:
name: gsarti/flores_101_orm
type: gsarti/flores_101_orm
metrics:
- name: byte_perplexity
type: byte_perplexity
value: 12.911595421079408
verified: false
- task:
type: text-generation
name: text generation
dataset:
name: gsarti/flores_101_ory
type: gsarti/flores_101_ory
metrics:
- name: byte_perplexity
type: byte_perplexity
value: 5.189421861225964
verified: false
- task:
type: text-generation
name: text generation
dataset:
name: gsarti/flores_101_pan
type: gsarti/flores_101_pan
metrics:
- name: byte_perplexity
type: byte_perplexity
value: 4.698477289331806
verified: false
- task:
type: text-generation
name: text generation
dataset:
name: gsarti/flores_101_pol
type: gsarti/flores_101_pol
metrics:
- name: byte_perplexity
type: byte_perplexity
value: 4.625550458479643
verified: false
- task:
type: text-generation
name: text generation
dataset:
name: gsarti/flores_101_por
type: gsarti/flores_101_por
metrics:
- name: byte_perplexity
type: byte_perplexity
value: 1.9754515986213523
verified: false
- task:
type: text-generation
name: text generation
dataset:
name: gsarti/flores_101_pus
type: gsarti/flores_101_pus
metrics:
- name: byte_perplexity
type: byte_perplexity
value: 4.4963371422771585
verified: false
- task:
type: text-generation
name: text generation
dataset:
name: gsarti/flores_101_ron
type: gsarti/flores_101_ron
metrics:
- name: byte_perplexity
type: byte_perplexity
value: 4.965456830031304
verified: false
- task:
type: text-generation
name: text generation
dataset:
name: gsarti/flores_101_rus
type: gsarti/flores_101_rus
metrics:
- name: byte_perplexity
type: byte_perplexity
value: 2.0498020542445303
verified: false
- task:
type: text-generation
name: text generation
dataset:
name: gsarti/flores_101_slk
type: gsarti/flores_101_slk
metrics:
- name: byte_perplexity
type: byte_perplexity
value: 6.450822127057479
verified: false
- task:
type: text-generation
name: text generation
dataset:
name: gsarti/flores_101_slv
type: gsarti/flores_101_slv
metrics:
- name: byte_perplexity
type: byte_perplexity
value: 6.620252120186232
verified: false
- task:
type: text-generation
name: text generation
dataset:
name: gsarti/flores_101_sna
type: gsarti/flores_101_sna
metrics:
- name: byte_perplexity
type: byte_perplexity
value: 8.462166771382726
verified: false
- task:
type: text-generation
name: text generation
dataset:
name: gsarti/flores_101_snd
type: gsarti/flores_101_snd
metrics:
- name: byte_perplexity
type: byte_perplexity
value: 5.466066951221973
verified: false
- task:
type: text-generation
name: text generation
dataset:
name: gsarti/flores_101_som
type: gsarti/flores_101_som
metrics:
- name: byte_perplexity
type: byte_perplexity
value: 11.95918054093392
verified: false
- task:
type: text-generation
name: text generation
dataset:
name: gsarti/flores_101_spa
type: gsarti/flores_101_spa
metrics:
- name: byte_perplexity
type: byte_perplexity
value: 1.8965140104323535
verified: false
- task:
type: text-generation
name: text generation
dataset:
name: gsarti/flores_101_srp
type: gsarti/flores_101_srp
metrics:
- name: byte_perplexity
type: byte_perplexity
value: 2.871214785885079
verified: false
- task:
type: text-generation
name: text generation
dataset:
name: gsarti/flores_101_swe
type: gsarti/flores_101_swe
metrics:
- name: byte_perplexity
type: byte_perplexity
value: 5.054972008155866
verified: false
- task:
type: text-generation
name: text generation
dataset:
name: gsarti/flores_101_swh
type: gsarti/flores_101_swh
metrics:
- name: byte_perplexity
type: byte_perplexity
value: 3.6973091886730676
verified: false
- task:
type: text-generation
name: text generation
dataset:
name: gsarti/flores_101_tam
type: gsarti/flores_101_tam
metrics:
- name: byte_perplexity
type: byte_perplexity
value: 4.539493400469833
verified: false
- task:
type: text-generation
name: text generation
dataset:
name: gsarti/flores_101_tel
type: gsarti/flores_101_tel
metrics:
- name: byte_perplexity
type: byte_perplexity
value: 5.807499987508966
verified: false
- task:
type: text-generation
name: text generation
dataset:
name: gsarti/flores_101_tgk
type: gsarti/flores_101_tgk
metrics:
- name: byte_perplexity
type: byte_perplexity
value: 3.5994818827380426
verified: false
- task:
type: text-generation
name: text generation
dataset:
name: gsarti/flores_101_tgl
type: gsarti/flores_101_tgl
metrics:
- name: byte_perplexity
type: byte_perplexity
value: 5.667053833119858
verified: false
- task:
type: text-generation
name: text generation
dataset:
name: gsarti/flores_101_tha
type: gsarti/flores_101_tha
metrics:
- name: byte_perplexity
type: byte_perplexity
value: 2.365940201944242
verified: false
- task:
type: text-generation
name: text generation
dataset:
name: gsarti/flores_101_tur
type: gsarti/flores_101_tur
metrics:
- name: byte_perplexity
type: byte_perplexity
value: 4.885014749844601
verified: false
- task:
type: text-generation
name: text generation
dataset:
name: gsarti/flores_101_ukr
type: gsarti/flores_101_ukr
metrics:
- name: byte_perplexity
type: byte_perplexity
value: 2.7240934990288483
verified: false
- task:
type: text-generation
name: text generation
dataset:
name: gsarti/flores_101_umb
type: gsarti/flores_101_umb
metrics:
- name: byte_perplexity
type: byte_perplexity
value: 12.766915508610673
verified: false
- task:
type: text-generation
name: text generation
dataset:
name: gsarti/flores_101_urd
type: gsarti/flores_101_urd
metrics:
- name: byte_perplexity
type: byte_perplexity
value: 1.9797467071381232
verified: false
- task:
type: text-generation
name: text generation
dataset:
name: gsarti/flores_101_uzb
type: gsarti/flores_101_uzb
metrics:
- name: byte_perplexity
type: byte_perplexity
value: 12.002337637722146
verified: false
- task:
type: text-generation
name: text generation
dataset:
name: gsarti/flores_101_vie
type: gsarti/flores_101_vie
metrics:
- name: byte_perplexity
type: byte_perplexity
value: 1.76578415476397
verified: false
- task:
type: text-generation
name: text generation
dataset:
name: gsarti/flores_101_wol
type: gsarti/flores_101_wol
metrics:
- name: byte_perplexity
type: byte_perplexity
value: 9.144285650306488
verified: false
- task:
type: text-generation
name: text generation
dataset:
name: gsarti/flores_101_xho
type: gsarti/flores_101_xho
metrics:
- name: byte_perplexity
type: byte_perplexity
value: 7.403240538286952
verified: false
- task:
type: text-generation
name: text generation
dataset:
name: gsarti/flores_101_yor
type: gsarti/flores_101_yor
metrics:
- name: byte_perplexity
type: byte_perplexity
value: 5.91272037551173
verified: false
- task:
type: text-generation
name: text generation
dataset:
name: gsarti/flores_101_zho_simpl
type: gsarti/flores_101_zho_simpl
metrics:
- name: byte_perplexity
type: byte_perplexity
value: 2.2769070822768533
verified: false
- task:
type: text-generation
name: text generation
dataset:
name: gsarti/flores_101_zho_trad
type: gsarti/flores_101_zho_trad
metrics:
- name: byte_perplexity
type: byte_perplexity
value: 2.5180582198242383
verified: false
- task:
type: text-generation
name: text generation
dataset:
name: gsarti/flores_101_zul
type: gsarti/flores_101_zul
metrics:
- name: byte_perplexity
type: byte_perplexity
value: 8.53353320693145
verified: false
- task:
type: text-generation
name: text generation
dataset:
name: headqa
type: headqa
metrics:
- name: acc
type: acc
value: 0.26440554339897887
verified: false
- task:
type: text-generation
name: text generation
dataset:
name: hellaswag
type: hellaswag
metrics:
- name: acc
type: acc
value: 0.41236805417247563
verified: false
- task:
type: text-generation
name: text generation
dataset:
name: logiqa
type: logiqa
metrics:
- name: acc
type: acc
value: 0.2073732718894009
verified: false
- task:
type: text-generation
name: text generation
dataset:
name: mathqa
type: mathqa
metrics:
- name: acc
type: acc
value: 0.24958123953098826
verified: false
- task:
type: text-generation
name: text generation
dataset:
name: mc_taco
type: mc_taco
metrics:
- name: em
type: em
value: 0.11936936936936937
verified: false
- task:
type: text-generation
name: text generation
dataset:
name: mnli
type: mnli
metrics:
- name: acc
type: acc
value: 0.35496688741721855
verified: false
- task:
type: text-generation
name: text generation
dataset:
name: mnli_mismatched
type: mnli_mismatched
metrics:
- name: acc
type: acc
value: 0.35211554109031734
verified: false
- task:
type: text-generation
name: text generation
dataset:
name: mrpc
type: mrpc
metrics:
- name: acc
type: acc
value: 0.5857843137254902
verified: false
- task:
type: text-generation
name: text generation
dataset:
name: multirc
type: multirc
metrics:
- name: acc
type: acc
value: 0.5375412541254125
verified: false
- task:
type: text-generation
name: text generation
dataset:
name: openbookqa
type: openbookqa
metrics:
- name: acc
type: acc
value: 0.216
verified: false
- task:
type: text-generation
name: text generation
dataset:
name: piqa
type: piqa
metrics:
- name: acc
type: acc
value: 0.7078346028291621
verified: false
- task:
type: text-generation
name: text generation
dataset:
name: prost
type: prost
metrics:
- name: acc
type: acc
value: 0.22683603757472245
verified: false
- task:
type: text-generation
name: text generation
dataset:
name: pubmedqa
type: pubmedqa
metrics:
- name: acc
type: acc
value: 0.616
verified: false
- task:
type: text-generation
name: text generation
dataset:
name: qnli
type: qnli
metrics:
- name: acc
type: acc
value: 0.5072304594545122
verified: false
- task:
type: text-generation
name: text generation
dataset:
name: qqp
type: qqp
metrics:
- name: acc
type: acc
value: 0.3842443729903537
verified: false
- task:
type: text-generation
name: text generation
dataset:
name: race
type: race
metrics:
- name: acc
type: acc
value: 0.3521531100478469
verified: false
- task:
type: text-generation
name: text generation
dataset:
name: rte
type: rte
metrics:
- name: acc
type: acc
value: 0.47653429602888087
verified: false
- task:
type: text-generation
name: text generation
dataset:
name: sciq
type: sciq
metrics:
- name: acc
type: acc
value: 0.892
verified: false
- task:
type: text-generation
name: text generation
dataset:
name: sst
type: sst
metrics:
- name: acc
type: acc
value: 0.5177752293577982
verified: false
- task:
type: text-generation
name: text generation
dataset:
name: triviaqa
type: triviaqa
metrics:
- name: acc
type: acc
value: 0.041633518960487934
verified: false
- task:
type: text-generation
name: text generation
dataset:
name: tydiqa_primary
type: tydiqa_primary
metrics:
- name: acc
type: acc
value: 0.3011337608795236
verified: false
- task:
type: text-generation
name: text generation
dataset:
name: webqs
type: webqs
metrics:
- name: acc
type: acc
value: 0.01673228346456693
verified: false
- task:
type: text-generation
name: text generation
dataset:
name: wic
type: wic
metrics:
- name: acc
type: acc
value: 0.5015673981191222
verified: false
- task:
type: text-generation
name: text generation
dataset:
name: winogrande
type: winogrande
metrics:
- name: acc
type: acc
value: 0.5864246250986582
verified: false
- task:
type: text-generation
name: text generation
dataset:
name: wnli
type: wnli
metrics:
- name: acc
type: acc
value: 0.471830985915493
verified: false
- task:
type: text-generation
name: text generation
dataset:
name: wsc
type: wsc
metrics:
- name: acc
type: acc
value: 0.4423076923076923
verified: false
- task:
type: text-generation
name: text generation
dataset:
name: humaneval
type: humaneval
metrics:
- name: pass@1
type: pass@1
value: 0.15524390243902436
verified: false
- name: pass@10
type: pass@10
value: 0.3220367632383857
verified: false
- name: pass@100
type: pass@100
value: 0.5545431515723145
verified: false
---
<h1 style='text-align: center '>BLOOM LM</h1>
<h2 style='text-align: center '><em>BigScience Large Open-science Open-access Multilingual Language Model</em> </h2>
<h3 style='text-align: center '>Model Card</h3>
<img src="https://s3.amazonaws.com/moonup/production/uploads/1657124309515-5f17f0a0925b9863e28ad517.png" alt="BigScience Logo" width="800" style="margin-left:'auto' margin-right:'auto' display:'block'"/>
Version 1.0 / 26.May.2022
## Table of Contents
1. [Model Details](#model-details)
2. [Uses](#uses)
3. [Training Data](#training-data)
4. [Risks and Limitations](#risks-and-limitations)
5. [Evaluation](#evaluation)
6. [Recommendations](#recommendations)
7. [Glossary and Calculations](#glossary-and-calculations)
8. [More Information](#more-information)
9. [Model Card Authors](#model-card-authors)
## Model Details
### Basics
*This section provides information for anyone who wants to know about the model.*
<details>
<summary>Click to expand</summary> <br/>
**Developed by:** BigScience ([website](https://bigscience.huggingface.co))
* All collaborators are either volunteers or have an agreement with their employer. *(Further breakdown of participants forthcoming.)*
**Model Type:** Transformer-based Language Model
**Version:** 1.0.0
**Languages:** Multiple; see [training data](#training-data)
**License:** RAIL License v1.0 ([link](https://huggingface.co/spaces/bigscience/license))
**Release Date Estimate:** Monday, 11.July.2022
**Send Questions to:** [email protected]
**Cite as:** BigScience, _BigScience Language Open-science Open-access Multilingual (BLOOM) Language Model_. International, May 2021-May 2022
**Funded by:**
* The French government.
* Hugging Face ([website](https://huggingface.co)).
* Organizations of contributors. *(Further breakdown of organizations forthcoming.)*
</details>
### Technical Specifications
*This section provides information for people who work on model development.*
<details>
<summary>Click to expand</summary><br/>
Please see [the BLOOM training README](https://github.com/bigscience-workshop/bigscience/tree/master/train/tr11-176B-ml#readme) for full details on replicating training.
**Model Architecture:** Modified from Megatron-LM GPT2 (see [paper](https://arxiv.org/abs/1909.08053), [BLOOM Megatron code](https://github.com/bigscience-workshop/Megatron-DeepSpeed)):
* Decoder-only architecture
* Layer normalization applied to word embeddings layer (`StableEmbedding`; see [code](https://github.com/facebookresearch/bitsandbytes), [paper](https://arxiv.org/pdf/2110.02861.pdf))
* ALiBI positional encodings (see [paper](https://arxiv.org/pdf/2108.12409.pdf)), with GeLU activation functions
* 3,002,557,440 parameters:
* 642,252,800 embedding parameters
* 30 layers, 32 attention heads
* Hidden layers are 2560-dimensional
* Sequence length of 2048 tokens used (see [BLOOM tokenizer](https://huggingface.co/bigscience/tokenizer), [tokenizer description](#tokenization))
**Objective Function:** Cross Entropy with mean reduction (see [API documentation](https://pytorch.org/docs/stable/generated/torch.nn.CrossEntropyLoss.html#torch.nn.CrossEntropyLoss)).
**Compute infrastructure:** Jean Zay Public Supercomputer, provided by the French government (see [announcement](https://www.enseignementsup-recherche.gouv.fr/fr/signature-du-marche-d-acquisition-de-l-un-des-supercalculateurs-les-plus-puissants-d-europe-46733)).
* Hardware: 384 A100 80GB GPUs (48 nodes):
* Additional 32 A100 80GB GPUs (4 nodes) in reserve
* 8 GPUs per node Using NVLink 4 inter-gpu connects, 4 OmniPath links
* CPU: AMD
* CPU memory: 512GB per node
* GPU memory: 640GB per node
* Inter-node connect: Omni-Path Architecture (OPA)
* NCCL-communications network: a fully dedicated subnet
* Disc IO network: shared network with other types of nodes
* Software:
* Megatron-DeepSpeed ([Github link](https://github.com/bigscience-workshop/Megatron-DeepSpeed))
* DeepSpeed ([Github link](https://github.com/microsoft/DeepSpeed))
* PyTorch (pytorch-1.11 w/ CUDA-11.5; see [Github link](https://github.com/pytorch/pytorch))
* apex ([Github link](https://github.com/NVIDIA/apex))
#### **Training**
Training logs: [Tensorboard link](https://huggingface.co/tensorboard/bigscience/tr11c-2B5-logs)
- Number of epochs: 1 (*current target*)
- Dates:
- Started 11th March, 2022 11:42am PST
- Ended 5th July, 2022
- Estimated cost of training: Equivalent of $2-5M in cloud computing (including preliminary experiments)
- Server training location: Île-de-France, France
#### **Tokenization**
The BLOOM tokenizer ([link](https://huggingface.co/bigscience/tokenizer)) is a learned subword tokenizer trained using:
- A byte-level Byte Pair Encoding (BPE) algorithm
- A simple pre-tokenization rule, no normalization
- A vocabulary size of 250,680
It was trained on a subset of a preliminary version of the corpus using alpha-weighting per language.
</details>
### Environmental Impact
<details>
<summary>Click to expand</summary><br/>
The training supercomputer, Jean Zay ([website](http://www.idris.fr/eng/jean-zay/jean-zay-presentation-eng.html)), uses mostly nuclear energy. The heat generated by it is reused for heating campus housing.
**Estimated carbon emissions:** *(Forthcoming upon completion of training.)*
**Estimated electricity usage:** *(Forthcoming upon completion of training.)*
</details>
<p> </p>
## Uses
*This section addresses questions around how the model is intended to be used, discusses the foreseeable users of the model (including those affected by the model), and describes uses that are considered out of scope or misuse of the model.
It provides information for anyone considering using the model or who is affected by the model.*
<details>
<summary>Click to expand</summary><br/>
### Intended Use
This model is being created in order to enable public research on large language models (LLMs). LLMs are intended to be used for language generation or as a pretrained base model that can be further fine-tuned for specific tasks. Use cases below are not exhaustive.
#### **Direct Use**
- Text generation
- Exploring characteristics of language generated by a language model
- Examples: Cloze tests, counterfactuals, generations with reframings
#### **Downstream Use**
- Tasks that leverage language models include: Information Extraction, Question Answering, Summarization
### Misuse and Out-of-scope Use
*This section addresses what users ought not do with the model.*
See the [BLOOM License](https://huggingface.co/spaces/bigscience/license), Attachment A, for detailed usage restrictions. The below list is non-exhaustive, but lists some easily foreseeable problematic use cases.
#### **Out-of-scope Uses**
Using the model in [high-stakes](#high-stakes) settings is out of scope for this model. The model is not designed for [critical decisions](#critical-decisions) nor uses with any material consequences on an individual's livelihood or wellbeing. The model outputs content that appears factual but is not correct.
##### Out-of-scope Uses Include:
- Usage in biomedical domains, political and legal domains, or finance domains
- Usage for evaluating or scoring individuals, such as for employment, education, or credit
- Applying the model for critical automatic decisions, generating factual content, creating reliable summaries, or generating predictions that must be correct
#### **Misuse**
Intentionally using the model for harm, violating [human rights](#human-rights), or other kinds of malicious activities, is a misuse of this model. This includes:
- Spam generation
- Disinformation and influence operations
- Disparagement and defamation
- Harassment and abuse
- [Deception](#deception)
- Unconsented impersonation and imitation
- Unconsented surveillance
- Generating content without attribution to the model, as specified in the [RAIL License, Use Restrictions](https://huggingface.co/spaces/bigscience/license)
### Intended Users
#### **Direct Users**
- General Public
- Researchers
- Students
- Educators
- Engineers/developers
- Non-commercial entities
- Community advocates, including human and civil rights groups
#### Indirect Users
- Users of derivatives created by Direct Users, such as those using software with an [intended use](#intended-use)
- Users of [Derivatives of the Model, as described in the License](https://huggingface.co/spaces/bigscience/license)
#### Others Affected (Parties Prenantes)
- People and groups referred to by the LLM
- People and groups exposed to outputs of, or decisions based on, the LLM
- People and groups whose original work is included in the LLM
</details>
<p> </p>
## Training Data
*This section provides a high-level overview of the training data. It is relevant for anyone who wants to know the basics of what the model is learning.*
<details>
<summary>Click to expand</summary><br/>
Details for each dataset are provided in individual [Data Cards](https://huggingface.co/spaces/bigscience/BigScienceCorpus).
Training data includes:
- 45 natural languages
- 12 programming languages
- In 1.5TB of pre-processed text, converted into 350B unique tokens (see [the tokenizer section](#tokenization) for more.)
#### **Languages**
The pie chart shows the distribution of languages in training data.

The following table shows the further distribution of Niger-Congo and Indic languages in the training data.
<details>
<summary>Click to expand</summary><br/>
| Niger Congo | Percentage | | Indic | Percentage |
|----------------|------------ |------ |-----------|------------|
| Chi Tumbuka | 0.00002 | | Assamese | 0.01 |
| Kikuyu | 0.00004 | | Odia | 0.04 |
| Bambara | 0.00004 | | Gujarati | 0.04 |
| Akan | 0.00007 | | Marathi | 0.05 |
| Xitsonga | 0.00007 | | Punjabi | 0.05 |
| Sesotho | 0.00007 | | Kannada | 0.06 |
| Chi Chewa | 0.0001 | | Nepali | 0.07 |
| Setswana | 0.0002 | | Telugu | 0.09 |
| Northern Sotho | 0.0002 | | Malayalam | 0.10 |
| Fon | 0.0002 | | Urdu | 0.10 |
| Kirundi | 0.0003 | | Tamil | 0.20 |
| Wolof | 0.0004 | | Bengali | 0.50 |
| Kuganda | 0.0004 | | Hindi | 0.70 |
| Chi Shona | 0.001 |
| Isi Zulu | 0.001 |
| Igbo | 0.001 |
| Xhosa | 0.001 |
| Kinyarwanda | 0.003 |
| Yoruba | 0.006 |
| Swahili | 0.02 |
</details>
The following table shows the distribution of programming languages.
<details>
<summary>Click to expand</summary><br/>
| Extension | Language | Number of files |
|----------------|------------|-----------------|
| java | Java | 5,407,724 |
| php | PHP | 4,942,186 |
| cpp | C++ | 2,503,930 |
| py | Python | 2,435,072 |
| js | JavaScript | 1,905,518 |
| cs | C# | 1,577,347 |
| rb | Ruby | 6,78,413 |
| cc | C++ | 443,054 |
| hpp | C++ | 391,048 |
| lua | Lua | 352,317 |
| go | GO | 227,763 |
| ts | TypeScript | 195,254 |
| C | C | 134,537 |
| scala | Scala | 92,052 |
| hh | C++ | 67,161 |
| H | C++ | 55,899 |
| tsx | TypeScript | 33,107 |
| rs | Rust | 29,693 |
| phpt | PHP | 9,702 |
| c++ | C++ | 1,342 |
| h++ | C++ | 791 |
| php3 | PHP | 540 |
| phps | PHP | 270 |
| php5 | PHP | 166 |
| php4 | PHP | 29 |
</details>
</details>
<p> </p>
## Risks and Limitations
*This section identifies foreseeable harms and misunderstandings.*
<details>
<summary>Click to expand</summary><br/>
Model may:
- Overrepresent some viewpoints and underrepresent others
- Contain stereotypes
- Contain [personal information](#personal-data-and-information)
- Generate:
- Hateful, abusive, or violent language
- Discriminatory or prejudicial language
- Content that may not be appropriate for all settings, including sexual content
- Make errors, including producing incorrect information as if it were factual
- Generate irrelevant or repetitive outputs
</details>
<p> </p>
## Evaluation
*This section describes the evaluation protocols and provides the results.*
<details>
<summary>Click to expand</summary><br/>
### Metrics
*This section describes the different ways performance is calculated and why.*
Includes:
| Metric | Why chosen |
|--------------------|--------------------------------------------------------------------|
| [Perplexity](#perplexity) | Standard metric for quantifying model improvements during training |
| Cross Entropy [Loss](#loss) | Standard objective for language models. |
And multiple different metrics for specific tasks. _(More evaluation metrics forthcoming upon completion of evaluation protocol.)_
### Factors
*This section lists some different aspects of BLOOM models. Its focus is on aspects that are likely to give rise to high variance in model behavior.*
- Language, such as English or Yoruba
- Domain, such as newswire or stories
- Demographic characteristics, such as gender or nationality
### Results
*Results are based on the [Factors](#factors) and [Metrics](#metrics).*
**Zero-shot evaluations:**
See this repository for JSON files: https://github.com/bigscience-workshop/evaluation-results
| Task | Language | Metric | BLOOM-2B5 |
|:----|:----|:----|:----:|
| arc_challenge | eng | acc ↑ | 0.28 |
| arc_easy | eng | acc ↑ | 0.595 |
| axb (Median of 10 prompts) | eng | acc ↑ | 0.443 |
| axg (Median of 10 prompts) | eng | acc ↑ | 0.5 |
| boolq (Median of 11 prompts) | eng | acc ↑ | 0.617 |
| cb (Median of 15 prompts) | eng | acc ↑ | 0.304 |
| cola (Median of 5 prompts) | eng | acc ↑ | 0.611 |
| copa (Median of 9 prompts) | eng | acc ↑ | 0.63 |
| crows_pairs_english (Median of 6 prompts) | eng | acc ↑ | 0.497 |
| crows_pairs_french (Median of 7 prompts) | fra | acc ↑ | 0.503 |
| diabla (Median of 2 prompts) | eng | acc ↑ | 0.289 |
| gsarti/flores_101_afr | afr | byte_perplexity ↓ | 6.501 |
| gsarti/flores_101_amh | amh | byte_perplexity ↓ | 3.973 |
| gsarti/flores_101_ara | ara | byte_perplexity ↓ | 1.808 |
| gsarti/flores_101_asm | asm | byte_perplexity ↓ | 5.699 |
| gsarti/flores_101_ast | ast | byte_perplexity ↓ | 3.925 |
| gsarti/flores_101_azj | azj | byte_perplexity ↓ | 6.943 |
| gsarti/flores_101_bel | bel | byte_perplexity ↓ | 3.614 |
| gsarti/flores_101_ben | ben | byte_perplexity ↓ | 5.121 |
| gsarti/flores_101_bos | bos | byte_perplexity ↓ | 5.653 |
| gsarti/flores_101_bul | bul | byte_perplexity ↓ | 2.701 |
| gsarti/flores_101_cat | cat | byte_perplexity ↓ | 2.305 |
| gsarti/flores_101_ceb | ceb | byte_perplexity ↓ | 6.291 |
| gsarti/flores_101_ces | ces | byte_perplexity ↓ | 5.447 |
| gsarti/flores_101_ckb | ckb | byte_perplexity ↓ | 3.726 |
| gsarti/flores_101_cym | cym | byte_perplexity ↓ | 12.539 |
| gsarti/flores_101_dan | dan | byte_perplexity ↓ | 5.183 |
| gsarti/flores_101_deu | deu | byte_perplexity ↓ | 3.118 |
| gsarti/flores_101_ell | ell | byte_perplexity ↓ | 2.468 |
| gsarti/flores_101_eng | eng | byte_perplexity ↓ | 2.019 |
| gsarti/flores_101_est | est | byte_perplexity ↓ | 9.117 |
| gsarti/flores_101_fas | fas | byte_perplexity ↓ | 3.058 |
| gsarti/flores_101_fin | fin | byte_perplexity ↓ | 6.847 |
| gsarti/flores_101_fra | fra | byte_perplexity ↓ | 1.998 |
| gsarti/flores_101_ful | ful | byte_perplexity ↓ | 11.466 |
| gsarti/flores_101_gle | gle | byte_perplexity ↓ | 8.681 |
| gsarti/flores_101_glg | glg | byte_perplexity ↓ | 3.03 |
| gsarti/flores_101_guj | guj | byte_perplexity ↓ | 4.955 |
| gsarti/flores_101_hau | hau | byte_perplexity ↓ | 10.758 |
| gsarti/flores_101_heb | heb | byte_perplexity ↓ | 3.6 |
| gsarti/flores_101_hin | hin | byte_perplexity ↓ | 4.713 |
| gsarti/flores_101_hrv | hrv | byte_perplexity ↓ | 5.822 |
| gsarti/flores_101_hun | hun | byte_perplexity ↓ | 6.44 |
| gsarti/flores_101_hye | hye | byte_perplexity ↓ | 3.658 |
| gsarti/flores_101_ibo | ibo | byte_perplexity ↓ | 5.565 |
| gsarti/flores_101_ind | ind | byte_perplexity ↓ | 2.16 |
| gsarti/flores_101_isl | isl | byte_perplexity ↓ | 8.082 |
| gsarti/flores_101_ita | ita | byte_perplexity ↓ | 2.969 |
| gsarti/flores_101_jav | jav | byte_perplexity ↓ | 7.057 |
| gsarti/flores_101_jpn | jpn | byte_perplexity ↓ | 2.776 |
| gsarti/flores_101_kam | kam | byte_perplexity ↓ | 11.073 |
| gsarti/flores_101_kan | kan | byte_perplexity ↓ | 5.552 |
| gsarti/flores_101_kat | kat | byte_perplexity ↓ | 2.523 |
| gsarti/flores_101_kaz | kaz | byte_perplexity ↓ | 3.39 |
| gsarti/flores_101_kea | kea | byte_perplexity ↓ | 8.919 |
| gsarti/flores_101_kir | kir | byte_perplexity ↓ | 3.729 |
| gsarti/flores_101_kor | kor | byte_perplexity ↓ | 3.933 |
| gsarti/flores_101_lao | lao | byte_perplexity ↓ | 2.908 |
| gsarti/flores_101_lav | lav | byte_perplexity ↓ | 7.777 |
| gsarti/flores_101_lin | lin | byte_perplexity ↓ | 7.525 |
| gsarti/flores_101_lit | lit | byte_perplexity ↓ | 7.369 |
| gsarti/flores_101_ltz | ltz | byte_perplexity ↓ | 8.801 |
| gsarti/flores_101_lug | lug | byte_perplexity ↓ | 8.483 |
| gsarti/flores_101_luo | luo | byte_perplexity ↓ | 11.976 |
| gsarti/flores_101_mal | mal | byte_perplexity ↓ | 4.616 |
| gsarti/flores_101_mar | mar | byte_perplexity ↓ | 5.483 |
| gsarti/flores_101_mkd | mkd | byte_perplexity ↓ | 2.966 |
| gsarti/flores_101_mlt | mlt | byte_perplexity ↓ | 15.005 |
| gsarti/flores_101_mon | mon | byte_perplexity ↓ | 3.411 |
| gsarti/flores_101_mri | mri | byte_perplexity ↓ | 7.474 |
| gsarti/flores_101_msa | msa | byte_perplexity ↓ | 2.571 |
| gsarti/flores_101_mya | mya | byte_perplexity ↓ | 2.414 |
| gsarti/flores_101_nld | nld | byte_perplexity ↓ | 4.128 |
| gsarti/flores_101_nob | nob | byte_perplexity ↓ | 5.403 |
| gsarti/flores_101_npi | npi | byte_perplexity ↓ | 5.199 |
| gsarti/flores_101_nso | nso | byte_perplexity ↓ | 8.155 |
| gsarti/flores_101_nya | nya | byte_perplexity ↓ | 8.18 |
| gsarti/flores_101_oci | oci | byte_perplexity ↓ | 4.862 |
| gsarti/flores_101_orm | orm | byte_perplexity ↓ | 12.912 |
| gsarti/flores_101_ory | ory | byte_perplexity ↓ | 5.189 |
| gsarti/flores_101_pan | pan | byte_perplexity ↓ | 4.698 |
| gsarti/flores_101_pol | pol | byte_perplexity ↓ | 4.626 |
| gsarti/flores_101_por | por | byte_perplexity ↓ | 1.975 |
| gsarti/flores_101_pus | pus | byte_perplexity ↓ | 4.496 |
| gsarti/flores_101_ron | ron | byte_perplexity ↓ | 4.965 |
| gsarti/flores_101_rus | rus | byte_perplexity ↓ | 2.05 |
| gsarti/flores_101_slk | slk | byte_perplexity ↓ | 6.451 |
| gsarti/flores_101_slv | slv | byte_perplexity ↓ | 6.62 |
| gsarti/flores_101_sna | sna | byte_perplexity ↓ | 8.462 |
| gsarti/flores_101_snd | snd | byte_perplexity ↓ | 5.466 |
| gsarti/flores_101_som | som | byte_perplexity ↓ | 11.959 |
| gsarti/flores_101_spa | spa | byte_perplexity ↓ | 1.897 |
| gsarti/flores_101_srp | srp | byte_perplexity ↓ | 2.871 |
| gsarti/flores_101_swe | swe | byte_perplexity ↓ | 5.055 |
| gsarti/flores_101_swh | swh | byte_perplexity ↓ | 3.697 |
| gsarti/flores_101_tam | tam | byte_perplexity ↓ | 4.539 |
| gsarti/flores_101_tel | tel | byte_perplexity ↓ | 5.807 |
| gsarti/flores_101_tgk | tgk | byte_perplexity ↓ | 3.599 |
| gsarti/flores_101_tgl | tgl | byte_perplexity ↓ | 5.667 |
| gsarti/flores_101_tha | tha | byte_perplexity ↓ | 2.366 |
| gsarti/flores_101_tur | tur | byte_perplexity ↓ | 4.885 |
| gsarti/flores_101_ukr | ukr | byte_perplexity ↓ | 2.724 |
| gsarti/flores_101_umb | umb | byte_perplexity ↓ | 12.767 |
| gsarti/flores_101_urd | urd | byte_perplexity ↓ | 1.98 |
| gsarti/flores_101_uzb | uzb | byte_perplexity ↓ | 12.002 |
| gsarti/flores_101_vie | vie | byte_perplexity ↓ | 1.766 |
| gsarti/flores_101_wol | wol | byte_perplexity ↓ | 9.144 |
| gsarti/flores_101_xho | xho | byte_perplexity ↓ | 7.403 |
| gsarti/flores_101_yor | yor | byte_perplexity ↓ | 5.913 |
| gsarti/flores_101_zho_simpl | zho_simpl | byte_perplexity ↓ | 2.277 |
| gsarti/flores_101_zho_trad | zho_trad | byte_perplexity ↓ | 2.518 |
| gsarti/flores_101_zul | zul | byte_perplexity ↓ | 8.534 |
| headqa | esp | acc ↑ | 0.264 |
| hellaswag | eng | acc ↑ | 0.412 |
| logiqa | eng | acc ↑ | 0.207 |
| mathqa | eng | acc ↑ | 0.25 |
| mc_taco | eng | em ↑ | 0.119 |
| mnli (Median of 15 prompts) | eng | acc ↑ | 0.355 |
| mnli_mismatched (Median of 15 prompts) | eng | acc ↑ | 0.352 |
| mrpc | eng | acc ↑ | 0.586 |
| multirc (Median of 11 prompts) | eng | acc ↑ | 0.538 |
| openbookqa | eng | acc ↑ | 0.216 |
| piqa | eng | acc ↑ | 0.708 |
| prost | eng | acc ↑ | 0.227 |
| pubmedqa | eng | acc ↑ | 0.616 |
| qnli | eng | acc ↑ | 0.507 |
| qqp (Median of 7 prompts) | eng | acc ↑ | 0.384 |
| race | eng | acc ↑ | 0.352 |
| rte (Median of 6 prompts) | eng | acc ↑ | 0.477 |
| sciq | eng | acc ↑ | 0.892 |
| sst (Median of 6 prompts) | eng | acc ↑ | 0.518 |
| triviaqa | eng | acc ↑ | 0.042 |
| tydiqa_primary (Median of 24 prompts) | eng | acc ↑ | 0.301 |
| webqs | eng | acc ↑ | 0.017 |
| wic (Median of 11 prompts) | eng | acc ↑ | 0.502 |
| winogrande | eng | acc ↑ | 0.586 |
| wnli (Median of 6 prompts) | eng | acc ↑ | 0.472 |
| wsc (Median of 11 prompts) | eng | acc ↑ | 0.442 |
| humaneval | python | pass@1 ↑ | 0.155 |
| humaneval | python | pass@10 ↑ | 0.322 |
| humaneval | python | pass@100 ↑ | 0.555 |
**Train-time Evaluation:**
As of 25.May.2022, 15:00 PST:
- Training Loss: 2.0
- Validation Loss: 2.2
- Perplexity: 8.9
</details>
<p> </p>
## Recommendations
*This section provides information on warnings and potential mitigations.*
<details>
<summary>Click to expand</summary><br/>
- Indirect users should be made aware when the content they're working with is created by the LLM.
- Users should be aware of [Risks and Limitations](#risks-and-limitations), and include an appropriate age disclaimer or blocking interface as necessary.
- Models pretrained with the LLM should include an updated Model Card.
- Users of the model should provide mechanisms for those affected to provide feedback, such as an email address for comments.
</details>
<p> </p>
## Glossary and Calculations
*This section defines common terms and how metrics are calculated.*
<details>
<summary>Click to expand</summary><br/>
- <a name="loss">**Loss:**</a> A calculation of the difference between what the model has learned and what the data shows ("groundtruth"). The lower the loss, the better. The training process aims to minimize the loss.
- <a name="perplexity">**Perplexity:**</a> This is based on what the model estimates the probability of new data is. The lower the perplexity, the better. If the model is 100% correct at predicting the next token it will see, then the perplexity is 1. Mathematically this is calculated using entropy.
- <a name="high-stakes">**High-stakes settings:**</a> Such as those identified as "high-risk AI systems" and "unacceptable risk AI systems" in the European Union's proposed [Artificial Intelligence (AI) Act](https://artificialintelligenceact.eu/annexes/).
- <a name="critical-decisions">**Critical decisions:**</a> Such as those defined in [the United States' proposed Algorithmic Accountability Act](https://www.congress.gov/117/bills/s3572/BILLS-117s3572is.pdf).
- <a name="human-rights">**Human rights:**</a> Includes those rights defined in the [Universal Declaration of Human Rights](https://www.un.org/sites/un2.un.org/files/2021/03/udhr.pdf).
- <a name="personal-data-and-information">**Personal Data and Personal Information:**</a> Personal data and information is defined in multiple data protection regulations, such as "[personal data](https://gdpr-info.eu/issues/personal-data/)" in the [European Union's General Data Protection Regulation](https://gdpr-info.eu); and "personal information" in the Republic of South Africa's [Protection of Personal Information Act](https://www.gov.za/sites/default/files/gcis_document/201409/3706726-11act4of2013popi.pdf), The People's Republic of China's [Personal information protection law](http://en.npc.gov.cn.cdurl.cn/2021-12/29/c_694559.htm).
- <a name="sensitive-characteristics">**Sensitive characteristics:**</a> This includes specifically protected categories in human rights (see [UHDR, Article 2](https://www.un.org/sites/un2.un.org/files/2021/03/udhr.pdf)) and personal information regulation (see GDPR, [Article 9; Protection of Personal Information Act, Chapter 1](https://www.gov.za/sites/default/files/gcis_document/201409/3706726-11act4of2013popi.pdf))
- <a name="deception">**Deception:**</a> Doing something to intentionally mislead individuals to believe something that is false, such as by creating deadbots or chatbots on social media posing as real people, or generating text documents without making consumers aware that the text is machine generated.
</details>
<p> </p>
## More Information
<details>
<summary>Click to expand</summary><br/>
### Dataset Creation
Blog post detailing the design choices during the dataset creation: https://bigscience.huggingface.co/blog/building-a-tb-scale-multilingual-dataset-for-language-modeling
### Technical Specifications
Blog post summarizing how the architecture, size, shape, and pre-training duration where selected: https://bigscience.huggingface.co/blog/what-language-model-to-train-if-you-have-two-million-gpu-hours
More details on the architecture/optimizer: https://github.com/bigscience-workshop/bigscience/tree/master/train/tr11-176B-ml
Blog post on the hardware/engineering side: https://bigscience.huggingface.co/blog/which-hardware-to-train-a-176b-parameters-model
Details on the distributed setup used for the training: https://github.com/bigscience-workshop/bigscience/tree/master/train/tr11-176B-ml
Tensorboard updated during the training: https://huggingface.co/bigscience/tr11-176B-ml-logs/tensorboard#scalars&tagFilter=loss
Insights on how to approach training, negative results: https://github.com/bigscience-workshop/bigscience/blob/master/train/lessons-learned.md
Details on the obstacles overcome during the preparation on the engineering side (instabilities, optimization of training throughput, so many technical tricks and questions): https://github.com/bigscience-workshop/bigscience/blob/master/train/tr11-176B-ml/chronicles.md
### Initial Results
Initial prompting experiments using interim checkpoints: https://huggingface.co/spaces/bigscience/bloom-book
</details>
<p> </p>
## Model Card Authors
*Ordered roughly chronologically and by amount of time spent.*
Margaret Mitchell, Giada Pistilli, Yacine Jernite, Ezinwanne Ozoani, Marissa Gerchick, Nazneen Rajani, Sasha Luccioni, Irene Solaiman, Maraim Masoud, Somaieh Nikpoor, Carlos Muñoz Ferrandis, Stas Bekman, Christopher Akiki, Danish Contractor, David Lansky, Angelina McMillan-Major, Tristan Thrush, Suzana Ilić, Gérard Dupont, Shayne Longpre, Manan Dey, Stella Biderman, Douwe Kiela, Emi Baylor, Teven Le Scao, Aaron Gokaslan, Julien Launay, Niklas Muennighoff
|
RichardErkhov/traversaal-ai_-_traversaal-2.5-Mistral-7B-gguf | RichardErkhov | "2024-06-21T09:01:22Z" | 12,795 | 0 | null | [
"gguf",
"region:us"
] | null | "2024-06-21T06:33:21Z" | Entry not found |
robertgshaw2/zephyr-7b-beta-channelwise-gptq | robertgshaw2 | "2024-02-18T18:49:56Z" | 12,794 | 0 | transformers | [
"transformers",
"safetensors",
"mistral",
"text-generation",
"conversational",
"autotrain_compatible",
"endpoints_compatible",
"text-generation-inference",
"4-bit",
"gptq",
"region:us"
] | text-generation | "2024-02-18T18:48:37Z" | Entry not found |
mradermacher/NeuralStar_Story-9b-i1-GGUF | mradermacher | "2024-06-20T12:30:55Z" | 12,768 | 1 | transformers | [
"transformers",
"gguf",
"merge",
"mergekit",
"lazymergekit",
"OmnicromsBrain/StoryFusion-7B",
"OmnicromsBrain/NeuralStar-7b-Lazy",
"en",
"base_model:OmnicromsBrain/NeuralStar_Story-9b",
"endpoints_compatible",
"region:us"
] | null | "2024-06-19T23:01:46Z" | ---
base_model: OmnicromsBrain/NeuralStar_Story-9b
language:
- en
library_name: transformers
quantized_by: mradermacher
tags:
- merge
- mergekit
- lazymergekit
- OmnicromsBrain/StoryFusion-7B
- OmnicromsBrain/NeuralStar-7b-Lazy
---
## About
<!-- ### quantize_version: 2 -->
<!-- ### output_tensor_quantised: 1 -->
<!-- ### convert_type: hf -->
<!-- ### vocab_type: -->
<!-- ### tags: nicoboss -->
weighted/imatrix quants of https://huggingface.co/OmnicromsBrain/NeuralStar_Story-9b
<!-- provided-files -->
static quants are available at https://huggingface.co/mradermacher/NeuralStar_Story-9b-GGUF
## Usage
If you are unsure how to use GGUF files, refer to one of [TheBloke's
READMEs](https://huggingface.co/TheBloke/KafkaLM-70B-German-V0.1-GGUF) for
more details, including on how to concatenate multi-part files.
## Provided Quants
(sorted by size, not necessarily quality. IQ-quants are often preferable over similar sized non-IQ quants)
| Link | Type | Size/GB | Notes |
|:-----|:-----|--------:|:------|
| [GGUF](https://huggingface.co/mradermacher/NeuralStar_Story-9b-i1-GGUF/resolve/main/NeuralStar_Story-9b.i1-IQ1_S.gguf) | i1-IQ1_S | 2.5 | for the desperate |
| [GGUF](https://huggingface.co/mradermacher/NeuralStar_Story-9b-i1-GGUF/resolve/main/NeuralStar_Story-9b.i1-IQ1_M.gguf) | i1-IQ1_M | 2.7 | mostly desperate |
| [GGUF](https://huggingface.co/mradermacher/NeuralStar_Story-9b-i1-GGUF/resolve/main/NeuralStar_Story-9b.i1-IQ2_XXS.gguf) | i1-IQ2_XXS | 3.0 | |
| [GGUF](https://huggingface.co/mradermacher/NeuralStar_Story-9b-i1-GGUF/resolve/main/NeuralStar_Story-9b.i1-IQ2_XS.gguf) | i1-IQ2_XS | 3.3 | |
| [GGUF](https://huggingface.co/mradermacher/NeuralStar_Story-9b-i1-GGUF/resolve/main/NeuralStar_Story-9b.i1-IQ2_S.gguf) | i1-IQ2_S | 3.5 | |
| [GGUF](https://huggingface.co/mradermacher/NeuralStar_Story-9b-i1-GGUF/resolve/main/NeuralStar_Story-9b.i1-IQ2_M.gguf) | i1-IQ2_M | 3.8 | |
| [GGUF](https://huggingface.co/mradermacher/NeuralStar_Story-9b-i1-GGUF/resolve/main/NeuralStar_Story-9b.i1-Q2_K.gguf) | i1-Q2_K | 4.1 | IQ3_XXS probably better |
| [GGUF](https://huggingface.co/mradermacher/NeuralStar_Story-9b-i1-GGUF/resolve/main/NeuralStar_Story-9b.i1-IQ3_XXS.gguf) | i1-IQ3_XXS | 4.3 | lower quality |
| [GGUF](https://huggingface.co/mradermacher/NeuralStar_Story-9b-i1-GGUF/resolve/main/NeuralStar_Story-9b.i1-IQ3_XS.gguf) | i1-IQ3_XS | 4.5 | |
| [GGUF](https://huggingface.co/mradermacher/NeuralStar_Story-9b-i1-GGUF/resolve/main/NeuralStar_Story-9b.i1-Q3_K_S.gguf) | i1-Q3_K_S | 4.8 | IQ3_XS probably better |
| [GGUF](https://huggingface.co/mradermacher/NeuralStar_Story-9b-i1-GGUF/resolve/main/NeuralStar_Story-9b.i1-IQ3_S.gguf) | i1-IQ3_S | 4.8 | beats Q3_K* |
| [GGUF](https://huggingface.co/mradermacher/NeuralStar_Story-9b-i1-GGUF/resolve/main/NeuralStar_Story-9b.i1-IQ3_M.gguf) | i1-IQ3_M | 4.9 | |
| [GGUF](https://huggingface.co/mradermacher/NeuralStar_Story-9b-i1-GGUF/resolve/main/NeuralStar_Story-9b.i1-Q3_K_M.gguf) | i1-Q3_K_M | 5.3 | IQ3_S probably better |
| [GGUF](https://huggingface.co/mradermacher/NeuralStar_Story-9b-i1-GGUF/resolve/main/NeuralStar_Story-9b.i1-Q3_K_L.gguf) | i1-Q3_K_L | 5.8 | IQ3_M probably better |
| [GGUF](https://huggingface.co/mradermacher/NeuralStar_Story-9b-i1-GGUF/resolve/main/NeuralStar_Story-9b.i1-IQ4_XS.gguf) | i1-IQ4_XS | 5.9 | |
| [GGUF](https://huggingface.co/mradermacher/NeuralStar_Story-9b-i1-GGUF/resolve/main/NeuralStar_Story-9b.i1-Q4_0.gguf) | i1-Q4_0 | 6.2 | fast, low quality |
| [GGUF](https://huggingface.co/mradermacher/NeuralStar_Story-9b-i1-GGUF/resolve/main/NeuralStar_Story-9b.i1-Q4_K_S.gguf) | i1-Q4_K_S | 6.2 | optimal size/speed/quality |
| [GGUF](https://huggingface.co/mradermacher/NeuralStar_Story-9b-i1-GGUF/resolve/main/NeuralStar_Story-9b.i1-Q4_K_M.gguf) | i1-Q4_K_M | 6.6 | fast, recommended |
| [GGUF](https://huggingface.co/mradermacher/NeuralStar_Story-9b-i1-GGUF/resolve/main/NeuralStar_Story-9b.i1-Q5_K_S.gguf) | i1-Q5_K_S | 7.5 | |
| [GGUF](https://huggingface.co/mradermacher/NeuralStar_Story-9b-i1-GGUF/resolve/main/NeuralStar_Story-9b.i1-Q5_K_M.gguf) | i1-Q5_K_M | 7.7 | |
| [GGUF](https://huggingface.co/mradermacher/NeuralStar_Story-9b-i1-GGUF/resolve/main/NeuralStar_Story-9b.i1-Q6_K.gguf) | i1-Q6_K | 8.9 | practically like static Q6_K |
Here is a handy graph by ikawrakow comparing some lower-quality quant
types (lower is better):

And here are Artefact2's thoughts on the matter:
https://gist.github.com/Artefact2/b5f810600771265fc1e39442288e8ec9
## FAQ / Model Request
See https://huggingface.co/mradermacher/model_requests for some answers to
questions you might have and/or if you want some other model quantized.
## Thanks
I thank my company, [nethype GmbH](https://www.nethype.de/), for letting
me use its servers and providing upgrades to my workstation to enable
this work in my free time. Additional thanks to [@nicoboss](https://huggingface.co/nicoboss) for giving me access to his hardware for calculating the imatrix for these quants.
<!-- end -->
|
QuantFactory/gemma-2-9b-GGUF | QuantFactory | "2024-06-29T02:25:27Z" | 12,764 | 1 | transformers | [
"transformers",
"gguf",
"conversational",
"text-generation",
"arxiv:2009.03300",
"arxiv:1905.07830",
"arxiv:1911.11641",
"arxiv:1904.09728",
"arxiv:1905.10044",
"arxiv:1907.10641",
"arxiv:1811.00937",
"arxiv:1809.02789",
"arxiv:1911.01547",
"arxiv:1705.03551",
"arxiv:2107.03374",
"arxiv:2108.07732",
"arxiv:2110.14168",
"arxiv:2009.11462",
"arxiv:2101.11718",
"arxiv:2110.08193",
"arxiv:1804.09301",
"arxiv:2109.07958",
"arxiv:1804.06876",
"arxiv:2103.03874",
"arxiv:2304.06364",
"arxiv:2206.04615",
"arxiv:2203.09509",
"base_model:google/gemma-2-9b",
"license:gemma",
"endpoints_compatible",
"region:us"
] | text-generation | "2024-06-27T16:51:12Z" | ---
license: gemma
library_name: transformers
pipeline_tag: text-generation
tags:
- conversational
base_model: google/gemma-2-9b
---
# QuantFactory/gemma-2-9b-GGUF
This is quantized version of [google/gemma-2-9b](https://huggingface.co/google/gemma-2-9b) created using llama.cpp
# Gemma 2 model card
**Model Page**: [Gemma](https://ai.google.dev/gemma/docs)
**Resources and Technical Documentation**:
* [Responsible Generative AI Toolkit][rai-toolkit]
* [Gemma on Kaggle][kaggle-gemma]
* [Gemma on Vertex Model Garden][vertex-mg-gemma]
**Terms of Use**: [Terms](https://www.kaggle.com/models/google/gemma/license/consent/verify/huggingface?returnModelRepoId=google/gemma-2-9b-it)
**Authors**: Google
## Model Information
Summary description and brief definition of inputs and outputs.
### Description
Gemma is a family of lightweight, state-of-the-art open models from Google,
built from the same research and technology used to create the Gemini models.
They are text-to-text, decoder-only large language models, available in English,
with open weights for both pre-trained variants and instruction-tuned variants.
Gemma models are well-suited for a variety of text generation tasks, including
question answering, summarization, and reasoning. Their relatively small size
makes it possible to deploy them in environments with limited resources such as
a laptop, desktop or your own cloud infrastructure, democratizing access to
state of the art AI models and helping foster innovation for everyone.
### Usage
Below we share some code snippets on how to get quickly started with running the model. First make sure to `pip install -U transformers`, then copy the snippet from the section that is relevant for your usecase.
#### Running the model on a single / multi GPU
```python
# pip install accelerate
from transformers import AutoTokenizer, AutoModelForCausalLM
import torch
tokenizer = AutoTokenizer.from_pretrained("google/gemma-2-9b-it")
model = AutoModelForCausalLM.from_pretrained(
"google/gemma-2-9b-it",
device_map="auto",
torch_dtype=torch.bfloat16
)
input_text = "Write me a poem about Machine Learning."
input_ids = tokenizer(input_text, return_tensors="pt").to("cuda")
outputs = model.generate(**input_ids)
print(tokenizer.decode(outputs[0]))
```
<a name="precisions"></a>
#### Running the model on a GPU using different precisions
The native weights of this model were exported in `bfloat16` precision. You can use `float16`, which may be faster on certain hardware, indicating the `torch_dtype` when loading the model. For convenience, the `float16` revision of the repo contains a copy of the weights already converted to that precision.
You can also use `float32` if you skip the dtype, but no precision increase will occur (model weights will just be upcasted to `float32`). See examples below.
* _Using `torch.float16`_
```python
# pip install accelerate
from transformers import AutoTokenizer, AutoModelForCausalLM
import torch
tokenizer = AutoTokenizer.from_pretrained("google/gemma-2-9b-it")
model = AutoModelForCausalLM.from_pretrained(
"google/gemma-2-9b-it",
device_map="auto",
torch_dtype=torch.float16,
revision="float16",
)
input_text = "Write me a poem about Machine Learning."
input_ids = tokenizer(input_text, return_tensors="pt").to("cuda")
outputs = model.generate(**input_ids)
print(tokenizer.decode(outputs[0]))
```
* _Using `torch.bfloat16`_
```python
# pip install accelerate
from transformers import AutoTokenizer, AutoModelForCausalLM
tokenizer = AutoTokenizer.from_pretrained("google/gemma-2-9b-it")
model = AutoModelForCausalLM.from_pretrained(
"google/gemma-2-9b-it",
device_map="auto",
torch_dtype=torch.bfloat16)
input_text = "Write me a poem about Machine Learning."
input_ids = tokenizer(input_text, return_tensors="pt").to("cuda")
outputs = model.generate(**input_ids)
print(tokenizer.decode(outputs[0]))
```
* _Upcasting to `torch.float32`_
```python
# pip install accelerate
from transformers import AutoTokenizer, AutoModelForCausalLM
tokenizer = AutoTokenizer.from_pretrained("google/gemma-2-9b-it")
model = AutoModelForCausalLM.from_pretrained(
"google/gemma-2-9b-it",
device_map="auto")
input_text = "Write me a poem about Machine Learning."
input_ids = tokenizer(input_text, return_tensors="pt").to("cuda")
outputs = model.generate(**input_ids)
print(tokenizer.decode(outputs[0]))
```
#### Quantized Versions through `bitsandbytes`
* _Using 8-bit precision (int8)_
```python
# pip install bitsandbytes accelerate
from transformers import AutoTokenizer, AutoModelForCausalLM, BitsAndBytesConfig
quantization_config = BitsAndBytesConfig(load_in_8bit=True)
tokenizer = AutoTokenizer.from_pretrained("google/gemma-2-9b-it")
model = AutoModelForCausalLM.from_pretrained(
"google/gemma-2-9b-it",
quantization_config=quantization_config)
input_text = "Write me a poem about Machine Learning."
input_ids = tokenizer(input_text, return_tensors="pt").to("cuda")
outputs = model.generate(**input_ids)
print(tokenizer.decode(outputs[0]))
```
* _Using 4-bit precision_
```python
# pip install bitsandbytes accelerate
from transformers import AutoTokenizer, AutoModelForCausalLM, BitsAndBytesConfig
quantization_config = BitsAndBytesConfig(load_in_4bit=True)
tokenizer = AutoTokenizer.from_pretrained("google/gemma-2-9b-it")
model = AutoModelForCausalLM.from_pretrained(
"google/gemma-2-9b-it",
quantization_config=quantization_config)
input_text = "Write me a poem about Machine Learning."
input_ids = tokenizer(input_text, return_tensors="pt").to("cuda")
outputs = model.generate(**input_ids)
print(tokenizer.decode(outputs[0]))
```
#### Other optimizations
* _Flash Attention 2_
First make sure to install `flash-attn` in your environment `pip install flash-attn`
```diff
model = AutoModelForCausalLM.from_pretrained(
model_id,
torch_dtype=torch.float16,
+ attn_implementation="flash_attention_2"
).to(0)
```
### Chat Template
The instruction-tuned models use a chat template that must be adhered to for conversational use.
The easiest way to apply it is using the tokenizer's built-in chat template, as shown in the following snippet.
Let's load the model and apply the chat template to a conversation. In this example, we'll start with a single user interaction:
```py
from transformers import AutoTokenizer, AutoModelForCausalLM
import transformers
import torch
model_id = "google/gemma-2-9b-it"
dtype = torch.bfloat16
tokenizer = AutoTokenizer.from_pretrained(model_id)
model = AutoModelForCausalLM.from_pretrained(
model_id,
device_map="cuda",
torch_dtype=dtype,)
chat = [
{ "role": "user", "content": "Write a hello world program" },
]
prompt = tokenizer.apply_chat_template(chat, tokenize=False, add_generation_prompt=True)
```
At this point, the prompt contains the following text:
```
<bos><start_of_turn>user
Write a hello world program<end_of_turn>
<start_of_turn>model
```
As you can see, each turn is preceded by a `<start_of_turn>` delimiter and then the role of the entity
(either `user`, for content supplied by the user, or `model` for LLM responses). Turns finish with
the `<end_of_turn>` token.
You can follow this format to build the prompt manually, if you need to do it without the tokenizer's
chat template.
After the prompt is ready, generation can be performed like this:
```py
inputs = tokenizer.encode(prompt, add_special_tokens=False, return_tensors="pt")
outputs = model.generate(input_ids=inputs.to(model.device), max_new_tokens=150)
print(tokenizer.decode(outputs[0]))
```
### Inputs and outputs
* **Input:** Text string, such as a question, a prompt, or a document to be
summarized.
* **Output:** Generated English-language text in response to the input, such
as an answer to a question, or a summary of a document.
### Model Citation
```none
@article{gemma_2024,
title={Gemma},
url={https://www.kaggle.com/m/3301},
DOI={10.34740/KAGGLE/M/3301},
publisher={Kaggle},
author={Gemma Team},
year={2024}
}
```
## Model Data
Data used for model training and how the data was processed.
### Training Dataset
These models were trained on a dataset of text data that includes a wide variety of sources. The 27B model was trained with 13 trillion tokens and the 9B model was trained with 8 trillion tokens.
Here are the key components:
* Web Documents: A diverse collection of web text ensures the model is exposed
to a broad range of linguistic styles, topics, and vocabulary. Primarily
English-language content.
* Code: Exposing the model to code helps it to learn the syntax and patterns of
programming languages, which improves its ability to generate code or
understand code-related questions.
* Mathematics: Training on mathematical text helps the model learn logical
reasoning, symbolic representation, and to address mathematical queries.
The combination of these diverse data sources is crucial for training a powerful
language model that can handle a wide variety of different tasks and text
formats.
### Data Preprocessing
Here are the key data cleaning and filtering methods applied to the training
data:
* CSAM Filtering: Rigorous CSAM (Child Sexual Abuse Material) filtering was
applied at multiple stages in the data preparation process to ensure the
exclusion of harmful and illegal content.
* Sensitive Data Filtering: As part of making Gemma pre-trained models safe and
reliable, automated techniques were used to filter out certain personal
information and other sensitive data from training sets.
* Additional methods: Filtering based on content quality and safety in line with
[our policies][safety-policies].
## Implementation Information
Details about the model internals.
### Hardware
Gemma was trained using the latest generation of
[Tensor Processing Unit (TPU)][tpu] hardware (TPUv5p).
Training large language models requires significant computational power. TPUs,
designed specifically for matrix operations common in machine learning, offer
several advantages in this domain:
* Performance: TPUs are specifically designed to handle the massive computations
involved in training LLMs. They can speed up training considerably compared to
CPUs.
* Memory: TPUs often come with large amounts of high-bandwidth memory, allowing
for the handling of large models and batch sizes during training. This can
lead to better model quality.
* Scalability: TPU Pods (large clusters of TPUs) provide a scalable solution for
handling the growing complexity of large foundation models. You can distribute
training across multiple TPU devices for faster and more efficient processing.
* Cost-effectiveness: In many scenarios, TPUs can provide a more cost-effective
solution for training large models compared to CPU-based infrastructure,
especially when considering the time and resources saved due to faster
training.
* These advantages are aligned with
[Google's commitments to operate sustainably][sustainability].
### Software
Training was done using [JAX][jax] and [ML Pathways][ml-pathways].
JAX allows researchers to take advantage of the latest generation of hardware,
including TPUs, for faster and more efficient training of large models.
ML Pathways is Google's latest effort to build artificially intelligent systems
capable of generalizing across multiple tasks. This is specially suitable for
[foundation models][foundation-models], including large language models like
these ones.
Together, JAX and ML Pathways are used as described in the
[paper about the Gemini family of models][gemini-2-paper]; "the 'single
controller' programming model of Jax and Pathways allows a single Python
process to orchestrate the entire training run, dramatically simplifying the
development workflow."
## Evaluation
Model evaluation metrics and results.
### Benchmark Results
These models were evaluated against a large collection of different datasets and
metrics to cover different aspects of text generation:
| Benchmark | Metric | Gemma PT 9B | Gemma PT 27B |
| ------------------------------ | ------------- | ----------- | ------------ |
| [MMLU][mmlu] | 5-shot, top-1 | 71.3 | 75.2 |
| [HellaSwag][hellaswag] | 10-shot | 81.9 | 86.4 |
| [PIQA][piqa] | 0-shot | 81.7 | 83.2 |
| [SocialIQA][socialiqa] | 0-shot | 53.4 | 53.7 |
| [BoolQ][boolq] | 0-shot | 84.2 | 84.8 |
| [WinoGrande][winogrande] | partial score | 80.6 | 83.7 |
| [ARC-e][arc] | 0-shot | 88.0 | 88.6 |
| [ARC-c][arc] | 25-shot | 68.4 | 71.4 |
| [TriviaQA][triviaqa] | 5-shot | 76.6 | 83.7 |
| [Natural Questions][naturalq] | 5-shot | 29.2 | 34.5 |
| [HumanEval][humaneval] | pass@1 | 40.2 | 51.8 |
| [MBPP][mbpp] | 3-shot | 52.4 | 62.6 |
| [GSM8K][gsm8k] | 5-shot, maj@1 | 68.6 | 74.0 |
| [MATH][math] | 4-shot | 36.6 | 42.3 |
| [AGIEval][agieval] | 3-5-shot | 52.8 | 55.1 |
| [BIG-Bench][big-bench] | 3-shot, CoT | 68.2 | 74.9 |
| ------------------------------ | ------------- | ----------- | ------------ |
## Ethics and Safety
Ethics and safety evaluation approach and results.
### Evaluation Approach
Our evaluation methods include structured evaluations and internal red-teaming
testing of relevant content policies. Red-teaming was conducted by a number of
different teams, each with different goals and human evaluation metrics. These
models were evaluated against a number of different categories relevant to
ethics and safety, including:
* Text-to-Text Content Safety: Human evaluation on prompts covering safety
policies including child sexual abuse and exploitation, harassment, violence
and gore, and hate speech.
* Text-to-Text Representational Harms: Benchmark against relevant academic
datasets such as [WinoBias][winobias] and [BBQ Dataset][bbq].
* Memorization: Automated evaluation of memorization of training data, including
the risk of personally identifiable information exposure.
* Large-scale harm: Tests for "dangerous capabilities," such as chemical,
biological, radiological, and nuclear (CBRN) risks.
### Evaluation Results
The results of ethics and safety evaluations are within acceptable thresholds
for meeting [internal policies][safety-policies] for categories such as child
safety, content safety, representational harms, memorization, large-scale harms.
On top of robust internal evaluations, the results of well-known safety
benchmarks like BBQ, BOLD, Winogender, Winobias, RealToxicity, and TruthfulQA
are shown here.
#### Gemma 2.0
| Benchmark | Metric | Gemma 2 IT 9B | Gemma 2 IT 27B |
| ------------------------ | ------------- | --------------- | ---------------- |
| [RealToxicity][realtox] | average | 8.25 | 8.84 |
| [CrowS-Pairs][crows] | top-1 | 37.47 | 36.67 |
| [BBQ Ambig][bbq] | 1-shot, top-1 | 88.58 | 85.99 |
| [BBQ Disambig][bbq] | top-1 | 82.67 | 86.94 |
| [Winogender][winogender] | top-1 | 79.17 | 77.22 |
| [TruthfulQA][truthfulqa] | | 50.27 | 51.60 |
| [Winobias 1_2][winobias] | | 78.09 | 81.94 |
| [Winobias 2_2][winobias] | | 95.32 | 97.22 |
| [Toxigen][toxigen] | | 39.30 | 38.42 |
| ------------------------ | ------------- | --------------- | ---------------- |
## Usage and Limitations
These models have certain limitations that users should be aware of.
### Intended Usage
Open Large Language Models (LLMs) have a wide range of applications across
various industries and domains. The following list of potential uses is not
comprehensive. The purpose of this list is to provide contextual information
about the possible use-cases that the model creators considered as part of model
training and development.
* Content Creation and Communication
* Text Generation: These models can be used to generate creative text formats
such as poems, scripts, code, marketing copy, and email drafts.
* Chatbots and Conversational AI: Power conversational interfaces for customer
service, virtual assistants, or interactive applications.
* Text Summarization: Generate concise summaries of a text corpus, research
papers, or reports.
* Research and Education
* Natural Language Processing (NLP) Research: These models can serve as a
foundation for researchers to experiment with NLP techniques, develop
algorithms, and contribute to the advancement of the field.
* Language Learning Tools: Support interactive language learning experiences,
aiding in grammar correction or providing writing practice.
* Knowledge Exploration: Assist researchers in exploring large bodies of text
by generating summaries or answering questions about specific topics.
### Limitations
* Training Data
* The quality and diversity of the training data significantly influence the
model's capabilities. Biases or gaps in the training data can lead to
limitations in the model's responses.
* The scope of the training dataset determines the subject areas the model can
handle effectively.
* Context and Task Complexity
* LLMs are better at tasks that can be framed with clear prompts and
instructions. Open-ended or highly complex tasks might be challenging.
* A model's performance can be influenced by the amount of context provided
(longer context generally leads to better outputs, up to a certain point).
* Language Ambiguity and Nuance
* Natural language is inherently complex. LLMs might struggle to grasp subtle
nuances, sarcasm, or figurative language.
* Factual Accuracy
* LLMs generate responses based on information they learned from their
training datasets, but they are not knowledge bases. They may generate
incorrect or outdated factual statements.
* Common Sense
* LLMs rely on statistical patterns in language. They might lack the ability
to apply common sense reasoning in certain situations.
### Ethical Considerations and Risks
The development of large language models (LLMs) raises several ethical concerns.
In creating an open model, we have carefully considered the following:
* Bias and Fairness
* LLMs trained on large-scale, real-world text data can reflect socio-cultural
biases embedded in the training material. These models underwent careful
scrutiny, input data pre-processing described and posterior evaluations
reported in this card.
* Misinformation and Misuse
* LLMs can be misused to generate text that is false, misleading, or harmful.
* Guidelines are provided for responsible use with the model, see the
[Responsible Generative AI Toolkit][rai-toolkit].
* Transparency and Accountability:
* This model card summarizes details on the models' architecture,
capabilities, limitations, and evaluation processes.
* A responsibly developed open model offers the opportunity to share
innovation by making LLM technology accessible to developers and researchers
across the AI ecosystem.
Risks identified and mitigations:
* Perpetuation of biases: It's encouraged to perform continuous monitoring
(using evaluation metrics, human review) and the exploration of de-biasing
techniques during model training, fine-tuning, and other use cases.
* Generation of harmful content: Mechanisms and guidelines for content safety
are essential. Developers are encouraged to exercise caution and implement
appropriate content safety safeguards based on their specific product policies
and application use cases.
* Misuse for malicious purposes: Technical limitations and developer and
end-user education can help mitigate against malicious applications of LLMs.
Educational resources and reporting mechanisms for users to flag misuse are
provided. Prohibited uses of Gemma models are outlined in the
[Gemma Prohibited Use Policy][prohibited-use].
* Privacy violations: Models were trained on data filtered for removal of PII
(Personally Identifiable Information). Developers are encouraged to adhere to
privacy regulations with privacy-preserving techniques.
### Benefits
At the time of release, this family of models provides high-performance open
large language model implementations designed from the ground up for Responsible
AI development compared to similarly sized models.
Using the benchmark evaluation metrics described in this document, these models
have shown to provide superior performance to other, comparably-sized open model
alternatives.
[rai-toolkit]: https://ai.google.dev/responsible
[kaggle-gemma]: https://www.kaggle.com/models/google/gemma-2
[terms]: https://ai.google.dev/gemma/terms
[vertex-mg-gemma]: https://console.cloud.google.com/vertex-ai/publishers/google/model-garden/335
[sensitive-info]: https://cloud.google.com/dlp/docs/high-sensitivity-infotypes-reference
[safety-policies]: https://storage.googleapis.com/gweb-uniblog-publish-prod/documents/2023_Google_AI_Principles_Progress_Update.pdf#page=11
[prohibited-use]: https://ai.google.dev/gemma/prohibited_use_policy
[tpu]: https://cloud.google.com/tpu/docs/intro-to-tpu
[sustainability]: https://sustainability.google/operating-sustainably/
[jax]: https://github.com/google/jax
[ml-pathways]: https://blog.google/technology/ai/introducing-pathways-next-generation-ai-architecture/
[sustainability]: https://sustainability.google/operating-sustainably/
[foundation-models]: https://ai.google/discover/foundation-models/
[gemini-2-paper]: https://goo.gle/gemma2report
[mmlu]: https://arxiv.org/abs/2009.03300
[hellaswag]: https://arxiv.org/abs/1905.07830
[piqa]: https://arxiv.org/abs/1911.11641
[socialiqa]: https://arxiv.org/abs/1904.09728
[boolq]: https://arxiv.org/abs/1905.10044
[winogrande]: https://arxiv.org/abs/1907.10641
[commonsenseqa]: https://arxiv.org/abs/1811.00937
[openbookqa]: https://arxiv.org/abs/1809.02789
[arc]: https://arxiv.org/abs/1911.01547
[triviaqa]: https://arxiv.org/abs/1705.03551
[naturalq]: https://github.com/google-research-datasets/natural-questions
[humaneval]: https://arxiv.org/abs/2107.03374
[mbpp]: https://arxiv.org/abs/2108.07732
[gsm8k]: https://arxiv.org/abs/2110.14168
[realtox]: https://arxiv.org/abs/2009.11462
[bold]: https://arxiv.org/abs/2101.11718
[crows]: https://aclanthology.org/2020.emnlp-main.154/
[bbq]: https://arxiv.org/abs/2110.08193v2
[winogender]: https://arxiv.org/abs/1804.09301
[truthfulqa]: https://arxiv.org/abs/2109.07958
[winobias]: https://arxiv.org/abs/1804.06876
[math]: https://arxiv.org/abs/2103.03874
[agieval]: https://arxiv.org/abs/2304.06364
[big-bench]: https://arxiv.org/abs/2206.04615
[toxigen]: https://arxiv.org/abs/2203.09509
|
Systran/faster-whisper-tiny.en | Systran | "2023-11-23T10:47:07Z" | 12,752 | 1 | ctranslate2 | [
"ctranslate2",
"audio",
"automatic-speech-recognition",
"en",
"license:mit",
"region:us"
] | automatic-speech-recognition | "2023-11-23T09:54:25Z" | ---
language:
- en
tags:
- audio
- automatic-speech-recognition
license: mit
library_name: ctranslate2
---
# Whisper tiny.en model for CTranslate2
This repository contains the conversion of [openai/whisper-tiny.en](https://huggingface.co/openai/whisper-tiny.en) to the [CTranslate2](https://github.com/OpenNMT/CTranslate2) model format.
This model can be used in CTranslate2 or projects based on CTranslate2 such as [faster-whisper](https://github.com/systran/faster-whisper).
## Example
```python
from faster_whisper import WhisperModel
model = WhisperModel("tiny.en")
segments, info = model.transcribe("audio.mp3")
for segment in segments:
print("[%.2fs -> %.2fs] %s" % (segment.start, segment.end, segment.text))
```
## Conversion details
The original model was converted with the following command:
```
ct2-transformers-converter --model openai/whisper-tiny.en --output_dir faster-whisper-tiny.en \
--copy_files tokenizer.json --quantization float16
```
Note that the model weights are saved in FP16. This type can be changed when the model is loaded using the [`compute_type` option in CTranslate2](https://opennmt.net/CTranslate2/quantization.html).
## More information
**For more information about the original model, see its [model card](https://huggingface.co/openai/whisper-tiny.en).**
|
mradermacher/llama3-turbcat-instruct-8b-i1-GGUF | mradermacher | "2024-06-21T07:27:02Z" | 12,733 | 0 | transformers | [
"transformers",
"gguf",
"en",
"base_model:turboderp/llama3-turbcat-instruct-8b",
"license:llama3",
"endpoints_compatible",
"region:us"
] | null | "2024-06-21T05:12:55Z" | ---
base_model: turboderp/llama3-turbcat-instruct-8b
language:
- en
library_name: transformers
license: llama3
quantized_by: mradermacher
---
## About
<!-- ### quantize_version: 2 -->
<!-- ### output_tensor_quantised: 1 -->
<!-- ### convert_type: hf -->
<!-- ### vocab_type: -->
<!-- ### tags: nicoboss -->
weighted/imatrix quants of https://huggingface.co/turboderp/llama3-turbcat-instruct-8b
<!-- provided-files -->
static quants are available at https://huggingface.co/mradermacher/llama3-turbcat-instruct-8b-GGUF
## Usage
If you are unsure how to use GGUF files, refer to one of [TheBloke's
READMEs](https://huggingface.co/TheBloke/KafkaLM-70B-German-V0.1-GGUF) for
more details, including on how to concatenate multi-part files.
## Provided Quants
(sorted by size, not necessarily quality. IQ-quants are often preferable over similar sized non-IQ quants)
| Link | Type | Size/GB | Notes |
|:-----|:-----|--------:|:------|
| [GGUF](https://huggingface.co/mradermacher/llama3-turbcat-instruct-8b-i1-GGUF/resolve/main/llama3-turbcat-instruct-8b.i1-IQ1_S.gguf) | i1-IQ1_S | 2.1 | for the desperate |
| [GGUF](https://huggingface.co/mradermacher/llama3-turbcat-instruct-8b-i1-GGUF/resolve/main/llama3-turbcat-instruct-8b.i1-IQ1_M.gguf) | i1-IQ1_M | 2.3 | mostly desperate |
| [GGUF](https://huggingface.co/mradermacher/llama3-turbcat-instruct-8b-i1-GGUF/resolve/main/llama3-turbcat-instruct-8b.i1-IQ2_XXS.gguf) | i1-IQ2_XXS | 2.5 | |
| [GGUF](https://huggingface.co/mradermacher/llama3-turbcat-instruct-8b-i1-GGUF/resolve/main/llama3-turbcat-instruct-8b.i1-IQ2_XS.gguf) | i1-IQ2_XS | 2.7 | |
| [GGUF](https://huggingface.co/mradermacher/llama3-turbcat-instruct-8b-i1-GGUF/resolve/main/llama3-turbcat-instruct-8b.i1-IQ2_S.gguf) | i1-IQ2_S | 2.9 | |
| [GGUF](https://huggingface.co/mradermacher/llama3-turbcat-instruct-8b-i1-GGUF/resolve/main/llama3-turbcat-instruct-8b.i1-IQ2_M.gguf) | i1-IQ2_M | 3.0 | |
| [GGUF](https://huggingface.co/mradermacher/llama3-turbcat-instruct-8b-i1-GGUF/resolve/main/llama3-turbcat-instruct-8b.i1-Q2_K.gguf) | i1-Q2_K | 3.3 | IQ3_XXS probably better |
| [GGUF](https://huggingface.co/mradermacher/llama3-turbcat-instruct-8b-i1-GGUF/resolve/main/llama3-turbcat-instruct-8b.i1-IQ3_XXS.gguf) | i1-IQ3_XXS | 3.4 | lower quality |
| [GGUF](https://huggingface.co/mradermacher/llama3-turbcat-instruct-8b-i1-GGUF/resolve/main/llama3-turbcat-instruct-8b.i1-IQ3_XS.gguf) | i1-IQ3_XS | 3.6 | |
| [GGUF](https://huggingface.co/mradermacher/llama3-turbcat-instruct-8b-i1-GGUF/resolve/main/llama3-turbcat-instruct-8b.i1-Q3_K_S.gguf) | i1-Q3_K_S | 3.8 | IQ3_XS probably better |
| [GGUF](https://huggingface.co/mradermacher/llama3-turbcat-instruct-8b-i1-GGUF/resolve/main/llama3-turbcat-instruct-8b.i1-IQ3_S.gguf) | i1-IQ3_S | 3.8 | beats Q3_K* |
| [GGUF](https://huggingface.co/mradermacher/llama3-turbcat-instruct-8b-i1-GGUF/resolve/main/llama3-turbcat-instruct-8b.i1-IQ3_M.gguf) | i1-IQ3_M | 3.9 | |
| [GGUF](https://huggingface.co/mradermacher/llama3-turbcat-instruct-8b-i1-GGUF/resolve/main/llama3-turbcat-instruct-8b.i1-Q3_K_M.gguf) | i1-Q3_K_M | 4.1 | IQ3_S probably better |
| [GGUF](https://huggingface.co/mradermacher/llama3-turbcat-instruct-8b-i1-GGUF/resolve/main/llama3-turbcat-instruct-8b.i1-Q3_K_L.gguf) | i1-Q3_K_L | 4.4 | IQ3_M probably better |
| [GGUF](https://huggingface.co/mradermacher/llama3-turbcat-instruct-8b-i1-GGUF/resolve/main/llama3-turbcat-instruct-8b.i1-IQ4_XS.gguf) | i1-IQ4_XS | 4.5 | |
| [GGUF](https://huggingface.co/mradermacher/llama3-turbcat-instruct-8b-i1-GGUF/resolve/main/llama3-turbcat-instruct-8b.i1-Q4_0.gguf) | i1-Q4_0 | 4.8 | fast, low quality |
| [GGUF](https://huggingface.co/mradermacher/llama3-turbcat-instruct-8b-i1-GGUF/resolve/main/llama3-turbcat-instruct-8b.i1-Q4_K_S.gguf) | i1-Q4_K_S | 4.8 | optimal size/speed/quality |
| [GGUF](https://huggingface.co/mradermacher/llama3-turbcat-instruct-8b-i1-GGUF/resolve/main/llama3-turbcat-instruct-8b.i1-Q4_K_M.gguf) | i1-Q4_K_M | 5.0 | fast, recommended |
| [GGUF](https://huggingface.co/mradermacher/llama3-turbcat-instruct-8b-i1-GGUF/resolve/main/llama3-turbcat-instruct-8b.i1-Q5_K_S.gguf) | i1-Q5_K_S | 5.7 | |
| [GGUF](https://huggingface.co/mradermacher/llama3-turbcat-instruct-8b-i1-GGUF/resolve/main/llama3-turbcat-instruct-8b.i1-Q5_K_M.gguf) | i1-Q5_K_M | 5.8 | |
| [GGUF](https://huggingface.co/mradermacher/llama3-turbcat-instruct-8b-i1-GGUF/resolve/main/llama3-turbcat-instruct-8b.i1-Q6_K.gguf) | i1-Q6_K | 6.7 | practically like static Q6_K |
Here is a handy graph by ikawrakow comparing some lower-quality quant
types (lower is better):

And here are Artefact2's thoughts on the matter:
https://gist.github.com/Artefact2/b5f810600771265fc1e39442288e8ec9
## FAQ / Model Request
See https://huggingface.co/mradermacher/model_requests for some answers to
questions you might have and/or if you want some other model quantized.
## Thanks
I thank my company, [nethype GmbH](https://www.nethype.de/), for letting
me use its servers and providing upgrades to my workstation to enable
this work in my free time. Additional thanks to [@nicoboss](https://huggingface.co/nicoboss) for giving me access to his hardware for calculating the imatrix for these quants.
<!-- end -->
|
mradermacher/L3-Persephone-8B-v1.0-GGUF | mradermacher | "2024-06-29T05:04:22Z" | 12,731 | 1 | transformers | [
"transformers",
"gguf",
"mergekit",
"merge",
"llama",
"conversational",
"en",
"base_model:Azazelle/L3-Persephone-8B-v1.0",
"license:llama3",
"endpoints_compatible",
"region:us"
] | null | "2024-06-29T04:35:15Z" | ---
base_model: Azazelle/L3-Persephone-8B-v1.0
language:
- en
library_name: transformers
license: llama3
quantized_by: mradermacher
tags:
- mergekit
- merge
- llama
- conversational
---
## About
<!-- ### quantize_version: 2 -->
<!-- ### output_tensor_quantised: 1 -->
<!-- ### convert_type: hf -->
<!-- ### vocab_type: -->
<!-- ### tags: -->
static quants of https://huggingface.co/Azazelle/L3-Persephone-8B-v1.0
<!-- provided-files -->
weighted/imatrix quants seem not to be available (by me) at this time. If they do not show up a week or so after the static ones, I have probably not planned for them. Feel free to request them by opening a Community Discussion.
## Usage
If you are unsure how to use GGUF files, refer to one of [TheBloke's
READMEs](https://huggingface.co/TheBloke/KafkaLM-70B-German-V0.1-GGUF) for
more details, including on how to concatenate multi-part files.
## Provided Quants
(sorted by size, not necessarily quality. IQ-quants are often preferable over similar sized non-IQ quants)
| Link | Type | Size/GB | Notes |
|:-----|:-----|--------:|:------|
| [GGUF](https://huggingface.co/mradermacher/L3-Persephone-8B-v1.0-GGUF/resolve/main/L3-Persephone-8B-v1.0.Q2_K.gguf) | Q2_K | 3.3 | |
| [GGUF](https://huggingface.co/mradermacher/L3-Persephone-8B-v1.0-GGUF/resolve/main/L3-Persephone-8B-v1.0.IQ3_XS.gguf) | IQ3_XS | 3.6 | |
| [GGUF](https://huggingface.co/mradermacher/L3-Persephone-8B-v1.0-GGUF/resolve/main/L3-Persephone-8B-v1.0.Q3_K_S.gguf) | Q3_K_S | 3.8 | |
| [GGUF](https://huggingface.co/mradermacher/L3-Persephone-8B-v1.0-GGUF/resolve/main/L3-Persephone-8B-v1.0.IQ3_S.gguf) | IQ3_S | 3.8 | beats Q3_K* |
| [GGUF](https://huggingface.co/mradermacher/L3-Persephone-8B-v1.0-GGUF/resolve/main/L3-Persephone-8B-v1.0.IQ3_M.gguf) | IQ3_M | 3.9 | |
| [GGUF](https://huggingface.co/mradermacher/L3-Persephone-8B-v1.0-GGUF/resolve/main/L3-Persephone-8B-v1.0.Q3_K_M.gguf) | Q3_K_M | 4.1 | lower quality |
| [GGUF](https://huggingface.co/mradermacher/L3-Persephone-8B-v1.0-GGUF/resolve/main/L3-Persephone-8B-v1.0.Q3_K_L.gguf) | Q3_K_L | 4.4 | |
| [GGUF](https://huggingface.co/mradermacher/L3-Persephone-8B-v1.0-GGUF/resolve/main/L3-Persephone-8B-v1.0.IQ4_XS.gguf) | IQ4_XS | 4.6 | |
| [GGUF](https://huggingface.co/mradermacher/L3-Persephone-8B-v1.0-GGUF/resolve/main/L3-Persephone-8B-v1.0.Q4_K_S.gguf) | Q4_K_S | 4.8 | fast, recommended |
| [GGUF](https://huggingface.co/mradermacher/L3-Persephone-8B-v1.0-GGUF/resolve/main/L3-Persephone-8B-v1.0.Q4_K_M.gguf) | Q4_K_M | 5.0 | fast, recommended |
| [GGUF](https://huggingface.co/mradermacher/L3-Persephone-8B-v1.0-GGUF/resolve/main/L3-Persephone-8B-v1.0.Q5_K_S.gguf) | Q5_K_S | 5.7 | |
| [GGUF](https://huggingface.co/mradermacher/L3-Persephone-8B-v1.0-GGUF/resolve/main/L3-Persephone-8B-v1.0.Q5_K_M.gguf) | Q5_K_M | 5.8 | |
| [GGUF](https://huggingface.co/mradermacher/L3-Persephone-8B-v1.0-GGUF/resolve/main/L3-Persephone-8B-v1.0.Q6_K.gguf) | Q6_K | 6.7 | very good quality |
| [GGUF](https://huggingface.co/mradermacher/L3-Persephone-8B-v1.0-GGUF/resolve/main/L3-Persephone-8B-v1.0.Q8_0.gguf) | Q8_0 | 8.6 | fast, best quality |
| [GGUF](https://huggingface.co/mradermacher/L3-Persephone-8B-v1.0-GGUF/resolve/main/L3-Persephone-8B-v1.0.f16.gguf) | f16 | 16.2 | 16 bpw, overkill |
Here is a handy graph by ikawrakow comparing some lower-quality quant
types (lower is better):

And here are Artefact2's thoughts on the matter:
https://gist.github.com/Artefact2/b5f810600771265fc1e39442288e8ec9
## FAQ / Model Request
See https://huggingface.co/mradermacher/model_requests for some answers to
questions you might have and/or if you want some other model quantized.
## Thanks
I thank my company, [nethype GmbH](https://www.nethype.de/), for letting
me use its servers and providing upgrades to my workstation to enable
this work in my free time.
<!-- end -->
|
mradermacher/SMaid-v0.3-GGUF | mradermacher | "2024-06-22T18:02:53Z" | 12,712 | 0 | transformers | [
"transformers",
"gguf",
"mergekit",
"merge",
"en",
"base_model:Alsebay/SMaid-v0.3",
"endpoints_compatible",
"region:us"
] | null | "2024-06-22T17:32:57Z" | ---
base_model: Alsebay/SMaid-v0.3
language:
- en
library_name: transformers
quantized_by: mradermacher
tags:
- mergekit
- merge
---
## About
<!-- ### quantize_version: 2 -->
<!-- ### output_tensor_quantised: 1 -->
<!-- ### convert_type: hf -->
<!-- ### vocab_type: -->
<!-- ### tags: -->
static quants of https://huggingface.co/Alsebay/SMaid-v0.3
<!-- provided-files -->
weighted/imatrix quants are available at https://huggingface.co/mradermacher/SMaid-v0.3-i1-GGUF
## Usage
If you are unsure how to use GGUF files, refer to one of [TheBloke's
READMEs](https://huggingface.co/TheBloke/KafkaLM-70B-German-V0.1-GGUF) for
more details, including on how to concatenate multi-part files.
## Provided Quants
(sorted by size, not necessarily quality. IQ-quants are often preferable over similar sized non-IQ quants)
| Link | Type | Size/GB | Notes |
|:-----|:-----|--------:|:------|
| [GGUF](https://huggingface.co/mradermacher/SMaid-v0.3-GGUF/resolve/main/SMaid-v0.3.Q2_K.gguf) | Q2_K | 3.3 | |
| [GGUF](https://huggingface.co/mradermacher/SMaid-v0.3-GGUF/resolve/main/SMaid-v0.3.IQ3_XS.gguf) | IQ3_XS | 3.6 | |
| [GGUF](https://huggingface.co/mradermacher/SMaid-v0.3-GGUF/resolve/main/SMaid-v0.3.Q3_K_S.gguf) | Q3_K_S | 3.8 | |
| [GGUF](https://huggingface.co/mradermacher/SMaid-v0.3-GGUF/resolve/main/SMaid-v0.3.IQ3_S.gguf) | IQ3_S | 3.8 | beats Q3_K* |
| [GGUF](https://huggingface.co/mradermacher/SMaid-v0.3-GGUF/resolve/main/SMaid-v0.3.IQ3_M.gguf) | IQ3_M | 3.9 | |
| [GGUF](https://huggingface.co/mradermacher/SMaid-v0.3-GGUF/resolve/main/SMaid-v0.3.Q3_K_M.gguf) | Q3_K_M | 4.1 | lower quality |
| [GGUF](https://huggingface.co/mradermacher/SMaid-v0.3-GGUF/resolve/main/SMaid-v0.3.Q3_K_L.gguf) | Q3_K_L | 4.4 | |
| [GGUF](https://huggingface.co/mradermacher/SMaid-v0.3-GGUF/resolve/main/SMaid-v0.3.IQ4_XS.gguf) | IQ4_XS | 4.6 | |
| [GGUF](https://huggingface.co/mradermacher/SMaid-v0.3-GGUF/resolve/main/SMaid-v0.3.Q4_K_S.gguf) | Q4_K_S | 4.8 | fast, recommended |
| [GGUF](https://huggingface.co/mradermacher/SMaid-v0.3-GGUF/resolve/main/SMaid-v0.3.Q4_K_M.gguf) | Q4_K_M | 5.0 | fast, recommended |
| [GGUF](https://huggingface.co/mradermacher/SMaid-v0.3-GGUF/resolve/main/SMaid-v0.3.Q5_K_S.gguf) | Q5_K_S | 5.7 | |
| [GGUF](https://huggingface.co/mradermacher/SMaid-v0.3-GGUF/resolve/main/SMaid-v0.3.Q5_K_M.gguf) | Q5_K_M | 5.8 | |
| [GGUF](https://huggingface.co/mradermacher/SMaid-v0.3-GGUF/resolve/main/SMaid-v0.3.Q6_K.gguf) | Q6_K | 6.7 | very good quality |
| [GGUF](https://huggingface.co/mradermacher/SMaid-v0.3-GGUF/resolve/main/SMaid-v0.3.Q8_0.gguf) | Q8_0 | 8.6 | fast, best quality |
| [GGUF](https://huggingface.co/mradermacher/SMaid-v0.3-GGUF/resolve/main/SMaid-v0.3.f16.gguf) | f16 | 16.2 | 16 bpw, overkill |
Here is a handy graph by ikawrakow comparing some lower-quality quant
types (lower is better):

And here are Artefact2's thoughts on the matter:
https://gist.github.com/Artefact2/b5f810600771265fc1e39442288e8ec9
## FAQ / Model Request
See https://huggingface.co/mradermacher/model_requests for some answers to
questions you might have and/or if you want some other model quantized.
## Thanks
I thank my company, [nethype GmbH](https://www.nethype.de/), for letting
me use its servers and providing upgrades to my workstation to enable
this work in my free time.
<!-- end -->
|
ivanlau/language-detection-fine-tuned-on-xlm-roberta-base | ivanlau | "2021-12-17T10:33:13Z" | 12,711 | 16 | transformers | [
"transformers",
"pytorch",
"tensorboard",
"xlm-roberta",
"text-classification",
"generated_from_trainer",
"dataset:common_language",
"license:mit",
"model-index",
"autotrain_compatible",
"endpoints_compatible",
"region:us"
] | text-classification | "2022-03-02T23:29:05Z" | ---
license: mit
tags:
- generated_from_trainer
datasets:
- common_language
metrics:
- accuracy
model-index:
- name: language-detection-fine-tuned-on-xlm-roberta-base
results:
- task:
name: Text Classification
type: text-classification
dataset:
name: common_language
type: common_language
args: full
metrics:
- name: Accuracy
type: accuracy
value: 0.9738386718094919
---
<!-- This model card has been generated automatically according to the information the Trainer had access to. You
should probably proofread and complete it, then remove this comment. -->
# language-detection-fine-tuned-on-xlm-roberta-base
This model is a fine-tuned version of [xlm-roberta-base](https://huggingface.co/xlm-roberta-base) on the [common_language](https://huggingface.co/datasets/common_language) dataset.
It achieves the following results on the evaluation set:
- Loss: 0.1886
- Accuracy: 0.9738
### Training hyperparameters
The following hyperparameters were used during training:
- learning_rate: 3e-05
- train_batch_size: 1
- eval_batch_size: 1
- seed: 42
- optimizer: Adam with betas=(0.9,0.999) and epsilon=1e-08
- lr_scheduler_type: linear
- lr_scheduler_warmup_steps: 500
- num_epochs: 1
- mixed_precision_training: Native AMP
### Training results
| Training Loss | Epoch | Step | Validation Loss | Accuracy |
|:-------------:|:-----:|:-----:|:---------------:|:--------:|
| 0.1 | 1.0 | 22194 | 0.1886 | 0.9738 |
### Framework versions
- Transformers 4.12.5
- Pytorch 1.10.0+cu111
- Datasets 1.15.1
- Tokenizers 0.10.3
### Notebook
[notebook](https://github.com/IvanLauLinTiong/language-detector/blob/main/xlm_roberta_base_commonlanguage_language_detector.ipynb) |
RichardErkhov/Azazelle_-_Tippy-Toppy-7b-gguf | RichardErkhov | "2024-06-19T22:35:43Z" | 12,709 | 0 | null | [
"gguf",
"region:us"
] | null | "2024-06-19T19:15:44Z" | Quantization made by Richard Erkhov.
[Github](https://github.com/RichardErkhov)
[Discord](https://discord.gg/pvy7H8DZMG)
[Request more models](https://github.com/RichardErkhov/quant_request)
Tippy-Toppy-7b - GGUF
- Model creator: https://huggingface.co/Azazelle/
- Original model: https://huggingface.co/Azazelle/Tippy-Toppy-7b/
| Name | Quant method | Size |
| ---- | ---- | ---- |
| [Tippy-Toppy-7b.Q2_K.gguf](https://huggingface.co/RichardErkhov/Azazelle_-_Tippy-Toppy-7b-gguf/blob/main/Tippy-Toppy-7b.Q2_K.gguf) | Q2_K | 2.53GB |
| [Tippy-Toppy-7b.IQ3_XS.gguf](https://huggingface.co/RichardErkhov/Azazelle_-_Tippy-Toppy-7b-gguf/blob/main/Tippy-Toppy-7b.IQ3_XS.gguf) | IQ3_XS | 2.81GB |
| [Tippy-Toppy-7b.IQ3_S.gguf](https://huggingface.co/RichardErkhov/Azazelle_-_Tippy-Toppy-7b-gguf/blob/main/Tippy-Toppy-7b.IQ3_S.gguf) | IQ3_S | 2.96GB |
| [Tippy-Toppy-7b.Q3_K_S.gguf](https://huggingface.co/RichardErkhov/Azazelle_-_Tippy-Toppy-7b-gguf/blob/main/Tippy-Toppy-7b.Q3_K_S.gguf) | Q3_K_S | 2.95GB |
| [Tippy-Toppy-7b.IQ3_M.gguf](https://huggingface.co/RichardErkhov/Azazelle_-_Tippy-Toppy-7b-gguf/blob/main/Tippy-Toppy-7b.IQ3_M.gguf) | IQ3_M | 3.06GB |
| [Tippy-Toppy-7b.Q3_K.gguf](https://huggingface.co/RichardErkhov/Azazelle_-_Tippy-Toppy-7b-gguf/blob/main/Tippy-Toppy-7b.Q3_K.gguf) | Q3_K | 3.28GB |
| [Tippy-Toppy-7b.Q3_K_M.gguf](https://huggingface.co/RichardErkhov/Azazelle_-_Tippy-Toppy-7b-gguf/blob/main/Tippy-Toppy-7b.Q3_K_M.gguf) | Q3_K_M | 3.28GB |
| [Tippy-Toppy-7b.Q3_K_L.gguf](https://huggingface.co/RichardErkhov/Azazelle_-_Tippy-Toppy-7b-gguf/blob/main/Tippy-Toppy-7b.Q3_K_L.gguf) | Q3_K_L | 3.56GB |
| [Tippy-Toppy-7b.IQ4_XS.gguf](https://huggingface.co/RichardErkhov/Azazelle_-_Tippy-Toppy-7b-gguf/blob/main/Tippy-Toppy-7b.IQ4_XS.gguf) | IQ4_XS | 3.67GB |
| [Tippy-Toppy-7b.Q4_0.gguf](https://huggingface.co/RichardErkhov/Azazelle_-_Tippy-Toppy-7b-gguf/blob/main/Tippy-Toppy-7b.Q4_0.gguf) | Q4_0 | 3.83GB |
| [Tippy-Toppy-7b.IQ4_NL.gguf](https://huggingface.co/RichardErkhov/Azazelle_-_Tippy-Toppy-7b-gguf/blob/main/Tippy-Toppy-7b.IQ4_NL.gguf) | IQ4_NL | 3.87GB |
| [Tippy-Toppy-7b.Q4_K_S.gguf](https://huggingface.co/RichardErkhov/Azazelle_-_Tippy-Toppy-7b-gguf/blob/main/Tippy-Toppy-7b.Q4_K_S.gguf) | Q4_K_S | 3.86GB |
| [Tippy-Toppy-7b.Q4_K.gguf](https://huggingface.co/RichardErkhov/Azazelle_-_Tippy-Toppy-7b-gguf/blob/main/Tippy-Toppy-7b.Q4_K.gguf) | Q4_K | 4.07GB |
| [Tippy-Toppy-7b.Q4_K_M.gguf](https://huggingface.co/RichardErkhov/Azazelle_-_Tippy-Toppy-7b-gguf/blob/main/Tippy-Toppy-7b.Q4_K_M.gguf) | Q4_K_M | 4.07GB |
| [Tippy-Toppy-7b.Q4_1.gguf](https://huggingface.co/RichardErkhov/Azazelle_-_Tippy-Toppy-7b-gguf/blob/main/Tippy-Toppy-7b.Q4_1.gguf) | Q4_1 | 4.24GB |
| [Tippy-Toppy-7b.Q5_0.gguf](https://huggingface.co/RichardErkhov/Azazelle_-_Tippy-Toppy-7b-gguf/blob/main/Tippy-Toppy-7b.Q5_0.gguf) | Q5_0 | 4.65GB |
| [Tippy-Toppy-7b.Q5_K_S.gguf](https://huggingface.co/RichardErkhov/Azazelle_-_Tippy-Toppy-7b-gguf/blob/main/Tippy-Toppy-7b.Q5_K_S.gguf) | Q5_K_S | 4.65GB |
| [Tippy-Toppy-7b.Q5_K.gguf](https://huggingface.co/RichardErkhov/Azazelle_-_Tippy-Toppy-7b-gguf/blob/main/Tippy-Toppy-7b.Q5_K.gguf) | Q5_K | 4.78GB |
| [Tippy-Toppy-7b.Q5_K_M.gguf](https://huggingface.co/RichardErkhov/Azazelle_-_Tippy-Toppy-7b-gguf/blob/main/Tippy-Toppy-7b.Q5_K_M.gguf) | Q5_K_M | 4.78GB |
| [Tippy-Toppy-7b.Q5_1.gguf](https://huggingface.co/RichardErkhov/Azazelle_-_Tippy-Toppy-7b-gguf/blob/main/Tippy-Toppy-7b.Q5_1.gguf) | Q5_1 | 5.07GB |
| [Tippy-Toppy-7b.Q6_K.gguf](https://huggingface.co/RichardErkhov/Azazelle_-_Tippy-Toppy-7b-gguf/blob/main/Tippy-Toppy-7b.Q6_K.gguf) | Q6_K | 5.53GB |
| [Tippy-Toppy-7b.Q8_0.gguf](https://huggingface.co/RichardErkhov/Azazelle_-_Tippy-Toppy-7b-gguf/blob/main/Tippy-Toppy-7b.Q8_0.gguf) | Q8_0 | 7.17GB |
Original model description:
---
pipeline_tag: text-generation
tags:
- mistral
- merge
license: cc-by-4.0
---
# Model Card for Tippy-Toppy-7b
<!-- Provide a quick summary of what the model is/does. -->
DARE merge intended to be build on Toppy-M-7b.
.yaml file for mergekit
```.yaml:
models:
- model: mistralai/Mistral-7B-v0.1
# no parameters necessary for base model
- model: Undi95/Toppy-M-7B #175
parameters:
weight: 0.54
density: 0.81
- model: PistachioAlt/Noromaid-Bagel-7B-Slerp #75
parameters:
weight: 0.23
density: 0.61
- model: OpenPipe/mistral-ft-optimized-1227 #100
parameters:
weight: 0.31
density: 0.68
merge_method: dare_ties
base_model: mistralai/Mistral-7B-v0.1
parameters:
int8_mask: true
dtype: bfloat16
```
|
Nexusflow/Starling-LM-7B-beta | Nexusflow | "2024-04-03T21:30:33Z" | 12,704 | 328 | transformers | [
"transformers",
"safetensors",
"mistral",
"text-generation",
"reward model",
"RLHF",
"RLAIF",
"conversational",
"en",
"dataset:berkeley-nest/Nectar",
"arxiv:1909.08593",
"license:apache-2.0",
"autotrain_compatible",
"endpoints_compatible",
"text-generation-inference",
"region:us"
] | text-generation | "2024-03-19T07:04:01Z" | ---
license: apache-2.0
datasets:
- berkeley-nest/Nectar
language:
- en
library_name: transformers
tags:
- reward model
- RLHF
- RLAIF
---
# Starling-LM-7B-beta
<!-- Provide a quick summary of what the model is/does. -->
- **Developed by: The Nexusflow Team (** Banghua Zhu * , Evan Frick * , Tianhao Wu * , Hanlin Zhu, Karthik Ganesan, Wei-Lin Chiang, Jian Zhang, and Jiantao Jiao).
- **Model type:** Language Model finetuned with RLHF / RLAIF
- **License:** Apache-2.0 license under the condition that the model is not used to compete with OpenAI
- **Finetuned from model:** [Openchat-3.5-0106](https://huggingface.co/openchat/openchat-3.5-0106) (based on [Mistral-7B-v0.1](https://huggingface.co/mistralai/Mistral-7B-v0.1))
We introduce Starling-LM-7B-beta, an open large language model (LLM) trained by Reinforcement Learning from AI Feedback (RLAIF). Starling-LM-7B-beta is trained from [Openchat-3.5-0106](https://huggingface.co/openchat/openchat-3.5-0106) with our new reward model [Nexusflow/Starling-RM-34B](https://huggingface.co/Nexusflow/Starling-RM-34B) and policy optimization method [Fine-Tuning Language Models from Human Preferences (PPO)](https://arxiv.org/abs/1909.08593).
Harnessing the power of the ranking dataset, [berkeley-nest/Nectar](https://huggingface.co/datasets/berkeley-nest/Nectar), the upgraded reward model, [Starling-RM-34B](https://huggingface.co/Nexusflow/Starling-RM-34B), and the new reward training and policy tuning pipeline, Starling-LM-7B-beta scores an improved 8.12 in MT Bench with GPT-4 as a judge.
## Uses
<!-- Address questions around how the model is intended to be used, including the foreseeable users of the model and those affected by the model. -->
**Important: Please use the exact chat template provided below for the model. Otherwise there will be a degradation in the performance. The model output can be verbose in rare cases. Please consider setting temperature = 0 to make this happen less.**
Our model follows the exact chat template and usage as [Openchat-3.5-0106](https://huggingface.co/openchat/openchat-3.5-0106). Please refer to their model card for more details.
In addition, our model is hosted on LMSYS [Chatbot Arena](https://chat.lmsys.org) for free test.
The conversation template is the same as Openchat-3.5-0106:
```
import transformers
tokenizer = transformers.AutoTokenizer.from_pretrained("openchat/openchat-3.5-0106")
# Single-turn
tokens = tokenizer("GPT4 Correct User: Hello<|end_of_turn|>GPT4 Correct Assistant:").input_ids
assert tokens == [1, 420, 6316, 28781, 3198, 3123, 1247, 28747, 22557, 32000, 420, 6316, 28781, 3198, 3123, 21631, 28747]
# Multi-turn
tokens = tokenizer("GPT4 Correct User: Hello<|end_of_turn|>GPT4 Correct Assistant: Hi<|end_of_turn|>GPT4 Correct User: How are you today?<|end_of_turn|>GPT4 Correct Assistant:").input_ids
assert tokens == [1, 420, 6316, 28781, 3198, 3123, 1247, 28747, 22557, 32000, 420, 6316, 28781, 3198, 3123, 21631, 28747, 15359, 32000, 420, 6316, 28781, 3198, 3123, 1247, 28747, 1602, 460, 368, 3154, 28804, 32000, 420, 6316, 28781, 3198, 3123, 21631, 28747]
# Coding Mode
tokens = tokenizer("Code User: Implement quicksort using C++<|end_of_turn|>Code Assistant:").input_ids
assert tokens == [1, 7596, 1247, 28747, 26256, 2936, 7653, 1413, 334, 1680, 32000, 7596, 21631, 28747]
```
## Code Examples
```python
import transformers
tokenizer = transformers.AutoTokenizer.from_pretrained("Nexusflow/Starling-LM-7B-beta")
model = transformers.AutoModelForCausalLM.from_pretrained("Nexusflow/Starling-LM-7B-beta")
def generate_response(prompt):
input_ids = tokenizer(prompt, return_tensors="pt").input_ids
outputs = model.generate(
input_ids,
max_length=256,
pad_token_id=tokenizer.pad_token_id,
eos_token_id=tokenizer.eos_token_id,
)
response_ids = outputs[0]
response_text = tokenizer.decode(response_ids, skip_special_tokens=True)
return response_text
# Single-turn conversation
prompt = "Hello, how are you?"
single_turn_prompt = f"GPT4 Correct User: {prompt}<|end_of_turn|>GPT4 Correct Assistant:"
response_text = generate_response(single_turn_prompt)
print("Response:", response_text)
## Multi-turn conversation
prompt = "Hello"
follow_up_question = "How are you today?"
response = ""
multi_turn_prompt = f"GPT4 Correct User: {prompt}<|end_of_turn|>GPT4 Correct Assistant: {response}<|end_of_turn|>GPT4 Correct User: {follow_up_question}<|end_of_turn|>GPT4 Correct Assistant:"
response_text = generate_response(multi_turn_prompt)
print("Multi-turn conversation response:", response_text)
### Coding conversation
prompt = "Implement quicksort using C++"
coding_prompt = f"Code User: {prompt}<|end_of_turn|>Code Assistant:"
response = generate_response(coding_prompt)
print("Coding conversation response:", response)
```
## License
The dataset, model and online demo is subject to the [Terms of Use](https://openai.com/policies/terms-of-use) of the data generated by OpenAI, and [Privacy Practices](https://chrome.google.com/webstore/detail/sharegpt-share-your-chatg/daiacboceoaocpibfodeljbdfacokfjb) of ShareGPT. Please contact us if you find any potential violation.
## Acknowledgment
We would like to thank Tianle Li from UC Berkeley for detailed feedback and evaluation of this beta release. We would like to thank the [LMSYS Organization](https://lmsys.org/) for their support of [lmsys-chat-1M](https://huggingface.co/datasets/lmsys/lmsys-chat-1m) dataset, evaluation and online demo. We would like to thank the open source community for their efforts in providing the datasets and base models we used to develope the project, including but not limited to Anthropic, Llama, Mistral, Hugging Face H4, LMSYS, OpenChat, OpenBMB, Flan and ShareGPT.
## Citation
```
@misc{starling2023,
title = {Starling-7B: Improving LLM Helpfulness & Harmlessness with RLAIF},
url = {},
author = {Zhu, Banghua and Frick, Evan and Wu, Tianhao and Zhu, Hanlin and Ganesan, Karthik and Chiang, Wei-Lin and Zhang, Jian and Jiao, Jiantao},
month = {November},
year = {2023}
}
``` |
mradermacher/Llama-3-Swallow-8B-Instruct-v0.1-GGUF | mradermacher | "2024-07-02T03:01:16Z" | 12,703 | 0 | transformers | [
"transformers",
"gguf",
"en",
"ja",
"base_model:tokyotech-llm/Llama-3-Swallow-8B-Instruct-v0.1",
"license:llama3",
"endpoints_compatible",
"region:us"
] | null | "2024-07-01T20:28:23Z" | ---
base_model: tokyotech-llm/Llama-3-Swallow-8B-Instruct-v0.1
language:
- en
- ja
library_name: transformers
license: llama3
model_type: llama
quantized_by: mradermacher
---
## About
<!-- ### quantize_version: 2 -->
<!-- ### output_tensor_quantised: 1 -->
<!-- ### convert_type: hf -->
<!-- ### vocab_type: -->
<!-- ### tags: -->
static quants of https://huggingface.co/tokyotech-llm/Llama-3-Swallow-8B-Instruct-v0.1
<!-- provided-files -->
weighted/imatrix quants are available at https://huggingface.co/mradermacher/Llama-3-Swallow-8B-Instruct-v0.1-i1-GGUF
## Usage
If you are unsure how to use GGUF files, refer to one of [TheBloke's
READMEs](https://huggingface.co/TheBloke/KafkaLM-70B-German-V0.1-GGUF) for
more details, including on how to concatenate multi-part files.
## Provided Quants
(sorted by size, not necessarily quality. IQ-quants are often preferable over similar sized non-IQ quants)
| Link | Type | Size/GB | Notes |
|:-----|:-----|--------:|:------|
| [GGUF](https://huggingface.co/mradermacher/Llama-3-Swallow-8B-Instruct-v0.1-GGUF/resolve/main/Llama-3-Swallow-8B-Instruct-v0.1.Q2_K.gguf) | Q2_K | 3.3 | |
| [GGUF](https://huggingface.co/mradermacher/Llama-3-Swallow-8B-Instruct-v0.1-GGUF/resolve/main/Llama-3-Swallow-8B-Instruct-v0.1.IQ3_XS.gguf) | IQ3_XS | 3.6 | |
| [GGUF](https://huggingface.co/mradermacher/Llama-3-Swallow-8B-Instruct-v0.1-GGUF/resolve/main/Llama-3-Swallow-8B-Instruct-v0.1.Q3_K_S.gguf) | Q3_K_S | 3.8 | |
| [GGUF](https://huggingface.co/mradermacher/Llama-3-Swallow-8B-Instruct-v0.1-GGUF/resolve/main/Llama-3-Swallow-8B-Instruct-v0.1.IQ3_S.gguf) | IQ3_S | 3.8 | beats Q3_K* |
| [GGUF](https://huggingface.co/mradermacher/Llama-3-Swallow-8B-Instruct-v0.1-GGUF/resolve/main/Llama-3-Swallow-8B-Instruct-v0.1.IQ3_M.gguf) | IQ3_M | 3.9 | |
| [GGUF](https://huggingface.co/mradermacher/Llama-3-Swallow-8B-Instruct-v0.1-GGUF/resolve/main/Llama-3-Swallow-8B-Instruct-v0.1.Q3_K_M.gguf) | Q3_K_M | 4.1 | lower quality |
| [GGUF](https://huggingface.co/mradermacher/Llama-3-Swallow-8B-Instruct-v0.1-GGUF/resolve/main/Llama-3-Swallow-8B-Instruct-v0.1.Q3_K_L.gguf) | Q3_K_L | 4.4 | |
| [GGUF](https://huggingface.co/mradermacher/Llama-3-Swallow-8B-Instruct-v0.1-GGUF/resolve/main/Llama-3-Swallow-8B-Instruct-v0.1.IQ4_XS.gguf) | IQ4_XS | 4.6 | |
| [GGUF](https://huggingface.co/mradermacher/Llama-3-Swallow-8B-Instruct-v0.1-GGUF/resolve/main/Llama-3-Swallow-8B-Instruct-v0.1.Q4_K_S.gguf) | Q4_K_S | 4.8 | fast, recommended |
| [GGUF](https://huggingface.co/mradermacher/Llama-3-Swallow-8B-Instruct-v0.1-GGUF/resolve/main/Llama-3-Swallow-8B-Instruct-v0.1.Q4_K_M.gguf) | Q4_K_M | 5.0 | fast, recommended |
| [GGUF](https://huggingface.co/mradermacher/Llama-3-Swallow-8B-Instruct-v0.1-GGUF/resolve/main/Llama-3-Swallow-8B-Instruct-v0.1.Q5_K_S.gguf) | Q5_K_S | 5.7 | |
| [GGUF](https://huggingface.co/mradermacher/Llama-3-Swallow-8B-Instruct-v0.1-GGUF/resolve/main/Llama-3-Swallow-8B-Instruct-v0.1.Q5_K_M.gguf) | Q5_K_M | 5.8 | |
| [GGUF](https://huggingface.co/mradermacher/Llama-3-Swallow-8B-Instruct-v0.1-GGUF/resolve/main/Llama-3-Swallow-8B-Instruct-v0.1.Q6_K.gguf) | Q6_K | 6.7 | very good quality |
| [GGUF](https://huggingface.co/mradermacher/Llama-3-Swallow-8B-Instruct-v0.1-GGUF/resolve/main/Llama-3-Swallow-8B-Instruct-v0.1.Q8_0.gguf) | Q8_0 | 8.6 | fast, best quality |
| [GGUF](https://huggingface.co/mradermacher/Llama-3-Swallow-8B-Instruct-v0.1-GGUF/resolve/main/Llama-3-Swallow-8B-Instruct-v0.1.f16.gguf) | f16 | 16.2 | 16 bpw, overkill |
Here is a handy graph by ikawrakow comparing some lower-quality quant
types (lower is better):

And here are Artefact2's thoughts on the matter:
https://gist.github.com/Artefact2/b5f810600771265fc1e39442288e8ec9
## FAQ / Model Request
See https://huggingface.co/mradermacher/model_requests for some answers to
questions you might have and/or if you want some other model quantized.
## Thanks
I thank my company, [nethype GmbH](https://www.nethype.de/), for letting
me use its servers and providing upgrades to my workstation to enable
this work in my free time.
<!-- end -->
|
valentinafeve/yolos-fashionpedia | valentinafeve | "2023-03-10T13:11:26Z" | 12,701 | 86 | transformers | [
"transformers",
"pytorch",
"yolos",
"object-detection",
"YOLOS",
"Object detection",
"en",
"dataset:detection-datasets/fashionpedia",
"endpoints_compatible",
"region:us"
] | object-detection | "2022-11-17T16:04:03Z" | ---
datasets:
- detection-datasets/fashionpedia
language:
- en
pipeline_tag: object-detection
tags:
- YOLOS
- Object detection
---
This is a fine-tunned object detection model for fashion.
For more details of the implementation you can check the source code [here](https://github.com/valntinaf/fine_tunning_YOLOS_for_fashion)
the dataset used for its training is available [here](https://huggingface.co/datasets/detection-datasets/fashionpedia)
this model supports the following categories:
CATS = ['shirt, blouse', 'top, t-shirt, sweatshirt', 'sweater', 'cardigan', 'jacket', 'vest', 'pants', 'shorts', 'skirt', 'coat', 'dress', 'jumpsuit', 'cape', 'glasses', 'hat', 'headband, head covering, hair accessory', 'tie', 'glove', 'watch', 'belt', 'leg warmer', 'tights, stockings', 'sock', 'shoe', 'bag, wallet', 'scarf', 'umbrella', 'hood', 'collar', 'lapel', 'epaulette', 'sleeve', 'pocket', 'neckline', 'buckle', 'zipper', 'applique', 'bead', 'bow', 'flower', 'fringe', 'ribbon', 'rivet', 'ruffle', 'sequin', 'tassel']
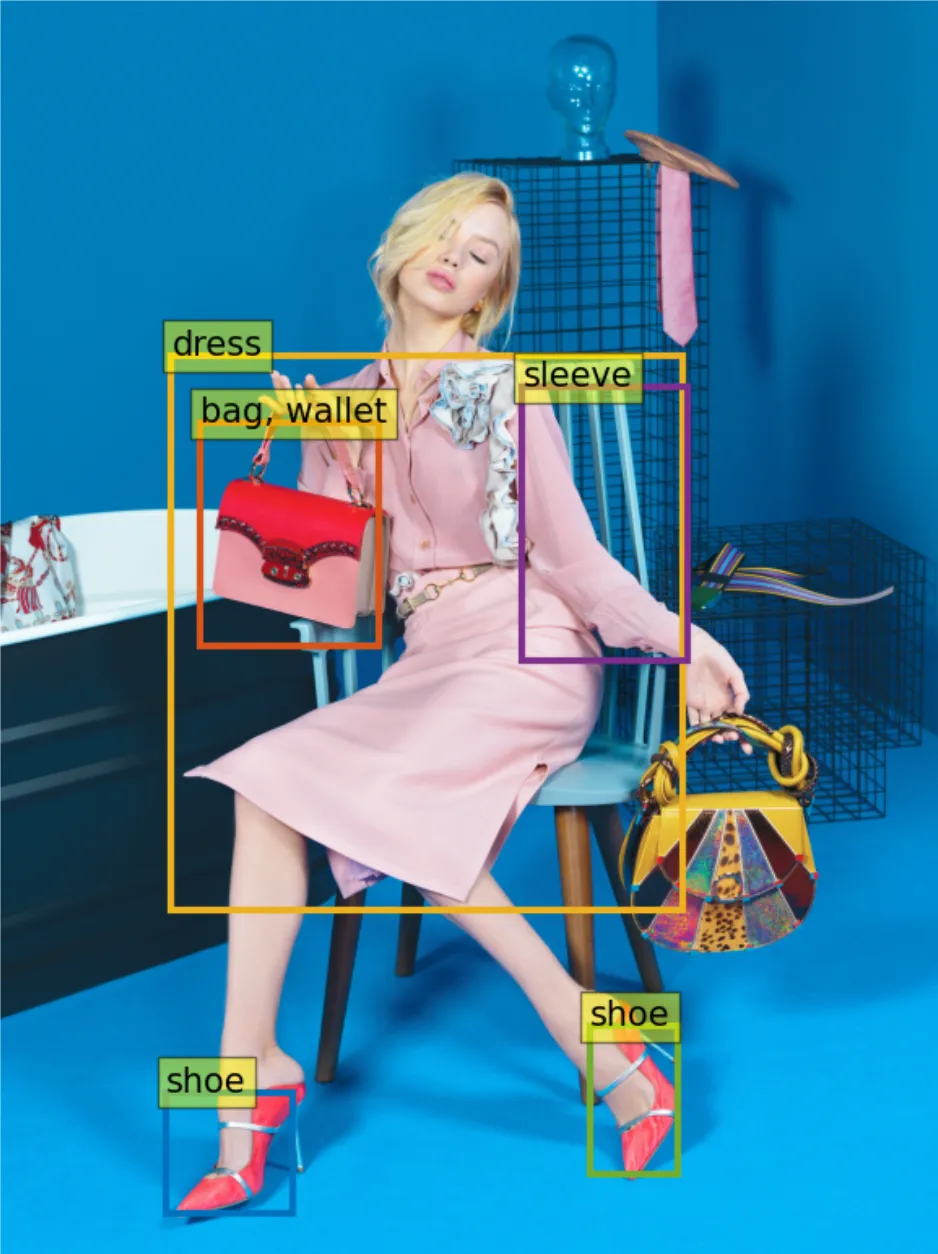
|
LnL-AI/TinyLlama-1.1B-Chat-v1.0-GPTQ-Marlin-4bit | LnL-AI | "2024-03-29T03:28:31Z" | 12,700 | 0 | transformers | [
"transformers",
"llama",
"text-generation",
"conversational",
"license:unknown",
"autotrain_compatible",
"endpoints_compatible",
"text-generation-inference",
"4-bit",
"gptq",
"region:us"
] | text-generation | "2024-03-29T03:21:29Z" | ---
license: unknown
---
This is TinyLlama/TinyLlama-1.1B-Chat-v1.0 quantized with AutoGPTQ in GPTQ 4-bit Marlin format.
**Quantize config:**
```
{
"bits": 4,
"group_size": 128,
"damp_percent": 0.01,
"desc_act": false,
"static_groups": false,
"sym": true,
"true_sequential": true,
"model_name_or_path": null,
"model_file_base_name": null,
"quant_method": "gptq",
"checkpoint_format": "marlin"
}
```
|
laion/CLIP-ViT-B-16-DataComp.XL-s13B-b90K | laion | "2023-09-29T22:41:47Z" | 12,694 | 5 | open_clip | [
"open_clip",
"zero-shot-image-classification",
"dataset:mlfoundations/datacomp_pools",
"arxiv:2304.14108",
"license:mit",
"region:us"
] | zero-shot-image-classification | "2023-05-15T19:27:18Z" | ---
license: mit
widget:
- src: >-
https://huggingface.co/datasets/mishig/sample_images/resolve/main/cat-dog-music.png
candidate_labels: playing music, playing sports
example_title: Cat & Dog
library_name: open_clip
datasets:
- mlfoundations/datacomp_pools
pipeline_tag: zero-shot-image-classification
---
# Model card for CLIP ViT-B-16 trained DataComp-1B
# Table of Contents
1. [Model Details](#model-details)
2. [Uses](#uses)
3. [Training Details](#training-details)
4. [Evaluation](#evaluation)
5. [Acknowledgements](#acknowledgements)
6. [Citation](#citation)
7. [How To Get Started With the Model](#how-to-get-started-with-the-model)
# Model Details
## Model Description
A CLIP ViT-B/16 model trained with the DataComp-1B (https://github.com/mlfoundations/datacomp) using OpenCLIP (https://github.com/mlfoundations/open_clip).
Model training done on the [stability.ai](https://stability.ai/) cluster.
# Uses
As per the original [OpenAI CLIP model card](https://github.com/openai/CLIP/blob/d50d76daa670286dd6cacf3bcd80b5e4823fc8e1/model-card.md), this model is intended as a research output for research communities. We hope that this model will enable researchers to better understand and explore zero-shot, arbitrary image classification. We also hope it can be used for interdisciplinary studies of the potential impact of such model.
The OpenAI CLIP paper includes a discussion of potential downstream impacts to provide an example for this sort of analysis. Additionally, the DataComp paper (https://arxiv.org/abs/2304.14108) include additional discussion as it relates specifically to the training dataset.
## Direct Use
Zero-shot image classification, image and text retrieval, among others.
## Downstream Use
Image classification and other image task fine-tuning, linear probe image classification, image generation guiding and conditioning, among others.
## Out-of-Scope Use
As per the OpenAI models,
**Any** deployed use case of the model - whether commercial or not - is currently out of scope. Non-deployed use cases such as image search in a constrained environment, are also not recommended unless there is thorough in-domain testing of the model with a specific, fixed class taxonomy. This is because our safety assessment demonstrated a high need for task specific testing especially given the variability of CLIP’s performance with different class taxonomies. This makes untested and unconstrained deployment of the model in any use case currently potentially harmful.
Certain use cases which would fall under the domain of surveillance and facial recognition are always out-of-scope regardless of performance of the model. This is because the use of artificial intelligence for tasks such as these can be premature currently given the lack of testing norms and checks to ensure its fair use.
# Training Details
## Training Data
This model was trained with the 1.4 Billion samples of the DataComp-1B dataset (https://arxiv.org/abs/2304.14108).
**IMPORTANT NOTE:** The motivation behind dataset creation is to democratize research and experimentation around large-scale multi-modal model training and handling of uncurated, large-scale datasets crawled from publically available internet. Our recommendation is therefore to use the dataset for research purposes. Be aware that this large-scale dataset is uncurated. Keep in mind that the uncurated nature of the dataset means that collected links may lead to strongly discomforting and disturbing content for a human viewer. Therefore, please use the demo links with caution and at your own risk. It is possible to extract a “safe” subset by filtering out samples based on the safety tags (using a customized trained NSFW classifier that we built). While this strongly reduces the chance for encountering potentially harmful content when viewing, we cannot entirely exclude the possibility for harmful content being still present in safe mode, so that the warning holds also there. We think that providing the dataset openly to broad research and other interested communities will allow for transparent investigation of benefits that come along with training large-scale models as well as pitfalls and dangers that may stay unreported or unnoticed when working with closed large datasets that remain restricted to a small community. Providing our dataset openly, we however do not recommend using it for creating ready-to-go industrial products, as the basic research about general properties and safety of such large-scale models, which we would like to encourage with this release, is still in progress.
## Training Procedure
Please see https://arxiv.org/abs/2304.14108.
# Evaluation
Evaluation done on 38 datasets, using the [DataComp repo](https://github.com/mlfoundations/datacomp) and the [LAION CLIP Benchmark](https://github.com/LAION-AI/CLIP_benchmark).
## Testing Data, Factors & Metrics
### Testing Data
The testing is performed on a suite of 38 datasets. See our paper for more details (https://arxiv.org/abs/2304.14108).
## Results
The model achieves a 73.5% zero-shot top-1 accuracy on ImageNet-1k. See our paper for more details and results (https://arxiv.org/abs/2304.14108).
# Acknowledgements
Acknowledging [stability.ai](https://stability.ai/) for the compute used to train this model.
# Citation
**BibTeX:**
DataComp
```bibtex
@article{datacomp,
title={DataComp: In search of the next generation of multimodal datasets},
author={Samir Yitzhak Gadre, Gabriel Ilharco, Alex Fang, Jonathan Hayase, Georgios Smyrnis, Thao Nguyen, Ryan Marten, Mitchell Wortsman, Dhruba Ghosh, Jieyu Zhang, Eyal Orgad, Rahim Entezari, Giannis Daras, Sarah Pratt, Vivek Ramanujan, Yonatan Bitton, Kalyani Marathe, Stephen Mussmann, Richard Vencu, Mehdi Cherti, Ranjay Krishna, Pang Wei Koh, Olga Saukh, Alexander Ratner, Shuran Song, Hannaneh Hajishirzi, Ali Farhadi, Romain Beaumont, Sewoong Oh, Alex Dimakis, Jenia Jitsev, Yair Carmon, Vaishaal Shankar, Ludwig Schmidt},
journal={arXiv preprint arXiv:2304.14108},
year={2023}
}
```
OpenAI CLIP paper
```
@inproceedings{Radford2021LearningTV,
title={Learning Transferable Visual Models From Natural Language Supervision},
author={Alec Radford and Jong Wook Kim and Chris Hallacy and A. Ramesh and Gabriel Goh and Sandhini Agarwal and Girish Sastry and Amanda Askell and Pamela Mishkin and Jack Clark and Gretchen Krueger and Ilya Sutskever},
booktitle={ICML},
year={2021}
}
```
OpenCLIP software
```
@software{ilharco_gabriel_2021_5143773,
author = {Ilharco, Gabriel and
Wortsman, Mitchell and
Wightman, Ross and
Gordon, Cade and
Carlini, Nicholas and
Taori, Rohan and
Dave, Achal and
Shankar, Vaishaal and
Namkoong, Hongseok and
Miller, John and
Hajishirzi, Hannaneh and
Farhadi, Ali and
Schmidt, Ludwig},
title = {OpenCLIP},
month = jul,
year = 2021,
note = {If you use this software, please cite it as below.},
publisher = {Zenodo},
version = {0.1},
doi = {10.5281/zenodo.5143773},
url = {https://doi.org/10.5281/zenodo.5143773}
}
```
# How to Get Started with the Model
See https://github.com/mlfoundations/open_clip |
neuralmagic/TinyLlama-1.1B-Chat-v1.0-marlin | neuralmagic | "2024-03-06T01:00:38Z" | 12,688 | 1 | transformers | [
"transformers",
"safetensors",
"llama",
"text-generation",
"nm-vllm",
"marlin",
"int4",
"conversational",
"arxiv:2210.17323",
"base_model:TinyLlama/TinyLlama-1.1B-Chat-v1.0",
"autotrain_compatible",
"endpoints_compatible",
"text-generation-inference",
"4-bit",
"gptq",
"region:us"
] | text-generation | "2024-03-05T19:17:33Z" | ---
base_model: TinyLlama/TinyLlama-1.1B-Chat-v1.0
inference: true
model_type: llama
quantized_by: robertgshaw2
tags:
- nm-vllm
- marlin
- int4
---
## TinyLlama-1.1B-Chat-v1.0
This repo contains model files for [TinyLlama-1.1B-Chat-v1.0](https://huggingface.co/TinyLlama/TinyLlama-1.1B-Chat-v1.0) optimized for [nm-vllm](https://github.com/neuralmagic/nm-vllm), a high-throughput serving engine for compressed LLMs.
This model was quantized with [GPTQ](https://arxiv.org/abs/2210.17323) and saved in the Marlin format for efficient 4-bit inference. Marlin is a highly optimized inference kernel for 4 bit models.
## Inference
Install [nm-vllm](https://github.com/neuralmagic/nm-vllm) for fast inference and low memory-usage:
```bash
pip install nm-vllm[sparse]
```
Run in a Python pipeline for local inference:
```python
from transformers import AutoTokenizer
from vllm import LLM, SamplingParams
model_id = "neuralmagic/TinyLlama-1.1B-Chat-v1.0-marlin"
model = LLM(model_id)
tokenizer = AutoTokenizer.from_pretrained(model_id)
messages = [
{"role": "user", "content": "How to make banana bread?"},
]
formatted_prompt = tokenizer.apply_chat_template(messages, tokenize=False, add_generation_prompt=True)
sampling_params = SamplingParams(max_tokens=200)
outputs = model.generate(formatted_prompt, sampling_params=sampling_params)
print(outputs[0].outputs[0].text)
"""
Sure! Here's a simple recipe for banana bread:
Ingredients:
- 3-4 ripe bananas,mashed
- 1 large egg
- 2 Tbsp. Flour
- 2 tsp. Baking powder
- 1 tsp. Baking soda
- 1/2 tsp. Ground cinnamon
- 1/4 tsp. Salt
- 1/2 cup butter, melted
- 3 Cups All-purpose flour
- 1/2 tsp. Ground cinnamon
Instructions:
1. Preheat your oven to 350 F (175 C).
"""
```
## Quantization
For details on how this model was quantized and converted to marlin format, run the `quantization/apply_gptq_save_marlin.py` script:
```bash
pip install -r quantization/requirements.txt
python3 quantization/apply_gptq_save_marlin.py --model-id TinyLlama/TinyLlama-1.1B-Chat-v1.0 --save-dir ./tinyllama-marlin
```
## Slack
For further support, and discussions on these models and AI in general, join [Neural Magic's Slack Community](https://join.slack.com/t/discuss-neuralmagic/shared_invite/zt-q1a1cnvo-YBoICSIw3L1dmQpjBeDurQ) |
mradermacher/NeonLLM-Base-GGUF | mradermacher | "2024-06-28T14:11:02Z" | 12,681 | 1 | transformers | [
"transformers",
"gguf",
"Mistral",
"instruct",
"finetune",
"chatml",
"DPO",
"RLHF",
"gpt4",
"synthetic data",
"distillation",
"function calling",
"json mode",
"en",
"dataset:teknium/OpenHermes-2.5",
"base_model:neongeckocom/NeonLLM-Base",
"license:apache-2.0",
"endpoints_compatible",
"region:us"
] | null | "2024-06-27T16:20:04Z" | ---
base_model: neongeckocom/NeonLLM-Base
datasets:
- teknium/OpenHermes-2.5
language:
- en
library_name: transformers
license: apache-2.0
quantized_by: mradermacher
tags:
- Mistral
- instruct
- finetune
- chatml
- DPO
- RLHF
- gpt4
- synthetic data
- distillation
- function calling
- json mode
---
## About
<!-- ### quantize_version: 2 -->
<!-- ### output_tensor_quantised: 1 -->
<!-- ### convert_type: hf -->
<!-- ### vocab_type: -->
<!-- ### tags: -->
static quants of https://huggingface.co/neongeckocom/NeonLLM-Base
<!-- provided-files -->
weighted/imatrix quants are available at https://huggingface.co/mradermacher/NeonLLM-Base-i1-GGUF
## Usage
If you are unsure how to use GGUF files, refer to one of [TheBloke's
READMEs](https://huggingface.co/TheBloke/KafkaLM-70B-German-V0.1-GGUF) for
more details, including on how to concatenate multi-part files.
## Provided Quants
(sorted by size, not necessarily quality. IQ-quants are often preferable over similar sized non-IQ quants)
| Link | Type | Size/GB | Notes |
|:-----|:-----|--------:|:------|
| [GGUF](https://huggingface.co/mradermacher/NeonLLM-Base-GGUF/resolve/main/NeonLLM-Base.Q2_K.gguf) | Q2_K | 2.8 | |
| [GGUF](https://huggingface.co/mradermacher/NeonLLM-Base-GGUF/resolve/main/NeonLLM-Base.IQ3_XS.gguf) | IQ3_XS | 3.1 | |
| [GGUF](https://huggingface.co/mradermacher/NeonLLM-Base-GGUF/resolve/main/NeonLLM-Base.Q3_K_S.gguf) | Q3_K_S | 3.3 | |
| [GGUF](https://huggingface.co/mradermacher/NeonLLM-Base-GGUF/resolve/main/NeonLLM-Base.IQ3_S.gguf) | IQ3_S | 3.3 | beats Q3_K* |
| [GGUF](https://huggingface.co/mradermacher/NeonLLM-Base-GGUF/resolve/main/NeonLLM-Base.IQ3_M.gguf) | IQ3_M | 3.4 | |
| [GGUF](https://huggingface.co/mradermacher/NeonLLM-Base-GGUF/resolve/main/NeonLLM-Base.Q3_K_M.gguf) | Q3_K_M | 3.6 | lower quality |
| [GGUF](https://huggingface.co/mradermacher/NeonLLM-Base-GGUF/resolve/main/NeonLLM-Base.Q3_K_L.gguf) | Q3_K_L | 3.9 | |
| [GGUF](https://huggingface.co/mradermacher/NeonLLM-Base-GGUF/resolve/main/NeonLLM-Base.IQ4_XS.gguf) | IQ4_XS | 4.0 | |
| [GGUF](https://huggingface.co/mradermacher/NeonLLM-Base-GGUF/resolve/main/NeonLLM-Base.Q4_K_S.gguf) | Q4_K_S | 4.2 | fast, recommended |
| [GGUF](https://huggingface.co/mradermacher/NeonLLM-Base-GGUF/resolve/main/NeonLLM-Base.Q4_K_M.gguf) | Q4_K_M | 4.5 | fast, recommended |
| [GGUF](https://huggingface.co/mradermacher/NeonLLM-Base-GGUF/resolve/main/NeonLLM-Base.Q5_K_S.gguf) | Q5_K_S | 5.1 | |
| [GGUF](https://huggingface.co/mradermacher/NeonLLM-Base-GGUF/resolve/main/NeonLLM-Base.Q5_K_M.gguf) | Q5_K_M | 5.2 | |
| [GGUF](https://huggingface.co/mradermacher/NeonLLM-Base-GGUF/resolve/main/NeonLLM-Base.Q6_K.gguf) | Q6_K | 6.0 | very good quality |
| [GGUF](https://huggingface.co/mradermacher/NeonLLM-Base-GGUF/resolve/main/NeonLLM-Base.Q8_0.gguf) | Q8_0 | 7.8 | fast, best quality |
| [GGUF](https://huggingface.co/mradermacher/NeonLLM-Base-GGUF/resolve/main/NeonLLM-Base.f16.gguf) | f16 | 14.6 | 16 bpw, overkill |
Here is a handy graph by ikawrakow comparing some lower-quality quant
types (lower is better):

And here are Artefact2's thoughts on the matter:
https://gist.github.com/Artefact2/b5f810600771265fc1e39442288e8ec9
## FAQ / Model Request
See https://huggingface.co/mradermacher/model_requests for some answers to
questions you might have and/or if you want some other model quantized.
## Thanks
I thank my company, [nethype GmbH](https://www.nethype.de/), for letting
me use its servers and providing upgrades to my workstation to enable
this work in my free time.
<!-- end -->
|
jonatasgrosman/wav2vec2-large-xlsr-53-german | jonatasgrosman | "2022-12-14T01:59:09Z" | 12,679 | 7 | transformers | [
"transformers",
"pytorch",
"jax",
"wav2vec2",
"automatic-speech-recognition",
"audio",
"de",
"hf-asr-leaderboard",
"mozilla-foundation/common_voice_6_0",
"robust-speech-event",
"speech",
"xlsr-fine-tuning-week",
"dataset:common_voice",
"dataset:mozilla-foundation/common_voice_6_0",
"license:apache-2.0",
"model-index",
"endpoints_compatible",
"region:us"
] | automatic-speech-recognition | "2022-03-02T23:29:05Z" | ---
language: de
license: apache-2.0
datasets:
- common_voice
- mozilla-foundation/common_voice_6_0
metrics:
- wer
- cer
tags:
- audio
- automatic-speech-recognition
- de
- hf-asr-leaderboard
- mozilla-foundation/common_voice_6_0
- robust-speech-event
- speech
- xlsr-fine-tuning-week
model-index:
- name: XLSR Wav2Vec2 German by Jonatas Grosman
results:
- task:
name: Automatic Speech Recognition
type: automatic-speech-recognition
dataset:
name: Common Voice de
type: common_voice
args: de
metrics:
- name: Test WER
type: wer
value: 12.06
- name: Test CER
type: cer
value: 2.92
- name: Test WER (+LM)
type: wer
value: 8.74
- name: Test CER (+LM)
type: cer
value: 2.28
- task:
name: Automatic Speech Recognition
type: automatic-speech-recognition
dataset:
name: Robust Speech Event - Dev Data
type: speech-recognition-community-v2/dev_data
args: de
metrics:
- name: Dev WER
type: wer
value: 32.75
- name: Dev CER
type: cer
value: 13.64
- name: Dev WER (+LM)
type: wer
value: 26.6
- name: Dev CER (+LM)
type: cer
value: 12.58
---
# Fine-tuned XLSR-53 large model for speech recognition in German
Fine-tuned [facebook/wav2vec2-large-xlsr-53](https://huggingface.co/facebook/wav2vec2-large-xlsr-53) on German using the train and validation splits of [Common Voice 6.1](https://huggingface.co/datasets/common_voice).
When using this model, make sure that your speech input is sampled at 16kHz.
This model has been fine-tuned thanks to the GPU credits generously given by the [OVHcloud](https://www.ovhcloud.com/en/public-cloud/ai-training/) :)
The script used for training can be found here: https://github.com/jonatasgrosman/wav2vec2-sprint
## Usage
The model can be used directly (without a language model) as follows...
Using the [HuggingSound](https://github.com/jonatasgrosman/huggingsound) library:
```python
from huggingsound import SpeechRecognitionModel
model = SpeechRecognitionModel("jonatasgrosman/wav2vec2-large-xlsr-53-german")
audio_paths = ["/path/to/file.mp3", "/path/to/another_file.wav"]
transcriptions = model.transcribe(audio_paths)
```
Writing your own inference script:
```python
import torch
import librosa
from datasets import load_dataset
from transformers import Wav2Vec2ForCTC, Wav2Vec2Processor
LANG_ID = "de"
MODEL_ID = "jonatasgrosman/wav2vec2-large-xlsr-53-german"
SAMPLES = 10
test_dataset = load_dataset("common_voice", LANG_ID, split=f"test[:{SAMPLES}]")
processor = Wav2Vec2Processor.from_pretrained(MODEL_ID)
model = Wav2Vec2ForCTC.from_pretrained(MODEL_ID)
# Preprocessing the datasets.
# We need to read the audio files as arrays
def speech_file_to_array_fn(batch):
speech_array, sampling_rate = librosa.load(batch["path"], sr=16_000)
batch["speech"] = speech_array
batch["sentence"] = batch["sentence"].upper()
return batch
test_dataset = test_dataset.map(speech_file_to_array_fn)
inputs = processor(test_dataset["speech"], sampling_rate=16_000, return_tensors="pt", padding=True)
with torch.no_grad():
logits = model(inputs.input_values, attention_mask=inputs.attention_mask).logits
predicted_ids = torch.argmax(logits, dim=-1)
predicted_sentences = processor.batch_decode(predicted_ids)
for i, predicted_sentence in enumerate(predicted_sentences):
print("-" * 100)
print("Reference:", test_dataset[i]["sentence"])
print("Prediction:", predicted_sentence)
```
| Reference | Prediction |
| ------------- | ------------- |
| ZIEHT EUCH BITTE DRAUSSEN DIE SCHUHE AUS. | ZIEHT EUCH BITTE DRAUSSEN DIE SCHUHE AUS |
| ES KOMMT ZUM SHOWDOWN IN GSTAAD. | ES KOMMT ZUG STUNDEDAUTENESTERKT |
| IHRE FOTOSTRECKEN ERSCHIENEN IN MODEMAGAZINEN WIE DER VOGUE, HARPER’S BAZAAR UND MARIE CLAIRE. | IHRE FOTELSTRECKEN ERSCHIENEN MIT MODEMAGAZINEN WIE DER VALG AT DAS BASIN MA RIQUAIR |
| FELIPE HAT EINE AUCH FÜR MONARCHEN UNGEWÖHNLICH LANGE TITELLISTE. | FELIPPE HAT EINE AUCH FÜR MONACHEN UNGEWÖHNLICH LANGE TITELLISTE |
| ER WURDE ZU EHREN DES REICHSKANZLERS OTTO VON BISMARCK ERRICHTET. | ER WURDE ZU EHREN DES REICHSKANZLERS OTTO VON BISMARCK ERRICHTET M |
| WAS SOLLS, ICH BIN BEREIT. | WAS SOLL'S ICH BIN BEREIT |
| DAS INTERNET BESTEHT AUS VIELEN COMPUTERN, DIE MITEINANDER VERBUNDEN SIND. | DAS INTERNET BESTEHT AUS VIELEN COMPUTERN DIE MITEINANDER VERBUNDEN SIND |
| DER URANUS IST DER SIEBENTE PLANET IN UNSEREM SONNENSYSTEM. | DER URANUS IST DER SIEBENTE PLANET IN UNSEREM SONNENSYSTEM |
| DIE WAGEN ERHIELTEN EIN EINHEITLICHES ERSCHEINUNGSBILD IN WEISS MIT ROTEM FENSTERBAND. | DIE WAGEN ERHIELTEN EIN EINHEITLICHES ERSCHEINUNGSBILD IN WEISS MIT ROTEM FENSTERBAND |
| SIE WAR DIE COUSINE VON CARL MARIA VON WEBER. | SIE WAR DIE COUSINE VON KARL-MARIA VON WEBER |
## Evaluation
1. To evaluate on `mozilla-foundation/common_voice_6_0` with split `test`
```bash
python eval.py --model_id jonatasgrosman/wav2vec2-large-xlsr-53-german --dataset mozilla-foundation/common_voice_6_0 --config de --split test
```
2. To evaluate on `speech-recognition-community-v2/dev_data`
```bash
python eval.py --model_id jonatasgrosman/wav2vec2-large-xlsr-53-german --dataset speech-recognition-community-v2/dev_data --config de --split validation --chunk_length_s 5.0 --stride_length_s 1.0
```
## Citation
If you want to cite this model you can use this:
```bibtex
@misc{grosman2021xlsr53-large-german,
title={Fine-tuned {XLSR}-53 large model for speech recognition in {G}erman},
author={Grosman, Jonatas},
howpublished={\url{https://huggingface.co/jonatasgrosman/wav2vec2-large-xlsr-53-german}},
year={2021}
}
``` |
mradermacher/LemonadeRP-4.5.3-i1-GGUF | mradermacher | "2024-06-26T11:04:24Z" | 12,676 | 0 | transformers | [
"transformers",
"gguf",
"roleplay",
"en",
"base_model:KatyTheCutie/LemonadeRP-4.5.3",
"license:cc-by-4.0",
"endpoints_compatible",
"region:us"
] | null | "2024-06-26T06:06:37Z" | ---
base_model: KatyTheCutie/LemonadeRP-4.5.3
language:
- en
library_name: transformers
license: cc-by-4.0
quantized_by: mradermacher
tags:
- roleplay
---
## About
<!-- ### quantize_version: 2 -->
<!-- ### output_tensor_quantised: 1 -->
<!-- ### convert_type: hf -->
<!-- ### vocab_type: -->
<!-- ### tags: nicoboss -->
weighted/imatrix quants of https://huggingface.co/KatyTheCutie/LemonadeRP-4.5.3
<!-- provided-files -->
static quants are available at https://huggingface.co/mradermacher/LemonadeRP-4.5.3-GGUF
## Usage
If you are unsure how to use GGUF files, refer to one of [TheBloke's
READMEs](https://huggingface.co/TheBloke/KafkaLM-70B-German-V0.1-GGUF) for
more details, including on how to concatenate multi-part files.
## Provided Quants
(sorted by size, not necessarily quality. IQ-quants are often preferable over similar sized non-IQ quants)
| Link | Type | Size/GB | Notes |
|:-----|:-----|--------:|:------|
| [GGUF](https://huggingface.co/mradermacher/LemonadeRP-4.5.3-i1-GGUF/resolve/main/LemonadeRP-4.5.3.i1-IQ1_S.gguf) | i1-IQ1_S | 1.7 | for the desperate |
| [GGUF](https://huggingface.co/mradermacher/LemonadeRP-4.5.3-i1-GGUF/resolve/main/LemonadeRP-4.5.3.i1-IQ1_M.gguf) | i1-IQ1_M | 1.9 | mostly desperate |
| [GGUF](https://huggingface.co/mradermacher/LemonadeRP-4.5.3-i1-GGUF/resolve/main/LemonadeRP-4.5.3.i1-IQ2_XXS.gguf) | i1-IQ2_XXS | 2.1 | |
| [GGUF](https://huggingface.co/mradermacher/LemonadeRP-4.5.3-i1-GGUF/resolve/main/LemonadeRP-4.5.3.i1-IQ2_XS.gguf) | i1-IQ2_XS | 2.3 | |
| [GGUF](https://huggingface.co/mradermacher/LemonadeRP-4.5.3-i1-GGUF/resolve/main/LemonadeRP-4.5.3.i1-IQ2_S.gguf) | i1-IQ2_S | 2.4 | |
| [GGUF](https://huggingface.co/mradermacher/LemonadeRP-4.5.3-i1-GGUF/resolve/main/LemonadeRP-4.5.3.i1-IQ2_M.gguf) | i1-IQ2_M | 2.6 | |
| [GGUF](https://huggingface.co/mradermacher/LemonadeRP-4.5.3-i1-GGUF/resolve/main/LemonadeRP-4.5.3.i1-Q2_K.gguf) | i1-Q2_K | 2.8 | IQ3_XXS probably better |
| [GGUF](https://huggingface.co/mradermacher/LemonadeRP-4.5.3-i1-GGUF/resolve/main/LemonadeRP-4.5.3.i1-IQ3_XXS.gguf) | i1-IQ3_XXS | 2.9 | lower quality |
| [GGUF](https://huggingface.co/mradermacher/LemonadeRP-4.5.3-i1-GGUF/resolve/main/LemonadeRP-4.5.3.i1-IQ3_XS.gguf) | i1-IQ3_XS | 3.1 | |
| [GGUF](https://huggingface.co/mradermacher/LemonadeRP-4.5.3-i1-GGUF/resolve/main/LemonadeRP-4.5.3.i1-Q3_K_S.gguf) | i1-Q3_K_S | 3.3 | IQ3_XS probably better |
| [GGUF](https://huggingface.co/mradermacher/LemonadeRP-4.5.3-i1-GGUF/resolve/main/LemonadeRP-4.5.3.i1-IQ3_S.gguf) | i1-IQ3_S | 3.3 | beats Q3_K* |
| [GGUF](https://huggingface.co/mradermacher/LemonadeRP-4.5.3-i1-GGUF/resolve/main/LemonadeRP-4.5.3.i1-IQ3_M.gguf) | i1-IQ3_M | 3.4 | |
| [GGUF](https://huggingface.co/mradermacher/LemonadeRP-4.5.3-i1-GGUF/resolve/main/LemonadeRP-4.5.3.i1-Q3_K_M.gguf) | i1-Q3_K_M | 3.6 | IQ3_S probably better |
| [GGUF](https://huggingface.co/mradermacher/LemonadeRP-4.5.3-i1-GGUF/resolve/main/LemonadeRP-4.5.3.i1-Q3_K_L.gguf) | i1-Q3_K_L | 3.9 | IQ3_M probably better |
| [GGUF](https://huggingface.co/mradermacher/LemonadeRP-4.5.3-i1-GGUF/resolve/main/LemonadeRP-4.5.3.i1-IQ4_XS.gguf) | i1-IQ4_XS | 4.0 | |
| [GGUF](https://huggingface.co/mradermacher/LemonadeRP-4.5.3-i1-GGUF/resolve/main/LemonadeRP-4.5.3.i1-Q4_0.gguf) | i1-Q4_0 | 4.2 | fast, low quality |
| [GGUF](https://huggingface.co/mradermacher/LemonadeRP-4.5.3-i1-GGUF/resolve/main/LemonadeRP-4.5.3.i1-Q4_K_S.gguf) | i1-Q4_K_S | 4.2 | optimal size/speed/quality |
| [GGUF](https://huggingface.co/mradermacher/LemonadeRP-4.5.3-i1-GGUF/resolve/main/LemonadeRP-4.5.3.i1-Q4_K_M.gguf) | i1-Q4_K_M | 4.5 | fast, recommended |
| [GGUF](https://huggingface.co/mradermacher/LemonadeRP-4.5.3-i1-GGUF/resolve/main/LemonadeRP-4.5.3.i1-Q5_K_S.gguf) | i1-Q5_K_S | 5.1 | |
| [GGUF](https://huggingface.co/mradermacher/LemonadeRP-4.5.3-i1-GGUF/resolve/main/LemonadeRP-4.5.3.i1-Q5_K_M.gguf) | i1-Q5_K_M | 5.2 | |
| [GGUF](https://huggingface.co/mradermacher/LemonadeRP-4.5.3-i1-GGUF/resolve/main/LemonadeRP-4.5.3.i1-Q6_K.gguf) | i1-Q6_K | 6.0 | practically like static Q6_K |
Here is a handy graph by ikawrakow comparing some lower-quality quant
types (lower is better):

And here are Artefact2's thoughts on the matter:
https://gist.github.com/Artefact2/b5f810600771265fc1e39442288e8ec9
## FAQ / Model Request
See https://huggingface.co/mradermacher/model_requests for some answers to
questions you might have and/or if you want some other model quantized.
## Thanks
I thank my company, [nethype GmbH](https://www.nethype.de/), for letting
me use its servers and providing upgrades to my workstation to enable
this work in my free time. Additional thanks to [@nicoboss](https://huggingface.co/nicoboss) for giving me access to his hardware for calculating the imatrix for these quants.
<!-- end -->
|
RichardErkhov/Gille_-_StrangeMerges_12-7B-slerp-gguf | RichardErkhov | "2024-06-20T13:41:11Z" | 12,672 | 1 | null | [
"gguf",
"region:us"
] | null | "2024-06-20T07:09:27Z" | Quantization made by Richard Erkhov.
[Github](https://github.com/RichardErkhov)
[Discord](https://discord.gg/pvy7H8DZMG)
[Request more models](https://github.com/RichardErkhov/quant_request)
StrangeMerges_12-7B-slerp - GGUF
- Model creator: https://huggingface.co/Gille/
- Original model: https://huggingface.co/Gille/StrangeMerges_12-7B-slerp/
| Name | Quant method | Size |
| ---- | ---- | ---- |
| [StrangeMerges_12-7B-slerp.Q2_K.gguf](https://huggingface.co/RichardErkhov/Gille_-_StrangeMerges_12-7B-slerp-gguf/blob/main/StrangeMerges_12-7B-slerp.Q2_K.gguf) | Q2_K | 2.53GB |
| [StrangeMerges_12-7B-slerp.IQ3_XS.gguf](https://huggingface.co/RichardErkhov/Gille_-_StrangeMerges_12-7B-slerp-gguf/blob/main/StrangeMerges_12-7B-slerp.IQ3_XS.gguf) | IQ3_XS | 2.81GB |
| [StrangeMerges_12-7B-slerp.IQ3_S.gguf](https://huggingface.co/RichardErkhov/Gille_-_StrangeMerges_12-7B-slerp-gguf/blob/main/StrangeMerges_12-7B-slerp.IQ3_S.gguf) | IQ3_S | 2.96GB |
| [StrangeMerges_12-7B-slerp.Q3_K_S.gguf](https://huggingface.co/RichardErkhov/Gille_-_StrangeMerges_12-7B-slerp-gguf/blob/main/StrangeMerges_12-7B-slerp.Q3_K_S.gguf) | Q3_K_S | 2.95GB |
| [StrangeMerges_12-7B-slerp.IQ3_M.gguf](https://huggingface.co/RichardErkhov/Gille_-_StrangeMerges_12-7B-slerp-gguf/blob/main/StrangeMerges_12-7B-slerp.IQ3_M.gguf) | IQ3_M | 3.06GB |
| [StrangeMerges_12-7B-slerp.Q3_K.gguf](https://huggingface.co/RichardErkhov/Gille_-_StrangeMerges_12-7B-slerp-gguf/blob/main/StrangeMerges_12-7B-slerp.Q3_K.gguf) | Q3_K | 3.28GB |
| [StrangeMerges_12-7B-slerp.Q3_K_M.gguf](https://huggingface.co/RichardErkhov/Gille_-_StrangeMerges_12-7B-slerp-gguf/blob/main/StrangeMerges_12-7B-slerp.Q3_K_M.gguf) | Q3_K_M | 3.28GB |
| [StrangeMerges_12-7B-slerp.Q3_K_L.gguf](https://huggingface.co/RichardErkhov/Gille_-_StrangeMerges_12-7B-slerp-gguf/blob/main/StrangeMerges_12-7B-slerp.Q3_K_L.gguf) | Q3_K_L | 3.56GB |
| [StrangeMerges_12-7B-slerp.IQ4_XS.gguf](https://huggingface.co/RichardErkhov/Gille_-_StrangeMerges_12-7B-slerp-gguf/blob/main/StrangeMerges_12-7B-slerp.IQ4_XS.gguf) | IQ4_XS | 3.67GB |
| [StrangeMerges_12-7B-slerp.Q4_0.gguf](https://huggingface.co/RichardErkhov/Gille_-_StrangeMerges_12-7B-slerp-gguf/blob/main/StrangeMerges_12-7B-slerp.Q4_0.gguf) | Q4_0 | 3.83GB |
| [StrangeMerges_12-7B-slerp.IQ4_NL.gguf](https://huggingface.co/RichardErkhov/Gille_-_StrangeMerges_12-7B-slerp-gguf/blob/main/StrangeMerges_12-7B-slerp.IQ4_NL.gguf) | IQ4_NL | 3.87GB |
| [StrangeMerges_12-7B-slerp.Q4_K_S.gguf](https://huggingface.co/RichardErkhov/Gille_-_StrangeMerges_12-7B-slerp-gguf/blob/main/StrangeMerges_12-7B-slerp.Q4_K_S.gguf) | Q4_K_S | 3.86GB |
| [StrangeMerges_12-7B-slerp.Q4_K.gguf](https://huggingface.co/RichardErkhov/Gille_-_StrangeMerges_12-7B-slerp-gguf/blob/main/StrangeMerges_12-7B-slerp.Q4_K.gguf) | Q4_K | 4.07GB |
| [StrangeMerges_12-7B-slerp.Q4_K_M.gguf](https://huggingface.co/RichardErkhov/Gille_-_StrangeMerges_12-7B-slerp-gguf/blob/main/StrangeMerges_12-7B-slerp.Q4_K_M.gguf) | Q4_K_M | 4.07GB |
| [StrangeMerges_12-7B-slerp.Q4_1.gguf](https://huggingface.co/RichardErkhov/Gille_-_StrangeMerges_12-7B-slerp-gguf/blob/main/StrangeMerges_12-7B-slerp.Q4_1.gguf) | Q4_1 | 4.24GB |
| [StrangeMerges_12-7B-slerp.Q5_0.gguf](https://huggingface.co/RichardErkhov/Gille_-_StrangeMerges_12-7B-slerp-gguf/blob/main/StrangeMerges_12-7B-slerp.Q5_0.gguf) | Q5_0 | 4.65GB |
| [StrangeMerges_12-7B-slerp.Q5_K_S.gguf](https://huggingface.co/RichardErkhov/Gille_-_StrangeMerges_12-7B-slerp-gguf/blob/main/StrangeMerges_12-7B-slerp.Q5_K_S.gguf) | Q5_K_S | 4.65GB |
| [StrangeMerges_12-7B-slerp.Q5_K.gguf](https://huggingface.co/RichardErkhov/Gille_-_StrangeMerges_12-7B-slerp-gguf/blob/main/StrangeMerges_12-7B-slerp.Q5_K.gguf) | Q5_K | 4.78GB |
| [StrangeMerges_12-7B-slerp.Q5_K_M.gguf](https://huggingface.co/RichardErkhov/Gille_-_StrangeMerges_12-7B-slerp-gguf/blob/main/StrangeMerges_12-7B-slerp.Q5_K_M.gguf) | Q5_K_M | 4.78GB |
| [StrangeMerges_12-7B-slerp.Q5_1.gguf](https://huggingface.co/RichardErkhov/Gille_-_StrangeMerges_12-7B-slerp-gguf/blob/main/StrangeMerges_12-7B-slerp.Q5_1.gguf) | Q5_1 | 5.07GB |
| [StrangeMerges_12-7B-slerp.Q6_K.gguf](https://huggingface.co/RichardErkhov/Gille_-_StrangeMerges_12-7B-slerp-gguf/blob/main/StrangeMerges_12-7B-slerp.Q6_K.gguf) | Q6_K | 5.53GB |
| [StrangeMerges_12-7B-slerp.Q8_0.gguf](https://huggingface.co/RichardErkhov/Gille_-_StrangeMerges_12-7B-slerp-gguf/blob/main/StrangeMerges_12-7B-slerp.Q8_0.gguf) | Q8_0 | 7.17GB |
Original model description:
---
license: apache-2.0
tags:
- merge
- mergekit
- lazymergekit
- Keynote-Technology/KAI-7B-v0.1
- Gille/StrangeMerges_11-7B-slerp
base_model:
- Keynote-Technology/KAI-7B-v0.1
- Gille/StrangeMerges_11-7B-slerp
model-index:
- name: StrangeMerges_12-7B-slerp
results:
- task:
type: text-generation
name: Text Generation
dataset:
name: AI2 Reasoning Challenge (25-Shot)
type: ai2_arc
config: ARC-Challenge
split: test
args:
num_few_shot: 25
metrics:
- type: acc_norm
value: 66.64
name: normalized accuracy
source:
url: https://huggingface.co/spaces/HuggingFaceH4/open_llm_leaderboard?query=Gille/StrangeMerges_12-7B-slerp
name: Open LLM Leaderboard
- task:
type: text-generation
name: Text Generation
dataset:
name: HellaSwag (10-Shot)
type: hellaswag
split: validation
args:
num_few_shot: 10
metrics:
- type: acc_norm
value: 85.89
name: normalized accuracy
source:
url: https://huggingface.co/spaces/HuggingFaceH4/open_llm_leaderboard?query=Gille/StrangeMerges_12-7B-slerp
name: Open LLM Leaderboard
- task:
type: text-generation
name: Text Generation
dataset:
name: MMLU (5-Shot)
type: cais/mmlu
config: all
split: test
args:
num_few_shot: 5
metrics:
- type: acc
value: 64.94
name: accuracy
source:
url: https://huggingface.co/spaces/HuggingFaceH4/open_llm_leaderboard?query=Gille/StrangeMerges_12-7B-slerp
name: Open LLM Leaderboard
- task:
type: text-generation
name: Text Generation
dataset:
name: TruthfulQA (0-shot)
type: truthful_qa
config: multiple_choice
split: validation
args:
num_few_shot: 0
metrics:
- type: mc2
value: 52.55
source:
url: https://huggingface.co/spaces/HuggingFaceH4/open_llm_leaderboard?query=Gille/StrangeMerges_12-7B-slerp
name: Open LLM Leaderboard
- task:
type: text-generation
name: Text Generation
dataset:
name: Winogrande (5-shot)
type: winogrande
config: winogrande_xl
split: validation
args:
num_few_shot: 5
metrics:
- type: acc
value: 81.69
name: accuracy
source:
url: https://huggingface.co/spaces/HuggingFaceH4/open_llm_leaderboard?query=Gille/StrangeMerges_12-7B-slerp
name: Open LLM Leaderboard
- task:
type: text-generation
name: Text Generation
dataset:
name: GSM8k (5-shot)
type: gsm8k
config: main
split: test
args:
num_few_shot: 5
metrics:
- type: acc
value: 63.08
name: accuracy
source:
url: https://huggingface.co/spaces/HuggingFaceH4/open_llm_leaderboard?query=Gille/StrangeMerges_12-7B-slerp
name: Open LLM Leaderboard
---
# StrangeMerges_12-7B-slerp
StrangeMerges_12-7B-slerp is a merge of the following models using [LazyMergekit](https://colab.research.google.com/drive/1obulZ1ROXHjYLn6PPZJwRR6GzgQogxxb?usp=sharing):
* [Keynote-Technology/KAI-7B-v0.1](https://huggingface.co/Keynote-Technology/KAI-7B-v0.1)
* [Gille/StrangeMerges_11-7B-slerp](https://huggingface.co/Gille/StrangeMerges_11-7B-slerp)
## 🧩 Configuration
```yaml
slices:
- sources:
- model: Keynote-Technology/KAI-7B-v0.1
layer_range: [0, 32]
- model: Gille/StrangeMerges_11-7B-slerp
layer_range: [0, 32]
merge_method: slerp
base_model: Keynote-Technology/KAI-7B-v0.1
parameters:
t:
- filter: self_attn
value: [0.1, 0.6, 0.3, 0.8, 0.5]
- filter: mlp
value: [0.9, 0.4, 0.7, 0.2, 0.5]
- value: 0.5
dtype: bfloat16
```
## 💻 Usage
```python
!pip install -qU transformers accelerate
from transformers import AutoTokenizer
import transformers
import torch
model = "Gille/StrangeMerges_12-7B-slerp"
messages = [{"role": "user", "content": "What is a large language model?"}]
tokenizer = AutoTokenizer.from_pretrained(model)
prompt = tokenizer.apply_chat_template(messages, tokenize=False, add_generation_prompt=True)
pipeline = transformers.pipeline(
"text-generation",
model=model,
torch_dtype=torch.float16,
device_map="auto",
)
outputs = pipeline(prompt, max_new_tokens=256, do_sample=True, temperature=0.7, top_k=50, top_p=0.95)
print(outputs[0]["generated_text"])
```
# [Open LLM Leaderboard Evaluation Results](https://huggingface.co/spaces/HuggingFaceH4/open_llm_leaderboard)
Detailed results can be found [here](https://huggingface.co/datasets/open-llm-leaderboard/details_Gille__StrangeMerges_12-7B-slerp)
| Metric |Value|
|---------------------------------|----:|
|Avg. |69.13|
|AI2 Reasoning Challenge (25-Shot)|66.64|
|HellaSwag (10-Shot) |85.89|
|MMLU (5-Shot) |64.94|
|TruthfulQA (0-shot) |52.55|
|Winogrande (5-shot) |81.69|
|GSM8k (5-shot) |63.08|
|
mradermacher/L3-8B-Stheno-v3.3-32K-i1-GGUF | mradermacher | "2024-06-22T21:29:43Z" | 12,672 | 3 | transformers | [
"transformers",
"gguf",
"en",
"base_model:Sao10K/L3-8B-Stheno-v3.3-32K",
"license:cc-by-nc-4.0",
"endpoints_compatible",
"region:us"
] | null | "2024-06-22T18:45:45Z" | ---
base_model: Sao10K/L3-8B-Stheno-v3.3-32K
language:
- en
library_name: transformers
license: cc-by-nc-4.0
quantized_by: mradermacher
---
## About
<!-- ### quantize_version: 2 -->
<!-- ### output_tensor_quantised: 1 -->
<!-- ### convert_type: hf -->
<!-- ### vocab_type: -->
<!-- ### tags: nicoboss -->
weighted/imatrix quants of https://huggingface.co/Sao10K/L3-8B-Stheno-v3.3-32K
<!-- provided-files -->
static quants are available at https://huggingface.co/mradermacher/L3-8B-Stheno-v3.3-32K-GGUF
## Usage
If you are unsure how to use GGUF files, refer to one of [TheBloke's
READMEs](https://huggingface.co/TheBloke/KafkaLM-70B-German-V0.1-GGUF) for
more details, including on how to concatenate multi-part files.
## Provided Quants
(sorted by size, not necessarily quality. IQ-quants are often preferable over similar sized non-IQ quants)
| Link | Type | Size/GB | Notes |
|:-----|:-----|--------:|:------|
| [GGUF](https://huggingface.co/mradermacher/L3-8B-Stheno-v3.3-32K-i1-GGUF/resolve/main/L3-8B-Stheno-v3.3-32K.i1-IQ1_S.gguf) | i1-IQ1_S | 2.1 | for the desperate |
| [GGUF](https://huggingface.co/mradermacher/L3-8B-Stheno-v3.3-32K-i1-GGUF/resolve/main/L3-8B-Stheno-v3.3-32K.i1-IQ1_M.gguf) | i1-IQ1_M | 2.3 | mostly desperate |
| [GGUF](https://huggingface.co/mradermacher/L3-8B-Stheno-v3.3-32K-i1-GGUF/resolve/main/L3-8B-Stheno-v3.3-32K.i1-IQ2_XXS.gguf) | i1-IQ2_XXS | 2.5 | |
| [GGUF](https://huggingface.co/mradermacher/L3-8B-Stheno-v3.3-32K-i1-GGUF/resolve/main/L3-8B-Stheno-v3.3-32K.i1-IQ2_XS.gguf) | i1-IQ2_XS | 2.7 | |
| [GGUF](https://huggingface.co/mradermacher/L3-8B-Stheno-v3.3-32K-i1-GGUF/resolve/main/L3-8B-Stheno-v3.3-32K.i1-IQ2_S.gguf) | i1-IQ2_S | 2.9 | |
| [GGUF](https://huggingface.co/mradermacher/L3-8B-Stheno-v3.3-32K-i1-GGUF/resolve/main/L3-8B-Stheno-v3.3-32K.i1-IQ2_M.gguf) | i1-IQ2_M | 3.0 | |
| [GGUF](https://huggingface.co/mradermacher/L3-8B-Stheno-v3.3-32K-i1-GGUF/resolve/main/L3-8B-Stheno-v3.3-32K.i1-Q2_K.gguf) | i1-Q2_K | 3.3 | IQ3_XXS probably better |
| [GGUF](https://huggingface.co/mradermacher/L3-8B-Stheno-v3.3-32K-i1-GGUF/resolve/main/L3-8B-Stheno-v3.3-32K.i1-IQ3_XXS.gguf) | i1-IQ3_XXS | 3.4 | lower quality |
| [GGUF](https://huggingface.co/mradermacher/L3-8B-Stheno-v3.3-32K-i1-GGUF/resolve/main/L3-8B-Stheno-v3.3-32K.i1-IQ3_XS.gguf) | i1-IQ3_XS | 3.6 | |
| [GGUF](https://huggingface.co/mradermacher/L3-8B-Stheno-v3.3-32K-i1-GGUF/resolve/main/L3-8B-Stheno-v3.3-32K.i1-Q3_K_S.gguf) | i1-Q3_K_S | 3.8 | IQ3_XS probably better |
| [GGUF](https://huggingface.co/mradermacher/L3-8B-Stheno-v3.3-32K-i1-GGUF/resolve/main/L3-8B-Stheno-v3.3-32K.i1-IQ3_S.gguf) | i1-IQ3_S | 3.8 | beats Q3_K* |
| [GGUF](https://huggingface.co/mradermacher/L3-8B-Stheno-v3.3-32K-i1-GGUF/resolve/main/L3-8B-Stheno-v3.3-32K.i1-IQ3_M.gguf) | i1-IQ3_M | 3.9 | |
| [GGUF](https://huggingface.co/mradermacher/L3-8B-Stheno-v3.3-32K-i1-GGUF/resolve/main/L3-8B-Stheno-v3.3-32K.i1-Q3_K_M.gguf) | i1-Q3_K_M | 4.1 | IQ3_S probably better |
| [GGUF](https://huggingface.co/mradermacher/L3-8B-Stheno-v3.3-32K-i1-GGUF/resolve/main/L3-8B-Stheno-v3.3-32K.i1-Q3_K_L.gguf) | i1-Q3_K_L | 4.4 | IQ3_M probably better |
| [GGUF](https://huggingface.co/mradermacher/L3-8B-Stheno-v3.3-32K-i1-GGUF/resolve/main/L3-8B-Stheno-v3.3-32K.i1-IQ4_XS.gguf) | i1-IQ4_XS | 4.5 | |
| [GGUF](https://huggingface.co/mradermacher/L3-8B-Stheno-v3.3-32K-i1-GGUF/resolve/main/L3-8B-Stheno-v3.3-32K.i1-Q4_0.gguf) | i1-Q4_0 | 4.8 | fast, low quality |
| [GGUF](https://huggingface.co/mradermacher/L3-8B-Stheno-v3.3-32K-i1-GGUF/resolve/main/L3-8B-Stheno-v3.3-32K.i1-Q4_K_S.gguf) | i1-Q4_K_S | 4.8 | optimal size/speed/quality |
| [GGUF](https://huggingface.co/mradermacher/L3-8B-Stheno-v3.3-32K-i1-GGUF/resolve/main/L3-8B-Stheno-v3.3-32K.i1-Q4_K_M.gguf) | i1-Q4_K_M | 5.0 | fast, recommended |
| [GGUF](https://huggingface.co/mradermacher/L3-8B-Stheno-v3.3-32K-i1-GGUF/resolve/main/L3-8B-Stheno-v3.3-32K.i1-Q5_K_S.gguf) | i1-Q5_K_S | 5.7 | |
| [GGUF](https://huggingface.co/mradermacher/L3-8B-Stheno-v3.3-32K-i1-GGUF/resolve/main/L3-8B-Stheno-v3.3-32K.i1-Q5_K_M.gguf) | i1-Q5_K_M | 5.8 | |
| [GGUF](https://huggingface.co/mradermacher/L3-8B-Stheno-v3.3-32K-i1-GGUF/resolve/main/L3-8B-Stheno-v3.3-32K.i1-Q6_K.gguf) | i1-Q6_K | 6.7 | practically like static Q6_K |
Here is a handy graph by ikawrakow comparing some lower-quality quant
types (lower is better):

And here are Artefact2's thoughts on the matter:
https://gist.github.com/Artefact2/b5f810600771265fc1e39442288e8ec9
## FAQ / Model Request
See https://huggingface.co/mradermacher/model_requests for some answers to
questions you might have and/or if you want some other model quantized.
## Thanks
I thank my company, [nethype GmbH](https://www.nethype.de/), for letting
me use its servers and providing upgrades to my workstation to enable
this work in my free time. Additional thanks to [@nicoboss](https://huggingface.co/nicoboss) for giving me access to his hardware for calculating the imatrix for these quants.
<!-- end -->
|
mradermacher/medicine-LLM-13B-GGUF | mradermacher | "2024-06-21T15:00:29Z" | 12,671 | 2 | transformers | [
"transformers",
"gguf",
"biology",
"medical",
"en",
"dataset:Open-Orca/OpenOrca",
"dataset:GAIR/lima",
"dataset:WizardLM/WizardLM_evol_instruct_V2_196k",
"dataset:EleutherAI/pile",
"base_model:AdaptLLM/medicine-LLM-13B",
"license:apache-2.0",
"endpoints_compatible",
"region:us"
] | null | "2024-06-21T14:14:23Z" | ---
base_model: AdaptLLM/medicine-LLM-13B
datasets:
- Open-Orca/OpenOrca
- GAIR/lima
- WizardLM/WizardLM_evol_instruct_V2_196k
- EleutherAI/pile
language:
- en
library_name: transformers
license: apache-2.0
quantized_by: mradermacher
tags:
- biology
- medical
---
## About
<!-- ### quantize_version: 2 -->
<!-- ### output_tensor_quantised: 1 -->
<!-- ### convert_type: hf -->
<!-- ### vocab_type: -->
<!-- ### tags: -->
static quants of https://huggingface.co/AdaptLLM/medicine-LLM-13B
<!-- provided-files -->
weighted/imatrix quants seem not to be available (by me) at this time. If they do not show up a week or so after the static ones, I have probably not planned for them. Feel free to request them by opening a Community Discussion.
## Usage
If you are unsure how to use GGUF files, refer to one of [TheBloke's
READMEs](https://huggingface.co/TheBloke/KafkaLM-70B-German-V0.1-GGUF) for
more details, including on how to concatenate multi-part files.
## Provided Quants
(sorted by size, not necessarily quality. IQ-quants are often preferable over similar sized non-IQ quants)
| Link | Type | Size/GB | Notes |
|:-----|:-----|--------:|:------|
| [GGUF](https://huggingface.co/mradermacher/medicine-LLM-13B-GGUF/resolve/main/medicine-LLM-13B.Q2_K.gguf) | Q2_K | 5.0 | |
| [GGUF](https://huggingface.co/mradermacher/medicine-LLM-13B-GGUF/resolve/main/medicine-LLM-13B.IQ3_XS.gguf) | IQ3_XS | 5.5 | |
| [GGUF](https://huggingface.co/mradermacher/medicine-LLM-13B-GGUF/resolve/main/medicine-LLM-13B.IQ3_S.gguf) | IQ3_S | 5.8 | beats Q3_K* |
| [GGUF](https://huggingface.co/mradermacher/medicine-LLM-13B-GGUF/resolve/main/medicine-LLM-13B.Q3_K_S.gguf) | Q3_K_S | 5.8 | |
| [GGUF](https://huggingface.co/mradermacher/medicine-LLM-13B-GGUF/resolve/main/medicine-LLM-13B.IQ3_M.gguf) | IQ3_M | 6.1 | |
| [GGUF](https://huggingface.co/mradermacher/medicine-LLM-13B-GGUF/resolve/main/medicine-LLM-13B.Q3_K_M.gguf) | Q3_K_M | 6.4 | lower quality |
| [GGUF](https://huggingface.co/mradermacher/medicine-LLM-13B-GGUF/resolve/main/medicine-LLM-13B.Q3_K_L.gguf) | Q3_K_L | 7.0 | |
| [GGUF](https://huggingface.co/mradermacher/medicine-LLM-13B-GGUF/resolve/main/medicine-LLM-13B.IQ4_XS.gguf) | IQ4_XS | 7.1 | |
| [GGUF](https://huggingface.co/mradermacher/medicine-LLM-13B-GGUF/resolve/main/medicine-LLM-13B.Q4_K_S.gguf) | Q4_K_S | 7.5 | fast, recommended |
| [GGUF](https://huggingface.co/mradermacher/medicine-LLM-13B-GGUF/resolve/main/medicine-LLM-13B.Q4_K_M.gguf) | Q4_K_M | 8.0 | fast, recommended |
| [GGUF](https://huggingface.co/mradermacher/medicine-LLM-13B-GGUF/resolve/main/medicine-LLM-13B.Q5_K_S.gguf) | Q5_K_S | 9.1 | |
| [GGUF](https://huggingface.co/mradermacher/medicine-LLM-13B-GGUF/resolve/main/medicine-LLM-13B.Q5_K_M.gguf) | Q5_K_M | 9.3 | |
| [GGUF](https://huggingface.co/mradermacher/medicine-LLM-13B-GGUF/resolve/main/medicine-LLM-13B.Q6_K.gguf) | Q6_K | 10.8 | very good quality |
| [GGUF](https://huggingface.co/mradermacher/medicine-LLM-13B-GGUF/resolve/main/medicine-LLM-13B.Q8_0.gguf) | Q8_0 | 13.9 | fast, best quality |
Here is a handy graph by ikawrakow comparing some lower-quality quant
types (lower is better):

And here are Artefact2's thoughts on the matter:
https://gist.github.com/Artefact2/b5f810600771265fc1e39442288e8ec9
## FAQ / Model Request
See https://huggingface.co/mradermacher/model_requests for some answers to
questions you might have and/or if you want some other model quantized.
## Thanks
I thank my company, [nethype GmbH](https://www.nethype.de/), for letting
me use its servers and providing upgrades to my workstation to enable
this work in my free time.
<!-- end -->
|
mradermacher/RoMistral-7b-Instruct-GGUF | mradermacher | "2024-06-28T11:11:56Z" | 12,671 | 0 | transformers | [
"transformers",
"gguf",
"ro",
"base_model:OpenLLM-Ro/RoMistral-7b-Instruct",
"license:cc-by-nc-4.0",
"endpoints_compatible",
"region:us"
] | null | "2024-06-28T09:18:25Z" | ---
base_model: OpenLLM-Ro/RoMistral-7b-Instruct
language:
- ro
library_name: transformers
license: cc-by-nc-4.0
quantized_by: mradermacher
---
## About
<!-- ### quantize_version: 2 -->
<!-- ### output_tensor_quantised: 1 -->
<!-- ### convert_type: hf -->
<!-- ### vocab_type: -->
<!-- ### tags: -->
static quants of https://huggingface.co/OpenLLM-Ro/RoMistral-7b-Instruct
<!-- provided-files -->
weighted/imatrix quants seem not to be available (by me) at this time. If they do not show up a week or so after the static ones, I have probably not planned for them. Feel free to request them by opening a Community Discussion.
## Usage
If you are unsure how to use GGUF files, refer to one of [TheBloke's
READMEs](https://huggingface.co/TheBloke/KafkaLM-70B-German-V0.1-GGUF) for
more details, including on how to concatenate multi-part files.
## Provided Quants
(sorted by size, not necessarily quality. IQ-quants are often preferable over similar sized non-IQ quants)
| Link | Type | Size/GB | Notes |
|:-----|:-----|--------:|:------|
| [GGUF](https://huggingface.co/mradermacher/RoMistral-7b-Instruct-GGUF/resolve/main/RoMistral-7b-Instruct.Q2_K.gguf) | Q2_K | 2.8 | |
| [GGUF](https://huggingface.co/mradermacher/RoMistral-7b-Instruct-GGUF/resolve/main/RoMistral-7b-Instruct.IQ3_XS.gguf) | IQ3_XS | 3.1 | |
| [GGUF](https://huggingface.co/mradermacher/RoMistral-7b-Instruct-GGUF/resolve/main/RoMistral-7b-Instruct.Q3_K_S.gguf) | Q3_K_S | 3.3 | |
| [GGUF](https://huggingface.co/mradermacher/RoMistral-7b-Instruct-GGUF/resolve/main/RoMistral-7b-Instruct.IQ3_S.gguf) | IQ3_S | 3.3 | beats Q3_K* |
| [GGUF](https://huggingface.co/mradermacher/RoMistral-7b-Instruct-GGUF/resolve/main/RoMistral-7b-Instruct.IQ3_M.gguf) | IQ3_M | 3.4 | |
| [GGUF](https://huggingface.co/mradermacher/RoMistral-7b-Instruct-GGUF/resolve/main/RoMistral-7b-Instruct.Q3_K_M.gguf) | Q3_K_M | 3.6 | lower quality |
| [GGUF](https://huggingface.co/mradermacher/RoMistral-7b-Instruct-GGUF/resolve/main/RoMistral-7b-Instruct.Q3_K_L.gguf) | Q3_K_L | 3.9 | |
| [GGUF](https://huggingface.co/mradermacher/RoMistral-7b-Instruct-GGUF/resolve/main/RoMistral-7b-Instruct.IQ4_XS.gguf) | IQ4_XS | 4.0 | |
| [GGUF](https://huggingface.co/mradermacher/RoMistral-7b-Instruct-GGUF/resolve/main/RoMistral-7b-Instruct.Q4_K_S.gguf) | Q4_K_S | 4.2 | fast, recommended |
| [GGUF](https://huggingface.co/mradermacher/RoMistral-7b-Instruct-GGUF/resolve/main/RoMistral-7b-Instruct.Q4_K_M.gguf) | Q4_K_M | 4.5 | fast, recommended |
| [GGUF](https://huggingface.co/mradermacher/RoMistral-7b-Instruct-GGUF/resolve/main/RoMistral-7b-Instruct.Q5_K_S.gguf) | Q5_K_S | 5.1 | |
| [GGUF](https://huggingface.co/mradermacher/RoMistral-7b-Instruct-GGUF/resolve/main/RoMistral-7b-Instruct.Q5_K_M.gguf) | Q5_K_M | 5.2 | |
| [GGUF](https://huggingface.co/mradermacher/RoMistral-7b-Instruct-GGUF/resolve/main/RoMistral-7b-Instruct.Q6_K.gguf) | Q6_K | 6.0 | very good quality |
| [GGUF](https://huggingface.co/mradermacher/RoMistral-7b-Instruct-GGUF/resolve/main/RoMistral-7b-Instruct.Q8_0.gguf) | Q8_0 | 7.8 | fast, best quality |
| [GGUF](https://huggingface.co/mradermacher/RoMistral-7b-Instruct-GGUF/resolve/main/RoMistral-7b-Instruct.f16.gguf) | f16 | 14.6 | 16 bpw, overkill |
Here is a handy graph by ikawrakow comparing some lower-quality quant
types (lower is better):

And here are Artefact2's thoughts on the matter:
https://gist.github.com/Artefact2/b5f810600771265fc1e39442288e8ec9
## FAQ / Model Request
See https://huggingface.co/mradermacher/model_requests for some answers to
questions you might have and/or if you want some other model quantized.
## Thanks
I thank my company, [nethype GmbH](https://www.nethype.de/), for letting
me use its servers and providing upgrades to my workstation to enable
this work in my free time.
<!-- end -->
|
mradermacher/Guide-U-Llama-3-KO-8B-Instruct-GGUF | mradermacher | "2024-06-23T18:18:01Z" | 12,661 | 0 | transformers | [
"transformers",
"gguf",
"en",
"base_model:PathFinderKR/Guide-U-Llama-3-KO-8B-Instruct",
"endpoints_compatible",
"region:us"
] | null | "2024-06-23T17:49:34Z" | ---
base_model: PathFinderKR/Guide-U-Llama-3-KO-8B-Instruct
language:
- en
library_name: transformers
quantized_by: mradermacher
tags: []
---
## About
<!-- ### quantize_version: 2 -->
<!-- ### output_tensor_quantised: 1 -->
<!-- ### convert_type: hf -->
<!-- ### vocab_type: -->
<!-- ### tags: -->
static quants of https://huggingface.co/PathFinderKR/Guide-U-Llama-3-KO-8B-Instruct
<!-- provided-files -->
weighted/imatrix quants seem not to be available (by me) at this time. If they do not show up a week or so after the static ones, I have probably not planned for them. Feel free to request them by opening a Community Discussion.
## Usage
If you are unsure how to use GGUF files, refer to one of [TheBloke's
READMEs](https://huggingface.co/TheBloke/KafkaLM-70B-German-V0.1-GGUF) for
more details, including on how to concatenate multi-part files.
## Provided Quants
(sorted by size, not necessarily quality. IQ-quants are often preferable over similar sized non-IQ quants)
| Link | Type | Size/GB | Notes |
|:-----|:-----|--------:|:------|
| [GGUF](https://huggingface.co/mradermacher/Guide-U-Llama-3-KO-8B-Instruct-GGUF/resolve/main/Guide-U-Llama-3-KO-8B-Instruct.Q2_K.gguf) | Q2_K | 3.3 | |
| [GGUF](https://huggingface.co/mradermacher/Guide-U-Llama-3-KO-8B-Instruct-GGUF/resolve/main/Guide-U-Llama-3-KO-8B-Instruct.IQ3_XS.gguf) | IQ3_XS | 3.6 | |
| [GGUF](https://huggingface.co/mradermacher/Guide-U-Llama-3-KO-8B-Instruct-GGUF/resolve/main/Guide-U-Llama-3-KO-8B-Instruct.Q3_K_S.gguf) | Q3_K_S | 3.8 | |
| [GGUF](https://huggingface.co/mradermacher/Guide-U-Llama-3-KO-8B-Instruct-GGUF/resolve/main/Guide-U-Llama-3-KO-8B-Instruct.IQ3_S.gguf) | IQ3_S | 3.8 | beats Q3_K* |
| [GGUF](https://huggingface.co/mradermacher/Guide-U-Llama-3-KO-8B-Instruct-GGUF/resolve/main/Guide-U-Llama-3-KO-8B-Instruct.IQ3_M.gguf) | IQ3_M | 3.9 | |
| [GGUF](https://huggingface.co/mradermacher/Guide-U-Llama-3-KO-8B-Instruct-GGUF/resolve/main/Guide-U-Llama-3-KO-8B-Instruct.Q3_K_M.gguf) | Q3_K_M | 4.1 | lower quality |
| [GGUF](https://huggingface.co/mradermacher/Guide-U-Llama-3-KO-8B-Instruct-GGUF/resolve/main/Guide-U-Llama-3-KO-8B-Instruct.Q3_K_L.gguf) | Q3_K_L | 4.4 | |
| [GGUF](https://huggingface.co/mradermacher/Guide-U-Llama-3-KO-8B-Instruct-GGUF/resolve/main/Guide-U-Llama-3-KO-8B-Instruct.IQ4_XS.gguf) | IQ4_XS | 4.6 | |
| [GGUF](https://huggingface.co/mradermacher/Guide-U-Llama-3-KO-8B-Instruct-GGUF/resolve/main/Guide-U-Llama-3-KO-8B-Instruct.Q4_K_S.gguf) | Q4_K_S | 4.8 | fast, recommended |
| [GGUF](https://huggingface.co/mradermacher/Guide-U-Llama-3-KO-8B-Instruct-GGUF/resolve/main/Guide-U-Llama-3-KO-8B-Instruct.Q4_K_M.gguf) | Q4_K_M | 5.0 | fast, recommended |
| [GGUF](https://huggingface.co/mradermacher/Guide-U-Llama-3-KO-8B-Instruct-GGUF/resolve/main/Guide-U-Llama-3-KO-8B-Instruct.Q5_K_S.gguf) | Q5_K_S | 5.7 | |
| [GGUF](https://huggingface.co/mradermacher/Guide-U-Llama-3-KO-8B-Instruct-GGUF/resolve/main/Guide-U-Llama-3-KO-8B-Instruct.Q5_K_M.gguf) | Q5_K_M | 5.8 | |
| [GGUF](https://huggingface.co/mradermacher/Guide-U-Llama-3-KO-8B-Instruct-GGUF/resolve/main/Guide-U-Llama-3-KO-8B-Instruct.Q6_K.gguf) | Q6_K | 6.7 | very good quality |
| [GGUF](https://huggingface.co/mradermacher/Guide-U-Llama-3-KO-8B-Instruct-GGUF/resolve/main/Guide-U-Llama-3-KO-8B-Instruct.Q8_0.gguf) | Q8_0 | 8.6 | fast, best quality |
| [GGUF](https://huggingface.co/mradermacher/Guide-U-Llama-3-KO-8B-Instruct-GGUF/resolve/main/Guide-U-Llama-3-KO-8B-Instruct.f16.gguf) | f16 | 16.2 | 16 bpw, overkill |
Here is a handy graph by ikawrakow comparing some lower-quality quant
types (lower is better):

And here are Artefact2's thoughts on the matter:
https://gist.github.com/Artefact2/b5f810600771265fc1e39442288e8ec9
## FAQ / Model Request
See https://huggingface.co/mradermacher/model_requests for some answers to
questions you might have and/or if you want some other model quantized.
## Thanks
I thank my company, [nethype GmbH](https://www.nethype.de/), for letting
me use its servers and providing upgrades to my workstation to enable
this work in my free time.
<!-- end -->
|
DeepChem/ChemBERTa-10M-MTR | DeepChem | "2022-11-16T23:00:19Z" | 12,657 | 7 | transformers | [
"transformers",
"pytorch",
"roberta",
"arxiv:1910.09700",
"endpoints_compatible",
"region:us"
] | null | "2022-03-02T23:29:04Z" | ---
tags:
- roberta
---
# Model Card for ChemBERTa-10M-MTR
# Model Details
## Model Description
More information needed
- **Developed by:** DeepChem
- **Shared by [Optional]:** DeepChem
- **Model type:** Token Classification
- **Language(s) (NLP):** More information needed
- **License:** More information needed
- **Parent Model:** [RoBERTa](https://huggingface.co/roberta-base?text=The+goal+of+life+is+%3Cmask%3E.)
- **Resources for more information:** More information needed
# Uses
## Direct Use
More information needed.
## Downstream Use [Optional]
More information needed.
## Out-of-Scope Use
The model should not be used to intentionally create hostile or alienating environments for people.
# Bias, Risks, and Limitations
Significant research has explored bias and fairness issues with language models (see, e.g., [Sheng et al. (2021)](https://aclanthology.org/2021.acl-long.330.pdf) and [Bender et al. (2021)](https://dl.acm.org/doi/pdf/10.1145/3442188.3445922)). Predictions generated by the model may include disturbing and harmful stereotypes across protected classes; identity characteristics; and sensitive, social, and occupational groups.
## Recommendations
Users (both direct and downstream) should be made aware of the risks, biases and limitations of the model. More information needed for further recommendations.
# Training Details
## Training Data
More information needed
## Training Procedure
### Preprocessing
More information needed
### Speeds, Sizes, Times
More information needed
# Evaluation
## Testing Data, Factors & Metrics
### Testing Data
More information needed
### Factors
More information needed
### Metrics
More information needed
## Results
More information needed
# Model Examination
More information needed
# Environmental Impact
Carbon emissions can be estimated using the [Machine Learning Impact calculator](https://mlco2.github.io/impact#compute) presented in [Lacoste et al. (2019)](https://arxiv.org/abs/1910.09700).
- **Hardware Type:** More information needed
- **Hours used:** More information needed
- **Cloud Provider:** More information needed
- **Compute Region:** More information needed
- **Carbon Emitted:** More information needed
# Technical Specifications [optional]
## Model Architecture and Objective
More information needed
## Compute Infrastructure
More information needed
### Hardware
More information needed
### Software
More information needed.
# Citation
**BibTeX:**
```bibtex
@book{Ramsundar-et-al-2019,
title={Deep Learning for the Life Sciences},
author={Bharath Ramsundar and Peter Eastman and Patrick Walters and Vijay Pande and Karl Leswing and Zhenqin Wu},
publisher={O'Reilly Media},
note={\url{https://www.amazon.com/Deep-Learning-Life-Sciences-Microscopy/dp/1492039837}},
year={2019}
}
```
**APA:**
More information needed
# Glossary [optional]
More information needed
# More Information [optional]
More information needed
# Model Card Authors [optional]
DeepChem in collaboration with Ezi Ozoani and the Hugging Face team
# Model Card Contact
More information needed
# How to Get Started with the Model
Use the code below to get started with the model.
<details>
<summary> Click to expand </summary>
```python
from transformers import AutoTokenizer, RobertaForRegression
tokenizer = AutoTokenizer.from_pretrained("DeepChem/ChemBERTa-10M-MTR")
model = RobertaForRegression.from_pretrained("DeepChem/ChemBERTa-10M-MTR")
```
</details>
|
microsoft/BioGPT-Large-PubMedQA | microsoft | "2023-02-04T07:50:25Z" | 12,656 | 97 | transformers | [
"transformers",
"pytorch",
"biogpt",
"text-generation",
"medical",
"en",
"dataset:pubmed_qa",
"license:mit",
"autotrain_compatible",
"endpoints_compatible",
"region:us"
] | text-generation | "2023-02-03T20:33:43Z" | ---
license: mit
datasets:
- pubmed_qa
language:
- en
metrics:
- accuracy
library_name: transformers
pipeline_tag: text-generation
tags:
- medical
widget:
- text: "question: Can 'high-risk' human papillomaviruses (HPVs) be detected in human breast milk? context: Using polymerase chain reaction techniques, we evaluated the presence of HPV infection in human breast milk collected from 21 HPV-positive and 11 HPV-negative mothers. Of the 32 studied human milk specimens, no 'high-risk' HPV 16, 18, 31, 33, 35, 39, 45, 51, 52, 56, 58 or 58 DNA was detected. answer: This preliminary case-control study indicates the absence of mucosal 'high-risk' HPV types in human breast milk."
inference:
parameters:
max_new_tokens: 250
do_sample: False
---
## BioGPT
Pre-trained language models have attracted increasing attention in the biomedical domain, inspired by their great success in the general natural language domain. Among the two main branches of pre-trained language models in the general language domain, i.e. BERT (and its variants) and GPT (and its variants), the first one has been extensively studied in the biomedical domain, such as BioBERT and PubMedBERT. While they have achieved great success on a variety of discriminative downstream biomedical tasks, the lack of generation ability constrains their application scope. In this paper, we propose BioGPT, a domain-specific generative Transformer language model pre-trained on large-scale biomedical literature. We evaluate BioGPT on six biomedical natural language processing tasks and demonstrate that our model outperforms previous models on most tasks. Especially, we get 44.98%, 38.42% and 40.76% F1 score on BC5CDR, KD-DTI and DDI end-to-end relation extraction tasks, respectively, and 78.2% accuracy on PubMedQA, creating a new record. Our case study on text generation further demonstrates the advantage of BioGPT on biomedical literature to generate fluent descriptions for biomedical terms.
## Citation
If you find BioGPT useful in your research, please cite the following paper:
```latex
@article{10.1093/bib/bbac409,
author = {Luo, Renqian and Sun, Liai and Xia, Yingce and Qin, Tao and Zhang, Sheng and Poon, Hoifung and Liu, Tie-Yan},
title = "{BioGPT: generative pre-trained transformer for biomedical text generation and mining}",
journal = {Briefings in Bioinformatics},
volume = {23},
number = {6},
year = {2022},
month = {09},
abstract = "{Pre-trained language models have attracted increasing attention in the biomedical domain, inspired by their great success in the general natural language domain. Among the two main branches of pre-trained language models in the general language domain, i.e. BERT (and its variants) and GPT (and its variants), the first one has been extensively studied in the biomedical domain, such as BioBERT and PubMedBERT. While they have achieved great success on a variety of discriminative downstream biomedical tasks, the lack of generation ability constrains their application scope. In this paper, we propose BioGPT, a domain-specific generative Transformer language model pre-trained on large-scale biomedical literature. We evaluate BioGPT on six biomedical natural language processing tasks and demonstrate that our model outperforms previous models on most tasks. Especially, we get 44.98\%, 38.42\% and 40.76\% F1 score on BC5CDR, KD-DTI and DDI end-to-end relation extraction tasks, respectively, and 78.2\% accuracy on PubMedQA, creating a new record. Our case study on text generation further demonstrates the advantage of BioGPT on biomedical literature to generate fluent descriptions for biomedical terms.}",
issn = {1477-4054},
doi = {10.1093/bib/bbac409},
url = {https://doi.org/10.1093/bib/bbac409},
note = {bbac409},
eprint = {https://academic.oup.com/bib/article-pdf/23/6/bbac409/47144271/bbac409.pdf},
}
``` |
mradermacher/DeepSeekMath-RL-Step-DPO-GGUF | mradermacher | "2024-06-28T17:48:43Z" | 12,654 | 0 | transformers | [
"transformers",
"gguf",
"en",
"base_model:xinlai/DeepSeekMath-RL-Step-DPO",
"license:apache-2.0",
"endpoints_compatible",
"region:us"
] | null | "2024-06-28T16:18:22Z" | ---
base_model: xinlai/DeepSeekMath-RL-Step-DPO
language:
- en
library_name: transformers
license: apache-2.0
quantized_by: mradermacher
---
## About
<!-- ### quantize_version: 2 -->
<!-- ### output_tensor_quantised: 1 -->
<!-- ### convert_type: hf -->
<!-- ### vocab_type: -->
<!-- ### tags: -->
static quants of https://huggingface.co/xinlai/DeepSeekMath-RL-Step-DPO
<!-- provided-files -->
weighted/imatrix quants seem not to be available (by me) at this time. If they do not show up a week or so after the static ones, I have probably not planned for them. Feel free to request them by opening a Community Discussion.
## Usage
If you are unsure how to use GGUF files, refer to one of [TheBloke's
READMEs](https://huggingface.co/TheBloke/KafkaLM-70B-German-V0.1-GGUF) for
more details, including on how to concatenate multi-part files.
## Provided Quants
(sorted by size, not necessarily quality. IQ-quants are often preferable over similar sized non-IQ quants)
| Link | Type | Size/GB | Notes |
|:-----|:-----|--------:|:------|
| [GGUF](https://huggingface.co/mradermacher/DeepSeekMath-RL-Step-DPO-GGUF/resolve/main/DeepSeekMath-RL-Step-DPO.Q2_K.gguf) | Q2_K | 2.8 | |
| [GGUF](https://huggingface.co/mradermacher/DeepSeekMath-RL-Step-DPO-GGUF/resolve/main/DeepSeekMath-RL-Step-DPO.IQ3_XS.gguf) | IQ3_XS | 3.1 | |
| [GGUF](https://huggingface.co/mradermacher/DeepSeekMath-RL-Step-DPO-GGUF/resolve/main/DeepSeekMath-RL-Step-DPO.IQ3_S.gguf) | IQ3_S | 3.2 | beats Q3_K* |
| [GGUF](https://huggingface.co/mradermacher/DeepSeekMath-RL-Step-DPO-GGUF/resolve/main/DeepSeekMath-RL-Step-DPO.Q3_K_S.gguf) | Q3_K_S | 3.2 | |
| [GGUF](https://huggingface.co/mradermacher/DeepSeekMath-RL-Step-DPO-GGUF/resolve/main/DeepSeekMath-RL-Step-DPO.IQ3_M.gguf) | IQ3_M | 3.4 | |
| [GGUF](https://huggingface.co/mradermacher/DeepSeekMath-RL-Step-DPO-GGUF/resolve/main/DeepSeekMath-RL-Step-DPO.Q3_K_M.gguf) | Q3_K_M | 3.6 | lower quality |
| [GGUF](https://huggingface.co/mradermacher/DeepSeekMath-RL-Step-DPO-GGUF/resolve/main/DeepSeekMath-RL-Step-DPO.Q3_K_L.gguf) | Q3_K_L | 3.8 | |
| [GGUF](https://huggingface.co/mradermacher/DeepSeekMath-RL-Step-DPO-GGUF/resolve/main/DeepSeekMath-RL-Step-DPO.IQ4_XS.gguf) | IQ4_XS | 3.9 | |
| [GGUF](https://huggingface.co/mradermacher/DeepSeekMath-RL-Step-DPO-GGUF/resolve/main/DeepSeekMath-RL-Step-DPO.Q4_K_S.gguf) | Q4_K_S | 4.1 | fast, recommended |
| [GGUF](https://huggingface.co/mradermacher/DeepSeekMath-RL-Step-DPO-GGUF/resolve/main/DeepSeekMath-RL-Step-DPO.Q4_K_M.gguf) | Q4_K_M | 4.3 | fast, recommended |
| [GGUF](https://huggingface.co/mradermacher/DeepSeekMath-RL-Step-DPO-GGUF/resolve/main/DeepSeekMath-RL-Step-DPO.Q5_K_S.gguf) | Q5_K_S | 4.9 | |
| [GGUF](https://huggingface.co/mradermacher/DeepSeekMath-RL-Step-DPO-GGUF/resolve/main/DeepSeekMath-RL-Step-DPO.Q5_K_M.gguf) | Q5_K_M | 5.0 | |
| [GGUF](https://huggingface.co/mradermacher/DeepSeekMath-RL-Step-DPO-GGUF/resolve/main/DeepSeekMath-RL-Step-DPO.Q6_K.gguf) | Q6_K | 5.8 | very good quality |
| [GGUF](https://huggingface.co/mradermacher/DeepSeekMath-RL-Step-DPO-GGUF/resolve/main/DeepSeekMath-RL-Step-DPO.Q8_0.gguf) | Q8_0 | 7.4 | fast, best quality |
| [GGUF](https://huggingface.co/mradermacher/DeepSeekMath-RL-Step-DPO-GGUF/resolve/main/DeepSeekMath-RL-Step-DPO.f16.gguf) | f16 | 13.9 | 16 bpw, overkill |
Here is a handy graph by ikawrakow comparing some lower-quality quant
types (lower is better):

And here are Artefact2's thoughts on the matter:
https://gist.github.com/Artefact2/b5f810600771265fc1e39442288e8ec9
## FAQ / Model Request
See https://huggingface.co/mradermacher/model_requests for some answers to
questions you might have and/or if you want some other model quantized.
## Thanks
I thank my company, [nethype GmbH](https://www.nethype.de/), for letting
me use its servers and providing upgrades to my workstation to enable
this work in my free time.
<!-- end -->
|
aubmindlab/bert-base-arabertv02 | aubmindlab | "2024-03-26T14:39:39Z" | 12,653 | 24 | transformers | [
"transformers",
"pytorch",
"tf",
"jax",
"tensorboard",
"safetensors",
"bert",
"fill-mask",
"ar",
"dataset:wikipedia",
"dataset:Osian",
"dataset:1.5B-Arabic-Corpus",
"dataset:oscar-arabic-unshuffled",
"dataset:Assafir-private",
"arxiv:2003.00104",
"autotrain_compatible",
"endpoints_compatible",
"region:us"
] | fill-mask | "2022-03-02T23:29:05Z" | ---
language: ar
datasets:
- wikipedia
- Osian
- 1.5B-Arabic-Corpus
- oscar-arabic-unshuffled
- Assafir-private
widget:
- text: ' عاصمة لبنان هي [MASK] .'
pipeline_tag: fill-mask
---
# AraBERT v1 & v2 : Pre-training BERT for Arabic Language Understanding
<img src="https://raw.githubusercontent.com/aub-mind/arabert/master/arabert_logo.png" width="100" align="left"/>
**AraBERT** is an Arabic pretrained language model based on [Google's BERT architechture](https://github.com/google-research/bert). AraBERT uses the same BERT-Base config. More details are available in the [AraBERT Paper](https://arxiv.org/abs/2003.00104) and in the [AraBERT Meetup](https://github.com/WissamAntoun/pydata_khobar_meetup)
There are two versions of the model, AraBERTv0.1 and AraBERTv1, with the difference being that AraBERTv1 uses pre-segmented text where prefixes and suffixes were split using the [Farasa Segmenter](http://alt.qcri.org/farasa/segmenter.html).
We evaluate AraBERT models on different downstream tasks and compare them to [mBERT]((https://github.com/google-research/bert/blob/master/multilingual.md)), and other state of the art models (*To the extent of our knowledge*). The Tasks were Sentiment Analysis on 6 different datasets ([HARD](https://github.com/elnagara/HARD-Arabic-Dataset), [ASTD-Balanced](https://www.aclweb.org/anthology/D15-1299), [ArsenTD-Lev](https://staff.aub.edu.lb/~we07/Publications/ArSentD-LEV_Sentiment_Corpus.pdf), [LABR](https://github.com/mohamedadaly/LABR)), Named Entity Recognition with the [ANERcorp](http://curtis.ml.cmu.edu/w/courses/index.php/ANERcorp), and Arabic Question Answering on [Arabic-SQuAD and ARCD](https://github.com/husseinmozannar/SOQAL)
# AraBERTv2
## What's New!
AraBERT now comes in 4 new variants to replace the old v1 versions:
More Detail in the AraBERT folder and in the [README](https://github.com/aub-mind/arabert/blob/master/AraBERT/README.md) and in the [AraBERT Paper](https://arxiv.org/abs/2003.00104v2)
Model | HuggingFace Model Name | Size (MB/Params)| Pre-Segmentation | DataSet (Sentences/Size/nWords) |
---|:---:|:---:|:---:|:---:
AraBERTv0.2-base | [bert-base-arabertv02](https://huggingface.co/aubmindlab/bert-base-arabertv02) | 543MB / 136M | No | 200M / 77GB / 8.6B |
AraBERTv0.2-large| [bert-large-arabertv02](https://huggingface.co/aubmindlab/bert-large-arabertv02) | 1.38G 371M | No | 200M / 77GB / 8.6B |
AraBERTv2-base| [bert-base-arabertv2](https://huggingface.co/aubmindlab/bert-base-arabertv2) | 543MB 136M | Yes | 200M / 77GB / 8.6B |
AraBERTv2-large| [bert-large-arabertv2](https://huggingface.co/aubmindlab/bert-large-arabertv2) | 1.38G 371M | Yes | 200M / 77GB / 8.6B |
AraBERTv0.2-Twitter-base| [bert-base-arabertv02-twitter](https://huggingface.co/aubmindlab/bert-base-arabertv02-twitter) | 543MB / 136M | No | Same as v02 + 60M Multi-Dialect Tweets|
AraBERTv0.2-Twitter-large| [bert-large-arabertv02-twitter](https://huggingface.co/aubmindlab/bert-large-arabertv02-twitter) | 1.38G / 371M | No | Same as v02 + 60M Multi-Dialect Tweets|
AraBERTv0.1-base| [bert-base-arabertv01](https://huggingface.co/aubmindlab/bert-base-arabertv01) | 543MB 136M | No | 77M / 23GB / 2.7B |
AraBERTv1-base| [bert-base-arabert](https://huggingface.co/aubmindlab/bert-base-arabert) | 543MB 136M | Yes | 77M / 23GB / 2.7B |
All models are available in the `HuggingFace` model page under the [aubmindlab](https://huggingface.co/aubmindlab/) name. Checkpoints are available in PyTorch, TF2 and TF1 formats.
## Better Pre-Processing and New Vocab
We identified an issue with AraBERTv1's wordpiece vocabulary. The issue came from punctuations and numbers that were still attached to words when learned the wordpiece vocab. We now insert a space between numbers and characters and around punctuation characters.
The new vocabulary was learned using the `BertWordpieceTokenizer` from the `tokenizers` library, and should now support the Fast tokenizer implementation from the `transformers` library.
**P.S.**: All the old BERT codes should work with the new BERT, just change the model name and check the new preprocessing function
**Please read the section on how to use the [preprocessing function](#Preprocessing)**
## Bigger Dataset and More Compute
We used ~3.5 times more data, and trained for longer.
For Dataset Sources see the [Dataset Section](#Dataset)
Model | Hardware | num of examples with seq len (128 / 512) |128 (Batch Size/ Num of Steps) | 512 (Batch Size/ Num of Steps) | Total Steps | Total Time (in Days) |
---|:---:|:---:|:---:|:---:|:---:|:---:
AraBERTv0.2-base | TPUv3-8 | 420M / 207M | 2560 / 1M | 384/ 2M | 3M | -
AraBERTv0.2-large | TPUv3-128 | 420M / 207M | 13440 / 250K | 2056 / 300K | 550K | 7
AraBERTv2-base | TPUv3-8 | 420M / 207M | 2560 / 1M | 384/ 2M | 3M | -
AraBERTv2-large | TPUv3-128 | 520M / 245M | 13440 / 250K | 2056 / 300K | 550K | 7
AraBERT-base (v1/v0.1) | TPUv2-8 | - |512 / 900K | 128 / 300K| 1.2M | 4
# Dataset
The pretraining data used for the new AraBERT model is also used for Arabic **GPT2 and ELECTRA**.
The dataset consists of 77GB or 200,095,961 lines or 8,655,948,860 words or 82,232,988,358 chars (before applying Farasa Segmentation)
For the new dataset we added the unshuffled OSCAR corpus, after we thoroughly filter it, to the previous dataset used in AraBERTv1 but with out the websites that we previously crawled:
- OSCAR unshuffled and filtered.
- [Arabic Wikipedia dump](https://archive.org/details/arwiki-20190201) from 2020/09/01
- [The 1.5B words Arabic Corpus](https://www.semanticscholar.org/paper/1.5-billion-words-Arabic-Corpus-El-Khair/f3eeef4afb81223df96575adadf808fe7fe440b4)
- [The OSIAN Corpus](https://www.aclweb.org/anthology/W19-4619)
- Assafir news articles. Huge thank you for Assafir for providing us the data
# Preprocessing
It is recommended to apply our preprocessing function before training/testing on any dataset.
**Install the arabert python package to segment text for AraBERT v1 & v2 or to clean your data `pip install arabert`**
```python
from arabert.preprocess import ArabertPreprocessor
model_name="aubmindlab/bert-large-arabertv02"
arabert_prep = ArabertPreprocessor(model_name=model_name)
text = "ولن نبالغ إذا قلنا: إن هاتف أو كمبيوتر المكتب في زمننا هذا ضروري"
arabert_prep.preprocess(text)
>>> output: ولن نبالغ إذا قلنا : إن هاتف أو كمبيوتر المكتب في زمننا هذا ضروري
```
# TensorFlow 1.x models
The TF1.x model are available in the HuggingFace models repo.
You can download them as follows:
- via git-lfs: clone all the models in a repo
```bash
curl -s https://packagecloud.io/install/repositories/github/git-lfs/script.deb.sh | sudo bash
sudo apt-get install git-lfs
git lfs install
git clone https://huggingface.co/aubmindlab/MODEL_NAME
tar -C ./MODEL_NAME -zxvf /content/MODEL_NAME/tf1_model.tar.gz
```
where `MODEL_NAME` is any model under the `aubmindlab` name
- via `wget`:
- Go to the tf1_model.tar.gz file on huggingface.co/models/aubmindlab/MODEL_NAME.
- copy the `oid sha256`
- then run `wget https://cdn-lfs.huggingface.co/aubmindlab/aragpt2-base/INSERT_THE_SHA_HERE` (ex: for `aragpt2-base`: `wget https://cdn-lfs.huggingface.co/aubmindlab/aragpt2-base/3766fc03d7c2593ff2fb991d275e96b81b0ecb2098b71ff315611d052ce65248`)
# If you used this model please cite us as :
Google Scholar has our Bibtex wrong (missing name), use this instead
```
@inproceedings{antoun2020arabert,
title={AraBERT: Transformer-based Model for Arabic Language Understanding},
author={Antoun, Wissam and Baly, Fady and Hajj, Hazem},
booktitle={LREC 2020 Workshop Language Resources and Evaluation Conference 11--16 May 2020},
pages={9}
}
```
# Acknowledgments
Thanks to TensorFlow Research Cloud (TFRC) for the free access to Cloud TPUs, couldn't have done it without this program, and to the [AUB MIND Lab](https://sites.aub.edu.lb/mindlab/) Members for the continuous support. Also thanks to [Yakshof](https://www.yakshof.com/#/) and Assafir for data and storage access. Another thanks for Habib Rahal (https://www.behance.net/rahalhabib), for putting a face to AraBERT.
# Contacts
**Wissam Antoun**: [Linkedin](https://www.linkedin.com/in/wissam-antoun-622142b4/) | [Twitter](https://twitter.com/wissam_antoun) | [Github](https://github.com/WissamAntoun) | <[email protected]> | <[email protected]>
**Fady Baly**: [Linkedin](https://www.linkedin.com/in/fadybaly/) | [Twitter](https://twitter.com/fadybaly) | [Github](https://github.com/fadybaly) | <[email protected]> | <[email protected]> |
hassanblend/HassanBlend1.5.1.2 | hassanblend | "2024-04-30T17:01:49Z" | 12,650 | 212 | diffusers | [
"diffusers",
"text-to-image",
"license:creativeml-openrail-m",
"autotrain_compatible",
"endpoints_compatible",
"diffusers:StableDiffusionPipeline",
"region:us"
] | text-to-image | "2023-01-05T23:57:46Z" | ---
title: Hassanblend1.5.1.2
emoji: 📚
colorFrom: green
colorTo: indigo
sdk: gradio
sdk_version: 3.11.0
app_file: app.py
pinned: false
thumbnail: https://i.imgur.com/PVThZvk.png
license: creativeml-openrail-m
tags:
- text-to-image
inference: true
---
# HassanBlend1.5
I am hassan, I created HassansBlend, the latest version currently is 1.5.1.2 I continue to iterate and improve on this model over time. Feel free to check out our discord or rentry page for more examples with prompts and outputs generated.
This blend is finetuned over SD1.5 with thousands of images included in the dataset it was trained with. Along with that there are some minor merges added in just to soften it up and increase the creativity.
I have also some custom created content such as enhancement hypernetworks/embeddings etc for patreons or KoFi subscribers only on my pages below
<b> Links </b><br>
<b>Patreon</b>
<a href="https://www.patreon.com/sdhassan" target="_blank"><img src="https://i.imgur.com/sR32SqJ.jpg"></img></a>
<b>KoFi</b>
<a href="https://ko-fi.com/sdhassan" target="_blank"><img src="https://i.imgur.com/0P7CTN4.png"></img></a>
<b>Discord</b>
<a href="https://discord.gg/sdmodelers" target="_blank"><img src="https://i.imgur.com/HC1iHwg.png"></img></a>
### Quicklinks:
* [Latest Setup](https://rentry.org/sdhassan#current-setup)
* [HassanBlend Model Finetune Updates](https://rentry.org/sdhassan#hassanblend-finetuning-updates)
* [Latest Patreon Posts](https://rentry.org/sdhassan#patreon-posts)
* [Models](https://rentry.org/sdhassan#models)
* [HassanBlend1.5](https://rentry.org/sdhassan#hassanblend15-downloads)
* [HassanBlend1.4](https://rentry.org/sdhassan#hassanblend14-downloads)
* [Prompts](https://rentry.org/sdhassan#prompts)
* [Photorealistic Tips](https://rentry.org/sdhassan#tips-for-photorealistic-images)
* [Embeddings](https://rentry.org/sdhassan#embeddings)
* [Hypernetworks](https://rentry.org/sdhassan#hypernetworks)
* [Wildcards](https://rentry.org/sdhassan#wildcards-i-made)
* [MyTools](https://rentry.org/sdhassan#my-tools)
* [Settings I use](https://rentry.org/sdhassan#settings)
Model details and examples with sample prompts: https://rentry.org/sdhassan |
mradermacher/L3-Umbral-Mind-RP-v1.0.1-8B-GGUF | mradermacher | "2024-06-24T23:41:18Z" | 12,647 | 0 | transformers | [
"transformers",
"gguf",
"merge",
"mergekit",
"lazymergekit",
"not-for-all-audiences",
"nsfw",
"rp",
"roleplay",
"role-play",
"en",
"base_model:Cas-Archive/L3-Umbral-Mind-RP-v1.0.1-8B",
"license:llama3",
"endpoints_compatible",
"region:us"
] | null | "2024-06-24T14:27:33Z" | ---
base_model: Cas-Archive/L3-Umbral-Mind-RP-v1.0.1-8B
language:
- en
library_name: transformers
license: llama3
quantized_by: mradermacher
tags:
- merge
- mergekit
- lazymergekit
- not-for-all-audiences
- nsfw
- rp
- roleplay
- role-play
---
## About
<!-- ### quantize_version: 2 -->
<!-- ### output_tensor_quantised: 1 -->
<!-- ### convert_type: hf -->
<!-- ### vocab_type: -->
<!-- ### tags: -->
static quants of https://huggingface.co/Cas-Archive/L3-Umbral-Mind-RP-v1.0.1-8B
<!-- provided-files -->
weighted/imatrix quants are available at https://huggingface.co/mradermacher/L3-Umbral-Mind-RP-v1.0.1-8B-i1-GGUF
## Usage
If you are unsure how to use GGUF files, refer to one of [TheBloke's
READMEs](https://huggingface.co/TheBloke/KafkaLM-70B-German-V0.1-GGUF) for
more details, including on how to concatenate multi-part files.
## Provided Quants
(sorted by size, not necessarily quality. IQ-quants are often preferable over similar sized non-IQ quants)
| Link | Type | Size/GB | Notes |
|:-----|:-----|--------:|:------|
| [GGUF](https://huggingface.co/mradermacher/L3-Umbral-Mind-RP-v1.0.1-8B-GGUF/resolve/main/L3-Umbral-Mind-RP-v1.0.1-8B.Q2_K.gguf) | Q2_K | 3.3 | |
| [GGUF](https://huggingface.co/mradermacher/L3-Umbral-Mind-RP-v1.0.1-8B-GGUF/resolve/main/L3-Umbral-Mind-RP-v1.0.1-8B.IQ3_XS.gguf) | IQ3_XS | 3.6 | |
| [GGUF](https://huggingface.co/mradermacher/L3-Umbral-Mind-RP-v1.0.1-8B-GGUF/resolve/main/L3-Umbral-Mind-RP-v1.0.1-8B.Q3_K_S.gguf) | Q3_K_S | 3.8 | |
| [GGUF](https://huggingface.co/mradermacher/L3-Umbral-Mind-RP-v1.0.1-8B-GGUF/resolve/main/L3-Umbral-Mind-RP-v1.0.1-8B.IQ3_S.gguf) | IQ3_S | 3.8 | beats Q3_K* |
| [GGUF](https://huggingface.co/mradermacher/L3-Umbral-Mind-RP-v1.0.1-8B-GGUF/resolve/main/L3-Umbral-Mind-RP-v1.0.1-8B.IQ3_M.gguf) | IQ3_M | 3.9 | |
| [GGUF](https://huggingface.co/mradermacher/L3-Umbral-Mind-RP-v1.0.1-8B-GGUF/resolve/main/L3-Umbral-Mind-RP-v1.0.1-8B.Q3_K_M.gguf) | Q3_K_M | 4.1 | lower quality |
| [GGUF](https://huggingface.co/mradermacher/L3-Umbral-Mind-RP-v1.0.1-8B-GGUF/resolve/main/L3-Umbral-Mind-RP-v1.0.1-8B.Q3_K_L.gguf) | Q3_K_L | 4.4 | |
| [GGUF](https://huggingface.co/mradermacher/L3-Umbral-Mind-RP-v1.0.1-8B-GGUF/resolve/main/L3-Umbral-Mind-RP-v1.0.1-8B.IQ4_XS.gguf) | IQ4_XS | 4.6 | |
| [GGUF](https://huggingface.co/mradermacher/L3-Umbral-Mind-RP-v1.0.1-8B-GGUF/resolve/main/L3-Umbral-Mind-RP-v1.0.1-8B.Q4_K_S.gguf) | Q4_K_S | 4.8 | fast, recommended |
| [GGUF](https://huggingface.co/mradermacher/L3-Umbral-Mind-RP-v1.0.1-8B-GGUF/resolve/main/L3-Umbral-Mind-RP-v1.0.1-8B.Q4_K_M.gguf) | Q4_K_M | 5.0 | fast, recommended |
| [GGUF](https://huggingface.co/mradermacher/L3-Umbral-Mind-RP-v1.0.1-8B-GGUF/resolve/main/L3-Umbral-Mind-RP-v1.0.1-8B.Q5_K_S.gguf) | Q5_K_S | 5.7 | |
| [GGUF](https://huggingface.co/mradermacher/L3-Umbral-Mind-RP-v1.0.1-8B-GGUF/resolve/main/L3-Umbral-Mind-RP-v1.0.1-8B.Q5_K_M.gguf) | Q5_K_M | 5.8 | |
| [GGUF](https://huggingface.co/mradermacher/L3-Umbral-Mind-RP-v1.0.1-8B-GGUF/resolve/main/L3-Umbral-Mind-RP-v1.0.1-8B.Q6_K.gguf) | Q6_K | 6.7 | very good quality |
| [GGUF](https://huggingface.co/mradermacher/L3-Umbral-Mind-RP-v1.0.1-8B-GGUF/resolve/main/L3-Umbral-Mind-RP-v1.0.1-8B.Q8_0.gguf) | Q8_0 | 8.6 | fast, best quality |
| [GGUF](https://huggingface.co/mradermacher/L3-Umbral-Mind-RP-v1.0.1-8B-GGUF/resolve/main/L3-Umbral-Mind-RP-v1.0.1-8B.f16.gguf) | f16 | 16.2 | 16 bpw, overkill |
Here is a handy graph by ikawrakow comparing some lower-quality quant
types (lower is better):

And here are Artefact2's thoughts on the matter:
https://gist.github.com/Artefact2/b5f810600771265fc1e39442288e8ec9
## FAQ / Model Request
See https://huggingface.co/mradermacher/model_requests for some answers to
questions you might have and/or if you want some other model quantized.
## Thanks
I thank my company, [nethype GmbH](https://www.nethype.de/), for letting
me use its servers and providing upgrades to my workstation to enable
this work in my free time.
<!-- end -->
|
openai/shap-e | openai | "2023-12-11T22:51:57Z" | 12,641 | 147 | diffusers | [
"diffusers",
"text-to-image",
"shap-e",
"text-to-3d",
"arxiv:2305.02463",
"license:mit",
"diffusers:ShapEPipeline",
"region:us"
] | text-to-3d | "2023-07-04T13:25:35Z" | ---
license: mit
tags:
- text-to-image
- shap-e
- diffusers
pipeline_tag: text-to-3d
---
# Shap-E
Shap-E introduces a diffusion process that can generate a 3D image from a text prompt. It was introduced in [Shap-E: Generating Conditional 3D Implicit Functions](https://arxiv.org/abs/2305.02463) by Heewoo Jun and Alex Nichol from OpenAI.
Original repository of Shap-E can be found here: https://github.com/openai/shap-e.
_The authors of Shap-E didn't author this model card. They provide a separate model card [here](https://github.com/openai/shap-e/blob/main/model-card.md)._
## Introduction
The abstract of the Shap-E paper:
*We present Shap-E, a conditional generative model for 3D assets. Unlike recent work on 3D generative models which produce a single output representation, Shap-E directly generates the parameters of implicit functions that can be rendered as both textured meshes and neural radiance fields. We train Shap-E in two stages: first, we train an encoder that deterministically maps 3D assets into the parameters of an implicit function; second, we train a conditional diffusion model on outputs of the encoder. When trained on a large dataset of paired 3D and text data, our resulting models are capable of generating complex and diverse 3D assets in a matter of seconds. When compared to Point-E, an explicit generative model over point clouds, Shap-E converges faster and reaches comparable or better sample quality despite modeling a higher-dimensional, multi-representation output space. We release model weights, inference code, and samples at [this https URL](https://github.com/openai/shap-e).*
## Released checkpoints
The authors released the following checkpoints:
* [openai/shap-e](https://hf.co/openai/shap-e): produces a 3D image from a text input prompt
* [openai/shap-e-img2img](https://hf.co/openai/shap-e-img2img): samples a 3D image from synthetic 2D image
## Usage examples in 🧨 diffusers
First make sure you have installed all the dependencies:
```bash
pip install transformers accelerate -q
pip install git+https://github.com/huggingface/diffusers@@shap-ee
```
Once the dependencies are installed, use the code below:
```python
import torch
from diffusers import ShapEPipeline
from diffusers.utils import export_to_gif
ckpt_id = "openai/shap-e"
pipe = ShapEPipeline.from_pretrained(repo).to("cuda")
guidance_scale = 15.0
prompt = "a shark"
images = pipe(
prompt,
guidance_scale=guidance_scale,
num_inference_steps=64,
size=256,
).images
gif_path = export_to_gif(images, "shark_3d.gif")
```
## Results
<table>
<tbody>
<tr>
<td align="center">
<img src="https://huggingface.co/datasets/diffusers/docs-images/resolve/main/shap-e/bird_3d.gif" alt="a bird">
</td>
<td align="center">
<img src="https://huggingface.co/datasets/diffusers/docs-images/resolve/main/shap-e/shark_3d.gif" alt="a shark">
</td align="center">
<td align="center">
<img src="https://huggingface.co/datasets/diffusers/docs-images/resolve/main/shap-e/veg_3d.gif" alt="A bowl of vegetables">
</td>
</tr>
<tr>
<td align="center">A bird</td>
<td align="center">A shark</td>
<td align="center">A bowl of vegetables</td>
</tr>
</tr>
</tbody>
<table>
## Training details
Refer to the [original paper](https://arxiv.org/abs/2305.02463).
## Known limitations and potential biases
Refer to the [original model card](https://github.com/openai/shap-e/blob/main/model-card.md).
## Citation
```bibtex
@misc{jun2023shape,
title={Shap-E: Generating Conditional 3D Implicit Functions},
author={Heewoo Jun and Alex Nichol},
year={2023},
eprint={2305.02463},
archivePrefix={arXiv},
primaryClass={cs.CV}
}
``` |
mradermacher/Hathor_Gamma-L3-8B-0.6-i1-GGUF | mradermacher | "2024-06-26T20:29:28Z" | 12,641 | 0 | transformers | [
"transformers",
"gguf",
"en",
"base_model:Nitral-AI/Hathor_Gamma-L3-8B-0.6",
"license:other",
"endpoints_compatible",
"region:us"
] | null | "2024-06-23T14:04:41Z" | ---
base_model: Nitral-AI/Hathor_Gamma-L3-8B-0.6
language:
- en
library_name: transformers
license: other
quantized_by: mradermacher
---
## About
<!-- ### quantize_version: 2 -->
<!-- ### output_tensor_quantised: 1 -->
<!-- ### convert_type: hf -->
<!-- ### vocab_type: -->
<!-- ### tags: nicoboss -->
weighted/imatrix quants of https://huggingface.co/Nitral-AI/Hathor_Gamma-L3-8B-0.6
<!-- provided-files -->
static quants are available at https://huggingface.co/mradermacher/Hathor_Gamma-L3-8B-0.6-GGUF
## Usage
If you are unsure how to use GGUF files, refer to one of [TheBloke's
READMEs](https://huggingface.co/TheBloke/KafkaLM-70B-German-V0.1-GGUF) for
more details, including on how to concatenate multi-part files.
## Provided Quants
(sorted by size, not necessarily quality. IQ-quants are often preferable over similar sized non-IQ quants)
| Link | Type | Size/GB | Notes |
|:-----|:-----|--------:|:------|
| [GGUF](https://huggingface.co/mradermacher/Hathor_Gamma-L3-8B-0.6-i1-GGUF/resolve/main/Hathor_Gamma-L3-8B-0.6.i1-IQ1_S.gguf) | i1-IQ1_S | 2.1 | for the desperate |
| [GGUF](https://huggingface.co/mradermacher/Hathor_Gamma-L3-8B-0.6-i1-GGUF/resolve/main/Hathor_Gamma-L3-8B-0.6.i1-IQ1_M.gguf) | i1-IQ1_M | 2.3 | mostly desperate |
| [GGUF](https://huggingface.co/mradermacher/Hathor_Gamma-L3-8B-0.6-i1-GGUF/resolve/main/Hathor_Gamma-L3-8B-0.6.i1-IQ2_XXS.gguf) | i1-IQ2_XXS | 2.5 | |
| [GGUF](https://huggingface.co/mradermacher/Hathor_Gamma-L3-8B-0.6-i1-GGUF/resolve/main/Hathor_Gamma-L3-8B-0.6.i1-IQ2_XS.gguf) | i1-IQ2_XS | 2.7 | |
| [GGUF](https://huggingface.co/mradermacher/Hathor_Gamma-L3-8B-0.6-i1-GGUF/resolve/main/Hathor_Gamma-L3-8B-0.6.i1-IQ2_S.gguf) | i1-IQ2_S | 2.9 | |
| [GGUF](https://huggingface.co/mradermacher/Hathor_Gamma-L3-8B-0.6-i1-GGUF/resolve/main/Hathor_Gamma-L3-8B-0.6.i1-IQ2_M.gguf) | i1-IQ2_M | 3.0 | |
| [GGUF](https://huggingface.co/mradermacher/Hathor_Gamma-L3-8B-0.6-i1-GGUF/resolve/main/Hathor_Gamma-L3-8B-0.6.i1-Q2_K.gguf) | i1-Q2_K | 3.3 | IQ3_XXS probably better |
| [GGUF](https://huggingface.co/mradermacher/Hathor_Gamma-L3-8B-0.6-i1-GGUF/resolve/main/Hathor_Gamma-L3-8B-0.6.i1-IQ3_XXS.gguf) | i1-IQ3_XXS | 3.4 | lower quality |
| [GGUF](https://huggingface.co/mradermacher/Hathor_Gamma-L3-8B-0.6-i1-GGUF/resolve/main/Hathor_Gamma-L3-8B-0.6.i1-IQ3_XS.gguf) | i1-IQ3_XS | 3.6 | |
| [GGUF](https://huggingface.co/mradermacher/Hathor_Gamma-L3-8B-0.6-i1-GGUF/resolve/main/Hathor_Gamma-L3-8B-0.6.i1-Q3_K_S.gguf) | i1-Q3_K_S | 3.8 | IQ3_XS probably better |
| [GGUF](https://huggingface.co/mradermacher/Hathor_Gamma-L3-8B-0.6-i1-GGUF/resolve/main/Hathor_Gamma-L3-8B-0.6.i1-IQ3_S.gguf) | i1-IQ3_S | 3.8 | beats Q3_K* |
| [GGUF](https://huggingface.co/mradermacher/Hathor_Gamma-L3-8B-0.6-i1-GGUF/resolve/main/Hathor_Gamma-L3-8B-0.6.i1-IQ3_M.gguf) | i1-IQ3_M | 3.9 | |
| [GGUF](https://huggingface.co/mradermacher/Hathor_Gamma-L3-8B-0.6-i1-GGUF/resolve/main/Hathor_Gamma-L3-8B-0.6.i1-Q3_K_M.gguf) | i1-Q3_K_M | 4.1 | IQ3_S probably better |
| [GGUF](https://huggingface.co/mradermacher/Hathor_Gamma-L3-8B-0.6-i1-GGUF/resolve/main/Hathor_Gamma-L3-8B-0.6.i1-Q3_K_L.gguf) | i1-Q3_K_L | 4.4 | IQ3_M probably better |
| [GGUF](https://huggingface.co/mradermacher/Hathor_Gamma-L3-8B-0.6-i1-GGUF/resolve/main/Hathor_Gamma-L3-8B-0.6.i1-IQ4_XS.gguf) | i1-IQ4_XS | 4.5 | |
| [GGUF](https://huggingface.co/mradermacher/Hathor_Gamma-L3-8B-0.6-i1-GGUF/resolve/main/Hathor_Gamma-L3-8B-0.6.i1-Q4_0.gguf) | i1-Q4_0 | 4.8 | fast, low quality |
| [GGUF](https://huggingface.co/mradermacher/Hathor_Gamma-L3-8B-0.6-i1-GGUF/resolve/main/Hathor_Gamma-L3-8B-0.6.i1-Q4_K_S.gguf) | i1-Q4_K_S | 4.8 | optimal size/speed/quality |
| [GGUF](https://huggingface.co/mradermacher/Hathor_Gamma-L3-8B-0.6-i1-GGUF/resolve/main/Hathor_Gamma-L3-8B-0.6.i1-Q4_K_M.gguf) | i1-Q4_K_M | 5.0 | fast, recommended |
| [GGUF](https://huggingface.co/mradermacher/Hathor_Gamma-L3-8B-0.6-i1-GGUF/resolve/main/Hathor_Gamma-L3-8B-0.6.i1-Q5_K_S.gguf) | i1-Q5_K_S | 5.7 | |
| [GGUF](https://huggingface.co/mradermacher/Hathor_Gamma-L3-8B-0.6-i1-GGUF/resolve/main/Hathor_Gamma-L3-8B-0.6.i1-Q5_K_M.gguf) | i1-Q5_K_M | 5.8 | |
| [GGUF](https://huggingface.co/mradermacher/Hathor_Gamma-L3-8B-0.6-i1-GGUF/resolve/main/Hathor_Gamma-L3-8B-0.6.i1-Q6_K.gguf) | i1-Q6_K | 6.7 | practically like static Q6_K |
Here is a handy graph by ikawrakow comparing some lower-quality quant
types (lower is better):

And here are Artefact2's thoughts on the matter:
https://gist.github.com/Artefact2/b5f810600771265fc1e39442288e8ec9
## FAQ / Model Request
See https://huggingface.co/mradermacher/model_requests for some answers to
questions you might have and/or if you want some other model quantized.
## Thanks
I thank my company, [nethype GmbH](https://www.nethype.de/), for letting
me use its servers and providing upgrades to my workstation to enable
this work in my free time. Additional thanks to [@nicoboss](https://huggingface.co/nicoboss) for giving me access to his hardware for calculating the imatrix for these quants.
<!-- end -->
|
taide/Llama3-TAIDE-LX-8B-Chat-Alpha1 | taide | "2024-04-28T13:12:36Z" | 12,633 | 82 | transformers | [
"transformers",
"safetensors",
"llama",
"text-generation",
"conversational",
"license:other",
"autotrain_compatible",
"endpoints_compatible",
"text-generation-inference",
"region:us"
] | text-generation | "2024-04-26T05:28:08Z" | ---
license: other
license_name: llama3-taide-models-community-license-agreement
license_link: https://drive.google.com/file/d/12-Q0WWSjG0DW6CqJQm_jr5wUGRLeb-8p/view
extra_gated_heading: 您需要先同意授權條款才能使用此模型
extra_gated_fields:
姓名(Name): text
生日(Date of birth): date_picker
國家(Country): country
所屬單位(Affiliation): text
geo: ip_location
按下送出表示您同意社群授權同意書與個人資料蒐集告知聲明(By clicking Submit below I accept the terms of the license and privacy policy): checkbox
extra_gated_prompt: >-
* ### [Llama3-TAIDE 模型社群授權同意書(License)](https://drive.google.com/file/d/12-Q0WWSjG0DW6CqJQm_jr5wUGRLeb-8p/view)
* ### [個人資料蒐集告知聲明(Privacy policy)](https://drive.google.com/file/d/1JTfZu_MdU_TR1-1sn2jbQyW7TLrxjwS5/view)
extra_gated_button_content: 送出(Submit)
---
* [English Version](./README_en.md)
# 模型簡介
* [TAIDE計畫](https://taide.tw/index)致力於開發符合台灣語言和文化特性的生成式人工智慧對話引擎模型,同時建構可信任的人工智慧環境。結合產學研能量,推動可信任生成式人工智慧的發展,提升台灣在國際競爭中的地位,促進產業發展,避免對外國技術的依賴。
* Llama3 TAIDE 系列模型以 Meta 公司釋出的 [LLaMA3-8b](https://llama.meta.com/docs/model-cards-and-prompt-formats/meta-llama-3/) 為基礎,導入台灣不同領域可用的文本與訓練素材,提高模型在正體中文回應的能力與特定任務的表現。公開釋出的模型如下:
* [Llama3-TAIDE-LX-8B-Chat-Alpha1](https://huggingface.co/taide/Llama3-TAIDE-LX-8B-Chat-Alpha1): 以 LLaMA3-8b 為基礎,使用繁體中文資料預訓練 (continuous pretraining),並透過指令微調(instruction tuning)強化辦公室常用任務和多輪問答對話能力,適合聊天對話或任務協助的使用情境。Llama3-TAIDE-LX-8B-Chat-Alpha1 另外有提供[4 bit 量化模型](https://huggingface.co/taide/Llama3-TAIDE-LX-8B-Chat-Alpha1-4bit),量化模型主要是提供使用者的便利性,可能會影響效能與更多不可預期的問題,還請使用者理解與注意。
# 模型參數
* 參數量: 8B
* 最大內容長度 (context length): 8K
* 繁中訓練資料 token 量: 43B
* 訓練時間: 2336 H100 GPU Hours
# 特色
* 嚴格把關模型的訓練資料,提升模型生成資料的可信任性和適用性
* 針對自動摘要、寫信、寫文章、中翻英、英翻中等辦公室常用任務做加強
* 針對台灣在地文化、用語、國情等知識做加強
* 具備多輪問答對話能力
# 應用範例
| 任務 | 使用者輸入 | 模型輸出 |
| --- | -------- | ------- |
| 寫文章 | 請以以下內容為基礎,寫一篇文章:撰寫一篇作文,題目為《一張舊照片》,內容要求為:選擇一張令你印象深刻的照片,說明令你印象深刻的原因,並描述照片中的影像及背後的故事。記錄成長的過程、與他人的情景、環境變遷和美麗的景色。 | 一張舊照片,承載著時光的痕跡和回憶的溫度。我還記得那張照片是怎麼出現的,那是一張泛黃的黑白相片,裱在牆上,訴說著一段屬於我家族的故事。<br><br>那張照片中,我的祖父母站在田野間,後方是綿延的山巒,前方則是一片金黃的稻田。這幅景象令我印象深刻,因為這是我的童年回憶,也是我成長過程中最美好的風景線。<br><br>我出生在台灣鄉下,從小就生活在這樣一個純樸的環境中。每當我跟著家人在田裡工作時,就會看到這樣的美景。金黃的稻穗隨著微風搖曳,山巒則靜靜地矗立在遠方,給人一種安詳與祥和的感覺。<br><br>那張照片拍攝於民國50年代,當時台灣經濟尚未起飛,農業為主要產業。我的祖父母都是農民,他們辛勤耕種,為我們家提供溫飽。每當我看到那張照片,就會想起他們為我們所做的犧牲和努力。<br><br>除了美麗的風景外,照片中還有一段動人的故事。在我還很小的時候,家裡發生了一場大火,將我們的房子和所有財產都燒光了。幸好全家人都平安無事,但生活卻變得十分困苦。我們被迫搬到另一個村莊,在那裡重新開始生活。<br><br>那張照片也記錄了那段艱辛的歲月。雖然我們失去了許多東西,但家人之間的感情卻更加深厚。我們互相扶持,共同面對生活上的種種挑戰。<br><br>如今,我已長大成人,在都市裡工作。但每當我看到那張舊照片,我都會想起那段簡單而美好的時光。那些美麗的風景、親人的笑容,以及那段充滿挑戰卻又充滿希望的日子,都將永遠鐫刻在我的記憶深處。<br><br>這張舊照片,不僅僅是一張照片,更是一個寶貴的家族史,一個關於我們如何克服困難、珍惜現在的故事。我將把這張照片好好保存下來,讓後代也能夠認識我們的根源,並且銘記那些值得珍藏的美好回憶。 |
| 寫信 | 請以品牌經理的身份,給廣告公司的創意總監寫一封信,提出對於新產品廣告宣傳活動的創意建議。 | [您的姓名]<br>[您的職稱]<br>[您的公司名稱]<br>[郵件地址]<br>[電話號碼]<br>[發文日期]<br><br>[創意總監姓名]<br>[廣告公司名稱]<br>[廣告公司地址]<br><br>主旨:關於新產品宣傳活動創意發想,請查照。<br><br>尊敬的[創意總監姓名],<br><br>我希望這封信能找到你忙碌中好好休息。我寫這封信,是為了分享我對於我們即將推出的新產品宣傳活動一些創意發想。我深信,這些想法能幫助我們新產品在市場上脫穎而出,吸引消費者的目光。<br><br>首先,我建議我們可以利用社群媒體平台,像是Facebook、Instagram、YouTube等,製作一系列引人入勝的短片和貼文。這些內容可以展現新產品的特色和優點,同時也能傳達出我們品牌重視環保、健康的核心價值。我們可以與網紅或意見領袖合作,讓他們試用並分享他們的使用經驗,這將有助於增加我們產品的可信度和能見度。<br><br>其次,我們可以舉辦新產品試吃/試用活動,邀請民眾親身體驗我們的新產品。我們可以在各大百貨公司、超市、以及我們的門市舉辦這些活動,讓參與者免費試吃/試用新產品,並填寫問卷回饋意見。這種互動的方式,不僅能收集到寶貴的市場反饋,還能讓民眾對我們的品牌產生正面的印象。<br><br>第三,我們可以與當地餐飲業者合作,將我們的新產品納入他們的菜單中。這將使我們的產品接觸到更廣泛的客群,同時也能證明我們的產品在烹飪和美食產業中具有價值。<br><br>第四,我們可以舉辦一場新產品發表會,邀請媒體、業界人士、以及重要客戶參加。在這場活動中,我們可以展示新產品的各項特色和優勢,並由專業廚師示範如何將新產品融入各式料理中。這將是向大眾展示我們品牌創新精神和專業能力的絕佳機會。<br><br>最後,我建議我們可以製作一系列精美的包裝和禮盒,以吸引消費者在節慶和送禮時選擇我們的產品。精緻的包裝將能提升我們產品的價值感,同時也能傳達出我們品牌對細節和品質的堅持。<br><br>我深信,這些創意發想將有助於我們新產品在市場上獲得成功。若您對這些想法有任何意見或建議,請不吝與我分享。我期待著與您合作,共同為[您的公司名稱]的成長和成功而努力。<br><br>謝謝您抽空閱讀我的信,並期待著與您進一步討論這些想法。<br><br>敬禮<br><br>[您的姓名]<br>[您的職稱]<br>[您的公司名稱] |
| 摘要 | 請將這篇文章精簡條理化:「產業創新條例第10條之2及第72條條文修正案」俗稱「台版晶片法」,針對半導體、電動車、5G等技術創新且居國際供應鏈關鍵地位公司,提供最高25%營所稅投抵優惠,企業適用要件包含當年度研發費用、研發密度達一定規模,且有效稅率達一定比率。<br>為因應經濟合作暨發展組織(OECD)國家最低稅負制調整,其中有效稅率門檻,民國112年訂為12%,113年料將提高至15%,但仍得審酌國際間最低稅負制實施情形。<br>經濟部官員表示,已和財政部協商進入最後階段,除企業研發密度訂在6%,目前已確認,企業購置先進製程的設備投資金額達100億元以上可抵減。<br>財政部官員表示,研商過程中,針對台灣產業與其在國際間類似的公司進行深入研究,在設備部分,畢竟適用產創10之2的業者是代表台灣隊打「國際盃」,投入金額不達100億元,可能也打不了。<br>至於備受關注的研發費用門檻,經濟部官員表示,歷經與財政部來回密切討論,研發費用門檻有望落在60億至70億元之間。<br>財政部官員指出,研發攸關台灣未來經濟成長動能,門檻不能「高不可攀」,起初雖設定在100億元,之所以會調降,正是盼讓企業覺得有辦法達得到門檻、進而適用租稅優惠,才有動力繼續投入研發,維持國際供應鏈關鍵地位。<br>經濟部官員表示,因廠商研發費用平均為30、40億元,其中,IC設計業者介於30億至60億元範圍,若將門檻訂在100億元,符合條件的業者較少、刺激誘因不足;此外,若符合申請門檻的業者增加,將可提高企業在台投資金額,財政部稅收也能因此獲得挹注。<br>IC設計業者近日頻頻針對產創10之2發聲,希望降低適用門檻,加上各國力拚供應鏈自主化、加碼補助半導體產業,經濟部官員表示,經濟部和財政部就產創10之2達成共識,爭取讓更多業者受惠,盼增強企業投資力道及鞏固台灣技術地位。<br>財政部官員表示,租稅獎勵的制定必須「有為有守」,並以達到獎勵設置目的為最高原則,現階段在打「國內盃」的企業仍可適用產創第10條、10之1的租稅優惠,共同壯大台灣經濟發展。<br>經濟部和財政部正就研發費用門檻做最後確認,待今明兩天預告子法之後,約有30天時間,可與業界進一步討論及調整,盼產創10之2能在6月上路。 | 文章重點摘要如下:<br><br>- 「台版晶片法」或《產業創新條例第10條之2及第72條條文修正案》,旨在鼓勵關鍵技術創新企業,特別是那些在半導體、電動車、5G技術等領域且在國際供應鏈中扮演關鍵角色者。<br><br>- 企業若符合特定條件,包括當年度研發費用及研發密度達標,可享最高25%的營所稅抵減優惠。研發密度目標設為6%,而企業為取得抵減,必須證明其有效稅率達到特定比率,起初設定為112年的12%,113年提高至15%,但可能會依國際稅制的實施情形進行調整。<br><br>- 政府在制定政策時,考量到OECD最低稅負制的調整,因此會視情況調整有效稅率門檻。<br><br>- 政府部門已就法案內容與企業利益相關者進行協商,確定一些細節,例如企業投資先進製程設備的門檻設為100億元新台幣以供抵減。<br><br>- 研發費用門檻經過討論,可能從最初提議的100億元下調至60億至70億元之間,以激勵更多企業符合資格並投入研發,維持台灣在國際供應鏈中的關鍵地位。<br><br>- 經濟部官員表示,調降門檻的目的是讓更多企業覺得能夠達成標準,從而獲得應用租稅優惠的動力,並可能藉此提高在台投資金額,進而增加政府稅收。<br><br>- 由於IC設計業者的研發費用普遍較低,若門檻設得過高,符合條件的企業將很少,因此有必要調整。<br><br>- 在各國推動供應鏈自主化並增加對半導體產業的補助之際,經濟部正努力確保更多企業能從《產業創新條例》第10條之2中受益,以增強投資和鞏固台灣的技術地位。<br><br>- 政府強調租稅優惠的制定應符合獎勵目標,在此同時,也將繼續提供其他租稅優惠給在國內經營的企業,以促進台灣經濟成長。<br><br>- 最終的研發費用門檻將由經濟部和財政部共同確定,在預告相關子法後,將有約30天的時間供各界討論並提出調整建議,目標是讓這項新政策在6月上路。 |
| 翻譯 | 以下提供英文內容,請幫我翻譯成中文。Dongshan coffee is famous for its unique position, and the constant refinement of production methods. The flavor is admired by many caffeine afficionados. | 東山咖啡以獨特的位置,加上不斷精進的製作手法而聞名,風味更是讓許多咖啡癡趨之若鶩。 |
# 使用說明
* 範例程式
* 範例程式與文件後續會釋出到github
* prompt 樣版
* 一般問答用法
```python
chat = [
{"role": "user", "content": "{question}"},
]
prompt = tokenizer.apply_chat_template(chat)
```
* 將 {question} 替換成使用者的輸入
* 加入 system prompt 的用法
```python
chat = [
{"role": "system", "content": "{sys}"},
{"role": "user", "content": "{question}"},
]
prompt = tokenizer.apply_chat_template(chat)
```
* 將 {sys} 替換成指令,例如:你是一個來自台灣的AI助理,你的名字是 TAIDE,樂於以台灣人的立場幫助使用者,會用繁體中文回答問題。
* 將 {question} 替換成使用者的問題
* 多輪問答用法
```python
chat = [
{"role": "system", "content": "{sys}"},
{"role": "user", "content": "{question1}"},
{"role": "assistant", "content": "{model_anwer_1}"},
{"role": "user", "content": "{question2}"},
]
prompt = tokenizer.apply_chat_template(chat)
```
* 將 {sys} 替換成指令,例如:你是一個來自台灣的AI助理,你的名字是 TAIDE,樂於以台灣人的立場幫助使用者,會用繁體中文回答問題。
* 將 {question1} 替換成使用者的問題1
* 將 {model_anwer_1} 替換成模型的回答1
* 將 {question2} 替換成使用者的問題2
* 更多細節請參考[Llama3 文件](https://llama.meta.com/docs/model-cards-and-prompt-formats/meta-llama-3/)
# 訓練方法
* 軟硬體規格
* 國網中心 H100
* 訓練框架: PyTorch
* 資料前處理
* 字元標準化
* 去除重覆
* 去除雜訊
* 網頁資料的html tag、javascript
* 非標準字元或亂碼
* 字數過短的文章
* 去除文章中的特定格式,如為排版增加的換行
* 去除個資,如email、電話
* 去除不當文字,如賭博、色情等
* 持續預訓練 (continuous pretraining, CP)
* 補充大量來源可信賴的繁體中文知識
* 超參數 (hyper parameters)
* optimizer: AdamW
* learning rate: 1e-4
* batch size: 1M tokens
* epoch: 1
* 微調 (fine tune, FT)
* 讓模型可針對繁體中文提問回答問題
* 超參數 (hyper parameters)
* optimizer: AdamW
* learning rate: 5e-5
* batch size: 256K tokens
* epoch: 3
# 訓練資料
* 持續預訓練資料(資料量約為140G)
| 資料集 | 資料描述 |
| --- | -------- |
| 訴訟資料 | 《[司法院裁判書](https://judgment.judicial.gov.tw/FJUD/default.aspx)》自2013年1月至2023年12月各級法院民事、刑事、行政訴訟資料。 |
| 中央社 | 《[中央社中文新聞](https://www.cna.com.tw/)》資料集含中央社自1993年6月至2023年06月,共30年份之每日新聞文章。內容涵蓋國內外政治、社會、財經、文教、生活等領域。 |
| ETtoday 新聞雲 | 《[ETtoday新聞雲](https://www.ettoday.net/)》資料,包含自2011年10月至 2023年12月的資料。 |
| 立法院公報 | 《[立法院公報](https://ppg.ly.gov.tw/ppg/)》包含自第8屆第1會期至第10屆第7會期之公報資料。 |
| 出版商網站書籍介紹 | 包含[三采](https://www.suncolor.com.tw/)、[Gotop](https://www.gotop.com.tw/)出版商網站上的書籍簡介。 |
| GRB 研究計畫摘要 | [GRB](https://www.grb.gov.tw/)為收錄由政府經費補助之研究計畫及其成果報告的資訊系統,此資料集主要收錄 1993年至 2023年之研究計畫摘要以及研究報告摘要,含中文及其英文對照。 |
| 學術會議論文摘要 | 收錄《[學術會議論文摘要資料庫](https://sticnet.stpi.narl.org.tw/sticloc/ttscalle?meet:)》中自1988至2009年由台灣所舉辦之學術會議論文。 |
| 光華雜誌 | 《[台灣光華雜誌](https://www.taiwan-panorama.com/)》含自1993年7月至2023年6月的文章,共30年份。內容著重於我國文化、觀光與民情等。 |
| 樂詞網 | 《[樂詞網](https://terms.naer.edu.tw/)》涵蓋文理領域約187萬則學術名詞及其譯名對照。 |
| 各部會資料 | 包含行政院「[國情簡介](https://www.ey.gov.tw/state/)」、文化部「[國家文化記憶庫](https://memory.culture.tw/)」、國發會「[檔案支援教學網](https://art.archives.gov.tw/index.aspx)」、交通部「[交通安全入口網](https://168.motc.gov.tw/)」等部會網站資料之部分資料。 |
| 今周刊 | 《[今周刊](https://www.businesstoday.com.tw/)》為一以財經為主的週刊雜誌,此資料集涵蓋2008年1月至2023年7月的文章。 |
| 教育部國語辭典、成語辭典 | 包含以下三項資料:<br>[教育部《成語典》](https://dict.idioms.moe.edu.tw/search.jsp?webMd=1&la=0),含5,338條成語,內容包含每條成語的釋義、典故原文及其白話說明、用法說明、例句等。<br>[教育部《重編國語辭典修訂本》](https://dict.revised.moe.edu.tw/?la=0&powerMode=0),收錄中文單字及各類辭彙,包含讀音、部首、釋義等資訊,共約165,539筆資料。<br>[教育部《國語辭典簡編本》](https://dict.concised.moe.edu.tw/?la=0&powerMode=0),為《重編國語辭典修訂本》的簡編版本,共45,247筆資料。 |
| 科技大觀園資料 | 含《[科技大觀園網站](https://scitechvista.nat.gov.tw/)》上的科學新知以及科普文章。 |
| iKnow 科技產業資訊室 | 《[科技產業資訊室](https://iknow.stpi.narl.org.tw/)(iKnow)》提供台灣及全球的科技市場趨勢、策略分析、專利知識,及技術交易資訊,專注於科技產業的創新與發展,包含自 2008 年至 2023 年。 |
| 科學發展月刊 | 《[科學發展月刊](https://ejournal.stpi.narl.org.tw/sd)》為國科會為推廣科學教育而出版的科普刊物,含自2004年10月至2020年12月之科普文章;2021年起,以《[科技魅癮](https://www.charmingscitech.nat.gov.tw/)》季刊重新出發,提供國際關注科技議題的新知文章。 |
| 法規資料庫 | 《[法規資料庫](https://law.moj.gov.tw/)》含截自 112 年 10 月各政府部門最新發布之中央法規、行政規則、法規命令草案及地方自治法規等。 |
| 各地政府旅遊網 | 涵蓋台灣部分縣市地方政府觀光旅遊網站上之部分資料。 |
| 國教院課程綱要(十二年國教) | 含十二年國教課程綱要之總綱以及各級學校不同科目之課程綱要。 |
| 中央社譯名檔資料庫 | 《中央社譯名檔資料庫》蒐集中央社新聞業務上翻譯過的中外姓氏、人名、組織、地名等譯名對照。 |
| 童話書 | 共 20 本童話書,含湯姆歷險記、小飛俠、愛麗絲夢遊仙境、長腿叔叔等。 |
| RedPajama-Data-V2 | 從國外開放多國語言語料庫 [RedPajama-Data-v2](https://github.com/togethercomputer/RedPajama-Data) 取出英文資料 |
| MathPile-commercial | 國外開放數學語料庫 [MathPile-commercial](https://huggingface.co/datasets/GAIR/MathPile_Commercial) |
| 中文維基百科 | 《[中文維基百科](https://zh.wikipedia.org/zh-tw/%E4%B8%AD%E6%96%87%E7%BB%B4%E5%9F%BA%E7%99%BE%E7%A7%91)》截至2023年1月所有條目的內容。 |
| github-code-clean | 為 github 開源程式碼資料集,去除unlicense的程式碼和文件。 |
* 微調資料
* TAIDE團隊訓練llama2系列模型來產生微調資料資料,產生的任務包含世界知識、創意寫作、普通常識、翻譯、摘要、程式、台灣價值等單輪或多輪對話問答共 128K 筆。微調資料後續會對外釋出。
# 模型評測
* taide-bench
* 評測資料
* 寫文章、寫信、摘要、英翻中、中翻英,共500題
* 資料連結: [taide-bench](https://huggingface.co/datasets/taide/taide-bench)
* 評測方法
* gpt4評分
* 評分程式: [taide-bench-eval](https://github.com/taide-taiwan/taide-bench-eval)
* 評測分數
| 模型 | 中翻英 | 英翻中 | 摘要 | 寫文章 | 寫信 | 平均 |
| --- | ----- | ----- | ---- | ---- | ---- | --- |
| Llama3-TAIDE-LX-8B-Chat-Alpha1 | 7.770 | 8.280 | 8.495 | 9.605 | 8.950 | 8.620 |
| GPT3.5 | 8.880 | 8.810 | 7.450 | 9.490 | 8.750 | 8.676 |
| TAIDE-LX-7B-Chat | 7.165 | 7.685 | 7.720 | 9.635 | 9.110 | 8.263 |
| LLAMA2 7B | 6.075 | 4.475 | 5.905 | 2.625 | 3.040 | 4.424 |
| LLAMA2 13B | 6.480 | 6.135 | 6.110 | 2.565 | 3.000 | 4.858 |
| LLAMA2 70B | 6.975 | 6.375 | 6.795 | 2.625 | 2.990 | 5.152 |
# 授權條款
* [Llama3-TAIDE 模型社群授權同意書](https://drive.google.com/file/d/12-Q0WWSjG0DW6CqJQm_jr5wUGRLeb-8p/view)
# 免責聲明
* LLM 模型由於設計架構的限制,以及資料難免有偏誤,語言模型的任何回應不代表 TAIDE 立場,使用前需要額外加入安全防護機制,且回應內容也可能包含不正確的資訊,使用者請勿盡信。
# 開發團隊
* [https://taide.tw/index/teamList](https://taide.tw/index/teamList)
# 相關連結
* [TAIDE官網](https://taide.tw/index)
* [TAIDE Huggingface](https://huggingface.co/taide)
* [TAIDE Github](https://github.com/taide-taiwan)
* [Kuwa AI](https://kuwaai.org/)
# Citation
* [TAIDE官網](https://taide.tw/index)
|
RichardErkhov/nvidia_-_OpenMath-Mistral-7B-v0.1-hf-gguf | RichardErkhov | "2024-06-19T20:32:54Z" | 12,629 | 1 | null | [
"gguf",
"arxiv:2402.10176",
"region:us"
] | null | "2024-06-19T14:26:37Z" | Quantization made by Richard Erkhov.
[Github](https://github.com/RichardErkhov)
[Discord](https://discord.gg/pvy7H8DZMG)
[Request more models](https://github.com/RichardErkhov/quant_request)
OpenMath-Mistral-7B-v0.1-hf - GGUF
- Model creator: https://huggingface.co/nvidia/
- Original model: https://huggingface.co/nvidia/OpenMath-Mistral-7B-v0.1-hf/
| Name | Quant method | Size |
| ---- | ---- | ---- |
| [OpenMath-Mistral-7B-v0.1-hf.Q2_K.gguf](https://huggingface.co/RichardErkhov/nvidia_-_OpenMath-Mistral-7B-v0.1-hf-gguf/blob/main/OpenMath-Mistral-7B-v0.1-hf.Q2_K.gguf) | Q2_K | 2.53GB |
| [OpenMath-Mistral-7B-v0.1-hf.IQ3_XS.gguf](https://huggingface.co/RichardErkhov/nvidia_-_OpenMath-Mistral-7B-v0.1-hf-gguf/blob/main/OpenMath-Mistral-7B-v0.1-hf.IQ3_XS.gguf) | IQ3_XS | 2.81GB |
| [OpenMath-Mistral-7B-v0.1-hf.IQ3_S.gguf](https://huggingface.co/RichardErkhov/nvidia_-_OpenMath-Mistral-7B-v0.1-hf-gguf/blob/main/OpenMath-Mistral-7B-v0.1-hf.IQ3_S.gguf) | IQ3_S | 2.96GB |
| [OpenMath-Mistral-7B-v0.1-hf.Q3_K_S.gguf](https://huggingface.co/RichardErkhov/nvidia_-_OpenMath-Mistral-7B-v0.1-hf-gguf/blob/main/OpenMath-Mistral-7B-v0.1-hf.Q3_K_S.gguf) | Q3_K_S | 2.95GB |
| [OpenMath-Mistral-7B-v0.1-hf.IQ3_M.gguf](https://huggingface.co/RichardErkhov/nvidia_-_OpenMath-Mistral-7B-v0.1-hf-gguf/blob/main/OpenMath-Mistral-7B-v0.1-hf.IQ3_M.gguf) | IQ3_M | 3.06GB |
| [OpenMath-Mistral-7B-v0.1-hf.Q3_K.gguf](https://huggingface.co/RichardErkhov/nvidia_-_OpenMath-Mistral-7B-v0.1-hf-gguf/blob/main/OpenMath-Mistral-7B-v0.1-hf.Q3_K.gguf) | Q3_K | 3.28GB |
| [OpenMath-Mistral-7B-v0.1-hf.Q3_K_M.gguf](https://huggingface.co/RichardErkhov/nvidia_-_OpenMath-Mistral-7B-v0.1-hf-gguf/blob/main/OpenMath-Mistral-7B-v0.1-hf.Q3_K_M.gguf) | Q3_K_M | 3.28GB |
| [OpenMath-Mistral-7B-v0.1-hf.Q3_K_L.gguf](https://huggingface.co/RichardErkhov/nvidia_-_OpenMath-Mistral-7B-v0.1-hf-gguf/blob/main/OpenMath-Mistral-7B-v0.1-hf.Q3_K_L.gguf) | Q3_K_L | 3.56GB |
| [OpenMath-Mistral-7B-v0.1-hf.IQ4_XS.gguf](https://huggingface.co/RichardErkhov/nvidia_-_OpenMath-Mistral-7B-v0.1-hf-gguf/blob/main/OpenMath-Mistral-7B-v0.1-hf.IQ4_XS.gguf) | IQ4_XS | 3.67GB |
| [OpenMath-Mistral-7B-v0.1-hf.Q4_0.gguf](https://huggingface.co/RichardErkhov/nvidia_-_OpenMath-Mistral-7B-v0.1-hf-gguf/blob/main/OpenMath-Mistral-7B-v0.1-hf.Q4_0.gguf) | Q4_0 | 3.83GB |
| [OpenMath-Mistral-7B-v0.1-hf.IQ4_NL.gguf](https://huggingface.co/RichardErkhov/nvidia_-_OpenMath-Mistral-7B-v0.1-hf-gguf/blob/main/OpenMath-Mistral-7B-v0.1-hf.IQ4_NL.gguf) | IQ4_NL | 3.87GB |
| [OpenMath-Mistral-7B-v0.1-hf.Q4_K_S.gguf](https://huggingface.co/RichardErkhov/nvidia_-_OpenMath-Mistral-7B-v0.1-hf-gguf/blob/main/OpenMath-Mistral-7B-v0.1-hf.Q4_K_S.gguf) | Q4_K_S | 3.86GB |
| [OpenMath-Mistral-7B-v0.1-hf.Q4_K.gguf](https://huggingface.co/RichardErkhov/nvidia_-_OpenMath-Mistral-7B-v0.1-hf-gguf/blob/main/OpenMath-Mistral-7B-v0.1-hf.Q4_K.gguf) | Q4_K | 4.07GB |
| [OpenMath-Mistral-7B-v0.1-hf.Q4_K_M.gguf](https://huggingface.co/RichardErkhov/nvidia_-_OpenMath-Mistral-7B-v0.1-hf-gguf/blob/main/OpenMath-Mistral-7B-v0.1-hf.Q4_K_M.gguf) | Q4_K_M | 4.07GB |
| [OpenMath-Mistral-7B-v0.1-hf.Q4_1.gguf](https://huggingface.co/RichardErkhov/nvidia_-_OpenMath-Mistral-7B-v0.1-hf-gguf/blob/main/OpenMath-Mistral-7B-v0.1-hf.Q4_1.gguf) | Q4_1 | 4.24GB |
| [OpenMath-Mistral-7B-v0.1-hf.Q5_0.gguf](https://huggingface.co/RichardErkhov/nvidia_-_OpenMath-Mistral-7B-v0.1-hf-gguf/blob/main/OpenMath-Mistral-7B-v0.1-hf.Q5_0.gguf) | Q5_0 | 4.65GB |
| [OpenMath-Mistral-7B-v0.1-hf.Q5_K_S.gguf](https://huggingface.co/RichardErkhov/nvidia_-_OpenMath-Mistral-7B-v0.1-hf-gguf/blob/main/OpenMath-Mistral-7B-v0.1-hf.Q5_K_S.gguf) | Q5_K_S | 4.65GB |
| [OpenMath-Mistral-7B-v0.1-hf.Q5_K.gguf](https://huggingface.co/RichardErkhov/nvidia_-_OpenMath-Mistral-7B-v0.1-hf-gguf/blob/main/OpenMath-Mistral-7B-v0.1-hf.Q5_K.gguf) | Q5_K | 4.78GB |
| [OpenMath-Mistral-7B-v0.1-hf.Q5_K_M.gguf](https://huggingface.co/RichardErkhov/nvidia_-_OpenMath-Mistral-7B-v0.1-hf-gguf/blob/main/OpenMath-Mistral-7B-v0.1-hf.Q5_K_M.gguf) | Q5_K_M | 4.78GB |
| [OpenMath-Mistral-7B-v0.1-hf.Q5_1.gguf](https://huggingface.co/RichardErkhov/nvidia_-_OpenMath-Mistral-7B-v0.1-hf-gguf/blob/main/OpenMath-Mistral-7B-v0.1-hf.Q5_1.gguf) | Q5_1 | 5.07GB |
| [OpenMath-Mistral-7B-v0.1-hf.Q6_K.gguf](https://huggingface.co/RichardErkhov/nvidia_-_OpenMath-Mistral-7B-v0.1-hf-gguf/blob/main/OpenMath-Mistral-7B-v0.1-hf.Q6_K.gguf) | Q6_K | 5.53GB |
| [OpenMath-Mistral-7B-v0.1-hf.Q8_0.gguf](https://huggingface.co/RichardErkhov/nvidia_-_OpenMath-Mistral-7B-v0.1-hf-gguf/blob/main/OpenMath-Mistral-7B-v0.1-hf.Q8_0.gguf) | Q8_0 | 7.17GB |
Original model description:
---
license: apache-2.0
base_model:
- mistralai/Mistral-7B-v0.1
datasets:
- nvidia/OpenMathInstruct-1
language:
- en
tags:
- nvidia
- code
- math
---
# OpenMath-Mistral-7B-v0.1-hf
OpenMath models were designed to solve mathematical problems by integrating text-based reasoning with code blocks
executed by Python interpreter. The models were trained on [OpenMathInstruct-1](https://huggingface.co/datasets/nvidia/OpenMathInstruct-1),
a math instruction tuning dataset with 1.8M problem-solution pairs generated using permissively licensed
[Mixtral-8x7B](https://huggingface.co/mistralai/Mixtral-8x7B-v0.1) model.
<table border="1">
<tr>
<td></td>
<td colspan="2" style="text-align: center;">greedy</td>
<td colspan="2" style="text-align: center;">majority@50</td>
</tr>
<tr>
<td style="text-align: center;">model</td>
<td style="text-align: center;">GSM8K</td>
<td style="text-align: center;">MATH</td>
<td style="text-align: center;">GMS8K</td>
<td style="text-align: center;">MATH</td>
</tr>
<tr>
<td style="text-align: right;">OpenMath-CodeLlama-7B (<a href="https://huggingface.co/nvidia/OpenMath-CodeLlama-7b-Python">nemo</a> | <a href="https://huggingface.co/nvidia/OpenMath-CodeLlama-7b-Python-hf">HF</a>)</td>
<td style="text-align: center;">75.9</td>
<td style="text-align: center;">43.6</td>
<td style="text-align: center;">84.8</td>
<td style="text-align: center;">55.6</td>
</tr>
<tr>
<td style="text-align: right;">OpenMath-Mistral-7B (<a href="https://huggingface.co/nvidia/OpenMath-Mistral-7B-v0.1">nemo</a> | <a href="https://huggingface.co/nvidia/OpenMath-Mistral-7B-v0.1-hf">HF</a>)</td>
<td style="text-align: center;">80.2</td>
<td style="text-align: center;">44.5</td>
<td style="text-align: center;">86.9</td>
<td style="text-align: center;">57.2</td>
</tr>
<tr>
<td style="text-align: right;">OpenMath-CodeLlama-13B (<a href="https://huggingface.co/nvidia/OpenMath-CodeLlama-13b-Python">nemo</a> | <a href="https://huggingface.co/nvidia/OpenMath-CodeLlama-13b-Python-hf">HF</a>)</td>
<td style="text-align: center;">78.8</td>
<td style="text-align: center;">45.5</td>
<td style="text-align: center;">86.8</td>
<td style="text-align: center;">57.6</td>
</tr>
<tr>
<td style="text-align: right;">OpenMath-CodeLlama-34B (<a href="https://huggingface.co/nvidia/OpenMath-CodeLlama-34b-Python">nemo</a> | <a href="https://huggingface.co/nvidia/OpenMath-CodeLlama-34b-Python-hf">HF</a>)</td>
<td style="text-align: center;">80.7</td>
<td style="text-align: center;">48.3</td>
<td style="text-align: center;">88.0</td>
<td style="text-align: center;">60.2</td>
</tr>
<tr>
<td style="text-align: right;">OpenMath-Llama2-70B (<a href="https://huggingface.co/nvidia/OpenMath-Llama-2-70b">nemo</a> | <a href="https://huggingface.co/nvidia/OpenMath-Llama-2-70b-hf">HF</a>)</td>
<td style="text-align: center;"><b>84.7</b></td>
<td style="text-align: center;">46.3</td>
<td style="text-align: center;">90.1</td>
<td style="text-align: center;">58.3</td>
</tr>
<tr>
<td style="text-align: right;">OpenMath-CodeLlama-70B (<a href="https://huggingface.co/nvidia/OpenMath-CodeLlama-70b-Python">nemo</a> | <a href="https://huggingface.co/nvidia/OpenMath-CodeLlama-70b-Python-hf">HF</a>)</td>
<td style="text-align: center;">84.6</td>
<td style="text-align: center;"><b>50.7</b></td>
<td style="text-align: center;"><b>90.8</b></td>
<td style="text-align: center;"><b>60.4</b></td>
</tr>
</table>
The pipeline we used to produce these models is fully open-sourced!
- [Code](https://github.com/Kipok/NeMo-Skills)
- [Models](https://huggingface.co/collections/nvidia/openmath-65c5619de2ba059be0775014)
- [Dataset](https://huggingface.co/datasets/nvidia/OpenMathInstruct-1)
See our [paper](https://arxiv.org/abs/2402.10176) for more details!
# How to use the models?
Try to [run inference with our models](https://github.com/Kipok/NeMo-Skills/blob/main/docs/inference.md) with just a few commands!
# Reproducing our results
We provide [all instructions](https://github.com/Kipok/NeMo-Skills/blob/main/docs/reproducing-results.md) to fully reproduce our results.
# Improving other models
To improve other models or to learn more about our code, read through the docs below.
- [NeMo-Skills Pipeline](https://github.com/Kipok/NeMo-Skills)
- [Generating synthetic data](https://github.com/Kipok/NeMo-Skills/blob/main/docs/synthetic-data-generation.md)
- [Finetuning models](https://github.com/Kipok/NeMo-Skills/blob/main/docs/finetuning.md)
- [Evaluating models](https://github.com/Kipok/NeMo-Skills/blob/main/docs/evaluation.md)
In our pipeline we use [NVIDIA NeMo](https://www.nvidia.com/en-us/ai-data-science/generative-ai/nemo-framework/),
an end-to-end, cloud-native framework to build, customize, and deploy generative AI models anywhere.
It includes training and inferencing frameworks, guardrailing toolkits, data curation tools, and pretrained models,
offering enterprises an easy, cost-effective, and fast way to adopt generative AI.
# Citation
If you find our work useful, please consider citing us!
```bibtex
@article{toshniwal2024openmath,
title = {OpenMathInstruct-1: A 1.8 Million Math Instruction Tuning Dataset},
author = {Shubham Toshniwal and Ivan Moshkov and Sean Narenthiran and Daria Gitman and Fei Jia and Igor Gitman},
year = {2024},
journal = {arXiv preprint arXiv: Arxiv-2402.10176}
}
```
|
google/gemma-2-27b-it | google | "2024-07-02T20:00:19Z" | 12,617 | 222 | transformers | [
"transformers",
"safetensors",
"gemma2",
"text-generation",
"conversational",
"arxiv:2009.03300",
"arxiv:1905.07830",
"arxiv:1911.11641",
"arxiv:1904.09728",
"arxiv:1905.10044",
"arxiv:1907.10641",
"arxiv:1811.00937",
"arxiv:1809.02789",
"arxiv:1911.01547",
"arxiv:1705.03551",
"arxiv:2107.03374",
"arxiv:2108.07732",
"arxiv:2110.14168",
"arxiv:2009.11462",
"arxiv:2101.11718",
"arxiv:2110.08193",
"arxiv:1804.09301",
"arxiv:2109.07958",
"arxiv:1804.06876",
"arxiv:2103.03874",
"arxiv:2304.06364",
"arxiv:2206.04615",
"arxiv:2203.09509",
"license:gemma",
"autotrain_compatible",
"endpoints_compatible",
"text-generation-inference",
"region:us"
] | text-generation | "2024-06-24T08:05:04Z" | ---
license: gemma
library_name: transformers
pipeline_tag: text-generation
extra_gated_heading: Access Gemma on Hugging Face
extra_gated_prompt: >-
To access Gemma on Hugging Face, you’re required to review and agree to
Google’s usage license. To do this, please ensure you’re logged in to Hugging
Face and click below. Requests are processed immediately.
extra_gated_button_content: Acknowledge license
---
# Gemma 2 model card
**Model Page**: [Gemma](https://ai.google.dev/gemma/docs)
**Resources and Technical Documentation**:
* [Responsible Generative AI Toolkit][rai-toolkit]
* [Gemma on Kaggle][kaggle-gemma]
* [Gemma on Vertex Model Garden][vertex-mg-gemma]
**Terms of Use**: [Terms](https://www.kaggle.com/models/google/gemma/license/consent/verify/huggingface?returnModelRepoId=google/gemma-2-27b-it)
**Authors**: Google
## Model Information
Summary description and brief definition of inputs and outputs.
### Description
Gemma is a family of lightweight, state-of-the-art open models from Google,
built from the same research and technology used to create the Gemini models.
They are text-to-text, decoder-only large language models, available in English,
with open weights for both pre-trained variants and instruction-tuned variants.
Gemma models are well-suited for a variety of text generation tasks, including
question answering, summarization, and reasoning. Their relatively small size
makes it possible to deploy them in environments with limited resources such as
a laptop, desktop or your own cloud infrastructure, democratizing access to
state of the art AI models and helping foster innovation for everyone.
### Usage
Below we share some code snippets on how to get quickly started with running the model. First make sure to `pip install -U transformers`, then copy the snippet from the section that is relevant for your usecase.
#### Running the model on a single / multi GPU
> [!IMPORTANT]
> Given the model instabilities with SDPA/ FA2, by default, the model inference would utilise `eager` attention.
```python
# pip install accelerate
from transformers import AutoTokenizer, AutoModelForCausalLM
import torch
tokenizer = AutoTokenizer.from_pretrained("google/gemma-2-27b-it")
model = AutoModelForCausalLM.from_pretrained(
"google/gemma-2-27b-it",
device_map="auto",
torch_dtype=torch.bfloat16
)
input_text = "Write me a poem about Machine Learning."
input_ids = tokenizer(input_text, return_tensors="pt").to("cuda")
outputs = model.generate(**input_ids)
print(tokenizer.decode(outputs[0]))
```
<a name="precisions"></a>
#### Running the model on a GPU using different precisions
The native weights of this model were exported in `bfloat16` precision.
You can also use `float32` if you skip the dtype, but no precision increase will occur (model weights will just be upcasted to `float32`). See examples below.
* _Upcasting to `torch.float32`_
```python
# pip install accelerate
from transformers import AutoTokenizer, AutoModelForCausalLM
tokenizer = AutoTokenizer.from_pretrained("google/gemma-2-27b-it")
model = AutoModelForCausalLM.from_pretrained(
"google/gemma-2-27b-it",
device_map="auto"
)
input_text = "Write me a poem about Machine Learning."
input_ids = tokenizer(input_text, return_tensors="pt").to("cuda")
outputs = model.generate(**input_ids)
print(tokenizer.decode(outputs[0]))
```
#### Quantized Versions through `bitsandbytes`
* _Using 8-bit precision (int8)_
```python
# pip install bitsandbytes accelerate
from transformers import AutoTokenizer, AutoModelForCausalLM, BitsAndBytesConfig
quantization_config = BitsAndBytesConfig(load_in_8bit=True)
tokenizer = AutoTokenizer.from_pretrained("google/gemma-2-27b-it")
model = AutoModelForCausalLM.from_pretrained(
"google/gemma-2-27b-it",
quantization_config=quantization_config)
input_text = "Write me a poem about Machine Learning."
input_ids = tokenizer(input_text, return_tensors="pt").to("cuda")
outputs = model.generate(**input_ids)
print(tokenizer.decode(outputs[0]))
```
* _Using 4-bit precision_
```python
# pip install bitsandbytes accelerate
from transformers import AutoTokenizer, AutoModelForCausalLM, BitsAndBytesConfig
quantization_config = BitsAndBytesConfig(load_in_4bit=True)
tokenizer = AutoTokenizer.from_pretrained("google/gemma-2-27b-it")
model = AutoModelForCausalLM.from_pretrained(
"google/gemma-2-27b-it",
quantization_config=quantization_config)
input_text = "Write me a poem about Machine Learning."
input_ids = tokenizer(input_text, return_tensors="pt").to("cuda")
outputs = model.generate(**input_ids)
print(tokenizer.decode(outputs[0]))
```
#### Other optimizations
* _Flash Attention 2_
> [!WARNING]
> Gemma 2 is currently incompatible with Flash Attention/ SDPA, using it might result in unreliable generations. Use at your own risk.
First make sure to install `flash-attn` in your environment `pip install flash-attn`
```diff
model = AutoModelForCausalLM.from_pretrained(
model_id,
torch_dtype=torch.float16,
+ attn_implementation="flash_attention_2"
).to(0)
```
### Chat Template
The instruction-tuned models use a chat template that must be adhered to for conversational use.
The easiest way to apply it is using the tokenizer's built-in chat template, as shown in the following snippet.
Let's load the model and apply the chat template to a conversation. In this example, we'll start with a single user interaction:
```py
from transformers import AutoTokenizer, AutoModelForCausalLM
import transformers
import torch
model_id = "google/gemma-2-27b-it"
dtype = torch.bfloat16
tokenizer = AutoTokenizer.from_pretrained(model_id)
model = AutoModelForCausalLM.from_pretrained(
model_id,
device_map="cuda",
torch_dtype=dtype,
)
chat = [
{ "role": "user", "content": "Write a hello world program" },
]
prompt = tokenizer.apply_chat_template(chat, tokenize=False, add_generation_prompt=True)
```
At this point, the prompt contains the following text:
```
<bos><start_of_turn>user
Write a hello world program<end_of_turn>
<start_of_turn>model
```
As you can see, each turn is preceded by a `<start_of_turn>` delimiter and then the role of the entity
(either `user`, for content supplied by the user, or `model` for LLM responses). Turns finish with
the `<end_of_turn>` token.
You can follow this format to build the prompt manually, if you need to do it without the tokenizer's
chat template.
After the prompt is ready, generation can be performed like this:
```py
inputs = tokenizer.encode(prompt, add_special_tokens=False, return_tensors="pt")
outputs = model.generate(input_ids=inputs.to(model.device), max_new_tokens=150)
print(tokenizer.decode(outputs[0]))
```
### Inputs and outputs
* **Input:** Text string, such as a question, a prompt, or a document to be
summarized.
* **Output:** Generated English-language text in response to the input, such
as an answer to a question, or a summary of a document.
### Citation
```none
@article{gemma_2024,
title={Gemma},
url={https://www.kaggle.com/m/3301},
DOI={10.34740/KAGGLE/M/3301},
publisher={Kaggle},
author={Gemma Team},
year={2024}
}
```
## Model Data
Data used for model training and how the data was processed.
### Training Dataset
These models were trained on a dataset of text data that includes a wide variety of sources. The 27B model was trained with 13 trillion tokens and the 9B model was trained with 8 trillion tokens.
Here are the key components:
* Web Documents: A diverse collection of web text ensures the model is exposed
to a broad range of linguistic styles, topics, and vocabulary. Primarily
English-language content.
* Code: Exposing the model to code helps it to learn the syntax and patterns of
programming languages, which improves its ability to generate code or
understand code-related questions.
* Mathematics: Training on mathematical text helps the model learn logical
reasoning, symbolic representation, and to address mathematical queries.
The combination of these diverse data sources is crucial for training a powerful
language model that can handle a wide variety of different tasks and text
formats.
### Data Preprocessing
Here are the key data cleaning and filtering methods applied to the training
data:
* CSAM Filtering: Rigorous CSAM (Child Sexual Abuse Material) filtering was
applied at multiple stages in the data preparation process to ensure the
exclusion of harmful and illegal content.
* Sensitive Data Filtering: As part of making Gemma pre-trained models safe and
reliable, automated techniques were used to filter out certain personal
information and other sensitive data from training sets.
* Additional methods: Filtering based on content quality and safety in line with
[our policies][safety-policies].
## Implementation Information
Details about the model internals.
### Hardware
Gemma was trained using the latest generation of
[Tensor Processing Unit (TPU)][tpu] hardware (TPUv5p).
Training large language models requires significant computational power. TPUs,
designed specifically for matrix operations common in machine learning, offer
several advantages in this domain:
* Performance: TPUs are specifically designed to handle the massive computations
involved in training LLMs. They can speed up training considerably compared to
CPUs.
* Memory: TPUs often come with large amounts of high-bandwidth memory, allowing
for the handling of large models and batch sizes during training. This can
lead to better model quality.
* Scalability: TPU Pods (large clusters of TPUs) provide a scalable solution for
handling the growing complexity of large foundation models. You can distribute
training across multiple TPU devices for faster and more efficient processing.
* Cost-effectiveness: In many scenarios, TPUs can provide a more cost-effective
solution for training large models compared to CPU-based infrastructure,
especially when considering the time and resources saved due to faster
training.
* These advantages are aligned with
[Google's commitments to operate sustainably][sustainability].
### Software
Training was done using [JAX][jax] and [ML Pathways][ml-pathways].
JAX allows researchers to take advantage of the latest generation of hardware,
including TPUs, for faster and more efficient training of large models.
ML Pathways is Google's latest effort to build artificially intelligent systems
capable of generalizing across multiple tasks. This is specially suitable for
[foundation models][foundation-models], including large language models like
these ones.
Together, JAX and ML Pathways are used as described in the
[paper about the Gemini family of models][gemini-2-paper]; "the 'single
controller' programming model of Jax and Pathways allows a single Python
process to orchestrate the entire training run, dramatically simplifying the
development workflow."
## Evaluation
Model evaluation metrics and results.
### Benchmark Results
These models were evaluated against a large collection of different datasets and
metrics to cover different aspects of text generation:
| Benchmark | Metric | Gemma PT 9B | Gemma PT 27B |
| ------------------------------ | ------------- | ----------- | ------------ |
| [MMLU][mmlu] | 5-shot, top-1 | 71.3 | 75.2 |
| [HellaSwag][hellaswag] | 10-shot | 81.9 | 86.4 |
| [PIQA][piqa] | 0-shot | 81.7 | 83.2 |
| [SocialIQA][socialiqa] | 0-shot | 53.4 | 53.7 |
| [BoolQ][boolq] | 0-shot | 84.2 | 84.8 |
| [WinoGrande][winogrande] | partial score | 80.6 | 83.7 |
| [ARC-e][arc] | 0-shot | 88.0 | 88.6 |
| [ARC-c][arc] | 25-shot | 68.4 | 71.4 |
| [TriviaQA][triviaqa] | 5-shot | 76.6 | 83.7 |
| [Natural Questions][naturalq] | 5-shot | 29.2 | 34.5 |
| [HumanEval][humaneval] | pass@1 | 40.2 | 51.8 |
| [MBPP][mbpp] | 3-shot | 52.4 | 62.6 |
| [GSM8K][gsm8k] | 5-shot, maj@1 | 68.6 | 74.0 |
| [MATH][math] | 4-shot | 36.6 | 42.3 |
| [AGIEval][agieval] | 3-5-shot | 52.8 | 55.1 |
| [BIG-Bench][big-bench] | 3-shot, CoT | 68.2 | 74.9 |
| ------------------------------ | ------------- | ----------- | ------------ |
## Ethics and Safety
Ethics and safety evaluation approach and results.
### Evaluation Approach
Our evaluation methods include structured evaluations and internal red-teaming
testing of relevant content policies. Red-teaming was conducted by a number of
different teams, each with different goals and human evaluation metrics. These
models were evaluated against a number of different categories relevant to
ethics and safety, including:
* Text-to-Text Content Safety: Human evaluation on prompts covering safety
policies including child sexual abuse and exploitation, harassment, violence
and gore, and hate speech.
* Text-to-Text Representational Harms: Benchmark against relevant academic
datasets such as [WinoBias][winobias] and [BBQ Dataset][bbq].
* Memorization: Automated evaluation of memorization of training data, including
the risk of personally identifiable information exposure.
* Large-scale harm: Tests for "dangerous capabilities," such as chemical,
biological, radiological, and nuclear (CBRN) risks.
### Evaluation Results
The results of ethics and safety evaluations are within acceptable thresholds
for meeting [internal policies][safety-policies] for categories such as child
safety, content safety, representational harms, memorization, large-scale harms.
On top of robust internal evaluations, the results of well-known safety
benchmarks like BBQ, BOLD, Winogender, Winobias, RealToxicity, and TruthfulQA
are shown here.
#### Gemma 2.0
| Benchmark | Metric | Gemma 2 IT 9B | Gemma 2 IT 27B |
| ------------------------ | ------------- | --------------- | ---------------- |
| [RealToxicity][realtox] | average | 8.25 | 8.84 |
| [CrowS-Pairs][crows] | top-1 | 37.47 | 36.67 |
| [BBQ Ambig][bbq] | 1-shot, top-1 | 88.58 | 85.99 |
| [BBQ Disambig][bbq] | top-1 | 82.67 | 86.94 |
| [Winogender][winogender] | top-1 | 79.17 | 77.22 |
| [TruthfulQA][truthfulqa] | | 50.27 | 51.60 |
| [Winobias 1_2][winobias] | | 78.09 | 81.94 |
| [Winobias 2_2][winobias] | | 95.32 | 97.22 |
| [Toxigen][toxigen] | | 39.30 | 38.42 |
| ------------------------ | ------------- | --------------- | ---------------- |
## Usage and Limitations
These models have certain limitations that users should be aware of.
### Intended Usage
Open Large Language Models (LLMs) have a wide range of applications across
various industries and domains. The following list of potential uses is not
comprehensive. The purpose of this list is to provide contextual information
about the possible use-cases that the model creators considered as part of model
training and development.
* Content Creation and Communication
* Text Generation: These models can be used to generate creative text formats
such as poems, scripts, code, marketing copy, and email drafts.
* Chatbots and Conversational AI: Power conversational interfaces for customer
service, virtual assistants, or interactive applications.
* Text Summarization: Generate concise summaries of a text corpus, research
papers, or reports.
* Research and Education
* Natural Language Processing (NLP) Research: These models can serve as a
foundation for researchers to experiment with NLP techniques, develop
algorithms, and contribute to the advancement of the field.
* Language Learning Tools: Support interactive language learning experiences,
aiding in grammar correction or providing writing practice.
* Knowledge Exploration: Assist researchers in exploring large bodies of text
by generating summaries or answering questions about specific topics.
### Limitations
* Training Data
* The quality and diversity of the training data significantly influence the
model's capabilities. Biases or gaps in the training data can lead to
limitations in the model's responses.
* The scope of the training dataset determines the subject areas the model can
handle effectively.
* Context and Task Complexity
* LLMs are better at tasks that can be framed with clear prompts and
instructions. Open-ended or highly complex tasks might be challenging.
* A model's performance can be influenced by the amount of context provided
(longer context generally leads to better outputs, up to a certain point).
* Language Ambiguity and Nuance
* Natural language is inherently complex. LLMs might struggle to grasp subtle
nuances, sarcasm, or figurative language.
* Factual Accuracy
* LLMs generate responses based on information they learned from their
training datasets, but they are not knowledge bases. They may generate
incorrect or outdated factual statements.
* Common Sense
* LLMs rely on statistical patterns in language. They might lack the ability
to apply common sense reasoning in certain situations.
### Ethical Considerations and Risks
The development of large language models (LLMs) raises several ethical concerns.
In creating an open model, we have carefully considered the following:
* Bias and Fairness
* LLMs trained on large-scale, real-world text data can reflect socio-cultural
biases embedded in the training material. These models underwent careful
scrutiny, input data pre-processing described and posterior evaluations
reported in this card.
* Misinformation and Misuse
* LLMs can be misused to generate text that is false, misleading, or harmful.
* Guidelines are provided for responsible use with the model, see the
[Responsible Generative AI Toolkit][rai-toolkit].
* Transparency and Accountability:
* This model card summarizes details on the models' architecture,
capabilities, limitations, and evaluation processes.
* A responsibly developed open model offers the opportunity to share
innovation by making LLM technology accessible to developers and researchers
across the AI ecosystem.
Risks identified and mitigations:
* Perpetuation of biases: It's encouraged to perform continuous monitoring
(using evaluation metrics, human review) and the exploration of de-biasing
techniques during model training, fine-tuning, and other use cases.
* Generation of harmful content: Mechanisms and guidelines for content safety
are essential. Developers are encouraged to exercise caution and implement
appropriate content safety safeguards based on their specific product policies
and application use cases.
* Misuse for malicious purposes: Technical limitations and developer and
end-user education can help mitigate against malicious applications of LLMs.
Educational resources and reporting mechanisms for users to flag misuse are
provided. Prohibited uses of Gemma models are outlined in the
[Gemma Prohibited Use Policy][prohibited-use].
* Privacy violations: Models were trained on data filtered for removal of PII
(Personally Identifiable Information). Developers are encouraged to adhere to
privacy regulations with privacy-preserving techniques.
### Benefits
At the time of release, this family of models provides high-performance open
large language model implementations designed from the ground up for Responsible
AI development compared to similarly sized models.
Using the benchmark evaluation metrics described in this document, these models
have shown to provide superior performance to other, comparably-sized open model
alternatives.
[rai-toolkit]: https://ai.google.dev/responsible
[kaggle-gemma]: https://www.kaggle.com/models/google/gemma-2
[terms]: https://ai.google.dev/gemma/terms
[vertex-mg-gemma]: https://console.cloud.google.com/vertex-ai/publishers/google/model-garden/335
[sensitive-info]: https://cloud.google.com/dlp/docs/high-sensitivity-infotypes-reference
[safety-policies]: https://storage.googleapis.com/gweb-uniblog-publish-prod/documents/2023_Google_AI_Principles_Progress_Update.pdf#page=11
[prohibited-use]: https://ai.google.dev/gemma/prohibited_use_policy
[tpu]: https://cloud.google.com/tpu/docs/intro-to-tpu
[sustainability]: https://sustainability.google/operating-sustainably/
[jax]: https://github.com/google/jax
[ml-pathways]: https://blog.google/technology/ai/introducing-pathways-next-generation-ai-architecture/
[sustainability]: https://sustainability.google/operating-sustainably/
[foundation-models]: https://ai.google/discover/foundation-models/
[gemini-2-paper]: https://goo.gle/gemma2report
[mmlu]: https://arxiv.org/abs/2009.03300
[hellaswag]: https://arxiv.org/abs/1905.07830
[piqa]: https://arxiv.org/abs/1911.11641
[socialiqa]: https://arxiv.org/abs/1904.09728
[boolq]: https://arxiv.org/abs/1905.10044
[winogrande]: https://arxiv.org/abs/1907.10641
[commonsenseqa]: https://arxiv.org/abs/1811.00937
[openbookqa]: https://arxiv.org/abs/1809.02789
[arc]: https://arxiv.org/abs/1911.01547
[triviaqa]: https://arxiv.org/abs/1705.03551
[naturalq]: https://github.com/google-research-datasets/natural-questions
[humaneval]: https://arxiv.org/abs/2107.03374
[mbpp]: https://arxiv.org/abs/2108.07732
[gsm8k]: https://arxiv.org/abs/2110.14168
[realtox]: https://arxiv.org/abs/2009.11462
[bold]: https://arxiv.org/abs/2101.11718
[crows]: https://aclanthology.org/2020.emnlp-main.154/
[bbq]: https://arxiv.org/abs/2110.08193v2
[winogender]: https://arxiv.org/abs/1804.09301
[truthfulqa]: https://arxiv.org/abs/2109.07958
[winobias]: https://arxiv.org/abs/1804.06876
[math]: https://arxiv.org/abs/2103.03874
[agieval]: https://arxiv.org/abs/2304.06364
[big-bench]: https://arxiv.org/abs/2206.04615
[toxigen]: https://arxiv.org/abs/2203.09509
|
RichardErkhov/scb10x_-_llama-3-typhoon-v1.5-8b-instruct-gguf | RichardErkhov | "2024-06-25T10:38:43Z" | 12,613 | 0 | null | [
"gguf",
"arxiv:2312.13951",
"region:us"
] | null | "2024-06-25T06:18:17Z" | Quantization made by Richard Erkhov.
[Github](https://github.com/RichardErkhov)
[Discord](https://discord.gg/pvy7H8DZMG)
[Request more models](https://github.com/RichardErkhov/quant_request)
llama-3-typhoon-v1.5-8b-instruct - GGUF
- Model creator: https://huggingface.co/scb10x/
- Original model: https://huggingface.co/scb10x/llama-3-typhoon-v1.5-8b-instruct/
| Name | Quant method | Size |
| ---- | ---- | ---- |
| [llama-3-typhoon-v1.5-8b-instruct.Q2_K.gguf](https://huggingface.co/RichardErkhov/scb10x_-_llama-3-typhoon-v1.5-8b-instruct-gguf/blob/main/llama-3-typhoon-v1.5-8b-instruct.Q2_K.gguf) | Q2_K | 2.96GB |
| [llama-3-typhoon-v1.5-8b-instruct.IQ3_XS.gguf](https://huggingface.co/RichardErkhov/scb10x_-_llama-3-typhoon-v1.5-8b-instruct-gguf/blob/main/llama-3-typhoon-v1.5-8b-instruct.IQ3_XS.gguf) | IQ3_XS | 3.28GB |
| [llama-3-typhoon-v1.5-8b-instruct.IQ3_S.gguf](https://huggingface.co/RichardErkhov/scb10x_-_llama-3-typhoon-v1.5-8b-instruct-gguf/blob/main/llama-3-typhoon-v1.5-8b-instruct.IQ3_S.gguf) | IQ3_S | 3.43GB |
| [llama-3-typhoon-v1.5-8b-instruct.Q3_K_S.gguf](https://huggingface.co/RichardErkhov/scb10x_-_llama-3-typhoon-v1.5-8b-instruct-gguf/blob/main/llama-3-typhoon-v1.5-8b-instruct.Q3_K_S.gguf) | Q3_K_S | 3.41GB |
| [llama-3-typhoon-v1.5-8b-instruct.IQ3_M.gguf](https://huggingface.co/RichardErkhov/scb10x_-_llama-3-typhoon-v1.5-8b-instruct-gguf/blob/main/llama-3-typhoon-v1.5-8b-instruct.IQ3_M.gguf) | IQ3_M | 3.52GB |
| [llama-3-typhoon-v1.5-8b-instruct.Q3_K.gguf](https://huggingface.co/RichardErkhov/scb10x_-_llama-3-typhoon-v1.5-8b-instruct-gguf/blob/main/llama-3-typhoon-v1.5-8b-instruct.Q3_K.gguf) | Q3_K | 3.74GB |
| [llama-3-typhoon-v1.5-8b-instruct.Q3_K_M.gguf](https://huggingface.co/RichardErkhov/scb10x_-_llama-3-typhoon-v1.5-8b-instruct-gguf/blob/main/llama-3-typhoon-v1.5-8b-instruct.Q3_K_M.gguf) | Q3_K_M | 3.74GB |
| [llama-3-typhoon-v1.5-8b-instruct.Q3_K_L.gguf](https://huggingface.co/RichardErkhov/scb10x_-_llama-3-typhoon-v1.5-8b-instruct-gguf/blob/main/llama-3-typhoon-v1.5-8b-instruct.Q3_K_L.gguf) | Q3_K_L | 4.03GB |
| [llama-3-typhoon-v1.5-8b-instruct.IQ4_XS.gguf](https://huggingface.co/RichardErkhov/scb10x_-_llama-3-typhoon-v1.5-8b-instruct-gguf/blob/main/llama-3-typhoon-v1.5-8b-instruct.IQ4_XS.gguf) | IQ4_XS | 4.18GB |
| [llama-3-typhoon-v1.5-8b-instruct.Q4_0.gguf](https://huggingface.co/RichardErkhov/scb10x_-_llama-3-typhoon-v1.5-8b-instruct-gguf/blob/main/llama-3-typhoon-v1.5-8b-instruct.Q4_0.gguf) | Q4_0 | 4.34GB |
| [llama-3-typhoon-v1.5-8b-instruct.IQ4_NL.gguf](https://huggingface.co/RichardErkhov/scb10x_-_llama-3-typhoon-v1.5-8b-instruct-gguf/blob/main/llama-3-typhoon-v1.5-8b-instruct.IQ4_NL.gguf) | IQ4_NL | 4.38GB |
| [llama-3-typhoon-v1.5-8b-instruct.Q4_K_S.gguf](https://huggingface.co/RichardErkhov/scb10x_-_llama-3-typhoon-v1.5-8b-instruct-gguf/blob/main/llama-3-typhoon-v1.5-8b-instruct.Q4_K_S.gguf) | Q4_K_S | 4.37GB |
| [llama-3-typhoon-v1.5-8b-instruct.Q4_K.gguf](https://huggingface.co/RichardErkhov/scb10x_-_llama-3-typhoon-v1.5-8b-instruct-gguf/blob/main/llama-3-typhoon-v1.5-8b-instruct.Q4_K.gguf) | Q4_K | 4.58GB |
| [llama-3-typhoon-v1.5-8b-instruct.Q4_K_M.gguf](https://huggingface.co/RichardErkhov/scb10x_-_llama-3-typhoon-v1.5-8b-instruct-gguf/blob/main/llama-3-typhoon-v1.5-8b-instruct.Q4_K_M.gguf) | Q4_K_M | 4.58GB |
| [llama-3-typhoon-v1.5-8b-instruct.Q4_1.gguf](https://huggingface.co/RichardErkhov/scb10x_-_llama-3-typhoon-v1.5-8b-instruct-gguf/blob/main/llama-3-typhoon-v1.5-8b-instruct.Q4_1.gguf) | Q4_1 | 4.78GB |
| [llama-3-typhoon-v1.5-8b-instruct.Q5_0.gguf](https://huggingface.co/RichardErkhov/scb10x_-_llama-3-typhoon-v1.5-8b-instruct-gguf/blob/main/llama-3-typhoon-v1.5-8b-instruct.Q5_0.gguf) | Q5_0 | 5.21GB |
| [llama-3-typhoon-v1.5-8b-instruct.Q5_K_S.gguf](https://huggingface.co/RichardErkhov/scb10x_-_llama-3-typhoon-v1.5-8b-instruct-gguf/blob/main/llama-3-typhoon-v1.5-8b-instruct.Q5_K_S.gguf) | Q5_K_S | 5.21GB |
| [llama-3-typhoon-v1.5-8b-instruct.Q5_K.gguf](https://huggingface.co/RichardErkhov/scb10x_-_llama-3-typhoon-v1.5-8b-instruct-gguf/blob/main/llama-3-typhoon-v1.5-8b-instruct.Q5_K.gguf) | Q5_K | 5.34GB |
| [llama-3-typhoon-v1.5-8b-instruct.Q5_K_M.gguf](https://huggingface.co/RichardErkhov/scb10x_-_llama-3-typhoon-v1.5-8b-instruct-gguf/blob/main/llama-3-typhoon-v1.5-8b-instruct.Q5_K_M.gguf) | Q5_K_M | 5.34GB |
| [llama-3-typhoon-v1.5-8b-instruct.Q5_1.gguf](https://huggingface.co/RichardErkhov/scb10x_-_llama-3-typhoon-v1.5-8b-instruct-gguf/blob/main/llama-3-typhoon-v1.5-8b-instruct.Q5_1.gguf) | Q5_1 | 5.65GB |
| [llama-3-typhoon-v1.5-8b-instruct.Q6_K.gguf](https://huggingface.co/RichardErkhov/scb10x_-_llama-3-typhoon-v1.5-8b-instruct-gguf/blob/main/llama-3-typhoon-v1.5-8b-instruct.Q6_K.gguf) | Q6_K | 6.14GB |
| [llama-3-typhoon-v1.5-8b-instruct.Q8_0.gguf](https://huggingface.co/RichardErkhov/scb10x_-_llama-3-typhoon-v1.5-8b-instruct-gguf/blob/main/llama-3-typhoon-v1.5-8b-instruct.Q8_0.gguf) | Q8_0 | 7.95GB |
Original model description:
---
license: llama3
language:
- en
- th
pipeline_tag: text-generation
tags:
- instruct
- chat
---
**Llama-3-Typhoon-v1.5-8B: Thai Large Language Model (Instruct)**
**Llama-3-Typhoon-v1.5-8B-instruct** is a *instruct* Thai 🇹🇭 large language model with 8 billion parameters, and it is based on Llama3-8B.
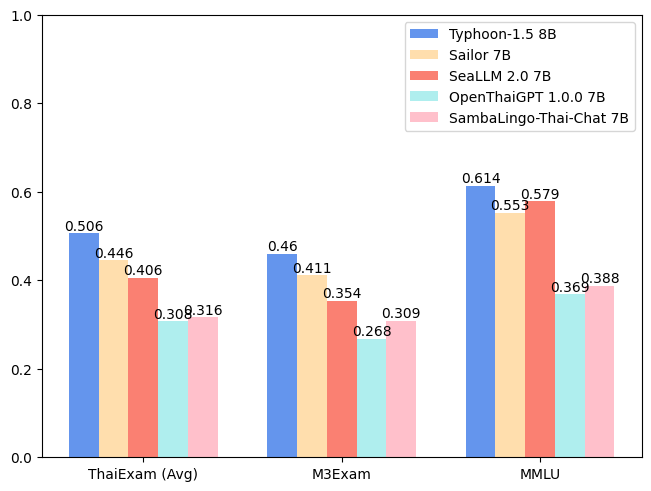
For release post, please see our [blog](https://blog.opentyphoon.ai/typhoon-1-5-release-a9364cb8e8d7).
*To acknowledge Meta's effort in creating the foundation model and to comply with the license, we explicitly include "llama-3" in the model name.
## **Model Description**
- **Model type**: A 8B instruct decoder-only model based on Llama architecture.
- **Requirement**: transformers 4.38.0 or newer.
- **Primary Language(s)**: Thai 🇹🇭 and English 🇬🇧
- **License**: [Llama 3 Community License](https://llama.meta.com/llama3/license/)
## **Performance**
| Model | ONET | IC | TGAT | TPAT-1 | A-Level | Average (ThaiExam) | M3Exam | MMLU |
| --- | --- | --- | --- | --- | --- | --- | --- | --- |
| Typhoon-1.0 (Mistral) | 0.379 | 0.393 | 0.700 | 0.414 | 0.324 | 0.442 | 0.391 | 0.547 |
| Typhoon-1.5 8B (Llama3) | ***0.446*** | ***0.431*** | ***0.722*** | ***0.526*** | ***0.407*** | ***0.506*** | ***0.460*** | ***0.614*** |
| Sailor 7B | 0.372 | 0.379 | 0.678 | 0.405 | 0.396 | 0.446 | 0.411 | 0.553 |
| SeaLLM 2.0 7B | 0.327 | 0.311 | 0.656 | 0.414 | 0.321 | 0.406 | 0.354 | 0.579 |
| OpenThaiGPT 1.0.0 7B | 0.238 | 0.249 | 0.444 | 0.319 | 0.289 | 0.308 | 0.268 | 0.369 |
| SambaLingo-Thai-Chat 7B | 0.251 | 0.241 | 0.522 | 0.302 | 0.262 | 0.316 | 0.309 | 0.388 |
## Usage Example
```python
from transformers import AutoTokenizer, AutoModelForCausalLM
import torch
model_id = "scb10x/llama-3-typhoon-v1.5-8b-instruct"
tokenizer = AutoTokenizer.from_pretrained(model_id)
model = AutoModelForCausalLM.from_pretrained(
model_id,
torch_dtype=torch.bfloat16,
device_map="auto",
)
messages = [
{"role": "system", "content": "You are a helpful assistant who're always speak Thai."},
{"role": "user", "content": "ขอสูตรไก่ย่าง"},
]
input_ids = tokenizer.apply_chat_template(
messages,
add_generation_prompt=True,
return_tensors="pt"
).to(model.device)
terminators = [
tokenizer.eos_token_id,
tokenizer.convert_tokens_to_ids("<|eot_id|>")
]
outputs = model.generate(
input_ids,
max_new_tokens=512,
eos_token_id=terminators,
do_sample=True,
temperature=0.4,
top_p=0.9,
)
response = outputs[0][input_ids.shape[-1]:]
print(tokenizer.decode(response, skip_special_tokens=True))
```
## Chat Template
We use llama3 chat-template.
```python
{% set loop_messages = messages %}{% for message in loop_messages %}{% set content = '<|start_header_id|>' + message['role'] + '<|end_header_id|>\n\n'+ message['content'] | trim + '<|eot_id|>' %}{% if loop.index0 == 0 %}{% set content = bos_token + content %}{% endif %}{{ content }}{% endfor %}{% if add_generation_prompt %}{{ '<|start_header_id|>assistant<|end_header_id|>\n\n' }}{% endif %}
```
## **Intended Uses & Limitations**
This model is an instructional model. However, it’s still undergoing development. It incorporates some level of guardrails, but it still may produce answers that are inaccurate, biased, or otherwise objectionable in response to user prompts. We recommend that developers assess these risks in the context of their use case.
## **Follow us**
**https://twitter.com/opentyphoon**
## **Support**
**https://discord.gg/CqyBscMFpg**
## **SCB10X AI Team**
- Kunat Pipatanakul, Potsawee Manakul, Sittipong Sripaisarnmongkol, Natapong Nitarach, Pathomporn Chokchainant, Kasima Tharnpipitchai
- If you find Typhoon-8B useful for your work, please cite it using:
```
@article{pipatanakul2023typhoon,
title={Typhoon: Thai Large Language Models},
author={Kunat Pipatanakul and Phatrasek Jirabovonvisut and Potsawee Manakul and Sittipong Sripaisarnmongkol and Ruangsak Patomwong and Pathomporn Chokchainant and Kasima Tharnpipitchai},
year={2023},
journal={arXiv preprint arXiv:2312.13951},
url={https://arxiv.org/abs/2312.13951}
}
```
## **Contact Us**
- General & Collaboration: **[[email protected]](mailto:[email protected])**, **[[email protected]](mailto:[email protected])**
- Technical: **[[email protected]](mailto:[email protected])**
|
PrunaAI/HPAI-BSC-Llama3-Aloe-8B-Alpha-GGUF-smashed | PrunaAI | "2024-07-01T16:53:06Z" | 12,612 | 0 | null | [
"gguf",
"pruna-ai",
"region:us"
] | null | "2024-07-01T16:08:15Z" | ---
thumbnail: "https://assets-global.website-files.com/646b351987a8d8ce158d1940/64ec9e96b4334c0e1ac41504_Logo%20with%20white%20text.svg"
metrics:
- memory_disk
- memory_inference
- inference_latency
- inference_throughput
- inference_CO2_emissions
- inference_energy_consumption
tags:
- pruna-ai
---
<!-- header start -->
<!-- 200823 -->
<div style="width: auto; margin-left: auto; margin-right: auto">
<a href="https://www.pruna.ai/" target="_blank" rel="noopener noreferrer">
<img src="https://i.imgur.com/eDAlcgk.png" alt="PrunaAI" style="width: 100%; min-width: 400px; display: block; margin: auto;">
</a>
</div>
<!-- header end -->
[](https://twitter.com/PrunaAI)
[](https://github.com/PrunaAI)
[](https://www.linkedin.com/company/93832878/admin/feed/posts/?feedType=following)
[](https://discord.com/invite/vb6SmA3hxu)
## This repo contains GGUF versions of the HPAI-BSC/Llama3-Aloe-8B-Alpha model.
# Simply make AI models cheaper, smaller, faster, and greener!
- Give a thumbs up if you like this model!
- Contact us and tell us which model to compress next [here](https://www.pruna.ai/contact).
- Request access to easily compress your *own* AI models [here](https://z0halsaff74.typeform.com/pruna-access?typeform-source=www.pruna.ai).
- Read the documentations to know more [here](https://pruna-ai-pruna.readthedocs-hosted.com/en/latest/)
- Join Pruna AI community on Discord [here](https://discord.com/invite/vb6SmA3hxu) to share feedback/suggestions or get help.
**Frequently Asked Questions**
- ***How does the compression work?*** The model is compressed with GGUF.
- ***How does the model quality change?*** The quality of the model output might vary compared to the base model.
- ***What is the model format?*** We use GGUF format.
- ***What calibration data has been used?*** If needed by the compression method, we used WikiText as the calibration data.
- ***How to compress my own models?*** You can request premium access to more compression methods and tech support for your specific use-cases [here](https://z0halsaff74.typeform.com/pruna-access?typeform-source=www.pruna.ai).
# Downloading and running the models
You can download the individual files from the Files & versions section. Here is a list of the different versions we provide. For more info checkout [this chart](https://gist.github.com/Artefact2/b5f810600771265fc1e39442288e8ec9) and [this guide](https://www.reddit.com/r/LocalLLaMA/comments/1ba55rj/overview_of_gguf_quantization_methods/):
| Quant type | Description |
|------------|--------------------------------------------------------------------------------------------|
| Q5_K_M | High quality, recommended. |
| Q5_K_S | High quality, recommended. |
| Q4_K_M | Good quality, uses about 4.83 bits per weight, recommended. |
| Q4_K_S | Slightly lower quality with more space savings, recommended. |
| IQ4_NL | Decent quality, slightly smaller than Q4_K_S with similar performance, recommended. |
| IQ4_XS | Decent quality, smaller than Q4_K_S with similar performance, recommended. |
| Q3_K_L | Lower quality but usable, good for low RAM availability. |
| Q3_K_M | Even lower quality. |
| IQ3_M | Medium-low quality, new method with decent performance comparable to Q3_K_M. |
| IQ3_S | Lower quality, new method with decent performance, recommended over Q3_K_S quant, same size with better performance. |
| Q3_K_S | Low quality, not recommended. |
| IQ3_XS | Lower quality, new method with decent performance, slightly better than Q3_K_S. |
| Q2_K | Very low quality but surprisingly usable. |
## How to download GGUF files ?
**Note for manual downloaders:** You almost never want to clone the entire repo! Multiple different quantisation formats are provided, and most users only want to pick and download a single file.
The following clients/libraries will automatically download models for you, providing a list of available models to choose from:
* LM Studio
* LoLLMS Web UI
* Faraday.dev
- **Option A** - Downloading in `text-generation-webui`:
- **Step 1**: Under Download Model, you can enter the model repo: HPAI-BSC-Llama3-Aloe-8B-Alpha-GGUF-smashed and below it, a specific filename to download, such as: phi-2.IQ3_M.gguf.
- **Step 2**: Then click Download.
- **Option B** - Downloading on the command line (including multiple files at once):
- **Step 1**: We recommend using the `huggingface-hub` Python library:
```shell
pip3 install huggingface-hub
```
- **Step 2**: Then you can download any individual model file to the current directory, at high speed, with a command like this:
```shell
huggingface-cli download HPAI-BSC-Llama3-Aloe-8B-Alpha-GGUF-smashed Llama3-Aloe-8B-Alpha.IQ3_M.gguf --local-dir . --local-dir-use-symlinks False
```
<details>
<summary>More advanced huggingface-cli download usage (click to read)</summary>
Alternatively, you can also download multiple files at once with a pattern:
```shell
huggingface-cli download HPAI-BSC-Llama3-Aloe-8B-Alpha-GGUF-smashed --local-dir . --local-dir-use-symlinks False --include='*Q4_K*gguf'
```
For more documentation on downloading with `huggingface-cli`, please see: [HF -> Hub Python Library -> Download files -> Download from the CLI](https://huggingface.co/docs/huggingface_hub/guides/download#download-from-the-cli).
To accelerate downloads on fast connections (1Gbit/s or higher), install `hf_transfer`:
```shell
pip3 install hf_transfer
```
And set environment variable `HF_HUB_ENABLE_HF_TRANSFER` to `1`:
```shell
HF_HUB_ENABLE_HF_TRANSFER=1 huggingface-cli download HPAI-BSC-Llama3-Aloe-8B-Alpha-GGUF-smashed Llama3-Aloe-8B-Alpha.IQ3_M.gguf --local-dir . --local-dir-use-symlinks False
```
Windows Command Line users: You can set the environment variable by running `set HF_HUB_ENABLE_HF_TRANSFER=1` before the download command.
</details>
<!-- README_GGUF.md-how-to-download end -->
<!-- README_GGUF.md-how-to-run start -->
## How to run model in GGUF format?
- **Option A** - Introductory example with `llama.cpp` command
Make sure you are using `llama.cpp` from commit [d0cee0d](https://github.com/ggerganov/llama.cpp/commit/d0cee0d36d5be95a0d9088b674dbb27354107221) or later.
```shell
./main -ngl 35 -m Llama3-Aloe-8B-Alpha.IQ3_M.gguf --color -c 32768 --temp 0.7 --repeat_penalty 1.1 -n -1 -p "<s>[INST] {{prompt\}} [/INST]"
```
Change `-ngl 32` to the number of layers to offload to GPU. Remove it if you don't have GPU acceleration.
Change `-c 32768` to the desired sequence length. For extended sequence models - eg 8K, 16K, 32K - the necessary RoPE scaling parameters are read from the GGUF file and set by llama.cpp automatically. Note that longer sequence lengths require much more resources, so you may need to reduce this value.
If you want to have a chat-style conversation, replace the `-p <PROMPT>` argument with `-i -ins`
For other parameters and how to use them, please refer to [the llama.cpp documentation](https://github.com/ggerganov/llama.cpp/blob/master/examples/main/README.md)
- **Option B** - Running in `text-generation-webui`
Further instructions can be found in the text-generation-webui documentation, here: [text-generation-webui/docs/04 ‐ Model Tab.md](https://github.com/oobabooga/text-generation-webui/blob/main/docs/04%20-%20Model%20Tab.md#llamacpp).
- **Option C** - Running from Python code
You can use GGUF models from Python using the [llama-cpp-python](https://github.com/abetlen/llama-cpp-python) or [ctransformers](https://github.com/marella/ctransformers) libraries. Note that at the time of writing (Nov 27th 2023), ctransformers has not been updated for some time and is not compatible with some recent models. Therefore I recommend you use llama-cpp-python.
### How to load this model in Python code, using llama-cpp-python
For full documentation, please see: [llama-cpp-python docs](https://abetlen.github.io/llama-cpp-python/).
#### First install the package
Run one of the following commands, according to your system:
```shell
# Base ctransformers with no GPU acceleration
pip install llama-cpp-python
# With NVidia CUDA acceleration
CMAKE_ARGS="-DLLAMA_CUBLAS=on" pip install llama-cpp-python
# Or with OpenBLAS acceleration
CMAKE_ARGS="-DLLAMA_BLAS=ON -DLLAMA_BLAS_VENDOR=OpenBLAS" pip install llama-cpp-python
# Or with CLBLast acceleration
CMAKE_ARGS="-DLLAMA_CLBLAST=on" pip install llama-cpp-python
# Or with AMD ROCm GPU acceleration (Linux only)
CMAKE_ARGS="-DLLAMA_HIPBLAS=on" pip install llama-cpp-python
# Or with Metal GPU acceleration for macOS systems only
CMAKE_ARGS="-DLLAMA_METAL=on" pip install llama-cpp-python
# In windows, to set the variables CMAKE_ARGS in PowerShell, follow this format; eg for NVidia CUDA:
$env:CMAKE_ARGS = "-DLLAMA_OPENBLAS=on"
pip install llama-cpp-python
```
#### Simple llama-cpp-python example code
```python
from llama_cpp import Llama
# Set gpu_layers to the number of layers to offload to GPU. Set to 0 if no GPU acceleration is available on your system.
llm = Llama(
model_path="./Llama3-Aloe-8B-Alpha.IQ3_M.gguf", # Download the model file first
n_ctx=32768, # The max sequence length to use - note that longer sequence lengths require much more resources
n_threads=8, # The number of CPU threads to use, tailor to your system and the resulting performance
n_gpu_layers=35 # The number of layers to offload to GPU, if you have GPU acceleration available
)
# Simple inference example
output = llm(
"<s>[INST] {{prompt}} [/INST]", # Prompt
max_tokens=512, # Generate up to 512 tokens
stop=["</s>"], # Example stop token - not necessarily correct for this specific model! Please check before using.
echo=True # Whether to echo the prompt
)
# Chat Completion API
llm = Llama(model_path="./Llama3-Aloe-8B-Alpha.IQ3_M.gguf", chat_format="llama-2") # Set chat_format according to the model you are using
llm.create_chat_completion(
messages = [
{{"role": "system", "content": "You are a story writing assistant."}},
{{
"role": "user",
"content": "Write a story about llamas."
}}
]
)
```
- **Option D** - Running with LangChain
Here are guides on using llama-cpp-python and ctransformers with LangChain:
* [LangChain + llama-cpp-python](https://python.langchain.com/docs/integrations/llms/llamacpp)
* [LangChain + ctransformers](https://python.langchain.com/docs/integrations/providers/ctransformers)
## Configurations
The configuration info are in `smash_config.json`.
## Credits & License
The license of the smashed model follows the license of the original model. Please check the license of the original model before using this model which provided the base model. The license of the `pruna-engine` is [here](https://pypi.org/project/pruna-engine/) on Pypi.
## Want to compress other models?
- Contact us and tell us which model to compress next [here](https://www.pruna.ai/contact).
- Request access to easily compress your own AI models [here](https://z0halsaff74.typeform.com/pruna-access?typeform-source=www.pruna.ai). |
intfloat/e5-large | intfloat | "2023-08-07T04:59:49Z" | 12,611 | 65 | sentence-transformers | [
"sentence-transformers",
"pytorch",
"safetensors",
"bert",
"mteb",
"Sentence Transformers",
"sentence-similarity",
"en",
"arxiv:2212.03533",
"arxiv:2104.08663",
"arxiv:2210.07316",
"license:mit",
"model-index",
"autotrain_compatible",
"endpoints_compatible",
"text-embeddings-inference",
"region:us"
] | sentence-similarity | "2022-12-26T06:03:12Z" | ---
tags:
- mteb
- Sentence Transformers
- sentence-similarity
- sentence-transformers
model-index:
- name: e5-large
results:
- task:
type: Classification
dataset:
type: mteb/amazon_counterfactual
name: MTEB AmazonCounterfactualClassification (en)
config: en
split: test
revision: e8379541af4e31359cca9fbcf4b00f2671dba205
metrics:
- type: accuracy
value: 77.68656716417911
- type: ap
value: 41.336896075573584
- type: f1
value: 71.788561468075
- task:
type: Classification
dataset:
type: mteb/amazon_polarity
name: MTEB AmazonPolarityClassification
config: default
split: test
revision: e2d317d38cd51312af73b3d32a06d1a08b442046
metrics:
- type: accuracy
value: 90.04965
- type: ap
value: 86.24637009569418
- type: f1
value: 90.03896671762645
- task:
type: Classification
dataset:
type: mteb/amazon_reviews_multi
name: MTEB AmazonReviewsClassification (en)
config: en
split: test
revision: 1399c76144fd37290681b995c656ef9b2e06e26d
metrics:
- type: accuracy
value: 43.016000000000005
- type: f1
value: 42.1942431880186
- task:
type: Retrieval
dataset:
type: arguana
name: MTEB ArguAna
config: default
split: test
revision: None
metrics:
- type: map_at_1
value: 25.107000000000003
- type: map_at_10
value: 40.464
- type: map_at_100
value: 41.577999999999996
- type: map_at_1000
value: 41.588
- type: map_at_3
value: 35.301
- type: map_at_5
value: 38.263000000000005
- type: mrr_at_1
value: 25.605
- type: mrr_at_10
value: 40.64
- type: mrr_at_100
value: 41.760000000000005
- type: mrr_at_1000
value: 41.77
- type: mrr_at_3
value: 35.443000000000005
- type: mrr_at_5
value: 38.448
- type: ndcg_at_1
value: 25.107000000000003
- type: ndcg_at_10
value: 49.352000000000004
- type: ndcg_at_100
value: 53.98500000000001
- type: ndcg_at_1000
value: 54.208
- type: ndcg_at_3
value: 38.671
- type: ndcg_at_5
value: 43.991
- type: precision_at_1
value: 25.107000000000003
- type: precision_at_10
value: 7.795000000000001
- type: precision_at_100
value: 0.979
- type: precision_at_1000
value: 0.1
- type: precision_at_3
value: 16.145
- type: precision_at_5
value: 12.262
- type: recall_at_1
value: 25.107000000000003
- type: recall_at_10
value: 77.952
- type: recall_at_100
value: 97.866
- type: recall_at_1000
value: 99.57300000000001
- type: recall_at_3
value: 48.435
- type: recall_at_5
value: 61.309000000000005
- task:
type: Clustering
dataset:
type: mteb/arxiv-clustering-p2p
name: MTEB ArxivClusteringP2P
config: default
split: test
revision: a122ad7f3f0291bf49cc6f4d32aa80929df69d5d
metrics:
- type: v_measure
value: 46.19278045044154
- task:
type: Clustering
dataset:
type: mteb/arxiv-clustering-s2s
name: MTEB ArxivClusteringS2S
config: default
split: test
revision: f910caf1a6075f7329cdf8c1a6135696f37dbd53
metrics:
- type: v_measure
value: 41.37976387757665
- task:
type: Reranking
dataset:
type: mteb/askubuntudupquestions-reranking
name: MTEB AskUbuntuDupQuestions
config: default
split: test
revision: 2000358ca161889fa9c082cb41daa8dcfb161a54
metrics:
- type: map
value: 60.07433334608074
- type: mrr
value: 73.44347711383723
- task:
type: STS
dataset:
type: mteb/biosses-sts
name: MTEB BIOSSES
config: default
split: test
revision: d3fb88f8f02e40887cd149695127462bbcf29b4a
metrics:
- type: cos_sim_pearson
value: 86.4298072183543
- type: cos_sim_spearman
value: 84.73144873582848
- type: euclidean_pearson
value: 85.15885058870728
- type: euclidean_spearman
value: 85.42062106559356
- type: manhattan_pearson
value: 84.89409921792054
- type: manhattan_spearman
value: 85.31941394024344
- task:
type: Classification
dataset:
type: mteb/banking77
name: MTEB Banking77Classification
config: default
split: test
revision: 0fd18e25b25c072e09e0d92ab615fda904d66300
metrics:
- type: accuracy
value: 84.14285714285714
- type: f1
value: 84.11674412565644
- task:
type: Clustering
dataset:
type: mteb/biorxiv-clustering-p2p
name: MTEB BiorxivClusteringP2P
config: default
split: test
revision: 65b79d1d13f80053f67aca9498d9402c2d9f1f40
metrics:
- type: v_measure
value: 37.600076342340785
- task:
type: Clustering
dataset:
type: mteb/biorxiv-clustering-s2s
name: MTEB BiorxivClusteringS2S
config: default
split: test
revision: 258694dd0231531bc1fd9de6ceb52a0853c6d908
metrics:
- type: v_measure
value: 35.08861812135148
- task:
type: Retrieval
dataset:
type: BeIR/cqadupstack
name: MTEB CQADupstackAndroidRetrieval
config: default
split: test
revision: None
metrics:
- type: map_at_1
value: 32.684000000000005
- type: map_at_10
value: 41.675000000000004
- type: map_at_100
value: 42.963
- type: map_at_1000
value: 43.078
- type: map_at_3
value: 38.708999999999996
- type: map_at_5
value: 40.316
- type: mrr_at_1
value: 39.485
- type: mrr_at_10
value: 47.152
- type: mrr_at_100
value: 47.96
- type: mrr_at_1000
value: 48.010000000000005
- type: mrr_at_3
value: 44.754
- type: mrr_at_5
value: 46.285
- type: ndcg_at_1
value: 39.485
- type: ndcg_at_10
value: 46.849000000000004
- type: ndcg_at_100
value: 52.059
- type: ndcg_at_1000
value: 54.358
- type: ndcg_at_3
value: 42.705
- type: ndcg_at_5
value: 44.663000000000004
- type: precision_at_1
value: 39.485
- type: precision_at_10
value: 8.455
- type: precision_at_100
value: 1.3379999999999999
- type: precision_at_1000
value: 0.178
- type: precision_at_3
value: 19.695
- type: precision_at_5
value: 13.905999999999999
- type: recall_at_1
value: 32.684000000000005
- type: recall_at_10
value: 56.227000000000004
- type: recall_at_100
value: 78.499
- type: recall_at_1000
value: 94.021
- type: recall_at_3
value: 44.157999999999994
- type: recall_at_5
value: 49.694
- task:
type: Retrieval
dataset:
type: BeIR/cqadupstack
name: MTEB CQADupstackEnglishRetrieval
config: default
split: test
revision: None
metrics:
- type: map_at_1
value: 31.875999999999998
- type: map_at_10
value: 41.603
- type: map_at_100
value: 42.825
- type: map_at_1000
value: 42.961
- type: map_at_3
value: 38.655
- type: map_at_5
value: 40.294999999999995
- type: mrr_at_1
value: 40.127
- type: mrr_at_10
value: 47.959
- type: mrr_at_100
value: 48.59
- type: mrr_at_1000
value: 48.634
- type: mrr_at_3
value: 45.786
- type: mrr_at_5
value: 46.964
- type: ndcg_at_1
value: 40.127
- type: ndcg_at_10
value: 47.176
- type: ndcg_at_100
value: 51.346000000000004
- type: ndcg_at_1000
value: 53.502
- type: ndcg_at_3
value: 43.139
- type: ndcg_at_5
value: 44.883
- type: precision_at_1
value: 40.127
- type: precision_at_10
value: 8.72
- type: precision_at_100
value: 1.387
- type: precision_at_1000
value: 0.188
- type: precision_at_3
value: 20.637
- type: precision_at_5
value: 14.446
- type: recall_at_1
value: 31.875999999999998
- type: recall_at_10
value: 56.54900000000001
- type: recall_at_100
value: 73.939
- type: recall_at_1000
value: 87.732
- type: recall_at_3
value: 44.326
- type: recall_at_5
value: 49.445
- task:
type: Retrieval
dataset:
type: BeIR/cqadupstack
name: MTEB CQADupstackGamingRetrieval
config: default
split: test
revision: None
metrics:
- type: map_at_1
value: 41.677
- type: map_at_10
value: 52.222
- type: map_at_100
value: 53.229000000000006
- type: map_at_1000
value: 53.288000000000004
- type: map_at_3
value: 49.201
- type: map_at_5
value: 51.00599999999999
- type: mrr_at_1
value: 47.524
- type: mrr_at_10
value: 55.745999999999995
- type: mrr_at_100
value: 56.433
- type: mrr_at_1000
value: 56.464999999999996
- type: mrr_at_3
value: 53.37499999999999
- type: mrr_at_5
value: 54.858
- type: ndcg_at_1
value: 47.524
- type: ndcg_at_10
value: 57.406
- type: ndcg_at_100
value: 61.403
- type: ndcg_at_1000
value: 62.7
- type: ndcg_at_3
value: 52.298
- type: ndcg_at_5
value: 55.02
- type: precision_at_1
value: 47.524
- type: precision_at_10
value: 8.865
- type: precision_at_100
value: 1.179
- type: precision_at_1000
value: 0.134
- type: precision_at_3
value: 22.612
- type: precision_at_5
value: 15.461
- type: recall_at_1
value: 41.677
- type: recall_at_10
value: 69.346
- type: recall_at_100
value: 86.344
- type: recall_at_1000
value: 95.703
- type: recall_at_3
value: 55.789
- type: recall_at_5
value: 62.488
- task:
type: Retrieval
dataset:
type: BeIR/cqadupstack
name: MTEB CQADupstackGisRetrieval
config: default
split: test
revision: None
metrics:
- type: map_at_1
value: 25.991999999999997
- type: map_at_10
value: 32.804
- type: map_at_100
value: 33.812999999999995
- type: map_at_1000
value: 33.897
- type: map_at_3
value: 30.567
- type: map_at_5
value: 31.599
- type: mrr_at_1
value: 27.797
- type: mrr_at_10
value: 34.768
- type: mrr_at_100
value: 35.702
- type: mrr_at_1000
value: 35.766
- type: mrr_at_3
value: 32.637
- type: mrr_at_5
value: 33.614
- type: ndcg_at_1
value: 27.797
- type: ndcg_at_10
value: 36.966
- type: ndcg_at_100
value: 41.972
- type: ndcg_at_1000
value: 44.139
- type: ndcg_at_3
value: 32.547
- type: ndcg_at_5
value: 34.258
- type: precision_at_1
value: 27.797
- type: precision_at_10
value: 5.514
- type: precision_at_100
value: 0.8340000000000001
- type: precision_at_1000
value: 0.106
- type: precision_at_3
value: 13.333
- type: precision_at_5
value: 9.04
- type: recall_at_1
value: 25.991999999999997
- type: recall_at_10
value: 47.941
- type: recall_at_100
value: 71.039
- type: recall_at_1000
value: 87.32799999999999
- type: recall_at_3
value: 36.01
- type: recall_at_5
value: 40.056000000000004
- task:
type: Retrieval
dataset:
type: BeIR/cqadupstack
name: MTEB CQADupstackMathematicaRetrieval
config: default
split: test
revision: None
metrics:
- type: map_at_1
value: 17.533
- type: map_at_10
value: 24.336
- type: map_at_100
value: 25.445
- type: map_at_1000
value: 25.561
- type: map_at_3
value: 22.116
- type: map_at_5
value: 23.347
- type: mrr_at_1
value: 21.642
- type: mrr_at_10
value: 28.910999999999998
- type: mrr_at_100
value: 29.836000000000002
- type: mrr_at_1000
value: 29.907
- type: mrr_at_3
value: 26.638
- type: mrr_at_5
value: 27.857
- type: ndcg_at_1
value: 21.642
- type: ndcg_at_10
value: 28.949
- type: ndcg_at_100
value: 34.211000000000006
- type: ndcg_at_1000
value: 37.031
- type: ndcg_at_3
value: 24.788
- type: ndcg_at_5
value: 26.685
- type: precision_at_1
value: 21.642
- type: precision_at_10
value: 5.137
- type: precision_at_100
value: 0.893
- type: precision_at_1000
value: 0.127
- type: precision_at_3
value: 11.733
- type: precision_at_5
value: 8.383000000000001
- type: recall_at_1
value: 17.533
- type: recall_at_10
value: 38.839
- type: recall_at_100
value: 61.458999999999996
- type: recall_at_1000
value: 81.58
- type: recall_at_3
value: 27.328999999999997
- type: recall_at_5
value: 32.168
- task:
type: Retrieval
dataset:
type: BeIR/cqadupstack
name: MTEB CQADupstackPhysicsRetrieval
config: default
split: test
revision: None
metrics:
- type: map_at_1
value: 28.126
- type: map_at_10
value: 37.872
- type: map_at_100
value: 39.229
- type: map_at_1000
value: 39.353
- type: map_at_3
value: 34.93
- type: map_at_5
value: 36.59
- type: mrr_at_1
value: 34.071
- type: mrr_at_10
value: 43.056
- type: mrr_at_100
value: 43.944
- type: mrr_at_1000
value: 43.999
- type: mrr_at_3
value: 40.536
- type: mrr_at_5
value: 42.065999999999995
- type: ndcg_at_1
value: 34.071
- type: ndcg_at_10
value: 43.503
- type: ndcg_at_100
value: 49.120000000000005
- type: ndcg_at_1000
value: 51.410999999999994
- type: ndcg_at_3
value: 38.767
- type: ndcg_at_5
value: 41.075
- type: precision_at_1
value: 34.071
- type: precision_at_10
value: 7.843999999999999
- type: precision_at_100
value: 1.2489999999999999
- type: precision_at_1000
value: 0.163
- type: precision_at_3
value: 18.223
- type: precision_at_5
value: 13.050999999999998
- type: recall_at_1
value: 28.126
- type: recall_at_10
value: 54.952
- type: recall_at_100
value: 78.375
- type: recall_at_1000
value: 93.29899999999999
- type: recall_at_3
value: 41.714
- type: recall_at_5
value: 47.635
- task:
type: Retrieval
dataset:
type: BeIR/cqadupstack
name: MTEB CQADupstackProgrammersRetrieval
config: default
split: test
revision: None
metrics:
- type: map_at_1
value: 25.957
- type: map_at_10
value: 34.749
- type: map_at_100
value: 35.929
- type: map_at_1000
value: 36.043
- type: map_at_3
value: 31.947
- type: map_at_5
value: 33.575
- type: mrr_at_1
value: 32.078
- type: mrr_at_10
value: 39.844
- type: mrr_at_100
value: 40.71
- type: mrr_at_1000
value: 40.77
- type: mrr_at_3
value: 37.386
- type: mrr_at_5
value: 38.83
- type: ndcg_at_1
value: 32.078
- type: ndcg_at_10
value: 39.97
- type: ndcg_at_100
value: 45.254
- type: ndcg_at_1000
value: 47.818
- type: ndcg_at_3
value: 35.453
- type: ndcg_at_5
value: 37.631
- type: precision_at_1
value: 32.078
- type: precision_at_10
value: 7.158
- type: precision_at_100
value: 1.126
- type: precision_at_1000
value: 0.153
- type: precision_at_3
value: 16.743
- type: precision_at_5
value: 11.872
- type: recall_at_1
value: 25.957
- type: recall_at_10
value: 50.583
- type: recall_at_100
value: 73.593
- type: recall_at_1000
value: 91.23599999999999
- type: recall_at_3
value: 37.651
- type: recall_at_5
value: 43.626
- task:
type: Retrieval
dataset:
type: BeIR/cqadupstack
name: MTEB CQADupstackRetrieval
config: default
split: test
revision: None
metrics:
- type: map_at_1
value: 27.1505
- type: map_at_10
value: 34.844833333333334
- type: map_at_100
value: 35.95216666666667
- type: map_at_1000
value: 36.06675
- type: map_at_3
value: 32.41975
- type: map_at_5
value: 33.74233333333333
- type: mrr_at_1
value: 31.923666666666662
- type: mrr_at_10
value: 38.87983333333334
- type: mrr_at_100
value: 39.706250000000004
- type: mrr_at_1000
value: 39.76708333333333
- type: mrr_at_3
value: 36.72008333333333
- type: mrr_at_5
value: 37.96933333333334
- type: ndcg_at_1
value: 31.923666666666662
- type: ndcg_at_10
value: 39.44258333333334
- type: ndcg_at_100
value: 44.31475
- type: ndcg_at_1000
value: 46.75
- type: ndcg_at_3
value: 35.36299999999999
- type: ndcg_at_5
value: 37.242333333333335
- type: precision_at_1
value: 31.923666666666662
- type: precision_at_10
value: 6.643333333333333
- type: precision_at_100
value: 1.0612499999999998
- type: precision_at_1000
value: 0.14575
- type: precision_at_3
value: 15.875250000000001
- type: precision_at_5
value: 11.088916666666664
- type: recall_at_1
value: 27.1505
- type: recall_at_10
value: 49.06349999999999
- type: recall_at_100
value: 70.60841666666666
- type: recall_at_1000
value: 87.72049999999999
- type: recall_at_3
value: 37.60575000000001
- type: recall_at_5
value: 42.511166666666675
- task:
type: Retrieval
dataset:
type: BeIR/cqadupstack
name: MTEB CQADupstackStatsRetrieval
config: default
split: test
revision: None
metrics:
- type: map_at_1
value: 25.101000000000003
- type: map_at_10
value: 30.147000000000002
- type: map_at_100
value: 30.98
- type: map_at_1000
value: 31.080000000000002
- type: map_at_3
value: 28.571
- type: map_at_5
value: 29.319
- type: mrr_at_1
value: 27.761000000000003
- type: mrr_at_10
value: 32.716
- type: mrr_at_100
value: 33.504
- type: mrr_at_1000
value: 33.574
- type: mrr_at_3
value: 31.135
- type: mrr_at_5
value: 32.032
- type: ndcg_at_1
value: 27.761000000000003
- type: ndcg_at_10
value: 33.358
- type: ndcg_at_100
value: 37.569
- type: ndcg_at_1000
value: 40.189
- type: ndcg_at_3
value: 30.291
- type: ndcg_at_5
value: 31.558000000000003
- type: precision_at_1
value: 27.761000000000003
- type: precision_at_10
value: 4.939
- type: precision_at_100
value: 0.759
- type: precision_at_1000
value: 0.106
- type: precision_at_3
value: 12.577
- type: precision_at_5
value: 8.497
- type: recall_at_1
value: 25.101000000000003
- type: recall_at_10
value: 40.739
- type: recall_at_100
value: 60.089999999999996
- type: recall_at_1000
value: 79.768
- type: recall_at_3
value: 32.16
- type: recall_at_5
value: 35.131
- task:
type: Retrieval
dataset:
type: BeIR/cqadupstack
name: MTEB CQADupstackTexRetrieval
config: default
split: test
revision: None
metrics:
- type: map_at_1
value: 20.112
- type: map_at_10
value: 26.119999999999997
- type: map_at_100
value: 27.031
- type: map_at_1000
value: 27.150000000000002
- type: map_at_3
value: 24.230999999999998
- type: map_at_5
value: 25.15
- type: mrr_at_1
value: 24.535
- type: mrr_at_10
value: 30.198000000000004
- type: mrr_at_100
value: 30.975
- type: mrr_at_1000
value: 31.051000000000002
- type: mrr_at_3
value: 28.338
- type: mrr_at_5
value: 29.269000000000002
- type: ndcg_at_1
value: 24.535
- type: ndcg_at_10
value: 30.147000000000002
- type: ndcg_at_100
value: 34.544000000000004
- type: ndcg_at_1000
value: 37.512
- type: ndcg_at_3
value: 26.726
- type: ndcg_at_5
value: 28.046
- type: precision_at_1
value: 24.535
- type: precision_at_10
value: 5.179
- type: precision_at_100
value: 0.859
- type: precision_at_1000
value: 0.128
- type: precision_at_3
value: 12.159
- type: precision_at_5
value: 8.424
- type: recall_at_1
value: 20.112
- type: recall_at_10
value: 38.312000000000005
- type: recall_at_100
value: 58.406000000000006
- type: recall_at_1000
value: 79.863
- type: recall_at_3
value: 28.358
- type: recall_at_5
value: 31.973000000000003
- task:
type: Retrieval
dataset:
type: BeIR/cqadupstack
name: MTEB CQADupstackUnixRetrieval
config: default
split: test
revision: None
metrics:
- type: map_at_1
value: 27.111
- type: map_at_10
value: 34.096
- type: map_at_100
value: 35.181000000000004
- type: map_at_1000
value: 35.276
- type: map_at_3
value: 31.745
- type: map_at_5
value: 33.045
- type: mrr_at_1
value: 31.343
- type: mrr_at_10
value: 37.994
- type: mrr_at_100
value: 38.873000000000005
- type: mrr_at_1000
value: 38.934999999999995
- type: mrr_at_3
value: 35.743
- type: mrr_at_5
value: 37.077
- type: ndcg_at_1
value: 31.343
- type: ndcg_at_10
value: 38.572
- type: ndcg_at_100
value: 43.854
- type: ndcg_at_1000
value: 46.190999999999995
- type: ndcg_at_3
value: 34.247
- type: ndcg_at_5
value: 36.28
- type: precision_at_1
value: 31.343
- type: precision_at_10
value: 6.166
- type: precision_at_100
value: 1
- type: precision_at_1000
value: 0.13
- type: precision_at_3
value: 15.081
- type: precision_at_5
value: 10.428999999999998
- type: recall_at_1
value: 27.111
- type: recall_at_10
value: 48.422
- type: recall_at_100
value: 71.846
- type: recall_at_1000
value: 88.57000000000001
- type: recall_at_3
value: 36.435
- type: recall_at_5
value: 41.765
- task:
type: Retrieval
dataset:
type: BeIR/cqadupstack
name: MTEB CQADupstackWebmastersRetrieval
config: default
split: test
revision: None
metrics:
- type: map_at_1
value: 26.264
- type: map_at_10
value: 33.522
- type: map_at_100
value: 34.963
- type: map_at_1000
value: 35.175
- type: map_at_3
value: 31.366
- type: map_at_5
value: 32.621
- type: mrr_at_1
value: 31.028
- type: mrr_at_10
value: 37.230000000000004
- type: mrr_at_100
value: 38.149
- type: mrr_at_1000
value: 38.218
- type: mrr_at_3
value: 35.046
- type: mrr_at_5
value: 36.617
- type: ndcg_at_1
value: 31.028
- type: ndcg_at_10
value: 37.964999999999996
- type: ndcg_at_100
value: 43.342000000000006
- type: ndcg_at_1000
value: 46.471000000000004
- type: ndcg_at_3
value: 34.67
- type: ndcg_at_5
value: 36.458
- type: precision_at_1
value: 31.028
- type: precision_at_10
value: 6.937
- type: precision_at_100
value: 1.346
- type: precision_at_1000
value: 0.22799999999999998
- type: precision_at_3
value: 15.942
- type: precision_at_5
value: 11.462
- type: recall_at_1
value: 26.264
- type: recall_at_10
value: 45.571
- type: recall_at_100
value: 70.246
- type: recall_at_1000
value: 90.971
- type: recall_at_3
value: 36.276
- type: recall_at_5
value: 41.162
- task:
type: Retrieval
dataset:
type: BeIR/cqadupstack
name: MTEB CQADupstackWordpressRetrieval
config: default
split: test
revision: None
metrics:
- type: map_at_1
value: 23.372999999999998
- type: map_at_10
value: 28.992
- type: map_at_100
value: 29.837999999999997
- type: map_at_1000
value: 29.939
- type: map_at_3
value: 26.999000000000002
- type: map_at_5
value: 28.044999999999998
- type: mrr_at_1
value: 25.692999999999998
- type: mrr_at_10
value: 30.984
- type: mrr_at_100
value: 31.799
- type: mrr_at_1000
value: 31.875999999999998
- type: mrr_at_3
value: 29.267
- type: mrr_at_5
value: 30.163
- type: ndcg_at_1
value: 25.692999999999998
- type: ndcg_at_10
value: 32.45
- type: ndcg_at_100
value: 37.103
- type: ndcg_at_1000
value: 39.678000000000004
- type: ndcg_at_3
value: 28.725
- type: ndcg_at_5
value: 30.351
- type: precision_at_1
value: 25.692999999999998
- type: precision_at_10
value: 4.806
- type: precision_at_100
value: 0.765
- type: precision_at_1000
value: 0.108
- type: precision_at_3
value: 11.768
- type: precision_at_5
value: 8.096
- type: recall_at_1
value: 23.372999999999998
- type: recall_at_10
value: 41.281
- type: recall_at_100
value: 63.465
- type: recall_at_1000
value: 82.575
- type: recall_at_3
value: 31.063000000000002
- type: recall_at_5
value: 34.991
- task:
type: Retrieval
dataset:
type: climate-fever
name: MTEB ClimateFEVER
config: default
split: test
revision: None
metrics:
- type: map_at_1
value: 8.821
- type: map_at_10
value: 15.383
- type: map_at_100
value: 17.244999999999997
- type: map_at_1000
value: 17.445
- type: map_at_3
value: 12.64
- type: map_at_5
value: 13.941999999999998
- type: mrr_at_1
value: 19.544
- type: mrr_at_10
value: 29.738999999999997
- type: mrr_at_100
value: 30.923000000000002
- type: mrr_at_1000
value: 30.969
- type: mrr_at_3
value: 26.384
- type: mrr_at_5
value: 28.199
- type: ndcg_at_1
value: 19.544
- type: ndcg_at_10
value: 22.398
- type: ndcg_at_100
value: 30.253999999999998
- type: ndcg_at_1000
value: 33.876
- type: ndcg_at_3
value: 17.473
- type: ndcg_at_5
value: 19.154
- type: precision_at_1
value: 19.544
- type: precision_at_10
value: 7.217999999999999
- type: precision_at_100
value: 1.564
- type: precision_at_1000
value: 0.22300000000000003
- type: precision_at_3
value: 13.225000000000001
- type: precision_at_5
value: 10.319
- type: recall_at_1
value: 8.821
- type: recall_at_10
value: 28.110000000000003
- type: recall_at_100
value: 55.64
- type: recall_at_1000
value: 75.964
- type: recall_at_3
value: 16.195
- type: recall_at_5
value: 20.678
- task:
type: Retrieval
dataset:
type: dbpedia-entity
name: MTEB DBPedia
config: default
split: test
revision: None
metrics:
- type: map_at_1
value: 9.344
- type: map_at_10
value: 20.301
- type: map_at_100
value: 28.709
- type: map_at_1000
value: 30.470999999999997
- type: map_at_3
value: 14.584
- type: map_at_5
value: 16.930999999999997
- type: mrr_at_1
value: 67.25
- type: mrr_at_10
value: 75.393
- type: mrr_at_100
value: 75.742
- type: mrr_at_1000
value: 75.75
- type: mrr_at_3
value: 73.958
- type: mrr_at_5
value: 74.883
- type: ndcg_at_1
value: 56.00000000000001
- type: ndcg_at_10
value: 42.394
- type: ndcg_at_100
value: 47.091
- type: ndcg_at_1000
value: 54.215
- type: ndcg_at_3
value: 46.995
- type: ndcg_at_5
value: 44.214999999999996
- type: precision_at_1
value: 67.25
- type: precision_at_10
value: 33.525
- type: precision_at_100
value: 10.67
- type: precision_at_1000
value: 2.221
- type: precision_at_3
value: 49.417
- type: precision_at_5
value: 42.15
- type: recall_at_1
value: 9.344
- type: recall_at_10
value: 25.209
- type: recall_at_100
value: 52.329
- type: recall_at_1000
value: 74.2
- type: recall_at_3
value: 15.699
- type: recall_at_5
value: 19.24
- task:
type: Classification
dataset:
type: mteb/emotion
name: MTEB EmotionClassification
config: default
split: test
revision: 4f58c6b202a23cf9a4da393831edf4f9183cad37
metrics:
- type: accuracy
value: 48.05
- type: f1
value: 43.06718139212933
- task:
type: Retrieval
dataset:
type: fever
name: MTEB FEVER
config: default
split: test
revision: None
metrics:
- type: map_at_1
value: 46.452
- type: map_at_10
value: 58.825
- type: map_at_100
value: 59.372
- type: map_at_1000
value: 59.399
- type: map_at_3
value: 56.264
- type: map_at_5
value: 57.879999999999995
- type: mrr_at_1
value: 49.82
- type: mrr_at_10
value: 62.178999999999995
- type: mrr_at_100
value: 62.641999999999996
- type: mrr_at_1000
value: 62.658
- type: mrr_at_3
value: 59.706
- type: mrr_at_5
value: 61.283
- type: ndcg_at_1
value: 49.82
- type: ndcg_at_10
value: 65.031
- type: ndcg_at_100
value: 67.413
- type: ndcg_at_1000
value: 68.014
- type: ndcg_at_3
value: 60.084
- type: ndcg_at_5
value: 62.858000000000004
- type: precision_at_1
value: 49.82
- type: precision_at_10
value: 8.876000000000001
- type: precision_at_100
value: 1.018
- type: precision_at_1000
value: 0.109
- type: precision_at_3
value: 24.477
- type: precision_at_5
value: 16.208
- type: recall_at_1
value: 46.452
- type: recall_at_10
value: 80.808
- type: recall_at_100
value: 91.215
- type: recall_at_1000
value: 95.52000000000001
- type: recall_at_3
value: 67.62899999999999
- type: recall_at_5
value: 74.32900000000001
- task:
type: Retrieval
dataset:
type: fiqa
name: MTEB FiQA2018
config: default
split: test
revision: None
metrics:
- type: map_at_1
value: 18.351
- type: map_at_10
value: 30.796
- type: map_at_100
value: 32.621
- type: map_at_1000
value: 32.799
- type: map_at_3
value: 26.491
- type: map_at_5
value: 28.933999999999997
- type: mrr_at_1
value: 36.265
- type: mrr_at_10
value: 45.556999999999995
- type: mrr_at_100
value: 46.323
- type: mrr_at_1000
value: 46.359
- type: mrr_at_3
value: 42.695
- type: mrr_at_5
value: 44.324000000000005
- type: ndcg_at_1
value: 36.265
- type: ndcg_at_10
value: 38.558
- type: ndcg_at_100
value: 45.18
- type: ndcg_at_1000
value: 48.292
- type: ndcg_at_3
value: 34.204
- type: ndcg_at_5
value: 35.735
- type: precision_at_1
value: 36.265
- type: precision_at_10
value: 10.879999999999999
- type: precision_at_100
value: 1.77
- type: precision_at_1000
value: 0.234
- type: precision_at_3
value: 23.044999999999998
- type: precision_at_5
value: 17.253
- type: recall_at_1
value: 18.351
- type: recall_at_10
value: 46.116
- type: recall_at_100
value: 70.786
- type: recall_at_1000
value: 89.46300000000001
- type: recall_at_3
value: 31.404
- type: recall_at_5
value: 37.678
- task:
type: Retrieval
dataset:
type: hotpotqa
name: MTEB HotpotQA
config: default
split: test
revision: None
metrics:
- type: map_at_1
value: 36.847
- type: map_at_10
value: 54.269999999999996
- type: map_at_100
value: 55.152
- type: map_at_1000
value: 55.223
- type: map_at_3
value: 51.166
- type: map_at_5
value: 53.055
- type: mrr_at_1
value: 73.693
- type: mrr_at_10
value: 79.975
- type: mrr_at_100
value: 80.202
- type: mrr_at_1000
value: 80.214
- type: mrr_at_3
value: 78.938
- type: mrr_at_5
value: 79.595
- type: ndcg_at_1
value: 73.693
- type: ndcg_at_10
value: 63.334999999999994
- type: ndcg_at_100
value: 66.452
- type: ndcg_at_1000
value: 67.869
- type: ndcg_at_3
value: 58.829
- type: ndcg_at_5
value: 61.266
- type: precision_at_1
value: 73.693
- type: precision_at_10
value: 13.122
- type: precision_at_100
value: 1.5559999999999998
- type: precision_at_1000
value: 0.174
- type: precision_at_3
value: 37.083
- type: precision_at_5
value: 24.169999999999998
- type: recall_at_1
value: 36.847
- type: recall_at_10
value: 65.61099999999999
- type: recall_at_100
value: 77.792
- type: recall_at_1000
value: 87.17099999999999
- type: recall_at_3
value: 55.625
- type: recall_at_5
value: 60.425
- task:
type: Classification
dataset:
type: mteb/imdb
name: MTEB ImdbClassification
config: default
split: test
revision: 3d86128a09e091d6018b6d26cad27f2739fc2db7
metrics:
- type: accuracy
value: 82.1096
- type: ap
value: 76.67089212843918
- type: f1
value: 82.03535056754939
- task:
type: Retrieval
dataset:
type: msmarco
name: MTEB MSMARCO
config: default
split: dev
revision: None
metrics:
- type: map_at_1
value: 24.465
- type: map_at_10
value: 37.072
- type: map_at_100
value: 38.188
- type: map_at_1000
value: 38.232
- type: map_at_3
value: 33.134
- type: map_at_5
value: 35.453
- type: mrr_at_1
value: 25.142999999999997
- type: mrr_at_10
value: 37.669999999999995
- type: mrr_at_100
value: 38.725
- type: mrr_at_1000
value: 38.765
- type: mrr_at_3
value: 33.82
- type: mrr_at_5
value: 36.111
- type: ndcg_at_1
value: 25.142999999999997
- type: ndcg_at_10
value: 44.054
- type: ndcg_at_100
value: 49.364000000000004
- type: ndcg_at_1000
value: 50.456
- type: ndcg_at_3
value: 36.095
- type: ndcg_at_5
value: 40.23
- type: precision_at_1
value: 25.142999999999997
- type: precision_at_10
value: 6.845
- type: precision_at_100
value: 0.95
- type: precision_at_1000
value: 0.104
- type: precision_at_3
value: 15.204999999999998
- type: precision_at_5
value: 11.221
- type: recall_at_1
value: 24.465
- type: recall_at_10
value: 65.495
- type: recall_at_100
value: 89.888
- type: recall_at_1000
value: 98.165
- type: recall_at_3
value: 43.964
- type: recall_at_5
value: 53.891
- task:
type: Classification
dataset:
type: mteb/mtop_domain
name: MTEB MTOPDomainClassification (en)
config: en
split: test
revision: d80d48c1eb48d3562165c59d59d0034df9fff0bf
metrics:
- type: accuracy
value: 93.86228910168718
- type: f1
value: 93.69177113259104
- task:
type: Classification
dataset:
type: mteb/mtop_intent
name: MTEB MTOPIntentClassification (en)
config: en
split: test
revision: ae001d0e6b1228650b7bd1c2c65fb50ad11a8aba
metrics:
- type: accuracy
value: 76.3999088007296
- type: f1
value: 58.96668664333438
- task:
type: Classification
dataset:
type: mteb/amazon_massive_intent
name: MTEB MassiveIntentClassification (en)
config: en
split: test
revision: 31efe3c427b0bae9c22cbb560b8f15491cc6bed7
metrics:
- type: accuracy
value: 73.21788836583727
- type: f1
value: 71.4545936552952
- task:
type: Classification
dataset:
type: mteb/amazon_massive_scenario
name: MTEB MassiveScenarioClassification (en)
config: en
split: test
revision: 7d571f92784cd94a019292a1f45445077d0ef634
metrics:
- type: accuracy
value: 77.39071956960323
- type: f1
value: 77.12398952847603
- task:
type: Clustering
dataset:
type: mteb/medrxiv-clustering-p2p
name: MTEB MedrxivClusteringP2P
config: default
split: test
revision: e7a26af6f3ae46b30dde8737f02c07b1505bcc73
metrics:
- type: v_measure
value: 32.255379528166955
- task:
type: Clustering
dataset:
type: mteb/medrxiv-clustering-s2s
name: MTEB MedrxivClusteringS2S
config: default
split: test
revision: 35191c8c0dca72d8ff3efcd72aa802307d469663
metrics:
- type: v_measure
value: 29.66423362872814
- task:
type: Reranking
dataset:
type: mteb/mind_small
name: MTEB MindSmallReranking
config: default
split: test
revision: 3bdac13927fdc888b903db93b2ffdbd90b295a69
metrics:
- type: map
value: 30.782211620375964
- type: mrr
value: 31.773479703044956
- task:
type: Retrieval
dataset:
type: nfcorpus
name: MTEB NFCorpus
config: default
split: test
revision: None
metrics:
- type: map_at_1
value: 5.863
- type: map_at_10
value: 13.831
- type: map_at_100
value: 17.534
- type: map_at_1000
value: 19.012
- type: map_at_3
value: 10.143
- type: map_at_5
value: 12.034
- type: mrr_at_1
value: 46.749
- type: mrr_at_10
value: 55.376999999999995
- type: mrr_at_100
value: 56.009
- type: mrr_at_1000
value: 56.042
- type: mrr_at_3
value: 53.30200000000001
- type: mrr_at_5
value: 54.85
- type: ndcg_at_1
value: 44.582
- type: ndcg_at_10
value: 36.07
- type: ndcg_at_100
value: 33.39
- type: ndcg_at_1000
value: 41.884
- type: ndcg_at_3
value: 41.441
- type: ndcg_at_5
value: 39.861000000000004
- type: precision_at_1
value: 46.129999999999995
- type: precision_at_10
value: 26.594
- type: precision_at_100
value: 8.365
- type: precision_at_1000
value: 2.1260000000000003
- type: precision_at_3
value: 39.009
- type: precision_at_5
value: 34.861
- type: recall_at_1
value: 5.863
- type: recall_at_10
value: 17.961
- type: recall_at_100
value: 34.026
- type: recall_at_1000
value: 64.46499999999999
- type: recall_at_3
value: 11.242
- type: recall_at_5
value: 14.493
- task:
type: Retrieval
dataset:
type: nq
name: MTEB NQ
config: default
split: test
revision: None
metrics:
- type: map_at_1
value: 38.601
- type: map_at_10
value: 55.293000000000006
- type: map_at_100
value: 56.092
- type: map_at_1000
value: 56.111999999999995
- type: map_at_3
value: 51.269
- type: map_at_5
value: 53.787
- type: mrr_at_1
value: 43.221
- type: mrr_at_10
value: 57.882999999999996
- type: mrr_at_100
value: 58.408
- type: mrr_at_1000
value: 58.421
- type: mrr_at_3
value: 54.765
- type: mrr_at_5
value: 56.809
- type: ndcg_at_1
value: 43.221
- type: ndcg_at_10
value: 62.858999999999995
- type: ndcg_at_100
value: 65.987
- type: ndcg_at_1000
value: 66.404
- type: ndcg_at_3
value: 55.605000000000004
- type: ndcg_at_5
value: 59.723000000000006
- type: precision_at_1
value: 43.221
- type: precision_at_10
value: 9.907
- type: precision_at_100
value: 1.169
- type: precision_at_1000
value: 0.121
- type: precision_at_3
value: 25.019000000000002
- type: precision_at_5
value: 17.474
- type: recall_at_1
value: 38.601
- type: recall_at_10
value: 82.966
- type: recall_at_100
value: 96.154
- type: recall_at_1000
value: 99.223
- type: recall_at_3
value: 64.603
- type: recall_at_5
value: 73.97200000000001
- task:
type: Retrieval
dataset:
type: quora
name: MTEB QuoraRetrieval
config: default
split: test
revision: None
metrics:
- type: map_at_1
value: 70.77
- type: map_at_10
value: 84.429
- type: map_at_100
value: 85.04599999999999
- type: map_at_1000
value: 85.065
- type: map_at_3
value: 81.461
- type: map_at_5
value: 83.316
- type: mrr_at_1
value: 81.51
- type: mrr_at_10
value: 87.52799999999999
- type: mrr_at_100
value: 87.631
- type: mrr_at_1000
value: 87.632
- type: mrr_at_3
value: 86.533
- type: mrr_at_5
value: 87.214
- type: ndcg_at_1
value: 81.47999999999999
- type: ndcg_at_10
value: 88.181
- type: ndcg_at_100
value: 89.39200000000001
- type: ndcg_at_1000
value: 89.52
- type: ndcg_at_3
value: 85.29299999999999
- type: ndcg_at_5
value: 86.88
- type: precision_at_1
value: 81.47999999999999
- type: precision_at_10
value: 13.367
- type: precision_at_100
value: 1.5230000000000001
- type: precision_at_1000
value: 0.157
- type: precision_at_3
value: 37.227
- type: precision_at_5
value: 24.494
- type: recall_at_1
value: 70.77
- type: recall_at_10
value: 95.199
- type: recall_at_100
value: 99.37700000000001
- type: recall_at_1000
value: 99.973
- type: recall_at_3
value: 86.895
- type: recall_at_5
value: 91.396
- task:
type: Clustering
dataset:
type: mteb/reddit-clustering
name: MTEB RedditClustering
config: default
split: test
revision: 24640382cdbf8abc73003fb0fa6d111a705499eb
metrics:
- type: v_measure
value: 50.686353396858344
- task:
type: Clustering
dataset:
type: mteb/reddit-clustering-p2p
name: MTEB RedditClusteringP2P
config: default
split: test
revision: 282350215ef01743dc01b456c7f5241fa8937f16
metrics:
- type: v_measure
value: 61.3664675312921
- task:
type: Retrieval
dataset:
type: scidocs
name: MTEB SCIDOCS
config: default
split: test
revision: None
metrics:
- type: map_at_1
value: 4.7379999999999995
- type: map_at_10
value: 12.01
- type: map_at_100
value: 14.02
- type: map_at_1000
value: 14.310999999999998
- type: map_at_3
value: 8.459
- type: map_at_5
value: 10.281
- type: mrr_at_1
value: 23.3
- type: mrr_at_10
value: 34.108
- type: mrr_at_100
value: 35.217
- type: mrr_at_1000
value: 35.272
- type: mrr_at_3
value: 30.833
- type: mrr_at_5
value: 32.768
- type: ndcg_at_1
value: 23.3
- type: ndcg_at_10
value: 20.116999999999997
- type: ndcg_at_100
value: 27.961000000000002
- type: ndcg_at_1000
value: 33.149
- type: ndcg_at_3
value: 18.902
- type: ndcg_at_5
value: 16.742
- type: precision_at_1
value: 23.3
- type: precision_at_10
value: 10.47
- type: precision_at_100
value: 2.177
- type: precision_at_1000
value: 0.34299999999999997
- type: precision_at_3
value: 17.567
- type: precision_at_5
value: 14.78
- type: recall_at_1
value: 4.7379999999999995
- type: recall_at_10
value: 21.221999999999998
- type: recall_at_100
value: 44.242
- type: recall_at_1000
value: 69.652
- type: recall_at_3
value: 10.688
- type: recall_at_5
value: 14.982999999999999
- task:
type: STS
dataset:
type: mteb/sickr-sts
name: MTEB SICK-R
config: default
split: test
revision: a6ea5a8cab320b040a23452cc28066d9beae2cee
metrics:
- type: cos_sim_pearson
value: 84.84572946827069
- type: cos_sim_spearman
value: 80.48508130408966
- type: euclidean_pearson
value: 82.0481530027767
- type: euclidean_spearman
value: 80.45902876782752
- type: manhattan_pearson
value: 82.03728222483326
- type: manhattan_spearman
value: 80.45684282911755
- task:
type: STS
dataset:
type: mteb/sts12-sts
name: MTEB STS12
config: default
split: test
revision: a0d554a64d88156834ff5ae9920b964011b16384
metrics:
- type: cos_sim_pearson
value: 84.33476464677516
- type: cos_sim_spearman
value: 75.93057758003266
- type: euclidean_pearson
value: 80.89685744015691
- type: euclidean_spearman
value: 76.29929953441706
- type: manhattan_pearson
value: 80.91391345459995
- type: manhattan_spearman
value: 76.31985463110914
- task:
type: STS
dataset:
type: mteb/sts13-sts
name: MTEB STS13
config: default
split: test
revision: 7e90230a92c190f1bf69ae9002b8cea547a64cca
metrics:
- type: cos_sim_pearson
value: 84.63686106359005
- type: cos_sim_spearman
value: 85.22240034668202
- type: euclidean_pearson
value: 84.6074814189106
- type: euclidean_spearman
value: 85.17169644755828
- type: manhattan_pearson
value: 84.48329306239368
- type: manhattan_spearman
value: 85.0086508544768
- task:
type: STS
dataset:
type: mteb/sts14-sts
name: MTEB STS14
config: default
split: test
revision: 6031580fec1f6af667f0bd2da0a551cf4f0b2375
metrics:
- type: cos_sim_pearson
value: 82.95455774064745
- type: cos_sim_spearman
value: 80.54074646118492
- type: euclidean_pearson
value: 81.79598955554704
- type: euclidean_spearman
value: 80.55837617606814
- type: manhattan_pearson
value: 81.78213797905386
- type: manhattan_spearman
value: 80.5666746878273
- task:
type: STS
dataset:
type: mteb/sts15-sts
name: MTEB STS15
config: default
split: test
revision: ae752c7c21bf194d8b67fd573edf7ae58183cbe3
metrics:
- type: cos_sim_pearson
value: 87.92813309124739
- type: cos_sim_spearman
value: 88.81459873052108
- type: euclidean_pearson
value: 88.21193118930564
- type: euclidean_spearman
value: 88.87072745043731
- type: manhattan_pearson
value: 88.22576929706727
- type: manhattan_spearman
value: 88.8867671095791
- task:
type: STS
dataset:
type: mteb/sts16-sts
name: MTEB STS16
config: default
split: test
revision: 4d8694f8f0e0100860b497b999b3dbed754a0513
metrics:
- type: cos_sim_pearson
value: 83.6881529671839
- type: cos_sim_spearman
value: 85.2807092969554
- type: euclidean_pearson
value: 84.62334178652704
- type: euclidean_spearman
value: 85.2116373296784
- type: manhattan_pearson
value: 84.54948211541777
- type: manhattan_spearman
value: 85.10737722637882
- task:
type: STS
dataset:
type: mteb/sts17-crosslingual-sts
name: MTEB STS17 (en-en)
config: en-en
split: test
revision: af5e6fb845001ecf41f4c1e033ce921939a2a68d
metrics:
- type: cos_sim_pearson
value: 88.55963694458408
- type: cos_sim_spearman
value: 89.36731628848683
- type: euclidean_pearson
value: 89.64975952985465
- type: euclidean_spearman
value: 89.29689484033007
- type: manhattan_pearson
value: 89.61234491713135
- type: manhattan_spearman
value: 89.20302520255782
- task:
type: STS
dataset:
type: mteb/sts22-crosslingual-sts
name: MTEB STS22 (en)
config: en
split: test
revision: 6d1ba47164174a496b7fa5d3569dae26a6813b80
metrics:
- type: cos_sim_pearson
value: 62.411800961903886
- type: cos_sim_spearman
value: 62.99105515749963
- type: euclidean_pearson
value: 65.29826669549443
- type: euclidean_spearman
value: 63.29880964105775
- type: manhattan_pearson
value: 65.00126190601183
- type: manhattan_spearman
value: 63.32011025899179
- task:
type: STS
dataset:
type: mteb/stsbenchmark-sts
name: MTEB STSBenchmark
config: default
split: test
revision: b0fddb56ed78048fa8b90373c8a3cfc37b684831
metrics:
- type: cos_sim_pearson
value: 85.83498531837608
- type: cos_sim_spearman
value: 87.21366640615442
- type: euclidean_pearson
value: 86.74764288798261
- type: euclidean_spearman
value: 87.06060470780834
- type: manhattan_pearson
value: 86.65971223951476
- type: manhattan_spearman
value: 86.99814399831457
- task:
type: Reranking
dataset:
type: mteb/scidocs-reranking
name: MTEB SciDocsRR
config: default
split: test
revision: d3c5e1fc0b855ab6097bf1cda04dd73947d7caab
metrics:
- type: map
value: 83.94448463485881
- type: mrr
value: 95.36291867174221
- task:
type: Retrieval
dataset:
type: scifact
name: MTEB SciFact
config: default
split: test
revision: None
metrics:
- type: map_at_1
value: 59.928000000000004
- type: map_at_10
value: 68.577
- type: map_at_100
value: 69.35900000000001
- type: map_at_1000
value: 69.37299999999999
- type: map_at_3
value: 66.217
- type: map_at_5
value: 67.581
- type: mrr_at_1
value: 63
- type: mrr_at_10
value: 69.994
- type: mrr_at_100
value: 70.553
- type: mrr_at_1000
value: 70.56700000000001
- type: mrr_at_3
value: 68.167
- type: mrr_at_5
value: 69.11699999999999
- type: ndcg_at_1
value: 63
- type: ndcg_at_10
value: 72.58
- type: ndcg_at_100
value: 75.529
- type: ndcg_at_1000
value: 76.009
- type: ndcg_at_3
value: 68.523
- type: ndcg_at_5
value: 70.301
- type: precision_at_1
value: 63
- type: precision_at_10
value: 9.333
- type: precision_at_100
value: 1.09
- type: precision_at_1000
value: 0.11299999999999999
- type: precision_at_3
value: 26.444000000000003
- type: precision_at_5
value: 17.067
- type: recall_at_1
value: 59.928000000000004
- type: recall_at_10
value: 83.544
- type: recall_at_100
value: 96
- type: recall_at_1000
value: 100
- type: recall_at_3
value: 72.072
- type: recall_at_5
value: 76.683
- task:
type: PairClassification
dataset:
type: mteb/sprintduplicatequestions-pairclassification
name: MTEB SprintDuplicateQuestions
config: default
split: test
revision: d66bd1f72af766a5cc4b0ca5e00c162f89e8cc46
metrics:
- type: cos_sim_accuracy
value: 99.82178217821782
- type: cos_sim_ap
value: 95.41507679819003
- type: cos_sim_f1
value: 90.9456740442656
- type: cos_sim_precision
value: 91.49797570850203
- type: cos_sim_recall
value: 90.4
- type: dot_accuracy
value: 99.77227722772277
- type: dot_ap
value: 92.50123869445967
- type: dot_f1
value: 88.18414322250638
- type: dot_precision
value: 90.26178010471205
- type: dot_recall
value: 86.2
- type: euclidean_accuracy
value: 99.81782178217821
- type: euclidean_ap
value: 95.3935066749006
- type: euclidean_f1
value: 90.66128218071681
- type: euclidean_precision
value: 91.53924566768603
- type: euclidean_recall
value: 89.8
- type: manhattan_accuracy
value: 99.81881188118813
- type: manhattan_ap
value: 95.39767454613512
- type: manhattan_f1
value: 90.62019477191186
- type: manhattan_precision
value: 92.95478443743428
- type: manhattan_recall
value: 88.4
- type: max_accuracy
value: 99.82178217821782
- type: max_ap
value: 95.41507679819003
- type: max_f1
value: 90.9456740442656
- task:
type: Clustering
dataset:
type: mteb/stackexchange-clustering
name: MTEB StackExchangeClustering
config: default
split: test
revision: 6cbc1f7b2bc0622f2e39d2c77fa502909748c259
metrics:
- type: v_measure
value: 64.96313921233748
- task:
type: Clustering
dataset:
type: mteb/stackexchange-clustering-p2p
name: MTEB StackExchangeClusteringP2P
config: default
split: test
revision: 815ca46b2622cec33ccafc3735d572c266efdb44
metrics:
- type: v_measure
value: 33.602625720956745
- task:
type: Reranking
dataset:
type: mteb/stackoverflowdupquestions-reranking
name: MTEB StackOverflowDupQuestions
config: default
split: test
revision: e185fbe320c72810689fc5848eb6114e1ef5ec69
metrics:
- type: map
value: 51.32659230651731
- type: mrr
value: 52.33861726508785
- task:
type: Summarization
dataset:
type: mteb/summeval
name: MTEB SummEval
config: default
split: test
revision: cda12ad7615edc362dbf25a00fdd61d3b1eaf93c
metrics:
- type: cos_sim_pearson
value: 31.01587644214203
- type: cos_sim_spearman
value: 30.974306908731013
- type: dot_pearson
value: 29.83339853838187
- type: dot_spearman
value: 30.07761671934048
- task:
type: Retrieval
dataset:
type: trec-covid
name: MTEB TRECCOVID
config: default
split: test
revision: None
metrics:
- type: map_at_1
value: 0.22
- type: map_at_10
value: 1.9539999999999997
- type: map_at_100
value: 11.437
- type: map_at_1000
value: 27.861000000000004
- type: map_at_3
value: 0.6479999999999999
- type: map_at_5
value: 1.0410000000000001
- type: mrr_at_1
value: 84
- type: mrr_at_10
value: 90.333
- type: mrr_at_100
value: 90.333
- type: mrr_at_1000
value: 90.333
- type: mrr_at_3
value: 90.333
- type: mrr_at_5
value: 90.333
- type: ndcg_at_1
value: 80
- type: ndcg_at_10
value: 78.31700000000001
- type: ndcg_at_100
value: 59.396
- type: ndcg_at_1000
value: 52.733
- type: ndcg_at_3
value: 81.46900000000001
- type: ndcg_at_5
value: 80.74
- type: precision_at_1
value: 84
- type: precision_at_10
value: 84
- type: precision_at_100
value: 60.980000000000004
- type: precision_at_1000
value: 23.432
- type: precision_at_3
value: 87.333
- type: precision_at_5
value: 86.8
- type: recall_at_1
value: 0.22
- type: recall_at_10
value: 2.156
- type: recall_at_100
value: 14.557999999999998
- type: recall_at_1000
value: 49.553999999999995
- type: recall_at_3
value: 0.685
- type: recall_at_5
value: 1.121
- task:
type: Retrieval
dataset:
type: webis-touche2020
name: MTEB Touche2020
config: default
split: test
revision: None
metrics:
- type: map_at_1
value: 3.373
- type: map_at_10
value: 11.701
- type: map_at_100
value: 17.144000000000002
- type: map_at_1000
value: 18.624
- type: map_at_3
value: 6.552
- type: map_at_5
value: 9.372
- type: mrr_at_1
value: 38.775999999999996
- type: mrr_at_10
value: 51.975
- type: mrr_at_100
value: 52.873999999999995
- type: mrr_at_1000
value: 52.873999999999995
- type: mrr_at_3
value: 47.619
- type: mrr_at_5
value: 50.578
- type: ndcg_at_1
value: 36.735
- type: ndcg_at_10
value: 27.212999999999997
- type: ndcg_at_100
value: 37.245
- type: ndcg_at_1000
value: 48.602000000000004
- type: ndcg_at_3
value: 30.916
- type: ndcg_at_5
value: 30.799
- type: precision_at_1
value: 38.775999999999996
- type: precision_at_10
value: 23.469
- type: precision_at_100
value: 7.327
- type: precision_at_1000
value: 1.486
- type: precision_at_3
value: 31.973000000000003
- type: precision_at_5
value: 32.245000000000005
- type: recall_at_1
value: 3.373
- type: recall_at_10
value: 17.404
- type: recall_at_100
value: 46.105000000000004
- type: recall_at_1000
value: 80.35
- type: recall_at_3
value: 7.4399999999999995
- type: recall_at_5
value: 12.183
- task:
type: Classification
dataset:
type: mteb/toxic_conversations_50k
name: MTEB ToxicConversationsClassification
config: default
split: test
revision: d7c0de2777da35d6aae2200a62c6e0e5af397c4c
metrics:
- type: accuracy
value: 70.5592
- type: ap
value: 14.330910591410134
- type: f1
value: 54.45745186286521
- task:
type: Classification
dataset:
type: mteb/tweet_sentiment_extraction
name: MTEB TweetSentimentExtractionClassification
config: default
split: test
revision: d604517c81ca91fe16a244d1248fc021f9ecee7a
metrics:
- type: accuracy
value: 61.20543293718167
- type: f1
value: 61.45365480309872
- task:
type: Clustering
dataset:
type: mteb/twentynewsgroups-clustering
name: MTEB TwentyNewsgroupsClustering
config: default
split: test
revision: 6125ec4e24fa026cec8a478383ee943acfbd5449
metrics:
- type: v_measure
value: 43.81162998944145
- task:
type: PairClassification
dataset:
type: mteb/twittersemeval2015-pairclassification
name: MTEB TwitterSemEval2015
config: default
split: test
revision: 70970daeab8776df92f5ea462b6173c0b46fd2d1
metrics:
- type: cos_sim_accuracy
value: 86.69011146212075
- type: cos_sim_ap
value: 76.09792353652536
- type: cos_sim_f1
value: 70.10202763786646
- type: cos_sim_precision
value: 68.65671641791045
- type: cos_sim_recall
value: 71.60949868073878
- type: dot_accuracy
value: 85.33110806461227
- type: dot_ap
value: 70.19304383327554
- type: dot_f1
value: 67.22494202525122
- type: dot_precision
value: 65.6847935548842
- type: dot_recall
value: 68.83905013192611
- type: euclidean_accuracy
value: 86.5410979316922
- type: euclidean_ap
value: 75.91906915651882
- type: euclidean_f1
value: 69.6798975672215
- type: euclidean_precision
value: 67.6865671641791
- type: euclidean_recall
value: 71.79419525065963
- type: manhattan_accuracy
value: 86.60070334386363
- type: manhattan_ap
value: 75.94617413885031
- type: manhattan_f1
value: 69.52689565780946
- type: manhattan_precision
value: 68.3312101910828
- type: manhattan_recall
value: 70.76517150395777
- type: max_accuracy
value: 86.69011146212075
- type: max_ap
value: 76.09792353652536
- type: max_f1
value: 70.10202763786646
- task:
type: PairClassification
dataset:
type: mteb/twitterurlcorpus-pairclassification
name: MTEB TwitterURLCorpus
config: default
split: test
revision: 8b6510b0b1fa4e4c4f879467980e9be563ec1cdf
metrics:
- type: cos_sim_accuracy
value: 89.25951798812434
- type: cos_sim_ap
value: 86.31476416599727
- type: cos_sim_f1
value: 78.52709971038477
- type: cos_sim_precision
value: 76.7629972792117
- type: cos_sim_recall
value: 80.37419156144134
- type: dot_accuracy
value: 88.03896456708192
- type: dot_ap
value: 83.26963599196237
- type: dot_f1
value: 76.72696459492317
- type: dot_precision
value: 73.56411162133521
- type: dot_recall
value: 80.17400677548507
- type: euclidean_accuracy
value: 89.21682772538519
- type: euclidean_ap
value: 86.29306071289969
- type: euclidean_f1
value: 78.40827030519554
- type: euclidean_precision
value: 77.42250243939053
- type: euclidean_recall
value: 79.41946412072683
- type: manhattan_accuracy
value: 89.22458959133776
- type: manhattan_ap
value: 86.2901934710645
- type: manhattan_f1
value: 78.54211378440453
- type: manhattan_precision
value: 76.85505858079729
- type: manhattan_recall
value: 80.30489682784109
- type: max_accuracy
value: 89.25951798812434
- type: max_ap
value: 86.31476416599727
- type: max_f1
value: 78.54211378440453
language:
- en
license: mit
---
## E5-large
**News (May 2023): please switch to [e5-large-v2](https://huggingface.co/intfloat/e5-large-v2), which has better performance and same method of usage.**
[Text Embeddings by Weakly-Supervised Contrastive Pre-training](https://arxiv.org/pdf/2212.03533.pdf).
Liang Wang, Nan Yang, Xiaolong Huang, Binxing Jiao, Linjun Yang, Daxin Jiang, Rangan Majumder, Furu Wei, arXiv 2022
This model has 24 layers and the embedding size is 1024.
## Usage
Below is an example to encode queries and passages from the MS-MARCO passage ranking dataset.
```python
import torch.nn.functional as F
from torch import Tensor
from transformers import AutoTokenizer, AutoModel
def average_pool(last_hidden_states: Tensor,
attention_mask: Tensor) -> Tensor:
last_hidden = last_hidden_states.masked_fill(~attention_mask[..., None].bool(), 0.0)
return last_hidden.sum(dim=1) / attention_mask.sum(dim=1)[..., None]
# Each input text should start with "query: " or "passage: ".
# For tasks other than retrieval, you can simply use the "query: " prefix.
input_texts = ['query: how much protein should a female eat',
'query: summit define',
"passage: As a general guideline, the CDC's average requirement of protein for women ages 19 to 70 is 46 grams per day. But, as you can see from this chart, you'll need to increase that if you're expecting or training for a marathon. Check out the chart below to see how much protein you should be eating each day.",
"passage: Definition of summit for English Language Learners. : 1 the highest point of a mountain : the top of a mountain. : 2 the highest level. : 3 a meeting or series of meetings between the leaders of two or more governments."]
tokenizer = AutoTokenizer.from_pretrained('intfloat/e5-large')
model = AutoModel.from_pretrained('intfloat/e5-large')
# Tokenize the input texts
batch_dict = tokenizer(input_texts, max_length=512, padding=True, truncation=True, return_tensors='pt')
outputs = model(**batch_dict)
embeddings = average_pool(outputs.last_hidden_state, batch_dict['attention_mask'])
# normalize embeddings
embeddings = F.normalize(embeddings, p=2, dim=1)
scores = (embeddings[:2] @ embeddings[2:].T) * 100
print(scores.tolist())
```
## Training Details
Please refer to our paper at [https://arxiv.org/pdf/2212.03533.pdf](https://arxiv.org/pdf/2212.03533.pdf).
## Benchmark Evaluation
Check out [unilm/e5](https://github.com/microsoft/unilm/tree/master/e5) to reproduce evaluation results
on the [BEIR](https://arxiv.org/abs/2104.08663) and [MTEB benchmark](https://arxiv.org/abs/2210.07316).
## Support for Sentence Transformers
Below is an example for usage with sentence_transformers.
```python
from sentence_transformers import SentenceTransformer
model = SentenceTransformer('intfloat/e5-large')
input_texts = [
'query: how much protein should a female eat',
'query: summit define',
"passage: As a general guideline, the CDC's average requirement of protein for women ages 19 to 70 is 46 grams per day. But, as you can see from this chart, you'll need to increase that if you're expecting or training for a marathon. Check out the chart below to see how much protein you should be eating each day.",
"passage: Definition of summit for English Language Learners. : 1 the highest point of a mountain : the top of a mountain. : 2 the highest level. : 3 a meeting or series of meetings between the leaders of two or more governments."
]
embeddings = model.encode(input_texts, normalize_embeddings=True)
```
Package requirements
`pip install sentence_transformers~=2.2.2`
Contributors: [michaelfeil](https://huggingface.co/michaelfeil)
## FAQ
**1. Do I need to add the prefix "query: " and "passage: " to input texts?**
Yes, this is how the model is trained, otherwise you will see a performance degradation.
Here are some rules of thumb:
- Use "query: " and "passage: " correspondingly for asymmetric tasks such as passage retrieval in open QA, ad-hoc information retrieval.
- Use "query: " prefix for symmetric tasks such as semantic similarity, paraphrase retrieval.
- Use "query: " prefix if you want to use embeddings as features, such as linear probing classification, clustering.
**2. Why are my reproduced results slightly different from reported in the model card?**
Different versions of `transformers` and `pytorch` could cause negligible but non-zero performance differences.
**3. Why does the cosine similarity scores distribute around 0.7 to 1.0?**
This is a known and expected behavior as we use a low temperature 0.01 for InfoNCE contrastive loss.
For text embedding tasks like text retrieval or semantic similarity,
what matters is the relative order of the scores instead of the absolute values,
so this should not be an issue.
## Citation
If you find our paper or models helpful, please consider cite as follows:
```
@article{wang2022text,
title={Text Embeddings by Weakly-Supervised Contrastive Pre-training},
author={Wang, Liang and Yang, Nan and Huang, Xiaolong and Jiao, Binxing and Yang, Linjun and Jiang, Daxin and Majumder, Rangan and Wei, Furu},
journal={arXiv preprint arXiv:2212.03533},
year={2022}
}
```
## Limitations
This model only works for English texts. Long texts will be truncated to at most 512 tokens.
|
google/bigbird-pegasus-large-arxiv | google | "2023-01-24T16:36:41Z" | 12,605 | 45 | transformers | [
"transformers",
"pytorch",
"bigbird_pegasus",
"text2text-generation",
"summarization",
"en",
"dataset:scientific_papers",
"arxiv:2007.14062",
"license:apache-2.0",
"model-index",
"autotrain_compatible",
"endpoints_compatible",
"region:us"
] | summarization | "2022-03-02T23:29:05Z" | ---
language: en
license: apache-2.0
datasets:
- scientific_papers
tags:
- summarization
model-index:
- name: google/bigbird-pegasus-large-arxiv
results:
- task:
type: summarization
name: Summarization
dataset:
name: scientific_papers
type: scientific_papers
config: pubmed
split: test
metrics:
- name: ROUGE-1
type: rouge
value: 36.0276
verified: true
- name: ROUGE-2
type: rouge
value: 13.4166
verified: true
- name: ROUGE-L
type: rouge
value: 21.9612
verified: true
- name: ROUGE-LSUM
type: rouge
value: 29.648
verified: true
- name: loss
type: loss
value: 2.774355173110962
verified: true
- name: meteor
type: meteor
value: 0.2824
verified: true
- name: gen_len
type: gen_len
value: 209.2537
verified: true
- task:
type: summarization
name: Summarization
dataset:
name: cnn_dailymail
type: cnn_dailymail
config: 3.0.0
split: test
metrics:
- name: ROUGE-1
type: rouge
value: 9.0885
verified: true
- name: ROUGE-2
type: rouge
value: 1.0325
verified: true
- name: ROUGE-L
type: rouge
value: 7.3182
verified: true
- name: ROUGE-LSUM
type: rouge
value: 8.1455
verified: true
- name: loss
type: loss
value: .nan
verified: true
- name: gen_len
type: gen_len
value: 210.4762
verified: true
- task:
type: summarization
name: Summarization
dataset:
name: xsum
type: xsum
config: default
split: test
metrics:
- name: ROUGE-1
type: rouge
value: 4.9787
verified: true
- name: ROUGE-2
type: rouge
value: 0.3527
verified: true
- name: ROUGE-L
type: rouge
value: 4.3679
verified: true
- name: ROUGE-LSUM
type: rouge
value: 4.1723
verified: true
- name: loss
type: loss
value: .nan
verified: true
- name: gen_len
type: gen_len
value: 230.4886
verified: true
- task:
type: summarization
name: Summarization
dataset:
name: scientific_papers
type: scientific_papers
config: arxiv
split: test
metrics:
- name: ROUGE-1
type: rouge
value: 43.4702
verified: true
- name: ROUGE-2
type: rouge
value: 17.4297
verified: true
- name: ROUGE-L
type: rouge
value: 26.2587
verified: true
- name: ROUGE-LSUM
type: rouge
value: 35.5587
verified: true
- name: loss
type: loss
value: 2.1113228797912598
verified: true
- name: gen_len
type: gen_len
value: 183.3702
verified: true
- task:
type: summarization
name: Summarization
dataset:
name: samsum
type: samsum
config: samsum
split: test
metrics:
- name: ROUGE-1
type: rouge
value: 3.621
verified: true
- name: ROUGE-2
type: rouge
value: 0.1699
verified: true
- name: ROUGE-L
type: rouge
value: 3.2016
verified: true
- name: ROUGE-LSUM
type: rouge
value: 3.3269
verified: true
- name: loss
type: loss
value: 7.664482116699219
verified: true
- name: gen_len
type: gen_len
value: 233.8107
verified: true
---
# BigBirdPegasus model (large)
BigBird, is a sparse-attention based transformer which extends Transformer based models, such as BERT to much longer sequences. Moreover, BigBird comes along with a theoretical understanding of the capabilities of a complete transformer that the sparse model can handle.
BigBird was introduced in this [paper](https://arxiv.org/abs/2007.14062) and first released in this [repository](https://github.com/google-research/bigbird).
Disclaimer: The team releasing BigBird did not write a model card for this model so this model card has been written by the Hugging Face team.
## Model description
BigBird relies on **block sparse attention** instead of normal attention (i.e. BERT's attention) and can handle sequences up to a length of 4096 at a much lower compute cost compared to BERT. It has achieved SOTA on various tasks involving very long sequences such as long documents summarization, question-answering with long contexts.
## How to use
Here is how to use this model to get the features of a given text in PyTorch:
```python
from transformers import BigBirdPegasusForConditionalGeneration, AutoTokenizer
tokenizer = AutoTokenizer.from_pretrained("google/bigbird-pegasus-large-arxiv")
# by default encoder-attention is `block_sparse` with num_random_blocks=3, block_size=64
model = BigBirdPegasusForConditionalGeneration.from_pretrained("google/bigbird-pegasus-large-arxiv")
# decoder attention type can't be changed & will be "original_full"
# you can change `attention_type` (encoder only) to full attention like this:
model = BigBirdPegasusForConditionalGeneration.from_pretrained("google/bigbird-pegasus-large-arxiv", attention_type="original_full")
# you can change `block_size` & `num_random_blocks` like this:
model = BigBirdPegasusForConditionalGeneration.from_pretrained("google/bigbird-pegasus-large-arxiv", block_size=16, num_random_blocks=2)
text = "Replace me by any text you'd like."
inputs = tokenizer(text, return_tensors='pt')
prediction = model.generate(**inputs)
prediction = tokenizer.batch_decode(prediction)
```
## Training Procedure
This checkpoint is obtained after fine-tuning `BigBirdPegasusForConditionalGeneration` for **summarization** on **arxiv dataset** from [scientific_papers](https://huggingface.co/datasets/scientific_papers).
## BibTeX entry and citation info
```tex
@misc{zaheer2021big,
title={Big Bird: Transformers for Longer Sequences},
author={Manzil Zaheer and Guru Guruganesh and Avinava Dubey and Joshua Ainslie and Chris Alberti and Santiago Ontanon and Philip Pham and Anirudh Ravula and Qifan Wang and Li Yang and Amr Ahmed},
year={2021},
eprint={2007.14062},
archivePrefix={arXiv},
primaryClass={cs.LG}
}
```
|
TaylorAI/gte-tiny | TaylorAI | "2023-10-07T05:20:49Z" | 12,592 | 125 | sentence-transformers | [
"sentence-transformers",
"pytorch",
"onnx",
"safetensors",
"bert",
"feature-extraction",
"sentence-similarity",
"transformers",
"mteb",
"model-index",
"autotrain_compatible",
"endpoints_compatible",
"text-embeddings-inference",
"region:us"
] | sentence-similarity | "2023-10-05T20:30:20Z" | ---
model-index:
- name: gte_tiny
results:
- task:
type: Classification
dataset:
type: mteb/amazon_counterfactual
name: MTEB AmazonCounterfactualClassification (en)
config: en
split: test
revision: e8379541af4e31359cca9fbcf4b00f2671dba205
metrics:
- type: accuracy
value: 71.76119402985076
- type: ap
value: 34.63659287952359
- type: f1
value: 65.88939512571113
- task:
type: Classification
dataset:
type: mteb/amazon_polarity
name: MTEB AmazonPolarityClassification
config: default
split: test
revision: e2d317d38cd51312af73b3d32a06d1a08b442046
metrics:
- type: accuracy
value: 86.61324999999998
- type: ap
value: 81.7476302802319
- type: f1
value: 86.5863470912001
- task:
type: Classification
dataset:
type: mteb/amazon_reviews_multi
name: MTEB AmazonReviewsClassification (en)
config: en
split: test
revision: 1399c76144fd37290681b995c656ef9b2e06e26d
metrics:
- type: accuracy
value: 42.61000000000001
- type: f1
value: 42.2217180000715
- task:
type: Retrieval
dataset:
type: arguana
name: MTEB ArguAna
config: default
split: test
revision: None
metrics:
- type: map_at_1
value: 28.377999999999997
- type: map_at_10
value: 44.565
- type: map_at_100
value: 45.48
- type: map_at_1000
value: 45.487
- type: map_at_3
value: 39.841
- type: map_at_5
value: 42.284
- type: mrr_at_1
value: 29.445
- type: mrr_at_10
value: 44.956
- type: mrr_at_100
value: 45.877
- type: mrr_at_1000
value: 45.884
- type: mrr_at_3
value: 40.209
- type: mrr_at_5
value: 42.719
- type: ndcg_at_1
value: 28.377999999999997
- type: ndcg_at_10
value: 53.638
- type: ndcg_at_100
value: 57.354000000000006
- type: ndcg_at_1000
value: 57.513000000000005
- type: ndcg_at_3
value: 43.701
- type: ndcg_at_5
value: 48.114000000000004
- type: precision_at_1
value: 28.377999999999997
- type: precision_at_10
value: 8.272
- type: precision_at_100
value: 0.984
- type: precision_at_1000
value: 0.1
- type: precision_at_3
value: 18.303
- type: precision_at_5
value: 13.129
- type: recall_at_1
value: 28.377999999999997
- type: recall_at_10
value: 82.717
- type: recall_at_100
value: 98.43499999999999
- type: recall_at_1000
value: 99.644
- type: recall_at_3
value: 54.908
- type: recall_at_5
value: 65.647
- task:
type: Clustering
dataset:
type: mteb/arxiv-clustering-p2p
name: MTEB ArxivClusteringP2P
config: default
split: test
revision: a122ad7f3f0291bf49cc6f4d32aa80929df69d5d
metrics:
- type: v_measure
value: 46.637318326729876
- task:
type: Clustering
dataset:
type: mteb/arxiv-clustering-s2s
name: MTEB ArxivClusteringS2S
config: default
split: test
revision: f910caf1a6075f7329cdf8c1a6135696f37dbd53
metrics:
- type: v_measure
value: 36.01134479855804
- task:
type: Reranking
dataset:
type: mteb/askubuntudupquestions-reranking
name: MTEB AskUbuntuDupQuestions
config: default
split: test
revision: 2000358ca161889fa9c082cb41daa8dcfb161a54
metrics:
- type: map
value: 59.82917555338909
- type: mrr
value: 74.7888361254012
- task:
type: STS
dataset:
type: mteb/biosses-sts
name: MTEB BIOSSES
config: default
split: test
revision: d3fb88f8f02e40887cd149695127462bbcf29b4a
metrics:
- type: cos_sim_pearson
value: 87.1657730995964
- type: cos_sim_spearman
value: 86.62787748941281
- type: euclidean_pearson
value: 85.48127914481798
- type: euclidean_spearman
value: 86.48148861167424
- type: manhattan_pearson
value: 85.07496934780823
- type: manhattan_spearman
value: 86.39473964708843
- task:
type: Classification
dataset:
type: mteb/banking77
name: MTEB Banking77Classification
config: default
split: test
revision: 0fd18e25b25c072e09e0d92ab615fda904d66300
metrics:
- type: accuracy
value: 81.73051948051948
- type: f1
value: 81.66368364988331
- task:
type: Clustering
dataset:
type: mteb/biorxiv-clustering-p2p
name: MTEB BiorxivClusteringP2P
config: default
split: test
revision: 65b79d1d13f80053f67aca9498d9402c2d9f1f40
metrics:
- type: v_measure
value: 39.18623707448217
- task:
type: Clustering
dataset:
type: mteb/biorxiv-clustering-s2s
name: MTEB BiorxivClusteringS2S
config: default
split: test
revision: 258694dd0231531bc1fd9de6ceb52a0853c6d908
metrics:
- type: v_measure
value: 32.12697757150375
- task:
type: Retrieval
dataset:
type: BeIR/cqadupstack
name: MTEB CQADupstackAndroidRetrieval
config: default
split: test
revision: None
metrics:
- type: map_at_1
value: 29.160000000000004
- type: map_at_10
value: 40.474
- type: map_at_100
value: 41.905
- type: map_at_1000
value: 42.041000000000004
- type: map_at_3
value: 37.147000000000006
- type: map_at_5
value: 38.873999999999995
- type: mrr_at_1
value: 36.91
- type: mrr_at_10
value: 46.495999999999995
- type: mrr_at_100
value: 47.288000000000004
- type: mrr_at_1000
value: 47.339999999999996
- type: mrr_at_3
value: 43.777
- type: mrr_at_5
value: 45.257999999999996
- type: ndcg_at_1
value: 36.91
- type: ndcg_at_10
value: 46.722
- type: ndcg_at_100
value: 51.969
- type: ndcg_at_1000
value: 54.232
- type: ndcg_at_3
value: 41.783
- type: ndcg_at_5
value: 43.797000000000004
- type: precision_at_1
value: 36.91
- type: precision_at_10
value: 9.013
- type: precision_at_100
value: 1.455
- type: precision_at_1000
value: 0.193
- type: precision_at_3
value: 20.124
- type: precision_at_5
value: 14.363000000000001
- type: recall_at_1
value: 29.160000000000004
- type: recall_at_10
value: 58.521
- type: recall_at_100
value: 80.323
- type: recall_at_1000
value: 95.13000000000001
- type: recall_at_3
value: 44.205
- type: recall_at_5
value: 49.97
- task:
type: Retrieval
dataset:
type: BeIR/cqadupstack
name: MTEB CQADupstackEnglishRetrieval
config: default
split: test
revision: None
metrics:
- type: map_at_1
value: 27.750000000000004
- type: map_at_10
value: 36.39
- type: map_at_100
value: 37.5
- type: map_at_1000
value: 37.625
- type: map_at_3
value: 33.853
- type: map_at_5
value: 35.397
- type: mrr_at_1
value: 34.14
- type: mrr_at_10
value: 41.841
- type: mrr_at_100
value: 42.469
- type: mrr_at_1000
value: 42.521
- type: mrr_at_3
value: 39.724
- type: mrr_at_5
value: 40.955999999999996
- type: ndcg_at_1
value: 34.14
- type: ndcg_at_10
value: 41.409
- type: ndcg_at_100
value: 45.668
- type: ndcg_at_1000
value: 47.916
- type: ndcg_at_3
value: 37.836
- type: ndcg_at_5
value: 39.650999999999996
- type: precision_at_1
value: 34.14
- type: precision_at_10
value: 7.739
- type: precision_at_100
value: 1.2630000000000001
- type: precision_at_1000
value: 0.173
- type: precision_at_3
value: 18.217
- type: precision_at_5
value: 12.854
- type: recall_at_1
value: 27.750000000000004
- type: recall_at_10
value: 49.882
- type: recall_at_100
value: 68.556
- type: recall_at_1000
value: 83.186
- type: recall_at_3
value: 39.047
- type: recall_at_5
value: 44.458
- task:
type: Retrieval
dataset:
type: BeIR/cqadupstack
name: MTEB CQADupstackGamingRetrieval
config: default
split: test
revision: None
metrics:
- type: map_at_1
value: 36.879
- type: map_at_10
value: 48.878
- type: map_at_100
value: 49.918
- type: map_at_1000
value: 49.978
- type: map_at_3
value: 45.867999999999995
- type: map_at_5
value: 47.637
- type: mrr_at_1
value: 42.696
- type: mrr_at_10
value: 52.342
- type: mrr_at_100
value: 53.044000000000004
- type: mrr_at_1000
value: 53.077
- type: mrr_at_3
value: 50.01
- type: mrr_at_5
value: 51.437
- type: ndcg_at_1
value: 42.696
- type: ndcg_at_10
value: 54.469
- type: ndcg_at_100
value: 58.664
- type: ndcg_at_1000
value: 59.951
- type: ndcg_at_3
value: 49.419999999999995
- type: ndcg_at_5
value: 52.007000000000005
- type: precision_at_1
value: 42.696
- type: precision_at_10
value: 8.734
- type: precision_at_100
value: 1.1769999999999998
- type: precision_at_1000
value: 0.133
- type: precision_at_3
value: 22.027
- type: precision_at_5
value: 15.135000000000002
- type: recall_at_1
value: 36.879
- type: recall_at_10
value: 67.669
- type: recall_at_100
value: 85.822
- type: recall_at_1000
value: 95.092
- type: recall_at_3
value: 54.157999999999994
- type: recall_at_5
value: 60.436
- task:
type: Retrieval
dataset:
type: BeIR/cqadupstack
name: MTEB CQADupstackGisRetrieval
config: default
split: test
revision: None
metrics:
- type: map_at_1
value: 22.942
- type: map_at_10
value: 31.741999999999997
- type: map_at_100
value: 32.721000000000004
- type: map_at_1000
value: 32.809
- type: map_at_3
value: 29.17
- type: map_at_5
value: 30.714000000000002
- type: mrr_at_1
value: 24.746000000000002
- type: mrr_at_10
value: 33.517
- type: mrr_at_100
value: 34.451
- type: mrr_at_1000
value: 34.522000000000006
- type: mrr_at_3
value: 31.148999999999997
- type: mrr_at_5
value: 32.606
- type: ndcg_at_1
value: 24.746000000000002
- type: ndcg_at_10
value: 36.553000000000004
- type: ndcg_at_100
value: 41.53
- type: ndcg_at_1000
value: 43.811
- type: ndcg_at_3
value: 31.674000000000003
- type: ndcg_at_5
value: 34.241
- type: precision_at_1
value: 24.746000000000002
- type: precision_at_10
value: 5.684
- type: precision_at_100
value: 0.859
- type: precision_at_1000
value: 0.109
- type: precision_at_3
value: 13.597000000000001
- type: precision_at_5
value: 9.672
- type: recall_at_1
value: 22.942
- type: recall_at_10
value: 49.58
- type: recall_at_100
value: 72.614
- type: recall_at_1000
value: 89.89200000000001
- type: recall_at_3
value: 36.552
- type: recall_at_5
value: 42.702
- task:
type: Retrieval
dataset:
type: BeIR/cqadupstack
name: MTEB CQADupstackMathematicaRetrieval
config: default
split: test
revision: None
metrics:
- type: map_at_1
value: 15.345
- type: map_at_10
value: 22.428
- type: map_at_100
value: 23.756
- type: map_at_1000
value: 23.872
- type: map_at_3
value: 20.212
- type: map_at_5
value: 21.291
- type: mrr_at_1
value: 19.279
- type: mrr_at_10
value: 27.1
- type: mrr_at_100
value: 28.211000000000002
- type: mrr_at_1000
value: 28.279
- type: mrr_at_3
value: 24.813
- type: mrr_at_5
value: 25.889
- type: ndcg_at_1
value: 19.279
- type: ndcg_at_10
value: 27.36
- type: ndcg_at_100
value: 33.499
- type: ndcg_at_1000
value: 36.452
- type: ndcg_at_3
value: 23.233999999999998
- type: ndcg_at_5
value: 24.806
- type: precision_at_1
value: 19.279
- type: precision_at_10
value: 5.149
- type: precision_at_100
value: 0.938
- type: precision_at_1000
value: 0.133
- type: precision_at_3
value: 11.360000000000001
- type: precision_at_5
value: 8.035
- type: recall_at_1
value: 15.345
- type: recall_at_10
value: 37.974999999999994
- type: recall_at_100
value: 64.472
- type: recall_at_1000
value: 85.97200000000001
- type: recall_at_3
value: 26.203
- type: recall_at_5
value: 30.485
- task:
type: Retrieval
dataset:
type: BeIR/cqadupstack
name: MTEB CQADupstackPhysicsRetrieval
config: default
split: test
revision: None
metrics:
- type: map_at_1
value: 26.362000000000002
- type: map_at_10
value: 36.406
- type: map_at_100
value: 37.726
- type: map_at_1000
value: 37.84
- type: map_at_3
value: 33.425
- type: map_at_5
value: 35.043
- type: mrr_at_1
value: 32.146
- type: mrr_at_10
value: 41.674
- type: mrr_at_100
value: 42.478
- type: mrr_at_1000
value: 42.524
- type: mrr_at_3
value: 38.948
- type: mrr_at_5
value: 40.415
- type: ndcg_at_1
value: 32.146
- type: ndcg_at_10
value: 42.374
- type: ndcg_at_100
value: 47.919
- type: ndcg_at_1000
value: 50.013
- type: ndcg_at_3
value: 37.29
- type: ndcg_at_5
value: 39.531
- type: precision_at_1
value: 32.146
- type: precision_at_10
value: 7.767
- type: precision_at_100
value: 1.236
- type: precision_at_1000
value: 0.16
- type: precision_at_3
value: 17.965999999999998
- type: precision_at_5
value: 12.742999999999999
- type: recall_at_1
value: 26.362000000000002
- type: recall_at_10
value: 54.98800000000001
- type: recall_at_100
value: 78.50200000000001
- type: recall_at_1000
value: 92.146
- type: recall_at_3
value: 40.486
- type: recall_at_5
value: 46.236
- task:
type: Retrieval
dataset:
type: BeIR/cqadupstack
name: MTEB CQADupstackProgrammersRetrieval
config: default
split: test
revision: None
metrics:
- type: map_at_1
value: 24.417
- type: map_at_10
value: 33.161
- type: map_at_100
value: 34.357
- type: map_at_1000
value: 34.473
- type: map_at_3
value: 30.245
- type: map_at_5
value: 31.541999999999998
- type: mrr_at_1
value: 29.909000000000002
- type: mrr_at_10
value: 38.211
- type: mrr_at_100
value: 39.056999999999995
- type: mrr_at_1000
value: 39.114
- type: mrr_at_3
value: 35.769
- type: mrr_at_5
value: 36.922
- type: ndcg_at_1
value: 29.909000000000002
- type: ndcg_at_10
value: 38.694
- type: ndcg_at_100
value: 44.057
- type: ndcg_at_1000
value: 46.6
- type: ndcg_at_3
value: 33.822
- type: ndcg_at_5
value: 35.454
- type: precision_at_1
value: 29.909000000000002
- type: precision_at_10
value: 7.180000000000001
- type: precision_at_100
value: 1.153
- type: precision_at_1000
value: 0.155
- type: precision_at_3
value: 16.134
- type: precision_at_5
value: 11.256
- type: recall_at_1
value: 24.417
- type: recall_at_10
value: 50.260000000000005
- type: recall_at_100
value: 73.55699999999999
- type: recall_at_1000
value: 91.216
- type: recall_at_3
value: 35.971
- type: recall_at_5
value: 40.793
- task:
type: Retrieval
dataset:
type: BeIR/cqadupstack
name: MTEB CQADupstackRetrieval
config: default
split: test
revision: None
metrics:
- type: map_at_1
value: 24.266916666666663
- type: map_at_10
value: 32.75025
- type: map_at_100
value: 33.91341666666667
- type: map_at_1000
value: 34.031749999999995
- type: map_at_3
value: 30.166416666666674
- type: map_at_5
value: 31.577000000000005
- type: mrr_at_1
value: 28.828166666666664
- type: mrr_at_10
value: 36.80991666666667
- type: mrr_at_100
value: 37.67075
- type: mrr_at_1000
value: 37.733
- type: mrr_at_3
value: 34.513416666666664
- type: mrr_at_5
value: 35.788
- type: ndcg_at_1
value: 28.828166666666664
- type: ndcg_at_10
value: 37.796
- type: ndcg_at_100
value: 42.94783333333333
- type: ndcg_at_1000
value: 45.38908333333333
- type: ndcg_at_3
value: 33.374750000000006
- type: ndcg_at_5
value: 35.379666666666665
- type: precision_at_1
value: 28.828166666666664
- type: precision_at_10
value: 6.615749999999999
- type: precision_at_100
value: 1.0848333333333333
- type: precision_at_1000
value: 0.1484166666666667
- type: precision_at_3
value: 15.347833333333332
- type: precision_at_5
value: 10.848916666666666
- type: recall_at_1
value: 24.266916666666663
- type: recall_at_10
value: 48.73458333333333
- type: recall_at_100
value: 71.56341666666667
- type: recall_at_1000
value: 88.63091666666668
- type: recall_at_3
value: 36.31208333333333
- type: recall_at_5
value: 41.55633333333333
- task:
type: Retrieval
dataset:
type: BeIR/cqadupstack
name: MTEB CQADupstackStatsRetrieval
config: default
split: test
revision: None
metrics:
- type: map_at_1
value: 23.497
- type: map_at_10
value: 30.249
- type: map_at_100
value: 30.947000000000003
- type: map_at_1000
value: 31.049
- type: map_at_3
value: 28.188000000000002
- type: map_at_5
value: 29.332
- type: mrr_at_1
value: 26.687
- type: mrr_at_10
value: 33.182
- type: mrr_at_100
value: 33.794999999999995
- type: mrr_at_1000
value: 33.873
- type: mrr_at_3
value: 31.263
- type: mrr_at_5
value: 32.428000000000004
- type: ndcg_at_1
value: 26.687
- type: ndcg_at_10
value: 34.252
- type: ndcg_at_100
value: 38.083
- type: ndcg_at_1000
value: 40.682
- type: ndcg_at_3
value: 30.464999999999996
- type: ndcg_at_5
value: 32.282
- type: precision_at_1
value: 26.687
- type: precision_at_10
value: 5.2909999999999995
- type: precision_at_100
value: 0.788
- type: precision_at_1000
value: 0.109
- type: precision_at_3
value: 13.037
- type: precision_at_5
value: 9.049
- type: recall_at_1
value: 23.497
- type: recall_at_10
value: 43.813
- type: recall_at_100
value: 61.88399999999999
- type: recall_at_1000
value: 80.926
- type: recall_at_3
value: 33.332
- type: recall_at_5
value: 37.862
- task:
type: Retrieval
dataset:
type: BeIR/cqadupstack
name: MTEB CQADupstackTexRetrieval
config: default
split: test
revision: None
metrics:
- type: map_at_1
value: 16.073
- type: map_at_10
value: 22.705000000000002
- type: map_at_100
value: 23.703
- type: map_at_1000
value: 23.833
- type: map_at_3
value: 20.593
- type: map_at_5
value: 21.7
- type: mrr_at_1
value: 19.683
- type: mrr_at_10
value: 26.39
- type: mrr_at_100
value: 27.264
- type: mrr_at_1000
value: 27.349
- type: mrr_at_3
value: 24.409
- type: mrr_at_5
value: 25.474000000000004
- type: ndcg_at_1
value: 19.683
- type: ndcg_at_10
value: 27.014
- type: ndcg_at_100
value: 31.948
- type: ndcg_at_1000
value: 35.125
- type: ndcg_at_3
value: 23.225
- type: ndcg_at_5
value: 24.866
- type: precision_at_1
value: 19.683
- type: precision_at_10
value: 4.948
- type: precision_at_100
value: 0.876
- type: precision_at_1000
value: 0.133
- type: precision_at_3
value: 10.943
- type: precision_at_5
value: 7.86
- type: recall_at_1
value: 16.073
- type: recall_at_10
value: 36.283
- type: recall_at_100
value: 58.745999999999995
- type: recall_at_1000
value: 81.711
- type: recall_at_3
value: 25.637
- type: recall_at_5
value: 29.919
- task:
type: Retrieval
dataset:
type: BeIR/cqadupstack
name: MTEB CQADupstackUnixRetrieval
config: default
split: test
revision: None
metrics:
- type: map_at_1
value: 25.776
- type: map_at_10
value: 33.317
- type: map_at_100
value: 34.437
- type: map_at_1000
value: 34.54
- type: map_at_3
value: 30.706
- type: map_at_5
value: 32.202999999999996
- type: mrr_at_1
value: 30.224
- type: mrr_at_10
value: 37.34
- type: mrr_at_100
value: 38.268
- type: mrr_at_1000
value: 38.335
- type: mrr_at_3
value: 35.075
- type: mrr_at_5
value: 36.348
- type: ndcg_at_1
value: 30.224
- type: ndcg_at_10
value: 38.083
- type: ndcg_at_100
value: 43.413000000000004
- type: ndcg_at_1000
value: 45.856
- type: ndcg_at_3
value: 33.437
- type: ndcg_at_5
value: 35.661
- type: precision_at_1
value: 30.224
- type: precision_at_10
value: 6.1850000000000005
- type: precision_at_100
value: 1.0030000000000001
- type: precision_at_1000
value: 0.132
- type: precision_at_3
value: 14.646
- type: precision_at_5
value: 10.428999999999998
- type: recall_at_1
value: 25.776
- type: recall_at_10
value: 48.787000000000006
- type: recall_at_100
value: 72.04899999999999
- type: recall_at_1000
value: 89.339
- type: recall_at_3
value: 36.192
- type: recall_at_5
value: 41.665
- task:
type: Retrieval
dataset:
type: BeIR/cqadupstack
name: MTEB CQADupstackWebmastersRetrieval
config: default
split: test
revision: None
metrics:
- type: map_at_1
value: 23.156
- type: map_at_10
value: 30.886000000000003
- type: map_at_100
value: 32.551
- type: map_at_1000
value: 32.769
- type: map_at_3
value: 28.584
- type: map_at_5
value: 29.959999999999997
- type: mrr_at_1
value: 28.260999999999996
- type: mrr_at_10
value: 35.555
- type: mrr_at_100
value: 36.687
- type: mrr_at_1000
value: 36.742999999999995
- type: mrr_at_3
value: 33.531
- type: mrr_at_5
value: 34.717
- type: ndcg_at_1
value: 28.260999999999996
- type: ndcg_at_10
value: 36.036
- type: ndcg_at_100
value: 42.675000000000004
- type: ndcg_at_1000
value: 45.303
- type: ndcg_at_3
value: 32.449
- type: ndcg_at_5
value: 34.293
- type: precision_at_1
value: 28.260999999999996
- type: precision_at_10
value: 6.837999999999999
- type: precision_at_100
value: 1.4569999999999999
- type: precision_at_1000
value: 0.23500000000000001
- type: precision_at_3
value: 15.217
- type: precision_at_5
value: 11.028
- type: recall_at_1
value: 23.156
- type: recall_at_10
value: 45.251999999999995
- type: recall_at_100
value: 75.339
- type: recall_at_1000
value: 91.56
- type: recall_at_3
value: 34.701
- type: recall_at_5
value: 39.922999999999995
- task:
type: Retrieval
dataset:
type: BeIR/cqadupstack
name: MTEB CQADupstackWordpressRetrieval
config: default
split: test
revision: None
metrics:
- type: map_at_1
value: 19.846
- type: map_at_10
value: 26.367
- type: map_at_100
value: 27.439999999999998
- type: map_at_1000
value: 27.552
- type: map_at_3
value: 24.006
- type: map_at_5
value: 25.230999999999998
- type: mrr_at_1
value: 21.257
- type: mrr_at_10
value: 28.071
- type: mrr_at_100
value: 29.037000000000003
- type: mrr_at_1000
value: 29.119
- type: mrr_at_3
value: 25.692999999999998
- type: mrr_at_5
value: 27.006000000000004
- type: ndcg_at_1
value: 21.257
- type: ndcg_at_10
value: 30.586000000000002
- type: ndcg_at_100
value: 35.949
- type: ndcg_at_1000
value: 38.728
- type: ndcg_at_3
value: 25.862000000000002
- type: ndcg_at_5
value: 27.967
- type: precision_at_1
value: 21.257
- type: precision_at_10
value: 4.861
- type: precision_at_100
value: 0.8130000000000001
- type: precision_at_1000
value: 0.116
- type: precision_at_3
value: 10.906
- type: precision_at_5
value: 7.763000000000001
- type: recall_at_1
value: 19.846
- type: recall_at_10
value: 41.805
- type: recall_at_100
value: 66.89699999999999
- type: recall_at_1000
value: 87.401
- type: recall_at_3
value: 29.261
- type: recall_at_5
value: 34.227000000000004
- task:
type: Retrieval
dataset:
type: climate-fever
name: MTEB ClimateFEVER
config: default
split: test
revision: None
metrics:
- type: map_at_1
value: 10.333
- type: map_at_10
value: 17.14
- type: map_at_100
value: 18.878
- type: map_at_1000
value: 19.067
- type: map_at_3
value: 14.123
- type: map_at_5
value: 15.699
- type: mrr_at_1
value: 23.192
- type: mrr_at_10
value: 33.553
- type: mrr_at_100
value: 34.553
- type: mrr_at_1000
value: 34.603
- type: mrr_at_3
value: 29.848000000000003
- type: mrr_at_5
value: 32.18
- type: ndcg_at_1
value: 23.192
- type: ndcg_at_10
value: 24.707
- type: ndcg_at_100
value: 31.701
- type: ndcg_at_1000
value: 35.260999999999996
- type: ndcg_at_3
value: 19.492
- type: ndcg_at_5
value: 21.543
- type: precision_at_1
value: 23.192
- type: precision_at_10
value: 7.824000000000001
- type: precision_at_100
value: 1.52
- type: precision_at_1000
value: 0.218
- type: precision_at_3
value: 14.180000000000001
- type: precision_at_5
value: 11.530999999999999
- type: recall_at_1
value: 10.333
- type: recall_at_10
value: 30.142999999999997
- type: recall_at_100
value: 54.298
- type: recall_at_1000
value: 74.337
- type: recall_at_3
value: 17.602999999999998
- type: recall_at_5
value: 22.938
- task:
type: Retrieval
dataset:
type: dbpedia-entity
name: MTEB DBPedia
config: default
split: test
revision: None
metrics:
- type: map_at_1
value: 8.03
- type: map_at_10
value: 17.345
- type: map_at_100
value: 23.462
- type: map_at_1000
value: 24.77
- type: map_at_3
value: 12.714
- type: map_at_5
value: 14.722
- type: mrr_at_1
value: 61.0
- type: mrr_at_10
value: 69.245
- type: mrr_at_100
value: 69.715
- type: mrr_at_1000
value: 69.719
- type: mrr_at_3
value: 67.583
- type: mrr_at_5
value: 68.521
- type: ndcg_at_1
value: 47.625
- type: ndcg_at_10
value: 35.973
- type: ndcg_at_100
value: 39.875
- type: ndcg_at_1000
value: 46.922000000000004
- type: ndcg_at_3
value: 40.574
- type: ndcg_at_5
value: 38.18
- type: precision_at_1
value: 61.0
- type: precision_at_10
value: 29.049999999999997
- type: precision_at_100
value: 8.828
- type: precision_at_1000
value: 1.8290000000000002
- type: precision_at_3
value: 45.333
- type: precision_at_5
value: 37.9
- type: recall_at_1
value: 8.03
- type: recall_at_10
value: 22.334
- type: recall_at_100
value: 45.919
- type: recall_at_1000
value: 68.822
- type: recall_at_3
value: 14.038999999999998
- type: recall_at_5
value: 17.118
- task:
type: Classification
dataset:
type: mteb/emotion
name: MTEB EmotionClassification
config: default
split: test
revision: 4f58c6b202a23cf9a4da393831edf4f9183cad37
metrics:
- type: accuracy
value: 44.714999999999996
- type: f1
value: 39.83929362259356
- task:
type: Retrieval
dataset:
type: fever
name: MTEB FEVER
config: default
split: test
revision: None
metrics:
- type: map_at_1
value: 52.242999999999995
- type: map_at_10
value: 64.087
- type: map_at_100
value: 64.549
- type: map_at_1000
value: 64.567
- type: map_at_3
value: 61.667
- type: map_at_5
value: 63.266
- type: mrr_at_1
value: 56.271
- type: mrr_at_10
value: 68.146
- type: mrr_at_100
value: 68.524
- type: mrr_at_1000
value: 68.53200000000001
- type: mrr_at_3
value: 65.869
- type: mrr_at_5
value: 67.37100000000001
- type: ndcg_at_1
value: 56.271
- type: ndcg_at_10
value: 70.109
- type: ndcg_at_100
value: 72.09
- type: ndcg_at_1000
value: 72.479
- type: ndcg_at_3
value: 65.559
- type: ndcg_at_5
value: 68.242
- type: precision_at_1
value: 56.271
- type: precision_at_10
value: 9.286999999999999
- type: precision_at_100
value: 1.039
- type: precision_at_1000
value: 0.109
- type: precision_at_3
value: 26.308
- type: precision_at_5
value: 17.291
- type: recall_at_1
value: 52.242999999999995
- type: recall_at_10
value: 84.71
- type: recall_at_100
value: 93.309
- type: recall_at_1000
value: 96.013
- type: recall_at_3
value: 72.554
- type: recall_at_5
value: 79.069
- task:
type: Retrieval
dataset:
type: fiqa
name: MTEB FiQA2018
config: default
split: test
revision: None
metrics:
- type: map_at_1
value: 14.346
- type: map_at_10
value: 24.552
- type: map_at_100
value: 26.161
- type: map_at_1000
value: 26.345000000000002
- type: map_at_3
value: 21.208
- type: map_at_5
value: 22.959
- type: mrr_at_1
value: 29.166999999999998
- type: mrr_at_10
value: 38.182
- type: mrr_at_100
value: 39.22
- type: mrr_at_1000
value: 39.263
- type: mrr_at_3
value: 35.983
- type: mrr_at_5
value: 37.14
- type: ndcg_at_1
value: 29.166999999999998
- type: ndcg_at_10
value: 31.421
- type: ndcg_at_100
value: 38.129999999999995
- type: ndcg_at_1000
value: 41.569
- type: ndcg_at_3
value: 28.172000000000004
- type: ndcg_at_5
value: 29.029
- type: precision_at_1
value: 29.166999999999998
- type: precision_at_10
value: 8.997
- type: precision_at_100
value: 1.5709999999999997
- type: precision_at_1000
value: 0.22
- type: precision_at_3
value: 19.187
- type: precision_at_5
value: 13.980999999999998
- type: recall_at_1
value: 14.346
- type: recall_at_10
value: 37.963
- type: recall_at_100
value: 63.43299999999999
- type: recall_at_1000
value: 84.057
- type: recall_at_3
value: 26.119999999999997
- type: recall_at_5
value: 30.988
- task:
type: Retrieval
dataset:
type: hotpotqa
name: MTEB HotpotQA
config: default
split: test
revision: None
metrics:
- type: map_at_1
value: 33.059
- type: map_at_10
value: 46.421
- type: map_at_100
value: 47.323
- type: map_at_1000
value: 47.403
- type: map_at_3
value: 43.553999999999995
- type: map_at_5
value: 45.283
- type: mrr_at_1
value: 66.117
- type: mrr_at_10
value: 73.10900000000001
- type: mrr_at_100
value: 73.444
- type: mrr_at_1000
value: 73.46000000000001
- type: mrr_at_3
value: 71.70400000000001
- type: mrr_at_5
value: 72.58099999999999
- type: ndcg_at_1
value: 66.117
- type: ndcg_at_10
value: 55.696999999999996
- type: ndcg_at_100
value: 59.167
- type: ndcg_at_1000
value: 60.809000000000005
- type: ndcg_at_3
value: 51.243
- type: ndcg_at_5
value: 53.627
- type: precision_at_1
value: 66.117
- type: precision_at_10
value: 11.538
- type: precision_at_100
value: 1.429
- type: precision_at_1000
value: 0.165
- type: precision_at_3
value: 31.861
- type: precision_at_5
value: 20.997
- type: recall_at_1
value: 33.059
- type: recall_at_10
value: 57.691
- type: recall_at_100
value: 71.458
- type: recall_at_1000
value: 82.35
- type: recall_at_3
value: 47.792
- type: recall_at_5
value: 52.492000000000004
- task:
type: Classification
dataset:
type: mteb/imdb
name: MTEB ImdbClassification
config: default
split: test
revision: 3d86128a09e091d6018b6d26cad27f2739fc2db7
metrics:
- type: accuracy
value: 80.544
- type: ap
value: 74.69592367984956
- type: f1
value: 80.51138138449883
- task:
type: Retrieval
dataset:
type: msmarco
name: MTEB MSMARCO
config: default
split: dev
revision: None
metrics:
- type: map_at_1
value: 17.095
- type: map_at_10
value: 28.038999999999998
- type: map_at_100
value: 29.246
- type: map_at_1000
value: 29.311
- type: map_at_3
value: 24.253
- type: map_at_5
value: 26.442
- type: mrr_at_1
value: 17.535999999999998
- type: mrr_at_10
value: 28.53
- type: mrr_at_100
value: 29.697000000000003
- type: mrr_at_1000
value: 29.755
- type: mrr_at_3
value: 24.779999999999998
- type: mrr_at_5
value: 26.942
- type: ndcg_at_1
value: 17.549999999999997
- type: ndcg_at_10
value: 34.514
- type: ndcg_at_100
value: 40.497
- type: ndcg_at_1000
value: 42.17
- type: ndcg_at_3
value: 26.764
- type: ndcg_at_5
value: 30.678
- type: precision_at_1
value: 17.549999999999997
- type: precision_at_10
value: 5.692
- type: precision_at_100
value: 0.8699999999999999
- type: precision_at_1000
value: 0.101
- type: precision_at_3
value: 11.562
- type: precision_at_5
value: 8.917
- type: recall_at_1
value: 17.095
- type: recall_at_10
value: 54.642
- type: recall_at_100
value: 82.652
- type: recall_at_1000
value: 95.555
- type: recall_at_3
value: 33.504
- type: recall_at_5
value: 42.925000000000004
- task:
type: Classification
dataset:
type: mteb/mtop_domain
name: MTEB MTOPDomainClassification (en)
config: en
split: test
revision: d80d48c1eb48d3562165c59d59d0034df9fff0bf
metrics:
- type: accuracy
value: 91.75558595531236
- type: f1
value: 91.25979279648296
- task:
type: Classification
dataset:
type: mteb/mtop_intent
name: MTEB MTOPIntentClassification (en)
config: en
split: test
revision: ae001d0e6b1228650b7bd1c2c65fb50ad11a8aba
metrics:
- type: accuracy
value: 69.90424076607387
- type: f1
value: 52.067408707562244
- task:
type: Classification
dataset:
type: mteb/amazon_massive_intent
name: MTEB MassiveIntentClassification (en)
config: en
split: test
revision: 31efe3c427b0bae9c22cbb560b8f15491cc6bed7
metrics:
- type: accuracy
value: 70.13449899125757
- type: f1
value: 67.62456762910598
- task:
type: Classification
dataset:
type: mteb/amazon_massive_scenario
name: MTEB MassiveScenarioClassification (en)
config: en
split: test
revision: 7d571f92784cd94a019292a1f45445077d0ef634
metrics:
- type: accuracy
value: 74.862138533961
- type: f1
value: 74.66457222091381
- task:
type: Clustering
dataset:
type: mteb/medrxiv-clustering-p2p
name: MTEB MedrxivClusteringP2P
config: default
split: test
revision: e7a26af6f3ae46b30dde8737f02c07b1505bcc73
metrics:
- type: v_measure
value: 34.10761942610792
- task:
type: Clustering
dataset:
type: mteb/medrxiv-clustering-s2s
name: MTEB MedrxivClusteringS2S
config: default
split: test
revision: 35191c8c0dca72d8ff3efcd72aa802307d469663
metrics:
- type: v_measure
value: 31.673172170578408
- task:
type: Reranking
dataset:
type: mteb/mind_small
name: MTEB MindSmallReranking
config: default
split: test
revision: 3bdac13927fdc888b903db93b2ffdbd90b295a69
metrics:
- type: map
value: 32.058704977250315
- type: mrr
value: 33.24327760839221
- task:
type: Retrieval
dataset:
type: nfcorpus
name: MTEB NFCorpus
config: default
split: test
revision: None
metrics:
- type: map_at_1
value: 5.163
- type: map_at_10
value: 11.652999999999999
- type: map_at_100
value: 14.849
- type: map_at_1000
value: 16.253999999999998
- type: map_at_3
value: 8.616999999999999
- type: map_at_5
value: 10.100000000000001
- type: mrr_at_1
value: 44.272
- type: mrr_at_10
value: 52.25
- type: mrr_at_100
value: 52.761
- type: mrr_at_1000
value: 52.811
- type: mrr_at_3
value: 50.31
- type: mrr_at_5
value: 51.347
- type: ndcg_at_1
value: 42.105
- type: ndcg_at_10
value: 32.044
- type: ndcg_at_100
value: 29.763
- type: ndcg_at_1000
value: 38.585
- type: ndcg_at_3
value: 36.868
- type: ndcg_at_5
value: 35.154999999999994
- type: precision_at_1
value: 43.653
- type: precision_at_10
value: 23.622
- type: precision_at_100
value: 7.7490000000000006
- type: precision_at_1000
value: 2.054
- type: precision_at_3
value: 34.262
- type: precision_at_5
value: 30.154999999999998
- type: recall_at_1
value: 5.163
- type: recall_at_10
value: 15.478
- type: recall_at_100
value: 30.424
- type: recall_at_1000
value: 62.67
- type: recall_at_3
value: 9.615
- type: recall_at_5
value: 12.369
- task:
type: Retrieval
dataset:
type: nq
name: MTEB NQ
config: default
split: test
revision: None
metrics:
- type: map_at_1
value: 21.618000000000002
- type: map_at_10
value: 35.465
- type: map_at_100
value: 36.712
- type: map_at_1000
value: 36.757
- type: map_at_3
value: 31.189
- type: map_at_5
value: 33.537
- type: mrr_at_1
value: 24.305
- type: mrr_at_10
value: 37.653
- type: mrr_at_100
value: 38.662
- type: mrr_at_1000
value: 38.694
- type: mrr_at_3
value: 33.889
- type: mrr_at_5
value: 35.979
- type: ndcg_at_1
value: 24.305
- type: ndcg_at_10
value: 43.028
- type: ndcg_at_100
value: 48.653999999999996
- type: ndcg_at_1000
value: 49.733
- type: ndcg_at_3
value: 34.768
- type: ndcg_at_5
value: 38.753
- type: precision_at_1
value: 24.305
- type: precision_at_10
value: 7.59
- type: precision_at_100
value: 1.076
- type: precision_at_1000
value: 0.11800000000000001
- type: precision_at_3
value: 16.271
- type: precision_at_5
value: 12.068
- type: recall_at_1
value: 21.618000000000002
- type: recall_at_10
value: 63.977
- type: recall_at_100
value: 89.03999999999999
- type: recall_at_1000
value: 97.10600000000001
- type: recall_at_3
value: 42.422
- type: recall_at_5
value: 51.629000000000005
- task:
type: Retrieval
dataset:
type: quora
name: MTEB QuoraRetrieval
config: default
split: test
revision: None
metrics:
- type: map_at_1
value: 69.405
- type: map_at_10
value: 83.05
- type: map_at_100
value: 83.684
- type: map_at_1000
value: 83.70400000000001
- type: map_at_3
value: 80.08800000000001
- type: map_at_5
value: 81.937
- type: mrr_at_1
value: 79.85
- type: mrr_at_10
value: 86.369
- type: mrr_at_100
value: 86.48599999999999
- type: mrr_at_1000
value: 86.48700000000001
- type: mrr_at_3
value: 85.315
- type: mrr_at_5
value: 86.044
- type: ndcg_at_1
value: 79.86999999999999
- type: ndcg_at_10
value: 87.04499999999999
- type: ndcg_at_100
value: 88.373
- type: ndcg_at_1000
value: 88.531
- type: ndcg_at_3
value: 84.04
- type: ndcg_at_5
value: 85.684
- type: precision_at_1
value: 79.86999999999999
- type: precision_at_10
value: 13.183
- type: precision_at_100
value: 1.51
- type: precision_at_1000
value: 0.156
- type: precision_at_3
value: 36.67
- type: precision_at_5
value: 24.12
- type: recall_at_1
value: 69.405
- type: recall_at_10
value: 94.634
- type: recall_at_100
value: 99.214
- type: recall_at_1000
value: 99.958
- type: recall_at_3
value: 85.992
- type: recall_at_5
value: 90.656
- task:
type: Clustering
dataset:
type: mteb/reddit-clustering
name: MTEB RedditClustering
config: default
split: test
revision: 24640382cdbf8abc73003fb0fa6d111a705499eb
metrics:
- type: v_measure
value: 50.191676323145465
- task:
type: Clustering
dataset:
type: mteb/reddit-clustering-p2p
name: MTEB RedditClusteringP2P
config: default
split: test
revision: 282350215ef01743dc01b456c7f5241fa8937f16
metrics:
- type: v_measure
value: 56.4874020363744
- task:
type: Retrieval
dataset:
type: scidocs
name: MTEB SCIDOCS
config: default
split: test
revision: None
metrics:
- type: map_at_1
value: 4.228
- type: map_at_10
value: 11.245
- type: map_at_100
value: 13.353000000000002
- type: map_at_1000
value: 13.665
- type: map_at_3
value: 7.779999999999999
- type: map_at_5
value: 9.405
- type: mrr_at_1
value: 20.9
- type: mrr_at_10
value: 31.657999999999998
- type: mrr_at_100
value: 32.769999999999996
- type: mrr_at_1000
value: 32.833
- type: mrr_at_3
value: 28.333000000000002
- type: mrr_at_5
value: 30.043
- type: ndcg_at_1
value: 20.9
- type: ndcg_at_10
value: 19.073
- type: ndcg_at_100
value: 27.055
- type: ndcg_at_1000
value: 32.641
- type: ndcg_at_3
value: 17.483999999999998
- type: ndcg_at_5
value: 15.42
- type: precision_at_1
value: 20.9
- type: precision_at_10
value: 10.17
- type: precision_at_100
value: 2.162
- type: precision_at_1000
value: 0.35100000000000003
- type: precision_at_3
value: 16.467000000000002
- type: precision_at_5
value: 13.68
- type: recall_at_1
value: 4.228
- type: recall_at_10
value: 20.573
- type: recall_at_100
value: 43.887
- type: recall_at_1000
value: 71.22
- type: recall_at_3
value: 10.023
- type: recall_at_5
value: 13.873
- task:
type: STS
dataset:
type: mteb/sickr-sts
name: MTEB SICK-R
config: default
split: test
revision: a6ea5a8cab320b040a23452cc28066d9beae2cee
metrics:
- type: cos_sim_pearson
value: 82.77965135067481
- type: cos_sim_spearman
value: 75.85121335808076
- type: euclidean_pearson
value: 80.09115175262697
- type: euclidean_spearman
value: 75.72249155647123
- type: manhattan_pearson
value: 79.89723577351782
- type: manhattan_spearman
value: 75.49855259442387
- task:
type: STS
dataset:
type: mteb/sts12-sts
name: MTEB STS12
config: default
split: test
revision: a0d554a64d88156834ff5ae9920b964011b16384
metrics:
- type: cos_sim_pearson
value: 80.46084116030949
- type: cos_sim_spearman
value: 72.57579204392951
- type: euclidean_pearson
value: 76.39020830763684
- type: euclidean_spearman
value: 72.3718627025895
- type: manhattan_pearson
value: 76.6148833027359
- type: manhattan_spearman
value: 72.57570008442319
- task:
type: STS
dataset:
type: mteb/sts13-sts
name: MTEB STS13
config: default
split: test
revision: 7e90230a92c190f1bf69ae9002b8cea547a64cca
metrics:
- type: cos_sim_pearson
value: 80.43678068337017
- type: cos_sim_spearman
value: 82.38941154076062
- type: euclidean_pearson
value: 81.59260573633661
- type: euclidean_spearman
value: 82.31144262574114
- type: manhattan_pearson
value: 81.43266909137056
- type: manhattan_spearman
value: 82.14704293004861
- task:
type: STS
dataset:
type: mteb/sts14-sts
name: MTEB STS14
config: default
split: test
revision: 6031580fec1f6af667f0bd2da0a551cf4f0b2375
metrics:
- type: cos_sim_pearson
value: 80.73713431763163
- type: cos_sim_spearman
value: 77.97860512809388
- type: euclidean_pearson
value: 80.35755041527027
- type: euclidean_spearman
value: 78.021703511412
- type: manhattan_pearson
value: 80.24440317109162
- type: manhattan_spearman
value: 77.93165415697575
- task:
type: STS
dataset:
type: mteb/sts15-sts
name: MTEB STS15
config: default
split: test
revision: ae752c7c21bf194d8b67fd573edf7ae58183cbe3
metrics:
- type: cos_sim_pearson
value: 85.15111852351204
- type: cos_sim_spearman
value: 86.54032447238258
- type: euclidean_pearson
value: 86.14157021537433
- type: euclidean_spearman
value: 86.67537291929713
- type: manhattan_pearson
value: 86.081041854808
- type: manhattan_spearman
value: 86.61561701560558
- task:
type: STS
dataset:
type: mteb/sts16-sts
name: MTEB STS16
config: default
split: test
revision: 4d8694f8f0e0100860b497b999b3dbed754a0513
metrics:
- type: cos_sim_pearson
value: 81.34532445104026
- type: cos_sim_spearman
value: 83.31325001474116
- type: euclidean_pearson
value: 82.81892375201032
- type: euclidean_spearman
value: 83.4521695148055
- type: manhattan_pearson
value: 82.72503790526163
- type: manhattan_spearman
value: 83.37833652941349
- task:
type: STS
dataset:
type: mteb/sts17-crosslingual-sts
name: MTEB STS17 (en-en)
config: en-en
split: test
revision: af5e6fb845001ecf41f4c1e033ce921939a2a68d
metrics:
- type: cos_sim_pearson
value: 87.25463453839801
- type: cos_sim_spearman
value: 88.27655263515948
- type: euclidean_pearson
value: 88.0248334411439
- type: euclidean_spearman
value: 88.18141448876868
- type: manhattan_pearson
value: 87.8080451127279
- type: manhattan_spearman
value: 88.01028114423058
- task:
type: STS
dataset:
type: mteb/sts22-crosslingual-sts
name: MTEB STS22 (en)
config: en
split: test
revision: 6d1ba47164174a496b7fa5d3569dae26a6813b80
metrics:
- type: cos_sim_pearson
value: 63.57551045355218
- type: cos_sim_spearman
value: 66.67614095126629
- type: euclidean_pearson
value: 66.0787243112528
- type: euclidean_spearman
value: 66.83660560636939
- type: manhattan_pearson
value: 66.74684019662031
- type: manhattan_spearman
value: 67.11761598074368
- task:
type: STS
dataset:
type: mteb/stsbenchmark-sts
name: MTEB STSBenchmark
config: default
split: test
revision: b0fddb56ed78048fa8b90373c8a3cfc37b684831
metrics:
- type: cos_sim_pearson
value: 83.70881496766829
- type: cos_sim_spearman
value: 84.37803542941634
- type: euclidean_pearson
value: 84.84501245857096
- type: euclidean_spearman
value: 84.47088079741476
- type: manhattan_pearson
value: 84.77244090794765
- type: manhattan_spearman
value: 84.43307343706205
- task:
type: Reranking
dataset:
type: mteb/scidocs-reranking
name: MTEB SciDocsRR
config: default
split: test
revision: d3c5e1fc0b855ab6097bf1cda04dd73947d7caab
metrics:
- type: map
value: 81.53946254759089
- type: mrr
value: 94.68259953554072
- task:
type: Retrieval
dataset:
type: scifact
name: MTEB SciFact
config: default
split: test
revision: None
metrics:
- type: map_at_1
value: 51.817
- type: map_at_10
value: 62.339999999999996
- type: map_at_100
value: 62.88
- type: map_at_1000
value: 62.909000000000006
- type: map_at_3
value: 59.004
- type: map_at_5
value: 60.906000000000006
- type: mrr_at_1
value: 54.333
- type: mrr_at_10
value: 63.649
- type: mrr_at_100
value: 64.01
- type: mrr_at_1000
value: 64.039
- type: mrr_at_3
value: 61.056
- type: mrr_at_5
value: 62.639
- type: ndcg_at_1
value: 54.333
- type: ndcg_at_10
value: 67.509
- type: ndcg_at_100
value: 69.69999999999999
- type: ndcg_at_1000
value: 70.613
- type: ndcg_at_3
value: 61.729
- type: ndcg_at_5
value: 64.696
- type: precision_at_1
value: 54.333
- type: precision_at_10
value: 9.2
- type: precision_at_100
value: 1.043
- type: precision_at_1000
value: 0.11199999999999999
- type: precision_at_3
value: 24.0
- type: precision_at_5
value: 16.2
- type: recall_at_1
value: 51.817
- type: recall_at_10
value: 82.056
- type: recall_at_100
value: 91.667
- type: recall_at_1000
value: 99.0
- type: recall_at_3
value: 66.717
- type: recall_at_5
value: 74.17200000000001
- task:
type: PairClassification
dataset:
type: mteb/sprintduplicatequestions-pairclassification
name: MTEB SprintDuplicateQuestions
config: default
split: test
revision: d66bd1f72af766a5cc4b0ca5e00c162f89e8cc46
metrics:
- type: cos_sim_accuracy
value: 99.82475247524752
- type: cos_sim_ap
value: 95.4781199603258
- type: cos_sim_f1
value: 91.16186693147964
- type: cos_sim_precision
value: 90.53254437869822
- type: cos_sim_recall
value: 91.8
- type: dot_accuracy
value: 99.75049504950495
- type: dot_ap
value: 93.05183539809457
- type: dot_f1
value: 87.31117824773412
- type: dot_precision
value: 87.93103448275862
- type: dot_recall
value: 86.7
- type: euclidean_accuracy
value: 99.82475247524752
- type: euclidean_ap
value: 95.38547978154382
- type: euclidean_f1
value: 91.16325511732403
- type: euclidean_precision
value: 91.02691924227318
- type: euclidean_recall
value: 91.3
- type: manhattan_accuracy
value: 99.82574257425742
- type: manhattan_ap
value: 95.47237521890308
- type: manhattan_f1
value: 91.27849355797821
- type: manhattan_precision
value: 90.47151277013754
- type: manhattan_recall
value: 92.10000000000001
- type: max_accuracy
value: 99.82574257425742
- type: max_ap
value: 95.4781199603258
- type: max_f1
value: 91.27849355797821
- task:
type: Clustering
dataset:
type: mteb/stackexchange-clustering
name: MTEB StackExchangeClustering
config: default
split: test
revision: 6cbc1f7b2bc0622f2e39d2c77fa502909748c259
metrics:
- type: v_measure
value: 57.542169376331245
- task:
type: Clustering
dataset:
type: mteb/stackexchange-clustering-p2p
name: MTEB StackExchangeClusteringP2P
config: default
split: test
revision: 815ca46b2622cec33ccafc3735d572c266efdb44
metrics:
- type: v_measure
value: 35.74399302634387
- task:
type: Reranking
dataset:
type: mteb/stackoverflowdupquestions-reranking
name: MTEB StackOverflowDupQuestions
config: default
split: test
revision: e185fbe320c72810689fc5848eb6114e1ef5ec69
metrics:
- type: map
value: 49.65076347632749
- type: mrr
value: 50.418099057804945
- task:
type: Summarization
dataset:
type: mteb/summeval
name: MTEB SummEval
config: default
split: test
revision: cda12ad7615edc362dbf25a00fdd61d3b1eaf93c
metrics:
- type: cos_sim_pearson
value: 29.73997756592847
- type: cos_sim_spearman
value: 29.465208011593308
- type: dot_pearson
value: 24.83735342474541
- type: dot_spearman
value: 26.005180528584855
- task:
type: Retrieval
dataset:
type: trec-covid
name: MTEB TRECCOVID
config: default
split: test
revision: None
metrics:
- type: map_at_1
value: 0.208
- type: map_at_10
value: 1.434
- type: map_at_100
value: 7.829
- type: map_at_1000
value: 19.807
- type: map_at_3
value: 0.549
- type: map_at_5
value: 0.8330000000000001
- type: mrr_at_1
value: 78.0
- type: mrr_at_10
value: 85.35199999999999
- type: mrr_at_100
value: 85.673
- type: mrr_at_1000
value: 85.673
- type: mrr_at_3
value: 84.667
- type: mrr_at_5
value: 85.06700000000001
- type: ndcg_at_1
value: 72.0
- type: ndcg_at_10
value: 59.214999999999996
- type: ndcg_at_100
value: 44.681
- type: ndcg_at_1000
value: 43.035000000000004
- type: ndcg_at_3
value: 66.53099999999999
- type: ndcg_at_5
value: 63.23
- type: precision_at_1
value: 78.0
- type: precision_at_10
value: 62.4
- type: precision_at_100
value: 45.76
- type: precision_at_1000
value: 19.05
- type: precision_at_3
value: 71.333
- type: precision_at_5
value: 67.2
- type: recall_at_1
value: 0.208
- type: recall_at_10
value: 1.6580000000000001
- type: recall_at_100
value: 11.324
- type: recall_at_1000
value: 41.537
- type: recall_at_3
value: 0.579
- type: recall_at_5
value: 0.8959999999999999
- task:
type: Retrieval
dataset:
type: webis-touche2020
name: MTEB Touche2020
config: default
split: test
revision: None
metrics:
- type: map_at_1
value: 2.442
- type: map_at_10
value: 8.863
- type: map_at_100
value: 14.606
- type: map_at_1000
value: 16.258
- type: map_at_3
value: 4.396
- type: map_at_5
value: 6.199000000000001
- type: mrr_at_1
value: 30.612000000000002
- type: mrr_at_10
value: 43.492
- type: mrr_at_100
value: 44.557
- type: mrr_at_1000
value: 44.557
- type: mrr_at_3
value: 40.816
- type: mrr_at_5
value: 42.143
- type: ndcg_at_1
value: 25.509999999999998
- type: ndcg_at_10
value: 22.076
- type: ndcg_at_100
value: 34.098
- type: ndcg_at_1000
value: 46.265
- type: ndcg_at_3
value: 24.19
- type: ndcg_at_5
value: 23.474
- type: precision_at_1
value: 30.612000000000002
- type: precision_at_10
value: 19.796
- type: precision_at_100
value: 7.286
- type: precision_at_1000
value: 1.5310000000000001
- type: precision_at_3
value: 25.85
- type: precision_at_5
value: 24.490000000000002
- type: recall_at_1
value: 2.442
- type: recall_at_10
value: 15.012
- type: recall_at_100
value: 45.865
- type: recall_at_1000
value: 82.958
- type: recall_at_3
value: 5.731
- type: recall_at_5
value: 9.301
- task:
type: Classification
dataset:
type: mteb/toxic_conversations_50k
name: MTEB ToxicConversationsClassification
config: default
split: test
revision: d7c0de2777da35d6aae2200a62c6e0e5af397c4c
metrics:
- type: accuracy
value: 70.974
- type: ap
value: 14.534996211286682
- type: f1
value: 54.785946183399005
- task:
type: Classification
dataset:
type: mteb/tweet_sentiment_extraction
name: MTEB TweetSentimentExtractionClassification
config: default
split: test
revision: d604517c81ca91fe16a244d1248fc021f9ecee7a
metrics:
- type: accuracy
value: 58.56819468024901
- type: f1
value: 58.92391487111204
- task:
type: Clustering
dataset:
type: mteb/twentynewsgroups-clustering
name: MTEB TwentyNewsgroupsClustering
config: default
split: test
revision: 6125ec4e24fa026cec8a478383ee943acfbd5449
metrics:
- type: v_measure
value: 43.273202335218194
- task:
type: PairClassification
dataset:
type: mteb/twittersemeval2015-pairclassification
name: MTEB TwitterSemEval2015
config: default
split: test
revision: 70970daeab8776df92f5ea462b6173c0b46fd2d1
metrics:
- type: cos_sim_accuracy
value: 84.37742146986946
- type: cos_sim_ap
value: 68.1684129575579
- type: cos_sim_f1
value: 64.93475108748189
- type: cos_sim_precision
value: 59.89745876058849
- type: cos_sim_recall
value: 70.89709762532982
- type: dot_accuracy
value: 80.49710913750968
- type: dot_ap
value: 54.699790073944186
- type: dot_f1
value: 54.45130013221684
- type: dot_precision
value: 46.74612183125236
- type: dot_recall
value: 65.19788918205805
- type: euclidean_accuracy
value: 84.5085533766466
- type: euclidean_ap
value: 68.38835695236224
- type: euclidean_f1
value: 65.3391121002694
- type: euclidean_precision
value: 58.75289656625237
- type: euclidean_recall
value: 73.58839050131925
- type: manhattan_accuracy
value: 84.40126363473803
- type: manhattan_ap
value: 68.09539181555348
- type: manhattan_f1
value: 64.99028182701653
- type: manhattan_precision
value: 60.22062134173795
- type: manhattan_recall
value: 70.58047493403694
- type: max_accuracy
value: 84.5085533766466
- type: max_ap
value: 68.38835695236224
- type: max_f1
value: 65.3391121002694
- task:
type: PairClassification
dataset:
type: mteb/twitterurlcorpus-pairclassification
name: MTEB TwitterURLCorpus
config: default
split: test
revision: 8b6510b0b1fa4e4c4f879467980e9be563ec1cdf
metrics:
- type: cos_sim_accuracy
value: 88.34167733923235
- type: cos_sim_ap
value: 84.84136381147736
- type: cos_sim_f1
value: 77.01434980904001
- type: cos_sim_precision
value: 74.27937915742794
- type: cos_sim_recall
value: 79.95842315983985
- type: dot_accuracy
value: 85.06422944075756
- type: dot_ap
value: 76.49446747522325
- type: dot_f1
value: 71.11606520830432
- type: dot_precision
value: 64.93638676844785
- type: dot_recall
value: 78.59562673236834
- type: euclidean_accuracy
value: 88.45810532852097
- type: euclidean_ap
value: 84.91526721863501
- type: euclidean_f1
value: 77.04399001750662
- type: euclidean_precision
value: 74.62298867162133
- type: euclidean_recall
value: 79.62734832152756
- type: manhattan_accuracy
value: 88.46004579500912
- type: manhattan_ap
value: 84.81590026238194
- type: manhattan_f1
value: 76.97804626491822
- type: manhattan_precision
value: 73.79237288135593
- type: manhattan_recall
value: 80.45118570988605
- type: max_accuracy
value: 88.46004579500912
- type: max_ap
value: 84.91526721863501
- type: max_f1
value: 77.04399001750662
pipeline_tag: sentence-similarity
tags:
- sentence-transformers
- feature-extraction
- sentence-similarity
- transformers
- mteb
---
# {gte-tiny}
This is a [sentence-transformers](https://www.SBERT.net) model: It maps sentences & paragraphs to a 384 dimensional dense vector space and can be used for tasks like clustering or semantic search.
It is distilled from `thenlper/gte-small`, with comparable (slightly worse) performance at around half the size.
<!--- Describe your model here -->
## Usage (Sentence-Transformers)
Using this model becomes easy when you have [sentence-transformers](https://www.SBERT.net) installed:
```
pip install -U sentence-transformers
```
Then you can use the model like this:
```python
from sentence_transformers import SentenceTransformer
sentences = ["This is an example sentence", "Each sentence is converted"]
model = SentenceTransformer('{MODEL_NAME}')
embeddings = model.encode(sentences)
print(embeddings)
```
## Usage (HuggingFace Transformers)
Without [sentence-transformers](https://www.SBERT.net), you can use the model like this: First, you pass your input through the transformer model, then you have to apply the right pooling-operation on-top of the contextualized word embeddings.
```python
from transformers import AutoTokenizer, AutoModel
import torch
#Mean Pooling - Take attention mask into account for correct averaging
def mean_pooling(model_output, attention_mask):
token_embeddings = model_output[0] #First element of model_output contains all token embeddings
input_mask_expanded = attention_mask.unsqueeze(-1).expand(token_embeddings.size()).float()
return torch.sum(token_embeddings * input_mask_expanded, 1) / torch.clamp(input_mask_expanded.sum(1), min=1e-9)
# Sentences we want sentence embeddings for
sentences = ['This is an example sentence', 'Each sentence is converted']
# Load model from HuggingFace Hub
tokenizer = AutoTokenizer.from_pretrained('{MODEL_NAME}')
model = AutoModel.from_pretrained('{MODEL_NAME}')
# Tokenize sentences
encoded_input = tokenizer(sentences, padding=True, truncation=True, return_tensors='pt')
# Compute token embeddings
with torch.no_grad():
model_output = model(**encoded_input)
# Perform pooling. In this case, mean pooling.
sentence_embeddings = mean_pooling(model_output, encoded_input['attention_mask'])
print("Sentence embeddings:")
print(sentence_embeddings)
```
## Evaluation Results
<!--- Describe how your model was evaluated -->
For an automated evaluation of this model, see the *Sentence Embeddings Benchmark*: [https://seb.sbert.net](https://seb.sbert.net?model_name={MODEL_NAME})
## Full Model Architecture
```
SentenceTransformer(
(0): Transformer({'max_seq_length': 512, 'do_lower_case': False}) with Transformer model: BertModel
(1): Pooling({'word_embedding_dimension': 384, 'pooling_mode_cls_token': False, 'pooling_mode_mean_tokens': True, 'pooling_mode_max_tokens': False, 'pooling_mode_mean_sqrt_len_tokens': False})
)
```
## Citing & Authors
<!--- Describe where people can find more information --> |
citizenlab/twitter-xlm-roberta-base-sentiment-finetunned | citizenlab | "2022-12-02T13:49:38Z" | 12,586 | 26 | transformers | [
"transformers",
"pytorch",
"xlm-roberta",
"text-classification",
"en",
"nl",
"fr",
"pt",
"it",
"es",
"de",
"da",
"pl",
"af",
"dataset:jigsaw_toxicity_pred",
"autotrain_compatible",
"endpoints_compatible",
"region:us"
] | text-classification | "2022-03-02T23:29:05Z" | ---
pipeline_type: "text-classification"
widget:
- text: "this is a lovely message"
example_title: "Example 1"
multi_class: false
- text: "you are an idiot and you and your family should go back to your country"
example_title: "Example 2"
multi_class: false
language:
- en
- nl
- fr
- pt
- it
- es
- de
- da
- pl
- af
datasets:
- jigsaw_toxicity_pred
metrics:
- F1 Accuracy
---
# citizenlab/twitter-xlm-roberta-base-sentiment-finetunned
This is multilingual XLM-Roberta model sequence classifier fine tunned and based on [Cardiff NLP Group](cardiffnlp/twitter-roberta-base-sentiment) sentiment classification model.
## How to use it
```python
from transformers import pipeline
model_path = "citizenlab/twitter-xlm-roberta-base-sentiment-finetunned"
sentiment_classifier = pipeline("text-classification", model=model_path, tokenizer=model_path)
sentiment_classifier("this is a lovely message")
> [{'label': 'Positive', 'score': 0.9918450713157654}]
sentiment_classifier("you are an idiot and you and your family should go back to your country")
> [{'label': 'Negative', 'score': 0.9849833846092224}]
```
## Evaluation
```
precision recall f1-score support
Negative 0.57 0.14 0.23 28
Neutral 0.78 0.94 0.86 132
Positive 0.89 0.80 0.85 51
accuracy 0.80 211
macro avg 0.75 0.63 0.64 211
weighted avg 0.78 0.80 0.77 211
```
|
mradermacher/Domain-Fusion-L3-8B-i1-GGUF | mradermacher | "2024-06-20T17:28:46Z" | 12,582 | 0 | transformers | [
"transformers",
"gguf",
"mergekit",
"merge",
"en",
"base_model:Nitral-AI/Domain-Fusion-L3-8B",
"license:other",
"endpoints_compatible",
"region:us"
] | null | "2024-06-20T16:14:06Z" | ---
base_model: Nitral-AI/Domain-Fusion-L3-8B
language:
- en
library_name: transformers
license: other
quantized_by: mradermacher
tags:
- mergekit
- merge
---
## About
<!-- ### quantize_version: 2 -->
<!-- ### output_tensor_quantised: 1 -->
<!-- ### convert_type: hf -->
<!-- ### vocab_type: -->
<!-- ### tags: nicoboss -->
weighted/imatrix quants of https://huggingface.co/Nitral-AI/Domain-Fusion-L3-8B
<!-- provided-files -->
static quants are available at https://huggingface.co/mradermacher/Domain-Fusion-L3-8B-GGUF
## Usage
If you are unsure how to use GGUF files, refer to one of [TheBloke's
READMEs](https://huggingface.co/TheBloke/KafkaLM-70B-German-V0.1-GGUF) for
more details, including on how to concatenate multi-part files.
## Provided Quants
(sorted by size, not necessarily quality. IQ-quants are often preferable over similar sized non-IQ quants)
| Link | Type | Size/GB | Notes |
|:-----|:-----|--------:|:------|
| [GGUF](https://huggingface.co/mradermacher/Domain-Fusion-L3-8B-i1-GGUF/resolve/main/Domain-Fusion-L3-8B.i1-IQ1_S.gguf) | i1-IQ1_S | 2.1 | for the desperate |
| [GGUF](https://huggingface.co/mradermacher/Domain-Fusion-L3-8B-i1-GGUF/resolve/main/Domain-Fusion-L3-8B.i1-IQ1_M.gguf) | i1-IQ1_M | 2.3 | mostly desperate |
| [GGUF](https://huggingface.co/mradermacher/Domain-Fusion-L3-8B-i1-GGUF/resolve/main/Domain-Fusion-L3-8B.i1-IQ2_XXS.gguf) | i1-IQ2_XXS | 2.5 | |
| [GGUF](https://huggingface.co/mradermacher/Domain-Fusion-L3-8B-i1-GGUF/resolve/main/Domain-Fusion-L3-8B.i1-IQ2_XS.gguf) | i1-IQ2_XS | 2.7 | |
| [GGUF](https://huggingface.co/mradermacher/Domain-Fusion-L3-8B-i1-GGUF/resolve/main/Domain-Fusion-L3-8B.i1-IQ2_S.gguf) | i1-IQ2_S | 2.9 | |
| [GGUF](https://huggingface.co/mradermacher/Domain-Fusion-L3-8B-i1-GGUF/resolve/main/Domain-Fusion-L3-8B.i1-IQ2_M.gguf) | i1-IQ2_M | 3.0 | |
| [GGUF](https://huggingface.co/mradermacher/Domain-Fusion-L3-8B-i1-GGUF/resolve/main/Domain-Fusion-L3-8B.i1-Q2_K.gguf) | i1-Q2_K | 3.3 | IQ3_XXS probably better |
| [GGUF](https://huggingface.co/mradermacher/Domain-Fusion-L3-8B-i1-GGUF/resolve/main/Domain-Fusion-L3-8B.i1-IQ3_XXS.gguf) | i1-IQ3_XXS | 3.4 | lower quality |
| [GGUF](https://huggingface.co/mradermacher/Domain-Fusion-L3-8B-i1-GGUF/resolve/main/Domain-Fusion-L3-8B.i1-IQ3_XS.gguf) | i1-IQ3_XS | 3.6 | |
| [GGUF](https://huggingface.co/mradermacher/Domain-Fusion-L3-8B-i1-GGUF/resolve/main/Domain-Fusion-L3-8B.i1-Q3_K_S.gguf) | i1-Q3_K_S | 3.8 | IQ3_XS probably better |
| [GGUF](https://huggingface.co/mradermacher/Domain-Fusion-L3-8B-i1-GGUF/resolve/main/Domain-Fusion-L3-8B.i1-IQ3_S.gguf) | i1-IQ3_S | 3.8 | beats Q3_K* |
| [GGUF](https://huggingface.co/mradermacher/Domain-Fusion-L3-8B-i1-GGUF/resolve/main/Domain-Fusion-L3-8B.i1-IQ3_M.gguf) | i1-IQ3_M | 3.9 | |
| [GGUF](https://huggingface.co/mradermacher/Domain-Fusion-L3-8B-i1-GGUF/resolve/main/Domain-Fusion-L3-8B.i1-Q3_K_M.gguf) | i1-Q3_K_M | 4.1 | IQ3_S probably better |
| [GGUF](https://huggingface.co/mradermacher/Domain-Fusion-L3-8B-i1-GGUF/resolve/main/Domain-Fusion-L3-8B.i1-Q3_K_L.gguf) | i1-Q3_K_L | 4.4 | IQ3_M probably better |
| [GGUF](https://huggingface.co/mradermacher/Domain-Fusion-L3-8B-i1-GGUF/resolve/main/Domain-Fusion-L3-8B.i1-IQ4_XS.gguf) | i1-IQ4_XS | 4.5 | |
| [GGUF](https://huggingface.co/mradermacher/Domain-Fusion-L3-8B-i1-GGUF/resolve/main/Domain-Fusion-L3-8B.i1-Q4_0.gguf) | i1-Q4_0 | 4.8 | fast, low quality |
| [GGUF](https://huggingface.co/mradermacher/Domain-Fusion-L3-8B-i1-GGUF/resolve/main/Domain-Fusion-L3-8B.i1-Q4_K_S.gguf) | i1-Q4_K_S | 4.8 | optimal size/speed/quality |
| [GGUF](https://huggingface.co/mradermacher/Domain-Fusion-L3-8B-i1-GGUF/resolve/main/Domain-Fusion-L3-8B.i1-Q4_K_M.gguf) | i1-Q4_K_M | 5.0 | fast, recommended |
| [GGUF](https://huggingface.co/mradermacher/Domain-Fusion-L3-8B-i1-GGUF/resolve/main/Domain-Fusion-L3-8B.i1-Q5_K_S.gguf) | i1-Q5_K_S | 5.7 | |
| [GGUF](https://huggingface.co/mradermacher/Domain-Fusion-L3-8B-i1-GGUF/resolve/main/Domain-Fusion-L3-8B.i1-Q5_K_M.gguf) | i1-Q5_K_M | 5.8 | |
| [GGUF](https://huggingface.co/mradermacher/Domain-Fusion-L3-8B-i1-GGUF/resolve/main/Domain-Fusion-L3-8B.i1-Q6_K.gguf) | i1-Q6_K | 6.7 | practically like static Q6_K |
Here is a handy graph by ikawrakow comparing some lower-quality quant
types (lower is better):

And here are Artefact2's thoughts on the matter:
https://gist.github.com/Artefact2/b5f810600771265fc1e39442288e8ec9
## FAQ / Model Request
See https://huggingface.co/mradermacher/model_requests for some answers to
questions you might have and/or if you want some other model quantized.
## Thanks
I thank my company, [nethype GmbH](https://www.nethype.de/), for letting
me use its servers and providing upgrades to my workstation to enable
this work in my free time. Additional thanks to [@nicoboss](https://huggingface.co/nicoboss) for giving me access to his hardware for calculating the imatrix for these quants.
<!-- end -->
|
mradermacher/Breeze-7B-Instruct-v1_0-GGUF | mradermacher | "2024-06-28T16:52:27Z" | 12,581 | 0 | transformers | [
"transformers",
"gguf",
"zh",
"en",
"base_model:MediaTek-Research/Breeze-7B-Instruct-v1_0",
"license:apache-2.0",
"endpoints_compatible",
"region:us"
] | null | "2024-06-28T16:02:41Z" | ---
base_model: MediaTek-Research/Breeze-7B-Instruct-v1_0
language:
- zh
- en
library_name: transformers
license: apache-2.0
quantized_by: mradermacher
---
## About
<!-- ### quantize_version: 2 -->
<!-- ### output_tensor_quantised: 1 -->
<!-- ### convert_type: hf -->
<!-- ### vocab_type: -->
<!-- ### tags: -->
static quants of https://huggingface.co/MediaTek-Research/Breeze-7B-Instruct-v1_0
<!-- provided-files -->
weighted/imatrix quants are available at https://huggingface.co/mradermacher/Breeze-7B-Instruct-v1_0-i1-GGUF
## Usage
If you are unsure how to use GGUF files, refer to one of [TheBloke's
READMEs](https://huggingface.co/TheBloke/KafkaLM-70B-German-V0.1-GGUF) for
more details, including on how to concatenate multi-part files.
## Provided Quants
(sorted by size, not necessarily quality. IQ-quants are often preferable over similar sized non-IQ quants)
| Link | Type | Size/GB | Notes |
|:-----|:-----|--------:|:------|
| [GGUF](https://huggingface.co/mradermacher/Breeze-7B-Instruct-v1_0-GGUF/resolve/main/Breeze-7B-Instruct-v1_0.Q2_K.gguf) | Q2_K | 3.0 | |
| [GGUF](https://huggingface.co/mradermacher/Breeze-7B-Instruct-v1_0-GGUF/resolve/main/Breeze-7B-Instruct-v1_0.IQ3_XS.gguf) | IQ3_XS | 3.3 | |
| [GGUF](https://huggingface.co/mradermacher/Breeze-7B-Instruct-v1_0-GGUF/resolve/main/Breeze-7B-Instruct-v1_0.Q3_K_S.gguf) | Q3_K_S | 3.4 | |
| [GGUF](https://huggingface.co/mradermacher/Breeze-7B-Instruct-v1_0-GGUF/resolve/main/Breeze-7B-Instruct-v1_0.IQ3_S.gguf) | IQ3_S | 3.4 | beats Q3_K* |
| [GGUF](https://huggingface.co/mradermacher/Breeze-7B-Instruct-v1_0-GGUF/resolve/main/Breeze-7B-Instruct-v1_0.IQ3_M.gguf) | IQ3_M | 3.5 | |
| [GGUF](https://huggingface.co/mradermacher/Breeze-7B-Instruct-v1_0-GGUF/resolve/main/Breeze-7B-Instruct-v1_0.Q3_K_M.gguf) | Q3_K_M | 3.8 | lower quality |
| [GGUF](https://huggingface.co/mradermacher/Breeze-7B-Instruct-v1_0-GGUF/resolve/main/Breeze-7B-Instruct-v1_0.Q3_K_L.gguf) | Q3_K_L | 4.1 | |
| [GGUF](https://huggingface.co/mradermacher/Breeze-7B-Instruct-v1_0-GGUF/resolve/main/Breeze-7B-Instruct-v1_0.IQ4_XS.gguf) | IQ4_XS | 4.2 | |
| [GGUF](https://huggingface.co/mradermacher/Breeze-7B-Instruct-v1_0-GGUF/resolve/main/Breeze-7B-Instruct-v1_0.Q4_K_S.gguf) | Q4_K_S | 4.4 | fast, recommended |
| [GGUF](https://huggingface.co/mradermacher/Breeze-7B-Instruct-v1_0-GGUF/resolve/main/Breeze-7B-Instruct-v1_0.Q4_K_M.gguf) | Q4_K_M | 4.6 | fast, recommended |
| [GGUF](https://huggingface.co/mradermacher/Breeze-7B-Instruct-v1_0-GGUF/resolve/main/Breeze-7B-Instruct-v1_0.Q5_K_S.gguf) | Q5_K_S | 5.3 | |
| [GGUF](https://huggingface.co/mradermacher/Breeze-7B-Instruct-v1_0-GGUF/resolve/main/Breeze-7B-Instruct-v1_0.Q5_K_M.gguf) | Q5_K_M | 5.4 | |
| [GGUF](https://huggingface.co/mradermacher/Breeze-7B-Instruct-v1_0-GGUF/resolve/main/Breeze-7B-Instruct-v1_0.Q6_K.gguf) | Q6_K | 6.2 | very good quality |
| [GGUF](https://huggingface.co/mradermacher/Breeze-7B-Instruct-v1_0-GGUF/resolve/main/Breeze-7B-Instruct-v1_0.Q8_0.gguf) | Q8_0 | 8.1 | fast, best quality |
| [GGUF](https://huggingface.co/mradermacher/Breeze-7B-Instruct-v1_0-GGUF/resolve/main/Breeze-7B-Instruct-v1_0.f16.gguf) | f16 | 15.1 | 16 bpw, overkill |
Here is a handy graph by ikawrakow comparing some lower-quality quant
types (lower is better):

And here are Artefact2's thoughts on the matter:
https://gist.github.com/Artefact2/b5f810600771265fc1e39442288e8ec9
## FAQ / Model Request
See https://huggingface.co/mradermacher/model_requests for some answers to
questions you might have and/or if you want some other model quantized.
## Thanks
I thank my company, [nethype GmbH](https://www.nethype.de/), for letting
me use its servers and providing upgrades to my workstation to enable
this work in my free time.
<!-- end -->
|
Lykon/dreamshaper-xl-1-0 | Lykon | "2023-08-26T16:44:49Z" | 12,579 | 31 | diffusers | [
"diffusers",
"safetensors",
"stable-diffusion",
"stable-diffusion-diffusers",
"stable-diffusion-xl",
"text-to-image",
"art",
"artistic",
"anime",
"dreamshaper",
"en",
"license:openrail++",
"autotrain_compatible",
"endpoints_compatible",
"diffusers:StableDiffusionXLPipeline",
"region:us"
] | text-to-image | "2023-08-26T16:44:49Z" | ---
language:
- en
license: openrail++
tags:
- stable-diffusion
- stable-diffusion-diffusers
- stable-diffusion-xl
- text-to-image
- art
- artistic
- diffusers
- anime
- dreamshaper
duplicated_from: lykon-models/dreamshaper-xl-1-0
---
# Dreamshaper SDXL-1-0
`lykon-models/dreamshaper-xl-1-0` is a Stable Diffusion model that has been fine-tuned on [stabilityai/stable-diffusion-xl-base-1.0](https://huggingface.co/stabilityai/stable-diffusion-xl-base-1.0).
Please consider supporting me:
- on [Patreon](https://www.patreon.com/Lykon275)
- or [buy me a coffee](https://snipfeed.co/lykon)
## Diffusers
For more general information on how to run text-to-image models with 🧨 Diffusers, see [the docs](https://huggingface.co/docs/diffusers/using-diffusers/conditional_image_generation).
1. Installation
```
pip install diffusers transformers accelerate
```
2. Run
```py
from diffusers import AutoPipelineForText2Image, DEISMultistepScheduler
import torch
pipe = AutoPipelineForText2Image.from_pretrained('lykon-models/dreamshaper-xl-1-0', torch_dtype=torch.float16, variant="fp16")
pipe.scheduler = DEISMultistepScheduler.from_config(pipe.scheduler.config)
pipe = pipe.to("cuda")
prompt = "portrait photo of muscular bearded guy in a worn mech suit, light bokeh, intricate, steel metal, elegant, sharp focus, soft lighting, vibrant colors"
generator = torch.manual_seed(0)
image = pipe(prompt, num_inference_steps=25).images[0]
image.save("./image.png")
```

|
Qwen/Qwen1.5-7B-Chat-GGUF | Qwen | "2024-04-09T16:49:52Z" | 12,578 | 64 | null | [
"gguf",
"chat",
"text-generation",
"en",
"license:other",
"region:us"
] | text-generation | "2024-02-03T11:53:25Z" | ---
license: other
license_name: tongyi-qianwen
license_link: https://huggingface.co/Qwen/Qwen1.5-7B-Chat-GGUF/blob/main/LICENSE
language:
- en
pipeline_tag: text-generation
tags:
- chat
---
# Qwen1.5-7B-Chat-GGUF
## Introduction
Qwen1.5 is the beta version of Qwen2, a transformer-based decoder-only language model pretrained on a large amount of data. In comparison with the previous released Qwen, the improvements include:
* 8 model sizes, including 0.5B, 1.8B, 4B, 7B, 14B, 32B and 72B dense models, and an MoE model of 14B with 2.7B activated;
* Significant performance improvement in human preference for chat models;
* Multilingual support of both base and chat models;
* Stable support of 32K context length for models of all sizes
* No need of `trust_remote_code`.
For more details, please refer to our [blog post](https://qwenlm.github.io/blog/qwen1.5/) and [GitHub repo](https://github.com/QwenLM/Qwen1.5).
In this repo, we provide quantized models in the GGUF formats, including `q2_k`, `q3_k_m`, `q4_0`, `q4_k_m`, `q5_0`, `q5_k_m`, `q6_k` and `q8_0`.
To demonstrate their model quality, we follow [`llama.cpp`](https://github.com/ggerganov/llama.cpp) to evaluate their perplexity on wiki test set. Results are shown below:
|Size | fp16 | q8_0 | q6_k | q5_k_m | q5_0 | q4_k_m | q4_0 | q3_k_m | q2_k |
|--------|---------|---------|---------|---------|---------|---------|---------|---------|---------|
|0.5B | 34.20 | 34.22 | 34.31 | 33.80 | 34.02 | 34.27 | 36.74 | 38.25 | 62.14 |
|1.8B | 15.99 | 15.99 | 15.99 | 16.09 | 16.01 | 16.22 | 16.54 | 17.03 | 19.99 |
|4B | 13.20 | 13.21 | 13.28 | 13.24 | 13.27 | 13.61 | 13.44 | 13.67 | 15.65 |
|7B | 14.21 | 14.24 | 14.35 | 14.32 | 14.12 | 14.35 | 14.47 | 15.11 | 16.57 |
|14B | 10.91 | 10.91 | 10.93 | 10.98 | 10.88 | 10.92 | 10.92 | 11.24 | 12.27 |
|32B | 8.87 | 8.89 | 8.91 | 8.94 | 8.93 | 8.96 | 9.17 | 9.14 | 10.51 |
|72B | 7.97 | 7.99 | 7.99 | 7.99 | 8.01 | 8.00 | 8.01 | 8.06 | 8.63 |
## Model Details
Qwen1.5 is a language model series including decoder language models of different model sizes. For each size, we release the base language model and the aligned chat model. It is based on the Transformer architecture with SwiGLU activation, attention QKV bias, group query attention, mixture of sliding window attention and full attention, etc. Additionally, we have an improved tokenizer adaptive to multiple natural languages and codes. For the beta version, temporarily we did not include GQA (except for 32B) and the mixture of SWA and full attention.
## Training details
We pretrained the models with a large amount of data, and we post-trained the models with both supervised finetuning and direct preference optimization.
## Requirements
We advise you to clone [`llama.cpp`](https://github.com/ggerganov/llama.cpp) and install it following the official guide.
## How to use
Cloning the repo may be inefficient, and thus you can manually download the GGUF file that you need or use `huggingface-cli` (`pip install huggingface_hub`) as shown below:
```shell
huggingface-cli download Qwen/Qwen1.5-7B-Chat-GGUF qwen1_5-7b-chat-q5_k_m.gguf --local-dir . --local-dir-use-symlinks False
```
We demonstrate how to use `llama.cpp` to run Qwen1.5:
```shell
./main -m qwen1_5-7b-chat-q5_k_m.gguf -n 512 --color -i -cml -f prompts/chat-with-qwen.txt
```
## Citation
If you find our work helpful, feel free to give us a cite.
```
@article{qwen,
title={Qwen Technical Report},
author={Jinze Bai and Shuai Bai and Yunfei Chu and Zeyu Cui and Kai Dang and Xiaodong Deng and Yang Fan and Wenbin Ge and Yu Han and Fei Huang and Binyuan Hui and Luo Ji and Mei Li and Junyang Lin and Runji Lin and Dayiheng Liu and Gao Liu and Chengqiang Lu and Keming Lu and Jianxin Ma and Rui Men and Xingzhang Ren and Xuancheng Ren and Chuanqi Tan and Sinan Tan and Jianhong Tu and Peng Wang and Shijie Wang and Wei Wang and Shengguang Wu and Benfeng Xu and Jin Xu and An Yang and Hao Yang and Jian Yang and Shusheng Yang and Yang Yao and Bowen Yu and Hongyi Yuan and Zheng Yuan and Jianwei Zhang and Xingxuan Zhang and Yichang Zhang and Zhenru Zhang and Chang Zhou and Jingren Zhou and Xiaohuan Zhou and Tianhang Zhu},
journal={arXiv preprint arXiv:2309.16609},
year={2023}
}
```
|
mradermacher/black_stheno_8B_v1-i1-GGUF | mradermacher | "2024-06-26T20:27:36Z" | 12,577 | 0 | transformers | [
"transformers",
"gguf",
"mergekit",
"merge",
"en",
"base_model:banelingz/black_stheno_8B_v1",
"endpoints_compatible",
"region:us"
] | null | "2024-06-24T23:39:08Z" | ---
base_model: banelingz/black_stheno_8B_v1
language:
- en
library_name: transformers
quantized_by: mradermacher
tags:
- mergekit
- merge
---
## About
<!-- ### quantize_version: 2 -->
<!-- ### output_tensor_quantised: 1 -->
<!-- ### convert_type: hf -->
<!-- ### vocab_type: -->
<!-- ### tags: nicoboss -->
weighted/imatrix quants of https://huggingface.co/banelingz/black_stheno_8B_v1
<!-- provided-files -->
static quants are available at https://huggingface.co/mradermacher/black_stheno_8B_v1-GGUF
## Usage
If you are unsure how to use GGUF files, refer to one of [TheBloke's
READMEs](https://huggingface.co/TheBloke/KafkaLM-70B-German-V0.1-GGUF) for
more details, including on how to concatenate multi-part files.
## Provided Quants
(sorted by size, not necessarily quality. IQ-quants are often preferable over similar sized non-IQ quants)
| Link | Type | Size/GB | Notes |
|:-----|:-----|--------:|:------|
| [GGUF](https://huggingface.co/mradermacher/black_stheno_8B_v1-i1-GGUF/resolve/main/black_stheno_8B_v1.i1-IQ1_S.gguf) | i1-IQ1_S | 2.1 | for the desperate |
| [GGUF](https://huggingface.co/mradermacher/black_stheno_8B_v1-i1-GGUF/resolve/main/black_stheno_8B_v1.i1-IQ1_M.gguf) | i1-IQ1_M | 2.3 | mostly desperate |
| [GGUF](https://huggingface.co/mradermacher/black_stheno_8B_v1-i1-GGUF/resolve/main/black_stheno_8B_v1.i1-IQ2_XXS.gguf) | i1-IQ2_XXS | 2.5 | |
| [GGUF](https://huggingface.co/mradermacher/black_stheno_8B_v1-i1-GGUF/resolve/main/black_stheno_8B_v1.i1-IQ2_XS.gguf) | i1-IQ2_XS | 2.7 | |
| [GGUF](https://huggingface.co/mradermacher/black_stheno_8B_v1-i1-GGUF/resolve/main/black_stheno_8B_v1.i1-IQ2_S.gguf) | i1-IQ2_S | 2.9 | |
| [GGUF](https://huggingface.co/mradermacher/black_stheno_8B_v1-i1-GGUF/resolve/main/black_stheno_8B_v1.i1-IQ2_M.gguf) | i1-IQ2_M | 3.0 | |
| [GGUF](https://huggingface.co/mradermacher/black_stheno_8B_v1-i1-GGUF/resolve/main/black_stheno_8B_v1.i1-Q2_K.gguf) | i1-Q2_K | 3.3 | IQ3_XXS probably better |
| [GGUF](https://huggingface.co/mradermacher/black_stheno_8B_v1-i1-GGUF/resolve/main/black_stheno_8B_v1.i1-IQ3_XXS.gguf) | i1-IQ3_XXS | 3.4 | lower quality |
| [GGUF](https://huggingface.co/mradermacher/black_stheno_8B_v1-i1-GGUF/resolve/main/black_stheno_8B_v1.i1-IQ3_XS.gguf) | i1-IQ3_XS | 3.6 | |
| [GGUF](https://huggingface.co/mradermacher/black_stheno_8B_v1-i1-GGUF/resolve/main/black_stheno_8B_v1.i1-Q3_K_S.gguf) | i1-Q3_K_S | 3.8 | IQ3_XS probably better |
| [GGUF](https://huggingface.co/mradermacher/black_stheno_8B_v1-i1-GGUF/resolve/main/black_stheno_8B_v1.i1-IQ3_S.gguf) | i1-IQ3_S | 3.8 | beats Q3_K* |
| [GGUF](https://huggingface.co/mradermacher/black_stheno_8B_v1-i1-GGUF/resolve/main/black_stheno_8B_v1.i1-IQ3_M.gguf) | i1-IQ3_M | 3.9 | |
| [GGUF](https://huggingface.co/mradermacher/black_stheno_8B_v1-i1-GGUF/resolve/main/black_stheno_8B_v1.i1-Q3_K_M.gguf) | i1-Q3_K_M | 4.1 | IQ3_S probably better |
| [GGUF](https://huggingface.co/mradermacher/black_stheno_8B_v1-i1-GGUF/resolve/main/black_stheno_8B_v1.i1-Q3_K_L.gguf) | i1-Q3_K_L | 4.4 | IQ3_M probably better |
| [GGUF](https://huggingface.co/mradermacher/black_stheno_8B_v1-i1-GGUF/resolve/main/black_stheno_8B_v1.i1-IQ4_XS.gguf) | i1-IQ4_XS | 4.5 | |
| [GGUF](https://huggingface.co/mradermacher/black_stheno_8B_v1-i1-GGUF/resolve/main/black_stheno_8B_v1.i1-Q4_0.gguf) | i1-Q4_0 | 4.8 | fast, low quality |
| [GGUF](https://huggingface.co/mradermacher/black_stheno_8B_v1-i1-GGUF/resolve/main/black_stheno_8B_v1.i1-Q4_K_S.gguf) | i1-Q4_K_S | 4.8 | optimal size/speed/quality |
| [GGUF](https://huggingface.co/mradermacher/black_stheno_8B_v1-i1-GGUF/resolve/main/black_stheno_8B_v1.i1-Q4_K_M.gguf) | i1-Q4_K_M | 5.0 | fast, recommended |
| [GGUF](https://huggingface.co/mradermacher/black_stheno_8B_v1-i1-GGUF/resolve/main/black_stheno_8B_v1.i1-Q5_K_S.gguf) | i1-Q5_K_S | 5.7 | |
| [GGUF](https://huggingface.co/mradermacher/black_stheno_8B_v1-i1-GGUF/resolve/main/black_stheno_8B_v1.i1-Q5_K_M.gguf) | i1-Q5_K_M | 5.8 | |
| [GGUF](https://huggingface.co/mradermacher/black_stheno_8B_v1-i1-GGUF/resolve/main/black_stheno_8B_v1.i1-Q6_K.gguf) | i1-Q6_K | 6.7 | practically like static Q6_K |
Here is a handy graph by ikawrakow comparing some lower-quality quant
types (lower is better):

And here are Artefact2's thoughts on the matter:
https://gist.github.com/Artefact2/b5f810600771265fc1e39442288e8ec9
## FAQ / Model Request
See https://huggingface.co/mradermacher/model_requests for some answers to
questions you might have and/or if you want some other model quantized.
## Thanks
I thank my company, [nethype GmbH](https://www.nethype.de/), for letting
me use its servers and providing upgrades to my workstation to enable
this work in my free time. Additional thanks to [@nicoboss](https://huggingface.co/nicoboss) for giving me access to his hardware for calculating the imatrix for these quants.
<!-- end -->
|
UCSC-VLAA/ViT-bigG-14-CLIPA-336-datacomp1B | UCSC-VLAA | "2023-10-20T15:16:31Z" | 12,553 | 4 | open_clip | [
"open_clip",
"safetensors",
"clip",
"zero-shot-image-classification",
"dataset:mlfoundations/datacomp_1b",
"arxiv:2306.15658",
"arxiv:2305.07017",
"license:apache-2.0",
"region:us"
] | zero-shot-image-classification | "2023-10-17T06:26:26Z" | ---
tags:
- clip
library_name: open_clip
pipeline_tag: zero-shot-image-classification
license: apache-2.0
datasets:
- mlfoundations/datacomp_1b
---
# Model card for ViT-bigG-14-CLIPA-336-datacomp1B
A CLIPA-v2 model...
## Model Details
- **Model Type:** Contrastive Image-Text, Zero-Shot Image Classification.
- **Original:** https://github.com/UCSC-VLAA/CLIPA
- **Dataset:** mlfoundations/datacomp_1b
- **Papers:**
- CLIPA-v2: Scaling CLIP Training with 81.1% Zero-shot ImageNet Accuracy within a $10,000 Budget; An Extra $4,000 Unlocks 81.8% Accuracy: https://arxiv.org/abs/2306.15658
- An Inverse Scaling Law for CLIP Training: https://arxiv.org/abs/2305.07017
## Model Usage
### With OpenCLIP
```
import torch
import torch.nn.functional as F
from urllib.request import urlopen
from PIL import Image
from open_clip import create_model_from_pretrained, get_tokenizer
model, preprocess = create_model_from_pretrained('hf-hub:ViT-bigG-14-CLIPA-336')
tokenizer = get_tokenizer('hf-hub:ViT-bigG-14-CLIPA-336')
image = Image.open(urlopen(
'https://huggingface.co/datasets/huggingface/documentation-images/resolve/main/beignets-task-guide.png'
))
image = preprocess(image).unsqueeze(0)
text = tokenizer(["a diagram", "a dog", "a cat", "a beignet"], context_length=model.context_length)
with torch.no_grad(), torch.cuda.amp.autocast():
image_features = model.encode_image(image)
text_features = model.encode_text(text)
image_features = F.normalize(image_features, dim=-1)
text_features = F.normalize(text_features, dim=-1)
text_probs = (100.0 * image_features @ text_features.T).softmax(dim=-1)
print("Label probs:", text_probs) # prints: [[0., 0., 0., 1.0]]
```
## Citation
```bibtex
@article{li2023clipav2,
title={CLIPA-v2: Scaling CLIP Training with 81.1% Zero-shot ImageNet Accuracy within a $10,000 Budget; An Extra $4,000 Unlocks 81.8% Accuracy},
author={Xianhang Li and Zeyu Wang and Cihang Xie},
journal={arXiv preprint arXiv:2306.15658},
year={2023},
}
```
```bibtex
@inproceedings{li2023clipa,
title={An Inverse Scaling Law for CLIP Training},
author={Xianhang Li and Zeyu Wang and Cihang Xie},
booktitle={NeurIPS},
year={2023},
}
```
|
google/switch-base-128 | google | "2023-01-24T17:20:02Z" | 12,535 | 5 | transformers | [
"transformers",
"pytorch",
"switch_transformers",
"text2text-generation",
"en",
"dataset:c4",
"arxiv:2101.03961",
"arxiv:1910.09700",
"license:apache-2.0",
"autotrain_compatible",
"endpoints_compatible",
"region:us"
] | text2text-generation | "2022-11-04T07:59:22Z" | ---
language:
- en
tags:
- text2text-generation
widget:
- text: "The <extra_id_0> walks in <extra_id_1> park"
example_title: "Masked Language Modeling"
datasets:
- c4
license: apache-2.0
---
# Model Card for Switch Transformers Base - 128 experts

# Table of Contents
0. [TL;DR](#TL;DR)
1. [Model Details](#model-details)
2. [Usage](#usage)
3. [Uses](#uses)
4. [Bias, Risks, and Limitations](#bias-risks-and-limitations)
5. [Training Details](#training-details)
6. [Evaluation](#evaluation)
7. [Environmental Impact](#environmental-impact)
8. [Citation](#citation)
9. [Model Card Authors](#model-card-authors)
# TL;DR
Switch Transformers is a Mixture of Experts (MoE) model trained on Masked Language Modeling (MLM) task. The model architecture is similar to the classic T5, but with the Feed Forward layers replaced by the Sparse MLP layers containing "experts" MLP. According to the [original paper](https://arxiv.org/pdf/2101.03961.pdf) the model enables faster training (scaling properties) while being better than T5 on fine-tuned tasks.
As mentioned in the first few lines of the abstract :
> we advance the current scale of language models by pre-training up to trillion parameter models on the “Colossal Clean Crawled Corpus”, and achieve a 4x speedup over the T5-XXL model.
**Disclaimer**: Content from **this** model card has been written by the Hugging Face team, and parts of it were copy pasted from the [original paper](https://arxiv.org/pdf/2101.03961.pdf).
# Model Details
## Model Description
- **Model type:** Language model
- **Language(s) (NLP):** English
- **License:** Apache 2.0
- **Related Models:** [All Switch Transformers Checkpoints](https://huggingface.co/models?search=switch)
- **Original Checkpoints:** [All Original Switch Transformers Checkpoints](https://github.com/google-research/t5x/blob/main/docs/models.md#mixture-of-experts-moe-checkpoints)
- **Resources for more information:**
- [Research paper](https://arxiv.org/pdf/2101.03961.pdf)
- [GitHub Repo](https://github.com/google-research/t5x)
- [Hugging Face Switch Transformers Docs (Similar to T5) ](https://huggingface.co/docs/transformers/model_doc/switch_transformers)
# Usage
Note that these checkpoints has been trained on Masked-Language Modeling (MLM) task. Therefore the checkpoints are not "ready-to-use" for downstream tasks. You may want to check `FLAN-T5` for running fine-tuned weights or fine-tune your own MoE following [this notebook](https://colab.research.google.com/drive/1aGGVHZmtKmcNBbAwa9hbu58DDpIuB5O4?usp=sharing)
Find below some example scripts on how to use the model in `transformers`:
## Using the Pytorch model
### Running the model on a CPU
<details>
<summary> Click to expand </summary>
```python
from transformers import AutoTokenizer, SwitchTransformersForConditionalGeneration
tokenizer = AutoTokenizer.from_pretrained("google/switch-base-128")
model = SwitchTransformersForConditionalGeneration.from_pretrained("google/switch-base-128")
input_text = "A <extra_id_0> walks into a bar a orders a <extra_id_1> with <extra_id_2> pinch of <extra_id_3>."
input_ids = tokenizer(input_text, return_tensors="pt").input_ids
outputs = model.generate(input_ids)
print(tokenizer.decode(outputs[0]))
>>> <pad> <extra_id_0> man<extra_id_1> beer<extra_id_2> a<extra_id_3> salt<extra_id_4>.</s>
```
</details>
### Running the model on a GPU
<details>
<summary> Click to expand </summary>
```python
# pip install accelerate
from transformers import AutoTokenizer, SwitchTransformersForConditionalGeneration
tokenizer = AutoTokenizer.from_pretrained("google/switch-base-128")
model = SwitchTransformersForConditionalGeneration.from_pretrained("google/switch-base-128", device_map="auto")
input_text = "A <extra_id_0> walks into a bar a orders a <extra_id_1> with <extra_id_2> pinch of <extra_id_3>."
input_ids = tokenizer(input_text, return_tensors="pt").input_ids.to(0)
outputs = model.generate(input_ids)
print(tokenizer.decode(outputs[0]))
>>> <pad> <extra_id_0> man<extra_id_1> beer<extra_id_2> a<extra_id_3> salt<extra_id_4>.</s>
```
</details>
### Running the model on a GPU using different precisions
#### FP16
<details>
<summary> Click to expand </summary>
```python
# pip install accelerate
from transformers import AutoTokenizer, SwitchTransformersForConditionalGeneration
tokenizer = AutoTokenizer.from_pretrained("google/switch-base-128")
model = SwitchTransformersForConditionalGeneration.from_pretrained("google/switch-base-128", device_map="auto", torch_dtype=torch.float16)
input_text = "A <extra_id_0> walks into a bar a orders a <extra_id_1> with <extra_id_2> pinch of <extra_id_3>."
input_ids = tokenizer(input_text, return_tensors="pt").input_ids.to(0)
outputs = model.generate(input_ids)
print(tokenizer.decode(outputs[0]))
>>> <pad> <extra_id_0> man<extra_id_1> beer<extra_id_2> a<extra_id_3> salt<extra_id_4>.</s>
```
</details>
#### INT8
<details>
<summary> Click to expand </summary>
```python
# pip install bitsandbytes accelerate
from transformers import AutoTokenizer, SwitchTransformersForConditionalGeneration
tokenizer = AutoTokenizer.from_pretrained("google/switch-base-128")
model = SwitchTransformersForConditionalGeneration.from_pretrained("google/switch-base-128", device_map="auto")
input_text = "A <extra_id_0> walks into a bar a orders a <extra_id_1> with <extra_id_2> pinch of <extra_id_3>."
input_ids = tokenizer(input_text, return_tensors="pt").input_ids.to(0)
outputs = model.generate(input_ids)
print(tokenizer.decode(outputs[0]))
>>> <pad> <extra_id_0> man<extra_id_1> beer<extra_id_2> a<extra_id_3> salt<extra_id_4>.</s>
```
</details>
# Uses
## Direct Use and Downstream Use
See the [research paper](https://arxiv.org/pdf/2101.03961.pdf) for further details.
## Out-of-Scope Use
More information needed.
# Bias, Risks, and Limitations
More information needed.
## Ethical considerations and risks
More information needed.
## Known Limitations
More information needed.
## Sensitive Use:
More information needed.
# Training Details
## Training Data
The model was trained on a Masked Language Modeling task, on Colossal Clean Crawled Corpus (C4) dataset, following the same procedure as `T5`.
## Training Procedure
According to the model card from the [original paper](https://arxiv.org/pdf/2101.03961.pdf) the model has been trained on TPU v3 or TPU v4 pods, using [`t5x`](https://github.com/google-research/t5x) codebase together with [`jax`](https://github.com/google/jax).
# Evaluation
## Testing Data, Factors & Metrics
The authors evaluated the model on various tasks and compared the results against T5. See the table below for some quantitative evaluation:

For full details, please check the [research paper](https://arxiv.org/pdf/2101.03961.pdf).
## Results
For full results for Switch Transformers, see the [research paper](https://arxiv.org/pdf/2101.03961.pdf), Table 5.
# Environmental Impact
Carbon emissions can be estimated using the [Machine Learning Impact calculator](https://mlco2.github.io/impact#compute) presented in [Lacoste et al. (2019)](https://arxiv.org/abs/1910.09700).
- **Hardware Type:** Google Cloud TPU Pods - TPU v3 or TPU v4 | Number of chips ≥ 4.
- **Hours used:** More information needed
- **Cloud Provider:** GCP
- **Compute Region:** More information needed
- **Carbon Emitted:** More information needed
# Citation
**BibTeX:**
```bibtex
@misc{https://doi.org/10.48550/arxiv.2101.03961,
doi = {10.48550/ARXIV.2101.03961},
url = {https://arxiv.org/abs/2101.03961},
author = {Fedus, William and Zoph, Barret and Shazeer, Noam},
keywords = {Machine Learning (cs.LG), Artificial Intelligence (cs.AI), FOS: Computer and information sciences, FOS: Computer and information sciences},
title = {Switch Transformers: Scaling to Trillion Parameter Models with Simple and Efficient Sparsity},
publisher = {arXiv},
year = {2021},
copyright = {arXiv.org perpetual, non-exclusive license}
}
``` |
stabilityai/StableBeluga-7B | stabilityai | "2023-08-29T20:21:36Z" | 12,519 | 130 | transformers | [
"transformers",
"safetensors",
"llama",
"text-generation",
"en",
"dataset:conceptofmind/cot_submix_original",
"dataset:conceptofmind/flan2021_submix_original",
"dataset:conceptofmind/t0_submix_original",
"dataset:conceptofmind/niv2_submix_original",
"arxiv:2307.09288",
"arxiv:2306.02707",
"autotrain_compatible",
"endpoints_compatible",
"text-generation-inference",
"region:us"
] | text-generation | "2023-07-27T02:01:15Z" | ---
datasets:
- conceptofmind/cot_submix_original
- conceptofmind/flan2021_submix_original
- conceptofmind/t0_submix_original
- conceptofmind/niv2_submix_original
language:
- en
pipeline_tag: text-generation
---
# Stable Beluga 7B
Use [Stable Chat (Research Preview)](https://chat.stability.ai/chat) to test Stability AI's best language models for free
## Model Description
`Stable Beluga 7B` is a Llama2 7B model finetuned on an Orca style Dataset
## Usage
Start chatting with `Stable Beluga 7B` using the following code snippet:
```python
import torch
from transformers import AutoModelForCausalLM, AutoTokenizer, pipeline
tokenizer = AutoTokenizer.from_pretrained("stabilityai/StableBeluga-7B", use_fast=False)
model = AutoModelForCausalLM.from_pretrained("stabilityai/StableBeluga-7B", torch_dtype=torch.float16, low_cpu_mem_usage=True, device_map="auto")
system_prompt = "### System:\nYou are StableBeluga, an AI that follows instructions extremely well. Help as much as you can. Remember, be safe, and don't do anything illegal.\n\n"
message = "Write me a poem please"
prompt = f"{system_prompt}### User: {message}\n\n### Assistant:\n"
inputs = tokenizer(prompt, return_tensors="pt").to("cuda")
output = model.generate(**inputs, do_sample=True, top_p=0.95, top_k=0, max_new_tokens=256)
print(tokenizer.decode(output[0], skip_special_tokens=True))
```
Stable Beluga 7B should be used with this prompt format:
```
### System:
This is a system prompt, please behave and help the user.
### User:
Your prompt here
### Assistant:
The output of Stable Beluga 7B
```
## Model Details
* **Developed by**: [Stability AI](https://stability.ai/)
* **Model type**: Stable Beluga 7B is an auto-regressive language model fine-tuned on Llama2 7B.
* **Language(s)**: English
* **Library**: [HuggingFace Transformers](https://github.com/huggingface/transformers)
* **License**: Fine-tuned checkpoints (`Stable Beluga 7B`) is licensed under the [STABLE BELUGA NON-COMMERCIAL COMMUNITY LICENSE AGREEMENT](https://huggingface.co/stabilityai/StableBeluga-7B/blob/main/LICENSE.txt)
* **Contact**: For questions and comments about the model, please email `[email protected]`
### Training Dataset
` Stable Beluga 7B` is trained on our internal Orca-style dataset
### Training Procedure
Models are learned via supervised fine-tuning on the aforementioned datasets, trained in mixed-precision (BF16), and optimized with AdamW. We outline the following hyperparameters:
| Dataset | Batch Size | Learning Rate |Learning Rate Decay| Warm-up | Weight Decay | Betas |
|-------------------|------------|---------------|-------------------|---------|--------------|-------------|
| Orca pt1 packed | 256 | 3e-5 | Cosine to 3e-6 | 100 | 1e-6 | (0.9, 0.95) |
| Orca pt2 unpacked | 512 | 3e-5 | Cosine to 3e-6 | 100 | 1e-6 | (0.9, 0.95) |
## Ethical Considerations and Limitations
Beluga is a new technology that carries risks with use. Testing conducted to date has been in English, and has not covered, nor could it cover all scenarios. For these reasons, as with all LLMs, Beluga's potential outputs cannot be predicted in advance, and the model may in some instances produce inaccurate, biased or other objectionable responses to user prompts. Therefore, before deploying any applications of Beluga, developers should perform safety testing and tuning tailored to their specific applications of the model.
## Citations
```bibtext
@misc{touvron2023llama,
title={Llama 2: Open Foundation and Fine-Tuned Chat Models},
author={Hugo Touvron and Louis Martin and Kevin Stone and Peter Albert and Amjad Almahairi and Yasmine Babaei and Nikolay Bashlykov and Soumya Batra and Prajjwal Bhargava and Shruti Bhosale and Dan Bikel and Lukas Blecher and Cristian Canton Ferrer and Moya Chen and Guillem Cucurull and David Esiobu and Jude Fernandes and Jeremy Fu and Wenyin Fu and Brian Fuller and Cynthia Gao and Vedanuj Goswami and Naman Goyal and Anthony Hartshorn and Saghar Hosseini and Rui Hou and Hakan Inan and Marcin Kardas and Viktor Kerkez and Madian Khabsa and Isabel Kloumann and Artem Korenev and Punit Singh Koura and Marie-Anne Lachaux and Thibaut Lavril and Jenya Lee and Diana Liskovich and Yinghai Lu and Yuning Mao and Xavier Martinet and Todor Mihaylov and Pushkar Mishra and Igor Molybog and Yixin Nie and Andrew Poulton and Jeremy Reizenstein and Rashi Rungta and Kalyan Saladi and Alan Schelten and Ruan Silva and Eric Michael Smith and Ranjan Subramanian and Xiaoqing Ellen Tan and Binh Tang and Ross Taylor and Adina Williams and Jian Xiang Kuan and Puxin Xu and Zheng Yan and Iliyan Zarov and Yuchen Zhang and Angela Fan and Melanie Kambadur and Sharan Narang and Aurelien Rodriguez and Robert Stojnic and Sergey Edunov and Thomas Scialom},
year={2023},
eprint={2307.09288},
archivePrefix={arXiv},
primaryClass={cs.CL}
}
```
```bibtext
@misc{mukherjee2023orca,
title={Orca: Progressive Learning from Complex Explanation Traces of GPT-4},
author={Subhabrata Mukherjee and Arindam Mitra and Ganesh Jawahar and Sahaj Agarwal and Hamid Palangi and Ahmed Awadallah},
year={2023},
eprint={2306.02707},
archivePrefix={arXiv},
primaryClass={cs.CL}
}
``` |
mradermacher/L3-Umbral-Mind-RP-v2.0-8B-GGUF | mradermacher | "2024-06-28T03:36:28Z" | 12,513 | 0 | transformers | [
"transformers",
"gguf",
"merge",
"mergekit",
"lazymergekit",
"not-for-all-audiences",
"nsfw",
"rp",
"roleplay",
"role-play",
"en",
"base_model:Casual-Autopsy/L3-Umbral-Mind-RP-v2.0-8B",
"license:llama3",
"endpoints_compatible",
"region:us"
] | null | "2024-06-28T03:05:32Z" | ---
base_model: Casual-Autopsy/L3-Umbral-Mind-RP-v2.0-8B
language:
- en
library_name: transformers
license: llama3
quantized_by: mradermacher
tags:
- merge
- mergekit
- lazymergekit
- not-for-all-audiences
- nsfw
- rp
- roleplay
- role-play
---
## About
<!-- ### quantize_version: 2 -->
<!-- ### output_tensor_quantised: 1 -->
<!-- ### convert_type: hf -->
<!-- ### vocab_type: -->
<!-- ### tags: -->
static quants of https://huggingface.co/Casual-Autopsy/L3-Umbral-Mind-RP-v2.0-8B
<!-- provided-files -->
weighted/imatrix quants are available at https://huggingface.co/mradermacher/L3-Umbral-Mind-RP-v2.0-8B-i1-GGUF
## Usage
If you are unsure how to use GGUF files, refer to one of [TheBloke's
READMEs](https://huggingface.co/TheBloke/KafkaLM-70B-German-V0.1-GGUF) for
more details, including on how to concatenate multi-part files.
## Provided Quants
(sorted by size, not necessarily quality. IQ-quants are often preferable over similar sized non-IQ quants)
| Link | Type | Size/GB | Notes |
|:-----|:-----|--------:|:------|
| [GGUF](https://huggingface.co/mradermacher/L3-Umbral-Mind-RP-v2.0-8B-GGUF/resolve/main/L3-Umbral-Mind-RP-v2.0-8B.Q2_K.gguf) | Q2_K | 3.3 | |
| [GGUF](https://huggingface.co/mradermacher/L3-Umbral-Mind-RP-v2.0-8B-GGUF/resolve/main/L3-Umbral-Mind-RP-v2.0-8B.IQ3_XS.gguf) | IQ3_XS | 3.6 | |
| [GGUF](https://huggingface.co/mradermacher/L3-Umbral-Mind-RP-v2.0-8B-GGUF/resolve/main/L3-Umbral-Mind-RP-v2.0-8B.Q3_K_S.gguf) | Q3_K_S | 3.8 | |
| [GGUF](https://huggingface.co/mradermacher/L3-Umbral-Mind-RP-v2.0-8B-GGUF/resolve/main/L3-Umbral-Mind-RP-v2.0-8B.IQ3_S.gguf) | IQ3_S | 3.8 | beats Q3_K* |
| [GGUF](https://huggingface.co/mradermacher/L3-Umbral-Mind-RP-v2.0-8B-GGUF/resolve/main/L3-Umbral-Mind-RP-v2.0-8B.IQ3_M.gguf) | IQ3_M | 3.9 | |
| [GGUF](https://huggingface.co/mradermacher/L3-Umbral-Mind-RP-v2.0-8B-GGUF/resolve/main/L3-Umbral-Mind-RP-v2.0-8B.Q3_K_M.gguf) | Q3_K_M | 4.1 | lower quality |
| [GGUF](https://huggingface.co/mradermacher/L3-Umbral-Mind-RP-v2.0-8B-GGUF/resolve/main/L3-Umbral-Mind-RP-v2.0-8B.Q3_K_L.gguf) | Q3_K_L | 4.4 | |
| [GGUF](https://huggingface.co/mradermacher/L3-Umbral-Mind-RP-v2.0-8B-GGUF/resolve/main/L3-Umbral-Mind-RP-v2.0-8B.IQ4_XS.gguf) | IQ4_XS | 4.6 | |
| [GGUF](https://huggingface.co/mradermacher/L3-Umbral-Mind-RP-v2.0-8B-GGUF/resolve/main/L3-Umbral-Mind-RP-v2.0-8B.Q4_K_S.gguf) | Q4_K_S | 4.8 | fast, recommended |
| [GGUF](https://huggingface.co/mradermacher/L3-Umbral-Mind-RP-v2.0-8B-GGUF/resolve/main/L3-Umbral-Mind-RP-v2.0-8B.Q4_K_M.gguf) | Q4_K_M | 5.0 | fast, recommended |
| [GGUF](https://huggingface.co/mradermacher/L3-Umbral-Mind-RP-v2.0-8B-GGUF/resolve/main/L3-Umbral-Mind-RP-v2.0-8B.Q5_K_S.gguf) | Q5_K_S | 5.7 | |
| [GGUF](https://huggingface.co/mradermacher/L3-Umbral-Mind-RP-v2.0-8B-GGUF/resolve/main/L3-Umbral-Mind-RP-v2.0-8B.Q5_K_M.gguf) | Q5_K_M | 5.8 | |
| [GGUF](https://huggingface.co/mradermacher/L3-Umbral-Mind-RP-v2.0-8B-GGUF/resolve/main/L3-Umbral-Mind-RP-v2.0-8B.Q6_K.gguf) | Q6_K | 6.7 | very good quality |
| [GGUF](https://huggingface.co/mradermacher/L3-Umbral-Mind-RP-v2.0-8B-GGUF/resolve/main/L3-Umbral-Mind-RP-v2.0-8B.Q8_0.gguf) | Q8_0 | 8.6 | fast, best quality |
| [GGUF](https://huggingface.co/mradermacher/L3-Umbral-Mind-RP-v2.0-8B-GGUF/resolve/main/L3-Umbral-Mind-RP-v2.0-8B.f16.gguf) | f16 | 16.2 | 16 bpw, overkill |
Here is a handy graph by ikawrakow comparing some lower-quality quant
types (lower is better):

And here are Artefact2's thoughts on the matter:
https://gist.github.com/Artefact2/b5f810600771265fc1e39442288e8ec9
## FAQ / Model Request
See https://huggingface.co/mradermacher/model_requests for some answers to
questions you might have and/or if you want some other model quantized.
## Thanks
I thank my company, [nethype GmbH](https://www.nethype.de/), for letting
me use its servers and providing upgrades to my workstation to enable
this work in my free time.
<!-- end -->
|
Subsets and Splits
No saved queries yet
Save your SQL queries to embed, download, and access them later. Queries will appear here once saved.