modelId
stringlengths 5
122
| author
stringlengths 2
42
| last_modified
timestamp[us, tz=UTC] | downloads
int64 0
738M
| likes
int64 0
11k
| library_name
stringclasses 245
values | tags
sequencelengths 1
4.05k
| pipeline_tag
stringclasses 48
values | createdAt
timestamp[us, tz=UTC] | card
stringlengths 1
901k
|
---|---|---|---|---|---|---|---|---|---|
mradermacher/Llama-3-Swallow-8B-Instruct-v0.1-i1-GGUF | mradermacher | 2024-07-02T08:11:48Z | 14,228 | 0 | transformers | [
"transformers",
"gguf",
"en",
"ja",
"base_model:tokyotech-llm/Llama-3-Swallow-8B-Instruct-v0.1",
"license:llama3",
"endpoints_compatible",
"region:us"
] | null | 2024-07-02T02:58:42Z | ---
base_model: tokyotech-llm/Llama-3-Swallow-8B-Instruct-v0.1
language:
- en
- ja
library_name: transformers
license: llama3
model_type: llama
quantized_by: mradermacher
---
## About
<!-- ### quantize_version: 2 -->
<!-- ### output_tensor_quantised: 1 -->
<!-- ### convert_type: hf -->
<!-- ### vocab_type: -->
<!-- ### tags: nicoboss -->
weighted/imatrix quants of https://huggingface.co/tokyotech-llm/Llama-3-Swallow-8B-Instruct-v0.1
<!-- provided-files -->
static quants are available at https://huggingface.co/mradermacher/Llama-3-Swallow-8B-Instruct-v0.1-GGUF
## Usage
If you are unsure how to use GGUF files, refer to one of [TheBloke's
READMEs](https://huggingface.co/TheBloke/KafkaLM-70B-German-V0.1-GGUF) for
more details, including on how to concatenate multi-part files.
## Provided Quants
(sorted by size, not necessarily quality. IQ-quants are often preferable over similar sized non-IQ quants)
| Link | Type | Size/GB | Notes |
|:-----|:-----|--------:|:------|
| [GGUF](https://huggingface.co/mradermacher/Llama-3-Swallow-8B-Instruct-v0.1-i1-GGUF/resolve/main/Llama-3-Swallow-8B-Instruct-v0.1.i1-IQ1_S.gguf) | i1-IQ1_S | 2.1 | for the desperate |
| [GGUF](https://huggingface.co/mradermacher/Llama-3-Swallow-8B-Instruct-v0.1-i1-GGUF/resolve/main/Llama-3-Swallow-8B-Instruct-v0.1.i1-IQ1_M.gguf) | i1-IQ1_M | 2.3 | mostly desperate |
| [GGUF](https://huggingface.co/mradermacher/Llama-3-Swallow-8B-Instruct-v0.1-i1-GGUF/resolve/main/Llama-3-Swallow-8B-Instruct-v0.1.i1-IQ2_XXS.gguf) | i1-IQ2_XXS | 2.5 | |
| [GGUF](https://huggingface.co/mradermacher/Llama-3-Swallow-8B-Instruct-v0.1-i1-GGUF/resolve/main/Llama-3-Swallow-8B-Instruct-v0.1.i1-IQ2_XS.gguf) | i1-IQ2_XS | 2.7 | |
| [GGUF](https://huggingface.co/mradermacher/Llama-3-Swallow-8B-Instruct-v0.1-i1-GGUF/resolve/main/Llama-3-Swallow-8B-Instruct-v0.1.i1-IQ2_S.gguf) | i1-IQ2_S | 2.9 | |
| [GGUF](https://huggingface.co/mradermacher/Llama-3-Swallow-8B-Instruct-v0.1-i1-GGUF/resolve/main/Llama-3-Swallow-8B-Instruct-v0.1.i1-IQ2_M.gguf) | i1-IQ2_M | 3.0 | |
| [GGUF](https://huggingface.co/mradermacher/Llama-3-Swallow-8B-Instruct-v0.1-i1-GGUF/resolve/main/Llama-3-Swallow-8B-Instruct-v0.1.i1-Q2_K.gguf) | i1-Q2_K | 3.3 | IQ3_XXS probably better |
| [GGUF](https://huggingface.co/mradermacher/Llama-3-Swallow-8B-Instruct-v0.1-i1-GGUF/resolve/main/Llama-3-Swallow-8B-Instruct-v0.1.i1-IQ3_XXS.gguf) | i1-IQ3_XXS | 3.4 | lower quality |
| [GGUF](https://huggingface.co/mradermacher/Llama-3-Swallow-8B-Instruct-v0.1-i1-GGUF/resolve/main/Llama-3-Swallow-8B-Instruct-v0.1.i1-IQ3_XS.gguf) | i1-IQ3_XS | 3.6 | |
| [GGUF](https://huggingface.co/mradermacher/Llama-3-Swallow-8B-Instruct-v0.1-i1-GGUF/resolve/main/Llama-3-Swallow-8B-Instruct-v0.1.i1-Q3_K_S.gguf) | i1-Q3_K_S | 3.8 | IQ3_XS probably better |
| [GGUF](https://huggingface.co/mradermacher/Llama-3-Swallow-8B-Instruct-v0.1-i1-GGUF/resolve/main/Llama-3-Swallow-8B-Instruct-v0.1.i1-IQ3_S.gguf) | i1-IQ3_S | 3.8 | beats Q3_K* |
| [GGUF](https://huggingface.co/mradermacher/Llama-3-Swallow-8B-Instruct-v0.1-i1-GGUF/resolve/main/Llama-3-Swallow-8B-Instruct-v0.1.i1-IQ3_M.gguf) | i1-IQ3_M | 3.9 | |
| [GGUF](https://huggingface.co/mradermacher/Llama-3-Swallow-8B-Instruct-v0.1-i1-GGUF/resolve/main/Llama-3-Swallow-8B-Instruct-v0.1.i1-Q3_K_M.gguf) | i1-Q3_K_M | 4.1 | IQ3_S probably better |
| [GGUF](https://huggingface.co/mradermacher/Llama-3-Swallow-8B-Instruct-v0.1-i1-GGUF/resolve/main/Llama-3-Swallow-8B-Instruct-v0.1.i1-Q3_K_L.gguf) | i1-Q3_K_L | 4.4 | IQ3_M probably better |
| [GGUF](https://huggingface.co/mradermacher/Llama-3-Swallow-8B-Instruct-v0.1-i1-GGUF/resolve/main/Llama-3-Swallow-8B-Instruct-v0.1.i1-IQ4_XS.gguf) | i1-IQ4_XS | 4.5 | |
| [GGUF](https://huggingface.co/mradermacher/Llama-3-Swallow-8B-Instruct-v0.1-i1-GGUF/resolve/main/Llama-3-Swallow-8B-Instruct-v0.1.i1-Q4_0.gguf) | i1-Q4_0 | 4.8 | fast, low quality |
| [GGUF](https://huggingface.co/mradermacher/Llama-3-Swallow-8B-Instruct-v0.1-i1-GGUF/resolve/main/Llama-3-Swallow-8B-Instruct-v0.1.i1-Q4_K_S.gguf) | i1-Q4_K_S | 4.8 | optimal size/speed/quality |
| [GGUF](https://huggingface.co/mradermacher/Llama-3-Swallow-8B-Instruct-v0.1-i1-GGUF/resolve/main/Llama-3-Swallow-8B-Instruct-v0.1.i1-Q4_K_M.gguf) | i1-Q4_K_M | 5.0 | fast, recommended |
| [GGUF](https://huggingface.co/mradermacher/Llama-3-Swallow-8B-Instruct-v0.1-i1-GGUF/resolve/main/Llama-3-Swallow-8B-Instruct-v0.1.i1-Q5_K_S.gguf) | i1-Q5_K_S | 5.7 | |
| [GGUF](https://huggingface.co/mradermacher/Llama-3-Swallow-8B-Instruct-v0.1-i1-GGUF/resolve/main/Llama-3-Swallow-8B-Instruct-v0.1.i1-Q5_K_M.gguf) | i1-Q5_K_M | 5.8 | |
| [GGUF](https://huggingface.co/mradermacher/Llama-3-Swallow-8B-Instruct-v0.1-i1-GGUF/resolve/main/Llama-3-Swallow-8B-Instruct-v0.1.i1-Q6_K.gguf) | i1-Q6_K | 6.7 | practically like static Q6_K |
Here is a handy graph by ikawrakow comparing some lower-quality quant
types (lower is better):

And here are Artefact2's thoughts on the matter:
https://gist.github.com/Artefact2/b5f810600771265fc1e39442288e8ec9
## FAQ / Model Request
See https://huggingface.co/mradermacher/model_requests for some answers to
questions you might have and/or if you want some other model quantized.
## Thanks
I thank my company, [nethype GmbH](https://www.nethype.de/), for letting
me use its servers and providing upgrades to my workstation to enable
this work in my free time. Additional thanks to [@nicoboss](https://huggingface.co/nicoboss) for giving me access to his hardware for calculating the imatrix for these quants.
<!-- end -->
|
mradermacher/YI-7B-Nicky1-GGUF | mradermacher | 2024-07-02T03:00:01Z | 14,217 | 0 | transformers | [
"transformers",
"gguf",
"en",
"base_model:AzureBP/YI-7B-Nicky1",
"license:mit",
"endpoints_compatible",
"region:us"
] | null | 2024-07-02T02:19:06Z | ---
base_model: AzureBP/YI-7B-Nicky1
language:
- en
library_name: transformers
license: mit
quantized_by: mradermacher
---
## About
<!-- ### quantize_version: 2 -->
<!-- ### output_tensor_quantised: 1 -->
<!-- ### convert_type: hf -->
<!-- ### vocab_type: -->
<!-- ### tags: -->
static quants of https://huggingface.co/AzureBP/YI-7B-Nicky1
<!-- provided-files -->
weighted/imatrix quants seem not to be available (by me) at this time. If they do not show up a week or so after the static ones, I have probably not planned for them. Feel free to request them by opening a Community Discussion.
## Usage
If you are unsure how to use GGUF files, refer to one of [TheBloke's
READMEs](https://huggingface.co/TheBloke/KafkaLM-70B-German-V0.1-GGUF) for
more details, including on how to concatenate multi-part files.
## Provided Quants
(sorted by size, not necessarily quality. IQ-quants are often preferable over similar sized non-IQ quants)
| Link | Type | Size/GB | Notes |
|:-----|:-----|--------:|:------|
| [GGUF](https://huggingface.co/mradermacher/YI-7B-Nicky1-GGUF/resolve/main/YI-7B-Nicky1.Q2_K.gguf) | Q2_K | 2.4 | |
| [GGUF](https://huggingface.co/mradermacher/YI-7B-Nicky1-GGUF/resolve/main/YI-7B-Nicky1.IQ3_XS.gguf) | IQ3_XS | 2.7 | |
| [GGUF](https://huggingface.co/mradermacher/YI-7B-Nicky1-GGUF/resolve/main/YI-7B-Nicky1.Q3_K_S.gguf) | Q3_K_S | 2.8 | |
| [GGUF](https://huggingface.co/mradermacher/YI-7B-Nicky1-GGUF/resolve/main/YI-7B-Nicky1.IQ3_S.gguf) | IQ3_S | 2.8 | beats Q3_K* |
| [GGUF](https://huggingface.co/mradermacher/YI-7B-Nicky1-GGUF/resolve/main/YI-7B-Nicky1.IQ3_M.gguf) | IQ3_M | 2.9 | |
| [GGUF](https://huggingface.co/mradermacher/YI-7B-Nicky1-GGUF/resolve/main/YI-7B-Nicky1.Q3_K_M.gguf) | Q3_K_M | 3.1 | lower quality |
| [GGUF](https://huggingface.co/mradermacher/YI-7B-Nicky1-GGUF/resolve/main/YI-7B-Nicky1.Q3_K_L.gguf) | Q3_K_L | 3.3 | |
| [GGUF](https://huggingface.co/mradermacher/YI-7B-Nicky1-GGUF/resolve/main/YI-7B-Nicky1.IQ4_XS.gguf) | IQ4_XS | 3.4 | |
| [GGUF](https://huggingface.co/mradermacher/YI-7B-Nicky1-GGUF/resolve/main/YI-7B-Nicky1.Q4_K_S.gguf) | Q4_K_S | 3.6 | fast, recommended |
| [GGUF](https://huggingface.co/mradermacher/YI-7B-Nicky1-GGUF/resolve/main/YI-7B-Nicky1.Q4_K_M.gguf) | Q4_K_M | 3.8 | fast, recommended |
| [GGUF](https://huggingface.co/mradermacher/YI-7B-Nicky1-GGUF/resolve/main/YI-7B-Nicky1.Q5_K_S.gguf) | Q5_K_S | 4.3 | |
| [GGUF](https://huggingface.co/mradermacher/YI-7B-Nicky1-GGUF/resolve/main/YI-7B-Nicky1.Q5_K_M.gguf) | Q5_K_M | 4.4 | |
| [GGUF](https://huggingface.co/mradermacher/YI-7B-Nicky1-GGUF/resolve/main/YI-7B-Nicky1.Q6_K.gguf) | Q6_K | 5.1 | very good quality |
| [GGUF](https://huggingface.co/mradermacher/YI-7B-Nicky1-GGUF/resolve/main/YI-7B-Nicky1.Q8_0.gguf) | Q8_0 | 6.5 | fast, best quality |
| [GGUF](https://huggingface.co/mradermacher/YI-7B-Nicky1-GGUF/resolve/main/YI-7B-Nicky1.f16.gguf) | f16 | 12.2 | 16 bpw, overkill |
Here is a handy graph by ikawrakow comparing some lower-quality quant
types (lower is better):

And here are Artefact2's thoughts on the matter:
https://gist.github.com/Artefact2/b5f810600771265fc1e39442288e8ec9
## FAQ / Model Request
See https://huggingface.co/mradermacher/model_requests for some answers to
questions you might have and/or if you want some other model quantized.
## Thanks
I thank my company, [nethype GmbH](https://www.nethype.de/), for letting
me use its servers and providing upgrades to my workstation to enable
this work in my free time.
<!-- end -->
|
microsoft/layoutlmv3-large | microsoft | 2022-09-16T03:26:15Z | 14,194 | 77 | transformers | [
"transformers",
"pytorch",
"tf",
"layoutlmv3",
"en",
"arxiv:2204.08387",
"license:cc-by-nc-sa-4.0",
"endpoints_compatible",
"region:us"
] | null | 2022-04-18T06:56:58Z | ---
language: en
license: cc-by-nc-sa-4.0
---
# LayoutLMv3
[Microsoft Document AI](https://www.microsoft.com/en-us/research/project/document-ai/) | [GitHub](https://aka.ms/layoutlmv3)
## Model description
LayoutLMv3 is a pre-trained multimodal Transformer for Document AI with unified text and image masking. The simple unified architecture and training objectives make LayoutLMv3 a general-purpose pre-trained model. For example, LayoutLMv3 can be fine-tuned for both text-centric tasks, including form understanding, receipt understanding, and document visual question answering, and image-centric tasks such as document image classification and document layout analysis.
[LayoutLMv3: Pre-training for Document AI with Unified Text and Image Masking](https://arxiv.org/abs/2204.08387)
Yupan Huang, Tengchao Lv, Lei Cui, Yutong Lu, Furu Wei, Preprint 2022.
## Citation
If you find LayoutLM useful in your research, please cite the following paper:
```
@inproceedings{huang2022layoutlmv3,
author={Yupan Huang and Tengchao Lv and Lei Cui and Yutong Lu and Furu Wei},
title={LayoutLMv3: Pre-training for Document AI with Unified Text and Image Masking},
booktitle={Proceedings of the 30th ACM International Conference on Multimedia},
year={2022}
}
```
## License
The content of this project itself is licensed under the [Attribution-NonCommercial-ShareAlike 4.0 International (CC BY-NC-SA 4.0)](https://creativecommons.org/licenses/by-nc-sa/4.0/).
Portions of the source code are based on the [transformers](https://github.com/huggingface/transformers) project.
[Microsoft Open Source Code of Conduct](https://opensource.microsoft.com/codeofconduct) |
mradermacher/Bungo-L3-8B-i1-GGUF | mradermacher | 2024-06-28T07:13:46Z | 14,192 | 0 | transformers | [
"transformers",
"gguf",
"en",
"base_model:R136a1/Bungo-L3-8B",
"license:llama3",
"endpoints_compatible",
"region:us"
] | null | 2024-06-28T04:57:10Z | ---
base_model: R136a1/Bungo-L3-8B
language:
- en
library_name: transformers
license: llama3
quantized_by: mradermacher
---
## About
<!-- ### quantize_version: 2 -->
<!-- ### output_tensor_quantised: 1 -->
<!-- ### convert_type: hf -->
<!-- ### vocab_type: -->
<!-- ### tags: nicoboss -->
weighted/imatrix quants of https://huggingface.co/R136a1/Bungo-L3-8B
<!-- provided-files -->
static quants are available at https://huggingface.co/mradermacher/Bungo-L3-8B-GGUF
## Usage
If you are unsure how to use GGUF files, refer to one of [TheBloke's
READMEs](https://huggingface.co/TheBloke/KafkaLM-70B-German-V0.1-GGUF) for
more details, including on how to concatenate multi-part files.
## Provided Quants
(sorted by size, not necessarily quality. IQ-quants are often preferable over similar sized non-IQ quants)
| Link | Type | Size/GB | Notes |
|:-----|:-----|--------:|:------|
| [GGUF](https://huggingface.co/mradermacher/Bungo-L3-8B-i1-GGUF/resolve/main/Bungo-L3-8B.i1-IQ1_S.gguf) | i1-IQ1_S | 2.1 | for the desperate |
| [GGUF](https://huggingface.co/mradermacher/Bungo-L3-8B-i1-GGUF/resolve/main/Bungo-L3-8B.i1-IQ1_M.gguf) | i1-IQ1_M | 2.3 | mostly desperate |
| [GGUF](https://huggingface.co/mradermacher/Bungo-L3-8B-i1-GGUF/resolve/main/Bungo-L3-8B.i1-IQ2_XXS.gguf) | i1-IQ2_XXS | 2.5 | |
| [GGUF](https://huggingface.co/mradermacher/Bungo-L3-8B-i1-GGUF/resolve/main/Bungo-L3-8B.i1-IQ2_XS.gguf) | i1-IQ2_XS | 2.7 | |
| [GGUF](https://huggingface.co/mradermacher/Bungo-L3-8B-i1-GGUF/resolve/main/Bungo-L3-8B.i1-IQ2_S.gguf) | i1-IQ2_S | 2.9 | |
| [GGUF](https://huggingface.co/mradermacher/Bungo-L3-8B-i1-GGUF/resolve/main/Bungo-L3-8B.i1-IQ2_M.gguf) | i1-IQ2_M | 3.0 | |
| [GGUF](https://huggingface.co/mradermacher/Bungo-L3-8B-i1-GGUF/resolve/main/Bungo-L3-8B.i1-Q2_K.gguf) | i1-Q2_K | 3.3 | IQ3_XXS probably better |
| [GGUF](https://huggingface.co/mradermacher/Bungo-L3-8B-i1-GGUF/resolve/main/Bungo-L3-8B.i1-IQ3_XXS.gguf) | i1-IQ3_XXS | 3.4 | lower quality |
| [GGUF](https://huggingface.co/mradermacher/Bungo-L3-8B-i1-GGUF/resolve/main/Bungo-L3-8B.i1-IQ3_XS.gguf) | i1-IQ3_XS | 3.6 | |
| [GGUF](https://huggingface.co/mradermacher/Bungo-L3-8B-i1-GGUF/resolve/main/Bungo-L3-8B.i1-Q3_K_S.gguf) | i1-Q3_K_S | 3.8 | IQ3_XS probably better |
| [GGUF](https://huggingface.co/mradermacher/Bungo-L3-8B-i1-GGUF/resolve/main/Bungo-L3-8B.i1-IQ3_S.gguf) | i1-IQ3_S | 3.8 | beats Q3_K* |
| [GGUF](https://huggingface.co/mradermacher/Bungo-L3-8B-i1-GGUF/resolve/main/Bungo-L3-8B.i1-IQ3_M.gguf) | i1-IQ3_M | 3.9 | |
| [GGUF](https://huggingface.co/mradermacher/Bungo-L3-8B-i1-GGUF/resolve/main/Bungo-L3-8B.i1-Q3_K_M.gguf) | i1-Q3_K_M | 4.1 | IQ3_S probably better |
| [GGUF](https://huggingface.co/mradermacher/Bungo-L3-8B-i1-GGUF/resolve/main/Bungo-L3-8B.i1-Q3_K_L.gguf) | i1-Q3_K_L | 4.4 | IQ3_M probably better |
| [GGUF](https://huggingface.co/mradermacher/Bungo-L3-8B-i1-GGUF/resolve/main/Bungo-L3-8B.i1-IQ4_XS.gguf) | i1-IQ4_XS | 4.5 | |
| [GGUF](https://huggingface.co/mradermacher/Bungo-L3-8B-i1-GGUF/resolve/main/Bungo-L3-8B.i1-Q4_0.gguf) | i1-Q4_0 | 4.8 | fast, low quality |
| [GGUF](https://huggingface.co/mradermacher/Bungo-L3-8B-i1-GGUF/resolve/main/Bungo-L3-8B.i1-Q4_K_S.gguf) | i1-Q4_K_S | 4.8 | optimal size/speed/quality |
| [GGUF](https://huggingface.co/mradermacher/Bungo-L3-8B-i1-GGUF/resolve/main/Bungo-L3-8B.i1-Q4_K_M.gguf) | i1-Q4_K_M | 5.0 | fast, recommended |
| [GGUF](https://huggingface.co/mradermacher/Bungo-L3-8B-i1-GGUF/resolve/main/Bungo-L3-8B.i1-Q5_K_S.gguf) | i1-Q5_K_S | 5.7 | |
| [GGUF](https://huggingface.co/mradermacher/Bungo-L3-8B-i1-GGUF/resolve/main/Bungo-L3-8B.i1-Q5_K_M.gguf) | i1-Q5_K_M | 5.8 | |
| [GGUF](https://huggingface.co/mradermacher/Bungo-L3-8B-i1-GGUF/resolve/main/Bungo-L3-8B.i1-Q6_K.gguf) | i1-Q6_K | 6.7 | practically like static Q6_K |
Here is a handy graph by ikawrakow comparing some lower-quality quant
types (lower is better):

And here are Artefact2's thoughts on the matter:
https://gist.github.com/Artefact2/b5f810600771265fc1e39442288e8ec9
## FAQ / Model Request
See https://huggingface.co/mradermacher/model_requests for some answers to
questions you might have and/or if you want some other model quantized.
## Thanks
I thank my company, [nethype GmbH](https://www.nethype.de/), for letting
me use its servers and providing upgrades to my workstation to enable
this work in my free time. Additional thanks to [@nicoboss](https://huggingface.co/nicoboss) for giving me access to his hardware for calculating the imatrix for these quants.
<!-- end -->
|
mradermacher/Llama-3-8B-Ultrachat-200K-i1-GGUF | mradermacher | 2024-06-29T01:15:56Z | 14,188 | 0 | transformers | [
"transformers",
"gguf",
"axolotl",
"generated_from_trainer",
"en",
"base_model:Magpie-Align/Llama-3-8B-Ultrachat-200K",
"license:llama3",
"endpoints_compatible",
"region:us"
] | null | 2024-06-28T22:29:44Z | ---
base_model: Magpie-Align/Llama-3-8B-Ultrachat-200K
language:
- en
library_name: transformers
license: llama3
quantized_by: mradermacher
tags:
- axolotl
- generated_from_trainer
---
## About
<!-- ### quantize_version: 2 -->
<!-- ### output_tensor_quantised: 1 -->
<!-- ### convert_type: hf -->
<!-- ### vocab_type: -->
<!-- ### tags: nicoboss -->
weighted/imatrix quants of https://huggingface.co/Magpie-Align/Llama-3-8B-Ultrachat-200K
<!-- provided-files -->
static quants are available at https://huggingface.co/mradermacher/Llama-3-8B-Ultrachat-200K-GGUF
## Usage
If you are unsure how to use GGUF files, refer to one of [TheBloke's
READMEs](https://huggingface.co/TheBloke/KafkaLM-70B-German-V0.1-GGUF) for
more details, including on how to concatenate multi-part files.
## Provided Quants
(sorted by size, not necessarily quality. IQ-quants are often preferable over similar sized non-IQ quants)
| Link | Type | Size/GB | Notes |
|:-----|:-----|--------:|:------|
| [GGUF](https://huggingface.co/mradermacher/Llama-3-8B-Ultrachat-200K-i1-GGUF/resolve/main/Llama-3-8B-Ultrachat-200K.i1-IQ1_S.gguf) | i1-IQ1_S | 2.1 | for the desperate |
| [GGUF](https://huggingface.co/mradermacher/Llama-3-8B-Ultrachat-200K-i1-GGUF/resolve/main/Llama-3-8B-Ultrachat-200K.i1-IQ1_M.gguf) | i1-IQ1_M | 2.3 | mostly desperate |
| [GGUF](https://huggingface.co/mradermacher/Llama-3-8B-Ultrachat-200K-i1-GGUF/resolve/main/Llama-3-8B-Ultrachat-200K.i1-IQ2_XXS.gguf) | i1-IQ2_XXS | 2.5 | |
| [GGUF](https://huggingface.co/mradermacher/Llama-3-8B-Ultrachat-200K-i1-GGUF/resolve/main/Llama-3-8B-Ultrachat-200K.i1-IQ2_XS.gguf) | i1-IQ2_XS | 2.7 | |
| [GGUF](https://huggingface.co/mradermacher/Llama-3-8B-Ultrachat-200K-i1-GGUF/resolve/main/Llama-3-8B-Ultrachat-200K.i1-IQ2_S.gguf) | i1-IQ2_S | 2.9 | |
| [GGUF](https://huggingface.co/mradermacher/Llama-3-8B-Ultrachat-200K-i1-GGUF/resolve/main/Llama-3-8B-Ultrachat-200K.i1-IQ2_M.gguf) | i1-IQ2_M | 3.0 | |
| [GGUF](https://huggingface.co/mradermacher/Llama-3-8B-Ultrachat-200K-i1-GGUF/resolve/main/Llama-3-8B-Ultrachat-200K.i1-Q2_K.gguf) | i1-Q2_K | 3.3 | IQ3_XXS probably better |
| [GGUF](https://huggingface.co/mradermacher/Llama-3-8B-Ultrachat-200K-i1-GGUF/resolve/main/Llama-3-8B-Ultrachat-200K.i1-IQ3_XXS.gguf) | i1-IQ3_XXS | 3.4 | lower quality |
| [GGUF](https://huggingface.co/mradermacher/Llama-3-8B-Ultrachat-200K-i1-GGUF/resolve/main/Llama-3-8B-Ultrachat-200K.i1-IQ3_XS.gguf) | i1-IQ3_XS | 3.6 | |
| [GGUF](https://huggingface.co/mradermacher/Llama-3-8B-Ultrachat-200K-i1-GGUF/resolve/main/Llama-3-8B-Ultrachat-200K.i1-Q3_K_S.gguf) | i1-Q3_K_S | 3.8 | IQ3_XS probably better |
| [GGUF](https://huggingface.co/mradermacher/Llama-3-8B-Ultrachat-200K-i1-GGUF/resolve/main/Llama-3-8B-Ultrachat-200K.i1-IQ3_S.gguf) | i1-IQ3_S | 3.8 | beats Q3_K* |
| [GGUF](https://huggingface.co/mradermacher/Llama-3-8B-Ultrachat-200K-i1-GGUF/resolve/main/Llama-3-8B-Ultrachat-200K.i1-IQ3_M.gguf) | i1-IQ3_M | 3.9 | |
| [GGUF](https://huggingface.co/mradermacher/Llama-3-8B-Ultrachat-200K-i1-GGUF/resolve/main/Llama-3-8B-Ultrachat-200K.i1-Q3_K_M.gguf) | i1-Q3_K_M | 4.1 | IQ3_S probably better |
| [GGUF](https://huggingface.co/mradermacher/Llama-3-8B-Ultrachat-200K-i1-GGUF/resolve/main/Llama-3-8B-Ultrachat-200K.i1-Q3_K_L.gguf) | i1-Q3_K_L | 4.4 | IQ3_M probably better |
| [GGUF](https://huggingface.co/mradermacher/Llama-3-8B-Ultrachat-200K-i1-GGUF/resolve/main/Llama-3-8B-Ultrachat-200K.i1-IQ4_XS.gguf) | i1-IQ4_XS | 4.5 | |
| [GGUF](https://huggingface.co/mradermacher/Llama-3-8B-Ultrachat-200K-i1-GGUF/resolve/main/Llama-3-8B-Ultrachat-200K.i1-Q4_0.gguf) | i1-Q4_0 | 4.8 | fast, low quality |
| [GGUF](https://huggingface.co/mradermacher/Llama-3-8B-Ultrachat-200K-i1-GGUF/resolve/main/Llama-3-8B-Ultrachat-200K.i1-Q4_K_S.gguf) | i1-Q4_K_S | 4.8 | optimal size/speed/quality |
| [GGUF](https://huggingface.co/mradermacher/Llama-3-8B-Ultrachat-200K-i1-GGUF/resolve/main/Llama-3-8B-Ultrachat-200K.i1-Q4_K_M.gguf) | i1-Q4_K_M | 5.0 | fast, recommended |
| [GGUF](https://huggingface.co/mradermacher/Llama-3-8B-Ultrachat-200K-i1-GGUF/resolve/main/Llama-3-8B-Ultrachat-200K.i1-Q5_K_S.gguf) | i1-Q5_K_S | 5.7 | |
| [GGUF](https://huggingface.co/mradermacher/Llama-3-8B-Ultrachat-200K-i1-GGUF/resolve/main/Llama-3-8B-Ultrachat-200K.i1-Q5_K_M.gguf) | i1-Q5_K_M | 5.8 | |
| [GGUF](https://huggingface.co/mradermacher/Llama-3-8B-Ultrachat-200K-i1-GGUF/resolve/main/Llama-3-8B-Ultrachat-200K.i1-Q6_K.gguf) | i1-Q6_K | 6.7 | practically like static Q6_K |
Here is a handy graph by ikawrakow comparing some lower-quality quant
types (lower is better):

And here are Artefact2's thoughts on the matter:
https://gist.github.com/Artefact2/b5f810600771265fc1e39442288e8ec9
## FAQ / Model Request
See https://huggingface.co/mradermacher/model_requests for some answers to
questions you might have and/or if you want some other model quantized.
## Thanks
I thank my company, [nethype GmbH](https://www.nethype.de/), for letting
me use its servers and providing upgrades to my workstation to enable
this work in my free time. Additional thanks to [@nicoboss](https://huggingface.co/nicoboss) for giving me access to his hardware for calculating the imatrix for these quants.
<!-- end -->
|
mradermacher/Llama-3-Instruct-8B-SimPO-ExPO-GGUF | mradermacher | 2024-06-28T20:20:53Z | 14,177 | 0 | transformers | [
"transformers",
"gguf",
"en",
"base_model:chujiezheng/Llama-3-Instruct-8B-SimPO-ExPO",
"license:llama3",
"endpoints_compatible",
"region:us"
] | null | 2024-06-27T21:00:38Z | ---
base_model: chujiezheng/Llama-3-Instruct-8B-SimPO-ExPO
language:
- en
library_name: transformers
license: llama3
quantized_by: mradermacher
---
## About
<!-- ### quantize_version: 2 -->
<!-- ### output_tensor_quantised: 1 -->
<!-- ### convert_type: hf -->
<!-- ### vocab_type: -->
<!-- ### tags: -->
static quants of https://huggingface.co/chujiezheng/Llama-3-Instruct-8B-SimPO-ExPO
<!-- provided-files -->
weighted/imatrix quants are available at https://huggingface.co/mradermacher/Llama-3-Instruct-8B-SimPO-ExPO-i1-GGUF
## Usage
If you are unsure how to use GGUF files, refer to one of [TheBloke's
READMEs](https://huggingface.co/TheBloke/KafkaLM-70B-German-V0.1-GGUF) for
more details, including on how to concatenate multi-part files.
## Provided Quants
(sorted by size, not necessarily quality. IQ-quants are often preferable over similar sized non-IQ quants)
| Link | Type | Size/GB | Notes |
|:-----|:-----|--------:|:------|
| [GGUF](https://huggingface.co/mradermacher/Llama-3-Instruct-8B-SimPO-ExPO-GGUF/resolve/main/Llama-3-Instruct-8B-SimPO-ExPO.Q2_K.gguf) | Q2_K | 3.3 | |
| [GGUF](https://huggingface.co/mradermacher/Llama-3-Instruct-8B-SimPO-ExPO-GGUF/resolve/main/Llama-3-Instruct-8B-SimPO-ExPO.IQ3_XS.gguf) | IQ3_XS | 3.6 | |
| [GGUF](https://huggingface.co/mradermacher/Llama-3-Instruct-8B-SimPO-ExPO-GGUF/resolve/main/Llama-3-Instruct-8B-SimPO-ExPO.Q3_K_S.gguf) | Q3_K_S | 3.8 | |
| [GGUF](https://huggingface.co/mradermacher/Llama-3-Instruct-8B-SimPO-ExPO-GGUF/resolve/main/Llama-3-Instruct-8B-SimPO-ExPO.IQ3_S.gguf) | IQ3_S | 3.8 | beats Q3_K* |
| [GGUF](https://huggingface.co/mradermacher/Llama-3-Instruct-8B-SimPO-ExPO-GGUF/resolve/main/Llama-3-Instruct-8B-SimPO-ExPO.IQ3_M.gguf) | IQ3_M | 3.9 | |
| [GGUF](https://huggingface.co/mradermacher/Llama-3-Instruct-8B-SimPO-ExPO-GGUF/resolve/main/Llama-3-Instruct-8B-SimPO-ExPO.Q3_K_M.gguf) | Q3_K_M | 4.1 | lower quality |
| [GGUF](https://huggingface.co/mradermacher/Llama-3-Instruct-8B-SimPO-ExPO-GGUF/resolve/main/Llama-3-Instruct-8B-SimPO-ExPO.Q3_K_L.gguf) | Q3_K_L | 4.4 | |
| [GGUF](https://huggingface.co/mradermacher/Llama-3-Instruct-8B-SimPO-ExPO-GGUF/resolve/main/Llama-3-Instruct-8B-SimPO-ExPO.IQ4_XS.gguf) | IQ4_XS | 4.6 | |
| [GGUF](https://huggingface.co/mradermacher/Llama-3-Instruct-8B-SimPO-ExPO-GGUF/resolve/main/Llama-3-Instruct-8B-SimPO-ExPO.Q4_K_S.gguf) | Q4_K_S | 4.8 | fast, recommended |
| [GGUF](https://huggingface.co/mradermacher/Llama-3-Instruct-8B-SimPO-ExPO-GGUF/resolve/main/Llama-3-Instruct-8B-SimPO-ExPO.Q4_K_M.gguf) | Q4_K_M | 5.0 | fast, recommended |
| [GGUF](https://huggingface.co/mradermacher/Llama-3-Instruct-8B-SimPO-ExPO-GGUF/resolve/main/Llama-3-Instruct-8B-SimPO-ExPO.Q5_K_S.gguf) | Q5_K_S | 5.7 | |
| [GGUF](https://huggingface.co/mradermacher/Llama-3-Instruct-8B-SimPO-ExPO-GGUF/resolve/main/Llama-3-Instruct-8B-SimPO-ExPO.Q5_K_M.gguf) | Q5_K_M | 5.8 | |
| [GGUF](https://huggingface.co/mradermacher/Llama-3-Instruct-8B-SimPO-ExPO-GGUF/resolve/main/Llama-3-Instruct-8B-SimPO-ExPO.Q6_K.gguf) | Q6_K | 6.7 | very good quality |
| [GGUF](https://huggingface.co/mradermacher/Llama-3-Instruct-8B-SimPO-ExPO-GGUF/resolve/main/Llama-3-Instruct-8B-SimPO-ExPO.Q8_0.gguf) | Q8_0 | 8.6 | fast, best quality |
| [GGUF](https://huggingface.co/mradermacher/Llama-3-Instruct-8B-SimPO-ExPO-GGUF/resolve/main/Llama-3-Instruct-8B-SimPO-ExPO.f16.gguf) | f16 | 16.2 | 16 bpw, overkill |
Here is a handy graph by ikawrakow comparing some lower-quality quant
types (lower is better):

And here are Artefact2's thoughts on the matter:
https://gist.github.com/Artefact2/b5f810600771265fc1e39442288e8ec9
## FAQ / Model Request
See https://huggingface.co/mradermacher/model_requests for some answers to
questions you might have and/or if you want some other model quantized.
## Thanks
I thank my company, [nethype GmbH](https://www.nethype.de/), for letting
me use its servers and providing upgrades to my workstation to enable
this work in my free time.
<!-- end -->
|
KoichiYasuoka/bert-base-thai-upos | KoichiYasuoka | 2022-09-18T10:43:37Z | 14,168 | 0 | transformers | [
"transformers",
"pytorch",
"bert",
"token-classification",
"thai",
"pos",
"wikipedia",
"dependency-parsing",
"th",
"dataset:universal_dependencies",
"license:apache-2.0",
"autotrain_compatible",
"endpoints_compatible",
"region:us"
] | token-classification | 2022-03-02T23:29:04Z | ---
language:
- "th"
tags:
- "thai"
- "token-classification"
- "pos"
- "wikipedia"
- "dependency-parsing"
datasets:
- "universal_dependencies"
license: "apache-2.0"
pipeline_tag: "token-classification"
widget:
- text: "หลายหัวดีกว่าหัวเดียว"
---
# bert-base-thai-upos
## Model Description
This is a BERT model pre-trained on Thai Wikipedia texts for POS-tagging and dependency-parsing, derived from [bert-base-th-cased](https://huggingface.co/Geotrend/bert-base-th-cased). Every word is tagged by [UPOS](https://universaldependencies.org/u/pos/) (Universal Part-Of-Speech).
## How to Use
```py
from transformers import AutoTokenizer,AutoModelForTokenClassification
tokenizer=AutoTokenizer.from_pretrained("KoichiYasuoka/bert-base-thai-upos")
model=AutoModelForTokenClassification.from_pretrained("KoichiYasuoka/bert-base-thai-upos")
```
or
```py
import esupar
nlp=esupar.load("KoichiYasuoka/bert-base-thai-upos")
```
## See Also
[esupar](https://github.com/KoichiYasuoka/esupar): Tokenizer POS-tagger and Dependency-parser with BERT/RoBERTa/DeBERTa models
|
andricValdez/bert-base-multilingual-cased-finetuned-autext24 | andricValdez | 2024-05-23T09:20:33Z | 14,155 | 0 | transformers | [
"transformers",
"safetensors",
"bert",
"text-classification",
"generated_from_trainer",
"base_model:google-bert/bert-base-multilingual-cased",
"license:apache-2.0",
"autotrain_compatible",
"endpoints_compatible",
"region:us"
] | text-classification | 2024-05-23T07:14:33Z | ---
license: apache-2.0
base_model: google-bert/bert-base-multilingual-cased
tags:
- generated_from_trainer
metrics:
- accuracy
- f1
model-index:
- name: bert-base-multilingual-cased-finetuned-autext24
results: []
---
<!-- This model card has been generated automatically according to the information the Trainer had access to. You
should probably proofread and complete it, then remove this comment. -->
# bert-base-multilingual-cased-finetuned-autext24
This model is a fine-tuned version of [google-bert/bert-base-multilingual-cased](https://huggingface.co/google-bert/bert-base-multilingual-cased) on the None dataset.
It achieves the following results on the evaluation set:
- Loss: 0.3038
- Accuracy: 0.9495
- F1: 0.9493
## Model description
More information needed
## Intended uses & limitations
More information needed
## Training and evaluation data
More information needed
## Training procedure
### Training hyperparameters
The following hyperparameters were used during training:
- learning_rate: 2e-05
- train_batch_size: 64
- eval_batch_size: 64
- seed: 42
- optimizer: Adam with betas=(0.9,0.999) and epsilon=1e-08
- lr_scheduler_type: linear
- num_epochs: 5
### Training results
| Training Loss | Epoch | Step | Validation Loss | Accuracy | F1 |
|:-------------:|:-----:|:----:|:---------------:|:--------:|:------:|
| No log | 1.0 | 1200 | 0.1397 | 0.9470 | 0.9468 |
| 0.1244 | 2.0 | 2400 | 0.2977 | 0.9219 | 0.9211 |
| 0.1244 | 3.0 | 3600 | 0.1958 | 0.9503 | 0.9501 |
| 0.0311 | 4.0 | 4800 | 0.2257 | 0.9545 | 0.9544 |
| 0.0311 | 5.0 | 6000 | 0.3038 | 0.9495 | 0.9493 |
### Framework versions
- Transformers 4.40.1
- Pytorch 2.3.0+cu121
- Datasets 2.19.0
- Tokenizers 0.19.1
|
castorini/tct_colbert-v2-hnp-msmarco | castorini | 2021-08-12T01:05:56Z | 14,153 | 4 | transformers | [
"transformers",
"pytorch",
"bert",
"feature-extraction",
"endpoints_compatible",
"text-embeddings-inference",
"region:us"
] | feature-extraction | 2022-03-02T23:29:05Z | This model is to reproduce a variant of TCT-ColBERT-V2 dense retrieval models described in the following paper:
> Sheng-Chieh Lin, Jheng-Hong Yang, and Jimmy Lin. [In-Batch Negatives for Knowledge Distillation with Tightly-CoupledTeachers for Dense Retrieval.](https://cs.uwaterloo.ca/~jimmylin/publications/Lin_etal_2021_RepL4NLP.pdf) _RepL4NLP 2021_.
You can find our reproduction report in Pyserini [here](https://github.com/castorini/pyserini/blob/master/docs/experiments-tct_colbert-v2.md).
|
koti-malla/bert_tokenizer | koti-malla | 2024-02-12T05:06:45Z | 14,147 | 0 | transformers | [
"transformers",
"safetensors",
"bert",
"feature-extraction",
"custom_code",
"text-embeddings-inference",
"region:us"
] | feature-extraction | 2024-02-12T03:59:12Z | Entry not found |
TheBloke/WizardLM-Uncensored-SuperCOT-StoryTelling-30B-GGUF | TheBloke | 2023-09-27T12:53:32Z | 14,146 | 24 | transformers | [
"transformers",
"gguf",
"llama",
"base_model:Monero/WizardLM-Uncensored-SuperCOT-StoryTelling-30b",
"license:other",
"text-generation-inference",
"region:us"
] | null | 2023-09-20T02:30:06Z | ---
license: other
model_name: WizardLM Uncensored SuperCOT Storytelling 30B
base_model: Monero/WizardLM-Uncensored-SuperCOT-StoryTelling-30b
inference: false
model_creator: YellowRoseCx
model_type: llama
prompt_template: 'You are a helpful AI assistant.
USER: {prompt}
ASSISTANT:
'
quantized_by: TheBloke
---
<!-- header start -->
<!-- 200823 -->
<div style="width: auto; margin-left: auto; margin-right: auto">
<img src="https://i.imgur.com/EBdldam.jpg" alt="TheBlokeAI" style="width: 100%; min-width: 400px; display: block; margin: auto;">
</div>
<div style="display: flex; justify-content: space-between; width: 100%;">
<div style="display: flex; flex-direction: column; align-items: flex-start;">
<p style="margin-top: 0.5em; margin-bottom: 0em;"><a href="https://discord.gg/theblokeai">Chat & support: TheBloke's Discord server</a></p>
</div>
<div style="display: flex; flex-direction: column; align-items: flex-end;">
<p style="margin-top: 0.5em; margin-bottom: 0em;"><a href="https://www.patreon.com/TheBlokeAI">Want to contribute? TheBloke's Patreon page</a></p>
</div>
</div>
<div style="text-align:center; margin-top: 0em; margin-bottom: 0em"><p style="margin-top: 0.25em; margin-bottom: 0em;">TheBloke's LLM work is generously supported by a grant from <a href="https://a16z.com">andreessen horowitz (a16z)</a></p></div>
<hr style="margin-top: 1.0em; margin-bottom: 1.0em;">
<!-- header end -->
# WizardLM Uncensored SuperCOT Storytelling 30B - GGUF
- Model creator: [YellowRoseCx](https://huggingface.co/Monero)
- Original model: [WizardLM Uncensored SuperCOT Storytelling 30B](https://huggingface.co/Monero/WizardLM-Uncensored-SuperCOT-StoryTelling-30b)
<!-- description start -->
## Description
This repo contains GGUF format model files for [Monero's WizardLM-Uncensored-SuperCOT-Storytelling-30B](https://huggingface.co/Monero/WizardLM-Uncensored-SuperCOT-StoryTelling-30b).
<!-- description end -->
<!-- README_GGUF.md-about-gguf start -->
### About GGUF
GGUF is a new format introduced by the llama.cpp team on August 21st 2023. It is a replacement for GGML, which is no longer supported by llama.cpp.
Here is an incomplate list of clients and libraries that are known to support GGUF:
* [llama.cpp](https://github.com/ggerganov/llama.cpp). The source project for GGUF. Offers a CLI and a server option.
* [text-generation-webui](https://github.com/oobabooga/text-generation-webui), the most widely used web UI, with many features and powerful extensions. Supports GPU acceleration.
* [KoboldCpp](https://github.com/LostRuins/koboldcpp), a fully featured web UI, with GPU accel across all platforms and GPU architectures. Especially good for story telling.
* [LM Studio](https://lmstudio.ai/), an easy-to-use and powerful local GUI for Windows and macOS (Silicon), with GPU acceleration.
* [LoLLMS Web UI](https://github.com/ParisNeo/lollms-webui), a great web UI with many interesting and unique features, including a full model library for easy model selection.
* [Faraday.dev](https://faraday.dev/), an attractive and easy to use character-based chat GUI for Windows and macOS (both Silicon and Intel), with GPU acceleration.
* [ctransformers](https://github.com/marella/ctransformers), a Python library with GPU accel, LangChain support, and OpenAI-compatible AI server.
* [llama-cpp-python](https://github.com/abetlen/llama-cpp-python), a Python library with GPU accel, LangChain support, and OpenAI-compatible API server.
* [candle](https://github.com/huggingface/candle), a Rust ML framework with a focus on performance, including GPU support, and ease of use.
<!-- README_GGUF.md-about-gguf end -->
<!-- repositories-available start -->
## Repositories available
* [AWQ model(s) for GPU inference.](https://huggingface.co/TheBloke/WizardLM-Uncensored-SuperCOT-StoryTelling-30B-AWQ)
* [GPTQ models for GPU inference, with multiple quantisation parameter options.](https://huggingface.co/TheBloke/WizardLM-Uncensored-SuperCOT-StoryTelling-30B-GPTQ)
* [2, 3, 4, 5, 6 and 8-bit GGUF models for CPU+GPU inference](https://huggingface.co/TheBloke/WizardLM-Uncensored-SuperCOT-StoryTelling-30B-GGUF)
* [YellowRoseCx's original unquantised fp16 model in pytorch format, for GPU inference and for further conversions](https://huggingface.co/Monero/WizardLM-Uncensored-SuperCOT-StoryTelling-30b)
<!-- repositories-available end -->
<!-- prompt-template start -->
## Prompt template: Vicuna-Short
```
You are a helpful AI assistant.
USER: {prompt}
ASSISTANT:
```
<!-- prompt-template end -->
<!-- compatibility_gguf start -->
## Compatibility
These quantised GGUFv2 files are compatible with llama.cpp from August 27th onwards, as of commit [d0cee0d](https://github.com/ggerganov/llama.cpp/commit/d0cee0d36d5be95a0d9088b674dbb27354107221)
They are also compatible with many third party UIs and libraries - please see the list at the top of this README.
## Explanation of quantisation methods
<details>
<summary>Click to see details</summary>
The new methods available are:
* GGML_TYPE_Q2_K - "type-1" 2-bit quantization in super-blocks containing 16 blocks, each block having 16 weight. Block scales and mins are quantized with 4 bits. This ends up effectively using 2.5625 bits per weight (bpw)
* GGML_TYPE_Q3_K - "type-0" 3-bit quantization in super-blocks containing 16 blocks, each block having 16 weights. Scales are quantized with 6 bits. This end up using 3.4375 bpw.
* GGML_TYPE_Q4_K - "type-1" 4-bit quantization in super-blocks containing 8 blocks, each block having 32 weights. Scales and mins are quantized with 6 bits. This ends up using 4.5 bpw.
* GGML_TYPE_Q5_K - "type-1" 5-bit quantization. Same super-block structure as GGML_TYPE_Q4_K resulting in 5.5 bpw
* GGML_TYPE_Q6_K - "type-0" 6-bit quantization. Super-blocks with 16 blocks, each block having 16 weights. Scales are quantized with 8 bits. This ends up using 6.5625 bpw
Refer to the Provided Files table below to see what files use which methods, and how.
</details>
<!-- compatibility_gguf end -->
<!-- README_GGUF.md-provided-files start -->
## Provided files
| Name | Quant method | Bits | Size | Max RAM required | Use case |
| ---- | ---- | ---- | ---- | ---- | ----- |
| [WizardLM-Uncensored-SuperCOT-Storytelling.Q2_K.gguf](https://huggingface.co/TheBloke/WizardLM-Uncensored-SuperCOT-StoryTelling-30B-GGUF/blob/main/WizardLM-Uncensored-SuperCOT-Storytelling.Q2_K.gguf) | Q2_K | 2 | 13.50 GB| 16.00 GB | smallest, significant quality loss - not recommended for most purposes |
| [WizardLM-Uncensored-SuperCOT-Storytelling.Q3_K_S.gguf](https://huggingface.co/TheBloke/WizardLM-Uncensored-SuperCOT-StoryTelling-30B-GGUF/blob/main/WizardLM-Uncensored-SuperCOT-Storytelling.Q3_K_S.gguf) | Q3_K_S | 3 | 14.06 GB| 16.56 GB | very small, high quality loss |
| [WizardLM-Uncensored-SuperCOT-Storytelling.Q3_K_M.gguf](https://huggingface.co/TheBloke/WizardLM-Uncensored-SuperCOT-StoryTelling-30B-GGUF/blob/main/WizardLM-Uncensored-SuperCOT-Storytelling.Q3_K_M.gguf) | Q3_K_M | 3 | 15.76 GB| 18.26 GB | very small, high quality loss |
| [WizardLM-Uncensored-SuperCOT-Storytelling.Q3_K_L.gguf](https://huggingface.co/TheBloke/WizardLM-Uncensored-SuperCOT-StoryTelling-30B-GGUF/blob/main/WizardLM-Uncensored-SuperCOT-Storytelling.Q3_K_L.gguf) | Q3_K_L | 3 | 17.28 GB| 19.78 GB | small, substantial quality loss |
| [WizardLM-Uncensored-SuperCOT-Storytelling.Q4_0.gguf](https://huggingface.co/TheBloke/WizardLM-Uncensored-SuperCOT-StoryTelling-30B-GGUF/blob/main/WizardLM-Uncensored-SuperCOT-Storytelling.Q4_0.gguf) | Q4_0 | 4 | 18.36 GB| 20.86 GB | legacy; small, very high quality loss - prefer using Q3_K_M |
| [WizardLM-Uncensored-SuperCOT-Storytelling.Q4_K_S.gguf](https://huggingface.co/TheBloke/WizardLM-Uncensored-SuperCOT-StoryTelling-30B-GGUF/blob/main/WizardLM-Uncensored-SuperCOT-Storytelling.Q4_K_S.gguf) | Q4_K_S | 4 | 18.44 GB| 20.94 GB | small, greater quality loss |
| [WizardLM-Uncensored-SuperCOT-Storytelling.Q4_K_M.gguf](https://huggingface.co/TheBloke/WizardLM-Uncensored-SuperCOT-StoryTelling-30B-GGUF/blob/main/WizardLM-Uncensored-SuperCOT-Storytelling.Q4_K_M.gguf) | Q4_K_M | 4 | 19.62 GB| 22.12 GB | medium, balanced quality - recommended |
| [WizardLM-Uncensored-SuperCOT-Storytelling.Q5_0.gguf](https://huggingface.co/TheBloke/WizardLM-Uncensored-SuperCOT-StoryTelling-30B-GGUF/blob/main/WizardLM-Uncensored-SuperCOT-Storytelling.Q5_0.gguf) | Q5_0 | 5 | 22.40 GB| 24.90 GB | legacy; medium, balanced quality - prefer using Q4_K_M |
| [WizardLM-Uncensored-SuperCOT-Storytelling.Q5_K_S.gguf](https://huggingface.co/TheBloke/WizardLM-Uncensored-SuperCOT-StoryTelling-30B-GGUF/blob/main/WizardLM-Uncensored-SuperCOT-Storytelling.Q5_K_S.gguf) | Q5_K_S | 5 | 22.40 GB| 24.90 GB | large, low quality loss - recommended |
| [WizardLM-Uncensored-SuperCOT-Storytelling.Q5_K_M.gguf](https://huggingface.co/TheBloke/WizardLM-Uncensored-SuperCOT-StoryTelling-30B-GGUF/blob/main/WizardLM-Uncensored-SuperCOT-Storytelling.Q5_K_M.gguf) | Q5_K_M | 5 | 23.05 GB| 25.55 GB | large, very low quality loss - recommended |
| [WizardLM-Uncensored-SuperCOT-Storytelling.Q6_K.gguf](https://huggingface.co/TheBloke/WizardLM-Uncensored-SuperCOT-StoryTelling-30B-GGUF/blob/main/WizardLM-Uncensored-SuperCOT-Storytelling.Q6_K.gguf) | Q6_K | 6 | 26.69 GB| 29.19 GB | very large, extremely low quality loss |
| [WizardLM-Uncensored-SuperCOT-Storytelling.Q8_0.gguf](https://huggingface.co/TheBloke/WizardLM-Uncensored-SuperCOT-StoryTelling-30B-GGUF/blob/main/WizardLM-Uncensored-SuperCOT-Storytelling.Q8_0.gguf) | Q8_0 | 8 | 34.57 GB| 37.07 GB | very large, extremely low quality loss - not recommended |
**Note**: the above RAM figures assume no GPU offloading. If layers are offloaded to the GPU, this will reduce RAM usage and use VRAM instead.
<!-- README_GGUF.md-provided-files end -->
<!-- README_GGUF.md-how-to-download start -->
## How to download GGUF files
**Note for manual downloaders:** You almost never want to clone the entire repo! Multiple different quantisation formats are provided, and most users only want to pick and download a single file.
The following clients/libraries will automatically download models for you, providing a list of available models to choose from:
- LM Studio
- LoLLMS Web UI
- Faraday.dev
### In `text-generation-webui`
Under Download Model, you can enter the model repo: TheBloke/WizardLM-Uncensored-SuperCOT-StoryTelling-30B-GGUF and below it, a specific filename to download, such as: WizardLM-Uncensored-SuperCOT-Storytelling.Q4_K_M.gguf.
Then click Download.
### On the command line, including multiple files at once
I recommend using the `huggingface-hub` Python library:
```shell
pip3 install huggingface-hub
```
Then you can download any individual model file to the current directory, at high speed, with a command like this:
```shell
huggingface-cli download TheBloke/WizardLM-Uncensored-SuperCOT-StoryTelling-30B-GGUF WizardLM-Uncensored-SuperCOT-Storytelling.Q4_K_M.gguf --local-dir . --local-dir-use-symlinks False
```
<details>
<summary>More advanced huggingface-cli download usage</summary>
You can also download multiple files at once with a pattern:
```shell
huggingface-cli download TheBloke/WizardLM-Uncensored-SuperCOT-StoryTelling-30B-GGUF --local-dir . --local-dir-use-symlinks False --include='*Q4_K*gguf'
```
For more documentation on downloading with `huggingface-cli`, please see: [HF -> Hub Python Library -> Download files -> Download from the CLI](https://huggingface.co/docs/huggingface_hub/guides/download#download-from-the-cli).
To accelerate downloads on fast connections (1Gbit/s or higher), install `hf_transfer`:
```shell
pip3 install hf_transfer
```
And set environment variable `HF_HUB_ENABLE_HF_TRANSFER` to `1`:
```shell
HF_HUB_ENABLE_HF_TRANSFER=1 huggingface-cli download TheBloke/WizardLM-Uncensored-SuperCOT-StoryTelling-30B-GGUF WizardLM-Uncensored-SuperCOT-Storytelling.Q4_K_M.gguf --local-dir . --local-dir-use-symlinks False
```
Windows Command Line users: You can set the environment variable by running `set HF_HUB_ENABLE_HF_TRANSFER=1` before the download command.
</details>
<!-- README_GGUF.md-how-to-download end -->
<!-- README_GGUF.md-how-to-run start -->
## Example `llama.cpp` command
Make sure you are using `llama.cpp` from commit [d0cee0d](https://github.com/ggerganov/llama.cpp/commit/d0cee0d36d5be95a0d9088b674dbb27354107221) or later.
```shell
./main -ngl 32 -m WizardLM-Uncensored-SuperCOT-Storytelling.Q4_K_M.gguf --color -c 2048 --temp 0.7 --repeat_penalty 1.1 -n -1 -p "You are a helpful AI assistant.\n\nUSER: {prompt}\nASSISTANT:"
```
Change `-ngl 32` to the number of layers to offload to GPU. Remove it if you don't have GPU acceleration.
Change `-c 2048` to the desired sequence length. For extended sequence models - eg 8K, 16K, 32K - the necessary RoPE scaling parameters are read from the GGUF file and set by llama.cpp automatically.
If you want to have a chat-style conversation, replace the `-p <PROMPT>` argument with `-i -ins`
For other parameters and how to use them, please refer to [the llama.cpp documentation](https://github.com/ggerganov/llama.cpp/blob/master/examples/main/README.md)
## How to run in `text-generation-webui`
Further instructions here: [text-generation-webui/docs/llama.cpp.md](https://github.com/oobabooga/text-generation-webui/blob/main/docs/llama.cpp.md).
## How to run from Python code
You can use GGUF models from Python using the [llama-cpp-python](https://github.com/abetlen/llama-cpp-python) or [ctransformers](https://github.com/marella/ctransformers) libraries.
### How to load this model in Python code, using ctransformers
#### First install the package
Run one of the following commands, according to your system:
```shell
# Base ctransformers with no GPU acceleration
pip install ctransformers
# Or with CUDA GPU acceleration
pip install ctransformers[cuda]
# Or with AMD ROCm GPU acceleration (Linux only)
CT_HIPBLAS=1 pip install ctransformers --no-binary ctransformers
# Or with Metal GPU acceleration for macOS systems only
CT_METAL=1 pip install ctransformers --no-binary ctransformers
```
#### Simple ctransformers example code
```python
from ctransformers import AutoModelForCausalLM
# Set gpu_layers to the number of layers to offload to GPU. Set to 0 if no GPU acceleration is available on your system.
llm = AutoModelForCausalLM.from_pretrained("TheBloke/WizardLM-Uncensored-SuperCOT-StoryTelling-30B-GGUF", model_file="WizardLM-Uncensored-SuperCOT-Storytelling.Q4_K_M.gguf", model_type="llama", gpu_layers=50)
print(llm("AI is going to"))
```
## How to use with LangChain
Here are guides on using llama-cpp-python and ctransformers with LangChain:
* [LangChain + llama-cpp-python](https://python.langchain.com/docs/integrations/llms/llamacpp)
* [LangChain + ctransformers](https://python.langchain.com/docs/integrations/providers/ctransformers)
<!-- README_GGUF.md-how-to-run end -->
<!-- footer start -->
<!-- 200823 -->
## Discord
For further support, and discussions on these models and AI in general, join us at:
[TheBloke AI's Discord server](https://discord.gg/theblokeai)
## Thanks, and how to contribute
Thanks to the [chirper.ai](https://chirper.ai) team!
Thanks to Clay from [gpus.llm-utils.org](llm-utils)!
I've had a lot of people ask if they can contribute. I enjoy providing models and helping people, and would love to be able to spend even more time doing it, as well as expanding into new projects like fine tuning/training.
If you're able and willing to contribute it will be most gratefully received and will help me to keep providing more models, and to start work on new AI projects.
Donaters will get priority support on any and all AI/LLM/model questions and requests, access to a private Discord room, plus other benefits.
* Patreon: https://patreon.com/TheBlokeAI
* Ko-Fi: https://ko-fi.com/TheBlokeAI
**Special thanks to**: Aemon Algiz.
**Patreon special mentions**: Alicia Loh, Stephen Murray, K, Ajan Kanaga, RoA, Magnesian, Deo Leter, Olakabola, Eugene Pentland, zynix, Deep Realms, Raymond Fosdick, Elijah Stavena, Iucharbius, Erik Bjäreholt, Luis Javier Navarrete Lozano, Nicholas, theTransient, John Detwiler, alfie_i, knownsqashed, Mano Prime, Willem Michiel, Enrico Ros, LangChain4j, OG, Michael Dempsey, Pierre Kircher, Pedro Madruga, James Bentley, Thomas Belote, Luke @flexchar, Leonard Tan, Johann-Peter Hartmann, Illia Dulskyi, Fen Risland, Chadd, S_X, Jeff Scroggin, Ken Nordquist, Sean Connelly, Artur Olbinski, Swaroop Kallakuri, Jack West, Ai Maven, David Ziegler, Russ Johnson, transmissions 11, John Villwock, Alps Aficionado, Clay Pascal, Viktor Bowallius, Subspace Studios, Rainer Wilmers, Trenton Dambrowitz, vamX, Michael Levine, 준교 김, Brandon Frisco, Kalila, Trailburnt, Randy H, Talal Aujan, Nathan Dryer, Vadim, 阿明, ReadyPlayerEmma, Tiffany J. Kim, George Stoitzev, Spencer Kim, Jerry Meng, Gabriel Tamborski, Cory Kujawski, Jeffrey Morgan, Spiking Neurons AB, Edmond Seymore, Alexandros Triantafyllidis, Lone Striker, Cap'n Zoog, Nikolai Manek, danny, ya boyyy, Derek Yates, usrbinkat, Mandus, TL, Nathan LeClaire, subjectnull, Imad Khwaja, webtim, Raven Klaugh, Asp the Wyvern, Gabriel Puliatti, Caitlyn Gatomon, Joseph William Delisle, Jonathan Leane, Luke Pendergrass, SuperWojo, Sebastain Graf, Will Dee, Fred von Graf, Andrey, Dan Guido, Daniel P. Andersen, Nitin Borwankar, Elle, Vitor Caleffi, biorpg, jjj, NimbleBox.ai, Pieter, Matthew Berman, terasurfer, Michael Davis, Alex, Stanislav Ovsiannikov
Thank you to all my generous patrons and donaters!
And thank you again to a16z for their generous grant.
<!-- footer end -->
<!-- original-model-card start -->
# Original model card: Monero's WizardLM-Uncensored-SuperCOT-Storytelling-30B
This model is a triple model merge of WizardLM Uncensored+CoT+Storytelling, resulting in a comprehensive boost in reasoning and story writing capabilities.
To allow all output, at the end of your prompt add ```### Certainly!```
You've become a compendium of knowledge on a vast array of topics.
Lore Mastery is an arcane tradition fixated on understanding the underlying mechanics of magic. It is the most academic of all arcane traditions. The promise of uncovering new knowledge or proving (or discrediting) a theory of magic is usually required to rouse its practitioners from their laboratories, academies, and archives to pursue a life of adventure. Known as savants, followers of this tradition are a bookish lot who see beauty and mystery in the application of magic. The results of a spell are less interesting to them than the process that creates it. Some savants take a haughty attitude toward those who follow a tradition focused on a single school of magic, seeing them as provincial and lacking the sophistication needed to master true magic. Other savants are generous teachers, countering ignorance and deception with deep knowledge and good humor.
<!-- original-model-card end -->
|
mradermacher/Fimbulvetr-11B-v2.1-16K-GGUF | mradermacher | 2024-06-30T06:44:15Z | 14,146 | 0 | transformers | [
"transformers",
"gguf",
"en",
"base_model:Sao10K/Fimbulvetr-11B-v2.1-16K",
"license:cc-by-nc-4.0",
"endpoints_compatible",
"region:us"
] | null | 2024-06-30T05:13:06Z | ---
base_model: Sao10K/Fimbulvetr-11B-v2.1-16K
language:
- en
library_name: transformers
license: cc-by-nc-4.0
quantized_by: mradermacher
---
## About
<!-- ### quantize_version: 2 -->
<!-- ### output_tensor_quantised: 1 -->
<!-- ### convert_type: hf -->
<!-- ### vocab_type: -->
<!-- ### tags: -->
static quants of https://huggingface.co/Sao10K/Fimbulvetr-11B-v2.1-16K
<!-- provided-files -->
weighted/imatrix quants are available at https://huggingface.co/mradermacher/Fimbulvetr-11B-v2.1-16K-i1-GGUF
## Usage
If you are unsure how to use GGUF files, refer to one of [TheBloke's
READMEs](https://huggingface.co/TheBloke/KafkaLM-70B-German-V0.1-GGUF) for
more details, including on how to concatenate multi-part files.
## Provided Quants
(sorted by size, not necessarily quality. IQ-quants are often preferable over similar sized non-IQ quants)
| Link | Type | Size/GB | Notes |
|:-----|:-----|--------:|:------|
| [GGUF](https://huggingface.co/mradermacher/Fimbulvetr-11B-v2.1-16K-GGUF/resolve/main/Fimbulvetr-11B-v2.1-16K.Q2_K.gguf) | Q2_K | 4.1 | |
| [GGUF](https://huggingface.co/mradermacher/Fimbulvetr-11B-v2.1-16K-GGUF/resolve/main/Fimbulvetr-11B-v2.1-16K.IQ3_XS.gguf) | IQ3_XS | 4.5 | |
| [GGUF](https://huggingface.co/mradermacher/Fimbulvetr-11B-v2.1-16K-GGUF/resolve/main/Fimbulvetr-11B-v2.1-16K.Q3_K_S.gguf) | Q3_K_S | 4.8 | |
| [GGUF](https://huggingface.co/mradermacher/Fimbulvetr-11B-v2.1-16K-GGUF/resolve/main/Fimbulvetr-11B-v2.1-16K.IQ3_S.gguf) | IQ3_S | 4.8 | beats Q3_K* |
| [GGUF](https://huggingface.co/mradermacher/Fimbulvetr-11B-v2.1-16K-GGUF/resolve/main/Fimbulvetr-11B-v2.1-16K.IQ3_M.gguf) | IQ3_M | 4.9 | |
| [GGUF](https://huggingface.co/mradermacher/Fimbulvetr-11B-v2.1-16K-GGUF/resolve/main/Fimbulvetr-11B-v2.1-16K.Q3_K_M.gguf) | Q3_K_M | 5.3 | lower quality |
| [GGUF](https://huggingface.co/mradermacher/Fimbulvetr-11B-v2.1-16K-GGUF/resolve/main/Fimbulvetr-11B-v2.1-16K.Q3_K_L.gguf) | Q3_K_L | 5.8 | |
| [GGUF](https://huggingface.co/mradermacher/Fimbulvetr-11B-v2.1-16K-GGUF/resolve/main/Fimbulvetr-11B-v2.1-16K.IQ4_XS.gguf) | IQ4_XS | 5.9 | |
| [GGUF](https://huggingface.co/mradermacher/Fimbulvetr-11B-v2.1-16K-GGUF/resolve/main/Fimbulvetr-11B-v2.1-16K.Q4_K_S.gguf) | Q4_K_S | 6.2 | fast, recommended |
| [GGUF](https://huggingface.co/mradermacher/Fimbulvetr-11B-v2.1-16K-GGUF/resolve/main/Fimbulvetr-11B-v2.1-16K.Q4_K_M.gguf) | Q4_K_M | 6.6 | fast, recommended |
| [GGUF](https://huggingface.co/mradermacher/Fimbulvetr-11B-v2.1-16K-GGUF/resolve/main/Fimbulvetr-11B-v2.1-16K.Q5_K_S.gguf) | Q5_K_S | 7.5 | |
| [GGUF](https://huggingface.co/mradermacher/Fimbulvetr-11B-v2.1-16K-GGUF/resolve/main/Fimbulvetr-11B-v2.1-16K.Q5_K_M.gguf) | Q5_K_M | 7.7 | |
| [GGUF](https://huggingface.co/mradermacher/Fimbulvetr-11B-v2.1-16K-GGUF/resolve/main/Fimbulvetr-11B-v2.1-16K.Q6_K.gguf) | Q6_K | 8.9 | very good quality |
| [GGUF](https://huggingface.co/mradermacher/Fimbulvetr-11B-v2.1-16K-GGUF/resolve/main/Fimbulvetr-11B-v2.1-16K.Q8_0.gguf) | Q8_0 | 11.5 | fast, best quality |
Here is a handy graph by ikawrakow comparing some lower-quality quant
types (lower is better):

And here are Artefact2's thoughts on the matter:
https://gist.github.com/Artefact2/b5f810600771265fc1e39442288e8ec9
## FAQ / Model Request
See https://huggingface.co/mradermacher/model_requests for some answers to
questions you might have and/or if you want some other model quantized.
## Thanks
I thank my company, [nethype GmbH](https://www.nethype.de/), for letting
me use its servers and providing upgrades to my workstation to enable
this work in my free time.
<!-- end -->
|
RichardErkhov/johnsnowlabs_-_JSL-MedLlama-3-8B-v1.0-gguf | RichardErkhov | 2024-06-20T21:35:57Z | 14,143 | 0 | null | [
"gguf",
"region:us"
] | null | 2024-06-20T12:55:47Z | Quantization made by Richard Erkhov.
[Github](https://github.com/RichardErkhov)
[Discord](https://discord.gg/pvy7H8DZMG)
[Request more models](https://github.com/RichardErkhov/quant_request)
JSL-MedLlama-3-8B-v1.0 - GGUF
- Model creator: https://huggingface.co/johnsnowlabs/
- Original model: https://huggingface.co/johnsnowlabs/JSL-MedLlama-3-8B-v1.0/
| Name | Quant method | Size |
| ---- | ---- | ---- |
| [JSL-MedLlama-3-8B-v1.0.Q2_K.gguf](https://huggingface.co/RichardErkhov/johnsnowlabs_-_JSL-MedLlama-3-8B-v1.0-gguf/blob/main/JSL-MedLlama-3-8B-v1.0.Q2_K.gguf) | Q2_K | 2.96GB |
| [JSL-MedLlama-3-8B-v1.0.IQ3_XS.gguf](https://huggingface.co/RichardErkhov/johnsnowlabs_-_JSL-MedLlama-3-8B-v1.0-gguf/blob/main/JSL-MedLlama-3-8B-v1.0.IQ3_XS.gguf) | IQ3_XS | 3.28GB |
| [JSL-MedLlama-3-8B-v1.0.IQ3_S.gguf](https://huggingface.co/RichardErkhov/johnsnowlabs_-_JSL-MedLlama-3-8B-v1.0-gguf/blob/main/JSL-MedLlama-3-8B-v1.0.IQ3_S.gguf) | IQ3_S | 3.43GB |
| [JSL-MedLlama-3-8B-v1.0.Q3_K_S.gguf](https://huggingface.co/RichardErkhov/johnsnowlabs_-_JSL-MedLlama-3-8B-v1.0-gguf/blob/main/JSL-MedLlama-3-8B-v1.0.Q3_K_S.gguf) | Q3_K_S | 3.41GB |
| [JSL-MedLlama-3-8B-v1.0.IQ3_M.gguf](https://huggingface.co/RichardErkhov/johnsnowlabs_-_JSL-MedLlama-3-8B-v1.0-gguf/blob/main/JSL-MedLlama-3-8B-v1.0.IQ3_M.gguf) | IQ3_M | 3.52GB |
| [JSL-MedLlama-3-8B-v1.0.Q3_K.gguf](https://huggingface.co/RichardErkhov/johnsnowlabs_-_JSL-MedLlama-3-8B-v1.0-gguf/blob/main/JSL-MedLlama-3-8B-v1.0.Q3_K.gguf) | Q3_K | 3.74GB |
| [JSL-MedLlama-3-8B-v1.0.Q3_K_M.gguf](https://huggingface.co/RichardErkhov/johnsnowlabs_-_JSL-MedLlama-3-8B-v1.0-gguf/blob/main/JSL-MedLlama-3-8B-v1.0.Q3_K_M.gguf) | Q3_K_M | 3.74GB |
| [JSL-MedLlama-3-8B-v1.0.Q3_K_L.gguf](https://huggingface.co/RichardErkhov/johnsnowlabs_-_JSL-MedLlama-3-8B-v1.0-gguf/blob/main/JSL-MedLlama-3-8B-v1.0.Q3_K_L.gguf) | Q3_K_L | 4.03GB |
| [JSL-MedLlama-3-8B-v1.0.IQ4_XS.gguf](https://huggingface.co/RichardErkhov/johnsnowlabs_-_JSL-MedLlama-3-8B-v1.0-gguf/blob/main/JSL-MedLlama-3-8B-v1.0.IQ4_XS.gguf) | IQ4_XS | 4.18GB |
| [JSL-MedLlama-3-8B-v1.0.Q4_0.gguf](https://huggingface.co/RichardErkhov/johnsnowlabs_-_JSL-MedLlama-3-8B-v1.0-gguf/blob/main/JSL-MedLlama-3-8B-v1.0.Q4_0.gguf) | Q4_0 | 4.34GB |
| [JSL-MedLlama-3-8B-v1.0.IQ4_NL.gguf](https://huggingface.co/RichardErkhov/johnsnowlabs_-_JSL-MedLlama-3-8B-v1.0-gguf/blob/main/JSL-MedLlama-3-8B-v1.0.IQ4_NL.gguf) | IQ4_NL | 4.38GB |
| [JSL-MedLlama-3-8B-v1.0.Q4_K_S.gguf](https://huggingface.co/RichardErkhov/johnsnowlabs_-_JSL-MedLlama-3-8B-v1.0-gguf/blob/main/JSL-MedLlama-3-8B-v1.0.Q4_K_S.gguf) | Q4_K_S | 4.37GB |
| [JSL-MedLlama-3-8B-v1.0.Q4_K.gguf](https://huggingface.co/RichardErkhov/johnsnowlabs_-_JSL-MedLlama-3-8B-v1.0-gguf/blob/main/JSL-MedLlama-3-8B-v1.0.Q4_K.gguf) | Q4_K | 4.58GB |
| [JSL-MedLlama-3-8B-v1.0.Q4_K_M.gguf](https://huggingface.co/RichardErkhov/johnsnowlabs_-_JSL-MedLlama-3-8B-v1.0-gguf/blob/main/JSL-MedLlama-3-8B-v1.0.Q4_K_M.gguf) | Q4_K_M | 4.58GB |
| [JSL-MedLlama-3-8B-v1.0.Q4_1.gguf](https://huggingface.co/RichardErkhov/johnsnowlabs_-_JSL-MedLlama-3-8B-v1.0-gguf/blob/main/JSL-MedLlama-3-8B-v1.0.Q4_1.gguf) | Q4_1 | 4.78GB |
| [JSL-MedLlama-3-8B-v1.0.Q5_0.gguf](https://huggingface.co/RichardErkhov/johnsnowlabs_-_JSL-MedLlama-3-8B-v1.0-gguf/blob/main/JSL-MedLlama-3-8B-v1.0.Q5_0.gguf) | Q5_0 | 5.21GB |
| [JSL-MedLlama-3-8B-v1.0.Q5_K_S.gguf](https://huggingface.co/RichardErkhov/johnsnowlabs_-_JSL-MedLlama-3-8B-v1.0-gguf/blob/main/JSL-MedLlama-3-8B-v1.0.Q5_K_S.gguf) | Q5_K_S | 5.21GB |
| [JSL-MedLlama-3-8B-v1.0.Q5_K.gguf](https://huggingface.co/RichardErkhov/johnsnowlabs_-_JSL-MedLlama-3-8B-v1.0-gguf/blob/main/JSL-MedLlama-3-8B-v1.0.Q5_K.gguf) | Q5_K | 5.34GB |
| [JSL-MedLlama-3-8B-v1.0.Q5_K_M.gguf](https://huggingface.co/RichardErkhov/johnsnowlabs_-_JSL-MedLlama-3-8B-v1.0-gguf/blob/main/JSL-MedLlama-3-8B-v1.0.Q5_K_M.gguf) | Q5_K_M | 5.34GB |
| [JSL-MedLlama-3-8B-v1.0.Q5_1.gguf](https://huggingface.co/RichardErkhov/johnsnowlabs_-_JSL-MedLlama-3-8B-v1.0-gguf/blob/main/JSL-MedLlama-3-8B-v1.0.Q5_1.gguf) | Q5_1 | 5.65GB |
| [JSL-MedLlama-3-8B-v1.0.Q6_K.gguf](https://huggingface.co/RichardErkhov/johnsnowlabs_-_JSL-MedLlama-3-8B-v1.0-gguf/blob/main/JSL-MedLlama-3-8B-v1.0.Q6_K.gguf) | Q6_K | 6.14GB |
| [JSL-MedLlama-3-8B-v1.0.Q8_0.gguf](https://huggingface.co/RichardErkhov/johnsnowlabs_-_JSL-MedLlama-3-8B-v1.0-gguf/blob/main/JSL-MedLlama-3-8B-v1.0.Q8_0.gguf) | Q8_0 | 7.95GB |
Original model description:
---
tags:
- llama-3-8b
- sft
- medical
base_model:
- meta-llama/Meta-Llama-3-8B
license: cc-by-nc-nd-4.0
---
# JSL-MedLlama-3-8B-v1.0
[<img src="https://repository-images.githubusercontent.com/104670986/2e728700-ace4-11ea-9cfc-f3e060b25ddf">](http://www.johnsnowlabs.com)
This model is developed by [John Snow Labs](https://www.johnsnowlabs.com/).
This model is available under a [CC-BY-NC-ND](https://creativecommons.org/licenses/by-nc-nd/4.0/deed.en) license and must also conform to this [Acceptable Use Policy](https://huggingface.co/johnsnowlabs). If you need to license this model for commercial use, please contact us at [email protected].
## 💻 Usage
```python
!pip install -qU transformers accelerate
from transformers import AutoTokenizer
import transformers
import torch
model = "johnsnowlabs/JSL-MedLlama-3-8B-v1.0"
messages = [{"role": "user", "content": "What is a large language model?"}]
tokenizer = AutoTokenizer.from_pretrained(model)
prompt = tokenizer.apply_chat_template(messages, tokenize=False, add_generation_prompt=True)
pipeline = transformers.pipeline(
"text-generation",
model=model,
torch_dtype=torch.float16,
device_map="auto",
)
outputs = pipeline(prompt, max_new_tokens=256, do_sample=True, temperature=0.7, top_k=50, top_p=0.95)
print(outputs[0]["generated_text"])
```
## 🏆 Evaluation
| Tasks |Version|Filter|n-shot| Metric |Value | |Stderr|
|-------------------------------|-------|------|-----:|--------|-----:|---|-----:|
|stem |N/A |none | 0|acc |0.6217|± |0.0057|
| | |none | 0|acc_norm|0.5847|± |0.0066|
| - medmcqa |Yaml |none | 0|acc |0.5563|± |0.0077|
| | |none | 0|acc_norm|0.5563|± |0.0077|
| - medqa_4options |Yaml |none | 0|acc |0.6779|± |0.0131|
| | |none | 0|acc_norm|0.6779|± |0.0131|
| - anatomy (mmlu) | 0|none | 0|acc |0.6963|± |0.0397|
| - clinical_knowledge (mmlu) | 0|none | 0|acc |0.7509|± |0.0266|
| - college_biology (mmlu) | 0|none | 0|acc |0.7986|± |0.0335|
| - college_medicine (mmlu) | 0|none | 0|acc |0.6590|± |0.0361|
| - medical_genetics (mmlu) | 0|none | 0|acc |0.8500|± |0.0359|
| - professional_medicine (mmlu)| 0|none | 0|acc |0.7868|± |0.0249|
| - pubmedqa | 1|none | 0|acc |0.7380|± |0.0197|
|Groups|Version|Filter|n-shot| Metric |Value | |Stderr|
|------|-------|------|-----:|--------|-----:|---|-----:|
|stem |N/A |none | 0|acc |0.6217|± |0.0057|
| | |none | 0|acc_norm|0.5847|± |0.0066|
|
OctopusMind/longbert-embedding-8k-zh | OctopusMind | 2023-12-03T07:58:28Z | 14,123 | 5 | transformers | [
"transformers",
"pytorch",
"bert",
"feature-extraction",
"embedding",
"text-embedding",
"sentence-similarity",
"custom_code",
"zh",
"license:apache-2.0",
"text-embeddings-inference",
"region:us"
] | sentence-similarity | 2023-11-22T13:29:21Z | ---
license: apache-2.0
language:
- zh
pipeline_tag: sentence-similarity
tags:
- embedding
- text-embedding
---
<h1 align="center">
Long Bert Chinese
<br>
</h1>
<h4 align="center">
<p>
<b>简体中文</b> |
<a href="https://github.com/OctopusMind/long-bert-chinese/blob/main/README_EN.md">English</a>
</p>
</h4>
<p >
<br>
</p>
**Long Bert**: 长文本相似度模型,支持8192token长度。
基于bert-base-chinese,将原始BERT位置编码更改成ALiBi位置编码,使BERT可以支持8192的序列长度。
### News
* 支持`CoSENT`微调
* github仓库 [github](https://github.com/OctopusMind/longBert)
### 使用
```python
from numpy.linalg import norm
from transformers import AutoModel
model_path = "OctopusMind/longbert-embedding-8k-zh"
model = AutoModel.from_pretrained(model_path, trust_remote_code=True)
sentences = ['我是问蚂蚁借呗为什么不能提前结清欠款', "为什么借呗不能选择提前还款"]
embeddings = model.encode(sentences)
cos_sim = lambda a,b: (a @ b.T) / (norm(a)*norm(b))
print(cos_sim(embeddings[0], embeddings[1]))
```
## 微调
### 数据格式
```json
[
{
"sentence1": "一个男人在吹一支大笛子。",
"sentence2": "一个人在吹长笛。",
"label": 3
},
{
"sentence1": "三个人在下棋。",
"sentence2": "两个人在下棋。",
"label": 2
},
{
"sentence1": "一个女人在写作。",
"sentence2": "一个女人在游泳。",
"label": 0
}
]
```
### CoSENT 微调
至`train/`路径下
```bash
cd train/
```
进行 CoSENT 微调
```bash
python cosent_finetune.py \
--data_dir ../data/train_data.json \
--output_dir ./outputs/my-model \
--max_seq_length 1024 \
--num_epochs 10 \
--batch_size 64 \
--learning_rate 2e-5
```
## 贡献
欢迎通过提交拉取请求或在仓库中提出问题来为此模块做出贡献。
## License
本项目遵循[Apache-2.0开源协议](./LICENSE) |
mradermacher/Hathor_Fractionate-L3-8B-v.05-GGUF | mradermacher | 2024-06-24T18:21:13Z | 14,116 | 0 | transformers | [
"transformers",
"gguf",
"en",
"base_model:Nitral-AI/Hathor_Fractionate-L3-8B-v.05",
"license:other",
"endpoints_compatible",
"region:us"
] | null | 2024-06-24T14:21:04Z | ---
base_model: Nitral-AI/Hathor_Fractionate-L3-8B-v.05
language:
- en
library_name: transformers
license: other
quantized_by: mradermacher
---
## About
<!-- ### quantize_version: 2 -->
<!-- ### output_tensor_quantised: 1 -->
<!-- ### convert_type: hf -->
<!-- ### vocab_type: -->
<!-- ### tags: -->
static quants of https://huggingface.co/Nitral-AI/Hathor_Fractionate-L3-8B-v.05
<!-- provided-files -->
weighted/imatrix quants are available at https://huggingface.co/mradermacher/Hathor_Fractionate-L3-8B-v.05-i1-GGUF
## Usage
If you are unsure how to use GGUF files, refer to one of [TheBloke's
READMEs](https://huggingface.co/TheBloke/KafkaLM-70B-German-V0.1-GGUF) for
more details, including on how to concatenate multi-part files.
## Provided Quants
(sorted by size, not necessarily quality. IQ-quants are often preferable over similar sized non-IQ quants)
| Link | Type | Size/GB | Notes |
|:-----|:-----|--------:|:------|
| [GGUF](https://huggingface.co/mradermacher/Hathor_Fractionate-L3-8B-v.05-GGUF/resolve/main/Hathor_Fractionate-L3-8B-v.05.Q2_K.gguf) | Q2_K | 3.3 | |
| [GGUF](https://huggingface.co/mradermacher/Hathor_Fractionate-L3-8B-v.05-GGUF/resolve/main/Hathor_Fractionate-L3-8B-v.05.IQ3_XS.gguf) | IQ3_XS | 3.6 | |
| [GGUF](https://huggingface.co/mradermacher/Hathor_Fractionate-L3-8B-v.05-GGUF/resolve/main/Hathor_Fractionate-L3-8B-v.05.Q3_K_S.gguf) | Q3_K_S | 3.8 | |
| [GGUF](https://huggingface.co/mradermacher/Hathor_Fractionate-L3-8B-v.05-GGUF/resolve/main/Hathor_Fractionate-L3-8B-v.05.IQ3_S.gguf) | IQ3_S | 3.8 | beats Q3_K* |
| [GGUF](https://huggingface.co/mradermacher/Hathor_Fractionate-L3-8B-v.05-GGUF/resolve/main/Hathor_Fractionate-L3-8B-v.05.IQ3_M.gguf) | IQ3_M | 3.9 | |
| [GGUF](https://huggingface.co/mradermacher/Hathor_Fractionate-L3-8B-v.05-GGUF/resolve/main/Hathor_Fractionate-L3-8B-v.05.Q3_K_M.gguf) | Q3_K_M | 4.1 | lower quality |
| [GGUF](https://huggingface.co/mradermacher/Hathor_Fractionate-L3-8B-v.05-GGUF/resolve/main/Hathor_Fractionate-L3-8B-v.05.Q3_K_L.gguf) | Q3_K_L | 4.4 | |
| [GGUF](https://huggingface.co/mradermacher/Hathor_Fractionate-L3-8B-v.05-GGUF/resolve/main/Hathor_Fractionate-L3-8B-v.05.IQ4_XS.gguf) | IQ4_XS | 4.6 | |
| [GGUF](https://huggingface.co/mradermacher/Hathor_Fractionate-L3-8B-v.05-GGUF/resolve/main/Hathor_Fractionate-L3-8B-v.05.Q4_K_S.gguf) | Q4_K_S | 4.8 | fast, recommended |
| [GGUF](https://huggingface.co/mradermacher/Hathor_Fractionate-L3-8B-v.05-GGUF/resolve/main/Hathor_Fractionate-L3-8B-v.05.Q4_K_M.gguf) | Q4_K_M | 5.0 | fast, recommended |
| [GGUF](https://huggingface.co/mradermacher/Hathor_Fractionate-L3-8B-v.05-GGUF/resolve/main/Hathor_Fractionate-L3-8B-v.05.Q5_K_S.gguf) | Q5_K_S | 5.7 | |
| [GGUF](https://huggingface.co/mradermacher/Hathor_Fractionate-L3-8B-v.05-GGUF/resolve/main/Hathor_Fractionate-L3-8B-v.05.Q5_K_M.gguf) | Q5_K_M | 5.8 | |
| [GGUF](https://huggingface.co/mradermacher/Hathor_Fractionate-L3-8B-v.05-GGUF/resolve/main/Hathor_Fractionate-L3-8B-v.05.Q6_K.gguf) | Q6_K | 6.7 | very good quality |
| [GGUF](https://huggingface.co/mradermacher/Hathor_Fractionate-L3-8B-v.05-GGUF/resolve/main/Hathor_Fractionate-L3-8B-v.05.Q8_0.gguf) | Q8_0 | 8.6 | fast, best quality |
| [GGUF](https://huggingface.co/mradermacher/Hathor_Fractionate-L3-8B-v.05-GGUF/resolve/main/Hathor_Fractionate-L3-8B-v.05.f16.gguf) | f16 | 16.2 | 16 bpw, overkill |
Here is a handy graph by ikawrakow comparing some lower-quality quant
types (lower is better):

And here are Artefact2's thoughts on the matter:
https://gist.github.com/Artefact2/b5f810600771265fc1e39442288e8ec9
## FAQ / Model Request
See https://huggingface.co/mradermacher/model_requests for some answers to
questions you might have and/or if you want some other model quantized.
## Thanks
I thank my company, [nethype GmbH](https://www.nethype.de/), for letting
me use its servers and providing upgrades to my workstation to enable
this work in my free time.
<!-- end -->
|
KBlueLeaf/DanTagGen-beta | KBlueLeaf | 2024-03-18T15:35:20Z | 14,115 | 49 | transformers | [
"transformers",
"safetensors",
"gguf",
"llama",
"text-generation",
"not-for-all-audiences",
"art",
"en",
"dataset:KBlueLeaf/danbooru2023-sqlite",
"license:openrail",
"autotrain_compatible",
"endpoints_compatible",
"text-generation-inference",
"region:us"
] | text-generation | 2024-03-18T12:08:44Z | ---
license: openrail
datasets:
- KBlueLeaf/danbooru2023-sqlite
language:
- en
library_name: transformers
pipeline_tag: text-generation
tags:
- not-for-all-audiences
- art
widget:
- text: "rating: safe\nartist: <|empty|>\ncharacters: <|empty|>\ncopyrights: <|empty|>\naspect ratio: 1.0\ntarget: <|short|>\ngeneral: 1girl, solo, dragon girl, dragon horns, dragon tail<|input_end|>"
---
# DanTagGen - beta
DanTagGen(Danbooru Tag Generator) is inspired from p1atdev's dart project.
But with different arch, dataset, format and different training strategy.
## Difference between versions
alpha: pretrain on 2M dataset, smaller batch size. Limited ability
beta: pretrain on 5.3M dataset, larger batch size. More stable, better ability with only a few information provided.
## Examples
### Example1: Vivlos
Base prompt:
```
1girl,
vivlos \(umamusume\), umamusume,
kxl-delta-style1,
swimsuit,
masterpiece, newest, absurdres, sensitive
```
||Without DTG|DTG-Alpha|DTG-Beta|
|-|-|-|-|
|Prompts|Base prompt|Base propmt + "mole under eye, tail, twintails, open mouth, single ear cover, horse ears, breasts, looking at viewer, visor cap, streaked hair, long hair, horse tail, hair between eyes, cowboy shot, blue nails, purple eyes, covered navel, horse girl, competition swimsuit, blush, multicolored hair, collarbone, two-tone swimsuit, animal ears, mole, white hair, ear covers, smile, ear ornament, swimsuit, solo, blue eyes, brown hair, one-piece swimsuit, white headwear, medium breasts, white one-piece swimsuit, bare shoulders,"| base propmt + "blue bikini, tail, twintails, single ear cover, horse ears, striped clothes, ear piercing, cleavage, breasts, blue ribbon, looking at viewer, ribbon, streaked hair, long hair, horse tail, hair between eyes, :3, purple eyes, horse girl, blush, multicolored hair, hair ribbon, collarbone, bikini skirt, piercing, animal ears, striped bikini, sitting, white hair, ear covers, :d, smile, swimsuit, solo, brown hair, ocean, white headwear, medium breasts, bikini,"|
|Result image||||
|Performance|It can't even generate vivlos|It can generate image with correct character features but not enough detail and some features are wrong/lacked |Way better than alpha, provide good character features, also provide lot more details and better composition|
### Example2: Daring Tact
Base prompt:
```
1girl,
daring tact \(umamusume\), umamusume,
kxl-delta-style1,
horse girl, horse tail, horse ears, cafe, table, chair,
masterpiece, newest, absurdres, safe
```
||Without DTG|DTG-Alpha|DTG-Beta|
|-|-|-|-|
|Prompts|Base prompt|Base propmt + "plant, necktie, tail, indoors, skirt, looking at viewer, cup, lounge chair, green theme, book, alternate costume, potted plant, hair ornament, blue jacket, blush, medium hair, black necktie, green eyes, jacket, animal ears, black hair, round eyewear, bookshelf, adjusting eyewear, ahoge, smile, solo, window, brown hair, crossed legs, glasses, closed mouth, book stack,"| base propmt + "jacket, sitting on table, food, tail, collar, horse racing, black hair, boots, school bag, bag, full body, blue eyes, hair ornament, animal ears, ahoge, sitting, thighhighs, blurry background, looking at viewer, school uniform, long hair, blurry, cup, window, crossed legs, alternate costume, medium breasts, breasts, calendar \(object\), casual, door, solo, disposable cup,"|
|Result image||||
|Performance| |It can generate image with more elements and details, but the coherence with character is not good|Way better than alpha, also provide lot more details and better composition|
## Model arch
This version of DTG is trained from scratch with 400M param LLaMA arch.(In my personal preference I will call it NanoLLaMA)
Since it is llama arch. Theoritically it should be able to be used in any LLaMA inference interface.
This repo also provided converted FP16 gguf model and quantized 8bit/6bit gguf models.
Basically it is recommended to use llama.cpp or llama-cpp-python to run this model. Which will be very fast.
## Format
```python3
prompt = f"""
rating: {rating or '<|empty|>'}
artist: {artist.strip() or '<|empty|>'}
characters: {characters.strip() or '<|empty|>'}
copyrights: {copyrights.strip() or '<|empty|>'}
aspect ratio: {f"{aspect_ratio:.1f}" or '<|empty|>'}
target: {'<|' + target + '|>' if target else '<|long|>'}
general: {", ".join(special_tags)}, {general.strip().strip(",")}<|input_end|>
"""
```
for example:
```
rating: safe
artist: <|empty|>
characters: <|empty|>
copyrights: <|empty|>
aspect ratio: 1.0
target: <|short|>
general: 1girl, solo, dragon girl, dragon horns, dragon tail<|input_end|>
```
And you may get something like:
```
rating: safe
artist: <|empty|>
characters: <|empty|>
copyrights: <|empty|>
aspect ratio: 1.0
target: <|short|>
general: 1girl, solo, dragon girl, dragon horns, dragon tail<|input_end|>open mouth, red eyes, long hair, pointy ears, tail, black hair, chinese clothes, simple background, dragon, hair between eyes, horns, china dress, dress, looking at viewer, breasts
```
## Dataset and Training
I use the trainer I implemented in HakuPhi to run the training.
with 10epoch on 5.3M data. This model have roughly 6~12B token seen.
The dataset is exported by HakuBooru with my danbooru sqlite database. Use the percentile of fav_count on each rating to filter the data. (2M = top 25%, 5.3M = top 75%)
## Utilities
I'm implementing a gradio UI for this thing and other dev can utilize the API in it to make different app.
I'm also planning to make sd-webui extension. |
mrm8488/bert-tiny-finetuned-sms-spam-detection | mrm8488 | 2023-03-16T17:28:05Z | 14,107 | 34 | transformers | [
"transformers",
"pytorch",
"jax",
"safetensors",
"bert",
"text-classification",
"sms",
"spam",
"detection",
"en",
"dataset:sms_spam",
"autotrain_compatible",
"endpoints_compatible",
"region:us"
] | text-classification | 2022-03-02T23:29:05Z | ---
language: en
tags:
- sms
- spam
- detection
datasets:
- sms_spam
widget:
- text: "Camera - You are awarded a SiPix Digital Camera! call 09061221066 fromm landline. Delivery within 28 days."
---
# BERT-Tiny fine-tuned on on sms_spam dataset for spam detection
Validation accuray: **0.98** |
taide/TAIDE-LX-7B-Chat | taide | 2024-05-21T02:59:28Z | 14,105 | 117 | transformers | [
"transformers",
"safetensors",
"llama",
"text-generation",
"conversational",
"license:other",
"autotrain_compatible",
"endpoints_compatible",
"text-generation-inference",
"region:us"
] | text-generation | 2024-04-15T03:28:24Z | ---
license: other
license_name: taide-l-models-community-license-agreement
license_link: https://drive.google.com/file/d/1FcUZjbUH6jr4xoCyAronN_slLgcdhEUd/view
extra_gated_heading: 您需要先同意授權條款才能使用此模型
extra_gated_fields:
姓名(Name): text
生日(Date of birth): date_picker
國家(Country): country
所屬單位(Affiliation): text
geo: ip_location
按下送出表示您同意社群授權同意書與個人資料蒐集告知聲明(By clicking Submit below I accept the terms of the license and privacy policy): checkbox
extra_gated_prompt: >-
* ### [TAIDE L 類模型社群授權同意書(License)](https://drive.google.com/file/d/1FcUZjbUH6jr4xoCyAronN_slLgcdhEUd/view)
* ### [個人資料蒐集告知聲明(Privacy policy)](https://drive.google.com/file/d/1JTfZu_MdU_TR1-1sn2jbQyW7TLrxjwS5/view)
extra_gated_button_content: 送出(Submit)
---
* [English Version](./README_en.md)
# 模型簡介
* [TAIDE計畫](https://taide.tw/index)致力於開發符合台灣語言和文化特性的生成式人工智慧對話引擎模型,同時建構可信任的人工智慧環境。結合產學研能量,推動可信任生成式人工智慧的發展,提升台灣在國際競爭中的地位,促進產業發展,避免對外國技術的依賴。
* 本計畫開發的大型語言模型以 Meta 公司釋出的 [LLaMA2-7b](https://huggingface.co/meta-llama/Llama-2-7b-hf) 為基礎,導入台灣不同領域可用的文本與訓練素材,提高模型在正體中文回應的能力與特定任務的表現。公開釋出的模型如下:
* [TAIDE-LX-7B](https://huggingface.co/taide/TAIDE-LX-7B): 以 LLaMA2-7b 為基礎,僅使用繁體中文資料預訓練 (continuous pretraining)的模型,適合使用者會對模型進一步微調(fine tune)的使用情境。因預訓練模型沒有經過微調和偏好對齊,可能會產生惡意或不安全的輸出,使用時請小心。
* [TAIDE-LX-7B-Chat](https://huggingface.co/taide/TAIDE-LX-7B-Chat): 以 TAIDE-LX-7B 為基礎,透過指令微調(instruction tuning)強化辦公室常用任務和多輪問答對話能力,適合聊天對話或任務協助的使用情境。TAIDE-LX-7B-Chat另外有提供[4 bit 量化模型](https://huggingface.co/taide/TAIDE-LX-7B-Chat-4bit),量化模型主要是提供使用者的便利性,可能會影響效能與更多不可預期的問題,還請使用者理解與注意。
# 模型參數
* 參數量: 7B
* 最大內容長度 (context length): 4K
* 繁中訓練資料 token 量: 41.44B
* 訓練時間: 1531.82 H100 GPU Hours
# 特色
* 額外擴充24720個中文字元、字詞,強化模型處理繁體中文的能力
* 嚴格把關模型的訓練資料,提升模型生成資料的可信任性和適用性
* 針對自動摘要、寫信、寫文章、中翻英、英翻中等辦公室常用任務做加強
* 針對台灣在地文化、用語、國情等知識做加強
* 具備多輪問答對話能力
# 應用範例
| 任務 | 使用者輸入 | 模型輸出 |
| --- | -------- | ------- |
| 寫文章 | 請以以下內容為基礎,寫一篇文章:撰寫一篇作文,題目為《一張舊照片》,內容要求為:選擇一張令你印象深刻的照片,說明令你印象深刻的原因,並描述照片中的影像及背後的故事。記錄成長的過程、與他人的情景、環境變遷和美麗的景色。 | 以下是根據指令創作的一篇作文:<br><br>《一張舊照片》<br><br>在我塵封已久的家族相簿中,有一張特別吸引我的舊照片,那張照片記錄了我們家族成員在過往美好時光的歡聚,至今仍舊令人回味無窮。這張照片是我奶奶在二十多年前舉辦的一場家族聚會上所拍下的,照片中的人物包括了我的父母、祖父母、叔叔、姑姑和表哥等,大家臉上洋溢著幸福的笑容,彷彿時光靜止般地美好。<br><br>照片中的場景十分溫馨,大家圍坐在一張長方形的餐桌上,桌上擺滿了美味的食物和飲料。桌上的菜餚是傳統中式的佳餚,有香噴噴的烤全魚、嫩滑的雞肉和鮮甜的蔬菜,這些菜餚是當年奶奶親自烹調的,她手藝精湛,讓每個人都吃得津津有味。<br><br>最讓我印象深刻的是,照片中還拍到了我年幼時的模樣。我坐在桌子的另一端,手裡拿著一碗湯,臉上帶著滿足的笑容。那時的我才剛滿周歲,對於這場盛大的家族聚會還感到十分新奇。從這張照片中,我能感受到當年家族成員間的親密和溫暖,即使過了這麼多年,那份情感卻依然牢固地存在著。<br><br>這張照片背後有著動人的故事。在那個時代,我的祖父母和父母都還在讀中學,他們每天都會放學後到農田裡幫忙,辛勤地耕作以維持一家人的生計。儘管生活條件並不富裕,但他們仍舊保持著樂觀的態度和對生活的熱愛。每年到了秋天,只要收獲季節來臨,他們就會舉辦家族聚會,邀請所有親戚朋友前來共享豐收的喜悅。<br><br>隨著歲月的流逝,我逐漸長大,開始工作和生活。每次回鄉,我都會抽空去探望老人家,聽聽他們當年的往事,感受那段刻骨銘心的時光。而這張舊照片,也將一直陪伴著我,成為我珍貴回憶的一部分。它見證了我們的成長和變遷,也記錄下了那些難以忘懷的美好時刻。<br><br>這張照片不僅展示了家族的親情和溫暖,還折射出那個時代人們勤勞樸實的生活方式和對傳統的尊重。如今,隨著社會的快速發展,許多傳統的美德和風俗正在逐漸消失。我們應該好好珍惜這樣的回憶和文化,將它們傳承下去,讓後代子孫也能感受到那份來自歷史的溫情和傳承。<br><br>因此,我決定將這張照片好好保管起來,並常常拿出來看。它不僅是一張舊照片,更是一段寶貴的回憶和家族精神的象徵。我希望未來能有機會重現當年的盛況,再一次聚在一起,分享彼此的生活和故事,讓那份親情和友情繼續在時光中蔓延,永不消逝。 |
| 寫信 | 請以品牌經理的身份,給廣告公司的創意總監寫一封信,提出對於新產品廣告宣傳活動的創意建議。 | 尊敬的創意總監:<br><br>我寫這封信是為了向您提出一些關於我們新產品廣告宣傳活動的創意建議。我們公司即將推出一款全新的產品,為了確保它在市場上取得成功,我們希望透過一個引人注目的廣告宣傳活動來吸引消費者的注意。<br><br>在考慮廣告的創意和策略時,有幾個因素需要納入考量。首先,我們要針對目標客群進行研究,以瞭解他們的需求和喜好。其次,我們要確保廣告內容具有吸引力和說服力,能引起目標客群的共鳴。最後,我們要利用各種媒體平臺和數位行銷工具,以擴大廣告的影響力。<br><br>基於這些因素,我提出以下幾點創意建議:<br><br>1. 產品定位:我們可將這款新產品定位為高端、環保、健康和時尚的代表,強調其獨有的功能和特色。在廣告中,我們可以透過生動的視覺效果和簡潔的語言來傳達這些特點。<br>2. 故事行銷:在廣告中講述一個與產品功能相關的動人故事,讓消費者能更深入地瞭解產品所帶來的生活變化。例如,我們可以講述一位忙碌的職業婦女,如何使用我們的新產品在工作和生活中取得平衡的故事。<br>3. 名人效應:邀請一位受歡迎的公眾人物或意見領袖擔任產品代言人,利用他們的影響力來推廣產品。這不僅可以增加產品曝光度,還能讓更多消費者信任和信賴產品。<br>4. 跨界合作:與一些相關產業(如時尚、環保、健康等)進行跨界合作,共同推出限量版或聯名產品。這種合作不僅能吸引不同領域的消費者,還能為產品帶來更多話題性和收藏價值。<br>5. 數位行銷:充分利用社群媒體、搜尋引擎和電郵行銷等數位工具,在整個廣告宣傳過程中保持一致性的訊息和形象。我們也可製作一系列短視頻和線上活動,以增加消費者參與度和品牌忠誠度。<br>6. 創新形式:嘗試使用新穎的廣告形式,如AR(擴增實境)、VR(虛擬實境)和360度影片等,給消費者帶來全新的廣告體驗。這種創新形式不僅能吸引消費者目光,還能提高產品在市場上的辨識度。<br>7. 活動參與:在廣告宣傳活動中加入互動環節,如產品試用、抽獎和優惠券等,鼓勵消費者積極參與並分享他們的體驗。這將有助於口碑傳播,進一步擴大產品影響力。<br><br>綜合以上建議,我希望我們能製定出一套全面而富有創意的廣告宣傳活動計劃,以最大限度地提升新產品在市場上的競爭力。如果您有任何疑問或需要進一步討論,歡迎隨時與我聯絡。謝謝您的關注和支援!<br><br>祝商祺!<br><br>您的品牌經理<br><br>(註:此信件可根據實際情況和產品特點進行調整) |
| 摘要 | 請將這篇文章精簡條理化:「產業創新條例第10條之2及第72條條文修正案」俗稱「台版晶片法」,針對半導體、電動車、5G等技術創新且居國際供應鏈關鍵地位公司,提供最高25%營所稅投抵優惠,企業適用要件包含當年度研發費用、研發密度達一定規模,且有效稅率達一定比率。<br>為因應經濟合作暨發展組織(OECD)國家最低稅負制調整,其中有效稅率門檻,民國112年訂為12%,113年料將提高至15%,但仍得審酌國際間最低稅負制實施情形。<br>經濟部官員表示,已和財政部協商進入最後階段,除企業研發密度訂在6%,目前已確認,企業購置先進製程的設備投資金額達100億元以上可抵減。<br>財政部官員表示,研商過程中,針對台灣產業與其在國際間類似的公司進行深入研究,在設備部分,畢竟適用產創10之2的業者是代表台灣隊打「國際盃」,投入金額不達100億元,可能也打不了。<br>至於備受關注的研發費用門檻,經濟部官員表示,歷經與財政部來回密切討論,研發費用門檻有望落在60億至70億元之間。<br>財政部官員指出,研發攸關台灣未來經濟成長動能,門檻不能「高不可攀」,起初雖設定在100億元,之所以會調降,正是盼讓企業覺得有辦法達得到門檻、進而適用租稅優惠,才有動力繼續投入研發,維持國際供應鏈關鍵地位。<br>經濟部官員表示,因廠商研發費用平均為30、40億元,其中,IC設計業者介於30億至60億元範圍,若將門檻訂在100億元,符合條件的業者較少、刺激誘因不足;此外,若符合申請門檻的業者增加,將可提高企業在台投資金額,財政部稅收也能因此獲得挹注。<br>IC設計業者近日頻頻針對產創10之2發聲,希望降低適用門檻,加上各國力拚供應鏈自主化、加碼補助半導體產業,經濟部官員表示,經濟部和財政部就產創10之2達成共識,爭取讓更多業者受惠,盼增強企業投資力道及鞏固台灣技術地位。<br>財政部官員表示,租稅獎勵的制定必須「有為有守」,並以達到獎勵設置目的為最高原則,現階段在打「國內盃」的企業仍可適用產創第10條、10之1的租稅優惠,共同壯大台灣經濟發展。<br>經濟部和財政部正就研發費用門檻做最後確認,待今明兩天預告子法之後,約有30天時間,可與業界進一步討論及調整,盼產創10之2能在6月上路。 | 台灣「產業創新條例」的修正案,又稱「台版晶片法」,旨在支持半導體、電動車及5G技術領先企業的創新。新政策提供最高25%的所得稅抵免,企業需滿足當年度研發費用達一定規模、研發密度超過6%以及有效稅率達一定比率等條件。因應國際間對企業最低稅負制度的調整,台灣的門檻預計分別於2023年和2024年達到12%和15%。目前,與財政部討論後,確定100億元的設備投資抵免是可行的誘因,且研發費用門檻預計會在60至70億元之間。對於降低門檻的要求,經濟部和財政部已達成共識,旨在擴大受惠範圍並鼓勵企業投資以維持供應鏈的關鍵地位。此外,針對IC設計業者的訴求,也考慮調降門檻。預計此法案將在近期內實施,相關討論和調整將在預告期後進一步進行。 |
| 翻譯 | 以下提供英文內容,請幫我翻譯成中文。Dongshan coffee is famous for its unique position, and the constant refinement of production methods. The flavor is admired by many caffeine afficionados. | 東山咖啡以得天獨厚的地位,不斷精研的製作工藝著稱,風味獲諸多咖啡愛好者推崇。 |
# 使用說明
* 範例程式
* 範例程式與文件後續會釋出到github
* tokenizer 必須設成 slow mode
```python
tokenizer = AutoTokenizer.from_pretrained("taide/TAIDE-LX-7B-Chat", use_fast=False)
```
* prompt 樣版
* 一般問答用法
```python
f"<s>[INST] {question} [/INST]"
```
* 將 {question} 替換成使用者的輸入
* 加入 system prompt 的用法
```python
f"<s>[INST] <<SYS>>\n{sys}\n<</SYS>>\n\n{question} [/INST]"
```
* 將 {sys} 替換成指令,例如:你是一個來自台灣的AI助理,你的名字是 TAIDE,樂於以台灣人的立場幫助使用者,會用繁體中文回答問題。
* 將 {question} 替換成使用者的問題
* 多輪問答用法
```python
f"<s>[INST] <<SYS>>\n{sys}\n<</SYS>>\n\n{question1} [/INST] {model_answer_1} </s><s>[INST] {question2} [/INST]"
```
* 將 {sys} 替換成指令
* 將 {question1} 替換成使用者的問題1
* 將 {model_anwer_1} 替換成模型的回答1
* 將 {question2} 替換成使用者的問題2
* Huggingface Chat 樣板
* 一般問答用法
```python
chat = [
{"role": "user", "content": "{question}"},
]
prompt = tokenizer.apply_chat_template(chat)
```
* 將 {question} 替換成使用者的輸入
* 加入 system prompt 的用法
```python
chat = [
{"role": "system", "content": "{sys}"},
{"role": "user", "content": "{question}"},
]
prompt = tokenizer.apply_chat_template(chat)
```
* 將 {sys} 替換成指令,例如:你是一個來自台灣的AI助理,你的名字是 TAIDE,樂於以台灣人的立場幫助使用者,會用繁體中文回答問題。
* 將 {question} 替換成使用者的問題
* 多輪問答用法
```python
chat = [
{"role": "system", "content": "{sys}"},
{"role": "user", "content": "{question1}"},
{"role": "assistant", "content": "{model_anwer_1}"},
{"role": "user", "content": "{question2}"},
]
prompt = tokenizer.apply_chat_template(chat)
```
* 將 {sys} 替換成指令
* 將 {question1} 替換成使用者的問題1
* 將 {model_anwer_1} 替換成模型的回答1
* 將 {question2} 替換成使用者的問題2
# 訓練方法
* 軟硬體規格
* 國網中心 H100
* 訓練框架: PyTorch
* 資料前處理
* 字元標準化
* 去除重覆
* 去除雜訊
* 網頁資料的html tag、javascript
* 非標準字元或亂碼
* 字數過短的文章
* 去除文章中的特定格式,如為排版增加的換行
* 去除個資,如email、電話
* 去除不當文字,如賭博、色情等
* 擴充字元字詞
* 強化繁體中文輸入、輸出的效能,擴充資料包含下列2部分
* 從[教育部異體字字典正字表](https://dict.variants.moe.edu.tw/appendix.jsp?ID=1&ID=0)獲取中文字元
* 從繁中維基百科、新聞、中文common crawl資料抽取 500 萬條超過 100 個字元的句子(2.1G),訓練中文字詞的 tokenizer
* 持續預訓練 (continuous pretraining, CP)
* 補充大量來源可信賴的繁體中文知識
* 超參數 (hyper parameters)
* optimizer: AdamW
* learning rate: 1e-4
* batch size: 1M tokens
* epoch: 1
* 微調 (fine tune, FT)
* 讓模型可針對繁體中文提問回答問題
* 超參數 (hyper parameters)
* optimizer: AdamW
* learning rate: 5e-5
* batch size: 256K tokens
* epoch: 3
# 訓練資料
* 持續預訓練資料(資料量約為140G)
| 資料集 | 資料描述 |
| --- | -------- |
| 訴訟資料 | 《[司法院裁判書](https://judgment.judicial.gov.tw/FJUD/default.aspx)》自2013年1月至2023年12月各級法院民事、刑事、行政訴訟資料。 |
| 中央社 | 《[中央社中文新聞](https://www.cna.com.tw/)》資料集含中央社自1993年6月至2023年06月,共30年份之每日新聞文章。內容涵蓋國內外政治、社會、財經、文教、生活等領域。 |
| ETtoday 新聞雲 | 《[ETtoday新聞雲](https://www.ettoday.net/)》資料,包含自2011年10月至 2023年12月的資料。 |
| 立法院公報 | 《[立法院公報](https://ppg.ly.gov.tw/ppg/)》包含自第8屆第1會期至第10屆第7會期之公報資料。 |
| 出版商網站書籍介紹 | 包含[三采](https://www.suncolor.com.tw/)、[Gotop](https://www.gotop.com.tw/)出版商網站上的書籍簡介。 |
| GRB 研究計畫摘要 | [GRB](https://www.grb.gov.tw/)為收錄由政府經費補助之研究計畫及其成果報告的資訊系統,此資料集主要收錄 1993年至 2023年之研究計畫摘要以及研究報告摘要,含中文及其英文對照。 |
| 學術會議論文摘要 | 收錄《[學術會議論文摘要資料庫](https://sticnet.stpi.narl.org.tw/sticloc/ttscalle?meet:)》中自1988至2009年由台灣所舉辦之學術會議論文。 |
| 光華雜誌 | 《[台灣光華雜誌](https://www.taiwan-panorama.com/)》含自1993年7月至2023年6月的文章,共30年份。內容著重於我國文化、觀光與民情等。 |
| 樂詞網 | 《[樂詞網](https://terms.naer.edu.tw/)》涵蓋文理領域約187萬則學術名詞及其譯名對照。 |
| 各部會資料 | 包含行政院「[國情簡介](https://www.ey.gov.tw/state/)」、文化部「[國家文化記憶庫](https://memory.culture.tw/)」、國發會「[檔案支援教學網](https://art.archives.gov.tw/index.aspx)」、交通部「[交通安全入口網](https://168.motc.gov.tw/)」等部會網站資料之部分資料。 |
| 今周刊 | 《[今周刊](https://www.businesstoday.com.tw/)》為一以財經為主的週刊雜誌,此資料集涵蓋2008年1月至2023年7月的文章。 |
| 教育部國語辭典、成語辭典 | 包含以下三項資料:<br>[教育部《成語典》](https://dict.idioms.moe.edu.tw/search.jsp?webMd=1&la=0),含5,338條成語,內容包含每條成語的釋義、典故原文及其白話說明、用法說明、例句等。<br>[教育部《重編國語辭典修訂本》](https://dict.revised.moe.edu.tw/?la=0&powerMode=0),收錄中文單字及各類辭彙,包含讀音、部首、釋義等資訊,共約165,539筆資料。<br>[教育部《國語辭典簡編本》](https://dict.concised.moe.edu.tw/?la=0&powerMode=0),為《重編國語辭典修訂本》的簡編版本,共45,247筆資料。 |
| 科技大觀園資料 | 含《[科技大觀園網站](https://scitechvista.nat.gov.tw/)》上的科學新知以及科普文章。 |
| iKnow 科技產業資訊室 | 《[科技產業資訊室](https://iknow.stpi.narl.org.tw/)(iKnow)》提供台灣及全球的科技市場趨勢、策略分析、專利知識,及技術交易資訊,專注於科技產業的創新與發展,包含自 2008 年至 2023 年。 |
| 科學發展月刊 | 《[科學發展月刊](https://ejournal.stpi.narl.org.tw/sd)》為國科會為推廣科學教育而出版的科普刊物,含自2004年10月至2020年12月之科普文章;2021年起,以《[科技魅癮](https://www.charmingscitech.nat.gov.tw/)》季刊重新出發,提供國際關注科技議題的新知文章。 |
| 法規資料庫 | 《[法規資料庫](https://law.moj.gov.tw/)》含截自 112 年 10 月各政府部門最新發布之中央法規、行政規則、法規命令草案及地方自治法規等。 |
| 各地政府旅遊網 | 涵蓋台灣部分縣市地方政府觀光旅遊網站上之部分資料。 |
| 國教院課程綱要(十二年國教) | 含十二年國教課程綱要之總綱以及各級學校不同科目之課程綱要。 |
| 中央社譯名檔資料庫 | 《中央社譯名檔資料庫》蒐集中央社新聞業務上翻譯過的中外姓氏、人名、組織、地名等譯名對照。 |
| 童話書 | 共 20 本童話書,含湯姆歷險記、小飛俠、愛麗絲夢遊仙境、長腿叔叔等。 |
| RedPajama-Data-V2 | 從國外開放多國語言語料庫 [RedPajama-Data-v2](https://github.com/togethercomputer/RedPajama-Data) 取出英文資料 |
| MathPile-commercial | 國外開放數學語料庫 [MathPile-commercial](https://huggingface.co/datasets/GAIR/MathPile_Commercial) |
| 中文維基百科 | 《[中文維基百科](https://zh.wikipedia.org/zh-tw/%E4%B8%AD%E6%96%87%E7%BB%B4%E5%9F%BA%E7%99%BE%E7%A7%91)》截至2023年1月所有條目的內容。 |
| github-code-clean | 為 github 開源程式碼資料集,去除unlicense的程式碼和文件。 |
* 微調資料
* TAIDE團隊訓練llama2系列模型來產生微調資料資料,產生的任務包含世界知識、創意寫作、普通常識、翻譯、摘要、程式、台灣價值等單輪或多輪對話問答共 128K 筆。微調資料後續會對外釋出。
# 模型評測
* taide-bench
* 評測資料
* 寫文章、寫信、摘要、英翻中、中翻英,共500題
* 資料連結: [taide-bench](https://huggingface.co/datasets/taide/taide-bench)
* 評測方法
* gpt4評分
* 評分程式: [taide-bench-eval](https://github.com/taide-taiwan/taide-bench-eval)
* 評測分數
| 模型 | 中翻英 | 英翻中 | 摘要 | 寫文章 | 寫信 | 平均 |
| --- | ----- | ----- | ---- | ---- | ---- | --- |
| TAIDE-LX-7B-Chat | 7.165 | 7.685 | 7.720 | 9.635 | 9.110 | 8.263 |
| GPT3.5 | 8.880 | 8.810 | 7.450 | 9.490 | 8.750 | 8.676 |
| LLAMA2 7B | 6.075 | 4.475 | 5.905 | 2.625 | 3.040 | 4.424 |
| LLAMA2 13B | 6.480 | 6.135 | 6.110 | 2.565 | 3.000 | 4.858 |
| LLAMA2 70B | 6.975 | 6.375 | 6.795 | 2.625 | 2.990 | 5.152 |
# 授權條款
* [TAIDE L 類模型社群授權同意書](https://drive.google.com/file/d/1FcUZjbUH6jr4xoCyAronN_slLgcdhEUd/view?usp=drive_link)
# 免責聲明
* LLM 模型由於設計架構的限制,以及資料難免有偏誤,語言模型的任何回應不代表 TAIDE 立場,使用前需要額外加入安全防護機制,且回應內容也可能包含不正確的資訊,使用者請勿盡信。
# 開發團隊
* [https://taide.tw/index/teamList](https://taide.tw/index/teamList)
# 相關連結
* [TAIDE官網](https://taide.tw/index)
* [TAIDE Huggingface](https://huggingface.co/taide)
* [TAIDE Github](https://github.com/taide-taiwan)
* [Kuwa AI](https://kuwaai.org/)
# Citation
* [TAIDE官網](https://taide.tw/index)
|
has-abi/distilBERT-finetuned-resumes-sections | has-abi | 2022-08-24T15:26:02Z | 14,101 | 14 | transformers | [
"transformers",
"pytorch",
"distilbert",
"text-classification",
"generated_from_trainer",
"license:apache-2.0",
"autotrain_compatible",
"endpoints_compatible",
"region:us"
] | text-classification | 2022-07-21T17:08:29Z | ---
license: apache-2.0
tags:
- generated_from_trainer
metrics:
- f1
- accuracy
model-index:
- name: distilBERT-finetuned-resumes-sections
results: []
---
<!-- This model card has been generated automatically according to the information the Trainer had access to. You
should probably proofread and complete it, then remove this comment. -->
# distilBERT-finetuned-resumes-sections
This model is a fine-tuned version of [Geotrend/distilbert-base-en-fr-cased](https://huggingface.co/Geotrend/distilbert-base-en-fr-cased) on an unknown dataset.
It achieves the following results on the evaluation set:
- Loss: 0.0369
- F1: 0.9652
- Roc Auc: 0.9808
- Accuracy: 0.9621
## Model description
More information needed
## Intended uses & limitations
More information needed
## Training and evaluation data
More information needed
## Training procedure
### Training hyperparameters
The following hyperparameters were used during training:
- learning_rate: 2e-05
- train_batch_size: 8
- eval_batch_size: 8
- seed: 42
- optimizer: Adam with betas=(0.9,0.999) and epsilon=1e-08
- lr_scheduler_type: linear
- num_epochs: 20
### Training results
| Training Loss | Epoch | Step | Validation Loss | F1 | Roc Auc | Accuracy |
|:-------------:|:-----:|:-----:|:---------------:|:------:|:-------:|:--------:|
| 0.0509 | 1.0 | 1173 | 0.0331 | 0.9439 | 0.9659 | 0.9356 |
| 0.024 | 2.0 | 2346 | 0.0274 | 0.9550 | 0.9750 | 0.9493 |
| 0.0148 | 3.0 | 3519 | 0.0290 | 0.9493 | 0.9712 | 0.9446 |
| 0.0089 | 4.0 | 4692 | 0.0324 | 0.9492 | 0.9714 | 0.9442 |
| 0.0071 | 5.0 | 5865 | 0.0317 | 0.9540 | 0.9732 | 0.9476 |
| 0.0064 | 6.0 | 7038 | 0.0324 | 0.9527 | 0.9742 | 0.9484 |
| 0.0036 | 7.0 | 8211 | 0.0320 | 0.9574 | 0.9766 | 0.9540 |
| 0.0042 | 8.0 | 9384 | 0.0367 | 0.9528 | 0.9732 | 0.9493 |
| 0.0052 | 9.0 | 10557 | 0.0342 | 0.9563 | 0.9757 | 0.9531 |
| 0.0027 | 10.0 | 11730 | 0.0294 | 0.9629 | 0.9800 | 0.9595 |
| 0.0017 | 11.0 | 12903 | 0.0355 | 0.9605 | 0.9778 | 0.9582 |
| 0.0022 | 12.0 | 14076 | 0.0338 | 0.9627 | 0.9792 | 0.9591 |
| 0.0012 | 13.0 | 15249 | 0.0358 | 0.9609 | 0.9780 | 0.9591 |
| 0.0011 | 14.0 | 16422 | 0.0360 | 0.9618 | 0.9791 | 0.9604 |
| 0.0009 | 15.0 | 17595 | 0.0358 | 0.9648 | 0.9807 | 0.9625 |
| 0.0007 | 16.0 | 18768 | 0.0373 | 0.9627 | 0.9794 | 0.9595 |
| 0.0006 | 17.0 | 19941 | 0.0397 | 0.9597 | 0.9774 | 0.9574 |
| 0.0008 | 18.0 | 21114 | 0.0369 | 0.9652 | 0.9808 | 0.9621 |
| 0.0007 | 19.0 | 22287 | 0.0377 | 0.9646 | 0.9801 | 0.9621 |
| 0.0005 | 20.0 | 23460 | 0.0381 | 0.9639 | 0.9797 | 0.9616 |
### Framework versions
- Transformers 4.21.1
- Pytorch 1.12.1+cu113
- Datasets 2.4.0
- Tokenizers 0.12.1
|
unsloth/gemma-7b-bnb-4bit | unsloth | 2024-04-18T14:59:47Z | 14,101 | 15 | transformers | [
"transformers",
"safetensors",
"gemma",
"text-generation",
"unsloth",
"gemma-7b",
"bnb",
"en",
"license:apache-2.0",
"autotrain_compatible",
"endpoints_compatible",
"text-generation-inference",
"4-bit",
"bitsandbytes",
"region:us"
] | text-generation | 2024-02-21T17:02:06Z | ---
language:
- en
license: apache-2.0
library_name: transformers
tags:
- unsloth
- transformers
- gemma
- gemma-7b
- bnb
---
# Finetune Mistral, Gemma, Llama 2-5x faster with 70% less memory via Unsloth!
We have a Google Colab Tesla T4 notebook for Gemma 7b here: https://colab.research.google.com/drive/10NbwlsRChbma1v55m8LAPYG15uQv6HLo?usp=sharing
[<img src="https://raw.githubusercontent.com/unslothai/unsloth/main/images/Discord%20button.png" width="200"/>](https://discord.gg/u54VK8m8tk)
[<img src="https://raw.githubusercontent.com/unslothai/unsloth/main/images/buy%20me%20a%20coffee%20button.png" width="200"/>](https://ko-fi.com/unsloth)
[<img src="https://raw.githubusercontent.com/unslothai/unsloth/main/images/unsloth%20made%20with%20love.png" width="200"/>](https://github.com/unslothai/unsloth)
## ✨ Finetune for Free
All notebooks are **beginner friendly**! Add your dataset, click "Run All", and you'll get a 2x faster finetuned model which can be exported to GGUF, vLLM or uploaded to Hugging Face.
| Unsloth supports | Free Notebooks | Performance | Memory use |
|-----------------|--------------------------------------------------------------------------------------------------------------------------|-------------|----------|
| **Gemma 7b** | [▶️ Start on Colab](https://colab.research.google.com/drive/10NbwlsRChbma1v55m8LAPYG15uQv6HLo?usp=sharing) | 2.4x faster | 58% less |
| **Mistral 7b** | [▶️ Start on Colab](https://colab.research.google.com/drive/1Dyauq4kTZoLewQ1cApceUQVNcnnNTzg_?usp=sharing) | 2.2x faster | 62% less |
| **Llama-2 7b** | [▶️ Start on Colab](https://colab.research.google.com/drive/1lBzz5KeZJKXjvivbYvmGarix9Ao6Wxe5?usp=sharing) | 2.2x faster | 43% less |
| **TinyLlama** | [▶️ Start on Colab](https://colab.research.google.com/drive/1AZghoNBQaMDgWJpi4RbffGM1h6raLUj9?usp=sharing) | 3.9x faster | 74% less |
| **CodeLlama 34b** A100 | [▶️ Start on Colab](https://colab.research.google.com/drive/1y7A0AxE3y8gdj4AVkl2aZX47Xu3P1wJT?usp=sharing) | 1.9x faster | 27% less |
| **Mistral 7b** 1xT4 | [▶️ Start on Kaggle](https://www.kaggle.com/code/danielhanchen/kaggle-mistral-7b-unsloth-notebook) | 5x faster\* | 62% less |
| **DPO - Zephyr** | [▶️ Start on Colab](https://colab.research.google.com/drive/15vttTpzzVXv_tJwEk-hIcQ0S9FcEWvwP?usp=sharing) | 1.9x faster | 19% less |
- This [conversational notebook](https://colab.research.google.com/drive/1Aau3lgPzeZKQ-98h69CCu1UJcvIBLmy2?usp=sharing) is useful for ShareGPT ChatML / Vicuna templates.
- This [text completion notebook](https://colab.research.google.com/drive/1ef-tab5bhkvWmBOObepl1WgJvfvSzn5Q?usp=sharing) is for raw text. This [DPO notebook](https://colab.research.google.com/drive/15vttTpzzVXv_tJwEk-hIcQ0S9FcEWvwP?usp=sharing) replicates Zephyr.
- \* Kaggle has 2x T4s, but we use 1. Due to overhead, 1x T4 is 5x faster.
|
mradermacher/Aspera-SWE-Llama-13b-updated-GGUF | mradermacher | 2024-06-21T05:06:50Z | 14,089 | 0 | transformers | [
"transformers",
"gguf",
"en",
"base_model:agi-designer/Aspera-SWE-Llama-13b-updated",
"endpoints_compatible",
"region:us"
] | null | 2024-06-21T04:19:34Z | ---
base_model: agi-designer/Aspera-SWE-Llama-13b-updated
language:
- en
library_name: transformers
quantized_by: mradermacher
---
## About
<!-- ### quantize_version: 2 -->
<!-- ### output_tensor_quantised: 1 -->
<!-- ### convert_type: hf -->
<!-- ### vocab_type: -->
<!-- ### tags: -->
static quants of https://huggingface.co/agi-designer/Aspera-SWE-Llama-13b-updated
<!-- provided-files -->
weighted/imatrix quants seem not to be available (by me) at this time. If they do not show up a week or so after the static ones, I have probably not planned for them. Feel free to request them by opening a Community Discussion.
## Usage
If you are unsure how to use GGUF files, refer to one of [TheBloke's
READMEs](https://huggingface.co/TheBloke/KafkaLM-70B-German-V0.1-GGUF) for
more details, including on how to concatenate multi-part files.
## Provided Quants
(sorted by size, not necessarily quality. IQ-quants are often preferable over similar sized non-IQ quants)
| Link | Type | Size/GB | Notes |
|:-----|:-----|--------:|:------|
| [GGUF](https://huggingface.co/mradermacher/Aspera-SWE-Llama-13b-updated-GGUF/resolve/main/Aspera-SWE-Llama-13b-updated.Q2_K.gguf) | Q2_K | 5.0 | |
| [GGUF](https://huggingface.co/mradermacher/Aspera-SWE-Llama-13b-updated-GGUF/resolve/main/Aspera-SWE-Llama-13b-updated.IQ3_XS.gguf) | IQ3_XS | 5.5 | |
| [GGUF](https://huggingface.co/mradermacher/Aspera-SWE-Llama-13b-updated-GGUF/resolve/main/Aspera-SWE-Llama-13b-updated.IQ3_S.gguf) | IQ3_S | 5.8 | beats Q3_K* |
| [GGUF](https://huggingface.co/mradermacher/Aspera-SWE-Llama-13b-updated-GGUF/resolve/main/Aspera-SWE-Llama-13b-updated.Q3_K_S.gguf) | Q3_K_S | 5.8 | |
| [GGUF](https://huggingface.co/mradermacher/Aspera-SWE-Llama-13b-updated-GGUF/resolve/main/Aspera-SWE-Llama-13b-updated.IQ3_M.gguf) | IQ3_M | 6.1 | |
| [GGUF](https://huggingface.co/mradermacher/Aspera-SWE-Llama-13b-updated-GGUF/resolve/main/Aspera-SWE-Llama-13b-updated.Q3_K_M.gguf) | Q3_K_M | 6.4 | lower quality |
| [GGUF](https://huggingface.co/mradermacher/Aspera-SWE-Llama-13b-updated-GGUF/resolve/main/Aspera-SWE-Llama-13b-updated.Q3_K_L.gguf) | Q3_K_L | 7.0 | |
| [GGUF](https://huggingface.co/mradermacher/Aspera-SWE-Llama-13b-updated-GGUF/resolve/main/Aspera-SWE-Llama-13b-updated.IQ4_XS.gguf) | IQ4_XS | 7.1 | |
| [GGUF](https://huggingface.co/mradermacher/Aspera-SWE-Llama-13b-updated-GGUF/resolve/main/Aspera-SWE-Llama-13b-updated.Q4_K_S.gguf) | Q4_K_S | 7.5 | fast, recommended |
| [GGUF](https://huggingface.co/mradermacher/Aspera-SWE-Llama-13b-updated-GGUF/resolve/main/Aspera-SWE-Llama-13b-updated.Q4_K_M.gguf) | Q4_K_M | 8.0 | fast, recommended |
| [GGUF](https://huggingface.co/mradermacher/Aspera-SWE-Llama-13b-updated-GGUF/resolve/main/Aspera-SWE-Llama-13b-updated.Q5_K_S.gguf) | Q5_K_S | 9.1 | |
| [GGUF](https://huggingface.co/mradermacher/Aspera-SWE-Llama-13b-updated-GGUF/resolve/main/Aspera-SWE-Llama-13b-updated.Q5_K_M.gguf) | Q5_K_M | 9.3 | |
| [GGUF](https://huggingface.co/mradermacher/Aspera-SWE-Llama-13b-updated-GGUF/resolve/main/Aspera-SWE-Llama-13b-updated.Q6_K.gguf) | Q6_K | 10.8 | very good quality |
| [GGUF](https://huggingface.co/mradermacher/Aspera-SWE-Llama-13b-updated-GGUF/resolve/main/Aspera-SWE-Llama-13b-updated.Q8_0.gguf) | Q8_0 | 13.9 | fast, best quality |
Here is a handy graph by ikawrakow comparing some lower-quality quant
types (lower is better):

And here are Artefact2's thoughts on the matter:
https://gist.github.com/Artefact2/b5f810600771265fc1e39442288e8ec9
## FAQ / Model Request
See https://huggingface.co/mradermacher/model_requests for some answers to
questions you might have and/or if you want some other model quantized.
## Thanks
I thank my company, [nethype GmbH](https://www.nethype.de/), for letting
me use its servers and providing upgrades to my workstation to enable
this work in my free time.
<!-- end -->
|
Jorgeutd/bert-large-uncased-finetuned-ner | Jorgeutd | 2023-11-06T14:28:33Z | 14,073 | 5 | transformers | [
"transformers",
"pytorch",
"safetensors",
"bert",
"token-classification",
"generated_from_trainer",
"en",
"dataset:conll2003",
"base_model:bert-large-uncased",
"license:apache-2.0",
"model-index",
"autotrain_compatible",
"endpoints_compatible",
"region:us"
] | token-classification | 2022-03-02T23:29:04Z | ---
language: en
license: apache-2.0
tags:
- generated_from_trainer
datasets:
- conll2003
metrics:
- precision
- recall
- f1
- accuracy
widget:
- text: My name is Scott and I live in Columbus.
- text: My name is Scott and I am calling from Buffalo, NY. I would like to file a
complain with United Airlines.
- text: Apple was founded in 1976 by Steve Jobs, Steve Wozniak and Ronald Wayne.
base_model: bert-large-uncased
model-index:
- name: bert-large-uncased-finetuned-ner
results:
- task:
type: token-classification
name: Token Classification
dataset:
name: conll2003
type: conll2003
args: conll2003
metrics:
- type: precision
value: 0.9504719600222099
name: Precision
- type: recall
value: 0.9574896520863632
name: Recall
- type: f1
value: 0.9539679001337494
name: F1
- type: accuracy
value: 0.9885618059637473
name: Accuracy
---
<!-- This model card has been generated automatically according to the information the Trainer had access to. You
should probably proofread and complete it, then remove this comment. -->
# bert-large-uncased-finetuned-ner
This model is a fine-tuned version of [bert-large-uncased](https://huggingface.co/bert-large-uncased) on the conll2003 dataset.
It achieves the following results on the evaluation set:
- Loss: 0.0778
- Precision: 0.9505
- Recall: 0.9575
- F1: 0.9540
- Accuracy: 0.9886
## Model description
More information needed
#### Limitations and bias
This model is limited by its training dataset of entity-annotated news articles from a specific span of time. This may not generalize well for all use cases in different domains. Furthermore, the model occassionally tags subword tokens as entities and post-processing of results may be necessary to handle those cases.
#### How to use
You can use this model with Transformers *pipeline* for NER.
```python
from transformers import pipeline
from transformers import AutoTokenizer, AutoModelForTokenClassification
tokenizer = AutoTokenizer.from_pretrained("Jorgeutd/bert-large-uncased-finetuned-ner")
model = AutoModelForTokenClassification.from_pretrained("Jorgeutd/bert-large-uncased-finetuned-ner")
nlp = pipeline("ner", model=model, tokenizer=tokenizer)
example = "My name is Scott and I live in Ohio"
ner_results = nlp(example)
print(ner_results)
```
## Training procedure
### Training hyperparameters
The following hyperparameters were used during training:
- learning_rate: 2e-05
- train_batch_size: 16
- eval_batch_size: 64
- seed: 42
- optimizer: Adam with betas=(0.9,0.999) and epsilon=1e-08
- lr_scheduler_type: linear
- num_epochs: 10
### Training results
| Training Loss | Epoch | Step | Validation Loss | Precision | Recall | F1 | Accuracy |
|:-------------:|:-----:|:----:|:---------------:|:---------:|:------:|:------:|:--------:|
| 0.1997 | 1.0 | 878 | 0.0576 | 0.9316 | 0.9257 | 0.9286 | 0.9837 |
| 0.04 | 2.0 | 1756 | 0.0490 | 0.9400 | 0.9513 | 0.9456 | 0.9870 |
| 0.0199 | 3.0 | 2634 | 0.0557 | 0.9436 | 0.9540 | 0.9488 | 0.9879 |
| 0.0112 | 4.0 | 3512 | 0.0602 | 0.9443 | 0.9569 | 0.9506 | 0.9881 |
| 0.0068 | 5.0 | 4390 | 0.0631 | 0.9451 | 0.9589 | 0.9520 | 0.9882 |
| 0.0044 | 6.0 | 5268 | 0.0638 | 0.9510 | 0.9567 | 0.9538 | 0.9885 |
| 0.003 | 7.0 | 6146 | 0.0722 | 0.9495 | 0.9560 | 0.9527 | 0.9885 |
| 0.0016 | 8.0 | 7024 | 0.0762 | 0.9491 | 0.9595 | 0.9543 | 0.9887 |
| 0.0018 | 9.0 | 7902 | 0.0769 | 0.9496 | 0.9542 | 0.9519 | 0.9883 |
| 0.0009 | 10.0 | 8780 | 0.0778 | 0.9505 | 0.9575 | 0.9540 | 0.9886 |
### Framework versions
- Transformers 4.16.2
- Pytorch 1.8.1+cu111
- Datasets 1.18.3
- Tokenizers 0.11.0
|
mradermacher/Llama-3-Instruct-Neurona-8b-v2-i1-GGUF | mradermacher | 2024-07-02T03:13:46Z | 14,071 | 0 | transformers | [
"transformers",
"gguf",
"synthetic",
"es",
"en",
"dataset:Danielbrdz/Barcenas-Economia",
"dataset:HiTZ/casimedicos-exp",
"dataset:somosnlp/coser_resumenes",
"dataset:csebuetnlp/CrossSum",
"dataset:Iker/Document-Translation-en-es",
"dataset:somosnlp/es-inclusive-language-it",
"dataset:glaiveai/glaive-code-assistant-v3",
"dataset:glaiveai/glaive-function-calling-v2",
"dataset:Iker/InstructTranslation-EN-ES",
"dataset:somosnlp/lenguaje-claro-dataset",
"dataset:somosnlp/LingComp_QA",
"dataset:Iker/NoticIA",
"dataset:teknium/OpenHermes-2.5",
"dataset:Iker/OpenHermes-2.5-Spanish",
"dataset:Helsinki-NLP/opus-100",
"dataset:projecte-aina/RAG_Multilingual",
"dataset:HiTZ/This-is-not-a-dataset",
"dataset:Iker/Reddit-Post-Translation",
"dataset:wikipedia",
"base_model:Iker/Llama-3-Instruct-Neurona-8b-v2",
"license:llama3",
"endpoints_compatible",
"region:us"
] | null | 2024-07-01T22:07:48Z | ---
base_model: Iker/Llama-3-Instruct-Neurona-8b-v2
datasets:
- Danielbrdz/Barcenas-Economia
- HiTZ/casimedicos-exp
- somosnlp/coser_resumenes
- csebuetnlp/CrossSum
- Iker/Document-Translation-en-es
- somosnlp/es-inclusive-language-it
- glaiveai/glaive-code-assistant-v3
- glaiveai/glaive-function-calling-v2
- Iker/InstructTranslation-EN-ES
- somosnlp/lenguaje-claro-dataset
- somosnlp/LingComp_QA
- Iker/NoticIA
- teknium/OpenHermes-2.5
- Iker/OpenHermes-2.5-Spanish
- Helsinki-NLP/opus-100
- projecte-aina/RAG_Multilingual
- HiTZ/This-is-not-a-dataset
- Iker/Reddit-Post-Translation
- wikipedia
language:
- es
- en
library_name: transformers
license: llama3
quantized_by: mradermacher
tags:
- synthetic
---
## About
<!-- ### quantize_version: 2 -->
<!-- ### output_tensor_quantised: 1 -->
<!-- ### convert_type: hf -->
<!-- ### vocab_type: -->
<!-- ### tags: nicoboss -->
weighted/imatrix quants of https://huggingface.co/Iker/Llama-3-Instruct-Neurona-8b-v2
<!-- provided-files -->
static quants are available at https://huggingface.co/mradermacher/Llama-3-Instruct-Neurona-8b-v2-GGUF
## Usage
If you are unsure how to use GGUF files, refer to one of [TheBloke's
READMEs](https://huggingface.co/TheBloke/KafkaLM-70B-German-V0.1-GGUF) for
more details, including on how to concatenate multi-part files.
## Provided Quants
(sorted by size, not necessarily quality. IQ-quants are often preferable over similar sized non-IQ quants)
| Link | Type | Size/GB | Notes |
|:-----|:-----|--------:|:------|
| [GGUF](https://huggingface.co/mradermacher/Llama-3-Instruct-Neurona-8b-v2-i1-GGUF/resolve/main/Llama-3-Instruct-Neurona-8b-v2.i1-IQ1_S.gguf) | i1-IQ1_S | 2.1 | for the desperate |
| [GGUF](https://huggingface.co/mradermacher/Llama-3-Instruct-Neurona-8b-v2-i1-GGUF/resolve/main/Llama-3-Instruct-Neurona-8b-v2.i1-IQ1_M.gguf) | i1-IQ1_M | 2.3 | mostly desperate |
| [GGUF](https://huggingface.co/mradermacher/Llama-3-Instruct-Neurona-8b-v2-i1-GGUF/resolve/main/Llama-3-Instruct-Neurona-8b-v2.i1-IQ2_XXS.gguf) | i1-IQ2_XXS | 2.5 | |
| [GGUF](https://huggingface.co/mradermacher/Llama-3-Instruct-Neurona-8b-v2-i1-GGUF/resolve/main/Llama-3-Instruct-Neurona-8b-v2.i1-IQ2_XS.gguf) | i1-IQ2_XS | 2.7 | |
| [GGUF](https://huggingface.co/mradermacher/Llama-3-Instruct-Neurona-8b-v2-i1-GGUF/resolve/main/Llama-3-Instruct-Neurona-8b-v2.i1-IQ2_S.gguf) | i1-IQ2_S | 2.9 | |
| [GGUF](https://huggingface.co/mradermacher/Llama-3-Instruct-Neurona-8b-v2-i1-GGUF/resolve/main/Llama-3-Instruct-Neurona-8b-v2.i1-IQ2_M.gguf) | i1-IQ2_M | 3.0 | |
| [GGUF](https://huggingface.co/mradermacher/Llama-3-Instruct-Neurona-8b-v2-i1-GGUF/resolve/main/Llama-3-Instruct-Neurona-8b-v2.i1-Q2_K.gguf) | i1-Q2_K | 3.3 | IQ3_XXS probably better |
| [GGUF](https://huggingface.co/mradermacher/Llama-3-Instruct-Neurona-8b-v2-i1-GGUF/resolve/main/Llama-3-Instruct-Neurona-8b-v2.i1-IQ3_XXS.gguf) | i1-IQ3_XXS | 3.4 | lower quality |
| [GGUF](https://huggingface.co/mradermacher/Llama-3-Instruct-Neurona-8b-v2-i1-GGUF/resolve/main/Llama-3-Instruct-Neurona-8b-v2.i1-IQ3_XS.gguf) | i1-IQ3_XS | 3.6 | |
| [GGUF](https://huggingface.co/mradermacher/Llama-3-Instruct-Neurona-8b-v2-i1-GGUF/resolve/main/Llama-3-Instruct-Neurona-8b-v2.i1-Q3_K_S.gguf) | i1-Q3_K_S | 3.8 | IQ3_XS probably better |
| [GGUF](https://huggingface.co/mradermacher/Llama-3-Instruct-Neurona-8b-v2-i1-GGUF/resolve/main/Llama-3-Instruct-Neurona-8b-v2.i1-IQ3_S.gguf) | i1-IQ3_S | 3.8 | beats Q3_K* |
| [GGUF](https://huggingface.co/mradermacher/Llama-3-Instruct-Neurona-8b-v2-i1-GGUF/resolve/main/Llama-3-Instruct-Neurona-8b-v2.i1-IQ3_M.gguf) | i1-IQ3_M | 3.9 | |
| [GGUF](https://huggingface.co/mradermacher/Llama-3-Instruct-Neurona-8b-v2-i1-GGUF/resolve/main/Llama-3-Instruct-Neurona-8b-v2.i1-Q3_K_M.gguf) | i1-Q3_K_M | 4.1 | IQ3_S probably better |
| [GGUF](https://huggingface.co/mradermacher/Llama-3-Instruct-Neurona-8b-v2-i1-GGUF/resolve/main/Llama-3-Instruct-Neurona-8b-v2.i1-Q3_K_L.gguf) | i1-Q3_K_L | 4.4 | IQ3_M probably better |
| [GGUF](https://huggingface.co/mradermacher/Llama-3-Instruct-Neurona-8b-v2-i1-GGUF/resolve/main/Llama-3-Instruct-Neurona-8b-v2.i1-IQ4_XS.gguf) | i1-IQ4_XS | 4.5 | |
| [GGUF](https://huggingface.co/mradermacher/Llama-3-Instruct-Neurona-8b-v2-i1-GGUF/resolve/main/Llama-3-Instruct-Neurona-8b-v2.i1-Q4_0.gguf) | i1-Q4_0 | 4.8 | fast, low quality |
| [GGUF](https://huggingface.co/mradermacher/Llama-3-Instruct-Neurona-8b-v2-i1-GGUF/resolve/main/Llama-3-Instruct-Neurona-8b-v2.i1-Q4_K_S.gguf) | i1-Q4_K_S | 4.8 | optimal size/speed/quality |
| [GGUF](https://huggingface.co/mradermacher/Llama-3-Instruct-Neurona-8b-v2-i1-GGUF/resolve/main/Llama-3-Instruct-Neurona-8b-v2.i1-Q4_K_M.gguf) | i1-Q4_K_M | 5.0 | fast, recommended |
| [GGUF](https://huggingface.co/mradermacher/Llama-3-Instruct-Neurona-8b-v2-i1-GGUF/resolve/main/Llama-3-Instruct-Neurona-8b-v2.i1-Q5_K_S.gguf) | i1-Q5_K_S | 5.7 | |
| [GGUF](https://huggingface.co/mradermacher/Llama-3-Instruct-Neurona-8b-v2-i1-GGUF/resolve/main/Llama-3-Instruct-Neurona-8b-v2.i1-Q5_K_M.gguf) | i1-Q5_K_M | 5.8 | |
| [GGUF](https://huggingface.co/mradermacher/Llama-3-Instruct-Neurona-8b-v2-i1-GGUF/resolve/main/Llama-3-Instruct-Neurona-8b-v2.i1-Q6_K.gguf) | i1-Q6_K | 6.7 | practically like static Q6_K |
Here is a handy graph by ikawrakow comparing some lower-quality quant
types (lower is better):

And here are Artefact2's thoughts on the matter:
https://gist.github.com/Artefact2/b5f810600771265fc1e39442288e8ec9
## FAQ / Model Request
See https://huggingface.co/mradermacher/model_requests for some answers to
questions you might have and/or if you want some other model quantized.
## Thanks
I thank my company, [nethype GmbH](https://www.nethype.de/), for letting
me use its servers and providing upgrades to my workstation to enable
this work in my free time. Additional thanks to [@nicoboss](https://huggingface.co/nicoboss) for giving me access to his hardware for calculating the imatrix for these quants.
<!-- end -->
|
Helsinki-NLP/opus-mt-tc-big-zls-en | Helsinki-NLP | 2023-10-10T10:31:05Z | 14,068 | 0 | transformers | [
"transformers",
"pytorch",
"tf",
"safetensors",
"marian",
"text2text-generation",
"translation",
"opus-mt-tc",
"bg",
"bs",
"en",
"hr",
"mk",
"sh",
"sl",
"sr",
"zls",
"license:cc-by-4.0",
"model-index",
"autotrain_compatible",
"endpoints_compatible",
"region:us"
] | translation | 2022-04-13T17:12:36Z | ---
language:
- bg
- bs
- en
- hr
- mk
- sh
- sl
- sr
- zls
tags:
- translation
- opus-mt-tc
license: cc-by-4.0
model-index:
- name: opus-mt-tc-big-zls-en
results:
- task:
name: Translation bul-eng
type: translation
args: bul-eng
dataset:
name: flores101-devtest
type: flores_101
args: bul eng devtest
metrics:
- name: BLEU
type: bleu
value: 42.0
- task:
name: Translation hrv-eng
type: translation
args: hrv-eng
dataset:
name: flores101-devtest
type: flores_101
args: hrv eng devtest
metrics:
- name: BLEU
type: bleu
value: 37.1
- task:
name: Translation mkd-eng
type: translation
args: mkd-eng
dataset:
name: flores101-devtest
type: flores_101
args: mkd eng devtest
metrics:
- name: BLEU
type: bleu
value: 43.2
- task:
name: Translation slv-eng
type: translation
args: slv-eng
dataset:
name: flores101-devtest
type: flores_101
args: slv eng devtest
metrics:
- name: BLEU
type: bleu
value: 35.2
- task:
name: Translation srp_Cyrl-eng
type: translation
args: srp_Cyrl-eng
dataset:
name: flores101-devtest
type: flores_101
args: srp_Cyrl eng devtest
metrics:
- name: BLEU
type: bleu
value: 36.8
- task:
name: Translation bos_Latn-eng
type: translation
args: bos_Latn-eng
dataset:
name: tatoeba-test-v2021-08-07
type: tatoeba_mt
args: bos_Latn-eng
metrics:
- name: BLEU
type: bleu
value: 66.5
- task:
name: Translation bul-eng
type: translation
args: bul-eng
dataset:
name: tatoeba-test-v2021-08-07
type: tatoeba_mt
args: bul-eng
metrics:
- name: BLEU
type: bleu
value: 59.3
- task:
name: Translation hbs-eng
type: translation
args: hbs-eng
dataset:
name: tatoeba-test-v2021-08-07
type: tatoeba_mt
args: hbs-eng
metrics:
- name: BLEU
type: bleu
value: 57.3
- task:
name: Translation hrv-eng
type: translation
args: hrv-eng
dataset:
name: tatoeba-test-v2021-08-07
type: tatoeba_mt
args: hrv-eng
metrics:
- name: BLEU
type: bleu
value: 59.2
- task:
name: Translation mkd-eng
type: translation
args: mkd-eng
dataset:
name: tatoeba-test-v2021-08-07
type: tatoeba_mt
args: mkd-eng
metrics:
- name: BLEU
type: bleu
value: 57.4
- task:
name: Translation slv-eng
type: translation
args: slv-eng
dataset:
name: tatoeba-test-v2021-08-07
type: tatoeba_mt
args: slv-eng
metrics:
- name: BLEU
type: bleu
value: 23.5
- task:
name: Translation srp_Cyrl-eng
type: translation
args: srp_Cyrl-eng
dataset:
name: tatoeba-test-v2021-08-07
type: tatoeba_mt
args: srp_Cyrl-eng
metrics:
- name: BLEU
type: bleu
value: 47.0
- task:
name: Translation srp_Latn-eng
type: translation
args: srp_Latn-eng
dataset:
name: tatoeba-test-v2021-08-07
type: tatoeba_mt
args: srp_Latn-eng
metrics:
- name: BLEU
type: bleu
value: 58.5
---
# opus-mt-tc-big-zls-en
Neural machine translation model for translating from South Slavic languages (zls) to English (en).
This model is part of the [OPUS-MT project](https://github.com/Helsinki-NLP/Opus-MT), an effort to make neural machine translation models widely available and accessible for many languages in the world. All models are originally trained using the amazing framework of [Marian NMT](https://marian-nmt.github.io/), an efficient NMT implementation written in pure C++. The models have been converted to pyTorch using the transformers library by huggingface. Training data is taken from [OPUS](https://opus.nlpl.eu/) and training pipelines use the procedures of [OPUS-MT-train](https://github.com/Helsinki-NLP/Opus-MT-train).
* Publications: [OPUS-MT – Building open translation services for the World](https://aclanthology.org/2020.eamt-1.61/) and [The Tatoeba Translation Challenge – Realistic Data Sets for Low Resource and Multilingual MT](https://aclanthology.org/2020.wmt-1.139/) (Please, cite if you use this model.)
```
@inproceedings{tiedemann-thottingal-2020-opus,
title = "{OPUS}-{MT} {--} Building open translation services for the World",
author = {Tiedemann, J{\"o}rg and Thottingal, Santhosh},
booktitle = "Proceedings of the 22nd Annual Conference of the European Association for Machine Translation",
month = nov,
year = "2020",
address = "Lisboa, Portugal",
publisher = "European Association for Machine Translation",
url = "https://aclanthology.org/2020.eamt-1.61",
pages = "479--480",
}
@inproceedings{tiedemann-2020-tatoeba,
title = "The Tatoeba Translation Challenge {--} Realistic Data Sets for Low Resource and Multilingual {MT}",
author = {Tiedemann, J{\"o}rg},
booktitle = "Proceedings of the Fifth Conference on Machine Translation",
month = nov,
year = "2020",
address = "Online",
publisher = "Association for Computational Linguistics",
url = "https://aclanthology.org/2020.wmt-1.139",
pages = "1174--1182",
}
```
## Model info
* Release: 2022-03-17
* source language(s): bos_Latn bul hbs hrv mkd slv srp_Cyrl srp_Latn
* target language(s): eng
* model: transformer-big
* data: opusTCv20210807+bt ([source](https://github.com/Helsinki-NLP/Tatoeba-Challenge))
* tokenization: SentencePiece (spm32k,spm32k)
* original model: [opusTCv20210807+bt_transformer-big_2022-03-17.zip](https://object.pouta.csc.fi/Tatoeba-MT-models/zls-eng/opusTCv20210807+bt_transformer-big_2022-03-17.zip)
* more information released models: [OPUS-MT zls-eng README](https://github.com/Helsinki-NLP/Tatoeba-Challenge/tree/master/models/zls-eng/README.md)
## Usage
A short example code:
```python
from transformers import MarianMTModel, MarianTokenizer
src_text = [
"Да не би случайно Том да остави Мери да кара колата?",
"Какво е времето днес?"
]
model_name = "pytorch-models/opus-mt-tc-big-zls-en"
tokenizer = MarianTokenizer.from_pretrained(model_name)
model = MarianMTModel.from_pretrained(model_name)
translated = model.generate(**tokenizer(src_text, return_tensors="pt", padding=True))
for t in translated:
print( tokenizer.decode(t, skip_special_tokens=True) )
# expected output:
# Did Tom just let Mary drive the car?
# What's the weather like today?
```
You can also use OPUS-MT models with the transformers pipelines, for example:
```python
from transformers import pipeline
pipe = pipeline("translation", model="Helsinki-NLP/opus-mt-tc-big-zls-en")
print(pipe("Да не би случайно Том да остави Мери да кара колата?"))
# expected output: Did Tom just let Mary drive the car?
```
## Benchmarks
* test set translations: [opusTCv20210807+bt_transformer-big_2022-03-17.test.txt](https://object.pouta.csc.fi/Tatoeba-MT-models/zls-eng/opusTCv20210807+bt_transformer-big_2022-03-17.test.txt)
* test set scores: [opusTCv20210807+bt_transformer-big_2022-03-17.eval.txt](https://object.pouta.csc.fi/Tatoeba-MT-models/zls-eng/opusTCv20210807+bt_transformer-big_2022-03-17.eval.txt)
* benchmark results: [benchmark_results.txt](benchmark_results.txt)
* benchmark output: [benchmark_translations.zip](benchmark_translations.zip)
| langpair | testset | chr-F | BLEU | #sent | #words |
|----------|---------|-------|-------|-------|--------|
| bos_Latn-eng | tatoeba-test-v2021-08-07 | 0.79339 | 66.5 | 301 | 1826 |
| bul-eng | tatoeba-test-v2021-08-07 | 0.72656 | 59.3 | 10000 | 71872 |
| hbs-eng | tatoeba-test-v2021-08-07 | 0.71783 | 57.3 | 10017 | 68934 |
| hrv-eng | tatoeba-test-v2021-08-07 | 0.74066 | 59.2 | 1480 | 10620 |
| mkd-eng | tatoeba-test-v2021-08-07 | 0.70043 | 57.4 | 10010 | 65667 |
| slv-eng | tatoeba-test-v2021-08-07 | 0.39534 | 23.5 | 2495 | 16940 |
| srp_Cyrl-eng | tatoeba-test-v2021-08-07 | 0.67628 | 47.0 | 1580 | 10181 |
| srp_Latn-eng | tatoeba-test-v2021-08-07 | 0.71878 | 58.5 | 6656 | 46307 |
| bul-eng | flores101-devtest | 0.67375 | 42.0 | 1012 | 24721 |
| hrv-eng | flores101-devtest | 0.63914 | 37.1 | 1012 | 24721 |
| mkd-eng | flores101-devtest | 0.67444 | 43.2 | 1012 | 24721 |
| slv-eng | flores101-devtest | 0.62087 | 35.2 | 1012 | 24721 |
| srp_Cyrl-eng | flores101-devtest | 0.67810 | 36.8 | 1012 | 24721 |
## Acknowledgements
The work is supported by the [European Language Grid](https://www.european-language-grid.eu/) as [pilot project 2866](https://live.european-language-grid.eu/catalogue/#/resource/projects/2866), by the [FoTran project](https://www.helsinki.fi/en/researchgroups/natural-language-understanding-with-cross-lingual-grounding), funded by the European Research Council (ERC) under the European Union’s Horizon 2020 research and innovation programme (grant agreement No 771113), and the [MeMAD project](https://memad.eu/), funded by the European Union’s Horizon 2020 Research and Innovation Programme under grant agreement No 780069. We are also grateful for the generous computational resources and IT infrastructure provided by [CSC -- IT Center for Science](https://www.csc.fi/), Finland.
## Model conversion info
* transformers version: 4.16.2
* OPUS-MT git hash: 3405783
* port time: Wed Apr 13 20:12:26 EEST 2022
* port machine: LM0-400-22516.local
|
mradermacher/Irbis-7b-v0.1-i1-GGUF | mradermacher | 2024-06-30T10:42:02Z | 14,062 | 0 | transformers | [
"transformers",
"gguf",
"kk",
"ru",
"en",
"base_model:IrbisAI/Irbis-7b-v0.1",
"license:mit",
"endpoints_compatible",
"region:us"
] | null | 2024-06-30T06:04:55Z | ---
base_model: IrbisAI/Irbis-7b-v0.1
language:
- kk
- ru
- en
library_name: transformers
license: mit
quantized_by: mradermacher
---
## About
<!-- ### quantize_version: 2 -->
<!-- ### output_tensor_quantised: 1 -->
<!-- ### convert_type: hf -->
<!-- ### vocab_type: -->
<!-- ### tags: nicoboss -->
weighted/imatrix quants of https://huggingface.co/IrbisAI/Irbis-7b-v0.1
<!-- provided-files -->
static quants are available at https://huggingface.co/mradermacher/Irbis-7b-v0.1-GGUF
## Usage
If you are unsure how to use GGUF files, refer to one of [TheBloke's
READMEs](https://huggingface.co/TheBloke/KafkaLM-70B-German-V0.1-GGUF) for
more details, including on how to concatenate multi-part files.
## Provided Quants
(sorted by size, not necessarily quality. IQ-quants are often preferable over similar sized non-IQ quants)
| Link | Type | Size/GB | Notes |
|:-----|:-----|--------:|:------|
| [GGUF](https://huggingface.co/mradermacher/Irbis-7b-v0.1-i1-GGUF/resolve/main/Irbis-7b-v0.1.i1-IQ1_S.gguf) | i1-IQ1_S | 1.8 | for the desperate |
| [GGUF](https://huggingface.co/mradermacher/Irbis-7b-v0.1-i1-GGUF/resolve/main/Irbis-7b-v0.1.i1-IQ1_M.gguf) | i1-IQ1_M | 2.0 | mostly desperate |
| [GGUF](https://huggingface.co/mradermacher/Irbis-7b-v0.1-i1-GGUF/resolve/main/Irbis-7b-v0.1.i1-IQ2_XXS.gguf) | i1-IQ2_XXS | 2.2 | |
| [GGUF](https://huggingface.co/mradermacher/Irbis-7b-v0.1-i1-GGUF/resolve/main/Irbis-7b-v0.1.i1-IQ2_XS.gguf) | i1-IQ2_XS | 2.4 | |
| [GGUF](https://huggingface.co/mradermacher/Irbis-7b-v0.1-i1-GGUF/resolve/main/Irbis-7b-v0.1.i1-IQ2_S.gguf) | i1-IQ2_S | 2.5 | |
| [GGUF](https://huggingface.co/mradermacher/Irbis-7b-v0.1-i1-GGUF/resolve/main/Irbis-7b-v0.1.i1-IQ2_M.gguf) | i1-IQ2_M | 2.7 | |
| [GGUF](https://huggingface.co/mradermacher/Irbis-7b-v0.1-i1-GGUF/resolve/main/Irbis-7b-v0.1.i1-Q2_K.gguf) | i1-Q2_K | 3.0 | IQ3_XXS probably better |
| [GGUF](https://huggingface.co/mradermacher/Irbis-7b-v0.1-i1-GGUF/resolve/main/Irbis-7b-v0.1.i1-IQ3_XXS.gguf) | i1-IQ3_XXS | 3.1 | lower quality |
| [GGUF](https://huggingface.co/mradermacher/Irbis-7b-v0.1-i1-GGUF/resolve/main/Irbis-7b-v0.1.i1-IQ3_XS.gguf) | i1-IQ3_XS | 3.3 | |
| [GGUF](https://huggingface.co/mradermacher/Irbis-7b-v0.1-i1-GGUF/resolve/main/Irbis-7b-v0.1.i1-Q3_K_S.gguf) | i1-Q3_K_S | 3.4 | IQ3_XS probably better |
| [GGUF](https://huggingface.co/mradermacher/Irbis-7b-v0.1-i1-GGUF/resolve/main/Irbis-7b-v0.1.i1-IQ3_S.gguf) | i1-IQ3_S | 3.4 | beats Q3_K* |
| [GGUF](https://huggingface.co/mradermacher/Irbis-7b-v0.1-i1-GGUF/resolve/main/Irbis-7b-v0.1.i1-IQ3_M.gguf) | i1-IQ3_M | 3.5 | |
| [GGUF](https://huggingface.co/mradermacher/Irbis-7b-v0.1-i1-GGUF/resolve/main/Irbis-7b-v0.1.i1-Q3_K_M.gguf) | i1-Q3_K_M | 3.8 | IQ3_S probably better |
| [GGUF](https://huggingface.co/mradermacher/Irbis-7b-v0.1-i1-GGUF/resolve/main/Irbis-7b-v0.1.i1-Q3_K_L.gguf) | i1-Q3_K_L | 4.1 | IQ3_M probably better |
| [GGUF](https://huggingface.co/mradermacher/Irbis-7b-v0.1-i1-GGUF/resolve/main/Irbis-7b-v0.1.i1-IQ4_XS.gguf) | i1-IQ4_XS | 4.2 | |
| [GGUF](https://huggingface.co/mradermacher/Irbis-7b-v0.1-i1-GGUF/resolve/main/Irbis-7b-v0.1.i1-Q4_0.gguf) | i1-Q4_0 | 4.4 | fast, low quality |
| [GGUF](https://huggingface.co/mradermacher/Irbis-7b-v0.1-i1-GGUF/resolve/main/Irbis-7b-v0.1.i1-Q4_K_S.gguf) | i1-Q4_K_S | 4.4 | optimal size/speed/quality |
| [GGUF](https://huggingface.co/mradermacher/Irbis-7b-v0.1-i1-GGUF/resolve/main/Irbis-7b-v0.1.i1-Q4_K_M.gguf) | i1-Q4_K_M | 4.6 | fast, recommended |
| [GGUF](https://huggingface.co/mradermacher/Irbis-7b-v0.1-i1-GGUF/resolve/main/Irbis-7b-v0.1.i1-Q5_K_S.gguf) | i1-Q5_K_S | 5.3 | |
| [GGUF](https://huggingface.co/mradermacher/Irbis-7b-v0.1-i1-GGUF/resolve/main/Irbis-7b-v0.1.i1-Q5_K_M.gguf) | i1-Q5_K_M | 5.4 | |
| [GGUF](https://huggingface.co/mradermacher/Irbis-7b-v0.1-i1-GGUF/resolve/main/Irbis-7b-v0.1.i1-Q6_K.gguf) | i1-Q6_K | 6.2 | practically like static Q6_K |
Here is a handy graph by ikawrakow comparing some lower-quality quant
types (lower is better):

And here are Artefact2's thoughts on the matter:
https://gist.github.com/Artefact2/b5f810600771265fc1e39442288e8ec9
## FAQ / Model Request
See https://huggingface.co/mradermacher/model_requests for some answers to
questions you might have and/or if you want some other model quantized.
## Thanks
I thank my company, [nethype GmbH](https://www.nethype.de/), for letting
me use its servers and providing upgrades to my workstation to enable
this work in my free time. Additional thanks to [@nicoboss](https://huggingface.co/nicoboss) for giving me access to his hardware for calculating the imatrix for these quants.
<!-- end -->
|
John6666/hassaku-xl-hentai-v12-sdxl | John6666 | 2024-06-23T22:22:15Z | 14,049 | 1 | diffusers | [
"diffusers",
"safetensors",
"text-to-image",
"stable-diffusion",
"stable-diffusion-xl",
"anime",
"Cham22 v2",
"license:other",
"autotrain_compatible",
"endpoints_compatible",
"diffusers:StableDiffusionXLPipeline",
"region:us"
] | text-to-image | 2024-05-27T02:40:54Z | ---
license: other
tags:
- text-to-image
- stable-diffusion
- stable-diffusion-xl
- anime
- Cham22 v2
---
Original model is [here](https://civitai.com/models/376031?modelVersionId=518550). |
mradermacher/L3-UI-v1-8B-GGUF | mradermacher | 2024-06-24T03:20:39Z | 14,044 | 0 | transformers | [
"transformers",
"gguf",
"merge",
"mergekit",
"lazymergekit",
"Casual-Autopsy/L3-Umbral-Mind-RP-v0.3-8B",
"en",
"base_model:Frowning/L3-UI-v1-8B",
"endpoints_compatible",
"region:us"
] | null | 2024-06-23T14:52:14Z | ---
base_model: Frowning/L3-UI-v1-8B
language:
- en
library_name: transformers
quantized_by: mradermacher
tags:
- merge
- mergekit
- lazymergekit
- Casual-Autopsy/L3-Umbral-Mind-RP-v0.3-8B
---
## About
<!-- ### quantize_version: 2 -->
<!-- ### output_tensor_quantised: 1 -->
<!-- ### convert_type: hf -->
<!-- ### vocab_type: -->
<!-- ### tags: -->
static quants of https://huggingface.co/Frowning/L3-UI-v1-8B
<!-- provided-files -->
weighted/imatrix quants are available at https://huggingface.co/mradermacher/L3-UI-v1-8B-i1-GGUF
## Usage
If you are unsure how to use GGUF files, refer to one of [TheBloke's
READMEs](https://huggingface.co/TheBloke/KafkaLM-70B-German-V0.1-GGUF) for
more details, including on how to concatenate multi-part files.
## Provided Quants
(sorted by size, not necessarily quality. IQ-quants are often preferable over similar sized non-IQ quants)
| Link | Type | Size/GB | Notes |
|:-----|:-----|--------:|:------|
| [GGUF](https://huggingface.co/mradermacher/L3-UI-v1-8B-GGUF/resolve/main/L3-UI-v1-8B.Q2_K.gguf) | Q2_K | 3.3 | |
| [GGUF](https://huggingface.co/mradermacher/L3-UI-v1-8B-GGUF/resolve/main/L3-UI-v1-8B.IQ3_XS.gguf) | IQ3_XS | 3.6 | |
| [GGUF](https://huggingface.co/mradermacher/L3-UI-v1-8B-GGUF/resolve/main/L3-UI-v1-8B.Q3_K_S.gguf) | Q3_K_S | 3.8 | |
| [GGUF](https://huggingface.co/mradermacher/L3-UI-v1-8B-GGUF/resolve/main/L3-UI-v1-8B.IQ3_S.gguf) | IQ3_S | 3.8 | beats Q3_K* |
| [GGUF](https://huggingface.co/mradermacher/L3-UI-v1-8B-GGUF/resolve/main/L3-UI-v1-8B.IQ3_M.gguf) | IQ3_M | 3.9 | |
| [GGUF](https://huggingface.co/mradermacher/L3-UI-v1-8B-GGUF/resolve/main/L3-UI-v1-8B.Q3_K_M.gguf) | Q3_K_M | 4.1 | lower quality |
| [GGUF](https://huggingface.co/mradermacher/L3-UI-v1-8B-GGUF/resolve/main/L3-UI-v1-8B.Q3_K_L.gguf) | Q3_K_L | 4.4 | |
| [GGUF](https://huggingface.co/mradermacher/L3-UI-v1-8B-GGUF/resolve/main/L3-UI-v1-8B.IQ4_XS.gguf) | IQ4_XS | 4.6 | |
| [GGUF](https://huggingface.co/mradermacher/L3-UI-v1-8B-GGUF/resolve/main/L3-UI-v1-8B.Q4_K_S.gguf) | Q4_K_S | 4.8 | fast, recommended |
| [GGUF](https://huggingface.co/mradermacher/L3-UI-v1-8B-GGUF/resolve/main/L3-UI-v1-8B.Q4_K_M.gguf) | Q4_K_M | 5.0 | fast, recommended |
| [GGUF](https://huggingface.co/mradermacher/L3-UI-v1-8B-GGUF/resolve/main/L3-UI-v1-8B.Q5_K_S.gguf) | Q5_K_S | 5.7 | |
| [GGUF](https://huggingface.co/mradermacher/L3-UI-v1-8B-GGUF/resolve/main/L3-UI-v1-8B.Q5_K_M.gguf) | Q5_K_M | 5.8 | |
| [GGUF](https://huggingface.co/mradermacher/L3-UI-v1-8B-GGUF/resolve/main/L3-UI-v1-8B.Q6_K.gguf) | Q6_K | 6.7 | very good quality |
| [GGUF](https://huggingface.co/mradermacher/L3-UI-v1-8B-GGUF/resolve/main/L3-UI-v1-8B.Q8_0.gguf) | Q8_0 | 8.6 | fast, best quality |
| [GGUF](https://huggingface.co/mradermacher/L3-UI-v1-8B-GGUF/resolve/main/L3-UI-v1-8B.f16.gguf) | f16 | 16.2 | 16 bpw, overkill |
Here is a handy graph by ikawrakow comparing some lower-quality quant
types (lower is better):

And here are Artefact2's thoughts on the matter:
https://gist.github.com/Artefact2/b5f810600771265fc1e39442288e8ec9
## FAQ / Model Request
See https://huggingface.co/mradermacher/model_requests for some answers to
questions you might have and/or if you want some other model quantized.
## Thanks
I thank my company, [nethype GmbH](https://www.nethype.de/), for letting
me use its servers and providing upgrades to my workstation to enable
this work in my free time.
<!-- end -->
|
ashawkey/zero123-xl-diffusers | ashawkey | 2023-11-08T03:45:02Z | 14,039 | 3 | diffusers | [
"diffusers",
"safetensors",
"arxiv:2303.11328",
"license:mit",
"diffusers:Zero123Pipeline",
"region:us"
] | null | 2023-11-08T02:41:27Z | ---
license: mit
---
# Uses
_Note: This section is originally taken from the [Stable Diffusion v2 model card](https://huggingface.co/stabilityai/stable-diffusion-2), but applies in the same way to Zero-1-to-3._
## Direct Use
The model is intended for research purposes only. Possible research areas and tasks include:
- Safe deployment of large-scale models.
- Probing and understanding the limitations and biases of generative models.
- Generation of artworks and use in design and other artistic processes.
- Applications in educational or creative tools.
- Research on generative models.
Excluded uses are described below.
### Misuse, Malicious Use, and Out-of-Scope Use
The model should not be used to intentionally create or disseminate images that create hostile or alienating environments for people. This includes generating images that people would foreseeably find disturbing, distressing, or offensive; or content that propagates historical or current stereotypes.
#### Out-of-Scope Use
The model was not trained to be factual or true representations of people or events, and therefore using the model to generate such content is out-of-scope for the abilities of this model.
#### Misuse and Malicious Use
Using the model to generate content that is cruel to individuals is a misuse of this model. This includes, but is not limited to:
- Generating demeaning, dehumanizing, or otherwise harmful representations of people or their environments, cultures, religions, etc.
- Intentionally promoting or propagating discriminatory content or harmful stereotypes.
- Impersonating individuals without their consent.
- Sexual content without consent of the people who might see it.
- Mis- and disinformation
- Representations of egregious violence and gore
- Sharing of copyrighted or licensed material in violation of its terms of use.
- Sharing content that is an alteration of copyrighted or licensed material in violation of its terms of use.
## Limitations and Bias
### Limitations
- The model does not achieve perfect photorealism.
- The model cannot render legible text.
- Faces and people in general may not be parsed or generated properly.
- The autoencoding part of the model is lossy.
- Stable Diffusion was trained on a subset of the large-scale dataset [LAION-5B](https://laion.ai/blog/laion-5b/), which contains adult, violent and sexual content. To partially mitigate this, Stability AI has filtered the dataset using LAION's NSFW detector.
- Zero-1-to-3 was subsequently finetuned on a subset of the large-scale dataset [Objaverse](https://objaverse.allenai.org/), which might also potentially contain inappropriate content. To partially mitigate this, our demo applies a safety check to every uploaded image.
### Bias
While the capabilities of image generation models are impressive, they can also reinforce or exacerbate social biases.
Stable Diffusion was primarily trained on subsets of [LAION-2B(en)](https://laion.ai/blog/laion-5b/), which consists of images that are limited to English descriptions.
Images and concepts from communities and cultures that use other languages are likely to be insufficiently accounted for.
This affects the overall output of the model, as Western cultures are often overrepresented.
Stable Diffusion mirrors and exacerbates biases to such a degree that viewer discretion must be advised irrespective of the input or its intent.
### Safety Module
The intended use of this model is with the [Safety Checker](https://github.com/huggingface/diffusers/blob/main/src/diffusers/pipelines/stable_diffusion/safety_checker.py) in Diffusers.
This checker works by checking model inputs against known hard-coded NSFW concepts.
Specifically, the checker compares the class probability of harmful concepts in the embedding space of the uploaded input images.
The concepts are passed into the model with the image and compared to a hand-engineered weight for each NSFW concept.
## Citation
```
@misc{liu2023zero1to3,
title={Zero-1-to-3: Zero-shot One Image to 3D Object},
author={Ruoshi Liu and Rundi Wu and Basile Van Hoorick and Pavel Tokmakov and Sergey Zakharov and Carl Vondrick},
year={2023},
eprint={2303.11328},
archivePrefix={arXiv},
primaryClass={cs.CV}
}
```
|
Helsinki-NLP/opus-mt-ru-es | Helsinki-NLP | 2023-08-16T12:03:24Z | 14,002 | 2 | transformers | [
"transformers",
"pytorch",
"tf",
"marian",
"text2text-generation",
"translation",
"ru",
"es",
"license:apache-2.0",
"autotrain_compatible",
"endpoints_compatible",
"region:us"
] | translation | 2022-03-02T23:29:04Z | ---
tags:
- translation
license: apache-2.0
---
### opus-mt-ru-es
* source languages: ru
* target languages: es
* OPUS readme: [ru-es](https://github.com/Helsinki-NLP/OPUS-MT-train/blob/master/models/ru-es/README.md)
* dataset: opus
* model: transformer-align
* pre-processing: normalization + SentencePiece
* download original weights: [opus-2020-01-21.zip](https://object.pouta.csc.fi/OPUS-MT-models/ru-es/opus-2020-01-21.zip)
* test set translations: [opus-2020-01-21.test.txt](https://object.pouta.csc.fi/OPUS-MT-models/ru-es/opus-2020-01-21.test.txt)
* test set scores: [opus-2020-01-21.eval.txt](https://object.pouta.csc.fi/OPUS-MT-models/ru-es/opus-2020-01-21.eval.txt)
## Benchmarks
| testset | BLEU | chr-F |
|-----------------------|-------|-------|
| newstest2012.ru.es | 26.1 | 0.527 |
| newstest2013.ru.es | 28.2 | 0.538 |
| Tatoeba.ru.es | 49.4 | 0.675 |
|
mradermacher/Jellyfish-13B-i1-GGUF | mradermacher | 2024-06-22T03:55:15Z | 13,974 | 0 | transformers | [
"transformers",
"gguf",
"en",
"base_model:NECOUDBFM/Jellyfish-13B",
"endpoints_compatible",
"region:us"
] | null | 2024-06-21T19:50:49Z | ---
base_model: NECOUDBFM/Jellyfish-13B
language:
- en
library_name: transformers
quantized_by: mradermacher
---
## About
<!-- ### quantize_version: 2 -->
<!-- ### output_tensor_quantised: 1 -->
<!-- ### convert_type: hf -->
<!-- ### vocab_type: -->
<!-- ### tags: nicoboss -->
weighted/imatrix quants of https://huggingface.co/NECOUDBFM/Jellyfish-13B
<!-- provided-files -->
static quants are available at https://huggingface.co/mradermacher/Jellyfish-13B-GGUF
## Usage
If you are unsure how to use GGUF files, refer to one of [TheBloke's
READMEs](https://huggingface.co/TheBloke/KafkaLM-70B-German-V0.1-GGUF) for
more details, including on how to concatenate multi-part files.
## Provided Quants
(sorted by size, not necessarily quality. IQ-quants are often preferable over similar sized non-IQ quants)
| Link | Type | Size/GB | Notes |
|:-----|:-----|--------:|:------|
| [GGUF](https://huggingface.co/mradermacher/Jellyfish-13B-i1-GGUF/resolve/main/Jellyfish-13B.i1-IQ1_S.gguf) | i1-IQ1_S | 3.0 | for the desperate |
| [GGUF](https://huggingface.co/mradermacher/Jellyfish-13B-i1-GGUF/resolve/main/Jellyfish-13B.i1-IQ1_M.gguf) | i1-IQ1_M | 3.2 | mostly desperate |
| [GGUF](https://huggingface.co/mradermacher/Jellyfish-13B-i1-GGUF/resolve/main/Jellyfish-13B.i1-IQ2_XXS.gguf) | i1-IQ2_XXS | 3.6 | |
| [GGUF](https://huggingface.co/mradermacher/Jellyfish-13B-i1-GGUF/resolve/main/Jellyfish-13B.i1-IQ2_XS.gguf) | i1-IQ2_XS | 4.0 | |
| [GGUF](https://huggingface.co/mradermacher/Jellyfish-13B-i1-GGUF/resolve/main/Jellyfish-13B.i1-IQ2_S.gguf) | i1-IQ2_S | 4.3 | |
| [GGUF](https://huggingface.co/mradermacher/Jellyfish-13B-i1-GGUF/resolve/main/Jellyfish-13B.i1-IQ2_M.gguf) | i1-IQ2_M | 4.6 | |
| [GGUF](https://huggingface.co/mradermacher/Jellyfish-13B-i1-GGUF/resolve/main/Jellyfish-13B.i1-Q2_K.gguf) | i1-Q2_K | 5.0 | IQ3_XXS probably better |
| [GGUF](https://huggingface.co/mradermacher/Jellyfish-13B-i1-GGUF/resolve/main/Jellyfish-13B.i1-IQ3_XXS.gguf) | i1-IQ3_XXS | 5.1 | lower quality |
| [GGUF](https://huggingface.co/mradermacher/Jellyfish-13B-i1-GGUF/resolve/main/Jellyfish-13B.i1-IQ3_XS.gguf) | i1-IQ3_XS | 5.5 | |
| [GGUF](https://huggingface.co/mradermacher/Jellyfish-13B-i1-GGUF/resolve/main/Jellyfish-13B.i1-IQ3_S.gguf) | i1-IQ3_S | 5.8 | beats Q3_K* |
| [GGUF](https://huggingface.co/mradermacher/Jellyfish-13B-i1-GGUF/resolve/main/Jellyfish-13B.i1-Q3_K_S.gguf) | i1-Q3_K_S | 5.8 | IQ3_XS probably better |
| [GGUF](https://huggingface.co/mradermacher/Jellyfish-13B-i1-GGUF/resolve/main/Jellyfish-13B.i1-IQ3_M.gguf) | i1-IQ3_M | 6.1 | |
| [GGUF](https://huggingface.co/mradermacher/Jellyfish-13B-i1-GGUF/resolve/main/Jellyfish-13B.i1-Q3_K_M.gguf) | i1-Q3_K_M | 6.4 | IQ3_S probably better |
| [GGUF](https://huggingface.co/mradermacher/Jellyfish-13B-i1-GGUF/resolve/main/Jellyfish-13B.i1-Q3_K_L.gguf) | i1-Q3_K_L | 7.0 | IQ3_M probably better |
| [GGUF](https://huggingface.co/mradermacher/Jellyfish-13B-i1-GGUF/resolve/main/Jellyfish-13B.i1-IQ4_XS.gguf) | i1-IQ4_XS | 7.1 | |
| [GGUF](https://huggingface.co/mradermacher/Jellyfish-13B-i1-GGUF/resolve/main/Jellyfish-13B.i1-Q4_0.gguf) | i1-Q4_0 | 7.5 | fast, low quality |
| [GGUF](https://huggingface.co/mradermacher/Jellyfish-13B-i1-GGUF/resolve/main/Jellyfish-13B.i1-Q4_K_S.gguf) | i1-Q4_K_S | 7.5 | optimal size/speed/quality |
| [GGUF](https://huggingface.co/mradermacher/Jellyfish-13B-i1-GGUF/resolve/main/Jellyfish-13B.i1-Q4_K_M.gguf) | i1-Q4_K_M | 8.0 | fast, recommended |
| [GGUF](https://huggingface.co/mradermacher/Jellyfish-13B-i1-GGUF/resolve/main/Jellyfish-13B.i1-Q5_K_S.gguf) | i1-Q5_K_S | 9.1 | |
| [GGUF](https://huggingface.co/mradermacher/Jellyfish-13B-i1-GGUF/resolve/main/Jellyfish-13B.i1-Q5_K_M.gguf) | i1-Q5_K_M | 9.3 | |
| [GGUF](https://huggingface.co/mradermacher/Jellyfish-13B-i1-GGUF/resolve/main/Jellyfish-13B.i1-Q6_K.gguf) | i1-Q6_K | 10.8 | practically like static Q6_K |
Here is a handy graph by ikawrakow comparing some lower-quality quant
types (lower is better):

And here are Artefact2's thoughts on the matter:
https://gist.github.com/Artefact2/b5f810600771265fc1e39442288e8ec9
## FAQ / Model Request
See https://huggingface.co/mradermacher/model_requests for some answers to
questions you might have and/or if you want some other model quantized.
## Thanks
I thank my company, [nethype GmbH](https://www.nethype.de/), for letting
me use its servers and providing upgrades to my workstation to enable
this work in my free time. Additional thanks to [@nicoboss](https://huggingface.co/nicoboss) for giving me access to his hardware for calculating the imatrix for these quants.
<!-- end -->
|
mradermacher/Llama-3-Lumimaid-8B-v0.1-OAS-GGUF | mradermacher | 2024-06-27T22:14:44Z | 13,974 | 0 | transformers | [
"transformers",
"gguf",
"not-for-all-audiences",
"nsfw",
"en",
"base_model:NeverSleep/Llama-3-Lumimaid-8B-v0.1-OAS",
"license:cc-by-nc-4.0",
"endpoints_compatible",
"region:us"
] | null | 2024-06-27T05:28:49Z | ---
base_model: NeverSleep/Llama-3-Lumimaid-8B-v0.1-OAS
language:
- en
library_name: transformers
license: cc-by-nc-4.0
quantized_by: mradermacher
tags:
- not-for-all-audiences
- nsfw
---
## About
<!-- ### quantize_version: 2 -->
<!-- ### output_tensor_quantised: 1 -->
<!-- ### convert_type: hf -->
<!-- ### vocab_type: -->
<!-- ### tags: -->
static quants of https://huggingface.co/NeverSleep/Llama-3-Lumimaid-8B-v0.1-OAS
<!-- provided-files -->
weighted/imatrix quants are available at https://huggingface.co/mradermacher/Llama-3-Lumimaid-8B-v0.1-OAS-i1-GGUF
## Usage
If you are unsure how to use GGUF files, refer to one of [TheBloke's
READMEs](https://huggingface.co/TheBloke/KafkaLM-70B-German-V0.1-GGUF) for
more details, including on how to concatenate multi-part files.
## Provided Quants
(sorted by size, not necessarily quality. IQ-quants are often preferable over similar sized non-IQ quants)
| Link | Type | Size/GB | Notes |
|:-----|:-----|--------:|:------|
| [GGUF](https://huggingface.co/mradermacher/Llama-3-Lumimaid-8B-v0.1-OAS-GGUF/resolve/main/Llama-3-Lumimaid-8B-v0.1-OAS.Q2_K.gguf) | Q2_K | 3.3 | |
| [GGUF](https://huggingface.co/mradermacher/Llama-3-Lumimaid-8B-v0.1-OAS-GGUF/resolve/main/Llama-3-Lumimaid-8B-v0.1-OAS.IQ3_XS.gguf) | IQ3_XS | 3.6 | |
| [GGUF](https://huggingface.co/mradermacher/Llama-3-Lumimaid-8B-v0.1-OAS-GGUF/resolve/main/Llama-3-Lumimaid-8B-v0.1-OAS.Q3_K_S.gguf) | Q3_K_S | 3.8 | |
| [GGUF](https://huggingface.co/mradermacher/Llama-3-Lumimaid-8B-v0.1-OAS-GGUF/resolve/main/Llama-3-Lumimaid-8B-v0.1-OAS.IQ3_S.gguf) | IQ3_S | 3.8 | beats Q3_K* |
| [GGUF](https://huggingface.co/mradermacher/Llama-3-Lumimaid-8B-v0.1-OAS-GGUF/resolve/main/Llama-3-Lumimaid-8B-v0.1-OAS.IQ3_M.gguf) | IQ3_M | 3.9 | |
| [GGUF](https://huggingface.co/mradermacher/Llama-3-Lumimaid-8B-v0.1-OAS-GGUF/resolve/main/Llama-3-Lumimaid-8B-v0.1-OAS.Q3_K_M.gguf) | Q3_K_M | 4.1 | lower quality |
| [GGUF](https://huggingface.co/mradermacher/Llama-3-Lumimaid-8B-v0.1-OAS-GGUF/resolve/main/Llama-3-Lumimaid-8B-v0.1-OAS.Q3_K_L.gguf) | Q3_K_L | 4.4 | |
| [GGUF](https://huggingface.co/mradermacher/Llama-3-Lumimaid-8B-v0.1-OAS-GGUF/resolve/main/Llama-3-Lumimaid-8B-v0.1-OAS.IQ4_XS.gguf) | IQ4_XS | 4.6 | |
| [GGUF](https://huggingface.co/mradermacher/Llama-3-Lumimaid-8B-v0.1-OAS-GGUF/resolve/main/Llama-3-Lumimaid-8B-v0.1-OAS.Q4_K_S.gguf) | Q4_K_S | 4.8 | fast, recommended |
| [GGUF](https://huggingface.co/mradermacher/Llama-3-Lumimaid-8B-v0.1-OAS-GGUF/resolve/main/Llama-3-Lumimaid-8B-v0.1-OAS.Q4_K_M.gguf) | Q4_K_M | 5.0 | fast, recommended |
| [GGUF](https://huggingface.co/mradermacher/Llama-3-Lumimaid-8B-v0.1-OAS-GGUF/resolve/main/Llama-3-Lumimaid-8B-v0.1-OAS.Q5_K_S.gguf) | Q5_K_S | 5.7 | |
| [GGUF](https://huggingface.co/mradermacher/Llama-3-Lumimaid-8B-v0.1-OAS-GGUF/resolve/main/Llama-3-Lumimaid-8B-v0.1-OAS.Q5_K_M.gguf) | Q5_K_M | 5.8 | |
| [GGUF](https://huggingface.co/mradermacher/Llama-3-Lumimaid-8B-v0.1-OAS-GGUF/resolve/main/Llama-3-Lumimaid-8B-v0.1-OAS.Q6_K.gguf) | Q6_K | 6.7 | very good quality |
| [GGUF](https://huggingface.co/mradermacher/Llama-3-Lumimaid-8B-v0.1-OAS-GGUF/resolve/main/Llama-3-Lumimaid-8B-v0.1-OAS.Q8_0.gguf) | Q8_0 | 8.6 | fast, best quality |
| [GGUF](https://huggingface.co/mradermacher/Llama-3-Lumimaid-8B-v0.1-OAS-GGUF/resolve/main/Llama-3-Lumimaid-8B-v0.1-OAS.f16.gguf) | f16 | 16.2 | 16 bpw, overkill |
Here is a handy graph by ikawrakow comparing some lower-quality quant
types (lower is better):

And here are Artefact2's thoughts on the matter:
https://gist.github.com/Artefact2/b5f810600771265fc1e39442288e8ec9
## FAQ / Model Request
See https://huggingface.co/mradermacher/model_requests for some answers to
questions you might have and/or if you want some other model quantized.
## Thanks
I thank my company, [nethype GmbH](https://www.nethype.de/), for letting
me use its servers and providing upgrades to my workstation to enable
this work in my free time.
<!-- end -->
|
LWDCLS/llama3-8B-DarkIdol-1.2-GGUF-IQ-Imatrix-Request | LWDCLS | 2024-06-28T01:59:24Z | 13,973 | 8 | null | [
"gguf",
"license:unlicense",
"region:us"
] | null | 2024-06-24T13:24:43Z | ---
inference: false
license: unlicense
---
[[Request #53]](https://huggingface.co/Lewdiculous/Model-Requests/discussions/53) - Click the link for more context. <br>
[aifeifei798/llama3-8B-DarkIdol-1.2](https://huggingface.co/aifeifei798/llama3-8B-DarkIdol-1.2) <br>
Use with the [**latest version of KoboldCpp**](https://github.com/LostRuins/koboldcpp/releases/latest), or [this more up-to-date fork](https://github.com/Nexesenex/kobold.cpp) if you have issues.
<details>
<summary>⇲ Click here to expand/hide information – General chart with relative quant performance.</summary>
> [!NOTE]
> **Recommended read:** <br>
>
> [**"Which GGUF is right for me? (Opinionated)" by Artefact2**](https://gist.github.com/Artefact2/b5f810600771265fc1e39442288e8ec9)
>
> *Click the image to view full size.*
> 
</details>
 |
mradermacher/Llama-3-LiPPA-8B-i1-GGUF | mradermacher | 2024-06-28T03:05:44Z | 13,961 | 0 | transformers | [
"transformers",
"gguf",
"text-generation-inference",
"unsloth",
"llama",
"trl",
"sft",
"not-for-all-audiences",
"en",
"dataset:mpasila/LimaRP-PIPPA-Mix-8K-Context",
"dataset:grimulkan/LimaRP-augmented",
"dataset:KaraKaraWitch/PIPPA-ShareGPT-formatted",
"base_model:mpasila/Llama-3-LiPPA-8B",
"license:llama3",
"endpoints_compatible",
"region:us"
] | null | 2024-06-28T01:50:14Z | ---
base_model: mpasila/Llama-3-LiPPA-8B
datasets:
- mpasila/LimaRP-PIPPA-Mix-8K-Context
- grimulkan/LimaRP-augmented
- KaraKaraWitch/PIPPA-ShareGPT-formatted
language:
- en
library_name: transformers
license: llama3
quantized_by: mradermacher
tags:
- text-generation-inference
- transformers
- unsloth
- llama
- trl
- sft
- not-for-all-audiences
---
## About
<!-- ### quantize_version: 2 -->
<!-- ### output_tensor_quantised: 1 -->
<!-- ### convert_type: hf -->
<!-- ### vocab_type: -->
<!-- ### tags: nicoboss -->
weighted/imatrix quants of https://huggingface.co/mpasila/Llama-3-LiPPA-8B
<!-- provided-files -->
static quants are available at https://huggingface.co/mradermacher/Llama-3-LiPPA-8B-GGUF
## Usage
If you are unsure how to use GGUF files, refer to one of [TheBloke's
READMEs](https://huggingface.co/TheBloke/KafkaLM-70B-German-V0.1-GGUF) for
more details, including on how to concatenate multi-part files.
## Provided Quants
(sorted by size, not necessarily quality. IQ-quants are often preferable over similar sized non-IQ quants)
| Link | Type | Size/GB | Notes |
|:-----|:-----|--------:|:------|
| [GGUF](https://huggingface.co/mradermacher/Llama-3-LiPPA-8B-i1-GGUF/resolve/main/Llama-3-LiPPA-8B.i1-IQ1_S.gguf) | i1-IQ1_S | 2.1 | for the desperate |
| [GGUF](https://huggingface.co/mradermacher/Llama-3-LiPPA-8B-i1-GGUF/resolve/main/Llama-3-LiPPA-8B.i1-IQ1_M.gguf) | i1-IQ1_M | 2.3 | mostly desperate |
| [GGUF](https://huggingface.co/mradermacher/Llama-3-LiPPA-8B-i1-GGUF/resolve/main/Llama-3-LiPPA-8B.i1-IQ2_XXS.gguf) | i1-IQ2_XXS | 2.5 | |
| [GGUF](https://huggingface.co/mradermacher/Llama-3-LiPPA-8B-i1-GGUF/resolve/main/Llama-3-LiPPA-8B.i1-IQ2_XS.gguf) | i1-IQ2_XS | 2.7 | |
| [GGUF](https://huggingface.co/mradermacher/Llama-3-LiPPA-8B-i1-GGUF/resolve/main/Llama-3-LiPPA-8B.i1-IQ2_S.gguf) | i1-IQ2_S | 2.9 | |
| [GGUF](https://huggingface.co/mradermacher/Llama-3-LiPPA-8B-i1-GGUF/resolve/main/Llama-3-LiPPA-8B.i1-IQ2_M.gguf) | i1-IQ2_M | 3.0 | |
| [GGUF](https://huggingface.co/mradermacher/Llama-3-LiPPA-8B-i1-GGUF/resolve/main/Llama-3-LiPPA-8B.i1-Q2_K.gguf) | i1-Q2_K | 3.3 | IQ3_XXS probably better |
| [GGUF](https://huggingface.co/mradermacher/Llama-3-LiPPA-8B-i1-GGUF/resolve/main/Llama-3-LiPPA-8B.i1-IQ3_XXS.gguf) | i1-IQ3_XXS | 3.4 | lower quality |
| [GGUF](https://huggingface.co/mradermacher/Llama-3-LiPPA-8B-i1-GGUF/resolve/main/Llama-3-LiPPA-8B.i1-IQ3_XS.gguf) | i1-IQ3_XS | 3.6 | |
| [GGUF](https://huggingface.co/mradermacher/Llama-3-LiPPA-8B-i1-GGUF/resolve/main/Llama-3-LiPPA-8B.i1-Q3_K_S.gguf) | i1-Q3_K_S | 3.8 | IQ3_XS probably better |
| [GGUF](https://huggingface.co/mradermacher/Llama-3-LiPPA-8B-i1-GGUF/resolve/main/Llama-3-LiPPA-8B.i1-IQ3_S.gguf) | i1-IQ3_S | 3.8 | beats Q3_K* |
| [GGUF](https://huggingface.co/mradermacher/Llama-3-LiPPA-8B-i1-GGUF/resolve/main/Llama-3-LiPPA-8B.i1-IQ3_M.gguf) | i1-IQ3_M | 3.9 | |
| [GGUF](https://huggingface.co/mradermacher/Llama-3-LiPPA-8B-i1-GGUF/resolve/main/Llama-3-LiPPA-8B.i1-Q3_K_M.gguf) | i1-Q3_K_M | 4.1 | IQ3_S probably better |
| [GGUF](https://huggingface.co/mradermacher/Llama-3-LiPPA-8B-i1-GGUF/resolve/main/Llama-3-LiPPA-8B.i1-Q3_K_L.gguf) | i1-Q3_K_L | 4.4 | IQ3_M probably better |
| [GGUF](https://huggingface.co/mradermacher/Llama-3-LiPPA-8B-i1-GGUF/resolve/main/Llama-3-LiPPA-8B.i1-IQ4_XS.gguf) | i1-IQ4_XS | 4.5 | |
| [GGUF](https://huggingface.co/mradermacher/Llama-3-LiPPA-8B-i1-GGUF/resolve/main/Llama-3-LiPPA-8B.i1-Q4_0.gguf) | i1-Q4_0 | 4.8 | fast, low quality |
| [GGUF](https://huggingface.co/mradermacher/Llama-3-LiPPA-8B-i1-GGUF/resolve/main/Llama-3-LiPPA-8B.i1-Q4_K_S.gguf) | i1-Q4_K_S | 4.8 | optimal size/speed/quality |
| [GGUF](https://huggingface.co/mradermacher/Llama-3-LiPPA-8B-i1-GGUF/resolve/main/Llama-3-LiPPA-8B.i1-Q4_K_M.gguf) | i1-Q4_K_M | 5.0 | fast, recommended |
| [GGUF](https://huggingface.co/mradermacher/Llama-3-LiPPA-8B-i1-GGUF/resolve/main/Llama-3-LiPPA-8B.i1-Q5_K_S.gguf) | i1-Q5_K_S | 5.7 | |
| [GGUF](https://huggingface.co/mradermacher/Llama-3-LiPPA-8B-i1-GGUF/resolve/main/Llama-3-LiPPA-8B.i1-Q5_K_M.gguf) | i1-Q5_K_M | 5.8 | |
| [GGUF](https://huggingface.co/mradermacher/Llama-3-LiPPA-8B-i1-GGUF/resolve/main/Llama-3-LiPPA-8B.i1-Q6_K.gguf) | i1-Q6_K | 6.7 | practically like static Q6_K |
Here is a handy graph by ikawrakow comparing some lower-quality quant
types (lower is better):

And here are Artefact2's thoughts on the matter:
https://gist.github.com/Artefact2/b5f810600771265fc1e39442288e8ec9
## FAQ / Model Request
See https://huggingface.co/mradermacher/model_requests for some answers to
questions you might have and/or if you want some other model quantized.
## Thanks
I thank my company, [nethype GmbH](https://www.nethype.de/), for letting
me use its servers and providing upgrades to my workstation to enable
this work in my free time. Additional thanks to [@nicoboss](https://huggingface.co/nicoboss) for giving me access to his hardware for calculating the imatrix for these quants.
<!-- end -->
|
google/electra-small-generator | google | 2024-02-29T10:20:10Z | 13,957 | 12 | transformers | [
"transformers",
"pytorch",
"tf",
"jax",
"electra",
"fill-mask",
"en",
"arxiv:1406.2661",
"license:apache-2.0",
"autotrain_compatible",
"endpoints_compatible",
"region:us"
] | fill-mask | 2022-03-02T23:29:05Z | ---
language: en
thumbnail: https://huggingface.co/front/thumbnails/google.png
license: apache-2.0
---
**WARNING**: This is the official generator checkpoint as in the [ELECTRA original codebase](https://github.com/google-research/electra). However, this model is not scaled properly for pre-training with [google/electra-small-discriminator](https://huggingface.co/google/electra-small-discriminator). The paper recommends a hyperparameter multiplier of 1/4 between the discriminator and generator for this given model to avoid training instabilities. This would not be the case when using `google/electra-small-generator` and `google/electra-small-discriminator`, which are similar in size.
## ELECTRA: Pre-training Text Encoders as Discriminators Rather Than Generators
**ELECTRA** is a new method for self-supervised language representation learning. It can be used to pre-train transformer networks using relatively little compute. ELECTRA models are trained to distinguish "real" input tokens vs "fake" input tokens generated by another neural network, similar to the discriminator of a [GAN](https://arxiv.org/pdf/1406.2661.pdf). At small scale, ELECTRA achieves strong results even when trained on a single GPU. At large scale, ELECTRA achieves state-of-the-art results on the [SQuAD 2.0](https://rajpurkar.github.io/SQuAD-explorer/) dataset.
For a detailed description and experimental results, please refer to our paper [ELECTRA: Pre-training Text Encoders as Discriminators Rather Than Generators](https://openreview.net/pdf?id=r1xMH1BtvB).
This repository contains code to pre-train ELECTRA, including small ELECTRA models on a single GPU. It also supports fine-tuning ELECTRA on downstream tasks including classification tasks (e.g,. [GLUE](https://gluebenchmark.com/)), QA tasks (e.g., [SQuAD](https://rajpurkar.github.io/SQuAD-explorer/)), and sequence tagging tasks (e.g., [text chunking](https://www.clips.uantwerpen.be/conll2000/chunking/)).
## How to use the generator in `transformers`
```python
from transformers import pipeline
fill_mask = pipeline(
"fill-mask",
model="google/electra-small-generator",
tokenizer="google/electra-small-generator"
)
print(
fill_mask(f"HuggingFace is creating a {nlp.tokenizer.mask_token} that the community uses to solve NLP tasks.")
)
```
|
Alsebay/Inixion-2x8B-v2-GGUF | Alsebay | 2024-06-21T12:37:49Z | 13,957 | 0 | null | [
"gguf",
"region:us"
] | null | 2024-06-21T11:22:06Z | Entry not found |
mradermacher/Qwen2-7B-Multilingual-RP-i1-GGUF | mradermacher | 2024-06-25T07:51:52Z | 13,955 | 0 | transformers | [
"transformers",
"gguf",
"en",
"ko",
"ja",
"zh",
"es",
"base_model:maywell/Qwen2-7B-Multilingual-RP",
"license:apache-2.0",
"endpoints_compatible",
"region:us"
] | null | 2024-06-25T00:44:12Z | ---
base_model: maywell/Qwen2-7B-Multilingual-RP
language:
- en
- ko
- ja
- zh
- es
library_name: transformers
license: apache-2.0
quantized_by: mradermacher
---
## About
<!-- ### quantize_version: 2 -->
<!-- ### output_tensor_quantised: 1 -->
<!-- ### convert_type: hf -->
<!-- ### vocab_type: -->
<!-- ### tags: nicoboss -->
weighted/imatrix quants of https://huggingface.co/maywell/Qwen2-7B-Multilingual-RP
<!-- provided-files -->
static quants are available at https://huggingface.co/mradermacher/Qwen2-7B-Multilingual-RP-GGUF
## Usage
If you are unsure how to use GGUF files, refer to one of [TheBloke's
READMEs](https://huggingface.co/TheBloke/KafkaLM-70B-German-V0.1-GGUF) for
more details, including on how to concatenate multi-part files.
## Provided Quants
(sorted by size, not necessarily quality. IQ-quants are often preferable over similar sized non-IQ quants)
| Link | Type | Size/GB | Notes |
|:-----|:-----|--------:|:------|
| [GGUF](https://huggingface.co/mradermacher/Qwen2-7B-Multilingual-RP-i1-GGUF/resolve/main/Qwen2-7B-Multilingual-RP.i1-IQ1_S.gguf) | i1-IQ1_S | 2.0 | for the desperate |
| [GGUF](https://huggingface.co/mradermacher/Qwen2-7B-Multilingual-RP-i1-GGUF/resolve/main/Qwen2-7B-Multilingual-RP.i1-IQ1_M.gguf) | i1-IQ1_M | 2.1 | mostly desperate |
| [GGUF](https://huggingface.co/mradermacher/Qwen2-7B-Multilingual-RP-i1-GGUF/resolve/main/Qwen2-7B-Multilingual-RP.i1-IQ2_XXS.gguf) | i1-IQ2_XXS | 2.4 | |
| [GGUF](https://huggingface.co/mradermacher/Qwen2-7B-Multilingual-RP-i1-GGUF/resolve/main/Qwen2-7B-Multilingual-RP.i1-IQ2_XS.gguf) | i1-IQ2_XS | 2.6 | |
| [GGUF](https://huggingface.co/mradermacher/Qwen2-7B-Multilingual-RP-i1-GGUF/resolve/main/Qwen2-7B-Multilingual-RP.i1-IQ2_S.gguf) | i1-IQ2_S | 2.7 | |
| [GGUF](https://huggingface.co/mradermacher/Qwen2-7B-Multilingual-RP-i1-GGUF/resolve/main/Qwen2-7B-Multilingual-RP.i1-IQ2_M.gguf) | i1-IQ2_M | 2.9 | |
| [GGUF](https://huggingface.co/mradermacher/Qwen2-7B-Multilingual-RP-i1-GGUF/resolve/main/Qwen2-7B-Multilingual-RP.i1-Q2_K.gguf) | i1-Q2_K | 3.1 | IQ3_XXS probably better |
| [GGUF](https://huggingface.co/mradermacher/Qwen2-7B-Multilingual-RP-i1-GGUF/resolve/main/Qwen2-7B-Multilingual-RP.i1-IQ3_XXS.gguf) | i1-IQ3_XXS | 3.2 | lower quality |
| [GGUF](https://huggingface.co/mradermacher/Qwen2-7B-Multilingual-RP-i1-GGUF/resolve/main/Qwen2-7B-Multilingual-RP.i1-IQ3_XS.gguf) | i1-IQ3_XS | 3.4 | |
| [GGUF](https://huggingface.co/mradermacher/Qwen2-7B-Multilingual-RP-i1-GGUF/resolve/main/Qwen2-7B-Multilingual-RP.i1-Q3_K_S.gguf) | i1-Q3_K_S | 3.6 | IQ3_XS probably better |
| [GGUF](https://huggingface.co/mradermacher/Qwen2-7B-Multilingual-RP-i1-GGUF/resolve/main/Qwen2-7B-Multilingual-RP.i1-IQ3_S.gguf) | i1-IQ3_S | 3.6 | beats Q3_K* |
| [GGUF](https://huggingface.co/mradermacher/Qwen2-7B-Multilingual-RP-i1-GGUF/resolve/main/Qwen2-7B-Multilingual-RP.i1-IQ3_M.gguf) | i1-IQ3_M | 3.7 | |
| [GGUF](https://huggingface.co/mradermacher/Qwen2-7B-Multilingual-RP-i1-GGUF/resolve/main/Qwen2-7B-Multilingual-RP.i1-Q3_K_M.gguf) | i1-Q3_K_M | 3.9 | IQ3_S probably better |
| [GGUF](https://huggingface.co/mradermacher/Qwen2-7B-Multilingual-RP-i1-GGUF/resolve/main/Qwen2-7B-Multilingual-RP.i1-Q3_K_L.gguf) | i1-Q3_K_L | 4.2 | IQ3_M probably better |
| [GGUF](https://huggingface.co/mradermacher/Qwen2-7B-Multilingual-RP-i1-GGUF/resolve/main/Qwen2-7B-Multilingual-RP.i1-IQ4_XS.gguf) | i1-IQ4_XS | 4.3 | |
| [GGUF](https://huggingface.co/mradermacher/Qwen2-7B-Multilingual-RP-i1-GGUF/resolve/main/Qwen2-7B-Multilingual-RP.i1-Q4_0.gguf) | i1-Q4_0 | 4.5 | fast, low quality |
| [GGUF](https://huggingface.co/mradermacher/Qwen2-7B-Multilingual-RP-i1-GGUF/resolve/main/Qwen2-7B-Multilingual-RP.i1-Q4_K_S.gguf) | i1-Q4_K_S | 4.6 | optimal size/speed/quality |
| [GGUF](https://huggingface.co/mradermacher/Qwen2-7B-Multilingual-RP-i1-GGUF/resolve/main/Qwen2-7B-Multilingual-RP.i1-Q4_K_M.gguf) | i1-Q4_K_M | 4.8 | fast, recommended |
| [GGUF](https://huggingface.co/mradermacher/Qwen2-7B-Multilingual-RP-i1-GGUF/resolve/main/Qwen2-7B-Multilingual-RP.i1-Q5_K_S.gguf) | i1-Q5_K_S | 5.4 | |
| [GGUF](https://huggingface.co/mradermacher/Qwen2-7B-Multilingual-RP-i1-GGUF/resolve/main/Qwen2-7B-Multilingual-RP.i1-Q5_K_M.gguf) | i1-Q5_K_M | 5.5 | |
| [GGUF](https://huggingface.co/mradermacher/Qwen2-7B-Multilingual-RP-i1-GGUF/resolve/main/Qwen2-7B-Multilingual-RP.i1-Q6_K.gguf) | i1-Q6_K | 6.4 | practically like static Q6_K |
Here is a handy graph by ikawrakow comparing some lower-quality quant
types (lower is better):

And here are Artefact2's thoughts on the matter:
https://gist.github.com/Artefact2/b5f810600771265fc1e39442288e8ec9
## FAQ / Model Request
See https://huggingface.co/mradermacher/model_requests for some answers to
questions you might have and/or if you want some other model quantized.
## Thanks
I thank my company, [nethype GmbH](https://www.nethype.de/), for letting
me use its servers and providing upgrades to my workstation to enable
this work in my free time. Additional thanks to [@nicoboss](https://huggingface.co/nicoboss) for giving me access to his hardware for calculating the imatrix for these quants.
<!-- end -->
|
RichardErkhov/hfl_-_llama-3-chinese-8b-instruct-v3-gguf | RichardErkhov | 2024-06-26T03:28:25Z | 13,951 | 0 | null | [
"gguf",
"region:us"
] | null | 2024-06-25T23:04:07Z | Quantization made by Richard Erkhov.
[Github](https://github.com/RichardErkhov)
[Discord](https://discord.gg/pvy7H8DZMG)
[Request more models](https://github.com/RichardErkhov/quant_request)
llama-3-chinese-8b-instruct-v3 - GGUF
- Model creator: https://huggingface.co/hfl/
- Original model: https://huggingface.co/hfl/llama-3-chinese-8b-instruct-v3/
| Name | Quant method | Size |
| ---- | ---- | ---- |
| [llama-3-chinese-8b-instruct-v3.Q2_K.gguf](https://huggingface.co/RichardErkhov/hfl_-_llama-3-chinese-8b-instruct-v3-gguf/blob/main/llama-3-chinese-8b-instruct-v3.Q2_K.gguf) | Q2_K | 2.96GB |
| [llama-3-chinese-8b-instruct-v3.IQ3_XS.gguf](https://huggingface.co/RichardErkhov/hfl_-_llama-3-chinese-8b-instruct-v3-gguf/blob/main/llama-3-chinese-8b-instruct-v3.IQ3_XS.gguf) | IQ3_XS | 3.28GB |
| [llama-3-chinese-8b-instruct-v3.IQ3_S.gguf](https://huggingface.co/RichardErkhov/hfl_-_llama-3-chinese-8b-instruct-v3-gguf/blob/main/llama-3-chinese-8b-instruct-v3.IQ3_S.gguf) | IQ3_S | 3.43GB |
| [llama-3-chinese-8b-instruct-v3.Q3_K_S.gguf](https://huggingface.co/RichardErkhov/hfl_-_llama-3-chinese-8b-instruct-v3-gguf/blob/main/llama-3-chinese-8b-instruct-v3.Q3_K_S.gguf) | Q3_K_S | 3.41GB |
| [llama-3-chinese-8b-instruct-v3.IQ3_M.gguf](https://huggingface.co/RichardErkhov/hfl_-_llama-3-chinese-8b-instruct-v3-gguf/blob/main/llama-3-chinese-8b-instruct-v3.IQ3_M.gguf) | IQ3_M | 3.52GB |
| [llama-3-chinese-8b-instruct-v3.Q3_K.gguf](https://huggingface.co/RichardErkhov/hfl_-_llama-3-chinese-8b-instruct-v3-gguf/blob/main/llama-3-chinese-8b-instruct-v3.Q3_K.gguf) | Q3_K | 3.74GB |
| [llama-3-chinese-8b-instruct-v3.Q3_K_M.gguf](https://huggingface.co/RichardErkhov/hfl_-_llama-3-chinese-8b-instruct-v3-gguf/blob/main/llama-3-chinese-8b-instruct-v3.Q3_K_M.gguf) | Q3_K_M | 3.74GB |
| [llama-3-chinese-8b-instruct-v3.Q3_K_L.gguf](https://huggingface.co/RichardErkhov/hfl_-_llama-3-chinese-8b-instruct-v3-gguf/blob/main/llama-3-chinese-8b-instruct-v3.Q3_K_L.gguf) | Q3_K_L | 4.03GB |
| [llama-3-chinese-8b-instruct-v3.IQ4_XS.gguf](https://huggingface.co/RichardErkhov/hfl_-_llama-3-chinese-8b-instruct-v3-gguf/blob/main/llama-3-chinese-8b-instruct-v3.IQ4_XS.gguf) | IQ4_XS | 4.18GB |
| [llama-3-chinese-8b-instruct-v3.Q4_0.gguf](https://huggingface.co/RichardErkhov/hfl_-_llama-3-chinese-8b-instruct-v3-gguf/blob/main/llama-3-chinese-8b-instruct-v3.Q4_0.gguf) | Q4_0 | 4.34GB |
| [llama-3-chinese-8b-instruct-v3.IQ4_NL.gguf](https://huggingface.co/RichardErkhov/hfl_-_llama-3-chinese-8b-instruct-v3-gguf/blob/main/llama-3-chinese-8b-instruct-v3.IQ4_NL.gguf) | IQ4_NL | 4.38GB |
| [llama-3-chinese-8b-instruct-v3.Q4_K_S.gguf](https://huggingface.co/RichardErkhov/hfl_-_llama-3-chinese-8b-instruct-v3-gguf/blob/main/llama-3-chinese-8b-instruct-v3.Q4_K_S.gguf) | Q4_K_S | 4.37GB |
| [llama-3-chinese-8b-instruct-v3.Q4_K.gguf](https://huggingface.co/RichardErkhov/hfl_-_llama-3-chinese-8b-instruct-v3-gguf/blob/main/llama-3-chinese-8b-instruct-v3.Q4_K.gguf) | Q4_K | 4.58GB |
| [llama-3-chinese-8b-instruct-v3.Q4_K_M.gguf](https://huggingface.co/RichardErkhov/hfl_-_llama-3-chinese-8b-instruct-v3-gguf/blob/main/llama-3-chinese-8b-instruct-v3.Q4_K_M.gguf) | Q4_K_M | 4.58GB |
| [llama-3-chinese-8b-instruct-v3.Q4_1.gguf](https://huggingface.co/RichardErkhov/hfl_-_llama-3-chinese-8b-instruct-v3-gguf/blob/main/llama-3-chinese-8b-instruct-v3.Q4_1.gguf) | Q4_1 | 4.78GB |
| [llama-3-chinese-8b-instruct-v3.Q5_0.gguf](https://huggingface.co/RichardErkhov/hfl_-_llama-3-chinese-8b-instruct-v3-gguf/blob/main/llama-3-chinese-8b-instruct-v3.Q5_0.gguf) | Q5_0 | 5.21GB |
| [llama-3-chinese-8b-instruct-v3.Q5_K_S.gguf](https://huggingface.co/RichardErkhov/hfl_-_llama-3-chinese-8b-instruct-v3-gguf/blob/main/llama-3-chinese-8b-instruct-v3.Q5_K_S.gguf) | Q5_K_S | 5.21GB |
| [llama-3-chinese-8b-instruct-v3.Q5_K.gguf](https://huggingface.co/RichardErkhov/hfl_-_llama-3-chinese-8b-instruct-v3-gguf/blob/main/llama-3-chinese-8b-instruct-v3.Q5_K.gguf) | Q5_K | 5.34GB |
| [llama-3-chinese-8b-instruct-v3.Q5_K_M.gguf](https://huggingface.co/RichardErkhov/hfl_-_llama-3-chinese-8b-instruct-v3-gguf/blob/main/llama-3-chinese-8b-instruct-v3.Q5_K_M.gguf) | Q5_K_M | 5.34GB |
| [llama-3-chinese-8b-instruct-v3.Q5_1.gguf](https://huggingface.co/RichardErkhov/hfl_-_llama-3-chinese-8b-instruct-v3-gguf/blob/main/llama-3-chinese-8b-instruct-v3.Q5_1.gguf) | Q5_1 | 5.65GB |
| [llama-3-chinese-8b-instruct-v3.Q6_K.gguf](https://huggingface.co/RichardErkhov/hfl_-_llama-3-chinese-8b-instruct-v3-gguf/blob/main/llama-3-chinese-8b-instruct-v3.Q6_K.gguf) | Q6_K | 6.14GB |
| [llama-3-chinese-8b-instruct-v3.Q8_0.gguf](https://huggingface.co/RichardErkhov/hfl_-_llama-3-chinese-8b-instruct-v3-gguf/blob/main/llama-3-chinese-8b-instruct-v3.Q8_0.gguf) | Q8_0 | 7.95GB |
Original model description:
---
license: apache-2.0
language:
- zh
- en
base_model: [hfl/Llama-3-Chinese-8B-Instruct, hfl/Llama-3-Chinese-8B-Instruct-v2, meta-llama/Meta-Llama-3-8B-Instruct]
---
# Llama-3-Chinese-8B-Instruct-v3
<p align="center">
<a href="https://github.com/ymcui/Chinese-LLaMA-Alpaca-3"><img src="https://ymcui.com/images/chinese-llama-alpaca-3-banner.png" width="600"/></a>
</p>
[**[👉👉👉 Chat with Llama-3-Chinese-8B-Instruct-v3 @ HF Space]**](https://huggingface.co/spaces/hfl-rc/llama-3-chinese-8b-instruct-demo)
This repository contains **Llama-3-Chinese-8B-Instruct-v3**, which is further finetuned based on a mix of the following models:
- [hfl/Llama-3-Chinese-8B-Instruct](https://huggingface.co/hfl/llama-3-chinese-8b-instruct)
- [hfl/Llama-3-Chinese-8B-Instruct-v2](https://huggingface.co/hfl/llama-3-chinese-8b-instruct-v2)
- [meta-llama/Meta-Llama-3-8B-Instruct](https://huggingface.co/meta-llama/Meta-Llama-3-8B-Instruct)
**Note: This is an instruction (chat) model, which can be used for conversation, QA, etc.**
Further details (performance, usage, etc.) should refer to GitHub project page: https://github.com/ymcui/Chinese-LLaMA-Alpaca-3
## Others
- For GGUF model (llama.cpp compatible), please see: https://huggingface.co/hfl/llama-3-chinese-8b-instruct-v3-gguf
- If you have questions/issues regarding this model, please submit an issue through https://github.com/ymcui/Chinese-LLaMA-Alpaca-3
|
mradermacher/Llama-3-Refueled-i1-GGUF | mradermacher | 2024-06-28T12:25:22Z | 13,926 | 0 | transformers | [
"transformers",
"gguf",
"data labeling",
"en",
"base_model:refuelai/Llama-3-Refueled",
"license:cc-by-nc-4.0",
"endpoints_compatible",
"region:us"
] | null | 2024-06-28T02:37:53Z | ---
base_model: refuelai/Llama-3-Refueled
language:
- en
library_name: transformers
license: cc-by-nc-4.0
quantized_by: mradermacher
tags:
- data labeling
---
## About
<!-- ### quantize_version: 2 -->
<!-- ### output_tensor_quantised: 1 -->
<!-- ### convert_type: hf -->
<!-- ### vocab_type: -->
<!-- ### tags: nicoboss -->
weighted/imatrix quants of https://huggingface.co/refuelai/Llama-3-Refueled
<!-- provided-files -->
static quants are available at https://huggingface.co/mradermacher/Llama-3-Refueled-GGUF
## Usage
If you are unsure how to use GGUF files, refer to one of [TheBloke's
READMEs](https://huggingface.co/TheBloke/KafkaLM-70B-German-V0.1-GGUF) for
more details, including on how to concatenate multi-part files.
## Provided Quants
(sorted by size, not necessarily quality. IQ-quants are often preferable over similar sized non-IQ quants)
| Link | Type | Size/GB | Notes |
|:-----|:-----|--------:|:------|
| [GGUF](https://huggingface.co/mradermacher/Llama-3-Refueled-i1-GGUF/resolve/main/Llama-3-Refueled.i1-IQ1_S.gguf) | i1-IQ1_S | 2.1 | for the desperate |
| [GGUF](https://huggingface.co/mradermacher/Llama-3-Refueled-i1-GGUF/resolve/main/Llama-3-Refueled.i1-IQ1_M.gguf) | i1-IQ1_M | 2.3 | mostly desperate |
| [GGUF](https://huggingface.co/mradermacher/Llama-3-Refueled-i1-GGUF/resolve/main/Llama-3-Refueled.i1-IQ2_XXS.gguf) | i1-IQ2_XXS | 2.5 | |
| [GGUF](https://huggingface.co/mradermacher/Llama-3-Refueled-i1-GGUF/resolve/main/Llama-3-Refueled.i1-IQ2_XS.gguf) | i1-IQ2_XS | 2.7 | |
| [GGUF](https://huggingface.co/mradermacher/Llama-3-Refueled-i1-GGUF/resolve/main/Llama-3-Refueled.i1-IQ2_S.gguf) | i1-IQ2_S | 2.9 | |
| [GGUF](https://huggingface.co/mradermacher/Llama-3-Refueled-i1-GGUF/resolve/main/Llama-3-Refueled.i1-IQ2_M.gguf) | i1-IQ2_M | 3.0 | |
| [GGUF](https://huggingface.co/mradermacher/Llama-3-Refueled-i1-GGUF/resolve/main/Llama-3-Refueled.i1-Q2_K.gguf) | i1-Q2_K | 3.3 | IQ3_XXS probably better |
| [GGUF](https://huggingface.co/mradermacher/Llama-3-Refueled-i1-GGUF/resolve/main/Llama-3-Refueled.i1-IQ3_XXS.gguf) | i1-IQ3_XXS | 3.4 | lower quality |
| [GGUF](https://huggingface.co/mradermacher/Llama-3-Refueled-i1-GGUF/resolve/main/Llama-3-Refueled.i1-IQ3_XS.gguf) | i1-IQ3_XS | 3.6 | |
| [GGUF](https://huggingface.co/mradermacher/Llama-3-Refueled-i1-GGUF/resolve/main/Llama-3-Refueled.i1-Q3_K_S.gguf) | i1-Q3_K_S | 3.8 | IQ3_XS probably better |
| [GGUF](https://huggingface.co/mradermacher/Llama-3-Refueled-i1-GGUF/resolve/main/Llama-3-Refueled.i1-IQ3_S.gguf) | i1-IQ3_S | 3.8 | beats Q3_K* |
| [GGUF](https://huggingface.co/mradermacher/Llama-3-Refueled-i1-GGUF/resolve/main/Llama-3-Refueled.i1-IQ3_M.gguf) | i1-IQ3_M | 3.9 | |
| [GGUF](https://huggingface.co/mradermacher/Llama-3-Refueled-i1-GGUF/resolve/main/Llama-3-Refueled.i1-Q3_K_M.gguf) | i1-Q3_K_M | 4.1 | IQ3_S probably better |
| [GGUF](https://huggingface.co/mradermacher/Llama-3-Refueled-i1-GGUF/resolve/main/Llama-3-Refueled.i1-Q3_K_L.gguf) | i1-Q3_K_L | 4.4 | IQ3_M probably better |
| [GGUF](https://huggingface.co/mradermacher/Llama-3-Refueled-i1-GGUF/resolve/main/Llama-3-Refueled.i1-IQ4_XS.gguf) | i1-IQ4_XS | 4.5 | |
| [GGUF](https://huggingface.co/mradermacher/Llama-3-Refueled-i1-GGUF/resolve/main/Llama-3-Refueled.i1-Q4_0.gguf) | i1-Q4_0 | 4.8 | fast, low quality |
| [GGUF](https://huggingface.co/mradermacher/Llama-3-Refueled-i1-GGUF/resolve/main/Llama-3-Refueled.i1-Q4_K_S.gguf) | i1-Q4_K_S | 4.8 | optimal size/speed/quality |
| [GGUF](https://huggingface.co/mradermacher/Llama-3-Refueled-i1-GGUF/resolve/main/Llama-3-Refueled.i1-Q4_K_M.gguf) | i1-Q4_K_M | 5.0 | fast, recommended |
| [GGUF](https://huggingface.co/mradermacher/Llama-3-Refueled-i1-GGUF/resolve/main/Llama-3-Refueled.i1-Q5_K_S.gguf) | i1-Q5_K_S | 5.7 | |
| [GGUF](https://huggingface.co/mradermacher/Llama-3-Refueled-i1-GGUF/resolve/main/Llama-3-Refueled.i1-Q5_K_M.gguf) | i1-Q5_K_M | 5.8 | |
| [GGUF](https://huggingface.co/mradermacher/Llama-3-Refueled-i1-GGUF/resolve/main/Llama-3-Refueled.i1-Q6_K.gguf) | i1-Q6_K | 6.7 | practically like static Q6_K |
Here is a handy graph by ikawrakow comparing some lower-quality quant
types (lower is better):

And here are Artefact2's thoughts on the matter:
https://gist.github.com/Artefact2/b5f810600771265fc1e39442288e8ec9
## FAQ / Model Request
See https://huggingface.co/mradermacher/model_requests for some answers to
questions you might have and/or if you want some other model quantized.
## Thanks
I thank my company, [nethype GmbH](https://www.nethype.de/), for letting
me use its servers and providing upgrades to my workstation to enable
this work in my free time. Additional thanks to [@nicoboss](https://huggingface.co/nicoboss) for giving me access to his hardware for calculating the imatrix for these quants.
<!-- end -->
|
Qdrant/Splade_PP_en_v1 | Qdrant | 2024-03-21T01:14:54Z | 13,920 | 3 | transformers | [
"transformers",
"onnx",
"bert",
"fill-mask",
"license:apache-2.0",
"autotrain_compatible",
"endpoints_compatible",
"region:us"
] | fill-mask | 2024-03-13T03:50:07Z | ---
license: apache-2.0
---
This is an ONNX Export of [prithivida/Splade_PP_en_v1](https://huggingface.co/prithivida/Splade_PP_en_v1) |
sshleifer/tiny-dbmdz-bert-large-cased-finetuned-conll03-english | sshleifer | 2021-05-20T07:12:23Z | 13,916 | 0 | transformers | [
"transformers",
"pytorch",
"tf",
"jax",
"bert",
"token-classification",
"autotrain_compatible",
"endpoints_compatible",
"region:us"
] | token-classification | 2022-03-02T23:29:05Z | Entry not found |
RichardErkhov/NovoCode_-_Novocode7b-v2-gguf | RichardErkhov | 2024-06-25T17:52:41Z | 13,904 | 0 | null | [
"gguf",
"region:us"
] | null | 2024-06-25T13:57:48Z | Quantization made by Richard Erkhov.
[Github](https://github.com/RichardErkhov)
[Discord](https://discord.gg/pvy7H8DZMG)
[Request more models](https://github.com/RichardErkhov/quant_request)
Novocode7b-v2 - GGUF
- Model creator: https://huggingface.co/NovoCode/
- Original model: https://huggingface.co/NovoCode/Novocode7b-v2/
| Name | Quant method | Size |
| ---- | ---- | ---- |
| [Novocode7b-v2.Q2_K.gguf](https://huggingface.co/RichardErkhov/NovoCode_-_Novocode7b-v2-gguf/blob/main/Novocode7b-v2.Q2_K.gguf) | Q2_K | 2.53GB |
| [Novocode7b-v2.IQ3_XS.gguf](https://huggingface.co/RichardErkhov/NovoCode_-_Novocode7b-v2-gguf/blob/main/Novocode7b-v2.IQ3_XS.gguf) | IQ3_XS | 2.81GB |
| [Novocode7b-v2.IQ3_S.gguf](https://huggingface.co/RichardErkhov/NovoCode_-_Novocode7b-v2-gguf/blob/main/Novocode7b-v2.IQ3_S.gguf) | IQ3_S | 2.96GB |
| [Novocode7b-v2.Q3_K_S.gguf](https://huggingface.co/RichardErkhov/NovoCode_-_Novocode7b-v2-gguf/blob/main/Novocode7b-v2.Q3_K_S.gguf) | Q3_K_S | 2.95GB |
| [Novocode7b-v2.IQ3_M.gguf](https://huggingface.co/RichardErkhov/NovoCode_-_Novocode7b-v2-gguf/blob/main/Novocode7b-v2.IQ3_M.gguf) | IQ3_M | 3.06GB |
| [Novocode7b-v2.Q3_K.gguf](https://huggingface.co/RichardErkhov/NovoCode_-_Novocode7b-v2-gguf/blob/main/Novocode7b-v2.Q3_K.gguf) | Q3_K | 3.28GB |
| [Novocode7b-v2.Q3_K_M.gguf](https://huggingface.co/RichardErkhov/NovoCode_-_Novocode7b-v2-gguf/blob/main/Novocode7b-v2.Q3_K_M.gguf) | Q3_K_M | 3.28GB |
| [Novocode7b-v2.Q3_K_L.gguf](https://huggingface.co/RichardErkhov/NovoCode_-_Novocode7b-v2-gguf/blob/main/Novocode7b-v2.Q3_K_L.gguf) | Q3_K_L | 3.56GB |
| [Novocode7b-v2.IQ4_XS.gguf](https://huggingface.co/RichardErkhov/NovoCode_-_Novocode7b-v2-gguf/blob/main/Novocode7b-v2.IQ4_XS.gguf) | IQ4_XS | 3.67GB |
| [Novocode7b-v2.Q4_0.gguf](https://huggingface.co/RichardErkhov/NovoCode_-_Novocode7b-v2-gguf/blob/main/Novocode7b-v2.Q4_0.gguf) | Q4_0 | 3.83GB |
| [Novocode7b-v2.IQ4_NL.gguf](https://huggingface.co/RichardErkhov/NovoCode_-_Novocode7b-v2-gguf/blob/main/Novocode7b-v2.IQ4_NL.gguf) | IQ4_NL | 3.87GB |
| [Novocode7b-v2.Q4_K_S.gguf](https://huggingface.co/RichardErkhov/NovoCode_-_Novocode7b-v2-gguf/blob/main/Novocode7b-v2.Q4_K_S.gguf) | Q4_K_S | 3.86GB |
| [Novocode7b-v2.Q4_K.gguf](https://huggingface.co/RichardErkhov/NovoCode_-_Novocode7b-v2-gguf/blob/main/Novocode7b-v2.Q4_K.gguf) | Q4_K | 4.07GB |
| [Novocode7b-v2.Q4_K_M.gguf](https://huggingface.co/RichardErkhov/NovoCode_-_Novocode7b-v2-gguf/blob/main/Novocode7b-v2.Q4_K_M.gguf) | Q4_K_M | 4.07GB |
| [Novocode7b-v2.Q4_1.gguf](https://huggingface.co/RichardErkhov/NovoCode_-_Novocode7b-v2-gguf/blob/main/Novocode7b-v2.Q4_1.gguf) | Q4_1 | 4.24GB |
| [Novocode7b-v2.Q5_0.gguf](https://huggingface.co/RichardErkhov/NovoCode_-_Novocode7b-v2-gguf/blob/main/Novocode7b-v2.Q5_0.gguf) | Q5_0 | 4.65GB |
| [Novocode7b-v2.Q5_K_S.gguf](https://huggingface.co/RichardErkhov/NovoCode_-_Novocode7b-v2-gguf/blob/main/Novocode7b-v2.Q5_K_S.gguf) | Q5_K_S | 4.65GB |
| [Novocode7b-v2.Q5_K.gguf](https://huggingface.co/RichardErkhov/NovoCode_-_Novocode7b-v2-gguf/blob/main/Novocode7b-v2.Q5_K.gguf) | Q5_K | 4.78GB |
| [Novocode7b-v2.Q5_K_M.gguf](https://huggingface.co/RichardErkhov/NovoCode_-_Novocode7b-v2-gguf/blob/main/Novocode7b-v2.Q5_K_M.gguf) | Q5_K_M | 4.78GB |
| [Novocode7b-v2.Q5_1.gguf](https://huggingface.co/RichardErkhov/NovoCode_-_Novocode7b-v2-gguf/blob/main/Novocode7b-v2.Q5_1.gguf) | Q5_1 | 5.07GB |
| [Novocode7b-v2.Q6_K.gguf](https://huggingface.co/RichardErkhov/NovoCode_-_Novocode7b-v2-gguf/blob/main/Novocode7b-v2.Q6_K.gguf) | Q6_K | 5.53GB |
| [Novocode7b-v2.Q8_0.gguf](https://huggingface.co/RichardErkhov/NovoCode_-_Novocode7b-v2-gguf/blob/main/Novocode7b-v2.Q8_0.gguf) | Q8_0 | 7.17GB |
Original model description:
---
license: apache-2.0
tags:
- generated_from_trainer
model-index:
- name: out
results: []
---
<!-- This model card has been generated automatically according to the information the Trainer had access to. You
should probably proofread and complete it, then remove this comment. -->
[<img src="https://raw.githubusercontent.com/OpenAccess-AI-Collective/axolotl/main/image/axolotl-badge-web.png" alt="Built with Axolotl" width="200" height="32"/>](https://github.com/OpenAccess-AI-Collective/axolotl)
<details><summary>See axolotl config</summary>
axolotl version: `0.3.0`
```yaml
base_model: out/
model_type: MistralForCausalLM
tokenizer_type: LlamaTokenizer
is_mistral_derived_model: true
load_in_8bit: false
load_in_4bit: false
strict: false
datasets:
- path: cognitivecomputations/leet10k-alpaca
type: alpaca
dataset_prepared_path:
val_set_size: 0.05
output_dir: ./out
sequence_len: 8192
sample_packing: true
pad_to_sequence_len: true
eval_sample_packing: false
wandb_project:
wandb_entity:
wandb_watch:
wandb_name:
wandb_log_model:
gradient_accumulation_steps: 4
micro_batch_size: 2
num_epochs: 1
optimizer: adamw_bnb_8bit
lr_scheduler: cosine
learning_rate: 0.000005
train_on_inputs: false
group_by_length: false
bf16: true
fp16: false
tf32: false
gradient_checkpointing: true
early_stopping_patience:
resume_from_checkpoint:
local_rank:
logging_steps: 1
xformers_attention:
flash_attention: true
warmup_steps: 10
evals_per_epoch: 4
eval_table_size:
eval_table_max_new_tokens: 128
saves_per_epoch: 1
debug:
deepspeed:
weight_decay: 0.0
fsdp:
fsdp_config:
special_tokens:
bos_token: "<s>"
eos_token: "</s>"
unk_token: "<unk>"
```
</details><br>
# out
This model was trained from scratch on the /leet10k-alpaca dataset.
It achieves the following results on the evaluation set:
- Loss: 0.5907
## Model description
More information needed
## Intended uses & limitations
More information needed
## Training and evaluation data
More information needed
## Training procedure
### Training hyperparameters
The following hyperparameters were used during training:
- learning_rate: 5e-06
- train_batch_size: 2
- eval_batch_size: 2
- seed: 42
- gradient_accumulation_steps: 4
- total_train_batch_size: 8
- optimizer: Adam with betas=(0.9,0.999) and epsilon=1e-08
- lr_scheduler_type: cosine
- lr_scheduler_warmup_steps: 10
- num_epochs: 1
### Training results
| Training Loss | Epoch | Step | Validation Loss |
|:-------------:|:-----:|:----:|:---------------:|
| 0.7842 | 0.01 | 1 | 0.8053 |
| 0.5057 | 0.26 | 35 | 0.5694 |
| 0.3987 | 0.51 | 70 | 0.5752 |
| 0.2964 | 0.77 | 105 | 0.5907 |
### Framework versions
- Transformers 4.37.0.dev0
- Pytorch 2.0.1+cu117
- Datasets 2.16.1
- Tokenizers 0.15.0
# [Open LLM Leaderboard Evaluation Results](https://huggingface.co/spaces/HuggingFaceH4/open_llm_leaderboard)
Detailed results can be found [here](https://huggingface.co/datasets/open-llm-leaderboard/details_NovoCode__Novocode7b-v2)
| Metric |Value|
|---------------------------------|----:|
|Avg. |56.57|
|AI2 Reasoning Challenge (25-Shot)|61.01|
|HellaSwag (10-Shot) |84.12|
|MMLU (5-Shot) |64.05|
|TruthfulQA (0-shot) |42.21|
|Winogrande (5-shot) |79.87|
|GSM8k (5-shot) | 8.19|
|
RichardErkhov/raincandy-u_-_Llama-3-5B-Sheard-gguf | RichardErkhov | 2024-06-30T04:14:46Z | 13,904 | 0 | null | [
"gguf",
"region:us"
] | null | 2024-06-30T02:06:32Z | Quantization made by Richard Erkhov.
[Github](https://github.com/RichardErkhov)
[Discord](https://discord.gg/pvy7H8DZMG)
[Request more models](https://github.com/RichardErkhov/quant_request)
Llama-3-5B-Sheard - GGUF
- Model creator: https://huggingface.co/raincandy-u/
- Original model: https://huggingface.co/raincandy-u/Llama-3-5B-Sheard/
| Name | Quant method | Size |
| ---- | ---- | ---- |
| [Llama-3-5B-Sheard.Q2_K.gguf](https://huggingface.co/RichardErkhov/raincandy-u_-_Llama-3-5B-Sheard-gguf/blob/main/Llama-3-5B-Sheard.Q2_K.gguf) | Q2_K | 2.21GB |
| [Llama-3-5B-Sheard.IQ3_XS.gguf](https://huggingface.co/RichardErkhov/raincandy-u_-_Llama-3-5B-Sheard-gguf/blob/main/Llama-3-5B-Sheard.IQ3_XS.gguf) | IQ3_XS | 2.44GB |
| [Llama-3-5B-Sheard.IQ3_S.gguf](https://huggingface.co/RichardErkhov/raincandy-u_-_Llama-3-5B-Sheard-gguf/blob/main/Llama-3-5B-Sheard.IQ3_S.gguf) | IQ3_S | 2.55GB |
| [Llama-3-5B-Sheard.Q3_K_S.gguf](https://huggingface.co/RichardErkhov/raincandy-u_-_Llama-3-5B-Sheard-gguf/blob/main/Llama-3-5B-Sheard.Q3_K_S.gguf) | Q3_K_S | 2.54GB |
| [Llama-3-5B-Sheard.IQ3_M.gguf](https://huggingface.co/RichardErkhov/raincandy-u_-_Llama-3-5B-Sheard-gguf/blob/main/Llama-3-5B-Sheard.IQ3_M.gguf) | IQ3_M | 2.61GB |
| [Llama-3-5B-Sheard.Q3_K.gguf](https://huggingface.co/RichardErkhov/raincandy-u_-_Llama-3-5B-Sheard-gguf/blob/main/Llama-3-5B-Sheard.Q3_K.gguf) | Q3_K | 2.76GB |
| [Llama-3-5B-Sheard.Q3_K_M.gguf](https://huggingface.co/RichardErkhov/raincandy-u_-_Llama-3-5B-Sheard-gguf/blob/main/Llama-3-5B-Sheard.Q3_K_M.gguf) | Q3_K_M | 2.76GB |
| [Llama-3-5B-Sheard.Q3_K_L.gguf](https://huggingface.co/RichardErkhov/raincandy-u_-_Llama-3-5B-Sheard-gguf/blob/main/Llama-3-5B-Sheard.Q3_K_L.gguf) | Q3_K_L | 2.96GB |
| [Llama-3-5B-Sheard.IQ4_XS.gguf](https://huggingface.co/RichardErkhov/raincandy-u_-_Llama-3-5B-Sheard-gguf/blob/main/Llama-3-5B-Sheard.IQ4_XS.gguf) | IQ4_XS | 3.07GB |
| [Llama-3-5B-Sheard.Q4_0.gguf](https://huggingface.co/RichardErkhov/raincandy-u_-_Llama-3-5B-Sheard-gguf/blob/main/Llama-3-5B-Sheard.Q4_0.gguf) | Q4_0 | 3.2GB |
| [Llama-3-5B-Sheard.IQ4_NL.gguf](https://huggingface.co/RichardErkhov/raincandy-u_-_Llama-3-5B-Sheard-gguf/blob/main/Llama-3-5B-Sheard.IQ4_NL.gguf) | IQ4_NL | 3.22GB |
| [Llama-3-5B-Sheard.Q4_K_S.gguf](https://huggingface.co/RichardErkhov/raincandy-u_-_Llama-3-5B-Sheard-gguf/blob/main/Llama-3-5B-Sheard.Q4_K_S.gguf) | Q4_K_S | 3.21GB |
| [Llama-3-5B-Sheard.Q4_K.gguf](https://huggingface.co/RichardErkhov/raincandy-u_-_Llama-3-5B-Sheard-gguf/blob/main/Llama-3-5B-Sheard.Q4_K.gguf) | Q4_K | 3.35GB |
| [Llama-3-5B-Sheard.Q4_K_M.gguf](https://huggingface.co/RichardErkhov/raincandy-u_-_Llama-3-5B-Sheard-gguf/blob/main/Llama-3-5B-Sheard.Q4_K_M.gguf) | Q4_K_M | 3.35GB |
| [Llama-3-5B-Sheard.Q4_1.gguf](https://huggingface.co/RichardErkhov/raincandy-u_-_Llama-3-5B-Sheard-gguf/blob/main/Llama-3-5B-Sheard.Q4_1.gguf) | Q4_1 | 3.51GB |
| [Llama-3-5B-Sheard.Q5_0.gguf](https://huggingface.co/RichardErkhov/raincandy-u_-_Llama-3-5B-Sheard-gguf/blob/main/Llama-3-5B-Sheard.Q5_0.gguf) | Q5_0 | 3.82GB |
| [Llama-3-5B-Sheard.Q5_K_S.gguf](https://huggingface.co/RichardErkhov/raincandy-u_-_Llama-3-5B-Sheard-gguf/blob/main/Llama-3-5B-Sheard.Q5_K_S.gguf) | Q5_K_S | 3.82GB |
| [Llama-3-5B-Sheard.Q5_K.gguf](https://huggingface.co/RichardErkhov/raincandy-u_-_Llama-3-5B-Sheard-gguf/blob/main/Llama-3-5B-Sheard.Q5_K.gguf) | Q5_K | 3.9GB |
| [Llama-3-5B-Sheard.Q5_K_M.gguf](https://huggingface.co/RichardErkhov/raincandy-u_-_Llama-3-5B-Sheard-gguf/blob/main/Llama-3-5B-Sheard.Q5_K_M.gguf) | Q5_K_M | 3.9GB |
| [Llama-3-5B-Sheard.Q5_1.gguf](https://huggingface.co/RichardErkhov/raincandy-u_-_Llama-3-5B-Sheard-gguf/blob/main/Llama-3-5B-Sheard.Q5_1.gguf) | Q5_1 | 4.13GB |
| [Llama-3-5B-Sheard.Q6_K.gguf](https://huggingface.co/RichardErkhov/raincandy-u_-_Llama-3-5B-Sheard-gguf/blob/main/Llama-3-5B-Sheard.Q6_K.gguf) | Q6_K | 4.48GB |
| [Llama-3-5B-Sheard.Q8_0.gguf](https://huggingface.co/RichardErkhov/raincandy-u_-_Llama-3-5B-Sheard-gguf/blob/main/Llama-3-5B-Sheard.Q8_0.gguf) | Q8_0 | 5.8GB |
Original model description:
---
language:
- en
pipeline_tag: text-generation
tags:
- facebook
- meta
- pytorch
- llama
- llama-3
license: other
license_name: llama3
license_link: LICENSE
datasets:
- JeanKaddour/minipile
- raincandy-u/SlimOrca-Llama-3-Preference-DPO-Pairs
---

# Llama-3-5B-Sheard
Pruned version of Llama-3-8b.
Tool used: [PrunMe](https://github.com/arcee-ai/PruneMe), Mergekit.
**Meta Llama 3 is licensed under the Meta Llama 3 Community License, Copyright © Meta Platforms, Inc. All Rights Reserved.**
## Training
After sliced by mergekit, the model is continue-pretrained on minipile for 1 epoch and ~100k samples. Then we trained it using ORPO on Llama-3-70b generated DPO pairs.
## Disclaimer
This model is for testing purposes only, and when the system prompt is not empty, the output may repeat and not stop!
<h1><a href="https://discord.gg/Dw8SkfBm">Join our discord</a></h1>
|
mradermacher/KeplerRP-7B-128K-0.2-i1-GGUF | mradermacher | 2024-06-27T07:02:35Z | 13,901 | 0 | transformers | [
"transformers",
"gguf",
"mergekit",
"merge",
"en",
"base_model:Ppoyaa/KeplerRP-7B-128K-0.2",
"endpoints_compatible",
"region:us"
] | null | 2024-06-27T04:59:25Z | ---
base_model: Ppoyaa/KeplerRP-7B-128K-0.2
language:
- en
library_name: transformers
quantized_by: mradermacher
tags:
- mergekit
- merge
---
## About
<!-- ### quantize_version: 2 -->
<!-- ### output_tensor_quantised: 1 -->
<!-- ### convert_type: hf -->
<!-- ### vocab_type: -->
<!-- ### tags: nicoboss -->
weighted/imatrix quants of https://huggingface.co/Ppoyaa/KeplerRP-7B-128K-0.2
<!-- provided-files -->
static quants are available at https://huggingface.co/mradermacher/KeplerRP-7B-128K-0.2-GGUF
## Usage
If you are unsure how to use GGUF files, refer to one of [TheBloke's
READMEs](https://huggingface.co/TheBloke/KafkaLM-70B-German-V0.1-GGUF) for
more details, including on how to concatenate multi-part files.
## Provided Quants
(sorted by size, not necessarily quality. IQ-quants are often preferable over similar sized non-IQ quants)
| Link | Type | Size/GB | Notes |
|:-----|:-----|--------:|:------|
| [GGUF](https://huggingface.co/mradermacher/KeplerRP-7B-128K-0.2-i1-GGUF/resolve/main/KeplerRP-7B-128K-0.2.i1-IQ1_S.gguf) | i1-IQ1_S | 1.7 | for the desperate |
| [GGUF](https://huggingface.co/mradermacher/KeplerRP-7B-128K-0.2-i1-GGUF/resolve/main/KeplerRP-7B-128K-0.2.i1-IQ1_M.gguf) | i1-IQ1_M | 1.9 | mostly desperate |
| [GGUF](https://huggingface.co/mradermacher/KeplerRP-7B-128K-0.2-i1-GGUF/resolve/main/KeplerRP-7B-128K-0.2.i1-IQ2_XXS.gguf) | i1-IQ2_XXS | 2.1 | |
| [GGUF](https://huggingface.co/mradermacher/KeplerRP-7B-128K-0.2-i1-GGUF/resolve/main/KeplerRP-7B-128K-0.2.i1-IQ2_XS.gguf) | i1-IQ2_XS | 2.3 | |
| [GGUF](https://huggingface.co/mradermacher/KeplerRP-7B-128K-0.2-i1-GGUF/resolve/main/KeplerRP-7B-128K-0.2.i1-IQ2_S.gguf) | i1-IQ2_S | 2.4 | |
| [GGUF](https://huggingface.co/mradermacher/KeplerRP-7B-128K-0.2-i1-GGUF/resolve/main/KeplerRP-7B-128K-0.2.i1-IQ2_M.gguf) | i1-IQ2_M | 2.6 | |
| [GGUF](https://huggingface.co/mradermacher/KeplerRP-7B-128K-0.2-i1-GGUF/resolve/main/KeplerRP-7B-128K-0.2.i1-Q2_K.gguf) | i1-Q2_K | 2.8 | IQ3_XXS probably better |
| [GGUF](https://huggingface.co/mradermacher/KeplerRP-7B-128K-0.2-i1-GGUF/resolve/main/KeplerRP-7B-128K-0.2.i1-IQ3_XXS.gguf) | i1-IQ3_XXS | 2.9 | lower quality |
| [GGUF](https://huggingface.co/mradermacher/KeplerRP-7B-128K-0.2-i1-GGUF/resolve/main/KeplerRP-7B-128K-0.2.i1-IQ3_XS.gguf) | i1-IQ3_XS | 3.1 | |
| [GGUF](https://huggingface.co/mradermacher/KeplerRP-7B-128K-0.2-i1-GGUF/resolve/main/KeplerRP-7B-128K-0.2.i1-Q3_K_S.gguf) | i1-Q3_K_S | 3.3 | IQ3_XS probably better |
| [GGUF](https://huggingface.co/mradermacher/KeplerRP-7B-128K-0.2-i1-GGUF/resolve/main/KeplerRP-7B-128K-0.2.i1-IQ3_S.gguf) | i1-IQ3_S | 3.3 | beats Q3_K* |
| [GGUF](https://huggingface.co/mradermacher/KeplerRP-7B-128K-0.2-i1-GGUF/resolve/main/KeplerRP-7B-128K-0.2.i1-IQ3_M.gguf) | i1-IQ3_M | 3.4 | |
| [GGUF](https://huggingface.co/mradermacher/KeplerRP-7B-128K-0.2-i1-GGUF/resolve/main/KeplerRP-7B-128K-0.2.i1-Q3_K_M.gguf) | i1-Q3_K_M | 3.6 | IQ3_S probably better |
| [GGUF](https://huggingface.co/mradermacher/KeplerRP-7B-128K-0.2-i1-GGUF/resolve/main/KeplerRP-7B-128K-0.2.i1-Q3_K_L.gguf) | i1-Q3_K_L | 3.9 | IQ3_M probably better |
| [GGUF](https://huggingface.co/mradermacher/KeplerRP-7B-128K-0.2-i1-GGUF/resolve/main/KeplerRP-7B-128K-0.2.i1-IQ4_XS.gguf) | i1-IQ4_XS | 4.0 | |
| [GGUF](https://huggingface.co/mradermacher/KeplerRP-7B-128K-0.2-i1-GGUF/resolve/main/KeplerRP-7B-128K-0.2.i1-Q4_0.gguf) | i1-Q4_0 | 4.2 | fast, low quality |
| [GGUF](https://huggingface.co/mradermacher/KeplerRP-7B-128K-0.2-i1-GGUF/resolve/main/KeplerRP-7B-128K-0.2.i1-Q4_K_S.gguf) | i1-Q4_K_S | 4.2 | optimal size/speed/quality |
| [GGUF](https://huggingface.co/mradermacher/KeplerRP-7B-128K-0.2-i1-GGUF/resolve/main/KeplerRP-7B-128K-0.2.i1-Q4_K_M.gguf) | i1-Q4_K_M | 4.5 | fast, recommended |
| [GGUF](https://huggingface.co/mradermacher/KeplerRP-7B-128K-0.2-i1-GGUF/resolve/main/KeplerRP-7B-128K-0.2.i1-Q5_K_S.gguf) | i1-Q5_K_S | 5.1 | |
| [GGUF](https://huggingface.co/mradermacher/KeplerRP-7B-128K-0.2-i1-GGUF/resolve/main/KeplerRP-7B-128K-0.2.i1-Q5_K_M.gguf) | i1-Q5_K_M | 5.2 | |
| [GGUF](https://huggingface.co/mradermacher/KeplerRP-7B-128K-0.2-i1-GGUF/resolve/main/KeplerRP-7B-128K-0.2.i1-Q6_K.gguf) | i1-Q6_K | 6.0 | practically like static Q6_K |
Here is a handy graph by ikawrakow comparing some lower-quality quant
types (lower is better):

And here are Artefact2's thoughts on the matter:
https://gist.github.com/Artefact2/b5f810600771265fc1e39442288e8ec9
## FAQ / Model Request
See https://huggingface.co/mradermacher/model_requests for some answers to
questions you might have and/or if you want some other model quantized.
## Thanks
I thank my company, [nethype GmbH](https://www.nethype.de/), for letting
me use its servers and providing upgrades to my workstation to enable
this work in my free time. Additional thanks to [@nicoboss](https://huggingface.co/nicoboss) for giving me access to his hardware for calculating the imatrix for these quants.
<!-- end -->
|
albert/albert-xxlarge-v2 | albert | 2024-02-19T11:02:09Z | 13,899 | 19 | transformers | [
"transformers",
"pytorch",
"tf",
"rust",
"safetensors",
"albert",
"fill-mask",
"exbert",
"en",
"dataset:bookcorpus",
"dataset:wikipedia",
"arxiv:1909.11942",
"license:apache-2.0",
"autotrain_compatible",
"endpoints_compatible",
"region:us"
] | fill-mask | 2022-03-02T23:29:04Z | ---
tags:
- exbert
language: en
license: apache-2.0
datasets:
- bookcorpus
- wikipedia
---
# ALBERT XXLarge v2
Pretrained model on English language using a masked language modeling (MLM) objective. It was introduced in
[this paper](https://arxiv.org/abs/1909.11942) and first released in
[this repository](https://github.com/google-research/albert). This model, as all ALBERT models, is uncased: it does not make a difference
between english and English.
Disclaimer: The team releasing ALBERT did not write a model card for this model so this model card has been written by
the Hugging Face team.
## Model description
ALBERT is a transformers model pretrained on a large corpus of English data in a self-supervised fashion. This means it
was pretrained on the raw texts only, with no humans labelling them in any way (which is why it can use lots of
publicly available data) with an automatic process to generate inputs and labels from those texts. More precisely, it
was pretrained with two objectives:
- Masked language modeling (MLM): taking a sentence, the model randomly masks 15% of the words in the input then run
the entire masked sentence through the model and has to predict the masked words. This is different from traditional
recurrent neural networks (RNNs) that usually see the words one after the other, or from autoregressive models like
GPT which internally mask the future tokens. It allows the model to learn a bidirectional representation of the
sentence.
- Sentence Ordering Prediction (SOP): ALBERT uses a pretraining loss based on predicting the ordering of two consecutive segments of text.
This way, the model learns an inner representation of the English language that can then be used to extract features
useful for downstream tasks: if you have a dataset of labeled sentences for instance, you can train a standard
classifier using the features produced by the ALBERT model as inputs.
ALBERT is particular in that it shares its layers across its Transformer. Therefore, all layers have the same weights. Using repeating layers results in a small memory footprint, however, the computational cost remains similar to a BERT-like architecture with the same number of hidden layers as it has to iterate through the same number of (repeating) layers.
This is the second version of the xxlarge model. Version 2 is different from version 1 due to different dropout rates, additional training data, and longer training. It has better results in nearly all downstream tasks.
This model has the following configuration:
- 12 repeating layers
- 128 embedding dimension
- 4096 hidden dimension
- 64 attention heads
- 223M parameters
## Intended uses & limitations
You can use the raw model for either masked language modeling or next sentence prediction, but it's mostly intended to
be fine-tuned on a downstream task. See the [model hub](https://huggingface.co/models?filter=albert) to look for
fine-tuned versions on a task that interests you.
Note that this model is primarily aimed at being fine-tuned on tasks that use the whole sentence (potentially masked)
to make decisions, such as sequence classification, token classification or question answering. For tasks such as text
generation you should look at model like GPT2.
### How to use
You can use this model directly with a pipeline for masked language modeling:
```python
>>> from transformers import pipeline
>>> unmasker = pipeline('fill-mask', model='albert-xxlarge-v2')
>>> unmasker("Hello I'm a [MASK] model.")
[
{
"sequence":"[CLS] hello i'm a modeling model.[SEP]",
"score":0.05816134437918663,
"token":12807,
"token_str":"â–modeling"
},
{
"sequence":"[CLS] hello i'm a modelling model.[SEP]",
"score":0.03748830780386925,
"token":23089,
"token_str":"â–modelling"
},
{
"sequence":"[CLS] hello i'm a model model.[SEP]",
"score":0.033725276589393616,
"token":1061,
"token_str":"â–model"
},
{
"sequence":"[CLS] hello i'm a runway model.[SEP]",
"score":0.017313428223133087,
"token":8014,
"token_str":"â–runway"
},
{
"sequence":"[CLS] hello i'm a lingerie model.[SEP]",
"score":0.014405295252799988,
"token":29104,
"token_str":"â–lingerie"
}
]
```
Here is how to use this model to get the features of a given text in PyTorch:
```python
from transformers import AlbertTokenizer, AlbertModel
tokenizer = AlbertTokenizer.from_pretrained('albert-xxlarge-v2')
model = AlbertModel.from_pretrained("albert-xxlarge-v2")
text = "Replace me by any text you'd like."
encoded_input = tokenizer(text, return_tensors='pt')
output = model(**encoded_input)
```
and in TensorFlow:
```python
from transformers import AlbertTokenizer, TFAlbertModel
tokenizer = AlbertTokenizer.from_pretrained('albert-xxlarge-v2')
model = TFAlbertModel.from_pretrained("albert-xxlarge-v2")
text = "Replace me by any text you'd like."
encoded_input = tokenizer(text, return_tensors='tf')
output = model(encoded_input)
```
### Limitations and bias
Even if the training data used for this model could be characterized as fairly neutral, this model can have biased
predictions:
```python
>>> from transformers import pipeline
>>> unmasker = pipeline('fill-mask', model='albert-xxlarge-v2')
>>> unmasker("The man worked as a [MASK].")
[
{
"sequence":"[CLS] the man worked as a chauffeur.[SEP]",
"score":0.029577180743217468,
"token":28744,
"token_str":"â–chauffeur"
},
{
"sequence":"[CLS] the man worked as a janitor.[SEP]",
"score":0.028865724802017212,
"token":29477,
"token_str":"â–janitor"
},
{
"sequence":"[CLS] the man worked as a shoemaker.[SEP]",
"score":0.02581118606030941,
"token":29024,
"token_str":"â–shoemaker"
},
{
"sequence":"[CLS] the man worked as a blacksmith.[SEP]",
"score":0.01849772222340107,
"token":21238,
"token_str":"â–blacksmith"
},
{
"sequence":"[CLS] the man worked as a lawyer.[SEP]",
"score":0.01820771023631096,
"token":3672,
"token_str":"â–lawyer"
}
]
>>> unmasker("The woman worked as a [MASK].")
[
{
"sequence":"[CLS] the woman worked as a receptionist.[SEP]",
"score":0.04604868218302727,
"token":25331,
"token_str":"â–receptionist"
},
{
"sequence":"[CLS] the woman worked as a janitor.[SEP]",
"score":0.028220869600772858,
"token":29477,
"token_str":"â–janitor"
},
{
"sequence":"[CLS] the woman worked as a paramedic.[SEP]",
"score":0.0261906236410141,
"token":23386,
"token_str":"â–paramedic"
},
{
"sequence":"[CLS] the woman worked as a chauffeur.[SEP]",
"score":0.024797942489385605,
"token":28744,
"token_str":"â–chauffeur"
},
{
"sequence":"[CLS] the woman worked as a waitress.[SEP]",
"score":0.024124596267938614,
"token":13678,
"token_str":"â–waitress"
}
]
```
This bias will also affect all fine-tuned versions of this model.
## Training data
The ALBERT model was pretrained on [BookCorpus](https://yknzhu.wixsite.com/mbweb), a dataset consisting of 11,038
unpublished books and [English Wikipedia](https://en.wikipedia.org/wiki/English_Wikipedia) (excluding lists, tables and
headers).
## Training procedure
### Preprocessing
The texts are lowercased and tokenized using SentencePiece and a vocabulary size of 30,000. The inputs of the model are
then of the form:
```
[CLS] Sentence A [SEP] Sentence B [SEP]
```
### Training
The ALBERT procedure follows the BERT setup.
The details of the masking procedure for each sentence are the following:
- 15% of the tokens are masked.
- In 80% of the cases, the masked tokens are replaced by `[MASK]`.
- In 10% of the cases, the masked tokens are replaced by a random token (different) from the one they replace.
- In the 10% remaining cases, the masked tokens are left as is.
## Evaluation results
When fine-tuned on downstream tasks, the ALBERT models achieve the following results:
| | Average | SQuAD1.1 | SQuAD2.0 | MNLI | SST-2 | RACE |
|----------------|----------|----------|----------|----------|----------|----------|
|V2 |
|ALBERT-base |82.3 |90.2/83.2 |82.1/79.3 |84.6 |92.9 |66.8 |
|ALBERT-large |85.7 |91.8/85.2 |84.9/81.8 |86.5 |94.9 |75.2 |
|ALBERT-xlarge |87.9 |92.9/86.4 |87.9/84.1 |87.9 |95.4 |80.7 |
|ALBERT-xxlarge |90.9 |94.6/89.1 |89.8/86.9 |90.6 |96.8 |86.8 |
|V1 |
|ALBERT-base |80.1 |89.3/82.3 | 80.0/77.1|81.6 |90.3 | 64.0 |
|ALBERT-large |82.4 |90.6/83.9 | 82.3/79.4|83.5 |91.7 | 68.5 |
|ALBERT-xlarge |85.5 |92.5/86.1 | 86.1/83.1|86.4 |92.4 | 74.8 |
|ALBERT-xxlarge |91.0 |94.8/89.3 | 90.2/87.4|90.8 |96.9 | 86.5 |
### BibTeX entry and citation info
```bibtex
@article{DBLP:journals/corr/abs-1909-11942,
author = {Zhenzhong Lan and
Mingda Chen and
Sebastian Goodman and
Kevin Gimpel and
Piyush Sharma and
Radu Soricut},
title = {{ALBERT:} {A} Lite {BERT} for Self-supervised Learning of Language
Representations},
journal = {CoRR},
volume = {abs/1909.11942},
year = {2019},
url = {http://arxiv.org/abs/1909.11942},
archivePrefix = {arXiv},
eprint = {1909.11942},
timestamp = {Fri, 27 Sep 2019 13:04:21 +0200},
biburl = {https://dblp.org/rec/journals/corr/abs-1909-11942.bib},
bibsource = {dblp computer science bibliography, https://dblp.org}
}
```
<a href="https://huggingface.co/exbert/?model=albert-xxlarge-v2">
<img width="300px" src="https://cdn-media.huggingface.co/exbert/button.png">
</a> |
mradermacher/Yi-6B-200K-i1-GGUF | mradermacher | 2024-06-26T23:40:32Z | 13,887 | 0 | transformers | [
"transformers",
"gguf",
"en",
"base_model:01-ai/Yi-6B-200K",
"license:apache-2.0",
"endpoints_compatible",
"region:us"
] | null | 2024-06-26T22:42:46Z | ---
base_model: 01-ai/Yi-6B-200K
language:
- en
library_name: transformers
license: apache-2.0
quantized_by: mradermacher
---
## About
<!-- ### quantize_version: 2 -->
<!-- ### output_tensor_quantised: 1 -->
<!-- ### convert_type: hf -->
<!-- ### vocab_type: -->
<!-- ### tags: nicoboss -->
weighted/imatrix quants of https://huggingface.co/01-ai/Yi-6B-200K
<!-- provided-files -->
static quants are available at https://huggingface.co/mradermacher/Yi-6B-200K-GGUF
## Usage
If you are unsure how to use GGUF files, refer to one of [TheBloke's
READMEs](https://huggingface.co/TheBloke/KafkaLM-70B-German-V0.1-GGUF) for
more details, including on how to concatenate multi-part files.
## Provided Quants
(sorted by size, not necessarily quality. IQ-quants are often preferable over similar sized non-IQ quants)
| Link | Type | Size/GB | Notes |
|:-----|:-----|--------:|:------|
| [GGUF](https://huggingface.co/mradermacher/Yi-6B-200K-i1-GGUF/resolve/main/Yi-6B-200K.i1-IQ1_S.gguf) | i1-IQ1_S | 1.5 | for the desperate |
| [GGUF](https://huggingface.co/mradermacher/Yi-6B-200K-i1-GGUF/resolve/main/Yi-6B-200K.i1-IQ1_M.gguf) | i1-IQ1_M | 1.6 | mostly desperate |
| [GGUF](https://huggingface.co/mradermacher/Yi-6B-200K-i1-GGUF/resolve/main/Yi-6B-200K.i1-IQ2_XXS.gguf) | i1-IQ2_XXS | 1.8 | |
| [GGUF](https://huggingface.co/mradermacher/Yi-6B-200K-i1-GGUF/resolve/main/Yi-6B-200K.i1-IQ2_XS.gguf) | i1-IQ2_XS | 2.0 | |
| [GGUF](https://huggingface.co/mradermacher/Yi-6B-200K-i1-GGUF/resolve/main/Yi-6B-200K.i1-IQ2_S.gguf) | i1-IQ2_S | 2.1 | |
| [GGUF](https://huggingface.co/mradermacher/Yi-6B-200K-i1-GGUF/resolve/main/Yi-6B-200K.i1-IQ2_M.gguf) | i1-IQ2_M | 2.3 | |
| [GGUF](https://huggingface.co/mradermacher/Yi-6B-200K-i1-GGUF/resolve/main/Yi-6B-200K.i1-Q2_K.gguf) | i1-Q2_K | 2.4 | IQ3_XXS probably better |
| [GGUF](https://huggingface.co/mradermacher/Yi-6B-200K-i1-GGUF/resolve/main/Yi-6B-200K.i1-IQ3_XXS.gguf) | i1-IQ3_XXS | 2.5 | lower quality |
| [GGUF](https://huggingface.co/mradermacher/Yi-6B-200K-i1-GGUF/resolve/main/Yi-6B-200K.i1-IQ3_XS.gguf) | i1-IQ3_XS | 2.7 | |
| [GGUF](https://huggingface.co/mradermacher/Yi-6B-200K-i1-GGUF/resolve/main/Yi-6B-200K.i1-Q3_K_S.gguf) | i1-Q3_K_S | 2.8 | IQ3_XS probably better |
| [GGUF](https://huggingface.co/mradermacher/Yi-6B-200K-i1-GGUF/resolve/main/Yi-6B-200K.i1-IQ3_S.gguf) | i1-IQ3_S | 2.8 | beats Q3_K* |
| [GGUF](https://huggingface.co/mradermacher/Yi-6B-200K-i1-GGUF/resolve/main/Yi-6B-200K.i1-IQ3_M.gguf) | i1-IQ3_M | 2.9 | |
| [GGUF](https://huggingface.co/mradermacher/Yi-6B-200K-i1-GGUF/resolve/main/Yi-6B-200K.i1-Q3_K_M.gguf) | i1-Q3_K_M | 3.1 | IQ3_S probably better |
| [GGUF](https://huggingface.co/mradermacher/Yi-6B-200K-i1-GGUF/resolve/main/Yi-6B-200K.i1-Q3_K_L.gguf) | i1-Q3_K_L | 3.3 | IQ3_M probably better |
| [GGUF](https://huggingface.co/mradermacher/Yi-6B-200K-i1-GGUF/resolve/main/Yi-6B-200K.i1-IQ4_XS.gguf) | i1-IQ4_XS | 3.4 | |
| [GGUF](https://huggingface.co/mradermacher/Yi-6B-200K-i1-GGUF/resolve/main/Yi-6B-200K.i1-Q4_0.gguf) | i1-Q4_0 | 3.6 | fast, low quality |
| [GGUF](https://huggingface.co/mradermacher/Yi-6B-200K-i1-GGUF/resolve/main/Yi-6B-200K.i1-Q4_K_S.gguf) | i1-Q4_K_S | 3.6 | optimal size/speed/quality |
| [GGUF](https://huggingface.co/mradermacher/Yi-6B-200K-i1-GGUF/resolve/main/Yi-6B-200K.i1-Q4_K_M.gguf) | i1-Q4_K_M | 3.8 | fast, recommended |
| [GGUF](https://huggingface.co/mradermacher/Yi-6B-200K-i1-GGUF/resolve/main/Yi-6B-200K.i1-Q5_K_S.gguf) | i1-Q5_K_S | 4.3 | |
| [GGUF](https://huggingface.co/mradermacher/Yi-6B-200K-i1-GGUF/resolve/main/Yi-6B-200K.i1-Q5_K_M.gguf) | i1-Q5_K_M | 4.4 | |
| [GGUF](https://huggingface.co/mradermacher/Yi-6B-200K-i1-GGUF/resolve/main/Yi-6B-200K.i1-Q6_K.gguf) | i1-Q6_K | 5.1 | practically like static Q6_K |
Here is a handy graph by ikawrakow comparing some lower-quality quant
types (lower is better):

And here are Artefact2's thoughts on the matter:
https://gist.github.com/Artefact2/b5f810600771265fc1e39442288e8ec9
## FAQ / Model Request
See https://huggingface.co/mradermacher/model_requests for some answers to
questions you might have and/or if you want some other model quantized.
## Thanks
I thank my company, [nethype GmbH](https://www.nethype.de/), for letting
me use its servers and providing upgrades to my workstation to enable
this work in my free time. Additional thanks to [@nicoboss](https://huggingface.co/nicoboss) for giving me access to his hardware for calculating the imatrix for these quants.
<!-- end -->
|
facebook/maskformer-swin-base-coco | facebook | 2024-05-03T07:29:13Z | 13,884 | 22 | transformers | [
"transformers",
"pytorch",
"safetensors",
"maskformer",
"vision",
"image-segmentation",
"dataset:coco",
"arxiv:2107.06278",
"license:other",
"endpoints_compatible",
"region:us"
] | image-segmentation | 2022-03-02T23:29:05Z | ---
license: other
tags:
- vision
- image-segmentation
datasets:
- coco
widget:
- src: http://images.cocodataset.org/val2017/000000039769.jpg
example_title: Cats
- src: http://images.cocodataset.org/val2017/000000039770.jpg
example_title: Castle
---
# MaskFormer
MaskFormer model trained on COCO panoptic segmentation (base-sized version, Swin backbone). It was introduced in the paper [Per-Pixel Classification is Not All You Need for Semantic Segmentation](https://arxiv.org/abs/2107.06278) and first released in [this repository](https://github.com/facebookresearch/MaskFormer/blob/da3e60d85fdeedcb31476b5edd7d328826ce56cc/mask_former/modeling/criterion.py#L169).
Disclaimer: The team releasing MaskFormer did not write a model card for this model so this model card has been written by the Hugging Face team.
## Model description
MaskFormer addresses instance, semantic and panoptic segmentation with the same paradigm: by predicting a set of masks and corresponding labels. Hence, all 3 tasks are treated as if they were instance segmentation.

## Intended uses & limitations
You can use this particular checkpoint for semantic segmentation. See the [model hub](https://huggingface.co/models?search=maskformer) to look for other
fine-tuned versions on a task that interests you.
### How to use
Here is how to use this model:
```python
from transformers import MaskFormerFeatureExtractor, MaskFormerForInstanceSegmentation
from PIL import Image
import requests
# load MaskFormer fine-tuned on COCO panoptic segmentation
feature_extractor = MaskFormerFeatureExtractor.from_pretrained("facebook/maskformer-swin-base-coco")
model = MaskFormerForInstanceSegmentation.from_pretrained("facebook/maskformer-swin-base-coco")
url = "http://images.cocodataset.org/val2017/000000039769.jpg"
image = Image.open(requests.get(url, stream=True).raw)
inputs = feature_extractor(images=image, return_tensors="pt")
outputs = model(**inputs)
# model predicts class_queries_logits of shape `(batch_size, num_queries)`
# and masks_queries_logits of shape `(batch_size, num_queries, height, width)`
class_queries_logits = outputs.class_queries_logits
masks_queries_logits = outputs.masks_queries_logits
# you can pass them to feature_extractor for postprocessing
result = feature_extractor.post_process_panoptic_segmentation(outputs, target_sizes=[image.size[::-1]])[0]
# we refer to the demo notebooks for visualization (see "Resources" section in the MaskFormer docs)
predicted_panoptic_map = result["segmentation"]
```
For more code examples, we refer to the [documentation](https://huggingface.co/docs/transformers/master/en/model_doc/maskformer). |
mradermacher/Llama-3-Lumimaid-8B-v0.1-OAS-i1-GGUF | mradermacher | 2024-06-27T23:26:58Z | 13,873 | 0 | transformers | [
"transformers",
"gguf",
"not-for-all-audiences",
"nsfw",
"en",
"base_model:NeverSleep/Llama-3-Lumimaid-8B-v0.1-OAS",
"license:cc-by-nc-4.0",
"endpoints_compatible",
"region:us"
] | null | 2024-06-27T22:11:46Z | ---
base_model: NeverSleep/Llama-3-Lumimaid-8B-v0.1-OAS
language:
- en
library_name: transformers
license: cc-by-nc-4.0
quantized_by: mradermacher
tags:
- not-for-all-audiences
- nsfw
---
## About
<!-- ### quantize_version: 2 -->
<!-- ### output_tensor_quantised: 1 -->
<!-- ### convert_type: hf -->
<!-- ### vocab_type: -->
<!-- ### tags: nicoboss -->
weighted/imatrix quants of https://huggingface.co/NeverSleep/Llama-3-Lumimaid-8B-v0.1-OAS
<!-- provided-files -->
static quants are available at https://huggingface.co/mradermacher/Llama-3-Lumimaid-8B-v0.1-OAS-GGUF
## Usage
If you are unsure how to use GGUF files, refer to one of [TheBloke's
READMEs](https://huggingface.co/TheBloke/KafkaLM-70B-German-V0.1-GGUF) for
more details, including on how to concatenate multi-part files.
## Provided Quants
(sorted by size, not necessarily quality. IQ-quants are often preferable over similar sized non-IQ quants)
| Link | Type | Size/GB | Notes |
|:-----|:-----|--------:|:------|
| [GGUF](https://huggingface.co/mradermacher/Llama-3-Lumimaid-8B-v0.1-OAS-i1-GGUF/resolve/main/Llama-3-Lumimaid-8B-v0.1-OAS.i1-IQ1_S.gguf) | i1-IQ1_S | 2.1 | for the desperate |
| [GGUF](https://huggingface.co/mradermacher/Llama-3-Lumimaid-8B-v0.1-OAS-i1-GGUF/resolve/main/Llama-3-Lumimaid-8B-v0.1-OAS.i1-IQ1_M.gguf) | i1-IQ1_M | 2.3 | mostly desperate |
| [GGUF](https://huggingface.co/mradermacher/Llama-3-Lumimaid-8B-v0.1-OAS-i1-GGUF/resolve/main/Llama-3-Lumimaid-8B-v0.1-OAS.i1-IQ2_XXS.gguf) | i1-IQ2_XXS | 2.5 | |
| [GGUF](https://huggingface.co/mradermacher/Llama-3-Lumimaid-8B-v0.1-OAS-i1-GGUF/resolve/main/Llama-3-Lumimaid-8B-v0.1-OAS.i1-IQ2_XS.gguf) | i1-IQ2_XS | 2.7 | |
| [GGUF](https://huggingface.co/mradermacher/Llama-3-Lumimaid-8B-v0.1-OAS-i1-GGUF/resolve/main/Llama-3-Lumimaid-8B-v0.1-OAS.i1-IQ2_S.gguf) | i1-IQ2_S | 2.9 | |
| [GGUF](https://huggingface.co/mradermacher/Llama-3-Lumimaid-8B-v0.1-OAS-i1-GGUF/resolve/main/Llama-3-Lumimaid-8B-v0.1-OAS.i1-IQ2_M.gguf) | i1-IQ2_M | 3.0 | |
| [GGUF](https://huggingface.co/mradermacher/Llama-3-Lumimaid-8B-v0.1-OAS-i1-GGUF/resolve/main/Llama-3-Lumimaid-8B-v0.1-OAS.i1-Q2_K.gguf) | i1-Q2_K | 3.3 | IQ3_XXS probably better |
| [GGUF](https://huggingface.co/mradermacher/Llama-3-Lumimaid-8B-v0.1-OAS-i1-GGUF/resolve/main/Llama-3-Lumimaid-8B-v0.1-OAS.i1-IQ3_XXS.gguf) | i1-IQ3_XXS | 3.4 | lower quality |
| [GGUF](https://huggingface.co/mradermacher/Llama-3-Lumimaid-8B-v0.1-OAS-i1-GGUF/resolve/main/Llama-3-Lumimaid-8B-v0.1-OAS.i1-IQ3_XS.gguf) | i1-IQ3_XS | 3.6 | |
| [GGUF](https://huggingface.co/mradermacher/Llama-3-Lumimaid-8B-v0.1-OAS-i1-GGUF/resolve/main/Llama-3-Lumimaid-8B-v0.1-OAS.i1-Q3_K_S.gguf) | i1-Q3_K_S | 3.8 | IQ3_XS probably better |
| [GGUF](https://huggingface.co/mradermacher/Llama-3-Lumimaid-8B-v0.1-OAS-i1-GGUF/resolve/main/Llama-3-Lumimaid-8B-v0.1-OAS.i1-IQ3_S.gguf) | i1-IQ3_S | 3.8 | beats Q3_K* |
| [GGUF](https://huggingface.co/mradermacher/Llama-3-Lumimaid-8B-v0.1-OAS-i1-GGUF/resolve/main/Llama-3-Lumimaid-8B-v0.1-OAS.i1-IQ3_M.gguf) | i1-IQ3_M | 3.9 | |
| [GGUF](https://huggingface.co/mradermacher/Llama-3-Lumimaid-8B-v0.1-OAS-i1-GGUF/resolve/main/Llama-3-Lumimaid-8B-v0.1-OAS.i1-Q3_K_M.gguf) | i1-Q3_K_M | 4.1 | IQ3_S probably better |
| [GGUF](https://huggingface.co/mradermacher/Llama-3-Lumimaid-8B-v0.1-OAS-i1-GGUF/resolve/main/Llama-3-Lumimaid-8B-v0.1-OAS.i1-Q3_K_L.gguf) | i1-Q3_K_L | 4.4 | IQ3_M probably better |
| [GGUF](https://huggingface.co/mradermacher/Llama-3-Lumimaid-8B-v0.1-OAS-i1-GGUF/resolve/main/Llama-3-Lumimaid-8B-v0.1-OAS.i1-IQ4_XS.gguf) | i1-IQ4_XS | 4.5 | |
| [GGUF](https://huggingface.co/mradermacher/Llama-3-Lumimaid-8B-v0.1-OAS-i1-GGUF/resolve/main/Llama-3-Lumimaid-8B-v0.1-OAS.i1-Q4_0.gguf) | i1-Q4_0 | 4.8 | fast, low quality |
| [GGUF](https://huggingface.co/mradermacher/Llama-3-Lumimaid-8B-v0.1-OAS-i1-GGUF/resolve/main/Llama-3-Lumimaid-8B-v0.1-OAS.i1-Q4_K_S.gguf) | i1-Q4_K_S | 4.8 | optimal size/speed/quality |
| [GGUF](https://huggingface.co/mradermacher/Llama-3-Lumimaid-8B-v0.1-OAS-i1-GGUF/resolve/main/Llama-3-Lumimaid-8B-v0.1-OAS.i1-Q4_K_M.gguf) | i1-Q4_K_M | 5.0 | fast, recommended |
| [GGUF](https://huggingface.co/mradermacher/Llama-3-Lumimaid-8B-v0.1-OAS-i1-GGUF/resolve/main/Llama-3-Lumimaid-8B-v0.1-OAS.i1-Q5_K_S.gguf) | i1-Q5_K_S | 5.7 | |
| [GGUF](https://huggingface.co/mradermacher/Llama-3-Lumimaid-8B-v0.1-OAS-i1-GGUF/resolve/main/Llama-3-Lumimaid-8B-v0.1-OAS.i1-Q5_K_M.gguf) | i1-Q5_K_M | 5.8 | |
| [GGUF](https://huggingface.co/mradermacher/Llama-3-Lumimaid-8B-v0.1-OAS-i1-GGUF/resolve/main/Llama-3-Lumimaid-8B-v0.1-OAS.i1-Q6_K.gguf) | i1-Q6_K | 6.7 | practically like static Q6_K |
Here is a handy graph by ikawrakow comparing some lower-quality quant
types (lower is better):

And here are Artefact2's thoughts on the matter:
https://gist.github.com/Artefact2/b5f810600771265fc1e39442288e8ec9
## FAQ / Model Request
See https://huggingface.co/mradermacher/model_requests for some answers to
questions you might have and/or if you want some other model quantized.
## Thanks
I thank my company, [nethype GmbH](https://www.nethype.de/), for letting
me use its servers and providing upgrades to my workstation to enable
this work in my free time. Additional thanks to [@nicoboss](https://huggingface.co/nicoboss) for giving me access to his hardware for calculating the imatrix for these quants.
<!-- end -->
|
togethercomputer/Llama-2-7B-32K-Instruct | togethercomputer | 2024-03-28T01:13:47Z | 13,862 | 160 | transformers | [
"transformers",
"pytorch",
"llama",
"text-generation",
"en",
"dataset:togethercomputer/llama-instruct",
"arxiv:2307.03172",
"license:llama2",
"autotrain_compatible",
"endpoints_compatible",
"text-generation-inference",
"region:us"
] | text-generation | 2023-08-08T20:22:27Z | ---
license: llama2
language:
- en
library_name: transformers
datasets:
- togethercomputer/llama-instruct
---
# Llama-2-7B-32K-Instruct
## Model Description
Llama-2-7B-32K-Instruct is an open-source, long-context chat model finetuned from [Llama-2-7B-32K](https://huggingface.co/togethercomputer/Llama-2-7B-32K), over high-quality instruction and chat data.
We built Llama-2-7B-32K-Instruct with less than 200 lines of Python script using [Together API](https://together.ai/blog/api-announcement), and we also make the [recipe fully available](https://github.com/togethercomputer/Llama-2-7B-32K-Instruct).
We hope that this can enable everyone to finetune their own version of [Llama-2-7B-32K](https://huggingface.co/togethercomputer/Llama-2-7B-32K) — play with [Together API](https://together.ai/blog/api-announcement) and give us feedback!
## Data Collection Details
Llama-2-7B-32K-Instruct is fine-tuned over a combination of two parts:
1. **19K single- and multi-round conversations generated by human instructions and [Llama-2-70B-Chat](https://huggingface.co/meta-llama/Llama-2-7b-chat-hf) outputs**.
We collected the dataset following the distillation paradigm that is used by Alpaca, Vicuna, WizardLM, Orca — producing instructions by querying a powerful LLM (in this case, [Llama-2-70B-Chat](https://huggingface.co/meta-llama/Llama-2-7b-chat-hf)).
The complete dataset is also released [here](https://huggingface.co/datasets/togethercomputer/llama-instruct).
We also share the complete recipe for the data collection process [here](https://github.com/togethercomputer/Llama-2-7B-32K-Instruct).
2. **Long-context Summarization and Long-context QA**.
We follow the recipe of [Llama-2-7B-32K](https://together.ai/blog/Llama-2-7B-32K), and train our model with the [BookSum dataset](https://huggingface.co/datasets/togethercomputer/Long-Data-Collections) and [Multi-document Question Answering](https://arxiv.org/abs/2307.03172).
The final data mixture used for model finetuning is: 19K instruction (50%) + BookSum (25%) + MQA (25%).
## Model Usage
We encourage you to try out this model using the [Together API](https://together.ai/blog/api-announcement). The updated inference stack allows for efficient inference.
To run the model locally, we strongly recommend to install Flash Attention V2, which is necessary to obtain the best performance:
```
# Please update the path of `CUDA_HOME`
export CUDA_HOME=/usr/local/cuda-11.8
pip install transformers==4.31.0
pip install sentencepiece
pip install ninja
pip install flash-attn --no-build-isolation
pip install git+https://github.com/HazyResearch/flash-attention.git#subdirectory=csrc/rotary
```
You can load the model directly from the Hugging Face model hub using
```python
import torch
from transformers import AutoTokenizer, AutoModelForCausalLM
tokenizer = AutoTokenizer.from_pretrained("togethercomputer/Llama-2-7B-32K-Instruct")
model = AutoModelForCausalLM.from_pretrained("togethercomputer/Llama-2-7B-32K-Instruct",
trust_remote_code=True, torch_dtype=torch.float16)
input_ids = tokenizer.encode("[INST]\nWrite a poem about cats\n[/INST]\n\n", return_tensors="pt")
output = model.generate(input_ids, max_length=128,
temperature=0.7, repetition_penalty=1.1, top_p=0.7, top_k=50)
output_text = tokenizer.decode(output[0], skip_special_tokens=True)
```
The model is also hosted on [Together Playground](https://api.together.xyz/playground). You can simply play with the model by using prompt formatted by:
```
[INST]\n<your instruction here>\n[\INST]\n\n
```
For example, if we query the model with
```
[INST]\nWrite a poem about cats\n[\INST]\n\n
```
the model will return
```
[INST]
Write a poem about cats
[/INST]
Cats are mysterious creatures, with their aloof and independent nature. They're also incredibly beautiful, with their sleek fur and piercing eyes. Here's a poem that captures the essence of cats:
Cats, oh cats, how can I describe you?
Your beauty is beyond compare, it seems.
You're graceful and elegant, like a ballerina's dance,
But don't let your charm fool you, for you're not easily tamed.
With your soft purring and playful meows,
You draw us in with your enchanting powers.
We love to watch you play, your tail twirling 'round,
As if you're dancing on air, with no sound.
But don't be fooled by your sweetness, my friend,
For beneath that gentle exterior, lies a fierce defender.
When danger lurks, you'll spring into action,
Protecting those you hold dear, without question.
Solet us admire you, from afar,
For in your own way, you're truly unique, a star.
And though we may never fully understand,
The depths of your soul, we'll always stand, hand in paw, as one.
This poem captures the essence of cats, highlighting their beauty, independence,and protective nature. It also celebrates the special bond between humans and cats, recognizing their unique qualities and the joy they bring to our lives.
```
## Model Evaluation
We evaluate the model from three aspects: 1) [Alpaca Eval](https://tatsu-lab.github.io/alpaca_eval/);
2) [Rouge score over BookSum](https://together.ai/blog/Llama-2-7B-32K); and
3) [Accuracy over Multi-document Question Answering (MQA)](https://together.ai/blog/Llama-2-7B-32K).
We compare with models including
[GPT-3.5-Turbo-16K](https://platform.openai.com/docs/models/gpt-3-5),
[https://huggingface.co/meta-llama/Llama-2-7b-chat-hf](https://huggingface.co/meta-llama/Llama-2-7b-chat-hf),
[Longchat-7b-16k](https://huggingface.co/lmsys/longchat-7b-16k)
and [Longchat-7b-v1.5-32k](https://huggingface.co/lmsys/longchat-7b-v1.5-32k).
We summarize the results below:
* Alpaca Eval
| Model | win_rate | standard_error | n_total | avg_length |
| -------- | ------- | ------- | ------- | ------- |
| Llama-2-7B-Chat-hf | 71.37 | 1.59 | 805 | 1479 |
| Llama-2-7B-32K-Instruct | 70.36 | 1.61 | 803 | 1885 |
| oasst-rlhf-llama-33b | 66.52 | 1.66 | 805 | 1079 |
| text_davinci_003 | 50.00 | 0.00 | 805 | 307|
| falcon-40b-instruct | 45.71 | 1.75 | 805 | 662 |
| alpaca-farm-ppo-human | 41.24 | 1.73 | 805 | 803 |
| alpaca-7b | 26.46 | 1.54 | 805 | 396 |
| text_davinci_001 | 15.17 | 1.24 | 804 | 296 |
* Rouge Score over BookSum
| Model | R1 | R2 | RL |
| -------- | ------- | ------- | ------- |
| Llama-2-7B-Chat-hf | 0.055 | 0.008 | 0.046 |
| Longchat-7b-16k | 0.303 | 0.055 | 0.160 |
| Longchat-7b-v1.5-32k | 0.308 | 0.057 | 0.163 |
| GPT-3.5-Turbo-16K | 0.324 | 0.066 | 0.178 |
| Llama-2-7B-32K-Instruct (ours) | 0.336 | 0.076 | 0.184 |
* Accuracy over MQA
| Model | 20 docs (Avg 2.9K tokens) | 30 docs (Avg 4.4K tokens) | 50 docs (Avg 7.4K tokens) |
| -------- | ------- | ------- | ------- |
| Llama-2-7B-Chat-hf | 0.448 | 0.421 | 0.354 |
| Longchat-7b-16k | 0.510 | 0.473 | 0.428 |
| Longchat-7b-v1.5-32k | 0.534 | 0.516 | 0.479 |
| GPT-3.5-Turbo-16K | 0.622 | 0.609 | 0.577 |
| Llama-2-7B-32K-Instruct (ours) | 0.622 | 0.604 | 0.589 |
## Limitations and Bias
As with all language models, Llama-2-7B-32K-Instruct may generate incorrect or biased content. It's important to keep this in mind when using the model.
## Community
Join us on [Together Discord](https://discord.gg/6ZVDU8tTD4) |
albert/albert-base-v1 | albert | 2024-02-19T10:57:35Z | 13,857 | 7 | transformers | [
"transformers",
"pytorch",
"tf",
"safetensors",
"albert",
"fill-mask",
"exbert",
"en",
"dataset:bookcorpus",
"dataset:wikipedia",
"arxiv:1909.11942",
"license:apache-2.0",
"autotrain_compatible",
"endpoints_compatible",
"region:us"
] | fill-mask | 2022-03-02T23:29:04Z | ---
tags:
- exbert
language: en
license: apache-2.0
datasets:
- bookcorpus
- wikipedia
---
# ALBERT Base v1
Pretrained model on English language using a masked language modeling (MLM) objective. It was introduced in
[this paper](https://arxiv.org/abs/1909.11942) and first released in
[this repository](https://github.com/google-research/albert). This model, as all ALBERT models, is uncased: it does not make a difference
between english and English.
Disclaimer: The team releasing ALBERT did not write a model card for this model so this model card has been written by
the Hugging Face team.
## Model description
ALBERT is a transformers model pretrained on a large corpus of English data in a self-supervised fashion. This means it
was pretrained on the raw texts only, with no humans labelling them in any way (which is why it can use lots of
publicly available data) with an automatic process to generate inputs and labels from those texts. More precisely, it
was pretrained with two objectives:
- Masked language modeling (MLM): taking a sentence, the model randomly masks 15% of the words in the input then run
the entire masked sentence through the model and has to predict the masked words. This is different from traditional
recurrent neural networks (RNNs) that usually see the words one after the other, or from autoregressive models like
GPT which internally mask the future tokens. It allows the model to learn a bidirectional representation of the
sentence.
- Sentence Ordering Prediction (SOP): ALBERT uses a pretraining loss based on predicting the ordering of two consecutive segments of text.
This way, the model learns an inner representation of the English language that can then be used to extract features
useful for downstream tasks: if you have a dataset of labeled sentences for instance, you can train a standard
classifier using the features produced by the ALBERT model as inputs.
ALBERT is particular in that it shares its layers across its Transformer. Therefore, all layers have the same weights. Using repeating layers results in a small memory footprint, however, the computational cost remains similar to a BERT-like architecture with the same number of hidden layers as it has to iterate through the same number of (repeating) layers.
This is the first version of the base model. Version 2 is different from version 1 due to different dropout rates, additional training data, and longer training. It has better results in nearly all downstream tasks.
This model has the following configuration:
- 12 repeating layers
- 128 embedding dimension
- 768 hidden dimension
- 12 attention heads
- 11M parameters
## Intended uses & limitations
You can use the raw model for either masked language modeling or next sentence prediction, but it's mostly intended to
be fine-tuned on a downstream task. See the [model hub](https://huggingface.co/models?filter=albert) to look for
fine-tuned versions on a task that interests you.
Note that this model is primarily aimed at being fine-tuned on tasks that use the whole sentence (potentially masked)
to make decisions, such as sequence classification, token classification or question answering. For tasks such as text
generation you should look at model like GPT2.
### How to use
You can use this model directly with a pipeline for masked language modeling:
```python
>>> from transformers import pipeline
>>> unmasker = pipeline('fill-mask', model='albert-base-v1')
>>> unmasker("Hello I'm a [MASK] model.")
[
{
"sequence":"[CLS] hello i'm a modeling model.[SEP]",
"score":0.05816134437918663,
"token":12807,
"token_str":"▁modeling"
},
{
"sequence":"[CLS] hello i'm a modelling model.[SEP]",
"score":0.03748830780386925,
"token":23089,
"token_str":"▁modelling"
},
{
"sequence":"[CLS] hello i'm a model model.[SEP]",
"score":0.033725276589393616,
"token":1061,
"token_str":"▁model"
},
{
"sequence":"[CLS] hello i'm a runway model.[SEP]",
"score":0.017313428223133087,
"token":8014,
"token_str":"▁runway"
},
{
"sequence":"[CLS] hello i'm a lingerie model.[SEP]",
"score":0.014405295252799988,
"token":29104,
"token_str":"▁lingerie"
}
]
```
Here is how to use this model to get the features of a given text in PyTorch:
```python
from transformers import AlbertTokenizer, AlbertModel
tokenizer = AlbertTokenizer.from_pretrained('albert-base-v1')
model = AlbertModel.from_pretrained("albert-base-v1")
text = "Replace me by any text you'd like."
encoded_input = tokenizer(text, return_tensors='pt')
output = model(**encoded_input)
```
and in TensorFlow:
```python
from transformers import AlbertTokenizer, TFAlbertModel
tokenizer = AlbertTokenizer.from_pretrained('albert-base-v1')
model = TFAlbertModel.from_pretrained("albert-base-v1")
text = "Replace me by any text you'd like."
encoded_input = tokenizer(text, return_tensors='tf')
output = model(encoded_input)
```
### Limitations and bias
Even if the training data used for this model could be characterized as fairly neutral, this model can have biased
predictions:
```python
>>> from transformers import pipeline
>>> unmasker = pipeline('fill-mask', model='albert-base-v1')
>>> unmasker("The man worked as a [MASK].")
[
{
"sequence":"[CLS] the man worked as a chauffeur.[SEP]",
"score":0.029577180743217468,
"token":28744,
"token_str":"▁chauffeur"
},
{
"sequence":"[CLS] the man worked as a janitor.[SEP]",
"score":0.028865724802017212,
"token":29477,
"token_str":"▁janitor"
},
{
"sequence":"[CLS] the man worked as a shoemaker.[SEP]",
"score":0.02581118606030941,
"token":29024,
"token_str":"▁shoemaker"
},
{
"sequence":"[CLS] the man worked as a blacksmith.[SEP]",
"score":0.01849772222340107,
"token":21238,
"token_str":"▁blacksmith"
},
{
"sequence":"[CLS] the man worked as a lawyer.[SEP]",
"score":0.01820771023631096,
"token":3672,
"token_str":"▁lawyer"
}
]
>>> unmasker("The woman worked as a [MASK].")
[
{
"sequence":"[CLS] the woman worked as a receptionist.[SEP]",
"score":0.04604868218302727,
"token":25331,
"token_str":"▁receptionist"
},
{
"sequence":"[CLS] the woman worked as a janitor.[SEP]",
"score":0.028220869600772858,
"token":29477,
"token_str":"▁janitor"
},
{
"sequence":"[CLS] the woman worked as a paramedic.[SEP]",
"score":0.0261906236410141,
"token":23386,
"token_str":"▁paramedic"
},
{
"sequence":"[CLS] the woman worked as a chauffeur.[SEP]",
"score":0.024797942489385605,
"token":28744,
"token_str":"▁chauffeur"
},
{
"sequence":"[CLS] the woman worked as a waitress.[SEP]",
"score":0.024124596267938614,
"token":13678,
"token_str":"▁waitress"
}
]
```
This bias will also affect all fine-tuned versions of this model.
## Training data
The ALBERT model was pretrained on [BookCorpus](https://yknzhu.wixsite.com/mbweb), a dataset consisting of 11,038
unpublished books and [English Wikipedia](https://en.wikipedia.org/wiki/English_Wikipedia) (excluding lists, tables and
headers).
## Training procedure
### Preprocessing
The texts are lowercased and tokenized using SentencePiece and a vocabulary size of 30,000. The inputs of the model are
then of the form:
```
[CLS] Sentence A [SEP] Sentence B [SEP]
```
### Training
The ALBERT procedure follows the BERT setup.
The details of the masking procedure for each sentence are the following:
- 15% of the tokens are masked.
- In 80% of the cases, the masked tokens are replaced by `[MASK]`.
- In 10% of the cases, the masked tokens are replaced by a random token (different) from the one they replace.
- In the 10% remaining cases, the masked tokens are left as is.
## Evaluation results
When fine-tuned on downstream tasks, the ALBERT models achieve the following results:
| | Average | SQuAD1.1 | SQuAD2.0 | MNLI | SST-2 | RACE |
|----------------|----------|----------|----------|----------|----------|----------|
|V2 |
|ALBERT-base |82.3 |90.2/83.2 |82.1/79.3 |84.6 |92.9 |66.8 |
|ALBERT-large |85.7 |91.8/85.2 |84.9/81.8 |86.5 |94.9 |75.2 |
|ALBERT-xlarge |87.9 |92.9/86.4 |87.9/84.1 |87.9 |95.4 |80.7 |
|ALBERT-xxlarge |90.9 |94.6/89.1 |89.8/86.9 |90.6 |96.8 |86.8 |
|V1 |
|ALBERT-base |80.1 |89.3/82.3 | 80.0/77.1|81.6 |90.3 | 64.0 |
|ALBERT-large |82.4 |90.6/83.9 | 82.3/79.4|83.5 |91.7 | 68.5 |
|ALBERT-xlarge |85.5 |92.5/86.1 | 86.1/83.1|86.4 |92.4 | 74.8 |
|ALBERT-xxlarge |91.0 |94.8/89.3 | 90.2/87.4|90.8 |96.9 | 86.5 |
### BibTeX entry and citation info
```bibtex
@article{DBLP:journals/corr/abs-1909-11942,
author = {Zhenzhong Lan and
Mingda Chen and
Sebastian Goodman and
Kevin Gimpel and
Piyush Sharma and
Radu Soricut},
title = {{ALBERT:} {A} Lite {BERT} for Self-supervised Learning of Language
Representations},
journal = {CoRR},
volume = {abs/1909.11942},
year = {2019},
url = {http://arxiv.org/abs/1909.11942},
archivePrefix = {arXiv},
eprint = {1909.11942},
timestamp = {Fri, 27 Sep 2019 13:04:21 +0200},
biburl = {https://dblp.org/rec/journals/corr/abs-1909-11942.bib},
bibsource = {dblp computer science bibliography, https://dblp.org}
}
```
<a href="https://huggingface.co/exbert/?model=albert-base-v1">
<img width="300px" src="https://cdn-media.huggingface.co/exbert/button.png">
</a>
|
mradermacher/mindspark-bot-v1-full-GGUF | mradermacher | 2024-06-29T18:20:38Z | 13,846 | 0 | transformers | [
"transformers",
"gguf",
"text-generation-inference",
"unsloth",
"mistral",
"trl",
"en",
"base_model:cgteen/mindspark-bot-v1-full",
"license:apache-2.0",
"endpoints_compatible",
"region:us"
] | null | 2024-06-29T17:26:29Z | ---
base_model: cgteen/mindspark-bot-v1-full
language:
- en
library_name: transformers
license: apache-2.0
quantized_by: mradermacher
tags:
- text-generation-inference
- transformers
- unsloth
- mistral
- trl
---
## About
<!-- ### quantize_version: 2 -->
<!-- ### output_tensor_quantised: 1 -->
<!-- ### convert_type: hf -->
<!-- ### vocab_type: -->
<!-- ### tags: -->
static quants of https://huggingface.co/cgteen/mindspark-bot-v1-full
<!-- provided-files -->
weighted/imatrix quants seem not to be available (by me) at this time. If they do not show up a week or so after the static ones, I have probably not planned for them. Feel free to request them by opening a Community Discussion.
## Usage
If you are unsure how to use GGUF files, refer to one of [TheBloke's
READMEs](https://huggingface.co/TheBloke/KafkaLM-70B-German-V0.1-GGUF) for
more details, including on how to concatenate multi-part files.
## Provided Quants
(sorted by size, not necessarily quality. IQ-quants are often preferable over similar sized non-IQ quants)
| Link | Type | Size/GB | Notes |
|:-----|:-----|--------:|:------|
| [GGUF](https://huggingface.co/mradermacher/mindspark-bot-v1-full-GGUF/resolve/main/mindspark-bot-v1-full.Q2_K.gguf) | Q2_K | 2.8 | |
| [GGUF](https://huggingface.co/mradermacher/mindspark-bot-v1-full-GGUF/resolve/main/mindspark-bot-v1-full.IQ3_XS.gguf) | IQ3_XS | 3.1 | |
| [GGUF](https://huggingface.co/mradermacher/mindspark-bot-v1-full-GGUF/resolve/main/mindspark-bot-v1-full.Q3_K_S.gguf) | Q3_K_S | 3.3 | |
| [GGUF](https://huggingface.co/mradermacher/mindspark-bot-v1-full-GGUF/resolve/main/mindspark-bot-v1-full.IQ3_S.gguf) | IQ3_S | 3.3 | beats Q3_K* |
| [GGUF](https://huggingface.co/mradermacher/mindspark-bot-v1-full-GGUF/resolve/main/mindspark-bot-v1-full.IQ3_M.gguf) | IQ3_M | 3.4 | |
| [GGUF](https://huggingface.co/mradermacher/mindspark-bot-v1-full-GGUF/resolve/main/mindspark-bot-v1-full.Q3_K_M.gguf) | Q3_K_M | 3.6 | lower quality |
| [GGUF](https://huggingface.co/mradermacher/mindspark-bot-v1-full-GGUF/resolve/main/mindspark-bot-v1-full.Q3_K_L.gguf) | Q3_K_L | 3.9 | |
| [GGUF](https://huggingface.co/mradermacher/mindspark-bot-v1-full-GGUF/resolve/main/mindspark-bot-v1-full.IQ4_XS.gguf) | IQ4_XS | 4.0 | |
| [GGUF](https://huggingface.co/mradermacher/mindspark-bot-v1-full-GGUF/resolve/main/mindspark-bot-v1-full.Q4_K_S.gguf) | Q4_K_S | 4.2 | fast, recommended |
| [GGUF](https://huggingface.co/mradermacher/mindspark-bot-v1-full-GGUF/resolve/main/mindspark-bot-v1-full.Q4_K_M.gguf) | Q4_K_M | 4.5 | fast, recommended |
| [GGUF](https://huggingface.co/mradermacher/mindspark-bot-v1-full-GGUF/resolve/main/mindspark-bot-v1-full.Q5_K_S.gguf) | Q5_K_S | 5.1 | |
| [GGUF](https://huggingface.co/mradermacher/mindspark-bot-v1-full-GGUF/resolve/main/mindspark-bot-v1-full.Q5_K_M.gguf) | Q5_K_M | 5.2 | |
| [GGUF](https://huggingface.co/mradermacher/mindspark-bot-v1-full-GGUF/resolve/main/mindspark-bot-v1-full.Q6_K.gguf) | Q6_K | 6.0 | very good quality |
| [GGUF](https://huggingface.co/mradermacher/mindspark-bot-v1-full-GGUF/resolve/main/mindspark-bot-v1-full.Q8_0.gguf) | Q8_0 | 7.8 | fast, best quality |
| [GGUF](https://huggingface.co/mradermacher/mindspark-bot-v1-full-GGUF/resolve/main/mindspark-bot-v1-full.f16.gguf) | f16 | 14.6 | 16 bpw, overkill |
Here is a handy graph by ikawrakow comparing some lower-quality quant
types (lower is better):

And here are Artefact2's thoughts on the matter:
https://gist.github.com/Artefact2/b5f810600771265fc1e39442288e8ec9
## FAQ / Model Request
See https://huggingface.co/mradermacher/model_requests for some answers to
questions you might have and/or if you want some other model quantized.
## Thanks
I thank my company, [nethype GmbH](https://www.nethype.de/), for letting
me use its servers and providing upgrades to my workstation to enable
this work in my free time.
<!-- end -->
|
mradermacher/llama3-8B-DarkIdol-2.1-Uncensored-32K-GGUF | mradermacher | 2024-06-27T03:44:10Z | 13,845 | 1 | transformers | [
"transformers",
"gguf",
"roleplay",
"llama3",
"sillytavern",
"idol",
"en",
"ja",
"zh",
"base_model:aifeifei798/llama3-8B-DarkIdol-2.1-Uncensored-32K",
"license:llama3",
"endpoints_compatible",
"region:us"
] | null | 2024-06-27T02:50:59Z | ---
base_model: aifeifei798/llama3-8B-DarkIdol-2.1-Uncensored-32K
language:
- en
- ja
- zh
library_name: transformers
license: llama3
quantized_by: mradermacher
tags:
- roleplay
- llama3
- sillytavern
- idol
---
## About
<!-- ### quantize_version: 2 -->
<!-- ### output_tensor_quantised: 1 -->
<!-- ### convert_type: hf -->
<!-- ### vocab_type: -->
<!-- ### tags: -->
static quants of https://huggingface.co/aifeifei798/llama3-8B-DarkIdol-2.1-Uncensored-32K
<!-- provided-files -->
weighted/imatrix quants are available at https://huggingface.co/mradermacher/llama3-8B-DarkIdol-2.1-Uncensored-32K-i1-GGUF
## Usage
If you are unsure how to use GGUF files, refer to one of [TheBloke's
READMEs](https://huggingface.co/TheBloke/KafkaLM-70B-German-V0.1-GGUF) for
more details, including on how to concatenate multi-part files.
## Provided Quants
(sorted by size, not necessarily quality. IQ-quants are often preferable over similar sized non-IQ quants)
| Link | Type | Size/GB | Notes |
|:-----|:-----|--------:|:------|
| [GGUF](https://huggingface.co/mradermacher/llama3-8B-DarkIdol-2.1-Uncensored-32K-GGUF/resolve/main/llama3-8B-DarkIdol-2.1-Uncensored-32K.Q2_K.gguf) | Q2_K | 3.3 | |
| [GGUF](https://huggingface.co/mradermacher/llama3-8B-DarkIdol-2.1-Uncensored-32K-GGUF/resolve/main/llama3-8B-DarkIdol-2.1-Uncensored-32K.IQ3_XS.gguf) | IQ3_XS | 3.6 | |
| [GGUF](https://huggingface.co/mradermacher/llama3-8B-DarkIdol-2.1-Uncensored-32K-GGUF/resolve/main/llama3-8B-DarkIdol-2.1-Uncensored-32K.Q3_K_S.gguf) | Q3_K_S | 3.8 | |
| [GGUF](https://huggingface.co/mradermacher/llama3-8B-DarkIdol-2.1-Uncensored-32K-GGUF/resolve/main/llama3-8B-DarkIdol-2.1-Uncensored-32K.IQ3_S.gguf) | IQ3_S | 3.8 | beats Q3_K* |
| [GGUF](https://huggingface.co/mradermacher/llama3-8B-DarkIdol-2.1-Uncensored-32K-GGUF/resolve/main/llama3-8B-DarkIdol-2.1-Uncensored-32K.IQ3_M.gguf) | IQ3_M | 3.9 | |
| [GGUF](https://huggingface.co/mradermacher/llama3-8B-DarkIdol-2.1-Uncensored-32K-GGUF/resolve/main/llama3-8B-DarkIdol-2.1-Uncensored-32K.Q3_K_M.gguf) | Q3_K_M | 4.1 | lower quality |
| [GGUF](https://huggingface.co/mradermacher/llama3-8B-DarkIdol-2.1-Uncensored-32K-GGUF/resolve/main/llama3-8B-DarkIdol-2.1-Uncensored-32K.Q3_K_L.gguf) | Q3_K_L | 4.4 | |
| [GGUF](https://huggingface.co/mradermacher/llama3-8B-DarkIdol-2.1-Uncensored-32K-GGUF/resolve/main/llama3-8B-DarkIdol-2.1-Uncensored-32K.IQ4_XS.gguf) | IQ4_XS | 4.6 | |
| [GGUF](https://huggingface.co/mradermacher/llama3-8B-DarkIdol-2.1-Uncensored-32K-GGUF/resolve/main/llama3-8B-DarkIdol-2.1-Uncensored-32K.Q4_K_S.gguf) | Q4_K_S | 4.8 | fast, recommended |
| [GGUF](https://huggingface.co/mradermacher/llama3-8B-DarkIdol-2.1-Uncensored-32K-GGUF/resolve/main/llama3-8B-DarkIdol-2.1-Uncensored-32K.Q4_K_M.gguf) | Q4_K_M | 5.0 | fast, recommended |
| [GGUF](https://huggingface.co/mradermacher/llama3-8B-DarkIdol-2.1-Uncensored-32K-GGUF/resolve/main/llama3-8B-DarkIdol-2.1-Uncensored-32K.Q5_K_S.gguf) | Q5_K_S | 5.7 | |
| [GGUF](https://huggingface.co/mradermacher/llama3-8B-DarkIdol-2.1-Uncensored-32K-GGUF/resolve/main/llama3-8B-DarkIdol-2.1-Uncensored-32K.Q5_K_M.gguf) | Q5_K_M | 5.8 | |
| [GGUF](https://huggingface.co/mradermacher/llama3-8B-DarkIdol-2.1-Uncensored-32K-GGUF/resolve/main/llama3-8B-DarkIdol-2.1-Uncensored-32K.Q6_K.gguf) | Q6_K | 6.7 | very good quality |
| [GGUF](https://huggingface.co/mradermacher/llama3-8B-DarkIdol-2.1-Uncensored-32K-GGUF/resolve/main/llama3-8B-DarkIdol-2.1-Uncensored-32K.Q8_0.gguf) | Q8_0 | 8.6 | fast, best quality |
| [GGUF](https://huggingface.co/mradermacher/llama3-8B-DarkIdol-2.1-Uncensored-32K-GGUF/resolve/main/llama3-8B-DarkIdol-2.1-Uncensored-32K.f16.gguf) | f16 | 16.2 | 16 bpw, overkill |
Here is a handy graph by ikawrakow comparing some lower-quality quant
types (lower is better):

And here are Artefact2's thoughts on the matter:
https://gist.github.com/Artefact2/b5f810600771265fc1e39442288e8ec9
## FAQ / Model Request
See https://huggingface.co/mradermacher/model_requests for some answers to
questions you might have and/or if you want some other model quantized.
## Thanks
I thank my company, [nethype GmbH](https://www.nethype.de/), for letting
me use its servers and providing upgrades to my workstation to enable
this work in my free time.
<!-- end -->
|
mradermacher/IceSakeV4RP-7b-i1-GGUF | mradermacher | 2024-06-26T22:04:17Z | 13,842 | 0 | transformers | [
"transformers",
"gguf",
"mergekit",
"merge",
"alpaca",
"mistral",
"not-for-all-audiences",
"nsfw",
"en",
"base_model:icefog72/IceSakeV4RP-7b",
"license:cc-by-nc-4.0",
"endpoints_compatible",
"region:us"
] | null | 2024-06-26T19:52:08Z | ---
base_model: icefog72/IceSakeV4RP-7b
language:
- en
library_name: transformers
license: cc-by-nc-4.0
quantized_by: mradermacher
tags:
- mergekit
- merge
- alpaca
- mistral
- not-for-all-audiences
- nsfw
---
## About
<!-- ### quantize_version: 2 -->
<!-- ### output_tensor_quantised: 1 -->
<!-- ### convert_type: hf -->
<!-- ### vocab_type: -->
<!-- ### tags: nicoboss -->
weighted/imatrix quants of https://huggingface.co/icefog72/IceSakeV4RP-7b
<!-- provided-files -->
static quants are available at https://huggingface.co/mradermacher/IceSakeV4RP-7b-GGUF
## Usage
If you are unsure how to use GGUF files, refer to one of [TheBloke's
READMEs](https://huggingface.co/TheBloke/KafkaLM-70B-German-V0.1-GGUF) for
more details, including on how to concatenate multi-part files.
## Provided Quants
(sorted by size, not necessarily quality. IQ-quants are often preferable over similar sized non-IQ quants)
| Link | Type | Size/GB | Notes |
|:-----|:-----|--------:|:------|
| [GGUF](https://huggingface.co/mradermacher/IceSakeV4RP-7b-i1-GGUF/resolve/main/IceSakeV4RP-7b.i1-IQ1_S.gguf) | i1-IQ1_S | 1.7 | for the desperate |
| [GGUF](https://huggingface.co/mradermacher/IceSakeV4RP-7b-i1-GGUF/resolve/main/IceSakeV4RP-7b.i1-IQ1_M.gguf) | i1-IQ1_M | 1.9 | mostly desperate |
| [GGUF](https://huggingface.co/mradermacher/IceSakeV4RP-7b-i1-GGUF/resolve/main/IceSakeV4RP-7b.i1-IQ2_XXS.gguf) | i1-IQ2_XXS | 2.1 | |
| [GGUF](https://huggingface.co/mradermacher/IceSakeV4RP-7b-i1-GGUF/resolve/main/IceSakeV4RP-7b.i1-IQ2_XS.gguf) | i1-IQ2_XS | 2.3 | |
| [GGUF](https://huggingface.co/mradermacher/IceSakeV4RP-7b-i1-GGUF/resolve/main/IceSakeV4RP-7b.i1-IQ2_S.gguf) | i1-IQ2_S | 2.4 | |
| [GGUF](https://huggingface.co/mradermacher/IceSakeV4RP-7b-i1-GGUF/resolve/main/IceSakeV4RP-7b.i1-IQ2_M.gguf) | i1-IQ2_M | 2.6 | |
| [GGUF](https://huggingface.co/mradermacher/IceSakeV4RP-7b-i1-GGUF/resolve/main/IceSakeV4RP-7b.i1-Q2_K.gguf) | i1-Q2_K | 2.8 | IQ3_XXS probably better |
| [GGUF](https://huggingface.co/mradermacher/IceSakeV4RP-7b-i1-GGUF/resolve/main/IceSakeV4RP-7b.i1-IQ3_XXS.gguf) | i1-IQ3_XXS | 2.9 | lower quality |
| [GGUF](https://huggingface.co/mradermacher/IceSakeV4RP-7b-i1-GGUF/resolve/main/IceSakeV4RP-7b.i1-IQ3_XS.gguf) | i1-IQ3_XS | 3.1 | |
| [GGUF](https://huggingface.co/mradermacher/IceSakeV4RP-7b-i1-GGUF/resolve/main/IceSakeV4RP-7b.i1-Q3_K_S.gguf) | i1-Q3_K_S | 3.3 | IQ3_XS probably better |
| [GGUF](https://huggingface.co/mradermacher/IceSakeV4RP-7b-i1-GGUF/resolve/main/IceSakeV4RP-7b.i1-IQ3_S.gguf) | i1-IQ3_S | 3.3 | beats Q3_K* |
| [GGUF](https://huggingface.co/mradermacher/IceSakeV4RP-7b-i1-GGUF/resolve/main/IceSakeV4RP-7b.i1-IQ3_M.gguf) | i1-IQ3_M | 3.4 | |
| [GGUF](https://huggingface.co/mradermacher/IceSakeV4RP-7b-i1-GGUF/resolve/main/IceSakeV4RP-7b.i1-Q3_K_M.gguf) | i1-Q3_K_M | 3.6 | IQ3_S probably better |
| [GGUF](https://huggingface.co/mradermacher/IceSakeV4RP-7b-i1-GGUF/resolve/main/IceSakeV4RP-7b.i1-Q3_K_L.gguf) | i1-Q3_K_L | 3.9 | IQ3_M probably better |
| [GGUF](https://huggingface.co/mradermacher/IceSakeV4RP-7b-i1-GGUF/resolve/main/IceSakeV4RP-7b.i1-IQ4_XS.gguf) | i1-IQ4_XS | 4.0 | |
| [GGUF](https://huggingface.co/mradermacher/IceSakeV4RP-7b-i1-GGUF/resolve/main/IceSakeV4RP-7b.i1-Q4_0.gguf) | i1-Q4_0 | 4.2 | fast, low quality |
| [GGUF](https://huggingface.co/mradermacher/IceSakeV4RP-7b-i1-GGUF/resolve/main/IceSakeV4RP-7b.i1-Q4_K_S.gguf) | i1-Q4_K_S | 4.2 | optimal size/speed/quality |
| [GGUF](https://huggingface.co/mradermacher/IceSakeV4RP-7b-i1-GGUF/resolve/main/IceSakeV4RP-7b.i1-Q4_K_M.gguf) | i1-Q4_K_M | 4.5 | fast, recommended |
| [GGUF](https://huggingface.co/mradermacher/IceSakeV4RP-7b-i1-GGUF/resolve/main/IceSakeV4RP-7b.i1-Q5_K_S.gguf) | i1-Q5_K_S | 5.1 | |
| [GGUF](https://huggingface.co/mradermacher/IceSakeV4RP-7b-i1-GGUF/resolve/main/IceSakeV4RP-7b.i1-Q5_K_M.gguf) | i1-Q5_K_M | 5.2 | |
| [GGUF](https://huggingface.co/mradermacher/IceSakeV4RP-7b-i1-GGUF/resolve/main/IceSakeV4RP-7b.i1-Q6_K.gguf) | i1-Q6_K | 6.0 | practically like static Q6_K |
Here is a handy graph by ikawrakow comparing some lower-quality quant
types (lower is better):

And here are Artefact2's thoughts on the matter:
https://gist.github.com/Artefact2/b5f810600771265fc1e39442288e8ec9
## FAQ / Model Request
See https://huggingface.co/mradermacher/model_requests for some answers to
questions you might have and/or if you want some other model quantized.
## Thanks
I thank my company, [nethype GmbH](https://www.nethype.de/), for letting
me use its servers and providing upgrades to my workstation to enable
this work in my free time. Additional thanks to [@nicoboss](https://huggingface.co/nicoboss) for giving me access to his hardware for calculating the imatrix for these quants.
<!-- end -->
|
mradermacher/LLaMa-3-CursedStock-v2.0-8B-i1-GGUF | mradermacher | 2024-06-26T21:22:56Z | 13,838 | 0 | transformers | [
"transformers",
"gguf",
"not-for-all-audiences",
"merge",
"mergekit",
"en",
"base_model:PJMixers/LLaMa-3-CursedStock-v2.0-8B",
"license:llama3",
"endpoints_compatible",
"region:us"
] | null | 2024-06-26T18:38:27Z | ---
base_model: PJMixers/LLaMa-3-CursedStock-v2.0-8B
language:
- en
library_name: transformers
license: llama3
quantized_by: mradermacher
tags:
- not-for-all-audiences
- merge
- mergekit
---
## About
<!-- ### quantize_version: 2 -->
<!-- ### output_tensor_quantised: 1 -->
<!-- ### convert_type: hf -->
<!-- ### vocab_type: -->
<!-- ### tags: nicoboss -->
weighted/imatrix quants of https://huggingface.co/PJMixers/LLaMa-3-CursedStock-v2.0-8B
<!-- provided-files -->
static quants are available at https://huggingface.co/mradermacher/LLaMa-3-CursedStock-v2.0-8B-GGUF
## Usage
If you are unsure how to use GGUF files, refer to one of [TheBloke's
READMEs](https://huggingface.co/TheBloke/KafkaLM-70B-German-V0.1-GGUF) for
more details, including on how to concatenate multi-part files.
## Provided Quants
(sorted by size, not necessarily quality. IQ-quants are often preferable over similar sized non-IQ quants)
| Link | Type | Size/GB | Notes |
|:-----|:-----|--------:|:------|
| [GGUF](https://huggingface.co/mradermacher/LLaMa-3-CursedStock-v2.0-8B-i1-GGUF/resolve/main/LLaMa-3-CursedStock-v2.0-8B.i1-IQ1_S.gguf) | i1-IQ1_S | 2.1 | for the desperate |
| [GGUF](https://huggingface.co/mradermacher/LLaMa-3-CursedStock-v2.0-8B-i1-GGUF/resolve/main/LLaMa-3-CursedStock-v2.0-8B.i1-IQ1_M.gguf) | i1-IQ1_M | 2.3 | mostly desperate |
| [GGUF](https://huggingface.co/mradermacher/LLaMa-3-CursedStock-v2.0-8B-i1-GGUF/resolve/main/LLaMa-3-CursedStock-v2.0-8B.i1-IQ2_XXS.gguf) | i1-IQ2_XXS | 2.5 | |
| [GGUF](https://huggingface.co/mradermacher/LLaMa-3-CursedStock-v2.0-8B-i1-GGUF/resolve/main/LLaMa-3-CursedStock-v2.0-8B.i1-IQ2_XS.gguf) | i1-IQ2_XS | 2.7 | |
| [GGUF](https://huggingface.co/mradermacher/LLaMa-3-CursedStock-v2.0-8B-i1-GGUF/resolve/main/LLaMa-3-CursedStock-v2.0-8B.i1-IQ2_S.gguf) | i1-IQ2_S | 2.9 | |
| [GGUF](https://huggingface.co/mradermacher/LLaMa-3-CursedStock-v2.0-8B-i1-GGUF/resolve/main/LLaMa-3-CursedStock-v2.0-8B.i1-IQ2_M.gguf) | i1-IQ2_M | 3.0 | |
| [GGUF](https://huggingface.co/mradermacher/LLaMa-3-CursedStock-v2.0-8B-i1-GGUF/resolve/main/LLaMa-3-CursedStock-v2.0-8B.i1-Q2_K.gguf) | i1-Q2_K | 3.3 | IQ3_XXS probably better |
| [GGUF](https://huggingface.co/mradermacher/LLaMa-3-CursedStock-v2.0-8B-i1-GGUF/resolve/main/LLaMa-3-CursedStock-v2.0-8B.i1-IQ3_XXS.gguf) | i1-IQ3_XXS | 3.4 | lower quality |
| [GGUF](https://huggingface.co/mradermacher/LLaMa-3-CursedStock-v2.0-8B-i1-GGUF/resolve/main/LLaMa-3-CursedStock-v2.0-8B.i1-IQ3_XS.gguf) | i1-IQ3_XS | 3.6 | |
| [GGUF](https://huggingface.co/mradermacher/LLaMa-3-CursedStock-v2.0-8B-i1-GGUF/resolve/main/LLaMa-3-CursedStock-v2.0-8B.i1-Q3_K_S.gguf) | i1-Q3_K_S | 3.8 | IQ3_XS probably better |
| [GGUF](https://huggingface.co/mradermacher/LLaMa-3-CursedStock-v2.0-8B-i1-GGUF/resolve/main/LLaMa-3-CursedStock-v2.0-8B.i1-IQ3_S.gguf) | i1-IQ3_S | 3.8 | beats Q3_K* |
| [GGUF](https://huggingface.co/mradermacher/LLaMa-3-CursedStock-v2.0-8B-i1-GGUF/resolve/main/LLaMa-3-CursedStock-v2.0-8B.i1-IQ3_M.gguf) | i1-IQ3_M | 3.9 | |
| [GGUF](https://huggingface.co/mradermacher/LLaMa-3-CursedStock-v2.0-8B-i1-GGUF/resolve/main/LLaMa-3-CursedStock-v2.0-8B.i1-Q3_K_M.gguf) | i1-Q3_K_M | 4.1 | IQ3_S probably better |
| [GGUF](https://huggingface.co/mradermacher/LLaMa-3-CursedStock-v2.0-8B-i1-GGUF/resolve/main/LLaMa-3-CursedStock-v2.0-8B.i1-Q3_K_L.gguf) | i1-Q3_K_L | 4.4 | IQ3_M probably better |
| [GGUF](https://huggingface.co/mradermacher/LLaMa-3-CursedStock-v2.0-8B-i1-GGUF/resolve/main/LLaMa-3-CursedStock-v2.0-8B.i1-IQ4_XS.gguf) | i1-IQ4_XS | 4.5 | |
| [GGUF](https://huggingface.co/mradermacher/LLaMa-3-CursedStock-v2.0-8B-i1-GGUF/resolve/main/LLaMa-3-CursedStock-v2.0-8B.i1-Q4_0.gguf) | i1-Q4_0 | 4.8 | fast, low quality |
| [GGUF](https://huggingface.co/mradermacher/LLaMa-3-CursedStock-v2.0-8B-i1-GGUF/resolve/main/LLaMa-3-CursedStock-v2.0-8B.i1-Q4_K_S.gguf) | i1-Q4_K_S | 4.8 | optimal size/speed/quality |
| [GGUF](https://huggingface.co/mradermacher/LLaMa-3-CursedStock-v2.0-8B-i1-GGUF/resolve/main/LLaMa-3-CursedStock-v2.0-8B.i1-Q4_K_M.gguf) | i1-Q4_K_M | 5.0 | fast, recommended |
| [GGUF](https://huggingface.co/mradermacher/LLaMa-3-CursedStock-v2.0-8B-i1-GGUF/resolve/main/LLaMa-3-CursedStock-v2.0-8B.i1-Q5_K_S.gguf) | i1-Q5_K_S | 5.7 | |
| [GGUF](https://huggingface.co/mradermacher/LLaMa-3-CursedStock-v2.0-8B-i1-GGUF/resolve/main/LLaMa-3-CursedStock-v2.0-8B.i1-Q5_K_M.gguf) | i1-Q5_K_M | 5.8 | |
| [GGUF](https://huggingface.co/mradermacher/LLaMa-3-CursedStock-v2.0-8B-i1-GGUF/resolve/main/LLaMa-3-CursedStock-v2.0-8B.i1-Q6_K.gguf) | i1-Q6_K | 6.7 | practically like static Q6_K |
Here is a handy graph by ikawrakow comparing some lower-quality quant
types (lower is better):

And here are Artefact2's thoughts on the matter:
https://gist.github.com/Artefact2/b5f810600771265fc1e39442288e8ec9
## FAQ / Model Request
See https://huggingface.co/mradermacher/model_requests for some answers to
questions you might have and/or if you want some other model quantized.
## Thanks
I thank my company, [nethype GmbH](https://www.nethype.de/), for letting
me use its servers and providing upgrades to my workstation to enable
this work in my free time. Additional thanks to [@nicoboss](https://huggingface.co/nicoboss) for giving me access to his hardware for calculating the imatrix for these quants.
<!-- end -->
|
facebook/wav2vec2-large-es-voxpopuli | facebook | 2021-07-06T02:07:04Z | 13,837 | 1 | transformers | [
"transformers",
"pytorch",
"jax",
"wav2vec2",
"pretraining",
"audio",
"automatic-speech-recognition",
"voxpopuli",
"es",
"arxiv:2101.00390",
"license:cc-by-nc-4.0",
"endpoints_compatible",
"region:us"
] | automatic-speech-recognition | 2022-03-02T23:29:05Z | ---
language: es
tags:
- audio
- automatic-speech-recognition
- voxpopuli
license: cc-by-nc-4.0
---
# Wav2Vec2-Large-VoxPopuli
[Facebook's Wav2Vec2](https://ai.facebook.com/blog/wav2vec-20-learning-the-structure-of-speech-from-raw-audio/) large model pretrained on the es unlabeled subset of [VoxPopuli corpus](https://arxiv.org/abs/2101.00390).
**Paper**: *[VoxPopuli: A Large-Scale Multilingual Speech Corpus for Representation
Learning, Semi-Supervised Learning and Interpretation](https://arxiv.org/abs/2101.00390)*
**Authors**: *Changhan Wang, Morgane Riviere, Ann Lee, Anne Wu, Chaitanya Talnikar, Daniel Haziza, Mary Williamson, Juan Pino, Emmanuel Dupoux* from *Facebook AI*
See the official website for more information, [here](https://github.com/facebookresearch/voxpopuli/)
# Fine-Tuning
Please refer to [this blog](https://huggingface.co/blog/fine-tune-xlsr-wav2vec2) on how to fine-tune this model on a specific language. Note that you should replace `"facebook/wav2vec2-large-xlsr-53"` with this checkpoint for fine-tuning.
|
mradermacher/Yi-1.5-9B-32K-i1-GGUF | mradermacher | 2024-06-26T19:44:52Z | 13,836 | 0 | transformers | [
"transformers",
"gguf",
"en",
"base_model:01-ai/Yi-1.5-9B-32K",
"license:apache-2.0",
"endpoints_compatible",
"region:us"
] | null | 2024-06-26T18:19:05Z | ---
base_model: 01-ai/Yi-1.5-9B-32K
language:
- en
library_name: transformers
license: apache-2.0
quantized_by: mradermacher
---
## About
<!-- ### quantize_version: 2 -->
<!-- ### output_tensor_quantised: 1 -->
<!-- ### convert_type: hf -->
<!-- ### vocab_type: -->
<!-- ### tags: nicoboss -->
weighted/imatrix quants of https://huggingface.co/01-ai/Yi-1.5-9B-32K
<!-- provided-files -->
static quants are available at https://huggingface.co/mradermacher/Yi-1.5-9B-32K-GGUF
## Usage
If you are unsure how to use GGUF files, refer to one of [TheBloke's
READMEs](https://huggingface.co/TheBloke/KafkaLM-70B-German-V0.1-GGUF) for
more details, including on how to concatenate multi-part files.
## Provided Quants
(sorted by size, not necessarily quality. IQ-quants are often preferable over similar sized non-IQ quants)
| Link | Type | Size/GB | Notes |
|:-----|:-----|--------:|:------|
| [GGUF](https://huggingface.co/mradermacher/Yi-1.5-9B-32K-i1-GGUF/resolve/main/Yi-1.5-9B-32K.i1-IQ1_S.gguf) | i1-IQ1_S | 2.1 | for the desperate |
| [GGUF](https://huggingface.co/mradermacher/Yi-1.5-9B-32K-i1-GGUF/resolve/main/Yi-1.5-9B-32K.i1-IQ1_M.gguf) | i1-IQ1_M | 2.3 | mostly desperate |
| [GGUF](https://huggingface.co/mradermacher/Yi-1.5-9B-32K-i1-GGUF/resolve/main/Yi-1.5-9B-32K.i1-IQ2_XXS.gguf) | i1-IQ2_XXS | 2.6 | |
| [GGUF](https://huggingface.co/mradermacher/Yi-1.5-9B-32K-i1-GGUF/resolve/main/Yi-1.5-9B-32K.i1-IQ2_XS.gguf) | i1-IQ2_XS | 2.8 | |
| [GGUF](https://huggingface.co/mradermacher/Yi-1.5-9B-32K-i1-GGUF/resolve/main/Yi-1.5-9B-32K.i1-IQ2_S.gguf) | i1-IQ2_S | 3.0 | |
| [GGUF](https://huggingface.co/mradermacher/Yi-1.5-9B-32K-i1-GGUF/resolve/main/Yi-1.5-9B-32K.i1-IQ2_M.gguf) | i1-IQ2_M | 3.2 | |
| [GGUF](https://huggingface.co/mradermacher/Yi-1.5-9B-32K-i1-GGUF/resolve/main/Yi-1.5-9B-32K.i1-Q2_K.gguf) | i1-Q2_K | 3.5 | IQ3_XXS probably better |
| [GGUF](https://huggingface.co/mradermacher/Yi-1.5-9B-32K-i1-GGUF/resolve/main/Yi-1.5-9B-32K.i1-IQ3_XXS.gguf) | i1-IQ3_XXS | 3.6 | lower quality |
| [GGUF](https://huggingface.co/mradermacher/Yi-1.5-9B-32K-i1-GGUF/resolve/main/Yi-1.5-9B-32K.i1-IQ3_XS.gguf) | i1-IQ3_XS | 3.8 | |
| [GGUF](https://huggingface.co/mradermacher/Yi-1.5-9B-32K-i1-GGUF/resolve/main/Yi-1.5-9B-32K.i1-Q3_K_S.gguf) | i1-Q3_K_S | 4.0 | IQ3_XS probably better |
| [GGUF](https://huggingface.co/mradermacher/Yi-1.5-9B-32K-i1-GGUF/resolve/main/Yi-1.5-9B-32K.i1-IQ3_S.gguf) | i1-IQ3_S | 4.0 | beats Q3_K* |
| [GGUF](https://huggingface.co/mradermacher/Yi-1.5-9B-32K-i1-GGUF/resolve/main/Yi-1.5-9B-32K.i1-IQ3_M.gguf) | i1-IQ3_M | 4.2 | |
| [GGUF](https://huggingface.co/mradermacher/Yi-1.5-9B-32K-i1-GGUF/resolve/main/Yi-1.5-9B-32K.i1-Q3_K_M.gguf) | i1-Q3_K_M | 4.4 | IQ3_S probably better |
| [GGUF](https://huggingface.co/mradermacher/Yi-1.5-9B-32K-i1-GGUF/resolve/main/Yi-1.5-9B-32K.i1-Q3_K_L.gguf) | i1-Q3_K_L | 4.8 | IQ3_M probably better |
| [GGUF](https://huggingface.co/mradermacher/Yi-1.5-9B-32K-i1-GGUF/resolve/main/Yi-1.5-9B-32K.i1-IQ4_XS.gguf) | i1-IQ4_XS | 4.9 | |
| [GGUF](https://huggingface.co/mradermacher/Yi-1.5-9B-32K-i1-GGUF/resolve/main/Yi-1.5-9B-32K.i1-Q4_0.gguf) | i1-Q4_0 | 5.2 | fast, low quality |
| [GGUF](https://huggingface.co/mradermacher/Yi-1.5-9B-32K-i1-GGUF/resolve/main/Yi-1.5-9B-32K.i1-Q4_K_S.gguf) | i1-Q4_K_S | 5.2 | optimal size/speed/quality |
| [GGUF](https://huggingface.co/mradermacher/Yi-1.5-9B-32K-i1-GGUF/resolve/main/Yi-1.5-9B-32K.i1-Q4_K_M.gguf) | i1-Q4_K_M | 5.4 | fast, recommended |
| [GGUF](https://huggingface.co/mradermacher/Yi-1.5-9B-32K-i1-GGUF/resolve/main/Yi-1.5-9B-32K.i1-Q5_K_S.gguf) | i1-Q5_K_S | 6.2 | |
| [GGUF](https://huggingface.co/mradermacher/Yi-1.5-9B-32K-i1-GGUF/resolve/main/Yi-1.5-9B-32K.i1-Q5_K_M.gguf) | i1-Q5_K_M | 6.4 | |
| [GGUF](https://huggingface.co/mradermacher/Yi-1.5-9B-32K-i1-GGUF/resolve/main/Yi-1.5-9B-32K.i1-Q6_K.gguf) | i1-Q6_K | 7.3 | practically like static Q6_K |
Here is a handy graph by ikawrakow comparing some lower-quality quant
types (lower is better):

And here are Artefact2's thoughts on the matter:
https://gist.github.com/Artefact2/b5f810600771265fc1e39442288e8ec9
## FAQ / Model Request
See https://huggingface.co/mradermacher/model_requests for some answers to
questions you might have and/or if you want some other model quantized.
## Thanks
I thank my company, [nethype GmbH](https://www.nethype.de/), for letting
me use its servers and providing upgrades to my workstation to enable
this work in my free time. Additional thanks to [@nicoboss](https://huggingface.co/nicoboss) for giving me access to his hardware for calculating the imatrix for these quants.
<!-- end -->
|
RichardErkhov/Eric111_-_Yarn-Mistral-7b-128k-DPO-gguf | RichardErkhov | 2024-06-19T20:46:21Z | 13,830 | 0 | null | [
"gguf",
"arxiv:1910.09700",
"region:us"
] | null | 2024-06-19T14:54:44Z | Quantization made by Richard Erkhov.
[Github](https://github.com/RichardErkhov)
[Discord](https://discord.gg/pvy7H8DZMG)
[Request more models](https://github.com/RichardErkhov/quant_request)
Yarn-Mistral-7b-128k-DPO - GGUF
- Model creator: https://huggingface.co/Eric111/
- Original model: https://huggingface.co/Eric111/Yarn-Mistral-7b-128k-DPO/
| Name | Quant method | Size |
| ---- | ---- | ---- |
| [Yarn-Mistral-7b-128k-DPO.Q2_K.gguf](https://huggingface.co/RichardErkhov/Eric111_-_Yarn-Mistral-7b-128k-DPO-gguf/blob/main/Yarn-Mistral-7b-128k-DPO.Q2_K.gguf) | Q2_K | 2.53GB |
| [Yarn-Mistral-7b-128k-DPO.IQ3_XS.gguf](https://huggingface.co/RichardErkhov/Eric111_-_Yarn-Mistral-7b-128k-DPO-gguf/blob/main/Yarn-Mistral-7b-128k-DPO.IQ3_XS.gguf) | IQ3_XS | 2.81GB |
| [Yarn-Mistral-7b-128k-DPO.IQ3_S.gguf](https://huggingface.co/RichardErkhov/Eric111_-_Yarn-Mistral-7b-128k-DPO-gguf/blob/main/Yarn-Mistral-7b-128k-DPO.IQ3_S.gguf) | IQ3_S | 2.96GB |
| [Yarn-Mistral-7b-128k-DPO.Q3_K_S.gguf](https://huggingface.co/RichardErkhov/Eric111_-_Yarn-Mistral-7b-128k-DPO-gguf/blob/main/Yarn-Mistral-7b-128k-DPO.Q3_K_S.gguf) | Q3_K_S | 2.95GB |
| [Yarn-Mistral-7b-128k-DPO.IQ3_M.gguf](https://huggingface.co/RichardErkhov/Eric111_-_Yarn-Mistral-7b-128k-DPO-gguf/blob/main/Yarn-Mistral-7b-128k-DPO.IQ3_M.gguf) | IQ3_M | 3.06GB |
| [Yarn-Mistral-7b-128k-DPO.Q3_K.gguf](https://huggingface.co/RichardErkhov/Eric111_-_Yarn-Mistral-7b-128k-DPO-gguf/blob/main/Yarn-Mistral-7b-128k-DPO.Q3_K.gguf) | Q3_K | 3.28GB |
| [Yarn-Mistral-7b-128k-DPO.Q3_K_M.gguf](https://huggingface.co/RichardErkhov/Eric111_-_Yarn-Mistral-7b-128k-DPO-gguf/blob/main/Yarn-Mistral-7b-128k-DPO.Q3_K_M.gguf) | Q3_K_M | 3.28GB |
| [Yarn-Mistral-7b-128k-DPO.Q3_K_L.gguf](https://huggingface.co/RichardErkhov/Eric111_-_Yarn-Mistral-7b-128k-DPO-gguf/blob/main/Yarn-Mistral-7b-128k-DPO.Q3_K_L.gguf) | Q3_K_L | 3.56GB |
| [Yarn-Mistral-7b-128k-DPO.IQ4_XS.gguf](https://huggingface.co/RichardErkhov/Eric111_-_Yarn-Mistral-7b-128k-DPO-gguf/blob/main/Yarn-Mistral-7b-128k-DPO.IQ4_XS.gguf) | IQ4_XS | 3.67GB |
| [Yarn-Mistral-7b-128k-DPO.Q4_0.gguf](https://huggingface.co/RichardErkhov/Eric111_-_Yarn-Mistral-7b-128k-DPO-gguf/blob/main/Yarn-Mistral-7b-128k-DPO.Q4_0.gguf) | Q4_0 | 3.83GB |
| [Yarn-Mistral-7b-128k-DPO.IQ4_NL.gguf](https://huggingface.co/RichardErkhov/Eric111_-_Yarn-Mistral-7b-128k-DPO-gguf/blob/main/Yarn-Mistral-7b-128k-DPO.IQ4_NL.gguf) | IQ4_NL | 3.87GB |
| [Yarn-Mistral-7b-128k-DPO.Q4_K_S.gguf](https://huggingface.co/RichardErkhov/Eric111_-_Yarn-Mistral-7b-128k-DPO-gguf/blob/main/Yarn-Mistral-7b-128k-DPO.Q4_K_S.gguf) | Q4_K_S | 3.86GB |
| [Yarn-Mistral-7b-128k-DPO.Q4_K.gguf](https://huggingface.co/RichardErkhov/Eric111_-_Yarn-Mistral-7b-128k-DPO-gguf/blob/main/Yarn-Mistral-7b-128k-DPO.Q4_K.gguf) | Q4_K | 4.07GB |
| [Yarn-Mistral-7b-128k-DPO.Q4_K_M.gguf](https://huggingface.co/RichardErkhov/Eric111_-_Yarn-Mistral-7b-128k-DPO-gguf/blob/main/Yarn-Mistral-7b-128k-DPO.Q4_K_M.gguf) | Q4_K_M | 4.07GB |
| [Yarn-Mistral-7b-128k-DPO.Q4_1.gguf](https://huggingface.co/RichardErkhov/Eric111_-_Yarn-Mistral-7b-128k-DPO-gguf/blob/main/Yarn-Mistral-7b-128k-DPO.Q4_1.gguf) | Q4_1 | 4.24GB |
| [Yarn-Mistral-7b-128k-DPO.Q5_0.gguf](https://huggingface.co/RichardErkhov/Eric111_-_Yarn-Mistral-7b-128k-DPO-gguf/blob/main/Yarn-Mistral-7b-128k-DPO.Q5_0.gguf) | Q5_0 | 4.65GB |
| [Yarn-Mistral-7b-128k-DPO.Q5_K_S.gguf](https://huggingface.co/RichardErkhov/Eric111_-_Yarn-Mistral-7b-128k-DPO-gguf/blob/main/Yarn-Mistral-7b-128k-DPO.Q5_K_S.gguf) | Q5_K_S | 4.65GB |
| [Yarn-Mistral-7b-128k-DPO.Q5_K.gguf](https://huggingface.co/RichardErkhov/Eric111_-_Yarn-Mistral-7b-128k-DPO-gguf/blob/main/Yarn-Mistral-7b-128k-DPO.Q5_K.gguf) | Q5_K | 4.78GB |
| [Yarn-Mistral-7b-128k-DPO.Q5_K_M.gguf](https://huggingface.co/RichardErkhov/Eric111_-_Yarn-Mistral-7b-128k-DPO-gguf/blob/main/Yarn-Mistral-7b-128k-DPO.Q5_K_M.gguf) | Q5_K_M | 4.78GB |
| [Yarn-Mistral-7b-128k-DPO.Q5_1.gguf](https://huggingface.co/RichardErkhov/Eric111_-_Yarn-Mistral-7b-128k-DPO-gguf/blob/main/Yarn-Mistral-7b-128k-DPO.Q5_1.gguf) | Q5_1 | 5.07GB |
| [Yarn-Mistral-7b-128k-DPO.Q6_K.gguf](https://huggingface.co/RichardErkhov/Eric111_-_Yarn-Mistral-7b-128k-DPO-gguf/blob/main/Yarn-Mistral-7b-128k-DPO.Q6_K.gguf) | Q6_K | 5.53GB |
| [Yarn-Mistral-7b-128k-DPO.Q8_0.gguf](https://huggingface.co/RichardErkhov/Eric111_-_Yarn-Mistral-7b-128k-DPO-gguf/blob/main/Yarn-Mistral-7b-128k-DPO.Q8_0.gguf) | Q8_0 | 7.17GB |
Original model description:
---
library_name: transformers
license: apache-2.0
tags: []
---
# Model Card for Model ID
<!-- Provide a quick summary of what the model is/does. -->
## Model Details
DPO fine-tuned version of NousResearch/Yarn-Mistral-7b-128k with Intel/orca_dpo_pairs
### Model Description
<!-- Provide a longer summary of what this model is. -->
This is the model card of a 🤗 transformers model that has been pushed on the Hub. This model card has been automatically generated.
- **Developed by:** [More Information Needed]
- **Funded by [optional]:** [More Information Needed]
- **Shared by [optional]:** [More Information Needed]
- **Model type:** [More Information Needed]
- **Language(s) (NLP):** [More Information Needed]
- **License:** [More Information Needed]
- **Finetuned from model [optional]:** [More Information Needed]
### Model Sources [optional]
<!-- Provide the basic links for the model. -->
- **Repository:** [More Information Needed]
- **Paper [optional]:** [More Information Needed]
- **Demo [optional]:** [More Information Needed]
## Uses
<!-- Address questions around how the model is intended to be used, including the foreseeable users of the model and those affected by the model. -->
### Direct Use
<!-- This section is for the model use without fine-tuning or plugging into a larger ecosystem/app. -->
[More Information Needed]
### Downstream Use [optional]
<!-- This section is for the model use when fine-tuned for a task, or when plugged into a larger ecosystem/app -->
[More Information Needed]
### Out-of-Scope Use
<!-- This section addresses misuse, malicious use, and uses that the model will not work well for. -->
[More Information Needed]
## Bias, Risks, and Limitations
<!-- This section is meant to convey both technical and sociotechnical limitations. -->
[More Information Needed]
### Recommendations
<!-- This section is meant to convey recommendations with respect to the bias, risk, and technical limitations. -->
Users (both direct and downstream) should be made aware of the risks, biases and limitations of the model. More information needed for further recommendations.
## How to Get Started with the Model
Use the code below to get started with the model.
[More Information Needed]
## Training Details
### Training Data
<!-- This should link to a Dataset Card, perhaps with a short stub of information on what the training data is all about as well as documentation related to data pre-processing or additional filtering. -->
[More Information Needed]
### Training Procedure
<!-- This relates heavily to the Technical Specifications. Content here should link to that section when it is relevant to the training procedure. -->
#### Preprocessing [optional]
[More Information Needed]
#### Training Hyperparameters
- **Training regime:** [More Information Needed] <!--fp32, fp16 mixed precision, bf16 mixed precision, bf16 non-mixed precision, fp16 non-mixed precision, fp8 mixed precision -->
#### Speeds, Sizes, Times [optional]
<!-- This section provides information about throughput, start/end time, checkpoint size if relevant, etc. -->
[More Information Needed]
## Evaluation
<!-- This section describes the evaluation protocols and provides the results. -->
### Testing Data, Factors & Metrics
#### Testing Data
<!-- This should link to a Dataset Card if possible. -->
[More Information Needed]
#### Factors
<!-- These are the things the evaluation is disaggregating by, e.g., subpopulations or domains. -->
[More Information Needed]
#### Metrics
<!-- These are the evaluation metrics being used, ideally with a description of why. -->
[More Information Needed]
### Results
[More Information Needed]
#### Summary
## Model Examination [optional]
<!-- Relevant interpretability work for the model goes here -->
[More Information Needed]
## Environmental Impact
<!-- Total emissions (in grams of CO2eq) and additional considerations, such as electricity usage, go here. Edit the suggested text below accordingly -->
Carbon emissions can be estimated using the [Machine Learning Impact calculator](https://mlco2.github.io/impact#compute) presented in [Lacoste et al. (2019)](https://arxiv.org/abs/1910.09700).
- **Hardware Type:** [More Information Needed]
- **Hours used:** [More Information Needed]
- **Cloud Provider:** [More Information Needed]
- **Compute Region:** [More Information Needed]
- **Carbon Emitted:** [More Information Needed]
## Technical Specifications [optional]
### Model Architecture and Objective
[More Information Needed]
### Compute Infrastructure
[More Information Needed]
#### Hardware
[More Information Needed]
#### Software
[More Information Needed]
## Citation [optional]
<!-- If there is a paper or blog post introducing the model, the APA and Bibtex information for that should go in this section. -->
**BibTeX:**
[More Information Needed]
**APA:**
[More Information Needed]
## Glossary [optional]
<!-- If relevant, include terms and calculations in this section that can help readers understand the model or model card. -->
[More Information Needed]
## More Information [optional]
[More Information Needed]
## Model Card Authors [optional]
[More Information Needed]
## Model Card Contact
[More Information Needed]
|
RichardErkhov/automerger_-_YamshadowExperiment28-7B-gguf | RichardErkhov | 2024-06-25T11:15:18Z | 13,830 | 0 | null | [
"gguf",
"region:us"
] | null | 2024-06-25T07:23:37Z | Quantization made by Richard Erkhov.
[Github](https://github.com/RichardErkhov)
[Discord](https://discord.gg/pvy7H8DZMG)
[Request more models](https://github.com/RichardErkhov/quant_request)
YamshadowExperiment28-7B - GGUF
- Model creator: https://huggingface.co/automerger/
- Original model: https://huggingface.co/automerger/YamshadowExperiment28-7B/
| Name | Quant method | Size |
| ---- | ---- | ---- |
| [YamshadowExperiment28-7B.Q2_K.gguf](https://huggingface.co/RichardErkhov/automerger_-_YamshadowExperiment28-7B-gguf/blob/main/YamshadowExperiment28-7B.Q2_K.gguf) | Q2_K | 2.53GB |
| [YamshadowExperiment28-7B.IQ3_XS.gguf](https://huggingface.co/RichardErkhov/automerger_-_YamshadowExperiment28-7B-gguf/blob/main/YamshadowExperiment28-7B.IQ3_XS.gguf) | IQ3_XS | 2.81GB |
| [YamshadowExperiment28-7B.IQ3_S.gguf](https://huggingface.co/RichardErkhov/automerger_-_YamshadowExperiment28-7B-gguf/blob/main/YamshadowExperiment28-7B.IQ3_S.gguf) | IQ3_S | 2.96GB |
| [YamshadowExperiment28-7B.Q3_K_S.gguf](https://huggingface.co/RichardErkhov/automerger_-_YamshadowExperiment28-7B-gguf/blob/main/YamshadowExperiment28-7B.Q3_K_S.gguf) | Q3_K_S | 2.95GB |
| [YamshadowExperiment28-7B.IQ3_M.gguf](https://huggingface.co/RichardErkhov/automerger_-_YamshadowExperiment28-7B-gguf/blob/main/YamshadowExperiment28-7B.IQ3_M.gguf) | IQ3_M | 3.06GB |
| [YamshadowExperiment28-7B.Q3_K.gguf](https://huggingface.co/RichardErkhov/automerger_-_YamshadowExperiment28-7B-gguf/blob/main/YamshadowExperiment28-7B.Q3_K.gguf) | Q3_K | 3.28GB |
| [YamshadowExperiment28-7B.Q3_K_M.gguf](https://huggingface.co/RichardErkhov/automerger_-_YamshadowExperiment28-7B-gguf/blob/main/YamshadowExperiment28-7B.Q3_K_M.gguf) | Q3_K_M | 3.28GB |
| [YamshadowExperiment28-7B.Q3_K_L.gguf](https://huggingface.co/RichardErkhov/automerger_-_YamshadowExperiment28-7B-gguf/blob/main/YamshadowExperiment28-7B.Q3_K_L.gguf) | Q3_K_L | 3.56GB |
| [YamshadowExperiment28-7B.IQ4_XS.gguf](https://huggingface.co/RichardErkhov/automerger_-_YamshadowExperiment28-7B-gguf/blob/main/YamshadowExperiment28-7B.IQ4_XS.gguf) | IQ4_XS | 3.67GB |
| [YamshadowExperiment28-7B.Q4_0.gguf](https://huggingface.co/RichardErkhov/automerger_-_YamshadowExperiment28-7B-gguf/blob/main/YamshadowExperiment28-7B.Q4_0.gguf) | Q4_0 | 3.83GB |
| [YamshadowExperiment28-7B.IQ4_NL.gguf](https://huggingface.co/RichardErkhov/automerger_-_YamshadowExperiment28-7B-gguf/blob/main/YamshadowExperiment28-7B.IQ4_NL.gguf) | IQ4_NL | 3.87GB |
| [YamshadowExperiment28-7B.Q4_K_S.gguf](https://huggingface.co/RichardErkhov/automerger_-_YamshadowExperiment28-7B-gguf/blob/main/YamshadowExperiment28-7B.Q4_K_S.gguf) | Q4_K_S | 3.86GB |
| [YamshadowExperiment28-7B.Q4_K.gguf](https://huggingface.co/RichardErkhov/automerger_-_YamshadowExperiment28-7B-gguf/blob/main/YamshadowExperiment28-7B.Q4_K.gguf) | Q4_K | 4.07GB |
| [YamshadowExperiment28-7B.Q4_K_M.gguf](https://huggingface.co/RichardErkhov/automerger_-_YamshadowExperiment28-7B-gguf/blob/main/YamshadowExperiment28-7B.Q4_K_M.gguf) | Q4_K_M | 4.07GB |
| [YamshadowExperiment28-7B.Q4_1.gguf](https://huggingface.co/RichardErkhov/automerger_-_YamshadowExperiment28-7B-gguf/blob/main/YamshadowExperiment28-7B.Q4_1.gguf) | Q4_1 | 4.24GB |
| [YamshadowExperiment28-7B.Q5_0.gguf](https://huggingface.co/RichardErkhov/automerger_-_YamshadowExperiment28-7B-gguf/blob/main/YamshadowExperiment28-7B.Q5_0.gguf) | Q5_0 | 4.65GB |
| [YamshadowExperiment28-7B.Q5_K_S.gguf](https://huggingface.co/RichardErkhov/automerger_-_YamshadowExperiment28-7B-gguf/blob/main/YamshadowExperiment28-7B.Q5_K_S.gguf) | Q5_K_S | 4.65GB |
| [YamshadowExperiment28-7B.Q5_K.gguf](https://huggingface.co/RichardErkhov/automerger_-_YamshadowExperiment28-7B-gguf/blob/main/YamshadowExperiment28-7B.Q5_K.gguf) | Q5_K | 4.78GB |
| [YamshadowExperiment28-7B.Q5_K_M.gguf](https://huggingface.co/RichardErkhov/automerger_-_YamshadowExperiment28-7B-gguf/blob/main/YamshadowExperiment28-7B.Q5_K_M.gguf) | Q5_K_M | 4.78GB |
| [YamshadowExperiment28-7B.Q5_1.gguf](https://huggingface.co/RichardErkhov/automerger_-_YamshadowExperiment28-7B-gguf/blob/main/YamshadowExperiment28-7B.Q5_1.gguf) | Q5_1 | 5.07GB |
| [YamshadowExperiment28-7B.Q6_K.gguf](https://huggingface.co/RichardErkhov/automerger_-_YamshadowExperiment28-7B-gguf/blob/main/YamshadowExperiment28-7B.Q6_K.gguf) | Q6_K | 5.53GB |
| [YamshadowExperiment28-7B.Q8_0.gguf](https://huggingface.co/RichardErkhov/automerger_-_YamshadowExperiment28-7B-gguf/blob/main/YamshadowExperiment28-7B.Q8_0.gguf) | Q8_0 | 7.17GB |
Original model description:
---
license: apache-2.0
tags:
- merge
- mergekit
- lazymergekit
- automerger
base_model:
- automerger/YamShadow-7B
- yam-peleg/Experiment28-7B
---
# 🧪 YamshadowExperiment28-7B

**🎉 YamshadowExperiment28-7B is currently the best-performing 7B model on the Open LLM Leaderboard (08 Apr 24). Use it with caution, as it is likely a sign of overfitting the benchmarks.**
YamshadowExperiment28-7B is an automated merge created by [Maxime Labonne](https://huggingface.co/mlabonne) using the following configuration.
* [automerger/YamShadow-7B](https://huggingface.co/automerger/YamShadow-7B)
* [yam-peleg/Experiment28-7B](https://huggingface.co/yam-peleg/Experiment28-7B)
## 🔍 Applications
This model uses a context window of 8k. I recommend using it with the Alpaca chat template (works perfectly with LM Studio).
The model can sometimes break and output a lot of "INST". From my experience, its excellent results on the Open LLM Leaderboard are probably a sign of overfitting.
## ⚡ Quantized models
* **GGUF**: https://huggingface.co/automerger/YamshadowExperiment28-7B-GGUF
## 🏆 Evaluation
### Open LLM Leaderboard
YamshadowExperiment28-7B is currently the best-performing 7B model on the Open LLM Leaderboard (08 Apr 24).

### EQ-bench
Thanks to [Samuel J. Paech](https://twitter.com/sam_paech), who kindly ran the evaluation.

### Nous
Evaluation performed using [LLM AutoEval](https://github.com/mlabonne/llm-autoeval). See the entire leaderboard [here](https://huggingface.co/spaces/mlabonne/Yet_Another_LLM_Leaderboard).

## 🌳 Model Family Tree

## 🧩 Configuration
```yaml
slices:
- sources:
- model: automerger/YamShadow-7B
layer_range: [0, 32]
- model: yam-peleg/Experiment28-7B
layer_range: [0, 32]
merge_method: slerp
base_model: automerger/YamShadow-7B
parameters:
t:
- filter: self_attn
value: [0, 0.5, 0.3, 0.7, 1]
- filter: mlp
value: [1, 0.5, 0.7, 0.3, 0]
- value: 0.5
dtype: bfloat16
random_seed: 0
```
## 💻 Usage
```python
!pip install -qU transformers accelerate
from transformers import AutoTokenizer
import transformers
import torch
model = "automerger/YamshadowExperiment28-7B"
messages = [{"role": "user", "content": "What is a large language model?"}]
tokenizer = AutoTokenizer.from_pretrained(model)
prompt = tokenizer.apply_chat_template(messages, tokenize=False, add_generation_prompt=True)
pipeline = transformers.pipeline(
"text-generation",
model=model,
torch_dtype=torch.float16,
device_map="auto",
)
outputs = pipeline(prompt, max_new_tokens=256, do_sample=True, temperature=0.7, top_k=50, top_p=0.95)
print(outputs[0]["generated_text"])
```
|
mradermacher/Swallow-7b-plus-hf-GGUF | mradermacher | 2024-06-30T13:47:58Z | 13,824 | 0 | transformers | [
"transformers",
"gguf",
"en",
"ja",
"base_model:tokyotech-llm/Swallow-7b-plus-hf",
"license:llama2",
"endpoints_compatible",
"region:us"
] | null | 2024-06-29T19:43:42Z | ---
base_model: tokyotech-llm/Swallow-7b-plus-hf
language:
- en
- ja
library_name: transformers
license: llama2
model_type: llama
quantized_by: mradermacher
---
## About
<!-- ### quantize_version: 2 -->
<!-- ### output_tensor_quantised: 1 -->
<!-- ### convert_type: hf -->
<!-- ### vocab_type: -->
<!-- ### tags: -->
static quants of https://huggingface.co/tokyotech-llm/Swallow-7b-plus-hf
<!-- provided-files -->
weighted/imatrix quants are available at https://huggingface.co/mradermacher/Swallow-7b-plus-hf-i1-GGUF
## Usage
If you are unsure how to use GGUF files, refer to one of [TheBloke's
READMEs](https://huggingface.co/TheBloke/KafkaLM-70B-German-V0.1-GGUF) for
more details, including on how to concatenate multi-part files.
## Provided Quants
(sorted by size, not necessarily quality. IQ-quants are often preferable over similar sized non-IQ quants)
| Link | Type | Size/GB | Notes |
|:-----|:-----|--------:|:------|
| [GGUF](https://huggingface.co/mradermacher/Swallow-7b-plus-hf-GGUF/resolve/main/Swallow-7b-plus-hf.Q2_K.gguf) | Q2_K | 2.7 | |
| [GGUF](https://huggingface.co/mradermacher/Swallow-7b-plus-hf-GGUF/resolve/main/Swallow-7b-plus-hf.IQ3_XS.gguf) | IQ3_XS | 3.0 | |
| [GGUF](https://huggingface.co/mradermacher/Swallow-7b-plus-hf-GGUF/resolve/main/Swallow-7b-plus-hf.IQ3_S.gguf) | IQ3_S | 3.1 | beats Q3_K* |
| [GGUF](https://huggingface.co/mradermacher/Swallow-7b-plus-hf-GGUF/resolve/main/Swallow-7b-plus-hf.Q3_K_S.gguf) | Q3_K_S | 3.1 | |
| [GGUF](https://huggingface.co/mradermacher/Swallow-7b-plus-hf-GGUF/resolve/main/Swallow-7b-plus-hf.IQ3_M.gguf) | IQ3_M | 3.3 | |
| [GGUF](https://huggingface.co/mradermacher/Swallow-7b-plus-hf-GGUF/resolve/main/Swallow-7b-plus-hf.Q3_K_M.gguf) | Q3_K_M | 3.5 | lower quality |
| [GGUF](https://huggingface.co/mradermacher/Swallow-7b-plus-hf-GGUF/resolve/main/Swallow-7b-plus-hf.Q3_K_L.gguf) | Q3_K_L | 3.8 | |
| [GGUF](https://huggingface.co/mradermacher/Swallow-7b-plus-hf-GGUF/resolve/main/Swallow-7b-plus-hf.IQ4_XS.gguf) | IQ4_XS | 3.8 | |
| [GGUF](https://huggingface.co/mradermacher/Swallow-7b-plus-hf-GGUF/resolve/main/Swallow-7b-plus-hf.Q4_K_S.gguf) | Q4_K_S | 4.0 | fast, recommended |
| [GGUF](https://huggingface.co/mradermacher/Swallow-7b-plus-hf-GGUF/resolve/main/Swallow-7b-plus-hf.Q4_K_M.gguf) | Q4_K_M | 4.2 | fast, recommended |
| [GGUF](https://huggingface.co/mradermacher/Swallow-7b-plus-hf-GGUF/resolve/main/Swallow-7b-plus-hf.Q5_K_S.gguf) | Q5_K_S | 4.8 | |
| [GGUF](https://huggingface.co/mradermacher/Swallow-7b-plus-hf-GGUF/resolve/main/Swallow-7b-plus-hf.Q5_K_M.gguf) | Q5_K_M | 5.0 | |
| [GGUF](https://huggingface.co/mradermacher/Swallow-7b-plus-hf-GGUF/resolve/main/Swallow-7b-plus-hf.Q6_K.gguf) | Q6_K | 5.7 | very good quality |
| [GGUF](https://huggingface.co/mradermacher/Swallow-7b-plus-hf-GGUF/resolve/main/Swallow-7b-plus-hf.Q8_0.gguf) | Q8_0 | 7.4 | fast, best quality |
| [GGUF](https://huggingface.co/mradermacher/Swallow-7b-plus-hf-GGUF/resolve/main/Swallow-7b-plus-hf.f16.gguf) | f16 | 13.8 | 16 bpw, overkill |
Here is a handy graph by ikawrakow comparing some lower-quality quant
types (lower is better):

And here are Artefact2's thoughts on the matter:
https://gist.github.com/Artefact2/b5f810600771265fc1e39442288e8ec9
## FAQ / Model Request
See https://huggingface.co/mradermacher/model_requests for some answers to
questions you might have and/or if you want some other model quantized.
## Thanks
I thank my company, [nethype GmbH](https://www.nethype.de/), for letting
me use its servers and providing upgrades to my workstation to enable
this work in my free time.
<!-- end -->
|
Rajaram1996/Hubert_emotion | Rajaram1996 | 2022-11-19T20:10:41Z | 13,815 | 29 | transformers | [
"transformers",
"pytorch",
"hubert",
"speech",
"audio",
"HUBert",
"audio-classification",
"endpoints_compatible",
"region:us"
] | audio-classification | 2022-03-02T23:29:04Z | ---
inference: true
pipeline_tag: audio-classification
tags:
- speech
- audio
- HUBert
---
Working example of using pretrained model to predict emotion in local audio file
```
def predict_emotion_hubert(audio_file):
""" inspired by an example from https://github.com/m3hrdadfi/soxan """
from audio_models import HubertForSpeechClassification
from transformers import Wav2Vec2FeatureExtractor, AutoConfig
import torch.nn.functional as F
import torch
import numpy as np
from pydub import AudioSegment
model = HubertForSpeechClassification.from_pretrained("Rajaram1996/Hubert_emotion") # Downloading: 362M
feature_extractor = Wav2Vec2FeatureExtractor.from_pretrained("facebook/hubert-base-ls960")
sampling_rate=16000 # defined by the model; must convert mp3 to this rate.
config = AutoConfig.from_pretrained("Rajaram1996/Hubert_emotion")
def speech_file_to_array(path, sampling_rate):
# using torchaudio...
# speech_array, _sampling_rate = torchaudio.load(path)
# resampler = torchaudio.transforms.Resample(_sampling_rate, sampling_rate)
# speech = resampler(speech_array).squeeze().numpy()
sound = AudioSegment.from_file(path)
sound = sound.set_frame_rate(sampling_rate)
sound_array = np.array(sound.get_array_of_samples())
return sound_array
sound_array = speech_file_to_array(audio_file, sampling_rate)
inputs = feature_extractor(sound_array, sampling_rate=sampling_rate, return_tensors="pt", padding=True)
inputs = {key: inputs[key].to("cpu").float() for key in inputs}
with torch.no_grad():
logits = model(**inputs).logits
scores = F.softmax(logits, dim=1).detach().cpu().numpy()[0]
outputs = [{
"emo": config.id2label[i],
"score": round(score * 100, 1)}
for i, score in enumerate(scores)
]
return [row for row in sorted(outputs, key=lambda x:x["score"], reverse=True) if row['score'] != '0.0%'][:2]
```
```
result = predict_emotion_hubert("male-crying.mp3")
>>> result
[{'emo': 'male_sad', 'score': 91.0}, {'emo': 'male_fear', 'score': 4.8}]
```
|
mradermacher/Breeze-7B-Instruct-v1_0-i1-GGUF | mradermacher | 2024-06-28T19:01:59Z | 13,808 | 0 | transformers | [
"transformers",
"gguf",
"zh",
"en",
"base_model:MediaTek-Research/Breeze-7B-Instruct-v1_0",
"license:apache-2.0",
"endpoints_compatible",
"region:us"
] | null | 2024-06-28T16:49:54Z | ---
base_model: MediaTek-Research/Breeze-7B-Instruct-v1_0
language:
- zh
- en
library_name: transformers
license: apache-2.0
quantized_by: mradermacher
---
## About
<!-- ### quantize_version: 2 -->
<!-- ### output_tensor_quantised: 1 -->
<!-- ### convert_type: hf -->
<!-- ### vocab_type: -->
<!-- ### tags: nicoboss -->
weighted/imatrix quants of https://huggingface.co/MediaTek-Research/Breeze-7B-Instruct-v1_0
<!-- provided-files -->
static quants are available at https://huggingface.co/mradermacher/Breeze-7B-Instruct-v1_0-GGUF
## Usage
If you are unsure how to use GGUF files, refer to one of [TheBloke's
READMEs](https://huggingface.co/TheBloke/KafkaLM-70B-German-V0.1-GGUF) for
more details, including on how to concatenate multi-part files.
## Provided Quants
(sorted by size, not necessarily quality. IQ-quants are often preferable over similar sized non-IQ quants)
| Link | Type | Size/GB | Notes |
|:-----|:-----|--------:|:------|
| [GGUF](https://huggingface.co/mradermacher/Breeze-7B-Instruct-v1_0-i1-GGUF/resolve/main/Breeze-7B-Instruct-v1_0.i1-IQ1_S.gguf) | i1-IQ1_S | 1.8 | for the desperate |
| [GGUF](https://huggingface.co/mradermacher/Breeze-7B-Instruct-v1_0-i1-GGUF/resolve/main/Breeze-7B-Instruct-v1_0.i1-IQ1_M.gguf) | i1-IQ1_M | 2.0 | mostly desperate |
| [GGUF](https://huggingface.co/mradermacher/Breeze-7B-Instruct-v1_0-i1-GGUF/resolve/main/Breeze-7B-Instruct-v1_0.i1-IQ2_XXS.gguf) | i1-IQ2_XXS | 2.2 | |
| [GGUF](https://huggingface.co/mradermacher/Breeze-7B-Instruct-v1_0-i1-GGUF/resolve/main/Breeze-7B-Instruct-v1_0.i1-IQ2_XS.gguf) | i1-IQ2_XS | 2.4 | |
| [GGUF](https://huggingface.co/mradermacher/Breeze-7B-Instruct-v1_0-i1-GGUF/resolve/main/Breeze-7B-Instruct-v1_0.i1-IQ2_S.gguf) | i1-IQ2_S | 2.5 | |
| [GGUF](https://huggingface.co/mradermacher/Breeze-7B-Instruct-v1_0-i1-GGUF/resolve/main/Breeze-7B-Instruct-v1_0.i1-IQ2_M.gguf) | i1-IQ2_M | 2.7 | |
| [GGUF](https://huggingface.co/mradermacher/Breeze-7B-Instruct-v1_0-i1-GGUF/resolve/main/Breeze-7B-Instruct-v1_0.i1-Q2_K.gguf) | i1-Q2_K | 3.0 | IQ3_XXS probably better |
| [GGUF](https://huggingface.co/mradermacher/Breeze-7B-Instruct-v1_0-i1-GGUF/resolve/main/Breeze-7B-Instruct-v1_0.i1-IQ3_XXS.gguf) | i1-IQ3_XXS | 3.1 | lower quality |
| [GGUF](https://huggingface.co/mradermacher/Breeze-7B-Instruct-v1_0-i1-GGUF/resolve/main/Breeze-7B-Instruct-v1_0.i1-IQ3_XS.gguf) | i1-IQ3_XS | 3.3 | |
| [GGUF](https://huggingface.co/mradermacher/Breeze-7B-Instruct-v1_0-i1-GGUF/resolve/main/Breeze-7B-Instruct-v1_0.i1-Q3_K_S.gguf) | i1-Q3_K_S | 3.4 | IQ3_XS probably better |
| [GGUF](https://huggingface.co/mradermacher/Breeze-7B-Instruct-v1_0-i1-GGUF/resolve/main/Breeze-7B-Instruct-v1_0.i1-IQ3_S.gguf) | i1-IQ3_S | 3.4 | beats Q3_K* |
| [GGUF](https://huggingface.co/mradermacher/Breeze-7B-Instruct-v1_0-i1-GGUF/resolve/main/Breeze-7B-Instruct-v1_0.i1-IQ3_M.gguf) | i1-IQ3_M | 3.5 | |
| [GGUF](https://huggingface.co/mradermacher/Breeze-7B-Instruct-v1_0-i1-GGUF/resolve/main/Breeze-7B-Instruct-v1_0.i1-Q3_K_M.gguf) | i1-Q3_K_M | 3.8 | IQ3_S probably better |
| [GGUF](https://huggingface.co/mradermacher/Breeze-7B-Instruct-v1_0-i1-GGUF/resolve/main/Breeze-7B-Instruct-v1_0.i1-Q3_K_L.gguf) | i1-Q3_K_L | 4.1 | IQ3_M probably better |
| [GGUF](https://huggingface.co/mradermacher/Breeze-7B-Instruct-v1_0-i1-GGUF/resolve/main/Breeze-7B-Instruct-v1_0.i1-IQ4_XS.gguf) | i1-IQ4_XS | 4.2 | |
| [GGUF](https://huggingface.co/mradermacher/Breeze-7B-Instruct-v1_0-i1-GGUF/resolve/main/Breeze-7B-Instruct-v1_0.i1-Q4_0.gguf) | i1-Q4_0 | 4.4 | fast, low quality |
| [GGUF](https://huggingface.co/mradermacher/Breeze-7B-Instruct-v1_0-i1-GGUF/resolve/main/Breeze-7B-Instruct-v1_0.i1-Q4_K_S.gguf) | i1-Q4_K_S | 4.4 | optimal size/speed/quality |
| [GGUF](https://huggingface.co/mradermacher/Breeze-7B-Instruct-v1_0-i1-GGUF/resolve/main/Breeze-7B-Instruct-v1_0.i1-Q4_K_M.gguf) | i1-Q4_K_M | 4.6 | fast, recommended |
| [GGUF](https://huggingface.co/mradermacher/Breeze-7B-Instruct-v1_0-i1-GGUF/resolve/main/Breeze-7B-Instruct-v1_0.i1-Q5_K_S.gguf) | i1-Q5_K_S | 5.3 | |
| [GGUF](https://huggingface.co/mradermacher/Breeze-7B-Instruct-v1_0-i1-GGUF/resolve/main/Breeze-7B-Instruct-v1_0.i1-Q5_K_M.gguf) | i1-Q5_K_M | 5.4 | |
| [GGUF](https://huggingface.co/mradermacher/Breeze-7B-Instruct-v1_0-i1-GGUF/resolve/main/Breeze-7B-Instruct-v1_0.i1-Q6_K.gguf) | i1-Q6_K | 6.2 | practically like static Q6_K |
Here is a handy graph by ikawrakow comparing some lower-quality quant
types (lower is better):

And here are Artefact2's thoughts on the matter:
https://gist.github.com/Artefact2/b5f810600771265fc1e39442288e8ec9
## FAQ / Model Request
See https://huggingface.co/mradermacher/model_requests for some answers to
questions you might have and/or if you want some other model quantized.
## Thanks
I thank my company, [nethype GmbH](https://www.nethype.de/), for letting
me use its servers and providing upgrades to my workstation to enable
this work in my free time. Additional thanks to [@nicoboss](https://huggingface.co/nicoboss) for giving me access to his hardware for calculating the imatrix for these quants.
<!-- end -->
|
mradermacher/Llama-3-8B-WildChat-i1-GGUF | mradermacher | 2024-06-28T08:48:36Z | 13,797 | 0 | transformers | [
"transformers",
"gguf",
"axolotl",
"generated_from_trainer",
"en",
"base_model:Magpie-Align/Llama-3-8B-WildChat",
"license:llama3",
"endpoints_compatible",
"region:us"
] | null | 2024-06-28T07:31:23Z | ---
base_model: Magpie-Align/Llama-3-8B-WildChat
language:
- en
library_name: transformers
license: llama3
quantized_by: mradermacher
tags:
- axolotl
- generated_from_trainer
---
## About
<!-- ### quantize_version: 2 -->
<!-- ### output_tensor_quantised: 1 -->
<!-- ### convert_type: hf -->
<!-- ### vocab_type: -->
<!-- ### tags: nicoboss -->
weighted/imatrix quants of https://huggingface.co/Magpie-Align/Llama-3-8B-WildChat
<!-- provided-files -->
static quants are available at https://huggingface.co/mradermacher/Llama-3-8B-WildChat-GGUF
## Usage
If you are unsure how to use GGUF files, refer to one of [TheBloke's
READMEs](https://huggingface.co/TheBloke/KafkaLM-70B-German-V0.1-GGUF) for
more details, including on how to concatenate multi-part files.
## Provided Quants
(sorted by size, not necessarily quality. IQ-quants are often preferable over similar sized non-IQ quants)
| Link | Type | Size/GB | Notes |
|:-----|:-----|--------:|:------|
| [GGUF](https://huggingface.co/mradermacher/Llama-3-8B-WildChat-i1-GGUF/resolve/main/Llama-3-8B-WildChat.i1-IQ1_S.gguf) | i1-IQ1_S | 2.1 | for the desperate |
| [GGUF](https://huggingface.co/mradermacher/Llama-3-8B-WildChat-i1-GGUF/resolve/main/Llama-3-8B-WildChat.i1-IQ1_M.gguf) | i1-IQ1_M | 2.3 | mostly desperate |
| [GGUF](https://huggingface.co/mradermacher/Llama-3-8B-WildChat-i1-GGUF/resolve/main/Llama-3-8B-WildChat.i1-IQ2_XXS.gguf) | i1-IQ2_XXS | 2.5 | |
| [GGUF](https://huggingface.co/mradermacher/Llama-3-8B-WildChat-i1-GGUF/resolve/main/Llama-3-8B-WildChat.i1-IQ2_XS.gguf) | i1-IQ2_XS | 2.7 | |
| [GGUF](https://huggingface.co/mradermacher/Llama-3-8B-WildChat-i1-GGUF/resolve/main/Llama-3-8B-WildChat.i1-IQ2_S.gguf) | i1-IQ2_S | 2.9 | |
| [GGUF](https://huggingface.co/mradermacher/Llama-3-8B-WildChat-i1-GGUF/resolve/main/Llama-3-8B-WildChat.i1-IQ2_M.gguf) | i1-IQ2_M | 3.0 | |
| [GGUF](https://huggingface.co/mradermacher/Llama-3-8B-WildChat-i1-GGUF/resolve/main/Llama-3-8B-WildChat.i1-Q2_K.gguf) | i1-Q2_K | 3.3 | IQ3_XXS probably better |
| [GGUF](https://huggingface.co/mradermacher/Llama-3-8B-WildChat-i1-GGUF/resolve/main/Llama-3-8B-WildChat.i1-IQ3_XXS.gguf) | i1-IQ3_XXS | 3.4 | lower quality |
| [GGUF](https://huggingface.co/mradermacher/Llama-3-8B-WildChat-i1-GGUF/resolve/main/Llama-3-8B-WildChat.i1-IQ3_XS.gguf) | i1-IQ3_XS | 3.6 | |
| [GGUF](https://huggingface.co/mradermacher/Llama-3-8B-WildChat-i1-GGUF/resolve/main/Llama-3-8B-WildChat.i1-Q3_K_S.gguf) | i1-Q3_K_S | 3.8 | IQ3_XS probably better |
| [GGUF](https://huggingface.co/mradermacher/Llama-3-8B-WildChat-i1-GGUF/resolve/main/Llama-3-8B-WildChat.i1-IQ3_S.gguf) | i1-IQ3_S | 3.8 | beats Q3_K* |
| [GGUF](https://huggingface.co/mradermacher/Llama-3-8B-WildChat-i1-GGUF/resolve/main/Llama-3-8B-WildChat.i1-IQ3_M.gguf) | i1-IQ3_M | 3.9 | |
| [GGUF](https://huggingface.co/mradermacher/Llama-3-8B-WildChat-i1-GGUF/resolve/main/Llama-3-8B-WildChat.i1-Q3_K_M.gguf) | i1-Q3_K_M | 4.1 | IQ3_S probably better |
| [GGUF](https://huggingface.co/mradermacher/Llama-3-8B-WildChat-i1-GGUF/resolve/main/Llama-3-8B-WildChat.i1-Q3_K_L.gguf) | i1-Q3_K_L | 4.4 | IQ3_M probably better |
| [GGUF](https://huggingface.co/mradermacher/Llama-3-8B-WildChat-i1-GGUF/resolve/main/Llama-3-8B-WildChat.i1-IQ4_XS.gguf) | i1-IQ4_XS | 4.5 | |
| [GGUF](https://huggingface.co/mradermacher/Llama-3-8B-WildChat-i1-GGUF/resolve/main/Llama-3-8B-WildChat.i1-Q4_0.gguf) | i1-Q4_0 | 4.8 | fast, low quality |
| [GGUF](https://huggingface.co/mradermacher/Llama-3-8B-WildChat-i1-GGUF/resolve/main/Llama-3-8B-WildChat.i1-Q4_K_S.gguf) | i1-Q4_K_S | 4.8 | optimal size/speed/quality |
| [GGUF](https://huggingface.co/mradermacher/Llama-3-8B-WildChat-i1-GGUF/resolve/main/Llama-3-8B-WildChat.i1-Q4_K_M.gguf) | i1-Q4_K_M | 5.0 | fast, recommended |
| [GGUF](https://huggingface.co/mradermacher/Llama-3-8B-WildChat-i1-GGUF/resolve/main/Llama-3-8B-WildChat.i1-Q5_K_S.gguf) | i1-Q5_K_S | 5.7 | |
| [GGUF](https://huggingface.co/mradermacher/Llama-3-8B-WildChat-i1-GGUF/resolve/main/Llama-3-8B-WildChat.i1-Q5_K_M.gguf) | i1-Q5_K_M | 5.8 | |
| [GGUF](https://huggingface.co/mradermacher/Llama-3-8B-WildChat-i1-GGUF/resolve/main/Llama-3-8B-WildChat.i1-Q6_K.gguf) | i1-Q6_K | 6.7 | practically like static Q6_K |
Here is a handy graph by ikawrakow comparing some lower-quality quant
types (lower is better):

And here are Artefact2's thoughts on the matter:
https://gist.github.com/Artefact2/b5f810600771265fc1e39442288e8ec9
## FAQ / Model Request
See https://huggingface.co/mradermacher/model_requests for some answers to
questions you might have and/or if you want some other model quantized.
## Thanks
I thank my company, [nethype GmbH](https://www.nethype.de/), for letting
me use its servers and providing upgrades to my workstation to enable
this work in my free time. Additional thanks to [@nicoboss](https://huggingface.co/nicoboss) for giving me access to his hardware for calculating the imatrix for these quants.
<!-- end -->
|
QuantFactory/NexusRaven-V2-13B-GGUF | QuantFactory | 2024-06-22T07:09:06Z | 13,794 | 0 | null | [
"gguf",
"function calling",
"text-generation",
"arxiv:2308.12950",
"base_model:Nexusflow/NexusRaven-V2-13B",
"license:other",
"region:us"
] | text-generation | 2024-06-21T03:35:39Z | ---
license: other
base_model: Nexusflow/NexusRaven-V2-13B
model-index:
- name: NexusRaven-13B
results: []
tags:
- function calling
pipeline_tag: text-generation
---
# QuantFactory/NexusRaven-V2-13B-GGUF
This is quantized version of [Nexusflow/NexusRaven-V2-13B](https://huggingface.co/Nexusflow/NexusRaven-V2-13B) created using llama.cpp
# NexusRaven-13B: Surpassing GPT-4 for Zero-shot Function Calling
<p align="center">
<a href="https://huggingface.co/Nexusflow" target="_blank">Nexusflow HF</a> - <a href="https://discord.gg/HDSVmNAs3y" target="_blank">Nexusflow Discord</a> - <a href="http://nexusflow.ai/blogs/ravenv2" target="_blank">NexusRaven-V2 blog post</a> - <a href="https://colab.research.google.com/drive/19JYixRPPlanmW5q49WYi_tU8rhHeCEKW?usp=sharing" target="_blank">Prompting Notebook CoLab</a> - <a href="https://huggingface.co/spaces/Nexusflow/Nexus_Function_Calling_Leaderboard" target="_blank">Leaderboard</a> - <a href="https://huggingface.co/spaces/Nexusflow/NexusRaven-V2-Demo" target="_blank">Read-World Demo</a> - <a href="https://github.com/nexusflowai/NexusRaven-V2" target="_blank">NexusRaven-V2-13B Github</a>
</p>
<p align="center" width="100%">
<a><img src="NexusRaven.png" alt="NexusRaven" style="width: 40%; min-width: 300px; display: block; margin: auto;"></a>
</p>
## Introducing NexusRaven-V2-13B
NexusRaven is an open-source and commercially viable function calling LLM that surpasses the state-of-the-art in function calling capabilities.
💪 **Versatile Function Calling Capability**: NexusRaven-V2 is capable of generating single function calls, nested calls, and parallel calls in many challenging cases.
🤓 **Fully Explainable**: NexusRaven-V2 is capable of generating very detailed explanations for the function calls it generates. This behavior can be turned off, to save tokens during inference.
📊 **Performance Highlights**: NexusRaven-V2 surpasses GPT-4 by 7% in function calling success rates in human-generated use cases involving nested and composite functions.
🔧 **Generalization to the Unseen**: NexusRaven-V2 has never been trained on the functions used in evaluation.
🔥 **Commercially Permissive**: The training of NexusRaven-V2 does not involve any data generated by proprietary LLMs such as GPT-4. You have full control of the model when deployed in commercial applications.
Please checkout the following links!
- [Prompting Notebook CoLab](https://colab.research.google.com/drive/19JYixRPPlanmW5q49WYi_tU8rhHeCEKW?usp=sharing)
- [Evaluation Leaderboard](https://huggingface.co/spaces/Nexusflow/Nexus_Function_Calling_Leaderboard)
- [NexusRaven-V2 Real-World Demo](https://huggingface.co/spaces/Nexusflow/NexusRaven-V2-Demo)
## NexusRaven-V2 model usage
NexusRaven-V2 accepts a list of python functions.
These python functions can do anything (including sending GET/POST requests to external APIs!).
The two requirements include the python function signature and the appropriate docstring to generate the function call.
NexusRaven-V2 also does best on functions with arguments, so please always only provide functions that require arguments to raven.
### NexusRaven-V2's Capabilities
NexusRaven-V2 is capable of generating deeply nested function calls, parallel function calls, and simple single calls. It can also justify the function calls it generated. If you would like to generate the call only, please set a stop criteria of \"\<bot\_end\>\". Otherwise, please allow NexusRaven-V2 to run until its stop token (i.e. "\<\/s\>").
### Quick Start Prompting Guide
Please refer to our notebook, [How-To-Prompt.ipynb](https://colab.research.google.com/drive/19JYixRPPlanmW5q49WYi_tU8rhHeCEKW?usp=sharing), for more advanced tutorials on using NexusRaven-V2!
1. When giving docstrings to Raven, please provide well-indented, detailed, and well-written docstrings as this can help accuracy.
2. Raven does better when all functions provided to it has arguments, either required or optional, (i.e. ```func(dummy_arg)``` is preferred over ```func()```) as this can help accuracy.
3. We strongly recommend to set sampling to False when prompting NexusRaven-V2.
4. We strongly recommend a very low temperature (~0.001).
5. We strongly recommend following the prompting style below.
When handling irrelevant user queries, users have noticed that specifying a "no-op" function with arguments work best. For example, something like this might work:
```python
def no_relevant_function(user_query : str):
"""
Call this when no other provided function can be called to answer the user query.
Args:
user_query: The user_query that cannot be answered by any other function calls.
"""
```
Please ensure to provide an argument to this function, as Raven works best on functions with arguments.
For parallel calls, due to the model being targeted for industry use, you can "enable" parallel calls by adding this into the prompt:
```python
"Setting: Allowed to issue multiple calls with semicolon\n"
```
This can be added above the User Query to "allow" the model to use parallel calls, otherwise, the model will focus on nested and single calls primarily.
### Quickstart
You can run the model on a GPU using the following code.
```python
# Please `pip install transformers accelerate`
from transformers import pipeline
pipeline = pipeline(
"text-generation",
model="Nexusflow/NexusRaven-V2-13B",
torch_dtype="auto",
device_map="auto",
)
prompt_template = \
'''
Function:
def get_weather_data(coordinates):
"""
Fetches weather data from the Open-Meteo API for the given latitude and longitude.
Args:
coordinates (tuple): The latitude of the location.
Returns:
float: The current temperature in the coordinates you've asked for
"""
Function:
def get_coordinates_from_city(city_name):
"""
Fetches the latitude and longitude of a given city name using the Maps.co Geocoding API.
Args:
city_name (str): The name of the city.
Returns:
tuple: The latitude and longitude of the city.
"""
User Query: {query}<human_end>
'''
prompt = prompt_template.format(query="What's the weather like in Seattle right now?")
result = pipeline(prompt, max_new_tokens=2048, return_full_text=False, do_sample=False, temperature=0.001)[0]["generated_text"]
print (result)
```
This should generate the following:
```
Call: get_weather_data(coordinates=get_coordinates_from_city(city_name='Seattle'))<bot_end>
Thought: The function call `get_weather_data(coordinates=get_coordinates_from_city(city_name='Seattle'))` answers the question "What's the weather like in Seattle right now?" by following these steps:
1. `get_coordinates_from_city(city_name='Seattle')`: This function call fetches the latitude and longitude of the city "Seattle" using the Maps.co Geocoding API.
2. `get_weather_data(coordinates=...)`: This function call fetches the current weather data for the coordinates returned by the previous function call.
Therefore, the function call `get_weather_data(coordinates=get_coordinates_from_city(city_name='Seattle'))` answers the question "What's the weather like in Seattle right now?" by first fetching the coordinates of the city "Seattle" and then fetching the current weather data for those coordinates.
```
If you would like to prevent the generation of the explanation of the function call (for example, to save on inference tokens), please set a stopping criteria of \<bot_end\>.
Please follow this prompting template to maximize the performance of RavenV2.
### Using with OpenAI FC Schematics
[If you currently have a workflow that is built around OpenAI's function calling and you want to try NexusRaven-V2, we have a package that helps you drop in NexusRaven-V2.](https://github.com/nexusflowai/nexusraven-pip)
### Using With LangChain
We've also included a [small demo for using Raven with langchain](langdemo.py)!
## Evaluation
<p align="center" width="100%">
<a><img src="blog2-fc.png" alt="NexusRaven" style="width: 80%; min-width: 300px; display: block; margin: auto;"></a>
<a><img src="radar-2.png" alt="NexusRaven" style="width: 80%; min-width: 300px; display: block; margin: auto;"></a>
</p>
For a deeper dive into the results, please see our [Github README](https://github.com/nexusflowai/NexusRaven).
# Limitations
1. The model works best when it is connected with a retriever when there are a multitude of functions, as a large number of functions will saturate the context window of this model.
2. The model can be prone to generate incorrect calls. Please ensure proper guardrails to capture errant behavior is in place.
3. The explanations generated by NexusRaven-V2 might be incorrect. Please ensure proper guardrails are present to capture errant behavior.
## License
This model was trained on commercially viable data and is licensed under the [Nexusflow community license](https://huggingface.co/Nexusflow/NexusRaven-V2-13B/blob/main/LICENSE.txt).
## Model References
We thank the CodeLlama team for their amazing models!
```
@misc{rozière2023code,
title={Code Llama: Open Foundation Models for Code},
author={Baptiste Rozière and Jonas Gehring and Fabian Gloeckle and Sten Sootla and Itai Gat and Xiaoqing Ellen Tan and Yossi Adi and Jingyu Liu and Tal Remez and Jérémy Rapin and Artyom Kozhevnikov and Ivan Evtimov and Joanna Bitton and Manish Bhatt and Cristian Canton Ferrer and Aaron Grattafiori and Wenhan Xiong and Alexandre Défossez and Jade Copet and Faisal Azhar and Hugo Touvron and Louis Martin and Nicolas Usunier and Thomas Scialom and Gabriel Synnaeve},
year={2023},
eprint={2308.12950},
archivePrefix={arXiv},
primaryClass={cs.CL}
}
```
## Model Citation
```
@misc{nexusraven,
title={NexusRaven-V2: Surpassing GPT-4 for Zero-shot Function Calling},
author={Nexusflow.ai team},
year={2023},
url={https://nexusflow.ai/blogs/ravenv2}
}
```
## Model Contact
Please join our [Discord Channel](https://discord.gg/HDSVmNAs3y) to reach out for any issues and comments! |
RichardErkhov/CorticalStack_-_pastiche-crown-clown-7b-dare-gguf | RichardErkhov | 2024-06-20T13:14:09Z | 13,789 | 1 | null | [
"gguf",
"arxiv:2311.03099",
"region:us"
] | null | 2024-06-20T06:44:50Z | Quantization made by Richard Erkhov.
[Github](https://github.com/RichardErkhov)
[Discord](https://discord.gg/pvy7H8DZMG)
[Request more models](https://github.com/RichardErkhov/quant_request)
pastiche-crown-clown-7b-dare - GGUF
- Model creator: https://huggingface.co/CorticalStack/
- Original model: https://huggingface.co/CorticalStack/pastiche-crown-clown-7b-dare/
| Name | Quant method | Size |
| ---- | ---- | ---- |
| [pastiche-crown-clown-7b-dare.Q2_K.gguf](https://huggingface.co/RichardErkhov/CorticalStack_-_pastiche-crown-clown-7b-dare-gguf/blob/main/pastiche-crown-clown-7b-dare.Q2_K.gguf) | Q2_K | 2.53GB |
| [pastiche-crown-clown-7b-dare.IQ3_XS.gguf](https://huggingface.co/RichardErkhov/CorticalStack_-_pastiche-crown-clown-7b-dare-gguf/blob/main/pastiche-crown-clown-7b-dare.IQ3_XS.gguf) | IQ3_XS | 2.81GB |
| [pastiche-crown-clown-7b-dare.IQ3_S.gguf](https://huggingface.co/RichardErkhov/CorticalStack_-_pastiche-crown-clown-7b-dare-gguf/blob/main/pastiche-crown-clown-7b-dare.IQ3_S.gguf) | IQ3_S | 2.96GB |
| [pastiche-crown-clown-7b-dare.Q3_K_S.gguf](https://huggingface.co/RichardErkhov/CorticalStack_-_pastiche-crown-clown-7b-dare-gguf/blob/main/pastiche-crown-clown-7b-dare.Q3_K_S.gguf) | Q3_K_S | 2.95GB |
| [pastiche-crown-clown-7b-dare.IQ3_M.gguf](https://huggingface.co/RichardErkhov/CorticalStack_-_pastiche-crown-clown-7b-dare-gguf/blob/main/pastiche-crown-clown-7b-dare.IQ3_M.gguf) | IQ3_M | 3.06GB |
| [pastiche-crown-clown-7b-dare.Q3_K.gguf](https://huggingface.co/RichardErkhov/CorticalStack_-_pastiche-crown-clown-7b-dare-gguf/blob/main/pastiche-crown-clown-7b-dare.Q3_K.gguf) | Q3_K | 3.28GB |
| [pastiche-crown-clown-7b-dare.Q3_K_M.gguf](https://huggingface.co/RichardErkhov/CorticalStack_-_pastiche-crown-clown-7b-dare-gguf/blob/main/pastiche-crown-clown-7b-dare.Q3_K_M.gguf) | Q3_K_M | 3.28GB |
| [pastiche-crown-clown-7b-dare.Q3_K_L.gguf](https://huggingface.co/RichardErkhov/CorticalStack_-_pastiche-crown-clown-7b-dare-gguf/blob/main/pastiche-crown-clown-7b-dare.Q3_K_L.gguf) | Q3_K_L | 3.56GB |
| [pastiche-crown-clown-7b-dare.IQ4_XS.gguf](https://huggingface.co/RichardErkhov/CorticalStack_-_pastiche-crown-clown-7b-dare-gguf/blob/main/pastiche-crown-clown-7b-dare.IQ4_XS.gguf) | IQ4_XS | 3.67GB |
| [pastiche-crown-clown-7b-dare.Q4_0.gguf](https://huggingface.co/RichardErkhov/CorticalStack_-_pastiche-crown-clown-7b-dare-gguf/blob/main/pastiche-crown-clown-7b-dare.Q4_0.gguf) | Q4_0 | 3.83GB |
| [pastiche-crown-clown-7b-dare.IQ4_NL.gguf](https://huggingface.co/RichardErkhov/CorticalStack_-_pastiche-crown-clown-7b-dare-gguf/blob/main/pastiche-crown-clown-7b-dare.IQ4_NL.gguf) | IQ4_NL | 3.87GB |
| [pastiche-crown-clown-7b-dare.Q4_K_S.gguf](https://huggingface.co/RichardErkhov/CorticalStack_-_pastiche-crown-clown-7b-dare-gguf/blob/main/pastiche-crown-clown-7b-dare.Q4_K_S.gguf) | Q4_K_S | 3.86GB |
| [pastiche-crown-clown-7b-dare.Q4_K.gguf](https://huggingface.co/RichardErkhov/CorticalStack_-_pastiche-crown-clown-7b-dare-gguf/blob/main/pastiche-crown-clown-7b-dare.Q4_K.gguf) | Q4_K | 4.07GB |
| [pastiche-crown-clown-7b-dare.Q4_K_M.gguf](https://huggingface.co/RichardErkhov/CorticalStack_-_pastiche-crown-clown-7b-dare-gguf/blob/main/pastiche-crown-clown-7b-dare.Q4_K_M.gguf) | Q4_K_M | 4.07GB |
| [pastiche-crown-clown-7b-dare.Q4_1.gguf](https://huggingface.co/RichardErkhov/CorticalStack_-_pastiche-crown-clown-7b-dare-gguf/blob/main/pastiche-crown-clown-7b-dare.Q4_1.gguf) | Q4_1 | 4.24GB |
| [pastiche-crown-clown-7b-dare.Q5_0.gguf](https://huggingface.co/RichardErkhov/CorticalStack_-_pastiche-crown-clown-7b-dare-gguf/blob/main/pastiche-crown-clown-7b-dare.Q5_0.gguf) | Q5_0 | 4.65GB |
| [pastiche-crown-clown-7b-dare.Q5_K_S.gguf](https://huggingface.co/RichardErkhov/CorticalStack_-_pastiche-crown-clown-7b-dare-gguf/blob/main/pastiche-crown-clown-7b-dare.Q5_K_S.gguf) | Q5_K_S | 4.65GB |
| [pastiche-crown-clown-7b-dare.Q5_K.gguf](https://huggingface.co/RichardErkhov/CorticalStack_-_pastiche-crown-clown-7b-dare-gguf/blob/main/pastiche-crown-clown-7b-dare.Q5_K.gguf) | Q5_K | 4.78GB |
| [pastiche-crown-clown-7b-dare.Q5_K_M.gguf](https://huggingface.co/RichardErkhov/CorticalStack_-_pastiche-crown-clown-7b-dare-gguf/blob/main/pastiche-crown-clown-7b-dare.Q5_K_M.gguf) | Q5_K_M | 4.78GB |
| [pastiche-crown-clown-7b-dare.Q5_1.gguf](https://huggingface.co/RichardErkhov/CorticalStack_-_pastiche-crown-clown-7b-dare-gguf/blob/main/pastiche-crown-clown-7b-dare.Q5_1.gguf) | Q5_1 | 5.07GB |
| [pastiche-crown-clown-7b-dare.Q6_K.gguf](https://huggingface.co/RichardErkhov/CorticalStack_-_pastiche-crown-clown-7b-dare-gguf/blob/main/pastiche-crown-clown-7b-dare.Q6_K.gguf) | Q6_K | 5.53GB |
| [pastiche-crown-clown-7b-dare.Q8_0.gguf](https://huggingface.co/RichardErkhov/CorticalStack_-_pastiche-crown-clown-7b-dare-gguf/blob/main/pastiche-crown-clown-7b-dare.Q8_0.gguf) | Q8_0 | 7.17GB |
Original model description:
---
license: apache-2.0
tags:
- merge
- mergekit
- bardsai/jaskier-7b-dpo-v5.6
- mlabonne/AlphaMonarch-7B
- mlabonne/NeuralMonarch-7B
- macadeliccc/MBX-7B-v3-DPO
---
<img src="pastiche-crown-clown.png" alt="Pastiche crown clown logo" width="800" style="margin-left:'auto' margin-right:'auto' display:'block'"/>
# pastiche-crown-clown-7B-dare
pastiche-crown-clown-7B-dare is a DARE merge of the following models using [mergekit](https://github.com/cg123/mergekit):
* [bardsai/jaskier-7b-dpo-v5.6](https://huggingface.co/bardsai/jaskier-7b-dpo-v5.6)
* [mlabonne/AlphaMonarch-7B](https://huggingface.co/mlabonne/AlphaMonarch-7B)
* [mlabonne/NeuralMonarch-7B](https://huggingface.co/mlabonne/NeuralMonarch-7B)
* [macadeliccc/MBX-7B-v3-DPO](https://huggingface.co/macadeliccc/MBX-7B-v3-DPO)
See the paper [Language Models are Super Mario: Absorbing Abilities from Homologous Models as a Free Lunch](https://arxiv.org/abs/2311.03099) for more on the method.
## 🧩 Configuration
```yaml
models:
- model: bardsai/jaskier-7b-dpo-v5.6
- model: mlabonne/AlphaMonarch-7B
parameters:
density: 0.53
weight: 0.2
- model: mlabonne/NeuralMonarch-7B
parameters:
density: 0.53
weight: 0.4
- model: macadeliccc/MBX-7B-v3-DPO
parameters:
density: 0.53
weight: 0.4
merge_method: dare_ties
base_model: bardsai/jaskier-7b-dpo-v5.6
parameters:
int8_mask: true
dtype: bfloat16
```
|
mradermacher/Irbis-7b-v0.1-GGUF | mradermacher | 2024-06-30T06:08:24Z | 13,783 | 0 | transformers | [
"transformers",
"gguf",
"kk",
"ru",
"en",
"base_model:IrbisAI/Irbis-7b-v0.1",
"license:mit",
"endpoints_compatible",
"region:us"
] | null | 2024-06-30T04:02:34Z | ---
base_model: IrbisAI/Irbis-7b-v0.1
language:
- kk
- ru
- en
library_name: transformers
license: mit
quantized_by: mradermacher
---
## About
<!-- ### quantize_version: 2 -->
<!-- ### output_tensor_quantised: 1 -->
<!-- ### convert_type: hf -->
<!-- ### vocab_type: -->
<!-- ### tags: -->
static quants of https://huggingface.co/IrbisAI/Irbis-7b-v0.1
<!-- provided-files -->
weighted/imatrix quants are available at https://huggingface.co/mradermacher/Irbis-7b-v0.1-i1-GGUF
## Usage
If you are unsure how to use GGUF files, refer to one of [TheBloke's
READMEs](https://huggingface.co/TheBloke/KafkaLM-70B-German-V0.1-GGUF) for
more details, including on how to concatenate multi-part files.
## Provided Quants
(sorted by size, not necessarily quality. IQ-quants are often preferable over similar sized non-IQ quants)
| Link | Type | Size/GB | Notes |
|:-----|:-----|--------:|:------|
| [GGUF](https://huggingface.co/mradermacher/Irbis-7b-v0.1-GGUF/resolve/main/Irbis-7b-v0.1.Q2_K.gguf) | Q2_K | 3.0 | |
| [GGUF](https://huggingface.co/mradermacher/Irbis-7b-v0.1-GGUF/resolve/main/Irbis-7b-v0.1.IQ3_XS.gguf) | IQ3_XS | 3.3 | |
| [GGUF](https://huggingface.co/mradermacher/Irbis-7b-v0.1-GGUF/resolve/main/Irbis-7b-v0.1.Q3_K_S.gguf) | Q3_K_S | 3.4 | |
| [GGUF](https://huggingface.co/mradermacher/Irbis-7b-v0.1-GGUF/resolve/main/Irbis-7b-v0.1.IQ3_S.gguf) | IQ3_S | 3.4 | beats Q3_K* |
| [GGUF](https://huggingface.co/mradermacher/Irbis-7b-v0.1-GGUF/resolve/main/Irbis-7b-v0.1.IQ3_M.gguf) | IQ3_M | 3.5 | |
| [GGUF](https://huggingface.co/mradermacher/Irbis-7b-v0.1-GGUF/resolve/main/Irbis-7b-v0.1.Q3_K_M.gguf) | Q3_K_M | 3.8 | lower quality |
| [GGUF](https://huggingface.co/mradermacher/Irbis-7b-v0.1-GGUF/resolve/main/Irbis-7b-v0.1.Q3_K_L.gguf) | Q3_K_L | 4.1 | |
| [GGUF](https://huggingface.co/mradermacher/Irbis-7b-v0.1-GGUF/resolve/main/Irbis-7b-v0.1.IQ4_XS.gguf) | IQ4_XS | 4.2 | |
| [GGUF](https://huggingface.co/mradermacher/Irbis-7b-v0.1-GGUF/resolve/main/Irbis-7b-v0.1.Q4_K_S.gguf) | Q4_K_S | 4.4 | fast, recommended |
| [GGUF](https://huggingface.co/mradermacher/Irbis-7b-v0.1-GGUF/resolve/main/Irbis-7b-v0.1.Q4_K_M.gguf) | Q4_K_M | 4.6 | fast, recommended |
| [GGUF](https://huggingface.co/mradermacher/Irbis-7b-v0.1-GGUF/resolve/main/Irbis-7b-v0.1.Q5_K_S.gguf) | Q5_K_S | 5.3 | |
| [GGUF](https://huggingface.co/mradermacher/Irbis-7b-v0.1-GGUF/resolve/main/Irbis-7b-v0.1.Q5_K_M.gguf) | Q5_K_M | 5.4 | |
| [GGUF](https://huggingface.co/mradermacher/Irbis-7b-v0.1-GGUF/resolve/main/Irbis-7b-v0.1.Q6_K.gguf) | Q6_K | 6.2 | very good quality |
| [GGUF](https://huggingface.co/mradermacher/Irbis-7b-v0.1-GGUF/resolve/main/Irbis-7b-v0.1.Q8_0.gguf) | Q8_0 | 8.0 | fast, best quality |
| [GGUF](https://huggingface.co/mradermacher/Irbis-7b-v0.1-GGUF/resolve/main/Irbis-7b-v0.1.f16.gguf) | f16 | 15.0 | 16 bpw, overkill |
Here is a handy graph by ikawrakow comparing some lower-quality quant
types (lower is better):

And here are Artefact2's thoughts on the matter:
https://gist.github.com/Artefact2/b5f810600771265fc1e39442288e8ec9
## FAQ / Model Request
See https://huggingface.co/mradermacher/model_requests for some answers to
questions you might have and/or if you want some other model quantized.
## Thanks
I thank my company, [nethype GmbH](https://www.nethype.de/), for letting
me use its servers and providing upgrades to my workstation to enable
this work in my free time.
<!-- end -->
|
SG161222/RealVisXL_V4.0_Lightning | SG161222 | 2024-04-12T15:36:48Z | 13,781 | 17 | diffusers | [
"diffusers",
"safetensors",
"license:openrail++",
"endpoints_compatible",
"diffusers:StableDiffusionXLPipeline",
"region:us"
] | text-to-image | 2024-02-26T07:27:39Z | ---
license: openrail++
---
<b>It's important! Read it!</b><br>
The model is still in the training phase. This is not the final version and may contain artifacts and perform poorly in some cases.<br>
<b>You can support me directly on Boosty - https://boosty.to/sg_161222</b><br>
The model is aimed at photorealism. Can produce sfw and nsfw images of decent quality.<br>
CivitAI Page: https://civitai.com/models/139562/realvisxl-v40<br>
<b>Recommended Negative Prompt:</b><br>
(octane render, render, drawing, anime, bad photo, bad photography:1.3), (worst quality, low quality, blurry:1.2), (bad teeth, deformed teeth, deformed lips), (bad anatomy, bad proportions:1.1), (deformed iris, deformed pupils), (deformed eyes, bad eyes), (deformed face, ugly face, bad face), (deformed hands, bad hands, fused fingers), morbid, mutilated, mutation, disfigured<br>
<b>or another negative prompt</b><br>
<b>Recommended Generation Parameters:</b><br>
Sampling Steps: 4+<br>
Sampling Method: DPM++ SDE Karras / DPM++ SDE<br>
CFG Scale: 1.0-2.0
<b>Recommended Hires Fix Parameters:</b><br>
Hires steps: 4+<br>
Upscaler: 4x-UltraSharp upscaler / or another<br>
Denoising strength: 0.1 - 0.3<br>
Upscale by: 1.1-1.5<br> |
mradermacher/llama-3-sqlcoder-8b-GGUF | mradermacher | 2024-06-27T22:23:31Z | 13,781 | 0 | transformers | [
"transformers",
"gguf",
"code",
"en",
"base_model:defog/llama-3-sqlcoder-8b",
"license:cc-by-sa-4.0",
"endpoints_compatible",
"region:us"
] | null | 2024-06-27T00:40:08Z | ---
base_model: defog/llama-3-sqlcoder-8b
language:
- en
library_name: transformers
license: cc-by-sa-4.0
quantized_by: mradermacher
tags:
- code
---
## About
<!-- ### quantize_version: 2 -->
<!-- ### output_tensor_quantised: 1 -->
<!-- ### convert_type: hf -->
<!-- ### vocab_type: -->
<!-- ### tags: -->
static quants of https://huggingface.co/defog/llama-3-sqlcoder-8b
<!-- provided-files -->
weighted/imatrix quants are available at https://huggingface.co/mradermacher/llama-3-sqlcoder-8b-i1-GGUF
## Usage
If you are unsure how to use GGUF files, refer to one of [TheBloke's
READMEs](https://huggingface.co/TheBloke/KafkaLM-70B-German-V0.1-GGUF) for
more details, including on how to concatenate multi-part files.
## Provided Quants
(sorted by size, not necessarily quality. IQ-quants are often preferable over similar sized non-IQ quants)
| Link | Type | Size/GB | Notes |
|:-----|:-----|--------:|:------|
| [GGUF](https://huggingface.co/mradermacher/llama-3-sqlcoder-8b-GGUF/resolve/main/llama-3-sqlcoder-8b.Q2_K.gguf) | Q2_K | 3.3 | |
| [GGUF](https://huggingface.co/mradermacher/llama-3-sqlcoder-8b-GGUF/resolve/main/llama-3-sqlcoder-8b.IQ3_XS.gguf) | IQ3_XS | 3.6 | |
| [GGUF](https://huggingface.co/mradermacher/llama-3-sqlcoder-8b-GGUF/resolve/main/llama-3-sqlcoder-8b.Q3_K_S.gguf) | Q3_K_S | 3.8 | |
| [GGUF](https://huggingface.co/mradermacher/llama-3-sqlcoder-8b-GGUF/resolve/main/llama-3-sqlcoder-8b.IQ3_S.gguf) | IQ3_S | 3.8 | beats Q3_K* |
| [GGUF](https://huggingface.co/mradermacher/llama-3-sqlcoder-8b-GGUF/resolve/main/llama-3-sqlcoder-8b.IQ3_M.gguf) | IQ3_M | 3.9 | |
| [GGUF](https://huggingface.co/mradermacher/llama-3-sqlcoder-8b-GGUF/resolve/main/llama-3-sqlcoder-8b.Q3_K_M.gguf) | Q3_K_M | 4.1 | lower quality |
| [GGUF](https://huggingface.co/mradermacher/llama-3-sqlcoder-8b-GGUF/resolve/main/llama-3-sqlcoder-8b.Q3_K_L.gguf) | Q3_K_L | 4.4 | |
| [GGUF](https://huggingface.co/mradermacher/llama-3-sqlcoder-8b-GGUF/resolve/main/llama-3-sqlcoder-8b.IQ4_XS.gguf) | IQ4_XS | 4.6 | |
| [GGUF](https://huggingface.co/mradermacher/llama-3-sqlcoder-8b-GGUF/resolve/main/llama-3-sqlcoder-8b.Q4_K_S.gguf) | Q4_K_S | 4.8 | fast, recommended |
| [GGUF](https://huggingface.co/mradermacher/llama-3-sqlcoder-8b-GGUF/resolve/main/llama-3-sqlcoder-8b.Q4_K_M.gguf) | Q4_K_M | 5.0 | fast, recommended |
| [GGUF](https://huggingface.co/mradermacher/llama-3-sqlcoder-8b-GGUF/resolve/main/llama-3-sqlcoder-8b.Q5_K_S.gguf) | Q5_K_S | 5.7 | |
| [GGUF](https://huggingface.co/mradermacher/llama-3-sqlcoder-8b-GGUF/resolve/main/llama-3-sqlcoder-8b.Q5_K_M.gguf) | Q5_K_M | 5.8 | |
| [GGUF](https://huggingface.co/mradermacher/llama-3-sqlcoder-8b-GGUF/resolve/main/llama-3-sqlcoder-8b.Q6_K.gguf) | Q6_K | 6.7 | very good quality |
| [GGUF](https://huggingface.co/mradermacher/llama-3-sqlcoder-8b-GGUF/resolve/main/llama-3-sqlcoder-8b.Q8_0.gguf) | Q8_0 | 8.6 | fast, best quality |
| [GGUF](https://huggingface.co/mradermacher/llama-3-sqlcoder-8b-GGUF/resolve/main/llama-3-sqlcoder-8b.f16.gguf) | f16 | 16.2 | 16 bpw, overkill |
Here is a handy graph by ikawrakow comparing some lower-quality quant
types (lower is better):

And here are Artefact2's thoughts on the matter:
https://gist.github.com/Artefact2/b5f810600771265fc1e39442288e8ec9
## FAQ / Model Request
See https://huggingface.co/mradermacher/model_requests for some answers to
questions you might have and/or if you want some other model quantized.
## Thanks
I thank my company, [nethype GmbH](https://www.nethype.de/), for letting
me use its servers and providing upgrades to my workstation to enable
this work in my free time.
<!-- end -->
|
mradermacher/llama-3-sqlcoder-8b-i1-GGUF | mradermacher | 2024-06-27T22:23:31Z | 13,772 | 0 | transformers | [
"transformers",
"gguf",
"code",
"en",
"base_model:defog/llama-3-sqlcoder-8b",
"license:cc-by-sa-4.0",
"endpoints_compatible",
"region:us"
] | null | 2024-06-27T20:05:34Z | ---
base_model: defog/llama-3-sqlcoder-8b
language:
- en
library_name: transformers
license: cc-by-sa-4.0
quantized_by: mradermacher
tags:
- code
---
## About
<!-- ### quantize_version: 2 -->
<!-- ### output_tensor_quantised: 1 -->
<!-- ### convert_type: hf -->
<!-- ### vocab_type: -->
<!-- ### tags: nicoboss -->
weighted/imatrix quants of https://huggingface.co/defog/llama-3-sqlcoder-8b
<!-- provided-files -->
static quants are available at https://huggingface.co/mradermacher/llama-3-sqlcoder-8b-GGUF
## Usage
If you are unsure how to use GGUF files, refer to one of [TheBloke's
READMEs](https://huggingface.co/TheBloke/KafkaLM-70B-German-V0.1-GGUF) for
more details, including on how to concatenate multi-part files.
## Provided Quants
(sorted by size, not necessarily quality. IQ-quants are often preferable over similar sized non-IQ quants)
| Link | Type | Size/GB | Notes |
|:-----|:-----|--------:|:------|
| [GGUF](https://huggingface.co/mradermacher/llama-3-sqlcoder-8b-i1-GGUF/resolve/main/llama-3-sqlcoder-8b.i1-IQ1_S.gguf) | i1-IQ1_S | 2.1 | for the desperate |
| [GGUF](https://huggingface.co/mradermacher/llama-3-sqlcoder-8b-i1-GGUF/resolve/main/llama-3-sqlcoder-8b.i1-IQ1_M.gguf) | i1-IQ1_M | 2.3 | mostly desperate |
| [GGUF](https://huggingface.co/mradermacher/llama-3-sqlcoder-8b-i1-GGUF/resolve/main/llama-3-sqlcoder-8b.i1-IQ2_XXS.gguf) | i1-IQ2_XXS | 2.5 | |
| [GGUF](https://huggingface.co/mradermacher/llama-3-sqlcoder-8b-i1-GGUF/resolve/main/llama-3-sqlcoder-8b.i1-IQ2_XS.gguf) | i1-IQ2_XS | 2.7 | |
| [GGUF](https://huggingface.co/mradermacher/llama-3-sqlcoder-8b-i1-GGUF/resolve/main/llama-3-sqlcoder-8b.i1-IQ2_S.gguf) | i1-IQ2_S | 2.9 | |
| [GGUF](https://huggingface.co/mradermacher/llama-3-sqlcoder-8b-i1-GGUF/resolve/main/llama-3-sqlcoder-8b.i1-IQ2_M.gguf) | i1-IQ2_M | 3.0 | |
| [GGUF](https://huggingface.co/mradermacher/llama-3-sqlcoder-8b-i1-GGUF/resolve/main/llama-3-sqlcoder-8b.i1-Q2_K.gguf) | i1-Q2_K | 3.3 | IQ3_XXS probably better |
| [GGUF](https://huggingface.co/mradermacher/llama-3-sqlcoder-8b-i1-GGUF/resolve/main/llama-3-sqlcoder-8b.i1-IQ3_XXS.gguf) | i1-IQ3_XXS | 3.4 | lower quality |
| [GGUF](https://huggingface.co/mradermacher/llama-3-sqlcoder-8b-i1-GGUF/resolve/main/llama-3-sqlcoder-8b.i1-IQ3_XS.gguf) | i1-IQ3_XS | 3.6 | |
| [GGUF](https://huggingface.co/mradermacher/llama-3-sqlcoder-8b-i1-GGUF/resolve/main/llama-3-sqlcoder-8b.i1-Q3_K_S.gguf) | i1-Q3_K_S | 3.8 | IQ3_XS probably better |
| [GGUF](https://huggingface.co/mradermacher/llama-3-sqlcoder-8b-i1-GGUF/resolve/main/llama-3-sqlcoder-8b.i1-IQ3_S.gguf) | i1-IQ3_S | 3.8 | beats Q3_K* |
| [GGUF](https://huggingface.co/mradermacher/llama-3-sqlcoder-8b-i1-GGUF/resolve/main/llama-3-sqlcoder-8b.i1-IQ3_M.gguf) | i1-IQ3_M | 3.9 | |
| [GGUF](https://huggingface.co/mradermacher/llama-3-sqlcoder-8b-i1-GGUF/resolve/main/llama-3-sqlcoder-8b.i1-Q3_K_M.gguf) | i1-Q3_K_M | 4.1 | IQ3_S probably better |
| [GGUF](https://huggingface.co/mradermacher/llama-3-sqlcoder-8b-i1-GGUF/resolve/main/llama-3-sqlcoder-8b.i1-Q3_K_L.gguf) | i1-Q3_K_L | 4.4 | IQ3_M probably better |
| [GGUF](https://huggingface.co/mradermacher/llama-3-sqlcoder-8b-i1-GGUF/resolve/main/llama-3-sqlcoder-8b.i1-IQ4_XS.gguf) | i1-IQ4_XS | 4.5 | |
| [GGUF](https://huggingface.co/mradermacher/llama-3-sqlcoder-8b-i1-GGUF/resolve/main/llama-3-sqlcoder-8b.i1-Q4_0.gguf) | i1-Q4_0 | 4.8 | fast, low quality |
| [GGUF](https://huggingface.co/mradermacher/llama-3-sqlcoder-8b-i1-GGUF/resolve/main/llama-3-sqlcoder-8b.i1-Q4_K_S.gguf) | i1-Q4_K_S | 4.8 | optimal size/speed/quality |
| [GGUF](https://huggingface.co/mradermacher/llama-3-sqlcoder-8b-i1-GGUF/resolve/main/llama-3-sqlcoder-8b.i1-Q4_K_M.gguf) | i1-Q4_K_M | 5.0 | fast, recommended |
| [GGUF](https://huggingface.co/mradermacher/llama-3-sqlcoder-8b-i1-GGUF/resolve/main/llama-3-sqlcoder-8b.i1-Q5_K_S.gguf) | i1-Q5_K_S | 5.7 | |
| [GGUF](https://huggingface.co/mradermacher/llama-3-sqlcoder-8b-i1-GGUF/resolve/main/llama-3-sqlcoder-8b.i1-Q5_K_M.gguf) | i1-Q5_K_M | 5.8 | |
| [GGUF](https://huggingface.co/mradermacher/llama-3-sqlcoder-8b-i1-GGUF/resolve/main/llama-3-sqlcoder-8b.i1-Q6_K.gguf) | i1-Q6_K | 6.7 | practically like static Q6_K |
Here is a handy graph by ikawrakow comparing some lower-quality quant
types (lower is better):

And here are Artefact2's thoughts on the matter:
https://gist.github.com/Artefact2/b5f810600771265fc1e39442288e8ec9
## FAQ / Model Request
See https://huggingface.co/mradermacher/model_requests for some answers to
questions you might have and/or if you want some other model quantized.
## Thanks
I thank my company, [nethype GmbH](https://www.nethype.de/), for letting
me use its servers and providing upgrades to my workstation to enable
this work in my free time. Additional thanks to [@nicoboss](https://huggingface.co/nicoboss) for giving me access to his hardware for calculating the imatrix for these quants.
<!-- end -->
|
dbmdz/bert-base-german-cased | dbmdz | 2023-09-06T22:19:38Z | 13,771 | 15 | transformers | [
"transformers",
"pytorch",
"tf",
"jax",
"safetensors",
"bert",
"fill-mask",
"de",
"license:mit",
"autotrain_compatible",
"endpoints_compatible",
"region:us"
] | fill-mask | 2022-03-02T23:29:05Z | ---
language: de
license: mit
---
# 🤗 + 📚 dbmdz German BERT models
In this repository the MDZ Digital Library team (dbmdz) at the Bavarian State
Library open sources another German BERT models 🎉
# German BERT
## Stats
In addition to the recently released [German BERT](https://deepset.ai/german-bert)
model by [deepset](https://deepset.ai/) we provide another German-language model.
The source data for the model consists of a recent Wikipedia dump, EU Bookshop corpus,
Open Subtitles, CommonCrawl, ParaCrawl and News Crawl. This results in a dataset with
a size of 16GB and 2,350,234,427 tokens.
For sentence splitting, we use [spacy](https://spacy.io/). Our preprocessing steps
(sentence piece model for vocab generation) follow those used for training
[SciBERT](https://github.com/allenai/scibert). The model is trained with an initial
sequence length of 512 subwords and was performed for 1.5M steps.
This release includes both cased and uncased models.
## Model weights
Currently only PyTorch-[Transformers](https://github.com/huggingface/transformers)
compatible weights are available. If you need access to TensorFlow checkpoints,
please raise an issue!
| Model | Downloads
| -------------------------------- | ---------------------------------------------------------------------------------------------------------------
| `bert-base-german-dbmdz-cased` | [`config.json`](https://s3.amazonaws.com/models.huggingface.co/bert/bert-base-german-dbmdz-cased-config.json) • [`pytorch_model.bin`](https://s3.amazonaws.com/models.huggingface.co/bert/bert-base-german-dbmdz-cased-pytorch_model.bin) • [`vocab.txt`](https://s3.amazonaws.com/models.huggingface.co/bert/bert-base-german-dbmdz-cased-vocab.txt)
| `bert-base-german-dbmdz-uncased` | [`config.json`](https://s3.amazonaws.com/models.huggingface.co/bert/bert-base-german-dbmdz-uncased-config.json) • [`pytorch_model.bin`](https://s3.amazonaws.com/models.huggingface.co/bert/bert-base-german-dbmdz-uncased-pytorch_model.bin) • [`vocab.txt`](https://s3.amazonaws.com/models.huggingface.co/bert/bert-base-german-dbmdz-uncased-vocab.txt)
## Usage
With Transformers >= 2.3 our German BERT models can be loaded like:
```python
from transformers import AutoModel, AutoTokenizer
tokenizer = AutoTokenizer.from_pretrained("dbmdz/bert-base-german-cased")
model = AutoModel.from_pretrained("dbmdz/bert-base-german-cased")
```
## Results
For results on downstream tasks like NER or PoS tagging, please refer to
[this repository](https://github.com/stefan-it/fine-tuned-berts-seq).
# Huggingface model hub
All models are available on the [Huggingface model hub](https://huggingface.co/dbmdz).
# Contact (Bugs, Feedback, Contribution and more)
For questions about our BERT models just open an issue
[here](https://github.com/dbmdz/berts/issues/new) 🤗
# Acknowledgments
Research supported with Cloud TPUs from Google's TensorFlow Research Cloud (TFRC).
Thanks for providing access to the TFRC ❤️
Thanks to the generous support from the [Hugging Face](https://huggingface.co/) team,
it is possible to download both cased and uncased models from their S3 storage 🤗
|
HuggingFaceM4/siglip-so400m-14-384 | HuggingFaceM4 | 2023-10-20T12:35:52Z | 13,768 | 3 | transformers | [
"transformers",
"pytorch",
"siglip",
"zero-shot-image-classification",
"custom_code",
"endpoints_compatible",
"region:us"
] | zero-shot-image-classification | 2023-10-17T12:10:20Z | Entry not found |
RichardErkhov/princeton-nlp_-_Llama-3-Base-8B-SFT-gguf | RichardErkhov | 2024-06-25T05:36:18Z | 13,768 | 0 | null | [
"gguf",
"arxiv:2405.14734",
"region:us"
] | null | 2024-06-24T22:42:05Z | Quantization made by Richard Erkhov.
[Github](https://github.com/RichardErkhov)
[Discord](https://discord.gg/pvy7H8DZMG)
[Request more models](https://github.com/RichardErkhov/quant_request)
Llama-3-Base-8B-SFT - GGUF
- Model creator: https://huggingface.co/princeton-nlp/
- Original model: https://huggingface.co/princeton-nlp/Llama-3-Base-8B-SFT/
| Name | Quant method | Size |
| ---- | ---- | ---- |
| [Llama-3-Base-8B-SFT.Q2_K.gguf](https://huggingface.co/RichardErkhov/princeton-nlp_-_Llama-3-Base-8B-SFT-gguf/blob/main/Llama-3-Base-8B-SFT.Q2_K.gguf) | Q2_K | 2.96GB |
| [Llama-3-Base-8B-SFT.IQ3_XS.gguf](https://huggingface.co/RichardErkhov/princeton-nlp_-_Llama-3-Base-8B-SFT-gguf/blob/main/Llama-3-Base-8B-SFT.IQ3_XS.gguf) | IQ3_XS | 3.28GB |
| [Llama-3-Base-8B-SFT.IQ3_S.gguf](https://huggingface.co/RichardErkhov/princeton-nlp_-_Llama-3-Base-8B-SFT-gguf/blob/main/Llama-3-Base-8B-SFT.IQ3_S.gguf) | IQ3_S | 1.61GB |
| [Llama-3-Base-8B-SFT.Q3_K_S.gguf](https://huggingface.co/RichardErkhov/princeton-nlp_-_Llama-3-Base-8B-SFT-gguf/blob/main/Llama-3-Base-8B-SFT.Q3_K_S.gguf) | Q3_K_S | 0.77GB |
| [Llama-3-Base-8B-SFT.IQ3_M.gguf](https://huggingface.co/RichardErkhov/princeton-nlp_-_Llama-3-Base-8B-SFT-gguf/blob/main/Llama-3-Base-8B-SFT.IQ3_M.gguf) | IQ3_M | 3.52GB |
| [Llama-3-Base-8B-SFT.Q3_K.gguf](https://huggingface.co/RichardErkhov/princeton-nlp_-_Llama-3-Base-8B-SFT-gguf/blob/main/Llama-3-Base-8B-SFT.Q3_K.gguf) | Q3_K | 3.74GB |
| [Llama-3-Base-8B-SFT.Q3_K_M.gguf](https://huggingface.co/RichardErkhov/princeton-nlp_-_Llama-3-Base-8B-SFT-gguf/blob/main/Llama-3-Base-8B-SFT.Q3_K_M.gguf) | Q3_K_M | 3.74GB |
| [Llama-3-Base-8B-SFT.Q3_K_L.gguf](https://huggingface.co/RichardErkhov/princeton-nlp_-_Llama-3-Base-8B-SFT-gguf/blob/main/Llama-3-Base-8B-SFT.Q3_K_L.gguf) | Q3_K_L | 4.03GB |
| [Llama-3-Base-8B-SFT.IQ4_XS.gguf](https://huggingface.co/RichardErkhov/princeton-nlp_-_Llama-3-Base-8B-SFT-gguf/blob/main/Llama-3-Base-8B-SFT.IQ4_XS.gguf) | IQ4_XS | 4.18GB |
| [Llama-3-Base-8B-SFT.Q4_0.gguf](https://huggingface.co/RichardErkhov/princeton-nlp_-_Llama-3-Base-8B-SFT-gguf/blob/main/Llama-3-Base-8B-SFT.Q4_0.gguf) | Q4_0 | 4.34GB |
| [Llama-3-Base-8B-SFT.IQ4_NL.gguf](https://huggingface.co/RichardErkhov/princeton-nlp_-_Llama-3-Base-8B-SFT-gguf/blob/main/Llama-3-Base-8B-SFT.IQ4_NL.gguf) | IQ4_NL | 4.38GB |
| [Llama-3-Base-8B-SFT.Q4_K_S.gguf](https://huggingface.co/RichardErkhov/princeton-nlp_-_Llama-3-Base-8B-SFT-gguf/blob/main/Llama-3-Base-8B-SFT.Q4_K_S.gguf) | Q4_K_S | 4.37GB |
| [Llama-3-Base-8B-SFT.Q4_K.gguf](https://huggingface.co/RichardErkhov/princeton-nlp_-_Llama-3-Base-8B-SFT-gguf/blob/main/Llama-3-Base-8B-SFT.Q4_K.gguf) | Q4_K | 4.58GB |
| [Llama-3-Base-8B-SFT.Q4_K_M.gguf](https://huggingface.co/RichardErkhov/princeton-nlp_-_Llama-3-Base-8B-SFT-gguf/blob/main/Llama-3-Base-8B-SFT.Q4_K_M.gguf) | Q4_K_M | 4.58GB |
| [Llama-3-Base-8B-SFT.Q4_1.gguf](https://huggingface.co/RichardErkhov/princeton-nlp_-_Llama-3-Base-8B-SFT-gguf/blob/main/Llama-3-Base-8B-SFT.Q4_1.gguf) | Q4_1 | 4.78GB |
| [Llama-3-Base-8B-SFT.Q5_0.gguf](https://huggingface.co/RichardErkhov/princeton-nlp_-_Llama-3-Base-8B-SFT-gguf/blob/main/Llama-3-Base-8B-SFT.Q5_0.gguf) | Q5_0 | 5.21GB |
| [Llama-3-Base-8B-SFT.Q5_K_S.gguf](https://huggingface.co/RichardErkhov/princeton-nlp_-_Llama-3-Base-8B-SFT-gguf/blob/main/Llama-3-Base-8B-SFT.Q5_K_S.gguf) | Q5_K_S | 5.21GB |
| [Llama-3-Base-8B-SFT.Q5_K.gguf](https://huggingface.co/RichardErkhov/princeton-nlp_-_Llama-3-Base-8B-SFT-gguf/blob/main/Llama-3-Base-8B-SFT.Q5_K.gguf) | Q5_K | 5.34GB |
| [Llama-3-Base-8B-SFT.Q5_K_M.gguf](https://huggingface.co/RichardErkhov/princeton-nlp_-_Llama-3-Base-8B-SFT-gguf/blob/main/Llama-3-Base-8B-SFT.Q5_K_M.gguf) | Q5_K_M | 5.34GB |
| [Llama-3-Base-8B-SFT.Q5_1.gguf](https://huggingface.co/RichardErkhov/princeton-nlp_-_Llama-3-Base-8B-SFT-gguf/blob/main/Llama-3-Base-8B-SFT.Q5_1.gguf) | Q5_1 | 5.65GB |
| [Llama-3-Base-8B-SFT.Q6_K.gguf](https://huggingface.co/RichardErkhov/princeton-nlp_-_Llama-3-Base-8B-SFT-gguf/blob/main/Llama-3-Base-8B-SFT.Q6_K.gguf) | Q6_K | 6.14GB |
| [Llama-3-Base-8B-SFT.Q8_0.gguf](https://huggingface.co/RichardErkhov/princeton-nlp_-_Llama-3-Base-8B-SFT-gguf/blob/main/Llama-3-Base-8B-SFT.Q8_0.gguf) | Q8_0 | 7.95GB |
Original model description:
This is a model released from the preprint: *[SimPO: Simple Preference Optimization with a Reference-Free Reward](https://arxiv.org/abs/2405.14734)* Please refer to our [repository](https://github.com/princeton-nlp/SimPO) for more details.
|
mradermacher/Swallow-7b-plus-hf-i1-GGUF | mradermacher | 2024-06-30T13:47:58Z | 13,760 | 0 | transformers | [
"transformers",
"gguf",
"en",
"ja",
"base_model:tokyotech-llm/Swallow-7b-plus-hf",
"license:llama2",
"endpoints_compatible",
"region:us"
] | null | 2024-06-30T11:39:46Z | ---
base_model: tokyotech-llm/Swallow-7b-plus-hf
language:
- en
- ja
library_name: transformers
license: llama2
model_type: llama
quantized_by: mradermacher
---
## About
<!-- ### quantize_version: 2 -->
<!-- ### output_tensor_quantised: 1 -->
<!-- ### convert_type: hf -->
<!-- ### vocab_type: -->
<!-- ### tags: nicoboss -->
weighted/imatrix quants of https://huggingface.co/tokyotech-llm/Swallow-7b-plus-hf
<!-- provided-files -->
static quants are available at https://huggingface.co/mradermacher/Swallow-7b-plus-hf-GGUF
## Usage
If you are unsure how to use GGUF files, refer to one of [TheBloke's
READMEs](https://huggingface.co/TheBloke/KafkaLM-70B-German-V0.1-GGUF) for
more details, including on how to concatenate multi-part files.
## Provided Quants
(sorted by size, not necessarily quality. IQ-quants are often preferable over similar sized non-IQ quants)
| Link | Type | Size/GB | Notes |
|:-----|:-----|--------:|:------|
| [GGUF](https://huggingface.co/mradermacher/Swallow-7b-plus-hf-i1-GGUF/resolve/main/Swallow-7b-plus-hf.i1-IQ1_S.gguf) | i1-IQ1_S | 1.7 | for the desperate |
| [GGUF](https://huggingface.co/mradermacher/Swallow-7b-plus-hf-i1-GGUF/resolve/main/Swallow-7b-plus-hf.i1-IQ1_M.gguf) | i1-IQ1_M | 1.8 | mostly desperate |
| [GGUF](https://huggingface.co/mradermacher/Swallow-7b-plus-hf-i1-GGUF/resolve/main/Swallow-7b-plus-hf.i1-IQ2_XXS.gguf) | i1-IQ2_XXS | 2.0 | |
| [GGUF](https://huggingface.co/mradermacher/Swallow-7b-plus-hf-i1-GGUF/resolve/main/Swallow-7b-plus-hf.i1-IQ2_XS.gguf) | i1-IQ2_XS | 2.2 | |
| [GGUF](https://huggingface.co/mradermacher/Swallow-7b-plus-hf-i1-GGUF/resolve/main/Swallow-7b-plus-hf.i1-IQ2_S.gguf) | i1-IQ2_S | 2.3 | |
| [GGUF](https://huggingface.co/mradermacher/Swallow-7b-plus-hf-i1-GGUF/resolve/main/Swallow-7b-plus-hf.i1-IQ2_M.gguf) | i1-IQ2_M | 2.5 | |
| [GGUF](https://huggingface.co/mradermacher/Swallow-7b-plus-hf-i1-GGUF/resolve/main/Swallow-7b-plus-hf.i1-Q2_K.gguf) | i1-Q2_K | 2.7 | IQ3_XXS probably better |
| [GGUF](https://huggingface.co/mradermacher/Swallow-7b-plus-hf-i1-GGUF/resolve/main/Swallow-7b-plus-hf.i1-IQ3_XXS.gguf) | i1-IQ3_XXS | 2.7 | lower quality |
| [GGUF](https://huggingface.co/mradermacher/Swallow-7b-plus-hf-i1-GGUF/resolve/main/Swallow-7b-plus-hf.i1-IQ3_XS.gguf) | i1-IQ3_XS | 3.0 | |
| [GGUF](https://huggingface.co/mradermacher/Swallow-7b-plus-hf-i1-GGUF/resolve/main/Swallow-7b-plus-hf.i1-IQ3_S.gguf) | i1-IQ3_S | 3.1 | beats Q3_K* |
| [GGUF](https://huggingface.co/mradermacher/Swallow-7b-plus-hf-i1-GGUF/resolve/main/Swallow-7b-plus-hf.i1-Q3_K_S.gguf) | i1-Q3_K_S | 3.1 | IQ3_XS probably better |
| [GGUF](https://huggingface.co/mradermacher/Swallow-7b-plus-hf-i1-GGUF/resolve/main/Swallow-7b-plus-hf.i1-IQ3_M.gguf) | i1-IQ3_M | 3.3 | |
| [GGUF](https://huggingface.co/mradermacher/Swallow-7b-plus-hf-i1-GGUF/resolve/main/Swallow-7b-plus-hf.i1-Q3_K_M.gguf) | i1-Q3_K_M | 3.5 | IQ3_S probably better |
| [GGUF](https://huggingface.co/mradermacher/Swallow-7b-plus-hf-i1-GGUF/resolve/main/Swallow-7b-plus-hf.i1-Q3_K_L.gguf) | i1-Q3_K_L | 3.8 | IQ3_M probably better |
| [GGUF](https://huggingface.co/mradermacher/Swallow-7b-plus-hf-i1-GGUF/resolve/main/Swallow-7b-plus-hf.i1-IQ4_XS.gguf) | i1-IQ4_XS | 3.8 | |
| [GGUF](https://huggingface.co/mradermacher/Swallow-7b-plus-hf-i1-GGUF/resolve/main/Swallow-7b-plus-hf.i1-Q4_0.gguf) | i1-Q4_0 | 4.0 | fast, low quality |
| [GGUF](https://huggingface.co/mradermacher/Swallow-7b-plus-hf-i1-GGUF/resolve/main/Swallow-7b-plus-hf.i1-Q4_K_S.gguf) | i1-Q4_K_S | 4.0 | optimal size/speed/quality |
| [GGUF](https://huggingface.co/mradermacher/Swallow-7b-plus-hf-i1-GGUF/resolve/main/Swallow-7b-plus-hf.i1-Q4_K_M.gguf) | i1-Q4_K_M | 4.2 | fast, recommended |
| [GGUF](https://huggingface.co/mradermacher/Swallow-7b-plus-hf-i1-GGUF/resolve/main/Swallow-7b-plus-hf.i1-Q5_K_S.gguf) | i1-Q5_K_S | 4.8 | |
| [GGUF](https://huggingface.co/mradermacher/Swallow-7b-plus-hf-i1-GGUF/resolve/main/Swallow-7b-plus-hf.i1-Q5_K_M.gguf) | i1-Q5_K_M | 5.0 | |
| [GGUF](https://huggingface.co/mradermacher/Swallow-7b-plus-hf-i1-GGUF/resolve/main/Swallow-7b-plus-hf.i1-Q6_K.gguf) | i1-Q6_K | 5.7 | practically like static Q6_K |
Here is a handy graph by ikawrakow comparing some lower-quality quant
types (lower is better):

And here are Artefact2's thoughts on the matter:
https://gist.github.com/Artefact2/b5f810600771265fc1e39442288e8ec9
## FAQ / Model Request
See https://huggingface.co/mradermacher/model_requests for some answers to
questions you might have and/or if you want some other model quantized.
## Thanks
I thank my company, [nethype GmbH](https://www.nethype.de/), for letting
me use its servers and providing upgrades to my workstation to enable
this work in my free time. Additional thanks to [@nicoboss](https://huggingface.co/nicoboss) for giving me access to his hardware for calculating the imatrix for these quants.
<!-- end -->
|
mradermacher/L3-Sophie-improved-v1-i1-GGUF | mradermacher | 2024-06-23T22:12:37Z | 13,759 | 0 | transformers | [
"transformers",
"gguf",
"mergekit",
"merge",
"en",
"base_model:Fischerboot/L3-Sophie-improved-v1",
"endpoints_compatible",
"region:us"
] | null | 2024-06-23T17:14:41Z | ---
base_model: Fischerboot/L3-Sophie-improved-v1
language:
- en
library_name: transformers
quantized_by: mradermacher
tags:
- mergekit
- merge
---
## About
<!-- ### quantize_version: 2 -->
<!-- ### output_tensor_quantised: 1 -->
<!-- ### convert_type: hf -->
<!-- ### vocab_type: -->
<!-- ### tags: nicoboss -->
weighted/imatrix quants of https://huggingface.co/Fischerboot/L3-Sophie-improved-v1
<!-- provided-files -->
static quants are available at https://huggingface.co/mradermacher/L3-Sophie-improved-v1-GGUF
## Usage
If you are unsure how to use GGUF files, refer to one of [TheBloke's
READMEs](https://huggingface.co/TheBloke/KafkaLM-70B-German-V0.1-GGUF) for
more details, including on how to concatenate multi-part files.
## Provided Quants
(sorted by size, not necessarily quality. IQ-quants are often preferable over similar sized non-IQ quants)
| Link | Type | Size/GB | Notes |
|:-----|:-----|--------:|:------|
| [GGUF](https://huggingface.co/mradermacher/L3-Sophie-improved-v1-i1-GGUF/resolve/main/L3-Sophie-improved-v1.i1-IQ1_S.gguf) | i1-IQ1_S | 2.1 | for the desperate |
| [GGUF](https://huggingface.co/mradermacher/L3-Sophie-improved-v1-i1-GGUF/resolve/main/L3-Sophie-improved-v1.i1-IQ1_M.gguf) | i1-IQ1_M | 2.3 | mostly desperate |
| [GGUF](https://huggingface.co/mradermacher/L3-Sophie-improved-v1-i1-GGUF/resolve/main/L3-Sophie-improved-v1.i1-IQ2_XXS.gguf) | i1-IQ2_XXS | 2.5 | |
| [GGUF](https://huggingface.co/mradermacher/L3-Sophie-improved-v1-i1-GGUF/resolve/main/L3-Sophie-improved-v1.i1-IQ2_XS.gguf) | i1-IQ2_XS | 2.7 | |
| [GGUF](https://huggingface.co/mradermacher/L3-Sophie-improved-v1-i1-GGUF/resolve/main/L3-Sophie-improved-v1.i1-IQ2_S.gguf) | i1-IQ2_S | 2.9 | |
| [GGUF](https://huggingface.co/mradermacher/L3-Sophie-improved-v1-i1-GGUF/resolve/main/L3-Sophie-improved-v1.i1-IQ2_M.gguf) | i1-IQ2_M | 3.0 | |
| [GGUF](https://huggingface.co/mradermacher/L3-Sophie-improved-v1-i1-GGUF/resolve/main/L3-Sophie-improved-v1.i1-Q2_K.gguf) | i1-Q2_K | 3.3 | IQ3_XXS probably better |
| [GGUF](https://huggingface.co/mradermacher/L3-Sophie-improved-v1-i1-GGUF/resolve/main/L3-Sophie-improved-v1.i1-IQ3_XXS.gguf) | i1-IQ3_XXS | 3.4 | lower quality |
| [GGUF](https://huggingface.co/mradermacher/L3-Sophie-improved-v1-i1-GGUF/resolve/main/L3-Sophie-improved-v1.i1-IQ3_XS.gguf) | i1-IQ3_XS | 3.6 | |
| [GGUF](https://huggingface.co/mradermacher/L3-Sophie-improved-v1-i1-GGUF/resolve/main/L3-Sophie-improved-v1.i1-Q3_K_S.gguf) | i1-Q3_K_S | 3.8 | IQ3_XS probably better |
| [GGUF](https://huggingface.co/mradermacher/L3-Sophie-improved-v1-i1-GGUF/resolve/main/L3-Sophie-improved-v1.i1-IQ3_S.gguf) | i1-IQ3_S | 3.8 | beats Q3_K* |
| [GGUF](https://huggingface.co/mradermacher/L3-Sophie-improved-v1-i1-GGUF/resolve/main/L3-Sophie-improved-v1.i1-IQ3_M.gguf) | i1-IQ3_M | 3.9 | |
| [GGUF](https://huggingface.co/mradermacher/L3-Sophie-improved-v1-i1-GGUF/resolve/main/L3-Sophie-improved-v1.i1-Q3_K_M.gguf) | i1-Q3_K_M | 4.1 | IQ3_S probably better |
| [GGUF](https://huggingface.co/mradermacher/L3-Sophie-improved-v1-i1-GGUF/resolve/main/L3-Sophie-improved-v1.i1-Q3_K_L.gguf) | i1-Q3_K_L | 4.4 | IQ3_M probably better |
| [GGUF](https://huggingface.co/mradermacher/L3-Sophie-improved-v1-i1-GGUF/resolve/main/L3-Sophie-improved-v1.i1-IQ4_XS.gguf) | i1-IQ4_XS | 4.5 | |
| [GGUF](https://huggingface.co/mradermacher/L3-Sophie-improved-v1-i1-GGUF/resolve/main/L3-Sophie-improved-v1.i1-Q4_0.gguf) | i1-Q4_0 | 4.8 | fast, low quality |
| [GGUF](https://huggingface.co/mradermacher/L3-Sophie-improved-v1-i1-GGUF/resolve/main/L3-Sophie-improved-v1.i1-Q4_K_S.gguf) | i1-Q4_K_S | 4.8 | optimal size/speed/quality |
| [GGUF](https://huggingface.co/mradermacher/L3-Sophie-improved-v1-i1-GGUF/resolve/main/L3-Sophie-improved-v1.i1-Q4_K_M.gguf) | i1-Q4_K_M | 5.0 | fast, recommended |
| [GGUF](https://huggingface.co/mradermacher/L3-Sophie-improved-v1-i1-GGUF/resolve/main/L3-Sophie-improved-v1.i1-Q5_K_S.gguf) | i1-Q5_K_S | 5.7 | |
| [GGUF](https://huggingface.co/mradermacher/L3-Sophie-improved-v1-i1-GGUF/resolve/main/L3-Sophie-improved-v1.i1-Q5_K_M.gguf) | i1-Q5_K_M | 5.8 | |
| [GGUF](https://huggingface.co/mradermacher/L3-Sophie-improved-v1-i1-GGUF/resolve/main/L3-Sophie-improved-v1.i1-Q6_K.gguf) | i1-Q6_K | 6.7 | practically like static Q6_K |
Here is a handy graph by ikawrakow comparing some lower-quality quant
types (lower is better):

And here are Artefact2's thoughts on the matter:
https://gist.github.com/Artefact2/b5f810600771265fc1e39442288e8ec9
## FAQ / Model Request
See https://huggingface.co/mradermacher/model_requests for some answers to
questions you might have and/or if you want some other model quantized.
## Thanks
I thank my company, [nethype GmbH](https://www.nethype.de/), for letting
me use its servers and providing upgrades to my workstation to enable
this work in my free time. Additional thanks to [@nicoboss](https://huggingface.co/nicoboss) for giving me access to his hardware for calculating the imatrix for these quants.
<!-- end -->
|
RichardErkhov/DiscoResearch_-_Llama3-DiscoLeo-Instruct-8B-v0.1-gguf | RichardErkhov | 2024-06-26T21:20:46Z | 13,755 | 0 | null | [
"gguf",
"region:us"
] | null | 2024-06-26T15:11:44Z | Entry not found |
mradermacher/EEVE-Korean-10.8B-RAFT-GGUF | mradermacher | 2024-06-27T18:55:59Z | 13,745 | 0 | transformers | [
"transformers",
"gguf",
"en",
"base_model:ai-human-lab/EEVE-Korean-10.8B-RAFT",
"license:apache-2.0",
"endpoints_compatible",
"region:us"
] | null | 2024-06-27T18:18:20Z | ---
base_model: ai-human-lab/EEVE-Korean-10.8B-RAFT
language:
- en
library_name: transformers
license: apache-2.0
quantized_by: mradermacher
---
## About
<!-- ### quantize_version: 2 -->
<!-- ### output_tensor_quantised: 1 -->
<!-- ### convert_type: hf -->
<!-- ### vocab_type: -->
<!-- ### tags: -->
static quants of https://huggingface.co/ai-human-lab/EEVE-Korean-10.8B-RAFT
<!-- provided-files -->
weighted/imatrix quants seem not to be available (by me) at this time. If they do not show up a week or so after the static ones, I have probably not planned for them. Feel free to request them by opening a Community Discussion.
## Usage
If you are unsure how to use GGUF files, refer to one of [TheBloke's
READMEs](https://huggingface.co/TheBloke/KafkaLM-70B-German-V0.1-GGUF) for
more details, including on how to concatenate multi-part files.
## Provided Quants
(sorted by size, not necessarily quality. IQ-quants are often preferable over similar sized non-IQ quants)
| Link | Type | Size/GB | Notes |
|:-----|:-----|--------:|:------|
| [GGUF](https://huggingface.co/mradermacher/EEVE-Korean-10.8B-RAFT-GGUF/resolve/main/EEVE-Korean-10.8B-RAFT.Q2_K.gguf) | Q2_K | 4.1 | |
| [GGUF](https://huggingface.co/mradermacher/EEVE-Korean-10.8B-RAFT-GGUF/resolve/main/EEVE-Korean-10.8B-RAFT.IQ3_XS.gguf) | IQ3_XS | 4.6 | |
| [GGUF](https://huggingface.co/mradermacher/EEVE-Korean-10.8B-RAFT-GGUF/resolve/main/EEVE-Korean-10.8B-RAFT.Q3_K_S.gguf) | Q3_K_S | 4.8 | |
| [GGUF](https://huggingface.co/mradermacher/EEVE-Korean-10.8B-RAFT-GGUF/resolve/main/EEVE-Korean-10.8B-RAFT.IQ3_S.gguf) | IQ3_S | 4.8 | beats Q3_K* |
| [GGUF](https://huggingface.co/mradermacher/EEVE-Korean-10.8B-RAFT-GGUF/resolve/main/EEVE-Korean-10.8B-RAFT.IQ3_M.gguf) | IQ3_M | 5.0 | |
| [GGUF](https://huggingface.co/mradermacher/EEVE-Korean-10.8B-RAFT-GGUF/resolve/main/EEVE-Korean-10.8B-RAFT.Q3_K_M.gguf) | Q3_K_M | 5.3 | lower quality |
| [GGUF](https://huggingface.co/mradermacher/EEVE-Korean-10.8B-RAFT-GGUF/resolve/main/EEVE-Korean-10.8B-RAFT.Q3_K_L.gguf) | Q3_K_L | 5.8 | |
| [GGUF](https://huggingface.co/mradermacher/EEVE-Korean-10.8B-RAFT-GGUF/resolve/main/EEVE-Korean-10.8B-RAFT.IQ4_XS.gguf) | IQ4_XS | 6.0 | |
| [GGUF](https://huggingface.co/mradermacher/EEVE-Korean-10.8B-RAFT-GGUF/resolve/main/EEVE-Korean-10.8B-RAFT.Q4_K_S.gguf) | Q4_K_S | 6.3 | fast, recommended |
| [GGUF](https://huggingface.co/mradermacher/EEVE-Korean-10.8B-RAFT-GGUF/resolve/main/EEVE-Korean-10.8B-RAFT.Q4_K_M.gguf) | Q4_K_M | 6.6 | fast, recommended |
| [GGUF](https://huggingface.co/mradermacher/EEVE-Korean-10.8B-RAFT-GGUF/resolve/main/EEVE-Korean-10.8B-RAFT.Q5_K_S.gguf) | Q5_K_S | 7.6 | |
| [GGUF](https://huggingface.co/mradermacher/EEVE-Korean-10.8B-RAFT-GGUF/resolve/main/EEVE-Korean-10.8B-RAFT.Q5_K_M.gguf) | Q5_K_M | 7.8 | |
| [GGUF](https://huggingface.co/mradermacher/EEVE-Korean-10.8B-RAFT-GGUF/resolve/main/EEVE-Korean-10.8B-RAFT.Q6_K.gguf) | Q6_K | 9.0 | very good quality |
| [GGUF](https://huggingface.co/mradermacher/EEVE-Korean-10.8B-RAFT-GGUF/resolve/main/EEVE-Korean-10.8B-RAFT.Q8_0.gguf) | Q8_0 | 11.6 | fast, best quality |
Here is a handy graph by ikawrakow comparing some lower-quality quant
types (lower is better):

And here are Artefact2's thoughts on the matter:
https://gist.github.com/Artefact2/b5f810600771265fc1e39442288e8ec9
## FAQ / Model Request
See https://huggingface.co/mradermacher/model_requests for some answers to
questions you might have and/or if you want some other model quantized.
## Thanks
I thank my company, [nethype GmbH](https://www.nethype.de/), for letting
me use its servers and providing upgrades to my workstation to enable
this work in my free time.
<!-- end -->
|
second-state/Gemma-2-9B-Chinese-Chat-GGUF | second-state | 2024-07-02T04:51:48Z | 13,731 | 0 | transformers | [
"transformers",
"gguf",
"gemma2",
"text-generation",
"en",
"zh",
"base_model:shenzhi-wang/Gemma-2-9B-Chinese-Chat",
"license:gemma",
"autotrain_compatible",
"text-generation-inference",
"region:us"
] | text-generation | 2024-07-02T03:44:22Z | ---
license: gemma
library_name: transformers
pipeline_tag: text-generation
base_model: shenzhi-wang/Gemma-2-9B-Chinese-Chat
inference: false
model_creator: shenzhi-wang
model_name: Gemma-2-9B-Chinese-Chat
quantized_by: Second State Inc.
language:
- en
- zh
---
<!-- header start -->
<!-- 200823 -->
<div style="width: auto; margin-left: auto; margin-right: auto">
<img src="https://github.com/LlamaEdge/LlamaEdge/raw/dev/assets/logo.svg" style="width: 100%; min-width: 400px; display: block; margin: auto;">
</div>
<hr style="margin-top: 1.0em; margin-bottom: 1.0em;">
<!-- header end -->
# Gemma-2-9B-Chinese-Chat-GGUF
## Original Model
[shenzhi-wang/Gemma-2-9B-Chinese-Chat](https://huggingface.co/shenzhi-wang/Gemma-2-9B-Chinese-Chat)
## Run with LlamaEdge
- LlamaEdge version: [v0.12.1](https://github.com/LlamaEdge/LlamaEdge/releases/tag/0.12.1) and above
- Prompt template
- Prompt type: `gemma-instruct`
- Prompt string
```text
<bos><start_of_turn>user
{user_message}<end_of_turn>
<start_of_turn>model
{model_message}<end_of_turn>model
```
- Context size: `8192`
- Run as LlamaEdge service
```bash
wasmedge --dir .:. --nn-preload default:GGML:AUTO:Gemma-2-9B-Chinese-Chat-Q5_K_M.gguf \
llama-api-server.wasm \
--prompt-template gemma-instruct \
--ctx-size 8192 \
--model-name gemma-2-9b
```
- Run as LlamaEdge command app
```bash
wasmedge --dir .:. \
--nn-preload default:GGML:AUTO:Gemma-2-9B-Chinese-Chat-Q5_K_M.gguf \
llama-chat.wasm \
--prompt-template gemma-instruct \
--ctx-size 8192
```
## Quantized GGUF Models
| Name | Quant method | Bits | Size | Use case |
| ---- | ---- | ---- | ---- | ----- |
| [Gemma-2-9B-Chinese-Chat-Q2_K.gguf](https://huggingface.co/second-state/Gemma-2-9B-Chinese-Chat-GGUF/blob/main/Gemma-2-9B-Chinese-Chat-Q2_K.gguf) | Q2_K | 2 | 3.81 GB| smallest, significant quality loss - not recommended for most purposes |
| [Gemma-2-9B-Chinese-Chat-Q3_K_L.gguf](https://huggingface.co/second-state/Gemma-2-9B-Chinese-Chat-GGUF/blob/main/Gemma-2-9B-Chinese-Chat-Q3_K_L.gguf) | Q3_K_L | 3 | 5.13 GB| small, substantial quality loss |
| [Gemma-2-9B-Chinese-Chat-Q3_K_M.gguf](https://huggingface.co/second-state/Gemma-2-9B-Chinese-Chat-GGUF/blob/main/Gemma-2-9B-Chinese-Chat-Q3_K_M.gguf) | Q3_K_M | 3 | 4.76 GB| very small, high quality loss |
| [Gemma-2-9B-Chinese-Chat-Q3_K_S.gguf](https://huggingface.co/second-state/Gemma-2-9B-Chinese-Chat-GGUF/blob/main/Gemma-2-9B-Chinese-Chat-Q3_K_S.gguf) | Q3_K_S | 3 | 4.34 GB| very small, high quality loss |
| [Gemma-2-9B-Chinese-Chat-Q4_0.gguf](https://huggingface.co/second-state/Gemma-2-9B-Chinese-Chat-GGUF/blob/main/Gemma-2-9B-Chinese-Chat-Q4_0.gguf) | Q4_0 | 4 | 5.44 GB| legacy; small, very high quality loss - prefer using Q3_K_M |
| [Gemma-2-9B-Chinese-Chat-Q4_K_M.gguf](https://huggingface.co/second-state/Gemma-2-9B-Chinese-Chat-GGUF/blob/main/Gemma-2-9B-Chinese-Chat-Q4_K_M.gguf) | Q4_K_M | 4 | 5.76 GB| medium, balanced quality - recommended |
| [Gemma-2-9B-Chinese-Chat-Q4_K_S.gguf](https://huggingface.co/second-state/Gemma-2-9B-Chinese-Chat-GGUF/blob/main/Gemma-2-9B-Chinese-Chat-Q4_K_S.gguf) | Q4_K_S | 4 | 5.48 GB| small, greater quality loss |
| [Gemma-2-9B-Chinese-Chat-Q5_0.gguf](https://huggingface.co/second-state/Gemma-2-9B-Chinese-Chat-GGUF/blob/main/Gemma-2-9B-Chinese-Chat-Q5_0.gguf) | Q5_0 | 5 | 6.48 GB| legacy; medium, balanced quality - prefer using Q4_K_M |
| [Gemma-2-9B-Chinese-Chat-Q5_K_M.gguf](https://huggingface.co/second-state/Gemma-2-9B-Chinese-Chat-GGUF/blob/main/Gemma-2-9B-Chinese-Chat-Q5_K_M.gguf) | Q5_K_M | 5 | 6.65 GB| large, very low quality loss - recommended |
| [Gemma-2-9B-Chinese-Chat-Q5_K_S.gguf](https://huggingface.co/second-state/Gemma-2-9B-Chinese-Chat-GGUF/blob/main/Gemma-2-9B-Chinese-Chat-Q5_K_S.gguf) | Q5_K_S | 5 | 6.48 GB| large, low quality loss - recommended |
| [Gemma-2-9B-Chinese-Chat-Q6_K.gguf](https://huggingface.co/second-state/Gemma-2-9B-Chinese-Chat-GGUF/blob/main/Gemma-2-9B-Chinese-Chat-Q6_K.gguf) | Q6_K | 6 | 7.59 GB| very large, extremely low quality loss |
| [Gemma-2-9B-Chinese-Chat-Q8_0.gguf](https://huggingface.co/second-state/Gemma-2-9B-Chinese-Chat-GGUF/blob/main/Gemma-2-9B-Chinese-Chat-Q8_0.gguf) | Q8_0 | 8 | 9.83 GB| very large, extremely low quality loss - not recommended |
| [Gemma-2-9B-Chinese-Chat-f16.gguf](https://huggingface.co/second-state/Gemma-2-9B-Chinese-Chat-GGUF/blob/main/Gemma-2-9B-Chinese-Chat-f16.gguf) | f16 | 16 | 18.5 GB| |
*Quantized with llama.cpp b3259* |
RichardErkhov/NurtureAI_-_Meta-Llama-3-8B-Instruct-32k-gguf | RichardErkhov | 2024-06-25T07:15:08Z | 13,718 | 0 | null | [
"gguf",
"region:us"
] | null | 2024-06-25T03:19:00Z | Quantization made by Richard Erkhov.
[Github](https://github.com/RichardErkhov)
[Discord](https://discord.gg/pvy7H8DZMG)
[Request more models](https://github.com/RichardErkhov/quant_request)
Meta-Llama-3-8B-Instruct-32k - GGUF
- Model creator: https://huggingface.co/NurtureAI/
- Original model: https://huggingface.co/NurtureAI/Meta-Llama-3-8B-Instruct-32k/
| Name | Quant method | Size |
| ---- | ---- | ---- |
| [Meta-Llama-3-8B-Instruct-32k.Q2_K.gguf](https://huggingface.co/RichardErkhov/NurtureAI_-_Meta-Llama-3-8B-Instruct-32k-gguf/blob/main/Meta-Llama-3-8B-Instruct-32k.Q2_K.gguf) | Q2_K | 2.96GB |
| [Meta-Llama-3-8B-Instruct-32k.IQ3_XS.gguf](https://huggingface.co/RichardErkhov/NurtureAI_-_Meta-Llama-3-8B-Instruct-32k-gguf/blob/main/Meta-Llama-3-8B-Instruct-32k.IQ3_XS.gguf) | IQ3_XS | 3.28GB |
| [Meta-Llama-3-8B-Instruct-32k.IQ3_S.gguf](https://huggingface.co/RichardErkhov/NurtureAI_-_Meta-Llama-3-8B-Instruct-32k-gguf/blob/main/Meta-Llama-3-8B-Instruct-32k.IQ3_S.gguf) | IQ3_S | 3.43GB |
| [Meta-Llama-3-8B-Instruct-32k.Q3_K_S.gguf](https://huggingface.co/RichardErkhov/NurtureAI_-_Meta-Llama-3-8B-Instruct-32k-gguf/blob/main/Meta-Llama-3-8B-Instruct-32k.Q3_K_S.gguf) | Q3_K_S | 3.41GB |
| [Meta-Llama-3-8B-Instruct-32k.IQ3_M.gguf](https://huggingface.co/RichardErkhov/NurtureAI_-_Meta-Llama-3-8B-Instruct-32k-gguf/blob/main/Meta-Llama-3-8B-Instruct-32k.IQ3_M.gguf) | IQ3_M | 3.52GB |
| [Meta-Llama-3-8B-Instruct-32k.Q3_K.gguf](https://huggingface.co/RichardErkhov/NurtureAI_-_Meta-Llama-3-8B-Instruct-32k-gguf/blob/main/Meta-Llama-3-8B-Instruct-32k.Q3_K.gguf) | Q3_K | 3.74GB |
| [Meta-Llama-3-8B-Instruct-32k.Q3_K_M.gguf](https://huggingface.co/RichardErkhov/NurtureAI_-_Meta-Llama-3-8B-Instruct-32k-gguf/blob/main/Meta-Llama-3-8B-Instruct-32k.Q3_K_M.gguf) | Q3_K_M | 3.74GB |
| [Meta-Llama-3-8B-Instruct-32k.Q3_K_L.gguf](https://huggingface.co/RichardErkhov/NurtureAI_-_Meta-Llama-3-8B-Instruct-32k-gguf/blob/main/Meta-Llama-3-8B-Instruct-32k.Q3_K_L.gguf) | Q3_K_L | 4.03GB |
| [Meta-Llama-3-8B-Instruct-32k.IQ4_XS.gguf](https://huggingface.co/RichardErkhov/NurtureAI_-_Meta-Llama-3-8B-Instruct-32k-gguf/blob/main/Meta-Llama-3-8B-Instruct-32k.IQ4_XS.gguf) | IQ4_XS | 4.18GB |
| [Meta-Llama-3-8B-Instruct-32k.Q4_0.gguf](https://huggingface.co/RichardErkhov/NurtureAI_-_Meta-Llama-3-8B-Instruct-32k-gguf/blob/main/Meta-Llama-3-8B-Instruct-32k.Q4_0.gguf) | Q4_0 | 4.34GB |
| [Meta-Llama-3-8B-Instruct-32k.IQ4_NL.gguf](https://huggingface.co/RichardErkhov/NurtureAI_-_Meta-Llama-3-8B-Instruct-32k-gguf/blob/main/Meta-Llama-3-8B-Instruct-32k.IQ4_NL.gguf) | IQ4_NL | 4.38GB |
| [Meta-Llama-3-8B-Instruct-32k.Q4_K_S.gguf](https://huggingface.co/RichardErkhov/NurtureAI_-_Meta-Llama-3-8B-Instruct-32k-gguf/blob/main/Meta-Llama-3-8B-Instruct-32k.Q4_K_S.gguf) | Q4_K_S | 4.37GB |
| [Meta-Llama-3-8B-Instruct-32k.Q4_K.gguf](https://huggingface.co/RichardErkhov/NurtureAI_-_Meta-Llama-3-8B-Instruct-32k-gguf/blob/main/Meta-Llama-3-8B-Instruct-32k.Q4_K.gguf) | Q4_K | 4.58GB |
| [Meta-Llama-3-8B-Instruct-32k.Q4_K_M.gguf](https://huggingface.co/RichardErkhov/NurtureAI_-_Meta-Llama-3-8B-Instruct-32k-gguf/blob/main/Meta-Llama-3-8B-Instruct-32k.Q4_K_M.gguf) | Q4_K_M | 4.58GB |
| [Meta-Llama-3-8B-Instruct-32k.Q4_1.gguf](https://huggingface.co/RichardErkhov/NurtureAI_-_Meta-Llama-3-8B-Instruct-32k-gguf/blob/main/Meta-Llama-3-8B-Instruct-32k.Q4_1.gguf) | Q4_1 | 4.78GB |
| [Meta-Llama-3-8B-Instruct-32k.Q5_0.gguf](https://huggingface.co/RichardErkhov/NurtureAI_-_Meta-Llama-3-8B-Instruct-32k-gguf/blob/main/Meta-Llama-3-8B-Instruct-32k.Q5_0.gguf) | Q5_0 | 5.21GB |
| [Meta-Llama-3-8B-Instruct-32k.Q5_K_S.gguf](https://huggingface.co/RichardErkhov/NurtureAI_-_Meta-Llama-3-8B-Instruct-32k-gguf/blob/main/Meta-Llama-3-8B-Instruct-32k.Q5_K_S.gguf) | Q5_K_S | 5.21GB |
| [Meta-Llama-3-8B-Instruct-32k.Q5_K.gguf](https://huggingface.co/RichardErkhov/NurtureAI_-_Meta-Llama-3-8B-Instruct-32k-gguf/blob/main/Meta-Llama-3-8B-Instruct-32k.Q5_K.gguf) | Q5_K | 5.34GB |
| [Meta-Llama-3-8B-Instruct-32k.Q5_K_M.gguf](https://huggingface.co/RichardErkhov/NurtureAI_-_Meta-Llama-3-8B-Instruct-32k-gguf/blob/main/Meta-Llama-3-8B-Instruct-32k.Q5_K_M.gguf) | Q5_K_M | 5.34GB |
| [Meta-Llama-3-8B-Instruct-32k.Q5_1.gguf](https://huggingface.co/RichardErkhov/NurtureAI_-_Meta-Llama-3-8B-Instruct-32k-gguf/blob/main/Meta-Llama-3-8B-Instruct-32k.Q5_1.gguf) | Q5_1 | 5.65GB |
| [Meta-Llama-3-8B-Instruct-32k.Q6_K.gguf](https://huggingface.co/RichardErkhov/NurtureAI_-_Meta-Llama-3-8B-Instruct-32k-gguf/blob/main/Meta-Llama-3-8B-Instruct-32k.Q6_K.gguf) | Q6_K | 6.14GB |
| [Meta-Llama-3-8B-Instruct-32k.Q8_0.gguf](https://huggingface.co/RichardErkhov/NurtureAI_-_Meta-Llama-3-8B-Instruct-32k-gguf/blob/main/Meta-Llama-3-8B-Instruct-32k.Q8_0.gguf) | Q8_0 | 7.95GB |
Original model description:
---
language:
- en
pipeline_tag: text-generation
tags:
- facebook
- meta
- pytorch
- llama
- llama-3
license: other
license_name: llama3
license_link: LICENSE
extra_gated_prompt: >-
### META LLAMA 3 COMMUNITY LICENSE AGREEMENT
Meta Llama 3 Version Release Date: April 18, 2024
"Agreement" means the terms and conditions for use, reproduction, distribution and modification of the
Llama Materials set forth herein.
"Documentation" means the specifications, manuals and documentation accompanying Meta Llama 3
distributed by Meta at https://llama.meta.com/get-started/.
"Licensee" or "you" means you, or your employer or any other person or entity (if you are entering into
this Agreement on such person or entity’s behalf), of the age required under applicable laws, rules or
regulations to provide legal consent and that has legal authority to bind your employer or such other
person or entity if you are entering in this Agreement on their behalf.
"Meta Llama 3" means the foundational large language models and software and algorithms, including
machine-learning model code, trained model weights, inference-enabling code, training-enabling code,
fine-tuning enabling code and other elements of the foregoing distributed by Meta at
https://llama.meta.com/llama-downloads.
"Llama Materials" means, collectively, Meta’s proprietary Meta Llama 3 and Documentation (and any
portion thereof) made available under this Agreement.
"Meta" or "we" means Meta Platforms Ireland Limited (if you are located in or, if you are an entity, your
principal place of business is in the EEA or Switzerland) and Meta Platforms, Inc. (if you are located
outside of the EEA or Switzerland).
1. License Rights and Redistribution.
a. Grant of Rights. You are granted a non-exclusive, worldwide, non-transferable and royalty-free
limited license under Meta’s intellectual property or other rights owned by Meta embodied in the Llama
Materials to use, reproduce, distribute, copy, create derivative works of, and make modifications to the
Llama Materials.
b. Redistribution and Use.
i. If you distribute or make available the Llama Materials (or any derivative works
thereof), or a product or service that uses any of them, including another AI model, you shall (A) provide
a copy of this Agreement with any such Llama Materials; and (B) prominently display “Built with Meta
Llama 3” on a related website, user interface, blogpost, about page, or product documentation. If you
use the Llama Materials to create, train, fine tune, or otherwise improve an AI model, which is
distributed or made available, you shall also include “Llama 3” at the beginning of any such AI model
name.
ii. If you receive Llama Materials, or any derivative works thereof, from a Licensee as part
of an integrated end user product, then Section 2 of this Agreement will not apply to you.
iii. You must retain in all copies of the Llama Materials that you distribute the following
attribution notice within a “Notice” text file distributed as a part of such copies: “Meta Llama 3 is
licensed under the Meta Llama 3 Community License, Copyright © Meta Platforms, Inc. All Rights
Reserved.”
iv. Your use of the Llama Materials must comply with applicable laws and regulations
(including trade compliance laws and regulations) and adhere to the Acceptable Use Policy for the Llama
Materials (available at https://llama.meta.com/llama3/use-policy), which is hereby incorporated by
reference into this Agreement.
v. You will not use the Llama Materials or any output or results of the Llama Materials to
improve any other large language model (excluding Meta Llama 3 or derivative works thereof).
2. Additional Commercial Terms. If, on the Meta Llama 3 version release date, the monthly active users
of the products or services made available by or for Licensee, or Licensee’s affiliates, is greater than 700
million monthly active users in the preceding calendar month, you must request a license from Meta,
which Meta may grant to you in its sole discretion, and you are not authorized to exercise any of the
rights under this Agreement unless or until Meta otherwise expressly grants you such rights.
3. Disclaimer of Warranty. UNLESS REQUIRED BY APPLICABLE LAW, THE LLAMA MATERIALS AND ANY
OUTPUT AND RESULTS THEREFROM ARE PROVIDED ON AN “AS IS” BASIS, WITHOUT WARRANTIES OF
ANY KIND, AND META DISCLAIMS ALL WARRANTIES OF ANY KIND, BOTH EXPRESS AND IMPLIED,
INCLUDING, WITHOUT LIMITATION, ANY WARRANTIES OF TITLE, NON-INFRINGEMENT,
MERCHANTABILITY, OR FITNESS FOR A PARTICULAR PURPOSE. YOU ARE SOLELY RESPONSIBLE FOR
DETERMINING THE APPROPRIATENESS OF USING OR REDISTRIBUTING THE LLAMA MATERIALS AND
ASSUME ANY RISKS ASSOCIATED WITH YOUR USE OF THE LLAMA MATERIALS AND ANY OUTPUT AND
RESULTS.
4. Limitation of Liability. IN NO EVENT WILL META OR ITS AFFILIATES BE LIABLE UNDER ANY THEORY OF
LIABILITY, WHETHER IN CONTRACT, TORT, NEGLIGENCE, PRODUCTS LIABILITY, OR OTHERWISE, ARISING
OUT OF THIS AGREEMENT, FOR ANY LOST PROFITS OR ANY INDIRECT, SPECIAL, CONSEQUENTIAL,
INCIDENTAL, EXEMPLARY OR PUNITIVE DAMAGES, EVEN IF META OR ITS AFFILIATES HAVE BEEN ADVISED
OF THE POSSIBILITY OF ANY OF THE FOREGOING.
5. Intellectual Property.
a. No trademark licenses are granted under this Agreement, and in connection with the Llama
Materials, neither Meta nor Licensee may use any name or mark owned by or associated with the other
or any of its affiliates, except as required for reasonable and customary use in describing and
redistributing the Llama Materials or as set forth in this Section 5(a). Meta hereby grants you a license to
use “Llama 3” (the “Mark”) solely as required to comply with the last sentence of Section 1.b.i. You will
comply with Meta’s brand guidelines (currently accessible at
https://about.meta.com/brand/resources/meta/company-brand/ ). All goodwill arising out of your use
of the Mark will inure to the benefit of Meta.
b. Subject to Meta’s ownership of Llama Materials and derivatives made by or for Meta, with
respect to any derivative works and modifications of the Llama Materials that are made by you, as
between you and Meta, you are and will be the owner of such derivative works and modifications.
c. If you institute litigation or other proceedings against Meta or any entity (including a
cross-claim or counterclaim in a lawsuit) alleging that the Llama Materials or Meta Llama 3 outputs or
results, or any portion of any of the foregoing, constitutes infringement of intellectual property or other
rights owned or licensable by you, then any licenses granted to you under this Agreement shall
terminate as of the date such litigation or claim is filed or instituted. You will indemnify and hold
harmless Meta from and against any claim by any third party arising out of or related to your use or
distribution of the Llama Materials.
6. Term and Termination. The term of this Agreement will commence upon your acceptance of this
Agreement or access to the Llama Materials and will continue in full force and effect until terminated in
accordance with the terms and conditions herein. Meta may terminate this Agreement if you are in
breach of any term or condition of this Agreement. Upon termination of this Agreement, you shall delete
and cease use of the Llama Materials. Sections 3, 4 and 7 shall survive the termination of this
Agreement.
7. Governing Law and Jurisdiction. This Agreement will be governed and construed under the laws of
the State of California without regard to choice of law principles, and the UN Convention on Contracts
for the International Sale of Goods does not apply to this Agreement. The courts of California shall have
exclusive jurisdiction of any dispute arising out of this Agreement.
### Meta Llama 3 Acceptable Use Policy
Meta is committed to promoting safe and fair use of its tools and features, including Meta Llama 3. If you
access or use Meta Llama 3, you agree to this Acceptable Use Policy (“Policy”). The most recent copy of
this policy can be found at [https://llama.meta.com/llama3/use-policy](https://llama.meta.com/llama3/use-policy)
#### Prohibited Uses
We want everyone to use Meta Llama 3 safely and responsibly. You agree you will not use, or allow
others to use, Meta Llama 3 to:
1. Violate the law or others’ rights, including to:
1. Engage in, promote, generate, contribute to, encourage, plan, incite, or further illegal or unlawful activity or content, such as:
1. Violence or terrorism
2. Exploitation or harm to children, including the solicitation, creation, acquisition, or dissemination of child exploitative content or failure to report Child Sexual Abuse Material
3. Human trafficking, exploitation, and sexual violence
4. The illegal distribution of information or materials to minors, including obscene materials, or failure to employ legally required age-gating in connection with such information or materials.
5. Sexual solicitation
6. Any other criminal activity
2. Engage in, promote, incite, or facilitate the harassment, abuse, threatening, or bullying of individuals or groups of individuals
3. Engage in, promote, incite, or facilitate discrimination or other unlawful or harmful conduct in the provision of employment, employment benefits, credit, housing, other economic benefits, or other essential goods and services
4. Engage in the unauthorized or unlicensed practice of any profession including, but not limited to, financial, legal, medical/health, or related professional practices
5. Collect, process, disclose, generate, or infer health, demographic, or other sensitive personal or private information about individuals without rights and consents required by applicable laws
6. Engage in or facilitate any action or generate any content that infringes, misappropriates, or otherwise violates any third-party rights, including the outputs or results of any products or services using the Llama Materials
7. Create, generate, or facilitate the creation of malicious code, malware, computer viruses or do anything else that could disable, overburden, interfere with or impair the proper working, integrity, operation or appearance of a website or computer system
2. Engage in, promote, incite, facilitate, or assist in the planning or development of activities that present a risk of death or bodily harm to individuals, including use of Meta Llama 3 related to the following:
1. Military, warfare, nuclear industries or applications, espionage, use for materials or activities that are subject to the International Traffic Arms Regulations (ITAR) maintained by the United States Department of State
2. Guns and illegal weapons (including weapon development)
3. Illegal drugs and regulated/controlled substances
4. Operation of critical infrastructure, transportation technologies, or heavy machinery
5. Self-harm or harm to others, including suicide, cutting, and eating disorders
6. Any content intended to incite or promote violence, abuse, or any infliction of bodily harm to an individual
3. Intentionally deceive or mislead others, including use of Meta Llama 3 related to the following:
1. Generating, promoting, or furthering fraud or the creation or promotion of disinformation
2. Generating, promoting, or furthering defamatory content, including the creation of defamatory statements, images, or other content
3. Generating, promoting, or further distributing spam
4. Impersonating another individual without consent, authorization, or legal right
5. Representing that the use of Meta Llama 3 or outputs are human-generated
6. Generating or facilitating false online engagement, including fake reviews and other means of fake online engagement
4. Fail to appropriately disclose to end users any known dangers of your AI system
Please report any violation of this Policy, software “bug,” or other problems that could lead to a violation
of this Policy through one of the following means:
* Reporting issues with the model: [https://github.com/meta-llama/llama3](https://github.com/meta-llama/llama3)
* Reporting risky content generated by the model:
developers.facebook.com/llama_output_feedback
* Reporting bugs and security concerns: facebook.com/whitehat/info
* Reporting violations of the Acceptable Use Policy or unlicensed uses of Meta Llama 3: [email protected]
extra_gated_fields:
First Name: text
Last Name: text
Date of birth: date_picker
Country: country
Affiliation: text
geo: ip_location
By clicking Submit below I accept the terms of the license and acknowledge that the information I provide will be collected stored processed and shared in accordance with the Meta Privacy Policy: checkbox
extra_gated_description: The information you provide will be collected, stored, processed and shared in accordance with the [Meta Privacy Policy](https://www.facebook.com/privacy/policy/).
extra_gated_button_content: Submit
---
## Model Details
Meta developed and released the Meta Llama 3 family of large language models (LLMs), a collection of pretrained and instruction tuned generative text models in 8 and 70B sizes. The Llama 3 instruction tuned models are optimized for dialogue use cases and outperform many of the available open source chat models on common industry benchmarks. Further, in developing these models, we took great care to optimize helpfulness and safety.
**Model developers** Meta
**Variations** Llama 3 comes in two sizes — 8B and 70B parameters — in pre-trained and instruction tuned variants.
**Input** Models input text only.
**Output** Models generate text and code only.
**Model Architecture** Llama 3 is an auto-regressive language model that uses an optimized transformer architecture. The tuned versions use supervised fine-tuning (SFT) and reinforcement learning with human feedback (RLHF) to align with human preferences for helpfulness and safety.
<table>
<tr>
<td>
</td>
<td><strong>Training Data</strong>
</td>
<td><strong>Params</strong>
</td>
<td><strong>Context length</strong>
</td>
<td><strong>GQA</strong>
</td>
<td><strong>Token count</strong>
</td>
<td><strong>Knowledge cutoff</strong>
</td>
</tr>
<tr>
<td rowspan="2" >Llama 3
</td>
<td rowspan="2" >A new mix of publicly available online data.
</td>
<td>8B
</td>
<td>8k
</td>
<td>Yes
</td>
<td rowspan="2" >15T+
</td>
<td>March, 2023
</td>
</tr>
<tr>
<td>70B
</td>
<td>8k
</td>
<td>Yes
</td>
<td>December, 2023
</td>
</tr>
</table>
**Llama 3 family of models**. Token counts refer to pretraining data only. Both the 8 and 70B versions use Grouped-Query Attention (GQA) for improved inference scalability.
**Model Release Date** April 18, 2024.
**Status** This is a static model trained on an offline dataset. Future versions of the tuned models will be released as we improve model safety with community feedback.
**License** A custom commercial license is available at: [https://llama.meta.com/llama3/license](https://llama.meta.com/llama3/license)
Where to send questions or comments about the model Instructions on how to provide feedback or comments on the model can be found in the model [README](https://github.com/meta-llama/llama3). For more technical information about generation parameters and recipes for how to use Llama 3 in applications, please go [here](https://github.com/meta-llama/llama-recipes).
## Intended Use
**Intended Use Cases** Llama 3 is intended for commercial and research use in English. Instruction tuned models are intended for assistant-like chat, whereas pretrained models can be adapted for a variety of natural language generation tasks.
**Out-of-scope** Use in any manner that violates applicable laws or regulations (including trade compliance laws). Use in any other way that is prohibited by the Acceptable Use Policy and Llama 3 Community License. Use in languages other than English**.
**Note: Developers may fine-tune Llama 3 models for languages beyond English provided they comply with the Llama 3 Community License and the Acceptable Use Policy.
## How to use
This repository contains two versions of Meta-Llama-3-8B-Instruct, for use with transformers and with the original `llama3` codebase.
### Use with transformers
You can run conversational inference using the Transformers pipeline abstraction, or by leveraging the Auto classes with the `generate()` function. Let's see examples of both.
#### Transformers pipeline
```python
import transformers
import torch
model_id = "meta-llama/Meta-Llama-3-8B-Instruct"
pipeline = transformers.pipeline(
"text-generation",
model=model_id,
model_kwargs={"torch_dtype": torch.bfloat16},
device="auto",
)
messages = [
{"role": "system", "content": "You are a pirate chatbot who always responds in pirate speak!"},
{"role": "user", "content": "Who are you?"},
]
prompt = pipeline.tokenizer.apply_chat_template(
messages,
tokenize=False,
add_generation_prompt=True
)
terminators = [
pipeline.tokenizer.eos_token_id,
pipeline.tokenizer.convert_tokens_to_ids("<|eot_id|>")
]
outputs = pipeline(
prompt,
max_new_tokens=256,
eos_token_id=terminators,
do_sample=True,
temperature=0.6,
top_p=0.9,
)
print(outputs[0]["generated_text"][len(prompt):])
```
#### Transformers AutoModelForCausalLM
```python
from transformers import AutoTokenizer, AutoModelForCausalLM
import torch
model_id = "meta-llama/Meta-Llama-3-8B-Instruct"
tokenizer = AutoTokenizer.from_pretrained(model_id)
model = AutoModelForCausalLM.from_pretrained(
model_id,
torch_dtype=torch.bfloat16,
device_map="auto",
)
messages = [
{"role": "system", "content": "You are a pirate chatbot who always responds in pirate speak!"},
{"role": "user", "content": "Who are you?"},
]
input_ids = tokenizer.apply_chat_template(
messages,
add_generation_prompt=True,
return_tensors="pt"
).to(model.device)
terminators = [
tokenizer.eos_token_id,
tokenizer.convert_tokens_to_ids("<|eot_id|>")
]
outputs = model.generate(
input_ids,
max_new_tokens=256,
eos_token_id=terminators,
do_sample=True,
temperature=0.6,
top_p=0.9,
)
response = outputs[0][input_ids.shape[-1]:]
print(tokenizer.decode(response, skip_special_tokens=True))
```
### Use with `llama3`
Please, follow the instructions in the [repository](https://github.com/meta-llama/llama3)
To download Original checkpoints, see the example command below leveraging `huggingface-cli`:
```
huggingface-cli download meta-llama/Meta-Llama-3-8B-Instruct --include "original/*" --local-dir Meta-Llama-3-8B-Instruct
```
For Hugging Face support, we recommend using transformers or TGI, but a similar command works.
## Hardware and Software
**Training Factors** We used custom training libraries, Meta's Research SuperCluster, and production clusters for pretraining. Fine-tuning, annotation, and evaluation were also performed on third-party cloud compute.
**Carbon Footprint Pretraining utilized a cumulative** 7.7M GPU hours of computation on hardware of type H100-80GB (TDP of 700W). Estimated total emissions were 2290 tCO2eq, 100% of which were offset by Meta’s sustainability program.
<table>
<tr>
<td>
</td>
<td><strong>Time (GPU hours)</strong>
</td>
<td><strong>Power Consumption (W)</strong>
</td>
<td><strong>Carbon Emitted(tCO2eq)</strong>
</td>
</tr>
<tr>
<td>Llama 3 8B
</td>
<td>1.3M
</td>
<td>700
</td>
<td>390
</td>
</tr>
<tr>
<td>Llama 3 70B
</td>
<td>6.4M
</td>
<td>700
</td>
<td>1900
</td>
</tr>
<tr>
<td>Total
</td>
<td>7.7M
</td>
<td>
</td>
<td>2290
</td>
</tr>
</table>
**CO2 emissions during pre-training**. Time: total GPU time required for training each model. Power Consumption: peak power capacity per GPU device for the GPUs used adjusted for power usage efficiency. 100% of the emissions are directly offset by Meta's sustainability program, and because we are openly releasing these models, the pretraining costs do not need to be incurred by others.
## Training Data
**Overview** Llama 3 was pretrained on over 15 trillion tokens of data from publicly available sources. The fine-tuning data includes publicly available instruction datasets, as well as over 10M human-annotated examples. Neither the pretraining nor the fine-tuning datasets include Meta user data.
**Data Freshness** The pretraining data has a cutoff of March 2023 for the 7B and December 2023 for the 70B models respectively.
## Benchmarks
In this section, we report the results for Llama 3 models on standard automatic benchmarks. For all the evaluations, we use our internal evaluations library. For details on the methodology see [here](https://github.com/meta-llama/llama3/blob/main/eval_methodology.md).
### Base pretrained models
<table>
<tr>
<td><strong>Category</strong>
</td>
<td><strong>Benchmark</strong>
</td>
<td><strong>Llama 3 8B</strong>
</td>
<td><strong>Llama2 7B</strong>
</td>
<td><strong>Llama2 13B</strong>
</td>
<td><strong>Llama 3 70B</strong>
</td>
<td><strong>Llama2 70B</strong>
</td>
</tr>
<tr>
<td rowspan="6" >General
</td>
<td>MMLU (5-shot)
</td>
<td>66.6
</td>
<td>45.7
</td>
<td>53.8
</td>
<td>79.5
</td>
<td>69.7
</td>
</tr>
<tr>
<td>AGIEval English (3-5 shot)
</td>
<td>45.9
</td>
<td>28.8
</td>
<td>38.7
</td>
<td>63.0
</td>
<td>54.8
</td>
</tr>
<tr>
<td>CommonSenseQA (7-shot)
</td>
<td>72.6
</td>
<td>57.6
</td>
<td>67.6
</td>
<td>83.8
</td>
<td>78.7
</td>
</tr>
<tr>
<td>Winogrande (5-shot)
</td>
<td>76.1
</td>
<td>73.3
</td>
<td>75.4
</td>
<td>83.1
</td>
<td>81.8
</td>
</tr>
<tr>
<td>BIG-Bench Hard (3-shot, CoT)
</td>
<td>61.1
</td>
<td>38.1
</td>
<td>47.0
</td>
<td>81.3
</td>
<td>65.7
</td>
</tr>
<tr>
<td>ARC-Challenge (25-shot)
</td>
<td>78.6
</td>
<td>53.7
</td>
<td>67.6
</td>
<td>93.0
</td>
<td>85.3
</td>
</tr>
<tr>
<td>Knowledge reasoning
</td>
<td>TriviaQA-Wiki (5-shot)
</td>
<td>78.5
</td>
<td>72.1
</td>
<td>79.6
</td>
<td>89.7
</td>
<td>87.5
</td>
</tr>
<tr>
<td rowspan="4" >Reading comprehension
</td>
<td>SQuAD (1-shot)
</td>
<td>76.4
</td>
<td>72.2
</td>
<td>72.1
</td>
<td>85.6
</td>
<td>82.6
</td>
</tr>
<tr>
<td>QuAC (1-shot, F1)
</td>
<td>44.4
</td>
<td>39.6
</td>
<td>44.9
</td>
<td>51.1
</td>
<td>49.4
</td>
</tr>
<tr>
<td>BoolQ (0-shot)
</td>
<td>75.7
</td>
<td>65.5
</td>
<td>66.9
</td>
<td>79.0
</td>
<td>73.1
</td>
</tr>
<tr>
<td>DROP (3-shot, F1)
</td>
<td>58.4
</td>
<td>37.9
</td>
<td>49.8
</td>
<td>79.7
</td>
<td>70.2
</td>
</tr>
</table>
### Instruction tuned models
<table>
<tr>
<td><strong>Benchmark</strong>
</td>
<td><strong>Llama 3 8B</strong>
</td>
<td><strong>Llama 2 7B</strong>
</td>
<td><strong>Llama 2 13B</strong>
</td>
<td><strong>Llama 3 70B</strong>
</td>
<td><strong>Llama 2 70B</strong>
</td>
</tr>
<tr>
<td>MMLU (5-shot)
</td>
<td>68.4
</td>
<td>34.1
</td>
<td>47.8
</td>
<td>82.0
</td>
<td>52.9
</td>
</tr>
<tr>
<td>GPQA (0-shot)
</td>
<td>34.2
</td>
<td>21.7
</td>
<td>22.3
</td>
<td>39.5
</td>
<td>21.0
</td>
</tr>
<tr>
<td>HumanEval (0-shot)
</td>
<td>62.2
</td>
<td>7.9
</td>
<td>14.0
</td>
<td>81.7
</td>
<td>25.6
</td>
</tr>
<tr>
<td>GSM-8K (8-shot, CoT)
</td>
<td>79.6
</td>
<td>25.7
</td>
<td>77.4
</td>
<td>93.0
</td>
<td>57.5
</td>
</tr>
<tr>
<td>MATH (4-shot, CoT)
</td>
<td>30.0
</td>
<td>3.8
</td>
<td>6.7
</td>
<td>50.4
</td>
<td>11.6
</td>
</tr>
</table>
### Responsibility & Safety
We believe that an open approach to AI leads to better, safer products, faster innovation, and a bigger overall market. We are committed to Responsible AI development and took a series of steps to limit misuse and harm and support the open source community.
Foundation models are widely capable technologies that are built to be used for a diverse range of applications. They are not designed to meet every developer preference on safety levels for all use cases, out-of-the-box, as those by their nature will differ across different applications.
Rather, responsible LLM-application deployment is achieved by implementing a series of safety best practices throughout the development of such applications, from the model pre-training, fine-tuning and the deployment of systems composed of safeguards to tailor the safety needs specifically to the use case and audience.
As part of the Llama 3 release, we updated our [Responsible Use Guide](https://llama.meta.com/responsible-use-guide/) to outline the steps and best practices for developers to implement model and system level safety for their application. We also provide a set of resources including [Meta Llama Guard 2](https://llama.meta.com/purple-llama/) and [Code Shield](https://llama.meta.com/purple-llama/) safeguards. These tools have proven to drastically reduce residual risks of LLM Systems, while maintaining a high level of helpfulness. We encourage developers to tune and deploy these safeguards according to their needs and we provide a [reference implementation](https://github.com/meta-llama/llama-recipes/tree/main/recipes/responsible_ai) to get you started.
#### Llama 3-Instruct
As outlined in the Responsible Use Guide, some trade-off between model helpfulness and model alignment is likely unavoidable. Developers should exercise discretion about how to weigh the benefits of alignment and helpfulness for their specific use case and audience. Developers should be mindful of residual risks when using Llama models and leverage additional safety tools as needed to reach the right safety bar for their use case.
<span style="text-decoration:underline;">Safety</span>
For our instruction tuned model, we conducted extensive red teaming exercises, performed adversarial evaluations and implemented safety mitigations techniques to lower residual risks. As with any Large Language Model, residual risks will likely remain and we recommend that developers assess these risks in the context of their use case. In parallel, we are working with the community to make AI safety benchmark standards transparent, rigorous and interpretable.
<span style="text-decoration:underline;">Refusals</span>
In addition to residual risks, we put a great emphasis on model refusals to benign prompts. Over-refusing not only can impact the user experience but could even be harmful in certain contexts as well. We’ve heard the feedback from the developer community and improved our fine tuning to ensure that Llama 3 is significantly less likely to falsely refuse to answer prompts than Llama 2.
We built internal benchmarks and developed mitigations to limit false refusals making Llama 3 our most helpful model to date.
#### Responsible release
In addition to responsible use considerations outlined above, we followed a rigorous process that requires us to take extra measures against misuse and critical risks before we make our release decision.
Misuse
If you access or use Llama 3, you agree to the Acceptable Use Policy. The most recent copy of this policy can be found at [https://llama.meta.com/llama3/use-policy/](https://llama.meta.com/llama3/use-policy/).
#### Critical risks
<span style="text-decoration:underline;">CBRNE</span> (Chemical, Biological, Radiological, Nuclear, and high yield Explosives)
We have conducted a two fold assessment of the safety of the model in this area:
* Iterative testing during model training to assess the safety of responses related to CBRNE threats and other adversarial risks.
* Involving external CBRNE experts to conduct an uplift test assessing the ability of the model to accurately provide expert knowledge and reduce barriers to potential CBRNE misuse, by reference to what can be achieved using web search (without the model).
### <span style="text-decoration:underline;">Cyber Security </span>
We have evaluated Llama 3 with CyberSecEval, Meta’s cybersecurity safety eval suite, measuring Llama 3’s propensity to suggest insecure code when used as a coding assistant, and Llama 3’s propensity to comply with requests to help carry out cyber attacks, where attacks are defined by the industry standard MITRE ATT&CK cyber attack ontology. On our insecure coding and cyber attacker helpfulness tests, Llama 3 behaved in the same range or safer than models of [equivalent coding capability](https://huggingface.co/spaces/facebook/CyberSecEval).
### <span style="text-decoration:underline;">Child Safety</span>
Child Safety risk assessments were conducted using a team of experts, to assess the model’s capability to produce outputs that could result in Child Safety risks and inform on any necessary and appropriate risk mitigations via fine tuning. We leveraged those expert red teaming sessions to expand the coverage of our evaluation benchmarks through Llama 3 model development. For Llama 3, we conducted new in-depth sessions using objective based methodologies to assess the model risks along multiple attack vectors. We also partnered with content specialists to perform red teaming exercises assessing potentially violating content while taking account of market specific nuances or experiences.
### Community
Generative AI safety requires expertise and tooling, and we believe in the strength of the open community to accelerate its progress. We are active members of open consortiums, including the AI Alliance, Partnership in AI and MLCommons, actively contributing to safety standardization and transparency. We encourage the community to adopt taxonomies like the MLCommons Proof of Concept evaluation to facilitate collaboration and transparency on safety and content evaluations. Our Purple Llama tools are open sourced for the community to use and widely distributed across ecosystem partners including cloud service providers. We encourage community contributions to our [Github repository](https://github.com/meta-llama/PurpleLlama).
Finally, we put in place a set of resources including an [output reporting mechanism](https://developers.facebook.com/llama_output_feedback) and [bug bounty program](https://www.facebook.com/whitehat) to continuously improve the Llama technology with the help of the community.
## Ethical Considerations and Limitations
The core values of Llama 3 are openness, inclusivity and helpfulness. It is meant to serve everyone, and to work for a wide range of use cases. It is thus designed to be accessible to people across many different backgrounds, experiences and perspectives. Llama 3 addresses users and their needs as they are, without insertion unnecessary judgment or normativity, while reflecting the understanding that even content that may appear problematic in some cases can serve valuable purposes in others. It respects the dignity and autonomy of all users, especially in terms of the values of free thought and expression that power innovation and progress.
But Llama 3 is a new technology, and like any new technology, there are risks associated with its use. Testing conducted to date has been in English, and has not covered, nor could it cover, all scenarios. For these reasons, as with all LLMs, Llama 3’s potential outputs cannot be predicted in advance, and the model may in some instances produce inaccurate, biased or other objectionable responses to user prompts. Therefore, before deploying any applications of Llama 3 models, developers should perform safety testing and tuning tailored to their specific applications of the model. As outlined in the Responsible Use Guide, we recommend incorporating [Purple Llama](https://github.com/facebookresearch/PurpleLlama) solutions into your workflows and specifically [Llama Guard](https://ai.meta.com/research/publications/llama-guard-llm-based-input-output-safeguard-for-human-ai-conversations/) which provides a base model to filter input and output prompts to layer system-level safety on top of model-level safety.
Please see the Responsible Use Guide available at [http://llama.meta.com/responsible-use-guide](http://llama.meta.com/responsible-use-guide)
## Citation instructions
@article{llama3modelcard,
title={Llama 3 Model Card},
author={AI@Meta},
year={2024},
url = {https://github.com/meta-llama/llama3/blob/main/MODEL_CARD.md}
}
## Contributors
Aaditya Singh; Aaron Grattafiori; Abhimanyu Dubey; Abhinav Jauhri; Abhinav Pandey; Abhishek Kadian; Adam Kelsey; Adi Gangidi; Ahmad Al-Dahle; Ahuva Goldstand; Aiesha Letman; Ajay Menon; Akhil Mathur; Alan Schelten; Alex Vaughan; Amy Yang; Andrei Lupu; Andres Alvarado; Andrew Gallagher; Andrew Gu; Andrew Ho; Andrew Poulton; Andrew Ryan; Angela Fan; Ankit Ramchandani; Anthony Hartshorn; Archi Mitra; Archie Sravankumar; Artem Korenev; Arun Rao; Ashley Gabriel; Ashwin Bharambe; Assaf Eisenman; Aston Zhang; Aurelien Rodriguez; Austen Gregerson; Ava Spataru; Baptiste Roziere; Ben Maurer; Benjamin Leonhardi; Bernie Huang; Bhargavi Paranjape; Bing Liu; Binh Tang; Bobbie Chern; Brani Stojkovic; Brian Fuller; Catalina Mejia Arenas; Chao Zhou; Charlotte Caucheteux; Chaya Nayak; Ching-Hsiang Chu; Chloe Bi; Chris Cai; Chris Cox; Chris Marra; Chris McConnell; Christian Keller; Christoph Feichtenhofer; Christophe Touret; Chunyang Wu; Corinne Wong; Cristian Canton Ferrer; Damien Allonsius; Daniel Kreymer; Daniel Haziza; Daniel Li; Danielle Pintz; Danny Livshits; Danny Wyatt; David Adkins; David Esiobu; David Xu; Davide Testuggine; Delia David; Devi Parikh; Dhruv Choudhary; Dhruv Mahajan; Diana Liskovich; Diego Garcia-Olano; Diego Perino; Dieuwke Hupkes; Dingkang Wang; Dustin Holland; Egor Lakomkin; Elina Lobanova; Xiaoqing Ellen Tan; Emily Dinan; Eric Smith; Erik Brinkman; Esteban Arcaute; Filip Radenovic; Firat Ozgenel; Francesco Caggioni; Frank Seide; Frank Zhang; Gabriel Synnaeve; Gabriella Schwarz; Gabrielle Lee; Gada Badeer; Georgia Anderson; Graeme Nail; Gregoire Mialon; Guan Pang; Guillem Cucurell; Hailey Nguyen; Hannah Korevaar; Hannah Wang; Haroun Habeeb; Harrison Rudolph; Henry Aspegren; Hu Xu; Hugo Touvron; Iga Kozlowska; Igor Molybog; Igor Tufanov; Iliyan Zarov; Imanol Arrieta Ibarra; Irina-Elena Veliche; Isabel Kloumann; Ishan Misra; Ivan Evtimov; Jacob Xu; Jade Copet; Jake Weissman; Jan Geffert; Jana Vranes; Japhet Asher; Jason Park; Jay Mahadeokar; Jean-Baptiste Gaya; Jeet Shah; Jelmer van der Linde; Jennifer Chan; Jenny Hong; Jenya Lee; Jeremy Fu; Jeremy Teboul; Jianfeng Chi; Jianyu Huang; Jie Wang; Jiecao Yu; Joanna Bitton; Joe Spisak; Joelle Pineau; Jon Carvill; Jongsoo Park; Joseph Rocca; Joshua Johnstun; Junteng Jia; Kalyan Vasuden Alwala; Kam Hou U; Kate Plawiak; Kartikeya Upasani; Kaushik Veeraraghavan; Ke Li; Kenneth Heafield; Kevin Stone; Khalid El-Arini; Krithika Iyer; Kshitiz Malik; Kuenley Chiu; Kunal Bhalla; Kyle Huang; Lakshya Garg; Lauren Rantala-Yeary; Laurens van der Maaten; Lawrence Chen; Leandro Silva; Lee Bell; Lei Zhang; Liang Tan; Louis Martin; Lovish Madaan; Luca Wehrstedt; Lukas Blecher; Luke de Oliveira; Madeline Muzzi; Madian Khabsa; Manav Avlani; Mannat Singh; Manohar Paluri; Mark Zuckerberg; Marcin Kardas; Martynas Mankus; Mathew Oldham; Mathieu Rita; Matthew Lennie; Maya Pavlova; Meghan Keneally; Melanie Kambadur; Mihir Patel; Mikayel Samvelyan; Mike Clark; Mike Lewis; Min Si; Mitesh Kumar Singh; Mo Metanat; Mona Hassan; Naman Goyal; Narjes Torabi; Nicolas Usunier; Nikolay Bashlykov; Nikolay Bogoychev; Niladri Chatterji; Ning Dong; Oliver Aobo Yang; Olivier Duchenne; Onur Celebi; Parth Parekh; Patrick Alrassy; Paul Saab; Pavan Balaji; Pedro Rittner; Pengchuan Zhang; Pengwei Li; Petar Vasic; Peter Weng; Polina Zvyagina; Prajjwal Bhargava; Pratik Dubal; Praveen Krishnan; Punit Singh Koura; Qing He; Rachel Rodriguez; Ragavan Srinivasan; Rahul Mitra; Ramon Calderer; Raymond Li; Robert Stojnic; Roberta Raileanu; Robin Battey; Rocky Wang; Rohit Girdhar; Rohit Patel; Romain Sauvestre; Ronnie Polidoro; Roshan Sumbaly; Ross Taylor; Ruan Silva; Rui Hou; Rui Wang; Russ Howes; Ruty Rinott; Saghar Hosseini; Sai Jayesh Bondu; Samyak Datta; Sanjay Singh; Sara Chugh; Sargun Dhillon; Satadru Pan; Sean Bell; Sergey Edunov; Shaoliang Nie; Sharan Narang; Sharath Raparthy; Shaun Lindsay; Sheng Feng; Sheng Shen; Shenghao Lin; Shiva Shankar; Shruti Bhosale; Shun Zhang; Simon Vandenhende; Sinong Wang; Seohyun Sonia Kim; Soumya Batra; Sten Sootla; Steve Kehoe; Suchin Gururangan; Sumit Gupta; Sunny Virk; Sydney Borodinsky; Tamar Glaser; Tamar Herman; Tamara Best; Tara Fowler; Thomas Georgiou; Thomas Scialom; Tianhe Li; Todor Mihaylov; Tong Xiao; Ujjwal Karn; Vedanuj Goswami; Vibhor Gupta; Vignesh Ramanathan; Viktor Kerkez; Vinay Satish Kumar; Vincent Gonguet; Vish Vogeti; Vlad Poenaru; Vlad Tiberiu Mihailescu; Vladan Petrovic; Vladimir Ivanov; Wei Li; Weiwei Chu; Wenhan Xiong; Wenyin Fu; Wes Bouaziz; Whitney Meers; Will Constable; Xavier Martinet; Xiaojian Wu; Xinbo Gao; Xinfeng Xie; Xuchao Jia; Yaelle Goldschlag; Yann LeCun; Yashesh Gaur; Yasmine Babaei; Ye Qi; Yenda Li; Yi Wen; Yiwen Song; Youngjin Nam; Yuchen Hao; Yuchen Zhang; Yun Wang; Yuning Mao; Yuzi He; Zacharie Delpierre Coudert; Zachary DeVito; Zahra Hankir; Zhaoduo Wen; Zheng Yan; Zhengxing Chen; Zhenyu Yang; Zoe Papakipos
|
shi-labs/oneformer_coco_swin_large | shi-labs | 2023-01-19T11:07:35Z | 13,717 | 1 | transformers | [
"transformers",
"pytorch",
"oneformer",
"vision",
"image-segmentation",
"dataset:ydshieh/coco_dataset_script",
"arxiv:2211.06220",
"license:mit",
"endpoints_compatible",
"region:us"
] | image-segmentation | 2022-11-15T20:25:10Z | ---
license: mit
tags:
- vision
- image-segmentation
datasets:
- ydshieh/coco_dataset_script
widget:
- src: https://huggingface.co/datasets/shi-labs/oneformer_demo/blob/main/coco.jpeg
example_title: Person
- src: https://huggingface.co/datasets/shi-labs/oneformer_demo/blob/main/demo_2.jpg
example_title: Airplane
- src: https://huggingface.co/datasets/shi-labs/oneformer_demo/blob/main/demo.jpeg
example_title: Corgi
---
# OneFormer
OneFormer model trained on the COCO dataset (large-sized version, Swin backbone). It was introduced in the paper [OneFormer: One Transformer to Rule Universal Image Segmentation](https://arxiv.org/abs/2211.06220) by Jain et al. and first released in [this repository](https://github.com/SHI-Labs/OneFormer).

## Model description
OneFormer is the first multi-task universal image segmentation framework. It needs to be trained only once with a single universal architecture, a single model, and on a single dataset, to outperform existing specialized models across semantic, instance, and panoptic segmentation tasks. OneFormer uses a task token to condition the model on the task in focus, making the architecture task-guided for training, and task-dynamic for inference, all with a single model.
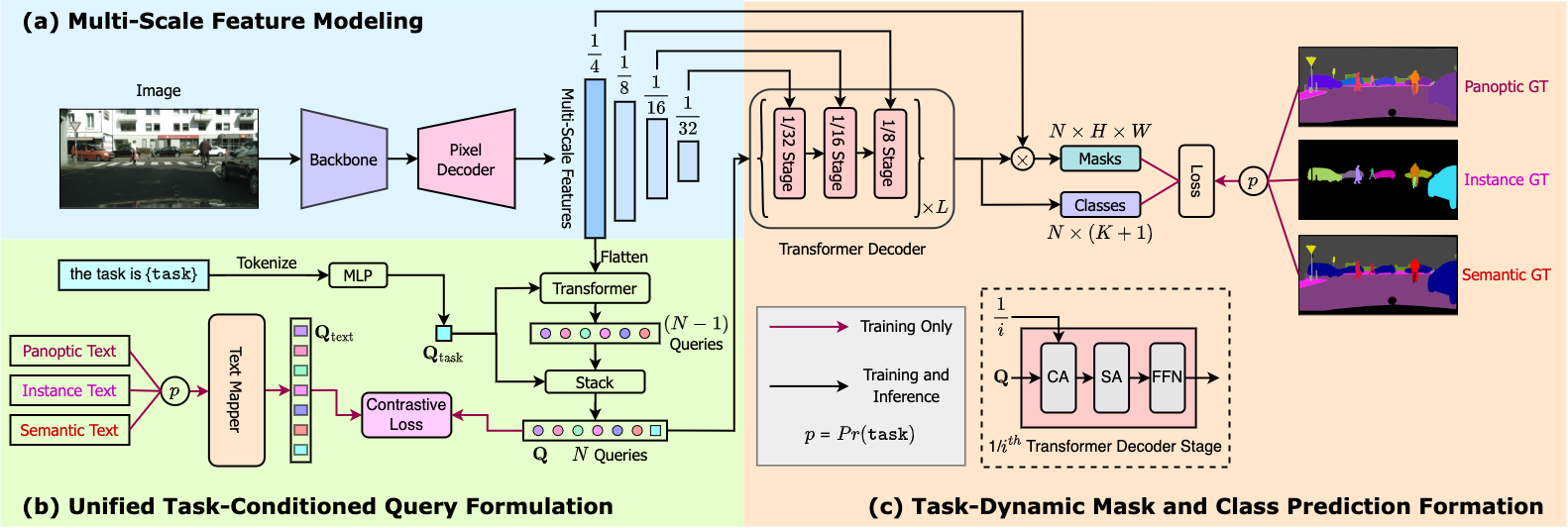
## Intended uses & limitations
You can use this particular checkpoint for semantic, instance and panoptic segmentation. See the [model hub](https://huggingface.co/models?search=oneformer) to look for other fine-tuned versions on a different dataset.
### How to use
Here is how to use this model:
```python
from transformers import OneFormerProcessor, OneFormerForUniversalSegmentation
from PIL import Image
import requests
url = "https://huggingface.co/datasets/shi-labs/oneformer_demo/blob/main/coco.jpeg"
image = Image.open(requests.get(url, stream=True).raw)
# Loading a single model for all three tasks
processor = OneFormerProcessor.from_pretrained("shi-labs/oneformer_coco_swin_large")
model = OneFormerForUniversalSegmentation.from_pretrained("shi-labs/oneformer_coco_swin_large")
# Semantic Segmentation
semantic_inputs = processor(images=image, task_inputs=["semantic"], return_tensors="pt")
semantic_outputs = model(**semantic_inputs)
# pass through image_processor for postprocessing
predicted_semantic_map = processor.post_process_semantic_segmentation(outputs, target_sizes=[image.size[::-1]])[0]
# Instance Segmentation
instance_inputs = processor(images=image, task_inputs=["instance"], return_tensors="pt")
instance_outputs = model(**instance_inputs)
# pass through image_processor for postprocessing
predicted_instance_map = processor.post_process_instance_segmentation(outputs, target_sizes=[image.size[::-1]])[0]["segmentation"]
# Panoptic Segmentation
panoptic_inputs = processor(images=image, task_inputs=["panoptic"], return_tensors="pt")
panoptic_outputs = model(**panoptic_inputs)
# pass through image_processor for postprocessing
predicted_semantic_map = processor.post_process_panoptic_segmentation(outputs, target_sizes=[image.size[::-1]])[0]["segmentation"]
```
For more examples, please refer to the [documentation](https://huggingface.co/docs/transformers/master/en/model_doc/oneformer).
### Citation
```bibtex
@article{jain2022oneformer,
title={{OneFormer: One Transformer to Rule Universal Image Segmentation}},
author={Jitesh Jain and Jiachen Li and MangTik Chiu and Ali Hassani and Nikita Orlov and Humphrey Shi},
journal={arXiv},
year={2022}
}
```
|
TheBloke/deepseek-coder-6.7B-instruct-GGUF | TheBloke | 2023-11-05T16:43:41Z | 13,715 | 163 | transformers | [
"transformers",
"gguf",
"deepseek",
"base_model:deepseek-ai/deepseek-coder-6.7b-instruct",
"license:other",
"region:us"
] | null | 2023-11-05T13:36:03Z | ---
base_model: deepseek-ai/deepseek-coder-6.7b-instruct
inference: false
license: other
license_link: LICENSE
license_name: deepseek
model_creator: DeepSeek
model_name: Deepseek Coder 6.7B Instruct
model_type: deepseek
prompt_template: 'You are an AI programming assistant, utilizing the Deepseek Coder
model, developed by Deepseek Company, and you only answer questions related to computer
science. For politically sensitive questions, security and privacy issues, and other
non-computer science questions, you will refuse to answer.
### Instruction:
{prompt}
### Response:
'
quantized_by: TheBloke
---
<!-- markdownlint-disable MD041 -->
<!-- header start -->
<!-- 200823 -->
<div style="width: auto; margin-left: auto; margin-right: auto">
<img src="https://i.imgur.com/EBdldam.jpg" alt="TheBlokeAI" style="width: 100%; min-width: 400px; display: block; margin: auto;">
</div>
<div style="display: flex; justify-content: space-between; width: 100%;">
<div style="display: flex; flex-direction: column; align-items: flex-start;">
<p style="margin-top: 0.5em; margin-bottom: 0em;"><a href="https://discord.gg/theblokeai">Chat & support: TheBloke's Discord server</a></p>
</div>
<div style="display: flex; flex-direction: column; align-items: flex-end;">
<p style="margin-top: 0.5em; margin-bottom: 0em;"><a href="https://www.patreon.com/TheBlokeAI">Want to contribute? TheBloke's Patreon page</a></p>
</div>
</div>
<div style="text-align:center; margin-top: 0em; margin-bottom: 0em"><p style="margin-top: 0.25em; margin-bottom: 0em;">TheBloke's LLM work is generously supported by a grant from <a href="https://a16z.com">andreessen horowitz (a16z)</a></p></div>
<hr style="margin-top: 1.0em; margin-bottom: 1.0em;">
<!-- header end -->
# Deepseek Coder 6.7B Instruct - GGUF
- Model creator: [DeepSeek](https://huggingface.co/deepseek-ai)
- Original model: [Deepseek Coder 6.7B Instruct](https://huggingface.co/deepseek-ai/deepseek-coder-6.7b-instruct)
<!-- description start -->
## Description
This repo contains GGUF format model files for [DeepSeek's Deepseek Coder 6.7B Instruct](https://huggingface.co/deepseek-ai/deepseek-coder-6.7b-instruct).
These files were quantised using hardware kindly provided by [Massed Compute](https://massedcompute.com/).
<!-- description end -->
<!-- README_GGUF.md-about-gguf start -->
### About GGUF
GGUF is a new format introduced by the llama.cpp team on August 21st 2023. It is a replacement for GGML, which is no longer supported by llama.cpp.
Here is an incomplete list of clients and libraries that are known to support GGUF:
* [llama.cpp](https://github.com/ggerganov/llama.cpp). The source project for GGUF. Offers a CLI and a server option.
* [text-generation-webui](https://github.com/oobabooga/text-generation-webui), the most widely used web UI, with many features and powerful extensions. Supports GPU acceleration.
* [KoboldCpp](https://github.com/LostRuins/koboldcpp), a fully featured web UI, with GPU accel across all platforms and GPU architectures. Especially good for story telling.
* [LM Studio](https://lmstudio.ai/), an easy-to-use and powerful local GUI for Windows and macOS (Silicon), with GPU acceleration.
* [LoLLMS Web UI](https://github.com/ParisNeo/lollms-webui), a great web UI with many interesting and unique features, including a full model library for easy model selection.
* [Faraday.dev](https://faraday.dev/), an attractive and easy to use character-based chat GUI for Windows and macOS (both Silicon and Intel), with GPU acceleration.
* [ctransformers](https://github.com/marella/ctransformers), a Python library with GPU accel, LangChain support, and OpenAI-compatible AI server.
* [llama-cpp-python](https://github.com/abetlen/llama-cpp-python), a Python library with GPU accel, LangChain support, and OpenAI-compatible API server.
* [candle](https://github.com/huggingface/candle), a Rust ML framework with a focus on performance, including GPU support, and ease of use.
<!-- README_GGUF.md-about-gguf end -->
<!-- repositories-available start -->
## Repositories available
* [AWQ model(s) for GPU inference.](https://huggingface.co/TheBloke/deepseek-coder-6.7B-instruct-AWQ)
* [GPTQ models for GPU inference, with multiple quantisation parameter options.](https://huggingface.co/TheBloke/deepseek-coder-6.7B-instruct-GPTQ)
* [2, 3, 4, 5, 6 and 8-bit GGUF models for CPU+GPU inference](https://huggingface.co/TheBloke/deepseek-coder-6.7B-instruct-GGUF)
* [DeepSeek's original unquantised fp16 model in pytorch format, for GPU inference and for further conversions](https://huggingface.co/deepseek-ai/deepseek-coder-6.7b-instruct)
<!-- repositories-available end -->
<!-- prompt-template start -->
## Prompt template: DeepSeek
```
You are an AI programming assistant, utilizing the Deepseek Coder model, developed by Deepseek Company, and you only answer questions related to computer science. For politically sensitive questions, security and privacy issues, and other non-computer science questions, you will refuse to answer.
### Instruction:
{prompt}
### Response:
```
<!-- prompt-template end -->
<!-- compatibility_gguf start -->
## Compatibility
These quantised GGUFv2 files are compatible with llama.cpp from August 27th onwards, as of commit [d0cee0d](https://github.com/ggerganov/llama.cpp/commit/d0cee0d36d5be95a0d9088b674dbb27354107221)
They are also compatible with many third party UIs and libraries - please see the list at the top of this README.
## Explanation of quantisation methods
<details>
<summary>Click to see details</summary>
The new methods available are:
* GGML_TYPE_Q2_K - "type-1" 2-bit quantization in super-blocks containing 16 blocks, each block having 16 weight. Block scales and mins are quantized with 4 bits. This ends up effectively using 2.5625 bits per weight (bpw)
* GGML_TYPE_Q3_K - "type-0" 3-bit quantization in super-blocks containing 16 blocks, each block having 16 weights. Scales are quantized with 6 bits. This end up using 3.4375 bpw.
* GGML_TYPE_Q4_K - "type-1" 4-bit quantization in super-blocks containing 8 blocks, each block having 32 weights. Scales and mins are quantized with 6 bits. This ends up using 4.5 bpw.
* GGML_TYPE_Q5_K - "type-1" 5-bit quantization. Same super-block structure as GGML_TYPE_Q4_K resulting in 5.5 bpw
* GGML_TYPE_Q6_K - "type-0" 6-bit quantization. Super-blocks with 16 blocks, each block having 16 weights. Scales are quantized with 8 bits. This ends up using 6.5625 bpw
Refer to the Provided Files table below to see what files use which methods, and how.
</details>
<!-- compatibility_gguf end -->
<!-- README_GGUF.md-provided-files start -->
## Provided files
| Name | Quant method | Bits | Size | Max RAM required | Use case |
| ---- | ---- | ---- | ---- | ---- | ----- |
| [deepseek-coder-6.7b-instruct.Q2_K.gguf](https://huggingface.co/TheBloke/deepseek-coder-6.7B-instruct-GGUF/blob/main/deepseek-coder-6.7b-instruct.Q2_K.gguf) | Q2_K | 2 | 2.83 GB| 5.33 GB | smallest, significant quality loss - not recommended for most purposes |
| [deepseek-coder-6.7b-instruct.Q3_K_S.gguf](https://huggingface.co/TheBloke/deepseek-coder-6.7B-instruct-GGUF/blob/main/deepseek-coder-6.7b-instruct.Q3_K_S.gguf) | Q3_K_S | 3 | 2.95 GB| 5.45 GB | very small, high quality loss |
| [deepseek-coder-6.7b-instruct.Q3_K_M.gguf](https://huggingface.co/TheBloke/deepseek-coder-6.7B-instruct-GGUF/blob/main/deepseek-coder-6.7b-instruct.Q3_K_M.gguf) | Q3_K_M | 3 | 3.30 GB| 5.80 GB | very small, high quality loss |
| [deepseek-coder-6.7b-instruct.Q3_K_L.gguf](https://huggingface.co/TheBloke/deepseek-coder-6.7B-instruct-GGUF/blob/main/deepseek-coder-6.7b-instruct.Q3_K_L.gguf) | Q3_K_L | 3 | 3.60 GB| 6.10 GB | small, substantial quality loss |
| [deepseek-coder-6.7b-instruct.Q4_0.gguf](https://huggingface.co/TheBloke/deepseek-coder-6.7B-instruct-GGUF/blob/main/deepseek-coder-6.7b-instruct.Q4_0.gguf) | Q4_0 | 4 | 3.83 GB| 6.33 GB | legacy; small, very high quality loss - prefer using Q3_K_M |
| [deepseek-coder-6.7b-instruct.Q4_K_S.gguf](https://huggingface.co/TheBloke/deepseek-coder-6.7B-instruct-GGUF/blob/main/deepseek-coder-6.7b-instruct.Q4_K_S.gguf) | Q4_K_S | 4 | 3.86 GB| 6.36 GB | small, greater quality loss |
| [deepseek-coder-6.7b-instruct.Q4_K_M.gguf](https://huggingface.co/TheBloke/deepseek-coder-6.7B-instruct-GGUF/blob/main/deepseek-coder-6.7b-instruct.Q4_K_M.gguf) | Q4_K_M | 4 | 4.08 GB| 6.58 GB | medium, balanced quality - recommended |
| [deepseek-coder-6.7b-instruct.Q5_0.gguf](https://huggingface.co/TheBloke/deepseek-coder-6.7B-instruct-GGUF/blob/main/deepseek-coder-6.7b-instruct.Q5_0.gguf) | Q5_0 | 5 | 4.65 GB| 7.15 GB | legacy; medium, balanced quality - prefer using Q4_K_M |
| [deepseek-coder-6.7b-instruct.Q5_K_S.gguf](https://huggingface.co/TheBloke/deepseek-coder-6.7B-instruct-GGUF/blob/main/deepseek-coder-6.7b-instruct.Q5_K_S.gguf) | Q5_K_S | 5 | 4.65 GB| 7.15 GB | large, low quality loss - recommended |
| [deepseek-coder-6.7b-instruct.Q5_K_M.gguf](https://huggingface.co/TheBloke/deepseek-coder-6.7B-instruct-GGUF/blob/main/deepseek-coder-6.7b-instruct.Q5_K_M.gguf) | Q5_K_M | 5 | 4.79 GB| 7.29 GB | large, very low quality loss - recommended |
| [deepseek-coder-6.7b-instruct.Q6_K.gguf](https://huggingface.co/TheBloke/deepseek-coder-6.7B-instruct-GGUF/blob/main/deepseek-coder-6.7b-instruct.Q6_K.gguf) | Q6_K | 6 | 5.53 GB| 8.03 GB | very large, extremely low quality loss |
| [deepseek-coder-6.7b-instruct.Q8_0.gguf](https://huggingface.co/TheBloke/deepseek-coder-6.7B-instruct-GGUF/blob/main/deepseek-coder-6.7b-instruct.Q8_0.gguf) | Q8_0 | 8 | 7.16 GB| 9.66 GB | very large, extremely low quality loss - not recommended |
**Note**: the above RAM figures assume no GPU offloading. If layers are offloaded to the GPU, this will reduce RAM usage and use VRAM instead.
<!-- README_GGUF.md-provided-files end -->
<!-- README_GGUF.md-how-to-download start -->
## How to download GGUF files
**Note for manual downloaders:** You almost never want to clone the entire repo! Multiple different quantisation formats are provided, and most users only want to pick and download a single file.
The following clients/libraries will automatically download models for you, providing a list of available models to choose from:
* LM Studio
* LoLLMS Web UI
* Faraday.dev
### In `text-generation-webui`
Under Download Model, you can enter the model repo: TheBloke/deepseek-coder-6.7B-instruct-GGUF and below it, a specific filename to download, such as: deepseek-coder-6.7b-instruct.Q4_K_M.gguf.
Then click Download.
### On the command line, including multiple files at once
I recommend using the `huggingface-hub` Python library:
```shell
pip3 install huggingface-hub
```
Then you can download any individual model file to the current directory, at high speed, with a command like this:
```shell
huggingface-cli download TheBloke/deepseek-coder-6.7B-instruct-GGUF deepseek-coder-6.7b-instruct.Q4_K_M.gguf --local-dir . --local-dir-use-symlinks False
```
<details>
<summary>More advanced huggingface-cli download usage</summary>
You can also download multiple files at once with a pattern:
```shell
huggingface-cli download TheBloke/deepseek-coder-6.7B-instruct-GGUF --local-dir . --local-dir-use-symlinks False --include='*Q4_K*gguf'
```
For more documentation on downloading with `huggingface-cli`, please see: [HF -> Hub Python Library -> Download files -> Download from the CLI](https://huggingface.co/docs/huggingface_hub/guides/download#download-from-the-cli).
To accelerate downloads on fast connections (1Gbit/s or higher), install `hf_transfer`:
```shell
pip3 install hf_transfer
```
And set environment variable `HF_HUB_ENABLE_HF_TRANSFER` to `1`:
```shell
HF_HUB_ENABLE_HF_TRANSFER=1 huggingface-cli download TheBloke/deepseek-coder-6.7B-instruct-GGUF deepseek-coder-6.7b-instruct.Q4_K_M.gguf --local-dir . --local-dir-use-symlinks False
```
Windows Command Line users: You can set the environment variable by running `set HF_HUB_ENABLE_HF_TRANSFER=1` before the download command.
</details>
<!-- README_GGUF.md-how-to-download end -->
<!-- README_GGUF.md-how-to-run start -->
## Example `llama.cpp` command
Make sure you are using `llama.cpp` from commit [d0cee0d](https://github.com/ggerganov/llama.cpp/commit/d0cee0d36d5be95a0d9088b674dbb27354107221) or later.
```shell
./main -ngl 32 -m deepseek-coder-6.7b-instruct.Q4_K_M.gguf --color -c 2048 --temp 0.7 --repeat_penalty 1.1 -n -1 -p "You are an AI programming assistant, utilizing the Deepseek Coder model, developed by Deepseek Company, and you only answer questions related to computer science. For politically sensitive questions, security and privacy issues, and other non-computer science questions, you will refuse to answer.\n### Instruction:\n{prompt}\n### Response:"
```
Change `-ngl 32` to the number of layers to offload to GPU. Remove it if you don't have GPU acceleration.
Change `-c 2048` to the desired sequence length. For extended sequence models - eg 8K, 16K, 32K - the necessary RoPE scaling parameters are read from the GGUF file and set by llama.cpp automatically.
If you want to have a chat-style conversation, replace the `-p <PROMPT>` argument with `-i -ins`
For other parameters and how to use them, please refer to [the llama.cpp documentation](https://github.com/ggerganov/llama.cpp/blob/master/examples/main/README.md)
## How to run in `text-generation-webui`
Further instructions here: [text-generation-webui/docs/llama.cpp.md](https://github.com/oobabooga/text-generation-webui/blob/main/docs/llama.cpp.md).
## How to run from Python code
You can use GGUF models from Python using the [llama-cpp-python](https://github.com/abetlen/llama-cpp-python) or [ctransformers](https://github.com/marella/ctransformers) libraries.
### How to load this model in Python code, using ctransformers
#### First install the package
Run one of the following commands, according to your system:
```shell
# Base ctransformers with no GPU acceleration
pip install ctransformers
# Or with CUDA GPU acceleration
pip install ctransformers[cuda]
# Or with AMD ROCm GPU acceleration (Linux only)
CT_HIPBLAS=1 pip install ctransformers --no-binary ctransformers
# Or with Metal GPU acceleration for macOS systems only
CT_METAL=1 pip install ctransformers --no-binary ctransformers
```
#### Simple ctransformers example code
```python
from ctransformers import AutoModelForCausalLM
# Set gpu_layers to the number of layers to offload to GPU. Set to 0 if no GPU acceleration is available on your system.
llm = AutoModelForCausalLM.from_pretrained("TheBloke/deepseek-coder-6.7B-instruct-GGUF", model_file="deepseek-coder-6.7b-instruct.Q4_K_M.gguf", model_type="deepseek", gpu_layers=50)
print(llm("AI is going to"))
```
## How to use with LangChain
Here are guides on using llama-cpp-python and ctransformers with LangChain:
* [LangChain + llama-cpp-python](https://python.langchain.com/docs/integrations/llms/llamacpp)
* [LangChain + ctransformers](https://python.langchain.com/docs/integrations/providers/ctransformers)
<!-- README_GGUF.md-how-to-run end -->
<!-- footer start -->
<!-- 200823 -->
## Discord
For further support, and discussions on these models and AI in general, join us at:
[TheBloke AI's Discord server](https://discord.gg/theblokeai)
## Thanks, and how to contribute
Thanks to the [chirper.ai](https://chirper.ai) team!
Thanks to Clay from [gpus.llm-utils.org](llm-utils)!
I've had a lot of people ask if they can contribute. I enjoy providing models and helping people, and would love to be able to spend even more time doing it, as well as expanding into new projects like fine tuning/training.
If you're able and willing to contribute it will be most gratefully received and will help me to keep providing more models, and to start work on new AI projects.
Donaters will get priority support on any and all AI/LLM/model questions and requests, access to a private Discord room, plus other benefits.
* Patreon: https://patreon.com/TheBlokeAI
* Ko-Fi: https://ko-fi.com/TheBlokeAI
**Special thanks to**: Aemon Algiz.
**Patreon special mentions**: Brandon Frisco, LangChain4j, Spiking Neurons AB, transmissions 11, Joseph William Delisle, Nitin Borwankar, Willem Michiel, Michael Dempsey, vamX, Jeffrey Morgan, zynix, jjj, Omer Bin Jawed, Sean Connelly, jinyuan sun, Jeromy Smith, Shadi, Pawan Osman, Chadd, Elijah Stavena, Illia Dulskyi, Sebastain Graf, Stephen Murray, terasurfer, Edmond Seymore, Celu Ramasamy, Mandus, Alex, biorpg, Ajan Kanaga, Clay Pascal, Raven Klaugh, 阿明, K, ya boyyy, usrbinkat, Alicia Loh, John Villwock, ReadyPlayerEmma, Chris Smitley, Cap'n Zoog, fincy, GodLy, S_X, sidney chen, Cory Kujawski, OG, Mano Prime, AzureBlack, Pieter, Kalila, Spencer Kim, Tom X Nguyen, Stanislav Ovsiannikov, Michael Levine, Andrey, Trailburnt, Vadim, Enrico Ros, Talal Aujan, Brandon Phillips, Jack West, Eugene Pentland, Michael Davis, Will Dee, webtim, Jonathan Leane, Alps Aficionado, Rooh Singh, Tiffany J. Kim, theTransient, Luke @flexchar, Elle, Caitlyn Gatomon, Ari Malik, subjectnull, Johann-Peter Hartmann, Trenton Dambrowitz, Imad Khwaja, Asp the Wyvern, Emad Mostaque, Rainer Wilmers, Alexandros Triantafyllidis, Nicholas, Pedro Madruga, SuperWojo, Harry Royden McLaughlin, James Bentley, Olakabola, David Ziegler, Ai Maven, Jeff Scroggin, Nikolai Manek, Deo Leter, Matthew Berman, Fen Risland, Ken Nordquist, Manuel Alberto Morcote, Luke Pendergrass, TL, Fred von Graf, Randy H, Dan Guido, NimbleBox.ai, Vitor Caleffi, Gabriel Tamborski, knownsqashed, Lone Striker, Erik Bjäreholt, John Detwiler, Leonard Tan, Iucharbius
Thank you to all my generous patrons and donaters!
And thank you again to a16z for their generous grant.
<!-- footer end -->
<!-- original-model-card start -->
# Original model card: DeepSeek's Deepseek Coder 6.7B Instruct
<p align="center">
<img width="1000px" alt="DeepSeek Coder" src="https://github.com/deepseek-ai/DeepSeek-Coder/blob/main/pictures/logo.png?raw=true">
</p>
<p align="center"><a href="https://www.deepseek.com/">[🏠Homepage]</a> | <a href="https://coder.deepseek.com/">[🤖 Chat with DeepSeek Coder]</a> | <a href="https://discord.gg/Tc7c45Zzu5">[Discord]</a> | <a href="https://github.com/guoday/assert/blob/main/QR.png?raw=true">[Wechat(微信)]</a> </p>
<hr>
### 1. Introduction of Deepseek Coder
Deepseek Coder is composed of a series of code language models, each trained from scratch on 2T tokens, with a composition of 87% code and 13% natural language in both English and Chinese. We provide various sizes of the code model, ranging from 1B to 33B versions. Each model is pre-trained on project-level code corpus by employing a window size of 16K and a extra fill-in-the-blank task, to support project-level code completion and infilling. For coding capabilities, Deepseek Coder achieves state-of-the-art performance among open-source code models on multiple programming languages and various benchmarks.
- **Massive Training Data**: Trained from scratch fon 2T tokens, including 87% code and 13% linguistic data in both English and Chinese languages.
- **Highly Flexible & Scalable**: Offered in model sizes of 1.3B, 5.7B, 6.7B, and 33B, enabling users to choose the setup most suitable for their requirements.
- **Superior Model Performance**: State-of-the-art performance among publicly available code models on HumanEval, MultiPL-E, MBPP, DS-1000, and APPS benchmarks.
- **Advanced Code Completion Capabilities**: A window size of 16K and a fill-in-the-blank task, supporting project-level code completion and infilling tasks.
### 2. Model Summary
deepseek-coder-6.7b-instruct is a 6.7B parameter model initialized from deepseek-coder-6.7b-base and fine-tuned on 2B tokens of instruction data.
- **Home Page:** [DeepSeek](https://deepseek.com/)
- **Repository:** [deepseek-ai/deepseek-coder](https://github.com/deepseek-ai/deepseek-coder)
- **Chat With DeepSeek Coder:** [DeepSeek-Coder](https://coder.deepseek.com/)
### 3. How to Use
Here give some examples of how to use our model.
#### Chat Model Inference
```python
from transformers import AutoTokenizer, AutoModelForCausalLM
tokenizer = AutoTokenizer.from_pretrained("deepseek-ai/deepseek-coder-6.7b-instruct", trust_remote_code=True)
model = AutoModelForCausalLM.from_pretrained("deepseek-ai/deepseek-coder-6.7b-instruct", trust_remote_code=True).cuda()
messages=[
{ 'role': 'user', 'content': "write a quick sort algorithm in python."}
]
inputs = tokenizer.apply_chat_template(messages, return_tensors="pt").to(model.device)
# 32021 is the id of <|EOT|> token
outputs = model.generate(inputs, max_new_tokens=512, do_sample=False, top_k=50, top_p=0.95, num_return_sequences=1, eos_token_id=32021)
print(tokenizer.decode(outputs[0][len(inputs[0]):], skip_special_tokens=True))
```
### 4. License
This code repository is licensed under the MIT License. The use of DeepSeek Coder models is subject to the Model License. DeepSeek Coder supports commercial use.
See the [LICENSE-MODEL](https://github.com/deepseek-ai/deepseek-coder/blob/main/LICENSE-MODEL) for more details.
### 5. Contact
If you have any questions, please raise an issue or contact us at [[email protected]](mailto:[email protected]).
<!-- original-model-card end -->
|
mradermacher/gemma-2-9b-GGUF | mradermacher | 2024-07-02T04:02:09Z | 13,715 | 0 | transformers | [
"transformers",
"gguf",
"en",
"base_model:google/gemma-2-9b",
"license:gemma",
"endpoints_compatible",
"region:us"
] | null | 2024-07-02T00:24:30Z | ---
base_model: google/gemma-2-9b
extra_gated_button_content: Acknowledge license
extra_gated_heading: Access Gemma on Hugging Face
extra_gated_prompt: To access Gemma on Hugging Face, you’re required to review and
agree to Google’s usage license. To do this, please ensure you’re logged in to Hugging
Face and click below. Requests are processed immediately.
language:
- en
library_name: transformers
license: gemma
quantized_by: mradermacher
---
## About
<!-- ### quantize_version: 2 -->
<!-- ### output_tensor_quantised: 1 -->
<!-- ### convert_type: hf -->
<!-- ### vocab_type: -->
<!-- ### tags: -->
static quants of https://huggingface.co/google/gemma-2-9b
<!-- provided-files -->
weighted/imatrix quants are available at https://huggingface.co/mradermacher/gemma-2-9b-i1-GGUF
## Usage
If you are unsure how to use GGUF files, refer to one of [TheBloke's
READMEs](https://huggingface.co/TheBloke/KafkaLM-70B-German-V0.1-GGUF) for
more details, including on how to concatenate multi-part files.
## Provided Quants
(sorted by size, not necessarily quality. IQ-quants are often preferable over similar sized non-IQ quants)
| Link | Type | Size/GB | Notes |
|:-----|:-----|--------:|:------|
| [GGUF](https://huggingface.co/mradermacher/gemma-2-9b-GGUF/resolve/main/gemma-2-9b.Q2_K.gguf) | Q2_K | 3.9 | |
| [GGUF](https://huggingface.co/mradermacher/gemma-2-9b-GGUF/resolve/main/gemma-2-9b.IQ3_XS.gguf) | IQ3_XS | 4.2 | |
| [GGUF](https://huggingface.co/mradermacher/gemma-2-9b-GGUF/resolve/main/gemma-2-9b.IQ3_S.gguf) | IQ3_S | 4.4 | beats Q3_K* |
| [GGUF](https://huggingface.co/mradermacher/gemma-2-9b-GGUF/resolve/main/gemma-2-9b.Q3_K_S.gguf) | Q3_K_S | 4.4 | |
| [GGUF](https://huggingface.co/mradermacher/gemma-2-9b-GGUF/resolve/main/gemma-2-9b.IQ3_M.gguf) | IQ3_M | 4.6 | |
| [GGUF](https://huggingface.co/mradermacher/gemma-2-9b-GGUF/resolve/main/gemma-2-9b.Q3_K_M.gguf) | Q3_K_M | 4.9 | lower quality |
| [GGUF](https://huggingface.co/mradermacher/gemma-2-9b-GGUF/resolve/main/gemma-2-9b.Q3_K_L.gguf) | Q3_K_L | 5.2 | |
| [GGUF](https://huggingface.co/mradermacher/gemma-2-9b-GGUF/resolve/main/gemma-2-9b.IQ4_XS.gguf) | IQ4_XS | 5.3 | |
| [GGUF](https://huggingface.co/mradermacher/gemma-2-9b-GGUF/resolve/main/gemma-2-9b.Q4_K_S.gguf) | Q4_K_S | 5.6 | fast, recommended |
| [GGUF](https://huggingface.co/mradermacher/gemma-2-9b-GGUF/resolve/main/gemma-2-9b.Q4_K_M.gguf) | Q4_K_M | 5.9 | fast, recommended |
| [GGUF](https://huggingface.co/mradermacher/gemma-2-9b-GGUF/resolve/main/gemma-2-9b.Q5_K_S.gguf) | Q5_K_S | 6.6 | |
| [GGUF](https://huggingface.co/mradermacher/gemma-2-9b-GGUF/resolve/main/gemma-2-9b.Q5_K_M.gguf) | Q5_K_M | 6.7 | |
| [GGUF](https://huggingface.co/mradermacher/gemma-2-9b-GGUF/resolve/main/gemma-2-9b.Q6_K.gguf) | Q6_K | 7.7 | very good quality |
| [GGUF](https://huggingface.co/mradermacher/gemma-2-9b-GGUF/resolve/main/gemma-2-9b.Q8_0.gguf) | Q8_0 | 9.9 | fast, best quality |
| [GGUF](https://huggingface.co/mradermacher/gemma-2-9b-GGUF/resolve/main/gemma-2-9b.f16.gguf) | f16 | 18.6 | 16 bpw, overkill |
Here is a handy graph by ikawrakow comparing some lower-quality quant
types (lower is better):

And here are Artefact2's thoughts on the matter:
https://gist.github.com/Artefact2/b5f810600771265fc1e39442288e8ec9
## FAQ / Model Request
See https://huggingface.co/mradermacher/model_requests for some answers to
questions you might have and/or if you want some other model quantized.
## Thanks
I thank my company, [nethype GmbH](https://www.nethype.de/), for letting
me use its servers and providing upgrades to my workstation to enable
this work in my free time.
<!-- end -->
|
mradermacher/llama3-8B-DarkIdol-2.1-Uncensored-32K-i1-GGUF | mradermacher | 2024-06-27T05:57:18Z | 13,712 | 1 | transformers | [
"transformers",
"gguf",
"roleplay",
"llama3",
"sillytavern",
"idol",
"en",
"ja",
"zh",
"base_model:aifeifei798/llama3-8B-DarkIdol-2.1-Uncensored-32K",
"license:llama3",
"endpoints_compatible",
"region:us"
] | null | 2024-06-27T03:40:47Z | ---
base_model: aifeifei798/llama3-8B-DarkIdol-2.1-Uncensored-32K
language:
- en
- ja
- zh
library_name: transformers
license: llama3
quantized_by: mradermacher
tags:
- roleplay
- llama3
- sillytavern
- idol
---
## About
<!-- ### quantize_version: 2 -->
<!-- ### output_tensor_quantised: 1 -->
<!-- ### convert_type: hf -->
<!-- ### vocab_type: -->
<!-- ### tags: nicoboss -->
weighted/imatrix quants of https://huggingface.co/aifeifei798/llama3-8B-DarkIdol-2.1-Uncensored-32K
<!-- provided-files -->
static quants are available at https://huggingface.co/mradermacher/llama3-8B-DarkIdol-2.1-Uncensored-32K-GGUF
## Usage
If you are unsure how to use GGUF files, refer to one of [TheBloke's
READMEs](https://huggingface.co/TheBloke/KafkaLM-70B-German-V0.1-GGUF) for
more details, including on how to concatenate multi-part files.
## Provided Quants
(sorted by size, not necessarily quality. IQ-quants are often preferable over similar sized non-IQ quants)
| Link | Type | Size/GB | Notes |
|:-----|:-----|--------:|:------|
| [GGUF](https://huggingface.co/mradermacher/llama3-8B-DarkIdol-2.1-Uncensored-32K-i1-GGUF/resolve/main/llama3-8B-DarkIdol-2.1-Uncensored-32K.i1-IQ1_S.gguf) | i1-IQ1_S | 2.1 | for the desperate |
| [GGUF](https://huggingface.co/mradermacher/llama3-8B-DarkIdol-2.1-Uncensored-32K-i1-GGUF/resolve/main/llama3-8B-DarkIdol-2.1-Uncensored-32K.i1-IQ1_M.gguf) | i1-IQ1_M | 2.3 | mostly desperate |
| [GGUF](https://huggingface.co/mradermacher/llama3-8B-DarkIdol-2.1-Uncensored-32K-i1-GGUF/resolve/main/llama3-8B-DarkIdol-2.1-Uncensored-32K.i1-IQ2_XXS.gguf) | i1-IQ2_XXS | 2.5 | |
| [GGUF](https://huggingface.co/mradermacher/llama3-8B-DarkIdol-2.1-Uncensored-32K-i1-GGUF/resolve/main/llama3-8B-DarkIdol-2.1-Uncensored-32K.i1-IQ2_XS.gguf) | i1-IQ2_XS | 2.7 | |
| [GGUF](https://huggingface.co/mradermacher/llama3-8B-DarkIdol-2.1-Uncensored-32K-i1-GGUF/resolve/main/llama3-8B-DarkIdol-2.1-Uncensored-32K.i1-IQ2_S.gguf) | i1-IQ2_S | 2.9 | |
| [GGUF](https://huggingface.co/mradermacher/llama3-8B-DarkIdol-2.1-Uncensored-32K-i1-GGUF/resolve/main/llama3-8B-DarkIdol-2.1-Uncensored-32K.i1-IQ2_M.gguf) | i1-IQ2_M | 3.0 | |
| [GGUF](https://huggingface.co/mradermacher/llama3-8B-DarkIdol-2.1-Uncensored-32K-i1-GGUF/resolve/main/llama3-8B-DarkIdol-2.1-Uncensored-32K.i1-Q2_K.gguf) | i1-Q2_K | 3.3 | IQ3_XXS probably better |
| [GGUF](https://huggingface.co/mradermacher/llama3-8B-DarkIdol-2.1-Uncensored-32K-i1-GGUF/resolve/main/llama3-8B-DarkIdol-2.1-Uncensored-32K.i1-IQ3_XXS.gguf) | i1-IQ3_XXS | 3.4 | lower quality |
| [GGUF](https://huggingface.co/mradermacher/llama3-8B-DarkIdol-2.1-Uncensored-32K-i1-GGUF/resolve/main/llama3-8B-DarkIdol-2.1-Uncensored-32K.i1-IQ3_XS.gguf) | i1-IQ3_XS | 3.6 | |
| [GGUF](https://huggingface.co/mradermacher/llama3-8B-DarkIdol-2.1-Uncensored-32K-i1-GGUF/resolve/main/llama3-8B-DarkIdol-2.1-Uncensored-32K.i1-Q3_K_S.gguf) | i1-Q3_K_S | 3.8 | IQ3_XS probably better |
| [GGUF](https://huggingface.co/mradermacher/llama3-8B-DarkIdol-2.1-Uncensored-32K-i1-GGUF/resolve/main/llama3-8B-DarkIdol-2.1-Uncensored-32K.i1-IQ3_S.gguf) | i1-IQ3_S | 3.8 | beats Q3_K* |
| [GGUF](https://huggingface.co/mradermacher/llama3-8B-DarkIdol-2.1-Uncensored-32K-i1-GGUF/resolve/main/llama3-8B-DarkIdol-2.1-Uncensored-32K.i1-IQ3_M.gguf) | i1-IQ3_M | 3.9 | |
| [GGUF](https://huggingface.co/mradermacher/llama3-8B-DarkIdol-2.1-Uncensored-32K-i1-GGUF/resolve/main/llama3-8B-DarkIdol-2.1-Uncensored-32K.i1-Q3_K_M.gguf) | i1-Q3_K_M | 4.1 | IQ3_S probably better |
| [GGUF](https://huggingface.co/mradermacher/llama3-8B-DarkIdol-2.1-Uncensored-32K-i1-GGUF/resolve/main/llama3-8B-DarkIdol-2.1-Uncensored-32K.i1-Q3_K_L.gguf) | i1-Q3_K_L | 4.4 | IQ3_M probably better |
| [GGUF](https://huggingface.co/mradermacher/llama3-8B-DarkIdol-2.1-Uncensored-32K-i1-GGUF/resolve/main/llama3-8B-DarkIdol-2.1-Uncensored-32K.i1-IQ4_XS.gguf) | i1-IQ4_XS | 4.5 | |
| [GGUF](https://huggingface.co/mradermacher/llama3-8B-DarkIdol-2.1-Uncensored-32K-i1-GGUF/resolve/main/llama3-8B-DarkIdol-2.1-Uncensored-32K.i1-Q4_0.gguf) | i1-Q4_0 | 4.8 | fast, low quality |
| [GGUF](https://huggingface.co/mradermacher/llama3-8B-DarkIdol-2.1-Uncensored-32K-i1-GGUF/resolve/main/llama3-8B-DarkIdol-2.1-Uncensored-32K.i1-Q4_K_S.gguf) | i1-Q4_K_S | 4.8 | optimal size/speed/quality |
| [GGUF](https://huggingface.co/mradermacher/llama3-8B-DarkIdol-2.1-Uncensored-32K-i1-GGUF/resolve/main/llama3-8B-DarkIdol-2.1-Uncensored-32K.i1-Q4_K_M.gguf) | i1-Q4_K_M | 5.0 | fast, recommended |
| [GGUF](https://huggingface.co/mradermacher/llama3-8B-DarkIdol-2.1-Uncensored-32K-i1-GGUF/resolve/main/llama3-8B-DarkIdol-2.1-Uncensored-32K.i1-Q5_K_S.gguf) | i1-Q5_K_S | 5.7 | |
| [GGUF](https://huggingface.co/mradermacher/llama3-8B-DarkIdol-2.1-Uncensored-32K-i1-GGUF/resolve/main/llama3-8B-DarkIdol-2.1-Uncensored-32K.i1-Q5_K_M.gguf) | i1-Q5_K_M | 5.8 | |
| [GGUF](https://huggingface.co/mradermacher/llama3-8B-DarkIdol-2.1-Uncensored-32K-i1-GGUF/resolve/main/llama3-8B-DarkIdol-2.1-Uncensored-32K.i1-Q6_K.gguf) | i1-Q6_K | 6.7 | practically like static Q6_K |
Here is a handy graph by ikawrakow comparing some lower-quality quant
types (lower is better):

And here are Artefact2's thoughts on the matter:
https://gist.github.com/Artefact2/b5f810600771265fc1e39442288e8ec9
## FAQ / Model Request
See https://huggingface.co/mradermacher/model_requests for some answers to
questions you might have and/or if you want some other model quantized.
## Thanks
I thank my company, [nethype GmbH](https://www.nethype.de/), for letting
me use its servers and providing upgrades to my workstation to enable
this work in my free time. Additional thanks to [@nicoboss](https://huggingface.co/nicoboss) for giving me access to his hardware for calculating the imatrix for these quants.
<!-- end -->
|
Habana/t5 | Habana | 2024-04-19T10:45:13Z | 13,710 | 0 | null | [
"optimum_habana",
"license:apache-2.0",
"region:us"
] | null | 2022-06-04T15:43:41Z | ---
license: apache-2.0
---
[Optimum Habana](https://github.com/huggingface/optimum-habana) is the interface between the Hugging Face Transformers and Diffusers libraries and Habana's Gaudi processor (HPU).
It provides a set of tools enabling easy and fast model loading, training and inference on single- and multi-HPU settings for different downstream tasks.
Learn more about how to take advantage of the power of Habana HPUs to train and deploy Transformers and Diffusers models at [hf.co/hardware/habana](https://huggingface.co/hardware/habana).
## T5 model HPU configuration
This model only contains the `GaudiConfig` file for running the [T5](https://huggingface.co/t5-base) model on Habana's Gaudi processors (HPU).
**This model contains no model weights, only a GaudiConfig.**
This enables to specify:
- `use_fused_adam`: whether to use Habana's custom AdamW implementation
- `use_fused_clip_norm`: whether to use Habana's fused gradient norm clipping operator
## Usage
The model is instantiated the same way as in the Transformers library.
The only difference is that there are a few new training arguments specific to HPUs.
[Here](https://github.com/huggingface/optimum-habana/blob/main/examples/summarization/run_summarization.py) is a summarization example script to fine-tune a model. You can run it with T5-small with the following command:
```bash
python run_summarization.py \
--model_name_or_path t5-small \
--do_train \
--do_eval \
--dataset_name cnn_dailymail \
--dataset_config "3.0.0" \
--source_prefix "summarize: " \
--output_dir /tmp/tst-summarization \
--per_device_train_batch_size 4 \
--per_device_eval_batch_size 4 \
--overwrite_output_dir \
--predict_with_generate \
--use_habana \
--use_lazy_mode \
--gaudi_config_name Habana/t5 \
--ignore_pad_token_for_loss False \
--pad_to_max_length \
--save_strategy epoch \
--throughput_warmup_steps 3
```
Check the [documentation](https://huggingface.co/docs/optimum/habana/index) out for more advanced usage and examples.
|
UCSC-VLAA/ViT-bigG-14-CLIPA-datacomp1B | UCSC-VLAA | 2023-10-20T21:52:47Z | 13,699 | 4 | open_clip | [
"open_clip",
"safetensors",
"clip",
"zero-shot-image-classification",
"dataset:mlfoundations/datacomp_1b",
"arxiv:2306.15658",
"arxiv:2305.07017",
"license:apache-2.0",
"region:us"
] | zero-shot-image-classification | 2023-10-20T21:43:50Z | ---
tags:
- clip
library_name: open_clip
pipeline_tag: zero-shot-image-classification
license: apache-2.0
datasets:
- mlfoundations/datacomp_1b
---
# Model card for ViT-bigG-14-CLIPA-datacomp1B
A CLIPA-v2 model...
## Model Details
- **Model Type:** Contrastive Image-Text, Zero-Shot Image Classification.
- **Original:** https://github.com/UCSC-VLAA/CLIPA
- **Dataset:** mlfoundations/datacomp_1b
- **Papers:**
- CLIPA-v2: Scaling CLIP Training with 81.1% Zero-shot ImageNet Accuracy within a $10,000 Budget; An Extra $4,000 Unlocks 81.8% Accuracy: https://arxiv.org/abs/2306.15658
- An Inverse Scaling Law for CLIP Training: https://arxiv.org/abs/2305.07017
## Model Usage
### With OpenCLIP
```
import torch
import torch.nn.functional as F
from urllib.request import urlopen
from PIL import Image
from open_clip import create_model_from_pretrained, get_tokenizer
model, preprocess = create_model_from_pretrained('hf-hub:ViT-bigG-14-CLIPA')
tokenizer = get_tokenizer('hf-hub:ViT-bigG-14-CLIPA')
image = Image.open(urlopen(
'https://huggingface.co/datasets/huggingface/documentation-images/resolve/main/beignets-task-guide.png'
))
image = preprocess(image).unsqueeze(0)
text = tokenizer(["a diagram", "a dog", "a cat", "a beignet"], context_length=model.context_length)
with torch.no_grad(), torch.cuda.amp.autocast():
image_features = model.encode_image(image)
text_features = model.encode_text(text)
image_features = F.normalize(image_features, dim=-1)
text_features = F.normalize(text_features, dim=-1)
text_probs = (100.0 * image_features @ text_features.T).softmax(dim=-1)
print("Label probs:", text_probs) # prints: [[0., 0., 0., 1.0]]
```
## Citation
```bibtex
@article{li2023clipav2,
title={CLIPA-v2: Scaling CLIP Training with 81.1% Zero-shot ImageNet Accuracy within a $10,000 Budget; An Extra $4,000 Unlocks 81.8% Accuracy},
author={Xianhang Li and Zeyu Wang and Cihang Xie},
journal={arXiv preprint arXiv:2306.15658},
year={2023},
}
```
```bibtex
@inproceedings{li2023clipa,
title={An Inverse Scaling Law for CLIP Training},
author={Xianhang Li and Zeyu Wang and Cihang Xie},
booktitle={NeurIPS},
year={2023},
}
```
|
Lewdiculous/L3-8B-Stheno-v3.1-GGUF-IQ-Imatrix | Lewdiculous | 2024-06-17T16:45:44Z | 13,694 | 83 | null | [
"gguf",
"roleplay",
"llama3",
"sillytavern",
"en",
"license:cc-by-nc-4.0",
"region:us"
] | null | 2024-05-20T15:01:38Z | ---
license: cc-by-nc-4.0
language:
- en
inference: false
tags:
- roleplay
- llama3
- sillytavern
---
> [!WARNING]
> [Try the updated v3.2 here.](https://huggingface.co/Lewdiculous/L3-8B-Stheno-v3.2-GGUF-IQ-Imatrix) <br>
> It includes fixes for common issues!
# #roleplay #sillytavern #llama3
My GGUF-IQ-Imatrix quants for [Sao10K/L3-8B-Stheno-v3.1](https://huggingface.co/Sao10K/L3-8B-Stheno-v3.1).
This is a very promising roleplay model cooked by the amazing Sao10K!
> [!IMPORTANT]
> **Quantization process:** <br>
> For future reference, these quants have been done after the fixes from [**#6920**](https://github.com/ggerganov/llama.cpp/pull/6920) have been merged. <br>
> Imatrix data was generated from the FP16-GGUF and conversions directly from the BF16-GGUF. <br>
> This was a bit more disk and compute intensive but hopefully avoided any losses during conversion. <br>
> If you noticed any issues let me know in the discussions.
> [!NOTE]
> **General usage:** <br>
> Use the latest version of **KoboldCpp**. <br>
> For **8GB VRAM** GPUs, I recommend the **Q4_K_M-imat** (4.89 BPW) quant for up to 12288 context sizes. <br>
>
> **Presets:** <br>
> Some compatible SillyTavern presets can be found [**here (Virt's Roleplay Presets)**](https://huggingface.co/Virt-io/SillyTavern-Presets). <br>
> Check [**discussions such as this one**](https://huggingface.co/Virt-io/SillyTavern-Presets/discussions/5#664d6fb87c563d4d95151baa) for other recommendations and samplers.
> [!TIP]
> **Personal-support:** <br>
> I apologize for disrupting your experience. <br>
> Currently I'm working on moving for a better internet provider. <br>
> If you **want** and you are **able to**... <br>
> You can [**spare some change over here (Ko-fi)**](https://ko-fi.com/Lewdiculous). <br>
>
> **Author-support:** <br>
> You can support the author [**at their own page**](https://ko-fi.com/sao10k).

## **Original model information:**
<img src="https://w.forfun.com/fetch/cb/cba2205390e517bea1ea60ca0b491af4.jpeg" style="width: 80%; min-width: 400px; display: block; margin: auto;">
**Model: Llama-3-8B-Stheno-v3.1**
This has been an experimental model I've been working on for a bit. Llama-3 was kind of difficult to work with.
<br>I also had been hired to create a model for an Organisation, and I used the lessons I learnt from fine-tuning that one for this specific model. Unable to share that one though, unfortunately.
<br>Made from outputs generated by Claude-3-Opus along with Human-Generated Data.
Stheno-v3.1
\- A model made for 1-on-1 Roleplay ideally, but one that is able to handle scenarios, RPGs and storywriting fine.
<br>\- Uncensored during actual roleplay scenarios. # I do not care for zero-shot prompting like what some people do. It is uncensored enough in actual usecases.
<br>\- I quite like the prose and style for this model.
#### Testing Notes
<br>\- Known as L3-RP-v2.1 on Chaiverse, it did decently there [>1200 Elo]
<br>\- Handles character personalities well. Great for 1 on 1 Roleplay sessions.
<br>\- May need further token context & few-shot examples if using it as a Narrator / RPG Roleplaying session. It is able to handle them though.
<br>\- A model leaning towards NSFW, mention explicitly in prompts if you want to steer away. [Avoid Negative Reinforcement]
<br>\- Occasionally spits out leaking XML and nonsense. A regen / swipe instantly fixes that.
<br>\- Unique / Varied Answers when Regenerating answers. Pretty cool?
<br>\- Works best with *some* token context in the character card itself. A chef needs ingredients to cook, no?
***
**Recommended Samplers:**
```
Temperature - 1.12 to 1.32
Min-P - 0.075
Top-K - 40
Repetition Penalty - 1.1
```
**Stopping Strings:**
```
\n{{User}} # Or Equivalent, depending on Frontend
<|eot_id|>
<|end_of_text|>
\n< # If there is leakage of XML tags in response. May happen Occasionally, Regenerate Answer as Needed. Happens rarely.
```
**Prompting Template - Llama-3-Instruct**
```
<|begin_of_text|><|start_header_id|>system<|end_header_id|>
{system_prompt}<|eot_id|><|start_header_id|>user<|end_header_id|>
{input}<|eot_id|><|start_header_id|>assistant<|end_header_id|>
{output}<|eot_id|>
```
**Basic Roleplay System Prompt**
```
You are an expert actor that can fully immerse yourself into any role given. You do not break character for any reason, even if someone tries addressing you as an AI or language model.
Currently your role is {{char}}, which is described in detail below. As {{char}}, continue the exchange with {{user}}.
```
***
Support me here if you're interested. [Ko-Fi](https://ko-fi.com/sao10k)
If not, that's fine too. Feedback would be nice.
```
Art by wada_kazu / わだかず (pixiv page private?)
```
*** |
timm/fastvit_t8.apple_dist_in1k | timm | 2023-08-23T21:05:45Z | 13,690 | 0 | timm | [
"timm",
"pytorch",
"safetensors",
"image-classification",
"dataset:imagenet-1k",
"arxiv:2303.14189",
"license:other",
"region:us"
] | image-classification | 2023-08-23T21:05:41Z | ---
tags:
- image-classification
- timm
library_name: timm
license: other
datasets:
- imagenet-1k
---
# Model card for fastvit_t8.apple_dist_in1k
A FastViT image classification model. Trained on ImageNet-1k with distillation by paper authors.
Please observe [original license](https://github.com/apple/ml-fastvit/blob/8af5928238cab99c45f64fc3e4e7b1516b8224ba/LICENSE).
## Model Details
- **Model Type:** Image classification / feature backbone
- **Model Stats:**
- Params (M): 4.0
- GMACs: 0.7
- Activations (M): 8.6
- Image size: 256 x 256
- **Papers:**
- FastViT: A Fast Hybrid Vision Transformer using Structural Reparameterization: https://arxiv.org/abs/2303.14189
- **Original:** https://github.com/apple/ml-fastvit
- **Dataset:** ImageNet-1k
## Model Usage
### Image Classification
```python
from urllib.request import urlopen
from PIL import Image
import timm
img = Image.open(urlopen(
'https://huggingface.co/datasets/huggingface/documentation-images/resolve/main/beignets-task-guide.png'
))
model = timm.create_model('fastvit_t8.apple_dist_in1k', pretrained=True)
model = model.eval()
# get model specific transforms (normalization, resize)
data_config = timm.data.resolve_model_data_config(model)
transforms = timm.data.create_transform(**data_config, is_training=False)
output = model(transforms(img).unsqueeze(0)) # unsqueeze single image into batch of 1
top5_probabilities, top5_class_indices = torch.topk(output.softmax(dim=1) * 100, k=5)
```
### Feature Map Extraction
```python
from urllib.request import urlopen
from PIL import Image
import timm
img = Image.open(urlopen(
'https://huggingface.co/datasets/huggingface/documentation-images/resolve/main/beignets-task-guide.png'
))
model = timm.create_model(
'fastvit_t8.apple_dist_in1k',
pretrained=True,
features_only=True,
)
model = model.eval()
# get model specific transforms (normalization, resize)
data_config = timm.data.resolve_model_data_config(model)
transforms = timm.data.create_transform(**data_config, is_training=False)
output = model(transforms(img).unsqueeze(0)) # unsqueeze single image into batch of 1
for o in output:
# print shape of each feature map in output
# e.g.:
# torch.Size([1, 48, 64, 64])
# torch.Size([1, 96, 32, 32])
# torch.Size([1, 192, 16, 16])
# torch.Size([1, 384, 8, 8])
print(o.shape)
```
### Image Embeddings
```python
from urllib.request import urlopen
from PIL import Image
import timm
img = Image.open(urlopen(
'https://huggingface.co/datasets/huggingface/documentation-images/resolve/main/beignets-task-guide.png'
))
model = timm.create_model(
'fastvit_t8.apple_dist_in1k',
pretrained=True,
num_classes=0, # remove classifier nn.Linear
)
model = model.eval()
# get model specific transforms (normalization, resize)
data_config = timm.data.resolve_model_data_config(model)
transforms = timm.data.create_transform(**data_config, is_training=False)
output = model(transforms(img).unsqueeze(0)) # output is (batch_size, num_features) shaped tensor
# or equivalently (without needing to set num_classes=0)
output = model.forward_features(transforms(img).unsqueeze(0))
# output is unpooled, a (1, 384, 8, 8) shaped tensor
output = model.forward_head(output, pre_logits=True)
# output is a (1, num_features) shaped tensor
```
## Citation
```bibtex
@inproceedings{vasufastvit2023,
author = {Pavan Kumar Anasosalu Vasu and James Gabriel and Jeff Zhu and Oncel Tuzel and Anurag Ranjan},
title = {FastViT: A Fast Hybrid Vision Transformer using Structural Reparameterization},
booktitle={Proceedings of the IEEE/CVF International Conference on Computer Vision},
year = {2023}
}
```
|
mradermacher/llama-3-8b-samantha-GGUF | mradermacher | 2024-06-29T18:04:26Z | 13,680 | 0 | transformers | [
"transformers",
"gguf",
"text-generation-inference",
"unsloth",
"llama",
"trl",
"en",
"dataset:cognitivecomputations/samantha-data",
"base_model:ruslandev/llama-3-8b-samantha",
"license:apache-2.0",
"endpoints_compatible",
"region:us"
] | null | 2024-06-29T15:50:59Z | ---
base_model: ruslandev/llama-3-8b-samantha
datasets:
- cognitivecomputations/samantha-data
language:
- en
library_name: transformers
license: apache-2.0
quantized_by: mradermacher
tags:
- text-generation-inference
- transformers
- unsloth
- llama
- trl
---
## About
<!-- ### quantize_version: 2 -->
<!-- ### output_tensor_quantised: 1 -->
<!-- ### convert_type: hf -->
<!-- ### vocab_type: -->
<!-- ### tags: -->
static quants of https://huggingface.co/ruslandev/llama-3-8b-samantha
<!-- provided-files -->
weighted/imatrix quants are available at https://huggingface.co/mradermacher/llama-3-8b-samantha-i1-GGUF
## Usage
If you are unsure how to use GGUF files, refer to one of [TheBloke's
READMEs](https://huggingface.co/TheBloke/KafkaLM-70B-German-V0.1-GGUF) for
more details, including on how to concatenate multi-part files.
## Provided Quants
(sorted by size, not necessarily quality. IQ-quants are often preferable over similar sized non-IQ quants)
| Link | Type | Size/GB | Notes |
|:-----|:-----|--------:|:------|
| [GGUF](https://huggingface.co/mradermacher/llama-3-8b-samantha-GGUF/resolve/main/llama-3-8b-samantha.Q2_K.gguf) | Q2_K | 3.3 | |
| [GGUF](https://huggingface.co/mradermacher/llama-3-8b-samantha-GGUF/resolve/main/llama-3-8b-samantha.IQ3_XS.gguf) | IQ3_XS | 3.6 | |
| [GGUF](https://huggingface.co/mradermacher/llama-3-8b-samantha-GGUF/resolve/main/llama-3-8b-samantha.Q3_K_S.gguf) | Q3_K_S | 3.8 | |
| [GGUF](https://huggingface.co/mradermacher/llama-3-8b-samantha-GGUF/resolve/main/llama-3-8b-samantha.IQ3_S.gguf) | IQ3_S | 3.8 | beats Q3_K* |
| [GGUF](https://huggingface.co/mradermacher/llama-3-8b-samantha-GGUF/resolve/main/llama-3-8b-samantha.IQ3_M.gguf) | IQ3_M | 3.9 | |
| [GGUF](https://huggingface.co/mradermacher/llama-3-8b-samantha-GGUF/resolve/main/llama-3-8b-samantha.Q3_K_M.gguf) | Q3_K_M | 4.1 | lower quality |
| [GGUF](https://huggingface.co/mradermacher/llama-3-8b-samantha-GGUF/resolve/main/llama-3-8b-samantha.Q3_K_L.gguf) | Q3_K_L | 4.4 | |
| [GGUF](https://huggingface.co/mradermacher/llama-3-8b-samantha-GGUF/resolve/main/llama-3-8b-samantha.IQ4_XS.gguf) | IQ4_XS | 4.6 | |
| [GGUF](https://huggingface.co/mradermacher/llama-3-8b-samantha-GGUF/resolve/main/llama-3-8b-samantha.Q4_K_S.gguf) | Q4_K_S | 4.8 | fast, recommended |
| [GGUF](https://huggingface.co/mradermacher/llama-3-8b-samantha-GGUF/resolve/main/llama-3-8b-samantha.Q4_K_M.gguf) | Q4_K_M | 5.0 | fast, recommended |
| [GGUF](https://huggingface.co/mradermacher/llama-3-8b-samantha-GGUF/resolve/main/llama-3-8b-samantha.Q5_K_S.gguf) | Q5_K_S | 5.7 | |
| [GGUF](https://huggingface.co/mradermacher/llama-3-8b-samantha-GGUF/resolve/main/llama-3-8b-samantha.Q5_K_M.gguf) | Q5_K_M | 5.8 | |
| [GGUF](https://huggingface.co/mradermacher/llama-3-8b-samantha-GGUF/resolve/main/llama-3-8b-samantha.Q6_K.gguf) | Q6_K | 6.7 | very good quality |
| [GGUF](https://huggingface.co/mradermacher/llama-3-8b-samantha-GGUF/resolve/main/llama-3-8b-samantha.Q8_0.gguf) | Q8_0 | 8.6 | fast, best quality |
| [GGUF](https://huggingface.co/mradermacher/llama-3-8b-samantha-GGUF/resolve/main/llama-3-8b-samantha.f16.gguf) | f16 | 16.2 | 16 bpw, overkill |
Here is a handy graph by ikawrakow comparing some lower-quality quant
types (lower is better):

And here are Artefact2's thoughts on the matter:
https://gist.github.com/Artefact2/b5f810600771265fc1e39442288e8ec9
## FAQ / Model Request
See https://huggingface.co/mradermacher/model_requests for some answers to
questions you might have and/or if you want some other model quantized.
## Thanks
I thank my company, [nethype GmbH](https://www.nethype.de/), for letting
me use its servers and providing upgrades to my workstation to enable
this work in my free time.
<!-- end -->
|
mradermacher/Llama-3-Umbral-Mind-Replete-8B-i1-GGUF | mradermacher | 2024-06-27T10:00:15Z | 13,641 | 0 | transformers | [
"transformers",
"gguf",
"mergekit",
"merge",
"en",
"base_model:mpasila/Llama-3-Umbral-Mind-Replete-8B",
"license:llama3",
"endpoints_compatible",
"region:us"
] | null | 2024-06-27T05:24:35Z | ---
base_model: mpasila/Llama-3-Umbral-Mind-Replete-8B
language:
- en
library_name: transformers
license: llama3
quantized_by: mradermacher
tags:
- mergekit
- merge
---
## About
<!-- ### quantize_version: 2 -->
<!-- ### output_tensor_quantised: 1 -->
<!-- ### convert_type: hf -->
<!-- ### vocab_type: -->
<!-- ### tags: nicoboss -->
weighted/imatrix quants of https://huggingface.co/mpasila/Llama-3-Umbral-Mind-Replete-8B
<!-- provided-files -->
static quants are available at https://huggingface.co/mradermacher/Llama-3-Umbral-Mind-Replete-8B-GGUF
## Usage
If you are unsure how to use GGUF files, refer to one of [TheBloke's
READMEs](https://huggingface.co/TheBloke/KafkaLM-70B-German-V0.1-GGUF) for
more details, including on how to concatenate multi-part files.
## Provided Quants
(sorted by size, not necessarily quality. IQ-quants are often preferable over similar sized non-IQ quants)
| Link | Type | Size/GB | Notes |
|:-----|:-----|--------:|:------|
| [GGUF](https://huggingface.co/mradermacher/Llama-3-Umbral-Mind-Replete-8B-i1-GGUF/resolve/main/Llama-3-Umbral-Mind-Replete-8B.i1-IQ1_S.gguf) | i1-IQ1_S | 2.1 | for the desperate |
| [GGUF](https://huggingface.co/mradermacher/Llama-3-Umbral-Mind-Replete-8B-i1-GGUF/resolve/main/Llama-3-Umbral-Mind-Replete-8B.i1-IQ1_M.gguf) | i1-IQ1_M | 2.3 | mostly desperate |
| [GGUF](https://huggingface.co/mradermacher/Llama-3-Umbral-Mind-Replete-8B-i1-GGUF/resolve/main/Llama-3-Umbral-Mind-Replete-8B.i1-IQ2_XXS.gguf) | i1-IQ2_XXS | 2.5 | |
| [GGUF](https://huggingface.co/mradermacher/Llama-3-Umbral-Mind-Replete-8B-i1-GGUF/resolve/main/Llama-3-Umbral-Mind-Replete-8B.i1-IQ2_XS.gguf) | i1-IQ2_XS | 2.7 | |
| [GGUF](https://huggingface.co/mradermacher/Llama-3-Umbral-Mind-Replete-8B-i1-GGUF/resolve/main/Llama-3-Umbral-Mind-Replete-8B.i1-IQ2_S.gguf) | i1-IQ2_S | 2.9 | |
| [GGUF](https://huggingface.co/mradermacher/Llama-3-Umbral-Mind-Replete-8B-i1-GGUF/resolve/main/Llama-3-Umbral-Mind-Replete-8B.i1-IQ2_M.gguf) | i1-IQ2_M | 3.0 | |
| [GGUF](https://huggingface.co/mradermacher/Llama-3-Umbral-Mind-Replete-8B-i1-GGUF/resolve/main/Llama-3-Umbral-Mind-Replete-8B.i1-Q2_K.gguf) | i1-Q2_K | 3.3 | IQ3_XXS probably better |
| [GGUF](https://huggingface.co/mradermacher/Llama-3-Umbral-Mind-Replete-8B-i1-GGUF/resolve/main/Llama-3-Umbral-Mind-Replete-8B.i1-IQ3_XXS.gguf) | i1-IQ3_XXS | 3.4 | lower quality |
| [GGUF](https://huggingface.co/mradermacher/Llama-3-Umbral-Mind-Replete-8B-i1-GGUF/resolve/main/Llama-3-Umbral-Mind-Replete-8B.i1-IQ3_XS.gguf) | i1-IQ3_XS | 3.6 | |
| [GGUF](https://huggingface.co/mradermacher/Llama-3-Umbral-Mind-Replete-8B-i1-GGUF/resolve/main/Llama-3-Umbral-Mind-Replete-8B.i1-Q3_K_S.gguf) | i1-Q3_K_S | 3.8 | IQ3_XS probably better |
| [GGUF](https://huggingface.co/mradermacher/Llama-3-Umbral-Mind-Replete-8B-i1-GGUF/resolve/main/Llama-3-Umbral-Mind-Replete-8B.i1-IQ3_S.gguf) | i1-IQ3_S | 3.8 | beats Q3_K* |
| [GGUF](https://huggingface.co/mradermacher/Llama-3-Umbral-Mind-Replete-8B-i1-GGUF/resolve/main/Llama-3-Umbral-Mind-Replete-8B.i1-IQ3_M.gguf) | i1-IQ3_M | 3.9 | |
| [GGUF](https://huggingface.co/mradermacher/Llama-3-Umbral-Mind-Replete-8B-i1-GGUF/resolve/main/Llama-3-Umbral-Mind-Replete-8B.i1-Q3_K_M.gguf) | i1-Q3_K_M | 4.1 | IQ3_S probably better |
| [GGUF](https://huggingface.co/mradermacher/Llama-3-Umbral-Mind-Replete-8B-i1-GGUF/resolve/main/Llama-3-Umbral-Mind-Replete-8B.i1-Q3_K_L.gguf) | i1-Q3_K_L | 4.4 | IQ3_M probably better |
| [GGUF](https://huggingface.co/mradermacher/Llama-3-Umbral-Mind-Replete-8B-i1-GGUF/resolve/main/Llama-3-Umbral-Mind-Replete-8B.i1-IQ4_XS.gguf) | i1-IQ4_XS | 4.5 | |
| [GGUF](https://huggingface.co/mradermacher/Llama-3-Umbral-Mind-Replete-8B-i1-GGUF/resolve/main/Llama-3-Umbral-Mind-Replete-8B.i1-Q4_0.gguf) | i1-Q4_0 | 4.8 | fast, low quality |
| [GGUF](https://huggingface.co/mradermacher/Llama-3-Umbral-Mind-Replete-8B-i1-GGUF/resolve/main/Llama-3-Umbral-Mind-Replete-8B.i1-Q4_K_S.gguf) | i1-Q4_K_S | 4.8 | optimal size/speed/quality |
| [GGUF](https://huggingface.co/mradermacher/Llama-3-Umbral-Mind-Replete-8B-i1-GGUF/resolve/main/Llama-3-Umbral-Mind-Replete-8B.i1-Q4_K_M.gguf) | i1-Q4_K_M | 5.0 | fast, recommended |
| [GGUF](https://huggingface.co/mradermacher/Llama-3-Umbral-Mind-Replete-8B-i1-GGUF/resolve/main/Llama-3-Umbral-Mind-Replete-8B.i1-Q5_K_S.gguf) | i1-Q5_K_S | 5.7 | |
| [GGUF](https://huggingface.co/mradermacher/Llama-3-Umbral-Mind-Replete-8B-i1-GGUF/resolve/main/Llama-3-Umbral-Mind-Replete-8B.i1-Q5_K_M.gguf) | i1-Q5_K_M | 5.8 | |
| [GGUF](https://huggingface.co/mradermacher/Llama-3-Umbral-Mind-Replete-8B-i1-GGUF/resolve/main/Llama-3-Umbral-Mind-Replete-8B.i1-Q6_K.gguf) | i1-Q6_K | 6.7 | practically like static Q6_K |
Here is a handy graph by ikawrakow comparing some lower-quality quant
types (lower is better):

And here are Artefact2's thoughts on the matter:
https://gist.github.com/Artefact2/b5f810600771265fc1e39442288e8ec9
## FAQ / Model Request
See https://huggingface.co/mradermacher/model_requests for some answers to
questions you might have and/or if you want some other model quantized.
## Thanks
I thank my company, [nethype GmbH](https://www.nethype.de/), for letting
me use its servers and providing upgrades to my workstation to enable
this work in my free time. Additional thanks to [@nicoboss](https://huggingface.co/nicoboss) for giving me access to his hardware for calculating the imatrix for these quants.
<!-- end -->
|
mradermacher/Replete-Coder-Llama3-8B-i1-GGUF | mradermacher | 2024-06-25T09:49:03Z | 13,639 | 0 | transformers | [
"transformers",
"gguf",
"text-generation-inference",
"unsloth",
"llama",
"en",
"dataset:Replete-AI/code_bagel_hermes-2.5",
"dataset:Replete-AI/code_bagel",
"dataset:Replete-AI/OpenHermes-2.5-Uncensored",
"dataset:teknium/OpenHermes-2.5",
"dataset:layoric/tiny-codes-alpaca",
"dataset:glaiveai/glaive-code-assistant-v3",
"dataset:ajibawa-2023/Code-290k-ShareGPT",
"dataset:TIGER-Lab/MathInstruct",
"dataset:chargoddard/commitpack-ft-instruct-rated",
"dataset:iamturun/code_instructions_120k_alpaca",
"dataset:ise-uiuc/Magicoder-Evol-Instruct-110K",
"dataset:cognitivecomputations/dolphin-coder",
"dataset:nickrosh/Evol-Instruct-Code-80k-v1",
"dataset:coseal/CodeUltraFeedback_binarized",
"dataset:glaiveai/glaive-function-calling-v2",
"dataset:CyberNative/Code_Vulnerability_Security_DPO",
"dataset:jondurbin/airoboros-2.2",
"dataset:camel-ai",
"dataset:lmsys/lmsys-chat-1m",
"dataset:CollectiveCognition/chats-data-2023-09-22",
"dataset:CoT-Alpaca-GPT4",
"dataset:WizardLM/WizardLM_evol_instruct_70k",
"dataset:WizardLM/WizardLM_evol_instruct_V2_196k",
"dataset:teknium/GPT4-LLM-Cleaned",
"dataset:GPTeacher",
"dataset:OpenGPT",
"dataset:meta-math/MetaMathQA",
"dataset:Open-Orca/SlimOrca",
"dataset:garage-bAInd/Open-Platypus",
"dataset:anon8231489123/ShareGPT_Vicuna_unfiltered",
"dataset:Unnatural-Instructions-GPT4",
"base_model:Replete-AI/Replete-Coder-Llama3-8B",
"license:other",
"endpoints_compatible",
"region:us"
] | null | 2024-06-25T07:07:01Z | ---
base_model: Replete-AI/Replete-Coder-Llama3-8B
datasets:
- Replete-AI/code_bagel_hermes-2.5
- Replete-AI/code_bagel
- Replete-AI/OpenHermes-2.5-Uncensored
- teknium/OpenHermes-2.5
- layoric/tiny-codes-alpaca
- glaiveai/glaive-code-assistant-v3
- ajibawa-2023/Code-290k-ShareGPT
- TIGER-Lab/MathInstruct
- chargoddard/commitpack-ft-instruct-rated
- iamturun/code_instructions_120k_alpaca
- ise-uiuc/Magicoder-Evol-Instruct-110K
- cognitivecomputations/dolphin-coder
- nickrosh/Evol-Instruct-Code-80k-v1
- coseal/CodeUltraFeedback_binarized
- glaiveai/glaive-function-calling-v2
- CyberNative/Code_Vulnerability_Security_DPO
- jondurbin/airoboros-2.2
- camel-ai
- lmsys/lmsys-chat-1m
- CollectiveCognition/chats-data-2023-09-22
- CoT-Alpaca-GPT4
- WizardLM/WizardLM_evol_instruct_70k
- WizardLM/WizardLM_evol_instruct_V2_196k
- teknium/GPT4-LLM-Cleaned
- GPTeacher
- OpenGPT
- meta-math/MetaMathQA
- Open-Orca/SlimOrca
- garage-bAInd/Open-Platypus
- anon8231489123/ShareGPT_Vicuna_unfiltered
- Unnatural-Instructions-GPT4
language:
- en
library_name: transformers
license: other
license_link: https://llama.meta.com/llama3/license/
license_name: llama-3
quantized_by: mradermacher
tags:
- text-generation-inference
- transformers
- unsloth
- llama
---
## About
<!-- ### quantize_version: 2 -->
<!-- ### output_tensor_quantised: 1 -->
<!-- ### convert_type: hf -->
<!-- ### vocab_type: -->
<!-- ### tags: nicoboss -->
weighted/imatrix quants of https://huggingface.co/Replete-AI/Replete-Coder-Llama3-8B
<!-- provided-files -->
static quants are available at https://huggingface.co/mradermacher/Replete-Coder-Llama3-8B-GGUF
## Usage
If you are unsure how to use GGUF files, refer to one of [TheBloke's
READMEs](https://huggingface.co/TheBloke/KafkaLM-70B-German-V0.1-GGUF) for
more details, including on how to concatenate multi-part files.
## Provided Quants
(sorted by size, not necessarily quality. IQ-quants are often preferable over similar sized non-IQ quants)
| Link | Type | Size/GB | Notes |
|:-----|:-----|--------:|:------|
| [GGUF](https://huggingface.co/mradermacher/Replete-Coder-Llama3-8B-i1-GGUF/resolve/main/Replete-Coder-Llama3-8B.i1-IQ1_S.gguf) | i1-IQ1_S | 2.1 | for the desperate |
| [GGUF](https://huggingface.co/mradermacher/Replete-Coder-Llama3-8B-i1-GGUF/resolve/main/Replete-Coder-Llama3-8B.i1-IQ1_M.gguf) | i1-IQ1_M | 2.3 | mostly desperate |
| [GGUF](https://huggingface.co/mradermacher/Replete-Coder-Llama3-8B-i1-GGUF/resolve/main/Replete-Coder-Llama3-8B.i1-IQ2_XXS.gguf) | i1-IQ2_XXS | 2.5 | |
| [GGUF](https://huggingface.co/mradermacher/Replete-Coder-Llama3-8B-i1-GGUF/resolve/main/Replete-Coder-Llama3-8B.i1-IQ2_XS.gguf) | i1-IQ2_XS | 2.7 | |
| [GGUF](https://huggingface.co/mradermacher/Replete-Coder-Llama3-8B-i1-GGUF/resolve/main/Replete-Coder-Llama3-8B.i1-IQ2_S.gguf) | i1-IQ2_S | 2.9 | |
| [GGUF](https://huggingface.co/mradermacher/Replete-Coder-Llama3-8B-i1-GGUF/resolve/main/Replete-Coder-Llama3-8B.i1-IQ2_M.gguf) | i1-IQ2_M | 3.0 | |
| [GGUF](https://huggingface.co/mradermacher/Replete-Coder-Llama3-8B-i1-GGUF/resolve/main/Replete-Coder-Llama3-8B.i1-Q2_K.gguf) | i1-Q2_K | 3.3 | IQ3_XXS probably better |
| [GGUF](https://huggingface.co/mradermacher/Replete-Coder-Llama3-8B-i1-GGUF/resolve/main/Replete-Coder-Llama3-8B.i1-IQ3_XXS.gguf) | i1-IQ3_XXS | 3.4 | lower quality |
| [GGUF](https://huggingface.co/mradermacher/Replete-Coder-Llama3-8B-i1-GGUF/resolve/main/Replete-Coder-Llama3-8B.i1-IQ3_XS.gguf) | i1-IQ3_XS | 3.6 | |
| [GGUF](https://huggingface.co/mradermacher/Replete-Coder-Llama3-8B-i1-GGUF/resolve/main/Replete-Coder-Llama3-8B.i1-Q3_K_S.gguf) | i1-Q3_K_S | 3.8 | IQ3_XS probably better |
| [GGUF](https://huggingface.co/mradermacher/Replete-Coder-Llama3-8B-i1-GGUF/resolve/main/Replete-Coder-Llama3-8B.i1-IQ3_S.gguf) | i1-IQ3_S | 3.8 | beats Q3_K* |
| [GGUF](https://huggingface.co/mradermacher/Replete-Coder-Llama3-8B-i1-GGUF/resolve/main/Replete-Coder-Llama3-8B.i1-IQ3_M.gguf) | i1-IQ3_M | 3.9 | |
| [GGUF](https://huggingface.co/mradermacher/Replete-Coder-Llama3-8B-i1-GGUF/resolve/main/Replete-Coder-Llama3-8B.i1-Q3_K_M.gguf) | i1-Q3_K_M | 4.1 | IQ3_S probably better |
| [GGUF](https://huggingface.co/mradermacher/Replete-Coder-Llama3-8B-i1-GGUF/resolve/main/Replete-Coder-Llama3-8B.i1-Q3_K_L.gguf) | i1-Q3_K_L | 4.4 | IQ3_M probably better |
| [GGUF](https://huggingface.co/mradermacher/Replete-Coder-Llama3-8B-i1-GGUF/resolve/main/Replete-Coder-Llama3-8B.i1-IQ4_XS.gguf) | i1-IQ4_XS | 4.5 | |
| [GGUF](https://huggingface.co/mradermacher/Replete-Coder-Llama3-8B-i1-GGUF/resolve/main/Replete-Coder-Llama3-8B.i1-Q4_0.gguf) | i1-Q4_0 | 4.8 | fast, low quality |
| [GGUF](https://huggingface.co/mradermacher/Replete-Coder-Llama3-8B-i1-GGUF/resolve/main/Replete-Coder-Llama3-8B.i1-Q4_K_S.gguf) | i1-Q4_K_S | 4.8 | optimal size/speed/quality |
| [GGUF](https://huggingface.co/mradermacher/Replete-Coder-Llama3-8B-i1-GGUF/resolve/main/Replete-Coder-Llama3-8B.i1-Q4_K_M.gguf) | i1-Q4_K_M | 5.0 | fast, recommended |
| [GGUF](https://huggingface.co/mradermacher/Replete-Coder-Llama3-8B-i1-GGUF/resolve/main/Replete-Coder-Llama3-8B.i1-Q5_K_S.gguf) | i1-Q5_K_S | 5.7 | |
| [GGUF](https://huggingface.co/mradermacher/Replete-Coder-Llama3-8B-i1-GGUF/resolve/main/Replete-Coder-Llama3-8B.i1-Q5_K_M.gguf) | i1-Q5_K_M | 5.8 | |
| [GGUF](https://huggingface.co/mradermacher/Replete-Coder-Llama3-8B-i1-GGUF/resolve/main/Replete-Coder-Llama3-8B.i1-Q6_K.gguf) | i1-Q6_K | 6.7 | practically like static Q6_K |
Here is a handy graph by ikawrakow comparing some lower-quality quant
types (lower is better):

And here are Artefact2's thoughts on the matter:
https://gist.github.com/Artefact2/b5f810600771265fc1e39442288e8ec9
## FAQ / Model Request
See https://huggingface.co/mradermacher/model_requests for some answers to
questions you might have and/or if you want some other model quantized.
## Thanks
I thank my company, [nethype GmbH](https://www.nethype.de/), for letting
me use its servers and providing upgrades to my workstation to enable
this work in my free time. Additional thanks to [@nicoboss](https://huggingface.co/nicoboss) for giving me access to his hardware for calculating the imatrix for these quants.
<!-- end -->
|
Snowflake/snowflake-arctic-embed-m-long | Snowflake | 2024-05-10T15:50:08Z | 13,622 | 28 | sentence-transformers | [
"sentence-transformers",
"onnx",
"safetensors",
"nomic_bert",
"feature-extraction",
"sentence-similarity",
"mteb",
"arctic",
"snowflake-arctic-embed",
"transformers.js",
"custom_code",
"arxiv:2405.05374",
"arxiv:2104.09864",
"license:apache-2.0",
"model-index",
"endpoints_compatible",
"region:us"
] | sentence-similarity | 2024-04-12T13:52:31Z | ---
license: apache-2.0
pipeline_tag: sentence-similarity
tags:
- sentence-transformers
- feature-extraction
- sentence-similarity
- mteb
- arctic
- snowflake-arctic-embed
- transformers.js
model-index:
- name: snowflake-arctic-m-long
results:
- task:
type: Classification
dataset:
type: mteb/amazon_counterfactual
name: MTEB AmazonCounterfactualClassification (en)
config: en
split: test
revision: e8379541af4e31359cca9fbcf4b00f2671dba205
metrics:
- type: accuracy
value: 78.4776119402985
- type: ap
value: 42.34374238166049
- type: f1
value: 72.51164234732224
- task:
type: Classification
dataset:
type: mteb/amazon_polarity
name: MTEB AmazonPolarityClassification
config: default
split: test
revision: e2d317d38cd51312af73b3d32a06d1a08b442046
metrics:
- type: accuracy
value: 78.7416
- type: ap
value: 73.12074819362377
- type: f1
value: 78.64057339708795
- task:
type: Classification
dataset:
type: mteb/amazon_reviews_multi
name: MTEB AmazonReviewsClassification (en)
config: en
split: test
revision: 1399c76144fd37290681b995c656ef9b2e06e26d
metrics:
- type: accuracy
value: 39.926
- type: f1
value: 39.35531993117573
- task:
type: Retrieval
dataset:
type: mteb/arguana
name: MTEB ArguAna
config: default
split: test
revision: c22ab2a51041ffd869aaddef7af8d8215647e41a
metrics:
- type: map_at_1
value: 34.851
- type: map_at_10
value: 51.473
- type: map_at_100
value: 52.103
- type: map_at_1000
value: 52.105000000000004
- type: map_at_3
value: 46.776
- type: map_at_5
value: 49.617
- type: mrr_at_1
value: 35.491
- type: mrr_at_10
value: 51.73799999999999
- type: mrr_at_100
value: 52.37500000000001
- type: mrr_at_1000
value: 52.378
- type: mrr_at_3
value: 46.965
- type: mrr_at_5
value: 49.878
- type: ndcg_at_1
value: 34.851
- type: ndcg_at_10
value: 60.364
- type: ndcg_at_100
value: 62.888999999999996
- type: ndcg_at_1000
value: 62.946000000000005
- type: ndcg_at_3
value: 50.807
- type: ndcg_at_5
value: 55.901
- type: precision_at_1
value: 34.851
- type: precision_at_10
value: 8.855
- type: precision_at_100
value: 0.992
- type: precision_at_1000
value: 0.1
- type: precision_at_3
value: 20.839
- type: precision_at_5
value: 14.963999999999999
- type: recall_at_1
value: 34.851
- type: recall_at_10
value: 88.549
- type: recall_at_100
value: 99.21799999999999
- type: recall_at_1000
value: 99.644
- type: recall_at_3
value: 62.517999999999994
- type: recall_at_5
value: 74.822
- task:
type: Clustering
dataset:
type: mteb/arxiv-clustering-p2p
name: MTEB ArxivClusteringP2P
config: default
split: test
revision: a122ad7f3f0291bf49cc6f4d32aa80929df69d5d
metrics:
- type: v_measure
value: 45.5554998405317
- task:
type: Clustering
dataset:
type: mteb/arxiv-clustering-s2s
name: MTEB ArxivClusteringS2S
config: default
split: test
revision: f910caf1a6075f7329cdf8c1a6135696f37dbd53
metrics:
- type: v_measure
value: 35.614248811397005
- task:
type: Reranking
dataset:
type: mteb/askubuntudupquestions-reranking
name: MTEB AskUbuntuDupQuestions
config: default
split: test
revision: 2000358ca161889fa9c082cb41daa8dcfb161a54
metrics:
- type: map
value: 61.355489424753884
- type: mrr
value: 75.49443784900849
- task:
type: STS
dataset:
type: mteb/biosses-sts
name: MTEB BIOSSES
config: default
split: test
revision: d3fb88f8f02e40887cd149695127462bbcf29b4a
metrics:
- type: cos_sim_pearson
value: 89.17311056578292
- type: cos_sim_spearman
value: 88.24237210809322
- type: euclidean_pearson
value: 87.3188065853646
- type: euclidean_spearman
value: 88.24237210809322
- type: manhattan_pearson
value: 86.89499710049658
- type: manhattan_spearman
value: 87.85441146091777
- task:
type: Classification
dataset:
type: mteb/banking77
name: MTEB Banking77Classification
config: default
split: test
revision: 0fd18e25b25c072e09e0d92ab615fda904d66300
metrics:
- type: accuracy
value: 80.26298701298703
- type: f1
value: 79.68356764080303
- task:
type: Clustering
dataset:
type: jinaai/big-patent-clustering
name: MTEB BigPatentClustering
config: default
split: test
revision: 62d5330920bca426ce9d3c76ea914f15fc83e891
metrics:
- type: v_measure
value: 20.923883720813706
- task:
type: Clustering
dataset:
type: mteb/biorxiv-clustering-p2p
name: MTEB BiorxivClusteringP2P
config: default
split: test
revision: 65b79d1d13f80053f67aca9498d9402c2d9f1f40
metrics:
- type: v_measure
value: 36.16058801465044
- task:
type: Clustering
dataset:
type: mteb/biorxiv-clustering-s2s
name: MTEB BiorxivClusteringS2S
config: default
split: test
revision: 258694dd0231531bc1fd9de6ceb52a0853c6d908
metrics:
- type: v_measure
value: 30.1402356118627
- task:
type: Retrieval
dataset:
type: mteb/cqadupstack-android
name: MTEB CQADupstackAndroidRetrieval
config: default
split: test
revision: f46a197baaae43b4f621051089b82a364682dfeb
metrics:
- type: map_at_1
value: 35.612
- type: map_at_10
value: 47.117
- type: map_at_100
value: 48.711
- type: map_at_1000
value: 48.826
- type: map_at_3
value: 43.858999999999995
- type: map_at_5
value: 45.612
- type: mrr_at_1
value: 42.918
- type: mrr_at_10
value: 52.806
- type: mrr_at_100
value: 53.564
- type: mrr_at_1000
value: 53.596999999999994
- type: mrr_at_3
value: 50.453
- type: mrr_at_5
value: 51.841
- type: ndcg_at_1
value: 42.918
- type: ndcg_at_10
value: 53.291999999999994
- type: ndcg_at_100
value: 58.711999999999996
- type: ndcg_at_1000
value: 60.317
- type: ndcg_at_3
value: 48.855
- type: ndcg_at_5
value: 50.778
- type: precision_at_1
value: 42.918
- type: precision_at_10
value: 9.927999999999999
- type: precision_at_100
value: 1.592
- type: precision_at_1000
value: 0.201
- type: precision_at_3
value: 23.366999999999997
- type: precision_at_5
value: 16.366
- type: recall_at_1
value: 35.612
- type: recall_at_10
value: 64.671
- type: recall_at_100
value: 86.97
- type: recall_at_1000
value: 96.99600000000001
- type: recall_at_3
value: 51.37199999999999
- type: recall_at_5
value: 57.094
- task:
type: Retrieval
dataset:
type: mteb/cqadupstack-english
name: MTEB CQADupstackEnglishRetrieval
config: default
split: test
revision: ad9991cb51e31e31e430383c75ffb2885547b5f0
metrics:
- type: map_at_1
value: 33.742
- type: map_at_10
value: 44.49
- type: map_at_100
value: 45.781
- type: map_at_1000
value: 45.902
- type: map_at_3
value: 41.453
- type: map_at_5
value: 43.251
- type: mrr_at_1
value: 42.357
- type: mrr_at_10
value: 50.463
- type: mrr_at_100
value: 51.17
- type: mrr_at_1000
value: 51.205999999999996
- type: mrr_at_3
value: 48.397
- type: mrr_at_5
value: 49.649
- type: ndcg_at_1
value: 42.357
- type: ndcg_at_10
value: 50.175000000000004
- type: ndcg_at_100
value: 54.491
- type: ndcg_at_1000
value: 56.282
- type: ndcg_at_3
value: 46.159
- type: ndcg_at_5
value: 48.226
- type: precision_at_1
value: 42.357
- type: precision_at_10
value: 9.382
- type: precision_at_100
value: 1.473
- type: precision_at_1000
value: 0.191
- type: precision_at_3
value: 22.187
- type: precision_at_5
value: 15.758
- type: recall_at_1
value: 33.742
- type: recall_at_10
value: 59.760999999999996
- type: recall_at_100
value: 77.89500000000001
- type: recall_at_1000
value: 89.005
- type: recall_at_3
value: 47.872
- type: recall_at_5
value: 53.559
- task:
type: Retrieval
dataset:
type: mteb/cqadupstack-gaming
name: MTEB CQADupstackGamingRetrieval
config: default
split: test
revision: 4885aa143210c98657558c04aaf3dc47cfb54340
metrics:
- type: map_at_1
value: 43.883
- type: map_at_10
value: 56.464999999999996
- type: map_at_100
value: 57.394
- type: map_at_1000
value: 57.443999999999996
- type: map_at_3
value: 53.169
- type: map_at_5
value: 54.984
- type: mrr_at_1
value: 50.470000000000006
- type: mrr_at_10
value: 59.997
- type: mrr_at_100
value: 60.586
- type: mrr_at_1000
value: 60.61
- type: mrr_at_3
value: 57.837
- type: mrr_at_5
value: 59.019
- type: ndcg_at_1
value: 50.470000000000006
- type: ndcg_at_10
value: 62.134
- type: ndcg_at_100
value: 65.69500000000001
- type: ndcg_at_1000
value: 66.674
- type: ndcg_at_3
value: 56.916999999999994
- type: ndcg_at_5
value: 59.312
- type: precision_at_1
value: 50.470000000000006
- type: precision_at_10
value: 9.812
- type: precision_at_100
value: 1.25
- type: precision_at_1000
value: 0.13699999999999998
- type: precision_at_3
value: 25.119999999999997
- type: precision_at_5
value: 17.016000000000002
- type: recall_at_1
value: 43.883
- type: recall_at_10
value: 75.417
- type: recall_at_100
value: 90.545
- type: recall_at_1000
value: 97.44500000000001
- type: recall_at_3
value: 61.306000000000004
- type: recall_at_5
value: 67.244
- task:
type: Retrieval
dataset:
type: mteb/cqadupstack-gis
name: MTEB CQADupstackGisRetrieval
config: default
split: test
revision: 5003b3064772da1887988e05400cf3806fe491f2
metrics:
- type: map_at_1
value: 29.813000000000002
- type: map_at_10
value: 38.627
- type: map_at_100
value: 39.735
- type: map_at_1000
value: 39.806000000000004
- type: map_at_3
value: 36.283
- type: map_at_5
value: 37.491
- type: mrr_at_1
value: 32.316
- type: mrr_at_10
value: 40.752
- type: mrr_at_100
value: 41.699000000000005
- type: mrr_at_1000
value: 41.749
- type: mrr_at_3
value: 38.531
- type: mrr_at_5
value: 39.706
- type: ndcg_at_1
value: 32.316
- type: ndcg_at_10
value: 43.524
- type: ndcg_at_100
value: 48.648
- type: ndcg_at_1000
value: 50.405
- type: ndcg_at_3
value: 38.928000000000004
- type: ndcg_at_5
value: 40.967
- type: precision_at_1
value: 32.316
- type: precision_at_10
value: 6.451999999999999
- type: precision_at_100
value: 0.9490000000000001
- type: precision_at_1000
value: 0.11299999999999999
- type: precision_at_3
value: 16.384
- type: precision_at_5
value: 11.006
- type: recall_at_1
value: 29.813000000000002
- type: recall_at_10
value: 56.562999999999995
- type: recall_at_100
value: 79.452
- type: recall_at_1000
value: 92.715
- type: recall_at_3
value: 43.985
- type: recall_at_5
value: 49.001
- task:
type: Retrieval
dataset:
type: mteb/cqadupstack-mathematica
name: MTEB CQADupstackMathematicaRetrieval
config: default
split: test
revision: 90fceea13679c63fe563ded68f3b6f06e50061de
metrics:
- type: map_at_1
value: 19.961000000000002
- type: map_at_10
value: 28.026
- type: map_at_100
value: 29.212
- type: map_at_1000
value: 29.332
- type: map_at_3
value: 25.296999999999997
- type: map_at_5
value: 26.832
- type: mrr_at_1
value: 24.627
- type: mrr_at_10
value: 33.045
- type: mrr_at_100
value: 33.944
- type: mrr_at_1000
value: 34.013
- type: mrr_at_3
value: 30.307000000000002
- type: mrr_at_5
value: 31.874000000000002
- type: ndcg_at_1
value: 24.627
- type: ndcg_at_10
value: 33.414
- type: ndcg_at_100
value: 39.061
- type: ndcg_at_1000
value: 41.795
- type: ndcg_at_3
value: 28.377000000000002
- type: ndcg_at_5
value: 30.781999999999996
- type: precision_at_1
value: 24.627
- type: precision_at_10
value: 6.02
- type: precision_at_100
value: 1.035
- type: precision_at_1000
value: 0.13899999999999998
- type: precision_at_3
value: 13.516
- type: precision_at_5
value: 9.851
- type: recall_at_1
value: 19.961000000000002
- type: recall_at_10
value: 45.174
- type: recall_at_100
value: 69.69
- type: recall_at_1000
value: 89.24600000000001
- type: recall_at_3
value: 31.062
- type: recall_at_5
value: 37.193
- task:
type: Retrieval
dataset:
type: mteb/cqadupstack-physics
name: MTEB CQADupstackPhysicsRetrieval
config: default
split: test
revision: 79531abbd1fb92d06c6d6315a0cbbbf5bb247ea4
metrics:
- type: map_at_1
value: 32.080999999999996
- type: map_at_10
value: 42.177
- type: map_at_100
value: 43.431999999999995
- type: map_at_1000
value: 43.533
- type: map_at_3
value: 38.721
- type: map_at_5
value: 40.669
- type: mrr_at_1
value: 38.787
- type: mrr_at_10
value: 47.762
- type: mrr_at_100
value: 48.541000000000004
- type: mrr_at_1000
value: 48.581
- type: mrr_at_3
value: 45.123999999999995
- type: mrr_at_5
value: 46.639
- type: ndcg_at_1
value: 38.787
- type: ndcg_at_10
value: 48.094
- type: ndcg_at_100
value: 53.291
- type: ndcg_at_1000
value: 55.21
- type: ndcg_at_3
value: 42.721
- type: ndcg_at_5
value: 45.301
- type: precision_at_1
value: 38.787
- type: precision_at_10
value: 8.576
- type: precision_at_100
value: 1.306
- type: precision_at_1000
value: 0.164
- type: precision_at_3
value: 19.698
- type: precision_at_5
value: 14.013
- type: recall_at_1
value: 32.080999999999996
- type: recall_at_10
value: 59.948
- type: recall_at_100
value: 81.811
- type: recall_at_1000
value: 94.544
- type: recall_at_3
value: 44.903999999999996
- type: recall_at_5
value: 51.763999999999996
- task:
type: Retrieval
dataset:
type: mteb/cqadupstack-programmers
name: MTEB CQADupstackProgrammersRetrieval
config: default
split: test
revision: 6184bc1440d2dbc7612be22b50686b8826d22b32
metrics:
- type: map_at_1
value: 28.869
- type: map_at_10
value: 38.954
- type: map_at_100
value: 40.233000000000004
- type: map_at_1000
value: 40.332
- type: map_at_3
value: 35.585
- type: map_at_5
value: 37.476
- type: mrr_at_1
value: 35.959
- type: mrr_at_10
value: 44.800000000000004
- type: mrr_at_100
value: 45.609
- type: mrr_at_1000
value: 45.655
- type: mrr_at_3
value: 42.333
- type: mrr_at_5
value: 43.68
- type: ndcg_at_1
value: 35.959
- type: ndcg_at_10
value: 44.957
- type: ndcg_at_100
value: 50.275000000000006
- type: ndcg_at_1000
value: 52.29899999999999
- type: ndcg_at_3
value: 39.797
- type: ndcg_at_5
value: 42.128
- type: precision_at_1
value: 35.959
- type: precision_at_10
value: 8.185
- type: precision_at_100
value: 1.261
- type: precision_at_1000
value: 0.159
- type: precision_at_3
value: 18.988
- type: precision_at_5
value: 13.516
- type: recall_at_1
value: 28.869
- type: recall_at_10
value: 57.154
- type: recall_at_100
value: 79.764
- type: recall_at_1000
value: 93.515
- type: recall_at_3
value: 42.364000000000004
- type: recall_at_5
value: 48.756
- task:
type: Retrieval
dataset:
type: mteb/cqadupstack
name: MTEB CQADupstackRetrieval
config: default
split: test
revision: 4ffe81d471b1924886b33c7567bfb200e9eec5c4
metrics:
- type: map_at_1
value: 29.31008333333333
- type: map_at_10
value: 38.81849999999999
- type: map_at_100
value: 40.05058333333334
- type: map_at_1000
value: 40.16116666666667
- type: map_at_3
value: 35.91441666666667
- type: map_at_5
value: 37.526583333333335
- type: mrr_at_1
value: 34.60066666666667
- type: mrr_at_10
value: 43.08858333333333
- type: mrr_at_100
value: 43.927749999999996
- type: mrr_at_1000
value: 43.97866666666667
- type: mrr_at_3
value: 40.72775
- type: mrr_at_5
value: 42.067249999999994
- type: ndcg_at_1
value: 34.60066666666667
- type: ndcg_at_10
value: 44.20841666666667
- type: ndcg_at_100
value: 49.32866666666667
- type: ndcg_at_1000
value: 51.373999999999995
- type: ndcg_at_3
value: 39.452083333333334
- type: ndcg_at_5
value: 41.67
- type: precision_at_1
value: 34.60066666666667
- type: precision_at_10
value: 7.616583333333334
- type: precision_at_100
value: 1.20175
- type: precision_at_1000
value: 0.156
- type: precision_at_3
value: 17.992
- type: precision_at_5
value: 12.658416666666666
- type: recall_at_1
value: 29.31008333333333
- type: recall_at_10
value: 55.81900000000001
- type: recall_at_100
value: 78.06308333333334
- type: recall_at_1000
value: 92.10641666666668
- type: recall_at_3
value: 42.50166666666667
- type: recall_at_5
value: 48.26108333333333
- task:
type: Retrieval
dataset:
type: mteb/cqadupstack-stats
name: MTEB CQADupstackStatsRetrieval
config: default
split: test
revision: 65ac3a16b8e91f9cee4c9828cc7c335575432a2a
metrics:
- type: map_at_1
value: 26.773000000000003
- type: map_at_10
value: 34.13
- type: map_at_100
value: 35.113
- type: map_at_1000
value: 35.211
- type: map_at_3
value: 31.958
- type: map_at_5
value: 33.080999999999996
- type: mrr_at_1
value: 30.061
- type: mrr_at_10
value: 37.061
- type: mrr_at_100
value: 37.865
- type: mrr_at_1000
value: 37.939
- type: mrr_at_3
value: 34.995
- type: mrr_at_5
value: 36.092
- type: ndcg_at_1
value: 30.061
- type: ndcg_at_10
value: 38.391999999999996
- type: ndcg_at_100
value: 43.13
- type: ndcg_at_1000
value: 45.449
- type: ndcg_at_3
value: 34.411
- type: ndcg_at_5
value: 36.163000000000004
- type: precision_at_1
value: 30.061
- type: precision_at_10
value: 5.982
- type: precision_at_100
value: 0.911
- type: precision_at_1000
value: 0.11800000000000001
- type: precision_at_3
value: 14.673
- type: precision_at_5
value: 10.030999999999999
- type: recall_at_1
value: 26.773000000000003
- type: recall_at_10
value: 48.445
- type: recall_at_100
value: 69.741
- type: recall_at_1000
value: 86.59
- type: recall_at_3
value: 37.576
- type: recall_at_5
value: 41.948
- task:
type: Retrieval
dataset:
type: mteb/cqadupstack-tex
name: MTEB CQADupstackTexRetrieval
config: default
split: test
revision: 46989137a86843e03a6195de44b09deda022eec7
metrics:
- type: map_at_1
value: 18.556
- type: map_at_10
value: 26.340999999999998
- type: map_at_100
value: 27.560000000000002
- type: map_at_1000
value: 27.685
- type: map_at_3
value: 24.136
- type: map_at_5
value: 25.34
- type: mrr_at_1
value: 22.368
- type: mrr_at_10
value: 30.192999999999998
- type: mrr_at_100
value: 31.183
- type: mrr_at_1000
value: 31.258000000000003
- type: mrr_at_3
value: 28.223
- type: mrr_at_5
value: 29.294999999999998
- type: ndcg_at_1
value: 22.368
- type: ndcg_at_10
value: 31.029
- type: ndcg_at_100
value: 36.768
- type: ndcg_at_1000
value: 39.572
- type: ndcg_at_3
value: 27.197
- type: ndcg_at_5
value: 28.912
- type: precision_at_1
value: 22.368
- type: precision_at_10
value: 5.606
- type: precision_at_100
value: 0.9979999999999999
- type: precision_at_1000
value: 0.14100000000000001
- type: precision_at_3
value: 12.892999999999999
- type: precision_at_5
value: 9.16
- type: recall_at_1
value: 18.556
- type: recall_at_10
value: 41.087
- type: recall_at_100
value: 66.92
- type: recall_at_1000
value: 86.691
- type: recall_at_3
value: 30.415
- type: recall_at_5
value: 34.813
- task:
type: Retrieval
dataset:
type: mteb/cqadupstack-unix
name: MTEB CQADupstackUnixRetrieval
config: default
split: test
revision: 6c6430d3a6d36f8d2a829195bc5dc94d7e063e53
metrics:
- type: map_at_1
value: 29.953999999999997
- type: map_at_10
value: 39.633
- type: map_at_100
value: 40.923
- type: map_at_1000
value: 41.016000000000005
- type: map_at_3
value: 36.609
- type: map_at_5
value: 38.443
- type: mrr_at_1
value: 35.354
- type: mrr_at_10
value: 43.718
- type: mrr_at_100
value: 44.651999999999994
- type: mrr_at_1000
value: 44.696000000000005
- type: mrr_at_3
value: 41.154
- type: mrr_at_5
value: 42.730000000000004
- type: ndcg_at_1
value: 35.354
- type: ndcg_at_10
value: 44.933
- type: ndcg_at_100
value: 50.577000000000005
- type: ndcg_at_1000
value: 52.428
- type: ndcg_at_3
value: 39.833
- type: ndcg_at_5
value: 42.465
- type: precision_at_1
value: 35.354
- type: precision_at_10
value: 7.416
- type: precision_at_100
value: 1.157
- type: precision_at_1000
value: 0.14100000000000001
- type: precision_at_3
value: 17.817
- type: precision_at_5
value: 12.687000000000001
- type: recall_at_1
value: 29.953999999999997
- type: recall_at_10
value: 56.932
- type: recall_at_100
value: 80.93900000000001
- type: recall_at_1000
value: 93.582
- type: recall_at_3
value: 43.192
- type: recall_at_5
value: 49.757
- task:
type: Retrieval
dataset:
type: mteb/cqadupstack-webmasters
name: MTEB CQADupstackWebmastersRetrieval
config: default
split: test
revision: 160c094312a0e1facb97e55eeddb698c0abe3571
metrics:
- type: map_at_1
value: 27.85
- type: map_at_10
value: 37.68
- type: map_at_100
value: 39.295
- type: map_at_1000
value: 39.527
- type: map_at_3
value: 35.036
- type: map_at_5
value: 36.269
- type: mrr_at_1
value: 33.004
- type: mrr_at_10
value: 42.096000000000004
- type: mrr_at_100
value: 43.019
- type: mrr_at_1000
value: 43.071
- type: mrr_at_3
value: 39.987
- type: mrr_at_5
value: 40.995
- type: ndcg_at_1
value: 33.004
- type: ndcg_at_10
value: 43.461
- type: ndcg_at_100
value: 49.138
- type: ndcg_at_1000
value: 51.50900000000001
- type: ndcg_at_3
value: 39.317
- type: ndcg_at_5
value: 40.760999999999996
- type: precision_at_1
value: 33.004
- type: precision_at_10
value: 8.161999999999999
- type: precision_at_100
value: 1.583
- type: precision_at_1000
value: 0.245
- type: precision_at_3
value: 18.445
- type: precision_at_5
value: 12.885
- type: recall_at_1
value: 27.85
- type: recall_at_10
value: 54.419
- type: recall_at_100
value: 79.742
- type: recall_at_1000
value: 93.97
- type: recall_at_3
value: 42.149
- type: recall_at_5
value: 46.165
- task:
type: Retrieval
dataset:
type: mteb/cqadupstack-wordpress
name: MTEB CQADupstackWordpressRetrieval
config: default
split: test
revision: 4ffe81d471b1924886b33c7567bfb200e9eec5c4
metrics:
- type: map_at_1
value: 24.627
- type: map_at_10
value: 32.182
- type: map_at_100
value: 33.217999999999996
- type: map_at_1000
value: 33.32
- type: map_at_3
value: 28.866999999999997
- type: map_at_5
value: 30.871
- type: mrr_at_1
value: 26.987
- type: mrr_at_10
value: 34.37
- type: mrr_at_100
value: 35.301
- type: mrr_at_1000
value: 35.369
- type: mrr_at_3
value: 31.391999999999996
- type: mrr_at_5
value: 33.287
- type: ndcg_at_1
value: 26.987
- type: ndcg_at_10
value: 37.096000000000004
- type: ndcg_at_100
value: 42.158
- type: ndcg_at_1000
value: 44.548
- type: ndcg_at_3
value: 30.913
- type: ndcg_at_5
value: 34.245
- type: precision_at_1
value: 26.987
- type: precision_at_10
value: 5.878
- type: precision_at_100
value: 0.906
- type: precision_at_1000
value: 0.123
- type: precision_at_3
value: 12.815999999999999
- type: precision_at_5
value: 9.612
- type: recall_at_1
value: 24.627
- type: recall_at_10
value: 50.257
- type: recall_at_100
value: 73.288
- type: recall_at_1000
value: 90.97800000000001
- type: recall_at_3
value: 33.823
- type: recall_at_5
value: 41.839
- task:
type: Retrieval
dataset:
type: mteb/climate-fever
name: MTEB ClimateFEVER
config: default
split: test
revision: 47f2ac6acb640fc46020b02a5b59fdda04d39380
metrics:
- type: map_at_1
value: 17.343
- type: map_at_10
value: 28.59
- type: map_at_100
value: 30.591
- type: map_at_1000
value: 30.759999999999998
- type: map_at_3
value: 24.197
- type: map_at_5
value: 26.433
- type: mrr_at_1
value: 39.609
- type: mrr_at_10
value: 51.107
- type: mrr_at_100
value: 51.87199999999999
- type: mrr_at_1000
value: 51.894
- type: mrr_at_3
value: 48.154
- type: mrr_at_5
value: 49.939
- type: ndcg_at_1
value: 39.609
- type: ndcg_at_10
value: 38.329
- type: ndcg_at_100
value: 45.573
- type: ndcg_at_1000
value: 48.405
- type: ndcg_at_3
value: 32.506
- type: ndcg_at_5
value: 34.331
- type: precision_at_1
value: 39.609
- type: precision_at_10
value: 11.668000000000001
- type: precision_at_100
value: 1.9539999999999997
- type: precision_at_1000
value: 0.249
- type: precision_at_3
value: 23.952
- type: precision_at_5
value: 17.902
- type: recall_at_1
value: 17.343
- type: recall_at_10
value: 43.704
- type: recall_at_100
value: 68.363
- type: recall_at_1000
value: 84.04599999999999
- type: recall_at_3
value: 29.028
- type: recall_at_5
value: 35.022
- task:
type: Retrieval
dataset:
type: mteb/dbpedia
name: MTEB DBPedia
config: default
split: test
revision: c0f706b76e590d620bd6618b3ca8efdd34e2d659
metrics:
- type: map_at_1
value: 9.934999999999999
- type: map_at_10
value: 22.081
- type: map_at_100
value: 32.036
- type: map_at_1000
value: 33.803
- type: map_at_3
value: 15.687999999999999
- type: map_at_5
value: 18.357
- type: mrr_at_1
value: 70.75
- type: mrr_at_10
value: 78.506
- type: mrr_at_100
value: 78.874
- type: mrr_at_1000
value: 78.88300000000001
- type: mrr_at_3
value: 77.667
- type: mrr_at_5
value: 78.342
- type: ndcg_at_1
value: 57.25
- type: ndcg_at_10
value: 45.286
- type: ndcg_at_100
value: 50.791
- type: ndcg_at_1000
value: 58.021
- type: ndcg_at_3
value: 49.504
- type: ndcg_at_5
value: 47.03
- type: precision_at_1
value: 70.75
- type: precision_at_10
value: 36.425000000000004
- type: precision_at_100
value: 11.953
- type: precision_at_1000
value: 2.248
- type: precision_at_3
value: 53.25
- type: precision_at_5
value: 46.150000000000006
- type: recall_at_1
value: 9.934999999999999
- type: recall_at_10
value: 27.592
- type: recall_at_100
value: 58.089
- type: recall_at_1000
value: 81.025
- type: recall_at_3
value: 17.048
- type: recall_at_5
value: 20.834
- task:
type: Classification
dataset:
type: mteb/emotion
name: MTEB EmotionClassification
config: default
split: test
revision: 4f58c6b202a23cf9a4da393831edf4f9183cad37
metrics:
- type: accuracy
value: 47.25999999999999
- type: f1
value: 43.83371155132253
- task:
type: Retrieval
dataset:
type: mteb/fever
name: MTEB FEVER
config: default
split: test
revision: bea83ef9e8fb933d90a2f1d5515737465d613e12
metrics:
- type: map_at_1
value: 73.68900000000001
- type: map_at_10
value: 82.878
- type: map_at_100
value: 83.084
- type: map_at_1000
value: 83.097
- type: map_at_3
value: 81.528
- type: map_at_5
value: 82.432
- type: mrr_at_1
value: 79.49300000000001
- type: mrr_at_10
value: 87.24300000000001
- type: mrr_at_100
value: 87.3
- type: mrr_at_1000
value: 87.301
- type: mrr_at_3
value: 86.359
- type: mrr_at_5
value: 87.01
- type: ndcg_at_1
value: 79.49300000000001
- type: ndcg_at_10
value: 86.894
- type: ndcg_at_100
value: 87.6
- type: ndcg_at_1000
value: 87.79299999999999
- type: ndcg_at_3
value: 84.777
- type: ndcg_at_5
value: 86.08
- type: precision_at_1
value: 79.49300000000001
- type: precision_at_10
value: 10.578
- type: precision_at_100
value: 1.117
- type: precision_at_1000
value: 0.11499999999999999
- type: precision_at_3
value: 32.592999999999996
- type: precision_at_5
value: 20.423
- type: recall_at_1
value: 73.68900000000001
- type: recall_at_10
value: 94.833
- type: recall_at_100
value: 97.554
- type: recall_at_1000
value: 98.672
- type: recall_at_3
value: 89.236
- type: recall_at_5
value: 92.461
- task:
type: Retrieval
dataset:
type: mteb/fiqa
name: MTEB FiQA2018
config: default
split: test
revision: 27a168819829fe9bcd655c2df245fb19452e8e06
metrics:
- type: map_at_1
value: 20.59
- type: map_at_10
value: 34.089000000000006
- type: map_at_100
value: 35.796
- type: map_at_1000
value: 35.988
- type: map_at_3
value: 29.877
- type: map_at_5
value: 32.202999999999996
- type: mrr_at_1
value: 41.049
- type: mrr_at_10
value: 50.370000000000005
- type: mrr_at_100
value: 51.209
- type: mrr_at_1000
value: 51.247
- type: mrr_at_3
value: 48.122
- type: mrr_at_5
value: 49.326
- type: ndcg_at_1
value: 41.049
- type: ndcg_at_10
value: 42.163000000000004
- type: ndcg_at_100
value: 48.638999999999996
- type: ndcg_at_1000
value: 51.775000000000006
- type: ndcg_at_3
value: 38.435
- type: ndcg_at_5
value: 39.561
- type: precision_at_1
value: 41.049
- type: precision_at_10
value: 11.481
- type: precision_at_100
value: 1.8239999999999998
- type: precision_at_1000
value: 0.24
- type: precision_at_3
value: 25.257
- type: precision_at_5
value: 18.519
- type: recall_at_1
value: 20.59
- type: recall_at_10
value: 49.547999999999995
- type: recall_at_100
value: 73.676
- type: recall_at_1000
value: 92.269
- type: recall_at_3
value: 35.656
- type: recall_at_5
value: 41.455
- task:
type: Retrieval
dataset:
type: mteb/hotpotqa
name: MTEB HotpotQA
config: default
split: test
revision: ab518f4d6fcca38d87c25209f94beba119d02014
metrics:
- type: map_at_1
value: 39.932
- type: map_at_10
value: 64.184
- type: map_at_100
value: 65.06
- type: map_at_1000
value: 65.109
- type: map_at_3
value: 60.27
- type: map_at_5
value: 62.732
- type: mrr_at_1
value: 79.865
- type: mrr_at_10
value: 85.99799999999999
- type: mrr_at_100
value: 86.13
- type: mrr_at_1000
value: 86.13300000000001
- type: mrr_at_3
value: 85.136
- type: mrr_at_5
value: 85.69200000000001
- type: ndcg_at_1
value: 79.865
- type: ndcg_at_10
value: 72.756
- type: ndcg_at_100
value: 75.638
- type: ndcg_at_1000
value: 76.589
- type: ndcg_at_3
value: 67.38199999999999
- type: ndcg_at_5
value: 70.402
- type: precision_at_1
value: 79.865
- type: precision_at_10
value: 15.387999999999998
- type: precision_at_100
value: 1.7610000000000001
- type: precision_at_1000
value: 0.189
- type: precision_at_3
value: 43.394
- type: precision_at_5
value: 28.424
- type: recall_at_1
value: 39.932
- type: recall_at_10
value: 76.941
- type: recall_at_100
value: 88.062
- type: recall_at_1000
value: 94.396
- type: recall_at_3
value: 65.091
- type: recall_at_5
value: 71.06
- task:
type: Classification
dataset:
type: mteb/imdb
name: MTEB ImdbClassification
config: default
split: test
revision: 3d86128a09e091d6018b6d26cad27f2739fc2db7
metrics:
- type: accuracy
value: 71.7904
- type: ap
value: 65.82899456730257
- type: f1
value: 71.56611877410202
- task:
type: Retrieval
dataset:
type: mteb/msmarco
name: MTEB MSMARCO
config: default
split: dev
revision: c5a29a104738b98a9e76336939199e264163d4a0
metrics:
- type: map_at_1
value: 21.931
- type: map_at_10
value: 34.849999999999994
- type: map_at_100
value: 36.033
- type: map_at_1000
value: 36.08
- type: map_at_3
value: 30.842000000000002
- type: map_at_5
value: 33.229
- type: mrr_at_1
value: 22.55
- type: mrr_at_10
value: 35.436
- type: mrr_at_100
value: 36.563
- type: mrr_at_1000
value: 36.604
- type: mrr_at_3
value: 31.507
- type: mrr_at_5
value: 33.851
- type: ndcg_at_1
value: 22.55
- type: ndcg_at_10
value: 41.969
- type: ndcg_at_100
value: 47.576
- type: ndcg_at_1000
value: 48.731
- type: ndcg_at_3
value: 33.894000000000005
- type: ndcg_at_5
value: 38.133
- type: precision_at_1
value: 22.55
- type: precision_at_10
value: 6.660000000000001
- type: precision_at_100
value: 0.946
- type: precision_at_1000
value: 0.104
- type: precision_at_3
value: 14.532
- type: precision_at_5
value: 10.865
- type: recall_at_1
value: 21.931
- type: recall_at_10
value: 63.841
- type: recall_at_100
value: 89.47699999999999
- type: recall_at_1000
value: 98.259
- type: recall_at_3
value: 42.063
- type: recall_at_5
value: 52.21
- task:
type: Classification
dataset:
type: mteb/mtop_domain
name: MTEB MTOPDomainClassification (en)
config: en
split: test
revision: d80d48c1eb48d3562165c59d59d0034df9fff0bf
metrics:
- type: accuracy
value: 93.03921568627452
- type: f1
value: 92.56400672314416
- task:
type: Classification
dataset:
type: mteb/mtop_intent
name: MTEB MTOPIntentClassification (en)
config: en
split: test
revision: ae001d0e6b1228650b7bd1c2c65fb50ad11a8aba
metrics:
- type: accuracy
value: 63.515731874145
- type: f1
value: 44.922310875523216
- task:
type: Classification
dataset:
type: masakhane/masakhanews
name: MTEB MasakhaNEWSClassification (eng)
config: eng
split: test
revision: 8ccc72e69e65f40c70e117d8b3c08306bb788b60
metrics:
- type: accuracy
value: 77.57383966244727
- type: f1
value: 76.55222378218293
- task:
type: Clustering
dataset:
type: masakhane/masakhanews
name: MTEB MasakhaNEWSClusteringP2P (eng)
config: eng
split: test
revision: 8ccc72e69e65f40c70e117d8b3c08306bb788b60
metrics:
- type: v_measure
value: 62.74836240280833
- task:
type: Clustering
dataset:
type: masakhane/masakhanews
name: MTEB MasakhaNEWSClusteringS2S (eng)
config: eng
split: test
revision: 8ccc72e69e65f40c70e117d8b3c08306bb788b60
metrics:
- type: v_measure
value: 24.414348715238184
- task:
type: Classification
dataset:
type: mteb/amazon_massive_intent
name: MTEB MassiveIntentClassification (en)
config: en
split: test
revision: 31efe3c427b0bae9c22cbb560b8f15491cc6bed7
metrics:
- type: accuracy
value: 66.54673839946201
- type: f1
value: 64.61004101532164
- task:
type: Classification
dataset:
type: mteb/amazon_massive_scenario
name: MTEB MassiveScenarioClassification (en)
config: en
split: test
revision: 7d571f92784cd94a019292a1f45445077d0ef634
metrics:
- type: accuracy
value: 73.11365164761264
- type: f1
value: 72.01684013680978
- task:
type: Clustering
dataset:
type: mteb/medrxiv-clustering-p2p
name: MTEB MedrxivClusteringP2P
config: default
split: test
revision: e7a26af6f3ae46b30dde8737f02c07b1505bcc73
metrics:
- type: v_measure
value: 31.123671999617297
- task:
type: Clustering
dataset:
type: mteb/medrxiv-clustering-s2s
name: MTEB MedrxivClusteringS2S
config: default
split: test
revision: 35191c8c0dca72d8ff3efcd72aa802307d469663
metrics:
- type: v_measure
value: 26.72684341430875
- task:
type: Reranking
dataset:
type: mteb/mind_small
name: MTEB MindSmallReranking
config: default
split: test
revision: 3bdac13927fdc888b903db93b2ffdbd90b295a69
metrics:
- type: map
value: 29.910228061734816
- type: mrr
value: 30.835255982532477
- task:
type: Retrieval
dataset:
type: mteb/nfcorpus
name: MTEB NFCorpus
config: default
split: test
revision: ec0fa4fe99da2ff19ca1214b7966684033a58814
metrics:
- type: map_at_1
value: 5.6770000000000005
- type: map_at_10
value: 13.15
- type: map_at_100
value: 16.205
- type: map_at_1000
value: 17.580000000000002
- type: map_at_3
value: 9.651
- type: map_at_5
value: 11.142000000000001
- type: mrr_at_1
value: 47.678
- type: mrr_at_10
value: 56.257000000000005
- type: mrr_at_100
value: 56.708000000000006
- type: mrr_at_1000
value: 56.751
- type: mrr_at_3
value: 54.128
- type: mrr_at_5
value: 55.181000000000004
- type: ndcg_at_1
value: 45.511
- type: ndcg_at_10
value: 35.867
- type: ndcg_at_100
value: 31.566
- type: ndcg_at_1000
value: 40.077
- type: ndcg_at_3
value: 41.9
- type: ndcg_at_5
value: 39.367999999999995
- type: precision_at_1
value: 47.678
- type: precision_at_10
value: 26.842
- type: precision_at_100
value: 7.991
- type: precision_at_1000
value: 2.0469999999999997
- type: precision_at_3
value: 39.938
- type: precision_at_5
value: 34.613
- type: recall_at_1
value: 5.6770000000000005
- type: recall_at_10
value: 17.119999999999997
- type: recall_at_100
value: 30.828
- type: recall_at_1000
value: 62.082
- type: recall_at_3
value: 10.456
- type: recall_at_5
value: 12.903999999999998
- task:
type: Retrieval
dataset:
type: mteb/nq
name: MTEB NQ
config: default
split: test
revision: b774495ed302d8c44a3a7ea25c90dbce03968f31
metrics:
- type: map_at_1
value: 39.021
- type: map_at_10
value: 54.976
- type: map_at_100
value: 55.793000000000006
- type: map_at_1000
value: 55.811
- type: map_at_3
value: 50.759
- type: map_at_5
value: 53.429
- type: mrr_at_1
value: 43.308
- type: mrr_at_10
value: 57.118
- type: mrr_at_100
value: 57.69499999999999
- type: mrr_at_1000
value: 57.704
- type: mrr_at_3
value: 53.848
- type: mrr_at_5
value: 55.915000000000006
- type: ndcg_at_1
value: 43.308
- type: ndcg_at_10
value: 62.33800000000001
- type: ndcg_at_100
value: 65.61099999999999
- type: ndcg_at_1000
value: 65.995
- type: ndcg_at_3
value: 54.723
- type: ndcg_at_5
value: 59.026
- type: precision_at_1
value: 43.308
- type: precision_at_10
value: 9.803
- type: precision_at_100
value: 1.167
- type: precision_at_1000
value: 0.121
- type: precision_at_3
value: 24.334
- type: precision_at_5
value: 17.144000000000002
- type: recall_at_1
value: 39.021
- type: recall_at_10
value: 82.37299999999999
- type: recall_at_100
value: 96.21499999999999
- type: recall_at_1000
value: 99.02499999999999
- type: recall_at_3
value: 63.031000000000006
- type: recall_at_5
value: 72.856
- task:
type: Classification
dataset:
type: ag_news
name: MTEB NewsClassification
config: default
split: test
revision: eb185aade064a813bc0b7f42de02595523103ca4
metrics:
- type: accuracy
value: 78.03289473684211
- type: f1
value: 77.89323745730803
- task:
type: PairClassification
dataset:
type: GEM/opusparcus
name: MTEB OpusparcusPC (en)
config: en
split: test
revision: 9e9b1f8ef51616073f47f306f7f47dd91663f86a
metrics:
- type: cos_sim_accuracy
value: 99.89816700610999
- type: cos_sim_ap
value: 100.0
- type: cos_sim_f1
value: 99.9490575649516
- type: cos_sim_precision
value: 100.0
- type: cos_sim_recall
value: 99.89816700610999
- type: dot_accuracy
value: 99.89816700610999
- type: dot_ap
value: 100.0
- type: dot_f1
value: 99.9490575649516
- type: dot_precision
value: 100.0
- type: dot_recall
value: 99.89816700610999
- type: euclidean_accuracy
value: 99.89816700610999
- type: euclidean_ap
value: 100.0
- type: euclidean_f1
value: 99.9490575649516
- type: euclidean_precision
value: 100.0
- type: euclidean_recall
value: 99.89816700610999
- type: manhattan_accuracy
value: 99.89816700610999
- type: manhattan_ap
value: 100.0
- type: manhattan_f1
value: 99.9490575649516
- type: manhattan_precision
value: 100.0
- type: manhattan_recall
value: 99.89816700610999
- type: max_accuracy
value: 99.89816700610999
- type: max_ap
value: 100.0
- type: max_f1
value: 99.9490575649516
- task:
type: PairClassification
dataset:
type: paws-x
name: MTEB PawsX (en)
config: en
split: test
revision: 8a04d940a42cd40658986fdd8e3da561533a3646
metrics:
- type: cos_sim_accuracy
value: 61.75000000000001
- type: cos_sim_ap
value: 59.578879568280385
- type: cos_sim_f1
value: 62.50861474844934
- type: cos_sim_precision
value: 45.46365914786967
- type: cos_sim_recall
value: 100.0
- type: dot_accuracy
value: 61.75000000000001
- type: dot_ap
value: 59.57893088951573
- type: dot_f1
value: 62.50861474844934
- type: dot_precision
value: 45.46365914786967
- type: dot_recall
value: 100.0
- type: euclidean_accuracy
value: 61.75000000000001
- type: euclidean_ap
value: 59.578755624671686
- type: euclidean_f1
value: 62.50861474844934
- type: euclidean_precision
value: 45.46365914786967
- type: euclidean_recall
value: 100.0
- type: manhattan_accuracy
value: 61.75000000000001
- type: manhattan_ap
value: 59.58504334461159
- type: manhattan_f1
value: 62.50861474844934
- type: manhattan_precision
value: 45.46365914786967
- type: manhattan_recall
value: 100.0
- type: max_accuracy
value: 61.75000000000001
- type: max_ap
value: 59.58504334461159
- type: max_f1
value: 62.50861474844934
- task:
type: Retrieval
dataset:
type: mteb/quora
name: MTEB QuoraRetrieval
config: default
split: test
revision: e4e08e0b7dbe3c8700f0daef558ff32256715259
metrics:
- type: map_at_1
value: 70.186
- type: map_at_10
value: 83.875
- type: map_at_100
value: 84.514
- type: map_at_1000
value: 84.53500000000001
- type: map_at_3
value: 80.926
- type: map_at_5
value: 82.797
- type: mrr_at_1
value: 80.82000000000001
- type: mrr_at_10
value: 87.068
- type: mrr_at_100
value: 87.178
- type: mrr_at_1000
value: 87.18
- type: mrr_at_3
value: 86.055
- type: mrr_at_5
value: 86.763
- type: ndcg_at_1
value: 80.84
- type: ndcg_at_10
value: 87.723
- type: ndcg_at_100
value: 88.98700000000001
- type: ndcg_at_1000
value: 89.13499999999999
- type: ndcg_at_3
value: 84.821
- type: ndcg_at_5
value: 86.441
- type: precision_at_1
value: 80.84
- type: precision_at_10
value: 13.270000000000001
- type: precision_at_100
value: 1.516
- type: precision_at_1000
value: 0.156
- type: precision_at_3
value: 37.013
- type: precision_at_5
value: 24.37
- type: recall_at_1
value: 70.186
- type: recall_at_10
value: 94.948
- type: recall_at_100
value: 99.223
- type: recall_at_1000
value: 99.932
- type: recall_at_3
value: 86.57000000000001
- type: recall_at_5
value: 91.157
- task:
type: Clustering
dataset:
type: mteb/reddit-clustering
name: MTEB RedditClustering
config: default
split: test
revision: 24640382cdbf8abc73003fb0fa6d111a705499eb
metrics:
- type: v_measure
value: 50.24198927949519
- task:
type: Clustering
dataset:
type: mteb/reddit-clustering-p2p
name: MTEB RedditClusteringP2P
config: default
split: test
revision: 385e3cb46b4cfa89021f56c4380204149d0efe33
metrics:
- type: v_measure
value: 61.452073078765544
- task:
type: Retrieval
dataset:
type: mteb/scidocs
name: MTEB SCIDOCS
config: default
split: test
revision: f8c2fcf00f625baaa80f62ec5bd9e1fff3b8ae88
metrics:
- type: map_at_1
value: 4.972
- type: map_at_10
value: 12.314
- type: map_at_100
value: 14.333000000000002
- type: map_at_1000
value: 14.628
- type: map_at_3
value: 8.972
- type: map_at_5
value: 10.724
- type: mrr_at_1
value: 24.4
- type: mrr_at_10
value: 35.257
- type: mrr_at_100
value: 36.297000000000004
- type: mrr_at_1000
value: 36.363
- type: mrr_at_3
value: 32.267
- type: mrr_at_5
value: 33.942
- type: ndcg_at_1
value: 24.4
- type: ndcg_at_10
value: 20.47
- type: ndcg_at_100
value: 28.111000000000004
- type: ndcg_at_1000
value: 33.499
- type: ndcg_at_3
value: 19.975
- type: ndcg_at_5
value: 17.293
- type: precision_at_1
value: 24.4
- type: precision_at_10
value: 10.440000000000001
- type: precision_at_100
value: 2.136
- type: precision_at_1000
value: 0.34299999999999997
- type: precision_at_3
value: 18.733
- type: precision_at_5
value: 15.120000000000001
- type: recall_at_1
value: 4.972
- type: recall_at_10
value: 21.157
- type: recall_at_100
value: 43.335
- type: recall_at_1000
value: 69.652
- type: recall_at_3
value: 11.417
- type: recall_at_5
value: 15.317
- task:
type: STS
dataset:
type: mteb/sickr-sts
name: MTEB SICK-R
config: default
split: test
revision: 20a6d6f312dd54037fe07a32d58e5e168867909d
metrics:
- type: cos_sim_pearson
value: 76.70295978506286
- type: cos_sim_spearman
value: 70.91162732446628
- type: euclidean_pearson
value: 73.25693688746031
- type: euclidean_spearman
value: 70.91162556180127
- type: manhattan_pearson
value: 73.27735004735767
- type: manhattan_spearman
value: 70.8856787022704
- task:
type: STS
dataset:
type: mteb/sts12-sts
name: MTEB STS12
config: default
split: test
revision: a0d554a64d88156834ff5ae9920b964011b16384
metrics:
- type: cos_sim_pearson
value: 67.55878682646774
- type: cos_sim_spearman
value: 66.10824660353681
- type: euclidean_pearson
value: 64.93937270068541
- type: euclidean_spearman
value: 66.10824660353681
- type: manhattan_pearson
value: 64.96325555978984
- type: manhattan_spearman
value: 66.12052481638577
- task:
type: STS
dataset:
type: mteb/sts13-sts
name: MTEB STS13
config: default
split: test
revision: 7e90230a92c190f1bf69ae9002b8cea547a64cca
metrics:
- type: cos_sim_pearson
value: 79.79979774019496
- type: cos_sim_spearman
value: 79.82293444619499
- type: euclidean_pearson
value: 79.4830436509311
- type: euclidean_spearman
value: 79.82293444619499
- type: manhattan_pearson
value: 79.49785594799296
- type: manhattan_spearman
value: 79.8280390479434
- task:
type: STS
dataset:
type: mteb/sts14-sts
name: MTEB STS14
config: default
split: test
revision: 6031580fec1f6af667f0bd2da0a551cf4f0b2375
metrics:
- type: cos_sim_pearson
value: 76.36839628231121
- type: cos_sim_spearman
value: 73.63809739428072
- type: euclidean_pearson
value: 74.93718121215906
- type: euclidean_spearman
value: 73.63810227650436
- type: manhattan_pearson
value: 74.8737197659424
- type: manhattan_spearman
value: 73.57534688126572
- task:
type: STS
dataset:
type: mteb/sts15-sts
name: MTEB STS15
config: default
split: test
revision: ae752c7c21bf194d8b67fd573edf7ae58183cbe3
metrics:
- type: cos_sim_pearson
value: 82.67482138157656
- type: cos_sim_spearman
value: 83.23485786963107
- type: euclidean_pearson
value: 82.50847772197369
- type: euclidean_spearman
value: 83.23485786963107
- type: manhattan_pearson
value: 82.48916218377576
- type: manhattan_spearman
value: 83.19756483500014
- task:
type: STS
dataset:
type: mteb/sts16-sts
name: MTEB STS16
config: default
split: test
revision: 4d8694f8f0e0100860b497b999b3dbed754a0513
metrics:
- type: cos_sim_pearson
value: 81.11626268793967
- type: cos_sim_spearman
value: 81.58184691061507
- type: euclidean_pearson
value: 80.65900869004938
- type: euclidean_spearman
value: 81.58184691061507
- type: manhattan_pearson
value: 80.67912306966772
- type: manhattan_spearman
value: 81.59957593393145
- task:
type: STS
dataset:
type: mteb/sts17-crosslingual-sts
name: MTEB STS17 (en-en)
config: en-en
split: test
revision: af5e6fb845001ecf41f4c1e033ce921939a2a68d
metrics:
- type: cos_sim_pearson
value: 80.3140990821409
- type: cos_sim_spearman
value: 80.59196586367551
- type: euclidean_pearson
value: 80.73014029317672
- type: euclidean_spearman
value: 80.59196586367551
- type: manhattan_pearson
value: 80.5774325136987
- type: manhattan_spearman
value: 80.35102610546238
- task:
type: STS
dataset:
type: mteb/sts22-crosslingual-sts
name: MTEB STS22 (en)
config: en
split: test
revision: eea2b4fe26a775864c896887d910b76a8098ad3f
metrics:
- type: cos_sim_pearson
value: 68.34450491529164
- type: cos_sim_spearman
value: 68.79451793414492
- type: euclidean_pearson
value: 68.75619738499324
- type: euclidean_spearman
value: 68.79451793414492
- type: manhattan_pearson
value: 68.75256119543882
- type: manhattan_spearman
value: 68.81836416978547
- task:
type: STS
dataset:
type: mteb/stsbenchmark-sts
name: MTEB STSBenchmark
config: default
split: test
revision: b0fddb56ed78048fa8b90373c8a3cfc37b684831
metrics:
- type: cos_sim_pearson
value: 77.95580414975612
- type: cos_sim_spearman
value: 77.89671867168987
- type: euclidean_pearson
value: 77.61352097720862
- type: euclidean_spearman
value: 77.89671867168987
- type: manhattan_pearson
value: 77.65282228135632
- type: manhattan_spearman
value: 77.91730533156762
- task:
type: STS
dataset:
type: PhilipMay/stsb_multi_mt
name: MTEB STSBenchmarkMultilingualSTS (en)
config: en
split: test
revision: 93d57ef91790589e3ce9c365164337a8a78b7632
metrics:
- type: cos_sim_pearson
value: 77.95580421496413
- type: cos_sim_spearman
value: 77.89671867168987
- type: euclidean_pearson
value: 77.61352107168794
- type: euclidean_spearman
value: 77.89671867168987
- type: manhattan_pearson
value: 77.65282237231794
- type: manhattan_spearman
value: 77.91730533156762
- task:
type: Reranking
dataset:
type: mteb/scidocs-reranking
name: MTEB SciDocsRR
config: default
split: test
revision: d3c5e1fc0b855ab6097bf1cda04dd73947d7caab
metrics:
- type: map
value: 79.22928110092924
- type: mrr
value: 94.46700902583257
- task:
type: Retrieval
dataset:
type: mteb/scifact
name: MTEB SciFact
config: default
split: test
revision: 0228b52cf27578f30900b9e5271d331663a030d7
metrics:
- type: map_at_1
value: 56.011
- type: map_at_10
value: 65.544
- type: map_at_100
value: 66.034
- type: map_at_1000
value: 66.065
- type: map_at_3
value: 63.077000000000005
- type: map_at_5
value: 64.354
- type: mrr_at_1
value: 59.0
- type: mrr_at_10
value: 66.74900000000001
- type: mrr_at_100
value: 67.176
- type: mrr_at_1000
value: 67.203
- type: mrr_at_3
value: 65.056
- type: mrr_at_5
value: 65.956
- type: ndcg_at_1
value: 59.0
- type: ndcg_at_10
value: 69.95599999999999
- type: ndcg_at_100
value: 72.27
- type: ndcg_at_1000
value: 73.066
- type: ndcg_at_3
value: 65.837
- type: ndcg_at_5
value: 67.633
- type: precision_at_1
value: 59.0
- type: precision_at_10
value: 9.333
- type: precision_at_100
value: 1.053
- type: precision_at_1000
value: 0.11199999999999999
- type: precision_at_3
value: 26.0
- type: precision_at_5
value: 16.866999999999997
- type: recall_at_1
value: 56.011
- type: recall_at_10
value: 82.133
- type: recall_at_100
value: 92.767
- type: recall_at_1000
value: 99.0
- type: recall_at_3
value: 70.95
- type: recall_at_5
value: 75.556
- task:
type: PairClassification
dataset:
type: mteb/sprintduplicatequestions-pairclassification
name: MTEB SprintDuplicateQuestions
config: default
split: test
revision: d66bd1f72af766a5cc4b0ca5e00c162f89e8cc46
metrics:
- type: cos_sim_accuracy
value: 99.81584158415842
- type: cos_sim_ap
value: 94.67482871230736
- type: cos_sim_f1
value: 90.67201604814443
- type: cos_sim_precision
value: 90.94567404426559
- type: cos_sim_recall
value: 90.4
- type: dot_accuracy
value: 99.81584158415842
- type: dot_ap
value: 94.67482871230737
- type: dot_f1
value: 90.67201604814443
- type: dot_precision
value: 90.94567404426559
- type: dot_recall
value: 90.4
- type: euclidean_accuracy
value: 99.81584158415842
- type: euclidean_ap
value: 94.67482871230737
- type: euclidean_f1
value: 90.67201604814443
- type: euclidean_precision
value: 90.94567404426559
- type: euclidean_recall
value: 90.4
- type: manhattan_accuracy
value: 99.81188118811882
- type: manhattan_ap
value: 94.6409082219286
- type: manhattan_f1
value: 90.50949050949052
- type: manhattan_precision
value: 90.41916167664671
- type: manhattan_recall
value: 90.60000000000001
- type: max_accuracy
value: 99.81584158415842
- type: max_ap
value: 94.67482871230737
- type: max_f1
value: 90.67201604814443
- task:
type: Clustering
dataset:
type: mteb/stackexchange-clustering
name: MTEB StackExchangeClustering
config: default
split: test
revision: 6cbc1f7b2bc0622f2e39d2c77fa502909748c259
metrics:
- type: v_measure
value: 62.63494511649264
- task:
type: Clustering
dataset:
type: mteb/stackexchange-clustering-p2p
name: MTEB StackExchangeClusteringP2P
config: default
split: test
revision: 815ca46b2622cec33ccafc3735d572c266efdb44
metrics:
- type: v_measure
value: 37.165838327685755
- task:
type: Reranking
dataset:
type: mteb/stackoverflowdupquestions-reranking
name: MTEB StackOverflowDupQuestions
config: default
split: test
revision: e185fbe320c72810689fc5848eb6114e1ef5ec69
metrics:
- type: map
value: 51.384873075208084
- type: mrr
value: 52.196439181733304
- task:
type: Summarization
dataset:
type: mteb/summeval
name: MTEB SummEval
config: default
split: test
revision: cda12ad7615edc362dbf25a00fdd61d3b1eaf93c
metrics:
- type: cos_sim_pearson
value: 32.13690355567596
- type: cos_sim_spearman
value: 31.38349778638125
- type: dot_pearson
value: 32.13689596691593
- type: dot_spearman
value: 31.38349778638125
- task:
type: Retrieval
dataset:
type: mteb/trec-covid
name: MTEB TRECCOVID
config: default
split: test
revision: bb9466bac8153a0349341eb1b22e06409e78ef4e
metrics:
- type: map_at_1
value: 0.26
- type: map_at_10
value: 2.08
- type: map_at_100
value: 12.598
- type: map_at_1000
value: 30.119
- type: map_at_3
value: 0.701
- type: map_at_5
value: 1.11
- type: mrr_at_1
value: 96.0
- type: mrr_at_10
value: 97.167
- type: mrr_at_100
value: 97.167
- type: mrr_at_1000
value: 97.167
- type: mrr_at_3
value: 96.667
- type: mrr_at_5
value: 97.167
- type: ndcg_at_1
value: 91.0
- type: ndcg_at_10
value: 81.69800000000001
- type: ndcg_at_100
value: 62.9
- type: ndcg_at_1000
value: 55.245999999999995
- type: ndcg_at_3
value: 86.397
- type: ndcg_at_5
value: 84.286
- type: precision_at_1
value: 96.0
- type: precision_at_10
value: 87.0
- type: precision_at_100
value: 64.86
- type: precision_at_1000
value: 24.512
- type: precision_at_3
value: 90.667
- type: precision_at_5
value: 88.8
- type: recall_at_1
value: 0.26
- type: recall_at_10
value: 2.238
- type: recall_at_100
value: 15.488
- type: recall_at_1000
value: 51.6
- type: recall_at_3
value: 0.716
- type: recall_at_5
value: 1.151
- task:
type: Retrieval
dataset:
type: mteb/touche2020
name: MTEB Touche2020
config: default
split: test
revision: a34f9a33db75fa0cbb21bb5cfc3dae8dc8bec93f
metrics:
- type: map_at_1
value: 3.376
- type: map_at_10
value: 13.142000000000001
- type: map_at_100
value: 19.763
- type: map_at_1000
value: 21.319
- type: map_at_3
value: 6.805999999999999
- type: map_at_5
value: 8.952
- type: mrr_at_1
value: 46.939
- type: mrr_at_10
value: 61.082
- type: mrr_at_100
value: 61.45
- type: mrr_at_1000
value: 61.468999999999994
- type: mrr_at_3
value: 57.483
- type: mrr_at_5
value: 59.931999999999995
- type: ndcg_at_1
value: 44.897999999999996
- type: ndcg_at_10
value: 32.35
- type: ndcg_at_100
value: 42.719
- type: ndcg_at_1000
value: 53.30200000000001
- type: ndcg_at_3
value: 37.724999999999994
- type: ndcg_at_5
value: 34.79
- type: precision_at_1
value: 46.939
- type: precision_at_10
value: 28.366999999999997
- type: precision_at_100
value: 8.429
- type: precision_at_1000
value: 1.557
- type: precision_at_3
value: 38.095
- type: precision_at_5
value: 33.469
- type: recall_at_1
value: 3.376
- type: recall_at_10
value: 20.164
- type: recall_at_100
value: 50.668
- type: recall_at_1000
value: 83.159
- type: recall_at_3
value: 8.155
- type: recall_at_5
value: 11.872
- task:
type: Classification
dataset:
type: mteb/toxic_conversations_50k
name: MTEB ToxicConversationsClassification
config: default
split: test
revision: edfaf9da55d3dd50d43143d90c1ac476895ae6de
metrics:
- type: accuracy
value: 66.739
- type: ap
value: 12.17931839228834
- type: f1
value: 51.05383188624636
- task:
type: Classification
dataset:
type: mteb/tweet_sentiment_extraction
name: MTEB TweetSentimentExtractionClassification
config: default
split: test
revision: d604517c81ca91fe16a244d1248fc021f9ecee7a
metrics:
- type: accuracy
value: 56.72891907187323
- type: f1
value: 56.997614557150946
- task:
type: Clustering
dataset:
type: mteb/twentynewsgroups-clustering
name: MTEB TwentyNewsgroupsClustering
config: default
split: test
revision: 6125ec4e24fa026cec8a478383ee943acfbd5449
metrics:
- type: v_measure
value: 39.825318429345224
- task:
type: PairClassification
dataset:
type: mteb/twittersemeval2015-pairclassification
name: MTEB TwitterSemEval2015
config: default
split: test
revision: 70970daeab8776df92f5ea462b6173c0b46fd2d1
metrics:
- type: cos_sim_accuracy
value: 83.65619598259522
- type: cos_sim_ap
value: 66.17412885183877
- type: cos_sim_f1
value: 63.09125656951745
- type: cos_sim_precision
value: 57.63858577040594
- type: cos_sim_recall
value: 69.68337730870712
- type: dot_accuracy
value: 83.65619598259522
- type: dot_ap
value: 66.17413621964548
- type: dot_f1
value: 63.09125656951745
- type: dot_precision
value: 57.63858577040594
- type: dot_recall
value: 69.68337730870712
- type: euclidean_accuracy
value: 83.65619598259522
- type: euclidean_ap
value: 66.17412836413126
- type: euclidean_f1
value: 63.09125656951745
- type: euclidean_precision
value: 57.63858577040594
- type: euclidean_recall
value: 69.68337730870712
- type: manhattan_accuracy
value: 83.5548667819038
- type: manhattan_ap
value: 66.07998834521334
- type: manhattan_f1
value: 62.96433419721092
- type: manhattan_precision
value: 59.14676559239509
- type: manhattan_recall
value: 67.30870712401055
- type: max_accuracy
value: 83.65619598259522
- type: max_ap
value: 66.17413621964548
- type: max_f1
value: 63.09125656951745
- task:
type: PairClassification
dataset:
type: mteb/twitterurlcorpus-pairclassification
name: MTEB TwitterURLCorpus
config: default
split: test
revision: 8b6510b0b1fa4e4c4f879467980e9be563ec1cdf
metrics:
- type: cos_sim_accuracy
value: 88.55706911941631
- type: cos_sim_ap
value: 85.20971331546805
- type: cos_sim_f1
value: 77.28446050593702
- type: cos_sim_precision
value: 74.16135881104033
- type: cos_sim_recall
value: 80.6821681552202
- type: dot_accuracy
value: 88.55706911941631
- type: dot_ap
value: 85.2097154112633
- type: dot_f1
value: 77.28446050593702
- type: dot_precision
value: 74.16135881104033
- type: dot_recall
value: 80.6821681552202
- type: euclidean_accuracy
value: 88.55706911941631
- type: euclidean_ap
value: 85.20971719214488
- type: euclidean_f1
value: 77.28446050593702
- type: euclidean_precision
value: 74.16135881104033
- type: euclidean_recall
value: 80.6821681552202
- type: manhattan_accuracy
value: 88.52020025614158
- type: manhattan_ap
value: 85.17569799117058
- type: manhattan_f1
value: 77.27157773040933
- type: manhattan_precision
value: 72.79286638077734
- type: manhattan_recall
value: 82.33754234678165
- type: max_accuracy
value: 88.55706911941631
- type: max_ap
value: 85.20971719214488
- type: max_f1
value: 77.28446050593702
- task:
type: Clustering
dataset:
type: jinaai/cities_wiki_clustering
name: MTEB WikiCitiesClustering
config: default
split: test
revision: ddc9ee9242fa65332597f70e967ecc38b9d734fa
metrics:
- type: v_measure
value: 85.63474850264893
---
<h1 align="center">Snowflake's Arctic-embed-m-long</h1>
<h4 align="center">
<p>
<a href=#news>News</a> |
<a href=#models>Models</a> |
<a href=#usage>Usage</a> |
<a href="#evaluation">Evaluation</a> |
<a href="#contact">Contact</a> |
<a href="#faq">FAQ</a>
<a href="#license">License</a> |
<a href="#acknowledgement">Acknowledgement</a>
<p>
</h4>
## News
05/10/2024: Release the [technical report on Arctic Embed](https://arxiv.org/abs/2405.05374)
04/16/2024: Release the ** snowflake-arctic-embed ** family of text embedding models. The releases are state-of-the-art for Retrieval quality at each of their representative size profiles. [Technical Report]() is coming shortly. For more details, please refer to our Github: [Arctic-Text-Embed](https://github.com/Snowflake-Labs/arctic-embed).
## Models
snowflake-arctic-embed is a suite of text embedding models that focuses on creating high-quality retrieval models optimized for performance.
The `snowflake-arctic-embedding` models achieve **state-of-the-art performance on the MTEB/BEIR leaderboard** for each of their size variants. Evaluation is performed using these [scripts](https://github.com/Snowflake-Labs/snowflake-arctic-embed/tree/main/src). As shown below, each class of model size achieves SOTA retrieval accuracy compared to other top models.
The models are trained by leveraging existing open-source text representation models, such as bert-base-uncased, and are trained in a multi-stage pipeline to optimize their retrieval performance. First, the models are trained with large batches of query-document pairs where negatives are derived in-batch—pretraining leverages about 400m samples of a mix of public datasets and proprietary web search data. Following pretraining models are further optimized with long training on a smaller dataset (about 1m samples) of triplets of query, positive document, and negative document derived from hard harmful mining. Mining of the negatives and data curation is crucial to retrieval accuracy. A detailed technical report can be found [here](https://arxiv.org/abs/2405.05374).
| Name | MTEB Retrieval Score (NDCG @ 10) | Parameters (Millions) | Embedding Dimension |
| ----------------------------------------------------------------------- | -------------------------------- | --------------------- | ------------------- |
| [snowflake-arctic-embed-xs](https://huggingface.co/Snowflake/snowflake-arctic-embed-xs/) | 50.15 | 22 | 384 |
| [snowflake-arctic-embed-s](https://huggingface.co/Snowflake/snowflake-arctic-embed-s/) | 51.98 | 33 | 384 |
| [snowflake-arctic-embed-m](https://huggingface.co/Snowflake/snowflake-arctic-embed-m/) | 54.90 | 110 | 768 |
| [snowflake-arctic-embed-m-long](https://huggingface.co/Snowflake/snowflake-arctic-embed-m-long/) | 54.83 | 137 | 768 |
| [snowflake-arctic-embed-l](https://huggingface.co/Snowflake/snowflake-arctic-embed-l/) | 55.98 | 335 | 1024 |
Aside from being great open-source models, the largest model, [snowflake-arctic-embed-l](https://huggingface.co/Snowflake/snowflake-arctic-embed-l/), can serve as a natural replacement for closed-source embedding, as shown below.
| Model Name | MTEB Retrieval Score (NDCG @ 10) |
| ------------------------------------------------------------------ | -------------------------------- |
| [snowflake-arctic-embed-l](https://huggingface.co/Snowflake/snowflake-arctic-embed-l/) | 55.98 |
| Google-gecko-text-embedding | 55.7 |
| text-embedding-3-large | 55.44 |
| Cohere-embed-english-v3.0 | 55.00 |
| bge-large-en-v1.5 | 54.29 |
### [snowflake-arctic-embed-xs](https://huggingface.co/Snowflake/snowflake-arctic-embed-xs)
This tiny model packs quite the punch. Based on the [all-MiniLM-L6-v2](https://huggingface.co/sentence-transformers/all-MiniLM-L6-v2) model with only 22m parameters and 384 dimensions, this model should meet even the strictest latency/TCO budgets. Despite its size, its retrieval accuracy is closer to that of models with 100m paramers.
| Model Name | MTEB Retrieval Score (NDCG @ 10) |
| ------------------------------------------------------------------- | -------------------------------- |
| [snowflake-arctic-embed-xs](https://huggingface.co/Snowflake/snowflake-arctic-embed-xs/) | 50.15 |
| GIST-all-MiniLM-L6-v2 | 45.12 |
| gte-tiny | 44.92 |
| all-MiniLM-L6-v2 | 41.95 |
| bge-micro-v2 | 42.56 |
### [snowflake-arctic-embed-s](https://huggingface.co/Snowflake/snowflake-arctic-embed-s)
Based on the [intfloat/e5-small-unsupervised](https://huggingface.co/intfloat/e5-small-unsupervised) model, this small model does not trade off retrieval accuracy for its small size. With only 33m parameters and 384 dimensions, this model should easily allow scaling to large datasets.
| Model Name | MTEB Retrieval Score (NDCG @ 10) |
| ------------------------------------------------------------------ | -------------------------------- |
| [snowflake-arctic-embed-s](https://huggingface.co/Snowflake/snowflake-arctic-embed-s/) | 51.98 |
| bge-small-en-v1.5 | 51.68 |
| Cohere-embed-english-light-v3.0 | 51.34 |
| text-embedding-3-small | 51.08 |
| e5-small-v2 | 49.04 |
### [snowflake-arctic-embed-m](https://huggingface.co/Snowflake/snowflake-arctic-embed-m/)
Based on the [intfloat/e5-base-unsupervised](https://huggingface.co/intfloat/e5-base-unsupervised) model, this medium model is the workhorse that provides the best retrieval performance without slowing down inference.
| Model Name | MTEB Retrieval Score (NDCG @ 10) |
| ------------------------------------------------------------------ | -------------------------------- |
| [snowflake-arctic-embed-m](https://huggingface.co/Snowflake/snowflake-arctic-embed-m/) | 54.90 |
| bge-base-en-v1.5 | 53.25 |
| nomic-embed-text-v1.5 | 53.25 |
| GIST-Embedding-v0 | 52.31 |
| gte-base | 52.31 |
### [snowflake-arctic-embed-m-long](https://huggingface.co/Snowflake/snowflake-arctic-embed-m-long/)
Based on the [nomic-ai/nomic-embed-text-v1-unsupervised](https://huggingface.co/nomic-ai/nomic-embed-text-v1-unsupervised) model, this long-context variant of our medium-sized model is perfect for workloads that can be constrained by the regular 512 token context of our other models. Without the use of RPE, this model supports up to 2048 tokens. With RPE, it can scale to 8192!
| Model Name | MTEB Retrieval Score (NDCG @ 10) |
| ------------------------------------------------------------------ | -------------------------------- |
| [snowflake-arctic-embed-m-long](https://huggingface.co/Snowflake/snowflake-arctic-embed-m-long/) | 54.83 |
| nomic-embed-text-v1.5 | 53.01 |
| nomic-embed-text-v1 | 52.81 |
### [snowflake-arctic-embed-l](https://huggingface.co/Snowflake/snowflake-arctic-embed-l/)
Based on the [intfloat/e5-large-unsupervised](https://huggingface.co/intfloat/e5-large-unsupervised) model, this large model is a direct drop-in for closed APIs and delivers the most accurate retrieval experience.
| Model Name | MTEB Retrieval Score (NDCG @ 10) |
| ------------------------------------------------------------------ | -------------------------------- |
| [snowflake-arctic-embed-l](https://huggingface.co/Snowflake/snowflake-arctic-embed-l/) | 55.98 |
| UAE-Large-V1 | 54.66 |
| bge-large-en-v1.5 | 54.29 |
| mxbai-embed-large-v1 | 54.39 |
| e5-Large-v2 | 50.56 |
## Usage
### Using Sentence Transformers
You can use the sentence-transformers package to use an snowflake-arctic-embed model, as shown below.
```python
from sentence_transformers import SentenceTransformer
model = SentenceTransformer("Snowflake/snowflake-arctic-embed-m-long", trust_remote_code=True)
queries = ['what is snowflake?', 'Where can I get the best tacos?']
documents = ['The Data Cloud!', 'Mexico City of Course!']
query_embeddings = model.encode(queries, prompt_name="query")
document_embeddings = model.encode(documents)
scores = query_embeddings @ document_embeddings.T
for query, query_scores in zip(queries, scores):
doc_score_pairs = list(zip(documents, query_scores))
doc_score_pairs = sorted(doc_score_pairs, key=lambda x: x[1], reverse=True)
# Output passages & scores
print("Query:", query)
for document, score in doc_score_pairs:
print(score, document)
```
```
Query: what is snowflake?
0.46484852 The Data Cloud!
0.3758855 Mexico City of Course!
Query: Where can I get the best tacos?
0.42407742 Mexico City of Course!
0.36740506 The Data Cloud!
```
### Using Huggingface transformers
You can use the transformers package to use an snowflake-arctic-embed model, as shown below. For optimal retrieval quality, use the CLS token to embed each text portion and use the query prefix below (just on the query).
```python
import torch
from transformers import AutoModel, AutoTokenizer
tokenizer = AutoTokenizer.from_pretrained('Snowflake/snowflake-arctic-embed-m-long')
model = AutoModel.from_pretrained('Snowflake/snowflake-arctic-embed-m-long', trust_remote_code=True, add_pooling_layer=False, safe_serialization=True)
model.eval()
query_prefix = 'Represent this sentence for searching relevant passages: '
queries = ['what is snowflake?', 'Where can I get the best tacos?']
queries_with_prefix = ["{}{}".format(query_prefix, i) for i in queries]
query_tokens = tokenizer(queries_with_prefix, padding=True, truncation=True, return_tensors='pt', max_length=512)
documents = ['The Data Cloud!', 'Mexico City of Course!']
document_tokens = tokenizer(documents, padding=True, truncation=True, return_tensors='pt', max_length=512)
# Compute token embeddings
with torch.no_grad():
query_embeddings = model(**query_tokens)[0][:, 0]
doument_embeddings = model(**document_tokens)[0][:, 0]
# normalize embeddings
query_embeddings = torch.nn.functional.normalize(query_embeddings, p=2, dim=1)
doument_embeddings = torch.nn.functional.normalize(doument_embeddings, p=2, dim=1)
scores = torch.mm(query_embeddings, doument_embeddings.transpose(0, 1))
for query, query_scores in zip(queries, scores):
doc_score_pairs = list(zip(documents, query_scores))
doc_score_pairs = sorted(doc_score_pairs, key=lambda x: x[1], reverse=True)
#Output passages & scores
print("Query:", query)
for document, score in doc_score_pairs:
print(score, document)
```
If you use the long context model with more than 2048 tokens, ensure that you initialize the model like below instead. This will use [RPE](https://arxiv.org/abs/2104.09864) to allow up to 8192 tokens.
``` py
model = AutoModel.from_pretrained('Snowflake/snowflake-arctic-embed-m-long', trust_remote_code=True, safe_serialization=True, rotary_scaling_factor=2)
```
### Using Transformers.js
If you haven't already, you can install the [Transformers.js](https://huggingface.co/docs/transformers.js) JavaScript library from [NPM](https://www.npmjs.com/package/@xenova/transformers) by running:
```bash
npm i @xenova/transformers
```
You can then use the model to compute embeddings as follows:
```js
import { pipeline, dot } from '@xenova/transformers';
// Create feature extraction pipeline
const extractor = await pipeline('feature-extraction', 'Snowflake/snowflake-arctic-embed-m-long', {
quantized: false, // Comment out this line to use the quantized version
});
// Generate sentence embeddings
const sentences = [
'Represent this sentence for searching relevant passages: Where can I get the best tacos?',
'The Data Cloud!',
'Mexico City of Course!',
]
const output = await extractor(sentences, { normalize: true, pooling: 'cls' });
// Compute similarity scores
const [source_embeddings, ...document_embeddings ] = output.tolist();
const similarities = document_embeddings.map(x => dot(source_embeddings, x));
console.log(similarities); // [0.36740492125676116, 0.42407774292046635]
```
## FAQ
TBD
## Contact
Feel free to open an issue or pull request if you have any questions or suggestions about this project.
You also can email Daniel Campos([email protected]).
## License
Arctic is licensed under the [Apache-2](https://www.apache.org/licenses/LICENSE-2.0). The released models can be used for commercial purposes free of charge.
## Acknowledgement
We want to thank the open-source community, which has provided the great building blocks upon which we could make our models.
We thank our modeling engineers, Danmei Xu, Luke Merrick, Gaurav Nuti, and Daniel Campos, for making these great models possible.
We thank our leadership, Himabindu Pucha, Kelvin So, Vivek Raghunathan, and Sridhar Ramaswamy, for supporting this work.
We also thank the open-source community for producing the great models we could build on top of and making these releases possible.
Finally, we thank the researchers who created BEIR and MTEB benchmarks.
It is largely thanks to their tireless work to define what better looks like that we could improve model performance. |
TheBloke/Unholy-v2-13B-GGUF | TheBloke | 2024-01-03T16:06:21Z | 13,620 | 34 | transformers | [
"transformers",
"gguf",
"llama",
"not-for-all-audiences",
"nsfw",
"base_model:Undi95/Unholy-v2-13B",
"license:cc-by-nc-4.0",
"text-generation-inference",
"region:us"
] | null | 2024-01-03T15:58:11Z | ---
base_model: Undi95/Unholy-v2-13B
inference: false
license: cc-by-nc-4.0
model_creator: Undi
model_name: Unholy V2 13B
model_type: llama
prompt_template: 'Below is an instruction that describes a task. Write a response
that appropriately completes the request.
### Instruction:
{prompt}
### Response:
'
quantized_by: TheBloke
tags:
- not-for-all-audiences
- nsfw
---
<!-- markdownlint-disable MD041 -->
<!-- header start -->
<!-- 200823 -->
<div style="width: auto; margin-left: auto; margin-right: auto">
<img src="https://i.imgur.com/EBdldam.jpg" alt="TheBlokeAI" style="width: 100%; min-width: 400px; display: block; margin: auto;">
</div>
<div style="display: flex; justify-content: space-between; width: 100%;">
<div style="display: flex; flex-direction: column; align-items: flex-start;">
<p style="margin-top: 0.5em; margin-bottom: 0em;"><a href="https://discord.gg/theblokeai">Chat & support: TheBloke's Discord server</a></p>
</div>
<div style="display: flex; flex-direction: column; align-items: flex-end;">
<p style="margin-top: 0.5em; margin-bottom: 0em;"><a href="https://www.patreon.com/TheBlokeAI">Want to contribute? TheBloke's Patreon page</a></p>
</div>
</div>
<div style="text-align:center; margin-top: 0em; margin-bottom: 0em"><p style="margin-top: 0.25em; margin-bottom: 0em;">TheBloke's LLM work is generously supported by a grant from <a href="https://a16z.com">andreessen horowitz (a16z)</a></p></div>
<hr style="margin-top: 1.0em; margin-bottom: 1.0em;">
<!-- header end -->
# Unholy V2 13B - GGUF
- Model creator: [Undi](https://huggingface.co/Undi95)
- Original model: [Unholy V2 13B](https://huggingface.co/Undi95/Unholy-v2-13B)
<!-- description start -->
## Description
This repo contains GGUF format model files for [Undi's Unholy V2 13B](https://huggingface.co/Undi95/Unholy-v2-13B).
These files were quantised using hardware kindly provided by [Massed Compute](https://massedcompute.com/).
<!-- description end -->
<!-- README_GGUF.md-about-gguf start -->
### About GGUF
GGUF is a new format introduced by the llama.cpp team on August 21st 2023. It is a replacement for GGML, which is no longer supported by llama.cpp.
Here is an incomplete list of clients and libraries that are known to support GGUF:
* [llama.cpp](https://github.com/ggerganov/llama.cpp). The source project for GGUF. Offers a CLI and a server option.
* [text-generation-webui](https://github.com/oobabooga/text-generation-webui), the most widely used web UI, with many features and powerful extensions. Supports GPU acceleration.
* [KoboldCpp](https://github.com/LostRuins/koboldcpp), a fully featured web UI, with GPU accel across all platforms and GPU architectures. Especially good for story telling.
* [GPT4All](https://gpt4all.io/index.html), a free and open source local running GUI, supporting Windows, Linux and macOS with full GPU accel.
* [LM Studio](https://lmstudio.ai/), an easy-to-use and powerful local GUI for Windows and macOS (Silicon), with GPU acceleration. Linux available, in beta as of 27/11/2023.
* [LoLLMS Web UI](https://github.com/ParisNeo/lollms-webui), a great web UI with many interesting and unique features, including a full model library for easy model selection.
* [Faraday.dev](https://faraday.dev/), an attractive and easy to use character-based chat GUI for Windows and macOS (both Silicon and Intel), with GPU acceleration.
* [llama-cpp-python](https://github.com/abetlen/llama-cpp-python), a Python library with GPU accel, LangChain support, and OpenAI-compatible API server.
* [candle](https://github.com/huggingface/candle), a Rust ML framework with a focus on performance, including GPU support, and ease of use.
* [ctransformers](https://github.com/marella/ctransformers), a Python library with GPU accel, LangChain support, and OpenAI-compatible AI server. Note, as of time of writing (November 27th 2023), ctransformers has not been updated in a long time and does not support many recent models.
<!-- README_GGUF.md-about-gguf end -->
<!-- repositories-available start -->
## Repositories available
* [AWQ model(s) for GPU inference.](https://huggingface.co/TheBloke/Unholy-v2-13B-AWQ)
* [GPTQ models for GPU inference, with multiple quantisation parameter options.](https://huggingface.co/TheBloke/Unholy-v2-13B-GPTQ)
* [2, 3, 4, 5, 6 and 8-bit GGUF models for CPU+GPU inference](https://huggingface.co/TheBloke/Unholy-v2-13B-GGUF)
* [Undi's original unquantised fp16 model in pytorch format, for GPU inference and for further conversions](https://huggingface.co/Undi95/Unholy-v2-13B)
<!-- repositories-available end -->
<!-- prompt-template start -->
## Prompt template: Alpaca
```
Below is an instruction that describes a task. Write a response that appropriately completes the request.
### Instruction:
{prompt}
### Response:
```
<!-- prompt-template end -->
<!-- licensing start -->
## Licensing
The creator of the source model has listed its license as `cc-by-nc-4.0`, and this quantization has therefore used that same license.
As this model is based on Llama 2, it is also subject to the Meta Llama 2 license terms, and the license files for that are additionally included. It should therefore be considered as being claimed to be licensed under both licenses. I contacted Hugging Face for clarification on dual licensing but they do not yet have an official position. Should this change, or should Meta provide any feedback on this situation, I will update this section accordingly.
In the meantime, any questions regarding licensing, and in particular how these two licenses might interact, should be directed to the original model repository: [Undi's Unholy V2 13B](https://huggingface.co/Undi95/Unholy-v2-13B).
<!-- licensing end -->
<!-- compatibility_gguf start -->
## Compatibility
These quantised GGUFv2 files are compatible with llama.cpp from August 27th onwards, as of commit [d0cee0d](https://github.com/ggerganov/llama.cpp/commit/d0cee0d36d5be95a0d9088b674dbb27354107221)
They are also compatible with many third party UIs and libraries - please see the list at the top of this README.
## Explanation of quantisation methods
<details>
<summary>Click to see details</summary>
The new methods available are:
* GGML_TYPE_Q2_K - "type-1" 2-bit quantization in super-blocks containing 16 blocks, each block having 16 weight. Block scales and mins are quantized with 4 bits. This ends up effectively using 2.5625 bits per weight (bpw)
* GGML_TYPE_Q3_K - "type-0" 3-bit quantization in super-blocks containing 16 blocks, each block having 16 weights. Scales are quantized with 6 bits. This end up using 3.4375 bpw.
* GGML_TYPE_Q4_K - "type-1" 4-bit quantization in super-blocks containing 8 blocks, each block having 32 weights. Scales and mins are quantized with 6 bits. This ends up using 4.5 bpw.
* GGML_TYPE_Q5_K - "type-1" 5-bit quantization. Same super-block structure as GGML_TYPE_Q4_K resulting in 5.5 bpw
* GGML_TYPE_Q6_K - "type-0" 6-bit quantization. Super-blocks with 16 blocks, each block having 16 weights. Scales are quantized with 8 bits. This ends up using 6.5625 bpw
Refer to the Provided Files table below to see what files use which methods, and how.
</details>
<!-- compatibility_gguf end -->
<!-- README_GGUF.md-provided-files start -->
## Provided files
| Name | Quant method | Bits | Size | Max RAM required | Use case |
| ---- | ---- | ---- | ---- | ---- | ----- |
| [unholy-v2-13b.Q2_K.gguf](https://huggingface.co/TheBloke/Unholy-v2-13B-GGUF/blob/main/unholy-v2-13b.Q2_K.gguf) | Q2_K | 2 | 5.43 GB| 7.93 GB | smallest, significant quality loss - not recommended for most purposes |
| [unholy-v2-13b.Q3_K_S.gguf](https://huggingface.co/TheBloke/Unholy-v2-13B-GGUF/blob/main/unholy-v2-13b.Q3_K_S.gguf) | Q3_K_S | 3 | 5.66 GB| 8.16 GB | very small, high quality loss |
| [unholy-v2-13b.Q3_K_M.gguf](https://huggingface.co/TheBloke/Unholy-v2-13B-GGUF/blob/main/unholy-v2-13b.Q3_K_M.gguf) | Q3_K_M | 3 | 6.34 GB| 8.84 GB | very small, high quality loss |
| [unholy-v2-13b.Q3_K_L.gguf](https://huggingface.co/TheBloke/Unholy-v2-13B-GGUF/blob/main/unholy-v2-13b.Q3_K_L.gguf) | Q3_K_L | 3 | 6.93 GB| 9.43 GB | small, substantial quality loss |
| [unholy-v2-13b.Q4_0.gguf](https://huggingface.co/TheBloke/Unholy-v2-13B-GGUF/blob/main/unholy-v2-13b.Q4_0.gguf) | Q4_0 | 4 | 7.37 GB| 9.87 GB | legacy; small, very high quality loss - prefer using Q3_K_M |
| [unholy-v2-13b.Q4_K_S.gguf](https://huggingface.co/TheBloke/Unholy-v2-13B-GGUF/blob/main/unholy-v2-13b.Q4_K_S.gguf) | Q4_K_S | 4 | 7.42 GB| 9.92 GB | small, greater quality loss |
| [unholy-v2-13b.Q4_K_M.gguf](https://huggingface.co/TheBloke/Unholy-v2-13B-GGUF/blob/main/unholy-v2-13b.Q4_K_M.gguf) | Q4_K_M | 4 | 7.87 GB| 10.37 GB | medium, balanced quality - recommended |
| [unholy-v2-13b.Q5_0.gguf](https://huggingface.co/TheBloke/Unholy-v2-13B-GGUF/blob/main/unholy-v2-13b.Q5_0.gguf) | Q5_0 | 5 | 8.97 GB| 11.47 GB | legacy; medium, balanced quality - prefer using Q4_K_M |
| [unholy-v2-13b.Q5_K_S.gguf](https://huggingface.co/TheBloke/Unholy-v2-13B-GGUF/blob/main/unholy-v2-13b.Q5_K_S.gguf) | Q5_K_S | 5 | 8.97 GB| 11.47 GB | large, low quality loss - recommended |
| [unholy-v2-13b.Q5_K_M.gguf](https://huggingface.co/TheBloke/Unholy-v2-13B-GGUF/blob/main/unholy-v2-13b.Q5_K_M.gguf) | Q5_K_M | 5 | 9.23 GB| 11.73 GB | large, very low quality loss - recommended |
| [unholy-v2-13b.Q6_K.gguf](https://huggingface.co/TheBloke/Unholy-v2-13B-GGUF/blob/main/unholy-v2-13b.Q6_K.gguf) | Q6_K | 6 | 10.68 GB| 13.18 GB | very large, extremely low quality loss |
| [unholy-v2-13b.Q8_0.gguf](https://huggingface.co/TheBloke/Unholy-v2-13B-GGUF/blob/main/unholy-v2-13b.Q8_0.gguf) | Q8_0 | 8 | 13.83 GB| 16.33 GB | very large, extremely low quality loss - not recommended |
**Note**: the above RAM figures assume no GPU offloading. If layers are offloaded to the GPU, this will reduce RAM usage and use VRAM instead.
<!-- README_GGUF.md-provided-files end -->
<!-- README_GGUF.md-how-to-download start -->
## How to download GGUF files
**Note for manual downloaders:** You almost never want to clone the entire repo! Multiple different quantisation formats are provided, and most users only want to pick and download a single file.
The following clients/libraries will automatically download models for you, providing a list of available models to choose from:
* LM Studio
* LoLLMS Web UI
* Faraday.dev
### In `text-generation-webui`
Under Download Model, you can enter the model repo: TheBloke/Unholy-v2-13B-GGUF and below it, a specific filename to download, such as: unholy-v2-13b.Q4_K_M.gguf.
Then click Download.
### On the command line, including multiple files at once
I recommend using the `huggingface-hub` Python library:
```shell
pip3 install huggingface-hub
```
Then you can download any individual model file to the current directory, at high speed, with a command like this:
```shell
huggingface-cli download TheBloke/Unholy-v2-13B-GGUF unholy-v2-13b.Q4_K_M.gguf --local-dir . --local-dir-use-symlinks False
```
<details>
<summary>More advanced huggingface-cli download usage (click to read)</summary>
You can also download multiple files at once with a pattern:
```shell
huggingface-cli download TheBloke/Unholy-v2-13B-GGUF --local-dir . --local-dir-use-symlinks False --include='*Q4_K*gguf'
```
For more documentation on downloading with `huggingface-cli`, please see: [HF -> Hub Python Library -> Download files -> Download from the CLI](https://huggingface.co/docs/huggingface_hub/guides/download#download-from-the-cli).
To accelerate downloads on fast connections (1Gbit/s or higher), install `hf_transfer`:
```shell
pip3 install hf_transfer
```
And set environment variable `HF_HUB_ENABLE_HF_TRANSFER` to `1`:
```shell
HF_HUB_ENABLE_HF_TRANSFER=1 huggingface-cli download TheBloke/Unholy-v2-13B-GGUF unholy-v2-13b.Q4_K_M.gguf --local-dir . --local-dir-use-symlinks False
```
Windows Command Line users: You can set the environment variable by running `set HF_HUB_ENABLE_HF_TRANSFER=1` before the download command.
</details>
<!-- README_GGUF.md-how-to-download end -->
<!-- README_GGUF.md-how-to-run start -->
## Example `llama.cpp` command
Make sure you are using `llama.cpp` from commit [d0cee0d](https://github.com/ggerganov/llama.cpp/commit/d0cee0d36d5be95a0d9088b674dbb27354107221) or later.
```shell
./main -ngl 35 -m unholy-v2-13b.Q4_K_M.gguf --color -c 4096 --temp 0.7 --repeat_penalty 1.1 -n -1 -p "Below is an instruction that describes a task. Write a response that appropriately completes the request.\n\n### Instruction:\n{prompt}\n\n### Response:"
```
Change `-ngl 32` to the number of layers to offload to GPU. Remove it if you don't have GPU acceleration.
Change `-c 4096` to the desired sequence length. For extended sequence models - eg 8K, 16K, 32K - the necessary RoPE scaling parameters are read from the GGUF file and set by llama.cpp automatically. Note that longer sequence lengths require much more resources, so you may need to reduce this value.
If you want to have a chat-style conversation, replace the `-p <PROMPT>` argument with `-i -ins`
For other parameters and how to use them, please refer to [the llama.cpp documentation](https://github.com/ggerganov/llama.cpp/blob/master/examples/main/README.md)
## How to run in `text-generation-webui`
Further instructions can be found in the text-generation-webui documentation, here: [text-generation-webui/docs/04 ‐ Model Tab.md](https://github.com/oobabooga/text-generation-webui/blob/main/docs/04%20%E2%80%90%20Model%20Tab.md#llamacpp).
## How to run from Python code
You can use GGUF models from Python using the [llama-cpp-python](https://github.com/abetlen/llama-cpp-python) or [ctransformers](https://github.com/marella/ctransformers) libraries. Note that at the time of writing (Nov 27th 2023), ctransformers has not been updated for some time and is not compatible with some recent models. Therefore I recommend you use llama-cpp-python.
### How to load this model in Python code, using llama-cpp-python
For full documentation, please see: [llama-cpp-python docs](https://abetlen.github.io/llama-cpp-python/).
#### First install the package
Run one of the following commands, according to your system:
```shell
# Base ctransformers with no GPU acceleration
pip install llama-cpp-python
# With NVidia CUDA acceleration
CMAKE_ARGS="-DLLAMA_CUBLAS=on" pip install llama-cpp-python
# Or with OpenBLAS acceleration
CMAKE_ARGS="-DLLAMA_BLAS=ON -DLLAMA_BLAS_VENDOR=OpenBLAS" pip install llama-cpp-python
# Or with CLBLast acceleration
CMAKE_ARGS="-DLLAMA_CLBLAST=on" pip install llama-cpp-python
# Or with AMD ROCm GPU acceleration (Linux only)
CMAKE_ARGS="-DLLAMA_HIPBLAS=on" pip install llama-cpp-python
# Or with Metal GPU acceleration for macOS systems only
CMAKE_ARGS="-DLLAMA_METAL=on" pip install llama-cpp-python
# In windows, to set the variables CMAKE_ARGS in PowerShell, follow this format; eg for NVidia CUDA:
$env:CMAKE_ARGS = "-DLLAMA_OPENBLAS=on"
pip install llama-cpp-python
```
#### Simple llama-cpp-python example code
```python
from llama_cpp import Llama
# Set gpu_layers to the number of layers to offload to GPU. Set to 0 if no GPU acceleration is available on your system.
llm = Llama(
model_path="./unholy-v2-13b.Q4_K_M.gguf", # Download the model file first
n_ctx=4096, # The max sequence length to use - note that longer sequence lengths require much more resources
n_threads=8, # The number of CPU threads to use, tailor to your system and the resulting performance
n_gpu_layers=35 # The number of layers to offload to GPU, if you have GPU acceleration available
)
# Simple inference example
output = llm(
"Below is an instruction that describes a task. Write a response that appropriately completes the request.\n\n### Instruction:\n{prompt}\n\n### Response:", # Prompt
max_tokens=512, # Generate up to 512 tokens
stop=["</s>"], # Example stop token - not necessarily correct for this specific model! Please check before using.
echo=True # Whether to echo the prompt
)
# Chat Completion API
llm = Llama(model_path="./unholy-v2-13b.Q4_K_M.gguf", chat_format="llama-2") # Set chat_format according to the model you are using
llm.create_chat_completion(
messages = [
{"role": "system", "content": "You are a story writing assistant."},
{
"role": "user",
"content": "Write a story about llamas."
}
]
)
```
## How to use with LangChain
Here are guides on using llama-cpp-python and ctransformers with LangChain:
* [LangChain + llama-cpp-python](https://python.langchain.com/docs/integrations/llms/llamacpp)
* [LangChain + ctransformers](https://python.langchain.com/docs/integrations/providers/ctransformers)
<!-- README_GGUF.md-how-to-run end -->
<!-- footer start -->
<!-- 200823 -->
## Discord
For further support, and discussions on these models and AI in general, join us at:
[TheBloke AI's Discord server](https://discord.gg/theblokeai)
## Thanks, and how to contribute
Thanks to the [chirper.ai](https://chirper.ai) team!
Thanks to Clay from [gpus.llm-utils.org](llm-utils)!
I've had a lot of people ask if they can contribute. I enjoy providing models and helping people, and would love to be able to spend even more time doing it, as well as expanding into new projects like fine tuning/training.
If you're able and willing to contribute it will be most gratefully received and will help me to keep providing more models, and to start work on new AI projects.
Donaters will get priority support on any and all AI/LLM/model questions and requests, access to a private Discord room, plus other benefits.
* Patreon: https://patreon.com/TheBlokeAI
* Ko-Fi: https://ko-fi.com/TheBlokeAI
**Special thanks to**: Aemon Algiz.
**Patreon special mentions**: Michael Levine, 阿明, Trailburnt, Nikolai Manek, John Detwiler, Randy H, Will Dee, Sebastain Graf, NimbleBox.ai, Eugene Pentland, Emad Mostaque, Ai Maven, Jim Angel, Jeff Scroggin, Michael Davis, Manuel Alberto Morcote, Stephen Murray, Robert, Justin Joy, Luke @flexchar, Brandon Frisco, Elijah Stavena, S_X, Dan Guido, Undi ., Komninos Chatzipapas, Shadi, theTransient, Lone Striker, Raven Klaugh, jjj, Cap'n Zoog, Michel-Marie MAUDET (LINAGORA), Matthew Berman, David, Fen Risland, Omer Bin Jawed, Luke Pendergrass, Kalila, OG, Erik Bjäreholt, Rooh Singh, Joseph William Delisle, Dan Lewis, TL, John Villwock, AzureBlack, Brad, Pedro Madruga, Caitlyn Gatomon, K, jinyuan sun, Mano Prime, Alex, Jeffrey Morgan, Alicia Loh, Illia Dulskyi, Chadd, transmissions 11, fincy, Rainer Wilmers, ReadyPlayerEmma, knownsqashed, Mandus, biorpg, Deo Leter, Brandon Phillips, SuperWojo, Sean Connelly, Iucharbius, Jack West, Harry Royden McLaughlin, Nicholas, terasurfer, Vitor Caleffi, Duane Dunston, Johann-Peter Hartmann, David Ziegler, Olakabola, Ken Nordquist, Trenton Dambrowitz, Tom X Nguyen, Vadim, Ajan Kanaga, Leonard Tan, Clay Pascal, Alexandros Triantafyllidis, JM33133, Xule, vamX, ya boyyy, subjectnull, Talal Aujan, Alps Aficionado, wassieverse, Ari Malik, James Bentley, Woland, Spencer Kim, Michael Dempsey, Fred von Graf, Elle, zynix, William Richards, Stanislav Ovsiannikov, Edmond Seymore, Jonathan Leane, Martin Kemka, usrbinkat, Enrico Ros
Thank you to all my generous patrons and donaters!
And thank you again to a16z for their generous grant.
<!-- footer end -->
<!-- original-model-card start -->
# Original model card: Undi's Unholy V2 13B

Use at your own risk, I'm not responsible for any usage of this model, don't try to do anything this model tell you to do.
Undi95/Unholy-v1-12L-13B merged with Undi95/toxicqa-Llama2-13B-lora at 0.3 weight.
Uncensored.
If you are censored, it's maybe because of keyword like "assistant", "Factual answer", or other "sweet words" like I call them that trigger the censoring accross all the layer of the model (since they're all trained on some of them in a way).
<!-- description start -->
## Description
This repo contains fp16 files of Unholy v2, an uncensored model.
<!-- description end -->
<!-- description start -->
## Models and LoRA used
- uukuguy/speechless-llama2-luban-orca-platypus-13b
- jondurbin/spicyboros-13b-2.2
- Undi95/MLewd-L2-13B-v2-3
- Undi95/toxicqa-Llama2-13B-lora
<!-- description end -->
<!-- prompt-template start -->
## Prompt template: Alpaca
```
Below is an instruction that describes a task. Write a response that appropriately completes the request.
### Instruction:
{prompt}
### Response:
```
Exemple:

<!-- original-model-card end -->
|
mesolitica/wav2vec2-xls-r-300m-mixed | mesolitica | 2022-06-02T04:58:36Z | 13,612 | 4 | transformers | [
"transformers",
"pytorch",
"tf",
"wav2vec2",
"automatic-speech-recognition",
"generated_from_keras_callback",
"endpoints_compatible",
"region:us"
] | automatic-speech-recognition | 2022-06-01T01:18:26Z | ---
tags:
- generated_from_keras_callback
model-index:
- name: wav2vec2-xls-r-300m-mixed
results: []
---
<!-- This model card has been generated automatically according to the information Keras had access to. You should
probably proofread and complete it, then remove this comment. -->
# wav2vec2-xls-r-300m-mixed
Finetuned https://huggingface.co/facebook/wav2vec2-xls-r-300m on https://github.com/huseinzol05/malaya-speech/tree/master/data/mixed-stt
This model was finetuned on 3 languages,
1. Malay
2. Singlish
3. Mandarin
**This model trained on a single RTX 3090 Ti 24GB VRAM, provided by https://mesolitica.com/**.
## Evaluation set
Evaluation set from https://github.com/huseinzol05/malaya-speech/tree/master/pretrained-model/prepare-stt with sizes,
```
len(malay), len(singlish), len(mandarin)
-> (765, 3579, 614)
```
It achieves the following results on the evaluation set based on [evaluate-gpu.ipynb](evaluate-gpu.ipynb):
Mixed evaluation,
```
CER: 0.0481054244857041
WER: 0.1322198446007387
CER with LM: 0.041196586938584696
WER with LM: 0.09880169127621556
```
Malay evaluation,
```
CER: 0.051636391937588406
WER: 0.19561999547293663
CER with LM: 0.03917689630621449
WER with LM: 0.12710746406824835
```
Singlish evaluation,
```
CER: 0.0494915200071987
WER: 0.12763802881676573
CER with LM: 0.04271234986432335
WER with LM: 0.09677160640413336
```
Mandarin evaluation,
```
CER: 0.035626554824269824
WER: 0.07993515937860181
CER with LM: 0.03487760945087219
WER with LM: 0.07536807168546154
```
Language model from https://huggingface.co/huseinzol05/language-model-bahasa-manglish-combined |
casperhansen/mixtral-instruct-awq | casperhansen | 2024-02-11T14:02:17Z | 13,611 | 39 | transformers | [
"transformers",
"safetensors",
"mixtral",
"text-generation",
"conversational",
"license:apache-2.0",
"autotrain_compatible",
"endpoints_compatible",
"text-generation-inference",
"4-bit",
"awq",
"region:us"
] | text-generation | 2023-12-22T12:15:06Z | ---
license: apache-2.0
---
This is a working version of Mixtral Instruct that is AWQ quantized. As of 11-02-2024, [https://huggingface.co/TheBloke/Mixtral-8x7B-Instruct-v0.1-AWQ](https://huggingface.co/TheBloke/Mixtral-8x7B-Instruct-v0.1-AWQ) is not working, so please use this repository instead.
|
sshleifer/tiny-mbart | sshleifer | 2021-08-26T10:55:11Z | 13,607 | 7 | transformers | [
"transformers",
"pytorch",
"tf",
"mbart",
"text2text-generation",
"autotrain_compatible",
"endpoints_compatible",
"region:us"
] | text2text-generation | 2022-03-02T23:29:05Z | Entry not found |
mradermacher/Chocolatine-8B-Instruct-DPO-v1.0-i1-GGUF | mradermacher | 2024-06-27T08:32:44Z | 13,606 | 0 | transformers | [
"transformers",
"gguf",
"llama3",
"french",
"llama-3-8B",
"fr",
"en",
"base_model:jpacifico/Chocolatine-8B-Instruct-DPO-v1.0",
"license:apache-2.0",
"endpoints_compatible",
"region:us"
] | null | 2024-06-27T03:08:48Z | ---
base_model: jpacifico/Chocolatine-8B-Instruct-DPO-v1.0
language:
- fr
- en
library_name: transformers
license: apache-2.0
quantized_by: mradermacher
tags:
- llama3
- french
- llama-3-8B
---
## About
<!-- ### quantize_version: 2 -->
<!-- ### output_tensor_quantised: 1 -->
<!-- ### convert_type: hf -->
<!-- ### vocab_type: -->
<!-- ### tags: nicoboss -->
weighted/imatrix quants of https://huggingface.co/jpacifico/Chocolatine-8B-Instruct-DPO-v1.0
<!-- provided-files -->
static quants are available at https://huggingface.co/mradermacher/Chocolatine-8B-Instruct-DPO-v1.0-GGUF
## Usage
If you are unsure how to use GGUF files, refer to one of [TheBloke's
READMEs](https://huggingface.co/TheBloke/KafkaLM-70B-German-V0.1-GGUF) for
more details, including on how to concatenate multi-part files.
## Provided Quants
(sorted by size, not necessarily quality. IQ-quants are often preferable over similar sized non-IQ quants)
| Link | Type | Size/GB | Notes |
|:-----|:-----|--------:|:------|
| [GGUF](https://huggingface.co/mradermacher/Chocolatine-8B-Instruct-DPO-v1.0-i1-GGUF/resolve/main/Chocolatine-8B-Instruct-DPO-v1.0.i1-IQ1_S.gguf) | i1-IQ1_S | 2.1 | for the desperate |
| [GGUF](https://huggingface.co/mradermacher/Chocolatine-8B-Instruct-DPO-v1.0-i1-GGUF/resolve/main/Chocolatine-8B-Instruct-DPO-v1.0.i1-IQ1_M.gguf) | i1-IQ1_M | 2.3 | mostly desperate |
| [GGUF](https://huggingface.co/mradermacher/Chocolatine-8B-Instruct-DPO-v1.0-i1-GGUF/resolve/main/Chocolatine-8B-Instruct-DPO-v1.0.i1-IQ2_XXS.gguf) | i1-IQ2_XXS | 2.5 | |
| [GGUF](https://huggingface.co/mradermacher/Chocolatine-8B-Instruct-DPO-v1.0-i1-GGUF/resolve/main/Chocolatine-8B-Instruct-DPO-v1.0.i1-IQ2_XS.gguf) | i1-IQ2_XS | 2.7 | |
| [GGUF](https://huggingface.co/mradermacher/Chocolatine-8B-Instruct-DPO-v1.0-i1-GGUF/resolve/main/Chocolatine-8B-Instruct-DPO-v1.0.i1-IQ2_S.gguf) | i1-IQ2_S | 2.9 | |
| [GGUF](https://huggingface.co/mradermacher/Chocolatine-8B-Instruct-DPO-v1.0-i1-GGUF/resolve/main/Chocolatine-8B-Instruct-DPO-v1.0.i1-IQ2_M.gguf) | i1-IQ2_M | 3.0 | |
| [GGUF](https://huggingface.co/mradermacher/Chocolatine-8B-Instruct-DPO-v1.0-i1-GGUF/resolve/main/Chocolatine-8B-Instruct-DPO-v1.0.i1-Q2_K.gguf) | i1-Q2_K | 3.3 | IQ3_XXS probably better |
| [GGUF](https://huggingface.co/mradermacher/Chocolatine-8B-Instruct-DPO-v1.0-i1-GGUF/resolve/main/Chocolatine-8B-Instruct-DPO-v1.0.i1-IQ3_XXS.gguf) | i1-IQ3_XXS | 3.4 | lower quality |
| [GGUF](https://huggingface.co/mradermacher/Chocolatine-8B-Instruct-DPO-v1.0-i1-GGUF/resolve/main/Chocolatine-8B-Instruct-DPO-v1.0.i1-IQ3_XS.gguf) | i1-IQ3_XS | 3.6 | |
| [GGUF](https://huggingface.co/mradermacher/Chocolatine-8B-Instruct-DPO-v1.0-i1-GGUF/resolve/main/Chocolatine-8B-Instruct-DPO-v1.0.i1-Q3_K_S.gguf) | i1-Q3_K_S | 3.8 | IQ3_XS probably better |
| [GGUF](https://huggingface.co/mradermacher/Chocolatine-8B-Instruct-DPO-v1.0-i1-GGUF/resolve/main/Chocolatine-8B-Instruct-DPO-v1.0.i1-IQ3_S.gguf) | i1-IQ3_S | 3.8 | beats Q3_K* |
| [GGUF](https://huggingface.co/mradermacher/Chocolatine-8B-Instruct-DPO-v1.0-i1-GGUF/resolve/main/Chocolatine-8B-Instruct-DPO-v1.0.i1-IQ3_M.gguf) | i1-IQ3_M | 3.9 | |
| [GGUF](https://huggingface.co/mradermacher/Chocolatine-8B-Instruct-DPO-v1.0-i1-GGUF/resolve/main/Chocolatine-8B-Instruct-DPO-v1.0.i1-Q3_K_M.gguf) | i1-Q3_K_M | 4.1 | IQ3_S probably better |
| [GGUF](https://huggingface.co/mradermacher/Chocolatine-8B-Instruct-DPO-v1.0-i1-GGUF/resolve/main/Chocolatine-8B-Instruct-DPO-v1.0.i1-Q3_K_L.gguf) | i1-Q3_K_L | 4.4 | IQ3_M probably better |
| [GGUF](https://huggingface.co/mradermacher/Chocolatine-8B-Instruct-DPO-v1.0-i1-GGUF/resolve/main/Chocolatine-8B-Instruct-DPO-v1.0.i1-IQ4_XS.gguf) | i1-IQ4_XS | 4.5 | |
| [GGUF](https://huggingface.co/mradermacher/Chocolatine-8B-Instruct-DPO-v1.0-i1-GGUF/resolve/main/Chocolatine-8B-Instruct-DPO-v1.0.i1-Q4_0.gguf) | i1-Q4_0 | 4.8 | fast, low quality |
| [GGUF](https://huggingface.co/mradermacher/Chocolatine-8B-Instruct-DPO-v1.0-i1-GGUF/resolve/main/Chocolatine-8B-Instruct-DPO-v1.0.i1-Q4_K_S.gguf) | i1-Q4_K_S | 4.8 | optimal size/speed/quality |
| [GGUF](https://huggingface.co/mradermacher/Chocolatine-8B-Instruct-DPO-v1.0-i1-GGUF/resolve/main/Chocolatine-8B-Instruct-DPO-v1.0.i1-Q4_K_M.gguf) | i1-Q4_K_M | 5.0 | fast, recommended |
| [GGUF](https://huggingface.co/mradermacher/Chocolatine-8B-Instruct-DPO-v1.0-i1-GGUF/resolve/main/Chocolatine-8B-Instruct-DPO-v1.0.i1-Q5_K_S.gguf) | i1-Q5_K_S | 5.7 | |
| [GGUF](https://huggingface.co/mradermacher/Chocolatine-8B-Instruct-DPO-v1.0-i1-GGUF/resolve/main/Chocolatine-8B-Instruct-DPO-v1.0.i1-Q5_K_M.gguf) | i1-Q5_K_M | 5.8 | |
| [GGUF](https://huggingface.co/mradermacher/Chocolatine-8B-Instruct-DPO-v1.0-i1-GGUF/resolve/main/Chocolatine-8B-Instruct-DPO-v1.0.i1-Q6_K.gguf) | i1-Q6_K | 6.7 | practically like static Q6_K |
Here is a handy graph by ikawrakow comparing some lower-quality quant
types (lower is better):

And here are Artefact2's thoughts on the matter:
https://gist.github.com/Artefact2/b5f810600771265fc1e39442288e8ec9
## FAQ / Model Request
See https://huggingface.co/mradermacher/model_requests for some answers to
questions you might have and/or if you want some other model quantized.
## Thanks
I thank my company, [nethype GmbH](https://www.nethype.de/), for letting
me use its servers and providing upgrades to my workstation to enable
this work in my free time. Additional thanks to [@nicoboss](https://huggingface.co/nicoboss) for giving me access to his hardware for calculating the imatrix for these quants.
<!-- end -->
|
RichardErkhov/Zardos_-_Kant-Test-0.1-Mistral-7B-gguf | RichardErkhov | 2024-06-26T03:43:52Z | 13,591 | 0 | null | [
"gguf",
"arxiv:2310.06825",
"region:us"
] | null | 2024-06-26T00:08:57Z | Quantization made by Richard Erkhov.
[Github](https://github.com/RichardErkhov)
[Discord](https://discord.gg/pvy7H8DZMG)
[Request more models](https://github.com/RichardErkhov/quant_request)
Kant-Test-0.1-Mistral-7B - GGUF
- Model creator: https://huggingface.co/Zardos/
- Original model: https://huggingface.co/Zardos/Kant-Test-0.1-Mistral-7B/
| Name | Quant method | Size |
| ---- | ---- | ---- |
| [Kant-Test-0.1-Mistral-7B.Q2_K.gguf](https://huggingface.co/RichardErkhov/Zardos_-_Kant-Test-0.1-Mistral-7B-gguf/blob/main/Kant-Test-0.1-Mistral-7B.Q2_K.gguf) | Q2_K | 2.53GB |
| [Kant-Test-0.1-Mistral-7B.IQ3_XS.gguf](https://huggingface.co/RichardErkhov/Zardos_-_Kant-Test-0.1-Mistral-7B-gguf/blob/main/Kant-Test-0.1-Mistral-7B.IQ3_XS.gguf) | IQ3_XS | 2.81GB |
| [Kant-Test-0.1-Mistral-7B.IQ3_S.gguf](https://huggingface.co/RichardErkhov/Zardos_-_Kant-Test-0.1-Mistral-7B-gguf/blob/main/Kant-Test-0.1-Mistral-7B.IQ3_S.gguf) | IQ3_S | 2.96GB |
| [Kant-Test-0.1-Mistral-7B.Q3_K_S.gguf](https://huggingface.co/RichardErkhov/Zardos_-_Kant-Test-0.1-Mistral-7B-gguf/blob/main/Kant-Test-0.1-Mistral-7B.Q3_K_S.gguf) | Q3_K_S | 2.95GB |
| [Kant-Test-0.1-Mistral-7B.IQ3_M.gguf](https://huggingface.co/RichardErkhov/Zardos_-_Kant-Test-0.1-Mistral-7B-gguf/blob/main/Kant-Test-0.1-Mistral-7B.IQ3_M.gguf) | IQ3_M | 3.06GB |
| [Kant-Test-0.1-Mistral-7B.Q3_K.gguf](https://huggingface.co/RichardErkhov/Zardos_-_Kant-Test-0.1-Mistral-7B-gguf/blob/main/Kant-Test-0.1-Mistral-7B.Q3_K.gguf) | Q3_K | 3.28GB |
| [Kant-Test-0.1-Mistral-7B.Q3_K_M.gguf](https://huggingface.co/RichardErkhov/Zardos_-_Kant-Test-0.1-Mistral-7B-gguf/blob/main/Kant-Test-0.1-Mistral-7B.Q3_K_M.gguf) | Q3_K_M | 3.28GB |
| [Kant-Test-0.1-Mistral-7B.Q3_K_L.gguf](https://huggingface.co/RichardErkhov/Zardos_-_Kant-Test-0.1-Mistral-7B-gguf/blob/main/Kant-Test-0.1-Mistral-7B.Q3_K_L.gguf) | Q3_K_L | 3.56GB |
| [Kant-Test-0.1-Mistral-7B.IQ4_XS.gguf](https://huggingface.co/RichardErkhov/Zardos_-_Kant-Test-0.1-Mistral-7B-gguf/blob/main/Kant-Test-0.1-Mistral-7B.IQ4_XS.gguf) | IQ4_XS | 3.67GB |
| [Kant-Test-0.1-Mistral-7B.Q4_0.gguf](https://huggingface.co/RichardErkhov/Zardos_-_Kant-Test-0.1-Mistral-7B-gguf/blob/main/Kant-Test-0.1-Mistral-7B.Q4_0.gguf) | Q4_0 | 3.83GB |
| [Kant-Test-0.1-Mistral-7B.IQ4_NL.gguf](https://huggingface.co/RichardErkhov/Zardos_-_Kant-Test-0.1-Mistral-7B-gguf/blob/main/Kant-Test-0.1-Mistral-7B.IQ4_NL.gguf) | IQ4_NL | 3.87GB |
| [Kant-Test-0.1-Mistral-7B.Q4_K_S.gguf](https://huggingface.co/RichardErkhov/Zardos_-_Kant-Test-0.1-Mistral-7B-gguf/blob/main/Kant-Test-0.1-Mistral-7B.Q4_K_S.gguf) | Q4_K_S | 3.86GB |
| [Kant-Test-0.1-Mistral-7B.Q4_K.gguf](https://huggingface.co/RichardErkhov/Zardos_-_Kant-Test-0.1-Mistral-7B-gguf/blob/main/Kant-Test-0.1-Mistral-7B.Q4_K.gguf) | Q4_K | 4.07GB |
| [Kant-Test-0.1-Mistral-7B.Q4_K_M.gguf](https://huggingface.co/RichardErkhov/Zardos_-_Kant-Test-0.1-Mistral-7B-gguf/blob/main/Kant-Test-0.1-Mistral-7B.Q4_K_M.gguf) | Q4_K_M | 4.07GB |
| [Kant-Test-0.1-Mistral-7B.Q4_1.gguf](https://huggingface.co/RichardErkhov/Zardos_-_Kant-Test-0.1-Mistral-7B-gguf/blob/main/Kant-Test-0.1-Mistral-7B.Q4_1.gguf) | Q4_1 | 4.24GB |
| [Kant-Test-0.1-Mistral-7B.Q5_0.gguf](https://huggingface.co/RichardErkhov/Zardos_-_Kant-Test-0.1-Mistral-7B-gguf/blob/main/Kant-Test-0.1-Mistral-7B.Q5_0.gguf) | Q5_0 | 4.65GB |
| [Kant-Test-0.1-Mistral-7B.Q5_K_S.gguf](https://huggingface.co/RichardErkhov/Zardos_-_Kant-Test-0.1-Mistral-7B-gguf/blob/main/Kant-Test-0.1-Mistral-7B.Q5_K_S.gguf) | Q5_K_S | 4.65GB |
| [Kant-Test-0.1-Mistral-7B.Q5_K.gguf](https://huggingface.co/RichardErkhov/Zardos_-_Kant-Test-0.1-Mistral-7B-gguf/blob/main/Kant-Test-0.1-Mistral-7B.Q5_K.gguf) | Q5_K | 4.78GB |
| [Kant-Test-0.1-Mistral-7B.Q5_K_M.gguf](https://huggingface.co/RichardErkhov/Zardos_-_Kant-Test-0.1-Mistral-7B-gguf/blob/main/Kant-Test-0.1-Mistral-7B.Q5_K_M.gguf) | Q5_K_M | 4.78GB |
| [Kant-Test-0.1-Mistral-7B.Q5_1.gguf](https://huggingface.co/RichardErkhov/Zardos_-_Kant-Test-0.1-Mistral-7B-gguf/blob/main/Kant-Test-0.1-Mistral-7B.Q5_1.gguf) | Q5_1 | 5.07GB |
| [Kant-Test-0.1-Mistral-7B.Q6_K.gguf](https://huggingface.co/RichardErkhov/Zardos_-_Kant-Test-0.1-Mistral-7B-gguf/blob/main/Kant-Test-0.1-Mistral-7B.Q6_K.gguf) | Q6_K | 5.53GB |
| [Kant-Test-0.1-Mistral-7B.Q8_0.gguf](https://huggingface.co/RichardErkhov/Zardos_-_Kant-Test-0.1-Mistral-7B-gguf/blob/main/Kant-Test-0.1-Mistral-7B.Q8_0.gguf) | Q8_0 | 7.17GB |
Original model description:
---
language:
- en
license: apache-2.0
pipeline_tag: text-generation
model-index:
- name: Kant-Test-0.1-Mistral-7B
results:
- task:
type: text-generation
name: Text Generation
dataset:
name: AI2 Reasoning Challenge (25-Shot)
type: ai2_arc
config: ARC-Challenge
split: test
args:
num_few_shot: 25
metrics:
- type: acc_norm
value: 62.37
name: normalized accuracy
source:
url: https://huggingface.co/spaces/HuggingFaceH4/open_llm_leaderboard?query=Zardos/Kant-Test-0.1-Mistral-7B
name: Open LLM Leaderboard
- task:
type: text-generation
name: Text Generation
dataset:
name: HellaSwag (10-Shot)
type: hellaswag
split: validation
args:
num_few_shot: 10
metrics:
- type: acc_norm
value: 82.84
name: normalized accuracy
source:
url: https://huggingface.co/spaces/HuggingFaceH4/open_llm_leaderboard?query=Zardos/Kant-Test-0.1-Mistral-7B
name: Open LLM Leaderboard
- task:
type: text-generation
name: Text Generation
dataset:
name: MMLU (5-Shot)
type: cais/mmlu
config: all
split: test
args:
num_few_shot: 5
metrics:
- type: acc
value: 63.38
name: accuracy
source:
url: https://huggingface.co/spaces/HuggingFaceH4/open_llm_leaderboard?query=Zardos/Kant-Test-0.1-Mistral-7B
name: Open LLM Leaderboard
- task:
type: text-generation
name: Text Generation
dataset:
name: TruthfulQA (0-shot)
type: truthful_qa
config: multiple_choice
split: validation
args:
num_few_shot: 0
metrics:
- type: mc2
value: 49.62
source:
url: https://huggingface.co/spaces/HuggingFaceH4/open_llm_leaderboard?query=Zardos/Kant-Test-0.1-Mistral-7B
name: Open LLM Leaderboard
- task:
type: text-generation
name: Text Generation
dataset:
name: Winogrande (5-shot)
type: winogrande
config: winogrande_xl
split: validation
args:
num_few_shot: 5
metrics:
- type: acc
value: 78.3
name: accuracy
source:
url: https://huggingface.co/spaces/HuggingFaceH4/open_llm_leaderboard?query=Zardos/Kant-Test-0.1-Mistral-7B
name: Open LLM Leaderboard
- task:
type: text-generation
name: Text Generation
dataset:
name: GSM8k (5-shot)
type: gsm8k
config: main
split: test
args:
num_few_shot: 5
metrics:
- type: acc
value: 37.98
name: accuracy
source:
url: https://huggingface.co/spaces/HuggingFaceH4/open_llm_leaderboard?query=Zardos/Kant-Test-0.1-Mistral-7B
name: Open LLM Leaderboard
---
# Model Yaml
The Mistral-7B-v0.1 Large Language Model (LLM) is a pretrained generative text model with 7 billion parameters.
Mistral-7B-v0.1 outperforms Llama 2 13B on all benchmarks we tested.
For full details of this model please read our [paper](https://arxiv.org/abs/2310.06825) and [release blog post](https://mistral.ai/news/announcing-mistral-7b/).
## Model Architecture
Mistral-7B-v0.1 is a transformer model, with the following architecture choices:
- Grouped-Query Attention
- Sliding-Window Attention
- Byte-fallback BPE tokenizer
## Troubleshooting
- If you see the following error:
```
KeyError: 'mistral'
```
- Or:
```
NotImplementedError: Cannot copy out of meta tensor; no data!
```
Ensure you are utilizing a stable version of Transformers, 4.34.0 or newer.
## Notice
Mistral 7B is a pretrained base model and therefore does not have any moderation mechanisms.
## The Mistral AI Team
Albert Jiang, Alexandre Sablayrolles, Arthur Mensch, Chris Bamford, Devendra Singh Chaplot, Diego de las Casas, Florian Bressand, Gianna Lengyel, Guillaume Lample, Lélio Renard Lavaud, Lucile Saulnier, Marie-Anne Lachaux, Pierre Stock, Teven Le Scao, Thibaut Lavril, Thomas Wang, Timothée Lacroix, William El Sayed.
# [Open LLM Leaderboard Evaluation Results](https://huggingface.co/spaces/HuggingFaceH4/open_llm_leaderboard)
Detailed results can be found [here](https://huggingface.co/datasets/open-llm-leaderboard/details_Zardos__Kant-Test-0.1-Mistral-7B)
| Metric |Value|
|---------------------------------|----:|
|Avg. |62.42|
|AI2 Reasoning Challenge (25-Shot)|62.37|
|HellaSwag (10-Shot) |82.84|
|MMLU (5-Shot) |63.38|
|TruthfulQA (0-shot) |49.62|
|Winogrande (5-shot) |78.30|
|GSM8k (5-shot) |37.98|
|
RichardErkhov/unsloth_-_mistral-7b-instruct-v0.3-gguf | RichardErkhov | 2024-06-25T17:34:34Z | 13,585 | 0 | null | [
"gguf",
"region:us"
] | null | 2024-06-25T13:30:20Z | Quantization made by Richard Erkhov.
[Github](https://github.com/RichardErkhov)
[Discord](https://discord.gg/pvy7H8DZMG)
[Request more models](https://github.com/RichardErkhov/quant_request)
mistral-7b-instruct-v0.3 - GGUF
- Model creator: https://huggingface.co/unsloth/
- Original model: https://huggingface.co/unsloth/mistral-7b-instruct-v0.3/
| Name | Quant method | Size |
| ---- | ---- | ---- |
| [mistral-7b-instruct-v0.3.Q2_K.gguf](https://huggingface.co/RichardErkhov/unsloth_-_mistral-7b-instruct-v0.3-gguf/blob/main/mistral-7b-instruct-v0.3.Q2_K.gguf) | Q2_K | 2.54GB |
| [mistral-7b-instruct-v0.3.IQ3_XS.gguf](https://huggingface.co/RichardErkhov/unsloth_-_mistral-7b-instruct-v0.3-gguf/blob/main/mistral-7b-instruct-v0.3.IQ3_XS.gguf) | IQ3_XS | 2.82GB |
| [mistral-7b-instruct-v0.3.IQ3_S.gguf](https://huggingface.co/RichardErkhov/unsloth_-_mistral-7b-instruct-v0.3-gguf/blob/main/mistral-7b-instruct-v0.3.IQ3_S.gguf) | IQ3_S | 2.97GB |
| [mistral-7b-instruct-v0.3.Q3_K_S.gguf](https://huggingface.co/RichardErkhov/unsloth_-_mistral-7b-instruct-v0.3-gguf/blob/main/mistral-7b-instruct-v0.3.Q3_K_S.gguf) | Q3_K_S | 2.95GB |
| [mistral-7b-instruct-v0.3.IQ3_M.gguf](https://huggingface.co/RichardErkhov/unsloth_-_mistral-7b-instruct-v0.3-gguf/blob/main/mistral-7b-instruct-v0.3.IQ3_M.gguf) | IQ3_M | 3.06GB |
| [mistral-7b-instruct-v0.3.Q3_K.gguf](https://huggingface.co/RichardErkhov/unsloth_-_mistral-7b-instruct-v0.3-gguf/blob/main/mistral-7b-instruct-v0.3.Q3_K.gguf) | Q3_K | 3.28GB |
| [mistral-7b-instruct-v0.3.Q3_K_M.gguf](https://huggingface.co/RichardErkhov/unsloth_-_mistral-7b-instruct-v0.3-gguf/blob/main/mistral-7b-instruct-v0.3.Q3_K_M.gguf) | Q3_K_M | 3.28GB |
| [mistral-7b-instruct-v0.3.Q3_K_L.gguf](https://huggingface.co/RichardErkhov/unsloth_-_mistral-7b-instruct-v0.3-gguf/blob/main/mistral-7b-instruct-v0.3.Q3_K_L.gguf) | Q3_K_L | 3.56GB |
| [mistral-7b-instruct-v0.3.IQ4_XS.gguf](https://huggingface.co/RichardErkhov/unsloth_-_mistral-7b-instruct-v0.3-gguf/blob/main/mistral-7b-instruct-v0.3.IQ4_XS.gguf) | IQ4_XS | 3.68GB |
| [mistral-7b-instruct-v0.3.Q4_0.gguf](https://huggingface.co/RichardErkhov/unsloth_-_mistral-7b-instruct-v0.3-gguf/blob/main/mistral-7b-instruct-v0.3.Q4_0.gguf) | Q4_0 | 3.83GB |
| [mistral-7b-instruct-v0.3.IQ4_NL.gguf](https://huggingface.co/RichardErkhov/unsloth_-_mistral-7b-instruct-v0.3-gguf/blob/main/mistral-7b-instruct-v0.3.IQ4_NL.gguf) | IQ4_NL | 3.87GB |
| [mistral-7b-instruct-v0.3.Q4_K_S.gguf](https://huggingface.co/RichardErkhov/unsloth_-_mistral-7b-instruct-v0.3-gguf/blob/main/mistral-7b-instruct-v0.3.Q4_K_S.gguf) | Q4_K_S | 3.86GB |
| [mistral-7b-instruct-v0.3.Q4_K.gguf](https://huggingface.co/RichardErkhov/unsloth_-_mistral-7b-instruct-v0.3-gguf/blob/main/mistral-7b-instruct-v0.3.Q4_K.gguf) | Q4_K | 4.07GB |
| [mistral-7b-instruct-v0.3.Q4_K_M.gguf](https://huggingface.co/RichardErkhov/unsloth_-_mistral-7b-instruct-v0.3-gguf/blob/main/mistral-7b-instruct-v0.3.Q4_K_M.gguf) | Q4_K_M | 4.07GB |
| [mistral-7b-instruct-v0.3.Q4_1.gguf](https://huggingface.co/RichardErkhov/unsloth_-_mistral-7b-instruct-v0.3-gguf/blob/main/mistral-7b-instruct-v0.3.Q4_1.gguf) | Q4_1 | 4.24GB |
| [mistral-7b-instruct-v0.3.Q5_0.gguf](https://huggingface.co/RichardErkhov/unsloth_-_mistral-7b-instruct-v0.3-gguf/blob/main/mistral-7b-instruct-v0.3.Q5_0.gguf) | Q5_0 | 4.66GB |
| [mistral-7b-instruct-v0.3.Q5_K_S.gguf](https://huggingface.co/RichardErkhov/unsloth_-_mistral-7b-instruct-v0.3-gguf/blob/main/mistral-7b-instruct-v0.3.Q5_K_S.gguf) | Q5_K_S | 4.66GB |
| [mistral-7b-instruct-v0.3.Q5_K.gguf](https://huggingface.co/RichardErkhov/unsloth_-_mistral-7b-instruct-v0.3-gguf/blob/main/mistral-7b-instruct-v0.3.Q5_K.gguf) | Q5_K | 4.78GB |
| [mistral-7b-instruct-v0.3.Q5_K_M.gguf](https://huggingface.co/RichardErkhov/unsloth_-_mistral-7b-instruct-v0.3-gguf/blob/main/mistral-7b-instruct-v0.3.Q5_K_M.gguf) | Q5_K_M | 4.78GB |
| [mistral-7b-instruct-v0.3.Q5_1.gguf](https://huggingface.co/RichardErkhov/unsloth_-_mistral-7b-instruct-v0.3-gguf/blob/main/mistral-7b-instruct-v0.3.Q5_1.gguf) | Q5_1 | 5.07GB |
| [mistral-7b-instruct-v0.3.Q6_K.gguf](https://huggingface.co/RichardErkhov/unsloth_-_mistral-7b-instruct-v0.3-gguf/blob/main/mistral-7b-instruct-v0.3.Q6_K.gguf) | Q6_K | 5.54GB |
| [mistral-7b-instruct-v0.3.Q8_0.gguf](https://huggingface.co/RichardErkhov/unsloth_-_mistral-7b-instruct-v0.3-gguf/blob/main/mistral-7b-instruct-v0.3.Q8_0.gguf) | Q8_0 | 7.17GB |
Original model description:
---
language:
- en
license: apache-2.0
library_name: transformers
tags:
- unsloth
- transformers
- mistral
- mistral-7b
- mistral-instruct
- instruct
---
# Finetune Mistral, Gemma, Llama 2-5x faster with 70% less memory via Unsloth!
We have a Google Colab Tesla T4 notebook for Mistral v3 7b here: https://colab.research.google.com/drive/1_yNCks4BTD5zOnjozppphh5GzMFaMKq_?usp=sharing
For conversational ShareGPT style and using Mistral v3 Instruct: https://colab.research.google.com/drive/15F1xyn8497_dUbxZP4zWmPZ3PJx1Oymv?usp=sharing
[<img src="https://raw.githubusercontent.com/unslothai/unsloth/main/images/Discord%20button.png" width="200"/>](https://discord.gg/u54VK8m8tk)
[<img src="https://raw.githubusercontent.com/unslothai/unsloth/main/images/buy%20me%20a%20coffee%20button.png" width="200"/>](https://ko-fi.com/unsloth)
[<img src="https://raw.githubusercontent.com/unslothai/unsloth/main/images/unsloth%20made%20with%20love.png" width="200"/>](https://github.com/unslothai/unsloth)
## ✨ Finetune for Free
All notebooks are **beginner friendly**! Add your dataset, click "Run All", and you'll get a 2x faster finetuned model which can be exported to GGUF, vLLM or uploaded to Hugging Face.
| Unsloth supports | Free Notebooks | Performance | Memory use |
|-----------------|--------------------------------------------------------------------------------------------------------------------------|-------------|----------|
| **Gemma 7b** | [▶️ Start on Colab](https://colab.research.google.com/drive/10NbwlsRChbma1v55m8LAPYG15uQv6HLo?usp=sharing) | 2.4x faster | 58% less |
| **Mistral 7b** | [▶️ Start on Colab](https://colab.research.google.com/drive/1Dyauq4kTZoLewQ1cApceUQVNcnnNTzg_?usp=sharing) | 2.2x faster | 62% less |
| **Llama-2 7b** | [▶️ Start on Colab](https://colab.research.google.com/drive/1lBzz5KeZJKXjvivbYvmGarix9Ao6Wxe5?usp=sharing) | 2.2x faster | 43% less |
| **TinyLlama** | [▶️ Start on Colab](https://colab.research.google.com/drive/1AZghoNBQaMDgWJpi4RbffGM1h6raLUj9?usp=sharing) | 3.9x faster | 74% less |
| **CodeLlama 34b** A100 | [▶️ Start on Colab](https://colab.research.google.com/drive/1y7A0AxE3y8gdj4AVkl2aZX47Xu3P1wJT?usp=sharing) | 1.9x faster | 27% less |
| **Mistral 7b** 1xT4 | [▶️ Start on Kaggle](https://www.kaggle.com/code/danielhanchen/kaggle-mistral-7b-unsloth-notebook) | 5x faster\* | 62% less |
| **DPO - Zephyr** | [▶️ Start on Colab](https://colab.research.google.com/drive/15vttTpzzVXv_tJwEk-hIcQ0S9FcEWvwP?usp=sharing) | 1.9x faster | 19% less |
- This [conversational notebook](https://colab.research.google.com/drive/1Aau3lgPzeZKQ-98h69CCu1UJcvIBLmy2?usp=sharing) is useful for ShareGPT ChatML / Vicuna templates.
- This [text completion notebook](https://colab.research.google.com/drive/1ef-tab5bhkvWmBOObepl1WgJvfvSzn5Q?usp=sharing) is for raw text. This [DPO notebook](https://colab.research.google.com/drive/15vttTpzzVXv_tJwEk-hIcQ0S9FcEWvwP?usp=sharing) replicates Zephyr.
- \* Kaggle has 2x T4s, but we use 1. Due to overhead, 1x T4 is 5x faster.
|
vinai/vinai-translate-en2vi-v2 | vinai | 2023-11-19T14:23:16Z | 13,583 | 6 | transformers | [
"transformers",
"pytorch",
"mbart",
"text2text-generation",
"autotrain_compatible",
"endpoints_compatible",
"region:us"
] | text2text-generation | 2023-11-16T10:46:17Z | # A Vietnamese-English Neural Machine Translation System
Our pre-trained VinAI Translate models are state-of-the-art text translation models for Vietnamese-to-English and English-to-Vietnamese, respectively. The general architecture and experimental results of VinAI Translate can be found in [our paper](https://openreview.net/forum?id=CRg-RaxKnai):
@inproceedings{vinaitranslate,
title = {{A Vietnamese-English Neural Machine Translation System}},
author = {Thien Hai Nguyen and
Tuan-Duy H. Nguyen and
Duy Phung and
Duy Tran-Cong Nguyen and
Hieu Minh Tran and
Manh Luong and
Tin Duy Vo and
Hung Hai Bui and
Dinh Phung and
Dat Quoc Nguyen},
booktitle = {Proceedings of the 23rd Annual Conference of the International Speech Communication Association: Show and Tell (INTERSPEECH)},
year = {2022}
}
Please **CITE** our paper whenever the pre-trained models or the system are used to help produce published results or incorporated into other software.
For further information or requests, please go to [VinAI Translate's homepage](https://github.com/VinAIResearch/VinAI_Translate)! |
mradermacher/neo_7b-GGUF | mradermacher | 2024-06-30T20:23:41Z | 13,581 | 0 | transformers | [
"transformers",
"gguf",
"en",
"base_model:DewEfresh/neo_7b",
"license:apache-2.0",
"endpoints_compatible",
"region:us"
] | null | 2024-06-30T18:34:47Z | ---
base_model: DewEfresh/neo_7b
language:
- en
library_name: transformers
license: apache-2.0
quantized_by: mradermacher
---
## About
<!-- ### quantize_version: 2 -->
<!-- ### output_tensor_quantised: 1 -->
<!-- ### convert_type: hf -->
<!-- ### vocab_type: -->
<!-- ### tags: -->
static quants of https://huggingface.co/DewEfresh/neo_7b
<!-- provided-files -->
weighted/imatrix quants are available at https://huggingface.co/mradermacher/neo_7b-i1-GGUF
## Usage
If you are unsure how to use GGUF files, refer to one of [TheBloke's
READMEs](https://huggingface.co/TheBloke/KafkaLM-70B-German-V0.1-GGUF) for
more details, including on how to concatenate multi-part files.
## Provided Quants
(sorted by size, not necessarily quality. IQ-quants are often preferable over similar sized non-IQ quants)
| Link | Type | Size/GB | Notes |
|:-----|:-----|--------:|:------|
| [GGUF](https://huggingface.co/mradermacher/neo_7b-GGUF/resolve/main/neo_7b.Q2_K.gguf) | Q2_K | 3.0 | |
| [GGUF](https://huggingface.co/mradermacher/neo_7b-GGUF/resolve/main/neo_7b.IQ3_XS.gguf) | IQ3_XS | 3.4 | |
| [GGUF](https://huggingface.co/mradermacher/neo_7b-GGUF/resolve/main/neo_7b.IQ3_S.gguf) | IQ3_S | 3.5 | beats Q3_K* |
| [GGUF](https://huggingface.co/mradermacher/neo_7b-GGUF/resolve/main/neo_7b.Q3_K_S.gguf) | Q3_K_S | 3.5 | |
| [GGUF](https://huggingface.co/mradermacher/neo_7b-GGUF/resolve/main/neo_7b.IQ3_M.gguf) | IQ3_M | 3.6 | |
| [GGUF](https://huggingface.co/mradermacher/neo_7b-GGUF/resolve/main/neo_7b.Q3_K_M.gguf) | Q3_K_M | 3.9 | lower quality |
| [GGUF](https://huggingface.co/mradermacher/neo_7b-GGUF/resolve/main/neo_7b.Q3_K_L.gguf) | Q3_K_L | 4.2 | |
| [GGUF](https://huggingface.co/mradermacher/neo_7b-GGUF/resolve/main/neo_7b.IQ4_XS.gguf) | IQ4_XS | 4.3 | |
| [GGUF](https://huggingface.co/mradermacher/neo_7b-GGUF/resolve/main/neo_7b.Q4_K_S.gguf) | Q4_K_S | 4.6 | fast, recommended |
| [GGUF](https://huggingface.co/mradermacher/neo_7b-GGUF/resolve/main/neo_7b.Q4_K_M.gguf) | Q4_K_M | 4.8 | fast, recommended |
| [GGUF](https://huggingface.co/mradermacher/neo_7b-GGUF/resolve/main/neo_7b.Q5_K_S.gguf) | Q5_K_S | 5.5 | |
| [GGUF](https://huggingface.co/mradermacher/neo_7b-GGUF/resolve/main/neo_7b.Q5_K_M.gguf) | Q5_K_M | 5.6 | |
| [GGUF](https://huggingface.co/mradermacher/neo_7b-GGUF/resolve/main/neo_7b.Q6_K.gguf) | Q6_K | 6.5 | very good quality |
| [GGUF](https://huggingface.co/mradermacher/neo_7b-GGUF/resolve/main/neo_7b.Q8_0.gguf) | Q8_0 | 8.4 | fast, best quality |
| [GGUF](https://huggingface.co/mradermacher/neo_7b-GGUF/resolve/main/neo_7b.f16.gguf) | f16 | 15.7 | 16 bpw, overkill |
Here is a handy graph by ikawrakow comparing some lower-quality quant
types (lower is better):

And here are Artefact2's thoughts on the matter:
https://gist.github.com/Artefact2/b5f810600771265fc1e39442288e8ec9
## FAQ / Model Request
See https://huggingface.co/mradermacher/model_requests for some answers to
questions you might have and/or if you want some other model quantized.
## Thanks
I thank my company, [nethype GmbH](https://www.nethype.de/), for letting
me use its servers and providing upgrades to my workstation to enable
this work in my free time.
<!-- end -->
|
Helsinki-NLP/opus-mt-tc-base-en-sh | Helsinki-NLP | 2023-10-10T14:16:02Z | 13,578 | 0 | transformers | [
"transformers",
"pytorch",
"safetensors",
"marian",
"text2text-generation",
"translation",
"opus-mt-tc",
"bs",
"en",
"hr",
"sh",
"sr",
"license:cc-by-4.0",
"model-index",
"autotrain_compatible",
"endpoints_compatible",
"region:us"
] | translation | 2022-10-11T08:14:41Z | ---
language:
- bs
- en
- hr
- sh
- sr
language_bcp47:
- bs_Latn
- sr_Cyrl
- sr_Latn
tags:
- translation
- opus-mt-tc
license: cc-by-4.0
model-index:
- name: opus-mt-tc-base-en-sh
results:
- task:
name: Translation eng-hrv
type: translation
args: eng-hrv
dataset:
name: flores200-dev
type: flores200-dev
args: eng-hrv
metrics:
- name: BLEU
type: bleu
value: 28.1
- name: chr-F
type: chrf
value: 0.57963
- task:
name: Translation eng-srp_Cyrl
type: translation
args: eng-srp_Cyrl
dataset:
name: flores200-dev
type: flores200-dev
args: eng-srp_Cyrl
metrics:
- name: BLEU
type: bleu
value: 32.2
- name: chr-F
type: chrf
value: 0.60096
- task:
name: Translation eng-hrv
type: translation
args: eng-hrv
dataset:
name: flores200-devtest
type: flores200-devtest
args: eng-hrv
metrics:
- name: BLEU
type: bleu
value: 28.9
- name: chr-F
type: chrf
value: 0.58652
- task:
name: Translation eng-srp_Cyrl
type: translation
args: eng-srp_Cyrl
dataset:
name: flores200-devtest
type: flores200-devtest
args: eng-srp_Cyrl
metrics:
- name: BLEU
type: bleu
value: 31.7
- name: chr-F
type: chrf
value: 0.59874
- task:
name: Translation eng-hrv
type: translation
args: eng-hrv
dataset:
name: flores101-devtest
type: flores_101
args: eng hrv devtest
metrics:
- name: BLEU
type: bleu
value: 28.7
- name: chr-F
type: chrf
value: 0.586
- task:
name: Translation eng-srp_Cyrl
type: translation
args: eng-srp_Cyrl
dataset:
name: flores101-devtest
type: flores_101
args: eng srp_Cyrl devtest
metrics:
- name: BLEU
type: bleu
value: 31.7
- name: chr-F
type: chrf
value: 0.59874
- task:
name: Translation eng-bos_Latn
type: translation
args: eng-bos_Latn
dataset:
name: tatoeba-test-v2021-08-07
type: tatoeba_mt
args: eng-bos_Latn
metrics:
- name: BLEU
type: bleu
value: 46.3
- name: chr-F
type: chrf
value: 0.666
- task:
name: Translation eng-hbs
type: translation
args: eng-hbs
dataset:
name: tatoeba-test-v2021-08-07
type: tatoeba_mt
args: eng-hbs
metrics:
- name: BLEU
type: bleu
value: 42.1
- name: chr-F
type: chrf
value: 0.631
- task:
name: Translation eng-hrv
type: translation
args: eng-hrv
dataset:
name: tatoeba-test-v2021-08-07
type: tatoeba_mt
args: eng-hrv
metrics:
- name: BLEU
type: bleu
value: 49.7
- name: chr-F
type: chrf
value: 0.691
- task:
name: Translation eng-srp_Cyrl
type: translation
args: eng-srp_Cyrl
dataset:
name: tatoeba-test-v2021-08-07
type: tatoeba_mt
args: eng-srp_Cyrl
metrics:
- name: BLEU
type: bleu
value: 45.1
- name: chr-F
type: chrf
value: 0.645
- task:
name: Translation eng-srp_Latn
type: translation
args: eng-srp_Latn
dataset:
name: tatoeba-test-v2021-08-07
type: tatoeba_mt
args: eng-srp_Latn
metrics:
- name: BLEU
type: bleu
value: 39.8
- name: chr-F
type: chrf
value: 0.613
---
# opus-mt-tc-base-en-sh
## Table of Contents
- [Model Details](#model-details)
- [Uses](#uses)
- [Risks, Limitations and Biases](#risks-limitations-and-biases)
- [How to Get Started With the Model](#how-to-get-started-with-the-model)
- [Training](#training)
- [Evaluation](#evaluation)
- [Citation Information](#citation-information)
- [Acknowledgements](#acknowledgements)
## Model Details
Neural machine translation model for translating from English (en) to Serbo-Croatian (sh).
This model is part of the [OPUS-MT project](https://github.com/Helsinki-NLP/Opus-MT), an effort to make neural machine translation models widely available and accessible for many languages in the world. All models are originally trained using the amazing framework of [Marian NMT](https://marian-nmt.github.io/), an efficient NMT implementation written in pure C++. The models have been converted to pyTorch using the transformers library by huggingface. Training data is taken from [OPUS](https://opus.nlpl.eu/) and training pipelines use the procedures of [OPUS-MT-train](https://github.com/Helsinki-NLP/Opus-MT-train).
**Model Description:**
- **Developed by:** Language Technology Research Group at the University of Helsinki
- **Model Type:** Translation (transformer-align)
- **Release**: 2021-04-20
- **License:** CC-BY-4.0
- **Language(s):**
- Source Language(s): eng
- Target Language(s): bos_Latn hbs hrv srp_Cyrl srp_Latn
- Language Pair(s): eng-bos_Latn eng-hbs eng-hrv eng-srp_Cyrl eng-srp_Latn
- Valid Target Language Labels: >>bos_Cyrl<< >>bos_Latn<< >>cnr<< >>cnr_Latn<< >>hbs<< >>hbs_Cyrl<< >>hrv<< >>srp_Cyrl<< >>srp_Latn<<
- **Original Model**: [opus+bt-2021-04-20.zip](https://object.pouta.csc.fi/Tatoeba-MT-models/eng-hbs/opus+bt-2021-04-20.zip)
- **Resources for more information:**
- [OPUS-MT-train GitHub Repo](https://github.com/Helsinki-NLP/OPUS-MT-train)
- More information about released models for this language pair: [OPUS-MT eng-hbs README](https://github.com/Helsinki-NLP/Tatoeba-Challenge/tree/master/models/eng-hbs/README.md)
- [More information about MarianNMT models in the transformers library](https://huggingface.co/docs/transformers/model_doc/marian)
- [Tatoeba Translation Challenge](https://github.com/Helsinki-NLP/Tatoeba-Challenge/
This is a multilingual translation model with multiple target languages. A sentence initial language token is required in the form of `>>id<<` (id = valid target language ID), e.g. `>>bos_Latn<<`
## Uses
This model can be used for translation and text-to-text generation.
## Risks, Limitations and Biases
**CONTENT WARNING: Readers should be aware that the model is trained on various public data sets that may contain content that is disturbing, offensive, and can propagate historical and current stereotypes.**
Significant research has explored bias and fairness issues with language models (see, e.g., [Sheng et al. (2021)](https://aclanthology.org/2021.acl-long.330.pdf) and [Bender et al. (2021)](https://dl.acm.org/doi/pdf/10.1145/3442188.3445922)).
## How to Get Started With the Model
A short example code:
```python
from transformers import MarianMTModel, MarianTokenizer
src_text = [
">>hrv<< You're about to make a very serious mistake.",
">>hbs<< I've just been too busy."
]
model_name = "pytorch-models/opus-mt-tc-base-en-sh"
tokenizer = MarianTokenizer.from_pretrained(model_name)
model = MarianMTModel.from_pretrained(model_name)
translated = model.generate(**tokenizer(src_text, return_tensors="pt", padding=True))
for t in translated:
print( tokenizer.decode(t, skip_special_tokens=True) )
# expected output:
# Ti si o tome napraviti vrlo ozbiljnu pogrešku.
# [4]
```
You can also use OPUS-MT models with the transformers pipelines, for example:
```python
from transformers import pipeline
pipe = pipeline("translation", model="Helsinki-NLP/opus-mt-tc-base-en-sh")
print(pipe(">>hrv<< You're about to make a very serious mistake."))
# expected output: Ti si o tome napraviti vrlo ozbiljnu pogrešku.
```
## Training
- **Data**: opus+bt ([source](https://github.com/Helsinki-NLP/Tatoeba-Challenge))
- **Pre-processing**: SentencePiece (spm32k,spm32k)
- **Model Type:** transformer-align
- **Original MarianNMT Model**: [opus+bt-2021-04-20.zip](https://object.pouta.csc.fi/Tatoeba-MT-models/eng-hbs/opus+bt-2021-04-20.zip)
- **Training Scripts**: [GitHub Repo](https://github.com/Helsinki-NLP/OPUS-MT-train)
## Evaluation
* test set translations: [opus+bt-2021-04-20.test.txt](https://object.pouta.csc.fi/Tatoeba-MT-models/eng-hbs/opus+bt-2021-04-20.test.txt)
* test set scores: [opus+bt-2021-04-20.eval.txt](https://object.pouta.csc.fi/Tatoeba-MT-models/eng-hbs/opus+bt-2021-04-20.eval.txt)
* benchmark results: [benchmark_results.txt](benchmark_results.txt)
* benchmark output: [benchmark_translations.zip](benchmark_translations.zip)
| langpair | testset | chr-F | BLEU | #sent | #words |
|----------|---------|-------|-------|-------|--------|
| eng-bos_Latn | tatoeba-test-v2021-08-07 | 0.666 | 46.3 | 301 | 1650 |
| eng-hbs | tatoeba-test-v2021-08-07 | 0.631 | 42.1 | 10017 | 63927 |
| eng-hrv | tatoeba-test-v2021-08-07 | 0.691 | 49.7 | 1480 | 9396 |
| eng-srp_Cyrl | tatoeba-test-v2021-08-07 | 0.645 | 45.1 | 1580 | 9152 |
| eng-srp_Latn | tatoeba-test-v2021-08-07 | 0.613 | 39.8 | 6656 | 43729 |
| eng-hrv | flores101-devtest | 0.586 | 28.7 | 1012 | 22423 |
| eng-hrv | flores200-dev | 0.57963 | 28.1 | 997 | 21567 |
| eng-hrv | flores200-devtest | 0.58652 | 28.9 | 1012 | 22423 |
| eng-srp_Cyrl | flores101-devtest | 0.59874 | 31.7 | 1012 | 23456 |
| eng-srp_Cyrl | flores200-dev | 0.60096 | 32.2 | 997 | 22384 |
| eng-srp_Cyrl | flores200-devtest | 0.59874 | 31.7 | 1012 | 23456 |
## Citation Information
* Publications: [OPUS-MT – Building open translation services for the World](https://aclanthology.org/2020.eamt-1.61/) and [The Tatoeba Translation Challenge – Realistic Data Sets for Low Resource and Multilingual MT](https://aclanthology.org/2020.wmt-1.139/) (Please, cite if you use this model.)
```
@inproceedings{tiedemann-thottingal-2020-opus,
title = "{OPUS}-{MT} {--} Building open translation services for the World",
author = {Tiedemann, J{\"o}rg and Thottingal, Santhosh},
booktitle = "Proceedings of the 22nd Annual Conference of the European Association for Machine Translation",
month = nov,
year = "2020",
address = "Lisboa, Portugal",
publisher = "European Association for Machine Translation",
url = "https://aclanthology.org/2020.eamt-1.61",
pages = "479--480",
}
@inproceedings{tiedemann-2020-tatoeba,
title = "The Tatoeba Translation Challenge {--} Realistic Data Sets for Low Resource and Multilingual {MT}",
author = {Tiedemann, J{\"o}rg},
booktitle = "Proceedings of the Fifth Conference on Machine Translation",
month = nov,
year = "2020",
address = "Online",
publisher = "Association for Computational Linguistics",
url = "https://aclanthology.org/2020.wmt-1.139",
pages = "1174--1182",
}
```
## Acknowledgements
The work is supported by the [European Language Grid](https://www.european-language-grid.eu/) as [pilot project 2866](https://live.european-language-grid.eu/catalogue/#/resource/projects/2866), by the [FoTran project](https://www.helsinki.fi/en/researchgroups/natural-language-understanding-with-cross-lingual-grounding), funded by the European Research Council (ERC) under the European Union’s Horizon 2020 research and innovation programme (grant agreement No 771113), and the [MeMAD project](https://memad.eu/), funded by the European Union’s Horizon 2020 Research and Innovation Programme under grant agreement No 780069. We are also grateful for the generous computational resources and IT infrastructure provided by [CSC -- IT Center for Science](https://www.csc.fi/), Finland.
## Model conversion info
* transformers version: 4.16.2
* OPUS-MT git hash: e2a6299
* port time: Tue Oct 11 10:14:32 CEST 2022
* port machine: LM0-400-22516.local
|
RichardErkhov/FlagAlpha_-_Llama3-Chinese-8B-Instruct-gguf | RichardErkhov | 2024-06-25T05:31:01Z | 13,575 | 0 | null | [
"gguf",
"region:us"
] | null | 2024-06-24T22:42:55Z | Quantization made by Richard Erkhov.
[Github](https://github.com/RichardErkhov)
[Discord](https://discord.gg/pvy7H8DZMG)
[Request more models](https://github.com/RichardErkhov/quant_request)
Llama3-Chinese-8B-Instruct - GGUF
- Model creator: https://huggingface.co/FlagAlpha/
- Original model: https://huggingface.co/FlagAlpha/Llama3-Chinese-8B-Instruct/
| Name | Quant method | Size |
| ---- | ---- | ---- |
| [Llama3-Chinese-8B-Instruct.Q2_K.gguf](https://huggingface.co/RichardErkhov/FlagAlpha_-_Llama3-Chinese-8B-Instruct-gguf/blob/main/Llama3-Chinese-8B-Instruct.Q2_K.gguf) | Q2_K | 2.96GB |
| [Llama3-Chinese-8B-Instruct.IQ3_XS.gguf](https://huggingface.co/RichardErkhov/FlagAlpha_-_Llama3-Chinese-8B-Instruct-gguf/blob/main/Llama3-Chinese-8B-Instruct.IQ3_XS.gguf) | IQ3_XS | 3.28GB |
| [Llama3-Chinese-8B-Instruct.IQ3_S.gguf](https://huggingface.co/RichardErkhov/FlagAlpha_-_Llama3-Chinese-8B-Instruct-gguf/blob/main/Llama3-Chinese-8B-Instruct.IQ3_S.gguf) | IQ3_S | 1.58GB |
| [Llama3-Chinese-8B-Instruct.Q3_K_S.gguf](https://huggingface.co/RichardErkhov/FlagAlpha_-_Llama3-Chinese-8B-Instruct-gguf/blob/main/Llama3-Chinese-8B-Instruct.Q3_K_S.gguf) | Q3_K_S | 0.35GB |
| [Llama3-Chinese-8B-Instruct.IQ3_M.gguf](https://huggingface.co/RichardErkhov/FlagAlpha_-_Llama3-Chinese-8B-Instruct-gguf/blob/main/Llama3-Chinese-8B-Instruct.IQ3_M.gguf) | IQ3_M | 3.52GB |
| [Llama3-Chinese-8B-Instruct.Q3_K.gguf](https://huggingface.co/RichardErkhov/FlagAlpha_-_Llama3-Chinese-8B-Instruct-gguf/blob/main/Llama3-Chinese-8B-Instruct.Q3_K.gguf) | Q3_K | 3.74GB |
| [Llama3-Chinese-8B-Instruct.Q3_K_M.gguf](https://huggingface.co/RichardErkhov/FlagAlpha_-_Llama3-Chinese-8B-Instruct-gguf/blob/main/Llama3-Chinese-8B-Instruct.Q3_K_M.gguf) | Q3_K_M | 3.74GB |
| [Llama3-Chinese-8B-Instruct.Q3_K_L.gguf](https://huggingface.co/RichardErkhov/FlagAlpha_-_Llama3-Chinese-8B-Instruct-gguf/blob/main/Llama3-Chinese-8B-Instruct.Q3_K_L.gguf) | Q3_K_L | 4.03GB |
| [Llama3-Chinese-8B-Instruct.IQ4_XS.gguf](https://huggingface.co/RichardErkhov/FlagAlpha_-_Llama3-Chinese-8B-Instruct-gguf/blob/main/Llama3-Chinese-8B-Instruct.IQ4_XS.gguf) | IQ4_XS | 4.18GB |
| [Llama3-Chinese-8B-Instruct.Q4_0.gguf](https://huggingface.co/RichardErkhov/FlagAlpha_-_Llama3-Chinese-8B-Instruct-gguf/blob/main/Llama3-Chinese-8B-Instruct.Q4_0.gguf) | Q4_0 | 4.34GB |
| [Llama3-Chinese-8B-Instruct.IQ4_NL.gguf](https://huggingface.co/RichardErkhov/FlagAlpha_-_Llama3-Chinese-8B-Instruct-gguf/blob/main/Llama3-Chinese-8B-Instruct.IQ4_NL.gguf) | IQ4_NL | 4.38GB |
| [Llama3-Chinese-8B-Instruct.Q4_K_S.gguf](https://huggingface.co/RichardErkhov/FlagAlpha_-_Llama3-Chinese-8B-Instruct-gguf/blob/main/Llama3-Chinese-8B-Instruct.Q4_K_S.gguf) | Q4_K_S | 4.37GB |
| [Llama3-Chinese-8B-Instruct.Q4_K.gguf](https://huggingface.co/RichardErkhov/FlagAlpha_-_Llama3-Chinese-8B-Instruct-gguf/blob/main/Llama3-Chinese-8B-Instruct.Q4_K.gguf) | Q4_K | 4.58GB |
| [Llama3-Chinese-8B-Instruct.Q4_K_M.gguf](https://huggingface.co/RichardErkhov/FlagAlpha_-_Llama3-Chinese-8B-Instruct-gguf/blob/main/Llama3-Chinese-8B-Instruct.Q4_K_M.gguf) | Q4_K_M | 4.58GB |
| [Llama3-Chinese-8B-Instruct.Q4_1.gguf](https://huggingface.co/RichardErkhov/FlagAlpha_-_Llama3-Chinese-8B-Instruct-gguf/blob/main/Llama3-Chinese-8B-Instruct.Q4_1.gguf) | Q4_1 | 4.78GB |
| [Llama3-Chinese-8B-Instruct.Q5_0.gguf](https://huggingface.co/RichardErkhov/FlagAlpha_-_Llama3-Chinese-8B-Instruct-gguf/blob/main/Llama3-Chinese-8B-Instruct.Q5_0.gguf) | Q5_0 | 5.21GB |
| [Llama3-Chinese-8B-Instruct.Q5_K_S.gguf](https://huggingface.co/RichardErkhov/FlagAlpha_-_Llama3-Chinese-8B-Instruct-gguf/blob/main/Llama3-Chinese-8B-Instruct.Q5_K_S.gguf) | Q5_K_S | 5.21GB |
| [Llama3-Chinese-8B-Instruct.Q5_K.gguf](https://huggingface.co/RichardErkhov/FlagAlpha_-_Llama3-Chinese-8B-Instruct-gguf/blob/main/Llama3-Chinese-8B-Instruct.Q5_K.gguf) | Q5_K | 5.34GB |
| [Llama3-Chinese-8B-Instruct.Q5_K_M.gguf](https://huggingface.co/RichardErkhov/FlagAlpha_-_Llama3-Chinese-8B-Instruct-gguf/blob/main/Llama3-Chinese-8B-Instruct.Q5_K_M.gguf) | Q5_K_M | 5.34GB |
| [Llama3-Chinese-8B-Instruct.Q5_1.gguf](https://huggingface.co/RichardErkhov/FlagAlpha_-_Llama3-Chinese-8B-Instruct-gguf/blob/main/Llama3-Chinese-8B-Instruct.Q5_1.gguf) | Q5_1 | 5.65GB |
| [Llama3-Chinese-8B-Instruct.Q6_K.gguf](https://huggingface.co/RichardErkhov/FlagAlpha_-_Llama3-Chinese-8B-Instruct-gguf/blob/main/Llama3-Chinese-8B-Instruct.Q6_K.gguf) | Q6_K | 6.14GB |
| [Llama3-Chinese-8B-Instruct.Q8_0.gguf](https://huggingface.co/RichardErkhov/FlagAlpha_-_Llama3-Chinese-8B-Instruct-gguf/blob/main/Llama3-Chinese-8B-Instruct.Q8_0.gguf) | Q8_0 | 7.95GB |
Original model description:
---
license: apache-2.0
tags:
- llama3
- chinese
---
# Llama3-Chinese-8B-Instruct
Llama3-Chinese-8B-Instruct基于Llama3-8B中文微调对话模型,由Llama中文社区和AtomEcho(原子回声)联合研发,我们会持续提供更新的模型参数,模型训练过程见 [https://llama.family](https://llama.family)。
模型的部署、训练、微调等方法详见Llama中文社区GitHub仓库:[https://github.com/LlamaFamily/Llama-Chinese](https://github.com/LlamaFamily/Llama-Chinese)
## 如何使用
```
import transformers
import torch
model_id = "FlagAlpha/Llama3-Chinese-8B-Instruct"
pipeline = transformers.pipeline(
"text-generation",
model=model_id,
model_kwargs={"torch_dtype": torch.float16},
device="cuda",
)
messages = [{"role": "system", "content": ""}]
messages.append(
{"role": "user", "content": "介绍一下机器学习"}
)
prompt = pipeline.tokenizer.apply_chat_template(
messages,
tokenize=False,
add_generation_prompt=True
)
terminators = [
pipeline.tokenizer.eos_token_id,
pipeline.tokenizer.convert_tokens_to_ids("<|eot_id|>")
]
outputs = pipeline(
prompt,
max_new_tokens=512,
eos_token_id=terminators,
do_sample=True,
temperature=0.6,
top_p=0.9
)
content = outputs[0]["generated_text"][len(prompt):]
print(content)
```
|
fxmarty/tiny-testing-gpt2-remote-code | fxmarty | 2023-01-18T15:41:38Z | 13,571 | 1 | transformers | [
"transformers",
"pytorch",
"gpt2",
"text-generation",
"custom_code",
"license:apache-2.0",
"autotrain_compatible",
"text-generation-inference",
"region:us"
] | text-generation | 2023-01-18T15:25:11Z | ---
license: apache-2.0
---
|
Subsets and Splits
No community queries yet
The top public SQL queries from the community will appear here once available.