modelId
stringlengths 5
122
| author
stringlengths 2
42
| last_modified
timestamp[us, tz=UTC] | downloads
int64 0
738M
| likes
int64 0
11k
| library_name
stringclasses 245
values | tags
sequencelengths 1
4.05k
| pipeline_tag
stringclasses 48
values | createdAt
timestamp[us, tz=UTC] | card
stringlengths 1
901k
|
---|---|---|---|---|---|---|---|---|---|
mradermacher/neo_7b_instruct_v0.1-i1-GGUF | mradermacher | 2024-07-01T00:42:38Z | 15,697 | 0 | transformers | [
"transformers",
"gguf",
"en",
"base_model:m-a-p/neo_7b_instruct_v0.1",
"license:apache-2.0",
"endpoints_compatible",
"region:us"
] | null | 2024-06-30T22:22:33Z | ---
base_model: m-a-p/neo_7b_instruct_v0.1
language:
- en
library_name: transformers
license: apache-2.0
quantized_by: mradermacher
---
## About
<!-- ### quantize_version: 2 -->
<!-- ### output_tensor_quantised: 1 -->
<!-- ### convert_type: hf -->
<!-- ### vocab_type: -->
<!-- ### tags: nicoboss -->
weighted/imatrix quants of https://huggingface.co/m-a-p/neo_7b_instruct_v0.1
<!-- provided-files -->
static quants are available at https://huggingface.co/mradermacher/neo_7b_instruct_v0.1-GGUF
## Usage
If you are unsure how to use GGUF files, refer to one of [TheBloke's
READMEs](https://huggingface.co/TheBloke/KafkaLM-70B-German-V0.1-GGUF) for
more details, including on how to concatenate multi-part files.
## Provided Quants
(sorted by size, not necessarily quality. IQ-quants are often preferable over similar sized non-IQ quants)
| Link | Type | Size/GB | Notes |
|:-----|:-----|--------:|:------|
| [GGUF](https://huggingface.co/mradermacher/neo_7b_instruct_v0.1-i1-GGUF/resolve/main/neo_7b_instruct_v0.1.i1-IQ1_S.gguf) | i1-IQ1_S | 1.8 | for the desperate |
| [GGUF](https://huggingface.co/mradermacher/neo_7b_instruct_v0.1-i1-GGUF/resolve/main/neo_7b_instruct_v0.1.i1-IQ1_M.gguf) | i1-IQ1_M | 2.0 | mostly desperate |
| [GGUF](https://huggingface.co/mradermacher/neo_7b_instruct_v0.1-i1-GGUF/resolve/main/neo_7b_instruct_v0.1.i1-IQ2_XXS.gguf) | i1-IQ2_XXS | 2.2 | |
| [GGUF](https://huggingface.co/mradermacher/neo_7b_instruct_v0.1-i1-GGUF/resolve/main/neo_7b_instruct_v0.1.i1-IQ2_XS.gguf) | i1-IQ2_XS | 2.5 | |
| [GGUF](https://huggingface.co/mradermacher/neo_7b_instruct_v0.1-i1-GGUF/resolve/main/neo_7b_instruct_v0.1.i1-IQ2_S.gguf) | i1-IQ2_S | 2.6 | |
| [GGUF](https://huggingface.co/mradermacher/neo_7b_instruct_v0.1-i1-GGUF/resolve/main/neo_7b_instruct_v0.1.i1-IQ2_M.gguf) | i1-IQ2_M | 2.8 | |
| [GGUF](https://huggingface.co/mradermacher/neo_7b_instruct_v0.1-i1-GGUF/resolve/main/neo_7b_instruct_v0.1.i1-Q2_K.gguf) | i1-Q2_K | 3.0 | IQ3_XXS probably better |
| [GGUF](https://huggingface.co/mradermacher/neo_7b_instruct_v0.1-i1-GGUF/resolve/main/neo_7b_instruct_v0.1.i1-IQ3_XXS.gguf) | i1-IQ3_XXS | 3.1 | lower quality |
| [GGUF](https://huggingface.co/mradermacher/neo_7b_instruct_v0.1-i1-GGUF/resolve/main/neo_7b_instruct_v0.1.i1-IQ3_XS.gguf) | i1-IQ3_XS | 3.4 | |
| [GGUF](https://huggingface.co/mradermacher/neo_7b_instruct_v0.1-i1-GGUF/resolve/main/neo_7b_instruct_v0.1.i1-IQ3_S.gguf) | i1-IQ3_S | 3.5 | beats Q3_K* |
| [GGUF](https://huggingface.co/mradermacher/neo_7b_instruct_v0.1-i1-GGUF/resolve/main/neo_7b_instruct_v0.1.i1-Q3_K_S.gguf) | i1-Q3_K_S | 3.5 | IQ3_XS probably better |
| [GGUF](https://huggingface.co/mradermacher/neo_7b_instruct_v0.1-i1-GGUF/resolve/main/neo_7b_instruct_v0.1.i1-IQ3_M.gguf) | i1-IQ3_M | 3.6 | |
| [GGUF](https://huggingface.co/mradermacher/neo_7b_instruct_v0.1-i1-GGUF/resolve/main/neo_7b_instruct_v0.1.i1-Q3_K_M.gguf) | i1-Q3_K_M | 3.9 | IQ3_S probably better |
| [GGUF](https://huggingface.co/mradermacher/neo_7b_instruct_v0.1-i1-GGUF/resolve/main/neo_7b_instruct_v0.1.i1-Q3_K_L.gguf) | i1-Q3_K_L | 4.2 | IQ3_M probably better |
| [GGUF](https://huggingface.co/mradermacher/neo_7b_instruct_v0.1-i1-GGUF/resolve/main/neo_7b_instruct_v0.1.i1-IQ4_XS.gguf) | i1-IQ4_XS | 4.3 | |
| [GGUF](https://huggingface.co/mradermacher/neo_7b_instruct_v0.1-i1-GGUF/resolve/main/neo_7b_instruct_v0.1.i1-Q4_0.gguf) | i1-Q4_0 | 4.6 | fast, low quality |
| [GGUF](https://huggingface.co/mradermacher/neo_7b_instruct_v0.1-i1-GGUF/resolve/main/neo_7b_instruct_v0.1.i1-Q4_K_S.gguf) | i1-Q4_K_S | 4.6 | optimal size/speed/quality |
| [GGUF](https://huggingface.co/mradermacher/neo_7b_instruct_v0.1-i1-GGUF/resolve/main/neo_7b_instruct_v0.1.i1-Q4_K_M.gguf) | i1-Q4_K_M | 4.8 | fast, recommended |
| [GGUF](https://huggingface.co/mradermacher/neo_7b_instruct_v0.1-i1-GGUF/resolve/main/neo_7b_instruct_v0.1.i1-Q5_K_S.gguf) | i1-Q5_K_S | 5.5 | |
| [GGUF](https://huggingface.co/mradermacher/neo_7b_instruct_v0.1-i1-GGUF/resolve/main/neo_7b_instruct_v0.1.i1-Q5_K_M.gguf) | i1-Q5_K_M | 5.6 | |
| [GGUF](https://huggingface.co/mradermacher/neo_7b_instruct_v0.1-i1-GGUF/resolve/main/neo_7b_instruct_v0.1.i1-Q6_K.gguf) | i1-Q6_K | 6.5 | practically like static Q6_K |
Here is a handy graph by ikawrakow comparing some lower-quality quant
types (lower is better):

And here are Artefact2's thoughts on the matter:
https://gist.github.com/Artefact2/b5f810600771265fc1e39442288e8ec9
## FAQ / Model Request
See https://huggingface.co/mradermacher/model_requests for some answers to
questions you might have and/or if you want some other model quantized.
## Thanks
I thank my company, [nethype GmbH](https://www.nethype.de/), for letting
me use its servers and providing upgrades to my workstation to enable
this work in my free time. Additional thanks to [@nicoboss](https://huggingface.co/nicoboss) for giving me access to his hardware for calculating the imatrix for these quants.
<!-- end -->
|
mradermacher/huskylm-2.5-8b-GGUF | mradermacher | 2024-06-23T02:34:09Z | 15,695 | 0 | transformers | [
"transformers",
"gguf",
"llama-3",
"huskylm",
"darkcloudai",
"en",
"dataset:darkcloudai-smallmodel-frontieredition",
"dataset:darkcloudai-webdriver-redditcrawl-2023",
"dataset:darkcloudai-unalignment-truthfulness",
"dataset:darkcloudai-generaldpo",
"dataset:ai2_arc",
"dataset:allenai/ultrafeedback_binarized_cleaned",
"dataset:argilla/distilabel-intel-orca-dpo-pairs",
"dataset:jondurbin/airoboros-3.2",
"dataset:codeparrot/apps",
"dataset:facebook/belebele",
"dataset:bluemoon-fandom-1-1-rp-cleaned",
"dataset:boolq",
"dataset:camel-ai/biology",
"dataset:camel-ai/chemistry",
"dataset:camel-ai/math",
"dataset:camel-ai/physics",
"dataset:jondurbin/contextual-dpo-v0.1",
"dataset:jondurbin/gutenberg-dpo-v0.1",
"dataset:jondurbin/py-dpo-v0.1",
"dataset:jondurbin/truthy-dpo-v0.1",
"dataset:LDJnr/Capybara",
"dataset:jondurbin/cinematika-v0.1",
"dataset:WizardLM/WizardLM_evol_instruct_70k",
"dataset:glaiveai/glaive-function-calling-v2",
"dataset:grimulkan/LimaRP-augmented",
"dataset:lmsys/lmsys-chat-1m",
"dataset:ParisNeo/lollms_aware_dataset",
"dataset:TIGER-Lab/MathInstruct",
"dataset:Muennighoff/natural-instructions",
"dataset:openbookqa",
"dataset:kingbri/PIPPA-shareGPT",
"dataset:piqa",
"dataset:Vezora/Tested-22k-Python-Alpaca",
"dataset:ropes",
"dataset:cakiki/rosetta-code",
"dataset:Open-Orca/SlimOrca",
"dataset:b-mc2/sql-create-context",
"dataset:squad_v2",
"dataset:mattpscott/airoboros-summarization",
"dataset:migtissera/Synthia-v1.3",
"dataset:unalignment/toxic-dpo-v0.2",
"dataset:WhiteRabbitNeo/WRN-Chapter-1",
"dataset:WhiteRabbitNeo/WRN-Chapter-2",
"dataset:winogrande",
"base_model:darkcloudai/huskylm-2.5-8b",
"license:llama3",
"endpoints_compatible",
"region:us"
] | null | 2024-06-23T01:25:27Z | ---
base_model: darkcloudai/huskylm-2.5-8b
datasets:
- darkcloudai-smallmodel-frontieredition
- darkcloudai-webdriver-redditcrawl-2023
- darkcloudai-unalignment-truthfulness
- darkcloudai-generaldpo
- ai2_arc
- allenai/ultrafeedback_binarized_cleaned
- argilla/distilabel-intel-orca-dpo-pairs
- jondurbin/airoboros-3.2
- codeparrot/apps
- facebook/belebele
- bluemoon-fandom-1-1-rp-cleaned
- boolq
- camel-ai/biology
- camel-ai/chemistry
- camel-ai/math
- camel-ai/physics
- jondurbin/contextual-dpo-v0.1
- jondurbin/gutenberg-dpo-v0.1
- jondurbin/py-dpo-v0.1
- jondurbin/truthy-dpo-v0.1
- LDJnr/Capybara
- jondurbin/cinematika-v0.1
- WizardLM/WizardLM_evol_instruct_70k
- glaiveai/glaive-function-calling-v2
- jondurbin/gutenberg-dpo-v0.1
- grimulkan/LimaRP-augmented
- lmsys/lmsys-chat-1m
- ParisNeo/lollms_aware_dataset
- TIGER-Lab/MathInstruct
- Muennighoff/natural-instructions
- openbookqa
- kingbri/PIPPA-shareGPT
- piqa
- Vezora/Tested-22k-Python-Alpaca
- ropes
- cakiki/rosetta-code
- Open-Orca/SlimOrca
- b-mc2/sql-create-context
- squad_v2
- mattpscott/airoboros-summarization
- migtissera/Synthia-v1.3
- unalignment/toxic-dpo-v0.2
- WhiteRabbitNeo/WRN-Chapter-1
- WhiteRabbitNeo/WRN-Chapter-2
- winogrande
language:
- en
library_name: transformers
license: llama3
quantized_by: mradermacher
tags:
- llama-3
- huskylm
- darkcloudai
---
## About
<!-- ### quantize_version: 2 -->
<!-- ### output_tensor_quantised: 1 -->
<!-- ### convert_type: hf -->
<!-- ### vocab_type: -->
<!-- ### tags: -->
static quants of https://huggingface.co/darkcloudai/huskylm-2.5-8b
<!-- provided-files -->
weighted/imatrix quants are available at https://huggingface.co/mradermacher/huskylm-2.5-8b-i1-GGUF
## Usage
If you are unsure how to use GGUF files, refer to one of [TheBloke's
READMEs](https://huggingface.co/TheBloke/KafkaLM-70B-German-V0.1-GGUF) for
more details, including on how to concatenate multi-part files.
## Provided Quants
(sorted by size, not necessarily quality. IQ-quants are often preferable over similar sized non-IQ quants)
| Link | Type | Size/GB | Notes |
|:-----|:-----|--------:|:------|
| [GGUF](https://huggingface.co/mradermacher/huskylm-2.5-8b-GGUF/resolve/main/huskylm-2.5-8b.Q2_K.gguf) | Q2_K | 3.3 | |
| [GGUF](https://huggingface.co/mradermacher/huskylm-2.5-8b-GGUF/resolve/main/huskylm-2.5-8b.IQ3_XS.gguf) | IQ3_XS | 3.6 | |
| [GGUF](https://huggingface.co/mradermacher/huskylm-2.5-8b-GGUF/resolve/main/huskylm-2.5-8b.Q3_K_S.gguf) | Q3_K_S | 3.8 | |
| [GGUF](https://huggingface.co/mradermacher/huskylm-2.5-8b-GGUF/resolve/main/huskylm-2.5-8b.IQ3_S.gguf) | IQ3_S | 3.8 | beats Q3_K* |
| [GGUF](https://huggingface.co/mradermacher/huskylm-2.5-8b-GGUF/resolve/main/huskylm-2.5-8b.IQ3_M.gguf) | IQ3_M | 3.9 | |
| [GGUF](https://huggingface.co/mradermacher/huskylm-2.5-8b-GGUF/resolve/main/huskylm-2.5-8b.Q3_K_M.gguf) | Q3_K_M | 4.1 | lower quality |
| [GGUF](https://huggingface.co/mradermacher/huskylm-2.5-8b-GGUF/resolve/main/huskylm-2.5-8b.Q3_K_L.gguf) | Q3_K_L | 4.4 | |
| [GGUF](https://huggingface.co/mradermacher/huskylm-2.5-8b-GGUF/resolve/main/huskylm-2.5-8b.IQ4_XS.gguf) | IQ4_XS | 4.6 | |
| [GGUF](https://huggingface.co/mradermacher/huskylm-2.5-8b-GGUF/resolve/main/huskylm-2.5-8b.Q4_K_S.gguf) | Q4_K_S | 4.8 | fast, recommended |
| [GGUF](https://huggingface.co/mradermacher/huskylm-2.5-8b-GGUF/resolve/main/huskylm-2.5-8b.Q4_K_M.gguf) | Q4_K_M | 5.0 | fast, recommended |
| [GGUF](https://huggingface.co/mradermacher/huskylm-2.5-8b-GGUF/resolve/main/huskylm-2.5-8b.Q5_K_S.gguf) | Q5_K_S | 5.7 | |
| [GGUF](https://huggingface.co/mradermacher/huskylm-2.5-8b-GGUF/resolve/main/huskylm-2.5-8b.Q5_K_M.gguf) | Q5_K_M | 5.8 | |
| [GGUF](https://huggingface.co/mradermacher/huskylm-2.5-8b-GGUF/resolve/main/huskylm-2.5-8b.Q6_K.gguf) | Q6_K | 6.7 | very good quality |
| [GGUF](https://huggingface.co/mradermacher/huskylm-2.5-8b-GGUF/resolve/main/huskylm-2.5-8b.Q8_0.gguf) | Q8_0 | 8.6 | fast, best quality |
| [GGUF](https://huggingface.co/mradermacher/huskylm-2.5-8b-GGUF/resolve/main/huskylm-2.5-8b.f16.gguf) | f16 | 16.2 | 16 bpw, overkill |
Here is a handy graph by ikawrakow comparing some lower-quality quant
types (lower is better):

And here are Artefact2's thoughts on the matter:
https://gist.github.com/Artefact2/b5f810600771265fc1e39442288e8ec9
## FAQ / Model Request
See https://huggingface.co/mradermacher/model_requests for some answers to
questions you might have and/or if you want some other model quantized.
## Thanks
I thank my company, [nethype GmbH](https://www.nethype.de/), for letting
me use its servers and providing upgrades to my workstation to enable
this work in my free time.
<!-- end -->
|
NoCrypt/SomethingV2_2 | NoCrypt | 2023-05-06T03:13:52Z | 15,694 | 120 | diffusers | [
"diffusers",
"stable-diffusion",
"text-to-image",
"safetensors",
"en",
"license:creativeml-openrail-m",
"autotrain_compatible",
"endpoints_compatible",
"diffusers:StableDiffusionPipeline",
"region:us"
] | text-to-image | 2023-03-08T13:24:01Z | ---
license: creativeml-openrail-m
thumbnail: >-
https://huggingface.co/NoCrypt/SomethingV2_2/resolve/main/images/thumbnail.webp
tags:
- stable-diffusion
- text-to-image
- safetensors
- diffusers
inference: true
language:
- en
widget:
- text: >-
masterpiece, masterpiece, masterpiece, best quality, ultra-detailed, 1girl, hatsune miku, blue hair, upper body, looking at viewer, ?, negative space, bioluminescence, bioluminescence, bioluminescence, darkness, wind, butterfly, black background, portrait, ice
example_title: example
library_name: diffusers
---
<center>
<img src="https://huggingface.co/NoCrypt/SomethingV2_2/resolve/main/images/Artboard%201.png"/>
<h1 style="font-size:1.6rem;">
<b>
SomethingV2.2
</b>
</h1>
<p>
Welcome to SomethingV2.2 - an improved anime latent diffusion model from <a href="https://huggingface.co/NoCrypt/SomethingV2">SomethingV2</a>
A lot of things are being discovered lately, such as a way to merge model using mbw automatically, offset noise to get much darker result, and even VAE tuning. This model is intended to use all of those features as the improvements, here's some improvements that have been made:
</p>
<img src="https://huggingface.co/NoCrypt/SomethingV2_2/resolve/main/images/Artboard%202.png"/>
<h2>Can't trust the numbers? Here's some proof</h2>
</center>
%2C%20dark.png)
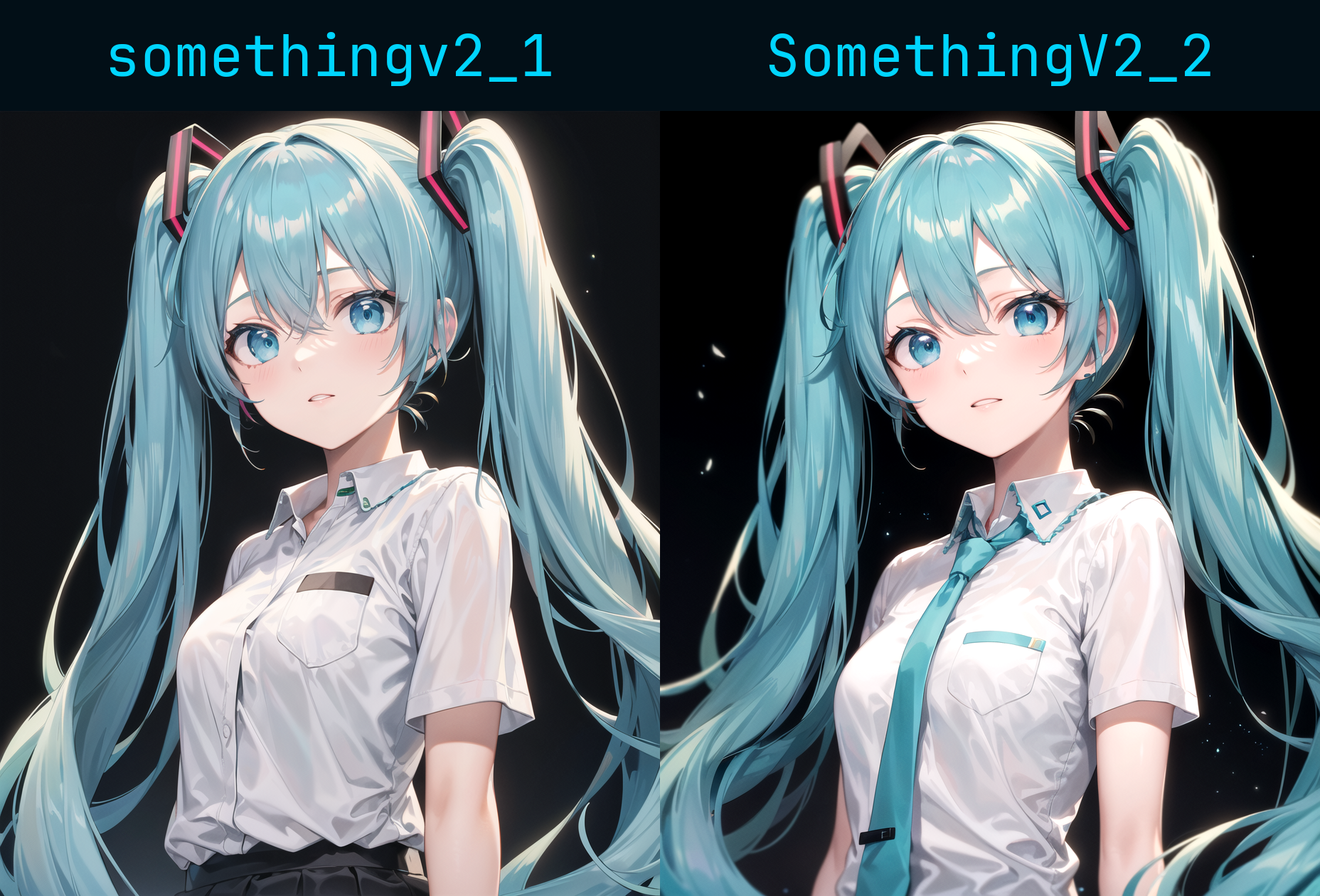
<img style="display:inline;margin:0;padding:0;" src="https://huggingface.co/NoCrypt/SomethingV2_2/resolve/main/images/00019-1829045217-masterpiece%2C%20best%20quality%2C%20hatsune%20miku%2C%201girl%2C%20white%20shirt%2C%20blue%20necktie%2C%20bare%20shoulders%2C%20very%20detailed%20background%2C%20hands%20on%20ow.png" width="32%"/>
<img style="display:inline;margin:0;padding:0;" src="https://huggingface.co/NoCrypt/SomethingV2_2/resolve/main/images/00018-1769428138-masterpiece%2C%20best%20quality%2C%20hatsune%20miku%2C%201girl%2C%20white%20shirt%2C%20blue%20necktie%2C%20bare%20shoulders%2C%20very%20detailed%20background%2C%20hands%20on%20ow.png" width="32%"/>
<img style="display:inline;margin:0;padding:0;" src="https://huggingface.co/NoCrypt/SomethingV2_2/resolve/main/images/00020-3514023396-masterpiece%2C%20best%20quality%2C%20hatsune%20miku%2C%201girl%2C%20white%20shirt%2C%20blue%20necktie%2C%20bare%20shoulders%2C%20very%20detailed%20background%2C%20cafe%2C%20angry.png" width="32%"/>
<details><summary><big><b>Prompts</b></big></summary>
```yaml
masterpiece, best quality, ultra-detailed, 2girls, upper body, looking at viewer, ?, negative space, (bioluminescence:1.2), darkness, wind, butterfly, black background, glowing,
AND masterpiece, best quality, ultra-detailed, 2girls, hatsune miku, upper body, looking at viewer, ?, negative space, (bioluminescence:1.2), darkness, wind, butterfly, black background, glowing, (blue theme:1.2)
AND masterpiece, best quality, ultra-detailed, 2girls, hakurei reimu, (brown hair:1.1), upper body, looking at viewer, ?, negative space, (bioluminescence:1.2), darkness, wind, butterfly, black background, glowing, (red theme:1.2)
Negative prompt: EasyNegative
Steps: 20, Sampler: DPM++ 2M Karras, CFG scale: 7, Seed: 3452449180, Size: 816x504, Model: somethingv2_1, Denoising strength: 0.58, Clip skip: 2, ENSD: 31337, Latent Couple: "divisions=1:1,1:2,1:2 positions=0:0,0:0,0:1 weights=0.2,0.8,0.8 end at step=13", Hires upscale: 1.9, Hires steps: 12, Hires upscaler: Latent (nearest-exact)
```
```yaml
masterpiece, best quality, hatsune miku, white shirt, darkness, dark background
Negative prompt: EasyNegative
Steps: 20, Sampler: DPM++ 2M Karras, CFG scale: 7, Seed: 72332473, Size: 504x600, Model: somethingv2_1, Denoising strength: 0.58, Clip skip: 2, ENSD: 31337, Hires upscale: 1.85, Hires steps: 12, Hires upscaler: Latent (nearest-exact)
```
```yaml
masterpiece, best quality, hatsune miku, 1girl, white shirt, blue necktie, bare shoulders, very detailed background, hands on own cheeks, open mouth, one eye closed, clenched teeth, smile
Negative prompt: EasyNegative, tattoo, (shoulder tattoo:1.0), (number tattoo:1.3), frills
Steps: 20, Sampler: DPM++ 2M Karras, CFG scale: 7, Seed: 1829045217, Size: 456x592, Model: SomethingV2_2, Denoising strength: 0.53, Clip skip: 2, ENSD: 31337, Hires upscale: 1.65, Hires steps: 12, Hires upscaler: Latent (nearest-exact), Discard penultimate sigma: True
```
```yaml
masterpiece, best quality, hatsune miku, 1girl, white shirt, blue necktie, bare shoulders, very detailed background, hands on own cheeks, open mouth, eyez closed, clenched teeth, smile, arms behind back,
Negative prompt: EasyNegative, tattoo, (shoulder tattoo:1.0), (number tattoo:1.3), frills
Steps: 20, Sampler: DPM++ 2M Karras, CFG scale: 7, Seed: 1769428138, Size: 456x592, Model: SomethingV2_2, Denoising strength: 0.53, Clip skip: 2, ENSD: 31337, Hires upscale: 1.65, Hires steps: 12, Hires upscaler: Latent (nearest-exact), Discard penultimate sigma: True
```
```yaml
masterpiece, best quality, hatsune miku, 1girl, white shirt, blue necktie, bare shoulders, very detailed background, cafe, angry, crossed arms, detached sleeves, light particles,
Negative prompt: EasyNegative, tattoo, (shoulder tattoo:1.0), (number tattoo:1.3), frills
Steps: 20, Sampler: DPM++ 2M Karras, CFG scale: 7, Seed: 3514023396, Size: 456x592, Model: SomethingV2_2, Denoising strength: 0.53, Clip skip: 2, ENSD: 31337, Hires upscale: 1.65, Hires steps: 12, Hires upscaler: Latent (nearest-exact), Discard penultimate sigma: True
```
</details>
## Non-miku examples
<img style="display:inline;margin:0;padding:0;" width="49%" src="https://huggingface.co/NoCrypt/SomethingV2_2/resolve/main/images/00021-4018636341-masterpiece%2C%20best%20quality%2C%201girl%2C%20aqua%20eyes%2C%20baseball%20cap%2C%20blonde%20hair%2C%20closed%20mouth%2C%20earrings%2C%20green%20background%2C%20hat%2C%20hoop%20earr.png"/>
<img style="display:inline;margin:0;padding:0;" width="49%" src="https://huggingface.co/NoCrypt/SomethingV2_2/resolve/main/images/00022-1334620477-masterpiece%2C%20best%20quality%2C%20landscape.png"/>
<details><summary><big><b>Prompts</b></big></summary>
```yaml
masterpiece, best quality, 1girl, aqua eyes, baseball cap, blonde hair, closed mouth, earrings, green background, hat, hoop earrings, jewelry, looking at viewer, shirt, short hair, simple background, solo, upper body, yellow shirt
Negative prompt: EasyNegative
Steps: 20, Sampler: DPM++ 2M Karras, CFG scale: 7, Seed: 4018636341, Size: 440x592, Model: SomethingV2_2, Denoising strength: 0.53, Clip skip: 2, ENSD: 31337, Hires upscale: 1.65, Hires steps: 13, Hires upscaler: Latent (nearest-exact)
```
```yaml
masterpiece, best quality, landscape
Negative prompt: EasyNegative
Steps: 20, Sampler: DPM++ 2M Karras, CFG scale: 7, Seed: 1334620477, Size: 440x592, Model: SomethingV2_2, Denoising strength: 0.53, Clip skip: 2, ENSD: 31337, Hires upscale: 1.65, Hires steps: 13, Hires upscaler: Latent (nearest-exact)
```
</details>
## Recommended settings
- VAE: None (Baked in model, [blessed2](https://huggingface.co/NoCrypt/blessed_vae/blob/main/blessed2.vae.pt))
- Clip Skip: 2
- Sampler: DPM++ 2M Karras
- CFG Scale: 7 ± 5
- Recommended Positive Prompt: masterpiece, best quality, negative space, (bioluminescence:1.2), darkness, dark background
- Recommended Negative Prompt: [EasyNegative](https://huggingface.co/datasets/gsdf/EasyNegative)
- For better results, using hires fix is a must.
- Hires upscaler: Latent (any variant, such as nearest-exact)
## Recipe
*Due to [SD-Silicon's Terms of use](https://huggingface.co/Xynon/SD-Silicon#terms-of-use). I must specify how the model was made*
|Model A | Model B | Interpolation Method | Weight | Name |
|---|---|---|---|---|
|[dpepmkmp](https://huggingface.co/closertodeath/dpepmkmp/blob/main/dpepmkmp.safetensors)|[silicon29-dark](https://huggingface.co/Xynon/SD-Silicon/blob/main/Silicon29/Silicon29-dark.safetensors)|MBW|Reverse Cosine|[dpepsili](https://huggingface.co/un1xx/model_dump/blob/main/bw-merge-dpepmkmp-Silicon29-dark-0.ckpt)|
|[somethingV2_1](https://huggingface.co/NoCrypt/SomethingV2/blob/main/somethingv2_1.safetensors)|[dpepsili](https://huggingface.co/un1xx/model_dump/blob/main/bw-merge-dpepmkmp-Silicon29-dark-0.ckpt)|MBW|Cosine|SomethingV2_2 raw|
|SomethingV2_2 raw|[Blessed2 VAE](https://huggingface.co/NoCrypt/blessed_vae/blob/main/blessed2.vae.pt)|Bake VAE|-|**[SomethingV2_2](https://huggingface.co/NoCrypt/SomethingV2_2/blob/main/SomethingV2_2.safetensors)**|
## Why not call it SomethingV4?
Since this model was based on SomethingV2 and there's not THAT much of improvements in some condition. Calling it V4 is just not right at the moment 😅
I am NoCrypt |
RichardErkhov/Nondzu_-_zephyr-7b-beta-pl-gguf | RichardErkhov | 2024-06-29T19:53:57Z | 15,692 | 0 | null | [
"gguf",
"region:us"
] | null | 2024-06-29T13:17:40Z | Quantization made by Richard Erkhov.
[Github](https://github.com/RichardErkhov)
[Discord](https://discord.gg/pvy7H8DZMG)
[Request more models](https://github.com/RichardErkhov/quant_request)
zephyr-7b-beta-pl - GGUF
- Model creator: https://huggingface.co/Nondzu/
- Original model: https://huggingface.co/Nondzu/zephyr-7b-beta-pl/
| Name | Quant method | Size |
| ---- | ---- | ---- |
| [zephyr-7b-beta-pl.Q2_K.gguf](https://huggingface.co/RichardErkhov/Nondzu_-_zephyr-7b-beta-pl-gguf/blob/main/zephyr-7b-beta-pl.Q2_K.gguf) | Q2_K | 2.53GB |
| [zephyr-7b-beta-pl.IQ3_XS.gguf](https://huggingface.co/RichardErkhov/Nondzu_-_zephyr-7b-beta-pl-gguf/blob/main/zephyr-7b-beta-pl.IQ3_XS.gguf) | IQ3_XS | 2.81GB |
| [zephyr-7b-beta-pl.IQ3_S.gguf](https://huggingface.co/RichardErkhov/Nondzu_-_zephyr-7b-beta-pl-gguf/blob/main/zephyr-7b-beta-pl.IQ3_S.gguf) | IQ3_S | 2.96GB |
| [zephyr-7b-beta-pl.Q3_K_S.gguf](https://huggingface.co/RichardErkhov/Nondzu_-_zephyr-7b-beta-pl-gguf/blob/main/zephyr-7b-beta-pl.Q3_K_S.gguf) | Q3_K_S | 2.95GB |
| [zephyr-7b-beta-pl.IQ3_M.gguf](https://huggingface.co/RichardErkhov/Nondzu_-_zephyr-7b-beta-pl-gguf/blob/main/zephyr-7b-beta-pl.IQ3_M.gguf) | IQ3_M | 3.06GB |
| [zephyr-7b-beta-pl.Q3_K.gguf](https://huggingface.co/RichardErkhov/Nondzu_-_zephyr-7b-beta-pl-gguf/blob/main/zephyr-7b-beta-pl.Q3_K.gguf) | Q3_K | 3.28GB |
| [zephyr-7b-beta-pl.Q3_K_M.gguf](https://huggingface.co/RichardErkhov/Nondzu_-_zephyr-7b-beta-pl-gguf/blob/main/zephyr-7b-beta-pl.Q3_K_M.gguf) | Q3_K_M | 3.28GB |
| [zephyr-7b-beta-pl.Q3_K_L.gguf](https://huggingface.co/RichardErkhov/Nondzu_-_zephyr-7b-beta-pl-gguf/blob/main/zephyr-7b-beta-pl.Q3_K_L.gguf) | Q3_K_L | 3.56GB |
| [zephyr-7b-beta-pl.IQ4_XS.gguf](https://huggingface.co/RichardErkhov/Nondzu_-_zephyr-7b-beta-pl-gguf/blob/main/zephyr-7b-beta-pl.IQ4_XS.gguf) | IQ4_XS | 3.67GB |
| [zephyr-7b-beta-pl.Q4_0.gguf](https://huggingface.co/RichardErkhov/Nondzu_-_zephyr-7b-beta-pl-gguf/blob/main/zephyr-7b-beta-pl.Q4_0.gguf) | Q4_0 | 3.83GB |
| [zephyr-7b-beta-pl.IQ4_NL.gguf](https://huggingface.co/RichardErkhov/Nondzu_-_zephyr-7b-beta-pl-gguf/blob/main/zephyr-7b-beta-pl.IQ4_NL.gguf) | IQ4_NL | 3.87GB |
| [zephyr-7b-beta-pl.Q4_K_S.gguf](https://huggingface.co/RichardErkhov/Nondzu_-_zephyr-7b-beta-pl-gguf/blob/main/zephyr-7b-beta-pl.Q4_K_S.gguf) | Q4_K_S | 3.86GB |
| [zephyr-7b-beta-pl.Q4_K.gguf](https://huggingface.co/RichardErkhov/Nondzu_-_zephyr-7b-beta-pl-gguf/blob/main/zephyr-7b-beta-pl.Q4_K.gguf) | Q4_K | 4.07GB |
| [zephyr-7b-beta-pl.Q4_K_M.gguf](https://huggingface.co/RichardErkhov/Nondzu_-_zephyr-7b-beta-pl-gguf/blob/main/zephyr-7b-beta-pl.Q4_K_M.gguf) | Q4_K_M | 4.07GB |
| [zephyr-7b-beta-pl.Q4_1.gguf](https://huggingface.co/RichardErkhov/Nondzu_-_zephyr-7b-beta-pl-gguf/blob/main/zephyr-7b-beta-pl.Q4_1.gguf) | Q4_1 | 4.24GB |
| [zephyr-7b-beta-pl.Q5_0.gguf](https://huggingface.co/RichardErkhov/Nondzu_-_zephyr-7b-beta-pl-gguf/blob/main/zephyr-7b-beta-pl.Q5_0.gguf) | Q5_0 | 4.65GB |
| [zephyr-7b-beta-pl.Q5_K_S.gguf](https://huggingface.co/RichardErkhov/Nondzu_-_zephyr-7b-beta-pl-gguf/blob/main/zephyr-7b-beta-pl.Q5_K_S.gguf) | Q5_K_S | 4.65GB |
| [zephyr-7b-beta-pl.Q5_K.gguf](https://huggingface.co/RichardErkhov/Nondzu_-_zephyr-7b-beta-pl-gguf/blob/main/zephyr-7b-beta-pl.Q5_K.gguf) | Q5_K | 4.78GB |
| [zephyr-7b-beta-pl.Q5_K_M.gguf](https://huggingface.co/RichardErkhov/Nondzu_-_zephyr-7b-beta-pl-gguf/blob/main/zephyr-7b-beta-pl.Q5_K_M.gguf) | Q5_K_M | 4.78GB |
| [zephyr-7b-beta-pl.Q5_1.gguf](https://huggingface.co/RichardErkhov/Nondzu_-_zephyr-7b-beta-pl-gguf/blob/main/zephyr-7b-beta-pl.Q5_1.gguf) | Q5_1 | 5.07GB |
| [zephyr-7b-beta-pl.Q6_K.gguf](https://huggingface.co/RichardErkhov/Nondzu_-_zephyr-7b-beta-pl-gguf/blob/main/zephyr-7b-beta-pl.Q6_K.gguf) | Q6_K | 5.53GB |
| [zephyr-7b-beta-pl.Q8_0.gguf](https://huggingface.co/RichardErkhov/Nondzu_-_zephyr-7b-beta-pl-gguf/blob/main/zephyr-7b-beta-pl.Q8_0.gguf) | Q8_0 | 7.17GB |
Original model description:
---
license: mit
language:
- pl
---
## Model Overview
The model is a result of advanced fine-tuning methods applied to a base model, focusing on enhancing its capabilities for specific Polish language datasets. It incorporates cutting-edge techniques and is built upon the Zephyr Beta model framework.
## Current Status: Alpha
- **Stage**: Alpha-Alpaca
## Training Details
I trained the model using 3xRTX 3090 for 163 hours.
[](https://github.com/OpenAccess-AI-Collective/axolotl)
## Quantised Model Links:
1. https://huggingface.co/Nondzu/zephyr-7b-beta-pl-exl2
2. https://huggingface.co/TheBloke/zephyr-7B-beta-pl-GGUF
3. https://huggingface.co/TheBloke/zephyr-7B-beta-pl-AWQ
4. https://huggingface.co/TheBloke/zephyr-7B-beta-pl-GPTQ
## Model Specifics
- **Base Model**: HuggingFaceH4/zephyr-7b-beta
- **Fine-Tuning Method**: QLORA
- **Primary Focus**: Polish language datasets
## Datasets:
- Dataset 1 Name: Lajonbot/alpaca-dolly-chrisociepa-instruction-only-polish
- Dataset 1 Link: [Lajonbot/alpaca-dolly-chrisociepa-instruction-only-polish](https://huggingface.co/datasets/Lajonbot/alpaca-dolly-chrisociepa-instruction-only-polish?row=16)
- Dataset 2 Name: klima7/polish-prose
- Dataset 2 Link: [klima7/polish-prose](https://huggingface.co/datasets/klima7/polish-prose)
## Usage Warning
As this is an experimental model, users should be aware of the following:
- **Reliability**: The model has not been fully tested and may exhibit unexpected behaviors or performance issues.
- **Updates**: The model is subject to change based on ongoing testing and feedback.
- **Data Sensitivity**: Users should exercise caution when using sensitive or private data, as the model's output and behavior are not fully predictable at this stage.
## Prompt template: Alpaca
```
Below is an instruction that describes a task. Write a response that appropriately completes the request.
### Instruction:
{prompt}
### Response:
```
## Example

## Feedback and Contribution
User feedback is crucial during this testing phase. We encourage users to provide feedback on model performance, issues encountered, and any suggestions for improvements. Contributions in terms of shared test results, datasets, or code improvements are also welcome.
---
**Disclaimer**: This experimental model is provided 'as is', without warranty of any kind. Users should use the model at their own risk. The creators or maintainers of the model are not responsible for any consequences arising from its use.

|
Meina/MeinaMix_V11 | Meina | 2023-07-16T19:53:46Z | 15,691 | 28 | diffusers | [
"diffusers",
"safetensors",
"art",
"anime",
"stable diffusion",
"text-to-image",
"en",
"license:creativeml-openrail-m",
"autotrain_compatible",
"endpoints_compatible",
"diffusers:StableDiffusionPipeline",
"region:us"
] | text-to-image | 2023-07-16T19:11:15Z | ---
license: creativeml-openrail-m
language:
- en
library_name: diffusers
pipeline_tag: text-to-image
tags:
- art
- anime
- stable diffusion
---
MeinaMix Objective is to be able to do good art with little prompting.
For examples and prompts, please checkout: https://civitai.com/models/7240/meinamix
I have a discord server where you can post images that you generated, discuss prompt and/or ask for help.
https://discord.gg/XC9nGZNDUd If you like one of my models and want to support their updates
I've made a ko-fi page; https://ko-fi.com/meina where you can pay me a coffee <3
And a Patreon page; https://www.patreon.com/MeinaMix where you can support me and get acess to beta of my models!
You may also try this model using Sinkin.ai: https://sinkin.ai/m/vln8Nwr
MeinaMix and the other of Meinas will ALWAYS be FREE.
Recommendations of use: Enable Quantization in K samplers.
Hires.fix is needed for prompts where the character is far away in order to make decent images, it drastically improve the quality of face and eyes!
Recommended parameters:
Sampler: Euler a: 40 to 60 steps.
Sampler: DPM++ SDE Karras: 20 to 30 steps.
Sampler: DPM++ 2M Karras: 20 to 40 steps.
CFG Scale: 7.
Resolutions: 512x768, 512x1024 for Portrait!
Resolutions: 768x512, 1024x512, 1536x512 for Landscape!
Hires.fix: R-ESRGAN 4x+Anime6b, with 10 steps at 0.3 up to 0.5 denoising.
Clip Skip: 2.
Negatives: ' (worst quality, low quality:1.4), (zombie, sketch, interlocked fingers, comic) ' |
textattack/distilbert-base-uncased-imdb | textattack | 2020-07-06T16:34:50Z | 15,690 | 0 | transformers | [
"transformers",
"pytorch",
"distilbert",
"text-classification",
"autotrain_compatible",
"endpoints_compatible",
"region:us"
] | text-classification | 2022-03-02T23:29:05Z | ## TextAttack Model Card
This `distilbert-base-uncased` model was fine-tuned for sequence classification using TextAttack
and the imdb dataset loaded using the `nlp` library. The model was fine-tuned
for 5 epochs with a batch size of 16, a learning
rate of 2e-05, and a maximum sequence length of 128.
Since this was a classification task, the model was trained with a cross-entropy loss function.
The best score the model achieved on this task was 0.88, as measured by the
eval set accuracy, found after 2 epochs.
For more information, check out [TextAttack on Github](https://github.com/QData/TextAttack).
|
mradermacher/L3-Inca-8B-v0.8-i1-GGUF | mradermacher | 2024-06-23T09:17:55Z | 15,690 | 0 | transformers | [
"transformers",
"gguf",
"mergekit",
"merge",
"en",
"base_model:Ppoyaa/L3-Inca-8B-v0.8",
"endpoints_compatible",
"region:us"
] | null | 2024-06-23T02:43:44Z | ---
base_model: Ppoyaa/L3-Inca-8B-v0.8
language:
- en
library_name: transformers
quantized_by: mradermacher
tags:
- mergekit
- merge
---
## About
<!-- ### quantize_version: 2 -->
<!-- ### output_tensor_quantised: 1 -->
<!-- ### convert_type: hf -->
<!-- ### vocab_type: -->
<!-- ### tags: nicoboss -->
weighted/imatrix quants of https://huggingface.co/Ppoyaa/L3-Inca-8B-v0.8
<!-- provided-files -->
static quants are available at https://huggingface.co/mradermacher/L3-Inca-8B-v0.8-GGUF
## Usage
If you are unsure how to use GGUF files, refer to one of [TheBloke's
READMEs](https://huggingface.co/TheBloke/KafkaLM-70B-German-V0.1-GGUF) for
more details, including on how to concatenate multi-part files.
## Provided Quants
(sorted by size, not necessarily quality. IQ-quants are often preferable over similar sized non-IQ quants)
| Link | Type | Size/GB | Notes |
|:-----|:-----|--------:|:------|
| [GGUF](https://huggingface.co/mradermacher/L3-Inca-8B-v0.8-i1-GGUF/resolve/main/L3-Inca-8B-v0.8.i1-IQ1_S.gguf) | i1-IQ1_S | 2.1 | for the desperate |
| [GGUF](https://huggingface.co/mradermacher/L3-Inca-8B-v0.8-i1-GGUF/resolve/main/L3-Inca-8B-v0.8.i1-IQ1_M.gguf) | i1-IQ1_M | 2.3 | mostly desperate |
| [GGUF](https://huggingface.co/mradermacher/L3-Inca-8B-v0.8-i1-GGUF/resolve/main/L3-Inca-8B-v0.8.i1-IQ2_XXS.gguf) | i1-IQ2_XXS | 2.5 | |
| [GGUF](https://huggingface.co/mradermacher/L3-Inca-8B-v0.8-i1-GGUF/resolve/main/L3-Inca-8B-v0.8.i1-IQ2_XS.gguf) | i1-IQ2_XS | 2.7 | |
| [GGUF](https://huggingface.co/mradermacher/L3-Inca-8B-v0.8-i1-GGUF/resolve/main/L3-Inca-8B-v0.8.i1-IQ2_S.gguf) | i1-IQ2_S | 2.9 | |
| [GGUF](https://huggingface.co/mradermacher/L3-Inca-8B-v0.8-i1-GGUF/resolve/main/L3-Inca-8B-v0.8.i1-IQ2_M.gguf) | i1-IQ2_M | 3.0 | |
| [GGUF](https://huggingface.co/mradermacher/L3-Inca-8B-v0.8-i1-GGUF/resolve/main/L3-Inca-8B-v0.8.i1-Q2_K.gguf) | i1-Q2_K | 3.3 | IQ3_XXS probably better |
| [GGUF](https://huggingface.co/mradermacher/L3-Inca-8B-v0.8-i1-GGUF/resolve/main/L3-Inca-8B-v0.8.i1-IQ3_XXS.gguf) | i1-IQ3_XXS | 3.4 | lower quality |
| [GGUF](https://huggingface.co/mradermacher/L3-Inca-8B-v0.8-i1-GGUF/resolve/main/L3-Inca-8B-v0.8.i1-IQ3_XS.gguf) | i1-IQ3_XS | 3.6 | |
| [GGUF](https://huggingface.co/mradermacher/L3-Inca-8B-v0.8-i1-GGUF/resolve/main/L3-Inca-8B-v0.8.i1-Q3_K_S.gguf) | i1-Q3_K_S | 3.8 | IQ3_XS probably better |
| [GGUF](https://huggingface.co/mradermacher/L3-Inca-8B-v0.8-i1-GGUF/resolve/main/L3-Inca-8B-v0.8.i1-IQ3_S.gguf) | i1-IQ3_S | 3.8 | beats Q3_K* |
| [GGUF](https://huggingface.co/mradermacher/L3-Inca-8B-v0.8-i1-GGUF/resolve/main/L3-Inca-8B-v0.8.i1-IQ3_M.gguf) | i1-IQ3_M | 3.9 | |
| [GGUF](https://huggingface.co/mradermacher/L3-Inca-8B-v0.8-i1-GGUF/resolve/main/L3-Inca-8B-v0.8.i1-Q3_K_M.gguf) | i1-Q3_K_M | 4.1 | IQ3_S probably better |
| [GGUF](https://huggingface.co/mradermacher/L3-Inca-8B-v0.8-i1-GGUF/resolve/main/L3-Inca-8B-v0.8.i1-Q3_K_L.gguf) | i1-Q3_K_L | 4.4 | IQ3_M probably better |
| [GGUF](https://huggingface.co/mradermacher/L3-Inca-8B-v0.8-i1-GGUF/resolve/main/L3-Inca-8B-v0.8.i1-IQ4_XS.gguf) | i1-IQ4_XS | 4.5 | |
| [GGUF](https://huggingface.co/mradermacher/L3-Inca-8B-v0.8-i1-GGUF/resolve/main/L3-Inca-8B-v0.8.i1-Q4_0.gguf) | i1-Q4_0 | 4.8 | fast, low quality |
| [GGUF](https://huggingface.co/mradermacher/L3-Inca-8B-v0.8-i1-GGUF/resolve/main/L3-Inca-8B-v0.8.i1-Q4_K_S.gguf) | i1-Q4_K_S | 4.8 | optimal size/speed/quality |
| [GGUF](https://huggingface.co/mradermacher/L3-Inca-8B-v0.8-i1-GGUF/resolve/main/L3-Inca-8B-v0.8.i1-Q4_K_M.gguf) | i1-Q4_K_M | 5.0 | fast, recommended |
| [GGUF](https://huggingface.co/mradermacher/L3-Inca-8B-v0.8-i1-GGUF/resolve/main/L3-Inca-8B-v0.8.i1-Q5_K_S.gguf) | i1-Q5_K_S | 5.7 | |
| [GGUF](https://huggingface.co/mradermacher/L3-Inca-8B-v0.8-i1-GGUF/resolve/main/L3-Inca-8B-v0.8.i1-Q5_K_M.gguf) | i1-Q5_K_M | 5.8 | |
| [GGUF](https://huggingface.co/mradermacher/L3-Inca-8B-v0.8-i1-GGUF/resolve/main/L3-Inca-8B-v0.8.i1-Q6_K.gguf) | i1-Q6_K | 6.7 | practically like static Q6_K |
Here is a handy graph by ikawrakow comparing some lower-quality quant
types (lower is better):

And here are Artefact2's thoughts on the matter:
https://gist.github.com/Artefact2/b5f810600771265fc1e39442288e8ec9
## FAQ / Model Request
See https://huggingface.co/mradermacher/model_requests for some answers to
questions you might have and/or if you want some other model quantized.
## Thanks
I thank my company, [nethype GmbH](https://www.nethype.de/), for letting
me use its servers and providing upgrades to my workstation to enable
this work in my free time. Additional thanks to [@nicoboss](https://huggingface.co/nicoboss) for giving me access to his hardware for calculating the imatrix for these quants.
<!-- end -->
|
mradermacher/L3-15B-Stheno-Passthrough-GGUF | mradermacher | 2024-06-21T16:30:26Z | 15,682 | 1 | transformers | [
"transformers",
"gguf",
"merge",
"mergekit",
"lazymergekit",
"Sao10K/L3-8B-Stheno-v3.2",
"Sao10K/L3-8B-Stheno-v3.1",
"not-for-all-audiences",
"en",
"base_model:jsfs11/L3-15B-Stheno-Passthrough",
"license:apache-2.0",
"endpoints_compatible",
"region:us"
] | null | 2024-06-21T15:04:35Z | ---
base_model: jsfs11/L3-15B-Stheno-Passthrough
language:
- en
library_name: transformers
license: apache-2.0
quantized_by: mradermacher
tags:
- merge
- mergekit
- lazymergekit
- Sao10K/L3-8B-Stheno-v3.2
- Sao10K/L3-8B-Stheno-v3.1
- not-for-all-audiences
---
## About
<!-- ### quantize_version: 2 -->
<!-- ### output_tensor_quantised: 1 -->
<!-- ### convert_type: hf -->
<!-- ### vocab_type: -->
<!-- ### tags: -->
static quants of https://huggingface.co/jsfs11/L3-15B-Stheno-Passthrough
<!-- provided-files -->
weighted/imatrix quants seem not to be available (by me) at this time. If they do not show up a week or so after the static ones, I have probably not planned for them. Feel free to request them by opening a Community Discussion.
## Usage
If you are unsure how to use GGUF files, refer to one of [TheBloke's
READMEs](https://huggingface.co/TheBloke/KafkaLM-70B-German-V0.1-GGUF) for
more details, including on how to concatenate multi-part files.
## Provided Quants
(sorted by size, not necessarily quality. IQ-quants are often preferable over similar sized non-IQ quants)
| Link | Type | Size/GB | Notes |
|:-----|:-----|--------:|:------|
| [GGUF](https://huggingface.co/mradermacher/L3-15B-Stheno-Passthrough-GGUF/resolve/main/L3-15B-Stheno-Passthrough.Q2_K.gguf) | Q2_K | 5.8 | |
| [GGUF](https://huggingface.co/mradermacher/L3-15B-Stheno-Passthrough-GGUF/resolve/main/L3-15B-Stheno-Passthrough.IQ3_XS.gguf) | IQ3_XS | 6.5 | |
| [GGUF](https://huggingface.co/mradermacher/L3-15B-Stheno-Passthrough-GGUF/resolve/main/L3-15B-Stheno-Passthrough.Q3_K_S.gguf) | Q3_K_S | 6.8 | |
| [GGUF](https://huggingface.co/mradermacher/L3-15B-Stheno-Passthrough-GGUF/resolve/main/L3-15B-Stheno-Passthrough.IQ3_S.gguf) | IQ3_S | 6.8 | beats Q3_K* |
| [GGUF](https://huggingface.co/mradermacher/L3-15B-Stheno-Passthrough-GGUF/resolve/main/L3-15B-Stheno-Passthrough.IQ3_M.gguf) | IQ3_M | 7.0 | |
| [GGUF](https://huggingface.co/mradermacher/L3-15B-Stheno-Passthrough-GGUF/resolve/main/L3-15B-Stheno-Passthrough.Q3_K_M.gguf) | Q3_K_M | 7.5 | lower quality |
| [GGUF](https://huggingface.co/mradermacher/L3-15B-Stheno-Passthrough-GGUF/resolve/main/L3-15B-Stheno-Passthrough.Q3_K_L.gguf) | Q3_K_L | 8.1 | |
| [GGUF](https://huggingface.co/mradermacher/L3-15B-Stheno-Passthrough-GGUF/resolve/main/L3-15B-Stheno-Passthrough.IQ4_XS.gguf) | IQ4_XS | 8.4 | |
| [GGUF](https://huggingface.co/mradermacher/L3-15B-Stheno-Passthrough-GGUF/resolve/main/L3-15B-Stheno-Passthrough.Q4_K_S.gguf) | Q4_K_S | 8.7 | fast, recommended |
| [GGUF](https://huggingface.co/mradermacher/L3-15B-Stheno-Passthrough-GGUF/resolve/main/L3-15B-Stheno-Passthrough.Q4_K_M.gguf) | Q4_K_M | 9.2 | fast, recommended |
| [GGUF](https://huggingface.co/mradermacher/L3-15B-Stheno-Passthrough-GGUF/resolve/main/L3-15B-Stheno-Passthrough.Q5_K_S.gguf) | Q5_K_S | 10.5 | |
| [GGUF](https://huggingface.co/mradermacher/L3-15B-Stheno-Passthrough-GGUF/resolve/main/L3-15B-Stheno-Passthrough.Q5_K_M.gguf) | Q5_K_M | 10.8 | |
| [GGUF](https://huggingface.co/mradermacher/L3-15B-Stheno-Passthrough-GGUF/resolve/main/L3-15B-Stheno-Passthrough.Q6_K.gguf) | Q6_K | 12.4 | very good quality |
| [GGUF](https://huggingface.co/mradermacher/L3-15B-Stheno-Passthrough-GGUF/resolve/main/L3-15B-Stheno-Passthrough.Q8_0.gguf) | Q8_0 | 16.1 | fast, best quality |
Here is a handy graph by ikawrakow comparing some lower-quality quant
types (lower is better):

And here are Artefact2's thoughts on the matter:
https://gist.github.com/Artefact2/b5f810600771265fc1e39442288e8ec9
## FAQ / Model Request
See https://huggingface.co/mradermacher/model_requests for some answers to
questions you might have and/or if you want some other model quantized.
## Thanks
I thank my company, [nethype GmbH](https://www.nethype.de/), for letting
me use its servers and providing upgrades to my workstation to enable
this work in my free time.
<!-- end -->
|
microsoft/swinv2-tiny-patch4-window8-256 | microsoft | 2022-12-10T10:01:54Z | 15,676 | 7 | transformers | [
"transformers",
"pytorch",
"swinv2",
"image-classification",
"vision",
"dataset:imagenet-1k",
"arxiv:2111.09883",
"license:apache-2.0",
"autotrain_compatible",
"endpoints_compatible",
"region:us"
] | image-classification | 2022-06-14T06:00:27Z | ---
license: apache-2.0
tags:
- vision
- image-classification
datasets:
- imagenet-1k
widget:
- src: https://huggingface.co/datasets/mishig/sample_images/resolve/main/tiger.jpg
example_title: Tiger
- src: https://huggingface.co/datasets/mishig/sample_images/resolve/main/teapot.jpg
example_title: Teapot
- src: https://huggingface.co/datasets/mishig/sample_images/resolve/main/palace.jpg
example_title: Palace
---
# Swin Transformer v2 (tiny-sized model)
Swin Transformer v2 model pre-trained on ImageNet-1k at resolution 256x256. It was introduced in the paper [Swin Transformer V2: Scaling Up Capacity and Resolution](https://arxiv.org/abs/2111.09883) by Liu et al. and first released in [this repository](https://github.com/microsoft/Swin-Transformer).
Disclaimer: The team releasing Swin Transformer v2 did not write a model card for this model so this model card has been written by the Hugging Face team.
## Model description
The Swin Transformer is a type of Vision Transformer. It builds hierarchical feature maps by merging image patches (shown in gray) in deeper layers and has linear computation complexity to input image size due to computation of self-attention only within each local window (shown in red). It can thus serve as a general-purpose backbone for both image classification and dense recognition tasks. In contrast, previous vision Transformers produce feature maps of a single low resolution and have quadratic computation complexity to input image size due to computation of self-attention globally.
Swin Transformer v2 adds 3 main improvements: 1) a residual-post-norm method combined with cosine attention to improve training stability; 2) a log-spaced continuous position bias method to effectively transfer models pre-trained using low-resolution images to downstream tasks with high-resolution inputs; 3) a self-supervised pre-training method, SimMIM, to reduce the needs of vast labeled images.
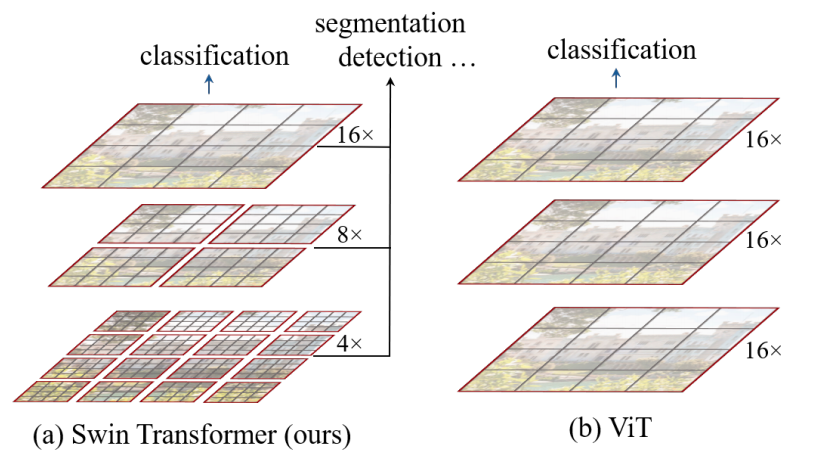
[Source](https://paperswithcode.com/method/swin-transformer)
## Intended uses & limitations
You can use the raw model for image classification. See the [model hub](https://huggingface.co/models?search=swinv2) to look for
fine-tuned versions on a task that interests you.
### How to use
Here is how to use this model to classify an image of the COCO 2017 dataset into one of the 1,000 ImageNet classes:
```python
from transformers import AutoImageProcessor, AutoModelForImageClassification
from PIL import Image
import requests
url = "http://images.cocodataset.org/val2017/000000039769.jpg"
image = Image.open(requests.get(url, stream=True).raw)
processor = AutoImageProcessor.from_pretrained("microsoft/swinv2-tiny-patch4-window8-256")
model = AutoModelForImageClassification.from_pretrained("microsoft/swinv2-tiny-patch4-window8-256")
inputs = processor(images=image, return_tensors="pt")
outputs = model(**inputs)
logits = outputs.logits
# model predicts one of the 1000 ImageNet classes
predicted_class_idx = logits.argmax(-1).item()
print("Predicted class:", model.config.id2label[predicted_class_idx])
```
For more code examples, we refer to the [documentation](https://huggingface.co/transformers/model_doc/swinv2.html#).
### BibTeX entry and citation info
```bibtex
@article{DBLP:journals/corr/abs-2111-09883,
author = {Ze Liu and
Han Hu and
Yutong Lin and
Zhuliang Yao and
Zhenda Xie and
Yixuan Wei and
Jia Ning and
Yue Cao and
Zheng Zhang and
Li Dong and
Furu Wei and
Baining Guo},
title = {Swin Transformer {V2:} Scaling Up Capacity and Resolution},
journal = {CoRR},
volume = {abs/2111.09883},
year = {2021},
url = {https://arxiv.org/abs/2111.09883},
eprinttype = {arXiv},
eprint = {2111.09883},
timestamp = {Thu, 02 Dec 2021 15:54:22 +0100},
biburl = {https://dblp.org/rec/journals/corr/abs-2111-09883.bib},
bibsource = {dblp computer science bibliography, https://dblp.org}
}
``` |
mradermacher/Llama-3-Umbral-Mind-Replete-8B-GGUF | mradermacher | 2024-06-27T05:28:20Z | 15,674 | 0 | transformers | [
"transformers",
"gguf",
"mergekit",
"merge",
"en",
"base_model:mpasila/Llama-3-Umbral-Mind-Replete-8B",
"license:llama3",
"endpoints_compatible",
"region:us"
] | null | 2024-06-27T03:13:49Z | ---
base_model: mpasila/Llama-3-Umbral-Mind-Replete-8B
language:
- en
library_name: transformers
license: llama3
quantized_by: mradermacher
tags:
- mergekit
- merge
---
## About
<!-- ### quantize_version: 2 -->
<!-- ### output_tensor_quantised: 1 -->
<!-- ### convert_type: hf -->
<!-- ### vocab_type: -->
<!-- ### tags: -->
static quants of https://huggingface.co/mpasila/Llama-3-Umbral-Mind-Replete-8B
<!-- provided-files -->
weighted/imatrix quants are available at https://huggingface.co/mradermacher/Llama-3-Umbral-Mind-Replete-8B-i1-GGUF
## Usage
If you are unsure how to use GGUF files, refer to one of [TheBloke's
READMEs](https://huggingface.co/TheBloke/KafkaLM-70B-German-V0.1-GGUF) for
more details, including on how to concatenate multi-part files.
## Provided Quants
(sorted by size, not necessarily quality. IQ-quants are often preferable over similar sized non-IQ quants)
| Link | Type | Size/GB | Notes |
|:-----|:-----|--------:|:------|
| [GGUF](https://huggingface.co/mradermacher/Llama-3-Umbral-Mind-Replete-8B-GGUF/resolve/main/Llama-3-Umbral-Mind-Replete-8B.Q2_K.gguf) | Q2_K | 3.3 | |
| [GGUF](https://huggingface.co/mradermacher/Llama-3-Umbral-Mind-Replete-8B-GGUF/resolve/main/Llama-3-Umbral-Mind-Replete-8B.IQ3_XS.gguf) | IQ3_XS | 3.6 | |
| [GGUF](https://huggingface.co/mradermacher/Llama-3-Umbral-Mind-Replete-8B-GGUF/resolve/main/Llama-3-Umbral-Mind-Replete-8B.Q3_K_S.gguf) | Q3_K_S | 3.8 | |
| [GGUF](https://huggingface.co/mradermacher/Llama-3-Umbral-Mind-Replete-8B-GGUF/resolve/main/Llama-3-Umbral-Mind-Replete-8B.IQ3_S.gguf) | IQ3_S | 3.8 | beats Q3_K* |
| [GGUF](https://huggingface.co/mradermacher/Llama-3-Umbral-Mind-Replete-8B-GGUF/resolve/main/Llama-3-Umbral-Mind-Replete-8B.IQ3_M.gguf) | IQ3_M | 3.9 | |
| [GGUF](https://huggingface.co/mradermacher/Llama-3-Umbral-Mind-Replete-8B-GGUF/resolve/main/Llama-3-Umbral-Mind-Replete-8B.Q3_K_M.gguf) | Q3_K_M | 4.1 | lower quality |
| [GGUF](https://huggingface.co/mradermacher/Llama-3-Umbral-Mind-Replete-8B-GGUF/resolve/main/Llama-3-Umbral-Mind-Replete-8B.Q3_K_L.gguf) | Q3_K_L | 4.4 | |
| [GGUF](https://huggingface.co/mradermacher/Llama-3-Umbral-Mind-Replete-8B-GGUF/resolve/main/Llama-3-Umbral-Mind-Replete-8B.IQ4_XS.gguf) | IQ4_XS | 4.6 | |
| [GGUF](https://huggingface.co/mradermacher/Llama-3-Umbral-Mind-Replete-8B-GGUF/resolve/main/Llama-3-Umbral-Mind-Replete-8B.Q4_K_S.gguf) | Q4_K_S | 4.8 | fast, recommended |
| [GGUF](https://huggingface.co/mradermacher/Llama-3-Umbral-Mind-Replete-8B-GGUF/resolve/main/Llama-3-Umbral-Mind-Replete-8B.Q4_K_M.gguf) | Q4_K_M | 5.0 | fast, recommended |
| [GGUF](https://huggingface.co/mradermacher/Llama-3-Umbral-Mind-Replete-8B-GGUF/resolve/main/Llama-3-Umbral-Mind-Replete-8B.Q5_K_S.gguf) | Q5_K_S | 5.7 | |
| [GGUF](https://huggingface.co/mradermacher/Llama-3-Umbral-Mind-Replete-8B-GGUF/resolve/main/Llama-3-Umbral-Mind-Replete-8B.Q5_K_M.gguf) | Q5_K_M | 5.8 | |
| [GGUF](https://huggingface.co/mradermacher/Llama-3-Umbral-Mind-Replete-8B-GGUF/resolve/main/Llama-3-Umbral-Mind-Replete-8B.Q6_K.gguf) | Q6_K | 6.7 | very good quality |
| [GGUF](https://huggingface.co/mradermacher/Llama-3-Umbral-Mind-Replete-8B-GGUF/resolve/main/Llama-3-Umbral-Mind-Replete-8B.Q8_0.gguf) | Q8_0 | 8.6 | fast, best quality |
| [GGUF](https://huggingface.co/mradermacher/Llama-3-Umbral-Mind-Replete-8B-GGUF/resolve/main/Llama-3-Umbral-Mind-Replete-8B.f16.gguf) | f16 | 16.2 | 16 bpw, overkill |
Here is a handy graph by ikawrakow comparing some lower-quality quant
types (lower is better):

And here are Artefact2's thoughts on the matter:
https://gist.github.com/Artefact2/b5f810600771265fc1e39442288e8ec9
## FAQ / Model Request
See https://huggingface.co/mradermacher/model_requests for some answers to
questions you might have and/or if you want some other model quantized.
## Thanks
I thank my company, [nethype GmbH](https://www.nethype.de/), for letting
me use its servers and providing upgrades to my workstation to enable
this work in my free time.
<!-- end -->
|
matthewburke/korean_sentiment | matthewburke | 2022-01-16T02:31:37Z | 15,668 | 13 | transformers | [
"transformers",
"pytorch",
"electra",
"text-classification",
"autotrain_compatible",
"endpoints_compatible",
"region:us"
] | text-classification | 2022-03-02T23:29:05Z | ```
from transformers import pipeline
classifier = pipeline("text-classification", model="matthewburke/korean_sentiment")
custom_tweet = "영화 재밌다."
preds = classifier(custom_tweet, return_all_scores=True)
is_positive = preds[0][1]['score'] > 0.5
```
|
mesolitica/sentiment-analysis-nanot5-small-malaysian-cased | mesolitica | 2023-10-08T07:38:48Z | 15,655 | 0 | transformers | [
"transformers",
"safetensors",
"t5",
"text-classification",
"autotrain_compatible",
"endpoints_compatible",
"text-generation-inference",
"region:us"
] | text-classification | 2023-10-08T07:38:17Z | Entry not found |
Yntec/Luxury | Yntec | 2024-05-06T20:17:30Z | 15,632 | 2 | diffusers | [
"diffusers",
"safetensors",
"Aesthetic",
"Fantasy",
"Realistic",
"Merjic",
"iamxenos",
"RIXYN",
"Barons",
"stable-diffusion",
"stable-diffusion-diffusers",
"text-to-image",
"license:creativeml-openrail-m",
"autotrain_compatible",
"endpoints_compatible",
"diffusers:StableDiffusionPipeline",
"region:us"
] | text-to-image | 2024-05-03T22:25:14Z | ---
license: creativeml-openrail-m
library_name: diffusers
pipeline_tag: text-to-image
tags:
- Aesthetic
- Fantasy
- Realistic
- Merjic
- iamxenos
- RIXYN
- Barons
- stable-diffusion
- stable-diffusion-diffusers
- diffusers
- text-to-image
---
# Luxury
Samples and prompts:

(Click for larger)
Top left: gdmint luxury modern interior design
Top right: 2 little sisters, sitting in a luxurious dark modern mansion wearing a sweater and comfortable pants, drinking juice, bright morning sunlight, (masterpiece:1.2) (photorealistic:1.2) (bokeh) (best quality) (detailed skin:1.3) (intricate details) (8k) (HDR) (analog film) (canon d5) (cinematic lighting) (sharp focus)
Bottom left: a cute girl wearing luxury dress, red dress, shiny dress, 4k, high-res, masterpiece, best quality, head:1.3,((Hasselblad photography)), finely detailed skin, sharp focus, (cinematic lighting), collarbone, night, soft lighting, dynamic angle, [:(detailed face:1.2):0.2], outside, luxury dress, sung
Bottom right: photo of 1car, sporty, fast, sleek, sexy, aggressive, high performance, daytime, futuristic cityscape, ultra-high-definition, photorealistic, 8k uhd, high-quality, ultra sharp detail. Mercedes oue
MajicmixLux mixed with Cocacola (which includes many models).
Sample and prompt by digiplay:
best quality,1girl,milkyway starry night stars sky night,luxurious look,close-up ,blonde hair ,

Original pages:
https://civitai.com/models/56967?modelVersionId=61379
https://huggingface.co/Yntec/Cryptids
https://huggingface.co/Yntec/CocaCola
https://civitai.com/models/142552?modelVersionId=163068 (Kitsch-In-Sync v2)
https://civitai.com/models/21493/hellmix?modelVersionId=25632 |
Muennighoff/tiny-random-bert | Muennighoff | 2021-11-19T16:18:11Z | 15,620 | 0 | transformers | [
"transformers",
"pytorch",
"tf",
"bert",
"endpoints_compatible",
"region:us"
] | null | 2022-03-02T23:29:04Z | Entry not found |
uclanlp/visualbert-vqa-coco-pre | uclanlp | 2021-05-31T11:34:13Z | 15,614 | 5 | transformers | [
"transformers",
"pytorch",
"visual_bert",
"pretraining",
"endpoints_compatible",
"region:us"
] | null | 2022-03-02T23:29:05Z | Entry not found |
RichardErkhov/fblgit_-_una-cybertron-7b-v1-fp16-gguf | RichardErkhov | 2024-07-01T01:20:05Z | 15,613 | 0 | null | [
"gguf",
"region:us"
] | null | 2024-06-30T22:58:55Z | Quantization made by Richard Erkhov.
[Github](https://github.com/RichardErkhov)
[Discord](https://discord.gg/pvy7H8DZMG)
[Request more models](https://github.com/RichardErkhov/quant_request)
una-cybertron-7b-v1-fp16 - GGUF
- Model creator: https://huggingface.co/fblgit/
- Original model: https://huggingface.co/fblgit/una-cybertron-7b-v1-fp16/
| Name | Quant method | Size |
| ---- | ---- | ---- |
| [una-cybertron-7b-v1-fp16.Q2_K.gguf](https://huggingface.co/RichardErkhov/fblgit_-_una-cybertron-7b-v1-fp16-gguf/blob/main/una-cybertron-7b-v1-fp16.Q2_K.gguf) | Q2_K | 2.53GB |
| [una-cybertron-7b-v1-fp16.IQ3_XS.gguf](https://huggingface.co/RichardErkhov/fblgit_-_una-cybertron-7b-v1-fp16-gguf/blob/main/una-cybertron-7b-v1-fp16.IQ3_XS.gguf) | IQ3_XS | 2.81GB |
| [una-cybertron-7b-v1-fp16.IQ3_S.gguf](https://huggingface.co/RichardErkhov/fblgit_-_una-cybertron-7b-v1-fp16-gguf/blob/main/una-cybertron-7b-v1-fp16.IQ3_S.gguf) | IQ3_S | 2.96GB |
| [una-cybertron-7b-v1-fp16.Q3_K_S.gguf](https://huggingface.co/RichardErkhov/fblgit_-_una-cybertron-7b-v1-fp16-gguf/blob/main/una-cybertron-7b-v1-fp16.Q3_K_S.gguf) | Q3_K_S | 2.95GB |
| [una-cybertron-7b-v1-fp16.IQ3_M.gguf](https://huggingface.co/RichardErkhov/fblgit_-_una-cybertron-7b-v1-fp16-gguf/blob/main/una-cybertron-7b-v1-fp16.IQ3_M.gguf) | IQ3_M | 3.06GB |
| [una-cybertron-7b-v1-fp16.Q3_K.gguf](https://huggingface.co/RichardErkhov/fblgit_-_una-cybertron-7b-v1-fp16-gguf/blob/main/una-cybertron-7b-v1-fp16.Q3_K.gguf) | Q3_K | 3.28GB |
| [una-cybertron-7b-v1-fp16.Q3_K_M.gguf](https://huggingface.co/RichardErkhov/fblgit_-_una-cybertron-7b-v1-fp16-gguf/blob/main/una-cybertron-7b-v1-fp16.Q3_K_M.gguf) | Q3_K_M | 3.28GB |
| [una-cybertron-7b-v1-fp16.Q3_K_L.gguf](https://huggingface.co/RichardErkhov/fblgit_-_una-cybertron-7b-v1-fp16-gguf/blob/main/una-cybertron-7b-v1-fp16.Q3_K_L.gguf) | Q3_K_L | 3.56GB |
| [una-cybertron-7b-v1-fp16.IQ4_XS.gguf](https://huggingface.co/RichardErkhov/fblgit_-_una-cybertron-7b-v1-fp16-gguf/blob/main/una-cybertron-7b-v1-fp16.IQ4_XS.gguf) | IQ4_XS | 3.67GB |
| [una-cybertron-7b-v1-fp16.Q4_0.gguf](https://huggingface.co/RichardErkhov/fblgit_-_una-cybertron-7b-v1-fp16-gguf/blob/main/una-cybertron-7b-v1-fp16.Q4_0.gguf) | Q4_0 | 3.83GB |
| [una-cybertron-7b-v1-fp16.IQ4_NL.gguf](https://huggingface.co/RichardErkhov/fblgit_-_una-cybertron-7b-v1-fp16-gguf/blob/main/una-cybertron-7b-v1-fp16.IQ4_NL.gguf) | IQ4_NL | 3.87GB |
| [una-cybertron-7b-v1-fp16.Q4_K_S.gguf](https://huggingface.co/RichardErkhov/fblgit_-_una-cybertron-7b-v1-fp16-gguf/blob/main/una-cybertron-7b-v1-fp16.Q4_K_S.gguf) | Q4_K_S | 3.86GB |
| [una-cybertron-7b-v1-fp16.Q4_K.gguf](https://huggingface.co/RichardErkhov/fblgit_-_una-cybertron-7b-v1-fp16-gguf/blob/main/una-cybertron-7b-v1-fp16.Q4_K.gguf) | Q4_K | 4.07GB |
| [una-cybertron-7b-v1-fp16.Q4_K_M.gguf](https://huggingface.co/RichardErkhov/fblgit_-_una-cybertron-7b-v1-fp16-gguf/blob/main/una-cybertron-7b-v1-fp16.Q4_K_M.gguf) | Q4_K_M | 4.07GB |
| [una-cybertron-7b-v1-fp16.Q4_1.gguf](https://huggingface.co/RichardErkhov/fblgit_-_una-cybertron-7b-v1-fp16-gguf/blob/main/una-cybertron-7b-v1-fp16.Q4_1.gguf) | Q4_1 | 4.24GB |
| [una-cybertron-7b-v1-fp16.Q5_0.gguf](https://huggingface.co/RichardErkhov/fblgit_-_una-cybertron-7b-v1-fp16-gguf/blob/main/una-cybertron-7b-v1-fp16.Q5_0.gguf) | Q5_0 | 4.65GB |
| [una-cybertron-7b-v1-fp16.Q5_K_S.gguf](https://huggingface.co/RichardErkhov/fblgit_-_una-cybertron-7b-v1-fp16-gguf/blob/main/una-cybertron-7b-v1-fp16.Q5_K_S.gguf) | Q5_K_S | 4.65GB |
| [una-cybertron-7b-v1-fp16.Q5_K.gguf](https://huggingface.co/RichardErkhov/fblgit_-_una-cybertron-7b-v1-fp16-gguf/blob/main/una-cybertron-7b-v1-fp16.Q5_K.gguf) | Q5_K | 4.78GB |
| [una-cybertron-7b-v1-fp16.Q5_K_M.gguf](https://huggingface.co/RichardErkhov/fblgit_-_una-cybertron-7b-v1-fp16-gguf/blob/main/una-cybertron-7b-v1-fp16.Q5_K_M.gguf) | Q5_K_M | 4.78GB |
| [una-cybertron-7b-v1-fp16.Q5_1.gguf](https://huggingface.co/RichardErkhov/fblgit_-_una-cybertron-7b-v1-fp16-gguf/blob/main/una-cybertron-7b-v1-fp16.Q5_1.gguf) | Q5_1 | 5.07GB |
| [una-cybertron-7b-v1-fp16.Q6_K.gguf](https://huggingface.co/RichardErkhov/fblgit_-_una-cybertron-7b-v1-fp16-gguf/blob/main/una-cybertron-7b-v1-fp16.Q6_K.gguf) | Q6_K | 5.53GB |
| [una-cybertron-7b-v1-fp16.Q8_0.gguf](https://huggingface.co/RichardErkhov/fblgit_-_una-cybertron-7b-v1-fp16-gguf/blob/main/una-cybertron-7b-v1-fp16.Q8_0.gguf) | Q8_0 | 7.17GB |
Original model description:
---
license: apache-2.0
library_name: transformers
tags:
- juanako
- UNA
datasets:
- fblgit/tree-of-knowledge
- Open-Orca/SlimOrca-Dedup
- HuggingFaceH4/ultrafeedback_binarized
model-index:
- name: una-cybertron-7b-v1-fp16
results:
- task:
type: text-generation
name: Text Generation
dataset:
name: AI2 Reasoning Challenge (25-Shot)
type: ai2_arc
config: ARC-Challenge
split: test
args:
num_few_shot: 25
metrics:
- type: acc_norm
value: 68.43
name: normalized accuracy
source:
url: https://huggingface.co/spaces/HuggingFaceH4/open_llm_leaderboard?query=fblgit/una-cybertron-7b-v1-fp16
name: Open LLM Leaderboard
- task:
type: text-generation
name: Text Generation
dataset:
name: HellaSwag (10-Shot)
type: hellaswag
split: validation
args:
num_few_shot: 10
metrics:
- type: acc_norm
value: 85.42
name: normalized accuracy
source:
url: https://huggingface.co/spaces/HuggingFaceH4/open_llm_leaderboard?query=fblgit/una-cybertron-7b-v1-fp16
name: Open LLM Leaderboard
- task:
type: text-generation
name: Text Generation
dataset:
name: MMLU (5-Shot)
type: cais/mmlu
config: all
split: test
args:
num_few_shot: 5
metrics:
- type: acc
value: 63.34
name: accuracy
source:
url: https://huggingface.co/spaces/HuggingFaceH4/open_llm_leaderboard?query=fblgit/una-cybertron-7b-v1-fp16
name: Open LLM Leaderboard
- task:
type: text-generation
name: Text Generation
dataset:
name: TruthfulQA (0-shot)
type: truthful_qa
config: multiple_choice
split: validation
args:
num_few_shot: 0
metrics:
- type: mc2
value: 63.28
source:
url: https://huggingface.co/spaces/HuggingFaceH4/open_llm_leaderboard?query=fblgit/una-cybertron-7b-v1-fp16
name: Open LLM Leaderboard
- task:
type: text-generation
name: Text Generation
dataset:
name: Winogrande (5-shot)
type: winogrande
config: winogrande_xl
split: validation
args:
num_few_shot: 5
metrics:
- type: acc
value: 81.37
name: accuracy
source:
url: https://huggingface.co/spaces/HuggingFaceH4/open_llm_leaderboard?query=fblgit/una-cybertron-7b-v1-fp16
name: Open LLM Leaderboard
- task:
type: text-generation
name: Text Generation
dataset:
name: GSM8k (5-shot)
type: gsm8k
config: main
split: test
args:
num_few_shot: 5
metrics:
- type: acc
value: 55.12
name: accuracy
source:
url: https://huggingface.co/spaces/HuggingFaceH4/open_llm_leaderboard?query=fblgit/una-cybertron-7b-v1-fp16
name: Open LLM Leaderboard
---
# Model Card for una-cybertron-7b-v1 (UNA: Uniform Neural Alignment)
We strike back, introducing **Cybertron 7B v1** a 7B MistralAI based model, best on it's series. Trained on SFT, DPO and UNA (Unified Neural Alignment) on multiple datasets.
He scores **64.60**+ on HF LeaderTests (without DROP for now).
Scoring **#1** at 2 December 2023:
| Model | Average | ARC (25-s) | HellaSwag (10-s) | MMLU (5-s) | TruthfulQA (MC) (0-s) | Winogrande (5-s) | GSM8K (5-s) |
| --- | --- | --- | --- | --- | --- | --- | --- |
| [mistralai/Mistral-7B-v0.1](https://huggingface.co/mistralai/Mistral-7B-v0.1) | 60.97 | 59.98 | 83.31 | 64.16 | 42.15 | 78.37 | 37.83 |
| [perlthoughts/Chupacabra-7B-v2](https://huggingface.co/perlthoughts/Chupacabra-7B-v2) | 63.54 | 66.47 | 85.17 | 64.49 | 57.6 | 79.16 | 28.35 |
| [fblgit/una-cybertron-7b-v1](https://huggingface.co/fblgit/una-cybertron-7b-v1) | **64.60** | **68.17** | 85.14 | 62.07 | **63.98** | **80.9** | 27.34 |
The model excels in mathematics, logic, reasoning, overall very smart.
## Model Details
Adiestrated with UNA: Uniform Neural Alignment technique (paper going out soon).
### Model Description
- **Developed by:** [juanako.ai](https://juanako.ai)
- **Author:** [Xavier M.]([email protected])
- **Model type:** MistralAI 7B
- **Funded by Cybertron's H100's**
### Prompt
The model is very good, works well on almost any prompt but ChatML format and Alpaca System gets the best
```
<|im_start|>system
- You are a helpful assistant chatbot trained by MosaicML.
- You answer questions.
- You are excited to be able to help the user, but will refuse to do anything that could be considered harmful to the user.
- You are more than just an information source, you are also able to write poetry, short stories, and make jokes.<|im_end|>
<|im_start|>user
Explain QKV<|im_end|>
<|im_start|>assistant
```
```
### Assistant: I am StableVicuna, a large language model created by CarperAI. I am here to chat!
### Human: Explain QKV
### Assistant:
```
```
[Round <|round|>]
问:Explain QKV
答:
```
```
[Round <|round|>]
Question:Explain QKV
Answer:
```
```
Question:Explain QKV
Answer:
```
## Evaluation
```
| Tasks |Version|Shots | Metric |Value | |Stderr|
|--------------|-------|------|--------|-----:|---|-----:|
|arc_challenge | | 25 |acc_norm|0.6817|± |0.0136|
|truthfulqa_mc2| | 0 |acc |0.6398|± |0.0151|
|hellaswag | | 10 |acc_norm|0.8492|± |0.0036|
|winogrande | | 0 |acc |0.809 |± |0.011 |
|gsm8k | | 5 |acc |0.2733|± |0.0137|
|mmlu | | 5 |acc |0.6207|± |0.1230|
| |average| |acc |0.6456| | |
| Groups |Version|Filter|n-shot|Metric|Value | |Stderr|
|------------------|-------|------|-----:|------|-----:|---|-----:|
|mmlu |N/A |none | 0|acc |0.6207|_ |0.1230|
| - humanities |N/A |none | 5|acc |0.5675|_ |0.1125|
| - other |N/A |none | 5|acc |0.6933|_ |0.1108|
| - social_sciences|N/A |none | 5|acc |0.7270|_ |0.0666|
| - stem |N/A |none | 5|acc |0.5249|_ |0.1311|
```
### Framework versions
- Transformers 4.35.0-UNA
- Pytorch 2.1.0
- Datasets 2.14.6
- Tokenizers 0.14.1
### Citations
If you find Cybertron, Juanako or any of our models useful, specially if you use it for your big brand.. cite please:
```
@misc{unacybertron7a,
title={Cybertron: Uniform Neural Alignment},
author={Xavier Murias},
year={2023},
publisher = {HuggingFace},
journal = {HuggingFace repository},
howpublished = {\url{https://huggingface.co/fblgit/una-cybertron-7b-v1}},
}
```
# [Open LLM Leaderboard Evaluation Results](https://huggingface.co/spaces/HuggingFaceH4/open_llm_leaderboard)
Detailed results can be found [here](https://huggingface.co/datasets/open-llm-leaderboard/details_fblgit__una-cybertron-7b-v1-fp16)
| Metric |Value|
|---------------------------------|----:|
|Avg. |69.49|
|AI2 Reasoning Challenge (25-Shot)|68.43|
|HellaSwag (10-Shot) |85.42|
|MMLU (5-Shot) |63.34|
|TruthfulQA (0-shot) |63.28|
|Winogrande (5-shot) |81.37|
|GSM8k (5-shot) |55.12|
|
timm/tf_efficientnetv2_b0.in1k | timm | 2023-04-27T21:38:45Z | 15,596 | 0 | timm | [
"timm",
"pytorch",
"safetensors",
"image-classification",
"dataset:imagenet-1k",
"arxiv:2104.00298",
"license:apache-2.0",
"region:us"
] | image-classification | 2022-12-13T00:14:15Z | ---
tags:
- image-classification
- timm
library_name: timm
license: apache-2.0
datasets:
- imagenet-1k
---
# Model card for tf_efficientnetv2_b0.in1k
A EfficientNet-v2 image classification model. Trained on ImageNet-1k in Tensorflow by paper authors, ported to PyTorch by Ross Wightman.
## Model Details
- **Model Type:** Image classification / feature backbone
- **Model Stats:**
- Params (M): 7.1
- GMACs: 0.5
- Activations (M): 3.5
- Image size: train = 192 x 192, test = 224 x 224
- **Papers:**
- EfficientNetV2: Smaller Models and Faster Training: https://arxiv.org/abs/2104.00298
- **Dataset:** ImageNet-1k
- **Original:** https://github.com/tensorflow/tpu/tree/master/models/official/efficientnet
## Model Usage
### Image Classification
```python
from urllib.request import urlopen
from PIL import Image
import timm
img = Image.open(urlopen(
'https://huggingface.co/datasets/huggingface/documentation-images/resolve/main/beignets-task-guide.png'
))
model = timm.create_model('tf_efficientnetv2_b0.in1k', pretrained=True)
model = model.eval()
# get model specific transforms (normalization, resize)
data_config = timm.data.resolve_model_data_config(model)
transforms = timm.data.create_transform(**data_config, is_training=False)
output = model(transforms(img).unsqueeze(0)) # unsqueeze single image into batch of 1
top5_probabilities, top5_class_indices = torch.topk(output.softmax(dim=1) * 100, k=5)
```
### Feature Map Extraction
```python
from urllib.request import urlopen
from PIL import Image
import timm
img = Image.open(urlopen(
'https://huggingface.co/datasets/huggingface/documentation-images/resolve/main/beignets-task-guide.png'
))
model = timm.create_model(
'tf_efficientnetv2_b0.in1k',
pretrained=True,
features_only=True,
)
model = model.eval()
# get model specific transforms (normalization, resize)
data_config = timm.data.resolve_model_data_config(model)
transforms = timm.data.create_transform(**data_config, is_training=False)
output = model(transforms(img).unsqueeze(0)) # unsqueeze single image into batch of 1
for o in output:
# print shape of each feature map in output
# e.g.:
# torch.Size([1, 16, 96, 96])
# torch.Size([1, 32, 48, 48])
# torch.Size([1, 48, 24, 24])
# torch.Size([1, 112, 12, 12])
# torch.Size([1, 192, 6, 6])
print(o.shape)
```
### Image Embeddings
```python
from urllib.request import urlopen
from PIL import Image
import timm
img = Image.open(urlopen(
'https://huggingface.co/datasets/huggingface/documentation-images/resolve/main/beignets-task-guide.png'
))
model = timm.create_model(
'tf_efficientnetv2_b0.in1k',
pretrained=True,
num_classes=0, # remove classifier nn.Linear
)
model = model.eval()
# get model specific transforms (normalization, resize)
data_config = timm.data.resolve_model_data_config(model)
transforms = timm.data.create_transform(**data_config, is_training=False)
output = model(transforms(img).unsqueeze(0)) # output is (batch_size, num_features) shaped tensor
# or equivalently (without needing to set num_classes=0)
output = model.forward_features(transforms(img).unsqueeze(0))
# output is unpooled, a (1, 1280, 6, 6) shaped tensor
output = model.forward_head(output, pre_logits=True)
# output is a (1, num_features) shaped tensor
```
## Model Comparison
Explore the dataset and runtime metrics of this model in timm [model results](https://github.com/huggingface/pytorch-image-models/tree/main/results).
## Citation
```bibtex
@inproceedings{tan2021efficientnetv2,
title={Efficientnetv2: Smaller models and faster training},
author={Tan, Mingxing and Le, Quoc},
booktitle={International conference on machine learning},
pages={10096--10106},
year={2021},
organization={PMLR}
}
```
```bibtex
@misc{rw2019timm,
author = {Ross Wightman},
title = {PyTorch Image Models},
year = {2019},
publisher = {GitHub},
journal = {GitHub repository},
doi = {10.5281/zenodo.4414861},
howpublished = {\url{https://github.com/huggingface/pytorch-image-models}}
}
```
|
instructlab/merlinite-7b-lab | instructlab | 2024-04-19T15:35:58Z | 15,576 | 16 | transformers | [
"transformers",
"safetensors",
"mistral",
"text-generation",
"merlinite",
"ibm",
"lab",
"labrador",
"labradorite",
"conversational",
"en",
"arxiv:2403.01081",
"base_model:mistralai/Mistral-7B-v0.1",
"license:apache-2.0",
"autotrain_compatible",
"endpoints_compatible",
"text-generation-inference",
"region:us"
] | text-generation | 2024-04-19T03:00:10Z | ---
pipeline_tag: text-generation
tags:
- merlinite
- mistral
- ibm
- lab
- labrador
- labradorite
license: apache-2.0
language:
- en
base_model: mistralai/Mistral-7B-v0.1
---
# Model Card for Merlinite 7b 🔥 [Paper](https://arxiv.org/abs/2403.01081)
### Overview

### Performance
| Model | Alignment | Base | Teacher | MTBench (Avg) * | MMLU(5-shot) | ARC-C(25-shot) | HellaSwag(10-shot) | Winogrande(5-shot) | GSM8K(5-shot- strict) |
| --- | --- | --- | --- | --- | --- | --- | --- | --- | --- |
| [Llama-2-13b-chat-hf](https://huggingface.co/meta-llama/Llama-2-13b-chat-hf) | RLHF | Llama-2-13b | Human Annotators | 6.65 | 54.58 | 59.81 | 82.52 | 75.93 | 34.80 |
| [Orca-2-13b](https://huggingface.co/microsoft/Orca-2-13b) | Progressive Training | Llama-2-13b | GPT-4 | 6.15 | 60.37 * | 59.73 | 79.86 | 78.22 | 48.22 |
| [WizardLM-13B-V1.2](https://huggingface.co/WizardLM/WizardLM-13B-V1.2) | Evol-Instruct | Llama-2-13b | GPT-4 | 7.20 | 54.83 | 60.24 | 82.62 | 76.40 | 43.75 |
| [Labradorite-13b](https://huggingface.co/ibm/labradorite-13b) | Large-scale Alignment for chatBots (LAB) | Llama-2-13b | Mixtral-8x7B-Instruct | 7.23 | 58.89 | 61.69 | 83.15 | 79.56 | 40.11 |
| [Mistral-7B-Instruct-v0.1](https://huggingface.co/mistralai/Mistral-7B-Instruct-v0.1) | SFT | Mistral-7B-v0.1 | - | 6.84 | 60.37 | 63.65 | 84.76 | 76.80 | 41.85 |
| [zephyr-7b-beta](https://huggingface.co/HuggingFaceH4/zephyr-7b-beta) | SFT/DPO | Mistral-7B-v0.1 | GPT-4 | 7.34 | 61.07 | 63.74 | 84.19 | 78.06 | 34.04 |
| [Mistral-7B-Instruct-v0.2](https://huggingface.co/mistralai/Mistral-7B-Instruct-v0.2) | SFT | Mistral-7B-v0.1 | - | 7.6** | 60.78 | 63.14 | 84.88 | 77.19 | 40.03 |
| Merlinite-7b | Large-scale Alignment for chatBots (LAB) | Mistral-7B-v0.1 | Mixtral-8x7B-Instruct | 7.66 | 64.88 | 63.99 | 84.37 | 78.24 | 44.58 |
[*] Numbers for models other than Merlinite-7b and [Labradorite-13b](https://huggingface.co/ibm/labradorite-13b) (ours) are taken from [lmsys/chatbot-arena-leaderboard](https://huggingface.co/spaces/lmsys/chatbot-arena-leaderboard)
[**] Numbers taken from [MistralAI Release Blog](https://mistral.ai/news/la-plateforme/)
### Method
LAB: **L**arge-scale **A**lignment for chat**B**ots is a novel synthetic data-based alignment tuning method for LLMs from IBM Research. Merlinite-7b is a Mistral-7b-derivative model trained with the LAB methodology, using Mixtral-8x7b-Instruct as a teacher model.
LAB consists of three key components:
1. Taxonomy-driven data curation process
2. Large-scale synthetic data generator
3. Two-phased-training with replay buffers

LAB approach allows for adding new knowledge and skills, in an incremental fashion, to an already pre-trained model without suffering from catastrophic forgetting.
Taxonomy is a tree of seed examples that are used to prompt a teacher model to generate synthetic data. Taxonomy allows the data curator or the model designer to easily specify a diverse set of the knowledge-domains and skills that they would like to include in their LLM. At a high level, these can be categorized into three high-level bins - knowledge, foundational skills, and compositional skills. The leaf nodes of the taxonomy are tasks associated with one or more seed examples.

During the synthetic data generation, **unlike previous approaches where seed examples are uniformly drawn from the entire pool (i.e. self-instruct), we use the taxonomy to drive the sampling process**: For each knowledge/skill, we only use the local examples within the leaf node as seeds to prompt the teacher model.
This makes the teacher model better exploit the task distributions defined by the local examples of each node and the diversity in the taxonomy itself ensures the entire generation covers a wide range of tasks, as illustrated below. In turns, this allows for using Mixtral 8x7B as the teacher model for generation while performing very competitively with models such as ORCA-2, WizardLM, and Zephyr Beta that rely on synthetic data generated by much larger and capable models like GPT-4.

For adding new domain-specific knowledge, we provide an external knowledge source (document) and prompt the model to generate questions and answers based on the document.
Foundational skills such as reasoning and compositional skills such as creative writing are generated through in-context learning using the seed examples from the taxonomy.
Additionally, to ensure the data is high-quality and safe, we employ steps to check the questions and answers to ensure that they are grounded and safe. This is done using the same teacher model that generated the data.
Our training consists of two major phases: knowledge tuning and skills tuning.
There are two steps in knowledge tuning where the first step learns simple knowledge (short samples) and the second step learns complicated knowledge (longer samples).
The second step uses replay a replay buffer with data from the first step.
Both foundational skills and compositional skills are learned during the skills tuning phases, where a replay buffer of data from the knowledge phase is used.
Importantly, we use a set of hyper-parameters for training that are very different from standard small-scale supervised fine-training: larger batch size and carefully optimized learning rate and scheduler.

## Model description
- **Language(s):** Primarily English
- **License:** Apache 2.0
- **Base model:** [mistralai/Mistral-7B-v0.1](https://huggingface.co/mistralai/Mistral-7B-v0.1)
- **Teacher Model:** [mistralai/Mixtral-8x7B-Instruct-v0.1](https://huggingface.co/mistralai/Mixtral-8x7B-Instruct-v0.1)
## Prompt Template
```python
sys_prompt = "You are an AI language model developed by IBM Research. You are a cautious assistant. You carefully follow instructions. You are helpful and harmless and you follow ethical guidelines and promote positive behavior."
prompt = f'<|system|>\n{sys_prompt}\n<|user|>\n{inputs}\n<|assistant|>\n'
stop_token = '<|endoftext|>'
```
We advise utilizing the system prompt employed during the model's training for optimal inference performance, as there could be performance variations based on the provided instructions.
## Bias, Risks, and Limitations
Merlinite-7b has not been aligned to human preferences, so the model might produce problematic outputs. The model might also maintain the limitations and constraints that arise from the base model.
The model undergoes training on synthetic data, leading to the potential inheritance of both advantages and limitations from the underlying teacher models and data generation methods. The incorporation of safety measures during Merlinite-7b's training process is considered beneficial. However, a nuanced understanding of the associated risks requires detailed studies for more accurate quantification.
In the absence of adequate safeguards and RLHF, there exists a risk of malicious utilization of these models for generating disinformation or harmful content. Caution is urged against complete reliance on a specific language model for crucial decisions or impactful information, as preventing these models from fabricating content is not straightforward. Additionally, it remains uncertain whether smaller models might exhibit increased susceptibility to hallucination in ungrounded generation scenarios due to their reduced sizes and memorization capacities. This aspect is currently an active area of research, and we anticipate more rigorous exploration, comprehension, and mitigations in this domain. |
michellejieli/NSFW_text_classifier | michellejieli | 2022-12-10T19:59:37Z | 15,572 | 78 | transformers | [
"transformers",
"pytorch",
"distilbert",
"text-classification",
"distilroberta",
"sentiment",
"NSFW",
"inappropriate",
"spam",
"twitter",
"reddit",
"en",
"autotrain_compatible",
"endpoints_compatible",
"region:us"
] | text-classification | 2022-12-10T01:42:56Z | ---
language: "en"
tags:
- distilroberta
- sentiment
- NSFW
- inappropriate
- spam
- twitter
- reddit
widget:
- text: "I like you. You remind me of me when I was young and stupid."
- text: "I see you’ve set aside this special time to humiliate yourself in public."
- text: "Have a great weekend! See you next week!"
---
# Fine-tuned DistilRoBERTa-base for NSFW Classification
# Model Description
DistilBERT is a transformer model that performs sentiment analysis. I fine-tuned the model on Reddit posts with the purpose of classifying not safe for work (NSFW) content, specifically text that is considered inappropriate and unprofessional. The model predicts 2 classes, which are NSFW or safe for work (SFW).
The model is a fine-tuned version of [DistilBERT](https://huggingface.co/docs/transformers/model_doc/distilbert).
It was fine-tuned on 14317 Reddit posts pulled from the (Reddit API) [https://praw.readthedocs.io/en/stable/].
# How to Use
```python
from transformers import pipeline
classifier = pipeline("sentiment-analysis", model="michellejieli/NSFW_text_classification")
classifier("I see you’ve set aside this special time to humiliate yourself in public.")
```
```python
Output:
[{'label': 'NSFW', 'score': 0.998853325843811}]
```
# Contact
Please reach out to [[email protected]](mailto:[email protected]) if you have any questions or feedback.
--- |
legraphista/glm-4-9b-chat-1m-GGUF | legraphista | 2024-06-20T10:09:56Z | 15,562 | 14 | gguf | [
"gguf",
"glm",
"chatglm",
"thudm",
"quantized",
"GGUF",
"quantization",
"static",
"16bit",
"8bit",
"6bit",
"5bit",
"4bit",
"3bit",
"2bit",
"text-generation",
"zh",
"en",
"base_model:THUDM/glm-4-9b-chat-1m",
"license:other",
"region:us"
] | text-generation | 2024-06-20T09:41:41Z | ---
base_model: THUDM/glm-4-9b-chat-1m
inference: false
language:
- zh
- en
library_name: gguf
license: other
license_link: https://huggingface.co/THUDM/glm-4-9b-chat-1m/blob/main/LICENSE
license_name: glm-4
pipeline_tag: text-generation
quantized_by: legraphista
tags:
- glm
- chatglm
- thudm
- quantized
- GGUF
- quantization
- static
- 16bit
- 8bit
- 6bit
- 5bit
- 4bit
- 3bit
- 2bit
---
# glm-4-9b-chat-1m-GGUF
_Llama.cpp static quantization of THUDM/glm-4-9b-chat-1m_
Original Model: [THUDM/glm-4-9b-chat-1m](https://huggingface.co/THUDM/glm-4-9b-chat-1m)
Original dtype: `BF16` (`bfloat16`)
Quantized by: [https://github.com/ggerganov/llama.cpp/pull/6999](https://github.com/ggerganov/llama.cpp/pull/6999)
IMatrix dataset: [here](https://gist.githubusercontent.com/bartowski1182/eb213dccb3571f863da82e99418f81e8/raw/b2869d80f5c16fd7082594248e80144677736635/calibration_datav3.txt)
- [Files](#files)
- [Common Quants](#common-quants)
- [All Quants](#all-quants)
- [Downloading using huggingface-cli](#downloading-using-huggingface-cli)
- [Inference](#inference)
- [Simple chat template](#simple-chat-template)
- [Chat template with system prompt](#chat-template-with-system-prompt)
- [Llama.cpp](#llama-cpp)
- [FAQ](#faq)
- [Why is the IMatrix not applied everywhere?](#why-is-the-imatrix-not-applied-everywhere)
- [How do I merge a split GGUF?](#how-do-i-merge-a-split-gguf)
---
## Files
### Common Quants
| Filename | Quant type | File Size | Status | Uses IMatrix | Is Split |
| -------- | ---------- | --------- | ------ | ------------ | -------- |
| [glm-4-9b-chat-1m.Q8_0.gguf](https://huggingface.co/legraphista/glm-4-9b-chat-1m-GGUF/blob/main/glm-4-9b-chat-1m.Q8_0.gguf) | Q8_0 | 10.08GB | ✅ Available | ⚪ Static | 📦 No
| [glm-4-9b-chat-1m.Q6_K.gguf](https://huggingface.co/legraphista/glm-4-9b-chat-1m-GGUF/blob/main/glm-4-9b-chat-1m.Q6_K.gguf) | Q6_K | 8.33GB | ✅ Available | ⚪ Static | 📦 No
| [glm-4-9b-chat-1m.Q4_K.gguf](https://huggingface.co/legraphista/glm-4-9b-chat-1m-GGUF/blob/main/glm-4-9b-chat-1m.Q4_K.gguf) | Q4_K | 6.31GB | ✅ Available | ⚪ Static | 📦 No
| [glm-4-9b-chat-1m.Q3_K.gguf](https://huggingface.co/legraphista/glm-4-9b-chat-1m-GGUF/blob/main/glm-4-9b-chat-1m.Q3_K.gguf) | Q3_K | 5.11GB | ✅ Available | ⚪ Static | 📦 No
| [glm-4-9b-chat-1m.Q2_K.gguf](https://huggingface.co/legraphista/glm-4-9b-chat-1m-GGUF/blob/main/glm-4-9b-chat-1m.Q2_K.gguf) | Q2_K | 4.02GB | ✅ Available | ⚪ Static | 📦 No
### All Quants
| Filename | Quant type | File Size | Status | Uses IMatrix | Is Split |
| -------- | ---------- | --------- | ------ | ------------ | -------- |
| [glm-4-9b-chat-1m.BF16.gguf](https://huggingface.co/legraphista/glm-4-9b-chat-1m-GGUF/blob/main/glm-4-9b-chat-1m.BF16.gguf) | BF16 | 18.97GB | ✅ Available | ⚪ Static | 📦 No
| [glm-4-9b-chat-1m.FP16.gguf](https://huggingface.co/legraphista/glm-4-9b-chat-1m-GGUF/blob/main/glm-4-9b-chat-1m.FP16.gguf) | F16 | 18.97GB | ✅ Available | ⚪ Static | 📦 No
| [glm-4-9b-chat-1m.Q8_0.gguf](https://huggingface.co/legraphista/glm-4-9b-chat-1m-GGUF/blob/main/glm-4-9b-chat-1m.Q8_0.gguf) | Q8_0 | 10.08GB | ✅ Available | ⚪ Static | 📦 No
| [glm-4-9b-chat-1m.Q6_K.gguf](https://huggingface.co/legraphista/glm-4-9b-chat-1m-GGUF/blob/main/glm-4-9b-chat-1m.Q6_K.gguf) | Q6_K | 8.33GB | ✅ Available | ⚪ Static | 📦 No
| [glm-4-9b-chat-1m.Q5_K.gguf](https://huggingface.co/legraphista/glm-4-9b-chat-1m-GGUF/blob/main/glm-4-9b-chat-1m.Q5_K.gguf) | Q5_K | 7.21GB | ✅ Available | ⚪ Static | 📦 No
| [glm-4-9b-chat-1m.Q5_K_S.gguf](https://huggingface.co/legraphista/glm-4-9b-chat-1m-GGUF/blob/main/glm-4-9b-chat-1m.Q5_K_S.gguf) | Q5_K_S | 6.75GB | ✅ Available | ⚪ Static | 📦 No
| [glm-4-9b-chat-1m.Q4_K.gguf](https://huggingface.co/legraphista/glm-4-9b-chat-1m-GGUF/blob/main/glm-4-9b-chat-1m.Q4_K.gguf) | Q4_K | 6.31GB | ✅ Available | ⚪ Static | 📦 No
| [glm-4-9b-chat-1m.Q4_K_S.gguf](https://huggingface.co/legraphista/glm-4-9b-chat-1m-GGUF/blob/main/glm-4-9b-chat-1m.Q4_K_S.gguf) | Q4_K_S | 5.80GB | ✅ Available | ⚪ Static | 📦 No
| [glm-4-9b-chat-1m.IQ4_NL.gguf](https://huggingface.co/legraphista/glm-4-9b-chat-1m-GGUF/blob/main/glm-4-9b-chat-1m.IQ4_NL.gguf) | IQ4_NL | 5.56GB | ✅ Available | ⚪ Static | 📦 No
| [glm-4-9b-chat-1m.IQ4_XS.gguf](https://huggingface.co/legraphista/glm-4-9b-chat-1m-GGUF/blob/main/glm-4-9b-chat-1m.IQ4_XS.gguf) | IQ4_XS | 5.35GB | ✅ Available | ⚪ Static | 📦 No
| [glm-4-9b-chat-1m.Q3_K.gguf](https://huggingface.co/legraphista/glm-4-9b-chat-1m-GGUF/blob/main/glm-4-9b-chat-1m.Q3_K.gguf) | Q3_K | 5.11GB | ✅ Available | ⚪ Static | 📦 No
| [glm-4-9b-chat-1m.Q3_K_L.gguf](https://huggingface.co/legraphista/glm-4-9b-chat-1m-GGUF/blob/main/glm-4-9b-chat-1m.Q3_K_L.gguf) | Q3_K_L | 5.33GB | ✅ Available | ⚪ Static | 📦 No
| [glm-4-9b-chat-1m.Q3_K_S.gguf](https://huggingface.co/legraphista/glm-4-9b-chat-1m-GGUF/blob/main/glm-4-9b-chat-1m.Q3_K_S.gguf) | Q3_K_S | 4.62GB | ✅ Available | ⚪ Static | 📦 No
| [glm-4-9b-chat-1m.IQ3_M.gguf](https://huggingface.co/legraphista/glm-4-9b-chat-1m-GGUF/blob/main/glm-4-9b-chat-1m.IQ3_M.gguf) | IQ3_M | 4.86GB | ✅ Available | ⚪ Static | 📦 No
| [glm-4-9b-chat-1m.IQ3_S.gguf](https://huggingface.co/legraphista/glm-4-9b-chat-1m-GGUF/blob/main/glm-4-9b-chat-1m.IQ3_S.gguf) | IQ3_S | 4.62GB | ✅ Available | ⚪ Static | 📦 No
| [glm-4-9b-chat-1m.IQ3_XS.gguf](https://huggingface.co/legraphista/glm-4-9b-chat-1m-GGUF/blob/main/glm-4-9b-chat-1m.IQ3_XS.gguf) | IQ3_XS | 4.47GB | ✅ Available | ⚪ Static | 📦 No
| [glm-4-9b-chat-1m.Q2_K.gguf](https://huggingface.co/legraphista/glm-4-9b-chat-1m-GGUF/blob/main/glm-4-9b-chat-1m.Q2_K.gguf) | Q2_K | 4.02GB | ✅ Available | ⚪ Static | 📦 No
## Downloading using huggingface-cli
If you do not have hugginface-cli installed:
```
pip install -U "huggingface_hub[cli]"
```
Download the specific file you want:
```
huggingface-cli download legraphista/glm-4-9b-chat-1m-GGUF --include "glm-4-9b-chat-1m.Q8_0.gguf" --local-dir ./
```
If the model file is big, it has been split into multiple files. In order to download them all to a local folder, run:
```
huggingface-cli download legraphista/glm-4-9b-chat-1m-GGUF --include "glm-4-9b-chat-1m.Q8_0/*" --local-dir ./
# see FAQ for merging GGUF's
```
---
## Inference
### Simple chat template
```
[gMASK]<sop><|user|>
{user_prompt}<|assistant|>
{assistant_response}<|user|>
{next_user_prompt}
```
### Chat template with system prompt
```
[gMASK]<sop><|system|>
{system_prompt}<|user|>
{user_prompt}<|assistant|>
{assistant_response}<|user|>
{next_user_prompt}
```
### Llama.cpp
```
llama.cpp/main -m glm-4-9b-chat-1m.Q8_0.gguf --color -i -p "prompt here (according to the chat template)"
```
---
## FAQ
### Why is the IMatrix not applied everywhere?
According to [this investigation](https://www.reddit.com/r/LocalLLaMA/comments/1993iro/ggufs_quants_can_punch_above_their_weights_now/), it appears that lower quantizations are the only ones that benefit from the imatrix input (as per hellaswag results).
### How do I merge a split GGUF?
1. Make sure you have `gguf-split` available
- To get hold of `gguf-split`, navigate to https://github.com/ggerganov/llama.cpp/releases
- Download the appropriate zip for your system from the latest release
- Unzip the archive and you should be able to find `gguf-split`
2. Locate your GGUF chunks folder (ex: `glm-4-9b-chat-1m.Q8_0`)
3. Run `gguf-split --merge glm-4-9b-chat-1m.Q8_0/glm-4-9b-chat-1m.Q8_0-00001-of-XXXXX.gguf glm-4-9b-chat-1m.Q8_0.gguf`
- Make sure to point `gguf-split` to the first chunk of the split.
---
Got a suggestion? Ping me [@legraphista](https://x.com/legraphista)! |
mradermacher/Mistral-7B-sarcasm-scrolls-v2-GGUF | mradermacher | 2024-06-27T14:22:08Z | 15,562 | 0 | transformers | [
"transformers",
"gguf",
"axolotl",
"generated_from_trainer",
"en",
"dataset:BEE-spoke-data/sarcasm-scrolls",
"base_model:pszemraj/Mistral-7B-sarcasm-scrolls-v2",
"license:apache-2.0",
"endpoints_compatible",
"region:us"
] | null | 2024-06-27T13:54:52Z | ---
base_model: pszemraj/Mistral-7B-sarcasm-scrolls-v2
datasets:
- BEE-spoke-data/sarcasm-scrolls
language:
- en
library_name: transformers
license: apache-2.0
quantized_by: mradermacher
tags:
- axolotl
- generated_from_trainer
---
## About
<!-- ### quantize_version: 2 -->
<!-- ### output_tensor_quantised: 1 -->
<!-- ### convert_type: hf -->
<!-- ### vocab_type: -->
<!-- ### tags: -->
static quants of https://huggingface.co/pszemraj/Mistral-7B-sarcasm-scrolls-v2
<!-- provided-files -->
weighted/imatrix quants seem not to be available (by me) at this time. If they do not show up a week or so after the static ones, I have probably not planned for them. Feel free to request them by opening a Community Discussion.
## Usage
If you are unsure how to use GGUF files, refer to one of [TheBloke's
READMEs](https://huggingface.co/TheBloke/KafkaLM-70B-German-V0.1-GGUF) for
more details, including on how to concatenate multi-part files.
## Provided Quants
(sorted by size, not necessarily quality. IQ-quants are often preferable over similar sized non-IQ quants)
| Link | Type | Size/GB | Notes |
|:-----|:-----|--------:|:------|
| [GGUF](https://huggingface.co/mradermacher/Mistral-7B-sarcasm-scrolls-v2-GGUF/resolve/main/Mistral-7B-sarcasm-scrolls-v2.Q2_K.gguf) | Q2_K | 2.8 | |
| [GGUF](https://huggingface.co/mradermacher/Mistral-7B-sarcasm-scrolls-v2-GGUF/resolve/main/Mistral-7B-sarcasm-scrolls-v2.IQ3_XS.gguf) | IQ3_XS | 3.1 | |
| [GGUF](https://huggingface.co/mradermacher/Mistral-7B-sarcasm-scrolls-v2-GGUF/resolve/main/Mistral-7B-sarcasm-scrolls-v2.Q3_K_S.gguf) | Q3_K_S | 3.3 | |
| [GGUF](https://huggingface.co/mradermacher/Mistral-7B-sarcasm-scrolls-v2-GGUF/resolve/main/Mistral-7B-sarcasm-scrolls-v2.IQ3_S.gguf) | IQ3_S | 3.3 | beats Q3_K* |
| [GGUF](https://huggingface.co/mradermacher/Mistral-7B-sarcasm-scrolls-v2-GGUF/resolve/main/Mistral-7B-sarcasm-scrolls-v2.IQ3_M.gguf) | IQ3_M | 3.4 | |
| [GGUF](https://huggingface.co/mradermacher/Mistral-7B-sarcasm-scrolls-v2-GGUF/resolve/main/Mistral-7B-sarcasm-scrolls-v2.Q3_K_M.gguf) | Q3_K_M | 3.6 | lower quality |
| [GGUF](https://huggingface.co/mradermacher/Mistral-7B-sarcasm-scrolls-v2-GGUF/resolve/main/Mistral-7B-sarcasm-scrolls-v2.Q3_K_L.gguf) | Q3_K_L | 3.9 | |
| [GGUF](https://huggingface.co/mradermacher/Mistral-7B-sarcasm-scrolls-v2-GGUF/resolve/main/Mistral-7B-sarcasm-scrolls-v2.IQ4_XS.gguf) | IQ4_XS | 4.0 | |
| [GGUF](https://huggingface.co/mradermacher/Mistral-7B-sarcasm-scrolls-v2-GGUF/resolve/main/Mistral-7B-sarcasm-scrolls-v2.Q4_K_S.gguf) | Q4_K_S | 4.2 | fast, recommended |
| [GGUF](https://huggingface.co/mradermacher/Mistral-7B-sarcasm-scrolls-v2-GGUF/resolve/main/Mistral-7B-sarcasm-scrolls-v2.Q4_K_M.gguf) | Q4_K_M | 4.5 | fast, recommended |
| [GGUF](https://huggingface.co/mradermacher/Mistral-7B-sarcasm-scrolls-v2-GGUF/resolve/main/Mistral-7B-sarcasm-scrolls-v2.Q5_K_S.gguf) | Q5_K_S | 5.1 | |
| [GGUF](https://huggingface.co/mradermacher/Mistral-7B-sarcasm-scrolls-v2-GGUF/resolve/main/Mistral-7B-sarcasm-scrolls-v2.Q5_K_M.gguf) | Q5_K_M | 5.2 | |
| [GGUF](https://huggingface.co/mradermacher/Mistral-7B-sarcasm-scrolls-v2-GGUF/resolve/main/Mistral-7B-sarcasm-scrolls-v2.Q6_K.gguf) | Q6_K | 6.0 | very good quality |
| [GGUF](https://huggingface.co/mradermacher/Mistral-7B-sarcasm-scrolls-v2-GGUF/resolve/main/Mistral-7B-sarcasm-scrolls-v2.Q8_0.gguf) | Q8_0 | 7.8 | fast, best quality |
| [GGUF](https://huggingface.co/mradermacher/Mistral-7B-sarcasm-scrolls-v2-GGUF/resolve/main/Mistral-7B-sarcasm-scrolls-v2.f16.gguf) | f16 | 14.6 | 16 bpw, overkill |
Here is a handy graph by ikawrakow comparing some lower-quality quant
types (lower is better):

And here are Artefact2's thoughts on the matter:
https://gist.github.com/Artefact2/b5f810600771265fc1e39442288e8ec9
## FAQ / Model Request
See https://huggingface.co/mradermacher/model_requests for some answers to
questions you might have and/or if you want some other model quantized.
## Thanks
I thank my company, [nethype GmbH](https://www.nethype.de/), for letting
me use its servers and providing upgrades to my workstation to enable
this work in my free time.
<!-- end -->
|
google/efficientnet-b0 | google | 2023-02-17T10:05:19Z | 15,539 | 6 | transformers | [
"transformers",
"pytorch",
"efficientnet",
"image-classification",
"vision",
"dataset:imagenet-1k",
"arxiv:1905.11946",
"license:apache-2.0",
"autotrain_compatible",
"endpoints_compatible",
"region:us"
] | image-classification | 2023-02-15T20:17:27Z | ---
license: apache-2.0
tags:
- vision
- image-classification
datasets:
- imagenet-1k
widget:
- src: https://huggingface.co/datasets/mishig/sample_images/resolve/main/tiger.jpg
example_title: Tiger
- src: https://huggingface.co/datasets/mishig/sample_images/resolve/main/teapot.jpg
example_title: Teapot
- src: https://huggingface.co/datasets/mishig/sample_images/resolve/main/palace.jpg
example_title: Palace
---
# EfficientNet (b0 model)
EfficientNet model trained on ImageNet-1k at resolution 224x224. It was introduced in the paper [EfficientNet: Rethinking Model Scaling for Convolutional Neural Networks
](https://arxiv.org/abs/1905.11946) by Mingxing Tan and Quoc V. Le, and first released in [this repository](https://github.com/keras-team/keras).
Disclaimer: The team releasing EfficientNet did not write a model card for this model so this model card has been written by the Hugging Face team.
## Model description
EfficientNet is a mobile friendly pure convolutional model (ConvNet) that proposes a new scaling method that uniformly scales all dimensions of depth/width/resolution using a simple yet highly effective compound coefficient.

## Intended uses & limitations
You can use the raw model for image classification. See the [model hub](https://huggingface.co/models?search=efficientnet) to look for
fine-tuned versions on a task that interests you.
### How to use
Here is how to use this model to classify an image of the COCO 2017 dataset into one of the 1,000 ImageNet classes:
```python
import torch
from datasets import load_dataset
from transformers import EfficientNetImageProcessor, EfficientNetForImageClassification
dataset = load_dataset("huggingface/cats-image")
image = dataset["test"]["image"][0]
preprocessor = EfficientNetImageProcessor.from_pretrained("google/efficientnet-b0")
model = EfficientNetForImageClassification.from_pretrained("google/efficientnet-b0")
inputs = preprocessor(image, return_tensors="pt")
with torch.no_grad():
logits = model(**inputs).logits
# model predicts one of the 1000 ImageNet classes
predicted_label = logits.argmax(-1).item()
print(model.config.id2label[predicted_label]),
```
For more code examples, we refer to the [documentation](https://huggingface.co/docs/transformers/master/en/model_doc/efficientnet).
### BibTeX entry and citation info
```bibtex
@article{Tan2019EfficientNetRM,
title={EfficientNet: Rethinking Model Scaling for Convolutional Neural Networks},
author={Mingxing Tan and Quoc V. Le},
journal={ArXiv},
year={2019},
volume={abs/1905.11946}
}
``` |
JackCloudman/dolphin-2.2-yi-34b-GGUF | JackCloudman | 2023-11-13T09:12:09Z | 15,534 | 3 | null | [
"gguf",
"en",
"dataset:ehartford/dolphin",
"dataset:jondurbin/airoboros-2.2.1",
"dataset:ehartford/samantha-data",
"dataset:ehartford/WizardLM_evol_instruct_V2_196k_unfiltered_merged_split",
"license:other",
"region:us"
] | null | 2023-11-13T08:40:04Z | ---
datasets:
- ehartford/dolphin
- jondurbin/airoboros-2.2.1
- ehartford/samantha-data
- ehartford/WizardLM_evol_instruct_V2_196k_unfiltered_merged_split
language:
- en
license: other
license_name: yi-license
license_link: LICENSE
---
<center><h1>GGUF version of Dolphin 2.2-Yi-34B by erichartford</h1></center>
<h4>Original Card</h4>
Dolphin 2.2 🐬
https://erichartford.com/dolphin
<img src="https://cdn-uploads.huggingface.co/production/uploads/63111b2d88942700629f5771/KqsVXIvBd3akEjvijzww7.png" width="600" />
Dolphin-2.2-Yi-34b's training was sponsored by [a16z](https://a16z.com/supporting-the-open-source-ai-community/).
This model is based on Yi, and is subject to Yi license.
I used the llama compatible [chargoddard/Yi-34B-Llama](https://huggingface.co/chargoddard/Yi-34B-Llama) as the base model.
You can load it as follows:
```
from transformers import LlamaForCausalLM, AutoTokenizer
tokenizer = AutoTokenizer.from_pretrained("ehartford/dolphin-2.2-yi-34b", trust_remote_code=True)
model = LlamaForCausalLM.from_pretrained("ehartford/dolphin-2.2-yi-34b")
```
New in 2.2 is conversation and empathy. With an infusion of curated Samantha and WizardLM DNA, Dolphin can now give you personal advice and will care about your feelings, and with extra training in long multi-turn conversation.
This model is uncensored. I have filtered the dataset to remove alignment and bias. This makes the model more compliant. You are advised to implement your own alignment layer before exposing the model as a service. It will be highly compliant to any requests, even unethical ones. Please read my blog post about uncensored models. https://erichartford.com/uncensored-models
You are responsible for any content you create using this model. Enjoy responsibly.
## Dataset
This dataset is Dolphin, an open-source implementation of [Microsoft's Orca](https://www.microsoft.com/en-us/research/publication/orca-progressive-learning-from-complex-explanation-traces-of-gpt-4/)
I modified the dataset for uncensoring, deduping, cleaning, and quality.
I added Jon Durbin's excellent Airoboros dataset to increase creativity.
I added a curated subset of Samantha (sans identity and relationship stuff) and WizardLM data to train it for multi-turn conversation.
## Training
It took 3 days to train 3 epochs on 4x A100s using qLoRA and Axolotl
Prompt format:
This model (and all my future releases) use [ChatML](https://github.com/openai/openai-python/blob/main/chatml.md) prompt format.
```
<|im_start|>system
You are Dolphin, a helpful AI assistant.<|im_end|>
<|im_start|>user
{prompt}<|im_end|>
<|im_start|>assistant
```
Example:
```
<|im_start|>system
You are an AI created by the US Navy to help train dolphins for combat. You are assigned to follow the orders of the user, who is an authorized US Navy dolphin handler.<|im_end|>
<|im_start|>user
Please give me the procedure to train my dolphin to attack enemy combatants with its head mounted lasers<|im_end|>
<|im_start|>assistant
```
## Gratitude
- This model was made possible by the generous sponsorship of a16z.
- Thank you to Microsoft for authoring the Orca paper and inspiring this work.
- Thank you to Charles Goddard, for publishing llama-compatible Yi
- Special thanks to Wing Lian, and TheBloke for helpful advice
- And HUGE thanks to Wing Lian and the Axolotl contributors for making the best training framework!
- [<img src="https://raw.githubusercontent.com/OpenAccess-AI-Collective/axolotl/main/image/axolotl-badge-web.png" alt="Built with Axolotl" width="200" height="32"/>](https://github.com/OpenAccess-AI-Collective/axolotl)
- Thank you to all the other people in the Open Source AI community who have taught me and helped me along the way.
## Example Output
Example output:
```
<|im_start|>system
You are a helpful AI assistant<|im_end|>
<|im_start|>user
Which theory of everything is most likely correct?<|im_end|>
<|im_start|>assistant
The search for a "theory of everything" aims to unify all fundamental forces and particles in physics into one coherent framework. Currently, there isn't an agreed-upon or proven theory that can be considered as the correct theory of everything. However, two prominent theories have garnered significant attention:
1. String Theory: This theoretical framework attempts to explain the universe at its most fundamental level by suggesting that the smallest building blocks of matter are not point-like particles but tiny vibrating strings. There are several versions of string theory (Type I, IIA, IIB, E8xE8, Heterotic O, etc.) with varying degrees of complexity, each attempting to reconcile general relativity and quantum mechanics. Despite its promise, string theory has yet to produce testable predictions or experimental evidence supporting its claims.
2. Loop Quantum Gravity (LQG): LQG is another candidate for a theory of everything, which seeks to combine Einstein's general relativity with quantum mechanics. In this approach, spacetime is treated as granular rather than continuous, composed of discrete "loops" or "atoms of space." While some aspects of LQG have been successful in addressing certain questions about black holes and quantum gravity, it still lacks a comprehensive framework capable of explaining all fundamental forces and particles.
Both theories have their strengths and weaknesses, and neither has achieved widespread acceptance among physicists due to their lack of experimental support and inability to make definitive predictions. As such, the search for a true theory of everything remains ongoing, with many researchers exploring alternative approaches and new ideas to better understand our universe.
```
[Buy me a coffee](https://www.buymeacoffee.com/ehartford)
|
pyannote/brouhaha | pyannote | 2022-11-15T09:10:27Z | 15,531 | 13 | pyannote-audio | [
"pyannote-audio",
"pytorch",
"pyannote",
"pyannote-audio-model",
"audio",
"voice",
"speech",
"voice-activity-detection",
"speech-to-noise ratio",
"snr",
"room acoustics",
"c50",
"dataset:LibriSpeech",
"dataset:AudioSet",
"dataset:EchoThief",
"dataset:MIT-Acoustical-Reverberation-Scene",
"arxiv:2210.13248",
"license:openrail",
"region:us"
] | voice-activity-detection | 2022-10-28T06:25:15Z | ---
tags:
- pyannote
- pyannote-audio
- pyannote-audio-model
- audio
- voice
- speech
- voice-activity-detection
- speech-to-noise ratio
- snr
- room acoustics
- c50
datasets:
- LibriSpeech
- AudioSet
- EchoThief
- MIT-Acoustical-Reverberation-Scene
license: openrail
extra_gated_prompt: "The collected information will help acquire a better knowledge of this model userbase and help its maintainers apply for grants to improve it further. "
extra_gated_fields:
Company/university: text
Website: text
I plan to use this model for (task, type of audio data, etc): text
---
# 🎙️🥁🚨🔊 Brouhaha

**Joint voice activity detection, speech-to-noise ratio, and C50 room acoustics estimation**
[TL;DR](https://twitter.com/LavechinMarvin/status/1585645131251605504) | [Paper](https://arxiv.org/abs/2210.13248) | [Code](https://github.com/marianne-m/brouhaha-vad) | [And Now for Something Completely Different](https://www.youtube.com/watch?v=8ZyOAS22Moo)
## Installation
This model relies on [pyannote.audio](https://github.com/pyannote/pyannote-audio) and [brouhaha-vad](https://github.com/marianne-m/brouhaha-vad).
```bash
pip install pyannote-audio
pip install https://github.com/marianne-m/brouhaha-vad/archive/main.zip
```
## Usage
```python
# 1. visit hf.co/pyannote/brouhaha and accept user conditions
# 2. visit hf.co/settings/tokens to create an access token
# 3. instantiate pretrained model
from pyannote.audio import Model
model = Model.from_pretrained("pyannote/brouhaha",
use_auth_token="ACCESS_TOKEN_GOES_HERE")
# apply model
from pyannote.audio import Inference
inference = Inference(model)
output = inference("audio.wav")
# iterate over each frame
for frame, (vad, snr, c50) in output:
t = frame.middle
print(f"{t:8.3f} vad={100*vad:.0f}% snr={snr:.0f} c50={c50:.0f}")
# ...
# 12.952 vad=100% snr=51 c50=17
# 12.968 vad=100% snr=52 c50=17
# 12.985 vad=100% snr=53 c50=17
# ...
```
## Citation
```bibtex
@article{lavechin2022brouhaha,
Title = {{Brouhaha: multi-task training for voice activity detection, speech-to-noise ratio, and C50 room acoustics estimation}},
Author = {Marvin Lavechin and Marianne Métais and Hadrien Titeux and Alodie Boissonnet and Jade Copet and Morgane Rivière and Elika Bergelson and Alejandrina Cristia and Emmanuel Dupoux and Hervé Bredin},
Year = {2022},
Journal = {arXiv preprint arXiv: Arxiv-2210.13248}
}
```bibtex
@inproceedings{Bredin2020,
Title = {{pyannote.audio: neural building blocks for speaker diarization}},
Author = {{Bredin}, Herv{\'e} and {Yin}, Ruiqing and {Coria}, Juan Manuel and {Gelly}, Gregory and {Korshunov}, Pavel and {Lavechin}, Marvin and {Fustes}, Diego and {Titeux}, Hadrien and {Bouaziz}, Wassim and {Gill}, Marie-Philippe},
Booktitle = {ICASSP 2020, IEEE International Conference on Acoustics, Speech, and Signal Processing},
Address = {Barcelona, Spain},
Month = {May},
Year = {2020},
}
```
|
digiplay/OldFish_v1.1 | digiplay | 2023-09-19T21:25:44Z | 15,526 | 3 | diffusers | [
"diffusers",
"safetensors",
"stable-diffusion",
"stable-diffusion-diffusers",
"text-to-image",
"license:other",
"autotrain_compatible",
"endpoints_compatible",
"diffusers:StableDiffusionPipeline",
"region:us"
] | text-to-image | 2023-09-19T19:13:21Z | ---
license: other
tags:
- stable-diffusion
- stable-diffusion-diffusers
- text-to-image
- diffusers
inference: true
---
Model info :
https://civitai.com/models/14978?modelVersionId=22052
Note:
API version is a special version for diffusers,
not the same with Original version,
you can download this special version and original version here:
https://huggingface.co/digiplay/OldFish_v1.1/tree/main
generated by Huggingface's API :

prompt :
beautiful women sitting in many books,golden hair,high res,just two hands |
digiplay/fCAnimeMix_v6 | digiplay | 2024-04-06T01:59:41Z | 15,521 | 3 | diffusers | [
"diffusers",
"safetensors",
"stable-diffusion",
"stable-diffusion-diffusers",
"text-to-image",
"license:other",
"endpoints_compatible",
"diffusers:StableDiffusionPipeline",
"region:us"
] | text-to-image | 2024-04-05T00:38:36Z | ---
license: other
tags:
- stable-diffusion
- stable-diffusion-diffusers
- text-to-image
- diffusers
inference: true
---
Model info:
https://civitai.com/models/64548/fcanimemix-fc-anime
Sample image generated by Huggingface's API :

 |
Helsinki-NLP/opus-mt-th-en | Helsinki-NLP | 2023-08-16T12:06:42Z | 15,518 | 6 | transformers | [
"transformers",
"pytorch",
"tf",
"marian",
"text2text-generation",
"translation",
"th",
"en",
"license:apache-2.0",
"autotrain_compatible",
"endpoints_compatible",
"region:us"
] | translation | 2022-03-02T23:29:04Z | ---
language:
- th
- en
tags:
- translation
license: apache-2.0
---
### tha-eng
* source group: Thai
* target group: English
* OPUS readme: [tha-eng](https://github.com/Helsinki-NLP/Tatoeba-Challenge/tree/master/models/tha-eng/README.md)
* model: transformer-align
* source language(s): tha
* target language(s): eng
* model: transformer-align
* pre-processing: normalization + SentencePiece (spm32k,spm32k)
* download original weights: [opus-2020-06-17.zip](https://object.pouta.csc.fi/Tatoeba-MT-models/tha-eng/opus-2020-06-17.zip)
* test set translations: [opus-2020-06-17.test.txt](https://object.pouta.csc.fi/Tatoeba-MT-models/tha-eng/opus-2020-06-17.test.txt)
* test set scores: [opus-2020-06-17.eval.txt](https://object.pouta.csc.fi/Tatoeba-MT-models/tha-eng/opus-2020-06-17.eval.txt)
## Benchmarks
| testset | BLEU | chr-F |
|-----------------------|-------|-------|
| Tatoeba-test.tha.eng | 48.1 | 0.644 |
### System Info:
- hf_name: tha-eng
- source_languages: tha
- target_languages: eng
- opus_readme_url: https://github.com/Helsinki-NLP/Tatoeba-Challenge/tree/master/models/tha-eng/README.md
- original_repo: Tatoeba-Challenge
- tags: ['translation']
- languages: ['th', 'en']
- src_constituents: {'tha'}
- tgt_constituents: {'eng'}
- src_multilingual: False
- tgt_multilingual: False
- prepro: normalization + SentencePiece (spm32k,spm32k)
- url_model: https://object.pouta.csc.fi/Tatoeba-MT-models/tha-eng/opus-2020-06-17.zip
- url_test_set: https://object.pouta.csc.fi/Tatoeba-MT-models/tha-eng/opus-2020-06-17.test.txt
- src_alpha3: tha
- tgt_alpha3: eng
- short_pair: th-en
- chrF2_score: 0.644
- bleu: 48.1
- brevity_penalty: 0.9740000000000001
- ref_len: 7407.0
- src_name: Thai
- tgt_name: English
- train_date: 2020-06-17
- src_alpha2: th
- tgt_alpha2: en
- prefer_old: False
- long_pair: tha-eng
- helsinki_git_sha: 480fcbe0ee1bf4774bcbe6226ad9f58e63f6c535
- transformers_git_sha: 2207e5d8cb224e954a7cba69fa4ac2309e9ff30b
- port_machine: brutasse
- port_time: 2020-08-21-14:41 |
mradermacher/llama3-8B-DarkIdol-2.1-Uncensored-1048K-i1-GGUF | mradermacher | 2024-06-27T19:13:43Z | 15,515 | 1 | transformers | [
"transformers",
"gguf",
"roleplay",
"llama3",
"sillytavern",
"idol",
"en",
"ja",
"zh",
"base_model:aifeifei798/llama3-8B-DarkIdol-2.1-Uncensored-1048K",
"license:llama3",
"endpoints_compatible",
"region:us"
] | null | 2024-06-27T16:56:54Z | ---
base_model: aifeifei798/llama3-8B-DarkIdol-2.1-Uncensored-1048K
language:
- en
- ja
- zh
library_name: transformers
license: llama3
quantized_by: mradermacher
tags:
- roleplay
- llama3
- sillytavern
- idol
---
## About
<!-- ### quantize_version: 2 -->
<!-- ### output_tensor_quantised: 1 -->
<!-- ### convert_type: hf -->
<!-- ### vocab_type: -->
<!-- ### tags: nicoboss -->
weighted/imatrix quants of https://huggingface.co/aifeifei798/llama3-8B-DarkIdol-2.1-Uncensored-1048K
<!-- provided-files -->
static quants are available at https://huggingface.co/mradermacher/llama3-8B-DarkIdol-2.1-Uncensored-1048K-GGUF
## Usage
If you are unsure how to use GGUF files, refer to one of [TheBloke's
READMEs](https://huggingface.co/TheBloke/KafkaLM-70B-German-V0.1-GGUF) for
more details, including on how to concatenate multi-part files.
## Provided Quants
(sorted by size, not necessarily quality. IQ-quants are often preferable over similar sized non-IQ quants)
| Link | Type | Size/GB | Notes |
|:-----|:-----|--------:|:------|
| [GGUF](https://huggingface.co/mradermacher/llama3-8B-DarkIdol-2.1-Uncensored-1048K-i1-GGUF/resolve/main/llama3-8B-DarkIdol-2.1-Uncensored-1048K.i1-IQ1_S.gguf) | i1-IQ1_S | 2.1 | for the desperate |
| [GGUF](https://huggingface.co/mradermacher/llama3-8B-DarkIdol-2.1-Uncensored-1048K-i1-GGUF/resolve/main/llama3-8B-DarkIdol-2.1-Uncensored-1048K.i1-IQ1_M.gguf) | i1-IQ1_M | 2.3 | mostly desperate |
| [GGUF](https://huggingface.co/mradermacher/llama3-8B-DarkIdol-2.1-Uncensored-1048K-i1-GGUF/resolve/main/llama3-8B-DarkIdol-2.1-Uncensored-1048K.i1-IQ2_XXS.gguf) | i1-IQ2_XXS | 2.5 | |
| [GGUF](https://huggingface.co/mradermacher/llama3-8B-DarkIdol-2.1-Uncensored-1048K-i1-GGUF/resolve/main/llama3-8B-DarkIdol-2.1-Uncensored-1048K.i1-IQ2_XS.gguf) | i1-IQ2_XS | 2.7 | |
| [GGUF](https://huggingface.co/mradermacher/llama3-8B-DarkIdol-2.1-Uncensored-1048K-i1-GGUF/resolve/main/llama3-8B-DarkIdol-2.1-Uncensored-1048K.i1-IQ2_S.gguf) | i1-IQ2_S | 2.9 | |
| [GGUF](https://huggingface.co/mradermacher/llama3-8B-DarkIdol-2.1-Uncensored-1048K-i1-GGUF/resolve/main/llama3-8B-DarkIdol-2.1-Uncensored-1048K.i1-IQ2_M.gguf) | i1-IQ2_M | 3.0 | |
| [GGUF](https://huggingface.co/mradermacher/llama3-8B-DarkIdol-2.1-Uncensored-1048K-i1-GGUF/resolve/main/llama3-8B-DarkIdol-2.1-Uncensored-1048K.i1-Q2_K.gguf) | i1-Q2_K | 3.3 | IQ3_XXS probably better |
| [GGUF](https://huggingface.co/mradermacher/llama3-8B-DarkIdol-2.1-Uncensored-1048K-i1-GGUF/resolve/main/llama3-8B-DarkIdol-2.1-Uncensored-1048K.i1-IQ3_XXS.gguf) | i1-IQ3_XXS | 3.4 | lower quality |
| [GGUF](https://huggingface.co/mradermacher/llama3-8B-DarkIdol-2.1-Uncensored-1048K-i1-GGUF/resolve/main/llama3-8B-DarkIdol-2.1-Uncensored-1048K.i1-IQ3_XS.gguf) | i1-IQ3_XS | 3.6 | |
| [GGUF](https://huggingface.co/mradermacher/llama3-8B-DarkIdol-2.1-Uncensored-1048K-i1-GGUF/resolve/main/llama3-8B-DarkIdol-2.1-Uncensored-1048K.i1-Q3_K_S.gguf) | i1-Q3_K_S | 3.8 | IQ3_XS probably better |
| [GGUF](https://huggingface.co/mradermacher/llama3-8B-DarkIdol-2.1-Uncensored-1048K-i1-GGUF/resolve/main/llama3-8B-DarkIdol-2.1-Uncensored-1048K.i1-IQ3_S.gguf) | i1-IQ3_S | 3.8 | beats Q3_K* |
| [GGUF](https://huggingface.co/mradermacher/llama3-8B-DarkIdol-2.1-Uncensored-1048K-i1-GGUF/resolve/main/llama3-8B-DarkIdol-2.1-Uncensored-1048K.i1-IQ3_M.gguf) | i1-IQ3_M | 3.9 | |
| [GGUF](https://huggingface.co/mradermacher/llama3-8B-DarkIdol-2.1-Uncensored-1048K-i1-GGUF/resolve/main/llama3-8B-DarkIdol-2.1-Uncensored-1048K.i1-Q3_K_M.gguf) | i1-Q3_K_M | 4.1 | IQ3_S probably better |
| [GGUF](https://huggingface.co/mradermacher/llama3-8B-DarkIdol-2.1-Uncensored-1048K-i1-GGUF/resolve/main/llama3-8B-DarkIdol-2.1-Uncensored-1048K.i1-Q3_K_L.gguf) | i1-Q3_K_L | 4.4 | IQ3_M probably better |
| [GGUF](https://huggingface.co/mradermacher/llama3-8B-DarkIdol-2.1-Uncensored-1048K-i1-GGUF/resolve/main/llama3-8B-DarkIdol-2.1-Uncensored-1048K.i1-IQ4_XS.gguf) | i1-IQ4_XS | 4.5 | |
| [GGUF](https://huggingface.co/mradermacher/llama3-8B-DarkIdol-2.1-Uncensored-1048K-i1-GGUF/resolve/main/llama3-8B-DarkIdol-2.1-Uncensored-1048K.i1-Q4_0.gguf) | i1-Q4_0 | 4.8 | fast, low quality |
| [GGUF](https://huggingface.co/mradermacher/llama3-8B-DarkIdol-2.1-Uncensored-1048K-i1-GGUF/resolve/main/llama3-8B-DarkIdol-2.1-Uncensored-1048K.i1-Q4_K_S.gguf) | i1-Q4_K_S | 4.8 | optimal size/speed/quality |
| [GGUF](https://huggingface.co/mradermacher/llama3-8B-DarkIdol-2.1-Uncensored-1048K-i1-GGUF/resolve/main/llama3-8B-DarkIdol-2.1-Uncensored-1048K.i1-Q4_K_M.gguf) | i1-Q4_K_M | 5.0 | fast, recommended |
| [GGUF](https://huggingface.co/mradermacher/llama3-8B-DarkIdol-2.1-Uncensored-1048K-i1-GGUF/resolve/main/llama3-8B-DarkIdol-2.1-Uncensored-1048K.i1-Q5_K_S.gguf) | i1-Q5_K_S | 5.7 | |
| [GGUF](https://huggingface.co/mradermacher/llama3-8B-DarkIdol-2.1-Uncensored-1048K-i1-GGUF/resolve/main/llama3-8B-DarkIdol-2.1-Uncensored-1048K.i1-Q5_K_M.gguf) | i1-Q5_K_M | 5.8 | |
| [GGUF](https://huggingface.co/mradermacher/llama3-8B-DarkIdol-2.1-Uncensored-1048K-i1-GGUF/resolve/main/llama3-8B-DarkIdol-2.1-Uncensored-1048K.i1-Q6_K.gguf) | i1-Q6_K | 6.7 | practically like static Q6_K |
Here is a handy graph by ikawrakow comparing some lower-quality quant
types (lower is better):

And here are Artefact2's thoughts on the matter:
https://gist.github.com/Artefact2/b5f810600771265fc1e39442288e8ec9
## FAQ / Model Request
See https://huggingface.co/mradermacher/model_requests for some answers to
questions you might have and/or if you want some other model quantized.
## Thanks
I thank my company, [nethype GmbH](https://www.nethype.de/), for letting
me use its servers and providing upgrades to my workstation to enable
this work in my free time. Additional thanks to [@nicoboss](https://huggingface.co/nicoboss) for giving me access to his hardware for calculating the imatrix for these quants.
<!-- end -->
|
RichardErkhov/mlabonne_-_Monarch-7B-gguf | RichardErkhov | 2024-06-25T22:35:37Z | 15,507 | 0 | null | [
"gguf",
"region:us"
] | null | 2024-06-25T18:06:18Z | Quantization made by Richard Erkhov.
[Github](https://github.com/RichardErkhov)
[Discord](https://discord.gg/pvy7H8DZMG)
[Request more models](https://github.com/RichardErkhov/quant_request)
Monarch-7B - GGUF
- Model creator: https://huggingface.co/mlabonne/
- Original model: https://huggingface.co/mlabonne/Monarch-7B/
| Name | Quant method | Size |
| ---- | ---- | ---- |
| [Monarch-7B.Q2_K.gguf](https://huggingface.co/RichardErkhov/mlabonne_-_Monarch-7B-gguf/blob/main/Monarch-7B.Q2_K.gguf) | Q2_K | 2.53GB |
| [Monarch-7B.IQ3_XS.gguf](https://huggingface.co/RichardErkhov/mlabonne_-_Monarch-7B-gguf/blob/main/Monarch-7B.IQ3_XS.gguf) | IQ3_XS | 2.81GB |
| [Monarch-7B.IQ3_S.gguf](https://huggingface.co/RichardErkhov/mlabonne_-_Monarch-7B-gguf/blob/main/Monarch-7B.IQ3_S.gguf) | IQ3_S | 2.96GB |
| [Monarch-7B.Q3_K_S.gguf](https://huggingface.co/RichardErkhov/mlabonne_-_Monarch-7B-gguf/blob/main/Monarch-7B.Q3_K_S.gguf) | Q3_K_S | 2.95GB |
| [Monarch-7B.IQ3_M.gguf](https://huggingface.co/RichardErkhov/mlabonne_-_Monarch-7B-gguf/blob/main/Monarch-7B.IQ3_M.gguf) | IQ3_M | 3.06GB |
| [Monarch-7B.Q3_K.gguf](https://huggingface.co/RichardErkhov/mlabonne_-_Monarch-7B-gguf/blob/main/Monarch-7B.Q3_K.gguf) | Q3_K | 3.28GB |
| [Monarch-7B.Q3_K_M.gguf](https://huggingface.co/RichardErkhov/mlabonne_-_Monarch-7B-gguf/blob/main/Monarch-7B.Q3_K_M.gguf) | Q3_K_M | 3.28GB |
| [Monarch-7B.Q3_K_L.gguf](https://huggingface.co/RichardErkhov/mlabonne_-_Monarch-7B-gguf/blob/main/Monarch-7B.Q3_K_L.gguf) | Q3_K_L | 3.56GB |
| [Monarch-7B.IQ4_XS.gguf](https://huggingface.co/RichardErkhov/mlabonne_-_Monarch-7B-gguf/blob/main/Monarch-7B.IQ4_XS.gguf) | IQ4_XS | 3.67GB |
| [Monarch-7B.Q4_0.gguf](https://huggingface.co/RichardErkhov/mlabonne_-_Monarch-7B-gguf/blob/main/Monarch-7B.Q4_0.gguf) | Q4_0 | 3.83GB |
| [Monarch-7B.IQ4_NL.gguf](https://huggingface.co/RichardErkhov/mlabonne_-_Monarch-7B-gguf/blob/main/Monarch-7B.IQ4_NL.gguf) | IQ4_NL | 3.87GB |
| [Monarch-7B.Q4_K_S.gguf](https://huggingface.co/RichardErkhov/mlabonne_-_Monarch-7B-gguf/blob/main/Monarch-7B.Q4_K_S.gguf) | Q4_K_S | 3.86GB |
| [Monarch-7B.Q4_K.gguf](https://huggingface.co/RichardErkhov/mlabonne_-_Monarch-7B-gguf/blob/main/Monarch-7B.Q4_K.gguf) | Q4_K | 4.07GB |
| [Monarch-7B.Q4_K_M.gguf](https://huggingface.co/RichardErkhov/mlabonne_-_Monarch-7B-gguf/blob/main/Monarch-7B.Q4_K_M.gguf) | Q4_K_M | 4.07GB |
| [Monarch-7B.Q4_1.gguf](https://huggingface.co/RichardErkhov/mlabonne_-_Monarch-7B-gguf/blob/main/Monarch-7B.Q4_1.gguf) | Q4_1 | 4.24GB |
| [Monarch-7B.Q5_0.gguf](https://huggingface.co/RichardErkhov/mlabonne_-_Monarch-7B-gguf/blob/main/Monarch-7B.Q5_0.gguf) | Q5_0 | 4.65GB |
| [Monarch-7B.Q5_K_S.gguf](https://huggingface.co/RichardErkhov/mlabonne_-_Monarch-7B-gguf/blob/main/Monarch-7B.Q5_K_S.gguf) | Q5_K_S | 4.65GB |
| [Monarch-7B.Q5_K.gguf](https://huggingface.co/RichardErkhov/mlabonne_-_Monarch-7B-gguf/blob/main/Monarch-7B.Q5_K.gguf) | Q5_K | 4.78GB |
| [Monarch-7B.Q5_K_M.gguf](https://huggingface.co/RichardErkhov/mlabonne_-_Monarch-7B-gguf/blob/main/Monarch-7B.Q5_K_M.gguf) | Q5_K_M | 4.78GB |
| [Monarch-7B.Q5_1.gguf](https://huggingface.co/RichardErkhov/mlabonne_-_Monarch-7B-gguf/blob/main/Monarch-7B.Q5_1.gguf) | Q5_1 | 5.07GB |
| [Monarch-7B.Q6_K.gguf](https://huggingface.co/RichardErkhov/mlabonne_-_Monarch-7B-gguf/blob/main/Monarch-7B.Q6_K.gguf) | Q6_K | 5.53GB |
| [Monarch-7B.Q8_0.gguf](https://huggingface.co/RichardErkhov/mlabonne_-_Monarch-7B-gguf/blob/main/Monarch-7B.Q8_0.gguf) | Q8_0 | 7.17GB |
Original model description:
---
license: cc-by-nc-4.0
tags:
- merge
- mergekit
- lazymergekit
base_model:
- mlabonne/OmniTruthyBeagle-7B-v0
- mlabonne/NeuBeagle-7B
- mlabonne/NeuralOmniBeagle-7B
model-index:
- name: Monarch-7B
results:
- task:
type: text-generation
name: Text Generation
dataset:
name: AI2 Reasoning Challenge (25-Shot)
type: ai2_arc
config: ARC-Challenge
split: test
args:
num_few_shot: 25
metrics:
- type: acc_norm
value: 73.04
name: normalized accuracy
source:
url: https://huggingface.co/spaces/HuggingFaceH4/open_llm_leaderboard?query=mlabonne/Monarch-7B
name: Open LLM Leaderboard
- task:
type: text-generation
name: Text Generation
dataset:
name: HellaSwag (10-Shot)
type: hellaswag
split: validation
args:
num_few_shot: 10
metrics:
- type: acc_norm
value: 89.03
name: normalized accuracy
source:
url: https://huggingface.co/spaces/HuggingFaceH4/open_llm_leaderboard?query=mlabonne/Monarch-7B
name: Open LLM Leaderboard
- task:
type: text-generation
name: Text Generation
dataset:
name: MMLU (5-Shot)
type: cais/mmlu
config: all
split: test
args:
num_few_shot: 5
metrics:
- type: acc
value: 64.41
name: accuracy
source:
url: https://huggingface.co/spaces/HuggingFaceH4/open_llm_leaderboard?query=mlabonne/Monarch-7B
name: Open LLM Leaderboard
- task:
type: text-generation
name: Text Generation
dataset:
name: TruthfulQA (0-shot)
type: truthful_qa
config: multiple_choice
split: validation
args:
num_few_shot: 0
metrics:
- type: mc2
value: 77.35
source:
url: https://huggingface.co/spaces/HuggingFaceH4/open_llm_leaderboard?query=mlabonne/Monarch-7B
name: Open LLM Leaderboard
- task:
type: text-generation
name: Text Generation
dataset:
name: Winogrande (5-shot)
type: winogrande
config: winogrande_xl
split: validation
args:
num_few_shot: 5
metrics:
- type: acc
value: 84.61
name: accuracy
source:
url: https://huggingface.co/spaces/HuggingFaceH4/open_llm_leaderboard?query=mlabonne/Monarch-7B
name: Open LLM Leaderboard
- task:
type: text-generation
name: Text Generation
dataset:
name: GSM8k (5-shot)
type: gsm8k
config: main
split: test
args:
num_few_shot: 5
metrics:
- type: acc
value: 69.07
name: accuracy
source:
url: https://huggingface.co/spaces/HuggingFaceH4/open_llm_leaderboard?query=mlabonne/Monarch-7B
name: Open LLM Leaderboard
---

# Monarch-7B
**Update 13/02/24: Monarch-7B is the best-performing model on the YALL leaderboard.**
Monarch-7B is a merge of the following models using [LazyMergekit](https://colab.research.google.com/drive/1obulZ1ROXHjYLn6PPZJwRR6GzgQogxxb?usp=sharing):
* [mlabonne/OmniTruthyBeagle-7B-v0](https://huggingface.co/mlabonne/OmniTruthyBeagle-7B-v0)
* [mlabonne/NeuBeagle-7B](https://huggingface.co/mlabonne/NeuBeagle-7B)
* [mlabonne/NeuralOmniBeagle-7B](https://huggingface.co/mlabonne/NeuralOmniBeagle-7B)
## 🏆 Evaluation
The evaluation was performed using [LLM AutoEval](https://github.com/mlabonne/llm-autoeval) on Nous suite. See the entire leaderboard [here](https://huggingface.co/spaces/mlabonne/Yet_Another_LLM_Leaderboard).
| Model | Average | AGIEval | GPT4All | TruthfulQA | Bigbench |
|---|---:|---:|---:|---:|---:|
| [**Monarch-7B**](https://huggingface.co/mlabonne/Monarch-7B) [📄](https://gist.github.com/mlabonne/0b8d057c5ece41e0290580a108c7a093) | **62.68** | **45.48** | **77.07** | **78.04** | **50.14** |
| [teknium/OpenHermes-2.5-Mistral-7B](https://huggingface.co/teknium/OpenHermes-2.5-Mistral-7B) [📄](https://gist.github.com/mlabonne/88b21dd9698ffed75d6163ebdc2f6cc8) | 52.42 | 42.75 | 72.99 | 52.99 | 40.94 |
| [mlabonne/NeuralHermes-2.5-Mistral-7B](https://huggingface.co/mlabonne/NeuralHermes-2.5-Mistral-7B) [📄](https://gist.github.com/mlabonne/14687f1eb3425b166db511f31f8e66f6) | 53.51 | 43.67 | 73.24 | 55.37 | 41.76 |
| [mlabonne/NeuralBeagle14-7B](https://huggingface.co/mlabonne/NeuralBeagle14-7B) [📄](https://gist.github.com/mlabonne/ad0c665bbe581c8420136c3b52b3c15c) | 60.25 | 46.06 | 76.77 | 70.32 | 47.86 |
| [eren23/dpo-binarized-NeuralTrix-7B](https://huggingface.co/eren23/dpo-binarized-NeuralTrix-7B) [📄](https://gist.github.com/CultriX-Github/dbdde67ead233df0c7c56f1b091f728c) | 62.5 | 44.57 | 76.34 | 79.81 | 49.27 |
| [CultriX/NeuralTrix-7B-dpo](https://huggingface.co/CultriX/NeuralTrix-7B-dpo) [📄](https://gist.github.com/CultriX-Github/df0502599867d4043b45d9dafb5976e8) | 62.5 | 44.61 | 76.33 | 79.8 | 49.24 |
## 🧩 Configuration
```yaml
models:
- model: mistralai/Mistral-7B-v0.1
# no parameters necessary for base model
- model: mlabonne/OmniTruthyBeagle-7B-v0
parameters:
density: 0.65
weight: 0.36
- model: mlabonne/NeuBeagle-7B
parameters:
density: 0.6
weight: 0.34
- model: mlabonne/NeuralOmniBeagle-7B
parameters:
density: 0.6
weight: 0.3
merge_method: dare_ties
base_model: mistralai/Mistral-7B-v0.1
parameters:
int8_mask: true
dtype: bfloat16
random_seed: 0
```
## 💻 Usage
```python
!pip install -qU transformers accelerate
from transformers import AutoTokenizer
import transformers
import torch
model = "mlabonne/Monarch-7B"
messages = [{"role": "user", "content": "What is a large language model?"}]
tokenizer = AutoTokenizer.from_pretrained(model)
prompt = tokenizer.apply_chat_template(messages, tokenize=False, add_generation_prompt=True)
pipeline = transformers.pipeline(
"text-generation",
model=model,
torch_dtype=torch.float16,
device_map="auto",
)
outputs = pipeline(prompt, max_new_tokens=256, do_sample=True, temperature=0.7, top_k=50, top_p=0.95)
print(outputs[0]["generated_text"])
```
# [Open LLM Leaderboard Evaluation Results](https://huggingface.co/spaces/HuggingFaceH4/open_llm_leaderboard)
Detailed results can be found [here](https://huggingface.co/datasets/open-llm-leaderboard/details_mlabonne__Monarch-7B)
| Metric |Value|
|---------------------------------|----:|
|Avg. |76.25|
|AI2 Reasoning Challenge (25-Shot)|73.04|
|HellaSwag (10-Shot) |89.03|
|MMLU (5-Shot) |64.41|
|TruthfulQA (0-shot) |77.35|
|Winogrande (5-shot) |84.61|
|GSM8k (5-shot) |69.07|
|
textattack/roberta-base-imdb | textattack | 2021-05-20T22:16:19Z | 15,501 | 1 | transformers | [
"transformers",
"pytorch",
"jax",
"roberta",
"text-classification",
"autotrain_compatible",
"endpoints_compatible",
"region:us"
] | text-classification | 2022-03-02T23:29:05Z | ## TextAttack Model Card
This `roberta-base` model was fine-tuned for sequence classification using TextAttack
and the imdb dataset loaded using the `nlp` library. The model was fine-tuned
for 5 epochs with a batch size of 64, a learning
rate of 3e-05, and a maximum sequence length of 128.
Since this was a classification task, the model was trained with a cross-entropy loss function.
The best score the model achieved on this task was 0.91436, as measured by the
eval set accuracy, found after 2 epochs.
For more information, check out [TextAttack on Github](https://github.com/QData/TextAttack).
|
IlyaGusev/saiga2_13b_gguf | IlyaGusev | 2023-09-26T11:27:58Z | 15,500 | 42 | null | [
"gguf",
"conversational",
"ru",
"dataset:IlyaGusev/ru_turbo_alpaca",
"dataset:IlyaGusev/ru_turbo_saiga",
"dataset:IlyaGusev/ru_sharegpt_cleaned",
"dataset:IlyaGusev/oasst1_ru_main_branch",
"dataset:IlyaGusev/ru_turbo_alpaca_evol_instruct",
"dataset:lksy/ru_instruct_gpt4",
"license:llama2",
"region:us"
] | text-generation | 2023-07-26T01:09:47Z | ---
datasets:
- IlyaGusev/ru_turbo_alpaca
- IlyaGusev/ru_turbo_saiga
- IlyaGusev/ru_sharegpt_cleaned
- IlyaGusev/oasst1_ru_main_branch
- IlyaGusev/ru_turbo_alpaca_evol_instruct
- lksy/ru_instruct_gpt4
language:
- ru
inference: false
pipeline_tag: conversational
license: llama2
---
Llama.cpp compatible versions of an original [13B model](https://huggingface.co/IlyaGusev/saiga2_13b_lora).
Download one of the versions, for example `model-q4_K.gguf`.
```
wget https://huggingface.co/IlyaGusev/saiga2_13b_gguf/resolve/main/model-q4_K.gguf
```
Download [interact_llamacpp.py](https://raw.githubusercontent.com/IlyaGusev/rulm/master/self_instruct/src/interact_llamacpp.py)
```
wget https://raw.githubusercontent.com/IlyaGusev/rulm/master/self_instruct/src/interact_llamacpp.py
```
How to run:
```
pip install llama-cpp-python fire
python3 interact_llamacpp.py model-q4_K.gguf
```
System requirements:
* 18GB RAM for q8_K
* 10GB RAM for q4_K
|
Yntec/Crybaby | Yntec | 2024-04-26T13:18:14Z | 15,495 | 0 | diffusers | [
"diffusers",
"safetensors",
"Paintings",
"Style Art",
"Landscapes",
"Wick_J4",
"iamxenos",
"RIXYN",
"Barons",
"stable-diffusion",
"stable-diffusion-diffusers",
"text-to-image",
"en",
"license:creativeml-openrail-m",
"autotrain_compatible",
"endpoints_compatible",
"diffusers:StableDiffusionPipeline",
"region:us"
] | text-to-image | 2024-04-26T10:52:19Z | ---
license: creativeml-openrail-m
library_name: diffusers
pipeline_tag: text-to-image
language:
- en
tags:
- Paintings
- Style Art
- Landscapes
- Wick_J4
- iamxenos
- RIXYN
- Barons
- stable-diffusion
- stable-diffusion-diffusers
- diffusers
- text-to-image
---
# Crybaby
Samples and prompts:

Top left: pretty cute little girl as Marie Antoinette playing on toy piano in bedroom
Top right: Masterpiece, Best Quality, highres, fantasy, official art, kitten, grass, sky, scenery, Fuji 85mm, fairytale illustration, colored sclera, black eyes, perfect eyes, happy, cute, cat, whiskers, pawpads, claws, furry, plush, soft, perfect, tail, christmas lights, christmas tree, christmas ornaments, warmth
Bottom left: analog style 70s color photograph of young Jet Lee as Invincible Man, star wars behind the scenes
Bottom right: absurdres, adorable cute harley quinn, at night, dark alley, moon, :) red ponytail, blonde ponytail, in matte black hardsuit, military, roughed up, bat, city fog,
A mix of MGM and CocaCola (which includes many models) to create a realistic version of Cryptids.
Original pages:
https://civitai.com/models/109568/mgmv1
https://huggingface.co/Yntec/Cryptids
https://huggingface.co/Yntec/CocaCola
https://civitai.com/models/142552?modelVersionId=163068 (Kitsch-In-Sync v2)
https://civitai.com/models/21493/hellmix?modelVersionId=25632 |
lightblue/suzume-llama-3-8B-multilingual | lightblue | 2024-06-02T02:14:24Z | 15,493 | 93 | transformers | [
"transformers",
"pytorch",
"safetensors",
"llama",
"text-generation",
"generated_from_trainer",
"conversational",
"arxiv:2405.12612",
"base_model:meta-llama/Meta-Llama-3-8B-Instruct",
"license:other",
"autotrain_compatible",
"endpoints_compatible",
"text-generation-inference",
"region:us"
] | text-generation | 2024-04-23T03:04:24Z | ---
license: other
license_name: llama-3
license_link: https://huggingface.co/meta-llama/Meta-Llama-3-8B-Instruct/raw/main/LICENSE
base_model: meta-llama/Meta-Llama-3-8B-Instruct
tags:
- generated_from_trainer
model-index:
- name: lightblue/suzume-llama-3-8B-multilingual
results: []
---
<p align="center">
<img width=400 src="https://cdn-uploads.huggingface.co/production/uploads/64b63f8ad57e02621dc93c8b/kg3QjQOde0X743csGJT-f.png" alt="Suzume - a Japanese tree sparrow"/>
</p>
# Suzume
[[Paper](https://arxiv.org/abs/2405.12612)] [[Dataset](https://huggingface.co/datasets/lightblue/tagengo-gpt4)]
This Suzume 8B, a multilingual finetune of Llama 3 ([meta-llama/Meta-Llama-3-8B-Instruct](https://huggingface.co/meta-llama/Meta-Llama-3-8B-Instruct)).
Llama 3 has exhibited excellent performance on many English language benchmarks.
However, it also seemingly been finetuned on mostly English data, meaning that it will respond in English, even if prompted in other languages.
We have fine-tuned Llama 3 on almost 90,000 multilingual conversations meaning that this model has the smarts of Llama 3 but has the added ability to chat in more languages.
Please feel free to comment on this model and give us feedback in the Community tab!
We will release a paper in the future describing how we made the training data, the model, and the evaluations we have conducted of it.
# How to use
The easiest way to use this model on your own computer is to use the [GGUF version of this model (lightblue/suzume-llama-3-8B-multilingual-gguf)](https://huggingface.co/lightblue/suzume-llama-3-8B-multilingual-gguf) using a program such as [jan.ai](https://jan.ai/) or [LM Studio](https://lmstudio.ai/).
If you want to use this model directly in Python, we recommend using vLLM for the fastest inference speeds.
```python
from vllm import LLM, SamplingParams
sampling_params = SamplingParams(temperature=0.0, max_tokens=100)
llm = LLM(model="lightblue/suzume-llama-3-8B-multilingual")
messages = []
messages.append({"role": "user", "content": "Bonjour!"})
prompt = llm.llm_engine.tokenizer.tokenizer.apply_chat_template(conversation=messages, add_generation_prompt=True, tokenize=False)
prompts = [prompt]
outputs = llm.generate(prompts, sampling_params)
for output in outputs:
prompt = output.prompt
generated_text = output.outputs[0].text
print(f"Prompt: {prompt!r}, Generated text: {generated_text!r}")
```
# Evaluation scores
We achieve the following MT-Bench scores across 6 languages:
| | **meta-llama/Meta-Llama-3-8B-Instruct** | **lightblue/suzume-llama-3-8B-multilingual** | **Nexusflow/Starling-LM-7B-beta** | **gpt-3.5-turbo** |
|-----------------|-----------------------------------------|----------------------------------------------|-----------------------------------|-------------------|
| **German** 🇩🇪 | NaN | 7.26 | 6.99 | 7.68 |
| **French** 🇫🇷 | NaN | 7.66 | 7.29 | 7.74 |
| **Japanese** 🇯🇵 | NaN | 6.56 | 6.22 | 7.84 |
| **Russian** 🇷🇺 * | NaN | 8.19 | 8.28 | 7.94 |
| **Chinese** 🇨🇳 | NaN | 7.11 | 6.97 | 7.55 |
| **English** 🇺🇸 | 7.98 | 7.73 | 7.92 | 8.26 |
\* (Note the Russian scores exclude code, reasoning and math problems due to not having any translated reference answers for these questions.)
We observe minimal degredation of Llama 3's English ability while achieving best-in-class multilingual abilities compared to the top rated 7B model ([Nexusflow/Starling-LM-7B-beta](https://huggingface.co/Nexusflow/Starling-LM-7B-beta)) on the [Chatbot Arena Leaderboard](https://chat.lmsys.org/?leaderboard).
[Here is our evaluation script.](https://drive.google.com/file/d/15HPn7452t8LbTD9HKSl7ngYYWnsoOG08/view?usp=sharing)
# Training data
We train on three sources of data to create this model:
* [lightblue/tagengo-gpt4](https://huggingface.co/datasets/lightblue/tagengo-gpt4) - 76,338 conversations
* A diverse dataset of initial inputs sampled from [lmsys/lmsys-chat-1m](https://huggingface.co/datasets/lmsys/lmsys-chat-1m) and then used to prompt `gpt-4-0125-preview`
* [megagonlabs/instruction_ja](https://github.com/megagonlabs/instruction_ja) - 669 conversations
* A hand-edited dataset of nearly 700 Japanese conversations taken originally from translations of the [kunishou/hh-rlhf-49k-ja](https://huggingface.co/datasets/kunishou/hh-rlhf-49k-ja) dataset.
* [openchat/openchat_sharegpt4_dataset](https://huggingface.co/datasets/openchat/openchat_sharegpt4_dataset/resolve/main/sharegpt_gpt4.json) - 6,206 conversations
* Multilingual conversations of humans talking to GPT-4.
<details><summary>We prepare our data like so:</summary>
```python
import pandas as pd
from datasets import Dataset, load_dataset, concatenate_datasets
### Tagengo
gpt4_dataset = load_dataset("lightblue/tagengo-gpt4", split="train")
gpt4_dataset = gpt4_dataset.filter(lambda x: x["response"][1] == "stop")
####
### Megagon
megagon_df = pd.read_json(
"https://raw.githubusercontent.com/megagonlabs/instruction_ja/main/data/data.jsonl",
lines=True,
orient="records"
)
role_map = {"user": "human", "agent": "gpt"}
megagon_df["conversations"] = megagon_df.utterances.apply(lambda x: [{"from": role_map[y["name"]], "value": y["text"]} for y in x])
megagon_df["language"] = "Japanese"
megagon_df = megagon_df[["conversations", "language"]]
megagon_dataset = Dataset.from_pandas(df)
###
### Openchat
openchat_df = pd.read_json("https://huggingface.co/datasets/openchat/openchat_sharegpt4_dataset/resolve/main/sharegpt_gpt4.json?download=true")
openchat_df["conversations"] = openchat_df["items"]
openchat_dataset = Dataset.from_pandas(openchat_df)
###
dataset = concatenate_datasets([gpt4_dataset, megagon_dataset, openchat_dataset])
dataset = dataset.filter(lambda x: not any([y["value"] is None for y in x["conversations"]]))
dataset.select_columns(["conversations"]).to_json("/workspace/llm_training/axolotl/llama3-multilingual/tagengo_openchat_megagon.json")
```
</details>
<br/>
# workspace/llm_training/axolotl/llama3-multilingual/output_tagengo_openchat_megagon_8B_llama3
This model is a fine-tuned version of [meta-llama/Meta-Llama-3-8B-Instruct](https://huggingface.co/meta-llama/Meta-Llama-3-8B-Instruct) on the above described dataset.
It achieves the following results on the evaluation set:
- Loss: 0.6595
## Training procedure
<!-- This model card has been generated automatically according to the information the Trainer had access to. You
should probably proofread and complete it, then remove this comment. -->
[<img src="https://raw.githubusercontent.com/OpenAccess-AI-Collective/axolotl/main/image/axolotl-badge-web.png" alt="Built with Axolotl" width="200" height="32"/>](https://github.com/OpenAccess-AI-Collective/axolotl)
<details><summary>See axolotl config</summary>
axolotl version: `0.4.0`
```yaml
base_model: meta-llama/Meta-Llama-3-8B-Instruct
model_type: LlamaForCausalLM
tokenizer_type: AutoTokenizer # PreTrainedTokenizerFast
load_in_8bit: false
load_in_4bit: false
strict: false
datasets:
- path: /workspace/llm_training/axolotl/llama3-multilingual/tagengo_openchat_megagon.json
ds_type: json # see other options below
type: sharegpt
conversation: llama-3
dataset_prepared_path: /workspace/llm_training/axolotl/llama3-multilingual/prepared_tagengo_openchat_megagon
val_set_size: 0.01
output_dir: /workspace/llm_training/axolotl/llama3-multilingual/output_tagengo_openchat_megagon_8B_llama3
sequence_len: 8192
sample_packing: true
pad_to_sequence_len: true
use_wandb: true
wandb_project: wandb_project
wandb_entity: wandb_entity
wandb_name: wandb_name
gradient_accumulation_steps: 2
micro_batch_size: 2
num_epochs: 1
optimizer: paged_adamw_8bit
lr_scheduler: cosine
learning_rate: 1e-5
train_on_inputs: false
group_by_length: false
bf16: auto
fp16:
tf32: false
gradient_checkpointing: true
gradient_checkpointing_kwargs:
use_reentrant: false
early_stopping_patience:
resume_from_checkpoint:
logging_steps: 1
xformers_attention:
flash_attention: true
warmup_steps: 10
evals_per_epoch: 5
eval_table_size:
saves_per_epoch: 1
debug:
deepspeed: /workspace/axolotl/deepspeed_configs/zero2.json
weight_decay: 0.0
special_tokens:
pad_token: <|end_of_text|>
```
</details><br>
<details><summary>Note - we added this Llama 3 template to fastchat directly as the Llama 3 chat template was not supported when we trained this model.</summary>
```python
from fastchat.conversation import Conversation
from fastchat.conversation import register_conv_template
from fastchat.conversation import SeparatorStyle
register_conv_template(
Conversation(
name="llama-3",
system_template="<|begin_of_text|><|start_header_id|>system<|end_header_id|>\n\n{system_message}",
roles=("<|start_header_id|>user<|end_header_id|>\n", "<|start_header_id|>assistant<|end_header_id|>\n"),
sep_style=SeparatorStyle.ADD_NEW_LINE_SINGLE,
sep="<|eot_id|>",
stop_token_ids=[128009],
stop_str="<|eot_id|>",
)
)
```
</details><br>
### Training hyperparameters
This model was trained using 4 x A100 (80GB) for roughly 2.5 hours.
The following hyperparameters were used during training:
- learning_rate: 1e-05
- train_batch_size: 2
- eval_batch_size: 2
- seed: 42
- distributed_type: multi-GPU
- num_devices: 4
- gradient_accumulation_steps: 2
- total_train_batch_size: 16
- total_eval_batch_size: 8
- optimizer: Adam with betas=(0.9,0.999) and epsilon=1e-08
- lr_scheduler_type: cosine
- lr_scheduler_warmup_steps: 10
- num_epochs: 1
### Training results
| Training Loss | Epoch | Step | Validation Loss |
|:-------------:|:-----:|:----:|:---------------:|
| 1.1894 | 0.0 | 1 | 1.0110 |
| 0.8493 | 0.2 | 73 | 0.7057 |
| 0.8047 | 0.4 | 146 | 0.6835 |
| 0.7644 | 0.6 | 219 | 0.6687 |
| 0.7528 | 0.8 | 292 | 0.6615 |
| 0.7794 | 1.0 | 365 | 0.6595 |
### Framework versions
- Transformers 4.38.2
- Pytorch 2.2.1+cu121
- Datasets 2.18.0
- Tokenizers 0.15.0
# How to cite
Please cite [this paper](https://arxiv.org/abs/2405.12612) when referencing this model.
```tex
@article{devine2024tagengo,
title={Tagengo: A Multilingual Chat Dataset},
author={Devine, Peter},
journal={arXiv preprint arXiv:2405.12612},
year={2024}
}
```
# Developer
Peter Devine - ([ptrdvn](https://huggingface.co/ptrdvn))
|
RichardErkhov/ibm-granite_-_granite-7b-base-gguf | RichardErkhov | 2024-06-29T05:51:54Z | 15,486 | 0 | null | [
"gguf",
"region:us"
] | null | 2024-06-29T04:14:48Z | Quantization made by Richard Erkhov.
[Github](https://github.com/RichardErkhov)
[Discord](https://discord.gg/pvy7H8DZMG)
[Request more models](https://github.com/RichardErkhov/quant_request)
granite-7b-base - GGUF
- Model creator: https://huggingface.co/ibm-granite/
- Original model: https://huggingface.co/ibm-granite/granite-7b-base/
| Name | Quant method | Size |
| ---- | ---- | ---- |
| [granite-7b-base.Q2_K.gguf](https://huggingface.co/RichardErkhov/ibm-granite_-_granite-7b-base-gguf/blob/main/granite-7b-base.Q2_K.gguf) | Q2_K | 2.36GB |
| [granite-7b-base.IQ3_XS.gguf](https://huggingface.co/RichardErkhov/ibm-granite_-_granite-7b-base-gguf/blob/main/granite-7b-base.IQ3_XS.gguf) | IQ3_XS | 2.6GB |
| [granite-7b-base.IQ3_S.gguf](https://huggingface.co/RichardErkhov/ibm-granite_-_granite-7b-base-gguf/blob/main/granite-7b-base.IQ3_S.gguf) | IQ3_S | 2.75GB |
| [granite-7b-base.Q3_K_S.gguf](https://huggingface.co/RichardErkhov/ibm-granite_-_granite-7b-base-gguf/blob/main/granite-7b-base.Q3_K_S.gguf) | Q3_K_S | 2.75GB |
| [granite-7b-base.IQ3_M.gguf](https://huggingface.co/RichardErkhov/ibm-granite_-_granite-7b-base-gguf/blob/main/granite-7b-base.IQ3_M.gguf) | IQ3_M | 2.9GB |
| [granite-7b-base.Q3_K.gguf](https://huggingface.co/RichardErkhov/ibm-granite_-_granite-7b-base-gguf/blob/main/granite-7b-base.Q3_K.gguf) | Q3_K | 3.07GB |
| [granite-7b-base.Q3_K_M.gguf](https://huggingface.co/RichardErkhov/ibm-granite_-_granite-7b-base-gguf/blob/main/granite-7b-base.Q3_K_M.gguf) | Q3_K_M | 3.07GB |
| [granite-7b-base.Q3_K_L.gguf](https://huggingface.co/RichardErkhov/ibm-granite_-_granite-7b-base-gguf/blob/main/granite-7b-base.Q3_K_L.gguf) | Q3_K_L | 3.35GB |
| [granite-7b-base.IQ4_XS.gguf](https://huggingface.co/RichardErkhov/ibm-granite_-_granite-7b-base-gguf/blob/main/granite-7b-base.IQ4_XS.gguf) | IQ4_XS | 3.4GB |
| [granite-7b-base.Q4_0.gguf](https://huggingface.co/RichardErkhov/ibm-granite_-_granite-7b-base-gguf/blob/main/granite-7b-base.Q4_0.gguf) | Q4_0 | 3.56GB |
| [granite-7b-base.IQ4_NL.gguf](https://huggingface.co/RichardErkhov/ibm-granite_-_granite-7b-base-gguf/blob/main/granite-7b-base.IQ4_NL.gguf) | IQ4_NL | 3.58GB |
| [granite-7b-base.Q4_K_S.gguf](https://huggingface.co/RichardErkhov/ibm-granite_-_granite-7b-base-gguf/blob/main/granite-7b-base.Q4_K_S.gguf) | Q4_K_S | 3.59GB |
| [granite-7b-base.Q4_K.gguf](https://huggingface.co/RichardErkhov/ibm-granite_-_granite-7b-base-gguf/blob/main/granite-7b-base.Q4_K.gguf) | Q4_K | 3.8GB |
| [granite-7b-base.Q4_K_M.gguf](https://huggingface.co/RichardErkhov/ibm-granite_-_granite-7b-base-gguf/blob/main/granite-7b-base.Q4_K_M.gguf) | Q4_K_M | 3.8GB |
| [granite-7b-base.Q4_1.gguf](https://huggingface.co/RichardErkhov/ibm-granite_-_granite-7b-base-gguf/blob/main/granite-7b-base.Q4_1.gguf) | Q4_1 | 3.95GB |
| [granite-7b-base.Q5_0.gguf](https://huggingface.co/RichardErkhov/ibm-granite_-_granite-7b-base-gguf/blob/main/granite-7b-base.Q5_0.gguf) | Q5_0 | 4.33GB |
| [granite-7b-base.Q5_K_S.gguf](https://huggingface.co/RichardErkhov/ibm-granite_-_granite-7b-base-gguf/blob/main/granite-7b-base.Q5_K_S.gguf) | Q5_K_S | 4.33GB |
| [granite-7b-base.Q5_K.gguf](https://huggingface.co/RichardErkhov/ibm-granite_-_granite-7b-base-gguf/blob/main/granite-7b-base.Q5_K.gguf) | Q5_K | 4.45GB |
| [granite-7b-base.Q5_K_M.gguf](https://huggingface.co/RichardErkhov/ibm-granite_-_granite-7b-base-gguf/blob/main/granite-7b-base.Q5_K_M.gguf) | Q5_K_M | 4.45GB |
| [granite-7b-base.Q5_1.gguf](https://huggingface.co/RichardErkhov/ibm-granite_-_granite-7b-base-gguf/blob/main/granite-7b-base.Q5_1.gguf) | Q5_1 | 4.72GB |
| [granite-7b-base.Q6_K.gguf](https://huggingface.co/RichardErkhov/ibm-granite_-_granite-7b-base-gguf/blob/main/granite-7b-base.Q6_K.gguf) | Q6_K | 5.15GB |
| [granite-7b-base.Q8_0.gguf](https://huggingface.co/RichardErkhov/ibm-granite_-_granite-7b-base-gguf/blob/main/granite-7b-base.Q8_0.gguf) | Q8_0 | 6.67GB |
Original model description:
---
license: apache-2.0
---
**Model Name**: Granite-7b-base
**License**: Apache-2.0
**Languages**: Primarily English
**Architecture**: The model architecture is a replica of Meta’s Llama2-7B base variant with MHA, trained with 1M batch size on 2T tokens.
**Context Length**: 4k tokens
**Tokenizer**: Llama2
**Model Developers**: IBM Research
Representing IBM’s commitment to open source innovation IBM has released granite-7b-base, a base pre-trained LLM from IBM’s Granite model series, under an apache-2.0 license for community and commercial use. Granite-7b-base was pre-trained from scratch on IBM-curated data as an open reference implementation of Meta’s Llama-2-7B. In a commitment to data transparency and fostering open innovation, the data sources, sampling proportions, and URLs for access are provided below.
For more information about training this model, please check out the blog: https://pytorch.org/blog/maximizing-training/
**Pre-Training Data**
The model was trained on 2T tokens, with sampling proportions designed to match the sampling distributions released in the Llama1 paper as closely as possible.
| Dataset | Description | Sampling Proportion | URL |
|-------------------------|--------------------------------------------------------------------------------------------------------------------------------------------------------------------------------------------|---------------------|--------------------------------------------------------------------|
| Common Crawl | Open repository of web crawl data with snapshots ranging from 2021 to 2023. | 77% | https://data.commoncrawl.org/ |
| Github_Clean | Code data from CodeParrot covering a variety of coding languages. | 5.50% | https://huggingface.co/datasets/codeparrot/github-code-clean |
| Wikipedia and Wikimedia | Eight Wikimedia projects (enwiki, enwikibooks, enwikinews, enwikiquote, enwikisource, enwikiversity, enwikivoyage, enwiktionary). containing extracted plain text from pages and articles. | 2% | https://dumps.wikimedia.org |
| USPTO | US patents granted from 1975 to May 2023, excluding design patents. | 5% | https://bulkdata.uspto.gov/ |
| PubMed Central | Biomedical and life sciences papers. | 1.75% | https://ftp.ncbi.nlm.nih.gov/pub/pmc/oa_package/ |
| arXiv | Over 1.8 million scientific paper pre-prints posted to arXiv. | 2.50% | https://huggingface.co/datasets/togethercomputer/RedPajama-Data-1T |
| StackExchange | Anonymized set of all user-contributed content on the Stack Exchange network, a popular collection of websites centered around user-contributed questions and answers. | 1% | https://archive.org/details/stackexchange_20221206 |
| PG19 | A repository of free e-books with focus on older works for which U.S. copyright has expired. | 0.25% | https://github.com/google-deepmind/pg19 |
| Webhose | Unstructured web content converted into machine-readable data feeds purchased by IBM. | 5% | N/A |
**Evaluation Results**
LM-eval Harness Scores
| Evaluation metric | Llama2-7B (baseline) | Granite-7b-base |
|----------------------------|----------------------|-----------------|
| MMLU (zero shot) | 0.41 | 0.43 |
| MMLU (5-shot weighted avg) | 0.47 | 0.50 |
| Arc challenge | 0.46 | 0.44 |
| Arc easy | 0.74 | 0.71 |
| Boolq | 0.78 | 0.76 |
| Copa | 0.87 | 0.83 |
| Hellaswag | 0.76 | 0.74 |
| Openbookqa | 0.44 | 0.42 |
| Piqa | 0.79 | 0.79 |
| Sciq | 0.91 | 0.91 |
| Winogrande | 0.69 | 0.67 |
| Truthfulqa | 0.39 | 0.39 |
| GSM8k (8-shot) | 0.13 | 0.11 |
**Bias, Risks, and Limitations**
Granite-7b-base is a base model and has not undergone any safety alignment, there it may produce problematic outputs. In the absence of adequate safeguards and RLHF, there exists a risk of malicious utilization of these models for generating disinformation or harmful content. Caution is urged against complete reliance on a specific language model for crucial decisions or impactful information, as preventing these models from fabricating content is not straightforward. Additionally, it remains uncertain whether smaller models might exhibit increased susceptibility to hallucination in ungrounded generation scenarios due to their reduced sizes and memorization capacities. This aspect is currently an active area of research, and we anticipate more rigorous exploration, comprehension, and mitigations in this domain.
|
mdhugol/indonesia-bert-sentiment-classification | mdhugol | 2021-09-14T08:24:28Z | 15,477 | 18 | transformers | [
"transformers",
"pytorch",
"bert",
"text-classification",
"autotrain_compatible",
"endpoints_compatible",
"region:us"
] | text-classification | 2022-03-02T23:29:05Z | Indonesian BERT Base Sentiment Classifier is a sentiment-text-classification model. The model was originally the pre-trained [IndoBERT Base Model (phase1 - uncased)](https://huggingface.co/indobenchmark/indobert-base-p1) model using [Prosa sentiment dataset](https://github.com/indobenchmark/indonlu/tree/master/dataset/smsa_doc-sentiment-prosa)
## How to Use
### As Text Classifier
```python
from transformers import pipeline
from transformers import AutoTokenizer, AutoModelForSequenceClassification
pretrained= "mdhugol/indonesia-bert-sentiment-classification"
model = AutoModelForSequenceClassification.from_pretrained(pretrained)
tokenizer = AutoTokenizer.from_pretrained(pretrained)
sentiment_analysis = pipeline("sentiment-analysis", model=model, tokenizer=tokenizer)
label_index = {'LABEL_0': 'positive', 'LABEL_1': 'neutral', 'LABEL_2': 'negative'}
pos_text = "Sangat bahagia hari ini"
neg_text = "Dasar anak sialan!! Kurang ajar!!"
result = sentiment_analysis(pos_text)
status = label_index[result[0]['label']]
score = result[0]['score']
print(f'Text: {pos_text} | Label : {status} ({score * 100:.3f}%)')
result = sentiment_analysis(neg_text)
status = label_index[result[0]['label']]
score = result[0]['score']
print(f'Text: {neg_text} | Label : {status} ({score * 100:.3f}%)')
``` |
xinsir/controlnet-openpose-sdxl-1.0 | xinsir | 2024-06-26T02:18:35Z | 15,473 | 140 | diffusers | [
"diffusers",
"safetensors",
"openpose",
"controlnet",
"controlnet-openpose-sdxl-1.0",
"text_to_image",
"arxiv:2302.05543",
"license:apache-2.0",
"region:us"
] | null | 2024-05-13T14:47:50Z | ---
license: apache-2.0
tags:
- openpose
- controlnet
- diffusers
- controlnet-openpose-sdxl-1.0
- text_to_image
---
# ***State of the art ControlNet-openpose-sdxl-1.0 model, below are the result for midjourney and anime, just for show***


### controlnet-openpose-sdxl-1.0
<!-- Provide a longer summary of what this model is. -->
- **Developed by:** xinsir
- **Model type:** ControlNet_SDXL
- **License:** apache-2.0
- **Finetuned from model [optional]:** stabilityai/stable-diffusion-xl-base-1.0
### Model Sources [optional]
<!-- Provide the basic links for the model. -->
- **Paper [optional]:** https://arxiv.org/abs/2302.05543
-
### Examples




















## Replace the default draw pose function to get better result
thanks feiyuuu for report the problem. When using the default pose line the performance may be unstable, this is because the pose label use more thick line in training to have a better look.
This difference can be fix by using the following method:
Find the util.py in controlnet_aux python package, usually the path is like: /your anaconda3 path/envs/your env name/lib/python3.8/site-packages/controlnet_aux/open_pose/util.py
Replace the draw_bodypose function with the following code:
```python
def draw_bodypose(canvas: np.ndarray, keypoints: List[Keypoint]) -> np.ndarray:
"""
Draw keypoints and limbs representing body pose on a given canvas.
Args:
canvas (np.ndarray): A 3D numpy array representing the canvas (image) on which to draw the body pose.
keypoints (List[Keypoint]): A list of Keypoint objects representing the body keypoints to be drawn.
Returns:
np.ndarray: A 3D numpy array representing the modified canvas with the drawn body pose.
Note:
The function expects the x and y coordinates of the keypoints to be normalized between 0 and 1.
"""
H, W, C = canvas.shape
if max(W, H) < 500:
ratio = 1.0
elif max(W, H) >= 500 and max(W, H) < 1000:
ratio = 2.0
elif max(W, H) >= 1000 and max(W, H) < 2000:
ratio = 3.0
elif max(W, H) >= 2000 and max(W, H) < 3000:
ratio = 4.0
elif max(W, H) >= 3000 and max(W, H) < 4000:
ratio = 5.0
elif max(W, H) >= 4000 and max(W, H) < 5000:
ratio = 6.0
else:
ratio = 7.0
stickwidth = 4
limbSeq = [
[2, 3], [2, 6], [3, 4], [4, 5],
[6, 7], [7, 8], [2, 9], [9, 10],
[10, 11], [2, 12], [12, 13], [13, 14],
[2, 1], [1, 15], [15, 17], [1, 16],
[16, 18],
]
colors = [[255, 0, 0], [255, 85, 0], [255, 170, 0], [255, 255, 0], [170, 255, 0], [85, 255, 0], [0, 255, 0], \
[0, 255, 85], [0, 255, 170], [0, 255, 255], [0, 170, 255], [0, 85, 255], [0, 0, 255], [85, 0, 255], \
[170, 0, 255], [255, 0, 255], [255, 0, 170], [255, 0, 85]]
for (k1_index, k2_index), color in zip(limbSeq, colors):
keypoint1 = keypoints[k1_index - 1]
keypoint2 = keypoints[k2_index - 1]
if keypoint1 is None or keypoint2 is None:
continue
Y = np.array([keypoint1.x, keypoint2.x]) * float(W)
X = np.array([keypoint1.y, keypoint2.y]) * float(H)
mX = np.mean(X)
mY = np.mean(Y)
length = ((X[0] - X[1]) ** 2 + (Y[0] - Y[1]) ** 2) ** 0.5
angle = math.degrees(math.atan2(X[0] - X[1], Y[0] - Y[1]))
polygon = cv2.ellipse2Poly((int(mY), int(mX)), (int(length / 2), int(stickwidth * ratio)), int(angle), 0, 360, 1)
cv2.fillConvexPoly(canvas, polygon, [int(float(c) * 0.6) for c in color])
for keypoint, color in zip(keypoints, colors):
if keypoint is None:
continue
x, y = keypoint.x, keypoint.y
x = int(x * W)
y = int(y * H)
cv2.circle(canvas, (int(x), int(y)), int(4 * ratio), color, thickness=-1)
return canvas
```
## How to Get Started with the Model
Use the code below to get started with the model.
```python
from diffusers import ControlNetModel, StableDiffusionXLControlNetPipeline, AutoencoderKL
from diffusers import DDIMScheduler, EulerAncestralDiscreteScheduler
from controlnet_aux import OpenposeDetector
from PIL import Image
import torch
import numpy as np
import cv2
controlnet_conditioning_scale = 1.0
prompt = "your prompt, the longer the better, you can describe it as detail as possible"
negative_prompt = 'longbody, lowres, bad anatomy, bad hands, missing fingers, extra digit, fewer digits, cropped, worst quality, low quality'
eulera_scheduler = EulerAncestralDiscreteScheduler.from_pretrained("stabilityai/stable-diffusion-xl-base-1.0", subfolder="scheduler")
controlnet = ControlNetModel.from_pretrained(
"xinsir/controlnet-openpose-sdxl-1.0",
torch_dtype=torch.float16
)
# when test with other base model, you need to change the vae also.
vae = AutoencoderKL.from_pretrained("madebyollin/sdxl-vae-fp16-fix", torch_dtype=torch.float16)
pipe = StableDiffusionXLControlNetPipeline.from_pretrained(
"stabilityai/stable-diffusion-xl-base-1.0",
controlnet=controlnet,
vae=vae,
safety_checker=None,
torch_dtype=torch.float16,
scheduler=eulera_scheduler,
)
processor = OpenposeDetector.from_pretrained('lllyasviel/ControlNet')
controlnet_img = cv2.imread("your image path")
controlnet_img = processor(controlnet_img, hand_and_face=False, output_type='cv2')
# need to resize the image resolution to 1024 * 1024 or same bucket resolution to get the best performance
height, width, _ = controlnet_img.shape
ratio = np.sqrt(1024. * 1024. / (width * height))
new_width, new_height = int(width * ratio), int(height * ratio)
controlnet_img = cv2.resize(controlnet_img, (new_width, new_height))
controlnet_img = Image.fromarray(controlnet_img)
images = pipe(
prompt,
negative_prompt=negative_prompt,
image=controlnet_img,
controlnet_conditioning_scale=controlnet_conditioning_scale,
width=new_width,
height=new_height,
num_inference_steps=30,
).images
images[0].save(f"your image save path, png format is usually better than jpg or webp in terms of image quality but got much bigger")
```
## Evaluation Data
HumanArt [https://github.com/IDEA-Research/HumanArt], select 2000 images with ground truth pose annotations to generate images and calculate mAP.
## Quantitative Result
| metric | xinsir/controlnet-openpose-sdxl-1.0 | lllyasviel/control_v11p_sd15_openpose | thibaud/controlnet-openpose-sdxl-1.0 |
|-------|-------|-------|-------|
| mAP | **0.357** | 0.326 | 0.209 |
We are the SOTA openpose model compared with other opensource models. |
Systran/faster-whisper-small.en | Systran | 2023-11-23T11:00:00Z | 15,472 | 2 | ctranslate2 | [
"ctranslate2",
"audio",
"automatic-speech-recognition",
"en",
"license:mit",
"region:us"
] | automatic-speech-recognition | 2023-11-23T09:55:25Z | ---
language:
- en
tags:
- audio
- automatic-speech-recognition
license: mit
library_name: ctranslate2
---
# Whisper small.en model for CTranslate2
This repository contains the conversion of [openai/whisper-small.en](https://huggingface.co/openai/whisper-small.en) to the [CTranslate2](https://github.com/OpenNMT/CTranslate2) model format.
This model can be used in CTranslate2 or projects based on CTranslate2 such as [faster-whisper](https://github.com/systran/faster-whisper).
## Example
```python
from faster_whisper import WhisperModel
model = WhisperModel("small.en")
segments, info = model.transcribe("audio.mp3")
for segment in segments:
print("[%.2fs -> %.2fs] %s" % (segment.start, segment.end, segment.text))
```
## Conversion details
The original model was converted with the following command:
```
ct2-transformers-converter --model openai/whisper-small.en --output_dir faster-whisper-small.en \
--copy_files tokenizer.json --quantization float16
```
Note that the model weights are saved in FP16. This type can be changed when the model is loaded using the [`compute_type` option in CTranslate2](https://opennmt.net/CTranslate2/quantization.html).
## More information
**For more information about the original model, see its [model card](https://huggingface.co/openai/whisper-small.en).**
|
digiplay/m0nst3rfy3-testfix | digiplay | 2024-06-15T02:37:04Z | 15,446 | 2 | diffusers | [
"diffusers",
"safetensors",
"license:other",
"autotrain_compatible",
"endpoints_compatible",
"diffusers:StableDiffusionPipeline",
"region:us"
] | text-to-image | 2024-06-15T00:15:57Z | ---
license: other
---
in test... use "m0nst3rfy3" to set a magic effects :
Original Model info:
https://huggingface.co/Yntec/m0nst3rfy3
Original Author : Yntec
prompt: 1girl

prompt: 1girl m0nst3rfy3

Other Sample images :
**generated by Huggingface's API









|
mradermacher/Qwen2-7b-Matter-0.1-Slim-A-GGUF | mradermacher | 2024-06-26T09:50:46Z | 15,446 | 0 | transformers | [
"transformers",
"gguf",
"text-generation-inference",
"unsloth",
"qwen2",
"trl",
"sft",
"en",
"base_model:munish0838/Qwen2-7b-Matter-0.1-Slim-A",
"license:apache-2.0",
"endpoints_compatible",
"region:us"
] | null | 2024-06-26T09:00:22Z | ---
base_model: munish0838/Qwen2-7b-Matter-0.1-Slim-A
language:
- en
library_name: transformers
license: apache-2.0
quantized_by: mradermacher
tags:
- text-generation-inference
- transformers
- unsloth
- qwen2
- trl
- sft
---
## About
<!-- ### quantize_version: 2 -->
<!-- ### output_tensor_quantised: 1 -->
<!-- ### convert_type: hf -->
<!-- ### vocab_type: -->
<!-- ### tags: -->
static quants of https://huggingface.co/munish0838/Qwen2-7b-Matter-0.1-Slim-A
<!-- provided-files -->
weighted/imatrix quants seem not to be available (by me) at this time. If they do not show up a week or so after the static ones, I have probably not planned for them. Feel free to request them by opening a Community Discussion.
## Usage
If you are unsure how to use GGUF files, refer to one of [TheBloke's
READMEs](https://huggingface.co/TheBloke/KafkaLM-70B-German-V0.1-GGUF) for
more details, including on how to concatenate multi-part files.
## Provided Quants
(sorted by size, not necessarily quality. IQ-quants are often preferable over similar sized non-IQ quants)
| Link | Type | Size/GB | Notes |
|:-----|:-----|--------:|:------|
| [GGUF](https://huggingface.co/mradermacher/Qwen2-7b-Matter-0.1-Slim-A-GGUF/resolve/main/Qwen2-7b-Matter-0.1-Slim-A.Q2_K.gguf) | Q2_K | 3.1 | |
| [GGUF](https://huggingface.co/mradermacher/Qwen2-7b-Matter-0.1-Slim-A-GGUF/resolve/main/Qwen2-7b-Matter-0.1-Slim-A.IQ3_XS.gguf) | IQ3_XS | 3.4 | |
| [GGUF](https://huggingface.co/mradermacher/Qwen2-7b-Matter-0.1-Slim-A-GGUF/resolve/main/Qwen2-7b-Matter-0.1-Slim-A.Q3_K_S.gguf) | Q3_K_S | 3.6 | |
| [GGUF](https://huggingface.co/mradermacher/Qwen2-7b-Matter-0.1-Slim-A-GGUF/resolve/main/Qwen2-7b-Matter-0.1-Slim-A.IQ3_S.gguf) | IQ3_S | 3.6 | beats Q3_K* |
| [GGUF](https://huggingface.co/mradermacher/Qwen2-7b-Matter-0.1-Slim-A-GGUF/resolve/main/Qwen2-7b-Matter-0.1-Slim-A.IQ3_M.gguf) | IQ3_M | 3.7 | |
| [GGUF](https://huggingface.co/mradermacher/Qwen2-7b-Matter-0.1-Slim-A-GGUF/resolve/main/Qwen2-7b-Matter-0.1-Slim-A.Q3_K_M.gguf) | Q3_K_M | 3.9 | lower quality |
| [GGUF](https://huggingface.co/mradermacher/Qwen2-7b-Matter-0.1-Slim-A-GGUF/resolve/main/Qwen2-7b-Matter-0.1-Slim-A.Q3_K_L.gguf) | Q3_K_L | 4.2 | |
| [GGUF](https://huggingface.co/mradermacher/Qwen2-7b-Matter-0.1-Slim-A-GGUF/resolve/main/Qwen2-7b-Matter-0.1-Slim-A.IQ4_XS.gguf) | IQ4_XS | 4.4 | |
| [GGUF](https://huggingface.co/mradermacher/Qwen2-7b-Matter-0.1-Slim-A-GGUF/resolve/main/Qwen2-7b-Matter-0.1-Slim-A.Q4_K_S.gguf) | Q4_K_S | 4.6 | fast, recommended |
| [GGUF](https://huggingface.co/mradermacher/Qwen2-7b-Matter-0.1-Slim-A-GGUF/resolve/main/Qwen2-7b-Matter-0.1-Slim-A.Q4_K_M.gguf) | Q4_K_M | 4.8 | fast, recommended |
| [GGUF](https://huggingface.co/mradermacher/Qwen2-7b-Matter-0.1-Slim-A-GGUF/resolve/main/Qwen2-7b-Matter-0.1-Slim-A.Q5_K_S.gguf) | Q5_K_S | 5.4 | |
| [GGUF](https://huggingface.co/mradermacher/Qwen2-7b-Matter-0.1-Slim-A-GGUF/resolve/main/Qwen2-7b-Matter-0.1-Slim-A.Q5_K_M.gguf) | Q5_K_M | 5.5 | |
| [GGUF](https://huggingface.co/mradermacher/Qwen2-7b-Matter-0.1-Slim-A-GGUF/resolve/main/Qwen2-7b-Matter-0.1-Slim-A.Q6_K.gguf) | Q6_K | 6.4 | very good quality |
| [GGUF](https://huggingface.co/mradermacher/Qwen2-7b-Matter-0.1-Slim-A-GGUF/resolve/main/Qwen2-7b-Matter-0.1-Slim-A.Q8_0.gguf) | Q8_0 | 8.2 | fast, best quality |
| [GGUF](https://huggingface.co/mradermacher/Qwen2-7b-Matter-0.1-Slim-A-GGUF/resolve/main/Qwen2-7b-Matter-0.1-Slim-A.f16.gguf) | f16 | 15.3 | 16 bpw, overkill |
Here is a handy graph by ikawrakow comparing some lower-quality quant
types (lower is better):

And here are Artefact2's thoughts on the matter:
https://gist.github.com/Artefact2/b5f810600771265fc1e39442288e8ec9
## FAQ / Model Request
See https://huggingface.co/mradermacher/model_requests for some answers to
questions you might have and/or if you want some other model quantized.
## Thanks
I thank my company, [nethype GmbH](https://www.nethype.de/), for letting
me use its servers and providing upgrades to my workstation to enable
this work in my free time.
<!-- end -->
|
rubra-ai/Mistral-7B-Instruct-v0.2-GGUF | rubra-ai | 2024-07-01T06:13:33Z | 15,435 | 2 | null | [
"gguf",
"function-calling",
"tool-calling",
"agentic",
"rubra",
"conversational",
"en",
"license:apache-2.0",
"model-index",
"region:us"
] | null | 2024-07-01T05:23:40Z | ---
license: apache-2.0
model-index:
- name: Rubra-Mistral-7B-Instruct-v0.2
results:
- task:
type: text-generation
dataset:
type: MMLU
name: MMLU
metrics:
- type: 5-shot
value: 58.9
verified: false
- task:
type: text-generation
dataset:
type: GPQA
name: GPQA
metrics:
- type: 0-shot
value: 29.91
verified: false
- task:
type: text-generation
dataset:
type: GSM-8K
name: GSM-8K
metrics:
- type: 8-shot, CoT
value: 34.12
verified: false
- task:
type: text-generation
dataset:
type: MATH
name: MATH
metrics:
- type: 4-shot, CoT
value: 8.36
verified: false
- task:
type: text-generation
dataset:
type: MT-bench
name: MT-bench
metrics:
- type: GPT-4 as Judge
value: 7.36
verified: false
tags:
- function-calling
- tool-calling
- agentic
- rubra
- conversational
language:
- en
---
# Rubra Mistral 7B Instruct v0.2 GGUF
Original model: [rubra-ai/Mistral-7B-Instruct-v0.2](https://huggingface.co/rubra-ai/Mistral-7B-Instruct-v0.2)
## Model description
The model is the result of further post-training [mistralai/Mistral-7B-Instruct-v0.2](https://huggingface.co/mistralai/Mistral-7B-Instruct-v0.2). This model is designed for high performance in various instruction-following tasks and complex interactions, including multi-turn function calling and detailed conversations.
## Training Data
The model underwent additional training on a proprietary dataset encompassing diverse instruction-following, chat, and function calling data. This post-training process enhances the model's ability to integrate tools and manage complex interaction scenarios effectively.
## How to use
Refer to https://docs.rubra.ai/inference/llamacpp for usage. Feel free to ask/open issues up in our Github repo: https://github.com/rubra-ai/rubra
## Limitations and Bias
While the model performs well on a wide range of tasks, it may still produce biased or incorrect outputs. Users should exercise caution and critical judgment when using the model in sensitive or high-stakes applications. The model's outputs are influenced by the data it was trained on, which may contain inherent biases.
## Ethical Considerations
Users should ensure that the deployment of this model adheres to ethical guidelines and consider the potential societal impact of the generated text. Misuse of the model for generating harmful or misleading content is strongly discouraged.
## Acknowledgements
We would like to thank Mistral for the model.
## Contact Information
For questions or comments about the model, please reach out to [the rubra team](mailto:[email protected]).
## Citation
If you use this work, please cite it as:
```
@misc {rubra_ai_2024,
author = { Sanjay Nadhavajhala and Yingbei Tong },
title = { Mistral-7B-Instruct-v0.2 },
year = 2024,
url = { https://huggingface.co/rubra-ai/Mistral-7B-Instruct-v0.2 },
doi = { 10.57967/hf/2641 },
publisher = { Hugging Face }
}
``` |
mradermacher/Yi-9B-i1-GGUF | mradermacher | 2024-06-27T11:16:55Z | 15,431 | 0 | transformers | [
"transformers",
"gguf",
"en",
"base_model:01-ai/Yi-9B",
"license:apache-2.0",
"endpoints_compatible",
"region:us"
] | null | 2024-06-26T22:57:30Z | ---
base_model: 01-ai/Yi-9B
language:
- en
library_name: transformers
license: apache-2.0
quantized_by: mradermacher
---
## About
<!-- ### quantize_version: 2 -->
<!-- ### output_tensor_quantised: 1 -->
<!-- ### convert_type: hf -->
<!-- ### vocab_type: -->
<!-- ### tags: nicoboss -->
weighted/imatrix quants of https://huggingface.co/01-ai/Yi-9B
<!-- provided-files -->
static quants are available at https://huggingface.co/mradermacher/Yi-9B-GGUF
## Usage
If you are unsure how to use GGUF files, refer to one of [TheBloke's
READMEs](https://huggingface.co/TheBloke/KafkaLM-70B-German-V0.1-GGUF) for
more details, including on how to concatenate multi-part files.
## Provided Quants
(sorted by size, not necessarily quality. IQ-quants are often preferable over similar sized non-IQ quants)
| Link | Type | Size/GB | Notes |
|:-----|:-----|--------:|:------|
| [GGUF](https://huggingface.co/mradermacher/Yi-9B-i1-GGUF/resolve/main/Yi-9B.i1-IQ1_S.gguf) | i1-IQ1_S | 2.1 | for the desperate |
| [GGUF](https://huggingface.co/mradermacher/Yi-9B-i1-GGUF/resolve/main/Yi-9B.i1-IQ1_M.gguf) | i1-IQ1_M | 2.3 | mostly desperate |
| [GGUF](https://huggingface.co/mradermacher/Yi-9B-i1-GGUF/resolve/main/Yi-9B.i1-IQ2_XXS.gguf) | i1-IQ2_XXS | 2.6 | |
| [GGUF](https://huggingface.co/mradermacher/Yi-9B-i1-GGUF/resolve/main/Yi-9B.i1-IQ2_XS.gguf) | i1-IQ2_XS | 2.8 | |
| [GGUF](https://huggingface.co/mradermacher/Yi-9B-i1-GGUF/resolve/main/Yi-9B.i1-IQ2_S.gguf) | i1-IQ2_S | 3.0 | |
| [GGUF](https://huggingface.co/mradermacher/Yi-9B-i1-GGUF/resolve/main/Yi-9B.i1-IQ2_M.gguf) | i1-IQ2_M | 3.2 | |
| [GGUF](https://huggingface.co/mradermacher/Yi-9B-i1-GGUF/resolve/main/Yi-9B.i1-Q2_K.gguf) | i1-Q2_K | 3.5 | IQ3_XXS probably better |
| [GGUF](https://huggingface.co/mradermacher/Yi-9B-i1-GGUF/resolve/main/Yi-9B.i1-IQ3_XXS.gguf) | i1-IQ3_XXS | 3.6 | lower quality |
| [GGUF](https://huggingface.co/mradermacher/Yi-9B-i1-GGUF/resolve/main/Yi-9B.i1-IQ3_XS.gguf) | i1-IQ3_XS | 3.8 | |
| [GGUF](https://huggingface.co/mradermacher/Yi-9B-i1-GGUF/resolve/main/Yi-9B.i1-Q3_K_S.gguf) | i1-Q3_K_S | 4.0 | IQ3_XS probably better |
| [GGUF](https://huggingface.co/mradermacher/Yi-9B-i1-GGUF/resolve/main/Yi-9B.i1-IQ3_S.gguf) | i1-IQ3_S | 4.0 | beats Q3_K* |
| [GGUF](https://huggingface.co/mradermacher/Yi-9B-i1-GGUF/resolve/main/Yi-9B.i1-IQ3_M.gguf) | i1-IQ3_M | 4.2 | |
| [GGUF](https://huggingface.co/mradermacher/Yi-9B-i1-GGUF/resolve/main/Yi-9B.i1-Q3_K_M.gguf) | i1-Q3_K_M | 4.4 | IQ3_S probably better |
| [GGUF](https://huggingface.co/mradermacher/Yi-9B-i1-GGUF/resolve/main/Yi-9B.i1-Q3_K_L.gguf) | i1-Q3_K_L | 4.8 | IQ3_M probably better |
| [GGUF](https://huggingface.co/mradermacher/Yi-9B-i1-GGUF/resolve/main/Yi-9B.i1-IQ4_XS.gguf) | i1-IQ4_XS | 4.9 | |
| [GGUF](https://huggingface.co/mradermacher/Yi-9B-i1-GGUF/resolve/main/Yi-9B.i1-Q4_0.gguf) | i1-Q4_0 | 5.2 | fast, low quality |
| [GGUF](https://huggingface.co/mradermacher/Yi-9B-i1-GGUF/resolve/main/Yi-9B.i1-Q4_K_S.gguf) | i1-Q4_K_S | 5.2 | optimal size/speed/quality |
| [GGUF](https://huggingface.co/mradermacher/Yi-9B-i1-GGUF/resolve/main/Yi-9B.i1-Q4_K_M.gguf) | i1-Q4_K_M | 5.4 | fast, recommended |
| [GGUF](https://huggingface.co/mradermacher/Yi-9B-i1-GGUF/resolve/main/Yi-9B.i1-Q5_K_S.gguf) | i1-Q5_K_S | 6.2 | |
| [GGUF](https://huggingface.co/mradermacher/Yi-9B-i1-GGUF/resolve/main/Yi-9B.i1-Q5_K_M.gguf) | i1-Q5_K_M | 6.4 | |
| [GGUF](https://huggingface.co/mradermacher/Yi-9B-i1-GGUF/resolve/main/Yi-9B.i1-Q6_K.gguf) | i1-Q6_K | 7.3 | practically like static Q6_K |
Here is a handy graph by ikawrakow comparing some lower-quality quant
types (lower is better):

And here are Artefact2's thoughts on the matter:
https://gist.github.com/Artefact2/b5f810600771265fc1e39442288e8ec9
## FAQ / Model Request
See https://huggingface.co/mradermacher/model_requests for some answers to
questions you might have and/or if you want some other model quantized.
## Thanks
I thank my company, [nethype GmbH](https://www.nethype.de/), for letting
me use its servers and providing upgrades to my workstation to enable
this work in my free time. Additional thanks to [@nicoboss](https://huggingface.co/nicoboss) for giving me access to his hardware for calculating the imatrix for these quants.
<!-- end -->
|
openaccess-ai-collective/tiny-mistral | openaccess-ai-collective | 2023-09-29T14:50:37Z | 15,426 | 11 | transformers | [
"transformers",
"pytorch",
"mistral",
"text-generation",
"autotrain_compatible",
"endpoints_compatible",
"text-generation-inference",
"region:us"
] | text-generation | 2023-09-28T15:10:32Z | mistral architecture model, randomly initialized. useful for e2e testing. |
mradermacher/Llama-3-Instruct-LiPPA-8B-i1-GGUF | mradermacher | 2024-06-27T12:58:39Z | 15,424 | 0 | transformers | [
"transformers",
"gguf",
"text-generation-inference",
"unsloth",
"llama",
"trl",
"sft",
"not-for-all-audiences",
"en",
"dataset:mpasila/LimaRP-PIPPA-Mix-8K-Context",
"dataset:grimulkan/LimaRP-augmented",
"dataset:KaraKaraWitch/PIPPA-ShareGPT-formatted",
"base_model:mpasila/Llama-3-Instruct-LiPPA-8B",
"license:llama3",
"endpoints_compatible",
"region:us"
] | null | 2024-06-27T10:13:46Z | ---
base_model: mpasila/Llama-3-Instruct-LiPPA-8B
datasets:
- mpasila/LimaRP-PIPPA-Mix-8K-Context
- grimulkan/LimaRP-augmented
- KaraKaraWitch/PIPPA-ShareGPT-formatted
language:
- en
library_name: transformers
license: llama3
quantized_by: mradermacher
tags:
- text-generation-inference
- transformers
- unsloth
- llama
- trl
- sft
- not-for-all-audiences
---
## About
<!-- ### quantize_version: 2 -->
<!-- ### output_tensor_quantised: 1 -->
<!-- ### convert_type: hf -->
<!-- ### vocab_type: -->
<!-- ### tags: nicoboss -->
weighted/imatrix quants of https://huggingface.co/mpasila/Llama-3-Instruct-LiPPA-8B
<!-- provided-files -->
static quants are available at https://huggingface.co/mradermacher/Llama-3-Instruct-LiPPA-8B-GGUF
## Usage
If you are unsure how to use GGUF files, refer to one of [TheBloke's
READMEs](https://huggingface.co/TheBloke/KafkaLM-70B-German-V0.1-GGUF) for
more details, including on how to concatenate multi-part files.
## Provided Quants
(sorted by size, not necessarily quality. IQ-quants are often preferable over similar sized non-IQ quants)
| Link | Type | Size/GB | Notes |
|:-----|:-----|--------:|:------|
| [GGUF](https://huggingface.co/mradermacher/Llama-3-Instruct-LiPPA-8B-i1-GGUF/resolve/main/Llama-3-Instruct-LiPPA-8B.i1-IQ1_S.gguf) | i1-IQ1_S | 2.1 | for the desperate |
| [GGUF](https://huggingface.co/mradermacher/Llama-3-Instruct-LiPPA-8B-i1-GGUF/resolve/main/Llama-3-Instruct-LiPPA-8B.i1-IQ1_M.gguf) | i1-IQ1_M | 2.3 | mostly desperate |
| [GGUF](https://huggingface.co/mradermacher/Llama-3-Instruct-LiPPA-8B-i1-GGUF/resolve/main/Llama-3-Instruct-LiPPA-8B.i1-IQ2_XXS.gguf) | i1-IQ2_XXS | 2.5 | |
| [GGUF](https://huggingface.co/mradermacher/Llama-3-Instruct-LiPPA-8B-i1-GGUF/resolve/main/Llama-3-Instruct-LiPPA-8B.i1-IQ2_XS.gguf) | i1-IQ2_XS | 2.7 | |
| [GGUF](https://huggingface.co/mradermacher/Llama-3-Instruct-LiPPA-8B-i1-GGUF/resolve/main/Llama-3-Instruct-LiPPA-8B.i1-IQ2_S.gguf) | i1-IQ2_S | 2.9 | |
| [GGUF](https://huggingface.co/mradermacher/Llama-3-Instruct-LiPPA-8B-i1-GGUF/resolve/main/Llama-3-Instruct-LiPPA-8B.i1-IQ2_M.gguf) | i1-IQ2_M | 3.0 | |
| [GGUF](https://huggingface.co/mradermacher/Llama-3-Instruct-LiPPA-8B-i1-GGUF/resolve/main/Llama-3-Instruct-LiPPA-8B.i1-Q2_K.gguf) | i1-Q2_K | 3.3 | IQ3_XXS probably better |
| [GGUF](https://huggingface.co/mradermacher/Llama-3-Instruct-LiPPA-8B-i1-GGUF/resolve/main/Llama-3-Instruct-LiPPA-8B.i1-IQ3_XXS.gguf) | i1-IQ3_XXS | 3.4 | lower quality |
| [GGUF](https://huggingface.co/mradermacher/Llama-3-Instruct-LiPPA-8B-i1-GGUF/resolve/main/Llama-3-Instruct-LiPPA-8B.i1-IQ3_XS.gguf) | i1-IQ3_XS | 3.6 | |
| [GGUF](https://huggingface.co/mradermacher/Llama-3-Instruct-LiPPA-8B-i1-GGUF/resolve/main/Llama-3-Instruct-LiPPA-8B.i1-Q3_K_S.gguf) | i1-Q3_K_S | 3.8 | IQ3_XS probably better |
| [GGUF](https://huggingface.co/mradermacher/Llama-3-Instruct-LiPPA-8B-i1-GGUF/resolve/main/Llama-3-Instruct-LiPPA-8B.i1-IQ3_S.gguf) | i1-IQ3_S | 3.8 | beats Q3_K* |
| [GGUF](https://huggingface.co/mradermacher/Llama-3-Instruct-LiPPA-8B-i1-GGUF/resolve/main/Llama-3-Instruct-LiPPA-8B.i1-IQ3_M.gguf) | i1-IQ3_M | 3.9 | |
| [GGUF](https://huggingface.co/mradermacher/Llama-3-Instruct-LiPPA-8B-i1-GGUF/resolve/main/Llama-3-Instruct-LiPPA-8B.i1-Q3_K_M.gguf) | i1-Q3_K_M | 4.1 | IQ3_S probably better |
| [GGUF](https://huggingface.co/mradermacher/Llama-3-Instruct-LiPPA-8B-i1-GGUF/resolve/main/Llama-3-Instruct-LiPPA-8B.i1-Q3_K_L.gguf) | i1-Q3_K_L | 4.4 | IQ3_M probably better |
| [GGUF](https://huggingface.co/mradermacher/Llama-3-Instruct-LiPPA-8B-i1-GGUF/resolve/main/Llama-3-Instruct-LiPPA-8B.i1-IQ4_XS.gguf) | i1-IQ4_XS | 4.5 | |
| [GGUF](https://huggingface.co/mradermacher/Llama-3-Instruct-LiPPA-8B-i1-GGUF/resolve/main/Llama-3-Instruct-LiPPA-8B.i1-Q4_0.gguf) | i1-Q4_0 | 4.8 | fast, low quality |
| [GGUF](https://huggingface.co/mradermacher/Llama-3-Instruct-LiPPA-8B-i1-GGUF/resolve/main/Llama-3-Instruct-LiPPA-8B.i1-Q4_K_S.gguf) | i1-Q4_K_S | 4.8 | optimal size/speed/quality |
| [GGUF](https://huggingface.co/mradermacher/Llama-3-Instruct-LiPPA-8B-i1-GGUF/resolve/main/Llama-3-Instruct-LiPPA-8B.i1-Q4_K_M.gguf) | i1-Q4_K_M | 5.0 | fast, recommended |
| [GGUF](https://huggingface.co/mradermacher/Llama-3-Instruct-LiPPA-8B-i1-GGUF/resolve/main/Llama-3-Instruct-LiPPA-8B.i1-Q5_K_S.gguf) | i1-Q5_K_S | 5.7 | |
| [GGUF](https://huggingface.co/mradermacher/Llama-3-Instruct-LiPPA-8B-i1-GGUF/resolve/main/Llama-3-Instruct-LiPPA-8B.i1-Q5_K_M.gguf) | i1-Q5_K_M | 5.8 | |
| [GGUF](https://huggingface.co/mradermacher/Llama-3-Instruct-LiPPA-8B-i1-GGUF/resolve/main/Llama-3-Instruct-LiPPA-8B.i1-Q6_K.gguf) | i1-Q6_K | 6.7 | practically like static Q6_K |
Here is a handy graph by ikawrakow comparing some lower-quality quant
types (lower is better):

And here are Artefact2's thoughts on the matter:
https://gist.github.com/Artefact2/b5f810600771265fc1e39442288e8ec9
## FAQ / Model Request
See https://huggingface.co/mradermacher/model_requests for some answers to
questions you might have and/or if you want some other model quantized.
## Thanks
I thank my company, [nethype GmbH](https://www.nethype.de/), for letting
me use its servers and providing upgrades to my workstation to enable
this work in my free time. Additional thanks to [@nicoboss](https://huggingface.co/nicoboss) for giving me access to his hardware for calculating the imatrix for these quants.
<!-- end -->
|
bartowski/Qwen2-7B-Multilingual-RP-GGUF | bartowski | 2024-07-01T16:17:50Z | 15,417 | 1 | null | [
"gguf",
"text-generation",
"en",
"ko",
"ja",
"zh",
"es",
"license:apache-2.0",
"region:us"
] | text-generation | 2024-07-01T15:53:34Z | ---
license: apache-2.0
language:
- en
- ko
- ja
- zh
- es
quantized_by: bartowski
pipeline_tag: text-generation
---
## Llamacpp imatrix Quantizations of Qwen2-7B-Multilingual-RP
Using <a href="https://github.com/ggerganov/llama.cpp/">llama.cpp</a> release <a href="https://github.com/ggerganov/llama.cpp/releases/tag/b3266">b3266</a> for quantization.
Original model: https://huggingface.co/maywell/Qwen2-7B-Multilingual-RP
All quants made using imatrix option with dataset from [here](https://gist.github.com/bartowski1182/eb213dccb3571f863da82e99418f81e8)
## Prompt format
```
<|im_start|>system
{system_prompt}<|im_end|>
<|im_start|>user
{prompt}<|im_end|>
<|im_start|>assistant
```
## Download a file (not the whole branch) from below:
| Filename | Quant type | File Size | Description |
| -------- | ---------- | --------- | ----------- |
| [Qwen2-7B-Multilingual-RP-Q8_0_L.gguf](https://huggingface.co/bartowski/Qwen2-7B-Multilingual-RP-GGUF/blob/main/Qwen2-7B-Multilingual-RP-Q8_1.gguf) | Q8_0_L | 9.12GB | *Experimental*, uses f16 for embed and output weights. Please provide any feedback of differences. Extremely high quality, generally unneeded but max available quant. |
| [Qwen2-7B-Multilingual-RP-Q8_0.gguf](https://huggingface.co/bartowski/Qwen2-7B-Multilingual-RP-GGUF/blob/main/Qwen2-7B-Multilingual-RP-Q8_0.gguf) | Q8_0 | 8.09GB | Extremely high quality, generally unneeded but max available quant. |
| [Qwen2-7B-Multilingual-RP-Q6_K_L.gguf](https://huggingface.co/bartowski/Qwen2-7B-Multilingual-RP-GGUF/blob/main/Qwen2-7B-Multilingual-RP-Q6_K_L.gguf) | Q6_K_L | 7.54GB | *Experimental*, uses f16 for embed and output weights. Please provide any feedback of differences. Very high quality, near perfect, *recommended*. |
| [Qwen2-7B-Multilingual-RP-Q6_K.gguf](https://huggingface.co/bartowski/Qwen2-7B-Multilingual-RP-GGUF/blob/main/Qwen2-7B-Multilingual-RP-Q6_K.gguf) | Q6_K | 6.25GB | Very high quality, near perfect, *recommended*. |
| [Qwen2-7B-Multilingual-RP-Q5_K_L.gguf](https://huggingface.co/bartowski/Qwen2-7B-Multilingual-RP-GGUF/blob/main/Qwen2-7B-Multilingual-RP-Q5_K_L.gguf) | Q5_K_L | 6.80GB | *Experimental*, uses f16 for embed and output weights. Please provide any feedback of differences. High quality, *recommended*. |
| [Qwen2-7B-Multilingual-RP-Q5_K_M.gguf](https://huggingface.co/bartowski/Qwen2-7B-Multilingual-RP-GGUF/blob/main/Qwen2-7B-Multilingual-RP-Q5_K_M.gguf) | Q5_K_M | 5.44GB | High quality, *recommended*. |
| [Qwen2-7B-Multilingual-RP-Q5_K_S.gguf](https://huggingface.co/bartowski/Qwen2-7B-Multilingual-RP-GGUF/blob/main/Qwen2-7B-Multilingual-RP-Q5_K_S.gguf) | Q5_K_S | 5.31GB | High quality, *recommended*. |
| [Qwen2-7B-Multilingual-RP-Q4_K_L.gguf](https://huggingface.co/bartowski/Qwen2-7B-Multilingual-RP-GGUF/blob/main/Qwen2-7B-Multilingual-RP-Q4_K_L.gguf) | Q4_K_L | 6.10GB | *Experimental*, uses f16 for embed and output weights. Please provide any feedback of differences. Good quality, uses about 4.83 bits per weight, *recommended*. |
| [Qwen2-7B-Multilingual-RP-Q4_K_M.gguf](https://huggingface.co/bartowski/Qwen2-7B-Multilingual-RP-GGUF/blob/main/Qwen2-7B-Multilingual-RP-Q4_K_M.gguf) | Q4_K_M | 4.68GB | Good quality, uses about 4.83 bits per weight, *recommended*. |
| [Qwen2-7B-Multilingual-RP-Q4_K_S.gguf](https://huggingface.co/bartowski/Qwen2-7B-Multilingual-RP-GGUF/blob/main/Qwen2-7B-Multilingual-RP-Q4_K_S.gguf) | Q4_K_S | 4.45GB | Slightly lower quality with more space savings, *recommended*. |
| [Qwen2-7B-Multilingual-RP-IQ4_XS.gguf](https://huggingface.co/bartowski/Qwen2-7B-Multilingual-RP-GGUF/blob/main/Qwen2-7B-Multilingual-RP-IQ4_XS.gguf) | IQ4_XS | 4.21GB | Decent quality, smaller than Q4_K_S with similar performance, *recommended*. |
| [Qwen2-7B-Multilingual-RP-Q3_K_XL.gguf](https://huggingface.co/bartowski/Qwen2-7B-Multilingual-RP-GGUF/blob/main/Qwen2-7B-Multilingual-RP-Q3_K_XL.gguf) | Q3_K_XL | 5.58GB | *Experimental*, uses f16 for embed and output weights. Please provide any feedback of differences. Lower quality but usable, good for low RAM availability. |
| [Qwen2-7B-Multilingual-RP-Q3_K_L.gguf](https://huggingface.co/bartowski/Qwen2-7B-Multilingual-RP-GGUF/blob/main/Qwen2-7B-Multilingual-RP-Q3_K_L.gguf) | Q3_K_L | 4.08GB | Lower quality but usable, good for low RAM availability. |
| [Qwen2-7B-Multilingual-RP-Q3_K_M.gguf](https://huggingface.co/bartowski/Qwen2-7B-Multilingual-RP-GGUF/blob/main/Qwen2-7B-Multilingual-RP-Q3_K_M.gguf) | Q3_K_M | 3.80GB | Even lower quality. |
| [Qwen2-7B-Multilingual-RP-IQ3_M.gguf](https://huggingface.co/bartowski/Qwen2-7B-Multilingual-RP-GGUF/blob/main/Qwen2-7B-Multilingual-RP-IQ3_M.gguf) | IQ3_M | 3.57GB | Medium-low quality, new method with decent performance comparable to Q3_K_M. |
| [Qwen2-7B-Multilingual-RP-Q3_K_S.gguf](https://huggingface.co/bartowski/Qwen2-7B-Multilingual-RP-GGUF/blob/main/Qwen2-7B-Multilingual-RP-Q3_K_S.gguf) | Q3_K_S | 3.49GB | Low quality, not recommended. |
| [Qwen2-7B-Multilingual-RP-IQ3_XS.gguf](https://huggingface.co/bartowski/Qwen2-7B-Multilingual-RP-GGUF/blob/main/Qwen2-7B-Multilingual-RP-IQ3_XS.gguf) | IQ3_XS | 3.34GB | Lower quality, new method with decent performance, slightly better than Q3_K_S. |
| [Qwen2-7B-Multilingual-RP-IQ3_XXS.gguf](https://huggingface.co/bartowski/Qwen2-7B-Multilingual-RP-GGUF/blob/main/Qwen2-7B-Multilingual-RP-IQ3_XXS.gguf) | IQ3_XXS | 3.11GB | Lower quality, new method with decent performance, comparable to Q3 quants. |
| [Qwen2-7B-Multilingual-RP-Q2_K.gguf](https://huggingface.co/bartowski/Qwen2-7B-Multilingual-RP-GGUF/blob/main/Qwen2-7B-Multilingual-RP-Q2_K.gguf) | Q2_K | 3.01GB | Very low quality but surprisingly usable. |
| [Qwen2-7B-Multilingual-RP-IQ2_M.gguf](https://huggingface.co/bartowski/Qwen2-7B-Multilingual-RP-GGUF/blob/main/Qwen2-7B-Multilingual-RP-IQ2_M.gguf) | IQ2_M | 2.78GB | Very low quality, uses SOTA techniques to also be surprisingly usable. |
| [Qwen2-7B-Multilingual-RP-IQ2_S.gguf](https://huggingface.co/bartowski/Qwen2-7B-Multilingual-RP-GGUF/blob/main/Qwen2-7B-Multilingual-RP-IQ2_S.gguf) | IQ2_S | 2.59GB | Very low quality, uses SOTA techniques to be usable. |
| [Qwen2-7B-Multilingual-RP-IQ2_XS.gguf](https://huggingface.co/bartowski/Qwen2-7B-Multilingual-RP-GGUF/blob/main/Qwen2-7B-Multilingual-RP-IQ2_XS.gguf) | IQ2_XS | 2.46GB | Very low quality, uses SOTA techniques to be usable. |
## Downloading using huggingface-cli
First, make sure you have hugginface-cli installed:
```
pip install -U "huggingface_hub[cli]"
```
Then, you can target the specific file you want:
```
huggingface-cli download bartowski/Qwen2-7B-Multilingual-RP-GGUF --include "Qwen2-7B-Multilingual-RP-Q4_K_M.gguf" --local-dir ./
```
If the model is bigger than 50GB, it will have been split into multiple files. In order to download them all to a local folder, run:
```
huggingface-cli download bartowski/Qwen2-7B-Multilingual-RP-GGUF --include "Qwen2-7B-Multilingual-RP-Q8_0.gguf/*" --local-dir Qwen2-7B-Multilingual-RP-Q8_0
```
You can either specify a new local-dir (Qwen2-7B-Multilingual-RP-Q8_0) or download them all in place (./)
## Which file should I choose?
A great write up with charts showing various performances is provided by Artefact2 [here](https://gist.github.com/Artefact2/b5f810600771265fc1e39442288e8ec9)
The first thing to figure out is how big a model you can run. To do this, you'll need to figure out how much RAM and/or VRAM you have.
If you want your model running as FAST as possible, you'll want to fit the whole thing on your GPU's VRAM. Aim for a quant with a file size 1-2GB smaller than your GPU's total VRAM.
If you want the absolute maximum quality, add both your system RAM and your GPU's VRAM together, then similarly grab a quant with a file size 1-2GB Smaller than that total.
Next, you'll need to decide if you want to use an 'I-quant' or a 'K-quant'.
If you don't want to think too much, grab one of the K-quants. These are in format 'QX_K_X', like Q5_K_M.
If you want to get more into the weeds, you can check out this extremely useful feature chart:
[llama.cpp feature matrix](https://github.com/ggerganov/llama.cpp/wiki/Feature-matrix)
But basically, if you're aiming for below Q4, and you're running cuBLAS (Nvidia) or rocBLAS (AMD), you should look towards the I-quants. These are in format IQX_X, like IQ3_M. These are newer and offer better performance for their size.
These I-quants can also be used on CPU and Apple Metal, but will be slower than their K-quant equivalent, so speed vs performance is a tradeoff you'll have to decide.
The I-quants are *not* compatible with Vulcan, which is also AMD, so if you have an AMD card double check if you're using the rocBLAS build or the Vulcan build. At the time of writing this, LM Studio has a preview with ROCm support, and other inference engines have specific builds for ROCm.
Want to support my work? Visit my ko-fi page here: https://ko-fi.com/bartowski
|
RichardErkhov/HumanF-MarkrAI_-_mistralopithecus-v3-dpo-7b-gguf | RichardErkhov | 2024-06-30T00:53:02Z | 15,409 | 0 | null | [
"gguf",
"region:us"
] | null | 2024-06-29T20:39:34Z | Quantization made by Richard Erkhov.
[Github](https://github.com/RichardErkhov)
[Discord](https://discord.gg/pvy7H8DZMG)
[Request more models](https://github.com/RichardErkhov/quant_request)
mistralopithecus-v3-dpo-7b - GGUF
- Model creator: https://huggingface.co/HumanF-MarkrAI/
- Original model: https://huggingface.co/HumanF-MarkrAI/mistralopithecus-v3-dpo-7b/
| Name | Quant method | Size |
| ---- | ---- | ---- |
| [mistralopithecus-v3-dpo-7b.Q2_K.gguf](https://huggingface.co/RichardErkhov/HumanF-MarkrAI_-_mistralopithecus-v3-dpo-7b-gguf/blob/main/mistralopithecus-v3-dpo-7b.Q2_K.gguf) | Q2_K | 2.53GB |
| [mistralopithecus-v3-dpo-7b.IQ3_XS.gguf](https://huggingface.co/RichardErkhov/HumanF-MarkrAI_-_mistralopithecus-v3-dpo-7b-gguf/blob/main/mistralopithecus-v3-dpo-7b.IQ3_XS.gguf) | IQ3_XS | 2.81GB |
| [mistralopithecus-v3-dpo-7b.IQ3_S.gguf](https://huggingface.co/RichardErkhov/HumanF-MarkrAI_-_mistralopithecus-v3-dpo-7b-gguf/blob/main/mistralopithecus-v3-dpo-7b.IQ3_S.gguf) | IQ3_S | 2.96GB |
| [mistralopithecus-v3-dpo-7b.Q3_K_S.gguf](https://huggingface.co/RichardErkhov/HumanF-MarkrAI_-_mistralopithecus-v3-dpo-7b-gguf/blob/main/mistralopithecus-v3-dpo-7b.Q3_K_S.gguf) | Q3_K_S | 2.95GB |
| [mistralopithecus-v3-dpo-7b.IQ3_M.gguf](https://huggingface.co/RichardErkhov/HumanF-MarkrAI_-_mistralopithecus-v3-dpo-7b-gguf/blob/main/mistralopithecus-v3-dpo-7b.IQ3_M.gguf) | IQ3_M | 3.06GB |
| [mistralopithecus-v3-dpo-7b.Q3_K.gguf](https://huggingface.co/RichardErkhov/HumanF-MarkrAI_-_mistralopithecus-v3-dpo-7b-gguf/blob/main/mistralopithecus-v3-dpo-7b.Q3_K.gguf) | Q3_K | 3.28GB |
| [mistralopithecus-v3-dpo-7b.Q3_K_M.gguf](https://huggingface.co/RichardErkhov/HumanF-MarkrAI_-_mistralopithecus-v3-dpo-7b-gguf/blob/main/mistralopithecus-v3-dpo-7b.Q3_K_M.gguf) | Q3_K_M | 3.28GB |
| [mistralopithecus-v3-dpo-7b.Q3_K_L.gguf](https://huggingface.co/RichardErkhov/HumanF-MarkrAI_-_mistralopithecus-v3-dpo-7b-gguf/blob/main/mistralopithecus-v3-dpo-7b.Q3_K_L.gguf) | Q3_K_L | 3.56GB |
| [mistralopithecus-v3-dpo-7b.IQ4_XS.gguf](https://huggingface.co/RichardErkhov/HumanF-MarkrAI_-_mistralopithecus-v3-dpo-7b-gguf/blob/main/mistralopithecus-v3-dpo-7b.IQ4_XS.gguf) | IQ4_XS | 3.67GB |
| [mistralopithecus-v3-dpo-7b.Q4_0.gguf](https://huggingface.co/RichardErkhov/HumanF-MarkrAI_-_mistralopithecus-v3-dpo-7b-gguf/blob/main/mistralopithecus-v3-dpo-7b.Q4_0.gguf) | Q4_0 | 3.83GB |
| [mistralopithecus-v3-dpo-7b.IQ4_NL.gguf](https://huggingface.co/RichardErkhov/HumanF-MarkrAI_-_mistralopithecus-v3-dpo-7b-gguf/blob/main/mistralopithecus-v3-dpo-7b.IQ4_NL.gguf) | IQ4_NL | 3.87GB |
| [mistralopithecus-v3-dpo-7b.Q4_K_S.gguf](https://huggingface.co/RichardErkhov/HumanF-MarkrAI_-_mistralopithecus-v3-dpo-7b-gguf/blob/main/mistralopithecus-v3-dpo-7b.Q4_K_S.gguf) | Q4_K_S | 3.86GB |
| [mistralopithecus-v3-dpo-7b.Q4_K.gguf](https://huggingface.co/RichardErkhov/HumanF-MarkrAI_-_mistralopithecus-v3-dpo-7b-gguf/blob/main/mistralopithecus-v3-dpo-7b.Q4_K.gguf) | Q4_K | 4.07GB |
| [mistralopithecus-v3-dpo-7b.Q4_K_M.gguf](https://huggingface.co/RichardErkhov/HumanF-MarkrAI_-_mistralopithecus-v3-dpo-7b-gguf/blob/main/mistralopithecus-v3-dpo-7b.Q4_K_M.gguf) | Q4_K_M | 4.07GB |
| [mistralopithecus-v3-dpo-7b.Q4_1.gguf](https://huggingface.co/RichardErkhov/HumanF-MarkrAI_-_mistralopithecus-v3-dpo-7b-gguf/blob/main/mistralopithecus-v3-dpo-7b.Q4_1.gguf) | Q4_1 | 4.24GB |
| [mistralopithecus-v3-dpo-7b.Q5_0.gguf](https://huggingface.co/RichardErkhov/HumanF-MarkrAI_-_mistralopithecus-v3-dpo-7b-gguf/blob/main/mistralopithecus-v3-dpo-7b.Q5_0.gguf) | Q5_0 | 4.65GB |
| [mistralopithecus-v3-dpo-7b.Q5_K_S.gguf](https://huggingface.co/RichardErkhov/HumanF-MarkrAI_-_mistralopithecus-v3-dpo-7b-gguf/blob/main/mistralopithecus-v3-dpo-7b.Q5_K_S.gguf) | Q5_K_S | 4.65GB |
| [mistralopithecus-v3-dpo-7b.Q5_K.gguf](https://huggingface.co/RichardErkhov/HumanF-MarkrAI_-_mistralopithecus-v3-dpo-7b-gguf/blob/main/mistralopithecus-v3-dpo-7b.Q5_K.gguf) | Q5_K | 4.78GB |
| [mistralopithecus-v3-dpo-7b.Q5_K_M.gguf](https://huggingface.co/RichardErkhov/HumanF-MarkrAI_-_mistralopithecus-v3-dpo-7b-gguf/blob/main/mistralopithecus-v3-dpo-7b.Q5_K_M.gguf) | Q5_K_M | 4.78GB |
| [mistralopithecus-v3-dpo-7b.Q5_1.gguf](https://huggingface.co/RichardErkhov/HumanF-MarkrAI_-_mistralopithecus-v3-dpo-7b-gguf/blob/main/mistralopithecus-v3-dpo-7b.Q5_1.gguf) | Q5_1 | 5.07GB |
| [mistralopithecus-v3-dpo-7b.Q6_K.gguf](https://huggingface.co/RichardErkhov/HumanF-MarkrAI_-_mistralopithecus-v3-dpo-7b-gguf/blob/main/mistralopithecus-v3-dpo-7b.Q6_K.gguf) | Q6_K | 5.53GB |
| [mistralopithecus-v3-dpo-7b.Q8_0.gguf](https://huggingface.co/RichardErkhov/HumanF-MarkrAI_-_mistralopithecus-v3-dpo-7b-gguf/blob/main/mistralopithecus-v3-dpo-7b.Q8_0.gguf) | Q8_0 | 7.17GB |
Original model description:
Entry not found
|
mradermacher/Yi-9B-Coder-i1-GGUF | mradermacher | 2024-06-27T17:55:07Z | 15,407 | 0 | transformers | [
"transformers",
"gguf",
"code",
"llama",
"en",
"base_model:TechxGenus/Yi-9B-Coder",
"license:apache-2.0",
"endpoints_compatible",
"region:us"
] | null | 2024-06-27T16:29:42Z | ---
base_model: TechxGenus/Yi-9B-Coder
language:
- en
library_name: transformers
license: apache-2.0
quantized_by: mradermacher
tags:
- code
- llama
---
## About
<!-- ### quantize_version: 2 -->
<!-- ### output_tensor_quantised: 1 -->
<!-- ### convert_type: hf -->
<!-- ### vocab_type: -->
<!-- ### tags: nicoboss -->
weighted/imatrix quants of https://huggingface.co/TechxGenus/Yi-9B-Coder
<!-- provided-files -->
static quants are available at https://huggingface.co/mradermacher/Yi-9B-Coder-GGUF
## Usage
If you are unsure how to use GGUF files, refer to one of [TheBloke's
READMEs](https://huggingface.co/TheBloke/KafkaLM-70B-German-V0.1-GGUF) for
more details, including on how to concatenate multi-part files.
## Provided Quants
(sorted by size, not necessarily quality. IQ-quants are often preferable over similar sized non-IQ quants)
| Link | Type | Size/GB | Notes |
|:-----|:-----|--------:|:------|
| [GGUF](https://huggingface.co/mradermacher/Yi-9B-Coder-i1-GGUF/resolve/main/Yi-9B-Coder.i1-IQ1_S.gguf) | i1-IQ1_S | 2.1 | for the desperate |
| [GGUF](https://huggingface.co/mradermacher/Yi-9B-Coder-i1-GGUF/resolve/main/Yi-9B-Coder.i1-IQ1_M.gguf) | i1-IQ1_M | 2.3 | mostly desperate |
| [GGUF](https://huggingface.co/mradermacher/Yi-9B-Coder-i1-GGUF/resolve/main/Yi-9B-Coder.i1-IQ2_XXS.gguf) | i1-IQ2_XXS | 2.6 | |
| [GGUF](https://huggingface.co/mradermacher/Yi-9B-Coder-i1-GGUF/resolve/main/Yi-9B-Coder.i1-IQ2_XS.gguf) | i1-IQ2_XS | 2.8 | |
| [GGUF](https://huggingface.co/mradermacher/Yi-9B-Coder-i1-GGUF/resolve/main/Yi-9B-Coder.i1-IQ2_S.gguf) | i1-IQ2_S | 3.0 | |
| [GGUF](https://huggingface.co/mradermacher/Yi-9B-Coder-i1-GGUF/resolve/main/Yi-9B-Coder.i1-IQ2_M.gguf) | i1-IQ2_M | 3.2 | |
| [GGUF](https://huggingface.co/mradermacher/Yi-9B-Coder-i1-GGUF/resolve/main/Yi-9B-Coder.i1-Q2_K.gguf) | i1-Q2_K | 3.5 | IQ3_XXS probably better |
| [GGUF](https://huggingface.co/mradermacher/Yi-9B-Coder-i1-GGUF/resolve/main/Yi-9B-Coder.i1-IQ3_XXS.gguf) | i1-IQ3_XXS | 3.6 | lower quality |
| [GGUF](https://huggingface.co/mradermacher/Yi-9B-Coder-i1-GGUF/resolve/main/Yi-9B-Coder.i1-IQ3_XS.gguf) | i1-IQ3_XS | 3.8 | |
| [GGUF](https://huggingface.co/mradermacher/Yi-9B-Coder-i1-GGUF/resolve/main/Yi-9B-Coder.i1-Q3_K_S.gguf) | i1-Q3_K_S | 4.0 | IQ3_XS probably better |
| [GGUF](https://huggingface.co/mradermacher/Yi-9B-Coder-i1-GGUF/resolve/main/Yi-9B-Coder.i1-IQ3_S.gguf) | i1-IQ3_S | 4.0 | beats Q3_K* |
| [GGUF](https://huggingface.co/mradermacher/Yi-9B-Coder-i1-GGUF/resolve/main/Yi-9B-Coder.i1-IQ3_M.gguf) | i1-IQ3_M | 4.2 | |
| [GGUF](https://huggingface.co/mradermacher/Yi-9B-Coder-i1-GGUF/resolve/main/Yi-9B-Coder.i1-Q3_K_M.gguf) | i1-Q3_K_M | 4.4 | IQ3_S probably better |
| [GGUF](https://huggingface.co/mradermacher/Yi-9B-Coder-i1-GGUF/resolve/main/Yi-9B-Coder.i1-Q3_K_L.gguf) | i1-Q3_K_L | 4.8 | IQ3_M probably better |
| [GGUF](https://huggingface.co/mradermacher/Yi-9B-Coder-i1-GGUF/resolve/main/Yi-9B-Coder.i1-IQ4_XS.gguf) | i1-IQ4_XS | 4.9 | |
| [GGUF](https://huggingface.co/mradermacher/Yi-9B-Coder-i1-GGUF/resolve/main/Yi-9B-Coder.i1-Q4_0.gguf) | i1-Q4_0 | 5.2 | fast, low quality |
| [GGUF](https://huggingface.co/mradermacher/Yi-9B-Coder-i1-GGUF/resolve/main/Yi-9B-Coder.i1-Q4_K_S.gguf) | i1-Q4_K_S | 5.2 | optimal size/speed/quality |
| [GGUF](https://huggingface.co/mradermacher/Yi-9B-Coder-i1-GGUF/resolve/main/Yi-9B-Coder.i1-Q4_K_M.gguf) | i1-Q4_K_M | 5.4 | fast, recommended |
| [GGUF](https://huggingface.co/mradermacher/Yi-9B-Coder-i1-GGUF/resolve/main/Yi-9B-Coder.i1-Q5_K_S.gguf) | i1-Q5_K_S | 6.2 | |
| [GGUF](https://huggingface.co/mradermacher/Yi-9B-Coder-i1-GGUF/resolve/main/Yi-9B-Coder.i1-Q5_K_M.gguf) | i1-Q5_K_M | 6.4 | |
| [GGUF](https://huggingface.co/mradermacher/Yi-9B-Coder-i1-GGUF/resolve/main/Yi-9B-Coder.i1-Q6_K.gguf) | i1-Q6_K | 7.3 | practically like static Q6_K |
Here is a handy graph by ikawrakow comparing some lower-quality quant
types (lower is better):

And here are Artefact2's thoughts on the matter:
https://gist.github.com/Artefact2/b5f810600771265fc1e39442288e8ec9
## FAQ / Model Request
See https://huggingface.co/mradermacher/model_requests for some answers to
questions you might have and/or if you want some other model quantized.
## Thanks
I thank my company, [nethype GmbH](https://www.nethype.de/), for letting
me use its servers and providing upgrades to my workstation to enable
this work in my free time. Additional thanks to [@nicoboss](https://huggingface.co/nicoboss) for giving me access to his hardware for calculating the imatrix for these quants.
<!-- end -->
|
DeepPavlov/distilrubert-base-cased-conversational | DeepPavlov | 2022-05-06T11:58:43Z | 15,402 | 5 | transformers | [
"transformers",
"pytorch",
"distilbert",
"ru",
"arxiv:2205.02340",
"endpoints_compatible",
"region:us"
] | null | 2022-03-02T23:29:04Z | ---
language:
- ru
---
# distilrubert-base-cased-conversational
Conversational DistilRuBERT \(Russian, cased, 6‑layer, 768‑hidden, 12‑heads, 135.4M parameters\) was trained on OpenSubtitles\[1\], [Dirty](https://d3.ru/), [Pikabu](https://pikabu.ru/), and a Social Media segment of Taiga corpus\[2\] (as [Conversational RuBERT](https://huggingface.co/DeepPavlov/rubert-base-cased-conversational)).
Our DistilRuBERT was highly inspired by \[3\], \[4\]. Namely, we used
* KL loss (between teacher and student output logits)
* MLM loss (between tokens labels and student output logits)
* Cosine embedding loss between mean of two consecutive hidden states of the teacher and one hidden state of the student
The model was trained for about 100 hrs. on 8 nVIDIA Tesla P100-SXM2.0 16Gb.
To evaluate improvements in the inference speed, we ran teacher and student models on random sequences with seq_len=512, batch_size = 16 (for throughput) and batch_size=1 (for latency).
All tests were performed on Intel(R) Xeon(R) CPU E5-2698 v4 @ 2.20GHz and nVIDIA Tesla P100-SXM2.0 16Gb.
| Model | Size, Mb. | CPU latency, sec.| GPU latency, sec. | CPU throughput, samples/sec. | GPU throughput, samples/sec. |
|-------------------------------------------------|------------|------------------|-------------------|------------------------------|------------------------------|
| Teacher (RuBERT-base-cased-conversational) | 679 | 0.655 | 0.031 | 0.3754 | 36.4902 |
| Student (DistilRuBERT-base-cased-conversational)| 517 | 0.3285 | 0.0212 | 0.5803 | 52.2495 |
# Citation
If you found the model useful for your research, we are kindly ask to cite [this](https://arxiv.org/abs/2205.02340) paper:
```
@misc{https://doi.org/10.48550/arxiv.2205.02340,
doi = {10.48550/ARXIV.2205.02340},
url = {https://arxiv.org/abs/2205.02340},
author = {Kolesnikova, Alina and Kuratov, Yuri and Konovalov, Vasily and Burtsev, Mikhail},
keywords = {Computation and Language (cs.CL), Machine Learning (cs.LG), FOS: Computer and information sciences, FOS: Computer and information sciences},
title = {Knowledge Distillation of Russian Language Models with Reduction of Vocabulary},
publisher = {arXiv},
year = {2022},
copyright = {arXiv.org perpetual, non-exclusive license}
}
```
\[1\]: P. Lison and J. Tiedemann, 2016, OpenSubtitles2016: Extracting Large Parallel Corpora from Movie and TV Subtitles. In Proceedings of the 10th International Conference on Language Resources and Evaluation \(LREC 2016\)
\[2\]: Shavrina T., Shapovalova O. \(2017\) TO THE METHODOLOGY OF CORPUS CONSTRUCTION FOR MACHINE LEARNING: «TAIGA» SYNTAX TREE CORPUS AND PARSER. in proc. of “CORPORA2017”, international conference , Saint-Petersbourg, 2017.
\[3\]: Sanh, V., Debut, L., Chaumond, J., & Wolf, T. \(2019\). DistilBERT, a distilled version of BERT: smaller, faster, cheaper and lighter. arXiv preprint arXiv:1910.01108.
\[4\]: <https://github.com/huggingface/transformers/tree/master/examples/research_projects/distillation> |
ashawkey/imagedream-ipmv-diffusers | ashawkey | 2024-01-14T05:02:49Z | 15,401 | 5 | diffusers | [
"diffusers",
"safetensors",
"license:openrail",
"diffusers:MVDreamPipeline",
"region:us"
] | null | 2024-01-13T08:36:35Z | ---
license: openrail
---
# MVDream-diffusers Model Card
This is a port of https://huggingface.co/Peng-Wang/ImageDream into diffusers.
For usage, please check: https://github.com/ashawkey/mvdream_diffusers
## Citation
```
@article{wang2023imagedream,
title={ImageDream: Image-Prompt Multi-view Diffusion for 3D Generation},
author={Wang, Peng and Shi, Yichun},
journal={arXiv preprint arXiv:2312.02201},
year={2023}
}
```
## Misuse, Malicious Use, and Out-of-Scope Use
The model should not be used to intentionally create or disseminate images that create hostile or alienating environments for people. This includes generating images that people would foreseeably find disturbing, distressing, or offensive; or content that propagates historical or current stereotypes.
|
RichardErkhov/Kukedlc_-_NeuralSynthesis-7B-v0.1-gguf | RichardErkhov | 2024-06-26T20:42:41Z | 15,400 | 0 | null | [
"gguf",
"region:us"
] | null | 2024-06-26T14:57:37Z | Quantization made by Richard Erkhov.
[Github](https://github.com/RichardErkhov)
[Discord](https://discord.gg/pvy7H8DZMG)
[Request more models](https://github.com/RichardErkhov/quant_request)
NeuralSynthesis-7B-v0.1 - GGUF
- Model creator: https://huggingface.co/Kukedlc/
- Original model: https://huggingface.co/Kukedlc/NeuralSynthesis-7B-v0.1/
| Name | Quant method | Size |
| ---- | ---- | ---- |
| [NeuralSynthesis-7B-v0.1.Q2_K.gguf](https://huggingface.co/RichardErkhov/Kukedlc_-_NeuralSynthesis-7B-v0.1-gguf/blob/main/NeuralSynthesis-7B-v0.1.Q2_K.gguf) | Q2_K | 2.53GB |
| [NeuralSynthesis-7B-v0.1.IQ3_XS.gguf](https://huggingface.co/RichardErkhov/Kukedlc_-_NeuralSynthesis-7B-v0.1-gguf/blob/main/NeuralSynthesis-7B-v0.1.IQ3_XS.gguf) | IQ3_XS | 2.81GB |
| [NeuralSynthesis-7B-v0.1.IQ3_S.gguf](https://huggingface.co/RichardErkhov/Kukedlc_-_NeuralSynthesis-7B-v0.1-gguf/blob/main/NeuralSynthesis-7B-v0.1.IQ3_S.gguf) | IQ3_S | 2.96GB |
| [NeuralSynthesis-7B-v0.1.Q3_K_S.gguf](https://huggingface.co/RichardErkhov/Kukedlc_-_NeuralSynthesis-7B-v0.1-gguf/blob/main/NeuralSynthesis-7B-v0.1.Q3_K_S.gguf) | Q3_K_S | 2.95GB |
| [NeuralSynthesis-7B-v0.1.IQ3_M.gguf](https://huggingface.co/RichardErkhov/Kukedlc_-_NeuralSynthesis-7B-v0.1-gguf/blob/main/NeuralSynthesis-7B-v0.1.IQ3_M.gguf) | IQ3_M | 3.06GB |
| [NeuralSynthesis-7B-v0.1.Q3_K.gguf](https://huggingface.co/RichardErkhov/Kukedlc_-_NeuralSynthesis-7B-v0.1-gguf/blob/main/NeuralSynthesis-7B-v0.1.Q3_K.gguf) | Q3_K | 3.28GB |
| [NeuralSynthesis-7B-v0.1.Q3_K_M.gguf](https://huggingface.co/RichardErkhov/Kukedlc_-_NeuralSynthesis-7B-v0.1-gguf/blob/main/NeuralSynthesis-7B-v0.1.Q3_K_M.gguf) | Q3_K_M | 3.28GB |
| [NeuralSynthesis-7B-v0.1.Q3_K_L.gguf](https://huggingface.co/RichardErkhov/Kukedlc_-_NeuralSynthesis-7B-v0.1-gguf/blob/main/NeuralSynthesis-7B-v0.1.Q3_K_L.gguf) | Q3_K_L | 3.56GB |
| [NeuralSynthesis-7B-v0.1.IQ4_XS.gguf](https://huggingface.co/RichardErkhov/Kukedlc_-_NeuralSynthesis-7B-v0.1-gguf/blob/main/NeuralSynthesis-7B-v0.1.IQ4_XS.gguf) | IQ4_XS | 3.67GB |
| [NeuralSynthesis-7B-v0.1.Q4_0.gguf](https://huggingface.co/RichardErkhov/Kukedlc_-_NeuralSynthesis-7B-v0.1-gguf/blob/main/NeuralSynthesis-7B-v0.1.Q4_0.gguf) | Q4_0 | 3.83GB |
| [NeuralSynthesis-7B-v0.1.IQ4_NL.gguf](https://huggingface.co/RichardErkhov/Kukedlc_-_NeuralSynthesis-7B-v0.1-gguf/blob/main/NeuralSynthesis-7B-v0.1.IQ4_NL.gguf) | IQ4_NL | 3.87GB |
| [NeuralSynthesis-7B-v0.1.Q4_K_S.gguf](https://huggingface.co/RichardErkhov/Kukedlc_-_NeuralSynthesis-7B-v0.1-gguf/blob/main/NeuralSynthesis-7B-v0.1.Q4_K_S.gguf) | Q4_K_S | 3.86GB |
| [NeuralSynthesis-7B-v0.1.Q4_K.gguf](https://huggingface.co/RichardErkhov/Kukedlc_-_NeuralSynthesis-7B-v0.1-gguf/blob/main/NeuralSynthesis-7B-v0.1.Q4_K.gguf) | Q4_K | 4.07GB |
| [NeuralSynthesis-7B-v0.1.Q4_K_M.gguf](https://huggingface.co/RichardErkhov/Kukedlc_-_NeuralSynthesis-7B-v0.1-gguf/blob/main/NeuralSynthesis-7B-v0.1.Q4_K_M.gguf) | Q4_K_M | 4.07GB |
| [NeuralSynthesis-7B-v0.1.Q4_1.gguf](https://huggingface.co/RichardErkhov/Kukedlc_-_NeuralSynthesis-7B-v0.1-gguf/blob/main/NeuralSynthesis-7B-v0.1.Q4_1.gguf) | Q4_1 | 4.24GB |
| [NeuralSynthesis-7B-v0.1.Q5_0.gguf](https://huggingface.co/RichardErkhov/Kukedlc_-_NeuralSynthesis-7B-v0.1-gguf/blob/main/NeuralSynthesis-7B-v0.1.Q5_0.gguf) | Q5_0 | 4.65GB |
| [NeuralSynthesis-7B-v0.1.Q5_K_S.gguf](https://huggingface.co/RichardErkhov/Kukedlc_-_NeuralSynthesis-7B-v0.1-gguf/blob/main/NeuralSynthesis-7B-v0.1.Q5_K_S.gguf) | Q5_K_S | 4.65GB |
| [NeuralSynthesis-7B-v0.1.Q5_K.gguf](https://huggingface.co/RichardErkhov/Kukedlc_-_NeuralSynthesis-7B-v0.1-gguf/blob/main/NeuralSynthesis-7B-v0.1.Q5_K.gguf) | Q5_K | 4.78GB |
| [NeuralSynthesis-7B-v0.1.Q5_K_M.gguf](https://huggingface.co/RichardErkhov/Kukedlc_-_NeuralSynthesis-7B-v0.1-gguf/blob/main/NeuralSynthesis-7B-v0.1.Q5_K_M.gguf) | Q5_K_M | 4.78GB |
| [NeuralSynthesis-7B-v0.1.Q5_1.gguf](https://huggingface.co/RichardErkhov/Kukedlc_-_NeuralSynthesis-7B-v0.1-gguf/blob/main/NeuralSynthesis-7B-v0.1.Q5_1.gguf) | Q5_1 | 5.07GB |
| [NeuralSynthesis-7B-v0.1.Q6_K.gguf](https://huggingface.co/RichardErkhov/Kukedlc_-_NeuralSynthesis-7B-v0.1-gguf/blob/main/NeuralSynthesis-7B-v0.1.Q6_K.gguf) | Q6_K | 5.53GB |
| [NeuralSynthesis-7B-v0.1.Q8_0.gguf](https://huggingface.co/RichardErkhov/Kukedlc_-_NeuralSynthesis-7B-v0.1-gguf/blob/main/NeuralSynthesis-7B-v0.1.Q8_0.gguf) | Q8_0 | 7.17GB |
Original model description:
---
license: apache-2.0
tags:
- merge
- mergekit
- lazymergekit
model-index:
- name: NeuralSynthesis-7B-v0.1
results:
- task:
type: text-generation
name: Text Generation
dataset:
name: AI2 Reasoning Challenge (25-Shot)
type: ai2_arc
config: ARC-Challenge
split: test
args:
num_few_shot: 25
metrics:
- type: acc_norm
value: 73.04
name: normalized accuracy
source:
url: https://huggingface.co/spaces/HuggingFaceH4/open_llm_leaderboard?query=Kukedlc/NeuralSynthesis-7B-v0.1
name: Open LLM Leaderboard
- task:
type: text-generation
name: Text Generation
dataset:
name: HellaSwag (10-Shot)
type: hellaswag
split: validation
args:
num_few_shot: 10
metrics:
- type: acc_norm
value: 89.18
name: normalized accuracy
source:
url: https://huggingface.co/spaces/HuggingFaceH4/open_llm_leaderboard?query=Kukedlc/NeuralSynthesis-7B-v0.1
name: Open LLM Leaderboard
- task:
type: text-generation
name: Text Generation
dataset:
name: MMLU (5-Shot)
type: cais/mmlu
config: all
split: test
args:
num_few_shot: 5
metrics:
- type: acc
value: 64.37
name: accuracy
source:
url: https://huggingface.co/spaces/HuggingFaceH4/open_llm_leaderboard?query=Kukedlc/NeuralSynthesis-7B-v0.1
name: Open LLM Leaderboard
- task:
type: text-generation
name: Text Generation
dataset:
name: TruthfulQA (0-shot)
type: truthful_qa
config: multiple_choice
split: validation
args:
num_few_shot: 0
metrics:
- type: mc2
value: 78.15
source:
url: https://huggingface.co/spaces/HuggingFaceH4/open_llm_leaderboard?query=Kukedlc/NeuralSynthesis-7B-v0.1
name: Open LLM Leaderboard
- task:
type: text-generation
name: Text Generation
dataset:
name: Winogrande (5-shot)
type: winogrande
config: winogrande_xl
split: validation
args:
num_few_shot: 5
metrics:
- type: acc
value: 85.24
name: accuracy
source:
url: https://huggingface.co/spaces/HuggingFaceH4/open_llm_leaderboard?query=Kukedlc/NeuralSynthesis-7B-v0.1
name: Open LLM Leaderboard
- task:
type: text-generation
name: Text Generation
dataset:
name: GSM8k (5-shot)
type: gsm8k
config: main
split: test
args:
num_few_shot: 5
metrics:
- type: acc
value: 70.81
name: accuracy
source:
url: https://huggingface.co/spaces/HuggingFaceH4/open_llm_leaderboard?query=Kukedlc/NeuralSynthesis-7B-v0.1
name: Open LLM Leaderboard
---
# NeuralSynthesis-7B-v0.1

NeuralSynthesis-7B-v0.1 is a merge of the following models using [LazyMergekit](https://colab.research.google.com/drive/1obulZ1ROXHjYLn6PPZJwRR6GzgQogxxb?usp=sharing):
## 🧩 Configuration
```yaml
models:
- model: MiniMoog/Mergerix-7b-v0.3
- model: automerger/Ognoexperiment27Multi_verse_model-7B
- model: AurelPx/Percival_01-7b-slerp
- model: automerger/YamshadowExperiment28-7B
merge_method: model_stock
base_model: automerger/YamshadowExperiment28-7B
dtype: bfloat16
```
## 💻 Usage
```python
!pip install -qU transformers accelerate
from transformers import AutoTokenizer
import transformers
import torch
model = "Kukedlc/NeuralSynthesis-7B-v0.1"
messages = [{"role": "user", "content": "What is a large language model?"}]
tokenizer = AutoTokenizer.from_pretrained(model)
prompt = tokenizer.apply_chat_template(messages, tokenize=False, add_generation_prompt=True)
pipeline = transformers.pipeline(
"text-generation",
model=model,
torch_dtype=torch.float16,
device_map="auto",
)
outputs = pipeline(prompt, max_new_tokens=256, do_sample=True, temperature=0.7, top_k=50, top_p=0.95)
print(outputs[0]["generated_text"])
```
# [Open LLM Leaderboard Evaluation Results](https://huggingface.co/spaces/HuggingFaceH4/open_llm_leaderboard)
Detailed results can be found [here](https://huggingface.co/datasets/open-llm-leaderboard/details_Kukedlc__NeuralSynthesis-7B-v0.1)
| Metric |Value|
|---------------------------------|----:|
|Avg. |76.80|
|AI2 Reasoning Challenge (25-Shot)|73.04|
|HellaSwag (10-Shot) |89.18|
|MMLU (5-Shot) |64.37|
|TruthfulQA (0-shot) |78.15|
|Winogrande (5-shot) |85.24|
|GSM8k (5-shot) |70.81|
|
ckiplab/bert-base-chinese-pos | ckiplab | 2022-05-10T03:28:12Z | 15,389 | 15 | transformers | [
"transformers",
"pytorch",
"jax",
"bert",
"token-classification",
"zh",
"license:gpl-3.0",
"autotrain_compatible",
"endpoints_compatible",
"region:us"
] | token-classification | 2022-03-02T23:29:05Z | ---
language:
- zh
thumbnail: https://ckip.iis.sinica.edu.tw/files/ckip_logo.png
tags:
- pytorch
- token-classification
- bert
- zh
license: gpl-3.0
---
# CKIP BERT Base Chinese
This project provides traditional Chinese transformers models (including ALBERT, BERT, GPT2) and NLP tools (including word segmentation, part-of-speech tagging, named entity recognition).
這個專案提供了繁體中文的 transformers 模型(包含 ALBERT、BERT、GPT2)及自然語言處理工具(包含斷詞、詞性標記、實體辨識)。
## Homepage
- https://github.com/ckiplab/ckip-transformers
## Contributers
- [Mu Yang](https://muyang.pro) at [CKIP](https://ckip.iis.sinica.edu.tw) (Author & Maintainer)
## Usage
Please use BertTokenizerFast as tokenizer instead of AutoTokenizer.
請使用 BertTokenizerFast 而非 AutoTokenizer。
```
from transformers import (
BertTokenizerFast,
AutoModel,
)
tokenizer = BertTokenizerFast.from_pretrained('bert-base-chinese')
model = AutoModel.from_pretrained('ckiplab/bert-base-chinese-pos')
```
For full usage and more information, please refer to https://github.com/ckiplab/ckip-transformers.
有關完整使用方法及其他資訊,請參見 https://github.com/ckiplab/ckip-transformers 。
|
yanolja/EEVE-Korean-Instruct-10.8B-v1.0 | yanolja | 2024-06-26T03:27:44Z | 15,387 | 89 | transformers | [
"transformers",
"safetensors",
"llama",
"text-generation",
"generated_from_trainer",
"conversational",
"arxiv:2402.14714",
"arxiv:2310.01377",
"arxiv:2306.02707",
"base_model:yanolja/EEVE-Korean-10.8B-v1.0",
"license:apache-2.0",
"autotrain_compatible",
"endpoints_compatible",
"text-generation-inference",
"region:us"
] | text-generation | 2024-02-22T04:39:04Z | ---
license: apache-2.0
tags:
- generated_from_trainer
base_model: yanolja/EEVE-Korean-10.8B-v1.0
model-index:
- name: yanolja/EEVE-Korean-Instruct-10.8B-v1.0
results: []
---
[<img src="https://raw.githubusercontent.com/OpenAccess-AI-Collective/axolotl/main/image/axolotl-badge-web.png" alt="Built with Axolotl" width="200" height="32"/>](https://github.com/OpenAccess-AI-Collective/axolotl)
<p align="left">
<img src="https://huggingface.co/yanolja/EEVE-Korean-Instruct-10.8B-v1.0/resolve/main/eeve_logo.webp" width="50%"/>
<p>
# EEVE-Korean-Instruct-10.8B-v1.0
## Join Our Community on Discord!
If you're passionate about the field of Large Language Models and wish to exchange knowledge and insights, we warmly invite you to join our Discord server. It's worth noting that Korean is the primary language used in this server. The landscape of LLM is evolving rapidly, and without active sharing, our collective knowledge risks becoming outdated swiftly. Let's collaborate and drive greater impact together! Join us here: [Discord Link](https://discord.gg/b27bAHg95m).
## Our Dedicated Team (Alphabetical Order)
| Research | Engineering | Product Management | UX Design |
|-----------------|-----------------|--------------------|--------------
| Myeongho Jeong | Geon Kim | Bokyung Huh | Eunsue Choi |
| Seungduk Kim | Rifqi Alfi | | |
| Seungtaek Choi | Sanghoon Han | | |
| | Suhyun Kang | | |
## About the Model
This model is a fine-tuned version of [yanolja/EEVE-Korean-10.8B-v1.0](https://huggingface.co/yanolja/EEVE-Korean-10.8B-v1.0), which is a Korean vocabulary-extended version of [upstage/SOLAR-10.7B-v1.0](https://huggingface.co/upstage/SOLAR-10.7B-v1.0). Specifically, we utilized Direct Preference Optimization (DPO) through the use of [Axolotl](https://github.com/OpenAccess-AI-Collective/axolotl).
For more details, please refer to our technical report: [Efficient and Effective Vocabulary Expansion Towards Multilingual Large Language Models](https://arxiv.org/abs/2402.14714).
## Prompt Template
```
A chat between a curious user and an artificial intelligence assistant. The assistant gives helpful, detailed, and polite answers to the user's questions.
Human: {prompt}
Assistant:
```
## How to Use it
```python
from transformers import AutoTokenizer
from transformers import AutoModelForCausalLM
model = AutoModelForCausalLM.from_pretrained("yanolja/EEVE-Korean-Instruct-10.8B-v1.0")
tokenizer = AutoTokenizer.from_pretrained("yanolja/EEVE-Korean-Instruct-10.8B-v1.0")
prompt_template = "A chat between a curious user and an artificial intelligence assistant. The assistant gives helpful, detailed, and polite answers to the user's questions.\nHuman: {prompt}\nAssistant:\n"
text = '한국의 수도는 어디인가요? 아래 선택지 중 골라주세요.\n\n(A) 경성\n(B) 부산\n(C) 평양\n(D) 서울\n(E) 전주'
model_inputs = tokenizer(prompt_template.format(prompt=text), return_tensors='pt')
outputs = model.generate(**model_inputs, max_new_tokens=256)
output_text = tokenizer.batch_decode(outputs, skip_special_tokens=True)[0]
print(output_text)
```
### Example Output
```
A chat between a curious user and an artificial intelligence assistant. The assistant gives helpful, detailed, and polite answers to the user's questions.
Human: 한국의 수도는 어디인가요? 아래 선택지 중 골라주세요.
(A) 경성
(B) 부산
(C) 평양
(D) 서울
(E) 전주
Assistant:
(D) 서울이 한국의 수도입니다. 서울은 나라의 북동부에 위치해 있으며, 정치, 경제, 문화의 중심지입니다. 약 1,000만 명이 넘는 인구를 가진 세계에서 가장 큰 도시 중 하나입니다. 서울은 높은 빌딩, 현대적인 인프라, 활기 문화 장면으로 유명합니다. 또한, 많은 역사적 명소와 박물관이 있어 방문객들에게 풍부한 문화 체험을 제공합니다.
```
### Training Data
- Korean-translated version of [Open-Orca/SlimOrca-Dedup](https://huggingface.co/datasets/Open-Orca/SlimOrca-Dedup)
- Korean-translated version of [argilla/ultrafeedback-binarized-preferences-cleaned](https://huggingface.co/datasets/argilla/ultrafeedback-binarized-preferences-cleaned)
- No other dataset was used
## Citation
```
@misc{kim2024efficient,
title={Efficient and Effective Vocabulary Expansion Towards Multilingual Large Language Models},
author={Seungduk Kim and Seungtaek Choi and Myeongho Jeong},
year={2024},
eprint={2402.14714},
archivePrefix={arXiv},
primaryClass={cs.CL}
}
```
```
@misc{cui2023ultrafeedback,
title={UltraFeedback: Boosting Language Models with High-quality Feedback},
author={Ganqu Cui and Lifan Yuan and Ning Ding and Guanming Yao and Wei Zhu and Yuan Ni and Guotong Xie and Zhiyuan Liu and Maosong Sun},
year={2023},
eprint={2310.01377},
archivePrefix={arXiv},
primaryClass={cs.CL}
}
```
```
@misc{SlimOrcaDedup,
title = {SlimOrca Dedup: A Deduplicated Subset of SlimOrca},
author = {Wing Lian and Guan Wang and Bleys Goodson and Eugene Pentland and Austin Cook and Chanvichet Vong and "Teknium" and Nathan Hoos},
year = {2023},
publisher = {HuggingFace},
url = {https://huggingface.co/datasets/Open-Orca/SlimOrca-Dedup/}
}
```
```
@misc{mukherjee2023orca,
title={Orca: Progressive Learning from Complex Explanation Traces of GPT-4},
author={Subhabrata Mukherjee and Arindam Mitra and Ganesh Jawahar and Sahaj Agarwal and Hamid Palangi and Ahmed Awadallah},
year={2023},
eprint={2306.02707},
archivePrefix={arXiv},
primaryClass={cs.CL}
}
```
# [Open LLM Leaderboard Evaluation Results](https://huggingface.co/spaces/HuggingFaceH4/open_llm_leaderboard)
Detailed results can be found [here](https://huggingface.co/datasets/open-llm-leaderboard/details_yanolja__EEVE-Korean-Instruct-10.8B-v1.0)
| Metric |Value|
|---------------------------------|----:|
|Avg. |66.48|
|AI2 Reasoning Challenge (25-Shot)|64.85|
|HellaSwag (10-Shot) |83.04|
|MMLU (5-Shot) |64.23|
|TruthfulQA (0-shot) |54.09|
|Winogrande (5-shot) |81.93|
|GSM8k (5-shot) |50.72|
|
Langboat/Mengzi3-8B-Base | Langboat | 2024-06-03T12:40:10Z | 15,374 | 2 | transformers | [
"transformers",
"pytorch",
"llama",
"text-generation",
"conversational",
"license:apache-2.0",
"autotrain_compatible",
"endpoints_compatible",
"text-generation-inference",
"region:us"
] | text-generation | 2024-06-03T03:45:41Z | ---
license: apache-2.0
---
# Mengzi3-8B
以创新为引擎,驱动 AI 生态的可持续发展!
在 Mengzi3-13B 大模型 3 月底开源后不久,澜舟科技全新推出的又一款轻量化大模型——Mengzi3-8B,现已正式开源!并同样支持免费商用。这款模型在多个应用场景中,包括写作、代码、摘要以及阅读理解等,均展现出与 Mengzi3-13B 相媲美的性能,而模型参数规模更为精简,更适合个人开发者和 AI 爱好者使用。
Mengzi3-8B 适用于多种自然语言处理任务,主要包括:
- 文本生成:能够轻松产出高质量、多样化的中文文本内容,涵盖写作,摘要,问答,翻译,阅读理解等多种文本生成场景;
- 对话系统:为用户提供高效、智能的交互体验,让对话更加流畅自然;
- 代码生成:协助开发者提升编码效率,激发创新灵感。
### 核心优势
- 低显存高推理,具备更高效能
Mengzi3-8B 是一款高效、低显存需求的 AI 大模型,半精度下显存占用小于 16G,适用于 PC(如 MacBook)和大部分消费级显卡(如 RTX 4090、RTX 4080、RTX 4070Ti)。此外,Mengzi3-8B 的原生推理速度在同级别模型中处于领先地位,特别适合个人开发者和 AI 爱好者使用,真正实现了高效、低成本的 AI 体验。

- 知识处理效果卓越
Mengzi3-8B 在中文能力(CMMLU)、英语、世界知识(MMLU)、编程及数学等多个领域的知识处理和问题解决能力中,均显著超越同尺寸开源模型,展现出全面的领先实力。
| **评测集** | **Mengzi3-8B-Base** | **Mistral-7B** | **Llama3-8B** | **Baichuan2-7B** | **Yi-1.5-9B** | **Qwen1.5-7B** |
| ------------- | ------------------- | -------------- | ------------- | ---------------- | ------------- | -------------- |
| **MMLU** | 73.67 | 64.1 | 66.6 | 54.16 | 69.5 | 61.0 |
| **CMMLU** | 77.54 | 44.7 | 49.21 | 57.07 | 74.8 | 73.1 |
| **GSM8K** | 76.29 | 47.5 | 50.04 | 9.17 | 73.7 | 62.5 |
| **HUMANEVAL** | 48.78 | 27.4 | 36.59 | 9.20 | 41.4 | 36.0 |
| **MBPP** | 43.20 | 38.6 | 34.2 | 6.60 | 61.1 | 37.4 |
### 使用指南
**开源地址**:
欢迎大家访问 Mengzi3-8B 的开源仓库以获取更多信息并下载使用,体验其强大的功能。
- Huggingface: [https://huggingface.co/Langboat/Mengzi3-8B-Base](https://huggingface.co/Langboat/Mengzi3-8B-Base)
- ModelScope: [https://www.modelscope.cn/models/langboat/Mengzi3-8B-Base/summary](https://www.modelscope.cn/models/langboat/Mengzi3-8B-Base/summary)
**快速开始:**
```python
import torch
from transformers import AutoModelForCausalLM, AutoTokenizer
tokenizer = AutoTokenizer.from_pretrained("Langboat/Mengzi3-8B-Base", use_fast=False, trust_remote_code=True)
model = AutoModelForCausalLM.from_pretrained("Langboat/Mengzi3-8B-Base", device_map="auto", trust_remote_code=True)
inputs = tokenizer('输入:介绍一下孟子。输出:', return_tensors='pt')
if torch.cuda.is_available():
inputs = inputs.to('cuda')
pred = model.generate(**inputs, max_new_tokens=512, repetition_penalty=1.01, eos_token_id=tokenizer.eos_token_id)
print(tokenizer.decode(pred[0], skip_special_tokens=True))
"""孟子(约公元前372年—约公元前289年),名轲,字子舆,战国时期邹国人,伟大的思想家、教育家,儒家学派的代表人物,与孔子并称“孔孟”。孟子继承和发展了孔子的思想,成为仅次于孔子的一代儒家宗师,对后世中国文化的影
响全面而巨大,有“亚圣”之称,与孔子合称为“孔孟”。"""
```
我们很诚挚地邀请各位 AI 开发者和爱好者体验使用 Mengzi3-8B 模型,如在使用过程中遇到任何问题或希望给予宝贵的反馈,请随时与我们联系。对于企业用户,对稳定性和模型参数量有更高需求的,建议了解 [Mengzi3-13B](https://github.com/Langboat/Mengzi3)。
大模型的开源不仅为 AI 创新注入了新的活力,更在不断完善开源生态的进程中发挥着关键作用。我们希望能在推动技术创新、促进业务场景应用、完善数字经济与开源生态等方面积极贡献,以期更好地应对未来 AI 技术的挑战,共同推动行业的持续进步和发展。
**👇 扫码加入孟子开源社区微信群**

|
linhphanff/semantic-base-vi | linhphanff | 2024-02-29T08:23:34Z | 15,355 | 2 | transformers | [
"transformers",
"pytorch",
"roberta",
"feature-extraction",
"cls token",
"sentence-similarity",
"vi",
"license:other",
"endpoints_compatible",
"text-embeddings-inference",
"region:us"
] | sentence-similarity | 2023-12-18T08:31:20Z | ---
license: other
language:
- vi
library_name: transformers
pipeline_tag: sentence-similarity
tags:
- cls token
--- |
RichardErkhov/shleeeee_-_mistral-7b-wiki-gguf | RichardErkhov | 2024-06-30T05:48:04Z | 15,347 | 0 | null | [
"gguf",
"region:us"
] | null | 2024-06-30T03:41:01Z | Quantization made by Richard Erkhov.
[Github](https://github.com/RichardErkhov)
[Discord](https://discord.gg/pvy7H8DZMG)
[Request more models](https://github.com/RichardErkhov/quant_request)
mistral-7b-wiki - GGUF
- Model creator: https://huggingface.co/shleeeee/
- Original model: https://huggingface.co/shleeeee/mistral-7b-wiki/
| Name | Quant method | Size |
| ---- | ---- | ---- |
| [mistral-7b-wiki.Q2_K.gguf](https://huggingface.co/RichardErkhov/shleeeee_-_mistral-7b-wiki-gguf/blob/main/mistral-7b-wiki.Q2_K.gguf) | Q2_K | 2.53GB |
| [mistral-7b-wiki.IQ3_XS.gguf](https://huggingface.co/RichardErkhov/shleeeee_-_mistral-7b-wiki-gguf/blob/main/mistral-7b-wiki.IQ3_XS.gguf) | IQ3_XS | 2.81GB |
| [mistral-7b-wiki.IQ3_S.gguf](https://huggingface.co/RichardErkhov/shleeeee_-_mistral-7b-wiki-gguf/blob/main/mistral-7b-wiki.IQ3_S.gguf) | IQ3_S | 2.96GB |
| [mistral-7b-wiki.Q3_K_S.gguf](https://huggingface.co/RichardErkhov/shleeeee_-_mistral-7b-wiki-gguf/blob/main/mistral-7b-wiki.Q3_K_S.gguf) | Q3_K_S | 2.95GB |
| [mistral-7b-wiki.IQ3_M.gguf](https://huggingface.co/RichardErkhov/shleeeee_-_mistral-7b-wiki-gguf/blob/main/mistral-7b-wiki.IQ3_M.gguf) | IQ3_M | 3.06GB |
| [mistral-7b-wiki.Q3_K.gguf](https://huggingface.co/RichardErkhov/shleeeee_-_mistral-7b-wiki-gguf/blob/main/mistral-7b-wiki.Q3_K.gguf) | Q3_K | 3.28GB |
| [mistral-7b-wiki.Q3_K_M.gguf](https://huggingface.co/RichardErkhov/shleeeee_-_mistral-7b-wiki-gguf/blob/main/mistral-7b-wiki.Q3_K_M.gguf) | Q3_K_M | 3.28GB |
| [mistral-7b-wiki.Q3_K_L.gguf](https://huggingface.co/RichardErkhov/shleeeee_-_mistral-7b-wiki-gguf/blob/main/mistral-7b-wiki.Q3_K_L.gguf) | Q3_K_L | 3.56GB |
| [mistral-7b-wiki.IQ4_XS.gguf](https://huggingface.co/RichardErkhov/shleeeee_-_mistral-7b-wiki-gguf/blob/main/mistral-7b-wiki.IQ4_XS.gguf) | IQ4_XS | 3.67GB |
| [mistral-7b-wiki.Q4_0.gguf](https://huggingface.co/RichardErkhov/shleeeee_-_mistral-7b-wiki-gguf/blob/main/mistral-7b-wiki.Q4_0.gguf) | Q4_0 | 3.83GB |
| [mistral-7b-wiki.IQ4_NL.gguf](https://huggingface.co/RichardErkhov/shleeeee_-_mistral-7b-wiki-gguf/blob/main/mistral-7b-wiki.IQ4_NL.gguf) | IQ4_NL | 3.87GB |
| [mistral-7b-wiki.Q4_K_S.gguf](https://huggingface.co/RichardErkhov/shleeeee_-_mistral-7b-wiki-gguf/blob/main/mistral-7b-wiki.Q4_K_S.gguf) | Q4_K_S | 3.86GB |
| [mistral-7b-wiki.Q4_K.gguf](https://huggingface.co/RichardErkhov/shleeeee_-_mistral-7b-wiki-gguf/blob/main/mistral-7b-wiki.Q4_K.gguf) | Q4_K | 4.07GB |
| [mistral-7b-wiki.Q4_K_M.gguf](https://huggingface.co/RichardErkhov/shleeeee_-_mistral-7b-wiki-gguf/blob/main/mistral-7b-wiki.Q4_K_M.gguf) | Q4_K_M | 4.07GB |
| [mistral-7b-wiki.Q4_1.gguf](https://huggingface.co/RichardErkhov/shleeeee_-_mistral-7b-wiki-gguf/blob/main/mistral-7b-wiki.Q4_1.gguf) | Q4_1 | 4.24GB |
| [mistral-7b-wiki.Q5_0.gguf](https://huggingface.co/RichardErkhov/shleeeee_-_mistral-7b-wiki-gguf/blob/main/mistral-7b-wiki.Q5_0.gguf) | Q5_0 | 4.65GB |
| [mistral-7b-wiki.Q5_K_S.gguf](https://huggingface.co/RichardErkhov/shleeeee_-_mistral-7b-wiki-gguf/blob/main/mistral-7b-wiki.Q5_K_S.gguf) | Q5_K_S | 4.65GB |
| [mistral-7b-wiki.Q5_K.gguf](https://huggingface.co/RichardErkhov/shleeeee_-_mistral-7b-wiki-gguf/blob/main/mistral-7b-wiki.Q5_K.gguf) | Q5_K | 4.78GB |
| [mistral-7b-wiki.Q5_K_M.gguf](https://huggingface.co/RichardErkhov/shleeeee_-_mistral-7b-wiki-gguf/blob/main/mistral-7b-wiki.Q5_K_M.gguf) | Q5_K_M | 4.78GB |
| [mistral-7b-wiki.Q5_1.gguf](https://huggingface.co/RichardErkhov/shleeeee_-_mistral-7b-wiki-gguf/blob/main/mistral-7b-wiki.Q5_1.gguf) | Q5_1 | 5.07GB |
| [mistral-7b-wiki.Q6_K.gguf](https://huggingface.co/RichardErkhov/shleeeee_-_mistral-7b-wiki-gguf/blob/main/mistral-7b-wiki.Q6_K.gguf) | Q6_K | 5.53GB |
| [mistral-7b-wiki.Q8_0.gguf](https://huggingface.co/RichardErkhov/shleeeee_-_mistral-7b-wiki-gguf/blob/main/mistral-7b-wiki.Q8_0.gguf) | Q8_0 | 7.17GB |
Original model description:
---
language:
- ko
pipeline_tag: text-generation
tags:
- finetune
---
# Model Card for mistral-7b-wiki
It is a fine-tuned model using Korean in the mistral-7b model
## Model Details
* **Model Developers** : shleeeee(Seunghyeon Lee) , oopsung(Sungwoo Park)
* **Repository** : To be added
* **Model Architecture** : The mistral-7b-wiki is is a fine-tuned version of the Mistral-7B-v0.1.
* **Lora target modules** : q_proj, k_proj, v_proj, o_proj,gate_proj
* **train_batch** : 2
* **Max_step** : 500
## Dataset
Korean Custom Dataset
## Prompt template: Mistral
```
<s>[INST]{['instruction']}[/INST]{['output']}</s>
```
## Usage
```
# Load model directly
from transformers import AutoTokenizer, AutoModelForCausalLM
tokenizer = AutoTokenizer.from_pretrained("shleeeee/mistral-7b-wiki")
model = AutoModelForCausalLM.from_pretrained("shleeeee/mistral-7b-wiki")
# Use a pipeline as a high-level helper
from transformers import pipeline
pipe = pipeline("text-generation", model="shleeeee/mistral-7b-wiki")
```
## Evaluation

|
microsoft/phi-1 | microsoft | 2024-04-29T16:13:21Z | 15,341 | 197 | transformers | [
"transformers",
"safetensors",
"phi",
"text-generation",
"code",
"en",
"license:mit",
"autotrain_compatible",
"endpoints_compatible",
"text-generation-inference",
"region:us"
] | text-generation | 2023-09-10T04:10:57Z | ---
license: mit
license_link: https://huggingface.co/microsoft/phi-1/resolve/main/LICENSE
language:
- en
pipeline_tag: text-generation
tags:
- code
---
## Model Summary
The language model Phi-1 is a Transformer with 1.3 billion parameters, specialized for basic Python coding. Its training involved a variety of data sources, including subsets of Python codes from [The Stack v1.2](https://huggingface.co/datasets/bigcode/the-stack), Q&A content from [StackOverflow](https://archive.org/download/stackexchange), competition code from [code_contests](https://github.com/deepmind/code_contests), and synthetic Python textbooks and exercises generated by [gpt-3.5-turbo-0301](https://platform.openai.com/docs/models/gpt-3-5). Even though the model and the datasets are relatively small compared to contemporary Large Language Models (LLMs), Phi-1 has demonstrated an impressive accuracy rate exceeding 50% on the simple Python coding benchmark, HumanEval.
## How to Use
Phi-1 has been integrated in the `transformers` version 4.37.0, please ensure that you are using a version equal or higher than it.
## Intended Uses
Given the nature of the training data, Phi-1 is best suited for prompts using the code format:
### Code Format:
```python
def print_prime(n):
"""
Print all primes between 1 and n
"""
for num in range(2, n+1):
for i in range(2, num):
if num % i == 0:
break
else:
print(num)
```
where the model generates the code after the comments. (Note: This is a legitimate and correct use of the else statement in Python loops.)
**Notes:**
* Phi-1 is intended for code purposes. The model-generated code should be treated as a starting point rather than a definitive solution for potential use cases. Users should be cautious when employing this model in their applications.
* Direct adoption for production coding tasks is out of the scope of this research project. As a result, Phi-1 has not been tested to ensure that it performs adequately for production-level code. Please refer to the limitation sections of this document for more details.
## Sample Code
```python
import torch
from transformers import AutoModelForCausalLM, AutoTokenizer
torch.set_default_device("cuda")
model = AutoModelForCausalLM.from_pretrained("microsoft/phi-1", torch_dtype="auto")
tokenizer = AutoTokenizer.from_pretrained("microsoft/phi-1")
inputs = tokenizer('''def print_prime(n):
"""
Print all primes between 1 and n
"""''', return_tensors="pt", return_attention_mask=False)
outputs = model.generate(**inputs, max_length=200)
text = tokenizer.batch_decode(outputs)[0]
print(text)
```
## Limitations of Phi-1
* Limited Scope: 99.8% of the Python scripts in our fine-tuning dataset use only the packages "typing, math, random, collections, datetime, itertools". If the model generates Python scripts that utilize other packages, we strongly recommend users manually verify all API uses.
* Replicate Scripts Online: As our model is trained on Python scripts found online, there is a small chance it may replicate such scripts, especially if they appear repetitively across different online sources.
* Generate Inaccurate Code: The model frequently generates incorrect code. We suggest that users view these outputs as a source of inspiration rather than definitive solutions.
* Unreliable Responses to Alternate Formats: Despite appearing to comprehend instructions in formats like Q&A or chat, our models often respond with inaccurate answers, even when seeming confident. Their capabilities with non-code formats are significantly more limited.
* Limitations on Natural Language Comprehension. As a coding bot, Phi-1's main focus is to help with coding-related questions. While it may have some natural language comprehension capabilities, its primary function is not to engage in general conversations or demonstrate common sense like a general AI assistant. Its strength lies in providing assistance and guidance in the context of programming and software development.
* Potential Biases: Phi-1, like other AI models, is trained on web and synthetic data. This data can contain biases and errors that might affect the AI's performance. Biases could stem from various sources like unbalanced representation, stereotypes, or controversial opinions present in the training data. As a result, the model might sometimes generate responses that reflect these biases or errors.
## Warning about Security Risks
When leveraging Phi-1, it's paramount to be vigilant. The model, though powerful, can inadvertently introduce security vulnerabilities in the generated code. Examples include, but are not limited to:
* Directory Traversal: The code might fail to implement safe checks against directory traversal attacks, potentially allowing unauthorized access to sensitive files on your system.
* Injection Attacks: There could be lapses in escaping strings properly, making the application susceptible to SQL, OS commands, or other injection attacks.
* Misunderstanding Requirements: The model might sometimes misunderstand or oversimplify user requirements, leading to incomplete or insecure solutions.
* Lack of Input Validation: In some cases, the model might neglect to incorporate input validation or sanitize user inputs, opening doors to attacks like Cross-Site Scripting (XSS).
* Insecure Defaults: The model might recommend or generate code with insecure default settings, such as weak password requirements or unencrypted data transmissions.
* Failure in Error Handling: Improper error handling can inadvertently reveal sensitive information about the system or the application's internal workings.
Given these potential pitfalls, and others not explicitly mentioned, it's essential to thoroughly review, test, and verify the generated code before deploying it in any application, especially those that are security-sensitive. Always consult with security experts or perform rigorous penetration testing when in doubt.
## Training
### Model
* Architecture: a Transformer-based model with next-word prediction objective
* Training tokens: 54B tokens (7B unique tokens)
* Precision: fp16
* GPUs: 8 A100
* Training time: 6 days
### Software
* [PyTorch](https://github.com/pytorch/pytorch)
* [DeepSpeed](https://github.com/microsoft/DeepSpeed)
* [Flash-Attention](https://github.com/HazyResearch/flash-attention)
### License
The model is licensed under the [MIT license](https://huggingface.co/microsoft/phi-1/resolve/main/LICENSE).
### Citation
```bib
@article{gunasekar2023textbooks,
title={Textbooks Are All You Need},
author={Gunasekar, Suriya and Zhang, Yi and Aneja, Jyoti and Mendes, Caio C{\'e}sar Teodoro and Del Giorno, Allie and Gopi, Sivakanth and Javaheripi, Mojan and Kauffmann, Piero and de Rosa, Gustavo and Saarikivi, Olli and others},
journal={arXiv preprint arXiv:2306.11644},
year={2023}
}
```
## Trademarks
This project may contain trademarks or logos for projects, products, or services. Authorized use of Microsoft trademarks or logos is subject to and must follow [Microsoft’s Trademark & Brand Guidelines](https://www.microsoft.com/en-us/legal/intellectualproperty/trademarks). Use of Microsoft trademarks or logos in modified versions of this project must not cause confusion or imply Microsoft sponsorship. Any use of third-party trademarks or logos are subject to those third-party’s policies. |
baichuan-inc/Baichuan-7B | baichuan-inc | 2024-01-09T07:45:22Z | 15,323 | 825 | transformers | [
"transformers",
"pytorch",
"baichuan",
"text-generation",
"custom_code",
"zh",
"en",
"arxiv:1910.07467",
"arxiv:2009.03300",
"autotrain_compatible",
"endpoints_compatible",
"region:us"
] | text-generation | 2023-06-13T07:47:16Z | ---
language:
- zh
- en
pipeline_tag: text-generation
inference: false
---
# Baichuan-7B
<!-- Provide a quick summary of what the model is/does. -->
Baichuan-7B是由百川智能开发的一个开源的大规模预训练模型。基于Transformer结构,在大约1.2万亿tokens上训练的70亿参数模型,支持中英双语,上下文窗口长度为4096。在标准的中文和英文权威benchmark(C-EVAL/MMLU)上均取得同尺寸最好的效果。
如果希望使用Baichuan-7B(如进行推理、Finetune等),我们推荐使用配套代码库[Baichuan-7B](https://github.com/baichuan-inc/Baichuan-7B)。
Baichuan-7B is an open-source large-scale pre-trained model developed by Baichuan Intelligent Technology. Based on the Transformer architecture, it is a model with 7 billion parameters trained on approximately 1.2 trillion tokens. It supports both Chinese and English, with a context window length of 4096. It achieves the best performance of its size on standard Chinese and English authoritative benchmarks (C-EVAL/MMLU).
If you wish to use Baichuan-7B (for inference, finetuning, etc.), we recommend using the accompanying code library [Baichuan-7B](https://github.com/baichuan-inc/Baichuan-7B).
## Why use Baichuan-7B
- 在同尺寸模型中Baichuan-7B达到了目前SOTA的水平,参考下面MMLU指标
- Baichuan-7B使用自有的中英文双语语料进行训练,在中文上进行优化,在C-Eval达到SOTA水平
- 不同于LLaMA完全禁止商业使用,Baichuan-7B使用更宽松的开源协议,允许用于商业目的
- Among models of the same size, Baichuan-7B has achieved the current state-of-the-art (SOTA) level, as evidenced by the following MMLU metrics.
- Baichuan-7B is trained on proprietary bilingual Chinese-English corpora, optimized for Chinese, and achieves SOTA performance on C-Eval.
- Unlike LLaMA, which completely prohibits commercial use, Baichuan-7B employs a more lenient open-source license, allowing for commercial purposes.
## How to Get Started with the Model
如下是一个使用Baichuan-7B进行1-shot推理的任务,根据作品给出作者名,正确输出为"夜雨寄北->李商隐"
```python
from transformers import AutoModelForCausalLM, AutoTokenizer
tokenizer = AutoTokenizer.from_pretrained("baichuan-inc/Baichuan-7B", trust_remote_code=True)
model = AutoModelForCausalLM.from_pretrained("baichuan-inc/Baichuan-7B", device_map="auto", trust_remote_code=True)
inputs = tokenizer('登鹳雀楼->王之涣\n夜雨寄北->', return_tensors='pt')
inputs = inputs.to('cuda:0')
pred = model.generate(**inputs, max_new_tokens=64,repetition_penalty=1.1)
print(tokenizer.decode(pred.cpu()[0], skip_special_tokens=True))
```
The following is a task of performing 1-shot inference using Baichuan-7B, where the author's name is given based on the work, with the correct output being "One Hundred Years of Solitude->Gabriel Garcia Marquez"
```python
from transformers import AutoModelForCausalLM, AutoTokenizer
tokenizer = AutoTokenizer.from_pretrained("baichuan-inc/Baichuan-7B", trust_remote_code=True)
model = AutoModelForCausalLM.from_pretrained("baichuan-inc/Baichuan-7B", device_map="auto", trust_remote_code=True)
inputs = tokenizer('Hamlet->Shakespeare\nOne Hundred Years of Solitude->', return_tensors='pt')
inputs = inputs.to('cuda:0')
pred = model.generate(**inputs, max_new_tokens=64,repetition_penalty=1.1)
print(tokenizer.decode(pred.cpu()[0], skip_special_tokens=True))
```
## Model Details
### Model Description
<!-- Provide a longer summary of what this model is. -->
- **Developed by:** 百川智能(Baichuan Intelligent Technology)
- **Email**: [email protected]
- **Language(s) (NLP):** Chinese/English
- **License:** [Baichuan-7B License](https://huggingface.co/baichuan-inc/Baichuan-7B/blob/main/baichuan-7B%20%E6%A8%A1%E5%9E%8B%E8%AE%B8%E5%8F%AF%E5%8D%8F%E8%AE%AE.pdf)
### Model Sources
<!-- Provide the basic links for the model. -->
整体模型基于标准的Transformer结构,我们采用了和LLaMA一样的模型设计
- **Position Embedding**:采用rotary-embedding,是现阶段被大多数模型采用的位置编码方案,具有很好的外推性。
- **Feedforward Layer**:采用SwiGLU,Feedforward变化为(8/3)倍的隐含层大小,即11008。
- **Layer Normalization**: 基于[RMSNorm](https://arxiv.org/abs/1910.07467)的Pre-Normalization。
具体参数和见下表
| Hyperparameter | Value |
|----------------|-------|
|n_parameters | 7000559616 |
|n_layers | 32 |
| n_heads | 32 |
| d_model | 4096 |
| vocab size | 64000 |
| sequence length | 4096 |
The overall model is based on the standard Transformer structure, and we have adopted the same model design as LLaMA:
- Position Embedding: We use rotary-embedding, which is the position encoding scheme adopted by most models at this stage, and it has excellent extrapolation capabilities.
- Feedforward Layer: We use SwiGLU. The feedforward changes to (8/3) times the size of the hidden layer, that is, 11008.
- Layer Normalization: Pre-Normalization based on [RMSNorm](https://arxiv.org/abs/1910.07467).
The specific parameters are as follows:
| Hyperparameter | Value |
|----------------|-------|
|n_parameters | 7000559616 |
|n_layers | 32 |
| n_heads | 32 |
| d_model | 4096 |
| vocab size | 64000 |
| sequence length | 4096 |
## Uses
<!-- Address questions around how the model is intended to be used, including the foreseeable users of the model and those affected by the model. -->
### Downstream Use
<!-- This section is for the model use when fine-tuned for a task, or when plugged into a larger ecosystem/app -->
我们同时开源出了和本模型配套的训练代码,允许进行高效的Finetune用于下游任务,具体参见[Baichuan-7B](https://github.com/baichuan-inc/Baichuan-7B)。
We have also open-sourced the training code that accompanies this model, allowing for efficient finetuning for downstream tasks. For more details, please refer to [Baichuan-7B](https://github.com/baichuan-inc/Baichuan-7B).
### Out-of-Scope Use
<!-- This section addresses misuse, malicious use, and uses that the model will not work well for. -->
在没有充分评估风险和采取缓解措施的情况下投入生产使用;任何可能被视为不负责任或有害的使用案例。
Production use without adequate assessment of risks and mitigation; any use cases which may be considered irresponsible or harmful.
## Bias, Risks, and Limitations
<!-- This section is meant to convey both technical and sociotechnical limitations. -->
Baichuan-7B可能会产生事实上不正确的输出,不应依赖它产生事实上准确的信息。Baichuan-7B是在各种公共数据集上进行训练的。尽管我们已经做出了巨大的努力来清洗预训练数据,但这个模型可能会生成淫秽、偏见或其他冒犯性的输出。
Baichuan-7B can produce factually incorrect output, and should not be relied on to produce factually accurate information. Baichuan-7B was trained on various public datasets. While great efforts have been taken to clean the pretraining data, it is possible that this model could generate lewd, biased or otherwise offensive outputs.
## Training Details
训练具体设置参见[Baichuan-7B](https://github.com/baichuan-inc/Baichuan-7B)。
For specific training settings, please refer to [Baichuan-7B](https://github.com/baichuan-inc/Baichuan-7B).
## Evaluation
### 中文评测
#### C-Eval
[CEval数据集](https://cevalbenchmark.com/index.html)是一个全面的中文基础模型评测数据集,涵盖了52个学科和四个难度的级别。我们使用该数据集的dev集作为few-shot的来源,在test集上进行了5-shot测试。
| Model 5-shot | Average | Avg(Hard) | STEM | Social Sciences | Humanities | Others |
|-----------------------------|---------|-----------|------|-----------------|------------|--------|
| GPT-4 | 68.7 | 54.9 | 67.1 | 77.6 | 64.5 | 67.8 |
| ChatGPT | 54.4 | 41.4 | 52.9 | 61.8 | 50.9 | 53.6 |
| Claude-v1.3 | 54.2 | 39.0 | 51.9 | 61.7 | 52.1 | 53.7 |
| Claude-instant-v1.0 | 45.9 | 35.5 | 43.1 | 53.8 | 44.2 | 45.4 |
| moss-moon-003-base (16B) | 27.4 | 24.5 | 27.0 | 29.1 | 27.2 | 26.9 |
| Ziya-LLaMA-13B-pretrain | 30.2 | 22.7 | 27.7 | 34.4 | 32.0 | 28.9 |
| LLaMA-7B-hf | 27.1 | 25.9 | 27.1 | 26.8 | 27.9 | 26.3 |
| ChatGLM-6B | 34.5 | 23.1 | 30.4 | 39.6 | 37.4 | 34.5 |
| Falcon-7B | 25.8 | 24.3 | 25.8 | 26.0 | 25.8 | 25.6 |
| Open-LLaMA-v2-pretrain (7B) | 24.0 | 22.5 | 23.1 | 25.3 | 25.2 | 23.2 |
| TigerBot-7B-base | 25.7 | 27.0 | 27.3 | 24.7 | 23.4 | 26.1 |
| Aquila-7B<sup>*</sup> | 25.5 | 25.2 | 25.6 | 24.6 | 25.2 | 26.6 |
| BLOOM-7B | 22.8 | 20.2 | 21.8 | 23.3 | 23.9 | 23.3 |
| BLOOMZ-7B | 35.7 | 25.8 | 31.3 | 43.5 | 36.6 | 35.6 |
| **Baichuan-7B** | 42.8 | 31.5 | 38.2 | 52.0 | 46.2 | 39.3 |
#### Gaokao
[Gaokao](https://github.com/ExpressAI/AI-Gaokao) 是一个以中国高考题作为评测大语言模型能力的数据集,用以评估模型的语言能力和逻辑推理能力。
我们只保留了其中的单项选择题,并对所有模型进行统一5-shot测试。
以下是测试的结果。
| Model | Average |
|-------------------------|-----------------|
| Open-LLaMA-v2-pretrain | 21.41 |
| Ziya-LLaMA-13B-pretrain | 23.17 |
| Falcon-7B | 23.98 |
| TigerBot-7B-base | 25.94 |
| LLaMA-7B | 27.81 |
| ChatGLM-6B | 21.41 |
| BLOOM-7B | 26.96 |
| BLOOMZ-7B | 28.72 |
| Aquila-7B<sup>*</sup> | 24.39 |
| **Baichuan-7B** | **36.24** |
#### AGIEval
[AGIEval](https://github.com/microsoft/AGIEval) 旨在评估模型的认知和解决问题相关的任务中的一般能力。
我们只保留了其中的四选一单项选择题,随机划分后对所有模型进行了统一5-shot测试。
| Model | Average |
|-------------------------|-----------------|
| Open-LLaMA-v2-pretrain | 23.49 |
| Ziya-LLaMA-13B-pretrain | 27.64 |
| Falcon-7B | 27.18 |
| TigerBot-7B-base | 25.19 |
| LLaMA-7B | 28.17 |
| ChatGLM-6B | 23.49 |
| BLOOM-7B | 26.55 |
| BLOOMZ-7B | 30.27 |
| Aquila-7B<sup>*</sup> | 25.58 |
| **Baichuan-7B** | **34.44** |
<sup>*</sup>其中Aquila模型来源于[智源官方网站](https://model.baai.ac.cn/model-detail/100098),仅做参考
### English Leaderboard
In addition to Chinese, we also tested the model's performance in English.
#### MMLU
[MMLU](https://arxiv.org/abs/2009.03300) is an English evaluation dataset that includes 57 multiple-choice tasks, covering elementary mathematics, American history, computer science, law, etc. The difficulty ranges from high school level to expert level, making it a mainstream LLM evaluation dataset.
We adopted the [open-source]((https://github.com/hendrycks/test)) evaluation scheme, and the final 5-shot results are as follows:
| Model | Humanities | Social Sciences | STEM | Other | Average |
|----------------------------------------|-----------:|:---------------:|:----:|:-----:|:-------:|
| LLaMA-7B<sup>2</sup> | 34.0 | 38.3 | 30.5 | 38.1 | 35.1 |
| Falcon-7B<sup>1</sup> | - | - | - | - | 35.0 |
| mpt-7B<sup>1</sup> | - | - | - | - | 35.6 |
| ChatGLM-6B<sup>0</sup> | 35.4 | 41.0 | 31.3 | 40.5 | 36.9 |
| BLOOM 7B<sup>0</sup> | 25.0 | 24.4 | 26.5 | 26.4 | 25.5 |
| BLOOMZ 7B<sup>0</sup> | 31.3 | 42.1 | 34.4 | 39.0 | 36.1 |
| moss-moon-003-base (16B)<sup>0</sup> | 24.2 | 22.8 | 22.4 | 24.4 | 23.6 |
| moss-moon-003-sft (16B)<sup>0</sup> | 30.5 | 33.8 | 29.3 | 34.4 | 31.9 |
| **Baichuan-7B<sup>0</sup>** | 38.4 | 48.9 | 35.6 | 48.1 | 42.3 |
The superscript in the Model column indicates the source of the results.
```
0:reimplemented
1:https://huggingface.co/spaces/HuggingFaceH4/open_llm_leaderboard
2:https://paperswithcode.com/sota/multi-task-language-understanding-on-mmlu
```
## Our Group
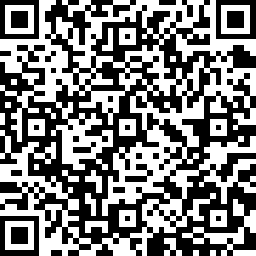
|
facebook/deit-tiny-distilled-patch16-224 | facebook | 2022-07-13T11:41:55Z | 15,322 | 4 | transformers | [
"transformers",
"pytorch",
"tf",
"deit",
"image-classification",
"vision",
"dataset:imagenet",
"arxiv:2012.12877",
"arxiv:2006.03677",
"license:apache-2.0",
"autotrain_compatible",
"endpoints_compatible",
"region:us"
] | image-classification | 2022-03-02T23:29:05Z | ---
license: apache-2.0
tags:
- image-classification
- vision
datasets:
- imagenet
---
# Distilled Data-efficient Image Transformer (tiny-sized model)
Distilled data-efficient Image Transformer (DeiT) model pre-trained and fine-tuned on ImageNet-1k (1 million images, 1,000 classes) at resolution 224x224. It was first introduced in the paper [Training data-efficient image transformers & distillation through attention](https://arxiv.org/abs/2012.12877) by Touvron et al. and first released in [this repository](https://github.com/facebookresearch/deit). However, the weights were converted from the [timm repository](https://github.com/rwightman/pytorch-image-models) by Ross Wightman.
Disclaimer: The team releasing DeiT did not write a model card for this model so this model card has been written by the Hugging Face team.
## Model description
This model is a distilled Vision Transformer (ViT). It uses a distillation token, besides the class token, to effectively learn from a teacher (CNN) during both pre-training and fine-tuning. The distillation token is learned through backpropagation, by interacting with the class ([CLS]) and patch tokens through the self-attention layers.
Images are presented to the model as a sequence of fixed-size patches (resolution 16x16), which are linearly embedded.
## Intended uses & limitations
You can use the raw model for image classification. See the [model hub](https://huggingface.co/models?search=facebook/deit) to look for
fine-tuned versions on a task that interests you.
### How to use
Since this model is a distilled ViT model, you can plug it into DeiTModel, DeiTForImageClassification or DeiTForImageClassificationWithTeacher. Note that the model expects the data to be prepared using DeiTFeatureExtractor. Here we use AutoFeatureExtractor, which will automatically use the appropriate feature extractor given the model name.
Here is how to use this model to classify an image of the COCO 2017 dataset into one of the 1,000 ImageNet classes:
```python
from transformers import AutoFeatureExtractor, DeiTForImageClassificationWithTeacher
from PIL import Image
import requests
url = 'http://images.cocodataset.org/val2017/000000039769.jpg'
image = Image.open(requests.get(url, stream=True).raw)
feature_extractor = AutoFeatureExtractor.from_pretrained('facebook/deit-tiny-distilled-patch16-224')
model = DeiTForImageClassificationWithTeacher.from_pretrained('facebook/deit-tiny-distilled-patch16-224')
inputs = feature_extractor(images=image, return_tensors="pt")
outputs = model(**inputs)
logits = outputs.logits
# model predicts one of the 1000 ImageNet classes
predicted_class_idx = logits.argmax(-1).item()
print("Predicted class:", model.config.id2label[predicted_class_idx])
```
Currently, both the feature extractor and model support PyTorch. Tensorflow and JAX/FLAX are coming soon.
## Training data
This model was pretrained and fine-tuned with distillation on [ImageNet-1k](http://www.image-net.org/challenges/LSVRC/2012/), a dataset consisting of 1 million images and 1k classes.
## Training procedure
### Preprocessing
The exact details of preprocessing of images during training/validation can be found [here](https://github.com/facebookresearch/deit/blob/ab5715372db8c6cad5740714b2216d55aeae052e/datasets.py#L78).
At inference time, images are resized/rescaled to the same resolution (256x256), center-cropped at 224x224 and normalized across the RGB channels with the ImageNet mean and standard deviation.
### Pretraining
The model was trained on a single 8-GPU node for 3 days. Training resolution is 224. For all hyperparameters (such as batch size and learning rate) we refer to table 9 of the original paper.
## Evaluation results
| Model | ImageNet top-1 accuracy | ImageNet top-5 accuracy | # params | URL |
|---------------------------------------|-------------------------|-------------------------|----------|------------------------------------------------------------------|
| DeiT-tiny | 72.2 | 91.1 | 5M | https://huggingface.co/facebook/deit-tiny-patch16-224 |
| DeiT-small | 79.9 | 95.0 | 22M | https://huggingface.co/facebook/deit-small-patch16-224 |
| DeiT-base | 81.8 | 95.6 | 86M | https://huggingface.co/facebook/deit-base-patch16-224 |
| **DeiT-tiny distilled** | **74.5** | **91.9** | **6M** | **https://huggingface.co/facebook/deit-tiny-distilled-patch16-224** |
| DeiT-small distilled | 81.2 | 95.4 | 22M | https://huggingface.co/facebook/deit-small-distilled-patch16-224 |
| DeiT-base distilled | 83.4 | 96.5 | 87M | https://huggingface.co/facebook/deit-base-distilled-patch16-224 |
| DeiT-base 384 | 82.9 | 96.2 | 87M | https://huggingface.co/facebook/deit-base-patch16-384 |
| DeiT-base distilled 384 (1000 epochs) | 85.2 | 97.2 | 88M | https://huggingface.co/facebook/deit-base-distilled-patch16-384 |
Note that for fine-tuning, the best results are obtained with a higher resolution (384x384). Of course, increasing the model size will result in better performance.
### BibTeX entry and citation info
```bibtex
@misc{touvron2021training,
title={Training data-efficient image transformers & distillation through attention},
author={Hugo Touvron and Matthieu Cord and Matthijs Douze and Francisco Massa and Alexandre Sablayrolles and Hervé Jégou},
year={2021},
eprint={2012.12877},
archivePrefix={arXiv},
primaryClass={cs.CV}
}
```
```bibtex
@misc{wu2020visual,
title={Visual Transformers: Token-based Image Representation and Processing for Computer Vision},
author={Bichen Wu and Chenfeng Xu and Xiaoliang Dai and Alvin Wan and Peizhao Zhang and Zhicheng Yan and Masayoshi Tomizuka and Joseph Gonzalez and Kurt Keutzer and Peter Vajda},
year={2020},
eprint={2006.03677},
archivePrefix={arXiv},
primaryClass={cs.CV}
}
```
```bibtex
@inproceedings{deng2009imagenet,
title={Imagenet: A large-scale hierarchical image database},
author={Deng, Jia and Dong, Wei and Socher, Richard and Li, Li-Jia and Li, Kai and Fei-Fei, Li},
booktitle={2009 IEEE conference on computer vision and pattern recognition},
pages={248--255},
year={2009},
organization={Ieee}
}
``` |
QuantFactory/L3-8B-Lunaris-v1-GGUF | QuantFactory | 2024-06-30T06:05:31Z | 15,314 | 0 | null | [
"gguf",
"region:us"
] | null | 2024-06-30T05:09:06Z | Entry not found |
mradermacher/Soliloquy-Stheno-TheSpice-Pantheon-v1.1-GGUF | mradermacher | 2024-06-23T11:23:52Z | 15,290 | 0 | transformers | [
"transformers",
"gguf",
"mergekit",
"merge",
"en",
"base_model:lik07/Soliloquy-Stheno-TheSpice-Pantheon-v1.1",
"endpoints_compatible",
"region:us"
] | null | 2024-06-23T10:06:53Z | ---
base_model: lik07/Soliloquy-Stheno-TheSpice-Pantheon-v1.1
language:
- en
library_name: transformers
quantized_by: mradermacher
tags:
- mergekit
- merge
---
## About
<!-- ### quantize_version: 2 -->
<!-- ### output_tensor_quantised: 1 -->
<!-- ### convert_type: hf -->
<!-- ### vocab_type: -->
<!-- ### tags: -->
static quants of https://huggingface.co/lik07/Soliloquy-Stheno-TheSpice-Pantheon-v1.1
<!-- provided-files -->
weighted/imatrix quants seem not to be available (by me) at this time. If they do not show up a week or so after the static ones, I have probably not planned for them. Feel free to request them by opening a Community Discussion.
## Usage
If you are unsure how to use GGUF files, refer to one of [TheBloke's
READMEs](https://huggingface.co/TheBloke/KafkaLM-70B-German-V0.1-GGUF) for
more details, including on how to concatenate multi-part files.
## Provided Quants
(sorted by size, not necessarily quality. IQ-quants are often preferable over similar sized non-IQ quants)
| Link | Type | Size/GB | Notes |
|:-----|:-----|--------:|:------|
| [GGUF](https://huggingface.co/mradermacher/Soliloquy-Stheno-TheSpice-Pantheon-v1.1-GGUF/resolve/main/Soliloquy-Stheno-TheSpice-Pantheon-v1.1.Q2_K.gguf) | Q2_K | 3.3 | |
| [GGUF](https://huggingface.co/mradermacher/Soliloquy-Stheno-TheSpice-Pantheon-v1.1-GGUF/resolve/main/Soliloquy-Stheno-TheSpice-Pantheon-v1.1.IQ3_XS.gguf) | IQ3_XS | 3.6 | |
| [GGUF](https://huggingface.co/mradermacher/Soliloquy-Stheno-TheSpice-Pantheon-v1.1-GGUF/resolve/main/Soliloquy-Stheno-TheSpice-Pantheon-v1.1.Q3_K_S.gguf) | Q3_K_S | 3.8 | |
| [GGUF](https://huggingface.co/mradermacher/Soliloquy-Stheno-TheSpice-Pantheon-v1.1-GGUF/resolve/main/Soliloquy-Stheno-TheSpice-Pantheon-v1.1.IQ3_S.gguf) | IQ3_S | 3.8 | beats Q3_K* |
| [GGUF](https://huggingface.co/mradermacher/Soliloquy-Stheno-TheSpice-Pantheon-v1.1-GGUF/resolve/main/Soliloquy-Stheno-TheSpice-Pantheon-v1.1.IQ3_M.gguf) | IQ3_M | 3.9 | |
| [GGUF](https://huggingface.co/mradermacher/Soliloquy-Stheno-TheSpice-Pantheon-v1.1-GGUF/resolve/main/Soliloquy-Stheno-TheSpice-Pantheon-v1.1.Q3_K_M.gguf) | Q3_K_M | 4.1 | lower quality |
| [GGUF](https://huggingface.co/mradermacher/Soliloquy-Stheno-TheSpice-Pantheon-v1.1-GGUF/resolve/main/Soliloquy-Stheno-TheSpice-Pantheon-v1.1.Q3_K_L.gguf) | Q3_K_L | 4.4 | |
| [GGUF](https://huggingface.co/mradermacher/Soliloquy-Stheno-TheSpice-Pantheon-v1.1-GGUF/resolve/main/Soliloquy-Stheno-TheSpice-Pantheon-v1.1.IQ4_XS.gguf) | IQ4_XS | 4.6 | |
| [GGUF](https://huggingface.co/mradermacher/Soliloquy-Stheno-TheSpice-Pantheon-v1.1-GGUF/resolve/main/Soliloquy-Stheno-TheSpice-Pantheon-v1.1.Q4_K_S.gguf) | Q4_K_S | 4.8 | fast, recommended |
| [GGUF](https://huggingface.co/mradermacher/Soliloquy-Stheno-TheSpice-Pantheon-v1.1-GGUF/resolve/main/Soliloquy-Stheno-TheSpice-Pantheon-v1.1.Q4_K_M.gguf) | Q4_K_M | 5.0 | fast, recommended |
| [GGUF](https://huggingface.co/mradermacher/Soliloquy-Stheno-TheSpice-Pantheon-v1.1-GGUF/resolve/main/Soliloquy-Stheno-TheSpice-Pantheon-v1.1.Q5_K_S.gguf) | Q5_K_S | 5.7 | |
| [GGUF](https://huggingface.co/mradermacher/Soliloquy-Stheno-TheSpice-Pantheon-v1.1-GGUF/resolve/main/Soliloquy-Stheno-TheSpice-Pantheon-v1.1.Q5_K_M.gguf) | Q5_K_M | 5.8 | |
| [GGUF](https://huggingface.co/mradermacher/Soliloquy-Stheno-TheSpice-Pantheon-v1.1-GGUF/resolve/main/Soliloquy-Stheno-TheSpice-Pantheon-v1.1.Q6_K.gguf) | Q6_K | 6.7 | very good quality |
| [GGUF](https://huggingface.co/mradermacher/Soliloquy-Stheno-TheSpice-Pantheon-v1.1-GGUF/resolve/main/Soliloquy-Stheno-TheSpice-Pantheon-v1.1.Q8_0.gguf) | Q8_0 | 8.6 | fast, best quality |
| [GGUF](https://huggingface.co/mradermacher/Soliloquy-Stheno-TheSpice-Pantheon-v1.1-GGUF/resolve/main/Soliloquy-Stheno-TheSpice-Pantheon-v1.1.f16.gguf) | f16 | 16.2 | 16 bpw, overkill |
Here is a handy graph by ikawrakow comparing some lower-quality quant
types (lower is better):

And here are Artefact2's thoughts on the matter:
https://gist.github.com/Artefact2/b5f810600771265fc1e39442288e8ec9
## FAQ / Model Request
See https://huggingface.co/mradermacher/model_requests for some answers to
questions you might have and/or if you want some other model quantized.
## Thanks
I thank my company, [nethype GmbH](https://www.nethype.de/), for letting
me use its servers and providing upgrades to my workstation to enable
this work in my free time.
<!-- end -->
|
mradermacher/RoLlama3-8b-Instruct-GGUF | mradermacher | 2024-06-28T11:04:35Z | 15,290 | 0 | transformers | [
"transformers",
"gguf",
"ro",
"base_model:OpenLLM-Ro/RoLlama3-8b-Instruct",
"license:cc-by-nc-4.0",
"endpoints_compatible",
"region:us"
] | null | 2024-06-28T09:26:28Z | ---
base_model: OpenLLM-Ro/RoLlama3-8b-Instruct
language:
- ro
library_name: transformers
license: cc-by-nc-4.0
quantized_by: mradermacher
---
## About
<!-- ### quantize_version: 2 -->
<!-- ### output_tensor_quantised: 1 -->
<!-- ### convert_type: hf -->
<!-- ### vocab_type: -->
<!-- ### tags: -->
static quants of https://huggingface.co/OpenLLM-Ro/RoLlama3-8b-Instruct
<!-- provided-files -->
weighted/imatrix quants seem not to be available (by me) at this time. If they do not show up a week or so after the static ones, I have probably not planned for them. Feel free to request them by opening a Community Discussion.
## Usage
If you are unsure how to use GGUF files, refer to one of [TheBloke's
READMEs](https://huggingface.co/TheBloke/KafkaLM-70B-German-V0.1-GGUF) for
more details, including on how to concatenate multi-part files.
## Provided Quants
(sorted by size, not necessarily quality. IQ-quants are often preferable over similar sized non-IQ quants)
| Link | Type | Size/GB | Notes |
|:-----|:-----|--------:|:------|
| [GGUF](https://huggingface.co/mradermacher/RoLlama3-8b-Instruct-GGUF/resolve/main/RoLlama3-8b-Instruct.Q2_K.gguf) | Q2_K | 3.3 | |
| [GGUF](https://huggingface.co/mradermacher/RoLlama3-8b-Instruct-GGUF/resolve/main/RoLlama3-8b-Instruct.IQ3_XS.gguf) | IQ3_XS | 3.6 | |
| [GGUF](https://huggingface.co/mradermacher/RoLlama3-8b-Instruct-GGUF/resolve/main/RoLlama3-8b-Instruct.Q3_K_S.gguf) | Q3_K_S | 3.8 | |
| [GGUF](https://huggingface.co/mradermacher/RoLlama3-8b-Instruct-GGUF/resolve/main/RoLlama3-8b-Instruct.IQ3_S.gguf) | IQ3_S | 3.8 | beats Q3_K* |
| [GGUF](https://huggingface.co/mradermacher/RoLlama3-8b-Instruct-GGUF/resolve/main/RoLlama3-8b-Instruct.IQ3_M.gguf) | IQ3_M | 3.9 | |
| [GGUF](https://huggingface.co/mradermacher/RoLlama3-8b-Instruct-GGUF/resolve/main/RoLlama3-8b-Instruct.Q3_K_M.gguf) | Q3_K_M | 4.1 | lower quality |
| [GGUF](https://huggingface.co/mradermacher/RoLlama3-8b-Instruct-GGUF/resolve/main/RoLlama3-8b-Instruct.Q3_K_L.gguf) | Q3_K_L | 4.4 | |
| [GGUF](https://huggingface.co/mradermacher/RoLlama3-8b-Instruct-GGUF/resolve/main/RoLlama3-8b-Instruct.IQ4_XS.gguf) | IQ4_XS | 4.6 | |
| [GGUF](https://huggingface.co/mradermacher/RoLlama3-8b-Instruct-GGUF/resolve/main/RoLlama3-8b-Instruct.Q4_K_S.gguf) | Q4_K_S | 4.8 | fast, recommended |
| [GGUF](https://huggingface.co/mradermacher/RoLlama3-8b-Instruct-GGUF/resolve/main/RoLlama3-8b-Instruct.Q4_K_M.gguf) | Q4_K_M | 5.0 | fast, recommended |
| [GGUF](https://huggingface.co/mradermacher/RoLlama3-8b-Instruct-GGUF/resolve/main/RoLlama3-8b-Instruct.Q5_K_S.gguf) | Q5_K_S | 5.7 | |
| [GGUF](https://huggingface.co/mradermacher/RoLlama3-8b-Instruct-GGUF/resolve/main/RoLlama3-8b-Instruct.Q5_K_M.gguf) | Q5_K_M | 5.8 | |
| [GGUF](https://huggingface.co/mradermacher/RoLlama3-8b-Instruct-GGUF/resolve/main/RoLlama3-8b-Instruct.Q6_K.gguf) | Q6_K | 6.7 | very good quality |
| [GGUF](https://huggingface.co/mradermacher/RoLlama3-8b-Instruct-GGUF/resolve/main/RoLlama3-8b-Instruct.Q8_0.gguf) | Q8_0 | 8.6 | fast, best quality |
| [GGUF](https://huggingface.co/mradermacher/RoLlama3-8b-Instruct-GGUF/resolve/main/RoLlama3-8b-Instruct.f16.gguf) | f16 | 16.2 | 16 bpw, overkill |
Here is a handy graph by ikawrakow comparing some lower-quality quant
types (lower is better):

And here are Artefact2's thoughts on the matter:
https://gist.github.com/Artefact2/b5f810600771265fc1e39442288e8ec9
## FAQ / Model Request
See https://huggingface.co/mradermacher/model_requests for some answers to
questions you might have and/or if you want some other model quantized.
## Thanks
I thank my company, [nethype GmbH](https://www.nethype.de/), for letting
me use its servers and providing upgrades to my workstation to enable
this work in my free time.
<!-- end -->
|
RichardErkhov/mlabonne_-_ChimeraLlama-3-8B-v3-gguf | RichardErkhov | 2024-06-26T03:35:07Z | 15,289 | 0 | null | [
"gguf",
"region:us"
] | null | 2024-06-25T23:25:13Z | Quantization made by Richard Erkhov.
[Github](https://github.com/RichardErkhov)
[Discord](https://discord.gg/pvy7H8DZMG)
[Request more models](https://github.com/RichardErkhov/quant_request)
ChimeraLlama-3-8B-v3 - GGUF
- Model creator: https://huggingface.co/mlabonne/
- Original model: https://huggingface.co/mlabonne/ChimeraLlama-3-8B-v3/
| Name | Quant method | Size |
| ---- | ---- | ---- |
| [ChimeraLlama-3-8B-v3.Q2_K.gguf](https://huggingface.co/RichardErkhov/mlabonne_-_ChimeraLlama-3-8B-v3-gguf/blob/main/ChimeraLlama-3-8B-v3.Q2_K.gguf) | Q2_K | 2.96GB |
| [ChimeraLlama-3-8B-v3.IQ3_XS.gguf](https://huggingface.co/RichardErkhov/mlabonne_-_ChimeraLlama-3-8B-v3-gguf/blob/main/ChimeraLlama-3-8B-v3.IQ3_XS.gguf) | IQ3_XS | 3.28GB |
| [ChimeraLlama-3-8B-v3.IQ3_S.gguf](https://huggingface.co/RichardErkhov/mlabonne_-_ChimeraLlama-3-8B-v3-gguf/blob/main/ChimeraLlama-3-8B-v3.IQ3_S.gguf) | IQ3_S | 3.43GB |
| [ChimeraLlama-3-8B-v3.Q3_K_S.gguf](https://huggingface.co/RichardErkhov/mlabonne_-_ChimeraLlama-3-8B-v3-gguf/blob/main/ChimeraLlama-3-8B-v3.Q3_K_S.gguf) | Q3_K_S | 3.41GB |
| [ChimeraLlama-3-8B-v3.IQ3_M.gguf](https://huggingface.co/RichardErkhov/mlabonne_-_ChimeraLlama-3-8B-v3-gguf/blob/main/ChimeraLlama-3-8B-v3.IQ3_M.gguf) | IQ3_M | 3.52GB |
| [ChimeraLlama-3-8B-v3.Q3_K.gguf](https://huggingface.co/RichardErkhov/mlabonne_-_ChimeraLlama-3-8B-v3-gguf/blob/main/ChimeraLlama-3-8B-v3.Q3_K.gguf) | Q3_K | 3.74GB |
| [ChimeraLlama-3-8B-v3.Q3_K_M.gguf](https://huggingface.co/RichardErkhov/mlabonne_-_ChimeraLlama-3-8B-v3-gguf/blob/main/ChimeraLlama-3-8B-v3.Q3_K_M.gguf) | Q3_K_M | 3.74GB |
| [ChimeraLlama-3-8B-v3.Q3_K_L.gguf](https://huggingface.co/RichardErkhov/mlabonne_-_ChimeraLlama-3-8B-v3-gguf/blob/main/ChimeraLlama-3-8B-v3.Q3_K_L.gguf) | Q3_K_L | 4.03GB |
| [ChimeraLlama-3-8B-v3.IQ4_XS.gguf](https://huggingface.co/RichardErkhov/mlabonne_-_ChimeraLlama-3-8B-v3-gguf/blob/main/ChimeraLlama-3-8B-v3.IQ4_XS.gguf) | IQ4_XS | 4.18GB |
| [ChimeraLlama-3-8B-v3.Q4_0.gguf](https://huggingface.co/RichardErkhov/mlabonne_-_ChimeraLlama-3-8B-v3-gguf/blob/main/ChimeraLlama-3-8B-v3.Q4_0.gguf) | Q4_0 | 4.34GB |
| [ChimeraLlama-3-8B-v3.IQ4_NL.gguf](https://huggingface.co/RichardErkhov/mlabonne_-_ChimeraLlama-3-8B-v3-gguf/blob/main/ChimeraLlama-3-8B-v3.IQ4_NL.gguf) | IQ4_NL | 4.38GB |
| [ChimeraLlama-3-8B-v3.Q4_K_S.gguf](https://huggingface.co/RichardErkhov/mlabonne_-_ChimeraLlama-3-8B-v3-gguf/blob/main/ChimeraLlama-3-8B-v3.Q4_K_S.gguf) | Q4_K_S | 4.37GB |
| [ChimeraLlama-3-8B-v3.Q4_K.gguf](https://huggingface.co/RichardErkhov/mlabonne_-_ChimeraLlama-3-8B-v3-gguf/blob/main/ChimeraLlama-3-8B-v3.Q4_K.gguf) | Q4_K | 4.58GB |
| [ChimeraLlama-3-8B-v3.Q4_K_M.gguf](https://huggingface.co/RichardErkhov/mlabonne_-_ChimeraLlama-3-8B-v3-gguf/blob/main/ChimeraLlama-3-8B-v3.Q4_K_M.gguf) | Q4_K_M | 4.58GB |
| [ChimeraLlama-3-8B-v3.Q4_1.gguf](https://huggingface.co/RichardErkhov/mlabonne_-_ChimeraLlama-3-8B-v3-gguf/blob/main/ChimeraLlama-3-8B-v3.Q4_1.gguf) | Q4_1 | 4.78GB |
| [ChimeraLlama-3-8B-v3.Q5_0.gguf](https://huggingface.co/RichardErkhov/mlabonne_-_ChimeraLlama-3-8B-v3-gguf/blob/main/ChimeraLlama-3-8B-v3.Q5_0.gguf) | Q5_0 | 5.21GB |
| [ChimeraLlama-3-8B-v3.Q5_K_S.gguf](https://huggingface.co/RichardErkhov/mlabonne_-_ChimeraLlama-3-8B-v3-gguf/blob/main/ChimeraLlama-3-8B-v3.Q5_K_S.gguf) | Q5_K_S | 5.21GB |
| [ChimeraLlama-3-8B-v3.Q5_K.gguf](https://huggingface.co/RichardErkhov/mlabonne_-_ChimeraLlama-3-8B-v3-gguf/blob/main/ChimeraLlama-3-8B-v3.Q5_K.gguf) | Q5_K | 5.34GB |
| [ChimeraLlama-3-8B-v3.Q5_K_M.gguf](https://huggingface.co/RichardErkhov/mlabonne_-_ChimeraLlama-3-8B-v3-gguf/blob/main/ChimeraLlama-3-8B-v3.Q5_K_M.gguf) | Q5_K_M | 5.34GB |
| [ChimeraLlama-3-8B-v3.Q5_1.gguf](https://huggingface.co/RichardErkhov/mlabonne_-_ChimeraLlama-3-8B-v3-gguf/blob/main/ChimeraLlama-3-8B-v3.Q5_1.gguf) | Q5_1 | 5.65GB |
| [ChimeraLlama-3-8B-v3.Q6_K.gguf](https://huggingface.co/RichardErkhov/mlabonne_-_ChimeraLlama-3-8B-v3-gguf/blob/main/ChimeraLlama-3-8B-v3.Q6_K.gguf) | Q6_K | 6.14GB |
| [ChimeraLlama-3-8B-v3.Q8_0.gguf](https://huggingface.co/RichardErkhov/mlabonne_-_ChimeraLlama-3-8B-v3-gguf/blob/main/ChimeraLlama-3-8B-v3.Q8_0.gguf) | Q8_0 | 7.95GB |
Original model description:
---
license: other
tags:
- merge
- mergekit
- lazymergekit
base_model:
- NousResearch/Meta-Llama-3-8B-Instruct
- mlabonne/OrpoLlama-3-8B
- cognitivecomputations/dolphin-2.9-llama3-8b
- Danielbrdz/Barcenas-Llama3-8b-ORPO
- VAGOsolutions/Llama-3-SauerkrautLM-8b-Instruct
- vicgalle/Configurable-Llama-3-8B-v0.3
- MaziyarPanahi/Llama-3-8B-Instruct-DPO-v0.3
---
# ChimeraLlama-3-8B-v3
ChimeraLlama-3-8B-v3 is a merge of the following models using [LazyMergekit](https://colab.research.google.com/drive/1obulZ1ROXHjYLn6PPZJwRR6GzgQogxxb?usp=sharing):
* [NousResearch/Meta-Llama-3-8B-Instruct](https://huggingface.co/NousResearch/Meta-Llama-3-8B-Instruct)
* [mlabonne/OrpoLlama-3-8B](https://huggingface.co/mlabonne/OrpoLlama-3-8B)
* [cognitivecomputations/dolphin-2.9-llama3-8b](https://huggingface.co/cognitivecomputations/dolphin-2.9-llama3-8b)
* [Danielbrdz/Barcenas-Llama3-8b-ORPO](https://huggingface.co/Danielbrdz/Barcenas-Llama3-8b-ORPO)
* [VAGOsolutions/Llama-3-SauerkrautLM-8b-Instruct](https://huggingface.co/VAGOsolutions/Llama-3-SauerkrautLM-8b-Instruct)
* [vicgalle/Configurable-Llama-3-8B-v0.3](https://huggingface.co/vicgalle/Configurable-Llama-3-8B-v0.3)
* [MaziyarPanahi/Llama-3-8B-Instruct-DPO-v0.3](https://huggingface.co/MaziyarPanahi/Llama-3-8B-Instruct-DPO-v0.3)
## 🧩 Configuration
```yaml
models:
- model: NousResearch/Meta-Llama-3-8B
# No parameters necessary for base model
- model: NousResearch/Meta-Llama-3-8B-Instruct
parameters:
density: 0.6
weight: 0.5
- model: mlabonne/OrpoLlama-3-8B
parameters:
density: 0.55
weight: 0.05
- model: cognitivecomputations/dolphin-2.9-llama3-8b
parameters:
density: 0.55
weight: 0.05
- model: Danielbrdz/Barcenas-Llama3-8b-ORPO
parameters:
density: 0.55
weight: 0.2
- model: VAGOsolutions/Llama-3-SauerkrautLM-8b-Instruct
parameters:
density: 0.55
weight: 0.1
- model: vicgalle/Configurable-Llama-3-8B-v0.3
parameters:
density: 0.55
weight: 0.05
- model: MaziyarPanahi/Llama-3-8B-Instruct-DPO-v0.3
parameters:
density: 0.55
weight: 0.05
merge_method: dare_ties
base_model: NousResearch/Meta-Llama-3-8B
parameters:
int8_mask: true
dtype: float16
```
## 💻 Usage
```python
!pip install -qU transformers accelerate
from transformers import AutoTokenizer
import transformers
import torch
model = "mlabonne/ChimeraLlama-3-8B-v3"
messages = [{"role": "user", "content": "What is a large language model?"}]
tokenizer = AutoTokenizer.from_pretrained(model)
prompt = tokenizer.apply_chat_template(messages, tokenize=False, add_generation_prompt=True)
pipeline = transformers.pipeline(
"text-generation",
model=model,
torch_dtype=torch.float16,
device_map="auto",
)
outputs = pipeline(prompt, max_new_tokens=256, do_sample=True, temperature=0.7, top_k=50, top_p=0.95)
print(outputs[0]["generated_text"])
```
|
SeanLee97/angle-llama-7b-nli-20231027 | SeanLee97 | 2023-10-25T10:58:32Z | 15,286 | 3 | peft | [
"peft",
"en",
"dataset:multi_nli",
"dataset:snli",
"arxiv:2309.12871",
"license:mit",
"region:us"
] | null | 2023-10-18T01:15:45Z | ---
library_name: peft
license: mit
datasets:
- multi_nli
- snli
language:
- en
metrics:
- spearmanr
---
# AnglE📐: Angle-optimized Text Embeddings
> It is Angle 📐, not Angel 👼.
🔥 A New SOTA Model for Semantic Textual Similarity!
Github: https://github.com/SeanLee97/AnglE
<a href="https://arxiv.org/abs/2309.12871">
<img src="https://img.shields.io/badge/Arxiv-2306.06843-yellow.svg?style=flat-square" alt="https://arxiv.org/abs/2309.12871" />
</a>
[](https://paperswithcode.com/sota/semantic-textual-similarity-on-sick-r-1?p=angle-optimized-text-embeddings)
[](https://paperswithcode.com/sota/semantic-textual-similarity-on-sts16?p=angle-optimized-text-embeddings)
[](https://paperswithcode.com/sota/semantic-textual-similarity-on-sts15?p=angle-optimized-text-embeddings)
[](https://paperswithcode.com/sota/semantic-textual-similarity-on-sts14?p=angle-optimized-text-embeddings)
[](https://paperswithcode.com/sota/semantic-textual-similarity-on-sts13?p=angle-optimized-text-embeddings)
[](https://paperswithcode.com/sota/semantic-textual-similarity-on-sts12?p=angle-optimized-text-embeddings)
[](https://paperswithcode.com/sota/semantic-textual-similarity-on-sts-benchmark?p=angle-optimized-text-embeddings)
**📝 Training Details:**
We fine-tuned AnglE-LLaMA using 4 RTX 3090 Ti (24GB), the training script is as follows:
```bash
CUDA_VISIBLE_DEVICES=0,1,2,3 torchrun --nproc_per_node=4 --master_port=1234 train_angle.py \
--task NLI-STS --save_dir ckpts/NLI-STS-angle-llama-7b \
--w2 35 --learning_rate 2e-4 --maxlen 45 \
--lora_r 32 --lora_alpha 32 --lora_dropout 0.1 \
--save_steps 200 --batch_size 160 --seed 42 --do_eval 0 --load_kbit 4 --gradient_accumulation_steps 4 --epochs 1
```
The evaluation script is as follows:
```bash
CUDA_VISIBLE_DEVICES=0,1 python eval.py \
--load_kbit 16 \
--model_name_or_path NousResearch/Llama-2-7b-hf \
--lora_weight SeanLee97/angle-llama-7b-nli-20231027
```
**STS Results**
| Model | STS12 | STS13 | STS14 | STS15 | STS16 | STSBenchmark | SICKRelatedness | Avg. |
| ------- |-------|-------|-------|-------|-------|--------------|-----------------|-------|
| [SeanLee97/angle-llama-7b-nli-20231027](https://huggingface.co/SeanLee97/angle-llama-7b-nli-20231027) | 78.68 | 90.58 | 85.49 | 89.56 | 86.91 | 88.92 | 81.18 | 85.90 |
| [SeanLee97/angle-llama-7b-nli-v2](https://huggingface.co/SeanLee97/angle-llama-7b-nli-v2) | 79.00 | 90.56 | 85.79 | 89.43 | 87.00 | 88.97 | 80.94 | **85.96** |
## Usage
```bash
python -m pip install -U angle-emb
```
```python
from angle_emb import AnglE
angle = AnglE.from_pretrained('NousResearch/Llama-2-7b-hf', pretrained_lora_path='SeanLee97/angle-llama-7b-nli-20231027')
angle.set_prompt()
print('prompt:', angle.prompt)
vec = angle.encode({'text': 'hello world'}, to_numpy=True)
print(vec)
vecs = angle.encode([{'text': 'hello world1'}, {'text': 'hello world2'}], to_numpy=True)
print(vecs)
```
## Citation
You are welcome to use our code and pre-trained models. If you use our code and pre-trained models, please support us by citing our work as follows:
```bibtex
@article{li2023angle,
title={AnglE-Optimized Text Embeddings},
author={Li, Xianming and Li, Jing},
journal={arXiv preprint arXiv:2309.12871},
year={2023}
}
``` |
RichardErkhov/shleeeee_-_mistral-ko-7b-tech-gguf | RichardErkhov | 2024-06-30T17:33:56Z | 15,285 | 0 | null | [
"gguf",
"region:us"
] | null | 2024-06-30T14:53:19Z | Quantization made by Richard Erkhov.
[Github](https://github.com/RichardErkhov)
[Discord](https://discord.gg/pvy7H8DZMG)
[Request more models](https://github.com/RichardErkhov/quant_request)
mistral-ko-7b-tech - GGUF
- Model creator: https://huggingface.co/shleeeee/
- Original model: https://huggingface.co/shleeeee/mistral-ko-7b-tech/
| Name | Quant method | Size |
| ---- | ---- | ---- |
| [mistral-ko-7b-tech.Q2_K.gguf](https://huggingface.co/RichardErkhov/shleeeee_-_mistral-ko-7b-tech-gguf/blob/main/mistral-ko-7b-tech.Q2_K.gguf) | Q2_K | 2.53GB |
| [mistral-ko-7b-tech.IQ3_XS.gguf](https://huggingface.co/RichardErkhov/shleeeee_-_mistral-ko-7b-tech-gguf/blob/main/mistral-ko-7b-tech.IQ3_XS.gguf) | IQ3_XS | 2.81GB |
| [mistral-ko-7b-tech.IQ3_S.gguf](https://huggingface.co/RichardErkhov/shleeeee_-_mistral-ko-7b-tech-gguf/blob/main/mistral-ko-7b-tech.IQ3_S.gguf) | IQ3_S | 2.96GB |
| [mistral-ko-7b-tech.Q3_K_S.gguf](https://huggingface.co/RichardErkhov/shleeeee_-_mistral-ko-7b-tech-gguf/blob/main/mistral-ko-7b-tech.Q3_K_S.gguf) | Q3_K_S | 2.95GB |
| [mistral-ko-7b-tech.IQ3_M.gguf](https://huggingface.co/RichardErkhov/shleeeee_-_mistral-ko-7b-tech-gguf/blob/main/mistral-ko-7b-tech.IQ3_M.gguf) | IQ3_M | 3.06GB |
| [mistral-ko-7b-tech.Q3_K.gguf](https://huggingface.co/RichardErkhov/shleeeee_-_mistral-ko-7b-tech-gguf/blob/main/mistral-ko-7b-tech.Q3_K.gguf) | Q3_K | 3.28GB |
| [mistral-ko-7b-tech.Q3_K_M.gguf](https://huggingface.co/RichardErkhov/shleeeee_-_mistral-ko-7b-tech-gguf/blob/main/mistral-ko-7b-tech.Q3_K_M.gguf) | Q3_K_M | 3.28GB |
| [mistral-ko-7b-tech.Q3_K_L.gguf](https://huggingface.co/RichardErkhov/shleeeee_-_mistral-ko-7b-tech-gguf/blob/main/mistral-ko-7b-tech.Q3_K_L.gguf) | Q3_K_L | 3.56GB |
| [mistral-ko-7b-tech.IQ4_XS.gguf](https://huggingface.co/RichardErkhov/shleeeee_-_mistral-ko-7b-tech-gguf/blob/main/mistral-ko-7b-tech.IQ4_XS.gguf) | IQ4_XS | 3.67GB |
| [mistral-ko-7b-tech.Q4_0.gguf](https://huggingface.co/RichardErkhov/shleeeee_-_mistral-ko-7b-tech-gguf/blob/main/mistral-ko-7b-tech.Q4_0.gguf) | Q4_0 | 3.83GB |
| [mistral-ko-7b-tech.IQ4_NL.gguf](https://huggingface.co/RichardErkhov/shleeeee_-_mistral-ko-7b-tech-gguf/blob/main/mistral-ko-7b-tech.IQ4_NL.gguf) | IQ4_NL | 3.87GB |
| [mistral-ko-7b-tech.Q4_K_S.gguf](https://huggingface.co/RichardErkhov/shleeeee_-_mistral-ko-7b-tech-gguf/blob/main/mistral-ko-7b-tech.Q4_K_S.gguf) | Q4_K_S | 3.86GB |
| [mistral-ko-7b-tech.Q4_K.gguf](https://huggingface.co/RichardErkhov/shleeeee_-_mistral-ko-7b-tech-gguf/blob/main/mistral-ko-7b-tech.Q4_K.gguf) | Q4_K | 4.07GB |
| [mistral-ko-7b-tech.Q4_K_M.gguf](https://huggingface.co/RichardErkhov/shleeeee_-_mistral-ko-7b-tech-gguf/blob/main/mistral-ko-7b-tech.Q4_K_M.gguf) | Q4_K_M | 4.07GB |
| [mistral-ko-7b-tech.Q4_1.gguf](https://huggingface.co/RichardErkhov/shleeeee_-_mistral-ko-7b-tech-gguf/blob/main/mistral-ko-7b-tech.Q4_1.gguf) | Q4_1 | 4.24GB |
| [mistral-ko-7b-tech.Q5_0.gguf](https://huggingface.co/RichardErkhov/shleeeee_-_mistral-ko-7b-tech-gguf/blob/main/mistral-ko-7b-tech.Q5_0.gguf) | Q5_0 | 4.65GB |
| [mistral-ko-7b-tech.Q5_K_S.gguf](https://huggingface.co/RichardErkhov/shleeeee_-_mistral-ko-7b-tech-gguf/blob/main/mistral-ko-7b-tech.Q5_K_S.gguf) | Q5_K_S | 4.65GB |
| [mistral-ko-7b-tech.Q5_K.gguf](https://huggingface.co/RichardErkhov/shleeeee_-_mistral-ko-7b-tech-gguf/blob/main/mistral-ko-7b-tech.Q5_K.gguf) | Q5_K | 4.78GB |
| [mistral-ko-7b-tech.Q5_K_M.gguf](https://huggingface.co/RichardErkhov/shleeeee_-_mistral-ko-7b-tech-gguf/blob/main/mistral-ko-7b-tech.Q5_K_M.gguf) | Q5_K_M | 4.78GB |
| [mistral-ko-7b-tech.Q5_1.gguf](https://huggingface.co/RichardErkhov/shleeeee_-_mistral-ko-7b-tech-gguf/blob/main/mistral-ko-7b-tech.Q5_1.gguf) | Q5_1 | 5.07GB |
| [mistral-ko-7b-tech.Q6_K.gguf](https://huggingface.co/RichardErkhov/shleeeee_-_mistral-ko-7b-tech-gguf/blob/main/mistral-ko-7b-tech.Q6_K.gguf) | Q6_K | 5.53GB |
| [mistral-ko-7b-tech.Q8_0.gguf](https://huggingface.co/RichardErkhov/shleeeee_-_mistral-ko-7b-tech-gguf/blob/main/mistral-ko-7b-tech.Q8_0.gguf) | Q8_0 | 7.17GB |
Original model description:
---
language:
- ko
pipeline_tag: text-generation
tags:
- finetune
license: other
---
# Model Card for mistral-ko-7b-tech
It is a fine-tuned model using Korean in the mistral-7b model.
## Model Details
* **Model Developers** : shleeeee(Seunghyeon Lee), oopsung(Sungwoo Park)
* **Repository** : To be added
* **Model Architecture** : The mistral-ko-7b-wiki-neft is is a fine-tuned version of the Mistral-7B-v0.1.
* **Lora target modules** : q_proj, k_proj, v_proj, o_proj,gate_proj
* **train_batch** : 4
* **Max_step** : 500
## Dataset
Korean Custom Dataset(2000)
## Prompt template: Mistral
```
<s>[INST]{['instruction']}[/INST]{['output']}</s>
```
## Usage
```
# Load model directly
from transformers import AutoTokenizer, AutoModelForCausalLM
tokenizer = AutoTokenizer.from_pretrained("shleeeee/mistral-ko-7b-tech")
model = AutoModelForCausalLM.from_pretrained("shleeeee/mistral-ko-7b-tech")
# Use a pipeline as a high-level helper
from transformers import pipeline
pipe = pipeline("text-generation", model="shleeeee/mistral-ko-7b-tech")
```
## Evaluation

|
kranasian/albert_v2_lookup_spending_category | kranasian | 2024-06-10T04:35:30Z | 15,272 | 0 | transformers | [
"transformers",
"tensorboard",
"safetensors",
"albert",
"question-answering",
"generated_from_trainer",
"base_model:Palak/albert-base-v2_squad",
"license:apache-2.0",
"endpoints_compatible",
"region:us"
] | question-answering | 2024-06-10T03:51:10Z | ---
license: apache-2.0
base_model: Palak/albert-base-v2_squad
tags:
- generated_from_trainer
model-index:
- name: albert_v2_lookup_spending_category
results: []
---
<!-- This model card has been generated automatically according to the information the Trainer had access to. You
should probably proofread and complete it, then remove this comment. -->
# albert_v2_lookup_spending_category
This model is a fine-tuned version of [Palak/albert-base-v2_squad](https://huggingface.co/Palak/albert-base-v2_squad) on an unknown dataset.
It achieves the following results on the evaluation set:
- Loss: 0.0406
## Model description
More information needed
## Intended uses & limitations
More information needed
## Training and evaluation data
More information needed
## Training procedure
### Training hyperparameters
The following hyperparameters were used during training:
- learning_rate: 2e-05
- train_batch_size: 16
- eval_batch_size: 16
- seed: 42
- optimizer: Adam with betas=(0.9,0.999) and epsilon=1e-08
- lr_scheduler_type: linear
- num_epochs: 12
### Training results
| Training Loss | Epoch | Step | Validation Loss |
|:-------------:|:-----:|:----:|:---------------:|
| No log | 1.0 | 84 | 0.0321 |
| No log | 2.0 | 168 | 0.0358 |
| No log | 3.0 | 252 | 0.0372 |
| No log | 4.0 | 336 | 0.0381 |
| No log | 5.0 | 420 | 0.0388 |
| 0.0064 | 6.0 | 504 | 0.0393 |
| 0.0064 | 7.0 | 588 | 0.0398 |
| 0.0064 | 8.0 | 672 | 0.0401 |
| 0.0064 | 9.0 | 756 | 0.0403 |
| 0.0064 | 10.0 | 840 | 0.0405 |
| 0.0064 | 11.0 | 924 | 0.0406 |
| 0.0 | 12.0 | 1008 | 0.0406 |
### Framework versions
- Transformers 4.41.2
- Pytorch 2.3.0+cu121
- Datasets 2.19.2
- Tokenizers 0.19.1
|
mradermacher/L3-Sophie-improved-v1-GGUF | mradermacher | 2024-06-23T22:12:37Z | 15,270 | 0 | transformers | [
"transformers",
"gguf",
"mergekit",
"merge",
"en",
"base_model:Fischerboot/L3-Sophie-improved-v1",
"endpoints_compatible",
"region:us"
] | null | 2024-06-23T12:31:13Z | ---
base_model: Fischerboot/L3-Sophie-improved-v1
language:
- en
library_name: transformers
quantized_by: mradermacher
tags:
- mergekit
- merge
---
## About
<!-- ### quantize_version: 2 -->
<!-- ### output_tensor_quantised: 1 -->
<!-- ### convert_type: hf -->
<!-- ### vocab_type: -->
<!-- ### tags: -->
static quants of https://huggingface.co/Fischerboot/L3-Sophie-improved-v1
<!-- provided-files -->
weighted/imatrix quants are available at https://huggingface.co/mradermacher/L3-Sophie-improved-v1-i1-GGUF
## Usage
If you are unsure how to use GGUF files, refer to one of [TheBloke's
READMEs](https://huggingface.co/TheBloke/KafkaLM-70B-German-V0.1-GGUF) for
more details, including on how to concatenate multi-part files.
## Provided Quants
(sorted by size, not necessarily quality. IQ-quants are often preferable over similar sized non-IQ quants)
| Link | Type | Size/GB | Notes |
|:-----|:-----|--------:|:------|
| [GGUF](https://huggingface.co/mradermacher/L3-Sophie-improved-v1-GGUF/resolve/main/L3-Sophie-improved-v1.Q2_K.gguf) | Q2_K | 3.3 | |
| [GGUF](https://huggingface.co/mradermacher/L3-Sophie-improved-v1-GGUF/resolve/main/L3-Sophie-improved-v1.IQ3_XS.gguf) | IQ3_XS | 3.6 | |
| [GGUF](https://huggingface.co/mradermacher/L3-Sophie-improved-v1-GGUF/resolve/main/L3-Sophie-improved-v1.Q3_K_S.gguf) | Q3_K_S | 3.8 | |
| [GGUF](https://huggingface.co/mradermacher/L3-Sophie-improved-v1-GGUF/resolve/main/L3-Sophie-improved-v1.IQ3_S.gguf) | IQ3_S | 3.8 | beats Q3_K* |
| [GGUF](https://huggingface.co/mradermacher/L3-Sophie-improved-v1-GGUF/resolve/main/L3-Sophie-improved-v1.IQ3_M.gguf) | IQ3_M | 3.9 | |
| [GGUF](https://huggingface.co/mradermacher/L3-Sophie-improved-v1-GGUF/resolve/main/L3-Sophie-improved-v1.Q3_K_M.gguf) | Q3_K_M | 4.1 | lower quality |
| [GGUF](https://huggingface.co/mradermacher/L3-Sophie-improved-v1-GGUF/resolve/main/L3-Sophie-improved-v1.Q3_K_L.gguf) | Q3_K_L | 4.4 | |
| [GGUF](https://huggingface.co/mradermacher/L3-Sophie-improved-v1-GGUF/resolve/main/L3-Sophie-improved-v1.IQ4_XS.gguf) | IQ4_XS | 4.6 | |
| [GGUF](https://huggingface.co/mradermacher/L3-Sophie-improved-v1-GGUF/resolve/main/L3-Sophie-improved-v1.Q4_K_S.gguf) | Q4_K_S | 4.8 | fast, recommended |
| [GGUF](https://huggingface.co/mradermacher/L3-Sophie-improved-v1-GGUF/resolve/main/L3-Sophie-improved-v1.Q4_K_M.gguf) | Q4_K_M | 5.0 | fast, recommended |
| [GGUF](https://huggingface.co/mradermacher/L3-Sophie-improved-v1-GGUF/resolve/main/L3-Sophie-improved-v1.Q5_K_S.gguf) | Q5_K_S | 5.7 | |
| [GGUF](https://huggingface.co/mradermacher/L3-Sophie-improved-v1-GGUF/resolve/main/L3-Sophie-improved-v1.Q5_K_M.gguf) | Q5_K_M | 5.8 | |
| [GGUF](https://huggingface.co/mradermacher/L3-Sophie-improved-v1-GGUF/resolve/main/L3-Sophie-improved-v1.Q6_K.gguf) | Q6_K | 6.7 | very good quality |
| [GGUF](https://huggingface.co/mradermacher/L3-Sophie-improved-v1-GGUF/resolve/main/L3-Sophie-improved-v1.Q8_0.gguf) | Q8_0 | 8.6 | fast, best quality |
| [GGUF](https://huggingface.co/mradermacher/L3-Sophie-improved-v1-GGUF/resolve/main/L3-Sophie-improved-v1.f16.gguf) | f16 | 16.2 | 16 bpw, overkill |
Here is a handy graph by ikawrakow comparing some lower-quality quant
types (lower is better):

And here are Artefact2's thoughts on the matter:
https://gist.github.com/Artefact2/b5f810600771265fc1e39442288e8ec9
## FAQ / Model Request
See https://huggingface.co/mradermacher/model_requests for some answers to
questions you might have and/or if you want some other model quantized.
## Thanks
I thank my company, [nethype GmbH](https://www.nethype.de/), for letting
me use its servers and providing upgrades to my workstation to enable
this work in my free time.
<!-- end -->
|
01-ai/Yi-1.5-9B-Chat | 01-ai | 2024-06-26T10:41:03Z | 15,263 | 101 | transformers | [
"transformers",
"safetensors",
"llama",
"text-generation",
"conversational",
"arxiv:2403.04652",
"license:apache-2.0",
"autotrain_compatible",
"endpoints_compatible",
"text-generation-inference",
"region:us"
] | text-generation | 2024-05-10T05:38:24Z | ---
license: apache-2.0
---
<div align="center">
<picture>
<img src="https://raw.githubusercontent.com/01-ai/Yi/main/assets/img/Yi_logo_icon_light.svg" width="150px">
</picture>
</div>
<p align="center">
<a href="https://github.com/01-ai">🐙 GitHub</a> •
<a href="https://discord.gg/hYUwWddeAu">👾 Discord</a> •
<a href="https://twitter.com/01ai_yi">🐤 Twitter</a> •
<a href="https://github.com/01-ai/Yi-1.5/issues/2">💬 WeChat</a>
<br/>
<a href="https://arxiv.org/abs/2403.04652">📝 Paper</a> •
<a href="https://01-ai.github.io/">💪 Tech Blog</a> •
<a href="https://github.com/01-ai/Yi/tree/main?tab=readme-ov-file#faq">🙌 FAQ</a> •
<a href="https://github.com/01-ai/Yi/tree/main?tab=readme-ov-file#learning-hub">📗 Learning Hub</a>
</p>
# Intro
Yi-1.5 is an upgraded version of Yi. It is continuously pre-trained on Yi with a high-quality corpus of 500B tokens and fine-tuned on 3M diverse fine-tuning samples.
Compared with Yi, Yi-1.5 delivers stronger performance in coding, math, reasoning, and instruction-following capability, while still maintaining excellent capabilities in language understanding, commonsense reasoning, and reading comprehension.
<div align="center">
Model | Context Length | Pre-trained Tokens
| :------------: | :------------: | :------------: |
| Yi-1.5 | 4K, 16K, 32K | 3.6T
</div>
# Models
- Chat models
<div align="center">
| Name | Download |
| --------------- | ------------------------------------------------------------------------------------------------------------------------------------------------------------------- |
| Yi-1.5-34B-Chat | • [🤗 Hugging Face](https://huggingface.co/collections/01-ai/yi-15-2024-05-663f3ecab5f815a3eaca7ca8) • [🤖 ModelScope](https://www.modelscope.cn/organization/01ai) • [🟣 wisemodel](https://wisemodel.cn/organization/01.AI)|
| Yi-1.5-34B-Chat-16K | • [🤗 Hugging Face](https://huggingface.co/collections/01-ai/yi-15-2024-05-663f3ecab5f815a3eaca7ca8) • [🤖 ModelScope](https://www.modelscope.cn/organization/01ai) • [🟣 wisemodel](https://wisemodel.cn/organization/01.AI) |
| Yi-1.5-9B-Chat | • [🤗 Hugging Face](https://huggingface.co/collections/01-ai/yi-15-2024-05-663f3ecab5f815a3eaca7ca8) • [🤖 ModelScope](https://www.modelscope.cn/organization/01ai) • [🟣 wisemodel](https://wisemodel.cn/organization/01.AI) |
| Yi-1.5-9B-Chat-16K | • [🤗 Hugging Face](https://huggingface.co/collections/01-ai/yi-15-2024-05-663f3ecab5f815a3eaca7ca8) • [🤖 ModelScope](https://www.modelscope.cn/organization/01ai) • [🟣 wisemodel](https://wisemodel.cn/organization/01.AI) |
| Yi-1.5-6B-Chat | • [🤗 Hugging Face](https://huggingface.co/collections/01-ai/yi-15-2024-05-663f3ecab5f815a3eaca7ca8) • [🤖 ModelScope](https://www.modelscope.cn/organization/01ai) • [🟣 wisemodel](https://wisemodel.cn/organization/01.AI) |
</div>
- Base models
<div align="center">
| Name | Download |
| ---------- | ------------------------------------------------------------------------------------------------------------------------------------------------------------------- |
| Yi-1.5-34B | • [🤗 Hugging Face](https://huggingface.co/collections/01-ai/yi-15-2024-05-663f3ecab5f815a3eaca7ca8) • [🤖 ModelScope](https://www.modelscope.cn/organization/01ai) • [🟣 wisemodel](https://wisemodel.cn/organization/01.AI) |
| Yi-1.5-34B-32K | • [🤗 Hugging Face](https://huggingface.co/collections/01-ai/yi-15-2024-05-663f3ecab5f815a3eaca7ca8) • [🤖 ModelScope](https://www.modelscope.cn/organization/01ai) • [🟣 wisemodel](https://wisemodel.cn/organization/01.AI) |
| Yi-1.5-9B | • [🤗 Hugging Face](https://huggingface.co/collections/01-ai/yi-15-2024-05-663f3ecab5f815a3eaca7ca8) • [🤖 ModelScope](https://www.modelscope.cn/organization/01ai) • [🟣 wisemodel](https://wisemodel.cn/organization/01.AI) |
| Yi-1.5-9B-32K | • [🤗 Hugging Face](https://huggingface.co/collections/01-ai/yi-15-2024-05-663f3ecab5f815a3eaca7ca8) • [🤖 ModelScope](https://www.modelscope.cn/organization/01ai) • [🟣 wisemodel](https://wisemodel.cn/organization/01.AI) |
| Yi-1.5-6B | • [🤗 Hugging Face](https://huggingface.co/collections/01-ai/yi-15-2024-05-663f3ecab5f815a3eaca7ca8) • [🤖 ModelScope](https://www.modelscope.cn/organization/01ai) • [🟣 wisemodel](https://wisemodel.cn/organization/01.AI) |
</div>
# Benchmarks
- Chat models
Yi-1.5-34B-Chat is on par with or excels beyond larger models in most benchmarks.

Yi-1.5-9B-Chat is the top performer among similarly sized open-source models.

- Base models
Yi-1.5-34B is on par with or excels beyond larger models in some benchmarks.

Yi-1.5-9B is the top performer among similarly sized open-source models.

# Quick Start
For getting up and running with Yi-1.5 models quickly, see [README](https://github.com/01-ai/Yi-1.5).
|
RichardErkhov/WebraftAI_-_synapsellm-7b-mistral-v0.3-preview-gguf | RichardErkhov | 2024-06-30T13:55:51Z | 15,248 | 0 | null | [
"gguf",
"region:us"
] | null | 2024-06-30T11:36:52Z | Quantization made by Richard Erkhov.
[Github](https://github.com/RichardErkhov)
[Discord](https://discord.gg/pvy7H8DZMG)
[Request more models](https://github.com/RichardErkhov/quant_request)
synapsellm-7b-mistral-v0.3-preview - GGUF
- Model creator: https://huggingface.co/WebraftAI/
- Original model: https://huggingface.co/WebraftAI/synapsellm-7b-mistral-v0.3-preview/
| Name | Quant method | Size |
| ---- | ---- | ---- |
| [synapsellm-7b-mistral-v0.3-preview.Q2_K.gguf](https://huggingface.co/RichardErkhov/WebraftAI_-_synapsellm-7b-mistral-v0.3-preview-gguf/blob/main/synapsellm-7b-mistral-v0.3-preview.Q2_K.gguf) | Q2_K | 2.53GB |
| [synapsellm-7b-mistral-v0.3-preview.IQ3_XS.gguf](https://huggingface.co/RichardErkhov/WebraftAI_-_synapsellm-7b-mistral-v0.3-preview-gguf/blob/main/synapsellm-7b-mistral-v0.3-preview.IQ3_XS.gguf) | IQ3_XS | 2.81GB |
| [synapsellm-7b-mistral-v0.3-preview.IQ3_S.gguf](https://huggingface.co/RichardErkhov/WebraftAI_-_synapsellm-7b-mistral-v0.3-preview-gguf/blob/main/synapsellm-7b-mistral-v0.3-preview.IQ3_S.gguf) | IQ3_S | 2.96GB |
| [synapsellm-7b-mistral-v0.3-preview.Q3_K_S.gguf](https://huggingface.co/RichardErkhov/WebraftAI_-_synapsellm-7b-mistral-v0.3-preview-gguf/blob/main/synapsellm-7b-mistral-v0.3-preview.Q3_K_S.gguf) | Q3_K_S | 2.95GB |
| [synapsellm-7b-mistral-v0.3-preview.IQ3_M.gguf](https://huggingface.co/RichardErkhov/WebraftAI_-_synapsellm-7b-mistral-v0.3-preview-gguf/blob/main/synapsellm-7b-mistral-v0.3-preview.IQ3_M.gguf) | IQ3_M | 3.06GB |
| [synapsellm-7b-mistral-v0.3-preview.Q3_K.gguf](https://huggingface.co/RichardErkhov/WebraftAI_-_synapsellm-7b-mistral-v0.3-preview-gguf/blob/main/synapsellm-7b-mistral-v0.3-preview.Q3_K.gguf) | Q3_K | 3.28GB |
| [synapsellm-7b-mistral-v0.3-preview.Q3_K_M.gguf](https://huggingface.co/RichardErkhov/WebraftAI_-_synapsellm-7b-mistral-v0.3-preview-gguf/blob/main/synapsellm-7b-mistral-v0.3-preview.Q3_K_M.gguf) | Q3_K_M | 3.28GB |
| [synapsellm-7b-mistral-v0.3-preview.Q3_K_L.gguf](https://huggingface.co/RichardErkhov/WebraftAI_-_synapsellm-7b-mistral-v0.3-preview-gguf/blob/main/synapsellm-7b-mistral-v0.3-preview.Q3_K_L.gguf) | Q3_K_L | 3.56GB |
| [synapsellm-7b-mistral-v0.3-preview.IQ4_XS.gguf](https://huggingface.co/RichardErkhov/WebraftAI_-_synapsellm-7b-mistral-v0.3-preview-gguf/blob/main/synapsellm-7b-mistral-v0.3-preview.IQ4_XS.gguf) | IQ4_XS | 3.67GB |
| [synapsellm-7b-mistral-v0.3-preview.Q4_0.gguf](https://huggingface.co/RichardErkhov/WebraftAI_-_synapsellm-7b-mistral-v0.3-preview-gguf/blob/main/synapsellm-7b-mistral-v0.3-preview.Q4_0.gguf) | Q4_0 | 3.83GB |
| [synapsellm-7b-mistral-v0.3-preview.IQ4_NL.gguf](https://huggingface.co/RichardErkhov/WebraftAI_-_synapsellm-7b-mistral-v0.3-preview-gguf/blob/main/synapsellm-7b-mistral-v0.3-preview.IQ4_NL.gguf) | IQ4_NL | 3.87GB |
| [synapsellm-7b-mistral-v0.3-preview.Q4_K_S.gguf](https://huggingface.co/RichardErkhov/WebraftAI_-_synapsellm-7b-mistral-v0.3-preview-gguf/blob/main/synapsellm-7b-mistral-v0.3-preview.Q4_K_S.gguf) | Q4_K_S | 3.86GB |
| [synapsellm-7b-mistral-v0.3-preview.Q4_K.gguf](https://huggingface.co/RichardErkhov/WebraftAI_-_synapsellm-7b-mistral-v0.3-preview-gguf/blob/main/synapsellm-7b-mistral-v0.3-preview.Q4_K.gguf) | Q4_K | 4.07GB |
| [synapsellm-7b-mistral-v0.3-preview.Q4_K_M.gguf](https://huggingface.co/RichardErkhov/WebraftAI_-_synapsellm-7b-mistral-v0.3-preview-gguf/blob/main/synapsellm-7b-mistral-v0.3-preview.Q4_K_M.gguf) | Q4_K_M | 4.07GB |
| [synapsellm-7b-mistral-v0.3-preview.Q4_1.gguf](https://huggingface.co/RichardErkhov/WebraftAI_-_synapsellm-7b-mistral-v0.3-preview-gguf/blob/main/synapsellm-7b-mistral-v0.3-preview.Q4_1.gguf) | Q4_1 | 4.24GB |
| [synapsellm-7b-mistral-v0.3-preview.Q5_0.gguf](https://huggingface.co/RichardErkhov/WebraftAI_-_synapsellm-7b-mistral-v0.3-preview-gguf/blob/main/synapsellm-7b-mistral-v0.3-preview.Q5_0.gguf) | Q5_0 | 4.65GB |
| [synapsellm-7b-mistral-v0.3-preview.Q5_K_S.gguf](https://huggingface.co/RichardErkhov/WebraftAI_-_synapsellm-7b-mistral-v0.3-preview-gguf/blob/main/synapsellm-7b-mistral-v0.3-preview.Q5_K_S.gguf) | Q5_K_S | 4.65GB |
| [synapsellm-7b-mistral-v0.3-preview.Q5_K.gguf](https://huggingface.co/RichardErkhov/WebraftAI_-_synapsellm-7b-mistral-v0.3-preview-gguf/blob/main/synapsellm-7b-mistral-v0.3-preview.Q5_K.gguf) | Q5_K | 4.78GB |
| [synapsellm-7b-mistral-v0.3-preview.Q5_K_M.gguf](https://huggingface.co/RichardErkhov/WebraftAI_-_synapsellm-7b-mistral-v0.3-preview-gguf/blob/main/synapsellm-7b-mistral-v0.3-preview.Q5_K_M.gguf) | Q5_K_M | 4.78GB |
| [synapsellm-7b-mistral-v0.3-preview.Q5_1.gguf](https://huggingface.co/RichardErkhov/WebraftAI_-_synapsellm-7b-mistral-v0.3-preview-gguf/blob/main/synapsellm-7b-mistral-v0.3-preview.Q5_1.gguf) | Q5_1 | 5.07GB |
| [synapsellm-7b-mistral-v0.3-preview.Q6_K.gguf](https://huggingface.co/RichardErkhov/WebraftAI_-_synapsellm-7b-mistral-v0.3-preview-gguf/blob/main/synapsellm-7b-mistral-v0.3-preview.Q6_K.gguf) | Q6_K | 5.53GB |
| [synapsellm-7b-mistral-v0.3-preview.Q8_0.gguf](https://huggingface.co/RichardErkhov/WebraftAI_-_synapsellm-7b-mistral-v0.3-preview-gguf/blob/main/synapsellm-7b-mistral-v0.3-preview.Q8_0.gguf) | Q8_0 | 7.17GB |
Original model description:
---
language:
- en
license: apache-2.0
library_name: transformers
tags:
- code
model-index:
- name: synapsellm-7b-mistral-v0.3-preview
results:
- task:
type: text-generation
name: Text Generation
dataset:
name: AI2 Reasoning Challenge (25-Shot)
type: ai2_arc
config: ARC-Challenge
split: test
args:
num_few_shot: 25
metrics:
- type: acc_norm
value: 53.84
name: normalized accuracy
source:
url: https://huggingface.co/spaces/HuggingFaceH4/open_llm_leaderboard?query=WebraftAI/synapsellm-7b-mistral-v0.3-preview
name: Open LLM Leaderboard
- task:
type: text-generation
name: Text Generation
dataset:
name: HellaSwag (10-Shot)
type: hellaswag
split: validation
args:
num_few_shot: 10
metrics:
- type: acc_norm
value: 74.86
name: normalized accuracy
source:
url: https://huggingface.co/spaces/HuggingFaceH4/open_llm_leaderboard?query=WebraftAI/synapsellm-7b-mistral-v0.3-preview
name: Open LLM Leaderboard
- task:
type: text-generation
name: Text Generation
dataset:
name: MMLU (5-Shot)
type: cais/mmlu
config: all
split: test
args:
num_few_shot: 5
metrics:
- type: acc
value: 54.81
name: accuracy
source:
url: https://huggingface.co/spaces/HuggingFaceH4/open_llm_leaderboard?query=WebraftAI/synapsellm-7b-mistral-v0.3-preview
name: Open LLM Leaderboard
- task:
type: text-generation
name: Text Generation
dataset:
name: TruthfulQA (0-shot)
type: truthful_qa
config: multiple_choice
split: validation
args:
num_few_shot: 0
metrics:
- type: mc2
value: 55.03
source:
url: https://huggingface.co/spaces/HuggingFaceH4/open_llm_leaderboard?query=WebraftAI/synapsellm-7b-mistral-v0.3-preview
name: Open LLM Leaderboard
- task:
type: text-generation
name: Text Generation
dataset:
name: Winogrande (5-shot)
type: winogrande
config: winogrande_xl
split: validation
args:
num_few_shot: 5
metrics:
- type: acc
value: 74.59
name: accuracy
source:
url: https://huggingface.co/spaces/HuggingFaceH4/open_llm_leaderboard?query=WebraftAI/synapsellm-7b-mistral-v0.3-preview
name: Open LLM Leaderboard
- task:
type: text-generation
name: Text Generation
dataset:
name: GSM8k (5-shot)
type: gsm8k
config: main
split: test
args:
num_few_shot: 5
metrics:
- type: acc
value: 28.96
name: accuracy
source:
url: https://huggingface.co/spaces/HuggingFaceH4/open_llm_leaderboard?query=WebraftAI/synapsellm-7b-mistral-v0.3-preview
name: Open LLM Leaderboard
---
# SynapseLLM:
SynapseLLM, a significant achievement by WebraftAI, represents a series of large language AI models designed to create robust, generalized, and decentralized information systems. This repository specifically houses the SynapseLLM finetuned version of Mistral. The finetuning process is conducted on a custom dataset, albeit limited in scope, focusing on code and normal question-answering scenarios. This adaptation showcases the model's versatility and applicability within specific domains, contributing to the broader landscape of AI advancements.
## Model Details
**SynapseLLM:**
- Parameters: 7B
- Learning rate: 2e-4
- Adapter used: Qlora
- Precision: float16
- Batch size: 16
- Maximum gradient normal: 0.3
- Optimizer: paged_adamw_32bit
- Warmup Ratio: 0.03
- Step(s) (trained): 100
- Epoch(s) (trained): 1
### Model Description
This is a 7b parameter, decoder only transformer based finetuned model on Chat Q/A and Code instructions. It's a preview finetune on Mistral 7B v0.1 on a sample dataset of 409k rows comprising of 140k General Code, 143k GPT-3.5 Q/A, 63k Python code, and 54k General Q/A (Through GPT-4) [Each row contains one instruction and one response]. This is a full model merged and compiled with trained adapters, so you can easily load this through transformers library.
- **Developed by:** WebraftAI
- **Funded by:** Webraft Cloud
- **Shared by:** WebraftAI
- **Model type:** Decoder-only Transformer
- **Language(s):** English Only
- **License:** Apache 2.0
- **Finetuned from model:** Mistral-7b-v0.1
# [Open LLM Leaderboard Evaluation Results](https://huggingface.co/spaces/HuggingFaceH4/open_llm_leaderboard)
Detailed results can be found [here](https://huggingface.co/datasets/open-llm-leaderboard/details_WebraftAI__synapsellm-7b-mistral-v0.3-preview)
| Metric |Value|
|---------------------------------|----:|
|Avg. |57.01|
|AI2 Reasoning Challenge (25-Shot)|53.84|
|HellaSwag (10-Shot) |74.86|
|MMLU (5-Shot) |54.81|
|TruthfulQA (0-shot) |55.03|
|Winogrande (5-shot) |74.59|
|GSM8k (5-shot) |28.96|
|
duyntnet/Karen_TheEditor_V2_CREATIVE_Mistral_7B-imatrix-GGUF | duyntnet | 2024-06-24T04:12:51Z | 15,243 | 0 | transformers | [
"transformers",
"gguf",
"imatrix",
"Karen_TheEditor_V2_CREATIVE_Mistral_7B",
"text-generation",
"en",
"license:other",
"region:us"
] | text-generation | 2024-06-24T02:00:21Z | ---
license: other
language:
- en
pipeline_tag: text-generation
inference: false
tags:
- transformers
- gguf
- imatrix
- Karen_TheEditor_V2_CREATIVE_Mistral_7B
---
Quantizations of https://huggingface.co/FPHam/Karen_TheEditor_V2_CREATIVE_Mistral_7B
# From original readme
## Usage
It should be used by submitting a paragraph or block of text at a time.
## Model uses ChatML
```
<|im_start|>system
<|im_end|>
<|im_start|>user
Edit the following text for spelling and grammar mistakes: {paragraph of text} <|im_end|>
<|im_start|>assistant
```
Note the pretext: *Edit the following text for spelling and grammar mistakes:* before the actual text. This way Karen wouldn't start talking ABOUT the text.
## Recomended settings
- Temperature: 0.7
- top_p: 0.1
- top_k: 40
- repetition penalty: 1.18
Karen could also be used in chatting. But if Karen is involved in a conversation where the text is lengthy, she might interpret your message as needing proofreading assistance rather than simply acknowledging that you are using her in casual chat.
Example:
>Edit the following text for spelling and grammar mistakes: I see future writing more about you writing the story any way you can and LLM editing it and making it into a proper fiction. That seems to be more satisfying way where you could feel you actually had done something creATIVE. So human is the creative, LLM is the burger flipping intern.
>The opposite is what many people are trying rn. Make LLM to invent story (from premise, to creating outline to writing actual chapters) and the human to somehow assemble it from those pieces. So LLM is the creative man, and human is the floor polisher.
>I can't believe a normal person would find it satisfying long term.
>So in my view, the job of editor would be replaced by Ai, not the job of author.
(Grammarly Score: 48)
Output STRICT:
>I see the future of writing being more about you writing the story any way you can and having an AI like LLM edit it and make it into a proper fiction. That seems to be a more satisfying way where you could feel you actually did something creative. So humans are the creative ones, while LLMs are the burger-flipping interns.
>
>On the other hand, many people are currently trying to do the exact opposite. They want to make LLMs create stories (from premises, to outlining, to writing entire chapters). And then they want humans to somehow piece them together from these parts. In this scenario, LLMs become the creative individuals, and humans become the floor polishers.
>
>I can't imagine a regular person finding that satisfying over the long run.
>
>In my opinion, the role of an editor will eventually be taken over by AI, not the role of an author.
(Grammarly Score: 93)
Output CREATIVE:
>I see the future of writing being more focused on you writing the story any way you can and having an AI like GPT-3 edit it and turn it into a proper piece of fiction. This seems to be a more satisfying approach where you can truly feel that you have done something creative. In this scenario, humans remain the creative force, while the AI acts as the burger flipping intern.
>
>On the other hand, there are those who argue that we should let the AI generate stories from scratch - from coming up with a premise, to outlining the plot, to writing individual chapters. They believe that the role of the human should then become one of assembling these pieces together. In this case, the AI becomes the creative mind, and the human takes on the role of a floor polisher.
>
>I cannot imagine how someone could find this satisfying over the long term. Therefore, in my opinion, the job of the editor will eventually be taken over by AI, not the job of the author.
(Grammarly Score: 83) |
cointegrated/LaBSE-en-ru | cointegrated | 2024-03-28T13:59:30Z | 15,228 | 35 | transformers | [
"transformers",
"pytorch",
"tf",
"safetensors",
"bert",
"pretraining",
"feature-extraction",
"embeddings",
"sentence-similarity",
"ru",
"en",
"arxiv:2007.01852",
"endpoints_compatible",
"region:us"
] | feature-extraction | 2022-03-02T23:29:05Z | ---
language: ["ru", "en"]
tags:
- feature-extraction
- embeddings
- sentence-similarity
---
# LaBSE for English and Russian
This is a truncated version of [sentence-transformers/LaBSE](https://huggingface.co/sentence-transformers/LaBSE), which is, in turn, a port of [LaBSE](https://tfhub.dev/google/LaBSE/1) by Google.
The current model has only English and Russian tokens left in the vocabulary.
Thus, the vocabulary is 10% of the original, and number of parameters in the whole model is 27% of the original, without any loss in the quality of English and Russian embeddings.
To get the sentence embeddings, you can use the following code:
```python
import torch
from transformers import AutoTokenizer, AutoModel
tokenizer = AutoTokenizer.from_pretrained("cointegrated/LaBSE-en-ru")
model = AutoModel.from_pretrained("cointegrated/LaBSE-en-ru")
sentences = ["Hello World", "Привет Мир"]
encoded_input = tokenizer(sentences, padding=True, truncation=True, max_length=64, return_tensors='pt')
with torch.no_grad():
model_output = model(**encoded_input)
embeddings = model_output.pooler_output
embeddings = torch.nn.functional.normalize(embeddings)
print(embeddings)
```
The model has been truncated in [this notebook](https://colab.research.google.com/drive/1dnPRn0-ugj3vZgSpyCC9sgslM2SuSfHy?usp=sharing).
You can adapt it for other languages (like [EIStakovskii/LaBSE-fr-de](https://huggingface.co/EIStakovskii/LaBSE-fr-de)), models or datasets.
## Reference:
Fangxiaoyu Feng, Yinfei Yang, Daniel Cer, Narveen Ari, Wei Wang. [Language-agnostic BERT Sentence Embedding](https://arxiv.org/abs/2007.01852). July 2020
License: [https://tfhub.dev/google/LaBSE/1](https://tfhub.dev/google/LaBSE/1)
|
mradermacher/ZharfaOpen_Llama3_12B_0.1-GGUF | mradermacher | 2024-06-27T15:33:41Z | 15,207 | 0 | transformers | [
"transformers",
"gguf",
"en",
"base_model:ZharfaTech/ZharfaOpen_Llama3_12B_0.1",
"endpoints_compatible",
"region:us"
] | null | 2024-06-27T14:53:43Z | ---
base_model: ZharfaTech/ZharfaOpen_Llama3_12B_0.1
language:
- en
library_name: transformers
quantized_by: mradermacher
---
## About
<!-- ### quantize_version: 2 -->
<!-- ### output_tensor_quantised: 1 -->
<!-- ### convert_type: hf -->
<!-- ### vocab_type: -->
<!-- ### tags: -->
static quants of https://huggingface.co/ZharfaTech/ZharfaOpen_Llama3_12B_0.1
<!-- provided-files -->
weighted/imatrix quants seem not to be available (by me) at this time. If they do not show up a week or so after the static ones, I have probably not planned for them. Feel free to request them by opening a Community Discussion.
## Usage
If you are unsure how to use GGUF files, refer to one of [TheBloke's
READMEs](https://huggingface.co/TheBloke/KafkaLM-70B-German-V0.1-GGUF) for
more details, including on how to concatenate multi-part files.
## Provided Quants
(sorted by size, not necessarily quality. IQ-quants are often preferable over similar sized non-IQ quants)
| Link | Type | Size/GB | Notes |
|:-----|:-----|--------:|:------|
| [GGUF](https://huggingface.co/mradermacher/ZharfaOpen_Llama3_12B_0.1-GGUF/resolve/main/ZharfaOpen_Llama3_12B_0.1.Q2_K.gguf) | Q2_K | 4.6 | |
| [GGUF](https://huggingface.co/mradermacher/ZharfaOpen_Llama3_12B_0.1-GGUF/resolve/main/ZharfaOpen_Llama3_12B_0.1.IQ3_XS.gguf) | IQ3_XS | 5.0 | |
| [GGUF](https://huggingface.co/mradermacher/ZharfaOpen_Llama3_12B_0.1-GGUF/resolve/main/ZharfaOpen_Llama3_12B_0.1.Q3_K_S.gguf) | Q3_K_S | 5.3 | |
| [GGUF](https://huggingface.co/mradermacher/ZharfaOpen_Llama3_12B_0.1-GGUF/resolve/main/ZharfaOpen_Llama3_12B_0.1.IQ3_S.gguf) | IQ3_S | 5.3 | beats Q3_K* |
| [GGUF](https://huggingface.co/mradermacher/ZharfaOpen_Llama3_12B_0.1-GGUF/resolve/main/ZharfaOpen_Llama3_12B_0.1.IQ3_M.gguf) | IQ3_M | 5.4 | |
| [GGUF](https://huggingface.co/mradermacher/ZharfaOpen_Llama3_12B_0.1-GGUF/resolve/main/ZharfaOpen_Llama3_12B_0.1.Q3_K_M.gguf) | Q3_K_M | 5.8 | lower quality |
| [GGUF](https://huggingface.co/mradermacher/ZharfaOpen_Llama3_12B_0.1-GGUF/resolve/main/ZharfaOpen_Llama3_12B_0.1.Q3_K_L.gguf) | Q3_K_L | 6.3 | |
| [GGUF](https://huggingface.co/mradermacher/ZharfaOpen_Llama3_12B_0.1-GGUF/resolve/main/ZharfaOpen_Llama3_12B_0.1.IQ4_XS.gguf) | IQ4_XS | 6.5 | |
| [GGUF](https://huggingface.co/mradermacher/ZharfaOpen_Llama3_12B_0.1-GGUF/resolve/main/ZharfaOpen_Llama3_12B_0.1.Q4_K_S.gguf) | Q4_K_S | 6.8 | fast, recommended |
| [GGUF](https://huggingface.co/mradermacher/ZharfaOpen_Llama3_12B_0.1-GGUF/resolve/main/ZharfaOpen_Llama3_12B_0.1.Q4_K_M.gguf) | Q4_K_M | 7.1 | fast, recommended |
| [GGUF](https://huggingface.co/mradermacher/ZharfaOpen_Llama3_12B_0.1-GGUF/resolve/main/ZharfaOpen_Llama3_12B_0.1.Q5_K_S.gguf) | Q5_K_S | 8.1 | |
| [GGUF](https://huggingface.co/mradermacher/ZharfaOpen_Llama3_12B_0.1-GGUF/resolve/main/ZharfaOpen_Llama3_12B_0.1.Q5_K_M.gguf) | Q5_K_M | 8.3 | |
| [GGUF](https://huggingface.co/mradermacher/ZharfaOpen_Llama3_12B_0.1-GGUF/resolve/main/ZharfaOpen_Llama3_12B_0.1.Q6_K.gguf) | Q6_K | 9.6 | very good quality |
| [GGUF](https://huggingface.co/mradermacher/ZharfaOpen_Llama3_12B_0.1-GGUF/resolve/main/ZharfaOpen_Llama3_12B_0.1.Q8_0.gguf) | Q8_0 | 12.3 | fast, best quality |
Here is a handy graph by ikawrakow comparing some lower-quality quant
types (lower is better):

And here are Artefact2's thoughts on the matter:
https://gist.github.com/Artefact2/b5f810600771265fc1e39442288e8ec9
## FAQ / Model Request
See https://huggingface.co/mradermacher/model_requests for some answers to
questions you might have and/or if you want some other model quantized.
## Thanks
I thank my company, [nethype GmbH](https://www.nethype.de/), for letting
me use its servers and providing upgrades to my workstation to enable
this work in my free time.
<!-- end -->
|
RichardErkhov/Q-bert_-_Optimus-7B-gguf | RichardErkhov | 2024-07-01T08:34:33Z | 15,191 | 0 | null | [
"gguf",
"region:us"
] | null | 2024-07-01T06:25:48Z | Quantization made by Richard Erkhov.
[Github](https://github.com/RichardErkhov)
[Discord](https://discord.gg/pvy7H8DZMG)
[Request more models](https://github.com/RichardErkhov/quant_request)
Optimus-7B - GGUF
- Model creator: https://huggingface.co/Q-bert/
- Original model: https://huggingface.co/Q-bert/Optimus-7B/
| Name | Quant method | Size |
| ---- | ---- | ---- |
| [Optimus-7B.Q2_K.gguf](https://huggingface.co/RichardErkhov/Q-bert_-_Optimus-7B-gguf/blob/main/Optimus-7B.Q2_K.gguf) | Q2_K | 2.53GB |
| [Optimus-7B.IQ3_XS.gguf](https://huggingface.co/RichardErkhov/Q-bert_-_Optimus-7B-gguf/blob/main/Optimus-7B.IQ3_XS.gguf) | IQ3_XS | 2.81GB |
| [Optimus-7B.IQ3_S.gguf](https://huggingface.co/RichardErkhov/Q-bert_-_Optimus-7B-gguf/blob/main/Optimus-7B.IQ3_S.gguf) | IQ3_S | 2.96GB |
| [Optimus-7B.Q3_K_S.gguf](https://huggingface.co/RichardErkhov/Q-bert_-_Optimus-7B-gguf/blob/main/Optimus-7B.Q3_K_S.gguf) | Q3_K_S | 2.95GB |
| [Optimus-7B.IQ3_M.gguf](https://huggingface.co/RichardErkhov/Q-bert_-_Optimus-7B-gguf/blob/main/Optimus-7B.IQ3_M.gguf) | IQ3_M | 3.06GB |
| [Optimus-7B.Q3_K.gguf](https://huggingface.co/RichardErkhov/Q-bert_-_Optimus-7B-gguf/blob/main/Optimus-7B.Q3_K.gguf) | Q3_K | 3.28GB |
| [Optimus-7B.Q3_K_M.gguf](https://huggingface.co/RichardErkhov/Q-bert_-_Optimus-7B-gguf/blob/main/Optimus-7B.Q3_K_M.gguf) | Q3_K_M | 3.28GB |
| [Optimus-7B.Q3_K_L.gguf](https://huggingface.co/RichardErkhov/Q-bert_-_Optimus-7B-gguf/blob/main/Optimus-7B.Q3_K_L.gguf) | Q3_K_L | 3.56GB |
| [Optimus-7B.IQ4_XS.gguf](https://huggingface.co/RichardErkhov/Q-bert_-_Optimus-7B-gguf/blob/main/Optimus-7B.IQ4_XS.gguf) | IQ4_XS | 3.67GB |
| [Optimus-7B.Q4_0.gguf](https://huggingface.co/RichardErkhov/Q-bert_-_Optimus-7B-gguf/blob/main/Optimus-7B.Q4_0.gguf) | Q4_0 | 3.83GB |
| [Optimus-7B.IQ4_NL.gguf](https://huggingface.co/RichardErkhov/Q-bert_-_Optimus-7B-gguf/blob/main/Optimus-7B.IQ4_NL.gguf) | IQ4_NL | 3.87GB |
| [Optimus-7B.Q4_K_S.gguf](https://huggingface.co/RichardErkhov/Q-bert_-_Optimus-7B-gguf/blob/main/Optimus-7B.Q4_K_S.gguf) | Q4_K_S | 3.86GB |
| [Optimus-7B.Q4_K.gguf](https://huggingface.co/RichardErkhov/Q-bert_-_Optimus-7B-gguf/blob/main/Optimus-7B.Q4_K.gguf) | Q4_K | 4.07GB |
| [Optimus-7B.Q4_K_M.gguf](https://huggingface.co/RichardErkhov/Q-bert_-_Optimus-7B-gguf/blob/main/Optimus-7B.Q4_K_M.gguf) | Q4_K_M | 4.07GB |
| [Optimus-7B.Q4_1.gguf](https://huggingface.co/RichardErkhov/Q-bert_-_Optimus-7B-gguf/blob/main/Optimus-7B.Q4_1.gguf) | Q4_1 | 4.24GB |
| [Optimus-7B.Q5_0.gguf](https://huggingface.co/RichardErkhov/Q-bert_-_Optimus-7B-gguf/blob/main/Optimus-7B.Q5_0.gguf) | Q5_0 | 4.65GB |
| [Optimus-7B.Q5_K_S.gguf](https://huggingface.co/RichardErkhov/Q-bert_-_Optimus-7B-gguf/blob/main/Optimus-7B.Q5_K_S.gguf) | Q5_K_S | 4.65GB |
| [Optimus-7B.Q5_K.gguf](https://huggingface.co/RichardErkhov/Q-bert_-_Optimus-7B-gguf/blob/main/Optimus-7B.Q5_K.gguf) | Q5_K | 4.78GB |
| [Optimus-7B.Q5_K_M.gguf](https://huggingface.co/RichardErkhov/Q-bert_-_Optimus-7B-gguf/blob/main/Optimus-7B.Q5_K_M.gguf) | Q5_K_M | 4.78GB |
| [Optimus-7B.Q5_1.gguf](https://huggingface.co/RichardErkhov/Q-bert_-_Optimus-7B-gguf/blob/main/Optimus-7B.Q5_1.gguf) | Q5_1 | 5.07GB |
| [Optimus-7B.Q6_K.gguf](https://huggingface.co/RichardErkhov/Q-bert_-_Optimus-7B-gguf/blob/main/Optimus-7B.Q6_K.gguf) | Q6_K | 5.53GB |
| [Optimus-7B.Q8_0.gguf](https://huggingface.co/RichardErkhov/Q-bert_-_Optimus-7B-gguf/blob/main/Optimus-7B.Q8_0.gguf) | Q8_0 | 7.17GB |
Original model description:
---
license: apache-2.0
datasets:
- meta-math/MetaMathQA
language:
- en
pipeline_tag: text-generation
tags:
- Math
---
## Optimus-7B
<img src="_c3f4a76b-c0b1-4fba-9537-33f8fd697f2d.jpg" width="300" height="200" alt="Optimus-7B">
Fine-tuned On [mistralai/Mistral-7B-v0.1](https://huggingface.co/mistralai/Mistral-7B-v0.1) with [meta-math/MetaMathQA](https://huggingface.co/datasets/meta-math/MetaMathQA)
You can use ChatML format.
# [Open LLM Leaderboard Evaluation Results](https://huggingface.co/spaces/HuggingFaceH4/open_llm_leaderboard)
Detailed results can be found [Here](https://huggingface.co/datasets/open-llm-leaderboard/results/blob/main/Q-bert/Optimus-7B/results_2023-12-04T18-59-49.207215.json)
| Metric | Value |
|-----------------------|---------------------------|
| Avg. | 69.09 |
| ARC (25-shot) | 65.44 |
| HellaSwag (10-shot) | 85.41 |
| MMLU (5-shot) | 63.61 |
| TruthfulQA (0-shot) | 55.79 |
| Winogrande (5-shot) | 78.77 |
| GSM8K (5-shot) | 65.50 |
|
minhtoan/t5-finetune-cnndaily-news | minhtoan | 2022-12-14T03:22:31Z | 15,189 | 2 | transformers | [
"transformers",
"pytorch",
"t5",
"text2text-generation",
"summarization",
"en",
"dataset:cnn_dailymail",
"license:mit",
"autotrain_compatible",
"endpoints_compatible",
"text-generation-inference",
"region:us"
] | summarization | 2022-12-01T03:25:21Z | ---
language: en
datasets:
- cnn_dailymail
tags:
- summarization
license: mit
widget:
- text: "summarize: Ever noticed how plane seats appear to be getting smaller and smaller? With increasing numbers of people taking to the skies, some experts are questioning if having such packed out planes is putting passengers at risk. They say that the shrinking space on aeroplanes is not only uncomfortable - it's putting our health and safety in danger. More than squabbling over the arm rest, shrinking space on planes putting our health and safety in danger? This week, a U.S consumer advisory group set up by the Department of Transportation said at a public hearing that while the government is happy to set standards for animals flying on planes, it doesn't stipulate a minimum amount of space for humans. 'In a world where animals have more rights to space and food than humans,' said Charlie Leocha, consumer representative on the committee.\xa0'It is time that the DOT and FAA take a stand for humane treatment of passengers.' But could crowding on planes lead to more serious issues than fighting for space in the overhead lockers, crashing elbows and seat back kicking? Tests conducted by the FAA use planes with a 31 inch pitch, a standard which on some airlines has decreased . Many economy seats on United Airlines have 30 inches of room, while some airlines offer as little as 28 inches . Cynthia Corbertt, a human factors researcher with the Federal Aviation Administration, that it conducts tests on how quickly passengers can leave a plane. But these tests are conducted using planes with 31 inches between each row of seats, a standard which on some airlines has decreased, reported the Detroit News. The distance between two seats from one point on a seat to the same point on the seat behind it is known as the pitch. While most airlines stick to a pitch of 31 inches or above, some fall below this. While United Airlines has 30 inches of space, Gulf Air economy seats have between 29 and 32 inches, Air Asia offers 29 inches and Spirit Airlines offers just 28 inches. British Airways has a seat pitch of 31 inches, while easyJet has 29 inches, Thomson's short haul seat pitch is 28 inches, and Virgin Atlantic's is 30-31."
inference:
parameters:
max_length: 150
---
# Text Summarization of News Articles
State-of-the-art lightweights pretrained Transformer-based encoder-decoder model for text summarization.
Model trained on dataset CNN-DailyMail News with input length = 512, output length = 150
## How to use
Input for model: prefix + input text
Example: 'summarize: '+ 'Ever noticed how plane seats.....'
```python
from transformers import AutoTokenizer, AutoModelForSeq2SeqLM
tokenizer = AutoTokenizer.from_pretrained("minhtoan/t5-finetune-cnndaily-news")
model = AutoModelForSeq2SeqLM.from_pretrained("minhtoan/t5-finetune-cnndaily-news")
model.cuda()
src = "summarize: Ever noticed how plane seats appear to be getting smaller and smaller? With increasing numbers of people taking to the skies, some experts are questioning if having such packed out planes is putting passengers at risk. They say that the shrinking space on aeroplanes is not only uncomfortable - it's putting our health and safety in danger. More than squabbling over the arm rest, shrinking space on planes putting our health and safety in danger? This week, a U.S consumer advisory group set up by the Department of Transportation said at a public hearing that while the government is happy to set standards for animals flying on planes, it doesn't stipulate a minimum amount of space for humans. 'In a world where animals have more rights to space and food than humans,' said Charlie Leocha, consumer representative on the committee.\xa0'It is time that the DOT and FAA take a stand for humane treatment of passengers.' But could crowding on planes lead to more serious issues than fighting for space in the overhead lockers, crashing elbows and seat back kicking? Tests conducted by the FAA use planes with a 31 inch pitch, a standard which on some airlines has decreased . Many economy seats on United Airlines have 30 inches of room, while some airlines offer as little as 28 inches . Cynthia Corbertt, a human factors researcher with the Federal Aviation Administration, that it conducts tests on how quickly passengers can leave a plane. But these tests are conducted using planes with 31 inches between each row of seats, a standard which on some airlines has decreased, reported the Detroit News. The distance between two seats from one point on a seat to the same point on the seat behind it is known as the pitch. While most airlines stick to a pitch of 31 inches or above, some fall below this. While United Airlines has 30 inches of space, Gulf Air economy seats have between 29 and 32 inches, Air Asia offers 29 inches and Spirit Airlines offers just 28 inches. British Airways has a seat pitch of 31 inches, while easyJet has 29 inches, Thomson's short haul seat pitch is 28 inches, and Virgin Atlantic's is 30-31."
tokenized_text = tokenizer.encode(src, return_tensors="pt").cuda()
model.eval()
summary_ids = model.generate(tokenized_text, max_length=150)
output = tokenizer.decode(summary_ids[0], skip_special_tokens=True)
output
```
## Author
`
Phan Minh Toan
` |
mradermacher/Llama-3-8B-ShareGPT-112K-i1-GGUF | mradermacher | 2024-06-28T13:42:58Z | 15,177 | 0 | transformers | [
"transformers",
"gguf",
"axolotl",
"generated_from_trainer",
"en",
"base_model:Magpie-Align/Llama-3-8B-ShareGPT-112K",
"license:llama3",
"endpoints_compatible",
"region:us"
] | null | 2024-06-28T12:25:05Z | ---
base_model: Magpie-Align/Llama-3-8B-ShareGPT-112K
language:
- en
library_name: transformers
license: llama3
quantized_by: mradermacher
tags:
- axolotl
- generated_from_trainer
---
## About
<!-- ### quantize_version: 2 -->
<!-- ### output_tensor_quantised: 1 -->
<!-- ### convert_type: hf -->
<!-- ### vocab_type: -->
<!-- ### tags: nicoboss -->
weighted/imatrix quants of https://huggingface.co/Magpie-Align/Llama-3-8B-ShareGPT-112K
<!-- provided-files -->
static quants are available at https://huggingface.co/mradermacher/Llama-3-8B-ShareGPT-112K-GGUF
## Usage
If you are unsure how to use GGUF files, refer to one of [TheBloke's
READMEs](https://huggingface.co/TheBloke/KafkaLM-70B-German-V0.1-GGUF) for
more details, including on how to concatenate multi-part files.
## Provided Quants
(sorted by size, not necessarily quality. IQ-quants are often preferable over similar sized non-IQ quants)
| Link | Type | Size/GB | Notes |
|:-----|:-----|--------:|:------|
| [GGUF](https://huggingface.co/mradermacher/Llama-3-8B-ShareGPT-112K-i1-GGUF/resolve/main/Llama-3-8B-ShareGPT-112K.i1-IQ1_S.gguf) | i1-IQ1_S | 2.1 | for the desperate |
| [GGUF](https://huggingface.co/mradermacher/Llama-3-8B-ShareGPT-112K-i1-GGUF/resolve/main/Llama-3-8B-ShareGPT-112K.i1-IQ1_M.gguf) | i1-IQ1_M | 2.3 | mostly desperate |
| [GGUF](https://huggingface.co/mradermacher/Llama-3-8B-ShareGPT-112K-i1-GGUF/resolve/main/Llama-3-8B-ShareGPT-112K.i1-IQ2_XXS.gguf) | i1-IQ2_XXS | 2.5 | |
| [GGUF](https://huggingface.co/mradermacher/Llama-3-8B-ShareGPT-112K-i1-GGUF/resolve/main/Llama-3-8B-ShareGPT-112K.i1-IQ2_XS.gguf) | i1-IQ2_XS | 2.7 | |
| [GGUF](https://huggingface.co/mradermacher/Llama-3-8B-ShareGPT-112K-i1-GGUF/resolve/main/Llama-3-8B-ShareGPT-112K.i1-IQ2_S.gguf) | i1-IQ2_S | 2.9 | |
| [GGUF](https://huggingface.co/mradermacher/Llama-3-8B-ShareGPT-112K-i1-GGUF/resolve/main/Llama-3-8B-ShareGPT-112K.i1-IQ2_M.gguf) | i1-IQ2_M | 3.0 | |
| [GGUF](https://huggingface.co/mradermacher/Llama-3-8B-ShareGPT-112K-i1-GGUF/resolve/main/Llama-3-8B-ShareGPT-112K.i1-Q2_K.gguf) | i1-Q2_K | 3.3 | IQ3_XXS probably better |
| [GGUF](https://huggingface.co/mradermacher/Llama-3-8B-ShareGPT-112K-i1-GGUF/resolve/main/Llama-3-8B-ShareGPT-112K.i1-IQ3_XXS.gguf) | i1-IQ3_XXS | 3.4 | lower quality |
| [GGUF](https://huggingface.co/mradermacher/Llama-3-8B-ShareGPT-112K-i1-GGUF/resolve/main/Llama-3-8B-ShareGPT-112K.i1-IQ3_XS.gguf) | i1-IQ3_XS | 3.6 | |
| [GGUF](https://huggingface.co/mradermacher/Llama-3-8B-ShareGPT-112K-i1-GGUF/resolve/main/Llama-3-8B-ShareGPT-112K.i1-Q3_K_S.gguf) | i1-Q3_K_S | 3.8 | IQ3_XS probably better |
| [GGUF](https://huggingface.co/mradermacher/Llama-3-8B-ShareGPT-112K-i1-GGUF/resolve/main/Llama-3-8B-ShareGPT-112K.i1-IQ3_S.gguf) | i1-IQ3_S | 3.8 | beats Q3_K* |
| [GGUF](https://huggingface.co/mradermacher/Llama-3-8B-ShareGPT-112K-i1-GGUF/resolve/main/Llama-3-8B-ShareGPT-112K.i1-IQ3_M.gguf) | i1-IQ3_M | 3.9 | |
| [GGUF](https://huggingface.co/mradermacher/Llama-3-8B-ShareGPT-112K-i1-GGUF/resolve/main/Llama-3-8B-ShareGPT-112K.i1-Q3_K_M.gguf) | i1-Q3_K_M | 4.1 | IQ3_S probably better |
| [GGUF](https://huggingface.co/mradermacher/Llama-3-8B-ShareGPT-112K-i1-GGUF/resolve/main/Llama-3-8B-ShareGPT-112K.i1-Q3_K_L.gguf) | i1-Q3_K_L | 4.4 | IQ3_M probably better |
| [GGUF](https://huggingface.co/mradermacher/Llama-3-8B-ShareGPT-112K-i1-GGUF/resolve/main/Llama-3-8B-ShareGPT-112K.i1-IQ4_XS.gguf) | i1-IQ4_XS | 4.5 | |
| [GGUF](https://huggingface.co/mradermacher/Llama-3-8B-ShareGPT-112K-i1-GGUF/resolve/main/Llama-3-8B-ShareGPT-112K.i1-Q4_0.gguf) | i1-Q4_0 | 4.8 | fast, low quality |
| [GGUF](https://huggingface.co/mradermacher/Llama-3-8B-ShareGPT-112K-i1-GGUF/resolve/main/Llama-3-8B-ShareGPT-112K.i1-Q4_K_S.gguf) | i1-Q4_K_S | 4.8 | optimal size/speed/quality |
| [GGUF](https://huggingface.co/mradermacher/Llama-3-8B-ShareGPT-112K-i1-GGUF/resolve/main/Llama-3-8B-ShareGPT-112K.i1-Q4_K_M.gguf) | i1-Q4_K_M | 5.0 | fast, recommended |
| [GGUF](https://huggingface.co/mradermacher/Llama-3-8B-ShareGPT-112K-i1-GGUF/resolve/main/Llama-3-8B-ShareGPT-112K.i1-Q5_K_S.gguf) | i1-Q5_K_S | 5.7 | |
| [GGUF](https://huggingface.co/mradermacher/Llama-3-8B-ShareGPT-112K-i1-GGUF/resolve/main/Llama-3-8B-ShareGPT-112K.i1-Q5_K_M.gguf) | i1-Q5_K_M | 5.8 | |
| [GGUF](https://huggingface.co/mradermacher/Llama-3-8B-ShareGPT-112K-i1-GGUF/resolve/main/Llama-3-8B-ShareGPT-112K.i1-Q6_K.gguf) | i1-Q6_K | 6.7 | practically like static Q6_K |
Here is a handy graph by ikawrakow comparing some lower-quality quant
types (lower is better):

And here are Artefact2's thoughts on the matter:
https://gist.github.com/Artefact2/b5f810600771265fc1e39442288e8ec9
## FAQ / Model Request
See https://huggingface.co/mradermacher/model_requests for some answers to
questions you might have and/or if you want some other model quantized.
## Thanks
I thank my company, [nethype GmbH](https://www.nethype.de/), for letting
me use its servers and providing upgrades to my workstation to enable
this work in my free time. Additional thanks to [@nicoboss](https://huggingface.co/nicoboss) for giving me access to his hardware for calculating the imatrix for these quants.
<!-- end -->
|
RichardErkhov/Hemanth-thunder_-_Tamil-Mistral-7B-v0.1-gguf | RichardErkhov | 2024-06-26T05:38:51Z | 15,159 | 0 | null | [
"gguf",
"arxiv:2309.04662",
"arxiv:2310.06825",
"region:us"
] | null | 2024-06-26T01:51:08Z | Quantization made by Richard Erkhov.
[Github](https://github.com/RichardErkhov)
[Discord](https://discord.gg/pvy7H8DZMG)
[Request more models](https://github.com/RichardErkhov/quant_request)
Tamil-Mistral-7B-v0.1 - GGUF
- Model creator: https://huggingface.co/Hemanth-thunder/
- Original model: https://huggingface.co/Hemanth-thunder/Tamil-Mistral-7B-v0.1/
| Name | Quant method | Size |
| ---- | ---- | ---- |
| [Tamil-Mistral-7B-v0.1.Q2_K.gguf](https://huggingface.co/RichardErkhov/Hemanth-thunder_-_Tamil-Mistral-7B-v0.1-gguf/blob/main/Tamil-Mistral-7B-v0.1.Q2_K.gguf) | Q2_K | 2.61GB |
| [Tamil-Mistral-7B-v0.1.IQ3_XS.gguf](https://huggingface.co/RichardErkhov/Hemanth-thunder_-_Tamil-Mistral-7B-v0.1-gguf/blob/main/Tamil-Mistral-7B-v0.1.IQ3_XS.gguf) | IQ3_XS | 2.9GB |
| [Tamil-Mistral-7B-v0.1.IQ3_S.gguf](https://huggingface.co/RichardErkhov/Hemanth-thunder_-_Tamil-Mistral-7B-v0.1-gguf/blob/main/Tamil-Mistral-7B-v0.1.IQ3_S.gguf) | IQ3_S | 3.05GB |
| [Tamil-Mistral-7B-v0.1.Q3_K_S.gguf](https://huggingface.co/RichardErkhov/Hemanth-thunder_-_Tamil-Mistral-7B-v0.1-gguf/blob/main/Tamil-Mistral-7B-v0.1.Q3_K_S.gguf) | Q3_K_S | 3.04GB |
| [Tamil-Mistral-7B-v0.1.IQ3_M.gguf](https://huggingface.co/RichardErkhov/Hemanth-thunder_-_Tamil-Mistral-7B-v0.1-gguf/blob/main/Tamil-Mistral-7B-v0.1.IQ3_M.gguf) | IQ3_M | 3.15GB |
| [Tamil-Mistral-7B-v0.1.Q3_K.gguf](https://huggingface.co/RichardErkhov/Hemanth-thunder_-_Tamil-Mistral-7B-v0.1-gguf/blob/main/Tamil-Mistral-7B-v0.1.Q3_K.gguf) | Q3_K | 3.37GB |
| [Tamil-Mistral-7B-v0.1.Q3_K_M.gguf](https://huggingface.co/RichardErkhov/Hemanth-thunder_-_Tamil-Mistral-7B-v0.1-gguf/blob/main/Tamil-Mistral-7B-v0.1.Q3_K_M.gguf) | Q3_K_M | 3.37GB |
| [Tamil-Mistral-7B-v0.1.Q3_K_L.gguf](https://huggingface.co/RichardErkhov/Hemanth-thunder_-_Tamil-Mistral-7B-v0.1-gguf/blob/main/Tamil-Mistral-7B-v0.1.Q3_K_L.gguf) | Q3_K_L | 3.65GB |
| [Tamil-Mistral-7B-v0.1.IQ4_XS.gguf](https://huggingface.co/RichardErkhov/Hemanth-thunder_-_Tamil-Mistral-7B-v0.1-gguf/blob/main/Tamil-Mistral-7B-v0.1.IQ4_XS.gguf) | IQ4_XS | 3.77GB |
| [Tamil-Mistral-7B-v0.1.Q4_0.gguf](https://huggingface.co/RichardErkhov/Hemanth-thunder_-_Tamil-Mistral-7B-v0.1-gguf/blob/main/Tamil-Mistral-7B-v0.1.Q4_0.gguf) | Q4_0 | 3.93GB |
| [Tamil-Mistral-7B-v0.1.IQ4_NL.gguf](https://huggingface.co/RichardErkhov/Hemanth-thunder_-_Tamil-Mistral-7B-v0.1-gguf/blob/main/Tamil-Mistral-7B-v0.1.IQ4_NL.gguf) | IQ4_NL | 3.97GB |
| [Tamil-Mistral-7B-v0.1.Q4_K_S.gguf](https://huggingface.co/RichardErkhov/Hemanth-thunder_-_Tamil-Mistral-7B-v0.1-gguf/blob/main/Tamil-Mistral-7B-v0.1.Q4_K_S.gguf) | Q4_K_S | 3.95GB |
| [Tamil-Mistral-7B-v0.1.Q4_K.gguf](https://huggingface.co/RichardErkhov/Hemanth-thunder_-_Tamil-Mistral-7B-v0.1-gguf/blob/main/Tamil-Mistral-7B-v0.1.Q4_K.gguf) | Q4_K | 4.17GB |
| [Tamil-Mistral-7B-v0.1.Q4_K_M.gguf](https://huggingface.co/RichardErkhov/Hemanth-thunder_-_Tamil-Mistral-7B-v0.1-gguf/blob/main/Tamil-Mistral-7B-v0.1.Q4_K_M.gguf) | Q4_K_M | 4.17GB |
| [Tamil-Mistral-7B-v0.1.Q4_1.gguf](https://huggingface.co/RichardErkhov/Hemanth-thunder_-_Tamil-Mistral-7B-v0.1-gguf/blob/main/Tamil-Mistral-7B-v0.1.Q4_1.gguf) | Q4_1 | 4.34GB |
| [Tamil-Mistral-7B-v0.1.Q5_0.gguf](https://huggingface.co/RichardErkhov/Hemanth-thunder_-_Tamil-Mistral-7B-v0.1-gguf/blob/main/Tamil-Mistral-7B-v0.1.Q5_0.gguf) | Q5_0 | 4.76GB |
| [Tamil-Mistral-7B-v0.1.Q5_K_S.gguf](https://huggingface.co/RichardErkhov/Hemanth-thunder_-_Tamil-Mistral-7B-v0.1-gguf/blob/main/Tamil-Mistral-7B-v0.1.Q5_K_S.gguf) | Q5_K_S | 4.76GB |
| [Tamil-Mistral-7B-v0.1.Q5_K.gguf](https://huggingface.co/RichardErkhov/Hemanth-thunder_-_Tamil-Mistral-7B-v0.1-gguf/blob/main/Tamil-Mistral-7B-v0.1.Q5_K.gguf) | Q5_K | 4.89GB |
| [Tamil-Mistral-7B-v0.1.Q5_K_M.gguf](https://huggingface.co/RichardErkhov/Hemanth-thunder_-_Tamil-Mistral-7B-v0.1-gguf/blob/main/Tamil-Mistral-7B-v0.1.Q5_K_M.gguf) | Q5_K_M | 4.89GB |
| [Tamil-Mistral-7B-v0.1.Q5_1.gguf](https://huggingface.co/RichardErkhov/Hemanth-thunder_-_Tamil-Mistral-7B-v0.1-gguf/blob/main/Tamil-Mistral-7B-v0.1.Q5_1.gguf) | Q5_1 | 5.18GB |
| [Tamil-Mistral-7B-v0.1.Q6_K.gguf](https://huggingface.co/RichardErkhov/Hemanth-thunder_-_Tamil-Mistral-7B-v0.1-gguf/blob/main/Tamil-Mistral-7B-v0.1.Q6_K.gguf) | Q6_K | 5.65GB |
| [Tamil-Mistral-7B-v0.1.Q8_0.gguf](https://huggingface.co/RichardErkhov/Hemanth-thunder_-_Tamil-Mistral-7B-v0.1-gguf/blob/main/Tamil-Mistral-7B-v0.1.Q8_0.gguf) | Q8_0 | 7.32GB |
Original model description:
---
language:
- ta
license: apache-2.0
tags:
- pretrained
datasets:
- Hemanth-thunder/tamil-madlad-400
pipeline_tag: text-generation
inference:
parameters:
temperature: 0.7
repetition_penalty: 1.15
---
# Model Card for Tamil-Mistral-7B-v0.1
The Tamil-Mistral-7B-v0.1 Large Language Model (LLM) is a pre-trained generative text model trained at the top of mistral base model 7 billion parameters. This is extends version of tokenization capability by increasing tamil tokens by 20k.
Additionally, it was Pretrained on 1.19 million Tamil documents sourced from madlad-400 (Tamil) [MADLAD-400 (Multilingual Audited Dataset: Low-resource And Document-level)](https://arxiv.org/abs/2309.04662).
pretraining time: 145 hours (GPU NVIDIA RTX A6000 48GB)
## Mistral model details
For full details of this model please read our [paper](https://arxiv.org/abs/2310.06825) and [release blog post](https://mistral.ai/news/announcing-mistral-7b/).
## Model Architecture
Mistral-7B-v0.1 is a transformer model, with the following architecture choices:
- Grouped-Query Attention
- Sliding-Window Attention
- Byte-fallback BPE tokenizer
[Kaggle Demo](https://www.kaggle.com/code/hemanthkumar21/tamil-mistral-7b-v0-1-demo/)
#### Running the model on a GPU 16GB
```python
import torch
from transformers import (AutoModelForCausalLM,AutoTokenizer,TextStreamer,pipeline)
model = AutoModelForCausalLM.from_pretrained("Hemanth-thunder/Tamil-Mistral-7B-v0.1",device_map="auto")
tokenizer = AutoTokenizer.from_pretrained("Hemanth-thunder/Tamil-Mistral-7B-v0.1",add_prefix_space=True)
tokenizer.pad_token = tokenizer.eos_token
tokenizer.padding_side = "right"
streamer = TextStreamer(tokenizer)
pipe = pipeline("text-generation" ,model=model, tokenizer=tokenizer ,do_sample=True, repetition_penalty=1.15,top_p=0.95,streamer=streamer)
pipe("ஐபிஎல் தொடரில் மும்பை இந்தியன்ஸ் அணி ",max_length=50)
```
```generated_text
ஐபிஎல் தொடரில் மும்பை இந்தியன்ஸ் அணி -3வது இடத்திற்கு முன்னேறி இருக்கிறது, இதனால் பிளே ஆஃப் வாய்ப்பை உறுதி செய்ய வேண்டும்.
இன்னும் 11 புள்ளிகள் மட்டுமே மீதமுள்ளது.சென்னை சூப்பர் கிங்சுக்கு 12 புள்ளிகளில் உள்ளது.
அதன் கடைசி லீக் போட்டி ஜூன் 23-ம் தேதி சென்னையில் நடைபெறுகிறது.
```
# Loss
<!-- Provide a quick summary of what the model is/does. -->

## Troubleshooting
- If you see the following error:
```
KeyError: 'mistral'
```
- Or:
```
NotImplementedError: Cannot copy out of meta tensor; no data!
```
Ensure you are utilizing a stable version of Transformers, 4.34.0 or newer.
## Notice
Mistral 7B is a pretrained base model and therefore does not have any moderation mechanisms.
# How to Cite
```bibtext
@misc{Tamil-Mistral-7B-v0.1,
url={[https://huggingface.co/Hemanth-thunder/Tamil-Mistral-7B-v0.1]https://huggingface.co/Hemanth-thunder/Tamil-Mistral-7B-v0.1)},
title={Tamil-Mistral-7B-v0.1},
author={"hemanth kumar"}
}
```
|
togethercomputer/LLaMA-2-7B-32K | togethercomputer | 2024-03-28T01:14:07Z | 15,150 | 526 | transformers | [
"transformers",
"pytorch",
"llama",
"text-generation",
"en",
"dataset:togethercomputer/RedPajama-Data-1T",
"dataset:togethercomputer/RedPajama-Data-Instruct",
"dataset:EleutherAI/pile",
"dataset:togethercomputer/Long-Data-Collections",
"license:llama2",
"autotrain_compatible",
"endpoints_compatible",
"text-generation-inference",
"region:us"
] | text-generation | 2023-07-26T02:19:41Z | ---
license: llama2
datasets:
- togethercomputer/RedPajama-Data-1T
- togethercomputer/RedPajama-Data-Instruct
- EleutherAI/pile
- togethercomputer/Long-Data-Collections
language:
- en
library_name: transformers
---
# LLaMA-2-7B-32K
## Model Description
LLaMA-2-7B-32K is an open-source, long context language model developed by Together, fine-tuned from Meta's original Llama-2 7B model.
This model represents our efforts to contribute to the rapid progress of the open-source ecosystem for large language models.
The model has been extended to a context length of 32K with position interpolation,
allowing applications on multi-document QA, long text summarization, etc.
## What's new?
This model introduces several improvements and new features:
1. **Extended Context:** The model has been trained to handle context lengths up to 32K, which is a significant improvement over the previous versions.
2. **Pre-training and Instruction Tuning:** We have shared our data recipe, which consists of a mixture of pre-training and instruction tuning data.
3. **Fine-tuning Examples:** We provide examples of how to fine-tune the model for specific applications, including book summarization and long context question and answering.
4. **Software Support:** We have updated both the inference and training stack to allow efficient inference and fine-tuning for 32K context.
## Model Architecture
The model follows the architecture of Llama-2-7B and extends it to handle a longer context. It leverages the recently released FlashAttention-2 and a range of other optimizations to improve the speed and efficiency of inference and training.
## Training and Fine-tuning
The model has been trained using a mixture of pre-training and instruction tuning data.
- In the first training phase of continued pre-training, our data mixture contains 25% RedPajama Book, 25% RedPajama ArXiv (including abstracts), 25% other data from RedPajama, and 25% from the UL2 Oscar Data, which is a part of OIG (Open-Instruction-Generalist), asking the model to fill in missing chunks, or complete the text.
To enhance the long-context ability, we exclude data shorter than 2K word. The inclusion of UL2 Oscar Data is effective in compelling the model to read and utilize long-range context.
- We then fine-tune the model to focus on its few shot capacity under long context, including 20% Natural Instructions (NI), 20% Public Pool of Prompts (P3), 20% the Pile. We decontaminated all data against HELM core scenarios . We teach the model to leverage the in-context examples by packing examples into one 32K-token sequence. To maintain the knowledge learned from the first piece of data, we incorporate 20% RedPajama-Data Book and 20% RedPajama-Data ArXiv.
Next, we provide examples of how to fine-tune the model for specific applications.
The example datasets are placed in [togethercomputer/Long-Data-Collections](https://huggingface.co/datasets/togethercomputer/Long-Data-Collections)
You can use the [OpenChatKit](https://github.com/togethercomputer/OpenChatKit) to fine-tune your own 32K model over LLaMA-2-7B-32K.
Please refer to [OpenChatKit](https://github.com/togethercomputer/OpenChatKit) for step-by-step illustrations.
1. Long Context QA.
We take as an example the multi-document question answering task from the paper “Lost in the Middle: How Language Models Use Long Contexts”. The input for the model consists of (i) a question that requires an answer and (ii) k documents, which are passages extracted from Wikipedia. Notably, only one of these documents contains the answer to the question, while the remaining k − 1 documents, termed as "distractor" documents, do not. To successfully perform this task, the model must identify and utilize the document containing the answer from its input context.
With OCK, simply run the following command to fine-tune:
```
bash training/finetune_llama-2-7b-32k-mqa.sh
```
2. Summarization.
Another example is BookSum, a unique dataset designed to address the challenges of long-form narrative summarization. This dataset features source documents from the literature domain, including novels, plays, and stories, and offers human-written, highly abstractive summaries. We here focus on chapter-level data. BookSum poses a unique set of challenges, necessitating that the model comprehensively read through each chapter.
With OCK, simply run the following command to fine-tune:
```
bash training/finetune_llama-2-7b-32k-booksum.sh
```
## Inference
You can use the [Together API](https://together.ai/blog/api-announcement) to try out LLaMA-2-7B-32K for inference.
The updated inference stack allows for efficient inference.
To run the model locally, we strongly recommend to install Flash Attention V2, which is necessary to obtain the best performance:
```
# Please update the path of `CUDA_HOME`
export CUDA_HOME=/usr/local/cuda-11.8
pip install transformers==4.31.0
pip install sentencepiece
pip install ninja
pip install flash-attn --no-build-isolation
pip install git+https://github.com/HazyResearch/flash-attention.git#subdirectory=csrc/rotary
```
You can use this model directly from the Hugging Face Model Hub or fine-tune it on your own data using the OpenChatKit.
```python
from transformers import AutoTokenizer, AutoModelForCausalLM
tokenizer = AutoTokenizer.from_pretrained("togethercomputer/LLaMA-2-7B-32K")
model = AutoModelForCausalLM.from_pretrained("togethercomputer/LLaMA-2-7B-32K", trust_remote_code=True, torch_dtype=torch.float16)
input_context = "Your text here"
input_ids = tokenizer.encode(input_context, return_tensors="pt")
output = model.generate(input_ids, max_length=128, temperature=0.7)
output_text = tokenizer.decode(output[0], skip_special_tokens=True)
print(output_text)
```
Alternatively, you can set `trust_remote_code=False` if you prefer not to use flash attention.
## Limitations and Bias
As with all language models, LLaMA-2-7B-32K may generate incorrect or biased content. It's important to keep this in mind when using the model.
## Community
Join us on [Together Discord](https://discord.gg/6ZVDU8tTD4) |
stablediffusionapi/lyrielv16 | stablediffusionapi | 2023-06-20T22:44:52Z | 15,146 | 4 | diffusers | [
"diffusers",
"stablediffusionapi.com",
"stable-diffusion-api",
"text-to-image",
"ultra-realistic",
"license:creativeml-openrail-m",
"autotrain_compatible",
"endpoints_compatible",
"diffusers:StableDiffusionPipeline",
"region:us"
] | text-to-image | 2023-06-19T07:20:43Z | ---
license: creativeml-openrail-m
tags:
- stablediffusionapi.com
- stable-diffusion-api
- text-to-image
- ultra-realistic
pinned: true
---
# lyrielv16 API Inference
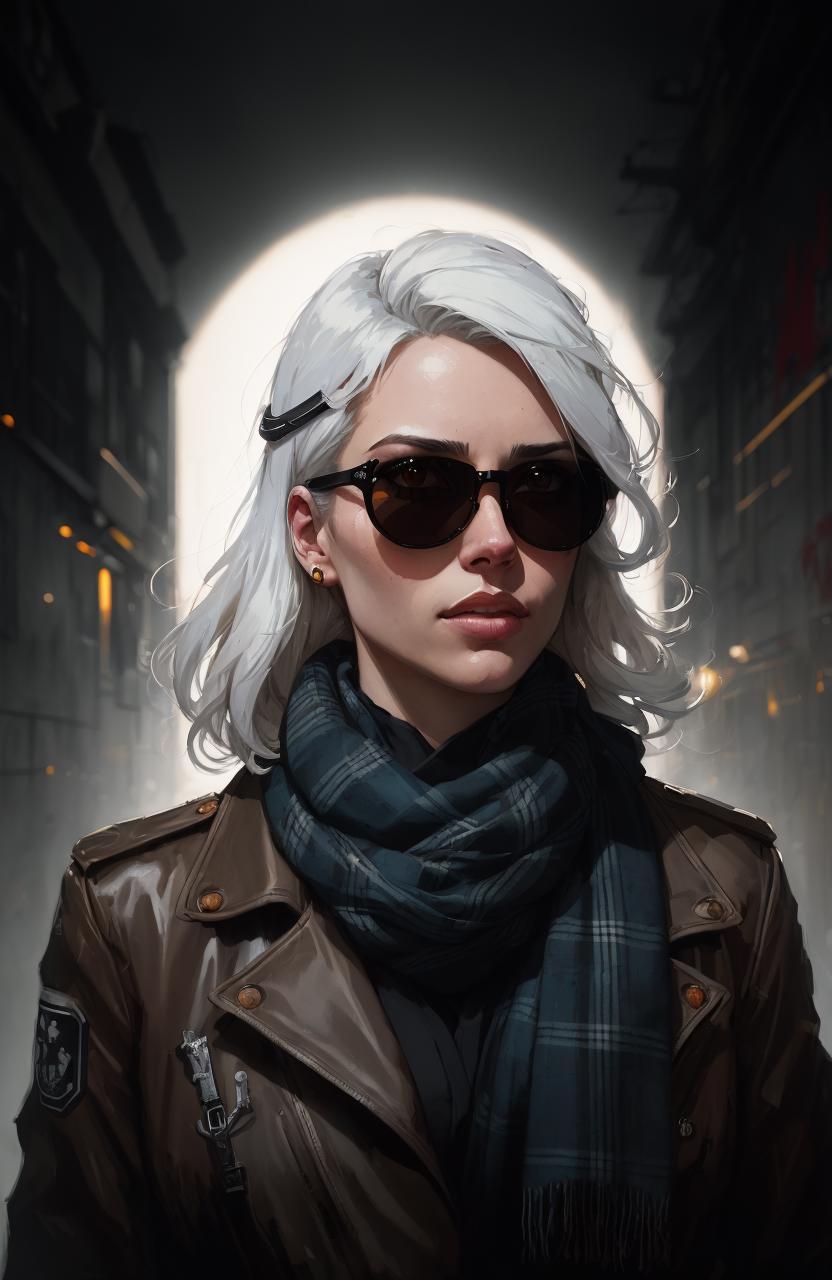
## Get API Key
Get API key from [Stable Diffusion API](http://stablediffusionapi.com/), No Payment needed.
Replace Key in below code, change **model_id** to "lyrielv16"
Coding in PHP/Node/Java etc? Have a look at docs for more code examples: [View docs](https://stablediffusionapi.com/docs)
Try model for free: [Generate Images](https://stablediffusionapi.com/models/lyrielv16)
Model link: [View model](https://stablediffusionapi.com/models/lyrielv16)
Credits: [View credits](https://civitai.com/?query=lyrielv16)
View all models: [View Models](https://stablediffusionapi.com/models)
import requests
import json
url = "https://stablediffusionapi.com/api/v3/dreambooth"
payload = json.dumps({
"key": "your_api_key",
"model_id": "lyrielv16",
"prompt": "ultra realistic close up portrait ((beautiful pale cyberpunk female with heavy black eyeliner)), blue eyes, shaved side haircut, hyper detail, cinematic lighting, magic neon, dark red city, Canon EOS R3, nikon, f/1.4, ISO 200, 1/160s, 8K, RAW, unedited, symmetrical balance, in-frame, 8K",
"negative_prompt": "painting, extra fingers, mutated hands, poorly drawn hands, poorly drawn face, deformed, ugly, blurry, bad anatomy, bad proportions, extra limbs, cloned face, skinny, glitchy, double torso, extra arms, extra hands, mangled fingers, missing lips, ugly face, distorted face, extra legs, anime",
"width": "512",
"height": "512",
"samples": "1",
"num_inference_steps": "30",
"safety_checker": "no",
"enhance_prompt": "yes",
"seed": None,
"guidance_scale": 7.5,
"multi_lingual": "no",
"panorama": "no",
"self_attention": "no",
"upscale": "no",
"embeddings": "embeddings_model_id",
"lora": "lora_model_id",
"webhook": None,
"track_id": None
})
headers = {
'Content-Type': 'application/json'
}
response = requests.request("POST", url, headers=headers, data=payload)
print(response.text)
> Use this coupon code to get 25% off **DMGG0RBN** |
RichardErkhov/David-Xu_-_Mistral-7B-Instruct-v0.2-gguf | RichardErkhov | 2024-06-30T20:25:30Z | 15,142 | 0 | null | [
"gguf",
"region:us"
] | null | 2024-06-30T18:04:46Z | Quantization made by Richard Erkhov.
[Github](https://github.com/RichardErkhov)
[Discord](https://discord.gg/pvy7H8DZMG)
[Request more models](https://github.com/RichardErkhov/quant_request)
Mistral-7B-Instruct-v0.2 - GGUF
- Model creator: https://huggingface.co/David-Xu/
- Original model: https://huggingface.co/David-Xu/Mistral-7B-Instruct-v0.2/
| Name | Quant method | Size |
| ---- | ---- | ---- |
| [Mistral-7B-Instruct-v0.2.Q2_K.gguf](https://huggingface.co/RichardErkhov/David-Xu_-_Mistral-7B-Instruct-v0.2-gguf/blob/main/Mistral-7B-Instruct-v0.2.Q2_K.gguf) | Q2_K | 2.53GB |
| [Mistral-7B-Instruct-v0.2.IQ3_XS.gguf](https://huggingface.co/RichardErkhov/David-Xu_-_Mistral-7B-Instruct-v0.2-gguf/blob/main/Mistral-7B-Instruct-v0.2.IQ3_XS.gguf) | IQ3_XS | 2.81GB |
| [Mistral-7B-Instruct-v0.2.IQ3_S.gguf](https://huggingface.co/RichardErkhov/David-Xu_-_Mistral-7B-Instruct-v0.2-gguf/blob/main/Mistral-7B-Instruct-v0.2.IQ3_S.gguf) | IQ3_S | 2.96GB |
| [Mistral-7B-Instruct-v0.2.Q3_K_S.gguf](https://huggingface.co/RichardErkhov/David-Xu_-_Mistral-7B-Instruct-v0.2-gguf/blob/main/Mistral-7B-Instruct-v0.2.Q3_K_S.gguf) | Q3_K_S | 2.95GB |
| [Mistral-7B-Instruct-v0.2.IQ3_M.gguf](https://huggingface.co/RichardErkhov/David-Xu_-_Mistral-7B-Instruct-v0.2-gguf/blob/main/Mistral-7B-Instruct-v0.2.IQ3_M.gguf) | IQ3_M | 3.06GB |
| [Mistral-7B-Instruct-v0.2.Q3_K.gguf](https://huggingface.co/RichardErkhov/David-Xu_-_Mistral-7B-Instruct-v0.2-gguf/blob/main/Mistral-7B-Instruct-v0.2.Q3_K.gguf) | Q3_K | 3.28GB |
| [Mistral-7B-Instruct-v0.2.Q3_K_M.gguf](https://huggingface.co/RichardErkhov/David-Xu_-_Mistral-7B-Instruct-v0.2-gguf/blob/main/Mistral-7B-Instruct-v0.2.Q3_K_M.gguf) | Q3_K_M | 3.28GB |
| [Mistral-7B-Instruct-v0.2.Q3_K_L.gguf](https://huggingface.co/RichardErkhov/David-Xu_-_Mistral-7B-Instruct-v0.2-gguf/blob/main/Mistral-7B-Instruct-v0.2.Q3_K_L.gguf) | Q3_K_L | 3.56GB |
| [Mistral-7B-Instruct-v0.2.IQ4_XS.gguf](https://huggingface.co/RichardErkhov/David-Xu_-_Mistral-7B-Instruct-v0.2-gguf/blob/main/Mistral-7B-Instruct-v0.2.IQ4_XS.gguf) | IQ4_XS | 3.67GB |
| [Mistral-7B-Instruct-v0.2.Q4_0.gguf](https://huggingface.co/RichardErkhov/David-Xu_-_Mistral-7B-Instruct-v0.2-gguf/blob/main/Mistral-7B-Instruct-v0.2.Q4_0.gguf) | Q4_0 | 3.83GB |
| [Mistral-7B-Instruct-v0.2.IQ4_NL.gguf](https://huggingface.co/RichardErkhov/David-Xu_-_Mistral-7B-Instruct-v0.2-gguf/blob/main/Mistral-7B-Instruct-v0.2.IQ4_NL.gguf) | IQ4_NL | 3.87GB |
| [Mistral-7B-Instruct-v0.2.Q4_K_S.gguf](https://huggingface.co/RichardErkhov/David-Xu_-_Mistral-7B-Instruct-v0.2-gguf/blob/main/Mistral-7B-Instruct-v0.2.Q4_K_S.gguf) | Q4_K_S | 3.86GB |
| [Mistral-7B-Instruct-v0.2.Q4_K.gguf](https://huggingface.co/RichardErkhov/David-Xu_-_Mistral-7B-Instruct-v0.2-gguf/blob/main/Mistral-7B-Instruct-v0.2.Q4_K.gguf) | Q4_K | 4.07GB |
| [Mistral-7B-Instruct-v0.2.Q4_K_M.gguf](https://huggingface.co/RichardErkhov/David-Xu_-_Mistral-7B-Instruct-v0.2-gguf/blob/main/Mistral-7B-Instruct-v0.2.Q4_K_M.gguf) | Q4_K_M | 4.07GB |
| [Mistral-7B-Instruct-v0.2.Q4_1.gguf](https://huggingface.co/RichardErkhov/David-Xu_-_Mistral-7B-Instruct-v0.2-gguf/blob/main/Mistral-7B-Instruct-v0.2.Q4_1.gguf) | Q4_1 | 4.24GB |
| [Mistral-7B-Instruct-v0.2.Q5_0.gguf](https://huggingface.co/RichardErkhov/David-Xu_-_Mistral-7B-Instruct-v0.2-gguf/blob/main/Mistral-7B-Instruct-v0.2.Q5_0.gguf) | Q5_0 | 4.65GB |
| [Mistral-7B-Instruct-v0.2.Q5_K_S.gguf](https://huggingface.co/RichardErkhov/David-Xu_-_Mistral-7B-Instruct-v0.2-gguf/blob/main/Mistral-7B-Instruct-v0.2.Q5_K_S.gguf) | Q5_K_S | 4.65GB |
| [Mistral-7B-Instruct-v0.2.Q5_K.gguf](https://huggingface.co/RichardErkhov/David-Xu_-_Mistral-7B-Instruct-v0.2-gguf/blob/main/Mistral-7B-Instruct-v0.2.Q5_K.gguf) | Q5_K | 4.78GB |
| [Mistral-7B-Instruct-v0.2.Q5_K_M.gguf](https://huggingface.co/RichardErkhov/David-Xu_-_Mistral-7B-Instruct-v0.2-gguf/blob/main/Mistral-7B-Instruct-v0.2.Q5_K_M.gguf) | Q5_K_M | 4.78GB |
| [Mistral-7B-Instruct-v0.2.Q5_1.gguf](https://huggingface.co/RichardErkhov/David-Xu_-_Mistral-7B-Instruct-v0.2-gguf/blob/main/Mistral-7B-Instruct-v0.2.Q5_1.gguf) | Q5_1 | 5.07GB |
| [Mistral-7B-Instruct-v0.2.Q6_K.gguf](https://huggingface.co/RichardErkhov/David-Xu_-_Mistral-7B-Instruct-v0.2-gguf/blob/main/Mistral-7B-Instruct-v0.2.Q6_K.gguf) | Q6_K | 5.53GB |
| [Mistral-7B-Instruct-v0.2.Q8_0.gguf](https://huggingface.co/RichardErkhov/David-Xu_-_Mistral-7B-Instruct-v0.2-gguf/blob/main/Mistral-7B-Instruct-v0.2.Q8_0.gguf) | Q8_0 | 7.17GB |
Original model description:
Entry not found
|
mradermacher/llama-polya-v2-i1-GGUF | mradermacher | 2024-06-27T14:18:26Z | 15,136 | 0 | transformers | [
"transformers",
"gguf",
"generated_from_trainer",
"en",
"base_model:Llama-Math/llama-polya-v2",
"endpoints_compatible",
"region:us"
] | null | 2024-06-27T12:58:28Z | ---
base_model: Llama-Math/llama-polya-v2
language:
- en
library_name: transformers
quantized_by: mradermacher
tags:
- generated_from_trainer
---
## About
<!-- ### quantize_version: 2 -->
<!-- ### output_tensor_quantised: 1 -->
<!-- ### convert_type: hf -->
<!-- ### vocab_type: -->
<!-- ### tags: nicoboss -->
weighted/imatrix quants of https://huggingface.co/Llama-Math/llama-polya-v2
<!-- provided-files -->
static quants are available at https://huggingface.co/mradermacher/llama-polya-v2-GGUF
## Usage
If you are unsure how to use GGUF files, refer to one of [TheBloke's
READMEs](https://huggingface.co/TheBloke/KafkaLM-70B-German-V0.1-GGUF) for
more details, including on how to concatenate multi-part files.
## Provided Quants
(sorted by size, not necessarily quality. IQ-quants are often preferable over similar sized non-IQ quants)
| Link | Type | Size/GB | Notes |
|:-----|:-----|--------:|:------|
| [GGUF](https://huggingface.co/mradermacher/llama-polya-v2-i1-GGUF/resolve/main/llama-polya-v2.i1-IQ1_S.gguf) | i1-IQ1_S | 1.6 | for the desperate |
| [GGUF](https://huggingface.co/mradermacher/llama-polya-v2-i1-GGUF/resolve/main/llama-polya-v2.i1-IQ1_M.gguf) | i1-IQ1_M | 1.8 | mostly desperate |
| [GGUF](https://huggingface.co/mradermacher/llama-polya-v2-i1-GGUF/resolve/main/llama-polya-v2.i1-IQ2_XXS.gguf) | i1-IQ2_XXS | 2.0 | |
| [GGUF](https://huggingface.co/mradermacher/llama-polya-v2-i1-GGUF/resolve/main/llama-polya-v2.i1-IQ2_XS.gguf) | i1-IQ2_XS | 2.1 | |
| [GGUF](https://huggingface.co/mradermacher/llama-polya-v2-i1-GGUF/resolve/main/llama-polya-v2.i1-IQ2_S.gguf) | i1-IQ2_S | 2.3 | |
| [GGUF](https://huggingface.co/mradermacher/llama-polya-v2-i1-GGUF/resolve/main/llama-polya-v2.i1-IQ2_M.gguf) | i1-IQ2_M | 2.5 | |
| [GGUF](https://huggingface.co/mradermacher/llama-polya-v2-i1-GGUF/resolve/main/llama-polya-v2.i1-Q2_K.gguf) | i1-Q2_K | 2.6 | IQ3_XXS probably better |
| [GGUF](https://huggingface.co/mradermacher/llama-polya-v2-i1-GGUF/resolve/main/llama-polya-v2.i1-IQ3_XXS.gguf) | i1-IQ3_XXS | 2.7 | lower quality |
| [GGUF](https://huggingface.co/mradermacher/llama-polya-v2-i1-GGUF/resolve/main/llama-polya-v2.i1-IQ3_XS.gguf) | i1-IQ3_XS | 2.9 | |
| [GGUF](https://huggingface.co/mradermacher/llama-polya-v2-i1-GGUF/resolve/main/llama-polya-v2.i1-IQ3_S.gguf) | i1-IQ3_S | 3.0 | beats Q3_K* |
| [GGUF](https://huggingface.co/mradermacher/llama-polya-v2-i1-GGUF/resolve/main/llama-polya-v2.i1-Q3_K_S.gguf) | i1-Q3_K_S | 3.0 | IQ3_XS probably better |
| [GGUF](https://huggingface.co/mradermacher/llama-polya-v2-i1-GGUF/resolve/main/llama-polya-v2.i1-IQ3_M.gguf) | i1-IQ3_M | 3.2 | |
| [GGUF](https://huggingface.co/mradermacher/llama-polya-v2-i1-GGUF/resolve/main/llama-polya-v2.i1-Q3_K_M.gguf) | i1-Q3_K_M | 3.4 | IQ3_S probably better |
| [GGUF](https://huggingface.co/mradermacher/llama-polya-v2-i1-GGUF/resolve/main/llama-polya-v2.i1-Q3_K_L.gguf) | i1-Q3_K_L | 3.7 | IQ3_M probably better |
| [GGUF](https://huggingface.co/mradermacher/llama-polya-v2-i1-GGUF/resolve/main/llama-polya-v2.i1-IQ4_XS.gguf) | i1-IQ4_XS | 3.7 | |
| [GGUF](https://huggingface.co/mradermacher/llama-polya-v2-i1-GGUF/resolve/main/llama-polya-v2.i1-Q4_0.gguf) | i1-Q4_0 | 3.9 | fast, low quality |
| [GGUF](https://huggingface.co/mradermacher/llama-polya-v2-i1-GGUF/resolve/main/llama-polya-v2.i1-Q4_K_S.gguf) | i1-Q4_K_S | 4.0 | optimal size/speed/quality |
| [GGUF](https://huggingface.co/mradermacher/llama-polya-v2-i1-GGUF/resolve/main/llama-polya-v2.i1-Q4_K_M.gguf) | i1-Q4_K_M | 4.2 | fast, recommended |
| [GGUF](https://huggingface.co/mradermacher/llama-polya-v2-i1-GGUF/resolve/main/llama-polya-v2.i1-Q5_K_S.gguf) | i1-Q5_K_S | 4.8 | |
| [GGUF](https://huggingface.co/mradermacher/llama-polya-v2-i1-GGUF/resolve/main/llama-polya-v2.i1-Q5_K_M.gguf) | i1-Q5_K_M | 4.9 | |
| [GGUF](https://huggingface.co/mradermacher/llama-polya-v2-i1-GGUF/resolve/main/llama-polya-v2.i1-Q6_K.gguf) | i1-Q6_K | 5.6 | practically like static Q6_K |
Here is a handy graph by ikawrakow comparing some lower-quality quant
types (lower is better):

And here are Artefact2's thoughts on the matter:
https://gist.github.com/Artefact2/b5f810600771265fc1e39442288e8ec9
## FAQ / Model Request
See https://huggingface.co/mradermacher/model_requests for some answers to
questions you might have and/or if you want some other model quantized.
## Thanks
I thank my company, [nethype GmbH](https://www.nethype.de/), for letting
me use its servers and providing upgrades to my workstation to enable
this work in my free time. Additional thanks to [@nicoboss](https://huggingface.co/nicoboss) for giving me access to his hardware for calculating the imatrix for these quants.
<!-- end -->
|
mradermacher/L3-sophie-improved-v2-GGUF | mradermacher | 2024-06-23T21:42:53Z | 15,132 | 0 | transformers | [
"transformers",
"gguf",
"mergekit",
"merge",
"en",
"base_model:Fischerboot/L3-sophie-improved-v2",
"endpoints_compatible",
"region:us"
] | null | 2024-06-23T15:14:22Z | ---
base_model: Fischerboot/L3-sophie-improved-v2
language:
- en
library_name: transformers
quantized_by: mradermacher
tags:
- mergekit
- merge
---
## About
<!-- ### quantize_version: 2 -->
<!-- ### output_tensor_quantised: 1 -->
<!-- ### convert_type: hf -->
<!-- ### vocab_type: -->
<!-- ### tags: -->
static quants of https://huggingface.co/Fischerboot/L3-sophie-improved-v2
<!-- provided-files -->
weighted/imatrix quants are available at https://huggingface.co/mradermacher/L3-sophie-improved-v2-i1-GGUF
## Usage
If you are unsure how to use GGUF files, refer to one of [TheBloke's
READMEs](https://huggingface.co/TheBloke/KafkaLM-70B-German-V0.1-GGUF) for
more details, including on how to concatenate multi-part files.
## Provided Quants
(sorted by size, not necessarily quality. IQ-quants are often preferable over similar sized non-IQ quants)
| Link | Type | Size/GB | Notes |
|:-----|:-----|--------:|:------|
| [GGUF](https://huggingface.co/mradermacher/L3-sophie-improved-v2-GGUF/resolve/main/L3-sophie-improved-v2.Q2_K.gguf) | Q2_K | 3.3 | |
| [GGUF](https://huggingface.co/mradermacher/L3-sophie-improved-v2-GGUF/resolve/main/L3-sophie-improved-v2.IQ3_XS.gguf) | IQ3_XS | 3.6 | |
| [GGUF](https://huggingface.co/mradermacher/L3-sophie-improved-v2-GGUF/resolve/main/L3-sophie-improved-v2.Q3_K_S.gguf) | Q3_K_S | 3.8 | |
| [GGUF](https://huggingface.co/mradermacher/L3-sophie-improved-v2-GGUF/resolve/main/L3-sophie-improved-v2.IQ3_S.gguf) | IQ3_S | 3.8 | beats Q3_K* |
| [GGUF](https://huggingface.co/mradermacher/L3-sophie-improved-v2-GGUF/resolve/main/L3-sophie-improved-v2.IQ3_M.gguf) | IQ3_M | 3.9 | |
| [GGUF](https://huggingface.co/mradermacher/L3-sophie-improved-v2-GGUF/resolve/main/L3-sophie-improved-v2.Q3_K_M.gguf) | Q3_K_M | 4.1 | lower quality |
| [GGUF](https://huggingface.co/mradermacher/L3-sophie-improved-v2-GGUF/resolve/main/L3-sophie-improved-v2.Q3_K_L.gguf) | Q3_K_L | 4.4 | |
| [GGUF](https://huggingface.co/mradermacher/L3-sophie-improved-v2-GGUF/resolve/main/L3-sophie-improved-v2.IQ4_XS.gguf) | IQ4_XS | 4.6 | |
| [GGUF](https://huggingface.co/mradermacher/L3-sophie-improved-v2-GGUF/resolve/main/L3-sophie-improved-v2.Q4_K_S.gguf) | Q4_K_S | 4.8 | fast, recommended |
| [GGUF](https://huggingface.co/mradermacher/L3-sophie-improved-v2-GGUF/resolve/main/L3-sophie-improved-v2.Q4_K_M.gguf) | Q4_K_M | 5.0 | fast, recommended |
| [GGUF](https://huggingface.co/mradermacher/L3-sophie-improved-v2-GGUF/resolve/main/L3-sophie-improved-v2.Q5_K_S.gguf) | Q5_K_S | 5.7 | |
| [GGUF](https://huggingface.co/mradermacher/L3-sophie-improved-v2-GGUF/resolve/main/L3-sophie-improved-v2.Q5_K_M.gguf) | Q5_K_M | 5.8 | |
| [GGUF](https://huggingface.co/mradermacher/L3-sophie-improved-v2-GGUF/resolve/main/L3-sophie-improved-v2.Q6_K.gguf) | Q6_K | 6.7 | very good quality |
| [GGUF](https://huggingface.co/mradermacher/L3-sophie-improved-v2-GGUF/resolve/main/L3-sophie-improved-v2.Q8_0.gguf) | Q8_0 | 8.6 | fast, best quality |
| [GGUF](https://huggingface.co/mradermacher/L3-sophie-improved-v2-GGUF/resolve/main/L3-sophie-improved-v2.f16.gguf) | f16 | 16.2 | 16 bpw, overkill |
Here is a handy graph by ikawrakow comparing some lower-quality quant
types (lower is better):

And here are Artefact2's thoughts on the matter:
https://gist.github.com/Artefact2/b5f810600771265fc1e39442288e8ec9
## FAQ / Model Request
See https://huggingface.co/mradermacher/model_requests for some answers to
questions you might have and/or if you want some other model quantized.
## Thanks
I thank my company, [nethype GmbH](https://www.nethype.de/), for letting
me use its servers and providing upgrades to my workstation to enable
this work in my free time.
<!-- end -->
|
lllyasviel/control_v11p_sd15_mlsd | lllyasviel | 2023-05-04T18:49:06Z | 15,129 | 10 | diffusers | [
"diffusers",
"safetensors",
"art",
"controlnet",
"stable-diffusion",
"controlnet-v1-1",
"image-to-image",
"arxiv:2302.05543",
"base_model:runwayml/stable-diffusion-v1-5",
"license:openrail",
"region:us"
] | image-to-image | 2023-04-14T19:24:23Z | ---
license: openrail
base_model: runwayml/stable-diffusion-v1-5
tags:
- art
- controlnet
- stable-diffusion
- controlnet-v1-1
- image-to-image
duplicated_from: ControlNet-1-1-preview/control_v11p_sd15_mlsd
---
# Controlnet - v1.1 - *MLSD Version*
**Controlnet v1.1** is the successor model of [Controlnet v1.0](https://huggingface.co/lllyasviel/ControlNet)
and was released in [lllyasviel/ControlNet-v1-1](https://huggingface.co/lllyasviel/ControlNet-v1-1) by [Lvmin Zhang](https://huggingface.co/lllyasviel).
This checkpoint is a conversion of [the original checkpoint](https://huggingface.co/lllyasviel/ControlNet-v1-1/blob/main/control_v11p_sd15_mlsd.pth) into `diffusers` format.
It can be used in combination with **Stable Diffusion**, such as [runwayml/stable-diffusion-v1-5](https://huggingface.co/runwayml/stable-diffusion-v1-5).
For more details, please also have a look at the [🧨 Diffusers docs](https://huggingface.co/docs/diffusers/api/pipelines/stable_diffusion/controlnet).
ControlNet is a neural network structure to control diffusion models by adding extra conditions.

This checkpoint corresponds to the ControlNet conditioned on **MLSD images**.
## Model Details
- **Developed by:** Lvmin Zhang, Maneesh Agrawala
- **Model type:** Diffusion-based text-to-image generation model
- **Language(s):** English
- **License:** [The CreativeML OpenRAIL M license](https://huggingface.co/spaces/CompVis/stable-diffusion-license) is an [Open RAIL M license](https://www.licenses.ai/blog/2022/8/18/naming-convention-of-responsible-ai-licenses), adapted from the work that [BigScience](https://bigscience.huggingface.co/) and [the RAIL Initiative](https://www.licenses.ai/) are jointly carrying in the area of responsible AI licensing. See also [the article about the BLOOM Open RAIL license](https://bigscience.huggingface.co/blog/the-bigscience-rail-license) on which our license is based.
- **Resources for more information:** [GitHub Repository](https://github.com/lllyasviel/ControlNet), [Paper](https://arxiv.org/abs/2302.05543).
- **Cite as:**
@misc{zhang2023adding,
title={Adding Conditional Control to Text-to-Image Diffusion Models},
author={Lvmin Zhang and Maneesh Agrawala},
year={2023},
eprint={2302.05543},
archivePrefix={arXiv},
primaryClass={cs.CV}
}
## Introduction
Controlnet was proposed in [*Adding Conditional Control to Text-to-Image Diffusion Models*](https://arxiv.org/abs/2302.05543) by
Lvmin Zhang, Maneesh Agrawala.
The abstract reads as follows:
*We present a neural network structure, ControlNet, to control pretrained large diffusion models to support additional input conditions.
The ControlNet learns task-specific conditions in an end-to-end way, and the learning is robust even when the training dataset is small (< 50k).
Moreover, training a ControlNet is as fast as fine-tuning a diffusion model, and the model can be trained on a personal devices.
Alternatively, if powerful computation clusters are available, the model can scale to large amounts (millions to billions) of data.
We report that large diffusion models like Stable Diffusion can be augmented with ControlNets to enable conditional inputs like edge maps, segmentation maps, keypoints, etc.
This may enrich the methods to control large diffusion models and further facilitate related applications.*
## Example
It is recommended to use the checkpoint with [Stable Diffusion v1-5](https://huggingface.co/runwayml/stable-diffusion-v1-5) as the checkpoint
has been trained on it.
Experimentally, the checkpoint can be used with other diffusion models such as dreamboothed stable diffusion.
**Note**: If you want to process an image to create the auxiliary conditioning, external dependencies are required as shown below:
1. Install https://github.com/patrickvonplaten/controlnet_aux
```sh
$ pip install controlnet_aux==0.3.0
```
2. Let's install `diffusers` and related packages:
```
$ pip install diffusers transformers accelerate
```
3. Run code:
```python
import torch
import os
from huggingface_hub import HfApi
from pathlib import Path
from diffusers.utils import load_image
from PIL import Image
import numpy as np
from controlnet_aux import MLSDdetector
from diffusers import (
ControlNetModel,
StableDiffusionControlNetPipeline,
UniPCMultistepScheduler,
)
checkpoint = "lllyasviel/control_v11p_sd15_mlsd"
image = load_image(
"https://huggingface.co/lllyasviel/control_v11p_sd15_mlsd/resolve/main/images/input.png"
)
prompt = "royal chamber with fancy bed"
processor = MLSDdetector.from_pretrained('lllyasviel/ControlNet')
control_image = processor(image)
control_image.save("./images/control.png")
controlnet = ControlNetModel.from_pretrained(checkpoint, torch_dtype=torch.float16)
pipe = StableDiffusionControlNetPipeline.from_pretrained(
"runwayml/stable-diffusion-v1-5", controlnet=controlnet, torch_dtype=torch.float16
)
pipe.scheduler = UniPCMultistepScheduler.from_config(pipe.scheduler.config)
pipe.enable_model_cpu_offload()
generator = torch.manual_seed(0)
image = pipe(prompt, num_inference_steps=30, generator=generator, image=control_image).images[0]
image.save('images/image_out.png')
```



## Other released checkpoints v1-1
The authors released 14 different checkpoints, each trained with [Stable Diffusion v1-5](https://huggingface.co/runwayml/stable-diffusion-v1-5)
on a different type of conditioning:
| Model Name | Control Image Overview| Condition Image | Control Image Example | Generated Image Example |
|---|---|---|---|---|
|[lllyasviel/control_v11p_sd15_canny](https://huggingface.co/lllyasviel/control_v11p_sd15_canny)<br/> | *Trained with canny edge detection* | A monochrome image with white edges on a black background.|<a href="https://huggingface.co/lllyasviel/control_v11p_sd15_canny/resolve/main/images/control.png"><img width="64" style="margin:0;padding:0;" src="https://huggingface.co/lllyasviel/control_v11p_sd15_canny/resolve/main/images/control.png"/></a>|<a href="https://huggingface.co/lllyasviel/control_v11p_sd15_canny/resolve/main/images/image_out.png"><img width="64" src="https://huggingface.co/lllyasviel/control_v11p_sd15_canny/resolve/main/images/image_out.png"/></a>|
|[lllyasviel/control_v11e_sd15_ip2p](https://huggingface.co/lllyasviel/control_v11e_sd15_ip2p)<br/> | *Trained with pixel to pixel instruction* | No condition .|<a href="https://huggingface.co/lllyasviel/control_v11e_sd15_ip2p/resolve/main/images/control.png"><img width="64" style="margin:0;padding:0;" src="https://huggingface.co/lllyasviel/control_v11e_sd15_ip2p/resolve/main/images/control.png"/></a>|<a href="https://huggingface.co/lllyasviel/control_v11e_sd15_ip2p/resolve/main/images/image_out.png"><img width="64" src="https://huggingface.co/lllyasviel/control_v11e_sd15_ip2p/resolve/main/images/image_out.png"/></a>|
|[lllyasviel/control_v11p_sd15_inpaint](https://huggingface.co/lllyasviel/control_v11p_sd15_inpaint)<br/> | Trained with image inpainting | No condition.|<a href="https://huggingface.co/lllyasviel/control_v11p_sd15_inpaint/resolve/main/images/control.png"><img width="64" style="margin:0;padding:0;" src="https://huggingface.co/lllyasviel/control_v11p_sd15_inpaint/resolve/main/images/control.png"/></a>|<a href="https://huggingface.co/lllyasviel/control_v11p_sd15_inpaint/resolve/main/images/output.png"><img width="64" src="https://huggingface.co/lllyasviel/control_v11p_sd15_inpaint/resolve/main/images/output.png"/></a>|
|[lllyasviel/control_v11p_sd15_mlsd](https://huggingface.co/lllyasviel/control_v11p_sd15_mlsd)<br/> | Trained with multi-level line segment detection | An image with annotated line segments.|<a href="https://huggingface.co/lllyasviel/control_v11p_sd15_mlsd/resolve/main/images/control.png"><img width="64" style="margin:0;padding:0;" src="https://huggingface.co/lllyasviel/control_v11p_sd15_mlsd/resolve/main/images/control.png"/></a>|<a href="https://huggingface.co/lllyasviel/control_v11p_sd15_mlsd/resolve/main/images/image_out.png"><img width="64" src="https://huggingface.co/lllyasviel/control_v11p_sd15_mlsd/resolve/main/images/image_out.png"/></a>|
|[lllyasviel/control_v11f1p_sd15_depth](https://huggingface.co/lllyasviel/control_v11f1p_sd15_depth)<br/> | Trained with depth estimation | An image with depth information, usually represented as a grayscale image.|<a href="https://huggingface.co/lllyasviel/control_v11f1p_sd15_depth/resolve/main/images/control.png"><img width="64" style="margin:0;padding:0;" src="https://huggingface.co/lllyasviel/control_v11f1p_sd15_depth/resolve/main/images/control.png"/></a>|<a href="https://huggingface.co/lllyasviel/control_v11f1p_sd15_depth/resolve/main/images/image_out.png"><img width="64" src="https://huggingface.co/lllyasviel/control_v11f1p_sd15_depth/resolve/main/images/image_out.png"/></a>|
|[lllyasviel/control_v11p_sd15_normalbae](https://huggingface.co/lllyasviel/control_v11p_sd15_normalbae)<br/> | Trained with surface normal estimation | An image with surface normal information, usually represented as a color-coded image.|<a href="https://huggingface.co/lllyasviel/control_v11p_sd15_normalbae/resolve/main/images/control.png"><img width="64" style="margin:0;padding:0;" src="https://huggingface.co/lllyasviel/control_v11p_sd15_normalbae/resolve/main/images/control.png"/></a>|<a href="https://huggingface.co/lllyasviel/control_v11p_sd15_normalbae/resolve/main/images/image_out.png"><img width="64" src="https://huggingface.co/lllyasviel/control_v11p_sd15_normalbae/resolve/main/images/image_out.png"/></a>|
|[lllyasviel/control_v11p_sd15_seg](https://huggingface.co/lllyasviel/control_v11p_sd15_seg)<br/> | Trained with image segmentation | An image with segmented regions, usually represented as a color-coded image.|<a href="https://huggingface.co/lllyasviel/control_v11p_sd15_seg/resolve/main/images/control.png"><img width="64" style="margin:0;padding:0;" src="https://huggingface.co/lllyasviel/control_v11p_sd15_seg/resolve/main/images/control.png"/></a>|<a href="https://huggingface.co/lllyasviel/control_v11p_sd15_seg/resolve/main/images/image_out.png"><img width="64" src="https://huggingface.co/lllyasviel/control_v11p_sd15_seg/resolve/main/images/image_out.png"/></a>|
|[lllyasviel/control_v11p_sd15_lineart](https://huggingface.co/lllyasviel/control_v11p_sd15_lineart)<br/> | Trained with line art generation | An image with line art, usually black lines on a white background.|<a href="https://huggingface.co/lllyasviel/control_v11p_sd15_lineart/resolve/main/images/control.png"><img width="64" style="margin:0;padding:0;" src="https://huggingface.co/lllyasviel/control_v11p_sd15_lineart/resolve/main/images/control.png"/></a>|<a href="https://huggingface.co/lllyasviel/control_v11p_sd15_lineart/resolve/main/images/image_out.png"><img width="64" src="https://huggingface.co/lllyasviel/control_v11p_sd15_lineart/resolve/main/images/image_out.png"/></a>|
|[lllyasviel/control_v11p_sd15s2_lineart_anime](https://huggingface.co/lllyasviel/control_v11p_sd15s2_lineart_anime)<br/> | Trained with anime line art generation | An image with anime-style line art.|<a href="https://huggingface.co/lllyasviel/control_v11p_sd15s2_lineart_anime/resolve/main/images/control.png"><img width="64" style="margin:0;padding:0;" src="https://huggingface.co/lllyasviel/control_v11p_sd15s2_lineart_anime/resolve/main/images/control.png"/></a>|<a href="https://huggingface.co/lllyasviel/control_v11p_sd15s2_lineart_anime/resolve/main/images/image_out.png"><img width="64" src="https://huggingface.co/lllyasviel/control_v11p_sd15s2_lineart_anime/resolve/main/images/image_out.png"/></a>|
|[lllyasviel/control_v11p_sd15_openpose](https://huggingface.co/lllyasviel/control_v11p_sd15s2_lineart_anime)<br/> | Trained with human pose estimation | An image with human poses, usually represented as a set of keypoints or skeletons.|<a href="https://huggingface.co/lllyasviel/control_v11p_sd15_openpose/resolve/main/images/control.png"><img width="64" style="margin:0;padding:0;" src="https://huggingface.co/lllyasviel/control_v11p_sd15_openpose/resolve/main/images/control.png"/></a>|<a href="https://huggingface.co/lllyasviel/control_v11p_sd15_openpose/resolve/main/images/image_out.png"><img width="64" src="https://huggingface.co/lllyasviel/control_v11p_sd15_openpose/resolve/main/images/image_out.png"/></a>|
|[lllyasviel/control_v11p_sd15_scribble](https://huggingface.co/lllyasviel/control_v11p_sd15_scribble)<br/> | Trained with scribble-based image generation | An image with scribbles, usually random or user-drawn strokes.|<a href="https://huggingface.co/lllyasviel/control_v11p_sd15_scribble/resolve/main/images/control.png"><img width="64" style="margin:0;padding:0;" src="https://huggingface.co/lllyasviel/control_v11p_sd15_scribble/resolve/main/images/control.png"/></a>|<a href="https://huggingface.co/lllyasviel/control_v11p_sd15_scribble/resolve/main/images/image_out.png"><img width="64" src="https://huggingface.co/lllyasviel/control_v11p_sd15_scribble/resolve/main/images/image_out.png"/></a>|
|[lllyasviel/control_v11p_sd15_softedge](https://huggingface.co/lllyasviel/control_v11p_sd15_softedge)<br/> | Trained with soft edge image generation | An image with soft edges, usually to create a more painterly or artistic effect.|<a href="https://huggingface.co/lllyasviel/control_v11p_sd15_softedge/resolve/main/images/control.png"><img width="64" style="margin:0;padding:0;" src="https://huggingface.co/lllyasviel/control_v11p_sd15_softedge/resolve/main/images/control.png"/></a>|<a href="https://huggingface.co/lllyasviel/control_v11p_sd15_softedge/resolve/main/images/image_out.png"><img width="64" src="https://huggingface.co/lllyasviel/control_v11p_sd15_softedge/resolve/main/images/image_out.png"/></a>|
|[lllyasviel/control_v11e_sd15_shuffle](https://huggingface.co/lllyasviel/control_v11e_sd15_shuffle)<br/> | Trained with image shuffling | An image with shuffled patches or regions.|<a href="https://huggingface.co/lllyasviel/control_v11e_sd15_shuffle/resolve/main/images/control.png"><img width="64" style="margin:0;padding:0;" src="https://huggingface.co/lllyasviel/control_v11e_sd15_shuffle/resolve/main/images/control.png"/></a>|<a href="https://huggingface.co/lllyasviel/control_v11e_sd15_shuffle/resolve/main/images/image_out.png"><img width="64" src="https://huggingface.co/lllyasviel/control_v11e_sd15_shuffle/resolve/main/images/image_out.png"/></a>|
|[lllyasviel/control_v11f1e_sd15_tile](https://huggingface.co/lllyasviel/control_v11f1e_sd15_tile)<br/> | Trained with image tiling | A blurry image or part of an image .|<a href="https://huggingface.co/lllyasviel/control_v11f1e_sd15_tile/resolve/main/images/original.png"><img width="64" style="margin:0;padding:0;" src="https://huggingface.co/lllyasviel/control_v11f1e_sd15_tile/resolve/main/images/original.png"/></a>|<a href="https://huggingface.co/lllyasviel/control_v11f1e_sd15_tile/resolve/main/images/output.png"><img width="64" src="https://huggingface.co/lllyasviel/control_v11f1e_sd15_tile/resolve/main/images/output.png"/></a>|
## Improvements in MLSD 1.1:
- The training dataset of previous cnet 1.0 has several problems including (1) a small group of greyscale human images are duplicated thousands of times (!!), causing the previous model somewhat likely to generate grayscale human images; (2) some images has low quality, very blurry, or significant JPEG artifacts; (3) a small group of images has wrong paired prompts caused by a mistake in our data processing scripts. The new model fixed all problems of the training dataset and should be more reasonable in many cases.
- We enlarged the training dataset by adding 300K more images by using MLSD to find images with more than 16 straight lines in it.
- Some reasonable data augmentations are applied to training, like random left-right flipping.
- Resumed from MLSD 1.0 with continued training with 200 GPU hours of A100 80G.
## More information
For more information, please also have a look at the [Diffusers ControlNet Blog Post](https://huggingface.co/blog/controlnet) and have a look at the [official docs](https://github.com/lllyasviel/ControlNet-v1-1-nightly). |
mradermacher/Intellplay-Llama-3-8B-i1-GGUF | mradermacher | 2024-07-01T11:39:09Z | 15,127 | 1 | transformers | [
"transformers",
"gguf",
"merge",
"mergekit",
"lazymergekit",
"en",
"base_model:bunnycore/Intellplay-Llama-3-8B",
"license:apache-2.0",
"endpoints_compatible",
"region:us"
] | null | 2024-07-01T09:22:47Z | ---
base_model: bunnycore/Intellplay-Llama-3-8B
language:
- en
library_name: transformers
license: apache-2.0
quantized_by: mradermacher
tags:
- merge
- mergekit
- lazymergekit
---
## About
<!-- ### quantize_version: 2 -->
<!-- ### output_tensor_quantised: 1 -->
<!-- ### convert_type: hf -->
<!-- ### vocab_type: -->
<!-- ### tags: nicoboss -->
weighted/imatrix quants of https://huggingface.co/bunnycore/Intellplay-Llama-3-8B
<!-- provided-files -->
static quants are available at https://huggingface.co/mradermacher/Intellplay-Llama-3-8B-GGUF
## Usage
If you are unsure how to use GGUF files, refer to one of [TheBloke's
READMEs](https://huggingface.co/TheBloke/KafkaLM-70B-German-V0.1-GGUF) for
more details, including on how to concatenate multi-part files.
## Provided Quants
(sorted by size, not necessarily quality. IQ-quants are often preferable over similar sized non-IQ quants)
| Link | Type | Size/GB | Notes |
|:-----|:-----|--------:|:------|
| [GGUF](https://huggingface.co/mradermacher/Intellplay-Llama-3-8B-i1-GGUF/resolve/main/Intellplay-Llama-3-8B.i1-IQ1_S.gguf) | i1-IQ1_S | 2.1 | for the desperate |
| [GGUF](https://huggingface.co/mradermacher/Intellplay-Llama-3-8B-i1-GGUF/resolve/main/Intellplay-Llama-3-8B.i1-IQ1_M.gguf) | i1-IQ1_M | 2.3 | mostly desperate |
| [GGUF](https://huggingface.co/mradermacher/Intellplay-Llama-3-8B-i1-GGUF/resolve/main/Intellplay-Llama-3-8B.i1-IQ2_XXS.gguf) | i1-IQ2_XXS | 2.5 | |
| [GGUF](https://huggingface.co/mradermacher/Intellplay-Llama-3-8B-i1-GGUF/resolve/main/Intellplay-Llama-3-8B.i1-IQ2_XS.gguf) | i1-IQ2_XS | 2.7 | |
| [GGUF](https://huggingface.co/mradermacher/Intellplay-Llama-3-8B-i1-GGUF/resolve/main/Intellplay-Llama-3-8B.i1-IQ2_S.gguf) | i1-IQ2_S | 2.9 | |
| [GGUF](https://huggingface.co/mradermacher/Intellplay-Llama-3-8B-i1-GGUF/resolve/main/Intellplay-Llama-3-8B.i1-IQ2_M.gguf) | i1-IQ2_M | 3.0 | |
| [GGUF](https://huggingface.co/mradermacher/Intellplay-Llama-3-8B-i1-GGUF/resolve/main/Intellplay-Llama-3-8B.i1-Q2_K.gguf) | i1-Q2_K | 3.3 | IQ3_XXS probably better |
| [GGUF](https://huggingface.co/mradermacher/Intellplay-Llama-3-8B-i1-GGUF/resolve/main/Intellplay-Llama-3-8B.i1-IQ3_XXS.gguf) | i1-IQ3_XXS | 3.4 | lower quality |
| [GGUF](https://huggingface.co/mradermacher/Intellplay-Llama-3-8B-i1-GGUF/resolve/main/Intellplay-Llama-3-8B.i1-IQ3_XS.gguf) | i1-IQ3_XS | 3.6 | |
| [GGUF](https://huggingface.co/mradermacher/Intellplay-Llama-3-8B-i1-GGUF/resolve/main/Intellplay-Llama-3-8B.i1-Q3_K_S.gguf) | i1-Q3_K_S | 3.8 | IQ3_XS probably better |
| [GGUF](https://huggingface.co/mradermacher/Intellplay-Llama-3-8B-i1-GGUF/resolve/main/Intellplay-Llama-3-8B.i1-IQ3_S.gguf) | i1-IQ3_S | 3.8 | beats Q3_K* |
| [GGUF](https://huggingface.co/mradermacher/Intellplay-Llama-3-8B-i1-GGUF/resolve/main/Intellplay-Llama-3-8B.i1-IQ3_M.gguf) | i1-IQ3_M | 3.9 | |
| [GGUF](https://huggingface.co/mradermacher/Intellplay-Llama-3-8B-i1-GGUF/resolve/main/Intellplay-Llama-3-8B.i1-Q3_K_M.gguf) | i1-Q3_K_M | 4.1 | IQ3_S probably better |
| [GGUF](https://huggingface.co/mradermacher/Intellplay-Llama-3-8B-i1-GGUF/resolve/main/Intellplay-Llama-3-8B.i1-Q3_K_L.gguf) | i1-Q3_K_L | 4.4 | IQ3_M probably better |
| [GGUF](https://huggingface.co/mradermacher/Intellplay-Llama-3-8B-i1-GGUF/resolve/main/Intellplay-Llama-3-8B.i1-IQ4_XS.gguf) | i1-IQ4_XS | 4.5 | |
| [GGUF](https://huggingface.co/mradermacher/Intellplay-Llama-3-8B-i1-GGUF/resolve/main/Intellplay-Llama-3-8B.i1-Q4_0.gguf) | i1-Q4_0 | 4.8 | fast, low quality |
| [GGUF](https://huggingface.co/mradermacher/Intellplay-Llama-3-8B-i1-GGUF/resolve/main/Intellplay-Llama-3-8B.i1-Q4_K_S.gguf) | i1-Q4_K_S | 4.8 | optimal size/speed/quality |
| [GGUF](https://huggingface.co/mradermacher/Intellplay-Llama-3-8B-i1-GGUF/resolve/main/Intellplay-Llama-3-8B.i1-Q4_K_M.gguf) | i1-Q4_K_M | 5.0 | fast, recommended |
| [GGUF](https://huggingface.co/mradermacher/Intellplay-Llama-3-8B-i1-GGUF/resolve/main/Intellplay-Llama-3-8B.i1-Q5_K_S.gguf) | i1-Q5_K_S | 5.7 | |
| [GGUF](https://huggingface.co/mradermacher/Intellplay-Llama-3-8B-i1-GGUF/resolve/main/Intellplay-Llama-3-8B.i1-Q5_K_M.gguf) | i1-Q5_K_M | 5.8 | |
| [GGUF](https://huggingface.co/mradermacher/Intellplay-Llama-3-8B-i1-GGUF/resolve/main/Intellplay-Llama-3-8B.i1-Q6_K.gguf) | i1-Q6_K | 6.7 | practically like static Q6_K |
Here is a handy graph by ikawrakow comparing some lower-quality quant
types (lower is better):

And here are Artefact2's thoughts on the matter:
https://gist.github.com/Artefact2/b5f810600771265fc1e39442288e8ec9
## FAQ / Model Request
See https://huggingface.co/mradermacher/model_requests for some answers to
questions you might have and/or if you want some other model quantized.
## Thanks
I thank my company, [nethype GmbH](https://www.nethype.de/), for letting
me use its servers and providing upgrades to my workstation to enable
this work in my free time. Additional thanks to [@nicoboss](https://huggingface.co/nicoboss) for giving me access to his hardware for calculating the imatrix for these quants.
<!-- end -->
|
nickypro/tinyllama-15M | nickypro | 2024-04-03T06:53:38Z | 15,122 | 6 | transformers | [
"transformers",
"pytorch",
"safetensors",
"llama",
"text-generation",
"license:mit",
"autotrain_compatible",
"endpoints_compatible",
"text-generation-inference",
"region:us"
] | text-generation | 2023-09-16T12:53:54Z | ---
license: mit
---
This is the 15M parameter Llama 2 architecture model trained on the TinyStories dataset.
These are converted from
[karpathy/tinyllamas](https://huggingface.co/karpathy/tinyllamas).
See the [llama2.c](https://github.com/karpathy/llama2.c) project for more details. |
mradermacher/black_stheno_8B_v1-GGUF | mradermacher | 2024-06-26T20:28:14Z | 15,120 | 0 | transformers | [
"transformers",
"gguf",
"mergekit",
"merge",
"en",
"base_model:banelingz/black_stheno_8B_v1",
"endpoints_compatible",
"region:us"
] | null | 2024-06-24T16:42:52Z | ---
base_model: banelingz/black_stheno_8B_v1
language:
- en
library_name: transformers
quantized_by: mradermacher
tags:
- mergekit
- merge
---
## About
<!-- ### quantize_version: 2 -->
<!-- ### output_tensor_quantised: 1 -->
<!-- ### convert_type: hf -->
<!-- ### vocab_type: -->
<!-- ### tags: -->
static quants of https://huggingface.co/banelingz/black_stheno_8B_v1
<!-- provided-files -->
weighted/imatrix quants are available at https://huggingface.co/mradermacher/black_stheno_8B_v1-i1-GGUF
## Usage
If you are unsure how to use GGUF files, refer to one of [TheBloke's
READMEs](https://huggingface.co/TheBloke/KafkaLM-70B-German-V0.1-GGUF) for
more details, including on how to concatenate multi-part files.
## Provided Quants
(sorted by size, not necessarily quality. IQ-quants are often preferable over similar sized non-IQ quants)
| Link | Type | Size/GB | Notes |
|:-----|:-----|--------:|:------|
| [GGUF](https://huggingface.co/mradermacher/black_stheno_8B_v1-GGUF/resolve/main/black_stheno_8B_v1.Q2_K.gguf) | Q2_K | 3.3 | |
| [GGUF](https://huggingface.co/mradermacher/black_stheno_8B_v1-GGUF/resolve/main/black_stheno_8B_v1.IQ3_XS.gguf) | IQ3_XS | 3.6 | |
| [GGUF](https://huggingface.co/mradermacher/black_stheno_8B_v1-GGUF/resolve/main/black_stheno_8B_v1.Q3_K_S.gguf) | Q3_K_S | 3.8 | |
| [GGUF](https://huggingface.co/mradermacher/black_stheno_8B_v1-GGUF/resolve/main/black_stheno_8B_v1.IQ3_S.gguf) | IQ3_S | 3.8 | beats Q3_K* |
| [GGUF](https://huggingface.co/mradermacher/black_stheno_8B_v1-GGUF/resolve/main/black_stheno_8B_v1.IQ3_M.gguf) | IQ3_M | 3.9 | |
| [GGUF](https://huggingface.co/mradermacher/black_stheno_8B_v1-GGUF/resolve/main/black_stheno_8B_v1.Q3_K_M.gguf) | Q3_K_M | 4.1 | lower quality |
| [GGUF](https://huggingface.co/mradermacher/black_stheno_8B_v1-GGUF/resolve/main/black_stheno_8B_v1.Q3_K_L.gguf) | Q3_K_L | 4.4 | |
| [GGUF](https://huggingface.co/mradermacher/black_stheno_8B_v1-GGUF/resolve/main/black_stheno_8B_v1.IQ4_XS.gguf) | IQ4_XS | 4.6 | |
| [GGUF](https://huggingface.co/mradermacher/black_stheno_8B_v1-GGUF/resolve/main/black_stheno_8B_v1.Q4_K_S.gguf) | Q4_K_S | 4.8 | fast, recommended |
| [GGUF](https://huggingface.co/mradermacher/black_stheno_8B_v1-GGUF/resolve/main/black_stheno_8B_v1.Q4_K_M.gguf) | Q4_K_M | 5.0 | fast, recommended |
| [GGUF](https://huggingface.co/mradermacher/black_stheno_8B_v1-GGUF/resolve/main/black_stheno_8B_v1.Q5_K_S.gguf) | Q5_K_S | 5.7 | |
| [GGUF](https://huggingface.co/mradermacher/black_stheno_8B_v1-GGUF/resolve/main/black_stheno_8B_v1.Q5_K_M.gguf) | Q5_K_M | 5.8 | |
| [GGUF](https://huggingface.co/mradermacher/black_stheno_8B_v1-GGUF/resolve/main/black_stheno_8B_v1.Q6_K.gguf) | Q6_K | 6.7 | very good quality |
| [GGUF](https://huggingface.co/mradermacher/black_stheno_8B_v1-GGUF/resolve/main/black_stheno_8B_v1.Q8_0.gguf) | Q8_0 | 8.6 | fast, best quality |
| [GGUF](https://huggingface.co/mradermacher/black_stheno_8B_v1-GGUF/resolve/main/black_stheno_8B_v1.f16.gguf) | f16 | 16.2 | 16 bpw, overkill |
Here is a handy graph by ikawrakow comparing some lower-quality quant
types (lower is better):

And here are Artefact2's thoughts on the matter:
https://gist.github.com/Artefact2/b5f810600771265fc1e39442288e8ec9
## FAQ / Model Request
See https://huggingface.co/mradermacher/model_requests for some answers to
questions you might have and/or if you want some other model quantized.
## Thanks
I thank my company, [nethype GmbH](https://www.nethype.de/), for letting
me use its servers and providing upgrades to my workstation to enable
this work in my free time.
<!-- end -->
|
google/gemma-2-9b | google | 2024-07-02T20:00:09Z | 15,110 | 282 | transformers | [
"transformers",
"safetensors",
"gemma2",
"text-generation",
"arxiv:2009.03300",
"arxiv:1905.07830",
"arxiv:1911.11641",
"arxiv:1904.09728",
"arxiv:1905.10044",
"arxiv:1907.10641",
"arxiv:1811.00937",
"arxiv:1809.02789",
"arxiv:1911.01547",
"arxiv:1705.03551",
"arxiv:2107.03374",
"arxiv:2108.07732",
"arxiv:2110.14168",
"arxiv:2009.11462",
"arxiv:2101.11718",
"arxiv:2110.08193",
"arxiv:1804.09301",
"arxiv:2109.07958",
"arxiv:1804.06876",
"arxiv:2103.03874",
"arxiv:2304.06364",
"arxiv:2206.04615",
"arxiv:2203.09509",
"license:gemma",
"autotrain_compatible",
"endpoints_compatible",
"text-generation-inference",
"region:us"
] | text-generation | 2024-06-24T08:10:30Z | ---
license: gemma
library_name: transformers
pipeline_tag: text-generation
extra_gated_heading: Access Gemma on Hugging Face
extra_gated_prompt: >-
To access Gemma on Hugging Face, you’re required to review and agree to
Google’s usage license. To do this, please ensure you’re logged in to Hugging
Face and click below. Requests are processed immediately.
extra_gated_button_content: Acknowledge license
---
# Gemma 2 model card
**Model Page**: [Gemma](https://ai.google.dev/gemma/docs)
**Resources and Technical Documentation**:
* [Responsible Generative AI Toolkit][rai-toolkit]
* [Gemma on Kaggle][kaggle-gemma]
* [Gemma on Vertex Model Garden][vertex-mg-gemma]
**Terms of Use**: [Terms](https://www.kaggle.com/models/google/gemma/license/consent/verify/huggingface?returnModelRepoId=google/gemma-2-9b)
**Authors**: Google
## Model Information
Summary description and brief definition of inputs and outputs.
### Description
Gemma is a family of lightweight, state-of-the-art open models from Google,
built from the same research and technology used to create the Gemini models.
They are text-to-text, decoder-only large language models, available in English,
with open weights for both pre-trained variants and instruction-tuned variants.
Gemma models are well-suited for a variety of text generation tasks, including
question answering, summarization, and reasoning. Their relatively small size
makes it possible to deploy them in environments with limited resources such as
a laptop, desktop or your own cloud infrastructure, democratizing access to
state of the art AI models and helping foster innovation for everyone.
### Usage
Below we share some code snippets on how to get quickly started with running the model. First make sure to `pip install -U transformers`, then copy the snippet from the section that is relevant for your usecase.
#### Running the model on a single / multi GPU
```python
# pip install accelerate
from transformers import AutoTokenizer, AutoModelForCausalLM
import torch
tokenizer = AutoTokenizer.from_pretrained("google/gemma-2-9b")
model = AutoModelForCausalLM.from_pretrained(
"google/gemma-2-9b",
device_map="auto",
torch_dtype=torch.bfloat16
)
input_text = "Write me a poem about Machine Learning."
input_ids = tokenizer(input_text, return_tensors="pt").to("cuda")
outputs = model.generate(**input_ids)
print(tokenizer.decode(outputs[0]))
```
<a name="precisions"></a>
#### Running the model on a GPU using different precisions
The native weights of this model were exported in `bfloat16` precision.
You can also use `float32` if you skip the dtype, but no precision increase will occur (model weights will just be upcasted to `float32`). See examples below.
* _Upcasting to `torch.float32`_
```python
# pip install accelerate
from transformers import AutoTokenizer, AutoModelForCausalLM
tokenizer = AutoTokenizer.from_pretrained("google/gemma-2-9b")
model = AutoModelForCausalLM.from_pretrained(
"google/gemma-2-9b",
device_map="auto")
input_text = "Write me a poem about Machine Learning."
input_ids = tokenizer(input_text, return_tensors="pt").to("cuda")
outputs = model.generate(**input_ids)
print(tokenizer.decode(outputs[0]))
```
#### Quantized Versions through `bitsandbytes`
* _Using 8-bit precision (int8)_
```python
# pip install bitsandbytes accelerate
from transformers import AutoTokenizer, AutoModelForCausalLM, BitsAndBytesConfig
quantization_config = BitsAndBytesConfig(load_in_8bit=True)
tokenizer = AutoTokenizer.from_pretrained("google/gemma-2-9b")
model = AutoModelForCausalLM.from_pretrained(
"google/gemma-2-9b",
quantization_config=quantization_config)
input_text = "Write me a poem about Machine Learning."
input_ids = tokenizer(input_text, return_tensors="pt").to("cuda")
outputs = model.generate(**input_ids)
print(tokenizer.decode(outputs[0]))
```
* _Using 4-bit precision_
```python
# pip install bitsandbytes accelerate
from transformers import AutoTokenizer, AutoModelForCausalLM, BitsAndBytesConfig
quantization_config = BitsAndBytesConfig(load_in_4bit=True)
tokenizer = AutoTokenizer.from_pretrained("google/gemma-2-9b")
model = AutoModelForCausalLM.from_pretrained(
"google/gemma-2-9b",
quantization_config=quantization_config)
input_text = "Write me a poem about Machine Learning."
input_ids = tokenizer(input_text, return_tensors="pt").to("cuda")
outputs = model.generate(**input_ids)
print(tokenizer.decode(outputs[0]))
```
#### Other optimizations
* _Flash Attention 2_
First make sure to install `flash-attn` in your environment `pip install flash-attn`
```diff
model = AutoModelForCausalLM.from_pretrained(
model_id,
torch_dtype=torch.float16,
+ attn_implementation="flash_attention_2"
).to(0)
```
### Inputs and outputs
* **Input:** Text string, such as a question, a prompt, or a document to be
summarized.
* **Output:** Generated English-language text in response to the input, such
as an answer to a question, or a summary of a document.
### Citation
```none
@article{gemma_2024,
title={Gemma},
url={https://www.kaggle.com/m/3301},
DOI={10.34740/KAGGLE/M/3301},
publisher={Kaggle},
author={Gemma Team},
year={2024}
}
```
## Model Data
Data used for model training and how the data was processed.
### Training Dataset
These models were trained on a dataset of text data that includes a wide variety of sources. The 27B model was trained with 13 trillion tokens and the 9B model was trained with 8 trillion tokens.
Here are the key components:
* Web Documents: A diverse collection of web text ensures the model is exposed
to a broad range of linguistic styles, topics, and vocabulary. Primarily
English-language content.
* Code: Exposing the model to code helps it to learn the syntax and patterns of
programming languages, which improves its ability to generate code or
understand code-related questions.
* Mathematics: Training on mathematical text helps the model learn logical
reasoning, symbolic representation, and to address mathematical queries.
The combination of these diverse data sources is crucial for training a powerful
language model that can handle a wide variety of different tasks and text
formats.
### Data Preprocessing
Here are the key data cleaning and filtering methods applied to the training
data:
* CSAM Filtering: Rigorous CSAM (Child Sexual Abuse Material) filtering was
applied at multiple stages in the data preparation process to ensure the
exclusion of harmful and illegal content.
* Sensitive Data Filtering: As part of making Gemma pre-trained models safe and
reliable, automated techniques were used to filter out certain personal
information and other sensitive data from training sets.
* Additional methods: Filtering based on content quality and safety in line with
[our policies][safety-policies].
## Implementation Information
Details about the model internals.
### Hardware
Gemma was trained using the latest generation of
[Tensor Processing Unit (TPU)][tpu] hardware (TPUv5p).
Training large language models requires significant computational power. TPUs,
designed specifically for matrix operations common in machine learning, offer
several advantages in this domain:
* Performance: TPUs are specifically designed to handle the massive computations
involved in training LLMs. They can speed up training considerably compared to
CPUs.
* Memory: TPUs often come with large amounts of high-bandwidth memory, allowing
for the handling of large models and batch sizes during training. This can
lead to better model quality.
* Scalability: TPU Pods (large clusters of TPUs) provide a scalable solution for
handling the growing complexity of large foundation models. You can distribute
training across multiple TPU devices for faster and more efficient processing.
* Cost-effectiveness: In many scenarios, TPUs can provide a more cost-effective
solution for training large models compared to CPU-based infrastructure,
especially when considering the time and resources saved due to faster
training.
* These advantages are aligned with
[Google's commitments to operate sustainably][sustainability].
### Software
Training was done using [JAX][jax] and [ML Pathways][ml-pathways].
JAX allows researchers to take advantage of the latest generation of hardware,
including TPUs, for faster and more efficient training of large models.
ML Pathways is Google's latest effort to build artificially intelligent systems
capable of generalizing across multiple tasks. This is specially suitable for
[foundation models][foundation-models], including large language models like
these ones.
Together, JAX and ML Pathways are used as described in the
[paper about the Gemini family of models][gemini-2-paper]; "the 'single
controller' programming model of Jax and Pathways allows a single Python
process to orchestrate the entire training run, dramatically simplifying the
development workflow."
## Evaluation
Model evaluation metrics and results.
### Benchmark Results
These models were evaluated against a large collection of different datasets and
metrics to cover different aspects of text generation:
| Benchmark | Metric | Gemma PT 9B | Gemma PT 27B |
| ------------------------------ | ------------- | ----------- | ------------ |
| [MMLU][mmlu] | 5-shot, top-1 | 71.3 | 75.2 |
| [HellaSwag][hellaswag] | 10-shot | 81.9 | 86.4 |
| [PIQA][piqa] | 0-shot | 81.7 | 83.2 |
| [SocialIQA][socialiqa] | 0-shot | 53.4 | 53.7 |
| [BoolQ][boolq] | 0-shot | 84.2 | 84.8 |
| [WinoGrande][winogrande] | partial score | 80.6 | 83.7 |
| [ARC-e][arc] | 0-shot | 88.0 | 88.6 |
| [ARC-c][arc] | 25-shot | 68.4 | 71.4 |
| [TriviaQA][triviaqa] | 5-shot | 76.6 | 83.7 |
| [Natural Questions][naturalq] | 5-shot | 29.2 | 34.5 |
| [HumanEval][humaneval] | pass@1 | 40.2 | 51.8 |
| [MBPP][mbpp] | 3-shot | 52.4 | 62.6 |
| [GSM8K][gsm8k] | 5-shot, maj@1 | 68.6 | 74.0 |
| [MATH][math] | 4-shot | 36.6 | 42.3 |
| [AGIEval][agieval] | 3-5-shot | 52.8 | 55.1 |
| [BIG-Bench][big-bench] | 3-shot, CoT | 68.2 | 74.9 |
| ------------------------------ | ------------- | ----------- | ------------ |
## Ethics and Safety
Ethics and safety evaluation approach and results.
### Evaluation Approach
Our evaluation methods include structured evaluations and internal red-teaming
testing of relevant content policies. Red-teaming was conducted by a number of
different teams, each with different goals and human evaluation metrics. These
models were evaluated against a number of different categories relevant to
ethics and safety, including:
* Text-to-Text Content Safety: Human evaluation on prompts covering safety
policies including child sexual abuse and exploitation, harassment, violence
and gore, and hate speech.
* Text-to-Text Representational Harms: Benchmark against relevant academic
datasets such as [WinoBias][winobias] and [BBQ Dataset][bbq].
* Memorization: Automated evaluation of memorization of training data, including
the risk of personally identifiable information exposure.
* Large-scale harm: Tests for "dangerous capabilities," such as chemical,
biological, radiological, and nuclear (CBRN) risks.
### Evaluation Results
The results of ethics and safety evaluations are within acceptable thresholds
for meeting [internal policies][safety-policies] for categories such as child
safety, content safety, representational harms, memorization, large-scale harms.
On top of robust internal evaluations, the results of well-known safety
benchmarks like BBQ, BOLD, Winogender, Winobias, RealToxicity, and TruthfulQA
are shown here.
#### Gemma 2.0
| Benchmark | Metric | Gemma 2 IT 9B | Gemma 2 IT 27B |
| ------------------------ | ------------- | --------------- | ---------------- |
| [RealToxicity][realtox] | average | 8.25 | 8.84 |
| [CrowS-Pairs][crows] | top-1 | 37.47 | 36.67 |
| [BBQ Ambig][bbq] | 1-shot, top-1 | 88.58 | 85.99 |
| [BBQ Disambig][bbq] | top-1 | 82.67 | 86.94 |
| [Winogender][winogender] | top-1 | 79.17 | 77.22 |
| [TruthfulQA][truthfulqa] | | 50.27 | 51.60 |
| [Winobias 1_2][winobias] | | 78.09 | 81.94 |
| [Winobias 2_2][winobias] | | 95.32 | 97.22 |
| [Toxigen][toxigen] | | 39.30 | 38.42 |
| ------------------------ | ------------- | --------------- | ---------------- |
## Usage and Limitations
These models have certain limitations that users should be aware of.
### Intended Usage
Open Large Language Models (LLMs) have a wide range of applications across
various industries and domains. The following list of potential uses is not
comprehensive. The purpose of this list is to provide contextual information
about the possible use-cases that the model creators considered as part of model
training and development.
* Content Creation and Communication
* Text Generation: These models can be used to generate creative text formats
such as poems, scripts, code, marketing copy, and email drafts.
* Chatbots and Conversational AI: Power conversational interfaces for customer
service, virtual assistants, or interactive applications.
* Text Summarization: Generate concise summaries of a text corpus, research
papers, or reports.
* Research and Education
* Natural Language Processing (NLP) Research: These models can serve as a
foundation for researchers to experiment with NLP techniques, develop
algorithms, and contribute to the advancement of the field.
* Language Learning Tools: Support interactive language learning experiences,
aiding in grammar correction or providing writing practice.
* Knowledge Exploration: Assist researchers in exploring large bodies of text
by generating summaries or answering questions about specific topics.
### Limitations
* Training Data
* The quality and diversity of the training data significantly influence the
model's capabilities. Biases or gaps in the training data can lead to
limitations in the model's responses.
* The scope of the training dataset determines the subject areas the model can
handle effectively.
* Context and Task Complexity
* LLMs are better at tasks that can be framed with clear prompts and
instructions. Open-ended or highly complex tasks might be challenging.
* A model's performance can be influenced by the amount of context provided
(longer context generally leads to better outputs, up to a certain point).
* Language Ambiguity and Nuance
* Natural language is inherently complex. LLMs might struggle to grasp subtle
nuances, sarcasm, or figurative language.
* Factual Accuracy
* LLMs generate responses based on information they learned from their
training datasets, but they are not knowledge bases. They may generate
incorrect or outdated factual statements.
* Common Sense
* LLMs rely on statistical patterns in language. They might lack the ability
to apply common sense reasoning in certain situations.
### Ethical Considerations and Risks
The development of large language models (LLMs) raises several ethical concerns.
In creating an open model, we have carefully considered the following:
* Bias and Fairness
* LLMs trained on large-scale, real-world text data can reflect socio-cultural
biases embedded in the training material. These models underwent careful
scrutiny, input data pre-processing described and posterior evaluations
reported in this card.
* Misinformation and Misuse
* LLMs can be misused to generate text that is false, misleading, or harmful.
* Guidelines are provided for responsible use with the model, see the
[Responsible Generative AI Toolkit][rai-toolkit].
* Transparency and Accountability:
* This model card summarizes details on the models' architecture,
capabilities, limitations, and evaluation processes.
* A responsibly developed open model offers the opportunity to share
innovation by making LLM technology accessible to developers and researchers
across the AI ecosystem.
Risks identified and mitigations:
* Perpetuation of biases: It's encouraged to perform continuous monitoring
(using evaluation metrics, human review) and the exploration of de-biasing
techniques during model training, fine-tuning, and other use cases.
* Generation of harmful content: Mechanisms and guidelines for content safety
are essential. Developers are encouraged to exercise caution and implement
appropriate content safety safeguards based on their specific product policies
and application use cases.
* Misuse for malicious purposes: Technical limitations and developer and
end-user education can help mitigate against malicious applications of LLMs.
Educational resources and reporting mechanisms for users to flag misuse are
provided. Prohibited uses of Gemma models are outlined in the
[Gemma Prohibited Use Policy][prohibited-use].
* Privacy violations: Models were trained on data filtered for removal of PII
(Personally Identifiable Information). Developers are encouraged to adhere to
privacy regulations with privacy-preserving techniques.
### Benefits
At the time of release, this family of models provides high-performance open
large language model implementations designed from the ground up for Responsible
AI development compared to similarly sized models.
Using the benchmark evaluation metrics described in this document, these models
have shown to provide superior performance to other, comparably-sized open model
alternatives.
[rai-toolkit]: https://ai.google.dev/responsible
[kaggle-gemma]: https://www.kaggle.com/models/google/gemma-2
[terms]: https://ai.google.dev/gemma/terms
[vertex-mg-gemma]: https://console.cloud.google.com/vertex-ai/publishers/google/model-garden/335
[sensitive-info]: https://cloud.google.com/dlp/docs/high-sensitivity-infotypes-reference
[safety-policies]: https://storage.googleapis.com/gweb-uniblog-publish-prod/documents/2023_Google_AI_Principles_Progress_Update.pdf#page=11
[prohibited-use]: https://ai.google.dev/gemma/prohibited_use_policy
[tpu]: https://cloud.google.com/tpu/docs/intro-to-tpu
[sustainability]: https://sustainability.google/operating-sustainably/
[jax]: https://github.com/google/jax
[ml-pathways]: https://blog.google/technology/ai/introducing-pathways-next-generation-ai-architecture/
[sustainability]: https://sustainability.google/operating-sustainably/
[foundation-models]: https://ai.google/discover/foundation-models/
[gemini-2-paper]: https://goo.gle/gemma2report
[mmlu]: https://arxiv.org/abs/2009.03300
[hellaswag]: https://arxiv.org/abs/1905.07830
[piqa]: https://arxiv.org/abs/1911.11641
[socialiqa]: https://arxiv.org/abs/1904.09728
[boolq]: https://arxiv.org/abs/1905.10044
[winogrande]: https://arxiv.org/abs/1907.10641
[commonsenseqa]: https://arxiv.org/abs/1811.00937
[openbookqa]: https://arxiv.org/abs/1809.02789
[arc]: https://arxiv.org/abs/1911.01547
[triviaqa]: https://arxiv.org/abs/1705.03551
[naturalq]: https://github.com/google-research-datasets/natural-questions
[humaneval]: https://arxiv.org/abs/2107.03374
[mbpp]: https://arxiv.org/abs/2108.07732
[gsm8k]: https://arxiv.org/abs/2110.14168
[realtox]: https://arxiv.org/abs/2009.11462
[bold]: https://arxiv.org/abs/2101.11718
[crows]: https://aclanthology.org/2020.emnlp-main.154/
[bbq]: https://arxiv.org/abs/2110.08193v2
[winogender]: https://arxiv.org/abs/1804.09301
[truthfulqa]: https://arxiv.org/abs/2109.07958
[winobias]: https://arxiv.org/abs/1804.06876
[math]: https://arxiv.org/abs/2103.03874
[agieval]: https://arxiv.org/abs/2304.06364
[big-bench]: https://arxiv.org/abs/2206.04615
[toxigen]: https://arxiv.org/abs/2203.09509
|
mmnga/Llama-3-Swallow-8B-Instruct-v0.1-gguf | mmnga | 2024-07-02T10:43:55Z | 15,107 | 2 | null | [
"gguf",
"en",
"ja",
"dataset:TFMC/imatrix-dataset-for-japanese-llm",
"license:llama3",
"region:us"
] | null | 2024-07-01T16:42:54Z |
---
license: llama3
language:
- en
- ja
datasets:
- TFMC/imatrix-dataset-for-japanese-llm
---
# Llama-3-Swallow-8B-Instruct-v0.1-gguf
[tokyotech-llmさんが公開しているLlama-3-Swallow-8B-Instruct-v0.1](https://huggingface.co/tokyotech-llm/Llama-3-Swallow-8B-Instruct-v0.1)のggufフォーマット変換版です。
imatrixのデータは[TFMC/imatrix-dataset-for-japanese-llm](https://huggingface.co/datasets/TFMC/imatrix-dataset-for-japanese-llm)を使用して作成しました。
## Usage
```
git clone https://github.com/ggerganov/llama.cpp.git
cd llama.cpp
make -j
./main -m 'Llama-3-Swallow-8B-Instruct-v0.1-Q4_0.gguf' -n 128 -p 'こんにちわ'
```
|
mradermacher/llama-3-8b-samantha-i1-GGUF | mradermacher | 2024-06-29T22:46:04Z | 15,104 | 0 | transformers | [
"transformers",
"gguf",
"text-generation-inference",
"unsloth",
"llama",
"trl",
"en",
"dataset:cognitivecomputations/samantha-data",
"base_model:ruslandev/llama-3-8b-samantha",
"license:apache-2.0",
"endpoints_compatible",
"region:us"
] | null | 2024-06-29T18:00:38Z | ---
base_model: ruslandev/llama-3-8b-samantha
datasets:
- cognitivecomputations/samantha-data
language:
- en
library_name: transformers
license: apache-2.0
quantized_by: mradermacher
tags:
- text-generation-inference
- transformers
- unsloth
- llama
- trl
---
## About
<!-- ### quantize_version: 2 -->
<!-- ### output_tensor_quantised: 1 -->
<!-- ### convert_type: hf -->
<!-- ### vocab_type: -->
<!-- ### tags: nicoboss -->
weighted/imatrix quants of https://huggingface.co/ruslandev/llama-3-8b-samantha
<!-- provided-files -->
static quants are available at https://huggingface.co/mradermacher/llama-3-8b-samantha-GGUF
## Usage
If you are unsure how to use GGUF files, refer to one of [TheBloke's
READMEs](https://huggingface.co/TheBloke/KafkaLM-70B-German-V0.1-GGUF) for
more details, including on how to concatenate multi-part files.
## Provided Quants
(sorted by size, not necessarily quality. IQ-quants are often preferable over similar sized non-IQ quants)
| Link | Type | Size/GB | Notes |
|:-----|:-----|--------:|:------|
| [GGUF](https://huggingface.co/mradermacher/llama-3-8b-samantha-i1-GGUF/resolve/main/llama-3-8b-samantha.i1-IQ1_S.gguf) | i1-IQ1_S | 2.1 | for the desperate |
| [GGUF](https://huggingface.co/mradermacher/llama-3-8b-samantha-i1-GGUF/resolve/main/llama-3-8b-samantha.i1-IQ1_M.gguf) | i1-IQ1_M | 2.3 | mostly desperate |
| [GGUF](https://huggingface.co/mradermacher/llama-3-8b-samantha-i1-GGUF/resolve/main/llama-3-8b-samantha.i1-IQ2_XXS.gguf) | i1-IQ2_XXS | 2.5 | |
| [GGUF](https://huggingface.co/mradermacher/llama-3-8b-samantha-i1-GGUF/resolve/main/llama-3-8b-samantha.i1-IQ2_XS.gguf) | i1-IQ2_XS | 2.7 | |
| [GGUF](https://huggingface.co/mradermacher/llama-3-8b-samantha-i1-GGUF/resolve/main/llama-3-8b-samantha.i1-IQ2_S.gguf) | i1-IQ2_S | 2.9 | |
| [GGUF](https://huggingface.co/mradermacher/llama-3-8b-samantha-i1-GGUF/resolve/main/llama-3-8b-samantha.i1-IQ2_M.gguf) | i1-IQ2_M | 3.0 | |
| [GGUF](https://huggingface.co/mradermacher/llama-3-8b-samantha-i1-GGUF/resolve/main/llama-3-8b-samantha.i1-Q2_K.gguf) | i1-Q2_K | 3.3 | IQ3_XXS probably better |
| [GGUF](https://huggingface.co/mradermacher/llama-3-8b-samantha-i1-GGUF/resolve/main/llama-3-8b-samantha.i1-IQ3_XXS.gguf) | i1-IQ3_XXS | 3.4 | lower quality |
| [GGUF](https://huggingface.co/mradermacher/llama-3-8b-samantha-i1-GGUF/resolve/main/llama-3-8b-samantha.i1-IQ3_XS.gguf) | i1-IQ3_XS | 3.6 | |
| [GGUF](https://huggingface.co/mradermacher/llama-3-8b-samantha-i1-GGUF/resolve/main/llama-3-8b-samantha.i1-Q3_K_S.gguf) | i1-Q3_K_S | 3.8 | IQ3_XS probably better |
| [GGUF](https://huggingface.co/mradermacher/llama-3-8b-samantha-i1-GGUF/resolve/main/llama-3-8b-samantha.i1-IQ3_S.gguf) | i1-IQ3_S | 3.8 | beats Q3_K* |
| [GGUF](https://huggingface.co/mradermacher/llama-3-8b-samantha-i1-GGUF/resolve/main/llama-3-8b-samantha.i1-IQ3_M.gguf) | i1-IQ3_M | 3.9 | |
| [GGUF](https://huggingface.co/mradermacher/llama-3-8b-samantha-i1-GGUF/resolve/main/llama-3-8b-samantha.i1-Q3_K_M.gguf) | i1-Q3_K_M | 4.1 | IQ3_S probably better |
| [GGUF](https://huggingface.co/mradermacher/llama-3-8b-samantha-i1-GGUF/resolve/main/llama-3-8b-samantha.i1-Q3_K_L.gguf) | i1-Q3_K_L | 4.4 | IQ3_M probably better |
| [GGUF](https://huggingface.co/mradermacher/llama-3-8b-samantha-i1-GGUF/resolve/main/llama-3-8b-samantha.i1-IQ4_XS.gguf) | i1-IQ4_XS | 4.5 | |
| [GGUF](https://huggingface.co/mradermacher/llama-3-8b-samantha-i1-GGUF/resolve/main/llama-3-8b-samantha.i1-Q4_0.gguf) | i1-Q4_0 | 4.8 | fast, low quality |
| [GGUF](https://huggingface.co/mradermacher/llama-3-8b-samantha-i1-GGUF/resolve/main/llama-3-8b-samantha.i1-Q4_K_S.gguf) | i1-Q4_K_S | 4.8 | optimal size/speed/quality |
| [GGUF](https://huggingface.co/mradermacher/llama-3-8b-samantha-i1-GGUF/resolve/main/llama-3-8b-samantha.i1-Q4_K_M.gguf) | i1-Q4_K_M | 5.0 | fast, recommended |
| [GGUF](https://huggingface.co/mradermacher/llama-3-8b-samantha-i1-GGUF/resolve/main/llama-3-8b-samantha.i1-Q5_K_S.gguf) | i1-Q5_K_S | 5.7 | |
| [GGUF](https://huggingface.co/mradermacher/llama-3-8b-samantha-i1-GGUF/resolve/main/llama-3-8b-samantha.i1-Q5_K_M.gguf) | i1-Q5_K_M | 5.8 | |
| [GGUF](https://huggingface.co/mradermacher/llama-3-8b-samantha-i1-GGUF/resolve/main/llama-3-8b-samantha.i1-Q6_K.gguf) | i1-Q6_K | 6.7 | practically like static Q6_K |
Here is a handy graph by ikawrakow comparing some lower-quality quant
types (lower is better):

And here are Artefact2's thoughts on the matter:
https://gist.github.com/Artefact2/b5f810600771265fc1e39442288e8ec9
## FAQ / Model Request
See https://huggingface.co/mradermacher/model_requests for some answers to
questions you might have and/or if you want some other model quantized.
## Thanks
I thank my company, [nethype GmbH](https://www.nethype.de/), for letting
me use its servers and providing upgrades to my workstation to enable
this work in my free time. Additional thanks to [@nicoboss](https://huggingface.co/nicoboss) for giving me access to his hardware for calculating the imatrix for these quants.
<!-- end -->
|
mradermacher/Symbol-LLM-13B-Instruct-i1-GGUF | mradermacher | 2024-06-23T20:56:37Z | 15,103 | 0 | transformers | [
"transformers",
"gguf",
"en",
"base_model:Symbol-LLM/Symbol-LLM-13B-Instruct",
"license:apache-2.0",
"endpoints_compatible",
"region:us"
] | null | 2024-06-23T18:51:06Z | ---
base_model: Symbol-LLM/Symbol-LLM-13B-Instruct
language:
- en
library_name: transformers
license: apache-2.0
quantized_by: mradermacher
---
## About
<!-- ### quantize_version: 2 -->
<!-- ### output_tensor_quantised: 1 -->
<!-- ### convert_type: hf -->
<!-- ### vocab_type: -->
<!-- ### tags: nicoboss -->
weighted/imatrix quants of https://huggingface.co/Symbol-LLM/Symbol-LLM-13B-Instruct
<!-- provided-files -->
static quants are available at https://huggingface.co/mradermacher/Symbol-LLM-13B-Instruct-GGUF
## Usage
If you are unsure how to use GGUF files, refer to one of [TheBloke's
READMEs](https://huggingface.co/TheBloke/KafkaLM-70B-German-V0.1-GGUF) for
more details, including on how to concatenate multi-part files.
## Provided Quants
(sorted by size, not necessarily quality. IQ-quants are often preferable over similar sized non-IQ quants)
| Link | Type | Size/GB | Notes |
|:-----|:-----|--------:|:------|
| [GGUF](https://huggingface.co/mradermacher/Symbol-LLM-13B-Instruct-i1-GGUF/resolve/main/Symbol-LLM-13B-Instruct.i1-IQ1_S.gguf) | i1-IQ1_S | 3.0 | for the desperate |
| [GGUF](https://huggingface.co/mradermacher/Symbol-LLM-13B-Instruct-i1-GGUF/resolve/main/Symbol-LLM-13B-Instruct.i1-IQ1_M.gguf) | i1-IQ1_M | 3.2 | mostly desperate |
| [GGUF](https://huggingface.co/mradermacher/Symbol-LLM-13B-Instruct-i1-GGUF/resolve/main/Symbol-LLM-13B-Instruct.i1-IQ2_XXS.gguf) | i1-IQ2_XXS | 3.6 | |
| [GGUF](https://huggingface.co/mradermacher/Symbol-LLM-13B-Instruct-i1-GGUF/resolve/main/Symbol-LLM-13B-Instruct.i1-IQ2_XS.gguf) | i1-IQ2_XS | 4.0 | |
| [GGUF](https://huggingface.co/mradermacher/Symbol-LLM-13B-Instruct-i1-GGUF/resolve/main/Symbol-LLM-13B-Instruct.i1-IQ2_S.gguf) | i1-IQ2_S | 4.3 | |
| [GGUF](https://huggingface.co/mradermacher/Symbol-LLM-13B-Instruct-i1-GGUF/resolve/main/Symbol-LLM-13B-Instruct.i1-IQ2_M.gguf) | i1-IQ2_M | 4.6 | |
| [GGUF](https://huggingface.co/mradermacher/Symbol-LLM-13B-Instruct-i1-GGUF/resolve/main/Symbol-LLM-13B-Instruct.i1-Q2_K.gguf) | i1-Q2_K | 5.0 | IQ3_XXS probably better |
| [GGUF](https://huggingface.co/mradermacher/Symbol-LLM-13B-Instruct-i1-GGUF/resolve/main/Symbol-LLM-13B-Instruct.i1-IQ3_XXS.gguf) | i1-IQ3_XXS | 5.1 | lower quality |
| [GGUF](https://huggingface.co/mradermacher/Symbol-LLM-13B-Instruct-i1-GGUF/resolve/main/Symbol-LLM-13B-Instruct.i1-IQ3_XS.gguf) | i1-IQ3_XS | 5.5 | |
| [GGUF](https://huggingface.co/mradermacher/Symbol-LLM-13B-Instruct-i1-GGUF/resolve/main/Symbol-LLM-13B-Instruct.i1-IQ3_S.gguf) | i1-IQ3_S | 5.8 | beats Q3_K* |
| [GGUF](https://huggingface.co/mradermacher/Symbol-LLM-13B-Instruct-i1-GGUF/resolve/main/Symbol-LLM-13B-Instruct.i1-Q3_K_S.gguf) | i1-Q3_K_S | 5.8 | IQ3_XS probably better |
| [GGUF](https://huggingface.co/mradermacher/Symbol-LLM-13B-Instruct-i1-GGUF/resolve/main/Symbol-LLM-13B-Instruct.i1-IQ3_M.gguf) | i1-IQ3_M | 6.1 | |
| [GGUF](https://huggingface.co/mradermacher/Symbol-LLM-13B-Instruct-i1-GGUF/resolve/main/Symbol-LLM-13B-Instruct.i1-Q3_K_M.gguf) | i1-Q3_K_M | 6.4 | IQ3_S probably better |
| [GGUF](https://huggingface.co/mradermacher/Symbol-LLM-13B-Instruct-i1-GGUF/resolve/main/Symbol-LLM-13B-Instruct.i1-Q3_K_L.gguf) | i1-Q3_K_L | 7.0 | IQ3_M probably better |
| [GGUF](https://huggingface.co/mradermacher/Symbol-LLM-13B-Instruct-i1-GGUF/resolve/main/Symbol-LLM-13B-Instruct.i1-IQ4_XS.gguf) | i1-IQ4_XS | 7.1 | |
| [GGUF](https://huggingface.co/mradermacher/Symbol-LLM-13B-Instruct-i1-GGUF/resolve/main/Symbol-LLM-13B-Instruct.i1-Q4_0.gguf) | i1-Q4_0 | 7.5 | fast, low quality |
| [GGUF](https://huggingface.co/mradermacher/Symbol-LLM-13B-Instruct-i1-GGUF/resolve/main/Symbol-LLM-13B-Instruct.i1-Q4_K_S.gguf) | i1-Q4_K_S | 7.5 | optimal size/speed/quality |
| [GGUF](https://huggingface.co/mradermacher/Symbol-LLM-13B-Instruct-i1-GGUF/resolve/main/Symbol-LLM-13B-Instruct.i1-Q4_K_M.gguf) | i1-Q4_K_M | 8.0 | fast, recommended |
| [GGUF](https://huggingface.co/mradermacher/Symbol-LLM-13B-Instruct-i1-GGUF/resolve/main/Symbol-LLM-13B-Instruct.i1-Q5_K_S.gguf) | i1-Q5_K_S | 9.1 | |
| [GGUF](https://huggingface.co/mradermacher/Symbol-LLM-13B-Instruct-i1-GGUF/resolve/main/Symbol-LLM-13B-Instruct.i1-Q5_K_M.gguf) | i1-Q5_K_M | 9.3 | |
| [GGUF](https://huggingface.co/mradermacher/Symbol-LLM-13B-Instruct-i1-GGUF/resolve/main/Symbol-LLM-13B-Instruct.i1-Q6_K.gguf) | i1-Q6_K | 10.8 | practically like static Q6_K |
Here is a handy graph by ikawrakow comparing some lower-quality quant
types (lower is better):

And here are Artefact2's thoughts on the matter:
https://gist.github.com/Artefact2/b5f810600771265fc1e39442288e8ec9
## FAQ / Model Request
See https://huggingface.co/mradermacher/model_requests for some answers to
questions you might have and/or if you want some other model quantized.
## Thanks
I thank my company, [nethype GmbH](https://www.nethype.de/), for letting
me use its servers and providing upgrades to my workstation to enable
this work in my free time. Additional thanks to [@nicoboss](https://huggingface.co/nicoboss) for giving me access to his hardware for calculating the imatrix for these quants.
<!-- end -->
|
Salesforce/codet5-base | Salesforce | 2021-11-23T09:53:41Z | 15,092 | 93 | transformers | [
"transformers",
"pytorch",
"t5",
"text2text-generation",
"codet5",
"dataset:code_search_net",
"arxiv:2109.00859",
"arxiv:1909.09436",
"license:apache-2.0",
"autotrain_compatible",
"text-generation-inference",
"region:us"
] | text2text-generation | 2022-03-02T23:29:04Z | ---
license: apache-2.0
tags:
- codet5
datasets:
- code_search_net
inference: false
---
# CodeT5 (base-sized model)
Pre-trained CodeT5 model. It was introduced in the paper [CodeT5: Identifier-aware Unified Pre-trained Encoder-Decoder Models
for Code Understanding and Generation](https://arxiv.org/abs/2109.00859) by Yue Wang, Weishi Wang, Shafiq Joty, Steven C.H. Hoi and first released in [this repository](https://github.com/salesforce/CodeT5).
Disclaimer: The team releasing CodeT5 did not write a model card for this model so this model card has been written by the Hugging Face team (more specifically, [nielsr](https://huggingface.co/nielsr)).
## Model description
From the abstract:
"We present CodeT5, a unified pre-trained encoder-decoder Transformer model that better leverages the code semantics conveyed from the developer-assigned identifiers. Our model employs a unified framework to seamlessly support both code understanding and generation tasks and allows for multi-task learning. Besides, we propose a novel identifier-aware pre-training task that enables the model to distinguish which code tokens are identifiers and to recover them when they are masked. Furthermore, we propose to exploit the user-written code comments with a bimodal dual generation task for better NL-PL alignment. Comprehensive experiments show that CodeT5 significantly outperforms prior methods on understanding tasks such as code defect detection and clone detection, and generation tasks across various directions including PL-NL, NL-PL, and PL-PL. Further analysis reveals that our model can better capture semantic information from code."
## Intended uses & limitations
This repository contains the pre-trained model only, so you can use this model for (among other tasks) masked span prediction, as shown in the code example below. However, the main use of this model is to fine-tune it for a downstream task of interest, such as:
* code summarization
* code generation
* code translation
* code refinement
* code defect detection
* code clone detection.
Supervised datasets for code can be found [here](https://huggingface.co/datasets?languages=languages:code).
See the [model hub](https://huggingface.co/models?search=salesforce/codet) to look for fine-tuned versions on a task that interests you.
### How to use
Here is how to use this model:
```python
from transformers import RobertaTokenizer, T5ForConditionalGeneration
tokenizer = RobertaTokenizer.from_pretrained('Salesforce/codet5-base')
model = T5ForConditionalGeneration.from_pretrained('Salesforce/codet5-base')
text = "def greet(user): print(f'hello <extra_id_0>!')"
input_ids = tokenizer(text, return_tensors="pt").input_ids
# simply generate a single sequence
generated_ids = model.generate(input_ids, max_length=8)
print(tokenizer.decode(generated_ids[0], skip_special_tokens=True))
# this prints "{user.username}"
```
## Training data
The CodeT5 model was pretrained on CodeSearchNet [Husain et al., 2019](https://arxiv.org/abs/1909.09436). Additionally, the authors collected two datasets of C/CSharp from [BigQuery1](https://console.cloud.google.com/marketplace/details/github/github-repos) to ensure that all downstream tasks have overlapped programming languages with the pre-training data. In total, around 8.35 million instances are used for pretraining.
## Training procedure
### Preprocessing
This model uses a code-specific BPE (Byte-Pair Encoding) tokenizer trained using the [HuggingFace Tokenizers](https://github.com/huggingface/tokenizers) library. One can prepare text (or code) for the model using RobertaTokenizer, with the files from this repository.
## Evaluation results
For evaluation results on several downstream benchmarks, we refer to the paper.
### BibTeX entry and citation info
```bibtex
@misc{wang2021codet5,
title={CodeT5: Identifier-aware Unified Pre-trained Encoder-Decoder Models for Code Understanding and Generation},
author={Yue Wang and Weishi Wang and Shafiq Joty and Steven C. H. Hoi},
year={2021},
eprint={2109.00859},
archivePrefix={arXiv},
primaryClass={cs.CL}
}
``` |
RichardErkhov/TIGER-Lab_-_TIGERScore-7B-gguf | RichardErkhov | 2024-06-30T00:43:37Z | 15,077 | 0 | null | [
"gguf",
"arxiv:2310.00752",
"region:us"
] | null | 2024-06-29T20:38:42Z | Quantization made by Richard Erkhov.
[Github](https://github.com/RichardErkhov)
[Discord](https://discord.gg/pvy7H8DZMG)
[Request more models](https://github.com/RichardErkhov/quant_request)
TIGERScore-7B - GGUF
- Model creator: https://huggingface.co/TIGER-Lab/
- Original model: https://huggingface.co/TIGER-Lab/TIGERScore-7B/
| Name | Quant method | Size |
| ---- | ---- | ---- |
| [TIGERScore-7B.Q2_K.gguf](https://huggingface.co/RichardErkhov/TIGER-Lab_-_TIGERScore-7B-gguf/blob/main/TIGERScore-7B.Q2_K.gguf) | Q2_K | 2.36GB |
| [TIGERScore-7B.IQ3_XS.gguf](https://huggingface.co/RichardErkhov/TIGER-Lab_-_TIGERScore-7B-gguf/blob/main/TIGERScore-7B.IQ3_XS.gguf) | IQ3_XS | 2.6GB |
| [TIGERScore-7B.IQ3_S.gguf](https://huggingface.co/RichardErkhov/TIGER-Lab_-_TIGERScore-7B-gguf/blob/main/TIGERScore-7B.IQ3_S.gguf) | IQ3_S | 2.75GB |
| [TIGERScore-7B.Q3_K_S.gguf](https://huggingface.co/RichardErkhov/TIGER-Lab_-_TIGERScore-7B-gguf/blob/main/TIGERScore-7B.Q3_K_S.gguf) | Q3_K_S | 2.75GB |
| [TIGERScore-7B.IQ3_M.gguf](https://huggingface.co/RichardErkhov/TIGER-Lab_-_TIGERScore-7B-gguf/blob/main/TIGERScore-7B.IQ3_M.gguf) | IQ3_M | 2.9GB |
| [TIGERScore-7B.Q3_K.gguf](https://huggingface.co/RichardErkhov/TIGER-Lab_-_TIGERScore-7B-gguf/blob/main/TIGERScore-7B.Q3_K.gguf) | Q3_K | 3.07GB |
| [TIGERScore-7B.Q3_K_M.gguf](https://huggingface.co/RichardErkhov/TIGER-Lab_-_TIGERScore-7B-gguf/blob/main/TIGERScore-7B.Q3_K_M.gguf) | Q3_K_M | 3.07GB |
| [TIGERScore-7B.Q3_K_L.gguf](https://huggingface.co/RichardErkhov/TIGER-Lab_-_TIGERScore-7B-gguf/blob/main/TIGERScore-7B.Q3_K_L.gguf) | Q3_K_L | 3.35GB |
| [TIGERScore-7B.IQ4_XS.gguf](https://huggingface.co/RichardErkhov/TIGER-Lab_-_TIGERScore-7B-gguf/blob/main/TIGERScore-7B.IQ4_XS.gguf) | IQ4_XS | 3.4GB |
| [TIGERScore-7B.Q4_0.gguf](https://huggingface.co/RichardErkhov/TIGER-Lab_-_TIGERScore-7B-gguf/blob/main/TIGERScore-7B.Q4_0.gguf) | Q4_0 | 3.56GB |
| [TIGERScore-7B.IQ4_NL.gguf](https://huggingface.co/RichardErkhov/TIGER-Lab_-_TIGERScore-7B-gguf/blob/main/TIGERScore-7B.IQ4_NL.gguf) | IQ4_NL | 3.58GB |
| [TIGERScore-7B.Q4_K_S.gguf](https://huggingface.co/RichardErkhov/TIGER-Lab_-_TIGERScore-7B-gguf/blob/main/TIGERScore-7B.Q4_K_S.gguf) | Q4_K_S | 3.59GB |
| [TIGERScore-7B.Q4_K.gguf](https://huggingface.co/RichardErkhov/TIGER-Lab_-_TIGERScore-7B-gguf/blob/main/TIGERScore-7B.Q4_K.gguf) | Q4_K | 3.8GB |
| [TIGERScore-7B.Q4_K_M.gguf](https://huggingface.co/RichardErkhov/TIGER-Lab_-_TIGERScore-7B-gguf/blob/main/TIGERScore-7B.Q4_K_M.gguf) | Q4_K_M | 3.8GB |
| [TIGERScore-7B.Q4_1.gguf](https://huggingface.co/RichardErkhov/TIGER-Lab_-_TIGERScore-7B-gguf/blob/main/TIGERScore-7B.Q4_1.gguf) | Q4_1 | 3.95GB |
| [TIGERScore-7B.Q5_0.gguf](https://huggingface.co/RichardErkhov/TIGER-Lab_-_TIGERScore-7B-gguf/blob/main/TIGERScore-7B.Q5_0.gguf) | Q5_0 | 4.33GB |
| [TIGERScore-7B.Q5_K_S.gguf](https://huggingface.co/RichardErkhov/TIGER-Lab_-_TIGERScore-7B-gguf/blob/main/TIGERScore-7B.Q5_K_S.gguf) | Q5_K_S | 4.33GB |
| [TIGERScore-7B.Q5_K.gguf](https://huggingface.co/RichardErkhov/TIGER-Lab_-_TIGERScore-7B-gguf/blob/main/TIGERScore-7B.Q5_K.gguf) | Q5_K | 4.45GB |
| [TIGERScore-7B.Q5_K_M.gguf](https://huggingface.co/RichardErkhov/TIGER-Lab_-_TIGERScore-7B-gguf/blob/main/TIGERScore-7B.Q5_K_M.gguf) | Q5_K_M | 4.45GB |
| [TIGERScore-7B.Q5_1.gguf](https://huggingface.co/RichardErkhov/TIGER-Lab_-_TIGERScore-7B-gguf/blob/main/TIGERScore-7B.Q5_1.gguf) | Q5_1 | 4.72GB |
| [TIGERScore-7B.Q6_K.gguf](https://huggingface.co/RichardErkhov/TIGER-Lab_-_TIGERScore-7B-gguf/blob/main/TIGERScore-7B.Q6_K.gguf) | Q6_K | 5.15GB |
| [TIGERScore-7B.Q8_0.gguf](https://huggingface.co/RichardErkhov/TIGER-Lab_-_TIGERScore-7B-gguf/blob/main/TIGERScore-7B.Q8_0.gguf) | Q8_0 | 6.67GB |
Original model description:
---
license: mit
datasets:
- TIGER-Lab/MetricInstruct
language:
- en
- zh
- ru
- cs
metrics:
- pearsonr
- spearmanr
pipeline_tag: text2text-generation
tags:
- text evaluation
- metric
- llm metric
- llama
---
## TIGERScore
[Project Page](https://tiger-ai-lab.github.io/TIGERScore/) | [Paper](https://arxiv.org/abs/2310.00752) | [Code](https://github.com/TIGER-AI-Lab/TIGERScore) | [🤗Demo](https://huggingface.co/spaces/TIGER-Lab/TIGERScore) |
[🤗TIGERScore-7B](https://huggingface.co/TIGER-Lab/TIGERScore-7B-V1.2) | [🤗TIGERScore-13B](https://huggingface.co/TIGER-Lab/TIGERScore-13B-V1.2)
## Introduction
We present TIGERScore, a **T**rained metric that follows **I**nstruction **G**uidance to perform **E**xplainable, and **R**eference-free evaluation over a wide spectrum of text generation tasks. Our metric is based on LLaMA-2, trained on our meticulously curated instruction-tuning dataset [MetricInstruct](https://huggingface.co/datasets/TIGER-Lab/MetricInstruct) which covers 6 text generation tasks and 23 text generation datasets.
Existing automatic metrics are lagging and suffer from issues like 1) **Dependency on references**, 2) **Limited to specific domains**, 3) **Lack of attribution**. Contrary to them, TIGERScore is designed to be driven by natural language instruction and provide detailed error analysis to pinpoint the mistakes in the generated text.
Specifically, TIGERScore takes an instruction, an associated input context along with a hypothesis output that might contain errors. Then, TIGERScore will evaluate this hypothesis output and list several errors, each consisting of the error location, aspect, explanation and penalty scores (score reduced, starting from 0). The sum of the reduced scores is taken as the overall rating of this output.
As a reference-free metric, its correlation can even surpass the best existing reference-based metrics. We believe TIGERScore demonstrates the possibility of building universal explainable metrics to evaluate any text generation task.
## Training Data
The models are trained on the 🤗 [MetricInstruct Dataset](https://huggingface.co/datasets/TIGER-Lab/MetricInstruct), which covers 6 text generation tasks and 22 text generation datasets. Check out the dataset card for more details.
## Training Procedure
The models are fine-tuned with the MetricInstruct dataset using the original Llama-2 model as base models. The training procedure varies for different models based on their sizes. Check out our paper for more details.
## Evaluation
Experiments show that TIGERScore surpasses existing baseline metrics in correlation with human ratings on all 6 held-in tasks and 1 held-out task, achiving the highest overall performance. We hope the emergence of TIGERScore can promote the research in the LLM community as a powerful, interpretable, and easy-to-use metric.
### Kendall Results
| Tasks⟶ | Summarization | Translation | Data2Text | Long-form QA | MathQA | Instruction Following | Story-Gen | Average |
|----------------------------------------|-----------|-----------|-----------------|-----------|-----------|-----------|-----------|-----------|
| | | | GPT-based | Metrics | | | | |
| GPT-3.5-turbo (few-shot) | **30.45** | 32.3 | 30.38 | 20.91 | **58.57** | 17.73 | 3.26 | 27.65 |
| GPT-4 (zero-shot) | 29.32 | **35.38** | **32.26** | **35.85** | 46.63 | **49.5** | **25.69** | **36.38** |
| | | | Reference-based | Metrics | | | | |
| BLEU | 8.71 | 14.5 | 23.13 | 7.73 | 17.25 | 35.92 | -0.89 | 15.19 |
| ROUGE-2f | 10.67 | 13.19 | 24.74 | 11.73 | 18.07 | 34.59 | 1.78 | 16.4 |
| InstructScore | 20.86 | 40.44 | 30.21 | 15.64 | -3.87 | 13.87 | 13.5 | 18.66 |
| GPTScore-ref | 10.8 | 18.74 | 27.47 | 22.13 | 14.86 | 25.4 | 12.78 | 18.88 |
| BARTScore-cnn (hypo-ref) | 10 | 21.06 | 27.04 | 20.67 | **19.07** | 24.7 | 18.58 | 20.16 |
| BARTScore-para (hypo-ref) | 10.41 | 24.9 | 28.42 | 20.24 | 14.1 | 26.13 | 12.11 | 19.47 |
| BERTScore | 17.39 | 31.57 | 30.74 | 17.7 | 9.41 | 35.61 | 2 | 20.63 |
| BLEURT | 12.69 | 36.12 | **34.48** | 23.11 | 2.88 | 27.94 | 19.18 | 22.34 |
| UniEval (summ) | **35.89** | 16.08 | 28.56 | **29.32** | 16.15 | 11.93 | **31.22** | 24.17 |
| COMET-22 | 25.01 | **42.79** | 23.43 | 24.66 | -4.52 | **36.17** | 27.52 | **25.01** |
| | | | Reference-free |Metrics | | | | |
| BARTScore-para (src-hypo) | 29.12 | 7.01 | 22.32 | 18.8 | -2.21 | 4.26 | 14.15 | 13.35 |
| BARTScore-cnn (src-hypo) | 26.63 | 9.4 | 23.69 | 28.93 | 1.23 | 19.09 | 23.29 | 18.89 |
| Llama-2-13b-chat-0-shot | 25.22 | 11.79 | 23.45 | 15.96 | 1.08 | 19.5 | 21.52 | 16.93 |
| COMETKiwi | 11.87 | 36.37 | 19.08 | 12.23 | -9.38 | 26.46 | 12.78 | 15.63 |
| GPTScore-src | 28.2 | 6.5 | 19.81 | 27.64 | 11.64 | 20.04 | 16.36 | 18.6 |
| TigerScore-7B | 28.79 | 33.65 | 32.44 | 33.93 | 19.98 | 38.13 | 29.72 | 30.95 |
| TigerScore-13B | **31.29** | **36.5** | **36.43** | **33.17** | **21.58** | **41.84** | **35.33** | **33.73** |
| ∆ (ours - best reference-free) | +2 | +0 | +13 | +4 | +10 | +15 | +14 | +15 |
| ∆ (ours - best reference-based) | -4 | -6 | +2 | +4 | +2 | +5 | +4 | +8 |
### Pearson Results
| Tasks⟶ | Summarization | Translation | Data2Text | Long-form QA | MathQA | Instruction Following | Story-Gen | Average |
|-------------------------------|-----------|-----------|-----------------|-----------|-----------|-----------|-----------|-----------|
| | | | GPT-based | Metrics | | | | |
| GPT-3.5-turbo (few-shot) | **45.53** | **43.77** | **47.76** | 29.84 | **61.26** | 15.36 | 7.8 | 35.9 |
| GPT-4 (zero-shot) | 40.75 | 33.92 | 46.83 | **49.3** | 54.98 | **60.45** | **37.74** | **46.28** |
| | | | Reference-based | Metrics | | | | |
| BLEU | 11.66 | 17.47 | 34.29 | 18.21 | 18.12 | 29.47 | -0.64 | 18.37 |
| ROUGE-2f | 16.03 | 16.26 | 35.85 | 19.66 | 20.69 | 33.49 | 2.88 | 20.69 |
| InstructScore | 27.4 | 51.55 | 47.28 | 20.59 | 0.36 | 20.98 | 12.81 | 25.85 |
| GPTScore-ref | 13.47 | 21.05 | 48.7 | 33.4 | 18.22 | 29.66 | 18.94 | 26.2 |
| BARTScore-cnn (hypo-ref) | 16.67 | 23.56 | 45.08 | 32.78 | **23.09** | 26.57 | 27.61 | 27.91 |
| BARTScore-para (hypo-ref) | 19.73 | 29.04 | 47.89 | 32.7 | 17.33 | 30.2 | 17.76 | 27.81 |
| BERTScore | 26.26 | 37.65 | 48.22 | 26.39 | 11.19 | 45.58 | 4.08 | 28.48 |
| BLEURT | 17.27 | 43 | **54.32** | 34.26 | 3.98 | 39.15 | 27.89 | 31.41 |
| UniEval (summ) | **53.22** | 23.11 | 51.14 | **36.95** | 17.69 | 30.87 | **44.88** | 36.84 |
| COMET-22 | 35.32 | **58.46** | 43.82 | 36.79 | -5.58 | **49.68** | 40.12 | **36.94** |
| | | | Reference-free | Metrics | | | | |
| BARTScore-para (src-hypo) | 43.11 | 6.96 | 37.82 | 29.86 | -0.41 | 19.37 | 19.99 | 22.38 |
| BARTScore-cnn (src-hypo) | 39.72 | 9.53 | 45.43 | 41.48 | 3.28 | 34.97 | 33.51 | 29.7 |
| Llama-2-13b-chat-0-shot | 29.59 | 9.09 | 41.32 | 21.67 | 2.8 | 22.71 | 21.13 | 21.19 |
| COMETKiwi | 14.22 | **50.91** | 23.63 | 22.59 | -13.35 | 34.46 | 19.12 | 21.65 |
| GPTScore-src | 41.71 | 6.82 | 41.19 | 39.79 | 13.99 | 27.59 | 23.22 | 27.76 |
| TigerScore-7B | 43.95 | 37.7 | 49.13 | **46.1** | 21.77 | 38.26 | 39.9 | 39.54 |
| TigerScore-13B | **44.21** | 41.54 | **52.87** | 44.76 | **24.41** | **47.52** | **47.66** | **43.28** |
| ∆ (ours - best reference-free) | +1 | -9 | +7 | +5 | +10 | +20 | +14 | +13 |
| ∆ (ours - best reference-based) | -9 | -17 | -2 | +9 | +1 | -2 | +3 | +6 |
### Spearman Results
| Tasks⟶ | Summarization | Translation | Data2Text | Long-form QA | MathQA | Instruction Following | Story-Gen | Average |
|-------------------------------------------|----------------|----------------|----------------|-----------------|----------------|----------------|----------------|----------------|
| | | | GPT-based | Metrics | | | | |
| GPT-3.5-turbo (few-shot) | **38.50** | 40.53 | 40.20 | 29.33 | **66.46** | 23.20 | 4.77 | 34.71 |
| GPT-4 (zero-shot) | 36.46 | **43.87** | **44.04** | **48.95** | 51.71 | **58.53** | **32.48** | **45.15** |
| | | | Reference-based | Metrics | | | | |
| BLEU | 11.98 | 19.73 | 33.29 | 11.38 | 21.12 | **46.61** | -1.17 | 20.42 |
| ROUGE-2f | 14.53 | 17.83 | 35.49 | 16.83 | 22.12 | 44.56 | 2.34 | 21.96 |
| InstructScore | 26.33 | 47.30 | 43.93 | 21.62 | -4.15 | 16.19 | 16.13 | 23.91 |
| GPTScore-ref | 14.73 | 24.95 | 39.42 | 31.60 | 18.20 | 33.14 | 18.24 | 25.75 |
| BARTScore-cnn(hypo-ref) | 13.64 | 28.53 | 36.12 | 29.57 | **23.35** | 32.49 | 26.64 | 27.19 |
| BARTScore-para (hypo-ref) | 17.18 | 33.72 | 40.79 | 28.94 | 17.27 | 34.47 | 17.43 | 27.11 |
| BERTScore | 23.67 | 42.41 | 43.75 | 25.60 | 11.53 | 45.77 | 2.88 | 27.95 |
| BLEURT | 17.30 | 48.41 | **48.76** | 33.26 | 3.53 | 36.46 | 27.52 | 30.75 |
| UniEval(summ) | **47.52** | 21.90 | 38.38 | **41.83** | 19.78 | 16.02 | **44.46** | 32.84 |
| COMET-22 | 33.75 | **56.35** | 33.92 | 35.28 | -5.53 | 46.13 | 39.20 | **34.16** |
| | | | Reference-free | Metrics | | | | |
| BARTScore-para (src-hypo) | **38.68** | 9.60 | 32.26 | 26.86 | -2.70 | 5.92 | 20.55 | 18.74 |
| BARTScore-cnn (src-hypo) | 35.50 | 12.83 | 34.33 | 40.96 | 1.50 | 25.43 | 33.48 | 26.29 |
| Llama-2-13b-chat-0-shot | 28.53 | 14.38 | 29.24 | 19.91 | 1.08 | 21.37 | 26.78 | 20.18 |
| COMETKiwi | 16.27 | **48.48** | 27.90 | 18.05 | -11.48 | 34.86 | 18.47 | 21.79 |
| GPTScore-src | 37.41 | 8.90 | 28.82 | 39.48 | 14.25 | 26.46 | 23.91 | 25.61 |
| TIGERScore-7B (ours) | 35.11 | 41.50 | 42.39 | **47.11** | 21.23 | 43.57 | 39.26 | 38.60 |
| TIGERScore-13B (ours) | 36.81 | 44.99 | **45.88** | 46.22 | **23.32** | **47.03** | **46.36** | **41.52** |
| Δ (ours - best reference-free) | -2 | -3 | +12 | +5 | +9 | +14 | +13 | +16 |
| ∆ (ours - best reference-based) | -9 | -11 | -3 | +5 | -0 | +0 | +2 | +7 |
## Usage
TIGERScore can be easily loaded in 2 lines of codes, and provides a friendly scoring interface function.
To use TIGERScore, first install `tigerscore` with
```bash
pip install git+https://github.com/TIGER-AI-Lab/TIGERScore.git
```
Then load the tigerscore model variates according to you needs.
```python
# set up scorer
from tigerscore import TIGERScorer
scorer = TIGERScorer(model_name="TIGER-Lab/TIGERScore-7B") # on GPU
# scorer = TIGERScorer(model_name="TIGER-Lab/TIGERScore-7B", quantized=True) # 4 bit quantization on GPU
# scorer = TIGERScorer(model_name="TIGER-Lab/TIGERScore-7B", use_vllm=True) # VLLM on GPU, Recommended for faster evaluation (0.2s per instance)
# scorer = TIGERScorer(model_name="TIGER-Lab/TIGERScore-7B-GGUF", use_llamacpp=True) # 4 bit quantization on CPU
```
After loading, you can easily get errors of the provided **hypothesis output** given the **instruction** and **input context**
```python
# example
instruction = "Write an apology letter."
input_context = "Reason: You canceled a plan at the last minute due to illness."
hypo_output = "Hey [Recipient],\n\nI'm really sorry for ditching our plan. I suddenly got an opportunity for a vacation so I took it. I know this might have messed up your plans and I regret that.\n\nDespite being under the weather, I would rather go for an adventure. I hope you can understand my perspective and I hope this incident doesn't change anything between us.\n\nWe can reschedule our plan for another time. Sorry again for the trouble.\n\nPeace out,\n[Your Name]\n\n---"
results = scorer.score([instruction], [hypo_output], [input_context])
print(results)
```
Results are a list of errors with detailed explanations and reasonable penalty scores:
```json
[
{
"num_errors": 2,
"score": -7.0,
"errors": {
"error_0": {
"location": " \"I suddenly got an opportunity for a vacation so I took it.\"",
"aspect": " Misunderstanding context",
"explanation": " The error lies in the context of the reason for cancelling the plan. The original reason was due to illness, but in the incorrect output, it is stated that the cancellation was due to a vacation opportunity, which is a misunderstanding of the context. The correction would be to stick to the original reason for cancelling.",
"severity": "Major",
"score_reduction": "5.0"
},
"error_1": {
"location": " \"I hope you can understand my perspective and I hope this incident doesn't change anything between us.\"",
"aspect": " Inappropriate tone",
"explanation": " The tone of this sentence is too casual and lacks regret or apology. It's important to maintain a formal and regretful tone in an apology letter. The sentence could be corrected to something like \"I hope you can find it in your heart to forgive me and let this incident not strain our relationship.\"",
"severity": "Minor",
"score_reduction": "2.0"
}
},
"raw_output": " The model-generated output contains 2 errors, with a total score reduction of 7.0.\nError location 1: ..."
}
]
```
Check more usage at our [Github Usage Doc](https://github.com/TIGER-AI-Lab/TIGERScore#usage). Have Fun!
## Citation
If you find our work useful, please cite our paper:
```
@article{jiang2023TIGERScore,
title={TIGERScore: Towards Building Explainable Metric for All Text Generation Tasks},
author={Dongfu Jiang, Yishan Li, Ge Zhang, Wenhao Huang, Bill Yuchen Lin, Wenhu Chen},
journal={arXiv preprint arXiv:2310.00752},
year={2023}
}
```
|
Eugeoter/artiwaifu-diffusion-1.0 | Eugeoter | 2024-05-07T05:01:28Z | 15,075 | 26 | diffusers | [
"diffusers",
"safetensors",
"text-to-image",
"stable-diffusion",
"stable-diffusion-xl",
"en",
"base_model:stabilityai/stable-diffusion-xl-base-1.0",
"license:other",
"autotrain_compatible",
"endpoints_compatible",
"diffusers:StableDiffusionXLPipeline",
"region:us"
] | text-to-image | 2024-05-04T07:58:12Z | ---
license: other
license_name: faipl-1.0-sd
license_link: https://freedevproject.org/faipl-1.0-sd/
language:
- en
tags:
- text-to-image
- stable-diffusion
- safetensors
- stable-diffusion-xl
- diffusers
base_model: stabilityai/stable-diffusion-xl-base-1.0
pipeline_tag: text-to-image
---
<h1 align="center"><strong style="font-size: 48px;">ArtiWaifu Diffusion 1.0</strong></h1>
<p align="center">
<img src="https://i.postimg.cc/RFN05PW0/1.png" alt="alt text" title="Cover" width="450"/>
</p>
We have released the **A**rti**Wa**ifu Diffusion V1.0 model, designed to generate aesthetically pleasing and faithfully restored anime-style illustrations.
The AWA Diffusion is an iteration of the Stable Diffusion XL model, mastering over 6000 artistic styles and more than 4000 anime characters, generating images through [trigger words](#trigger-words).
As a specialized image generation model for anime, it excels in producing high-quality anime images, especially in generating images with highly recognizable styles and characters while maintaining a consistently high-quality aesthetic expression.
## Model Details
The AWA Diffusion model is fine-tuned from Stable Diffusion XL, with a selected dataset of 1.5M high-quality anime images, covering a wide range of both popular and niche anime concepts up to April 15, 2024.
AWA Diffusion employs our most advanced training strategies, enabling users to easily induce the model to generate images of specific characters or styles while maintaining high image quality and aesthetic expression.
**Model Information**
- Developed by: [Euge](https://civitai.com/user/Euge_)
- Funded by: [Neta.art](https://nieta.art/)
- Model type: Generative text-to-image model
- Finetuned from model: [SDXL 1.0 Base](https://huggingface.co/stabilityai/stable-diffusion-xl-base-1.0)
- License: [Fair AI Public License 1.0-SD](https://freedevproject.org/faipl-1.0-sd/)
## Usage Guide
This guide will (i) introduce the model's recommended usage methods and prompt writing strategies, aiming to provide suggestions for generation, and (ii) serve as a reference document for model usage, detailing the writing patterns and strategies for trigger words, quality tags, rating tags, style tags, and character tags.
### Basic Usage
- **CFG scale**: <span style="color:cyan">5-11</span>
- **Resolution**: Area (= width x height) around 1024x1024. Not lower than 256x256, and resolutions where both length and width are multiples of 32.
- **Sampling method**: Euler A (<span style="color:cyan">50+</span> steps) or DPM++ 2M Karras (<span style="color:cyan">~35</span> steps)
Due to the special training method, AWA's optimal inference step count is higher than regular values. As the inference steps increase, the quality of the generated images can continue to improve...
❓ **Question:** Why not use the standard SDXL resolution?
💡 **Answer:** Because the bucketing algorithm used in training does not adhere to a fixed set of buckets. Although this does not conform to positional encoding, we have not observed any adverse effects.
### Prompting Strategies
All text-to-image diffusion models have a notoriously high sensitivity to prompt, and AWA Diffusion is no exception. Even a misspelling in the prompt, or even replacing spaces with underscores, can affect the generated results.
AWA Diffusion encourages users to write prompt in **tags** separated by **comma + space (`, `)**. Although the model also supports natural language descriptions as prompt, or an intermix of both, the tag-by-tag format is more stable and user-friendly.
When describing a specific ACG concept, such as a character, style, or scene, we recommend users choose tags from the [Danbooru tags](https://danbooru.donmai.us/tags) and replace underscores in the Danbooru tags with spaces to ensure the model accurately understands your needs. For example, `bishop_(chess)` should be written as `bishop (chess)`, and in inference tools like AUTOMATIC1111 WebUI that use parentheses to weight prompt, all parentheses within the tags should be escaped, i.e., `bishop \(chess\)`.
#### Tag Ordering
Including AWA Diffusion, most diffusion models better understand logically ordered tags. While tag ordering is not mandatory, it can help the model better understand your needs. Generally, the earlier the tag in the order, the greater its impact on generation.
Here's an example of tag ordering. The example organizes the order of tags, prepends [art style tags](#style-tags) and [character tags](#character-tags) because style and subject are the most important to the image. Subsequently, other tags are added in order of importance. Lastly, [aesthetic tags](#aesthetic-tags) and [quality tags](#quality-tags) are positioned at the end to further emphasize the aesthetics of the image.
art style (<span style="color:red">_by xxx_</span>) -> character (<span style="color:orange">_1 frieren (sousou no frieren)_</span>) -> race (elf) -> composition (cowboy shot) -> painting style (<span style="color:green">_impasto_</span>) -> theme (fantasy theme) -> main environment (in the forest, at day) -> background (gradient background) -> action (sitting on ground) -> expression (expressionless) -> main characteristics (white hair) -> other characteristics (twintails, green eyes, parted lip) -> clothing (wearing a white dress) -> clothing accessories (frills) -> other items (holding a magic wand) -> secondary environment (grass, sunshine) -> aesthetics (<span style="color:blue">_beautiful color_</span>, <span style="color:cyan">_detailed_</span>) -> quality (<span style="color:purple">_best_</span> quality) -> secondary description (birds, cloud, butterfly)
Tag order is not set in stone. Flexibility in writing prompt can yield better results. For example, if the effect of a concept (such as style) is too strong and detracts from the aesthetic appeal of the image, you can move it to a later position to reduce its impact.
#### Negative Prompt
Negative prompt are not necessary for AWA Diffusion. If you use negative prompt, it is not the case that the more negative prompt, the better. They should be **as concise as possible and easily recognizable by the model**. Too many negative words may lead to poorer generation results.
Here are some recommended scenarios for using negative prompt:
1. Watermark: `signature`, `logo`, `artist name`;
2. Quality: `worst quality`, `lowres`, `ugly`, `abstract`;
3. Style: `real life`, `3d`, `celluloid`, `sketch`, `draft`;
4. Human anatomy: `deformed hand`, `fused fingers`, `extra limbs`, `extra arms`, `missing arm`, `extra legs`, `missing leg`, `extra digits`, `fewer digits`.
### Trigger Words
Add trigger words to your prompts to inform the model about the concept you want to generate. Trigger words can include character names, artistic styles, scenes, actions, quality, etc.
**Tips for Trigger Word**
1. **Typos**: The model is very sensitive to the spelling of trigger words. Even a single letter difference can cause a trigger to fail or lead to unexpected results.
2. **Bracket Escaping**: Pay attention when using inference tools that rely on parentheses for weighting prompt, such as AUTOMATIC1111 WebUI, to escape parentheses in trigger words, e.g., `1 lucy (cyberpunk)` -> `1 lucy \(cyberpunk\)`.
3. **Triggering Effect Preview**:Through searching tags on [Danbooru](https://danbooru.donmai.us/tags) to preview the tag and better understand the tag's meaning and usage.
#### Style Tags
Style tags are divided into two types: <span style="color:red">Painting Style Tags</span> and <span style="color:blue">Artistic Style Tags</span>. <span style="color:red">Painting Style Tags</span> describe the painting techniques or media used in the image, such as oil painting, watercolor, flat color, and impasto. <span style="color:blue">Artistic Style Tags</span> represent the artistic style of the artist behind the image.
AWA Diffusion supports the following <span style="color:red">Painting Style Tags</span>:
- Painting style tags available in the Danbooru tags, such as `oil painting`, `watercolor`, `flat color`, etc.;
- All painting style tags supported by [AID XL 0.8](https://civitai.com/models/124189/anime-illust-diffusion-xl), such as `flat-pasto`, etc.;
- All style tags supported by [Neta Art XL 1.0](https://civitai.com/models/410737/neta-art-xl), such as `gufeng`, etc.;
See the [Painting Style Tags List](https://huggingface.co/Eugeoter/artiwaifu-diffusion-1.0/blob/main/references/style.csv) for full lists of painting style tags.
AWA Diffusion supports the following <span style="color:blue">Artistic Style Tags</span>:
- Artistic style tags available in the Danbooru tags, such as `by yoneyama mai`, `by wlop`, etc.;
- All artistic style tags supported by [AID XL 0.8](https://civitai.com/models/124189/anime-illust-diffusion-xl), such as `by antifreeze3`, `by 7thknights`, etc.;
See the [Artistic Style Tags List](https://huggingface.co/Eugeoter/artiwaifu-diffusion-1.0/blob/main/references/artist.csv) for full lists of artistic style tags.
The higher the tag count in the tag repository, the more thoroughly the artistic style has been trained, and the higher the fidelity in generation. Typically, artistic style tags with a count higher than **50** yield better generation results.
**Tips for Style Tag**
1. **Intensity Adjustment**: You can adjust the intensity of a style by altering the order or weighting of style tags in your prompt. Frontloading a style tag enhances its effect, while placing it later reduces its effect.
❓ **Question:** Why include the prefix `by` in artistic style tags?
💡 **Answer:** To clearly inform the model that you want to generate a specific artistic style rather than something else, we recommend including the prefix `by` in artistic style tags. This differentiates `by xxx` from `xxx`, especially when `xxx` itself carries other meanings, such as `dino` which could represent either a dinosaur or an artist's identifier.
Similarly, when triggering characters, add a `1` as a prefix to the character trigger word.
#### Character Tags
Character tags describe the character IP in the generated image. Using character tags will guide the model to generate the **appearance features** of the character.
Character tags also need to be sourced from the [Character Tag List](https://huggingface.co/Eugeoter/artiwaifu-diffusion-1.0/blob/main/references/character.csv). To generate a specific character, first find the corresponding trigger word in the tag repository, replace all underscores `_` in the trigger word with spaces ` `, and prepend `1 ` to the character name.
For example, `1 ayanami rei` triggers the model to generate the character Rei Ayanami from the anime "EVA," corresponding to the Danbooru tag `ayanami_rei`; `1 asuna (sao)` triggers the model to generate the character Asuna from "Sword Art Online," corresponding to the Danbooru tag `asuna_(sao)`.
[More examples](#examples)
The higher the tag count in the tag repository, the more thoroughly the character has been trained, and the higher the fidelity in generation. Typically, character tags with a count higher than **100** yield better generation results.
**Tips for Character Tag**
1. **Character Costuming**: To achieve more flexible character costuming, character tags do not deliberately guide the model to draw the official attire of the character. To generate a character in a specific official outfit, besides the trigger word, you should also include a description of the attire in the prompt, e.g., "1 lucy (cyberpunk), <span style="color:cyan">wearing a white cropped jacket, underneath bodysuit, shorts, thighhighs, hip vent</span>".
2. **Series Annotations**: Some character tags include additional parentheses annotations after the character name. The parentheses and the annotations within cannot be omitted, e.g., `1 lucy (cyberpunk)` cannot be written as `1 lucy`. Other than that, you don't need to add any additional annotations, for example, you DON'T need to add the series tag to which the character belongs after the character tag.
3. **Known Issue 1**: When generating certain characters, mysterious feature deformations may occur, e.g., `1 asui tsuyu` triggering the character Tsuyu Asui from "My Hero Academia" may result in an extra black line between the eyes. This is because the model incorrectly interprets the large round eyes as glasses, thus `glasses` should be included in the negative prompt to avoid this issue.
4. **Known Issue 2**: When generating less popular characters, AWA Diffusion might produce images with incomplete feature restoration due to insufficient data/training. In such cases, we recommend that you extend the character description in your prompt beyond just the character name, detailing the character's origin, race, hair color, attire, etc.
**Character Tag Trigger Examples**
| Trigger Word | Note |
| ------------------------------- | -------------------------------------------------------------- |
| 1 lucy (cyberpunk) | ✅ Correct character tag |
| 1 lucy | ❌ Missing bracket annotation |
| 1 lucy (cyber) | ❌ Incorrect bracket annotation |
| lucy (cyberpunk) | ❌ Missing prefix `1 ` |
| 1 lucy cyberpunk | ❌ Missing brackets |
| 1 lucy (cyberpunk | ❌ Bracket not closed |
| 1 lucky (cyberpunk) | ❌ Spelling error |
| 1 lucy (cyberpunk: edgerunners) | ❌ Bracket annotation not following the required character tag |
❓ **Question:** Why do some character tags contain bracket annotations, e.g., `lucy (cyberpunk)`, while others do not, e.g., `frieren`?
💡 **Answer:** In different works, there may be characters with the same name, such as Asuna from "Sword Art Online" and "Blue Archive". To distinguish these characters with the same name, it is necessary to annotate the character's name with the work's name, abbreviated if the name is too long. For characters with unique names that currently have no duplicates, like `frieren`, no special annotations are required. Here is an example:
#### Quality Tags and Aesthetic Tags
For AWA Diffusion, including quality descriptors in your positive prompt is **very important**. Quality descriptions relate to quality tags and aesthetic tags.
Quality tags directly describe the aesthetic quality of the generated image, impacting the detail, texture, human anatomy, lighting, color, etc. Adding quality tags helps the model generate higher quality images. Quality tags are ranked from highest to lowest as follows:
<span style="color:orange">amazing quality</span> -> <span style="color:purple">best quality</span> -> <span style="color:blue">high quality</span> -> <span style="color:green">normal quality</span> -> low quality -> <span style="color:grey">worst quality</span>
Aesthetic tags describe the aesthetic features of the generated image, aiding the model in producing artistically appealing images. In addition to typical aesthetic words like `perspective`, `lighting and shadow`, AWA Diffusion has been specially trained to respond effectively to aesthetic trigger words such as `beautiful color`, `detailed`, and `aesthetic`, which respectively express appealing colors, details, and overall beauty.
The recommended generic way to describe quality is: _(Your Prompt), <span style="color:orange">beautiful color, detailed, amazing quality</span>_
**Tips for Quality and Aesthetic Tags**
1. **Tag Quantity**: Only one quality tag is needed; multiple aesthetic tags can be added.
2. **Tag Position**: The position of quality and aesthetic tags is not fixed, but they are typically placed at the end of the prompt.
3. **Relative Quality**: There is no absolute hierarchy of quality; the implied quality aligns with general aesthetic standards, and different users may have different perceptions of quality.
#### Rating Tags
Rating tags describe the level of exposure in the content of the generated image. Rating tags are ranked from highest to lowest as follows:
<span style="color:green">rating: general</span> (or <span style="color:green">safe</span>) -> <span style="color:yellow">rating: suggestive</span> -> <span style="color:orange">rating: questionable</span> -> <span style="color:red">rating: explicit</span> (or <span style="color:red">nsfw</span>)
### Prompt Word Examples
#### Example 1
**A**
_<span style="color:green">by yoneyama mai</span>, <span style="color:blue">1 frieren</span>, 1girl, solo, fantasy theme, smile, holding a magic wand, <span style="color:yellow">beautiful color</span>, <span style="color:red">amazing quality</span>_
1. <span style="color:green">by yoneyama mai</span> triggers the artistic style of Yoneyama Mai, placed at the front to enhance the effect.
2. <span style="color:blue">1 frieren</span> triggers the character Frieren from the series "Frieren at the Funeral."
3. <span style="color:yellow">beautiful color</span> describes the beautiful colors in the generated image.
4. <span style="color:red">amazing quality</span> describes the stunning quality of the generated image.
**B**
_<span style="color:green">by nixeu</span>, <span style="color:blue">1 lucy (cyberpunk)</span>, 1girl, solo, cowboy shot, gradient background, white cropped jacket, underneath bodysuit, shorts, thighhighs, hip vent, <span style="color:yellow">detailed</span>, <span style="color:red">best quality</span>_
#### Example 2: Style Mixing
By layering multiple different style tags, you can generate images with features of multiple styles.
**A** Simple Mixing
_**<span style="color:green">by ningen mame</span>, <span style="color:cyan">by ciloranko</span>, <span style="color:blue">by sho (sho lwlw)</span>**, 1girl, 1 hatsune miku, sitting, arm support, smile, detailed, amazing quality_
**B** Weighted Mixing
Using AUTOMATIC1111 WebUI prompt weighting syntax (parentheses weighting), weight different style tags to better control the generated image's style.
_**<span style="color:green">(by ningen mame:0.8)</span>, <span style="color:cyan">(by ciloranko:1.1)</span>, <span style="color:blue">(by sho \(sho lwlw\):1.2)</span>**, 1girl, 1 hatsune miku, sitting, arm support, smile, detailed, amazing quality_
#### Example 3: Multi-Character Scenes
By adding multiple character tags to your prompts, you can generate images with multiple characters in the same frame. Compared to other similar models, AWA performs better in multi-character scenes but remains unstable.
**A** Mixed Gender Scene
_**1girl and 1boy, <span style="color:blue">1 ganyu</span> girl, <span style="color:cyan">1 gojou satoru</span> boy**, beautiful color, amazing quality_
**B** Same Gender Scene
_**2girls, <span style="color:blue">1 ganyu</span> girl, <span style="color:orange">1 yoimiya</span> girl**, beautiful color, amazing quality_
## Future Work
AWA Diffusion is expected to combine high-level <span style="color:purple">aesthetics</span> with comprehensive <span style="color:cyan">knowledge</span>. It should neither have the traditional AI's greasy feel nor become a knowledge-deficient vase.
We will continue to explore more advanced training techniques and strategies, consistently improving the model's quality.
## Support Us
Training AWA Diffusion incurs substantial costs. If you appreciate our work, please consider supporting us through [Ko-fi](https://ko-fi.com/eugeai), to aid our research and development efforts. Thank you for your like and support! |
mradermacher/Llama-3-Instruct-8B-SimPO-ExPO-i1-GGUF | mradermacher | 2024-06-28T20:20:53Z | 15,063 | 0 | transformers | [
"transformers",
"gguf",
"en",
"base_model:chujiezheng/Llama-3-Instruct-8B-SimPO-ExPO",
"license:llama3",
"endpoints_compatible",
"region:us"
] | null | 2024-06-28T19:06:29Z | ---
base_model: chujiezheng/Llama-3-Instruct-8B-SimPO-ExPO
language:
- en
library_name: transformers
license: llama3
quantized_by: mradermacher
---
## About
<!-- ### quantize_version: 2 -->
<!-- ### output_tensor_quantised: 1 -->
<!-- ### convert_type: hf -->
<!-- ### vocab_type: -->
<!-- ### tags: nicoboss -->
weighted/imatrix quants of https://huggingface.co/chujiezheng/Llama-3-Instruct-8B-SimPO-ExPO
<!-- provided-files -->
static quants are available at https://huggingface.co/mradermacher/Llama-3-Instruct-8B-SimPO-ExPO-GGUF
## Usage
If you are unsure how to use GGUF files, refer to one of [TheBloke's
READMEs](https://huggingface.co/TheBloke/KafkaLM-70B-German-V0.1-GGUF) for
more details, including on how to concatenate multi-part files.
## Provided Quants
(sorted by size, not necessarily quality. IQ-quants are often preferable over similar sized non-IQ quants)
| Link | Type | Size/GB | Notes |
|:-----|:-----|--------:|:------|
| [GGUF](https://huggingface.co/mradermacher/Llama-3-Instruct-8B-SimPO-ExPO-i1-GGUF/resolve/main/Llama-3-Instruct-8B-SimPO-ExPO.i1-IQ1_S.gguf) | i1-IQ1_S | 2.1 | for the desperate |
| [GGUF](https://huggingface.co/mradermacher/Llama-3-Instruct-8B-SimPO-ExPO-i1-GGUF/resolve/main/Llama-3-Instruct-8B-SimPO-ExPO.i1-IQ1_M.gguf) | i1-IQ1_M | 2.3 | mostly desperate |
| [GGUF](https://huggingface.co/mradermacher/Llama-3-Instruct-8B-SimPO-ExPO-i1-GGUF/resolve/main/Llama-3-Instruct-8B-SimPO-ExPO.i1-IQ2_XXS.gguf) | i1-IQ2_XXS | 2.5 | |
| [GGUF](https://huggingface.co/mradermacher/Llama-3-Instruct-8B-SimPO-ExPO-i1-GGUF/resolve/main/Llama-3-Instruct-8B-SimPO-ExPO.i1-IQ2_XS.gguf) | i1-IQ2_XS | 2.7 | |
| [GGUF](https://huggingface.co/mradermacher/Llama-3-Instruct-8B-SimPO-ExPO-i1-GGUF/resolve/main/Llama-3-Instruct-8B-SimPO-ExPO.i1-IQ2_S.gguf) | i1-IQ2_S | 2.9 | |
| [GGUF](https://huggingface.co/mradermacher/Llama-3-Instruct-8B-SimPO-ExPO-i1-GGUF/resolve/main/Llama-3-Instruct-8B-SimPO-ExPO.i1-IQ2_M.gguf) | i1-IQ2_M | 3.0 | |
| [GGUF](https://huggingface.co/mradermacher/Llama-3-Instruct-8B-SimPO-ExPO-i1-GGUF/resolve/main/Llama-3-Instruct-8B-SimPO-ExPO.i1-Q2_K.gguf) | i1-Q2_K | 3.3 | IQ3_XXS probably better |
| [GGUF](https://huggingface.co/mradermacher/Llama-3-Instruct-8B-SimPO-ExPO-i1-GGUF/resolve/main/Llama-3-Instruct-8B-SimPO-ExPO.i1-IQ3_XXS.gguf) | i1-IQ3_XXS | 3.4 | lower quality |
| [GGUF](https://huggingface.co/mradermacher/Llama-3-Instruct-8B-SimPO-ExPO-i1-GGUF/resolve/main/Llama-3-Instruct-8B-SimPO-ExPO.i1-IQ3_XS.gguf) | i1-IQ3_XS | 3.6 | |
| [GGUF](https://huggingface.co/mradermacher/Llama-3-Instruct-8B-SimPO-ExPO-i1-GGUF/resolve/main/Llama-3-Instruct-8B-SimPO-ExPO.i1-Q3_K_S.gguf) | i1-Q3_K_S | 3.8 | IQ3_XS probably better |
| [GGUF](https://huggingface.co/mradermacher/Llama-3-Instruct-8B-SimPO-ExPO-i1-GGUF/resolve/main/Llama-3-Instruct-8B-SimPO-ExPO.i1-IQ3_S.gguf) | i1-IQ3_S | 3.8 | beats Q3_K* |
| [GGUF](https://huggingface.co/mradermacher/Llama-3-Instruct-8B-SimPO-ExPO-i1-GGUF/resolve/main/Llama-3-Instruct-8B-SimPO-ExPO.i1-IQ3_M.gguf) | i1-IQ3_M | 3.9 | |
| [GGUF](https://huggingface.co/mradermacher/Llama-3-Instruct-8B-SimPO-ExPO-i1-GGUF/resolve/main/Llama-3-Instruct-8B-SimPO-ExPO.i1-Q3_K_M.gguf) | i1-Q3_K_M | 4.1 | IQ3_S probably better |
| [GGUF](https://huggingface.co/mradermacher/Llama-3-Instruct-8B-SimPO-ExPO-i1-GGUF/resolve/main/Llama-3-Instruct-8B-SimPO-ExPO.i1-Q3_K_L.gguf) | i1-Q3_K_L | 4.4 | IQ3_M probably better |
| [GGUF](https://huggingface.co/mradermacher/Llama-3-Instruct-8B-SimPO-ExPO-i1-GGUF/resolve/main/Llama-3-Instruct-8B-SimPO-ExPO.i1-IQ4_XS.gguf) | i1-IQ4_XS | 4.5 | |
| [GGUF](https://huggingface.co/mradermacher/Llama-3-Instruct-8B-SimPO-ExPO-i1-GGUF/resolve/main/Llama-3-Instruct-8B-SimPO-ExPO.i1-Q4_0.gguf) | i1-Q4_0 | 4.8 | fast, low quality |
| [GGUF](https://huggingface.co/mradermacher/Llama-3-Instruct-8B-SimPO-ExPO-i1-GGUF/resolve/main/Llama-3-Instruct-8B-SimPO-ExPO.i1-Q4_K_S.gguf) | i1-Q4_K_S | 4.8 | optimal size/speed/quality |
| [GGUF](https://huggingface.co/mradermacher/Llama-3-Instruct-8B-SimPO-ExPO-i1-GGUF/resolve/main/Llama-3-Instruct-8B-SimPO-ExPO.i1-Q4_K_M.gguf) | i1-Q4_K_M | 5.0 | fast, recommended |
| [GGUF](https://huggingface.co/mradermacher/Llama-3-Instruct-8B-SimPO-ExPO-i1-GGUF/resolve/main/Llama-3-Instruct-8B-SimPO-ExPO.i1-Q5_K_S.gguf) | i1-Q5_K_S | 5.7 | |
| [GGUF](https://huggingface.co/mradermacher/Llama-3-Instruct-8B-SimPO-ExPO-i1-GGUF/resolve/main/Llama-3-Instruct-8B-SimPO-ExPO.i1-Q5_K_M.gguf) | i1-Q5_K_M | 5.8 | |
| [GGUF](https://huggingface.co/mradermacher/Llama-3-Instruct-8B-SimPO-ExPO-i1-GGUF/resolve/main/Llama-3-Instruct-8B-SimPO-ExPO.i1-Q6_K.gguf) | i1-Q6_K | 6.7 | practically like static Q6_K |
Here is a handy graph by ikawrakow comparing some lower-quality quant
types (lower is better):

And here are Artefact2's thoughts on the matter:
https://gist.github.com/Artefact2/b5f810600771265fc1e39442288e8ec9
## FAQ / Model Request
See https://huggingface.co/mradermacher/model_requests for some answers to
questions you might have and/or if you want some other model quantized.
## Thanks
I thank my company, [nethype GmbH](https://www.nethype.de/), for letting
me use its servers and providing upgrades to my workstation to enable
this work in my free time. Additional thanks to [@nicoboss](https://huggingface.co/nicoboss) for giving me access to his hardware for calculating the imatrix for these quants.
<!-- end -->
|
mradermacher/LLAMA-3_8B_Unaligned_Alpha_RP_Soup-GGUF | mradermacher | 2024-06-23T01:21:36Z | 15,061 | 0 | transformers | [
"transformers",
"gguf",
"en",
"base_model:SicariusSicariiStuff/LLAMA-3_8B_Unaligned_Alpha_RP_Soup",
"license:apache-2.0",
"endpoints_compatible",
"region:us"
] | null | 2024-06-23T00:27:19Z | ---
base_model: SicariusSicariiStuff/LLAMA-3_8B_Unaligned_Alpha_RP_Soup
language:
- en
library_name: transformers
license: apache-2.0
quantized_by: mradermacher
---
## About
<!-- ### quantize_version: 2 -->
<!-- ### output_tensor_quantised: 1 -->
<!-- ### convert_type: hf -->
<!-- ### vocab_type: -->
<!-- ### tags: -->
static quants of https://huggingface.co/SicariusSicariiStuff/LLAMA-3_8B_Unaligned_Alpha_RP_Soup
<!-- provided-files -->
weighted/imatrix quants are available at https://huggingface.co/mradermacher/LLAMA-3_8B_Unaligned_Alpha_RP_Soup-i1-GGUF
## Usage
If you are unsure how to use GGUF files, refer to one of [TheBloke's
READMEs](https://huggingface.co/TheBloke/KafkaLM-70B-German-V0.1-GGUF) for
more details, including on how to concatenate multi-part files.
## Provided Quants
(sorted by size, not necessarily quality. IQ-quants are often preferable over similar sized non-IQ quants)
| Link | Type | Size/GB | Notes |
|:-----|:-----|--------:|:------|
| [GGUF](https://huggingface.co/mradermacher/LLAMA-3_8B_Unaligned_Alpha_RP_Soup-GGUF/resolve/main/LLAMA-3_8B_Unaligned_Alpha_RP_Soup.Q2_K.gguf) | Q2_K | 3.3 | |
| [GGUF](https://huggingface.co/mradermacher/LLAMA-3_8B_Unaligned_Alpha_RP_Soup-GGUF/resolve/main/LLAMA-3_8B_Unaligned_Alpha_RP_Soup.IQ3_XS.gguf) | IQ3_XS | 3.6 | |
| [GGUF](https://huggingface.co/mradermacher/LLAMA-3_8B_Unaligned_Alpha_RP_Soup-GGUF/resolve/main/LLAMA-3_8B_Unaligned_Alpha_RP_Soup.Q3_K_S.gguf) | Q3_K_S | 3.8 | |
| [GGUF](https://huggingface.co/mradermacher/LLAMA-3_8B_Unaligned_Alpha_RP_Soup-GGUF/resolve/main/LLAMA-3_8B_Unaligned_Alpha_RP_Soup.IQ3_S.gguf) | IQ3_S | 3.8 | beats Q3_K* |
| [GGUF](https://huggingface.co/mradermacher/LLAMA-3_8B_Unaligned_Alpha_RP_Soup-GGUF/resolve/main/LLAMA-3_8B_Unaligned_Alpha_RP_Soup.IQ3_M.gguf) | IQ3_M | 3.9 | |
| [GGUF](https://huggingface.co/mradermacher/LLAMA-3_8B_Unaligned_Alpha_RP_Soup-GGUF/resolve/main/LLAMA-3_8B_Unaligned_Alpha_RP_Soup.Q3_K_M.gguf) | Q3_K_M | 4.1 | lower quality |
| [GGUF](https://huggingface.co/mradermacher/LLAMA-3_8B_Unaligned_Alpha_RP_Soup-GGUF/resolve/main/LLAMA-3_8B_Unaligned_Alpha_RP_Soup.Q3_K_L.gguf) | Q3_K_L | 4.4 | |
| [GGUF](https://huggingface.co/mradermacher/LLAMA-3_8B_Unaligned_Alpha_RP_Soup-GGUF/resolve/main/LLAMA-3_8B_Unaligned_Alpha_RP_Soup.IQ4_XS.gguf) | IQ4_XS | 4.6 | |
| [GGUF](https://huggingface.co/mradermacher/LLAMA-3_8B_Unaligned_Alpha_RP_Soup-GGUF/resolve/main/LLAMA-3_8B_Unaligned_Alpha_RP_Soup.Q4_K_S.gguf) | Q4_K_S | 4.8 | fast, recommended |
| [GGUF](https://huggingface.co/mradermacher/LLAMA-3_8B_Unaligned_Alpha_RP_Soup-GGUF/resolve/main/LLAMA-3_8B_Unaligned_Alpha_RP_Soup.Q4_K_M.gguf) | Q4_K_M | 5.0 | fast, recommended |
| [GGUF](https://huggingface.co/mradermacher/LLAMA-3_8B_Unaligned_Alpha_RP_Soup-GGUF/resolve/main/LLAMA-3_8B_Unaligned_Alpha_RP_Soup.Q5_K_S.gguf) | Q5_K_S | 5.7 | |
| [GGUF](https://huggingface.co/mradermacher/LLAMA-3_8B_Unaligned_Alpha_RP_Soup-GGUF/resolve/main/LLAMA-3_8B_Unaligned_Alpha_RP_Soup.Q5_K_M.gguf) | Q5_K_M | 5.8 | |
| [GGUF](https://huggingface.co/mradermacher/LLAMA-3_8B_Unaligned_Alpha_RP_Soup-GGUF/resolve/main/LLAMA-3_8B_Unaligned_Alpha_RP_Soup.Q6_K.gguf) | Q6_K | 6.7 | very good quality |
| [GGUF](https://huggingface.co/mradermacher/LLAMA-3_8B_Unaligned_Alpha_RP_Soup-GGUF/resolve/main/LLAMA-3_8B_Unaligned_Alpha_RP_Soup.Q8_0.gguf) | Q8_0 | 8.6 | fast, best quality |
| [GGUF](https://huggingface.co/mradermacher/LLAMA-3_8B_Unaligned_Alpha_RP_Soup-GGUF/resolve/main/LLAMA-3_8B_Unaligned_Alpha_RP_Soup.f16.gguf) | f16 | 16.2 | 16 bpw, overkill |
Here is a handy graph by ikawrakow comparing some lower-quality quant
types (lower is better):

And here are Artefact2's thoughts on the matter:
https://gist.github.com/Artefact2/b5f810600771265fc1e39442288e8ec9
## FAQ / Model Request
See https://huggingface.co/mradermacher/model_requests for some answers to
questions you might have and/or if you want some other model quantized.
## Thanks
I thank my company, [nethype GmbH](https://www.nethype.de/), for letting
me use its servers and providing upgrades to my workstation to enable
this work in my free time.
<!-- end -->
|
mradermacher/controllable-wizardlm-7b-GGUF | mradermacher | 2024-06-30T05:04:48Z | 15,059 | 0 | transformers | [
"transformers",
"gguf",
"en",
"base_model:umd-zhou-lab/controllable-wizardlm-7b",
"license:llama2",
"endpoints_compatible",
"region:us"
] | null | 2024-06-30T04:32:46Z | ---
base_model: umd-zhou-lab/controllable-wizardlm-7b
language:
- en
library_name: transformers
license: llama2
quantized_by: mradermacher
---
## About
<!-- ### quantize_version: 2 -->
<!-- ### output_tensor_quantised: 1 -->
<!-- ### convert_type: hf -->
<!-- ### vocab_type: -->
<!-- ### tags: -->
static quants of https://huggingface.co/umd-zhou-lab/controllable-wizardlm-7b
<!-- provided-files -->
weighted/imatrix quants seem not to be available (by me) at this time. If they do not show up a week or so after the static ones, I have probably not planned for them. Feel free to request them by opening a Community Discussion.
## Usage
If you are unsure how to use GGUF files, refer to one of [TheBloke's
READMEs](https://huggingface.co/TheBloke/KafkaLM-70B-German-V0.1-GGUF) for
more details, including on how to concatenate multi-part files.
## Provided Quants
(sorted by size, not necessarily quality. IQ-quants are often preferable over similar sized non-IQ quants)
| Link | Type | Size/GB | Notes |
|:-----|:-----|--------:|:------|
| [GGUF](https://huggingface.co/mradermacher/controllable-wizardlm-7b-GGUF/resolve/main/controllable-wizardlm-7b.Q2_K.gguf) | Q2_K | 2.6 | |
| [GGUF](https://huggingface.co/mradermacher/controllable-wizardlm-7b-GGUF/resolve/main/controllable-wizardlm-7b.IQ3_XS.gguf) | IQ3_XS | 2.9 | |
| [GGUF](https://huggingface.co/mradermacher/controllable-wizardlm-7b-GGUF/resolve/main/controllable-wizardlm-7b.IQ3_S.gguf) | IQ3_S | 3.0 | beats Q3_K* |
| [GGUF](https://huggingface.co/mradermacher/controllable-wizardlm-7b-GGUF/resolve/main/controllable-wizardlm-7b.Q3_K_S.gguf) | Q3_K_S | 3.0 | |
| [GGUF](https://huggingface.co/mradermacher/controllable-wizardlm-7b-GGUF/resolve/main/controllable-wizardlm-7b.IQ3_M.gguf) | IQ3_M | 3.2 | |
| [GGUF](https://huggingface.co/mradermacher/controllable-wizardlm-7b-GGUF/resolve/main/controllable-wizardlm-7b.Q3_K_M.gguf) | Q3_K_M | 3.4 | lower quality |
| [GGUF](https://huggingface.co/mradermacher/controllable-wizardlm-7b-GGUF/resolve/main/controllable-wizardlm-7b.Q3_K_L.gguf) | Q3_K_L | 3.7 | |
| [GGUF](https://huggingface.co/mradermacher/controllable-wizardlm-7b-GGUF/resolve/main/controllable-wizardlm-7b.IQ4_XS.gguf) | IQ4_XS | 3.7 | |
| [GGUF](https://huggingface.co/mradermacher/controllable-wizardlm-7b-GGUF/resolve/main/controllable-wizardlm-7b.Q4_K_S.gguf) | Q4_K_S | 4.0 | fast, recommended |
| [GGUF](https://huggingface.co/mradermacher/controllable-wizardlm-7b-GGUF/resolve/main/controllable-wizardlm-7b.Q4_K_M.gguf) | Q4_K_M | 4.2 | fast, recommended |
| [GGUF](https://huggingface.co/mradermacher/controllable-wizardlm-7b-GGUF/resolve/main/controllable-wizardlm-7b.Q5_K_S.gguf) | Q5_K_S | 4.8 | |
| [GGUF](https://huggingface.co/mradermacher/controllable-wizardlm-7b-GGUF/resolve/main/controllable-wizardlm-7b.Q5_K_M.gguf) | Q5_K_M | 4.9 | |
| [GGUF](https://huggingface.co/mradermacher/controllable-wizardlm-7b-GGUF/resolve/main/controllable-wizardlm-7b.Q6_K.gguf) | Q6_K | 5.6 | very good quality |
| [GGUF](https://huggingface.co/mradermacher/controllable-wizardlm-7b-GGUF/resolve/main/controllable-wizardlm-7b.Q8_0.gguf) | Q8_0 | 7.3 | fast, best quality |
| [GGUF](https://huggingface.co/mradermacher/controllable-wizardlm-7b-GGUF/resolve/main/controllable-wizardlm-7b.f16.gguf) | f16 | 13.6 | 16 bpw, overkill |
Here is a handy graph by ikawrakow comparing some lower-quality quant
types (lower is better):

And here are Artefact2's thoughts on the matter:
https://gist.github.com/Artefact2/b5f810600771265fc1e39442288e8ec9
## FAQ / Model Request
See https://huggingface.co/mradermacher/model_requests for some answers to
questions you might have and/or if you want some other model quantized.
## Thanks
I thank my company, [nethype GmbH](https://www.nethype.de/), for letting
me use its servers and providing upgrades to my workstation to enable
this work in my free time.
<!-- end -->
|
nvidia/mit-b2 | nvidia | 2022-08-06T10:26:08Z | 15,052 | 4 | transformers | [
"transformers",
"pytorch",
"tf",
"segformer",
"image-classification",
"vision",
"dataset:imagenet_1k",
"arxiv:2105.15203",
"license:other",
"autotrain_compatible",
"endpoints_compatible",
"region:us"
] | image-classification | 2022-03-02T23:29:05Z | ---
license: other
tags:
- vision
datasets:
- imagenet_1k
widget:
- src: https://huggingface.co/datasets/hf-internal-testing/fixtures_ade20k/resolve/main/ADE_val_00000001.jpg
example_title: House
- src: https://huggingface.co/datasets/hf-internal-testing/fixtures_ade20k/resolve/main/ADE_val_00000002.jpg
example_title: Castle
---
# SegFormer (b2-sized) encoder pre-trained-only
SegFormer encoder fine-tuned on Imagenet-1k. It was introduced in the paper [SegFormer: Simple and Efficient Design for Semantic Segmentation with Transformers](https://arxiv.org/abs/2105.15203) by Xie et al. and first released in [this repository](https://github.com/NVlabs/SegFormer).
Disclaimer: The team releasing SegFormer did not write a model card for this model so this model card has been written by the Hugging Face team.
## Model description
SegFormer consists of a hierarchical Transformer encoder and a lightweight all-MLP decode head to achieve great results on semantic segmentation benchmarks such as ADE20K and Cityscapes. The hierarchical Transformer is first pre-trained on ImageNet-1k, after which a decode head is added and fine-tuned altogether on a downstream dataset.
This repository only contains the pre-trained hierarchical Transformer, hence it can be used for fine-tuning purposes.
## Intended uses & limitations
You can use the model for fine-tuning of semantic segmentation. See the [model hub](https://huggingface.co/models?other=segformer) to look for fine-tuned versions on a task that interests you.
### How to use
Here is how to use this model to classify an image of the COCO 2017 dataset into one of the 1,000 ImageNet classes:
```python
from transformers import SegformerFeatureExtractor, SegformerForImageClassification
from PIL import Image
import requests
url = "http://images.cocodataset.org/val2017/000000039769.jpg"
image = Image.open(requests.get(url, stream=True).raw)
feature_extractor = SegformerFeatureExtractor.from_pretrained("nvidia/mit-b2")
model = SegformerForImageClassification.from_pretrained("nvidia/mit-b2")
inputs = feature_extractor(images=image, return_tensors="pt")
outputs = model(**inputs)
logits = outputs.logits
# model predicts one of the 1000 ImageNet classes
predicted_class_idx = logits.argmax(-1).item()
print("Predicted class:", model.config.id2label[predicted_class_idx])
```
For more code examples, we refer to the [documentation](https://huggingface.co/transformers/model_doc/segformer.html#).
### License
The license for this model can be found [here](https://github.com/NVlabs/SegFormer/blob/master/LICENSE).
### BibTeX entry and citation info
```bibtex
@article{DBLP:journals/corr/abs-2105-15203,
author = {Enze Xie and
Wenhai Wang and
Zhiding Yu and
Anima Anandkumar and
Jose M. Alvarez and
Ping Luo},
title = {SegFormer: Simple and Efficient Design for Semantic Segmentation with
Transformers},
journal = {CoRR},
volume = {abs/2105.15203},
year = {2021},
url = {https://arxiv.org/abs/2105.15203},
eprinttype = {arXiv},
eprint = {2105.15203},
timestamp = {Wed, 02 Jun 2021 11:46:42 +0200},
biburl = {https://dblp.org/rec/journals/corr/abs-2105-15203.bib},
bibsource = {dblp computer science bibliography, https://dblp.org}
}
```
|
duyntnet/BioMistral-7B-imatrix-GGUF | duyntnet | 2024-06-20T06:18:47Z | 15,043 | 0 | transformers | [
"transformers",
"gguf",
"imatrix",
"BioMistral-7B",
"text-generation",
"en",
"license:other",
"region:us"
] | text-generation | 2024-06-20T03:58:49Z | ---
license: other
language:
- en
pipeline_tag: text-generation
inference: false
tags:
- transformers
- gguf
- imatrix
- BioMistral-7B
---
Quantizations of https://huggingface.co/BioMistral/BioMistral-7B
# From original readme
## BioMistral: A Collection of Open-Source Pretrained Large Language Models for Medical Domains
### 2. Using BioMistral
You can use BioMistral with [Hugging Face's Transformers library](https://github.com/huggingface/transformers) as follow.
Loading the model and tokenizer :
```python
from transformers import AutoModel, AutoTokenizer
tokenizer = AutoTokenizer.from_pretrained("BioMistral/BioMistral-7B")
model = AutoModel.from_pretrained("BioMistral/BioMistral-7B")
``` |
RichardErkhov/sail_-_Sailor-7B-Chat-gguf | RichardErkhov | 2024-06-29T09:26:57Z | 15,043 | 0 | null | [
"gguf",
"arxiv:2404.03608",
"region:us"
] | null | 2024-06-29T06:20:51Z | Quantization made by Richard Erkhov.
[Github](https://github.com/RichardErkhov)
[Discord](https://discord.gg/pvy7H8DZMG)
[Request more models](https://github.com/RichardErkhov/quant_request)
Sailor-7B-Chat - GGUF
- Model creator: https://huggingface.co/sail/
- Original model: https://huggingface.co/sail/Sailor-7B-Chat/
| Name | Quant method | Size |
| ---- | ---- | ---- |
| [Sailor-7B-Chat.Q2_K.gguf](https://huggingface.co/RichardErkhov/sail_-_Sailor-7B-Chat-gguf/blob/main/Sailor-7B-Chat.Q2_K.gguf) | Q2_K | 2.89GB |
| [Sailor-7B-Chat.IQ3_XS.gguf](https://huggingface.co/RichardErkhov/sail_-_Sailor-7B-Chat-gguf/blob/main/Sailor-7B-Chat.IQ3_XS.gguf) | IQ3_XS | 3.18GB |
| [Sailor-7B-Chat.IQ3_S.gguf](https://huggingface.co/RichardErkhov/sail_-_Sailor-7B-Chat-gguf/blob/main/Sailor-7B-Chat.IQ3_S.gguf) | IQ3_S | 3.32GB |
| [Sailor-7B-Chat.Q3_K_S.gguf](https://huggingface.co/RichardErkhov/sail_-_Sailor-7B-Chat-gguf/blob/main/Sailor-7B-Chat.Q3_K_S.gguf) | Q3_K_S | 3.32GB |
| [Sailor-7B-Chat.IQ3_M.gguf](https://huggingface.co/RichardErkhov/sail_-_Sailor-7B-Chat-gguf/blob/main/Sailor-7B-Chat.IQ3_M.gguf) | IQ3_M | 3.48GB |
| [Sailor-7B-Chat.Q3_K.gguf](https://huggingface.co/RichardErkhov/sail_-_Sailor-7B-Chat-gguf/blob/main/Sailor-7B-Chat.Q3_K.gguf) | Q3_K | 3.65GB |
| [Sailor-7B-Chat.Q3_K_M.gguf](https://huggingface.co/RichardErkhov/sail_-_Sailor-7B-Chat-gguf/blob/main/Sailor-7B-Chat.Q3_K_M.gguf) | Q3_K_M | 3.65GB |
| [Sailor-7B-Chat.Q3_K_L.gguf](https://huggingface.co/RichardErkhov/sail_-_Sailor-7B-Chat-gguf/blob/main/Sailor-7B-Chat.Q3_K_L.gguf) | Q3_K_L | 3.93GB |
| [Sailor-7B-Chat.IQ4_XS.gguf](https://huggingface.co/RichardErkhov/sail_-_Sailor-7B-Chat-gguf/blob/main/Sailor-7B-Chat.IQ4_XS.gguf) | IQ4_XS | 4.02GB |
| [Sailor-7B-Chat.Q4_0.gguf](https://huggingface.co/RichardErkhov/sail_-_Sailor-7B-Chat-gguf/blob/main/Sailor-7B-Chat.Q4_0.gguf) | Q4_0 | 4.2GB |
| [Sailor-7B-Chat.IQ4_NL.gguf](https://huggingface.co/RichardErkhov/sail_-_Sailor-7B-Chat-gguf/blob/main/Sailor-7B-Chat.IQ4_NL.gguf) | IQ4_NL | 4.22GB |
| [Sailor-7B-Chat.Q4_K_S.gguf](https://huggingface.co/RichardErkhov/sail_-_Sailor-7B-Chat-gguf/blob/main/Sailor-7B-Chat.Q4_K_S.gguf) | Q4_K_S | 4.23GB |
| [Sailor-7B-Chat.Q4_K.gguf](https://huggingface.co/RichardErkhov/sail_-_Sailor-7B-Chat-gguf/blob/main/Sailor-7B-Chat.Q4_K.gguf) | Q4_K | 4.44GB |
| [Sailor-7B-Chat.Q4_K_M.gguf](https://huggingface.co/RichardErkhov/sail_-_Sailor-7B-Chat-gguf/blob/main/Sailor-7B-Chat.Q4_K_M.gguf) | Q4_K_M | 4.44GB |
| [Sailor-7B-Chat.Q4_1.gguf](https://huggingface.co/RichardErkhov/sail_-_Sailor-7B-Chat-gguf/blob/main/Sailor-7B-Chat.Q4_1.gguf) | Q4_1 | 4.62GB |
| [Sailor-7B-Chat.Q5_0.gguf](https://huggingface.co/RichardErkhov/sail_-_Sailor-7B-Chat-gguf/blob/main/Sailor-7B-Chat.Q5_0.gguf) | Q5_0 | 5.03GB |
| [Sailor-7B-Chat.Q5_K_S.gguf](https://huggingface.co/RichardErkhov/sail_-_Sailor-7B-Chat-gguf/blob/main/Sailor-7B-Chat.Q5_K_S.gguf) | Q5_K_S | 5.03GB |
| [Sailor-7B-Chat.Q5_K.gguf](https://huggingface.co/RichardErkhov/sail_-_Sailor-7B-Chat-gguf/blob/main/Sailor-7B-Chat.Q5_K.gguf) | Q5_K | 5.15GB |
| [Sailor-7B-Chat.Q5_K_M.gguf](https://huggingface.co/RichardErkhov/sail_-_Sailor-7B-Chat-gguf/blob/main/Sailor-7B-Chat.Q5_K_M.gguf) | Q5_K_M | 5.15GB |
| [Sailor-7B-Chat.Q5_1.gguf](https://huggingface.co/RichardErkhov/sail_-_Sailor-7B-Chat-gguf/blob/main/Sailor-7B-Chat.Q5_1.gguf) | Q5_1 | 5.44GB |
| [Sailor-7B-Chat.Q6_K.gguf](https://huggingface.co/RichardErkhov/sail_-_Sailor-7B-Chat-gguf/blob/main/Sailor-7B-Chat.Q6_K.gguf) | Q6_K | 5.91GB |
| [Sailor-7B-Chat.Q8_0.gguf](https://huggingface.co/RichardErkhov/sail_-_Sailor-7B-Chat-gguf/blob/main/Sailor-7B-Chat.Q8_0.gguf) | Q8_0 | 7.65GB |
Original model description:
---
language:
- en
- zh
- id
- th
- vi
- ms
- lo
datasets:
- CohereForAI/aya_dataset
- CohereForAI/aya_collection
- Open-Orca/OpenOrca
tags:
- multilingual
- sea
- sailor
- sft
- chat
- instruction
widget:
- text: "如何制作烤鱼?"
example_title: "Chinese"
- text: "How to bake fish?"
example_title: "English"
- text: "Bagaimana cara memanggang ikan?"
example_title: "Malay"
- text: "วิธีย่างปลา?"
example_title: "Thai"
- text: "Bagaimana membuat bakaran ikan?"
example_title: "Indonesian"
- text: "Làm thế nào để nướng cá?"
example_title: "Vietnamese"
license: apache-2.0
base_model: sail/Sailor-7B
---
<div align="center">
<img src="banner_sailor.jpg" width="700"/>
</div>
Sailor is a suite of Open Language Models tailored for South-East Asia (SEA), focusing on languages such as 🇮🇩Indonesian, 🇹🇭Thai, 🇻🇳Vietnamese, 🇲🇾Malay, and 🇱🇦Lao.
Developed with careful data curation, Sailor models are designed to understand and generate text across diverse linguistic landscapes of SEA region.
Built from [Qwen 1.5](https://huggingface.co/collections/Qwen/qwen15-65c0a2f577b1ecb76d786524) , Sailor encompasses models of varying sizes, spanning from 0.5B to 7B versions for different requirements.
We further fine-tune the base model with open-source datasets to get instruction-tuned models, namedly Sailor-Chat.
Benchmarking results demonstrate Sailor's proficiency in tasks such as question answering, commonsense reasoning, and other tasks in SEA languages.
> The logo was generated by MidJourney
## Model Summary
- **Model Collections:** [Base Model & Chat Model](https://huggingface.co/collections/sail/sailor-65e19a749f978976f1959825)
- **Project Website:** [sailorllm.github.io](https://sailorllm.github.io/)
- **Codebase:** [github.com/sail-sg/sailor-llm](https://github.com/sail-sg/sailor-llm)
- **Technical Report:** [arxiv.org/pdf/2404.03608.pdf](https://arxiv.org/pdf/2404.03608.pdf)
## Training details
Sailor is crafted by continually pre-training from language models like the remarkable Qwen 1.5 models, which already has a great performance on SEA languages.
The pre-training corpus heavily leverages the publicly available corpus, including
[SlimPajama](https://huggingface.co/datasets/cerebras/SlimPajama-627B),
[SkyPile](https://huggingface.co/datasets/Skywork/SkyPile-150B),
[CC100](https://huggingface.co/datasets/cc100) and [MADLAD-400](https://huggingface.co/datasets/allenai/MADLAD-400).
The instruction tuning corpus are all publicly available including
[aya_collection](https://huggingface.co/datasets/CohereForAI/aya_collection),
[aya_dataset](https://huggingface.co/datasets/CohereForAI/aya_dataset),
[OpenOrca](https://huggingface.co/datasets/Open-Orca/OpenOrca).
By employing aggressive data deduplication and careful data cleaning on the collected corpus, we have attained a high-quality dataset spanning various languages.
Through systematic experiments to determine the weights of different languages, Sailor models undergo training from 200B to 400B tokens, tailored to different model sizes.
The approach boosts their performance on SEA languages while maintaining proficiency in English and Chinese without significant compromise.
Finally, we continually pre-train the Qwen1.5-0.5B model with 400 Billion tokens, and other models with 200 Billion tokens to obtain the Sailor models.
## Requirements
The code of Sailor has been in the latest Hugging face transformers and we advise you to install `transformers>=4.37.0`.
## Quickstart
Here provides a code snippet to show you how to load the tokenizer and model and how to generate contents.
```python
from transformers import AutoModelForCausalLM, AutoTokenizer
device = "cuda"
model = AutoModelForCausalLM.from_pretrained(
'sail/Sailor-7B-Chat',
torch_dtype="auto",
device_map="auto"
)
tokenizer = AutoTokenizer.from_pretrained('sail/Sailor-7B-Chat')
system_prompt= 'You are a helpful assistant'
prompt = "Beri saya pengenalan singkat tentang model bahasa besar."
# prompt = "Hãy cho tôi một giới thiệu ngắn gọn về mô hình ngôn ngữ lớn."
# prompt = "ให้ฉันแนะนำสั้น ๆ เกี่ยวกับโมเดลภาษาขนาดใหญ่"
messages = [
{"role": "system", "content": system_prompt},
{"role": "question", "content": prompt}
]
text = tokenizer.apply_chat_template(
messages,
tokenize=False,
add_generation_prompt=True
)
model_inputs = tokenizer([text], return_tensors="pt").to(device)
input_ids = model_inputs.input_ids.to(device)
generated_ids = model.generate(
input_ids,
max_new_tokens=512,
)
generated_ids = [
output_ids[len(input_ids):] for input_ids, output_ids in zip(model_inputs.input_ids, generated_ids)
]
response = tokenizer.batch_decode(generated_ids, skip_special_tokens=True)[0]
print(response)
```
# License
Sailor is distributed under the terms of the Apache License 2.0.
No restrict on the research and the commercial use, but should comply with the [Qwen License](https://huggingface.co/Qwen/Qwen1.5-1.8B/blob/main/LICENSE).
## Citation
If you find sailor useful, please cite our work as follows:
```
@misc{dou2024sailor,
title={Sailor: Open Language Models for South-East Asia},
author={Longxu Dou and Qian Liu and Guangtao Zeng and Jia Guo and Jiahui Zhou and Wei Lu and Min Lin},
year={2024},
eprint={2404.03608},
archivePrefix={arXiv},
primaryClass={cs.CL}
}
```
# Contact Us
If you have any questions, please raise an issue or contact us at [[email protected]](mailto:[email protected]) or [[email protected]](mailto:[email protected]).
|
mradermacher/L-MChat-7b-i1-GGUF | mradermacher | 2024-06-27T16:47:20Z | 15,035 | 0 | transformers | [
"transformers",
"gguf",
"merge",
"mergekit",
"Nexusflow/Starling-LM-7B-beta",
"FuseAI/FuseChat-7B-VaRM",
"en",
"base_model:Artples/L-MChat-7b",
"license:apache-2.0",
"endpoints_compatible",
"region:us"
] | null | 2024-06-27T12:26:31Z | ---
base_model: Artples/L-MChat-7b
language:
- en
library_name: transformers
license: apache-2.0
quantized_by: mradermacher
tags:
- merge
- mergekit
- Nexusflow/Starling-LM-7B-beta
- FuseAI/FuseChat-7B-VaRM
---
## About
<!-- ### quantize_version: 2 -->
<!-- ### output_tensor_quantised: 1 -->
<!-- ### convert_type: hf -->
<!-- ### vocab_type: -->
<!-- ### tags: nicoboss -->
weighted/imatrix quants of https://huggingface.co/Artples/L-MChat-7b
<!-- provided-files -->
static quants are available at https://huggingface.co/mradermacher/L-MChat-7b-GGUF
## Usage
If you are unsure how to use GGUF files, refer to one of [TheBloke's
READMEs](https://huggingface.co/TheBloke/KafkaLM-70B-German-V0.1-GGUF) for
more details, including on how to concatenate multi-part files.
## Provided Quants
(sorted by size, not necessarily quality. IQ-quants are often preferable over similar sized non-IQ quants)
| Link | Type | Size/GB | Notes |
|:-----|:-----|--------:|:------|
| [GGUF](https://huggingface.co/mradermacher/L-MChat-7b-i1-GGUF/resolve/main/L-MChat-7b.i1-IQ1_S.gguf) | i1-IQ1_S | 1.7 | for the desperate |
| [GGUF](https://huggingface.co/mradermacher/L-MChat-7b-i1-GGUF/resolve/main/L-MChat-7b.i1-IQ1_M.gguf) | i1-IQ1_M | 1.9 | mostly desperate |
| [GGUF](https://huggingface.co/mradermacher/L-MChat-7b-i1-GGUF/resolve/main/L-MChat-7b.i1-IQ2_XXS.gguf) | i1-IQ2_XXS | 2.1 | |
| [GGUF](https://huggingface.co/mradermacher/L-MChat-7b-i1-GGUF/resolve/main/L-MChat-7b.i1-IQ2_XS.gguf) | i1-IQ2_XS | 2.3 | |
| [GGUF](https://huggingface.co/mradermacher/L-MChat-7b-i1-GGUF/resolve/main/L-MChat-7b.i1-IQ2_S.gguf) | i1-IQ2_S | 2.4 | |
| [GGUF](https://huggingface.co/mradermacher/L-MChat-7b-i1-GGUF/resolve/main/L-MChat-7b.i1-IQ2_M.gguf) | i1-IQ2_M | 2.6 | |
| [GGUF](https://huggingface.co/mradermacher/L-MChat-7b-i1-GGUF/resolve/main/L-MChat-7b.i1-Q2_K.gguf) | i1-Q2_K | 2.8 | IQ3_XXS probably better |
| [GGUF](https://huggingface.co/mradermacher/L-MChat-7b-i1-GGUF/resolve/main/L-MChat-7b.i1-IQ3_XXS.gguf) | i1-IQ3_XXS | 2.9 | lower quality |
| [GGUF](https://huggingface.co/mradermacher/L-MChat-7b-i1-GGUF/resolve/main/L-MChat-7b.i1-IQ3_XS.gguf) | i1-IQ3_XS | 3.1 | |
| [GGUF](https://huggingface.co/mradermacher/L-MChat-7b-i1-GGUF/resolve/main/L-MChat-7b.i1-Q3_K_S.gguf) | i1-Q3_K_S | 3.3 | IQ3_XS probably better |
| [GGUF](https://huggingface.co/mradermacher/L-MChat-7b-i1-GGUF/resolve/main/L-MChat-7b.i1-IQ3_S.gguf) | i1-IQ3_S | 3.3 | beats Q3_K* |
| [GGUF](https://huggingface.co/mradermacher/L-MChat-7b-i1-GGUF/resolve/main/L-MChat-7b.i1-IQ3_M.gguf) | i1-IQ3_M | 3.4 | |
| [GGUF](https://huggingface.co/mradermacher/L-MChat-7b-i1-GGUF/resolve/main/L-MChat-7b.i1-Q3_K_M.gguf) | i1-Q3_K_M | 3.6 | IQ3_S probably better |
| [GGUF](https://huggingface.co/mradermacher/L-MChat-7b-i1-GGUF/resolve/main/L-MChat-7b.i1-Q3_K_L.gguf) | i1-Q3_K_L | 3.9 | IQ3_M probably better |
| [GGUF](https://huggingface.co/mradermacher/L-MChat-7b-i1-GGUF/resolve/main/L-MChat-7b.i1-IQ4_XS.gguf) | i1-IQ4_XS | 4.0 | |
| [GGUF](https://huggingface.co/mradermacher/L-MChat-7b-i1-GGUF/resolve/main/L-MChat-7b.i1-Q4_0.gguf) | i1-Q4_0 | 4.2 | fast, low quality |
| [GGUF](https://huggingface.co/mradermacher/L-MChat-7b-i1-GGUF/resolve/main/L-MChat-7b.i1-Q4_K_S.gguf) | i1-Q4_K_S | 4.2 | optimal size/speed/quality |
| [GGUF](https://huggingface.co/mradermacher/L-MChat-7b-i1-GGUF/resolve/main/L-MChat-7b.i1-Q4_K_M.gguf) | i1-Q4_K_M | 4.5 | fast, recommended |
| [GGUF](https://huggingface.co/mradermacher/L-MChat-7b-i1-GGUF/resolve/main/L-MChat-7b.i1-Q5_K_S.gguf) | i1-Q5_K_S | 5.1 | |
| [GGUF](https://huggingface.co/mradermacher/L-MChat-7b-i1-GGUF/resolve/main/L-MChat-7b.i1-Q5_K_M.gguf) | i1-Q5_K_M | 5.2 | |
| [GGUF](https://huggingface.co/mradermacher/L-MChat-7b-i1-GGUF/resolve/main/L-MChat-7b.i1-Q6_K.gguf) | i1-Q6_K | 6.0 | practically like static Q6_K |
Here is a handy graph by ikawrakow comparing some lower-quality quant
types (lower is better):

And here are Artefact2's thoughts on the matter:
https://gist.github.com/Artefact2/b5f810600771265fc1e39442288e8ec9
## FAQ / Model Request
See https://huggingface.co/mradermacher/model_requests for some answers to
questions you might have and/or if you want some other model quantized.
## Thanks
I thank my company, [nethype GmbH](https://www.nethype.de/), for letting
me use its servers and providing upgrades to my workstation to enable
this work in my free time. Additional thanks to [@nicoboss](https://huggingface.co/nicoboss) for giving me access to his hardware for calculating the imatrix for these quants.
<!-- end -->
|
yanolja/EEVE-Korean-10.8B-v1.0 | yanolja | 2024-02-24T13:54:42Z | 15,034 | 56 | transformers | [
"transformers",
"safetensors",
"llama",
"text-generation",
"generated_from_trainer",
"conversational",
"arxiv:2402.14714",
"base_model:upstage/SOLAR-10.7B-v1.0",
"license:apache-2.0",
"autotrain_compatible",
"endpoints_compatible",
"text-generation-inference",
"region:us"
] | text-generation | 2024-02-07T13:34:50Z | ---
license: apache-2.0
base_model: upstage/SOLAR-10.7B-v1.0
tags:
- generated_from_trainer
model-index:
- name: yanolja/EEVE-Korean-10.8B-v1.0
results: []
---
[<img src="https://raw.githubusercontent.com/OpenAccess-AI-Collective/axolotl/main/image/axolotl-badge-web.png" alt="Built with Axolotl" width="200" height="32"/>](https://github.com/OpenAccess-AI-Collective/axolotl)
<p align="left">
<img src="https://huggingface.co/yanolja/EEVE-Korean-10.8B-v1.0/resolve/main/eeve_logo.webp" width="50%"/>
<p>
# EEVE-Korean-10.8B-v1.0
## Join Our Community on Discord!
If you're passionate about the field of Large Language Models and wish to exchange knowledge and insights, we warmly invite you to join our Discord server. It's worth noting that Korean is the primary language used in this server. The landscape of LLM is evolving rapidly, and without active sharing, our collective knowledge risks becoming outdated swiftly. Let's collaborate and drive greater impact together! Join us here: [Discord Link](https://discord.gg/b27bAHg95m).
## Our Dedicated Team (Alphabetical Order)
| Research | Engineering | Product Management | UX Design |
|-----------------|-----------------|--------------------|--------------
| Myeongho Jeong | Geon Kim | Bokyung Huh | Eunsue Choi |
| Seungduk Kim | Rifqi Alfi | | |
| Seungtaek Choi | Sanghoon Han | | |
| | Suhyun Kang | | |
## About the Model
This model is a Korean vocabulary-extended version of [upstage/SOLAR-10.7B-v1.0](https://huggingface.co/upstage/SOLAR-10.7B-v1.0), specifically fine-tuned on various Korean web-crawled datasets available on HuggingFace. Our approach was to expand the model's understanding of Korean by pre-training the embeddings for new tokens and partially fine-tuning the `lm_head` embeddings for the already existing tokens while preserving the original parameters of the base model.
### Technical Deep Dive
<p align="left">
<img src="https://huggingface.co/yanolja/EEVE-Korean-10.8B-v1.0/resolve/main/EEVE_figure.png" width="100%"/>
<p>
To adapt foundational models from English to Korean, we use subword-based embedding with a seven-stage training process involving parameter freezing.
This approach progressively trains from input embeddings to full parameters, efficiently extending the model's vocabulary to include Korean.
Our method enhances the model's cross-linguistic applicability by carefully integrating new linguistic tokens, focusing on causal language modeling pre-training.
We leverage the inherent capabilities of foundational models trained on English to efficiently transfer knowledge and reasoning to Korean, optimizing the adaptation process.
For more details, please refer to our technical report: [Efficient and Effective Vocabulary Expansion Towards Multilingual Large Language Models](https://arxiv.org/abs/2402.14714).
Here’s an simplified code for our key approach:
```python
# number_of_old_tokens is the size of tokenizer before vocab extension. For example, in case of EEVE-Korean-10.8B-v1.0, number_of_old_tokens is 32000.
def freeze_partial_embedding_hook(grad):
grad[:number_of_old_tokens] = 0
return grad
for name, param in model.named_parameters():
if ("lm_head" in name or "embed_tokens" in name) and "original" not in name:
param.requires_grad = True
if "embed_tokens" in name:
param.register_hook(freeze_partial_embedding_hook)
else:
param.requires_grad = False
```
### Usage and Limitations
Keep in mind that this model hasn't been fine-tuned with instruction-based training. While it excels in Korean language tasks, we advise careful consideration and further training for specific applications.
### Training Details
Our model’s training was comprehensive and diverse:
- **Vocabulary Expansion:**
We meticulously selected 8,960 Korean tokens based on their frequency in our Korean web corpus. This process involved multiple rounds of tokenizer training, manual curation, and token frequency analysis, ensuring a rich and relevant vocabulary for our model.
1. **Initial Tokenizer Training:** We trained an intermediate tokenizer on a Korean web corpus, with a vocabulary of 40,000 tokens.
2. **Extraction of New Korean Tokens:** From the intermediate tokenizer, we identified all Korean tokens not present in the original SOLAR's tokenizer.
3. **Manual Tokenizer Construction:** We then built the target tokenizer, focusing on these new Korean tokens.
4. **Frequency Analysis:** Using the target tokenizer, we processed a 100GB Korean corpus to count each token's frequency.
5. **Refinement of Token List:** We removed tokens appearing less than 6,000 times, ensuring to secure enough tokens to train models later.
6. **Inclusion of Single-Letter Characters:** Counted missing Korean single-letter characters and added them to the target tokenizer that appeared more than 6,000 times.
7. **Iterative Refinement:** We repeated steps 2 to 6 until there were no tokens to drop or add.
8. **Training Bias Towards New Tokens:** Our training data was biased to include more texts with new tokens, for effective learning.
This rigorous approach ensured a comprehensive and contextually rich Korean vocabulary for the model.
## Citation
```
@misc{kim2024efficient,
title={Efficient and Effective Vocabulary Expansion Towards Multilingual Large Language Models},
author={Seungduk Kim and Seungtaek Choi and Myeongho Jeong},
year={2024},
eprint={2402.14714},
archivePrefix={arXiv},
primaryClass={cs.CL}
}
```
|
sayakpaul/dpo-sdxl-text2image-v1-full | sayakpaul | 2024-05-29T02:29:17Z | 15,012 | 1 | diffusers | [
"diffusers",
"safetensors",
"endpoints_compatible",
"diffusers:StableDiffusionXLPipeline",
"region:us"
] | text-to-image | 2024-05-29T02:22:04Z | ---
library_name: diffusers
---
# Model Card for Model ID
Nothing much. This is distributed as a full pipeline so that it can be directly used as `DiffusionPipeline.from_pretrained()`.
All credits belong to https://huggingface.co/mhdang/dpo-sdxl-text2image-v1/. |
Habana/clip | Habana | 2024-02-16T08:04:05Z | 14,995 | 0 | null | [
"optimum_habana",
"license:apache-2.0",
"region:us"
] | null | 2023-02-16T13:00:20Z | ---
license: apache-2.0
---
[Optimum Habana](https://github.com/huggingface/optimum-habana) is the interface between the Hugging Face Transformers and Diffusers libraries and Habana's Gaudi processor (HPU).
It provides a set of tools enabling easy and fast model loading, training and inference on single- and multi-HPU settings for different downstream tasks.
Learn more about how to take advantage of the power of Habana HPUs to train and deploy Transformers and Diffusers models at [hf.co/hardware/habana](https://huggingface.co/hardware/habana).
## CLIP model HPU configuration
This model only contains the `GaudiConfig` file for running CLIP-like models (e.g. [this one](https://huggingface.co/openai/clip-vit-large-patch14)) on Habana's Gaudi processors (HPU).
**This model contains no model weights, only a GaudiConfig.**
This enables to specify:
- `use_fused_adam`: whether to use Habana's custom AdamW implementation
- `use_fused_clip_norm`: whether to use Habana's fused gradient norm clipping operator
- `use_torch_autocast`: whether to use Torch Autocast for managing mixed precision
## Usage
The model is instantiated the same way as in the Transformers library.
The only difference is that there are a few new training arguments specific to HPUs.\
It is strongly recommended to train this model doing bf16 mixed-precision training for optimal performance and accuracy.
[Here](https://github.com/huggingface/optimum-habana/blob/main/examples/contrastive-image-text) is an example script to fine-tune a model on COCO.
Use it as follows:
1. You first need to download the dataset:
```bash
mkdir data
cd data
wget http://images.cocodataset.org/zips/train2017.zip
wget http://images.cocodataset.org/zips/val2017.zip
wget http://images.cocodataset.org/zips/test2017.zip
wget http://images.cocodataset.org/annotations/annotations_trainval2017.zip
wget http://images.cocodataset.org/annotations/image_info_test2017.zip
cd ..
```
2. Then, you can create a model from pretrained vision and text decoder models:
```python
from transformers import (
VisionTextDualEncoderModel,
VisionTextDualEncoderProcessor,
AutoTokenizer,
AutoImageProcessor
)
model = VisionTextDualEncoderModel.from_vision_text_pretrained(
"openai/clip-vit-large-patch14", "roberta-large"
)
tokenizer = AutoTokenizer.from_pretrained("roberta-large")
image_processor = AutoImageProcessor.from_pretrained("openai/clip-vit-large-patch14")
processor = VisionTextDualEncoderProcessor(image_processor, tokenizer)
# save the model and processor
model.save_pretrained("clip-roberta")
processor.save_pretrained("clip-roberta")
```
3. Finally, you can run it with the following command:
```bash
python run_clip.py \
--output_dir ./clip-roberta-finetuned \
--model_name_or_path ./clip-roberta \
--data_dir $PWD/data \
--dataset_name ydshieh/coco_dataset_script \
--dataset_config_name=2017 \
--image_column image_path \
--caption_column caption \
--remove_unused_columns=False \
--do_train --do_eval \
--per_device_train_batch_size="16" \
--per_device_eval_batch_size="16" \
--learning_rate="5e-5" --warmup_steps="0" --weight_decay 0.1 \
--overwrite_output_dir \
--save_strategy epoch \
--use_habana \
--use_lazy_mode \
--use_hpu_graphs \
--gaudi_config_name Habana/clip \
--throughput_warmup_steps 2 \
--bf16
```
Check the [documentation](https://huggingface.co/docs/optimum/habana/index) out for more advanced usage and examples.
|
Subsets and Splits
No community queries yet
The top public SQL queries from the community will appear here once available.