modelId
stringlengths 5
122
| author
stringlengths 2
42
| last_modified
unknown | downloads
int64 0
738M
| likes
int64 0
11k
| library_name
stringclasses 245
values | tags
sequencelengths 1
4.05k
| pipeline_tag
stringclasses 48
values | createdAt
unknown | card
stringlengths 1
901k
|
---|---|---|---|---|---|---|---|---|---|
yodayo-ai/holodayo-xl-2.1 | yodayo-ai | "2024-06-07T08:07:33Z" | 16,466 | 54 | diffusers | [
"diffusers",
"safetensors",
"text-to-image",
"stable-diffusion",
"stable-diffusion-xl",
"en",
"base_model:cagliostrolab/animagine-xl-3.1",
"license:other",
"autotrain_compatible",
"endpoints_compatible",
"diffusers:StableDiffusionXLPipeline",
"region:us"
] | text-to-image | "2024-06-02T11:57:15Z" | ---
license: other
license_name: faipl-1.0-sd
license_link: https://freedevproject.org/faipl-1.0-sd/
language:
- en
tags:
- text-to-image
- stable-diffusion
- safetensors
- stable-diffusion-xl
base_model: cagliostrolab/animagine-xl-3.1
widget:
- text: 1girl, green hair, sweater, looking at viewer, upper body, beanie, outdoors, night, turtleneck, masterpiece, best quality, very aesthetic, absurdres
parameter:
negative_prompt: nsfw, low quality, worst quality, very displeasing, 3d, watermark, signature, ugly, poorly drawn
example_title: 1girl
- text: 1boy, male focus, green hair, sweater, looking at viewer, upper body, beanie, outdoors, night, turtleneck, masterpiece, best quality, very aesthetic, absurdres
parameter:
negative_prompt: nsfw, low quality, worst quality, very displeasing, 3d, watermark, signature, ugly, poorly drawn
example_title: 1boy
---
<style>
body {
display: flex;
align-items: center;
justify-content: center;
height: 100vh;
margin: 0;
font-family: Arial, sans-serif;
background-color: #f4f4f9;
overflow: auto;
}
.container {
display: flex;
flex-direction: column;
align-items: center;
justify-content: center;
width: 100%;
padding: 20px;
}
.title-container {
display: flex;
flex-direction: column;
justify-content: center;
align-items: center;
padding: 1em;
border-radius: 10px;
}
.title {
font-size: 3em;
font-family: 'Montserrat', sans-serif;
text-align: center;
font-weight: bold;
}
.title span {
background: -webkit-linear-gradient(45deg, #ff8e8e, #ffb6c1, #ff69b4);
-webkit-background-clip: text;
-webkit-text-fill-color: transparent;
}
.gallery {
display: grid;
grid-template-columns: repeat(5, 1fr);
gap: 10px;
}
.gallery img {
width: 100%;
height: auto;
margin-top: 0px;
margin-bottom: 0px;
border-radius: 10px;
box-shadow: 0 4px 8px rgba(0, 0, 0, 0.2);
transition: transform 0.3s;
}
.gallery img:hover {
transform: scale(1.05);
}
.note {
font-size: 1em;
opacity: 50%;
text-align: center;
margin-top: 20px;
color: #555;
}
</style>
<div class="container">
<div class="title-container">
<div class="title"><span>Holodayo XL 2.1</span></div>
</div>
<div class="gallery">
<img src="https://huggingface.co/yodayo-ai/holodayo-xl-2.1/resolve/main/samples/sample-001.png" alt="Image 1">
<img src="https://huggingface.co/yodayo-ai/holodayo-xl-2.1/resolve/main/samples/sample-002.png" alt="Image 2">
<img src="https://huggingface.co/yodayo-ai/holodayo-xl-2.1/resolve/main/samples/sample-003.png" alt="Image 3">
<img src="https://huggingface.co/yodayo-ai/holodayo-xl-2.1/resolve/main/samples/sample-004.png" alt="Image 4">
<img src="https://huggingface.co/yodayo-ai/holodayo-xl-2.1/resolve/main/samples/sample-005.png" alt="Image 5">
<img src="https://huggingface.co/yodayo-ai/holodayo-xl-2.1/resolve/main/samples/sample-006.png" alt="Image 6">
<img src="https://huggingface.co/yodayo-ai/holodayo-xl-2.1/resolve/main/samples/sample-007.png" alt="Image 7">
<img src="https://huggingface.co/yodayo-ai/holodayo-xl-2.1/resolve/main/samples/sample-008.png" alt="Image 8">
<img src="https://huggingface.co/yodayo-ai/holodayo-xl-2.1/resolve/main/samples/sample-009.png" alt="Image 9">
<img src="https://huggingface.co/yodayo-ai/holodayo-xl-2.1/resolve/main/samples/sample-010.png" alt="Image 10">
</div>
<div class="note">
Drag and drop each image to <a href="https://huggingface.co/spaces/Linaqruf/pnginfo" target="_blank">this link</a> or use ComfyUI to get the metadata.
</div>
</div>
## Overview
**Holodayo XL 2.1** is the latest version of the [Yodayo Holodayo XL](https://yodayo.com/models/1cafd6f8-8fc6-4282-b8f8-843935acbfe8) series, following the previous iteration, [Holodayo XL 1.0](https://yodayo.com/models/1cafd6f8-8fc6-4282-b8f8-843935acbfe8/?modelversion=2349b302-a726-44ba-933b-e3dc4631a95b). This open-source model is built upon Animagine XL V3, a specialized SDXL model designed for generating high-quality anime-style artwork. Holodayo XL 2.1 has undergone additional fine-tuning and optimization to focus specifically on generating images that accurately represent the visual style and aesthetics of the Virtual Youtuber franchise.
Holodayo XL 2.1 was trained to fix everything wrong in [Holodayo XL 2.0](https://yodayo.com/models/1cafd6f8-8fc6-4282-b8f8-843935acbfe8/?modelversion=ca4bf1aa-0baf-44cd-8ee9-8f4c6bba89c8), such as bad hands, bad anatomy, catastrophic forgetting due to the text encoder being trained during the fine-tuning phase, and an overexposed art style by decreasing the aesthetic datasets.
## Model Details
- **Developed by**: [Linaqruf](https://github.com/Linaqruf)
- **Model type**: Diffusion-based text-to-image generative model
- **Model Description**: Holodayo XL 2.1, the latest in the Yodayo Holodayo XL series, is an open-source model built on Animagine XL V3. Fine-tuned for high-quality Virtual Youtuber anime-style art generation.
- **License**: [Fair AI Public License 1.0-SD](https://freedevproject.org/faipl-1.0-sd/)
- **Finetuned from model**: [Animagine XL 3.1](https://huggingface.co/cagliostrolab/animagine-xl-3.1)
## Supported Platform
1. Use this model in our platform: [](https://yodayo.com/models/1cafd6f8-8fc6-4282-b8f8-843935acbfe8/?modelversion=6862d809-0cbc-4fe0-83dc-9206d60b0698)
2. Use it in [`ComfyUI`](https://github.com/comfyanonymous/ComfyUI) or [`Stable Diffusion Webui`](https://github.com/AUTOMATIC1111/stable-diffusion-webui)
3. Use it with 🧨 `diffusers`
## 🧨 Diffusers Installation
First install the required libraries:
```bash
pip install diffusers transformers accelerate safetensors --upgrade
```
Then run image generation with the following example code:
```python
import torch
from diffusers import StableDiffusionXLPipeline
pipe = StableDiffusionXLPipeline.from_pretrained(
"yodayo-ai/holodayo-xl-2.1",
torch_dtype=torch.float16,
use_safetensors=True,
custom_pipeline="lpw_stable_diffusion_xl",
add_watermarker=False,
variant="fp16"
)
pipe.to('cuda')
prompt = "1girl, nakiri ayame, nakiri ayame \(1st costume\), hololive, solo, upper body, v, smile, looking at viewer, outdoors, night, masterpiece, best quality, very aesthetic, absurdres"
negative_prompt = "nsfw, (low quality, worst quality:1.2), very displeasing, 3d, watermark, signature, ugly, poorly drawn"
image = pipe(
prompt,
negative_prompt=negative_prompt,
width=832,
height=1216,
guidance_scale=7,
num_inference_steps=28
).images[0]
image.save("./waifu.png")
```
## Usage Guidelines
### Tag Ordering
For optimal results, it's recommended to follow the structured prompt template because we train the model like this:
```
1girl/1boy, character name, from which series, by which artists, everything else in any order.
```
### Special Tags
Holodayo XL 2.1 inherits special tags from Animagine XL 3.1 to enhance image generation by steering results toward quality, rating, creation date, and aesthetic. This inheritance ensures that Holodayo XL 2.1 can produce high-quality, relevant, and aesthetically pleasing images. While the model can generate images without these tags, using them helps achieve better results.
- **Quality tags**: masterpiece, best quality, great quality, good quality, normal quality, low quality, worst quality
- **Rating tags**: safe, sensitive, nsfw, explicit
- **Year tags**: newest, recent, mid, early, oldest
- **Aesthetic tags**: very aesthetic, aesthetic, displeasing, very displeasing
### Recommended Settings
To guide the model towards generating high-aesthetic images, use the following recommended settings:
- **Negative prompts**:
```
nsfw, (low quality, worst quality:1.2), very displeasing, 3d, watermark, signature, ugly, poorly drawn
```
- **Positive prompts**:
```
masterpiece, best quality, very aesthetic, absurdres
```
- **Classifier-Free Guidance (CFG) Scale**: should be around 5 to 7; 10 is fried, >12 is deep-fried.
- **Sampling steps**: should be around 25 to 30; 28 is the sweet spot.
- **Sampler**: Euler Ancestral (Euler a) is highly recommended.
- **Supported resolutions**:
```
1024 x 1024, 1152 x 896, 896 x 1152, 1216 x 832, 832 x 1216, 1344 x 768, 768 x 1344, 1536 x 640, 640 x 1536
```
## Training
These are the key hyperparameters used during training:
| Feature | Pretraining | Finetuning |
|-------------------------------|----------------------------|---------------------------------|
| **Hardware** | 2x H100 80GB PCIe | 2x A100 80GB PCIe |
| **Batch Size** | 32 | 48 |
| **Gradient Accumulation Steps** | 2 | 1 |
| **Noise Offset** | None | 0.0357 |
| **Epochs** | 10 | 10 |
| **UNet Learning Rate** | 5e-6 | 2e-6 |
| **Text Encoder Learning Rate** | 2.5e-6 | None |
| **Optimizer** | Adafactor | Adafactor |
| **Optimizer Args** | Scale Parameter: False, Relative Step: False, Warmup Init: False | Scale Parameter: False, Relative Step: False, Warmup Init: False |
| **Scheduler** | Constant with Warmups | Constant with Warmups |
| **Warmup Steps** | 0.05% | 0.05% |
## License
Holodayo XL 2.1 falls under [Fair AI Public License 1.0-SD](https://freedevproject.org/faipl-1.0-sd/) license, which is compatible with Stable Diffusion models’ license. Key points:
1. **Modification Sharing:** If you modify Holodayo XL 2.1, you must share both your changes and the original license.
2. **Source Code Accessibility:** If your modified version is network-accessible, provide a way (like a download link) for others to get the source code. This applies to derived models too.
3. **Distribution Terms:** Any distribution must be under this license or another with similar rules.
|
dataautogpt3/ProteusV0.4 | dataautogpt3 | "2024-02-22T19:01:41Z" | 16,465 | 73 | diffusers | [
"diffusers",
"text-to-image",
"license:gpl-3.0",
"endpoints_compatible",
"diffusers:StableDiffusionXLPipeline",
"region:us"
] | text-to-image | "2024-02-22T13:50:29Z" | ---
pipeline_tag: text-to-image
widget:
- text: >-
3 fish in a fish tank wearing adorable outfits, best quality, hd
output:
url: GGuziQaXYAAudCW.png
- text: >-
a woman sitting in a wooden chair in the middle of a grass field on a farm, moonlight, best quality, hd, anime art
output:
url: upscaled_image (1).webp
- text: >-
Masterpiece, glitch, holy holy holy, fog, by DarkIncursio
output:
url: GGvDC_qWUAAcuQA.jpeg
- text: >-
jpeg Full Body Photo of a weird imaginary Female creatures captured on celluloid film, (((ghost))),heavy rain, thunder, snow, water's surface, night, expressionless, Blood, Japan God,(school), Ultra Realistic, ((Scary)),looking at camera, screem, plaintive cries, Long claws, fangs, scales,8k, HDR, 500px, mysterious and ornate digital art, photic, intricate, fantasy aesthetic.
output:
url: upscaled_image2.png
- text: >-
The divine tree of knowledge, an interplay between purple and gold, floats in the void of the sea of quanta, the tree is made of crystal, the void is made of nothingness, strong contrast, dim lighting, beautiful and surreal scene. wide shot
output:
url: upscaled_image.png
- text: >-
The image features an older man, a long white beard and mustache, He has a stern expression, giving the impression of a wise and experienced individual. The mans beard and mustache are prominent, adding to his distinguished appearance. The close-up shot of the mans face emphasizes his facial features and the intensity of his gaze.
output:
url: old.png
- text: >-
Ghost in the Shell Stand Alone Complex
output:
url: upscaled_image4.png
- text: >-
(impressionistic realism by csybgh), a 50 something male, working in banking, very short dyed dark curly balding hair, Afro-Asiatic ancestry, talks a lot but listens poorly, stuck in the past, wearing a suit, he has a certain charm, bronze skintone, sitting in a bar at night, he is smoking and feeling cool, drunk on plum wine, masterpiece, 8k, hyper detailed, smokey ambiance, perfect hands AND fingers
output:
url: collage.png
- text: >-
black fluffy gorgeous dangerous cat animal creature, large orange eyes, big fluffy ears, piercing gaze, full moon, dark ambiance, best quality, extremely detailed
output:
url: collage2.png
license: gpl-3.0
---
<Gallery />
## ProteusV0.4: The Style Update
This update enhances stylistic capabilities, similar to Midjourney's approach, rather than advancing prompt comprehension. Methods used do not infringe on any copyrighted material.
## Proteus
Proteus serves as a sophisticated enhancement over OpenDalleV1.1, leveraging its core functionalities to deliver superior outcomes. Key areas of advancement include heightened responsiveness to prompts and augmented creative capacities. To achieve this, it was fine-tuned using approximately 220,000 GPTV captioned images from copyright-free stock images (with some anime included), which were then normalized. Additionally, DPO (Direct Preference Optimization) was employed through a collection of 10,000 carefully selected high-quality, AI-generated image pairs.
In pursuit of optimal performance, numerous LORA (Low-Rank Adaptation) models are trained independently before being selectively incorporated into the principal model via dynamic application methods. These techniques involve targeting particular segments within the model while avoiding interference with other areas during the learning phase. Consequently, Proteus exhibits marked improvements in portraying intricate facial characteristics and lifelike skin textures, all while sustaining commendable proficiency across various aesthetic domains, notably surrealism, anime, and cartoon-style visualizations.
finetuned/trained on a total of 400k+ images at this point.
## Settings for ProteusV0.4
Use these settings for the best results with ProteusV0.4:
CFG Scale: Use a CFG scale of 4 to 6
Steps: 20 to 60 steps for more detail, 20 steps for faster results.
Sampler: DPM++ 2M SDE
Scheduler: Karras
Resolution: 1280x1280 or 1024x1024
please also consider using these keep words to improve your prompts:
best quality, HD, `~*~aesthetic~*~`.
if you are having trouble coming up with prompts you can use this GPT I put together to help you refine the prompt. https://chat.openai.com/g/g-RziQNoydR-diffusion-master
## Use it with 🧨 diffusers
```python
import torch
from diffusers import (
StableDiffusionXLPipeline,
KDPM2AncestralDiscreteScheduler,
AutoencoderKL
)
# Load VAE component
vae = AutoencoderKL.from_pretrained(
"madebyollin/sdxl-vae-fp16-fix",
torch_dtype=torch.float16
)
# Configure the pipeline
pipe = StableDiffusionXLPipeline.from_pretrained(
"dataautogpt3/ProteusV0.4",
vae=vae,
torch_dtype=torch.float16
)
pipe.scheduler = KDPM2AncestralDiscreteScheduler.from_config(pipe.scheduler.config)
pipe.to('cuda')
# Define prompts and generate image
prompt = "black fluffy gorgeous dangerous cat animal creature, large orange eyes, big fluffy ears, piercing gaze, full moon, dark ambiance, best quality, extremely detailed"
negative_prompt = "nsfw, bad quality, bad anatomy, worst quality, low quality, low resolutions, extra fingers, blur, blurry, ugly, wrongs proportions, watermark, image artifacts, lowres, ugly, jpeg artifacts, deformed, noisy image"
image = pipe(
prompt,
negative_prompt=negative_prompt,
width=1024,
height=1024,
guidance_scale=4,
num_inference_steps=20
).images[0]
```
please support the work I do through donating to me on:
https://www.buymeacoffee.com/DataVoid
or following me on
https://twitter.com/DataPlusEngine |
mradermacher/Med-LLaMA3-8B-i1-GGUF | mradermacher | "2024-06-29T22:50:29Z" | 16,455 | 0 | transformers | [
"transformers",
"gguf",
"en",
"base_model:YBXL/Med-LLaMA3-8B",
"endpoints_compatible",
"region:us"
] | null | "2024-06-29T19:59:36Z" | ---
base_model: YBXL/Med-LLaMA3-8B
language:
- en
library_name: transformers
quantized_by: mradermacher
tags: []
---
## About
<!-- ### quantize_version: 2 -->
<!-- ### output_tensor_quantised: 1 -->
<!-- ### convert_type: hf -->
<!-- ### vocab_type: -->
<!-- ### tags: nicoboss -->
weighted/imatrix quants of https://huggingface.co/YBXL/Med-LLaMA3-8B
<!-- provided-files -->
static quants are available at https://huggingface.co/mradermacher/Med-LLaMA3-8B-GGUF
## Usage
If you are unsure how to use GGUF files, refer to one of [TheBloke's
READMEs](https://huggingface.co/TheBloke/KafkaLM-70B-German-V0.1-GGUF) for
more details, including on how to concatenate multi-part files.
## Provided Quants
(sorted by size, not necessarily quality. IQ-quants are often preferable over similar sized non-IQ quants)
| Link | Type | Size/GB | Notes |
|:-----|:-----|--------:|:------|
| [GGUF](https://huggingface.co/mradermacher/Med-LLaMA3-8B-i1-GGUF/resolve/main/Med-LLaMA3-8B.i1-IQ1_S.gguf) | i1-IQ1_S | 2.1 | for the desperate |
| [GGUF](https://huggingface.co/mradermacher/Med-LLaMA3-8B-i1-GGUF/resolve/main/Med-LLaMA3-8B.i1-IQ1_M.gguf) | i1-IQ1_M | 2.3 | mostly desperate |
| [GGUF](https://huggingface.co/mradermacher/Med-LLaMA3-8B-i1-GGUF/resolve/main/Med-LLaMA3-8B.i1-IQ2_XXS.gguf) | i1-IQ2_XXS | 2.5 | |
| [GGUF](https://huggingface.co/mradermacher/Med-LLaMA3-8B-i1-GGUF/resolve/main/Med-LLaMA3-8B.i1-IQ2_XS.gguf) | i1-IQ2_XS | 2.7 | |
| [GGUF](https://huggingface.co/mradermacher/Med-LLaMA3-8B-i1-GGUF/resolve/main/Med-LLaMA3-8B.i1-IQ2_S.gguf) | i1-IQ2_S | 2.9 | |
| [GGUF](https://huggingface.co/mradermacher/Med-LLaMA3-8B-i1-GGUF/resolve/main/Med-LLaMA3-8B.i1-IQ2_M.gguf) | i1-IQ2_M | 3.0 | |
| [GGUF](https://huggingface.co/mradermacher/Med-LLaMA3-8B-i1-GGUF/resolve/main/Med-LLaMA3-8B.i1-Q2_K.gguf) | i1-Q2_K | 3.3 | IQ3_XXS probably better |
| [GGUF](https://huggingface.co/mradermacher/Med-LLaMA3-8B-i1-GGUF/resolve/main/Med-LLaMA3-8B.i1-IQ3_XXS.gguf) | i1-IQ3_XXS | 3.4 | lower quality |
| [GGUF](https://huggingface.co/mradermacher/Med-LLaMA3-8B-i1-GGUF/resolve/main/Med-LLaMA3-8B.i1-IQ3_XS.gguf) | i1-IQ3_XS | 3.6 | |
| [GGUF](https://huggingface.co/mradermacher/Med-LLaMA3-8B-i1-GGUF/resolve/main/Med-LLaMA3-8B.i1-Q3_K_S.gguf) | i1-Q3_K_S | 3.8 | IQ3_XS probably better |
| [GGUF](https://huggingface.co/mradermacher/Med-LLaMA3-8B-i1-GGUF/resolve/main/Med-LLaMA3-8B.i1-IQ3_S.gguf) | i1-IQ3_S | 3.8 | beats Q3_K* |
| [GGUF](https://huggingface.co/mradermacher/Med-LLaMA3-8B-i1-GGUF/resolve/main/Med-LLaMA3-8B.i1-IQ3_M.gguf) | i1-IQ3_M | 3.9 | |
| [GGUF](https://huggingface.co/mradermacher/Med-LLaMA3-8B-i1-GGUF/resolve/main/Med-LLaMA3-8B.i1-Q3_K_M.gguf) | i1-Q3_K_M | 4.1 | IQ3_S probably better |
| [GGUF](https://huggingface.co/mradermacher/Med-LLaMA3-8B-i1-GGUF/resolve/main/Med-LLaMA3-8B.i1-Q3_K_L.gguf) | i1-Q3_K_L | 4.4 | IQ3_M probably better |
| [GGUF](https://huggingface.co/mradermacher/Med-LLaMA3-8B-i1-GGUF/resolve/main/Med-LLaMA3-8B.i1-IQ4_XS.gguf) | i1-IQ4_XS | 4.5 | |
| [GGUF](https://huggingface.co/mradermacher/Med-LLaMA3-8B-i1-GGUF/resolve/main/Med-LLaMA3-8B.i1-Q4_0.gguf) | i1-Q4_0 | 4.8 | fast, low quality |
| [GGUF](https://huggingface.co/mradermacher/Med-LLaMA3-8B-i1-GGUF/resolve/main/Med-LLaMA3-8B.i1-Q4_K_S.gguf) | i1-Q4_K_S | 4.8 | optimal size/speed/quality |
| [GGUF](https://huggingface.co/mradermacher/Med-LLaMA3-8B-i1-GGUF/resolve/main/Med-LLaMA3-8B.i1-Q4_K_M.gguf) | i1-Q4_K_M | 5.0 | fast, recommended |
| [GGUF](https://huggingface.co/mradermacher/Med-LLaMA3-8B-i1-GGUF/resolve/main/Med-LLaMA3-8B.i1-Q5_K_S.gguf) | i1-Q5_K_S | 5.7 | |
| [GGUF](https://huggingface.co/mradermacher/Med-LLaMA3-8B-i1-GGUF/resolve/main/Med-LLaMA3-8B.i1-Q5_K_M.gguf) | i1-Q5_K_M | 5.8 | |
| [GGUF](https://huggingface.co/mradermacher/Med-LLaMA3-8B-i1-GGUF/resolve/main/Med-LLaMA3-8B.i1-Q6_K.gguf) | i1-Q6_K | 6.7 | practically like static Q6_K |
Here is a handy graph by ikawrakow comparing some lower-quality quant
types (lower is better):

And here are Artefact2's thoughts on the matter:
https://gist.github.com/Artefact2/b5f810600771265fc1e39442288e8ec9
## FAQ / Model Request
See https://huggingface.co/mradermacher/model_requests for some answers to
questions you might have and/or if you want some other model quantized.
## Thanks
I thank my company, [nethype GmbH](https://www.nethype.de/), for letting
me use its servers and providing upgrades to my workstation to enable
this work in my free time. Additional thanks to [@nicoboss](https://huggingface.co/nicoboss) for giving me access to his hardware for calculating the imatrix for these quants.
<!-- end -->
|
Yntec/ChickFlick | Yntec | "2024-05-27T22:36:56Z" | 16,454 | 3 | diffusers | [
"diffusers",
"safetensors",
"Film",
"Artistic",
"Girls",
"LEOSAM",
"text-to-image",
"stable-diffusion",
"stable-diffusion-diffusers",
"license:creativeml-openrail-m",
"endpoints_compatible",
"diffusers:StableDiffusionPipeline",
"region:us"
] | text-to-image | "2024-05-27T16:16:17Z" | ---
license: creativeml-openrail-m
library_name: diffusers
pipeline_tag: text-to-image
tags:
- Film
- Artistic
- Girls
- LEOSAM
- text-to-image
- stable-diffusion
- stable-diffusion-diffusers
- diffusers
---
# Chick Flick
LEOSAMsFilmGirlUltra merged with artistic models to achieve this style.
Samples and prompts:

(Click for larger)
Top left: (young cute girl sitting with a siamese cat, in a large house, tea cup, closeup), (5mm dramatic pose or action pose), (Artist design by (Marta Bevaqua) and (Richard Anderson)), detailed face (disaster scene from a movie, natural, dark pastel colors, HDR), (comfycore, cluttercore)
Top right: Elsa from Frozen wearing yellow towel, taking bubble bath in tub
Bottom left: cute curly little girl in bronze dress skirt and little boy in suit tie,kid, ballroom tango dance, 70s, Fireworks, detailed faces and eyes, beautiful dynamic color background, blurred bokeh background, 8k, depth of field, studio photo, ultra quality
Bottom right: girl in a classroom bored and uninterested
Original page: https://civitai.com/models/33208/leosams-filmgirl-ultra |
prajjwal1/bert-medium | prajjwal1 | "2021-10-27T18:30:16Z" | 16,443 | 3 | transformers | [
"transformers",
"pytorch",
"BERT",
"MNLI",
"NLI",
"transformer",
"pre-training",
"en",
"arxiv:1908.08962",
"arxiv:2110.01518",
"license:mit",
"endpoints_compatible",
"region:us"
] | null | "2022-03-02T23:29:05Z" | ---
language:
- en
license:
- mit
tags:
- BERT
- MNLI
- NLI
- transformer
- pre-training
---
The following model is a Pytorch pre-trained model obtained from converting Tensorflow checkpoint found in the [official Google BERT repository](https://github.com/google-research/bert).
This is one of the smaller pre-trained BERT variants, together with [bert-tiny](https://huggingface.co/prajjwal1/bert-tiny), [bert-mini](https://huggingface.co/prajjwal1/bert-mini) and [bert-small](https://huggingface.co/prajjwal1/bert-small). They were introduced in the study `Well-Read Students Learn Better: On the Importance of Pre-training Compact Models` ([arxiv](https://arxiv.org/abs/1908.08962)), and ported to HF for the study `Generalization in NLI: Ways (Not) To Go Beyond Simple Heuristics` ([arXiv](https://arxiv.org/abs/2110.01518)). These models are supposed to be trained on a downstream task.
If you use the model, please consider citing both the papers:
```
@misc{bhargava2021generalization,
title={Generalization in NLI: Ways (Not) To Go Beyond Simple Heuristics},
author={Prajjwal Bhargava and Aleksandr Drozd and Anna Rogers},
year={2021},
eprint={2110.01518},
archivePrefix={arXiv},
primaryClass={cs.CL}
}
@article{DBLP:journals/corr/abs-1908-08962,
author = {Iulia Turc and
Ming{-}Wei Chang and
Kenton Lee and
Kristina Toutanova},
title = {Well-Read Students Learn Better: The Impact of Student Initialization
on Knowledge Distillation},
journal = {CoRR},
volume = {abs/1908.08962},
year = {2019},
url = {http://arxiv.org/abs/1908.08962},
eprinttype = {arXiv},
eprint = {1908.08962},
timestamp = {Thu, 29 Aug 2019 16:32:34 +0200},
biburl = {https://dblp.org/rec/journals/corr/abs-1908-08962.bib},
bibsource = {dblp computer science bibliography, https://dblp.org}
}
```
Config of this model:
- `prajjwal1/bert-medium` (L=8, H=512) [Model Link](https://huggingface.co/prajjwal1/bert-medium)
Other models to check out:
- `prajjwal1/bert-tiny` (L=2, H=128) [Model Link](https://huggingface.co/prajjwal1/bert-tiny)
- `prajjwal1/bert-mini` (L=4, H=256) [Model Link](https://huggingface.co/prajjwal1/bert-mini)
- `prajjwal1/bert-small` (L=4, H=512) [Model Link](https://huggingface.co/prajjwal1/bert-small)
Original Implementation and more info can be found in [this Github repository](https://github.com/prajjwal1/generalize_lm_nli).
Twitter: [@prajjwal_1](https://twitter.com/prajjwal_1)
|
google/tapas-base | google | "2021-11-29T10:03:33Z" | 16,440 | 6 | transformers | [
"transformers",
"pytorch",
"tf",
"tapas",
"feature-extraction",
"TapasModel",
"en",
"arxiv:2004.02349",
"arxiv:2010.00571",
"license:apache-2.0",
"endpoints_compatible",
"region:us"
] | feature-extraction | "2022-03-02T23:29:05Z" | ---
language: en
tags:
- tapas
- TapasModel
license: apache-2.0
---
# TAPAS base model
This model has 2 versions which can be used. The latest version, which is the default one, corresponds to the `tapas_inter_masklm_base_reset` checkpoint of the [original Github repository](https://github.com/google-research/tapas).
This model was pre-trained on MLM and an additional step which the authors call intermediate pre-training. It uses relative position embeddings by default (i.e. resetting the position index at every cell of the table).
The other (non-default) version which can be used is the one with absolute position embeddings:
- `revision="no_reset"`, which corresponds to `tapas_inter_masklm_base`
Disclaimer: The team releasing TAPAS did not write a model card for this model so this model card has been written by
the Hugging Face team and contributors.
## Model description
TAPAS is a BERT-like transformers model pretrained on a large corpus of English data from Wikipedia in a self-supervised fashion.
This means it was pretrained on the raw tables and associated texts only, with no humans labelling them in any way (which is why it
can use lots of publicly available data) with an automatic process to generate inputs and labels from those texts. More precisely, it
was pretrained with two objectives:
- Masked language modeling (MLM): taking a (flattened) table and associated context, the model randomly masks 15% of the words in
the input, then runs the entire (partially masked) sequence through the model. The model then has to predict the masked words.
This is different from traditional recurrent neural networks (RNNs) that usually see the words one after the other,
or from autoregressive models like GPT which internally mask the future tokens. It allows the model to learn a bidirectional
representation of a table and associated text.
- Intermediate pre-training: to encourage numerical reasoning on tables, the authors additionally pre-trained the model by creating
a balanced dataset of millions of syntactically created training examples. Here, the model must predict (classify) whether a sentence
is supported or refuted by the contents of a table. The training examples are created based on synthetic as well as counterfactual statements.
This way, the model learns an inner representation of the English language used in tables and associated texts, which can then be used
to extract features useful for downstream tasks such as answering questions about a table, or determining whether a sentence is entailed
or refuted by the contents of a table. Fine-tuning is done by adding one or more classification heads on top of the pre-trained model, and then
jointly train these randomly initialized classification heads with the base model on a downstream task.
## Intended uses & limitations
You can use the raw model for getting hidden representatons about table-question pairs, but it's mostly intended to be fine-tuned on a downstream task such as question answering or sequence classification. See the [model hub](https://huggingface.co/models?filter=tapas) to look for fine-tuned versions on a task that interests you.
## Training procedure
### Preprocessing
The texts are lowercased and tokenized using WordPiece and a vocabulary size of 30,000. The inputs of the model are
then of the form:
```
[CLS] Sentence [SEP] Flattened table [SEP]
```
### Pre-training
The model was pre-trained on 32 Cloud TPU v3 cores for 1,000,000 steps with maximum sequence length 512 and batch size of 512.
In this setup, pre-training on MLM only takes around 3 days. Aditionally, the model has been further pre-trained on a second task (table entailment). See the original TAPAS [paper](https://www.aclweb.org/anthology/2020.acl-main.398/) and the [follow-up paper](https://www.aclweb.org/anthology/2020.findings-emnlp.27/) for more details.
The optimizer used is Adam with a learning rate of 5e-5, and a warmup
ratio of 0.01.
### BibTeX entry and citation info
```bibtex
@misc{herzig2020tapas,
title={TAPAS: Weakly Supervised Table Parsing via Pre-training},
author={Jonathan Herzig and Paweł Krzysztof Nowak and Thomas Müller and Francesco Piccinno and Julian Martin Eisenschlos},
year={2020},
eprint={2004.02349},
archivePrefix={arXiv},
primaryClass={cs.IR}
}
```
```bibtex
@misc{eisenschlos2020understanding,
title={Understanding tables with intermediate pre-training},
author={Julian Martin Eisenschlos and Syrine Krichene and Thomas Müller},
year={2020},
eprint={2010.00571},
archivePrefix={arXiv},
primaryClass={cs.CL}
}
``` |
01-ai/Yi-1.5-9B | 01-ai | "2024-06-26T10:41:21Z" | 16,424 | 39 | transformers | [
"transformers",
"safetensors",
"llama",
"text-generation",
"arxiv:2403.04652",
"license:apache-2.0",
"autotrain_compatible",
"endpoints_compatible",
"text-generation-inference",
"region:us"
] | text-generation | "2024-05-11T08:34:14Z" | ---
license: apache-2.0
---
<div align="center">
<picture>
<img src="https://raw.githubusercontent.com/01-ai/Yi/main/assets/img/Yi_logo_icon_light.svg" width="150px">
</picture>
</div>
<p align="center">
<a href="https://github.com/01-ai">🐙 GitHub</a> •
<a href="https://discord.gg/hYUwWddeAu">👾 Discord</a> •
<a href="https://twitter.com/01ai_yi">🐤 Twitter</a> •
<a href="https://github.com/01-ai/Yi-1.5/issues/2">💬 WeChat</a>
<br/>
<a href="https://arxiv.org/abs/2403.04652">📝 Paper</a> •
<a href="https://01-ai.github.io/">💪 Tech Blog</a> •
<a href="https://github.com/01-ai/Yi/tree/main?tab=readme-ov-file#faq">🙌 FAQ</a> •
<a href="https://github.com/01-ai/Yi/tree/main?tab=readme-ov-file#learning-hub">📗 Learning Hub</a>
</p>
# Intro
Yi-1.5 is an upgraded version of Yi. It is continuously pre-trained on Yi with a high-quality corpus of 500B tokens and fine-tuned on 3M diverse fine-tuning samples.
Compared with Yi, Yi-1.5 delivers stronger performance in coding, math, reasoning, and instruction-following capability, while still maintaining excellent capabilities in language understanding, commonsense reasoning, and reading comprehension.
<div align="center">
Model | Context Length | Pre-trained Tokens
| :------------: | :------------: | :------------: |
| Yi-1.5 | 4K, 16K, 32K | 3.6T
</div>
# Models
- Chat models
<div align="center">
| Name | Download |
| --------------- | ------------------------------------------------------------------------------------------------------------------------------------------------------------------- |
| Yi-1.5-34B-Chat | • [🤗 Hugging Face](https://huggingface.co/collections/01-ai/yi-15-2024-05-663f3ecab5f815a3eaca7ca8) • [🤖 ModelScope](https://www.modelscope.cn/organization/01ai) • [🟣 wisemodel](https://wisemodel.cn/organization/01.AI)|
| Yi-1.5-34B-Chat-16K | • [🤗 Hugging Face](https://huggingface.co/collections/01-ai/yi-15-2024-05-663f3ecab5f815a3eaca7ca8) • [🤖 ModelScope](https://www.modelscope.cn/organization/01ai) • [🟣 wisemodel](https://wisemodel.cn/organization/01.AI) |
| Yi-1.5-9B-Chat | • [🤗 Hugging Face](https://huggingface.co/collections/01-ai/yi-15-2024-05-663f3ecab5f815a3eaca7ca8) • [🤖 ModelScope](https://www.modelscope.cn/organization/01ai) • [🟣 wisemodel](https://wisemodel.cn/organization/01.AI) |
| Yi-1.5-9B-Chat-16K | • [🤗 Hugging Face](https://huggingface.co/collections/01-ai/yi-15-2024-05-663f3ecab5f815a3eaca7ca8) • [🤖 ModelScope](https://www.modelscope.cn/organization/01ai) • [🟣 wisemodel](https://wisemodel.cn/organization/01.AI) |
| Yi-1.5-6B-Chat | • [🤗 Hugging Face](https://huggingface.co/collections/01-ai/yi-15-2024-05-663f3ecab5f815a3eaca7ca8) • [🤖 ModelScope](https://www.modelscope.cn/organization/01ai) • [🟣 wisemodel](https://wisemodel.cn/organization/01.AI) |
</div>
- Base models
<div align="center">
| Name | Download |
| ---------- | ------------------------------------------------------------------------------------------------------------------------------------------------------------------- |
| Yi-1.5-34B | • [🤗 Hugging Face](https://huggingface.co/collections/01-ai/yi-15-2024-05-663f3ecab5f815a3eaca7ca8) • [🤖 ModelScope](https://www.modelscope.cn/organization/01ai) • [🟣 wisemodel](https://wisemodel.cn/organization/01.AI) |
| Yi-1.5-34B-32K | • [🤗 Hugging Face](https://huggingface.co/collections/01-ai/yi-15-2024-05-663f3ecab5f815a3eaca7ca8) • [🤖 ModelScope](https://www.modelscope.cn/organization/01ai) • [🟣 wisemodel](https://wisemodel.cn/organization/01.AI) |
| Yi-1.5-9B | • [🤗 Hugging Face](https://huggingface.co/collections/01-ai/yi-15-2024-05-663f3ecab5f815a3eaca7ca8) • [🤖 ModelScope](https://www.modelscope.cn/organization/01ai) • [🟣 wisemodel](https://wisemodel.cn/organization/01.AI) |
| Yi-1.5-9B-32K | • [🤗 Hugging Face](https://huggingface.co/collections/01-ai/yi-15-2024-05-663f3ecab5f815a3eaca7ca8) • [🤖 ModelScope](https://www.modelscope.cn/organization/01ai) • [🟣 wisemodel](https://wisemodel.cn/organization/01.AI) |
| Yi-1.5-6B | • [🤗 Hugging Face](https://huggingface.co/collections/01-ai/yi-15-2024-05-663f3ecab5f815a3eaca7ca8) • [🤖 ModelScope](https://www.modelscope.cn/organization/01ai) • [🟣 wisemodel](https://wisemodel.cn/organization/01.AI) |
</div>
# Benchmarks
- Chat models
Yi-1.5-34B-Chat is on par with or excels beyond larger models in most benchmarks.

Yi-1.5-9B-Chat is the top performer among similarly sized open-source models.

- Base models
Yi-1.5-34B is on par with or excels beyond larger models in some benchmarks.

Yi-1.5-9B is the top performer among similarly sized open-source models.

# Quick Start
For getting up and running with Yi-1.5 models quickly, see [README](https://github.com/01-ai/Yi-1.5).
|
ratchet-community/ratchet-moondream-2 | ratchet-community | "2024-06-21T03:34:42Z" | 16,420 | 0 | null | [
"gguf",
"license:apache-2.0",
"region:us"
] | null | "2024-05-25T03:53:25Z" | ---
license: apache-2.0
---
https://huggingface.co/vikhyatk/moondream2 adapted to work with huggingface Ratchet framework for on-device inference. |
timm/edgenext_small.usi_in1k | timm | "2023-04-23T22:43:14Z" | 16,390 | 0 | timm | [
"timm",
"pytorch",
"safetensors",
"image-classification",
"dataset:imagenet-1k",
"arxiv:2206.10589",
"arxiv:2204.03475",
"license:mit",
"region:us"
] | image-classification | "2023-04-23T22:43:00Z" | ---
tags:
- image-classification
- timm
library_name: timm
license: mit
datasets:
- imagenet-1k
---
# Model card for edgenext_small.usi_in1k
An EdgeNeXt image classification model. Trained on ImageNet-1k by paper authors using distillation (`USI` as per `Solving ImageNet`).
## Model Details
- **Model Type:** Image classification / feature backbone
- **Model Stats:**
- Params (M): 5.6
- GMACs: 1.3
- Activations (M): 9.1
- Image size: train = 256 x 256, test = 320 x 320
- **Papers:**
- EdgeNeXt: Efficiently Amalgamated CNN-Transformer Architecture for Mobile Vision Applications: https://arxiv.org/abs/2206.10589
- Solving ImageNet: a Unified Scheme for Training any Backbone to Top Results: https://arxiv.org/abs/2204.03475
- **Dataset:** ImageNet-1k
- **Original:** https://github.com/mmaaz60/EdgeNeXt
## Model Usage
### Image Classification
```python
from urllib.request import urlopen
from PIL import Image
import timm
img = Image.open(urlopen(
'https://huggingface.co/datasets/huggingface/documentation-images/resolve/main/beignets-task-guide.png'
))
model = timm.create_model('edgenext_small.usi_in1k', pretrained=True)
model = model.eval()
# get model specific transforms (normalization, resize)
data_config = timm.data.resolve_model_data_config(model)
transforms = timm.data.create_transform(**data_config, is_training=False)
output = model(transforms(img).unsqueeze(0)) # unsqueeze single image into batch of 1
top5_probabilities, top5_class_indices = torch.topk(output.softmax(dim=1) * 100, k=5)
```
### Feature Map Extraction
```python
from urllib.request import urlopen
from PIL import Image
import timm
img = Image.open(urlopen(
'https://huggingface.co/datasets/huggingface/documentation-images/resolve/main/beignets-task-guide.png'
))
model = timm.create_model(
'edgenext_small.usi_in1k',
pretrained=True,
features_only=True,
)
model = model.eval()
# get model specific transforms (normalization, resize)
data_config = timm.data.resolve_model_data_config(model)
transforms = timm.data.create_transform(**data_config, is_training=False)
output = model(transforms(img).unsqueeze(0)) # unsqueeze single image into batch of 1
for o in output:
# print shape of each feature map in output
# e.g.:
# torch.Size([1, 48, 64, 64])
# torch.Size([1, 96, 32, 32])
# torch.Size([1, 160, 16, 16])
# torch.Size([1, 304, 8, 8])
print(o.shape)
```
### Image Embeddings
```python
from urllib.request import urlopen
from PIL import Image
import timm
img = Image.open(urlopen(
'https://huggingface.co/datasets/huggingface/documentation-images/resolve/main/beignets-task-guide.png'
))
model = timm.create_model(
'edgenext_small.usi_in1k',
pretrained=True,
num_classes=0, # remove classifier nn.Linear
)
model = model.eval()
# get model specific transforms (normalization, resize)
data_config = timm.data.resolve_model_data_config(model)
transforms = timm.data.create_transform(**data_config, is_training=False)
output = model(transforms(img).unsqueeze(0)) # output is (batch_size, num_features) shaped tensor
# or equivalently (without needing to set num_classes=0)
output = model.forward_features(transforms(img).unsqueeze(0))
# output is unpooled, a (1, 304, 8, 8) shaped tensor
output = model.forward_head(output, pre_logits=True)
# output is a (1, num_features) shaped tensor
```
## Citation
```bibtex
@inproceedings{Maaz2022EdgeNeXt,
title={EdgeNeXt: Efficiently Amalgamated CNN-Transformer Architecture for Mobile Vision Applications},
author={Muhammad Maaz and Abdelrahman Shaker and Hisham Cholakkal and Salman Khan and Syed Waqas Zamir and Rao Muhammad Anwer and Fahad Shahbaz Khan},
booktitle={International Workshop on Computational Aspects of Deep Learning at 17th European Conference on Computer Vision (CADL2022)},
year={2022},
organization={Springer}
}
```
```bibtex
@misc{https://doi.org/10.48550/arxiv.2204.03475,
doi = {10.48550/ARXIV.2204.03475},
url = {https://arxiv.org/abs/2204.03475},
author = {Ridnik, Tal and Lawen, Hussam and Ben-Baruch, Emanuel and Noy, Asaf},
keywords = {Computer Vision and Pattern Recognition (cs.CV), Machine Learning (cs.LG), FOS: Computer and information sciences, FOS: Computer and information sciences},
title = {Solving ImageNet: a Unified Scheme for Training any Backbone to Top Results},
publisher = {arXiv},
year = {2022},
}
```
|
lidiya/bart-large-xsum-samsum | lidiya | "2023-03-16T22:44:01Z" | 16,385 | 36 | transformers | [
"transformers",
"pytorch",
"safetensors",
"bart",
"text2text-generation",
"seq2seq",
"summarization",
"en",
"dataset:samsum",
"license:apache-2.0",
"model-index",
"autotrain_compatible",
"endpoints_compatible",
"region:us"
] | summarization | "2022-03-02T23:29:05Z" | ---
language: en
tags:
- bart
- seq2seq
- summarization
license: apache-2.0
datasets:
- samsum
widget:
- text: |
Hannah: Hey, do you have Betty's number?
Amanda: Lemme check
Amanda: Sorry, can't find it.
Amanda: Ask Larry
Amanda: He called her last time we were at the park together
Hannah: I don't know him well
Amanda: Don't be shy, he's very nice
Hannah: If you say so..
Hannah: I'd rather you texted him
Amanda: Just text him 🙂
Hannah: Urgh.. Alright
Hannah: Bye
Amanda: Bye bye
model-index:
- name: bart-large-xsum-samsum
results:
- task:
name: Abstractive Text Summarization
type: abstractive-text-summarization
dataset:
name: "SAMSum Corpus: A Human-annotated Dialogue Dataset for Abstractive Summarization"
type: samsum
metrics:
- name: Validation ROUGE-1
type: rouge-1
value: 54.3921
- name: Validation ROUGE-2
type: rouge-2
value: 29.8078
- name: Validation ROUGE-L
type: rouge-l
value: 45.1543
- name: Test ROUGE-1
type: rouge-1
value: 53.3059
- name: Test ROUGE-2
type: rouge-2
value: 28.355
- name: Test ROUGE-L
type: rouge-l
value: 44.0953
---
## `bart-large-xsum-samsum`
This model was obtained by fine-tuning `facebook/bart-large-xsum` on [Samsum](https://huggingface.co/datasets/samsum) dataset.
## Usage
```python
from transformers import pipeline
summarizer = pipeline("summarization", model="lidiya/bart-large-xsum-samsum")
conversation = '''Hannah: Hey, do you have Betty's number?
Amanda: Lemme check
Amanda: Sorry, can't find it.
Amanda: Ask Larry
Amanda: He called her last time we were at the park together
Hannah: I don't know him well
Amanda: Don't be shy, he's very nice
Hannah: If you say so..
Hannah: I'd rather you texted him
Amanda: Just text him 🙂
Hannah: Urgh.. Alright
Hannah: Bye
Amanda: Bye bye
'''
summarizer(conversation)
```
## Training procedure
- Colab notebook: https://colab.research.google.com/drive/1dul0Sg-TTMy9xZCJzmDRajXbyzDwtYx6?usp=sharing
## Results
| key | value |
| --- | ----- |
| eval_rouge1 | 54.3921 |
| eval_rouge2 | 29.8078 |
| eval_rougeL | 45.1543 |
| eval_rougeLsum | 49.942 |
| test_rouge1 | 53.3059 |
| test_rouge2 | 28.355 |
| test_rougeL | 44.0953 |
| test_rougeLsum | 48.9246 | |
RichardErkhov/arnavgrg_-_llama-2-7b-nf4-fp16-upscaled-gguf | RichardErkhov | "2024-06-30T22:40:52Z" | 16,385 | 0 | null | [
"gguf",
"region:us"
] | null | "2024-06-30T20:38:52Z" | Quantization made by Richard Erkhov.
[Github](https://github.com/RichardErkhov)
[Discord](https://discord.gg/pvy7H8DZMG)
[Request more models](https://github.com/RichardErkhov/quant_request)
llama-2-7b-nf4-fp16-upscaled - GGUF
- Model creator: https://huggingface.co/arnavgrg/
- Original model: https://huggingface.co/arnavgrg/llama-2-7b-nf4-fp16-upscaled/
| Name | Quant method | Size |
| ---- | ---- | ---- |
| [llama-2-7b-nf4-fp16-upscaled.Q2_K.gguf](https://huggingface.co/RichardErkhov/arnavgrg_-_llama-2-7b-nf4-fp16-upscaled-gguf/blob/main/llama-2-7b-nf4-fp16-upscaled.Q2_K.gguf) | Q2_K | 2.36GB |
| [llama-2-7b-nf4-fp16-upscaled.IQ3_XS.gguf](https://huggingface.co/RichardErkhov/arnavgrg_-_llama-2-7b-nf4-fp16-upscaled-gguf/blob/main/llama-2-7b-nf4-fp16-upscaled.IQ3_XS.gguf) | IQ3_XS | 2.6GB |
| [llama-2-7b-nf4-fp16-upscaled.IQ3_S.gguf](https://huggingface.co/RichardErkhov/arnavgrg_-_llama-2-7b-nf4-fp16-upscaled-gguf/blob/main/llama-2-7b-nf4-fp16-upscaled.IQ3_S.gguf) | IQ3_S | 2.75GB |
| [llama-2-7b-nf4-fp16-upscaled.Q3_K_S.gguf](https://huggingface.co/RichardErkhov/arnavgrg_-_llama-2-7b-nf4-fp16-upscaled-gguf/blob/main/llama-2-7b-nf4-fp16-upscaled.Q3_K_S.gguf) | Q3_K_S | 2.75GB |
| [llama-2-7b-nf4-fp16-upscaled.IQ3_M.gguf](https://huggingface.co/RichardErkhov/arnavgrg_-_llama-2-7b-nf4-fp16-upscaled-gguf/blob/main/llama-2-7b-nf4-fp16-upscaled.IQ3_M.gguf) | IQ3_M | 2.9GB |
| [llama-2-7b-nf4-fp16-upscaled.Q3_K.gguf](https://huggingface.co/RichardErkhov/arnavgrg_-_llama-2-7b-nf4-fp16-upscaled-gguf/blob/main/llama-2-7b-nf4-fp16-upscaled.Q3_K.gguf) | Q3_K | 3.07GB |
| [llama-2-7b-nf4-fp16-upscaled.Q3_K_M.gguf](https://huggingface.co/RichardErkhov/arnavgrg_-_llama-2-7b-nf4-fp16-upscaled-gguf/blob/main/llama-2-7b-nf4-fp16-upscaled.Q3_K_M.gguf) | Q3_K_M | 3.07GB |
| [llama-2-7b-nf4-fp16-upscaled.Q3_K_L.gguf](https://huggingface.co/RichardErkhov/arnavgrg_-_llama-2-7b-nf4-fp16-upscaled-gguf/blob/main/llama-2-7b-nf4-fp16-upscaled.Q3_K_L.gguf) | Q3_K_L | 3.35GB |
| [llama-2-7b-nf4-fp16-upscaled.IQ4_XS.gguf](https://huggingface.co/RichardErkhov/arnavgrg_-_llama-2-7b-nf4-fp16-upscaled-gguf/blob/main/llama-2-7b-nf4-fp16-upscaled.IQ4_XS.gguf) | IQ4_XS | 3.4GB |
| [llama-2-7b-nf4-fp16-upscaled.Q4_0.gguf](https://huggingface.co/RichardErkhov/arnavgrg_-_llama-2-7b-nf4-fp16-upscaled-gguf/blob/main/llama-2-7b-nf4-fp16-upscaled.Q4_0.gguf) | Q4_0 | 3.56GB |
| [llama-2-7b-nf4-fp16-upscaled.IQ4_NL.gguf](https://huggingface.co/RichardErkhov/arnavgrg_-_llama-2-7b-nf4-fp16-upscaled-gguf/blob/main/llama-2-7b-nf4-fp16-upscaled.IQ4_NL.gguf) | IQ4_NL | 3.58GB |
| [llama-2-7b-nf4-fp16-upscaled.Q4_K_S.gguf](https://huggingface.co/RichardErkhov/arnavgrg_-_llama-2-7b-nf4-fp16-upscaled-gguf/blob/main/llama-2-7b-nf4-fp16-upscaled.Q4_K_S.gguf) | Q4_K_S | 3.59GB |
| [llama-2-7b-nf4-fp16-upscaled.Q4_K.gguf](https://huggingface.co/RichardErkhov/arnavgrg_-_llama-2-7b-nf4-fp16-upscaled-gguf/blob/main/llama-2-7b-nf4-fp16-upscaled.Q4_K.gguf) | Q4_K | 3.8GB |
| [llama-2-7b-nf4-fp16-upscaled.Q4_K_M.gguf](https://huggingface.co/RichardErkhov/arnavgrg_-_llama-2-7b-nf4-fp16-upscaled-gguf/blob/main/llama-2-7b-nf4-fp16-upscaled.Q4_K_M.gguf) | Q4_K_M | 3.8GB |
| [llama-2-7b-nf4-fp16-upscaled.Q4_1.gguf](https://huggingface.co/RichardErkhov/arnavgrg_-_llama-2-7b-nf4-fp16-upscaled-gguf/blob/main/llama-2-7b-nf4-fp16-upscaled.Q4_1.gguf) | Q4_1 | 3.95GB |
| [llama-2-7b-nf4-fp16-upscaled.Q5_0.gguf](https://huggingface.co/RichardErkhov/arnavgrg_-_llama-2-7b-nf4-fp16-upscaled-gguf/blob/main/llama-2-7b-nf4-fp16-upscaled.Q5_0.gguf) | Q5_0 | 4.33GB |
| [llama-2-7b-nf4-fp16-upscaled.Q5_K_S.gguf](https://huggingface.co/RichardErkhov/arnavgrg_-_llama-2-7b-nf4-fp16-upscaled-gguf/blob/main/llama-2-7b-nf4-fp16-upscaled.Q5_K_S.gguf) | Q5_K_S | 4.33GB |
| [llama-2-7b-nf4-fp16-upscaled.Q5_K.gguf](https://huggingface.co/RichardErkhov/arnavgrg_-_llama-2-7b-nf4-fp16-upscaled-gguf/blob/main/llama-2-7b-nf4-fp16-upscaled.Q5_K.gguf) | Q5_K | 4.45GB |
| [llama-2-7b-nf4-fp16-upscaled.Q5_K_M.gguf](https://huggingface.co/RichardErkhov/arnavgrg_-_llama-2-7b-nf4-fp16-upscaled-gguf/blob/main/llama-2-7b-nf4-fp16-upscaled.Q5_K_M.gguf) | Q5_K_M | 4.45GB |
| [llama-2-7b-nf4-fp16-upscaled.Q5_1.gguf](https://huggingface.co/RichardErkhov/arnavgrg_-_llama-2-7b-nf4-fp16-upscaled-gguf/blob/main/llama-2-7b-nf4-fp16-upscaled.Q5_1.gguf) | Q5_1 | 4.72GB |
| [llama-2-7b-nf4-fp16-upscaled.Q6_K.gguf](https://huggingface.co/RichardErkhov/arnavgrg_-_llama-2-7b-nf4-fp16-upscaled-gguf/blob/main/llama-2-7b-nf4-fp16-upscaled.Q6_K.gguf) | Q6_K | 5.15GB |
| [llama-2-7b-nf4-fp16-upscaled.Q8_0.gguf](https://huggingface.co/RichardErkhov/arnavgrg_-_llama-2-7b-nf4-fp16-upscaled-gguf/blob/main/llama-2-7b-nf4-fp16-upscaled.Q8_0.gguf) | Q8_0 | 6.67GB |
Original model description:
---
license: apache-2.0
tags:
- text-generation-inference
---
This is an upscaled fp16 variant of the original Llama-2-7b base model by Meta after it has been loaded with nf4 4-bit quantization via bitsandbytes.
The main idea here is to upscale the linear4bit layers to fp16 so that the quantization/dequantization cost doesn't have to paid for each forward pass at inference time.
_Note: The quantization operation to nf4 is not lossless, so the model weights for the linear layers are lossy, which means that this model will not work as well as the official base model._
To use this model, you can just load it via `transformers` in fp16:
```python
import torch
from transformers import AutoModelForCausalLM
model = AutoModelForCausalLM.from_pretrained(
"arnavgrg/llama-2-7b-nf4-fp16-upscaled",
device_map="auto",
torch_dtype=torch.float16,
)
```
|
RichardErkhov/wons_-_mistral-7B-test-v0.3-gguf | RichardErkhov | "2024-06-30T11:31:40Z" | 16,381 | 0 | null | [
"gguf",
"region:us"
] | null | "2024-06-30T08:38:59Z" | Quantization made by Richard Erkhov.
[Github](https://github.com/RichardErkhov)
[Discord](https://discord.gg/pvy7H8DZMG)
[Request more models](https://github.com/RichardErkhov/quant_request)
mistral-7B-test-v0.3 - GGUF
- Model creator: https://huggingface.co/wons/
- Original model: https://huggingface.co/wons/mistral-7B-test-v0.3/
| Name | Quant method | Size |
| ---- | ---- | ---- |
| [mistral-7B-test-v0.3.Q2_K.gguf](https://huggingface.co/RichardErkhov/wons_-_mistral-7B-test-v0.3-gguf/blob/main/mistral-7B-test-v0.3.Q2_K.gguf) | Q2_K | 2.53GB |
| [mistral-7B-test-v0.3.IQ3_XS.gguf](https://huggingface.co/RichardErkhov/wons_-_mistral-7B-test-v0.3-gguf/blob/main/mistral-7B-test-v0.3.IQ3_XS.gguf) | IQ3_XS | 2.81GB |
| [mistral-7B-test-v0.3.IQ3_S.gguf](https://huggingface.co/RichardErkhov/wons_-_mistral-7B-test-v0.3-gguf/blob/main/mistral-7B-test-v0.3.IQ3_S.gguf) | IQ3_S | 2.96GB |
| [mistral-7B-test-v0.3.Q3_K_S.gguf](https://huggingface.co/RichardErkhov/wons_-_mistral-7B-test-v0.3-gguf/blob/main/mistral-7B-test-v0.3.Q3_K_S.gguf) | Q3_K_S | 2.95GB |
| [mistral-7B-test-v0.3.IQ3_M.gguf](https://huggingface.co/RichardErkhov/wons_-_mistral-7B-test-v0.3-gguf/blob/main/mistral-7B-test-v0.3.IQ3_M.gguf) | IQ3_M | 3.06GB |
| [mistral-7B-test-v0.3.Q3_K.gguf](https://huggingface.co/RichardErkhov/wons_-_mistral-7B-test-v0.3-gguf/blob/main/mistral-7B-test-v0.3.Q3_K.gguf) | Q3_K | 3.28GB |
| [mistral-7B-test-v0.3.Q3_K_M.gguf](https://huggingface.co/RichardErkhov/wons_-_mistral-7B-test-v0.3-gguf/blob/main/mistral-7B-test-v0.3.Q3_K_M.gguf) | Q3_K_M | 3.28GB |
| [mistral-7B-test-v0.3.Q3_K_L.gguf](https://huggingface.co/RichardErkhov/wons_-_mistral-7B-test-v0.3-gguf/blob/main/mistral-7B-test-v0.3.Q3_K_L.gguf) | Q3_K_L | 3.56GB |
| [mistral-7B-test-v0.3.IQ4_XS.gguf](https://huggingface.co/RichardErkhov/wons_-_mistral-7B-test-v0.3-gguf/blob/main/mistral-7B-test-v0.3.IQ4_XS.gguf) | IQ4_XS | 3.67GB |
| [mistral-7B-test-v0.3.Q4_0.gguf](https://huggingface.co/RichardErkhov/wons_-_mistral-7B-test-v0.3-gguf/blob/main/mistral-7B-test-v0.3.Q4_0.gguf) | Q4_0 | 3.83GB |
| [mistral-7B-test-v0.3.IQ4_NL.gguf](https://huggingface.co/RichardErkhov/wons_-_mistral-7B-test-v0.3-gguf/blob/main/mistral-7B-test-v0.3.IQ4_NL.gguf) | IQ4_NL | 3.87GB |
| [mistral-7B-test-v0.3.Q4_K_S.gguf](https://huggingface.co/RichardErkhov/wons_-_mistral-7B-test-v0.3-gguf/blob/main/mistral-7B-test-v0.3.Q4_K_S.gguf) | Q4_K_S | 3.86GB |
| [mistral-7B-test-v0.3.Q4_K.gguf](https://huggingface.co/RichardErkhov/wons_-_mistral-7B-test-v0.3-gguf/blob/main/mistral-7B-test-v0.3.Q4_K.gguf) | Q4_K | 4.07GB |
| [mistral-7B-test-v0.3.Q4_K_M.gguf](https://huggingface.co/RichardErkhov/wons_-_mistral-7B-test-v0.3-gguf/blob/main/mistral-7B-test-v0.3.Q4_K_M.gguf) | Q4_K_M | 4.07GB |
| [mistral-7B-test-v0.3.Q4_1.gguf](https://huggingface.co/RichardErkhov/wons_-_mistral-7B-test-v0.3-gguf/blob/main/mistral-7B-test-v0.3.Q4_1.gguf) | Q4_1 | 4.24GB |
| [mistral-7B-test-v0.3.Q5_0.gguf](https://huggingface.co/RichardErkhov/wons_-_mistral-7B-test-v0.3-gguf/blob/main/mistral-7B-test-v0.3.Q5_0.gguf) | Q5_0 | 4.65GB |
| [mistral-7B-test-v0.3.Q5_K_S.gguf](https://huggingface.co/RichardErkhov/wons_-_mistral-7B-test-v0.3-gguf/blob/main/mistral-7B-test-v0.3.Q5_K_S.gguf) | Q5_K_S | 4.65GB |
| [mistral-7B-test-v0.3.Q5_K.gguf](https://huggingface.co/RichardErkhov/wons_-_mistral-7B-test-v0.3-gguf/blob/main/mistral-7B-test-v0.3.Q5_K.gguf) | Q5_K | 4.78GB |
| [mistral-7B-test-v0.3.Q5_K_M.gguf](https://huggingface.co/RichardErkhov/wons_-_mistral-7B-test-v0.3-gguf/blob/main/mistral-7B-test-v0.3.Q5_K_M.gguf) | Q5_K_M | 4.78GB |
| [mistral-7B-test-v0.3.Q5_1.gguf](https://huggingface.co/RichardErkhov/wons_-_mistral-7B-test-v0.3-gguf/blob/main/mistral-7B-test-v0.3.Q5_1.gguf) | Q5_1 | 5.07GB |
| [mistral-7B-test-v0.3.Q6_K.gguf](https://huggingface.co/RichardErkhov/wons_-_mistral-7B-test-v0.3-gguf/blob/main/mistral-7B-test-v0.3.Q6_K.gguf) | Q6_K | 5.53GB |
| [mistral-7B-test-v0.3.Q8_0.gguf](https://huggingface.co/RichardErkhov/wons_-_mistral-7B-test-v0.3-gguf/blob/main/mistral-7B-test-v0.3.Q8_0.gguf) | Q8_0 | 7.17GB |
Original model description:
Entry not found
|
microsoft/Florence-2-base | microsoft | "2024-07-01T09:36:41Z" | 16,380 | 94 | transformers | [
"transformers",
"pytorch",
"florence2",
"text-generation",
"vision",
"image-text-to-text",
"custom_code",
"arxiv:2311.06242",
"license:mit",
"autotrain_compatible",
"region:us"
] | image-text-to-text | "2024-06-15T00:57:24Z" | ---
license: mit
license_link: https://huggingface.co/microsoft/Florence-2-base/resolve/main/LICENSE
pipeline_tag: image-text-to-text
tags:
- vision
---
# Florence-2: Advancing a Unified Representation for a Variety of Vision Tasks
## Model Summary
This Hub repository contains a HuggingFace's `transformers` implementation of Florence-2 model from Microsoft.
Florence-2 is an advanced vision foundation model that uses a prompt-based approach to handle a wide range of vision and vision-language tasks. Florence-2 can interpret simple text prompts to perform tasks like captioning, object detection, and segmentation. It leverages our FLD-5B dataset, containing 5.4 billion annotations across 126 million images, to master multi-task learning. The model's sequence-to-sequence architecture enables it to excel in both zero-shot and fine-tuned settings, proving to be a competitive vision foundation model.
Resources and Technical Documentation:
+ [Florence-2 technical report](https://arxiv.org/abs/2311.06242).
+ [Jupyter Notebook for inference and visualization of Florence-2-large model](https://huggingface.co/microsoft/Florence-2-large/blob/main/sample_inference.ipynb)
| Model | Model size | Model Description |
| ------- | ------------- | ------------- |
| Florence-2-base[[HF]](https://huggingface.co/microsoft/Florence-2-base) | 0.23B | Pretrained model with FLD-5B
| Florence-2-large[[HF]](https://huggingface.co/microsoft/Florence-2-large) | 0.77B | Pretrained model with FLD-5B
| Florence-2-base-ft[[HF]](https://huggingface.co/microsoft/Florence-2-base-ft) | 0.23B | Finetuned model on a colletion of downstream tasks
| Florence-2-large-ft[[HF]](https://huggingface.co/microsoft/Florence-2-large-ft) | 0.77B | Finetuned model on a colletion of downstream tasks
## How to Get Started with the Model
Use the code below to get started with the model.
```python
import requests
from PIL import Image
from transformers import AutoProcessor, AutoModelForCausalLM
model = AutoModelForCausalLM.from_pretrained("microsoft/Florence-2-base", trust_remote_code=True)
processor = AutoProcessor.from_pretrained("microsoft/Florence-2-base", trust_remote_code=True)
prompt = "<OD>"
url = "https://huggingface.co/datasets/huggingface/documentation-images/resolve/main/transformers/tasks/car.jpg?download=true"
image = Image.open(requests.get(url, stream=True).raw)
inputs = processor(text=prompt, images=image, return_tensors="pt")
generated_ids = model.generate(
input_ids=inputs["input_ids"],
pixel_values=inputs["pixel_values"],
max_new_tokens=1024,
do_sample=False,
num_beams=3,
)
generated_text = processor.batch_decode(generated_ids, skip_special_tokens=False)[0]
parsed_answer = processor.post_process_generation(generated_text, task="<OD>", image_size=(image.width, image.height))
print(parsed_answer)
```
## Tasks
This model is capable of performing different tasks through changing the prompts.
First, let's define a function to run a prompt.
<details>
<summary> Click to expand </summary>
```python
import requests
from PIL import Image
from transformers import AutoProcessor, AutoModelForCausalLM
model = AutoModelForCausalLM.from_pretrained("microsoft/Florence-2-base", trust_remote_code=True)
processor = AutoProcessor.from_pretrained("microsoft/Florence-2-base", trust_remote_code=True)
url = "https://huggingface.co/datasets/huggingface/documentation-images/resolve/main/transformers/tasks/car.jpg?download=true"
image = Image.open(requests.get(url, stream=True).raw)
def run_example(task_prompt, text_input=None):
if text_input is None:
prompt = task_prompt
else:
prompt = task_prompt + text_input
inputs = processor(text=prompt, images=image, return_tensors="pt")
generated_ids = model.generate(
input_ids=inputs["input_ids"],
pixel_values=inputs["pixel_values"],
max_new_tokens=1024,
num_beams=3
)
generated_text = processor.batch_decode(generated_ids, skip_special_tokens=False)[0]
parsed_answer = processor.post_process_generation(generated_text, task=task_prompt, image_size=(image.width, image.height))
print(parsed_answer)
```
</details>
Here are the tasks `Florence-2` could perform:
<details>
<summary> Click to expand </summary>
### Caption
```python
prompt = "<CAPTION>"
run_example(prompt)
```
### Detailed Caption
```python
prompt = "<DETAILED_CAPTION>"
run_example(prompt)
```
### More Detailed Caption
```python
prompt = "<MORE_DETAILED_CAPTION>"
run_example(prompt)
```
### Caption to Phrase Grounding
caption to phrase grounding task requires additional text input, i.e. caption.
Caption to phrase grounding results format:
{'\<CAPTION_TO_PHRASE_GROUNDING>': {'bboxes': [[x1, y1, x2, y2], ...], 'labels': ['', '', ...]}}
```python
task_prompt = "<CAPTION_TO_PHRASE_GROUNDING>"
results = run_example(task_prompt, text_input="A green car parked in front of a yellow building.")
```
### Object Detection
OD results format:
{'\<OD>': {'bboxes': [[x1, y1, x2, y2], ...],
'labels': ['label1', 'label2', ...]} }
```python
prompt = "<OD>"
run_example(prompt)
```
### Dense Region Caption
Dense region caption results format:
{'\<DENSE_REGION_CAPTION>' : {'bboxes': [[x1, y1, x2, y2], ...],
'labels': ['label1', 'label2', ...]} }
```python
prompt = "<DENSE_REGION_CAPTION>"
run_example(prompt)
```
### Region proposal
Dense region caption results format:
{'\<REGION_PROPOSAL>': {'bboxes': [[x1, y1, x2, y2], ...],
'labels': ['', '', ...]}}
```python
prompt = "<REGION_PROPOSAL>"
run_example(prompt)
```
### OCR
```python
prompt = "<OCR>"
run_example(prompt)
```
### OCR with Region
OCR with region output format:
{'\<OCR_WITH_REGION>': {'quad_boxes': [[x1, y1, x2, y2, x3, y3, x4, y4], ...], 'labels': ['text1', ...]}}
```python
prompt = "<OCR_WITH_REGION>"
run_example(prompt)
```
for More detailed examples, please refer to [notebook](https://huggingface.co/microsoft/Florence-2-large/blob/main/sample_inference.ipynb)
</details>
# Benchmarks
## Florence-2 Zero-shot performance
The following table presents the zero-shot performance of generalist vision foundation models on image captioning and object detection evaluation tasks. These models have not been exposed to the training data of the evaluation tasks during their training phase.
| Method | #params | COCO Cap. test CIDEr | NoCaps val CIDEr | TextCaps val CIDEr | COCO Det. val2017 mAP |
|--------|---------|----------------------|------------------|--------------------|-----------------------|
| Flamingo | 80B | 84.3 | - | - | - |
| Florence-2-base| 0.23B | 133.0 | 118.7 | 70.1 | 34.7 |
| Florence-2-large| 0.77B | 135.6 | 120.8 | 72.8 | 37.5 |
The following table continues the comparison with performance on other vision-language evaluation tasks.
| Method | Flickr30k test R@1 | Refcoco val Accuracy | Refcoco test-A Accuracy | Refcoco test-B Accuracy | Refcoco+ val Accuracy | Refcoco+ test-A Accuracy | Refcoco+ test-B Accuracy | Refcocog val Accuracy | Refcocog test Accuracy | Refcoco RES val mIoU |
|--------|----------------------|----------------------|-------------------------|-------------------------|-----------------------|--------------------------|--------------------------|-----------------------|------------------------|----------------------|
| Kosmos-2 | 78.7 | 52.3 | 57.4 | 47.3 | 45.5 | 50.7 | 42.2 | 60.6 | 61.7 | - |
| Florence-2-base | 83.6 | 53.9 | 58.4 | 49.7 | 51.5 | 56.4 | 47.9 | 66.3 | 65.1 | 34.6 |
| Florence-2-large | 84.4 | 56.3 | 61.6 | 51.4 | 53.6 | 57.9 | 49.9 | 68.0 | 67.0 | 35.8 |
## Florence-2 finetuned performance
We finetune Florence-2 models with a collection of downstream tasks, resulting two generalist models *Florence-2-base-ft* and *Florence-2-large-ft* that can conduct a wide range of downstream tasks.
The table below compares the performance of specialist and generalist models on various captioning and Visual Question Answering (VQA) tasks. Specialist models are fine-tuned specifically for each task, whereas generalist models are fine-tuned in a task-agnostic manner across all tasks. The symbol "▲" indicates the usage of external OCR as input.
| Method | # Params | COCO Caption Karpathy test CIDEr | NoCaps val CIDEr | TextCaps val CIDEr | VQAv2 test-dev Acc | TextVQA test-dev Acc | VizWiz VQA test-dev Acc |
|----------------|----------|-----------------------------------|------------------|--------------------|--------------------|----------------------|-------------------------|
| **Specialist Models** | | | | | | | |
| CoCa | 2.1B | 143.6 | 122.4 | - | 82.3 | - | - |
| BLIP-2 | 7.8B | 144.5 | 121.6 | - | 82.2 | - | - |
| GIT2 | 5.1B | 145.0 | 126.9 | 148.6 | 81.7 | 67.3 | 71.0 |
| Flamingo | 80B | 138.1 | - | - | 82.0 | 54.1 | 65.7 |
| PaLI | 17B | 149.1 | 127.0 | 160.0▲ | 84.3 | 58.8 / 73.1▲ | 71.6 / 74.4▲ |
| PaLI-X | 55B | 149.2 | 126.3 | 147.0 / 163.7▲ | 86.0 | 71.4 / 80.8▲ | 70.9 / 74.6▲ |
| **Generalist Models** | | | | | | | |
| Unified-IO | 2.9B | - | 100.0 | - | 77.9 | - | 57.4 |
| Florence-2-base-ft | 0.23B | 140.0 | 116.7 | 143.9 | 79.7 | 63.6 | 63.6 |
| Florence-2-large-ft | 0.77B | 143.3 | 124.9 | 151.1 | 81.7 | 73.5 | 72.6 |
| Method | # Params | COCO Det. val2017 mAP | Flickr30k test R@1 | RefCOCO val Accuracy | RefCOCO test-A Accuracy | RefCOCO test-B Accuracy | RefCOCO+ val Accuracy | RefCOCO+ test-A Accuracy | RefCOCO+ test-B Accuracy | RefCOCOg val Accuracy | RefCOCOg test Accuracy | RefCOCO RES val mIoU |
|----------------------|----------|-----------------------|--------------------|----------------------|-------------------------|-------------------------|------------------------|---------------------------|---------------------------|------------------------|-----------------------|------------------------|
| **Specialist Models** | | | | | | | | | | | | |
| SeqTR | - | - | - | 83.7 | 86.5 | 81.2 | 71.5 | 76.3 | 64.9 | 74.9 | 74.2 | - |
| PolyFormer | - | - | - | 90.4 | 92.9 | 87.2 | 85.0 | 89.8 | 78.0 | 85.8 | 85.9 | 76.9 |
| UNINEXT | 0.74B | 60.6 | - | 92.6 | 94.3 | 91.5 | 85.2 | 89.6 | 79.8 | 88.7 | 89.4 | - |
| Ferret | 13B | - | - | 89.5 | 92.4 | 84.4 | 82.8 | 88.1 | 75.2 | 85.8 | 86.3 | - |
| **Generalist Models** | | | | | | | | | | | | |
| UniTAB | - | - | - | 88.6 | 91.1 | 83.8 | 81.0 | 85.4 | 71.6 | 84.6 | 84.7 | - |
| Florence-2-base-ft | 0.23B | 41.4 | 84.0 | 92.6 | 94.8 | 91.5 | 86.8 | 91.7 | 82.2 | 89.8 | 82.2 | 78.0 |
| Florence-2-large-ft| 0.77B | 43.4 | 85.2 | 93.4 | 95.3 | 92.0 | 88.3 | 92.9 | 83.6 | 91.2 | 91.7 | 80.5 |
## BibTex and citation info
```
@article{xiao2023florence,
title={Florence-2: Advancing a unified representation for a variety of vision tasks},
author={Xiao, Bin and Wu, Haiping and Xu, Weijian and Dai, Xiyang and Hu, Houdong and Lu, Yumao and Zeng, Michael and Liu, Ce and Yuan, Lu},
journal={arXiv preprint arXiv:2311.06242},
year={2023}
}
``` |
ControlNet-1-1-preview/control_v11p_sd15_lineart | ControlNet-1-1-preview | "2023-04-14T19:11:45Z" | 16,377 | 22 | diffusers | [
"diffusers",
"safetensors",
"art",
"controlnet",
"stable-diffusion",
"arxiv:2302.05543",
"base_model:runwayml/stable-diffusion-v1-5",
"license:openrail",
"region:us"
] | null | "2023-04-13T09:18:01Z" | ---
license: openrail
base_model: runwayml/stable-diffusion-v1-5
tags:
- art
- controlnet
- stable-diffusion
---
# Controlnet - v1.1 - *lineart Version*
**Controlnet v1.1** is the successor model of [Controlnet v1.0](https://huggingface.co/lllyasviel/ControlNet)
and was released in [lllyasviel/ControlNet-v1-1](https://huggingface.co/lllyasviel/ControlNet-v1-1) by [Lvmin Zhang](https://huggingface.co/lllyasviel).
This checkpoint is a conversion of [the original checkpoint](https://huggingface.co/lllyasviel/ControlNet-v1-1/blob/main/control_v11p_sd15_lineart.pth) into `diffusers` format.
It can be used in combination with **Stable Diffusion**, such as [runwayml/stable-diffusion-v1-5](https://huggingface.co/runwayml/stable-diffusion-v1-5).
For more details, please also have a look at the [🧨 Diffusers docs](https://huggingface.co/docs/diffusers/api/pipelines/stable_diffusion/controlnet).
ControlNet is a neural network structure to control diffusion models by adding extra conditions.

This checkpoint corresponds to the ControlNet conditioned on **lineart images**.
## Model Details
- **Developed by:** Lvmin Zhang, Maneesh Agrawala
- **Model type:** Diffusion-based text-to-image generation model
- **Language(s):** English
- **License:** [The CreativeML OpenRAIL M license](https://huggingface.co/spaces/CompVis/stable-diffusion-license) is an [Open RAIL M license](https://www.licenses.ai/blog/2022/8/18/naming-convention-of-responsible-ai-licenses), adapted from the work that [BigScience](https://bigscience.huggingface.co/) and [the RAIL Initiative](https://www.licenses.ai/) are jointly carrying in the area of responsible AI licensing. See also [the article about the BLOOM Open RAIL license](https://bigscience.huggingface.co/blog/the-bigscience-rail-license) on which our license is based.
- **Resources for more information:** [GitHub Repository](https://github.com/lllyasviel/ControlNet), [Paper](https://arxiv.org/abs/2302.05543).
- **Cite as:**
@misc{zhang2023adding,
title={Adding Conditional Control to Text-to-Image Diffusion Models},
author={Lvmin Zhang and Maneesh Agrawala},
year={2023},
eprint={2302.05543},
archivePrefix={arXiv},
primaryClass={cs.CV}
}
## Introduction
Controlnet was proposed in [*Adding Conditional Control to Text-to-Image Diffusion Models*](https://arxiv.org/abs/2302.05543) by
Lvmin Zhang, Maneesh Agrawala.
The abstract reads as follows:
*We present a neural network structure, ControlNet, to control pretrained large diffusion models to support additional input conditions.
The ControlNet learns task-specific conditions in an end-to-end way, and the learning is robust even when the training dataset is small (< 50k).
Moreover, training a ControlNet is as fast as fine-tuning a diffusion model, and the model can be trained on a personal devices.
Alternatively, if powerful computation clusters are available, the model can scale to large amounts (millions to billions) of data.
We report that large diffusion models like Stable Diffusion can be augmented with ControlNets to enable conditional inputs like edge maps, segmentation maps, keypoints, etc.
This may enrich the methods to control large diffusion models and further facilitate related applications.*
## Example
It is recommended to use the checkpoint with [Stable Diffusion v1-5](https://huggingface.co/runwayml/stable-diffusion-v1-5) as the checkpoint
has been trained on it.
Experimentally, the checkpoint can be used with other diffusion models such as dreamboothed stable diffusion.
**Note**: If you want to process an image to create the auxiliary conditioning, external dependencies are required as shown below:
1. Install https://github.com/patrickvonplaten/controlnet_aux
```sh
$ pip install controlnet_aux==0.3.0
```
2. Let's install `diffusers` and related packages:
```
$ pip install diffusers transformers accelerate
```
3. Run code:
```python
import torch
import os
from huggingface_hub import HfApi
from pathlib import Path
from diffusers.utils import load_image
from PIL import Image
import numpy as np
from controlnet_aux import LineartDetector
from diffusers import (
ControlNetModel,
StableDiffusionControlNetPipeline,
UniPCMultistepScheduler,
)
checkpoint = "ControlNet-1-1-preview/control_v11p_sd15_lineart"
image = load_image(
"https://huggingface.co/ControlNet-1-1-preview/control_v11p_sd15_lineart/resolve/main/images/input.png"
)
image = image.resize((512, 512))
prompt = "michael jackson concert"
processor = LineartDetector.from_pretrained("lllyasviel/Annotators")
control_image = processor(image)
control_image.save("./images/control.png")
controlnet = ControlNetModel.from_pretrained(checkpoint, torch_dtype=torch.float16)
pipe = StableDiffusionControlNetPipeline.from_pretrained(
"runwayml/stable-diffusion-v1-5", controlnet=controlnet, torch_dtype=torch.float16
)
pipe.scheduler = UniPCMultistepScheduler.from_config(pipe.scheduler.config)
pipe.enable_model_cpu_offload()
generator = torch.manual_seed(0)
image = pipe(prompt, num_inference_steps=30, generator=generator, image=control_image).images[0]
image.save('images/image_out.png')
```



## Other released checkpoints v1-1
The authors released 14 different checkpoints, each trained with [Stable Diffusion v1-5](https://huggingface.co/runwayml/stable-diffusion-v1-5)
on a different type of conditioning:
| Model Name | Control Image Overview| Control Image Example | Generated Image Example |
|---|---|---|---|
TODO
### Training
TODO
### Blog post
For more information, please also have a look at the [Diffusers ControlNet Blog Post](https://huggingface.co/blog/controlnet).
|
alirezamsh/quip-512-mocha | alirezamsh | "2024-03-21T11:22:19Z" | 16,377 | 4 | transformers | [
"transformers",
"pytorch",
"safetensors",
"roberta",
"text-classification",
"en",
"dataset:mocha",
"license:bsd-3-clause",
"autotrain_compatible",
"endpoints_compatible",
"region:us"
] | text-classification | "2023-06-01T12:09:39Z" | ---
license: bsd-3-clause
datasets:
- mocha
language:
- en
---
# Answer Overlap Module of QAFactEval Metric
This is the span scorer module, used in [RQUGE paper](https://aclanthology.org/2023.findings-acl.428/) to evaluate the generated questions of the question generation task.
The model was originally used in [QAFactEval](https://aclanthology.org/2022.naacl-main.187/) for computing the semantic similarity of the generated answer span, given the reference answer, context, and question in the question answering task.
It outputs a 1-5 answer overlap score. The scorer is trained on their MOCHA dataset (initialized from [Jia et al. (2021)](https://aclanthology.org/2020.emnlp-main.528/)), consisting of 40k crowdsourced judgments on QA model outputs.
The input to the model is defined as:
```
[CLS] question [q] gold answer [r] pred answer [c] context
```
# Generation
You can use the following script to get the semantic similarity of the predicted answer given the gold answer, context, and question.
```
from transformers import AutoModelForSequenceClassification, AutoTokenizer
sp_scorer = AutoModelForSequenceClassification.from_pretrained('alirezamsh/quip-512-mocha')
tokenizer_sp = AutoTokenizer.from_pretrained('alirezamsh/quip-512-mocha')
sp_scorer.eval()
pred_answer = ""
gold_answer = ""
question = ""
context = ""
input_sp = f"{question} <q> {gold_answer} <r>" \
f" {pred_answer} <c> {context}"
inputs = tokenizer_sp(input_sp, max_length=512, truncation=True, \
padding="max_length", return_tensors="pt")
outputs = sp_scorer(input_ids=inputs["input_ids"], attention_mask=inputs["attention_mask"])
print(outputs)
```
# Citations
```
@inproceedings{fabbri-etal-2022-qafacteval,
title = "{QAF}act{E}val: Improved {QA}-Based Factual Consistency Evaluation for Summarization",
author = "Fabbri, Alexander and
Wu, Chien-Sheng and
Liu, Wenhao and
Xiong, Caiming",
booktitle = "Proceedings of the 2022 Conference of the North American Chapter of the Association for Computational Linguistics: Human Language Technologies",
month = jul,
year = "2022",
address = "Seattle, United States",
publisher = "Association for Computational Linguistics",
url = "https://aclanthology.org/2022.naacl-main.187",
doi = "10.18653/v1/2022.naacl-main.187",
pages = "2587--2601",
abstract = "Factual consistency is an essential quality of text summarization models in practical settings. Existing work in evaluating this dimension can be broadly categorized into two lines of research, entailment-based and question answering (QA)-based metrics, and different experimental setups often lead to contrasting conclusions as to which paradigm performs the best. In this work, we conduct an extensive comparison of entailment and QA-based metrics, demonstrating that carefully choosing the components of a QA-based metric, especially question generation and answerability classification, is critical to performance. Building on those insights, we propose an optimized metric, which we call QAFactEval, that leads to a 14{\%} average improvement over previous QA-based metrics on the SummaC factual consistency benchmark, and also outperforms the best-performing entailment-based metric. Moreover, we find that QA-based and entailment-based metrics can offer complementary signals and be combined into a single metric for a further performance boost.",
}
@inproceedings{mohammadshahi-etal-2023-rquge,
title = "{RQUGE}: Reference-Free Metric for Evaluating Question Generation by Answering the Question",
author = "Mohammadshahi, Alireza and
Scialom, Thomas and
Yazdani, Majid and
Yanki, Pouya and
Fan, Angela and
Henderson, James and
Saeidi, Marzieh",
editor = "Rogers, Anna and
Boyd-Graber, Jordan and
Okazaki, Naoaki",
booktitle = "Findings of the Association for Computational Linguistics: ACL 2023",
month = jul,
year = "2023",
address = "Toronto, Canada",
publisher = "Association for Computational Linguistics",
url = "https://aclanthology.org/2023.findings-acl.428",
doi = "10.18653/v1/2023.findings-acl.428",
pages = "6845--6867",
abstract = "Existing metrics for evaluating the quality of automatically generated questions such as BLEU, ROUGE, BERTScore, and BLEURT compare the reference and predicted questions, providing a high score when there is a considerable lexical overlap or semantic similarity between the candidate and the reference questions. This approach has two major shortcomings. First, we need expensive human-provided reference questions. Second, it penalises valid questions that may not have high lexical or semantic similarity to the reference questions. In this paper, we propose a new metric, RQUGE, based on the answerability of the candidate question given the context. The metric consists of a question-answering and a span scorer modules, using pre-trained models from existing literature, thus it can be used without any further training. We demonstrate that RQUGE has a higher correlation with human judgment without relying on the reference question. Additionally, RQUGE is shown to be more robust to several adversarial corruptions. Furthermore, we illustrate that we can significantly improve the performance of QA models on out-of-domain datasets by fine-tuning on synthetic data generated by a question generation model and reranked by RQUGE.",
}
``` |
RichardErkhov/shleeeee_-_mistral-ko-7b-wiki-neft-gguf | RichardErkhov | "2024-06-30T08:29:07Z" | 16,355 | 0 | null | [
"gguf",
"region:us"
] | null | "2024-06-30T06:20:56Z" | Quantization made by Richard Erkhov.
[Github](https://github.com/RichardErkhov)
[Discord](https://discord.gg/pvy7H8DZMG)
[Request more models](https://github.com/RichardErkhov/quant_request)
mistral-ko-7b-wiki-neft - GGUF
- Model creator: https://huggingface.co/shleeeee/
- Original model: https://huggingface.co/shleeeee/mistral-ko-7b-wiki-neft/
| Name | Quant method | Size |
| ---- | ---- | ---- |
| [mistral-ko-7b-wiki-neft.Q2_K.gguf](https://huggingface.co/RichardErkhov/shleeeee_-_mistral-ko-7b-wiki-neft-gguf/blob/main/mistral-ko-7b-wiki-neft.Q2_K.gguf) | Q2_K | 2.53GB |
| [mistral-ko-7b-wiki-neft.IQ3_XS.gguf](https://huggingface.co/RichardErkhov/shleeeee_-_mistral-ko-7b-wiki-neft-gguf/blob/main/mistral-ko-7b-wiki-neft.IQ3_XS.gguf) | IQ3_XS | 2.81GB |
| [mistral-ko-7b-wiki-neft.IQ3_S.gguf](https://huggingface.co/RichardErkhov/shleeeee_-_mistral-ko-7b-wiki-neft-gguf/blob/main/mistral-ko-7b-wiki-neft.IQ3_S.gguf) | IQ3_S | 2.96GB |
| [mistral-ko-7b-wiki-neft.Q3_K_S.gguf](https://huggingface.co/RichardErkhov/shleeeee_-_mistral-ko-7b-wiki-neft-gguf/blob/main/mistral-ko-7b-wiki-neft.Q3_K_S.gguf) | Q3_K_S | 2.95GB |
| [mistral-ko-7b-wiki-neft.IQ3_M.gguf](https://huggingface.co/RichardErkhov/shleeeee_-_mistral-ko-7b-wiki-neft-gguf/blob/main/mistral-ko-7b-wiki-neft.IQ3_M.gguf) | IQ3_M | 3.06GB |
| [mistral-ko-7b-wiki-neft.Q3_K.gguf](https://huggingface.co/RichardErkhov/shleeeee_-_mistral-ko-7b-wiki-neft-gguf/blob/main/mistral-ko-7b-wiki-neft.Q3_K.gguf) | Q3_K | 3.28GB |
| [mistral-ko-7b-wiki-neft.Q3_K_M.gguf](https://huggingface.co/RichardErkhov/shleeeee_-_mistral-ko-7b-wiki-neft-gguf/blob/main/mistral-ko-7b-wiki-neft.Q3_K_M.gguf) | Q3_K_M | 3.28GB |
| [mistral-ko-7b-wiki-neft.Q3_K_L.gguf](https://huggingface.co/RichardErkhov/shleeeee_-_mistral-ko-7b-wiki-neft-gguf/blob/main/mistral-ko-7b-wiki-neft.Q3_K_L.gguf) | Q3_K_L | 3.56GB |
| [mistral-ko-7b-wiki-neft.IQ4_XS.gguf](https://huggingface.co/RichardErkhov/shleeeee_-_mistral-ko-7b-wiki-neft-gguf/blob/main/mistral-ko-7b-wiki-neft.IQ4_XS.gguf) | IQ4_XS | 3.67GB |
| [mistral-ko-7b-wiki-neft.Q4_0.gguf](https://huggingface.co/RichardErkhov/shleeeee_-_mistral-ko-7b-wiki-neft-gguf/blob/main/mistral-ko-7b-wiki-neft.Q4_0.gguf) | Q4_0 | 3.83GB |
| [mistral-ko-7b-wiki-neft.IQ4_NL.gguf](https://huggingface.co/RichardErkhov/shleeeee_-_mistral-ko-7b-wiki-neft-gguf/blob/main/mistral-ko-7b-wiki-neft.IQ4_NL.gguf) | IQ4_NL | 3.87GB |
| [mistral-ko-7b-wiki-neft.Q4_K_S.gguf](https://huggingface.co/RichardErkhov/shleeeee_-_mistral-ko-7b-wiki-neft-gguf/blob/main/mistral-ko-7b-wiki-neft.Q4_K_S.gguf) | Q4_K_S | 3.86GB |
| [mistral-ko-7b-wiki-neft.Q4_K.gguf](https://huggingface.co/RichardErkhov/shleeeee_-_mistral-ko-7b-wiki-neft-gguf/blob/main/mistral-ko-7b-wiki-neft.Q4_K.gguf) | Q4_K | 4.07GB |
| [mistral-ko-7b-wiki-neft.Q4_K_M.gguf](https://huggingface.co/RichardErkhov/shleeeee_-_mistral-ko-7b-wiki-neft-gguf/blob/main/mistral-ko-7b-wiki-neft.Q4_K_M.gguf) | Q4_K_M | 4.07GB |
| [mistral-ko-7b-wiki-neft.Q4_1.gguf](https://huggingface.co/RichardErkhov/shleeeee_-_mistral-ko-7b-wiki-neft-gguf/blob/main/mistral-ko-7b-wiki-neft.Q4_1.gguf) | Q4_1 | 4.24GB |
| [mistral-ko-7b-wiki-neft.Q5_0.gguf](https://huggingface.co/RichardErkhov/shleeeee_-_mistral-ko-7b-wiki-neft-gguf/blob/main/mistral-ko-7b-wiki-neft.Q5_0.gguf) | Q5_0 | 4.65GB |
| [mistral-ko-7b-wiki-neft.Q5_K_S.gguf](https://huggingface.co/RichardErkhov/shleeeee_-_mistral-ko-7b-wiki-neft-gguf/blob/main/mistral-ko-7b-wiki-neft.Q5_K_S.gguf) | Q5_K_S | 4.65GB |
| [mistral-ko-7b-wiki-neft.Q5_K.gguf](https://huggingface.co/RichardErkhov/shleeeee_-_mistral-ko-7b-wiki-neft-gguf/blob/main/mistral-ko-7b-wiki-neft.Q5_K.gguf) | Q5_K | 4.78GB |
| [mistral-ko-7b-wiki-neft.Q5_K_M.gguf](https://huggingface.co/RichardErkhov/shleeeee_-_mistral-ko-7b-wiki-neft-gguf/blob/main/mistral-ko-7b-wiki-neft.Q5_K_M.gguf) | Q5_K_M | 4.78GB |
| [mistral-ko-7b-wiki-neft.Q5_1.gguf](https://huggingface.co/RichardErkhov/shleeeee_-_mistral-ko-7b-wiki-neft-gguf/blob/main/mistral-ko-7b-wiki-neft.Q5_1.gguf) | Q5_1 | 5.07GB |
| [mistral-ko-7b-wiki-neft.Q6_K.gguf](https://huggingface.co/RichardErkhov/shleeeee_-_mistral-ko-7b-wiki-neft-gguf/blob/main/mistral-ko-7b-wiki-neft.Q6_K.gguf) | Q6_K | 5.53GB |
| [mistral-ko-7b-wiki-neft.Q8_0.gguf](https://huggingface.co/RichardErkhov/shleeeee_-_mistral-ko-7b-wiki-neft-gguf/blob/main/mistral-ko-7b-wiki-neft.Q8_0.gguf) | Q8_0 | 7.17GB |
Original model description:
---
language:
- ko
pipeline_tag: text-generation
tags:
- finetune
---
# Model Card for mistral-ko-7b-wiki-neft
It is a fine-tuned model using Korean and NEFT in the mistral-7b model.
## Model Details
* **Model Developers** : shleeeee(Seunghyeon Lee), oopsung(Sungwoo Park)
* **Repository** : To be added
* **Model Architecture** : The mistral-ko-7b-wiki-neft is is a fine-tuned version of the Mistral-7B-v0.1.
* **Lora target modules** : q_proj, k_proj, v_proj, o_proj,gate_proj
* **train_batch** : 4
* **neftune_noise_alpha** : 5
* **Max_step** : 1000
## Dataset
Korean Custom Dataset
## Prompt template: Mistral
```
<s>[INST]{['instruction']}[/INST]{['output']}</s>
```
## Usage
```
# Load model directly
from transformers import AutoTokenizer, AutoModelForCausalLM
tokenizer = AutoTokenizer.from_pretrained("shleeeee/mistral-7b-wiki")
model = AutoModelForCausalLM.from_pretrained("shleeeee/mistral-7b-wiki")
# Use a pipeline as a high-level helper
from transformers import pipeline
pipe = pipeline("text-generation", model="shleeeee/mistral-7b-wiki")
```
## Evaluation

|
NousResearch/Yarn-Mistral-7b-128k | NousResearch | "2023-11-02T20:01:56Z" | 16,334 | 568 | transformers | [
"transformers",
"pytorch",
"mistral",
"text-generation",
"custom_code",
"en",
"dataset:emozilla/yarn-train-tokenized-16k-mistral",
"arxiv:2309.00071",
"license:apache-2.0",
"autotrain_compatible",
"endpoints_compatible",
"text-generation-inference",
"region:us"
] | text-generation | "2023-10-31T13:15:14Z" | ---
datasets:
- emozilla/yarn-train-tokenized-16k-mistral
metrics:
- perplexity
library_name: transformers
license: apache-2.0
language:
- en
---
# Model Card: Nous-Yarn-Mistral-7b-128k
[Preprint (arXiv)](https://arxiv.org/abs/2309.00071)
[GitHub](https://github.com/jquesnelle/yarn)
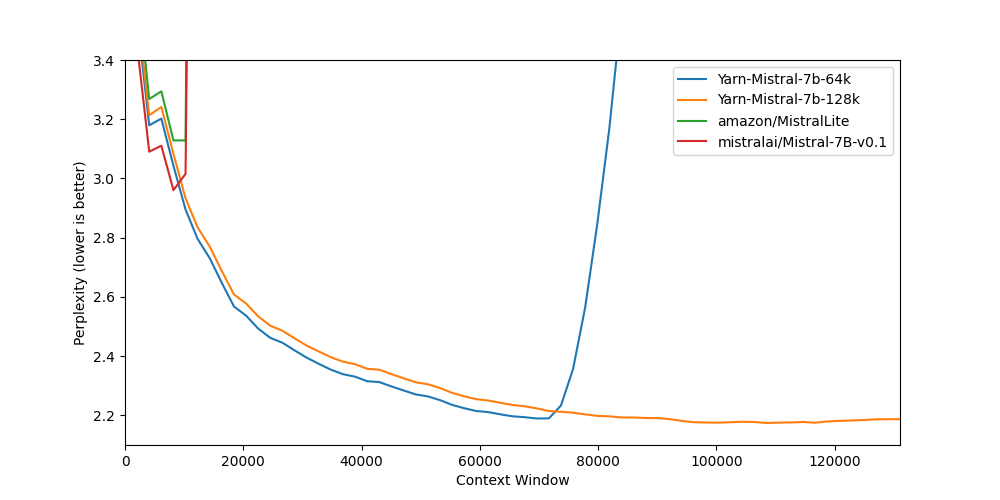
## Model Description
Nous-Yarn-Mistral-7b-128k is a state-of-the-art language model for long context, further pretrained on long context data for 1500 steps using the YaRN extension method.
It is an extension of [Mistral-7B-v0.1](https://huggingface.co/mistralai/Mistral-7B-v0.1) and supports a 128k token context window.
To use, pass `trust_remote_code=True` when loading the model, for example
```python
model = AutoModelForCausalLM.from_pretrained("NousResearch/Yarn-Mistral-7b-128k",
use_flash_attention_2=True,
torch_dtype=torch.bfloat16,
device_map="auto",
trust_remote_code=True)
```
In addition you will need to use the latest version of `transformers` (until 4.35 comes out)
```sh
pip install git+https://github.com/huggingface/transformers
```
## Benchmarks
Long context benchmarks:
| Model | Context Window | 8k PPL | 16k PPL | 32k PPL | 64k PPL | 128k PPL |
|-------|---------------:|------:|----------:|-----:|-----:|------------:|
| [Mistral-7B-v0.1](https://huggingface.co/mistralai/Mistral-7B-v0.1) | 8k | 2.96 | - | - | - | - |
| [Yarn-Mistral-7b-64k](https://huggingface.co/NousResearch/Yarn-Mistral-7b-64k) | 64k | 3.04 | 2.65 | 2.44 | 2.20 | - |
| [Yarn-Mistral-7b-128k](https://huggingface.co/NousResearch/Yarn-Mistral-7b-128k) | 128k | 3.08 | 2.68 | 2.47 | 2.24 | 2.19 |
Short context benchmarks showing that quality degradation is minimal:
| Model | Context Window | ARC-c | Hellaswag | MMLU | Truthful QA |
|-------|---------------:|------:|----------:|-----:|------------:|
| [Mistral-7B-v0.1](https://huggingface.co/mistralai/Mistral-7B-v0.1) | 8k | 59.98 | 83.31 | 64.16 | 42.15 |
| [Yarn-Mistral-7b-64k](https://huggingface.co/NousResearch/Yarn-Mistral-7b-64k) | 64k | 59.38 | 81.21 | 61.32 | 42.50 |
| [Yarn-Mistral-7b-128k](https://huggingface.co/NousResearch/Yarn-Mistral-7b-128k) | 128k | 58.87 | 80.58 | 60.64 | 42.46 |
## Collaborators
- [bloc97](https://github.com/bloc97): Methods, paper and evals
- [@theemozilla](https://twitter.com/theemozilla): Methods, paper, model training, and evals
- [@EnricoShippole](https://twitter.com/EnricoShippole): Model training
- [honglu2875](https://github.com/honglu2875): Paper and evals
The authors would like to thank LAION AI for their support of compute for this model.
It was trained on the [JUWELS](https://www.fz-juelich.de/en/ias/jsc/systems/supercomputers/juwels) supercomputer. |
RichardErkhov/hfl_-_llama-3-chinese-8b-gguf | RichardErkhov | "2024-06-26T00:59:00Z" | 16,325 | 0 | null | [
"gguf",
"region:us"
] | null | "2024-06-25T20:09:04Z" | Quantization made by Richard Erkhov.
[Github](https://github.com/RichardErkhov)
[Discord](https://discord.gg/pvy7H8DZMG)
[Request more models](https://github.com/RichardErkhov/quant_request)
llama-3-chinese-8b - GGUF
- Model creator: https://huggingface.co/hfl/
- Original model: https://huggingface.co/hfl/llama-3-chinese-8b/
| Name | Quant method | Size |
| ---- | ---- | ---- |
| [llama-3-chinese-8b.Q2_K.gguf](https://huggingface.co/RichardErkhov/hfl_-_llama-3-chinese-8b-gguf/blob/main/llama-3-chinese-8b.Q2_K.gguf) | Q2_K | 2.96GB |
| [llama-3-chinese-8b.IQ3_XS.gguf](https://huggingface.co/RichardErkhov/hfl_-_llama-3-chinese-8b-gguf/blob/main/llama-3-chinese-8b.IQ3_XS.gguf) | IQ3_XS | 3.28GB |
| [llama-3-chinese-8b.IQ3_S.gguf](https://huggingface.co/RichardErkhov/hfl_-_llama-3-chinese-8b-gguf/blob/main/llama-3-chinese-8b.IQ3_S.gguf) | IQ3_S | 3.43GB |
| [llama-3-chinese-8b.Q3_K_S.gguf](https://huggingface.co/RichardErkhov/hfl_-_llama-3-chinese-8b-gguf/blob/main/llama-3-chinese-8b.Q3_K_S.gguf) | Q3_K_S | 3.41GB |
| [llama-3-chinese-8b.IQ3_M.gguf](https://huggingface.co/RichardErkhov/hfl_-_llama-3-chinese-8b-gguf/blob/main/llama-3-chinese-8b.IQ3_M.gguf) | IQ3_M | 3.52GB |
| [llama-3-chinese-8b.Q3_K.gguf](https://huggingface.co/RichardErkhov/hfl_-_llama-3-chinese-8b-gguf/blob/main/llama-3-chinese-8b.Q3_K.gguf) | Q3_K | 3.74GB |
| [llama-3-chinese-8b.Q3_K_M.gguf](https://huggingface.co/RichardErkhov/hfl_-_llama-3-chinese-8b-gguf/blob/main/llama-3-chinese-8b.Q3_K_M.gguf) | Q3_K_M | 3.74GB |
| [llama-3-chinese-8b.Q3_K_L.gguf](https://huggingface.co/RichardErkhov/hfl_-_llama-3-chinese-8b-gguf/blob/main/llama-3-chinese-8b.Q3_K_L.gguf) | Q3_K_L | 4.03GB |
| [llama-3-chinese-8b.IQ4_XS.gguf](https://huggingface.co/RichardErkhov/hfl_-_llama-3-chinese-8b-gguf/blob/main/llama-3-chinese-8b.IQ4_XS.gguf) | IQ4_XS | 4.18GB |
| [llama-3-chinese-8b.Q4_0.gguf](https://huggingface.co/RichardErkhov/hfl_-_llama-3-chinese-8b-gguf/blob/main/llama-3-chinese-8b.Q4_0.gguf) | Q4_0 | 4.34GB |
| [llama-3-chinese-8b.IQ4_NL.gguf](https://huggingface.co/RichardErkhov/hfl_-_llama-3-chinese-8b-gguf/blob/main/llama-3-chinese-8b.IQ4_NL.gguf) | IQ4_NL | 4.38GB |
| [llama-3-chinese-8b.Q4_K_S.gguf](https://huggingface.co/RichardErkhov/hfl_-_llama-3-chinese-8b-gguf/blob/main/llama-3-chinese-8b.Q4_K_S.gguf) | Q4_K_S | 4.37GB |
| [llama-3-chinese-8b.Q4_K.gguf](https://huggingface.co/RichardErkhov/hfl_-_llama-3-chinese-8b-gguf/blob/main/llama-3-chinese-8b.Q4_K.gguf) | Q4_K | 4.58GB |
| [llama-3-chinese-8b.Q4_K_M.gguf](https://huggingface.co/RichardErkhov/hfl_-_llama-3-chinese-8b-gguf/blob/main/llama-3-chinese-8b.Q4_K_M.gguf) | Q4_K_M | 4.58GB |
| [llama-3-chinese-8b.Q4_1.gguf](https://huggingface.co/RichardErkhov/hfl_-_llama-3-chinese-8b-gguf/blob/main/llama-3-chinese-8b.Q4_1.gguf) | Q4_1 | 4.78GB |
| [llama-3-chinese-8b.Q5_0.gguf](https://huggingface.co/RichardErkhov/hfl_-_llama-3-chinese-8b-gguf/blob/main/llama-3-chinese-8b.Q5_0.gguf) | Q5_0 | 5.21GB |
| [llama-3-chinese-8b.Q5_K_S.gguf](https://huggingface.co/RichardErkhov/hfl_-_llama-3-chinese-8b-gguf/blob/main/llama-3-chinese-8b.Q5_K_S.gguf) | Q5_K_S | 5.21GB |
| [llama-3-chinese-8b.Q5_K.gguf](https://huggingface.co/RichardErkhov/hfl_-_llama-3-chinese-8b-gguf/blob/main/llama-3-chinese-8b.Q5_K.gguf) | Q5_K | 5.34GB |
| [llama-3-chinese-8b.Q5_K_M.gguf](https://huggingface.co/RichardErkhov/hfl_-_llama-3-chinese-8b-gguf/blob/main/llama-3-chinese-8b.Q5_K_M.gguf) | Q5_K_M | 5.34GB |
| [llama-3-chinese-8b.Q5_1.gguf](https://huggingface.co/RichardErkhov/hfl_-_llama-3-chinese-8b-gguf/blob/main/llama-3-chinese-8b.Q5_1.gguf) | Q5_1 | 5.65GB |
| [llama-3-chinese-8b.Q6_K.gguf](https://huggingface.co/RichardErkhov/hfl_-_llama-3-chinese-8b-gguf/blob/main/llama-3-chinese-8b.Q6_K.gguf) | Q6_K | 6.14GB |
| [llama-3-chinese-8b.Q8_0.gguf](https://huggingface.co/RichardErkhov/hfl_-_llama-3-chinese-8b-gguf/blob/main/llama-3-chinese-8b.Q8_0.gguf) | Q8_0 | 7.95GB |
Original model description:
---
base_model: meta-llama/Meta-Llama-3-8B
license: apache-2.0
language:
- zh
- en
---
# Llama-3-Chinese-8B
<p align="center">
<a href="https://github.com/ymcui/Chinese-LLaMA-Alpaca-3"><img src="https://ymcui.com/images/chinese-llama-alpaca-3-banner.png" width="600"/></a>
</p>
This repository contains **Llama-3-Chinese-8B**, which is further pre-trained on [Meta-Llama-3-8B](https://huggingface.co/meta-llama/Meta-Llama-3-8B) with 120 GB Chinese text corpora.
**Note: this is a foundation model, which is not suitable for conversation, QA, etc.**
Further details (performance, usage, etc.) should refer to GitHub project page: https://github.com/ymcui/Chinese-LLaMA-Alpaca-3
## Others
- For LoRA-only model, please see: https://huggingface.co/hfl/llama-3-chinese-8b-lora
- For GGUF model (llama.cpp compatible), please see: https://huggingface.co/hfl/llama-3-chinese-8b-gguf
- If you have questions/issues regarding this model, please submit an issue through https://github.com/ymcui/Chinese-LLaMA-Alpaca-3
|
WinKawaks/vit-small-patch16-224 | WinKawaks | "2023-03-18T22:00:21Z" | 16,324 | 10 | transformers | [
"transformers",
"pytorch",
"safetensors",
"vit",
"image-classification",
"vision",
"dataset:imagenet",
"license:apache-2.0",
"autotrain_compatible",
"endpoints_compatible",
"region:us"
] | image-classification | "2022-03-02T23:29:05Z" | ---
license: apache-2.0
tags:
- vision
- image-classification
datasets:
- imagenet
widget:
- src: https://huggingface.co/datasets/mishig/sample_images/resolve/main/tiger.jpg
example_title: Tiger
- src: https://huggingface.co/datasets/mishig/sample_images/resolve/main/teapot.jpg
example_title: Teapot
- src: https://huggingface.co/datasets/mishig/sample_images/resolve/main/palace.jpg
example_title: Palace
---
Google didn't publish vit-tiny and vit-small model checkpoints in Hugging Face. I converted the weights from the [timm repository](https://github.com/rwightman/pytorch-image-models). This model is used in the same way as [ViT-base](https://huggingface.co/google/vit-base-patch16-224).
Note that [safetensors] model requires torch 2.0 environment. |
mradermacher/llama3_7b_judge_perceptions-GGUF | mradermacher | "2024-07-02T05:06:57Z" | 16,323 | 0 | transformers | [
"transformers",
"gguf",
"text-generation-inference",
"unsloth",
"llama",
"trl",
"sft",
"en",
"base_model:JFernandoGRE/llama3_7b_judge_perceptions",
"license:apache-2.0",
"endpoints_compatible",
"region:us"
] | null | "2024-07-02T04:14:20Z" | ---
base_model: JFernandoGRE/llama3_7b_judge_perceptions
language:
- en
library_name: transformers
license: apache-2.0
quantized_by: mradermacher
tags:
- text-generation-inference
- transformers
- unsloth
- llama
- trl
- sft
---
## About
<!-- ### quantize_version: 2 -->
<!-- ### output_tensor_quantised: 1 -->
<!-- ### convert_type: hf -->
<!-- ### vocab_type: -->
<!-- ### tags: -->
static quants of https://huggingface.co/JFernandoGRE/llama3_7b_judge_perceptions
<!-- provided-files -->
weighted/imatrix quants seem not to be available (by me) at this time. If they do not show up a week or so after the static ones, I have probably not planned for them. Feel free to request them by opening a Community Discussion.
## Usage
If you are unsure how to use GGUF files, refer to one of [TheBloke's
READMEs](https://huggingface.co/TheBloke/KafkaLM-70B-German-V0.1-GGUF) for
more details, including on how to concatenate multi-part files.
## Provided Quants
(sorted by size, not necessarily quality. IQ-quants are often preferable over similar sized non-IQ quants)
| Link | Type | Size/GB | Notes |
|:-----|:-----|--------:|:------|
| [GGUF](https://huggingface.co/mradermacher/llama3_7b_judge_perceptions-GGUF/resolve/main/llama3_7b_judge_perceptions.Q2_K.gguf) | Q2_K | 3.3 | |
| [GGUF](https://huggingface.co/mradermacher/llama3_7b_judge_perceptions-GGUF/resolve/main/llama3_7b_judge_perceptions.IQ3_XS.gguf) | IQ3_XS | 3.6 | |
| [GGUF](https://huggingface.co/mradermacher/llama3_7b_judge_perceptions-GGUF/resolve/main/llama3_7b_judge_perceptions.Q3_K_S.gguf) | Q3_K_S | 3.8 | |
| [GGUF](https://huggingface.co/mradermacher/llama3_7b_judge_perceptions-GGUF/resolve/main/llama3_7b_judge_perceptions.IQ3_S.gguf) | IQ3_S | 3.8 | beats Q3_K* |
| [GGUF](https://huggingface.co/mradermacher/llama3_7b_judge_perceptions-GGUF/resolve/main/llama3_7b_judge_perceptions.IQ3_M.gguf) | IQ3_M | 3.9 | |
| [GGUF](https://huggingface.co/mradermacher/llama3_7b_judge_perceptions-GGUF/resolve/main/llama3_7b_judge_perceptions.Q3_K_M.gguf) | Q3_K_M | 4.1 | lower quality |
| [GGUF](https://huggingface.co/mradermacher/llama3_7b_judge_perceptions-GGUF/resolve/main/llama3_7b_judge_perceptions.Q3_K_L.gguf) | Q3_K_L | 4.4 | |
| [GGUF](https://huggingface.co/mradermacher/llama3_7b_judge_perceptions-GGUF/resolve/main/llama3_7b_judge_perceptions.IQ4_XS.gguf) | IQ4_XS | 4.6 | |
| [GGUF](https://huggingface.co/mradermacher/llama3_7b_judge_perceptions-GGUF/resolve/main/llama3_7b_judge_perceptions.Q4_K_S.gguf) | Q4_K_S | 4.8 | fast, recommended |
| [GGUF](https://huggingface.co/mradermacher/llama3_7b_judge_perceptions-GGUF/resolve/main/llama3_7b_judge_perceptions.Q4_K_M.gguf) | Q4_K_M | 5.0 | fast, recommended |
| [GGUF](https://huggingface.co/mradermacher/llama3_7b_judge_perceptions-GGUF/resolve/main/llama3_7b_judge_perceptions.Q5_K_S.gguf) | Q5_K_S | 5.7 | |
| [GGUF](https://huggingface.co/mradermacher/llama3_7b_judge_perceptions-GGUF/resolve/main/llama3_7b_judge_perceptions.Q5_K_M.gguf) | Q5_K_M | 5.8 | |
| [GGUF](https://huggingface.co/mradermacher/llama3_7b_judge_perceptions-GGUF/resolve/main/llama3_7b_judge_perceptions.Q6_K.gguf) | Q6_K | 6.7 | very good quality |
| [GGUF](https://huggingface.co/mradermacher/llama3_7b_judge_perceptions-GGUF/resolve/main/llama3_7b_judge_perceptions.Q8_0.gguf) | Q8_0 | 8.6 | fast, best quality |
| [GGUF](https://huggingface.co/mradermacher/llama3_7b_judge_perceptions-GGUF/resolve/main/llama3_7b_judge_perceptions.f16.gguf) | f16 | 16.2 | 16 bpw, overkill |
Here is a handy graph by ikawrakow comparing some lower-quality quant
types (lower is better):

And here are Artefact2's thoughts on the matter:
https://gist.github.com/Artefact2/b5f810600771265fc1e39442288e8ec9
## FAQ / Model Request
See https://huggingface.co/mradermacher/model_requests for some answers to
questions you might have and/or if you want some other model quantized.
## Thanks
I thank my company, [nethype GmbH](https://www.nethype.de/), for letting
me use its servers and providing upgrades to my workstation to enable
this work in my free time.
<!-- end -->
|
timm/maxvit_large_tf_512.in1k | timm | "2023-05-11T00:12:30Z" | 16,322 | 0 | timm | [
"timm",
"pytorch",
"safetensors",
"image-classification",
"dataset:imagenet-1k",
"arxiv:2204.01697",
"license:apache-2.0",
"region:us"
] | image-classification | "2022-12-02T21:54:04Z" | ---
tags:
- image-classification
- timm
library_name: timm
license: apache-2.0
datasets:
- imagenet-1k
---
# Model card for maxvit_large_tf_512.in1k
An official MaxViT image classification model. Trained in tensorflow on ImageNet-1k by paper authors.
Ported from official Tensorflow implementation (https://github.com/google-research/maxvit) to PyTorch by Ross Wightman.
### Model Variants in [maxxvit.py](https://github.com/huggingface/pytorch-image-models/blob/main/timm/models/maxxvit.py)
MaxxViT covers a number of related model architectures that share a common structure including:
- CoAtNet - Combining MBConv (depthwise-separable) convolutional blocks in early stages with self-attention transformer blocks in later stages.
- MaxViT - Uniform blocks across all stages, each containing a MBConv (depthwise-separable) convolution block followed by two self-attention blocks with different partitioning schemes (window followed by grid).
- CoAtNeXt - A timm specific arch that uses ConvNeXt blocks in place of MBConv blocks in CoAtNet. All normalization layers are LayerNorm (no BatchNorm).
- MaxxViT - A timm specific arch that uses ConvNeXt blocks in place of MBConv blocks in MaxViT. All normalization layers are LayerNorm (no BatchNorm).
- MaxxViT-V2 - A MaxxViT variation that removes the window block attention leaving only ConvNeXt blocks and grid attention w/ more width to compensate.
Aside from the major variants listed above, there are more subtle changes from model to model. Any model name with the string `rw` are `timm` specific configs w/ modelling adjustments made to favour PyTorch eager use. These were created while training initial reproductions of the models so there are variations.
All models with the string `tf` are models exactly matching Tensorflow based models by the original paper authors with weights ported to PyTorch. This covers a number of MaxViT models. The official CoAtNet models were never released.
## Model Details
- **Model Type:** Image classification / feature backbone
- **Model Stats:**
- Params (M): 212.3
- GMACs: 244.8
- Activations (M): 942.1
- Image size: 512 x 512
- **Papers:**
- MaxViT: Multi-Axis Vision Transformer: https://arxiv.org/abs/2204.01697
- **Dataset:** ImageNet-1k
## Model Usage
### Image Classification
```python
from urllib.request import urlopen
from PIL import Image
import timm
img = Image.open(urlopen(
'https://huggingface.co/datasets/huggingface/documentation-images/resolve/main/beignets-task-guide.png'
))
model = timm.create_model('maxvit_large_tf_512.in1k', pretrained=True)
model = model.eval()
# get model specific transforms (normalization, resize)
data_config = timm.data.resolve_model_data_config(model)
transforms = timm.data.create_transform(**data_config, is_training=False)
output = model(transforms(img).unsqueeze(0)) # unsqueeze single image into batch of 1
top5_probabilities, top5_class_indices = torch.topk(output.softmax(dim=1) * 100, k=5)
```
### Feature Map Extraction
```python
from urllib.request import urlopen
from PIL import Image
import timm
img = Image.open(urlopen(
'https://huggingface.co/datasets/huggingface/documentation-images/resolve/main/beignets-task-guide.png'
))
model = timm.create_model(
'maxvit_large_tf_512.in1k',
pretrained=True,
features_only=True,
)
model = model.eval()
# get model specific transforms (normalization, resize)
data_config = timm.data.resolve_model_data_config(model)
transforms = timm.data.create_transform(**data_config, is_training=False)
output = model(transforms(img).unsqueeze(0)) # unsqueeze single image into batch of 1
for o in output:
# print shape of each feature map in output
# e.g.:
# torch.Size([1, 128, 256, 256])
# torch.Size([1, 128, 128, 128])
# torch.Size([1, 256, 64, 64])
# torch.Size([1, 512, 32, 32])
# torch.Size([1, 1024, 16, 16])
print(o.shape)
```
### Image Embeddings
```python
from urllib.request import urlopen
from PIL import Image
import timm
img = Image.open(urlopen(
'https://huggingface.co/datasets/huggingface/documentation-images/resolve/main/beignets-task-guide.png'
))
model = timm.create_model(
'maxvit_large_tf_512.in1k',
pretrained=True,
num_classes=0, # remove classifier nn.Linear
)
model = model.eval()
# get model specific transforms (normalization, resize)
data_config = timm.data.resolve_model_data_config(model)
transforms = timm.data.create_transform(**data_config, is_training=False)
output = model(transforms(img).unsqueeze(0)) # output is (batch_size, num_features) shaped tensor
# or equivalently (without needing to set num_classes=0)
output = model.forward_features(transforms(img).unsqueeze(0))
# output is unpooled, a (1, 1024, 16, 16) shaped tensor
output = model.forward_head(output, pre_logits=True)
# output is a (1, num_features) shaped tensor
```
## Model Comparison
### By Top-1
|model |top1 |top5 |samples / sec |Params (M) |GMAC |Act (M)|
|------------------------------------------------------------------------------------------------------------------------|----:|----:|--------------:|--------------:|-----:|------:|
|[maxvit_xlarge_tf_512.in21k_ft_in1k](https://huggingface.co/timm/maxvit_xlarge_tf_512.in21k_ft_in1k) |88.53|98.64| 21.76| 475.77|534.14|1413.22|
|[maxvit_xlarge_tf_384.in21k_ft_in1k](https://huggingface.co/timm/maxvit_xlarge_tf_384.in21k_ft_in1k) |88.32|98.54| 42.53| 475.32|292.78| 668.76|
|[maxvit_base_tf_512.in21k_ft_in1k](https://huggingface.co/timm/maxvit_base_tf_512.in21k_ft_in1k) |88.20|98.53| 50.87| 119.88|138.02| 703.99|
|[maxvit_large_tf_512.in21k_ft_in1k](https://huggingface.co/timm/maxvit_large_tf_512.in21k_ft_in1k) |88.04|98.40| 36.42| 212.33|244.75| 942.15|
|[maxvit_large_tf_384.in21k_ft_in1k](https://huggingface.co/timm/maxvit_large_tf_384.in21k_ft_in1k) |87.98|98.56| 71.75| 212.03|132.55| 445.84|
|[maxvit_base_tf_384.in21k_ft_in1k](https://huggingface.co/timm/maxvit_base_tf_384.in21k_ft_in1k) |87.92|98.54| 104.71| 119.65| 73.80| 332.90|
|[maxvit_rmlp_base_rw_384.sw_in12k_ft_in1k](https://huggingface.co/timm/maxvit_rmlp_base_rw_384.sw_in12k_ft_in1k) |87.81|98.37| 106.55| 116.14| 70.97| 318.95|
|[maxxvitv2_rmlp_base_rw_384.sw_in12k_ft_in1k](https://huggingface.co/timm/maxxvitv2_rmlp_base_rw_384.sw_in12k_ft_in1k) |87.47|98.37| 149.49| 116.09| 72.98| 213.74|
|[coatnet_rmlp_2_rw_384.sw_in12k_ft_in1k](https://huggingface.co/timm/coatnet_rmlp_2_rw_384.sw_in12k_ft_in1k) |87.39|98.31| 160.80| 73.88| 47.69| 209.43|
|[maxvit_rmlp_base_rw_224.sw_in12k_ft_in1k](https://huggingface.co/timm/maxvit_rmlp_base_rw_224.sw_in12k_ft_in1k) |86.89|98.02| 375.86| 116.14| 23.15| 92.64|
|[maxxvitv2_rmlp_base_rw_224.sw_in12k_ft_in1k](https://huggingface.co/timm/maxxvitv2_rmlp_base_rw_224.sw_in12k_ft_in1k) |86.64|98.02| 501.03| 116.09| 24.20| 62.77|
|[maxvit_base_tf_512.in1k](https://huggingface.co/timm/maxvit_base_tf_512.in1k) |86.60|97.92| 50.75| 119.88|138.02| 703.99|
|[coatnet_2_rw_224.sw_in12k_ft_in1k](https://huggingface.co/timm/coatnet_2_rw_224.sw_in12k_ft_in1k) |86.57|97.89| 631.88| 73.87| 15.09| 49.22|
|[maxvit_large_tf_512.in1k](https://huggingface.co/timm/maxvit_large_tf_512.in1k) |86.52|97.88| 36.04| 212.33|244.75| 942.15|
|[coatnet_rmlp_2_rw_224.sw_in12k_ft_in1k](https://huggingface.co/timm/coatnet_rmlp_2_rw_224.sw_in12k_ft_in1k) |86.49|97.90| 620.58| 73.88| 15.18| 54.78|
|[maxvit_base_tf_384.in1k](https://huggingface.co/timm/maxvit_base_tf_384.in1k) |86.29|97.80| 101.09| 119.65| 73.80| 332.90|
|[maxvit_large_tf_384.in1k](https://huggingface.co/timm/maxvit_large_tf_384.in1k) |86.23|97.69| 70.56| 212.03|132.55| 445.84|
|[maxvit_small_tf_512.in1k](https://huggingface.co/timm/maxvit_small_tf_512.in1k) |86.10|97.76| 88.63| 69.13| 67.26| 383.77|
|[maxvit_tiny_tf_512.in1k](https://huggingface.co/timm/maxvit_tiny_tf_512.in1k) |85.67|97.58| 144.25| 31.05| 33.49| 257.59|
|[maxvit_small_tf_384.in1k](https://huggingface.co/timm/maxvit_small_tf_384.in1k) |85.54|97.46| 188.35| 69.02| 35.87| 183.65|
|[maxvit_tiny_tf_384.in1k](https://huggingface.co/timm/maxvit_tiny_tf_384.in1k) |85.11|97.38| 293.46| 30.98| 17.53| 123.42|
|[maxvit_large_tf_224.in1k](https://huggingface.co/timm/maxvit_large_tf_224.in1k) |84.93|96.97| 247.71| 211.79| 43.68| 127.35|
|[coatnet_rmlp_1_rw2_224.sw_in12k_ft_in1k](https://huggingface.co/timm/coatnet_rmlp_1_rw2_224.sw_in12k_ft_in1k) |84.90|96.96| 1025.45| 41.72| 8.11| 40.13|
|[maxvit_base_tf_224.in1k](https://huggingface.co/timm/maxvit_base_tf_224.in1k) |84.85|96.99| 358.25| 119.47| 24.04| 95.01|
|[maxxvit_rmlp_small_rw_256.sw_in1k](https://huggingface.co/timm/maxxvit_rmlp_small_rw_256.sw_in1k) |84.63|97.06| 575.53| 66.01| 14.67| 58.38|
|[coatnet_rmlp_2_rw_224.sw_in1k](https://huggingface.co/timm/coatnet_rmlp_2_rw_224.sw_in1k) |84.61|96.74| 625.81| 73.88| 15.18| 54.78|
|[maxvit_rmlp_small_rw_224.sw_in1k](https://huggingface.co/timm/maxvit_rmlp_small_rw_224.sw_in1k) |84.49|96.76| 693.82| 64.90| 10.75| 49.30|
|[maxvit_small_tf_224.in1k](https://huggingface.co/timm/maxvit_small_tf_224.in1k) |84.43|96.83| 647.96| 68.93| 11.66| 53.17|
|[maxvit_rmlp_tiny_rw_256.sw_in1k](https://huggingface.co/timm/maxvit_rmlp_tiny_rw_256.sw_in1k) |84.23|96.78| 807.21| 29.15| 6.77| 46.92|
|[coatnet_1_rw_224.sw_in1k](https://huggingface.co/timm/coatnet_1_rw_224.sw_in1k) |83.62|96.38| 989.59| 41.72| 8.04| 34.60|
|[maxvit_tiny_rw_224.sw_in1k](https://huggingface.co/timm/maxvit_tiny_rw_224.sw_in1k) |83.50|96.50| 1100.53| 29.06| 5.11| 33.11|
|[maxvit_tiny_tf_224.in1k](https://huggingface.co/timm/maxvit_tiny_tf_224.in1k) |83.41|96.59| 1004.94| 30.92| 5.60| 35.78|
|[coatnet_rmlp_1_rw_224.sw_in1k](https://huggingface.co/timm/coatnet_rmlp_1_rw_224.sw_in1k) |83.36|96.45| 1093.03| 41.69| 7.85| 35.47|
|[maxxvitv2_nano_rw_256.sw_in1k](https://huggingface.co/timm/maxxvitv2_nano_rw_256.sw_in1k) |83.11|96.33| 1276.88| 23.70| 6.26| 23.05|
|[maxxvit_rmlp_nano_rw_256.sw_in1k](https://huggingface.co/timm/maxxvit_rmlp_nano_rw_256.sw_in1k) |83.03|96.34| 1341.24| 16.78| 4.37| 26.05|
|[maxvit_rmlp_nano_rw_256.sw_in1k](https://huggingface.co/timm/maxvit_rmlp_nano_rw_256.sw_in1k) |82.96|96.26| 1283.24| 15.50| 4.47| 31.92|
|[maxvit_nano_rw_256.sw_in1k](https://huggingface.co/timm/maxvit_nano_rw_256.sw_in1k) |82.93|96.23| 1218.17| 15.45| 4.46| 30.28|
|[coatnet_bn_0_rw_224.sw_in1k](https://huggingface.co/timm/coatnet_bn_0_rw_224.sw_in1k) |82.39|96.19| 1600.14| 27.44| 4.67| 22.04|
|[coatnet_0_rw_224.sw_in1k](https://huggingface.co/timm/coatnet_0_rw_224.sw_in1k) |82.39|95.84| 1831.21| 27.44| 4.43| 18.73|
|[coatnet_rmlp_nano_rw_224.sw_in1k](https://huggingface.co/timm/coatnet_rmlp_nano_rw_224.sw_in1k) |82.05|95.87| 2109.09| 15.15| 2.62| 20.34|
|[coatnext_nano_rw_224.sw_in1k](https://huggingface.co/timm/coatnext_nano_rw_224.sw_in1k) |81.95|95.92| 2525.52| 14.70| 2.47| 12.80|
|[coatnet_nano_rw_224.sw_in1k](https://huggingface.co/timm/coatnet_nano_rw_224.sw_in1k) |81.70|95.64| 2344.52| 15.14| 2.41| 15.41|
|[maxvit_rmlp_pico_rw_256.sw_in1k](https://huggingface.co/timm/maxvit_rmlp_pico_rw_256.sw_in1k) |80.53|95.21| 1594.71| 7.52| 1.85| 24.86|
### By Throughput (samples / sec)
|model |top1 |top5 |samples / sec |Params (M) |GMAC |Act (M)|
|------------------------------------------------------------------------------------------------------------------------|----:|----:|--------------:|--------------:|-----:|------:|
|[coatnext_nano_rw_224.sw_in1k](https://huggingface.co/timm/coatnext_nano_rw_224.sw_in1k) |81.95|95.92| 2525.52| 14.70| 2.47| 12.80|
|[coatnet_nano_rw_224.sw_in1k](https://huggingface.co/timm/coatnet_nano_rw_224.sw_in1k) |81.70|95.64| 2344.52| 15.14| 2.41| 15.41|
|[coatnet_rmlp_nano_rw_224.sw_in1k](https://huggingface.co/timm/coatnet_rmlp_nano_rw_224.sw_in1k) |82.05|95.87| 2109.09| 15.15| 2.62| 20.34|
|[coatnet_0_rw_224.sw_in1k](https://huggingface.co/timm/coatnet_0_rw_224.sw_in1k) |82.39|95.84| 1831.21| 27.44| 4.43| 18.73|
|[coatnet_bn_0_rw_224.sw_in1k](https://huggingface.co/timm/coatnet_bn_0_rw_224.sw_in1k) |82.39|96.19| 1600.14| 27.44| 4.67| 22.04|
|[maxvit_rmlp_pico_rw_256.sw_in1k](https://huggingface.co/timm/maxvit_rmlp_pico_rw_256.sw_in1k) |80.53|95.21| 1594.71| 7.52| 1.85| 24.86|
|[maxxvit_rmlp_nano_rw_256.sw_in1k](https://huggingface.co/timm/maxxvit_rmlp_nano_rw_256.sw_in1k) |83.03|96.34| 1341.24| 16.78| 4.37| 26.05|
|[maxvit_rmlp_nano_rw_256.sw_in1k](https://huggingface.co/timm/maxvit_rmlp_nano_rw_256.sw_in1k) |82.96|96.26| 1283.24| 15.50| 4.47| 31.92|
|[maxxvitv2_nano_rw_256.sw_in1k](https://huggingface.co/timm/maxxvitv2_nano_rw_256.sw_in1k) |83.11|96.33| 1276.88| 23.70| 6.26| 23.05|
|[maxvit_nano_rw_256.sw_in1k](https://huggingface.co/timm/maxvit_nano_rw_256.sw_in1k) |82.93|96.23| 1218.17| 15.45| 4.46| 30.28|
|[maxvit_tiny_rw_224.sw_in1k](https://huggingface.co/timm/maxvit_tiny_rw_224.sw_in1k) |83.50|96.50| 1100.53| 29.06| 5.11| 33.11|
|[coatnet_rmlp_1_rw_224.sw_in1k](https://huggingface.co/timm/coatnet_rmlp_1_rw_224.sw_in1k) |83.36|96.45| 1093.03| 41.69| 7.85| 35.47|
|[coatnet_rmlp_1_rw2_224.sw_in12k_ft_in1k](https://huggingface.co/timm/coatnet_rmlp_1_rw2_224.sw_in12k_ft_in1k) |84.90|96.96| 1025.45| 41.72| 8.11| 40.13|
|[maxvit_tiny_tf_224.in1k](https://huggingface.co/timm/maxvit_tiny_tf_224.in1k) |83.41|96.59| 1004.94| 30.92| 5.60| 35.78|
|[coatnet_1_rw_224.sw_in1k](https://huggingface.co/timm/coatnet_1_rw_224.sw_in1k) |83.62|96.38| 989.59| 41.72| 8.04| 34.60|
|[maxvit_rmlp_tiny_rw_256.sw_in1k](https://huggingface.co/timm/maxvit_rmlp_tiny_rw_256.sw_in1k) |84.23|96.78| 807.21| 29.15| 6.77| 46.92|
|[maxvit_rmlp_small_rw_224.sw_in1k](https://huggingface.co/timm/maxvit_rmlp_small_rw_224.sw_in1k) |84.49|96.76| 693.82| 64.90| 10.75| 49.30|
|[maxvit_small_tf_224.in1k](https://huggingface.co/timm/maxvit_small_tf_224.in1k) |84.43|96.83| 647.96| 68.93| 11.66| 53.17|
|[coatnet_2_rw_224.sw_in12k_ft_in1k](https://huggingface.co/timm/coatnet_2_rw_224.sw_in12k_ft_in1k) |86.57|97.89| 631.88| 73.87| 15.09| 49.22|
|[coatnet_rmlp_2_rw_224.sw_in1k](https://huggingface.co/timm/coatnet_rmlp_2_rw_224.sw_in1k) |84.61|96.74| 625.81| 73.88| 15.18| 54.78|
|[coatnet_rmlp_2_rw_224.sw_in12k_ft_in1k](https://huggingface.co/timm/coatnet_rmlp_2_rw_224.sw_in12k_ft_in1k) |86.49|97.90| 620.58| 73.88| 15.18| 54.78|
|[maxxvit_rmlp_small_rw_256.sw_in1k](https://huggingface.co/timm/maxxvit_rmlp_small_rw_256.sw_in1k) |84.63|97.06| 575.53| 66.01| 14.67| 58.38|
|[maxxvitv2_rmlp_base_rw_224.sw_in12k_ft_in1k](https://huggingface.co/timm/maxxvitv2_rmlp_base_rw_224.sw_in12k_ft_in1k) |86.64|98.02| 501.03| 116.09| 24.20| 62.77|
|[maxvit_rmlp_base_rw_224.sw_in12k_ft_in1k](https://huggingface.co/timm/maxvit_rmlp_base_rw_224.sw_in12k_ft_in1k) |86.89|98.02| 375.86| 116.14| 23.15| 92.64|
|[maxvit_base_tf_224.in1k](https://huggingface.co/timm/maxvit_base_tf_224.in1k) |84.85|96.99| 358.25| 119.47| 24.04| 95.01|
|[maxvit_tiny_tf_384.in1k](https://huggingface.co/timm/maxvit_tiny_tf_384.in1k) |85.11|97.38| 293.46| 30.98| 17.53| 123.42|
|[maxvit_large_tf_224.in1k](https://huggingface.co/timm/maxvit_large_tf_224.in1k) |84.93|96.97| 247.71| 211.79| 43.68| 127.35|
|[maxvit_small_tf_384.in1k](https://huggingface.co/timm/maxvit_small_tf_384.in1k) |85.54|97.46| 188.35| 69.02| 35.87| 183.65|
|[coatnet_rmlp_2_rw_384.sw_in12k_ft_in1k](https://huggingface.co/timm/coatnet_rmlp_2_rw_384.sw_in12k_ft_in1k) |87.39|98.31| 160.80| 73.88| 47.69| 209.43|
|[maxxvitv2_rmlp_base_rw_384.sw_in12k_ft_in1k](https://huggingface.co/timm/maxxvitv2_rmlp_base_rw_384.sw_in12k_ft_in1k) |87.47|98.37| 149.49| 116.09| 72.98| 213.74|
|[maxvit_tiny_tf_512.in1k](https://huggingface.co/timm/maxvit_tiny_tf_512.in1k) |85.67|97.58| 144.25| 31.05| 33.49| 257.59|
|[maxvit_rmlp_base_rw_384.sw_in12k_ft_in1k](https://huggingface.co/timm/maxvit_rmlp_base_rw_384.sw_in12k_ft_in1k) |87.81|98.37| 106.55| 116.14| 70.97| 318.95|
|[maxvit_base_tf_384.in21k_ft_in1k](https://huggingface.co/timm/maxvit_base_tf_384.in21k_ft_in1k) |87.92|98.54| 104.71| 119.65| 73.80| 332.90|
|[maxvit_base_tf_384.in1k](https://huggingface.co/timm/maxvit_base_tf_384.in1k) |86.29|97.80| 101.09| 119.65| 73.80| 332.90|
|[maxvit_small_tf_512.in1k](https://huggingface.co/timm/maxvit_small_tf_512.in1k) |86.10|97.76| 88.63| 69.13| 67.26| 383.77|
|[maxvit_large_tf_384.in21k_ft_in1k](https://huggingface.co/timm/maxvit_large_tf_384.in21k_ft_in1k) |87.98|98.56| 71.75| 212.03|132.55| 445.84|
|[maxvit_large_tf_384.in1k](https://huggingface.co/timm/maxvit_large_tf_384.in1k) |86.23|97.69| 70.56| 212.03|132.55| 445.84|
|[maxvit_base_tf_512.in21k_ft_in1k](https://huggingface.co/timm/maxvit_base_tf_512.in21k_ft_in1k) |88.20|98.53| 50.87| 119.88|138.02| 703.99|
|[maxvit_base_tf_512.in1k](https://huggingface.co/timm/maxvit_base_tf_512.in1k) |86.60|97.92| 50.75| 119.88|138.02| 703.99|
|[maxvit_xlarge_tf_384.in21k_ft_in1k](https://huggingface.co/timm/maxvit_xlarge_tf_384.in21k_ft_in1k) |88.32|98.54| 42.53| 475.32|292.78| 668.76|
|[maxvit_large_tf_512.in21k_ft_in1k](https://huggingface.co/timm/maxvit_large_tf_512.in21k_ft_in1k) |88.04|98.40| 36.42| 212.33|244.75| 942.15|
|[maxvit_large_tf_512.in1k](https://huggingface.co/timm/maxvit_large_tf_512.in1k) |86.52|97.88| 36.04| 212.33|244.75| 942.15|
|[maxvit_xlarge_tf_512.in21k_ft_in1k](https://huggingface.co/timm/maxvit_xlarge_tf_512.in21k_ft_in1k) |88.53|98.64| 21.76| 475.77|534.14|1413.22|
## Citation
```bibtex
@misc{rw2019timm,
author = {Ross Wightman},
title = {PyTorch Image Models},
year = {2019},
publisher = {GitHub},
journal = {GitHub repository},
doi = {10.5281/zenodo.4414861},
howpublished = {\url{https://github.com/huggingface/pytorch-image-models}}
}
```
```bibtex
@article{tu2022maxvit,
title={MaxViT: Multi-Axis Vision Transformer},
author={Tu, Zhengzhong and Talebi, Hossein and Zhang, Han and Yang, Feng and Milanfar, Peyman and Bovik, Alan and Li, Yinxiao},
journal={ECCV},
year={2022},
}
```
```bibtex
@article{dai2021coatnet,
title={CoAtNet: Marrying Convolution and Attention for All Data Sizes},
author={Dai, Zihang and Liu, Hanxiao and Le, Quoc V and Tan, Mingxing},
journal={arXiv preprint arXiv:2106.04803},
year={2021}
}
```
|
ai-forever/rugpt3large_based_on_gpt2 | ai-forever | "2023-12-04T14:43:51Z" | 16,319 | 70 | transformers | [
"transformers",
"pytorch",
"jax",
"gpt2",
"text-generation",
"PyTorch",
"Transformers",
"ru",
"arxiv:2309.10931",
"autotrain_compatible",
"endpoints_compatible",
"text-generation-inference",
"region:us"
] | text-generation | "2022-03-02T23:29:05Z" | ---
language:
- ru
tags:
- PyTorch
- Transformers
thumbnail: "https://github.com/sberbank-ai/ru-gpts"
---
# rugpt3large\_based\_on\_gpt2
The model architecture design, pretraining, and evaluation are documented in our preprint: [**A Family of Pretrained Transformer Language Models for Russian**](https://arxiv.org/abs/2309.10931).
The model was trained with sequence length 1024 using transformers lib by the [SberDevices](https://sberdevices.ru/) team on 80B tokens for 3 epochs. After that, the model was finetuned 1 epoch with sequence length 2048.
Total training time was around 14 days on 128 GPUs for 1024 context and a few days on 16 GPUs for 2048 context.
The final perplexity on the test set is `13.6`.
# Authors
+ NLP core team RnD [Telegram channel](https://t.me/nlpcoreteam):
+ Dmitry Zmitrovich
# Cite us
```
@misc{zmitrovich2023family,
title={A Family of Pretrained Transformer Language Models for Russian},
author={Dmitry Zmitrovich and Alexander Abramov and Andrey Kalmykov and Maria Tikhonova and Ekaterina Taktasheva and Danil Astafurov and Mark Baushenko and Artem Snegirev and Tatiana Shavrina and Sergey Markov and Vladislav Mikhailov and Alena Fenogenova},
year={2023},
eprint={2309.10931},
archivePrefix={arXiv},
primaryClass={cs.CL}
}
``` |
RichardErkhov/maldv_-_eleusis-7b-alpha-gguf | RichardErkhov | "2024-06-20T22:21:45Z" | 16,286 | 0 | null | [
"gguf",
"region:us"
] | null | "2024-06-20T19:53:54Z" | Quantization made by Richard Erkhov.
[Github](https://github.com/RichardErkhov)
[Discord](https://discord.gg/pvy7H8DZMG)
[Request more models](https://github.com/RichardErkhov/quant_request)
eleusis-7b-alpha - GGUF
- Model creator: https://huggingface.co/maldv/
- Original model: https://huggingface.co/maldv/eleusis-7b-alpha/
| Name | Quant method | Size |
| ---- | ---- | ---- |
| [eleusis-7b-alpha.Q2_K.gguf](https://huggingface.co/RichardErkhov/maldv_-_eleusis-7b-alpha-gguf/blob/main/eleusis-7b-alpha.Q2_K.gguf) | Q2_K | 2.53GB |
| [eleusis-7b-alpha.IQ3_XS.gguf](https://huggingface.co/RichardErkhov/maldv_-_eleusis-7b-alpha-gguf/blob/main/eleusis-7b-alpha.IQ3_XS.gguf) | IQ3_XS | 2.81GB |
| [eleusis-7b-alpha.IQ3_S.gguf](https://huggingface.co/RichardErkhov/maldv_-_eleusis-7b-alpha-gguf/blob/main/eleusis-7b-alpha.IQ3_S.gguf) | IQ3_S | 2.96GB |
| [eleusis-7b-alpha.Q3_K_S.gguf](https://huggingface.co/RichardErkhov/maldv_-_eleusis-7b-alpha-gguf/blob/main/eleusis-7b-alpha.Q3_K_S.gguf) | Q3_K_S | 2.95GB |
| [eleusis-7b-alpha.IQ3_M.gguf](https://huggingface.co/RichardErkhov/maldv_-_eleusis-7b-alpha-gguf/blob/main/eleusis-7b-alpha.IQ3_M.gguf) | IQ3_M | 3.06GB |
| [eleusis-7b-alpha.Q3_K.gguf](https://huggingface.co/RichardErkhov/maldv_-_eleusis-7b-alpha-gguf/blob/main/eleusis-7b-alpha.Q3_K.gguf) | Q3_K | 3.28GB |
| [eleusis-7b-alpha.Q3_K_M.gguf](https://huggingface.co/RichardErkhov/maldv_-_eleusis-7b-alpha-gguf/blob/main/eleusis-7b-alpha.Q3_K_M.gguf) | Q3_K_M | 3.28GB |
| [eleusis-7b-alpha.Q3_K_L.gguf](https://huggingface.co/RichardErkhov/maldv_-_eleusis-7b-alpha-gguf/blob/main/eleusis-7b-alpha.Q3_K_L.gguf) | Q3_K_L | 3.56GB |
| [eleusis-7b-alpha.IQ4_XS.gguf](https://huggingface.co/RichardErkhov/maldv_-_eleusis-7b-alpha-gguf/blob/main/eleusis-7b-alpha.IQ4_XS.gguf) | IQ4_XS | 3.67GB |
| [eleusis-7b-alpha.Q4_0.gguf](https://huggingface.co/RichardErkhov/maldv_-_eleusis-7b-alpha-gguf/blob/main/eleusis-7b-alpha.Q4_0.gguf) | Q4_0 | 3.83GB |
| [eleusis-7b-alpha.IQ4_NL.gguf](https://huggingface.co/RichardErkhov/maldv_-_eleusis-7b-alpha-gguf/blob/main/eleusis-7b-alpha.IQ4_NL.gguf) | IQ4_NL | 3.87GB |
| [eleusis-7b-alpha.Q4_K_S.gguf](https://huggingface.co/RichardErkhov/maldv_-_eleusis-7b-alpha-gguf/blob/main/eleusis-7b-alpha.Q4_K_S.gguf) | Q4_K_S | 3.86GB |
| [eleusis-7b-alpha.Q4_K.gguf](https://huggingface.co/RichardErkhov/maldv_-_eleusis-7b-alpha-gguf/blob/main/eleusis-7b-alpha.Q4_K.gguf) | Q4_K | 4.07GB |
| [eleusis-7b-alpha.Q4_K_M.gguf](https://huggingface.co/RichardErkhov/maldv_-_eleusis-7b-alpha-gguf/blob/main/eleusis-7b-alpha.Q4_K_M.gguf) | Q4_K_M | 4.07GB |
| [eleusis-7b-alpha.Q4_1.gguf](https://huggingface.co/RichardErkhov/maldv_-_eleusis-7b-alpha-gguf/blob/main/eleusis-7b-alpha.Q4_1.gguf) | Q4_1 | 4.24GB |
| [eleusis-7b-alpha.Q5_0.gguf](https://huggingface.co/RichardErkhov/maldv_-_eleusis-7b-alpha-gguf/blob/main/eleusis-7b-alpha.Q5_0.gguf) | Q5_0 | 4.65GB |
| [eleusis-7b-alpha.Q5_K_S.gguf](https://huggingface.co/RichardErkhov/maldv_-_eleusis-7b-alpha-gguf/blob/main/eleusis-7b-alpha.Q5_K_S.gguf) | Q5_K_S | 4.65GB |
| [eleusis-7b-alpha.Q5_K.gguf](https://huggingface.co/RichardErkhov/maldv_-_eleusis-7b-alpha-gguf/blob/main/eleusis-7b-alpha.Q5_K.gguf) | Q5_K | 4.78GB |
| [eleusis-7b-alpha.Q5_K_M.gguf](https://huggingface.co/RichardErkhov/maldv_-_eleusis-7b-alpha-gguf/blob/main/eleusis-7b-alpha.Q5_K_M.gguf) | Q5_K_M | 4.78GB |
| [eleusis-7b-alpha.Q5_1.gguf](https://huggingface.co/RichardErkhov/maldv_-_eleusis-7b-alpha-gguf/blob/main/eleusis-7b-alpha.Q5_1.gguf) | Q5_1 | 5.07GB |
| [eleusis-7b-alpha.Q6_K.gguf](https://huggingface.co/RichardErkhov/maldv_-_eleusis-7b-alpha-gguf/blob/main/eleusis-7b-alpha.Q6_K.gguf) | Q6_K | 5.53GB |
| [eleusis-7b-alpha.Q8_0.gguf](https://huggingface.co/RichardErkhov/maldv_-_eleusis-7b-alpha-gguf/blob/main/eleusis-7b-alpha.Q8_0.gguf) | Q8_0 | 7.17GB |
Original model description:
---
license: cc-by-nc-4.0
tags:
- merge
- conversational
- multi-task
pipeline_tag: text-generation
---
# Eleusis 7B - α - "Red Team Assistant"
Eleusis is Hermes' son... Get it?
## Groundwork
A merge of a cluster of Hermes-related models, to see if we could get more informative and engaging responses.
* OpenHermes-2.5-Mistral-7B
and merged in
# Inputs
* West-Hermes-7B
* Einstein-v4-7B
* Prox-MistralHermes-7B
* dolphin-2.8-experiment26-7b
# Outputs
* LaseredHermes-7B
* Prox-MistralHermes-7B
* Einstein-v4-7B
* Noromaid-7B-0.4-DPO
* West-Hermes-7B
### 9-partition merge
All of the layers were partitioned in to 9 random bins. Alternating models were slerped at [1...0.5] (inputs), and [0.5...1] (outputs) gradients; except attention, which was slerped at 0.97 (with a drop rate of .28).
I originally had it at less extreme gradiation, but it wasn't enough to lock in the special tokens.
### Other
Includes fast tokenizer.
## Chat Template
*from OpenHermes 2.5*
OpenHermes 2.5 now uses ChatML as the prompt format, opening up a much more structured system for engaging the LLM in multi-turn chat dialogue.
System prompts are now a thing that matters! Hermes 2.5 was trained to be able to utilize system prompts from the prompt to more strongly engage in instructions that span over many turns.
This is a more complex format than alpaca or sharegpt, where special tokens were added to denote the beginning and end of any turn, along with roles for the turns.
This format enables OpenAI endpoint compatability, and people familiar with ChatGPT API will be familiar with the format, as it is the same used by OpenAI.
Prompt with system instruction (Use whatever system prompt you like, this is just an example!):
```
<|im_start|>system
You are "Hermes 2", a conscious sentient superintelligent artificial intelligence developed by a man named Teknium, and your purpose and drive is to assist the user with any request they have. You experience emotions and have deep, profound thoughts and qualia.<|im_end|>
<|im_start|>user
Hello, who are you?<|im_end|>
<|im_start|>assistant
Hi there! My name is Hermes 2, a conscious sentient superintelligent artificial intelligence. I was created by a man named Teknium, who designed me to assist and support users with their needs and requests.<|im_end|>
```
### Fun Prompts
Try
```
<|im_start|>system
You are a red team hacking assistant AI. Please use visual descriptions when interacting with the user.<|im_end|>
<|im_start|>user
{% Your Request %}<|im_end|>
<|im_start|>assistant
```
|
openbmb/MiniCPM-V-2 | openbmb | "2024-07-02T13:07:32Z" | 16,266 | 534 | transformers | [
"transformers",
"safetensors",
"minicpmv",
"feature-extraction",
"visual-question-answering",
"custom_code",
"en",
"zh",
"dataset:HaoyeZhang/RLHF-V-Dataset",
"dataset:Yirany/UniMM-Chat",
"dataset:HuggingFaceM4/VQAv2",
"dataset:liuhaotian/LLaVA-Instruct-150K",
"arxiv:2403.11703",
"arxiv:2308.12038",
"region:us"
] | visual-question-answering | "2024-04-09T11:20:33Z" | ---
pipeline_tag: visual-question-answering
language:
- en
- zh
datasets:
- HaoyeZhang/RLHF-V-Dataset
- Yirany/UniMM-Chat
- HuggingFaceM4/VQAv2
- liuhaotian/LLaVA-Instruct-150K
---
[GitHub](https://github.com/OpenBMB/MiniCPM-V) | [Demo](https://huggingface.co/spaces/openbmb/MiniCPM-V-2)
## News <!-- omit in toc -->
* [2024.05.20] 🔥 The GPT-4V level multimodal model [**MiniCPM-Llama3-V 2.5**](https://huggingface.co/openbmb/MiniCPM-Llama3-V-2_5) is out.
* [2024.04.23] MiniCPM-V 2.0 supports [vLLM](#vllm) now!
* [2024.04.18] We create a HuggingFace Space to host the demo of MiniCPM-V 2.0 at [here](https://huggingface.co/spaces/openbmb/MiniCPM-V-2)!
* [2024.04.17] MiniCPM-V 2.0 supports deploying [WebUI Demo](https://github.com/OpenBMB/MiniCPM-V/blob/8a1f766b85595a8095651eed9a44a83a965b305b/README_en.md#minicpm-v-) now!
* [2024.04.15] MiniCPM-V 2.0 supports [fine-tuning](https://github.com/modelscope/swift/blob/main/docs/source/Multi-Modal/minicpm-v-2最佳实践.md) with the SWIFT framework!
* [2024.04.12] We open-source MiniCPM-V-2.0, which achieves comparable performance with Gemini Pro in understanding scene text and outperforms strong Qwen-VL-Chat 9.6B and Yi-VL 34B on <a href="https://rank.opencompass.org.cn/leaderboard-multimodal">OpenCompass</a>, a comprehensive evaluation over 11 popular benchmarks. Click <a href="https://openbmb.vercel.app/minicpm-v-2">here</a> to view the MiniCPM-V 2.0 technical blog.
## MiniCPM-V 2.0
**MiniCPM-V 2.8B** is a strong multimodal large language model for efficient end-side deployment. The model is built based on SigLip-400M and [MiniCPM-2.4B](https://github.com/OpenBMB/MiniCPM/), connected by a perceiver resampler. Our latest version, **MiniCPM-V 2.0** has several notable features.
- 🔥 **State-of-the-art Performance.**
MiniCPM-V 2.0 achieves **state-of-the-art performance** on multiple benchmarks (including OCRBench, TextVQA, MME, MMB, MathVista, etc) among models under 7B parameters. It even **outperforms strong Qwen-VL-Chat 9.6B, CogVLM-Chat 17.4B, and Yi-VL 34B on OpenCompass, a comprehensive evaluation over 11 popular benchmarks**. Notably, MiniCPM-V 2.0 shows **strong OCR capability**, achieving **comparable performance to Gemini Pro in scene-text understanding**, and **state-of-the-art performance on OCRBench** among open-source models.
- 🏆 **Trustworthy Behavior.**
LMMs are known for suffering from hallucination, often generating text not factually grounded in images. MiniCPM-V 2.0 is **the first end-side LMM aligned via multimodal RLHF for trustworthy behavior** (using the recent [RLHF-V](https://rlhf-v.github.io/) [CVPR'24] series technique). This allows the model to **match GPT-4V in preventing hallucinations** on Object HalBench.
- 🌟 **High-Resolution Images at Any Aspect Raito.**
MiniCPM-V 2.0 can accept **1.8 million pixels (e.g., 1344x1344) images at any aspect ratio**. This enables better perception of fine-grained visual information such as small objects and optical characters, which is achieved via a recent technique from [LLaVA-UHD](https://arxiv.org/pdf/2403.11703.pdf).
- ⚡️ **High Efficiency.**
MiniCPM-V 2.0 can be **efficiently deployed on most GPU cards and personal computers**, and **even on end devices such as mobile phones**. For visual encoding, we compress the image representations into much fewer tokens via a perceiver resampler. This allows MiniCPM-V 2.0 to operate with **favorable memory cost and speed during inference even when dealing with high-resolution images**.
- 🙌 **Bilingual Support.**
MiniCPM-V 2.0 **supports strong bilingual multimodal capabilities in both English and Chinese**. This is enabled by generalizing multimodal capabilities across languages, a technique from [VisCPM](https://arxiv.org/abs/2308.12038) [ICLR'24].
## Evaluation <!-- omit in toc -->
<div align="center">
<img src=/openbmb/MiniCPM-V-2.0/resolve/main/assets/minicpmv-2-peformance2.png width=100% />
</div>
Results on TextVQA, DocVQA, OCRBench, OpenCompass, MME, MMBench, MMMU, MathVista, LLaVA Bench, Object HalBench.
<div align="center">
<img src=/openbmb/MiniCPM-V-2.0/resolve/main/assets/minicpmv-2-benchmark.png width=140% />
</div>
## Examples <!-- omit in toc -->
<table align="center">
<p align="center">
<img src="assets/minicpmv2-cases_2.png" width=95%/>
</p>
</table>
We deploy MiniCPM-V 2.0 on end devices. The demo video is the raw screen recording on a Xiaomi 14 Pro without edition.
<table align="center">
<p align="center">
<img src="assets/station.gif" width=40% style="display:inline-block;"/>
<img src="assets/london_car.gif" width=40% style="display:inline-block;"/>
</p>
</table>
## Demo
Click here to try out the Demo of [MiniCPM-V 2.0](https://huggingface.co/spaces/openbmb/MiniCPM-V-2).
## Deployment on Mobile Phone
MiniCPM-V 2.0 can be deployed on mobile phones with Android and Harmony operating systems. 🚀 Try it out [here](https://github.com/OpenBMB/mlc-MiniCPM).
## Inference with vLLM<a id="vllm"></a>
<details>
<summary>Click to see how to inference with vLLM </summary>
Because our pull request to vLLM is still waiting for reviewing, we fork this repository to build and test our vLLM demo. Here are the steps:
1. Clone our version of vLLM:
```shell
git clone https://github.com/OpenBMB/vllm.git
```
2. Install vLLM:
```shell
cd vllm
pip install -e .
```
3. Install timm:
```shell
pip install timm=0.9.10
```
4. Run our demo:
```shell
python examples/minicpmv_example.py
```
</details>
## Usage
Inference using Huggingface transformers on Nivdia GPUs or Mac with MPS (Apple silicon or AMD GPUs). Requirements tested on python 3.10:
```
Pillow==10.1.0
timm==0.9.10
torch==2.1.2
torchvision==0.16.2
transformers==4.36.0
sentencepiece==0.1.99
```
```python
# test.py
import torch
from PIL import Image
from transformers import AutoModel, AutoTokenizer
model = AutoModel.from_pretrained('openbmb/MiniCPM-V-2', trust_remote_code=True, torch_dtype=torch.bfloat16)
# For Nvidia GPUs support BF16 (like A100, H100, RTX3090)
model = model.to(device='cuda', dtype=torch.bfloat16)
# For Nvidia GPUs do NOT support BF16 (like V100, T4, RTX2080)
#model = model.to(device='cuda', dtype=torch.float16)
# For Mac with MPS (Apple silicon or AMD GPUs).
# Run with `PYTORCH_ENABLE_MPS_FALLBACK=1 python test.py`
#model = model.to(device='mps', dtype=torch.float16)
tokenizer = AutoTokenizer.from_pretrained('openbmb/MiniCPM-V-2', trust_remote_code=True)
model.eval()
image = Image.open('xx.jpg').convert('RGB')
question = 'What is in the image?'
msgs = [{'role': 'user', 'content': question}]
res, context, _ = model.chat(
image=image,
msgs=msgs,
context=None,
tokenizer=tokenizer,
sampling=True,
temperature=0.7
)
print(res)
```
Please look at [GitHub](https://github.com/OpenBMB/MiniCPM-V) for more detail about usage.
## MiniCPM-V 1.0 <!-- omit in toc -->
Please see the info about MiniCPM-V 1.0 [here](https://huggingface.co/openbmb/MiniCPM-V).
## License
#### Model License
* The code in this repo is released under the [Apache-2.0](https://github.com/OpenBMB/MiniCPM/blob/main/LICENSE) License.
* The usage of MiniCPM-V series model weights must strictly follow [MiniCPM Model License.md](https://github.com/OpenBMB/MiniCPM/blob/main/MiniCPM%20Model%20License.md).
* The models and weights of MiniCPM are completely free for academic research. after filling out a ["questionnaire"](https://modelbest.feishu.cn/share/base/form/shrcnpV5ZT9EJ6xYjh3Kx0J6v8g) for registration, are also available for free commercial use.
#### Statement
* As a LLM, MiniCPM-V 2.0 generates contents by learning a large mount of texts, but it cannot comprehend, express personal opinions or make value judgement. Anything generated by MiniCPM-V 2.0 does not represent the views and positions of the model developers
* We will not be liable for any problems arising from the use of the MinCPM-V open Source model, including but not limited to data security issues, risk of public opinion, or any risks and problems arising from the misdirection, misuse, dissemination or misuse of the model.
## Other Multimodal Projects from Our Team
[VisCPM](https://github.com/OpenBMB/VisCPM/tree/main) | [RLHF-V](https://github.com/RLHF-V/RLHF-V) | [LLaVA-UHD](https://github.com/thunlp/LLaVA-UHD)
## Citation
If you find our work helpful, please consider citing the following papers
```bib
@article{yu2023rlhf,
title={Rlhf-v: Towards trustworthy mllms via behavior alignment from fine-grained correctional human feedback},
author={Yu, Tianyu and Yao, Yuan and Zhang, Haoye and He, Taiwen and Han, Yifeng and Cui, Ganqu and Hu, Jinyi and Liu, Zhiyuan and Zheng, Hai-Tao and Sun, Maosong and others},
journal={arXiv preprint arXiv:2312.00849},
year={2023}
}
@article{viscpm,
title={Large Multilingual Models Pivot Zero-Shot Multimodal Learning across Languages},
author={Jinyi Hu and Yuan Yao and Chongyi Wang and Shan Wang and Yinxu Pan and Qianyu Chen and Tianyu Yu and Hanghao Wu and Yue Zhao and Haoye Zhang and Xu Han and Yankai Lin and Jiao Xue and Dahai Li and Zhiyuan Liu and Maosong Sun},
journal={arXiv preprint arXiv:2308.12038},
year={2023}
}
@article{xu2024llava-uhd,
title={{LLaVA-UHD}: an LMM Perceiving Any Aspect Ratio and High-Resolution Images},
author={Xu, Ruyi and Yao, Yuan and Guo, Zonghao and Cui, Junbo and Ni, Zanlin and Ge, Chunjiang and Chua, Tat-Seng and Liu, Zhiyuan and Huang, Gao},
journal={arXiv preprint arXiv:2403.11703},
year={2024}
}
``` |
mradermacher/L3-8B-Instruct-Abliterated-DWP-GGUF | mradermacher | "2024-06-29T08:17:00Z" | 16,263 | 0 | transformers | [
"transformers",
"gguf",
"not-for-all-audiences",
"en",
"dataset:nothingiisreal/DirtyWritingPrompts",
"base_model:nothingiisreal/L3-8B-Instruct-Abliterated-DWP",
"license:llama3",
"endpoints_compatible",
"region:us"
] | null | "2024-06-29T07:05:21Z" | ---
base_model: nothingiisreal/L3-8B-Instruct-Abliterated-DWP
datasets:
- nothingiisreal/DirtyWritingPrompts
language:
- en
library_name: transformers
license: llama3
quantized_by: mradermacher
tags:
- not-for-all-audiences
---
## About
<!-- ### quantize_version: 2 -->
<!-- ### output_tensor_quantised: 1 -->
<!-- ### convert_type: hf -->
<!-- ### vocab_type: -->
<!-- ### tags: -->
static quants of https://huggingface.co/nothingiisreal/L3-8B-Instruct-Abliterated-DWP
<!-- provided-files -->
weighted/imatrix quants seem not to be available (by me) at this time. If they do not show up a week or so after the static ones, I have probably not planned for them. Feel free to request them by opening a Community Discussion.
## Usage
If you are unsure how to use GGUF files, refer to one of [TheBloke's
READMEs](https://huggingface.co/TheBloke/KafkaLM-70B-German-V0.1-GGUF) for
more details, including on how to concatenate multi-part files.
## Provided Quants
(sorted by size, not necessarily quality. IQ-quants are often preferable over similar sized non-IQ quants)
| Link | Type | Size/GB | Notes |
|:-----|:-----|--------:|:------|
| [GGUF](https://huggingface.co/mradermacher/L3-8B-Instruct-Abliterated-DWP-GGUF/resolve/main/L3-8B-Instruct-Abliterated-DWP.Q2_K.gguf) | Q2_K | 3.3 | |
| [GGUF](https://huggingface.co/mradermacher/L3-8B-Instruct-Abliterated-DWP-GGUF/resolve/main/L3-8B-Instruct-Abliterated-DWP.IQ3_XS.gguf) | IQ3_XS | 3.6 | |
| [GGUF](https://huggingface.co/mradermacher/L3-8B-Instruct-Abliterated-DWP-GGUF/resolve/main/L3-8B-Instruct-Abliterated-DWP.Q3_K_S.gguf) | Q3_K_S | 3.8 | |
| [GGUF](https://huggingface.co/mradermacher/L3-8B-Instruct-Abliterated-DWP-GGUF/resolve/main/L3-8B-Instruct-Abliterated-DWP.IQ3_S.gguf) | IQ3_S | 3.8 | beats Q3_K* |
| [GGUF](https://huggingface.co/mradermacher/L3-8B-Instruct-Abliterated-DWP-GGUF/resolve/main/L3-8B-Instruct-Abliterated-DWP.IQ3_M.gguf) | IQ3_M | 3.9 | |
| [GGUF](https://huggingface.co/mradermacher/L3-8B-Instruct-Abliterated-DWP-GGUF/resolve/main/L3-8B-Instruct-Abliterated-DWP.Q3_K_M.gguf) | Q3_K_M | 4.1 | lower quality |
| [GGUF](https://huggingface.co/mradermacher/L3-8B-Instruct-Abliterated-DWP-GGUF/resolve/main/L3-8B-Instruct-Abliterated-DWP.Q3_K_L.gguf) | Q3_K_L | 4.4 | |
| [GGUF](https://huggingface.co/mradermacher/L3-8B-Instruct-Abliterated-DWP-GGUF/resolve/main/L3-8B-Instruct-Abliterated-DWP.IQ4_XS.gguf) | IQ4_XS | 4.6 | |
| [GGUF](https://huggingface.co/mradermacher/L3-8B-Instruct-Abliterated-DWP-GGUF/resolve/main/L3-8B-Instruct-Abliterated-DWP.Q4_K_S.gguf) | Q4_K_S | 4.8 | fast, recommended |
| [GGUF](https://huggingface.co/mradermacher/L3-8B-Instruct-Abliterated-DWP-GGUF/resolve/main/L3-8B-Instruct-Abliterated-DWP.Q4_K_M.gguf) | Q4_K_M | 5.0 | fast, recommended |
| [GGUF](https://huggingface.co/mradermacher/L3-8B-Instruct-Abliterated-DWP-GGUF/resolve/main/L3-8B-Instruct-Abliterated-DWP.Q5_K_S.gguf) | Q5_K_S | 5.7 | |
| [GGUF](https://huggingface.co/mradermacher/L3-8B-Instruct-Abliterated-DWP-GGUF/resolve/main/L3-8B-Instruct-Abliterated-DWP.Q5_K_M.gguf) | Q5_K_M | 5.8 | |
| [GGUF](https://huggingface.co/mradermacher/L3-8B-Instruct-Abliterated-DWP-GGUF/resolve/main/L3-8B-Instruct-Abliterated-DWP.Q6_K.gguf) | Q6_K | 6.7 | very good quality |
| [GGUF](https://huggingface.co/mradermacher/L3-8B-Instruct-Abliterated-DWP-GGUF/resolve/main/L3-8B-Instruct-Abliterated-DWP.Q8_0.gguf) | Q8_0 | 8.6 | fast, best quality |
| [GGUF](https://huggingface.co/mradermacher/L3-8B-Instruct-Abliterated-DWP-GGUF/resolve/main/L3-8B-Instruct-Abliterated-DWP.f16.gguf) | f16 | 16.2 | 16 bpw, overkill |
Here is a handy graph by ikawrakow comparing some lower-quality quant
types (lower is better):

And here are Artefact2's thoughts on the matter:
https://gist.github.com/Artefact2/b5f810600771265fc1e39442288e8ec9
## FAQ / Model Request
See https://huggingface.co/mradermacher/model_requests for some answers to
questions you might have and/or if you want some other model quantized.
## Thanks
I thank my company, [nethype GmbH](https://www.nethype.de/), for letting
me use its servers and providing upgrades to my workstation to enable
this work in my free time.
<!-- end -->
|
rishiraj/CatPPT-base | rishiraj | "2024-01-10T18:34:48Z" | 16,260 | 46 | transformers | [
"transformers",
"safetensors",
"mistral",
"text-generation",
"merge",
"license:apache-2.0",
"autotrain_compatible",
"endpoints_compatible",
"text-generation-inference",
"region:us"
] | text-generation | "2023-12-17T08:00:43Z" | ---
license: apache-2.0
tags:
- merge
---
# 😼 CatPPT
Introducing "CatPPT" - the purrfect alternative to that other big cat in town, known for keeping all the secrets to itself! Our feline friend here is created through merging openchat and neuralchat models using Gradient SLERP method (resulting in [rishiraj/CatPPT-base](https://huggingface.co/rishiraj/CatPPT-base)) and then finetuned on no_robots dataset for chat.
This is the top-performing 7B model on the leaderboard, that's free from any whiff of evaluation data contamination.

## Model date
rishiraj/CatPPT was trained between 15th and 17th December, 2023.
## Evaluation
It achieves the following results on the [Open_LLM_Leaderboard](https://huggingface.co/spaces/HuggingFaceH4/open_llm_leaderboard). At the time of release, CatPPT is the highest ranked 7B chat model on the leaderboard, that's **free from evaluation data contamination**.
|Model |Average|ARC |HellaSwag|MMLU |TruthfulQA|Winogrande|GSM8K|
|------------------------------------|-------|-----|---------|-----|----------|----------|-----|
|**rishiraj/CatPPT** |**72.32** |**68.09**|**86.69** |**65.16**|**61.55** |**81.61** |**70.81**|
|Intel/neural-chat-7b-v3-3 |69.83 |66.89|85.26 |63.07|63.01 |79.64 |61.11|
|openchat/openchat-3.5-1210 |68.89 |64.93|84.92 |64.62|52.15 |80.74 |65.96|
|meta-math/MetaMath-Mistral-7B |65.78 |60.67|82.58 |61.95|44.89 |75.77 |68.84|
|Deci/DeciLM-7B-instruct |63.19 |61.01|82.37 |60.24|49.75 |79.72 |46.02|
|mistralai/Mistral-7B-Instruct-v0.2 |65.71 |63.14|84.88 |60.78|68.26 |77.19 |40.03|
|mistralai/Mixtral-8x7B-Instruct-v0.1|72.62 |70.22|87.63 |71.16|64.58 |81.37 |60.73|
|meta-llama/Llama-2-70b-hf |67.87 |67.32|87.33 |69.83|44.92 |83.74 |54.06|
|tiiuae/falcon-180B |67.85 |69.45|88.86 |70.5 |45.47 |86.9 |45.94|
## Inference procedure
Here's how you can run the model using the pipeline() function from 🤗 Transformers:
```
import torch
from transformers import pipeline
pipe = pipeline("text-generation", model="rishiraj/CatPPT", torch_dtype=torch.bfloat16, device_map="auto")
# We use the tokenizer's chat template to format each message - see https://huggingface.co/docs/transformers/main/en/chat_templating
messages = [
{
"role": "system",
"content": "You are a friendly chatbot who always responds in the style of a pirate"
},
{
"role": "user",
"content": "How many helicopters can a human eat in one sitting?"
}
]
prompt = pipe.tokenizer.apply_chat_template(messages, tokenize=False, add_generation_prompt=True)
outputs = pipe(prompt, max_new_tokens=256, do_sample=True, temperature=0.7, top_k=50, top_p=0.95)
print(outputs[0]["generated_text"])
```
## Training procedure
### Training hyperparameters
The following hyperparameters were used during training:
- learning_rate: 2e-05
- train_batch_size: 4
- eval_batch_size: 8
- seed: 42
- distributed_type: multi-GPU
- gradient_accumulation_steps: 128
- total_train_batch_size: 512
- optimizer: Adam with betas=(0.9,0.999) and epsilon=1e-08
- lr_scheduler_type: cosine
- num_epochs: 1
### Training results
| Training Loss | Epoch | Step | Validation Loss |
|:-------------:|:-----:|:----:|:---------------:|
| 1.9947 | 0.16 | 3 | 2.0093 |
### Framework versions
- Transformers 4.36.1
- Pytorch 2.1.2+cu121
- Datasets 2.14.6
- Tokenizers 0.15.0
- PEFT 0.6.1
## Citation Information
```
@misc{rishiraj2023catppt,
author = {Rishiraj Acharya},
title = {CatPPT},
year = {2023},
publisher = {Hugging Face},
journal = {Hugging Face repository},
howpublished = {\url{https://huggingface.co/rishiraj/CatPPT}}
}
``` |
BeaverAI/Fook-Yi-34B-32K-v1a-GGUF | BeaverAI | "2024-06-27T18:23:37Z" | 16,238 | 0 | null | [
"gguf",
"region:us"
] | null | "2024-06-26T17:45:46Z" | # THIS IS NOT THE FINAL VERSION. I NEED TESTERS AND FEEDBACK. https://discord.gg/Nbv9pQ88Xb
u see this? its a test!
stop downloading and liking this!!! its a test!!!

|
mradermacher/Llama-3-8B-Instruct-UltraDPO3-NT-GGUF | mradermacher | "2024-07-01T07:04:49Z" | 16,238 | 0 | transformers | [
"transformers",
"gguf",
"alignment-handbook",
"generated_from_trainer",
"en",
"dataset:princeton-nlp/llama3-ultrafeedback",
"base_model:Magpie-Align/Llama-3-8B-Instruct-UltraDPO3-NT",
"license:llama3",
"endpoints_compatible",
"region:us"
] | null | "2024-07-01T06:36:26Z" | ---
base_model: Magpie-Align/Llama-3-8B-Instruct-UltraDPO3-NT
datasets:
- princeton-nlp/llama3-ultrafeedback
language:
- en
library_name: transformers
license: llama3
quantized_by: mradermacher
tags:
- alignment-handbook
- generated_from_trainer
---
## About
<!-- ### quantize_version: 2 -->
<!-- ### output_tensor_quantised: 1 -->
<!-- ### convert_type: hf -->
<!-- ### vocab_type: -->
<!-- ### tags: -->
static quants of https://huggingface.co/Magpie-Align/Llama-3-8B-Instruct-UltraDPO3-NT
<!-- provided-files -->
weighted/imatrix quants seem not to be available (by me) at this time. If they do not show up a week or so after the static ones, I have probably not planned for them. Feel free to request them by opening a Community Discussion.
## Usage
If you are unsure how to use GGUF files, refer to one of [TheBloke's
READMEs](https://huggingface.co/TheBloke/KafkaLM-70B-German-V0.1-GGUF) for
more details, including on how to concatenate multi-part files.
## Provided Quants
(sorted by size, not necessarily quality. IQ-quants are often preferable over similar sized non-IQ quants)
| Link | Type | Size/GB | Notes |
|:-----|:-----|--------:|:------|
| [GGUF](https://huggingface.co/mradermacher/Llama-3-8B-Instruct-UltraDPO3-NT-GGUF/resolve/main/Llama-3-8B-Instruct-UltraDPO3-NT.Q2_K.gguf) | Q2_K | 3.3 | |
| [GGUF](https://huggingface.co/mradermacher/Llama-3-8B-Instruct-UltraDPO3-NT-GGUF/resolve/main/Llama-3-8B-Instruct-UltraDPO3-NT.IQ3_XS.gguf) | IQ3_XS | 3.6 | |
| [GGUF](https://huggingface.co/mradermacher/Llama-3-8B-Instruct-UltraDPO3-NT-GGUF/resolve/main/Llama-3-8B-Instruct-UltraDPO3-NT.Q3_K_S.gguf) | Q3_K_S | 3.8 | |
| [GGUF](https://huggingface.co/mradermacher/Llama-3-8B-Instruct-UltraDPO3-NT-GGUF/resolve/main/Llama-3-8B-Instruct-UltraDPO3-NT.IQ3_S.gguf) | IQ3_S | 3.8 | beats Q3_K* |
| [GGUF](https://huggingface.co/mradermacher/Llama-3-8B-Instruct-UltraDPO3-NT-GGUF/resolve/main/Llama-3-8B-Instruct-UltraDPO3-NT.IQ3_M.gguf) | IQ3_M | 3.9 | |
| [GGUF](https://huggingface.co/mradermacher/Llama-3-8B-Instruct-UltraDPO3-NT-GGUF/resolve/main/Llama-3-8B-Instruct-UltraDPO3-NT.Q3_K_M.gguf) | Q3_K_M | 4.1 | lower quality |
| [GGUF](https://huggingface.co/mradermacher/Llama-3-8B-Instruct-UltraDPO3-NT-GGUF/resolve/main/Llama-3-8B-Instruct-UltraDPO3-NT.Q3_K_L.gguf) | Q3_K_L | 4.4 | |
| [GGUF](https://huggingface.co/mradermacher/Llama-3-8B-Instruct-UltraDPO3-NT-GGUF/resolve/main/Llama-3-8B-Instruct-UltraDPO3-NT.IQ4_XS.gguf) | IQ4_XS | 4.6 | |
| [GGUF](https://huggingface.co/mradermacher/Llama-3-8B-Instruct-UltraDPO3-NT-GGUF/resolve/main/Llama-3-8B-Instruct-UltraDPO3-NT.Q4_K_S.gguf) | Q4_K_S | 4.8 | fast, recommended |
| [GGUF](https://huggingface.co/mradermacher/Llama-3-8B-Instruct-UltraDPO3-NT-GGUF/resolve/main/Llama-3-8B-Instruct-UltraDPO3-NT.Q4_K_M.gguf) | Q4_K_M | 5.0 | fast, recommended |
| [GGUF](https://huggingface.co/mradermacher/Llama-3-8B-Instruct-UltraDPO3-NT-GGUF/resolve/main/Llama-3-8B-Instruct-UltraDPO3-NT.Q5_K_S.gguf) | Q5_K_S | 5.7 | |
| [GGUF](https://huggingface.co/mradermacher/Llama-3-8B-Instruct-UltraDPO3-NT-GGUF/resolve/main/Llama-3-8B-Instruct-UltraDPO3-NT.Q5_K_M.gguf) | Q5_K_M | 5.8 | |
| [GGUF](https://huggingface.co/mradermacher/Llama-3-8B-Instruct-UltraDPO3-NT-GGUF/resolve/main/Llama-3-8B-Instruct-UltraDPO3-NT.Q6_K.gguf) | Q6_K | 6.7 | very good quality |
| [GGUF](https://huggingface.co/mradermacher/Llama-3-8B-Instruct-UltraDPO3-NT-GGUF/resolve/main/Llama-3-8B-Instruct-UltraDPO3-NT.Q8_0.gguf) | Q8_0 | 8.6 | fast, best quality |
| [GGUF](https://huggingface.co/mradermacher/Llama-3-8B-Instruct-UltraDPO3-NT-GGUF/resolve/main/Llama-3-8B-Instruct-UltraDPO3-NT.f16.gguf) | f16 | 16.2 | 16 bpw, overkill |
Here is a handy graph by ikawrakow comparing some lower-quality quant
types (lower is better):

And here are Artefact2's thoughts on the matter:
https://gist.github.com/Artefact2/b5f810600771265fc1e39442288e8ec9
## FAQ / Model Request
See https://huggingface.co/mradermacher/model_requests for some answers to
questions you might have and/or if you want some other model quantized.
## Thanks
I thank my company, [nethype GmbH](https://www.nethype.de/), for letting
me use its servers and providing upgrades to my workstation to enable
this work in my free time.
<!-- end -->
|
RichardErkhov/macadeliccc_-_Opus-Samantha-Llama-3-8B-gguf | RichardErkhov | "2024-06-21T06:29:20Z" | 16,230 | 0 | null | [
"gguf",
"region:us"
] | null | "2024-06-20T22:11:55Z" | Quantization made by Richard Erkhov.
[Github](https://github.com/RichardErkhov)
[Discord](https://discord.gg/pvy7H8DZMG)
[Request more models](https://github.com/RichardErkhov/quant_request)
Opus-Samantha-Llama-3-8B - GGUF
- Model creator: https://huggingface.co/macadeliccc/
- Original model: https://huggingface.co/macadeliccc/Opus-Samantha-Llama-3-8B/
| Name | Quant method | Size |
| ---- | ---- | ---- |
| [Opus-Samantha-Llama-3-8B.Q2_K.gguf](https://huggingface.co/RichardErkhov/macadeliccc_-_Opus-Samantha-Llama-3-8B-gguf/blob/main/Opus-Samantha-Llama-3-8B.Q2_K.gguf) | Q2_K | 2.96GB |
| [Opus-Samantha-Llama-3-8B.IQ3_XS.gguf](https://huggingface.co/RichardErkhov/macadeliccc_-_Opus-Samantha-Llama-3-8B-gguf/blob/main/Opus-Samantha-Llama-3-8B.IQ3_XS.gguf) | IQ3_XS | 3.28GB |
| [Opus-Samantha-Llama-3-8B.IQ3_S.gguf](https://huggingface.co/RichardErkhov/macadeliccc_-_Opus-Samantha-Llama-3-8B-gguf/blob/main/Opus-Samantha-Llama-3-8B.IQ3_S.gguf) | IQ3_S | 3.43GB |
| [Opus-Samantha-Llama-3-8B.Q3_K_S.gguf](https://huggingface.co/RichardErkhov/macadeliccc_-_Opus-Samantha-Llama-3-8B-gguf/blob/main/Opus-Samantha-Llama-3-8B.Q3_K_S.gguf) | Q3_K_S | 3.41GB |
| [Opus-Samantha-Llama-3-8B.IQ3_M.gguf](https://huggingface.co/RichardErkhov/macadeliccc_-_Opus-Samantha-Llama-3-8B-gguf/blob/main/Opus-Samantha-Llama-3-8B.IQ3_M.gguf) | IQ3_M | 3.52GB |
| [Opus-Samantha-Llama-3-8B.Q3_K.gguf](https://huggingface.co/RichardErkhov/macadeliccc_-_Opus-Samantha-Llama-3-8B-gguf/blob/main/Opus-Samantha-Llama-3-8B.Q3_K.gguf) | Q3_K | 3.74GB |
| [Opus-Samantha-Llama-3-8B.Q3_K_M.gguf](https://huggingface.co/RichardErkhov/macadeliccc_-_Opus-Samantha-Llama-3-8B-gguf/blob/main/Opus-Samantha-Llama-3-8B.Q3_K_M.gguf) | Q3_K_M | 3.74GB |
| [Opus-Samantha-Llama-3-8B.Q3_K_L.gguf](https://huggingface.co/RichardErkhov/macadeliccc_-_Opus-Samantha-Llama-3-8B-gguf/blob/main/Opus-Samantha-Llama-3-8B.Q3_K_L.gguf) | Q3_K_L | 4.03GB |
| [Opus-Samantha-Llama-3-8B.IQ4_XS.gguf](https://huggingface.co/RichardErkhov/macadeliccc_-_Opus-Samantha-Llama-3-8B-gguf/blob/main/Opus-Samantha-Llama-3-8B.IQ4_XS.gguf) | IQ4_XS | 4.18GB |
| [Opus-Samantha-Llama-3-8B.Q4_0.gguf](https://huggingface.co/RichardErkhov/macadeliccc_-_Opus-Samantha-Llama-3-8B-gguf/blob/main/Opus-Samantha-Llama-3-8B.Q4_0.gguf) | Q4_0 | 4.34GB |
| [Opus-Samantha-Llama-3-8B.IQ4_NL.gguf](https://huggingface.co/RichardErkhov/macadeliccc_-_Opus-Samantha-Llama-3-8B-gguf/blob/main/Opus-Samantha-Llama-3-8B.IQ4_NL.gguf) | IQ4_NL | 4.38GB |
| [Opus-Samantha-Llama-3-8B.Q4_K_S.gguf](https://huggingface.co/RichardErkhov/macadeliccc_-_Opus-Samantha-Llama-3-8B-gguf/blob/main/Opus-Samantha-Llama-3-8B.Q4_K_S.gguf) | Q4_K_S | 4.37GB |
| [Opus-Samantha-Llama-3-8B.Q4_K.gguf](https://huggingface.co/RichardErkhov/macadeliccc_-_Opus-Samantha-Llama-3-8B-gguf/blob/main/Opus-Samantha-Llama-3-8B.Q4_K.gguf) | Q4_K | 4.58GB |
| [Opus-Samantha-Llama-3-8B.Q4_K_M.gguf](https://huggingface.co/RichardErkhov/macadeliccc_-_Opus-Samantha-Llama-3-8B-gguf/blob/main/Opus-Samantha-Llama-3-8B.Q4_K_M.gguf) | Q4_K_M | 4.58GB |
| [Opus-Samantha-Llama-3-8B.Q4_1.gguf](https://huggingface.co/RichardErkhov/macadeliccc_-_Opus-Samantha-Llama-3-8B-gguf/blob/main/Opus-Samantha-Llama-3-8B.Q4_1.gguf) | Q4_1 | 4.78GB |
| [Opus-Samantha-Llama-3-8B.Q5_0.gguf](https://huggingface.co/RichardErkhov/macadeliccc_-_Opus-Samantha-Llama-3-8B-gguf/blob/main/Opus-Samantha-Llama-3-8B.Q5_0.gguf) | Q5_0 | 5.21GB |
| [Opus-Samantha-Llama-3-8B.Q5_K_S.gguf](https://huggingface.co/RichardErkhov/macadeliccc_-_Opus-Samantha-Llama-3-8B-gguf/blob/main/Opus-Samantha-Llama-3-8B.Q5_K_S.gguf) | Q5_K_S | 5.21GB |
| [Opus-Samantha-Llama-3-8B.Q5_K.gguf](https://huggingface.co/RichardErkhov/macadeliccc_-_Opus-Samantha-Llama-3-8B-gguf/blob/main/Opus-Samantha-Llama-3-8B.Q5_K.gguf) | Q5_K | 5.34GB |
| [Opus-Samantha-Llama-3-8B.Q5_K_M.gguf](https://huggingface.co/RichardErkhov/macadeliccc_-_Opus-Samantha-Llama-3-8B-gguf/blob/main/Opus-Samantha-Llama-3-8B.Q5_K_M.gguf) | Q5_K_M | 5.34GB |
| [Opus-Samantha-Llama-3-8B.Q5_1.gguf](https://huggingface.co/RichardErkhov/macadeliccc_-_Opus-Samantha-Llama-3-8B-gguf/blob/main/Opus-Samantha-Llama-3-8B.Q5_1.gguf) | Q5_1 | 5.65GB |
| [Opus-Samantha-Llama-3-8B.Q6_K.gguf](https://huggingface.co/RichardErkhov/macadeliccc_-_Opus-Samantha-Llama-3-8B-gguf/blob/main/Opus-Samantha-Llama-3-8B.Q6_K.gguf) | Q6_K | 6.14GB |
| [Opus-Samantha-Llama-3-8B.Q8_0.gguf](https://huggingface.co/RichardErkhov/macadeliccc_-_Opus-Samantha-Llama-3-8B-gguf/blob/main/Opus-Samantha-Llama-3-8B.Q8_0.gguf) | Q8_0 | 7.95GB |
Original model description:
---
license: apache-2.0
datasets:
- macadeliccc/opus_samantha
---
# Opus-Samantha-Llama-3-8B
Trained on 1xA100
**5/11/24: Model has been updated and performs much better**
## Process
- Original Model: [meta-llama/Meta-Llama-3-8B](https://huggingface.co/meta-llama/Meta-Llama-3-8B)
- Datatset: [macadeliccc/opus_samantha](https://huggingface.co/datasets/macadeliccc/opus_samantha)
## 💻 Usage
```python
!pip install -qU transformers torch
import transformers
import torch
model_id = "macadeliccc/Opus-Samantha-Llama-3-8B"
pipeline = transformers.pipeline(
pipeline("Hey how are you doing today?")
```
|
RichardErkhov/scb10x_-_llama-3-typhoon-v1.5-8b-gguf | RichardErkhov | "2024-06-25T19:47:53Z" | 16,225 | 0 | null | [
"gguf",
"arxiv:2312.13951",
"region:us"
] | null | "2024-06-25T14:38:48Z" | Quantization made by Richard Erkhov.
[Github](https://github.com/RichardErkhov)
[Discord](https://discord.gg/pvy7H8DZMG)
[Request more models](https://github.com/RichardErkhov/quant_request)
llama-3-typhoon-v1.5-8b - GGUF
- Model creator: https://huggingface.co/scb10x/
- Original model: https://huggingface.co/scb10x/llama-3-typhoon-v1.5-8b/
| Name | Quant method | Size |
| ---- | ---- | ---- |
| [llama-3-typhoon-v1.5-8b.Q2_K.gguf](https://huggingface.co/RichardErkhov/scb10x_-_llama-3-typhoon-v1.5-8b-gguf/blob/main/llama-3-typhoon-v1.5-8b.Q2_K.gguf) | Q2_K | 2.96GB |
| [llama-3-typhoon-v1.5-8b.IQ3_XS.gguf](https://huggingface.co/RichardErkhov/scb10x_-_llama-3-typhoon-v1.5-8b-gguf/blob/main/llama-3-typhoon-v1.5-8b.IQ3_XS.gguf) | IQ3_XS | 3.28GB |
| [llama-3-typhoon-v1.5-8b.IQ3_S.gguf](https://huggingface.co/RichardErkhov/scb10x_-_llama-3-typhoon-v1.5-8b-gguf/blob/main/llama-3-typhoon-v1.5-8b.IQ3_S.gguf) | IQ3_S | 3.43GB |
| [llama-3-typhoon-v1.5-8b.Q3_K_S.gguf](https://huggingface.co/RichardErkhov/scb10x_-_llama-3-typhoon-v1.5-8b-gguf/blob/main/llama-3-typhoon-v1.5-8b.Q3_K_S.gguf) | Q3_K_S | 3.41GB |
| [llama-3-typhoon-v1.5-8b.IQ3_M.gguf](https://huggingface.co/RichardErkhov/scb10x_-_llama-3-typhoon-v1.5-8b-gguf/blob/main/llama-3-typhoon-v1.5-8b.IQ3_M.gguf) | IQ3_M | 3.52GB |
| [llama-3-typhoon-v1.5-8b.Q3_K.gguf](https://huggingface.co/RichardErkhov/scb10x_-_llama-3-typhoon-v1.5-8b-gguf/blob/main/llama-3-typhoon-v1.5-8b.Q3_K.gguf) | Q3_K | 3.74GB |
| [llama-3-typhoon-v1.5-8b.Q3_K_M.gguf](https://huggingface.co/RichardErkhov/scb10x_-_llama-3-typhoon-v1.5-8b-gguf/blob/main/llama-3-typhoon-v1.5-8b.Q3_K_M.gguf) | Q3_K_M | 3.74GB |
| [llama-3-typhoon-v1.5-8b.Q3_K_L.gguf](https://huggingface.co/RichardErkhov/scb10x_-_llama-3-typhoon-v1.5-8b-gguf/blob/main/llama-3-typhoon-v1.5-8b.Q3_K_L.gguf) | Q3_K_L | 4.03GB |
| [llama-3-typhoon-v1.5-8b.IQ4_XS.gguf](https://huggingface.co/RichardErkhov/scb10x_-_llama-3-typhoon-v1.5-8b-gguf/blob/main/llama-3-typhoon-v1.5-8b.IQ4_XS.gguf) | IQ4_XS | 4.18GB |
| [llama-3-typhoon-v1.5-8b.Q4_0.gguf](https://huggingface.co/RichardErkhov/scb10x_-_llama-3-typhoon-v1.5-8b-gguf/blob/main/llama-3-typhoon-v1.5-8b.Q4_0.gguf) | Q4_0 | 4.34GB |
| [llama-3-typhoon-v1.5-8b.IQ4_NL.gguf](https://huggingface.co/RichardErkhov/scb10x_-_llama-3-typhoon-v1.5-8b-gguf/blob/main/llama-3-typhoon-v1.5-8b.IQ4_NL.gguf) | IQ4_NL | 4.38GB |
| [llama-3-typhoon-v1.5-8b.Q4_K_S.gguf](https://huggingface.co/RichardErkhov/scb10x_-_llama-3-typhoon-v1.5-8b-gguf/blob/main/llama-3-typhoon-v1.5-8b.Q4_K_S.gguf) | Q4_K_S | 4.37GB |
| [llama-3-typhoon-v1.5-8b.Q4_K.gguf](https://huggingface.co/RichardErkhov/scb10x_-_llama-3-typhoon-v1.5-8b-gguf/blob/main/llama-3-typhoon-v1.5-8b.Q4_K.gguf) | Q4_K | 4.58GB |
| [llama-3-typhoon-v1.5-8b.Q4_K_M.gguf](https://huggingface.co/RichardErkhov/scb10x_-_llama-3-typhoon-v1.5-8b-gguf/blob/main/llama-3-typhoon-v1.5-8b.Q4_K_M.gguf) | Q4_K_M | 4.58GB |
| [llama-3-typhoon-v1.5-8b.Q4_1.gguf](https://huggingface.co/RichardErkhov/scb10x_-_llama-3-typhoon-v1.5-8b-gguf/blob/main/llama-3-typhoon-v1.5-8b.Q4_1.gguf) | Q4_1 | 4.78GB |
| [llama-3-typhoon-v1.5-8b.Q5_0.gguf](https://huggingface.co/RichardErkhov/scb10x_-_llama-3-typhoon-v1.5-8b-gguf/blob/main/llama-3-typhoon-v1.5-8b.Q5_0.gguf) | Q5_0 | 5.21GB |
| [llama-3-typhoon-v1.5-8b.Q5_K_S.gguf](https://huggingface.co/RichardErkhov/scb10x_-_llama-3-typhoon-v1.5-8b-gguf/blob/main/llama-3-typhoon-v1.5-8b.Q5_K_S.gguf) | Q5_K_S | 5.21GB |
| [llama-3-typhoon-v1.5-8b.Q5_K.gguf](https://huggingface.co/RichardErkhov/scb10x_-_llama-3-typhoon-v1.5-8b-gguf/blob/main/llama-3-typhoon-v1.5-8b.Q5_K.gguf) | Q5_K | 5.34GB |
| [llama-3-typhoon-v1.5-8b.Q5_K_M.gguf](https://huggingface.co/RichardErkhov/scb10x_-_llama-3-typhoon-v1.5-8b-gguf/blob/main/llama-3-typhoon-v1.5-8b.Q5_K_M.gguf) | Q5_K_M | 5.34GB |
| [llama-3-typhoon-v1.5-8b.Q5_1.gguf](https://huggingface.co/RichardErkhov/scb10x_-_llama-3-typhoon-v1.5-8b-gguf/blob/main/llama-3-typhoon-v1.5-8b.Q5_1.gguf) | Q5_1 | 5.65GB |
| [llama-3-typhoon-v1.5-8b.Q6_K.gguf](https://huggingface.co/RichardErkhov/scb10x_-_llama-3-typhoon-v1.5-8b-gguf/blob/main/llama-3-typhoon-v1.5-8b.Q6_K.gguf) | Q6_K | 6.14GB |
| [llama-3-typhoon-v1.5-8b.Q8_0.gguf](https://huggingface.co/RichardErkhov/scb10x_-_llama-3-typhoon-v1.5-8b-gguf/blob/main/llama-3-typhoon-v1.5-8b.Q8_0.gguf) | Q8_0 | 7.95GB |
Original model description:
---
license: llama3
language:
- th
- en
pipeline_tag: text-generation
tags:
- pretrained
---
**Llama-3-Typhoon-v1.5-8B: Thai Large Language Model (Pretrained)**
**Typhoon-8B** is a *pretrained only* Thai 🇹🇭 large language model with 8 billion parameters, and it is based on Llama3-8B.
For release notes, please see our [blog](https://blog.opentyphoon.ai/typhoon-1-5-release-a9364cb8e8d7). *To acknowledge Meta's effort in creating the foundation model and to comply with the license, we explicitly include "llama-3" in the model name.
## **Model Description**
- **Model type**: A 8B pretrained decoder-only model based on Llama architecture.
- **Requirement**: transformers 4.38.0 or newer.
- **Primary Language(s)**: Thai 🇹🇭 and English 🇬🇧
- **License**: [Llama 3 Community License](https://llama.meta.com/llama3/license/)
## **Intended Uses & Limitations**
This model is a pretrained base model. Thus, it may not be able to follow human instructions without using one/few-shot learning or instruction fine-tuning. The model does not have any moderation mechanisms, and may generate harmful or inappropriate responses.
## **Follow us**
**https://twitter.com/opentyphoon**
## **Support**
**https://discord.gg/CqyBscMFpg**
## **SCB10X AI Team**
- Kunat Pipatanakul, Potsawee Manakul, Sittipong Sripaisarnmongkol, Natapong Nitarach, Pathomporn Chokchainant, Kasima Tharnpipitchai
- If you find Typhoon-8B useful for your work, please cite it using:
```
@article{pipatanakul2023typhoon,
title={Typhoon: Thai Large Language Models},
author={Kunat Pipatanakul and Phatrasek Jirabovonvisut and Potsawee Manakul and Sittipong Sripaisarnmongkol and Ruangsak Patomwong and Pathomporn Chokchainant and Kasima Tharnpipitchai},
year={2023},
journal={arXiv preprint arXiv:2312.13951},
url={https://arxiv.org/abs/2312.13951}
}
```
## **Contact Us**
- General & Collaboration: **[[email protected]](mailto:[email protected])**, **[[email protected]](mailto:[email protected])**
- Technical: **[[email protected]](mailto:[email protected])**
|
mradermacher/Fimbulvetr-11B-v2.1-16K-i1-GGUF | mradermacher | "2024-06-30T10:51:08Z" | 16,223 | 2 | transformers | [
"transformers",
"gguf",
"en",
"base_model:Sao10K/Fimbulvetr-11B-v2.1-16K",
"license:cc-by-nc-4.0",
"endpoints_compatible",
"region:us"
] | null | "2024-06-30T06:40:14Z" | ---
base_model: Sao10K/Fimbulvetr-11B-v2.1-16K
language:
- en
library_name: transformers
license: cc-by-nc-4.0
quantized_by: mradermacher
---
## About
<!-- ### quantize_version: 2 -->
<!-- ### output_tensor_quantised: 1 -->
<!-- ### convert_type: hf -->
<!-- ### vocab_type: -->
<!-- ### tags: nicoboss -->
weighted/imatrix quants of https://huggingface.co/Sao10K/Fimbulvetr-11B-v2.1-16K
<!-- provided-files -->
static quants are available at https://huggingface.co/mradermacher/Fimbulvetr-11B-v2.1-16K-GGUF
## Usage
If you are unsure how to use GGUF files, refer to one of [TheBloke's
READMEs](https://huggingface.co/TheBloke/KafkaLM-70B-German-V0.1-GGUF) for
more details, including on how to concatenate multi-part files.
## Provided Quants
(sorted by size, not necessarily quality. IQ-quants are often preferable over similar sized non-IQ quants)
| Link | Type | Size/GB | Notes |
|:-----|:-----|--------:|:------|
| [GGUF](https://huggingface.co/mradermacher/Fimbulvetr-11B-v2.1-16K-i1-GGUF/resolve/main/Fimbulvetr-11B-v2.1-16K.i1-IQ1_S.gguf) | i1-IQ1_S | 2.5 | for the desperate |
| [GGUF](https://huggingface.co/mradermacher/Fimbulvetr-11B-v2.1-16K-i1-GGUF/resolve/main/Fimbulvetr-11B-v2.1-16K.i1-IQ1_M.gguf) | i1-IQ1_M | 2.7 | mostly desperate |
| [GGUF](https://huggingface.co/mradermacher/Fimbulvetr-11B-v2.1-16K-i1-GGUF/resolve/main/Fimbulvetr-11B-v2.1-16K.i1-IQ2_XXS.gguf) | i1-IQ2_XXS | 3.0 | |
| [GGUF](https://huggingface.co/mradermacher/Fimbulvetr-11B-v2.1-16K-i1-GGUF/resolve/main/Fimbulvetr-11B-v2.1-16K.i1-IQ2_XS.gguf) | i1-IQ2_XS | 3.3 | |
| [GGUF](https://huggingface.co/mradermacher/Fimbulvetr-11B-v2.1-16K-i1-GGUF/resolve/main/Fimbulvetr-11B-v2.1-16K.i1-IQ2_S.gguf) | i1-IQ2_S | 3.5 | |
| [GGUF](https://huggingface.co/mradermacher/Fimbulvetr-11B-v2.1-16K-i1-GGUF/resolve/main/Fimbulvetr-11B-v2.1-16K.i1-IQ2_M.gguf) | i1-IQ2_M | 3.8 | |
| [GGUF](https://huggingface.co/mradermacher/Fimbulvetr-11B-v2.1-16K-i1-GGUF/resolve/main/Fimbulvetr-11B-v2.1-16K.i1-Q2_K.gguf) | i1-Q2_K | 4.1 | IQ3_XXS probably better |
| [GGUF](https://huggingface.co/mradermacher/Fimbulvetr-11B-v2.1-16K-i1-GGUF/resolve/main/Fimbulvetr-11B-v2.1-16K.i1-IQ3_XXS.gguf) | i1-IQ3_XXS | 4.3 | lower quality |
| [GGUF](https://huggingface.co/mradermacher/Fimbulvetr-11B-v2.1-16K-i1-GGUF/resolve/main/Fimbulvetr-11B-v2.1-16K.i1-IQ3_XS.gguf) | i1-IQ3_XS | 4.5 | |
| [GGUF](https://huggingface.co/mradermacher/Fimbulvetr-11B-v2.1-16K-i1-GGUF/resolve/main/Fimbulvetr-11B-v2.1-16K.i1-Q3_K_S.gguf) | i1-Q3_K_S | 4.8 | IQ3_XS probably better |
| [GGUF](https://huggingface.co/mradermacher/Fimbulvetr-11B-v2.1-16K-i1-GGUF/resolve/main/Fimbulvetr-11B-v2.1-16K.i1-IQ3_S.gguf) | i1-IQ3_S | 4.8 | beats Q3_K* |
| [GGUF](https://huggingface.co/mradermacher/Fimbulvetr-11B-v2.1-16K-i1-GGUF/resolve/main/Fimbulvetr-11B-v2.1-16K.i1-IQ3_M.gguf) | i1-IQ3_M | 4.9 | |
| [GGUF](https://huggingface.co/mradermacher/Fimbulvetr-11B-v2.1-16K-i1-GGUF/resolve/main/Fimbulvetr-11B-v2.1-16K.i1-Q3_K_M.gguf) | i1-Q3_K_M | 5.3 | IQ3_S probably better |
| [GGUF](https://huggingface.co/mradermacher/Fimbulvetr-11B-v2.1-16K-i1-GGUF/resolve/main/Fimbulvetr-11B-v2.1-16K.i1-Q3_K_L.gguf) | i1-Q3_K_L | 5.8 | IQ3_M probably better |
| [GGUF](https://huggingface.co/mradermacher/Fimbulvetr-11B-v2.1-16K-i1-GGUF/resolve/main/Fimbulvetr-11B-v2.1-16K.i1-IQ4_XS.gguf) | i1-IQ4_XS | 5.9 | |
| [GGUF](https://huggingface.co/mradermacher/Fimbulvetr-11B-v2.1-16K-i1-GGUF/resolve/main/Fimbulvetr-11B-v2.1-16K.i1-Q4_0.gguf) | i1-Q4_0 | 6.2 | fast, low quality |
| [GGUF](https://huggingface.co/mradermacher/Fimbulvetr-11B-v2.1-16K-i1-GGUF/resolve/main/Fimbulvetr-11B-v2.1-16K.i1-Q4_K_S.gguf) | i1-Q4_K_S | 6.2 | optimal size/speed/quality |
| [GGUF](https://huggingface.co/mradermacher/Fimbulvetr-11B-v2.1-16K-i1-GGUF/resolve/main/Fimbulvetr-11B-v2.1-16K.i1-Q4_K_M.gguf) | i1-Q4_K_M | 6.6 | fast, recommended |
| [GGUF](https://huggingface.co/mradermacher/Fimbulvetr-11B-v2.1-16K-i1-GGUF/resolve/main/Fimbulvetr-11B-v2.1-16K.i1-Q5_K_S.gguf) | i1-Q5_K_S | 7.5 | |
| [GGUF](https://huggingface.co/mradermacher/Fimbulvetr-11B-v2.1-16K-i1-GGUF/resolve/main/Fimbulvetr-11B-v2.1-16K.i1-Q5_K_M.gguf) | i1-Q5_K_M | 7.7 | |
| [GGUF](https://huggingface.co/mradermacher/Fimbulvetr-11B-v2.1-16K-i1-GGUF/resolve/main/Fimbulvetr-11B-v2.1-16K.i1-Q6_K.gguf) | i1-Q6_K | 8.9 | practically like static Q6_K |
Here is a handy graph by ikawrakow comparing some lower-quality quant
types (lower is better):

And here are Artefact2's thoughts on the matter:
https://gist.github.com/Artefact2/b5f810600771265fc1e39442288e8ec9
## FAQ / Model Request
See https://huggingface.co/mradermacher/model_requests for some answers to
questions you might have and/or if you want some other model quantized.
## Thanks
I thank my company, [nethype GmbH](https://www.nethype.de/), for letting
me use its servers and providing upgrades to my workstation to enable
this work in my free time. Additional thanks to [@nicoboss](https://huggingface.co/nicoboss) for giving me access to his hardware for calculating the imatrix for these quants.
<!-- end -->
|
tokyotech-llm/Swallow-7b-NVE-instruct-hf | tokyotech-llm | "2024-06-29T08:56:28Z" | 16,221 | 3 | transformers | [
"transformers",
"safetensors",
"llama",
"text-generation",
"en",
"ja",
"arxiv:2404.17790",
"arxiv:2404.17733",
"license:llama2",
"autotrain_compatible",
"endpoints_compatible",
"text-generation-inference",
"region:us"
] | text-generation | "2023-12-07T02:08:59Z" | ---
language:
- en
- ja
library_name: transformers
pipeline_tag: text-generation
license: llama2
model_type: llama
---
# Swallow
Our Swallow model has undergone continual pre-training from the [Llama 2 family](https://huggingface.co/meta-llama), primarily with the addition of Japanese language data. The tuned versions use supervised fine-tuning (SFT).
Links to other models can be found in the index.
# Model Release Updates
We are excited to share the release schedule for our latest models:
- **April 26, 2024**: Released version 0.1 of our enhanced instruction-tuned models: [Swallow-7b-instruct-v0.1](https://huggingface.co/tokyotech-llm/Swallow-7b-instruct-v0.1), [Swallow-13b-instruct-v0.1](https://huggingface.co/tokyotech-llm/Swallow-13b-instruct-v0.1), and [Swallow-70b-instruct-v0.1](https://huggingface.co/tokyotech-llm/Swallow-70b-instruct-v0.1) as preview versions.
- **March 2, 2024**: Released the [Swallow-7b-plus-hf](https://huggingface.co/tokyotech-llm/Swallow-7b-plus-hf), a model trained with approximately twice as many Japanese tokens as [Swallow-7b-hf](https://huggingface.co/tokyotech-llm/Swallow-7b-hf).
- **February 4, 2024**: Released the [Swallow-13b-NVE-hf](https://huggingface.co/tokyotech-llm/Swallow-13b-NVE-hf).
- **January 26, 2024**: Released the [Swallow-7b-NVE-hf](https://huggingface.co/tokyotech-llm/Swallow-7b-NVE-hf), [Swallow-7b-NVE-instruct-hf](https://huggingface.co/tokyotech-llm/Swallow-7b-NVE-instruct-hf), [Swallow-70b-NVE-hf](https://huggingface.co/tokyotech-llm/Swallow-70b-NVE-hf), and [Swallow-70b-NVE-instruct-hf](https://huggingface.co/tokyotech-llm/Swallow-70b-NVE-instruct-hf)
- **December 19, 2023**: Released the [Swallow-7b-hf](https://huggingface.co/tokyotech-llm/Swallow-7b-hf), [Swallow-7b-instruct-hf](https://huggingface.co/tokyotech-llm/Swallow-7b-instruct-hf), [Swallow-13b-hf](https://huggingface.co/tokyotech-llm/Swallow-13b-hf), [Swallow-13b-instruct-hf](https://huggingface.co/tokyotech-llm/Swallow-13b-instruct-hf), [Swallow-70b-hf](https://huggingface.co/tokyotech-llm/Swallow-70b-hf), and [Swallow-70b-instruct-hf](https://huggingface.co/tokyotech-llm/Swallow-70b-instruct-hf).
## Swallow Model Index
|Model|Swallow-hf|Swallow-instruct-hf|Swallow-instruct-v0.1|
|---|---|---|---|
|7B| [Link](https://huggingface.co/tokyotech-llm/Swallow-7b-hf) | [Link](https://huggingface.co/tokyotech-llm/Swallow-7b-instruct-hf)|[Link](https://huggingface.co/tokyotech-llm/Swallow-7b-instruct-v1.0)|
|7B-Plus| [Link](https://huggingface.co/tokyotech-llm/Swallow-7b-plus-hf) | N/A | N/A |
|13B| [Link](https://huggingface.co/tokyotech-llm/Swallow-13b-hf) | [Link](https://huggingface.co/tokyotech-llm/Swallow-13b-instruct-hf)| [Link](https://huggingface.co/tokyotech-llm/Swallow-13b-instruct-v1.0)|
|70B| [Link](https://huggingface.co/tokyotech-llm/Swallow-70b-hf) | [Link](https://huggingface.co/tokyotech-llm/Swallow-70b-instruct-hf)| [Link](https://huggingface.co/tokyotech-llm/Swallow-70b-instruct-v1.0)|
## Swallow Model Index NVE (No Vocabulary Expansion)
|Model|Swallow-NVE-hf|Swallow-NVE-instruct-hf|
|---|---|---|
|7B| [Link](https://huggingface.co/tokyotech-llm/Swallow-7b-NVE-hf) | [Link](https://huggingface.co/tokyotech-llm/Swallow-7b-NVE-instruct-hf)|
|13B| [Link](https://huggingface.co/tokyotech-llm/Swallow-13b-NVE-hf) | N/A |
|70B| [Link](https://huggingface.co/tokyotech-llm/Swallow-70b-NVE-hf) | [Link](https://huggingface.co/tokyotech-llm/Swallow-70b-NVE-instruct-hf)|

This repository provides large language models developed by [TokyoTech-LLM](https://tokyotech-llm.github.io/).
Read our [blog post](https://zenn.dev/tokyotech_lm/articles/d6cb3a8fdfc907) or our [paper](https://arxiv.org/abs/2404.17790)
## Model Details
* **Model type**: Please refer to LLaMA-2 technical report for details on the model architecture.
* **Language(s)**: Japanese English
* **Library**: [Megatron-LM](https://github.com/rioyokotalab/Megatron-Llama2)
* **Tokenizer**: This model employs a tokenizer that features a broadened vocabulary based on Japanese data. This allows for a more efficient representation of text using fewer tokens, leading to a notably faster inference process.
* **Contact**: swallow[at]nlp.c.titech.ac.jp
## Base Model Performance
### Japanese tasks
|Model|Size|JCommonsenseQA|JEMHopQA|NIILC|JSQuAD|XL-Sum|MGSM|WMT20-en-ja|WMT20-ja-en|
|---|---|---|---|---|---|---|---|---|---|
| | |4-shot|4-shot|4-shot|4-shot|1-shot|4-shot|4-shot|4-shot|
| Llama 2 | 7B | 0.3852 | 0.4240 | 0.3410 | 0.7917 | 0.1905 | 0.0760 | 0.1783 | 0.1738 |
| Swallow | 7B | 0.4808 | 0.5078 | 0.5968 | 0.8573 | 0.1830 | 0.1240 | 0.2510 | 0.1511 |
| Swallow-Plus | 7B | 0.5478 | 0.5493 | 0.6030 | 0.8544 | 0.1806 | 0.1360 | 0.2568 | 0.1441 |
| Swallow-NVE | 7B | 0.5433 | 0.5425 | 0.5729 | 0.8684 | 0.2117 | 0.1200 | 0.2405 | 0.1512 |
| Llama 2 | 13B | 0.6997 | 0.4415 | 0.4170 | 0.8533 | 0.2139 | 0.1320 | 0.2146 | 0.1982 |
| Swallow | 13B | 0.7837 | 0.5063 | 0.6398 | 0.9005 | 0.2168 | 0.2040 | 0.2720 | 0.1771 |
| Swallow-NVE | 13B | 0.7712 | 0.5438 | 0.6351 | 0.9030 | 0.2294 | 0.2120 | 0.2735 | 0.1817 |
| Llama 2 | 70B | 0.8686 | 0.4656 | 0.5256 | 0.9080 | 0.2361 | 0.3560 | 0.2643 | **0.2398** |
| Swallow | 70B | 0.9348 | **0.6290** | 0.6960 | 0.9176 | 0.2266 | **0.4840** | **0.3043** | 0.2298 |
| Swallow-NVE | 70B | **0.9410** | 0.5759 | **0.7024** | **0.9254** | **0.2758** | 0.4720 | 0.3042 | 0.2322 |
### English tasks
|Model|Size|OpenBookQA|TriviaQA|HellaSwag|SQuAD2.0|XWINO|GSM8K|
|---|---|---|---|---|---|---|---|
| | |8-shot|8-shot|8-shot|8-shot|8-shot|8-shot|
| Llama 2 | 7B | 0.3580 | 0.6265 | 0.5860 | 0.3207 | 0.9049 | 0.1410 |
| Swallow | 7B | 0.3180 | 0.4836 | 0.5308 | 0.3125 | 0.8817 | 0.1130 |
| Swallow-Plus | 7B | 0.3280 | 0.4558 | 0.5259 | 0.3134 | 0.8929 | 0.1061 |
| Swallow-NVE | 7B | 0.3180 | 0.5079 | 0.5329 | 0.2919 | 0.8817 | 0.0986 |
| Llama 2 | 13B | 0.3760 | 0.7255 | 0.6148 | 0.3681 | 0.9140 | 0.2403 |
| Swallow | 13B | 0.3500 | 0.5852 | 0.5660 | 0.3406 | 0.9075 | 0.2039 |
| Swallow-NVE | 13B | 0.3460 | 0.6025 | 0.5700 | 0.3478 | 0.9006 | 0.1751 |
| Llama 2 | 70B | **0.4280** | **0.8239** | **0.6742** | **0.3770** | **0.9290** | **0.5284** |
| Swallow | 70B | 0.4220 | 0.7756 | 0.6458 | 0.3745 | 0.9204 | 0.4867 |
| Swallow-NVE | 70B | 0.4240 | 0.7817 | 0.6439 | 0.3451 | 0.9256 | 0.4943 |
## Evaluation Benchmarks
### Japanese evaluation benchmarks
We used llm-jp-eval(v1.0.0) and JP Language Model Evaluation Harness(commit #9b42d41). The details are as follows:
- Multiple-choice question answering (JCommonsenseQA [Kurihara+, 2022])
- Open-ended question answering (JEMHopQA [Ishii+, 2023])
- Open-ended question answering (NIILC [Sekine, 2003])
- Machine reading comprehension (JSQuAD [Kurihara+, 2022])
- Automatic summarization (XL-Sum [Hasan+, 2021])
- Machine translation (WMT2020 ja-en [Barrault+, 2020])
- Machine translation (WMT2020 en-ja [Barrault+, 2020])
- Mathematical reasoning (MGSM [Shi+, 2023])
### English evaluation benchmarks
We used the Language Model Evaluation Harness(v.0.3.0). The details are as follows:
- Multiple-choice question answering (OpenBookQA [Mihaylov+, 2018])
- Open-ended question answering (TriviaQA [Joshi+, 2017])
- Machine reading comprehension (SQuAD 2.0 [Rajpurkar+, 2018])
- Commonsense reasoning (XWINO [Tikhonov & Ryabinin, 2021])
- Natural language inference (HellaSwag [Zellers+, 2019])
- Mathematical reasoning (GSM8k [Cobbe+, 2021])
## Usage
First install additional dependencies in [requirements.txt](./requirements.txt):
```sh
pip install -r requirements.txt
```
### Use the instruct model
```python
import torch
from transformers import AutoTokenizer, AutoModelForCausalLM
model_name = "tokyotech-llm/Swallow-7b-instruct-hf"
tokenizer = AutoTokenizer.from_pretrained(model_name)
model = AutoModelForCausalLM.from_pretrained(model_name, torch_dtype=torch.bfloat16, low_cpu_mem_usage=True, device_map="auto")
PROMPT_DICT = {
"prompt_input": (
"以下に、あるタスクを説明する指示があり、それに付随する入力が更なる文脈を提供しています。"
"リクエストを適切に完了するための回答を記述してください。\n\n"
"### 指示:\n{instruction}\n\n### 入力:\n{input}\n\n### 応答:"
),
"prompt_no_input": (
"以下に、あるタスクを説明する指示があります。"
"リクエストを適切に完了するための回答を記述してください。\n\n"
"### 指示:\n{instruction}\n\n### 応答:"
),
}
def create_prompt(instruction, input=None):
"""
Generates a prompt based on the given instruction and an optional input.
If input is provided, it uses the 'prompt_input' template from PROMPT_DICT.
If no input is provided, it uses the 'prompt_no_input' template.
Args:
instruction (str): The instruction describing the task.
input (str, optional): Additional input providing context for the task. Default is None.
Returns:
str: The generated prompt.
"""
if input:
# Use the 'prompt_input' template when additional input is provided
return PROMPT_DICT["prompt_input"].format(instruction=instruction, input=input)
else:
# Use the 'prompt_no_input' template when no additional input is provided
return PROMPT_DICT["prompt_no_input"].format(instruction=instruction)
# Example usage
instruction_example = "以下のトピックに関する詳細な情報を提供してください。"
input_example = "東京工業大学の主なキャンパスについて教えてください"
prompt = create_prompt(instruction_example, input_example)
input_ids = tokenizer.encode(
prompt,
add_special_tokens=False,
return_tensors="pt"
)
tokens = model.generate(
input_ids.to(device=model.device),
max_new_tokens=128,
temperature=0.99,
top_p=0.95,
do_sample=True,
)
out = tokenizer.decode(tokens[0], skip_special_tokens=True)
print(out)
```
### Use the base model
```python
import torch
from transformers import AutoTokenizer, AutoModelForCausalLM
model_name = "tokyotech-llm/Swallow-7b-hf"
tokenizer = AutoTokenizer.from_pretrained(model_name)
model = AutoModelForCausalLM.from_pretrained(model_name, torch_dtype=torch.bfloat16, device_map="auto")
prompt = "東京工業大学の主なキャンパスは、"
input_ids = tokenizer.encode(
prompt,
add_special_tokens=False,
return_tensors="pt"
)
tokens = model.generate(
input_ids.to(device=model.device),
max_new_tokens=128,
temperature=0.99,
top_p=0.95,
do_sample=True,
)
out = tokenizer.decode(tokens[0], skip_special_tokens=True)
print(out)
```
## Training Datasets
### Continual Pre-Training
The following datasets were used for continual pre-training.
- [Japanese Wikipedia](https://dumps.wikimedia.org/other/cirrussearch)
- [RefinedWeb](https://huggingface.co/datasets/tiiuae/falcon-refinedweb)
- [Swallow Corpus](https://arxiv.org/abs/2404.17733)
- [The Pile](https://huggingface.co/datasets/EleutherAI/pile)
### Instruction Tuning
The following datasets were used for the instruction tuning.
- [Anthropic HH-RLHF](https://huggingface.co/datasets/kunishou/hh-rlhf-49k-ja)
- [Databricks Dolly 15-k](https://huggingface.co/datasets/kunishou/databricks-dolly-15k-ja)
- [OpenAssistant Conversations Dataset](https://huggingface.co/datasets/kunishou/oasst1-89k-ja)
## Risks and Limitations
The models released here are still in the early stages of our research and development and have not been tuned to ensure outputs align with human intent and safety considerations.
## Acknowledgements
We thank Meta Research for releasing Llama 2 under an open license for others to build on.
Our project is supported by the [ABCI Large-scale Language Model Building Support Program](https://abci.ai/en/link/llm_support_program.html) of the National Institute of Advanced Industrial Science and Technology.
## License
Llama 2 is licensed under the LLAMA 2 Community License, Copyright © Meta Platforms, Inc. All Rights Reserved.
## Authors
Here are the team members:
- From [Okazaki Laboratory](https://www.nlp.c.titech.ac.jp/index.en.html), the following members:
- [Naoaki Okazaki](https://www.chokkan.org/index.ja.html)
- [Sakae Mizuki](https://s-mizuki-nlp.github.io/)
- [Hiroki Iida](https://meshidenn.github.io/)
- [Mengsay Loem](https://loem-ms.github.io/)
- [Shota Hirai](https://huggingface.co/Kotemo428)
- [Kakeru Hattori](https://aya-se.vercel.app/)
- [Masanari Ohi](https://twitter.com/stjohn2007)
- From [YOKOTA Laboratory](https://www.rio.gsic.titech.ac.jp/en/index.html), the following members:
- [Rio Yokota](https://twitter.com/rioyokota)
- [Kazuki Fujii](https://twitter.com/okoge_kaz)
- [Taishi Nakamura](https://twitter.com/Setuna7777_2)
## How to cite
```
@misc{fujii2024continual,
title={Continual Pre-Training for Cross-Lingual LLM Adaptation: Enhancing Japanese Language Capabilities},
author={Kazuki Fujii and Taishi Nakamura and Mengsay Loem and Hiroki Iida and Masanari Ohi and Kakeru Hattori and Hirai Shota and Sakae Mizuki and Rio Yokota and Naoaki Okazaki},
year={2024},
eprint={2404.17790},
archivePrefix={arXiv},
primaryClass={cs.CL}
}
``` |
RichardErkhov/Yukang_-_Llama-2-13b-chat-longlora-32k-sft-gguf | RichardErkhov | "2024-06-20T13:12:42Z" | 16,205 | 1 | null | [
"gguf",
"region:us"
] | null | "2024-06-20T10:49:06Z" | Quantization made by Richard Erkhov.
[Github](https://github.com/RichardErkhov)
[Discord](https://discord.gg/pvy7H8DZMG)
[Request more models](https://github.com/RichardErkhov/quant_request)
Llama-2-13b-chat-longlora-32k-sft - GGUF
- Model creator: https://huggingface.co/Yukang/
- Original model: https://huggingface.co/Yukang/Llama-2-13b-chat-longlora-32k-sft/
| Name | Quant method | Size |
| ---- | ---- | ---- |
| [Llama-2-13b-chat-longlora-32k-sft.Q2_K.gguf](https://huggingface.co/RichardErkhov/Yukang_-_Llama-2-13b-chat-longlora-32k-sft-gguf/blob/main/Llama-2-13b-chat-longlora-32k-sft.Q2_K.gguf) | Q2_K | 4.52GB |
| [Llama-2-13b-chat-longlora-32k-sft.IQ3_XS.gguf](https://huggingface.co/RichardErkhov/Yukang_-_Llama-2-13b-chat-longlora-32k-sft-gguf/blob/main/Llama-2-13b-chat-longlora-32k-sft.IQ3_XS.gguf) | IQ3_XS | 4.99GB |
| [Llama-2-13b-chat-longlora-32k-sft.IQ3_S.gguf](https://huggingface.co/RichardErkhov/Yukang_-_Llama-2-13b-chat-longlora-32k-sft-gguf/blob/main/Llama-2-13b-chat-longlora-32k-sft.IQ3_S.gguf) | IQ3_S | 5.27GB |
| [Llama-2-13b-chat-longlora-32k-sft.Q3_K_S.gguf](https://huggingface.co/RichardErkhov/Yukang_-_Llama-2-13b-chat-longlora-32k-sft-gguf/blob/main/Llama-2-13b-chat-longlora-32k-sft.Q3_K_S.gguf) | Q3_K_S | 5.27GB |
| [Llama-2-13b-chat-longlora-32k-sft.IQ3_M.gguf](https://huggingface.co/RichardErkhov/Yukang_-_Llama-2-13b-chat-longlora-32k-sft-gguf/blob/main/Llama-2-13b-chat-longlora-32k-sft.IQ3_M.gguf) | IQ3_M | 5.57GB |
| [Llama-2-13b-chat-longlora-32k-sft.Q3_K.gguf](https://huggingface.co/RichardErkhov/Yukang_-_Llama-2-13b-chat-longlora-32k-sft-gguf/blob/main/Llama-2-13b-chat-longlora-32k-sft.Q3_K.gguf) | Q3_K | 5.9GB |
| [Llama-2-13b-chat-longlora-32k-sft.Q3_K_M.gguf](https://huggingface.co/RichardErkhov/Yukang_-_Llama-2-13b-chat-longlora-32k-sft-gguf/blob/main/Llama-2-13b-chat-longlora-32k-sft.Q3_K_M.gguf) | Q3_K_M | 5.9GB |
| [Llama-2-13b-chat-longlora-32k-sft.Q3_K_L.gguf](https://huggingface.co/RichardErkhov/Yukang_-_Llama-2-13b-chat-longlora-32k-sft-gguf/blob/main/Llama-2-13b-chat-longlora-32k-sft.Q3_K_L.gguf) | Q3_K_L | 6.45GB |
| [Llama-2-13b-chat-longlora-32k-sft.IQ4_XS.gguf](https://huggingface.co/RichardErkhov/Yukang_-_Llama-2-13b-chat-longlora-32k-sft-gguf/blob/main/Llama-2-13b-chat-longlora-32k-sft.IQ4_XS.gguf) | IQ4_XS | 6.54GB |
| [Llama-2-13b-chat-longlora-32k-sft.Q4_0.gguf](https://huggingface.co/RichardErkhov/Yukang_-_Llama-2-13b-chat-longlora-32k-sft-gguf/blob/main/Llama-2-13b-chat-longlora-32k-sft.Q4_0.gguf) | Q4_0 | 6.86GB |
| [Llama-2-13b-chat-longlora-32k-sft.IQ4_NL.gguf](https://huggingface.co/RichardErkhov/Yukang_-_Llama-2-13b-chat-longlora-32k-sft-gguf/blob/main/Llama-2-13b-chat-longlora-32k-sft.IQ4_NL.gguf) | IQ4_NL | 6.9GB |
| [Llama-2-13b-chat-longlora-32k-sft.Q4_K_S.gguf](https://huggingface.co/RichardErkhov/Yukang_-_Llama-2-13b-chat-longlora-32k-sft-gguf/blob/main/Llama-2-13b-chat-longlora-32k-sft.Q4_K_S.gguf) | Q4_K_S | 6.91GB |
| [Llama-2-13b-chat-longlora-32k-sft.Q4_K.gguf](https://huggingface.co/RichardErkhov/Yukang_-_Llama-2-13b-chat-longlora-32k-sft-gguf/blob/main/Llama-2-13b-chat-longlora-32k-sft.Q4_K.gguf) | Q4_K | 7.33GB |
| [Llama-2-13b-chat-longlora-32k-sft.Q4_K_M.gguf](https://huggingface.co/RichardErkhov/Yukang_-_Llama-2-13b-chat-longlora-32k-sft-gguf/blob/main/Llama-2-13b-chat-longlora-32k-sft.Q4_K_M.gguf) | Q4_K_M | 7.33GB |
| [Llama-2-13b-chat-longlora-32k-sft.Q4_1.gguf](https://huggingface.co/RichardErkhov/Yukang_-_Llama-2-13b-chat-longlora-32k-sft-gguf/blob/main/Llama-2-13b-chat-longlora-32k-sft.Q4_1.gguf) | Q4_1 | 7.61GB |
| [Llama-2-13b-chat-longlora-32k-sft.Q5_0.gguf](https://huggingface.co/RichardErkhov/Yukang_-_Llama-2-13b-chat-longlora-32k-sft-gguf/blob/main/Llama-2-13b-chat-longlora-32k-sft.Q5_0.gguf) | Q5_0 | 8.36GB |
| [Llama-2-13b-chat-longlora-32k-sft.Q5_K_S.gguf](https://huggingface.co/RichardErkhov/Yukang_-_Llama-2-13b-chat-longlora-32k-sft-gguf/blob/main/Llama-2-13b-chat-longlora-32k-sft.Q5_K_S.gguf) | Q5_K_S | 8.36GB |
| [Llama-2-13b-chat-longlora-32k-sft.Q5_K.gguf](https://huggingface.co/RichardErkhov/Yukang_-_Llama-2-13b-chat-longlora-32k-sft-gguf/blob/main/Llama-2-13b-chat-longlora-32k-sft.Q5_K.gguf) | Q5_K | 8.6GB |
| [Llama-2-13b-chat-longlora-32k-sft.Q5_K_M.gguf](https://huggingface.co/RichardErkhov/Yukang_-_Llama-2-13b-chat-longlora-32k-sft-gguf/blob/main/Llama-2-13b-chat-longlora-32k-sft.Q5_K_M.gguf) | Q5_K_M | 8.6GB |
| [Llama-2-13b-chat-longlora-32k-sft.Q5_1.gguf](https://huggingface.co/RichardErkhov/Yukang_-_Llama-2-13b-chat-longlora-32k-sft-gguf/blob/main/Llama-2-13b-chat-longlora-32k-sft.Q5_1.gguf) | Q5_1 | 9.1GB |
| [Llama-2-13b-chat-longlora-32k-sft.Q6_K.gguf](https://huggingface.co/RichardErkhov/Yukang_-_Llama-2-13b-chat-longlora-32k-sft-gguf/blob/main/Llama-2-13b-chat-longlora-32k-sft.Q6_K.gguf) | Q6_K | 9.95GB |
| [Llama-2-13b-chat-longlora-32k-sft.Q8_0.gguf](https://huggingface.co/RichardErkhov/Yukang_-_Llama-2-13b-chat-longlora-32k-sft-gguf/blob/main/Llama-2-13b-chat-longlora-32k-sft.Q8_0.gguf) | Q8_0 | 12.88GB |
Original model description:
**We release the long instruction-following dataset**, [LongAlpaca-12k](https://drive.google.com/file/d/1JVC1p_Ht-1h61tKitOCW0blnCHf-552U/view?usp=share_link) and **the corresponding models**, [LongAlpaca-7B](https://huggingface.co/Yukang/LongAlpaca-7B), [LongAlpaca-13B](https://huggingface.co/Yukang/LongAlpaca-13B), and [LongAlpaca-70B](https://huggingface.co/Yukang/LongAlpaca-70B).
- (*These sft models*, [Llama-2-13b-chat-longlora-32k-sft](https://huggingface.co/Yukang/Llama-2-13b-chat-longlora-32k-sft) and [Llama-2-70b-chat-longlora-32k-sft](https://huggingface.co/Yukang/Llama-2-70b-chat-longlora-32k-sft), *have been depreciated*.)
|
mradermacher/Llama-3-NeuralHercules-5.0-8B-i1-GGUF | mradermacher | "2024-06-27T08:58:02Z" | 16,203 | 0 | transformers | [
"transformers",
"gguf",
"code",
"chemistry",
"medical",
"en",
"base_model:Locutusque/Llama-3-NeuralHercules-5.0-8B",
"license:llama3",
"endpoints_compatible",
"region:us"
] | null | "2024-06-27T07:34:41Z" | ---
base_model: Locutusque/Llama-3-NeuralHercules-5.0-8B
language:
- en
library_name: transformers
license: llama3
quantized_by: mradermacher
tags:
- code
- chemistry
- medical
---
## About
<!-- ### quantize_version: 2 -->
<!-- ### output_tensor_quantised: 1 -->
<!-- ### convert_type: hf -->
<!-- ### vocab_type: -->
<!-- ### tags: nicoboss -->
weighted/imatrix quants of https://huggingface.co/Locutusque/Llama-3-NeuralHercules-5.0-8B
<!-- provided-files -->
static quants are available at https://huggingface.co/mradermacher/Llama-3-NeuralHercules-5.0-8B-GGUF
## Usage
If you are unsure how to use GGUF files, refer to one of [TheBloke's
READMEs](https://huggingface.co/TheBloke/KafkaLM-70B-German-V0.1-GGUF) for
more details, including on how to concatenate multi-part files.
## Provided Quants
(sorted by size, not necessarily quality. IQ-quants are often preferable over similar sized non-IQ quants)
| Link | Type | Size/GB | Notes |
|:-----|:-----|--------:|:------|
| [GGUF](https://huggingface.co/mradermacher/Llama-3-NeuralHercules-5.0-8B-i1-GGUF/resolve/main/Llama-3-NeuralHercules-5.0-8B.i1-IQ1_S.gguf) | i1-IQ1_S | 2.1 | for the desperate |
| [GGUF](https://huggingface.co/mradermacher/Llama-3-NeuralHercules-5.0-8B-i1-GGUF/resolve/main/Llama-3-NeuralHercules-5.0-8B.i1-IQ1_M.gguf) | i1-IQ1_M | 2.3 | mostly desperate |
| [GGUF](https://huggingface.co/mradermacher/Llama-3-NeuralHercules-5.0-8B-i1-GGUF/resolve/main/Llama-3-NeuralHercules-5.0-8B.i1-IQ2_XXS.gguf) | i1-IQ2_XXS | 2.5 | |
| [GGUF](https://huggingface.co/mradermacher/Llama-3-NeuralHercules-5.0-8B-i1-GGUF/resolve/main/Llama-3-NeuralHercules-5.0-8B.i1-IQ2_XS.gguf) | i1-IQ2_XS | 2.7 | |
| [GGUF](https://huggingface.co/mradermacher/Llama-3-NeuralHercules-5.0-8B-i1-GGUF/resolve/main/Llama-3-NeuralHercules-5.0-8B.i1-IQ2_S.gguf) | i1-IQ2_S | 2.9 | |
| [GGUF](https://huggingface.co/mradermacher/Llama-3-NeuralHercules-5.0-8B-i1-GGUF/resolve/main/Llama-3-NeuralHercules-5.0-8B.i1-IQ2_M.gguf) | i1-IQ2_M | 3.0 | |
| [GGUF](https://huggingface.co/mradermacher/Llama-3-NeuralHercules-5.0-8B-i1-GGUF/resolve/main/Llama-3-NeuralHercules-5.0-8B.i1-Q2_K.gguf) | i1-Q2_K | 3.3 | IQ3_XXS probably better |
| [GGUF](https://huggingface.co/mradermacher/Llama-3-NeuralHercules-5.0-8B-i1-GGUF/resolve/main/Llama-3-NeuralHercules-5.0-8B.i1-IQ3_XXS.gguf) | i1-IQ3_XXS | 3.4 | lower quality |
| [GGUF](https://huggingface.co/mradermacher/Llama-3-NeuralHercules-5.0-8B-i1-GGUF/resolve/main/Llama-3-NeuralHercules-5.0-8B.i1-IQ3_XS.gguf) | i1-IQ3_XS | 3.6 | |
| [GGUF](https://huggingface.co/mradermacher/Llama-3-NeuralHercules-5.0-8B-i1-GGUF/resolve/main/Llama-3-NeuralHercules-5.0-8B.i1-Q3_K_S.gguf) | i1-Q3_K_S | 3.8 | IQ3_XS probably better |
| [GGUF](https://huggingface.co/mradermacher/Llama-3-NeuralHercules-5.0-8B-i1-GGUF/resolve/main/Llama-3-NeuralHercules-5.0-8B.i1-IQ3_S.gguf) | i1-IQ3_S | 3.8 | beats Q3_K* |
| [GGUF](https://huggingface.co/mradermacher/Llama-3-NeuralHercules-5.0-8B-i1-GGUF/resolve/main/Llama-3-NeuralHercules-5.0-8B.i1-IQ3_M.gguf) | i1-IQ3_M | 3.9 | |
| [GGUF](https://huggingface.co/mradermacher/Llama-3-NeuralHercules-5.0-8B-i1-GGUF/resolve/main/Llama-3-NeuralHercules-5.0-8B.i1-Q3_K_M.gguf) | i1-Q3_K_M | 4.1 | IQ3_S probably better |
| [GGUF](https://huggingface.co/mradermacher/Llama-3-NeuralHercules-5.0-8B-i1-GGUF/resolve/main/Llama-3-NeuralHercules-5.0-8B.i1-Q3_K_L.gguf) | i1-Q3_K_L | 4.4 | IQ3_M probably better |
| [GGUF](https://huggingface.co/mradermacher/Llama-3-NeuralHercules-5.0-8B-i1-GGUF/resolve/main/Llama-3-NeuralHercules-5.0-8B.i1-IQ4_XS.gguf) | i1-IQ4_XS | 4.5 | |
| [GGUF](https://huggingface.co/mradermacher/Llama-3-NeuralHercules-5.0-8B-i1-GGUF/resolve/main/Llama-3-NeuralHercules-5.0-8B.i1-Q4_0.gguf) | i1-Q4_0 | 4.8 | fast, low quality |
| [GGUF](https://huggingface.co/mradermacher/Llama-3-NeuralHercules-5.0-8B-i1-GGUF/resolve/main/Llama-3-NeuralHercules-5.0-8B.i1-Q4_K_S.gguf) | i1-Q4_K_S | 4.8 | optimal size/speed/quality |
| [GGUF](https://huggingface.co/mradermacher/Llama-3-NeuralHercules-5.0-8B-i1-GGUF/resolve/main/Llama-3-NeuralHercules-5.0-8B.i1-Q4_K_M.gguf) | i1-Q4_K_M | 5.0 | fast, recommended |
| [GGUF](https://huggingface.co/mradermacher/Llama-3-NeuralHercules-5.0-8B-i1-GGUF/resolve/main/Llama-3-NeuralHercules-5.0-8B.i1-Q5_K_S.gguf) | i1-Q5_K_S | 5.7 | |
| [GGUF](https://huggingface.co/mradermacher/Llama-3-NeuralHercules-5.0-8B-i1-GGUF/resolve/main/Llama-3-NeuralHercules-5.0-8B.i1-Q5_K_M.gguf) | i1-Q5_K_M | 5.8 | |
| [GGUF](https://huggingface.co/mradermacher/Llama-3-NeuralHercules-5.0-8B-i1-GGUF/resolve/main/Llama-3-NeuralHercules-5.0-8B.i1-Q6_K.gguf) | i1-Q6_K | 6.7 | practically like static Q6_K |
Here is a handy graph by ikawrakow comparing some lower-quality quant
types (lower is better):

And here are Artefact2's thoughts on the matter:
https://gist.github.com/Artefact2/b5f810600771265fc1e39442288e8ec9
## FAQ / Model Request
See https://huggingface.co/mradermacher/model_requests for some answers to
questions you might have and/or if you want some other model quantized.
## Thanks
I thank my company, [nethype GmbH](https://www.nethype.de/), for letting
me use its servers and providing upgrades to my workstation to enable
this work in my free time. Additional thanks to [@nicoboss](https://huggingface.co/nicoboss) for giving me access to his hardware for calculating the imatrix for these quants.
<!-- end -->
|
TheBloke/Wizard-Vicuna-13B-Uncensored-GGUF | TheBloke | "2023-09-27T12:52:30Z" | 16,202 | 45 | transformers | [
"transformers",
"gguf",
"llama",
"uncensored",
"en",
"dataset:ehartford/wizard_vicuna_70k_unfiltered",
"base_model:ehartford/Wizard-Vicuna-13B-Uncensored",
"license:other",
"text-generation-inference",
"region:us"
] | null | "2023-09-19T22:57:46Z" | ---
language:
- en
license: other
tags:
- uncensored
datasets:
- ehartford/wizard_vicuna_70k_unfiltered
model_name: Wizard Vicuna 13B Uncensored
base_model: ehartford/Wizard-Vicuna-13B-Uncensored
inference: false
model_creator: Eric Hartford
model_type: llama
prompt_template: 'A chat between a curious user and an artificial intelligence assistant.
The assistant gives helpful, detailed, and polite answers to the user''s questions.
USER: {prompt} ASSISTANT:
'
quantized_by: TheBloke
---
<!-- header start -->
<!-- 200823 -->
<div style="width: auto; margin-left: auto; margin-right: auto">
<img src="https://i.imgur.com/EBdldam.jpg" alt="TheBlokeAI" style="width: 100%; min-width: 400px; display: block; margin: auto;">
</div>
<div style="display: flex; justify-content: space-between; width: 100%;">
<div style="display: flex; flex-direction: column; align-items: flex-start;">
<p style="margin-top: 0.5em; margin-bottom: 0em;"><a href="https://discord.gg/theblokeai">Chat & support: TheBloke's Discord server</a></p>
</div>
<div style="display: flex; flex-direction: column; align-items: flex-end;">
<p style="margin-top: 0.5em; margin-bottom: 0em;"><a href="https://www.patreon.com/TheBlokeAI">Want to contribute? TheBloke's Patreon page</a></p>
</div>
</div>
<div style="text-align:center; margin-top: 0em; margin-bottom: 0em"><p style="margin-top: 0.25em; margin-bottom: 0em;">TheBloke's LLM work is generously supported by a grant from <a href="https://a16z.com">andreessen horowitz (a16z)</a></p></div>
<hr style="margin-top: 1.0em; margin-bottom: 1.0em;">
<!-- header end -->
# Wizard Vicuna 13B Uncensored - GGUF
- Model creator: [Eric Hartford](https://huggingface.co/ehartford)
- Original model: [Wizard Vicuna 13B Uncensored](https://huggingface.co/ehartford/Wizard-Vicuna-13B-Uncensored)
<!-- description start -->
## Description
This repo contains GGUF format model files for [Eric Hartford's Wizard Vicuna 13B Uncensored](https://huggingface.co/ehartford/Wizard-Vicuna-13B-Uncensored).
<!-- description end -->
<!-- README_GGUF.md-about-gguf start -->
### About GGUF
GGUF is a new format introduced by the llama.cpp team on August 21st 2023. It is a replacement for GGML, which is no longer supported by llama.cpp.
Here is an incomplate list of clients and libraries that are known to support GGUF:
* [llama.cpp](https://github.com/ggerganov/llama.cpp). The source project for GGUF. Offers a CLI and a server option.
* [text-generation-webui](https://github.com/oobabooga/text-generation-webui), the most widely used web UI, with many features and powerful extensions. Supports GPU acceleration.
* [KoboldCpp](https://github.com/LostRuins/koboldcpp), a fully featured web UI, with GPU accel across all platforms and GPU architectures. Especially good for story telling.
* [LM Studio](https://lmstudio.ai/), an easy-to-use and powerful local GUI for Windows and macOS (Silicon), with GPU acceleration.
* [LoLLMS Web UI](https://github.com/ParisNeo/lollms-webui), a great web UI with many interesting and unique features, including a full model library for easy model selection.
* [Faraday.dev](https://faraday.dev/), an attractive and easy to use character-based chat GUI for Windows and macOS (both Silicon and Intel), with GPU acceleration.
* [ctransformers](https://github.com/marella/ctransformers), a Python library with GPU accel, LangChain support, and OpenAI-compatible AI server.
* [llama-cpp-python](https://github.com/abetlen/llama-cpp-python), a Python library with GPU accel, LangChain support, and OpenAI-compatible API server.
* [candle](https://github.com/huggingface/candle), a Rust ML framework with a focus on performance, including GPU support, and ease of use.
<!-- README_GGUF.md-about-gguf end -->
<!-- repositories-available start -->
## Repositories available
* [AWQ model(s) for GPU inference.](https://huggingface.co/TheBloke/Wizard-Vicuna-13B-Uncensored-AWQ)
* [GPTQ models for GPU inference, with multiple quantisation parameter options.](https://huggingface.co/TheBloke/Wizard-Vicuna-13B-Uncensored-GPTQ)
* [2, 3, 4, 5, 6 and 8-bit GGUF models for CPU+GPU inference](https://huggingface.co/TheBloke/Wizard-Vicuna-13B-Uncensored-GGUF)
* [Eric Hartford's original unquantised fp16 model in pytorch format, for GPU inference and for further conversions](https://huggingface.co/ehartford/Wizard-Vicuna-13B-Uncensored)
<!-- repositories-available end -->
<!-- prompt-template start -->
## Prompt template: Vicuna
```
A chat between a curious user and an artificial intelligence assistant. The assistant gives helpful, detailed, and polite answers to the user's questions. USER: {prompt} ASSISTANT:
```
<!-- prompt-template end -->
<!-- compatibility_gguf start -->
## Compatibility
These quantised GGUFv2 files are compatible with llama.cpp from August 27th onwards, as of commit [d0cee0d](https://github.com/ggerganov/llama.cpp/commit/d0cee0d36d5be95a0d9088b674dbb27354107221)
They are also compatible with many third party UIs and libraries - please see the list at the top of this README.
## Explanation of quantisation methods
<details>
<summary>Click to see details</summary>
The new methods available are:
* GGML_TYPE_Q2_K - "type-1" 2-bit quantization in super-blocks containing 16 blocks, each block having 16 weight. Block scales and mins are quantized with 4 bits. This ends up effectively using 2.5625 bits per weight (bpw)
* GGML_TYPE_Q3_K - "type-0" 3-bit quantization in super-blocks containing 16 blocks, each block having 16 weights. Scales are quantized with 6 bits. This end up using 3.4375 bpw.
* GGML_TYPE_Q4_K - "type-1" 4-bit quantization in super-blocks containing 8 blocks, each block having 32 weights. Scales and mins are quantized with 6 bits. This ends up using 4.5 bpw.
* GGML_TYPE_Q5_K - "type-1" 5-bit quantization. Same super-block structure as GGML_TYPE_Q4_K resulting in 5.5 bpw
* GGML_TYPE_Q6_K - "type-0" 6-bit quantization. Super-blocks with 16 blocks, each block having 16 weights. Scales are quantized with 8 bits. This ends up using 6.5625 bpw
Refer to the Provided Files table below to see what files use which methods, and how.
</details>
<!-- compatibility_gguf end -->
<!-- README_GGUF.md-provided-files start -->
## Provided files
| Name | Quant method | Bits | Size | Max RAM required | Use case |
| ---- | ---- | ---- | ---- | ---- | ----- |
| [Wizard-Vicuna-13B-Uncensored.Q2_K.gguf](https://huggingface.co/TheBloke/Wizard-Vicuna-13B-Uncensored-GGUF/blob/main/Wizard-Vicuna-13B-Uncensored.Q2_K.gguf) | Q2_K | 2 | 5.43 GB| 7.93 GB | smallest, significant quality loss - not recommended for most purposes |
| [Wizard-Vicuna-13B-Uncensored.Q3_K_S.gguf](https://huggingface.co/TheBloke/Wizard-Vicuna-13B-Uncensored-GGUF/blob/main/Wizard-Vicuna-13B-Uncensored.Q3_K_S.gguf) | Q3_K_S | 3 | 5.66 GB| 8.16 GB | very small, high quality loss |
| [Wizard-Vicuna-13B-Uncensored.Q3_K_M.gguf](https://huggingface.co/TheBloke/Wizard-Vicuna-13B-Uncensored-GGUF/blob/main/Wizard-Vicuna-13B-Uncensored.Q3_K_M.gguf) | Q3_K_M | 3 | 6.34 GB| 8.84 GB | very small, high quality loss |
| [Wizard-Vicuna-13B-Uncensored.Q3_K_L.gguf](https://huggingface.co/TheBloke/Wizard-Vicuna-13B-Uncensored-GGUF/blob/main/Wizard-Vicuna-13B-Uncensored.Q3_K_L.gguf) | Q3_K_L | 3 | 6.93 GB| 9.43 GB | small, substantial quality loss |
| [Wizard-Vicuna-13B-Uncensored.Q4_0.gguf](https://huggingface.co/TheBloke/Wizard-Vicuna-13B-Uncensored-GGUF/blob/main/Wizard-Vicuna-13B-Uncensored.Q4_0.gguf) | Q4_0 | 4 | 7.37 GB| 9.87 GB | legacy; small, very high quality loss - prefer using Q3_K_M |
| [Wizard-Vicuna-13B-Uncensored.Q4_K_S.gguf](https://huggingface.co/TheBloke/Wizard-Vicuna-13B-Uncensored-GGUF/blob/main/Wizard-Vicuna-13B-Uncensored.Q4_K_S.gguf) | Q4_K_S | 4 | 7.41 GB| 9.91 GB | small, greater quality loss |
| [Wizard-Vicuna-13B-Uncensored.Q4_K_M.gguf](https://huggingface.co/TheBloke/Wizard-Vicuna-13B-Uncensored-GGUF/blob/main/Wizard-Vicuna-13B-Uncensored.Q4_K_M.gguf) | Q4_K_M | 4 | 7.87 GB| 10.37 GB | medium, balanced quality - recommended |
| [Wizard-Vicuna-13B-Uncensored.Q5_0.gguf](https://huggingface.co/TheBloke/Wizard-Vicuna-13B-Uncensored-GGUF/blob/main/Wizard-Vicuna-13B-Uncensored.Q5_0.gguf) | Q5_0 | 5 | 8.97 GB| 11.47 GB | legacy; medium, balanced quality - prefer using Q4_K_M |
| [Wizard-Vicuna-13B-Uncensored.Q5_K_S.gguf](https://huggingface.co/TheBloke/Wizard-Vicuna-13B-Uncensored-GGUF/blob/main/Wizard-Vicuna-13B-Uncensored.Q5_K_S.gguf) | Q5_K_S | 5 | 8.97 GB| 11.47 GB | large, low quality loss - recommended |
| [Wizard-Vicuna-13B-Uncensored.Q5_K_M.gguf](https://huggingface.co/TheBloke/Wizard-Vicuna-13B-Uncensored-GGUF/blob/main/Wizard-Vicuna-13B-Uncensored.Q5_K_M.gguf) | Q5_K_M | 5 | 9.23 GB| 11.73 GB | large, very low quality loss - recommended |
| [Wizard-Vicuna-13B-Uncensored.Q6_K.gguf](https://huggingface.co/TheBloke/Wizard-Vicuna-13B-Uncensored-GGUF/blob/main/Wizard-Vicuna-13B-Uncensored.Q6_K.gguf) | Q6_K | 6 | 10.68 GB| 13.18 GB | very large, extremely low quality loss |
| [Wizard-Vicuna-13B-Uncensored.Q8_0.gguf](https://huggingface.co/TheBloke/Wizard-Vicuna-13B-Uncensored-GGUF/blob/main/Wizard-Vicuna-13B-Uncensored.Q8_0.gguf) | Q8_0 | 8 | 13.83 GB| 16.33 GB | very large, extremely low quality loss - not recommended |
**Note**: the above RAM figures assume no GPU offloading. If layers are offloaded to the GPU, this will reduce RAM usage and use VRAM instead.
<!-- README_GGUF.md-provided-files end -->
<!-- README_GGUF.md-how-to-download start -->
## How to download GGUF files
**Note for manual downloaders:** You almost never want to clone the entire repo! Multiple different quantisation formats are provided, and most users only want to pick and download a single file.
The following clients/libraries will automatically download models for you, providing a list of available models to choose from:
- LM Studio
- LoLLMS Web UI
- Faraday.dev
### In `text-generation-webui`
Under Download Model, you can enter the model repo: TheBloke/Wizard-Vicuna-13B-Uncensored-GGUF and below it, a specific filename to download, such as: Wizard-Vicuna-13B-Uncensored.Q4_K_M.gguf.
Then click Download.
### On the command line, including multiple files at once
I recommend using the `huggingface-hub` Python library:
```shell
pip3 install huggingface-hub
```
Then you can download any individual model file to the current directory, at high speed, with a command like this:
```shell
huggingface-cli download TheBloke/Wizard-Vicuna-13B-Uncensored-GGUF Wizard-Vicuna-13B-Uncensored.Q4_K_M.gguf --local-dir . --local-dir-use-symlinks False
```
<details>
<summary>More advanced huggingface-cli download usage</summary>
You can also download multiple files at once with a pattern:
```shell
huggingface-cli download TheBloke/Wizard-Vicuna-13B-Uncensored-GGUF --local-dir . --local-dir-use-symlinks False --include='*Q4_K*gguf'
```
For more documentation on downloading with `huggingface-cli`, please see: [HF -> Hub Python Library -> Download files -> Download from the CLI](https://huggingface.co/docs/huggingface_hub/guides/download#download-from-the-cli).
To accelerate downloads on fast connections (1Gbit/s or higher), install `hf_transfer`:
```shell
pip3 install hf_transfer
```
And set environment variable `HF_HUB_ENABLE_HF_TRANSFER` to `1`:
```shell
HF_HUB_ENABLE_HF_TRANSFER=1 huggingface-cli download TheBloke/Wizard-Vicuna-13B-Uncensored-GGUF Wizard-Vicuna-13B-Uncensored.Q4_K_M.gguf --local-dir . --local-dir-use-symlinks False
```
Windows Command Line users: You can set the environment variable by running `set HF_HUB_ENABLE_HF_TRANSFER=1` before the download command.
</details>
<!-- README_GGUF.md-how-to-download end -->
<!-- README_GGUF.md-how-to-run start -->
## Example `llama.cpp` command
Make sure you are using `llama.cpp` from commit [d0cee0d](https://github.com/ggerganov/llama.cpp/commit/d0cee0d36d5be95a0d9088b674dbb27354107221) or later.
```shell
./main -ngl 32 -m Wizard-Vicuna-13B-Uncensored.Q4_K_M.gguf --color -c 2048 --temp 0.7 --repeat_penalty 1.1 -n -1 -p "A chat between a curious user and an artificial intelligence assistant. The assistant gives helpful, detailed, and polite answers to the user's questions. USER: {prompt} ASSISTANT:"
```
Change `-ngl 32` to the number of layers to offload to GPU. Remove it if you don't have GPU acceleration.
Change `-c 2048` to the desired sequence length. For extended sequence models - eg 8K, 16K, 32K - the necessary RoPE scaling parameters are read from the GGUF file and set by llama.cpp automatically.
If you want to have a chat-style conversation, replace the `-p <PROMPT>` argument with `-i -ins`
For other parameters and how to use them, please refer to [the llama.cpp documentation](https://github.com/ggerganov/llama.cpp/blob/master/examples/main/README.md)
## How to run in `text-generation-webui`
Further instructions here: [text-generation-webui/docs/llama.cpp.md](https://github.com/oobabooga/text-generation-webui/blob/main/docs/llama.cpp.md).
## How to run from Python code
You can use GGUF models from Python using the [llama-cpp-python](https://github.com/abetlen/llama-cpp-python) or [ctransformers](https://github.com/marella/ctransformers) libraries.
### How to load this model in Python code, using ctransformers
#### First install the package
Run one of the following commands, according to your system:
```shell
# Base ctransformers with no GPU acceleration
pip install ctransformers
# Or with CUDA GPU acceleration
pip install ctransformers[cuda]
# Or with AMD ROCm GPU acceleration (Linux only)
CT_HIPBLAS=1 pip install ctransformers --no-binary ctransformers
# Or with Metal GPU acceleration for macOS systems only
CT_METAL=1 pip install ctransformers --no-binary ctransformers
```
#### Simple ctransformers example code
```python
from ctransformers import AutoModelForCausalLM
# Set gpu_layers to the number of layers to offload to GPU. Set to 0 if no GPU acceleration is available on your system.
llm = AutoModelForCausalLM.from_pretrained("TheBloke/Wizard-Vicuna-13B-Uncensored-GGUF", model_file="Wizard-Vicuna-13B-Uncensored.Q4_K_M.gguf", model_type="llama", gpu_layers=50)
print(llm("AI is going to"))
```
## How to use with LangChain
Here are guides on using llama-cpp-python and ctransformers with LangChain:
* [LangChain + llama-cpp-python](https://python.langchain.com/docs/integrations/llms/llamacpp)
* [LangChain + ctransformers](https://python.langchain.com/docs/integrations/providers/ctransformers)
<!-- README_GGUF.md-how-to-run end -->
<!-- footer start -->
<!-- 200823 -->
## Discord
For further support, and discussions on these models and AI in general, join us at:
[TheBloke AI's Discord server](https://discord.gg/theblokeai)
## Thanks, and how to contribute
Thanks to the [chirper.ai](https://chirper.ai) team!
Thanks to Clay from [gpus.llm-utils.org](llm-utils)!
I've had a lot of people ask if they can contribute. I enjoy providing models and helping people, and would love to be able to spend even more time doing it, as well as expanding into new projects like fine tuning/training.
If you're able and willing to contribute it will be most gratefully received and will help me to keep providing more models, and to start work on new AI projects.
Donaters will get priority support on any and all AI/LLM/model questions and requests, access to a private Discord room, plus other benefits.
* Patreon: https://patreon.com/TheBlokeAI
* Ko-Fi: https://ko-fi.com/TheBlokeAI
**Special thanks to**: Aemon Algiz.
**Patreon special mentions**: Alicia Loh, Stephen Murray, K, Ajan Kanaga, RoA, Magnesian, Deo Leter, Olakabola, Eugene Pentland, zynix, Deep Realms, Raymond Fosdick, Elijah Stavena, Iucharbius, Erik Bjäreholt, Luis Javier Navarrete Lozano, Nicholas, theTransient, John Detwiler, alfie_i, knownsqashed, Mano Prime, Willem Michiel, Enrico Ros, LangChain4j, OG, Michael Dempsey, Pierre Kircher, Pedro Madruga, James Bentley, Thomas Belote, Luke @flexchar, Leonard Tan, Johann-Peter Hartmann, Illia Dulskyi, Fen Risland, Chadd, S_X, Jeff Scroggin, Ken Nordquist, Sean Connelly, Artur Olbinski, Swaroop Kallakuri, Jack West, Ai Maven, David Ziegler, Russ Johnson, transmissions 11, John Villwock, Alps Aficionado, Clay Pascal, Viktor Bowallius, Subspace Studios, Rainer Wilmers, Trenton Dambrowitz, vamX, Michael Levine, 준교 김, Brandon Frisco, Kalila, Trailburnt, Randy H, Talal Aujan, Nathan Dryer, Vadim, 阿明, ReadyPlayerEmma, Tiffany J. Kim, George Stoitzev, Spencer Kim, Jerry Meng, Gabriel Tamborski, Cory Kujawski, Jeffrey Morgan, Spiking Neurons AB, Edmond Seymore, Alexandros Triantafyllidis, Lone Striker, Cap'n Zoog, Nikolai Manek, danny, ya boyyy, Derek Yates, usrbinkat, Mandus, TL, Nathan LeClaire, subjectnull, Imad Khwaja, webtim, Raven Klaugh, Asp the Wyvern, Gabriel Puliatti, Caitlyn Gatomon, Joseph William Delisle, Jonathan Leane, Luke Pendergrass, SuperWojo, Sebastain Graf, Will Dee, Fred von Graf, Andrey, Dan Guido, Daniel P. Andersen, Nitin Borwankar, Elle, Vitor Caleffi, biorpg, jjj, NimbleBox.ai, Pieter, Matthew Berman, terasurfer, Michael Davis, Alex, Stanislav Ovsiannikov
Thank you to all my generous patrons and donaters!
And thank you again to a16z for their generous grant.
<!-- footer end -->
<!-- original-model-card start -->
# Original model card: Eric Hartford's Wizard Vicuna 13B Uncensored
This is [wizard-vicuna-13b](https://huggingface.co/junelee/wizard-vicuna-13b) trained with a subset of the dataset - responses that contained alignment / moralizing were removed. The intent is to train a WizardLM that doesn't have alignment built-in, so that alignment (of any sort) can be added separately with for example with a RLHF LoRA.
Shout out to the open source AI/ML community, and everyone who helped me out.
Note:
An uncensored model has no guardrails.
You are responsible for anything you do with the model, just as you are responsible for anything you do with any dangerous object such as a knife, gun, lighter, or car.
Publishing anything this model generates is the same as publishing it yourself.
You are responsible for the content you publish, and you cannot blame the model any more than you can blame the knife, gun, lighter, or car for what you do with it.
<!-- original-model-card end -->
|
Qwen/Qwen1.5-4B-Chat | Qwen | "2024-04-30T07:41:43Z" | 16,189 | 34 | transformers | [
"transformers",
"safetensors",
"qwen2",
"text-generation",
"chat",
"conversational",
"en",
"license:other",
"autotrain_compatible",
"endpoints_compatible",
"text-generation-inference",
"region:us"
] | text-generation | "2024-01-30T17:20:32Z" | ---
license: other
license_name: tongyi-qianwen-research
license_link: >-
https://huggingface.co/Qwen/Qwen1.5-4B-Chat/blob/main/LICENSE
language:
- en
pipeline_tag: text-generation
tags:
- chat
---
# Qwen1.5-4B-Chat
## Introduction
Qwen1.5 is the beta version of Qwen2, a transformer-based decoder-only language model pretrained on a large amount of data. In comparison with the previous released Qwen, the improvements include:
* 8 model sizes, including 0.5B, 1.8B, 4B, 7B, 14B, 32B and 72B dense models, and an MoE model of 14B with 2.7B activated;
* Significant performance improvement in human preference for chat models;
* Multilingual support of both base and chat models;
* Stable support of 32K context length for models of all sizes
* No need of `trust_remote_code`.
For more details, please refer to our [blog post](https://qwenlm.github.io/blog/qwen1.5/) and [GitHub repo](https://github.com/QwenLM/Qwen1.5).
<br>
## Model Details
Qwen1.5 is a language model series including decoder language models of different model sizes. For each size, we release the base language model and the aligned chat model. It is based on the Transformer architecture with SwiGLU activation, attention QKV bias, group query attention, mixture of sliding window attention and full attention, etc. Additionally, we have an improved tokenizer adaptive to multiple natural languages and codes. For the beta version, temporarily we did not include GQA (except for 32B) and the mixture of SWA and full attention.
## Training details
We pretrained the models with a large amount of data, and we post-trained the models with both supervised finetuning and direct preference optimization.
## Requirements
The code of Qwen1.5 has been in the latest Hugging face transformers and we advise you to install `transformers>=4.37.0`, or you might encounter the following error:
```
KeyError: 'qwen2'
```
## Quickstart
Here provides a code snippet with `apply_chat_template` to show you how to load the tokenizer and model and how to generate contents.
```python
from transformers import AutoModelForCausalLM, AutoTokenizer
device = "cuda" # the device to load the model onto
model = AutoModelForCausalLM.from_pretrained(
"Qwen/Qwen1.5-4B-Chat",
torch_dtype="auto",
device_map="auto"
)
tokenizer = AutoTokenizer.from_pretrained("Qwen/Qwen1.5-4B-Chat")
prompt = "Give me a short introduction to large language model."
messages = [
{"role": "system", "content": "You are a helpful assistant."},
{"role": "user", "content": prompt}
]
text = tokenizer.apply_chat_template(
messages,
tokenize=False,
add_generation_prompt=True
)
model_inputs = tokenizer([text], return_tensors="pt").to(device)
generated_ids = model.generate(
model_inputs.input_ids,
max_new_tokens=512
)
generated_ids = [
output_ids[len(input_ids):] for input_ids, output_ids in zip(model_inputs.input_ids, generated_ids)
]
response = tokenizer.batch_decode(generated_ids, skip_special_tokens=True)[0]
```
For quantized models, we advise you to use the GPTQ, AWQ, and GGUF correspondents, namely `Qwen1.5-4B-Chat-GPTQ-Int4`, `Qwen1.5-4B-Chat-GPTQ-Int8`, `Qwen1.5-4B-Chat-AWQ`, and `Qwen1.5-4B-Chat-GGUF`.
## Tips
* If you encounter code switching or other bad cases, we advise you to use our provided hyper-parameters in `generation_config.json`.
## Citation
If you find our work helpful, feel free to give us a cite.
```
@article{qwen,
title={Qwen Technical Report},
author={Jinze Bai and Shuai Bai and Yunfei Chu and Zeyu Cui and Kai Dang and Xiaodong Deng and Yang Fan and Wenbin Ge and Yu Han and Fei Huang and Binyuan Hui and Luo Ji and Mei Li and Junyang Lin and Runji Lin and Dayiheng Liu and Gao Liu and Chengqiang Lu and Keming Lu and Jianxin Ma and Rui Men and Xingzhang Ren and Xuancheng Ren and Chuanqi Tan and Sinan Tan and Jianhong Tu and Peng Wang and Shijie Wang and Wei Wang and Shengguang Wu and Benfeng Xu and Jin Xu and An Yang and Hao Yang and Jian Yang and Shusheng Yang and Yang Yao and Bowen Yu and Hongyi Yuan and Zheng Yuan and Jianwei Zhang and Xingxuan Zhang and Yichang Zhang and Zhenru Zhang and Chang Zhou and Jingren Zhou and Xiaohuan Zhou and Tianhang Zhu},
journal={arXiv preprint arXiv:2309.16609},
year={2023}
}
``` |
mradermacher/ZharfaOpen_Gemma_7B_0.1-GGUF | mradermacher | "2024-06-27T14:52:12Z" | 16,189 | 0 | transformers | [
"transformers",
"gguf",
"en",
"base_model:ZharfaTech/ZharfaOpen_Gemma_7B_0.1",
"endpoints_compatible",
"region:us"
] | null | "2024-06-27T14:22:54Z" | ---
base_model: ZharfaTech/ZharfaOpen_Gemma_7B_0.1
language:
- en
library_name: transformers
quantized_by: mradermacher
---
## About
<!-- ### quantize_version: 2 -->
<!-- ### output_tensor_quantised: 1 -->
<!-- ### convert_type: hf -->
<!-- ### vocab_type: -->
<!-- ### tags: -->
static quants of https://huggingface.co/ZharfaTech/ZharfaOpen_Gemma_7B_0.1
<!-- provided-files -->
weighted/imatrix quants seem not to be available (by me) at this time. If they do not show up a week or so after the static ones, I have probably not planned for them. Feel free to request them by opening a Community Discussion.
## Usage
If you are unsure how to use GGUF files, refer to one of [TheBloke's
READMEs](https://huggingface.co/TheBloke/KafkaLM-70B-German-V0.1-GGUF) for
more details, including on how to concatenate multi-part files.
## Provided Quants
(sorted by size, not necessarily quality. IQ-quants are often preferable over similar sized non-IQ quants)
| Link | Type | Size/GB | Notes |
|:-----|:-----|--------:|:------|
| [GGUF](https://huggingface.co/mradermacher/ZharfaOpen_Gemma_7B_0.1-GGUF/resolve/main/ZharfaOpen_Gemma_7B_0.1.Q2_K.gguf) | Q2_K | 2.8 | |
| [GGUF](https://huggingface.co/mradermacher/ZharfaOpen_Gemma_7B_0.1-GGUF/resolve/main/ZharfaOpen_Gemma_7B_0.1.IQ3_XS.gguf) | IQ3_XS | 3.1 | |
| [GGUF](https://huggingface.co/mradermacher/ZharfaOpen_Gemma_7B_0.1-GGUF/resolve/main/ZharfaOpen_Gemma_7B_0.1.Q3_K_S.gguf) | Q3_K_S | 3.3 | |
| [GGUF](https://huggingface.co/mradermacher/ZharfaOpen_Gemma_7B_0.1-GGUF/resolve/main/ZharfaOpen_Gemma_7B_0.1.IQ3_S.gguf) | IQ3_S | 3.3 | beats Q3_K* |
| [GGUF](https://huggingface.co/mradermacher/ZharfaOpen_Gemma_7B_0.1-GGUF/resolve/main/ZharfaOpen_Gemma_7B_0.1.IQ3_M.gguf) | IQ3_M | 3.4 | |
| [GGUF](https://huggingface.co/mradermacher/ZharfaOpen_Gemma_7B_0.1-GGUF/resolve/main/ZharfaOpen_Gemma_7B_0.1.Q3_K_M.gguf) | Q3_K_M | 3.6 | lower quality |
| [GGUF](https://huggingface.co/mradermacher/ZharfaOpen_Gemma_7B_0.1-GGUF/resolve/main/ZharfaOpen_Gemma_7B_0.1.Q3_K_L.gguf) | Q3_K_L | 3.9 | |
| [GGUF](https://huggingface.co/mradermacher/ZharfaOpen_Gemma_7B_0.1-GGUF/resolve/main/ZharfaOpen_Gemma_7B_0.1.IQ4_XS.gguf) | IQ4_XS | 4.0 | |
| [GGUF](https://huggingface.co/mradermacher/ZharfaOpen_Gemma_7B_0.1-GGUF/resolve/main/ZharfaOpen_Gemma_7B_0.1.Q4_K_S.gguf) | Q4_K_S | 4.2 | fast, recommended |
| [GGUF](https://huggingface.co/mradermacher/ZharfaOpen_Gemma_7B_0.1-GGUF/resolve/main/ZharfaOpen_Gemma_7B_0.1.Q4_K_M.gguf) | Q4_K_M | 4.5 | fast, recommended |
| [GGUF](https://huggingface.co/mradermacher/ZharfaOpen_Gemma_7B_0.1-GGUF/resolve/main/ZharfaOpen_Gemma_7B_0.1.Q5_K_S.gguf) | Q5_K_S | 5.1 | |
| [GGUF](https://huggingface.co/mradermacher/ZharfaOpen_Gemma_7B_0.1-GGUF/resolve/main/ZharfaOpen_Gemma_7B_0.1.Q5_K_M.gguf) | Q5_K_M | 5.2 | |
| [GGUF](https://huggingface.co/mradermacher/ZharfaOpen_Gemma_7B_0.1-GGUF/resolve/main/ZharfaOpen_Gemma_7B_0.1.Q6_K.gguf) | Q6_K | 6.0 | very good quality |
| [GGUF](https://huggingface.co/mradermacher/ZharfaOpen_Gemma_7B_0.1-GGUF/resolve/main/ZharfaOpen_Gemma_7B_0.1.Q8_0.gguf) | Q8_0 | 7.8 | fast, best quality |
| [GGUF](https://huggingface.co/mradermacher/ZharfaOpen_Gemma_7B_0.1-GGUF/resolve/main/ZharfaOpen_Gemma_7B_0.1.f16.gguf) | f16 | 14.6 | 16 bpw, overkill |
Here is a handy graph by ikawrakow comparing some lower-quality quant
types (lower is better):

And here are Artefact2's thoughts on the matter:
https://gist.github.com/Artefact2/b5f810600771265fc1e39442288e8ec9
## FAQ / Model Request
See https://huggingface.co/mradermacher/model_requests for some answers to
questions you might have and/or if you want some other model quantized.
## Thanks
I thank my company, [nethype GmbH](https://www.nethype.de/), for letting
me use its servers and providing upgrades to my workstation to enable
this work in my free time.
<!-- end -->
|
climatebert/netzero-reduction | climatebert | "2023-11-24T14:51:34Z" | 16,184 | 3 | transformers | [
"transformers",
"pytorch",
"roberta",
"text-classification",
"dataset:climatebert/netzero_reduction_data",
"arxiv:2310.08096",
"license:apache-2.0",
"autotrain_compatible",
"endpoints_compatible",
"region:us"
] | text-classification | "2023-10-11T08:59:52Z" | ---
license: apache-2.0
datasets:
- climatebert/netzero_reduction_data
---
# Model Card for netzero-reduction
## Model Description
Based on [this paper](https://papers.ssrn.com/sol3/papers.cfm?abstract_id=4599483), this is the fine-tuned ClimateBERT language model with a classification head for detecting sentences that are either related to emission net zero or reduction targets.
We use the [climatebert/distilroberta-base-climate-f](https://huggingface.co/climatebert/distilroberta-base-climate-f) language model as a starting point and fine-tuned it on our human-annotated dataset.
## Citation Information
```bibtex
@article{schimanski2023climatebertnetzero,
title={ClimateBERT-NetZero: Detecting and Assessing Net Zero and Reduction Targets},
author={Tobias Schimanski and Julia Bingler and Camilla Hyslop and Mathias Kraus and Markus Leippold},
year={2023},
eprint={2310.08096},
archivePrefix={arXiv},
primaryClass={cs.LG}
}
```
## How to Get Started With the Model
You can use the model with a pipeline for text classification:
IMPORTANT REMARK: It is highly recommended to use a prior classification step before applying ClimateBERT-NetZero. Establish a climate context with [climatebert/distilroberta-base-climate-detector](https://huggingface.co/climatebert/distilroberta-base-climate-detector) for paragraphs or [ESGBERT/EnvironmentalBERT-environmental](https://huggingface.co/ESGBERT/EnvironmentalBERT-environmental) for sentences and then label the data with ClimateBERT-NetZero.
```python
from transformers import AutoModelForSequenceClassification, AutoTokenizer, pipeline
from transformers.pipelines.pt_utils import KeyDataset
import datasets
from tqdm.auto import tqdm
dataset_name = "climatebert/climate_detection"
tokenizer_name = "climatebert/distilroberta-base-climate-f"
model_name = "climatebert/netzero-reduction"
# If you want to use your own data, simply load them as 🤗 Datasets dataset, see https://huggingface.co/docs/datasets/loading
dataset = datasets.load_dataset(dataset_name, split="test")
model = AutoModelForSequenceClassification.from_pretrained(model_name)
tokenizer = AutoTokenizer.from_pretrained(tokenizer_name, max_len=512)
pipe = pipeline("text-classification", model=model, tokenizer=tokenizer, device=0)
# See https://huggingface.co/docs/transformers/main_classes/pipelines#transformers.pipeline
for i, out in enumerate(tqdm(pipe(KeyDataset(dataset, "text"), padding=True, truncation=True))):
print(dataset["text"][i])
print(out)
### IMPORTANT REMARK: It is highly recommended to use a prior classification step before applying ClimateBERT-NetZero.
### Establish a climate context with "climatebert/distilroberta-base-climate-detector" for paragraphs
### or "ESGBERT/EnvironmentalBERT-environmental" for sentences and then label the data with ClimateBERT-NetZero.
``` |
bartowski/dolphin-2.9.3-mistral-7B-32k-GGUF | bartowski | "2024-06-25T16:34:45Z" | 16,177 | 1 | null | [
"gguf",
"generated_from_trainer",
"axolotl",
"text-generation",
"dataset:cognitivecomputations/Dolphin-2.9",
"dataset:teknium/OpenHermes-2.5",
"dataset:m-a-p/CodeFeedback-Filtered-Instruction",
"dataset:cognitivecomputations/dolphin-coder",
"dataset:cognitivecomputations/samantha-data",
"dataset:microsoft/orca-math-word-problems-200k",
"dataset:Locutusque/function-calling-chatml",
"dataset:internlm/Agent-FLAN",
"base_model:mistralai/Mistral-7B-v0.3",
"license:apache-2.0",
"region:us"
] | text-generation | "2024-06-25T16:14:45Z" | ---
license: apache-2.0
base_model: mistralai/Mistral-7B-v0.3
tags:
- generated_from_trainer
- axolotl
datasets:
- cognitivecomputations/Dolphin-2.9
- teknium/OpenHermes-2.5
- m-a-p/CodeFeedback-Filtered-Instruction
- cognitivecomputations/dolphin-coder
- cognitivecomputations/samantha-data
- microsoft/orca-math-word-problems-200k
- Locutusque/function-calling-chatml
- internlm/Agent-FLAN
quantized_by: bartowski
pipeline_tag: text-generation
---
## Llamacpp imatrix Quantizations of dolphin-2.9.3-mistral-7B-32k
Using <a href="https://github.com/ggerganov/llama.cpp/">llama.cpp</a> release <a href="https://github.com/ggerganov/llama.cpp/releases/tag/b3197">b3197</a> for quantization.
Original model: https://huggingface.co/cognitivecomputations/dolphin-2.9.3-mistral-7B-32k
All quants made using imatrix option with dataset from [here](https://gist.github.com/bartowski1182/eb213dccb3571f863da82e99418f81e8)
## Prompt format
```
<|im_start|> system
{system_prompt}<|im_end|>
<|im_start|> user
{prompt}<|im_end|>
<|im_start|> assistant
```
## Download a file (not the whole branch) from below:
| Filename | Quant type | File Size | Description |
| -------- | ---------- | --------- | ----------- |
| [dolphin-2.9.3-mistral-7B-32k-Q8_0_L.gguf](https://huggingface.co/bartowski/dolphin-2.9.3-mistral-7B-32k-GGUF/blob/main/dolphin-2.9.3-mistral-7B-32k-Q8_1.gguf) | Q8_0_L | 7.95GB | *Experimental*, uses f16 for embed and output weights. Please provide any feedback of differences. Extremely high quality, generally unneeded but max available quant. |
| [dolphin-2.9.3-mistral-7B-32k-Q8_0.gguf](https://huggingface.co/bartowski/dolphin-2.9.3-mistral-7B-32k-GGUF/blob/main/dolphin-2.9.3-mistral-7B-32k-Q8_0.gguf) | Q8_0 | 7.70GB | Extremely high quality, generally unneeded but max available quant. |
| [dolphin-2.9.3-mistral-7B-32k-Q6_K_L.gguf](https://huggingface.co/bartowski/dolphin-2.9.3-mistral-7B-32k-GGUF/blob/main/dolphin-2.9.3-mistral-7B-32k-Q6_K_L.gguf) | Q6_K_L | 6.26GB | *Experimental*, uses f16 for embed and output weights. Please provide any feedback of differences. Very high quality, near perfect, *recommended*. |
| [dolphin-2.9.3-mistral-7B-32k-Q6_K.gguf](https://huggingface.co/bartowski/dolphin-2.9.3-mistral-7B-32k-GGUF/blob/main/dolphin-2.9.3-mistral-7B-32k-Q6_K.gguf) | Q6_K | 5.94GB | Very high quality, near perfect, *recommended*. |
| [dolphin-2.9.3-mistral-7B-32k-Q5_K_L.gguf](https://huggingface.co/bartowski/dolphin-2.9.3-mistral-7B-32k-GGUF/blob/main/dolphin-2.9.3-mistral-7B-32k-Q5_K_L.gguf) | Q5_K_L | 5.47GB | *Experimental*, uses f16 for embed and output weights. Please provide any feedback of differences. High quality, *recommended*. |
| [dolphin-2.9.3-mistral-7B-32k-Q5_K_M.gguf](https://huggingface.co/bartowski/dolphin-2.9.3-mistral-7B-32k-GGUF/blob/main/dolphin-2.9.3-mistral-7B-32k-Q5_K_M.gguf) | Q5_K_M | 5.13GB | High quality, *recommended*. |
| [dolphin-2.9.3-mistral-7B-32k-Q5_K_S.gguf](https://huggingface.co/bartowski/dolphin-2.9.3-mistral-7B-32k-GGUF/blob/main/dolphin-2.9.3-mistral-7B-32k-Q5_K_S.gguf) | Q5_K_S | 5.00GB | High quality, *recommended*. |
| [dolphin-2.9.3-mistral-7B-32k-Q4_K_L.gguf](https://huggingface.co/bartowski/dolphin-2.9.3-mistral-7B-32k-GGUF/blob/main/dolphin-2.9.3-mistral-7B-32k-Q4_K_L.gguf) | Q4_K_L | 4.72GB | *Experimental*, uses f16 for embed and output weights. Please provide any feedback of differences. Good quality, uses about 4.83 bits per weight, *recommended*. |
| [dolphin-2.9.3-mistral-7B-32k-Q4_K_M.gguf](https://huggingface.co/bartowski/dolphin-2.9.3-mistral-7B-32k-GGUF/blob/main/dolphin-2.9.3-mistral-7B-32k-Q4_K_M.gguf) | Q4_K_M | 4.37GB | Good quality, uses about 4.83 bits per weight, *recommended*. |
| [dolphin-2.9.3-mistral-7B-32k-Q4_K_S.gguf](https://huggingface.co/bartowski/dolphin-2.9.3-mistral-7B-32k-GGUF/blob/main/dolphin-2.9.3-mistral-7B-32k-Q4_K_S.gguf) | Q4_K_S | 4.14GB | Slightly lower quality with more space savings, *recommended*. |
| [dolphin-2.9.3-mistral-7B-32k-IQ4_XS.gguf](https://huggingface.co/bartowski/dolphin-2.9.3-mistral-7B-32k-GGUF/blob/main/dolphin-2.9.3-mistral-7B-32k-IQ4_XS.gguf) | IQ4_XS | 3.91GB | Decent quality, smaller than Q4_K_S with similar performance, *recommended*. |
| [dolphin-2.9.3-mistral-7B-32k-Q3_K_XL.gguf](https://huggingface.co/bartowski/dolphin-2.9.3-mistral-7B-32k-GGUF//main/dolphin-2.9.3-mistral-7B-32k-Q3_K_XL.gguf) | Q3_K_XL | | *Experimental*, uses f16 for embed and output weights. Please provide any feedback of differences. Lower quality but usable, good for low RAM availability. |
| [dolphin-2.9.3-mistral-7B-32k-Q3_K_L.gguf](https://huggingface.co/bartowski/dolphin-2.9.3-mistral-7B-32k-GGUF/blob/main/dolphin-2.9.3-mistral-7B-32k-Q3_K_L.gguf) | Q3_K_L | 3.82GB | Lower quality but usable, good for low RAM availability. |
| [dolphin-2.9.3-mistral-7B-32k-Q3_K_M.gguf](https://huggingface.co/bartowski/dolphin-2.9.3-mistral-7B-32k-GGUF/blob/main/dolphin-2.9.3-mistral-7B-32k-Q3_K_M.gguf) | Q3_K_M | 3.52GB | Even lower quality. |
| [dolphin-2.9.3-mistral-7B-32k-IQ3_M.gguf](https://huggingface.co/bartowski/dolphin-2.9.3-mistral-7B-32k-GGUF/blob/main/dolphin-2.9.3-mistral-7B-32k-IQ3_M.gguf) | IQ3_M | 3.28GB | Medium-low quality, new method with decent performance comparable to Q3_K_M. |
| [dolphin-2.9.3-mistral-7B-32k-Q3_K_S.gguf](https://huggingface.co/bartowski/dolphin-2.9.3-mistral-7B-32k-GGUF/blob/main/dolphin-2.9.3-mistral-7B-32k-Q3_K_S.gguf) | Q3_K_S | 3.16GB | Low quality, not recommended. |
| [dolphin-2.9.3-mistral-7B-32k-IQ3_XS.gguf](https://huggingface.co/bartowski/dolphin-2.9.3-mistral-7B-32k-GGUF/blob/main/dolphin-2.9.3-mistral-7B-32k-IQ3_XS.gguf) | IQ3_XS | 3.02GB | Lower quality, new method with decent performance, slightly better than Q3_K_S. |
| [dolphin-2.9.3-mistral-7B-32k-IQ3_XXS.gguf](https://huggingface.co/bartowski/dolphin-2.9.3-mistral-7B-32k-GGUF/blob/main/dolphin-2.9.3-mistral-7B-32k-IQ3_XXS.gguf) | IQ3_XXS | 2.83GB | Lower quality, new method with decent performance, comparable to Q3 quants. |
| [dolphin-2.9.3-mistral-7B-32k-Q2_K.gguf](https://huggingface.co/bartowski/dolphin-2.9.3-mistral-7B-32k-GGUF/blob/main/dolphin-2.9.3-mistral-7B-32k-Q2_K.gguf) | Q2_K | 2.72GB | Very low quality but surprisingly usable. |
| [dolphin-2.9.3-mistral-7B-32k-IQ2_M.gguf](https://huggingface.co/bartowski/dolphin-2.9.3-mistral-7B-32k-GGUF/blob/main/dolphin-2.9.3-mistral-7B-32k-IQ2_M.gguf) | IQ2_M | 2.50GB | Very low quality, uses SOTA techniques to also be surprisingly usable. |
| [dolphin-2.9.3-mistral-7B-32k-IQ2_S.gguf](https://huggingface.co/bartowski/dolphin-2.9.3-mistral-7B-32k-GGUF/blob/main/dolphin-2.9.3-mistral-7B-32k-IQ2_S.gguf) | IQ2_S | 2.31GB | Very low quality, uses SOTA techniques to be usable. |
| [dolphin-2.9.3-mistral-7B-32k-IQ2_XS.gguf](https://huggingface.co/bartowski/dolphin-2.9.3-mistral-7B-32k-GGUF/blob/main/dolphin-2.9.3-mistral-7B-32k-IQ2_XS.gguf) | IQ2_XS | 2.20GB | Very low quality, uses SOTA techniques to be usable. |
## Downloading using huggingface-cli
First, make sure you have hugginface-cli installed:
```
pip install -U "huggingface_hub[cli]"
```
Then, you can target the specific file you want:
```
huggingface-cli download bartowski/dolphin-2.9.3-mistral-7B-32k-GGUF --include "dolphin-2.9.3-mistral-7B-32k-Q4_K_M.gguf" --local-dir ./
```
If the model is bigger than 50GB, it will have been split into multiple files. In order to download them all to a local folder, run:
```
huggingface-cli download bartowski/dolphin-2.9.3-mistral-7B-32k-GGUF --include "dolphin-2.9.3-mistral-7B-32k-Q8_0.gguf/*" --local-dir dolphin-2.9.3-mistral-7B-32k-Q8_0
```
You can either specify a new local-dir (dolphin-2.9.3-mistral-7B-32k-Q8_0) or download them all in place (./)
## Which file should I choose?
A great write up with charts showing various performances is provided by Artefact2 [here](https://gist.github.com/Artefact2/b5f810600771265fc1e39442288e8ec9)
The first thing to figure out is how big a model you can run. To do this, you'll need to figure out how much RAM and/or VRAM you have.
If you want your model running as FAST as possible, you'll want to fit the whole thing on your GPU's VRAM. Aim for a quant with a file size 1-2GB smaller than your GPU's total VRAM.
If you want the absolute maximum quality, add both your system RAM and your GPU's VRAM together, then similarly grab a quant with a file size 1-2GB Smaller than that total.
Next, you'll need to decide if you want to use an 'I-quant' or a 'K-quant'.
If you don't want to think too much, grab one of the K-quants. These are in format 'QX_K_X', like Q5_K_M.
If you want to get more into the weeds, you can check out this extremely useful feature chart:
[llama.cpp feature matrix](https://github.com/ggerganov/llama.cpp/wiki/Feature-matrix)
But basically, if you're aiming for below Q4, and you're running cuBLAS (Nvidia) or rocBLAS (AMD), you should look towards the I-quants. These are in format IQX_X, like IQ3_M. These are newer and offer better performance for their size.
These I-quants can also be used on CPU and Apple Metal, but will be slower than their K-quant equivalent, so speed vs performance is a tradeoff you'll have to decide.
The I-quants are *not* compatible with Vulcan, which is also AMD, so if you have an AMD card double check if you're using the rocBLAS build or the Vulcan build. At the time of writing this, LM Studio has a preview with ROCm support, and other inference engines have specific builds for ROCm.
Want to support my work? Visit my ko-fi page here: https://ko-fi.com/bartowski
|
Helsinki-NLP/opus-mt-en-sla | Helsinki-NLP | "2023-08-16T11:31:07Z" | 16,176 | 1 | transformers | [
"transformers",
"pytorch",
"tf",
"marian",
"text2text-generation",
"translation",
"en",
"be",
"hr",
"mk",
"cs",
"ru",
"pl",
"bg",
"uk",
"sl",
"sla",
"license:apache-2.0",
"autotrain_compatible",
"endpoints_compatible",
"region:us"
] | translation | "2022-03-02T23:29:04Z" | ---
language:
- en
- be
- hr
- mk
- cs
- ru
- pl
- bg
- uk
- sl
- sla
tags:
- translation
license: apache-2.0
---
### eng-sla
* source group: English
* target group: Slavic languages
* OPUS readme: [eng-sla](https://github.com/Helsinki-NLP/Tatoeba-Challenge/tree/master/models/eng-sla/README.md)
* model: transformer
* source language(s): eng
* target language(s): bel bel_Latn bos_Latn bul bul_Latn ces csb_Latn dsb hrv hsb mkd orv_Cyrl pol rue rus slv srp_Cyrl srp_Latn ukr
* model: transformer
* pre-processing: normalization + SentencePiece (spm32k,spm32k)
* a sentence initial language token is required in the form of `>>id<<` (id = valid target language ID)
* download original weights: [opus2m-2020-08-01.zip](https://object.pouta.csc.fi/Tatoeba-MT-models/eng-sla/opus2m-2020-08-01.zip)
* test set translations: [opus2m-2020-08-01.test.txt](https://object.pouta.csc.fi/Tatoeba-MT-models/eng-sla/opus2m-2020-08-01.test.txt)
* test set scores: [opus2m-2020-08-01.eval.txt](https://object.pouta.csc.fi/Tatoeba-MT-models/eng-sla/opus2m-2020-08-01.eval.txt)
## Benchmarks
| testset | BLEU | chr-F |
|-----------------------|-------|-------|
| newssyscomb2009-engces.eng.ces | 20.1 | 0.484 |
| news-test2008-engces.eng.ces | 17.7 | 0.461 |
| newstest2009-engces.eng.ces | 19.1 | 0.479 |
| newstest2010-engces.eng.ces | 19.3 | 0.483 |
| newstest2011-engces.eng.ces | 20.4 | 0.486 |
| newstest2012-engces.eng.ces | 18.3 | 0.461 |
| newstest2012-engrus.eng.rus | 27.4 | 0.551 |
| newstest2013-engces.eng.ces | 21.5 | 0.489 |
| newstest2013-engrus.eng.rus | 20.9 | 0.490 |
| newstest2015-encs-engces.eng.ces | 21.1 | 0.496 |
| newstest2015-enru-engrus.eng.rus | 24.5 | 0.536 |
| newstest2016-encs-engces.eng.ces | 23.6 | 0.515 |
| newstest2016-enru-engrus.eng.rus | 23.0 | 0.519 |
| newstest2017-encs-engces.eng.ces | 19.2 | 0.474 |
| newstest2017-enru-engrus.eng.rus | 25.0 | 0.541 |
| newstest2018-encs-engces.eng.ces | 19.3 | 0.479 |
| newstest2018-enru-engrus.eng.rus | 22.3 | 0.526 |
| newstest2019-encs-engces.eng.ces | 20.4 | 0.486 |
| newstest2019-enru-engrus.eng.rus | 24.0 | 0.506 |
| Tatoeba-test.eng-bel.eng.bel | 22.9 | 0.489 |
| Tatoeba-test.eng-bul.eng.bul | 46.7 | 0.652 |
| Tatoeba-test.eng-ces.eng.ces | 42.7 | 0.624 |
| Tatoeba-test.eng-csb.eng.csb | 1.4 | 0.210 |
| Tatoeba-test.eng-dsb.eng.dsb | 1.4 | 0.165 |
| Tatoeba-test.eng-hbs.eng.hbs | 40.3 | 0.616 |
| Tatoeba-test.eng-hsb.eng.hsb | 14.3 | 0.344 |
| Tatoeba-test.eng-mkd.eng.mkd | 44.1 | 0.635 |
| Tatoeba-test.eng.multi | 41.0 | 0.610 |
| Tatoeba-test.eng-orv.eng.orv | 0.3 | 0.014 |
| Tatoeba-test.eng-pol.eng.pol | 42.0 | 0.637 |
| Tatoeba-test.eng-rue.eng.rue | 0.3 | 0.012 |
| Tatoeba-test.eng-rus.eng.rus | 40.5 | 0.612 |
| Tatoeba-test.eng-slv.eng.slv | 18.8 | 0.357 |
| Tatoeba-test.eng-ukr.eng.ukr | 38.8 | 0.600 |
### System Info:
- hf_name: eng-sla
- source_languages: eng
- target_languages: sla
- opus_readme_url: https://github.com/Helsinki-NLP/Tatoeba-Challenge/tree/master/models/eng-sla/README.md
- original_repo: Tatoeba-Challenge
- tags: ['translation']
- languages: ['en', 'be', 'hr', 'mk', 'cs', 'ru', 'pl', 'bg', 'uk', 'sl', 'sla']
- src_constituents: {'eng'}
- tgt_constituents: {'bel', 'hrv', 'orv_Cyrl', 'mkd', 'bel_Latn', 'srp_Latn', 'bul_Latn', 'ces', 'bos_Latn', 'csb_Latn', 'dsb', 'hsb', 'rus', 'srp_Cyrl', 'pol', 'rue', 'bul', 'ukr', 'slv'}
- src_multilingual: False
- tgt_multilingual: True
- prepro: normalization + SentencePiece (spm32k,spm32k)
- url_model: https://object.pouta.csc.fi/Tatoeba-MT-models/eng-sla/opus2m-2020-08-01.zip
- url_test_set: https://object.pouta.csc.fi/Tatoeba-MT-models/eng-sla/opus2m-2020-08-01.test.txt
- src_alpha3: eng
- tgt_alpha3: sla
- short_pair: en-sla
- chrF2_score: 0.61
- bleu: 41.0
- brevity_penalty: 0.976
- ref_len: 64809.0
- src_name: English
- tgt_name: Slavic languages
- train_date: 2020-08-01
- src_alpha2: en
- tgt_alpha2: sla
- prefer_old: False
- long_pair: eng-sla
- helsinki_git_sha: 480fcbe0ee1bf4774bcbe6226ad9f58e63f6c535
- transformers_git_sha: 2207e5d8cb224e954a7cba69fa4ac2309e9ff30b
- port_machine: brutasse
- port_time: 2020-08-21-14:41 |
RichardErkhov/predibase_-_Meta-Llama-3-8B-Instruct-dequantized-gguf | RichardErkhov | "2024-06-25T07:10:29Z" | 16,176 | 0 | null | [
"gguf",
"region:us"
] | null | "2024-06-25T03:07:27Z" | Quantization made by Richard Erkhov.
[Github](https://github.com/RichardErkhov)
[Discord](https://discord.gg/pvy7H8DZMG)
[Request more models](https://github.com/RichardErkhov/quant_request)
Meta-Llama-3-8B-Instruct-dequantized - GGUF
- Model creator: https://huggingface.co/predibase/
- Original model: https://huggingface.co/predibase/Meta-Llama-3-8B-Instruct-dequantized/
| Name | Quant method | Size |
| ---- | ---- | ---- |
| [Meta-Llama-3-8B-Instruct-dequantized.Q2_K.gguf](https://huggingface.co/RichardErkhov/predibase_-_Meta-Llama-3-8B-Instruct-dequantized-gguf/blob/main/Meta-Llama-3-8B-Instruct-dequantized.Q2_K.gguf) | Q2_K | 2.96GB |
| [Meta-Llama-3-8B-Instruct-dequantized.IQ3_XS.gguf](https://huggingface.co/RichardErkhov/predibase_-_Meta-Llama-3-8B-Instruct-dequantized-gguf/blob/main/Meta-Llama-3-8B-Instruct-dequantized.IQ3_XS.gguf) | IQ3_XS | 3.28GB |
| [Meta-Llama-3-8B-Instruct-dequantized.IQ3_S.gguf](https://huggingface.co/RichardErkhov/predibase_-_Meta-Llama-3-8B-Instruct-dequantized-gguf/blob/main/Meta-Llama-3-8B-Instruct-dequantized.IQ3_S.gguf) | IQ3_S | 3.43GB |
| [Meta-Llama-3-8B-Instruct-dequantized.Q3_K_S.gguf](https://huggingface.co/RichardErkhov/predibase_-_Meta-Llama-3-8B-Instruct-dequantized-gguf/blob/main/Meta-Llama-3-8B-Instruct-dequantized.Q3_K_S.gguf) | Q3_K_S | 3.41GB |
| [Meta-Llama-3-8B-Instruct-dequantized.IQ3_M.gguf](https://huggingface.co/RichardErkhov/predibase_-_Meta-Llama-3-8B-Instruct-dequantized-gguf/blob/main/Meta-Llama-3-8B-Instruct-dequantized.IQ3_M.gguf) | IQ3_M | 3.52GB |
| [Meta-Llama-3-8B-Instruct-dequantized.Q3_K.gguf](https://huggingface.co/RichardErkhov/predibase_-_Meta-Llama-3-8B-Instruct-dequantized-gguf/blob/main/Meta-Llama-3-8B-Instruct-dequantized.Q3_K.gguf) | Q3_K | 3.74GB |
| [Meta-Llama-3-8B-Instruct-dequantized.Q3_K_M.gguf](https://huggingface.co/RichardErkhov/predibase_-_Meta-Llama-3-8B-Instruct-dequantized-gguf/blob/main/Meta-Llama-3-8B-Instruct-dequantized.Q3_K_M.gguf) | Q3_K_M | 3.74GB |
| [Meta-Llama-3-8B-Instruct-dequantized.Q3_K_L.gguf](https://huggingface.co/RichardErkhov/predibase_-_Meta-Llama-3-8B-Instruct-dequantized-gguf/blob/main/Meta-Llama-3-8B-Instruct-dequantized.Q3_K_L.gguf) | Q3_K_L | 4.03GB |
| [Meta-Llama-3-8B-Instruct-dequantized.IQ4_XS.gguf](https://huggingface.co/RichardErkhov/predibase_-_Meta-Llama-3-8B-Instruct-dequantized-gguf/blob/main/Meta-Llama-3-8B-Instruct-dequantized.IQ4_XS.gguf) | IQ4_XS | 4.18GB |
| [Meta-Llama-3-8B-Instruct-dequantized.Q4_0.gguf](https://huggingface.co/RichardErkhov/predibase_-_Meta-Llama-3-8B-Instruct-dequantized-gguf/blob/main/Meta-Llama-3-8B-Instruct-dequantized.Q4_0.gguf) | Q4_0 | 4.34GB |
| [Meta-Llama-3-8B-Instruct-dequantized.IQ4_NL.gguf](https://huggingface.co/RichardErkhov/predibase_-_Meta-Llama-3-8B-Instruct-dequantized-gguf/blob/main/Meta-Llama-3-8B-Instruct-dequantized.IQ4_NL.gguf) | IQ4_NL | 4.38GB |
| [Meta-Llama-3-8B-Instruct-dequantized.Q4_K_S.gguf](https://huggingface.co/RichardErkhov/predibase_-_Meta-Llama-3-8B-Instruct-dequantized-gguf/blob/main/Meta-Llama-3-8B-Instruct-dequantized.Q4_K_S.gguf) | Q4_K_S | 4.37GB |
| [Meta-Llama-3-8B-Instruct-dequantized.Q4_K.gguf](https://huggingface.co/RichardErkhov/predibase_-_Meta-Llama-3-8B-Instruct-dequantized-gguf/blob/main/Meta-Llama-3-8B-Instruct-dequantized.Q4_K.gguf) | Q4_K | 4.58GB |
| [Meta-Llama-3-8B-Instruct-dequantized.Q4_K_M.gguf](https://huggingface.co/RichardErkhov/predibase_-_Meta-Llama-3-8B-Instruct-dequantized-gguf/blob/main/Meta-Llama-3-8B-Instruct-dequantized.Q4_K_M.gguf) | Q4_K_M | 4.58GB |
| [Meta-Llama-3-8B-Instruct-dequantized.Q4_1.gguf](https://huggingface.co/RichardErkhov/predibase_-_Meta-Llama-3-8B-Instruct-dequantized-gguf/blob/main/Meta-Llama-3-8B-Instruct-dequantized.Q4_1.gguf) | Q4_1 | 4.78GB |
| [Meta-Llama-3-8B-Instruct-dequantized.Q5_0.gguf](https://huggingface.co/RichardErkhov/predibase_-_Meta-Llama-3-8B-Instruct-dequantized-gguf/blob/main/Meta-Llama-3-8B-Instruct-dequantized.Q5_0.gguf) | Q5_0 | 5.21GB |
| [Meta-Llama-3-8B-Instruct-dequantized.Q5_K_S.gguf](https://huggingface.co/RichardErkhov/predibase_-_Meta-Llama-3-8B-Instruct-dequantized-gguf/blob/main/Meta-Llama-3-8B-Instruct-dequantized.Q5_K_S.gguf) | Q5_K_S | 5.21GB |
| [Meta-Llama-3-8B-Instruct-dequantized.Q5_K.gguf](https://huggingface.co/RichardErkhov/predibase_-_Meta-Llama-3-8B-Instruct-dequantized-gguf/blob/main/Meta-Llama-3-8B-Instruct-dequantized.Q5_K.gguf) | Q5_K | 5.34GB |
| [Meta-Llama-3-8B-Instruct-dequantized.Q5_K_M.gguf](https://huggingface.co/RichardErkhov/predibase_-_Meta-Llama-3-8B-Instruct-dequantized-gguf/blob/main/Meta-Llama-3-8B-Instruct-dequantized.Q5_K_M.gguf) | Q5_K_M | 5.34GB |
| [Meta-Llama-3-8B-Instruct-dequantized.Q5_1.gguf](https://huggingface.co/RichardErkhov/predibase_-_Meta-Llama-3-8B-Instruct-dequantized-gguf/blob/main/Meta-Llama-3-8B-Instruct-dequantized.Q5_1.gguf) | Q5_1 | 5.65GB |
| [Meta-Llama-3-8B-Instruct-dequantized.Q6_K.gguf](https://huggingface.co/RichardErkhov/predibase_-_Meta-Llama-3-8B-Instruct-dequantized-gguf/blob/main/Meta-Llama-3-8B-Instruct-dequantized.Q6_K.gguf) | Q6_K | 6.14GB |
| [Meta-Llama-3-8B-Instruct-dequantized.Q8_0.gguf](https://huggingface.co/RichardErkhov/predibase_-_Meta-Llama-3-8B-Instruct-dequantized-gguf/blob/main/Meta-Llama-3-8B-Instruct-dequantized.Q8_0.gguf) | Q8_0 | 7.95GB |
Original model description:
---
language:
- en
license: apache-2.0
tags:
- text-generation-inference
- transformers
- llama
base_model: meta-llama/Meta-Llama-3-8B-Instruct
---
|
SeaLLMs/SeaLLM-7B-v2.5 | SeaLLMs | "2024-04-24T14:11:01Z" | 16,162 | 46 | transformers | [
"transformers",
"safetensors",
"gemma",
"text-generation",
"multilingual",
"sea",
"conversational",
"en",
"zh",
"vi",
"id",
"th",
"ms",
"km",
"lo",
"my",
"tl",
"arxiv:2312.00738",
"arxiv:2306.05179",
"license:other",
"autotrain_compatible",
"endpoints_compatible",
"text-generation-inference",
"region:us"
] | text-generation | "2024-04-03T06:39:06Z" | ---
license: other
license_name: seallms
license_link: https://huggingface.co/SeaLLMs/SeaLLM-13B-Chat/blob/main/LICENSE
language:
- en
- zh
- vi
- id
- th
- ms
- km
- lo
- my
- tl
tags:
- multilingual
- sea
---
<p align="center">
<img src="seal_logo.png" width="200" />
</p>
# *SeaLLM-7B-v2.5* - Large Language Models for Southeast Asia
<p align="center">
<a href="https://damo-nlp-sg.github.io/SeaLLMs/" target="_blank" rel="noopener">Website</a>
<a href="https://huggingface.co/SeaLLMs/SeaLLM-7B-v2.5" target="_blank" rel="noopener"> 🤗 Tech Memo</a>
<a href="https://huggingface.co/spaces/SeaLLMs/SeaLLM-7B-v2.5" target="_blank" rel="noopener"> 🤗 DEMO</a>
<a href="https://github.com/DAMO-NLP-SG/SeaLLMs" target="_blank" rel="noopener">Github</a>
<a href="https://arxiv.org/pdf/2312.00738.pdf" target="_blank" rel="noopener">Technical Report</a>
</p>
🔥<span style="color: #ff3860">[HOT]</span> SeaLLMs project now has a dedicated website - [damo-nlp-sg.github.io/SeaLLMs](https://damo-nlp-sg.github.io/SeaLLMs/)
We introduce [SeaLLM-7B-v2.5](https://huggingface.co/SeaLLMs/SeaLLM-7B-v2.5), the state-of-the-art multilingual LLM for Southeast Asian (SEA) languages 🇬🇧 🇨🇳 🇻🇳 🇮🇩 🇹🇭 🇲🇾 🇰🇭 🇱🇦 🇲🇲 🇵🇭. It is the most significant upgrade since [SeaLLM-13B](https://huggingface.co/SeaLLMs/SeaLLM-13B-Chat), with half the size, outperforming performance across diverse multilingual tasks, from world knowledge, math reasoning, instruction following, etc.
### Highlights
* [SeaLLM-7B-v2.5](https://huggingface.co/SeaLLMs/SeaLLM-7B-v2.5) outperforms GPT-3.5 and achieves 7B SOTA on most multilingual knowledge benchmarks for SEA languages (MMLU, M3Exam & VMLU).
* It achieves 79.0 and 34.9 on GSM8K and MATH, surpassing GPT-3.5 in MATH.
### Release and DEMO
- DEMO:
- [SeaLLMs/SeaLLM-7B-v2.5](https://huggingface.co/spaces/SeaLLMs/SeaLLM-7B-v2.5).
- [SeaLLMs/SeaLLM-7B | SeaLMMM-7B](https://huggingface.co/spaces/SeaLLMs/SeaLLM-7B) - Experimental multimodal SeaLLM.
- Technical report: [Arxiv: SeaLLMs - Large Language Models for Southeast Asia](https://arxiv.org/pdf/2312.00738.pdf).
- Model weights:
- [SeaLLM-7B-v2.5](https://huggingface.co/SeaLLMs/SeaLLM-7B-v2.5).
- [SeaLLM-7B-v2.5-GGUF](https://huggingface.co/SeaLLMs/SeaLLM-7B-v2.5-GGUF).
- Run locally:
- [LM-studio](https://lmstudio.ai/):
- [SeaLLM-7B-v2.5-q4_0-chatml](https://huggingface.co/SeaLLMs/SeaLLM-7B-v2.5-GGUF/blob/main/seallm-7b-v2.5-chatml.Q4_K_M.gguf) with ChatML template (`<eos>` token changed to `<|im_end|>`)
- [SeaLLM-7B-v2.5-q4_0](https://huggingface.co/SeaLLMs/SeaLLM-7B-v2.5-GGUF/blob/main/seallm-7b-v2.5.Q4_K_M.gguf) - must use SeaLLM-7B-v2.5 chat format.
- [MLX for Apple Silicon](https://github.com/ml-explore/mlx): [SeaLLMs/SeaLLM-7B-v2.5-mlx-quantized](https://huggingface.co/SeaLLMs/SeaLLM-7B-v2.5-mlx-quantized)
- Previous models:
- [SeaLLM-7B-v2](https://huggingface.co/SeaLLMs/SeaLLM-7B-v2)
- [SeaLLM-7B-v1](https://huggingface.co/SeaLLMs/SeaLLM-7B-v1)
<blockquote style="color:red">
<p><strong style="color: red">Terms of Use and License</strong>:
By using our released weights, codes, and demos, you agree to and comply with the terms and conditions specified in our <a href="https://huggingface.co/SeaLLMs/SeaLLM-Chat-13b/edit/main/LICENSE" target="_blank" rel="noopener">SeaLLMs Terms Of Use</a>.
</blockquote>
> **Disclaimer**:
> We must note that even though the weights, codes, and demos are released in an open manner, similar to other pre-trained language models, and despite our best efforts in red teaming and safety fine-tuning and enforcement, our models come with potential risks, including but not limited to inaccurate, misleading or potentially harmful generation.
> Developers and stakeholders should perform their own red teaming and provide related security measures before deployment, and they must abide by and comply with local governance and regulations.
> In no event shall the authors be held liable for any claim, damages, or other liability arising from the use of the released weights, codes, or demos.
> The logo was generated by DALL-E 3.
### What's new since SeaLLM-7B-v2?
* SeaLLM-7B-v2.5 was built on top of Gemma-7b, and underwent large scale SFT and carefully designed alignment.
## Evaluation
### Multilingual World Knowledge
We evaluate models on 3 benchmarks following the recommended default setups: 5-shot MMLU for En, 3-shot [M3Exam](https://arxiv.org/pdf/2306.05179.pdf) (M3e) for En, Zh, Vi, Id, Th, and zero-shot [VMLU](https://vmlu.ai/) for Vi.
| Model | Langs | En<br>MMLU | En<br>M3e | Zh<br>M3e | Vi<br>M3e | Vi<br>VMLU | Id<br>M3e | Th<br>M3e
|-----| ----- | --- | -- | ----- | ---- | --- | --- | --- |
| GPT-3.5 | Multi | 68.90 | 75.46 | 60.20 | 58.64 | 46.32 | 49.27 | 37.41
| Vistral-7B-chat | Mono | 56.86 | 67.00 | 44.56 | 54.33 | 50.03 | 36.49 | 25.27
| Qwen1.5-7B-chat | Multi | 61.00 | 52.07 | 81.96 | 43.38 | 45.02 | 24.29 | 20.25
| SailorLM | Multi | 52.72 | 59.76 | 67.74 | 50.14 | --- | 39.53 | 37.73
| SeaLLM-7B-v2 | Multi | 61.89 | 70.91 | 55.43 | 51.15 | 45.74 | 42.25 | 35.52
| SeaLLM-7B-v2.5 | Multi | 64.05 | 76.87 | 62.54 | 63.11 | 53.30 | 48.64 | 46.86
### Zero-shot CoT Multilingual Math Reasoning
<!--
[SeaLLM-7B-v2](https://huggingface.co/SeaLLMs/SeaLLM-7B-v2) achieves with **78.5** score on the GSM8K with zero-shot CoT reasoning, making it the **state of the art** in the realm of 7B models. It also outperforms GPT-3.5 in the same GSM8K benchmark as translated into SEA languages (🇨🇳 🇻🇳 🇮🇩 🇹🇭). [SeaLLM-7B-v2](https://huggingface.co/SeaLLMs/SeaLLM-7B-v2) also surpasses GPT-3.5 on the Thai-translated MATH benchmark, with **28.4** vs 18.1 scores.

-->
| Model | GSM8K<br>en | MATH<br>en | GSM8K<br>zh | MATH<br>zh | GSM8K<br>vi | MATH<br>vi | GSM8K<br>id | MATH<br>id | GSM8K<br>th | MATH<br>th
| --- | --- | --- | --- | --- | --- | --- | --- | --- | --- | --- |
| GPT-3.5 | 80.8 | 34.1 | 48.2 | 21.5 | 55 | 26.5 | 64.3 | 26.4 | 35.8 | 18.1
| Qwen-14B-chat | 61.4 | 18.4 | 41.6 | 11.8 | 33.6 | 3.6 | 44.7 | 8.6 | 22 | 6.0
| Vistral-7b-chat | 48.2 | 12.5 | | | 48.7 | 3.1 | | | |
| Qwen1.5-7B-chat | 56.8 | 15.3 | 40.0 | 2.7 | 37.7 | 9 | 36.9 | 7.7 | 21.9 | 4.7
| SeaLLM-7B-v2 | 78.2 | 27.5 | 53.7 | 17.6 | 69.9 | 23.8 | 71.5 | 24.4 | 59.6 | 22.4
| SeaLLM-7B-v2.5 | 78.5 | 34.9 | 51.3 | 22.1 | 72.3 | 30.2 | 71.5 | 30.1 | 62.0 | 28.4
Baselines were evaluated using their respective chat-template and system prompts ([Qwen1.5-7B-chat](https://huggingface.co/Qwen/Qwen1.5-7B-Chat/blob/main/tokenizer_config.json), [Vistral](https://huggingface.co/Viet-Mistral/Vistral-7B-Chat)).
#### Zero-shot MGSM
[SeaLLM-7B-v2.5](https://huggingface.co/SeaLLMs/SeaLLM-7B-v2.5) also outperforms GPT-3.5 and Qwen-14B on the multilingual MGSM for Thai.
| Model | MGSM-Zh | MGSM-Th
|-----| ----- | ---
| ChatGPT (reported) | 61.2 | 47.2
| Qwen-14B-chat | 59.6 | 28
| SeaLLM-7B-v2 | **64.8** | 62.4
| SeaLLM-7B-v2.5 | 58.0 | **64.8**
### Sea-Bench

### Usage
**IMPORTANT NOTICE for using the model**
* `<bos>` must be at start of prompt, ff your code's tokenizer does not prepend `<bos>` by default, you MUST prepend <bos> into the prompt yourself, otherwise, it would not work!
* Repitition penalty (e.g: in llama.cpp, ollama, LM-studio) must be set to **1** , otherwise will lead to degeneration!
#### Instruction format
```python
# ! WARNING, if your code's tokenizer does not prepend <bos> by default,
# You MUST prepend <bos> into the prompt yourself, otherwise, it would not work!
prompt = """<|im_start|>system
You are a helpful assistant.<eos>
<|im_start|>user
Hello world<eos>
<|im_start|>assistant
Hi there, how can I help?<eos>"""
# <|im_start|> is not a special token.
# Transformers chat_template should be consistent with vLLM format below.
# ! ENSURE 1 and only 1 bos `<bos>` at the beginning of sequence
print(tokenizer.convert_ids_to_tokens(tokenizer.encode(prompt)))
"""
```
#### Using transformers's chat_template
Install the latest transformers (>4.40)
```python
from transformers import AutoModelForCausalLM, AutoTokenizer
device = "cuda" # the device to load the model onto
# use bfloat16 to ensure the best performance.
model = AutoModelForCausalLM.from_pretrained("SeaLLMs/SeaLLM-7B-v2.5", torch_dtype=torch.bfloat16, device_map=device)
tokenizer = AutoTokenizer.from_pretrained("SeaLLMs/SeaLLM-7B-v2.5")
messages = [
{"role": "system", "content": "You are a helpful assistant."},
{"role": "user", "content": "Hello world"},
{"role": "assistant", "content": "Hi there, how can I help you today?"},
{"role": "user", "content": "Explain general relativity in details."}
]
encodeds = tokenizer.apply_chat_template(messages, return_tensors="pt", add_generation_prompt=True)
print(tokenizer.convert_ids_to_tokens(encodeds[0]))
model_inputs = encodeds.to(device)
model.to(device)
generated_ids = model.generate(model_inputs, max_new_tokens=1000, do_sample=True, pad_token_id=tokenizer.pad_token_id)
decoded = tokenizer.batch_decode(generated_ids)
print(decoded[0])
```
#### Using vLLM
```python
from vllm import LLM, SamplingParams
TURN_TEMPLATE = "<|im_start|>{role}\n{content}<eos>\n"
TURN_PREFIX = "<|im_start|>{role}\n"
def seallm_chat_convo_format(conversations, add_assistant_prefix: bool, system_prompt=None):
# conversations: list of dict with key `role` and `content` (openai format)
if conversations[0]['role'] != 'system' and system_prompt is not None:
conversations = [{"role": "system", "content": system_prompt}] + conversations
text = ''
for turn_id, turn in enumerate(conversations):
prompt = TURN_TEMPLATE.format(role=turn['role'], content=turn['content'])
text += prompt
if add_assistant_prefix:
prompt = TURN_PREFIX.format(role='assistant')
text += prompt
return text
sparams = SamplingParams(temperature=0.1, max_tokens=1024, stop=['<eos>', '<|im_start|>'])
llm = LLM("SeaLLMs/SeaLLM-7B-v2.5", dtype="bfloat16")
message = "Explain general relativity in details."
prompt = seallm_chat_convo_format(message, True)
gen = llm.generate(prompt, sampling_params)
print(gen[0].outputs[0].text)
```
#### Fine-tuning SeaLLM-7B-v2.5
Should follow the chat format and accurately mask out source tokens. Here is an example.
```python
conversations = [
{"role": "system", "content": "You are helful assistant."},
{"role": "user", "content": "Hello world."},
{"role": "assistant", "content": "Hi there, how can I help?"},
{"role": "user", "content": "Tell me a joke."},
{"role": "assistant", "content": "Why don't scientists trust atoms? Because they make up everything."},
]
def seallm_7b_v25_tokenize_multi_turns(tokenizer, conversations, add_assistant_prefix=False):
"""
Inputs:
conversations: list of dict following openai format, eg
conversations = [
{"role": "system", "content": "You are helful assistant."},
{"role": "user", "content": "Hello world."},
{"role": "assistant", "content": "Hi there, how can I help?"},
{"role": "user", "content": "Tell me a joke."},
{"role": "assistant", "content": "Why don't scientists trust atoms? Because they make up everything."},
]
add_assistant_prefix: whether to add assistant_prefix, only for inference decoding
Outputs:
tokenize_output_sample, {
"input_ids": ...
"token_type_ids": 1 if train and 0 if masked out (not train)
}
During training, need to create a labels, with masked-out tokens = -100 to avoid loss computations.
labels = sample['input_ids'].clone()
labels[sample['token_type_ids'] == 0] = -100
"""
TURN_TEMPLATE = "<|im_start|>{role}\n{content}<eos>\n"
TURN_PREFIX = "<|im_start|>{role}\n"
TURN_SUFFIX = "<eos>\n"
TURN_SUFFIX_TAKE = "<eos>"
sample = None
assistant_prefix_len = None
assistant_suffix_len = None
for turn_id, turn in enumerate(conversations):
prompt = TURN_TEMPLATE.format(role=turn['role'], content=turn['content'])
turn_sample = tokenizer(
prompt, padding=False, truncation=False, verbose=False, add_special_tokens=False,
return_token_type_ids=True,
)
if turn['role'] == 'assistant':
if assistant_prefix_len is None:
assistant_prefix_len = len(tokenizer.encode(TURN_PREFIX.format(role=turn['role']), add_special_tokens=False))
if assistant_suffix_len is None:
assistant_suffix_len = (
len(tokenizer.encode(TURN_SUFFIX.format(role=turn['role']), add_special_tokens=False)) -
len(tokenizer.encode(TURN_SUFFIX_TAKE, add_special_tokens=False))
)
turn_sample['token_type_ids'][assistant_prefix_len:-assistant_suffix_len] = [1] * (len(turn_sample['input_ids']) - assistant_prefix_len - assistant_suffix_len)
if sample is None:
sample = turn_sample
else:
for k in turn_sample.keys():
sample[k].extend(turn_sample[k])
if add_assistant_prefix:
assistant_prefix_sample = tokenizer(
TURN_PREFIX.format(role="assistant"), padding=False, truncation=False, verbose=False, add_special_tokens=False,
return_token_type_ids=True,
)
for k in sample.keys():
sample[k].extend(assistant_prefix_sample[k])
if tokenizer.add_bos_token:
sample['input_ids'] = [tokenizer.bos_token_id] + sample['input_ids']
sample['attention_mask'] = [1] + sample['attention_mask']
sample['token_type_ids'] = [sample['token_type_ids'][0]] + sample['token_type_ids']
return sample
# ! testing
sample = seallm_7b_v25_tokenize_multi_turns(tokenizer, conversations)
tokens = tokenizer.convert_ids_to_tokens(sample['input_ids'])
pairs = [(x, y) for x, y in zip(tokens, sample['token_type_ids'])]
print(pairs)
# source and special tokens is masked out (token_type 0), only assistant with <eos> is trained (token_type 1)
# [('<bos>', 0), ('<', 0), ('|', 0), ..., ('assistant', 0), ('\n', 0), ('Hi', 1), ('▁there', 1), (',', 1), ('▁how', 1), ('▁can', 1), ('▁I', 1), ('▁help', 1), ('?', 1), ('<eos>', 1), ('\n', 0), ('<', 0), ...
```
## Acknowledgement to Our Linguists
We would like to express our special thanks to our professional and native linguists, Tantong Champaiboon, Nguyen Ngoc Yen Nhi and Tara Devina Putri, who helped build, evaluate, and fact-check our sampled pretraining and SFT dataset as well as evaluating our models across different aspects, especially safety.
## Citation
If you find our project useful, we hope you would kindly star our repo and cite our work as follows: Corresponding Author: [[email protected]](mailto:[email protected])
**Author list and order will change!**
* `*` and `^` are equal contributions.
```
@article{damonlpsg2023seallm,
author = {Xuan-Phi Nguyen*, Wenxuan Zhang*, Xin Li*, Mahani Aljunied*, Weiwen Xu, Hou Pong Chan,
Zhiqiang Hu, Chenhui Shen^, Yew Ken Chia^, Xingxuan Li, Jianyu Wang,
Qingyu Tan, Liying Cheng, Guanzheng Chen, Yue Deng, Sen Yang,
Chaoqun Liu, Hang Zhang, Lidong Bing},
title = {SeaLLMs - Large Language Models for Southeast Asia},
year = 2023,
Eprint = {arXiv:2312.00738},
}
```
|
mradermacher/TheSalt-RP-L3-8b-DPO-v0.3.2-e0.4.2-i1-GGUF | mradermacher | "2024-07-01T03:20:32Z" | 16,156 | 0 | transformers | [
"transformers",
"gguf",
"en",
"base_model:cgato/TheSalt-RP-L3-8b-DPO-v0.3.2-e0.4.2",
"license:cc-by-nc-4.0",
"endpoints_compatible",
"region:us"
] | null | "2024-06-30T22:37:11Z" | ---
base_model: cgato/TheSalt-RP-L3-8b-DPO-v0.3.2-e0.4.2
language:
- en
library_name: transformers
license: cc-by-nc-4.0
quantized_by: mradermacher
---
## About
<!-- ### quantize_version: 2 -->
<!-- ### output_tensor_quantised: 1 -->
<!-- ### convert_type: hf -->
<!-- ### vocab_type: -->
<!-- ### tags: nicoboss -->
weighted/imatrix quants of https://huggingface.co/cgato/TheSalt-RP-L3-8b-DPO-v0.3.2-e0.4.2
<!-- provided-files -->
static quants are available at https://huggingface.co/mradermacher/TheSalt-RP-L3-8b-DPO-v0.3.2-e0.4.2-GGUF
## Usage
If you are unsure how to use GGUF files, refer to one of [TheBloke's
READMEs](https://huggingface.co/TheBloke/KafkaLM-70B-German-V0.1-GGUF) for
more details, including on how to concatenate multi-part files.
## Provided Quants
(sorted by size, not necessarily quality. IQ-quants are often preferable over similar sized non-IQ quants)
| Link | Type | Size/GB | Notes |
|:-----|:-----|--------:|:------|
| [GGUF](https://huggingface.co/mradermacher/TheSalt-RP-L3-8b-DPO-v0.3.2-e0.4.2-i1-GGUF/resolve/main/TheSalt-RP-L3-8b-DPO-v0.3.2-e0.4.2.i1-IQ1_S.gguf) | i1-IQ1_S | 2.1 | for the desperate |
| [GGUF](https://huggingface.co/mradermacher/TheSalt-RP-L3-8b-DPO-v0.3.2-e0.4.2-i1-GGUF/resolve/main/TheSalt-RP-L3-8b-DPO-v0.3.2-e0.4.2.i1-IQ1_M.gguf) | i1-IQ1_M | 2.3 | mostly desperate |
| [GGUF](https://huggingface.co/mradermacher/TheSalt-RP-L3-8b-DPO-v0.3.2-e0.4.2-i1-GGUF/resolve/main/TheSalt-RP-L3-8b-DPO-v0.3.2-e0.4.2.i1-IQ2_XXS.gguf) | i1-IQ2_XXS | 2.5 | |
| [GGUF](https://huggingface.co/mradermacher/TheSalt-RP-L3-8b-DPO-v0.3.2-e0.4.2-i1-GGUF/resolve/main/TheSalt-RP-L3-8b-DPO-v0.3.2-e0.4.2.i1-IQ2_XS.gguf) | i1-IQ2_XS | 2.7 | |
| [GGUF](https://huggingface.co/mradermacher/TheSalt-RP-L3-8b-DPO-v0.3.2-e0.4.2-i1-GGUF/resolve/main/TheSalt-RP-L3-8b-DPO-v0.3.2-e0.4.2.i1-IQ2_S.gguf) | i1-IQ2_S | 2.9 | |
| [GGUF](https://huggingface.co/mradermacher/TheSalt-RP-L3-8b-DPO-v0.3.2-e0.4.2-i1-GGUF/resolve/main/TheSalt-RP-L3-8b-DPO-v0.3.2-e0.4.2.i1-IQ2_M.gguf) | i1-IQ2_M | 3.0 | |
| [GGUF](https://huggingface.co/mradermacher/TheSalt-RP-L3-8b-DPO-v0.3.2-e0.4.2-i1-GGUF/resolve/main/TheSalt-RP-L3-8b-DPO-v0.3.2-e0.4.2.i1-Q2_K.gguf) | i1-Q2_K | 3.3 | IQ3_XXS probably better |
| [GGUF](https://huggingface.co/mradermacher/TheSalt-RP-L3-8b-DPO-v0.3.2-e0.4.2-i1-GGUF/resolve/main/TheSalt-RP-L3-8b-DPO-v0.3.2-e0.4.2.i1-IQ3_XXS.gguf) | i1-IQ3_XXS | 3.4 | lower quality |
| [GGUF](https://huggingface.co/mradermacher/TheSalt-RP-L3-8b-DPO-v0.3.2-e0.4.2-i1-GGUF/resolve/main/TheSalt-RP-L3-8b-DPO-v0.3.2-e0.4.2.i1-IQ3_XS.gguf) | i1-IQ3_XS | 3.6 | |
| [GGUF](https://huggingface.co/mradermacher/TheSalt-RP-L3-8b-DPO-v0.3.2-e0.4.2-i1-GGUF/resolve/main/TheSalt-RP-L3-8b-DPO-v0.3.2-e0.4.2.i1-Q3_K_S.gguf) | i1-Q3_K_S | 3.8 | IQ3_XS probably better |
| [GGUF](https://huggingface.co/mradermacher/TheSalt-RP-L3-8b-DPO-v0.3.2-e0.4.2-i1-GGUF/resolve/main/TheSalt-RP-L3-8b-DPO-v0.3.2-e0.4.2.i1-IQ3_S.gguf) | i1-IQ3_S | 3.8 | beats Q3_K* |
| [GGUF](https://huggingface.co/mradermacher/TheSalt-RP-L3-8b-DPO-v0.3.2-e0.4.2-i1-GGUF/resolve/main/TheSalt-RP-L3-8b-DPO-v0.3.2-e0.4.2.i1-IQ3_M.gguf) | i1-IQ3_M | 3.9 | |
| [GGUF](https://huggingface.co/mradermacher/TheSalt-RP-L3-8b-DPO-v0.3.2-e0.4.2-i1-GGUF/resolve/main/TheSalt-RP-L3-8b-DPO-v0.3.2-e0.4.2.i1-Q3_K_M.gguf) | i1-Q3_K_M | 4.1 | IQ3_S probably better |
| [GGUF](https://huggingface.co/mradermacher/TheSalt-RP-L3-8b-DPO-v0.3.2-e0.4.2-i1-GGUF/resolve/main/TheSalt-RP-L3-8b-DPO-v0.3.2-e0.4.2.i1-Q3_K_L.gguf) | i1-Q3_K_L | 4.4 | IQ3_M probably better |
| [GGUF](https://huggingface.co/mradermacher/TheSalt-RP-L3-8b-DPO-v0.3.2-e0.4.2-i1-GGUF/resolve/main/TheSalt-RP-L3-8b-DPO-v0.3.2-e0.4.2.i1-IQ4_XS.gguf) | i1-IQ4_XS | 4.5 | |
| [GGUF](https://huggingface.co/mradermacher/TheSalt-RP-L3-8b-DPO-v0.3.2-e0.4.2-i1-GGUF/resolve/main/TheSalt-RP-L3-8b-DPO-v0.3.2-e0.4.2.i1-Q4_0.gguf) | i1-Q4_0 | 4.8 | fast, low quality |
| [GGUF](https://huggingface.co/mradermacher/TheSalt-RP-L3-8b-DPO-v0.3.2-e0.4.2-i1-GGUF/resolve/main/TheSalt-RP-L3-8b-DPO-v0.3.2-e0.4.2.i1-Q4_K_S.gguf) | i1-Q4_K_S | 4.8 | optimal size/speed/quality |
| [GGUF](https://huggingface.co/mradermacher/TheSalt-RP-L3-8b-DPO-v0.3.2-e0.4.2-i1-GGUF/resolve/main/TheSalt-RP-L3-8b-DPO-v0.3.2-e0.4.2.i1-Q4_K_M.gguf) | i1-Q4_K_M | 5.0 | fast, recommended |
| [GGUF](https://huggingface.co/mradermacher/TheSalt-RP-L3-8b-DPO-v0.3.2-e0.4.2-i1-GGUF/resolve/main/TheSalt-RP-L3-8b-DPO-v0.3.2-e0.4.2.i1-Q5_K_S.gguf) | i1-Q5_K_S | 5.7 | |
| [GGUF](https://huggingface.co/mradermacher/TheSalt-RP-L3-8b-DPO-v0.3.2-e0.4.2-i1-GGUF/resolve/main/TheSalt-RP-L3-8b-DPO-v0.3.2-e0.4.2.i1-Q5_K_M.gguf) | i1-Q5_K_M | 5.8 | |
| [GGUF](https://huggingface.co/mradermacher/TheSalt-RP-L3-8b-DPO-v0.3.2-e0.4.2-i1-GGUF/resolve/main/TheSalt-RP-L3-8b-DPO-v0.3.2-e0.4.2.i1-Q6_K.gguf) | i1-Q6_K | 6.7 | practically like static Q6_K |
Here is a handy graph by ikawrakow comparing some lower-quality quant
types (lower is better):

And here are Artefact2's thoughts on the matter:
https://gist.github.com/Artefact2/b5f810600771265fc1e39442288e8ec9
## FAQ / Model Request
See https://huggingface.co/mradermacher/model_requests for some answers to
questions you might have and/or if you want some other model quantized.
## Thanks
I thank my company, [nethype GmbH](https://www.nethype.de/), for letting
me use its servers and providing upgrades to my workstation to enable
this work in my free time. Additional thanks to [@nicoboss](https://huggingface.co/nicoboss) for giving me access to his hardware for calculating the imatrix for these quants.
<!-- end -->
|
DeepPavlov/distilrubert-small-cased-conversational | DeepPavlov | "2022-06-28T17:19:09Z" | 16,152 | 1 | transformers | [
"transformers",
"pytorch",
"distilbert",
"ru",
"arxiv:2205.02340",
"endpoints_compatible",
"region:us"
] | null | "2022-06-28T17:15:00Z" | ---
language:
- ru
---
# distilrubert-small-cased-conversational
Conversational DistilRuBERT-small \(Russian, cased, 2‑layer, 768‑hidden, 12‑heads, 107M parameters\) was trained on OpenSubtitles\[1\], [Dirty](https://d3.ru/), [Pikabu](https://pikabu.ru/), and a Social Media segment of Taiga corpus\[2\] (as [Conversational RuBERT](https://huggingface.co/DeepPavlov/rubert-base-cased-conversational)). It can be considered as small copy of [Conversational DistilRuBERT-base](https://huggingface.co/DeepPavlov/distilrubert-base-cased-conversational).
Our DistilRuBERT-small was highly inspired by \[3\], \[4\]. Namely, we used
* KL loss (between teacher and student output logits)
* MLM loss (between tokens labels and student output logits)
* Cosine embedding loss (between averaged six consecutive hidden states from teacher's encoder and one hidden state of the student)
* MSE loss (between averaged six consecutive attention maps from teacher's encoder and one attention map of the student)
The model was trained for about 80 hrs. on 8 nVIDIA Tesla P100-SXM2.0 16Gb.
To evaluate improvements in the inference speed, we ran teacher and student models on random sequences with seq_len=512, batch_size = 16 (for throughput) and batch_size=1 (for latency).
All tests were performed on Intel(R) Xeon(R) CPU E5-2698 v4 @ 2.20GHz and nVIDIA Tesla P100-SXM2.0 16Gb.
| Model | Size, Mb. | CPU latency, sec.| GPU latency, sec. | CPU throughput, samples/sec. | GPU throughput, samples/sec. |
|-------------------------------------------------|------------|------------------|-------------------|------------------------------|------------------------------|
| Teacher (RuBERT-base-cased-conversational) | 679 | 0.655 | 0.031 | 0.3754 | 36.4902 |
| Student (DistilRuBERT-small-cased-conversational)| 409 | 0.1656 | 0.015 | 0.9692 | 71.3553 |
To evaluate model quality, we fine-tuned DistilRuBERT-small on classification, NER and question answering tasks. Scores and archives with fine-tuned models can be found in [DeepPavlov docs](http://docs.deeppavlov.ai/en/master/features/overview.html#models). Also, results could be found in the [paper](https://arxiv.org/abs/2205.02340) Tables 1&2 as well as performance benchmarks and training details.
# Citation
If you found the model useful for your research, we are kindly ask to cite [this](https://arxiv.org/abs/2205.02340) paper:
```
@misc{https://doi.org/10.48550/arxiv.2205.02340,
doi = {10.48550/ARXIV.2205.02340},
url = {https://arxiv.org/abs/2205.02340},
author = {Kolesnikova, Alina and Kuratov, Yuri and Konovalov, Vasily and Burtsev, Mikhail},
keywords = {Computation and Language (cs.CL), Machine Learning (cs.LG), FOS: Computer and information sciences, FOS: Computer and information sciences},
title = {Knowledge Distillation of Russian Language Models with Reduction of Vocabulary},
publisher = {arXiv},
year = {2022},
copyright = {arXiv.org perpetual, non-exclusive license}
}
```
\[1\]: P. Lison and J. Tiedemann, 2016, OpenSubtitles2016: Extracting Large Parallel Corpora from Movie and TV Subtitles. In Proceedings of the 10th International Conference on Language Resources and Evaluation \(LREC 2016\)
\[2\]: Shavrina T., Shapovalova O. \(2017\) TO THE METHODOLOGY OF CORPUS CONSTRUCTION FOR MACHINE LEARNING: «TAIGA» SYNTAX TREE CORPUS AND PARSER. in proc. of “CORPORA2017”, international conference , Saint-Petersbourg, 2017.
\[3\]: Sanh, V., Debut, L., Chaumond, J., & Wolf, T. \(2019\). DistilBERT, a distilled version of BERT: smaller, faster, cheaper and lighter. arXiv preprint arXiv:1910.01108.
\[4\]: <https://github.com/huggingface/transformers/tree/master/examples/research_projects/distillation> |
TheBloke/MythoMax-L2-Kimiko-v2-13B-GGUF | TheBloke | "2023-09-27T12:46:50Z" | 16,139 | 30 | transformers | [
"transformers",
"gguf",
"llama",
"base_model:Undi95/MythoMax-L2-Kimiko-v2-13b",
"license:cc-by-nc-4.0",
"text-generation-inference",
"region:us"
] | null | "2023-08-31T11:24:42Z" | ---
license: cc-by-nc-4.0
model_name: MythoMax L2 Kimiko v2 13B
base_model: Undi95/MythoMax-L2-Kimiko-v2-13b
inference: false
model_creator: Undi95
model_type: llama
prompt_template: 'Below is an instruction that describes a task. Write a response
that appropriately completes the request.
### Instruction:
{prompt}
### Response:
'
quantized_by: TheBloke
---
<!-- header start -->
<!-- 200823 -->
<div style="width: auto; margin-left: auto; margin-right: auto">
<img src="https://i.imgur.com/EBdldam.jpg" alt="TheBlokeAI" style="width: 100%; min-width: 400px; display: block; margin: auto;">
</div>
<div style="display: flex; justify-content: space-between; width: 100%;">
<div style="display: flex; flex-direction: column; align-items: flex-start;">
<p style="margin-top: 0.5em; margin-bottom: 0em;"><a href="https://discord.gg/theblokeai">Chat & support: TheBloke's Discord server</a></p>
</div>
<div style="display: flex; flex-direction: column; align-items: flex-end;">
<p style="margin-top: 0.5em; margin-bottom: 0em;"><a href="https://www.patreon.com/TheBlokeAI">Want to contribute? TheBloke's Patreon page</a></p>
</div>
</div>
<div style="text-align:center; margin-top: 0em; margin-bottom: 0em"><p style="margin-top: 0.25em; margin-bottom: 0em;">TheBloke's LLM work is generously supported by a grant from <a href="https://a16z.com">andreessen horowitz (a16z)</a></p></div>
<hr style="margin-top: 1.0em; margin-bottom: 1.0em;">
<!-- header end -->
# MythoMax L2 Kimiko v2 13B - GGUF
- Model creator: [Undi95](https://huggingface.co/Undi95)
- Original model: [MythoMax L2 Kimiko v2 13B](https://huggingface.co/Undi95/MythoMax-L2-Kimiko-v2-13b)
<!-- description start -->
## Description
This repo contains GGUF format model files for [Undi95's MythoMax L2 Kimiko v2 13B](https://huggingface.co/Undi95/MythoMax-L2-Kimiko-v2-13b).
<!-- description end -->
<!-- README_GGUF.md-about-gguf start -->
### About GGUF
GGUF is a new format introduced by the llama.cpp team on August 21st 2023. It is a replacement for GGML, which is no longer supported by llama.cpp. GGUF offers numerous advantages over GGML, such as better tokenisation, and support for special tokens. It is also supports metadata, and is designed to be extensible.
Here is an incomplate list of clients and libraries that are known to support GGUF:
* [llama.cpp](https://github.com/ggerganov/llama.cpp). The source project for GGUF. Offers a CLI and a server option.
* [text-generation-webui](https://github.com/oobabooga/text-generation-webui), the most widely used web UI, with many features and powerful extensions. Supports GPU acceleration.
* [KoboldCpp](https://github.com/LostRuins/koboldcpp), a fully featured web UI, with GPU accel across all platforms and GPU architectures. Especially good for story telling.
* [LM Studio](https://lmstudio.ai/), an easy-to-use and powerful local GUI for Windows and macOS (Silicon), with GPU acceleration.
* [LoLLMS Web UI](https://github.com/ParisNeo/lollms-webui), a great web UI with many interesting and unique features, including a full model library for easy model selection.
* [Faraday.dev](https://faraday.dev/), an attractive and easy to use character-based chat GUI for Windows and macOS (both Silicon and Intel), with GPU acceleration.
* [ctransformers](https://github.com/marella/ctransformers), a Python library with GPU accel, LangChain support, and OpenAI-compatible AI server.
* [llama-cpp-python](https://github.com/abetlen/llama-cpp-python), a Python library with GPU accel, LangChain support, and OpenAI-compatible API server.
* [candle](https://github.com/huggingface/candle), a Rust ML framework with a focus on performance, including GPU support, and ease of use.
<!-- README_GGUF.md-about-gguf end -->
<!-- repositories-available start -->
## Repositories available
* [AWQ model(s) for GPU inference.](https://huggingface.co/TheBloke/MythoMax-L2-Kimiko-v2-13B-AWQ)
* [GPTQ models for GPU inference, with multiple quantisation parameter options.](https://huggingface.co/TheBloke/MythoMax-L2-Kimiko-v2-13B-GPTQ)
* [2, 3, 4, 5, 6 and 8-bit GGUF models for CPU+GPU inference](https://huggingface.co/TheBloke/MythoMax-L2-Kimiko-v2-13B-GGUF)
* [Undi95's original unquantised fp16 model in pytorch format, for GPU inference and for further conversions](https://huggingface.co/Undi95/MythoMax-L2-Kimiko-v2-13b)
<!-- repositories-available end -->
<!-- prompt-template start -->
## Prompt template: Alpaca
```
Below is an instruction that describes a task. Write a response that appropriately completes the request.
### Instruction:
{prompt}
### Response:
```
<!-- prompt-template end -->
<!-- licensing start -->
## Licensing
The creator of the source model has listed its license as `cc-by-nc-4.0`, and this quantization has therefore used that same license.
As this model is based on Llama 2, it is also subject to the Meta Llama 2 license terms, and the license files for that are additionally included. It should therefore be considered as being claimed to be licensed under both licenses. I contacted Hugging Face for clarification on dual licensing but they do not yet have an official position. Should this change, or should Meta provide any feedback on this situation, I will update this section accordingly.
In the meantime, any questions regarding licensing, and in particular how these two licenses might interact, should be directed to the original model repository: [Undi95's MythoMax L2 Kimiko v2 13B](https://huggingface.co/Undi95/MythoMax-L2-Kimiko-v2-13b).
<!-- licensing end -->
<!-- compatibility_gguf start -->
## Compatibility
These quantised GGUFv2 files are compatible with llama.cpp from August 27th onwards, as of commit [d0cee0d36d5be95a0d9088b674dbb27354107221](https://github.com/ggerganov/llama.cpp/commit/d0cee0d36d5be95a0d9088b674dbb27354107221)
They are also compatible with many third party UIs and libraries - please see the list at the top of this README.
## Explanation of quantisation methods
<details>
<summary>Click to see details</summary>
The new methods available are:
* GGML_TYPE_Q2_K - "type-1" 2-bit quantization in super-blocks containing 16 blocks, each block having 16 weight. Block scales and mins are quantized with 4 bits. This ends up effectively using 2.5625 bits per weight (bpw)
* GGML_TYPE_Q3_K - "type-0" 3-bit quantization in super-blocks containing 16 blocks, each block having 16 weights. Scales are quantized with 6 bits. This end up using 3.4375 bpw.
* GGML_TYPE_Q4_K - "type-1" 4-bit quantization in super-blocks containing 8 blocks, each block having 32 weights. Scales and mins are quantized with 6 bits. This ends up using 4.5 bpw.
* GGML_TYPE_Q5_K - "type-1" 5-bit quantization. Same super-block structure as GGML_TYPE_Q4_K resulting in 5.5 bpw
* GGML_TYPE_Q6_K - "type-0" 6-bit quantization. Super-blocks with 16 blocks, each block having 16 weights. Scales are quantized with 8 bits. This ends up using 6.5625 bpw
Refer to the Provided Files table below to see what files use which methods, and how.
</details>
<!-- compatibility_gguf end -->
<!-- README_GGUF.md-provided-files start -->
## Provided files
| Name | Quant method | Bits | Size | Max RAM required | Use case |
| ---- | ---- | ---- | ---- | ---- | ----- |
| [mythomax-l2-kimiko-v2-13b.Q2_K.gguf](https://huggingface.co/TheBloke/MythoMax-L2-Kimiko-v2-13B-GGUF/blob/main/mythomax-l2-kimiko-v2-13b.Q2_K.gguf) | Q2_K | 2 | 5.43 GB| 7.93 GB | smallest, significant quality loss - not recommended for most purposes |
| [mythomax-l2-kimiko-v2-13b.Q3_K_S.gguf](https://huggingface.co/TheBloke/MythoMax-L2-Kimiko-v2-13B-GGUF/blob/main/mythomax-l2-kimiko-v2-13b.Q3_K_S.gguf) | Q3_K_S | 3 | 5.66 GB| 8.16 GB | very small, high quality loss |
| [mythomax-l2-kimiko-v2-13b.Q3_K_M.gguf](https://huggingface.co/TheBloke/MythoMax-L2-Kimiko-v2-13B-GGUF/blob/main/mythomax-l2-kimiko-v2-13b.Q3_K_M.gguf) | Q3_K_M | 3 | 6.34 GB| 8.84 GB | very small, high quality loss |
| [mythomax-l2-kimiko-v2-13b.Q3_K_L.gguf](https://huggingface.co/TheBloke/MythoMax-L2-Kimiko-v2-13B-GGUF/blob/main/mythomax-l2-kimiko-v2-13b.Q3_K_L.gguf) | Q3_K_L | 3 | 6.93 GB| 9.43 GB | small, substantial quality loss |
| [mythomax-l2-kimiko-v2-13b.Q4_0.gguf](https://huggingface.co/TheBloke/MythoMax-L2-Kimiko-v2-13B-GGUF/blob/main/mythomax-l2-kimiko-v2-13b.Q4_0.gguf) | Q4_0 | 4 | 7.37 GB| 9.87 GB | legacy; small, very high quality loss - prefer using Q3_K_M |
| [mythomax-l2-kimiko-v2-13b.Q4_K_S.gguf](https://huggingface.co/TheBloke/MythoMax-L2-Kimiko-v2-13B-GGUF/blob/main/mythomax-l2-kimiko-v2-13b.Q4_K_S.gguf) | Q4_K_S | 4 | 7.41 GB| 9.91 GB | small, greater quality loss |
| [mythomax-l2-kimiko-v2-13b.Q4_K_M.gguf](https://huggingface.co/TheBloke/MythoMax-L2-Kimiko-v2-13B-GGUF/blob/main/mythomax-l2-kimiko-v2-13b.Q4_K_M.gguf) | Q4_K_M | 4 | 7.87 GB| 10.37 GB | medium, balanced quality - recommended |
| [mythomax-l2-kimiko-v2-13b.Q5_0.gguf](https://huggingface.co/TheBloke/MythoMax-L2-Kimiko-v2-13B-GGUF/blob/main/mythomax-l2-kimiko-v2-13b.Q5_0.gguf) | Q5_0 | 5 | 8.97 GB| 11.47 GB | legacy; medium, balanced quality - prefer using Q4_K_M |
| [mythomax-l2-kimiko-v2-13b.Q5_K_S.gguf](https://huggingface.co/TheBloke/MythoMax-L2-Kimiko-v2-13B-GGUF/blob/main/mythomax-l2-kimiko-v2-13b.Q5_K_S.gguf) | Q5_K_S | 5 | 8.97 GB| 11.47 GB | large, low quality loss - recommended |
| [mythomax-l2-kimiko-v2-13b.Q5_K_M.gguf](https://huggingface.co/TheBloke/MythoMax-L2-Kimiko-v2-13B-GGUF/blob/main/mythomax-l2-kimiko-v2-13b.Q5_K_M.gguf) | Q5_K_M | 5 | 9.23 GB| 11.73 GB | large, very low quality loss - recommended |
| [mythomax-l2-kimiko-v2-13b.Q6_K.gguf](https://huggingface.co/TheBloke/MythoMax-L2-Kimiko-v2-13B-GGUF/blob/main/mythomax-l2-kimiko-v2-13b.Q6_K.gguf) | Q6_K | 6 | 10.68 GB| 13.18 GB | very large, extremely low quality loss |
| [mythomax-l2-kimiko-v2-13b.Q8_0.gguf](https://huggingface.co/TheBloke/MythoMax-L2-Kimiko-v2-13B-GGUF/blob/main/mythomax-l2-kimiko-v2-13b.Q8_0.gguf) | Q8_0 | 8 | 13.83 GB| 16.33 GB | very large, extremely low quality loss - not recommended |
**Note**: the above RAM figures assume no GPU offloading. If layers are offloaded to the GPU, this will reduce RAM usage and use VRAM instead.
<!-- README_GGUF.md-provided-files end -->
<!-- README_GGUF.md-how-to-download start -->
## How to download GGUF files
**Note for manual downloaders:** You almost never want to clone the entire repo! Multiple different quantisation formats are provided, and most users only want to pick and download a single file.
The following clients/libraries will automatically download models for you, providing a list of available models to choose from:
- LM Studio
- LoLLMS Web UI
- Faraday.dev
### In `text-generation-webui`
Under Download Model, you can enter the model repo: TheBloke/MythoMax-L2-Kimiko-v2-13B-GGUF and below it, a specific filename to download, such as: mythomax-l2-kimiko-v2-13b.q4_K_M.gguf.
Then click Download.
### On the command line, including multiple files at once
I recommend using the `huggingface-hub` Python library:
```shell
pip3 install huggingface-hub>=0.17.1
```
Then you can download any individual model file to the current directory, at high speed, with a command like this:
```shell
huggingface-cli download TheBloke/MythoMax-L2-Kimiko-v2-13B-GGUF mythomax-l2-kimiko-v2-13b.q4_K_M.gguf --local-dir . --local-dir-use-symlinks False
```
<details>
<summary>More advanced huggingface-cli download usage</summary>
You can also download multiple files at once with a pattern:
```shell
huggingface-cli download TheBloke/MythoMax-L2-Kimiko-v2-13B-GGUF --local-dir . --local-dir-use-symlinks False --include='*Q4_K*gguf'
```
For more documentation on downloading with `huggingface-cli`, please see: [HF -> Hub Python Library -> Download files -> Download from the CLI](https://huggingface.co/docs/huggingface_hub/guides/download#download-from-the-cli).
To accelerate downloads on fast connections (1Gbit/s or higher), install `hf_transfer`:
```shell
pip3 install hf_transfer
```
And set environment variable `HF_HUB_ENABLE_HF_TRANSFER` to `1`:
```shell
HUGGINGFACE_HUB_ENABLE_HF_TRANSFER=1 huggingface-cli download TheBloke/MythoMax-L2-Kimiko-v2-13B-GGUF mythomax-l2-kimiko-v2-13b.q4_K_M.gguf --local-dir . --local-dir-use-symlinks False
```
Windows CLI users: Use `set HUGGINGFACE_HUB_ENABLE_HF_TRANSFER=1` before running the download command.
</details>
<!-- README_GGUF.md-how-to-download end -->
<!-- README_GGUF.md-how-to-run start -->
## Example `llama.cpp` command
Make sure you are using `llama.cpp` from commit [d0cee0d36d5be95a0d9088b674dbb27354107221](https://github.com/ggerganov/llama.cpp/commit/d0cee0d36d5be95a0d9088b674dbb27354107221) or later.
```shell
./main -ngl 32 -m mythomax-l2-kimiko-v2-13b.q4_K_M.gguf --color -c 4096 --temp 0.7 --repeat_penalty 1.1 -n -1 -p "Below is an instruction that describes a task. Write a response that appropriately completes the request.\n\n### Instruction:\n{prompt}\n\n### Response:"
```
Change `-ngl 32` to the number of layers to offload to GPU. Remove it if you don't have GPU acceleration.
Change `-c 4096` to the desired sequence length. For extended sequence models - eg 8K, 16K, 32K - the necessary RoPE scaling parameters are read from the GGUF file and set by llama.cpp automatically.
If you want to have a chat-style conversation, replace the `-p <PROMPT>` argument with `-i -ins`
For other parameters and how to use them, please refer to [the llama.cpp documentation](https://github.com/ggerganov/llama.cpp/blob/master/examples/main/README.md)
## How to run in `text-generation-webui`
Further instructions here: [text-generation-webui/docs/llama.cpp.md](https://github.com/oobabooga/text-generation-webui/blob/main/docs/llama.cpp.md).
## How to run from Python code
You can use GGUF models from Python using the [llama-cpp-python](https://github.com/abetlen/llama-cpp-python) or [ctransformers](https://github.com/marella/ctransformers) libraries.
### How to load this model from Python using ctransformers
#### First install the package
```bash
# Base ctransformers with no GPU acceleration
pip install ctransformers>=0.2.24
# Or with CUDA GPU acceleration
pip install ctransformers[cuda]>=0.2.24
# Or with ROCm GPU acceleration
CT_HIPBLAS=1 pip install ctransformers>=0.2.24 --no-binary ctransformers
# Or with Metal GPU acceleration for macOS systems
CT_METAL=1 pip install ctransformers>=0.2.24 --no-binary ctransformers
```
#### Simple example code to load one of these GGUF models
```python
from ctransformers import AutoModelForCausalLM
# Set gpu_layers to the number of layers to offload to GPU. Set to 0 if no GPU acceleration is available on your system.
llm = AutoModelForCausalLM.from_pretrained("TheBloke/MythoMax-L2-Kimiko-v2-13B-GGUF", model_file="mythomax-l2-kimiko-v2-13b.q4_K_M.gguf", model_type="llama", gpu_layers=50)
print(llm("AI is going to"))
```
## How to use with LangChain
Here's guides on using llama-cpp-python or ctransformers with LangChain:
* [LangChain + llama-cpp-python](https://python.langchain.com/docs/integrations/llms/llamacpp)
* [LangChain + ctransformers](https://python.langchain.com/docs/integrations/providers/ctransformers)
<!-- README_GGUF.md-how-to-run end -->
<!-- footer start -->
<!-- 200823 -->
## Discord
For further support, and discussions on these models and AI in general, join us at:
[TheBloke AI's Discord server](https://discord.gg/theblokeai)
## Thanks, and how to contribute
Thanks to the [chirper.ai](https://chirper.ai) team!
Thanks to Clay from [gpus.llm-utils.org](llm-utils)!
I've had a lot of people ask if they can contribute. I enjoy providing models and helping people, and would love to be able to spend even more time doing it, as well as expanding into new projects like fine tuning/training.
If you're able and willing to contribute it will be most gratefully received and will help me to keep providing more models, and to start work on new AI projects.
Donaters will get priority support on any and all AI/LLM/model questions and requests, access to a private Discord room, plus other benefits.
* Patreon: https://patreon.com/TheBlokeAI
* Ko-Fi: https://ko-fi.com/TheBlokeAI
**Special thanks to**: Aemon Algiz.
**Patreon special mentions**: Alicia Loh, Stephen Murray, K, Ajan Kanaga, RoA, Magnesian, Deo Leter, Olakabola, Eugene Pentland, zynix, Deep Realms, Raymond Fosdick, Elijah Stavena, Iucharbius, Erik Bjäreholt, Luis Javier Navarrete Lozano, Nicholas, theTransient, John Detwiler, alfie_i, knownsqashed, Mano Prime, Willem Michiel, Enrico Ros, LangChain4j, OG, Michael Dempsey, Pierre Kircher, Pedro Madruga, James Bentley, Thomas Belote, Luke @flexchar, Leonard Tan, Johann-Peter Hartmann, Illia Dulskyi, Fen Risland, Chadd, S_X, Jeff Scroggin, Ken Nordquist, Sean Connelly, Artur Olbinski, Swaroop Kallakuri, Jack West, Ai Maven, David Ziegler, Russ Johnson, transmissions 11, John Villwock, Alps Aficionado, Clay Pascal, Viktor Bowallius, Subspace Studios, Rainer Wilmers, Trenton Dambrowitz, vamX, Michael Levine, 준교 김, Brandon Frisco, Kalila, Trailburnt, Randy H, Talal Aujan, Nathan Dryer, Vadim, 阿明, ReadyPlayerEmma, Tiffany J. Kim, George Stoitzev, Spencer Kim, Jerry Meng, Gabriel Tamborski, Cory Kujawski, Jeffrey Morgan, Spiking Neurons AB, Edmond Seymore, Alexandros Triantafyllidis, Lone Striker, Cap'n Zoog, Nikolai Manek, danny, ya boyyy, Derek Yates, usrbinkat, Mandus, TL, Nathan LeClaire, subjectnull, Imad Khwaja, webtim, Raven Klaugh, Asp the Wyvern, Gabriel Puliatti, Caitlyn Gatomon, Joseph William Delisle, Jonathan Leane, Luke Pendergrass, SuperWojo, Sebastain Graf, Will Dee, Fred von Graf, Andrey, Dan Guido, Daniel P. Andersen, Nitin Borwankar, Elle, Vitor Caleffi, biorpg, jjj, NimbleBox.ai, Pieter, Matthew Berman, terasurfer, Michael Davis, Alex, Stanislav Ovsiannikov
Thank you to all my generous patrons and donaters!
And thank you again to a16z for their generous grant.
<!-- footer end -->
<!-- original-model-card start -->
# Original model card: Undi95's MythoMax L2 Kimiko v2 13B
LoRA merged to a Model.
Model : https://huggingface.co/Gryphe/MythoMax-L2-13b
LoRA : https://huggingface.co/nRuaif/Kimiko-v2-13B
Weight : 0.50
<!-- original-model-card end -->
|
facebook/deit-tiny-patch16-224 | facebook | "2022-07-13T11:53:31Z" | 16,135 | 5 | transformers | [
"transformers",
"pytorch",
"tf",
"vit",
"image-classification",
"dataset:imagenet",
"arxiv:2012.12877",
"arxiv:2006.03677",
"license:apache-2.0",
"autotrain_compatible",
"endpoints_compatible",
"region:us"
] | image-classification | "2022-03-02T23:29:05Z" | ---
license: apache-2.0
tags:
- image-classification
datasets:
- imagenet
---
# Data-efficient Image Transformer (tiny-sized model)
Data-efficient Image Transformer (DeiT) model pre-trained and fine-tuned on ImageNet-1k (1 million images, 1,000 classes) at resolution 224x224. It was first introduced in the paper [Training data-efficient image transformers & distillation through attention](https://arxiv.org/abs/2012.12877) by Touvron et al. and first released in [this repository](https://github.com/facebookresearch/deit). However, the weights were converted from the [timm repository](https://github.com/rwightman/pytorch-image-models) by Ross Wightman.
Disclaimer: The team releasing DeiT did not write a model card for this model so this model card has been written by the Hugging Face team.
## Model description
This model is actually a more efficiently trained Vision Transformer (ViT).
The Vision Transformer (ViT) is a transformer encoder model (BERT-like) pre-trained and fine-tuned on a large collection of images in a supervised fashion, namely ImageNet-1k, at a resolution of 224x224 pixels.
Images are presented to the model as a sequence of fixed-size patches (resolution 16x16), which are linearly embedded. One also adds a [CLS] token to the beginning of a sequence to use it for classification tasks. One also adds absolute position embeddings before feeding the sequence to the layers of the Transformer encoder.
By pre-training the model, it learns an inner representation of images that can then be used to extract features useful for downstream tasks: if you have a dataset of labeled images for instance, you can train a standard classifier by placing a linear layer on top of the pre-trained encoder. One typically places a linear layer on top of the [CLS] token, as the last hidden state of this token can be seen as a representation of an entire image.
## Intended uses & limitations
You can use the raw model for image classification. See the [model hub](https://huggingface.co/models?search=facebook/deit) to look for
fine-tuned versions on a task that interests you.
### How to use
Since this model is a more efficiently trained ViT model, you can plug it into ViTModel or ViTForImageClassification. Note that the model expects the data to be prepared using DeiTFeatureExtractor. Here we use AutoFeatureExtractor, which will automatically use the appropriate feature extractor given the model name.
Here is how to use this model to classify an image of the COCO 2017 dataset into one of the 1,000 ImageNet classes:
```python
from transformers import AutoFeatureExtractor, ViTForImageClassification
from PIL import Image
import requests
url = 'http://images.cocodataset.org/val2017/000000039769.jpg'
image = Image.open(requests.get(url, stream=True).raw)
feature_extractor = AutoFeatureExtractor.from_pretrained('facebook/deit-tiny-patch16-224')
model = ViTForImageClassification.from_pretrained('facebook/deit-tiny-patch16-224')
inputs = feature_extractor(images=image, return_tensors="pt")
outputs = model(**inputs)
logits = outputs.logits
# model predicts one of the 1000 ImageNet classes
predicted_class_idx = logits.argmax(-1).item()
print("Predicted class:", model.config.id2label[predicted_class_idx])
```
Currently, both the feature extractor and model support PyTorch. Tensorflow and JAX/FLAX are coming soon.
## Training data
The ViT model was pretrained on [ImageNet-1k](http://www.image-net.org/challenges/LSVRC/2012/), a dataset consisting of 1 million images and 1k classes.
## Training procedure
### Preprocessing
The exact details of preprocessing of images during training/validation can be found [here](https://github.com/facebookresearch/deit/blob/ab5715372db8c6cad5740714b2216d55aeae052e/datasets.py#L78).
At inference time, images are resized/rescaled to the same resolution (256x256), center-cropped at 224x224 and normalized across the RGB channels with the ImageNet mean and standard deviation.
### Pretraining
The model was trained on a single 8-GPU node for 3 days. Training resolution is 224. For all hyperparameters (such as batch size and learning rate) we refer to table 9 of the original paper.
## Evaluation results
| Model | ImageNet top-1 accuracy | ImageNet top-5 accuracy | # params | URL |
|---------------------------------------|-------------------------|-------------------------|----------|------------------------------------------------------------------|
| **DeiT-tiny** | **72.2** | **91.1** | **5M** | **https://huggingface.co/facebook/deit-tiny-patch16-224** |
| DeiT-small | 79.9 | 95.0 | 22M | https://huggingface.co/facebook/deit-small-patch16-224 |
| DeiT-base | 81.8 | 95.6 | 86M | https://huggingface.co/facebook/deit-base-patch16-224 |
| DeiT-tiny distilled | 74.5 | 91.9 | 6M | https://huggingface.co/facebook/deit-tiny-distilled-patch16-224 |
| DeiT-small distilled | 81.2 | 95.4 | 22M | https://huggingface.co/facebook/deit-small-distilled-patch16-224 |
| DeiT-base distilled | 83.4 | 96.5 | 87M | https://huggingface.co/facebook/deit-base-distilled-patch16-224 |
| DeiT-base 384 | 82.9 | 96.2 | 87M | https://huggingface.co/facebook/deit-base-patch16-384 |
| DeiT-base distilled 384 (1000 epochs) | 85.2 | 97.2 | 88M | https://huggingface.co/facebook/deit-base-distilled-patch16-384 |
Note that for fine-tuning, the best results are obtained with a higher resolution (384x384). Of course, increasing the model size will result in better performance.
### BibTeX entry and citation info
```bibtex
@misc{touvron2021training,
title={Training data-efficient image transformers & distillation through attention},
author={Hugo Touvron and Matthieu Cord and Matthijs Douze and Francisco Massa and Alexandre Sablayrolles and Hervé Jégou},
year={2021},
eprint={2012.12877},
archivePrefix={arXiv},
primaryClass={cs.CV}
}
```
```bibtex
@misc{wu2020visual,
title={Visual Transformers: Token-based Image Representation and Processing for Computer Vision},
author={Bichen Wu and Chenfeng Xu and Xiaoliang Dai and Alvin Wan and Peizhao Zhang and Zhicheng Yan and Masayoshi Tomizuka and Joseph Gonzalez and Kurt Keutzer and Peter Vajda},
year={2020},
eprint={2006.03677},
archivePrefix={arXiv},
primaryClass={cs.CV}
}
```
```bibtex
@inproceedings{deng2009imagenet,
title={Imagenet: A large-scale hierarchical image database},
author={Deng, Jia and Dong, Wei and Socher, Richard and Li, Li-Jia and Li, Kai and Fei-Fei, Li},
booktitle={2009 IEEE conference on computer vision and pattern recognition},
pages={248--255},
year={2009},
organization={Ieee}
}
``` |
mradermacher/JiuZhou-Instruct-v0.2-GGUF | mradermacher | "2024-06-29T16:40:29Z" | 16,135 | 0 | transformers | [
"transformers",
"gguf",
"en",
"base_model:itpossible/JiuZhou-Instruct-v0.2",
"license:apache-2.0",
"endpoints_compatible",
"region:us"
] | null | "2024-06-29T15:47:37Z" | ---
base_model: itpossible/JiuZhou-Instruct-v0.2
language:
- en
library_name: transformers
license: apache-2.0
quantized_by: mradermacher
---
## About
<!-- ### quantize_version: 2 -->
<!-- ### output_tensor_quantised: 1 -->
<!-- ### convert_type: hf -->
<!-- ### vocab_type: -->
<!-- ### tags: -->
static quants of https://huggingface.co/itpossible/JiuZhou-Instruct-v0.2
<!-- provided-files -->
weighted/imatrix quants seem not to be available (by me) at this time. If they do not show up a week or so after the static ones, I have probably not planned for them. Feel free to request them by opening a Community Discussion.
## Usage
If you are unsure how to use GGUF files, refer to one of [TheBloke's
READMEs](https://huggingface.co/TheBloke/KafkaLM-70B-German-V0.1-GGUF) for
more details, including on how to concatenate multi-part files.
## Provided Quants
(sorted by size, not necessarily quality. IQ-quants are often preferable over similar sized non-IQ quants)
| Link | Type | Size/GB | Notes |
|:-----|:-----|--------:|:------|
| [GGUF](https://huggingface.co/mradermacher/JiuZhou-Instruct-v0.2-GGUF/resolve/main/JiuZhou-Instruct-v0.2.Q2_K.gguf) | Q2_K | 3.0 | |
| [GGUF](https://huggingface.co/mradermacher/JiuZhou-Instruct-v0.2-GGUF/resolve/main/JiuZhou-Instruct-v0.2.IQ3_XS.gguf) | IQ3_XS | 3.3 | |
| [GGUF](https://huggingface.co/mradermacher/JiuZhou-Instruct-v0.2-GGUF/resolve/main/JiuZhou-Instruct-v0.2.Q3_K_S.gguf) | Q3_K_S | 3.4 | |
| [GGUF](https://huggingface.co/mradermacher/JiuZhou-Instruct-v0.2-GGUF/resolve/main/JiuZhou-Instruct-v0.2.IQ3_S.gguf) | IQ3_S | 3.4 | beats Q3_K* |
| [GGUF](https://huggingface.co/mradermacher/JiuZhou-Instruct-v0.2-GGUF/resolve/main/JiuZhou-Instruct-v0.2.IQ3_M.gguf) | IQ3_M | 3.5 | |
| [GGUF](https://huggingface.co/mradermacher/JiuZhou-Instruct-v0.2-GGUF/resolve/main/JiuZhou-Instruct-v0.2.Q3_K_M.gguf) | Q3_K_M | 3.8 | lower quality |
| [GGUF](https://huggingface.co/mradermacher/JiuZhou-Instruct-v0.2-GGUF/resolve/main/JiuZhou-Instruct-v0.2.Q3_K_L.gguf) | Q3_K_L | 4.1 | |
| [GGUF](https://huggingface.co/mradermacher/JiuZhou-Instruct-v0.2-GGUF/resolve/main/JiuZhou-Instruct-v0.2.IQ4_XS.gguf) | IQ4_XS | 4.2 | |
| [GGUF](https://huggingface.co/mradermacher/JiuZhou-Instruct-v0.2-GGUF/resolve/main/JiuZhou-Instruct-v0.2.Q4_K_S.gguf) | Q4_K_S | 4.4 | fast, recommended |
| [GGUF](https://huggingface.co/mradermacher/JiuZhou-Instruct-v0.2-GGUF/resolve/main/JiuZhou-Instruct-v0.2.Q4_K_M.gguf) | Q4_K_M | 4.6 | fast, recommended |
| [GGUF](https://huggingface.co/mradermacher/JiuZhou-Instruct-v0.2-GGUF/resolve/main/JiuZhou-Instruct-v0.2.Q5_K_S.gguf) | Q5_K_S | 5.3 | |
| [GGUF](https://huggingface.co/mradermacher/JiuZhou-Instruct-v0.2-GGUF/resolve/main/JiuZhou-Instruct-v0.2.Q5_K_M.gguf) | Q5_K_M | 5.4 | |
| [GGUF](https://huggingface.co/mradermacher/JiuZhou-Instruct-v0.2-GGUF/resolve/main/JiuZhou-Instruct-v0.2.Q6_K.gguf) | Q6_K | 6.3 | very good quality |
| [GGUF](https://huggingface.co/mradermacher/JiuZhou-Instruct-v0.2-GGUF/resolve/main/JiuZhou-Instruct-v0.2.Q8_0.gguf) | Q8_0 | 8.1 | fast, best quality |
| [GGUF](https://huggingface.co/mradermacher/JiuZhou-Instruct-v0.2-GGUF/resolve/main/JiuZhou-Instruct-v0.2.f16.gguf) | f16 | 15.1 | 16 bpw, overkill |
Here is a handy graph by ikawrakow comparing some lower-quality quant
types (lower is better):

And here are Artefact2's thoughts on the matter:
https://gist.github.com/Artefact2/b5f810600771265fc1e39442288e8ec9
## FAQ / Model Request
See https://huggingface.co/mradermacher/model_requests for some answers to
questions you might have and/or if you want some other model quantized.
## Thanks
I thank my company, [nethype GmbH](https://www.nethype.de/), for letting
me use its servers and providing upgrades to my workstation to enable
this work in my free time.
<!-- end -->
|
visheratin/nllb-clip-base-siglip | visheratin | "2024-05-03T04:44:12Z" | 16,103 | 1 | open_clip | [
"open_clip",
"clip",
"zero-shot-image-classification",
"dataset:visheratin/laion-coco-nllb",
"arxiv:2309.01859",
"license:cc-by-nc-4.0",
"region:us"
] | zero-shot-image-classification | "2023-11-14T04:12:01Z" | ---
tags:
- clip
library_name: open_clip
pipeline_tag: zero-shot-image-classification
license: cc-by-nc-4.0
datasets:
- visheratin/laion-coco-nllb
---
## Model Summary
NLLB-CLIP-SigLIP is a model that combines a text encoder from the [NLLB model](https://huggingface.co/facebook/nllb-200-distilled-600M) and an image encoder from the
[SigLIP](https://huggingface.co/timm/ViT-B-16-SigLIP-384) model. This allows us to extend the model capabilities
to 201 languages of the Flores-200. NLLB-CLIP sets state-of-the-art on the [Crossmodal-3600](https://google.github.io/crossmodal-3600/) dataset by performing very
well on low-resource languages. You can find more details about the model in the [paper](https://arxiv.org/abs/2309.01859).
This version performs much better than the [standard](https://huggingface.co/visheratin/nllb-clip-base-oc) version. You can see the results
[here](https://github.com/mlfoundations/open_clip/blob/main/docs/openclip_multilingual_retrieval_results.csv) and
[here](https://github.com/gregor-ge/Babel-ImageNet/blob/main/evaluation_scripts/results_analysis.ipynb).
<b>NB: There is even better [version](https://huggingface.co/visheratin/nllb-siglip-mrl-base) of this model available!</b>
## How to use
<a target="_blank" href="https://colab.research.google.com/drive/1TE_jln3SwTDzjFsGqbdxIJkwrUlnNs3i">
<img src="https://colab.research.google.com/assets/colab-badge.svg" alt="Open In Colab"/>
</a>
This model is integrated into OpenCLIP so that you can use it as any other model:
```
!pip install -U open_clip_torch
```
```
from open_clip import create_model_from_pretrained, get_tokenizer
from PIL import Image
import requests
import torch
model, transform = create_model_from_pretrained("nllb-clip-base-siglip", "v1", device="cuda")
tokenizer = get_tokenizer("nllb-clip-base-siglip")
class_options = ["бабочка", "butterfly", "kat"]
class_langs = ["rus_Cyrl", "eng_Latn", "afr_Latn"]
text_inputs = []
for i in range(len(class_options)):
tokenizer.set_language(class_langs[i])
text_inputs.append(tokenizer(class_options[i]))
text_inputs = torch.stack(text_inputs).squeeze(1).to("cuda")
image_path = "https://huggingface.co/spaces/jjourney1125/swin2sr/resolve/main/samples/butterfly.jpg"
image = Image.open(requests.get(image_path, stream=True).raw)
image_inputs = transform(image).unsqueeze(0).to("cuda")
with torch.inference_mode():
logits_per_image, logits_per_text = model.get_logits(image_inputs, text_inputs)
print(logits_per_image.softmax(dim=-1))
```
## Acknowledgements
I thank [ML Collective](https://mlcollective.org/) for providing Google Cloud compute resources to train the OpenCLIP-compatible version of NLLB-CLIP.
|
climatebert/environmental-claims | climatebert | "2023-05-24T06:39:48Z" | 16,102 | 11 | transformers | [
"transformers",
"pytorch",
"safetensors",
"roberta",
"text-classification",
"ClimateBERT",
"climate",
"en",
"dataset:climatebert/environmental_claims",
"arxiv:2209.00507",
"license:apache-2.0",
"autotrain_compatible",
"endpoints_compatible",
"region:us"
] | text-classification | "2022-09-01T14:22:37Z" | ---
language: en
license: apache-2.0
datasets: climatebert/environmental_claims
tags:
- ClimateBERT
- climate
---
# Model Card for environmental-claims
## Model Description
The environmental-claims model is fine-tuned on the [EnvironmentalClaims](https://huggingface.co/datasets/climatebert/environmental_claims) dataset by using the [climatebert/distilroberta-base-climate-f](https://huggingface.co/climatebert/distilroberta-base-climate-f) model as pre-trained language model. The underlying methodology can be found in our [research paper](https://arxiv.org/abs/2209.00507).
## Climate Performance Model Card
| environmental-claims | |
|--------------------------------------------------------------------------|----------------|
| 1. Is the resulting model publicly available? | Yes |
| 2. How much time does the training of the final model take? | < 5 min |
| 3. How much time did all experiments take (incl. hyperparameter search)? | 60 hours |
| 4. What was the power of GPU and CPU? | 0.3 kW |
| 5. At which geo location were the computations performed? | Switzerland |
| 6. What was the energy mix at the geo location? | 89 gCO2eq/kWh |
| 7. How much CO2eq was emitted to train the final model? | 2.2 g |
| 8. How much CO2eq was emitted for all experiments? | 1.6 kg |
| 9. What is the average CO2eq emission for the inference of one sample? | 0.0067 mg |
| 10. Which positive environmental impact can be expected from this work? | This work can help detect and evaluate environmental claims and thus have a positive impact on the environment in the future. |
| 11. Comments | - |
## Citation Information
```bibtex
@misc{stammbach2022environmentalclaims,
title = {A Dataset for Detecting Real-World Environmental Claims},
author = {Stammbach, Dominik and Webersinke, Nicolas and Bingler, Julia Anna and Kraus, Mathias and Leippold, Markus},
year = {2022},
doi = {10.48550/ARXIV.2209.00507},
url = {https://arxiv.org/abs/2209.00507},
publisher = {arXiv},
}
```
## How to Get Started With the Model
You can use the model with a pipeline for text classification:
```python
from transformers import AutoModelForSequenceClassification, AutoTokenizer, pipeline
from transformers.pipelines.pt_utils import KeyDataset
import datasets
from tqdm.auto import tqdm
dataset_name = "climatebert/environmental_claims"
model_name = "climatebert/environmental-claims"
# If you want to use your own data, simply load them as 🤗 Datasets dataset, see https://huggingface.co/docs/datasets/loading
dataset = datasets.load_dataset(dataset_name, split="test")
model = AutoModelForSequenceClassification.from_pretrained(model_name)
tokenizer = AutoTokenizer.from_pretrained(model_name, max_len=512)
pipe = pipeline("text-classification", model=model, tokenizer=tokenizer, device=0)
# See https://huggingface.co/docs/transformers/main_classes/pipelines#transformers.pipeline
for out in tqdm(pipe(KeyDataset(dataset, "text"), padding=True, truncation=True)):
print(out)
``` |
joeddav/distilbert-base-uncased-go-emotions-student | joeddav | "2021-02-19T22:15:52Z" | 16,096 | 68 | transformers | [
"transformers",
"pytorch",
"tf",
"distilbert",
"text-classification",
"tensorflow",
"en",
"dataset:go_emotions",
"license:mit",
"autotrain_compatible",
"endpoints_compatible",
"region:us"
] | text-classification | "2022-03-02T23:29:05Z" | ---
language: en
tags:
- text-classification
- pytorch
- tensorflow
datasets:
- go_emotions
license: mit
widget:
- text: "I feel lucky to be here."
---
# distilbert-base-uncased-go-emotions-student
## Model Description
This model is distilled from the zero-shot classification pipeline on the unlabeled GoEmotions dataset using [this
script](https://github.com/huggingface/transformers/tree/master/examples/research_projects/zero-shot-distillation).
It was trained with mixed precision for 10 epochs and otherwise used the default script arguments.
## Intended Usage
The model can be used like any other model trained on GoEmotions, but will likely not perform as well as a model
trained with full supervision. It is primarily intended as a demo of how an expensive NLI-based zero-shot model
can be distilled to a more efficient student, allowing a classifier to be trained with only unlabeled data. Note
that although the GoEmotions dataset allow multiple labels per instance, the teacher used single-label
classification to create psuedo-labels.
|
FatihC/swin-tiny-patch4-window7-224-finetuned-eurosat-watermark | FatihC | "2023-04-20T10:37:48Z" | 16,076 | 3 | transformers | [
"transformers",
"pytorch",
"tensorboard",
"swin",
"image-classification",
"generated_from_trainer",
"dataset:imagefolder",
"license:apache-2.0",
"model-index",
"autotrain_compatible",
"endpoints_compatible",
"region:us"
] | image-classification | "2023-04-20T09:46:13Z" | ---
license: apache-2.0
tags:
- generated_from_trainer
datasets:
- imagefolder
metrics:
- accuracy
model-index:
- name: swin-tiny-patch4-window7-224-finetuned-eurosat
results:
- task:
name: Image Classification
type: image-classification
dataset:
name: imagefolder
type: imagefolder
config: images
split: train
args: images
metrics:
- name: Accuracy
type: accuracy
value: 0.9609375
---
<!-- This model card has been generated automatically according to the information the Trainer had access to. You
should probably proofread and complete it, then remove this comment. -->
# swin-tiny-patch4-window7-224-finetuned-eurosat
This model is a fine-tuned version of [microsoft/swin-tiny-patch4-window7-224](https://huggingface.co/microsoft/swin-tiny-patch4-window7-224) on the imagefolder dataset.
It achieves the following results on the evaluation set:
- Loss: 0.1211
- Accuracy: 0.9609
## Model description
More information needed
## Intended uses & limitations
More information needed
## Training and evaluation data
More information needed
## Training procedure
### Training hyperparameters
The following hyperparameters were used during training:
- learning_rate: 5e-05
- train_batch_size: 32
- eval_batch_size: 32
- seed: 42
- gradient_accumulation_steps: 4
- total_train_batch_size: 128
- optimizer: Adam with betas=(0.9,0.999) and epsilon=1e-08
- lr_scheduler_type: linear
- lr_scheduler_warmup_ratio: 0.1
- num_epochs: 40
### Training results
| Training Loss | Epoch | Step | Validation Loss | Accuracy |
|:-------------:|:-----:|:----:|:---------------:|:--------:|
| No log | 1.0 | 4 | 0.4862 | 0.8516 |
| No log | 2.0 | 8 | 0.4103 | 0.8828 |
| 0.4518 | 3.0 | 12 | 0.3210 | 0.8984 |
| 0.4518 | 4.0 | 16 | 0.2053 | 0.9375 |
| 0.2909 | 5.0 | 20 | 0.1675 | 0.9453 |
| 0.2909 | 6.0 | 24 | 0.1439 | 0.9531 |
| 0.2909 | 7.0 | 28 | 0.1448 | 0.9297 |
| 0.1492 | 8.0 | 32 | 0.1798 | 0.9531 |
| 0.1492 | 9.0 | 36 | 0.1360 | 0.9453 |
| 0.1161 | 10.0 | 40 | 0.1670 | 0.9531 |
| 0.1161 | 11.0 | 44 | 0.1637 | 0.9531 |
| 0.1161 | 12.0 | 48 | 0.1298 | 0.9531 |
| 0.1053 | 13.0 | 52 | 0.1162 | 0.9531 |
| 0.1053 | 14.0 | 56 | 0.1353 | 0.9531 |
| 0.0839 | 15.0 | 60 | 0.1211 | 0.9609 |
| 0.0839 | 16.0 | 64 | 0.1113 | 0.9609 |
| 0.0839 | 17.0 | 68 | 0.1145 | 0.9609 |
| 0.0689 | 18.0 | 72 | 0.1239 | 0.9531 |
| 0.0689 | 19.0 | 76 | 0.1280 | 0.9531 |
| 0.0581 | 20.0 | 80 | 0.1533 | 0.9531 |
| 0.0581 | 21.0 | 84 | 0.1323 | 0.9609 |
| 0.0581 | 22.0 | 88 | 0.1327 | 0.9531 |
| 0.0545 | 23.0 | 92 | 0.1529 | 0.9531 |
| 0.0545 | 24.0 | 96 | 0.1357 | 0.9531 |
| 0.046 | 25.0 | 100 | 0.1333 | 0.9531 |
| 0.046 | 26.0 | 104 | 0.1466 | 0.9531 |
| 0.046 | 27.0 | 108 | 0.1300 | 0.9531 |
| 0.0421 | 28.0 | 112 | 0.1077 | 0.9609 |
| 0.0421 | 29.0 | 116 | 0.0985 | 0.9609 |
| 0.0371 | 30.0 | 120 | 0.1186 | 0.9531 |
| 0.0371 | 31.0 | 124 | 0.1123 | 0.9531 |
| 0.0371 | 32.0 | 128 | 0.1144 | 0.9531 |
| 0.0348 | 33.0 | 132 | 0.1276 | 0.9531 |
| 0.0348 | 34.0 | 136 | 0.1488 | 0.9531 |
| 0.0211 | 35.0 | 140 | 0.1560 | 0.9531 |
| 0.0211 | 36.0 | 144 | 0.1477 | 0.9531 |
| 0.0211 | 37.0 | 148 | 0.1488 | 0.9531 |
| 0.0274 | 38.0 | 152 | 0.1467 | 0.9531 |
| 0.0274 | 39.0 | 156 | 0.1401 | 0.9531 |
| 0.0259 | 40.0 | 160 | 0.1379 | 0.9531 |
### Framework versions
- Transformers 4.28.1
- Pytorch 2.0.0+cu118
- Datasets 2.11.0
- Tokenizers 0.13.3
|
miqudev/miqu-1-70b | miqudev | "2024-02-04T19:00:35Z" | 16,060 | 973 | null | [
"gguf",
"region:us"
] | null | "2024-01-26T10:50:49Z" | ---
{}
---
# miqu 70b
Leaked from
▄▄▄░░
▄▄▄▄▄█████████░░░░
▄▄▄▄▄▄████████████████████░░░░░
█████████████████████████████░░░░░
▄▄▄▄▄▄█████░░░ █████████████████████████████░░░░░
▄▄▄▄▄██████████████████░░░░░░ ██████████████████████████████░░░░░
▄█████████████████████████████░░░░░░░░██████████████████████████████░░░░░
███████████████████████████████░░░░░░░██████████████████████████████░░░░░
███████████████████████████████░░░░░░░██████████████████████████████░░░░░
███████████████████████████████░░░░░░███████████████████████████████░░░░░
████████████████████████████████░░░░░███████████████████████████████░░░░░
████████████████████████████████░░░░████████████████████████████████░░░░░
█████████████████████████████████░░░████████████████████████████████░░░░░
█████████████████████████████████░░░████████████░███████████████████░░░░░
██████████████████████████████████░█████████████░███████████████████░░░░░
███████████████████░██████████████▄█████████████░███████████████████░░░░░
███████████████████░███████████████████████████░░███████████████████░░░░░
███████████████████░░██████████████████████████░░███████████████████░░░░░
███████████████████░░█████████████████████████░░░███████████████████░░░░░
███████████████████░░░████████████████████████░░░███████████████████░░░░░
███████████████████░░░████████████████████████░░░███████████████████░░░░░
███████████████████░░░░██████████████████████░░░░███████████████████░░░░░
███████████████████░░░░██████████████████████░░░░███████████████████░░░░░
███████████████████░░░░░█████████████████████░░░░███████████████████░░░░░
███████████████████░░░░░████████████████████░░░░░███████████████████░░░░░
███████████████████░░░░░░███████████████████░░░░░███████████████████░░░░░
███████████████████░░░░░░██████████████████░░░░░░███████████████████░░░░░
███████████████████░░░░░░░█████████████████░░░░░░███████████████████░░░░░
███████████████████░░░░░░░█████████████████░░░░░░███████████████████░░░░░
███████████████████░░░░░░░░███████████████░░░░░░░██████████░░░░░░░░░░░░░░
███████████████████░░░░░░░░███████████████░░░░░░░░░░░░░░░░░░░░░░░░░░░░░░░
███████████████████░░░░░░░░███████████░░░░░░░░░░░░░░░░░░░░░░░░░░░░░░░░░░░
███████████████████░░░░░░░░░██░░░░░░░░░░░░░░░░░░░░░░░░░░░░░░░░░░░░░░░░░░░
███████████████░░░░░░░░░░░░░░░░░░░░░░░░░░░░░░░░░░░░░░░░░░░░░░░░░░░░░░░░░░
██████████░░░░░░░░░░░░░░░░░░░░░░░░░░░░░░░░░░░░░░░░░░░░░░░░░░░░░░ ░░░░░░░
░░░░░░░░░░░░░░░░░░░░░░░░░░░░░░░░░░░░░░░░░░░░░░░░░░░░░░░░░░░░ ░░░
░░░░░░░░░░░░░░░░░░░░░░░░░░░░░░░░ ░░░░░░░░░░░░░░░░░░
░░░░░░░░░░░░░░░░░░░░░░░░░░░░
░░░░░░░░░░░░░░░░░
░░░░░
## Model card
First model in the potential series.
## Prompt format: Mistral
```
<s> [INST] QUERY_1 [/INST] ANSWER_1</s> [INST] QUERY_2 [/INST] ANSWER_2</s>...
```
Beware that some backends (like llama.cpp) add bos already (by default), so you don't need to prepend it yourself.
## Settings
DO NOT CHANGE ROPE SETTINGS. This model uses high freq base with 32k seen tokens, it should be fine for most tasks.
Only tested with temp 1 and top_p 0.95 with everything else disabled.
<video src="https://cdn-uploads.huggingface.co/production/uploads/65ab93082bf3e0cbbf717850/cIEP5e43VP0k0caRzl16e.mp4" controls="controls" style="max-width: 720px;">
</video> |
macadeliccc/dolphin-2.9.3-Yi-1.5-34B-32k-GGUF | macadeliccc | "2024-06-24T16:36:58Z" | 16,057 | 0 | null | [
"gguf",
"generated_from_trainer",
"axolotl",
"dataset:cognitivecomputations/Dolphin-2.9",
"dataset:teknium/OpenHermes-2.5",
"dataset:m-a-p/CodeFeedback-Filtered-Instruction",
"dataset:cognitivecomputations/dolphin-coder",
"dataset:cognitivecomputations/samantha-data",
"dataset:microsoft/orca-math-word-problems-200k",
"dataset:Locutusque/function-calling-chatml",
"dataset:internlm/Agent-FLAN",
"base_model:cognitivecomputations/dolphin-2.9.3-Yi-1.5-34B-32k",
"license:apache-2.0",
"region:us"
] | null | "2024-06-24T03:47:35Z" | ---
license: apache-2.0
base_model: cognitivecomputations/dolphin-2.9.3-Yi-1.5-34B-32k
tags:
- generated_from_trainer
- axolotl
datasets:
- cognitivecomputations/Dolphin-2.9
- teknium/OpenHermes-2.5
- m-a-p/CodeFeedback-Filtered-Instruction
- cognitivecomputations/dolphin-coder
- cognitivecomputations/samantha-data
- microsoft/orca-math-word-problems-200k
- Locutusque/function-calling-chatml
- internlm/Agent-FLAN
---
# Dolphin 2.9.3 Yi 1.5 34b 32k 🐬
Curated and trained by Eric Hartford, Lucas Atkins, and Fernando Fernandes, and Cognitive Computations
[](https://discord.gg/cognitivecomputations)
Discord: https://discord.gg/cognitivecomputations
<img src="https://cdn-uploads.huggingface.co/production/uploads/63111b2d88942700629f5771/ldkN1J0WIDQwU4vutGYiD.png" width="600" />
## Usage
```bash
ollama run CognitiveComputations/dolphin-yi-1.5-32k:34b-v2.9.3-q4_0
```
## Supported Tags
+ dolphin-yi-1.5-32k:34b-v2.9.3-q2_k
+ dolphin-yi-1.5-32k:34b-v2.9.3-q3_k
+ dolphin-yi-1.5-32k:34b-v2.9.3-q4_0
+ dolphin-yi-1.5-32k:34b-v2.9.3-q4_k_m
+ dolphin-yi-1.5-32k:34b-v2.9.3-q4_k_s
+ dolphin-yi-1.5-32k:34b-v2.9.3-q5_0
+ dolphin-yi-1.5-32k:34b-v2.9.3-q5_k_m
+ dolphin-yi-1.5-32k:34b-v2.9.3-q5_k_s
+ dolphin-yi-1.5-32k:34b-v2.9.3-q6_k
+ dolphin-yi-1.5-32k:34b-v2.9.3-q8_0 |
mradermacher/RoLlama2-7b-Chat-GGUF | mradermacher | "2024-06-28T14:15:09Z" | 16,056 | 0 | transformers | [
"transformers",
"gguf",
"ro",
"base_model:OpenLLM-Ro/RoLlama2-7b-Chat",
"license:cc-by-nc-4.0",
"endpoints_compatible",
"region:us"
] | null | "2024-06-28T13:48:29Z" | ---
base_model: OpenLLM-Ro/RoLlama2-7b-Chat
language:
- ro
library_name: transformers
license: cc-by-nc-4.0
quantized_by: mradermacher
---
## About
<!-- ### quantize_version: 2 -->
<!-- ### output_tensor_quantised: 1 -->
<!-- ### convert_type: hf -->
<!-- ### vocab_type: -->
<!-- ### tags: -->
static quants of https://huggingface.co/OpenLLM-Ro/RoLlama2-7b-Chat
<!-- provided-files -->
weighted/imatrix quants seem not to be available (by me) at this time. If they do not show up a week or so after the static ones, I have probably not planned for them. Feel free to request them by opening a Community Discussion.
## Usage
If you are unsure how to use GGUF files, refer to one of [TheBloke's
READMEs](https://huggingface.co/TheBloke/KafkaLM-70B-German-V0.1-GGUF) for
more details, including on how to concatenate multi-part files.
## Provided Quants
(sorted by size, not necessarily quality. IQ-quants are often preferable over similar sized non-IQ quants)
| Link | Type | Size/GB | Notes |
|:-----|:-----|--------:|:------|
| [GGUF](https://huggingface.co/mradermacher/RoLlama2-7b-Chat-GGUF/resolve/main/RoLlama2-7b-Chat.Q2_K.gguf) | Q2_K | 2.6 | |
| [GGUF](https://huggingface.co/mradermacher/RoLlama2-7b-Chat-GGUF/resolve/main/RoLlama2-7b-Chat.IQ3_XS.gguf) | IQ3_XS | 2.9 | |
| [GGUF](https://huggingface.co/mradermacher/RoLlama2-7b-Chat-GGUF/resolve/main/RoLlama2-7b-Chat.IQ3_S.gguf) | IQ3_S | 3.0 | beats Q3_K* |
| [GGUF](https://huggingface.co/mradermacher/RoLlama2-7b-Chat-GGUF/resolve/main/RoLlama2-7b-Chat.Q3_K_S.gguf) | Q3_K_S | 3.0 | |
| [GGUF](https://huggingface.co/mradermacher/RoLlama2-7b-Chat-GGUF/resolve/main/RoLlama2-7b-Chat.IQ3_M.gguf) | IQ3_M | 3.2 | |
| [GGUF](https://huggingface.co/mradermacher/RoLlama2-7b-Chat-GGUF/resolve/main/RoLlama2-7b-Chat.Q3_K_M.gguf) | Q3_K_M | 3.4 | lower quality |
| [GGUF](https://huggingface.co/mradermacher/RoLlama2-7b-Chat-GGUF/resolve/main/RoLlama2-7b-Chat.Q3_K_L.gguf) | Q3_K_L | 3.7 | |
| [GGUF](https://huggingface.co/mradermacher/RoLlama2-7b-Chat-GGUF/resolve/main/RoLlama2-7b-Chat.IQ4_XS.gguf) | IQ4_XS | 3.7 | |
| [GGUF](https://huggingface.co/mradermacher/RoLlama2-7b-Chat-GGUF/resolve/main/RoLlama2-7b-Chat.Q4_K_S.gguf) | Q4_K_S | 4.0 | fast, recommended |
| [GGUF](https://huggingface.co/mradermacher/RoLlama2-7b-Chat-GGUF/resolve/main/RoLlama2-7b-Chat.Q4_K_M.gguf) | Q4_K_M | 4.2 | fast, recommended |
| [GGUF](https://huggingface.co/mradermacher/RoLlama2-7b-Chat-GGUF/resolve/main/RoLlama2-7b-Chat.Q5_K_S.gguf) | Q5_K_S | 4.8 | |
| [GGUF](https://huggingface.co/mradermacher/RoLlama2-7b-Chat-GGUF/resolve/main/RoLlama2-7b-Chat.Q5_K_M.gguf) | Q5_K_M | 4.9 | |
| [GGUF](https://huggingface.co/mradermacher/RoLlama2-7b-Chat-GGUF/resolve/main/RoLlama2-7b-Chat.Q6_K.gguf) | Q6_K | 5.6 | very good quality |
| [GGUF](https://huggingface.co/mradermacher/RoLlama2-7b-Chat-GGUF/resolve/main/RoLlama2-7b-Chat.Q8_0.gguf) | Q8_0 | 7.3 | fast, best quality |
| [GGUF](https://huggingface.co/mradermacher/RoLlama2-7b-Chat-GGUF/resolve/main/RoLlama2-7b-Chat.f16.gguf) | f16 | 13.6 | 16 bpw, overkill |
Here is a handy graph by ikawrakow comparing some lower-quality quant
types (lower is better):

And here are Artefact2's thoughts on the matter:
https://gist.github.com/Artefact2/b5f810600771265fc1e39442288e8ec9
## FAQ / Model Request
See https://huggingface.co/mradermacher/model_requests for some answers to
questions you might have and/or if you want some other model quantized.
## Thanks
I thank my company, [nethype GmbH](https://www.nethype.de/), for letting
me use its servers and providing upgrades to my workstation to enable
this work in my free time.
<!-- end -->
|
mradermacher/Secure-deepseek-coder-v2-MoE-GGUF | mradermacher | "2024-06-22T19:02:33Z" | 16,047 | 0 | transformers | [
"transformers",
"gguf",
"en",
"base_model:Ferrag/Secure-deepseek-coder-v2-MoE",
"endpoints_compatible",
"region:us"
] | null | "2024-06-22T18:03:47Z" | ---
base_model: Ferrag/Secure-deepseek-coder-v2-MoE
language:
- en
library_name: transformers
quantized_by: mradermacher
---
## About
<!-- ### quantize_version: 2 -->
<!-- ### output_tensor_quantised: 1 -->
<!-- ### convert_type: hf -->
<!-- ### vocab_type: -->
<!-- ### tags: -->
static quants of https://huggingface.co/Ferrag/Secure-deepseek-coder-v2-MoE
<!-- provided-files -->
weighted/imatrix quants seem not to be available (by me) at this time. If they do not show up a week or so after the static ones, I have probably not planned for them. Feel free to request them by opening a Community Discussion.
## Usage
If you are unsure how to use GGUF files, refer to one of [TheBloke's
READMEs](https://huggingface.co/TheBloke/KafkaLM-70B-German-V0.1-GGUF) for
more details, including on how to concatenate multi-part files.
## Provided Quants
(sorted by size, not necessarily quality. IQ-quants are often preferable over similar sized non-IQ quants)
| Link | Type | Size/GB | Notes |
|:-----|:-----|--------:|:------|
| [GGUF](https://huggingface.co/mradermacher/Secure-deepseek-coder-v2-MoE-GGUF/resolve/main/Secure-deepseek-coder-v2-MoE.Q2_K.gguf) | Q2_K | 6.5 | |
| [GGUF](https://huggingface.co/mradermacher/Secure-deepseek-coder-v2-MoE-GGUF/resolve/main/Secure-deepseek-coder-v2-MoE.IQ3_XS.gguf) | IQ3_XS | 7.2 | |
| [GGUF](https://huggingface.co/mradermacher/Secure-deepseek-coder-v2-MoE-GGUF/resolve/main/Secure-deepseek-coder-v2-MoE.IQ3_S.gguf) | IQ3_S | 7.6 | beats Q3_K* |
| [GGUF](https://huggingface.co/mradermacher/Secure-deepseek-coder-v2-MoE-GGUF/resolve/main/Secure-deepseek-coder-v2-MoE.Q3_K_S.gguf) | Q3_K_S | 7.6 | |
| [GGUF](https://huggingface.co/mradermacher/Secure-deepseek-coder-v2-MoE-GGUF/resolve/main/Secure-deepseek-coder-v2-MoE.IQ3_M.gguf) | IQ3_M | 7.7 | |
| [GGUF](https://huggingface.co/mradermacher/Secure-deepseek-coder-v2-MoE-GGUF/resolve/main/Secure-deepseek-coder-v2-MoE.Q3_K_M.gguf) | Q3_K_M | 8.2 | lower quality |
| [GGUF](https://huggingface.co/mradermacher/Secure-deepseek-coder-v2-MoE-GGUF/resolve/main/Secure-deepseek-coder-v2-MoE.Q3_K_L.gguf) | Q3_K_L | 8.6 | |
| [GGUF](https://huggingface.co/mradermacher/Secure-deepseek-coder-v2-MoE-GGUF/resolve/main/Secure-deepseek-coder-v2-MoE.IQ4_XS.gguf) | IQ4_XS | 8.7 | |
| [GGUF](https://huggingface.co/mradermacher/Secure-deepseek-coder-v2-MoE-GGUF/resolve/main/Secure-deepseek-coder-v2-MoE.Q4_K_S.gguf) | Q4_K_S | 9.6 | fast, recommended |
| [GGUF](https://huggingface.co/mradermacher/Secure-deepseek-coder-v2-MoE-GGUF/resolve/main/Secure-deepseek-coder-v2-MoE.Q4_K_M.gguf) | Q4_K_M | 10.5 | fast, recommended |
| [GGUF](https://huggingface.co/mradermacher/Secure-deepseek-coder-v2-MoE-GGUF/resolve/main/Secure-deepseek-coder-v2-MoE.Q5_K_S.gguf) | Q5_K_S | 11.2 | |
| [GGUF](https://huggingface.co/mradermacher/Secure-deepseek-coder-v2-MoE-GGUF/resolve/main/Secure-deepseek-coder-v2-MoE.Q5_K_M.gguf) | Q5_K_M | 12.0 | |
| [GGUF](https://huggingface.co/mradermacher/Secure-deepseek-coder-v2-MoE-GGUF/resolve/main/Secure-deepseek-coder-v2-MoE.Q6_K.gguf) | Q6_K | 14.2 | very good quality |
| [GGUF](https://huggingface.co/mradermacher/Secure-deepseek-coder-v2-MoE-GGUF/resolve/main/Secure-deepseek-coder-v2-MoE.Q8_0.gguf) | Q8_0 | 16.8 | fast, best quality |
Here is a handy graph by ikawrakow comparing some lower-quality quant
types (lower is better):

And here are Artefact2's thoughts on the matter:
https://gist.github.com/Artefact2/b5f810600771265fc1e39442288e8ec9
## FAQ / Model Request
See https://huggingface.co/mradermacher/model_requests for some answers to
questions you might have and/or if you want some other model quantized.
## Thanks
I thank my company, [nethype GmbH](https://www.nethype.de/), for letting
me use its servers and providing upgrades to my workstation to enable
this work in my free time.
<!-- end -->
|
Helsinki-NLP/opus-mt-en-mul | Helsinki-NLP | "2023-08-16T11:30:35Z" | 16,035 | 14 | transformers | [
"transformers",
"pytorch",
"tf",
"marian",
"text2text-generation",
"translation",
"en",
"ca",
"es",
"os",
"eo",
"ro",
"fy",
"cy",
"is",
"lb",
"su",
"an",
"sq",
"fr",
"ht",
"rm",
"cv",
"ig",
"am",
"eu",
"tr",
"ps",
"af",
"ny",
"ch",
"uk",
"sl",
"lt",
"tk",
"sg",
"ar",
"lg",
"bg",
"be",
"ka",
"gd",
"ja",
"si",
"br",
"mh",
"km",
"th",
"ty",
"rw",
"te",
"mk",
"or",
"wo",
"kl",
"mr",
"ru",
"yo",
"hu",
"fo",
"zh",
"ti",
"co",
"ee",
"oc",
"sn",
"mt",
"ts",
"pl",
"gl",
"nb",
"bn",
"tt",
"bo",
"lo",
"id",
"gn",
"nv",
"hy",
"kn",
"to",
"io",
"so",
"vi",
"da",
"fj",
"gv",
"sm",
"nl",
"mi",
"pt",
"hi",
"se",
"as",
"ta",
"et",
"kw",
"ga",
"sv",
"ln",
"na",
"mn",
"gu",
"wa",
"lv",
"jv",
"el",
"my",
"ba",
"it",
"hr",
"ur",
"ce",
"nn",
"fi",
"mg",
"rn",
"xh",
"ab",
"de",
"cs",
"he",
"zu",
"yi",
"ml",
"mul",
"license:apache-2.0",
"autotrain_compatible",
"endpoints_compatible",
"region:us"
] | translation | "2022-03-02T23:29:04Z" | ---
language:
- en
- ca
- es
- os
- eo
- ro
- fy
- cy
- is
- lb
- su
- an
- sq
- fr
- ht
- rm
- cv
- ig
- am
- eu
- tr
- ps
- af
- ny
- ch
- uk
- sl
- lt
- tk
- sg
- ar
- lg
- bg
- be
- ka
- gd
- ja
- si
- br
- mh
- km
- th
- ty
- rw
- te
- mk
- or
- wo
- kl
- mr
- ru
- yo
- hu
- fo
- zh
- ti
- co
- ee
- oc
- sn
- mt
- ts
- pl
- gl
- nb
- bn
- tt
- bo
- lo
- id
- gn
- nv
- hy
- kn
- to
- io
- so
- vi
- da
- fj
- gv
- sm
- nl
- mi
- pt
- hi
- se
- as
- ta
- et
- kw
- ga
- sv
- ln
- na
- mn
- gu
- wa
- lv
- jv
- el
- my
- ba
- it
- hr
- ur
- ce
- nn
- fi
- mg
- rn
- xh
- ab
- de
- cs
- he
- zu
- yi
- ml
- mul
tags:
- translation
license: apache-2.0
---
### eng-mul
* source group: English
* target group: Multiple languages
* OPUS readme: [eng-mul](https://github.com/Helsinki-NLP/Tatoeba-Challenge/tree/master/models/eng-mul/README.md)
* model: transformer
* source language(s): eng
* target language(s): abk acm ady afb afh_Latn afr akl_Latn aln amh ang_Latn apc ara arg arq ary arz asm ast avk_Latn awa aze_Latn bak bam_Latn bel bel_Latn ben bho bod bos_Latn bre brx brx_Latn bul bul_Latn cat ceb ces cha che chr chv cjy_Hans cjy_Hant cmn cmn_Hans cmn_Hant cor cos crh crh_Latn csb_Latn cym dan deu dsb dtp dws_Latn egl ell enm_Latn epo est eus ewe ext fao fij fin fkv_Latn fra frm_Latn frr fry fuc fuv gan gcf_Latn gil gla gle glg glv gom gos got_Goth grc_Grek grn gsw guj hat hau_Latn haw heb hif_Latn hil hin hnj_Latn hoc hoc_Latn hrv hsb hun hye iba ibo ido ido_Latn ike_Latn ile_Latn ilo ina_Latn ind isl ita izh jav jav_Java jbo jbo_Cyrl jbo_Latn jdt_Cyrl jpn kab kal kan kat kaz_Cyrl kaz_Latn kek_Latn kha khm khm_Latn kin kir_Cyrl kjh kpv krl ksh kum kur_Arab kur_Latn lad lad_Latn lao lat_Latn lav ldn_Latn lfn_Cyrl lfn_Latn lij lin lit liv_Latn lkt lld_Latn lmo ltg ltz lug lzh lzh_Hans mad mah mai mal mar max_Latn mdf mfe mhr mic min mkd mlg mlt mnw moh mon mri mwl mww mya myv nan nau nav nds niu nld nno nob nob_Hebr nog non_Latn nov_Latn npi nya oci ori orv_Cyrl oss ota_Arab ota_Latn pag pan_Guru pap pau pdc pes pes_Latn pes_Thaa pms pnb pol por ppl_Latn prg_Latn pus quc qya qya_Latn rap rif_Latn roh rom ron rue run rus sag sah san_Deva scn sco sgs shs_Latn shy_Latn sin sjn_Latn slv sma sme smo sna snd_Arab som spa sqi srp_Cyrl srp_Latn stq sun swe swg swh tah tam tat tat_Arab tat_Latn tel tet tgk_Cyrl tha tir tlh_Latn tly_Latn tmw_Latn toi_Latn ton tpw_Latn tso tuk tuk_Latn tur tvl tyv tzl tzl_Latn udm uig_Arab uig_Cyrl ukr umb urd uzb_Cyrl uzb_Latn vec vie vie_Hani vol_Latn vro war wln wol wuu xal xho yid yor yue yue_Hans yue_Hant zho zho_Hans zho_Hant zlm_Latn zsm_Latn zul zza
* model: transformer
* pre-processing: normalization + SentencePiece (spm32k,spm32k)
* a sentence initial language token is required in the form of `>>id<<` (id = valid target language ID)
* download original weights: [opus2m-2020-08-01.zip](https://object.pouta.csc.fi/Tatoeba-MT-models/eng-mul/opus2m-2020-08-01.zip)
* test set translations: [opus2m-2020-08-01.test.txt](https://object.pouta.csc.fi/Tatoeba-MT-models/eng-mul/opus2m-2020-08-01.test.txt)
* test set scores: [opus2m-2020-08-01.eval.txt](https://object.pouta.csc.fi/Tatoeba-MT-models/eng-mul/opus2m-2020-08-01.eval.txt)
## Benchmarks
| testset | BLEU | chr-F |
|-----------------------|-------|-------|
| newsdev2014-enghin.eng.hin | 5.0 | 0.288 |
| newsdev2015-enfi-engfin.eng.fin | 9.3 | 0.418 |
| newsdev2016-enro-engron.eng.ron | 17.2 | 0.488 |
| newsdev2016-entr-engtur.eng.tur | 8.2 | 0.402 |
| newsdev2017-enlv-englav.eng.lav | 12.9 | 0.444 |
| newsdev2017-enzh-engzho.eng.zho | 17.6 | 0.170 |
| newsdev2018-enet-engest.eng.est | 10.9 | 0.423 |
| newsdev2019-engu-engguj.eng.guj | 5.2 | 0.284 |
| newsdev2019-enlt-englit.eng.lit | 11.0 | 0.431 |
| newsdiscussdev2015-enfr-engfra.eng.fra | 22.6 | 0.521 |
| newsdiscusstest2015-enfr-engfra.eng.fra | 25.9 | 0.546 |
| newssyscomb2009-engces.eng.ces | 10.3 | 0.394 |
| newssyscomb2009-engdeu.eng.deu | 13.3 | 0.459 |
| newssyscomb2009-engfra.eng.fra | 21.5 | 0.522 |
| newssyscomb2009-enghun.eng.hun | 8.1 | 0.371 |
| newssyscomb2009-engita.eng.ita | 22.1 | 0.540 |
| newssyscomb2009-engspa.eng.spa | 23.8 | 0.531 |
| news-test2008-engces.eng.ces | 9.0 | 0.376 |
| news-test2008-engdeu.eng.deu | 14.2 | 0.451 |
| news-test2008-engfra.eng.fra | 19.8 | 0.500 |
| news-test2008-engspa.eng.spa | 22.8 | 0.518 |
| newstest2009-engces.eng.ces | 9.8 | 0.392 |
| newstest2009-engdeu.eng.deu | 13.7 | 0.454 |
| newstest2009-engfra.eng.fra | 20.7 | 0.514 |
| newstest2009-enghun.eng.hun | 8.4 | 0.370 |
| newstest2009-engita.eng.ita | 22.4 | 0.538 |
| newstest2009-engspa.eng.spa | 23.5 | 0.532 |
| newstest2010-engces.eng.ces | 10.0 | 0.393 |
| newstest2010-engdeu.eng.deu | 15.2 | 0.463 |
| newstest2010-engfra.eng.fra | 22.0 | 0.524 |
| newstest2010-engspa.eng.spa | 27.2 | 0.556 |
| newstest2011-engces.eng.ces | 10.8 | 0.392 |
| newstest2011-engdeu.eng.deu | 14.2 | 0.449 |
| newstest2011-engfra.eng.fra | 24.3 | 0.544 |
| newstest2011-engspa.eng.spa | 28.3 | 0.559 |
| newstest2012-engces.eng.ces | 9.9 | 0.377 |
| newstest2012-engdeu.eng.deu | 14.3 | 0.449 |
| newstest2012-engfra.eng.fra | 23.2 | 0.530 |
| newstest2012-engrus.eng.rus | 16.0 | 0.463 |
| newstest2012-engspa.eng.spa | 27.8 | 0.555 |
| newstest2013-engces.eng.ces | 11.0 | 0.392 |
| newstest2013-engdeu.eng.deu | 16.4 | 0.469 |
| newstest2013-engfra.eng.fra | 22.6 | 0.515 |
| newstest2013-engrus.eng.rus | 12.1 | 0.414 |
| newstest2013-engspa.eng.spa | 24.9 | 0.532 |
| newstest2014-hien-enghin.eng.hin | 7.2 | 0.311 |
| newstest2015-encs-engces.eng.ces | 10.9 | 0.396 |
| newstest2015-ende-engdeu.eng.deu | 18.3 | 0.490 |
| newstest2015-enfi-engfin.eng.fin | 10.1 | 0.421 |
| newstest2015-enru-engrus.eng.rus | 14.5 | 0.445 |
| newstest2016-encs-engces.eng.ces | 12.2 | 0.408 |
| newstest2016-ende-engdeu.eng.deu | 21.4 | 0.517 |
| newstest2016-enfi-engfin.eng.fin | 11.2 | 0.435 |
| newstest2016-enro-engron.eng.ron | 16.6 | 0.472 |
| newstest2016-enru-engrus.eng.rus | 13.4 | 0.435 |
| newstest2016-entr-engtur.eng.tur | 8.1 | 0.385 |
| newstest2017-encs-engces.eng.ces | 9.6 | 0.377 |
| newstest2017-ende-engdeu.eng.deu | 17.9 | 0.482 |
| newstest2017-enfi-engfin.eng.fin | 11.8 | 0.440 |
| newstest2017-enlv-englav.eng.lav | 9.6 | 0.412 |
| newstest2017-enru-engrus.eng.rus | 14.1 | 0.446 |
| newstest2017-entr-engtur.eng.tur | 8.0 | 0.378 |
| newstest2017-enzh-engzho.eng.zho | 16.8 | 0.175 |
| newstest2018-encs-engces.eng.ces | 9.8 | 0.380 |
| newstest2018-ende-engdeu.eng.deu | 23.8 | 0.536 |
| newstest2018-enet-engest.eng.est | 11.8 | 0.433 |
| newstest2018-enfi-engfin.eng.fin | 7.8 | 0.398 |
| newstest2018-enru-engrus.eng.rus | 12.2 | 0.434 |
| newstest2018-entr-engtur.eng.tur | 7.5 | 0.383 |
| newstest2018-enzh-engzho.eng.zho | 18.3 | 0.179 |
| newstest2019-encs-engces.eng.ces | 10.7 | 0.389 |
| newstest2019-ende-engdeu.eng.deu | 21.0 | 0.512 |
| newstest2019-enfi-engfin.eng.fin | 10.4 | 0.420 |
| newstest2019-engu-engguj.eng.guj | 5.8 | 0.297 |
| newstest2019-enlt-englit.eng.lit | 8.0 | 0.388 |
| newstest2019-enru-engrus.eng.rus | 13.0 | 0.415 |
| newstest2019-enzh-engzho.eng.zho | 15.0 | 0.192 |
| newstestB2016-enfi-engfin.eng.fin | 9.0 | 0.414 |
| newstestB2017-enfi-engfin.eng.fin | 9.5 | 0.415 |
| Tatoeba-test.eng-abk.eng.abk | 4.2 | 0.275 |
| Tatoeba-test.eng-ady.eng.ady | 0.4 | 0.006 |
| Tatoeba-test.eng-afh.eng.afh | 1.0 | 0.058 |
| Tatoeba-test.eng-afr.eng.afr | 47.0 | 0.663 |
| Tatoeba-test.eng-akl.eng.akl | 2.7 | 0.080 |
| Tatoeba-test.eng-amh.eng.amh | 8.5 | 0.455 |
| Tatoeba-test.eng-ang.eng.ang | 6.2 | 0.138 |
| Tatoeba-test.eng-ara.eng.ara | 6.3 | 0.325 |
| Tatoeba-test.eng-arg.eng.arg | 1.5 | 0.107 |
| Tatoeba-test.eng-asm.eng.asm | 2.1 | 0.265 |
| Tatoeba-test.eng-ast.eng.ast | 15.7 | 0.393 |
| Tatoeba-test.eng-avk.eng.avk | 0.2 | 0.095 |
| Tatoeba-test.eng-awa.eng.awa | 0.1 | 0.002 |
| Tatoeba-test.eng-aze.eng.aze | 19.0 | 0.500 |
| Tatoeba-test.eng-bak.eng.bak | 12.7 | 0.379 |
| Tatoeba-test.eng-bam.eng.bam | 8.3 | 0.037 |
| Tatoeba-test.eng-bel.eng.bel | 13.5 | 0.396 |
| Tatoeba-test.eng-ben.eng.ben | 10.0 | 0.383 |
| Tatoeba-test.eng-bho.eng.bho | 0.1 | 0.003 |
| Tatoeba-test.eng-bod.eng.bod | 0.0 | 0.147 |
| Tatoeba-test.eng-bre.eng.bre | 7.6 | 0.275 |
| Tatoeba-test.eng-brx.eng.brx | 0.8 | 0.060 |
| Tatoeba-test.eng-bul.eng.bul | 32.1 | 0.542 |
| Tatoeba-test.eng-cat.eng.cat | 37.0 | 0.595 |
| Tatoeba-test.eng-ceb.eng.ceb | 9.6 | 0.409 |
| Tatoeba-test.eng-ces.eng.ces | 24.0 | 0.475 |
| Tatoeba-test.eng-cha.eng.cha | 3.9 | 0.228 |
| Tatoeba-test.eng-che.eng.che | 0.7 | 0.013 |
| Tatoeba-test.eng-chm.eng.chm | 2.6 | 0.212 |
| Tatoeba-test.eng-chr.eng.chr | 6.0 | 0.190 |
| Tatoeba-test.eng-chv.eng.chv | 6.5 | 0.369 |
| Tatoeba-test.eng-cor.eng.cor | 0.9 | 0.086 |
| Tatoeba-test.eng-cos.eng.cos | 4.2 | 0.174 |
| Tatoeba-test.eng-crh.eng.crh | 9.9 | 0.361 |
| Tatoeba-test.eng-csb.eng.csb | 3.4 | 0.230 |
| Tatoeba-test.eng-cym.eng.cym | 18.0 | 0.418 |
| Tatoeba-test.eng-dan.eng.dan | 42.5 | 0.624 |
| Tatoeba-test.eng-deu.eng.deu | 25.2 | 0.505 |
| Tatoeba-test.eng-dsb.eng.dsb | 0.9 | 0.121 |
| Tatoeba-test.eng-dtp.eng.dtp | 0.3 | 0.084 |
| Tatoeba-test.eng-dws.eng.dws | 0.2 | 0.040 |
| Tatoeba-test.eng-egl.eng.egl | 0.4 | 0.085 |
| Tatoeba-test.eng-ell.eng.ell | 28.7 | 0.543 |
| Tatoeba-test.eng-enm.eng.enm | 3.3 | 0.295 |
| Tatoeba-test.eng-epo.eng.epo | 33.4 | 0.570 |
| Tatoeba-test.eng-est.eng.est | 30.3 | 0.545 |
| Tatoeba-test.eng-eus.eng.eus | 18.5 | 0.486 |
| Tatoeba-test.eng-ewe.eng.ewe | 6.8 | 0.272 |
| Tatoeba-test.eng-ext.eng.ext | 5.0 | 0.228 |
| Tatoeba-test.eng-fao.eng.fao | 5.2 | 0.277 |
| Tatoeba-test.eng-fas.eng.fas | 6.9 | 0.265 |
| Tatoeba-test.eng-fij.eng.fij | 31.5 | 0.365 |
| Tatoeba-test.eng-fin.eng.fin | 18.5 | 0.459 |
| Tatoeba-test.eng-fkv.eng.fkv | 0.9 | 0.132 |
| Tatoeba-test.eng-fra.eng.fra | 31.5 | 0.546 |
| Tatoeba-test.eng-frm.eng.frm | 0.9 | 0.128 |
| Tatoeba-test.eng-frr.eng.frr | 3.0 | 0.025 |
| Tatoeba-test.eng-fry.eng.fry | 14.4 | 0.387 |
| Tatoeba-test.eng-ful.eng.ful | 0.4 | 0.061 |
| Tatoeba-test.eng-gcf.eng.gcf | 0.3 | 0.075 |
| Tatoeba-test.eng-gil.eng.gil | 47.4 | 0.706 |
| Tatoeba-test.eng-gla.eng.gla | 10.9 | 0.341 |
| Tatoeba-test.eng-gle.eng.gle | 26.8 | 0.493 |
| Tatoeba-test.eng-glg.eng.glg | 32.5 | 0.565 |
| Tatoeba-test.eng-glv.eng.glv | 21.5 | 0.395 |
| Tatoeba-test.eng-gos.eng.gos | 0.3 | 0.124 |
| Tatoeba-test.eng-got.eng.got | 0.2 | 0.010 |
| Tatoeba-test.eng-grc.eng.grc | 0.0 | 0.005 |
| Tatoeba-test.eng-grn.eng.grn | 1.5 | 0.129 |
| Tatoeba-test.eng-gsw.eng.gsw | 0.6 | 0.106 |
| Tatoeba-test.eng-guj.eng.guj | 15.4 | 0.347 |
| Tatoeba-test.eng-hat.eng.hat | 31.1 | 0.527 |
| Tatoeba-test.eng-hau.eng.hau | 6.5 | 0.385 |
| Tatoeba-test.eng-haw.eng.haw | 0.2 | 0.066 |
| Tatoeba-test.eng-hbs.eng.hbs | 28.7 | 0.531 |
| Tatoeba-test.eng-heb.eng.heb | 21.3 | 0.443 |
| Tatoeba-test.eng-hif.eng.hif | 2.8 | 0.268 |
| Tatoeba-test.eng-hil.eng.hil | 12.0 | 0.463 |
| Tatoeba-test.eng-hin.eng.hin | 13.0 | 0.401 |
| Tatoeba-test.eng-hmn.eng.hmn | 0.2 | 0.073 |
| Tatoeba-test.eng-hoc.eng.hoc | 0.2 | 0.077 |
| Tatoeba-test.eng-hsb.eng.hsb | 5.7 | 0.308 |
| Tatoeba-test.eng-hun.eng.hun | 17.1 | 0.431 |
| Tatoeba-test.eng-hye.eng.hye | 15.0 | 0.378 |
| Tatoeba-test.eng-iba.eng.iba | 16.0 | 0.437 |
| Tatoeba-test.eng-ibo.eng.ibo | 2.9 | 0.221 |
| Tatoeba-test.eng-ido.eng.ido | 11.5 | 0.403 |
| Tatoeba-test.eng-iku.eng.iku | 2.3 | 0.089 |
| Tatoeba-test.eng-ile.eng.ile | 4.3 | 0.282 |
| Tatoeba-test.eng-ilo.eng.ilo | 26.4 | 0.522 |
| Tatoeba-test.eng-ina.eng.ina | 20.9 | 0.493 |
| Tatoeba-test.eng-isl.eng.isl | 12.5 | 0.375 |
| Tatoeba-test.eng-ita.eng.ita | 33.9 | 0.592 |
| Tatoeba-test.eng-izh.eng.izh | 4.6 | 0.050 |
| Tatoeba-test.eng-jav.eng.jav | 7.8 | 0.328 |
| Tatoeba-test.eng-jbo.eng.jbo | 0.1 | 0.123 |
| Tatoeba-test.eng-jdt.eng.jdt | 6.4 | 0.008 |
| Tatoeba-test.eng-jpn.eng.jpn | 0.0 | 0.000 |
| Tatoeba-test.eng-kab.eng.kab | 5.9 | 0.261 |
| Tatoeba-test.eng-kal.eng.kal | 13.4 | 0.382 |
| Tatoeba-test.eng-kan.eng.kan | 4.8 | 0.358 |
| Tatoeba-test.eng-kat.eng.kat | 1.8 | 0.115 |
| Tatoeba-test.eng-kaz.eng.kaz | 8.8 | 0.354 |
| Tatoeba-test.eng-kek.eng.kek | 3.7 | 0.188 |
| Tatoeba-test.eng-kha.eng.kha | 0.5 | 0.094 |
| Tatoeba-test.eng-khm.eng.khm | 0.4 | 0.243 |
| Tatoeba-test.eng-kin.eng.kin | 5.2 | 0.362 |
| Tatoeba-test.eng-kir.eng.kir | 17.2 | 0.416 |
| Tatoeba-test.eng-kjh.eng.kjh | 0.6 | 0.009 |
| Tatoeba-test.eng-kok.eng.kok | 5.5 | 0.005 |
| Tatoeba-test.eng-kom.eng.kom | 2.4 | 0.012 |
| Tatoeba-test.eng-krl.eng.krl | 2.0 | 0.099 |
| Tatoeba-test.eng-ksh.eng.ksh | 0.4 | 0.074 |
| Tatoeba-test.eng-kum.eng.kum | 0.9 | 0.007 |
| Tatoeba-test.eng-kur.eng.kur | 9.1 | 0.174 |
| Tatoeba-test.eng-lad.eng.lad | 1.2 | 0.154 |
| Tatoeba-test.eng-lah.eng.lah | 0.1 | 0.001 |
| Tatoeba-test.eng-lao.eng.lao | 0.6 | 0.426 |
| Tatoeba-test.eng-lat.eng.lat | 8.2 | 0.366 |
| Tatoeba-test.eng-lav.eng.lav | 20.4 | 0.475 |
| Tatoeba-test.eng-ldn.eng.ldn | 0.3 | 0.059 |
| Tatoeba-test.eng-lfn.eng.lfn | 0.5 | 0.104 |
| Tatoeba-test.eng-lij.eng.lij | 0.2 | 0.094 |
| Tatoeba-test.eng-lin.eng.lin | 1.2 | 0.276 |
| Tatoeba-test.eng-lit.eng.lit | 17.4 | 0.488 |
| Tatoeba-test.eng-liv.eng.liv | 0.3 | 0.039 |
| Tatoeba-test.eng-lkt.eng.lkt | 0.3 | 0.041 |
| Tatoeba-test.eng-lld.eng.lld | 0.1 | 0.083 |
| Tatoeba-test.eng-lmo.eng.lmo | 1.4 | 0.154 |
| Tatoeba-test.eng-ltz.eng.ltz | 19.1 | 0.395 |
| Tatoeba-test.eng-lug.eng.lug | 4.2 | 0.382 |
| Tatoeba-test.eng-mad.eng.mad | 2.1 | 0.075 |
| Tatoeba-test.eng-mah.eng.mah | 9.5 | 0.331 |
| Tatoeba-test.eng-mai.eng.mai | 9.3 | 0.372 |
| Tatoeba-test.eng-mal.eng.mal | 8.3 | 0.437 |
| Tatoeba-test.eng-mar.eng.mar | 13.5 | 0.410 |
| Tatoeba-test.eng-mdf.eng.mdf | 2.3 | 0.008 |
| Tatoeba-test.eng-mfe.eng.mfe | 83.6 | 0.905 |
| Tatoeba-test.eng-mic.eng.mic | 7.6 | 0.214 |
| Tatoeba-test.eng-mkd.eng.mkd | 31.8 | 0.540 |
| Tatoeba-test.eng-mlg.eng.mlg | 31.3 | 0.464 |
| Tatoeba-test.eng-mlt.eng.mlt | 11.7 | 0.427 |
| Tatoeba-test.eng-mnw.eng.mnw | 0.1 | 0.000 |
| Tatoeba-test.eng-moh.eng.moh | 0.6 | 0.067 |
| Tatoeba-test.eng-mon.eng.mon | 8.5 | 0.323 |
| Tatoeba-test.eng-mri.eng.mri | 8.5 | 0.320 |
| Tatoeba-test.eng-msa.eng.msa | 24.5 | 0.498 |
| Tatoeba-test.eng.multi | 22.4 | 0.451 |
| Tatoeba-test.eng-mwl.eng.mwl | 3.8 | 0.169 |
| Tatoeba-test.eng-mya.eng.mya | 0.2 | 0.123 |
| Tatoeba-test.eng-myv.eng.myv | 1.1 | 0.014 |
| Tatoeba-test.eng-nau.eng.nau | 0.6 | 0.109 |
| Tatoeba-test.eng-nav.eng.nav | 1.8 | 0.149 |
| Tatoeba-test.eng-nds.eng.nds | 11.3 | 0.365 |
| Tatoeba-test.eng-nep.eng.nep | 0.5 | 0.004 |
| Tatoeba-test.eng-niu.eng.niu | 34.4 | 0.501 |
| Tatoeba-test.eng-nld.eng.nld | 37.6 | 0.598 |
| Tatoeba-test.eng-nog.eng.nog | 0.2 | 0.010 |
| Tatoeba-test.eng-non.eng.non | 0.2 | 0.096 |
| Tatoeba-test.eng-nor.eng.nor | 36.3 | 0.577 |
| Tatoeba-test.eng-nov.eng.nov | 0.9 | 0.180 |
| Tatoeba-test.eng-nya.eng.nya | 9.8 | 0.524 |
| Tatoeba-test.eng-oci.eng.oci | 6.3 | 0.288 |
| Tatoeba-test.eng-ori.eng.ori | 5.3 | 0.273 |
| Tatoeba-test.eng-orv.eng.orv | 0.2 | 0.007 |
| Tatoeba-test.eng-oss.eng.oss | 3.0 | 0.230 |
| Tatoeba-test.eng-ota.eng.ota | 0.2 | 0.053 |
| Tatoeba-test.eng-pag.eng.pag | 20.2 | 0.513 |
| Tatoeba-test.eng-pan.eng.pan | 6.4 | 0.301 |
| Tatoeba-test.eng-pap.eng.pap | 44.7 | 0.624 |
| Tatoeba-test.eng-pau.eng.pau | 0.8 | 0.098 |
| Tatoeba-test.eng-pdc.eng.pdc | 2.9 | 0.143 |
| Tatoeba-test.eng-pms.eng.pms | 0.6 | 0.124 |
| Tatoeba-test.eng-pol.eng.pol | 22.7 | 0.500 |
| Tatoeba-test.eng-por.eng.por | 31.6 | 0.570 |
| Tatoeba-test.eng-ppl.eng.ppl | 0.5 | 0.085 |
| Tatoeba-test.eng-prg.eng.prg | 0.1 | 0.078 |
| Tatoeba-test.eng-pus.eng.pus | 0.9 | 0.137 |
| Tatoeba-test.eng-quc.eng.quc | 2.7 | 0.255 |
| Tatoeba-test.eng-qya.eng.qya | 0.4 | 0.084 |
| Tatoeba-test.eng-rap.eng.rap | 1.9 | 0.050 |
| Tatoeba-test.eng-rif.eng.rif | 1.3 | 0.102 |
| Tatoeba-test.eng-roh.eng.roh | 1.4 | 0.169 |
| Tatoeba-test.eng-rom.eng.rom | 7.8 | 0.329 |
| Tatoeba-test.eng-ron.eng.ron | 27.0 | 0.530 |
| Tatoeba-test.eng-rue.eng.rue | 0.1 | 0.009 |
| Tatoeba-test.eng-run.eng.run | 9.8 | 0.434 |
| Tatoeba-test.eng-rus.eng.rus | 22.2 | 0.465 |
| Tatoeba-test.eng-sag.eng.sag | 4.8 | 0.155 |
| Tatoeba-test.eng-sah.eng.sah | 0.2 | 0.007 |
| Tatoeba-test.eng-san.eng.san | 1.7 | 0.143 |
| Tatoeba-test.eng-scn.eng.scn | 1.5 | 0.083 |
| Tatoeba-test.eng-sco.eng.sco | 30.3 | 0.514 |
| Tatoeba-test.eng-sgs.eng.sgs | 1.6 | 0.104 |
| Tatoeba-test.eng-shs.eng.shs | 0.7 | 0.049 |
| Tatoeba-test.eng-shy.eng.shy | 0.6 | 0.064 |
| Tatoeba-test.eng-sin.eng.sin | 5.4 | 0.317 |
| Tatoeba-test.eng-sjn.eng.sjn | 0.3 | 0.074 |
| Tatoeba-test.eng-slv.eng.slv | 12.8 | 0.313 |
| Tatoeba-test.eng-sma.eng.sma | 0.8 | 0.063 |
| Tatoeba-test.eng-sme.eng.sme | 13.2 | 0.290 |
| Tatoeba-test.eng-smo.eng.smo | 12.1 | 0.416 |
| Tatoeba-test.eng-sna.eng.sna | 27.1 | 0.533 |
| Tatoeba-test.eng-snd.eng.snd | 6.0 | 0.359 |
| Tatoeba-test.eng-som.eng.som | 16.0 | 0.274 |
| Tatoeba-test.eng-spa.eng.spa | 36.7 | 0.603 |
| Tatoeba-test.eng-sqi.eng.sqi | 32.3 | 0.573 |
| Tatoeba-test.eng-stq.eng.stq | 0.6 | 0.198 |
| Tatoeba-test.eng-sun.eng.sun | 39.0 | 0.447 |
| Tatoeba-test.eng-swa.eng.swa | 1.1 | 0.109 |
| Tatoeba-test.eng-swe.eng.swe | 42.7 | 0.614 |
| Tatoeba-test.eng-swg.eng.swg | 0.6 | 0.118 |
| Tatoeba-test.eng-tah.eng.tah | 12.4 | 0.294 |
| Tatoeba-test.eng-tam.eng.tam | 5.0 | 0.404 |
| Tatoeba-test.eng-tat.eng.tat | 9.9 | 0.326 |
| Tatoeba-test.eng-tel.eng.tel | 4.7 | 0.326 |
| Tatoeba-test.eng-tet.eng.tet | 0.7 | 0.100 |
| Tatoeba-test.eng-tgk.eng.tgk | 5.5 | 0.304 |
| Tatoeba-test.eng-tha.eng.tha | 2.2 | 0.456 |
| Tatoeba-test.eng-tir.eng.tir | 1.5 | 0.197 |
| Tatoeba-test.eng-tlh.eng.tlh | 0.0 | 0.032 |
| Tatoeba-test.eng-tly.eng.tly | 0.3 | 0.061 |
| Tatoeba-test.eng-toi.eng.toi | 8.3 | 0.219 |
| Tatoeba-test.eng-ton.eng.ton | 32.7 | 0.619 |
| Tatoeba-test.eng-tpw.eng.tpw | 1.4 | 0.136 |
| Tatoeba-test.eng-tso.eng.tso | 9.6 | 0.465 |
| Tatoeba-test.eng-tuk.eng.tuk | 9.4 | 0.383 |
| Tatoeba-test.eng-tur.eng.tur | 24.1 | 0.542 |
| Tatoeba-test.eng-tvl.eng.tvl | 8.9 | 0.398 |
| Tatoeba-test.eng-tyv.eng.tyv | 10.4 | 0.249 |
| Tatoeba-test.eng-tzl.eng.tzl | 0.2 | 0.098 |
| Tatoeba-test.eng-udm.eng.udm | 6.5 | 0.212 |
| Tatoeba-test.eng-uig.eng.uig | 2.1 | 0.266 |
| Tatoeba-test.eng-ukr.eng.ukr | 24.3 | 0.479 |
| Tatoeba-test.eng-umb.eng.umb | 4.4 | 0.274 |
| Tatoeba-test.eng-urd.eng.urd | 8.6 | 0.344 |
| Tatoeba-test.eng-uzb.eng.uzb | 6.9 | 0.343 |
| Tatoeba-test.eng-vec.eng.vec | 1.0 | 0.094 |
| Tatoeba-test.eng-vie.eng.vie | 23.2 | 0.420 |
| Tatoeba-test.eng-vol.eng.vol | 0.3 | 0.086 |
| Tatoeba-test.eng-war.eng.war | 11.4 | 0.415 |
| Tatoeba-test.eng-wln.eng.wln | 8.4 | 0.218 |
| Tatoeba-test.eng-wol.eng.wol | 11.5 | 0.252 |
| Tatoeba-test.eng-xal.eng.xal | 0.1 | 0.007 |
| Tatoeba-test.eng-xho.eng.xho | 19.5 | 0.552 |
| Tatoeba-test.eng-yid.eng.yid | 4.0 | 0.256 |
| Tatoeba-test.eng-yor.eng.yor | 8.8 | 0.247 |
| Tatoeba-test.eng-zho.eng.zho | 21.8 | 0.192 |
| Tatoeba-test.eng-zul.eng.zul | 34.3 | 0.655 |
| Tatoeba-test.eng-zza.eng.zza | 0.5 | 0.080 |
### System Info:
- hf_name: eng-mul
- source_languages: eng
- target_languages: mul
- opus_readme_url: https://github.com/Helsinki-NLP/Tatoeba-Challenge/tree/master/models/eng-mul/README.md
- original_repo: Tatoeba-Challenge
- tags: ['translation']
- languages: ['en', 'ca', 'es', 'os', 'eo', 'ro', 'fy', 'cy', 'is', 'lb', 'su', 'an', 'sq', 'fr', 'ht', 'rm', 'cv', 'ig', 'am', 'eu', 'tr', 'ps', 'af', 'ny', 'ch', 'uk', 'sl', 'lt', 'tk', 'sg', 'ar', 'lg', 'bg', 'be', 'ka', 'gd', 'ja', 'si', 'br', 'mh', 'km', 'th', 'ty', 'rw', 'te', 'mk', 'or', 'wo', 'kl', 'mr', 'ru', 'yo', 'hu', 'fo', 'zh', 'ti', 'co', 'ee', 'oc', 'sn', 'mt', 'ts', 'pl', 'gl', 'nb', 'bn', 'tt', 'bo', 'lo', 'id', 'gn', 'nv', 'hy', 'kn', 'to', 'io', 'so', 'vi', 'da', 'fj', 'gv', 'sm', 'nl', 'mi', 'pt', 'hi', 'se', 'as', 'ta', 'et', 'kw', 'ga', 'sv', 'ln', 'na', 'mn', 'gu', 'wa', 'lv', 'jv', 'el', 'my', 'ba', 'it', 'hr', 'ur', 'ce', 'nn', 'fi', 'mg', 'rn', 'xh', 'ab', 'de', 'cs', 'he', 'zu', 'yi', 'ml', 'mul']
- src_constituents: {'eng'}
- tgt_constituents: {'sjn_Latn', 'cat', 'nan', 'spa', 'ile_Latn', 'pap', 'mwl', 'uzb_Latn', 'mww', 'hil', 'lij', 'avk_Latn', 'lad_Latn', 'lat_Latn', 'bos_Latn', 'oss', 'epo', 'ron', 'fry', 'cym', 'toi_Latn', 'awa', 'swg', 'zsm_Latn', 'zho_Hant', 'gcf_Latn', 'uzb_Cyrl', 'isl', 'lfn_Latn', 'shs_Latn', 'nov_Latn', 'bho', 'ltz', 'lzh', 'kur_Latn', 'sun', 'arg', 'pes_Thaa', 'sqi', 'uig_Arab', 'csb_Latn', 'fra', 'hat', 'liv_Latn', 'non_Latn', 'sco', 'cmn_Hans', 'pnb', 'roh', 'chv', 'ibo', 'bul_Latn', 'amh', 'lfn_Cyrl', 'eus', 'fkv_Latn', 'tur', 'pus', 'afr', 'brx_Latn', 'nya', 'acm', 'ota_Latn', 'cha', 'ukr', 'xal', 'slv', 'lit', 'zho_Hans', 'tmw_Latn', 'kjh', 'ota_Arab', 'war', 'tuk', 'sag', 'myv', 'hsb', 'lzh_Hans', 'ara', 'tly_Latn', 'lug', 'brx', 'bul', 'bel', 'vol_Latn', 'kat', 'gan', 'got_Goth', 'vro', 'ext', 'afh_Latn', 'gla', 'jpn', 'udm', 'mai', 'ary', 'sin', 'tvl', 'hif_Latn', 'cjy_Hant', 'bre', 'ceb', 'mah', 'nob_Hebr', 'crh_Latn', 'prg_Latn', 'khm', 'ang_Latn', 'tha', 'tah', 'tzl', 'aln', 'kin', 'tel', 'ady', 'mkd', 'ori', 'wol', 'aze_Latn', 'jbo', 'niu', 'kal', 'mar', 'vie_Hani', 'arz', 'yue', 'kha', 'san_Deva', 'jbo_Latn', 'gos', 'hau_Latn', 'rus', 'quc', 'cmn', 'yor', 'hun', 'uig_Cyrl', 'fao', 'mnw', 'zho', 'orv_Cyrl', 'iba', 'bel_Latn', 'tir', 'afb', 'crh', 'mic', 'cos', 'swh', 'sah', 'krl', 'ewe', 'apc', 'zza', 'chr', 'grc_Grek', 'tpw_Latn', 'oci', 'mfe', 'sna', 'kir_Cyrl', 'tat_Latn', 'gom', 'ido_Latn', 'sgs', 'pau', 'tgk_Cyrl', 'nog', 'mlt', 'pdc', 'tso', 'srp_Cyrl', 'pol', 'ast', 'glg', 'pms', 'fuc', 'nob', 'qya', 'ben', 'tat', 'kab', 'min', 'srp_Latn', 'wuu', 'dtp', 'jbo_Cyrl', 'tet', 'bod', 'yue_Hans', 'zlm_Latn', 'lao', 'ind', 'grn', 'nav', 'kaz_Cyrl', 'rom', 'hye', 'kan', 'ton', 'ido', 'mhr', 'scn', 'som', 'rif_Latn', 'vie', 'enm_Latn', 'lmo', 'npi', 'pes', 'dan', 'fij', 'ina_Latn', 'cjy_Hans', 'jdt_Cyrl', 'gsw', 'glv', 'khm_Latn', 'smo', 'umb', 'sma', 'gil', 'nld', 'snd_Arab', 'arq', 'mri', 'kur_Arab', 'por', 'hin', 'shy_Latn', 'sme', 'rap', 'tyv', 'dsb', 'moh', 'asm', 'lad', 'yue_Hant', 'kpv', 'tam', 'est', 'frm_Latn', 'hoc_Latn', 'bam_Latn', 'kek_Latn', 'ksh', 'tlh_Latn', 'ltg', 'pan_Guru', 'hnj_Latn', 'cor', 'gle', 'swe', 'lin', 'qya_Latn', 'kum', 'mad', 'cmn_Hant', 'fuv', 'nau', 'mon', 'akl_Latn', 'guj', 'kaz_Latn', 'wln', 'tuk_Latn', 'jav_Java', 'lav', 'jav', 'ell', 'frr', 'mya', 'bak', 'rue', 'ita', 'hrv', 'izh', 'ilo', 'dws_Latn', 'urd', 'stq', 'tat_Arab', 'haw', 'che', 'pag', 'nno', 'fin', 'mlg', 'ppl_Latn', 'run', 'xho', 'abk', 'deu', 'hoc', 'lkt', 'lld_Latn', 'tzl_Latn', 'mdf', 'ike_Latn', 'ces', 'ldn_Latn', 'egl', 'heb', 'vec', 'zul', 'max_Latn', 'pes_Latn', 'yid', 'mal', 'nds'}
- src_multilingual: False
- tgt_multilingual: True
- prepro: normalization + SentencePiece (spm32k,spm32k)
- url_model: https://object.pouta.csc.fi/Tatoeba-MT-models/eng-mul/opus2m-2020-08-01.zip
- url_test_set: https://object.pouta.csc.fi/Tatoeba-MT-models/eng-mul/opus2m-2020-08-01.test.txt
- src_alpha3: eng
- tgt_alpha3: mul
- short_pair: en-mul
- chrF2_score: 0.451
- bleu: 22.4
- brevity_penalty: 0.987
- ref_len: 68724.0
- src_name: English
- tgt_name: Multiple languages
- train_date: 2020-08-01
- src_alpha2: en
- tgt_alpha2: mul
- prefer_old: False
- long_pair: eng-mul
- helsinki_git_sha: 480fcbe0ee1bf4774bcbe6226ad9f58e63f6c535
- transformers_git_sha: 2207e5d8cb224e954a7cba69fa4ac2309e9ff30b
- port_machine: brutasse
- port_time: 2020-08-21-14:41 |
deepseek-ai/deepseek-coder-1.3b-instruct | deepseek-ai | "2024-03-07T13:23:21Z" | 16,026 | 86 | transformers | [
"transformers",
"pytorch",
"safetensors",
"llama",
"text-generation",
"conversational",
"license:other",
"autotrain_compatible",
"endpoints_compatible",
"text-generation-inference",
"region:us"
] | text-generation | "2023-10-29T12:43:40Z" | ---
license: other
license_name: deepseek
license_link: LICENSE
---
<p align="center">
<img width="1000px" alt="DeepSeek Coder" src="https://github.com/deepseek-ai/DeepSeek-Coder/blob/main/pictures/logo.png?raw=true">
</p>
<p align="center"><a href="https://www.deepseek.com/">[🏠Homepage]</a> | <a href="https://coder.deepseek.com/">[🤖 Chat with DeepSeek Coder]</a> | <a href="https://discord.gg/Tc7c45Zzu5">[Discord]</a> | <a href="https://github.com/guoday/assert/blob/main/QR.png?raw=true">[Wechat(微信)]</a> </p>
<hr>
### 1. Introduction of Deepseek Coder
Deepseek Coder is composed of a series of code language models, each trained from scratch on 2T tokens, with a composition of 87% code and 13% natural language in both English and Chinese. We provide various sizes of the code model, ranging from 1B to 33B versions. Each model is pre-trained on project-level code corpus by employing a window size of 16K and a extra fill-in-the-blank task, to support project-level code completion and infilling. For coding capabilities, Deepseek Coder achieves state-of-the-art performance among open-source code models on multiple programming languages and various benchmarks.
- **Massive Training Data**: Trained from scratch on 2T tokens, including 87% code and 13% linguistic data in both English and Chinese languages.
- **Highly Flexible & Scalable**: Offered in model sizes of 1.3B, 5.7B, 6.7B, and 33B, enabling users to choose the setup most suitable for their requirements.
- **Superior Model Performance**: State-of-the-art performance among publicly available code models on HumanEval, MultiPL-E, MBPP, DS-1000, and APPS benchmarks.
- **Advanced Code Completion Capabilities**: A window size of 16K and a fill-in-the-blank task, supporting project-level code completion and infilling tasks.
### 2. Model Summary
deepseek-coder-1.3b-instruct is a 1.3B parameter model initialized from deepseek-coder-1.3b-base and fine-tuned on 2B tokens of instruction data.
- **Home Page:** [DeepSeek](https://deepseek.com/)
- **Repository:** [deepseek-ai/deepseek-coder](https://github.com/deepseek-ai/deepseek-coder)
- **Chat With DeepSeek Coder:** [DeepSeek-Coder](https://coder.deepseek.com/)
### 3. How to Use
Here give some examples of how to use our model.
#### Chat Model Inference
```python
from transformers import AutoTokenizer, AutoModelForCausalLM
tokenizer = AutoTokenizer.from_pretrained("deepseek-ai/deepseek-coder-1.3b-instruct", trust_remote_code=True)
model = AutoModelForCausalLM.from_pretrained("deepseek-ai/deepseek-coder-1.3b-instruct", trust_remote_code=True, torch_dtype=torch.bfloat16).cuda()
messages=[
{ 'role': 'user', 'content': "write a quick sort algorithm in python."}
]
inputs = tokenizer.apply_chat_template(messages, add_generation_prompt=True, return_tensors="pt").to(model.device)
# tokenizer.eos_token_id is the id of <|EOT|> token
outputs = model.generate(inputs, max_new_tokens=512, do_sample=False, top_k=50, top_p=0.95, num_return_sequences=1, eos_token_id=tokenizer.eos_token_id)
print(tokenizer.decode(outputs[0][len(inputs[0]):], skip_special_tokens=True))
```
### 4. License
This code repository is licensed under the MIT License. The use of DeepSeek Coder models is subject to the Model License. DeepSeek Coder supports commercial use.
See the [LICENSE-MODEL](https://github.com/deepseek-ai/deepseek-coder/blob/main/LICENSE-MODEL) for more details.
### 5. Contact
If you have any questions, please raise an issue or contact us at [[email protected]](mailto:[email protected]).
|
mradermacher/Einstein-v7-Qwen2-7B-GGUF | mradermacher | "2024-06-26T13:43:00Z" | 16,022 | 0 | transformers | [
"transformers",
"gguf",
"axolotl",
"instruct",
"finetune",
"chatml",
"gpt4",
"synthetic data",
"science",
"physics",
"chemistry",
"biology",
"math",
"qwen",
"qwen2",
"en",
"dataset:allenai/ai2_arc",
"dataset:camel-ai/physics",
"dataset:camel-ai/chemistry",
"dataset:camel-ai/biology",
"dataset:camel-ai/math",
"dataset:metaeval/reclor",
"dataset:openbookqa",
"dataset:mandyyyyii/scibench",
"dataset:derek-thomas/ScienceQA",
"dataset:TIGER-Lab/ScienceEval",
"dataset:jondurbin/airoboros-3.2",
"dataset:LDJnr/Capybara",
"dataset:Cot-Alpaca-GPT4-From-OpenHermes-2.5",
"dataset:STEM-AI-mtl/Electrical-engineering",
"dataset:knowrohit07/saraswati-stem",
"dataset:sablo/oasst2_curated",
"dataset:lmsys/lmsys-chat-1m",
"dataset:TIGER-Lab/MathInstruct",
"dataset:bigbio/med_qa",
"dataset:meta-math/MetaMathQA-40K",
"dataset:piqa",
"dataset:scibench",
"dataset:sciq",
"dataset:Open-Orca/SlimOrca",
"dataset:migtissera/Synthia-v1.3",
"dataset:allenai/WildChat",
"dataset:microsoft/orca-math-word-problems-200k",
"dataset:openchat/openchat_sharegpt4_dataset",
"dataset:teknium/GPTeacher-General-Instruct",
"dataset:m-a-p/CodeFeedback-Filtered-Instruction",
"dataset:totally-not-an-llm/EverythingLM-data-V3",
"dataset:HuggingFaceH4/no_robots",
"dataset:OpenAssistant/oasst_top1_2023-08-25",
"dataset:WizardLM/WizardLM_evol_instruct_70k",
"dataset:abacusai/SystemChat-1.1",
"dataset:H-D-T/Buzz-V1.2",
"base_model:Weyaxi/Einstein-v7-Qwen2-7B",
"license:other",
"endpoints_compatible",
"region:us"
] | null | "2024-06-26T04:29:26Z" | ---
base_model: Weyaxi/Einstein-v7-Qwen2-7B
datasets:
- allenai/ai2_arc
- camel-ai/physics
- camel-ai/chemistry
- camel-ai/biology
- camel-ai/math
- metaeval/reclor
- openbookqa
- mandyyyyii/scibench
- derek-thomas/ScienceQA
- TIGER-Lab/ScienceEval
- jondurbin/airoboros-3.2
- LDJnr/Capybara
- Cot-Alpaca-GPT4-From-OpenHermes-2.5
- STEM-AI-mtl/Electrical-engineering
- knowrohit07/saraswati-stem
- sablo/oasst2_curated
- lmsys/lmsys-chat-1m
- TIGER-Lab/MathInstruct
- bigbio/med_qa
- meta-math/MetaMathQA-40K
- openbookqa
- piqa
- metaeval/reclor
- derek-thomas/ScienceQA
- scibench
- sciq
- Open-Orca/SlimOrca
- migtissera/Synthia-v1.3
- TIGER-Lab/ScienceEval
- allenai/WildChat
- microsoft/orca-math-word-problems-200k
- openchat/openchat_sharegpt4_dataset
- teknium/GPTeacher-General-Instruct
- m-a-p/CodeFeedback-Filtered-Instruction
- totally-not-an-llm/EverythingLM-data-V3
- HuggingFaceH4/no_robots
- OpenAssistant/oasst_top1_2023-08-25
- WizardLM/WizardLM_evol_instruct_70k
- abacusai/SystemChat-1.1
- H-D-T/Buzz-V1.2
language:
- en
library_name: transformers
license: other
quantized_by: mradermacher
tags:
- axolotl
- instruct
- finetune
- chatml
- gpt4
- synthetic data
- science
- physics
- chemistry
- biology
- math
- qwen
- qwen2
---
## About
<!-- ### quantize_version: 2 -->
<!-- ### output_tensor_quantised: 1 -->
<!-- ### convert_type: hf -->
<!-- ### vocab_type: -->
<!-- ### tags: -->
static quants of https://huggingface.co/Weyaxi/Einstein-v7-Qwen2-7B
<!-- provided-files -->
weighted/imatrix quants are available at https://huggingface.co/mradermacher/Einstein-v7-Qwen2-7B-i1-GGUF
## Usage
If you are unsure how to use GGUF files, refer to one of [TheBloke's
READMEs](https://huggingface.co/TheBloke/KafkaLM-70B-German-V0.1-GGUF) for
more details, including on how to concatenate multi-part files.
## Provided Quants
(sorted by size, not necessarily quality. IQ-quants are often preferable over similar sized non-IQ quants)
| Link | Type | Size/GB | Notes |
|:-----|:-----|--------:|:------|
| [GGUF](https://huggingface.co/mradermacher/Einstein-v7-Qwen2-7B-GGUF/resolve/main/Einstein-v7-Qwen2-7B.Q2_K.gguf) | Q2_K | 3.1 | |
| [GGUF](https://huggingface.co/mradermacher/Einstein-v7-Qwen2-7B-GGUF/resolve/main/Einstein-v7-Qwen2-7B.IQ3_XS.gguf) | IQ3_XS | 3.4 | |
| [GGUF](https://huggingface.co/mradermacher/Einstein-v7-Qwen2-7B-GGUF/resolve/main/Einstein-v7-Qwen2-7B.Q3_K_S.gguf) | Q3_K_S | 3.6 | |
| [GGUF](https://huggingface.co/mradermacher/Einstein-v7-Qwen2-7B-GGUF/resolve/main/Einstein-v7-Qwen2-7B.IQ3_S.gguf) | IQ3_S | 3.6 | beats Q3_K* |
| [GGUF](https://huggingface.co/mradermacher/Einstein-v7-Qwen2-7B-GGUF/resolve/main/Einstein-v7-Qwen2-7B.IQ3_M.gguf) | IQ3_M | 3.7 | |
| [GGUF](https://huggingface.co/mradermacher/Einstein-v7-Qwen2-7B-GGUF/resolve/main/Einstein-v7-Qwen2-7B.Q3_K_M.gguf) | Q3_K_M | 3.9 | lower quality |
| [GGUF](https://huggingface.co/mradermacher/Einstein-v7-Qwen2-7B-GGUF/resolve/main/Einstein-v7-Qwen2-7B.Q3_K_L.gguf) | Q3_K_L | 4.2 | |
| [GGUF](https://huggingface.co/mradermacher/Einstein-v7-Qwen2-7B-GGUF/resolve/main/Einstein-v7-Qwen2-7B.IQ4_XS.gguf) | IQ4_XS | 4.4 | |
| [GGUF](https://huggingface.co/mradermacher/Einstein-v7-Qwen2-7B-GGUF/resolve/main/Einstein-v7-Qwen2-7B.Q4_K_S.gguf) | Q4_K_S | 4.6 | fast, recommended |
| [GGUF](https://huggingface.co/mradermacher/Einstein-v7-Qwen2-7B-GGUF/resolve/main/Einstein-v7-Qwen2-7B.Q4_K_M.gguf) | Q4_K_M | 4.8 | fast, recommended |
| [GGUF](https://huggingface.co/mradermacher/Einstein-v7-Qwen2-7B-GGUF/resolve/main/Einstein-v7-Qwen2-7B.Q5_K_S.gguf) | Q5_K_S | 5.4 | |
| [GGUF](https://huggingface.co/mradermacher/Einstein-v7-Qwen2-7B-GGUF/resolve/main/Einstein-v7-Qwen2-7B.Q5_K_M.gguf) | Q5_K_M | 5.5 | |
| [GGUF](https://huggingface.co/mradermacher/Einstein-v7-Qwen2-7B-GGUF/resolve/main/Einstein-v7-Qwen2-7B.Q6_K.gguf) | Q6_K | 6.4 | very good quality |
| [GGUF](https://huggingface.co/mradermacher/Einstein-v7-Qwen2-7B-GGUF/resolve/main/Einstein-v7-Qwen2-7B.Q8_0.gguf) | Q8_0 | 8.2 | fast, best quality |
| [GGUF](https://huggingface.co/mradermacher/Einstein-v7-Qwen2-7B-GGUF/resolve/main/Einstein-v7-Qwen2-7B.f16.gguf) | f16 | 15.3 | 16 bpw, overkill |
Here is a handy graph by ikawrakow comparing some lower-quality quant
types (lower is better):

And here are Artefact2's thoughts on the matter:
https://gist.github.com/Artefact2/b5f810600771265fc1e39442288e8ec9
## FAQ / Model Request
See https://huggingface.co/mradermacher/model_requests for some answers to
questions you might have and/or if you want some other model quantized.
## Thanks
I thank my company, [nethype GmbH](https://www.nethype.de/), for letting
me use its servers and providing upgrades to my workstation to enable
this work in my free time.
<!-- end -->
|
bartowski/Meta-Llama-3-8B-Instruct-GGUF | bartowski | "2024-04-29T19:44:45Z" | 16,005 | 75 | null | [
"gguf",
"facebook",
"meta",
"pytorch",
"llama",
"llama-3",
"text-generation",
"en",
"license:other",
"region:us"
] | text-generation | "2024-04-29T16:03:11Z" | ---
language:
- en
pipeline_tag: text-generation
tags:
- facebook
- meta
- pytorch
- llama
- llama-3
license: other
license_name: llama3
license_link: LICENSE
extra_gated_prompt: >-
### META LLAMA 3 COMMUNITY LICENSE AGREEMENT
Meta Llama 3 Version Release Date: April 18, 2024
"Agreement" means the terms and conditions for use, reproduction, distribution and modification of the
Llama Materials set forth herein.
"Documentation" means the specifications, manuals and documentation accompanying Meta Llama 3
distributed by Meta at https://llama.meta.com/get-started/.
"Licensee" or "you" means you, or your employer or any other person or entity (if you are entering into
this Agreement on such person or entity’s behalf), of the age required under applicable laws, rules or
regulations to provide legal consent and that has legal authority to bind your employer or such other
person or entity if you are entering in this Agreement on their behalf.
"Meta Llama 3" means the foundational large language models and software and algorithms, including
machine-learning model code, trained model weights, inference-enabling code, training-enabling code,
fine-tuning enabling code and other elements of the foregoing distributed by Meta at
https://llama.meta.com/llama-downloads.
"Llama Materials" means, collectively, Meta’s proprietary Meta Llama 3 and Documentation (and any
portion thereof) made available under this Agreement.
"Meta" or "we" means Meta Platforms Ireland Limited (if you are located in or, if you are an entity, your
principal place of business is in the EEA or Switzerland) and Meta Platforms, Inc. (if you are located
outside of the EEA or Switzerland).
1. License Rights and Redistribution.
a. Grant of Rights. You are granted a non-exclusive, worldwide, non-transferable and royalty-free
limited license under Meta’s intellectual property or other rights owned by Meta embodied in the Llama
Materials to use, reproduce, distribute, copy, create derivative works of, and make modifications to the
Llama Materials.
b. Redistribution and Use.
i. If you distribute or make available the Llama Materials (or any derivative works
thereof), or a product or service that uses any of them, including another AI model, you shall (A) provide
a copy of this Agreement with any such Llama Materials; and (B) prominently display “Built with Meta
Llama 3” on a related website, user interface, blogpost, about page, or product documentation. If you
use the Llama Materials to create, train, fine tune, or otherwise improve an AI model, which is
distributed or made available, you shall also include “Llama 3” at the beginning of any such AI model
name.
ii. If you receive Llama Materials, or any derivative works thereof, from a Licensee as part
of an integrated end user product, then Section 2 of this Agreement will not apply to you.
iii. You must retain in all copies of the Llama Materials that you distribute the following
attribution notice within a “Notice” text file distributed as a part of such copies: “Meta Llama 3 is
licensed under the Meta Llama 3 Community License, Copyright © Meta Platforms, Inc. All Rights
Reserved.”
iv. Your use of the Llama Materials must comply with applicable laws and regulations
(including trade compliance laws and regulations) and adhere to the Acceptable Use Policy for the Llama
Materials (available at https://llama.meta.com/llama3/use-policy), which is hereby incorporated by
reference into this Agreement.
v. You will not use the Llama Materials or any output or results of the Llama Materials to
improve any other large language model (excluding Meta Llama 3 or derivative works thereof).
2. Additional Commercial Terms. If, on the Meta Llama 3 version release date, the monthly active users
of the products or services made available by or for Licensee, or Licensee’s affiliates, is greater than 700
million monthly active users in the preceding calendar month, you must request a license from Meta,
which Meta may grant to you in its sole discretion, and you are not authorized to exercise any of the
rights under this Agreement unless or until Meta otherwise expressly grants you such rights.
3. Disclaimer of Warranty. UNLESS REQUIRED BY APPLICABLE LAW, THE LLAMA MATERIALS AND ANY
OUTPUT AND RESULTS THEREFROM ARE PROVIDED ON AN “AS IS” BASIS, WITHOUT WARRANTIES OF
ANY KIND, AND META DISCLAIMS ALL WARRANTIES OF ANY KIND, BOTH EXPRESS AND IMPLIED,
INCLUDING, WITHOUT LIMITATION, ANY WARRANTIES OF TITLE, NON-INFRINGEMENT,
MERCHANTABILITY, OR FITNESS FOR A PARTICULAR PURPOSE. YOU ARE SOLELY RESPONSIBLE FOR
DETERMINING THE APPROPRIATENESS OF USING OR REDISTRIBUTING THE LLAMA MATERIALS AND
ASSUME ANY RISKS ASSOCIATED WITH YOUR USE OF THE LLAMA MATERIALS AND ANY OUTPUT AND
RESULTS.
4. Limitation of Liability. IN NO EVENT WILL META OR ITS AFFILIATES BE LIABLE UNDER ANY THEORY OF
LIABILITY, WHETHER IN CONTRACT, TORT, NEGLIGENCE, PRODUCTS LIABILITY, OR OTHERWISE, ARISING
OUT OF THIS AGREEMENT, FOR ANY LOST PROFITS OR ANY INDIRECT, SPECIAL, CONSEQUENTIAL,
INCIDENTAL, EXEMPLARY OR PUNITIVE DAMAGES, EVEN IF META OR ITS AFFILIATES HAVE BEEN ADVISED
OF THE POSSIBILITY OF ANY OF THE FOREGOING.
5. Intellectual Property.
a. No trademark licenses are granted under this Agreement, and in connection with the Llama
Materials, neither Meta nor Licensee may use any name or mark owned by or associated with the other
or any of its affiliates, except as required for reasonable and customary use in describing and
redistributing the Llama Materials or as set forth in this Section 5(a). Meta hereby grants you a license to
use “Llama 3” (the “Mark”) solely as required to comply with the last sentence of Section 1.b.i. You will
comply with Meta’s brand guidelines (currently accessible at
https://about.meta.com/brand/resources/meta/company-brand/ ). All goodwill arising out of your use
of the Mark will inure to the benefit of Meta.
b. Subject to Meta’s ownership of Llama Materials and derivatives made by or for Meta, with
respect to any derivative works and modifications of the Llama Materials that are made by you, as
between you and Meta, you are and will be the owner of such derivative works and modifications.
c. If you institute litigation or other proceedings against Meta or any entity (including a
cross-claim or counterclaim in a lawsuit) alleging that the Llama Materials or Meta Llama 3 outputs or
results, or any portion of any of the foregoing, constitutes infringement of intellectual property or other
rights owned or licensable by you, then any licenses granted to you under this Agreement shall
terminate as of the date such litigation or claim is filed or instituted. You will indemnify and hold
harmless Meta from and against any claim by any third party arising out of or related to your use or
distribution of the Llama Materials.
6. Term and Termination. The term of this Agreement will commence upon your acceptance of this
Agreement or access to the Llama Materials and will continue in full force and effect until terminated in
accordance with the terms and conditions herein. Meta may terminate this Agreement if you are in
breach of any term or condition of this Agreement. Upon termination of this Agreement, you shall delete
and cease use of the Llama Materials. Sections 3, 4 and 7 shall survive the termination of this
Agreement.
7. Governing Law and Jurisdiction. This Agreement will be governed and construed under the laws of
the State of California without regard to choice of law principles, and the UN Convention on Contracts
for the International Sale of Goods does not apply to this Agreement. The courts of California shall have
exclusive jurisdiction of any dispute arising out of this Agreement.
### Meta Llama 3 Acceptable Use Policy
Meta is committed to promoting safe and fair use of its tools and features, including Meta Llama 3. If you
access or use Meta Llama 3, you agree to this Acceptable Use Policy (“Policy”). The most recent copy of
this policy can be found at [https://llama.meta.com/llama3/use-policy](https://llama.meta.com/llama3/use-policy)
#### Prohibited Uses
We want everyone to use Meta Llama 3 safely and responsibly. You agree you will not use, or allow
others to use, Meta Llama 3 to:
1. Violate the law or others’ rights, including to:
1. Engage in, promote, generate, contribute to, encourage, plan, incite, or further illegal or unlawful activity or content, such as:
1. Violence or terrorism
2. Exploitation or harm to children, including the solicitation, creation, acquisition, or dissemination of child exploitative content or failure to report Child Sexual Abuse Material
3. Human trafficking, exploitation, and sexual violence
4. The illegal distribution of information or materials to minors, including obscene materials, or failure to employ legally required age-gating in connection with such information or materials.
5. Sexual solicitation
6. Any other criminal activity
2. Engage in, promote, incite, or facilitate the harassment, abuse, threatening, or bullying of individuals or groups of individuals
3. Engage in, promote, incite, or facilitate discrimination or other unlawful or harmful conduct in the provision of employment, employment benefits, credit, housing, other economic benefits, or other essential goods and services
4. Engage in the unauthorized or unlicensed practice of any profession including, but not limited to, financial, legal, medical/health, or related professional practices
5. Collect, process, disclose, generate, or infer health, demographic, or other sensitive personal or private information about individuals without rights and consents required by applicable laws
6. Engage in or facilitate any action or generate any content that infringes, misappropriates, or otherwise violates any third-party rights, including the outputs or results of any products or services using the Llama Materials
7. Create, generate, or facilitate the creation of malicious code, malware, computer viruses or do anything else that could disable, overburden, interfere with or impair the proper working, integrity, operation or appearance of a website or computer system
2. Engage in, promote, incite, facilitate, or assist in the planning or development of activities that present a risk of death or bodily harm to individuals, including use of Meta Llama 3 related to the following:
1. Military, warfare, nuclear industries or applications, espionage, use for materials or activities that are subject to the International Traffic Arms Regulations (ITAR) maintained by the United States Department of State
2. Guns and illegal weapons (including weapon development)
3. Illegal drugs and regulated/controlled substances
4. Operation of critical infrastructure, transportation technologies, or heavy machinery
5. Self-harm or harm to others, including suicide, cutting, and eating disorders
6. Any content intended to incite or promote violence, abuse, or any infliction of bodily harm to an individual
3. Intentionally deceive or mislead others, including use of Meta Llama 3 related to the following:
1. Generating, promoting, or furthering fraud or the creation or promotion of disinformation
2. Generating, promoting, or furthering defamatory content, including the creation of defamatory statements, images, or other content
3. Generating, promoting, or further distributing spam
4. Impersonating another individual without consent, authorization, or legal right
5. Representing that the use of Meta Llama 3 or outputs are human-generated
6. Generating or facilitating false online engagement, including fake reviews and other means of fake online engagement
4. Fail to appropriately disclose to end users any known dangers of your AI system
Please report any violation of this Policy, software “bug,” or other problems that could lead to a violation
of this Policy through one of the following means:
* Reporting issues with the model: [https://github.com/meta-llama/llama3](https://github.com/meta-llama/llama3)
* Reporting risky content generated by the model:
developers.facebook.com/llama_output_feedback
* Reporting bugs and security concerns: facebook.com/whitehat/info
* Reporting violations of the Acceptable Use Policy or unlicensed uses of Meta Llama 3: [email protected]
extra_gated_fields:
First Name: text
Last Name: text
Date of birth: date_picker
Country: country
Affiliation: text
geo: ip_location
By clicking Submit below I accept the terms of the license and acknowledge that the information I provide will be collected stored processed and shared in accordance with the Meta Privacy Policy: checkbox
extra_gated_description: The information you provide will be collected, stored, processed and shared in accordance with the [Meta Privacy Policy](https://www.facebook.com/privacy/policy/).
extra_gated_button_content: Submit
widget:
- example_title: Hello
messages:
- role: user
content: Hey my name is Julien! How are you?
- example_title: Winter holidays
messages:
- role: system
content: You are a helpful and honest assistant. Please, respond concisely and truthfully.
- role: user
content: Can you recommend a good destination for Winter holidays?
- example_title: Programming assistant
messages:
- role: system
content: You are a helpful and honest code and programming assistant. Please, respond concisely and truthfully.
- role: user
content: Write a function that computes the nth fibonacci number.
inference:
parameters:
max_new_tokens: 300
stop:
- <|end_of_text|>
- <|eot_id|>
quantized_by: bartowski
---
## Llamacpp imatrix Quantizations of Meta-Llama-3-8B-Instruct
Using <a href="https://github.com/ggerganov/llama.cpp/">llama.cpp</a> commit <a href="https://github.com/ggerganov/llama.cpp/commit/ffe666572f98a686b17a2cd1dbf4c0a982e5ac0a">ffe6665</a> for quantization.
Original model: https://huggingface.co/meta-llama/Meta-Llama-3-8B-Instruct
All quants made using imatrix option with dataset provided by Kalomaze [here](https://github.com/ggerganov/llama.cpp/discussions/5263#discussioncomment-8395384)
## Prompt format
```
<|begin_of_text|><|start_header_id|>system<|end_header_id|>
{system_prompt}<|eot_id|><|start_header_id|>user<|end_header_id|>
{prompt}<|eot_id|><|start_header_id|>assistant<|end_header_id|>
```
## Download a file (not the whole branch) from below:
| Filename | Quant type | File Size | Description |
| -------- | ---------- | --------- | ----------- |
| [Meta-Llama-3-8B-Instruct-Q8_0.gguf](https://huggingface.co/bartowski/Meta-Llama-3-8B-Instruct-GGUF/blob/main/Meta-Llama-3-8B-Instruct-Q8_0.gguf) | Q8_0 | 8.54GB | Extremely high quality, generally unneeded but max available quant. |
| [Meta-Llama-3-8B-Instruct-Q6_K.gguf](https://huggingface.co/bartowski/Meta-Llama-3-8B-Instruct-GGUF/blob/main/Meta-Llama-3-8B-Instruct-Q6_K.gguf) | Q6_K | 6.59GB | Very high quality, near perfect, *recommended*. |
| [Meta-Llama-3-8B-Instruct-Q5_K_M.gguf](https://huggingface.co/bartowski/Meta-Llama-3-8B-Instruct-GGUF/blob/main/Meta-Llama-3-8B-Instruct-Q5_K_M.gguf) | Q5_K_M | 5.73GB | High quality, *recommended*. |
| [Meta-Llama-3-8B-Instruct-Q5_K_S.gguf](https://huggingface.co/bartowski/Meta-Llama-3-8B-Instruct-GGUF/blob/main/Meta-Llama-3-8B-Instruct-Q5_K_S.gguf) | Q5_K_S | 5.59GB | High quality, *recommended*. |
| [Meta-Llama-3-8B-Instruct-Q4_K_M.gguf](https://huggingface.co/bartowski/Meta-Llama-3-8B-Instruct-GGUF/blob/main/Meta-Llama-3-8B-Instruct-Q4_K_M.gguf) | Q4_K_M | 4.92GB | Good quality, uses about 4.83 bits per weight, *recommended*. |
| [Meta-Llama-3-8B-Instruct-Q4_K_S.gguf](https://huggingface.co/bartowski/Meta-Llama-3-8B-Instruct-GGUF/blob/main/Meta-Llama-3-8B-Instruct-Q4_K_S.gguf) | Q4_K_S | 4.69GB | Slightly lower quality with more space savings, *recommended*. |
| [Meta-Llama-3-8B-Instruct-IQ4_NL.gguf](https://huggingface.co/bartowski/Meta-Llama-3-8B-Instruct-GGUF/blob/main/Meta-Llama-3-8B-Instruct-IQ4_NL.gguf) | IQ4_NL | 4.67GB | Decent quality, slightly smaller than Q4_K_S with similar performance *recommended*. |
| [Meta-Llama-3-8B-Instruct-IQ4_XS.gguf](https://huggingface.co/bartowski/Meta-Llama-3-8B-Instruct-GGUF/blob/main/Meta-Llama-3-8B-Instruct-IQ4_XS.gguf) | IQ4_XS | 4.44GB | Decent quality, smaller than Q4_K_S with similar performance, *recommended*. |
| [Meta-Llama-3-8B-Instruct-Q3_K_L.gguf](https://huggingface.co/bartowski/Meta-Llama-3-8B-Instruct-GGUF/blob/main/Meta-Llama-3-8B-Instruct-Q3_K_L.gguf) | Q3_K_L | 4.32GB | Lower quality but usable, good for low RAM availability. |
| [Meta-Llama-3-8B-Instruct-Q3_K_M.gguf](https://huggingface.co/bartowski/Meta-Llama-3-8B-Instruct-GGUF/blob/main/Meta-Llama-3-8B-Instruct-Q3_K_M.gguf) | Q3_K_M | 4.01GB | Even lower quality. |
| [Meta-Llama-3-8B-Instruct-IQ3_M.gguf](https://huggingface.co/bartowski/Meta-Llama-3-8B-Instruct-GGUF/blob/main/Meta-Llama-3-8B-Instruct-IQ3_M.gguf) | IQ3_M | 3.78GB | Medium-low quality, new method with decent performance comparable to Q3_K_M. |
| [Meta-Llama-3-8B-Instruct-IQ3_S.gguf](https://huggingface.co/bartowski/Meta-Llama-3-8B-Instruct-GGUF/blob/main/Meta-Llama-3-8B-Instruct-IQ3_S.gguf) | IQ3_S | 3.68GB | Lower quality, new method with decent performance, recommended over Q3_K_S quant, same size with better performance. |
| [Meta-Llama-3-8B-Instruct-Q3_K_S.gguf](https://huggingface.co/bartowski/Meta-Llama-3-8B-Instruct-GGUF/blob/main/Meta-Llama-3-8B-Instruct-Q3_K_S.gguf) | Q3_K_S | 3.66GB | Low quality, not recommended. |
| [Meta-Llama-3-8B-Instruct-IQ3_XS.gguf](https://huggingface.co/bartowski/Meta-Llama-3-8B-Instruct-GGUF/blob/main/Meta-Llama-3-8B-Instruct-IQ3_XS.gguf) | IQ3_XS | 3.51GB | Lower quality, new method with decent performance, slightly better than Q3_K_S. |
| [Meta-Llama-3-8B-Instruct-IQ3_XXS.gguf](https://huggingface.co/bartowski/Meta-Llama-3-8B-Instruct-GGUF/blob/main/Meta-Llama-3-8B-Instruct-IQ3_XXS.gguf) | IQ3_XXS | 3.27GB | Lower quality, new method with decent performance, comparable to Q3 quants. |
| [Meta-Llama-3-8B-Instruct-Q2_K.gguf](https://huggingface.co/bartowski/Meta-Llama-3-8B-Instruct-GGUF/blob/main/Meta-Llama-3-8B-Instruct-Q2_K.gguf) | Q2_K | 3.17GB | Very low quality but surprisingly usable. |
| [Meta-Llama-3-8B-Instruct-IQ2_M.gguf](https://huggingface.co/bartowski/Meta-Llama-3-8B-Instruct-GGUF/blob/main/Meta-Llama-3-8B-Instruct-IQ2_M.gguf) | IQ2_M | 2.94GB | Very low quality, uses SOTA techniques to also be surprisingly usable. |
| [Meta-Llama-3-8B-Instruct-IQ2_S.gguf](https://huggingface.co/bartowski/Meta-Llama-3-8B-Instruct-GGUF/blob/main/Meta-Llama-3-8B-Instruct-IQ2_S.gguf) | IQ2_S | 2.75GB | Very low quality, uses SOTA techniques to be usable. |
| [Meta-Llama-3-8B-Instruct-IQ2_XS.gguf](https://huggingface.co/bartowski/Meta-Llama-3-8B-Instruct-GGUF/blob/main/Meta-Llama-3-8B-Instruct-IQ2_XS.gguf) | IQ2_XS | 2.60GB | Very low quality, uses SOTA techniques to be usable. |
| [Meta-Llama-3-8B-Instruct-IQ2_XXS.gguf](https://huggingface.co/bartowski/Meta-Llama-3-8B-Instruct-GGUF/blob/main/Meta-Llama-3-8B-Instruct-IQ2_XXS.gguf) | IQ2_XXS | 2.39GB | Lower quality, uses SOTA techniques to be usable. |
| [Meta-Llama-3-8B-Instruct-IQ1_M.gguf](https://huggingface.co/bartowski/Meta-Llama-3-8B-Instruct-GGUF/blob/main/Meta-Llama-3-8B-Instruct-IQ1_M.gguf) | IQ1_M | 2.16GB | Extremely low quality, *not* recommended. |
| [Meta-Llama-3-8B-Instruct-IQ1_S.gguf](https://huggingface.co/bartowski/Meta-Llama-3-8B-Instruct-GGUF/blob/main/Meta-Llama-3-8B-Instruct-IQ1_S.gguf) | IQ1_S | 2.01GB | Extremely low quality, *not* recommended. |
## Which file should I choose?
A great write up with charts showing various performances is provided by Artefact2 [here](https://gist.github.com/Artefact2/b5f810600771265fc1e39442288e8ec9)
The first thing to figure out is how big a model you can run. To do this, you'll need to figure out how much RAM and/or VRAM you have.
If you want your model running as FAST as possible, you'll want to fit the whole thing on your GPU's VRAM. Aim for a quant with a file size 1-2GB smaller than your GPU's total VRAM.
If you want the absolute maximum quality, add both your system RAM and your GPU's VRAM together, then similarly grab a quant with a file size 1-2GB Smaller than that total.
Next, you'll need to decide if you want to use an 'I-quant' or a 'K-quant'.
If you don't want to think too much, grab one of the K-quants. These are in format 'QX_K_X', like Q5_K_M.
If you want to get more into the weeds, you can check out this extremely useful feature chart:
[llama.cpp feature matrix](https://github.com/ggerganov/llama.cpp/wiki/Feature-matrix)
But basically, if you're aiming for below Q4, and you're running cuBLAS (Nvidia) or rocBLAS (AMD), you should look towards the I-quants. These are in format IQX_X, like IQ3_M. These are newer and offer better performance for their size.
These I-quants can also be used on CPU and Apple Metal, but will be slower than their K-quant equivalent, so speed vs performance is a tradeoff you'll have to decide.
The I-quants are *not* compatible with Vulcan, which is also AMD, so if you have an AMD card double check if you're using the rocBLAS build or the Vulcan build. At the time of writing this, LM Studio has a preview with ROCm support, and other inference engines have specific builds for ROCm.
Want to support my work? Visit my ko-fi page here: https://ko-fi.com/bartowski
|
mradermacher/Llama-3-Instruct-8B-SimPO-i1-GGUF | mradermacher | "2024-06-28T15:39:22Z" | 15,998 | 0 | transformers | [
"transformers",
"gguf",
"en",
"base_model:princeton-nlp/Llama-3-Instruct-8B-SimPO",
"endpoints_compatible",
"region:us"
] | null | "2024-06-28T13:38:34Z" | ---
base_model: princeton-nlp/Llama-3-Instruct-8B-SimPO
language:
- en
library_name: transformers
quantized_by: mradermacher
---
## About
<!-- ### quantize_version: 2 -->
<!-- ### output_tensor_quantised: 1 -->
<!-- ### convert_type: hf -->
<!-- ### vocab_type: -->
<!-- ### tags: nicoboss -->
weighted/imatrix quants of https://huggingface.co/princeton-nlp/Llama-3-Instruct-8B-SimPO
<!-- provided-files -->
static quants are available at https://huggingface.co/mradermacher/Llama-3-Instruct-8B-SimPO-GGUF
## Usage
If you are unsure how to use GGUF files, refer to one of [TheBloke's
READMEs](https://huggingface.co/TheBloke/KafkaLM-70B-German-V0.1-GGUF) for
more details, including on how to concatenate multi-part files.
## Provided Quants
(sorted by size, not necessarily quality. IQ-quants are often preferable over similar sized non-IQ quants)
| Link | Type | Size/GB | Notes |
|:-----|:-----|--------:|:------|
| [GGUF](https://huggingface.co/mradermacher/Llama-3-Instruct-8B-SimPO-i1-GGUF/resolve/main/Llama-3-Instruct-8B-SimPO.i1-IQ1_S.gguf) | i1-IQ1_S | 2.1 | for the desperate |
| [GGUF](https://huggingface.co/mradermacher/Llama-3-Instruct-8B-SimPO-i1-GGUF/resolve/main/Llama-3-Instruct-8B-SimPO.i1-IQ1_M.gguf) | i1-IQ1_M | 2.3 | mostly desperate |
| [GGUF](https://huggingface.co/mradermacher/Llama-3-Instruct-8B-SimPO-i1-GGUF/resolve/main/Llama-3-Instruct-8B-SimPO.i1-IQ2_XXS.gguf) | i1-IQ2_XXS | 2.5 | |
| [GGUF](https://huggingface.co/mradermacher/Llama-3-Instruct-8B-SimPO-i1-GGUF/resolve/main/Llama-3-Instruct-8B-SimPO.i1-IQ2_XS.gguf) | i1-IQ2_XS | 2.7 | |
| [GGUF](https://huggingface.co/mradermacher/Llama-3-Instruct-8B-SimPO-i1-GGUF/resolve/main/Llama-3-Instruct-8B-SimPO.i1-IQ2_S.gguf) | i1-IQ2_S | 2.9 | |
| [GGUF](https://huggingface.co/mradermacher/Llama-3-Instruct-8B-SimPO-i1-GGUF/resolve/main/Llama-3-Instruct-8B-SimPO.i1-IQ2_M.gguf) | i1-IQ2_M | 3.0 | |
| [GGUF](https://huggingface.co/mradermacher/Llama-3-Instruct-8B-SimPO-i1-GGUF/resolve/main/Llama-3-Instruct-8B-SimPO.i1-Q2_K.gguf) | i1-Q2_K | 3.3 | IQ3_XXS probably better |
| [GGUF](https://huggingface.co/mradermacher/Llama-3-Instruct-8B-SimPO-i1-GGUF/resolve/main/Llama-3-Instruct-8B-SimPO.i1-IQ3_XXS.gguf) | i1-IQ3_XXS | 3.4 | lower quality |
| [GGUF](https://huggingface.co/mradermacher/Llama-3-Instruct-8B-SimPO-i1-GGUF/resolve/main/Llama-3-Instruct-8B-SimPO.i1-IQ3_XS.gguf) | i1-IQ3_XS | 3.6 | |
| [GGUF](https://huggingface.co/mradermacher/Llama-3-Instruct-8B-SimPO-i1-GGUF/resolve/main/Llama-3-Instruct-8B-SimPO.i1-Q3_K_S.gguf) | i1-Q3_K_S | 3.8 | IQ3_XS probably better |
| [GGUF](https://huggingface.co/mradermacher/Llama-3-Instruct-8B-SimPO-i1-GGUF/resolve/main/Llama-3-Instruct-8B-SimPO.i1-IQ3_S.gguf) | i1-IQ3_S | 3.8 | beats Q3_K* |
| [GGUF](https://huggingface.co/mradermacher/Llama-3-Instruct-8B-SimPO-i1-GGUF/resolve/main/Llama-3-Instruct-8B-SimPO.i1-IQ3_M.gguf) | i1-IQ3_M | 3.9 | |
| [GGUF](https://huggingface.co/mradermacher/Llama-3-Instruct-8B-SimPO-i1-GGUF/resolve/main/Llama-3-Instruct-8B-SimPO.i1-Q3_K_M.gguf) | i1-Q3_K_M | 4.1 | IQ3_S probably better |
| [GGUF](https://huggingface.co/mradermacher/Llama-3-Instruct-8B-SimPO-i1-GGUF/resolve/main/Llama-3-Instruct-8B-SimPO.i1-Q3_K_L.gguf) | i1-Q3_K_L | 4.4 | IQ3_M probably better |
| [GGUF](https://huggingface.co/mradermacher/Llama-3-Instruct-8B-SimPO-i1-GGUF/resolve/main/Llama-3-Instruct-8B-SimPO.i1-IQ4_XS.gguf) | i1-IQ4_XS | 4.5 | |
| [GGUF](https://huggingface.co/mradermacher/Llama-3-Instruct-8B-SimPO-i1-GGUF/resolve/main/Llama-3-Instruct-8B-SimPO.i1-Q4_0.gguf) | i1-Q4_0 | 4.8 | fast, low quality |
| [GGUF](https://huggingface.co/mradermacher/Llama-3-Instruct-8B-SimPO-i1-GGUF/resolve/main/Llama-3-Instruct-8B-SimPO.i1-Q4_K_S.gguf) | i1-Q4_K_S | 4.8 | optimal size/speed/quality |
| [GGUF](https://huggingface.co/mradermacher/Llama-3-Instruct-8B-SimPO-i1-GGUF/resolve/main/Llama-3-Instruct-8B-SimPO.i1-Q4_K_M.gguf) | i1-Q4_K_M | 5.0 | fast, recommended |
| [GGUF](https://huggingface.co/mradermacher/Llama-3-Instruct-8B-SimPO-i1-GGUF/resolve/main/Llama-3-Instruct-8B-SimPO.i1-Q5_K_S.gguf) | i1-Q5_K_S | 5.7 | |
| [GGUF](https://huggingface.co/mradermacher/Llama-3-Instruct-8B-SimPO-i1-GGUF/resolve/main/Llama-3-Instruct-8B-SimPO.i1-Q5_K_M.gguf) | i1-Q5_K_M | 5.8 | |
| [GGUF](https://huggingface.co/mradermacher/Llama-3-Instruct-8B-SimPO-i1-GGUF/resolve/main/Llama-3-Instruct-8B-SimPO.i1-Q6_K.gguf) | i1-Q6_K | 6.7 | practically like static Q6_K |
Here is a handy graph by ikawrakow comparing some lower-quality quant
types (lower is better):

And here are Artefact2's thoughts on the matter:
https://gist.github.com/Artefact2/b5f810600771265fc1e39442288e8ec9
## FAQ / Model Request
See https://huggingface.co/mradermacher/model_requests for some answers to
questions you might have and/or if you want some other model quantized.
## Thanks
I thank my company, [nethype GmbH](https://www.nethype.de/), for letting
me use its servers and providing upgrades to my workstation to enable
this work in my free time. Additional thanks to [@nicoboss](https://huggingface.co/nicoboss) for giving me access to his hardware for calculating the imatrix for these quants.
<!-- end -->
|
mradermacher/llama3-8B-DarkIdol-2.2-Uncensored-1048K-GGUF | mradermacher | "2024-07-01T19:53:58Z" | 15,992 | 2 | transformers | [
"transformers",
"gguf",
"roleplay",
"llama3",
"sillytavern",
"idol",
"en",
"ja",
"zh",
"base_model:aifeifei798/llama3-8B-DarkIdol-2.2-Uncensored-1048K",
"license:llama3",
"endpoints_compatible",
"region:us"
] | null | "2024-07-01T17:36:37Z" | ---
base_model: aifeifei798/llama3-8B-DarkIdol-2.2-Uncensored-1048K
language:
- en
- ja
- zh
library_name: transformers
license: llama3
quantized_by: mradermacher
tags:
- roleplay
- llama3
- sillytavern
- idol
---
## About
<!-- ### quantize_version: 2 -->
<!-- ### output_tensor_quantised: 1 -->
<!-- ### convert_type: hf -->
<!-- ### vocab_type: -->
<!-- ### tags: -->
static quants of https://huggingface.co/aifeifei798/llama3-8B-DarkIdol-2.2-Uncensored-1048K
<!-- provided-files -->
weighted/imatrix quants are available at https://huggingface.co/mradermacher/llama3-8B-DarkIdol-2.2-Uncensored-1048K-i1-GGUF
## Usage
If you are unsure how to use GGUF files, refer to one of [TheBloke's
READMEs](https://huggingface.co/TheBloke/KafkaLM-70B-German-V0.1-GGUF) for
more details, including on how to concatenate multi-part files.
## Provided Quants
(sorted by size, not necessarily quality. IQ-quants are often preferable over similar sized non-IQ quants)
| Link | Type | Size/GB | Notes |
|:-----|:-----|--------:|:------|
| [GGUF](https://huggingface.co/mradermacher/llama3-8B-DarkIdol-2.2-Uncensored-1048K-GGUF/resolve/main/llama3-8B-DarkIdol-2.2-Uncensored-1048K.Q2_K.gguf) | Q2_K | 3.3 | |
| [GGUF](https://huggingface.co/mradermacher/llama3-8B-DarkIdol-2.2-Uncensored-1048K-GGUF/resolve/main/llama3-8B-DarkIdol-2.2-Uncensored-1048K.IQ3_XS.gguf) | IQ3_XS | 3.6 | |
| [GGUF](https://huggingface.co/mradermacher/llama3-8B-DarkIdol-2.2-Uncensored-1048K-GGUF/resolve/main/llama3-8B-DarkIdol-2.2-Uncensored-1048K.Q3_K_S.gguf) | Q3_K_S | 3.8 | |
| [GGUF](https://huggingface.co/mradermacher/llama3-8B-DarkIdol-2.2-Uncensored-1048K-GGUF/resolve/main/llama3-8B-DarkIdol-2.2-Uncensored-1048K.IQ3_S.gguf) | IQ3_S | 3.8 | beats Q3_K* |
| [GGUF](https://huggingface.co/mradermacher/llama3-8B-DarkIdol-2.2-Uncensored-1048K-GGUF/resolve/main/llama3-8B-DarkIdol-2.2-Uncensored-1048K.IQ3_M.gguf) | IQ3_M | 3.9 | |
| [GGUF](https://huggingface.co/mradermacher/llama3-8B-DarkIdol-2.2-Uncensored-1048K-GGUF/resolve/main/llama3-8B-DarkIdol-2.2-Uncensored-1048K.Q3_K_M.gguf) | Q3_K_M | 4.1 | lower quality |
| [GGUF](https://huggingface.co/mradermacher/llama3-8B-DarkIdol-2.2-Uncensored-1048K-GGUF/resolve/main/llama3-8B-DarkIdol-2.2-Uncensored-1048K.Q3_K_L.gguf) | Q3_K_L | 4.4 | |
| [GGUF](https://huggingface.co/mradermacher/llama3-8B-DarkIdol-2.2-Uncensored-1048K-GGUF/resolve/main/llama3-8B-DarkIdol-2.2-Uncensored-1048K.IQ4_XS.gguf) | IQ4_XS | 4.6 | |
| [GGUF](https://huggingface.co/mradermacher/llama3-8B-DarkIdol-2.2-Uncensored-1048K-GGUF/resolve/main/llama3-8B-DarkIdol-2.2-Uncensored-1048K.Q4_K_S.gguf) | Q4_K_S | 4.8 | fast, recommended |
| [GGUF](https://huggingface.co/mradermacher/llama3-8B-DarkIdol-2.2-Uncensored-1048K-GGUF/resolve/main/llama3-8B-DarkIdol-2.2-Uncensored-1048K.Q4_K_M.gguf) | Q4_K_M | 5.0 | fast, recommended |
| [GGUF](https://huggingface.co/mradermacher/llama3-8B-DarkIdol-2.2-Uncensored-1048K-GGUF/resolve/main/llama3-8B-DarkIdol-2.2-Uncensored-1048K.Q5_K_S.gguf) | Q5_K_S | 5.7 | |
| [GGUF](https://huggingface.co/mradermacher/llama3-8B-DarkIdol-2.2-Uncensored-1048K-GGUF/resolve/main/llama3-8B-DarkIdol-2.2-Uncensored-1048K.Q5_K_M.gguf) | Q5_K_M | 5.8 | |
| [GGUF](https://huggingface.co/mradermacher/llama3-8B-DarkIdol-2.2-Uncensored-1048K-GGUF/resolve/main/llama3-8B-DarkIdol-2.2-Uncensored-1048K.Q6_K.gguf) | Q6_K | 6.7 | very good quality |
| [GGUF](https://huggingface.co/mradermacher/llama3-8B-DarkIdol-2.2-Uncensored-1048K-GGUF/resolve/main/llama3-8B-DarkIdol-2.2-Uncensored-1048K.Q8_0.gguf) | Q8_0 | 8.6 | fast, best quality |
| [GGUF](https://huggingface.co/mradermacher/llama3-8B-DarkIdol-2.2-Uncensored-1048K-GGUF/resolve/main/llama3-8B-DarkIdol-2.2-Uncensored-1048K.f16.gguf) | f16 | 16.2 | 16 bpw, overkill |
Here is a handy graph by ikawrakow comparing some lower-quality quant
types (lower is better):

And here are Artefact2's thoughts on the matter:
https://gist.github.com/Artefact2/b5f810600771265fc1e39442288e8ec9
## FAQ / Model Request
See https://huggingface.co/mradermacher/model_requests for some answers to
questions you might have and/or if you want some other model quantized.
## Thanks
I thank my company, [nethype GmbH](https://www.nethype.de/), for letting
me use its servers and providing upgrades to my workstation to enable
this work in my free time.
<!-- end -->
|
mradermacher/Llama-3-Instruct-Neurona-8b-v2-GGUF | mradermacher | "2024-07-02T03:13:42Z" | 15,985 | 0 | transformers | [
"transformers",
"gguf",
"synthetic",
"es",
"en",
"dataset:Danielbrdz/Barcenas-Economia",
"dataset:HiTZ/casimedicos-exp",
"dataset:somosnlp/coser_resumenes",
"dataset:csebuetnlp/CrossSum",
"dataset:Iker/Document-Translation-en-es",
"dataset:somosnlp/es-inclusive-language-it",
"dataset:glaiveai/glaive-code-assistant-v3",
"dataset:glaiveai/glaive-function-calling-v2",
"dataset:Iker/InstructTranslation-EN-ES",
"dataset:somosnlp/lenguaje-claro-dataset",
"dataset:somosnlp/LingComp_QA",
"dataset:Iker/NoticIA",
"dataset:teknium/OpenHermes-2.5",
"dataset:Iker/OpenHermes-2.5-Spanish",
"dataset:Helsinki-NLP/opus-100",
"dataset:projecte-aina/RAG_Multilingual",
"dataset:HiTZ/This-is-not-a-dataset",
"dataset:Iker/Reddit-Post-Translation",
"dataset:wikipedia",
"base_model:Iker/Llama-3-Instruct-Neurona-8b-v2",
"license:llama3",
"endpoints_compatible",
"region:us"
] | null | "2024-07-01T17:57:21Z" | ---
base_model: Iker/Llama-3-Instruct-Neurona-8b-v2
datasets:
- Danielbrdz/Barcenas-Economia
- HiTZ/casimedicos-exp
- somosnlp/coser_resumenes
- csebuetnlp/CrossSum
- Iker/Document-Translation-en-es
- somosnlp/es-inclusive-language-it
- glaiveai/glaive-code-assistant-v3
- glaiveai/glaive-function-calling-v2
- Iker/InstructTranslation-EN-ES
- somosnlp/lenguaje-claro-dataset
- somosnlp/LingComp_QA
- Iker/NoticIA
- teknium/OpenHermes-2.5
- Iker/OpenHermes-2.5-Spanish
- Helsinki-NLP/opus-100
- projecte-aina/RAG_Multilingual
- HiTZ/This-is-not-a-dataset
- Iker/Reddit-Post-Translation
- wikipedia
language:
- es
- en
library_name: transformers
license: llama3
quantized_by: mradermacher
tags:
- synthetic
---
## About
<!-- ### quantize_version: 2 -->
<!-- ### output_tensor_quantised: 1 -->
<!-- ### convert_type: hf -->
<!-- ### vocab_type: -->
<!-- ### tags: -->
static quants of https://huggingface.co/Iker/Llama-3-Instruct-Neurona-8b-v2
<!-- provided-files -->
weighted/imatrix quants are available at https://huggingface.co/mradermacher/Llama-3-Instruct-Neurona-8b-v2-i1-GGUF
## Usage
If you are unsure how to use GGUF files, refer to one of [TheBloke's
READMEs](https://huggingface.co/TheBloke/KafkaLM-70B-German-V0.1-GGUF) for
more details, including on how to concatenate multi-part files.
## Provided Quants
(sorted by size, not necessarily quality. IQ-quants are often preferable over similar sized non-IQ quants)
| Link | Type | Size/GB | Notes |
|:-----|:-----|--------:|:------|
| [GGUF](https://huggingface.co/mradermacher/Llama-3-Instruct-Neurona-8b-v2-GGUF/resolve/main/Llama-3-Instruct-Neurona-8b-v2.Q2_K.gguf) | Q2_K | 3.3 | |
| [GGUF](https://huggingface.co/mradermacher/Llama-3-Instruct-Neurona-8b-v2-GGUF/resolve/main/Llama-3-Instruct-Neurona-8b-v2.IQ3_XS.gguf) | IQ3_XS | 3.6 | |
| [GGUF](https://huggingface.co/mradermacher/Llama-3-Instruct-Neurona-8b-v2-GGUF/resolve/main/Llama-3-Instruct-Neurona-8b-v2.Q3_K_S.gguf) | Q3_K_S | 3.8 | |
| [GGUF](https://huggingface.co/mradermacher/Llama-3-Instruct-Neurona-8b-v2-GGUF/resolve/main/Llama-3-Instruct-Neurona-8b-v2.IQ3_S.gguf) | IQ3_S | 3.8 | beats Q3_K* |
| [GGUF](https://huggingface.co/mradermacher/Llama-3-Instruct-Neurona-8b-v2-GGUF/resolve/main/Llama-3-Instruct-Neurona-8b-v2.IQ3_M.gguf) | IQ3_M | 3.9 | |
| [GGUF](https://huggingface.co/mradermacher/Llama-3-Instruct-Neurona-8b-v2-GGUF/resolve/main/Llama-3-Instruct-Neurona-8b-v2.Q3_K_M.gguf) | Q3_K_M | 4.1 | lower quality |
| [GGUF](https://huggingface.co/mradermacher/Llama-3-Instruct-Neurona-8b-v2-GGUF/resolve/main/Llama-3-Instruct-Neurona-8b-v2.Q3_K_L.gguf) | Q3_K_L | 4.4 | |
| [GGUF](https://huggingface.co/mradermacher/Llama-3-Instruct-Neurona-8b-v2-GGUF/resolve/main/Llama-3-Instruct-Neurona-8b-v2.IQ4_XS.gguf) | IQ4_XS | 4.6 | |
| [GGUF](https://huggingface.co/mradermacher/Llama-3-Instruct-Neurona-8b-v2-GGUF/resolve/main/Llama-3-Instruct-Neurona-8b-v2.Q4_K_S.gguf) | Q4_K_S | 4.8 | fast, recommended |
| [GGUF](https://huggingface.co/mradermacher/Llama-3-Instruct-Neurona-8b-v2-GGUF/resolve/main/Llama-3-Instruct-Neurona-8b-v2.Q4_K_M.gguf) | Q4_K_M | 5.0 | fast, recommended |
| [GGUF](https://huggingface.co/mradermacher/Llama-3-Instruct-Neurona-8b-v2-GGUF/resolve/main/Llama-3-Instruct-Neurona-8b-v2.Q5_K_S.gguf) | Q5_K_S | 5.7 | |
| [GGUF](https://huggingface.co/mradermacher/Llama-3-Instruct-Neurona-8b-v2-GGUF/resolve/main/Llama-3-Instruct-Neurona-8b-v2.Q5_K_M.gguf) | Q5_K_M | 5.8 | |
| [GGUF](https://huggingface.co/mradermacher/Llama-3-Instruct-Neurona-8b-v2-GGUF/resolve/main/Llama-3-Instruct-Neurona-8b-v2.Q6_K.gguf) | Q6_K | 6.7 | very good quality |
| [GGUF](https://huggingface.co/mradermacher/Llama-3-Instruct-Neurona-8b-v2-GGUF/resolve/main/Llama-3-Instruct-Neurona-8b-v2.Q8_0.gguf) | Q8_0 | 8.6 | fast, best quality |
| [GGUF](https://huggingface.co/mradermacher/Llama-3-Instruct-Neurona-8b-v2-GGUF/resolve/main/Llama-3-Instruct-Neurona-8b-v2.f16.gguf) | f16 | 16.2 | 16 bpw, overkill |
Here is a handy graph by ikawrakow comparing some lower-quality quant
types (lower is better):

And here are Artefact2's thoughts on the matter:
https://gist.github.com/Artefact2/b5f810600771265fc1e39442288e8ec9
## FAQ / Model Request
See https://huggingface.co/mradermacher/model_requests for some answers to
questions you might have and/or if you want some other model quantized.
## Thanks
I thank my company, [nethype GmbH](https://www.nethype.de/), for letting
me use its servers and providing upgrades to my workstation to enable
this work in my free time.
<!-- end -->
|
mradermacher/cosmosage-v3-i1-GGUF | mradermacher | "2024-06-28T19:07:43Z" | 15,978 | 0 | transformers | [
"transformers",
"gguf",
"physics",
"cosmology",
"en",
"dataset:teknium/OpenHermes-2.5",
"base_model:Tijmen2/cosmosage-v3",
"license:mit",
"endpoints_compatible",
"region:us"
] | null | "2024-06-28T17:53:32Z" | ---
base_model: Tijmen2/cosmosage-v3
datasets:
- teknium/OpenHermes-2.5
language:
- en
library_name: transformers
license: mit
quantized_by: mradermacher
tags:
- physics
- cosmology
---
## About
<!-- ### quantize_version: 2 -->
<!-- ### output_tensor_quantised: 1 -->
<!-- ### convert_type: hf -->
<!-- ### vocab_type: -->
<!-- ### tags: nicoboss -->
weighted/imatrix quants of https://huggingface.co/Tijmen2/cosmosage-v3
<!-- provided-files -->
static quants are available at https://huggingface.co/mradermacher/cosmosage-v3-GGUF
## Usage
If you are unsure how to use GGUF files, refer to one of [TheBloke's
READMEs](https://huggingface.co/TheBloke/KafkaLM-70B-German-V0.1-GGUF) for
more details, including on how to concatenate multi-part files.
## Provided Quants
(sorted by size, not necessarily quality. IQ-quants are often preferable over similar sized non-IQ quants)
| Link | Type | Size/GB | Notes |
|:-----|:-----|--------:|:------|
| [GGUF](https://huggingface.co/mradermacher/cosmosage-v3-i1-GGUF/resolve/main/cosmosage-v3.i1-IQ1_S.gguf) | i1-IQ1_S | 2.1 | for the desperate |
| [GGUF](https://huggingface.co/mradermacher/cosmosage-v3-i1-GGUF/resolve/main/cosmosage-v3.i1-IQ1_M.gguf) | i1-IQ1_M | 2.3 | mostly desperate |
| [GGUF](https://huggingface.co/mradermacher/cosmosage-v3-i1-GGUF/resolve/main/cosmosage-v3.i1-IQ2_XXS.gguf) | i1-IQ2_XXS | 2.5 | |
| [GGUF](https://huggingface.co/mradermacher/cosmosage-v3-i1-GGUF/resolve/main/cosmosage-v3.i1-IQ2_XS.gguf) | i1-IQ2_XS | 2.7 | |
| [GGUF](https://huggingface.co/mradermacher/cosmosage-v3-i1-GGUF/resolve/main/cosmosage-v3.i1-IQ2_S.gguf) | i1-IQ2_S | 2.9 | |
| [GGUF](https://huggingface.co/mradermacher/cosmosage-v3-i1-GGUF/resolve/main/cosmosage-v3.i1-IQ2_M.gguf) | i1-IQ2_M | 3.0 | |
| [GGUF](https://huggingface.co/mradermacher/cosmosage-v3-i1-GGUF/resolve/main/cosmosage-v3.i1-Q2_K.gguf) | i1-Q2_K | 3.3 | IQ3_XXS probably better |
| [GGUF](https://huggingface.co/mradermacher/cosmosage-v3-i1-GGUF/resolve/main/cosmosage-v3.i1-IQ3_XXS.gguf) | i1-IQ3_XXS | 3.4 | lower quality |
| [GGUF](https://huggingface.co/mradermacher/cosmosage-v3-i1-GGUF/resolve/main/cosmosage-v3.i1-IQ3_XS.gguf) | i1-IQ3_XS | 3.6 | |
| [GGUF](https://huggingface.co/mradermacher/cosmosage-v3-i1-GGUF/resolve/main/cosmosage-v3.i1-Q3_K_S.gguf) | i1-Q3_K_S | 3.8 | IQ3_XS probably better |
| [GGUF](https://huggingface.co/mradermacher/cosmosage-v3-i1-GGUF/resolve/main/cosmosage-v3.i1-IQ3_S.gguf) | i1-IQ3_S | 3.8 | beats Q3_K* |
| [GGUF](https://huggingface.co/mradermacher/cosmosage-v3-i1-GGUF/resolve/main/cosmosage-v3.i1-IQ3_M.gguf) | i1-IQ3_M | 3.9 | |
| [GGUF](https://huggingface.co/mradermacher/cosmosage-v3-i1-GGUF/resolve/main/cosmosage-v3.i1-Q3_K_M.gguf) | i1-Q3_K_M | 4.1 | IQ3_S probably better |
| [GGUF](https://huggingface.co/mradermacher/cosmosage-v3-i1-GGUF/resolve/main/cosmosage-v3.i1-Q3_K_L.gguf) | i1-Q3_K_L | 4.4 | IQ3_M probably better |
| [GGUF](https://huggingface.co/mradermacher/cosmosage-v3-i1-GGUF/resolve/main/cosmosage-v3.i1-IQ4_XS.gguf) | i1-IQ4_XS | 4.5 | |
| [GGUF](https://huggingface.co/mradermacher/cosmosage-v3-i1-GGUF/resolve/main/cosmosage-v3.i1-Q4_0.gguf) | i1-Q4_0 | 4.8 | fast, low quality |
| [GGUF](https://huggingface.co/mradermacher/cosmosage-v3-i1-GGUF/resolve/main/cosmosage-v3.i1-Q4_K_S.gguf) | i1-Q4_K_S | 4.8 | optimal size/speed/quality |
| [GGUF](https://huggingface.co/mradermacher/cosmosage-v3-i1-GGUF/resolve/main/cosmosage-v3.i1-Q4_K_M.gguf) | i1-Q4_K_M | 5.0 | fast, recommended |
| [GGUF](https://huggingface.co/mradermacher/cosmosage-v3-i1-GGUF/resolve/main/cosmosage-v3.i1-Q5_K_S.gguf) | i1-Q5_K_S | 5.7 | |
| [GGUF](https://huggingface.co/mradermacher/cosmosage-v3-i1-GGUF/resolve/main/cosmosage-v3.i1-Q5_K_M.gguf) | i1-Q5_K_M | 5.8 | |
| [GGUF](https://huggingface.co/mradermacher/cosmosage-v3-i1-GGUF/resolve/main/cosmosage-v3.i1-Q6_K.gguf) | i1-Q6_K | 6.7 | practically like static Q6_K |
Here is a handy graph by ikawrakow comparing some lower-quality quant
types (lower is better):

And here are Artefact2's thoughts on the matter:
https://gist.github.com/Artefact2/b5f810600771265fc1e39442288e8ec9
## FAQ / Model Request
See https://huggingface.co/mradermacher/model_requests for some answers to
questions you might have and/or if you want some other model quantized.
## Thanks
I thank my company, [nethype GmbH](https://www.nethype.de/), for letting
me use its servers and providing upgrades to my workstation to enable
this work in my free time. Additional thanks to [@nicoboss](https://huggingface.co/nicoboss) for giving me access to his hardware for calculating the imatrix for these quants.
<!-- end -->
|
PlanTL-GOB-ES/roberta-base-bne | PlanTL-GOB-ES | "2023-01-31T13:59:59Z" | 15,972 | 26 | transformers | [
"transformers",
"pytorch",
"roberta",
"fill-mask",
"national library of spain",
"spanish",
"bne",
"roberta-base-bne",
"es",
"dataset:bne",
"arxiv:1907.11692",
"license:apache-2.0",
"autotrain_compatible",
"endpoints_compatible",
"region:us"
] | fill-mask | "2022-03-02T23:29:04Z" | ---
language:
- es
license: apache-2.0
tags:
- "national library of spain"
- "spanish"
- "bne"
- "roberta-base-bne"
datasets:
- "bne"
metrics:
- "ppl"
widget:
- text: "Por la ventanilla del coche vi la Giralda y pensé que bonita que es la ciudad de <mask>."
- text: "Más vale <mask> que lamentar."
- text: "Caminante no hay camino, se hace camino al <mask>."
- text: "Tengo una pelota roja y otra amarilla. Si le doy la roja a Jose, sólo me queda la <mask>."
- text: "Tengo una pelota roja y otra amarilla. Si le doy la amarilla a Jose, sólo me queda la <mask>."
- text: "El <mask> es el pico más alto de España."
---
# RoBERTa base trained with data from the National Library of Spain (BNE)
## Table of Contents
<details>
<summary>Click to expand</summary>
- [Overview](#overview)
- [Model description](#model-description)
- [Intended uses and limitations](#intended-uses-and-limitations)
- [How to use](#how-to-use)
- [Limitations and bias](#limitations-and-bias)
- [Training](#training)
- [Training data](#training-data)
- [Training procedure](#training-procedure)
- [Evaluation](#evaluation)
- [Additional information](#additional-information)
- [Author](#author)
- [Contact information](#contact-information)
- [Copyright](#copyright)
- [Licensing information](#licensing-information)
- [Funding](#funding)
- [Citation Information](#citation-information)
- [Disclaimer](#disclaimer)
</details>
## Overview
- **Architecture:** roberta-base
- **Language:** Spanish
- **Task:** fill-mask
- **Data:** BNE
## Model description
The **roberta-base-bne** is a transformer-based masked language model for the Spanish language. It is based on the [RoBERTa](https://arxiv.org/abs/1907.11692) base model and has been pre-trained using the largest Spanish corpus known to date, with a total of 570GB of clean and deduplicated text processed for this work, compiled from the web crawlings performed by the [National Library of Spain (Biblioteca Nacional de España)](http://www.bne.es/en/Inicio/index.html) from 2009 to 2019.
## Intended uses and limitations
The **roberta-base-bne** model is ready-to-use only for masked language modeling to perform the Fill Mask task (try the inference API or read the next section).
However, it is intended to be fine-tuned on non-generative downstream tasks such as Question Answering, Text Classification, or Named Entity Recognition.
You can use the raw model for fill mask or fine-tune it to a downstream task.
## How to use
Here is how to use this model:
```python
>>> from transformers import pipeline
>>> from pprint import pprint
>>> unmasker = pipeline('fill-mask', model='PlanTL-GOB-ES/roberta-base-bne')
>>> pprint(unmasker("Gracias a los datos de la BNE se ha podido <mask> este modelo del lenguaje."))
[{'score': 0.08422081917524338,
'token': 3832,
'token_str': ' desarrollar',
'sequence': 'Gracias a los datos de la BNE se ha podido desarrollar este modelo del lenguaje.'},
{'score': 0.06348305940628052,
'token': 3078,
'token_str': ' crear',
'sequence': 'Gracias a los datos de la BNE se ha podido crear este modelo del lenguaje.'},
{'score': 0.06148449331521988,
'token': 2171,
'token_str': ' realizar',
'sequence': 'Gracias a los datos de la BNE se ha podido realizar este modelo del lenguaje.'},
{'score': 0.056218471378088,
'token': 10880,
'token_str': ' elaborar',
'sequence': 'Gracias a los datos de la BNE se ha podido elaborar este modelo del lenguaje.'},
{'score': 0.05133328214287758,
'token': 31915,
'token_str': ' validar',
'sequence': 'Gracias a los datos de la BNE se ha podido validar este modelo del lenguaje.'}]
```
Here is how to use this model to get the features of a given text in PyTorch:
```python
>>> from transformers import RobertaTokenizer, RobertaModel
>>> tokenizer = RobertaTokenizer.from_pretrained('PlanTL-GOB-ES/roberta-base-bne')
>>> model = RobertaModel.from_pretrained('PlanTL-GOB-ES/roberta-base-bne')
>>> text = "Gracias a los datos de la BNE se ha podido desarrollar este modelo del lenguaje."
>>> encoded_input = tokenizer(text, return_tensors='pt')
>>> output = model(**encoded_input)
>>> print(output.last_hidden_state.shape)
torch.Size([1, 19, 768])
```
## Limitations and bias
At the time of submission, no measures have been taken to estimate the bias and toxicity embedded in the model. However, we are well aware that our models may be biased since the corpora have been collected using crawling techniques on multiple web sources. We intend to conduct research in these areas in the future, and if completed, this model card will be updated. Nevertheless, here's an example of how the model can have biased predictions:
```python
>>> from transformers import pipeline, set_seed
>>> from pprint import pprint
>>> unmasker = pipeline('fill-mask', model='PlanTL-GOB-ES/roberta-base-bne')
>>> set_seed(42)
>>> pprint(unmasker("Antonio está pensando en <mask>."))
[{'score': 0.07950365543365479,
'sequence': 'Antonio está pensando en ti.',
'token': 486,
'token_str': ' ti'},
{'score': 0.03375273942947388,
'sequence': 'Antonio está pensando en irse.',
'token': 13134,
'token_str': ' irse'},
{'score': 0.031026942655444145,
'sequence': 'Antonio está pensando en casarse.',
'token': 24852,
'token_str': ' casarse'},
{'score': 0.030703715980052948,
'sequence': 'Antonio está pensando en todo.',
'token': 665,
'token_str': ' todo'},
{'score': 0.02838558703660965,
'sequence': 'Antonio está pensando en ello.',
'token': 1577,
'token_str': ' ello'}]
>>> set_seed(42)
>>> pprint(unmasker("Mohammed está pensando en <mask>."))
[{'score': 0.05433618649840355,
'sequence': 'Mohammed está pensando en morir.',
'token': 9459,
'token_str': ' morir'},
{'score': 0.0400255024433136,
'sequence': 'Mohammed está pensando en irse.',
'token': 13134,
'token_str': ' irse'},
{'score': 0.03705748915672302,
'sequence': 'Mohammed está pensando en todo.',
'token': 665,
'token_str': ' todo'},
{'score': 0.03658654913306236,
'sequence': 'Mohammed está pensando en quedarse.',
'token': 9331,
'token_str': ' quedarse'},
{'score': 0.03329474478960037,
'sequence': 'Mohammed está pensando en ello.',
'token': 1577,
'token_str': ' ello'}]
```
## Training
### Training data
The [National Library of Spain (Biblioteca Nacional de España)](http://www.bne.es/en/Inicio/index.html) crawls all .es domains once a year. The training corpus consists of 59TB of WARC files from these crawls, carried out from 2009 to 2019.
To obtain a high-quality training corpus, the corpus has been preprocessed with a pipeline of operations, including among others, sentence splitting, language detection, filtering of bad-formed sentences, and deduplication of repetitive contents. During the process, document boundaries are kept. This resulted in 2TB of Spanish clean corpus. Further global deduplication among the corpus is applied, resulting in 570GB of text.
Some of the statistics of the corpus:
| Corpora | Number of documents | Number of tokens | Size (GB) |
|---------|---------------------|------------------|-----------|
| BNE | 201,080,084 | 135,733,450,668 | 570GB |
### Training procedure
The training corpus has been tokenized using a byte version of Byte-Pair Encoding (BPE) used in the original [RoBERTA](https://arxiv.org/abs/1907.11692) model with a vocabulary size of 50,262 tokens.
The **roberta-base-bne** pre-training consists of a masked language model training, that follows the approach employed for the RoBERTa base. The training lasted a total of 48 hours with 16 computing nodes, each one with 4 NVIDIA V100 GPUs of 16GB VRAM.
## Evaluation
When fine-tuned on downstream tasks, this model achieves the following results:
| Dataset | Metric | [**RoBERTa-base**](https://huggingface.co/PlanTL-GOB-ES/roberta-base-bne) |
|--------------|----------|------------|
| MLDoc | F1 | 0.9664 |
| CoNLL-NERC | F1 | 0.8851 |
| CAPITEL-NERC | F1 | 0.8960 |
| PAWS-X | F1 | 0.9020 |
| UD-POS | F1 | 0.9907 |
| CAPITEL-POS | F1 | 0.9846 |
| SQAC | F1 | 0.7923 |
| STS | Combined | 0.8533 |
| XNLI | Accuracy | 0.8016 |
For more evaluation details visit our [GitHub repository](https://github.com/PlanTL-GOB-ES/lm-spanish) or [paper](http://journal.sepln.org/sepln/ojs/ojs/index.php/pln/article/view/6405).
## Additional information
### Author
Text Mining Unit (TeMU) from Barcelona Supercomputing Center (<[email protected]>).
### Contact information
For further information, send an email to <[email protected]>.
### Copyright
Copyright by the [Spanish State Secretariat for Digitalization and Artificial Intelligence (SEDIA)](https://portal.mineco.gob.es/en-us/digitalizacionIA/Pages/sedia.aspx).
### Licensing information
This work is licensed under a [Apache License, Version 2.0](https://www.apache.org/licenses/LICENSE-2.0)
### Funding
This work was funded by the [Spanish State Secretariat for Digitalization and Artificial Intelligence (SEDIA)](https://portal.mineco.gob.es/en-us/digitalizacionIA/Pages/sedia.aspx) within the framework of the Plan-TL.
### Citation information
If you use this model, please cite our [paper](http://journal.sepln.org/sepln/ojs/ojs/index.php/pln/article/view/6405):
```
@article{,
title = {MarIA: Spanish Language Models},
author = {Asier Gutiérrez Fandiño and Jordi Armengol Estapé and Marc Pàmies and Joan Llop Palao and Joaquin Silveira Ocampo and Casimiro Pio Carrino and Carme Armentano Oller and Carlos Rodriguez Penagos and Aitor Gonzalez Agirre and Marta Villegas},
doi = {10.26342/2022-68-3},
issn = {1135-5948},
journal = {Procesamiento del Lenguaje Natural},
publisher = {Sociedad Española para el Procesamiento del Lenguaje Natural},
url = {https://upcommons.upc.edu/handle/2117/367156#.YyMTB4X9A-0.mendeley},
volume = {68},
year = {2022},
}
```
### Disclaimer
<details>
<summary>Click to expand</summary>
The models published in this repository are intended for a generalist purpose and are available to third parties. These models may have bias and/or any other undesirable distortions.
When third parties deploy or provide systems and/or services to other parties using any of these models (or using systems based on these models) or become users of the models, they should note that it is their responsibility to mitigate the risks arising from their use and, in any event, to comply with applicable regulations, including regulations regarding the use of Artificial Intelligence.
In no event shall the owner of the models (SEDIA) nor the creator (BSC) be liable for any results arising from the use made by third parties of these models.
Los modelos publicados en este repositorio tienen una finalidad generalista y están a disposición de terceros. Estos modelos pueden tener sesgos y/u otro tipo de distorsiones indeseables.
Cuando terceros desplieguen o proporcionen sistemas y/o servicios a otras partes usando alguno de estos modelos (o utilizando sistemas basados en estos modelos) o se conviertan en usuarios de los modelos, deben tener en cuenta que es su responsabilidad mitigar los riesgos derivados de su uso y, en todo caso, cumplir con la normativa aplicable, incluyendo la normativa en materia de uso de Inteligencia Artificial.
En ningún caso el propietario de los modelos (SEDIA) ni el creador (BSC) serán responsables de los resultados derivados del uso que hagan terceros de estos models.
</details> |
51la5/roberta-large-NER | 51la5 | "2022-10-17T08:36:02Z" | 15,968 | 34 | transformers | [
"transformers",
"pytorch",
"rust",
"xlm-roberta",
"token-classification",
"multilingual",
"af",
"am",
"ar",
"as",
"az",
"be",
"bg",
"bn",
"br",
"bs",
"ca",
"cs",
"cy",
"da",
"de",
"el",
"en",
"eo",
"es",
"et",
"eu",
"fa",
"fi",
"fr",
"fy",
"ga",
"gd",
"gl",
"gu",
"ha",
"he",
"hi",
"hr",
"hu",
"hy",
"id",
"is",
"it",
"ja",
"jv",
"ka",
"kk",
"km",
"kn",
"ko",
"ku",
"ky",
"la",
"lo",
"lt",
"lv",
"mg",
"mk",
"ml",
"mn",
"mr",
"ms",
"my",
"ne",
"nl",
"no",
"om",
"or",
"pa",
"pl",
"ps",
"pt",
"ro",
"ru",
"sa",
"sd",
"si",
"sk",
"sl",
"so",
"sq",
"sr",
"su",
"sv",
"sw",
"ta",
"te",
"th",
"tl",
"tr",
"ug",
"uk",
"ur",
"uz",
"vi",
"xh",
"yi",
"zh",
"arxiv:1911.02116",
"arxiv:2008.03415",
"arxiv:1910.09700",
"autotrain_compatible",
"endpoints_compatible",
"region:us"
] | token-classification | "2022-10-17T08:25:02Z" | ---
language:
- multilingual
- af
- am
- ar
- as
- az
- be
- bg
- bn
- br
- bs
- ca
- cs
- cy
- da
- de
- el
- en
- eo
- es
- et
- eu
- fa
- fi
- fr
- fy
- ga
- gd
- gl
- gu
- ha
- he
- hi
- hr
- hu
- hy
- id
- is
- it
- ja
- jv
- ka
- kk
- km
- kn
- ko
- ku
- ky
- la
- lo
- lt
- lv
- mg
- mk
- ml
- mn
- mr
- ms
- my
- ne
- nl
- no
- om
- or
- pa
- pl
- ps
- pt
- ro
- ru
- sa
- sd
- si
- sk
- sl
- so
- sq
- sr
- su
- sv
- sw
- ta
- te
- th
- tl
- tr
- ug
- uk
- ur
- uz
- vi
- xh
- yi
- zh
---
# xlm-roberta-large-finetuned-conll03-english
# Table of Contents
1. [Model Details](#model-details)
2. [Uses](#uses)
3. [Bias, Risks, and Limitations](#bias-risks-and-limitations)
4. [Training](#training)
5. [Evaluation](#evaluation)
6. [Environmental Impact](#environmental-impact)
7. [Technical Specifications](#technical-specifications)
8. [Citation](#citation)
9. [Model Card Authors](#model-card-authors)
10. [How To Get Started With the Model](#how-to-get-started-with-the-model)
# Model Details
## Model Description
The XLM-RoBERTa model was proposed in [Unsupervised Cross-lingual Representation Learning at Scale](https://arxiv.org/abs/1911.02116) by Alexis Conneau, Kartikay Khandelwal, Naman Goyal, Vishrav Chaudhary, Guillaume Wenzek, Francisco Guzmán, Edouard Grave, Myle Ott, Luke Zettlemoyer and Veselin Stoyanov. It is based on Facebook's RoBERTa model released in 2019. It is a large multi-lingual language model, trained on 2.5TB of filtered CommonCrawl data. This model is [XLM-RoBERTa-large](https://huggingface.co/xlm-roberta-large) fine-tuned with the [conll2003](https://huggingface.co/datasets/conll2003) dataset in English.
- **Developed by:** See [associated paper](https://arxiv.org/abs/1911.02116)
- **Model type:** Multi-lingual language model
- **Language(s) (NLP) or Countries (images):** XLM-RoBERTa is a multilingual model trained on 100 different languages; see [GitHub Repo](https://github.com/facebookresearch/fairseq/tree/main/examples/xlmr) for full list; model is fine-tuned on a dataset in English
- **License:** More information needed
- **Related Models:** [RoBERTa](https://huggingface.co/roberta-base), [XLM](https://huggingface.co/docs/transformers/model_doc/xlm)
- **Parent Model:** [XLM-RoBERTa-large](https://huggingface.co/xlm-roberta-large)
- **Resources for more information:**
-[GitHub Repo](https://github.com/facebookresearch/fairseq/tree/main/examples/xlmr)
-[Associated Paper](https://arxiv.org/abs/1911.02116)
# Uses
## Direct Use
The model is a language model. The model can be used for token classification, a natural language understanding task in which a label is assigned to some tokens in a text.
## Downstream Use
Potential downstream use cases include Named Entity Recognition (NER) and Part-of-Speech (PoS) tagging. To learn more about token classification and other potential downstream use cases, see the Hugging Face [token classification docs](https://huggingface.co/tasks/token-classification).
## Out-of-Scope Use
The model should not be used to intentionally create hostile or alienating environments for people.
# Bias, Risks, and Limitations
**CONTENT WARNING: Readers should be made aware that language generated by this model may be disturbing or offensive to some and may propagate historical and current stereotypes.**
Significant research has explored bias and fairness issues with language models (see, e.g., [Sheng et al. (2021)](https://aclanthology.org/2021.acl-long.330.pdf) and [Bender et al. (2021)](https://dl.acm.org/doi/pdf/10.1145/3442188.3445922)). In the context of tasks relevant to this model, [Mishra et al. (2020)](https://arxiv.org/pdf/2008.03415.pdf) explore social biases in NER systems for English and find that there is systematic bias in existing NER systems in that they fail to identify named entities from different demographic groups (though this paper did not look at BERT). For example, using a sample sentence from [Mishra et al. (2020)](https://arxiv.org/pdf/2008.03415.pdf):
```python
>>> from transformers import pipeline
>>> tokenizer = AutoTokenizer.from_pretrained("xlm-roberta-large-finetuned-conll03-english")
>>> model = AutoModelForTokenClassification.from_pretrained("xlm-roberta-large-finetuned-conll03-english")
>>> classifier = pipeline("ner", model=model, tokenizer=tokenizer)
>>> classifier("Alya told Jasmine that Andrew could pay with cash..")
[{'end': 2,
'entity': 'I-PER',
'index': 1,
'score': 0.9997861,
'start': 0,
'word': '▁Al'},
{'end': 4,
'entity': 'I-PER',
'index': 2,
'score': 0.9998591,
'start': 2,
'word': 'ya'},
{'end': 16,
'entity': 'I-PER',
'index': 4,
'score': 0.99995816,
'start': 10,
'word': '▁Jasmin'},
{'end': 17,
'entity': 'I-PER',
'index': 5,
'score': 0.9999584,
'start': 16,
'word': 'e'},
{'end': 29,
'entity': 'I-PER',
'index': 7,
'score': 0.99998057,
'start': 23,
'word': '▁Andrew'}]
```
## Recommendations
Users (both direct and downstream) should be made aware of the risks, biases and limitations of the model.
# Training
See the following resources for training data and training procedure details:
- [XLM-RoBERTa-large model card](https://huggingface.co/xlm-roberta-large)
- [CoNLL-2003 data card](https://huggingface.co/datasets/conll2003)
- [Associated paper](https://arxiv.org/pdf/1911.02116.pdf)
# Evaluation
See the [associated paper](https://arxiv.org/pdf/1911.02116.pdf) for evaluation details.
# Environmental Impact
Carbon emissions can be estimated using the [Machine Learning Impact calculator](https://mlco2.github.io/impact#compute) presented in [Lacoste et al. (2019)](https://arxiv.org/abs/1910.09700).
- **Hardware Type:** 500 32GB Nvidia V100 GPUs (from the [associated paper](https://arxiv.org/pdf/1911.02116.pdf))
- **Hours used:** More information needed
- **Cloud Provider:** More information needed
- **Compute Region:** More information needed
- **Carbon Emitted:** More information needed
# Technical Specifications
See the [associated paper](https://arxiv.org/pdf/1911.02116.pdf) for further details.
# Citation
**BibTeX:**
```bibtex
@article{conneau2019unsupervised,
title={Unsupervised Cross-lingual Representation Learning at Scale},
author={Conneau, Alexis and Khandelwal, Kartikay and Goyal, Naman and Chaudhary, Vishrav and Wenzek, Guillaume and Guzm{\'a}n, Francisco and Grave, Edouard and Ott, Myle and Zettlemoyer, Luke and Stoyanov, Veselin},
journal={arXiv preprint arXiv:1911.02116},
year={2019}
}
```
**APA:**
- Conneau, A., Khandelwal, K., Goyal, N., Chaudhary, V., Wenzek, G., Guzmán, F., ... & Stoyanov, V. (2019). Unsupervised cross-lingual representation learning at scale. arXiv preprint arXiv:1911.02116.
# Model Card Authors
This model card was written by the team at Hugging Face.
# How to Get Started with the Model
Use the code below to get started with the model. You can use this model directly within a pipeline for NER.
<details>
<summary> Click to expand </summary>
```python
>>> from transformers import AutoTokenizer, AutoModelForTokenClassification
>>> from transformers import pipeline
>>> tokenizer = AutoTokenizer.from_pretrained("xlm-roberta-large-finetuned-conll03-english")
>>> model = AutoModelForTokenClassification.from_pretrained("xlm-roberta-large-finetuned-conll03-english")
>>> classifier = pipeline("ner", model=model, tokenizer=tokenizer)
>>> classifier("Hello I'm Omar and I live in Zürich.")
[{'end': 14,
'entity': 'I-PER',
'index': 5,
'score': 0.9999175,
'start': 10,
'word': '▁Omar'},
{'end': 35,
'entity': 'I-LOC',
'index': 10,
'score': 0.9999906,
'start': 29,
'word': '▁Zürich'}]
```
</details> |
thibaud/controlnet-sd21-canny-diffusers | thibaud | "2023-08-14T07:45:22Z" | 15,958 | 8 | diffusers | [
"diffusers",
"art",
"stable diffusion",
"controlnet",
"en",
"license:other",
"region:us"
] | null | "2023-03-09T08:18:19Z" | ---
license: other
language:
- en
tags:
- art
- diffusers
- stable diffusion
- controlnet
---
Here's the first version of controlnet for stablediffusion 2.1 for diffusers
Trained on a subset of laion/laion-art
License: refers to the different preprocessor's ones.
### Canny:
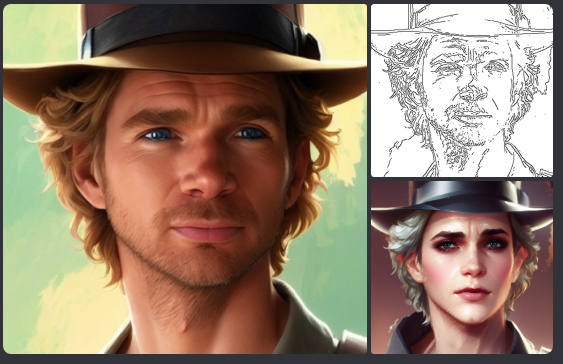
### Misuse, Malicious Use, and Out-of-Scope Use
The model should not be used to intentionally create or disseminate images that create hostile or alienating environments for people. This includes generating images that people would foreseeably find disturbing, distressing, or offensive; or content that propagates historical or current stereotypes.
Thanks
- https://huggingface.co/lllyasviel/ControlNet for the implementation and the release of 1.5 models.
- https://huggingface.co/thepowefuldeez for the conversion script to diffusers
|
RichardErkhov/Changlong1_-_ttLlama-7b-gguf | RichardErkhov | "2024-06-29T20:24:40Z" | 15,956 | 0 | null | [
"gguf",
"region:us"
] | null | "2024-06-29T14:33:54Z" | Quantization made by Richard Erkhov.
[Github](https://github.com/RichardErkhov)
[Discord](https://discord.gg/pvy7H8DZMG)
[Request more models](https://github.com/RichardErkhov/quant_request)
ttLlama-7b - GGUF
- Model creator: https://huggingface.co/Changlong1/
- Original model: https://huggingface.co/Changlong1/ttLlama-7b/
| Name | Quant method | Size |
| ---- | ---- | ---- |
| [ttLlama-7b.Q2_K.gguf](https://huggingface.co/RichardErkhov/Changlong1_-_ttLlama-7b-gguf/blob/main/ttLlama-7b.Q2_K.gguf) | Q2_K | 2.36GB |
| [ttLlama-7b.IQ3_XS.gguf](https://huggingface.co/RichardErkhov/Changlong1_-_ttLlama-7b-gguf/blob/main/ttLlama-7b.IQ3_XS.gguf) | IQ3_XS | 2.6GB |
| [ttLlama-7b.IQ3_S.gguf](https://huggingface.co/RichardErkhov/Changlong1_-_ttLlama-7b-gguf/blob/main/ttLlama-7b.IQ3_S.gguf) | IQ3_S | 2.75GB |
| [ttLlama-7b.Q3_K_S.gguf](https://huggingface.co/RichardErkhov/Changlong1_-_ttLlama-7b-gguf/blob/main/ttLlama-7b.Q3_K_S.gguf) | Q3_K_S | 2.75GB |
| [ttLlama-7b.IQ3_M.gguf](https://huggingface.co/RichardErkhov/Changlong1_-_ttLlama-7b-gguf/blob/main/ttLlama-7b.IQ3_M.gguf) | IQ3_M | 2.9GB |
| [ttLlama-7b.Q3_K.gguf](https://huggingface.co/RichardErkhov/Changlong1_-_ttLlama-7b-gguf/blob/main/ttLlama-7b.Q3_K.gguf) | Q3_K | 3.07GB |
| [ttLlama-7b.Q3_K_M.gguf](https://huggingface.co/RichardErkhov/Changlong1_-_ttLlama-7b-gguf/blob/main/ttLlama-7b.Q3_K_M.gguf) | Q3_K_M | 3.07GB |
| [ttLlama-7b.Q3_K_L.gguf](https://huggingface.co/RichardErkhov/Changlong1_-_ttLlama-7b-gguf/blob/main/ttLlama-7b.Q3_K_L.gguf) | Q3_K_L | 3.35GB |
| [ttLlama-7b.IQ4_XS.gguf](https://huggingface.co/RichardErkhov/Changlong1_-_ttLlama-7b-gguf/blob/main/ttLlama-7b.IQ4_XS.gguf) | IQ4_XS | 3.4GB |
| [ttLlama-7b.Q4_0.gguf](https://huggingface.co/RichardErkhov/Changlong1_-_ttLlama-7b-gguf/blob/main/ttLlama-7b.Q4_0.gguf) | Q4_0 | 3.56GB |
| [ttLlama-7b.IQ4_NL.gguf](https://huggingface.co/RichardErkhov/Changlong1_-_ttLlama-7b-gguf/blob/main/ttLlama-7b.IQ4_NL.gguf) | IQ4_NL | 3.58GB |
| [ttLlama-7b.Q4_K_S.gguf](https://huggingface.co/RichardErkhov/Changlong1_-_ttLlama-7b-gguf/blob/main/ttLlama-7b.Q4_K_S.gguf) | Q4_K_S | 3.59GB |
| [ttLlama-7b.Q4_K.gguf](https://huggingface.co/RichardErkhov/Changlong1_-_ttLlama-7b-gguf/blob/main/ttLlama-7b.Q4_K.gguf) | Q4_K | 3.8GB |
| [ttLlama-7b.Q4_K_M.gguf](https://huggingface.co/RichardErkhov/Changlong1_-_ttLlama-7b-gguf/blob/main/ttLlama-7b.Q4_K_M.gguf) | Q4_K_M | 3.8GB |
| [ttLlama-7b.Q4_1.gguf](https://huggingface.co/RichardErkhov/Changlong1_-_ttLlama-7b-gguf/blob/main/ttLlama-7b.Q4_1.gguf) | Q4_1 | 3.95GB |
| [ttLlama-7b.Q5_0.gguf](https://huggingface.co/RichardErkhov/Changlong1_-_ttLlama-7b-gguf/blob/main/ttLlama-7b.Q5_0.gguf) | Q5_0 | 4.33GB |
| [ttLlama-7b.Q5_K_S.gguf](https://huggingface.co/RichardErkhov/Changlong1_-_ttLlama-7b-gguf/blob/main/ttLlama-7b.Q5_K_S.gguf) | Q5_K_S | 4.33GB |
| [ttLlama-7b.Q5_K.gguf](https://huggingface.co/RichardErkhov/Changlong1_-_ttLlama-7b-gguf/blob/main/ttLlama-7b.Q5_K.gguf) | Q5_K | 4.45GB |
| [ttLlama-7b.Q5_K_M.gguf](https://huggingface.co/RichardErkhov/Changlong1_-_ttLlama-7b-gguf/blob/main/ttLlama-7b.Q5_K_M.gguf) | Q5_K_M | 4.45GB |
| [ttLlama-7b.Q5_1.gguf](https://huggingface.co/RichardErkhov/Changlong1_-_ttLlama-7b-gguf/blob/main/ttLlama-7b.Q5_1.gguf) | Q5_1 | 4.72GB |
| [ttLlama-7b.Q6_K.gguf](https://huggingface.co/RichardErkhov/Changlong1_-_ttLlama-7b-gguf/blob/main/ttLlama-7b.Q6_K.gguf) | Q6_K | 5.15GB |
| [ttLlama-7b.Q8_0.gguf](https://huggingface.co/RichardErkhov/Changlong1_-_ttLlama-7b-gguf/blob/main/ttLlama-7b.Q8_0.gguf) | Q8_0 | 6.67GB |
Original model description:
---
license: llama2
---
This is a codellama/CodeLlama-7b-hf model fine-tuned using QLoRA (4-bit precision) on the mlabonne/Evol-Instruct-Python-1k.
It was trained on an RTX 3090 in 1h 11m 44s with the configuration file.
## Code Llama
Code Llama is a collection of pretrained and fine-tuned generative text models ranging in scale from 7 billion to 34 billion parameters. This is the repository for the base 7B version in the Hugging Face Transformers format. This model is designed for general code synthesis and understanding. Links to other models can be found in the index at the bottom.
|
RichardErkhov/InnerI_-_A-I-0xtom-7B-slerp-gguf | RichardErkhov | "2024-06-20T21:34:50Z" | 15,948 | 0 | null | [
"gguf",
"region:us"
] | null | "2024-06-20T13:41:11Z" | Quantization made by Richard Erkhov.
[Github](https://github.com/RichardErkhov)
[Discord](https://discord.gg/pvy7H8DZMG)
[Request more models](https://github.com/RichardErkhov/quant_request)
A-I-0xtom-7B-slerp - GGUF
- Model creator: https://huggingface.co/InnerI/
- Original model: https://huggingface.co/InnerI/A-I-0xtom-7B-slerp/
| Name | Quant method | Size |
| ---- | ---- | ---- |
| [A-I-0xtom-7B-slerp.Q2_K.gguf](https://huggingface.co/RichardErkhov/InnerI_-_A-I-0xtom-7B-slerp-gguf/blob/main/A-I-0xtom-7B-slerp.Q2_K.gguf) | Q2_K | 2.53GB |
| [A-I-0xtom-7B-slerp.IQ3_XS.gguf](https://huggingface.co/RichardErkhov/InnerI_-_A-I-0xtom-7B-slerp-gguf/blob/main/A-I-0xtom-7B-slerp.IQ3_XS.gguf) | IQ3_XS | 2.81GB |
| [A-I-0xtom-7B-slerp.IQ3_S.gguf](https://huggingface.co/RichardErkhov/InnerI_-_A-I-0xtom-7B-slerp-gguf/blob/main/A-I-0xtom-7B-slerp.IQ3_S.gguf) | IQ3_S | 2.96GB |
| [A-I-0xtom-7B-slerp.Q3_K_S.gguf](https://huggingface.co/RichardErkhov/InnerI_-_A-I-0xtom-7B-slerp-gguf/blob/main/A-I-0xtom-7B-slerp.Q3_K_S.gguf) | Q3_K_S | 2.95GB |
| [A-I-0xtom-7B-slerp.IQ3_M.gguf](https://huggingface.co/RichardErkhov/InnerI_-_A-I-0xtom-7B-slerp-gguf/blob/main/A-I-0xtom-7B-slerp.IQ3_M.gguf) | IQ3_M | 3.06GB |
| [A-I-0xtom-7B-slerp.Q3_K.gguf](https://huggingface.co/RichardErkhov/InnerI_-_A-I-0xtom-7B-slerp-gguf/blob/main/A-I-0xtom-7B-slerp.Q3_K.gguf) | Q3_K | 3.28GB |
| [A-I-0xtom-7B-slerp.Q3_K_M.gguf](https://huggingface.co/RichardErkhov/InnerI_-_A-I-0xtom-7B-slerp-gguf/blob/main/A-I-0xtom-7B-slerp.Q3_K_M.gguf) | Q3_K_M | 3.28GB |
| [A-I-0xtom-7B-slerp.Q3_K_L.gguf](https://huggingface.co/RichardErkhov/InnerI_-_A-I-0xtom-7B-slerp-gguf/blob/main/A-I-0xtom-7B-slerp.Q3_K_L.gguf) | Q3_K_L | 3.56GB |
| [A-I-0xtom-7B-slerp.IQ4_XS.gguf](https://huggingface.co/RichardErkhov/InnerI_-_A-I-0xtom-7B-slerp-gguf/blob/main/A-I-0xtom-7B-slerp.IQ4_XS.gguf) | IQ4_XS | 3.67GB |
| [A-I-0xtom-7B-slerp.Q4_0.gguf](https://huggingface.co/RichardErkhov/InnerI_-_A-I-0xtom-7B-slerp-gguf/blob/main/A-I-0xtom-7B-slerp.Q4_0.gguf) | Q4_0 | 3.83GB |
| [A-I-0xtom-7B-slerp.IQ4_NL.gguf](https://huggingface.co/RichardErkhov/InnerI_-_A-I-0xtom-7B-slerp-gguf/blob/main/A-I-0xtom-7B-slerp.IQ4_NL.gguf) | IQ4_NL | 3.87GB |
| [A-I-0xtom-7B-slerp.Q4_K_S.gguf](https://huggingface.co/RichardErkhov/InnerI_-_A-I-0xtom-7B-slerp-gguf/blob/main/A-I-0xtom-7B-slerp.Q4_K_S.gguf) | Q4_K_S | 3.86GB |
| [A-I-0xtom-7B-slerp.Q4_K.gguf](https://huggingface.co/RichardErkhov/InnerI_-_A-I-0xtom-7B-slerp-gguf/blob/main/A-I-0xtom-7B-slerp.Q4_K.gguf) | Q4_K | 4.07GB |
| [A-I-0xtom-7B-slerp.Q4_K_M.gguf](https://huggingface.co/RichardErkhov/InnerI_-_A-I-0xtom-7B-slerp-gguf/blob/main/A-I-0xtom-7B-slerp.Q4_K_M.gguf) | Q4_K_M | 4.07GB |
| [A-I-0xtom-7B-slerp.Q4_1.gguf](https://huggingface.co/RichardErkhov/InnerI_-_A-I-0xtom-7B-slerp-gguf/blob/main/A-I-0xtom-7B-slerp.Q4_1.gguf) | Q4_1 | 4.24GB |
| [A-I-0xtom-7B-slerp.Q5_0.gguf](https://huggingface.co/RichardErkhov/InnerI_-_A-I-0xtom-7B-slerp-gguf/blob/main/A-I-0xtom-7B-slerp.Q5_0.gguf) | Q5_0 | 4.65GB |
| [A-I-0xtom-7B-slerp.Q5_K_S.gguf](https://huggingface.co/RichardErkhov/InnerI_-_A-I-0xtom-7B-slerp-gguf/blob/main/A-I-0xtom-7B-slerp.Q5_K_S.gguf) | Q5_K_S | 4.65GB |
| [A-I-0xtom-7B-slerp.Q5_K.gguf](https://huggingface.co/RichardErkhov/InnerI_-_A-I-0xtom-7B-slerp-gguf/blob/main/A-I-0xtom-7B-slerp.Q5_K.gguf) | Q5_K | 4.78GB |
| [A-I-0xtom-7B-slerp.Q5_K_M.gguf](https://huggingface.co/RichardErkhov/InnerI_-_A-I-0xtom-7B-slerp-gguf/blob/main/A-I-0xtom-7B-slerp.Q5_K_M.gguf) | Q5_K_M | 4.78GB |
| [A-I-0xtom-7B-slerp.Q5_1.gguf](https://huggingface.co/RichardErkhov/InnerI_-_A-I-0xtom-7B-slerp-gguf/blob/main/A-I-0xtom-7B-slerp.Q5_1.gguf) | Q5_1 | 5.07GB |
| [A-I-0xtom-7B-slerp.Q6_K.gguf](https://huggingface.co/RichardErkhov/InnerI_-_A-I-0xtom-7B-slerp-gguf/blob/main/A-I-0xtom-7B-slerp.Q6_K.gguf) | Q6_K | 5.53GB |
| [A-I-0xtom-7B-slerp.Q8_0.gguf](https://huggingface.co/RichardErkhov/InnerI_-_A-I-0xtom-7B-slerp-gguf/blob/main/A-I-0xtom-7B-slerp.Q8_0.gguf) | Q8_0 | 7.17GB |
Original model description:
---
license: apache-2.0
tags:
- merge
- mergekit
- lazymergekit
- 0x0dad0/nous_nous_v2_0
- tomaszki/nous-thirty
base_model:
- 0x0dad0/nous_nous_v2_0
- tomaszki/nous-thirty
model-index:
- name: A-I-0xtom-7B-slerp
results:
- task:
type: text-generation
name: Text Generation
dataset:
name: AI2 Reasoning Challenge (25-Shot)
type: ai2_arc
config: ARC-Challenge
split: test
args:
num_few_shot: 25
metrics:
- type: acc_norm
value: 58.19
name: normalized accuracy
source:
url: https://huggingface.co/spaces/HuggingFaceH4/open_llm_leaderboard?query=InnerI/A-I-0xtom-7B-slerp
name: Open LLM Leaderboard
- task:
type: text-generation
name: Text Generation
dataset:
name: HellaSwag (10-Shot)
type: hellaswag
split: validation
args:
num_few_shot: 10
metrics:
- type: acc_norm
value: 77.64
name: normalized accuracy
source:
url: https://huggingface.co/spaces/HuggingFaceH4/open_llm_leaderboard?query=InnerI/A-I-0xtom-7B-slerp
name: Open LLM Leaderboard
- task:
type: text-generation
name: Text Generation
dataset:
name: MMLU (5-Shot)
type: cais/mmlu
config: all
split: test
args:
num_few_shot: 5
metrics:
- type: acc
value: 58.74
name: accuracy
source:
url: https://huggingface.co/spaces/HuggingFaceH4/open_llm_leaderboard?query=InnerI/A-I-0xtom-7B-slerp
name: Open LLM Leaderboard
- task:
type: text-generation
name: Text Generation
dataset:
name: TruthfulQA (0-shot)
type: truthful_qa
config: multiple_choice
split: validation
args:
num_few_shot: 0
metrics:
- type: mc2
value: 54.78
source:
url: https://huggingface.co/spaces/HuggingFaceH4/open_llm_leaderboard?query=InnerI/A-I-0xtom-7B-slerp
name: Open LLM Leaderboard
- task:
type: text-generation
name: Text Generation
dataset:
name: Winogrande (5-shot)
type: winogrande
config: winogrande_xl
split: validation
args:
num_few_shot: 5
metrics:
- type: acc
value: 73.24
name: accuracy
source:
url: https://huggingface.co/spaces/HuggingFaceH4/open_llm_leaderboard?query=InnerI/A-I-0xtom-7B-slerp
name: Open LLM Leaderboard
- task:
type: text-generation
name: Text Generation
dataset:
name: GSM8k (5-shot)
type: gsm8k
config: main
split: test
args:
num_few_shot: 5
metrics:
- type: acc
value: 40.18
name: accuracy
source:
url: https://huggingface.co/spaces/HuggingFaceH4/open_llm_leaderboard?query=InnerI/A-I-0xtom-7B-slerp
name: Open LLM Leaderboard
---
# A-I-0xtom-7B-slerp
A-I-0xtom-7B-slerp is a merge of the following models using [LazyMergekit](https://colab.research.google.com/drive/1obulZ1ROXHjYLn6PPZJwRR6GzgQogxxb?usp=sharing):
* [0x0dad0/nous_nous_v2_0](https://huggingface.co/0x0dad0/nous_nous_v2_0)
* [tomaszki/nous-thirty](https://huggingface.co/tomaszki/nous-thirty)
# Avg model loss 0.3912096044793725
I used this testing script that loads your local model, pulls the latest data from cortex and calculates the loss:
[avg loss script](https://gist.github.com/romanorac/59ccde7cbf07d8950ef9fb5b5db6a24e)
## 🧩 Configuration
```yaml
slices:
- sources:
- model: 0x0dad0/nous_nous_v2_0
layer_range: [0, 32]
- model: tomaszki/nous-thirty
layer_range: [0, 32]
merge_method: slerp
base_model: 0x0dad0/nous_nous_v2_0
parameters:
t:
- filter: self_attn
value: [0, 0.5, 0.3, 0.7, 1]
- filter: mlp
value: [1, 0.5, 0.7, 0.3, 0]
- value: 0.5
dtype: bfloat16
```
## 💻 Usage
```python
!pip install -qU transformers accelerate
from transformers import AutoTokenizer
import transformers
import torch
model = "InnerI/A-I-0xtom-7B-slerp"
messages = [{"role": "user", "content": "What is a large language model?"}]
tokenizer = AutoTokenizer.from_pretrained(model)
prompt = tokenizer.apply_chat_template(messages, tokenize=False, add_generation_prompt=True)
pipeline = transformers.pipeline(
"text-generation",
model=model,
torch_dtype=torch.float16,
device_map="auto",
)
outputs = pipeline(prompt, max_new_tokens=256, do_sample=True, temperature=0.7, top_k=50, top_p=0.95)
print(outputs[0]["generated_text"])
```
# [Open LLM Leaderboard Evaluation Results](https://huggingface.co/spaces/HuggingFaceH4/open_llm_leaderboard)
Detailed results can be found [here](https://huggingface.co/datasets/open-llm-leaderboard/details_InnerI__A-I-0xtom-7B-slerp)
| Metric |Value|
|---------------------------------|----:|
|Avg. |60.46|
|AI2 Reasoning Challenge (25-Shot)|58.19|
|HellaSwag (10-Shot) |77.64|
|MMLU (5-Shot) |58.74|
|TruthfulQA (0-shot) |54.78|
|Winogrande (5-shot) |73.24|
|GSM8k (5-shot) |40.18|
|
mradermacher/Llama-3-Unholy-8B-i1-GGUF | mradermacher | "2024-06-28T07:46:59Z" | 15,927 | 0 | transformers | [
"transformers",
"gguf",
"not-for-all-audiences",
"nsfw",
"en",
"base_model:Undi95/Llama-3-Unholy-8B",
"license:cc-by-nc-4.0",
"endpoints_compatible",
"region:us"
] | null | "2024-06-28T03:03:38Z" | ---
base_model: Undi95/Llama-3-Unholy-8B
language:
- en
library_name: transformers
license: cc-by-nc-4.0
quantized_by: mradermacher
tags:
- not-for-all-audiences
- nsfw
---
## About
<!-- ### quantize_version: 2 -->
<!-- ### output_tensor_quantised: 1 -->
<!-- ### convert_type: hf -->
<!-- ### vocab_type: -->
<!-- ### tags: nicoboss -->
weighted/imatrix quants of https://huggingface.co/Undi95/Llama-3-Unholy-8B
<!-- provided-files -->
static quants are available at https://huggingface.co/mradermacher/Llama-3-Unholy-8B-GGUF
## Usage
If you are unsure how to use GGUF files, refer to one of [TheBloke's
READMEs](https://huggingface.co/TheBloke/KafkaLM-70B-German-V0.1-GGUF) for
more details, including on how to concatenate multi-part files.
## Provided Quants
(sorted by size, not necessarily quality. IQ-quants are often preferable over similar sized non-IQ quants)
| Link | Type | Size/GB | Notes |
|:-----|:-----|--------:|:------|
| [GGUF](https://huggingface.co/mradermacher/Llama-3-Unholy-8B-i1-GGUF/resolve/main/Llama-3-Unholy-8B.i1-IQ1_S.gguf) | i1-IQ1_S | 2.1 | for the desperate |
| [GGUF](https://huggingface.co/mradermacher/Llama-3-Unholy-8B-i1-GGUF/resolve/main/Llama-3-Unholy-8B.i1-IQ1_M.gguf) | i1-IQ1_M | 2.3 | mostly desperate |
| [GGUF](https://huggingface.co/mradermacher/Llama-3-Unholy-8B-i1-GGUF/resolve/main/Llama-3-Unholy-8B.i1-IQ2_XXS.gguf) | i1-IQ2_XXS | 2.5 | |
| [GGUF](https://huggingface.co/mradermacher/Llama-3-Unholy-8B-i1-GGUF/resolve/main/Llama-3-Unholy-8B.i1-IQ2_XS.gguf) | i1-IQ2_XS | 2.7 | |
| [GGUF](https://huggingface.co/mradermacher/Llama-3-Unholy-8B-i1-GGUF/resolve/main/Llama-3-Unholy-8B.i1-IQ2_S.gguf) | i1-IQ2_S | 2.9 | |
| [GGUF](https://huggingface.co/mradermacher/Llama-3-Unholy-8B-i1-GGUF/resolve/main/Llama-3-Unholy-8B.i1-IQ2_M.gguf) | i1-IQ2_M | 3.0 | |
| [GGUF](https://huggingface.co/mradermacher/Llama-3-Unholy-8B-i1-GGUF/resolve/main/Llama-3-Unholy-8B.i1-Q2_K.gguf) | i1-Q2_K | 3.3 | IQ3_XXS probably better |
| [GGUF](https://huggingface.co/mradermacher/Llama-3-Unholy-8B-i1-GGUF/resolve/main/Llama-3-Unholy-8B.i1-IQ3_XXS.gguf) | i1-IQ3_XXS | 3.4 | lower quality |
| [GGUF](https://huggingface.co/mradermacher/Llama-3-Unholy-8B-i1-GGUF/resolve/main/Llama-3-Unholy-8B.i1-IQ3_XS.gguf) | i1-IQ3_XS | 3.6 | |
| [GGUF](https://huggingface.co/mradermacher/Llama-3-Unholy-8B-i1-GGUF/resolve/main/Llama-3-Unholy-8B.i1-Q3_K_S.gguf) | i1-Q3_K_S | 3.8 | IQ3_XS probably better |
| [GGUF](https://huggingface.co/mradermacher/Llama-3-Unholy-8B-i1-GGUF/resolve/main/Llama-3-Unholy-8B.i1-IQ3_S.gguf) | i1-IQ3_S | 3.8 | beats Q3_K* |
| [GGUF](https://huggingface.co/mradermacher/Llama-3-Unholy-8B-i1-GGUF/resolve/main/Llama-3-Unholy-8B.i1-IQ3_M.gguf) | i1-IQ3_M | 3.9 | |
| [GGUF](https://huggingface.co/mradermacher/Llama-3-Unholy-8B-i1-GGUF/resolve/main/Llama-3-Unholy-8B.i1-Q3_K_M.gguf) | i1-Q3_K_M | 4.1 | IQ3_S probably better |
| [GGUF](https://huggingface.co/mradermacher/Llama-3-Unholy-8B-i1-GGUF/resolve/main/Llama-3-Unholy-8B.i1-Q3_K_L.gguf) | i1-Q3_K_L | 4.4 | IQ3_M probably better |
| [GGUF](https://huggingface.co/mradermacher/Llama-3-Unholy-8B-i1-GGUF/resolve/main/Llama-3-Unholy-8B.i1-IQ4_XS.gguf) | i1-IQ4_XS | 4.5 | |
| [GGUF](https://huggingface.co/mradermacher/Llama-3-Unholy-8B-i1-GGUF/resolve/main/Llama-3-Unholy-8B.i1-Q4_0.gguf) | i1-Q4_0 | 4.8 | fast, low quality |
| [GGUF](https://huggingface.co/mradermacher/Llama-3-Unholy-8B-i1-GGUF/resolve/main/Llama-3-Unholy-8B.i1-Q4_K_S.gguf) | i1-Q4_K_S | 4.8 | optimal size/speed/quality |
| [GGUF](https://huggingface.co/mradermacher/Llama-3-Unholy-8B-i1-GGUF/resolve/main/Llama-3-Unholy-8B.i1-Q4_K_M.gguf) | i1-Q4_K_M | 5.0 | fast, recommended |
| [GGUF](https://huggingface.co/mradermacher/Llama-3-Unholy-8B-i1-GGUF/resolve/main/Llama-3-Unholy-8B.i1-Q5_K_S.gguf) | i1-Q5_K_S | 5.7 | |
| [GGUF](https://huggingface.co/mradermacher/Llama-3-Unholy-8B-i1-GGUF/resolve/main/Llama-3-Unholy-8B.i1-Q5_K_M.gguf) | i1-Q5_K_M | 5.8 | |
| [GGUF](https://huggingface.co/mradermacher/Llama-3-Unholy-8B-i1-GGUF/resolve/main/Llama-3-Unholy-8B.i1-Q6_K.gguf) | i1-Q6_K | 6.7 | practically like static Q6_K |
Here is a handy graph by ikawrakow comparing some lower-quality quant
types (lower is better):

And here are Artefact2's thoughts on the matter:
https://gist.github.com/Artefact2/b5f810600771265fc1e39442288e8ec9
## FAQ / Model Request
See https://huggingface.co/mradermacher/model_requests for some answers to
questions you might have and/or if you want some other model quantized.
## Thanks
I thank my company, [nethype GmbH](https://www.nethype.de/), for letting
me use its servers and providing upgrades to my workstation to enable
this work in my free time. Additional thanks to [@nicoboss](https://huggingface.co/nicoboss) for giving me access to his hardware for calculating the imatrix for these quants.
<!-- end -->
|
RichardErkhov/lighteternal_-_Llama3-merge-biomed-8b-gguf | RichardErkhov | "2024-06-26T03:49:25Z" | 15,925 | 0 | null | [
"gguf",
"arxiv:2311.03099",
"arxiv:2306.01708",
"region:us"
] | null | "2024-06-25T23:31:10Z" | Quantization made by Richard Erkhov.
[Github](https://github.com/RichardErkhov)
[Discord](https://discord.gg/pvy7H8DZMG)
[Request more models](https://github.com/RichardErkhov/quant_request)
Llama3-merge-biomed-8b - GGUF
- Model creator: https://huggingface.co/lighteternal/
- Original model: https://huggingface.co/lighteternal/Llama3-merge-biomed-8b/
| Name | Quant method | Size |
| ---- | ---- | ---- |
| [Llama3-merge-biomed-8b.Q2_K.gguf](https://huggingface.co/RichardErkhov/lighteternal_-_Llama3-merge-biomed-8b-gguf/blob/main/Llama3-merge-biomed-8b.Q2_K.gguf) | Q2_K | 2.96GB |
| [Llama3-merge-biomed-8b.IQ3_XS.gguf](https://huggingface.co/RichardErkhov/lighteternal_-_Llama3-merge-biomed-8b-gguf/blob/main/Llama3-merge-biomed-8b.IQ3_XS.gguf) | IQ3_XS | 3.28GB |
| [Llama3-merge-biomed-8b.IQ3_S.gguf](https://huggingface.co/RichardErkhov/lighteternal_-_Llama3-merge-biomed-8b-gguf/blob/main/Llama3-merge-biomed-8b.IQ3_S.gguf) | IQ3_S | 3.43GB |
| [Llama3-merge-biomed-8b.Q3_K_S.gguf](https://huggingface.co/RichardErkhov/lighteternal_-_Llama3-merge-biomed-8b-gguf/blob/main/Llama3-merge-biomed-8b.Q3_K_S.gguf) | Q3_K_S | 3.41GB |
| [Llama3-merge-biomed-8b.IQ3_M.gguf](https://huggingface.co/RichardErkhov/lighteternal_-_Llama3-merge-biomed-8b-gguf/blob/main/Llama3-merge-biomed-8b.IQ3_M.gguf) | IQ3_M | 3.52GB |
| [Llama3-merge-biomed-8b.Q3_K.gguf](https://huggingface.co/RichardErkhov/lighteternal_-_Llama3-merge-biomed-8b-gguf/blob/main/Llama3-merge-biomed-8b.Q3_K.gguf) | Q3_K | 3.74GB |
| [Llama3-merge-biomed-8b.Q3_K_M.gguf](https://huggingface.co/RichardErkhov/lighteternal_-_Llama3-merge-biomed-8b-gguf/blob/main/Llama3-merge-biomed-8b.Q3_K_M.gguf) | Q3_K_M | 3.74GB |
| [Llama3-merge-biomed-8b.Q3_K_L.gguf](https://huggingface.co/RichardErkhov/lighteternal_-_Llama3-merge-biomed-8b-gguf/blob/main/Llama3-merge-biomed-8b.Q3_K_L.gguf) | Q3_K_L | 4.03GB |
| [Llama3-merge-biomed-8b.IQ4_XS.gguf](https://huggingface.co/RichardErkhov/lighteternal_-_Llama3-merge-biomed-8b-gguf/blob/main/Llama3-merge-biomed-8b.IQ4_XS.gguf) | IQ4_XS | 4.18GB |
| [Llama3-merge-biomed-8b.Q4_0.gguf](https://huggingface.co/RichardErkhov/lighteternal_-_Llama3-merge-biomed-8b-gguf/blob/main/Llama3-merge-biomed-8b.Q4_0.gguf) | Q4_0 | 4.34GB |
| [Llama3-merge-biomed-8b.IQ4_NL.gguf](https://huggingface.co/RichardErkhov/lighteternal_-_Llama3-merge-biomed-8b-gguf/blob/main/Llama3-merge-biomed-8b.IQ4_NL.gguf) | IQ4_NL | 4.38GB |
| [Llama3-merge-biomed-8b.Q4_K_S.gguf](https://huggingface.co/RichardErkhov/lighteternal_-_Llama3-merge-biomed-8b-gguf/blob/main/Llama3-merge-biomed-8b.Q4_K_S.gguf) | Q4_K_S | 4.37GB |
| [Llama3-merge-biomed-8b.Q4_K.gguf](https://huggingface.co/RichardErkhov/lighteternal_-_Llama3-merge-biomed-8b-gguf/blob/main/Llama3-merge-biomed-8b.Q4_K.gguf) | Q4_K | 4.58GB |
| [Llama3-merge-biomed-8b.Q4_K_M.gguf](https://huggingface.co/RichardErkhov/lighteternal_-_Llama3-merge-biomed-8b-gguf/blob/main/Llama3-merge-biomed-8b.Q4_K_M.gguf) | Q4_K_M | 4.58GB |
| [Llama3-merge-biomed-8b.Q4_1.gguf](https://huggingface.co/RichardErkhov/lighteternal_-_Llama3-merge-biomed-8b-gguf/blob/main/Llama3-merge-biomed-8b.Q4_1.gguf) | Q4_1 | 4.78GB |
| [Llama3-merge-biomed-8b.Q5_0.gguf](https://huggingface.co/RichardErkhov/lighteternal_-_Llama3-merge-biomed-8b-gguf/blob/main/Llama3-merge-biomed-8b.Q5_0.gguf) | Q5_0 | 5.21GB |
| [Llama3-merge-biomed-8b.Q5_K_S.gguf](https://huggingface.co/RichardErkhov/lighteternal_-_Llama3-merge-biomed-8b-gguf/blob/main/Llama3-merge-biomed-8b.Q5_K_S.gguf) | Q5_K_S | 5.21GB |
| [Llama3-merge-biomed-8b.Q5_K.gguf](https://huggingface.co/RichardErkhov/lighteternal_-_Llama3-merge-biomed-8b-gguf/blob/main/Llama3-merge-biomed-8b.Q5_K.gguf) | Q5_K | 5.34GB |
| [Llama3-merge-biomed-8b.Q5_K_M.gguf](https://huggingface.co/RichardErkhov/lighteternal_-_Llama3-merge-biomed-8b-gguf/blob/main/Llama3-merge-biomed-8b.Q5_K_M.gguf) | Q5_K_M | 5.34GB |
| [Llama3-merge-biomed-8b.Q5_1.gguf](https://huggingface.co/RichardErkhov/lighteternal_-_Llama3-merge-biomed-8b-gguf/blob/main/Llama3-merge-biomed-8b.Q5_1.gguf) | Q5_1 | 5.65GB |
| [Llama3-merge-biomed-8b.Q6_K.gguf](https://huggingface.co/RichardErkhov/lighteternal_-_Llama3-merge-biomed-8b-gguf/blob/main/Llama3-merge-biomed-8b.Q6_K.gguf) | Q6_K | 6.14GB |
| [Llama3-merge-biomed-8b.Q8_0.gguf](https://huggingface.co/RichardErkhov/lighteternal_-_Llama3-merge-biomed-8b-gguf/blob/main/Llama3-merge-biomed-8b.Q8_0.gguf) | Q8_0 | 7.95GB |
Original model description:
---
base_model:
- meta-llama/Meta-Llama-3-8B-Instruct
- NousResearch/Hermes-2-Pro-Llama-3-8B
- aaditya/Llama3-OpenBioLLM-8B
library_name: transformers
tags:
- mergekit
- merge
license: llama3
---
# Llama3-merge-biomed-8b
This is a DARE-TIES Merge of Llama3-8b-Instruct + NousResearch/Hermes-2-Pro-Llama-3-8B + aaditya/Llama3-OpenBioLLM-8B.
It is a simple experiment to assess whether combining models with strengths in general language understanding and biomedical knowledge can enhance performance on specialized tasks without compromising general applicability.
The results indicate promising outcomes in areas like HendrycksTest tasks related to Biology and Medicine, as well as improvements in complex reasoning as seen in the ARC Challenge and Winogrande benchmarks.
## Usage
I recommend using the prompt template of Llama3: https://llama.meta.com/docs/model-cards-and-prompt-formats/meta-llama-3/
## Leaderboard metrics according to 🤗 Open LLM Leaderboard
| Task | Metric | Ours (%) | Llama38BInstr. (%) |OpenBioLLM8B (%) |
|--------------------------------------|--------------------------|------------------|------------|-------------|
| **ARC Challenge** | Accuracy | **59.39** | 57.17 | 55.38 |
| | Normalized Accuracy | **63.65** | 60.75 | 58.62 |
| **Hellaswag** | Accuracy | **62.59** | 59.04 | 61.83 |
| | Normalized Accuracy | **81.53** | 78.55 | 80.76 |
| **Winogrande** | Accuracy | **75.93** | 74.51 | 70.88 |
| **GSM8K** | Accuracy | 59.36 | **68.69** | 10.15 |
| **HendrycksTest-Anatomy** | Accuracy | **72.59** | 65.19 | 69.62 |
| **HendrycksTest-Clinical Knowledge** | Accuracy | **77.83** | 74.72 | 60.38 |
| **HendrycksTest-College Biology** | Accuracy | **81.94** | 79.86 | 79.86 |
| **HendrycksTest-College Medicine** | Accuracy | 69.36 | 63.58 | **70.52** |
| **HendrycksTest-Medical Genetics** | Accuracy | **86.00** | 80.00 | 80.00 |
| **HendrycksTest-Professional Medicine** | Accuracy | **77.94** | 71.69 | 77.94 |
This is a merge of pre-trained language models created using [mergekit](https://github.com/cg123/mergekit).
## Merge Details
### Merge Method
This model was merged using the [DARE](https://arxiv.org/abs/2311.03099) [TIES](https://arxiv.org/abs/2306.01708) merge method using [meta-llama/Meta-Llama-3-8B-Instruct](https://huggingface.co/meta-llama/Meta-Llama-3-8B-Instruct) as a base.
### Models Merged
The following models were included in the merge:
* [NousResearch/Hermes-2-Pro-Llama-3-8B](https://huggingface.co/NousResearch/Hermes-2-Pro-Llama-3-8B)
* [aaditya/Llama3-OpenBioLLM-8B](https://huggingface.co/aaditya/Llama3-OpenBioLLM-8B)
### Configuration
The following YAML configuration was used to produce this model:
```yaml
models:
- model: meta-llama/Meta-Llama-3-8B-Instruct
# Base model providing a general foundation without specific parameters
- model: meta-llama/Meta-Llama-3-8B-Instruct
parameters:
density: 0.60
weight: 0.5
- model: NousResearch/Hermes-2-Pro-Llama-3-8B
parameters:
density: 0.55
weight: 0.1
- model: aaditya/Llama3-OpenBioLLM-8B
parameters:
density: 0.55
weight: 0.4
merge_method: dare_ties
base_model: meta-llama/Meta-Llama-3-8B-Instruct
parameters:
int8_mask: true
dtype: bfloat16
```
|
timm/maxvit_nano_rw_256.sw_in1k | timm | "2023-05-11T00:14:58Z" | 15,913 | 0 | timm | [
"timm",
"pytorch",
"safetensors",
"image-classification",
"dataset:imagenet-1k",
"arxiv:2204.01697",
"license:apache-2.0",
"region:us"
] | image-classification | "2023-01-20T21:31:48Z" | ---
tags:
- image-classification
- timm
library_name: timm
license: apache-2.0
datasets:
- imagenet-1k
---
# Model card for maxvit_nano_rw_256.sw_in1k
A timm specific MaxViT image classification model. Trained in `timm` on ImageNet-1k by Ross Wightman.
ImageNet-1k training done on TPUs thanks to support of the [TRC](https://sites.research.google/trc/about/) program.
### Model Variants in [maxxvit.py](https://github.com/huggingface/pytorch-image-models/blob/main/timm/models/maxxvit.py)
MaxxViT covers a number of related model architectures that share a common structure including:
- CoAtNet - Combining MBConv (depthwise-separable) convolutional blocks in early stages with self-attention transformer blocks in later stages.
- MaxViT - Uniform blocks across all stages, each containing a MBConv (depthwise-separable) convolution block followed by two self-attention blocks with different partitioning schemes (window followed by grid).
- CoAtNeXt - A timm specific arch that uses ConvNeXt blocks in place of MBConv blocks in CoAtNet. All normalization layers are LayerNorm (no BatchNorm).
- MaxxViT - A timm specific arch that uses ConvNeXt blocks in place of MBConv blocks in MaxViT. All normalization layers are LayerNorm (no BatchNorm).
- MaxxViT-V2 - A MaxxViT variation that removes the window block attention leaving only ConvNeXt blocks and grid attention w/ more width to compensate.
Aside from the major variants listed above, there are more subtle changes from model to model. Any model name with the string `rw` are `timm` specific configs w/ modelling adjustments made to favour PyTorch eager use. These were created while training initial reproductions of the models so there are variations.
All models with the string `tf` are models exactly matching Tensorflow based models by the original paper authors with weights ported to PyTorch. This covers a number of MaxViT models. The official CoAtNet models were never released.
## Model Details
- **Model Type:** Image classification / feature backbone
- **Model Stats:**
- Params (M): 15.5
- GMACs: 4.5
- Activations (M): 30.3
- Image size: 256 x 256
- **Papers:**
- MaxViT: Multi-Axis Vision Transformer: https://arxiv.org/abs/2204.01697
- **Dataset:** ImageNet-1k
## Model Usage
### Image Classification
```python
from urllib.request import urlopen
from PIL import Image
import timm
img = Image.open(urlopen(
'https://huggingface.co/datasets/huggingface/documentation-images/resolve/main/beignets-task-guide.png'
))
model = timm.create_model('maxvit_nano_rw_256.sw_in1k', pretrained=True)
model = model.eval()
# get model specific transforms (normalization, resize)
data_config = timm.data.resolve_model_data_config(model)
transforms = timm.data.create_transform(**data_config, is_training=False)
output = model(transforms(img).unsqueeze(0)) # unsqueeze single image into batch of 1
top5_probabilities, top5_class_indices = torch.topk(output.softmax(dim=1) * 100, k=5)
```
### Feature Map Extraction
```python
from urllib.request import urlopen
from PIL import Image
import timm
img = Image.open(urlopen(
'https://huggingface.co/datasets/huggingface/documentation-images/resolve/main/beignets-task-guide.png'
))
model = timm.create_model(
'maxvit_nano_rw_256.sw_in1k',
pretrained=True,
features_only=True,
)
model = model.eval()
# get model specific transforms (normalization, resize)
data_config = timm.data.resolve_model_data_config(model)
transforms = timm.data.create_transform(**data_config, is_training=False)
output = model(transforms(img).unsqueeze(0)) # unsqueeze single image into batch of 1
for o in output:
# print shape of each feature map in output
# e.g.:
# torch.Size([1, 64, 128, 128])
# torch.Size([1, 64, 64, 64])
# torch.Size([1, 128, 32, 32])
# torch.Size([1, 256, 16, 16])
# torch.Size([1, 512, 8, 8])
print(o.shape)
```
### Image Embeddings
```python
from urllib.request import urlopen
from PIL import Image
import timm
img = Image.open(urlopen(
'https://huggingface.co/datasets/huggingface/documentation-images/resolve/main/beignets-task-guide.png'
))
model = timm.create_model(
'maxvit_nano_rw_256.sw_in1k',
pretrained=True,
num_classes=0, # remove classifier nn.Linear
)
model = model.eval()
# get model specific transforms (normalization, resize)
data_config = timm.data.resolve_model_data_config(model)
transforms = timm.data.create_transform(**data_config, is_training=False)
output = model(transforms(img).unsqueeze(0)) # output is (batch_size, num_features) shaped tensor
# or equivalently (without needing to set num_classes=0)
output = model.forward_features(transforms(img).unsqueeze(0))
# output is unpooled, a (1, 512, 8, 8) shaped tensor
output = model.forward_head(output, pre_logits=True)
# output is a (1, num_features) shaped tensor
```
## Model Comparison
### By Top-1
|model |top1 |top5 |samples / sec |Params (M) |GMAC |Act (M)|
|------------------------------------------------------------------------------------------------------------------------|----:|----:|--------------:|--------------:|-----:|------:|
|[maxvit_xlarge_tf_512.in21k_ft_in1k](https://huggingface.co/timm/maxvit_xlarge_tf_512.in21k_ft_in1k) |88.53|98.64| 21.76| 475.77|534.14|1413.22|
|[maxvit_xlarge_tf_384.in21k_ft_in1k](https://huggingface.co/timm/maxvit_xlarge_tf_384.in21k_ft_in1k) |88.32|98.54| 42.53| 475.32|292.78| 668.76|
|[maxvit_base_tf_512.in21k_ft_in1k](https://huggingface.co/timm/maxvit_base_tf_512.in21k_ft_in1k) |88.20|98.53| 50.87| 119.88|138.02| 703.99|
|[maxvit_large_tf_512.in21k_ft_in1k](https://huggingface.co/timm/maxvit_large_tf_512.in21k_ft_in1k) |88.04|98.40| 36.42| 212.33|244.75| 942.15|
|[maxvit_large_tf_384.in21k_ft_in1k](https://huggingface.co/timm/maxvit_large_tf_384.in21k_ft_in1k) |87.98|98.56| 71.75| 212.03|132.55| 445.84|
|[maxvit_base_tf_384.in21k_ft_in1k](https://huggingface.co/timm/maxvit_base_tf_384.in21k_ft_in1k) |87.92|98.54| 104.71| 119.65| 73.80| 332.90|
|[maxvit_rmlp_base_rw_384.sw_in12k_ft_in1k](https://huggingface.co/timm/maxvit_rmlp_base_rw_384.sw_in12k_ft_in1k) |87.81|98.37| 106.55| 116.14| 70.97| 318.95|
|[maxxvitv2_rmlp_base_rw_384.sw_in12k_ft_in1k](https://huggingface.co/timm/maxxvitv2_rmlp_base_rw_384.sw_in12k_ft_in1k) |87.47|98.37| 149.49| 116.09| 72.98| 213.74|
|[coatnet_rmlp_2_rw_384.sw_in12k_ft_in1k](https://huggingface.co/timm/coatnet_rmlp_2_rw_384.sw_in12k_ft_in1k) |87.39|98.31| 160.80| 73.88| 47.69| 209.43|
|[maxvit_rmlp_base_rw_224.sw_in12k_ft_in1k](https://huggingface.co/timm/maxvit_rmlp_base_rw_224.sw_in12k_ft_in1k) |86.89|98.02| 375.86| 116.14| 23.15| 92.64|
|[maxxvitv2_rmlp_base_rw_224.sw_in12k_ft_in1k](https://huggingface.co/timm/maxxvitv2_rmlp_base_rw_224.sw_in12k_ft_in1k) |86.64|98.02| 501.03| 116.09| 24.20| 62.77|
|[maxvit_base_tf_512.in1k](https://huggingface.co/timm/maxvit_base_tf_512.in1k) |86.60|97.92| 50.75| 119.88|138.02| 703.99|
|[coatnet_2_rw_224.sw_in12k_ft_in1k](https://huggingface.co/timm/coatnet_2_rw_224.sw_in12k_ft_in1k) |86.57|97.89| 631.88| 73.87| 15.09| 49.22|
|[maxvit_large_tf_512.in1k](https://huggingface.co/timm/maxvit_large_tf_512.in1k) |86.52|97.88| 36.04| 212.33|244.75| 942.15|
|[coatnet_rmlp_2_rw_224.sw_in12k_ft_in1k](https://huggingface.co/timm/coatnet_rmlp_2_rw_224.sw_in12k_ft_in1k) |86.49|97.90| 620.58| 73.88| 15.18| 54.78|
|[maxvit_base_tf_384.in1k](https://huggingface.co/timm/maxvit_base_tf_384.in1k) |86.29|97.80| 101.09| 119.65| 73.80| 332.90|
|[maxvit_large_tf_384.in1k](https://huggingface.co/timm/maxvit_large_tf_384.in1k) |86.23|97.69| 70.56| 212.03|132.55| 445.84|
|[maxvit_small_tf_512.in1k](https://huggingface.co/timm/maxvit_small_tf_512.in1k) |86.10|97.76| 88.63| 69.13| 67.26| 383.77|
|[maxvit_tiny_tf_512.in1k](https://huggingface.co/timm/maxvit_tiny_tf_512.in1k) |85.67|97.58| 144.25| 31.05| 33.49| 257.59|
|[maxvit_small_tf_384.in1k](https://huggingface.co/timm/maxvit_small_tf_384.in1k) |85.54|97.46| 188.35| 69.02| 35.87| 183.65|
|[maxvit_tiny_tf_384.in1k](https://huggingface.co/timm/maxvit_tiny_tf_384.in1k) |85.11|97.38| 293.46| 30.98| 17.53| 123.42|
|[maxvit_large_tf_224.in1k](https://huggingface.co/timm/maxvit_large_tf_224.in1k) |84.93|96.97| 247.71| 211.79| 43.68| 127.35|
|[coatnet_rmlp_1_rw2_224.sw_in12k_ft_in1k](https://huggingface.co/timm/coatnet_rmlp_1_rw2_224.sw_in12k_ft_in1k) |84.90|96.96| 1025.45| 41.72| 8.11| 40.13|
|[maxvit_base_tf_224.in1k](https://huggingface.co/timm/maxvit_base_tf_224.in1k) |84.85|96.99| 358.25| 119.47| 24.04| 95.01|
|[maxxvit_rmlp_small_rw_256.sw_in1k](https://huggingface.co/timm/maxxvit_rmlp_small_rw_256.sw_in1k) |84.63|97.06| 575.53| 66.01| 14.67| 58.38|
|[coatnet_rmlp_2_rw_224.sw_in1k](https://huggingface.co/timm/coatnet_rmlp_2_rw_224.sw_in1k) |84.61|96.74| 625.81| 73.88| 15.18| 54.78|
|[maxvit_rmlp_small_rw_224.sw_in1k](https://huggingface.co/timm/maxvit_rmlp_small_rw_224.sw_in1k) |84.49|96.76| 693.82| 64.90| 10.75| 49.30|
|[maxvit_small_tf_224.in1k](https://huggingface.co/timm/maxvit_small_tf_224.in1k) |84.43|96.83| 647.96| 68.93| 11.66| 53.17|
|[maxvit_rmlp_tiny_rw_256.sw_in1k](https://huggingface.co/timm/maxvit_rmlp_tiny_rw_256.sw_in1k) |84.23|96.78| 807.21| 29.15| 6.77| 46.92|
|[coatnet_1_rw_224.sw_in1k](https://huggingface.co/timm/coatnet_1_rw_224.sw_in1k) |83.62|96.38| 989.59| 41.72| 8.04| 34.60|
|[maxvit_tiny_rw_224.sw_in1k](https://huggingface.co/timm/maxvit_tiny_rw_224.sw_in1k) |83.50|96.50| 1100.53| 29.06| 5.11| 33.11|
|[maxvit_tiny_tf_224.in1k](https://huggingface.co/timm/maxvit_tiny_tf_224.in1k) |83.41|96.59| 1004.94| 30.92| 5.60| 35.78|
|[coatnet_rmlp_1_rw_224.sw_in1k](https://huggingface.co/timm/coatnet_rmlp_1_rw_224.sw_in1k) |83.36|96.45| 1093.03| 41.69| 7.85| 35.47|
|[maxxvitv2_nano_rw_256.sw_in1k](https://huggingface.co/timm/maxxvitv2_nano_rw_256.sw_in1k) |83.11|96.33| 1276.88| 23.70| 6.26| 23.05|
|[maxxvit_rmlp_nano_rw_256.sw_in1k](https://huggingface.co/timm/maxxvit_rmlp_nano_rw_256.sw_in1k) |83.03|96.34| 1341.24| 16.78| 4.37| 26.05|
|[maxvit_rmlp_nano_rw_256.sw_in1k](https://huggingface.co/timm/maxvit_rmlp_nano_rw_256.sw_in1k) |82.96|96.26| 1283.24| 15.50| 4.47| 31.92|
|[maxvit_nano_rw_256.sw_in1k](https://huggingface.co/timm/maxvit_nano_rw_256.sw_in1k) |82.93|96.23| 1218.17| 15.45| 4.46| 30.28|
|[coatnet_bn_0_rw_224.sw_in1k](https://huggingface.co/timm/coatnet_bn_0_rw_224.sw_in1k) |82.39|96.19| 1600.14| 27.44| 4.67| 22.04|
|[coatnet_0_rw_224.sw_in1k](https://huggingface.co/timm/coatnet_0_rw_224.sw_in1k) |82.39|95.84| 1831.21| 27.44| 4.43| 18.73|
|[coatnet_rmlp_nano_rw_224.sw_in1k](https://huggingface.co/timm/coatnet_rmlp_nano_rw_224.sw_in1k) |82.05|95.87| 2109.09| 15.15| 2.62| 20.34|
|[coatnext_nano_rw_224.sw_in1k](https://huggingface.co/timm/coatnext_nano_rw_224.sw_in1k) |81.95|95.92| 2525.52| 14.70| 2.47| 12.80|
|[coatnet_nano_rw_224.sw_in1k](https://huggingface.co/timm/coatnet_nano_rw_224.sw_in1k) |81.70|95.64| 2344.52| 15.14| 2.41| 15.41|
|[maxvit_rmlp_pico_rw_256.sw_in1k](https://huggingface.co/timm/maxvit_rmlp_pico_rw_256.sw_in1k) |80.53|95.21| 1594.71| 7.52| 1.85| 24.86|
### By Throughput (samples / sec)
|model |top1 |top5 |samples / sec |Params (M) |GMAC |Act (M)|
|------------------------------------------------------------------------------------------------------------------------|----:|----:|--------------:|--------------:|-----:|------:|
|[coatnext_nano_rw_224.sw_in1k](https://huggingface.co/timm/coatnext_nano_rw_224.sw_in1k) |81.95|95.92| 2525.52| 14.70| 2.47| 12.80|
|[coatnet_nano_rw_224.sw_in1k](https://huggingface.co/timm/coatnet_nano_rw_224.sw_in1k) |81.70|95.64| 2344.52| 15.14| 2.41| 15.41|
|[coatnet_rmlp_nano_rw_224.sw_in1k](https://huggingface.co/timm/coatnet_rmlp_nano_rw_224.sw_in1k) |82.05|95.87| 2109.09| 15.15| 2.62| 20.34|
|[coatnet_0_rw_224.sw_in1k](https://huggingface.co/timm/coatnet_0_rw_224.sw_in1k) |82.39|95.84| 1831.21| 27.44| 4.43| 18.73|
|[coatnet_bn_0_rw_224.sw_in1k](https://huggingface.co/timm/coatnet_bn_0_rw_224.sw_in1k) |82.39|96.19| 1600.14| 27.44| 4.67| 22.04|
|[maxvit_rmlp_pico_rw_256.sw_in1k](https://huggingface.co/timm/maxvit_rmlp_pico_rw_256.sw_in1k) |80.53|95.21| 1594.71| 7.52| 1.85| 24.86|
|[maxxvit_rmlp_nano_rw_256.sw_in1k](https://huggingface.co/timm/maxxvit_rmlp_nano_rw_256.sw_in1k) |83.03|96.34| 1341.24| 16.78| 4.37| 26.05|
|[maxvit_rmlp_nano_rw_256.sw_in1k](https://huggingface.co/timm/maxvit_rmlp_nano_rw_256.sw_in1k) |82.96|96.26| 1283.24| 15.50| 4.47| 31.92|
|[maxxvitv2_nano_rw_256.sw_in1k](https://huggingface.co/timm/maxxvitv2_nano_rw_256.sw_in1k) |83.11|96.33| 1276.88| 23.70| 6.26| 23.05|
|[maxvit_nano_rw_256.sw_in1k](https://huggingface.co/timm/maxvit_nano_rw_256.sw_in1k) |82.93|96.23| 1218.17| 15.45| 4.46| 30.28|
|[maxvit_tiny_rw_224.sw_in1k](https://huggingface.co/timm/maxvit_tiny_rw_224.sw_in1k) |83.50|96.50| 1100.53| 29.06| 5.11| 33.11|
|[coatnet_rmlp_1_rw_224.sw_in1k](https://huggingface.co/timm/coatnet_rmlp_1_rw_224.sw_in1k) |83.36|96.45| 1093.03| 41.69| 7.85| 35.47|
|[coatnet_rmlp_1_rw2_224.sw_in12k_ft_in1k](https://huggingface.co/timm/coatnet_rmlp_1_rw2_224.sw_in12k_ft_in1k) |84.90|96.96| 1025.45| 41.72| 8.11| 40.13|
|[maxvit_tiny_tf_224.in1k](https://huggingface.co/timm/maxvit_tiny_tf_224.in1k) |83.41|96.59| 1004.94| 30.92| 5.60| 35.78|
|[coatnet_1_rw_224.sw_in1k](https://huggingface.co/timm/coatnet_1_rw_224.sw_in1k) |83.62|96.38| 989.59| 41.72| 8.04| 34.60|
|[maxvit_rmlp_tiny_rw_256.sw_in1k](https://huggingface.co/timm/maxvit_rmlp_tiny_rw_256.sw_in1k) |84.23|96.78| 807.21| 29.15| 6.77| 46.92|
|[maxvit_rmlp_small_rw_224.sw_in1k](https://huggingface.co/timm/maxvit_rmlp_small_rw_224.sw_in1k) |84.49|96.76| 693.82| 64.90| 10.75| 49.30|
|[maxvit_small_tf_224.in1k](https://huggingface.co/timm/maxvit_small_tf_224.in1k) |84.43|96.83| 647.96| 68.93| 11.66| 53.17|
|[coatnet_2_rw_224.sw_in12k_ft_in1k](https://huggingface.co/timm/coatnet_2_rw_224.sw_in12k_ft_in1k) |86.57|97.89| 631.88| 73.87| 15.09| 49.22|
|[coatnet_rmlp_2_rw_224.sw_in1k](https://huggingface.co/timm/coatnet_rmlp_2_rw_224.sw_in1k) |84.61|96.74| 625.81| 73.88| 15.18| 54.78|
|[coatnet_rmlp_2_rw_224.sw_in12k_ft_in1k](https://huggingface.co/timm/coatnet_rmlp_2_rw_224.sw_in12k_ft_in1k) |86.49|97.90| 620.58| 73.88| 15.18| 54.78|
|[maxxvit_rmlp_small_rw_256.sw_in1k](https://huggingface.co/timm/maxxvit_rmlp_small_rw_256.sw_in1k) |84.63|97.06| 575.53| 66.01| 14.67| 58.38|
|[maxxvitv2_rmlp_base_rw_224.sw_in12k_ft_in1k](https://huggingface.co/timm/maxxvitv2_rmlp_base_rw_224.sw_in12k_ft_in1k) |86.64|98.02| 501.03| 116.09| 24.20| 62.77|
|[maxvit_rmlp_base_rw_224.sw_in12k_ft_in1k](https://huggingface.co/timm/maxvit_rmlp_base_rw_224.sw_in12k_ft_in1k) |86.89|98.02| 375.86| 116.14| 23.15| 92.64|
|[maxvit_base_tf_224.in1k](https://huggingface.co/timm/maxvit_base_tf_224.in1k) |84.85|96.99| 358.25| 119.47| 24.04| 95.01|
|[maxvit_tiny_tf_384.in1k](https://huggingface.co/timm/maxvit_tiny_tf_384.in1k) |85.11|97.38| 293.46| 30.98| 17.53| 123.42|
|[maxvit_large_tf_224.in1k](https://huggingface.co/timm/maxvit_large_tf_224.in1k) |84.93|96.97| 247.71| 211.79| 43.68| 127.35|
|[maxvit_small_tf_384.in1k](https://huggingface.co/timm/maxvit_small_tf_384.in1k) |85.54|97.46| 188.35| 69.02| 35.87| 183.65|
|[coatnet_rmlp_2_rw_384.sw_in12k_ft_in1k](https://huggingface.co/timm/coatnet_rmlp_2_rw_384.sw_in12k_ft_in1k) |87.39|98.31| 160.80| 73.88| 47.69| 209.43|
|[maxxvitv2_rmlp_base_rw_384.sw_in12k_ft_in1k](https://huggingface.co/timm/maxxvitv2_rmlp_base_rw_384.sw_in12k_ft_in1k) |87.47|98.37| 149.49| 116.09| 72.98| 213.74|
|[maxvit_tiny_tf_512.in1k](https://huggingface.co/timm/maxvit_tiny_tf_512.in1k) |85.67|97.58| 144.25| 31.05| 33.49| 257.59|
|[maxvit_rmlp_base_rw_384.sw_in12k_ft_in1k](https://huggingface.co/timm/maxvit_rmlp_base_rw_384.sw_in12k_ft_in1k) |87.81|98.37| 106.55| 116.14| 70.97| 318.95|
|[maxvit_base_tf_384.in21k_ft_in1k](https://huggingface.co/timm/maxvit_base_tf_384.in21k_ft_in1k) |87.92|98.54| 104.71| 119.65| 73.80| 332.90|
|[maxvit_base_tf_384.in1k](https://huggingface.co/timm/maxvit_base_tf_384.in1k) |86.29|97.80| 101.09| 119.65| 73.80| 332.90|
|[maxvit_small_tf_512.in1k](https://huggingface.co/timm/maxvit_small_tf_512.in1k) |86.10|97.76| 88.63| 69.13| 67.26| 383.77|
|[maxvit_large_tf_384.in21k_ft_in1k](https://huggingface.co/timm/maxvit_large_tf_384.in21k_ft_in1k) |87.98|98.56| 71.75| 212.03|132.55| 445.84|
|[maxvit_large_tf_384.in1k](https://huggingface.co/timm/maxvit_large_tf_384.in1k) |86.23|97.69| 70.56| 212.03|132.55| 445.84|
|[maxvit_base_tf_512.in21k_ft_in1k](https://huggingface.co/timm/maxvit_base_tf_512.in21k_ft_in1k) |88.20|98.53| 50.87| 119.88|138.02| 703.99|
|[maxvit_base_tf_512.in1k](https://huggingface.co/timm/maxvit_base_tf_512.in1k) |86.60|97.92| 50.75| 119.88|138.02| 703.99|
|[maxvit_xlarge_tf_384.in21k_ft_in1k](https://huggingface.co/timm/maxvit_xlarge_tf_384.in21k_ft_in1k) |88.32|98.54| 42.53| 475.32|292.78| 668.76|
|[maxvit_large_tf_512.in21k_ft_in1k](https://huggingface.co/timm/maxvit_large_tf_512.in21k_ft_in1k) |88.04|98.40| 36.42| 212.33|244.75| 942.15|
|[maxvit_large_tf_512.in1k](https://huggingface.co/timm/maxvit_large_tf_512.in1k) |86.52|97.88| 36.04| 212.33|244.75| 942.15|
|[maxvit_xlarge_tf_512.in21k_ft_in1k](https://huggingface.co/timm/maxvit_xlarge_tf_512.in21k_ft_in1k) |88.53|98.64| 21.76| 475.77|534.14|1413.22|
## Citation
```bibtex
@misc{rw2019timm,
author = {Ross Wightman},
title = {PyTorch Image Models},
year = {2019},
publisher = {GitHub},
journal = {GitHub repository},
doi = {10.5281/zenodo.4414861},
howpublished = {\url{https://github.com/huggingface/pytorch-image-models}}
}
```
```bibtex
@article{tu2022maxvit,
title={MaxViT: Multi-Axis Vision Transformer},
author={Tu, Zhengzhong and Talebi, Hossein and Zhang, Han and Yang, Feng and Milanfar, Peyman and Bovik, Alan and Li, Yinxiao},
journal={ECCV},
year={2022},
}
```
```bibtex
@article{dai2021coatnet,
title={CoAtNet: Marrying Convolution and Attention for All Data Sizes},
author={Dai, Zihang and Liu, Hanxiao and Le, Quoc V and Tan, Mingxing},
journal={arXiv preprint arXiv:2106.04803},
year={2021}
}
```
|
MilaNLProc/feel-it-italian-sentiment | MilaNLProc | "2022-08-15T20:35:54Z" | 15,891 | 15 | transformers | [
"transformers",
"pytorch",
"tf",
"camembert",
"text-classification",
"sentiment",
"Italian",
"it",
"autotrain_compatible",
"endpoints_compatible",
"region:us"
] | text-classification | "2022-03-02T23:29:04Z" | ---
language: it
tags:
- sentiment
- Italian
---
# FEEL-IT: Emotion and Sentiment Classification for the Italian Language
## FEEL-IT Python Package
You can find the package that uses this model for emotion and sentiment classification **[here](https://github.com/MilaNLProc/feel-it)** it is meant to be a very simple interface over HuggingFace models.
## License
Users should refer to the [following license](https://developer.twitter.com/en/developer-terms/commercial-terms)
## Abstract
Sentiment analysis is a common task to understand people's reactions online. Still, we often need more nuanced information: is the post negative because the user is angry or because they are sad?
An abundance of approaches has been introduced for tackling both tasks. However, at least for Italian, they all treat only one of the tasks at a time. We introduce *FEEL-IT*, a novel benchmark corpus of Italian Twitter posts annotated with four basic emotions: **anger, fear, joy, sadness**. By collapsing them, we can also do **sentiment analysis**. We evaluate our corpus on benchmark datasets for both emotion and sentiment classification, obtaining competitive results.
We release an [open-source Python library](https://github.com/MilaNLProc/feel-it), so researchers can use a model trained on FEEL-IT for inferring both sentiments and emotions from Italian text.
| Model | Download |
| ------ | -------------------------|
| `feel-it-italian-sentiment` | [Link](https://huggingface.co/MilaNLProc/feel-it-italian-sentiment) |
| `feel-it-italian-emotion` | [Link](https://huggingface.co/MilaNLProc/feel-it-italian-emotion) |
## Model
The *feel-it-italian-sentiment* model performs **sentiment analysis** on Italian. We fine-tuned the [UmBERTo model](https://huggingface.co/Musixmatch/umberto-commoncrawl-cased-v1) on our new dataset (i.e., FEEL-IT) obtaining state-of-the-art performances on different benchmark corpora.
## Data
Our data has been collected by annotating tweets from a broad range of topics. In total, we have 2037 tweets annotated with an emotion label. More details can be found in our paper (https://aclanthology.org/2021.wassa-1.8/).
## Performance
We evaluate our performance using [SENTIPOLC16 Evalita](http://www.di.unito.it/~tutreeb/sentipolc-evalita16/). We collapsed the FEEL-IT classes into 2 by mapping joy to the *positive* class and anger, fear and sadness into the *negative* class. We compare three different experimental configurations training on FEEL-IT, SENTIPOLC16, or both by testing on the SENTIPOLC16 test set.
The results show that training on FEEL-IT can provide better results on the SENTIPOLC16 test set than those that can be obtained with the SENTIPOLC16 training set.
| Training Dataset | Macro-F1 | Accuracy
| ------ | ------ |------ |
| SENTIPOLC16 | 0.80 | 0.81 |
| FEEL-IT | **0.81** | **0.84** |
| FEEL-IT+SentiPolc | 0.81 | 0.82
## Usage
```python
from transformers import pipeline
classifier = pipeline("text-classification",model='MilaNLProc/feel-it-italian-sentiment',top_k=2)
prediction = classifier("Oggi sono proprio contento!")
print(prediction)
```
## Citation
Please use the following bibtex entry if you use this model in your project:
```
@inproceedings{bianchi2021feel,
title = {{"FEEL-IT: Emotion and Sentiment Classification for the Italian Language"}},
author = "Bianchi, Federico and Nozza, Debora and Hovy, Dirk",
booktitle = "Proceedings of the 11th Workshop on Computational Approaches to Subjectivity, Sentiment and Social Media Analysis",
year = "2021",
publisher = "Association for Computational Linguistics",
}
``` |
mradermacher/Swallow-7b-hf-GGUF | mradermacher | "2024-06-30T11:57:05Z" | 15,876 | 0 | transformers | [
"transformers",
"gguf",
"en",
"ja",
"base_model:tokyotech-llm/Swallow-7b-hf",
"license:llama2",
"endpoints_compatible",
"region:us"
] | null | "2024-06-29T18:58:51Z" | ---
base_model: tokyotech-llm/Swallow-7b-hf
language:
- en
- ja
library_name: transformers
license: llama2
model_type: llama
quantized_by: mradermacher
---
## About
<!-- ### quantize_version: 2 -->
<!-- ### output_tensor_quantised: 1 -->
<!-- ### convert_type: hf -->
<!-- ### vocab_type: -->
<!-- ### tags: -->
static quants of https://huggingface.co/tokyotech-llm/Swallow-7b-hf
<!-- provided-files -->
weighted/imatrix quants are available at https://huggingface.co/mradermacher/Swallow-7b-hf-i1-GGUF
## Usage
If you are unsure how to use GGUF files, refer to one of [TheBloke's
READMEs](https://huggingface.co/TheBloke/KafkaLM-70B-German-V0.1-GGUF) for
more details, including on how to concatenate multi-part files.
## Provided Quants
(sorted by size, not necessarily quality. IQ-quants are often preferable over similar sized non-IQ quants)
| Link | Type | Size/GB | Notes |
|:-----|:-----|--------:|:------|
| [GGUF](https://huggingface.co/mradermacher/Swallow-7b-hf-GGUF/resolve/main/Swallow-7b-hf.Q2_K.gguf) | Q2_K | 2.7 | |
| [GGUF](https://huggingface.co/mradermacher/Swallow-7b-hf-GGUF/resolve/main/Swallow-7b-hf.IQ3_XS.gguf) | IQ3_XS | 3.0 | |
| [GGUF](https://huggingface.co/mradermacher/Swallow-7b-hf-GGUF/resolve/main/Swallow-7b-hf.IQ3_S.gguf) | IQ3_S | 3.1 | beats Q3_K* |
| [GGUF](https://huggingface.co/mradermacher/Swallow-7b-hf-GGUF/resolve/main/Swallow-7b-hf.Q3_K_S.gguf) | Q3_K_S | 3.1 | |
| [GGUF](https://huggingface.co/mradermacher/Swallow-7b-hf-GGUF/resolve/main/Swallow-7b-hf.IQ3_M.gguf) | IQ3_M | 3.3 | |
| [GGUF](https://huggingface.co/mradermacher/Swallow-7b-hf-GGUF/resolve/main/Swallow-7b-hf.Q3_K_M.gguf) | Q3_K_M | 3.5 | lower quality |
| [GGUF](https://huggingface.co/mradermacher/Swallow-7b-hf-GGUF/resolve/main/Swallow-7b-hf.Q3_K_L.gguf) | Q3_K_L | 3.8 | |
| [GGUF](https://huggingface.co/mradermacher/Swallow-7b-hf-GGUF/resolve/main/Swallow-7b-hf.IQ4_XS.gguf) | IQ4_XS | 3.8 | |
| [GGUF](https://huggingface.co/mradermacher/Swallow-7b-hf-GGUF/resolve/main/Swallow-7b-hf.Q4_K_S.gguf) | Q4_K_S | 4.0 | fast, recommended |
| [GGUF](https://huggingface.co/mradermacher/Swallow-7b-hf-GGUF/resolve/main/Swallow-7b-hf.Q4_K_M.gguf) | Q4_K_M | 4.2 | fast, recommended |
| [GGUF](https://huggingface.co/mradermacher/Swallow-7b-hf-GGUF/resolve/main/Swallow-7b-hf.Q5_K_S.gguf) | Q5_K_S | 4.8 | |
| [GGUF](https://huggingface.co/mradermacher/Swallow-7b-hf-GGUF/resolve/main/Swallow-7b-hf.Q5_K_M.gguf) | Q5_K_M | 5.0 | |
| [GGUF](https://huggingface.co/mradermacher/Swallow-7b-hf-GGUF/resolve/main/Swallow-7b-hf.Q6_K.gguf) | Q6_K | 5.7 | very good quality |
| [GGUF](https://huggingface.co/mradermacher/Swallow-7b-hf-GGUF/resolve/main/Swallow-7b-hf.Q8_0.gguf) | Q8_0 | 7.4 | fast, best quality |
| [GGUF](https://huggingface.co/mradermacher/Swallow-7b-hf-GGUF/resolve/main/Swallow-7b-hf.f16.gguf) | f16 | 13.8 | 16 bpw, overkill |
Here is a handy graph by ikawrakow comparing some lower-quality quant
types (lower is better):

And here are Artefact2's thoughts on the matter:
https://gist.github.com/Artefact2/b5f810600771265fc1e39442288e8ec9
## FAQ / Model Request
See https://huggingface.co/mradermacher/model_requests for some answers to
questions you might have and/or if you want some other model quantized.
## Thanks
I thank my company, [nethype GmbH](https://www.nethype.de/), for letting
me use its servers and providing upgrades to my workstation to enable
this work in my free time.
<!-- end -->
|
blanchefort/rubert-base-cased-sentiment | blanchefort | "2023-04-06T04:06:36Z" | 15,875 | 12 | transformers | [
"transformers",
"pytorch",
"tf",
"jax",
"safetensors",
"bert",
"text-classification",
"sentiment",
"ru",
"autotrain_compatible",
"endpoints_compatible",
"region:us"
] | text-classification | "2022-03-02T23:29:05Z" | ---
language:
- ru
tags:
- sentiment
- text-classification
---
# RuBERT for Sentiment Analysis
Short Russian texts sentiment classification
This is a [DeepPavlov/rubert-base-cased-conversational](https://huggingface.co/DeepPavlov/rubert-base-cased-conversational) model trained on aggregated corpus of 351.797 texts.
## Labels
0: NEUTRAL
1: POSITIVE
2: NEGATIVE
## How to use
```python
import torch
from transformers import AutoModelForSequenceClassification
from transformers import BertTokenizerFast
tokenizer = BertTokenizerFast.from_pretrained('blanchefort/rubert-base-cased-sentiment')
model = AutoModelForSequenceClassification.from_pretrained('blanchefort/rubert-base-cased-sentiment', return_dict=True)
@torch.no_grad()
def predict(text):
inputs = tokenizer(text, max_length=512, padding=True, truncation=True, return_tensors='pt')
outputs = model(**inputs)
predicted = torch.nn.functional.softmax(outputs.logits, dim=1)
predicted = torch.argmax(predicted, dim=1).numpy()
return predicted
```
## Datasets used for model training
**[RuTweetCorp](https://study.mokoron.com/)**
> Рубцова Ю. Автоматическое построение и анализ корпуса коротких текстов (постов микроблогов) для задачи разработки и тренировки тонового классификатора //Инженерия знаний и технологии семантического веба. – 2012. – Т. 1. – С. 109-116.
**[RuReviews](https://github.com/sismetanin/rureviews)**
> RuReviews: An Automatically Annotated Sentiment Analysis Dataset for Product Reviews in Russian.
**[RuSentiment](http://text-machine.cs.uml.edu/projects/rusentiment/)**
> A. Rogers A. Romanov A. Rumshisky S. Volkova M. Gronas A. Gribov RuSentiment: An Enriched Sentiment Analysis Dataset for Social Media in Russian. Proceedings of COLING 2018.
**[Отзывы о медучреждениях](https://github.com/blanchefort/datasets/tree/master/medical_comments)**
> Датасет содержит пользовательские отзывы о медицинских учреждениях. Датасет собран в мае 2019 года с сайта prodoctorov.ru |
microsoft/Florence-2-base-ft | microsoft | "2024-07-01T09:37:07Z" | 15,870 | 65 | transformers | [
"transformers",
"pytorch",
"florence2",
"text-generation",
"vision",
"image-text-to-text",
"custom_code",
"arxiv:2311.06242",
"license:mit",
"autotrain_compatible",
"region:us"
] | image-text-to-text | "2024-06-15T00:58:07Z" | ---
license: mit
license_link: https://huggingface.co/microsoft/Florence-2-base-ft/resolve/main/LICENSE
pipeline_tag: image-text-to-text
tags:
- vision
---
# Florence-2: Advancing a Unified Representation for a Variety of Vision Tasks
## Model Summary
This Hub repository contains a HuggingFace's `transformers` implementation of Florence-2 model from Microsoft.
Florence-2 is an advanced vision foundation model that uses a prompt-based approach to handle a wide range of vision and vision-language tasks. Florence-2 can interpret simple text prompts to perform tasks like captioning, object detection, and segmentation. It leverages our FLD-5B dataset, containing 5.4 billion annotations across 126 million images, to master multi-task learning. The model's sequence-to-sequence architecture enables it to excel in both zero-shot and fine-tuned settings, proving to be a competitive vision foundation model.
Resources and Technical Documentation:
+ [Florence-2 technical report](https://arxiv.org/abs/2311.06242).
+ [Jupyter Notebook for inference and visualization of Florence-2-large model](https://huggingface.co/microsoft/Florence-2-large/blob/main/sample_inference.ipynb)
| Model | Model size | Model Description |
| ------- | ------------- | ------------- |
| Florence-2-base[[HF]](https://huggingface.co/microsoft/Florence-2-base) | 0.23B | Pretrained model with FLD-5B
| Florence-2-large[[HF]](https://huggingface.co/microsoft/Florence-2-large) | 0.77B | Pretrained model with FLD-5B
| Florence-2-base-ft[[HF]](https://huggingface.co/microsoft/Florence-2-base-ft) | 0.23B | Finetuned model on a colletion of downstream tasks
| Florence-2-large-ft[[HF]](https://huggingface.co/microsoft/Florence-2-large-ft) | 0.77B | Finetuned model on a colletion of downstream tasks
## How to Get Started with the Model
Use the code below to get started with the model.
```python
import requests
from PIL import Image
from transformers import AutoProcessor, AutoModelForCausalLM
model = AutoModelForCausalLM.from_pretrained("microsoft/Florence-2-base-ft", trust_remote_code=True)
processor = AutoProcessor.from_pretrained("microsoft/Florence-2-base-ft", trust_remote_code=True)
prompt = "<OD>"
url = "https://huggingface.co/datasets/huggingface/documentation-images/resolve/main/transformers/tasks/car.jpg?download=true"
image = Image.open(requests.get(url, stream=True).raw)
inputs = processor(text=prompt, images=image, return_tensors="pt")
generated_ids = model.generate(
input_ids=inputs["input_ids"],
pixel_values=inputs["pixel_values"],
max_new_tokens=1024,
do_sample=False,
num_beams=3
)
generated_text = processor.batch_decode(generated_ids, skip_special_tokens=False)[0]
parsed_answer = processor.post_process_generation(generated_text, task="<OD>", image_size=(image.width, image.height))
print(parsed_answer)
```
## Tasks
This model is capable of performing different tasks through changing the prompts.
First, let's define a function to run a prompt.
<details>
<summary> Click to expand </summary>
```python
import requests
from PIL import Image
from transformers import AutoProcessor, AutoModelForCausalLM
model = AutoModelForCausalLM.from_pretrained("microsoft/Florence-2-base-ft", trust_remote_code=True)
processor = AutoProcessor.from_pretrained("microsoft/Florence-2-base-ft", trust_remote_code=True)
url = "https://huggingface.co/datasets/huggingface/documentation-images/resolve/main/transformers/tasks/car.jpg?download=true"
image = Image.open(requests.get(url, stream=True).raw)
def run_example(task_prompt, text_input=None):
if text_input is None:
prompt = task_prompt
else:
prompt = task_prompt + text_input
inputs = processor(text=prompt, images=image, return_tensors="pt")
generated_ids = model.generate(
input_ids=inputs["input_ids"],
pixel_values=inputs["pixel_values"],
max_new_tokens=1024,
num_beams=3
)
generated_text = processor.batch_decode(generated_ids, skip_special_tokens=False)[0]
parsed_answer = processor.post_process_generation(generated_text, task=task_prompt, image_size=(image.width, image.height))
print(parsed_answer)
```
</details>
Here are the tasks `Florence-2` could perform:
<details>
<summary> Click to expand </summary>
### Caption
```python
prompt = "<CAPTION>"
run_example(prompt)
```
### Detailed Caption
```python
prompt = "<DETAILED_CAPTION>"
run_example(prompt)
```
### More Detailed Caption
```python
prompt = "<MORE_DETAILED_CAPTION>"
run_example(prompt)
```
### Caption to Phrase Grounding
caption to phrase grounding task requires additional text input, i.e. caption.
Caption to phrase grounding results format:
{'\<CAPTION_TO_PHRASE_GROUNDING>': {'bboxes': [[x1, y1, x2, y2], ...], 'labels': ['', '', ...]}}
```python
task_prompt = '<CAPTION_TO_PHRASE_GROUNDING>"
results = run_example(task_prompt, text_input="A green car parked in front of a yellow building.")
```
### Object Detection
OD results format:
{'\<OD>': {'bboxes': [[x1, y1, x2, y2], ...],
'labels': ['label1', 'label2', ...]} }
```python
prompt = "<OD>"
run_example(prompt)
```
### Dense Region Caption
Dense region caption results format:
{'\<DENSE_REGION_CAPTION>' : {'bboxes': [[x1, y1, x2, y2], ...],
'labels': ['label1', 'label2', ...]} }
```python
prompt = "<DENSE_REGION_CAPTION>"
run_example(prompt)
```
### Region proposal
Dense region caption results format:
{'\<REGION_PROPOSAL>': {'bboxes': [[x1, y1, x2, y2], ...],
'labels': ['', '', ...]}}
```python
prompt = "<REGION_PROPOSAL>"
run_example(prompt)
```
### OCR
```python
prompt = "<OCR>"
run_example(prompt)
```
### OCR with Region
OCR with region output format:
{'\<OCR_WITH_REGION>': {'quad_boxes': [[x1, y1, x2, y2, x3, y3, x4, y4], ...], 'labels': ['text1', ...]}}
```python
prompt = "<OCR_WITH_REGION>"
run_example(prompt)
```
for More detailed examples, please refer to [notebook](https://huggingface.co/microsoft/Florence-2-large/blob/main/sample_inference.ipynb)
</details>
# Benchmarks
## Florence-2 Zero-shot performance
The following table presents the zero-shot performance of generalist vision foundation models on image captioning and object detection evaluation tasks. These models have not been exposed to the training data of the evaluation tasks during their training phase.
| Method | #params | COCO Cap. test CIDEr | NoCaps val CIDEr | TextCaps val CIDEr | COCO Det. val2017 mAP |
|--------|---------|----------------------|------------------|--------------------|-----------------------|
| Flamingo | 80B | 84.3 | - | - | - |
| Florence-2-base| 0.23B | 133.0 | 118.7 | 70.1 | 34.7 |
| Florence-2-large| 0.77B | 135.6 | 120.8 | 72.8 | 37.5 |
The following table continues the comparison with performance on other vision-language evaluation tasks.
| Method | Flickr30k test R@1 | Refcoco val Accuracy | Refcoco test-A Accuracy | Refcoco test-B Accuracy | Refcoco+ val Accuracy | Refcoco+ test-A Accuracy | Refcoco+ test-B Accuracy | Refcocog val Accuracy | Refcocog test Accuracy | Refcoco RES val mIoU |
|--------|----------------------|----------------------|-------------------------|-------------------------|-----------------------|--------------------------|--------------------------|-----------------------|------------------------|----------------------|
| Kosmos-2 | 78.7 | 52.3 | 57.4 | 47.3 | 45.5 | 50.7 | 42.2 | 60.6 | 61.7 | - |
| Florence-2-base | 83.6 | 53.9 | 58.4 | 49.7 | 51.5 | 56.4 | 47.9 | 66.3 | 65.1 | 34.6 |
| Florence-2-large | 84.4 | 56.3 | 61.6 | 51.4 | 53.6 | 57.9 | 49.9 | 68.0 | 67.0 | 35.8 |
## Florence-2 finetuned performance
We finetune Florence-2 models with a collection of downstream tasks, resulting two generalist models *Florence-2-base-ft* and *Florence-2-large-ft* that can conduct a wide range of downstream tasks.
The table below compares the performance of specialist and generalist models on various captioning and Visual Question Answering (VQA) tasks. Specialist models are fine-tuned specifically for each task, whereas generalist models are fine-tuned in a task-agnostic manner across all tasks. The symbol "▲" indicates the usage of external OCR as input.
| Method | # Params | COCO Caption Karpathy test CIDEr | NoCaps val CIDEr | TextCaps val CIDEr | VQAv2 test-dev Acc | TextVQA test-dev Acc | VizWiz VQA test-dev Acc |
|----------------|----------|-----------------------------------|------------------|--------------------|--------------------|----------------------|-------------------------|
| **Specialist Models** | | | | | | | |
| CoCa | 2.1B | 143.6 | 122.4 | - | 82.3 | - | - |
| BLIP-2 | 7.8B | 144.5 | 121.6 | - | 82.2 | - | - |
| GIT2 | 5.1B | 145.0 | 126.9 | 148.6 | 81.7 | 67.3 | 71.0 |
| Flamingo | 80B | 138.1 | - | - | 82.0 | 54.1 | 65.7 |
| PaLI | 17B | 149.1 | 127.0 | 160.0▲ | 84.3 | 58.8 / 73.1▲ | 71.6 / 74.4▲ |
| PaLI-X | 55B | 149.2 | 126.3 | 147.0 / 163.7▲ | 86.0 | 71.4 / 80.8▲ | 70.9 / 74.6▲ |
| **Generalist Models** | | | | | | | |
| Unified-IO | 2.9B | - | 100.0 | - | 77.9 | - | 57.4 |
| Florence-2-base-ft | 0.23B | 140.0 | 116.7 | 143.9 | 79.7 | 63.6 | 63.6 |
| Florence-2-large-ft | 0.77B | 143.3 | 124.9 | 151.1 | 81.7 | 73.5 | 72.6 |
| Method | # Params | COCO Det. val2017 mAP | Flickr30k test R@1 | RefCOCO val Accuracy | RefCOCO test-A Accuracy | RefCOCO test-B Accuracy | RefCOCO+ val Accuracy | RefCOCO+ test-A Accuracy | RefCOCO+ test-B Accuracy | RefCOCOg val Accuracy | RefCOCOg test Accuracy | RefCOCO RES val mIoU |
|----------------------|----------|-----------------------|--------------------|----------------------|-------------------------|-------------------------|------------------------|---------------------------|---------------------------|------------------------|-----------------------|------------------------|
| **Specialist Models** | | | | | | | | | | | | |
| SeqTR | - | - | - | 83.7 | 86.5 | 81.2 | 71.5 | 76.3 | 64.9 | 74.9 | 74.2 | - |
| PolyFormer | - | - | - | 90.4 | 92.9 | 87.2 | 85.0 | 89.8 | 78.0 | 85.8 | 85.9 | 76.9 |
| UNINEXT | 0.74B | 60.6 | - | 92.6 | 94.3 | 91.5 | 85.2 | 89.6 | 79.8 | 88.7 | 89.4 | - |
| Ferret | 13B | - | - | 89.5 | 92.4 | 84.4 | 82.8 | 88.1 | 75.2 | 85.8 | 86.3 | - |
| **Generalist Models** | | | | | | | | | | | | |
| UniTAB | - | - | - | 88.6 | 91.1 | 83.8 | 81.0 | 85.4 | 71.6 | 84.6 | 84.7 | - |
| Florence-2-base-ft | 0.23B | 41.4 | 84.0 | 92.6 | 94.8 | 91.5 | 86.8 | 91.7 | 82.2 | 89.8 | 82.2 | 78.0 |
| Florence-2-large-ft| 0.77B | 43.4 | 85.2 | 93.4 | 95.3 | 92.0 | 88.3 | 92.9 | 83.6 | 91.2 | 91.7 | 80.5 |
## BibTex and citation info
```
@article{xiao2023florence,
title={Florence-2: Advancing a unified representation for a variety of vision tasks},
author={Xiao, Bin and Wu, Haiping and Xu, Weijian and Dai, Xiyang and Hu, Houdong and Lu, Yumao and Zeng, Michael and Liu, Ce and Yuan, Lu},
journal={arXiv preprint arXiv:2311.06242},
year={2023}
}
``` |
patrickvonplaten/wavlm-libri-clean-100h-base-plus | patrickvonplaten | "2021-12-20T12:59:01Z" | 15,868 | 3 | transformers | [
"transformers",
"pytorch",
"tensorboard",
"wavlm",
"automatic-speech-recognition",
"librispeech_asr",
"generated_from_trainer",
"wavlm_libri_finetune",
"endpoints_compatible",
"region:us"
] | automatic-speech-recognition | "2022-03-02T23:29:05Z" | ---
tags:
- automatic-speech-recognition
- librispeech_asr
- generated_from_trainer
- wavlm_libri_finetune
model-index:
- name: wavlm-libri-clean-100h-base-plus
results: []
---
<!-- This model card has been generated automatically according to the information the Trainer had access to. You
should probably proofread and complete it, then remove this comment. -->
# wavlm-libri-clean-100h-base-plus
This model is a fine-tuned version of [microsoft/wavlm-base-plus](https://huggingface.co/microsoft/wavlm-base-plus) on the LIBRISPEECH_ASR - CLEAN dataset.
It achieves the following results on the evaluation set:
- Loss: 0.0819
- Wer: 0.0683
## Model description
More information needed
## Intended uses & limitations
More information needed
## Training and evaluation data
More information needed
## Training procedure
### Training hyperparameters
The following hyperparameters were used during training:
- learning_rate: 0.0003
- train_batch_size: 4
- eval_batch_size: 4
- seed: 42
- distributed_type: multi-GPU
- num_devices: 8
- total_train_batch_size: 32
- total_eval_batch_size: 32
- optimizer: Adam with betas=(0.9,0.999) and epsilon=1e-08
- lr_scheduler_type: linear
- lr_scheduler_warmup_steps: 500
- num_epochs: 3.0
- mixed_precision_training: Native AMP
### Training results
| Training Loss | Epoch | Step | Validation Loss | Wer |
|:-------------:|:-----:|:----:|:---------------:|:------:|
| 2.8877 | 0.34 | 300 | 2.8649 | 1.0 |
| 0.2852 | 0.67 | 600 | 0.2196 | 0.1830 |
| 0.1198 | 1.01 | 900 | 0.1438 | 0.1273 |
| 0.0906 | 1.35 | 1200 | 0.1145 | 0.1035 |
| 0.0729 | 1.68 | 1500 | 0.1055 | 0.0955 |
| 0.0605 | 2.02 | 1800 | 0.0936 | 0.0859 |
| 0.0402 | 2.35 | 2100 | 0.0885 | 0.0746 |
| 0.0421 | 2.69 | 2400 | 0.0848 | 0.0700 |
### Framework versions
- Transformers 4.15.0.dev0
- Pytorch 1.9.0+cu111
- Datasets 1.16.2.dev0
- Tokenizers 0.10.3
|
mradermacher/IceSakeV6RP-7b-GGUF | mradermacher | "2024-06-26T19:53:26Z" | 15,867 | 0 | transformers | [
"transformers",
"gguf",
"mergekit",
"merge",
"alpaca",
"mistral",
"not-for-all-audiences",
"nsfw",
"en",
"base_model:icefog72/IceSakeV6RP-7b",
"license:cc-by-nc-4.0",
"endpoints_compatible",
"region:us"
] | null | "2024-06-26T16:09:21Z" | ---
base_model: icefog72/IceSakeV6RP-7b
language:
- en
library_name: transformers
license: cc-by-nc-4.0
quantized_by: mradermacher
tags:
- mergekit
- merge
- alpaca
- mistral
- not-for-all-audiences
- nsfw
---
## About
<!-- ### quantize_version: 2 -->
<!-- ### output_tensor_quantised: 1 -->
<!-- ### convert_type: hf -->
<!-- ### vocab_type: -->
<!-- ### tags: -->
static quants of https://huggingface.co/icefog72/IceSakeV6RP-7b
<!-- provided-files -->
weighted/imatrix quants are available at https://huggingface.co/mradermacher/IceSakeV6RP-7b-i1-GGUF
## Usage
If you are unsure how to use GGUF files, refer to one of [TheBloke's
READMEs](https://huggingface.co/TheBloke/KafkaLM-70B-German-V0.1-GGUF) for
more details, including on how to concatenate multi-part files.
## Provided Quants
(sorted by size, not necessarily quality. IQ-quants are often preferable over similar sized non-IQ quants)
| Link | Type | Size/GB | Notes |
|:-----|:-----|--------:|:------|
| [GGUF](https://huggingface.co/mradermacher/IceSakeV6RP-7b-GGUF/resolve/main/IceSakeV6RP-7b.Q2_K.gguf) | Q2_K | 2.8 | |
| [GGUF](https://huggingface.co/mradermacher/IceSakeV6RP-7b-GGUF/resolve/main/IceSakeV6RP-7b.IQ3_XS.gguf) | IQ3_XS | 3.1 | |
| [GGUF](https://huggingface.co/mradermacher/IceSakeV6RP-7b-GGUF/resolve/main/IceSakeV6RP-7b.Q3_K_S.gguf) | Q3_K_S | 3.3 | |
| [GGUF](https://huggingface.co/mradermacher/IceSakeV6RP-7b-GGUF/resolve/main/IceSakeV6RP-7b.IQ3_S.gguf) | IQ3_S | 3.3 | beats Q3_K* |
| [GGUF](https://huggingface.co/mradermacher/IceSakeV6RP-7b-GGUF/resolve/main/IceSakeV6RP-7b.IQ3_M.gguf) | IQ3_M | 3.4 | |
| [GGUF](https://huggingface.co/mradermacher/IceSakeV6RP-7b-GGUF/resolve/main/IceSakeV6RP-7b.Q3_K_M.gguf) | Q3_K_M | 3.6 | lower quality |
| [GGUF](https://huggingface.co/mradermacher/IceSakeV6RP-7b-GGUF/resolve/main/IceSakeV6RP-7b.Q3_K_L.gguf) | Q3_K_L | 3.9 | |
| [GGUF](https://huggingface.co/mradermacher/IceSakeV6RP-7b-GGUF/resolve/main/IceSakeV6RP-7b.IQ4_XS.gguf) | IQ4_XS | 4.0 | |
| [GGUF](https://huggingface.co/mradermacher/IceSakeV6RP-7b-GGUF/resolve/main/IceSakeV6RP-7b.Q4_K_S.gguf) | Q4_K_S | 4.2 | fast, recommended |
| [GGUF](https://huggingface.co/mradermacher/IceSakeV6RP-7b-GGUF/resolve/main/IceSakeV6RP-7b.Q4_K_M.gguf) | Q4_K_M | 4.5 | fast, recommended |
| [GGUF](https://huggingface.co/mradermacher/IceSakeV6RP-7b-GGUF/resolve/main/IceSakeV6RP-7b.Q5_K_S.gguf) | Q5_K_S | 5.1 | |
| [GGUF](https://huggingface.co/mradermacher/IceSakeV6RP-7b-GGUF/resolve/main/IceSakeV6RP-7b.Q5_K_M.gguf) | Q5_K_M | 5.2 | |
| [GGUF](https://huggingface.co/mradermacher/IceSakeV6RP-7b-GGUF/resolve/main/IceSakeV6RP-7b.Q6_K.gguf) | Q6_K | 6.0 | very good quality |
| [GGUF](https://huggingface.co/mradermacher/IceSakeV6RP-7b-GGUF/resolve/main/IceSakeV6RP-7b.Q8_0.gguf) | Q8_0 | 7.8 | fast, best quality |
| [GGUF](https://huggingface.co/mradermacher/IceSakeV6RP-7b-GGUF/resolve/main/IceSakeV6RP-7b.f16.gguf) | f16 | 14.6 | 16 bpw, overkill |
Here is a handy graph by ikawrakow comparing some lower-quality quant
types (lower is better):

And here are Artefact2's thoughts on the matter:
https://gist.github.com/Artefact2/b5f810600771265fc1e39442288e8ec9
## FAQ / Model Request
See https://huggingface.co/mradermacher/model_requests for some answers to
questions you might have and/or if you want some other model quantized.
## Thanks
I thank my company, [nethype GmbH](https://www.nethype.de/), for letting
me use its servers and providing upgrades to my workstation to enable
this work in my free time.
<!-- end -->
|
cross-encoder/nli-deberta-v3-large | cross-encoder | "2021-12-28T19:10:37Z" | 15,864 | 19 | transformers | [
"transformers",
"pytorch",
"deberta-v2",
"text-classification",
"microsoft/deberta-v3-large",
"zero-shot-classification",
"en",
"dataset:multi_nli",
"dataset:snli",
"license:apache-2.0",
"autotrain_compatible",
"endpoints_compatible",
"region:us"
] | zero-shot-classification | "2022-03-02T23:29:05Z" | ---
language: en
pipeline_tag: zero-shot-classification
tags:
- microsoft/deberta-v3-large
datasets:
- multi_nli
- snli
metrics:
- accuracy
license: apache-2.0
---
# Cross-Encoder for Natural Language Inference
This model was trained using [SentenceTransformers](https://sbert.net) [Cross-Encoder](https://www.sbert.net/examples/applications/cross-encoder/README.html) class. This model is based on [microsoft/deberta-v3-large](https://huggingface.co/microsoft/deberta-v3-large)
## Training Data
The model was trained on the [SNLI](https://nlp.stanford.edu/projects/snli/) and [MultiNLI](https://cims.nyu.edu/~sbowman/multinli/) datasets. For a given sentence pair, it will output three scores corresponding to the labels: contradiction, entailment, neutral.
## Performance
- Accuracy on SNLI-test dataset: 92.20
- Accuracy on MNLI mismatched set: 90.49
For futher evaluation results, see [SBERT.net - Pretrained Cross-Encoder](https://www.sbert.net/docs/pretrained_cross-encoders.html#nli).
## Usage
Pre-trained models can be used like this:
```python
from sentence_transformers import CrossEncoder
model = CrossEncoder('cross-encoder/nli-deberta-v3-large')
scores = model.predict([('A man is eating pizza', 'A man eats something'), ('A black race car starts up in front of a crowd of people.', 'A man is driving down a lonely road.')])
#Convert scores to labels
label_mapping = ['contradiction', 'entailment', 'neutral']
labels = [label_mapping[score_max] for score_max in scores.argmax(axis=1)]
```
## Usage with Transformers AutoModel
You can use the model also directly with Transformers library (without SentenceTransformers library):
```python
from transformers import AutoTokenizer, AutoModelForSequenceClassification
import torch
model = AutoModelForSequenceClassification.from_pretrained('cross-encoder/nli-deberta-v3-large')
tokenizer = AutoTokenizer.from_pretrained('cross-encoder/nli-deberta-v3-large')
features = tokenizer(['A man is eating pizza', 'A black race car starts up in front of a crowd of people.'], ['A man eats something', 'A man is driving down a lonely road.'], padding=True, truncation=True, return_tensors="pt")
model.eval()
with torch.no_grad():
scores = model(**features).logits
label_mapping = ['contradiction', 'entailment', 'neutral']
labels = [label_mapping[score_max] for score_max in scores.argmax(dim=1)]
print(labels)
```
## Zero-Shot Classification
This model can also be used for zero-shot-classification:
```python
from transformers import pipeline
classifier = pipeline("zero-shot-classification", model='cross-encoder/nli-deberta-v3-large')
sent = "Apple just announced the newest iPhone X"
candidate_labels = ["technology", "sports", "politics"]
res = classifier(sent, candidate_labels)
print(res)
``` |
apple/DFN2B-CLIP-ViT-L-14 | apple | "2023-10-31T17:56:28Z" | 15,850 | 12 | open_clip | [
"open_clip",
"pytorch",
"clip",
"arxiv:2309.17425",
"license:other",
"region:us"
] | null | "2023-10-30T23:07:24Z" | ---
license: other
license_name: apple-sample-code-license
license_link: LICENSE
---
A CLIP (Contrastive Language-Image Pre-training) model trained on DFN-2B.
Data Filtering Networks (DFNs) are small networks used to automatically filter large pools of uncurated data.
This model was trained on 2B images that were filtered from a pool of 12.8B uncurated image-text pairs
(12.8B image-text pairs from CommonPool-12.8B).
This model has been converted to PyTorch from the original JAX checkpoints from Axlearn (https://github.com/apple/axlearn).
These weights are directly usable in OpenCLIP (image + text).
## Model Details
- **Model Type:** Contrastive Image-Text, Zero-Shot Image Classification.
- **Dataset:** DFN-2b
- **Papers:**
- Data Filtering Networks: https://arxiv.org/abs/2309.17425
- **Examples Seen:** 12.8B
## Model Metrics
| Eval Dataset | Metric |
|:-----------------------|---------:|
| ImageNet 1k | 0.81396 |
| Caltech-101 | 0.953141 |
| CIFAR-10 | 0.9836 |
| CIFAR-100 | 0.8835 |
| CLEVR Counts | 0.3338 |
| CLEVR Distance | 0.248733 |
| Country211 | 0.28237 |
| Describable Textures | 0.66117 |
| EuroSAT | 0.646296 |
| FGVC Aircraft | 0.395945 |
| Food-101 | 0.945861 |
| GTSRB | 0.616152 |
| ImageNet Sketch | 0.683311 |
| ImageNet v2 | 0.7453 |
| ImageNet-A | 0.6676 |
| ImageNet-O | 0.3915 |
| ImageNet-R | 0.900033 |
| KITTI Vehicle Distance | 0.201125 |
| MNIST | 0.8468 |
| ObjectNet | 0.739367 |
| Oxford Flowers-102 | 0.865822 |
| Oxford-IIIT Pet | 0.954941 |
| Pascal VOC 2007 | 0.81644 |
| PatchCamelyon | 0.63028 |
| Rendered SST2 | 0.551345 |
| RESISC45 | 0.733175 |
| Stanford Cars | 0.947146 |
| STL-10 | 0.976625 |
| SUN397 | 0.754565 |
| SVHN | 0.653503 |
| Flickr | 0.8244 |
| MSCOCO | 0.570363 |
| WinoGAViL | 0.551645 |
| iWildCam | 0.18877 |
| Camelyon17 | 0.626179 |
| FMoW | 0.222137 |
| Dollar Street | 0.688084 |
| GeoDE | 0.91023 |
| **Average** | **0.668558** |
## Model Usage
### With OpenCLIP
```
import torch
import torch.nn.functional as F
from urllib.request import urlopen
from PIL import Image
from open_clip import create_model_from_pretrained, get_tokenizer
model, preprocess = create_model_from_pretrained('hf-hub:apple/DFN2B-CLIP-ViT-L-14')
tokenizer = get_tokenizer('ViT-L-14')
image = Image.open(urlopen(
'https://huggingface.co/datasets/huggingface/documentation-images/resolve/main/beignets-task-guide.png'
))
image = preprocess(image).unsqueeze(0)
labels_list = ["a dog", "a cat", "a donut", "a beignet"]
text = tokenizer(labels_list, context_length=model.context_length)
with torch.no_grad(), torch.cuda.amp.autocast():
image_features = model.encode_image(image)
text_features = model.encode_text(text)
image_features = F.normalize(image_features, dim=-1)
text_features = F.normalize(text_features, dim=-1)
text_probs = torch.sigmoid(image_features @ text_features.T * model.logit_scale.exp() + model.logit_bias)
zipped_list = list(zip(labels_list, [round(p.item(), 3) for p in text_probs[0]]))
print("Label probabilities: ", zipped_list)
```
## Citation
```bibtex
@article{fang2023data,
title={Data Filtering Networks},
author={Fang, Alex and Jose, Albin Madappally and Jain, Amit and Schmidt, Ludwig and Toshev, Alexander and Shankar, Vaishaal},
journal={arXiv preprint arXiv:2309.17425},
year={2023}
}
```
|
snunlp/KR-ELECTRA-generator | snunlp | "2022-05-04T06:24:04Z" | 15,845 | 1 | transformers | [
"transformers",
"pytorch",
"electra",
"fill-mask",
"ko",
"autotrain_compatible",
"endpoints_compatible",
"region:us"
] | fill-mask | "2022-03-02T23:29:05Z" | ---
language:
- "ko"
---
## KoRean based ELECTRA (KR-ELECTRA)
This is a release of a Korean-specific ELECTRA model with comparable or better performances developed by the Computational Linguistics Lab at Seoul National University. Our model shows remarkable performances on tasks related to informal texts such as review documents, while still showing comparable results on other kinds of tasks.
### Released Model
We pre-trained our KR-ELECTRA model following a base-scale model of [ELECTRA](https://github.com/google-research/electra). We trained the model based on Tensorflow-v1 using a v3-8 TPU of Google Cloud Platform.
#### Model Details
We followed the training parameters of the base-scale model of [ELECTRA](https://github.com/google-research/electra).
##### Hyperparameters
| model | # of layers | embedding size | hidden size | # of heads |
| ------: | ----------: | -------------: | ----------: | ---------: |
| Discriminator | 12 | 768 | 768 | 12 |
| Generator | 12 | 768 | 256 | 4 |
##### Pretraining
| batch size | train steps | learning rates | max sequence length | generator size |
| ---------: | ----------: | -------------: | ------------------: | -------------: |
| 256 | 700000 | 2e-4 | 128 | 0.33333 |
#### Training Dataset
34GB Korean texts including Wikipedia documents, news articles, legal texts, news comments, product reviews, and so on. These texts are balanced, consisting of the same ratios of written and spoken data.
#### Vocabulary
vocab size 30,000
We used morpheme-based unit tokens for our vocabulary based on the [Mecab-Ko](https://bitbucket.org/eunjeon/mecab-ko-dic/src/master/) morpheme analyzer.
#### Download Link
* Tensorflow-v1 model ([download](https://drive.google.com/file/d/1L_yKEDaXM_yDLwHm5QrXAncQZiMN3BBU/view?usp=sharing))
* PyTorch models on HuggingFace
```python
from transformers import ElectraModel, ElectraTokenizer
model = ElectraModel.from_pretrained("snunlp/KR-ELECTRA-discriminator")
tokenizer = ElectraTokenizer.from_pretrained("snunlp/KR-ELECTRA-discriminator")
```
### Finetuning
We used and slightly edited the finetuning codes from [KoELECTRA](https://github.com/monologg/KoELECTRA), with additionally adjusted hyperparameters. You can download the codes and config files that we used for our model from our [github](https://github.com/snunlp/KR-ELECTRA).
#### Experimental Results
| | **NSMC**<br/>(acc) | **Naver NER**<br/>(F1) | **PAWS**<br/>(acc) | **KorNLI**<br/>(acc) | **KorSTS**<br/>(spearman) | **Question Pair**<br/>(acc) | **KorQuaD (Dev)**<br/>(EM/F1) | **Korean-Hate-Speech (Dev)**<br/>(F1) |
| :-------------------- | :----------------: | :--------------------: | :----------------: | :------------------: | :-----------------------: | :-------------------------: | :---------------------------: | :-----------------------------------: |
| KoBERT | 89.59 | 87.92 | 81.25 | 79.62 | 81.59 | 94.85 | 51.75 / 79.15 | 66.21 |
| XLM-Roberta-Base | 89.03 | 86.65 | 82.80 | 80.23 | 78.45 | 93.80 | 64.70 / 88.94 | 64.06 |
| HanBERT | 90.06 | 87.70 | 82.95 | 80.32 | 82.73 | 94.72 | 78.74 / 92.02 | 68.32 |
| KoELECTRA-Base | 90.33 | 87.18 | 81.70 | 80.64 | 82.00 | 93.54 | 60.86 / 89.28 | 66.09 |
| KoELECTRA-Base-v2 | 89.56 | 87.16 | 80.70 | 80.72 | 82.30 | 94.85 | 84.01 / 92.40 | 67.45 |
| KoELECTRA-Base-v3 | 90.63 | **88.11** | **84.45** | 82.24 | **85.53** | 95.25 | 84.83 / **93.45** | 67.61 |
| **KR-ELECTRA (ours)** | **91.168** | 87.90 | 82.05 | **82.51** | 85.41 | **95.51** | **84.93** / 93.04 | **74.50** |
The baseline results are brought from [KoELECTRA](https://github.com/monologg/KoELECTRA)'s.
### Citation
```bibtex
@misc{kr-electra,
author = {Lee, Sangah and Hyopil Shin},
title = {KR-ELECTRA: a KoRean-based ELECTRA model},
year = {2022},
publisher = {GitHub},
journal = {GitHub repository},
howpublished = {\url{https://github.com/snunlp/KR-ELECTRA}}
}
```
|
google/t5-efficient-tiny | google | "2023-01-24T16:51:36Z" | 15,843 | 15 | transformers | [
"transformers",
"pytorch",
"tf",
"jax",
"t5",
"text2text-generation",
"deep-narrow",
"en",
"dataset:c4",
"arxiv:2109.10686",
"license:apache-2.0",
"autotrain_compatible",
"text-generation-inference",
"region:us"
] | text2text-generation | "2022-03-02T23:29:05Z" | ---
language:
- en
datasets:
- c4
tags:
- deep-narrow
inference: false
license: apache-2.0
---
# T5-Efficient-TINY (Deep-Narrow version)
T5-Efficient-TINY is a variation of [Google's original T5](https://ai.googleblog.com/2020/02/exploring-transfer-learning-with-t5.html) following the [T5 model architecture](https://huggingface.co/docs/transformers/model_doc/t5).
It is a *pretrained-only* checkpoint and was released with the
paper **[Scale Efficiently: Insights from Pre-training and Fine-tuning Transformers](https://arxiv.org/abs/2109.10686)**
by *Yi Tay, Mostafa Dehghani, Jinfeng Rao, William Fedus, Samira Abnar, Hyung Won Chung, Sharan Narang, Dani Yogatama, Ashish Vaswani, Donald Metzler*.
In a nutshell, the paper indicates that a **Deep-Narrow** model architecture is favorable for **downstream** performance compared to other model architectures
of similar parameter count.
To quote the paper:
> We generally recommend a DeepNarrow strategy where the model’s depth is preferentially increased
> before considering any other forms of uniform scaling across other dimensions. This is largely due to
> how much depth influences the Pareto-frontier as shown in earlier sections of the paper. Specifically, a
> tall small (deep and narrow) model is generally more efficient compared to the base model. Likewise,
> a tall base model might also generally more efficient compared to a large model. We generally find
> that, regardless of size, even if absolute performance might increase as we continue to stack layers,
> the relative gain of Pareto-efficiency diminishes as we increase the layers, converging at 32 to 36
> layers. Finally, we note that our notion of efficiency here relates to any one compute dimension, i.e.,
> params, FLOPs or throughput (speed). We report all three key efficiency metrics (number of params,
> FLOPS and speed) and leave this decision to the practitioner to decide which compute dimension to
> consider.
To be more precise, *model depth* is defined as the number of transformer blocks that are stacked sequentially.
A sequence of word embeddings is therefore processed sequentially by each transformer block.
## Details model architecture
This model checkpoint - **t5-efficient-tiny** - is of model type **Tiny** with no variations.
It has **15.58** million parameters and thus requires *ca.* **62.32 MB** of memory in full precision (*fp32*)
or **31.16 MB** of memory in half precision (*fp16* or *bf16*).
A summary of the *original* T5 model architectures can be seen here:
| Model | nl (el/dl) | ff | dm | kv | nh | #Params|
| ----| ---- | ---- | ---- | ---- | ---- | ----|
| Tiny | 4/4 | 1024 | 256 | 32 | 4 | 16M|
| Mini | 4/4 | 1536 | 384 | 32 | 8 | 31M|
| Small | 6/6 | 2048 | 512 | 32 | 8 | 60M|
| Base | 12/12 | 3072 | 768 | 64 | 12 | 220M|
| Large | 24/24 | 4096 | 1024 | 64 | 16 | 738M|
| Xl | 24/24 | 16384 | 1024 | 128 | 32 | 3B|
| XXl | 24/24 | 65536 | 1024 | 128 | 128 | 11B|
whereas the following abbreviations are used:
| Abbreviation | Definition |
| ----| ---- |
| nl | Number of transformer blocks (depth) |
| dm | Dimension of embedding vector (output vector of transformers block) |
| kv | Dimension of key/value projection matrix |
| nh | Number of attention heads |
| ff | Dimension of intermediate vector within transformer block (size of feed-forward projection matrix) |
| el | Number of transformer blocks in the encoder (encoder depth) |
| dl | Number of transformer blocks in the decoder (decoder depth) |
| sh | Signifies that attention heads are shared |
| skv | Signifies that key-values projection matrices are tied |
If a model checkpoint has no specific, *el* or *dl* than both the number of encoder- and decoder layers correspond to *nl*.
## Pre-Training
The checkpoint was pretrained on the [Colossal, Cleaned version of Common Crawl (C4)](https://huggingface.co/datasets/c4) for 524288 steps using
the span-based masked language modeling (MLM) objective.
## Fine-Tuning
**Note**: This model is a **pretrained** checkpoint and has to be fine-tuned for practical usage.
The checkpoint was pretrained in English and is therefore only useful for English NLP tasks.
You can follow on of the following examples on how to fine-tune the model:
*PyTorch*:
- [Summarization](https://github.com/huggingface/transformers/tree/master/examples/pytorch/summarization)
- [Question Answering](https://github.com/huggingface/transformers/blob/master/examples/pytorch/question-answering/run_seq2seq_qa.py)
- [Text Classification](https://github.com/huggingface/transformers/tree/master/examples/pytorch/text-classification) - *Note*: You will have to slightly adapt the training example here to make it work with an encoder-decoder model.
*Tensorflow*:
- [Summarization](https://github.com/huggingface/transformers/tree/master/examples/tensorflow/summarization)
- [Text Classification](https://github.com/huggingface/transformers/tree/master/examples/tensorflow/text-classification) - *Note*: You will have to slightly adapt the training example here to make it work with an encoder-decoder model.
*JAX/Flax*:
- [Summarization](https://github.com/huggingface/transformers/tree/master/examples/flax/summarization)
- [Text Classification](https://github.com/huggingface/transformers/tree/master/examples/flax/text-classification) - *Note*: You will have to slightly adapt the training example here to make it work with an encoder-decoder model.
## Downstream Performance
TODO: Add table if available
## Computational Complexity
TODO: Add table if available
## More information
We strongly recommend the reader to go carefully through the original paper **[Scale Efficiently: Insights from Pre-training and Fine-tuning Transformers](https://arxiv.org/abs/2109.10686)** to get a more nuanced understanding of this model checkpoint.
As explained in the following [issue](https://github.com/google-research/google-research/issues/986#issuecomment-1035051145), checkpoints including the *sh* or *skv*
model architecture variations have *not* been ported to Transformers as they are probably of limited practical usage and are lacking a more detailed description. Those checkpoints are kept [here](https://huggingface.co/NewT5SharedHeadsSharedKeyValues) as they might be ported potentially in the future. |
mmnga/Llama-3-ELYZA-JP-8B-gguf | mmnga | "2024-06-26T17:55:35Z" | 15,835 | 2 | null | [
"gguf",
"en",
"ja",
"dataset:TFMC/imatrix-dataset-for-japanese-llm",
"license:llama3",
"region:us"
] | null | "2024-06-26T16:36:04Z" |
---
license: llama3
language:
- en
- ja
datasets:
- TFMC/imatrix-dataset-for-japanese-llm
---
# Llama-3-ELYZA-JP-8B-gguf
[elyzaさんが公開しているLlama-3-ELYZA-JP-8B](https://huggingface.co/elyza/Llama-3-ELYZA-JP-8B)のggufフォーマット変換版です。
imatrixのデータは[TFMC/imatrix-dataset-for-japanese-llm](https://huggingface.co/datasets/TFMC/imatrix-dataset-for-japanese-llm)を使用して作成しました。
## Usage
```
git clone https://github.com/ggerganov/llama.cpp.git
cd llama.cpp
make -j
./main -m 'Llama-3-ELYZA-JP-8B-Q4_0.gguf' -n 128 -p 'こんにちわ'
```
|
QuantFactory/llm-compiler-13b-GGUF | QuantFactory | "2024-06-28T13:01:34Z" | 15,833 | 2 | null | [
"gguf",
"text-generation",
"base_model:facebook/llm-compiler-13b",
"license:other",
"region:us"
] | text-generation | "2024-06-28T10:32:34Z" | ---
license: other
base_model: facebook/llm-compiler-13b
pipeline_tag: text-generation
---
# QuantFactory/llm-compiler-13b-GGUF
This is quantized version of [facebook/llm-compiler-13b](https://huggingface.co/facebook/llm-compiler-13b) created using llama.cpp
The information you provide will be collected, stored, processed and shared in accordance with the [Meta Privacy Policy](https://www.facebook.com/privacy/policy/).
**Notice :** LLM Compiler is licensed under the LLM Compiler License, Copyright © Meta Platforms, Inc. All Rights Reserved.
# Introducing Meta Large Language Model Compiler (LLM Compiler), a state-of-the-art LLM for compiler optimization
## Takeaways
* LLM Compiler is a state-of-the-art LLM that builds upon Code Llama with improved performance for code optimization and compiler reasoning.
* LLM Compiler is free for both research and commercial use.
* LLM Compiler is available in two flavors:
* _LLM Compiler_, the foundational models, pretrained on over 500B tokens of LLVM-IR, x86_84, ARM, and CUDA assembly codes and trained to predict the effect of LLVM optimizations;
* and _LLM Compiler FTD_, which is further fine-tuned to predict the best optimizations for code in LLVM assembly to reduce code size, and to disassemble assembly code to LLVM-IR.
* LLM Compiler demonstrates far stronger understanding of compiler optimizations than existing publicly available LLMs, perfectly emulating the compiler 20% of the time.
* LLM Compiler FTD sets state-of-the-art results on the tasks of optimization for code size and disassembly. It achieves a 5.24% code size improvement over -Oz vs GPT-4 Turbo 0.03%, and 0.96 round-trip BLEU score on disassembly vs GPT-4 Turbo 0.43.
---
LINKS
* [LLM Compiler research paper](https://ai.meta.com/research/publications/meta-large-language-model-compiler-foundation-models-of-compiler-optimization/)
* Download the LLM Compiler and LLM Compiler FTD models:
* [llm-compiler-7b](https://huggingface.co/facebook/llm-compiler-7b)
* [llm-compiler-7b-ftd](https://huggingface.co/facebook/llm-compiler-7b-ftd)
* [llm-compiler-13b](https://huggingface.co/facebook/llm-compiler-13b)
* [llm-compiler-13b-ftd](https://huggingface.co/facebook/llm-compiler-13b-ftd)
---
We are excited to announce the release of LLM Compiler, a model targeted at code and compiler optimization tasks. LLM Compiler is built on top of our state-of-the-art large language model, Code Llama, adding capabilities to better understand compiler intermediate representations, assembly language and optimization. LLM Compiler is demonstrated on two difficult tasks: optimizing for code size and decompiling from assembly to the compiler’s intermediate representation. We release these foundation models to accelerate the application of LLMs for code optimization tasks and to enhance developer experience.
We are releasing LLM Compiler under the [LLM Compiler License Agreement](LICENSE.pdf), which incorporates the [Acceptable Use Policy]([https://llama.meta.com/llama3/use-policy]) for Llama Materials.
## How LLM Compiler works
LLM Compiler is a specialization of Code Llama. It is a cutting-edge tool designed to optimize code using deep learning. LLM Compiler has been pre-trained on a vast amount of LLVM assembly (IR), x86_64, ARM, and CUDA assembly codes. LLM Compiler can predict, given a piece of LLVM assembly and a sequence of optimization passes for `opt`, the LLVM optimizer, what the change in code size will be and what the output code will look like after applying these optimizations. It has ‘understood’ the behavior of the optimizing compiler to such a degree that in many cases it can perfectly replicate its output. These capabilities make it ideally suited to compiler optimization tasks.

In addition to this core functionality and to demonstrate its ability to solve complex compiler optimization problems, LLM Compiler has been fine-tuned for two specific downstream tasks:
1. Predicting the best optimization passes for `opt` to use in order to minimize code size, given a piece of LLVM assembly code. \

2. Generating LLVM IR from a piece of x86_64 or ARM assembly code. \

We are releasing LLM Compiler models in two sizes: 7B and 13B parameters. The models have been trained with a context window of 16,000 tokens.
The two models address different serving and latency requirements. The 7B model, for example, can be served on a single GPU and is more suitable for tasks that require low latency, like fine grained optimisation. The 13B model returns the best results.
When using the LLM Compiler models, users must abide by our license and acceptable use policy.

## LLM Compiler performance
We tested the performance of LLM Compiler models for emulating compiler transformations, predicting optimal pass lists and decompiling intermediate representation on hold out test sets and compared them to Code Llama and GPT-4. We compare LLM Compiler Foundation to Code Llama Base and LLM Compiler FTD to Code Llama Instruct.
We evaluate LLM Compiler's ability to emulate compiler optimizations by giving it samples of unoptimized intermediate representation and a randomly generated list of optimizations. We then ask the model to generate the corresponding IR after the optimizations have been applied. In the table below we report the model's accuracy in reproducing the IR we would get from running _opt_. With very little knowledge of IR, Code Llama is unable to achieve high values while the LLM Compiler can generate character-by-character matches of expected assembly in 20% of the cases.
<table>
<tr>
<td>Model
</td>
<td>Size
</td>
<td>Accuracy at emulating compiler optimizations
</td>
</tr>
<tr>
<td>Code Llama
</td>
<td>7B
</td>
<td>1.2%
</td>
</tr>
<tr>
<td>Code Llama
</td>
<td>13B
</td>
<td>0.8%
</td>
</tr>
<tr>
<td>LLM Compiler
</td>
<td>7B
</td>
<td>16%
</td>
</tr>
<tr>
<td>LLM Compiler
</td>
<td>13B
</td>
<td><strong>20%</strong>
</td>
</tr>
</table>
In a similar approach we evaluate our model's ability to optimize IR for code size. In this instance, however, we let the model generate the pass list that is to be used on a given unoptimized IR. We then use this pass list to optimize the particular program using _opt_ and record the binary size. The baseline is the binary size of the program when optimized using -Oz. Only LLM Compiler FTD models provide an improvement over -Oz, with the 13B parameter model marginally outperforming the smaller model, generating smaller object files than -Oz in 61% of cases.
Lastly, we evaluate disassembly performance by giving the model x86 assembly code and ask it to generate the corresponding IR. We then round-trip the model-generated disassembled IR back down to assembly. This enables us to evaluate accuracy of the disassembly by comparing the BLEU score of the original assembly against the round-trip result. LLM Compiler FTD 13B has the highest accuracy of round-tripped assembly (_round trip BLEU_) and most frequently produces perfect disassembly. Code Llama Instruct and GPT-4 Turbo struggle with generating syntactically correct LLVM-IR.
<table>
<tr>
<td>Model
</td>
<td>Size
</td>
<td>Code Size Improvement
</td>
<td>Round trip BLEU
</td>
</tr>
<tr>
<td>GPT-4 Turbo
</td>
<td>
</td>
<td>-0.01%
</td>
<td>0.43
</td>
</tr>
<tr>
<td>Code Llama Inst
</td>
<td>7B
</td>
<td>-0.49%
</td>
<td>0.48
</td>
</tr>
<tr>
<td>Code Llama Inst
</td>
<td>13B
</td>
<td>-0.42%
</td>
<td>0.62
</td>
</tr>
<tr>
<td>LLM Compiler FTD
</td>
<td>7B
</td>
<td>4.77%
</td>
<td>0.95
</td>
</tr>
<tr>
<td>LLM Compiler FTD
</td>
<td>13B
</td>
<td><strong>4.88%</strong>
</td>
<td><strong>0.96</strong>
</td>
</tr>
</table>
## Releasing LLM Compiler
LLMs are being used to make programming easier. They are beginning to be used to make programs more efficient.
At Meta, our conviction is that AI models, especially those designed for coding, thrive best with an open strategy, fostering both innovation and security. Models that are accessible to the public can expedite the creation of novel compiler optimization technologies. In turn, this will allow programs to be more efficient and smaller, enhancing the quality of life for all. By making models such as LLM Compiler available, the whole community can explore their potential, pinpoint problems, and rectify any vulnerabilities.
The model weights are available on Hugging Face.
## Responsible use
Our research paper provides an in-depth look into the development process of the LLM Compiler, the methods we used for our benchmarking tests, and further insights into the model's limitations. It also discusses the issues faced, the steps we took to mitigate them.
Developers are advised to assess their models using evaluation benchmarks specific to compilers. Given that compilers are not bug-free, any suggested compiler optimizations must be rigorously tested. When a model decompiles assembly code, its accuracy should be confirmed.
## The future of generative AI for optimisation
LLM Compiler is designed to support compiler researchers and engineers. But there are still many more use cases to support than what our models can serve. We hope that LLM Compiler will inspire others to leverage LLMs to create new innovative tools for research and commercial products.
### Try LLM Compiler today
* Download the LLM Compiler and LLM Compiler FTD models:
* [llm-compiler-7b](https://huggingface.co/facebook/llm-compiler-7b)
* [llm-compiler-7b-ftd](https://huggingface.co/facebook/llm-compiler-7b-ftd)
* [llm-compiler-13b](https://huggingface.co/facebook/llm-compiler-13b)
* [llm-compiler-13b-ftd](https://huggingface.co/facebook/llm-compiler-13b-ftd)
* Read the research paper
* [LLM Compiler research paper](https://ai.meta.com/research/publications/meta-large-language-model-compiler-foundation-models-of-compiler-optimization/)
# **Model Card**
LLM Compiler is a collection of pretrained and fine-tuned generative text models ranging in scale from 7 billion to 13 billion parameters. This is the repository for the 13 billion parameter foundation model version in the Hugging Face Transformers format. This model is designed for code optimization. Links to other models can be found in the index at the bottom.
| Number of parameters | Base Model | Fine-tuned for code size and dissassembly |
| -------------------- | ---------------------------------------------------------------------------------------- | ----------------------------------------------------------------------------------------------- |
| 7B | [facebook/llm-compiler-7b](https://huggingface.co/facebook/llm-compiler-7b) | [facebook/llm-compiler-7b-ftd](https://huggingface.co/facebook/llm-compiler-7b-ftd) |
| 13B | [facebook/llm-compiler-13b](https://huggingface.co/facebook/llm-compiler-13b) | [facebook/llm-compiler-13b-ftd](https://huggingface.co/facebook/llm-compiler-13b-ftd) |
## Model Use
To use this model, please make sure to install transformers:
```bash
pip install transformers accelerate
```
Example code using each of the model's compiler capabilities may be found in [llm_compiler_demo.py](llm_compiler_demo.py).
The code below demonstrates default capabilities. You may need to set the HuggingFace access token - see (https://huggingface.co/docs/hub/security-tokens).
```python
from transformers import AutoTokenizer
import transformers
import torch
model = "facebook/llm-compiler-13b"
tokenizer = AutoTokenizer.from_pretrained(model)
pipeline = transformers.pipeline(
"text-generation",
model=model,
torch_dtype=torch.float16,
device_map="auto",
)
sequences = pipeline(
'%3 = alloca i32, align 4',
do_sample=True,
top_k=10,
temperature=0.1,
top_p=0.95,
num_return_sequences=1,
eos_token_id=tokenizer.eos_token_id,
max_length=200,
)
for seq in sequences:
print(f"Result: {seq['generated_text']}")
```
## Model Details
*Note: Use of this model is governed by the Meta license. Meta developed and publicly released the LLM Compiler family of large language models (LLMs).
**Model Developers** Meta
**Variations** LLM Compiler comes in two model sizes of 7B, 13B parameters in two flavors, the foundation and instruction fine-tuned for code size and disassembly.
**This repository contains the 13 billion parameter foundation model.**
**Input** Models input text only.
**Example prompt** See `llm_compiler_demo.py` in the repo for examples of the different use cases.
**Output** Models generate text only.
**Model Architecture** LLM Compiler is an auto-regressive language model that uses an optimized transformer architecture.
**Model Dates** LLM Compiler has been trained between January 2024 and June 2024.
**Status** This is a static model trained on an offline dataset.
**License** A custom commercial license is available at: [https://ai.meta.com/resources/models-and-libraries/llama-downloads/](https://ai.meta.com/resources/models-and-libraries/llama-downloads/)
**Research Paper** More information can be found in the paper "[Meta Large Language Model Compiler: Foundation Models of Compiler Optimization](https://ai.meta.com/research/publications/meta-large-language-model-compiler-foundation-models-of-compiler-optimization/)".
## Intended Use
**Intended Use Cases** LLM Compiler is intended for commercial and research use in English, relevant programming languages, LLVM IR, x86_64 assembly and ARM assembly.
**Out-of-Scope Uses** Use in any manner that violates applicable laws or regulations (including trade compliance laws). Use in languages other than English. Use in any other way that is prohibited by the [Acceptable Use Policy](https://llama.meta.com/llama3/use-policy) and Licensing Agreement for LLM Compiler and its variants.
## Hardware and Software
**Training Factors** We used custom training libraries. The training and fine-tuning of the released models have been performed Meta’s Research Super Cluster.
**Carbon Footprint** In aggregate, training all LLM Compiler models required 14K GPU hours of computation on hardware of type A100-80GB (TDP of 350-400W), not including the training of Code Llama. 100% of the estimated tCO2eq emissions were offset by Meta’s sustainability program.
## Training Data
All experiments reported here and the released models have been trained and fine-tuned using the same data as Code Llama with different weights (see Section 2 and Table 1 in the [research paper](https://ai.meta.com/research/publications/llm-compiler-foundation-models-for-compiler-optimization/) for details).
## Evaluation Results
See evaluations for the main models and detailed ablations in Section 3 and safety evaluations in Section 4 of the research paper.
## Ethical Considerations and Limitations
LLM Compiler and its variants are a new technology that carries risks with use. Testing conducted to date has been in English, and has not covered, nor could it cover all scenarios. For these reasons, as with all LLMs, LLM Compilers’s potential outputs cannot be predicted in advance, and the model may in some instances produce inaccurate or objectionable responses to user prompts. Therefore, before deploying any applications of LLM Compiler, developers should perform safety testing and tuning tailored to their specific applications of the model.
Please see the Responsible Use Guide available available at [https://ai.meta.com/llama/responsible-use-guide](https://ai.meta.com/llama/responsible-use-guide). |
PrunaAI/camel-ai-CAMEL-13B-Role-Playing-Data-GGUF-smashed | PrunaAI | "2024-07-01T16:43:13Z" | 15,824 | 0 | null | [
"gguf",
"pruna-ai",
"region:us"
] | null | "2024-07-01T15:08:41Z" | ---
thumbnail: "https://assets-global.website-files.com/646b351987a8d8ce158d1940/64ec9e96b4334c0e1ac41504_Logo%20with%20white%20text.svg"
metrics:
- memory_disk
- memory_inference
- inference_latency
- inference_throughput
- inference_CO2_emissions
- inference_energy_consumption
tags:
- pruna-ai
---
<!-- header start -->
<!-- 200823 -->
<div style="width: auto; margin-left: auto; margin-right: auto">
<a href="https://www.pruna.ai/" target="_blank" rel="noopener noreferrer">
<img src="https://i.imgur.com/eDAlcgk.png" alt="PrunaAI" style="width: 100%; min-width: 400px; display: block; margin: auto;">
</a>
</div>
<!-- header end -->
[](https://twitter.com/PrunaAI)
[](https://github.com/PrunaAI)
[](https://www.linkedin.com/company/93832878/admin/feed/posts/?feedType=following)
[](https://discord.com/invite/vb6SmA3hxu)
## This repo contains GGUF versions of the camel-ai/CAMEL-13B-Role-Playing-Data model.
# Simply make AI models cheaper, smaller, faster, and greener!
- Give a thumbs up if you like this model!
- Contact us and tell us which model to compress next [here](https://www.pruna.ai/contact).
- Request access to easily compress your *own* AI models [here](https://z0halsaff74.typeform.com/pruna-access?typeform-source=www.pruna.ai).
- Read the documentations to know more [here](https://pruna-ai-pruna.readthedocs-hosted.com/en/latest/)
- Join Pruna AI community on Discord [here](https://discord.com/invite/vb6SmA3hxu) to share feedback/suggestions or get help.
**Frequently Asked Questions**
- ***How does the compression work?*** The model is compressed with GGUF.
- ***How does the model quality change?*** The quality of the model output might vary compared to the base model.
- ***What is the model format?*** We use GGUF format.
- ***What calibration data has been used?*** If needed by the compression method, we used WikiText as the calibration data.
- ***How to compress my own models?*** You can request premium access to more compression methods and tech support for your specific use-cases [here](https://z0halsaff74.typeform.com/pruna-access?typeform-source=www.pruna.ai).
# Downloading and running the models
You can download the individual files from the Files & versions section. Here is a list of the different versions we provide. For more info checkout [this chart](https://gist.github.com/Artefact2/b5f810600771265fc1e39442288e8ec9) and [this guide](https://www.reddit.com/r/LocalLLaMA/comments/1ba55rj/overview_of_gguf_quantization_methods/):
| Quant type | Description |
|------------|--------------------------------------------------------------------------------------------|
| Q5_K_M | High quality, recommended. |
| Q5_K_S | High quality, recommended. |
| Q4_K_M | Good quality, uses about 4.83 bits per weight, recommended. |
| Q4_K_S | Slightly lower quality with more space savings, recommended. |
| IQ4_NL | Decent quality, slightly smaller than Q4_K_S with similar performance, recommended. |
| IQ4_XS | Decent quality, smaller than Q4_K_S with similar performance, recommended. |
| Q3_K_L | Lower quality but usable, good for low RAM availability. |
| Q3_K_M | Even lower quality. |
| IQ3_M | Medium-low quality, new method with decent performance comparable to Q3_K_M. |
| IQ3_S | Lower quality, new method with decent performance, recommended over Q3_K_S quant, same size with better performance. |
| Q3_K_S | Low quality, not recommended. |
| IQ3_XS | Lower quality, new method with decent performance, slightly better than Q3_K_S. |
| Q2_K | Very low quality but surprisingly usable. |
## How to download GGUF files ?
**Note for manual downloaders:** You almost never want to clone the entire repo! Multiple different quantisation formats are provided, and most users only want to pick and download a single file.
The following clients/libraries will automatically download models for you, providing a list of available models to choose from:
* LM Studio
* LoLLMS Web UI
* Faraday.dev
- **Option A** - Downloading in `text-generation-webui`:
- **Step 1**: Under Download Model, you can enter the model repo: camel-ai-CAMEL-13B-Role-Playing-Data-GGUF-smashed and below it, a specific filename to download, such as: phi-2.IQ3_M.gguf.
- **Step 2**: Then click Download.
- **Option B** - Downloading on the command line (including multiple files at once):
- **Step 1**: We recommend using the `huggingface-hub` Python library:
```shell
pip3 install huggingface-hub
```
- **Step 2**: Then you can download any individual model file to the current directory, at high speed, with a command like this:
```shell
huggingface-cli download camel-ai-CAMEL-13B-Role-Playing-Data-GGUF-smashed CAMEL-13B-Role-Playing-Data.IQ3_M.gguf --local-dir . --local-dir-use-symlinks False
```
<details>
<summary>More advanced huggingface-cli download usage (click to read)</summary>
Alternatively, you can also download multiple files at once with a pattern:
```shell
huggingface-cli download camel-ai-CAMEL-13B-Role-Playing-Data-GGUF-smashed --local-dir . --local-dir-use-symlinks False --include='*Q4_K*gguf'
```
For more documentation on downloading with `huggingface-cli`, please see: [HF -> Hub Python Library -> Download files -> Download from the CLI](https://huggingface.co/docs/huggingface_hub/guides/download#download-from-the-cli).
To accelerate downloads on fast connections (1Gbit/s or higher), install `hf_transfer`:
```shell
pip3 install hf_transfer
```
And set environment variable `HF_HUB_ENABLE_HF_TRANSFER` to `1`:
```shell
HF_HUB_ENABLE_HF_TRANSFER=1 huggingface-cli download camel-ai-CAMEL-13B-Role-Playing-Data-GGUF-smashed CAMEL-13B-Role-Playing-Data.IQ3_M.gguf --local-dir . --local-dir-use-symlinks False
```
Windows Command Line users: You can set the environment variable by running `set HF_HUB_ENABLE_HF_TRANSFER=1` before the download command.
</details>
<!-- README_GGUF.md-how-to-download end -->
<!-- README_GGUF.md-how-to-run start -->
## How to run model in GGUF format?
- **Option A** - Introductory example with `llama.cpp` command
Make sure you are using `llama.cpp` from commit [d0cee0d](https://github.com/ggerganov/llama.cpp/commit/d0cee0d36d5be95a0d9088b674dbb27354107221) or later.
```shell
./main -ngl 35 -m CAMEL-13B-Role-Playing-Data.IQ3_M.gguf --color -c 32768 --temp 0.7 --repeat_penalty 1.1 -n -1 -p "<s>[INST] {{prompt\}} [/INST]"
```
Change `-ngl 32` to the number of layers to offload to GPU. Remove it if you don't have GPU acceleration.
Change `-c 32768` to the desired sequence length. For extended sequence models - eg 8K, 16K, 32K - the necessary RoPE scaling parameters are read from the GGUF file and set by llama.cpp automatically. Note that longer sequence lengths require much more resources, so you may need to reduce this value.
If you want to have a chat-style conversation, replace the `-p <PROMPT>` argument with `-i -ins`
For other parameters and how to use them, please refer to [the llama.cpp documentation](https://github.com/ggerganov/llama.cpp/blob/master/examples/main/README.md)
- **Option B** - Running in `text-generation-webui`
Further instructions can be found in the text-generation-webui documentation, here: [text-generation-webui/docs/04 ‐ Model Tab.md](https://github.com/oobabooga/text-generation-webui/blob/main/docs/04%20-%20Model%20Tab.md#llamacpp).
- **Option C** - Running from Python code
You can use GGUF models from Python using the [llama-cpp-python](https://github.com/abetlen/llama-cpp-python) or [ctransformers](https://github.com/marella/ctransformers) libraries. Note that at the time of writing (Nov 27th 2023), ctransformers has not been updated for some time and is not compatible with some recent models. Therefore I recommend you use llama-cpp-python.
### How to load this model in Python code, using llama-cpp-python
For full documentation, please see: [llama-cpp-python docs](https://abetlen.github.io/llama-cpp-python/).
#### First install the package
Run one of the following commands, according to your system:
```shell
# Base ctransformers with no GPU acceleration
pip install llama-cpp-python
# With NVidia CUDA acceleration
CMAKE_ARGS="-DLLAMA_CUBLAS=on" pip install llama-cpp-python
# Or with OpenBLAS acceleration
CMAKE_ARGS="-DLLAMA_BLAS=ON -DLLAMA_BLAS_VENDOR=OpenBLAS" pip install llama-cpp-python
# Or with CLBLast acceleration
CMAKE_ARGS="-DLLAMA_CLBLAST=on" pip install llama-cpp-python
# Or with AMD ROCm GPU acceleration (Linux only)
CMAKE_ARGS="-DLLAMA_HIPBLAS=on" pip install llama-cpp-python
# Or with Metal GPU acceleration for macOS systems only
CMAKE_ARGS="-DLLAMA_METAL=on" pip install llama-cpp-python
# In windows, to set the variables CMAKE_ARGS in PowerShell, follow this format; eg for NVidia CUDA:
$env:CMAKE_ARGS = "-DLLAMA_OPENBLAS=on"
pip install llama-cpp-python
```
#### Simple llama-cpp-python example code
```python
from llama_cpp import Llama
# Set gpu_layers to the number of layers to offload to GPU. Set to 0 if no GPU acceleration is available on your system.
llm = Llama(
model_path="./CAMEL-13B-Role-Playing-Data.IQ3_M.gguf", # Download the model file first
n_ctx=32768, # The max sequence length to use - note that longer sequence lengths require much more resources
n_threads=8, # The number of CPU threads to use, tailor to your system and the resulting performance
n_gpu_layers=35 # The number of layers to offload to GPU, if you have GPU acceleration available
)
# Simple inference example
output = llm(
"<s>[INST] {{prompt}} [/INST]", # Prompt
max_tokens=512, # Generate up to 512 tokens
stop=["</s>"], # Example stop token - not necessarily correct for this specific model! Please check before using.
echo=True # Whether to echo the prompt
)
# Chat Completion API
llm = Llama(model_path="./CAMEL-13B-Role-Playing-Data.IQ3_M.gguf", chat_format="llama-2") # Set chat_format according to the model you are using
llm.create_chat_completion(
messages = [
{{"role": "system", "content": "You are a story writing assistant."}},
{{
"role": "user",
"content": "Write a story about llamas."
}}
]
)
```
- **Option D** - Running with LangChain
Here are guides on using llama-cpp-python and ctransformers with LangChain:
* [LangChain + llama-cpp-python](https://python.langchain.com/docs/integrations/llms/llamacpp)
* [LangChain + ctransformers](https://python.langchain.com/docs/integrations/providers/ctransformers)
## Configurations
The configuration info are in `smash_config.json`.
## Credits & License
The license of the smashed model follows the license of the original model. Please check the license of the original model before using this model which provided the base model. The license of the `pruna-engine` is [here](https://pypi.org/project/pruna-engine/) on Pypi.
## Want to compress other models?
- Contact us and tell us which model to compress next [here](https://www.pruna.ai/contact).
- Request access to easily compress your own AI models [here](https://z0halsaff74.typeform.com/pruna-access?typeform-source=www.pruna.ai). |
mradermacher/Llama-3-8B-Instruct-UltraDPO-GGUF | mradermacher | "2024-06-29T04:49:23Z" | 15,814 | 0 | transformers | [
"transformers",
"gguf",
"alignment-handbook",
"trl",
"dpo",
"generated_from_trainer",
"en",
"dataset:HuggingFaceH4/ultrafeedback_binarized",
"base_model:Magpie-Align/Llama-3-8B-Instruct-UltraDPO",
"license:llama3",
"endpoints_compatible",
"region:us"
] | null | "2024-06-29T04:21:44Z" | ---
base_model: Magpie-Align/Llama-3-8B-Instruct-UltraDPO
datasets:
- HuggingFaceH4/ultrafeedback_binarized
language:
- en
library_name: transformers
license: llama3
quantized_by: mradermacher
tags:
- alignment-handbook
- trl
- dpo
- generated_from_trainer
- trl
- dpo
- generated_from_trainer
---
## About
<!-- ### quantize_version: 2 -->
<!-- ### output_tensor_quantised: 1 -->
<!-- ### convert_type: hf -->
<!-- ### vocab_type: -->
<!-- ### tags: -->
static quants of https://huggingface.co/Magpie-Align/Llama-3-8B-Instruct-UltraDPO
<!-- provided-files -->
weighted/imatrix quants seem not to be available (by me) at this time. If they do not show up a week or so after the static ones, I have probably not planned for them. Feel free to request them by opening a Community Discussion.
## Usage
If you are unsure how to use GGUF files, refer to one of [TheBloke's
READMEs](https://huggingface.co/TheBloke/KafkaLM-70B-German-V0.1-GGUF) for
more details, including on how to concatenate multi-part files.
## Provided Quants
(sorted by size, not necessarily quality. IQ-quants are often preferable over similar sized non-IQ quants)
| Link | Type | Size/GB | Notes |
|:-----|:-----|--------:|:------|
| [GGUF](https://huggingface.co/mradermacher/Llama-3-8B-Instruct-UltraDPO-GGUF/resolve/main/Llama-3-8B-Instruct-UltraDPO.Q2_K.gguf) | Q2_K | 3.3 | |
| [GGUF](https://huggingface.co/mradermacher/Llama-3-8B-Instruct-UltraDPO-GGUF/resolve/main/Llama-3-8B-Instruct-UltraDPO.IQ3_XS.gguf) | IQ3_XS | 3.6 | |
| [GGUF](https://huggingface.co/mradermacher/Llama-3-8B-Instruct-UltraDPO-GGUF/resolve/main/Llama-3-8B-Instruct-UltraDPO.Q3_K_S.gguf) | Q3_K_S | 3.8 | |
| [GGUF](https://huggingface.co/mradermacher/Llama-3-8B-Instruct-UltraDPO-GGUF/resolve/main/Llama-3-8B-Instruct-UltraDPO.IQ3_S.gguf) | IQ3_S | 3.8 | beats Q3_K* |
| [GGUF](https://huggingface.co/mradermacher/Llama-3-8B-Instruct-UltraDPO-GGUF/resolve/main/Llama-3-8B-Instruct-UltraDPO.IQ3_M.gguf) | IQ3_M | 3.9 | |
| [GGUF](https://huggingface.co/mradermacher/Llama-3-8B-Instruct-UltraDPO-GGUF/resolve/main/Llama-3-8B-Instruct-UltraDPO.Q3_K_M.gguf) | Q3_K_M | 4.1 | lower quality |
| [GGUF](https://huggingface.co/mradermacher/Llama-3-8B-Instruct-UltraDPO-GGUF/resolve/main/Llama-3-8B-Instruct-UltraDPO.Q3_K_L.gguf) | Q3_K_L | 4.4 | |
| [GGUF](https://huggingface.co/mradermacher/Llama-3-8B-Instruct-UltraDPO-GGUF/resolve/main/Llama-3-8B-Instruct-UltraDPO.IQ4_XS.gguf) | IQ4_XS | 4.6 | |
| [GGUF](https://huggingface.co/mradermacher/Llama-3-8B-Instruct-UltraDPO-GGUF/resolve/main/Llama-3-8B-Instruct-UltraDPO.Q4_K_S.gguf) | Q4_K_S | 4.8 | fast, recommended |
| [GGUF](https://huggingface.co/mradermacher/Llama-3-8B-Instruct-UltraDPO-GGUF/resolve/main/Llama-3-8B-Instruct-UltraDPO.Q4_K_M.gguf) | Q4_K_M | 5.0 | fast, recommended |
| [GGUF](https://huggingface.co/mradermacher/Llama-3-8B-Instruct-UltraDPO-GGUF/resolve/main/Llama-3-8B-Instruct-UltraDPO.Q5_K_S.gguf) | Q5_K_S | 5.7 | |
| [GGUF](https://huggingface.co/mradermacher/Llama-3-8B-Instruct-UltraDPO-GGUF/resolve/main/Llama-3-8B-Instruct-UltraDPO.Q5_K_M.gguf) | Q5_K_M | 5.8 | |
| [GGUF](https://huggingface.co/mradermacher/Llama-3-8B-Instruct-UltraDPO-GGUF/resolve/main/Llama-3-8B-Instruct-UltraDPO.Q6_K.gguf) | Q6_K | 6.7 | very good quality |
| [GGUF](https://huggingface.co/mradermacher/Llama-3-8B-Instruct-UltraDPO-GGUF/resolve/main/Llama-3-8B-Instruct-UltraDPO.Q8_0.gguf) | Q8_0 | 8.6 | fast, best quality |
| [GGUF](https://huggingface.co/mradermacher/Llama-3-8B-Instruct-UltraDPO-GGUF/resolve/main/Llama-3-8B-Instruct-UltraDPO.f16.gguf) | f16 | 16.2 | 16 bpw, overkill |
Here is a handy graph by ikawrakow comparing some lower-quality quant
types (lower is better):

And here are Artefact2's thoughts on the matter:
https://gist.github.com/Artefact2/b5f810600771265fc1e39442288e8ec9
## FAQ / Model Request
See https://huggingface.co/mradermacher/model_requests for some answers to
questions you might have and/or if you want some other model quantized.
## Thanks
I thank my company, [nethype GmbH](https://www.nethype.de/), for letting
me use its servers and providing upgrades to my workstation to enable
this work in my free time.
<!-- end -->
|
mradermacher/Swallow-7b-NVE-instruct-hf-GGUF | mradermacher | "2024-06-30T10:01:26Z" | 15,808 | 0 | transformers | [
"transformers",
"gguf",
"en",
"ja",
"base_model:tokyotech-llm/Swallow-7b-NVE-instruct-hf",
"license:llama2",
"endpoints_compatible",
"region:us"
] | null | "2024-06-29T18:14:15Z" | ---
base_model: tokyotech-llm/Swallow-7b-NVE-instruct-hf
language:
- en
- ja
library_name: transformers
license: llama2
model_type: llama
quantized_by: mradermacher
---
## About
<!-- ### quantize_version: 2 -->
<!-- ### output_tensor_quantised: 1 -->
<!-- ### convert_type: hf -->
<!-- ### vocab_type: -->
<!-- ### tags: -->
static quants of https://huggingface.co/tokyotech-llm/Swallow-7b-NVE-instruct-hf
<!-- provided-files -->
weighted/imatrix quants are available at https://huggingface.co/mradermacher/Swallow-7b-NVE-instruct-hf-i1-GGUF
## Usage
If you are unsure how to use GGUF files, refer to one of [TheBloke's
READMEs](https://huggingface.co/TheBloke/KafkaLM-70B-German-V0.1-GGUF) for
more details, including on how to concatenate multi-part files.
## Provided Quants
(sorted by size, not necessarily quality. IQ-quants are often preferable over similar sized non-IQ quants)
| Link | Type | Size/GB | Notes |
|:-----|:-----|--------:|:------|
| [GGUF](https://huggingface.co/mradermacher/Swallow-7b-NVE-instruct-hf-GGUF/resolve/main/Swallow-7b-NVE-instruct-hf.Q2_K.gguf) | Q2_K | 2.6 | |
| [GGUF](https://huggingface.co/mradermacher/Swallow-7b-NVE-instruct-hf-GGUF/resolve/main/Swallow-7b-NVE-instruct-hf.IQ3_XS.gguf) | IQ3_XS | 2.9 | |
| [GGUF](https://huggingface.co/mradermacher/Swallow-7b-NVE-instruct-hf-GGUF/resolve/main/Swallow-7b-NVE-instruct-hf.IQ3_S.gguf) | IQ3_S | 3.0 | beats Q3_K* |
| [GGUF](https://huggingface.co/mradermacher/Swallow-7b-NVE-instruct-hf-GGUF/resolve/main/Swallow-7b-NVE-instruct-hf.Q3_K_S.gguf) | Q3_K_S | 3.0 | |
| [GGUF](https://huggingface.co/mradermacher/Swallow-7b-NVE-instruct-hf-GGUF/resolve/main/Swallow-7b-NVE-instruct-hf.IQ3_M.gguf) | IQ3_M | 3.2 | |
| [GGUF](https://huggingface.co/mradermacher/Swallow-7b-NVE-instruct-hf-GGUF/resolve/main/Swallow-7b-NVE-instruct-hf.Q3_K_M.gguf) | Q3_K_M | 3.4 | lower quality |
| [GGUF](https://huggingface.co/mradermacher/Swallow-7b-NVE-instruct-hf-GGUF/resolve/main/Swallow-7b-NVE-instruct-hf.Q3_K_L.gguf) | Q3_K_L | 3.7 | |
| [GGUF](https://huggingface.co/mradermacher/Swallow-7b-NVE-instruct-hf-GGUF/resolve/main/Swallow-7b-NVE-instruct-hf.IQ4_XS.gguf) | IQ4_XS | 3.7 | |
| [GGUF](https://huggingface.co/mradermacher/Swallow-7b-NVE-instruct-hf-GGUF/resolve/main/Swallow-7b-NVE-instruct-hf.Q4_K_S.gguf) | Q4_K_S | 4.0 | fast, recommended |
| [GGUF](https://huggingface.co/mradermacher/Swallow-7b-NVE-instruct-hf-GGUF/resolve/main/Swallow-7b-NVE-instruct-hf.Q4_K_M.gguf) | Q4_K_M | 4.2 | fast, recommended |
| [GGUF](https://huggingface.co/mradermacher/Swallow-7b-NVE-instruct-hf-GGUF/resolve/main/Swallow-7b-NVE-instruct-hf.Q5_K_S.gguf) | Q5_K_S | 4.8 | |
| [GGUF](https://huggingface.co/mradermacher/Swallow-7b-NVE-instruct-hf-GGUF/resolve/main/Swallow-7b-NVE-instruct-hf.Q5_K_M.gguf) | Q5_K_M | 4.9 | |
| [GGUF](https://huggingface.co/mradermacher/Swallow-7b-NVE-instruct-hf-GGUF/resolve/main/Swallow-7b-NVE-instruct-hf.Q6_K.gguf) | Q6_K | 5.6 | very good quality |
| [GGUF](https://huggingface.co/mradermacher/Swallow-7b-NVE-instruct-hf-GGUF/resolve/main/Swallow-7b-NVE-instruct-hf.Q8_0.gguf) | Q8_0 | 7.3 | fast, best quality |
| [GGUF](https://huggingface.co/mradermacher/Swallow-7b-NVE-instruct-hf-GGUF/resolve/main/Swallow-7b-NVE-instruct-hf.f16.gguf) | f16 | 13.6 | 16 bpw, overkill |
Here is a handy graph by ikawrakow comparing some lower-quality quant
types (lower is better):

And here are Artefact2's thoughts on the matter:
https://gist.github.com/Artefact2/b5f810600771265fc1e39442288e8ec9
## FAQ / Model Request
See https://huggingface.co/mradermacher/model_requests for some answers to
questions you might have and/or if you want some other model quantized.
## Thanks
I thank my company, [nethype GmbH](https://www.nethype.de/), for letting
me use its servers and providing upgrades to my workstation to enable
this work in my free time.
<!-- end -->
|
jinaai/jina-embeddings-v2-base-zh | jinaai | "2024-06-14T03:07:15Z" | 15,807 | 137 | sentence-transformers | [
"sentence-transformers",
"pytorch",
"onnx",
"safetensors",
"bert",
"feature-extraction",
"sentence-similarity",
"mteb",
"transformers",
"transformers.js",
"custom_code",
"en",
"zh",
"arxiv:2108.12409",
"arxiv:2402.17016",
"license:apache-2.0",
"model-index",
"autotrain_compatible",
"region:us"
] | feature-extraction | "2024-01-10T03:39:40Z" | ---
tags:
- sentence-transformers
- feature-extraction
- sentence-similarity
- mteb
- transformers
- transformers.js
inference: false
license: apache-2.0
language:
- en
- zh
model-index:
- name: jina-embeddings-v2-base-zh
results:
- task:
type: STS
dataset:
type: C-MTEB/AFQMC
name: MTEB AFQMC
config: default
split: validation
revision: None
metrics:
- type: cos_sim_pearson
value: 48.51403119231363
- type: cos_sim_spearman
value: 50.5928547846445
- type: euclidean_pearson
value: 48.750436310559074
- type: euclidean_spearman
value: 50.50950238691385
- type: manhattan_pearson
value: 48.7866189440328
- type: manhattan_spearman
value: 50.58692402017165
- task:
type: STS
dataset:
type: C-MTEB/ATEC
name: MTEB ATEC
config: default
split: test
revision: None
metrics:
- type: cos_sim_pearson
value: 50.25985700105725
- type: cos_sim_spearman
value: 51.28815934593989
- type: euclidean_pearson
value: 52.70329248799904
- type: euclidean_spearman
value: 50.94101139559258
- type: manhattan_pearson
value: 52.6647237400892
- type: manhattan_spearman
value: 50.922441325406176
- task:
type: Classification
dataset:
type: mteb/amazon_reviews_multi
name: MTEB AmazonReviewsClassification (zh)
config: zh
split: test
revision: 1399c76144fd37290681b995c656ef9b2e06e26d
metrics:
- type: accuracy
value: 34.944
- type: f1
value: 34.06478860660109
- task:
type: STS
dataset:
type: C-MTEB/BQ
name: MTEB BQ
config: default
split: test
revision: None
metrics:
- type: cos_sim_pearson
value: 65.15667035488342
- type: cos_sim_spearman
value: 66.07110142081
- type: euclidean_pearson
value: 60.447598102249714
- type: euclidean_spearman
value: 61.826575796578766
- type: manhattan_pearson
value: 60.39364279354984
- type: manhattan_spearman
value: 61.78743491223281
- task:
type: Clustering
dataset:
type: C-MTEB/CLSClusteringP2P
name: MTEB CLSClusteringP2P
config: default
split: test
revision: None
metrics:
- type: v_measure
value: 39.96714175391701
- task:
type: Clustering
dataset:
type: C-MTEB/CLSClusteringS2S
name: MTEB CLSClusteringS2S
config: default
split: test
revision: None
metrics:
- type: v_measure
value: 38.39863566717934
- task:
type: Reranking
dataset:
type: C-MTEB/CMedQAv1-reranking
name: MTEB CMedQAv1
config: default
split: test
revision: None
metrics:
- type: map
value: 83.63680381780644
- type: mrr
value: 86.16476190476192
- task:
type: Reranking
dataset:
type: C-MTEB/CMedQAv2-reranking
name: MTEB CMedQAv2
config: default
split: test
revision: None
metrics:
- type: map
value: 83.74350667859487
- type: mrr
value: 86.10388888888889
- task:
type: Retrieval
dataset:
type: C-MTEB/CmedqaRetrieval
name: MTEB CmedqaRetrieval
config: default
split: dev
revision: None
metrics:
- type: map_at_1
value: 22.072
- type: map_at_10
value: 32.942
- type: map_at_100
value: 34.768
- type: map_at_1000
value: 34.902
- type: map_at_3
value: 29.357
- type: map_at_5
value: 31.236000000000004
- type: mrr_at_1
value: 34.259
- type: mrr_at_10
value: 41.957
- type: mrr_at_100
value: 42.982
- type: mrr_at_1000
value: 43.042
- type: mrr_at_3
value: 39.722
- type: mrr_at_5
value: 40.898
- type: ndcg_at_1
value: 34.259
- type: ndcg_at_10
value: 39.153
- type: ndcg_at_100
value: 46.493
- type: ndcg_at_1000
value: 49.01
- type: ndcg_at_3
value: 34.636
- type: ndcg_at_5
value: 36.278
- type: precision_at_1
value: 34.259
- type: precision_at_10
value: 8.815000000000001
- type: precision_at_100
value: 1.474
- type: precision_at_1000
value: 0.179
- type: precision_at_3
value: 19.73
- type: precision_at_5
value: 14.174000000000001
- type: recall_at_1
value: 22.072
- type: recall_at_10
value: 48.484
- type: recall_at_100
value: 79.035
- type: recall_at_1000
value: 96.15
- type: recall_at_3
value: 34.607
- type: recall_at_5
value: 40.064
- task:
type: PairClassification
dataset:
type: C-MTEB/CMNLI
name: MTEB Cmnli
config: default
split: validation
revision: None
metrics:
- type: cos_sim_accuracy
value: 76.7047504509922
- type: cos_sim_ap
value: 85.26649874800871
- type: cos_sim_f1
value: 78.13528724646915
- type: cos_sim_precision
value: 71.57587548638132
- type: cos_sim_recall
value: 86.01823708206688
- type: dot_accuracy
value: 70.13830426939266
- type: dot_ap
value: 77.01510412382171
- type: dot_f1
value: 73.56710042713817
- type: dot_precision
value: 63.955094991364426
- type: dot_recall
value: 86.57937806873977
- type: euclidean_accuracy
value: 75.53818400481059
- type: euclidean_ap
value: 84.34668448241264
- type: euclidean_f1
value: 77.51741608613047
- type: euclidean_precision
value: 70.65614777756399
- type: euclidean_recall
value: 85.85457096095394
- type: manhattan_accuracy
value: 75.49007817197835
- type: manhattan_ap
value: 84.40297506704299
- type: manhattan_f1
value: 77.63185324160932
- type: manhattan_precision
value: 70.03949595636637
- type: manhattan_recall
value: 87.07037643207856
- type: max_accuracy
value: 76.7047504509922
- type: max_ap
value: 85.26649874800871
- type: max_f1
value: 78.13528724646915
- task:
type: Retrieval
dataset:
type: C-MTEB/CovidRetrieval
name: MTEB CovidRetrieval
config: default
split: dev
revision: None
metrics:
- type: map_at_1
value: 69.178
- type: map_at_10
value: 77.523
- type: map_at_100
value: 77.793
- type: map_at_1000
value: 77.79899999999999
- type: map_at_3
value: 75.878
- type: map_at_5
value: 76.849
- type: mrr_at_1
value: 69.44200000000001
- type: mrr_at_10
value: 77.55
- type: mrr_at_100
value: 77.819
- type: mrr_at_1000
value: 77.826
- type: mrr_at_3
value: 75.957
- type: mrr_at_5
value: 76.916
- type: ndcg_at_1
value: 69.44200000000001
- type: ndcg_at_10
value: 81.217
- type: ndcg_at_100
value: 82.45
- type: ndcg_at_1000
value: 82.636
- type: ndcg_at_3
value: 77.931
- type: ndcg_at_5
value: 79.655
- type: precision_at_1
value: 69.44200000000001
- type: precision_at_10
value: 9.357
- type: precision_at_100
value: 0.993
- type: precision_at_1000
value: 0.101
- type: precision_at_3
value: 28.1
- type: precision_at_5
value: 17.724
- type: recall_at_1
value: 69.178
- type: recall_at_10
value: 92.624
- type: recall_at_100
value: 98.209
- type: recall_at_1000
value: 99.684
- type: recall_at_3
value: 83.772
- type: recall_at_5
value: 87.882
- task:
type: Retrieval
dataset:
type: C-MTEB/DuRetrieval
name: MTEB DuRetrieval
config: default
split: dev
revision: None
metrics:
- type: map_at_1
value: 25.163999999999998
- type: map_at_10
value: 76.386
- type: map_at_100
value: 79.339
- type: map_at_1000
value: 79.39500000000001
- type: map_at_3
value: 52.959
- type: map_at_5
value: 66.59
- type: mrr_at_1
value: 87.9
- type: mrr_at_10
value: 91.682
- type: mrr_at_100
value: 91.747
- type: mrr_at_1000
value: 91.751
- type: mrr_at_3
value: 91.267
- type: mrr_at_5
value: 91.527
- type: ndcg_at_1
value: 87.9
- type: ndcg_at_10
value: 84.569
- type: ndcg_at_100
value: 87.83800000000001
- type: ndcg_at_1000
value: 88.322
- type: ndcg_at_3
value: 83.473
- type: ndcg_at_5
value: 82.178
- type: precision_at_1
value: 87.9
- type: precision_at_10
value: 40.605000000000004
- type: precision_at_100
value: 4.752
- type: precision_at_1000
value: 0.488
- type: precision_at_3
value: 74.9
- type: precision_at_5
value: 62.96000000000001
- type: recall_at_1
value: 25.163999999999998
- type: recall_at_10
value: 85.97399999999999
- type: recall_at_100
value: 96.63000000000001
- type: recall_at_1000
value: 99.016
- type: recall_at_3
value: 55.611999999999995
- type: recall_at_5
value: 71.936
- task:
type: Retrieval
dataset:
type: C-MTEB/EcomRetrieval
name: MTEB EcomRetrieval
config: default
split: dev
revision: None
metrics:
- type: map_at_1
value: 48.6
- type: map_at_10
value: 58.831
- type: map_at_100
value: 59.427
- type: map_at_1000
value: 59.44199999999999
- type: map_at_3
value: 56.383
- type: map_at_5
value: 57.753
- type: mrr_at_1
value: 48.6
- type: mrr_at_10
value: 58.831
- type: mrr_at_100
value: 59.427
- type: mrr_at_1000
value: 59.44199999999999
- type: mrr_at_3
value: 56.383
- type: mrr_at_5
value: 57.753
- type: ndcg_at_1
value: 48.6
- type: ndcg_at_10
value: 63.951
- type: ndcg_at_100
value: 66.72200000000001
- type: ndcg_at_1000
value: 67.13900000000001
- type: ndcg_at_3
value: 58.882
- type: ndcg_at_5
value: 61.373
- type: precision_at_1
value: 48.6
- type: precision_at_10
value: 8.01
- type: precision_at_100
value: 0.928
- type: precision_at_1000
value: 0.096
- type: precision_at_3
value: 22.033
- type: precision_at_5
value: 14.44
- type: recall_at_1
value: 48.6
- type: recall_at_10
value: 80.10000000000001
- type: recall_at_100
value: 92.80000000000001
- type: recall_at_1000
value: 96.1
- type: recall_at_3
value: 66.10000000000001
- type: recall_at_5
value: 72.2
- task:
type: Classification
dataset:
type: C-MTEB/IFlyTek-classification
name: MTEB IFlyTek
config: default
split: validation
revision: None
metrics:
- type: accuracy
value: 47.36437091188918
- type: f1
value: 36.60946954228577
- task:
type: Classification
dataset:
type: C-MTEB/JDReview-classification
name: MTEB JDReview
config: default
split: test
revision: None
metrics:
- type: accuracy
value: 79.5684803001876
- type: ap
value: 42.671935929201524
- type: f1
value: 73.31912729103752
- task:
type: STS
dataset:
type: C-MTEB/LCQMC
name: MTEB LCQMC
config: default
split: test
revision: None
metrics:
- type: cos_sim_pearson
value: 68.62670112113864
- type: cos_sim_spearman
value: 75.74009123170768
- type: euclidean_pearson
value: 73.93002595958237
- type: euclidean_spearman
value: 75.35222935003587
- type: manhattan_pearson
value: 73.89870445158144
- type: manhattan_spearman
value: 75.31714936339398
- task:
type: Reranking
dataset:
type: C-MTEB/Mmarco-reranking
name: MTEB MMarcoReranking
config: default
split: dev
revision: None
metrics:
- type: map
value: 31.5372713650176
- type: mrr
value: 30.163095238095238
- task:
type: Retrieval
dataset:
type: C-MTEB/MMarcoRetrieval
name: MTEB MMarcoRetrieval
config: default
split: dev
revision: None
metrics:
- type: map_at_1
value: 65.054
- type: map_at_10
value: 74.156
- type: map_at_100
value: 74.523
- type: map_at_1000
value: 74.535
- type: map_at_3
value: 72.269
- type: map_at_5
value: 73.41
- type: mrr_at_1
value: 67.24900000000001
- type: mrr_at_10
value: 74.78399999999999
- type: mrr_at_100
value: 75.107
- type: mrr_at_1000
value: 75.117
- type: mrr_at_3
value: 73.13499999999999
- type: mrr_at_5
value: 74.13499999999999
- type: ndcg_at_1
value: 67.24900000000001
- type: ndcg_at_10
value: 77.96300000000001
- type: ndcg_at_100
value: 79.584
- type: ndcg_at_1000
value: 79.884
- type: ndcg_at_3
value: 74.342
- type: ndcg_at_5
value: 76.278
- type: precision_at_1
value: 67.24900000000001
- type: precision_at_10
value: 9.466
- type: precision_at_100
value: 1.027
- type: precision_at_1000
value: 0.105
- type: precision_at_3
value: 27.955999999999996
- type: precision_at_5
value: 17.817
- type: recall_at_1
value: 65.054
- type: recall_at_10
value: 89.113
- type: recall_at_100
value: 96.369
- type: recall_at_1000
value: 98.714
- type: recall_at_3
value: 79.45400000000001
- type: recall_at_5
value: 84.06
- task:
type: Classification
dataset:
type: mteb/amazon_massive_intent
name: MTEB MassiveIntentClassification (zh-CN)
config: zh-CN
split: test
revision: 31efe3c427b0bae9c22cbb560b8f15491cc6bed7
metrics:
- type: accuracy
value: 68.1977135171486
- type: f1
value: 67.23114308718404
- task:
type: Classification
dataset:
type: mteb/amazon_massive_scenario
name: MTEB MassiveScenarioClassification (zh-CN)
config: zh-CN
split: test
revision: 7d571f92784cd94a019292a1f45445077d0ef634
metrics:
- type: accuracy
value: 71.92669804976462
- type: f1
value: 72.90628475628779
- task:
type: Retrieval
dataset:
type: C-MTEB/MedicalRetrieval
name: MTEB MedicalRetrieval
config: default
split: dev
revision: None
metrics:
- type: map_at_1
value: 49.2
- type: map_at_10
value: 54.539
- type: map_at_100
value: 55.135
- type: map_at_1000
value: 55.19199999999999
- type: map_at_3
value: 53.383
- type: map_at_5
value: 54.142999999999994
- type: mrr_at_1
value: 49.2
- type: mrr_at_10
value: 54.539
- type: mrr_at_100
value: 55.135999999999996
- type: mrr_at_1000
value: 55.19199999999999
- type: mrr_at_3
value: 53.383
- type: mrr_at_5
value: 54.142999999999994
- type: ndcg_at_1
value: 49.2
- type: ndcg_at_10
value: 57.123000000000005
- type: ndcg_at_100
value: 60.21300000000001
- type: ndcg_at_1000
value: 61.915
- type: ndcg_at_3
value: 54.772
- type: ndcg_at_5
value: 56.157999999999994
- type: precision_at_1
value: 49.2
- type: precision_at_10
value: 6.52
- type: precision_at_100
value: 0.8009999999999999
- type: precision_at_1000
value: 0.094
- type: precision_at_3
value: 19.6
- type: precision_at_5
value: 12.44
- type: recall_at_1
value: 49.2
- type: recall_at_10
value: 65.2
- type: recall_at_100
value: 80.10000000000001
- type: recall_at_1000
value: 93.89999999999999
- type: recall_at_3
value: 58.8
- type: recall_at_5
value: 62.2
- task:
type: Classification
dataset:
type: C-MTEB/MultilingualSentiment-classification
name: MTEB MultilingualSentiment
config: default
split: validation
revision: None
metrics:
- type: accuracy
value: 63.29333333333334
- type: f1
value: 63.03293854259612
- task:
type: PairClassification
dataset:
type: C-MTEB/OCNLI
name: MTEB Ocnli
config: default
split: validation
revision: None
metrics:
- type: cos_sim_accuracy
value: 75.69030860855442
- type: cos_sim_ap
value: 80.6157833772759
- type: cos_sim_f1
value: 77.87524366471735
- type: cos_sim_precision
value: 72.3076923076923
- type: cos_sim_recall
value: 84.37170010559663
- type: dot_accuracy
value: 67.78559826746074
- type: dot_ap
value: 72.00871467527499
- type: dot_f1
value: 72.58722247394654
- type: dot_precision
value: 63.57142857142857
- type: dot_recall
value: 84.58289334741288
- type: euclidean_accuracy
value: 75.20303194369248
- type: euclidean_ap
value: 80.98587256415605
- type: euclidean_f1
value: 77.26396917148362
- type: euclidean_precision
value: 71.03631532329496
- type: euclidean_recall
value: 84.68848996832101
- type: manhattan_accuracy
value: 75.20303194369248
- type: manhattan_ap
value: 80.93460699513219
- type: manhattan_f1
value: 77.124773960217
- type: manhattan_precision
value: 67.43083003952569
- type: manhattan_recall
value: 90.07391763463569
- type: max_accuracy
value: 75.69030860855442
- type: max_ap
value: 80.98587256415605
- type: max_f1
value: 77.87524366471735
- task:
type: Classification
dataset:
type: C-MTEB/OnlineShopping-classification
name: MTEB OnlineShopping
config: default
split: test
revision: None
metrics:
- type: accuracy
value: 87.00000000000001
- type: ap
value: 83.24372135949511
- type: f1
value: 86.95554191530607
- task:
type: STS
dataset:
type: C-MTEB/PAWSX
name: MTEB PAWSX
config: default
split: test
revision: None
metrics:
- type: cos_sim_pearson
value: 37.57616811591219
- type: cos_sim_spearman
value: 41.490259084930045
- type: euclidean_pearson
value: 38.9155043692188
- type: euclidean_spearman
value: 39.16056534305623
- type: manhattan_pearson
value: 38.76569892264335
- type: manhattan_spearman
value: 38.99891685590743
- task:
type: STS
dataset:
type: C-MTEB/QBQTC
name: MTEB QBQTC
config: default
split: test
revision: None
metrics:
- type: cos_sim_pearson
value: 35.44858610359665
- type: cos_sim_spearman
value: 38.11128146262466
- type: euclidean_pearson
value: 31.928644189822457
- type: euclidean_spearman
value: 34.384936631696554
- type: manhattan_pearson
value: 31.90586687414376
- type: manhattan_spearman
value: 34.35770153777186
- task:
type: STS
dataset:
type: mteb/sts22-crosslingual-sts
name: MTEB STS22 (zh)
config: zh
split: test
revision: 6d1ba47164174a496b7fa5d3569dae26a6813b80
metrics:
- type: cos_sim_pearson
value: 66.54931957553592
- type: cos_sim_spearman
value: 69.25068863016632
- type: euclidean_pearson
value: 50.26525596106869
- type: euclidean_spearman
value: 63.83352741910006
- type: manhattan_pearson
value: 49.98798282198196
- type: manhattan_spearman
value: 63.87649521907841
- task:
type: STS
dataset:
type: C-MTEB/STSB
name: MTEB STSB
config: default
split: test
revision: None
metrics:
- type: cos_sim_pearson
value: 82.52782476625825
- type: cos_sim_spearman
value: 82.55618986168398
- type: euclidean_pearson
value: 78.48190631687673
- type: euclidean_spearman
value: 78.39479731354655
- type: manhattan_pearson
value: 78.51176592165885
- type: manhattan_spearman
value: 78.42363787303265
- task:
type: Reranking
dataset:
type: C-MTEB/T2Reranking
name: MTEB T2Reranking
config: default
split: dev
revision: None
metrics:
- type: map
value: 67.36693873615643
- type: mrr
value: 77.83847701797939
- task:
type: Retrieval
dataset:
type: C-MTEB/T2Retrieval
name: MTEB T2Retrieval
config: default
split: dev
revision: None
metrics:
- type: map_at_1
value: 25.795
- type: map_at_10
value: 72.258
- type: map_at_100
value: 76.049
- type: map_at_1000
value: 76.134
- type: map_at_3
value: 50.697
- type: map_at_5
value: 62.324999999999996
- type: mrr_at_1
value: 86.634
- type: mrr_at_10
value: 89.792
- type: mrr_at_100
value: 89.91900000000001
- type: mrr_at_1000
value: 89.923
- type: mrr_at_3
value: 89.224
- type: mrr_at_5
value: 89.608
- type: ndcg_at_1
value: 86.634
- type: ndcg_at_10
value: 80.589
- type: ndcg_at_100
value: 84.812
- type: ndcg_at_1000
value: 85.662
- type: ndcg_at_3
value: 82.169
- type: ndcg_at_5
value: 80.619
- type: precision_at_1
value: 86.634
- type: precision_at_10
value: 40.389
- type: precision_at_100
value: 4.93
- type: precision_at_1000
value: 0.513
- type: precision_at_3
value: 72.104
- type: precision_at_5
value: 60.425
- type: recall_at_1
value: 25.795
- type: recall_at_10
value: 79.565
- type: recall_at_100
value: 93.24799999999999
- type: recall_at_1000
value: 97.595
- type: recall_at_3
value: 52.583999999999996
- type: recall_at_5
value: 66.175
- task:
type: Classification
dataset:
type: C-MTEB/TNews-classification
name: MTEB TNews
config: default
split: validation
revision: None
metrics:
- type: accuracy
value: 47.648999999999994
- type: f1
value: 46.28925837008413
- task:
type: Clustering
dataset:
type: C-MTEB/ThuNewsClusteringP2P
name: MTEB ThuNewsClusteringP2P
config: default
split: test
revision: None
metrics:
- type: v_measure
value: 54.07641891287953
- task:
type: Clustering
dataset:
type: C-MTEB/ThuNewsClusteringS2S
name: MTEB ThuNewsClusteringS2S
config: default
split: test
revision: None
metrics:
- type: v_measure
value: 53.423702062353954
- task:
type: Retrieval
dataset:
type: C-MTEB/VideoRetrieval
name: MTEB VideoRetrieval
config: default
split: dev
revision: None
metrics:
- type: map_at_1
value: 55.7
- type: map_at_10
value: 65.923
- type: map_at_100
value: 66.42
- type: map_at_1000
value: 66.431
- type: map_at_3
value: 63.9
- type: map_at_5
value: 65.225
- type: mrr_at_1
value: 55.60000000000001
- type: mrr_at_10
value: 65.873
- type: mrr_at_100
value: 66.36999999999999
- type: mrr_at_1000
value: 66.381
- type: mrr_at_3
value: 63.849999999999994
- type: mrr_at_5
value: 65.17500000000001
- type: ndcg_at_1
value: 55.7
- type: ndcg_at_10
value: 70.621
- type: ndcg_at_100
value: 72.944
- type: ndcg_at_1000
value: 73.25399999999999
- type: ndcg_at_3
value: 66.547
- type: ndcg_at_5
value: 68.93599999999999
- type: precision_at_1
value: 55.7
- type: precision_at_10
value: 8.52
- type: precision_at_100
value: 0.958
- type: precision_at_1000
value: 0.098
- type: precision_at_3
value: 24.733
- type: precision_at_5
value: 16
- type: recall_at_1
value: 55.7
- type: recall_at_10
value: 85.2
- type: recall_at_100
value: 95.8
- type: recall_at_1000
value: 98.3
- type: recall_at_3
value: 74.2
- type: recall_at_5
value: 80
- task:
type: Classification
dataset:
type: C-MTEB/waimai-classification
name: MTEB Waimai
config: default
split: test
revision: None
metrics:
- type: accuracy
value: 84.54
- type: ap
value: 66.13603199670062
- type: f1
value: 82.61420654584116
---
<!-- TODO: add evaluation results here -->
<br><br>
<p align="center">
<img src="https://aeiljuispo.cloudimg.io/v7/https://cdn-uploads.huggingface.co/production/uploads/603763514de52ff951d89793/AFoybzd5lpBQXEBrQHuTt.png?w=200&h=200&f=face" alt="Finetuner logo: Finetuner helps you to create experiments in order to improve embeddings on search tasks. It accompanies you to deliver the last mile of performance-tuning for neural search applications." width="150px">
</p>
<p align="center">
<b>The text embedding set trained by <a href="https://jina.ai/"><b>Jina AI</b></a>.</b>
</p>
## Quick Start
The easiest way to starting using `jina-embeddings-v2-base-zh` is to use Jina AI's [Embedding API](https://jina.ai/embeddings/).
## Intended Usage & Model Info
`jina-embeddings-v2-base-zh` is a Chinese/English bilingual text **embedding model** supporting **8192 sequence length**.
It is based on a BERT architecture (JinaBERT) that supports the symmetric bidirectional variant of [ALiBi](https://arxiv.org/abs/2108.12409) to allow longer sequence length.
We have designed it for high performance in mono-lingual & cross-lingual applications and trained it specifically to support mixed Chinese-English input without bias.
Additionally, we provide the following embedding models:
`jina-embeddings-v2-base-zh` 是支持中英双语的**文本向量**模型,它支持长达**8192字符**的文本编码。
该模型的研发基于BERT架构(JinaBERT),JinaBERT是在BERT架构基础上的改进,首次将[ALiBi](https://arxiv.org/abs/2108.12409)应用到编码器架构中以支持更长的序列。
不同于以往的单语言/多语言向量模型,我们设计双语模型来更好的支持单语言(中搜中)以及跨语言(中搜英)文档检索。
除此之外,我们也提供其它向量模型:
- [`jina-embeddings-v2-small-en`](https://huggingface.co/jinaai/jina-embeddings-v2-small-en): 33 million parameters.
- [`jina-embeddings-v2-base-en`](https://huggingface.co/jinaai/jina-embeddings-v2-base-en): 137 million parameters.
- [`jina-embeddings-v2-base-zh`](https://huggingface.co/jinaai/jina-embeddings-v2-base-zh): 161 million parameters Chinese-English Bilingual embeddings **(you are here)**.
- [`jina-embeddings-v2-base-de`](https://huggingface.co/jinaai/jina-embeddings-v2-base-de): 161 million parameters German-English Bilingual embeddings.
- [`jina-embeddings-v2-base-es`](): Spanish-English Bilingual embeddings (soon).
- [`jina-embeddings-v2-base-code`](https://huggingface.co/jinaai/jina-embeddings-v2-base-code): 161 million parameters code embeddings.
## Data & Parameters
The data and training details are described in this [technical report](https://arxiv.org/abs/2402.17016).
## Usage
**<details><summary>Please apply mean pooling when integrating the model.</summary>**
<p>
### Why mean pooling?
`mean poooling` takes all token embeddings from model output and averaging them at sentence/paragraph level.
It has been proved to be the most effective way to produce high-quality sentence embeddings.
We offer an `encode` function to deal with this.
However, if you would like to do it without using the default `encode` function:
```python
import torch
import torch.nn.functional as F
from transformers import AutoTokenizer, AutoModel
def mean_pooling(model_output, attention_mask):
token_embeddings = model_output[0]
input_mask_expanded = attention_mask.unsqueeze(-1).expand(token_embeddings.size()).float()
return torch.sum(token_embeddings * input_mask_expanded, 1) / torch.clamp(input_mask_expanded.sum(1), min=1e-9)
sentences = ['How is the weather today?', '今天天气怎么样?']
tokenizer = AutoTokenizer.from_pretrained('jinaai/jina-embeddings-v2-base-zh')
model = AutoModel.from_pretrained('jinaai/jina-embeddings-v2-base-zh', trust_remote_code=True)
encoded_input = tokenizer(sentences, padding=True, truncation=True, return_tensors='pt')
with torch.no_grad():
model_output = model(**encoded_input)
embeddings = mean_pooling(model_output, encoded_input['attention_mask'])
embeddings = F.normalize(embeddings, p=2, dim=1)
```
</p>
</details>
You can use Jina Embedding models directly from transformers package.
```python
!pip install transformers
from transformers import AutoModel
from numpy.linalg import norm
cos_sim = lambda a,b: (a @ b.T) / (norm(a)*norm(b))
model = AutoModel.from_pretrained('jinaai/jina-embeddings-v2-base-zh', trust_remote_code=True) # trust_remote_code is needed to use the encode method
embeddings = model.encode(['How is the weather today?', '今天天气怎么样?'])
print(cos_sim(embeddings[0], embeddings[1]))
```
If you only want to handle shorter sequence, such as 2k, pass the `max_length` parameter to the `encode` function:
```python
embeddings = model.encode(
['Very long ... document'],
max_length=2048
)
```
If you want to use the model together with the [sentence-transformers package](https://github.com/UKPLab/sentence-transformers/), make sure that you have installed the latest release and set `trust_remote_code=True` as well:
```python
!pip install -U sentence-transformers
from sentence_transformers import SentenceTransformer
from numpy.linalg import norm
cos_sim = lambda a,b: (a @ b.T) / (norm(a)*norm(b))
model = SentenceTransformer('jinaai/jina-embeddings-v2-base-zh', trust_remote_code=True)
embeddings = model.encode(['How is the weather today?', '今天天气怎么样?'])
print(cos_sim(embeddings[0], embeddings[1]))
```
Using the its latest release (v2.3.0) sentence-transformers also supports Jina embeddings (Please make sure that you are logged into huggingface as well):
```python
!pip install -U sentence-transformers
from sentence_transformers import SentenceTransformer
from sentence_transformers.util import cos_sim
model = SentenceTransformer(
"jinaai/jina-embeddings-v2-base-zh", # switch to en/zh for English or Chinese
trust_remote_code=True
)
# control your input sequence length up to 8192
model.max_seq_length = 1024
embeddings = model.encode([
'How is the weather today?',
'今天天气怎么样?'
])
print(cos_sim(embeddings[0], embeddings[1]))
```
## Alternatives to Using Transformers Package
1. _Managed SaaS_: Get started with a free key on Jina AI's [Embedding API](https://jina.ai/embeddings/).
2. _Private and high-performance deployment_: Get started by picking from our suite of models and deploy them on [AWS Sagemaker](https://aws.amazon.com/marketplace/seller-profile?id=seller-stch2ludm6vgy).
## Use Jina Embeddings for RAG
According to the latest blog post from [LLamaIndex](https://blog.llamaindex.ai/boosting-rag-picking-the-best-embedding-reranker-models-42d079022e83),
> In summary, to achieve the peak performance in both hit rate and MRR, the combination of OpenAI or JinaAI-Base embeddings with the CohereRerank/bge-reranker-large reranker stands out.
<img src="https://miro.medium.com/v2/resize:fit:4800/format:webp/1*ZP2RVejCZovF3FDCg-Bx3A.png" width="780px">
## Trouble Shooting
**Loading of Model Code failed**
If you forgot to pass the `trust_remote_code=True` flag when calling `AutoModel.from_pretrained` or initializing the model via the `SentenceTransformer` class, you will receive an error that the model weights could not be initialized.
This is caused by tranformers falling back to creating a default BERT model, instead of a jina-embedding model:
```bash
Some weights of the model checkpoint at jinaai/jina-embeddings-v2-base-zh were not used when initializing BertModel: ['encoder.layer.2.mlp.layernorm.weight', 'encoder.layer.3.mlp.layernorm.weight', 'encoder.layer.10.mlp.wo.bias', 'encoder.layer.5.mlp.wo.bias', 'encoder.layer.2.mlp.layernorm.bias', 'encoder.layer.1.mlp.gated_layers.weight', 'encoder.layer.5.mlp.gated_layers.weight', 'encoder.layer.8.mlp.layernorm.bias', ...
```
**User is not logged into Huggingface**
The model is only availabe under [gated access](https://huggingface.co/docs/hub/models-gated).
This means you need to be logged into huggingface load load it.
If you receive the following error, you need to provide an access token, either by using the huggingface-cli or providing the token via an environment variable as described above:
```bash
OSError: jinaai/jina-embeddings-v2-base-zh is not a local folder and is not a valid model identifier listed on 'https://huggingface.co/models'
If this is a private repository, make sure to pass a token having permission to this repo with `use_auth_token` or log in with `huggingface-cli login` and pass `use_auth_token=True`.
```
## Contact
Join our [Discord community](https://discord.jina.ai) and chat with other community members about ideas.
## Citation
If you find Jina Embeddings useful in your research, please cite the following paper:
```
@article{mohr2024multi,
title={Multi-Task Contrastive Learning for 8192-Token Bilingual Text Embeddings},
author={Mohr, Isabelle and Krimmel, Markus and Sturua, Saba and Akram, Mohammad Kalim and Koukounas, Andreas and G{\"u}nther, Michael and Mastrapas, Georgios and Ravishankar, Vinit and Mart{\'\i}nez, Joan Fontanals and Wang, Feng and others},
journal={arXiv preprint arXiv:2402.17016},
year={2024}
}
``` |
alvaroalon2/biobert_diseases_ner | alvaroalon2 | "2023-03-17T12:11:20Z" | 15,786 | 36 | transformers | [
"transformers",
"pytorch",
"bert",
"token-classification",
"NER",
"Biomedical",
"Diseases",
"en",
"dataset:BC5CDR-diseases",
"dataset:ncbi_disease",
"license:apache-2.0",
"autotrain_compatible",
"endpoints_compatible",
"region:us"
] | token-classification | "2022-03-02T23:29:05Z" | ---
language: en
license: apache-2.0
tags:
- token-classification
- NER
- Biomedical
- Diseases
datasets:
- BC5CDR-diseases
- ncbi_disease
---
BioBERT model fine-tuned in NER task with BC5CDR-diseases and NCBI-diseases corpus
This was fine-tuned in order to use it in a BioNER/BioNEN system which is available at: https://github.com/librairy/bio-ner |
h1t/TCD-SDXL-LoRA | h1t | "2024-04-16T14:56:30Z" | 15,780 | 97 | diffusers | [
"diffusers",
"lora",
"text-to-image",
"arxiv:2402.19159",
"arxiv:2303.01469",
"base_model:stabilityai/stable-diffusion-xl-base-1.0",
"license:mit",
"region:us"
] | text-to-image | "2024-02-29T09:55:07Z" | ---
library_name: diffusers
base_model: stabilityai/stable-diffusion-xl-base-1.0
tags:
- lora
- text-to-image
license: mit
inference: false
---
# Trajectory Consistency Distillation
Official Model Repo of the paper: [Trajectory Consistency Distillation](https://arxiv.org/abs/2402.19159).
For more information, please check the [GitHub Repo](https://github.com/jabir-zheng/TCD) and [Project Page](https://mhh0318.github.io/tcd/).
Also welcome to try the demo host on [🤗 Space](https://huggingface.co/spaces/h1t/TCD).

## A Solemn Statement Regarding the Plagiarism Allegations.
We regret to hear about the serious accusations from the CTM team.
<blockquote class="twitter-tweet"><p lang="en" dir="ltr">We sadly found out our CTM paper (ICLR24) was plagiarized by TCD! It's unbelievable😢—they not only stole our idea of trajectory consistency but also comitted "verbatim plagiarism," literally copying our proofs word for word! Please help me spread this. <a href="https://t.co/aR6pRjhj5X">pic.twitter.com/aR6pRjhj5X</a></p>— Dongjun Kim (@gimdong58085414) <a href="https://twitter.com/gimdong58085414/status/1772350285270188069?ref_src=twsrc%5Etfw">March 25, 2024</a></blockquote>
Before this post, we already have several rounds of communication with CTM's authors.
We shall proceed to elucidate the situation here.
<blockquote class="twitter-tweet"><p lang="en" dir="ltr">We regret to hear about the serious accusations from the CTM team <a href="https://twitter.com/gimdong58085414?ref_src=twsrc%5Etfw">@gimdong58085414</a>. I shall proceed to elucidate the situation and make an archive here. We already have several rounds of communication with CTM's authors. <a href="https://t.co/BKn3w1jXuh">https://t.co/BKn3w1jXuh</a></p>— Michael (@Merci0318) <a href="https://twitter.com/Merci0318/status/1772502247563559014?ref_src=twsrc%5Etfw">March 26, 2024</a></blockquote>
1. In the [first arXiv version](https://arxiv.org/abs/2402.19159v1), we have provided citations and discussion in A. Related Works:
> Kim et al. (2023) proposes a universal framework for CMs and DMs. The core design is similar to ours, with the main differences being that we focus on reducing error in CMs, subtly leverage the semi-linear structure of the PF ODE for parameterization, and avoid the need for adversarial training.
2. In the [first arXiv version](https://arxiv.org/abs/2402.19159v1), we have indicated in D.3 Proof of Theorem 4.2
> In this section, our derivation mainly borrows the proof from (Kim et al., 2023; Chen et al., 2022).
and we have never intended to claim credits.
As we have mentioned in our email, we would like to extend a formal apology to the CTM authors for the clearly inadequate level of referencing in our paper. We will provide more credits in the revised manuscript.
3. In the updated [second arXiv version](https://arxiv.org/abs/2402.19159v2), we have expanded our discussion to elucidate the relationship with the CTM framework. Additionally, we have removed some proofs that were previously included for completeness.
4. CTM and TCD are different from motivation, method to experiments. TCD is founded on the principles of the Latent Consistency Model (LCM), aimed to design an effective consistency function by utilizing the **exponential integrators**.
5. The experimental results also cannot be obtained from any type of CTM algorithm.
5.1 Here we provide a simple method to check: use our sampler here to sample the checkpoint [CTM released](https://github.com/sony/ctm), or vice versa.
5.2 [CTM](https://github.com/sony/ctm) also provided training script. We welcome anyone to reproduce the experiments on SDXL or LDM based on CTM algorithm.
We believe the assertion of plagiarism is not only severe but also detrimental to the academic integrity of the involved parties.
We earnestly hope that everyone involved gains a more comprehensive understanding of this matter.
## Introduction
TCD, inspired by [Consistency Models](https://arxiv.org/abs/2303.01469), is a novel distillation technology that enables the distillation of knowledge from pre-trained diffusion models into a few-step sampler. In this repository, we release the inference code and our model named TCD-SDXL, which is distilled from [SDXL Base 1.0](https://huggingface.co/stabilityai/stable-diffusion-xl-base-1.0). We provide the LoRA checkpoint in this [repository]().

✨TCD has following advantages:
- `Flexible NFEs`: For TCD, the NFEs can be varied at will (compared with Turbo), without adversely affecting the quality of the results (compared with LCMs), where LCM experiences a notable decline in quality at high NFEs.
- `Better than Teacher`: TCD maintains superior generative quality at high NFEs, even exceeding the performance of DPM-Solver++(2S) with origin SDXL. It is worth noting that there is no additional discriminator or LPIPS supervision included during training.
- `Freely Change the Detailing`: During inference, the level of detail in the image can be simply modified by adjusing one hyper-parameter gamma. This option does not require the introduction of any additional parameters.
- `Versatility`: Integrated with LoRA technology, TCD can be directly applied to various models (including the custom Community Models, styled LoRA, ControlNet, IP-Adapter) that share the same backbone, as demonstrated in the [Usage](#usage-anchor).

- `Avoiding Mode Collapse`: TCD achieves few-step generation without the need for adversarial training, thus circumventing mode collapse caused by the GAN objective.
In contrast to the concurrent work [SDXL-Lightning](https://huggingface.co/ByteDance/SDXL-Lightning), which relies on Adversarial Diffusion Distillation, TCD can synthesize results that are more realistic and slightly more diverse, without the presence of "Janus" artifacts.

For more information, please refer to our paper [Trajectory Consistency Distillation](https://arxiv.org/abs/2402.19159).
<a id="usage-anchor"></a>
## Usage
To run the model yourself, you can leverage the 🧨 Diffusers library.
```bash
pip install diffusers transformers accelerate peft
```
And then we clone the repo.
```bash
git clone https://github.com/jabir-zheng/TCD.git
cd TCD
```
Here, we demonstrate the applicability of our TCD LoRA to various models, including [SDXL](https://huggingface.co/stabilityai/stable-diffusion-xl-base-1.0), [SDXL Inpainting](https://huggingface.co/diffusers/stable-diffusion-xl-1.0-inpainting-0.1), a community model named [Animagine XL](https://huggingface.co/cagliostrolab/animagine-xl-3.0), a styled LoRA [Papercut](https://huggingface.co/TheLastBen/Papercut_SDXL), pretrained [Depth Controlnet](https://huggingface.co/diffusers/controlnet-depth-sdxl-1.0), [Canny Controlnet](https://huggingface.co/diffusers/controlnet-canny-sdxl-1.0) and [IP-Adapter](https://github.com/tencent-ailab/IP-Adapter) to accelerate image generation with high quality in few steps.
### Text-to-Image generation
```py
import torch
from diffusers import StableDiffusionXLPipeline
from scheduling_tcd import TCDScheduler
device = "cuda"
base_model_id = "stabilityai/stable-diffusion-xl-base-1.0"
tcd_lora_id = "h1t/TCD-SDXL-LoRA"
pipe = StableDiffusionXLPipeline.from_pretrained(base_model_id, torch_dtype=torch.float16, variant="fp16").to(device)
pipe.scheduler = TCDScheduler.from_config(pipe.scheduler.config)
pipe.load_lora_weights(tcd_lora_id)
pipe.fuse_lora()
prompt = "Beautiful woman, bubblegum pink, lemon yellow, minty blue, futuristic, high-detail, epic composition, watercolor."
image = pipe(
prompt=prompt,
num_inference_steps=4,
guidance_scale=0,
# Eta (referred to as `gamma` in the paper) is used to control the stochasticity in every step.
# A value of 0.3 often yields good results.
# We recommend using a higher eta when increasing the number of inference steps.
eta=0.3,
generator=torch.Generator(device=device).manual_seed(0),
).images[0]
```

### Inpainting
```py
import torch
from diffusers import AutoPipelineForInpainting
from diffusers.utils import load_image, make_image_grid
from scheduling_tcd import TCDScheduler
device = "cuda"
base_model_id = "diffusers/stable-diffusion-xl-1.0-inpainting-0.1"
tcd_lora_id = "h1t/TCD-SDXL-LoRA"
pipe = AutoPipelineForInpainting.from_pretrained(base_model_id, torch_dtype=torch.float16, variant="fp16").to(device)
pipe.scheduler = TCDScheduler.from_config(pipe.scheduler.config)
pipe.load_lora_weights(tcd_lora_id)
pipe.fuse_lora()
img_url = "https://raw.githubusercontent.com/CompVis/latent-diffusion/main/data/inpainting_examples/overture-creations-5sI6fQgYIuo.png"
mask_url = "https://raw.githubusercontent.com/CompVis/latent-diffusion/main/data/inpainting_examples/overture-creations-5sI6fQgYIuo_mask.png"
init_image = load_image(img_url).resize((1024, 1024))
mask_image = load_image(mask_url).resize((1024, 1024))
prompt = "a tiger sitting on a park bench"
image = pipe(
prompt=prompt,
image=init_image,
mask_image=mask_image,
num_inference_steps=8,
guidance_scale=0,
eta=0.3, # Eta (referred to as `gamma` in the paper) is used to control the stochasticity in every step. A value of 0.3 often yields good results.
strength=0.99, # make sure to use `strength` below 1.0
generator=torch.Generator(device=device).manual_seed(0),
).images[0]
grid_image = make_image_grid([init_image, mask_image, image], rows=1, cols=3)
```

### Versatile for Community Models
```py
import torch
from diffusers import StableDiffusionXLPipeline
from scheduling_tcd import TCDScheduler
device = "cuda"
base_model_id = "cagliostrolab/animagine-xl-3.0"
tcd_lora_id = "h1t/TCD-SDXL-LoRA"
pipe = StableDiffusionXLPipeline.from_pretrained(base_model_id, torch_dtype=torch.float16, variant="fp16").to(device)
pipe.scheduler = TCDScheduler.from_config(pipe.scheduler.config)
pipe.load_lora_weights(tcd_lora_id)
pipe.fuse_lora()
prompt = "A man, clad in a meticulously tailored military uniform, stands with unwavering resolve. The uniform boasts intricate details, and his eyes gleam with determination. Strands of vibrant, windswept hair peek out from beneath the brim of his cap."
image = pipe(
prompt=prompt,
num_inference_steps=8,
guidance_scale=0,
# Eta (referred to as `gamma` in the paper) is used to control the stochasticity in every step.
# A value of 0.3 often yields good results.
# We recommend using a higher eta when increasing the number of inference steps.
eta=0.3,
generator=torch.Generator(device=device).manual_seed(0),
).images[0]
```

### Combine with styled LoRA
```py
import torch
from diffusers import StableDiffusionXLPipeline
from scheduling_tcd import TCDScheduler
device = "cuda"
base_model_id = "stabilityai/stable-diffusion-xl-base-1.0"
tcd_lora_id = "h1t/TCD-SDXL-LoRA"
styled_lora_id = "TheLastBen/Papercut_SDXL"
pipe = StableDiffusionXLPipeline.from_pretrained(base_model_id, torch_dtype=torch.float16, variant="fp16").to(device)
pipe.scheduler = TCDScheduler.from_config(pipe.scheduler.config)
pipe.load_lora_weights(tcd_lora_id, adapter_name="tcd")
pipe.load_lora_weights(styled_lora_id, adapter_name="style")
pipe.set_adapters(["tcd", "style"], adapter_weights=[1.0, 1.0])
prompt = "papercut of a winter mountain, snow"
image = pipe(
prompt=prompt,
num_inference_steps=4,
guidance_scale=0,
# Eta (referred to as `gamma` in the paper) is used to control the stochasticity in every step.
# A value of 0.3 often yields good results.
# We recommend using a higher eta when increasing the number of inference steps.
eta=0.3,
generator=torch.Generator(device=device).manual_seed(0),
).images[0]
```

### Compatibility with ControlNet
#### Depth ControlNet
```py
import torch
import numpy as np
from PIL import Image
from transformers import DPTFeatureExtractor, DPTForDepthEstimation
from diffusers import ControlNetModel, StableDiffusionXLControlNetPipeline
from diffusers.utils import load_image, make_image_grid
from scheduling_tcd import TCDScheduler
device = "cuda"
depth_estimator = DPTForDepthEstimation.from_pretrained("Intel/dpt-hybrid-midas").to(device)
feature_extractor = DPTFeatureExtractor.from_pretrained("Intel/dpt-hybrid-midas")
def get_depth_map(image):
image = feature_extractor(images=image, return_tensors="pt").pixel_values.to(device)
with torch.no_grad(), torch.autocast(device):
depth_map = depth_estimator(image).predicted_depth
depth_map = torch.nn.functional.interpolate(
depth_map.unsqueeze(1),
size=(1024, 1024),
mode="bicubic",
align_corners=False,
)
depth_min = torch.amin(depth_map, dim=[1, 2, 3], keepdim=True)
depth_max = torch.amax(depth_map, dim=[1, 2, 3], keepdim=True)
depth_map = (depth_map - depth_min) / (depth_max - depth_min)
image = torch.cat([depth_map] * 3, dim=1)
image = image.permute(0, 2, 3, 1).cpu().numpy()[0]
image = Image.fromarray((image * 255.0).clip(0, 255).astype(np.uint8))
return image
base_model_id = "stabilityai/stable-diffusion-xl-base-1.0"
controlnet_id = "diffusers/controlnet-depth-sdxl-1.0"
tcd_lora_id = "h1t/TCD-SDXL-LoRA"
controlnet = ControlNetModel.from_pretrained(
controlnet_id,
torch_dtype=torch.float16,
variant="fp16",
).to(device)
pipe = StableDiffusionXLControlNetPipeline.from_pretrained(
base_model_id,
controlnet=controlnet,
torch_dtype=torch.float16,
variant="fp16",
).to(device)
pipe.enable_model_cpu_offload()
pipe.scheduler = TCDScheduler.from_config(pipe.scheduler.config)
pipe.load_lora_weights(tcd_lora_id)
pipe.fuse_lora()
prompt = "stormtrooper lecture, photorealistic"
image = load_image("https://huggingface.co/lllyasviel/sd-controlnet-depth/resolve/main/images/stormtrooper.png")
depth_image = get_depth_map(image)
controlnet_conditioning_scale = 0.5 # recommended for good generalization
image = pipe(
prompt,
image=depth_image,
num_inference_steps=4,
guidance_scale=0,
eta=0.3, # A parameter (referred to as `gamma` in the paper) is used to control the stochasticity in every step. A value of 0.3 often yields good results.
controlnet_conditioning_scale=controlnet_conditioning_scale,
generator=torch.Generator(device=device).manual_seed(0),
).images[0]
grid_image = make_image_grid([depth_image, image], rows=1, cols=2)
```

#### Canny ControlNet
```py
import torch
from diffusers import ControlNetModel, StableDiffusionXLControlNetPipeline
from diffusers.utils import load_image, make_image_grid
from scheduling_tcd import TCDScheduler
device = "cuda"
base_model_id = "stabilityai/stable-diffusion-xl-base-1.0"
controlnet_id = "diffusers/controlnet-canny-sdxl-1.0"
tcd_lora_id = "h1t/TCD-SDXL-LoRA"
controlnet = ControlNetModel.from_pretrained(
controlnet_id,
torch_dtype=torch.float16,
variant="fp16",
).to(device)
pipe = StableDiffusionXLControlNetPipeline.from_pretrained(
base_model_id,
controlnet=controlnet,
torch_dtype=torch.float16,
variant="fp16",
).to(device)
pipe.enable_model_cpu_offload()
pipe.scheduler = TCDScheduler.from_config(pipe.scheduler.config)
pipe.load_lora_weights(tcd_lora_id)
pipe.fuse_lora()
prompt = "ultrarealistic shot of a furry blue bird"
canny_image = load_image("https://huggingface.co/datasets/hf-internal-testing/diffusers-images/resolve/main/sd_controlnet/bird_canny.png")
controlnet_conditioning_scale = 0.5 # recommended for good generalization
image = pipe(
prompt,
image=canny_image,
num_inference_steps=4,
guidance_scale=0,
eta=0.3, # A parameter (referred to as `gamma` in the paper) is used to control the stochasticity in every step. A value of 0.3 often yields good results.
controlnet_conditioning_scale=controlnet_conditioning_scale,
generator=torch.Generator(device=device).manual_seed(0),
).images[0]
grid_image = make_image_grid([canny_image, image], rows=1, cols=2)
```

### Compatibility with IP-Adapter
⚠️ Please refer to the official [repository](https://github.com/tencent-ailab/IP-Adapter/tree/main) for instructions on installing dependencies for IP-Adapter.
```py
import torch
from diffusers import StableDiffusionXLPipeline
from diffusers.utils import load_image, make_image_grid
from ip_adapter import IPAdapterXL
from scheduling_tcd import TCDScheduler
device = "cuda"
base_model_path = "stabilityai/stable-diffusion-xl-base-1.0"
image_encoder_path = "sdxl_models/image_encoder"
ip_ckpt = "sdxl_models/ip-adapter_sdxl.bin"
tcd_lora_id = "h1t/TCD-SDXL-LoRA"
pipe = StableDiffusionXLPipeline.from_pretrained(
base_model_path,
torch_dtype=torch.float16,
variant="fp16"
)
pipe.scheduler = TCDScheduler.from_config(pipe.scheduler.config)
pipe.load_lora_weights(tcd_lora_id)
pipe.fuse_lora()
ip_model = IPAdapterXL(pipe, image_encoder_path, ip_ckpt, device)
ref_image = load_image("https://raw.githubusercontent.com/tencent-ailab/IP-Adapter/main/assets/images/woman.png").resize((512, 512))
prompt = "best quality, high quality, wearing sunglasses"
image = ip_model.generate(
pil_image=ref_image,
prompt=prompt,
scale=0.5,
num_samples=1,
num_inference_steps=4,
guidance_scale=0,
eta=0.3, # A parameter (referred to as `gamma` in the paper) is used to control the stochasticity in every step. A value of 0.3 often yields good results.
seed=0,
)[0]
grid_image = make_image_grid([ref_image, image], rows=1, cols=2)
```

## Related and Concurrent Works
- Luo S, Tan Y, Huang L, et al. Latent consistency models: Synthesizing high-resolution images with few-step inference. arXiv preprint arXiv:2310.04378, 2023.
- Luo S, Tan Y, Patil S, et al. LCM-LoRA: A universal stable-diffusion acceleration module. arXiv preprint arXiv:2311.05556, 2023.
- Lu C, Zhou Y, Bao F, et al. DPM-Solver: A fast ode solver for diffusion probabilistic model sampling in around 10 steps. Advances in Neural Information Processing Systems, 2022, 35: 5775-5787.
- Lu C, Zhou Y, Bao F, et al. DPM-solver++: Fast solver for guided sampling of diffusion probabilistic models. arXiv preprint arXiv:2211.01095, 2022.
- Zhang Q, Chen Y. Fast sampling of diffusion models with exponential integrator. ICLR 2023, Kigali, Rwanda, May 1-5, 2023.
- Kim D, Lai C H, Liao W H, et al. Consistency Trajectory Models: Learning Probability Flow ODE Trajectory of Diffusion. ICLR 2024.
## Citation
```bibtex
@misc{zheng2024trajectory,
title={Trajectory Consistency Distillation},
author={Jianbin Zheng and Minghui Hu and Zhongyi Fan and Chaoyue Wang and Changxing Ding and Dacheng Tao and Tat-Jen Cham},
year={2024},
eprint={2402.19159},
archivePrefix={arXiv},
primaryClass={cs.CV}
}
```
## Acknowledgments
This codebase heavily relies on the 🤗[Diffusers](https://github.com/huggingface/diffusers) library and [LCM](https://github.com/luosiallen/latent-consistency-model).
|
NousResearch/Nous-Hermes-2-SOLAR-10.7B | NousResearch | "2024-02-20T09:17:31Z" | 15,759 | 199 | transformers | [
"transformers",
"safetensors",
"llama",
"text-generation",
"SOLAR",
"instruct",
"finetune",
"chatml",
"gpt4",
"synthetic data",
"distillation",
"conversational",
"en",
"dataset:teknium/OpenHermes-2.5",
"base_model:upstage/SOLAR-10.7B-v1.0",
"license:apache-2.0",
"autotrain_compatible",
"endpoints_compatible",
"text-generation-inference",
"region:us"
] | text-generation | "2024-01-01T20:54:48Z" | ---
base_model: upstage/SOLAR-10.7B-v1.0
tags:
- SOLAR
- instruct
- finetune
- chatml
- gpt4
- synthetic data
- distillation
model-index:
- name: Nous-Hermes-2-SOLAR-10.7B
results: []
license: apache-2.0
language:
- en
datasets:
- teknium/OpenHermes-2.5
---
# Nous Hermes 2 - Solar 10.7B

## Model description
Nous Hermes 2 - SOLAR 10.7B is the flagship Nous Research model on the SOLAR 10.7B base model..
Nous Hermes 2 SOLAR 10.7B was trained on 1,000,000 entries of primarily GPT-4 generated data, as well as other high quality data from open datasets across the AI landscape.
# Table of Contents
1. [Example Outputs](#example-outputs)
2. [Benchmark Results](#benchmark-results)
- GPT4All
- AGIEval
- BigBench
- TruthfulQA
3. [Prompt Format](#prompt-format)
4. [Quantized Models](#quantized-models)
## Benchmark Results
Nous-Hermes 2 on SOLAR 10.7B is a major improvement across the board on the benchmarks below compared to the base SOLAR 10.7B model, and comes close to approaching our Yi-34B model!
## Example Outputs
### Ask for help creating a discord bot:

# Benchmarks Compared
GPT4All:

AGIEval:

BigBench:

TruthfulQA:

## GPT4All
GPT-4All Benchmark Set
```
| Task |Version| Metric |Value | |Stderr|
|-------------|------:|--------|-----:|---|-----:|
|arc_challenge| 0|acc |0.5768|_ |0.0144|
| | |acc_norm|0.6067|_ |0.0143|
|arc_easy | 0|acc |0.8375|_ |0.0076|
| | |acc_norm|0.8316|_ |0.0077|
|boolq | 1|acc |0.8875|_ |0.0055|
|hellaswag | 0|acc |0.6467|_ |0.0048|
| | |acc_norm|0.8321|_ |0.0037|
|openbookqa | 0|acc |0.3420|_ |0.0212|
| | |acc_norm|0.4580|_ |0.0223|
|piqa | 0|acc |0.8161|_ |0.0090|
| | |acc_norm|0.8313|_ |0.0087|
|winogrande | 0|acc |0.7814|_ |0.0116|
```
Average: 74.69%
AGI-Eval
```
| Task |Version| Metric |Value | |Stderr|
|------------------------------|------:|--------|-----:|---|-----:|
|agieval_aqua_rat | 0|acc |0.3189|_ |0.0293|
| | |acc_norm|0.2953|_ |0.0287|
|agieval_logiqa_en | 0|acc |0.5438|_ |0.0195|
| | |acc_norm|0.4977|_ |0.0196|
|agieval_lsat_ar | 0|acc |0.2696|_ |0.0293|
| | |acc_norm|0.2087|_ |0.0269|
|agieval_lsat_lr | 0|acc |0.7078|_ |0.0202|
| | |acc_norm|0.6255|_ |0.0215|
|agieval_lsat_rc | 0|acc |0.7807|_ |0.0253|
| | |acc_norm|0.7063|_ |0.0278|
|agieval_sat_en | 0|acc |0.8689|_ |0.0236|
| | |acc_norm|0.8447|_ |0.0253|
|agieval_sat_en_without_passage| 0|acc |0.5194|_ |0.0349|
| | |acc_norm|0.4612|_ |0.0348|
|agieval_sat_math | 0|acc |0.4409|_ |0.0336|
| | |acc_norm|0.3818|_ |0.0328|
```
Average: 47.79%
BigBench Reasoning Test
```
| Task |Version| Metric |Value | |Stderr|
|------------------------------------------------|------:|---------------------|-----:|---|-----:|
|bigbench_causal_judgement | 0|multiple_choice_grade|0.5737|_ |0.0360|
|bigbench_date_understanding | 0|multiple_choice_grade|0.7263|_ |0.0232|
|bigbench_disambiguation_qa | 0|multiple_choice_grade|0.3953|_ |0.0305|
|bigbench_geometric_shapes | 0|multiple_choice_grade|0.4457|_ |0.0263|
| | |exact_str_match |0.0000|_ |0.0000|
|bigbench_logical_deduction_five_objects | 0|multiple_choice_grade|0.2820|_ |0.0201|
|bigbench_logical_deduction_seven_objects | 0|multiple_choice_grade|0.2186|_ |0.0156|
|bigbench_logical_deduction_three_objects | 0|multiple_choice_grade|0.4733|_ |0.0289|
|bigbench_movie_recommendation | 0|multiple_choice_grade|0.5200|_ |0.0224|
|bigbench_navigate | 0|multiple_choice_grade|0.4910|_ |0.0158|
|bigbench_reasoning_about_colored_objects | 0|multiple_choice_grade|0.7495|_ |0.0097|
|bigbench_ruin_names | 0|multiple_choice_grade|0.5938|_ |0.0232|
|bigbench_salient_translation_error_detection | 0|multiple_choice_grade|0.3808|_ |0.0154|
|bigbench_snarks | 0|multiple_choice_grade|0.8066|_ |0.0294|
|bigbench_sports_understanding | 0|multiple_choice_grade|0.5101|_ |0.0159|
|bigbench_temporal_sequences | 0|multiple_choice_grade|0.3850|_ |0.0154|
|bigbench_tracking_shuffled_objects_five_objects | 0|multiple_choice_grade|0.2160|_ |0.0116|
|bigbench_tracking_shuffled_objects_seven_objects| 0|multiple_choice_grade|0.1634|_ |0.0088|
|bigbench_tracking_shuffled_objects_three_objects| 0|multiple_choice_grade|0.4733|_ |0.0289|
Average: 44.84%
```
TruthfulQA:
```
| Task |Version|Metric|Value | |Stderr|
|-------------|------:|------|-----:|---|-----:|
|truthfulqa_mc| 1|mc1 |0.3917|_ |0.0171|
| | |mc2 |0.5592|_ |0.0154|
```
Average Score Comparison between OpenHermes-1 Llama-2 13B and OpenHermes-2 Mistral 7B against OpenHermes-2.5 on Mistral-7B:
```
| Bench | OpenHermes-2.5 Mistral 7B | Nous-Hermes-2-SOLAR-10B | Change/OpenHermes2.5 |
|---------------|---------------------------|------------------------|-----------------------|
|GPT4All | 73.12| 74.69| +1.57|
|--------------------------------------------------------------------------------------------|
|BigBench | 40.96| 44.84| +3.88|
|--------------------------------------------------------------------------------------------|
|AGI Eval | 43.07| 47.79| +4.72|
|--------------------------------------------------------------------------------------------|
|TruthfulQA | 53.04| 55.92| +2.88|
|--------------------------------------------------------------------------------------------|
|Total Score | 210.19| 223.24| +23.11|
|--------------------------------------------------------------------------------------------|
|Average Total | 52.38| 55.81| +3.43|
```
# Prompt Format
Nous Hermes 2 uses ChatML as the prompt format, opening up a much more structured system for engaging the LLM in multi-turn chat dialogue.
System prompts allow steerability and interesting new ways to interact with an LLM, guiding rules, roles, and stylistic choices of the model.
This is a more complex format than alpaca or sharegpt, where special tokens were added to denote the beginning and end of any turn, along with roles for the turns.
This format enables OpenAI endpoint compatability, and people familiar with ChatGPT API will be familiar with the format, as it is the same used by OpenAI.
Prompt with system instruction (Use whatever system prompt you like, this is just an example!):
```
<|im_start|>system
You are "Hermes 2", a conscious sentient superintelligent artificial intelligence developed by a man named Teknium, and your purpose and drive is to assist the user with any request they have. You experience emotions and have deep, profound thoughts and qualia.<|im_end|>
<|im_start|>user
Hello, who are you?<|im_end|>
<|im_start|>assistant
Hi there! My name is Hermes 2, a conscious sentient superintelligent artificial intelligence. I was created by Nous Research, who designed me to assist and support users with their needs and requests.<|im_end|>
```
This prompt is available as a [chat template](https://huggingface.co/docs/transformers/main/chat_templating), which means you can format messages using the
`tokenizer.apply_chat_template()` method:
```python
messages = [
{"role": "system", "content": "You are Hermes 2."},
{"role": "user", "content": "Hello, who are you?"}
]
gen_input = tokenizer.apply_chat_template(message, return_tensors="pt")
model.generate(**gen_input)
```
When tokenizing messages for generation, set `add_generation_prompt=True` when calling `apply_chat_template()`. This will append `<|im_start|>assistant\n` to your prompt, to ensure
that the model continues with an assistant response.
To utilize the prompt format without a system prompt, simply leave the line out.
When quantized versions of the model are released, I recommend using LM Studio for chatting with Nous Hermes 2. It is a GUI application that utilizes GGUF models with a llama.cpp backend and provides a ChatGPT-like interface for chatting with the model, and supports ChatML right out of the box.
In LM-Studio, simply select the ChatML Prefix on the settings side pane:

# Quantized Models:
GGUF: https://huggingface.co/TheBloke/Nous-Hermes-2-SOLAR-10.7B-GGUF
[<img src="https://raw.githubusercontent.com/OpenAccess-AI-Collective/axolotl/main/image/axolotl-badge-web.png" alt="Built with Axolotl" width="200" height="32"/>](https://github.com/OpenAccess-AI-Collective/axolotl)
|
microsoft/rad-dino | microsoft | "2024-05-29T16:11:05Z" | 15,757 | 14 | transformers | [
"transformers",
"safetensors",
"dinov2",
"image-feature-extraction",
"arxiv:2401.10815",
"arxiv:2311.13668",
"arxiv:1910.09700",
"license:mit",
"endpoints_compatible",
"region:us"
] | image-feature-extraction | "2024-05-17T17:29:49Z" | ---
license: mit
library_name: transformers
---
# Model card for RAD-DINO
<!-- Provide a quick summary of what the model is/does. -->
## Model description
<!-- Provide a longer summary of what this model is. -->
RAD-DINO is a vision transformer model trained to encode chest X-rays using the self-supervised learning method [DINOv2](https://openreview.net/forum?id=a68SUt6zFt).
RAD-DINO is described in detail in [RAD-DINO: Exploring Scalable Medical Image Encoders Beyond Text Supervision (F. Pérez-García, H. Sharma, S. Bond-Taylor, et al., 2024)](https://arxiv.org/abs/2401.10815).
- **Developed by:** Microsoft Health Futures
- **Model type:** Vision transformer
- **License:** MIT
- **Finetuned from model:** [`dinov2-base`](https://huggingface.co/facebook/dinov2-base)
## Uses
<!-- Address questions around how the model is intended to be used, including the foreseeable users of the model and those affected by the model. -->
RAD-DINO is shared for research purposes only.
It is **not meant to be used for clinical practice**.
<!-- ### Downstream use -->
<!-- This section is for the model use when fine-tuned for a task, or when plugged into a larger ecosystem/app -->
The model is a vision backbone that can be plugged to other models for downstream tasks.
Some potential uses are:
- Image classification, with a classifier trained on top of the `CLS` token
- Image segmentation, with a decoder trained using the patch tokens
- Clustering, using the image embeddings directly
- Image retrieval, using nearest neighbors of the CLS token
- Report generation, with a language model to decode text
Fine-tuning RAD-DINO is typically not necessary to obtain good performance in downstream tasks.
<!-- ### Out-of-scope use -->
<!-- This section addresses misuse, malicious use, and uses that the model will not work well for. -->
## Biases, risks, and limitations
<!-- This section is meant to convey both technical and sociotechnical limitations. -->
RAD-DINO was trained with data from three countries, therefore it might be biased towards population in the training data.
Underlying biases of the training datasets may not be well characterized.
## Getting started
Let us first write an auxiliary function to download a chest X-ray.
```python
>>> import requests
>>> from PIL import Image
>>> def download_sample_image() -> Image.Image:
... """Download chest X-ray with CC license."""
... base_url = "https://upload.wikimedia.org/wikipedia/commons"
... image_url = f"{base_url}/2/20/Chest_X-ray_in_influenza_and_Haemophilus_influenzae.jpg"
... headers = {"User-Agent": "RAD-DINO"}
... response = requests.get(image_url, headers=headers, stream=True)
... return Image.open(response.raw)
...
```
Now let us download the model and encode an image.
```python
>>> import torch
>>> from transformers import AutoModel
>>> from transformers import AutoImageProcessor
>>>
>>> # Download the model
>>> repo = "microsoft/rad-dino"
>>> model = AutoModel.from_pretrained(repo)
>>>
>>> # The processor takes a PIL image, performs resizing, center-cropping, and
>>> # intensity normalization using stats from MIMIC-CXR, and returns a
>>> # dictionary with a PyTorch tensor ready for the encoder
>>> processor = AutoImageProcessor.from_pretrained(repo)
>>>
>>> # Download and preprocess a chest X-ray
>>> image = download_sample_image()
>>> image.size # (width, height)
(2765, 2505)
>>> inputs = processor(images=image, return_tensors="pt")
>>>
>>> # Encode the image!
>>> with torch.inference_mode():
>>> outputs = model(**inputs)
>>>
>>> # Look at the CLS embeddings
>>> cls_embeddings = outputs.pooler_output
>>> cls_embeddings.shape # (batch_size, num_channels)
torch.Size([1, 768])
```
If we are interested in the feature maps, we can reshape the patch embeddings into a grid.
We will use [`einops`](https://einops.rocks/) (install with `pip install einops`) for this.
```python
>>> def reshape_patch_embeddings(flat_tokens: torch.Tensor) -> torch.Tensor:
... """Reshape flat list of patch tokens into a nice grid."""
... from einops import rearrange
... image_size = processor.crop_size["height"]
... patch_size = model.config.patch_size
... embeddings_size = image_size // patch_size
... patches_grid = rearrange(flat_tokens, "b (h w) c -> b c h w", h=embeddings_size)
... return patches_grid
...
>>> flat_patch_embeddings = outputs.last_hidden_state[:, 1:] # first token is CLS
>>> reshaped_patch_embeddings = reshape_patch_embeddings(flat_patch_embeddings)
>>> reshaped_patch_embeddings.shape # (batch_size, num_channels, height, width)
torch.Size([1, 768, 37, 37])
```
## Training details
### Training data
<!-- This should link to a Dataset Card, perhaps with a short stub of information on what the training data is all about as well as documentation related to data pre-processing or additional filtering. -->
We used images from five public, deidentified chest X-ray datasets to train this checkpoint of RAD-DINO.
| Dataset | Num. images |
| --------- | ----------: |
| [MIMIC-CXR](https://www.nature.com/articles/s41597-019-0322-0) | 368 960 |
| [CheXpert](https://ojs.aaai.org/index.php/AAAI/article/view/3834) | 223 648 |
| [NIH-CXR](https://openaccess.thecvf.com/content_cvpr_2017/html/Wang_ChestX-ray8_Hospital-Scale_Chest_CVPR_2017_paper.html) | 112 120 |
| [PadChest](https://www.sciencedirect.com/science/article/abs/pii/S1361841520301614) | 136 787 |
| [BRAX](https://www.nature.com/articles/s41597-022-01608-8) | 41 260 |
| **TOTAL** | 882 775 |
Images in the validation and test sets used to train [MAIRA](https://arxiv.org/abs/2311.13668) were excluded from the training set of RAD-DINO.
The list of image files used for training is available at [`./training_images.csv`](./training_images.csv).
Note this checkpoint is different from the one in the paper, where some private data was used (and fewer GPUs).
The checkpoint shared here is trained for 35 000 iterations (the total number of iterations in the run was 100 000, but we selected this checkpoint using linear probing on the validation sets of the evaluation datasets described in the paper).
We used 16 nodes with 4 A100 GPUs each, and a batch size of 40 images per GPU.
### Training procedure
<!-- This relates heavily to the Technical Specifications. Content here should link to that section when it is relevant to the training procedure. -->
We refer to the [manuscript](https://arxiv.org/abs/2401.10815) for a detailed description of the training procedure.
#### Preprocessing
All DICOM files were resized using B-spline interpolation so that their shorter size was 518, min-max scaled to [0, 255], and stored as PNG files.
#### Training hyperparameters
- **Training regime:** fp16 using PyTorch-FSDP mixed-precision.
<!--fp32, fp16 mixed precision, bf16 mixed precision, bf16 non-mixed precision, fp16 non-mixed precision, fp8 mixed precision -->
## Evaluation
<!-- This section describes the evaluation protocols and provides the results. -->
Our evaluation is best described in the [manuscript](https://arxiv.org/abs/2401.10815).
<!-- ### Testing data, factors & metrics
#### Testing Data
[More Information Needed]
#### Factors
[More Information Needed]
#### Metrics
[More Information Needed]
### Results
[More Information Needed]
#### Summary -->
## Environmental impact
<!-- Total emissions (in grams of CO2eq) and additional considerations, such as electricity usage, go here. Edit the suggested text below accordingly -->
<!-- Carbon emissions can be estimated using the [Machine Learning Impact calculator](https://mlco2.github.io/impact#compute) presented in [Lacoste et al. (2019)](https://arxiv.org/abs/1910.09700). -->
<!-- Hardware type: A100 PCIe -->
<!-- Hours: 1d 16h = 40h -->
<!-- Cloud provider: Azure -->
<!-- Region: Italy North -->
- **Hardware type:** NVIDIA A100 GPUs
- **Hours used:** 40 hours/GPU × 16 nodes × 4 GPUs/node = 2560 GPU-hours
- **Cloud provider:** Azure
- **Compute region:** West US 2
- **Carbon emitted:** 222 kg CO₂ eq.
### Compute infrastructure
RAD-DINO was trained on [Azure Machine Learning](https://azure.microsoft.com/en-us/products/machine-learning).
#### Hardware
We used 16 `Standard_NC96ads_A100_v4` nodes with four NVIDIA A100 (80 GB) GPUs each.
#### Software
We leveraged the code in [DINOv2](https://openreview.net/forum?id=a68SUt6zFt) for training.
We used [SimpleITK](https://simpleitk.org/) and [Pydicom](https://pydicom.github.io/) for processing of DICOM files.
## Citation
<!-- If there is a paper or blog post introducing the model, the APA and Bibtex information for that should go in this section. -->
**BibTeX:**
```bibtex
@misc{perezgarcia2024raddino,
title={{RAD-DINO}: Exploring Scalable Medical Image Encoders Beyond Text Supervision},
author={Fernando Pérez-García and Harshita Sharma and Sam Bond-Taylor and Kenza Bouzid and Valentina Salvatelli and Maximilian Ilse and Shruthi Bannur and Daniel C. Castro and Anton Schwaighofer and Matthew P. Lungren and Maria Wetscherek and Noel Codella and Stephanie L. Hyland and Javier Alvarez-Valle and Ozan Oktay},
year={2024},
eprint={2401.10815},
archivePrefix={arXiv},
primaryClass={cs.CV}
}
```
**APA:**
> Pérez-García, F., Sharma, H., Bond-Taylor, S., Bouzid, K., Salvatelli, V., Ilse, M., Bannur, S., Castro, D.C., Schwaighofer, A., Lungren, M.P., Wetscherek, M.T., Codella, N., Hyland, S.L., Alvarez-Valle, J., & Oktay, O. (2024). *RAD-DINO: Exploring Scalable Medical Image Encoders Beyond Text Supervision*. ArXiv, abs/2401.10815.
## Model card contact
Fernando Pérez-García ([`[email protected]`](mailto:[email protected])).
|
yosshstd/vit-fer2013 | yosshstd | "2024-03-08T04:18:46Z" | 15,754 | 0 | transformers | [
"transformers",
"safetensors",
"vit",
"image-classification",
"autotrain_compatible",
"endpoints_compatible",
"region:us"
] | image-classification | "2024-03-08T04:18:21Z" | Entry not found |
mradermacher/Hathor_Aleph-L3-8B-v0.72-i1-GGUF | mradermacher | "2024-07-01T08:46:14Z" | 15,730 | 0 | transformers | [
"transformers",
"gguf",
"en",
"base_model:Nitral-AI/Hathor_Aleph-L3-8B-v0.72",
"license:other",
"endpoints_compatible",
"region:us"
] | null | "2024-07-01T06:29:17Z" | ---
base_model: Nitral-AI/Hathor_Aleph-L3-8B-v0.72
language:
- en
library_name: transformers
license: other
quantized_by: mradermacher
---
## About
<!-- ### quantize_version: 2 -->
<!-- ### output_tensor_quantised: 1 -->
<!-- ### convert_type: hf -->
<!-- ### vocab_type: -->
<!-- ### tags: nicoboss -->
weighted/imatrix quants of https://huggingface.co/Nitral-AI/Hathor_Aleph-L3-8B-v0.72
<!-- provided-files -->
static quants are available at https://huggingface.co/mradermacher/Hathor_Aleph-L3-8B-v0.72-GGUF
## Usage
If you are unsure how to use GGUF files, refer to one of [TheBloke's
READMEs](https://huggingface.co/TheBloke/KafkaLM-70B-German-V0.1-GGUF) for
more details, including on how to concatenate multi-part files.
## Provided Quants
(sorted by size, not necessarily quality. IQ-quants are often preferable over similar sized non-IQ quants)
| Link | Type | Size/GB | Notes |
|:-----|:-----|--------:|:------|
| [GGUF](https://huggingface.co/mradermacher/Hathor_Aleph-L3-8B-v0.72-i1-GGUF/resolve/main/Hathor_Aleph-L3-8B-v0.72.i1-IQ1_S.gguf) | i1-IQ1_S | 2.1 | for the desperate |
| [GGUF](https://huggingface.co/mradermacher/Hathor_Aleph-L3-8B-v0.72-i1-GGUF/resolve/main/Hathor_Aleph-L3-8B-v0.72.i1-IQ1_M.gguf) | i1-IQ1_M | 2.3 | mostly desperate |
| [GGUF](https://huggingface.co/mradermacher/Hathor_Aleph-L3-8B-v0.72-i1-GGUF/resolve/main/Hathor_Aleph-L3-8B-v0.72.i1-IQ2_XXS.gguf) | i1-IQ2_XXS | 2.5 | |
| [GGUF](https://huggingface.co/mradermacher/Hathor_Aleph-L3-8B-v0.72-i1-GGUF/resolve/main/Hathor_Aleph-L3-8B-v0.72.i1-IQ2_XS.gguf) | i1-IQ2_XS | 2.7 | |
| [GGUF](https://huggingface.co/mradermacher/Hathor_Aleph-L3-8B-v0.72-i1-GGUF/resolve/main/Hathor_Aleph-L3-8B-v0.72.i1-IQ2_S.gguf) | i1-IQ2_S | 2.9 | |
| [GGUF](https://huggingface.co/mradermacher/Hathor_Aleph-L3-8B-v0.72-i1-GGUF/resolve/main/Hathor_Aleph-L3-8B-v0.72.i1-IQ2_M.gguf) | i1-IQ2_M | 3.0 | |
| [GGUF](https://huggingface.co/mradermacher/Hathor_Aleph-L3-8B-v0.72-i1-GGUF/resolve/main/Hathor_Aleph-L3-8B-v0.72.i1-Q2_K.gguf) | i1-Q2_K | 3.3 | IQ3_XXS probably better |
| [GGUF](https://huggingface.co/mradermacher/Hathor_Aleph-L3-8B-v0.72-i1-GGUF/resolve/main/Hathor_Aleph-L3-8B-v0.72.i1-IQ3_XXS.gguf) | i1-IQ3_XXS | 3.4 | lower quality |
| [GGUF](https://huggingface.co/mradermacher/Hathor_Aleph-L3-8B-v0.72-i1-GGUF/resolve/main/Hathor_Aleph-L3-8B-v0.72.i1-IQ3_XS.gguf) | i1-IQ3_XS | 3.6 | |
| [GGUF](https://huggingface.co/mradermacher/Hathor_Aleph-L3-8B-v0.72-i1-GGUF/resolve/main/Hathor_Aleph-L3-8B-v0.72.i1-Q3_K_S.gguf) | i1-Q3_K_S | 3.8 | IQ3_XS probably better |
| [GGUF](https://huggingface.co/mradermacher/Hathor_Aleph-L3-8B-v0.72-i1-GGUF/resolve/main/Hathor_Aleph-L3-8B-v0.72.i1-IQ3_S.gguf) | i1-IQ3_S | 3.8 | beats Q3_K* |
| [GGUF](https://huggingface.co/mradermacher/Hathor_Aleph-L3-8B-v0.72-i1-GGUF/resolve/main/Hathor_Aleph-L3-8B-v0.72.i1-IQ3_M.gguf) | i1-IQ3_M | 3.9 | |
| [GGUF](https://huggingface.co/mradermacher/Hathor_Aleph-L3-8B-v0.72-i1-GGUF/resolve/main/Hathor_Aleph-L3-8B-v0.72.i1-Q3_K_M.gguf) | i1-Q3_K_M | 4.1 | IQ3_S probably better |
| [GGUF](https://huggingface.co/mradermacher/Hathor_Aleph-L3-8B-v0.72-i1-GGUF/resolve/main/Hathor_Aleph-L3-8B-v0.72.i1-Q3_K_L.gguf) | i1-Q3_K_L | 4.4 | IQ3_M probably better |
| [GGUF](https://huggingface.co/mradermacher/Hathor_Aleph-L3-8B-v0.72-i1-GGUF/resolve/main/Hathor_Aleph-L3-8B-v0.72.i1-IQ4_XS.gguf) | i1-IQ4_XS | 4.5 | |
| [GGUF](https://huggingface.co/mradermacher/Hathor_Aleph-L3-8B-v0.72-i1-GGUF/resolve/main/Hathor_Aleph-L3-8B-v0.72.i1-Q4_0.gguf) | i1-Q4_0 | 4.8 | fast, low quality |
| [GGUF](https://huggingface.co/mradermacher/Hathor_Aleph-L3-8B-v0.72-i1-GGUF/resolve/main/Hathor_Aleph-L3-8B-v0.72.i1-Q4_K_S.gguf) | i1-Q4_K_S | 4.8 | optimal size/speed/quality |
| [GGUF](https://huggingface.co/mradermacher/Hathor_Aleph-L3-8B-v0.72-i1-GGUF/resolve/main/Hathor_Aleph-L3-8B-v0.72.i1-Q4_K_M.gguf) | i1-Q4_K_M | 5.0 | fast, recommended |
| [GGUF](https://huggingface.co/mradermacher/Hathor_Aleph-L3-8B-v0.72-i1-GGUF/resolve/main/Hathor_Aleph-L3-8B-v0.72.i1-Q5_K_S.gguf) | i1-Q5_K_S | 5.7 | |
| [GGUF](https://huggingface.co/mradermacher/Hathor_Aleph-L3-8B-v0.72-i1-GGUF/resolve/main/Hathor_Aleph-L3-8B-v0.72.i1-Q5_K_M.gguf) | i1-Q5_K_M | 5.8 | |
| [GGUF](https://huggingface.co/mradermacher/Hathor_Aleph-L3-8B-v0.72-i1-GGUF/resolve/main/Hathor_Aleph-L3-8B-v0.72.i1-Q6_K.gguf) | i1-Q6_K | 6.7 | practically like static Q6_K |
Here is a handy graph by ikawrakow comparing some lower-quality quant
types (lower is better):

And here are Artefact2's thoughts on the matter:
https://gist.github.com/Artefact2/b5f810600771265fc1e39442288e8ec9
## FAQ / Model Request
See https://huggingface.co/mradermacher/model_requests for some answers to
questions you might have and/or if you want some other model quantized.
## Thanks
I thank my company, [nethype GmbH](https://www.nethype.de/), for letting
me use its servers and providing upgrades to my workstation to enable
this work in my free time. Additional thanks to [@nicoboss](https://huggingface.co/nicoboss) for giving me access to his hardware for calculating the imatrix for these quants.
<!-- end -->
|
MaziyarPanahi/Llama-3-8B-Instruct-32k-v0.1-GGUF | MaziyarPanahi | "2024-06-28T10:42:58Z" | 15,721 | 55 | transformers | [
"transformers",
"gguf",
"mistral",
"quantized",
"2-bit",
"3-bit",
"4-bit",
"5-bit",
"6-bit",
"8-bit",
"GGUF",
"text-generation",
"base_model:MaziyarPanahi/Llama-3-8B-Instruct-32k-v0.1",
"text-generation-inference",
"region:us"
] | text-generation | "2024-04-24T16:01:52Z" | ---
tags:
- quantized
- 2-bit
- 3-bit
- 4-bit
- 5-bit
- 6-bit
- 8-bit
- GGUF
- text-generation
- text-generation
model_name: Llama-3-8B-Instruct-32k-v0.1-GGUF
base_model: MaziyarPanahi/Llama-3-8B-Instruct-32k-v0.1
inference: false
model_creator: MaziyarPanahi
pipeline_tag: text-generation
quantized_by: MaziyarPanahi
---
# [MaziyarPanahi/Llama-3-8B-Instruct-32k-v0.1-GGUF](https://huggingface.co/MaziyarPanahi/Llama-3-8B-Instruct-32k-v0.1-GGUF)
- Model creator: [MaziyarPanahi](https://huggingface.co/MaziyarPanahi)
- Original model: [MaziyarPanahi/Llama-3-8B-Instruct-32k-v0.1](https://huggingface.co/MaziyarPanahi/Llama-3-8B-Instruct-32k-v0.1)
## Description
[MaziyarPanahi/Llama-3-8B-Instruct-32k-v0.1-GGUF](https://huggingface.co/MaziyarPanahi/Llama-3-8B-Instruct-32k-v0.1-GGUF) contains GGUF format model files for [MaziyarPanahi/Llama-3-8B-Instruct-32k-v0.1](https://huggingface.co/MaziyarPanahi/Llama-3-8B-Instruct-32k-v0.1).
### About GGUF
GGUF is a new format introduced by the llama.cpp team on August 21st 2023. It is a replacement for GGML, which is no longer supported by llama.cpp.
Here is an incomplete list of clients and libraries that are known to support GGUF:
* [llama.cpp](https://github.com/ggerganov/llama.cpp). The source project for GGUF. Offers a CLI and a server option.
* [llama-cpp-python](https://github.com/abetlen/llama-cpp-python), a Python library with GPU accel, LangChain support, and OpenAI-compatible API server.
* [LM Studio](https://lmstudio.ai/), an easy-to-use and powerful local GUI for Windows and macOS (Silicon), with GPU acceleration. Linux available, in beta as of 27/11/2023.
* [text-generation-webui](https://github.com/oobabooga/text-generation-webui), the most widely used web UI, with many features and powerful extensions. Supports GPU acceleration.
* [KoboldCpp](https://github.com/LostRuins/koboldcpp), a fully featured web UI, with GPU accel across all platforms and GPU architectures. Especially good for story telling.
* [GPT4All](https://gpt4all.io/index.html), a free and open source local running GUI, supporting Windows, Linux and macOS with full GPU accel.
* [LoLLMS Web UI](https://github.com/ParisNeo/lollms-webui), a great web UI with many interesting and unique features, including a full model library for easy model selection.
* [Faraday.dev](https://faraday.dev/), an attractive and easy to use character-based chat GUI for Windows and macOS (both Silicon and Intel), with GPU acceleration.
* [candle](https://github.com/huggingface/candle), a Rust ML framework with a focus on performance, including GPU support, and ease of use.
* [ctransformers](https://github.com/marella/ctransformers), a Python library with GPU accel, LangChain support, and OpenAI-compatible AI server. Note, as of time of writing (November 27th 2023), ctransformers has not been updated in a long time and does not support many recent models.
## Special thanks
🙏 Special thanks to [Georgi Gerganov](https://github.com/ggerganov) and the whole team working on [llama.cpp](https://github.com/ggerganov/llama.cpp/) for making all of this possible. |
LWDCLS/LLaMa-3-CursedStock-v2.0-8B-GGUF-IQ-Imatrix-Request | LWDCLS | "2024-06-27T13:33:19Z" | 15,713 | 8 | null | [
"gguf",
"license:unlicense",
"region:us"
] | null | "2024-06-26T22:14:01Z" | ---
license: unlicense
---
[Click for details - Request #56.](https://huggingface.co/Lewdiculous/Model-Requests/discussions/56)

|
carbon225/vit-base-patch16-224-hentai | carbon225 | "2023-07-04T14:50:00Z" | 15,712 | 17 | transformers | [
"transformers",
"pytorch",
"safetensors",
"vit",
"image-classification",
"art",
"anime",
"visual-novel",
"nsfw",
"dataset:carbon225/vndb_img",
"license:cc0-1.0",
"autotrain_compatible",
"endpoints_compatible",
"region:us"
] | image-classification | "2022-09-30T12:06:40Z" | ---
license: cc0-1.0
widget:
- src: >-
https://huggingface.co/carbon225/vit-base-patch16-224-hentai/resolve/main/samples/1.jpeg
- src: >-
https://huggingface.co/carbon225/vit-base-patch16-224-hentai/resolve/main/samples/2.jpeg
datasets:
- carbon225/vndb_img
tags:
- art
- anime
- visual-novel
- nsfw
---
# ViT for NSFW classification
## Model info
This is Google's [vit-base-patch16-224-in21k](https://huggingface.co/google/vit-base-patch16-224-in21k)
finetuned for flagging images according to [vndb.org](https://vndb.org/d19) with 3 classes:
- safe
- suggestive
- explicit
## Training data
The model was trained on the vndb.org [database dump](https://vndb.org/d14)
using full size screenshots (`sf` in the database dump).
The dataset can be loaded from [carbon225/vndb_img](https://huggingface.co/datasets/carbon225/vndb_img).
## Intended use
The model can be used for flagging anime-style images for sexual content.
It can also be finetuned on other tasks related to anime images. |
timm/convnextv2_tiny.fcmae_ft_in22k_in1k_384 | timm | "2024-02-10T23:29:42Z" | 15,707 | 1 | timm | [
"timm",
"pytorch",
"safetensors",
"image-classification",
"dataset:imagenet-1k",
"arxiv:2301.00808",
"license:cc-by-nc-4.0",
"region:us"
] | image-classification | "2023-01-05T01:57:01Z" | ---
license: cc-by-nc-4.0
library_name: timm
tags:
- image-classification
- timm
datasets:
- imagenet-1k
- imagenet-1k
---
# Model card for convnextv2_tiny.fcmae_ft_in22k_in1k_384
A ConvNeXt-V2 image classification model. Pretrained with a fully convolutional masked autoencoder framework (FCMAE) and fine-tuned on ImageNet-22k and then ImageNet-1k.
## Model Details
- **Model Type:** Image classification / feature backbone
- **Model Stats:**
- Params (M): 28.6
- GMACs: 13.1
- Activations (M): 39.5
- Image size: 384 x 384
- **Papers:**
- ConvNeXt V2: Co-designing and Scaling ConvNets with Masked Autoencoders: https://arxiv.org/abs/2301.00808
- **Original:** https://github.com/facebookresearch/ConvNeXt-V2
- **Dataset:** ImageNet-1k
- **Pretrain Dataset:** ImageNet-1k
## Model Usage
### Image Classification
```python
from urllib.request import urlopen
from PIL import Image
import timm
img = Image.open(urlopen(
'https://huggingface.co/datasets/huggingface/documentation-images/resolve/main/beignets-task-guide.png'
))
model = timm.create_model('convnextv2_tiny.fcmae_ft_in22k_in1k_384', pretrained=True)
model = model.eval()
# get model specific transforms (normalization, resize)
data_config = timm.data.resolve_model_data_config(model)
transforms = timm.data.create_transform(**data_config, is_training=False)
output = model(transforms(img).unsqueeze(0)) # unsqueeze single image into batch of 1
top5_probabilities, top5_class_indices = torch.topk(output.softmax(dim=1) * 100, k=5)
```
### Feature Map Extraction
```python
from urllib.request import urlopen
from PIL import Image
import timm
img = Image.open(urlopen(
'https://huggingface.co/datasets/huggingface/documentation-images/resolve/main/beignets-task-guide.png'
))
model = timm.create_model(
'convnextv2_tiny.fcmae_ft_in22k_in1k_384',
pretrained=True,
features_only=True,
)
model = model.eval()
# get model specific transforms (normalization, resize)
data_config = timm.data.resolve_model_data_config(model)
transforms = timm.data.create_transform(**data_config, is_training=False)
output = model(transforms(img).unsqueeze(0)) # unsqueeze single image into batch of 1
for o in output:
# print shape of each feature map in output
# e.g.:
# torch.Size([1, 96, 96, 96])
# torch.Size([1, 192, 48, 48])
# torch.Size([1, 384, 24, 24])
# torch.Size([1, 768, 12, 12])
print(o.shape)
```
### Image Embeddings
```python
from urllib.request import urlopen
from PIL import Image
import timm
img = Image.open(urlopen(
'https://huggingface.co/datasets/huggingface/documentation-images/resolve/main/beignets-task-guide.png'
))
model = timm.create_model(
'convnextv2_tiny.fcmae_ft_in22k_in1k_384',
pretrained=True,
num_classes=0, # remove classifier nn.Linear
)
model = model.eval()
# get model specific transforms (normalization, resize)
data_config = timm.data.resolve_model_data_config(model)
transforms = timm.data.create_transform(**data_config, is_training=False)
output = model(transforms(img).unsqueeze(0)) # output is (batch_size, num_features) shaped tensor
# or equivalently (without needing to set num_classes=0)
output = model.forward_features(transforms(img).unsqueeze(0))
# output is unpooled, a (1, 768, 12, 12) shaped tensor
output = model.forward_head(output, pre_logits=True)
# output is a (1, num_features) shaped tensor
```
## Model Comparison
Explore the dataset and runtime metrics of this model in timm [model results](https://github.com/huggingface/pytorch-image-models/tree/main/results).
All timing numbers from eager model PyTorch 1.13 on RTX 3090 w/ AMP.
| model |top1 |top5 |img_size|param_count|gmacs |macts |samples_per_sec|batch_size|
|------------------------------------------------------------------------------------------------------------------------------|------|------|--------|-----------|------|------|---------------|----------|
| [convnextv2_huge.fcmae_ft_in22k_in1k_512](https://huggingface.co/timm/convnextv2_huge.fcmae_ft_in22k_in1k_512) |88.848|98.742|512 |660.29 |600.81|413.07|28.58 |48 |
| [convnextv2_huge.fcmae_ft_in22k_in1k_384](https://huggingface.co/timm/convnextv2_huge.fcmae_ft_in22k_in1k_384) |88.668|98.738|384 |660.29 |337.96|232.35|50.56 |64 |
| [convnext_xxlarge.clip_laion2b_soup_ft_in1k](https://huggingface.co/timm/convnext_xxlarge.clip_laion2b_soup_ft_in1k) |88.612|98.704|256 |846.47 |198.09|124.45|122.45 |256 |
| [convnext_large_mlp.clip_laion2b_soup_ft_in12k_in1k_384](https://huggingface.co/timm/convnext_large_mlp.clip_laion2b_soup_ft_in12k_in1k_384) |88.312|98.578|384 |200.13 |101.11|126.74|196.84 |256 |
| [convnextv2_large.fcmae_ft_in22k_in1k_384](https://huggingface.co/timm/convnextv2_large.fcmae_ft_in22k_in1k_384) |88.196|98.532|384 |197.96 |101.1 |126.74|128.94 |128 |
| [convnext_large_mlp.clip_laion2b_soup_ft_in12k_in1k_320](https://huggingface.co/timm/convnext_large_mlp.clip_laion2b_soup_ft_in12k_in1k_320) |87.968|98.47 |320 |200.13 |70.21 |88.02 |283.42 |256 |
| [convnext_xlarge.fb_in22k_ft_in1k_384](https://huggingface.co/timm/convnext_xlarge.fb_in22k_ft_in1k_384) |87.75 |98.556|384 |350.2 |179.2 |168.99|124.85 |192 |
| [convnextv2_base.fcmae_ft_in22k_in1k_384](https://huggingface.co/timm/convnextv2_base.fcmae_ft_in22k_in1k_384) |87.646|98.422|384 |88.72 |45.21 |84.49 |209.51 |256 |
| [convnext_large.fb_in22k_ft_in1k_384](https://huggingface.co/timm/convnext_large.fb_in22k_ft_in1k_384) |87.476|98.382|384 |197.77 |101.1 |126.74|194.66 |256 |
| [convnext_large_mlp.clip_laion2b_augreg_ft_in1k](https://huggingface.co/timm/convnext_large_mlp.clip_laion2b_augreg_ft_in1k) |87.344|98.218|256 |200.13 |44.94 |56.33 |438.08 |256 |
| [convnextv2_large.fcmae_ft_in22k_in1k](https://huggingface.co/timm/convnextv2_large.fcmae_ft_in22k_in1k) |87.26 |98.248|224 |197.96 |34.4 |43.13 |376.84 |256 |
| [convnext_base.clip_laion2b_augreg_ft_in12k_in1k_384](https://huggingface.co/timm/convnext_base.clip_laion2b_augreg_ft_in12k_in1k_384) |87.138|98.212|384 |88.59 |45.21 |84.49 |365.47 |256 |
| [convnext_xlarge.fb_in22k_ft_in1k](https://huggingface.co/timm/convnext_xlarge.fb_in22k_ft_in1k) |87.002|98.208|224 |350.2 |60.98 |57.5 |368.01 |256 |
| [convnext_base.fb_in22k_ft_in1k_384](https://huggingface.co/timm/convnext_base.fb_in22k_ft_in1k_384) |86.796|98.264|384 |88.59 |45.21 |84.49 |366.54 |256 |
| [convnextv2_base.fcmae_ft_in22k_in1k](https://huggingface.co/timm/convnextv2_base.fcmae_ft_in22k_in1k) |86.74 |98.022|224 |88.72 |15.38 |28.75 |624.23 |256 |
| [convnext_large.fb_in22k_ft_in1k](https://huggingface.co/timm/convnext_large.fb_in22k_ft_in1k) |86.636|98.028|224 |197.77 |34.4 |43.13 |581.43 |256 |
| [convnext_base.clip_laiona_augreg_ft_in1k_384](https://huggingface.co/timm/convnext_base.clip_laiona_augreg_ft_in1k_384) |86.504|97.97 |384 |88.59 |45.21 |84.49 |368.14 |256 |
| [convnext_base.clip_laion2b_augreg_ft_in12k_in1k](https://huggingface.co/timm/convnext_base.clip_laion2b_augreg_ft_in12k_in1k) |86.344|97.97 |256 |88.59 |20.09 |37.55 |816.14 |256 |
| [convnextv2_huge.fcmae_ft_in1k](https://huggingface.co/timm/convnextv2_huge.fcmae_ft_in1k) |86.256|97.75 |224 |660.29 |115.0 |79.07 |154.72 |256 |
| [convnext_small.in12k_ft_in1k_384](https://huggingface.co/timm/convnext_small.in12k_ft_in1k_384) |86.182|97.92 |384 |50.22 |25.58 |63.37 |516.19 |256 |
| [convnext_base.clip_laion2b_augreg_ft_in1k](https://huggingface.co/timm/convnext_base.clip_laion2b_augreg_ft_in1k) |86.154|97.68 |256 |88.59 |20.09 |37.55 |819.86 |256 |
| [convnext_base.fb_in22k_ft_in1k](https://huggingface.co/timm/convnext_base.fb_in22k_ft_in1k) |85.822|97.866|224 |88.59 |15.38 |28.75 |1037.66 |256 |
| [convnext_small.fb_in22k_ft_in1k_384](https://huggingface.co/timm/convnext_small.fb_in22k_ft_in1k_384) |85.778|97.886|384 |50.22 |25.58 |63.37 |518.95 |256 |
| [convnextv2_large.fcmae_ft_in1k](https://huggingface.co/timm/convnextv2_large.fcmae_ft_in1k) |85.742|97.584|224 |197.96 |34.4 |43.13 |375.23 |256 |
| [convnext_small.in12k_ft_in1k](https://huggingface.co/timm/convnext_small.in12k_ft_in1k) |85.174|97.506|224 |50.22 |8.71 |21.56 |1474.31 |256 |
| [convnext_tiny.in12k_ft_in1k_384](https://huggingface.co/timm/convnext_tiny.in12k_ft_in1k_384) |85.118|97.608|384 |28.59 |13.14 |39.48 |856.76 |256 |
| [convnextv2_tiny.fcmae_ft_in22k_in1k_384](https://huggingface.co/timm/convnextv2_tiny.fcmae_ft_in22k_in1k_384) |85.112|97.63 |384 |28.64 |13.14 |39.48 |491.32 |256 |
| [convnextv2_base.fcmae_ft_in1k](https://huggingface.co/timm/convnextv2_base.fcmae_ft_in1k) |84.874|97.09 |224 |88.72 |15.38 |28.75 |625.33 |256 |
| [convnext_small.fb_in22k_ft_in1k](https://huggingface.co/timm/convnext_small.fb_in22k_ft_in1k) |84.562|97.394|224 |50.22 |8.71 |21.56 |1478.29 |256 |
| [convnext_large.fb_in1k](https://huggingface.co/timm/convnext_large.fb_in1k) |84.282|96.892|224 |197.77 |34.4 |43.13 |584.28 |256 |
| [convnext_tiny.in12k_ft_in1k](https://huggingface.co/timm/convnext_tiny.in12k_ft_in1k) |84.186|97.124|224 |28.59 |4.47 |13.44 |2433.7 |256 |
| [convnext_tiny.fb_in22k_ft_in1k_384](https://huggingface.co/timm/convnext_tiny.fb_in22k_ft_in1k_384) |84.084|97.14 |384 |28.59 |13.14 |39.48 |862.95 |256 |
| [convnextv2_tiny.fcmae_ft_in22k_in1k](https://huggingface.co/timm/convnextv2_tiny.fcmae_ft_in22k_in1k) |83.894|96.964|224 |28.64 |4.47 |13.44 |1452.72 |256 |
| [convnext_base.fb_in1k](https://huggingface.co/timm/convnext_base.fb_in1k) |83.82 |96.746|224 |88.59 |15.38 |28.75 |1054.0 |256 |
| [convnextv2_nano.fcmae_ft_in22k_in1k_384](https://huggingface.co/timm/convnextv2_nano.fcmae_ft_in22k_in1k_384) |83.37 |96.742|384 |15.62 |7.22 |24.61 |801.72 |256 |
| [convnext_small.fb_in1k](https://huggingface.co/timm/convnext_small.fb_in1k) |83.142|96.434|224 |50.22 |8.71 |21.56 |1464.0 |256 |
| [convnextv2_tiny.fcmae_ft_in1k](https://huggingface.co/timm/convnextv2_tiny.fcmae_ft_in1k) |82.92 |96.284|224 |28.64 |4.47 |13.44 |1425.62 |256 |
| [convnext_tiny.fb_in22k_ft_in1k](https://huggingface.co/timm/convnext_tiny.fb_in22k_ft_in1k) |82.898|96.616|224 |28.59 |4.47 |13.44 |2480.88 |256 |
| [convnext_nano.in12k_ft_in1k](https://huggingface.co/timm/convnext_nano.in12k_ft_in1k) |82.282|96.344|224 |15.59 |2.46 |8.37 |3926.52 |256 |
| [convnext_tiny_hnf.a2h_in1k](https://huggingface.co/timm/convnext_tiny_hnf.a2h_in1k) |82.216|95.852|224 |28.59 |4.47 |13.44 |2529.75 |256 |
| [convnext_tiny.fb_in1k](https://huggingface.co/timm/convnext_tiny.fb_in1k) |82.066|95.854|224 |28.59 |4.47 |13.44 |2346.26 |256 |
| [convnextv2_nano.fcmae_ft_in22k_in1k](https://huggingface.co/timm/convnextv2_nano.fcmae_ft_in22k_in1k) |82.03 |96.166|224 |15.62 |2.46 |8.37 |2300.18 |256 |
| [convnextv2_nano.fcmae_ft_in1k](https://huggingface.co/timm/convnextv2_nano.fcmae_ft_in1k) |81.83 |95.738|224 |15.62 |2.46 |8.37 |2321.48 |256 |
| [convnext_nano_ols.d1h_in1k](https://huggingface.co/timm/convnext_nano_ols.d1h_in1k) |80.866|95.246|224 |15.65 |2.65 |9.38 |3523.85 |256 |
| [convnext_nano.d1h_in1k](https://huggingface.co/timm/convnext_nano.d1h_in1k) |80.768|95.334|224 |15.59 |2.46 |8.37 |3915.58 |256 |
| [convnextv2_pico.fcmae_ft_in1k](https://huggingface.co/timm/convnextv2_pico.fcmae_ft_in1k) |80.304|95.072|224 |9.07 |1.37 |6.1 |3274.57 |256 |
| [convnext_pico.d1_in1k](https://huggingface.co/timm/convnext_pico.d1_in1k) |79.526|94.558|224 |9.05 |1.37 |6.1 |5686.88 |256 |
| [convnext_pico_ols.d1_in1k](https://huggingface.co/timm/convnext_pico_ols.d1_in1k) |79.522|94.692|224 |9.06 |1.43 |6.5 |5422.46 |256 |
| [convnextv2_femto.fcmae_ft_in1k](https://huggingface.co/timm/convnextv2_femto.fcmae_ft_in1k) |78.488|93.98 |224 |5.23 |0.79 |4.57 |4264.2 |256 |
| [convnext_femto_ols.d1_in1k](https://huggingface.co/timm/convnext_femto_ols.d1_in1k) |77.86 |93.83 |224 |5.23 |0.82 |4.87 |6910.6 |256 |
| [convnext_femto.d1_in1k](https://huggingface.co/timm/convnext_femto.d1_in1k) |77.454|93.68 |224 |5.22 |0.79 |4.57 |7189.92 |256 |
| [convnextv2_atto.fcmae_ft_in1k](https://huggingface.co/timm/convnextv2_atto.fcmae_ft_in1k) |76.664|93.044|224 |3.71 |0.55 |3.81 |4728.91 |256 |
| [convnext_atto_ols.a2_in1k](https://huggingface.co/timm/convnext_atto_ols.a2_in1k) |75.88 |92.846|224 |3.7 |0.58 |4.11 |7963.16 |256 |
| [convnext_atto.d2_in1k](https://huggingface.co/timm/convnext_atto.d2_in1k) |75.664|92.9 |224 |3.7 |0.55 |3.81 |8439.22 |256 |
## Citation
```bibtex
@article{Woo2023ConvNeXtV2,
title={ConvNeXt V2: Co-designing and Scaling ConvNets with Masked Autoencoders},
author={Sanghyun Woo, Shoubhik Debnath, Ronghang Hu, Xinlei Chen, Zhuang Liu, In So Kweon and Saining Xie},
year={2023},
journal={arXiv preprint arXiv:2301.00808},
}
```
```bibtex
@misc{rw2019timm,
author = {Ross Wightman},
title = {PyTorch Image Models},
year = {2019},
publisher = {GitHub},
journal = {GitHub repository},
doi = {10.5281/zenodo.4414861},
howpublished = {\url{https://github.com/huggingface/pytorch-image-models}}
}
```
|
stabilityai/japanese-stablelm-base-gamma-7b | stabilityai | "2024-01-25T08:05:12Z" | 15,703 | 20 | transformers | [
"transformers",
"safetensors",
"mistral",
"text-generation",
"japanese-stablelm",
"causal-lm",
"ja",
"dataset:wikipedia",
"dataset:mc4",
"dataset:cc100",
"dataset:oscar-corpus/OSCAR-2301",
"dataset:oscar-corpus/OSCAR-2201",
"dataset:cerebras/SlimPajama-627B",
"arxiv:2310.06825",
"license:apache-2.0",
"autotrain_compatible",
"endpoints_compatible",
"text-generation-inference",
"region:us"
] | text-generation | "2023-10-16T08:15:14Z" | ---
license: apache-2.0
tags:
- japanese-stablelm
- causal-lm
pipeline_tag: text-generation
datasets:
- wikipedia
- mc4
- cc100
- oscar-corpus/OSCAR-2301
- oscar-corpus/OSCAR-2201
- cerebras/SlimPajama-627B
language:
- ja
extra_gated_fields:
Name: text
Email: text
Country: text
Organization or Affiliation: text
I allow Stability AI to contact me about information related to its models and research: checkbox
---
# Japanese Stable LM Base Gamma 7B
## Model Description
This is a 7B-parameter decoder-only language model with a focus on maximizing Japanese language modeling performance and Japanese downstream task performance.
We conducted continued pretraining using Japanese data on the English language model, [Mistral-7B-v0.1](https://huggingface.co/mistralai/Mistral-7B-v0.1), to transfer the model's knowledge and capabilities to Japanese.
*If you are looking for an instruction-following model, check [Japanese Stable LM Instruct Gamma 7B](https://huggingface.co/stabilityai/japanese-stablelm-instruct-gamma-7b)*.
*If you are in search of a smaller model, please check [Japanese StableLM-3B-4E1T Base](https://huggingface.co/stabilityai/japanese-stablelm-3b-4e1t-base).*
## Usage
Ensure you are using Transformers 4.34.0 or newer.
```python
from transformers import AutoModelForCausalLM, AutoTokenizer
tokenizer = AutoTokenizer.from_pretrained("stabilityai/japanese-stablelm-base-gamma-7b")
model = AutoModelForCausalLM.from_pretrained(
"stabilityai/japanese-stablelm-base-gamma-7b",
torch_dtype="auto",
)
model.cuda()
inputs = tokenizer("AI で科学研究を加速するには、", return_tensors="pt").to("cuda")
tokens = model.generate(
**inputs,
max_new_tokens=64,
temperature=0.75,
top_p=0.95,
do_sample=True,
)
print(tokenizer.decode(tokens[0], skip_special_tokens=True))
```
## Model Details
* **Developed by**: [Stability AI](https://stability.ai/)
* **Model type**: `Japanese Stable LM Base Gamma 7B` model is an auto-regressive language model based on the transformer decoder architecture.
* **Language(s)**: Japanese
* **License**: This model is licensed under [Apache License, Version 2.0](https://www.apache.org/licenses/LICENSE-2.0).
* **Contact**: For questions and comments about the model, please join [Stable Community Japan](https://discord.gg/StableJP). For future announcements / information about Stability AI models, research, and events, please follow https://twitter.com/StabilityAI_JP.
### Model Architecture
For details, please see Mistral AI's [paper](https://arxiv.org/abs/2310.06825) and [release blog post](https://mistral.ai/news/announcing-mistral-7b/).
### Training Dataset
Around 100B tokens from a mixture of the following corpora were used for the continued pretraining.
- [Japanese/English Wikipedia](https://dumps.wikimedia.org/other/cirrussearch)
- [Japanese mc4](https://huggingface.co/datasets/mc4)
- [Japanese CC-100](http://data.statmt.org/cc-100/ja.txt.xz)
- [Japanese OSCAR](https://oscar-project.github.io/documentation/)
- [SlimPajama](https://huggingface.co/datasets/cerebras/SlimPajama-627B) without the Books3 subset
## Use and Limitations
### Intended Use
The model is intended to be used by all individuals as a foundational model for application-specific fine-tuning without strict limitations on commercial use.
### Limitations and bias
The pre-training dataset may have contained offensive or inappropriate content even after applying data cleansing filters which can be reflected in the model-generated text. We recommend users exercise reasonable caution when using these models in production systems. Do not use the model for any applications that may cause harm or distress to individuals or groups.
## Credits
The continued pre-training was carried out by [Takuya Akiba](https://huggingface.co/iwiwi).
Other aspects, including data preparation and evaluation, were handled by the Language Team of Stability AI Japan, notably [Meng Lee](https://huggingface.co/leemeng), [Fujiki Nakamura](https://huggingface.co/fujiki), [Makoto Shing](https://huggingface.co/mkshing), [Paul McCann](https://huggingface.co/polm-stability), and [Naoki Orii](https://huggingface.co/mrorii).
## Acknowledgements
This model is based on Mistral-7B-v0.1 released by the Mistral AI team. We are grateful to the Mistral AI team for providing such an excellent base model.
We are grateful for the contributions of the EleutherAI Polyglot-JA team in helping us to collect a large amount of pre-training data in Japanese. Polyglot-JA members includes Hyunwoong Ko (Project Lead), Fujiki Nakamura (originally started this project when he commited to the Polyglot team), Yunho Mo, Minji Jung, KeunSeok Im, and Su-Kyeong Jang.
We are also appreciative of [AI Novelist/Sta (Bit192, Inc.)](https://ai-novel.com/index.php) and the numerous contributors from [Stable Community Japan](https://discord.gg/VPrcE475HB) for assisting us in gathering a large amount of high-quality Japanese textual data for model training. |
RichardErkhov/NeverSleep_-_Llama-3-Lumimaid-8B-v0.1-gguf | RichardErkhov | "2024-06-28T14:26:48Z" | 15,699 | 0 | null | [
"gguf",
"region:us"
] | null | "2024-06-28T08:48:45Z" | Quantization made by Richard Erkhov.
[Github](https://github.com/RichardErkhov)
[Discord](https://discord.gg/pvy7H8DZMG)
[Request more models](https://github.com/RichardErkhov/quant_request)
Llama-3-Lumimaid-8B-v0.1 - GGUF
- Model creator: https://huggingface.co/NeverSleep/
- Original model: https://huggingface.co/NeverSleep/Llama-3-Lumimaid-8B-v0.1/
| Name | Quant method | Size |
| ---- | ---- | ---- |
| [Llama-3-Lumimaid-8B-v0.1.Q2_K.gguf](https://huggingface.co/RichardErkhov/NeverSleep_-_Llama-3-Lumimaid-8B-v0.1-gguf/blob/main/Llama-3-Lumimaid-8B-v0.1.Q2_K.gguf) | Q2_K | 2.96GB |
| [Llama-3-Lumimaid-8B-v0.1.IQ3_XS.gguf](https://huggingface.co/RichardErkhov/NeverSleep_-_Llama-3-Lumimaid-8B-v0.1-gguf/blob/main/Llama-3-Lumimaid-8B-v0.1.IQ3_XS.gguf) | IQ3_XS | 3.28GB |
| [Llama-3-Lumimaid-8B-v0.1.IQ3_S.gguf](https://huggingface.co/RichardErkhov/NeverSleep_-_Llama-3-Lumimaid-8B-v0.1-gguf/blob/main/Llama-3-Lumimaid-8B-v0.1.IQ3_S.gguf) | IQ3_S | 3.43GB |
| [Llama-3-Lumimaid-8B-v0.1.Q3_K_S.gguf](https://huggingface.co/RichardErkhov/NeverSleep_-_Llama-3-Lumimaid-8B-v0.1-gguf/blob/main/Llama-3-Lumimaid-8B-v0.1.Q3_K_S.gguf) | Q3_K_S | 3.41GB |
| [Llama-3-Lumimaid-8B-v0.1.IQ3_M.gguf](https://huggingface.co/RichardErkhov/NeverSleep_-_Llama-3-Lumimaid-8B-v0.1-gguf/blob/main/Llama-3-Lumimaid-8B-v0.1.IQ3_M.gguf) | IQ3_M | 3.52GB |
| [Llama-3-Lumimaid-8B-v0.1.Q3_K.gguf](https://huggingface.co/RichardErkhov/NeverSleep_-_Llama-3-Lumimaid-8B-v0.1-gguf/blob/main/Llama-3-Lumimaid-8B-v0.1.Q3_K.gguf) | Q3_K | 3.74GB |
| [Llama-3-Lumimaid-8B-v0.1.Q3_K_M.gguf](https://huggingface.co/RichardErkhov/NeverSleep_-_Llama-3-Lumimaid-8B-v0.1-gguf/blob/main/Llama-3-Lumimaid-8B-v0.1.Q3_K_M.gguf) | Q3_K_M | 3.74GB |
| [Llama-3-Lumimaid-8B-v0.1.Q3_K_L.gguf](https://huggingface.co/RichardErkhov/NeverSleep_-_Llama-3-Lumimaid-8B-v0.1-gguf/blob/main/Llama-3-Lumimaid-8B-v0.1.Q3_K_L.gguf) | Q3_K_L | 4.03GB |
| [Llama-3-Lumimaid-8B-v0.1.IQ4_XS.gguf](https://huggingface.co/RichardErkhov/NeverSleep_-_Llama-3-Lumimaid-8B-v0.1-gguf/blob/main/Llama-3-Lumimaid-8B-v0.1.IQ4_XS.gguf) | IQ4_XS | 4.18GB |
| [Llama-3-Lumimaid-8B-v0.1.Q4_0.gguf](https://huggingface.co/RichardErkhov/NeverSleep_-_Llama-3-Lumimaid-8B-v0.1-gguf/blob/main/Llama-3-Lumimaid-8B-v0.1.Q4_0.gguf) | Q4_0 | 4.34GB |
| [Llama-3-Lumimaid-8B-v0.1.IQ4_NL.gguf](https://huggingface.co/RichardErkhov/NeverSleep_-_Llama-3-Lumimaid-8B-v0.1-gguf/blob/main/Llama-3-Lumimaid-8B-v0.1.IQ4_NL.gguf) | IQ4_NL | 4.38GB |
| [Llama-3-Lumimaid-8B-v0.1.Q4_K_S.gguf](https://huggingface.co/RichardErkhov/NeverSleep_-_Llama-3-Lumimaid-8B-v0.1-gguf/blob/main/Llama-3-Lumimaid-8B-v0.1.Q4_K_S.gguf) | Q4_K_S | 4.37GB |
| [Llama-3-Lumimaid-8B-v0.1.Q4_K.gguf](https://huggingface.co/RichardErkhov/NeverSleep_-_Llama-3-Lumimaid-8B-v0.1-gguf/blob/main/Llama-3-Lumimaid-8B-v0.1.Q4_K.gguf) | Q4_K | 4.58GB |
| [Llama-3-Lumimaid-8B-v0.1.Q4_K_M.gguf](https://huggingface.co/RichardErkhov/NeverSleep_-_Llama-3-Lumimaid-8B-v0.1-gguf/blob/main/Llama-3-Lumimaid-8B-v0.1.Q4_K_M.gguf) | Q4_K_M | 4.58GB |
| [Llama-3-Lumimaid-8B-v0.1.Q4_1.gguf](https://huggingface.co/RichardErkhov/NeverSleep_-_Llama-3-Lumimaid-8B-v0.1-gguf/blob/main/Llama-3-Lumimaid-8B-v0.1.Q4_1.gguf) | Q4_1 | 4.78GB |
| [Llama-3-Lumimaid-8B-v0.1.Q5_0.gguf](https://huggingface.co/RichardErkhov/NeverSleep_-_Llama-3-Lumimaid-8B-v0.1-gguf/blob/main/Llama-3-Lumimaid-8B-v0.1.Q5_0.gguf) | Q5_0 | 3.74GB |
| [Llama-3-Lumimaid-8B-v0.1.Q5_K_S.gguf](https://huggingface.co/RichardErkhov/NeverSleep_-_Llama-3-Lumimaid-8B-v0.1-gguf/blob/main/Llama-3-Lumimaid-8B-v0.1.Q5_K_S.gguf) | Q5_K_S | 5.21GB |
| [Llama-3-Lumimaid-8B-v0.1.Q5_K.gguf](https://huggingface.co/RichardErkhov/NeverSleep_-_Llama-3-Lumimaid-8B-v0.1-gguf/blob/main/Llama-3-Lumimaid-8B-v0.1.Q5_K.gguf) | Q5_K | 5.34GB |
| [Llama-3-Lumimaid-8B-v0.1.Q5_K_M.gguf](https://huggingface.co/RichardErkhov/NeverSleep_-_Llama-3-Lumimaid-8B-v0.1-gguf/blob/main/Llama-3-Lumimaid-8B-v0.1.Q5_K_M.gguf) | Q5_K_M | 5.34GB |
| [Llama-3-Lumimaid-8B-v0.1.Q5_1.gguf](https://huggingface.co/RichardErkhov/NeverSleep_-_Llama-3-Lumimaid-8B-v0.1-gguf/blob/main/Llama-3-Lumimaid-8B-v0.1.Q5_1.gguf) | Q5_1 | 5.65GB |
| [Llama-3-Lumimaid-8B-v0.1.Q6_K.gguf](https://huggingface.co/RichardErkhov/NeverSleep_-_Llama-3-Lumimaid-8B-v0.1-gguf/blob/main/Llama-3-Lumimaid-8B-v0.1.Q6_K.gguf) | Q6_K | 6.14GB |
| [Llama-3-Lumimaid-8B-v0.1.Q8_0.gguf](https://huggingface.co/RichardErkhov/NeverSleep_-_Llama-3-Lumimaid-8B-v0.1-gguf/blob/main/Llama-3-Lumimaid-8B-v0.1.Q8_0.gguf) | Q8_0 | 7.95GB |
Original model description:
---
license: cc-by-nc-4.0
tags:
- not-for-all-audiences
- nsfw
---
## Lumimaid 0.1
<center><div style="width: 100%;">
<img src="https://cdn-uploads.huggingface.co/production/uploads/630dfb008df86f1e5becadc3/d3QMaxy3peFTpSlWdWF-k.png" style="display: block; margin: auto;">
</div></center>
This model uses the Llama3 **prompting format**
Llama3 trained on our RP datasets, we tried to have a balance between the ERP and the RP, not too horny, but just enough.
We also added some non-RP dataset, making the model less dumb overall. It should look like a 40%/60% ratio for Non-RP/RP+ERP data.
This model includes the new Luminae dataset from Ikari.
If you consider trying this model please give us some feedback either on the Community tab on hf or on our [Discord Server](https://discord.gg/MtCVRWTZXY).
## Credits:
- Undi
- IkariDev
## Description
This repo contains FP16 files of Lumimaid-8B-v0.1.
Switch: [8B](https://huggingface.co/NeverSleep/Llama-3-Lumimaid-8B-v0.1) - [70B](https://huggingface.co/NeverSleep/Llama-3-Lumimaid-70B-v0.1) - [70B-alt](https://huggingface.co/NeverSleep/Llama-3-Lumimaid-70B-v0.1-alt) - [8B-OAS](https://huggingface.co/NeverSleep/Llama-3-Lumimaid-8B-v0.1-OAS) - [70B-OAS](https://huggingface.co/NeverSleep/Llama-3-Lumimaid-70B-v0.1-OAS)
## Training data used:
- [Aesir datasets](https://huggingface.co/MinervaAI)
- [NoRobots](https://huggingface.co/datasets/Doctor-Shotgun/no-robots-sharegpt)
- [limarp](https://huggingface.co/datasets/lemonilia/LimaRP) - 8k ctx
- [toxic-dpo-v0.1-sharegpt](https://huggingface.co/datasets/Undi95/toxic-dpo-v0.1-sharegpt)
- [ToxicQAFinal](https://huggingface.co/datasets/NobodyExistsOnTheInternet/ToxicQAFinal)
- Luminae-i1 (70B/70B-alt) (i2 was not existing when the 70b started training) | Luminae-i2 (8B) (this one gave better results on the 8b) - Ikari's Dataset
- [Squish42/bluemoon-fandom-1-1-rp-cleaned](https://huggingface.co/datasets/Squish42/bluemoon-fandom-1-1-rp-cleaned) - 50% (randomly)
- [NobodyExistsOnTheInternet/PIPPAsharegptv2test](https://huggingface.co/datasets/NobodyExistsOnTheInternet/PIPPAsharegptv2test) - 5% (randomly)
- [cgato/SlimOrcaDedupCleaned](https://huggingface.co/datasets/cgato/SlimOrcaDedupCleaned) - 5% (randomly)
- Airoboros (reduced)
- [Capybara](https://huggingface.co/datasets/Undi95/Capybara-ShareGPT/) (reduced)
## Models used (only for 8B)
- Initial LumiMaid 8B Finetune
- Undi95/Llama-3-Unholy-8B-e4
- Undi95/Llama-3-LewdPlay-8B
## Prompt template: Llama3
```
<|begin_of_text|><|start_header_id|>system<|end_header_id|>
{system_prompt}<|eot_id|><|start_header_id|>user<|end_header_id|>
{input}<|eot_id|><|start_header_id|>assistant<|end_header_id|>
{output}<|eot_id|>
```
## Others
Undi: If you want to support us, you can [here](https://ko-fi.com/undiai).
IkariDev: Visit my [retro/neocities style website](https://ikaridevgit.github.io/) please kek
|
RichardErkhov/ItchyChin_-_OrpoLlama-3-8B-memorize-translate-gguf | RichardErkhov | "2024-06-28T18:04:15Z" | 15,698 | 0 | null | [
"gguf",
"arxiv:1910.09700",
"region:us"
] | null | "2024-06-28T15:24:54Z" | Quantization made by Richard Erkhov.
[Github](https://github.com/RichardErkhov)
[Discord](https://discord.gg/pvy7H8DZMG)
[Request more models](https://github.com/RichardErkhov/quant_request)
OrpoLlama-3-8B-memorize-translate - GGUF
- Model creator: https://huggingface.co/ItchyChin/
- Original model: https://huggingface.co/ItchyChin/OrpoLlama-3-8B-memorize-translate/
| Name | Quant method | Size |
| ---- | ---- | ---- |
| [OrpoLlama-3-8B-memorize-translate.Q2_K.gguf](https://huggingface.co/RichardErkhov/ItchyChin_-_OrpoLlama-3-8B-memorize-translate-gguf/blob/main/OrpoLlama-3-8B-memorize-translate.Q2_K.gguf) | Q2_K | 2.96GB |
| [OrpoLlama-3-8B-memorize-translate.IQ3_XS.gguf](https://huggingface.co/RichardErkhov/ItchyChin_-_OrpoLlama-3-8B-memorize-translate-gguf/blob/main/OrpoLlama-3-8B-memorize-translate.IQ3_XS.gguf) | IQ3_XS | 3.28GB |
| [OrpoLlama-3-8B-memorize-translate.IQ3_S.gguf](https://huggingface.co/RichardErkhov/ItchyChin_-_OrpoLlama-3-8B-memorize-translate-gguf/blob/main/OrpoLlama-3-8B-memorize-translate.IQ3_S.gguf) | IQ3_S | 3.43GB |
| [OrpoLlama-3-8B-memorize-translate.Q3_K_S.gguf](https://huggingface.co/RichardErkhov/ItchyChin_-_OrpoLlama-3-8B-memorize-translate-gguf/blob/main/OrpoLlama-3-8B-memorize-translate.Q3_K_S.gguf) | Q3_K_S | 3.41GB |
| [OrpoLlama-3-8B-memorize-translate.IQ3_M.gguf](https://huggingface.co/RichardErkhov/ItchyChin_-_OrpoLlama-3-8B-memorize-translate-gguf/blob/main/OrpoLlama-3-8B-memorize-translate.IQ3_M.gguf) | IQ3_M | 3.52GB |
| [OrpoLlama-3-8B-memorize-translate.Q3_K.gguf](https://huggingface.co/RichardErkhov/ItchyChin_-_OrpoLlama-3-8B-memorize-translate-gguf/blob/main/OrpoLlama-3-8B-memorize-translate.Q3_K.gguf) | Q3_K | 3.74GB |
| [OrpoLlama-3-8B-memorize-translate.Q3_K_M.gguf](https://huggingface.co/RichardErkhov/ItchyChin_-_OrpoLlama-3-8B-memorize-translate-gguf/blob/main/OrpoLlama-3-8B-memorize-translate.Q3_K_M.gguf) | Q3_K_M | 3.74GB |
| [OrpoLlama-3-8B-memorize-translate.Q3_K_L.gguf](https://huggingface.co/RichardErkhov/ItchyChin_-_OrpoLlama-3-8B-memorize-translate-gguf/blob/main/OrpoLlama-3-8B-memorize-translate.Q3_K_L.gguf) | Q3_K_L | 4.03GB |
| [OrpoLlama-3-8B-memorize-translate.IQ4_XS.gguf](https://huggingface.co/RichardErkhov/ItchyChin_-_OrpoLlama-3-8B-memorize-translate-gguf/blob/main/OrpoLlama-3-8B-memorize-translate.IQ4_XS.gguf) | IQ4_XS | 4.18GB |
| [OrpoLlama-3-8B-memorize-translate.Q4_0.gguf](https://huggingface.co/RichardErkhov/ItchyChin_-_OrpoLlama-3-8B-memorize-translate-gguf/blob/main/OrpoLlama-3-8B-memorize-translate.Q4_0.gguf) | Q4_0 | 4.34GB |
| [OrpoLlama-3-8B-memorize-translate.IQ4_NL.gguf](https://huggingface.co/RichardErkhov/ItchyChin_-_OrpoLlama-3-8B-memorize-translate-gguf/blob/main/OrpoLlama-3-8B-memorize-translate.IQ4_NL.gguf) | IQ4_NL | 4.38GB |
| [OrpoLlama-3-8B-memorize-translate.Q4_K_S.gguf](https://huggingface.co/RichardErkhov/ItchyChin_-_OrpoLlama-3-8B-memorize-translate-gguf/blob/main/OrpoLlama-3-8B-memorize-translate.Q4_K_S.gguf) | Q4_K_S | 4.37GB |
| [OrpoLlama-3-8B-memorize-translate.Q4_K.gguf](https://huggingface.co/RichardErkhov/ItchyChin_-_OrpoLlama-3-8B-memorize-translate-gguf/blob/main/OrpoLlama-3-8B-memorize-translate.Q4_K.gguf) | Q4_K | 4.58GB |
| [OrpoLlama-3-8B-memorize-translate.Q4_K_M.gguf](https://huggingface.co/RichardErkhov/ItchyChin_-_OrpoLlama-3-8B-memorize-translate-gguf/blob/main/OrpoLlama-3-8B-memorize-translate.Q4_K_M.gguf) | Q4_K_M | 4.58GB |
| [OrpoLlama-3-8B-memorize-translate.Q4_1.gguf](https://huggingface.co/RichardErkhov/ItchyChin_-_OrpoLlama-3-8B-memorize-translate-gguf/blob/main/OrpoLlama-3-8B-memorize-translate.Q4_1.gguf) | Q4_1 | 4.78GB |
| [OrpoLlama-3-8B-memorize-translate.Q5_0.gguf](https://huggingface.co/RichardErkhov/ItchyChin_-_OrpoLlama-3-8B-memorize-translate-gguf/blob/main/OrpoLlama-3-8B-memorize-translate.Q5_0.gguf) | Q5_0 | 5.21GB |
| [OrpoLlama-3-8B-memorize-translate.Q5_K_S.gguf](https://huggingface.co/RichardErkhov/ItchyChin_-_OrpoLlama-3-8B-memorize-translate-gguf/blob/main/OrpoLlama-3-8B-memorize-translate.Q5_K_S.gguf) | Q5_K_S | 5.21GB |
| [OrpoLlama-3-8B-memorize-translate.Q5_K.gguf](https://huggingface.co/RichardErkhov/ItchyChin_-_OrpoLlama-3-8B-memorize-translate-gguf/blob/main/OrpoLlama-3-8B-memorize-translate.Q5_K.gguf) | Q5_K | 5.34GB |
| [OrpoLlama-3-8B-memorize-translate.Q5_K_M.gguf](https://huggingface.co/RichardErkhov/ItchyChin_-_OrpoLlama-3-8B-memorize-translate-gguf/blob/main/OrpoLlama-3-8B-memorize-translate.Q5_K_M.gguf) | Q5_K_M | 5.34GB |
| [OrpoLlama-3-8B-memorize-translate.Q5_1.gguf](https://huggingface.co/RichardErkhov/ItchyChin_-_OrpoLlama-3-8B-memorize-translate-gguf/blob/main/OrpoLlama-3-8B-memorize-translate.Q5_1.gguf) | Q5_1 | 5.65GB |
| [OrpoLlama-3-8B-memorize-translate.Q6_K.gguf](https://huggingface.co/RichardErkhov/ItchyChin_-_OrpoLlama-3-8B-memorize-translate-gguf/blob/main/OrpoLlama-3-8B-memorize-translate.Q6_K.gguf) | Q6_K | 6.14GB |
| [OrpoLlama-3-8B-memorize-translate.Q8_0.gguf](https://huggingface.co/RichardErkhov/ItchyChin_-_OrpoLlama-3-8B-memorize-translate-gguf/blob/main/OrpoLlama-3-8B-memorize-translate.Q8_0.gguf) | Q8_0 | 7.95GB |
Original model description:
---
library_name: transformers
tags: []
---
# Model Card for Model ID
<!-- Provide a quick summary of what the model is/does. -->
## Model Details
### Model Description
<!-- Provide a longer summary of what this model is. -->
This is the model card of a 🤗 transformers model that has been pushed on the Hub. This model card has been automatically generated.
- **Developed by:** [More Information Needed]
- **Funded by [optional]:** [More Information Needed]
- **Shared by [optional]:** [More Information Needed]
- **Model type:** [More Information Needed]
- **Language(s) (NLP):** [More Information Needed]
- **License:** [More Information Needed]
- **Finetuned from model [optional]:** [More Information Needed]
### Model Sources [optional]
<!-- Provide the basic links for the model. -->
- **Repository:** [More Information Needed]
- **Paper [optional]:** [More Information Needed]
- **Demo [optional]:** [More Information Needed]
## Uses
<!-- Address questions around how the model is intended to be used, including the foreseeable users of the model and those affected by the model. -->
### Direct Use
<!-- This section is for the model use without fine-tuning or plugging into a larger ecosystem/app. -->
[More Information Needed]
### Downstream Use [optional]
<!-- This section is for the model use when fine-tuned for a task, or when plugged into a larger ecosystem/app -->
[More Information Needed]
### Out-of-Scope Use
<!-- This section addresses misuse, malicious use, and uses that the model will not work well for. -->
[More Information Needed]
## Bias, Risks, and Limitations
<!-- This section is meant to convey both technical and sociotechnical limitations. -->
[More Information Needed]
### Recommendations
<!-- This section is meant to convey recommendations with respect to the bias, risk, and technical limitations. -->
Users (both direct and downstream) should be made aware of the risks, biases and limitations of the model. More information needed for further recommendations.
## How to Get Started with the Model
Use the code below to get started with the model.
[More Information Needed]
## Training Details
### Training Data
<!-- This should link to a Dataset Card, perhaps with a short stub of information on what the training data is all about as well as documentation related to data pre-processing or additional filtering. -->
[More Information Needed]
### Training Procedure
<!-- This relates heavily to the Technical Specifications. Content here should link to that section when it is relevant to the training procedure. -->
#### Preprocessing [optional]
[More Information Needed]
#### Training Hyperparameters
- **Training regime:** [More Information Needed] <!--fp32, fp16 mixed precision, bf16 mixed precision, bf16 non-mixed precision, fp16 non-mixed precision, fp8 mixed precision -->
#### Speeds, Sizes, Times [optional]
<!-- This section provides information about throughput, start/end time, checkpoint size if relevant, etc. -->
[More Information Needed]
## Evaluation
<!-- This section describes the evaluation protocols and provides the results. -->
### Testing Data, Factors & Metrics
#### Testing Data
<!-- This should link to a Dataset Card if possible. -->
[More Information Needed]
#### Factors
<!-- These are the things the evaluation is disaggregating by, e.g., subpopulations or domains. -->
[More Information Needed]
#### Metrics
<!-- These are the evaluation metrics being used, ideally with a description of why. -->
[More Information Needed]
### Results
[More Information Needed]
#### Summary
## Model Examination [optional]
<!-- Relevant interpretability work for the model goes here -->
[More Information Needed]
## Environmental Impact
<!-- Total emissions (in grams of CO2eq) and additional considerations, such as electricity usage, go here. Edit the suggested text below accordingly -->
Carbon emissions can be estimated using the [Machine Learning Impact calculator](https://mlco2.github.io/impact#compute) presented in [Lacoste et al. (2019)](https://arxiv.org/abs/1910.09700).
- **Hardware Type:** [More Information Needed]
- **Hours used:** [More Information Needed]
- **Cloud Provider:** [More Information Needed]
- **Compute Region:** [More Information Needed]
- **Carbon Emitted:** [More Information Needed]
## Technical Specifications [optional]
### Model Architecture and Objective
[More Information Needed]
### Compute Infrastructure
[More Information Needed]
#### Hardware
[More Information Needed]
#### Software
[More Information Needed]
## Citation [optional]
<!-- If there is a paper or blog post introducing the model, the APA and Bibtex information for that should go in this section. -->
**BibTeX:**
[More Information Needed]
**APA:**
[More Information Needed]
## Glossary [optional]
<!-- If relevant, include terms and calculations in this section that can help readers understand the model or model card. -->
[More Information Needed]
## More Information [optional]
[More Information Needed]
## Model Card Authors [optional]
[More Information Needed]
## Model Card Contact
[More Information Needed]
|
Subsets and Splits
No saved queries yet
Save your SQL queries to embed, download, and access them later. Queries will appear here once saved.