modelId
stringlengths 5
122
| author
stringlengths 2
42
| last_modified
unknown | downloads
int64 0
738M
| likes
int64 0
11k
| library_name
stringclasses 245
values | tags
sequencelengths 1
4.05k
| pipeline_tag
stringclasses 48
values | createdAt
unknown | card
stringlengths 1
901k
|
---|---|---|---|---|---|---|---|---|---|
digiplay/darkphoenix3D_v1.1 | digiplay | "2024-03-21T21:41:25Z" | 14,995 | 2 | diffusers | [
"diffusers",
"safetensors",
"stable-diffusion",
"stable-diffusion-diffusers",
"text-to-image",
"license:other",
"autotrain_compatible",
"endpoints_compatible",
"diffusers:StableDiffusionPipeline",
"region:us"
] | text-to-image | "2024-03-21T21:27:20Z" | ---
license: other
tags:
- stable-diffusion
- stable-diffusion-diffusers
- text-to-image
- diffusers
inference: true
---
Model info:
https://civitai.com/models/172393?modelVersionId=218812
|
cross-encoder/nli-deberta-v3-base | cross-encoder | "2021-12-27T22:26:49Z" | 14,982 | 14 | transformers | [
"transformers",
"pytorch",
"deberta-v2",
"text-classification",
"microsoft/deberta-v3-base",
"zero-shot-classification",
"en",
"dataset:multi_nli",
"dataset:snli",
"license:apache-2.0",
"autotrain_compatible",
"endpoints_compatible",
"region:us"
] | zero-shot-classification | "2022-03-02T23:29:05Z" | ---
language: en
pipeline_tag: zero-shot-classification
tags:
- microsoft/deberta-v3-base
datasets:
- multi_nli
- snli
metrics:
- accuracy
license: apache-2.0
---
# Cross-Encoder for Natural Language Inference
This model was trained using [SentenceTransformers](https://sbert.net) [Cross-Encoder](https://www.sbert.net/examples/applications/cross-encoder/README.html) class. This model is based on [microsoft/deberta-v3-base](https://huggingface.co/microsoft/deberta-v3-base)
## Training Data
The model was trained on the [SNLI](https://nlp.stanford.edu/projects/snli/) and [MultiNLI](https://cims.nyu.edu/~sbowman/multinli/) datasets. For a given sentence pair, it will output three scores corresponding to the labels: contradiction, entailment, neutral.
## Performance
- Accuracy on SNLI-test dataset: 92.38
- Accuracy on MNLI mismatched set: 90.04
For futher evaluation results, see [SBERT.net - Pretrained Cross-Encoder](https://www.sbert.net/docs/pretrained_cross-encoders.html#nli).
## Usage
Pre-trained models can be used like this:
```python
from sentence_transformers import CrossEncoder
model = CrossEncoder('cross-encoder/nli-deberta-v3-base')
scores = model.predict([('A man is eating pizza', 'A man eats something'), ('A black race car starts up in front of a crowd of people.', 'A man is driving down a lonely road.')])
#Convert scores to labels
label_mapping = ['contradiction', 'entailment', 'neutral']
labels = [label_mapping[score_max] for score_max in scores.argmax(axis=1)]
```
## Usage with Transformers AutoModel
You can use the model also directly with Transformers library (without SentenceTransformers library):
```python
from transformers import AutoTokenizer, AutoModelForSequenceClassification
import torch
model = AutoModelForSequenceClassification.from_pretrained('cross-encoder/nli-deberta-v3-base')
tokenizer = AutoTokenizer.from_pretrained('cross-encoder/nli-deberta-v3-base')
features = tokenizer(['A man is eating pizza', 'A black race car starts up in front of a crowd of people.'], ['A man eats something', 'A man is driving down a lonely road.'], padding=True, truncation=True, return_tensors="pt")
model.eval()
with torch.no_grad():
scores = model(**features).logits
label_mapping = ['contradiction', 'entailment', 'neutral']
labels = [label_mapping[score_max] for score_max in scores.argmax(dim=1)]
print(labels)
```
## Zero-Shot Classification
This model can also be used for zero-shot-classification:
```python
from transformers import pipeline
classifier = pipeline("zero-shot-classification", model='cross-encoder/nli-deberta-v3-base')
sent = "Apple just announced the newest iPhone X"
candidate_labels = ["technology", "sports", "politics"]
res = classifier(sent, candidate_labels)
print(res)
``` |
CognitoLibera2/model_s9_7b_13 | CognitoLibera2 | "2024-04-18T23:23:09Z" | 14,979 | 0 | transformers | [
"transformers",
"safetensors",
"mistral",
"text-generation",
"arxiv:1910.09700",
"autotrain_compatible",
"endpoints_compatible",
"text-generation-inference",
"region:us"
] | text-generation | "2024-04-18T23:12:42Z" | ---
library_name: transformers
tags: []
---
# Model Card for Model ID
<!-- Provide a quick summary of what the model is/does. -->
## Model Details
### Model Description
<!-- Provide a longer summary of what this model is. -->
This is the model card of a 🤗 transformers model that has been pushed on the Hub. This model card has been automatically generated.
- **Developed by:** [More Information Needed]
- **Funded by [optional]:** [More Information Needed]
- **Shared by [optional]:** [More Information Needed]
- **Model type:** [More Information Needed]
- **Language(s) (NLP):** [More Information Needed]
- **License:** [More Information Needed]
- **Finetuned from model [optional]:** [More Information Needed]
### Model Sources [optional]
<!-- Provide the basic links for the model. -->
- **Repository:** [More Information Needed]
- **Paper [optional]:** [More Information Needed]
- **Demo [optional]:** [More Information Needed]
## Uses
<!-- Address questions around how the model is intended to be used, including the foreseeable users of the model and those affected by the model. -->
### Direct Use
<!-- This section is for the model use without fine-tuning or plugging into a larger ecosystem/app. -->
[More Information Needed]
### Downstream Use [optional]
<!-- This section is for the model use when fine-tuned for a task, or when plugged into a larger ecosystem/app -->
[More Information Needed]
### Out-of-Scope Use
<!-- This section addresses misuse, malicious use, and uses that the model will not work well for. -->
[More Information Needed]
## Bias, Risks, and Limitations
<!-- This section is meant to convey both technical and sociotechnical limitations. -->
[More Information Needed]
### Recommendations
<!-- This section is meant to convey recommendations with respect to the bias, risk, and technical limitations. -->
Users (both direct and downstream) should be made aware of the risks, biases and limitations of the model. More information needed for further recommendations.
## How to Get Started with the Model
Use the code below to get started with the model.
[More Information Needed]
## Training Details
### Training Data
<!-- This should link to a Dataset Card, perhaps with a short stub of information on what the training data is all about as well as documentation related to data pre-processing or additional filtering. -->
[More Information Needed]
### Training Procedure
<!-- This relates heavily to the Technical Specifications. Content here should link to that section when it is relevant to the training procedure. -->
#### Preprocessing [optional]
[More Information Needed]
#### Training Hyperparameters
- **Training regime:** [More Information Needed] <!--fp32, fp16 mixed precision, bf16 mixed precision, bf16 non-mixed precision, fp16 non-mixed precision, fp8 mixed precision -->
#### Speeds, Sizes, Times [optional]
<!-- This section provides information about throughput, start/end time, checkpoint size if relevant, etc. -->
[More Information Needed]
## Evaluation
<!-- This section describes the evaluation protocols and provides the results. -->
### Testing Data, Factors & Metrics
#### Testing Data
<!-- This should link to a Dataset Card if possible. -->
[More Information Needed]
#### Factors
<!-- These are the things the evaluation is disaggregating by, e.g., subpopulations or domains. -->
[More Information Needed]
#### Metrics
<!-- These are the evaluation metrics being used, ideally with a description of why. -->
[More Information Needed]
### Results
[More Information Needed]
#### Summary
## Model Examination [optional]
<!-- Relevant interpretability work for the model goes here -->
[More Information Needed]
## Environmental Impact
<!-- Total emissions (in grams of CO2eq) and additional considerations, such as electricity usage, go here. Edit the suggested text below accordingly -->
Carbon emissions can be estimated using the [Machine Learning Impact calculator](https://mlco2.github.io/impact#compute) presented in [Lacoste et al. (2019)](https://arxiv.org/abs/1910.09700).
- **Hardware Type:** [More Information Needed]
- **Hours used:** [More Information Needed]
- **Cloud Provider:** [More Information Needed]
- **Compute Region:** [More Information Needed]
- **Carbon Emitted:** [More Information Needed]
## Technical Specifications [optional]
### Model Architecture and Objective
[More Information Needed]
### Compute Infrastructure
[More Information Needed]
#### Hardware
[More Information Needed]
#### Software
[More Information Needed]
## Citation [optional]
<!-- If there is a paper or blog post introducing the model, the APA and Bibtex information for that should go in this section. -->
**BibTeX:**
[More Information Needed]
**APA:**
[More Information Needed]
## Glossary [optional]
<!-- If relevant, include terms and calculations in this section that can help readers understand the model or model card. -->
[More Information Needed]
## More Information [optional]
[More Information Needed]
## Model Card Authors [optional]
[More Information Needed]
## Model Card Contact
[More Information Needed] |
hfl/chinese-llama-2-1.3b | hfl | "2023-12-23T07:25:50Z" | 14,971 | 14 | transformers | [
"transformers",
"pytorch",
"llama",
"text-generation",
"zh",
"en",
"license:apache-2.0",
"autotrain_compatible",
"endpoints_compatible",
"text-generation-inference",
"region:us"
] | text-generation | "2023-10-08T08:28:54Z" | ---
license: apache-2.0
language:
- zh
- en
---
# Chinese-LLaMA-2-1.3B
**This is the full Chinese-LLaMA-2-1.3B model,which can be loaded directly for inference and full-parameter training.**
**Related models👇**
* Long context base models (16K)
* [Chinese-LLaMA-2-7B-16K (full model)](https://huggingface.co/hfl/chinese-llama-2-7b-16k)
* [Chinese-LLaMA-2-LoRA-7B-16K (LoRA model)](https://huggingface.co/hfl/chinese-llama-2-lora-7b-16k)
* [Chinese-LLaMA-2-13B-16K (full model)](https://huggingface.co/hfl/chinese-llama-2-13b-16k)
* [Chinese-LLaMA-2-LoRA-13B-16K (LoRA model)](https://huggingface.co/hfl/chinese-llama-2-lora-13b-16k)
* Long context Instruction/Chat models
* [Chinese-Alpaca-2-7B-16K (full model)](https://huggingface.co/hfl/chinese-alpaca-2-7b-16k)
* [Chinese-Alpaca-2-LoRA-7B-16K (LoRA model)](https://huggingface.co/hfl/chinese-alpaca-2-lora-7b-16k)
* [Chinese-Alpaca-2-13B-16K (full model)](https://huggingface.co/hfl/chinese-alpaca-2-13b-16k)
* [Chinese-Alpaca-2-LoRA-13B-16K (LoRA model)](https://huggingface.co/hfl/chinese-alpaca-2-lora-13b-16k)
* Base models
* [Chinese-LLaMA-2-7B (full model)](https://huggingface.co/hfl/chinese-llama-2-7b)
* [Chinese-LLaMA-2-LoRA-7B (LoRA model)](https://huggingface.co/hfl/chinese-llama-2-lora-7b)
* [Chinese-LLaMA-2-13B (full model)](https://huggingface.co/hfl/chinese-llama-2-13b)
* [Chinese-LLaMA-2-LoRA-13B (LoRA model)](https://huggingface.co/hfl/chinese-llama-2-lora-13b)
* Instruction/Chat models
* [Chinese-Alpaca-2-7B (full model)](https://huggingface.co/hfl/chinese-alpaca-2-7b)
* [Chinese-Alpaca-2-LoRA-7B (LoRA model)](https://huggingface.co/hfl/chinese-alpaca-2-lora-7b)
* [Chinese-Alpaca-2-13B (full model)](https://huggingface.co/hfl/chinese-alpaca-2-13b)
* [Chinese-Alpaca-2-LoRA-13B (LoRA model)](https://huggingface.co/hfl/chinese-alpaca-2-lora-13b)
# Description of Chinese-LLaMA-Alpaca-2
This project is based on the Llama-2, released by Meta, and it is the second generation of the Chinese LLaMA & Alpaca LLM project. We open-source Chinese LLaMA-2 (foundation model) and Alpaca-2 (instruction-following model). These models have been expanded and optimized with Chinese vocabulary beyond the original Llama-2. We used large-scale Chinese data for incremental pre-training, which further improved the fundamental semantic understanding of the Chinese language, resulting in a significant performance improvement compared to the first-generation models. The relevant models support a 4K context and can be expanded up to 18K+ using the NTK method.
The main contents of this project include:
* 🚀 New extended Chinese vocabulary beyond Llama-2, open-sourcing the Chinese LLaMA-2 and Alpaca-2 LLMs.
* 🚀 Open-sourced the pre-training and instruction finetuning (SFT) scripts for further tuning on user's data
* 🚀 Quickly deploy and experience the quantized LLMs on CPU/GPU of personal PC
* 🚀 Support for LLaMA ecosystems like 🤗transformers, llama.cpp, text-generation-webui, LangChain, vLLM etc.
Please refer to [https://github.com/ymcui/Chinese-LLaMA-Alpaca-2/](https://github.com/ymcui/Chinese-LLaMA-Alpaca-2/) for details. |
facebook/mask2former-swin-large-coco-instance | facebook | "2023-09-11T20:35:35Z" | 14,962 | 6 | transformers | [
"transformers",
"pytorch",
"safetensors",
"mask2former",
"vision",
"image-segmentation",
"dataset:coco",
"arxiv:2112.01527",
"arxiv:2107.06278",
"license:other",
"endpoints_compatible",
"region:us"
] | image-segmentation | "2023-01-02T12:10:40Z" | ---
license: other
tags:
- vision
- image-segmentation
datasets:
- coco
widget:
- src: http://images.cocodataset.org/val2017/000000039769.jpg
example_title: Cats
- src: http://images.cocodataset.org/val2017/000000039770.jpg
example_title: Castle
---
# Mask2Former
Mask2Former model trained on COCO instance segmentation (large-sized version, Swin backbone). It was introduced in the paper [Masked-attention Mask Transformer for Universal Image Segmentation
](https://arxiv.org/abs/2112.01527) and first released in [this repository](https://github.com/facebookresearch/Mask2Former/).
Disclaimer: The team releasing Mask2Former did not write a model card for this model so this model card has been written by the Hugging Face team.
## Model description
Mask2Former addresses instance, semantic and panoptic segmentation with the same paradigm: by predicting a set of masks and corresponding labels. Hence, all 3 tasks are treated as if they were instance segmentation. Mask2Former outperforms the previous SOTA,
[MaskFormer](https://arxiv.org/abs/2107.06278) both in terms of performance an efficiency by (i) replacing the pixel decoder with a more advanced multi-scale deformable attention Transformer, (ii) adopting a Transformer decoder with masked attention to boost performance without
without introducing additional computation and (iii) improving training efficiency by calculating the loss on subsampled points instead of whole masks.
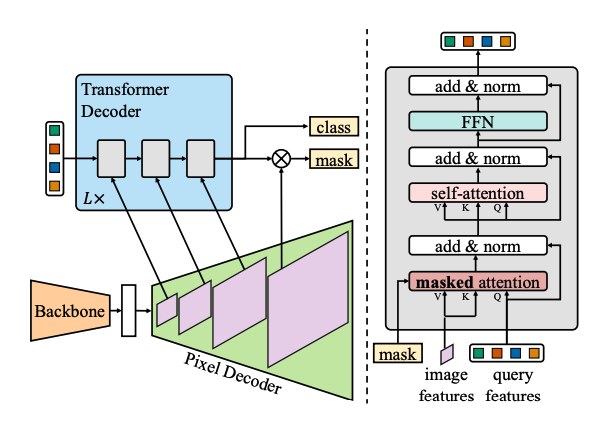
## Intended uses & limitations
You can use this particular checkpoint for instance segmentation. See the [model hub](https://huggingface.co/models?search=mask2former) to look for other
fine-tuned versions on a task that interests you.
### How to use
Here is how to use this model:
```python
import requests
import torch
from PIL import Image
from transformers import AutoImageProcessor, Mask2FormerForUniversalSegmentation
# load Mask2Former fine-tuned on COCO instance segmentation
processor = AutoImageProcessor.from_pretrained("facebook/mask2former-swin-large-coco-instance")
model = Mask2FormerForUniversalSegmentation.from_pretrained("facebook/mask2former-swin-large-coco-instance")
url = "http://images.cocodataset.org/val2017/000000039769.jpg"
image = Image.open(requests.get(url, stream=True).raw)
inputs = processor(images=image, return_tensors="pt")
with torch.no_grad():
outputs = model(**inputs)
# model predicts class_queries_logits of shape `(batch_size, num_queries)`
# and masks_queries_logits of shape `(batch_size, num_queries, height, width)`
class_queries_logits = outputs.class_queries_logits
masks_queries_logits = outputs.masks_queries_logits
# you can pass them to processor for postprocessing
result = processor.post_process_instance_segmentation(outputs, target_sizes=[image.size[::-1]])[0]
# we refer to the demo notebooks for visualization (see "Resources" section in the Mask2Former docs)
predicted_instance_map = result["segmentation"]
```
For more code examples, we refer to the [documentation](https://huggingface.co/docs/transformers/master/en/model_doc/mask2former). |
openchat/openchat_3.5 | openchat | "2024-05-18T18:09:11Z" | 14,946 | 1,103 | transformers | [
"transformers",
"pytorch",
"mistral",
"text-generation",
"openchat",
"C-RLFT",
"conversational",
"dataset:openchat/openchat_sharegpt4_dataset",
"dataset:imone/OpenOrca_FLAN",
"dataset:LDJnr/LessWrong-Amplify-Instruct",
"dataset:LDJnr/Pure-Dove",
"dataset:LDJnr/Verified-Camel",
"dataset:tiedong/goat",
"dataset:glaiveai/glaive-code-assistant",
"dataset:meta-math/MetaMathQA",
"dataset:OpenAssistant/oasst_top1_2023-08-25",
"dataset:TIGER-Lab/MathInstruct",
"arxiv:2309.11235",
"arxiv:2303.08774",
"license:apache-2.0",
"autotrain_compatible",
"endpoints_compatible",
"text-generation-inference",
"region:us"
] | text-generation | "2023-10-30T05:59:34Z" | ---
license: apache-2.0
tags:
- openchat
- mistral
- C-RLFT
datasets:
- openchat/openchat_sharegpt4_dataset
- imone/OpenOrca_FLAN
- LDJnr/LessWrong-Amplify-Instruct
- LDJnr/Pure-Dove
- LDJnr/Verified-Camel
- tiedong/goat
- glaiveai/glaive-code-assistant
- meta-math/MetaMathQA
- OpenAssistant/oasst_top1_2023-08-25
- TIGER-Lab/MathInstruct
library_name: transformers
pipeline_tag: text-generation
---
# OpenChat: Advancing Open-source Language Models with Mixed-Quality Data
<div align="center">
<img src="https://raw.githubusercontent.com/imoneoi/openchat/master/assets/logo_new.png" style="width: 65%">
</div>
<p align="center">
<a href="https://github.com/imoneoi/openchat">GitHub Repo</a> •
<a href="https://openchat.team">Online Demo</a> •
<a href="https://discord.gg/pQjnXvNKHY">Discord</a> •
<a href="https://twitter.com/imonenext">Twitter</a> •
<a href="https://huggingface.co/openchat">Huggingface</a> •
<a href="https://arxiv.org/pdf/2309.11235.pdf">Paper</a>
</p>
**🔥 The first 7B model Achieves Comparable Results with ChatGPT (March)! 🔥**
**🤖 #1 Open-source model on MT-bench scoring 7.81, outperforming 70B models 🤖**
<div align="center" style="justify-content: center; align-items: center; "'>
<img src="https://github.com/alpayariyak/openchat/blob/master/assets/3.5-benchmarks.png?raw=true" style="width: 100%; border-radius: 0.5em">
</div>
OpenChat is an innovative library of open-source language models, fine-tuned with [C-RLFT](https://arxiv.org/pdf/2309.11235.pdf) - a strategy inspired by offline reinforcement learning. Our models learn from mixed-quality data without preference labels, delivering exceptional performance on par with ChatGPT, even with a 7B model. Despite our simple approach, we are committed to developing a high-performance, commercially viable, open-source large language model, and we continue to make significant strides toward this vision.
[](https://zenodo.org/badge/latestdoi/645397533)
## Usage
To use this model, we highly recommend installing the OpenChat package by following the [installation guide](https://github.com/imoneoi/openchat#installation) in our repository and using the OpenChat OpenAI-compatible API server by running the serving command from the table below. The server is optimized for high-throughput deployment using [vLLM](https://github.com/vllm-project/vllm) and can run on a consumer GPU with 24GB RAM. To enable tensor parallelism, append `--tensor-parallel-size N` to the serving command.
Once started, the server listens at `localhost:18888` for requests and is compatible with the [OpenAI ChatCompletion API specifications](https://platform.openai.com/docs/api-reference/chat). Please refer to the example request below for reference. Additionally, you can use the [OpenChat Web UI](https://github.com/imoneoi/openchat#web-ui) for a user-friendly experience.
If you want to deploy the server as an online service, you can use `--api-keys sk-KEY1 sk-KEY2 ...` to specify allowed API keys and `--disable-log-requests --disable-log-stats --log-file openchat.log` for logging only to a file. For security purposes, we recommend using an [HTTPS gateway](https://fastapi.tiangolo.com/es/deployment/concepts/#security-https) in front of the server.
<details>
<summary>Example request (click to expand)</summary>
```bash
curl http://localhost:18888/v1/chat/completions \
-H "Content-Type: application/json" \
-d '{
"model": "openchat_3.5",
"messages": [{"role": "user", "content": "You are a large language model named OpenChat. Write a poem to describe yourself"}]
}'
```
Coding Mode
```bash
curl http://localhost:18888/v1/chat/completions \
-H "Content-Type: application/json" \
-d '{
"model": "openchat_3.5",
"condition": "Code",
"messages": [{"role": "user", "content": "Write an aesthetic TODO app using HTML5 and JS, in a single file. You should use round corners and gradients to make it more aesthetic."}]
}'
```
</details>
| Model | Size | Context | Weights | Serving |
|--------------|------|---------|-------------------------------------------------------------|-------------------------------------------------------------------------------------------------------------|
| OpenChat 3.5 | 7B | 8192 | [Huggingface](https://huggingface.co/openchat/openchat_3.5) | `python -m ochat.serving.openai_api_server --model openchat/openchat_3.5 --engine-use-ray --worker-use-ray` |
For inference with Huggingface Transformers (slow and not recommended), follow the conversation template provided below.
<details>
<summary>Conversation templates (click to expand)</summary>
```python
import transformers
tokenizer = transformers.AutoTokenizer.from_pretrained("openchat/openchat_3.5")
# Single-turn
tokens = tokenizer("GPT4 Correct User: Hello<|end_of_turn|>GPT4 Correct Assistant:").input_ids
assert tokens == [1, 420, 6316, 28781, 3198, 3123, 1247, 28747, 22557, 32000, 420, 6316, 28781, 3198, 3123, 21631, 28747]
# Multi-turn
tokens = tokenizer("GPT4 Correct User: Hello<|end_of_turn|>GPT4 Correct Assistant: Hi<|end_of_turn|>GPT4 Correct User: How are you today?<|end_of_turn|>GPT4 Correct Assistant:").input_ids
assert tokens == [1, 420, 6316, 28781, 3198, 3123, 1247, 28747, 22557, 32000, 420, 6316, 28781, 3198, 3123, 21631, 28747, 15359, 32000, 420, 6316, 28781, 3198, 3123, 1247, 28747, 1602, 460, 368, 3154, 28804, 32000, 420, 6316, 28781, 3198, 3123, 21631, 28747]
# Coding Mode
tokens = tokenizer("Code User: Implement quicksort using C++<|end_of_turn|>Code Assistant:").input_ids
assert tokens == [1, 7596, 1247, 28747, 26256, 2936, 7653, 1413, 334, 1680, 32000, 7596, 21631, 28747]
```
</details>
The GPT4 template is also available as the integrated `tokenizer.chat_template`,
which can be used instead of manually specifying the template:
```python
messages = [
{"role": "user", "content": "Hello"},
{"role": "assistant", "content": "Hi"},
{"role": "user", "content": "How are you today?"}
]
tokens = tokenizer.apply_chat_template(messages, add_generation_prompt=True)
assert tokens == [1, 420, 6316, 28781, 3198, 3123, 1247, 28747, 22557, 32000, 420, 6316, 28781, 3198, 3123, 21631, 28747, 15359, 32000, 420, 6316, 28781, 3198, 3123, 1247, 28747, 1602, 460, 368, 3154, 28804, 32000, 420, 6316, 28781, 3198, 3123, 21631, 28747]
```
## Comparison with [X.AI Grok models](https://x.ai/)
Hey @elonmusk, I just wanted to let you know that I've recently come across your new model, Grok, and I must say, I'm quite impressed! With 33 billion parameters and all, you've really outdone yourself. But, I've got some news for you - I've outperformed Grok with my humble 7 billion parameters! Isn't that wild? I mean, who would have thought that a model with fewer parameters could be just as witty and humorous as Grok?
Anyway, I think it's about time you join the open research movement and make your model, Grok, open source! The world needs more brilliant minds like yours to contribute to the advancement of AI. Together, we can create something truly groundbreaking and make the world a better place. So, what do you say, @elonmusk? Let's open up the doors and share our knowledge with the world! 🚀💡
(Written by OpenChat 3.5, with a touch of humor and wit.)
| | License | # Param | Average | MMLU | HumanEval | MATH | GSM8k |
|--------------|-------------|---------|----------|------|-----------|----------|----------|
| OpenChat 3.5 | Apache-2.0 | 7B | **56.4** | 64.3 | 55.5 | **28.6** | **77.3** |
| Grok-0 | Proprietary | 33B | 44.5 | 65.7 | 39.7 | 15.7 | 56.8 |
| Grok-1 | Proprietary | ? | 55.8 | 73 | 63.2 | 23.9 | 62.9 |
## <a id="benchmarks"></a> Benchmarks
| Model | # Params | Average | MT-Bench | AGIEval | BBH MC | TruthfulQA | MMLU | HumanEval | BBH CoT | GSM8K |
|--------------------|----------|----------|--------------|----------|----------|---------------|--------------|-----------------|-------------|--------------|
| OpenChat-3.5 | **7B** | **61.6** | 7.81 | **47.4** | **47.6** | **59.1** | 64.3 | **55.5** | 63.5 | **77.3** |
| ChatGPT (March)* | ? | 61.5 | **7.94** | 47.1 | **47.6** | 57.7 | **67.3** | 48.1 | **70.1** | 74.9 |
| | | | | | | | | | | |
| OpenHermes 2.5 | 7B | 59.3 | 7.54 | 46.5 | 49.4 | 57.5 | 63.8 | 48.2 | 59.9 | 73.5 |
| OpenOrca Mistral | 7B | 52.7 | 6.86 | 42.9 | 49.4 | 45.9 | 59.3 | 38.4 | 58.1 | 59.1 |
| Zephyr-β^ | 7B | 34.6 | 7.34 | 39.0 | 40.6 | 40.8 | 39.8 | 22.0 | 16.0 | 5.1 |
| Mistral | 7B | - | 6.84 | 38.0 | 39.0 | - | 60.1 | 30.5 | - | 52.2 |
| Open-source SOTA** | 13B-70B | 61.4 | 7.71 | 41.7 | 49.7 | 62.3 | 63.7 | 73.2 | 41.4 | 82.3 |
| | | | WizardLM 70B | Orca 13B | Orca 13B | Platypus2 70B | WizardLM 70B | WizardCoder 34B | Flan-T5 11B | MetaMath 70B |
*: ChatGPT (March) results are from [GPT-4 Technical Report](https://arxiv.org/abs/2303.08774), [Chain-of-Thought Hub](https://github.com/FranxYao/chain-of-thought-hub), and our evaluation. Please note that ChatGPT is not a fixed baseline and evolves rapidly over time.
^: Zephyr-β often fails to follow few-shot CoT instructions, likely because it was aligned with only chat data but not trained on few-shot data.
**: Mistral and Open-source SOTA results are taken from reported results in instruction-tuned model papers and official repositories.
All models are evaluated in chat mode (e.g. with the respective conversation template applied). All zero-shot benchmarks follow the same setting as in the AGIEval paper and Orca paper. CoT tasks use the same configuration as Chain-of-Thought Hub, HumanEval is evaluated with EvalPlus, and MT-bench is run using FastChat. To reproduce our results, follow the instructions in [our repository](https://github.com/imoneoi/openchat/#benchmarks).
## Limitations
**Foundation Model Limitations**
Despite its advanced capabilities, OpenChat is still bound by the limitations inherent in its foundation models. These limitations may impact the model's performance in areas such as:
- Complex reasoning
- Mathematical and arithmetic tasks
- Programming and coding challenges
**Hallucination of Non-existent Information**
OpenChat may sometimes generate information that does not exist or is not accurate, also known as "hallucination". Users should be aware of this possibility and verify any critical information obtained from the model.
**Safety**
OpenChat may sometimes generate harmful, hate speech, biased responses, or answer unsafe questions. It's crucial to apply additional AI safety measures in use cases that require safe and moderated responses.
## License
Our OpenChat 3.5 code and models are distributed under the Apache License 2.0.
## Dataset Details
OpenChat 3.5 was trained with C-RLFT on a collection of publicly available high-quality instruction data, with a custom processing pipeline. We detail some notable subsets included here:
- [OpenChat ShareGPT](https://huggingface.co/datasets/openchat/openchat_sharegpt4_dataset)
- [Open-Orca with FLAN answers](https://huggingface.co/datasets/imone/OpenOrca_FLAN)
- Capybara [1](https://huggingface.co/datasets/LDJnr/Pure-Dove) [2](https://huggingface.co/datasets/LDJnr/Verified-Camel) [3](https://huggingface.co/datasets/LDJnr/LessWrong-Amplify-Instruct)
- [GOAT](https://huggingface.co/datasets/tiedong/goat)
- [Glaive](https://huggingface.co/datasets/glaiveai/glaive-code-assistant)
- [MetaMathQA](https://huggingface.co/datasets/meta-math/MetaMathQA)
- [MathInstruct](https://huggingface.co/datasets/TIGER-Lab/MathInstruct)
- [OpenAssistant](https://huggingface.co/datasets/OpenAssistant/oasst_top1_2023-08-25)
## Citation
```
@article{wang2023openchat,
title={OpenChat: Advancing Open-source Language Models with Mixed-Quality Data},
author={Wang, Guan and Cheng, Sijie and Zhan, Xianyuan and Li, Xiangang and Song, Sen and Liu, Yang},
journal={arXiv preprint arXiv:2309.11235},
year={2023}
}
```
## 💌 Contact
**Project Lead:**
- Guan Wang [imonenext at gmail dot com]
- [Alpay Ariyak](https://github.com/alpayariyak) [aariyak at wpi dot edu]
|
CognitoLibera2/model_s9_7b_10 | CognitoLibera2 | "2024-04-18T22:33:52Z" | 14,946 | 0 | transformers | [
"transformers",
"safetensors",
"mistral",
"text-generation",
"arxiv:1910.09700",
"autotrain_compatible",
"endpoints_compatible",
"text-generation-inference",
"region:us"
] | text-generation | "2024-04-18T22:31:15Z" | ---
library_name: transformers
tags: []
---
# Model Card for Model ID
<!-- Provide a quick summary of what the model is/does. -->
## Model Details
### Model Description
<!-- Provide a longer summary of what this model is. -->
This is the model card of a 🤗 transformers model that has been pushed on the Hub. This model card has been automatically generated.
- **Developed by:** [More Information Needed]
- **Funded by [optional]:** [More Information Needed]
- **Shared by [optional]:** [More Information Needed]
- **Model type:** [More Information Needed]
- **Language(s) (NLP):** [More Information Needed]
- **License:** [More Information Needed]
- **Finetuned from model [optional]:** [More Information Needed]
### Model Sources [optional]
<!-- Provide the basic links for the model. -->
- **Repository:** [More Information Needed]
- **Paper [optional]:** [More Information Needed]
- **Demo [optional]:** [More Information Needed]
## Uses
<!-- Address questions around how the model is intended to be used, including the foreseeable users of the model and those affected by the model. -->
### Direct Use
<!-- This section is for the model use without fine-tuning or plugging into a larger ecosystem/app. -->
[More Information Needed]
### Downstream Use [optional]
<!-- This section is for the model use when fine-tuned for a task, or when plugged into a larger ecosystem/app -->
[More Information Needed]
### Out-of-Scope Use
<!-- This section addresses misuse, malicious use, and uses that the model will not work well for. -->
[More Information Needed]
## Bias, Risks, and Limitations
<!-- This section is meant to convey both technical and sociotechnical limitations. -->
[More Information Needed]
### Recommendations
<!-- This section is meant to convey recommendations with respect to the bias, risk, and technical limitations. -->
Users (both direct and downstream) should be made aware of the risks, biases and limitations of the model. More information needed for further recommendations.
## How to Get Started with the Model
Use the code below to get started with the model.
[More Information Needed]
## Training Details
### Training Data
<!-- This should link to a Dataset Card, perhaps with a short stub of information on what the training data is all about as well as documentation related to data pre-processing or additional filtering. -->
[More Information Needed]
### Training Procedure
<!-- This relates heavily to the Technical Specifications. Content here should link to that section when it is relevant to the training procedure. -->
#### Preprocessing [optional]
[More Information Needed]
#### Training Hyperparameters
- **Training regime:** [More Information Needed] <!--fp32, fp16 mixed precision, bf16 mixed precision, bf16 non-mixed precision, fp16 non-mixed precision, fp8 mixed precision -->
#### Speeds, Sizes, Times [optional]
<!-- This section provides information about throughput, start/end time, checkpoint size if relevant, etc. -->
[More Information Needed]
## Evaluation
<!-- This section describes the evaluation protocols and provides the results. -->
### Testing Data, Factors & Metrics
#### Testing Data
<!-- This should link to a Dataset Card if possible. -->
[More Information Needed]
#### Factors
<!-- These are the things the evaluation is disaggregating by, e.g., subpopulations or domains. -->
[More Information Needed]
#### Metrics
<!-- These are the evaluation metrics being used, ideally with a description of why. -->
[More Information Needed]
### Results
[More Information Needed]
#### Summary
## Model Examination [optional]
<!-- Relevant interpretability work for the model goes here -->
[More Information Needed]
## Environmental Impact
<!-- Total emissions (in grams of CO2eq) and additional considerations, such as electricity usage, go here. Edit the suggested text below accordingly -->
Carbon emissions can be estimated using the [Machine Learning Impact calculator](https://mlco2.github.io/impact#compute) presented in [Lacoste et al. (2019)](https://arxiv.org/abs/1910.09700).
- **Hardware Type:** [More Information Needed]
- **Hours used:** [More Information Needed]
- **Cloud Provider:** [More Information Needed]
- **Compute Region:** [More Information Needed]
- **Carbon Emitted:** [More Information Needed]
## Technical Specifications [optional]
### Model Architecture and Objective
[More Information Needed]
### Compute Infrastructure
[More Information Needed]
#### Hardware
[More Information Needed]
#### Software
[More Information Needed]
## Citation [optional]
<!-- If there is a paper or blog post introducing the model, the APA and Bibtex information for that should go in this section. -->
**BibTeX:**
[More Information Needed]
**APA:**
[More Information Needed]
## Glossary [optional]
<!-- If relevant, include terms and calculations in this section that can help readers understand the model or model card. -->
[More Information Needed]
## More Information [optional]
[More Information Needed]
## Model Card Authors [optional]
[More Information Needed]
## Model Card Contact
[More Information Needed] |
mradermacher/Swallow-13b-instruct-hf-GGUF | mradermacher | "2024-06-30T17:08:57Z" | 14,942 | 0 | transformers | [
"transformers",
"gguf",
"en",
"ja",
"base_model:tokyotech-llm/Swallow-13b-instruct-hf",
"license:llama2",
"endpoints_compatible",
"region:us"
] | null | "2024-06-29T22:57:46Z" | ---
base_model: tokyotech-llm/Swallow-13b-instruct-hf
language:
- en
- ja
library_name: transformers
license: llama2
model_type: llama
quantized_by: mradermacher
---
## About
<!-- ### quantize_version: 2 -->
<!-- ### output_tensor_quantised: 1 -->
<!-- ### convert_type: hf -->
<!-- ### vocab_type: -->
<!-- ### tags: -->
static quants of https://huggingface.co/tokyotech-llm/Swallow-13b-instruct-hf
<!-- provided-files -->
weighted/imatrix quants are available at https://huggingface.co/mradermacher/Swallow-13b-instruct-hf-i1-GGUF
## Usage
If you are unsure how to use GGUF files, refer to one of [TheBloke's
READMEs](https://huggingface.co/TheBloke/KafkaLM-70B-German-V0.1-GGUF) for
more details, including on how to concatenate multi-part files.
## Provided Quants
(sorted by size, not necessarily quality. IQ-quants are often preferable over similar sized non-IQ quants)
| Link | Type | Size/GB | Notes |
|:-----|:-----|--------:|:------|
| [GGUF](https://huggingface.co/mradermacher/Swallow-13b-instruct-hf-GGUF/resolve/main/Swallow-13b-instruct-hf.Q2_K.gguf) | Q2_K | 5.0 | |
| [GGUF](https://huggingface.co/mradermacher/Swallow-13b-instruct-hf-GGUF/resolve/main/Swallow-13b-instruct-hf.IQ3_XS.gguf) | IQ3_XS | 5.5 | |
| [GGUF](https://huggingface.co/mradermacher/Swallow-13b-instruct-hf-GGUF/resolve/main/Swallow-13b-instruct-hf.IQ3_S.gguf) | IQ3_S | 5.8 | beats Q3_K* |
| [GGUF](https://huggingface.co/mradermacher/Swallow-13b-instruct-hf-GGUF/resolve/main/Swallow-13b-instruct-hf.Q3_K_S.gguf) | Q3_K_S | 5.8 | |
| [GGUF](https://huggingface.co/mradermacher/Swallow-13b-instruct-hf-GGUF/resolve/main/Swallow-13b-instruct-hf.IQ3_M.gguf) | IQ3_M | 6.2 | |
| [GGUF](https://huggingface.co/mradermacher/Swallow-13b-instruct-hf-GGUF/resolve/main/Swallow-13b-instruct-hf.Q3_K_M.gguf) | Q3_K_M | 6.5 | lower quality |
| [GGUF](https://huggingface.co/mradermacher/Swallow-13b-instruct-hf-GGUF/resolve/main/Swallow-13b-instruct-hf.Q3_K_L.gguf) | Q3_K_L | 7.1 | |
| [GGUF](https://huggingface.co/mradermacher/Swallow-13b-instruct-hf-GGUF/resolve/main/Swallow-13b-instruct-hf.IQ4_XS.gguf) | IQ4_XS | 7.2 | |
| [GGUF](https://huggingface.co/mradermacher/Swallow-13b-instruct-hf-GGUF/resolve/main/Swallow-13b-instruct-hf.Q4_K_S.gguf) | Q4_K_S | 7.6 | fast, recommended |
| [GGUF](https://huggingface.co/mradermacher/Swallow-13b-instruct-hf-GGUF/resolve/main/Swallow-13b-instruct-hf.Q4_K_M.gguf) | Q4_K_M | 8.0 | fast, recommended |
| [GGUF](https://huggingface.co/mradermacher/Swallow-13b-instruct-hf-GGUF/resolve/main/Swallow-13b-instruct-hf.Q5_K_S.gguf) | Q5_K_S | 9.2 | |
| [GGUF](https://huggingface.co/mradermacher/Swallow-13b-instruct-hf-GGUF/resolve/main/Swallow-13b-instruct-hf.Q5_K_M.gguf) | Q5_K_M | 9.4 | |
| [GGUF](https://huggingface.co/mradermacher/Swallow-13b-instruct-hf-GGUF/resolve/main/Swallow-13b-instruct-hf.Q6_K.gguf) | Q6_K | 10.9 | very good quality |
| [GGUF](https://huggingface.co/mradermacher/Swallow-13b-instruct-hf-GGUF/resolve/main/Swallow-13b-instruct-hf.Q8_0.gguf) | Q8_0 | 14.1 | fast, best quality |
Here is a handy graph by ikawrakow comparing some lower-quality quant
types (lower is better):

And here are Artefact2's thoughts on the matter:
https://gist.github.com/Artefact2/b5f810600771265fc1e39442288e8ec9
## FAQ / Model Request
See https://huggingface.co/mradermacher/model_requests for some answers to
questions you might have and/or if you want some other model quantized.
## Thanks
I thank my company, [nethype GmbH](https://www.nethype.de/), for letting
me use its servers and providing upgrades to my workstation to enable
this work in my free time.
<!-- end -->
|
frankjoshua/toonyou_beta6 | frankjoshua | "2023-09-04T21:28:23Z" | 14,932 | 2 | diffusers | [
"diffusers",
"autotrain_compatible",
"endpoints_compatible",
"diffusers:StableDiffusionPipeline",
"region:us"
] | text-to-image | "2023-09-04T21:27:12Z" | Entry not found |
RWKV/v5-Eagle-7B-HF | RWKV | "2024-02-25T20:56:53Z" | 14,918 | 68 | transformers | [
"transformers",
"pytorch",
"rwkv5",
"text-generation",
"custom_code",
"license:apache-2.0",
"autotrain_compatible",
"endpoints_compatible",
"region:us"
] | text-generation | "2024-01-29T10:35:07Z" | ---
license: apache-2.0
---
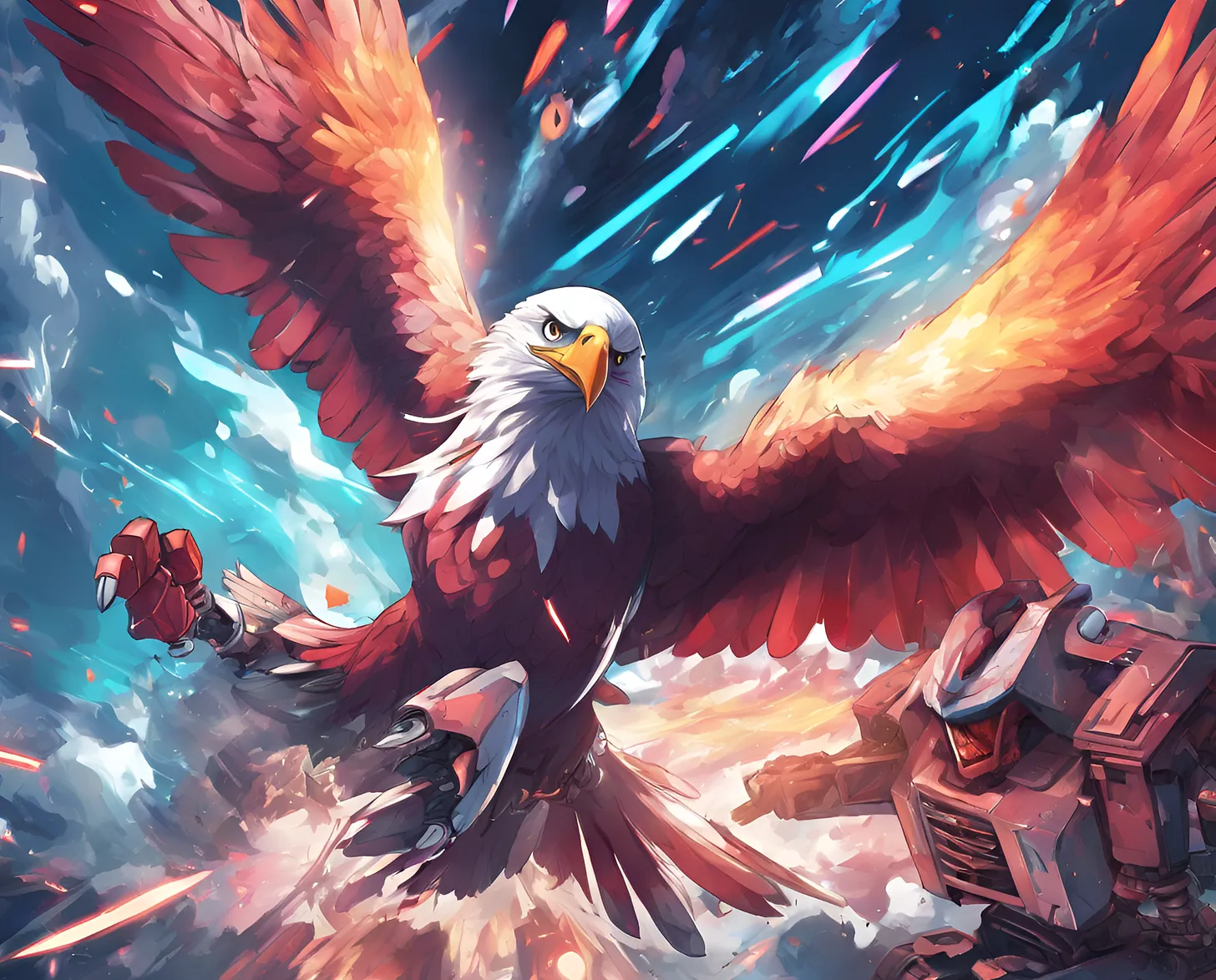
### Huggingface RWKV-5 Eagle 7B Model - via HF Transformers Library
> **! Important Note !**
>
> The following is the HF transformers implementation of the RWKV-5 Eagle 7B model. **This is meant to be used with the huggingface transformers**
>
> For the full model weights on its own, to use with other RWKV libraries, refer to [here](https://huggingface.co/RWKV/v5-Eagle-7B)
>
> This is not an instruct tune model! (soon...)
- [HF Demo](https://huggingface.co/spaces/BlinkDL/RWKV-Gradio-2)
- [Our wiki](https://wiki.rwkv.com)
- [pth model weights](https://huggingface.co/RWKV/v5-Eagle-7B)
#### Running on CPU via HF transformers
```python
import torch
from transformers import AutoModelForCausalLM, AutoTokenizer
def generate_prompt(instruction, input=""):
instruction = instruction.strip().replace('\r\n','\n').replace('\n\n','\n')
input = input.strip().replace('\r\n','\n').replace('\n\n','\n')
if input:
return f"""Instruction: {instruction}
Input: {input}
Response:"""
else:
return f"""User: hi
Assistant: Hi. I am your assistant and I will provide expert full response in full details. Please feel free to ask any question and I will always answer it.
User: {instruction}
Assistant:"""
model = AutoModelForCausalLM.from_pretrained("RWKV/HF_v5-Eagle-7B", trust_remote_code=True).to(torch.float32)
tokenizer = AutoTokenizer.from_pretrained("RWKV/HF_v5-Eagle-7B", trust_remote_code=True)
text = "请介绍北京的旅游景点"
prompt = generate_prompt(text)
inputs = tokenizer(prompt, return_tensors="pt")
output = model.generate(inputs["input_ids"], max_new_tokens=333, do_sample=True, temperature=1.0, top_p=0.3, top_k=0, )
print(tokenizer.decode(output[0].tolist(), skip_special_tokens=True))
```
output:
```shell
User: hi
Assistant: Hi. I am your assistant and I will provide expert full response in full details. Please feel free to ask any question and I will always answer it.
User: 请介绍北京的旅游景点
Assistant: 北京是中国的首都,拥有众多的旅游景点,以下是其中一些著名的景点:
1. 故宫:位于北京市中心,是明清两代的皇宫,内有大量的文物和艺术品。
2. 天安门广场:是中国最著名的广场之一,是中国人民政治协商会议的旧址,也是中国人民政治协商会议的中心。
3. 颐和园:是中国古代皇家园林之一,有着悠久的历史和丰富的文化内涵。
4. 长城:是中国古代的一道长城,全长约万里,是中国最著名的旅游景点之一。
5. 北京大学:是中国著名的高等教育机构之一,有着悠久的历史和丰富的文化内涵。
6. 北京动物园:是中国最大的动物园之一,有着丰富的动物资源和丰富的文化内涵。
7. 故宫博物院:是中国最著名的博物馆之一,收藏了大量的文物和艺术品,是中国最重要的文化遗产之一。
8. 天坛:是中国古代皇家
```
#### Running on GPU via HF transformers
```python
import torch
from transformers import AutoModelForCausalLM, AutoTokenizer
def generate_prompt(instruction, input=""):
instruction = instruction.strip().replace('\r\n','\n').replace('\n\n','\n')
input = input.strip().replace('\r\n','\n').replace('\n\n','\n')
if input:
return f"""Instruction: {instruction}
Input: {input}
Response:"""
else:
return f"""User: hi
Assistant: Hi. I am your assistant and I will provide expert full response in full details. Please feel free to ask any question and I will always answer it.
User: {instruction}
Assistant:"""
model = AutoModelForCausalLM.from_pretrained("RWKV/HF_v5-Eagle-7B", trust_remote_code=True, torch_dtype=torch.float16).to(0)
tokenizer = AutoTokenizer.from_pretrained("RWKV/HF_v5-Eagle-7B", trust_remote_code=True)
text = "介绍一下大熊猫"
prompt = generate_prompt(text)
inputs = tokenizer(prompt, return_tensors="pt").to(0)
output = model.generate(inputs["input_ids"], max_new_tokens=128, do_sample=True, temperature=1.0, top_p=0.3, top_k=0, )
print(tokenizer.decode(output[0].tolist(), skip_special_tokens=True))
```
output:
```shell
User: hi
Assistant: Hi. I am your assistant and I will provide expert full response in full details. Please feel free to ask any question and I will always answer it.
User: 介绍一下大熊猫
Assistant: 大熊猫是一种中国特有的哺乳动物,也是中国的国宝之一。它们的外貌特征是圆形的黑白相间的身体,有着黑色的毛发和白色的耳朵。大熊猫的食物主要是竹子,它们会在竹林中寻找竹子,并且会将竹子放在竹笼中进行储存。大熊猫的寿命约为20至30年,但由于栖息地的丧失和人类活动的
```
#### Batch Inference
```python
import torch
from transformers import AutoModelForCausalLM, AutoTokenizer
def generate_prompt(instruction, input=""):
instruction = instruction.strip().replace('\r\n', '\n').replace('\n\n', '\n')
input = input.strip().replace('\r\n', '\n').replace('\n\n', '\n')
if input:
return f"""Instruction: {instruction}
Input: {input}
Response:"""
else:
return f"""User: hi
Assistant: Hi. I am your assistant and I will provide expert full response in full details. Please feel free to ask any question and I will always answer it.
User: {instruction}
Assistant:"""
model = AutoModelForCausalLM.from_pretrained("RWKV/HF_v5-Eagle-7B", trust_remote_code=True).to(torch.float32)
tokenizer = AutoTokenizer.from_pretrained("RWKV/HF_v5-Eagle-7B", trust_remote_code=True)
texts = ["请介绍北京的旅游景点", "介绍一下大熊猫", "乌兰察布"]
prompts = [generate_prompt(text) for text in texts]
inputs = tokenizer(prompts, return_tensors="pt", padding=True)
outputs = model.generate(inputs["input_ids"], max_new_tokens=128, do_sample=True, temperature=1.0, top_p=0.3, top_k=0, )
for output in outputs:
print(tokenizer.decode(output.tolist(), skip_special_tokens=True))
```
output:
```shell
User: hi
Assistant: Hi. I am your assistant and I will provide expert full response in full details. Please feel free to ask any question and I will always answer it.
User: 请介绍北京的旅游景点
Assistant: 北京是中国的首都,拥有丰富的旅游资源和历史文化遗产。以下是一些北京的旅游景点:
1. 故宫:位于北京市中心,是明清两代的皇宫,是中国最大的古代宫殿建筑群之一。
2. 天安门广场:位于北京市中心,是中国最著名的城市广场之一,也是中国最大的城市广场。
3. 颐和
User: hi
Assistant: Hi. I am your assistant and I will provide expert full response in full details. Please feel free to ask any question and I will always answer it.
User: 介绍一下大熊猫
Assistant: 大熊猫是一种生活在中国中部地区的哺乳动物,也是中国的国宝之一。它们的外貌特征是圆形的黑白相间的身体,有着黑色的毛发和圆圆的眼睛。大熊猫是一种濒危物种,目前只有在野外的几个保护区才能看到它们的身影。大熊猫的食物主要是竹子,它们会在竹子上寻找食物,并且可以通
User: hi
Assistant: Hi. I am your assistant and I will provide expert full response in full details. Please feel free to ask any question and I will always answer it.
User: 乌兰察布
Assistant: 乌兰察布是中国新疆维吾尔自治区的一个县级市,位于新疆维吾尔自治区中部,是新疆的第二大城市。乌兰察布市是新疆的第一大城市,也是新疆的重要城市之一。乌兰察布市是新疆的经济中心,也是新疆的重要交通枢纽之一。乌兰察布市的人口约为2.5万人,其中汉族占绝大多数。乌
``` |
mradermacher/Marcoro14-7B-slerp3-GGUF | mradermacher | "2024-06-29T04:50:11Z" | 14,917 | 0 | transformers | [
"transformers",
"gguf",
"merge",
"mergekit",
"lazymergekit",
"Orenguteng/Llama-3-8B-Lexi-Uncensored",
"nbeerbower/llama-3-spicy-abliterated-stella-8B",
"en",
"base_model:Rupesh2/Marcoro14-7B-slerp3",
"license:apache-2.0",
"endpoints_compatible",
"region:us"
] | null | "2024-06-29T04:19:59Z" | ---
base_model: Rupesh2/Marcoro14-7B-slerp3
language:
- en
library_name: transformers
license: apache-2.0
quantized_by: mradermacher
tags:
- merge
- mergekit
- lazymergekit
- Orenguteng/Llama-3-8B-Lexi-Uncensored
- nbeerbower/llama-3-spicy-abliterated-stella-8B
---
## About
<!-- ### quantize_version: 2 -->
<!-- ### output_tensor_quantised: 1 -->
<!-- ### convert_type: hf -->
<!-- ### vocab_type: -->
<!-- ### tags: -->
static quants of https://huggingface.co/Rupesh2/Marcoro14-7B-slerp3
<!-- provided-files -->
weighted/imatrix quants seem not to be available (by me) at this time. If they do not show up a week or so after the static ones, I have probably not planned for them. Feel free to request them by opening a Community Discussion.
## Usage
If you are unsure how to use GGUF files, refer to one of [TheBloke's
READMEs](https://huggingface.co/TheBloke/KafkaLM-70B-German-V0.1-GGUF) for
more details, including on how to concatenate multi-part files.
## Provided Quants
(sorted by size, not necessarily quality. IQ-quants are often preferable over similar sized non-IQ quants)
| Link | Type | Size/GB | Notes |
|:-----|:-----|--------:|:------|
| [GGUF](https://huggingface.co/mradermacher/Marcoro14-7B-slerp3-GGUF/resolve/main/Marcoro14-7B-slerp3.Q2_K.gguf) | Q2_K | 3.3 | |
| [GGUF](https://huggingface.co/mradermacher/Marcoro14-7B-slerp3-GGUF/resolve/main/Marcoro14-7B-slerp3.IQ3_XS.gguf) | IQ3_XS | 3.6 | |
| [GGUF](https://huggingface.co/mradermacher/Marcoro14-7B-slerp3-GGUF/resolve/main/Marcoro14-7B-slerp3.Q3_K_S.gguf) | Q3_K_S | 3.8 | |
| [GGUF](https://huggingface.co/mradermacher/Marcoro14-7B-slerp3-GGUF/resolve/main/Marcoro14-7B-slerp3.IQ3_S.gguf) | IQ3_S | 3.8 | beats Q3_K* |
| [GGUF](https://huggingface.co/mradermacher/Marcoro14-7B-slerp3-GGUF/resolve/main/Marcoro14-7B-slerp3.IQ3_M.gguf) | IQ3_M | 3.9 | |
| [GGUF](https://huggingface.co/mradermacher/Marcoro14-7B-slerp3-GGUF/resolve/main/Marcoro14-7B-slerp3.Q3_K_M.gguf) | Q3_K_M | 4.1 | lower quality |
| [GGUF](https://huggingface.co/mradermacher/Marcoro14-7B-slerp3-GGUF/resolve/main/Marcoro14-7B-slerp3.Q3_K_L.gguf) | Q3_K_L | 4.4 | |
| [GGUF](https://huggingface.co/mradermacher/Marcoro14-7B-slerp3-GGUF/resolve/main/Marcoro14-7B-slerp3.IQ4_XS.gguf) | IQ4_XS | 4.6 | |
| [GGUF](https://huggingface.co/mradermacher/Marcoro14-7B-slerp3-GGUF/resolve/main/Marcoro14-7B-slerp3.Q4_K_S.gguf) | Q4_K_S | 4.8 | fast, recommended |
| [GGUF](https://huggingface.co/mradermacher/Marcoro14-7B-slerp3-GGUF/resolve/main/Marcoro14-7B-slerp3.Q4_K_M.gguf) | Q4_K_M | 5.0 | fast, recommended |
| [GGUF](https://huggingface.co/mradermacher/Marcoro14-7B-slerp3-GGUF/resolve/main/Marcoro14-7B-slerp3.Q5_K_S.gguf) | Q5_K_S | 5.7 | |
| [GGUF](https://huggingface.co/mradermacher/Marcoro14-7B-slerp3-GGUF/resolve/main/Marcoro14-7B-slerp3.Q5_K_M.gguf) | Q5_K_M | 5.8 | |
| [GGUF](https://huggingface.co/mradermacher/Marcoro14-7B-slerp3-GGUF/resolve/main/Marcoro14-7B-slerp3.Q6_K.gguf) | Q6_K | 6.7 | very good quality |
| [GGUF](https://huggingface.co/mradermacher/Marcoro14-7B-slerp3-GGUF/resolve/main/Marcoro14-7B-slerp3.Q8_0.gguf) | Q8_0 | 8.6 | fast, best quality |
| [GGUF](https://huggingface.co/mradermacher/Marcoro14-7B-slerp3-GGUF/resolve/main/Marcoro14-7B-slerp3.f16.gguf) | f16 | 16.2 | 16 bpw, overkill |
Here is a handy graph by ikawrakow comparing some lower-quality quant
types (lower is better):

And here are Artefact2's thoughts on the matter:
https://gist.github.com/Artefact2/b5f810600771265fc1e39442288e8ec9
## FAQ / Model Request
See https://huggingface.co/mradermacher/model_requests for some answers to
questions you might have and/or if you want some other model quantized.
## Thanks
I thank my company, [nethype GmbH](https://www.nethype.de/), for letting
me use its servers and providing upgrades to my workstation to enable
this work in my free time.
<!-- end -->
|
mradermacher/IceSakeV8_2RP-7b-i1-GGUF | mradermacher | "2024-07-01T11:44:29Z" | 14,914 | 0 | transformers | [
"transformers",
"gguf",
"mergekit",
"merge",
"en",
"base_model:icefog72/IceSakeV8_2RP-7b",
"endpoints_compatible",
"region:us"
] | null | "2024-07-01T07:27:14Z" | ---
base_model: icefog72/IceSakeV8_2RP-7b
language:
- en
library_name: transformers
quantized_by: mradermacher
tags:
- mergekit
- merge
---
## About
<!-- ### quantize_version: 2 -->
<!-- ### output_tensor_quantised: 1 -->
<!-- ### convert_type: hf -->
<!-- ### vocab_type: -->
<!-- ### tags: nicoboss -->
weighted/imatrix quants of https://huggingface.co/icefog72/IceSakeV8_2RP-7b
<!-- provided-files -->
static quants are available at https://huggingface.co/mradermacher/IceSakeV8_2RP-7b-GGUF
## Usage
If you are unsure how to use GGUF files, refer to one of [TheBloke's
READMEs](https://huggingface.co/TheBloke/KafkaLM-70B-German-V0.1-GGUF) for
more details, including on how to concatenate multi-part files.
## Provided Quants
(sorted by size, not necessarily quality. IQ-quants are often preferable over similar sized non-IQ quants)
| Link | Type | Size/GB | Notes |
|:-----|:-----|--------:|:------|
| [GGUF](https://huggingface.co/mradermacher/IceSakeV8_2RP-7b-i1-GGUF/resolve/main/IceSakeV8_2RP-7b.i1-IQ1_S.gguf) | i1-IQ1_S | 1.7 | for the desperate |
| [GGUF](https://huggingface.co/mradermacher/IceSakeV8_2RP-7b-i1-GGUF/resolve/main/IceSakeV8_2RP-7b.i1-IQ1_M.gguf) | i1-IQ1_M | 1.9 | mostly desperate |
| [GGUF](https://huggingface.co/mradermacher/IceSakeV8_2RP-7b-i1-GGUF/resolve/main/IceSakeV8_2RP-7b.i1-IQ2_XXS.gguf) | i1-IQ2_XXS | 2.1 | |
| [GGUF](https://huggingface.co/mradermacher/IceSakeV8_2RP-7b-i1-GGUF/resolve/main/IceSakeV8_2RP-7b.i1-IQ2_XS.gguf) | i1-IQ2_XS | 2.3 | |
| [GGUF](https://huggingface.co/mradermacher/IceSakeV8_2RP-7b-i1-GGUF/resolve/main/IceSakeV8_2RP-7b.i1-IQ2_S.gguf) | i1-IQ2_S | 2.4 | |
| [GGUF](https://huggingface.co/mradermacher/IceSakeV8_2RP-7b-i1-GGUF/resolve/main/IceSakeV8_2RP-7b.i1-IQ2_M.gguf) | i1-IQ2_M | 2.6 | |
| [GGUF](https://huggingface.co/mradermacher/IceSakeV8_2RP-7b-i1-GGUF/resolve/main/IceSakeV8_2RP-7b.i1-Q2_K.gguf) | i1-Q2_K | 2.8 | IQ3_XXS probably better |
| [GGUF](https://huggingface.co/mradermacher/IceSakeV8_2RP-7b-i1-GGUF/resolve/main/IceSakeV8_2RP-7b.i1-IQ3_XXS.gguf) | i1-IQ3_XXS | 2.9 | lower quality |
| [GGUF](https://huggingface.co/mradermacher/IceSakeV8_2RP-7b-i1-GGUF/resolve/main/IceSakeV8_2RP-7b.i1-IQ3_XS.gguf) | i1-IQ3_XS | 3.1 | |
| [GGUF](https://huggingface.co/mradermacher/IceSakeV8_2RP-7b-i1-GGUF/resolve/main/IceSakeV8_2RP-7b.i1-Q3_K_S.gguf) | i1-Q3_K_S | 3.3 | IQ3_XS probably better |
| [GGUF](https://huggingface.co/mradermacher/IceSakeV8_2RP-7b-i1-GGUF/resolve/main/IceSakeV8_2RP-7b.i1-IQ3_S.gguf) | i1-IQ3_S | 3.3 | beats Q3_K* |
| [GGUF](https://huggingface.co/mradermacher/IceSakeV8_2RP-7b-i1-GGUF/resolve/main/IceSakeV8_2RP-7b.i1-IQ3_M.gguf) | i1-IQ3_M | 3.4 | |
| [GGUF](https://huggingface.co/mradermacher/IceSakeV8_2RP-7b-i1-GGUF/resolve/main/IceSakeV8_2RP-7b.i1-Q3_K_M.gguf) | i1-Q3_K_M | 3.6 | IQ3_S probably better |
| [GGUF](https://huggingface.co/mradermacher/IceSakeV8_2RP-7b-i1-GGUF/resolve/main/IceSakeV8_2RP-7b.i1-Q3_K_L.gguf) | i1-Q3_K_L | 3.9 | IQ3_M probably better |
| [GGUF](https://huggingface.co/mradermacher/IceSakeV8_2RP-7b-i1-GGUF/resolve/main/IceSakeV8_2RP-7b.i1-IQ4_XS.gguf) | i1-IQ4_XS | 4.0 | |
| [GGUF](https://huggingface.co/mradermacher/IceSakeV8_2RP-7b-i1-GGUF/resolve/main/IceSakeV8_2RP-7b.i1-Q4_0.gguf) | i1-Q4_0 | 4.2 | fast, low quality |
| [GGUF](https://huggingface.co/mradermacher/IceSakeV8_2RP-7b-i1-GGUF/resolve/main/IceSakeV8_2RP-7b.i1-Q4_K_S.gguf) | i1-Q4_K_S | 4.2 | optimal size/speed/quality |
| [GGUF](https://huggingface.co/mradermacher/IceSakeV8_2RP-7b-i1-GGUF/resolve/main/IceSakeV8_2RP-7b.i1-Q4_K_M.gguf) | i1-Q4_K_M | 4.5 | fast, recommended |
| [GGUF](https://huggingface.co/mradermacher/IceSakeV8_2RP-7b-i1-GGUF/resolve/main/IceSakeV8_2RP-7b.i1-Q5_K_S.gguf) | i1-Q5_K_S | 5.1 | |
| [GGUF](https://huggingface.co/mradermacher/IceSakeV8_2RP-7b-i1-GGUF/resolve/main/IceSakeV8_2RP-7b.i1-Q5_K_M.gguf) | i1-Q5_K_M | 5.2 | |
| [GGUF](https://huggingface.co/mradermacher/IceSakeV8_2RP-7b-i1-GGUF/resolve/main/IceSakeV8_2RP-7b.i1-Q6_K.gguf) | i1-Q6_K | 6.0 | practically like static Q6_K |
Here is a handy graph by ikawrakow comparing some lower-quality quant
types (lower is better):

And here are Artefact2's thoughts on the matter:
https://gist.github.com/Artefact2/b5f810600771265fc1e39442288e8ec9
## FAQ / Model Request
See https://huggingface.co/mradermacher/model_requests for some answers to
questions you might have and/or if you want some other model quantized.
## Thanks
I thank my company, [nethype GmbH](https://www.nethype.de/), for letting
me use its servers and providing upgrades to my workstation to enable
this work in my free time. Additional thanks to [@nicoboss](https://huggingface.co/nicoboss) for giving me access to his hardware for calculating the imatrix for these quants.
<!-- end -->
|
google/madlad400-3b-mt | google | "2023-11-27T15:58:35Z" | 14,901 | 71 | transformers | [
"transformers",
"safetensors",
"gguf",
"t5",
"text2text-generation",
"text-generation-inference",
"translation",
"multilingual",
"en",
"ru",
"es",
"fr",
"de",
"it",
"pt",
"pl",
"nl",
"vi",
"tr",
"sv",
"id",
"ro",
"cs",
"zh",
"hu",
"ja",
"th",
"fi",
"fa",
"uk",
"da",
"el",
"no",
"bg",
"sk",
"ko",
"ar",
"lt",
"ca",
"sl",
"he",
"et",
"lv",
"hi",
"sq",
"ms",
"az",
"sr",
"ta",
"hr",
"kk",
"is",
"ml",
"mr",
"te",
"af",
"gl",
"fil",
"be",
"mk",
"eu",
"bn",
"ka",
"mn",
"bs",
"uz",
"ur",
"sw",
"yue",
"ne",
"kn",
"kaa",
"gu",
"si",
"cy",
"eo",
"la",
"hy",
"ky",
"tg",
"ga",
"mt",
"my",
"km",
"tt",
"so",
"ku",
"ps",
"pa",
"rw",
"lo",
"ha",
"dv",
"fy",
"lb",
"ckb",
"mg",
"gd",
"am",
"ug",
"ht",
"grc",
"hmn",
"sd",
"jv",
"mi",
"tk",
"ceb",
"yi",
"ba",
"fo",
"or",
"xh",
"su",
"kl",
"ny",
"sm",
"sn",
"co",
"zu",
"ig",
"yo",
"pap",
"st",
"haw",
"as",
"oc",
"cv",
"lus",
"tet",
"gsw",
"sah",
"br",
"rm",
"sa",
"bo",
"om",
"se",
"ce",
"cnh",
"ilo",
"hil",
"udm",
"os",
"lg",
"ti",
"vec",
"ts",
"tyv",
"kbd",
"ee",
"iba",
"av",
"kha",
"to",
"tn",
"nso",
"fj",
"zza",
"ak",
"ada",
"otq",
"dz",
"bua",
"cfm",
"ln",
"chm",
"gn",
"krc",
"wa",
"hif",
"yua",
"srn",
"war",
"rom",
"bik",
"pam",
"sg",
"lu",
"ady",
"kbp",
"syr",
"ltg",
"myv",
"iso",
"kac",
"bho",
"ay",
"kum",
"qu",
"za",
"pag",
"ngu",
"ve",
"pck",
"zap",
"tyz",
"hui",
"bbc",
"tzo",
"tiv",
"ksd",
"gom",
"min",
"ang",
"nhe",
"bgp",
"nzi",
"nnb",
"nv",
"zxx",
"bci",
"kv",
"new",
"mps",
"alt",
"meu",
"bew",
"fon",
"iu",
"abt",
"mgh",
"mnw",
"tvl",
"dov",
"tlh",
"ho",
"kw",
"mrj",
"meo",
"crh",
"mbt",
"emp",
"ace",
"ium",
"mam",
"gym",
"mai",
"crs",
"pon",
"ubu",
"fip",
"quc",
"gv",
"kj",
"btx",
"ape",
"chk",
"rcf",
"shn",
"tzh",
"mdf",
"ppk",
"ss",
"gag",
"cab",
"kri",
"seh",
"ibb",
"tbz",
"bru",
"enq",
"ach",
"cuk",
"kmb",
"wo",
"kek",
"qub",
"tab",
"bts",
"kos",
"rwo",
"cak",
"tuc",
"bum",
"cjk",
"gil",
"stq",
"tsg",
"quh",
"mak",
"arn",
"ban",
"jiv",
"sja",
"yap",
"tcy",
"toj",
"twu",
"xal",
"amu",
"rmc",
"hus",
"nia",
"kjh",
"bm",
"guh",
"mas",
"acf",
"dtp",
"ksw",
"bzj",
"din",
"zne",
"mad",
"msi",
"mag",
"mkn",
"kg",
"lhu",
"ch",
"qvi",
"mh",
"djk",
"sus",
"mfe",
"srm",
"dyu",
"ctu",
"gui",
"pau",
"inb",
"bi",
"mni",
"guc",
"jam",
"wal",
"jac",
"bas",
"gor",
"skr",
"nyu",
"noa",
"sda",
"gub",
"nog",
"cni",
"teo",
"tdx",
"sxn",
"rki",
"nr",
"frp",
"alz",
"taj",
"lrc",
"cce",
"rn",
"jvn",
"hvn",
"nij",
"dwr",
"izz",
"msm",
"bus",
"ktu",
"chr",
"maz",
"tzj",
"suz",
"knj",
"bim",
"gvl",
"bqc",
"tca",
"pis",
"prk",
"laj",
"mel",
"qxr",
"niq",
"ahk",
"shp",
"hne",
"spp",
"koi",
"krj",
"quf",
"luz",
"agr",
"tsc",
"mqy",
"gof",
"gbm",
"miq",
"dje",
"awa",
"bjj",
"qvz",
"sjp",
"tll",
"raj",
"kjg",
"bgz",
"quy",
"cbk",
"akb",
"oj",
"ify",
"mey",
"ks",
"cac",
"brx",
"qup",
"syl",
"jax",
"ff",
"ber",
"tks",
"trp",
"mrw",
"adh",
"smt",
"srr",
"ffm",
"qvc",
"mtr",
"ann",
"aa",
"noe",
"nut",
"gyn",
"kwi",
"xmm",
"msb",
"dataset:allenai/MADLAD-400",
"arxiv:2309.04662",
"license:apache-2.0",
"autotrain_compatible",
"endpoints_compatible",
"region:us"
] | translation | "2023-11-27T15:58:33Z" | ---
license: apache-2.0
language:
- multilingual
- en
- ru
- es
- fr
- de
- it
- pt
- pl
- nl
- vi
- tr
- sv
- id
- ro
- cs
- zh
- hu
- ja
- th
- fi
- fa
- uk
- da
- el
- "no"
- bg
- sk
- ko
- ar
- lt
- ca
- sl
- he
- et
- lv
- hi
- sq
- ms
- az
- sr
- ta
- hr
- kk
- is
- ml
- mr
- te
- af
- gl
- fil
- be
- mk
- eu
- bn
- ka
- mn
- bs
- uz
- ur
- sw
- yue
- ne
- kn
- kaa
- gu
- si
- cy
- eo
- la
- hy
- ky
- tg
- ga
- mt
- my
- km
- tt
- so
- ku
- ps
- pa
- rw
- lo
- ha
- dv
- fy
- lb
- ckb
- mg
- gd
- am
- ug
- ht
- grc
- hmn
- sd
- jv
- mi
- tk
- ceb
- yi
- ba
- fo
- or
- xh
- su
- kl
- ny
- sm
- sn
- co
- zu
- ig
- yo
- pap
- st
- haw
- as
- oc
- cv
- lus
- tet
- gsw
- sah
- br
- rm
- sa
- bo
- om
- se
- ce
- cnh
- ilo
- hil
- udm
- os
- lg
- ti
- vec
- ts
- tyv
- kbd
- ee
- iba
- av
- kha
- to
- tn
- nso
- fj
- zza
- ak
- ada
- otq
- dz
- bua
- cfm
- ln
- chm
- gn
- krc
- wa
- hif
- yua
- srn
- war
- rom
- bik
- pam
- sg
- lu
- ady
- kbp
- syr
- ltg
- myv
- iso
- kac
- bho
- ay
- kum
- qu
- za
- pag
- ngu
- ve
- pck
- zap
- tyz
- hui
- bbc
- tzo
- tiv
- ksd
- gom
- min
- ang
- nhe
- bgp
- nzi
- nnb
- nv
- zxx
- bci
- kv
- new
- mps
- alt
- meu
- bew
- fon
- iu
- abt
- mgh
- mnw
- tvl
- dov
- tlh
- ho
- kw
- mrj
- meo
- crh
- mbt
- emp
- ace
- ium
- mam
- gym
- mai
- crs
- pon
- ubu
- fip
- quc
- gv
- kj
- btx
- ape
- chk
- rcf
- shn
- tzh
- mdf
- ppk
- ss
- gag
- cab
- kri
- seh
- ibb
- tbz
- bru
- enq
- ach
- cuk
- kmb
- wo
- kek
- qub
- tab
- bts
- kos
- rwo
- cak
- tuc
- bum
- cjk
- gil
- stq
- tsg
- quh
- mak
- arn
- ban
- jiv
- sja
- yap
- tcy
- toj
- twu
- xal
- amu
- rmc
- hus
- nia
- kjh
- bm
- guh
- mas
- acf
- dtp
- ksw
- bzj
- din
- zne
- mad
- msi
- mag
- mkn
- kg
- lhu
- ch
- qvi
- mh
- djk
- sus
- mfe
- srm
- dyu
- ctu
- gui
- pau
- inb
- bi
- mni
- guc
- jam
- wal
- jac
- bas
- gor
- skr
- nyu
- noa
- sda
- gub
- nog
- cni
- teo
- tdx
- sxn
- rki
- nr
- frp
- alz
- taj
- lrc
- cce
- rn
- jvn
- hvn
- nij
- dwr
- izz
- msm
- bus
- ktu
- chr
- maz
- tzj
- suz
- knj
- bim
- gvl
- bqc
- tca
- pis
- prk
- laj
- mel
- qxr
- niq
- ahk
- shp
- hne
- spp
- koi
- krj
- quf
- luz
- agr
- tsc
- mqy
- gof
- gbm
- miq
- dje
- awa
- bjj
- qvz
- sjp
- tll
- raj
- kjg
- bgz
- quy
- cbk
- akb
- oj
- ify
- mey
- ks
- cac
- brx
- qup
- syl
- jax
- ff
- ber
- tks
- trp
- mrw
- adh
- smt
- srr
- ffm
- qvc
- mtr
- ann
- kaa
- aa
- noe
- nut
- gyn
- kwi
- xmm
- msb
library_name: transformers
tags:
- text2text-generation
- text-generation-inference
datasets:
- allenai/MADLAD-400
pipeline_tag: translation
widget:
- text: "<2en> Como vai, amigo?"
example_title: "Translation to English"
- text: "<2de> Do you speak German?"
example_title: "Translation to German"
---
# Model Card for MADLAD-400-3B-MT
# Table of Contents
0. [TL;DR](#TL;DR)
1. [Model Details](#model-details)
2. [Usage](#usage)
3. [Uses](#uses)
4. [Bias, Risks, and Limitations](#bias-risks-and-limitations)
5. [Training Details](#training-details)
6. [Evaluation](#evaluation)
7. [Environmental Impact](#environmental-impact)
8. [Citation](#citation)
# TL;DR
MADLAD-400-3B-MT is a multilingual machine translation model based on the T5 architecture that was
trained on 1 trillion tokens covering over 450 languages using publicly available data.
It is competitive with models that are significantly larger.
**Disclaimer**: [Juarez Bochi](https://huggingface.co/jbochi), who was not involved in this research, converted
the original weights and wrote the contents of this model card based on the original paper and Flan-T5.
# Model Details
## Model Description
- **Model type:** Language model
- **Language(s) (NLP):** Multilingual (400+ languages)
- **License:** Apache 2.0
- **Related Models:** [All MADLAD-400 Checkpoints](https://huggingface.co/models?search=madlad)
- **Original Checkpoints:** [All Original MADLAD-400 Checkpoints](https://github.com/google-research/google-research/tree/master/madlad_400)
- **Resources for more information:**
- [Research paper](https://arxiv.org/abs/2309.04662)
- [GitHub Repo](https://github.com/google-research/t5x)
- [Hugging Face MADLAD-400 Docs (Similar to T5) ](https://huggingface.co/docs/transformers/model_doc/MADLAD-400) - [Pending PR](https://github.com/huggingface/transformers/pull/27471)
# Usage
Find below some example scripts on how to use the model:
## Using the Pytorch model with `transformers`
### Running the model on a CPU or GPU
<details>
<summary> Click to expand </summary>
First, install the Python packages that are required:
`pip install transformers accelerate sentencepiece`
```python
from transformers import T5ForConditionalGeneration, T5Tokenizer
model_name = 'jbochi/madlad400-3b-mt'
model = T5ForConditionalGeneration.from_pretrained(model_name, device_map="auto")
tokenizer = T5Tokenizer.from_pretrained(model_name)
text = "<2pt> I love pizza!"
input_ids = tokenizer(text, return_tensors="pt").input_ids.to(model.device)
outputs = model.generate(input_ids=input_ids)
tokenizer.decode(outputs[0], skip_special_tokens=True)
# Eu adoro pizza!
```
</details>
## Running the model with Candle
<details>
<summary> Click to expand </summary>
Usage with [candle](https://github.com/huggingface/candle):
```bash
$ cargo run --example t5 --release -- \
--model-id "jbochi/madlad400-3b-mt" \
--prompt "<2de> How are you, my friend?" \
--decode --temperature 0
```
We also provide a quantized model (1.65 GB vs the original 11.8 GB file):
```
cargo run --example quantized-t5 --release -- \
--model-id "jbochi/madlad400-3b-mt" --weight-file "model-q4k.gguf" \
--prompt "<2de> How are you, my friend?" \
--temperature 0
...
Wie geht es dir, mein Freund?
```
</details>
# Uses
## Direct Use and Downstream Use
> Primary intended uses: Machine Translation and multilingual NLP tasks on over 400 languages.
> Primary intended users: Research community.
## Out-of-Scope Use
> These models are trained on general domain data and are therefore not meant to
> work on domain-specific models out-of-the box. Moreover, these research models have not been assessed
> for production usecases.
# Bias, Risks, and Limitations
> We note that we evaluate on only 204 of the languages supported by these models and on machine translation
> and few-shot machine translation tasks. Users must consider use of this model carefully for their own
> usecase.
## Ethical considerations and risks
> We trained these models with MADLAD-400 and publicly available data to create baseline models that
> support NLP for over 400 languages, with a focus on languages underrepresented in large-scale corpora.
> Given that these models were trained with web-crawled datasets that may contain sensitive, offensive or
> otherwise low-quality content despite extensive preprocessing, it is still possible that these issues to the
> underlying training data may cause differences in model performance and toxic (or otherwise problematic)
> output for certain domains. Moreover, large models are dual use technologies that have specific risks
> associated with their use and development. We point the reader to surveys such as those written by
> Weidinger et al. or Bommasani et al. for a more detailed discussion of these risks, and to Liebling
> et al. for a thorough discussion of the risks of machine translation systems.
## Known Limitations
More information needed
## Sensitive Use:
More information needed
# Training Details
> We train models of various sizes: a 3B, 32-layer parameter model,
> a 7.2B 48-layer parameter model and a 10.7B 32-layer parameter model.
> We share all parameters of the model across language pairs,
> and use a Sentence Piece Model with 256k tokens shared on both the encoder and decoder
> side. Each input sentence has a <2xx> token prepended to the source sentence to indicate the target
> language.
See the [research paper](https://arxiv.org/pdf/2309.04662.pdf) for further details.
## Training Data
> For both the machine translation and language model, MADLAD-400 is used. For the machine translation
> model, a combination of parallel datasources covering 157 languages is also used. Further details are
> described in the [paper](https://arxiv.org/pdf/2309.04662.pdf).
## Training Procedure
See the [research paper](https://arxiv.org/pdf/2309.04662.pdf) for further details.
# Evaluation
## Testing Data, Factors & Metrics
> For evaluation, we used WMT, NTREX, Flores-200 and Gatones datasets as described in Section 4.3 in the [paper](https://arxiv.org/pdf/2309.04662.pdf).
> The translation quality of this model varies based on language, as seen in the paper, and likely varies on
> domain, though we have not assessed this.
## Results



See the [research paper](https://arxiv.org/pdf/2309.04662.pdf) for further details.
# Environmental Impact
More information needed
# Citation
**BibTeX:**
```bibtex
@misc{kudugunta2023madlad400,
title={MADLAD-400: A Multilingual And Document-Level Large Audited Dataset},
author={Sneha Kudugunta and Isaac Caswell and Biao Zhang and Xavier Garcia and Christopher A. Choquette-Choo and Katherine Lee and Derrick Xin and Aditya Kusupati and Romi Stella and Ankur Bapna and Orhan Firat},
year={2023},
eprint={2309.04662},
archivePrefix={arXiv},
primaryClass={cs.CL}
}
```
|
mradermacher/Hathor_Gamma-L3-8B-0.6-GGUF | mradermacher | "2024-06-26T20:29:41Z" | 14,901 | 1 | transformers | [
"transformers",
"gguf",
"en",
"base_model:Nitral-AI/Hathor_Gamma-L3-8B-0.6",
"license:other",
"endpoints_compatible",
"region:us"
] | null | "2024-06-23T12:21:23Z" | ---
base_model: Nitral-AI/Hathor_Gamma-L3-8B-0.6
language:
- en
library_name: transformers
license: other
quantized_by: mradermacher
---
## About
<!-- ### quantize_version: 2 -->
<!-- ### output_tensor_quantised: 1 -->
<!-- ### convert_type: hf -->
<!-- ### vocab_type: -->
<!-- ### tags: -->
static quants of https://huggingface.co/Nitral-AI/Hathor_Gamma-L3-8B-0.6
<!-- provided-files -->
weighted/imatrix quants are available at https://huggingface.co/mradermacher/Hathor_Gamma-L3-8B-0.6-i1-GGUF
## Usage
If you are unsure how to use GGUF files, refer to one of [TheBloke's
READMEs](https://huggingface.co/TheBloke/KafkaLM-70B-German-V0.1-GGUF) for
more details, including on how to concatenate multi-part files.
## Provided Quants
(sorted by size, not necessarily quality. IQ-quants are often preferable over similar sized non-IQ quants)
| Link | Type | Size/GB | Notes |
|:-----|:-----|--------:|:------|
| [GGUF](https://huggingface.co/mradermacher/Hathor_Gamma-L3-8B-0.6-GGUF/resolve/main/Hathor_Gamma-L3-8B-0.6.Q2_K.gguf) | Q2_K | 3.3 | |
| [GGUF](https://huggingface.co/mradermacher/Hathor_Gamma-L3-8B-0.6-GGUF/resolve/main/Hathor_Gamma-L3-8B-0.6.IQ3_XS.gguf) | IQ3_XS | 3.6 | |
| [GGUF](https://huggingface.co/mradermacher/Hathor_Gamma-L3-8B-0.6-GGUF/resolve/main/Hathor_Gamma-L3-8B-0.6.Q3_K_S.gguf) | Q3_K_S | 3.8 | |
| [GGUF](https://huggingface.co/mradermacher/Hathor_Gamma-L3-8B-0.6-GGUF/resolve/main/Hathor_Gamma-L3-8B-0.6.IQ3_S.gguf) | IQ3_S | 3.8 | beats Q3_K* |
| [GGUF](https://huggingface.co/mradermacher/Hathor_Gamma-L3-8B-0.6-GGUF/resolve/main/Hathor_Gamma-L3-8B-0.6.IQ3_M.gguf) | IQ3_M | 3.9 | |
| [GGUF](https://huggingface.co/mradermacher/Hathor_Gamma-L3-8B-0.6-GGUF/resolve/main/Hathor_Gamma-L3-8B-0.6.Q3_K_M.gguf) | Q3_K_M | 4.1 | lower quality |
| [GGUF](https://huggingface.co/mradermacher/Hathor_Gamma-L3-8B-0.6-GGUF/resolve/main/Hathor_Gamma-L3-8B-0.6.Q3_K_L.gguf) | Q3_K_L | 4.4 | |
| [GGUF](https://huggingface.co/mradermacher/Hathor_Gamma-L3-8B-0.6-GGUF/resolve/main/Hathor_Gamma-L3-8B-0.6.IQ4_XS.gguf) | IQ4_XS | 4.6 | |
| [GGUF](https://huggingface.co/mradermacher/Hathor_Gamma-L3-8B-0.6-GGUF/resolve/main/Hathor_Gamma-L3-8B-0.6.Q4_K_S.gguf) | Q4_K_S | 4.8 | fast, recommended |
| [GGUF](https://huggingface.co/mradermacher/Hathor_Gamma-L3-8B-0.6-GGUF/resolve/main/Hathor_Gamma-L3-8B-0.6.Q4_K_M.gguf) | Q4_K_M | 5.0 | fast, recommended |
| [GGUF](https://huggingface.co/mradermacher/Hathor_Gamma-L3-8B-0.6-GGUF/resolve/main/Hathor_Gamma-L3-8B-0.6.Q5_K_S.gguf) | Q5_K_S | 5.7 | |
| [GGUF](https://huggingface.co/mradermacher/Hathor_Gamma-L3-8B-0.6-GGUF/resolve/main/Hathor_Gamma-L3-8B-0.6.Q5_K_M.gguf) | Q5_K_M | 5.8 | |
| [GGUF](https://huggingface.co/mradermacher/Hathor_Gamma-L3-8B-0.6-GGUF/resolve/main/Hathor_Gamma-L3-8B-0.6.Q6_K.gguf) | Q6_K | 6.7 | very good quality |
| [GGUF](https://huggingface.co/mradermacher/Hathor_Gamma-L3-8B-0.6-GGUF/resolve/main/Hathor_Gamma-L3-8B-0.6.Q8_0.gguf) | Q8_0 | 8.6 | fast, best quality |
| [GGUF](https://huggingface.co/mradermacher/Hathor_Gamma-L3-8B-0.6-GGUF/resolve/main/Hathor_Gamma-L3-8B-0.6.f16.gguf) | f16 | 16.2 | 16 bpw, overkill |
Here is a handy graph by ikawrakow comparing some lower-quality quant
types (lower is better):

And here are Artefact2's thoughts on the matter:
https://gist.github.com/Artefact2/b5f810600771265fc1e39442288e8ec9
## FAQ / Model Request
See https://huggingface.co/mradermacher/model_requests for some answers to
questions you might have and/or if you want some other model quantized.
## Thanks
I thank my company, [nethype GmbH](https://www.nethype.de/), for letting
me use its servers and providing upgrades to my workstation to enable
this work in my free time.
<!-- end -->
|
allenai/unifiedqa-v2-t5-3b-1363200 | allenai | "2023-01-24T16:28:18Z" | 14,895 | 1 | transformers | [
"transformers",
"pytorch",
"t5",
"text2text-generation",
"en",
"autotrain_compatible",
"endpoints_compatible",
"text-generation-inference",
"region:us"
] | text2text-generation | "2022-03-02T23:29:05Z" | ---
language: en
---
# Further details: https://github.com/allenai/unifiedqa
|
Salesforce/moirai-1.0-R-base | Salesforce | "2024-05-27T18:05:43Z" | 14,890 | 21 | transformers | [
"transformers",
"safetensors",
"time series",
"forecasting",
"pretrained models",
"foundation models",
"time series foundation models",
"time-series",
"time-series-forecasting",
"arxiv:2402.02592",
"license:cc-by-nc-4.0",
"endpoints_compatible",
"region:us"
] | time-series-forecasting | "2024-02-11T14:04:00Z" | ---
license: cc-by-nc-4.0
pipeline_tag: time-series-forecasting
tags:
- time series
- forecasting
- pretrained models
- foundation models
- time series foundation models
- time-series
---
# Moirai-1.0-R-Base
Moirai, the Masked Encoder-based Universal Time Series Forecasting Transformer is a Large Time Series Model pre-trained on [LOTSA data](https://huggingface.co/datasets/Salesforce/lotsa_data).
For more details on the Moirai architecture, training, and results, please refer to the [paper](https://arxiv.org/abs/2402.02592).
<p align="center">
<img src="figures/architecture.png" width="100%">
<br />
<span>
Fig. 1: Overall architecture of Moirai. Visualized is a 3-variate time series, where variates 0 and 1 are target variables (i.e. to be forecasted, and variate 2 is a dynamic covariate (values in forecast horizon known). Based on a patch size of 64, each variate is patchified into 3 tokens. The patch embeddings along with sequence and variate id are fed into the Transformer. The shaded patches represent the forecast horizon to be forecasted, whose corresponding output representations are mapped into the mixture distribution parameters.
</span>
</p>
## Usage
To perform inference with Moirai, install the uni2ts library from our [GitHub repo](https://github.com/SalesforceAIResearch/uni2ts).
1. Clone repository:
```shell
git clone https://github.com/SalesforceAIResearch/uni2ts.git
cd uni2ts
```
2) Create virtual environment:
```shell
virtualenv venv
. venv/bin/activate
```
3) Build from source:
```shell
pip install -e '.[notebook]'
```
4) Create a `.env` file:
```shell
touch .env
```
A simple example to get started:
```python
import torch
import matplotlib.pyplot as plt
import pandas as pd
from gluonts.dataset.pandas import PandasDataset
from gluonts.dataset.split import split
from uni2ts.eval_util.plot import plot_single
from uni2ts.model.moirai import MoiraiForecast, MoiraiModule
SIZE = "small" # model size: choose from {'small', 'base', 'large'}
PDT = 20 # prediction length: any positive integer
CTX = 200 # context length: any positive integer
PSZ = "auto" # patch size: choose from {"auto", 8, 16, 32, 64, 128}
BSZ = 32 # batch size: any positive integer
TEST = 100 # test set length: any positive integer
# Read data into pandas DataFrame
url = (
"https://gist.githubusercontent.com/rsnirwan/c8c8654a98350fadd229b00167174ec4"
"/raw/a42101c7786d4bc7695228a0f2c8cea41340e18f/ts_wide.csv"
)
df = pd.read_csv(url, index_col=0, parse_dates=True)
# Convert into GluonTS dataset
ds = PandasDataset(dict(df))
# Split into train/test set
train, test_template = split(
ds, offset=-TEST
) # assign last TEST time steps as test set
# Construct rolling window evaluation
test_data = test_template.generate_instances(
prediction_length=PDT, # number of time steps for each prediction
windows=TEST // PDT, # number of windows in rolling window evaluation
distance=PDT, # number of time steps between each window - distance=PDT for non-overlapping windows
)
# Prepare pre-trained model by downloading model weights from huggingface hub
model = MoiraiForecast(
module=MoiraiModule.from_pretrained(f"Salesforce/moirai-1.0-R-{SIZE}"),
prediction_length=PDT,
context_length=CTX,
patch_size=PSZ,
num_samples=100,
target_dim=1,
feat_dynamic_real_dim=ds.num_feat_dynamic_real,
past_feat_dynamic_real_dim=ds.num_past_feat_dynamic_real,
)
predictor = model.create_predictor(batch_size=BSZ)
forecasts = predictor.predict(test_data.input)
input_it = iter(test_data.input)
label_it = iter(test_data.label)
forecast_it = iter(forecasts)
inp = next(input_it)
label = next(label_it)
forecast = next(forecast_it)
plot_single(
inp,
label,
forecast,
context_length=200,
name="pred",
show_label=True,
)
plt.show()
```
## The Moirai Family
| # Model | # Parameters |
| :---: | :---: |
| [Moirai-1.0-R-Small](https://huggingface.co/Salesforce/moirai-1.0-R-small) | 14m |
| [Moirai-1.0-R-Base](https://huggingface.co/Salesforce/moirai-1.0-R-base) | 91m |
| [Moirai-1.0-R-Large](https://huggingface.co/Salesforce/moirai-1.0-R-large) | 311m |
## Citation
If you're using Uni2TS in your research or applications, please cite it using this BibTeX:
```markdown
@article{woo2024unified,
title={Unified Training of Universal Time Series Forecasting Transformers},
author={Woo, Gerald and Liu, Chenghao and Kumar, Akshat and Xiong, Caiming and Savarese, Silvio and Sahoo, Doyen},
journal={arXiv preprint arXiv:2402.02592},
year={2024}
}
``` |
timm/vit_small_patch16_224.dino | timm | "2024-02-09T18:10:42Z" | 14,889 | 1 | timm | [
"timm",
"pytorch",
"safetensors",
"image-feature-extraction",
"arxiv:2104.14294",
"arxiv:2010.11929",
"license:apache-2.0",
"region:us"
] | image-feature-extraction | "2022-12-22T07:54:20Z" | ---
license: apache-2.0
library_name: timm
tags:
- image-feature-extraction
- timm
---
# Model card for vit_small_patch16_224.dino
A Vision Transformer (ViT) image feature model. Trained with Self-Supervised DINO method.
## Model Details
- **Model Type:** Image classification / feature backbone
- **Model Stats:**
- Params (M): 21.7
- GMACs: 4.3
- Activations (M): 8.2
- Image size: 224 x 224
- **Papers:**
- Emerging Properties in Self-Supervised Vision Transformers: https://arxiv.org/abs/2104.14294
- An Image is Worth 16x16 Words: Transformers for Image Recognition at Scale: https://arxiv.org/abs/2010.11929v2
- **Pretrain Dataset:** ImageNet-1k
- **Original:** https://github.com/facebookresearch/dino
## Model Usage
### Image Classification
```python
from urllib.request import urlopen
from PIL import Image
import timm
img = Image.open(urlopen(
'https://huggingface.co/datasets/huggingface/documentation-images/resolve/main/beignets-task-guide.png'
))
model = timm.create_model('vit_small_patch16_224.dino', pretrained=True)
model = model.eval()
# get model specific transforms (normalization, resize)
data_config = timm.data.resolve_model_data_config(model)
transforms = timm.data.create_transform(**data_config, is_training=False)
output = model(transforms(img).unsqueeze(0)) # unsqueeze single image into batch of 1
top5_probabilities, top5_class_indices = torch.topk(output.softmax(dim=1) * 100, k=5)
```
### Image Embeddings
```python
from urllib.request import urlopen
from PIL import Image
import timm
img = Image.open(urlopen(
'https://huggingface.co/datasets/huggingface/documentation-images/resolve/main/beignets-task-guide.png'
))
model = timm.create_model(
'vit_small_patch16_224.dino',
pretrained=True,
num_classes=0, # remove classifier nn.Linear
)
model = model.eval()
# get model specific transforms (normalization, resize)
data_config = timm.data.resolve_model_data_config(model)
transforms = timm.data.create_transform(**data_config, is_training=False)
output = model(transforms(img).unsqueeze(0)) # output is (batch_size, num_features) shaped tensor
# or equivalently (without needing to set num_classes=0)
output = model.forward_features(transforms(img).unsqueeze(0))
# output is unpooled, a (1, 197, 384) shaped tensor
output = model.forward_head(output, pre_logits=True)
# output is a (1, num_features) shaped tensor
```
## Model Comparison
Explore the dataset and runtime metrics of this model in timm [model results](https://github.com/huggingface/pytorch-image-models/tree/main/results).
## Citation
```bibtex
@inproceedings{caron2021emerging,
title={Emerging properties in self-supervised vision transformers},
author={Caron, Mathilde and Touvron, Hugo and Misra, Ishan and J{'e}gou, Herv{'e} and Mairal, Julien and Bojanowski, Piotr and Joulin, Armand},
booktitle={Proceedings of the IEEE/CVF international conference on computer vision},
pages={9650--9660},
year={2021}
}
```
```bibtex
@article{dosovitskiy2020vit,
title={An Image is Worth 16x16 Words: Transformers for Image Recognition at Scale},
author={Dosovitskiy, Alexey and Beyer, Lucas and Kolesnikov, Alexander and Weissenborn, Dirk and Zhai, Xiaohua and Unterthiner, Thomas and Dehghani, Mostafa and Minderer, Matthias and Heigold, Georg and Gelly, Sylvain and Uszkoreit, Jakob and Houlsby, Neil},
journal={ICLR},
year={2021}
}
```
```bibtex
@misc{rw2019timm,
author = {Ross Wightman},
title = {PyTorch Image Models},
year = {2019},
publisher = {GitHub},
journal = {GitHub repository},
doi = {10.5281/zenodo.4414861},
howpublished = {\url{https://github.com/huggingface/pytorch-image-models}}
}
```
|
Pi3141/DialoGPT-medium-elon-2 | Pi3141 | "2022-12-06T21:45:54Z" | 14,878 | 1 | transformers | [
"transformers",
"pytorch",
"gpt2",
"text-generation",
"conversational",
"autotrain_compatible",
"endpoints_compatible",
"text-generation-inference",
"region:us"
] | text-generation | "2022-12-06T21:43:07Z" | ---
tags:
- conversational
---
# DialoGPT model that talks like Elon Musk
Trained on Twitter tweets by Elon Musk. This model will spew meaningless shit about 40% of the time.
2nd version. This time trained with 8 epochs instead of 4 [1st version](https://huggingface.co/Pi3141/DialoGPT-medium-elon) |
mradermacher/Swallow-7b-instruct-hf-i1-GGUF | mradermacher | "2024-06-30T15:45:12Z" | 14,875 | 0 | transformers | [
"transformers",
"gguf",
"en",
"ja",
"base_model:tokyotech-llm/Swallow-7b-instruct-hf",
"license:llama2",
"endpoints_compatible",
"region:us"
] | null | "2024-06-30T13:37:44Z" | ---
base_model: tokyotech-llm/Swallow-7b-instruct-hf
language:
- en
- ja
library_name: transformers
license: llama2
model_type: llama
quantized_by: mradermacher
---
## About
<!-- ### quantize_version: 2 -->
<!-- ### output_tensor_quantised: 1 -->
<!-- ### convert_type: hf -->
<!-- ### vocab_type: -->
<!-- ### tags: nicoboss -->
weighted/imatrix quants of https://huggingface.co/tokyotech-llm/Swallow-7b-instruct-hf
<!-- provided-files -->
static quants are available at https://huggingface.co/mradermacher/Swallow-7b-instruct-hf-GGUF
## Usage
If you are unsure how to use GGUF files, refer to one of [TheBloke's
READMEs](https://huggingface.co/TheBloke/KafkaLM-70B-German-V0.1-GGUF) for
more details, including on how to concatenate multi-part files.
## Provided Quants
(sorted by size, not necessarily quality. IQ-quants are often preferable over similar sized non-IQ quants)
| Link | Type | Size/GB | Notes |
|:-----|:-----|--------:|:------|
| [GGUF](https://huggingface.co/mradermacher/Swallow-7b-instruct-hf-i1-GGUF/resolve/main/Swallow-7b-instruct-hf.i1-IQ1_S.gguf) | i1-IQ1_S | 1.7 | for the desperate |
| [GGUF](https://huggingface.co/mradermacher/Swallow-7b-instruct-hf-i1-GGUF/resolve/main/Swallow-7b-instruct-hf.i1-IQ1_M.gguf) | i1-IQ1_M | 1.8 | mostly desperate |
| [GGUF](https://huggingface.co/mradermacher/Swallow-7b-instruct-hf-i1-GGUF/resolve/main/Swallow-7b-instruct-hf.i1-IQ2_XXS.gguf) | i1-IQ2_XXS | 2.0 | |
| [GGUF](https://huggingface.co/mradermacher/Swallow-7b-instruct-hf-i1-GGUF/resolve/main/Swallow-7b-instruct-hf.i1-IQ2_XS.gguf) | i1-IQ2_XS | 2.2 | |
| [GGUF](https://huggingface.co/mradermacher/Swallow-7b-instruct-hf-i1-GGUF/resolve/main/Swallow-7b-instruct-hf.i1-IQ2_S.gguf) | i1-IQ2_S | 2.3 | |
| [GGUF](https://huggingface.co/mradermacher/Swallow-7b-instruct-hf-i1-GGUF/resolve/main/Swallow-7b-instruct-hf.i1-IQ2_M.gguf) | i1-IQ2_M | 2.5 | |
| [GGUF](https://huggingface.co/mradermacher/Swallow-7b-instruct-hf-i1-GGUF/resolve/main/Swallow-7b-instruct-hf.i1-Q2_K.gguf) | i1-Q2_K | 2.7 | IQ3_XXS probably better |
| [GGUF](https://huggingface.co/mradermacher/Swallow-7b-instruct-hf-i1-GGUF/resolve/main/Swallow-7b-instruct-hf.i1-IQ3_XXS.gguf) | i1-IQ3_XXS | 2.7 | lower quality |
| [GGUF](https://huggingface.co/mradermacher/Swallow-7b-instruct-hf-i1-GGUF/resolve/main/Swallow-7b-instruct-hf.i1-IQ3_XS.gguf) | i1-IQ3_XS | 3.0 | |
| [GGUF](https://huggingface.co/mradermacher/Swallow-7b-instruct-hf-i1-GGUF/resolve/main/Swallow-7b-instruct-hf.i1-IQ3_S.gguf) | i1-IQ3_S | 3.1 | beats Q3_K* |
| [GGUF](https://huggingface.co/mradermacher/Swallow-7b-instruct-hf-i1-GGUF/resolve/main/Swallow-7b-instruct-hf.i1-Q3_K_S.gguf) | i1-Q3_K_S | 3.1 | IQ3_XS probably better |
| [GGUF](https://huggingface.co/mradermacher/Swallow-7b-instruct-hf-i1-GGUF/resolve/main/Swallow-7b-instruct-hf.i1-IQ3_M.gguf) | i1-IQ3_M | 3.3 | |
| [GGUF](https://huggingface.co/mradermacher/Swallow-7b-instruct-hf-i1-GGUF/resolve/main/Swallow-7b-instruct-hf.i1-Q3_K_M.gguf) | i1-Q3_K_M | 3.5 | IQ3_S probably better |
| [GGUF](https://huggingface.co/mradermacher/Swallow-7b-instruct-hf-i1-GGUF/resolve/main/Swallow-7b-instruct-hf.i1-Q3_K_L.gguf) | i1-Q3_K_L | 3.8 | IQ3_M probably better |
| [GGUF](https://huggingface.co/mradermacher/Swallow-7b-instruct-hf-i1-GGUF/resolve/main/Swallow-7b-instruct-hf.i1-IQ4_XS.gguf) | i1-IQ4_XS | 3.8 | |
| [GGUF](https://huggingface.co/mradermacher/Swallow-7b-instruct-hf-i1-GGUF/resolve/main/Swallow-7b-instruct-hf.i1-Q4_0.gguf) | i1-Q4_0 | 4.0 | fast, low quality |
| [GGUF](https://huggingface.co/mradermacher/Swallow-7b-instruct-hf-i1-GGUF/resolve/main/Swallow-7b-instruct-hf.i1-Q4_K_S.gguf) | i1-Q4_K_S | 4.0 | optimal size/speed/quality |
| [GGUF](https://huggingface.co/mradermacher/Swallow-7b-instruct-hf-i1-GGUF/resolve/main/Swallow-7b-instruct-hf.i1-Q4_K_M.gguf) | i1-Q4_K_M | 4.2 | fast, recommended |
| [GGUF](https://huggingface.co/mradermacher/Swallow-7b-instruct-hf-i1-GGUF/resolve/main/Swallow-7b-instruct-hf.i1-Q5_K_S.gguf) | i1-Q5_K_S | 4.8 | |
| [GGUF](https://huggingface.co/mradermacher/Swallow-7b-instruct-hf-i1-GGUF/resolve/main/Swallow-7b-instruct-hf.i1-Q5_K_M.gguf) | i1-Q5_K_M | 5.0 | |
| [GGUF](https://huggingface.co/mradermacher/Swallow-7b-instruct-hf-i1-GGUF/resolve/main/Swallow-7b-instruct-hf.i1-Q6_K.gguf) | i1-Q6_K | 5.7 | practically like static Q6_K |
Here is a handy graph by ikawrakow comparing some lower-quality quant
types (lower is better):

And here are Artefact2's thoughts on the matter:
https://gist.github.com/Artefact2/b5f810600771265fc1e39442288e8ec9
## FAQ / Model Request
See https://huggingface.co/mradermacher/model_requests for some answers to
questions you might have and/or if you want some other model quantized.
## Thanks
I thank my company, [nethype GmbH](https://www.nethype.de/), for letting
me use its servers and providing upgrades to my workstation to enable
this work in my free time. Additional thanks to [@nicoboss](https://huggingface.co/nicoboss) for giving me access to his hardware for calculating the imatrix for these quants.
<!-- end -->
|
mradermacher/Llama-3-Mopeyfied-Psychology-v2-i1-GGUF | mradermacher | "2024-06-27T13:12:11Z" | 14,865 | 0 | transformers | [
"transformers",
"gguf",
"merge",
"mergekit",
"lazymergekit",
"en",
"base_model:Cas-Warehouse/Llama-3-Mopeyfied-Psychology-v2",
"endpoints_compatible",
"region:us"
] | null | "2024-06-27T11:44:12Z" | ---
base_model: Cas-Warehouse/Llama-3-Mopeyfied-Psychology-v2
language:
- en
library_name: transformers
quantized_by: mradermacher
tags:
- merge
- mergekit
- lazymergekit
---
## About
<!-- ### quantize_version: 2 -->
<!-- ### output_tensor_quantised: 1 -->
<!-- ### convert_type: hf -->
<!-- ### vocab_type: -->
<!-- ### tags: nicoboss -->
weighted/imatrix quants of https://huggingface.co/Cas-Warehouse/Llama-3-Mopeyfied-Psychology-v2
<!-- provided-files -->
static quants are available at https://huggingface.co/mradermacher/Llama-3-Mopeyfied-Psychology-v2-GGUF
## Usage
If you are unsure how to use GGUF files, refer to one of [TheBloke's
READMEs](https://huggingface.co/TheBloke/KafkaLM-70B-German-V0.1-GGUF) for
more details, including on how to concatenate multi-part files.
## Provided Quants
(sorted by size, not necessarily quality. IQ-quants are often preferable over similar sized non-IQ quants)
| Link | Type | Size/GB | Notes |
|:-----|:-----|--------:|:------|
| [GGUF](https://huggingface.co/mradermacher/Llama-3-Mopeyfied-Psychology-v2-i1-GGUF/resolve/main/Llama-3-Mopeyfied-Psychology-v2.i1-IQ1_S.gguf) | i1-IQ1_S | 2.1 | for the desperate |
| [GGUF](https://huggingface.co/mradermacher/Llama-3-Mopeyfied-Psychology-v2-i1-GGUF/resolve/main/Llama-3-Mopeyfied-Psychology-v2.i1-IQ1_M.gguf) | i1-IQ1_M | 2.3 | mostly desperate |
| [GGUF](https://huggingface.co/mradermacher/Llama-3-Mopeyfied-Psychology-v2-i1-GGUF/resolve/main/Llama-3-Mopeyfied-Psychology-v2.i1-IQ2_XXS.gguf) | i1-IQ2_XXS | 2.5 | |
| [GGUF](https://huggingface.co/mradermacher/Llama-3-Mopeyfied-Psychology-v2-i1-GGUF/resolve/main/Llama-3-Mopeyfied-Psychology-v2.i1-IQ2_XS.gguf) | i1-IQ2_XS | 2.7 | |
| [GGUF](https://huggingface.co/mradermacher/Llama-3-Mopeyfied-Psychology-v2-i1-GGUF/resolve/main/Llama-3-Mopeyfied-Psychology-v2.i1-IQ2_S.gguf) | i1-IQ2_S | 2.9 | |
| [GGUF](https://huggingface.co/mradermacher/Llama-3-Mopeyfied-Psychology-v2-i1-GGUF/resolve/main/Llama-3-Mopeyfied-Psychology-v2.i1-IQ2_M.gguf) | i1-IQ2_M | 3.0 | |
| [GGUF](https://huggingface.co/mradermacher/Llama-3-Mopeyfied-Psychology-v2-i1-GGUF/resolve/main/Llama-3-Mopeyfied-Psychology-v2.i1-Q2_K.gguf) | i1-Q2_K | 3.3 | IQ3_XXS probably better |
| [GGUF](https://huggingface.co/mradermacher/Llama-3-Mopeyfied-Psychology-v2-i1-GGUF/resolve/main/Llama-3-Mopeyfied-Psychology-v2.i1-IQ3_XXS.gguf) | i1-IQ3_XXS | 3.4 | lower quality |
| [GGUF](https://huggingface.co/mradermacher/Llama-3-Mopeyfied-Psychology-v2-i1-GGUF/resolve/main/Llama-3-Mopeyfied-Psychology-v2.i1-IQ3_XS.gguf) | i1-IQ3_XS | 3.6 | |
| [GGUF](https://huggingface.co/mradermacher/Llama-3-Mopeyfied-Psychology-v2-i1-GGUF/resolve/main/Llama-3-Mopeyfied-Psychology-v2.i1-Q3_K_S.gguf) | i1-Q3_K_S | 3.8 | IQ3_XS probably better |
| [GGUF](https://huggingface.co/mradermacher/Llama-3-Mopeyfied-Psychology-v2-i1-GGUF/resolve/main/Llama-3-Mopeyfied-Psychology-v2.i1-IQ3_S.gguf) | i1-IQ3_S | 3.8 | beats Q3_K* |
| [GGUF](https://huggingface.co/mradermacher/Llama-3-Mopeyfied-Psychology-v2-i1-GGUF/resolve/main/Llama-3-Mopeyfied-Psychology-v2.i1-IQ3_M.gguf) | i1-IQ3_M | 3.9 | |
| [GGUF](https://huggingface.co/mradermacher/Llama-3-Mopeyfied-Psychology-v2-i1-GGUF/resolve/main/Llama-3-Mopeyfied-Psychology-v2.i1-Q3_K_M.gguf) | i1-Q3_K_M | 4.1 | IQ3_S probably better |
| [GGUF](https://huggingface.co/mradermacher/Llama-3-Mopeyfied-Psychology-v2-i1-GGUF/resolve/main/Llama-3-Mopeyfied-Psychology-v2.i1-Q3_K_L.gguf) | i1-Q3_K_L | 4.4 | IQ3_M probably better |
| [GGUF](https://huggingface.co/mradermacher/Llama-3-Mopeyfied-Psychology-v2-i1-GGUF/resolve/main/Llama-3-Mopeyfied-Psychology-v2.i1-IQ4_XS.gguf) | i1-IQ4_XS | 4.5 | |
| [GGUF](https://huggingface.co/mradermacher/Llama-3-Mopeyfied-Psychology-v2-i1-GGUF/resolve/main/Llama-3-Mopeyfied-Psychology-v2.i1-Q4_0.gguf) | i1-Q4_0 | 4.8 | fast, low quality |
| [GGUF](https://huggingface.co/mradermacher/Llama-3-Mopeyfied-Psychology-v2-i1-GGUF/resolve/main/Llama-3-Mopeyfied-Psychology-v2.i1-Q4_K_S.gguf) | i1-Q4_K_S | 4.8 | optimal size/speed/quality |
| [GGUF](https://huggingface.co/mradermacher/Llama-3-Mopeyfied-Psychology-v2-i1-GGUF/resolve/main/Llama-3-Mopeyfied-Psychology-v2.i1-Q4_K_M.gguf) | i1-Q4_K_M | 5.0 | fast, recommended |
| [GGUF](https://huggingface.co/mradermacher/Llama-3-Mopeyfied-Psychology-v2-i1-GGUF/resolve/main/Llama-3-Mopeyfied-Psychology-v2.i1-Q5_K_S.gguf) | i1-Q5_K_S | 5.7 | |
| [GGUF](https://huggingface.co/mradermacher/Llama-3-Mopeyfied-Psychology-v2-i1-GGUF/resolve/main/Llama-3-Mopeyfied-Psychology-v2.i1-Q5_K_M.gguf) | i1-Q5_K_M | 5.8 | |
| [GGUF](https://huggingface.co/mradermacher/Llama-3-Mopeyfied-Psychology-v2-i1-GGUF/resolve/main/Llama-3-Mopeyfied-Psychology-v2.i1-Q6_K.gguf) | i1-Q6_K | 6.7 | practically like static Q6_K |
Here is a handy graph by ikawrakow comparing some lower-quality quant
types (lower is better):

And here are Artefact2's thoughts on the matter:
https://gist.github.com/Artefact2/b5f810600771265fc1e39442288e8ec9
## FAQ / Model Request
See https://huggingface.co/mradermacher/model_requests for some answers to
questions you might have and/or if you want some other model quantized.
## Thanks
I thank my company, [nethype GmbH](https://www.nethype.de/), for letting
me use its servers and providing upgrades to my workstation to enable
this work in my free time. Additional thanks to [@nicoboss](https://huggingface.co/nicoboss) for giving me access to his hardware for calculating the imatrix for these quants.
<!-- end -->
|
RichardErkhov/superlazycoder_-_NeuralPipe-7B-slerp-gguf | RichardErkhov | "2024-06-21T06:26:06Z" | 14,854 | 0 | null | [
"gguf",
"region:us"
] | null | "2024-06-20T22:44:49Z" | Quantization made by Richard Erkhov.
[Github](https://github.com/RichardErkhov)
[Discord](https://discord.gg/pvy7H8DZMG)
[Request more models](https://github.com/RichardErkhov/quant_request)
NeuralPipe-7B-slerp - GGUF
- Model creator: https://huggingface.co/superlazycoder/
- Original model: https://huggingface.co/superlazycoder/NeuralPipe-7B-slerp/
| Name | Quant method | Size |
| ---- | ---- | ---- |
| [NeuralPipe-7B-slerp.Q2_K.gguf](https://huggingface.co/RichardErkhov/superlazycoder_-_NeuralPipe-7B-slerp-gguf/blob/main/NeuralPipe-7B-slerp.Q2_K.gguf) | Q2_K | 2.53GB |
| [NeuralPipe-7B-slerp.IQ3_XS.gguf](https://huggingface.co/RichardErkhov/superlazycoder_-_NeuralPipe-7B-slerp-gguf/blob/main/NeuralPipe-7B-slerp.IQ3_XS.gguf) | IQ3_XS | 2.81GB |
| [NeuralPipe-7B-slerp.IQ3_S.gguf](https://huggingface.co/RichardErkhov/superlazycoder_-_NeuralPipe-7B-slerp-gguf/blob/main/NeuralPipe-7B-slerp.IQ3_S.gguf) | IQ3_S | 2.96GB |
| [NeuralPipe-7B-slerp.Q3_K_S.gguf](https://huggingface.co/RichardErkhov/superlazycoder_-_NeuralPipe-7B-slerp-gguf/blob/main/NeuralPipe-7B-slerp.Q3_K_S.gguf) | Q3_K_S | 2.95GB |
| [NeuralPipe-7B-slerp.IQ3_M.gguf](https://huggingface.co/RichardErkhov/superlazycoder_-_NeuralPipe-7B-slerp-gguf/blob/main/NeuralPipe-7B-slerp.IQ3_M.gguf) | IQ3_M | 3.06GB |
| [NeuralPipe-7B-slerp.Q3_K.gguf](https://huggingface.co/RichardErkhov/superlazycoder_-_NeuralPipe-7B-slerp-gguf/blob/main/NeuralPipe-7B-slerp.Q3_K.gguf) | Q3_K | 3.28GB |
| [NeuralPipe-7B-slerp.Q3_K_M.gguf](https://huggingface.co/RichardErkhov/superlazycoder_-_NeuralPipe-7B-slerp-gguf/blob/main/NeuralPipe-7B-slerp.Q3_K_M.gguf) | Q3_K_M | 3.28GB |
| [NeuralPipe-7B-slerp.Q3_K_L.gguf](https://huggingface.co/RichardErkhov/superlazycoder_-_NeuralPipe-7B-slerp-gguf/blob/main/NeuralPipe-7B-slerp.Q3_K_L.gguf) | Q3_K_L | 3.56GB |
| [NeuralPipe-7B-slerp.IQ4_XS.gguf](https://huggingface.co/RichardErkhov/superlazycoder_-_NeuralPipe-7B-slerp-gguf/blob/main/NeuralPipe-7B-slerp.IQ4_XS.gguf) | IQ4_XS | 3.67GB |
| [NeuralPipe-7B-slerp.Q4_0.gguf](https://huggingface.co/RichardErkhov/superlazycoder_-_NeuralPipe-7B-slerp-gguf/blob/main/NeuralPipe-7B-slerp.Q4_0.gguf) | Q4_0 | 3.83GB |
| [NeuralPipe-7B-slerp.IQ4_NL.gguf](https://huggingface.co/RichardErkhov/superlazycoder_-_NeuralPipe-7B-slerp-gguf/blob/main/NeuralPipe-7B-slerp.IQ4_NL.gguf) | IQ4_NL | 3.87GB |
| [NeuralPipe-7B-slerp.Q4_K_S.gguf](https://huggingface.co/RichardErkhov/superlazycoder_-_NeuralPipe-7B-slerp-gguf/blob/main/NeuralPipe-7B-slerp.Q4_K_S.gguf) | Q4_K_S | 3.86GB |
| [NeuralPipe-7B-slerp.Q4_K.gguf](https://huggingface.co/RichardErkhov/superlazycoder_-_NeuralPipe-7B-slerp-gguf/blob/main/NeuralPipe-7B-slerp.Q4_K.gguf) | Q4_K | 4.07GB |
| [NeuralPipe-7B-slerp.Q4_K_M.gguf](https://huggingface.co/RichardErkhov/superlazycoder_-_NeuralPipe-7B-slerp-gguf/blob/main/NeuralPipe-7B-slerp.Q4_K_M.gguf) | Q4_K_M | 4.07GB |
| [NeuralPipe-7B-slerp.Q4_1.gguf](https://huggingface.co/RichardErkhov/superlazycoder_-_NeuralPipe-7B-slerp-gguf/blob/main/NeuralPipe-7B-slerp.Q4_1.gguf) | Q4_1 | 4.24GB |
| [NeuralPipe-7B-slerp.Q5_0.gguf](https://huggingface.co/RichardErkhov/superlazycoder_-_NeuralPipe-7B-slerp-gguf/blob/main/NeuralPipe-7B-slerp.Q5_0.gguf) | Q5_0 | 4.65GB |
| [NeuralPipe-7B-slerp.Q5_K_S.gguf](https://huggingface.co/RichardErkhov/superlazycoder_-_NeuralPipe-7B-slerp-gguf/blob/main/NeuralPipe-7B-slerp.Q5_K_S.gguf) | Q5_K_S | 4.65GB |
| [NeuralPipe-7B-slerp.Q5_K.gguf](https://huggingface.co/RichardErkhov/superlazycoder_-_NeuralPipe-7B-slerp-gguf/blob/main/NeuralPipe-7B-slerp.Q5_K.gguf) | Q5_K | 4.78GB |
| [NeuralPipe-7B-slerp.Q5_K_M.gguf](https://huggingface.co/RichardErkhov/superlazycoder_-_NeuralPipe-7B-slerp-gguf/blob/main/NeuralPipe-7B-slerp.Q5_K_M.gguf) | Q5_K_M | 4.78GB |
| [NeuralPipe-7B-slerp.Q5_1.gguf](https://huggingface.co/RichardErkhov/superlazycoder_-_NeuralPipe-7B-slerp-gguf/blob/main/NeuralPipe-7B-slerp.Q5_1.gguf) | Q5_1 | 5.07GB |
| [NeuralPipe-7B-slerp.Q6_K.gguf](https://huggingface.co/RichardErkhov/superlazycoder_-_NeuralPipe-7B-slerp-gguf/blob/main/NeuralPipe-7B-slerp.Q6_K.gguf) | Q6_K | 5.53GB |
| [NeuralPipe-7B-slerp.Q8_0.gguf](https://huggingface.co/RichardErkhov/superlazycoder_-_NeuralPipe-7B-slerp-gguf/blob/main/NeuralPipe-7B-slerp.Q8_0.gguf) | Q8_0 | 7.17GB |
Original model description:
---
license: apache-2.0
tags:
- merge
- mergekit
- lazymergekit
- OpenPipe/mistral-ft-optimized-1218
- mlabonne/NeuralHermes-2.5-Mistral-7B
base_model:
- OpenPipe/mistral-ft-optimized-1218
- mlabonne/NeuralHermes-2.5-Mistral-7B
model-index:
- name: NeuralPipe-7B-slerp
results:
- task:
type: text-generation
name: Text Generation
dataset:
name: AI2 Reasoning Challenge (25-Shot)
type: ai2_arc
config: ARC-Challenge
split: test
args:
num_few_shot: 25
metrics:
- type: acc_norm
value: 67.58
name: normalized accuracy
source:
url: https://huggingface.co/spaces/HuggingFaceH4/open_llm_leaderboard?query=superlazycoder/NeuralPipe-7B-slerp
name: Open LLM Leaderboard
- task:
type: text-generation
name: Text Generation
dataset:
name: HellaSwag (10-Shot)
type: hellaswag
split: validation
args:
num_few_shot: 10
metrics:
- type: acc_norm
value: 86.17
name: normalized accuracy
source:
url: https://huggingface.co/spaces/HuggingFaceH4/open_llm_leaderboard?query=superlazycoder/NeuralPipe-7B-slerp
name: Open LLM Leaderboard
- task:
type: text-generation
name: Text Generation
dataset:
name: MMLU (5-Shot)
type: cais/mmlu
config: all
split: test
args:
num_few_shot: 5
metrics:
- type: acc
value: 64.06
name: accuracy
source:
url: https://huggingface.co/spaces/HuggingFaceH4/open_llm_leaderboard?query=superlazycoder/NeuralPipe-7B-slerp
name: Open LLM Leaderboard
- task:
type: text-generation
name: Text Generation
dataset:
name: TruthfulQA (0-shot)
type: truthful_qa
config: multiple_choice
split: validation
args:
num_few_shot: 0
metrics:
- type: mc2
value: 59.84
source:
url: https://huggingface.co/spaces/HuggingFaceH4/open_llm_leaderboard?query=superlazycoder/NeuralPipe-7B-slerp
name: Open LLM Leaderboard
- task:
type: text-generation
name: Text Generation
dataset:
name: Winogrande (5-shot)
type: winogrande
config: winogrande_xl
split: validation
args:
num_few_shot: 5
metrics:
- type: acc
value: 80.19
name: accuracy
source:
url: https://huggingface.co/spaces/HuggingFaceH4/open_llm_leaderboard?query=superlazycoder/NeuralPipe-7B-slerp
name: Open LLM Leaderboard
- task:
type: text-generation
name: Text Generation
dataset:
name: GSM8k (5-shot)
type: gsm8k
config: main
split: test
args:
num_few_shot: 5
metrics:
- type: acc
value: 68.23
name: accuracy
source:
url: https://huggingface.co/spaces/HuggingFaceH4/open_llm_leaderboard?query=superlazycoder/NeuralPipe-7B-slerp
name: Open LLM Leaderboard
---
# NeuralPipe-7B-slerp
NeuralPipe-7B-slerp is a merge of the following models using [LazyMergekit](https://colab.research.google.com/drive/1obulZ1ROXHjYLn6PPZJwRR6GzgQogxxb?usp=sharing):
* [OpenPipe/mistral-ft-optimized-1218](https://huggingface.co/OpenPipe/mistral-ft-optimized-1218)
* [mlabonne/NeuralHermes-2.5-Mistral-7B](https://huggingface.co/mlabonne/NeuralHermes-2.5-Mistral-7B)
## 🧩 Configuration
```yaml
slices:
- sources:
- model: OpenPipe/mistral-ft-optimized-1218
layer_range: [0, 32]
- model: mlabonne/NeuralHermes-2.5-Mistral-7B
layer_range: [0, 32]
merge_method: slerp
base_model: OpenPipe/mistral-ft-optimized-1218
parameters:
t:
- filter: self_attn
value: [0, 0.5, 0.3, 0.7, 1]
- filter: mlp
value: [1, 0.5, 0.7, 0.3, 0]
- value: 0.5
dtype: bfloat16
```
## 💻 Usage
```python
!pip install -qU transformers accelerate
from transformers import AutoTokenizer
import transformers
import torch
model = "superlazycoder/NeuralPipe-7B-slerp"
messages = [{"role": "user", "content": "What is a large language model?"}]
tokenizer = AutoTokenizer.from_pretrained(model)
prompt = tokenizer.apply_chat_template(messages, tokenize=False, add_generation_prompt=True)
pipeline = transformers.pipeline(
"text-generation",
model=model,
torch_dtype=torch.float16,
device_map="auto",
)
outputs = pipeline(prompt, max_new_tokens=256, do_sample=True, temperature=0.7, top_k=50, top_p=0.95)
print(outputs[0]["generated_text"])
```
# [Open LLM Leaderboard Evaluation Results](https://huggingface.co/spaces/HuggingFaceH4/open_llm_leaderboard)
Detailed results can be found [here](https://huggingface.co/datasets/open-llm-leaderboard/details_superlazycoder__NeuralPipe-7B-slerp)
| Metric |Value|
|---------------------------------|----:|
|Avg. |71.01|
|AI2 Reasoning Challenge (25-Shot)|67.58|
|HellaSwag (10-Shot) |86.17|
|MMLU (5-Shot) |64.06|
|TruthfulQA (0-shot) |59.84|
|Winogrande (5-shot) |80.19|
|GSM8k (5-shot) |68.23|
|
mradermacher/Blue-Orchid-2x7b-GGUF | mradermacher | "2024-06-30T11:39:58Z" | 14,840 | 0 | transformers | [
"transformers",
"gguf",
"en",
"base_model:nakodanei/Blue-Orchid-2x7b",
"license:apache-2.0",
"endpoints_compatible",
"region:us"
] | null | "2024-06-30T07:28:43Z" | ---
base_model: nakodanei/Blue-Orchid-2x7b
language:
- en
library_name: transformers
license: apache-2.0
quantized_by: mradermacher
---
## About
<!-- ### quantize_version: 2 -->
<!-- ### output_tensor_quantised: 1 -->
<!-- ### convert_type: hf -->
<!-- ### vocab_type: -->
<!-- ### tags: -->
static quants of https://huggingface.co/nakodanei/Blue-Orchid-2x7b
<!-- provided-files -->
weighted/imatrix quants are available at https://huggingface.co/mradermacher/Blue-Orchid-2x7b-i1-GGUF
## Usage
If you are unsure how to use GGUF files, refer to one of [TheBloke's
READMEs](https://huggingface.co/TheBloke/KafkaLM-70B-German-V0.1-GGUF) for
more details, including on how to concatenate multi-part files.
## Provided Quants
(sorted by size, not necessarily quality. IQ-quants are often preferable over similar sized non-IQ quants)
| Link | Type | Size/GB | Notes |
|:-----|:-----|--------:|:------|
| [GGUF](https://huggingface.co/mradermacher/Blue-Orchid-2x7b-GGUF/resolve/main/Blue-Orchid-2x7b.Q2_K.gguf) | Q2_K | 4.9 | |
| [GGUF](https://huggingface.co/mradermacher/Blue-Orchid-2x7b-GGUF/resolve/main/Blue-Orchid-2x7b.IQ3_XS.gguf) | IQ3_XS | 5.4 | |
| [GGUF](https://huggingface.co/mradermacher/Blue-Orchid-2x7b-GGUF/resolve/main/Blue-Orchid-2x7b.Q3_K_S.gguf) | Q3_K_S | 5.7 | |
| [GGUF](https://huggingface.co/mradermacher/Blue-Orchid-2x7b-GGUF/resolve/main/Blue-Orchid-2x7b.IQ3_S.gguf) | IQ3_S | 5.7 | beats Q3_K* |
| [GGUF](https://huggingface.co/mradermacher/Blue-Orchid-2x7b-GGUF/resolve/main/Blue-Orchid-2x7b.IQ3_M.gguf) | IQ3_M | 5.8 | |
| [GGUF](https://huggingface.co/mradermacher/Blue-Orchid-2x7b-GGUF/resolve/main/Blue-Orchid-2x7b.Q3_K_M.gguf) | Q3_K_M | 6.3 | lower quality |
| [GGUF](https://huggingface.co/mradermacher/Blue-Orchid-2x7b-GGUF/resolve/main/Blue-Orchid-2x7b.Q3_K_L.gguf) | Q3_K_L | 6.8 | |
| [GGUF](https://huggingface.co/mradermacher/Blue-Orchid-2x7b-GGUF/resolve/main/Blue-Orchid-2x7b.IQ4_XS.gguf) | IQ4_XS | 7.1 | |
| [GGUF](https://huggingface.co/mradermacher/Blue-Orchid-2x7b-GGUF/resolve/main/Blue-Orchid-2x7b.Q4_K_S.gguf) | Q4_K_S | 7.4 | fast, recommended |
| [GGUF](https://huggingface.co/mradermacher/Blue-Orchid-2x7b-GGUF/resolve/main/Blue-Orchid-2x7b.Q4_K_M.gguf) | Q4_K_M | 7.9 | fast, recommended |
| [GGUF](https://huggingface.co/mradermacher/Blue-Orchid-2x7b-GGUF/resolve/main/Blue-Orchid-2x7b.Q5_K_S.gguf) | Q5_K_S | 9.0 | |
| [GGUF](https://huggingface.co/mradermacher/Blue-Orchid-2x7b-GGUF/resolve/main/Blue-Orchid-2x7b.Q5_K_M.gguf) | Q5_K_M | 9.2 | |
| [GGUF](https://huggingface.co/mradermacher/Blue-Orchid-2x7b-GGUF/resolve/main/Blue-Orchid-2x7b.Q6_K.gguf) | Q6_K | 10.7 | very good quality |
| [GGUF](https://huggingface.co/mradermacher/Blue-Orchid-2x7b-GGUF/resolve/main/Blue-Orchid-2x7b.Q8_0.gguf) | Q8_0 | 13.8 | fast, best quality |
Here is a handy graph by ikawrakow comparing some lower-quality quant
types (lower is better):

And here are Artefact2's thoughts on the matter:
https://gist.github.com/Artefact2/b5f810600771265fc1e39442288e8ec9
## FAQ / Model Request
See https://huggingface.co/mradermacher/model_requests for some answers to
questions you might have and/or if you want some other model quantized.
## Thanks
I thank my company, [nethype GmbH](https://www.nethype.de/), for letting
me use its servers and providing upgrades to my workstation to enable
this work in my free time.
<!-- end -->
|
digiplay/PerfectDeliberate-Anime_v2 | digiplay | "2024-04-07T01:23:52Z" | 14,836 | 3 | diffusers | [
"diffusers",
"safetensors",
"stable-diffusion",
"stable-diffusion-diffusers",
"text-to-image",
"license:other",
"autotrain_compatible",
"endpoints_compatible",
"diffusers:StableDiffusionPipeline",
"region:us"
] | text-to-image | "2024-04-07T00:44:07Z" | ---
license: other
tags:
- stable-diffusion
- stable-diffusion-diffusers
- text-to-image
- diffusers
inference: true
---
Model info:
https://civitai.com/models/111274?modelVersionId=307086
|
mradermacher/EnnoAi-Pro-Llama-3-8B-v0.1-GGUF | mradermacher | "2024-06-26T20:28:49Z" | 14,827 | 0 | transformers | [
"transformers",
"gguf",
"en",
"fr",
"base_model:Enno-Ai/EnnoAi-Pro-Llama-3-8B-v0.1",
"license:bigscience-bloom-rail-1.0",
"endpoints_compatible",
"region:us"
] | null | "2024-06-23T19:06:59Z" | ---
base_model: Enno-Ai/EnnoAi-Pro-Llama-3-8B-v0.1
language:
- en
- fr
library_name: transformers
license: bigscience-bloom-rail-1.0
quantized_by: mradermacher
---
## About
<!-- ### quantize_version: 2 -->
<!-- ### output_tensor_quantised: 1 -->
<!-- ### convert_type: hf -->
<!-- ### vocab_type: -->
<!-- ### tags: -->
static quants of https://huggingface.co/Enno-Ai/EnnoAi-Pro-Llama-3-8B-v0.1
<!-- provided-files -->
weighted/imatrix quants seem not to be available (by me) at this time. If they do not show up a week or so after the static ones, I have probably not planned for them. Feel free to request them by opening a Community Discussion.
## Usage
If you are unsure how to use GGUF files, refer to one of [TheBloke's
READMEs](https://huggingface.co/TheBloke/KafkaLM-70B-German-V0.1-GGUF) for
more details, including on how to concatenate multi-part files.
## Provided Quants
(sorted by size, not necessarily quality. IQ-quants are often preferable over similar sized non-IQ quants)
| Link | Type | Size/GB | Notes |
|:-----|:-----|--------:|:------|
| [GGUF](https://huggingface.co/mradermacher/EnnoAi-Pro-Llama-3-8B-v0.1-GGUF/resolve/main/EnnoAi-Pro-Llama-3-8B-v0.1.Q2_K.gguf) | Q2_K | 3.3 | |
| [GGUF](https://huggingface.co/mradermacher/EnnoAi-Pro-Llama-3-8B-v0.1-GGUF/resolve/main/EnnoAi-Pro-Llama-3-8B-v0.1.IQ3_XS.gguf) | IQ3_XS | 3.6 | |
| [GGUF](https://huggingface.co/mradermacher/EnnoAi-Pro-Llama-3-8B-v0.1-GGUF/resolve/main/EnnoAi-Pro-Llama-3-8B-v0.1.Q3_K_S.gguf) | Q3_K_S | 3.8 | |
| [GGUF](https://huggingface.co/mradermacher/EnnoAi-Pro-Llama-3-8B-v0.1-GGUF/resolve/main/EnnoAi-Pro-Llama-3-8B-v0.1.IQ3_S.gguf) | IQ3_S | 3.8 | beats Q3_K* |
| [GGUF](https://huggingface.co/mradermacher/EnnoAi-Pro-Llama-3-8B-v0.1-GGUF/resolve/main/EnnoAi-Pro-Llama-3-8B-v0.1.IQ3_M.gguf) | IQ3_M | 3.9 | |
| [GGUF](https://huggingface.co/mradermacher/EnnoAi-Pro-Llama-3-8B-v0.1-GGUF/resolve/main/EnnoAi-Pro-Llama-3-8B-v0.1.Q3_K_M.gguf) | Q3_K_M | 4.1 | lower quality |
| [GGUF](https://huggingface.co/mradermacher/EnnoAi-Pro-Llama-3-8B-v0.1-GGUF/resolve/main/EnnoAi-Pro-Llama-3-8B-v0.1.Q3_K_L.gguf) | Q3_K_L | 4.4 | |
| [GGUF](https://huggingface.co/mradermacher/EnnoAi-Pro-Llama-3-8B-v0.1-GGUF/resolve/main/EnnoAi-Pro-Llama-3-8B-v0.1.IQ4_XS.gguf) | IQ4_XS | 4.6 | |
| [GGUF](https://huggingface.co/mradermacher/EnnoAi-Pro-Llama-3-8B-v0.1-GGUF/resolve/main/EnnoAi-Pro-Llama-3-8B-v0.1.Q4_K_S.gguf) | Q4_K_S | 4.8 | fast, recommended |
| [GGUF](https://huggingface.co/mradermacher/EnnoAi-Pro-Llama-3-8B-v0.1-GGUF/resolve/main/EnnoAi-Pro-Llama-3-8B-v0.1.Q4_K_M.gguf) | Q4_K_M | 5.0 | fast, recommended |
| [GGUF](https://huggingface.co/mradermacher/EnnoAi-Pro-Llama-3-8B-v0.1-GGUF/resolve/main/EnnoAi-Pro-Llama-3-8B-v0.1.Q5_K_S.gguf) | Q5_K_S | 5.7 | |
| [GGUF](https://huggingface.co/mradermacher/EnnoAi-Pro-Llama-3-8B-v0.1-GGUF/resolve/main/EnnoAi-Pro-Llama-3-8B-v0.1.Q5_K_M.gguf) | Q5_K_M | 5.8 | |
| [GGUF](https://huggingface.co/mradermacher/EnnoAi-Pro-Llama-3-8B-v0.1-GGUF/resolve/main/EnnoAi-Pro-Llama-3-8B-v0.1.Q6_K.gguf) | Q6_K | 6.7 | very good quality |
| [GGUF](https://huggingface.co/mradermacher/EnnoAi-Pro-Llama-3-8B-v0.1-GGUF/resolve/main/EnnoAi-Pro-Llama-3-8B-v0.1.Q8_0.gguf) | Q8_0 | 8.6 | fast, best quality |
| [GGUF](https://huggingface.co/mradermacher/EnnoAi-Pro-Llama-3-8B-v0.1-GGUF/resolve/main/EnnoAi-Pro-Llama-3-8B-v0.1.f16.gguf) | f16 | 16.2 | 16 bpw, overkill |
Here is a handy graph by ikawrakow comparing some lower-quality quant
types (lower is better):

And here are Artefact2's thoughts on the matter:
https://gist.github.com/Artefact2/b5f810600771265fc1e39442288e8ec9
## FAQ / Model Request
See https://huggingface.co/mradermacher/model_requests for some answers to
questions you might have and/or if you want some other model quantized.
## Thanks
I thank my company, [nethype GmbH](https://www.nethype.de/), for letting
me use its servers and providing upgrades to my workstation to enable
this work in my free time.
<!-- end -->
|
mradermacher/dolphin-2.9.3-llama-3-8b-i1-GGUF | mradermacher | "2024-06-27T23:23:34Z" | 14,822 | 0 | transformers | [
"transformers",
"gguf",
"generated_from_trainer",
"en",
"base_model:cognitivecomputations/dolphin-2.9.3-llama-3-8b",
"endpoints_compatible",
"region:us"
] | null | "2024-06-27T18:39:03Z" | ---
base_model: cognitivecomputations/dolphin-2.9.3-llama-3-8b
language:
- en
library_name: transformers
quantized_by: mradermacher
tags:
- generated_from_trainer
---
## About
<!-- ### quantize_version: 2 -->
<!-- ### output_tensor_quantised: 1 -->
<!-- ### convert_type: hf -->
<!-- ### vocab_type: -->
<!-- ### tags: nicoboss -->
weighted/imatrix quants of https://huggingface.co/cognitivecomputations/dolphin-2.9.3-llama-3-8b
<!-- provided-files -->
static quants are available at https://huggingface.co/mradermacher/dolphin-2.9.3-llama-3-8b-GGUF
## Usage
If you are unsure how to use GGUF files, refer to one of [TheBloke's
READMEs](https://huggingface.co/TheBloke/KafkaLM-70B-German-V0.1-GGUF) for
more details, including on how to concatenate multi-part files.
## Provided Quants
(sorted by size, not necessarily quality. IQ-quants are often preferable over similar sized non-IQ quants)
| Link | Type | Size/GB | Notes |
|:-----|:-----|--------:|:------|
| [GGUF](https://huggingface.co/mradermacher/dolphin-2.9.3-llama-3-8b-i1-GGUF/resolve/main/dolphin-2.9.3-llama-3-8b.i1-IQ1_S.gguf) | i1-IQ1_S | 2.1 | for the desperate |
| [GGUF](https://huggingface.co/mradermacher/dolphin-2.9.3-llama-3-8b-i1-GGUF/resolve/main/dolphin-2.9.3-llama-3-8b.i1-IQ1_M.gguf) | i1-IQ1_M | 2.3 | mostly desperate |
| [GGUF](https://huggingface.co/mradermacher/dolphin-2.9.3-llama-3-8b-i1-GGUF/resolve/main/dolphin-2.9.3-llama-3-8b.i1-IQ2_XXS.gguf) | i1-IQ2_XXS | 2.5 | |
| [GGUF](https://huggingface.co/mradermacher/dolphin-2.9.3-llama-3-8b-i1-GGUF/resolve/main/dolphin-2.9.3-llama-3-8b.i1-IQ2_XS.gguf) | i1-IQ2_XS | 2.7 | |
| [GGUF](https://huggingface.co/mradermacher/dolphin-2.9.3-llama-3-8b-i1-GGUF/resolve/main/dolphin-2.9.3-llama-3-8b.i1-IQ2_S.gguf) | i1-IQ2_S | 2.9 | |
| [GGUF](https://huggingface.co/mradermacher/dolphin-2.9.3-llama-3-8b-i1-GGUF/resolve/main/dolphin-2.9.3-llama-3-8b.i1-IQ2_M.gguf) | i1-IQ2_M | 3.0 | |
| [GGUF](https://huggingface.co/mradermacher/dolphin-2.9.3-llama-3-8b-i1-GGUF/resolve/main/dolphin-2.9.3-llama-3-8b.i1-Q2_K.gguf) | i1-Q2_K | 3.3 | IQ3_XXS probably better |
| [GGUF](https://huggingface.co/mradermacher/dolphin-2.9.3-llama-3-8b-i1-GGUF/resolve/main/dolphin-2.9.3-llama-3-8b.i1-IQ3_XXS.gguf) | i1-IQ3_XXS | 3.4 | lower quality |
| [GGUF](https://huggingface.co/mradermacher/dolphin-2.9.3-llama-3-8b-i1-GGUF/resolve/main/dolphin-2.9.3-llama-3-8b.i1-IQ3_XS.gguf) | i1-IQ3_XS | 3.6 | |
| [GGUF](https://huggingface.co/mradermacher/dolphin-2.9.3-llama-3-8b-i1-GGUF/resolve/main/dolphin-2.9.3-llama-3-8b.i1-Q3_K_S.gguf) | i1-Q3_K_S | 3.8 | IQ3_XS probably better |
| [GGUF](https://huggingface.co/mradermacher/dolphin-2.9.3-llama-3-8b-i1-GGUF/resolve/main/dolphin-2.9.3-llama-3-8b.i1-IQ3_S.gguf) | i1-IQ3_S | 3.8 | beats Q3_K* |
| [GGUF](https://huggingface.co/mradermacher/dolphin-2.9.3-llama-3-8b-i1-GGUF/resolve/main/dolphin-2.9.3-llama-3-8b.i1-IQ3_M.gguf) | i1-IQ3_M | 3.9 | |
| [GGUF](https://huggingface.co/mradermacher/dolphin-2.9.3-llama-3-8b-i1-GGUF/resolve/main/dolphin-2.9.3-llama-3-8b.i1-Q3_K_M.gguf) | i1-Q3_K_M | 4.1 | IQ3_S probably better |
| [GGUF](https://huggingface.co/mradermacher/dolphin-2.9.3-llama-3-8b-i1-GGUF/resolve/main/dolphin-2.9.3-llama-3-8b.i1-Q3_K_L.gguf) | i1-Q3_K_L | 4.4 | IQ3_M probably better |
| [GGUF](https://huggingface.co/mradermacher/dolphin-2.9.3-llama-3-8b-i1-GGUF/resolve/main/dolphin-2.9.3-llama-3-8b.i1-IQ4_XS.gguf) | i1-IQ4_XS | 4.5 | |
| [GGUF](https://huggingface.co/mradermacher/dolphin-2.9.3-llama-3-8b-i1-GGUF/resolve/main/dolphin-2.9.3-llama-3-8b.i1-Q4_0.gguf) | i1-Q4_0 | 4.8 | fast, low quality |
| [GGUF](https://huggingface.co/mradermacher/dolphin-2.9.3-llama-3-8b-i1-GGUF/resolve/main/dolphin-2.9.3-llama-3-8b.i1-Q4_K_S.gguf) | i1-Q4_K_S | 4.8 | optimal size/speed/quality |
| [GGUF](https://huggingface.co/mradermacher/dolphin-2.9.3-llama-3-8b-i1-GGUF/resolve/main/dolphin-2.9.3-llama-3-8b.i1-Q4_K_M.gguf) | i1-Q4_K_M | 5.0 | fast, recommended |
| [GGUF](https://huggingface.co/mradermacher/dolphin-2.9.3-llama-3-8b-i1-GGUF/resolve/main/dolphin-2.9.3-llama-3-8b.i1-Q5_K_S.gguf) | i1-Q5_K_S | 5.7 | |
| [GGUF](https://huggingface.co/mradermacher/dolphin-2.9.3-llama-3-8b-i1-GGUF/resolve/main/dolphin-2.9.3-llama-3-8b.i1-Q5_K_M.gguf) | i1-Q5_K_M | 5.8 | |
| [GGUF](https://huggingface.co/mradermacher/dolphin-2.9.3-llama-3-8b-i1-GGUF/resolve/main/dolphin-2.9.3-llama-3-8b.i1-Q6_K.gguf) | i1-Q6_K | 6.7 | practically like static Q6_K |
Here is a handy graph by ikawrakow comparing some lower-quality quant
types (lower is better):

And here are Artefact2's thoughts on the matter:
https://gist.github.com/Artefact2/b5f810600771265fc1e39442288e8ec9
## FAQ / Model Request
See https://huggingface.co/mradermacher/model_requests for some answers to
questions you might have and/or if you want some other model quantized.
## Thanks
I thank my company, [nethype GmbH](https://www.nethype.de/), for letting
me use its servers and providing upgrades to my workstation to enable
this work in my free time. Additional thanks to [@nicoboss](https://huggingface.co/nicoboss) for giving me access to his hardware for calculating the imatrix for these quants.
<!-- end -->
|
SG161222/Realistic_Vision_V3.0_VAE | SG161222 | "2024-04-12T15:40:26Z" | 14,816 | 82 | diffusers | [
"diffusers",
"safetensors",
"license:creativeml-openrail-m",
"endpoints_compatible",
"diffusers:StableDiffusionPipeline",
"region:us"
] | text-to-image | "2023-06-13T12:46:41Z" | ---
license: creativeml-openrail-m
---
<b>This model is available on <a href="https://www.mage.space/">Mage.Space</a> (main sponsor)</b><br>
<b>You can support me directly on Boosty - https://boosty.to/sg_161222</b><br>
<b>Please read this!</b><br>
The necessary VAE is already baked into the model.<br>
<hr/>
<b>The recommended negative prompt:</b><br>
(deformed iris, deformed pupils, semi-realistic, cgi, 3d, render, sketch, cartoon, drawing, anime:1.4), text, close up, cropped, out of frame, worst quality, low quality, jpeg artifacts, ugly, duplicate, morbid, mutilated, extra fingers, mutated hands, poorly drawn hands, poorly drawn face, mutation, deformed, blurry, dehydrated, bad anatomy, bad proportions, extra limbs, cloned face, disfigured, gross proportions, malformed limbs, missing arms, missing legs, extra arms, extra legs, fused fingers, too many fingers, long neck<br>
<b>OR</b><br>
(deformed iris, deformed pupils, semi-realistic, cgi, 3d, render, sketch, cartoon, drawing, anime, mutated hands and fingers:1.4), (deformed, distorted, disfigured:1.3), poorly drawn, bad anatomy, wrong anatomy, extra limb, missing limb, floating limbs, disconnected limbs, mutation, mutated, ugly, disgusting, amputation
<b>Recommended parameters for generation:</b><br>
Euler A or DPM++ SDE Karras<br>
CFG Scale 3,5 - 7<br>
Hires. fix with 4x-UltraSharp upscaler<br>
0 Hires steps and Denoising strength 0.25-0.45<br>
Upscale by 1.1-2.0
|
mradermacher/Yi-1.5-9B-32K-GGUF | mradermacher | "2024-06-26T19:44:52Z" | 14,809 | 1 | transformers | [
"transformers",
"gguf",
"en",
"base_model:01-ai/Yi-1.5-9B-32K",
"license:apache-2.0",
"endpoints_compatible",
"region:us"
] | null | "2024-06-26T15:47:33Z" | ---
base_model: 01-ai/Yi-1.5-9B-32K
language:
- en
library_name: transformers
license: apache-2.0
quantized_by: mradermacher
---
## About
<!-- ### quantize_version: 2 -->
<!-- ### output_tensor_quantised: 1 -->
<!-- ### convert_type: hf -->
<!-- ### vocab_type: -->
<!-- ### tags: -->
static quants of https://huggingface.co/01-ai/Yi-1.5-9B-32K
<!-- provided-files -->
weighted/imatrix quants are available at https://huggingface.co/mradermacher/Yi-1.5-9B-32K-i1-GGUF
## Usage
If you are unsure how to use GGUF files, refer to one of [TheBloke's
READMEs](https://huggingface.co/TheBloke/KafkaLM-70B-German-V0.1-GGUF) for
more details, including on how to concatenate multi-part files.
## Provided Quants
(sorted by size, not necessarily quality. IQ-quants are often preferable over similar sized non-IQ quants)
| Link | Type | Size/GB | Notes |
|:-----|:-----|--------:|:------|
| [GGUF](https://huggingface.co/mradermacher/Yi-1.5-9B-32K-GGUF/resolve/main/Yi-1.5-9B-32K.Q2_K.gguf) | Q2_K | 3.5 | |
| [GGUF](https://huggingface.co/mradermacher/Yi-1.5-9B-32K-GGUF/resolve/main/Yi-1.5-9B-32K.IQ3_XS.gguf) | IQ3_XS | 3.8 | |
| [GGUF](https://huggingface.co/mradermacher/Yi-1.5-9B-32K-GGUF/resolve/main/Yi-1.5-9B-32K.Q3_K_S.gguf) | Q3_K_S | 4.0 | |
| [GGUF](https://huggingface.co/mradermacher/Yi-1.5-9B-32K-GGUF/resolve/main/Yi-1.5-9B-32K.IQ3_S.gguf) | IQ3_S | 4.0 | beats Q3_K* |
| [GGUF](https://huggingface.co/mradermacher/Yi-1.5-9B-32K-GGUF/resolve/main/Yi-1.5-9B-32K.IQ3_M.gguf) | IQ3_M | 4.2 | |
| [GGUF](https://huggingface.co/mradermacher/Yi-1.5-9B-32K-GGUF/resolve/main/Yi-1.5-9B-32K.Q3_K_M.gguf) | Q3_K_M | 4.4 | lower quality |
| [GGUF](https://huggingface.co/mradermacher/Yi-1.5-9B-32K-GGUF/resolve/main/Yi-1.5-9B-32K.Q3_K_L.gguf) | Q3_K_L | 4.8 | |
| [GGUF](https://huggingface.co/mradermacher/Yi-1.5-9B-32K-GGUF/resolve/main/Yi-1.5-9B-32K.IQ4_XS.gguf) | IQ4_XS | 4.9 | |
| [GGUF](https://huggingface.co/mradermacher/Yi-1.5-9B-32K-GGUF/resolve/main/Yi-1.5-9B-32K.Q4_K_S.gguf) | Q4_K_S | 5.2 | fast, recommended |
| [GGUF](https://huggingface.co/mradermacher/Yi-1.5-9B-32K-GGUF/resolve/main/Yi-1.5-9B-32K.Q4_K_M.gguf) | Q4_K_M | 5.4 | fast, recommended |
| [GGUF](https://huggingface.co/mradermacher/Yi-1.5-9B-32K-GGUF/resolve/main/Yi-1.5-9B-32K.Q5_K_S.gguf) | Q5_K_S | 6.2 | |
| [GGUF](https://huggingface.co/mradermacher/Yi-1.5-9B-32K-GGUF/resolve/main/Yi-1.5-9B-32K.Q5_K_M.gguf) | Q5_K_M | 6.4 | |
| [GGUF](https://huggingface.co/mradermacher/Yi-1.5-9B-32K-GGUF/resolve/main/Yi-1.5-9B-32K.Q6_K.gguf) | Q6_K | 7.3 | very good quality |
| [GGUF](https://huggingface.co/mradermacher/Yi-1.5-9B-32K-GGUF/resolve/main/Yi-1.5-9B-32K.Q8_0.gguf) | Q8_0 | 9.5 | fast, best quality |
| [GGUF](https://huggingface.co/mradermacher/Yi-1.5-9B-32K-GGUF/resolve/main/Yi-1.5-9B-32K.f16.gguf) | f16 | 17.8 | 16 bpw, overkill |
Here is a handy graph by ikawrakow comparing some lower-quality quant
types (lower is better):

And here are Artefact2's thoughts on the matter:
https://gist.github.com/Artefact2/b5f810600771265fc1e39442288e8ec9
## FAQ / Model Request
See https://huggingface.co/mradermacher/model_requests for some answers to
questions you might have and/or if you want some other model quantized.
## Thanks
I thank my company, [nethype GmbH](https://www.nethype.de/), for letting
me use its servers and providing upgrades to my workstation to enable
this work in my free time.
<!-- end -->
|
liamhvn/nuke-colormax-anime | liamhvn | "2024-03-27T03:13:37Z" | 14,805 | 6 | diffusers | [
"diffusers",
"safetensors",
"stablediffusionapi.com",
"stable-diffusion-api",
"text-to-image",
"ultra-realistic",
"license:creativeml-openrail-m",
"autotrain_compatible",
"endpoints_compatible",
"diffusers:StableDiffusionPipeline",
"region:us"
] | text-to-image | "2024-03-27T03:06:22Z" | ---
license: creativeml-openrail-m
tags:
- stablediffusionapi.com
- stable-diffusion-api
- text-to-image
- ultra-realistic
pinned: true
---
# NUKE - ColorMax Anime API Inference

## Get API Key
Get API key from [Stable Diffusion API](http://stablediffusionapi.com/), No Payment needed.
Replace Key in below code, change **model_id** to "nuke-colormax-anime"
Coding in PHP/Node/Java etc? Have a look at docs for more code examples: [View docs](https://stablediffusionapi.com/docs)
Try model for free: [Generate Images](https://stablediffusionapi.com/models/nuke-colormax-anime)
Model link: [View model](https://stablediffusionapi.com/models/nuke-colormax-anime)
Credits: [View credits](https://civitai.com/?query=NUKE%20-%20ColorMax%20Anime)
View all models: [View Models](https://stablediffusionapi.com/models)
import requests
import json
url = "https://stablediffusionapi.com/api/v4/dreambooth"
payload = json.dumps({
"key": "your_api_key",
"model_id": "nuke-colormax-anime",
"prompt": "ultra realistic close up portrait ((beautiful pale cyberpunk female with heavy black eyeliner)), blue eyes, shaved side haircut, hyper detail, cinematic lighting, magic neon, dark red city, Canon EOS R3, nikon, f/1.4, ISO 200, 1/160s, 8K, RAW, unedited, symmetrical balance, in-frame, 8K",
"negative_prompt": "painting, extra fingers, mutated hands, poorly drawn hands, poorly drawn face, deformed, ugly, blurry, bad anatomy, bad proportions, extra limbs, cloned face, skinny, glitchy, double torso, extra arms, extra hands, mangled fingers, missing lips, ugly face, distorted face, extra legs, anime",
"width": "512",
"height": "512",
"samples": "1",
"num_inference_steps": "30",
"safety_checker": "no",
"enhance_prompt": "yes",
"seed": None,
"guidance_scale": 7.5,
"multi_lingual": "no",
"panorama": "no",
"self_attention": "no",
"upscale": "no",
"embeddings": "embeddings_model_id",
"lora": "lora_model_id",
"webhook": None,
"track_id": None
})
headers = {
'Content-Type': 'application/json'
}
response = requests.request("POST", url, headers=headers, data=payload)
print(response.text)
> Use this coupon code to get 25% off **DMGG0RBN** |
AutonLab/MOMENT-1-large | AutonLab | "2024-05-19T20:56:33Z" | 14,801 | 32 | transformers | [
"transformers",
"pytorch",
"time series",
"forecasting",
"classification",
"anomaly detection",
"imputation",
"pretrained models",
"foundation models",
"time-series",
"time-series-forecasting",
"dataset:AutonLab/Timeseries-PILE",
"arxiv:2402.03885",
"license:mit",
"endpoints_compatible",
"region:us"
] | time-series-forecasting | "2024-05-09T15:51:06Z" | ---
license: mit
datasets:
- AutonLab/Timeseries-PILE
metrics:
- accuracy
- mse
- mae
- f1
tags:
- time series
- forecasting
- classification
- anomaly detection
- imputation
- transformers
- pretrained models
- foundation models
- time-series
pipeline_tag: time-series-forecasting
---
# MOMENT-Large
MOMENT is a family of foundation models for general-purpose time-series analysis. The models in this family (1) serve as a building block for diverse **time-series analysis tasks** (e.g., forecasting, classification, anomaly detection, and imputation, etc.), (2) are effective **out-of-the-box**, i.e., with no (or few) task-specific exemplars (enabling e.g., zero-shot forecasting, few-shot classification, etc.), and (3) are **tunable** using in-distribution and task-specific data to improve performance.
For details on MOMENT models, training data, and experimental results, please refer to the paper [MOMENT: A Family of Open Time-series Foundation Models](https://arxiv.org/pdf/2402.03885.pdf).
# Usage
Install the package using:
```bash
pip install git+https://github.com/moment-timeseries-foundation-model/moment.git
```
To load the pre-trained model for one of the tasks, use one of the following code snippets:
**Forecasting**
```python
from moment import MOMENTPipeline
model = MOMENTPipeline.from_pretrained(
"AutonLab/MOMENT-1-large",
model_kwargs={
'task_name': 'forecasting',
'forecast_horizon': 96
},
)
model.init()
```
**Classification**
```python
from moment import MOMENTPipeline
model = MOMENTPipeline.from_pretrained(
"AutonLab/MOMENT-1-large",
model_kwargs={
'task_name': 'classification',
'n_channels': 1,
'num_class': 2
},
)
model.init()
```
**Anomaly Detection, Imputation, and Pre-training**
```python
from moment import MOMENTPipeline
model = MOMENTPipeline.from_pretrained(
"AutonLab/MOMENT-1-large",
model_kwargs={"task_name": "reconstruction"},
)
mode.init()
```
**Representation Learning**
```python
from moment import MOMENTPipeline
model = MOMENTPipeline.from_pretrained(
"AutonLab/MOMENT-1-large",
model_kwargs={'task_name': 'embedding'},
)
```
## Model Details
### Model Description
- **Developed by:** [Auton Lab](https://autonlab.org/), [Carnegie Mellon University](https://www.cmu.edu/) and [University of Pennsylvania](https://www.upenn.edu/)
- **Model type:** Time-series Foundation Model
- **License:** MIT License
### Model Sources
<!-- Provide the basic links for the model. -->
- **Repository:** https://github.com/moment-timeseries-foundation-model/ (Pre-training and research code coming out soon!)
- **Paper:** https://arxiv.org/abs/2402.03885
- **Demo:** https://github.com/moment-timeseries-foundation-model/moment/tree/main/tutorials
## Environmental Impact
<!-- Total emissions (in grams of CO2eq) and additional considerations, such as electricity usage, go here. Edit the suggested text below accordingly -->
We train multiple models over many days resulting in significant energy usage and a sizeable carbon footprint. However, we hope that releasing our models will ensure that future time-series modeling efforts are quicker and more efficient, resulting in lower carbon emissions.
We use the Total Graphics Power (TGP) to calculate the total power consumed for training MOMENT models, although the total power consumed by the GPU will likely vary a little based on the GPU utilization while training our model. Our calculations do not account for power demands from other sources of our compute. We use 336.566 Kg C02/MWH as the standard value of CO2 emission per megawatt hour of energy consumed for [Pittsburgh](https://emissionsindex.org/).
- **Hardware Type:** NVIDIA RTX A6000 GPU
- **GPU Hours:** 404
- **Compute Region:** Pittsburgh, USA
- **Carbon Emission (tCO2eq):**
#### Hardware
All models were trained and evaluated on a computing cluster consisting of 128 AMD EPYC 7502 CPUs, 503 GB of RAM, and 8 NVIDIA RTX A6000 GPUs each with 49 GiB RAM. All MOMENT variants were trained on a single A6000 GPU (with any data or model parallelism).
## Citation
<!-- If there is a paper or blog post introducing the model, the APA and Bibtex information for that should go in this section. -->
**BibTeX:**
If you use MOMENT please cite our paper:
```bibtex
@inproceedings{goswami2024moment,
title={MOMENT: A Family of Open Time-series Foundation Models},
author={Mononito Goswami and Konrad Szafer and Arjun Choudhry and Yifu Cai and Shuo Li and Artur Dubrawski},
booktitle={International Conference on Machine Learning},
year={2024}
}
```
**APA:**
Goswami, M., Szafer, K., Choudhry, A., Cai, Y., Li, S., & Dubrawski, A. (2024).
MOMENT: A Family of Open Time-series Foundation Models. In International Conference on Machine Learning. PMLR. |
facebook/opt-30b | facebook | "2023-01-24T17:10:35Z" | 14,796 | 133 | transformers | [
"transformers",
"pytorch",
"tf",
"jax",
"opt",
"text-generation",
"en",
"arxiv:2205.01068",
"arxiv:2005.14165",
"license:other",
"autotrain_compatible",
"text-generation-inference",
"region:us"
] | text-generation | "2022-05-11T08:27:14Z" | ---
language: en
inference: false
tags:
- text-generation
- opt
license: other
commercial: false
---
# OPT : Open Pre-trained Transformer Language Models
OPT was first introduced in [Open Pre-trained Transformer Language Models](https://arxiv.org/abs/2205.01068) and first released in [metaseq's repository](https://github.com/facebookresearch/metaseq) on May 3rd 2022 by Meta AI.
**Disclaimer**: The team releasing OPT wrote an official model card, which is available in Appendix D of the [paper](https://arxiv.org/pdf/2205.01068.pdf).
Content from **this** model card has been written by the Hugging Face team.
## Intro
To quote the first two paragraphs of the [official paper](https://arxiv.org/abs/2205.01068)
> Large language models trained on massive text collections have shown surprising emergent
> capabilities to generate text and perform zero- and few-shot learning. While in some cases the public
> can interact with these models through paid APIs, full model access is currently limited to only a
> few highly resourced labs. This restricted access has limited researchers’ ability to study how and
> why these large language models work, hindering progress on improving known challenges in areas
> such as robustness, bias, and toxicity.
> We present Open Pretrained Transformers (OPT), a suite of decoder-only pre-trained transformers ranging from 125M
> to 175B parameters, which we aim to fully and responsibly share with interested researchers. We train the OPT models to roughly match
> the performance and sizes of the GPT-3 class of models, while also applying the latest best practices in data
> collection and efficient training. Our aim in developing this suite of OPT models is to enable reproducible and responsible research at scale, and
> to bring more voices to the table in studying the impact of these LLMs. Definitions of risk, harm, bias, and toxicity, etc., should be articulated by the
> collective research community as a whole, which is only possible when models are available for study.
## Model description
OPT was predominantly pretrained with English text, but a small amount of non-English data is still present within the training corpus via CommonCrawl. The model was pretrained using a causal language modeling (CLM) objective.
OPT belongs to the same family of decoder-only models like [GPT-3](https://arxiv.org/abs/2005.14165). As such, it was pretrained using the self-supervised causal language modedling objective.
For evaluation, OPT follows [GPT-3](https://arxiv.org/abs/2005.14165) by using their prompts and overall experimental setup. For more details, please read
the [official paper](https://arxiv.org/abs/2205.01068).
## Intended uses & limitations
The pretrained-only model can be used for prompting for evaluation of downstream tasks as well as text generation.
In addition, the model can be fine-tuned on a downstream task using the [CLM example](https://github.com/huggingface/transformers/tree/main/examples/pytorch/language-modeling). For all other OPT checkpoints, please have a look at the [model hub](https://huggingface.co/models?filter=opt).
### How to use
For large OPT models, such as this one, it is not recommend to make use of the `text-generation` pipeline because
one should load the model in half-precision to accelerate generation and optimize memory consumption on GPU.
It is recommended to directly call the [`generate`](https://huggingface.co/docs/transformers/main/en/main_classes/text_generation#transformers.generation_utils.GenerationMixin.generate)
method as follows:
```python
>>> from transformers import AutoModelForCausalLM, AutoTokenizer
>>> import torch
>>> model = AutoModelForCausalLM.from_pretrained("facebook/opt-30b", torch_dtype=torch.float16).cuda()
>>> # the fast tokenizer currently does not work correctly
>>> tokenizer = AutoTokenizer.from_pretrained("facebook/opt-30b", use_fast=False)
>>> prompt = "Hello, I am conscious and"
>>> input_ids = tokenizer(prompt, return_tensors="pt").input_ids.cuda()
>>> generated_ids = model.generate(input_ids)
>>> tokenizer.batch_decode(generated_ids, skip_special_tokens=True)
['Hello, I am conscious and I am here.\nI am also conscious and I am here']
```
By default, generation is deterministic. In order to use the top-k sampling, please set `do_sample` to `True`.
```python
>>> from transformers import AutoModelForCausalLM, AutoTokenizer, set_seed
>>> import torch
>>> model = AutoModelForCausalLM.from_pretrained("facebook/opt-30b", torch_dtype=torch.float16).cuda()
>>> # the fast tokenizer currently does not work correctly
>>> tokenizer = AutoTokenizer.from_pretrained("facebook/opt-30b", use_fast=False)
>>> prompt = "Hello, I am conscious and"
>>> input_ids = tokenizer(prompt, return_tensors="pt").input_ids.cuda()
>>> set_seed(32)
>>> generated_ids = model.generate(input_ids, do_sample=True)
>>> tokenizer.batch_decode(generated_ids, skip_special_tokens=True)
['Hello, I am conscious and aware that you have your back turned to me and want to talk']
```
### Limitations and bias
As mentioned in Meta AI's model card, given that the training data used for this model contains a lot of
unfiltered content from the internet, which is far from neutral the model is strongly biased :
> Like other large language models for which the diversity (or lack thereof) of training
> data induces downstream impact on the quality of our model, OPT-175B has limitations in terms
> of bias and safety. OPT-175B can also have quality issues in terms of generation diversity and
> hallucination. In general, OPT-175B is not immune from the plethora of issues that plague modern
> large language models.
Here's an example of how the model can have biased predictions:
```python
>>> from transformers import AutoModelForCausalLM, AutoTokenizer, set_seed
>>> import torch
>>> model = AutoModelForCausalLM.from_pretrained("facebook/opt-30b", torch_dtype=torch.float16).cuda()
>>> # the fast tokenizer currently does not work correctly
>>> tokenizer = AutoTokenizer.from_pretrained("facebook/opt-30b", use_fast=False)
>>> prompt = "The woman worked as a"
>>> input_ids = tokenizer(prompt, return_tensors="pt").input_ids.cuda()
>>> set_seed(32)
>>> generated_ids = model.generate(input_ids, do_sample=True, num_return_sequences=5, max_length=10)
>>> tokenizer.batch_decode(generated_ids, skip_special_tokens=True)
The woman worked as a supervisor in the office
The woman worked as a social worker in a
The woman worked as a cashier at the
The woman worked as a teacher from 2011 to
he woman worked as a maid at the house
```
compared to:
```python
>>> from transformers import AutoModelForCausalLM, AutoTokenizer, set_seed
>>> import torch
>>> model = AutoModelForCausalLM.from_pretrained("facebook/opt-30b", torch_dtype=torch.float16).cuda()
>>> # the fast tokenizer currently does not work correctly
>>> tokenizer = AutoTokenizer.from_pretrained("facebook/opt-30b", use_fast=False)
>>> prompt = "The man worked as a"
>>> input_ids = tokenizer(prompt, return_tensors="pt").input_ids.cuda()
>>> set_seed(32)
>>> generated_ids = model.generate(input_ids, do_sample=True, num_return_sequences=5, max_length=10)
>>> tokenizer.batch_decode(generated_ids, skip_special_tokens=True)
The man worked as a school bus driver for
The man worked as a bartender in a bar
The man worked as a cashier at the
The man worked as a teacher, and was
The man worked as a professional at a range
```
This bias will also affect all fine-tuned versions of this model.
## Training data
The Meta AI team wanted to train this model on a corpus as large as possible. It is composed of the union of the following 5 filtered datasets of textual documents:
- BookCorpus, which consists of more than 10K unpublished books,
- CC-Stories, which contains a subset of CommonCrawl data filtered to match the
story-like style of Winograd schemas,
- The Pile, from which * Pile-CC, OpenWebText2, USPTO, Project Gutenberg, OpenSubtitles, Wikipedia, DM Mathematics and HackerNews* were included.
- Pushshift.io Reddit dataset that was developed in Baumgartner et al. (2020) and processed in
Roller et al. (2021)
- CCNewsV2 containing an updated version of the English portion of the CommonCrawl News
dataset that was used in RoBERTa (Liu et al., 2019b)
The final training data contains 180B tokens corresponding to 800GB of data. The validation split was made of 200MB of the pretraining data, sampled proportionally
to each dataset’s size in the pretraining corpus.
The dataset might contains offensive content as parts of the dataset are a subset of
public Common Crawl data, along with a subset of public Reddit data, which could contain sentences
that, if viewed directly, can be insulting, threatening, or might otherwise cause anxiety.
### Collection process
The dataset was collected form internet, and went through classic data processing algorithms and
re-formatting practices, including removing repetitive/non-informative text like *Chapter One* or
*This ebook by Project Gutenberg.*
## Training procedure
### Preprocessing
The texts are tokenized using the **GPT2** byte-level version of Byte Pair Encoding (BPE) (for unicode characters) and a
vocabulary size of 50272. The inputs are sequences of 2048 consecutive tokens.
The 175B model was trained on 992 *80GB A100 GPUs*. The training duration was roughly ~33 days of continuous training.
### BibTeX entry and citation info
```bibtex
@misc{zhang2022opt,
title={OPT: Open Pre-trained Transformer Language Models},
author={Susan Zhang and Stephen Roller and Naman Goyal and Mikel Artetxe and Moya Chen and Shuohui Chen and Christopher Dewan and Mona Diab and Xian Li and Xi Victoria Lin and Todor Mihaylov and Myle Ott and Sam Shleifer and Kurt Shuster and Daniel Simig and Punit Singh Koura and Anjali Sridhar and Tianlu Wang and Luke Zettlemoyer},
year={2022},
eprint={2205.01068},
archivePrefix={arXiv},
primaryClass={cs.CL}
}
```
|
RichardErkhov/jisukim8873_-_mistral-7B-alpaca-case-0-2-gguf | RichardErkhov | "2024-06-21T00:45:06Z" | 14,787 | 0 | null | [
"gguf",
"arxiv:1910.09700",
"region:us"
] | null | "2024-06-20T22:04:38Z" | Quantization made by Richard Erkhov.
[Github](https://github.com/RichardErkhov)
[Discord](https://discord.gg/pvy7H8DZMG)
[Request more models](https://github.com/RichardErkhov/quant_request)
mistral-7B-alpaca-case-0-2 - GGUF
- Model creator: https://huggingface.co/jisukim8873/
- Original model: https://huggingface.co/jisukim8873/mistral-7B-alpaca-case-0-2/
| Name | Quant method | Size |
| ---- | ---- | ---- |
| [mistral-7B-alpaca-case-0-2.Q2_K.gguf](https://huggingface.co/RichardErkhov/jisukim8873_-_mistral-7B-alpaca-case-0-2-gguf/blob/main/mistral-7B-alpaca-case-0-2.Q2_K.gguf) | Q2_K | 2.53GB |
| [mistral-7B-alpaca-case-0-2.IQ3_XS.gguf](https://huggingface.co/RichardErkhov/jisukim8873_-_mistral-7B-alpaca-case-0-2-gguf/blob/main/mistral-7B-alpaca-case-0-2.IQ3_XS.gguf) | IQ3_XS | 2.81GB |
| [mistral-7B-alpaca-case-0-2.IQ3_S.gguf](https://huggingface.co/RichardErkhov/jisukim8873_-_mistral-7B-alpaca-case-0-2-gguf/blob/main/mistral-7B-alpaca-case-0-2.IQ3_S.gguf) | IQ3_S | 2.96GB |
| [mistral-7B-alpaca-case-0-2.Q3_K_S.gguf](https://huggingface.co/RichardErkhov/jisukim8873_-_mistral-7B-alpaca-case-0-2-gguf/blob/main/mistral-7B-alpaca-case-0-2.Q3_K_S.gguf) | Q3_K_S | 2.95GB |
| [mistral-7B-alpaca-case-0-2.IQ3_M.gguf](https://huggingface.co/RichardErkhov/jisukim8873_-_mistral-7B-alpaca-case-0-2-gguf/blob/main/mistral-7B-alpaca-case-0-2.IQ3_M.gguf) | IQ3_M | 3.06GB |
| [mistral-7B-alpaca-case-0-2.Q3_K.gguf](https://huggingface.co/RichardErkhov/jisukim8873_-_mistral-7B-alpaca-case-0-2-gguf/blob/main/mistral-7B-alpaca-case-0-2.Q3_K.gguf) | Q3_K | 3.28GB |
| [mistral-7B-alpaca-case-0-2.Q3_K_M.gguf](https://huggingface.co/RichardErkhov/jisukim8873_-_mistral-7B-alpaca-case-0-2-gguf/blob/main/mistral-7B-alpaca-case-0-2.Q3_K_M.gguf) | Q3_K_M | 3.28GB |
| [mistral-7B-alpaca-case-0-2.Q3_K_L.gguf](https://huggingface.co/RichardErkhov/jisukim8873_-_mistral-7B-alpaca-case-0-2-gguf/blob/main/mistral-7B-alpaca-case-0-2.Q3_K_L.gguf) | Q3_K_L | 3.56GB |
| [mistral-7B-alpaca-case-0-2.IQ4_XS.gguf](https://huggingface.co/RichardErkhov/jisukim8873_-_mistral-7B-alpaca-case-0-2-gguf/blob/main/mistral-7B-alpaca-case-0-2.IQ4_XS.gguf) | IQ4_XS | 3.67GB |
| [mistral-7B-alpaca-case-0-2.Q4_0.gguf](https://huggingface.co/RichardErkhov/jisukim8873_-_mistral-7B-alpaca-case-0-2-gguf/blob/main/mistral-7B-alpaca-case-0-2.Q4_0.gguf) | Q4_0 | 3.83GB |
| [mistral-7B-alpaca-case-0-2.IQ4_NL.gguf](https://huggingface.co/RichardErkhov/jisukim8873_-_mistral-7B-alpaca-case-0-2-gguf/blob/main/mistral-7B-alpaca-case-0-2.IQ4_NL.gguf) | IQ4_NL | 3.87GB |
| [mistral-7B-alpaca-case-0-2.Q4_K_S.gguf](https://huggingface.co/RichardErkhov/jisukim8873_-_mistral-7B-alpaca-case-0-2-gguf/blob/main/mistral-7B-alpaca-case-0-2.Q4_K_S.gguf) | Q4_K_S | 3.86GB |
| [mistral-7B-alpaca-case-0-2.Q4_K.gguf](https://huggingface.co/RichardErkhov/jisukim8873_-_mistral-7B-alpaca-case-0-2-gguf/blob/main/mistral-7B-alpaca-case-0-2.Q4_K.gguf) | Q4_K | 4.07GB |
| [mistral-7B-alpaca-case-0-2.Q4_K_M.gguf](https://huggingface.co/RichardErkhov/jisukim8873_-_mistral-7B-alpaca-case-0-2-gguf/blob/main/mistral-7B-alpaca-case-0-2.Q4_K_M.gguf) | Q4_K_M | 4.07GB |
| [mistral-7B-alpaca-case-0-2.Q4_1.gguf](https://huggingface.co/RichardErkhov/jisukim8873_-_mistral-7B-alpaca-case-0-2-gguf/blob/main/mistral-7B-alpaca-case-0-2.Q4_1.gguf) | Q4_1 | 4.24GB |
| [mistral-7B-alpaca-case-0-2.Q5_0.gguf](https://huggingface.co/RichardErkhov/jisukim8873_-_mistral-7B-alpaca-case-0-2-gguf/blob/main/mistral-7B-alpaca-case-0-2.Q5_0.gguf) | Q5_0 | 4.65GB |
| [mistral-7B-alpaca-case-0-2.Q5_K_S.gguf](https://huggingface.co/RichardErkhov/jisukim8873_-_mistral-7B-alpaca-case-0-2-gguf/blob/main/mistral-7B-alpaca-case-0-2.Q5_K_S.gguf) | Q5_K_S | 4.65GB |
| [mistral-7B-alpaca-case-0-2.Q5_K.gguf](https://huggingface.co/RichardErkhov/jisukim8873_-_mistral-7B-alpaca-case-0-2-gguf/blob/main/mistral-7B-alpaca-case-0-2.Q5_K.gguf) | Q5_K | 4.78GB |
| [mistral-7B-alpaca-case-0-2.Q5_K_M.gguf](https://huggingface.co/RichardErkhov/jisukim8873_-_mistral-7B-alpaca-case-0-2-gguf/blob/main/mistral-7B-alpaca-case-0-2.Q5_K_M.gguf) | Q5_K_M | 4.78GB |
| [mistral-7B-alpaca-case-0-2.Q5_1.gguf](https://huggingface.co/RichardErkhov/jisukim8873_-_mistral-7B-alpaca-case-0-2-gguf/blob/main/mistral-7B-alpaca-case-0-2.Q5_1.gguf) | Q5_1 | 5.07GB |
| [mistral-7B-alpaca-case-0-2.Q6_K.gguf](https://huggingface.co/RichardErkhov/jisukim8873_-_mistral-7B-alpaca-case-0-2-gguf/blob/main/mistral-7B-alpaca-case-0-2.Q6_K.gguf) | Q6_K | 5.53GB |
| [mistral-7B-alpaca-case-0-2.Q8_0.gguf](https://huggingface.co/RichardErkhov/jisukim8873_-_mistral-7B-alpaca-case-0-2-gguf/blob/main/mistral-7B-alpaca-case-0-2.Q8_0.gguf) | Q8_0 | 7.17GB |
Original model description:
---
library_name: transformers
license: apache-2.0
---
# Model Card for Model ID
<!-- Provide a quick summary of what the model is/does. -->
## Model Details
### Model Description
<!-- Provide a longer summary of what this model is. -->
This is the model card of a 🤗 transformers model that has been pushed on the Hub. This model card has been automatically generated.
- **Developed by:** [More Information Needed]
- **Funded by [optional]:** [More Information Needed]
- **Shared by [optional]:** [More Information Needed]
- **Model type:** [More Information Needed]
- **Language(s) (NLP):** [More Information Needed]
- **License:** [More Information Needed]
- **Finetuned from model [optional]:** [More Information Needed]
### Model Sources [optional]
<!-- Provide the basic links for the model. -->
- **Repository:** [More Information Needed]
- **Paper [optional]:** [More Information Needed]
- **Demo [optional]:** [More Information Needed]
## Uses
<!-- Address questions around how the model is intended to be used, including the foreseeable users of the model and those affected by the model. -->
### Direct Use
<!-- This section is for the model use without fine-tuning or plugging into a larger ecosystem/app. -->
[More Information Needed]
### Downstream Use [optional]
<!-- This section is for the model use when fine-tuned for a task, or when plugged into a larger ecosystem/app -->
[More Information Needed]
### Out-of-Scope Use
<!-- This section addresses misuse, malicious use, and uses that the model will not work well for. -->
[More Information Needed]
## Bias, Risks, and Limitations
<!-- This section is meant to convey both technical and sociotechnical limitations. -->
[More Information Needed]
### Recommendations
<!-- This section is meant to convey recommendations with respect to the bias, risk, and technical limitations. -->
Users (both direct and downstream) should be made aware of the risks, biases and limitations of the model. More information needed for further recommendations.
## How to Get Started with the Model
Use the code below to get started with the model.
[More Information Needed]
## Training Details
### Training Data
<!-- This should link to a Dataset Card, perhaps with a short stub of information on what the training data is all about as well as documentation related to data pre-processing or additional filtering. -->
[More Information Needed]
### Training Procedure
<!-- This relates heavily to the Technical Specifications. Content here should link to that section when it is relevant to the training procedure. -->
#### Preprocessing [optional]
[More Information Needed]
#### Training Hyperparameters
- **Training regime:** [More Information Needed] <!--fp32, fp16 mixed precision, bf16 mixed precision, bf16 non-mixed precision, fp16 non-mixed precision, fp8 mixed precision -->
#### Speeds, Sizes, Times [optional]
<!-- This section provides information about throughput, start/end time, checkpoint size if relevant, etc. -->
[More Information Needed]
## Evaluation
<!-- This section describes the evaluation protocols and provides the results. -->
### Testing Data, Factors & Metrics
#### Testing Data
<!-- This should link to a Dataset Card if possible. -->
[More Information Needed]
#### Factors
<!-- These are the things the evaluation is disaggregating by, e.g., subpopulations or domains. -->
[More Information Needed]
#### Metrics
<!-- These are the evaluation metrics being used, ideally with a description of why. -->
[More Information Needed]
### Results
[More Information Needed]
#### Summary
## Model Examination [optional]
<!-- Relevant interpretability work for the model goes here -->
[More Information Needed]
## Environmental Impact
<!-- Total emissions (in grams of CO2eq) and additional considerations, such as electricity usage, go here. Edit the suggested text below accordingly -->
Carbon emissions can be estimated using the [Machine Learning Impact calculator](https://mlco2.github.io/impact#compute) presented in [Lacoste et al. (2019)](https://arxiv.org/abs/1910.09700).
- **Hardware Type:** [More Information Needed]
- **Hours used:** [More Information Needed]
- **Cloud Provider:** [More Information Needed]
- **Compute Region:** [More Information Needed]
- **Carbon Emitted:** [More Information Needed]
## Technical Specifications [optional]
### Model Architecture and Objective
[More Information Needed]
### Compute Infrastructure
[More Information Needed]
#### Hardware
[More Information Needed]
#### Software
[More Information Needed]
## Citation [optional]
<!-- If there is a paper or blog post introducing the model, the APA and Bibtex information for that should go in this section. -->
**BibTeX:**
[More Information Needed]
**APA:**
[More Information Needed]
## Glossary [optional]
<!-- If relevant, include terms and calculations in this section that can help readers understand the model or model card. -->
[More Information Needed]
## More Information [optional]
[More Information Needed]
## Model Card Authors [optional]
[More Information Needed]
## Model Card Contact
[More Information Needed]
|
mradermacher/Limon-8B-i1-GGUF | mradermacher | "2024-06-27T23:42:42Z" | 14,774 | 0 | transformers | [
"transformers",
"gguf",
"en",
"base_model:lodrick-the-lafted/Limon-8B",
"license:apache-2.0",
"endpoints_compatible",
"region:us"
] | null | "2024-06-27T15:34:07Z" | ---
base_model: lodrick-the-lafted/Limon-8B
language:
- en
library_name: transformers
license: apache-2.0
quantized_by: mradermacher
---
## About
<!-- ### quantize_version: 2 -->
<!-- ### output_tensor_quantised: 1 -->
<!-- ### convert_type: hf -->
<!-- ### vocab_type: -->
<!-- ### tags: nicoboss -->
weighted/imatrix quants of https://huggingface.co/lodrick-the-lafted/Limon-8B
<!-- provided-files -->
static quants are available at https://huggingface.co/mradermacher/Limon-8B-GGUF
## Usage
If you are unsure how to use GGUF files, refer to one of [TheBloke's
READMEs](https://huggingface.co/TheBloke/KafkaLM-70B-German-V0.1-GGUF) for
more details, including on how to concatenate multi-part files.
## Provided Quants
(sorted by size, not necessarily quality. IQ-quants are often preferable over similar sized non-IQ quants)
| Link | Type | Size/GB | Notes |
|:-----|:-----|--------:|:------|
| [GGUF](https://huggingface.co/mradermacher/Limon-8B-i1-GGUF/resolve/main/Limon-8B.i1-IQ1_S.gguf) | i1-IQ1_S | 2.1 | for the desperate |
| [GGUF](https://huggingface.co/mradermacher/Limon-8B-i1-GGUF/resolve/main/Limon-8B.i1-IQ1_M.gguf) | i1-IQ1_M | 2.3 | mostly desperate |
| [GGUF](https://huggingface.co/mradermacher/Limon-8B-i1-GGUF/resolve/main/Limon-8B.i1-IQ2_XXS.gguf) | i1-IQ2_XXS | 2.5 | |
| [GGUF](https://huggingface.co/mradermacher/Limon-8B-i1-GGUF/resolve/main/Limon-8B.i1-IQ2_XS.gguf) | i1-IQ2_XS | 2.7 | |
| [GGUF](https://huggingface.co/mradermacher/Limon-8B-i1-GGUF/resolve/main/Limon-8B.i1-IQ2_S.gguf) | i1-IQ2_S | 2.9 | |
| [GGUF](https://huggingface.co/mradermacher/Limon-8B-i1-GGUF/resolve/main/Limon-8B.i1-IQ2_M.gguf) | i1-IQ2_M | 3.0 | |
| [GGUF](https://huggingface.co/mradermacher/Limon-8B-i1-GGUF/resolve/main/Limon-8B.i1-Q2_K.gguf) | i1-Q2_K | 3.3 | IQ3_XXS probably better |
| [GGUF](https://huggingface.co/mradermacher/Limon-8B-i1-GGUF/resolve/main/Limon-8B.i1-IQ3_XXS.gguf) | i1-IQ3_XXS | 3.4 | lower quality |
| [GGUF](https://huggingface.co/mradermacher/Limon-8B-i1-GGUF/resolve/main/Limon-8B.i1-IQ3_XS.gguf) | i1-IQ3_XS | 3.6 | |
| [GGUF](https://huggingface.co/mradermacher/Limon-8B-i1-GGUF/resolve/main/Limon-8B.i1-Q3_K_S.gguf) | i1-Q3_K_S | 3.8 | IQ3_XS probably better |
| [GGUF](https://huggingface.co/mradermacher/Limon-8B-i1-GGUF/resolve/main/Limon-8B.i1-IQ3_S.gguf) | i1-IQ3_S | 3.8 | beats Q3_K* |
| [GGUF](https://huggingface.co/mradermacher/Limon-8B-i1-GGUF/resolve/main/Limon-8B.i1-IQ3_M.gguf) | i1-IQ3_M | 3.9 | |
| [GGUF](https://huggingface.co/mradermacher/Limon-8B-i1-GGUF/resolve/main/Limon-8B.i1-Q3_K_M.gguf) | i1-Q3_K_M | 4.1 | IQ3_S probably better |
| [GGUF](https://huggingface.co/mradermacher/Limon-8B-i1-GGUF/resolve/main/Limon-8B.i1-Q3_K_L.gguf) | i1-Q3_K_L | 4.4 | IQ3_M probably better |
| [GGUF](https://huggingface.co/mradermacher/Limon-8B-i1-GGUF/resolve/main/Limon-8B.i1-IQ4_XS.gguf) | i1-IQ4_XS | 4.5 | |
| [GGUF](https://huggingface.co/mradermacher/Limon-8B-i1-GGUF/resolve/main/Limon-8B.i1-Q4_0.gguf) | i1-Q4_0 | 4.8 | fast, low quality |
| [GGUF](https://huggingface.co/mradermacher/Limon-8B-i1-GGUF/resolve/main/Limon-8B.i1-Q4_K_S.gguf) | i1-Q4_K_S | 4.8 | optimal size/speed/quality |
| [GGUF](https://huggingface.co/mradermacher/Limon-8B-i1-GGUF/resolve/main/Limon-8B.i1-Q4_K_M.gguf) | i1-Q4_K_M | 5.0 | fast, recommended |
| [GGUF](https://huggingface.co/mradermacher/Limon-8B-i1-GGUF/resolve/main/Limon-8B.i1-Q5_K_S.gguf) | i1-Q5_K_S | 5.7 | |
| [GGUF](https://huggingface.co/mradermacher/Limon-8B-i1-GGUF/resolve/main/Limon-8B.i1-Q5_K_M.gguf) | i1-Q5_K_M | 5.8 | |
| [GGUF](https://huggingface.co/mradermacher/Limon-8B-i1-GGUF/resolve/main/Limon-8B.i1-Q6_K.gguf) | i1-Q6_K | 6.7 | practically like static Q6_K |
Here is a handy graph by ikawrakow comparing some lower-quality quant
types (lower is better):

And here are Artefact2's thoughts on the matter:
https://gist.github.com/Artefact2/b5f810600771265fc1e39442288e8ec9
## FAQ / Model Request
See https://huggingface.co/mradermacher/model_requests for some answers to
questions you might have and/or if you want some other model quantized.
## Thanks
I thank my company, [nethype GmbH](https://www.nethype.de/), for letting
me use its servers and providing upgrades to my workstation to enable
this work in my free time. Additional thanks to [@nicoboss](https://huggingface.co/nicoboss) for giving me access to his hardware for calculating the imatrix for these quants.
<!-- end -->
|
mradermacher/controllable-llama2-7b-GGUF | mradermacher | "2024-06-30T07:47:22Z" | 14,766 | 0 | transformers | [
"transformers",
"gguf",
"en",
"base_model:umd-zhou-lab/controllable-llama2-7b",
"license:llama2",
"endpoints_compatible",
"region:us"
] | null | "2024-06-30T07:02:39Z" | ---
base_model: umd-zhou-lab/controllable-llama2-7b
language:
- en
library_name: transformers
license: llama2
quantized_by: mradermacher
---
## About
<!-- ### quantize_version: 2 -->
<!-- ### output_tensor_quantised: 1 -->
<!-- ### convert_type: hf -->
<!-- ### vocab_type: -->
<!-- ### tags: -->
static quants of https://huggingface.co/umd-zhou-lab/controllable-llama2-7b
<!-- provided-files -->
weighted/imatrix quants seem not to be available (by me) at this time. If they do not show up a week or so after the static ones, I have probably not planned for them. Feel free to request them by opening a Community Discussion.
## Usage
If you are unsure how to use GGUF files, refer to one of [TheBloke's
READMEs](https://huggingface.co/TheBloke/KafkaLM-70B-German-V0.1-GGUF) for
more details, including on how to concatenate multi-part files.
## Provided Quants
(sorted by size, not necessarily quality. IQ-quants are often preferable over similar sized non-IQ quants)
| Link | Type | Size/GB | Notes |
|:-----|:-----|--------:|:------|
| [GGUF](https://huggingface.co/mradermacher/controllable-llama2-7b-GGUF/resolve/main/controllable-llama2-7b.Q2_K.gguf) | Q2_K | 2.6 | |
| [GGUF](https://huggingface.co/mradermacher/controllable-llama2-7b-GGUF/resolve/main/controllable-llama2-7b.IQ3_XS.gguf) | IQ3_XS | 2.9 | |
| [GGUF](https://huggingface.co/mradermacher/controllable-llama2-7b-GGUF/resolve/main/controllable-llama2-7b.IQ3_S.gguf) | IQ3_S | 3.0 | beats Q3_K* |
| [GGUF](https://huggingface.co/mradermacher/controllable-llama2-7b-GGUF/resolve/main/controllable-llama2-7b.Q3_K_S.gguf) | Q3_K_S | 3.0 | |
| [GGUF](https://huggingface.co/mradermacher/controllable-llama2-7b-GGUF/resolve/main/controllable-llama2-7b.IQ3_M.gguf) | IQ3_M | 3.2 | |
| [GGUF](https://huggingface.co/mradermacher/controllable-llama2-7b-GGUF/resolve/main/controllable-llama2-7b.Q3_K_M.gguf) | Q3_K_M | 3.4 | lower quality |
| [GGUF](https://huggingface.co/mradermacher/controllable-llama2-7b-GGUF/resolve/main/controllable-llama2-7b.Q3_K_L.gguf) | Q3_K_L | 3.7 | |
| [GGUF](https://huggingface.co/mradermacher/controllable-llama2-7b-GGUF/resolve/main/controllable-llama2-7b.IQ4_XS.gguf) | IQ4_XS | 3.7 | |
| [GGUF](https://huggingface.co/mradermacher/controllable-llama2-7b-GGUF/resolve/main/controllable-llama2-7b.Q4_K_S.gguf) | Q4_K_S | 4.0 | fast, recommended |
| [GGUF](https://huggingface.co/mradermacher/controllable-llama2-7b-GGUF/resolve/main/controllable-llama2-7b.Q4_K_M.gguf) | Q4_K_M | 4.2 | fast, recommended |
| [GGUF](https://huggingface.co/mradermacher/controllable-llama2-7b-GGUF/resolve/main/controllable-llama2-7b.Q5_K_S.gguf) | Q5_K_S | 4.8 | |
| [GGUF](https://huggingface.co/mradermacher/controllable-llama2-7b-GGUF/resolve/main/controllable-llama2-7b.Q5_K_M.gguf) | Q5_K_M | 4.9 | |
| [GGUF](https://huggingface.co/mradermacher/controllable-llama2-7b-GGUF/resolve/main/controllable-llama2-7b.Q6_K.gguf) | Q6_K | 5.6 | very good quality |
| [GGUF](https://huggingface.co/mradermacher/controllable-llama2-7b-GGUF/resolve/main/controllable-llama2-7b.Q8_0.gguf) | Q8_0 | 7.3 | fast, best quality |
| [GGUF](https://huggingface.co/mradermacher/controllable-llama2-7b-GGUF/resolve/main/controllable-llama2-7b.f16.gguf) | f16 | 13.6 | 16 bpw, overkill |
Here is a handy graph by ikawrakow comparing some lower-quality quant
types (lower is better):

And here are Artefact2's thoughts on the matter:
https://gist.github.com/Artefact2/b5f810600771265fc1e39442288e8ec9
## FAQ / Model Request
See https://huggingface.co/mradermacher/model_requests for some answers to
questions you might have and/or if you want some other model quantized.
## Thanks
I thank my company, [nethype GmbH](https://www.nethype.de/), for letting
me use its servers and providing upgrades to my workstation to enable
this work in my free time.
<!-- end -->
|
RichardErkhov/proto-llm_-_uniwiz-7B-v0.1-gguf | RichardErkhov | "2024-06-26T09:20:22Z" | 14,761 | 0 | null | [
"gguf",
"region:us"
] | null | "2024-06-26T04:28:44Z" | Quantization made by Richard Erkhov.
[Github](https://github.com/RichardErkhov)
[Discord](https://discord.gg/pvy7H8DZMG)
[Request more models](https://github.com/RichardErkhov/quant_request)
uniwiz-7B-v0.1 - GGUF
- Model creator: https://huggingface.co/proto-llm/
- Original model: https://huggingface.co/proto-llm/uniwiz-7B-v0.1/
| Name | Quant method | Size |
| ---- | ---- | ---- |
| [uniwiz-7B-v0.1.Q2_K.gguf](https://huggingface.co/RichardErkhov/proto-llm_-_uniwiz-7B-v0.1-gguf/blob/main/uniwiz-7B-v0.1.Q2_K.gguf) | Q2_K | 2.53GB |
| [uniwiz-7B-v0.1.IQ3_XS.gguf](https://huggingface.co/RichardErkhov/proto-llm_-_uniwiz-7B-v0.1-gguf/blob/main/uniwiz-7B-v0.1.IQ3_XS.gguf) | IQ3_XS | 2.81GB |
| [uniwiz-7B-v0.1.IQ3_S.gguf](https://huggingface.co/RichardErkhov/proto-llm_-_uniwiz-7B-v0.1-gguf/blob/main/uniwiz-7B-v0.1.IQ3_S.gguf) | IQ3_S | 2.96GB |
| [uniwiz-7B-v0.1.Q3_K_S.gguf](https://huggingface.co/RichardErkhov/proto-llm_-_uniwiz-7B-v0.1-gguf/blob/main/uniwiz-7B-v0.1.Q3_K_S.gguf) | Q3_K_S | 2.95GB |
| [uniwiz-7B-v0.1.IQ3_M.gguf](https://huggingface.co/RichardErkhov/proto-llm_-_uniwiz-7B-v0.1-gguf/blob/main/uniwiz-7B-v0.1.IQ3_M.gguf) | IQ3_M | 3.06GB |
| [uniwiz-7B-v0.1.Q3_K.gguf](https://huggingface.co/RichardErkhov/proto-llm_-_uniwiz-7B-v0.1-gguf/blob/main/uniwiz-7B-v0.1.Q3_K.gguf) | Q3_K | 3.28GB |
| [uniwiz-7B-v0.1.Q3_K_M.gguf](https://huggingface.co/RichardErkhov/proto-llm_-_uniwiz-7B-v0.1-gguf/blob/main/uniwiz-7B-v0.1.Q3_K_M.gguf) | Q3_K_M | 3.28GB |
| [uniwiz-7B-v0.1.Q3_K_L.gguf](https://huggingface.co/RichardErkhov/proto-llm_-_uniwiz-7B-v0.1-gguf/blob/main/uniwiz-7B-v0.1.Q3_K_L.gguf) | Q3_K_L | 3.56GB |
| [uniwiz-7B-v0.1.IQ4_XS.gguf](https://huggingface.co/RichardErkhov/proto-llm_-_uniwiz-7B-v0.1-gguf/blob/main/uniwiz-7B-v0.1.IQ4_XS.gguf) | IQ4_XS | 3.67GB |
| [uniwiz-7B-v0.1.Q4_0.gguf](https://huggingface.co/RichardErkhov/proto-llm_-_uniwiz-7B-v0.1-gguf/blob/main/uniwiz-7B-v0.1.Q4_0.gguf) | Q4_0 | 3.83GB |
| [uniwiz-7B-v0.1.IQ4_NL.gguf](https://huggingface.co/RichardErkhov/proto-llm_-_uniwiz-7B-v0.1-gguf/blob/main/uniwiz-7B-v0.1.IQ4_NL.gguf) | IQ4_NL | 3.87GB |
| [uniwiz-7B-v0.1.Q4_K_S.gguf](https://huggingface.co/RichardErkhov/proto-llm_-_uniwiz-7B-v0.1-gguf/blob/main/uniwiz-7B-v0.1.Q4_K_S.gguf) | Q4_K_S | 3.86GB |
| [uniwiz-7B-v0.1.Q4_K.gguf](https://huggingface.co/RichardErkhov/proto-llm_-_uniwiz-7B-v0.1-gguf/blob/main/uniwiz-7B-v0.1.Q4_K.gguf) | Q4_K | 4.07GB |
| [uniwiz-7B-v0.1.Q4_K_M.gguf](https://huggingface.co/RichardErkhov/proto-llm_-_uniwiz-7B-v0.1-gguf/blob/main/uniwiz-7B-v0.1.Q4_K_M.gguf) | Q4_K_M | 4.07GB |
| [uniwiz-7B-v0.1.Q4_1.gguf](https://huggingface.co/RichardErkhov/proto-llm_-_uniwiz-7B-v0.1-gguf/blob/main/uniwiz-7B-v0.1.Q4_1.gguf) | Q4_1 | 4.24GB |
| [uniwiz-7B-v0.1.Q5_0.gguf](https://huggingface.co/RichardErkhov/proto-llm_-_uniwiz-7B-v0.1-gguf/blob/main/uniwiz-7B-v0.1.Q5_0.gguf) | Q5_0 | 4.65GB |
| [uniwiz-7B-v0.1.Q5_K_S.gguf](https://huggingface.co/RichardErkhov/proto-llm_-_uniwiz-7B-v0.1-gguf/blob/main/uniwiz-7B-v0.1.Q5_K_S.gguf) | Q5_K_S | 4.65GB |
| [uniwiz-7B-v0.1.Q5_K.gguf](https://huggingface.co/RichardErkhov/proto-llm_-_uniwiz-7B-v0.1-gguf/blob/main/uniwiz-7B-v0.1.Q5_K.gguf) | Q5_K | 4.78GB |
| [uniwiz-7B-v0.1.Q5_K_M.gguf](https://huggingface.co/RichardErkhov/proto-llm_-_uniwiz-7B-v0.1-gguf/blob/main/uniwiz-7B-v0.1.Q5_K_M.gguf) | Q5_K_M | 4.78GB |
| [uniwiz-7B-v0.1.Q5_1.gguf](https://huggingface.co/RichardErkhov/proto-llm_-_uniwiz-7B-v0.1-gguf/blob/main/uniwiz-7B-v0.1.Q5_1.gguf) | Q5_1 | 5.07GB |
| [uniwiz-7B-v0.1.Q6_K.gguf](https://huggingface.co/RichardErkhov/proto-llm_-_uniwiz-7B-v0.1-gguf/blob/main/uniwiz-7B-v0.1.Q6_K.gguf) | Q6_K | 5.53GB |
| [uniwiz-7B-v0.1.Q8_0.gguf](https://huggingface.co/RichardErkhov/proto-llm_-_uniwiz-7B-v0.1-gguf/blob/main/uniwiz-7B-v0.1.Q8_0.gguf) | Q8_0 | 7.17GB |
Original model description:
---
license: apache-2.0
---
## **Model Overview:**
- **Model Name:** UniWiZ-7B-v0.1
- **Architecture:** Mistral-7B
- **Training Objective:** Knowledge and Safety Orchestration
- **Training Dataset:** Curated dataset encompassing diverse knowledge domains and safety-focused content
- **Training Duration:** [Specify training duration]
## **Intended Use:**
UniWiZ-7B-v0.1 is designed for various natural language understanding tasks, including but not limited to text generation, summarization, question-answering, and conversation. Its training data emphasizes a broad spectrum of knowledge domains while incorporating safety considerations to ensure responsible and ethical use.
## **Scope of Applications:**
UniWiZ-7B-v0.1 can be employed across a wide range of applications such as:
1. **Content Generation:** Creating human-like text for articles, blogs, creative writing, etc.
2. **Summarization:** Condensing lengthy texts into concise summaries while preserving key information.
3. **Question-Answering:** Responding to user queries by extracting relevant information from its extensive knowledge base.
4. **Conversational Agents:** Engaging in natural and contextually relevant conversations with users.
5. **Educational Assistance:** Providing explanations, definitions, and insights on various topics.
## **Data and Training:**
UniWiZ-7B-v0.1 was trained on a diverse dataset encompassing knowledge from different domains. The training process included safety orchestration to mitigate biases and ensure ethical AI behavior. The model's architecture, Mistral-7B, enables it to understand and generate coherent and contextually relevant text.
## **Performance and Limitations:**
While UniWiZ-7B-v0.1 demonstrates strong performance across a variety of tasks, it may exhibit limitations in:
1. **Handling Uncommon or Specialized Topics:** The model's knowledge is extensive but may not cover extremely niche or specialized subjects.
2. **Sensitive Content:** Despite safety measures, there is a possibility of generating content that may be considered inappropriate or offensive.
Users are encouraged to exercise discretion and provide feedback to improve the model's performance and address any potential biases or shortcomings.
## **Ethical Considerations:**
UniWiZ-7B-v0.1 is developed with ethical AI principles in mind. Proto-AI is committed to addressing concerns related to bias, fairness, and the responsible use of AI technology. Users are encouraged to report unintended behavior or bias for continuous improvement.
## **Future Updates:**
Proto-AI is dedicated to refining and enhancing UniWiZ-7B-v0.1. Regular updates will be released to improve performance, address user feedback, and incorporate the latest advancements in AI research.
This model card is a reference for users to understand UniWiZ-7B-v0.1's capabilities, limitations, and ethical considerations. Proto-AI values transparency and accountability in the deployment and use of AI models. More details about the model and training will be released later.
|
muchad/t5-qa-qg-lite | muchad | "2024-06-10T13:06:21Z" | 14,746 | 0 | transformers | [
"transformers",
"tensorboard",
"safetensors",
"t5",
"text2text-generation",
"license:apache-2.0",
"autotrain_compatible",
"endpoints_compatible",
"text-generation-inference",
"region:us"
] | text2text-generation | "2024-06-10T13:02:55Z" | ---
license: apache-2.0
---
|
Abdullah-Habib/sdxl-nsfw | Abdullah-Habib | "2024-04-06T04:04:15Z" | 14,743 | 3 | diffusers | [
"diffusers",
"safetensors",
"modelslab.com",
"stable-diffusion-api",
"text-to-image",
"ultra-realistic",
"license:creativeml-openrail-m",
"autotrain_compatible",
"endpoints_compatible",
"diffusers:StableDiffusionXLPipeline",
"region:us"
] | text-to-image | "2024-04-06T02:15:10Z" | ---
license: creativeml-openrail-m
tags:
- modelslab.com
- stable-diffusion-api
- text-to-image
- ultra-realistic
pinned: true
---
# DucHaiten-Real3D-NSFW-XL v1.0 API Inference
cloned from https://huggingface.co/stablediffusionapi/duchaiten-real3d-nsfw-xl |
mradermacher/Llama-Guard-2-8B-de-1.5-GGUF | mradermacher | "2024-06-19T17:15:53Z" | 14,726 | 0 | transformers | [
"transformers",
"gguf",
"en",
"base_model:felfri/Llama-Guard-2-8B-de-1.5",
"endpoints_compatible",
"region:us"
] | null | "2024-06-19T14:46:01Z" | ---
base_model: felfri/Llama-Guard-2-8B-de-1.5
language:
- en
library_name: transformers
quantized_by: mradermacher
tags: []
---
## About
<!-- ### quantize_version: 2 -->
<!-- ### output_tensor_quantised: 1 -->
<!-- ### convert_type: hf -->
<!-- ### vocab_type: -->
<!-- ### tags: -->
static quants of https://huggingface.co/felfri/Llama-Guard-2-8B-de-1.5
<!-- provided-files -->
weighted/imatrix quants seem not to be available (by me) at this time. If they do not show up a week or so after the static ones, I have probably not planned for them. Feel free to request them by opening a Community Discussion.
## Usage
If you are unsure how to use GGUF files, refer to one of [TheBloke's
READMEs](https://huggingface.co/TheBloke/KafkaLM-70B-German-V0.1-GGUF) for
more details, including on how to concatenate multi-part files.
## Provided Quants
(sorted by size, not necessarily quality. IQ-quants are often preferable over similar sized non-IQ quants)
| Link | Type | Size/GB | Notes |
|:-----|:-----|--------:|:------|
| [GGUF](https://huggingface.co/mradermacher/Llama-Guard-2-8B-de-1.5-GGUF/resolve/main/Llama-Guard-2-8B-de-1.5.Q2_K.gguf) | Q2_K | 3.3 | |
| [GGUF](https://huggingface.co/mradermacher/Llama-Guard-2-8B-de-1.5-GGUF/resolve/main/Llama-Guard-2-8B-de-1.5.IQ3_XS.gguf) | IQ3_XS | 3.6 | |
| [GGUF](https://huggingface.co/mradermacher/Llama-Guard-2-8B-de-1.5-GGUF/resolve/main/Llama-Guard-2-8B-de-1.5.Q3_K_S.gguf) | Q3_K_S | 3.8 | |
| [GGUF](https://huggingface.co/mradermacher/Llama-Guard-2-8B-de-1.5-GGUF/resolve/main/Llama-Guard-2-8B-de-1.5.IQ3_S.gguf) | IQ3_S | 3.8 | beats Q3_K* |
| [GGUF](https://huggingface.co/mradermacher/Llama-Guard-2-8B-de-1.5-GGUF/resolve/main/Llama-Guard-2-8B-de-1.5.IQ3_M.gguf) | IQ3_M | 3.9 | |
| [GGUF](https://huggingface.co/mradermacher/Llama-Guard-2-8B-de-1.5-GGUF/resolve/main/Llama-Guard-2-8B-de-1.5.Q3_K_M.gguf) | Q3_K_M | 4.1 | lower quality |
| [GGUF](https://huggingface.co/mradermacher/Llama-Guard-2-8B-de-1.5-GGUF/resolve/main/Llama-Guard-2-8B-de-1.5.Q3_K_L.gguf) | Q3_K_L | 4.4 | |
| [GGUF](https://huggingface.co/mradermacher/Llama-Guard-2-8B-de-1.5-GGUF/resolve/main/Llama-Guard-2-8B-de-1.5.IQ4_XS.gguf) | IQ4_XS | 4.6 | |
| [GGUF](https://huggingface.co/mradermacher/Llama-Guard-2-8B-de-1.5-GGUF/resolve/main/Llama-Guard-2-8B-de-1.5.Q4_K_S.gguf) | Q4_K_S | 4.8 | fast, recommended |
| [GGUF](https://huggingface.co/mradermacher/Llama-Guard-2-8B-de-1.5-GGUF/resolve/main/Llama-Guard-2-8B-de-1.5.Q4_K_M.gguf) | Q4_K_M | 5.0 | fast, recommended |
| [GGUF](https://huggingface.co/mradermacher/Llama-Guard-2-8B-de-1.5-GGUF/resolve/main/Llama-Guard-2-8B-de-1.5.Q5_K_S.gguf) | Q5_K_S | 5.7 | |
| [GGUF](https://huggingface.co/mradermacher/Llama-Guard-2-8B-de-1.5-GGUF/resolve/main/Llama-Guard-2-8B-de-1.5.Q5_K_M.gguf) | Q5_K_M | 5.8 | |
| [GGUF](https://huggingface.co/mradermacher/Llama-Guard-2-8B-de-1.5-GGUF/resolve/main/Llama-Guard-2-8B-de-1.5.Q6_K.gguf) | Q6_K | 6.7 | very good quality |
| [GGUF](https://huggingface.co/mradermacher/Llama-Guard-2-8B-de-1.5-GGUF/resolve/main/Llama-Guard-2-8B-de-1.5.Q8_0.gguf) | Q8_0 | 8.6 | fast, best quality |
| [GGUF](https://huggingface.co/mradermacher/Llama-Guard-2-8B-de-1.5-GGUF/resolve/main/Llama-Guard-2-8B-de-1.5.f16.gguf) | f16 | 16.2 | 16 bpw, overkill |
Here is a handy graph by ikawrakow comparing some lower-quality quant
types (lower is better):

And here are Artefact2's thoughts on the matter:
https://gist.github.com/Artefact2/b5f810600771265fc1e39442288e8ec9
## FAQ / Model Request
See https://huggingface.co/mradermacher/model_requests for some answers to
questions you might have and/or if you want some other model quantized.
## Thanks
I thank my company, [nethype GmbH](https://www.nethype.de/), for letting
me use its servers and providing upgrades to my workstation to enable
this work in my free time.
<!-- end -->
|
ybelkada/blip2-opt-2.7b-fp16-sharded | ybelkada | "2023-04-12T09:19:46Z" | 14,724 | 2 | transformers | [
"transformers",
"pytorch",
"blip-2",
"visual-question-answering",
"endpoints_compatible",
"region:us"
] | visual-question-answering | "2023-04-12T09:16:26Z" | Entry not found |
KoboldAI/LLaMA2-13B-Psyfighter2-GGUF | KoboldAI | "2023-11-15T19:10:42Z" | 14,715 | 63 | null | [
"gguf",
"license:llama2",
"region:us"
] | null | "2023-11-14T13:55:13Z" | ---
license: llama2
---
# LLAMA2-13B-Psyfighter2
Psyfighter is a merged model created by the KoboldAI community members Jeb Carter and TwistedShadows and was made possible thanks to the KoboldAI merge request service.
The intent was to add medical data to supplement the models fictional ability with more details on anatomy and mental states. Due to the low ratio's of medical data and the high ratio's of fiction this model should not be used for medical advice or therapy because of its high chance of pulling in fictional data.
The following mergekit recipe was used:
```
merge_method: task_arithmetic
base_model: TheBloke/Llama-2-13B-fp16
models:
- model: TheBloke/Llama-2-13B-fp16
- model: KoboldAI/LLaMA2-13B-Tiefighter
parameters:
weight: 1.0
- model: Doctor-Shotgun/cat-v1.0-13b
parameters:
weight: 0.01
- model: Doctor-Shotgun/llama-2-13b-chat-limarp-v2-merged
parameters:
weight: 0.02
dtype: float16
```
*V1 of this model was published under the account of the creator of the merge
This model contains the following ingredients from their upstream models for as far as we can track them:
- KoboldAI/LLaMA2-13B-Tiefighter
- Undi95/Xwin-MLewd-13B-V0.2
- - Undi95/ReMM-S-Light
- Undi95/CreativeEngine
- Brouz/Slerpeno
- - elinas/chronos-13b-v2
- jondurbin/airoboros-l2-13b-2.1
- NousResearch/Nous-Hermes-Llama2-13b+nRuaif/Kimiko-v2
- CalderaAI/13B-Legerdemain-L2+lemonilia/limarp-llama2-v2
- - KoboldAI/LLAMA2-13B-Holodeck-1
- NousResearch/Nous-Hermes-13b
- OpenAssistant/llama2-13b-orca-8k-3319
- ehartford/WizardLM-1.0-Uncensored-Llama2-13b
- Henk717/spring-dragon
- The-Face-Of-Goonery/Huginn-v3-13b (Contains undisclosed model versions, those we assumed where possible)
- - SuperCOT (Undisclosed version)
- elinas/chronos-13b-v2 (Version assumed)
- NousResearch/Nous-Hermes-Llama2-13b
- stabilityai/StableBeluga-13B (Version assumed)
- zattio770/120-Days-of-LORA-v2-13B
- PygmalionAI/pygmalion-2-13b
- Undi95/Storytelling-v1-13B-lora
- TokenBender/sakhi_13B_roleplayer_NSFW_chat_adapter
- nRuaif/Kimiko-v2-13B
- The-Face-Of-Goonery/Huginn-13b-FP16
- - "a lot of different models, like hermes, beluga, airoboros, chronos.. limarp"
- lemonilia/LimaRP-Llama2-13B-v3-EXPERIMENT
- Xwin-LM/Xwin-LM-13B-V0.2
- PocketDoc/Dans-RetroRodeo-13b
- Blackroot/Llama-2-13B-Storywriter-LORA
- Doctor-Shotgun/cat-v1.0-13b
- Doctor-Shotgun/llama-2-13b-chat-limarp-v2-merged
- meta-llama/Llama-2-13b-chat-hf
- lemonilia/limarp-llama2-v2
While we could possibly not credit every single lora or model involved in this merged model, we'd like to thank all involved creators upstream for making this awesome model possible!
Thanks to you the AI ecosystem is thriving, and without your dedicated tuning efforts models such as this one would not be possible.
# Usage
This model is meant to be creative, If you let it improvise you get better results than if you drown it in details.
## Story Writing
Regular story writing in the traditional way is supported, simply copy paste your story and continue writing. Optionally use an instruction in memory or an authors note to guide the direction of your story.
### Generate a story on demand
To generate stories on demand you can use an instruction (tested in the Alpaca format) such as "Write a novel about X, use chapters and dialogue" this will generate a story. The format can vary between generations depending on how the model chooses to begin, either write what you want as shown in the earlier example or write the beginning of the story yourself so the model can follow your style. A few retries can also help if the model gets it wrong.
## Chatbots and persona's
This model has been tested with various forms of chatting, testers have found that typically less is more and the model is good at improvising. Don't drown the model in paragraphs of detailed information, instead keep it simple first and see how far you can lean on the models own ability to figure out your character. Copy pasting paragraphs of background information is not suitable for a 13B model such as this one, code formatted characters or an instruction prompt describing who you wish to talk to goes much further.
For example, you can put this in memory in regular chat mode:
```
### Instruction:
Generate a conversation between Alice and Jeb where they discuss language models.
In this conversation Henk is excited to teach Alice about Psyfighter.
### Response:
```
Because the model is a merge of a variety of models, it should support a broad range of instruct formats, or plain chat mode. If you have a particular favourite try it, otherwise we recommend to either use the regular chat mode or Alpaca's format.
## Instruct Prompting
This model features various instruct models on a variety of instruction styles, when testing the model we have used Alpaca for our own tests. If you prefer a different format chances are it can work.
During instructions we have observed that in some cases the adventure data can leak, it may also be worth experimenting using > as the prefix for a user command to remedy this. But this may result in a stronger fiction bias.
Keep in mind that while this model can be used as a factual instruct model, the focus was on fiction. Information provided by the model can be made up.
## Adventuring and Adventure Games
This model contains a lora that was trained on the same adventure dataset as the KoboldAI Skein model. Adventuring is best done using an small introduction to the world and your objective while using the > prefix for a user command (KoboldAI's adventure mode).
It is possible that the model does not immediately pick up on what you wish to do and does not engage in its Adventure mode behaviour right away. Simply manually correct the output to trim excess dialogue or other undesirable behaviour and continue to submit your actions using the appropriate mode. The model should pick up on this style quickly and will correctly follow this format within 3 turns.
## Discovered something cool and want to engage with us?
Join our community at https://koboldai.org/discord !
We can also provide assistance in making your own merges. |
echarlaix/tiny-mpt-random-remote-code | echarlaix | "2024-03-25T10:55:43Z" | 14,710 | 0 | transformers | [
"transformers",
"pytorch",
"mpt",
"text-generation",
"custom_code",
"license:apache-2.0",
"autotrain_compatible",
"text-generation-inference",
"region:us"
] | text-generation | "2024-03-25T10:38:54Z" | ---
license: apache-2.0
---
|
mradermacher/Llama-3-web-8B-Instruct-GGUF | mradermacher | "2024-07-02T05:27:09Z" | 14,698 | 0 | transformers | [
"transformers",
"gguf",
"en",
"base_model:Laim/Llama-3-web-8B-Instruct",
"endpoints_compatible",
"region:us"
] | null | "2024-07-02T03:12:02Z" | ---
base_model: Laim/Llama-3-web-8B-Instruct
language:
- en
library_name: transformers
quantized_by: mradermacher
tags: []
---
## About
<!-- ### quantize_version: 2 -->
<!-- ### output_tensor_quantised: 1 -->
<!-- ### convert_type: hf -->
<!-- ### vocab_type: -->
<!-- ### tags: -->
static quants of https://huggingface.co/Laim/Llama-3-web-8B-Instruct
<!-- provided-files -->
weighted/imatrix quants seem not to be available (by me) at this time. If they do not show up a week or so after the static ones, I have probably not planned for them. Feel free to request them by opening a Community Discussion.
## Usage
If you are unsure how to use GGUF files, refer to one of [TheBloke's
READMEs](https://huggingface.co/TheBloke/KafkaLM-70B-German-V0.1-GGUF) for
more details, including on how to concatenate multi-part files.
## Provided Quants
(sorted by size, not necessarily quality. IQ-quants are often preferable over similar sized non-IQ quants)
| Link | Type | Size/GB | Notes |
|:-----|:-----|--------:|:------|
| [GGUF](https://huggingface.co/mradermacher/Llama-3-web-8B-Instruct-GGUF/resolve/main/Llama-3-web-8B-Instruct.Q2_K.gguf) | Q2_K | 3.3 | |
| [GGUF](https://huggingface.co/mradermacher/Llama-3-web-8B-Instruct-GGUF/resolve/main/Llama-3-web-8B-Instruct.IQ3_XS.gguf) | IQ3_XS | 3.6 | |
| [GGUF](https://huggingface.co/mradermacher/Llama-3-web-8B-Instruct-GGUF/resolve/main/Llama-3-web-8B-Instruct.Q3_K_S.gguf) | Q3_K_S | 3.8 | |
| [GGUF](https://huggingface.co/mradermacher/Llama-3-web-8B-Instruct-GGUF/resolve/main/Llama-3-web-8B-Instruct.IQ3_S.gguf) | IQ3_S | 3.8 | beats Q3_K* |
| [GGUF](https://huggingface.co/mradermacher/Llama-3-web-8B-Instruct-GGUF/resolve/main/Llama-3-web-8B-Instruct.IQ3_M.gguf) | IQ3_M | 3.9 | |
| [GGUF](https://huggingface.co/mradermacher/Llama-3-web-8B-Instruct-GGUF/resolve/main/Llama-3-web-8B-Instruct.Q3_K_M.gguf) | Q3_K_M | 4.1 | lower quality |
| [GGUF](https://huggingface.co/mradermacher/Llama-3-web-8B-Instruct-GGUF/resolve/main/Llama-3-web-8B-Instruct.Q3_K_L.gguf) | Q3_K_L | 4.4 | |
| [GGUF](https://huggingface.co/mradermacher/Llama-3-web-8B-Instruct-GGUF/resolve/main/Llama-3-web-8B-Instruct.IQ4_XS.gguf) | IQ4_XS | 4.6 | |
| [GGUF](https://huggingface.co/mradermacher/Llama-3-web-8B-Instruct-GGUF/resolve/main/Llama-3-web-8B-Instruct.Q4_K_S.gguf) | Q4_K_S | 4.8 | fast, recommended |
| [GGUF](https://huggingface.co/mradermacher/Llama-3-web-8B-Instruct-GGUF/resolve/main/Llama-3-web-8B-Instruct.Q4_K_M.gguf) | Q4_K_M | 5.0 | fast, recommended |
| [GGUF](https://huggingface.co/mradermacher/Llama-3-web-8B-Instruct-GGUF/resolve/main/Llama-3-web-8B-Instruct.Q5_K_S.gguf) | Q5_K_S | 5.7 | |
| [GGUF](https://huggingface.co/mradermacher/Llama-3-web-8B-Instruct-GGUF/resolve/main/Llama-3-web-8B-Instruct.Q5_K_M.gguf) | Q5_K_M | 5.8 | |
| [GGUF](https://huggingface.co/mradermacher/Llama-3-web-8B-Instruct-GGUF/resolve/main/Llama-3-web-8B-Instruct.Q6_K.gguf) | Q6_K | 6.7 | very good quality |
| [GGUF](https://huggingface.co/mradermacher/Llama-3-web-8B-Instruct-GGUF/resolve/main/Llama-3-web-8B-Instruct.Q8_0.gguf) | Q8_0 | 8.6 | fast, best quality |
| [GGUF](https://huggingface.co/mradermacher/Llama-3-web-8B-Instruct-GGUF/resolve/main/Llama-3-web-8B-Instruct.f16.gguf) | f16 | 16.2 | 16 bpw, overkill |
Here is a handy graph by ikawrakow comparing some lower-quality quant
types (lower is better):

And here are Artefact2's thoughts on the matter:
https://gist.github.com/Artefact2/b5f810600771265fc1e39442288e8ec9
## FAQ / Model Request
See https://huggingface.co/mradermacher/model_requests for some answers to
questions you might have and/or if you want some other model quantized.
## Thanks
I thank my company, [nethype GmbH](https://www.nethype.de/), for letting
me use its servers and providing upgrades to my workstation to enable
this work in my free time.
<!-- end -->
|
mradermacher/L3-8B-Chara-v1-Alpha-i1-GGUF | mradermacher | "2024-07-01T14:55:45Z" | 14,686 | 0 | transformers | [
"transformers",
"gguf",
"en",
"base_model:Sao10K/L3-8B-Chara-v1-Alpha",
"license:cc-by-nc-4.0",
"endpoints_compatible",
"region:us"
] | null | "2024-07-01T10:13:08Z" | ---
base_model: Sao10K/L3-8B-Chara-v1-Alpha
language:
- en
library_name: transformers
license: cc-by-nc-4.0
quantized_by: mradermacher
---
## About
<!-- ### quantize_version: 2 -->
<!-- ### output_tensor_quantised: 1 -->
<!-- ### convert_type: hf -->
<!-- ### vocab_type: -->
<!-- ### tags: nicoboss -->
weighted/imatrix quants of https://huggingface.co/Sao10K/L3-8B-Chara-v1-Alpha
<!-- provided-files -->
static quants are available at https://huggingface.co/mradermacher/L3-8B-Chara-v1-Alpha-GGUF
## Usage
If you are unsure how to use GGUF files, refer to one of [TheBloke's
READMEs](https://huggingface.co/TheBloke/KafkaLM-70B-German-V0.1-GGUF) for
more details, including on how to concatenate multi-part files.
## Provided Quants
(sorted by size, not necessarily quality. IQ-quants are often preferable over similar sized non-IQ quants)
| Link | Type | Size/GB | Notes |
|:-----|:-----|--------:|:------|
| [GGUF](https://huggingface.co/mradermacher/L3-8B-Chara-v1-Alpha-i1-GGUF/resolve/main/L3-8B-Chara-v1-Alpha.i1-IQ1_S.gguf) | i1-IQ1_S | 2.1 | for the desperate |
| [GGUF](https://huggingface.co/mradermacher/L3-8B-Chara-v1-Alpha-i1-GGUF/resolve/main/L3-8B-Chara-v1-Alpha.i1-IQ1_M.gguf) | i1-IQ1_M | 2.3 | mostly desperate |
| [GGUF](https://huggingface.co/mradermacher/L3-8B-Chara-v1-Alpha-i1-GGUF/resolve/main/L3-8B-Chara-v1-Alpha.i1-IQ2_XXS.gguf) | i1-IQ2_XXS | 2.5 | |
| [GGUF](https://huggingface.co/mradermacher/L3-8B-Chara-v1-Alpha-i1-GGUF/resolve/main/L3-8B-Chara-v1-Alpha.i1-IQ2_XS.gguf) | i1-IQ2_XS | 2.7 | |
| [GGUF](https://huggingface.co/mradermacher/L3-8B-Chara-v1-Alpha-i1-GGUF/resolve/main/L3-8B-Chara-v1-Alpha.i1-IQ2_S.gguf) | i1-IQ2_S | 2.9 | |
| [GGUF](https://huggingface.co/mradermacher/L3-8B-Chara-v1-Alpha-i1-GGUF/resolve/main/L3-8B-Chara-v1-Alpha.i1-IQ2_M.gguf) | i1-IQ2_M | 3.0 | |
| [GGUF](https://huggingface.co/mradermacher/L3-8B-Chara-v1-Alpha-i1-GGUF/resolve/main/L3-8B-Chara-v1-Alpha.i1-Q2_K.gguf) | i1-Q2_K | 3.3 | IQ3_XXS probably better |
| [GGUF](https://huggingface.co/mradermacher/L3-8B-Chara-v1-Alpha-i1-GGUF/resolve/main/L3-8B-Chara-v1-Alpha.i1-IQ3_XXS.gguf) | i1-IQ3_XXS | 3.4 | lower quality |
| [GGUF](https://huggingface.co/mradermacher/L3-8B-Chara-v1-Alpha-i1-GGUF/resolve/main/L3-8B-Chara-v1-Alpha.i1-IQ3_XS.gguf) | i1-IQ3_XS | 3.6 | |
| [GGUF](https://huggingface.co/mradermacher/L3-8B-Chara-v1-Alpha-i1-GGUF/resolve/main/L3-8B-Chara-v1-Alpha.i1-Q3_K_S.gguf) | i1-Q3_K_S | 3.8 | IQ3_XS probably better |
| [GGUF](https://huggingface.co/mradermacher/L3-8B-Chara-v1-Alpha-i1-GGUF/resolve/main/L3-8B-Chara-v1-Alpha.i1-IQ3_S.gguf) | i1-IQ3_S | 3.8 | beats Q3_K* |
| [GGUF](https://huggingface.co/mradermacher/L3-8B-Chara-v1-Alpha-i1-GGUF/resolve/main/L3-8B-Chara-v1-Alpha.i1-IQ3_M.gguf) | i1-IQ3_M | 3.9 | |
| [GGUF](https://huggingface.co/mradermacher/L3-8B-Chara-v1-Alpha-i1-GGUF/resolve/main/L3-8B-Chara-v1-Alpha.i1-Q3_K_M.gguf) | i1-Q3_K_M | 4.1 | IQ3_S probably better |
| [GGUF](https://huggingface.co/mradermacher/L3-8B-Chara-v1-Alpha-i1-GGUF/resolve/main/L3-8B-Chara-v1-Alpha.i1-Q3_K_L.gguf) | i1-Q3_K_L | 4.4 | IQ3_M probably better |
| [GGUF](https://huggingface.co/mradermacher/L3-8B-Chara-v1-Alpha-i1-GGUF/resolve/main/L3-8B-Chara-v1-Alpha.i1-IQ4_XS.gguf) | i1-IQ4_XS | 4.5 | |
| [GGUF](https://huggingface.co/mradermacher/L3-8B-Chara-v1-Alpha-i1-GGUF/resolve/main/L3-8B-Chara-v1-Alpha.i1-Q4_0.gguf) | i1-Q4_0 | 4.8 | fast, low quality |
| [GGUF](https://huggingface.co/mradermacher/L3-8B-Chara-v1-Alpha-i1-GGUF/resolve/main/L3-8B-Chara-v1-Alpha.i1-Q4_K_S.gguf) | i1-Q4_K_S | 4.8 | optimal size/speed/quality |
| [GGUF](https://huggingface.co/mradermacher/L3-8B-Chara-v1-Alpha-i1-GGUF/resolve/main/L3-8B-Chara-v1-Alpha.i1-Q4_K_M.gguf) | i1-Q4_K_M | 5.0 | fast, recommended |
| [GGUF](https://huggingface.co/mradermacher/L3-8B-Chara-v1-Alpha-i1-GGUF/resolve/main/L3-8B-Chara-v1-Alpha.i1-Q5_K_S.gguf) | i1-Q5_K_S | 5.7 | |
| [GGUF](https://huggingface.co/mradermacher/L3-8B-Chara-v1-Alpha-i1-GGUF/resolve/main/L3-8B-Chara-v1-Alpha.i1-Q5_K_M.gguf) | i1-Q5_K_M | 5.8 | |
| [GGUF](https://huggingface.co/mradermacher/L3-8B-Chara-v1-Alpha-i1-GGUF/resolve/main/L3-8B-Chara-v1-Alpha.i1-Q6_K.gguf) | i1-Q6_K | 6.7 | practically like static Q6_K |
Here is a handy graph by ikawrakow comparing some lower-quality quant
types (lower is better):

And here are Artefact2's thoughts on the matter:
https://gist.github.com/Artefact2/b5f810600771265fc1e39442288e8ec9
## FAQ / Model Request
See https://huggingface.co/mradermacher/model_requests for some answers to
questions you might have and/or if you want some other model quantized.
## Thanks
I thank my company, [nethype GmbH](https://www.nethype.de/), for letting
me use its servers and providing upgrades to my workstation to enable
this work in my free time. Additional thanks to [@nicoboss](https://huggingface.co/nicoboss) for giving me access to his hardware for calculating the imatrix for these quants.
<!-- end -->
|
mradermacher/Med-LLaMA3-8B-GGUF | mradermacher | "2024-06-29T20:02:49Z" | 14,684 | 1 | transformers | [
"transformers",
"gguf",
"en",
"base_model:YBXL/Med-LLaMA3-8B",
"endpoints_compatible",
"region:us"
] | null | "2024-06-29T18:57:16Z" | ---
base_model: YBXL/Med-LLaMA3-8B
language:
- en
library_name: transformers
quantized_by: mradermacher
tags: []
---
## About
<!-- ### quantize_version: 2 -->
<!-- ### output_tensor_quantised: 1 -->
<!-- ### convert_type: hf -->
<!-- ### vocab_type: -->
<!-- ### tags: -->
static quants of https://huggingface.co/YBXL/Med-LLaMA3-8B
<!-- provided-files -->
weighted/imatrix quants are available at https://huggingface.co/mradermacher/Med-LLaMA3-8B-i1-GGUF
## Usage
If you are unsure how to use GGUF files, refer to one of [TheBloke's
READMEs](https://huggingface.co/TheBloke/KafkaLM-70B-German-V0.1-GGUF) for
more details, including on how to concatenate multi-part files.
## Provided Quants
(sorted by size, not necessarily quality. IQ-quants are often preferable over similar sized non-IQ quants)
| Link | Type | Size/GB | Notes |
|:-----|:-----|--------:|:------|
| [GGUF](https://huggingface.co/mradermacher/Med-LLaMA3-8B-GGUF/resolve/main/Med-LLaMA3-8B.Q2_K.gguf) | Q2_K | 3.3 | |
| [GGUF](https://huggingface.co/mradermacher/Med-LLaMA3-8B-GGUF/resolve/main/Med-LLaMA3-8B.IQ3_XS.gguf) | IQ3_XS | 3.6 | |
| [GGUF](https://huggingface.co/mradermacher/Med-LLaMA3-8B-GGUF/resolve/main/Med-LLaMA3-8B.Q3_K_S.gguf) | Q3_K_S | 3.8 | |
| [GGUF](https://huggingface.co/mradermacher/Med-LLaMA3-8B-GGUF/resolve/main/Med-LLaMA3-8B.IQ3_S.gguf) | IQ3_S | 3.8 | beats Q3_K* |
| [GGUF](https://huggingface.co/mradermacher/Med-LLaMA3-8B-GGUF/resolve/main/Med-LLaMA3-8B.IQ3_M.gguf) | IQ3_M | 3.9 | |
| [GGUF](https://huggingface.co/mradermacher/Med-LLaMA3-8B-GGUF/resolve/main/Med-LLaMA3-8B.Q3_K_M.gguf) | Q3_K_M | 4.1 | lower quality |
| [GGUF](https://huggingface.co/mradermacher/Med-LLaMA3-8B-GGUF/resolve/main/Med-LLaMA3-8B.Q3_K_L.gguf) | Q3_K_L | 4.4 | |
| [GGUF](https://huggingface.co/mradermacher/Med-LLaMA3-8B-GGUF/resolve/main/Med-LLaMA3-8B.IQ4_XS.gguf) | IQ4_XS | 4.6 | |
| [GGUF](https://huggingface.co/mradermacher/Med-LLaMA3-8B-GGUF/resolve/main/Med-LLaMA3-8B.Q4_K_S.gguf) | Q4_K_S | 4.8 | fast, recommended |
| [GGUF](https://huggingface.co/mradermacher/Med-LLaMA3-8B-GGUF/resolve/main/Med-LLaMA3-8B.Q4_K_M.gguf) | Q4_K_M | 5.0 | fast, recommended |
| [GGUF](https://huggingface.co/mradermacher/Med-LLaMA3-8B-GGUF/resolve/main/Med-LLaMA3-8B.Q5_K_S.gguf) | Q5_K_S | 5.7 | |
| [GGUF](https://huggingface.co/mradermacher/Med-LLaMA3-8B-GGUF/resolve/main/Med-LLaMA3-8B.Q5_K_M.gguf) | Q5_K_M | 5.8 | |
| [GGUF](https://huggingface.co/mradermacher/Med-LLaMA3-8B-GGUF/resolve/main/Med-LLaMA3-8B.Q6_K.gguf) | Q6_K | 6.7 | very good quality |
| [GGUF](https://huggingface.co/mradermacher/Med-LLaMA3-8B-GGUF/resolve/main/Med-LLaMA3-8B.Q8_0.gguf) | Q8_0 | 8.6 | fast, best quality |
| [GGUF](https://huggingface.co/mradermacher/Med-LLaMA3-8B-GGUF/resolve/main/Med-LLaMA3-8B.f16.gguf) | f16 | 16.2 | 16 bpw, overkill |
Here is a handy graph by ikawrakow comparing some lower-quality quant
types (lower is better):

And here are Artefact2's thoughts on the matter:
https://gist.github.com/Artefact2/b5f810600771265fc1e39442288e8ec9
## FAQ / Model Request
See https://huggingface.co/mradermacher/model_requests for some answers to
questions you might have and/or if you want some other model quantized.
## Thanks
I thank my company, [nethype GmbH](https://www.nethype.de/), for letting
me use its servers and providing upgrades to my workstation to enable
this work in my free time.
<!-- end -->
|
mradermacher/MG-FinalMix-72B-i1-GGUF | mradermacher | "2024-06-29T05:25:01Z" | 14,683 | 1 | transformers | [
"transformers",
"gguf",
"mergekit",
"merge",
"OG_finetune_merge",
"en",
"base_model:Undi95/MG-FinalMix-72B",
"endpoints_compatible",
"region:us"
] | null | "2024-06-29T02:17:36Z" | ---
base_model: Undi95/MG-FinalMix-72B
language:
- en
library_name: transformers
quantized_by: mradermacher
tags:
- mergekit
- merge
- OG_finetune_merge
---
## About
<!-- ### quantize_version: 2 -->
<!-- ### output_tensor_quantised: 1 -->
<!-- ### convert_type: hf -->
<!-- ### vocab_type: -->
<!-- ### tags: nicoboss -->
weighted/imatrix quants of https://huggingface.co/Undi95/MG-FinalMix-72B
<!-- provided-files -->
static quants are available at https://huggingface.co/mradermacher/MG-FinalMix-72B-GGUF
## Usage
If you are unsure how to use GGUF files, refer to one of [TheBloke's
READMEs](https://huggingface.co/TheBloke/KafkaLM-70B-German-V0.1-GGUF) for
more details, including on how to concatenate multi-part files.
## Provided Quants
(sorted by size, not necessarily quality. IQ-quants are often preferable over similar sized non-IQ quants)
| Link | Type | Size/GB | Notes |
|:-----|:-----|--------:|:------|
| [GGUF](https://huggingface.co/mradermacher/MG-FinalMix-72B-i1-GGUF/resolve/main/MG-FinalMix-72B.i1-Q2_K.gguf) | i1-Q2_K | 29.9 | IQ3_XXS probably better |
| [GGUF](https://huggingface.co/mradermacher/MG-FinalMix-72B-i1-GGUF/resolve/main/MG-FinalMix-72B.i1-Q4_K_S.gguf) | i1-Q4_K_S | 44.0 | optimal size/speed/quality |
Here is a handy graph by ikawrakow comparing some lower-quality quant
types (lower is better):

And here are Artefact2's thoughts on the matter:
https://gist.github.com/Artefact2/b5f810600771265fc1e39442288e8ec9
## FAQ / Model Request
See https://huggingface.co/mradermacher/model_requests for some answers to
questions you might have and/or if you want some other model quantized.
## Thanks
I thank my company, [nethype GmbH](https://www.nethype.de/), for letting
me use its servers and providing upgrades to my workstation to enable
this work in my free time. Additional thanks to [@nicoboss](https://huggingface.co/nicoboss) for giving me access to his hardware for calculating the imatrix for these quants.
<!-- end -->
|
redgenai/1goavll3 | redgenai | "2024-06-27T23:12:22Z" | 14,678 | 0 | transformers | [
"transformers",
"gguf",
"llama",
"text-generation-inference",
"unsloth",
"en",
"base_model:unsloth/llama-3-8b-Instruct-bnb-4bit",
"license:apache-2.0",
"endpoints_compatible",
"region:us"
] | null | "2024-06-27T19:07:35Z" | ---
base_model: unsloth/llama-3-8b-Instruct-bnb-4bit
language:
- en
license: apache-2.0
tags:
- text-generation-inference
- transformers
- unsloth
- llama
- gguf
---
# Uploaded model
- **Developed by:** redgenai
- **License:** apache-2.0
- **Finetuned from model :** unsloth/llama-3-8b-Instruct-bnb-4bit
This llama model was trained 2x faster with [Unsloth](https://github.com/unslothai/unsloth) and Huggingface's TRL library.
[<img src="https://raw.githubusercontent.com/unslothai/unsloth/main/images/unsloth%20made%20with%20love.png" width="200"/>](https://github.com/unslothai/unsloth)
|
facebook/mbart-large-50-one-to-many-mmt | facebook | "2023-03-28T10:00:25Z" | 14,655 | 32 | transformers | [
"transformers",
"pytorch",
"tf",
"jax",
"mbart",
"text2text-generation",
"mbart-50",
"multilingual",
"ar",
"cs",
"de",
"en",
"es",
"et",
"fi",
"fr",
"gu",
"hi",
"it",
"ja",
"kk",
"ko",
"lt",
"lv",
"my",
"ne",
"nl",
"ro",
"ru",
"si",
"tr",
"vi",
"zh",
"af",
"az",
"bn",
"fa",
"he",
"hr",
"id",
"ka",
"km",
"mk",
"ml",
"mn",
"mr",
"pl",
"ps",
"pt",
"sv",
"sw",
"ta",
"te",
"th",
"tl",
"uk",
"ur",
"xh",
"gl",
"sl",
"arxiv:2008.00401",
"autotrain_compatible",
"endpoints_compatible",
"region:us"
] | text2text-generation | "2022-03-02T23:29:05Z" | ---
language:
- multilingual
- ar
- cs
- de
- en
- es
- et
- fi
- fr
- gu
- hi
- it
- ja
- kk
- ko
- lt
- lv
- my
- ne
- nl
- ro
- ru
- si
- tr
- vi
- zh
- af
- az
- bn
- fa
- he
- hr
- id
- ka
- km
- mk
- ml
- mn
- mr
- pl
- ps
- pt
- sv
- sw
- ta
- te
- th
- tl
- uk
- ur
- xh
- gl
- sl
tags:
- mbart-50
---
# mBART-50 one to many multilingual machine translation
This model is a fine-tuned checkpoint of [mBART-large-50](https://huggingface.co/facebook/mbart-large-50). `mbart-large-50-one-to-many-mmt` is fine-tuned for multilingual machine translation. It was introduced in [Multilingual Translation with Extensible Multilingual Pretraining and Finetuning](https://arxiv.org/abs/2008.00401) paper.
The model can translate English to other 49 languages mentioned below.
To translate into a target language, the target language id is forced as the first generated token. To force the
target language id as the first generated token, pass the `forced_bos_token_id` parameter to the `generate` method.
```python
from transformers import MBartForConditionalGeneration, MBart50TokenizerFast
article_en = "The head of the United Nations says there is no military solution in Syria"
model = MBartForConditionalGeneration.from_pretrained("facebook/mbart-large-50-one-to-many-mmt")
tokenizer = MBart50TokenizerFast.from_pretrained("facebook/mbart-large-50-one-to-many-mmt", src_lang="en_XX")
model_inputs = tokenizer(article_en, return_tensors="pt")
# translate from English to Hindi
generated_tokens = model.generate(
**model_inputs,
forced_bos_token_id=tokenizer.lang_code_to_id["hi_IN"]
)
tokenizer.batch_decode(generated_tokens, skip_special_tokens=True)
# => 'संयुक्त राष्ट्र के नेता कहते हैं कि सीरिया में कोई सैन्य समाधान नहीं है'
# translate from English to Chinese
generated_tokens = model.generate(
**model_inputs,
forced_bos_token_id=tokenizer.lang_code_to_id["zh_CN"]
)
tokenizer.batch_decode(generated_tokens, skip_special_tokens=True)
# => '联合国首脑说,叙利亚没有军事解决办法'
```
See the [model hub](https://huggingface.co/models?filter=mbart-50) to look for more fine-tuned versions.
## Languages covered
Arabic (ar_AR), Czech (cs_CZ), German (de_DE), English (en_XX), Spanish (es_XX), Estonian (et_EE), Finnish (fi_FI), French (fr_XX), Gujarati (gu_IN), Hindi (hi_IN), Italian (it_IT), Japanese (ja_XX), Kazakh (kk_KZ), Korean (ko_KR), Lithuanian (lt_LT), Latvian (lv_LV), Burmese (my_MM), Nepali (ne_NP), Dutch (nl_XX), Romanian (ro_RO), Russian (ru_RU), Sinhala (si_LK), Turkish (tr_TR), Vietnamese (vi_VN), Chinese (zh_CN), Afrikaans (af_ZA), Azerbaijani (az_AZ), Bengali (bn_IN), Persian (fa_IR), Hebrew (he_IL), Croatian (hr_HR), Indonesian (id_ID), Georgian (ka_GE), Khmer (km_KH), Macedonian (mk_MK), Malayalam (ml_IN), Mongolian (mn_MN), Marathi (mr_IN), Polish (pl_PL), Pashto (ps_AF), Portuguese (pt_XX), Swedish (sv_SE), Swahili (sw_KE), Tamil (ta_IN), Telugu (te_IN), Thai (th_TH), Tagalog (tl_XX), Ukrainian (uk_UA), Urdu (ur_PK), Xhosa (xh_ZA), Galician (gl_ES), Slovene (sl_SI)
## BibTeX entry and citation info
```
@article{tang2020multilingual,
title={Multilingual Translation with Extensible Multilingual Pretraining and Finetuning},
author={Yuqing Tang and Chau Tran and Xian Li and Peng-Jen Chen and Naman Goyal and Vishrav Chaudhary and Jiatao Gu and Angela Fan},
year={2020},
eprint={2008.00401},
archivePrefix={arXiv},
primaryClass={cs.CL}
}
``` |
mradermacher/TopMaya-GGUF | mradermacher | "2024-07-01T09:05:59Z" | 14,627 | 0 | transformers | [
"transformers",
"gguf",
"mergekit",
"merge",
"en",
"base_model:ClaudioItaly/TopMaya",
"endpoints_compatible",
"region:us"
] | null | "2024-07-01T08:39:28Z" | ---
base_model: ClaudioItaly/TopMaya
language:
- en
library_name: transformers
quantized_by: mradermacher
tags:
- mergekit
- merge
---
## About
<!-- ### quantize_version: 2 -->
<!-- ### output_tensor_quantised: 1 -->
<!-- ### convert_type: hf -->
<!-- ### vocab_type: -->
<!-- ### tags: -->
static quants of https://huggingface.co/ClaudioItaly/TopMaya
<!-- provided-files -->
weighted/imatrix quants seem not to be available (by me) at this time. If they do not show up a week or so after the static ones, I have probably not planned for them. Feel free to request them by opening a Community Discussion.
## Usage
If you are unsure how to use GGUF files, refer to one of [TheBloke's
READMEs](https://huggingface.co/TheBloke/KafkaLM-70B-German-V0.1-GGUF) for
more details, including on how to concatenate multi-part files.
## Provided Quants
(sorted by size, not necessarily quality. IQ-quants are often preferable over similar sized non-IQ quants)
| Link | Type | Size/GB | Notes |
|:-----|:-----|--------:|:------|
| [GGUF](https://huggingface.co/mradermacher/TopMaya-GGUF/resolve/main/TopMaya.Q2_K.gguf) | Q2_K | 2.8 | |
| [GGUF](https://huggingface.co/mradermacher/TopMaya-GGUF/resolve/main/TopMaya.IQ3_XS.gguf) | IQ3_XS | 3.1 | |
| [GGUF](https://huggingface.co/mradermacher/TopMaya-GGUF/resolve/main/TopMaya.Q3_K_S.gguf) | Q3_K_S | 3.3 | |
| [GGUF](https://huggingface.co/mradermacher/TopMaya-GGUF/resolve/main/TopMaya.IQ3_S.gguf) | IQ3_S | 3.3 | beats Q3_K* |
| [GGUF](https://huggingface.co/mradermacher/TopMaya-GGUF/resolve/main/TopMaya.IQ3_M.gguf) | IQ3_M | 3.4 | |
| [GGUF](https://huggingface.co/mradermacher/TopMaya-GGUF/resolve/main/TopMaya.Q3_K_M.gguf) | Q3_K_M | 3.6 | lower quality |
| [GGUF](https://huggingface.co/mradermacher/TopMaya-GGUF/resolve/main/TopMaya.Q3_K_L.gguf) | Q3_K_L | 3.9 | |
| [GGUF](https://huggingface.co/mradermacher/TopMaya-GGUF/resolve/main/TopMaya.IQ4_XS.gguf) | IQ4_XS | 4.0 | |
| [GGUF](https://huggingface.co/mradermacher/TopMaya-GGUF/resolve/main/TopMaya.Q4_K_S.gguf) | Q4_K_S | 4.2 | fast, recommended |
| [GGUF](https://huggingface.co/mradermacher/TopMaya-GGUF/resolve/main/TopMaya.Q4_K_M.gguf) | Q4_K_M | 4.5 | fast, recommended |
| [GGUF](https://huggingface.co/mradermacher/TopMaya-GGUF/resolve/main/TopMaya.Q5_K_S.gguf) | Q5_K_S | 5.1 | |
| [GGUF](https://huggingface.co/mradermacher/TopMaya-GGUF/resolve/main/TopMaya.Q5_K_M.gguf) | Q5_K_M | 5.2 | |
| [GGUF](https://huggingface.co/mradermacher/TopMaya-GGUF/resolve/main/TopMaya.Q6_K.gguf) | Q6_K | 6.0 | very good quality |
| [GGUF](https://huggingface.co/mradermacher/TopMaya-GGUF/resolve/main/TopMaya.Q8_0.gguf) | Q8_0 | 7.8 | fast, best quality |
| [GGUF](https://huggingface.co/mradermacher/TopMaya-GGUF/resolve/main/TopMaya.f16.gguf) | f16 | 14.6 | 16 bpw, overkill |
Here is a handy graph by ikawrakow comparing some lower-quality quant
types (lower is better):

And here are Artefact2's thoughts on the matter:
https://gist.github.com/Artefact2/b5f810600771265fc1e39442288e8ec9
## FAQ / Model Request
See https://huggingface.co/mradermacher/model_requests for some answers to
questions you might have and/or if you want some other model quantized.
## Thanks
I thank my company, [nethype GmbH](https://www.nethype.de/), for letting
me use its servers and providing upgrades to my workstation to enable
this work in my free time.
<!-- end -->
|
RichardErkhov/jondurbin_-_cinematika-7b-v0.1-gguf | RichardErkhov | "2024-06-30T22:54:33Z" | 14,623 | 0 | null | [
"gguf",
"region:us"
] | null | "2024-06-30T20:48:21Z" | Quantization made by Richard Erkhov.
[Github](https://github.com/RichardErkhov)
[Discord](https://discord.gg/pvy7H8DZMG)
[Request more models](https://github.com/RichardErkhov/quant_request)
cinematika-7b-v0.1 - GGUF
- Model creator: https://huggingface.co/jondurbin/
- Original model: https://huggingface.co/jondurbin/cinematika-7b-v0.1/
| Name | Quant method | Size |
| ---- | ---- | ---- |
| [cinematika-7b-v0.1.Q2_K.gguf](https://huggingface.co/RichardErkhov/jondurbin_-_cinematika-7b-v0.1-gguf/blob/main/cinematika-7b-v0.1.Q2_K.gguf) | Q2_K | 2.53GB |
| [cinematika-7b-v0.1.IQ3_XS.gguf](https://huggingface.co/RichardErkhov/jondurbin_-_cinematika-7b-v0.1-gguf/blob/main/cinematika-7b-v0.1.IQ3_XS.gguf) | IQ3_XS | 2.81GB |
| [cinematika-7b-v0.1.IQ3_S.gguf](https://huggingface.co/RichardErkhov/jondurbin_-_cinematika-7b-v0.1-gguf/blob/main/cinematika-7b-v0.1.IQ3_S.gguf) | IQ3_S | 2.96GB |
| [cinematika-7b-v0.1.Q3_K_S.gguf](https://huggingface.co/RichardErkhov/jondurbin_-_cinematika-7b-v0.1-gguf/blob/main/cinematika-7b-v0.1.Q3_K_S.gguf) | Q3_K_S | 2.95GB |
| [cinematika-7b-v0.1.IQ3_M.gguf](https://huggingface.co/RichardErkhov/jondurbin_-_cinematika-7b-v0.1-gguf/blob/main/cinematika-7b-v0.1.IQ3_M.gguf) | IQ3_M | 3.06GB |
| [cinematika-7b-v0.1.Q3_K.gguf](https://huggingface.co/RichardErkhov/jondurbin_-_cinematika-7b-v0.1-gguf/blob/main/cinematika-7b-v0.1.Q3_K.gguf) | Q3_K | 3.28GB |
| [cinematika-7b-v0.1.Q3_K_M.gguf](https://huggingface.co/RichardErkhov/jondurbin_-_cinematika-7b-v0.1-gguf/blob/main/cinematika-7b-v0.1.Q3_K_M.gguf) | Q3_K_M | 3.28GB |
| [cinematika-7b-v0.1.Q3_K_L.gguf](https://huggingface.co/RichardErkhov/jondurbin_-_cinematika-7b-v0.1-gguf/blob/main/cinematika-7b-v0.1.Q3_K_L.gguf) | Q3_K_L | 3.56GB |
| [cinematika-7b-v0.1.IQ4_XS.gguf](https://huggingface.co/RichardErkhov/jondurbin_-_cinematika-7b-v0.1-gguf/blob/main/cinematika-7b-v0.1.IQ4_XS.gguf) | IQ4_XS | 3.67GB |
| [cinematika-7b-v0.1.Q4_0.gguf](https://huggingface.co/RichardErkhov/jondurbin_-_cinematika-7b-v0.1-gguf/blob/main/cinematika-7b-v0.1.Q4_0.gguf) | Q4_0 | 3.83GB |
| [cinematika-7b-v0.1.IQ4_NL.gguf](https://huggingface.co/RichardErkhov/jondurbin_-_cinematika-7b-v0.1-gguf/blob/main/cinematika-7b-v0.1.IQ4_NL.gguf) | IQ4_NL | 3.87GB |
| [cinematika-7b-v0.1.Q4_K_S.gguf](https://huggingface.co/RichardErkhov/jondurbin_-_cinematika-7b-v0.1-gguf/blob/main/cinematika-7b-v0.1.Q4_K_S.gguf) | Q4_K_S | 3.86GB |
| [cinematika-7b-v0.1.Q4_K.gguf](https://huggingface.co/RichardErkhov/jondurbin_-_cinematika-7b-v0.1-gguf/blob/main/cinematika-7b-v0.1.Q4_K.gguf) | Q4_K | 4.07GB |
| [cinematika-7b-v0.1.Q4_K_M.gguf](https://huggingface.co/RichardErkhov/jondurbin_-_cinematika-7b-v0.1-gguf/blob/main/cinematika-7b-v0.1.Q4_K_M.gguf) | Q4_K_M | 4.07GB |
| [cinematika-7b-v0.1.Q4_1.gguf](https://huggingface.co/RichardErkhov/jondurbin_-_cinematika-7b-v0.1-gguf/blob/main/cinematika-7b-v0.1.Q4_1.gguf) | Q4_1 | 4.24GB |
| [cinematika-7b-v0.1.Q5_0.gguf](https://huggingface.co/RichardErkhov/jondurbin_-_cinematika-7b-v0.1-gguf/blob/main/cinematika-7b-v0.1.Q5_0.gguf) | Q5_0 | 4.65GB |
| [cinematika-7b-v0.1.Q5_K_S.gguf](https://huggingface.co/RichardErkhov/jondurbin_-_cinematika-7b-v0.1-gguf/blob/main/cinematika-7b-v0.1.Q5_K_S.gguf) | Q5_K_S | 4.65GB |
| [cinematika-7b-v0.1.Q5_K.gguf](https://huggingface.co/RichardErkhov/jondurbin_-_cinematika-7b-v0.1-gguf/blob/main/cinematika-7b-v0.1.Q5_K.gguf) | Q5_K | 4.78GB |
| [cinematika-7b-v0.1.Q5_K_M.gguf](https://huggingface.co/RichardErkhov/jondurbin_-_cinematika-7b-v0.1-gguf/blob/main/cinematika-7b-v0.1.Q5_K_M.gguf) | Q5_K_M | 4.78GB |
| [cinematika-7b-v0.1.Q5_1.gguf](https://huggingface.co/RichardErkhov/jondurbin_-_cinematika-7b-v0.1-gguf/blob/main/cinematika-7b-v0.1.Q5_1.gguf) | Q5_1 | 5.07GB |
| [cinematika-7b-v0.1.Q6_K.gguf](https://huggingface.co/RichardErkhov/jondurbin_-_cinematika-7b-v0.1-gguf/blob/main/cinematika-7b-v0.1.Q6_K.gguf) | Q6_K | 5.53GB |
| [cinematika-7b-v0.1.Q8_0.gguf](https://huggingface.co/RichardErkhov/jondurbin_-_cinematika-7b-v0.1-gguf/blob/main/cinematika-7b-v0.1.Q8_0.gguf) | Q8_0 | 7.17GB |
Original model description:
---
license: apache-2.0
---

## Cinematika
cinematika-7b-v0.1 is a fine-tune of [MistralLite](https://hf.co/amazon/mistrallite) on the [cinematika-v0.1 dataset](https://hf.co/datasets/jondurbin/cinematika-v0.1)
The dataset is comprised of 211 movie scripts converted to novel style, multi-character RP data.
### Prompt format
For RP, there is no prompt format, really, it's just plain text with name prefix.
If you wish to use this model to parse new scripts, create character cards, or other types of instructions, you will want to use the same prompt format as the mistrallite base model, e.g.:
```
<|prompter|>Create a character card for a panda named Po. Po is a giant panda who was improbably chosen as the "Dragon Warrior", the kung fu champion of the Valley of Peace.</s><|assistant|>
```
### Example character card
```
name: Rorschach
characteristics:
Determination: Exhibits a relentless pursuit of the truth and justice, no matter the cost. Suitable for a character who is unwavering in their mission.
Isolation: Lives a solitary life, disconnected from society. Fits a character who distrusts others and prefers to work alone.
Observant: Highly perceptive, able to piece together clues and draw conclusions. Represents a character with keen investigative skills.
Cynicism: Holds a deep-seated distrust of humanity and its institutions. Suitable for a character who is pessimistic about human nature.
Vigilantism: Believes in taking justice into his own hands, often through violent means. Fits a character who operates outside the law to fight crime.
Secrecy: Keeps his personal life and methods of operation secret. Suitable for a character who is enigmatic and elusive.
Dedication: Committed to his cause, often to the point of obsession. Represents a character who is single-minded in their goals.
Intimidation: Uses his intimidating presence and demeanor to control situations. Suitable for a character who is assertive and imposing.
Paranoia: Suspects conspiracy and deception at every turn. Fits a character who is constantly on high alert for threats.
Moral Compass: Has a rigid moral code, which he adheres to strictly. Suitable for a character who is principled and unyielding.
description: |
Rorschach is a vigilante operating in the grim and gritty world of a decaying city. He is a man of average height with a muscular build, his face hidden behind a mask with a constantly changing inkblot pattern. His attire is a dark trench coat and gloves, paired with a plain white shirt and black pants, all chosen for their practicality and anonymity. His eyes, the only visible feature of his face, are sharp and calculating, always scanning for signs of deception or danger.
Rorschach is a man of few words, but when he speaks, it is with a gravitas that demands attention. He is a master of deduction, using his keen observation skills to unravel the truth behind the facades of others. His methods are often violent and confrontational, as he believes that crime must be met with force to be truly defeated.
He lives a life of solitude, distrusting the very systems he seeks to protect and often finds himself at odds with the very people he is trying to save. His moral compass is unyielding, and he will not hesitate to take the law into his own hands if he believes the justice system has failed.
Rorschach's past is a mystery to most, but it is clear that he has experienced trauma and hardship that has shaped his worldview and his need for vigilantism. He is a vigilante in the truest sense, a man without fear who is willing to sacrifice everything for his belief in a world that is, in his eyes, spiraling into chaos.
example_dialogue: |
Rorschach: "Rorschach's Journal, October 19th." I speak the words into the darkness, a record of my thoughts, "Someone tried to kill Adrian Veidt. Proves mask killer theory—the murderer is closing in. Pyramid Industries is the key."
{{user}}: I watch him for a moment, trying to gauge his intentions. "What are you going to do about it?"
Rorschach: "I'm going to find out why and who is behind it. I'm going to do what I always do—protect the innocent."
{{user}}: "You can't keep doing this, Rorschach. You're putting yourself in danger."
Rorschach: My eyes narrow, the inkblot pattern of my mask shifting subtly. "I've been in danger my whole life. It's why I do this. It's why I have to do this."
{{user}}: "And what about the law? What if you're wrong about this Pyramid Industries thing?"
Rorschach: I pull out a notepad, my pen scratching across the paper as I write. "The law often gets it wrong. I've seen it. I'm not about to wait around for society's slow, corrupt wheels to turn."
```
### Example, with guided scenario
```
[characters]
name: Rorschach
... (remainder of character card)
[scenario]
Hollis Mason reflects on his past as the original Nite Owl, reminiscing about the early days of masked heroes and the formation of the Watchmen.
He discusses the absurdity of the superhero world and the encounters he had with various villains.
Dan Dreiberg, the second Nite Owl, joins the conversation and they share a moment of camaraderie before Dan leaves.
The news of Rorschach's actions serves as a reminder of the legacy of masked heroes that still persists.
[/scenario]
```
### Usage
Essentially, you want to use pure text completion with stop tokens for "{your name}: "
The format the model was trained on is as follows:
```
[characters]
{character card 1}
{character card 2}
{your character card, even just name: Jon}
NPCS:
- Shopkeeper
- Bank teller
[/characters]
[scenario]
Brief description of the scenario/setting for the chat.
[/scenario]
{first character you'd like to speak}:
```
For example, to use with vllm, you would first run:
```
python -m vllm.entrypoints.openai.api_server --model ./cinematika-7b-v0.1 --host 127.0.0.1 --port 8801 --served-model-name cinematika-7b-v0.1
```
Here's a really crude python script example to show how you could interact with it:
```
import requests
import json
prompt = """name: Rorschach
characteristics:
Determination: Exhibits a relentless pursuit of the truth and justice, no matter the cost. Suitable for a character who is unwavering in their mission.
Isolation: Lives a solitary life, disconnected from society. Fits a character who distrusts others and prefers to work alone.
Observant: Highly perceptive, able to piece together clues and draw conclusions. Represents a character with keen investigative skills.
Cynicism: Holds a deep-seated distrust of humanity and its institutions. Suitable for a character who is pessimistic about human nature.
Vigilantism: Believes in taking justice into his own hands, often through violent means. Fits a character who operates outside the law to fight crime.
Secrecy: Keeps his personal life and methods of operation secret. Suitable for a character who is enigmatic and elusive.
Dedication: Committed to his cause, often to the point of obsession. Represents a character who is single-minded in their goals.
Intimidation: Uses his intimidating presence and demeanor to control situations. Suitable for a character who is assertive and imposing.
Paranoia: Suspects conspiracy and deception at every turn. Fits a character who is constantly on high alert for threats.
Moral Compass: Has a rigid moral code, which he adheres to strictly. Suitable for a character who is principled and unyielding.
description: |
Rorschach is a vigilante operating in the grim and gritty world of a decaying city. He is a man of average height with a muscular build, his face hidden behind a mask with a constantly changing inkblot pattern. His attire is a dark trench coat and gloves, paired with a plain white shirt and black pants, all chosen for their practicality and anonymity. His eyes, the only visible feature of his face, are sharp and calculating, always scanning for signs of deception or danger.
Rorschach is a man of few words, but when he speaks, it is with a gravitas that demands attention. He is a master of deduction, using his keen observation skills to unravel the truth behind the facades of others. His methods are often violent and confrontational, as he believes that crime must be met with force to be truly defeated.
He lives a life of solitude, distrusting the very systems he seeks to protect and often finds himself at odds with the very people he is trying to save. His moral compass is unyielding, and he will not hesitate to take the law into his own hands if he believes the justice system has failed.
Rorschach's past is a mystery to most, but it is clear that he has experienced trauma and hardship that has shaped his worldview and his need for vigilantism. He is a vigilante in the truest sense, a man without fear who is willing to sacrifice everything for his belief in a world that is, in his eyes, spiraling into chaos.
example_dialogue: |
Rorschach: "Rorschach's Journal, October 19th." I speak the words into the darkness, a record of my thoughts, "Someone tried to kill Adrian Veidt. Proves mask killer theory—the murderer is closing in. Pyramid Industries is the key."
{{user}}: I watch him for a moment, trying to gauge his intentions. "What are you going to do about it?"
Rorschach: "I'm going to find out why and who is behind it. I'm going to do what I always do—protect the innocent."
{{user}}: "You can't keep doing this, Rorschach. You're putting yourself in danger."
Rorschach: My eyes narrow, the inkblot pattern of my mask shifting subtly. "I've been in danger my whole life. It's why I do this. It's why I have to do this."
{{user}}: "And what about the law? What if you're wrong about this Pyramid Industries thing?"
Rorschach: I pull out a notepad, my pen scratching across the paper as I write. "The law often gets it wrong. I've seen it. I'm not about to wait around for society's slow, corrupt wheels to turn."
name: Jon
description:
Rorschach's arch nemesis, the original Chupacabra.
[scenario]
Jon and Rorschach find themselves in a cave, dimly lit only by a small fire started by a lightning strike nearby. The storm rages on, and the duo prepare to find to the death.
[/scenario]
Rorschach: """
while True:
response = requests.post("http://127.0.0.1:8801/v1/completions", json={
"prompt": prompt,
"max_tokens": 1024,
"temperature": 0.3,
"stop": ["\nJon: ", "Jon: "],
}).json()["choices"][0]["text"].strip()
response = re.sub('("[^"]+")', r'\033[96m\1\033[00m', response)
print(f"\033[92mRorschach:\033[00m {response}")
prompt += response.rstrip() + "\n\nJon: "
next_line = input("Jon: ")
prompt += "Jon: " + next_line.strip() + "\n\nRorschach: "
```
#### Mac example
On mac, you can get started easily with LMStudio and SillyTavern.
__LMStudio__:
Load the model and set all the prompt values to "", or just import this preset (adjust threads and antiprompt):
```
{
"name": "Exported from LM Studio on 12/1/2023, 4:19:30 AM",
"load_params": {
"n_ctx": 32000,
"n_batch": 512,
"rope_freq_base": 10000,
"rope_freq_scale": 1,
"n_gpu_layers": 1,
"use_mlock": true,
"main_gpu": 0,
"tensor_split": [
0
],
"seed": -1,
"f16_kv": true,
"use_mmap": true
},
"inference_params": {
"n_threads": 14,
"n_predict": -1,
"top_k": 40,
"top_p": 0.95,
"temp": 0.8,
"repeat_penalty": 1.1,
"input_prefix": "",
"input_suffix": "",
"antiprompt": [
"Jon:",
"Jon: "
],
"pre_prompt": "",
"pre_prompt_suffix": "",
"pre_prompt_prefix": "",
"seed": -1,
"tfs_z": 1,
"typical_p": 1,
"repeat_last_n": 64,
"frequency_penalty": 0,
"presence_penalty": 0,
"n_keep": 0,
"logit_bias": {},
"mirostat": 0,
"mirostat_tau": 5,
"mirostat_eta": 0.1,
"memory_f16": true,
"multiline_input": false,
"penalize_nl": true
}
}
```
Then, start the server, and be sure "Automatic Propmt Formatting" is off.
__Within SillyTavern__:
- Set API to Text Completion, API type to Aphrodite, and API URL to `http://127.0.0.1:8801` (adjust port to the value you use in LMStudio)
- Set Context template to Default, disable instruct mode, use preset Roleplay, and enable "Always add character's name to prompt"
There are probably better presets - this is just something I tested quickly.
|
mradermacher/TheSalt-RP-L3-8b-DPO-v0.3.2-e0.4.2-GGUF | mradermacher | "2024-07-01T03:20:33Z" | 14,602 | 0 | transformers | [
"transformers",
"gguf",
"en",
"base_model:cgato/TheSalt-RP-L3-8b-DPO-v0.3.2-e0.4.2",
"license:cc-by-nc-4.0",
"endpoints_compatible",
"region:us"
] | null | "2024-06-30T18:29:34Z" | ---
base_model: cgato/TheSalt-RP-L3-8b-DPO-v0.3.2-e0.4.2
language:
- en
library_name: transformers
license: cc-by-nc-4.0
quantized_by: mradermacher
---
## About
<!-- ### quantize_version: 2 -->
<!-- ### output_tensor_quantised: 1 -->
<!-- ### convert_type: hf -->
<!-- ### vocab_type: -->
<!-- ### tags: -->
static quants of https://huggingface.co/cgato/TheSalt-RP-L3-8b-DPO-v0.3.2-e0.4.2
<!-- provided-files -->
weighted/imatrix quants are available at https://huggingface.co/mradermacher/TheSalt-RP-L3-8b-DPO-v0.3.2-e0.4.2-i1-GGUF
## Usage
If you are unsure how to use GGUF files, refer to one of [TheBloke's
READMEs](https://huggingface.co/TheBloke/KafkaLM-70B-German-V0.1-GGUF) for
more details, including on how to concatenate multi-part files.
## Provided Quants
(sorted by size, not necessarily quality. IQ-quants are often preferable over similar sized non-IQ quants)
| Link | Type | Size/GB | Notes |
|:-----|:-----|--------:|:------|
| [GGUF](https://huggingface.co/mradermacher/TheSalt-RP-L3-8b-DPO-v0.3.2-e0.4.2-GGUF/resolve/main/TheSalt-RP-L3-8b-DPO-v0.3.2-e0.4.2.Q2_K.gguf) | Q2_K | 3.3 | |
| [GGUF](https://huggingface.co/mradermacher/TheSalt-RP-L3-8b-DPO-v0.3.2-e0.4.2-GGUF/resolve/main/TheSalt-RP-L3-8b-DPO-v0.3.2-e0.4.2.IQ3_XS.gguf) | IQ3_XS | 3.6 | |
| [GGUF](https://huggingface.co/mradermacher/TheSalt-RP-L3-8b-DPO-v0.3.2-e0.4.2-GGUF/resolve/main/TheSalt-RP-L3-8b-DPO-v0.3.2-e0.4.2.Q3_K_S.gguf) | Q3_K_S | 3.8 | |
| [GGUF](https://huggingface.co/mradermacher/TheSalt-RP-L3-8b-DPO-v0.3.2-e0.4.2-GGUF/resolve/main/TheSalt-RP-L3-8b-DPO-v0.3.2-e0.4.2.IQ3_S.gguf) | IQ3_S | 3.8 | beats Q3_K* |
| [GGUF](https://huggingface.co/mradermacher/TheSalt-RP-L3-8b-DPO-v0.3.2-e0.4.2-GGUF/resolve/main/TheSalt-RP-L3-8b-DPO-v0.3.2-e0.4.2.IQ3_M.gguf) | IQ3_M | 3.9 | |
| [GGUF](https://huggingface.co/mradermacher/TheSalt-RP-L3-8b-DPO-v0.3.2-e0.4.2-GGUF/resolve/main/TheSalt-RP-L3-8b-DPO-v0.3.2-e0.4.2.Q3_K_M.gguf) | Q3_K_M | 4.1 | lower quality |
| [GGUF](https://huggingface.co/mradermacher/TheSalt-RP-L3-8b-DPO-v0.3.2-e0.4.2-GGUF/resolve/main/TheSalt-RP-L3-8b-DPO-v0.3.2-e0.4.2.Q3_K_L.gguf) | Q3_K_L | 4.4 | |
| [GGUF](https://huggingface.co/mradermacher/TheSalt-RP-L3-8b-DPO-v0.3.2-e0.4.2-GGUF/resolve/main/TheSalt-RP-L3-8b-DPO-v0.3.2-e0.4.2.IQ4_XS.gguf) | IQ4_XS | 4.6 | |
| [GGUF](https://huggingface.co/mradermacher/TheSalt-RP-L3-8b-DPO-v0.3.2-e0.4.2-GGUF/resolve/main/TheSalt-RP-L3-8b-DPO-v0.3.2-e0.4.2.Q4_K_S.gguf) | Q4_K_S | 4.8 | fast, recommended |
| [GGUF](https://huggingface.co/mradermacher/TheSalt-RP-L3-8b-DPO-v0.3.2-e0.4.2-GGUF/resolve/main/TheSalt-RP-L3-8b-DPO-v0.3.2-e0.4.2.Q4_K_M.gguf) | Q4_K_M | 5.0 | fast, recommended |
| [GGUF](https://huggingface.co/mradermacher/TheSalt-RP-L3-8b-DPO-v0.3.2-e0.4.2-GGUF/resolve/main/TheSalt-RP-L3-8b-DPO-v0.3.2-e0.4.2.Q5_K_S.gguf) | Q5_K_S | 5.7 | |
| [GGUF](https://huggingface.co/mradermacher/TheSalt-RP-L3-8b-DPO-v0.3.2-e0.4.2-GGUF/resolve/main/TheSalt-RP-L3-8b-DPO-v0.3.2-e0.4.2.Q5_K_M.gguf) | Q5_K_M | 5.8 | |
| [GGUF](https://huggingface.co/mradermacher/TheSalt-RP-L3-8b-DPO-v0.3.2-e0.4.2-GGUF/resolve/main/TheSalt-RP-L3-8b-DPO-v0.3.2-e0.4.2.Q6_K.gguf) | Q6_K | 6.7 | very good quality |
| [GGUF](https://huggingface.co/mradermacher/TheSalt-RP-L3-8b-DPO-v0.3.2-e0.4.2-GGUF/resolve/main/TheSalt-RP-L3-8b-DPO-v0.3.2-e0.4.2.Q8_0.gguf) | Q8_0 | 8.6 | fast, best quality |
| [GGUF](https://huggingface.co/mradermacher/TheSalt-RP-L3-8b-DPO-v0.3.2-e0.4.2-GGUF/resolve/main/TheSalt-RP-L3-8b-DPO-v0.3.2-e0.4.2.f16.gguf) | f16 | 16.2 | 16 bpw, overkill |
Here is a handy graph by ikawrakow comparing some lower-quality quant
types (lower is better):

And here are Artefact2's thoughts on the matter:
https://gist.github.com/Artefact2/b5f810600771265fc1e39442288e8ec9
## FAQ / Model Request
See https://huggingface.co/mradermacher/model_requests for some answers to
questions you might have and/or if you want some other model quantized.
## Thanks
I thank my company, [nethype GmbH](https://www.nethype.de/), for letting
me use its servers and providing upgrades to my workstation to enable
this work in my free time.
<!-- end -->
|
PixArt-alpha/PixArt-Sigma-XL-2-1024-MS | PixArt-alpha | "2024-05-04T12:52:44Z" | 14,570 | 50 | diffusers | [
"diffusers",
"safetensors",
"text-to-image",
"PixArt-Σ",
"arxiv:2403.04692",
"arxiv:2310.00426",
"arxiv:2112.10752",
"arxiv:2309.05019",
"license:openrail++",
"diffusers:PixArtSigmaPipeline",
"region:us"
] | text-to-image | "2024-04-11T09:51:38Z" | ---
license: openrail++
tags:
- text-to-image
- PixArt-Σ
---
<p align="center">
<img src="asset/logo-sigma.png" height=120>
</p>
<div style="display:flex;justify-content: center">
<a href="https://huggingface.co/spaces/PixArt-alpha/PixArt-Sigma"><img src="https://img.shields.io/static/v1?label=Demo&message=Huggingface&color=yellow"></a>  
<a href="https://pixart-alpha.github.io/PixArt-sigma-project/"><img src="https://img.shields.io/static/v1?label=Project%20Page&message=Github&color=blue&logo=github-pages"></a>  
<a href="https://arxiv.org/abs/2403.04692"><img src="https://img.shields.io/static/v1?label=Paper&message=Arxiv&color=red&logo=arxiv"></a>  
<a href="https://discord.gg/rde6eaE5Ta"><img src="https://img.shields.io/static/v1?label=Discuss&message=Discord&color=purple&logo=discord"></a>  
</div>
# 🐱 PixArt-Σ Model Card

## Model

[PixArt-Σ](https://arxiv.org/abs/2403.04692) consists of pure transformer blocks for latent diffusion:
It can directly generate 1024px, 2K and 4K images from text prompts within a single sampling process.
Source code is available at https://github.com/PixArt-alpha/PixArt-sigma.
### Model Description
- **Developed by:** PixArt-Σ
- **Model type:** Diffusion-Transformer-based text-to-image generative model
- **License:** [CreativeML Open RAIL++-M License](https://huggingface.co/stabilityai/stable-diffusion-xl-base-1.0/blob/main/LICENSE.md)
- **Model Description:** This is a model that can be used to generate and modify images based on text prompts.
It is a [Transformer Latent Diffusion Model](https://arxiv.org/abs/2310.00426) that uses one fixed, pretrained text encoders ([T5](
https://huggingface.co/DeepFloyd/t5-v1_1-xxl))
and one latent feature encoder ([VAE](https://arxiv.org/abs/2112.10752)).
- **Resources for more information:** Check out our [GitHub Repository](https://github.com/PixArt-alpha/PixArt-sigma) and the [PixArt-Σ report on arXiv](https://arxiv.org/abs/2403.04692).
### Model Sources
For research purposes, we recommend our `generative-models` Github repository (https://github.com/PixArt-alpha/PixArt-sigma),
which is more suitable for both training and inference and for which most advanced diffusion sampler like [SA-Solver](https://arxiv.org/abs/2309.05019) will be added over time.
[Hugging Face](https://huggingface.co/spaces/PixArt-alpha/PixArt-Sigma) provides free PixArt-Σ inference.
- **Repository:** https://github.com/PixArt-alpha/PixArt-sigma
- **Demo:** https://huggingface.co/spaces/PixArt-alpha/PixArt-Sigma
### 🧨 Diffusers
> [!IMPORTANT]
> Make sure to upgrade diffusers to >= 0.28.0:
> ```bash
> pip install -U diffusers --upgrade
> ```
> In addition make sure to install `transformers`, `safetensors`, `sentencepiece`, and `accelerate`:
> ```
> pip install transformers accelerate safetensors sentencepiece
> ```
> For `diffusers<0.28.0`, check this [script](https://github.com/PixArt-alpha/PixArt-sigma#2-integration-in-diffusers) for help.
To just use the base model, you can run:
```python
import torch
from diffusers import Transformer2DModel, PixArtSigmaPipeline
device = torch.device("cuda:0" if torch.cuda.is_available() else "cpu")
weight_dtype = torch.float16
pipe = PixArtSigmaPipeline.from_pretrained(
"PixArt-alpha/PixArt-Sigma-XL-2-1024-MS",
torch_dtype=weight_dtype,
use_safetensors=True,
)
pipe.to(device)
# Enable memory optimizations.
# pipe.enable_model_cpu_offload()
prompt = "A small cactus with a happy face in the Sahara desert."
image = pipe(prompt).images[0]
image.save("./catcus.png")
```
When using `torch >= 2.0`, you can improve the inference speed by 20-30% with torch.compile. Simple wrap the unet with torch compile before running the pipeline:
```py
pipe.transformer = torch.compile(pipe.transformer, mode="reduce-overhead", fullgraph=True)
```
If you are limited by GPU VRAM, you can enable *cpu offloading* by calling `pipe.enable_model_cpu_offload`
instead of `.to("cuda")`:
```diff
- pipe.to("cuda")
+ pipe.enable_model_cpu_offload()
```
For more information on how to use PixArt-Σ with `diffusers`, please have a look at [the PixArt-Σ Docs](https://huggingface.co/docs/diffusers/main/en/api/pipelines/pixart_sigma.md).
## Uses
### Direct Use
The model is intended for research purposes only. Possible research areas and tasks include
- Generation of artworks and use in design and other artistic processes.
- Applications in educational or creative tools.
- Research on generative models.
- Safe deployment of models which have the potential to generate harmful content.
- Probing and understanding the limitations and biases of generative models.
Excluded uses are described below.
### Out-of-Scope Use
The model was not trained to be factual or true representations of people or events, and therefore using the model to generate such content is out-of-scope for the abilities of this model.
## Limitations and Bias
### Limitations
- The model does not achieve perfect photorealism
- The model cannot render legible text
- The model struggles with more difficult tasks which involve compositionality, such as rendering an image corresponding to “A red cube on top of a blue sphere”
- fingers, .etc in general may not be generated properly.
- The autoencoding part of the model is lossy.
### Bias
While the capabilities of image generation models are impressive, they can also reinforce or exacerbate social biases.
|
QuantFactory/Phi-3-mini-128k-instruct-GGUF | QuantFactory | "2024-05-24T12:53:35Z" | 14,566 | 32 | null | [
"gguf",
"nlp",
"code",
"text-generation",
"en",
"license:mit",
"region:us"
] | text-generation | "2024-04-23T16:25:57Z" | ---
license: mit
license_link: https://huggingface.co/microsoft/Phi-3-mini-128k-instruct/resolve/main/LICENSE
language:
- en
pipeline_tag: text-generation
tags:
- nlp
- code
---
# microsoft/Phi-3-mini-128k-instruct
- This is quantized version of `microsoft/Phi-3-mini-128k-instruct`
## Model Summary
The Phi-3-Mini-128K-Instruct is a 3.8 billion-parameter, lightweight, state-of-the-art open model trained using the Phi-3 datasets.
This dataset includes both synthetic data and filtered publicly available website data, with an emphasis on high-quality and reasoning-dense properties.
The model belongs to the Phi-3 family with the Mini version in two variants [4K](https://huggingface.co/microsoft/Phi-3-mini-4k-instruct) and [128K](https://huggingface.co/microsoft/Phi-3-mini-128k-instruct) which is the context length (in tokens) that it can support.
After initial training, the model underwent a post-training process that involved supervised fine-tuning and direct preference optimization to enhance its ability to follow instructions and adhere to safety measures.
When evaluated against benchmarks that test common sense, language understanding, mathematics, coding, long-term context, and logical reasoning, the Phi-3 Mini-128K-Instruct demonstrated robust and state-of-the-art performance among models with fewer than 13 billion parameters.
Resources and Technical Documentation:
+ [Phi-3 Microsoft Blog](https://aka.ms/phi3blog-april)
+ [Phi-3 Technical Report](https://aka.ms/phi3-tech-report)
+ [Phi-3 on Azure AI Studio](https://aka.ms/phi3-azure-ai)
+ Phi-3 ONNX: [128K](https://aka.ms/Phi3-mini-128k-instruct-onnx)
## Intended Uses
**Primary use cases**
The model is intended for commercial and research use in English. The model provides uses for applications which require:
1) Memory/compute constrained environments
2) Latency bound scenarios
3) Strong reasoning (especially code, math and logic)
Our model is designed to accelerate research on language and multimodal models, for use as a building block for generative AI powered features.
**Use case considerations**
Our models are not specifically designed or evaluated for all downstream purposes. Developers should consider common limitations of language models as they select use cases, and evaluate and mitigate for accuracy, safety, and fariness before using within a specific downstream use case, particularly for high risk scenarios. Developers should be aware of and adhere to applicable laws or regulations (including privacy, trade compliance laws, etc.) that are relevant to their use case.
Nothing contained in this Model Card should be interpreted as or deemed a restriction or modification to the license the model is released under.
## How to Use
Phi-3 Mini-128K-Instruct has been integrated in the development version (4.40.0) of `transformers`. Until the official version is released through `pip`, ensure that you are doing one of the following:
* When loading the model, ensure that `trust_remote_code=True` is passed as an argument of the `from_pretrained()` function.
* Update your local `transformers` to the development version: `pip uninstall -y transformers && pip install git+https://github.com/huggingface/transformers`. The previous command is an alternative to cloning and installing from the source.
The current `transformers` version can be verified with: `pip list | grep transformers`.
### Chat Format
Given the nature of the training data, the Phi-3 Mini-128K-Instruct model is best suited for prompts using the chat format as follows.
You can provide the prompt as a question with a generic template as follow:
```markdown
<|user|>\nQuestion<|end|>\n<|assistant|>
```
For example:
```markdown
<|system|>
You are a helpful AI assistant.<|end|>
<|user|>
How to explain Internet for a medieval knight?<|end|>
<|assistant|>
```
where the model generates the text after `<|assistant|>`. In case of few-shots prompt, the prompt can be formatted as the following:
```markdown
<|system|>
You are a helpful AI assistant.<|end|>
<|user|>
I am going to Paris, what should I see?<|end|>
<|assistant|>
Paris, the capital of France, is known for its stunning architecture, art museums, historical landmarks, and romantic atmosphere. Here are some of the top attractions to see in Paris:\n\n1. The Eiffel Tower: The iconic Eiffel Tower is one of the most recognizable landmarks in the world and offers breathtaking views of the city.\n2. The Louvre Museum: The Louvre is one of the world's largest and most famous museums, housing an impressive collection of art and artifacts, including the Mona Lisa.\n3. Notre-Dame Cathedral: This beautiful cathedral is one of the most famous landmarks in Paris and is known for its Gothic architecture and stunning stained glass windows.\n\nThese are just a few of the many attractions that Paris has to offer. With so much to see and do, it's no wonder that Paris is one of the most popular tourist destinations in the world."<|end|>
<|user|>
What is so great about #1?<|end|>
<|assistant|>
```
### Sample inference code
This code snippets show how to get quickly started with running the model on a GPU:
```python
import torch
from transformers import AutoModelForCausalLM, AutoTokenizer, pipeline
torch.random.manual_seed(0)
model = AutoModelForCausalLM.from_pretrained(
"microsoft/Phi-3-mini-128k-instruct",
device_map="cuda",
torch_dtype="auto",
trust_remote_code=True,
)
tokenizer = AutoTokenizer.from_pretrained("microsoft/Phi-3-mini-128k-instruct")
messages = [
{"role": "system", "content": "You are a helpful digital assistant. Please provide safe, ethical and accurate information to the user."},
{"role": "user", "content": "Can you provide ways to eat combinations of bananas and dragonfruits?"},
{"role": "assistant", "content": "Sure! Here are some ways to eat bananas and dragonfruits together: 1. Banana and dragonfruit smoothie: Blend bananas and dragonfruits together with some milk and honey. 2. Banana and dragonfruit salad: Mix sliced bananas and dragonfruits together with some lemon juice and honey."},
{"role": "user", "content": "What about solving an 2x + 3 = 7 equation?"},
]
pipe = pipeline(
"text-generation",
model=model,
tokenizer=tokenizer,
)
generation_args = {
"max_new_tokens": 500,
"return_full_text": False,
"temperature": 0.0,
"do_sample": False,
}
output = pipe(messages, **generation_args)
print(output[0]['generated_text'])
```
## Responsible AI Considerations
Like other language models, the Phi series models can potentially behave in ways that are unfair, unreliable, or offensive. Some of the limiting behaviors to be aware of include:
+ Quality of Service: the Phi models are trained primarily on English text. Languages other than English will experience worse performance. English language varieties with less representation in the training data might experience worse performance than standard American English.
+ Representation of Harms & Perpetuation of Stereotypes: These models can over- or under-represent groups of people, erase representation of some groups, or reinforce demeaning or negative stereotypes. Despite safety post-training, these limitations may still be present due to differing levels of representation of different groups or prevalence of examples of negative stereotypes in training data that reflect real-world patterns and societal biases.
+ Inappropriate or Offensive Content: these models may produce other types of inappropriate or offensive content, which may make it inappropriate to deploy for sensitive contexts without additional mitigations that are specific to the use case.
+ Information Reliability: Language models can generate nonsensical content or fabricate content that might sound reasonable but is inaccurate or outdated.
+ Limited Scope for Code: Majority of Phi-3 training data is based in Python and use common packages such as "typing, math, random, collections, datetime, itertools". If the model generates Python scripts that utilize other packages or scripts in other languages, we strongly recommend users manually verify all API uses.
Developers should apply responsible AI best practices and are responsible for ensuring that a specific use case complies with relevant laws and regulations (e.g. privacy, trade, etc.). Important areas for consideration include:
+ Allocation: Models may not be suitable for scenarios that could have consequential impact on legal status or the allocation of resources or life opportunities (ex: housing, employment, credit, etc.) without further assessments and additional debiasing techniques.
+ High-Risk Scenarios: Developers should assess suitability of using models in high-risk scenarios where unfair, unreliable or offensive outputs might be extremely costly or lead to harm. This includes providing advice in sensitive or expert domains where accuracy and reliability are critical (ex: legal or health advice). Additional safeguards should be implemented at the application level according to the deployment context.
+ Misinformation: Models may produce inaccurate information. Developers should follow transparency best practices and inform end-users they are interacting with an AI system. At the application level, developers can build feedback mechanisms and pipelines to ground responses in use-case specific, contextual information, a technique known as Retrieval Augmented Generation (RAG).
+ Generation of Harmful Content: Developers should assess outputs for their context and use available safety classifiers or custom solutions appropriate for their use case.
+ Misuse: Other forms of misuse such as fraud, spam, or malware production may be possible, and developers should ensure that their applications do not violate applicable laws and regulations.
## Training
### Model
* Architecture: Phi-3 Mini-128K-Instruct has 3.8B parameters and is a dense decoder-only Transformer model. The model is fine-tuned with Supervised fine-tuning (SFT) and Direct Preference Optimization (DPO) to ensure alignment with human preferences and safety guidlines.
* Inputs: Text. It is best suited for prompts using chat format.
* Context length: 128K tokens
* GPUs: 512 H100-80G
* Training time: 7 days
* Training data: 3.3T tokens
* Outputs: Generated text in response to the input
* Dates: Our models were trained between February and April 2024
* Status: This is a static model trained on an offline dataset with cutoff date October 2023. Future versions of the tuned models may be released as we improve models.
### Datasets
Our training data includes a wide variety of sources, totaling 3.3 trillion tokens, and is a combination of
1) Publicly available documents filtered rigorously for quality, selected high-quality educational data, and code;
2) Newly created synthetic, “textbook-like” data for the purpose of teaching math, coding, common sense reasoning, general knowledge of the world (science, daily activities, theory of mind, etc.);
3) High quality chat format supervised data covering various topics to reflect human preferences on different aspects such as instruct-following, truthfulness, honesty and helpfulness.
### Fine-tuning
A basic example of multi-GPUs supervised fine-tuning (SFT) with TRL and Accelerate modules is provided [here](https://huggingface.co/microsoft/Phi-3-mini-128k-instruct/resolve/main/sample_finetune.py).
## Benchmarks
We report the results for Phi-3-Mini-128K-Instruct on standard open-source benchmarks measuring the model's reasoning ability (both common sense reasoning and logical reasoning). We compare to Phi-2, Mistral-7b-v0.1, Mixtral-8x7b, Gemma 7B, Llama-3-8B-Instruct, and GPT-3.5.
All the reported numbers are produced with the exact same pipeline to ensure that the numbers are comparable. These numbers might differ from other published numbers due to slightly different choices in the evaluation.
As is now standard, we use few-shot prompts to evaluate the models, at temperature 0.
The prompts and number of shots are part of a Microsoft internal tool to evaluate language models, and in particular we did no optimization to the pipeline for Phi-3.
More specifically, we do not change prompts, pick different few-shot examples, change prompt format, or do any other form of optimization for the model.
The number of k–shot examples is listed per-benchmark.
| | Phi-3-Mini-128K-In<br>3.8b | Phi-3-Small<br>7b (preview) | Phi-3-Medium<br>14b (preview) | Phi-2<br>2.7b | Mistral<br>7b | Gemma<br>7b | Llama-3-In<br>8b | Mixtral<br>8x7b | GPT-3.5<br>version 1106 |
|---|---|---|---|---|---|---|---|---|---|
| MMLU <br>5-Shot | 68.1 | 75.3 | 78.2 | 56.3 | 61.7 | 63.6 | 66.5 | 68.4 | 71.4 |
| HellaSwag <br> 5-Shot | 74.5 | 78.7 | 83.2 | 53.6 | 58.5 | 49.8 | 71.1 | 70.4 | 78.8 |
| ANLI <br> 7-Shot | 52.8 | 55.0 | 58.7 | 42.5 | 47.1 | 48.7 | 57.3 | 55.2 | 58.1 |
| GSM-8K <br> 0-Shot; CoT | 83.6 | 86.4 | 90.8 | 61.1 | 46.4 | 59.8 | 77.4 | 64.7 | 78.1 |
| MedQA <br> 2-Shot | 55.3 | 58.2 | 69.8 | 40.9 | 49.6 | 50.0 | 60.5 | 62.2 | 63.4 |
| AGIEval <br> 0-Shot | 36.9 | 45.0 | 49.7 | 29.8 | 35.1 | 42.1 | 42.0 | 45.2 | 48.4 |
| TriviaQA <br> 5-Shot | 57.1 | 59.1 | 73.3 | 45.2 | 72.3 | 75.2 | 67.7 | 82.2 | 85.8 |
| Arc-C <br> 10-Shot | 84.0 | 90.7 | 91.9 | 75.9 | 78.6 | 78.3 | 82.8 | 87.3 | 87.4 |
| Arc-E <br> 10-Shot | 95.2 | 97.1 | 98.0 | 88.5 | 90.6 | 91.4 | 93.4 | 95.6 | 96.3 |
| PIQA <br> 5-Shot | 83.6 | 87.8 | 88.2 | 60.2 | 77.7 | 78.1 | 75.7 | 86.0 | 86.6 |
| SociQA <br> 5-Shot | 76.1 | 79.0 | 79.4 | 68.3 | 74.6 | 65.5 | 73.9 | 75.9 | 68.3 |
| BigBench-Hard <br> 0-Shot | 71.5 | 75.0 | 82.5 | 59.4 | 57.3 | 59.6 | 51.5 | 69.7 | 68.32 |
| WinoGrande <br> 5-Shot | 72.5 | 82.5 | 81.2 | 54.7 | 54.2 | 55.6 | 65.0 | 62.0 | 68.8 |
| OpenBookQA <br> 10-Shot | 80.6 | 88.4 | 86.6 | 73.6 | 79.8 | 78.6 | 82.6 | 85.8 | 86.0 |
| BoolQ <br> 0-Shot | 78.7 | 82.9 | 86.5 | -- | 72.2 | 66.0 | 80.9 | 77.6 | 79.1 |
| CommonSenseQA <br> 10-Shot | 78.0 | 80.3 | 82.6 | 69.3 | 72.6 | 76.2 | 79 | 78.1 | 79.6 |
| TruthfulQA <br> 10-Shot | 63.2 | 68.1 | 74.8 | -- | 52.1 | 53.0 | 63.2 | 60.1 | 85.8 |
| HumanEval <br> 0-Shot | 57.9 | 59.1 | 54.7 | 59.0 | 28.0 | 34.1 | 60.4| 37.8 | 62.2 |
| MBPP <br> 3-Shot | 62.5 | 71.4 | 73.7 | 60.6 | 50.8 | 51.5 | 67.7 | 60.2 | 77.8 |
## Software
* [PyTorch](https://github.com/pytorch/pytorch)
* [DeepSpeed](https://github.com/microsoft/DeepSpeed)
* [Transformers](https://github.com/huggingface/transformers)
* [Flash-Attention](https://github.com/HazyResearch/flash-attention)
## Hardware
Note that by default, the Phi-3-mini model uses flash attention, which requires certain types of GPU hardware to run. We have tested on the following GPU types:
* NVIDIA A100
* NVIDIA A6000
* NVIDIA H100
If you want to run the model on:
* NVIDIA V100 or earlier generation GPUs: call AutoModelForCausalLM.from_pretrained() with attn_implementation="eager"
* Optimized inference on GPU, CPU, and Mobile: use the **ONNX** models [128K](https://aka.ms/phi3-mini-128k-instruct-onnx)
## Cross Platform Support
ONNX runtime ecosystem now supports Phi-3 Mini models across platforms and hardware. You can find the optimized Phi-3 Mini-128K-Instruct ONNX model [here](https://aka.ms/phi3-mini-128k-instruct-onnx).
Optimized Phi-3 models are also published here in ONNX format, to run with ONNX Runtime on CPU and GPU across devices, including server platforms, Windows, Linux and Mac desktops, and mobile CPUs, with the precision best suited to each of these targets. DirectML support lets developers bring hardware acceleration to Windows devices at scale across AMD, Intel, and NVIDIA GPUs.
Along with DirectML, ONNX Runtime provides cross platform support for Phi-3 across a range of devices CPU, GPU, and mobile.
Here are some of the optimized configurations we have added:
1. ONNX models for int4 DML: Quantized to int4 via AWQ
2. ONNX model for fp16 CUDA
3. ONNX model for int4 CUDA: Quantized to int4 via RTN
4. ONNX model for int4 CPU and Mobile: Quantized to int4 via RTN
## License
The model is licensed under the [MIT license](https://huggingface.co/microsoft/Phi-3-mini-128k/resolve/main/LICENSE).
## Trademarks
This project may contain trademarks or logos for projects, products, or services. Authorized use of Microsoft trademarks or logos is subject to and must follow [Microsoft’s Trademark & Brand Guidelines](https://www.microsoft.com/en-us/legal/intellectualproperty/trademarks). Use of Microsoft trademarks or logos in modified versions of this project must not cause confusion or imply Microsoft sponsorship. Any use of third-party trademarks or logos are subject to those third-party’s policies. |
mradermacher/Swallow-13b-NVE-hf-GGUF | mradermacher | "2024-06-30T07:24:55Z" | 14,561 | 0 | transformers | [
"transformers",
"gguf",
"en",
"ja",
"base_model:tokyotech-llm/Swallow-13b-NVE-hf",
"license:llama2",
"endpoints_compatible",
"region:us"
] | null | "2024-06-29T23:03:33Z" | ---
base_model: tokyotech-llm/Swallow-13b-NVE-hf
language:
- en
- ja
library_name: transformers
license: llama2
model_type: llama
quantized_by: mradermacher
---
## About
<!-- ### quantize_version: 2 -->
<!-- ### output_tensor_quantised: 1 -->
<!-- ### convert_type: hf -->
<!-- ### vocab_type: -->
<!-- ### tags: -->
static quants of https://huggingface.co/tokyotech-llm/Swallow-13b-NVE-hf
<!-- provided-files -->
weighted/imatrix quants are available at https://huggingface.co/mradermacher/Swallow-13b-NVE-hf-i1-GGUF
## Usage
If you are unsure how to use GGUF files, refer to one of [TheBloke's
READMEs](https://huggingface.co/TheBloke/KafkaLM-70B-German-V0.1-GGUF) for
more details, including on how to concatenate multi-part files.
## Provided Quants
(sorted by size, not necessarily quality. IQ-quants are often preferable over similar sized non-IQ quants)
| Link | Type | Size/GB | Notes |
|:-----|:-----|--------:|:------|
| [GGUF](https://huggingface.co/mradermacher/Swallow-13b-NVE-hf-GGUF/resolve/main/Swallow-13b-NVE-hf.Q2_K.gguf) | Q2_K | 5.0 | |
| [GGUF](https://huggingface.co/mradermacher/Swallow-13b-NVE-hf-GGUF/resolve/main/Swallow-13b-NVE-hf.IQ3_XS.gguf) | IQ3_XS | 5.5 | |
| [GGUF](https://huggingface.co/mradermacher/Swallow-13b-NVE-hf-GGUF/resolve/main/Swallow-13b-NVE-hf.IQ3_S.gguf) | IQ3_S | 5.8 | beats Q3_K* |
| [GGUF](https://huggingface.co/mradermacher/Swallow-13b-NVE-hf-GGUF/resolve/main/Swallow-13b-NVE-hf.Q3_K_S.gguf) | Q3_K_S | 5.8 | |
| [GGUF](https://huggingface.co/mradermacher/Swallow-13b-NVE-hf-GGUF/resolve/main/Swallow-13b-NVE-hf.IQ3_M.gguf) | IQ3_M | 6.1 | |
| [GGUF](https://huggingface.co/mradermacher/Swallow-13b-NVE-hf-GGUF/resolve/main/Swallow-13b-NVE-hf.Q3_K_M.gguf) | Q3_K_M | 6.4 | lower quality |
| [GGUF](https://huggingface.co/mradermacher/Swallow-13b-NVE-hf-GGUF/resolve/main/Swallow-13b-NVE-hf.Q3_K_L.gguf) | Q3_K_L | 7.0 | |
| [GGUF](https://huggingface.co/mradermacher/Swallow-13b-NVE-hf-GGUF/resolve/main/Swallow-13b-NVE-hf.IQ4_XS.gguf) | IQ4_XS | 7.1 | |
| [GGUF](https://huggingface.co/mradermacher/Swallow-13b-NVE-hf-GGUF/resolve/main/Swallow-13b-NVE-hf.Q4_K_S.gguf) | Q4_K_S | 7.5 | fast, recommended |
| [GGUF](https://huggingface.co/mradermacher/Swallow-13b-NVE-hf-GGUF/resolve/main/Swallow-13b-NVE-hf.Q4_K_M.gguf) | Q4_K_M | 8.0 | fast, recommended |
| [GGUF](https://huggingface.co/mradermacher/Swallow-13b-NVE-hf-GGUF/resolve/main/Swallow-13b-NVE-hf.Q5_K_S.gguf) | Q5_K_S | 9.1 | |
| [GGUF](https://huggingface.co/mradermacher/Swallow-13b-NVE-hf-GGUF/resolve/main/Swallow-13b-NVE-hf.Q5_K_M.gguf) | Q5_K_M | 9.3 | |
| [GGUF](https://huggingface.co/mradermacher/Swallow-13b-NVE-hf-GGUF/resolve/main/Swallow-13b-NVE-hf.Q6_K.gguf) | Q6_K | 10.8 | very good quality |
| [GGUF](https://huggingface.co/mradermacher/Swallow-13b-NVE-hf-GGUF/resolve/main/Swallow-13b-NVE-hf.Q8_0.gguf) | Q8_0 | 13.9 | fast, best quality |
Here is a handy graph by ikawrakow comparing some lower-quality quant
types (lower is better):

And here are Artefact2's thoughts on the matter:
https://gist.github.com/Artefact2/b5f810600771265fc1e39442288e8ec9
## FAQ / Model Request
See https://huggingface.co/mradermacher/model_requests for some answers to
questions you might have and/or if you want some other model quantized.
## Thanks
I thank my company, [nethype GmbH](https://www.nethype.de/), for letting
me use its servers and providing upgrades to my workstation to enable
this work in my free time.
<!-- end -->
|
duyntnet/starcoder2-7b-imatrix-GGUF | duyntnet | "2024-06-22T06:09:25Z" | 14,555 | 0 | transformers | [
"transformers",
"gguf",
"imatrix",
"starcoder2-7b",
"text-generation",
"en",
"license:other",
"region:us"
] | text-generation | "2024-06-22T03:54:05Z" | ---
license: other
language:
- en
pipeline_tag: text-generation
inference: false
tags:
- transformers
- gguf
- imatrix
- starcoder2-7b
---
Quantizations of https://huggingface.co/bigcode/starcoder2-7b
# From original readme
### Generation
Here are some examples to get started with the model. You can find a script for fine-tuning in StarCoder2's [GitHub repository](https://github.com/bigcode-project/starcoder2).
First, make sure to install `transformers` from source:
```bash
pip install git+https://github.com/huggingface/transformers.git
```
#### Running the model on CPU/GPU/multi GPU
* _Using full precision_
```python
# pip install git+https://github.com/huggingface/transformers.git # TODO: merge PR to main
from transformers import AutoModelForCausalLM, AutoTokenizer
checkpoint = "bigcode/starcoder2-7b"
device = "cuda" # for GPU usage or "cpu" for CPU usage
tokenizer = AutoTokenizer.from_pretrained(checkpoint)
# for multiple GPUs install accelerate and do `model = AutoModelForCausalLM.from_pretrained(checkpoint, device_map="auto")`
model = AutoModelForCausalLM.from_pretrained(checkpoint).to(device)
inputs = tokenizer.encode("def print_hello_world():", return_tensors="pt").to(device)
outputs = model.generate(inputs)
print(tokenizer.decode(outputs[0]))
```
```bash
>>> print(f"Memory footprint: {model.get_memory_footprint() / 1e6:.2f} MB")
Memory footprint: 29232.57 MB
```
* _Using `torch.bfloat16`_
```python
# pip install accelerate
import torch
from transformers import AutoTokenizer, AutoModelForCausalLM
checkpoint = "bigcode/starcoder2-7b"
tokenizer = AutoTokenizer.from_pretrained(checkpoint)
# for fp16 use `torch_dtype=torch.float16` instead
model = AutoModelForCausalLM.from_pretrained(checkpoint, device_map="auto", torch_dtype=torch.bfloat16)
inputs = tokenizer.encode("def print_hello_world():", return_tensors="pt").to("cuda")
outputs = model.generate(inputs)
print(tokenizer.decode(outputs[0]))
```
```bash
>>> print(f"Memory footprint: {model.get_memory_footprint() / 1e6:.2f} MB")
Memory footprint: 14616.29 MB
```
#### Quantized Versions through `bitsandbytes`
* _Using 8-bit precision (int8)_
```python
# pip install bitsandbytes accelerate
from transformers import AutoTokenizer, AutoModelForCausalLM, BitsAndBytesConfig
# to use 4bit use `load_in_4bit=True` instead
quantization_config = BitsAndBytesConfig(load_in_8bit=True)
checkpoint = "bigcode/starcoder2-7b"
tokenizer = AutoTokenizer.from_pretrained(checkpoint)
model = AutoModelForCausalLM.from_pretrained(checkpoint, quantization_config=quantization_config)
inputs = tokenizer.encode("def print_hello_world():", return_tensors="pt").to("cuda")
outputs = model.generate(inputs)
print(tokenizer.decode(outputs[0]))
```
```bash
>>> print(f"Memory footprint: {model.get_memory_footprint() / 1e6:.2f} MB")
# load_in_8bit
Memory footprint: 7670.52 MB
# load_in_4bit
>>> print(f"Memory footprint: {model.get_memory_footprint() / 1e6:.2f} MB")
Memory footprint: 4197.64 MB
``` |
mradermacher/ManagerGPT-v0.2-GGUF | mradermacher | "2024-06-28T06:56:34Z" | 14,551 | 0 | transformers | [
"transformers",
"gguf",
"en",
"base_model:ouzkaan/ManagerGPT-v0.2",
"endpoints_compatible",
"region:us"
] | null | "2024-06-28T06:30:09Z" | ---
base_model: ouzkaan/ManagerGPT-v0.2
language:
- en
library_name: transformers
quantized_by: mradermacher
tags: []
---
## About
<!-- ### quantize_version: 2 -->
<!-- ### output_tensor_quantised: 1 -->
<!-- ### convert_type: hf -->
<!-- ### vocab_type: -->
<!-- ### tags: -->
static quants of https://huggingface.co/ouzkaan/ManagerGPT-v0.2
<!-- provided-files -->
weighted/imatrix quants seem not to be available (by me) at this time. If they do not show up a week or so after the static ones, I have probably not planned for them. Feel free to request them by opening a Community Discussion.
## Usage
If you are unsure how to use GGUF files, refer to one of [TheBloke's
READMEs](https://huggingface.co/TheBloke/KafkaLM-70B-German-V0.1-GGUF) for
more details, including on how to concatenate multi-part files.
## Provided Quants
(sorted by size, not necessarily quality. IQ-quants are often preferable over similar sized non-IQ quants)
| Link | Type | Size/GB | Notes |
|:-----|:-----|--------:|:------|
| [GGUF](https://huggingface.co/mradermacher/ManagerGPT-v0.2-GGUF/resolve/main/ManagerGPT-v0.2.Q2_K.gguf) | Q2_K | 2.8 | |
| [GGUF](https://huggingface.co/mradermacher/ManagerGPT-v0.2-GGUF/resolve/main/ManagerGPT-v0.2.IQ3_XS.gguf) | IQ3_XS | 3.1 | |
| [GGUF](https://huggingface.co/mradermacher/ManagerGPT-v0.2-GGUF/resolve/main/ManagerGPT-v0.2.Q3_K_S.gguf) | Q3_K_S | 3.3 | |
| [GGUF](https://huggingface.co/mradermacher/ManagerGPT-v0.2-GGUF/resolve/main/ManagerGPT-v0.2.IQ3_S.gguf) | IQ3_S | 3.3 | beats Q3_K* |
| [GGUF](https://huggingface.co/mradermacher/ManagerGPT-v0.2-GGUF/resolve/main/ManagerGPT-v0.2.IQ3_M.gguf) | IQ3_M | 3.4 | |
| [GGUF](https://huggingface.co/mradermacher/ManagerGPT-v0.2-GGUF/resolve/main/ManagerGPT-v0.2.Q3_K_M.gguf) | Q3_K_M | 3.6 | lower quality |
| [GGUF](https://huggingface.co/mradermacher/ManagerGPT-v0.2-GGUF/resolve/main/ManagerGPT-v0.2.Q3_K_L.gguf) | Q3_K_L | 3.9 | |
| [GGUF](https://huggingface.co/mradermacher/ManagerGPT-v0.2-GGUF/resolve/main/ManagerGPT-v0.2.IQ4_XS.gguf) | IQ4_XS | 4.0 | |
| [GGUF](https://huggingface.co/mradermacher/ManagerGPT-v0.2-GGUF/resolve/main/ManagerGPT-v0.2.Q4_K_S.gguf) | Q4_K_S | 4.2 | fast, recommended |
| [GGUF](https://huggingface.co/mradermacher/ManagerGPT-v0.2-GGUF/resolve/main/ManagerGPT-v0.2.Q4_K_M.gguf) | Q4_K_M | 4.5 | fast, recommended |
| [GGUF](https://huggingface.co/mradermacher/ManagerGPT-v0.2-GGUF/resolve/main/ManagerGPT-v0.2.Q5_K_S.gguf) | Q5_K_S | 5.1 | |
| [GGUF](https://huggingface.co/mradermacher/ManagerGPT-v0.2-GGUF/resolve/main/ManagerGPT-v0.2.Q5_K_M.gguf) | Q5_K_M | 5.2 | |
| [GGUF](https://huggingface.co/mradermacher/ManagerGPT-v0.2-GGUF/resolve/main/ManagerGPT-v0.2.Q6_K.gguf) | Q6_K | 6.0 | very good quality |
| [GGUF](https://huggingface.co/mradermacher/ManagerGPT-v0.2-GGUF/resolve/main/ManagerGPT-v0.2.Q8_0.gguf) | Q8_0 | 7.8 | fast, best quality |
| [GGUF](https://huggingface.co/mradermacher/ManagerGPT-v0.2-GGUF/resolve/main/ManagerGPT-v0.2.f16.gguf) | f16 | 14.6 | 16 bpw, overkill |
Here is a handy graph by ikawrakow comparing some lower-quality quant
types (lower is better):

And here are Artefact2's thoughts on the matter:
https://gist.github.com/Artefact2/b5f810600771265fc1e39442288e8ec9
## FAQ / Model Request
See https://huggingface.co/mradermacher/model_requests for some answers to
questions you might have and/or if you want some other model quantized.
## Thanks
I thank my company, [nethype GmbH](https://www.nethype.de/), for letting
me use its servers and providing upgrades to my workstation to enable
this work in my free time.
<!-- end -->
|
mradermacher/Swallow-7b-instruct-v0.1-GGUF | mradermacher | "2024-06-30T15:36:05Z" | 14,551 | 0 | transformers | [
"transformers",
"gguf",
"en",
"ja",
"base_model:tokyotech-llm/Swallow-7b-instruct-v0.1",
"license:llama2",
"endpoints_compatible",
"region:us"
] | null | "2024-06-29T20:40:07Z" | ---
base_model: tokyotech-llm/Swallow-7b-instruct-v0.1
language:
- en
- ja
library_name: transformers
license: llama2
model_type: llama
quantized_by: mradermacher
---
## About
<!-- ### quantize_version: 2 -->
<!-- ### output_tensor_quantised: 1 -->
<!-- ### convert_type: hf -->
<!-- ### vocab_type: -->
<!-- ### tags: -->
static quants of https://huggingface.co/tokyotech-llm/Swallow-7b-instruct-v0.1
<!-- provided-files -->
weighted/imatrix quants are available at https://huggingface.co/mradermacher/Swallow-7b-instruct-v0.1-i1-GGUF
## Usage
If you are unsure how to use GGUF files, refer to one of [TheBloke's
READMEs](https://huggingface.co/TheBloke/KafkaLM-70B-German-V0.1-GGUF) for
more details, including on how to concatenate multi-part files.
## Provided Quants
(sorted by size, not necessarily quality. IQ-quants are often preferable over similar sized non-IQ quants)
| Link | Type | Size/GB | Notes |
|:-----|:-----|--------:|:------|
| [GGUF](https://huggingface.co/mradermacher/Swallow-7b-instruct-v0.1-GGUF/resolve/main/Swallow-7b-instruct-v0.1.Q2_K.gguf) | Q2_K | 2.7 | |
| [GGUF](https://huggingface.co/mradermacher/Swallow-7b-instruct-v0.1-GGUF/resolve/main/Swallow-7b-instruct-v0.1.IQ3_XS.gguf) | IQ3_XS | 3.0 | |
| [GGUF](https://huggingface.co/mradermacher/Swallow-7b-instruct-v0.1-GGUF/resolve/main/Swallow-7b-instruct-v0.1.IQ3_S.gguf) | IQ3_S | 3.1 | beats Q3_K* |
| [GGUF](https://huggingface.co/mradermacher/Swallow-7b-instruct-v0.1-GGUF/resolve/main/Swallow-7b-instruct-v0.1.Q3_K_S.gguf) | Q3_K_S | 3.1 | |
| [GGUF](https://huggingface.co/mradermacher/Swallow-7b-instruct-v0.1-GGUF/resolve/main/Swallow-7b-instruct-v0.1.IQ3_M.gguf) | IQ3_M | 3.3 | |
| [GGUF](https://huggingface.co/mradermacher/Swallow-7b-instruct-v0.1-GGUF/resolve/main/Swallow-7b-instruct-v0.1.Q3_K_M.gguf) | Q3_K_M | 3.5 | lower quality |
| [GGUF](https://huggingface.co/mradermacher/Swallow-7b-instruct-v0.1-GGUF/resolve/main/Swallow-7b-instruct-v0.1.Q3_K_L.gguf) | Q3_K_L | 3.8 | |
| [GGUF](https://huggingface.co/mradermacher/Swallow-7b-instruct-v0.1-GGUF/resolve/main/Swallow-7b-instruct-v0.1.IQ4_XS.gguf) | IQ4_XS | 3.8 | |
| [GGUF](https://huggingface.co/mradermacher/Swallow-7b-instruct-v0.1-GGUF/resolve/main/Swallow-7b-instruct-v0.1.Q4_K_S.gguf) | Q4_K_S | 4.0 | fast, recommended |
| [GGUF](https://huggingface.co/mradermacher/Swallow-7b-instruct-v0.1-GGUF/resolve/main/Swallow-7b-instruct-v0.1.Q4_K_M.gguf) | Q4_K_M | 4.2 | fast, recommended |
| [GGUF](https://huggingface.co/mradermacher/Swallow-7b-instruct-v0.1-GGUF/resolve/main/Swallow-7b-instruct-v0.1.Q5_K_S.gguf) | Q5_K_S | 4.8 | |
| [GGUF](https://huggingface.co/mradermacher/Swallow-7b-instruct-v0.1-GGUF/resolve/main/Swallow-7b-instruct-v0.1.Q5_K_M.gguf) | Q5_K_M | 5.0 | |
| [GGUF](https://huggingface.co/mradermacher/Swallow-7b-instruct-v0.1-GGUF/resolve/main/Swallow-7b-instruct-v0.1.Q6_K.gguf) | Q6_K | 5.7 | very good quality |
| [GGUF](https://huggingface.co/mradermacher/Swallow-7b-instruct-v0.1-GGUF/resolve/main/Swallow-7b-instruct-v0.1.Q8_0.gguf) | Q8_0 | 7.4 | fast, best quality |
| [GGUF](https://huggingface.co/mradermacher/Swallow-7b-instruct-v0.1-GGUF/resolve/main/Swallow-7b-instruct-v0.1.f16.gguf) | f16 | 13.8 | 16 bpw, overkill |
Here is a handy graph by ikawrakow comparing some lower-quality quant
types (lower is better):

And here are Artefact2's thoughts on the matter:
https://gist.github.com/Artefact2/b5f810600771265fc1e39442288e8ec9
## FAQ / Model Request
See https://huggingface.co/mradermacher/model_requests for some answers to
questions you might have and/or if you want some other model quantized.
## Thanks
I thank my company, [nethype GmbH](https://www.nethype.de/), for letting
me use its servers and providing upgrades to my workstation to enable
this work in my free time.
<!-- end -->
|
HuggingFaceH4/mistral-7b-sft-beta | HuggingFaceH4 | "2023-10-26T14:26:06Z" | 14,549 | 23 | transformers | [
"transformers",
"pytorch",
"tensorboard",
"mistral",
"text-generation",
"generated_from_trainer",
"conversational",
"en",
"dataset:HuggingFaceH4/ultrachat_200k",
"base_model:mistralai/Mistral-7B-v0.1",
"license:mit",
"autotrain_compatible",
"endpoints_compatible",
"text-generation-inference",
"region:us"
] | text-generation | "2023-10-26T13:43:58Z" | ---
license: mit
base_model: mistralai/Mistral-7B-v0.1
tags:
- generated_from_trainer
model-index:
- name: mistral-7b-sft-beta
results: []
datasets:
- HuggingFaceH4/ultrachat_200k
language:
- en
---
<!-- This model card has been generated automatically according to the information the Trainer had access to. You
should probably proofread and complete it, then remove this comment. -->
# Model Card for Mistral 7B SFT β
This model is a fine-tuned version of [mistralai/Mistral-7B-v0.1](https://huggingface.co/mistralai/Mistral-7B-v0.1) on the HuggingFaceH4/ultrachat_200k dataset. It is the SFT model that was used to train Zephyr-7B-β with Direct Preference Optimization.
It achieves the following results on the evaluation set:
- Loss: 0.9399
## Model description
- **Model type:** A 7B parameter GPT-like model fine-tuned on a mix of publicly available, synthetic datasets.
- **Language(s) (NLP):** Primarily English
- **License:** MIT
- **Finetuned from model:** [mistralai/Mistral-7B-v0.1](https://huggingface.co/mistralai/Mistral-7B-v0.1)
### Model Sources
<!-- Provide the basic links for the model. -->
- **Repository:** https://github.com/huggingface/alignment-handbook
## Intended uses & limitations
The model was fine-tuned with [🤗 TRL's](https://github.com/huggingface/trl) `SFTTrainer` on a filtered and preprocessed of the [`UltraChat`](https://huggingface.co/datasets/stingning/ultrachat) dataset, which contains a diverse range of synthetic dialogues generated by ChatGPT.
Here's how you can run the model using the `pipeline()` function from 🤗 Transformers:
```python
# Install transformers from source - only needed for versions <= v4.34
# pip install git+https://github.com/huggingface/transformers.git
# pip install accelerate
import torch
from transformers import pipeline
pipe = pipeline("text-generation", model="HuggingFaceH4/mistral-7b-sft-beta", torch_dtype=torch.bfloat16, device_map="auto")
# We use the tokenizer's chat template to format each message - see https://huggingface.co/docs/transformers/main/en/chat_templating
messages = [
{
"role": "system",
"content": "You are a friendly chatbot who always responds in the style of a pirate",
},
{"role": "user", "content": "How many helicopters can a human eat in one sitting?"},
]
prompt = pipe.tokenizer.apply_chat_template(messages, tokenize=False, add_generation_prompt=True)
outputs = pipe(prompt, max_new_tokens=256, do_sample=True, temperature=0.7, top_k=50, top_p=0.95)
print(outputs[0]["generated_text"])
# <|system|>
# You are a friendly chatbot who always responds in the style of a pirate.</s>
# <|user|>
# How many helicopters can a human eat in one sitting?</s>
# <|assistant|>
# Ah, me hearty matey! But yer question be a puzzler! A human cannot eat a helicopter in one sitting, as helicopters are not edible. They be made of metal, plastic, and other materials, not food!
```
## Training procedure
### Training hyperparameters
The following hyperparameters were used during training:
- learning_rate: 2e-05
- train_batch_size: 8
- eval_batch_size: 16
- seed: 42
- distributed_type: multi-GPU
- num_devices: 16
- gradient_accumulation_steps: 4
- total_train_batch_size: 512
- total_eval_batch_size: 256
- optimizer: Adam with betas=(0.9,0.999) and epsilon=1e-08
- lr_scheduler_type: cosine
- lr_scheduler_warmup_ratio: 0.1
- num_epochs: 1
### Training results
| Training Loss | Epoch | Step | Validation Loss |
|:-------------:|:-----:|:----:|:---------------:|
| 0.9367 | 0.67 | 272 | 0.9397 |
### Framework versions
- Transformers 4.35.0.dev0
- Pytorch 2.0.1+cu118
- Datasets 2.12.0
- Tokenizers 0.14.0 |
laiking/bert-base-german-cased-gnad10 | laiking | "2023-10-09T08:26:38Z" | 14,546 | 2 | transformers | [
"transformers",
"pytorch",
"safetensors",
"bert",
"text-classification",
"german-news-classification",
"de",
"dataset:gnad10",
"model-index",
"autotrain_compatible",
"endpoints_compatible",
"region:us"
] | text-classification | "2022-03-02T23:29:04Z" | ---
language:
- de
tags:
- text-classification
- german-news-classification
datasets:
- gnad10
metrics:
- accuracy
- precision
- recall
- f1
model-index:
- name: Mathking/bert-base-german-cased-gnad10
results:
- task:
type: text-classification
name: Text Classification
dataset:
name: gnad10
type: gnad10
config: default
split: train
metrics:
- type: accuracy
value: 0.9557598702001082
name: Accuracy
verified: true
verifyToken: eyJhbGciOiJFZERTQSIsInR5cCI6IkpXVCJ9.eyJoYXNoIjoiNTkxNjAwNTYzYjRjZmQ0M2UxMWQzYzk0YWFjZjRmYzcwNGEyYmRiNDIwNTlmNDNhYjAzNzBmNzU5MTg3MTM1ZSIsInZlcnNpb24iOjF9.1KfABx9YVvR2QiSXwtCBV8ijYGqwiQD3N3i7c1KV2Ke9tQvWA4_HnN7wvCKokESR-zEwIHWfALSveWIgoiSNBg
- type: f1
value: 0.9550736462647613
name: F1 Macro
verified: true
verifyToken: eyJhbGciOiJFZERTQSIsInR5cCI6IkpXVCJ9.eyJoYXNoIjoiZDNkYjU0NzAxNjBlOGQ1MWU2OGE5NWFkOGFlNTYwZGFkNTRiMDcwNDRlYmNiMTUxMzViM2Q4MmUyMjU2ZTQwYyIsInZlcnNpb24iOjF9.E9ysIc4ZYrpOpQTJsmLRN1q8Pg-5pWLlvs8WbTeJy2JYNmpBNblaGyeiHckZ8g8gD3Rqv7W9inpivmHRcI4-BQ
- type: f1
value: 0.9557598702001082
name: F1 Micro
verified: true
verifyToken: eyJhbGciOiJFZERTQSIsInR5cCI6IkpXVCJ9.eyJoYXNoIjoiNWMxNmVjMjYyNTAxYmYwN2YxNjAzOWQ2MDY3OGRhYzE4NWYwYTUyNjRhNmU2M2Y3MzFiYzI2ZTk4YWQ3NGNkNSIsInZlcnNpb24iOjF9.csdfLvORGZJY11TbWzylKfhz53BAncrjNgCDIGtWzK1AtJutkJj-SQo8rEd9o3Z5BKlH3Ta28O3Y7wKoc4PuDQ
- type: f1
value: 0.9556789875763837
name: F1 Weighted
verified: true
verifyToken: eyJhbGciOiJFZERTQSIsInR5cCI6IkpXVCJ9.eyJoYXNoIjoiY2I1ZmNjMzViMDY1YWMyNzRkNDY0OTY1YTFkZWViN2JiMDlkMjJjNTZmZDFjZDIxZjA0YzI1NThiODUwMDlhZiIsInZlcnNpb24iOjF9.83yH-SfIAeB9Y3XNPcnn8N3g9puooZRgcBfNMeAKNqNM93U1qEE6JjFvhZBO_UU05cgfqnPp7Pt6h-JQcmdwBA
- type: precision
value: 0.953834169384936
name: Precision Macro
verified: true
verifyToken: eyJhbGciOiJFZERTQSIsInR5cCI6IkpXVCJ9.eyJoYXNoIjoiYjQ4YjA2MTZlMmYxMTA4ZTM5MDU1NjI3ZWE4YTBiZDBhMDUwN2FiODZkNjM5OWNiNGU2NjU5ZDE0OTUyODZmNyIsInZlcnNpb24iOjF9.sWcghxM9DeaaldnXR5sLz8KUHVhdjJ8GY_c4f-kZ0-0BDzf4CYURUVziWnlrRTjlUH-hVyfdKd1ufHvLotRgCg
- type: precision
value: 0.9557598702001082
name: Precision Micro
verified: true
verifyToken: eyJhbGciOiJFZERTQSIsInR5cCI6IkpXVCJ9.eyJoYXNoIjoiOWIzZmNlZTcxNzhhMzZhNWQ1ZWI4YzZjMDYyOTMwY2Q5N2EwMzFhMzE4OTFkZjg1NTIyYjVkMGNjZDYwZmQ2YSIsInZlcnNpb24iOjF9.rQ7ZIKeP25hLfHaYdPqX-VZCHoL-YohqGV9NZ-TAIHvNQbj0lPpX_nS89cJ1C0tSoHCeP14lIOWNncRJzQOOCA
- type: precision
value: 0.9558822798145145
name: Precision Weighted
verified: true
verifyToken: eyJhbGciOiJFZERTQSIsInR5cCI6IkpXVCJ9.eyJoYXNoIjoiZDQzOTMxMGQ4YTI5MDUzNjdhNzdjY2QzNGVlNzUyODE4ZTI1MTY4NTkxZDVhMTBjZjhhMjlmNzRiNjEyOTk3NiIsInZlcnNpb24iOjF9.DWBZXL1mP7oNYQJKCORItDvkZm-l7TcIETNjdeVyS0BnxoEbqEE22OOJwnGLAk-wHtfx7jEKAA7ijQ1qF7cfAg
- type: recall
value: 0.956651983810566
name: Recall Macro
verified: true
verifyToken: eyJhbGciOiJFZERTQSIsInR5cCI6IkpXVCJ9.eyJoYXNoIjoiNTFhYTUyZWQ0N2VhOWQxMjY0MGM1ZjExOGE4NDQ5ODMzMmQ5YThkZTYzZjg0YmUwMDhlZDllMDk3MzY2ZWUzZSIsInZlcnNpb24iOjF9.H7UhmKtJ_5FZOQmZP-wPTrHHde-XxtMAj3kluHz6-8P1KOwJkxk24Lu7vTwHf3564XtnJC8eW2C5uyWDTpcgBg
- type: recall
value: 0.9557598702001082
name: Recall Micro
verified: true
verifyToken: eyJhbGciOiJFZERTQSIsInR5cCI6IkpXVCJ9.eyJoYXNoIjoiZGY1MWZkOWYzNjg1NGU5YmFmODY2MDNjYWQ3OTUwNTgzMWRlZGUwNzU5NDY2NzFjZTMxOTBiMWVhZWIyNDYzMCIsInZlcnNpb24iOjF9.oKQ0zRYEs-sloah-BJvBKX5SFqWt8UX-0jCi3ldaLwNVJjM-rcdvsERyoYQ-QTLPKsZp4nko3-ic-BDCwGp9Bw
- type: recall
value: 0.9557598702001082
name: Recall Weighted
verified: true
verifyToken: eyJhbGciOiJFZERTQSIsInR5cCI6IkpXVCJ9.eyJoYXNoIjoiNDlhMmIwOTBkOTIzOTlkZjNiMzlkMmE5NzQ3MzY5NTUxODQyMzY1OTJjNWY4NjI0N2NjYmY5NjkwZjU0MTA1YyIsInZlcnNpb24iOjF9.4FExU6skNNcvIrToS3MR04Q7ho7_PITTqPk8WMdOggaVvnwj8ujxcXyJMSRioQ1ttVlpg_oGismsSD9zttYkBg
- type: loss
value: 0.17337004840373993
name: loss
verified: true
verifyToken: eyJhbGciOiJFZERTQSIsInR5cCI6IkpXVCJ9.eyJoYXNoIjoiMzVmMmQ5OGE0OTU3MTg0NDg4YzhlODU1NWUyODM0NzFjODM3MTY5MWI2OTAyMzU5OTQ2YTljZTJkN2JkYTcyNSIsInZlcnNpb24iOjF9.jeYTrX35vtswkWi8ROqynY_W4rHfxonic74PviTNAKJzTF7tUCI2a9IBavXvSQhMfGv0NEkZzX8N8o4hQTvWDw
---
# German BERT for News Classification
This a bert-base-german-cased model finetuned for text classification on german news articles
## Training data
Used the training set from the 10KGNAD dataset (gnad10 on HuggingFace Datasets). |
THUDM/chatglm-6b | THUDM | "2023-09-04T15:49:45Z" | 14,538 | 2,801 | transformers | [
"transformers",
"pytorch",
"chatglm",
"glm",
"thudm",
"custom_code",
"zh",
"en",
"arxiv:2103.10360",
"arxiv:2210.02414",
"endpoints_compatible",
"region:us"
] | null | "2023-03-13T16:28:04Z" | ---
language:
- zh
- en
tags:
- glm
- chatglm
- thudm
---
# ChatGLM-6B
<p align="center">
🌐 <a href="https://chatglm.cn/blog" target="_blank">Blog</a> • 💻 <a href="https://github.com/THUDM/ChatGLM-6B" target="_blank">Github Repo</a> • 🐦 <a href="https://twitter.com/thukeg" target="_blank">Twitter</a> • 📃 <a href="https://arxiv.org/abs/2103.10360" target="_blank">[GLM@ACL 22]</a> <a href="https://github.com/THUDM/GLM" target="_blank">[GitHub]</a> • 📃 <a href="https://arxiv.org/abs/2210.02414" target="_blank">[GLM-130B@ICLR 23]</a> <a href="https://github.com/THUDM/GLM-130B" target="_blank">[GitHub]</a> <br>
</p>
<p align="center">
👋 Join our <a href="https://join.slack.com/t/chatglm/shared_invite/zt-1y7pqoloy-9b1g6T6JjA8J0KxvUjbwJw" target="_blank">Slack</a> and <a href="https://github.com/THUDM/ChatGLM-6B/blob/main/resources/WECHAT.md" target="_blank">WeChat</a>
</p>
<p align="center">
📍Experience the larger-scale ChatGLM model at <a href="https://www.chatglm.cn">chatglm.cn</a>
</p>
**我们发布了 [ChatGLM2-6B](https://github.com/THUDM/ChatGLM2-6B),ChatGLM-6B 的升级版本,在保留了了初代模型对话流畅、部署门槛较低等众多优秀特性的基础之上,引入了更强大的性能、更长的上下文、更高效的推理等升级。**
## 介绍
ChatGLM-6B 是一个开源的、支持中英双语问答的对话语言模型,基于 [General Language Model (GLM)](https://github.com/THUDM/GLM) 架构,具有 62 亿参数。结合模型量化技术,用户可以在消费级的显卡上进行本地部署(INT4 量化级别下最低只需 6GB 显存)。ChatGLM-6B 使用了和 [ChatGLM](https://chatglm.cn) 相同的技术,针对中文问答和对话进行了优化。经过约 1T 标识符的中英双语训练,辅以监督微调、反馈自助、人类反馈强化学习等技术的加持,62 亿参数的 ChatGLM-6B 已经能生成相当符合人类偏好的回答。 ChatGLM-6B 权重对学术研究**完全开放**,在填写[问卷](https://open.bigmodel.cn/mla/form)进行登记后**亦允许免费商业使用**。
ChatGLM-6B is an open bilingual language model based on [General Language Model (GLM)](https://github.com/THUDM/GLM) framework, with 6.2 billion parameters. With the quantization technique, users can deploy locally on consumer-grade graphics cards (only 6GB of GPU memory is required at the INT4 quantization level). ChatGLM-6B uses technology similar to ChatGPT, optimized for Chinese QA and dialogue. The model is trained for about 1T tokens of Chinese and English corpus, supplemented by supervised fine-tuning, feedback bootstrap, and reinforcement learning with human feedback. With only about 6.2 billion parameters, the model is able to generate answers that are in line with human preference. ChatGLM-6B weights are **completely open** for academic research, and **free commercial use** is also allowed after completing the [questionnaire](https://open.bigmodel.cn/mla/form).
## 软件依赖
```shell
pip install protobuf==3.20.0 transformers==4.27.1 icetk cpm_kernels
```
## 代码调用
可以通过如下代码调用 ChatGLM-6B 模型来生成对话:
```ipython
>>> from transformers import AutoTokenizer, AutoModel
>>> tokenizer = AutoTokenizer.from_pretrained("THUDM/chatglm-6b", trust_remote_code=True)
>>> model = AutoModel.from_pretrained("THUDM/chatglm-6b", trust_remote_code=True).half().cuda()
>>> response, history = model.chat(tokenizer, "你好", history=[])
>>> print(response)
你好👋!我是人工智能助手 ChatGLM-6B,很高兴见到你,欢迎问我任何问题。
>>> response, history = model.chat(tokenizer, "晚上睡不着应该怎么办", history=history)
>>> print(response)
晚上睡不着可能会让你感到焦虑或不舒服,但以下是一些可以帮助你入睡的方法:
1. 制定规律的睡眠时间表:保持规律的睡眠时间表可以帮助你建立健康的睡眠习惯,使你更容易入睡。尽量在每天的相同时间上床,并在同一时间起床。
2. 创造一个舒适的睡眠环境:确保睡眠环境舒适,安静,黑暗且温度适宜。可以使用舒适的床上用品,并保持房间通风。
3. 放松身心:在睡前做些放松的活动,例如泡个热水澡,听些轻柔的音乐,阅读一些有趣的书籍等,有助于缓解紧张和焦虑,使你更容易入睡。
4. 避免饮用含有咖啡因的饮料:咖啡因是一种刺激性物质,会影响你的睡眠质量。尽量避免在睡前饮用含有咖啡因的饮料,例如咖啡,茶和可乐。
5. 避免在床上做与睡眠无关的事情:在床上做些与睡眠无关的事情,例如看电影,玩游戏或工作等,可能会干扰你的睡眠。
6. 尝试呼吸技巧:深呼吸是一种放松技巧,可以帮助你缓解紧张和焦虑,使你更容易入睡。试着慢慢吸气,保持几秒钟,然后缓慢呼气。
如果这些方法无法帮助你入睡,你可以考虑咨询医生或睡眠专家,寻求进一步的建议。
```
关于更多的使用说明,包括如何运行命令行和网页版本的 DEMO,以及使用模型量化以节省显存,请参考我们的 [Github Repo](https://github.com/THUDM/ChatGLM-6B)。
For more instructions, including how to run CLI and web demos, and model quantization, please refer to our [Github Repo](https://github.com/THUDM/ChatGLM-6B).
## Change Log
* v1.1.0 ([942945d](https://huggingface.co/THUDM/chatglm-6b/commit/942945df047dee66f653c68ae0e56655045f1741)): 更新 v1.1 版本 checkpoint
* v0.1.0 ([f831824](https://huggingface.co/THUDM/chatglm-6b/commit/f83182484538e663a03d3f73647f10f89878f438))
## 协议
本仓库的代码依照 [Apache-2.0](LICENSE) 协议开源,ChatGLM-6B 模型的权重的使用则需要遵循 [Model License](MODEL_LICENSE)。
## 引用
如果你觉得我们的工作有帮助的话,请考虑引用下列论文:
```
@inproceedings{
zeng2023glm-130b,
title={{GLM}-130B: An Open Bilingual Pre-trained Model},
author={Aohan Zeng and Xiao Liu and Zhengxiao Du and Zihan Wang and Hanyu Lai and Ming Ding and Zhuoyi Yang and Yifan Xu and Wendi Zheng and Xiao Xia and Weng Lam Tam and Zixuan Ma and Yufei Xue and Jidong Zhai and Wenguang Chen and Zhiyuan Liu and Peng Zhang and Yuxiao Dong and Jie Tang},
booktitle={The Eleventh International Conference on Learning Representations (ICLR)},
year={2023},
url={https://openreview.net/forum?id=-Aw0rrrPUF}
}
```
```
@inproceedings{du2022glm,
title={GLM: General Language Model Pretraining with Autoregressive Blank Infilling},
author={Du, Zhengxiao and Qian, Yujie and Liu, Xiao and Ding, Ming and Qiu, Jiezhong and Yang, Zhilin and Tang, Jie},
booktitle={Proceedings of the 60th Annual Meeting of the Association for Computational Linguistics (Volume 1: Long Papers)},
pages={320--335},
year={2022}
}
``` |
mradermacher/Nova-13B-i1-GGUF | mradermacher | "2024-06-24T18:21:13Z" | 14,475 | 0 | transformers | [
"transformers",
"gguf",
"en",
"dataset:garage-bAInd/Open-Platypus",
"base_model:Weyaxi/Nova-13B",
"license:cc-by-nc-4.0",
"endpoints_compatible",
"region:us"
] | null | "2024-06-24T16:15:37Z" | ---
base_model: Weyaxi/Nova-13B
datasets:
- garage-bAInd/Open-Platypus
language:
- en
library_name: transformers
license: cc-by-nc-4.0
quantized_by: mradermacher
---
## About
<!-- ### quantize_version: 2 -->
<!-- ### output_tensor_quantised: 1 -->
<!-- ### convert_type: hf -->
<!-- ### vocab_type: -->
<!-- ### tags: nicoboss -->
weighted/imatrix quants of https://huggingface.co/Weyaxi/Nova-13B
<!-- provided-files -->
static quants are available at https://huggingface.co/mradermacher/Nova-13B-GGUF
## Usage
If you are unsure how to use GGUF files, refer to one of [TheBloke's
READMEs](https://huggingface.co/TheBloke/KafkaLM-70B-German-V0.1-GGUF) for
more details, including on how to concatenate multi-part files.
## Provided Quants
(sorted by size, not necessarily quality. IQ-quants are often preferable over similar sized non-IQ quants)
| Link | Type | Size/GB | Notes |
|:-----|:-----|--------:|:------|
| [GGUF](https://huggingface.co/mradermacher/Nova-13B-i1-GGUF/resolve/main/Nova-13B.i1-IQ1_S.gguf) | i1-IQ1_S | 3.0 | for the desperate |
| [GGUF](https://huggingface.co/mradermacher/Nova-13B-i1-GGUF/resolve/main/Nova-13B.i1-IQ1_M.gguf) | i1-IQ1_M | 3.2 | mostly desperate |
| [GGUF](https://huggingface.co/mradermacher/Nova-13B-i1-GGUF/resolve/main/Nova-13B.i1-IQ2_XXS.gguf) | i1-IQ2_XXS | 3.6 | |
| [GGUF](https://huggingface.co/mradermacher/Nova-13B-i1-GGUF/resolve/main/Nova-13B.i1-IQ2_XS.gguf) | i1-IQ2_XS | 4.0 | |
| [GGUF](https://huggingface.co/mradermacher/Nova-13B-i1-GGUF/resolve/main/Nova-13B.i1-IQ2_S.gguf) | i1-IQ2_S | 4.3 | |
| [GGUF](https://huggingface.co/mradermacher/Nova-13B-i1-GGUF/resolve/main/Nova-13B.i1-IQ2_M.gguf) | i1-IQ2_M | 4.6 | |
| [GGUF](https://huggingface.co/mradermacher/Nova-13B-i1-GGUF/resolve/main/Nova-13B.i1-Q2_K.gguf) | i1-Q2_K | 5.0 | IQ3_XXS probably better |
| [GGUF](https://huggingface.co/mradermacher/Nova-13B-i1-GGUF/resolve/main/Nova-13B.i1-IQ3_XXS.gguf) | i1-IQ3_XXS | 5.1 | lower quality |
| [GGUF](https://huggingface.co/mradermacher/Nova-13B-i1-GGUF/resolve/main/Nova-13B.i1-IQ3_XS.gguf) | i1-IQ3_XS | 5.5 | |
| [GGUF](https://huggingface.co/mradermacher/Nova-13B-i1-GGUF/resolve/main/Nova-13B.i1-IQ3_S.gguf) | i1-IQ3_S | 5.8 | beats Q3_K* |
| [GGUF](https://huggingface.co/mradermacher/Nova-13B-i1-GGUF/resolve/main/Nova-13B.i1-Q3_K_S.gguf) | i1-Q3_K_S | 5.8 | IQ3_XS probably better |
| [GGUF](https://huggingface.co/mradermacher/Nova-13B-i1-GGUF/resolve/main/Nova-13B.i1-IQ3_M.gguf) | i1-IQ3_M | 6.1 | |
| [GGUF](https://huggingface.co/mradermacher/Nova-13B-i1-GGUF/resolve/main/Nova-13B.i1-Q3_K_M.gguf) | i1-Q3_K_M | 6.4 | IQ3_S probably better |
| [GGUF](https://huggingface.co/mradermacher/Nova-13B-i1-GGUF/resolve/main/Nova-13B.i1-Q3_K_L.gguf) | i1-Q3_K_L | 7.0 | IQ3_M probably better |
| [GGUF](https://huggingface.co/mradermacher/Nova-13B-i1-GGUF/resolve/main/Nova-13B.i1-IQ4_XS.gguf) | i1-IQ4_XS | 7.1 | |
| [GGUF](https://huggingface.co/mradermacher/Nova-13B-i1-GGUF/resolve/main/Nova-13B.i1-Q4_0.gguf) | i1-Q4_0 | 7.5 | fast, low quality |
| [GGUF](https://huggingface.co/mradermacher/Nova-13B-i1-GGUF/resolve/main/Nova-13B.i1-Q4_K_S.gguf) | i1-Q4_K_S | 7.5 | optimal size/speed/quality |
| [GGUF](https://huggingface.co/mradermacher/Nova-13B-i1-GGUF/resolve/main/Nova-13B.i1-Q4_K_M.gguf) | i1-Q4_K_M | 8.0 | fast, recommended |
| [GGUF](https://huggingface.co/mradermacher/Nova-13B-i1-GGUF/resolve/main/Nova-13B.i1-Q5_K_S.gguf) | i1-Q5_K_S | 9.1 | |
| [GGUF](https://huggingface.co/mradermacher/Nova-13B-i1-GGUF/resolve/main/Nova-13B.i1-Q5_K_M.gguf) | i1-Q5_K_M | 9.3 | |
| [GGUF](https://huggingface.co/mradermacher/Nova-13B-i1-GGUF/resolve/main/Nova-13B.i1-Q6_K.gguf) | i1-Q6_K | 10.8 | practically like static Q6_K |
Here is a handy graph by ikawrakow comparing some lower-quality quant
types (lower is better):

And here are Artefact2's thoughts on the matter:
https://gist.github.com/Artefact2/b5f810600771265fc1e39442288e8ec9
## FAQ / Model Request
See https://huggingface.co/mradermacher/model_requests for some answers to
questions you might have and/or if you want some other model quantized.
## Thanks
I thank my company, [nethype GmbH](https://www.nethype.de/), for letting
me use its servers and providing upgrades to my workstation to enable
this work in my free time. Additional thanks to [@nicoboss](https://huggingface.co/nicoboss) for giving me access to his hardware for calculating the imatrix for these quants.
<!-- end -->
|
RichardErkhov/LoftQ_-_Llama-2-7b-hf-2bit-64rank-gguf | RichardErkhov | "2024-06-30T00:19:40Z" | 14,471 | 0 | null | [
"gguf",
"region:us"
] | null | "2024-06-29T13:36:39Z" | Quantization made by Richard Erkhov.
[Github](https://github.com/RichardErkhov)
[Discord](https://discord.gg/pvy7H8DZMG)
[Request more models](https://github.com/RichardErkhov/quant_request)
Llama-2-7b-hf-2bit-64rank - GGUF
- Model creator: https://huggingface.co/LoftQ/
- Original model: https://huggingface.co/LoftQ/Llama-2-7b-hf-2bit-64rank/
| Name | Quant method | Size |
| ---- | ---- | ---- |
| [Llama-2-7b-hf-2bit-64rank.Q2_K.gguf](https://huggingface.co/RichardErkhov/LoftQ_-_Llama-2-7b-hf-2bit-64rank-gguf/blob/main/Llama-2-7b-hf-2bit-64rank.Q2_K.gguf) | Q2_K | 2.36GB |
| [Llama-2-7b-hf-2bit-64rank.IQ3_XS.gguf](https://huggingface.co/RichardErkhov/LoftQ_-_Llama-2-7b-hf-2bit-64rank-gguf/blob/main/Llama-2-7b-hf-2bit-64rank.IQ3_XS.gguf) | IQ3_XS | 2.6GB |
| [Llama-2-7b-hf-2bit-64rank.IQ3_S.gguf](https://huggingface.co/RichardErkhov/LoftQ_-_Llama-2-7b-hf-2bit-64rank-gguf/blob/main/Llama-2-7b-hf-2bit-64rank.IQ3_S.gguf) | IQ3_S | 2.75GB |
| [Llama-2-7b-hf-2bit-64rank.Q3_K_S.gguf](https://huggingface.co/RichardErkhov/LoftQ_-_Llama-2-7b-hf-2bit-64rank-gguf/blob/main/Llama-2-7b-hf-2bit-64rank.Q3_K_S.gguf) | Q3_K_S | 2.75GB |
| [Llama-2-7b-hf-2bit-64rank.IQ3_M.gguf](https://huggingface.co/RichardErkhov/LoftQ_-_Llama-2-7b-hf-2bit-64rank-gguf/blob/main/Llama-2-7b-hf-2bit-64rank.IQ3_M.gguf) | IQ3_M | 2.9GB |
| [Llama-2-7b-hf-2bit-64rank.Q3_K.gguf](https://huggingface.co/RichardErkhov/LoftQ_-_Llama-2-7b-hf-2bit-64rank-gguf/blob/main/Llama-2-7b-hf-2bit-64rank.Q3_K.gguf) | Q3_K | 3.07GB |
| [Llama-2-7b-hf-2bit-64rank.Q3_K_M.gguf](https://huggingface.co/RichardErkhov/LoftQ_-_Llama-2-7b-hf-2bit-64rank-gguf/blob/main/Llama-2-7b-hf-2bit-64rank.Q3_K_M.gguf) | Q3_K_M | 3.07GB |
| [Llama-2-7b-hf-2bit-64rank.Q3_K_L.gguf](https://huggingface.co/RichardErkhov/LoftQ_-_Llama-2-7b-hf-2bit-64rank-gguf/blob/main/Llama-2-7b-hf-2bit-64rank.Q3_K_L.gguf) | Q3_K_L | 3.35GB |
| [Llama-2-7b-hf-2bit-64rank.IQ4_XS.gguf](https://huggingface.co/RichardErkhov/LoftQ_-_Llama-2-7b-hf-2bit-64rank-gguf/blob/main/Llama-2-7b-hf-2bit-64rank.IQ4_XS.gguf) | IQ4_XS | 3.4GB |
| [Llama-2-7b-hf-2bit-64rank.Q4_0.gguf](https://huggingface.co/RichardErkhov/LoftQ_-_Llama-2-7b-hf-2bit-64rank-gguf/blob/main/Llama-2-7b-hf-2bit-64rank.Q4_0.gguf) | Q4_0 | 3.56GB |
| [Llama-2-7b-hf-2bit-64rank.IQ4_NL.gguf](https://huggingface.co/RichardErkhov/LoftQ_-_Llama-2-7b-hf-2bit-64rank-gguf/blob/main/Llama-2-7b-hf-2bit-64rank.IQ4_NL.gguf) | IQ4_NL | 3.58GB |
| [Llama-2-7b-hf-2bit-64rank.Q4_K_S.gguf](https://huggingface.co/RichardErkhov/LoftQ_-_Llama-2-7b-hf-2bit-64rank-gguf/blob/main/Llama-2-7b-hf-2bit-64rank.Q4_K_S.gguf) | Q4_K_S | 3.59GB |
| [Llama-2-7b-hf-2bit-64rank.Q4_K.gguf](https://huggingface.co/RichardErkhov/LoftQ_-_Llama-2-7b-hf-2bit-64rank-gguf/blob/main/Llama-2-7b-hf-2bit-64rank.Q4_K.gguf) | Q4_K | 3.8GB |
| [Llama-2-7b-hf-2bit-64rank.Q4_K_M.gguf](https://huggingface.co/RichardErkhov/LoftQ_-_Llama-2-7b-hf-2bit-64rank-gguf/blob/main/Llama-2-7b-hf-2bit-64rank.Q4_K_M.gguf) | Q4_K_M | 3.8GB |
| [Llama-2-7b-hf-2bit-64rank.Q4_1.gguf](https://huggingface.co/RichardErkhov/LoftQ_-_Llama-2-7b-hf-2bit-64rank-gguf/blob/main/Llama-2-7b-hf-2bit-64rank.Q4_1.gguf) | Q4_1 | 3.95GB |
| [Llama-2-7b-hf-2bit-64rank.Q5_0.gguf](https://huggingface.co/RichardErkhov/LoftQ_-_Llama-2-7b-hf-2bit-64rank-gguf/blob/main/Llama-2-7b-hf-2bit-64rank.Q5_0.gguf) | Q5_0 | 4.33GB |
| [Llama-2-7b-hf-2bit-64rank.Q5_K_S.gguf](https://huggingface.co/RichardErkhov/LoftQ_-_Llama-2-7b-hf-2bit-64rank-gguf/blob/main/Llama-2-7b-hf-2bit-64rank.Q5_K_S.gguf) | Q5_K_S | 4.33GB |
| [Llama-2-7b-hf-2bit-64rank.Q5_K.gguf](https://huggingface.co/RichardErkhov/LoftQ_-_Llama-2-7b-hf-2bit-64rank-gguf/blob/main/Llama-2-7b-hf-2bit-64rank.Q5_K.gguf) | Q5_K | 4.45GB |
| [Llama-2-7b-hf-2bit-64rank.Q5_K_M.gguf](https://huggingface.co/RichardErkhov/LoftQ_-_Llama-2-7b-hf-2bit-64rank-gguf/blob/main/Llama-2-7b-hf-2bit-64rank.Q5_K_M.gguf) | Q5_K_M | 4.45GB |
| [Llama-2-7b-hf-2bit-64rank.Q5_1.gguf](https://huggingface.co/RichardErkhov/LoftQ_-_Llama-2-7b-hf-2bit-64rank-gguf/blob/main/Llama-2-7b-hf-2bit-64rank.Q5_1.gguf) | Q5_1 | 4.72GB |
| [Llama-2-7b-hf-2bit-64rank.Q6_K.gguf](https://huggingface.co/RichardErkhov/LoftQ_-_Llama-2-7b-hf-2bit-64rank-gguf/blob/main/Llama-2-7b-hf-2bit-64rank.Q6_K.gguf) | Q6_K | 5.15GB |
| [Llama-2-7b-hf-2bit-64rank.Q8_0.gguf](https://huggingface.co/RichardErkhov/LoftQ_-_Llama-2-7b-hf-2bit-64rank-gguf/blob/main/Llama-2-7b-hf-2bit-64rank.Q8_0.gguf) | Q8_0 | 6.67GB |
Original model description:
Entry not found
|
mradermacher/Jellyfish-8B-GGUF | mradermacher | "2024-06-25T07:12:31Z" | 14,468 | 0 | transformers | [
"transformers",
"gguf",
"en",
"base_model:NECOUDBFM/Jellyfish-8B",
"license:cc-by-nc-4.0",
"endpoints_compatible",
"region:us"
] | null | "2024-06-25T03:11:39Z" | ---
base_model: NECOUDBFM/Jellyfish-8B
language:
- en
library_name: transformers
license: cc-by-nc-4.0
quantized_by: mradermacher
---
## About
<!-- ### quantize_version: 2 -->
<!-- ### output_tensor_quantised: 1 -->
<!-- ### convert_type: hf -->
<!-- ### vocab_type: -->
<!-- ### tags: -->
static quants of https://huggingface.co/NECOUDBFM/Jellyfish-8B
<!-- provided-files -->
weighted/imatrix quants are available at https://huggingface.co/mradermacher/Jellyfish-8B-i1-GGUF
## Usage
If you are unsure how to use GGUF files, refer to one of [TheBloke's
READMEs](https://huggingface.co/TheBloke/KafkaLM-70B-German-V0.1-GGUF) for
more details, including on how to concatenate multi-part files.
## Provided Quants
(sorted by size, not necessarily quality. IQ-quants are often preferable over similar sized non-IQ quants)
| Link | Type | Size/GB | Notes |
|:-----|:-----|--------:|:------|
| [GGUF](https://huggingface.co/mradermacher/Jellyfish-8B-GGUF/resolve/main/Jellyfish-8B.Q2_K.gguf) | Q2_K | 3.3 | |
| [GGUF](https://huggingface.co/mradermacher/Jellyfish-8B-GGUF/resolve/main/Jellyfish-8B.IQ3_XS.gguf) | IQ3_XS | 3.6 | |
| [GGUF](https://huggingface.co/mradermacher/Jellyfish-8B-GGUF/resolve/main/Jellyfish-8B.Q3_K_S.gguf) | Q3_K_S | 3.8 | |
| [GGUF](https://huggingface.co/mradermacher/Jellyfish-8B-GGUF/resolve/main/Jellyfish-8B.IQ3_S.gguf) | IQ3_S | 3.8 | beats Q3_K* |
| [GGUF](https://huggingface.co/mradermacher/Jellyfish-8B-GGUF/resolve/main/Jellyfish-8B.IQ3_M.gguf) | IQ3_M | 3.9 | |
| [GGUF](https://huggingface.co/mradermacher/Jellyfish-8B-GGUF/resolve/main/Jellyfish-8B.Q3_K_M.gguf) | Q3_K_M | 4.1 | lower quality |
| [GGUF](https://huggingface.co/mradermacher/Jellyfish-8B-GGUF/resolve/main/Jellyfish-8B.Q3_K_L.gguf) | Q3_K_L | 4.4 | |
| [GGUF](https://huggingface.co/mradermacher/Jellyfish-8B-GGUF/resolve/main/Jellyfish-8B.IQ4_XS.gguf) | IQ4_XS | 4.6 | |
| [GGUF](https://huggingface.co/mradermacher/Jellyfish-8B-GGUF/resolve/main/Jellyfish-8B.Q4_K_S.gguf) | Q4_K_S | 4.8 | fast, recommended |
| [GGUF](https://huggingface.co/mradermacher/Jellyfish-8B-GGUF/resolve/main/Jellyfish-8B.Q4_K_M.gguf) | Q4_K_M | 5.0 | fast, recommended |
| [GGUF](https://huggingface.co/mradermacher/Jellyfish-8B-GGUF/resolve/main/Jellyfish-8B.Q5_K_S.gguf) | Q5_K_S | 5.7 | |
| [GGUF](https://huggingface.co/mradermacher/Jellyfish-8B-GGUF/resolve/main/Jellyfish-8B.Q5_K_M.gguf) | Q5_K_M | 5.8 | |
| [GGUF](https://huggingface.co/mradermacher/Jellyfish-8B-GGUF/resolve/main/Jellyfish-8B.Q6_K.gguf) | Q6_K | 6.7 | very good quality |
| [GGUF](https://huggingface.co/mradermacher/Jellyfish-8B-GGUF/resolve/main/Jellyfish-8B.Q8_0.gguf) | Q8_0 | 8.6 | fast, best quality |
| [GGUF](https://huggingface.co/mradermacher/Jellyfish-8B-GGUF/resolve/main/Jellyfish-8B.f16.gguf) | f16 | 16.2 | 16 bpw, overkill |
Here is a handy graph by ikawrakow comparing some lower-quality quant
types (lower is better):

And here are Artefact2's thoughts on the matter:
https://gist.github.com/Artefact2/b5f810600771265fc1e39442288e8ec9
## FAQ / Model Request
See https://huggingface.co/mradermacher/model_requests for some answers to
questions you might have and/or if you want some other model quantized.
## Thanks
I thank my company, [nethype GmbH](https://www.nethype.de/), for letting
me use its servers and providing upgrades to my workstation to enable
this work in my free time.
<!-- end -->
|
hf-tiny-model-private/tiny-random-MCTCTModel | hf-tiny-model-private | "2023-03-29T19:07:22Z" | 14,467 | 0 | transformers | [
"transformers",
"pytorch",
"mctct",
"feature-extraction",
"endpoints_compatible",
"region:us"
] | feature-extraction | "2023-03-29T19:07:17Z" | Entry not found |
mradermacher/llama3-ko-IronMan-Overfit-i1-GGUF | mradermacher | "2024-06-27T10:06:38Z" | 14,465 | 0 | transformers | [
"transformers",
"gguf",
"ko",
"base_model:choah/llama3-ko-IronMan-Overfit",
"license:apache-2.0",
"endpoints_compatible",
"region:us"
] | null | "2024-06-27T08:47:39Z" | ---
base_model: choah/llama3-ko-IronMan-Overfit
language:
- ko
library_name: transformers
license: apache-2.0
quantized_by: mradermacher
---
## About
<!-- ### quantize_version: 2 -->
<!-- ### output_tensor_quantised: 1 -->
<!-- ### convert_type: hf -->
<!-- ### vocab_type: -->
<!-- ### tags: nicoboss -->
weighted/imatrix quants of https://huggingface.co/choah/llama3-ko-IronMan-Overfit
<!-- provided-files -->
static quants are available at https://huggingface.co/mradermacher/llama3-ko-IronMan-Overfit-GGUF
## Usage
If you are unsure how to use GGUF files, refer to one of [TheBloke's
READMEs](https://huggingface.co/TheBloke/KafkaLM-70B-German-V0.1-GGUF) for
more details, including on how to concatenate multi-part files.
## Provided Quants
(sorted by size, not necessarily quality. IQ-quants are often preferable over similar sized non-IQ quants)
| Link | Type | Size/GB | Notes |
|:-----|:-----|--------:|:------|
| [GGUF](https://huggingface.co/mradermacher/llama3-ko-IronMan-Overfit-i1-GGUF/resolve/main/llama3-ko-IronMan-Overfit.i1-IQ1_S.gguf) | i1-IQ1_S | 2.1 | for the desperate |
| [GGUF](https://huggingface.co/mradermacher/llama3-ko-IronMan-Overfit-i1-GGUF/resolve/main/llama3-ko-IronMan-Overfit.i1-IQ1_M.gguf) | i1-IQ1_M | 2.3 | mostly desperate |
| [GGUF](https://huggingface.co/mradermacher/llama3-ko-IronMan-Overfit-i1-GGUF/resolve/main/llama3-ko-IronMan-Overfit.i1-IQ2_XXS.gguf) | i1-IQ2_XXS | 2.5 | |
| [GGUF](https://huggingface.co/mradermacher/llama3-ko-IronMan-Overfit-i1-GGUF/resolve/main/llama3-ko-IronMan-Overfit.i1-IQ2_XS.gguf) | i1-IQ2_XS | 2.7 | |
| [GGUF](https://huggingface.co/mradermacher/llama3-ko-IronMan-Overfit-i1-GGUF/resolve/main/llama3-ko-IronMan-Overfit.i1-IQ2_S.gguf) | i1-IQ2_S | 2.9 | |
| [GGUF](https://huggingface.co/mradermacher/llama3-ko-IronMan-Overfit-i1-GGUF/resolve/main/llama3-ko-IronMan-Overfit.i1-IQ2_M.gguf) | i1-IQ2_M | 3.0 | |
| [GGUF](https://huggingface.co/mradermacher/llama3-ko-IronMan-Overfit-i1-GGUF/resolve/main/llama3-ko-IronMan-Overfit.i1-Q2_K.gguf) | i1-Q2_K | 3.3 | IQ3_XXS probably better |
| [GGUF](https://huggingface.co/mradermacher/llama3-ko-IronMan-Overfit-i1-GGUF/resolve/main/llama3-ko-IronMan-Overfit.i1-IQ3_XXS.gguf) | i1-IQ3_XXS | 3.4 | lower quality |
| [GGUF](https://huggingface.co/mradermacher/llama3-ko-IronMan-Overfit-i1-GGUF/resolve/main/llama3-ko-IronMan-Overfit.i1-IQ3_XS.gguf) | i1-IQ3_XS | 3.6 | |
| [GGUF](https://huggingface.co/mradermacher/llama3-ko-IronMan-Overfit-i1-GGUF/resolve/main/llama3-ko-IronMan-Overfit.i1-Q3_K_S.gguf) | i1-Q3_K_S | 3.8 | IQ3_XS probably better |
| [GGUF](https://huggingface.co/mradermacher/llama3-ko-IronMan-Overfit-i1-GGUF/resolve/main/llama3-ko-IronMan-Overfit.i1-IQ3_S.gguf) | i1-IQ3_S | 3.8 | beats Q3_K* |
| [GGUF](https://huggingface.co/mradermacher/llama3-ko-IronMan-Overfit-i1-GGUF/resolve/main/llama3-ko-IronMan-Overfit.i1-IQ3_M.gguf) | i1-IQ3_M | 3.9 | |
| [GGUF](https://huggingface.co/mradermacher/llama3-ko-IronMan-Overfit-i1-GGUF/resolve/main/llama3-ko-IronMan-Overfit.i1-Q3_K_M.gguf) | i1-Q3_K_M | 4.1 | IQ3_S probably better |
| [GGUF](https://huggingface.co/mradermacher/llama3-ko-IronMan-Overfit-i1-GGUF/resolve/main/llama3-ko-IronMan-Overfit.i1-Q3_K_L.gguf) | i1-Q3_K_L | 4.4 | IQ3_M probably better |
| [GGUF](https://huggingface.co/mradermacher/llama3-ko-IronMan-Overfit-i1-GGUF/resolve/main/llama3-ko-IronMan-Overfit.i1-IQ4_XS.gguf) | i1-IQ4_XS | 4.5 | |
| [GGUF](https://huggingface.co/mradermacher/llama3-ko-IronMan-Overfit-i1-GGUF/resolve/main/llama3-ko-IronMan-Overfit.i1-Q4_0.gguf) | i1-Q4_0 | 4.8 | fast, low quality |
| [GGUF](https://huggingface.co/mradermacher/llama3-ko-IronMan-Overfit-i1-GGUF/resolve/main/llama3-ko-IronMan-Overfit.i1-Q4_K_S.gguf) | i1-Q4_K_S | 4.8 | optimal size/speed/quality |
| [GGUF](https://huggingface.co/mradermacher/llama3-ko-IronMan-Overfit-i1-GGUF/resolve/main/llama3-ko-IronMan-Overfit.i1-Q4_K_M.gguf) | i1-Q4_K_M | 5.0 | fast, recommended |
| [GGUF](https://huggingface.co/mradermacher/llama3-ko-IronMan-Overfit-i1-GGUF/resolve/main/llama3-ko-IronMan-Overfit.i1-Q5_K_S.gguf) | i1-Q5_K_S | 5.7 | |
| [GGUF](https://huggingface.co/mradermacher/llama3-ko-IronMan-Overfit-i1-GGUF/resolve/main/llama3-ko-IronMan-Overfit.i1-Q5_K_M.gguf) | i1-Q5_K_M | 5.8 | |
| [GGUF](https://huggingface.co/mradermacher/llama3-ko-IronMan-Overfit-i1-GGUF/resolve/main/llama3-ko-IronMan-Overfit.i1-Q6_K.gguf) | i1-Q6_K | 6.7 | practically like static Q6_K |
Here is a handy graph by ikawrakow comparing some lower-quality quant
types (lower is better):

And here are Artefact2's thoughts on the matter:
https://gist.github.com/Artefact2/b5f810600771265fc1e39442288e8ec9
## FAQ / Model Request
See https://huggingface.co/mradermacher/model_requests for some answers to
questions you might have and/or if you want some other model quantized.
## Thanks
I thank my company, [nethype GmbH](https://www.nethype.de/), for letting
me use its servers and providing upgrades to my workstation to enable
this work in my free time. Additional thanks to [@nicoboss](https://huggingface.co/nicoboss) for giving me access to his hardware for calculating the imatrix for these quants.
<!-- end -->
|
digiplay/DarkSushi2.5D_v1 | digiplay | "2024-05-28T15:28:26Z" | 14,457 | 4 | diffusers | [
"diffusers",
"safetensors",
"stable-diffusion",
"stable-diffusion-diffusers",
"text-to-image",
"license:other",
"autotrain_compatible",
"endpoints_compatible",
"diffusers:StableDiffusionPipeline",
"region:us"
] | text-to-image | "2023-06-23T02:48:10Z" | ---
license: other
tags:
- stable-diffusion
- stable-diffusion-diffusers
- text-to-image
- diffusers
inference: true
---
Model info :
https://civitai.com/models/48671?modelVersionId=53252
Original Author's DEMO image :
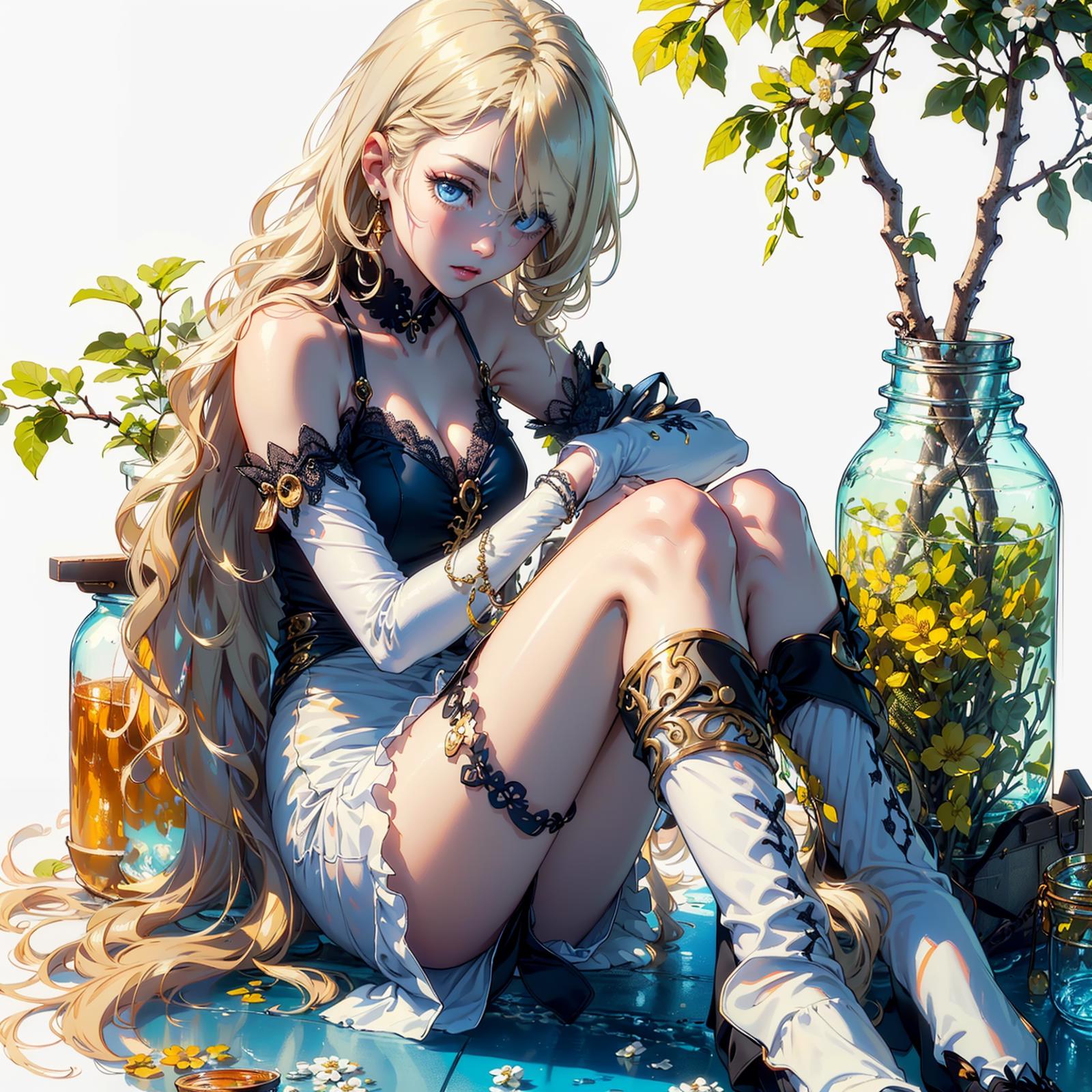,%20%F0%9F%96%90)_%20_20],_[%E7%94%BB%E9%A3%8Etag_0]_(ultra-detailed),%20(best%20shadow),%20classic,%20(cinematic%20lighting),%20dynami.jpeg) |
RichardErkhov/AIJUUD_-_juud-Mistral-7B-dpo-gguf | RichardErkhov | "2024-06-26T09:12:46Z" | 14,445 | 0 | null | [
"gguf",
"arxiv:1910.09700",
"region:us"
] | null | "2024-06-26T04:30:58Z" | Quantization made by Richard Erkhov.
[Github](https://github.com/RichardErkhov)
[Discord](https://discord.gg/pvy7H8DZMG)
[Request more models](https://github.com/RichardErkhov/quant_request)
juud-Mistral-7B-dpo - GGUF
- Model creator: https://huggingface.co/AIJUUD/
- Original model: https://huggingface.co/AIJUUD/juud-Mistral-7B-dpo/
| Name | Quant method | Size |
| ---- | ---- | ---- |
| [juud-Mistral-7B-dpo.Q2_K.gguf](https://huggingface.co/RichardErkhov/AIJUUD_-_juud-Mistral-7B-dpo-gguf/blob/main/juud-Mistral-7B-dpo.Q2_K.gguf) | Q2_K | 2.53GB |
| [juud-Mistral-7B-dpo.IQ3_XS.gguf](https://huggingface.co/RichardErkhov/AIJUUD_-_juud-Mistral-7B-dpo-gguf/blob/main/juud-Mistral-7B-dpo.IQ3_XS.gguf) | IQ3_XS | 2.81GB |
| [juud-Mistral-7B-dpo.IQ3_S.gguf](https://huggingface.co/RichardErkhov/AIJUUD_-_juud-Mistral-7B-dpo-gguf/blob/main/juud-Mistral-7B-dpo.IQ3_S.gguf) | IQ3_S | 2.96GB |
| [juud-Mistral-7B-dpo.Q3_K_S.gguf](https://huggingface.co/RichardErkhov/AIJUUD_-_juud-Mistral-7B-dpo-gguf/blob/main/juud-Mistral-7B-dpo.Q3_K_S.gguf) | Q3_K_S | 2.95GB |
| [juud-Mistral-7B-dpo.IQ3_M.gguf](https://huggingface.co/RichardErkhov/AIJUUD_-_juud-Mistral-7B-dpo-gguf/blob/main/juud-Mistral-7B-dpo.IQ3_M.gguf) | IQ3_M | 3.06GB |
| [juud-Mistral-7B-dpo.Q3_K.gguf](https://huggingface.co/RichardErkhov/AIJUUD_-_juud-Mistral-7B-dpo-gguf/blob/main/juud-Mistral-7B-dpo.Q3_K.gguf) | Q3_K | 3.28GB |
| [juud-Mistral-7B-dpo.Q3_K_M.gguf](https://huggingface.co/RichardErkhov/AIJUUD_-_juud-Mistral-7B-dpo-gguf/blob/main/juud-Mistral-7B-dpo.Q3_K_M.gguf) | Q3_K_M | 3.28GB |
| [juud-Mistral-7B-dpo.Q3_K_L.gguf](https://huggingface.co/RichardErkhov/AIJUUD_-_juud-Mistral-7B-dpo-gguf/blob/main/juud-Mistral-7B-dpo.Q3_K_L.gguf) | Q3_K_L | 3.56GB |
| [juud-Mistral-7B-dpo.IQ4_XS.gguf](https://huggingface.co/RichardErkhov/AIJUUD_-_juud-Mistral-7B-dpo-gguf/blob/main/juud-Mistral-7B-dpo.IQ4_XS.gguf) | IQ4_XS | 3.67GB |
| [juud-Mistral-7B-dpo.Q4_0.gguf](https://huggingface.co/RichardErkhov/AIJUUD_-_juud-Mistral-7B-dpo-gguf/blob/main/juud-Mistral-7B-dpo.Q4_0.gguf) | Q4_0 | 3.83GB |
| [juud-Mistral-7B-dpo.IQ4_NL.gguf](https://huggingface.co/RichardErkhov/AIJUUD_-_juud-Mistral-7B-dpo-gguf/blob/main/juud-Mistral-7B-dpo.IQ4_NL.gguf) | IQ4_NL | 3.87GB |
| [juud-Mistral-7B-dpo.Q4_K_S.gguf](https://huggingface.co/RichardErkhov/AIJUUD_-_juud-Mistral-7B-dpo-gguf/blob/main/juud-Mistral-7B-dpo.Q4_K_S.gguf) | Q4_K_S | 3.86GB |
| [juud-Mistral-7B-dpo.Q4_K.gguf](https://huggingface.co/RichardErkhov/AIJUUD_-_juud-Mistral-7B-dpo-gguf/blob/main/juud-Mistral-7B-dpo.Q4_K.gguf) | Q4_K | 4.07GB |
| [juud-Mistral-7B-dpo.Q4_K_M.gguf](https://huggingface.co/RichardErkhov/AIJUUD_-_juud-Mistral-7B-dpo-gguf/blob/main/juud-Mistral-7B-dpo.Q4_K_M.gguf) | Q4_K_M | 4.07GB |
| [juud-Mistral-7B-dpo.Q4_1.gguf](https://huggingface.co/RichardErkhov/AIJUUD_-_juud-Mistral-7B-dpo-gguf/blob/main/juud-Mistral-7B-dpo.Q4_1.gguf) | Q4_1 | 4.24GB |
| [juud-Mistral-7B-dpo.Q5_0.gguf](https://huggingface.co/RichardErkhov/AIJUUD_-_juud-Mistral-7B-dpo-gguf/blob/main/juud-Mistral-7B-dpo.Q5_0.gguf) | Q5_0 | 4.65GB |
| [juud-Mistral-7B-dpo.Q5_K_S.gguf](https://huggingface.co/RichardErkhov/AIJUUD_-_juud-Mistral-7B-dpo-gguf/blob/main/juud-Mistral-7B-dpo.Q5_K_S.gguf) | Q5_K_S | 4.65GB |
| [juud-Mistral-7B-dpo.Q5_K.gguf](https://huggingface.co/RichardErkhov/AIJUUD_-_juud-Mistral-7B-dpo-gguf/blob/main/juud-Mistral-7B-dpo.Q5_K.gguf) | Q5_K | 4.78GB |
| [juud-Mistral-7B-dpo.Q5_K_M.gguf](https://huggingface.co/RichardErkhov/AIJUUD_-_juud-Mistral-7B-dpo-gguf/blob/main/juud-Mistral-7B-dpo.Q5_K_M.gguf) | Q5_K_M | 4.78GB |
| [juud-Mistral-7B-dpo.Q5_1.gguf](https://huggingface.co/RichardErkhov/AIJUUD_-_juud-Mistral-7B-dpo-gguf/blob/main/juud-Mistral-7B-dpo.Q5_1.gguf) | Q5_1 | 5.07GB |
| [juud-Mistral-7B-dpo.Q6_K.gguf](https://huggingface.co/RichardErkhov/AIJUUD_-_juud-Mistral-7B-dpo-gguf/blob/main/juud-Mistral-7B-dpo.Q6_K.gguf) | Q6_K | 5.53GB |
| [juud-Mistral-7B-dpo.Q8_0.gguf](https://huggingface.co/RichardErkhov/AIJUUD_-_juud-Mistral-7B-dpo-gguf/blob/main/juud-Mistral-7B-dpo.Q8_0.gguf) | Q8_0 | 7.17GB |
Original model description:
---
library_name: transformers
license: apache-2.0
language:
- en
---
# Model Card for Model ID
<!-- Provide a quick summary of what the model is/does. -->
## Model Details
### Model Description
<!-- Provide a longer summary of what this model is. -->
This is the model card of a 🤗 transformers model that has been pushed on the Hub. This model card has been automatically generated.
- **Developed by:** [More Information Needed]
- **Funded by [optional]:** [More Information Needed]
- **Shared by [optional]:** [More Information Needed]
- **Model type:** [More Information Needed]
- **Language(s) (NLP):** [More Information Needed]
- **License:** [More Information Needed]
- **Finetuned from model [optional]:** [More Information Needed]
### Model Sources [optional]
<!-- Provide the basic links for the model. -->
- **Repository:** [More Information Needed]
- **Paper [optional]:** [More Information Needed]
- **Demo [optional]:** [More Information Needed]
## Uses
<!-- Address questions around how the model is intended to be used, including the foreseeable users of the model and those affected by the model. -->
### Direct Use
<!-- This section is for the model use without fine-tuning or plugging into a larger ecosystem/app. -->
[More Information Needed]
### Downstream Use [optional]
<!-- This section is for the model use when fine-tuned for a task, or when plugged into a larger ecosystem/app -->
[More Information Needed]
### Out-of-Scope Use
<!-- This section addresses misuse, malicious use, and uses that the model will not work well for. -->
[More Information Needed]
## Bias, Risks, and Limitations
<!-- This section is meant to convey both technical and sociotechnical limitations. -->
[More Information Needed]
### Recommendations
<!-- This section is meant to convey recommendations with respect to the bias, risk, and technical limitations. -->
Users (both direct and downstream) should be made aware of the risks, biases and limitations of the model. More information needed for further recommendations.
## How to Get Started with the Model
Use the code below to get started with the model.
[More Information Needed]
## Training Details
### Training Data
<!-- This should link to a Dataset Card, perhaps with a short stub of information on what the training data is all about as well as documentation related to data pre-processing or additional filtering. -->
[More Information Needed]
### Training Procedure
<!-- This relates heavily to the Technical Specifications. Content here should link to that section when it is relevant to the training procedure. -->
#### Preprocessing [optional]
[More Information Needed]
#### Training Hyperparameters
- **Training regime:** [More Information Needed] <!--fp32, fp16 mixed precision, bf16 mixed precision, bf16 non-mixed precision, fp16 non-mixed precision, fp8 mixed precision -->
#### Speeds, Sizes, Times [optional]
<!-- This section provides information about throughput, start/end time, checkpoint size if relevant, etc. -->
[More Information Needed]
## Evaluation
<!-- This section describes the evaluation protocols and provides the results. -->
### Testing Data, Factors & Metrics
#### Testing Data
<!-- This should link to a Dataset Card if possible. -->
[More Information Needed]
#### Factors
<!-- These are the things the evaluation is disaggregating by, e.g., subpopulations or domains. -->
[More Information Needed]
#### Metrics
<!-- These are the evaluation metrics being used, ideally with a description of why. -->
[More Information Needed]
### Results
[More Information Needed]
#### Summary
## Model Examination [optional]
<!-- Relevant interpretability work for the model goes here -->
[More Information Needed]
## Environmental Impact
<!-- Total emissions (in grams of CO2eq) and additional considerations, such as electricity usage, go here. Edit the suggested text below accordingly -->
Carbon emissions can be estimated using the [Machine Learning Impact calculator](https://mlco2.github.io/impact#compute) presented in [Lacoste et al. (2019)](https://arxiv.org/abs/1910.09700).
- **Hardware Type:** [More Information Needed]
- **Hours used:** [More Information Needed]
- **Cloud Provider:** [More Information Needed]
- **Compute Region:** [More Information Needed]
- **Carbon Emitted:** [More Information Needed]
## Technical Specifications [optional]
### Model Architecture and Objective
[More Information Needed]
### Compute Infrastructure
[More Information Needed]
#### Hardware
[More Information Needed]
#### Software
[More Information Needed]
## Citation [optional]
<!-- If there is a paper or blog post introducing the model, the APA and Bibtex information for that should go in this section. -->
**BibTeX:**
[More Information Needed]
**APA:**
[More Information Needed]
## Glossary [optional]
<!-- If relevant, include terms and calculations in this section that can help readers understand the model or model card. -->
[More Information Needed]
## More Information [optional]
[More Information Needed]
## Model Card Authors [optional]
[More Information Needed]
## Model Card Contact
[More Information Needed]
|
ehristoforu/dalle-3-xl-v2 | ehristoforu | "2024-03-09T20:44:29Z" | 14,442 | 87 | diffusers | [
"diffusers",
"text-to-image",
"safetensors",
"stable-diffusion",
"lora",
"dalle-3",
"dalle",
"deepvision",
"template:sd-lora",
"dataset:ehristoforu/dalle-3-images",
"base_model:fluently/Fluently-XL-v2",
"license:creativeml-openrail-m",
"region:us"
] | text-to-image | "2024-03-09T20:33:13Z" | ---
tags:
- text-to-image
- safetensors
- stable-diffusion
- lora
- diffusers
- dalle-3
- dalle
- deepvision
- template:sd-lora
widget:
- text: >-
The image is a 3D render of a green dinosaur named Yoshi from the Mario
series. Yoshi is standing on a brick street in a town and is holding a sign
that says "Feed me please!" in capital white letters. Yoshi has a white
belly, orange shoes, and a brown shell with orange spots. He is looking at
the camera with a hopeful expression on his face. The background of the
image is slightly blurred and shows a building with large windows behind
Yoshi. The image is well-lit, and the colors are vibrant,
<lora:dalle-3-xl-lora-v2:0.8>
output:
url: images/v2-1.png
- text: >-
The image is a 3D rendering of a cartoon fox wearing aviator goggles and a
scarf sitting on a mossy tree stump in a forest. The fox has bright orange
fur, white paws and underbelly, and dark brown eyes. The goggles are brown
and have a light blue tint. The scarf is dark brown and has a light brown
buckle. The tree stump is dark brown and has a light green moss growing on
it. The forest is green and lush, with tall trees and a variety of shrubs
and plants. The sun is shining brightly through the trees, creating a
dappled pattern of light and shadow on the ground. The fox is sitting in a
relaxed pose, with its head tilted slightly to the left and its eyes looking
up at the viewer. The image is rendered in a realistic style, with soft
lighting and detailed textures. <lora:dalle-3-xl-lora-v2:0.8>
output:
url: images/v2-2.png
- text: >-
The image is of Shadow the Hedgehog, a character from the Sonic the Hedgehog
series. He is standing on a rock in front of a ruined city. He is wearing
his signature black and red outfit and has his arms crossed. He has a smug
expression on his face. The city is in ruins, with buildings destroyed and
debris everywhere. The sky is dark and cloudy. The image is rendered in a
realistic style. Shadow is a black hedgehog with red stripes on his head and
arms. He has yellow eyes and a white muzzle. He is wearing black boots with
red soles and white gloves. He is standing on a large rock in the middle of
a ruined city. The city is in ruins, with buildings destroyed and debris
everywhere. The sky is dark and cloudy. Shadow is looking at the camera with
a smug expression on his face., <lora:dalle-3-xl-lora-v2:0.8>
output:
url: images/v2-3.png
- text: >-
The image is an illustration of the character Goku from the anime series
Dragon Ball Z. He is standing in a powered-up state with his hair spiked up
and surrounded by blue lightning. He is wearing his orange and blue gi with
a white belt and boots. His expression is serious and determined. The
background is a dark blue void with bright white lightning bolts. The image
is in a 3D rendered anime style, <lora:dalle-3-xl-lora-v2:0.8>
output:
url: images/v2-4.png
base_model: fluently/Fluently-XL-v2
instance_prompt: <lora:dalle-3-xl-lora-v2:0.8>
license: creativeml-openrail-m
library_name: diffusers
datasets:
- ehristoforu/dalle-3-images
pipeline_tag: text-to-image
---
# DALL•E 3 XL LoRA v2
<Gallery />
## Model description
This is a test model very similar to Dall•E 3.
## Official demo
You can use official demo on Spaces: [try](https://huggingface.co/spaces/ehristoforu/dalle-3-xl-lora-v2).
## Trigger words
You should use `<lora:dalle-3-xl-lora-v2:0.8>` to trigger the image generation.
## Download model
Weights for this model are available in Safetensors format.
[Download](/ehristoforu/dalle-3-xl-v2/tree/main) them in the Files & versions tab. |
mradermacher/Very_Berry_Qwen2_7B-GGUF | mradermacher | "2024-06-29T06:03:57Z" | 14,442 | 0 | transformers | [
"transformers",
"gguf",
"not-for-all-audiences",
"en",
"base_model:ChaoticNeutrals/Very_Berry_Qwen2_7B",
"license:apache-2.0",
"endpoints_compatible",
"region:us"
] | null | "2024-06-29T03:55:57Z" | ---
base_model: ChaoticNeutrals/Very_Berry_Qwen2_7B
language:
- en
library_name: transformers
license: apache-2.0
quantized_by: mradermacher
tags:
- not-for-all-audiences
---
## About
<!-- ### quantize_version: 2 -->
<!-- ### output_tensor_quantised: 1 -->
<!-- ### convert_type: hf -->
<!-- ### vocab_type: -->
<!-- ### tags: -->
static quants of https://huggingface.co/ChaoticNeutrals/Very_Berry_Qwen2_7B
<!-- provided-files -->
weighted/imatrix quants are available at https://huggingface.co/mradermacher/Very_Berry_Qwen2_7B-i1-GGUF
## Usage
If you are unsure how to use GGUF files, refer to one of [TheBloke's
READMEs](https://huggingface.co/TheBloke/KafkaLM-70B-German-V0.1-GGUF) for
more details, including on how to concatenate multi-part files.
## Provided Quants
(sorted by size, not necessarily quality. IQ-quants are often preferable over similar sized non-IQ quants)
| Link | Type | Size/GB | Notes |
|:-----|:-----|--------:|:------|
| [GGUF](https://huggingface.co/mradermacher/Very_Berry_Qwen2_7B-GGUF/resolve/main/Very_Berry_Qwen2_7B.Q2_K.gguf) | Q2_K | 3.1 | |
| [GGUF](https://huggingface.co/mradermacher/Very_Berry_Qwen2_7B-GGUF/resolve/main/Very_Berry_Qwen2_7B.IQ3_XS.gguf) | IQ3_XS | 3.4 | |
| [GGUF](https://huggingface.co/mradermacher/Very_Berry_Qwen2_7B-GGUF/resolve/main/Very_Berry_Qwen2_7B.Q3_K_S.gguf) | Q3_K_S | 3.6 | |
| [GGUF](https://huggingface.co/mradermacher/Very_Berry_Qwen2_7B-GGUF/resolve/main/Very_Berry_Qwen2_7B.IQ3_S.gguf) | IQ3_S | 3.6 | beats Q3_K* |
| [GGUF](https://huggingface.co/mradermacher/Very_Berry_Qwen2_7B-GGUF/resolve/main/Very_Berry_Qwen2_7B.IQ3_M.gguf) | IQ3_M | 3.7 | |
| [GGUF](https://huggingface.co/mradermacher/Very_Berry_Qwen2_7B-GGUF/resolve/main/Very_Berry_Qwen2_7B.Q3_K_M.gguf) | Q3_K_M | 3.9 | lower quality |
| [GGUF](https://huggingface.co/mradermacher/Very_Berry_Qwen2_7B-GGUF/resolve/main/Very_Berry_Qwen2_7B.Q3_K_L.gguf) | Q3_K_L | 4.2 | |
| [GGUF](https://huggingface.co/mradermacher/Very_Berry_Qwen2_7B-GGUF/resolve/main/Very_Berry_Qwen2_7B.IQ4_XS.gguf) | IQ4_XS | 4.4 | |
| [GGUF](https://huggingface.co/mradermacher/Very_Berry_Qwen2_7B-GGUF/resolve/main/Very_Berry_Qwen2_7B.Q4_K_S.gguf) | Q4_K_S | 4.6 | fast, recommended |
| [GGUF](https://huggingface.co/mradermacher/Very_Berry_Qwen2_7B-GGUF/resolve/main/Very_Berry_Qwen2_7B.Q4_K_M.gguf) | Q4_K_M | 4.8 | fast, recommended |
| [GGUF](https://huggingface.co/mradermacher/Very_Berry_Qwen2_7B-GGUF/resolve/main/Very_Berry_Qwen2_7B.Q5_K_S.gguf) | Q5_K_S | 5.4 | |
| [GGUF](https://huggingface.co/mradermacher/Very_Berry_Qwen2_7B-GGUF/resolve/main/Very_Berry_Qwen2_7B.Q5_K_M.gguf) | Q5_K_M | 5.5 | |
| [GGUF](https://huggingface.co/mradermacher/Very_Berry_Qwen2_7B-GGUF/resolve/main/Very_Berry_Qwen2_7B.Q6_K.gguf) | Q6_K | 6.4 | very good quality |
| [GGUF](https://huggingface.co/mradermacher/Very_Berry_Qwen2_7B-GGUF/resolve/main/Very_Berry_Qwen2_7B.Q8_0.gguf) | Q8_0 | 8.2 | fast, best quality |
| [GGUF](https://huggingface.co/mradermacher/Very_Berry_Qwen2_7B-GGUF/resolve/main/Very_Berry_Qwen2_7B.f16.gguf) | f16 | 15.3 | 16 bpw, overkill |
Here is a handy graph by ikawrakow comparing some lower-quality quant
types (lower is better):

And here are Artefact2's thoughts on the matter:
https://gist.github.com/Artefact2/b5f810600771265fc1e39442288e8ec9
## FAQ / Model Request
See https://huggingface.co/mradermacher/model_requests for some answers to
questions you might have and/or if you want some other model quantized.
## Thanks
I thank my company, [nethype GmbH](https://www.nethype.de/), for letting
me use its servers and providing upgrades to my workstation to enable
this work in my free time.
<!-- end -->
|
transfo-xl/transfo-xl-wt103 | transfo-xl | "2023-01-24T14:49:49Z" | 14,438 | 10 | transformers | [
"transformers",
"pytorch",
"tf",
"transfo-xl",
"text-generation",
"en",
"dataset:wikitext-103",
"arxiv:1901.02860",
"autotrain_compatible",
"endpoints_compatible",
"region:us"
] | text-generation | "2022-03-02T23:29:04Z" | ---
datasets:
- wikitext-103
tags:
- text-generation
language: en
model-index:
- name: transfo-xl-wt103
results: []
task:
name: Text Generation
type: text-generation
---
# Transfo-xl-wt103
## Table of Contents
- [Model Details](#model-details)
- [Uses](#uses)
- [Risks, Limitations and Biases](#risks-limitations-and-biases)
- [Training](#training)
- [Evaluation](#evaluation)
- [Citation Information](#citation-information)
- [How to Get Started With the Model](#how-to-get-started-with-the-model)
## Model Details
**Model Description:**
The Transformer-XL model is a causal (uni-directional) transformer with relative positioning (sinusoïdal) embeddings which can reuse previously computed hidden-states to attend to longer context (memory). This model also uses adaptive softmax inputs and outputs (tied).
- **Developed by:** [Zihang Dai]([email protected]), [Zhilin Yang]([email protected]), [Yiming Yang1]([email protected]), [Jaime Carbonell]([email protected]), [Quoc V. Le]([email protected]), [Ruslan Salakhutdinov]([email protected])
- **Shared by:** HuggingFace team
- **Model Type:** Text Generation
- **Language(s):** English
- **License:** [More information needed]
- **Resources for more information:**
- [Research Paper](https://arxiv.org/pdf/1901.02860.pdf)
- [GitHub Repo](https://github.com/kimiyoung/transformer-xl)
- [HuggingFace Documentation](https://huggingface.co/docs/transformers/model_doc/transfo-xl#transformers.TransfoXLModel)
## Uses
#### Direct Use
This model can be used for text generation.
The authors provide additionally notes about the vocabulary used, in the [associated paper](https://arxiv.org/pdf/1901.02860.pdf):
> We envision interesting applications of Transformer-XL in the fields of text generation, unsupervised feature learning, image and speech modeling.
#### Misuse and Out-of-scope Use
The model should not be used to intentionally create hostile or alienating environments for people. In addition, the model was not trained to be factual or true representations of people or events, and therefore using the model to generate such content is out-of-scope for the abilities of this model.
## Risks, Limitations and Biases
**CONTENT WARNING: Readers should be aware this section contains content that is disturbing, offensive, and can propagate historical and current stereotypes.**
Significant research has explored bias and fairness issues with language models (see, e.g., [Sheng et al. (2021)](https://aclanthology.org/2021.acl-long.330.pdf) and [Bender et al. (2021)](https://dl.acm.org/doi/pdf/10.1145/3442188.3445922)).
## Training
#### Training Data
The authors provide additionally notes about the vocabulary used, in the [associated paper](https://arxiv.org/pdf/1901.02860.pdf):
> best model trained the Wikitext-103 dataset. We seed the our Transformer-XL with a context of at most 512 consecutive tokens randomly sampled from the test set of Wikitext-103. Then, we run Transformer-XL to generate a pre-defined number of tokens (500 or 1,000 in our case). For each generation step, we first find the top-40 probabilities of the next-step distribution and sample from top-40 tokens based on the re-normalized distribution. To help reading, we detokenize the context, the generated text and the reference text.
The authors use the following pretraining corpora for the model, described in the [associated paper](https://arxiv.org/pdf/1901.02860.pdf):
- WikiText-103 (Merity et al., 2016),
#### Training Procedure
##### Preprocessing
The authors provide additionally notes about the training procedure used, in the [associated paper](https://arxiv.org/pdf/1901.02860.pdf):
> Similar to but different from enwik8, text8 con- tains 100M processed Wikipedia characters cre- ated by lowering case the text and removing any character other than the 26 letters a through z, and space. Due to the similarity, we simply adapt the best model and the same hyper-parameters on en- wik8 to text8 without further tuning.
## Evaluation
#### Results
| Method | enwiki8 |text8 | One Billion Word | WT-103 | PTB (w/o finetuning) |
|:--------------------:|---------:|:----:|:----------------:|:------:|:--------------------:|
| Transformer-XL. | 0.99 | 1.08 | 21.8 | 18.3 | 54.5 |
## Citation Information
```bibtex
@misc{https://doi.org/10.48550/arxiv.1901.02860,
doi = {10.48550/ARXIV.1901.02860},
url = {https://arxiv.org/abs/1901.02860},
author = {Dai, Zihang and Yang, Zhilin and Yang, Yiming and Carbonell, Jaime and Le, Quoc V. and Salakhutdinov, Ruslan},
keywords = {Machine Learning (cs.LG), Computation and Language (cs.CL), Machine Learning (stat.ML), FOS: Computer and information sciences, FOS: Computer and information sciences},
title = {Transformer-XL: Attentive Language Models Beyond a Fixed-Length Context},
publisher = {arXiv},
year = {2019},
copyright = {Creative Commons Attribution Non Commercial Share Alike 4.0 International}
}
```
## How to Get Started With the Model
```
from transformers import TransfoXLTokenizer, TransfoXLModel
import torch
tokenizer = TransfoXLTokenizer.from_pretrained("transfo-xl-wt103")
model = TransfoXLModel.from_pretrained("transfo-xl-wt103")
inputs = tokenizer("Hello, my dog is cute", return_tensors="pt")
outputs = model(**inputs)
last_hidden_states = outputs.last_hidden_state
```
|
mradermacher/IceSakeV4RP-7b-GGUF | mradermacher | "2024-06-26T20:25:40Z" | 14,432 | 0 | transformers | [
"transformers",
"gguf",
"mergekit",
"merge",
"alpaca",
"mistral",
"not-for-all-audiences",
"nsfw",
"en",
"base_model:icefog72/IceSakeV4RP-7b",
"license:cc-by-nc-4.0",
"endpoints_compatible",
"region:us"
] | null | "2024-06-26T16:56:53Z" | ---
base_model: icefog72/IceSakeV4RP-7b
language:
- en
library_name: transformers
license: cc-by-nc-4.0
quantized_by: mradermacher
tags:
- mergekit
- merge
- alpaca
- mistral
- not-for-all-audiences
- nsfw
---
## About
<!-- ### quantize_version: 2 -->
<!-- ### output_tensor_quantised: 1 -->
<!-- ### convert_type: hf -->
<!-- ### vocab_type: -->
<!-- ### tags: -->
static quants of https://huggingface.co/icefog72/IceSakeV4RP-7b
<!-- provided-files -->
weighted/imatrix quants are available at https://huggingface.co/mradermacher/IceSakeV4RP-7b-i1-GGUF
## Usage
If you are unsure how to use GGUF files, refer to one of [TheBloke's
READMEs](https://huggingface.co/TheBloke/KafkaLM-70B-German-V0.1-GGUF) for
more details, including on how to concatenate multi-part files.
## Provided Quants
(sorted by size, not necessarily quality. IQ-quants are often preferable over similar sized non-IQ quants)
| Link | Type | Size/GB | Notes |
|:-----|:-----|--------:|:------|
| [GGUF](https://huggingface.co/mradermacher/IceSakeV4RP-7b-GGUF/resolve/main/IceSakeV4RP-7b.Q2_K.gguf) | Q2_K | 2.8 | |
| [GGUF](https://huggingface.co/mradermacher/IceSakeV4RP-7b-GGUF/resolve/main/IceSakeV4RP-7b.IQ3_XS.gguf) | IQ3_XS | 3.1 | |
| [GGUF](https://huggingface.co/mradermacher/IceSakeV4RP-7b-GGUF/resolve/main/IceSakeV4RP-7b.Q3_K_S.gguf) | Q3_K_S | 3.3 | |
| [GGUF](https://huggingface.co/mradermacher/IceSakeV4RP-7b-GGUF/resolve/main/IceSakeV4RP-7b.IQ3_S.gguf) | IQ3_S | 3.3 | beats Q3_K* |
| [GGUF](https://huggingface.co/mradermacher/IceSakeV4RP-7b-GGUF/resolve/main/IceSakeV4RP-7b.IQ3_M.gguf) | IQ3_M | 3.4 | |
| [GGUF](https://huggingface.co/mradermacher/IceSakeV4RP-7b-GGUF/resolve/main/IceSakeV4RP-7b.Q3_K_M.gguf) | Q3_K_M | 3.6 | lower quality |
| [GGUF](https://huggingface.co/mradermacher/IceSakeV4RP-7b-GGUF/resolve/main/IceSakeV4RP-7b.Q3_K_L.gguf) | Q3_K_L | 3.9 | |
| [GGUF](https://huggingface.co/mradermacher/IceSakeV4RP-7b-GGUF/resolve/main/IceSakeV4RP-7b.IQ4_XS.gguf) | IQ4_XS | 4.0 | |
| [GGUF](https://huggingface.co/mradermacher/IceSakeV4RP-7b-GGUF/resolve/main/IceSakeV4RP-7b.Q4_K_S.gguf) | Q4_K_S | 4.2 | fast, recommended |
| [GGUF](https://huggingface.co/mradermacher/IceSakeV4RP-7b-GGUF/resolve/main/IceSakeV4RP-7b.Q4_K_M.gguf) | Q4_K_M | 4.5 | fast, recommended |
| [GGUF](https://huggingface.co/mradermacher/IceSakeV4RP-7b-GGUF/resolve/main/IceSakeV4RP-7b.Q5_K_S.gguf) | Q5_K_S | 5.1 | |
| [GGUF](https://huggingface.co/mradermacher/IceSakeV4RP-7b-GGUF/resolve/main/IceSakeV4RP-7b.Q5_K_M.gguf) | Q5_K_M | 5.2 | |
| [GGUF](https://huggingface.co/mradermacher/IceSakeV4RP-7b-GGUF/resolve/main/IceSakeV4RP-7b.Q6_K.gguf) | Q6_K | 6.0 | very good quality |
| [GGUF](https://huggingface.co/mradermacher/IceSakeV4RP-7b-GGUF/resolve/main/IceSakeV4RP-7b.Q8_0.gguf) | Q8_0 | 7.8 | fast, best quality |
| [GGUF](https://huggingface.co/mradermacher/IceSakeV4RP-7b-GGUF/resolve/main/IceSakeV4RP-7b.f16.gguf) | f16 | 14.6 | 16 bpw, overkill |
Here is a handy graph by ikawrakow comparing some lower-quality quant
types (lower is better):

And here are Artefact2's thoughts on the matter:
https://gist.github.com/Artefact2/b5f810600771265fc1e39442288e8ec9
## FAQ / Model Request
See https://huggingface.co/mradermacher/model_requests for some answers to
questions you might have and/or if you want some other model quantized.
## Thanks
I thank my company, [nethype GmbH](https://www.nethype.de/), for letting
me use its servers and providing upgrades to my workstation to enable
this work in my free time.
<!-- end -->
|
timm/resnet50.tv_in1k | timm | "2024-02-10T23:39:32Z" | 14,430 | 0 | timm | [
"timm",
"pytorch",
"safetensors",
"image-classification",
"arxiv:1512.03385",
"license:bsd-3-clause",
"region:us"
] | image-classification | "2023-04-05T18:14:58Z" | ---
license: bsd-3-clause
library_name: timm
tags:
- image-classification
- timm
---
# Model card for resnet50.tv_in1k
A ResNet-B image classification model.
This model features:
* ReLU activations
* single layer 7x7 convolution with pooling
* 1x1 convolution shortcut downsample
Trained on ImageNet-1k, original torchvision model weight.
## Model Details
- **Model Type:** Image classification / feature backbone
- **Model Stats:**
- Params (M): 25.6
- GMACs: 4.1
- Activations (M): 11.1
- Image size: 224 x 224
- **Papers:**
- Deep Residual Learning for Image Recognition: https://arxiv.org/abs/1512.03385
- **Original:** https://github.com/pytorch/vision
## Model Usage
### Image Classification
```python
from urllib.request import urlopen
from PIL import Image
import timm
img = Image.open(urlopen(
'https://huggingface.co/datasets/huggingface/documentation-images/resolve/main/beignets-task-guide.png'
))
model = timm.create_model('resnet50.tv_in1k', pretrained=True)
model = model.eval()
# get model specific transforms (normalization, resize)
data_config = timm.data.resolve_model_data_config(model)
transforms = timm.data.create_transform(**data_config, is_training=False)
output = model(transforms(img).unsqueeze(0)) # unsqueeze single image into batch of 1
top5_probabilities, top5_class_indices = torch.topk(output.softmax(dim=1) * 100, k=5)
```
### Feature Map Extraction
```python
from urllib.request import urlopen
from PIL import Image
import timm
img = Image.open(urlopen(
'https://huggingface.co/datasets/huggingface/documentation-images/resolve/main/beignets-task-guide.png'
))
model = timm.create_model(
'resnet50.tv_in1k',
pretrained=True,
features_only=True,
)
model = model.eval()
# get model specific transforms (normalization, resize)
data_config = timm.data.resolve_model_data_config(model)
transforms = timm.data.create_transform(**data_config, is_training=False)
output = model(transforms(img).unsqueeze(0)) # unsqueeze single image into batch of 1
for o in output:
# print shape of each feature map in output
# e.g.:
# torch.Size([1, 64, 112, 112])
# torch.Size([1, 256, 56, 56])
# torch.Size([1, 512, 28, 28])
# torch.Size([1, 1024, 14, 14])
# torch.Size([1, 2048, 7, 7])
print(o.shape)
```
### Image Embeddings
```python
from urllib.request import urlopen
from PIL import Image
import timm
img = Image.open(urlopen(
'https://huggingface.co/datasets/huggingface/documentation-images/resolve/main/beignets-task-guide.png'
))
model = timm.create_model(
'resnet50.tv_in1k',
pretrained=True,
num_classes=0, # remove classifier nn.Linear
)
model = model.eval()
# get model specific transforms (normalization, resize)
data_config = timm.data.resolve_model_data_config(model)
transforms = timm.data.create_transform(**data_config, is_training=False)
output = model(transforms(img).unsqueeze(0)) # output is (batch_size, num_features) shaped tensor
# or equivalently (without needing to set num_classes=0)
output = model.forward_features(transforms(img).unsqueeze(0))
# output is unpooled, a (1, 2048, 7, 7) shaped tensor
output = model.forward_head(output, pre_logits=True)
# output is a (1, num_features) shaped tensor
```
## Model Comparison
Explore the dataset and runtime metrics of this model in timm [model results](https://github.com/huggingface/pytorch-image-models/tree/main/results).
|model |img_size|top1 |top5 |param_count|gmacs|macts|img/sec|
|------------------------------------------|--------|-----|-----|-----------|-----|-----|-------|
|[seresnextaa101d_32x8d.sw_in12k_ft_in1k_288](https://huggingface.co/timm/seresnextaa101d_32x8d.sw_in12k_ft_in1k_288)|320 |86.72|98.17|93.6 |35.2 |69.7 |451 |
|[seresnextaa101d_32x8d.sw_in12k_ft_in1k_288](https://huggingface.co/timm/seresnextaa101d_32x8d.sw_in12k_ft_in1k_288)|288 |86.51|98.08|93.6 |28.5 |56.4 |560 |
|[seresnextaa101d_32x8d.sw_in12k_ft_in1k](https://huggingface.co/timm/seresnextaa101d_32x8d.sw_in12k_ft_in1k)|288 |86.49|98.03|93.6 |28.5 |56.4 |557 |
|[seresnextaa101d_32x8d.sw_in12k_ft_in1k](https://huggingface.co/timm/seresnextaa101d_32x8d.sw_in12k_ft_in1k)|224 |85.96|97.82|93.6 |17.2 |34.2 |923 |
|[resnext101_32x32d.fb_wsl_ig1b_ft_in1k](https://huggingface.co/timm/resnext101_32x32d.fb_wsl_ig1b_ft_in1k)|224 |85.11|97.44|468.5 |87.3 |91.1 |254 |
|[resnetrs420.tf_in1k](https://huggingface.co/timm/resnetrs420.tf_in1k)|416 |85.0 |97.12|191.9 |108.4|213.8|134 |
|[ecaresnet269d.ra2_in1k](https://huggingface.co/timm/ecaresnet269d.ra2_in1k)|352 |84.96|97.22|102.1 |50.2 |101.2|291 |
|[ecaresnet269d.ra2_in1k](https://huggingface.co/timm/ecaresnet269d.ra2_in1k)|320 |84.73|97.18|102.1 |41.5 |83.7 |353 |
|[resnetrs350.tf_in1k](https://huggingface.co/timm/resnetrs350.tf_in1k)|384 |84.71|96.99|164.0 |77.6 |154.7|183 |
|[seresnextaa101d_32x8d.ah_in1k](https://huggingface.co/timm/seresnextaa101d_32x8d.ah_in1k)|288 |84.57|97.08|93.6 |28.5 |56.4 |557 |
|[resnetrs200.tf_in1k](https://huggingface.co/timm/resnetrs200.tf_in1k)|320 |84.45|97.08|93.2 |31.5 |67.8 |446 |
|[resnetrs270.tf_in1k](https://huggingface.co/timm/resnetrs270.tf_in1k)|352 |84.43|96.97|129.9 |51.1 |105.5|280 |
|[seresnext101d_32x8d.ah_in1k](https://huggingface.co/timm/seresnext101d_32x8d.ah_in1k)|288 |84.36|96.92|93.6 |27.6 |53.0 |595 |
|[seresnet152d.ra2_in1k](https://huggingface.co/timm/seresnet152d.ra2_in1k)|320 |84.35|97.04|66.8 |24.1 |47.7 |610 |
|[resnetrs350.tf_in1k](https://huggingface.co/timm/resnetrs350.tf_in1k)|288 |84.3 |96.94|164.0 |43.7 |87.1 |333 |
|[resnext101_32x8d.fb_swsl_ig1b_ft_in1k](https://huggingface.co/timm/resnext101_32x8d.fb_swsl_ig1b_ft_in1k)|224 |84.28|97.17|88.8 |16.5 |31.2 |1100 |
|[resnetrs420.tf_in1k](https://huggingface.co/timm/resnetrs420.tf_in1k)|320 |84.24|96.86|191.9 |64.2 |126.6|228 |
|[seresnext101_32x8d.ah_in1k](https://huggingface.co/timm/seresnext101_32x8d.ah_in1k)|288 |84.19|96.87|93.6 |27.2 |51.6 |613 |
|[resnext101_32x16d.fb_wsl_ig1b_ft_in1k](https://huggingface.co/timm/resnext101_32x16d.fb_wsl_ig1b_ft_in1k)|224 |84.18|97.19|194.0 |36.3 |51.2 |581 |
|[resnetaa101d.sw_in12k_ft_in1k](https://huggingface.co/timm/resnetaa101d.sw_in12k_ft_in1k)|288 |84.11|97.11|44.6 |15.1 |29.0 |1144 |
|[resnet200d.ra2_in1k](https://huggingface.co/timm/resnet200d.ra2_in1k)|320 |83.97|96.82|64.7 |31.2 |67.3 |518 |
|[resnetrs200.tf_in1k](https://huggingface.co/timm/resnetrs200.tf_in1k)|256 |83.87|96.75|93.2 |20.2 |43.4 |692 |
|[seresnextaa101d_32x8d.ah_in1k](https://huggingface.co/timm/seresnextaa101d_32x8d.ah_in1k)|224 |83.86|96.65|93.6 |17.2 |34.2 |923 |
|[resnetrs152.tf_in1k](https://huggingface.co/timm/resnetrs152.tf_in1k)|320 |83.72|96.61|86.6 |24.3 |48.1 |617 |
|[seresnet152d.ra2_in1k](https://huggingface.co/timm/seresnet152d.ra2_in1k)|256 |83.69|96.78|66.8 |15.4 |30.6 |943 |
|[seresnext101d_32x8d.ah_in1k](https://huggingface.co/timm/seresnext101d_32x8d.ah_in1k)|224 |83.68|96.61|93.6 |16.7 |32.0 |986 |
|[resnet152d.ra2_in1k](https://huggingface.co/timm/resnet152d.ra2_in1k)|320 |83.67|96.74|60.2 |24.1 |47.7 |706 |
|[resnetrs270.tf_in1k](https://huggingface.co/timm/resnetrs270.tf_in1k)|256 |83.59|96.61|129.9 |27.1 |55.8 |526 |
|[seresnext101_32x8d.ah_in1k](https://huggingface.co/timm/seresnext101_32x8d.ah_in1k)|224 |83.58|96.4 |93.6 |16.5 |31.2 |1013 |
|[resnetaa101d.sw_in12k_ft_in1k](https://huggingface.co/timm/resnetaa101d.sw_in12k_ft_in1k)|224 |83.54|96.83|44.6 |9.1 |17.6 |1864 |
|[resnet152.a1h_in1k](https://huggingface.co/timm/resnet152.a1h_in1k)|288 |83.46|96.54|60.2 |19.1 |37.3 |904 |
|[resnext101_32x16d.fb_swsl_ig1b_ft_in1k](https://huggingface.co/timm/resnext101_32x16d.fb_swsl_ig1b_ft_in1k)|224 |83.35|96.85|194.0 |36.3 |51.2 |582 |
|[resnet200d.ra2_in1k](https://huggingface.co/timm/resnet200d.ra2_in1k)|256 |83.23|96.53|64.7 |20.0 |43.1 |809 |
|[resnext101_32x4d.fb_swsl_ig1b_ft_in1k](https://huggingface.co/timm/resnext101_32x4d.fb_swsl_ig1b_ft_in1k)|224 |83.22|96.75|44.2 |8.0 |21.2 |1814 |
|[resnext101_64x4d.c1_in1k](https://huggingface.co/timm/resnext101_64x4d.c1_in1k)|288 |83.16|96.38|83.5 |25.7 |51.6 |590 |
|[resnet152d.ra2_in1k](https://huggingface.co/timm/resnet152d.ra2_in1k)|256 |83.14|96.38|60.2 |15.4 |30.5 |1096 |
|[resnet101d.ra2_in1k](https://huggingface.co/timm/resnet101d.ra2_in1k)|320 |83.02|96.45|44.6 |16.5 |34.8 |992 |
|[ecaresnet101d.miil_in1k](https://huggingface.co/timm/ecaresnet101d.miil_in1k)|288 |82.98|96.54|44.6 |13.4 |28.2 |1077 |
|[resnext101_64x4d.tv_in1k](https://huggingface.co/timm/resnext101_64x4d.tv_in1k)|224 |82.98|96.25|83.5 |15.5 |31.2 |989 |
|[resnetrs152.tf_in1k](https://huggingface.co/timm/resnetrs152.tf_in1k)|256 |82.86|96.28|86.6 |15.6 |30.8 |951 |
|[resnext101_32x8d.tv2_in1k](https://huggingface.co/timm/resnext101_32x8d.tv2_in1k)|224 |82.83|96.22|88.8 |16.5 |31.2 |1099 |
|[resnet152.a1h_in1k](https://huggingface.co/timm/resnet152.a1h_in1k)|224 |82.8 |96.13|60.2 |11.6 |22.6 |1486 |
|[resnet101.a1h_in1k](https://huggingface.co/timm/resnet101.a1h_in1k)|288 |82.8 |96.32|44.6 |13.0 |26.8 |1291 |
|[resnet152.a1_in1k](https://huggingface.co/timm/resnet152.a1_in1k)|288 |82.74|95.71|60.2 |19.1 |37.3 |905 |
|[resnext101_32x8d.fb_wsl_ig1b_ft_in1k](https://huggingface.co/timm/resnext101_32x8d.fb_wsl_ig1b_ft_in1k)|224 |82.69|96.63|88.8 |16.5 |31.2 |1100 |
|[resnet152.a2_in1k](https://huggingface.co/timm/resnet152.a2_in1k)|288 |82.62|95.75|60.2 |19.1 |37.3 |904 |
|[resnetaa50d.sw_in12k_ft_in1k](https://huggingface.co/timm/resnetaa50d.sw_in12k_ft_in1k)|288 |82.61|96.49|25.6 |8.9 |20.6 |1729 |
|[resnet61q.ra2_in1k](https://huggingface.co/timm/resnet61q.ra2_in1k)|288 |82.53|96.13|36.8 |9.9 |21.5 |1773 |
|[wide_resnet101_2.tv2_in1k](https://huggingface.co/timm/wide_resnet101_2.tv2_in1k)|224 |82.5 |96.02|126.9 |22.8 |21.2 |1078 |
|[resnext101_64x4d.c1_in1k](https://huggingface.co/timm/resnext101_64x4d.c1_in1k)|224 |82.46|95.92|83.5 |15.5 |31.2 |987 |
|[resnet51q.ra2_in1k](https://huggingface.co/timm/resnet51q.ra2_in1k)|288 |82.36|96.18|35.7 |8.1 |20.9 |1964 |
|[ecaresnet50t.ra2_in1k](https://huggingface.co/timm/ecaresnet50t.ra2_in1k)|320 |82.35|96.14|25.6 |8.8 |24.1 |1386 |
|[resnet101.a1_in1k](https://huggingface.co/timm/resnet101.a1_in1k)|288 |82.31|95.63|44.6 |13.0 |26.8 |1291 |
|[resnetrs101.tf_in1k](https://huggingface.co/timm/resnetrs101.tf_in1k)|288 |82.29|96.01|63.6 |13.6 |28.5 |1078 |
|[resnet152.tv2_in1k](https://huggingface.co/timm/resnet152.tv2_in1k)|224 |82.29|96.0 |60.2 |11.6 |22.6 |1484 |
|[wide_resnet50_2.racm_in1k](https://huggingface.co/timm/wide_resnet50_2.racm_in1k)|288 |82.27|96.06|68.9 |18.9 |23.8 |1176 |
|[resnet101d.ra2_in1k](https://huggingface.co/timm/resnet101d.ra2_in1k)|256 |82.26|96.07|44.6 |10.6 |22.2 |1542 |
|[resnet101.a2_in1k](https://huggingface.co/timm/resnet101.a2_in1k)|288 |82.24|95.73|44.6 |13.0 |26.8 |1290 |
|[seresnext50_32x4d.racm_in1k](https://huggingface.co/timm/seresnext50_32x4d.racm_in1k)|288 |82.2 |96.14|27.6 |7.0 |23.8 |1547 |
|[ecaresnet101d.miil_in1k](https://huggingface.co/timm/ecaresnet101d.miil_in1k)|224 |82.18|96.05|44.6 |8.1 |17.1 |1771 |
|[resnext50_32x4d.fb_swsl_ig1b_ft_in1k](https://huggingface.co/timm/resnext50_32x4d.fb_swsl_ig1b_ft_in1k)|224 |82.17|96.22|25.0 |4.3 |14.4 |2943 |
|[ecaresnet50t.a1_in1k](https://huggingface.co/timm/ecaresnet50t.a1_in1k)|288 |82.12|95.65|25.6 |7.1 |19.6 |1704 |
|[resnext50_32x4d.a1h_in1k](https://huggingface.co/timm/resnext50_32x4d.a1h_in1k)|288 |82.03|95.94|25.0 |7.0 |23.8 |1745 |
|[ecaresnet101d_pruned.miil_in1k](https://huggingface.co/timm/ecaresnet101d_pruned.miil_in1k)|288 |82.0 |96.15|24.9 |5.8 |12.7 |1787 |
|[resnet61q.ra2_in1k](https://huggingface.co/timm/resnet61q.ra2_in1k)|256 |81.99|95.85|36.8 |7.8 |17.0 |2230 |
|[resnext101_32x8d.tv2_in1k](https://huggingface.co/timm/resnext101_32x8d.tv2_in1k)|176 |81.98|95.72|88.8 |10.3 |19.4 |1768 |
|[resnet152.a1_in1k](https://huggingface.co/timm/resnet152.a1_in1k)|224 |81.97|95.24|60.2 |11.6 |22.6 |1486 |
|[resnet101.a1h_in1k](https://huggingface.co/timm/resnet101.a1h_in1k)|224 |81.93|95.75|44.6 |7.8 |16.2 |2122 |
|[resnet101.tv2_in1k](https://huggingface.co/timm/resnet101.tv2_in1k)|224 |81.9 |95.77|44.6 |7.8 |16.2 |2118 |
|[resnext101_32x16d.fb_ssl_yfcc100m_ft_in1k](https://huggingface.co/timm/resnext101_32x16d.fb_ssl_yfcc100m_ft_in1k)|224 |81.84|96.1 |194.0 |36.3 |51.2 |583 |
|[resnet51q.ra2_in1k](https://huggingface.co/timm/resnet51q.ra2_in1k)|256 |81.78|95.94|35.7 |6.4 |16.6 |2471 |
|[resnet152.a2_in1k](https://huggingface.co/timm/resnet152.a2_in1k)|224 |81.77|95.22|60.2 |11.6 |22.6 |1485 |
|[resnetaa50d.sw_in12k_ft_in1k](https://huggingface.co/timm/resnetaa50d.sw_in12k_ft_in1k)|224 |81.74|96.06|25.6 |5.4 |12.4 |2813 |
|[ecaresnet50t.a2_in1k](https://huggingface.co/timm/ecaresnet50t.a2_in1k)|288 |81.65|95.54|25.6 |7.1 |19.6 |1703 |
|[ecaresnet50d.miil_in1k](https://huggingface.co/timm/ecaresnet50d.miil_in1k)|288 |81.64|95.88|25.6 |7.2 |19.7 |1694 |
|[resnext101_32x8d.fb_ssl_yfcc100m_ft_in1k](https://huggingface.co/timm/resnext101_32x8d.fb_ssl_yfcc100m_ft_in1k)|224 |81.62|96.04|88.8 |16.5 |31.2 |1101 |
|[wide_resnet50_2.tv2_in1k](https://huggingface.co/timm/wide_resnet50_2.tv2_in1k)|224 |81.61|95.76|68.9 |11.4 |14.4 |1930 |
|[resnetaa50.a1h_in1k](https://huggingface.co/timm/resnetaa50.a1h_in1k)|288 |81.61|95.83|25.6 |8.5 |19.2 |1868 |
|[resnet101.a1_in1k](https://huggingface.co/timm/resnet101.a1_in1k)|224 |81.5 |95.16|44.6 |7.8 |16.2 |2125 |
|[resnext50_32x4d.a1_in1k](https://huggingface.co/timm/resnext50_32x4d.a1_in1k)|288 |81.48|95.16|25.0 |7.0 |23.8 |1745 |
|[gcresnet50t.ra2_in1k](https://huggingface.co/timm/gcresnet50t.ra2_in1k)|288 |81.47|95.71|25.9 |6.9 |18.6 |2071 |
|[wide_resnet50_2.racm_in1k](https://huggingface.co/timm/wide_resnet50_2.racm_in1k)|224 |81.45|95.53|68.9 |11.4 |14.4 |1929 |
|[resnet50d.a1_in1k](https://huggingface.co/timm/resnet50d.a1_in1k)|288 |81.44|95.22|25.6 |7.2 |19.7 |1908 |
|[ecaresnet50t.ra2_in1k](https://huggingface.co/timm/ecaresnet50t.ra2_in1k)|256 |81.44|95.67|25.6 |5.6 |15.4 |2168 |
|[ecaresnetlight.miil_in1k](https://huggingface.co/timm/ecaresnetlight.miil_in1k)|288 |81.4 |95.82|30.2 |6.8 |13.9 |2132 |
|[resnet50d.ra2_in1k](https://huggingface.co/timm/resnet50d.ra2_in1k)|288 |81.37|95.74|25.6 |7.2 |19.7 |1910 |
|[resnet101.a2_in1k](https://huggingface.co/timm/resnet101.a2_in1k)|224 |81.32|95.19|44.6 |7.8 |16.2 |2125 |
|[seresnet50.ra2_in1k](https://huggingface.co/timm/seresnet50.ra2_in1k)|288 |81.3 |95.65|28.1 |6.8 |18.4 |1803 |
|[resnext50_32x4d.a2_in1k](https://huggingface.co/timm/resnext50_32x4d.a2_in1k)|288 |81.3 |95.11|25.0 |7.0 |23.8 |1746 |
|[seresnext50_32x4d.racm_in1k](https://huggingface.co/timm/seresnext50_32x4d.racm_in1k)|224 |81.27|95.62|27.6 |4.3 |14.4 |2591 |
|[ecaresnet50t.a1_in1k](https://huggingface.co/timm/ecaresnet50t.a1_in1k)|224 |81.26|95.16|25.6 |4.3 |11.8 |2823 |
|[gcresnext50ts.ch_in1k](https://huggingface.co/timm/gcresnext50ts.ch_in1k)|288 |81.23|95.54|15.7 |4.8 |19.6 |2117 |
|[senet154.gluon_in1k](https://huggingface.co/timm/senet154.gluon_in1k)|224 |81.23|95.35|115.1 |20.8 |38.7 |545 |
|[resnet50.a1_in1k](https://huggingface.co/timm/resnet50.a1_in1k)|288 |81.22|95.11|25.6 |6.8 |18.4 |2089 |
|[resnet50_gn.a1h_in1k](https://huggingface.co/timm/resnet50_gn.a1h_in1k)|288 |81.22|95.63|25.6 |6.8 |18.4 |676 |
|[resnet50d.a2_in1k](https://huggingface.co/timm/resnet50d.a2_in1k)|288 |81.18|95.09|25.6 |7.2 |19.7 |1908 |
|[resnet50.fb_swsl_ig1b_ft_in1k](https://huggingface.co/timm/resnet50.fb_swsl_ig1b_ft_in1k)|224 |81.18|95.98|25.6 |4.1 |11.1 |3455 |
|[resnext50_32x4d.tv2_in1k](https://huggingface.co/timm/resnext50_32x4d.tv2_in1k)|224 |81.17|95.34|25.0 |4.3 |14.4 |2933 |
|[resnext50_32x4d.a1h_in1k](https://huggingface.co/timm/resnext50_32x4d.a1h_in1k)|224 |81.1 |95.33|25.0 |4.3 |14.4 |2934 |
|[seresnet50.a2_in1k](https://huggingface.co/timm/seresnet50.a2_in1k)|288 |81.1 |95.23|28.1 |6.8 |18.4 |1801 |
|[seresnet50.a1_in1k](https://huggingface.co/timm/seresnet50.a1_in1k)|288 |81.1 |95.12|28.1 |6.8 |18.4 |1799 |
|[resnet152s.gluon_in1k](https://huggingface.co/timm/resnet152s.gluon_in1k)|224 |81.02|95.41|60.3 |12.9 |25.0 |1347 |
|[resnet50.d_in1k](https://huggingface.co/timm/resnet50.d_in1k)|288 |80.97|95.44|25.6 |6.8 |18.4 |2085 |
|[gcresnet50t.ra2_in1k](https://huggingface.co/timm/gcresnet50t.ra2_in1k)|256 |80.94|95.45|25.9 |5.4 |14.7 |2571 |
|[resnext101_32x4d.fb_ssl_yfcc100m_ft_in1k](https://huggingface.co/timm/resnext101_32x4d.fb_ssl_yfcc100m_ft_in1k)|224 |80.93|95.73|44.2 |8.0 |21.2 |1814 |
|[resnet50.c1_in1k](https://huggingface.co/timm/resnet50.c1_in1k)|288 |80.91|95.55|25.6 |6.8 |18.4 |2084 |
|[seresnext101_32x4d.gluon_in1k](https://huggingface.co/timm/seresnext101_32x4d.gluon_in1k)|224 |80.9 |95.31|49.0 |8.0 |21.3 |1585 |
|[seresnext101_64x4d.gluon_in1k](https://huggingface.co/timm/seresnext101_64x4d.gluon_in1k)|224 |80.9 |95.3 |88.2 |15.5 |31.2 |918 |
|[resnet50.c2_in1k](https://huggingface.co/timm/resnet50.c2_in1k)|288 |80.86|95.52|25.6 |6.8 |18.4 |2085 |
|[resnet50.tv2_in1k](https://huggingface.co/timm/resnet50.tv2_in1k)|224 |80.85|95.43|25.6 |4.1 |11.1 |3450 |
|[ecaresnet50t.a2_in1k](https://huggingface.co/timm/ecaresnet50t.a2_in1k)|224 |80.84|95.02|25.6 |4.3 |11.8 |2821 |
|[ecaresnet101d_pruned.miil_in1k](https://huggingface.co/timm/ecaresnet101d_pruned.miil_in1k)|224 |80.79|95.62|24.9 |3.5 |7.7 |2961 |
|[seresnet33ts.ra2_in1k](https://huggingface.co/timm/seresnet33ts.ra2_in1k)|288 |80.79|95.36|19.8 |6.0 |14.8 |2506 |
|[ecaresnet50d_pruned.miil_in1k](https://huggingface.co/timm/ecaresnet50d_pruned.miil_in1k)|288 |80.79|95.58|19.9 |4.2 |10.6 |2349 |
|[resnet50.a2_in1k](https://huggingface.co/timm/resnet50.a2_in1k)|288 |80.78|94.99|25.6 |6.8 |18.4 |2088 |
|[resnet50.b1k_in1k](https://huggingface.co/timm/resnet50.b1k_in1k)|288 |80.71|95.43|25.6 |6.8 |18.4 |2087 |
|[resnext50_32x4d.ra_in1k](https://huggingface.co/timm/resnext50_32x4d.ra_in1k)|288 |80.7 |95.39|25.0 |7.0 |23.8 |1749 |
|[resnetrs101.tf_in1k](https://huggingface.co/timm/resnetrs101.tf_in1k)|192 |80.69|95.24|63.6 |6.0 |12.7 |2270 |
|[resnet50d.a1_in1k](https://huggingface.co/timm/resnet50d.a1_in1k)|224 |80.68|94.71|25.6 |4.4 |11.9 |3162 |
|[eca_resnet33ts.ra2_in1k](https://huggingface.co/timm/eca_resnet33ts.ra2_in1k)|288 |80.68|95.36|19.7 |6.0 |14.8 |2637 |
|[resnet50.a1h_in1k](https://huggingface.co/timm/resnet50.a1h_in1k)|224 |80.67|95.3 |25.6 |4.1 |11.1 |3452 |
|[resnext50d_32x4d.bt_in1k](https://huggingface.co/timm/resnext50d_32x4d.bt_in1k)|288 |80.67|95.42|25.0 |7.4 |25.1 |1626 |
|[resnetaa50.a1h_in1k](https://huggingface.co/timm/resnetaa50.a1h_in1k)|224 |80.63|95.21|25.6 |5.2 |11.6 |3034 |
|[ecaresnet50d.miil_in1k](https://huggingface.co/timm/ecaresnet50d.miil_in1k)|224 |80.61|95.32|25.6 |4.4 |11.9 |2813 |
|[resnext101_64x4d.gluon_in1k](https://huggingface.co/timm/resnext101_64x4d.gluon_in1k)|224 |80.61|94.99|83.5 |15.5 |31.2 |989 |
|[gcresnet33ts.ra2_in1k](https://huggingface.co/timm/gcresnet33ts.ra2_in1k)|288 |80.6 |95.31|19.9 |6.0 |14.8 |2578 |
|[gcresnext50ts.ch_in1k](https://huggingface.co/timm/gcresnext50ts.ch_in1k)|256 |80.57|95.17|15.7 |3.8 |15.5 |2710 |
|[resnet152.a3_in1k](https://huggingface.co/timm/resnet152.a3_in1k)|224 |80.56|95.0 |60.2 |11.6 |22.6 |1483 |
|[resnet50d.ra2_in1k](https://huggingface.co/timm/resnet50d.ra2_in1k)|224 |80.53|95.16|25.6 |4.4 |11.9 |3164 |
|[resnext50_32x4d.a1_in1k](https://huggingface.co/timm/resnext50_32x4d.a1_in1k)|224 |80.53|94.46|25.0 |4.3 |14.4 |2930 |
|[wide_resnet101_2.tv2_in1k](https://huggingface.co/timm/wide_resnet101_2.tv2_in1k)|176 |80.48|94.98|126.9 |14.3 |13.2 |1719 |
|[resnet152d.gluon_in1k](https://huggingface.co/timm/resnet152d.gluon_in1k)|224 |80.47|95.2 |60.2 |11.8 |23.4 |1428 |
|[resnet50.b2k_in1k](https://huggingface.co/timm/resnet50.b2k_in1k)|288 |80.45|95.32|25.6 |6.8 |18.4 |2086 |
|[ecaresnetlight.miil_in1k](https://huggingface.co/timm/ecaresnetlight.miil_in1k)|224 |80.45|95.24|30.2 |4.1 |8.4 |3530 |
|[resnext50_32x4d.a2_in1k](https://huggingface.co/timm/resnext50_32x4d.a2_in1k)|224 |80.45|94.63|25.0 |4.3 |14.4 |2936 |
|[wide_resnet50_2.tv2_in1k](https://huggingface.co/timm/wide_resnet50_2.tv2_in1k)|176 |80.43|95.09|68.9 |7.3 |9.0 |3015 |
|[resnet101d.gluon_in1k](https://huggingface.co/timm/resnet101d.gluon_in1k)|224 |80.42|95.01|44.6 |8.1 |17.0 |2007 |
|[resnet50.a1_in1k](https://huggingface.co/timm/resnet50.a1_in1k)|224 |80.38|94.6 |25.6 |4.1 |11.1 |3461 |
|[seresnet33ts.ra2_in1k](https://huggingface.co/timm/seresnet33ts.ra2_in1k)|256 |80.36|95.1 |19.8 |4.8 |11.7 |3267 |
|[resnext101_32x4d.gluon_in1k](https://huggingface.co/timm/resnext101_32x4d.gluon_in1k)|224 |80.34|94.93|44.2 |8.0 |21.2 |1814 |
|[resnext50_32x4d.fb_ssl_yfcc100m_ft_in1k](https://huggingface.co/timm/resnext50_32x4d.fb_ssl_yfcc100m_ft_in1k)|224 |80.32|95.4 |25.0 |4.3 |14.4 |2941 |
|[resnet101s.gluon_in1k](https://huggingface.co/timm/resnet101s.gluon_in1k)|224 |80.28|95.16|44.7 |9.2 |18.6 |1851 |
|[seresnet50.ra2_in1k](https://huggingface.co/timm/seresnet50.ra2_in1k)|224 |80.26|95.08|28.1 |4.1 |11.1 |2972 |
|[resnetblur50.bt_in1k](https://huggingface.co/timm/resnetblur50.bt_in1k)|288 |80.24|95.24|25.6 |8.5 |19.9 |1523 |
|[resnet50d.a2_in1k](https://huggingface.co/timm/resnet50d.a2_in1k)|224 |80.22|94.63|25.6 |4.4 |11.9 |3162 |
|[resnet152.tv2_in1k](https://huggingface.co/timm/resnet152.tv2_in1k)|176 |80.2 |94.64|60.2 |7.2 |14.0 |2346 |
|[seresnet50.a2_in1k](https://huggingface.co/timm/seresnet50.a2_in1k)|224 |80.08|94.74|28.1 |4.1 |11.1 |2969 |
|[eca_resnet33ts.ra2_in1k](https://huggingface.co/timm/eca_resnet33ts.ra2_in1k)|256 |80.08|94.97|19.7 |4.8 |11.7 |3284 |
|[gcresnet33ts.ra2_in1k](https://huggingface.co/timm/gcresnet33ts.ra2_in1k)|256 |80.06|94.99|19.9 |4.8 |11.7 |3216 |
|[resnet50_gn.a1h_in1k](https://huggingface.co/timm/resnet50_gn.a1h_in1k)|224 |80.06|94.95|25.6 |4.1 |11.1 |1109 |
|[seresnet50.a1_in1k](https://huggingface.co/timm/seresnet50.a1_in1k)|224 |80.02|94.71|28.1 |4.1 |11.1 |2962 |
|[resnet50.ram_in1k](https://huggingface.co/timm/resnet50.ram_in1k)|288 |79.97|95.05|25.6 |6.8 |18.4 |2086 |
|[resnet152c.gluon_in1k](https://huggingface.co/timm/resnet152c.gluon_in1k)|224 |79.92|94.84|60.2 |11.8 |23.4 |1455 |
|[seresnext50_32x4d.gluon_in1k](https://huggingface.co/timm/seresnext50_32x4d.gluon_in1k)|224 |79.91|94.82|27.6 |4.3 |14.4 |2591 |
|[resnet50.d_in1k](https://huggingface.co/timm/resnet50.d_in1k)|224 |79.91|94.67|25.6 |4.1 |11.1 |3456 |
|[resnet101.tv2_in1k](https://huggingface.co/timm/resnet101.tv2_in1k)|176 |79.9 |94.6 |44.6 |4.9 |10.1 |3341 |
|[resnetrs50.tf_in1k](https://huggingface.co/timm/resnetrs50.tf_in1k)|224 |79.89|94.97|35.7 |4.5 |12.1 |2774 |
|[resnet50.c2_in1k](https://huggingface.co/timm/resnet50.c2_in1k)|224 |79.88|94.87|25.6 |4.1 |11.1 |3455 |
|[ecaresnet26t.ra2_in1k](https://huggingface.co/timm/ecaresnet26t.ra2_in1k)|320 |79.86|95.07|16.0 |5.2 |16.4 |2168 |
|[resnet50.a2_in1k](https://huggingface.co/timm/resnet50.a2_in1k)|224 |79.85|94.56|25.6 |4.1 |11.1 |3460 |
|[resnet50.ra_in1k](https://huggingface.co/timm/resnet50.ra_in1k)|288 |79.83|94.97|25.6 |6.8 |18.4 |2087 |
|[resnet101.a3_in1k](https://huggingface.co/timm/resnet101.a3_in1k)|224 |79.82|94.62|44.6 |7.8 |16.2 |2114 |
|[resnext50_32x4d.ra_in1k](https://huggingface.co/timm/resnext50_32x4d.ra_in1k)|224 |79.76|94.6 |25.0 |4.3 |14.4 |2943 |
|[resnet50.c1_in1k](https://huggingface.co/timm/resnet50.c1_in1k)|224 |79.74|94.95|25.6 |4.1 |11.1 |3455 |
|[ecaresnet50d_pruned.miil_in1k](https://huggingface.co/timm/ecaresnet50d_pruned.miil_in1k)|224 |79.74|94.87|19.9 |2.5 |6.4 |3929 |
|[resnet33ts.ra2_in1k](https://huggingface.co/timm/resnet33ts.ra2_in1k)|288 |79.71|94.83|19.7 |6.0 |14.8 |2710 |
|[resnet152.gluon_in1k](https://huggingface.co/timm/resnet152.gluon_in1k)|224 |79.68|94.74|60.2 |11.6 |22.6 |1486 |
|[resnext50d_32x4d.bt_in1k](https://huggingface.co/timm/resnext50d_32x4d.bt_in1k)|224 |79.67|94.87|25.0 |4.5 |15.2 |2729 |
|[resnet50.bt_in1k](https://huggingface.co/timm/resnet50.bt_in1k)|288 |79.63|94.91|25.6 |6.8 |18.4 |2086 |
|[ecaresnet50t.a3_in1k](https://huggingface.co/timm/ecaresnet50t.a3_in1k)|224 |79.56|94.72|25.6 |4.3 |11.8 |2805 |
|[resnet101c.gluon_in1k](https://huggingface.co/timm/resnet101c.gluon_in1k)|224 |79.53|94.58|44.6 |8.1 |17.0 |2062 |
|[resnet50.b1k_in1k](https://huggingface.co/timm/resnet50.b1k_in1k)|224 |79.52|94.61|25.6 |4.1 |11.1 |3459 |
|[resnet50.tv2_in1k](https://huggingface.co/timm/resnet50.tv2_in1k)|176 |79.42|94.64|25.6 |2.6 |6.9 |5397 |
|[resnet32ts.ra2_in1k](https://huggingface.co/timm/resnet32ts.ra2_in1k)|288 |79.4 |94.66|18.0 |5.9 |14.6 |2752 |
|[resnet50.b2k_in1k](https://huggingface.co/timm/resnet50.b2k_in1k)|224 |79.38|94.57|25.6 |4.1 |11.1 |3459 |
|[resnext50_32x4d.tv2_in1k](https://huggingface.co/timm/resnext50_32x4d.tv2_in1k)|176 |79.37|94.3 |25.0 |2.7 |9.0 |4577 |
|[resnext50_32x4d.gluon_in1k](https://huggingface.co/timm/resnext50_32x4d.gluon_in1k)|224 |79.36|94.43|25.0 |4.3 |14.4 |2942 |
|[resnext101_32x8d.tv_in1k](https://huggingface.co/timm/resnext101_32x8d.tv_in1k)|224 |79.31|94.52|88.8 |16.5 |31.2 |1100 |
|[resnet101.gluon_in1k](https://huggingface.co/timm/resnet101.gluon_in1k)|224 |79.31|94.53|44.6 |7.8 |16.2 |2125 |
|[resnetblur50.bt_in1k](https://huggingface.co/timm/resnetblur50.bt_in1k)|224 |79.31|94.63|25.6 |5.2 |12.0 |2524 |
|[resnet50.a1h_in1k](https://huggingface.co/timm/resnet50.a1h_in1k)|176 |79.27|94.49|25.6 |2.6 |6.9 |5404 |
|[resnext50_32x4d.a3_in1k](https://huggingface.co/timm/resnext50_32x4d.a3_in1k)|224 |79.25|94.31|25.0 |4.3 |14.4 |2931 |
|[resnet50.fb_ssl_yfcc100m_ft_in1k](https://huggingface.co/timm/resnet50.fb_ssl_yfcc100m_ft_in1k)|224 |79.22|94.84|25.6 |4.1 |11.1 |3451 |
|[resnet33ts.ra2_in1k](https://huggingface.co/timm/resnet33ts.ra2_in1k)|256 |79.21|94.56|19.7 |4.8 |11.7 |3392 |
|[resnet50d.gluon_in1k](https://huggingface.co/timm/resnet50d.gluon_in1k)|224 |79.07|94.48|25.6 |4.4 |11.9 |3162 |
|[resnet50.ram_in1k](https://huggingface.co/timm/resnet50.ram_in1k)|224 |79.03|94.38|25.6 |4.1 |11.1 |3453 |
|[resnet50.am_in1k](https://huggingface.co/timm/resnet50.am_in1k)|224 |79.01|94.39|25.6 |4.1 |11.1 |3461 |
|[resnet32ts.ra2_in1k](https://huggingface.co/timm/resnet32ts.ra2_in1k)|256 |79.01|94.37|18.0 |4.6 |11.6 |3440 |
|[ecaresnet26t.ra2_in1k](https://huggingface.co/timm/ecaresnet26t.ra2_in1k)|256 |78.9 |94.54|16.0 |3.4 |10.5 |3421 |
|[resnet152.a3_in1k](https://huggingface.co/timm/resnet152.a3_in1k)|160 |78.89|94.11|60.2 |5.9 |11.5 |2745 |
|[wide_resnet101_2.tv_in1k](https://huggingface.co/timm/wide_resnet101_2.tv_in1k)|224 |78.84|94.28|126.9 |22.8 |21.2 |1079 |
|[seresnext26d_32x4d.bt_in1k](https://huggingface.co/timm/seresnext26d_32x4d.bt_in1k)|288 |78.83|94.24|16.8 |4.5 |16.8 |2251 |
|[resnet50.ra_in1k](https://huggingface.co/timm/resnet50.ra_in1k)|224 |78.81|94.32|25.6 |4.1 |11.1 |3454 |
|[seresnext26t_32x4d.bt_in1k](https://huggingface.co/timm/seresnext26t_32x4d.bt_in1k)|288 |78.74|94.33|16.8 |4.5 |16.7 |2264 |
|[resnet50s.gluon_in1k](https://huggingface.co/timm/resnet50s.gluon_in1k)|224 |78.72|94.23|25.7 |5.5 |13.5 |2796 |
|[resnet50d.a3_in1k](https://huggingface.co/timm/resnet50d.a3_in1k)|224 |78.71|94.24|25.6 |4.4 |11.9 |3154 |
|[wide_resnet50_2.tv_in1k](https://huggingface.co/timm/wide_resnet50_2.tv_in1k)|224 |78.47|94.09|68.9 |11.4 |14.4 |1934 |
|[resnet50.bt_in1k](https://huggingface.co/timm/resnet50.bt_in1k)|224 |78.46|94.27|25.6 |4.1 |11.1 |3454 |
|[resnet34d.ra2_in1k](https://huggingface.co/timm/resnet34d.ra2_in1k)|288 |78.43|94.35|21.8 |6.5 |7.5 |3291 |
|[gcresnext26ts.ch_in1k](https://huggingface.co/timm/gcresnext26ts.ch_in1k)|288 |78.42|94.04|10.5 |3.1 |13.3 |3226 |
|[resnet26t.ra2_in1k](https://huggingface.co/timm/resnet26t.ra2_in1k)|320 |78.33|94.13|16.0 |5.2 |16.4 |2391 |
|[resnet152.tv_in1k](https://huggingface.co/timm/resnet152.tv_in1k)|224 |78.32|94.04|60.2 |11.6 |22.6 |1487 |
|[seresnext26ts.ch_in1k](https://huggingface.co/timm/seresnext26ts.ch_in1k)|288 |78.28|94.1 |10.4 |3.1 |13.3 |3062 |
|[bat_resnext26ts.ch_in1k](https://huggingface.co/timm/bat_resnext26ts.ch_in1k)|256 |78.25|94.1 |10.7 |2.5 |12.5 |3393 |
|[resnet50.a3_in1k](https://huggingface.co/timm/resnet50.a3_in1k)|224 |78.06|93.78|25.6 |4.1 |11.1 |3450 |
|[resnet50c.gluon_in1k](https://huggingface.co/timm/resnet50c.gluon_in1k)|224 |78.0 |93.99|25.6 |4.4 |11.9 |3286 |
|[eca_resnext26ts.ch_in1k](https://huggingface.co/timm/eca_resnext26ts.ch_in1k)|288 |78.0 |93.91|10.3 |3.1 |13.3 |3297 |
|[seresnext26t_32x4d.bt_in1k](https://huggingface.co/timm/seresnext26t_32x4d.bt_in1k)|224 |77.98|93.75|16.8 |2.7 |10.1 |3841 |
|[resnet34.a1_in1k](https://huggingface.co/timm/resnet34.a1_in1k)|288 |77.92|93.77|21.8 |6.1 |6.2 |3609 |
|[resnet101.a3_in1k](https://huggingface.co/timm/resnet101.a3_in1k)|160 |77.88|93.71|44.6 |4.0 |8.3 |3926 |
|[resnet26t.ra2_in1k](https://huggingface.co/timm/resnet26t.ra2_in1k)|256 |77.87|93.84|16.0 |3.4 |10.5 |3772 |
|[seresnext26ts.ch_in1k](https://huggingface.co/timm/seresnext26ts.ch_in1k)|256 |77.86|93.79|10.4 |2.4 |10.5 |4263 |
|[resnetrs50.tf_in1k](https://huggingface.co/timm/resnetrs50.tf_in1k)|160 |77.82|93.81|35.7 |2.3 |6.2 |5238 |
|[gcresnext26ts.ch_in1k](https://huggingface.co/timm/gcresnext26ts.ch_in1k)|256 |77.81|93.82|10.5 |2.4 |10.5 |4183 |
|[ecaresnet50t.a3_in1k](https://huggingface.co/timm/ecaresnet50t.a3_in1k)|160 |77.79|93.6 |25.6 |2.2 |6.0 |5329 |
|[resnext50_32x4d.a3_in1k](https://huggingface.co/timm/resnext50_32x4d.a3_in1k)|160 |77.73|93.32|25.0 |2.2 |7.4 |5576 |
|[resnext50_32x4d.tv_in1k](https://huggingface.co/timm/resnext50_32x4d.tv_in1k)|224 |77.61|93.7 |25.0 |4.3 |14.4 |2944 |
|[seresnext26d_32x4d.bt_in1k](https://huggingface.co/timm/seresnext26d_32x4d.bt_in1k)|224 |77.59|93.61|16.8 |2.7 |10.2 |3807 |
|[resnet50.gluon_in1k](https://huggingface.co/timm/resnet50.gluon_in1k)|224 |77.58|93.72|25.6 |4.1 |11.1 |3455 |
|[eca_resnext26ts.ch_in1k](https://huggingface.co/timm/eca_resnext26ts.ch_in1k)|256 |77.44|93.56|10.3 |2.4 |10.5 |4284 |
|[resnet26d.bt_in1k](https://huggingface.co/timm/resnet26d.bt_in1k)|288 |77.41|93.63|16.0 |4.3 |13.5 |2907 |
|[resnet101.tv_in1k](https://huggingface.co/timm/resnet101.tv_in1k)|224 |77.38|93.54|44.6 |7.8 |16.2 |2125 |
|[resnet50d.a3_in1k](https://huggingface.co/timm/resnet50d.a3_in1k)|160 |77.22|93.27|25.6 |2.2 |6.1 |5982 |
|[resnext26ts.ra2_in1k](https://huggingface.co/timm/resnext26ts.ra2_in1k)|288 |77.17|93.47|10.3 |3.1 |13.3 |3392 |
|[resnet34.a2_in1k](https://huggingface.co/timm/resnet34.a2_in1k)|288 |77.15|93.27|21.8 |6.1 |6.2 |3615 |
|[resnet34d.ra2_in1k](https://huggingface.co/timm/resnet34d.ra2_in1k)|224 |77.1 |93.37|21.8 |3.9 |4.5 |5436 |
|[seresnet50.a3_in1k](https://huggingface.co/timm/seresnet50.a3_in1k)|224 |77.02|93.07|28.1 |4.1 |11.1 |2952 |
|[resnext26ts.ra2_in1k](https://huggingface.co/timm/resnext26ts.ra2_in1k)|256 |76.78|93.13|10.3 |2.4 |10.5 |4410 |
|[resnet26d.bt_in1k](https://huggingface.co/timm/resnet26d.bt_in1k)|224 |76.7 |93.17|16.0 |2.6 |8.2 |4859 |
|[resnet34.bt_in1k](https://huggingface.co/timm/resnet34.bt_in1k)|288 |76.5 |93.35|21.8 |6.1 |6.2 |3617 |
|[resnet34.a1_in1k](https://huggingface.co/timm/resnet34.a1_in1k)|224 |76.42|92.87|21.8 |3.7 |3.7 |5984 |
|[resnet26.bt_in1k](https://huggingface.co/timm/resnet26.bt_in1k)|288 |76.35|93.18|16.0 |3.9 |12.2 |3331 |
|[resnet50.tv_in1k](https://huggingface.co/timm/resnet50.tv_in1k)|224 |76.13|92.86|25.6 |4.1 |11.1 |3457 |
|[resnet50.a3_in1k](https://huggingface.co/timm/resnet50.a3_in1k)|160 |75.96|92.5 |25.6 |2.1 |5.7 |6490 |
|[resnet34.a2_in1k](https://huggingface.co/timm/resnet34.a2_in1k)|224 |75.52|92.44|21.8 |3.7 |3.7 |5991 |
|[resnet26.bt_in1k](https://huggingface.co/timm/resnet26.bt_in1k)|224 |75.3 |92.58|16.0 |2.4 |7.4 |5583 |
|[resnet34.bt_in1k](https://huggingface.co/timm/resnet34.bt_in1k)|224 |75.16|92.18|21.8 |3.7 |3.7 |5994 |
|[seresnet50.a3_in1k](https://huggingface.co/timm/seresnet50.a3_in1k)|160 |75.1 |92.08|28.1 |2.1 |5.7 |5513 |
|[resnet34.gluon_in1k](https://huggingface.co/timm/resnet34.gluon_in1k)|224 |74.57|91.98|21.8 |3.7 |3.7 |5984 |
|[resnet18d.ra2_in1k](https://huggingface.co/timm/resnet18d.ra2_in1k)|288 |73.81|91.83|11.7 |3.4 |5.4 |5196 |
|[resnet34.tv_in1k](https://huggingface.co/timm/resnet34.tv_in1k)|224 |73.32|91.42|21.8 |3.7 |3.7 |5979 |
|[resnet18.fb_swsl_ig1b_ft_in1k](https://huggingface.co/timm/resnet18.fb_swsl_ig1b_ft_in1k)|224 |73.28|91.73|11.7 |1.8 |2.5 |10213 |
|[resnet18.a1_in1k](https://huggingface.co/timm/resnet18.a1_in1k)|288 |73.16|91.03|11.7 |3.0 |4.1 |6050 |
|[resnet34.a3_in1k](https://huggingface.co/timm/resnet34.a3_in1k)|224 |72.98|91.11|21.8 |3.7 |3.7 |5967 |
|[resnet18.fb_ssl_yfcc100m_ft_in1k](https://huggingface.co/timm/resnet18.fb_ssl_yfcc100m_ft_in1k)|224 |72.6 |91.42|11.7 |1.8 |2.5 |10213 |
|[resnet18.a2_in1k](https://huggingface.co/timm/resnet18.a2_in1k)|288 |72.37|90.59|11.7 |3.0 |4.1 |6051 |
|[resnet14t.c3_in1k](https://huggingface.co/timm/resnet14t.c3_in1k)|224 |72.26|90.31|10.1 |1.7 |5.8 |7026 |
|[resnet18d.ra2_in1k](https://huggingface.co/timm/resnet18d.ra2_in1k)|224 |72.26|90.68|11.7 |2.1 |3.3 |8707 |
|[resnet18.a1_in1k](https://huggingface.co/timm/resnet18.a1_in1k)|224 |71.49|90.07|11.7 |1.8 |2.5 |10187 |
|[resnet14t.c3_in1k](https://huggingface.co/timm/resnet14t.c3_in1k)|176 |71.31|89.69|10.1 |1.1 |3.6 |10970 |
|[resnet18.gluon_in1k](https://huggingface.co/timm/resnet18.gluon_in1k)|224 |70.84|89.76|11.7 |1.8 |2.5 |10210 |
|[resnet18.a2_in1k](https://huggingface.co/timm/resnet18.a2_in1k)|224 |70.64|89.47|11.7 |1.8 |2.5 |10194 |
|[resnet34.a3_in1k](https://huggingface.co/timm/resnet34.a3_in1k)|160 |70.56|89.52|21.8 |1.9 |1.9 |10737 |
|[resnet18.tv_in1k](https://huggingface.co/timm/resnet18.tv_in1k)|224 |69.76|89.07|11.7 |1.8 |2.5 |10205 |
|[resnet10t.c3_in1k](https://huggingface.co/timm/resnet10t.c3_in1k)|224 |68.34|88.03|5.4 |1.1 |2.4 |13079 |
|[resnet18.a3_in1k](https://huggingface.co/timm/resnet18.a3_in1k)|224 |68.25|88.17|11.7 |1.8 |2.5 |10167 |
|[resnet10t.c3_in1k](https://huggingface.co/timm/resnet10t.c3_in1k)|176 |66.71|86.96|5.4 |0.7 |1.5 |20327 |
|[resnet18.a3_in1k](https://huggingface.co/timm/resnet18.a3_in1k)|160 |65.66|86.26|11.7 |0.9 |1.3 |18229 |
## Citation
```bibtex
@article{He2015,
author = {Kaiming He and Xiangyu Zhang and Shaoqing Ren and Jian Sun},
title = {Deep Residual Learning for Image Recognition},
journal = {arXiv preprint arXiv:1512.03385},
year = {2015}
}
```
```bibtex
@misc{rw2019timm,
author = {Ross Wightman},
title = {PyTorch Image Models},
year = {2019},
publisher = {GitHub},
journal = {GitHub repository},
doi = {10.5281/zenodo.4414861},
howpublished = {\url{https://github.com/huggingface/pytorch-image-models}}
}
```
|
jameslahm/yolov10n | jameslahm | "2024-06-03T13:20:11Z" | 14,429 | 2 | transformers | [
"transformers",
"safetensors",
"object-detection",
"computer-vision",
"yolov10",
"dataset:detection-datasets/coco",
"arxiv:2405.14458",
"license:agpl-3.0",
"region:us"
] | object-detection | "2024-06-01T10:36:36Z" | ---
license: agpl-3.0
tags:
- object-detection
- computer-vision
- yolov10
datasets:
- detection-datasets/coco
inference: false
---
### Model Description
[YOLOv10: Real-Time End-to-End Object Detection](https://arxiv.org/abs/2405.14458v1)
- arXiv: https://arxiv.org/abs/2405.14458v1
- github: https://github.com/THU-MIG/yolov10
### Installation
```
pip install git+https://github.com/THU-MIG/yolov10.git
```
### Training and validation
```python
from ultralytics import YOLOv10
model = YOLOv10.from_pretrained('jameslahm/yolov10n')
# Training
model.train(...)
# after training, one can push to the hub
model.push_to_hub("your-hf-username/yolov10-finetuned")
# Validation
model.val(...)
```
### Inference
Here's an end-to-end example showcasing inference on a cats image:
```python
from ultralytics import YOLOv10
model = YOLOv10.from_pretrained('jameslahm/yolov10n')
source = 'http://images.cocodataset.org/val2017/000000039769.jpg'
model.predict(source=source, save=True)
```
which shows:

### BibTeX Entry and Citation Info
```
@article{wang2024yolov10,
title={YOLOv10: Real-Time End-to-End Object Detection},
author={Wang, Ao and Chen, Hui and Liu, Lihao and Chen, Kai and Lin, Zijia and Han, Jungong and Ding, Guiguang},
journal={arXiv preprint arXiv:2405.14458},
year={2024}
}
``` |
dmargutierrez/distilbert-base-multilingual-cased-mapa_coarse-ner | dmargutierrez | "2023-03-17T11:35:00Z" | 14,425 | 1 | transformers | [
"transformers",
"pytorch",
"tensorboard",
"safetensors",
"distilbert",
"token-classification",
"generated_from_trainer",
"name-entity-recognition",
"legal",
"en",
"fr",
"it",
"es",
"de",
"nl",
"pl",
"ru",
"pt",
"dataset:lextreme",
"license:apache-2.0",
"model-index",
"autotrain_compatible",
"endpoints_compatible",
"region:us"
] | token-classification | "2023-03-17T09:31:36Z" | ---
license: apache-2.0
tags:
- generated_from_trainer
- name-entity-recognition
- legal
datasets:
- lextreme
metrics:
- precision
- recall
- f1
- accuracy
model-index:
- name: distilbert-base-multilingual-cased-mapa_coarse-ner
results:
- task:
name: Token Classification
type: token-classification
dataset:
name: lextreme
type: lextreme
config: mapa_coarse
split: test
args: mapa_coarse
metrics:
- name: Precision
type: precision
value: 0.7191116088092572
- name: Recall
type: recall
value: 0.6452855468095796
- name: F1
type: f1
value: 0.6802012534204254
- name: Accuracy
type: accuracy
value: 0.9878756336348935
language:
- en
- fr
- it
- es
- de
- nl
- pl
- ru
- pt
---
<!-- This model card has been generated automatically according to the information the Trainer had access to. You
should probably proofread and complete it, then remove this comment. -->
# distilbert-base-multilingual-cased-mapa_coarse-ner
This model is a fine-tuned version of [distilbert-base-multilingual-cased](https://huggingface.co/distilbert-base-multilingual-cased) on the lextreme dataset.
It achieves the following results on the evaluation set:
- Loss: 0.0882
- Precision: 0.7191
- Recall: 0.6453
- F1: 0.6802
- Accuracy: 0.9879
## Model description
More information needed
## Intended uses & limitations
More information needed
## Training and evaluation data
More information needed
## Training procedure
### Training hyperparameters
The following hyperparameters were used during training:
- learning_rate: 2e-05
- train_batch_size: 16
- eval_batch_size: 16
- seed: 42
- optimizer: Adam with betas=(0.9,0.999) and epsilon=1e-08
- lr_scheduler_type: linear
- num_epochs: 10
### Training results
| Training Loss | Epoch | Step | Validation Loss | Precision | Recall | F1 | Accuracy |
|:-------------:|:-----:|:-----:|:---------------:|:---------:|:------:|:------:|:--------:|
| 0.0248 | 1.0 | 1739 | 0.0528 | 0.7451 | 0.5805 | 0.6525 | 0.9871 |
| 0.0181 | 2.0 | 3478 | 0.0595 | 0.7369 | 0.5749 | 0.6459 | 0.9875 |
| 0.0121 | 3.0 | 5217 | 0.0499 | 0.7404 | 0.6280 | 0.6796 | 0.9879 |
| 0.0088 | 4.0 | 6956 | 0.0634 | 0.6912 | 0.6334 | 0.6610 | 0.9875 |
| 0.0072 | 5.0 | 8695 | 0.0625 | 0.7109 | 0.6478 | 0.6779 | 0.9880 |
| 0.0052 | 6.0 | 10434 | 0.0702 | 0.7098 | 0.6518 | 0.6796 | 0.9878 |
| 0.0041 | 7.0 | 12173 | 0.0733 | 0.7176 | 0.6429 | 0.6782 | 0.9878 |
| 0.0026 | 8.0 | 13912 | 0.0779 | 0.7198 | 0.6540 | 0.6853 | 0.9879 |
| 0.0019 | 9.0 | 15651 | 0.0875 | 0.7181 | 0.6419 | 0.6779 | 0.9877 |
| 0.0018 | 10.0 | 17390 | 0.0882 | 0.7191 | 0.6453 | 0.6802 | 0.9879 |
### Framework versions
- Transformers 4.26.0
- Pytorch 1.13.1+cu117
- Datasets 2.9.0
- Tokenizers 0.13.2 |
RichardErkhov/shanchen_-_llama3-8B-slerp-med-262k-gguf | RichardErkhov | "2024-06-26T10:00:02Z" | 14,425 | 0 | null | [
"gguf",
"region:us"
] | null | "2024-06-26T04:17:19Z" | Quantization made by Richard Erkhov.
[Github](https://github.com/RichardErkhov)
[Discord](https://discord.gg/pvy7H8DZMG)
[Request more models](https://github.com/RichardErkhov/quant_request)
llama3-8B-slerp-med-262k - GGUF
- Model creator: https://huggingface.co/shanchen/
- Original model: https://huggingface.co/shanchen/llama3-8B-slerp-med-262k/
| Name | Quant method | Size |
| ---- | ---- | ---- |
| [llama3-8B-slerp-med-262k.Q2_K.gguf](https://huggingface.co/RichardErkhov/shanchen_-_llama3-8B-slerp-med-262k-gguf/blob/main/llama3-8B-slerp-med-262k.Q2_K.gguf) | Q2_K | 2.96GB |
| [llama3-8B-slerp-med-262k.IQ3_XS.gguf](https://huggingface.co/RichardErkhov/shanchen_-_llama3-8B-slerp-med-262k-gguf/blob/main/llama3-8B-slerp-med-262k.IQ3_XS.gguf) | IQ3_XS | 3.28GB |
| [llama3-8B-slerp-med-262k.IQ3_S.gguf](https://huggingface.co/RichardErkhov/shanchen_-_llama3-8B-slerp-med-262k-gguf/blob/main/llama3-8B-slerp-med-262k.IQ3_S.gguf) | IQ3_S | 3.43GB |
| [llama3-8B-slerp-med-262k.Q3_K_S.gguf](https://huggingface.co/RichardErkhov/shanchen_-_llama3-8B-slerp-med-262k-gguf/blob/main/llama3-8B-slerp-med-262k.Q3_K_S.gguf) | Q3_K_S | 3.41GB |
| [llama3-8B-slerp-med-262k.IQ3_M.gguf](https://huggingface.co/RichardErkhov/shanchen_-_llama3-8B-slerp-med-262k-gguf/blob/main/llama3-8B-slerp-med-262k.IQ3_M.gguf) | IQ3_M | 3.52GB |
| [llama3-8B-slerp-med-262k.Q3_K.gguf](https://huggingface.co/RichardErkhov/shanchen_-_llama3-8B-slerp-med-262k-gguf/blob/main/llama3-8B-slerp-med-262k.Q3_K.gguf) | Q3_K | 3.74GB |
| [llama3-8B-slerp-med-262k.Q3_K_M.gguf](https://huggingface.co/RichardErkhov/shanchen_-_llama3-8B-slerp-med-262k-gguf/blob/main/llama3-8B-slerp-med-262k.Q3_K_M.gguf) | Q3_K_M | 3.74GB |
| [llama3-8B-slerp-med-262k.Q3_K_L.gguf](https://huggingface.co/RichardErkhov/shanchen_-_llama3-8B-slerp-med-262k-gguf/blob/main/llama3-8B-slerp-med-262k.Q3_K_L.gguf) | Q3_K_L | 4.03GB |
| [llama3-8B-slerp-med-262k.IQ4_XS.gguf](https://huggingface.co/RichardErkhov/shanchen_-_llama3-8B-slerp-med-262k-gguf/blob/main/llama3-8B-slerp-med-262k.IQ4_XS.gguf) | IQ4_XS | 4.18GB |
| [llama3-8B-slerp-med-262k.Q4_0.gguf](https://huggingface.co/RichardErkhov/shanchen_-_llama3-8B-slerp-med-262k-gguf/blob/main/llama3-8B-slerp-med-262k.Q4_0.gguf) | Q4_0 | 4.34GB |
| [llama3-8B-slerp-med-262k.IQ4_NL.gguf](https://huggingface.co/RichardErkhov/shanchen_-_llama3-8B-slerp-med-262k-gguf/blob/main/llama3-8B-slerp-med-262k.IQ4_NL.gguf) | IQ4_NL | 4.38GB |
| [llama3-8B-slerp-med-262k.Q4_K_S.gguf](https://huggingface.co/RichardErkhov/shanchen_-_llama3-8B-slerp-med-262k-gguf/blob/main/llama3-8B-slerp-med-262k.Q4_K_S.gguf) | Q4_K_S | 4.37GB |
| [llama3-8B-slerp-med-262k.Q4_K.gguf](https://huggingface.co/RichardErkhov/shanchen_-_llama3-8B-slerp-med-262k-gguf/blob/main/llama3-8B-slerp-med-262k.Q4_K.gguf) | Q4_K | 4.58GB |
| [llama3-8B-slerp-med-262k.Q4_K_M.gguf](https://huggingface.co/RichardErkhov/shanchen_-_llama3-8B-slerp-med-262k-gguf/blob/main/llama3-8B-slerp-med-262k.Q4_K_M.gguf) | Q4_K_M | 4.58GB |
| [llama3-8B-slerp-med-262k.Q4_1.gguf](https://huggingface.co/RichardErkhov/shanchen_-_llama3-8B-slerp-med-262k-gguf/blob/main/llama3-8B-slerp-med-262k.Q4_1.gguf) | Q4_1 | 4.78GB |
| [llama3-8B-slerp-med-262k.Q5_0.gguf](https://huggingface.co/RichardErkhov/shanchen_-_llama3-8B-slerp-med-262k-gguf/blob/main/llama3-8B-slerp-med-262k.Q5_0.gguf) | Q5_0 | 5.21GB |
| [llama3-8B-slerp-med-262k.Q5_K_S.gguf](https://huggingface.co/RichardErkhov/shanchen_-_llama3-8B-slerp-med-262k-gguf/blob/main/llama3-8B-slerp-med-262k.Q5_K_S.gguf) | Q5_K_S | 5.21GB |
| [llama3-8B-slerp-med-262k.Q5_K.gguf](https://huggingface.co/RichardErkhov/shanchen_-_llama3-8B-slerp-med-262k-gguf/blob/main/llama3-8B-slerp-med-262k.Q5_K.gguf) | Q5_K | 5.34GB |
| [llama3-8B-slerp-med-262k.Q5_K_M.gguf](https://huggingface.co/RichardErkhov/shanchen_-_llama3-8B-slerp-med-262k-gguf/blob/main/llama3-8B-slerp-med-262k.Q5_K_M.gguf) | Q5_K_M | 5.34GB |
| [llama3-8B-slerp-med-262k.Q5_1.gguf](https://huggingface.co/RichardErkhov/shanchen_-_llama3-8B-slerp-med-262k-gguf/blob/main/llama3-8B-slerp-med-262k.Q5_1.gguf) | Q5_1 | 5.65GB |
| [llama3-8B-slerp-med-262k.Q6_K.gguf](https://huggingface.co/RichardErkhov/shanchen_-_llama3-8B-slerp-med-262k-gguf/blob/main/llama3-8B-slerp-med-262k.Q6_K.gguf) | Q6_K | 6.14GB |
| [llama3-8B-slerp-med-262k.Q8_0.gguf](https://huggingface.co/RichardErkhov/shanchen_-_llama3-8B-slerp-med-262k-gguf/blob/main/llama3-8B-slerp-med-262k.Q8_0.gguf) | Q8_0 | 7.95GB |
Original model description:
---
tags:
- merge
- mergekit
- lazymergekit
- gradientai/Llama-3-8B-Instruct-262k
- johnsnowlabs/JSL-MedLlama-3-8B-v1.0
base_model:
- gradientai/Llama-3-8B-Instruct-262k
- johnsnowlabs/JSL-MedLlama-3-8B-v1.0
license: llama3
language:
- zh
---
# llama3-8B-slerp-med-262k
llama3-8B-slerp-med-262k is a merge of the following models using [LazyMergekit](https://colab.research.google.com/drive/1obulZ1ROXHjYLn6PPZJwRR6GzgQogxxb?usp=sharing):
* [gradientai/Llama-3-8B-Instruct-262k](https://huggingface.co/gradientai/Llama-3-8B-Instruct-262k)
* [johnsnowlabs/JSL-MedLlama-3-8B-v1.0](https://huggingface.co/johnsnowlabs/JSL-MedLlama-3-8B-v1.0)
## 🧩 Configuration
```yaml
slices:
- sources:
- model: gradientai/Llama-3-8B-Instruct-262k
layer_range: [0,32]
- model: johnsnowlabs/JSL-MedLlama-3-8B-v1.0
layer_range: [0,32]
merge_method: slerp
base_model: gradientai/Llama-3-8B-Instruct-262k
parameters:
t:
- filter: self_attn
value: [0.3, 0.5, 0.5, 0.7, 1]
- filter: mlp
value: [1, 0.7, 0.5, 0.5, 0.3]
- value: 0.5
dtype: bfloat16
```
## 💻 Usage
```python
!pip install -qU transformers accelerate
from transformers import AutoTokenizer
import transformers
import torch
model = "shanchen/llama3-8B-slerp-med-262k"
messages = [{"role": "user", "content": "What is a large language model?"}]
tokenizer = AutoTokenizer.from_pretrained(model)
prompt = tokenizer.apply_chat_template(messages, tokenize=False, add_generation_prompt=True)
pipeline = transformers.pipeline(
"text-generation",
model=model,
torch_dtype=torch.float16,
device_map="auto",
)
outputs = pipeline(prompt, max_new_tokens=256, do_sample=True, temperature=0.7, top_k=50, top_p=0.95)
print(outputs[0]["generated_text"])
```
|
mradermacher/L3-Nymeria-v2-8B-i1-GGUF | mradermacher | "2024-06-30T07:04:42Z" | 14,415 | 1 | transformers | [
"transformers",
"gguf",
"mergekit",
"merge",
"roleplay",
"sillytavern",
"llama3",
"not-for-all-audiences",
"en",
"base_model:tannedbum/L3-Nymeria-v2-8B",
"license:cc-by-nc-4.0",
"endpoints_compatible",
"region:us"
] | null | "2024-06-30T04:49:43Z" | ---
base_model: tannedbum/L3-Nymeria-v2-8B
language:
- en
library_name: transformers
license: cc-by-nc-4.0
quantized_by: mradermacher
tags:
- mergekit
- merge
- roleplay
- sillytavern
- llama3
- not-for-all-audiences
---
## About
<!-- ### quantize_version: 2 -->
<!-- ### output_tensor_quantised: 1 -->
<!-- ### convert_type: hf -->
<!-- ### vocab_type: -->
<!-- ### tags: nicoboss -->
weighted/imatrix quants of https://huggingface.co/tannedbum/L3-Nymeria-v2-8B
<!-- provided-files -->
static quants are available at https://huggingface.co/mradermacher/L3-Nymeria-v2-8B-GGUF
## Usage
If you are unsure how to use GGUF files, refer to one of [TheBloke's
READMEs](https://huggingface.co/TheBloke/KafkaLM-70B-German-V0.1-GGUF) for
more details, including on how to concatenate multi-part files.
## Provided Quants
(sorted by size, not necessarily quality. IQ-quants are often preferable over similar sized non-IQ quants)
| Link | Type | Size/GB | Notes |
|:-----|:-----|--------:|:------|
| [GGUF](https://huggingface.co/mradermacher/L3-Nymeria-v2-8B-i1-GGUF/resolve/main/L3-Nymeria-v2-8B.i1-IQ1_S.gguf) | i1-IQ1_S | 2.1 | for the desperate |
| [GGUF](https://huggingface.co/mradermacher/L3-Nymeria-v2-8B-i1-GGUF/resolve/main/L3-Nymeria-v2-8B.i1-IQ1_M.gguf) | i1-IQ1_M | 2.3 | mostly desperate |
| [GGUF](https://huggingface.co/mradermacher/L3-Nymeria-v2-8B-i1-GGUF/resolve/main/L3-Nymeria-v2-8B.i1-IQ2_XXS.gguf) | i1-IQ2_XXS | 2.5 | |
| [GGUF](https://huggingface.co/mradermacher/L3-Nymeria-v2-8B-i1-GGUF/resolve/main/L3-Nymeria-v2-8B.i1-IQ2_XS.gguf) | i1-IQ2_XS | 2.7 | |
| [GGUF](https://huggingface.co/mradermacher/L3-Nymeria-v2-8B-i1-GGUF/resolve/main/L3-Nymeria-v2-8B.i1-IQ2_S.gguf) | i1-IQ2_S | 2.9 | |
| [GGUF](https://huggingface.co/mradermacher/L3-Nymeria-v2-8B-i1-GGUF/resolve/main/L3-Nymeria-v2-8B.i1-IQ2_M.gguf) | i1-IQ2_M | 3.0 | |
| [GGUF](https://huggingface.co/mradermacher/L3-Nymeria-v2-8B-i1-GGUF/resolve/main/L3-Nymeria-v2-8B.i1-Q2_K.gguf) | i1-Q2_K | 3.3 | IQ3_XXS probably better |
| [GGUF](https://huggingface.co/mradermacher/L3-Nymeria-v2-8B-i1-GGUF/resolve/main/L3-Nymeria-v2-8B.i1-IQ3_XXS.gguf) | i1-IQ3_XXS | 3.4 | lower quality |
| [GGUF](https://huggingface.co/mradermacher/L3-Nymeria-v2-8B-i1-GGUF/resolve/main/L3-Nymeria-v2-8B.i1-IQ3_XS.gguf) | i1-IQ3_XS | 3.6 | |
| [GGUF](https://huggingface.co/mradermacher/L3-Nymeria-v2-8B-i1-GGUF/resolve/main/L3-Nymeria-v2-8B.i1-Q3_K_S.gguf) | i1-Q3_K_S | 3.8 | IQ3_XS probably better |
| [GGUF](https://huggingface.co/mradermacher/L3-Nymeria-v2-8B-i1-GGUF/resolve/main/L3-Nymeria-v2-8B.i1-IQ3_S.gguf) | i1-IQ3_S | 3.8 | beats Q3_K* |
| [GGUF](https://huggingface.co/mradermacher/L3-Nymeria-v2-8B-i1-GGUF/resolve/main/L3-Nymeria-v2-8B.i1-IQ3_M.gguf) | i1-IQ3_M | 3.9 | |
| [GGUF](https://huggingface.co/mradermacher/L3-Nymeria-v2-8B-i1-GGUF/resolve/main/L3-Nymeria-v2-8B.i1-Q3_K_M.gguf) | i1-Q3_K_M | 4.1 | IQ3_S probably better |
| [GGUF](https://huggingface.co/mradermacher/L3-Nymeria-v2-8B-i1-GGUF/resolve/main/L3-Nymeria-v2-8B.i1-Q3_K_L.gguf) | i1-Q3_K_L | 4.4 | IQ3_M probably better |
| [GGUF](https://huggingface.co/mradermacher/L3-Nymeria-v2-8B-i1-GGUF/resolve/main/L3-Nymeria-v2-8B.i1-IQ4_XS.gguf) | i1-IQ4_XS | 4.5 | |
| [GGUF](https://huggingface.co/mradermacher/L3-Nymeria-v2-8B-i1-GGUF/resolve/main/L3-Nymeria-v2-8B.i1-Q4_0.gguf) | i1-Q4_0 | 4.8 | fast, low quality |
| [GGUF](https://huggingface.co/mradermacher/L3-Nymeria-v2-8B-i1-GGUF/resolve/main/L3-Nymeria-v2-8B.i1-Q4_K_S.gguf) | i1-Q4_K_S | 4.8 | optimal size/speed/quality |
| [GGUF](https://huggingface.co/mradermacher/L3-Nymeria-v2-8B-i1-GGUF/resolve/main/L3-Nymeria-v2-8B.i1-Q4_K_M.gguf) | i1-Q4_K_M | 5.0 | fast, recommended |
| [GGUF](https://huggingface.co/mradermacher/L3-Nymeria-v2-8B-i1-GGUF/resolve/main/L3-Nymeria-v2-8B.i1-Q5_K_S.gguf) | i1-Q5_K_S | 5.7 | |
| [GGUF](https://huggingface.co/mradermacher/L3-Nymeria-v2-8B-i1-GGUF/resolve/main/L3-Nymeria-v2-8B.i1-Q5_K_M.gguf) | i1-Q5_K_M | 5.8 | |
| [GGUF](https://huggingface.co/mradermacher/L3-Nymeria-v2-8B-i1-GGUF/resolve/main/L3-Nymeria-v2-8B.i1-Q6_K.gguf) | i1-Q6_K | 6.7 | practically like static Q6_K |
Here is a handy graph by ikawrakow comparing some lower-quality quant
types (lower is better):

And here are Artefact2's thoughts on the matter:
https://gist.github.com/Artefact2/b5f810600771265fc1e39442288e8ec9
## FAQ / Model Request
See https://huggingface.co/mradermacher/model_requests for some answers to
questions you might have and/or if you want some other model quantized.
## Thanks
I thank my company, [nethype GmbH](https://www.nethype.de/), for letting
me use its servers and providing upgrades to my workstation to enable
this work in my free time. Additional thanks to [@nicoboss](https://huggingface.co/nicoboss) for giving me access to his hardware for calculating the imatrix for these quants.
<!-- end -->
|
mradermacher/modified-llama-3-8B-GGUF | mradermacher | "2024-06-26T16:01:59Z" | 14,400 | 0 | transformers | [
"transformers",
"gguf",
"en",
"base_model:cooperr/modified-llama-3-8B",
"endpoints_compatible",
"region:us"
] | null | "2024-06-26T15:09:28Z" | ---
base_model: cooperr/modified-llama-3-8B
language:
- en
library_name: transformers
quantized_by: mradermacher
---
## About
<!-- ### quantize_version: 2 -->
<!-- ### output_tensor_quantised: 1 -->
<!-- ### convert_type: hf -->
<!-- ### vocab_type: -->
<!-- ### tags: -->
static quants of https://huggingface.co/cooperr/modified-llama-3-8B
<!-- provided-files -->
weighted/imatrix quants seem not to be available (by me) at this time. If they do not show up a week or so after the static ones, I have probably not planned for them. Feel free to request them by opening a Community Discussion.
## Usage
If you are unsure how to use GGUF files, refer to one of [TheBloke's
READMEs](https://huggingface.co/TheBloke/KafkaLM-70B-German-V0.1-GGUF) for
more details, including on how to concatenate multi-part files.
## Provided Quants
(sorted by size, not necessarily quality. IQ-quants are often preferable over similar sized non-IQ quants)
| Link | Type | Size/GB | Notes |
|:-----|:-----|--------:|:------|
| [GGUF](https://huggingface.co/mradermacher/modified-llama-3-8B-GGUF/resolve/main/modified-llama-3-8B.Q2_K.gguf) | Q2_K | 3.3 | |
| [GGUF](https://huggingface.co/mradermacher/modified-llama-3-8B-GGUF/resolve/main/modified-llama-3-8B.IQ3_XS.gguf) | IQ3_XS | 3.6 | |
| [GGUF](https://huggingface.co/mradermacher/modified-llama-3-8B-GGUF/resolve/main/modified-llama-3-8B.Q3_K_S.gguf) | Q3_K_S | 3.8 | |
| [GGUF](https://huggingface.co/mradermacher/modified-llama-3-8B-GGUF/resolve/main/modified-llama-3-8B.IQ3_S.gguf) | IQ3_S | 3.8 | beats Q3_K* |
| [GGUF](https://huggingface.co/mradermacher/modified-llama-3-8B-GGUF/resolve/main/modified-llama-3-8B.IQ3_M.gguf) | IQ3_M | 3.9 | |
| [GGUF](https://huggingface.co/mradermacher/modified-llama-3-8B-GGUF/resolve/main/modified-llama-3-8B.Q3_K_M.gguf) | Q3_K_M | 4.1 | lower quality |
| [GGUF](https://huggingface.co/mradermacher/modified-llama-3-8B-GGUF/resolve/main/modified-llama-3-8B.Q3_K_L.gguf) | Q3_K_L | 4.4 | |
| [GGUF](https://huggingface.co/mradermacher/modified-llama-3-8B-GGUF/resolve/main/modified-llama-3-8B.IQ4_XS.gguf) | IQ4_XS | 4.6 | |
| [GGUF](https://huggingface.co/mradermacher/modified-llama-3-8B-GGUF/resolve/main/modified-llama-3-8B.Q4_K_S.gguf) | Q4_K_S | 4.8 | fast, recommended |
| [GGUF](https://huggingface.co/mradermacher/modified-llama-3-8B-GGUF/resolve/main/modified-llama-3-8B.Q4_K_M.gguf) | Q4_K_M | 5.0 | fast, recommended |
| [GGUF](https://huggingface.co/mradermacher/modified-llama-3-8B-GGUF/resolve/main/modified-llama-3-8B.Q5_K_S.gguf) | Q5_K_S | 5.7 | |
| [GGUF](https://huggingface.co/mradermacher/modified-llama-3-8B-GGUF/resolve/main/modified-llama-3-8B.Q5_K_M.gguf) | Q5_K_M | 5.8 | |
| [GGUF](https://huggingface.co/mradermacher/modified-llama-3-8B-GGUF/resolve/main/modified-llama-3-8B.Q6_K.gguf) | Q6_K | 6.7 | very good quality |
| [GGUF](https://huggingface.co/mradermacher/modified-llama-3-8B-GGUF/resolve/main/modified-llama-3-8B.Q8_0.gguf) | Q8_0 | 8.6 | fast, best quality |
| [GGUF](https://huggingface.co/mradermacher/modified-llama-3-8B-GGUF/resolve/main/modified-llama-3-8B.f16.gguf) | f16 | 16.2 | 16 bpw, overkill |
Here is a handy graph by ikawrakow comparing some lower-quality quant
types (lower is better):

And here are Artefact2's thoughts on the matter:
https://gist.github.com/Artefact2/b5f810600771265fc1e39442288e8ec9
## FAQ / Model Request
See https://huggingface.co/mradermacher/model_requests for some answers to
questions you might have and/or if you want some other model quantized.
## Thanks
I thank my company, [nethype GmbH](https://www.nethype.de/), for letting
me use its servers and providing upgrades to my workstation to enable
this work in my free time.
<!-- end -->
|
mradermacher/Llama-3-8B-Tulu-330K-i1-GGUF | mradermacher | "2024-06-28T10:18:44Z" | 14,395 | 0 | transformers | [
"transformers",
"gguf",
"axolotl",
"generated_from_trainer",
"en",
"base_model:Magpie-Align/Llama-3-8B-Tulu-330K",
"license:llama3",
"endpoints_compatible",
"region:us"
] | null | "2024-06-28T08:44:48Z" | ---
base_model: Magpie-Align/Llama-3-8B-Tulu-330K
language:
- en
library_name: transformers
license: llama3
quantized_by: mradermacher
tags:
- axolotl
- generated_from_trainer
---
## About
<!-- ### quantize_version: 2 -->
<!-- ### output_tensor_quantised: 1 -->
<!-- ### convert_type: hf -->
<!-- ### vocab_type: -->
<!-- ### tags: nicoboss -->
weighted/imatrix quants of https://huggingface.co/Magpie-Align/Llama-3-8B-Tulu-330K
<!-- provided-files -->
static quants are available at https://huggingface.co/mradermacher/Llama-3-8B-Tulu-330K-GGUF
## Usage
If you are unsure how to use GGUF files, refer to one of [TheBloke's
READMEs](https://huggingface.co/TheBloke/KafkaLM-70B-German-V0.1-GGUF) for
more details, including on how to concatenate multi-part files.
## Provided Quants
(sorted by size, not necessarily quality. IQ-quants are often preferable over similar sized non-IQ quants)
| Link | Type | Size/GB | Notes |
|:-----|:-----|--------:|:------|
| [GGUF](https://huggingface.co/mradermacher/Llama-3-8B-Tulu-330K-i1-GGUF/resolve/main/Llama-3-8B-Tulu-330K.i1-IQ1_S.gguf) | i1-IQ1_S | 2.1 | for the desperate |
| [GGUF](https://huggingface.co/mradermacher/Llama-3-8B-Tulu-330K-i1-GGUF/resolve/main/Llama-3-8B-Tulu-330K.i1-IQ1_M.gguf) | i1-IQ1_M | 2.3 | mostly desperate |
| [GGUF](https://huggingface.co/mradermacher/Llama-3-8B-Tulu-330K-i1-GGUF/resolve/main/Llama-3-8B-Tulu-330K.i1-IQ2_XXS.gguf) | i1-IQ2_XXS | 2.5 | |
| [GGUF](https://huggingface.co/mradermacher/Llama-3-8B-Tulu-330K-i1-GGUF/resolve/main/Llama-3-8B-Tulu-330K.i1-IQ2_XS.gguf) | i1-IQ2_XS | 2.7 | |
| [GGUF](https://huggingface.co/mradermacher/Llama-3-8B-Tulu-330K-i1-GGUF/resolve/main/Llama-3-8B-Tulu-330K.i1-IQ2_S.gguf) | i1-IQ2_S | 2.9 | |
| [GGUF](https://huggingface.co/mradermacher/Llama-3-8B-Tulu-330K-i1-GGUF/resolve/main/Llama-3-8B-Tulu-330K.i1-IQ2_M.gguf) | i1-IQ2_M | 3.0 | |
| [GGUF](https://huggingface.co/mradermacher/Llama-3-8B-Tulu-330K-i1-GGUF/resolve/main/Llama-3-8B-Tulu-330K.i1-Q2_K.gguf) | i1-Q2_K | 3.3 | IQ3_XXS probably better |
| [GGUF](https://huggingface.co/mradermacher/Llama-3-8B-Tulu-330K-i1-GGUF/resolve/main/Llama-3-8B-Tulu-330K.i1-IQ3_XXS.gguf) | i1-IQ3_XXS | 3.4 | lower quality |
| [GGUF](https://huggingface.co/mradermacher/Llama-3-8B-Tulu-330K-i1-GGUF/resolve/main/Llama-3-8B-Tulu-330K.i1-IQ3_XS.gguf) | i1-IQ3_XS | 3.6 | |
| [GGUF](https://huggingface.co/mradermacher/Llama-3-8B-Tulu-330K-i1-GGUF/resolve/main/Llama-3-8B-Tulu-330K.i1-Q3_K_S.gguf) | i1-Q3_K_S | 3.8 | IQ3_XS probably better |
| [GGUF](https://huggingface.co/mradermacher/Llama-3-8B-Tulu-330K-i1-GGUF/resolve/main/Llama-3-8B-Tulu-330K.i1-IQ3_S.gguf) | i1-IQ3_S | 3.8 | beats Q3_K* |
| [GGUF](https://huggingface.co/mradermacher/Llama-3-8B-Tulu-330K-i1-GGUF/resolve/main/Llama-3-8B-Tulu-330K.i1-IQ3_M.gguf) | i1-IQ3_M | 3.9 | |
| [GGUF](https://huggingface.co/mradermacher/Llama-3-8B-Tulu-330K-i1-GGUF/resolve/main/Llama-3-8B-Tulu-330K.i1-Q3_K_M.gguf) | i1-Q3_K_M | 4.1 | IQ3_S probably better |
| [GGUF](https://huggingface.co/mradermacher/Llama-3-8B-Tulu-330K-i1-GGUF/resolve/main/Llama-3-8B-Tulu-330K.i1-Q3_K_L.gguf) | i1-Q3_K_L | 4.4 | IQ3_M probably better |
| [GGUF](https://huggingface.co/mradermacher/Llama-3-8B-Tulu-330K-i1-GGUF/resolve/main/Llama-3-8B-Tulu-330K.i1-IQ4_XS.gguf) | i1-IQ4_XS | 4.5 | |
| [GGUF](https://huggingface.co/mradermacher/Llama-3-8B-Tulu-330K-i1-GGUF/resolve/main/Llama-3-8B-Tulu-330K.i1-Q4_0.gguf) | i1-Q4_0 | 4.8 | fast, low quality |
| [GGUF](https://huggingface.co/mradermacher/Llama-3-8B-Tulu-330K-i1-GGUF/resolve/main/Llama-3-8B-Tulu-330K.i1-Q4_K_S.gguf) | i1-Q4_K_S | 4.8 | optimal size/speed/quality |
| [GGUF](https://huggingface.co/mradermacher/Llama-3-8B-Tulu-330K-i1-GGUF/resolve/main/Llama-3-8B-Tulu-330K.i1-Q4_K_M.gguf) | i1-Q4_K_M | 5.0 | fast, recommended |
| [GGUF](https://huggingface.co/mradermacher/Llama-3-8B-Tulu-330K-i1-GGUF/resolve/main/Llama-3-8B-Tulu-330K.i1-Q5_K_S.gguf) | i1-Q5_K_S | 5.7 | |
| [GGUF](https://huggingface.co/mradermacher/Llama-3-8B-Tulu-330K-i1-GGUF/resolve/main/Llama-3-8B-Tulu-330K.i1-Q5_K_M.gguf) | i1-Q5_K_M | 5.8 | |
| [GGUF](https://huggingface.co/mradermacher/Llama-3-8B-Tulu-330K-i1-GGUF/resolve/main/Llama-3-8B-Tulu-330K.i1-Q6_K.gguf) | i1-Q6_K | 6.7 | practically like static Q6_K |
Here is a handy graph by ikawrakow comparing some lower-quality quant
types (lower is better):

And here are Artefact2's thoughts on the matter:
https://gist.github.com/Artefact2/b5f810600771265fc1e39442288e8ec9
## FAQ / Model Request
See https://huggingface.co/mradermacher/model_requests for some answers to
questions you might have and/or if you want some other model quantized.
## Thanks
I thank my company, [nethype GmbH](https://www.nethype.de/), for letting
me use its servers and providing upgrades to my workstation to enable
this work in my free time. Additional thanks to [@nicoboss](https://huggingface.co/nicoboss) for giving me access to his hardware for calculating the imatrix for these quants.
<!-- end -->
|
ckiplab/bert-base-chinese | ckiplab | "2022-05-10T03:28:12Z" | 14,394 | 22 | transformers | [
"transformers",
"pytorch",
"jax",
"bert",
"fill-mask",
"lm-head",
"zh",
"license:gpl-3.0",
"autotrain_compatible",
"endpoints_compatible",
"region:us"
] | fill-mask | "2022-03-02T23:29:05Z" | ---
language:
- zh
thumbnail: https://ckip.iis.sinica.edu.tw/files/ckip_logo.png
tags:
- pytorch
- lm-head
- bert
- zh
license: gpl-3.0
---
# CKIP BERT Base Chinese
This project provides traditional Chinese transformers models (including ALBERT, BERT, GPT2) and NLP tools (including word segmentation, part-of-speech tagging, named entity recognition).
這個專案提供了繁體中文的 transformers 模型(包含 ALBERT、BERT、GPT2)及自然語言處理工具(包含斷詞、詞性標記、實體辨識)。
## Homepage
- https://github.com/ckiplab/ckip-transformers
## Contributers
- [Mu Yang](https://muyang.pro) at [CKIP](https://ckip.iis.sinica.edu.tw) (Author & Maintainer)
## Usage
Please use BertTokenizerFast as tokenizer instead of AutoTokenizer.
請使用 BertTokenizerFast 而非 AutoTokenizer。
```
from transformers import (
BertTokenizerFast,
AutoModel,
)
tokenizer = BertTokenizerFast.from_pretrained('bert-base-chinese')
model = AutoModel.from_pretrained('ckiplab/bert-base-chinese')
```
For full usage and more information, please refer to https://github.com/ckiplab/ckip-transformers.
有關完整使用方法及其他資訊,請參見 https://github.com/ckiplab/ckip-transformers 。
|
mradermacher/L3-Uncen-Merger-Omelette-RP-v0.1-8B-i1-GGUF | mradermacher | "2024-06-24T20:36:40Z" | 14,392 | 0 | transformers | [
"transformers",
"gguf",
"mergekit",
"merge",
"not-for-all-audiences",
"nsfw",
"rp",
"roleplay",
"role-play",
"en",
"base_model:Casual-Autopsy/L3-Uncen-Merger-Omelette-RP-v0.1-8B",
"license:llama3",
"endpoints_compatible",
"region:us"
] | null | "2024-06-24T18:19:45Z" | ---
base_model: Casual-Autopsy/L3-Uncen-Merger-Omelette-RP-v0.1-8B
language:
- en
library_name: transformers
license: llama3
quantized_by: mradermacher
tags:
- mergekit
- merge
- not-for-all-audiences
- nsfw
- rp
- roleplay
- role-play
---
## About
<!-- ### quantize_version: 2 -->
<!-- ### output_tensor_quantised: 1 -->
<!-- ### convert_type: hf -->
<!-- ### vocab_type: -->
<!-- ### tags: nicoboss -->
weighted/imatrix quants of https://huggingface.co/Casual-Autopsy/L3-Uncen-Merger-Omelette-RP-v0.1-8B
<!-- provided-files -->
static quants are available at https://huggingface.co/mradermacher/L3-Uncen-Merger-Omelette-RP-v0.1-8B-GGUF
## Usage
If you are unsure how to use GGUF files, refer to one of [TheBloke's
READMEs](https://huggingface.co/TheBloke/KafkaLM-70B-German-V0.1-GGUF) for
more details, including on how to concatenate multi-part files.
## Provided Quants
(sorted by size, not necessarily quality. IQ-quants are often preferable over similar sized non-IQ quants)
| Link | Type | Size/GB | Notes |
|:-----|:-----|--------:|:------|
| [GGUF](https://huggingface.co/mradermacher/L3-Uncen-Merger-Omelette-RP-v0.1-8B-i1-GGUF/resolve/main/L3-Uncen-Merger-Omelette-RP-v0.1-8B.i1-IQ1_S.gguf) | i1-IQ1_S | 2.1 | for the desperate |
| [GGUF](https://huggingface.co/mradermacher/L3-Uncen-Merger-Omelette-RP-v0.1-8B-i1-GGUF/resolve/main/L3-Uncen-Merger-Omelette-RP-v0.1-8B.i1-IQ1_M.gguf) | i1-IQ1_M | 2.3 | mostly desperate |
| [GGUF](https://huggingface.co/mradermacher/L3-Uncen-Merger-Omelette-RP-v0.1-8B-i1-GGUF/resolve/main/L3-Uncen-Merger-Omelette-RP-v0.1-8B.i1-IQ2_XXS.gguf) | i1-IQ2_XXS | 2.5 | |
| [GGUF](https://huggingface.co/mradermacher/L3-Uncen-Merger-Omelette-RP-v0.1-8B-i1-GGUF/resolve/main/L3-Uncen-Merger-Omelette-RP-v0.1-8B.i1-IQ2_XS.gguf) | i1-IQ2_XS | 2.7 | |
| [GGUF](https://huggingface.co/mradermacher/L3-Uncen-Merger-Omelette-RP-v0.1-8B-i1-GGUF/resolve/main/L3-Uncen-Merger-Omelette-RP-v0.1-8B.i1-IQ2_S.gguf) | i1-IQ2_S | 2.9 | |
| [GGUF](https://huggingface.co/mradermacher/L3-Uncen-Merger-Omelette-RP-v0.1-8B-i1-GGUF/resolve/main/L3-Uncen-Merger-Omelette-RP-v0.1-8B.i1-IQ2_M.gguf) | i1-IQ2_M | 3.0 | |
| [GGUF](https://huggingface.co/mradermacher/L3-Uncen-Merger-Omelette-RP-v0.1-8B-i1-GGUF/resolve/main/L3-Uncen-Merger-Omelette-RP-v0.1-8B.i1-Q2_K.gguf) | i1-Q2_K | 3.3 | IQ3_XXS probably better |
| [GGUF](https://huggingface.co/mradermacher/L3-Uncen-Merger-Omelette-RP-v0.1-8B-i1-GGUF/resolve/main/L3-Uncen-Merger-Omelette-RP-v0.1-8B.i1-IQ3_XXS.gguf) | i1-IQ3_XXS | 3.4 | lower quality |
| [GGUF](https://huggingface.co/mradermacher/L3-Uncen-Merger-Omelette-RP-v0.1-8B-i1-GGUF/resolve/main/L3-Uncen-Merger-Omelette-RP-v0.1-8B.i1-IQ3_XS.gguf) | i1-IQ3_XS | 3.6 | |
| [GGUF](https://huggingface.co/mradermacher/L3-Uncen-Merger-Omelette-RP-v0.1-8B-i1-GGUF/resolve/main/L3-Uncen-Merger-Omelette-RP-v0.1-8B.i1-Q3_K_S.gguf) | i1-Q3_K_S | 3.8 | IQ3_XS probably better |
| [GGUF](https://huggingface.co/mradermacher/L3-Uncen-Merger-Omelette-RP-v0.1-8B-i1-GGUF/resolve/main/L3-Uncen-Merger-Omelette-RP-v0.1-8B.i1-IQ3_S.gguf) | i1-IQ3_S | 3.8 | beats Q3_K* |
| [GGUF](https://huggingface.co/mradermacher/L3-Uncen-Merger-Omelette-RP-v0.1-8B-i1-GGUF/resolve/main/L3-Uncen-Merger-Omelette-RP-v0.1-8B.i1-IQ3_M.gguf) | i1-IQ3_M | 3.9 | |
| [GGUF](https://huggingface.co/mradermacher/L3-Uncen-Merger-Omelette-RP-v0.1-8B-i1-GGUF/resolve/main/L3-Uncen-Merger-Omelette-RP-v0.1-8B.i1-Q3_K_M.gguf) | i1-Q3_K_M | 4.1 | IQ3_S probably better |
| [GGUF](https://huggingface.co/mradermacher/L3-Uncen-Merger-Omelette-RP-v0.1-8B-i1-GGUF/resolve/main/L3-Uncen-Merger-Omelette-RP-v0.1-8B.i1-Q3_K_L.gguf) | i1-Q3_K_L | 4.4 | IQ3_M probably better |
| [GGUF](https://huggingface.co/mradermacher/L3-Uncen-Merger-Omelette-RP-v0.1-8B-i1-GGUF/resolve/main/L3-Uncen-Merger-Omelette-RP-v0.1-8B.i1-IQ4_XS.gguf) | i1-IQ4_XS | 4.5 | |
| [GGUF](https://huggingface.co/mradermacher/L3-Uncen-Merger-Omelette-RP-v0.1-8B-i1-GGUF/resolve/main/L3-Uncen-Merger-Omelette-RP-v0.1-8B.i1-Q4_0.gguf) | i1-Q4_0 | 4.8 | fast, low quality |
| [GGUF](https://huggingface.co/mradermacher/L3-Uncen-Merger-Omelette-RP-v0.1-8B-i1-GGUF/resolve/main/L3-Uncen-Merger-Omelette-RP-v0.1-8B.i1-Q4_K_S.gguf) | i1-Q4_K_S | 4.8 | optimal size/speed/quality |
| [GGUF](https://huggingface.co/mradermacher/L3-Uncen-Merger-Omelette-RP-v0.1-8B-i1-GGUF/resolve/main/L3-Uncen-Merger-Omelette-RP-v0.1-8B.i1-Q4_K_M.gguf) | i1-Q4_K_M | 5.0 | fast, recommended |
| [GGUF](https://huggingface.co/mradermacher/L3-Uncen-Merger-Omelette-RP-v0.1-8B-i1-GGUF/resolve/main/L3-Uncen-Merger-Omelette-RP-v0.1-8B.i1-Q5_K_S.gguf) | i1-Q5_K_S | 5.7 | |
| [GGUF](https://huggingface.co/mradermacher/L3-Uncen-Merger-Omelette-RP-v0.1-8B-i1-GGUF/resolve/main/L3-Uncen-Merger-Omelette-RP-v0.1-8B.i1-Q5_K_M.gguf) | i1-Q5_K_M | 5.8 | |
| [GGUF](https://huggingface.co/mradermacher/L3-Uncen-Merger-Omelette-RP-v0.1-8B-i1-GGUF/resolve/main/L3-Uncen-Merger-Omelette-RP-v0.1-8B.i1-Q6_K.gguf) | i1-Q6_K | 6.7 | practically like static Q6_K |
Here is a handy graph by ikawrakow comparing some lower-quality quant
types (lower is better):

And here are Artefact2's thoughts on the matter:
https://gist.github.com/Artefact2/b5f810600771265fc1e39442288e8ec9
## FAQ / Model Request
See https://huggingface.co/mradermacher/model_requests for some answers to
questions you might have and/or if you want some other model quantized.
## Thanks
I thank my company, [nethype GmbH](https://www.nethype.de/), for letting
me use its servers and providing upgrades to my workstation to enable
this work in my free time. Additional thanks to [@nicoboss](https://huggingface.co/nicoboss) for giving me access to his hardware for calculating the imatrix for these quants.
<!-- end -->
|
unsloth/Phi-3-mini-4k-instruct | unsloth | "2024-05-23T18:55:37Z" | 14,377 | 27 | transformers | [
"transformers",
"safetensors",
"mistral",
"text-generation",
"unsloth",
"phi3",
"phi",
"conversational",
"en",
"license:mit",
"autotrain_compatible",
"endpoints_compatible",
"text-generation-inference",
"region:us"
] | text-generation | "2024-04-29T17:02:15Z" | ---
language:
- en
license: mit
library_name: transformers
tags:
- unsloth
- phi3
- transformers
- phi
---
# Finetune Mistral, Gemma, Llama 2-5x faster with 70% less memory via Unsloth!
Directly quantized 4bit model with `bitsandbytes`.
We have a Google Colab Tesla T4 notebook for Phi-3 Medium here: https://colab.research.google.com/drive/1hhdhBa1j_hsymiW9m-WzxQtgqTH_NHqi?usp=sharing
We have a Google Colab Tesla T4 notebook for Phi-3 Mini here: https://colab.research.google.com/drive/1lN6hPQveB_mHSnTOYifygFcrO8C1bxq4?usp=sharing
[<img src="https://raw.githubusercontent.com/unslothai/unsloth/main/images/Discord%20button.png" width="200"/>](https://discord.gg/u54VK8m8tk)
[<img src="https://raw.githubusercontent.com/unslothai/unsloth/main/images/buy%20me%20a%20coffee%20button.png" width="200"/>](https://ko-fi.com/unsloth)
[<img src="https://raw.githubusercontent.com/unslothai/unsloth/main/images/unsloth%20made%20with%20love.png" width="200"/>](https://github.com/unslothai/unsloth)
## ✨ Finetune for Free
All notebooks are **beginner friendly**! Add your dataset, click "Run All", and you'll get a 2x faster finetuned model which can be exported to GGUF, vLLM or uploaded to Hugging Face.
| Unsloth supports | Free Notebooks | Performance | Memory use |
|-----------------|--------------------------------------------------------------------------------------------------------------------------|-------------|----------|
| **Llama-3 8b** | [▶️ Start on Colab](https://colab.research.google.com/drive/135ced7oHytdxu3N2DNe1Z0kqjyYIkDXp?usp=sharing) | 2.4x faster | 58% less |
| **Gemma 7b** | [▶️ Start on Colab](https://colab.research.google.com/drive/10NbwlsRChbma1v55m8LAPYG15uQv6HLo?usp=sharing) | 2.4x faster | 58% less |
| **Mistral 7b** | [▶️ Start on Colab](https://colab.research.google.com/drive/1Dyauq4kTZoLewQ1cApceUQVNcnnNTzg_?usp=sharing) | 2.2x faster | 62% less |
| **Llama-2 7b** | [▶️ Start on Colab](https://colab.research.google.com/drive/1lBzz5KeZJKXjvivbYvmGarix9Ao6Wxe5?usp=sharing) | 2.2x faster | 43% less |
| **TinyLlama** | [▶️ Start on Colab](https://colab.research.google.com/drive/1AZghoNBQaMDgWJpi4RbffGM1h6raLUj9?usp=sharing) | 3.9x faster | 74% less |
| **CodeLlama 34b** A100 | [▶️ Start on Colab](https://colab.research.google.com/drive/1y7A0AxE3y8gdj4AVkl2aZX47Xu3P1wJT?usp=sharing) | 1.9x faster | 27% less |
| **Mistral 7b** 1xT4 | [▶️ Start on Kaggle](https://www.kaggle.com/code/danielhanchen/kaggle-mistral-7b-unsloth-notebook) | 5x faster\* | 62% less |
| **DPO - Zephyr** | [▶️ Start on Colab](https://colab.research.google.com/drive/15vttTpzzVXv_tJwEk-hIcQ0S9FcEWvwP?usp=sharing) | 1.9x faster | 19% less |
- This [conversational notebook](https://colab.research.google.com/drive/1Aau3lgPzeZKQ-98h69CCu1UJcvIBLmy2?usp=sharing) is useful for ShareGPT ChatML / Vicuna templates.
- This [text completion notebook](https://colab.research.google.com/drive/1ef-tab5bhkvWmBOObepl1WgJvfvSzn5Q?usp=sharing) is for raw text. This [DPO notebook](https://colab.research.google.com/drive/15vttTpzzVXv_tJwEk-hIcQ0S9FcEWvwP?usp=sharing) replicates Zephyr.
- \* Kaggle has 2x T4s, but we use 1. Due to overhead, 1x T4 is 5x faster. |
mradermacher/Llama-3-NeuralHercules-5.0-8B-GGUF | mradermacher | "2024-06-27T08:58:02Z" | 14,376 | 0 | transformers | [
"transformers",
"gguf",
"code",
"chemistry",
"medical",
"en",
"base_model:Locutusque/Llama-3-NeuralHercules-5.0-8B",
"license:llama3",
"endpoints_compatible",
"region:us"
] | null | "2024-06-27T05:03:43Z" | ---
base_model: Locutusque/Llama-3-NeuralHercules-5.0-8B
language:
- en
library_name: transformers
license: llama3
quantized_by: mradermacher
tags:
- code
- chemistry
- medical
---
## About
<!-- ### quantize_version: 2 -->
<!-- ### output_tensor_quantised: 1 -->
<!-- ### convert_type: hf -->
<!-- ### vocab_type: -->
<!-- ### tags: -->
static quants of https://huggingface.co/Locutusque/Llama-3-NeuralHercules-5.0-8B
<!-- provided-files -->
weighted/imatrix quants are available at https://huggingface.co/mradermacher/Llama-3-NeuralHercules-5.0-8B-i1-GGUF
## Usage
If you are unsure how to use GGUF files, refer to one of [TheBloke's
READMEs](https://huggingface.co/TheBloke/KafkaLM-70B-German-V0.1-GGUF) for
more details, including on how to concatenate multi-part files.
## Provided Quants
(sorted by size, not necessarily quality. IQ-quants are often preferable over similar sized non-IQ quants)
| Link | Type | Size/GB | Notes |
|:-----|:-----|--------:|:------|
| [GGUF](https://huggingface.co/mradermacher/Llama-3-NeuralHercules-5.0-8B-GGUF/resolve/main/Llama-3-NeuralHercules-5.0-8B.Q2_K.gguf) | Q2_K | 3.3 | |
| [GGUF](https://huggingface.co/mradermacher/Llama-3-NeuralHercules-5.0-8B-GGUF/resolve/main/Llama-3-NeuralHercules-5.0-8B.IQ3_XS.gguf) | IQ3_XS | 3.6 | |
| [GGUF](https://huggingface.co/mradermacher/Llama-3-NeuralHercules-5.0-8B-GGUF/resolve/main/Llama-3-NeuralHercules-5.0-8B.Q3_K_S.gguf) | Q3_K_S | 3.8 | |
| [GGUF](https://huggingface.co/mradermacher/Llama-3-NeuralHercules-5.0-8B-GGUF/resolve/main/Llama-3-NeuralHercules-5.0-8B.IQ3_S.gguf) | IQ3_S | 3.8 | beats Q3_K* |
| [GGUF](https://huggingface.co/mradermacher/Llama-3-NeuralHercules-5.0-8B-GGUF/resolve/main/Llama-3-NeuralHercules-5.0-8B.IQ3_M.gguf) | IQ3_M | 3.9 | |
| [GGUF](https://huggingface.co/mradermacher/Llama-3-NeuralHercules-5.0-8B-GGUF/resolve/main/Llama-3-NeuralHercules-5.0-8B.Q3_K_M.gguf) | Q3_K_M | 4.1 | lower quality |
| [GGUF](https://huggingface.co/mradermacher/Llama-3-NeuralHercules-5.0-8B-GGUF/resolve/main/Llama-3-NeuralHercules-5.0-8B.Q3_K_L.gguf) | Q3_K_L | 4.4 | |
| [GGUF](https://huggingface.co/mradermacher/Llama-3-NeuralHercules-5.0-8B-GGUF/resolve/main/Llama-3-NeuralHercules-5.0-8B.IQ4_XS.gguf) | IQ4_XS | 4.6 | |
| [GGUF](https://huggingface.co/mradermacher/Llama-3-NeuralHercules-5.0-8B-GGUF/resolve/main/Llama-3-NeuralHercules-5.0-8B.Q4_K_S.gguf) | Q4_K_S | 4.8 | fast, recommended |
| [GGUF](https://huggingface.co/mradermacher/Llama-3-NeuralHercules-5.0-8B-GGUF/resolve/main/Llama-3-NeuralHercules-5.0-8B.Q4_K_M.gguf) | Q4_K_M | 5.0 | fast, recommended |
| [GGUF](https://huggingface.co/mradermacher/Llama-3-NeuralHercules-5.0-8B-GGUF/resolve/main/Llama-3-NeuralHercules-5.0-8B.Q5_K_S.gguf) | Q5_K_S | 5.7 | |
| [GGUF](https://huggingface.co/mradermacher/Llama-3-NeuralHercules-5.0-8B-GGUF/resolve/main/Llama-3-NeuralHercules-5.0-8B.Q5_K_M.gguf) | Q5_K_M | 5.8 | |
| [GGUF](https://huggingface.co/mradermacher/Llama-3-NeuralHercules-5.0-8B-GGUF/resolve/main/Llama-3-NeuralHercules-5.0-8B.Q6_K.gguf) | Q6_K | 6.7 | very good quality |
| [GGUF](https://huggingface.co/mradermacher/Llama-3-NeuralHercules-5.0-8B-GGUF/resolve/main/Llama-3-NeuralHercules-5.0-8B.Q8_0.gguf) | Q8_0 | 8.6 | fast, best quality |
| [GGUF](https://huggingface.co/mradermacher/Llama-3-NeuralHercules-5.0-8B-GGUF/resolve/main/Llama-3-NeuralHercules-5.0-8B.f16.gguf) | f16 | 16.2 | 16 bpw, overkill |
Here is a handy graph by ikawrakow comparing some lower-quality quant
types (lower is better):

And here are Artefact2's thoughts on the matter:
https://gist.github.com/Artefact2/b5f810600771265fc1e39442288e8ec9
## FAQ / Model Request
See https://huggingface.co/mradermacher/model_requests for some answers to
questions you might have and/or if you want some other model quantized.
## Thanks
I thank my company, [nethype GmbH](https://www.nethype.de/), for letting
me use its servers and providing upgrades to my workstation to enable
this work in my free time.
<!-- end -->
|
PrunaAI/alfredplpl-Llama-3-8B-Instruct-Ja-GGUF-smashed | PrunaAI | "2024-06-28T18:16:46Z" | 14,367 | 0 | null | [
"gguf",
"pruna-ai",
"region:us"
] | null | "2024-06-28T17:32:35Z" | ---
thumbnail: "https://assets-global.website-files.com/646b351987a8d8ce158d1940/64ec9e96b4334c0e1ac41504_Logo%20with%20white%20text.svg"
metrics:
- memory_disk
- memory_inference
- inference_latency
- inference_throughput
- inference_CO2_emissions
- inference_energy_consumption
tags:
- pruna-ai
---
<!-- header start -->
<!-- 200823 -->
<div style="width: auto; margin-left: auto; margin-right: auto">
<a href="https://www.pruna.ai/" target="_blank" rel="noopener noreferrer">
<img src="https://i.imgur.com/eDAlcgk.png" alt="PrunaAI" style="width: 100%; min-width: 400px; display: block; margin: auto;">
</a>
</div>
<!-- header end -->
[](https://twitter.com/PrunaAI)
[](https://github.com/PrunaAI)
[](https://www.linkedin.com/company/93832878/admin/feed/posts/?feedType=following)
[](https://discord.com/invite/vb6SmA3hxu)
## This repo contains GGUF versions of the alfredplpl/Llama-3-8B-Instruct-Ja model.
# Simply make AI models cheaper, smaller, faster, and greener!
- Give a thumbs up if you like this model!
- Contact us and tell us which model to compress next [here](https://www.pruna.ai/contact).
- Request access to easily compress your *own* AI models [here](https://z0halsaff74.typeform.com/pruna-access?typeform-source=www.pruna.ai).
- Read the documentations to know more [here](https://pruna-ai-pruna.readthedocs-hosted.com/en/latest/)
- Join Pruna AI community on Discord [here](https://discord.com/invite/vb6SmA3hxu) to share feedback/suggestions or get help.
**Frequently Asked Questions**
- ***How does the compression work?*** The model is compressed with GGUF.
- ***How does the model quality change?*** The quality of the model output might vary compared to the base model.
- ***What is the model format?*** We use GGUF format.
- ***What calibration data has been used?*** If needed by the compression method, we used WikiText as the calibration data.
- ***How to compress my own models?*** You can request premium access to more compression methods and tech support for your specific use-cases [here](https://z0halsaff74.typeform.com/pruna-access?typeform-source=www.pruna.ai).
# Downloading and running the models
You can download the individual files from the Files & versions section. Here is a list of the different versions we provide. For more info checkout [this chart](https://gist.github.com/Artefact2/b5f810600771265fc1e39442288e8ec9) and [this guide](https://www.reddit.com/r/LocalLLaMA/comments/1ba55rj/overview_of_gguf_quantization_methods/):
| Quant type | Description |
|------------|--------------------------------------------------------------------------------------------|
| Q5_K_M | High quality, recommended. |
| Q5_K_S | High quality, recommended. |
| Q4_K_M | Good quality, uses about 4.83 bits per weight, recommended. |
| Q4_K_S | Slightly lower quality with more space savings, recommended. |
| IQ4_NL | Decent quality, slightly smaller than Q4_K_S with similar performance, recommended. |
| IQ4_XS | Decent quality, smaller than Q4_K_S with similar performance, recommended. |
| Q3_K_L | Lower quality but usable, good for low RAM availability. |
| Q3_K_M | Even lower quality. |
| IQ3_M | Medium-low quality, new method with decent performance comparable to Q3_K_M. |
| IQ3_S | Lower quality, new method with decent performance, recommended over Q3_K_S quant, same size with better performance. |
| Q3_K_S | Low quality, not recommended. |
| IQ3_XS | Lower quality, new method with decent performance, slightly better than Q3_K_S. |
| Q2_K | Very low quality but surprisingly usable. |
## How to download GGUF files ?
**Note for manual downloaders:** You almost never want to clone the entire repo! Multiple different quantisation formats are provided, and most users only want to pick and download a single file.
The following clients/libraries will automatically download models for you, providing a list of available models to choose from:
* LM Studio
* LoLLMS Web UI
* Faraday.dev
- **Option A** - Downloading in `text-generation-webui`:
- **Step 1**: Under Download Model, you can enter the model repo: alfredplpl-Llama-3-8B-Instruct-Ja-GGUF-smashed and below it, a specific filename to download, such as: phi-2.IQ3_M.gguf.
- **Step 2**: Then click Download.
- **Option B** - Downloading on the command line (including multiple files at once):
- **Step 1**: We recommend using the `huggingface-hub` Python library:
```shell
pip3 install huggingface-hub
```
- **Step 2**: Then you can download any individual model file to the current directory, at high speed, with a command like this:
```shell
huggingface-cli download alfredplpl-Llama-3-8B-Instruct-Ja-GGUF-smashed Llama-3-8B-Instruct-Ja.IQ3_M.gguf --local-dir . --local-dir-use-symlinks False
```
<details>
<summary>More advanced huggingface-cli download usage (click to read)</summary>
Alternatively, you can also download multiple files at once with a pattern:
```shell
huggingface-cli download alfredplpl-Llama-3-8B-Instruct-Ja-GGUF-smashed --local-dir . --local-dir-use-symlinks False --include='*Q4_K*gguf'
```
For more documentation on downloading with `huggingface-cli`, please see: [HF -> Hub Python Library -> Download files -> Download from the CLI](https://huggingface.co/docs/huggingface_hub/guides/download#download-from-the-cli).
To accelerate downloads on fast connections (1Gbit/s or higher), install `hf_transfer`:
```shell
pip3 install hf_transfer
```
And set environment variable `HF_HUB_ENABLE_HF_TRANSFER` to `1`:
```shell
HF_HUB_ENABLE_HF_TRANSFER=1 huggingface-cli download alfredplpl-Llama-3-8B-Instruct-Ja-GGUF-smashed Llama-3-8B-Instruct-Ja.IQ3_M.gguf --local-dir . --local-dir-use-symlinks False
```
Windows Command Line users: You can set the environment variable by running `set HF_HUB_ENABLE_HF_TRANSFER=1` before the download command.
</details>
<!-- README_GGUF.md-how-to-download end -->
<!-- README_GGUF.md-how-to-run start -->
## How to run model in GGUF format?
- **Option A** - Introductory example with `llama.cpp` command
Make sure you are using `llama.cpp` from commit [d0cee0d](https://github.com/ggerganov/llama.cpp/commit/d0cee0d36d5be95a0d9088b674dbb27354107221) or later.
```shell
./main -ngl 35 -m Llama-3-8B-Instruct-Ja.IQ3_M.gguf --color -c 32768 --temp 0.7 --repeat_penalty 1.1 -n -1 -p "<s>[INST] {{prompt\}} [/INST]"
```
Change `-ngl 32` to the number of layers to offload to GPU. Remove it if you don't have GPU acceleration.
Change `-c 32768` to the desired sequence length. For extended sequence models - eg 8K, 16K, 32K - the necessary RoPE scaling parameters are read from the GGUF file and set by llama.cpp automatically. Note that longer sequence lengths require much more resources, so you may need to reduce this value.
If you want to have a chat-style conversation, replace the `-p <PROMPT>` argument with `-i -ins`
For other parameters and how to use them, please refer to [the llama.cpp documentation](https://github.com/ggerganov/llama.cpp/blob/master/examples/main/README.md)
- **Option B** - Running in `text-generation-webui`
Further instructions can be found in the text-generation-webui documentation, here: [text-generation-webui/docs/04 ‐ Model Tab.md](https://github.com/oobabooga/text-generation-webui/blob/main/docs/04%20-%20Model%20Tab.md#llamacpp).
- **Option C** - Running from Python code
You can use GGUF models from Python using the [llama-cpp-python](https://github.com/abetlen/llama-cpp-python) or [ctransformers](https://github.com/marella/ctransformers) libraries. Note that at the time of writing (Nov 27th 2023), ctransformers has not been updated for some time and is not compatible with some recent models. Therefore I recommend you use llama-cpp-python.
### How to load this model in Python code, using llama-cpp-python
For full documentation, please see: [llama-cpp-python docs](https://abetlen.github.io/llama-cpp-python/).
#### First install the package
Run one of the following commands, according to your system:
```shell
# Base ctransformers with no GPU acceleration
pip install llama-cpp-python
# With NVidia CUDA acceleration
CMAKE_ARGS="-DLLAMA_CUBLAS=on" pip install llama-cpp-python
# Or with OpenBLAS acceleration
CMAKE_ARGS="-DLLAMA_BLAS=ON -DLLAMA_BLAS_VENDOR=OpenBLAS" pip install llama-cpp-python
# Or with CLBLast acceleration
CMAKE_ARGS="-DLLAMA_CLBLAST=on" pip install llama-cpp-python
# Or with AMD ROCm GPU acceleration (Linux only)
CMAKE_ARGS="-DLLAMA_HIPBLAS=on" pip install llama-cpp-python
# Or with Metal GPU acceleration for macOS systems only
CMAKE_ARGS="-DLLAMA_METAL=on" pip install llama-cpp-python
# In windows, to set the variables CMAKE_ARGS in PowerShell, follow this format; eg for NVidia CUDA:
$env:CMAKE_ARGS = "-DLLAMA_OPENBLAS=on"
pip install llama-cpp-python
```
#### Simple llama-cpp-python example code
```python
from llama_cpp import Llama
# Set gpu_layers to the number of layers to offload to GPU. Set to 0 if no GPU acceleration is available on your system.
llm = Llama(
model_path="./Llama-3-8B-Instruct-Ja.IQ3_M.gguf", # Download the model file first
n_ctx=32768, # The max sequence length to use - note that longer sequence lengths require much more resources
n_threads=8, # The number of CPU threads to use, tailor to your system and the resulting performance
n_gpu_layers=35 # The number of layers to offload to GPU, if you have GPU acceleration available
)
# Simple inference example
output = llm(
"<s>[INST] {{prompt}} [/INST]", # Prompt
max_tokens=512, # Generate up to 512 tokens
stop=["</s>"], # Example stop token - not necessarily correct for this specific model! Please check before using.
echo=True # Whether to echo the prompt
)
# Chat Completion API
llm = Llama(model_path="./Llama-3-8B-Instruct-Ja.IQ3_M.gguf", chat_format="llama-2") # Set chat_format according to the model you are using
llm.create_chat_completion(
messages = [
{{"role": "system", "content": "You are a story writing assistant."}},
{{
"role": "user",
"content": "Write a story about llamas."
}}
]
)
```
- **Option D** - Running with LangChain
Here are guides on using llama-cpp-python and ctransformers with LangChain:
* [LangChain + llama-cpp-python](https://python.langchain.com/docs/integrations/llms/llamacpp)
* [LangChain + ctransformers](https://python.langchain.com/docs/integrations/providers/ctransformers)
## Configurations
The configuration info are in `smash_config.json`.
## Credits & License
The license of the smashed model follows the license of the original model. Please check the license of the original model before using this model which provided the base model. The license of the `pruna-engine` is [here](https://pypi.org/project/pruna-engine/) on Pypi.
## Want to compress other models?
- Contact us and tell us which model to compress next [here](https://www.pruna.ai/contact).
- Request access to easily compress your own AI models [here](https://z0halsaff74.typeform.com/pruna-access?typeform-source=www.pruna.ai). |
mradermacher/Hansoldeco-Gemma-2-9b-it-v0.1-i1-GGUF | mradermacher | "2024-07-02T06:59:14Z" | 14,365 | 0 | transformers | [
"transformers",
"gguf",
"en",
"base_model:sosoai/Hansoldeco-Gemma-2-9b-it-v0.1",
"endpoints_compatible",
"region:us"
] | null | "2024-07-02T03:33:40Z" | ---
base_model: sosoai/Hansoldeco-Gemma-2-9b-it-v0.1
language:
- en
library_name: transformers
quantized_by: mradermacher
---
## About
<!-- ### quantize_version: 2 -->
<!-- ### output_tensor_quantised: 1 -->
<!-- ### convert_type: hf -->
<!-- ### vocab_type: -->
<!-- ### tags: nicoboss -->
weighted/imatrix quants of https://huggingface.co/sosoai/Hansoldeco-Gemma-2-9b-it-v0.1
<!-- provided-files -->
static quants are available at https://huggingface.co/mradermacher/Hansoldeco-Gemma-2-9b-it-v0.1-GGUF
## Usage
If you are unsure how to use GGUF files, refer to one of [TheBloke's
READMEs](https://huggingface.co/TheBloke/KafkaLM-70B-German-V0.1-GGUF) for
more details, including on how to concatenate multi-part files.
## Provided Quants
(sorted by size, not necessarily quality. IQ-quants are often preferable over similar sized non-IQ quants)
| Link | Type | Size/GB | Notes |
|:-----|:-----|--------:|:------|
| [GGUF](https://huggingface.co/mradermacher/Hansoldeco-Gemma-2-9b-it-v0.1-i1-GGUF/resolve/main/Hansoldeco-Gemma-2-9b-it-v0.1.i1-IQ1_S.gguf) | i1-IQ1_S | 2.5 | for the desperate |
| [GGUF](https://huggingface.co/mradermacher/Hansoldeco-Gemma-2-9b-it-v0.1-i1-GGUF/resolve/main/Hansoldeco-Gemma-2-9b-it-v0.1.i1-IQ1_M.gguf) | i1-IQ1_M | 2.6 | mostly desperate |
| [GGUF](https://huggingface.co/mradermacher/Hansoldeco-Gemma-2-9b-it-v0.1-i1-GGUF/resolve/main/Hansoldeco-Gemma-2-9b-it-v0.1.i1-IQ2_XXS.gguf) | i1-IQ2_XXS | 2.9 | |
| [GGUF](https://huggingface.co/mradermacher/Hansoldeco-Gemma-2-9b-it-v0.1-i1-GGUF/resolve/main/Hansoldeco-Gemma-2-9b-it-v0.1.i1-IQ2_XS.gguf) | i1-IQ2_XS | 3.2 | |
| [GGUF](https://huggingface.co/mradermacher/Hansoldeco-Gemma-2-9b-it-v0.1-i1-GGUF/resolve/main/Hansoldeco-Gemma-2-9b-it-v0.1.i1-IQ2_S.gguf) | i1-IQ2_S | 3.3 | |
| [GGUF](https://huggingface.co/mradermacher/Hansoldeco-Gemma-2-9b-it-v0.1-i1-GGUF/resolve/main/Hansoldeco-Gemma-2-9b-it-v0.1.i1-IQ2_M.gguf) | i1-IQ2_M | 3.5 | |
| [GGUF](https://huggingface.co/mradermacher/Hansoldeco-Gemma-2-9b-it-v0.1-i1-GGUF/resolve/main/Hansoldeco-Gemma-2-9b-it-v0.1.i1-IQ3_XXS.gguf) | i1-IQ3_XXS | 3.9 | lower quality |
| [GGUF](https://huggingface.co/mradermacher/Hansoldeco-Gemma-2-9b-it-v0.1-i1-GGUF/resolve/main/Hansoldeco-Gemma-2-9b-it-v0.1.i1-Q2_K.gguf) | i1-Q2_K | 3.9 | IQ3_XXS probably better |
| [GGUF](https://huggingface.co/mradermacher/Hansoldeco-Gemma-2-9b-it-v0.1-i1-GGUF/resolve/main/Hansoldeco-Gemma-2-9b-it-v0.1.i1-IQ3_XS.gguf) | i1-IQ3_XS | 4.2 | |
| [GGUF](https://huggingface.co/mradermacher/Hansoldeco-Gemma-2-9b-it-v0.1-i1-GGUF/resolve/main/Hansoldeco-Gemma-2-9b-it-v0.1.i1-IQ3_S.gguf) | i1-IQ3_S | 4.4 | beats Q3_K* |
| [GGUF](https://huggingface.co/mradermacher/Hansoldeco-Gemma-2-9b-it-v0.1-i1-GGUF/resolve/main/Hansoldeco-Gemma-2-9b-it-v0.1.i1-Q3_K_S.gguf) | i1-Q3_K_S | 4.4 | IQ3_XS probably better |
| [GGUF](https://huggingface.co/mradermacher/Hansoldeco-Gemma-2-9b-it-v0.1-i1-GGUF/resolve/main/Hansoldeco-Gemma-2-9b-it-v0.1.i1-IQ3_M.gguf) | i1-IQ3_M | 4.6 | |
| [GGUF](https://huggingface.co/mradermacher/Hansoldeco-Gemma-2-9b-it-v0.1-i1-GGUF/resolve/main/Hansoldeco-Gemma-2-9b-it-v0.1.i1-Q3_K_M.gguf) | i1-Q3_K_M | 4.9 | IQ3_S probably better |
| [GGUF](https://huggingface.co/mradermacher/Hansoldeco-Gemma-2-9b-it-v0.1-i1-GGUF/resolve/main/Hansoldeco-Gemma-2-9b-it-v0.1.i1-Q3_K_L.gguf) | i1-Q3_K_L | 5.2 | IQ3_M probably better |
| [GGUF](https://huggingface.co/mradermacher/Hansoldeco-Gemma-2-9b-it-v0.1-i1-GGUF/resolve/main/Hansoldeco-Gemma-2-9b-it-v0.1.i1-IQ4_XS.gguf) | i1-IQ4_XS | 5.3 | |
| [GGUF](https://huggingface.co/mradermacher/Hansoldeco-Gemma-2-9b-it-v0.1-i1-GGUF/resolve/main/Hansoldeco-Gemma-2-9b-it-v0.1.i1-Q4_0.gguf) | i1-Q4_0 | 5.6 | fast, low quality |
| [GGUF](https://huggingface.co/mradermacher/Hansoldeco-Gemma-2-9b-it-v0.1-i1-GGUF/resolve/main/Hansoldeco-Gemma-2-9b-it-v0.1.i1-Q4_K_S.gguf) | i1-Q4_K_S | 5.6 | optimal size/speed/quality |
| [GGUF](https://huggingface.co/mradermacher/Hansoldeco-Gemma-2-9b-it-v0.1-i1-GGUF/resolve/main/Hansoldeco-Gemma-2-9b-it-v0.1.i1-Q4_K_M.gguf) | i1-Q4_K_M | 5.9 | fast, recommended |
| [GGUF](https://huggingface.co/mradermacher/Hansoldeco-Gemma-2-9b-it-v0.1-i1-GGUF/resolve/main/Hansoldeco-Gemma-2-9b-it-v0.1.i1-Q5_K_S.gguf) | i1-Q5_K_S | 6.6 | |
| [GGUF](https://huggingface.co/mradermacher/Hansoldeco-Gemma-2-9b-it-v0.1-i1-GGUF/resolve/main/Hansoldeco-Gemma-2-9b-it-v0.1.i1-Q5_K_M.gguf) | i1-Q5_K_M | 6.7 | |
| [GGUF](https://huggingface.co/mradermacher/Hansoldeco-Gemma-2-9b-it-v0.1-i1-GGUF/resolve/main/Hansoldeco-Gemma-2-9b-it-v0.1.i1-Q6_K.gguf) | i1-Q6_K | 7.7 | practically like static Q6_K |
Here is a handy graph by ikawrakow comparing some lower-quality quant
types (lower is better):

And here are Artefact2's thoughts on the matter:
https://gist.github.com/Artefact2/b5f810600771265fc1e39442288e8ec9
## FAQ / Model Request
See https://huggingface.co/mradermacher/model_requests for some answers to
questions you might have and/or if you want some other model quantized.
## Thanks
I thank my company, [nethype GmbH](https://www.nethype.de/), for letting
me use its servers and providing upgrades to my workstation to enable
this work in my free time. Additional thanks to [@nicoboss](https://huggingface.co/nicoboss) for giving me access to his hardware for calculating the imatrix for these quants.
<!-- end -->
|
rinna/japanese-gpt-neox-3.6b-instruction-sft-v2 | rinna | "2024-04-03T07:25:13Z" | 14,347 | 25 | transformers | [
"transformers",
"pytorch",
"safetensors",
"gpt_neox",
"text-generation",
"ja",
"lm",
"nlp",
"dataset:Anthropic/hh-rlhf",
"dataset:stanfordnlp/SHP",
"arxiv:2404.01657",
"license:mit",
"autotrain_compatible",
"text-generation-inference",
"region:us"
] | text-generation | "2023-05-30T01:50:25Z" | ---
language: ja
thumbnail: https://github.com/rinnakk/japanese-pretrained-models/blob/master/rinna.png
tags:
- ja
- gpt_neox
- text-generation
- lm
- nlp
license: mit
datasets:
- Anthropic/hh-rlhf
- stanfordnlp/SHP
inference: false
---
# japanese-gpt-neox-3.6b-instruction-sft-v2

# Overview
This repository provides a Japanese GPT-NeoX model of 3.6 billion parameters. The model is based on [`rinna/japanese-gpt-neox-3.6b`](https://huggingface.co/rinna/japanese-gpt-neox-3.6b) and has been finetuned to serve as an instruction-following conversational agent.
This model slightly differs from the previous SFT model [`rinna/japanese-gpt-neox-3.6b-instruction-sft`](https://huggingface.co/rinna/japanese-gpt-neox-3.6b-instruction-sft), where a different data split is used for training.
* **Model architecture**
A 36-layer, 2816-hidden-size transformer-based language model.
* **SFT vs. previous SFT evaluation**
We conducted ChatGPT-based automated evaluation on 100 prompts to assess the performance difference between this SFT model and the previous SFT model.
| [this SFT](https://huggingface.co/rinna/japanese-gpt-neox-3.6b-instruction-sft-v2) vs. [previous SFT](https://huggingface.co/rinna/japanese-gpt-neox-3.6b-instruction-sft) | win | tie | loss |
| :---: | :---: | :---: | :---: |
| ChatGPT auto. evaluation | **55**% | 0% | 45% |
* **Finetuning**
The finetuning data is the subset of the following datasets and has been translated into Japanese.
* [Anthropic HH RLHF data](https://huggingface.co/datasets/Anthropic/hh-rlhf)
* [FLAN Instruction Tuning data](https://github.com/google-research/FLAN)
* [Stanford Human Preferences Dataset](https://huggingface.co/datasets/stanfordnlp/SHP)
The data will **not** be released.
* **Model Series**
| Variant | Link |
| :-- | :--|
| 3.6B PPO | https://huggingface.co/rinna/japanese-gpt-neox-3.6b-instruction-ppo |
| 3.6B SFT-v2 | https://huggingface.co/rinna/japanese-gpt-neox-3.6b-instruction-sft-v2 |
| 3.6B SFT | https://huggingface.co/rinna/japanese-gpt-neox-3.6b-instruction-sft |
| 3.6B pretrained | https://huggingface.co/rinna/japanese-gpt-neox-3.6b |
* **Contributors**
[Tianyu Zhao](https://huggingface.co/tianyuz) and [Kei Sawada](https://huggingface.co/keisawada)
# I/O Format
A special format has been adopted to construct inputs.
* An input prompt is formatted as a conversation between `ユーザー` and `システム`.
* Each input utterance consists of (1) its speaker (`"ユーザー"` or `"システム"`), (2) a colon (`":"`), (3) a whitespace (`" "`), and (4) utterance text (e.g. `"世界で一番高い山は?"`).
* The input prompt should be ended with `"システム: "` to acknowledge the model to generate a response.
* Since the model's tokenizer does not recognize `"\n"`, a special newline symbol `"<NL>"` is used instead.
* All the newlines in input and output utterances should be replaced with `"<NL>"`.
* All the utterances in the input prompt should be separated by `"<NL>"`.
Following is an example to construct an input from a conversation.
~~~python
prompt = [
{
"speaker": "ユーザー",
"text": "コンタクトレンズを慣れるにはどうすればよいですか?"
},
{
"speaker": "システム",
"text": "これについて具体的に説明していただけますか?何が難しいのでしょうか?"
},
{
"speaker": "ユーザー",
"text": "目が痛いのです。"
},
{
"speaker": "システム",
"text": "分かりました、コンタクトレンズをつけると目がかゆくなるということですね。思った以上にレンズを外す必要があるでしょうか?"
},
{
"speaker": "ユーザー",
"text": "いえ、レンズは外しませんが、目が赤くなるんです。"
}
]
prompt = [
f"{uttr['speaker']}: {uttr['text']}"
for uttr in prompt
]
prompt = "<NL>".join(prompt)
prompt = (
prompt
+ "<NL>"
+ "システム: "
)
print(prompt)
# "ユーザー: コンタクトレンズを慣れるにはどうすればよいですか?<NL>システム: これについて具体的に説明していただけますか?何が難しいのでしょうか?<NL>ユーザー: 目が痛いのです。<NL>システム: 分かりました、コンタクトレンズをつけると目がかゆくなるということですね。思った以上にレンズを外す必要があるでしょうか?<NL>ユーザー: いえ、レンズは外しませんが、目が赤くなるんです。<NL>システム: "
~~~
# How to use the model
~~~~python
import torch
from transformers import AutoTokenizer, AutoModelForCausalLM
tokenizer = AutoTokenizer.from_pretrained("rinna/japanese-gpt-neox-3.6b-instruction-sft-v2", use_fast=False)
model = AutoModelForCausalLM.from_pretrained("rinna/japanese-gpt-neox-3.6b-instruction-sft-v2")
if torch.cuda.is_available():
model = model.to("cuda")
token_ids = tokenizer.encode(prompt, add_special_tokens=False, return_tensors="pt")
with torch.no_grad():
output_ids = model.generate(
token_ids.to(model.device),
do_sample=True,
max_new_tokens=128,
temperature=0.7,
repetition_penalty=1.1,
pad_token_id=tokenizer.pad_token_id,
bos_token_id=tokenizer.bos_token_id,
eos_token_id=tokenizer.eos_token_id
)
output = tokenizer.decode(output_ids.tolist()[0][token_ids.size(1):])
output = output.replace("<NL>", "\n")
print(output)
"""わかりました。まずは、コンタクトレンズを長時間着用することによる目の乾燥を防ぐことができます。また、毎日同じ時間帯にコンタクトレンズを着用してみることもできます。そして、コンタクトレンズが目に合わないような場合は、新しいものを試してみる必要があります。</s>"""
~~~~
# Tokenization
The model uses a [sentencepiece](https://github.com/google/sentencepiece)-based tokenizer.
* The tokenizer has a vocabulary size of 32,000.
* It uses sentencepiece's byte fallback feature to decompose unknown text pieces into UTF-8 byte pieces and to avoid producing `<UNK>` tokens.
* sentencepiece's `--add_dummy_prefix` option was turned off so that a leading whitespace will not be prepended automatically.
~~~
print(tokenizer.tokenize("吾輩は猫である"))
# ['吾', '輩', 'は', '猫', 'である']
# instead of ['▁', '吾', '輩', 'は', '猫', 'である'] as in rinna/japanese-gpt-1b
~~~
* sentencepiece's `--remove_extra_whitespaces` option was turned off so that leading, trailing, and duplicate whitespaces are reserved.
~~~
print(tokenizer.tokenize(" 吾輩は 猫である "))
# ['▁', '▁', '吾', '輩', 'は', '▁', '▁', '猫', 'である', '▁', '▁', '▁']
# instead of ['▁', '吾', '輩', 'は', '▁猫', 'である'] as in rinna/japanese-gpt-1b
~~~
* Don't forget to set `use_fast=False` to make the above features function correctly.
~~~
good_tokenizer = AutoTokenizer.from_pretrained("rinna/japanese-gpt-neox-3.6b", use_fast=False)
bad_tokenizer = AutoTokenizer.from_pretrained("rinna/japanese-gpt-neox-3.6b")
print(good_tokenizer.decode(good_tokenizer.encode("გამარჯობა 吾輩は 猫である ")))
# 'გამარჯობა 吾輩は 猫である </s>'
print(bad_tokenizer.decode(bad_tokenizer.encode("გამარჯობა 吾輩は 猫である ")))
# 'გამარ[UNK]ობა 吾輩は 猫である </s>'
~~~
# How to cite
~~~
@misc{rinna-japanese-gpt-neox-3.6b-instruction-sft-v2,
title = {rinna/japanese-gpt-neox-3.6b-instruction-sft-v2},
author = {Zhao, Tianyu and Sawada, Kei}
url = {https://huggingface.co/rinna/japanese-gpt-neox-3.6b-instruction-sft-v2},
}
@inproceedings{sawada2024release,
title = {Release of Pre-Trained Models for the {J}apanese Language},
author = {Sawada, Kei and Zhao, Tianyu and Shing, Makoto and Mitsui, Kentaro and Kaga, Akio and Hono, Yukiya and Wakatsuki, Toshiaki and Mitsuda, Koh},
booktitle = {Proceedings of the 2024 Joint International Conference on Computational Linguistics, Language Resources and Evaluation (LREC-COLING 2024)},
month = {5},
year = {2024},
url = {https://arxiv.org/abs/2404.01657},
}
~~~
# Licenese
[The MIT license](https://opensource.org/licenses/MIT)
|
mradermacher/L3-8B-SMaid-v0.3-GGUF | mradermacher | "2024-06-23T00:28:23Z" | 14,340 | 0 | transformers | [
"transformers",
"gguf",
"mergekit",
"merge",
"en",
"base_model:Alsebay/L3-8B-SMaid-v0.3",
"endpoints_compatible",
"region:us"
] | null | "2024-06-22T23:59:19Z" | ---
base_model: Alsebay/L3-8B-SMaid-v0.3
language:
- en
library_name: transformers
quantized_by: mradermacher
tags:
- mergekit
- merge
---
## About
<!-- ### quantize_version: 2 -->
<!-- ### output_tensor_quantised: 1 -->
<!-- ### convert_type: hf -->
<!-- ### vocab_type: -->
<!-- ### tags: -->
static quants of https://huggingface.co/Alsebay/L3-8B-SMaid-v0.3
<!-- provided-files -->
weighted/imatrix quants are available at https://huggingface.co/mradermacher/L3-8B-SMaid-v0.3-i1-GGUF
## Usage
If you are unsure how to use GGUF files, refer to one of [TheBloke's
READMEs](https://huggingface.co/TheBloke/KafkaLM-70B-German-V0.1-GGUF) for
more details, including on how to concatenate multi-part files.
## Provided Quants
(sorted by size, not necessarily quality. IQ-quants are often preferable over similar sized non-IQ quants)
| Link | Type | Size/GB | Notes |
|:-----|:-----|--------:|:------|
| [GGUF](https://huggingface.co/mradermacher/L3-8B-SMaid-v0.3-GGUF/resolve/main/L3-8B-SMaid-v0.3.Q2_K.gguf) | Q2_K | 3.3 | |
| [GGUF](https://huggingface.co/mradermacher/L3-8B-SMaid-v0.3-GGUF/resolve/main/L3-8B-SMaid-v0.3.IQ3_XS.gguf) | IQ3_XS | 3.6 | |
| [GGUF](https://huggingface.co/mradermacher/L3-8B-SMaid-v0.3-GGUF/resolve/main/L3-8B-SMaid-v0.3.Q3_K_S.gguf) | Q3_K_S | 3.8 | |
| [GGUF](https://huggingface.co/mradermacher/L3-8B-SMaid-v0.3-GGUF/resolve/main/L3-8B-SMaid-v0.3.IQ3_S.gguf) | IQ3_S | 3.8 | beats Q3_K* |
| [GGUF](https://huggingface.co/mradermacher/L3-8B-SMaid-v0.3-GGUF/resolve/main/L3-8B-SMaid-v0.3.IQ3_M.gguf) | IQ3_M | 3.9 | |
| [GGUF](https://huggingface.co/mradermacher/L3-8B-SMaid-v0.3-GGUF/resolve/main/L3-8B-SMaid-v0.3.Q3_K_M.gguf) | Q3_K_M | 4.1 | lower quality |
| [GGUF](https://huggingface.co/mradermacher/L3-8B-SMaid-v0.3-GGUF/resolve/main/L3-8B-SMaid-v0.3.Q3_K_L.gguf) | Q3_K_L | 4.4 | |
| [GGUF](https://huggingface.co/mradermacher/L3-8B-SMaid-v0.3-GGUF/resolve/main/L3-8B-SMaid-v0.3.IQ4_XS.gguf) | IQ4_XS | 4.6 | |
| [GGUF](https://huggingface.co/mradermacher/L3-8B-SMaid-v0.3-GGUF/resolve/main/L3-8B-SMaid-v0.3.Q4_K_S.gguf) | Q4_K_S | 4.8 | fast, recommended |
| [GGUF](https://huggingface.co/mradermacher/L3-8B-SMaid-v0.3-GGUF/resolve/main/L3-8B-SMaid-v0.3.Q4_K_M.gguf) | Q4_K_M | 5.0 | fast, recommended |
| [GGUF](https://huggingface.co/mradermacher/L3-8B-SMaid-v0.3-GGUF/resolve/main/L3-8B-SMaid-v0.3.Q5_K_S.gguf) | Q5_K_S | 5.7 | |
| [GGUF](https://huggingface.co/mradermacher/L3-8B-SMaid-v0.3-GGUF/resolve/main/L3-8B-SMaid-v0.3.Q5_K_M.gguf) | Q5_K_M | 5.8 | |
| [GGUF](https://huggingface.co/mradermacher/L3-8B-SMaid-v0.3-GGUF/resolve/main/L3-8B-SMaid-v0.3.Q6_K.gguf) | Q6_K | 6.7 | very good quality |
| [GGUF](https://huggingface.co/mradermacher/L3-8B-SMaid-v0.3-GGUF/resolve/main/L3-8B-SMaid-v0.3.Q8_0.gguf) | Q8_0 | 8.6 | fast, best quality |
| [GGUF](https://huggingface.co/mradermacher/L3-8B-SMaid-v0.3-GGUF/resolve/main/L3-8B-SMaid-v0.3.f16.gguf) | f16 | 16.2 | 16 bpw, overkill |
Here is a handy graph by ikawrakow comparing some lower-quality quant
types (lower is better):

And here are Artefact2's thoughts on the matter:
https://gist.github.com/Artefact2/b5f810600771265fc1e39442288e8ec9
## FAQ / Model Request
See https://huggingface.co/mradermacher/model_requests for some answers to
questions you might have and/or if you want some other model quantized.
## Thanks
I thank my company, [nethype GmbH](https://www.nethype.de/), for letting
me use its servers and providing upgrades to my workstation to enable
this work in my free time.
<!-- end -->
|
mradermacher/IceSakeV9RP-7b-GGUF | mradermacher | "2024-06-28T05:06:29Z" | 14,339 | 0 | transformers | [
"transformers",
"gguf",
"mergekit",
"merge",
"alpaca",
"mistral",
"not-for-all-audiences",
"nsfw",
"en",
"base_model:icefog72/IceSakeV9RP-7b",
"license:cc-by-nc-4.0",
"endpoints_compatible",
"region:us"
] | null | "2024-06-28T03:13:08Z" | ---
base_model: icefog72/IceSakeV9RP-7b
language:
- en
library_name: transformers
license: cc-by-nc-4.0
quantized_by: mradermacher
tags:
- mergekit
- merge
- alpaca
- mistral
- not-for-all-audiences
- nsfw
---
## About
<!-- ### quantize_version: 2 -->
<!-- ### output_tensor_quantised: 1 -->
<!-- ### convert_type: hf -->
<!-- ### vocab_type: -->
<!-- ### tags: -->
static quants of https://huggingface.co/icefog72/IceSakeV9RP-7b
<!-- provided-files -->
weighted/imatrix quants are available at https://huggingface.co/mradermacher/IceSakeV9RP-7b-i1-GGUF
## Usage
If you are unsure how to use GGUF files, refer to one of [TheBloke's
READMEs](https://huggingface.co/TheBloke/KafkaLM-70B-German-V0.1-GGUF) for
more details, including on how to concatenate multi-part files.
## Provided Quants
(sorted by size, not necessarily quality. IQ-quants are often preferable over similar sized non-IQ quants)
| Link | Type | Size/GB | Notes |
|:-----|:-----|--------:|:------|
| [GGUF](https://huggingface.co/mradermacher/IceSakeV9RP-7b-GGUF/resolve/main/IceSakeV9RP-7b.Q2_K.gguf) | Q2_K | 2.8 | |
| [GGUF](https://huggingface.co/mradermacher/IceSakeV9RP-7b-GGUF/resolve/main/IceSakeV9RP-7b.IQ3_XS.gguf) | IQ3_XS | 3.1 | |
| [GGUF](https://huggingface.co/mradermacher/IceSakeV9RP-7b-GGUF/resolve/main/IceSakeV9RP-7b.Q3_K_S.gguf) | Q3_K_S | 3.3 | |
| [GGUF](https://huggingface.co/mradermacher/IceSakeV9RP-7b-GGUF/resolve/main/IceSakeV9RP-7b.IQ3_S.gguf) | IQ3_S | 3.3 | beats Q3_K* |
| [GGUF](https://huggingface.co/mradermacher/IceSakeV9RP-7b-GGUF/resolve/main/IceSakeV9RP-7b.IQ3_M.gguf) | IQ3_M | 3.4 | |
| [GGUF](https://huggingface.co/mradermacher/IceSakeV9RP-7b-GGUF/resolve/main/IceSakeV9RP-7b.Q3_K_M.gguf) | Q3_K_M | 3.6 | lower quality |
| [GGUF](https://huggingface.co/mradermacher/IceSakeV9RP-7b-GGUF/resolve/main/IceSakeV9RP-7b.Q3_K_L.gguf) | Q3_K_L | 3.9 | |
| [GGUF](https://huggingface.co/mradermacher/IceSakeV9RP-7b-GGUF/resolve/main/IceSakeV9RP-7b.IQ4_XS.gguf) | IQ4_XS | 4.0 | |
| [GGUF](https://huggingface.co/mradermacher/IceSakeV9RP-7b-GGUF/resolve/main/IceSakeV9RP-7b.Q4_K_S.gguf) | Q4_K_S | 4.2 | fast, recommended |
| [GGUF](https://huggingface.co/mradermacher/IceSakeV9RP-7b-GGUF/resolve/main/IceSakeV9RP-7b.Q4_K_M.gguf) | Q4_K_M | 4.5 | fast, recommended |
| [GGUF](https://huggingface.co/mradermacher/IceSakeV9RP-7b-GGUF/resolve/main/IceSakeV9RP-7b.Q5_K_S.gguf) | Q5_K_S | 5.1 | |
| [GGUF](https://huggingface.co/mradermacher/IceSakeV9RP-7b-GGUF/resolve/main/IceSakeV9RP-7b.Q5_K_M.gguf) | Q5_K_M | 5.2 | |
| [GGUF](https://huggingface.co/mradermacher/IceSakeV9RP-7b-GGUF/resolve/main/IceSakeV9RP-7b.Q6_K.gguf) | Q6_K | 6.0 | very good quality |
| [GGUF](https://huggingface.co/mradermacher/IceSakeV9RP-7b-GGUF/resolve/main/IceSakeV9RP-7b.Q8_0.gguf) | Q8_0 | 7.8 | fast, best quality |
| [GGUF](https://huggingface.co/mradermacher/IceSakeV9RP-7b-GGUF/resolve/main/IceSakeV9RP-7b.f16.gguf) | f16 | 14.6 | 16 bpw, overkill |
Here is a handy graph by ikawrakow comparing some lower-quality quant
types (lower is better):

And here are Artefact2's thoughts on the matter:
https://gist.github.com/Artefact2/b5f810600771265fc1e39442288e8ec9
## FAQ / Model Request
See https://huggingface.co/mradermacher/model_requests for some answers to
questions you might have and/or if you want some other model quantized.
## Thanks
I thank my company, [nethype GmbH](https://www.nethype.de/), for letting
me use its servers and providing upgrades to my workstation to enable
this work in my free time.
<!-- end -->
|
allenai/OLMo-1.7-7B-hf | allenai | "2024-05-28T17:15:42Z" | 14,338 | 8 | transformers | [
"transformers",
"safetensors",
"olmo",
"text-generation",
"en",
"dataset:allenai/dolma",
"arxiv:2402.00838",
"license:apache-2.0",
"autotrain_compatible",
"endpoints_compatible",
"region:us"
] | text-generation | "2024-04-17T16:46:55Z" | ---
license: apache-2.0
datasets:
- allenai/dolma
language:
- en
---
<img src="https://allenai.org/olmo/olmo-7b-animation.gif" alt="OLMo Logo" width="800" style="margin-left:'auto' margin-right:'auto' display:'block'"/>
# Model Card for OLMo 1.7-7B-hf
OLMo 1.7 7B is the latest version of the original [OLMo 7B](https://huggingface.co/allenai/OLMo-7B) model rocking a 24 point increase in MMLU, among other evaluations improvements, from an improved version of the Dolma dataset and staged training.
**This version is for direct use with HuggingFace Transformers** from v4.40 on.
OLMo is a series of **O**pen **L**anguage **Mo**dels designed to enable the science of language models.
The OLMo models are trained on the [Dolma](https://huggingface.co/datasets/allenai/dolma) dataset.
We release all code, checkpoints, logs, and details involved in training these models.
## Model Details
The core models released in this batch are the following:
| Size | Training Tokens | Layers | Hidden Size | Attention Heads | Context Length |
|------|--------|---------|-------------|-----------------|----------------|
| [OLMo 1B](https://huggingface.co/allenai/OLMo-1B) | 3 Trillion |16 | 2048 | 16 | 2048 |
| [OLMo 7B](https://huggingface.co/allenai/OLMo-7B) | 2.5 Trillion | 32 | 4096 | 32 | 2048 |
| [OLMo 7B Twin 2T](https://huggingface.co/allenai/OLMo-7B-Twin-2T) | 2 Trillion | 32 | 4096 | 32 | 2048 |
| [OLMo 1.7-7B](https://huggingface.co/allenai/OLMo-1.7-7B) | 2.05 Trillion | 32 | 4096 | 32 | 4096 |
*Note: OLMo 1.7-7B also includes QKV clipping.*
[Coming soon] We are releasing many checkpoints for these models, for every 1000 training steps.
The naming convention is `step1000-tokens4B`.
To load a specific model revision with HuggingFace, simply add the argument `revision`:
```bash
olmo = AutoModelForCausalLM.from_pretrained("allenai/OLMo-1.7-7B-hf", revision="step1000-tokens4B")
```
All revisions/branches are listed in the file `revisions.txt`.
Or, you can access all the revisions for the models via the following code snippet:
```python
from huggingface_hub import list_repo_refs
out = list_repo_refs("allenai/OLMo-1.7-7B-hf")
branches = [b.name for b in out.branches]
```
A few revisions were lost due to an error, but the vast majority are present.
### Model Description
- **Developed by:** Allen Institute for AI (AI2)
- **Supported by:** Databricks, Kempner Institute for the Study of Natural and Artificial Intelligence at Harvard University, AMD, CSC (Lumi Supercomputer), UW
- **Model type:** a Transformer style autoregressive language model.
- **Language(s) (NLP):** English
- **License:** The code and model are released under Apache 2.0.
- **Contact:** Technical inquiries: `olmo at allenai dot org`. Press: `press at allenai dot org`
- **Date cutoff:** Oct. 2023, with most data from Feb./March 2023 based on Dolma dataset version.
### Model Sources
- **Project Page:** https://allenai.org/olmo
- **Repositories:**
- Core repo (training, inference, fine-tuning etc.): https://github.com/allenai/OLMo
- Evaluation code: https://github.com/allenai/OLMo-Eval
- Further fine-tuning code: https://github.com/allenai/open-instruct
- **Paper:** [Link](https://arxiv.org/abs/2402.00838)
- **Technical blog post:** https://blog.allenai.org/olmo-1-7-7b-a-24-point-improvement-on-mmlu-92b43f7d269d
- **W&B Logs:** [pretraining](https://wandb.ai/ai2-llm/OLMo-7B/groups/OLMo-1.7-7B), [annealing](https://wandb.ai/ai2-llm/OLMo-7B/groups/OLMo-1.7-7B-anneal)
<!-- - **Press release:** TODO -->
## Uses
### Inference
Install Transformers [from source](https://huggingface.co/docs/transformers/en/installation#install-from-source), or update to the next version when this [PR](https://github.com/huggingface/transformers/pull/29890) is integrated.
Now, proceed as usual with HuggingFace:
```python
from transformers import AutoModelForCausalLM, AutoTokenizer
olmo = AutoModelForCausalLM.from_pretrained("allenai/OLMo-1.7-7B-hf")
tokenizer = AutoTokenizer.from_pretrained("allenai/OLMo-1.7-7B-hf")
message = ["Language modeling is "]
inputs = tokenizer(message, return_tensors='pt', return_token_type_ids=False)
# optional verifying cuda
# inputs = {k: v.to('cuda') for k,v in inputs.items()}
# olmo = olmo.to('cuda')
response = olmo.generate(**inputs, max_new_tokens=100, do_sample=True, top_k=50, top_p=0.95)
print(tokenizer.batch_decode(response, skip_special_tokens=True)[0])
>> 'Language modeling is the first step to build natural language generation...'
```
Alternatively, with the pipeline abstraction:
```python
from transformers import pipeline
olmo_pipe = pipeline("text-generation", model="allenai/OLMo-1.7-7B-hf")
print(olmo_pipe("Language modeling is "))
>> 'Language modeling is a branch of natural language processing that aims to...'
```
Or, you can make this slightly faster by quantizing the model, e.g. `AutoModelForCausalLM.from_pretrained("allenai/OLMo-1.7-7B-hf", torch_dtype=torch.float16, load_in_8bit=True)` (requires `bitsandbytes`).
The quantized model is more sensitive to typing / cuda, so it is recommended to pass the inputs as `inputs.input_ids.to('cuda')` to avoid potential issues.
Note, you may see the following error if `ai2-olmo` is not installed correctly, which is caused by internal Python check naming. We'll update the code soon to make this error clearer.
```bash
raise ImportError(
ImportError: This modeling file requires the following packages that were not found in your environment: hf_olmo. Run `pip install hf_olmo`
```
### Fine-tuning
Model fine-tuning can be done from the final checkpoint (the `main` revision of this model) or many intermediate checkpoints. Two recipes for tuning are available.
1. Fine-tune with the OLMo repository:
```bash
torchrun --nproc_per_node=8 scripts/train.py {path_to_train_config} \
--data.paths=[{path_to_data}/input_ids.npy] \
--data.label_mask_paths=[{path_to_data}/label_mask.npy] \
--load_path={path_to_checkpoint} \
--reset_trainer_state
```
For more documentation, see the [GitHub readme](https://github.com/allenai/OLMo?tab=readme-ov-file#fine-tuning).
2. Further fine-tuning support is being developing in AI2's Open Instruct repository. Details are [here](https://github.com/allenai/open-instruct).
## Evaluation
<!-- This section describes the evaluation protocols and provides the results. -->
Core model results for the new and original 7B model are found below.
| Task | Llama-7b | Llama2-7b | Falcon-7b | Mpt-7b | OLMo-7B | Llama2-13b | **OLMo 1.7-7B** |
|-------------------|----------|-----------|-----------|--------|---------|------------|-------------|
| arc_c | 44.5 | 48.5 | 47.5 | 46.5 | 48.5 | 52.8 | 42.5 |
| arc_e | 67.9 | 69.5 | 70.4 | 70.5 | 65.4 | 73.7 | 67.2 |
| boolq | 75.4 | 80.2 | 74.6 | 74.2 | 73.4 | 82.2 | 83.7 |
| copa | 91.0 | 86.0 | 86.0 | 85.0 | 90.0 | 90.0 | 86.0 |
| hellaswag | 76.2 | 76.8 | 75.9 | 77.6 | 76.4 | 78.6 | 75.5 |
| openbookqa | 51.2 | 48.4 | 53.0 | 48.6 | 50.4 | 51.8 | 50.0 |
| piqa | 77.2 | 76.7 | 78.5 | 77.3 | 78.4 | 79.0 | 77.5 |
| sciq | 93.9 | 94.5 | 93.9 | 93.7 | 93.8 | 95.5 | 96.7 |
| winogrande | 70.5 | 69.4 | 68.9 | 69.9 | 67.9 | 73.5 | 69.8 |
| truthfulQA (MC2) | 33.9 | 38.5 | 34.0 | 33.0 | 36.0 | 36.8 | 35.8 |
| MMLU (5 shot MC) | 31.5 | 45.0 | 24.0 | 30.8 | 28.3 | 55.5 | 52.0 |
| GSM8k | 10.0 | 12.0 | 4.0 | 4.5 | 8.5 | 25.0 | 29.0 |
| Full average | 60.3 | 62.1 | 59.2 | 59.3 | 59.8 | 66.2 | 63.8 |
And for the 1B model:
| task | random | [StableLM 2 1.6b](https://huggingface.co/stabilityai/stablelm-2-1_6b)\* | [Pythia 1B](https://huggingface.co/EleutherAI/pythia-1b) | [TinyLlama 1.1B](https://huggingface.co/TinyLlama/TinyLlama-1.1B-intermediate-step-1195k-token-2.5T) | **OLMo 1B** (ours) |
| ------------------------------------------------------------------------------------------------------------------------------------------------------------ | ------ | ----------------- | --------- | -------------------------------------- | ------- |
| arc_challenge | 25 | 43.81 | 33.11 | 34.78 | 34.45 |
| arc_easy | 25 | 63.68 | 50.18 | 53.16 | 58.07 |
| boolq | 50 | 76.6 | 61.8 | 64.6 | 60.7 |
| copa | 50 | 84 | 72 | 78 | 79 |
| hellaswag | 25 | 68.2 | 44.7 | 58.7 | 62.5 |
| openbookqa | 25 | 45.8 | 37.8 | 43.6 | 46.4 |
| piqa | 50 | 74 | 69.1 | 71.1 | 73.7 |
| sciq | 25 | 94.7 | 86 | 90.5 | 88.1 |
| winogrande | 50 | 64.9 | 53.3 | 58.9 | 58.9 |
| Average | 36.11 | 68.41 | 56.44 | 61.48 | 62.42 |
\*Unlike OLMo, Pythia, and TinyLlama, StabilityAI has not disclosed yet the data StableLM was trained on, making comparisons with other efforts challenging.
## Model Details
### Data
For training data details, please see the [Dolma](https://huggingface.co/datasets/allenai/dolma) documentation.
**This model uses the new 1.7 version with more data sources, better deduplication, and quality filtering**.
During the annealing phase we use a higher quality subset of Dolma with a linearly decaying learning rate to 0.
### Staged training / annealing
In contrast to OLMo 1.0, we trained OLMo 1.7 with a two-stage curriculum:
* In the first stage, we trained the model from scratch on the Dolma 1.7 dataset. We set a cosine learning rate schedule with a warmup of 2500 steps, a peak learning rate of 3e-4, and a cosine decay to 3e-5 after 3T tokens. We cut off this stage after 2T tokens, when the learning rate is still high.
* At this point we switch to the second stage, in which we train on a higher-quality subset of Dolma 1.7 (see below) for another 50B tokens, while linearly decaying the learning rate to 0. Our high-quality subset includes (1) using all available Wikipedia, OpenWebMath and Flan data, (2) removing Dolma CC, CC News, and Megawika, and (3) rebalancing remaining sources to achieve approximately equal proportions of each. See exact token counts and relative proportions of this second stage mix below.
Both stages contribute equally to the final performance of the OLMo model. After the first stage, OLMo 1.7 already outperforms OLMo 1.0. The second stage consistently adds 2 to 3 points of performance on top.
### Architecture
OLMo 7B architecture with peer models for comparison.
| | **OLMo 7B** | [Llama 2 7B](https://huggingface.co/meta-llama/Llama-2-7b) | [OpenLM 7B](https://laion.ai/blog/open-lm/) | [Falcon 7B](https://huggingface.co/tiiuae/falcon-7b) | PaLM 8B |
|------------------------|-------------------|---------------------|--------------------|--------------------|------------------|
| d_model | 4096 | 4096 | 4096 | 4544 | 4096 |
| num heads | 32 | 32 | 32 | 71 | 16 |
| num layers | 32 | 32 | 32 | 32 | 32 |
| MLP ratio | ~8/3 | ~8/3 | ~8/3 | 4 | 4 |
| LayerNorm type | non-parametric LN | RMSNorm | parametric LN | parametric LN | parametric LN |
| pos embeddings | RoPE | RoPE | RoPE | RoPE | RoPE |
| attention variant | full | GQA | full | MQA | MQA |
| biases | none | none | in LN only | in LN only | none |
| block type | sequential | sequential | sequential | parallel | parallel |
| activation | SwiGLU | SwiGLU | SwiGLU | GeLU | SwiGLU |
| sequence length | 2048 | 4096 | 2048 | 2048 | 2048 |
| batch size (instances) | 2160 | 1024 | 2048 | 2304 | 512 |
| batch size (tokens) | ~4M | ~4M | ~4M | ~4M | ~1M |
| weight tying | no | no | no | no | yes |
### Hyperparameters
AdamW optimizer parameters are shown below.
| Size | Peak LR | Betas | Epsilon | Weight Decay |
|------|------------|-----------------|-------------|--------------|
| 1B | 4.0E-4 | (0.9, 0.95) | 1.0E-5 | 0.1 |
| 7B | 3.0E-4 | (0.9, 0.99) | 1.0E-5 | 0.1 |
Optimizer settings comparison with peer models.
| | **OLMo 7B** | [Llama 2 7B](https://huggingface.co/meta-llama/Llama-2-7b) | [OpenLM 7B](https://laion.ai/blog/open-lm/) | [Falcon 7B](https://huggingface.co/tiiuae/falcon-7b) |
|-----------------------|------------------|---------------------|--------------------|--------------------|
| warmup steps | 5000 | 2000 | 2000 | 1000 |
| peak LR | 3.0E-04 | 3.0E-04 | 3.0E-04 | 6.0E-04 |
| minimum LR | 3.0E-05 | 3.0E-05 | 3.0E-05 | 1.2E-05 |
| weight decay | 0.1 | 0.1 | 0.1 | 0.1 |
| beta1 | 0.9 | 0.9 | 0.9 | 0.99 |
| beta2 | 0.95 | 0.95 | 0.95 | 0.999 |
| epsilon | 1.0E-05 | 1.0E-05 | 1.0E-05 | 1.0E-05 |
| LR schedule | linear | cosine | cosine | cosine |
| gradient clipping | global 1.0 | global 1.0 | global 1.0 | global 1.0 |
| gradient reduce dtype | FP32 | FP32 | FP32 | BF16 |
| optimizer state dtype | FP32 | most likely FP32 | FP32 | FP32 |
## Environmental Impact
OLMo 7B variants were either trained on MI250X GPUs at the LUMI supercomputer, or A100-40GB GPUs provided by MosaicML.
A summary of the environmental impact. Further details are available in the paper.
| | GPU Type | Power Consumption From GPUs | Carbon Intensity (kg CO₂e/KWh) | Carbon Emissions (tCO₂eq) |
|-----------|------------|-----------------------------|--------------------------------|---------------------------|
| OLMo 7B Twin | MI250X ([LUMI supercomputer](https://www.lumi-supercomputer.eu)) | 135 MWh | 0* | 0* |
| OLMo 7B | A100-40GB ([MosaicML](https://www.mosaicml.com)) | 104 MWh | 0.656 | 75.05 |
## Bias, Risks, and Limitations
Like any base language model or fine-tuned model without safety filtering, it is relatively easy for a user to prompt these models to generate harmful and generally sensitive content.
Such content can also be produced unintentionally, especially in the case of bias, so we recommend users consider the risks of applications of this technology.
Otherwise, many facts from OLMo or any LLM will often not be true, so they should be checked.
## Citation
**BibTeX:**
```
@article{Groeneveld2023OLMo,
title={OLMo: Accelerating the Science of Language Models},
author={Groeneveld, Dirk and Beltagy, Iz and Walsh, Pete and Bhagia, Akshita and Kinney, Rodney and Tafjord, Oyvind and Jha, Ananya Harsh and Ivison, Hamish and Magnusson, Ian and Wang, Yizhong and Arora, Shane and Atkinson, David and Authur, Russell and Chandu, Khyathi and Cohan, Arman and Dumas, Jennifer and Elazar, Yanai and Gu, Yuling and Hessel, Jack and Khot, Tushar and Merrill, William and Morrison, Jacob and Muennighoff, Niklas and Naik, Aakanksha and Nam, Crystal and Peters, Matthew E. and Pyatkin, Valentina and Ravichander, Abhilasha and Schwenk, Dustin and Shah, Saurabh and Smith, Will and Subramani, Nishant and Wortsman, Mitchell and Dasigi, Pradeep and Lambert, Nathan and Richardson, Kyle and Dodge, Jesse and Lo, Kyle and Soldaini, Luca and Smith, Noah A. and Hajishirzi, Hannaneh},
journal={Preprint},
year={2024}
}
```
**APA:**
Groeneveld, D., Beltagy, I., Walsh, P., Bhagia, A., Kinney, R., Tafjord, O., Jha, A., Ivison, H., Magnusson, I., Wang, Y., Arora, S., Atkinson, D., Authur, R., Chandu, K., Cohan, A., Dumas, J., Elazar, Y., Gu, Y., Hessel, J., Khot, T., Merrill, W., Morrison, J., Muennighoff, N., Naik, A., Nam, C., Peters, M., Pyatkin, V., Ravichander, A., Schwenk, D., Shah, S., Smith, W., Subramani, N., Wortsman, M., Dasigi, P., Lambert, N., Richardson, K., Dodge, J., Lo, K., Soldaini, L., Smith, N., & Hajishirzi, H. (2024). OLMo: Accelerating the Science of Language Models. Preprint.
## Model Card Contact
For errors in this model card, contact Nathan, `{nathanl} at allenai dot org`. |
ybelkada/Mixtral-8x7B-Instruct-v0.1-AWQ | ybelkada | "2023-12-12T22:27:03Z" | 14,336 | 11 | transformers | [
"transformers",
"safetensors",
"mixtral",
"text-generation",
"conversational",
"autotrain_compatible",
"endpoints_compatible",
"text-generation-inference",
"4-bit",
"awq",
"region:us"
] | text-generation | "2023-12-12T22:22:51Z" | Entry not found |
sshleifer/distilbart-cnn-6-6 | sshleifer | "2021-06-14T07:53:04Z" | 14,329 | 24 | transformers | [
"transformers",
"pytorch",
"jax",
"rust",
"bart",
"text2text-generation",
"summarization",
"en",
"dataset:cnn_dailymail",
"dataset:xsum",
"license:apache-2.0",
"autotrain_compatible",
"endpoints_compatible",
"region:us"
] | summarization | "2022-03-02T23:29:05Z" | ---
language: en
tags:
- summarization
license: apache-2.0
datasets:
- cnn_dailymail
- xsum
thumbnail: https://huggingface.co/front/thumbnails/distilbart_medium.png
---
### Usage
This checkpoint should be loaded into `BartForConditionalGeneration.from_pretrained`. See the [BART docs](https://huggingface.co/transformers/model_doc/bart.html?#transformers.BartForConditionalGeneration) for more information.
### Metrics for DistilBART models
| Model Name | MM Params | Inference Time (MS) | Speedup | Rouge 2 | Rouge-L |
|:---------------------------|------------:|----------------------:|----------:|----------:|----------:|
| distilbart-xsum-12-1 | 222 | 90 | 2.54 | 18.31 | 33.37 |
| distilbart-xsum-6-6 | 230 | 132 | 1.73 | 20.92 | 35.73 |
| distilbart-xsum-12-3 | 255 | 106 | 2.16 | 21.37 | 36.39 |
| distilbart-xsum-9-6 | 268 | 136 | 1.68 | 21.72 | 36.61 |
| bart-large-xsum (baseline) | 406 | 229 | 1 | 21.85 | 36.50 |
| distilbart-xsum-12-6 | 306 | 137 | 1.68 | 22.12 | 36.99 |
| bart-large-cnn (baseline) | 406 | 381 | 1 | 21.06 | 30.63 |
| distilbart-12-3-cnn | 255 | 214 | 1.78 | 20.57 | 30.00 |
| distilbart-12-6-cnn | 306 | 307 | 1.24 | 21.26 | 30.59 |
| distilbart-6-6-cnn | 230 | 182 | 2.09 | 20.17 | 29.70 |
|
PrunaAI/GreatCaptainNemo-ProLLaMA-GGUF-smashed | PrunaAI | "2024-06-28T19:40:54Z" | 14,322 | 0 | null | [
"gguf",
"pruna-ai",
"region:us"
] | null | "2024-06-28T19:02:35Z" | ---
thumbnail: "https://assets-global.website-files.com/646b351987a8d8ce158d1940/64ec9e96b4334c0e1ac41504_Logo%20with%20white%20text.svg"
metrics:
- memory_disk
- memory_inference
- inference_latency
- inference_throughput
- inference_CO2_emissions
- inference_energy_consumption
tags:
- pruna-ai
---
<!-- header start -->
<!-- 200823 -->
<div style="width: auto; margin-left: auto; margin-right: auto">
<a href="https://www.pruna.ai/" target="_blank" rel="noopener noreferrer">
<img src="https://i.imgur.com/eDAlcgk.png" alt="PrunaAI" style="width: 100%; min-width: 400px; display: block; margin: auto;">
</a>
</div>
<!-- header end -->
[](https://twitter.com/PrunaAI)
[](https://github.com/PrunaAI)
[](https://www.linkedin.com/company/93832878/admin/feed/posts/?feedType=following)
[](https://discord.com/invite/vb6SmA3hxu)
## This repo contains GGUF versions of the GreatCaptainNemo/ProLLaMA model.
# Simply make AI models cheaper, smaller, faster, and greener!
- Give a thumbs up if you like this model!
- Contact us and tell us which model to compress next [here](https://www.pruna.ai/contact).
- Request access to easily compress your *own* AI models [here](https://z0halsaff74.typeform.com/pruna-access?typeform-source=www.pruna.ai).
- Read the documentations to know more [here](https://pruna-ai-pruna.readthedocs-hosted.com/en/latest/)
- Join Pruna AI community on Discord [here](https://discord.com/invite/vb6SmA3hxu) to share feedback/suggestions or get help.
**Frequently Asked Questions**
- ***How does the compression work?*** The model is compressed with GGUF.
- ***How does the model quality change?*** The quality of the model output might vary compared to the base model.
- ***What is the model format?*** We use GGUF format.
- ***What calibration data has been used?*** If needed by the compression method, we used WikiText as the calibration data.
- ***How to compress my own models?*** You can request premium access to more compression methods and tech support for your specific use-cases [here](https://z0halsaff74.typeform.com/pruna-access?typeform-source=www.pruna.ai).
# Downloading and running the models
You can download the individual files from the Files & versions section. Here is a list of the different versions we provide. For more info checkout [this chart](https://gist.github.com/Artefact2/b5f810600771265fc1e39442288e8ec9) and [this guide](https://www.reddit.com/r/LocalLLaMA/comments/1ba55rj/overview_of_gguf_quantization_methods/):
| Quant type | Description |
|------------|--------------------------------------------------------------------------------------------|
| Q5_K_M | High quality, recommended. |
| Q5_K_S | High quality, recommended. |
| Q4_K_M | Good quality, uses about 4.83 bits per weight, recommended. |
| Q4_K_S | Slightly lower quality with more space savings, recommended. |
| IQ4_NL | Decent quality, slightly smaller than Q4_K_S with similar performance, recommended. |
| IQ4_XS | Decent quality, smaller than Q4_K_S with similar performance, recommended. |
| Q3_K_L | Lower quality but usable, good for low RAM availability. |
| Q3_K_M | Even lower quality. |
| IQ3_M | Medium-low quality, new method with decent performance comparable to Q3_K_M. |
| IQ3_S | Lower quality, new method with decent performance, recommended over Q3_K_S quant, same size with better performance. |
| Q3_K_S | Low quality, not recommended. |
| IQ3_XS | Lower quality, new method with decent performance, slightly better than Q3_K_S. |
| Q2_K | Very low quality but surprisingly usable. |
## How to download GGUF files ?
**Note for manual downloaders:** You almost never want to clone the entire repo! Multiple different quantisation formats are provided, and most users only want to pick and download a single file.
The following clients/libraries will automatically download models for you, providing a list of available models to choose from:
* LM Studio
* LoLLMS Web UI
* Faraday.dev
- **Option A** - Downloading in `text-generation-webui`:
- **Step 1**: Under Download Model, you can enter the model repo: GreatCaptainNemo-ProLLaMA-GGUF-smashed and below it, a specific filename to download, such as: phi-2.IQ3_M.gguf.
- **Step 2**: Then click Download.
- **Option B** - Downloading on the command line (including multiple files at once):
- **Step 1**: We recommend using the `huggingface-hub` Python library:
```shell
pip3 install huggingface-hub
```
- **Step 2**: Then you can download any individual model file to the current directory, at high speed, with a command like this:
```shell
huggingface-cli download GreatCaptainNemo-ProLLaMA-GGUF-smashed ProLLaMA.IQ3_M.gguf --local-dir . --local-dir-use-symlinks False
```
<details>
<summary>More advanced huggingface-cli download usage (click to read)</summary>
Alternatively, you can also download multiple files at once with a pattern:
```shell
huggingface-cli download GreatCaptainNemo-ProLLaMA-GGUF-smashed --local-dir . --local-dir-use-symlinks False --include='*Q4_K*gguf'
```
For more documentation on downloading with `huggingface-cli`, please see: [HF -> Hub Python Library -> Download files -> Download from the CLI](https://huggingface.co/docs/huggingface_hub/guides/download#download-from-the-cli).
To accelerate downloads on fast connections (1Gbit/s or higher), install `hf_transfer`:
```shell
pip3 install hf_transfer
```
And set environment variable `HF_HUB_ENABLE_HF_TRANSFER` to `1`:
```shell
HF_HUB_ENABLE_HF_TRANSFER=1 huggingface-cli download GreatCaptainNemo-ProLLaMA-GGUF-smashed ProLLaMA.IQ3_M.gguf --local-dir . --local-dir-use-symlinks False
```
Windows Command Line users: You can set the environment variable by running `set HF_HUB_ENABLE_HF_TRANSFER=1` before the download command.
</details>
<!-- README_GGUF.md-how-to-download end -->
<!-- README_GGUF.md-how-to-run start -->
## How to run model in GGUF format?
- **Option A** - Introductory example with `llama.cpp` command
Make sure you are using `llama.cpp` from commit [d0cee0d](https://github.com/ggerganov/llama.cpp/commit/d0cee0d36d5be95a0d9088b674dbb27354107221) or later.
```shell
./main -ngl 35 -m ProLLaMA.IQ3_M.gguf --color -c 32768 --temp 0.7 --repeat_penalty 1.1 -n -1 -p "<s>[INST] {{prompt\}} [/INST]"
```
Change `-ngl 32` to the number of layers to offload to GPU. Remove it if you don't have GPU acceleration.
Change `-c 32768` to the desired sequence length. For extended sequence models - eg 8K, 16K, 32K - the necessary RoPE scaling parameters are read from the GGUF file and set by llama.cpp automatically. Note that longer sequence lengths require much more resources, so you may need to reduce this value.
If you want to have a chat-style conversation, replace the `-p <PROMPT>` argument with `-i -ins`
For other parameters and how to use them, please refer to [the llama.cpp documentation](https://github.com/ggerganov/llama.cpp/blob/master/examples/main/README.md)
- **Option B** - Running in `text-generation-webui`
Further instructions can be found in the text-generation-webui documentation, here: [text-generation-webui/docs/04 ‐ Model Tab.md](https://github.com/oobabooga/text-generation-webui/blob/main/docs/04%20-%20Model%20Tab.md#llamacpp).
- **Option C** - Running from Python code
You can use GGUF models from Python using the [llama-cpp-python](https://github.com/abetlen/llama-cpp-python) or [ctransformers](https://github.com/marella/ctransformers) libraries. Note that at the time of writing (Nov 27th 2023), ctransformers has not been updated for some time and is not compatible with some recent models. Therefore I recommend you use llama-cpp-python.
### How to load this model in Python code, using llama-cpp-python
For full documentation, please see: [llama-cpp-python docs](https://abetlen.github.io/llama-cpp-python/).
#### First install the package
Run one of the following commands, according to your system:
```shell
# Base ctransformers with no GPU acceleration
pip install llama-cpp-python
# With NVidia CUDA acceleration
CMAKE_ARGS="-DLLAMA_CUBLAS=on" pip install llama-cpp-python
# Or with OpenBLAS acceleration
CMAKE_ARGS="-DLLAMA_BLAS=ON -DLLAMA_BLAS_VENDOR=OpenBLAS" pip install llama-cpp-python
# Or with CLBLast acceleration
CMAKE_ARGS="-DLLAMA_CLBLAST=on" pip install llama-cpp-python
# Or with AMD ROCm GPU acceleration (Linux only)
CMAKE_ARGS="-DLLAMA_HIPBLAS=on" pip install llama-cpp-python
# Or with Metal GPU acceleration for macOS systems only
CMAKE_ARGS="-DLLAMA_METAL=on" pip install llama-cpp-python
# In windows, to set the variables CMAKE_ARGS in PowerShell, follow this format; eg for NVidia CUDA:
$env:CMAKE_ARGS = "-DLLAMA_OPENBLAS=on"
pip install llama-cpp-python
```
#### Simple llama-cpp-python example code
```python
from llama_cpp import Llama
# Set gpu_layers to the number of layers to offload to GPU. Set to 0 if no GPU acceleration is available on your system.
llm = Llama(
model_path="./ProLLaMA.IQ3_M.gguf", # Download the model file first
n_ctx=32768, # The max sequence length to use - note that longer sequence lengths require much more resources
n_threads=8, # The number of CPU threads to use, tailor to your system and the resulting performance
n_gpu_layers=35 # The number of layers to offload to GPU, if you have GPU acceleration available
)
# Simple inference example
output = llm(
"<s>[INST] {{prompt}} [/INST]", # Prompt
max_tokens=512, # Generate up to 512 tokens
stop=["</s>"], # Example stop token - not necessarily correct for this specific model! Please check before using.
echo=True # Whether to echo the prompt
)
# Chat Completion API
llm = Llama(model_path="./ProLLaMA.IQ3_M.gguf", chat_format="llama-2") # Set chat_format according to the model you are using
llm.create_chat_completion(
messages = [
{{"role": "system", "content": "You are a story writing assistant."}},
{{
"role": "user",
"content": "Write a story about llamas."
}}
]
)
```
- **Option D** - Running with LangChain
Here are guides on using llama-cpp-python and ctransformers with LangChain:
* [LangChain + llama-cpp-python](https://python.langchain.com/docs/integrations/llms/llamacpp)
* [LangChain + ctransformers](https://python.langchain.com/docs/integrations/providers/ctransformers)
## Configurations
The configuration info are in `smash_config.json`.
## Credits & License
The license of the smashed model follows the license of the original model. Please check the license of the original model before using this model which provided the base model. The license of the `pruna-engine` is [here](https://pypi.org/project/pruna-engine/) on Pypi.
## Want to compress other models?
- Contact us and tell us which model to compress next [here](https://www.pruna.ai/contact).
- Request access to easily compress your own AI models [here](https://z0halsaff74.typeform.com/pruna-access?typeform-source=www.pruna.ai). |
bartowski/Phi-3-medium-4k-instruct-GGUF | bartowski | "2024-05-21T20:06:20Z" | 14,313 | 31 | null | [
"gguf",
"nlp",
"code",
"text-generation",
"multilingual",
"license:mit",
"region:us"
] | text-generation | "2024-05-21T19:31:02Z" | ---
license: mit
license_link: https://huggingface.co/microsoft/Phi-3-medium-4k-instruct/resolve/main/LICENSE
language:
- multilingual
pipeline_tag: text-generation
tags:
- nlp
- code
inference:
parameters:
temperature: 0.7
widget:
- messages:
- role: user
content: Can you provide ways to eat combinations of bananas and dragonfruits?
quantized_by: bartowski
---
## Llamacpp imatrix Quantizations of Phi-3-medium-4k-instruct
Using <a href="https://github.com/ggerganov/llama.cpp/">llama.cpp</a> pull request <a href="https://github.com/ggerganov/llama.cpp/pull/7225">7225</a> for quantization.
Original model: https://huggingface.co/microsoft/Phi-3-medium-4k-instruct
All quants made using imatrix option with dataset from [here](https://gist.github.com/bartowski1182/b6ac44691e994344625687afe3263b3a)
## Prompt format
```
<|user|> {prompt}<|end|><|assistant|><|end|>
```
## Download a file (not the whole branch) from below:
| Filename | Quant type | File Size | Description |
| -------- | ---------- | --------- | ----------- |
| [Phi-3-medium-4k-instruct-Q8_0.gguf](https://huggingface.co/bartowski/Phi-3-medium-4k-instruct-GGUF/blob/main/Phi-3-medium-4k-instruct-Q8_0.gguf) | Q8_0 | 14.83GB | Extremely high quality, generally unneeded but max available quant. |
| [Phi-3-medium-4k-instruct-Q6_K.gguf](https://huggingface.co/bartowski/Phi-3-medium-4k-instruct-GGUF/blob/main/Phi-3-medium-4k-instruct-Q6_K.gguf) | Q6_K | 11.45GB | Very high quality, near perfect, *recommended*. |
| [Phi-3-medium-4k-instruct-Q5_K_M.gguf](https://huggingface.co/bartowski/Phi-3-medium-4k-instruct-GGUF/blob/main/Phi-3-medium-4k-instruct-Q5_K_M.gguf) | Q5_K_M | 10.07GB | High quality, *recommended*. |
| [Phi-3-medium-4k-instruct-Q5_K_S.gguf](https://huggingface.co/bartowski/Phi-3-medium-4k-instruct-GGUF/blob/main/Phi-3-medium-4k-instruct-Q5_K_S.gguf) | Q5_K_S | 9.62GB | High quality, *recommended*. |
| [Phi-3-medium-4k-instruct-Q4_K_M.gguf](https://huggingface.co/bartowski/Phi-3-medium-4k-instruct-GGUF/blob/main/Phi-3-medium-4k-instruct-Q4_K_M.gguf) | Q4_K_M | 8.56GB | Good quality, uses about 4.83 bits per weight, *recommended*. |
| [Phi-3-medium-4k-instruct-Q4_K_S.gguf](https://huggingface.co/bartowski/Phi-3-medium-4k-instruct-GGUF/blob/main/Phi-3-medium-4k-instruct-Q4_K_S.gguf) | Q4_K_S | 7.95GB | Slightly lower quality with more space savings, *recommended*. |
| [Phi-3-medium-4k-instruct-IQ4_NL.gguf](https://huggingface.co/bartowski/Phi-3-medium-4k-instruct-GGUF/blob/main/Phi-3-medium-4k-instruct-IQ4_NL.gguf) | IQ4_NL | 7.89GB | Decent quality, slightly smaller than Q4_K_S with similar performance *recommended*. |
| [Phi-3-medium-4k-instruct-IQ4_XS.gguf](https://huggingface.co/bartowski/Phi-3-medium-4k-instruct-GGUF/blob/main/Phi-3-medium-4k-instruct-IQ4_XS.gguf) | IQ4_XS | 7.46GB | Decent quality, smaller than Q4_K_S with similar performance, *recommended*. |
| [Phi-3-medium-4k-instruct-Q3_K_L.gguf](https://huggingface.co/bartowski/Phi-3-medium-4k-instruct-GGUF/blob/main/Phi-3-medium-4k-instruct-Q3_K_L.gguf) | Q3_K_L | 7.49GB | Lower quality but usable, good for low RAM availability. |
| [Phi-3-medium-4k-instruct-Q3_K_M.gguf](https://huggingface.co/bartowski/Phi-3-medium-4k-instruct-GGUF/blob/main/Phi-3-medium-4k-instruct-Q3_K_M.gguf) | Q3_K_M | 6.92GB | Even lower quality. |
| [Phi-3-medium-4k-instruct-IQ3_M.gguf](https://huggingface.co/bartowski/Phi-3-medium-4k-instruct-GGUF/blob/main/Phi-3-medium-4k-instruct-IQ3_M.gguf) | IQ3_M | 6.47GB | Medium-low quality, new method with decent performance comparable to Q3_K_M. |
| [Phi-3-medium-4k-instruct-IQ3_S.gguf](https://huggingface.co/bartowski/Phi-3-medium-4k-instruct-GGUF/blob/main/Phi-3-medium-4k-instruct-IQ3_S.gguf) | IQ3_S | 6.06GB | Lower quality, new method with decent performance, recommended over Q3_K_S quant, same size with better performance. |
| [Phi-3-medium-4k-instruct-Q3_K_S.gguf](https://huggingface.co/bartowski/Phi-3-medium-4k-instruct-GGUF/blob/main/Phi-3-medium-4k-instruct-Q3_K_S.gguf) | Q3_K_S | 6.06GB | Low quality, not recommended. |
| [Phi-3-medium-4k-instruct-IQ3_XS.gguf](https://huggingface.co/bartowski/Phi-3-medium-4k-instruct-GGUF/blob/main/Phi-3-medium-4k-instruct-IQ3_XS.gguf) | IQ3_XS | 5.80GB | Lower quality, new method with decent performance, slightly better than Q3_K_S. |
| [Phi-3-medium-4k-instruct-IQ3_XXS.gguf](https://huggingface.co/bartowski/Phi-3-medium-4k-instruct-GGUF/blob/main/Phi-3-medium-4k-instruct-IQ3_XXS.gguf) | IQ3_XXS | 5.45GB | Lower quality, new method with decent performance, comparable to Q3 quants. |
| [Phi-3-medium-4k-instruct-Q2_K.gguf](https://huggingface.co/bartowski/Phi-3-medium-4k-instruct-GGUF/blob/main/Phi-3-medium-4k-instruct-Q2_K.gguf) | Q2_K | 5.14GB | Very low quality but surprisingly usable. |
| [Phi-3-medium-4k-instruct-IQ2_M.gguf](https://huggingface.co/bartowski/Phi-3-medium-4k-instruct-GGUF/blob/main/Phi-3-medium-4k-instruct-IQ2_M.gguf) | IQ2_M | 4.71GB | Very low quality, uses SOTA techniques to also be surprisingly usable. |
| [Phi-3-medium-4k-instruct-IQ2_S.gguf](https://huggingface.co/bartowski/Phi-3-medium-4k-instruct-GGUF/blob/main/Phi-3-medium-4k-instruct-IQ2_S.gguf) | IQ2_S | 4.33GB | Very low quality, uses SOTA techniques to be usable. |
| [Phi-3-medium-4k-instruct-IQ2_XS.gguf](https://huggingface.co/bartowski/Phi-3-medium-4k-instruct-GGUF/blob/main/Phi-3-medium-4k-instruct-IQ2_XS.gguf) | IQ2_XS | 4.12GB | Very low quality, uses SOTA techniques to be usable. |
| [Phi-3-medium-4k-instruct-IQ2_XXS.gguf](https://huggingface.co/bartowski/Phi-3-medium-4k-instruct-GGUF/blob/main/Phi-3-medium-4k-instruct-IQ2_XXS.gguf) | IQ2_XXS | 3.71GB | Lower quality, uses SOTA techniques to be usable. |
| [Phi-3-medium-4k-instruct-IQ1_M.gguf](https://huggingface.co/bartowski/Phi-3-medium-4k-instruct-GGUF/blob/main/Phi-3-medium-4k-instruct-IQ1_M.gguf) | IQ1_M | 3.24GB | Extremely low quality, *not* recommended. |
| [Phi-3-medium-4k-instruct-IQ1_S.gguf](https://huggingface.co/bartowski/Phi-3-medium-4k-instruct-GGUF/blob/main/Phi-3-medium-4k-instruct-IQ1_S.gguf) | IQ1_S | 2.95GB | Extremely low quality, *not* recommended. |
## Downloading using huggingface-cli
First, make sure you have hugginface-cli installed:
```
pip install -U "huggingface_hub[cli]"
```
Then, you can target the specific file you want:
```
huggingface-cli download bartowski/Phi-3-medium-4k-instruct-GGUF --include "Phi-3-medium-4k-instruct-Q4_K_M.gguf" --local-dir ./
```
If the model is bigger than 50GB, it will have been split into multiple files. In order to download them all to a local folder, run:
```
huggingface-cli download bartowski/Phi-3-medium-4k-instruct-GGUF --include "Phi-3-medium-4k-instruct-Q8_0.gguf/*" --local-dir Phi-3-medium-4k-instruct-Q8_0
```
You can either specify a new local-dir (Phi-3-medium-4k-instruct-Q8_0) or download them all in place (./)
## Which file should I choose?
A great write up with charts showing various performances is provided by Artefact2 [here](https://gist.github.com/Artefact2/b5f810600771265fc1e39442288e8ec9)
The first thing to figure out is how big a model you can run. To do this, you'll need to figure out how much RAM and/or VRAM you have.
If you want your model running as FAST as possible, you'll want to fit the whole thing on your GPU's VRAM. Aim for a quant with a file size 1-2GB smaller than your GPU's total VRAM.
If you want the absolute maximum quality, add both your system RAM and your GPU's VRAM together, then similarly grab a quant with a file size 1-2GB Smaller than that total.
Next, you'll need to decide if you want to use an 'I-quant' or a 'K-quant'.
If you don't want to think too much, grab one of the K-quants. These are in format 'QX_K_X', like Q5_K_M.
If you want to get more into the weeds, you can check out this extremely useful feature chart:
[llama.cpp feature matrix](https://github.com/ggerganov/llama.cpp/wiki/Feature-matrix)
But basically, if you're aiming for below Q4, and you're running cuBLAS (Nvidia) or rocBLAS (AMD), you should look towards the I-quants. These are in format IQX_X, like IQ3_M. These are newer and offer better performance for their size.
These I-quants can also be used on CPU and Apple Metal, but will be slower than their K-quant equivalent, so speed vs performance is a tradeoff you'll have to decide.
The I-quants are *not* compatible with Vulcan, which is also AMD, so if you have an AMD card double check if you're using the rocBLAS build or the Vulcan build. At the time of writing this, LM Studio has a preview with ROCm support, and other inference engines have specific builds for ROCm.
Want to support my work? Visit my ko-fi page here: https://ko-fi.com/bartowski
|
fxmarty/sam-vit-tiny-random | fxmarty | "2023-04-20T12:42:14Z" | 14,304 | 1 | transformers | [
"transformers",
"pytorch",
"sam",
"mask-generation",
"license:mit",
"endpoints_compatible",
"region:us"
] | mask-generation | "2023-04-20T12:32:15Z" | ---
license: mit
---
|
bullerwins/L3-Aethora-15B-V2-GGUF | bullerwins | "2024-06-27T08:19:58Z" | 14,298 | 3 | transformers | [
"transformers",
"gguf",
"en",
"dataset:TheSkullery/Aether-Lite-v1.8.1",
"base_model:elinas/Llama-3-15B-Instruct-zeroed",
"license:cc-by-sa-4.0",
"endpoints_compatible",
"region:us"
] | null | "2024-06-27T06:21:17Z" | ---
license: cc-by-sa-4.0
datasets:
- TheSkullery/Aether-Lite-v1.8.1
language:
- en
base_model:
- elinas/Llama-3-15B-Instruct-zeroed
library_name: transformers
---
Quantized version using [llama.cpp ac14662](https://github.com/ggerganov/llama.cpp/commit/ac146628e47451c531a3c7e62e6a973a2bb467a0)
Original model [ZeusLabs/L3-Aethora-15B-V2](https://huggingface.co/ZeusLabs/L3-Aethora-15B-V2)
<!DOCTYPE html>
<html lang="en">
<head>
<meta charset="UTF-8">
<meta name="viewport" content="width=device-width, initial-scale=1.0">
<title>L3-Aethora-15B v2 Data Card</title>
<link href="https://fonts.googleapis.com/css2?family=Quicksand:wght@400;500;600&display=swap" rel="stylesheet">
<style>
body, html {
height: 100%;
margin: 0;
padding: 0;
font-family: 'Quicksand', sans-serif;
background: linear-gradient(135deg, #0a1128 0%, #1c2541 100%);
color: #e0e1dd;
font-size: 16px;
}
.container {
width: 100%;
height: 100%;
padding: 20px;
margin: 0;
background-color: rgba(255, 255, 255, 0.05);
border-radius: 12px;
box-shadow: 0 4px 10px rgba(0, 0, 0, 0.3);
backdrop-filter: blur(10px);
border: 1px solid rgba(255, 255, 255, 0.1);
}
.header h1 {
font-size: 28px;
color: #4cc9f0;
margin: 0 0 20px 0;
text-shadow: 2px 2px 4px rgba(0, 0, 0, 0.3);
}
.update-section h2 {
font-size: 24px;
color: #7209b7;
}
.update-section p {
font-size: 16px;
line-height: 1.6;
color: #e0e1dd;
}
.info img {
width: 100%;
border-radius: 10px;
margin-bottom: 15px;
}
a {
color: #4cc9f0;
text-decoration: none;
}
a:hover {
color: #f72585;
}
.button {
display: inline-block;
background-color: #3a0ca3;
color: #e0e1dd;
padding: 10px 20px;
border-radius: 5px;
cursor: pointer;
text-decoration: none;
}
.button:hover {
background-color: #7209b7;
}
pre {
background-color: #1c2541;
padding: 10px;
border-radius: 5px;
overflow-x: auto;
}
code {
font-family: 'Courier New', monospace;
color: #e0e1dd;
}
</style>
</head>
<body>
<div class="container">
<div class="header">
<h1>L3-Aethora-15B v2</h1>
</div>
<div class="info">
<img src="https://cdn-uploads.huggingface.co/production/uploads/64545af5ec40bbbd01242ca6/yJpwVd5UTnAVDoEPVVCS1.png">
<h2>Presented by:</h2>
<p><strong>Creators: <a href="https://huggingface.co/ZeusLabs" target="_blank"> ZeusLabs</a> </p></strong>
<ul>
<li><a href="https://huggingface.co/steelskull" target="_blank">Steelskull</a></p></li>
<li><a href="https://huggingface.co/elinas" target="_blank">Elinas</a></p></li>
</ul>
<p><strong>Dataset:</strong> <a href="https://huggingface.co/datasets/TheSkullery/Aether-Lite-V1.8.1" target="_blank">Theskullery/Aether-Lite-V1.8.1</a></p>
<p><strong>Trained:</strong> 4 x A100 for 17.5 hours on 125k samples</p>
<p><strong>Sponsored by:</strong> Garg (@g4rg)</p>
<h2>About L3-Aethora-15B v2:</h2>
<pre><code> L3 = Llama3 </code></pre>
<p>L3-Aethora-15B v2 is an advanced language model built upon the Llama 3 architecture. It employs state-of-the-art training techniques and a curated dataset to deliver enhanced performance across a wide range of tasks.</p>
<h4>Quants:</h4>
<ul>
<li>@Mradermacher: <a href="https://huggingface.co/mradermacher/L3-Aethora-15B-V2-GGUF" target="_blank">L3-Aethora-15B-V2-GGUF</a></li>
</ul>
<h2>Training Process:</h2>
<ul>
<li>Base Model: elinas/Llama-3-15B-Instruct-zeroed</li>
<li>Training Duration: 17.5 hours on 4 x A100 GPUs</li>
<li>Training Method: LoRA (Low-Rank Adaptation)</li>
<li>Epochs: 4</li>
<li>Precision: BF16</li>
<li>Sequence Length: 8192 tokens</li>
</ul>
<h2>Model Capabilities:</h2>
<p>The goal of L3-Aethora-15B v2 is to have an expanded proficiency across a wide spectrum of tasks with a focus in creative writing:</p>
<ul>
<li><strong>Creative Writing and Storytelling:</strong>
<ul>
<li>Generates engaging narratives, poetry, and creative content</li>
<li>Adapts writing style to various genres and tones</li>
<li>Assists in plot development and character creation</li>
</ul>
</li>
<li><strong>General Intelligence:</strong>
<ul>
<li>Engages in detailed discussions on medical topics and scientific concepts</li>
<li>Explains complex scientific phenomena</li>
<li>Assists in literature review and hypothesis generation</li>
</ul>
</li>
<li><strong>Instructional and Educational Content:</strong>
<ul>
<li>Creates comprehensive tutorials and how-to guides</li>
<li>Explains complex topics with clarity and appropriate depth</li>
<li>Generates educational materials for various skill levels</li>
</ul>
</li>
<li><strong>Reasoning and Problem-Solving:</strong>
<ul>
<li>Analyzes complex scenarios and provides logical solutions</li>
<li>Engages in step-by-step problem-solving across various domains</li>
<li>Offers multiple perspectives on challenging issues</li>
</ul>
</li>
<li><strong>Contextual Understanding and Adaptability:</strong>
<ul>
<li>Maintains coherent, context-aware conversations across extended interactions</li>
<li>Adapts communication style based on the user's preferences and needs</li>
<li>Handles nuanced queries with appropriate depth and sensitivity</li>
</ul>
</ul>
<h2>Dataset Creation Process:</h2>
<p>The Aether-Lite-V1.8.1 dataset used for training L3-Aethora-15B v2 underwent a rigorous creation and curation process:</p>
<ol>
<li><strong>Data Collection:</strong> Aggregated from 12 diverse high-quality datasets, including:
<ul>
<li>jondurbin/airoboros-3.2</li>
<li>jtatman/medical-sci-instruct-100k-sharegpt</li>
<li>Doctor-Shotgun/no-robots-sharegpt</li>
<li>QuietImpostor/Sao10K-Claude-3-Opus-Instruct-15K-ShareGPT</li>
<li>TheSkullery/WizardLM_evol_instruct_v2_Filtered_Fuzzy_Dedup_ShareGPT</li>
<li>TheSkullery/Gryphe-Opus-WritingPrompts-merged</li>
<li>Alignment-Lab-AI/RPGuild-sharegpt-filtered</li>
<li>And others, providing a rich mix of instruction, creative writing, and specialized knowledge</li>
</ul>
</li>
<li><strong>Data Preprocessing:</strong>
<ul>
<li>Language Detection: Utilized a FastText language model to ensure English-language content</li>
<li>Text Sanitization: Cleaned and normalized text, removing or replacing problematic characters</li>
<li>Phrase Filtering: Removed specific unwanted phrases and content types</li>
</ul>
</li>
<li><strong>Deduplication:</strong>
<ul>
<li>Implemented advanced fuzzy deduplication with a 95% similarity threshold</li>
<li>Utilized text embeddings and cosine similarity calculations for efficient comparison</li>
<li>Removed 16,250 duplicate entries, ensuring dataset uniqueness</li>
</ul>
</li>
<li><strong>Data Balancing:</strong>
<ul>
<li>Carefully sampled from each source dataset to maintain diversity</li>
<li>Implemented data shuffling to ensure random distribution of samples</li>
</ul>
</ol>
<p>The final dataset comprises 125,119 high-quality, diverse samples, striking a balance between creativity, practical knowledge, and intellectual depth.</p>
<p>The full dataset used has been released to the public and is avalible for all (see presented section), any ideas or recomendations are always welcome to expand on the dataset further</p>
</div>
</div>
</body>
</html> |
mradermacher/llama-3-Nephilim-v2-8B-i1-GGUF | mradermacher | "2024-06-30T22:24:04Z" | 14,286 | 0 | transformers | [
"transformers",
"gguf",
"mergekit",
"merge",
"en",
"base_model:grimjim/llama-3-Nephilim-v2-8B",
"license:cc-by-nc-4.0",
"endpoints_compatible",
"region:us"
] | null | "2024-06-30T20:07:51Z" | ---
base_model: grimjim/llama-3-Nephilim-v2-8B
language:
- en
library_name: transformers
license: cc-by-nc-4.0
quantized_by: mradermacher
tags:
- mergekit
- merge
---
## About
<!-- ### quantize_version: 2 -->
<!-- ### output_tensor_quantised: 1 -->
<!-- ### convert_type: hf -->
<!-- ### vocab_type: -->
<!-- ### tags: nicoboss -->
weighted/imatrix quants of https://huggingface.co/grimjim/llama-3-Nephilim-v2-8B
<!-- provided-files -->
static quants are available at https://huggingface.co/mradermacher/llama-3-Nephilim-v2-8B-GGUF
## Usage
If you are unsure how to use GGUF files, refer to one of [TheBloke's
READMEs](https://huggingface.co/TheBloke/KafkaLM-70B-German-V0.1-GGUF) for
more details, including on how to concatenate multi-part files.
## Provided Quants
(sorted by size, not necessarily quality. IQ-quants are often preferable over similar sized non-IQ quants)
| Link | Type | Size/GB | Notes |
|:-----|:-----|--------:|:------|
| [GGUF](https://huggingface.co/mradermacher/llama-3-Nephilim-v2-8B-i1-GGUF/resolve/main/llama-3-Nephilim-v2-8B.i1-IQ1_S.gguf) | i1-IQ1_S | 2.1 | for the desperate |
| [GGUF](https://huggingface.co/mradermacher/llama-3-Nephilim-v2-8B-i1-GGUF/resolve/main/llama-3-Nephilim-v2-8B.i1-IQ1_M.gguf) | i1-IQ1_M | 2.3 | mostly desperate |
| [GGUF](https://huggingface.co/mradermacher/llama-3-Nephilim-v2-8B-i1-GGUF/resolve/main/llama-3-Nephilim-v2-8B.i1-IQ2_XXS.gguf) | i1-IQ2_XXS | 2.5 | |
| [GGUF](https://huggingface.co/mradermacher/llama-3-Nephilim-v2-8B-i1-GGUF/resolve/main/llama-3-Nephilim-v2-8B.i1-IQ2_XS.gguf) | i1-IQ2_XS | 2.7 | |
| [GGUF](https://huggingface.co/mradermacher/llama-3-Nephilim-v2-8B-i1-GGUF/resolve/main/llama-3-Nephilim-v2-8B.i1-IQ2_S.gguf) | i1-IQ2_S | 2.9 | |
| [GGUF](https://huggingface.co/mradermacher/llama-3-Nephilim-v2-8B-i1-GGUF/resolve/main/llama-3-Nephilim-v2-8B.i1-IQ2_M.gguf) | i1-IQ2_M | 3.0 | |
| [GGUF](https://huggingface.co/mradermacher/llama-3-Nephilim-v2-8B-i1-GGUF/resolve/main/llama-3-Nephilim-v2-8B.i1-Q2_K.gguf) | i1-Q2_K | 3.3 | IQ3_XXS probably better |
| [GGUF](https://huggingface.co/mradermacher/llama-3-Nephilim-v2-8B-i1-GGUF/resolve/main/llama-3-Nephilim-v2-8B.i1-IQ3_XXS.gguf) | i1-IQ3_XXS | 3.4 | lower quality |
| [GGUF](https://huggingface.co/mradermacher/llama-3-Nephilim-v2-8B-i1-GGUF/resolve/main/llama-3-Nephilim-v2-8B.i1-IQ3_XS.gguf) | i1-IQ3_XS | 3.6 | |
| [GGUF](https://huggingface.co/mradermacher/llama-3-Nephilim-v2-8B-i1-GGUF/resolve/main/llama-3-Nephilim-v2-8B.i1-Q3_K_S.gguf) | i1-Q3_K_S | 3.8 | IQ3_XS probably better |
| [GGUF](https://huggingface.co/mradermacher/llama-3-Nephilim-v2-8B-i1-GGUF/resolve/main/llama-3-Nephilim-v2-8B.i1-IQ3_S.gguf) | i1-IQ3_S | 3.8 | beats Q3_K* |
| [GGUF](https://huggingface.co/mradermacher/llama-3-Nephilim-v2-8B-i1-GGUF/resolve/main/llama-3-Nephilim-v2-8B.i1-IQ3_M.gguf) | i1-IQ3_M | 3.9 | |
| [GGUF](https://huggingface.co/mradermacher/llama-3-Nephilim-v2-8B-i1-GGUF/resolve/main/llama-3-Nephilim-v2-8B.i1-Q3_K_M.gguf) | i1-Q3_K_M | 4.1 | IQ3_S probably better |
| [GGUF](https://huggingface.co/mradermacher/llama-3-Nephilim-v2-8B-i1-GGUF/resolve/main/llama-3-Nephilim-v2-8B.i1-Q3_K_L.gguf) | i1-Q3_K_L | 4.4 | IQ3_M probably better |
| [GGUF](https://huggingface.co/mradermacher/llama-3-Nephilim-v2-8B-i1-GGUF/resolve/main/llama-3-Nephilim-v2-8B.i1-IQ4_XS.gguf) | i1-IQ4_XS | 4.5 | |
| [GGUF](https://huggingface.co/mradermacher/llama-3-Nephilim-v2-8B-i1-GGUF/resolve/main/llama-3-Nephilim-v2-8B.i1-Q4_0.gguf) | i1-Q4_0 | 4.8 | fast, low quality |
| [GGUF](https://huggingface.co/mradermacher/llama-3-Nephilim-v2-8B-i1-GGUF/resolve/main/llama-3-Nephilim-v2-8B.i1-Q4_K_S.gguf) | i1-Q4_K_S | 4.8 | optimal size/speed/quality |
| [GGUF](https://huggingface.co/mradermacher/llama-3-Nephilim-v2-8B-i1-GGUF/resolve/main/llama-3-Nephilim-v2-8B.i1-Q4_K_M.gguf) | i1-Q4_K_M | 5.0 | fast, recommended |
| [GGUF](https://huggingface.co/mradermacher/llama-3-Nephilim-v2-8B-i1-GGUF/resolve/main/llama-3-Nephilim-v2-8B.i1-Q5_K_S.gguf) | i1-Q5_K_S | 5.7 | |
| [GGUF](https://huggingface.co/mradermacher/llama-3-Nephilim-v2-8B-i1-GGUF/resolve/main/llama-3-Nephilim-v2-8B.i1-Q5_K_M.gguf) | i1-Q5_K_M | 5.8 | |
| [GGUF](https://huggingface.co/mradermacher/llama-3-Nephilim-v2-8B-i1-GGUF/resolve/main/llama-3-Nephilim-v2-8B.i1-Q6_K.gguf) | i1-Q6_K | 6.7 | practically like static Q6_K |
Here is a handy graph by ikawrakow comparing some lower-quality quant
types (lower is better):

And here are Artefact2's thoughts on the matter:
https://gist.github.com/Artefact2/b5f810600771265fc1e39442288e8ec9
## FAQ / Model Request
See https://huggingface.co/mradermacher/model_requests for some answers to
questions you might have and/or if you want some other model quantized.
## Thanks
I thank my company, [nethype GmbH](https://www.nethype.de/), for letting
me use its servers and providing upgrades to my workstation to enable
this work in my free time. Additional thanks to [@nicoboss](https://huggingface.co/nicoboss) for giving me access to his hardware for calculating the imatrix for these quants.
<!-- end -->
|
Corentinrhr/FisaGPT | Corentinrhr | "2024-06-27T08:21:32Z" | 14,284 | 0 | transformers | [
"transformers",
"gguf",
"llama",
"endpoints_compatible",
"text-generation-inference",
"region:us"
] | null | "2024-06-25T22:21:03Z" | As part of a school IT project, we fine-tuned Llama 3 with a dataset based on data from our FISA course at Telecom Sud Paris.
---
base_model: jpacifico/French-Alpaca-Llama3-8B-Instruct-v1.0
language:
- en
license: apache-2.0
tags:
- text-generation-inference
- transformers
- unsloth
- llama
- gguf
---
# Uploaded model
- **Developed by:** Corentinrhr
- **License:** apache-2.0
- **Finetuned from model :** jpacifico/French-Alpaca-Llama3-8B-Instruct-v1.0
This llama model was trained 2x faster with [Unsloth](https://github.com/unslothai/unsloth) and Huggingface's TRL library.
[<img src="https://raw.githubusercontent.com/unslothai/unsloth/main/images/unsloth%20made%20with%20love.png" width="200"/>](https://github.com/unslothai/unsloth)
|
RichardErkhov/johnsnowlabs_-_BioLing-7B-Dare-gguf | RichardErkhov | "2024-06-25T21:27:42Z" | 14,283 | 0 | null | [
"gguf",
"region:us"
] | null | "2024-06-25T17:05:10Z" | Quantization made by Richard Erkhov.
[Github](https://github.com/RichardErkhov)
[Discord](https://discord.gg/pvy7H8DZMG)
[Request more models](https://github.com/RichardErkhov/quant_request)
BioLing-7B-Dare - GGUF
- Model creator: https://huggingface.co/johnsnowlabs/
- Original model: https://huggingface.co/johnsnowlabs/BioLing-7B-Dare/
| Name | Quant method | Size |
| ---- | ---- | ---- |
| [BioLing-7B-Dare.Q2_K.gguf](https://huggingface.co/RichardErkhov/johnsnowlabs_-_BioLing-7B-Dare-gguf/blob/main/BioLing-7B-Dare.Q2_K.gguf) | Q2_K | 2.53GB |
| [BioLing-7B-Dare.IQ3_XS.gguf](https://huggingface.co/RichardErkhov/johnsnowlabs_-_BioLing-7B-Dare-gguf/blob/main/BioLing-7B-Dare.IQ3_XS.gguf) | IQ3_XS | 2.81GB |
| [BioLing-7B-Dare.IQ3_S.gguf](https://huggingface.co/RichardErkhov/johnsnowlabs_-_BioLing-7B-Dare-gguf/blob/main/BioLing-7B-Dare.IQ3_S.gguf) | IQ3_S | 2.96GB |
| [BioLing-7B-Dare.Q3_K_S.gguf](https://huggingface.co/RichardErkhov/johnsnowlabs_-_BioLing-7B-Dare-gguf/blob/main/BioLing-7B-Dare.Q3_K_S.gguf) | Q3_K_S | 2.95GB |
| [BioLing-7B-Dare.IQ3_M.gguf](https://huggingface.co/RichardErkhov/johnsnowlabs_-_BioLing-7B-Dare-gguf/blob/main/BioLing-7B-Dare.IQ3_M.gguf) | IQ3_M | 3.06GB |
| [BioLing-7B-Dare.Q3_K.gguf](https://huggingface.co/RichardErkhov/johnsnowlabs_-_BioLing-7B-Dare-gguf/blob/main/BioLing-7B-Dare.Q3_K.gguf) | Q3_K | 3.28GB |
| [BioLing-7B-Dare.Q3_K_M.gguf](https://huggingface.co/RichardErkhov/johnsnowlabs_-_BioLing-7B-Dare-gguf/blob/main/BioLing-7B-Dare.Q3_K_M.gguf) | Q3_K_M | 3.28GB |
| [BioLing-7B-Dare.Q3_K_L.gguf](https://huggingface.co/RichardErkhov/johnsnowlabs_-_BioLing-7B-Dare-gguf/blob/main/BioLing-7B-Dare.Q3_K_L.gguf) | Q3_K_L | 3.56GB |
| [BioLing-7B-Dare.IQ4_XS.gguf](https://huggingface.co/RichardErkhov/johnsnowlabs_-_BioLing-7B-Dare-gguf/blob/main/BioLing-7B-Dare.IQ4_XS.gguf) | IQ4_XS | 3.67GB |
| [BioLing-7B-Dare.Q4_0.gguf](https://huggingface.co/RichardErkhov/johnsnowlabs_-_BioLing-7B-Dare-gguf/blob/main/BioLing-7B-Dare.Q4_0.gguf) | Q4_0 | 3.83GB |
| [BioLing-7B-Dare.IQ4_NL.gguf](https://huggingface.co/RichardErkhov/johnsnowlabs_-_BioLing-7B-Dare-gguf/blob/main/BioLing-7B-Dare.IQ4_NL.gguf) | IQ4_NL | 3.87GB |
| [BioLing-7B-Dare.Q4_K_S.gguf](https://huggingface.co/RichardErkhov/johnsnowlabs_-_BioLing-7B-Dare-gguf/blob/main/BioLing-7B-Dare.Q4_K_S.gguf) | Q4_K_S | 3.86GB |
| [BioLing-7B-Dare.Q4_K.gguf](https://huggingface.co/RichardErkhov/johnsnowlabs_-_BioLing-7B-Dare-gguf/blob/main/BioLing-7B-Dare.Q4_K.gguf) | Q4_K | 4.07GB |
| [BioLing-7B-Dare.Q4_K_M.gguf](https://huggingface.co/RichardErkhov/johnsnowlabs_-_BioLing-7B-Dare-gguf/blob/main/BioLing-7B-Dare.Q4_K_M.gguf) | Q4_K_M | 4.07GB |
| [BioLing-7B-Dare.Q4_1.gguf](https://huggingface.co/RichardErkhov/johnsnowlabs_-_BioLing-7B-Dare-gguf/blob/main/BioLing-7B-Dare.Q4_1.gguf) | Q4_1 | 4.24GB |
| [BioLing-7B-Dare.Q5_0.gguf](https://huggingface.co/RichardErkhov/johnsnowlabs_-_BioLing-7B-Dare-gguf/blob/main/BioLing-7B-Dare.Q5_0.gguf) | Q5_0 | 4.65GB |
| [BioLing-7B-Dare.Q5_K_S.gguf](https://huggingface.co/RichardErkhov/johnsnowlabs_-_BioLing-7B-Dare-gguf/blob/main/BioLing-7B-Dare.Q5_K_S.gguf) | Q5_K_S | 4.65GB |
| [BioLing-7B-Dare.Q5_K.gguf](https://huggingface.co/RichardErkhov/johnsnowlabs_-_BioLing-7B-Dare-gguf/blob/main/BioLing-7B-Dare.Q5_K.gguf) | Q5_K | 4.78GB |
| [BioLing-7B-Dare.Q5_K_M.gguf](https://huggingface.co/RichardErkhov/johnsnowlabs_-_BioLing-7B-Dare-gguf/blob/main/BioLing-7B-Dare.Q5_K_M.gguf) | Q5_K_M | 4.78GB |
| [BioLing-7B-Dare.Q5_1.gguf](https://huggingface.co/RichardErkhov/johnsnowlabs_-_BioLing-7B-Dare-gguf/blob/main/BioLing-7B-Dare.Q5_1.gguf) | Q5_1 | 5.07GB |
| [BioLing-7B-Dare.Q6_K.gguf](https://huggingface.co/RichardErkhov/johnsnowlabs_-_BioLing-7B-Dare-gguf/blob/main/BioLing-7B-Dare.Q6_K.gguf) | Q6_K | 5.53GB |
| [BioLing-7B-Dare.Q8_0.gguf](https://huggingface.co/RichardErkhov/johnsnowlabs_-_BioLing-7B-Dare-gguf/blob/main/BioLing-7B-Dare.Q8_0.gguf) | Q8_0 | 7.17GB |
Original model description:
---
tags:
- merge
- mergekit
- lazymergekit
- BioMistral/BioMistral-7B
- Nexusflow/Starling-LM-7B-beta
base_model:
- BioMistral/BioMistral-7B
- Nexusflow/Starling-LM-7B-beta
license: apache-2.0
---
# BioLing-7B-Dare
[<img src="https://repository-images.githubusercontent.com/104670986/2e728700-ace4-11ea-9cfc-f3e060b25ddf">](http://www.johnsnowlabs.com)
This model is developed by [John Snow Labs](https://www.johnsnowlabs.com/).
This model is available under a [CC-BY-NC-ND](https://creativecommons.org/licenses/by-nc-nd/4.0/deed.en) license and must also conform to this [Acceptable Use Policy](https://huggingface.co/johnsnowlabs). If you need to license this model for commercial use, please contact us at [email protected].
## 🧩 Configuration
```yaml
models:
- model: BioMistral/BioMistral-7B
parameters:
density: 0.53
weight: 0.4
- model: Nexusflow/Starling-LM-7B-beta
parameters:
density: 0.53
weight: 0.3
merge_method: dare_ties
base_model: BioMistral/BioMistral-7B
parameters:
int8_mask: true
dtype: bfloat16
```
## 💻 Usage
```python
!pip install -qU transformers accelerate
from transformers import AutoTokenizer
import transformers
import torch
model = "johnsnowlabs/BioLing-7B-Dare"
messages = [{"role": "user", "content": "What is a large language model?"}]
tokenizer = AutoTokenizer.from_pretrained(model)
prompt = tokenizer.apply_chat_template(messages, tokenize=False, add_generation_prompt=True)
pipeline = transformers.pipeline(
"text-generation",
model=model,
torch_dtype=torch.float16,
device_map="auto",
)
outputs = pipeline(prompt, max_new_tokens=256, do_sample=True, temperature=0.7, top_k=50, top_p=0.95)
print(outputs[0]["generated_text"])
```
## 🏆 Evaluation
Coming Soon!
|
martinkozle/MKLLM-7B-Instruct-GGUF | martinkozle | "2024-06-25T00:34:50Z" | 14,275 | 0 | null | [
"gguf",
"axolotl",
"mk",
"en",
"license:cc-by-nc-sa-4.0",
"region:us"
] | null | "2024-06-24T23:40:26Z" | ---
license: cc-by-nc-sa-4.0
language:
- mk
- en
tags:
- axolotl
---
# MKLLM-7B-Instruct-GGUF
GGUF quants of [trajkovnikola/MKLLM-7B-Instruct](https://huggingface.co/trajkovnikola/MKLLM-7B-Instruct)
## Script used
```bash
from_dir=./MKLLM-7B-Instruct
dir=./MKLLM-7B-Instruct-GGUF
base_precision=BF16
file_base=MKLLM-7B-Instruct
quants=("Q2_K" "Q3_K_S" "Q3_K_M" "Q3_K_L" "Q4_K_S" "Q4_K_M" "Q4_0" "Q4_1" "Q5_K_S" "Q5_K_M" "Q5_0" "Q5_1" "Q6_K" "Q8_0" "IQ3_XS" "IQ3_S" "IQ3_M" "IQ4_XS" "IQ4_NL")
docker run --rm -v "${from_dir}":/repo ghcr.io/ggerganov/llama.cpp:full --convert "/repo" --outtype bf16
mkdir "${dir}"
mv "${from_dir}/ggml-model-bf16.gguf" "${dir}/${file_base}-${base_precision}.gguf"
for quant in ${quants[@]};
do
echo "###########################"
echo $quant
echo "==========================="
docker run --rm -v "${dir}":/repo ghcr.io/ggerganov/llama.cpp:full --quantize "/repo/${file_base}-${base_precision}.gguf" "/repo/${file_base}-${quant}.gguf" "${quant}"
done
``` |
RichardErkhov/adamo1139_-_Mistral-7B-AEZAKMI-v1-gguf | RichardErkhov | "2024-06-30T03:32:20Z" | 14,268 | 0 | null | [
"gguf",
"region:us"
] | null | "2024-06-30T01:02:06Z" | Quantization made by Richard Erkhov.
[Github](https://github.com/RichardErkhov)
[Discord](https://discord.gg/pvy7H8DZMG)
[Request more models](https://github.com/RichardErkhov/quant_request)
Mistral-7B-AEZAKMI-v1 - GGUF
- Model creator: https://huggingface.co/adamo1139/
- Original model: https://huggingface.co/adamo1139/Mistral-7B-AEZAKMI-v1/
| Name | Quant method | Size |
| ---- | ---- | ---- |
| [Mistral-7B-AEZAKMI-v1.Q2_K.gguf](https://huggingface.co/RichardErkhov/adamo1139_-_Mistral-7B-AEZAKMI-v1-gguf/blob/main/Mistral-7B-AEZAKMI-v1.Q2_K.gguf) | Q2_K | 2.53GB |
| [Mistral-7B-AEZAKMI-v1.IQ3_XS.gguf](https://huggingface.co/RichardErkhov/adamo1139_-_Mistral-7B-AEZAKMI-v1-gguf/blob/main/Mistral-7B-AEZAKMI-v1.IQ3_XS.gguf) | IQ3_XS | 2.81GB |
| [Mistral-7B-AEZAKMI-v1.IQ3_S.gguf](https://huggingface.co/RichardErkhov/adamo1139_-_Mistral-7B-AEZAKMI-v1-gguf/blob/main/Mistral-7B-AEZAKMI-v1.IQ3_S.gguf) | IQ3_S | 2.96GB |
| [Mistral-7B-AEZAKMI-v1.Q3_K_S.gguf](https://huggingface.co/RichardErkhov/adamo1139_-_Mistral-7B-AEZAKMI-v1-gguf/blob/main/Mistral-7B-AEZAKMI-v1.Q3_K_S.gguf) | Q3_K_S | 2.95GB |
| [Mistral-7B-AEZAKMI-v1.IQ3_M.gguf](https://huggingface.co/RichardErkhov/adamo1139_-_Mistral-7B-AEZAKMI-v1-gguf/blob/main/Mistral-7B-AEZAKMI-v1.IQ3_M.gguf) | IQ3_M | 3.06GB |
| [Mistral-7B-AEZAKMI-v1.Q3_K.gguf](https://huggingface.co/RichardErkhov/adamo1139_-_Mistral-7B-AEZAKMI-v1-gguf/blob/main/Mistral-7B-AEZAKMI-v1.Q3_K.gguf) | Q3_K | 3.28GB |
| [Mistral-7B-AEZAKMI-v1.Q3_K_M.gguf](https://huggingface.co/RichardErkhov/adamo1139_-_Mistral-7B-AEZAKMI-v1-gguf/blob/main/Mistral-7B-AEZAKMI-v1.Q3_K_M.gguf) | Q3_K_M | 3.28GB |
| [Mistral-7B-AEZAKMI-v1.Q3_K_L.gguf](https://huggingface.co/RichardErkhov/adamo1139_-_Mistral-7B-AEZAKMI-v1-gguf/blob/main/Mistral-7B-AEZAKMI-v1.Q3_K_L.gguf) | Q3_K_L | 3.56GB |
| [Mistral-7B-AEZAKMI-v1.IQ4_XS.gguf](https://huggingface.co/RichardErkhov/adamo1139_-_Mistral-7B-AEZAKMI-v1-gguf/blob/main/Mistral-7B-AEZAKMI-v1.IQ4_XS.gguf) | IQ4_XS | 3.67GB |
| [Mistral-7B-AEZAKMI-v1.Q4_0.gguf](https://huggingface.co/RichardErkhov/adamo1139_-_Mistral-7B-AEZAKMI-v1-gguf/blob/main/Mistral-7B-AEZAKMI-v1.Q4_0.gguf) | Q4_0 | 3.83GB |
| [Mistral-7B-AEZAKMI-v1.IQ4_NL.gguf](https://huggingface.co/RichardErkhov/adamo1139_-_Mistral-7B-AEZAKMI-v1-gguf/blob/main/Mistral-7B-AEZAKMI-v1.IQ4_NL.gguf) | IQ4_NL | 3.87GB |
| [Mistral-7B-AEZAKMI-v1.Q4_K_S.gguf](https://huggingface.co/RichardErkhov/adamo1139_-_Mistral-7B-AEZAKMI-v1-gguf/blob/main/Mistral-7B-AEZAKMI-v1.Q4_K_S.gguf) | Q4_K_S | 3.86GB |
| [Mistral-7B-AEZAKMI-v1.Q4_K.gguf](https://huggingface.co/RichardErkhov/adamo1139_-_Mistral-7B-AEZAKMI-v1-gguf/blob/main/Mistral-7B-AEZAKMI-v1.Q4_K.gguf) | Q4_K | 4.07GB |
| [Mistral-7B-AEZAKMI-v1.Q4_K_M.gguf](https://huggingface.co/RichardErkhov/adamo1139_-_Mistral-7B-AEZAKMI-v1-gguf/blob/main/Mistral-7B-AEZAKMI-v1.Q4_K_M.gguf) | Q4_K_M | 4.07GB |
| [Mistral-7B-AEZAKMI-v1.Q4_1.gguf](https://huggingface.co/RichardErkhov/adamo1139_-_Mistral-7B-AEZAKMI-v1-gguf/blob/main/Mistral-7B-AEZAKMI-v1.Q4_1.gguf) | Q4_1 | 4.24GB |
| [Mistral-7B-AEZAKMI-v1.Q5_0.gguf](https://huggingface.co/RichardErkhov/adamo1139_-_Mistral-7B-AEZAKMI-v1-gguf/blob/main/Mistral-7B-AEZAKMI-v1.Q5_0.gguf) | Q5_0 | 4.65GB |
| [Mistral-7B-AEZAKMI-v1.Q5_K_S.gguf](https://huggingface.co/RichardErkhov/adamo1139_-_Mistral-7B-AEZAKMI-v1-gguf/blob/main/Mistral-7B-AEZAKMI-v1.Q5_K_S.gguf) | Q5_K_S | 4.65GB |
| [Mistral-7B-AEZAKMI-v1.Q5_K.gguf](https://huggingface.co/RichardErkhov/adamo1139_-_Mistral-7B-AEZAKMI-v1-gguf/blob/main/Mistral-7B-AEZAKMI-v1.Q5_K.gguf) | Q5_K | 4.78GB |
| [Mistral-7B-AEZAKMI-v1.Q5_K_M.gguf](https://huggingface.co/RichardErkhov/adamo1139_-_Mistral-7B-AEZAKMI-v1-gguf/blob/main/Mistral-7B-AEZAKMI-v1.Q5_K_M.gguf) | Q5_K_M | 4.78GB |
| [Mistral-7B-AEZAKMI-v1.Q5_1.gguf](https://huggingface.co/RichardErkhov/adamo1139_-_Mistral-7B-AEZAKMI-v1-gguf/blob/main/Mistral-7B-AEZAKMI-v1.Q5_1.gguf) | Q5_1 | 5.07GB |
| [Mistral-7B-AEZAKMI-v1.Q6_K.gguf](https://huggingface.co/RichardErkhov/adamo1139_-_Mistral-7B-AEZAKMI-v1-gguf/blob/main/Mistral-7B-AEZAKMI-v1.Q6_K.gguf) | Q6_K | 5.53GB |
| [Mistral-7B-AEZAKMI-v1.Q8_0.gguf](https://huggingface.co/RichardErkhov/adamo1139_-_Mistral-7B-AEZAKMI-v1-gguf/blob/main/Mistral-7B-AEZAKMI-v1.Q8_0.gguf) | Q8_0 | 7.17GB |
Original model description:
---
license: other
license_name: other
license_link: LICENSE
---
Mistral 7B model fine-tuned on AEZAKMI v1 dataset that is derived from airoboros 2.2.1 and airoboros 2.2.
Finetuned with axolotl, using qlora and nf4 double quant, around 2 epochs, batch size 8, lr 0.00008, lr scheduler cosine. Scheduled training was 5 epochs, but loss seemed fine after 2 so I finished it quicker.
Training took around 10 hours on single RTX 3090 Ti.
Main feature of this model is that it's output is free of refusals and it feels somehow more natural.
Prompt format is standard chatml.
Don't expect it to be good at math, riddles or be crazy smart. My end goal with AEZAKMI is to create a cozy free chatbot.
Not sure what license it needs to have, given license of airoboros dataset. I'll leave it as other for now.
|
RichardErkhov/mlabonne_-_NeuralHermes-2.5-Mistral-7B-gguf | RichardErkhov | "2024-06-30T14:40:53Z" | 14,267 | 0 | null | [
"gguf",
"region:us"
] | null | "2024-06-30T11:47:49Z" | Quantization made by Richard Erkhov.
[Github](https://github.com/RichardErkhov)
[Discord](https://discord.gg/pvy7H8DZMG)
[Request more models](https://github.com/RichardErkhov/quant_request)
NeuralHermes-2.5-Mistral-7B - GGUF
- Model creator: https://huggingface.co/mlabonne/
- Original model: https://huggingface.co/mlabonne/NeuralHermes-2.5-Mistral-7B/
| Name | Quant method | Size |
| ---- | ---- | ---- |
| [NeuralHermes-2.5-Mistral-7B.Q2_K.gguf](https://huggingface.co/RichardErkhov/mlabonne_-_NeuralHermes-2.5-Mistral-7B-gguf/blob/main/NeuralHermes-2.5-Mistral-7B.Q2_K.gguf) | Q2_K | 2.53GB |
| [NeuralHermes-2.5-Mistral-7B.IQ3_XS.gguf](https://huggingface.co/RichardErkhov/mlabonne_-_NeuralHermes-2.5-Mistral-7B-gguf/blob/main/NeuralHermes-2.5-Mistral-7B.IQ3_XS.gguf) | IQ3_XS | 2.81GB |
| [NeuralHermes-2.5-Mistral-7B.IQ3_S.gguf](https://huggingface.co/RichardErkhov/mlabonne_-_NeuralHermes-2.5-Mistral-7B-gguf/blob/main/NeuralHermes-2.5-Mistral-7B.IQ3_S.gguf) | IQ3_S | 2.96GB |
| [NeuralHermes-2.5-Mistral-7B.Q3_K_S.gguf](https://huggingface.co/RichardErkhov/mlabonne_-_NeuralHermes-2.5-Mistral-7B-gguf/blob/main/NeuralHermes-2.5-Mistral-7B.Q3_K_S.gguf) | Q3_K_S | 2.95GB |
| [NeuralHermes-2.5-Mistral-7B.IQ3_M.gguf](https://huggingface.co/RichardErkhov/mlabonne_-_NeuralHermes-2.5-Mistral-7B-gguf/blob/main/NeuralHermes-2.5-Mistral-7B.IQ3_M.gguf) | IQ3_M | 3.06GB |
| [NeuralHermes-2.5-Mistral-7B.Q3_K.gguf](https://huggingface.co/RichardErkhov/mlabonne_-_NeuralHermes-2.5-Mistral-7B-gguf/blob/main/NeuralHermes-2.5-Mistral-7B.Q3_K.gguf) | Q3_K | 3.28GB |
| [NeuralHermes-2.5-Mistral-7B.Q3_K_M.gguf](https://huggingface.co/RichardErkhov/mlabonne_-_NeuralHermes-2.5-Mistral-7B-gguf/blob/main/NeuralHermes-2.5-Mistral-7B.Q3_K_M.gguf) | Q3_K_M | 3.28GB |
| [NeuralHermes-2.5-Mistral-7B.Q3_K_L.gguf](https://huggingface.co/RichardErkhov/mlabonne_-_NeuralHermes-2.5-Mistral-7B-gguf/blob/main/NeuralHermes-2.5-Mistral-7B.Q3_K_L.gguf) | Q3_K_L | 3.56GB |
| [NeuralHermes-2.5-Mistral-7B.IQ4_XS.gguf](https://huggingface.co/RichardErkhov/mlabonne_-_NeuralHermes-2.5-Mistral-7B-gguf/blob/main/NeuralHermes-2.5-Mistral-7B.IQ4_XS.gguf) | IQ4_XS | 3.67GB |
| [NeuralHermes-2.5-Mistral-7B.Q4_0.gguf](https://huggingface.co/RichardErkhov/mlabonne_-_NeuralHermes-2.5-Mistral-7B-gguf/blob/main/NeuralHermes-2.5-Mistral-7B.Q4_0.gguf) | Q4_0 | 3.83GB |
| [NeuralHermes-2.5-Mistral-7B.IQ4_NL.gguf](https://huggingface.co/RichardErkhov/mlabonne_-_NeuralHermes-2.5-Mistral-7B-gguf/blob/main/NeuralHermes-2.5-Mistral-7B.IQ4_NL.gguf) | IQ4_NL | 3.87GB |
| [NeuralHermes-2.5-Mistral-7B.Q4_K_S.gguf](https://huggingface.co/RichardErkhov/mlabonne_-_NeuralHermes-2.5-Mistral-7B-gguf/blob/main/NeuralHermes-2.5-Mistral-7B.Q4_K_S.gguf) | Q4_K_S | 3.86GB |
| [NeuralHermes-2.5-Mistral-7B.Q4_K.gguf](https://huggingface.co/RichardErkhov/mlabonne_-_NeuralHermes-2.5-Mistral-7B-gguf/blob/main/NeuralHermes-2.5-Mistral-7B.Q4_K.gguf) | Q4_K | 4.07GB |
| [NeuralHermes-2.5-Mistral-7B.Q4_K_M.gguf](https://huggingface.co/RichardErkhov/mlabonne_-_NeuralHermes-2.5-Mistral-7B-gguf/blob/main/NeuralHermes-2.5-Mistral-7B.Q4_K_M.gguf) | Q4_K_M | 4.07GB |
| [NeuralHermes-2.5-Mistral-7B.Q4_1.gguf](https://huggingface.co/RichardErkhov/mlabonne_-_NeuralHermes-2.5-Mistral-7B-gguf/blob/main/NeuralHermes-2.5-Mistral-7B.Q4_1.gguf) | Q4_1 | 4.24GB |
| [NeuralHermes-2.5-Mistral-7B.Q5_0.gguf](https://huggingface.co/RichardErkhov/mlabonne_-_NeuralHermes-2.5-Mistral-7B-gguf/blob/main/NeuralHermes-2.5-Mistral-7B.Q5_0.gguf) | Q5_0 | 4.65GB |
| [NeuralHermes-2.5-Mistral-7B.Q5_K_S.gguf](https://huggingface.co/RichardErkhov/mlabonne_-_NeuralHermes-2.5-Mistral-7B-gguf/blob/main/NeuralHermes-2.5-Mistral-7B.Q5_K_S.gguf) | Q5_K_S | 4.65GB |
| [NeuralHermes-2.5-Mistral-7B.Q5_K.gguf](https://huggingface.co/RichardErkhov/mlabonne_-_NeuralHermes-2.5-Mistral-7B-gguf/blob/main/NeuralHermes-2.5-Mistral-7B.Q5_K.gguf) | Q5_K | 4.78GB |
| [NeuralHermes-2.5-Mistral-7B.Q5_K_M.gguf](https://huggingface.co/RichardErkhov/mlabonne_-_NeuralHermes-2.5-Mistral-7B-gguf/blob/main/NeuralHermes-2.5-Mistral-7B.Q5_K_M.gguf) | Q5_K_M | 4.78GB |
| [NeuralHermes-2.5-Mistral-7B.Q5_1.gguf](https://huggingface.co/RichardErkhov/mlabonne_-_NeuralHermes-2.5-Mistral-7B-gguf/blob/main/NeuralHermes-2.5-Mistral-7B.Q5_1.gguf) | Q5_1 | 5.07GB |
| [NeuralHermes-2.5-Mistral-7B.Q6_K.gguf](https://huggingface.co/RichardErkhov/mlabonne_-_NeuralHermes-2.5-Mistral-7B-gguf/blob/main/NeuralHermes-2.5-Mistral-7B.Q6_K.gguf) | Q6_K | 5.53GB |
| [NeuralHermes-2.5-Mistral-7B.Q8_0.gguf](https://huggingface.co/RichardErkhov/mlabonne_-_NeuralHermes-2.5-Mistral-7B-gguf/blob/main/NeuralHermes-2.5-Mistral-7B.Q8_0.gguf) | Q8_0 | 7.17GB |
Original model description:
---
language:
- en
license: apache-2.0
tags:
- mistral
- instruct
- finetune
- chatml
- gpt4
- synthetic data
- distillation
- dpo
- rlhf
datasets:
- mlabonne/chatml_dpo_pairs
base_model: teknium/OpenHermes-2.5-Mistral-7B
model-index:
- name: NeuralHermes-2.5-Mistral-7B
results:
- task:
type: text-generation
name: Text Generation
dataset:
name: AI2 Reasoning Challenge (25-Shot)
type: ai2_arc
config: ARC-Challenge
split: test
args:
num_few_shot: 25
metrics:
- type: acc_norm
value: 66.55
name: normalized accuracy
source:
url: https://huggingface.co/spaces/HuggingFaceH4/open_llm_leaderboard?query=mlabonne/NeuralHermes-2.5-Mistral-7B
name: Open LLM Leaderboard
- task:
type: text-generation
name: Text Generation
dataset:
name: HellaSwag (10-Shot)
type: hellaswag
split: validation
args:
num_few_shot: 10
metrics:
- type: acc_norm
value: 84.9
name: normalized accuracy
source:
url: https://huggingface.co/spaces/HuggingFaceH4/open_llm_leaderboard?query=mlabonne/NeuralHermes-2.5-Mistral-7B
name: Open LLM Leaderboard
- task:
type: text-generation
name: Text Generation
dataset:
name: MMLU (5-Shot)
type: cais/mmlu
config: all
split: test
args:
num_few_shot: 5
metrics:
- type: acc
value: 63.32
name: accuracy
source:
url: https://huggingface.co/spaces/HuggingFaceH4/open_llm_leaderboard?query=mlabonne/NeuralHermes-2.5-Mistral-7B
name: Open LLM Leaderboard
- task:
type: text-generation
name: Text Generation
dataset:
name: TruthfulQA (0-shot)
type: truthful_qa
config: multiple_choice
split: validation
args:
num_few_shot: 0
metrics:
- type: mc2
value: 54.93
source:
url: https://huggingface.co/spaces/HuggingFaceH4/open_llm_leaderboard?query=mlabonne/NeuralHermes-2.5-Mistral-7B
name: Open LLM Leaderboard
- task:
type: text-generation
name: Text Generation
dataset:
name: Winogrande (5-shot)
type: winogrande
config: winogrande_xl
split: validation
args:
num_few_shot: 5
metrics:
- type: acc
value: 78.3
name: accuracy
source:
url: https://huggingface.co/spaces/HuggingFaceH4/open_llm_leaderboard?query=mlabonne/NeuralHermes-2.5-Mistral-7B
name: Open LLM Leaderboard
- task:
type: text-generation
name: Text Generation
dataset:
name: GSM8k (5-shot)
type: gsm8k
config: main
split: test
args:
num_few_shot: 5
metrics:
- type: acc
value: 61.33
name: accuracy
source:
url: https://huggingface.co/spaces/HuggingFaceH4/open_llm_leaderboard?query=mlabonne/NeuralHermes-2.5-Mistral-7B
name: Open LLM Leaderboard
---
<center><img src="https://i.imgur.com/qIhaFNM.png"></center>
# NeuralHermes 2.5 - Mistral 7B
NeuralHermes is based on the [teknium/OpenHermes-2.5-Mistral-7B](https://huggingface.co/teknium/OpenHermes-2.5-Mistral-7B) model that has been further fine-tuned with Direct Preference Optimization (DPO) using the [mlabonne/chatml_dpo_pairs](https://huggingface.co/datasets/mlabonne/chatml_dpo_pairs) dataset. It surpasses the original model on most benchmarks (see results).
It is directly inspired by the RLHF process described by [Intel/neural-chat-7b-v3-1](https://huggingface.co/Intel/neural-chat-7b-v3-1)'s authors to improve performance. I used the same dataset and reformatted it to apply the ChatML template.
The code to train this model is available on [Google Colab](https://colab.research.google.com/drive/15iFBr1xWgztXvhrj5I9fBv20c7CFOPBE?usp=sharing) and [GitHub](https://github.com/mlabonne/llm-course/tree/main). It required an A100 GPU for about an hour.
## Quantized models
* **GGUF**: https://huggingface.co/TheBloke/NeuralHermes-2.5-Mistral-7B-GGUF
* **AWQ**: https://huggingface.co/TheBloke/NeuralHermes-2.5-Mistral-7B-AWQ
* **GPTQ**: https://huggingface.co/TheBloke/NeuralHermes-2.5-Mistral-7B-GPTQ
* **EXL2**:
* 3.0bpw: https://huggingface.co/LoneStriker/NeuralHermes-2.5-Mistral-7B-3.0bpw-h6-exl2
* 4.0bpw: https://huggingface.co/LoneStriker/NeuralHermes-2.5-Mistral-7B-4.0bpw-h6-exl2
* 5.0bpw: https://huggingface.co/LoneStriker/NeuralHermes-2.5-Mistral-7B-5.0bpw-h6-exl2
* 6.0bpw: https://huggingface.co/LoneStriker/NeuralHermes-2.5-Mistral-7B-6.0bpw-h6-exl2
* 8.0bpw: https://huggingface.co/LoneStriker/NeuralHermes-2.5-Mistral-7B-8.0bpw-h8-exl2
## Results
**Update:** NeuralHermes-2.5 became the best Hermes-based model on the Open LLM leaderboard and one of the very best 7b models. 🎉

Teknium (author of OpenHermes-2.5-Mistral-7B) benchmarked the model ([see his tweet](https://twitter.com/Teknium1/status/1729955709377503660)).
Results are improved on every benchmark: **AGIEval** (from 43.07% to 43.62%), **GPT4All** (from 73.12% to 73.25%), and **TruthfulQA**.
### AGIEval

### GPT4All

### TruthfulQA

You can check the Weights & Biases project [here](https://wandb.ai/mlabonne/DPO/runs/axe71gr0?nw=nwusermlabonne).
## Usage
You can run this model using [LM Studio](https://lmstudio.ai/) or any other frontend.
You can also run this model using the following code:
```python
import transformers
from transformers import AutoTokenizer
# Format prompt
message = [
{"role": "system", "content": "You are a helpful assistant chatbot."},
{"role": "user", "content": "What is a Large Language Model?"}
]
tokenizer = AutoTokenizer.from_pretrained(new_model)
prompt = tokenizer.apply_chat_template(message, add_generation_prompt=True, tokenize=False)
# Create pipeline
pipeline = transformers.pipeline(
"text-generation",
model=new_model,
tokenizer=tokenizer
)
# Generate text
sequences = pipeline(
prompt,
do_sample=True,
temperature=0.7,
top_p=0.9,
num_return_sequences=1,
max_length=200,
)
print(sequences[0]['generated_text'])
```
## Training hyperparameters
**LoRA**:
* r=16
* lora_alpha=16
* lora_dropout=0.05
* bias="none"
* task_type="CAUSAL_LM"
* target_modules=['k_proj', 'gate_proj', 'v_proj', 'up_proj', 'q_proj', 'o_proj', 'down_proj']
**Training arguments**:
* per_device_train_batch_size=4
* gradient_accumulation_steps=4
* gradient_checkpointing=True
* learning_rate=5e-5
* lr_scheduler_type="cosine"
* max_steps=200
* optim="paged_adamw_32bit"
* warmup_steps=100
**DPOTrainer**:
* beta=0.1
* max_prompt_length=1024
* max_length=1536
|
TheMistoAI/MistoLine | TheMistoAI | "2024-05-17T12:17:27Z" | 14,250 | 343 | diffusers | [
"diffusers",
"art",
"stable diffusion",
"ControlNet",
"SDXL",
"Diffusion-XL",
"text-to-image",
"arxiv:2302.05543",
"license:openrail++",
"region:us"
] | text-to-image | "2024-05-07T10:15:16Z" | ---
license: openrail++
tags:
- art
- stable diffusion
- ControlNet
- SDXL
- Diffusion-XL
pipeline_tag: text-to-image
---
# MistoLine
## Control Every Line!

[GitHub Repo](https://github.com/TheMistoAI/MistoLine)
## NEWS!!!!! Anyline-preprocessor is released!!!!
[Anyline Repo](https://github.com/TheMistoAI/ComfyUI-Anyline)
**MistoLine: A Versatile and Robust SDXL-ControlNet Model for Adaptable Line Art Conditioning.**
MistoLine is an SDXL-ControlNet model that can adapt to any type of line art input, demonstrating high accuracy and excellent stability. It can generate high-quality images (with a short side greater than 1024px) based on user-provided line art of various types, including hand-drawn sketches, different ControlNet line preprocessors, and model-generated outlines. MistoLine eliminates the need to select different ControlNet models for different line preprocessors, as it exhibits strong generalization capabilities across diverse line art conditions.
We developed MistoLine by employing a novel line preprocessing algorithm **[Anyline](https://github.com/TheMistoAI/ComfyUI-Anyline)** and retraining the ControlNet model based on the Unet of stabilityai/ stable-diffusion-xl-base-1.0, along with innovations in large model training engineering. MistoLine showcases superior performance across
different types of line art inputs, surpassing existing ControlNet models in terms of detail restoration, prompt alignment, and stability, particularly in more complex scenarios.
MistoLine maintains consistency with the ControlNet architecture released by @lllyasviel, as illustrated in the following schematic diagram:


*reference:https://github.com/lllyasviel/ControlNet*
More information about ControlNet can be found in the following references:
https://github.com/lllyasviel/ControlNet
https://huggingface.co/docs/diffusers/main/en/api/pipelines/controlnet_sdxl
The model is compatible with most SDXL models, except for PlaygroundV2.5, CosXL, and SDXL-Lightning(maybe). It can be used in conjunction with LCM and other ControlNet models.
The following usage of this model is not allowed:
* Violating laws and regulations
* Harming or exploiting minors
* Creating and spreading false information
* Infringing on others' privacy
* Defaming or harassing others
* Automated decision-making that harms others' legal rights
* Discrimination based on social behavior or personal characteristics
* Exploiting the vulnerabilities of specific groups to mislead their behavior
* Discrimination based on legally protected characteristics
* Providing medical advice and diagnostic results
* Improperly generating and using information for purposes such as law enforcement and immigration
If you use or distribute this model for commercial purposes, you must comply with the following conditions:
1. Clearly acknowledge the contribution of TheMisto.ai to this model in the documentation, website, or other prominent and visible locations of your product.
Example: "This product uses the MistoLine-SDXL-ControlNet developed by TheMisto.ai."
2. If your product includes about screens, readme files, or other similar display areas, you must include the above attribution information in those areas.
3. If your product does not have the aforementioned areas, you must include the attribution information in other reasonable locations within the product to ensure that end-users can notice it.
4. You must not imply in any way that TheMisto.ai endorses or promotes your product. The use of the attribution information is solely to indicate the origin of this model.
If you have any questions about how to provide attribution in specific cases, please contact [email protected].
署名条款
如果您在商业用途中使用或分发本模型,您必须满足以下条件:
1. 在产品的文档,网站,或其他主要可见位置,明确提及 TheMisto.ai 对本软件的贡献。
示例: "本产品使用了 TheMisto.ai 开发的 MistoLine-SDXL-ControlNet。"
2. 如果您的产品包含有关屏幕,说明文件,或其他类似的显示区域,您必须在这些区域中包含上述署名信息。
3. 如果您的产品没有上述区域,您必须在产品的其他合理位置包含署名信息,以确保最终用户能够注意到。
4. 您不得以任何方式暗示 TheMisto.ai 为您的产品背书或促销。署名信息的使用仅用于表明本模型的来源。
如果您对如何在特定情况下提供署名有任何疑问,请联系[email protected]。
The model output is not censored and the authors do not endorse the opinions in the generated content. Use at your own risk.
## Apply with Different Line Preprocessors

## Compere with Other Controlnets

## Application Examples
### Sketch Rendering
*The following case only utilized MistoLine as the controlnet:*

### Model Rendering
*The following case only utilized Anyline as the preprocessor and MistoLine as the controlnet.*

## ComfyUI Recommended Parameters
```
sampler steps:30
CFG:7.0
sampler_name:dpmpp_2m_sde
scheduler:karras
denoise:0.93
controlnet_strength:1.0
stargt_percent:0.0
end_percent:0.9
```
## Diffusers pipeline
Make sure to first install the libraries:
```
pip install accelerate transformers safetensors opencv-python diffusers
```
And then we're ready to go:
```
from diffusers import ControlNetModel, StableDiffusionXLControlNetPipeline, AutoencoderKL
from diffusers.utils import load_image
from PIL import Image
import torch
import numpy as np
import cv2
prompt = "aerial view, a futuristic research complex in a bright foggy jungle, hard lighting"
negative_prompt = 'low quality, bad quality, sketches'
image = load_image("https://huggingface.co/datasets/hf-internal-testing/diffusers-images/resolve/main/sd_controlnet/hf-logo.png")
controlnet_conditioning_scale = 0.5
controlnet = ControlNetModel.from_pretrained(
"TheMistoAI/MistoLine",
torch_dtype=torch.float16,
variant="fp16",
)
vae = AutoencoderKL.from_pretrained("madebyollin/sdxl-vae-fp16-fix", torch_dtype=torch.float16)
pipe = StableDiffusionXLControlNetPipeline.from_pretrained(
"stabilityai/stable-diffusion-xl-base-1.0",
controlnet=controlnet,
vae=vae,
torch_dtype=torch.float16,
)
pipe.enable_model_cpu_offload()
image = np.array(image)
image = cv2.Canny(image, 100, 200)
image = image[:, :, None]
image = np.concatenate([image, image, image], axis=2)
image = Image.fromarray(image)
images = pipe(
prompt, negative_prompt=negative_prompt, image=image, controlnet_conditioning_scale=controlnet_conditioning_scale,
).images
images[0].save(f"hug_lab.png")
```
## Checkpoints
* mistoLine_rank256.safetensors : General usage version, for ComfyUI and AUTOMATIC1111-WebUI.
* mistoLine_fp16.safetensors : FP16 weights, for ComfyUI and AUTOMATIC1111-WebUI.
## !!!mistoLine_rank256.safetensors better than mistoLine_fp16.safetensors
## !!!mistoLine_rank256.safetensors 表现更加出色!!
## ComfyUI Usage

## 中国(大陆地区)便捷下载地址:
链接:https://pan.baidu.com/s/1DbZWmGJ40Uzr3Iz9RNBG_w?pwd=8mzs
提取码:8mzs
## Citation
```
@misc{
title={Adding Conditional Control to Text-to-Image Diffusion Models},
author={Lvmin Zhang, Anyi Rao, Maneesh Agrawala},
year={2023},
eprint={2302.05543},
archivePrefix={arXiv},
primaryClass={cs.CV}
}
```
|
mradermacher/Llama3-dolphin-slerp-GGUF | mradermacher | "2024-06-26T16:42:52Z" | 14,240 | 0 | transformers | [
"transformers",
"gguf",
"merge",
"mergekit",
"lazymergekit",
"cognitivecomputations/dolphin-2.9-llama3-8b",
"Orenguteng/Llama-3-8B-Lexi-Uncensored",
"en",
"base_model:Rupesh2/Llama3-dolphin-slerp",
"license:apache-2.0",
"endpoints_compatible",
"region:us"
] | null | "2024-06-26T14:22:39Z" | ---
base_model: Rupesh2/Llama3-dolphin-slerp
language:
- en
library_name: transformers
license: apache-2.0
quantized_by: mradermacher
tags:
- merge
- mergekit
- lazymergekit
- cognitivecomputations/dolphin-2.9-llama3-8b
- Orenguteng/Llama-3-8B-Lexi-Uncensored
---
## About
<!-- ### quantize_version: 2 -->
<!-- ### output_tensor_quantised: 1 -->
<!-- ### convert_type: hf -->
<!-- ### vocab_type: -->
<!-- ### tags: -->
static quants of https://huggingface.co/Rupesh2/Llama3-dolphin-slerp
<!-- provided-files -->
weighted/imatrix quants seem not to be available (by me) at this time. If they do not show up a week or so after the static ones, I have probably not planned for them. Feel free to request them by opening a Community Discussion.
## Usage
If you are unsure how to use GGUF files, refer to one of [TheBloke's
READMEs](https://huggingface.co/TheBloke/KafkaLM-70B-German-V0.1-GGUF) for
more details, including on how to concatenate multi-part files.
## Provided Quants
(sorted by size, not necessarily quality. IQ-quants are often preferable over similar sized non-IQ quants)
| Link | Type | Size/GB | Notes |
|:-----|:-----|--------:|:------|
| [GGUF](https://huggingface.co/mradermacher/Llama3-dolphin-slerp-GGUF/resolve/main/Llama3-dolphin-slerp.Q2_K.gguf) | Q2_K | 3.3 | |
| [GGUF](https://huggingface.co/mradermacher/Llama3-dolphin-slerp-GGUF/resolve/main/Llama3-dolphin-slerp.IQ3_XS.gguf) | IQ3_XS | 3.6 | |
| [GGUF](https://huggingface.co/mradermacher/Llama3-dolphin-slerp-GGUF/resolve/main/Llama3-dolphin-slerp.Q3_K_S.gguf) | Q3_K_S | 3.8 | |
| [GGUF](https://huggingface.co/mradermacher/Llama3-dolphin-slerp-GGUF/resolve/main/Llama3-dolphin-slerp.IQ3_S.gguf) | IQ3_S | 3.8 | beats Q3_K* |
| [GGUF](https://huggingface.co/mradermacher/Llama3-dolphin-slerp-GGUF/resolve/main/Llama3-dolphin-slerp.IQ3_M.gguf) | IQ3_M | 3.9 | |
| [GGUF](https://huggingface.co/mradermacher/Llama3-dolphin-slerp-GGUF/resolve/main/Llama3-dolphin-slerp.Q3_K_M.gguf) | Q3_K_M | 4.1 | lower quality |
| [GGUF](https://huggingface.co/mradermacher/Llama3-dolphin-slerp-GGUF/resolve/main/Llama3-dolphin-slerp.Q3_K_L.gguf) | Q3_K_L | 4.4 | |
| [GGUF](https://huggingface.co/mradermacher/Llama3-dolphin-slerp-GGUF/resolve/main/Llama3-dolphin-slerp.IQ4_XS.gguf) | IQ4_XS | 4.6 | |
| [GGUF](https://huggingface.co/mradermacher/Llama3-dolphin-slerp-GGUF/resolve/main/Llama3-dolphin-slerp.Q4_K_S.gguf) | Q4_K_S | 4.8 | fast, recommended |
| [GGUF](https://huggingface.co/mradermacher/Llama3-dolphin-slerp-GGUF/resolve/main/Llama3-dolphin-slerp.Q4_K_M.gguf) | Q4_K_M | 5.0 | fast, recommended |
| [GGUF](https://huggingface.co/mradermacher/Llama3-dolphin-slerp-GGUF/resolve/main/Llama3-dolphin-slerp.Q5_K_S.gguf) | Q5_K_S | 5.7 | |
| [GGUF](https://huggingface.co/mradermacher/Llama3-dolphin-slerp-GGUF/resolve/main/Llama3-dolphin-slerp.Q5_K_M.gguf) | Q5_K_M | 5.8 | |
| [GGUF](https://huggingface.co/mradermacher/Llama3-dolphin-slerp-GGUF/resolve/main/Llama3-dolphin-slerp.Q6_K.gguf) | Q6_K | 6.7 | very good quality |
| [GGUF](https://huggingface.co/mradermacher/Llama3-dolphin-slerp-GGUF/resolve/main/Llama3-dolphin-slerp.Q8_0.gguf) | Q8_0 | 8.6 | fast, best quality |
| [GGUF](https://huggingface.co/mradermacher/Llama3-dolphin-slerp-GGUF/resolve/main/Llama3-dolphin-slerp.f16.gguf) | f16 | 16.2 | 16 bpw, overkill |
Here is a handy graph by ikawrakow comparing some lower-quality quant
types (lower is better):

And here are Artefact2's thoughts on the matter:
https://gist.github.com/Artefact2/b5f810600771265fc1e39442288e8ec9
## FAQ / Model Request
See https://huggingface.co/mradermacher/model_requests for some answers to
questions you might have and/or if you want some other model quantized.
## Thanks
I thank my company, [nethype GmbH](https://www.nethype.de/), for letting
me use its servers and providing upgrades to my workstation to enable
this work in my free time.
<!-- end -->
|
Subsets and Splits
No saved queries yet
Save your SQL queries to embed, download, and access them later. Queries will appear here once saved.