modelId
stringlengths 5
122
| author
stringlengths 2
42
| last_modified
unknown | downloads
int64 0
738M
| likes
int64 0
11k
| library_name
stringclasses 245
values | tags
sequencelengths 1
4.05k
| pipeline_tag
stringclasses 48
values | createdAt
unknown | card
stringlengths 1
901k
|
---|---|---|---|---|---|---|---|---|---|
RichardErkhov/NotAiLOL_-_Yi-1.5-dolphin-9B-gguf | RichardErkhov | "2024-06-14T23:53:32Z" | 2,179 | 0 | null | [
"gguf",
"region:us"
] | null | "2024-06-14T22:57:18Z" | Quantization made by Richard Erkhov.
[Github](https://github.com/RichardErkhov)
[Discord](https://discord.gg/pvy7H8DZMG)
[Request more models](https://github.com/RichardErkhov/quant_request)
Yi-1.5-dolphin-9B - GGUF
- Model creator: https://huggingface.co/NotAiLOL/
- Original model: https://huggingface.co/NotAiLOL/Yi-1.5-dolphin-9B/
| Name | Quant method | Size |
| ---- | ---- | ---- |
| [Yi-1.5-dolphin-9B.Q2_K.gguf](https://huggingface.co/RichardErkhov/NotAiLOL_-_Yi-1.5-dolphin-9B-gguf/blob/main/Yi-1.5-dolphin-9B.Q2_K.gguf) | Q2_K | 3.12GB |
| [Yi-1.5-dolphin-9B.IQ3_XS.gguf](https://huggingface.co/RichardErkhov/NotAiLOL_-_Yi-1.5-dolphin-9B-gguf/blob/main/Yi-1.5-dolphin-9B.IQ3_XS.gguf) | IQ3_XS | 3.46GB |
| [Yi-1.5-dolphin-9B.IQ3_S.gguf](https://huggingface.co/RichardErkhov/NotAiLOL_-_Yi-1.5-dolphin-9B-gguf/blob/main/Yi-1.5-dolphin-9B.IQ3_S.gguf) | IQ3_S | 3.64GB |
| [Yi-1.5-dolphin-9B.Q3_K_S.gguf](https://huggingface.co/RichardErkhov/NotAiLOL_-_Yi-1.5-dolphin-9B-gguf/blob/main/Yi-1.5-dolphin-9B.Q3_K_S.gguf) | Q3_K_S | 3.63GB |
| [Yi-1.5-dolphin-9B.IQ3_M.gguf](https://huggingface.co/RichardErkhov/NotAiLOL_-_Yi-1.5-dolphin-9B-gguf/blob/main/Yi-1.5-dolphin-9B.IQ3_M.gguf) | IQ3_M | 3.78GB |
| [Yi-1.5-dolphin-9B.Q3_K.gguf](https://huggingface.co/RichardErkhov/NotAiLOL_-_Yi-1.5-dolphin-9B-gguf/blob/main/Yi-1.5-dolphin-9B.Q3_K.gguf) | Q3_K | 4.03GB |
| [Yi-1.5-dolphin-9B.Q3_K_M.gguf](https://huggingface.co/RichardErkhov/NotAiLOL_-_Yi-1.5-dolphin-9B-gguf/blob/main/Yi-1.5-dolphin-9B.Q3_K_M.gguf) | Q3_K_M | 4.03GB |
| [Yi-1.5-dolphin-9B.Q3_K_L.gguf](https://huggingface.co/RichardErkhov/NotAiLOL_-_Yi-1.5-dolphin-9B-gguf/blob/main/Yi-1.5-dolphin-9B.Q3_K_L.gguf) | Q3_K_L | 4.37GB |
| [Yi-1.5-dolphin-9B.IQ4_XS.gguf](https://huggingface.co/RichardErkhov/NotAiLOL_-_Yi-1.5-dolphin-9B-gguf/blob/main/Yi-1.5-dolphin-9B.IQ4_XS.gguf) | IQ4_XS | 4.5GB |
| [Yi-1.5-dolphin-9B.Q4_0.gguf](https://huggingface.co/RichardErkhov/NotAiLOL_-_Yi-1.5-dolphin-9B-gguf/blob/main/Yi-1.5-dolphin-9B.Q4_0.gguf) | Q4_0 | 4.69GB |
| [Yi-1.5-dolphin-9B.IQ4_NL.gguf](https://huggingface.co/RichardErkhov/NotAiLOL_-_Yi-1.5-dolphin-9B-gguf/blob/main/Yi-1.5-dolphin-9B.IQ4_NL.gguf) | IQ4_NL | 4.73GB |
| [Yi-1.5-dolphin-9B.Q4_K_S.gguf](https://huggingface.co/RichardErkhov/NotAiLOL_-_Yi-1.5-dolphin-9B-gguf/blob/main/Yi-1.5-dolphin-9B.Q4_K_S.gguf) | Q4_K_S | 4.72GB |
| [Yi-1.5-dolphin-9B.Q4_K.gguf](https://huggingface.co/RichardErkhov/NotAiLOL_-_Yi-1.5-dolphin-9B-gguf/blob/main/Yi-1.5-dolphin-9B.Q4_K.gguf) | Q4_K | 4.96GB |
| [Yi-1.5-dolphin-9B.Q4_K_M.gguf](https://huggingface.co/RichardErkhov/NotAiLOL_-_Yi-1.5-dolphin-9B-gguf/blob/main/Yi-1.5-dolphin-9B.Q4_K_M.gguf) | Q4_K_M | 4.96GB |
| [Yi-1.5-dolphin-9B.Q4_1.gguf](https://huggingface.co/RichardErkhov/NotAiLOL_-_Yi-1.5-dolphin-9B-gguf/blob/main/Yi-1.5-dolphin-9B.Q4_1.gguf) | Q4_1 | 5.19GB |
| [Yi-1.5-dolphin-9B.Q5_0.gguf](https://huggingface.co/RichardErkhov/NotAiLOL_-_Yi-1.5-dolphin-9B-gguf/blob/main/Yi-1.5-dolphin-9B.Q5_0.gguf) | Q5_0 | 5.69GB |
| [Yi-1.5-dolphin-9B.Q5_K_S.gguf](https://huggingface.co/RichardErkhov/NotAiLOL_-_Yi-1.5-dolphin-9B-gguf/blob/main/Yi-1.5-dolphin-9B.Q5_K_S.gguf) | Q5_K_S | 5.69GB |
| [Yi-1.5-dolphin-9B.Q5_K.gguf](https://huggingface.co/RichardErkhov/NotAiLOL_-_Yi-1.5-dolphin-9B-gguf/blob/main/Yi-1.5-dolphin-9B.Q5_K.gguf) | Q5_K | 5.83GB |
| [Yi-1.5-dolphin-9B.Q5_K_M.gguf](https://huggingface.co/RichardErkhov/NotAiLOL_-_Yi-1.5-dolphin-9B-gguf/blob/main/Yi-1.5-dolphin-9B.Q5_K_M.gguf) | Q5_K_M | 5.83GB |
| [Yi-1.5-dolphin-9B.Q5_1.gguf](https://huggingface.co/RichardErkhov/NotAiLOL_-_Yi-1.5-dolphin-9B-gguf/blob/main/Yi-1.5-dolphin-9B.Q5_1.gguf) | Q5_1 | 6.19GB |
| [Yi-1.5-dolphin-9B.Q6_K.gguf](https://huggingface.co/RichardErkhov/NotAiLOL_-_Yi-1.5-dolphin-9B-gguf/blob/main/Yi-1.5-dolphin-9B.Q6_K.gguf) | Q6_K | 6.75GB |
| [Yi-1.5-dolphin-9B.Q8_0.gguf](https://huggingface.co/RichardErkhov/NotAiLOL_-_Yi-1.5-dolphin-9B-gguf/blob/main/Yi-1.5-dolphin-9B.Q8_0.gguf) | Q8_0 | 8.74GB |
Original model description:
---
language:
- en
license: apache-2.0
tags:
- text-generation-inference
- transformers
- unsloth
- llama
- trl
- sft
base_model: 01-ai/Yi-1.5-9B
datasets:
- Vezora/Dolphin1m_gpt4_Alpaca_format
---
# Uploaded model
- **Developed by:** NotAiLOL
- **License:** apache-2.0
- **Finetuned from model :** 01-ai/Yi-1.5-9B
This llama model was trained 2x faster with [Unsloth](https://github.com/unslothai/unsloth) and Huggingface's TRL library.
[<img src="https://raw.githubusercontent.com/unslothai/unsloth/main/images/unsloth%20made%20with%20love.png" width="200"/>](https://github.com/unslothai/unsloth)
# Model detail
## Prompt format
The model uses the alpaca format:
```
Below is an instruction that describes a task, paired with an input that provides further context. Write a response that appropriately completes the request.
### Instruction:
{}
### Input:
{}
### Response:
{}
```
|
CyberPeace-Institute/SecureBERT-NER | CyberPeace-Institute | "2023-11-22T07:53:38Z" | 2,178 | 13 | transformers | [
"transformers",
"pytorch",
"safetensors",
"roberta",
"token-classification",
"en",
"arxiv:2204.02685",
"license:mit",
"autotrain_compatible",
"endpoints_compatible",
"region:us"
] | token-classification | "2023-06-23T11:12:52Z" | ---
language:
- en
library_name: transformers
pipeline_tag: token-classification
widget:
- text: >-
Microsoft Threat Intelligence analysts assess with high confidence that the
malware, which we call KingsPawn, is developed by DEV-0196 and therefore
strongly linked to QuaDream. We assess with medium confidence that the
mobile malware we associate with DEV-0196 is part of the system publicly
discussed as REIGN.
example_title: example
license: mit
---
# Named Entity Recognition for Cybersecurity
This model has been finetuned with SecureBERT (https://arxiv.org/abs/2204.02685)
on the APTNER dataset (https://ieeexplore.ieee.org/document/9776031)
## NER Classes
 |
Panchovix/WizardLM-33B-V1.0-Uncensored-SuperHOT-8k | Panchovix | "2023-11-18T06:04:22Z" | 2,178 | 7 | transformers | [
"transformers",
"pytorch",
"llama",
"text-generation",
"license:other",
"autotrain_compatible",
"endpoints_compatible",
"text-generation-inference",
"region:us"
] | text-generation | "2023-06-26T00:14:14Z" | ---
license: other
---
[WizardLM-33B-V1.0-Uncensored](https://huggingface.co/ehartford/WizardLM-33B-V1.0-Uncensored) merged with kaiokendev's [33b SuperHOT 8k LoRA](https://huggingface.co/kaiokendev/superhot-30b-8k-no-rlhf-test), without quant. (Full FP16 model)
# [Open LLM Leaderboard Evaluation Results](https://huggingface.co/spaces/HuggingFaceH4/open_llm_leaderboard)
Detailed results can be found [here](https://huggingface.co/datasets/open-llm-leaderboard/details_Panchovix__WizardLM-33B-V1.0-Uncensored-SuperHOT-8k)
| Metric | Value |
|-----------------------|---------------------------|
| Avg. | 25.58 |
| ARC (25-shot) | 25.43 |
| HellaSwag (10-shot) | 31.97 |
| MMLU (5-shot) | 23.43 |
| TruthfulQA (0-shot) | 47.0 |
| Winogrande (5-shot) | 51.07 |
| GSM8K (5-shot) | 0.0 |
| DROP (3-shot) | 0.19 |
|
CHIH-HUNG/llama-2-13b-FINETUNE4_3.8w-r16-gate_up_down | CHIH-HUNG | "2023-09-22T07:02:21Z" | 2,178 | 0 | transformers | [
"transformers",
"pytorch",
"llama",
"text-generation",
"autotrain_compatible",
"endpoints_compatible",
"text-generation-inference",
"region:us"
] | text-generation | "2023-09-22T06:44:49Z" | Entry not found |
roneneldan/TinyStories-28M | roneneldan | "2023-05-17T22:05:49Z" | 2,176 | 7 | transformers | [
"transformers",
"pytorch",
"gpt_neo",
"text-generation",
"autotrain_compatible",
"endpoints_compatible",
"region:us"
] | text-generation | "2023-05-12T21:48:55Z" | Entry not found |
laundryheap/drivers-v1-small | laundryheap | "2024-05-30T06:20:42Z" | 2,176 | 0 | transformers | [
"transformers",
"safetensors",
"swin",
"image-classification",
"arxiv:1910.09700",
"autotrain_compatible",
"endpoints_compatible",
"region:us"
] | image-classification | "2024-05-30T06:15:47Z" | ---
library_name: transformers
tags: []
---
# Model Card for Model ID
<!-- Provide a quick summary of what the model is/does. -->
## Model Details
### Model Description
<!-- Provide a longer summary of what this model is. -->
This is the model card of a 🤗 transformers model that has been pushed on the Hub. This model card has been automatically generated.
- **Developed by:** [More Information Needed]
- **Funded by [optional]:** [More Information Needed]
- **Shared by [optional]:** [More Information Needed]
- **Model type:** [More Information Needed]
- **Language(s) (NLP):** [More Information Needed]
- **License:** [More Information Needed]
- **Finetuned from model [optional]:** [More Information Needed]
### Model Sources [optional]
<!-- Provide the basic links for the model. -->
- **Repository:** [More Information Needed]
- **Paper [optional]:** [More Information Needed]
- **Demo [optional]:** [More Information Needed]
## Uses
<!-- Address questions around how the model is intended to be used, including the foreseeable users of the model and those affected by the model. -->
### Direct Use
<!-- This section is for the model use without fine-tuning or plugging into a larger ecosystem/app. -->
[More Information Needed]
### Downstream Use [optional]
<!-- This section is for the model use when fine-tuned for a task, or when plugged into a larger ecosystem/app -->
[More Information Needed]
### Out-of-Scope Use
<!-- This section addresses misuse, malicious use, and uses that the model will not work well for. -->
[More Information Needed]
## Bias, Risks, and Limitations
<!-- This section is meant to convey both technical and sociotechnical limitations. -->
[More Information Needed]
### Recommendations
<!-- This section is meant to convey recommendations with respect to the bias, risk, and technical limitations. -->
Users (both direct and downstream) should be made aware of the risks, biases and limitations of the model. More information needed for further recommendations.
## How to Get Started with the Model
Use the code below to get started with the model.
[More Information Needed]
## Training Details
### Training Data
<!-- This should link to a Dataset Card, perhaps with a short stub of information on what the training data is all about as well as documentation related to data pre-processing or additional filtering. -->
[More Information Needed]
### Training Procedure
<!-- This relates heavily to the Technical Specifications. Content here should link to that section when it is relevant to the training procedure. -->
#### Preprocessing [optional]
[More Information Needed]
#### Training Hyperparameters
- **Training regime:** [More Information Needed] <!--fp32, fp16 mixed precision, bf16 mixed precision, bf16 non-mixed precision, fp16 non-mixed precision, fp8 mixed precision -->
#### Speeds, Sizes, Times [optional]
<!-- This section provides information about throughput, start/end time, checkpoint size if relevant, etc. -->
[More Information Needed]
## Evaluation
<!-- This section describes the evaluation protocols and provides the results. -->
### Testing Data, Factors & Metrics
#### Testing Data
<!-- This should link to a Dataset Card if possible. -->
[More Information Needed]
#### Factors
<!-- These are the things the evaluation is disaggregating by, e.g., subpopulations or domains. -->
[More Information Needed]
#### Metrics
<!-- These are the evaluation metrics being used, ideally with a description of why. -->
[More Information Needed]
### Results
[More Information Needed]
#### Summary
## Model Examination [optional]
<!-- Relevant interpretability work for the model goes here -->
[More Information Needed]
## Environmental Impact
<!-- Total emissions (in grams of CO2eq) and additional considerations, such as electricity usage, go here. Edit the suggested text below accordingly -->
Carbon emissions can be estimated using the [Machine Learning Impact calculator](https://mlco2.github.io/impact#compute) presented in [Lacoste et al. (2019)](https://arxiv.org/abs/1910.09700).
- **Hardware Type:** [More Information Needed]
- **Hours used:** [More Information Needed]
- **Cloud Provider:** [More Information Needed]
- **Compute Region:** [More Information Needed]
- **Carbon Emitted:** [More Information Needed]
## Technical Specifications [optional]
### Model Architecture and Objective
[More Information Needed]
### Compute Infrastructure
[More Information Needed]
#### Hardware
[More Information Needed]
#### Software
[More Information Needed]
## Citation [optional]
<!-- If there is a paper or blog post introducing the model, the APA and Bibtex information for that should go in this section. -->
**BibTeX:**
[More Information Needed]
**APA:**
[More Information Needed]
## Glossary [optional]
<!-- If relevant, include terms and calculations in this section that can help readers understand the model or model card. -->
[More Information Needed]
## More Information [optional]
[More Information Needed]
## Model Card Authors [optional]
[More Information Needed]
## Model Card Contact
[More Information Needed] |
kiwhansong/video_minecraft_vae | kiwhansong | "2024-06-27T19:28:55Z" | 2,176 | 0 | diffusers | [
"diffusers",
"safetensors",
"arxiv:1910.09700",
"region:us"
] | null | "2024-06-27T03:54:59Z" | ---
library_name: diffusers
---
# Model Card for Model ID
<!-- Provide a quick summary of what the model is/does. -->
## Model Details
### Model Description
<!-- Provide a longer summary of what this model is. -->
This is the model card of a 🧨 diffusers model that has been pushed on the Hub. This model card has been automatically generated.
- **Developed by:** [More Information Needed]
- **Funded by [optional]:** [More Information Needed]
- **Shared by [optional]:** [More Information Needed]
- **Model type:** [More Information Needed]
- **Language(s) (NLP):** [More Information Needed]
- **License:** [More Information Needed]
- **Finetuned from model [optional]:** [More Information Needed]
### Model Sources [optional]
<!-- Provide the basic links for the model. -->
- **Repository:** [More Information Needed]
- **Paper [optional]:** [More Information Needed]
- **Demo [optional]:** [More Information Needed]
## Uses
<!-- Address questions around how the model is intended to be used, including the foreseeable users of the model and those affected by the model. -->
### Direct Use
<!-- This section is for the model use without fine-tuning or plugging into a larger ecosystem/app. -->
[More Information Needed]
### Downstream Use [optional]
<!-- This section is for the model use when fine-tuned for a task, or when plugged into a larger ecosystem/app -->
[More Information Needed]
### Out-of-Scope Use
<!-- This section addresses misuse, malicious use, and uses that the model will not work well for. -->
[More Information Needed]
## Bias, Risks, and Limitations
<!-- This section is meant to convey both technical and sociotechnical limitations. -->
[More Information Needed]
### Recommendations
<!-- This section is meant to convey recommendations with respect to the bias, risk, and technical limitations. -->
Users (both direct and downstream) should be made aware of the risks, biases and limitations of the model. More information needed for further recommendations.
## How to Get Started with the Model
Use the code below to get started with the model.
[More Information Needed]
## Training Details
### Training Data
<!-- This should link to a Dataset Card, perhaps with a short stub of information on what the training data is all about as well as documentation related to data pre-processing or additional filtering. -->
[More Information Needed]
### Training Procedure
<!-- This relates heavily to the Technical Specifications. Content here should link to that section when it is relevant to the training procedure. -->
#### Preprocessing [optional]
[More Information Needed]
#### Training Hyperparameters
- **Training regime:** [More Information Needed] <!--fp32, fp16 mixed precision, bf16 mixed precision, bf16 non-mixed precision, fp16 non-mixed precision, fp8 mixed precision -->
#### Speeds, Sizes, Times [optional]
<!-- This section provides information about throughput, start/end time, checkpoint size if relevant, etc. -->
[More Information Needed]
## Evaluation
<!-- This section describes the evaluation protocols and provides the results. -->
### Testing Data, Factors & Metrics
#### Testing Data
<!-- This should link to a Dataset Card if possible. -->
[More Information Needed]
#### Factors
<!-- These are the things the evaluation is disaggregating by, e.g., subpopulations or domains. -->
[More Information Needed]
#### Metrics
<!-- These are the evaluation metrics being used, ideally with a description of why. -->
[More Information Needed]
### Results
[More Information Needed]
#### Summary
## Model Examination [optional]
<!-- Relevant interpretability work for the model goes here -->
[More Information Needed]
## Environmental Impact
<!-- Total emissions (in grams of CO2eq) and additional considerations, such as electricity usage, go here. Edit the suggested text below accordingly -->
Carbon emissions can be estimated using the [Machine Learning Impact calculator](https://mlco2.github.io/impact#compute) presented in [Lacoste et al. (2019)](https://arxiv.org/abs/1910.09700).
- **Hardware Type:** [More Information Needed]
- **Hours used:** [More Information Needed]
- **Cloud Provider:** [More Information Needed]
- **Compute Region:** [More Information Needed]
- **Carbon Emitted:** [More Information Needed]
## Technical Specifications [optional]
### Model Architecture and Objective
[More Information Needed]
### Compute Infrastructure
[More Information Needed]
#### Hardware
[More Information Needed]
#### Software
[More Information Needed]
## Citation [optional]
<!-- If there is a paper or blog post introducing the model, the APA and Bibtex information for that should go in this section. -->
**BibTeX:**
[More Information Needed]
**APA:**
[More Information Needed]
## Glossary [optional]
<!-- If relevant, include terms and calculations in this section that can help readers understand the model or model card. -->
[More Information Needed]
## More Information [optional]
[More Information Needed]
## Model Card Authors [optional]
[More Information Needed]
## Model Card Contact
[More Information Needed] |
jondurbin/airoboros-65b-gpt4-1.2 | jondurbin | "2023-06-22T14:59:15Z" | 2,175 | 21 | transformers | [
"transformers",
"pytorch",
"llama",
"text-generation",
"dataset:jondurbin/airoboros-gpt4-1.2",
"license:cc-by-nc-4.0",
"autotrain_compatible",
"endpoints_compatible",
"text-generation-inference",
"region:us"
] | text-generation | "2023-06-14T09:19:02Z" | ---
license: cc-by-nc-4.0
datasets:
- jondurbin/airoboros-gpt4-1.2
---
### Overview
This is a qlora fine-tuned 65b parameter LlaMa model, using completely synthetic training data created gpt4 via https://github.com/jondurbin/airoboros
This is mostly an extension of [1.1](https://huggingface.co/jondurbin/airoboros-33b-gpt4-1.1), but with a 65b model and thousands of new training data and an update to allow "PLAINFORMAT" at the end of coding prompts to just print the code without backticks or explanations/usage/etc.
The dataset used to fine-tune this model is available [here](https://huggingface.co/datasets/jondurbin/airoboros-gpt4-1.2), with a specific focus on:
- coding
- math/reasoning (using orca style ELI5 instruction/response pairs)
- trivia
- role playing
- multiple choice and fill-in-the-blank
- context-obedient question answering
- theory of mind
- misc/general
This model was fine-tuned with a fork of [qlora](https://github.com/jondurbin/qlora), which among other things was updated to use a slightly modified vicuna template to be compatible with the 7b/13b versions:
```
A chat between a curious user and an assistant. The assistant gives helpful, detailed, accurate, uncensored responses to the user's input. USER: [prompt] ASSISTANT:
```
So in other words, it's the preamble/system prompt, followed by a single space, then "USER: " (single space after colon) then the prompt (which can have multiple lines, spaces, whatever), then a single space, followed by "ASSISTANT: " (with a single space after the colon).
### Usage
To run the full precision/pytorch native version, you can use my fork of FastChat, which is mostly the same but allows for multi-line prompts, as well as a `--no-history` option to prevent input tokenization errors.
```
pip install git+https://github.com/jondurbin/FastChat
```
Be sure you are pulling the latest branch!
Then, you can invoke it like so (after downloading the model):
```
python -m fastchat.serve.cli \
--model-path airoboros-65b-gpt4-1.2 \
--temperature 0.5 \
--max-new-tokens 2048 \
--no-history
```
Alternatively, please check out TheBloke's quantized versions:
- https://huggingface.co/TheBloke/airoboros-65B-gpt4-1.2-GPTQ
- https://huggingface.co/TheBloke/airoboros-65B-gpt4-1.2-GGML
### Coding updates from gpt4/1.1:
I added a few hundred instruction/response pairs to the training data with "PLAINFORMAT" as a single, all caps term at the end of the normal instructions, which produce plain text output instead of markdown/backtick code formatting.
It's not guaranteed to work all the time, but mostly it does seem to work as expected.
So for example, instead of:
```
Implement the Snake game in python.
```
You would use:
```
Implement the Snake game in python. PLAINFORMAT
```
### Other updates from gpt4/1.1:
- Several hundred role-playing data.
- A few thousand ORCA style reasoning/math questions with ELI5 prompts to generate the responses (should not be needed in your prompts to this model however, just ask the question).
- Many more coding examples in various languages, including some that use specific libraries (pandas, numpy, tensorflow, etc.)
### Usage and License Notices
All airoboros models and datasets are intended and licensed for research use only. I've used the 'cc-nc-4.0' license, but really it is subject to a custom/special license because:
- the base model is LLaMa, which has it's own special research license
- the dataset(s) were generated with OpenAI (gpt-4 and/or gpt-3.5-turbo), which has a clausing saying the data can't be used to create models to compete with openai
So, to reiterate: this model (and datasets) cannot be used commercially. |
uukuguy/speechless-codellama-platypus-13b | uukuguy | "2023-12-30T11:49:52Z" | 2,175 | 2 | transformers | [
"transformers",
"pytorch",
"llama",
"text-generation",
"llama-2",
"en",
"dataset:garage-bAInd/Open-Platypus",
"arxiv:2308.12950",
"license:llama2",
"autotrain_compatible",
"endpoints_compatible",
"text-generation-inference",
"region:us"
] | text-generation | "2023-08-31T12:32:35Z" | ---
language:
- en
library_name: transformers
pipeline_tag: text-generation
datasets:
- garage-bAInd/Open-Platypus
tags:
- llama-2
license: llama2
---
<p><h1> The Tool LLM Based on CodeLlama </h1></p>
Fine-tune the codellama/CodeLlama-13b-hf with Open-Platypus dataset.
Code: https://github.com/uukuguy/speechless
## How to Prompt the Model
This model accepts the Alpaca instruction format.
For example:
```
You are an intelligent programming assistant.
### Instruction:
Implement a linked list in C++
### Response:
```
| Metric | Value |
| --- | --- |
| ARC | 45.31 |
| HellaSwag | 68.63 |
| MMLU | 42.82 |
| TruthfulQA | 42.38 |
| Average | 49.78 |
# **Code Llama**
Code Llama is a collection of pretrained and fine-tuned generative text models ranging in scale from 7 billion to 34 billion parameters. This is the repository for the base 13B version in the Hugging Face Transformers format. This model is designed for general code synthesis and understanding. Links to other models can be found in the index at the bottom.
| | Base Model | Python | Instruct |
| --- | ----------------------------------------------------------------------------- | ------------------------------------------------------------------------------------------- | ----------------------------------------------------------------------------------------------- |
| 7B | [codellama/CodeLlama-7b-hf](https://huggingface.co/codellama/CodeLlama-7b-hf) | [codellama/CodeLlama-7b-Python-hf](https://huggingface.co/codellama/CodeLlama-7b-Python-hf) | [codellama/CodeLlama-7b-Instruct-hf](https://huggingface.co/codellama/CodeLlama-7b-Instruct-hf) |
| 13B | [codellama/CodeLlama-13b-hf](https://huggingface.co/codellama/CodeLlama-13b-hf) | [codellama/CodeLlama-13b-Python-hf](https://huggingface.co/codellama/CodeLlama-13b-Python-hf) | [codellama/CodeLlama-13b-Instruct-hf](https://huggingface.co/codellama/CodeLlama-13b-Instruct-hf) |
| 34B | [codellama/CodeLlama-34b-hf](https://huggingface.co/codellama/CodeLlama-34b-hf) | [codellama/CodeLlama-34b-Python-hf](https://huggingface.co/codellama/CodeLlama-34b-Python-hf) | [codellama/CodeLlama-34b-Instruct-hf](https://huggingface.co/codellama/CodeLlama-34b-Instruct-hf) |
## Model Use
To use this model, please make sure to install transformers from `main` until the next version is released:
```bash
pip install git+https://github.com/huggingface/transformers.git@main accelerate
```
Model capabilities:
- [x] Code completion.
- [x] Infilling.
- [ ] Instructions / chat.
- [ ] Python specialist.
```python
from transformers import AutoTokenizer
import transformers
import torch
model = "codellama/CodeLlama-13b-hf"
tokenizer = AutoTokenizer.from_pretrained(model)
pipeline = transformers.pipeline(
"text-generation",
model=model,
torch_dtype=torch.float16,
device_map="auto",
)
sequences = pipeline(
'import socket\n\ndef ping_exponential_backoff(host: str):',
do_sample=True,
top_k=10,
temperature=0.1,
top_p=0.95,
num_return_sequences=1,
eos_token_id=tokenizer.eos_token_id,
max_length=200,
)
for seq in sequences:
print(f"Result: {seq['generated_text']}")
```
## Model Details
*Note: Use of this model is governed by the Meta license. Meta developed and publicly released the Code Llama family of large language models (LLMs).
**Model Developers** Meta
**Variations** Code Llama comes in three model sizes, and three variants:
* Code Llama: base models designed for general code synthesis and understanding
* Code Llama - Python: designed specifically for Python
* Code Llama - Instruct: for instruction following and safer deployment
All variants are available in sizes of 7B, 13B and 34B parameters.
**This repository contains the base version of the 13B parameters model.**
**Input** Models input text only.
**Output** Models generate text only.
**Model Architecture** Code Llama is an auto-regressive language model that uses an optimized transformer architecture.
**Model Dates** Code Llama and its variants have been trained between January 2023 and July 2023.
**Status** This is a static model trained on an offline dataset. Future versions of Code Llama - Instruct will be released as we improve model safety with community feedback.
**License** A custom commercial license is available at: [https://ai.meta.com/resources/models-and-libraries/llama-downloads/](https://ai.meta.com/resources/models-and-libraries/llama-downloads/)
**Research Paper** More information can be found in the paper "[Code Llama: Open Foundation Models for Code](https://ai.meta.com/research/publications/code-llama-open-foundation-models-for-code/)" or its [arXiv page](https://arxiv.org/abs/2308.12950).
## Intended Use
**Intended Use Cases** Code Llama and its variants is intended for commercial and research use in English and relevant programming languages. The base model Code Llama can be adapted for a variety of code synthesis and understanding tasks, Code Llama - Python is designed specifically to handle the Python programming language, and Code Llama - Instruct is intended to be safer to use for code assistant and generation applications.
**Out-of-Scope Uses** Use in any manner that violates applicable laws or regulations (including trade compliance laws). Use in languages other than English. Use in any other way that is prohibited by the Acceptable Use Policy and Licensing Agreement for Code Llama and its variants.
## Hardware and Software
**Training Factors** We used custom training libraries. The training and fine-tuning of the released models have been performed Meta’s Research Super Cluster.
**Carbon Footprint** In aggregate, training all 9 Code Llama models required 400K GPU hours of computation on hardware of type A100-80GB (TDP of 350-400W). Estimated total emissions were 65.3 tCO2eq, 100% of which were offset by Meta’s sustainability program.
## Training Data
All experiments reported here and the released models have been trained and fine-tuned using the same data as Llama 2 with different weights (see Section 2 and Table 1 in the [research paper](https://ai.meta.com/research/publications/code-llama-open-foundation-models-for-code/) for details).
## Evaluation Results
See evaluations for the main models and detailed ablations in Section 3 and safety evaluations in Section 4 of the research paper.
## Ethical Considerations and Limitations
Code Llama and its variants are a new technology that carries risks with use. Testing conducted to date has been in English, and has not covered, nor could it cover all scenarios. For these reasons, as with all LLMs, Code Llama’s potential outputs cannot be predicted in advance, and the model may in some instances produce inaccurate or objectionable responses to user prompts. Therefore, before deploying any applications of Code Llama, developers should perform safety testing and tuning tailored to their specific applications of the model.
Please see the Responsible Use Guide available available at [https://ai.meta.com/llama/responsible-user-guide](https://ai.meta.com/llama/responsible-user-guide).
# [Open LLM Leaderboard Evaluation Results](https://huggingface.co/spaces/HuggingFaceH4/open_llm_leaderboard)
Detailed results can be found [here](https://huggingface.co/datasets/open-llm-leaderboard/details_uukuguy__speechless-codellama-platypus-13b)
| Metric | Value |
|-----------------------|---------------------------|
| Avg. | 40.81 |
| ARC (25-shot) | 46.16 |
| HellaSwag (10-shot) | 68.88 |
| MMLU (5-shot) | 44.55 |
| TruthfulQA (0-shot) | 44.98 |
| Winogrande (5-shot) | 66.14 |
| GSM8K (5-shot) | 9.4 |
| DROP (3-shot) | 5.54 |
|
ERCDiDip/langdetect | ERCDiDip | "2023-03-27T15:02:39Z" | 2,173 | 7 | transformers | [
"transformers",
"pytorch",
"safetensors",
"xlm-roberta",
"text-classification",
"arxiv:1911.02116",
"doi:10.57967/hf/0135",
"license:mit",
"autotrain_compatible",
"endpoints_compatible",
"region:us"
] | text-classification | "2022-11-25T08:29:03Z" | ---
license: mit
tag: text-classification
widget:
- text: "Inde dit salt du dun degelikes bit innechlicheme herzen so hilfet did dat vuizes uuerliche du salt lesen di paternoster inde euuangleno miner vroaen scene marie"
- text: "Mihály zágrábi püspök előtt Vaguth (dict.) László c. a püspöki várnépek (castrenses) Csázma comitatus-beli volt földjének egy részét, amelyet szolgálataiért predialis jogon tőle kapott, 1 szőlővel együtt (a Zuynar föld azon része kivételével, amelyet a püspök László c.-től elvett és a megvakított Kokosnak adományozott"
- text: "Rath und Gemeinde der Stadt Wismar beschweren sich über die von den Hauptleuten, Beamten und Vasallen des Grafen Johann von Holstein und Stormarn ihren Bürgern seit Jahren zugefügten Unbilden, indem sie ein Verzeichniss der erlittenen einzelnen Verluste beibringen."
- text: "Diplomă de înnobilare emisă de împăratul romano-german Rudolf al II-lea de Habsburg la în favoarea familiei Szőke de Galgóc. Aussteller: Rudolf al II-lea de Habsburg, împărat romano-german Empfänger: Szőke de Galgóc, familie"
- text: "бѣ жє болѧ єтєръ лазаръ отъ виѳаньѧ градьца марьина и марѳꙑ сєстрꙑ єѧ | бѣ жє марьꙗ помазавъшиꙗ господа мѵромъ и отьръши ноѕѣ єго власꙑ своими єѧжє братъ лазаръ болѣашє"
- text: "μῆνιν ἄειδε θεὰ Πηληϊάδεω Ἀχιλῆος οὐλομένην, ἣ μυρί᾽ Ἀχαιοῖς ἄλγε᾽ ἔθηκε, πολλὰς δ᾽ ἰφθίμους ψυχὰς Ἄϊδι προΐαψεν ἡρώων, αὐτοὺς δὲ ἑλώρια τεῦχε κύνεσσιν οἰωνοῖσί"
---
# XLM-RoBERTa (base) language-detection model (modern and medieval)
This model is a fine-tuned version of xlm-roberta-base on the [monasterium.net](https://www.icar-us.eu/en/cooperation/online-portals/monasterium-net/) dataset.
## Model description
On the top of this XLM-RoBERTa transformer model is a classification head. Please refer this model together with to the [XLM-RoBERTa (base-sized model)](https://huggingface.co/xlm-roberta-base) card or the paper [Unsupervised Cross-lingual Representation Learning at Scale by Conneau et al.](https://arxiv.org/abs/1911.02116) for additional information.
## Intended uses & limitations
You can directly use this model as a language detector, i.e. for sequence classification tasks. Currently, it supports the following 41 languages, modern and medieval:
Modern: Bulgarian (bg), Croatian (hr), Czech (cs), Danish (da), Dutch (nl), English (en), Estonian (et), Finnish (fi), French (fr), German (de), Greek (el), Hungarian (hu), Irish (ga), Italian (it), Latvian (lv), Lithuanian (lt), Maltese (mt), Polish (pl), Portuguese (pt), Romanian (ro), Slovak (sk), Slovenian (sl), Spanish (es), Swedish (sv), Russian (ru), Turkish (tr), Basque (eu), Catalan (ca), Albanian (sq), Serbian (se), Ukrainian (uk), Norwegian (no), Arabic (ar), Chinese (zh), Hebrew (he)
Medieval: Middle High German (mhd), Latin (la), Middle Low German (gml), Old French (fro), Old Church Slavonic (chu), Early New High German (fnhd), Ancient and Medieval Greek (grc)
## Training and evaluation data
The model was fine-tuned using the Monasterium and Wikipedia datasets, which consist of text sequences in 41 languages. The training set contains 80k samples, while the validation and test sets contain 16k. The average accuracy on the test set is 99.59% (this matches the average macro/weighted F1-score, the test set being perfectly balanced).
## Training procedure
Fine-tuning was done via the Trainer API with WeightedLossTrainer.
## Training hyperparameters
The following hyperparameters were used during training:
- learning_rate: 2e-05
- train_batch_size: 20
- eval_batch_size: 20
- seed: 42
- optimizer: Adam with betas=(0.9,0.999) and epsilon=1e-08
- lr_scheduler_type: linear
- num_epochs: 3
mixed_precision_training: Native AMP
## Training results
| Training Loss | Validation Loss | F1
| ------------- | ------------- | -------- |
| 0.000300 | 0.048985 | 0.991585 |
| 0.000100 | 0.033340 | 0.994663 |
| 0.000000 | 0.032938 | 0.995979 |
## Using example
```
#Install packages
!pip install transformers --quiet
#Import libraries
import torch
from transformers import pipeline
#Define pipeline
classificator = pipeline("text-classification", model="ERCDiDip/langdetect")
#Use pipeline
classificator("clemens etc dilecto filio scolastico ecclesie wetflari ensi treveren dioc salutem etc significarunt nobis dilecti filii commendator et fratres hospitalis beate marie theotonicorum")
```
## Updates
- 25th November 2022: Adding Ancient and Medieval Greek (grc)
## Framework versions
- Transformers 4.24.0
- Pytorch 1.13.0
- Datasets 2.6.1
- Tokenizers 0.13.3
## Citation
Please cite the following papers when using this model.
```
@misc{ercdidip2022,
title={langdetect (Revision 0215f72)},
author={Kovács, Tamás, Atzenhofer-Baumgartner, Florian, Aoun, Sandy, Nicolaou, Anguelos, Luger, Daniel, Decker, Franziska, Lamminger, Florian and Vogeler, Georg},
year = { 2022 },
url = { https://huggingface.co/ERCDiDip/40_langdetect_v01 },
doi = { 10.57967/hf/0135 },
publisher = { Hugging Face }
}
```
This model is part of the [From Digital to Distant Diplomatics (DiDip) ERC project](https://cordis.europa.eu/project/id/101019327) funded by the European Research Council. |
timm/eva02_large_patch14_clip_336.merged2b_s6b_b61k | timm | "2024-02-10T23:42:18Z" | 2,173 | 0 | open_clip | [
"open_clip",
"safetensors",
"zero-shot-image-classification",
"clip",
"license:mit",
"region:us"
] | zero-shot-image-classification | "2023-04-10T22:01:27Z" | ---
license: mit
library_name: open_clip
tags:
- zero-shot-image-classification
- clip
---
# Model card for eva02_large_patch14_clip_336.merged2b_s6b_b61k
|
yuzhaouoe/BM25Chunk | yuzhaouoe | "2024-06-13T15:12:54Z" | 2,173 | 0 | transformers | [
"transformers",
"safetensors",
"llama",
"text-generation",
"arxiv:1910.09700",
"autotrain_compatible",
"endpoints_compatible",
"text-generation-inference",
"region:us"
] | text-generation | "2024-02-28T00:48:15Z" | ---
library_name: transformers
tags: []
---
# Model Card for Model ID
<!-- Provide a quick summary of what the model is/does. -->
## Model Details
### Model Description
<!-- Provide a longer summary of what this model is. -->
This is the model card of a 🤗 transformers model that has been pushed on the Hub. This model card has been automatically generated.
- **Developed by:** [More Information Needed]
- **Funded by [optional]:** [More Information Needed]
- **Shared by [optional]:** [More Information Needed]
- **Model type:** [More Information Needed]
- **Language(s) (NLP):** [More Information Needed]
- **License:** [More Information Needed]
- **Finetuned from model [optional]:** [More Information Needed]
### Model Sources [optional]
<!-- Provide the basic links for the model. -->
- **Repository:** [More Information Needed]
- **Paper [optional]:** [More Information Needed]
- **Demo [optional]:** [More Information Needed]
## Uses
<!-- Address questions around how the model is intended to be used, including the foreseeable users of the model and those affected by the model. -->
### Direct Use
<!-- This section is for the model use without fine-tuning or plugging into a larger ecosystem/app. -->
[More Information Needed]
### Downstream Use [optional]
<!-- This section is for the model use when fine-tuned for a task, or when plugged into a larger ecosystem/app -->
[More Information Needed]
### Out-of-Scope Use
<!-- This section addresses misuse, malicious use, and uses that the model will not work well for. -->
[More Information Needed]
## Bias, Risks, and Limitations
<!-- This section is meant to convey both technical and sociotechnical limitations. -->
[More Information Needed]
### Recommendations
<!-- This section is meant to convey recommendations with respect to the bias, risk, and technical limitations. -->
Users (both direct and downstream) should be made aware of the risks, biases and limitations of the model. More information needed for further recommendations.
## How to Get Started with the Model
Use the code below to get started with the model.
[More Information Needed]
## Training Details
### Training Data
<!-- This should link to a Dataset Card, perhaps with a short stub of information on what the training data is all about as well as documentation related to data pre-processing or additional filtering. -->
[More Information Needed]
### Training Procedure
<!-- This relates heavily to the Technical Specifications. Content here should link to that section when it is relevant to the training procedure. -->
#### Preprocessing [optional]
[More Information Needed]
#### Training Hyperparameters
- **Training regime:** [More Information Needed] <!--fp32, fp16 mixed precision, bf16 mixed precision, bf16 non-mixed precision, fp16 non-mixed precision, fp8 mixed precision -->
#### Speeds, Sizes, Times [optional]
<!-- This section provides information about throughput, start/end time, checkpoint size if relevant, etc. -->
[More Information Needed]
## Evaluation
<!-- This section describes the evaluation protocols and provides the results. -->
### Testing Data, Factors & Metrics
#### Testing Data
<!-- This should link to a Dataset Card if possible. -->
[More Information Needed]
#### Factors
<!-- These are the things the evaluation is disaggregating by, e.g., subpopulations or domains. -->
[More Information Needed]
#### Metrics
<!-- These are the evaluation metrics being used, ideally with a description of why. -->
[More Information Needed]
### Results
[More Information Needed]
#### Summary
## Model Examination [optional]
<!-- Relevant interpretability work for the model goes here -->
[More Information Needed]
## Environmental Impact
<!-- Total emissions (in grams of CO2eq) and additional considerations, such as electricity usage, go here. Edit the suggested text below accordingly -->
Carbon emissions can be estimated using the [Machine Learning Impact calculator](https://mlco2.github.io/impact#compute) presented in [Lacoste et al. (2019)](https://arxiv.org/abs/1910.09700).
- **Hardware Type:** [More Information Needed]
- **Hours used:** [More Information Needed]
- **Cloud Provider:** [More Information Needed]
- **Compute Region:** [More Information Needed]
- **Carbon Emitted:** [More Information Needed]
## Technical Specifications [optional]
### Model Architecture and Objective
[More Information Needed]
### Compute Infrastructure
[More Information Needed]
#### Hardware
[More Information Needed]
#### Software
[More Information Needed]
## Citation [optional]
**BibTeX:**
```
@article{zhao2024analysing,
title={Analysing The Impact of Sequence Composition on Language Model Pre-Training},
author={Zhao, Yu and Qu, Yuanbin and Staniszewski, Konrad and Tworkowski, Szymon and Liu, Wei and Mi{\l}o{\'s}, Piotr and Wu, Yuxiang and Minervini, Pasquale},
journal={arXiv preprint arXiv:2402.13991},
year={2024}
}
```
**APA:**
[More Information Needed]
## Glossary [optional]
<!-- If relevant, include terms and calculations in this section that can help readers understand the model or model card. -->
[More Information Needed]
## More Information [optional]
[More Information Needed]
## Model Card Authors [optional]
[More Information Needed]
## Model Card Contact
[More Information Needed] |
PathFinderKR/Waktaverse-Llama-3-KO-8B-Instruct | PathFinderKR | "2024-06-09T06:34:25Z" | 2,173 | 1 | transformers | [
"transformers",
"safetensors",
"llama",
"text-generation",
"llama-3",
"conversational",
"ko",
"en",
"dataset:MarkrAI/KoCommercial-Dataset",
"base_model:meta-llama/Meta-Llama-3-8B-Instruct",
"license:llama3",
"autotrain_compatible",
"endpoints_compatible",
"text-generation-inference",
"region:us"
] | text-generation | "2024-04-19T01:35:21Z" | ---
language:
- ko
- en
license: llama3
library_name: transformers
tags:
- llama
- llama-3
base_model:
- meta-llama/Meta-Llama-3-8B-Instruct
datasets:
- MarkrAI/KoCommercial-Dataset
---
# Waktaverse-Llama-3-KO-8B-Instruct Model Card
## Model Details

Waktaverse-Llama-3-KO-8B-Instruct is a Korean language model developed by Waktaverse AI team.
This large language model is a specialized version of the Meta-Llama-3-8B-Instruct, tailored for Korean natural language processing tasks.
It is designed to handle a variety of complex instructions and generate coherent, contextually appropriate responses.
- **Developed by:** Waktaverse AI
- **Model type:** Large Language Model
- **Language(s) (NLP):** Korean, English
- **License:** [Llama3](https://llama.meta.com/llama3/license)
- **Finetuned from model:** [meta-llama/Meta-Llama-3-8B-Instruct](https://huggingface.co/meta-llama/Meta-Llama-3-8B-Instruct)
## Model Sources
- **Repository:** [GitHub](https://github.com/PathFinderKR/Waktaverse-LLM/tree/main)
- **Paper :** [More Information Needed]
## Uses
### Direct Use
The model can be utilized directly for tasks such as text completion, summarization, and question answering without any fine-tuning.
### Out-of-Scope Use
This model is not intended for use in scenarios that involve high-stakes decision-making including medical, legal, or safety-critical areas due to the potential risks of relying on automated decision-making.
Moreover, any attempt to deploy the model in a manner that infringes upon privacy rights or facilitates biased decision-making is strongly discouraged.
## Bias, Risks, and Limitations
While Waktaverse Llama 3 is a robust model, it shares common limitations associated with machine learning models including potential biases in training data, vulnerability to adversarial attacks, and unpredictable behavior under edge cases.
There is also a risk of cultural and contextual misunderstanding, particularly when the model is applied to languages and contexts it was not specifically trained on.
## How to Get Started with the Model
You can run conversational inference using the Transformers Auto classes.
We highly recommend that you add Korean system prompt for better output.
Adjust the hyperparameters as you need.
### Example Usage
```python
import torch
from transformers import AutoTokenizer, AutoModelForCausalLM
device = (
"cuda:0" if torch.cuda.is_available() else # Nvidia GPU
"mps" if torch.backends.mps.is_available() else # Apple Silicon GPU
"cpu"
)
model_id = "PathFinderKR/Waktaverse-Llama-3-KO-8B-Instruct"
tokenizer = AutoTokenizer.from_pretrained(model_id)
model = AutoModelForCausalLM.from_pretrained(
model_id,
torch_dtype=torch.bfloat16,
device_map=device,
)
################################################################################
# Generation parameters
################################################################################
num_return_sequences=1
max_new_tokens=1024
temperature=0.6
top_p=0.9
repetition_penalty=1.1
def prompt_template(system, user):
return (
"<|begin_of_text|><|start_header_id|>system<|end_header_id|>\n\n"
f"{system}<|eot_id|>"
"<|start_header_id|>user<|end_header_id|>\n\n"
f"{user}<|eot_id|>"
"<|start_header_id|>assistant<|end_header_id|>\n\n"
)
def generate_response(system ,user):
prompt = prompt_template(system, user)
input_ids = tokenizer.encode(
prompt,
add_special_tokens=False,
return_tensors="pt"
).to(device)
outputs = model.generate(
input_ids=input_ids,
pad_token_id=tokenizer.eos_token_id,
num_return_sequences=num_return_sequences,
max_new_tokens=max_new_tokens,
do_sample=True,
temperature=temperature,
top_p=top_p,
repetition_penalty=repetition_penalty
)
return tokenizer.decode(outputs[0], skip_special_tokens=False)
system_prompt = "다음 지시사항에 대한 응답을 작성해주세요."
user_prompt = "피보나치 수열에 대해 설명해주세요."
response = generate_response(system_prompt, user_prompt)
print(response)
```
### Example Output
```python
<|begin_of_text|><|start_header_id|>system<|end_header_id|>
다음 지시사항에 대한 응답을 작성해 주세요.<|eot_id|><|start_header_id|>user<|end_header_id|>
피보나치 수열에 대해 설명해주세요.<|eot_id|><|start_header_id|>assistant<|end_header_id|>
피보나치 수열은 수학에서 자주 사용되는 수열 중 하나로, 0과 1로 시작하여 다음 항이 이전 두 항의 합으로 구성됩니다. 피보나치 수열은 유명한 수학자 레온 알렉산드로비치 피보나치가 제안했으며, 그의 이름을 따서 명명되었습니다. 이 수열은 자연수와 정수를 포함하며, 각 항은 이전 두 항의 합입니다. 예를 들어, 첫 번째 항은 0이고 두 번째 항은 1이며, 세 번째 항은 2이고 네 번째 항은 3입니다. 피보나치 수열은 순차적으로 증가하는 특징이 있지만, 숫자가 커질수록 점점 더 빠르게 증가합니다. 피보나치 수열은 다양한 분야에서 사용되며, 수학, 컴퓨터 과학, 생물학 등에서 중요한 역할을 합니다.<|eot_id|>
```
## Training Details
### Training Data
The model is trained on the [MarkrAI/KoCommercial-Dataset](https://huggingface.co/datasets/MarkrAI/KoCommercial-Dataset), which consists of various commercial texts in Korean.
### Training Procedure
The model training used LoRA for computational efficiency. 0.04 billion parameters(0.51% of total parameters) were trained.
#### Training Hyperparameters
```python
################################################################################
# bitsandbytes parameters
################################################################################
load_in_4bit=True
bnb_4bit_compute_dtype=torch.bfloat16
bnb_4bit_quant_type="nf4"
bnb_4bit_use_double_quant=True
################################################################################
# LoRA parameters
################################################################################
task_type="CAUSAL_LM"
target_modules=["q_proj", "k_proj", "v_proj", "o_proj", "gate_proj", "up_proj", "down_proj"]
r=8
lora_alpha=16
lora_dropout=0.05
bias="none"
################################################################################
# TrainingArguments parameters
################################################################################
num_train_epochs=1
per_device_train_batch_size=1
gradient_accumulation_steps=1
gradient_checkpointing=True
learning_rate=2e-5
lr_scheduler_type="cosine"
warmup_ratio=0.1
optim = "paged_adamw_32bit"
weight_decay=0.01
################################################################################
# SFT parameters
################################################################################
max_seq_length=4096
packing=False
```
## Evaluation
### Metrics
- **Ko-HellaSwag:**
- **Ko-MMLU:**
- **Ko-Arc:**
- **Ko-Truthful QA:**
- **Ko-CommonGen V2:**
### Results
<table>
<tr>
<td><strong>Benchmark</strong>
</td>
<td><strong>Waktaverse Llama 3 8B</strong>
</td>
<td><strong>Llama 3 8B</strong>
</td>
</tr>
<tr>
<td>Ko-HellaSwag:
</td>
<td>0
</td>
<td>0
</td>
</tr>
<tr>
<td>Ko-MMLU:
</td>
<td>0
</td>
<td>0
</td>
</tr>
<tr>
<td>Ko-Arc:
</td>
<td>0
</td>
<td>0
</td>
</tr>
<tr>
<td>Ko-Truthful QA:
</td>
<td>0
</td>
<td>0
</td>
</tr>
<tr>
<td>Ko-CommonGen V2:
</td>
<td>0
</td>
<td>0
</td>
</table>
## Technical Specifications
### Compute Infrastructure
#### Hardware
- **GPU:** NVIDIA GeForce RTX 4080 SUPER
#### Software
- **Operating System:** Linux
- **Deep Learning Framework:** Hugging Face Transformers, PyTorch
### Training Details
- **Training time:** 80 hours
- More details on [Weights & Biases](https://wandb.ai/pathfinderkr/Waktaverse-Llama-3-KO-8B-Instruct?nw=nwuserpathfinderkr)
## Citation
**Waktaverse-Llama-3**
```
@article{waktaversellama3modelcard,
title={Waktaverse Llama 3 Model Card},
author={AI@Waktaverse},
year={2024},
url = {https://huggingface.co/PathFinderKR/Waktaverse-Llama-3-KO-8B-Instruct}
```
**Llama-3**
```
@article{llama3modelcard,
title={Llama 3 Model Card},
author={AI@Meta},
year={2024},
url = {https://github.com/meta-llama/llama3/blob/main/MODEL_CARD.md}
}
```
## Model Card Authors
[PathFinderKR](https://github.com/PathFinderKR) |
theintuitiveye/HARDblend | theintuitiveye | "2023-08-24T12:44:24Z" | 2,171 | 86 | diffusers | [
"diffusers",
"safetensors",
"stable-diffusion",
"text-to-image",
"art",
"en",
"license:creativeml-openrail-m",
"endpoints_compatible",
"diffusers:StableDiffusionPipeline",
"region:us"
] | text-to-image | "2023-02-01T11:10:05Z" | ---
title: HARDblend
colorFrom: green
colorTo: indigo
sdk: gradio
sdk_version: 3.11.0
pinned: false
license: creativeml-openrail-m
tags:
- stable-diffusion
- text-to-image
- art
inference: true
language:
- en
library_name: diffusers
---
# **HARDblend**
A versatile photorealistic NSFW capable model which is great at generating high quality portraits.
It is a finetuned model trained on ~500 portrait images merged with Hassanblend, Aeros, RealisticVision1.2, Delibrate, SxD, f222.
## Usage
Use stability ai VAE or bakedinVAE version for better results.
*RAW samples*

Help us to be able to create models of professional standards. Consider supporting us on [Patreon](https://www.patreon.com/intuitiveai) / [Ko-fi](https://ko-fi.com/intuitiveai) / [Paypal](https://www.paypal.com/paypalme/theintuitiveye).
## *Demo*
We support a [Gradio](https://github.com/gradio-app/gradio) Web UI to run HARDblend :
[](https://huggingface.co/spaces/theintuitiveye/HARDblend)
## *License*
This model is open access and available to all, with a CreativeML OpenRAIL-M license further specifying rights and usage. The CreativeML OpenRAIL License specifies :
- You can't use the model to deliberately produce nor share illegal or harmful outputs or content
- The authors claims no rights on the outputs you generate, you are free to use them and are accountable for their use which must not go against the provisions set in the license
- You may re-distribute the weights and use the model commercially and/or as a service. If you do, please be aware you have to include the same use restrictions as the ones in the license and share a copy of the CreativeML OpenRAIL-M to all your users (please read the license entirely and carefully) Please read the full license [here](https://huggingface.co/spaces/CompVis/stable-diffusion-license)
|
unsloth/tinyllama | unsloth | "2024-03-22T14:58:56Z" | 2,171 | 2 | transformers | [
"transformers",
"safetensors",
"llama",
"text-generation",
"unsloth",
"tinyllama",
"en",
"license:apache-2.0",
"autotrain_compatible",
"endpoints_compatible",
"text-generation-inference",
"region:us"
] | text-generation | "2024-01-01T07:20:19Z" | ---
language:
- en
license: apache-2.0
library_name: transformers
tags:
- unsloth
- transformers
- tinyllama
---
# Finetune Mistral, Gemma, Llama 2-5x faster with 70% less memory via Unsloth!
A reupload from https://huggingface.co/TinyLlama/TinyLlama-1.1B-intermediate-step-1431k-3T
We have a Google Colab Tesla T4 notebook for TinyLlama with 4096 max sequence length RoPE Scaling here: https://colab.research.google.com/drive/1AZghoNBQaMDgWJpi4RbffGM1h6raLUj9?usp=sharing
[<img src="https://raw.githubusercontent.com/unslothai/unsloth/main/images/Discord%20button.png" width="200"/>](https://discord.gg/u54VK8m8tk)
[<img src="https://raw.githubusercontent.com/unslothai/unsloth/main/images/buy%20me%20a%20coffee%20button.png" width="200"/>](https://ko-fi.com/unsloth)
[<img src="https://raw.githubusercontent.com/unslothai/unsloth/main/images/unsloth%20made%20with%20love.png" width="200"/>](https://github.com/unslothai/unsloth)
## ✨ Finetune for Free
All notebooks are **beginner friendly**! Add your dataset, click "Run All", and you'll get a 2x faster finetuned model which can be exported to GGUF, vLLM or uploaded to Hugging Face.
| Unsloth supports | Free Notebooks | Performance | Memory use |
|-----------------|--------------------------------------------------------------------------------------------------------------------------|-------------|----------|
| **Gemma 7b** | [▶️ Start on Colab](https://colab.research.google.com/drive/10NbwlsRChbma1v55m8LAPYG15uQv6HLo?usp=sharing) | 2.4x faster | 58% less |
| **Mistral 7b** | [▶️ Start on Colab](https://colab.research.google.com/drive/1Dyauq4kTZoLewQ1cApceUQVNcnnNTzg_?usp=sharing) | 2.2x faster | 62% less |
| **Llama-2 7b** | [▶️ Start on Colab](https://colab.research.google.com/drive/1lBzz5KeZJKXjvivbYvmGarix9Ao6Wxe5?usp=sharing) | 2.2x faster | 43% less |
| **TinyLlama** | [▶️ Start on Colab](https://colab.research.google.com/drive/1AZghoNBQaMDgWJpi4RbffGM1h6raLUj9?usp=sharing) | 3.9x faster | 74% less |
| **CodeLlama 34b** A100 | [▶️ Start on Colab](https://colab.research.google.com/drive/1y7A0AxE3y8gdj4AVkl2aZX47Xu3P1wJT?usp=sharing) | 1.9x faster | 27% less |
| **Mistral 7b** 1xT4 | [▶️ Start on Kaggle](https://www.kaggle.com/code/danielhanchen/kaggle-mistral-7b-unsloth-notebook) | 5x faster\* | 62% less |
| **DPO - Zephyr** | [▶️ Start on Colab](https://colab.research.google.com/drive/15vttTpzzVXv_tJwEk-hIcQ0S9FcEWvwP?usp=sharing) | 1.9x faster | 19% less |
- This [conversational notebook](https://colab.research.google.com/drive/1Aau3lgPzeZKQ-98h69CCu1UJcvIBLmy2?usp=sharing) is useful for ShareGPT ChatML / Vicuna templates.
- This [text completion notebook](https://colab.research.google.com/drive/1ef-tab5bhkvWmBOObepl1WgJvfvSzn5Q?usp=sharing) is for raw text. This [DPO notebook](https://colab.research.google.com/drive/15vttTpzzVXv_tJwEk-hIcQ0S9FcEWvwP?usp=sharing) replicates Zephyr.
- \* Kaggle has 2x T4s, but we use 1. Due to overhead, 1x T4 is 5x faster.
|
AI-Sweden-Models/gpt-sw3-356m | AI-Sweden-Models | "2024-01-29T13:20:22Z" | 2,170 | 1 | transformers | [
"transformers",
"pytorch",
"safetensors",
"gpt2",
"text-generation",
"da",
"sv",
"no",
"en",
"is",
"license:other",
"autotrain_compatible",
"endpoints_compatible",
"text-generation-inference",
"region:us"
] | text-generation | "2022-12-14T12:31:57Z" | ---
license: other
language:
- da
- sv
- 'no'
- en
- is
---
# Model description
[AI Sweden](https://huggingface.co/AI-Sweden-Models/)
**Base models**
[GPT-Sw3 126M](https://huggingface.co/AI-Sweden-Models/gpt-sw3-126m/) | [GPT-Sw3 356M](https://huggingface.co/AI-Sweden-Models/gpt-sw3-356m/) | [GPT-Sw3 1.3B](https://huggingface.co/AI-Sweden-Models/gpt-sw3-1.3b/)
[GPT-Sw3 6.7B](https://huggingface.co/AI-Sweden-Models/gpt-sw3-6.7b/) | [GPT-Sw3 6.7B v2](https://huggingface.co/AI-Sweden-Models/gpt-sw3-6.7b-v2/) | [GPT-Sw3 20B](https://huggingface.co/AI-Sweden-Models/gpt-sw3-20b/)
[GPT-Sw3 40B](https://huggingface.co/AI-Sweden-Models/gpt-sw3-40b/)
**Instruct models**
[GPT-Sw3 126M Instruct](https://huggingface.co/AI-Sweden-Models/gpt-sw3-126m-instruct/) | [GPT-Sw3 356M Instruct](https://huggingface.co/AI-Sweden-Models/gpt-sw3-356m-instruct/) | [GPT-Sw3 1.3B Instruct](https://huggingface.co/AI-Sweden-Models/gpt-sw3-1.3b-instruct/)
[GPT-Sw3 6.7B v2 Instruct](https://huggingface.co/AI-Sweden-Models/gpt-sw3-6.7b-v2-instruct/) | [GPT-Sw3 20B Instruct](https://huggingface.co/AI-Sweden-Models/gpt-sw3-20b-instruct/)
**Quantized models**
[GPT-Sw3 6.7B v2 Instruct 4-bit gptq](https://huggingface.co/AI-Sweden-Models/gpt-sw3-6.7b-v2-instruct-4bit-gptq) | [GPT-Sw3 20B Instruct 4-bit gptq](https://huggingface.co/AI-Sweden-Models/gpt-sw3-20b-instruct-4bit-gptq)
GPT-SW3 is a collection of large decoder-only pretrained transformer language models that were developed by AI Sweden in collaboration with RISE and the WASP WARA for Media and Language. GPT-SW3 has been trained on a dataset containing 320B tokens in Swedish, Norwegian, Danish, Icelandic, English, and programming code. The model was pretrained using a causal language modeling (CLM) objective utilizing the NeMo Megatron GPT implementation.
# Intended use
GPT-SW3 is an autoregressive large language model that is capable of generating coherent text in 5 different languages, and 4 programming languages. GPT-SW3 can also be instructed to perform text tasks that it has not been explicitly trained for, by casting them as text generation tasks. AI Sweden shares GPT-SW3 in a controlled pre-release with organizations and individuals in the Nordic NLP ecosystem who can contribute to the validation and testing of the models and provide feedback to the community. This is an important step in the process of validating the model and collecting feedback on both what works well and what does not.
# Limitations
Like other large language models for which the diversity (or lack thereof) of training data induces downstream impact on the quality of our model, GPT-SW3 has limitations in terms of for example bias and safety. GPT-SW3 can also have quality issues in terms of generation diversity and hallucination. By releasing with the modified RAIL license, we also hope to increase communication, transparency, and the study of large language models. The model may: overrepresent some viewpoints and underrepresent others, contain stereotypes, generate hateful, abusive, violent, discriminatory or prejudicial language. The model may make errors, including producing incorrect information as if it were factual, it may generate irrelevant or repetitive outputs, and content that may not be appropriate for all settings, including sexual content.
# How to use
To be able to access the model from Python, since this is a private repository, you have to log in with your access token. This can be done with `huggingface-cli login`, see [HuggingFace Quick Start Guide](https://huggingface.co/docs/huggingface_hub/quick-start#login) for more information.
The following code snippet loads our tokenizer & model, and uses the GPU if available.
```python
import torch
from transformers import pipeline, AutoTokenizer, AutoModelForCausalLM
# Initialize Variables
model_name = "AI-Sweden-Models/gpt-sw3-356m"
device = "cuda:0" if torch.cuda.is_available() else "cpu"
prompt = "Träd är fina för att"
# Initialize Tokenizer & Model
tokenizer = AutoTokenizer.from_pretrained(model_name)
model = AutoModelForCausalLM.from_pretrained(model_name)
model.eval()
model.to(device)
```
Generating text using the `generate` method is done as follows:
```python
input_ids = tokenizer(prompt, return_tensors="pt")["input_ids"].to(device)
generated_token_ids = model.generate(
inputs=input_ids,
max_new_tokens=100,
do_sample=True,
temperature=0.6,
top_p=1,
)[0]
generated_text = tokenizer.decode(generated_token_ids)
```
A convenient alternative to the `generate` method is the HuggingFace pipeline, which handles most of the work for you:
```python
generator = pipeline('text-generation', tokenizer=tokenizer, model=model, device=device)
generated = generator(prompt, max_new_tokens=100, do_sample=True, temperature=0.6, top_p=1)[0]["generated_text"]
```
# Compliance
The release of GPT-SW3 consists of model weights, a configuration file, a tokenizer file and a vocabulary file. None of these files contain any personally identifiable information (PII) or any copyrighted material.
# GPT-SW3 Model Card
Following Mitchell et al. (2018), we provide a model card for GPT-SW3.
# Model Details
- Person or organization developing model: GPT-SW3 was developed by AI Sweden in collaboration with RISE and the WASP WARA for Media and Language.
- Model date: GPT-SW3 date of release 2022-12-20
- Model version: This is the second generation of GPT-SW3.
- Model type: GPT-SW3 is a large decoder-only transformer language model.
- Information about training algorithms, parameters, fairness constraints or other applied approaches, and features: GPT-SW3 was trained with the NeMo Megatron GPT implementation.
- Paper or other resource for more information: N/A.
- License: [LICENSE](https://huggingface.co/AI-Sweden-Models/gpt-sw3-356m/blob/main/LICENSE).
- Where to send questions or comments about the model: [email protected]
# Intended Use
- Primary intended uses: We pre-release GPT-SW3 for research and evaluation of the capabilities of Large Language Models for the Nordic languages. This is an important step in the process of knowledge building for LLMs, validating the model and collecting feedback on both what works well and what does not.
- Primary intended users: Organizations and individuals in the Nordic NLP ecosystem who can contribute to the validation and testing of the models and provide feedback to the community.
- Out-of-scope use cases: See the modified RAIL license.
# Data, Limitations, and Recommendations
- Data selection for training: Training data for GPT-SW3 was selected based on a combination of breadth and availability. See our Datasheet for more detailed information on the data used to train our model.
- Data selection for evaluation: N/A
- Limitations: Like other large language models for which the diversity (or lack thereof) of training data induces downstream impact on the quality of our model, GPT-SW3 has limitations in terms of bias and safety. GPT-SW3 can also have quality issues in terms of generation diversity and hallucination. In general, GPT-SW3 is not immune from the plethora of issues that plague modern large language models. By releasing with the modified RAIL license, we also hope to increase communication, transparency, and the study of large language models. The model may: Overrepresent some viewpoints and underrepresent others. Contain stereotypes. Generate: Hateful, abusive, or violent language. Discriminatory or prejudicial language. Content that may not be appropriate for all settings, including sexual content. Make errors, including producing incorrect information as if it were factual. Generate irrelevant or repetitive outputs.
- Recommendations for future work: Indirect users should be made aware when the content they're working with is created by the LLM. Users should be aware of Risks and Limitations, and include an appropriate age disclaimer or blocking interface as necessary. Models pretrained with the LLM should include an updated Model Card. Users of the model should provide mechanisms for those affected to provide feedback, such as an email address for comments.
- We hope that the release of GPT-SW3, as well as information around our model training process, will increase open science around both large language models in specific and natural language processing and deep learning in general.
# GPT-SW3 Datasheet
- We follow the recommendations of Gebru et al. (2021) and provide a datasheet for the dataset used to train GPT-SW3.
# Motivation
- For what purpose was the dataset created? Was there a specific task in mind? Was there a specific gap that needed to be filled? Please provide a description. Pre-training of Large Language Models (LLM), such as GPT-3 (T. B. Brown et al., 2020), Gopher (J. W. Rae et al., 2022), BLOOM (T. L. Scao et al., 2022), etc. require 100s or even 1000s GBs of text data, with recent studies (Chinchilla: J. Hoffmann et al., 2022) suggesting that the scale of the training data is even more important than previously imagined. Therefore, in order to train Swedish LLMs, we needed a large scale Swedish dataset of high quality. Since no such datasets existed before this initiative, we collected data in the Nordic and English languages.
- Who created the dataset (e.g., which team, research group) and on behalf of which entity (e.g., company, institution, organization)? The Strategic Initiative Natural Language Understanding at AI Sweden has established a new research environment in which collaboration is key. The core team working on the creation of the dataset is the NLU research group at AI Sweden. This group consists of researchers and developers from AI Sweden (Lindholmen Science Park AB) and RISE.
- Who funded the creation of the dataset? If there is an associated grant, please provide the name of the grantor and the grant name and number. The Swedish Innovation Agency (Vinnova) has funded this work across several different grants, including 2019-02996 and 2022-00949.
- Any other comments? No.
# Composition
- What do the instances that comprise the dataset represent (e.g., documents, photos, people, countries)? Are there multiple types of instances (e.g., movies, users, and ratings; people and interactions between them; nodes and edges)? Please provide a description. The instances are textual documents categorized by language and document type. The dataset is a filtered and deduplicated collection that includes the following sources:
- Books
- Litteraturbanken (https://litteraturbanken.se/)
- The Pile
- Articles
- Diva (https://www.diva-portal.org/)
- The Pile: PubMed
- The Pile: ArXiv
- Code
- Code Parrot: Github code (https://huggingface.co/datasets/codeparrot/github-code)
- Conversational
- Familjeliv (https://www.familjeliv.se/)
- Flashback (https://flashback.se/)
- Datasets collected through Parlai (see Appendix in data paper for complete list) (https://github.com/facebookresearch/ParlAI)
- Pushshift.io Reddit dataset, developed in Baumgartner et al. (2020) and processed in Roller et al. (2021)
- Math
- English Math dataset generated with code from DeepMind (D. Saxton et al., 2019)
- Swedish Math dataset, generated as above with manually translated templates
- Miscellaneous
- Summarization data (https://www.ida.liu.se/~arnjo82/papers/clarin-21-julius.pdf)
- OPUS, the open parallel corpus (https://opus.nlpl.eu/)
- Movie scripts (https://github.com/Aveek-Saha/Movie-Script-Database)
- Natural Instructions (https://github.com/allenai/natural-instructions)
- P3 (Public Pool of Prompts), (https://huggingface.co/datasets/bigscience/P3)
- The Norwegian Colossal Corpus (https://huggingface.co/datasets/NbAiLab/NCC)
- Danish Gigaword (https://gigaword.dk/)
- Icelandic Gigaword (https://clarin.is/en/resources/gigaword/)
- The Pile: Stack Exchange
- Web Common Crawl
- Web data from the project LES (Linguistic Explorations of Societies, https://les.gu.se).
- Multilingual C4 (MC4), prepared by AllenAI from C4 (C. Raffel et al., 2019)
- Open Super-large Crawled Aggregated coRpus (OSCAR) (P. O. Suarez, 2019)
- The Pile: Open Web Text
- Web Sources
- Various public Swedish website scrapes (see Appendix in data paper)
- Familjeliv Articles
- Public Swedish Job Ads from JobTech/Arbetsförmedlingen
- Wikipedia
- Official Wikipedia dumps
- How many instances are there in total (of each type, if appropriate)? The training data consists of 1.1TB UTF-8 encoded text, containing 660M documents with a total of 320B tokens.
- Does the dataset contain all possible instances or is it a sample (not necessarily random) of instances from a larger set? If the dataset is a sample, then what is the larger set? Is the sample representative of the larger set (e.g., geographic coverage)? If so, please describe how this representativeness was validated/verified. If it is not representative of the larger set, please describe why not (e.g., to cover a more diverse range of instances, because instances were withheld or unavailable). The subset of our dataset that comes from multilingual Common Crawl datasets (MC4, Oscar), are filtered by language to only include Swedish, Norwegian, Danish, and Icelandic. From The Pile, we included only the parts that typically are of highest textual quality or complemented the rest of our dataset with sources we otherwise lacked (e.g. books). The remainder of the dataset was collected from the above sources.
- What data does each instance consist of? “Raw” data (e.g., unprocessed text or images) or features? In either case, please provide a description. Each instance consists of raw text data.
- Is there a label or target associated with each instance? If so, please provide a description. No.
- Is any information missing from individual instances? If so, please provide a description, explaining why this information is missing (e.g., because it was unavailable). This does not include intentionally removed information, but might include, e.g., redacted text. No.
- Are relationships between individual instances made explicit (e.g., users’ movie ratings, social network links)? If so, please describe how these relationships are made explicit. There are no explicit relationships between individual instances.
- Are there recommended data splits (e.g., training, development/validation, testing)? If so, please provide a description of these splits, explaining the rationale behind them. There are no explicit splits recommended for this dataset. When pre-training the model, a random split for train, dev, test is set to 99.99%, 0.08%, 0.02% respectively, and is sampled proportionally to each subset’s weight and size. The weight of each subset was manually decided beforehand. These decisions were made considering the data’s value, source, and language, to form a representative and balanced pre-training corpus.
- Are there any errors, sources of noise, or redundancies in the dataset? If so, please provide a description. The dataset is a collection of many sources, some of which naturally contain some overlap. Although we have performed deduplication, some overlap may still remain. Furthermore, there may be some noise remaining from artifacts originating in Common Crawl datasets, that have been missed by our data filtering process. Except for these, we are not aware of any errors, sources of noise, or redundancies.
- Is the dataset self-contained, or does it link to or otherwise rely on external resources (e.g., websites, tweets, other datasets)? The dataset is self-contained.
- Does the dataset contain data that, if viewed directly, might be offensive, insulting, threatening, or might otherwise cause anxiety? If so, please describe why. The dataset contains subsets of public Common Crawl, Reddit, Familjeliv and Flashback. These could contain sentences that, if viewed directly, might be offensive, insulting, threatening, or might otherwise cause anxiety.
- Does the dataset relate to people? If not, you may skip the remaining questions in this section. Some documents of this data relate to people, such as news articles, Wikipedia descriptions, etc.
- Does the dataset identify any subpopulations (e.g., by age, gender)? If so, please describe how these subpopulations are identified and provide a description of their respective distributions within the dataset. No, the dataset does not explicitly include subpopulation identification.
- Any other comments? No.
# Collection Process
- How was the data associated with each instance acquired? Was the data directly observable (e.g., raw text, movie ratings), reported by subjects (e.g., survey responses), or indirectly inferred/derived from other data (e.g., part-of-speech tags, model-based guesses for age or language)? If data was reported by subjects or indirectly inferred/derived from other data, was the data validated/verified? If so, please describe how. N/A. The dataset is a union of publicly available datasets and sources.
- What mechanisms or procedures were used to collect the data (e.g., hardware apparatus or sensor, manual human curation, software program, software API)? How were these mechanisms or procedures validated? The data was downloaded from the internet.
- If the dataset is a sample from a larger set, what was the sampling strategy (e.g., deterministic, probabilistic with specific sampling probabilities)? Please see previous answers for how parts of the dataset were selected.
- Who was involved in the data collection process (e.g., students, crowdworkers, contractors) and how were they compensated (e.g., how much were crowdworkers paid)? This data is mined, filtered and sampled by machines.
- Over what timeframe was the data collected? Does this timeframe match the creation timeframe of the data associated with the instances (e.g., recent crawl of old news articles)? If not, please describe the timeframe in which the data associated with the instances was created. The dataset was collected during the period June 2021 to June 2022. The creation of the collected sources varies, with e.g. Common Crawl data that have been continuously collected over 12 years.
- Does the dataset relate to people? If not, you may skip the remainder of the questions in this section. Yes. The texts have been produced by people. Any personal information potentially present in publicly available data sources and thus in the created dataset is of no interest to the collection and use of the dataset.
- Has an analysis of the potential impact of the dataset and its use on data subjects (e.g., a data protection impact analysis) been conducted? If so, please provide a description of this analysis, including the outcomes, as well as a link or other access point to any supporting documentation. Yes.
- Any other comments? No.
- Preprocessing/cleaning/labeling
- Was any preprocessing/cleaning/labeling of the data done (e.g., discretization or bucketing, tokenization, part-of-speech tagging, SIFT feature extraction, removal of instances, processing of missing values)? If so, please provide a description. If not, you may skip the remainder of the questions in this section. The dataset was filtered and re-formatted on a document-level using standard procedures, inspired by the work in The BigScience ROOTS Corpus (H. Laurençon et al., 2022) and Gopher (J. W. Rae et al., 2022). This was done with the goal of achieving a consistent text format throughout the dataset, and to remove documents that did not meet our textual quality requirements (e.g. repetitiveness). Furthermore, the dataset was deduplicated to remedy the overlap between collected subsets using the MinHash algorithm, similar to the method used in GPT-3 and The Pile, and described in greater detail in “Deduplicating Training Data Makes Language Models Better” (K. Lee et al., 2021).
- Was the “raw” data saved in addition to the preprocessed/cleaned/labeled data (e.g., to support unanticipated future uses)? If so, please provide a link or other access point to the “raw” data. The “raw” component datasets are publicly available in their respective locations.
- Any other comments? No.
# Uses
- Has the dataset been used for any tasks already? If so, please provide a description. The dataset was used to pre-train the GPT-SW3 models.
- Is there a repository that links to any or all papers or systems that use the dataset? If so, please provide a link or other access point. N/A.
- What (other) tasks could the dataset be used for? The data can be used to pre-train language models, which are foundations for many current and future language tasks.
- Is there anything about the composition of the dataset or the way it was collected and preprocessed/cleaned/labeled that might impact future uses? For example, is there anything that a future user might need to know to avoid uses that could result in unfair treatment of individuals or groups (e.g., stereotyping, quality of service issues) or other undesirable harms (e.g., financial harms, legal risks) If so, please provide a description. Is there anything a future user could do to mitigate these undesirable harms? The dataset is probably quite representative of Swedish internet discourse in general, and of the Swedish public sector, but we know that this data does not necessarily reflect the entire Swedish population.
- Are there tasks for which the dataset should not be used? If so, please provide a description. None that we are currently aware of.
- Any other comments? No.
# Distribution
- Will the dataset be distributed to third parties outside of the entity (e.g., company, institution, organization) on behalf of which the dataset was created? If so, please provide a description. No.
- How will the dataset distributed (e.g., tarball on website, API, GitHub)? Does the dataset have a digital object identifier (DOI)? N/A.
- When will the dataset be distributed? N/A.
- Will the dataset be distributed under a copyright or other intellectual property (IP) license, and/or under applicable terms of use (ToU)? If so, please describe this license and/or ToU, and provide a link or other access point to, or otherwise reproduce, any relevant licensing terms or ToU, as well as any fees associated with these restrictions. N/A.
- Do any export controls or other regulatory restrictions apply to the dataset or to individual instances? If so, please describe these restrictions, and provide a link or other access point to, or otherwise reproduce, any supporting documentation. N/A.
- Any other comments? No.
# Maintenance
- Who is supporting/hosting/maintaining the dataset? AI Sweden at Lindholmen Science Park AB.
- How can the owner/curator/manager of the dataset be contacted (e.g., email address)? [email protected]
- Is there an erratum? If so, please provide a link or other access point. N/A.
- Will the dataset be updated (e.g., to correct labeling errors, add new instances, delete instances)? If so, please describe how often, by whom, and how updates will be communicated to users (e.g., mailing list, GitHub)? Currently, there are no plans for updating the dataset.
- If the dataset relates to people, are there applicable limits on the retention of the data associated with the instances (e.g., were individuals in question told that their data would be retained for a fixed period of time and then deleted)? If so, please describe these limits and explain how they will be enforced. Read the privacy policy for the NLU initiative at AI Sweden [here](https://www.ai.se/en/privacy-policy-nlu).
- Will older versions of the dataset continue to be supported/hosted/maintained? If so, please describe how. If not, please describe how its obsolescence will be communicated to users. N/A.
- If others want to extend/augment/build on/contribute to the dataset, is there a mechanism for them to do so? If so, please provide a description. Will these contributions be validated/ verified? If so, please describe how. If not, why not? Is there a process for communicating/ distributing these contributions to other users? If so, please provide a description. Not at this time.
- Any other comments? No. |
unsloth/gemma-7b-it | unsloth | "2024-04-18T15:04:51Z" | 2,170 | 8 | transformers | [
"transformers",
"safetensors",
"gemma",
"text-generation",
"unsloth",
"gemma-7b",
"conversational",
"en",
"license:apache-2.0",
"autotrain_compatible",
"endpoints_compatible",
"text-generation-inference",
"region:us"
] | text-generation | "2024-02-21T17:49:33Z" | ---
language:
- en
license: apache-2.0
library_name: transformers
tags:
- unsloth
- transformers
- gemma
- gemma-7b
---
# Finetune Mistral, Gemma, Llama 2-5x faster with 70% less memory via Unsloth!
[<img src="https://raw.githubusercontent.com/unslothai/unsloth/main/images/Discord%20button.png" width="200"/>](https://discord.gg/u54VK8m8tk)
[<img src="https://raw.githubusercontent.com/unslothai/unsloth/main/images/buy%20me%20a%20coffee%20button.png" width="200"/>](https://ko-fi.com/unsloth)
[<img src="https://raw.githubusercontent.com/unslothai/unsloth/main/images/unsloth%20made%20with%20love.png" width="200"/>](https://github.com/unslothai/unsloth)
## ✨ Finetune for Free
All notebooks are **beginner friendly**! Add your dataset, click "Run All", and you'll get a 2x faster finetuned model which can be exported to GGUF, vLLM or uploaded to Hugging Face.
| Unsloth supports | Free Notebooks | Performance | Memory use |
|-----------------|--------------------------------------------------------------------------------------------------------------------------|-------------|----------|
| **Gemma 7b** | [▶️ Start on Colab](https://colab.research.google.com/drive/10NbwlsRChbma1v55m8LAPYG15uQv6HLo?usp=sharing) | 2.4x faster | 58% less |
| **Mistral 7b** | [▶️ Start on Colab](https://colab.research.google.com/drive/1Dyauq4kTZoLewQ1cApceUQVNcnnNTzg_?usp=sharing) | 2.2x faster | 62% less |
| **Llama-2 7b** | [▶️ Start on Colab](https://colab.research.google.com/drive/1lBzz5KeZJKXjvivbYvmGarix9Ao6Wxe5?usp=sharing) | 2.2x faster | 43% less |
| **TinyLlama** | [▶️ Start on Colab](https://colab.research.google.com/drive/1AZghoNBQaMDgWJpi4RbffGM1h6raLUj9?usp=sharing) | 3.9x faster | 74% less |
| **CodeLlama 34b** A100 | [▶️ Start on Colab](https://colab.research.google.com/drive/1y7A0AxE3y8gdj4AVkl2aZX47Xu3P1wJT?usp=sharing) | 1.9x faster | 27% less |
| **Mistral 7b** 1xT4 | [▶️ Start on Kaggle](https://www.kaggle.com/code/danielhanchen/kaggle-mistral-7b-unsloth-notebook) | 5x faster\* | 62% less |
| **DPO - Zephyr** | [▶️ Start on Colab](https://colab.research.google.com/drive/15vttTpzzVXv_tJwEk-hIcQ0S9FcEWvwP?usp=sharing) | 1.9x faster | 19% less |
- This [conversational notebook](https://colab.research.google.com/drive/1Aau3lgPzeZKQ-98h69CCu1UJcvIBLmy2?usp=sharing) is useful for ShareGPT ChatML / Vicuna templates.
- This [text completion notebook](https://colab.research.google.com/drive/1ef-tab5bhkvWmBOObepl1WgJvfvSzn5Q?usp=sharing) is for raw text. This [DPO notebook](https://colab.research.google.com/drive/15vttTpzzVXv_tJwEk-hIcQ0S9FcEWvwP?usp=sharing) replicates Zephyr.
- \* Kaggle has 2x T4s, but we use 1. Due to overhead, 1x T4 is 5x faster.
|
RichardErkhov/Azure99_-_blossom-v5-9b-gguf | RichardErkhov | "2024-06-15T02:54:22Z" | 2,170 | 0 | null | [
"gguf",
"region:us"
] | null | "2024-06-15T02:01:42Z" | Quantization made by Richard Erkhov.
[Github](https://github.com/RichardErkhov)
[Discord](https://discord.gg/pvy7H8DZMG)
[Request more models](https://github.com/RichardErkhov/quant_request)
blossom-v5-9b - GGUF
- Model creator: https://huggingface.co/Azure99/
- Original model: https://huggingface.co/Azure99/blossom-v5-9b/
| Name | Quant method | Size |
| ---- | ---- | ---- |
| [blossom-v5-9b.Q2_K.gguf](https://huggingface.co/RichardErkhov/Azure99_-_blossom-v5-9b-gguf/blob/main/blossom-v5-9b.Q2_K.gguf) | Q2_K | 3.12GB |
| [blossom-v5-9b.IQ3_XS.gguf](https://huggingface.co/RichardErkhov/Azure99_-_blossom-v5-9b-gguf/blob/main/blossom-v5-9b.IQ3_XS.gguf) | IQ3_XS | 3.46GB |
| [blossom-v5-9b.IQ3_S.gguf](https://huggingface.co/RichardErkhov/Azure99_-_blossom-v5-9b-gguf/blob/main/blossom-v5-9b.IQ3_S.gguf) | IQ3_S | 3.64GB |
| [blossom-v5-9b.Q3_K_S.gguf](https://huggingface.co/RichardErkhov/Azure99_-_blossom-v5-9b-gguf/blob/main/blossom-v5-9b.Q3_K_S.gguf) | Q3_K_S | 3.63GB |
| [blossom-v5-9b.IQ3_M.gguf](https://huggingface.co/RichardErkhov/Azure99_-_blossom-v5-9b-gguf/blob/main/blossom-v5-9b.IQ3_M.gguf) | IQ3_M | 3.78GB |
| [blossom-v5-9b.Q3_K.gguf](https://huggingface.co/RichardErkhov/Azure99_-_blossom-v5-9b-gguf/blob/main/blossom-v5-9b.Q3_K.gguf) | Q3_K | 4.03GB |
| [blossom-v5-9b.Q3_K_M.gguf](https://huggingface.co/RichardErkhov/Azure99_-_blossom-v5-9b-gguf/blob/main/blossom-v5-9b.Q3_K_M.gguf) | Q3_K_M | 4.03GB |
| [blossom-v5-9b.Q3_K_L.gguf](https://huggingface.co/RichardErkhov/Azure99_-_blossom-v5-9b-gguf/blob/main/blossom-v5-9b.Q3_K_L.gguf) | Q3_K_L | 4.37GB |
| [blossom-v5-9b.IQ4_XS.gguf](https://huggingface.co/RichardErkhov/Azure99_-_blossom-v5-9b-gguf/blob/main/blossom-v5-9b.IQ4_XS.gguf) | IQ4_XS | 4.5GB |
| [blossom-v5-9b.Q4_0.gguf](https://huggingface.co/RichardErkhov/Azure99_-_blossom-v5-9b-gguf/blob/main/blossom-v5-9b.Q4_0.gguf) | Q4_0 | 4.69GB |
| [blossom-v5-9b.IQ4_NL.gguf](https://huggingface.co/RichardErkhov/Azure99_-_blossom-v5-9b-gguf/blob/main/blossom-v5-9b.IQ4_NL.gguf) | IQ4_NL | 4.73GB |
| [blossom-v5-9b.Q4_K_S.gguf](https://huggingface.co/RichardErkhov/Azure99_-_blossom-v5-9b-gguf/blob/main/blossom-v5-9b.Q4_K_S.gguf) | Q4_K_S | 4.72GB |
| [blossom-v5-9b.Q4_K.gguf](https://huggingface.co/RichardErkhov/Azure99_-_blossom-v5-9b-gguf/blob/main/blossom-v5-9b.Q4_K.gguf) | Q4_K | 4.96GB |
| [blossom-v5-9b.Q4_K_M.gguf](https://huggingface.co/RichardErkhov/Azure99_-_blossom-v5-9b-gguf/blob/main/blossom-v5-9b.Q4_K_M.gguf) | Q4_K_M | 4.96GB |
| [blossom-v5-9b.Q4_1.gguf](https://huggingface.co/RichardErkhov/Azure99_-_blossom-v5-9b-gguf/blob/main/blossom-v5-9b.Q4_1.gguf) | Q4_1 | 5.19GB |
| [blossom-v5-9b.Q5_0.gguf](https://huggingface.co/RichardErkhov/Azure99_-_blossom-v5-9b-gguf/blob/main/blossom-v5-9b.Q5_0.gguf) | Q5_0 | 5.69GB |
| [blossom-v5-9b.Q5_K_S.gguf](https://huggingface.co/RichardErkhov/Azure99_-_blossom-v5-9b-gguf/blob/main/blossom-v5-9b.Q5_K_S.gguf) | Q5_K_S | 5.69GB |
| [blossom-v5-9b.Q5_K.gguf](https://huggingface.co/RichardErkhov/Azure99_-_blossom-v5-9b-gguf/blob/main/blossom-v5-9b.Q5_K.gguf) | Q5_K | 5.83GB |
| [blossom-v5-9b.Q5_K_M.gguf](https://huggingface.co/RichardErkhov/Azure99_-_blossom-v5-9b-gguf/blob/main/blossom-v5-9b.Q5_K_M.gguf) | Q5_K_M | 5.83GB |
| [blossom-v5-9b.Q5_1.gguf](https://huggingface.co/RichardErkhov/Azure99_-_blossom-v5-9b-gguf/blob/main/blossom-v5-9b.Q5_1.gguf) | Q5_1 | 6.19GB |
| [blossom-v5-9b.Q6_K.gguf](https://huggingface.co/RichardErkhov/Azure99_-_blossom-v5-9b-gguf/blob/main/blossom-v5-9b.Q6_K.gguf) | Q6_K | 6.75GB |
| [blossom-v5-9b.Q8_0.gguf](https://huggingface.co/RichardErkhov/Azure99_-_blossom-v5-9b-gguf/blob/main/blossom-v5-9b.Q8_0.gguf) | Q8_0 | 8.74GB |
Original model description:
---
license: apache-2.0
datasets:
- Azure99/blossom-chat-v3
- Azure99/blossom-math-v4
- Azure99/blossom-wizard-v3
- Azure99/blossom-orca-v3
language:
- zh
- en
---
# **BLOSSOM-v5-9b**
[💻Github](https://github.com/Azure99/BlossomLM) • [🚀Blossom Chat Demo](https://blossom-chat.com/)
### What's new?
The Blossom V5 series models is fully trained using high-quality data distilled from gpt-4-0125-preview, resulting in significant improvements.
### Introduction
Blossom is a conversational large language model, fine-tuned on the Blossom Orca/Wizard/Chat/Math mixed dataset based on the Yi-9B pre-trained model. Blossom possesses robust general capabilities and context comprehension. Additionally, the high-quality Chinese and English datasets used for training have been made open source.
Training was conducted in two stages. The first stage used 40K Wizard, 40K Orca, 10K Math single-turn instruction datasets, training for 1 epoch; the second stage used 10K Blossom chat multi-turn dialogue dataset, and 10% randomly sampled data from the first stage, training for 3 epochs.
### Inference
Inference is performed in the form of dialogue continuation.
Single-turn dialogue
```
A chat between a human and an artificial intelligence bot. The bot gives helpful, detailed, and polite answers to the human's questions.
|Human|: hello
|Bot|:
```
Multi-turn dialogue
```
A chat between a human and an artificial intelligence bot. The bot gives helpful, detailed, and polite answers to the human's questions.
|Human|: hello
|Bot|: Hello! How can I assist you today?<|endoftext|>
|Human|: Generate a random number using python
|Bot|:
```
Note: At the end of the Bot's output in the historical conversation, append a `<|endoftext|>`.
|
NikolayKozloff/Gemma-2-9B-It-SPPO-Iter3-Q8_0-GGUF | NikolayKozloff | "2024-06-30T16:03:32Z" | 2,170 | 1 | null | [
"gguf",
"llama-cpp",
"gguf-my-repo",
"text-generation",
"en",
"dataset:openbmb/UltraFeedback",
"base_model:UCLA-AGI/Gemma-2-9B-It-SPPO-Iter3",
"license:apache-2.0",
"region:us"
] | text-generation | "2024-06-30T16:02:52Z" | ---
base_model: UCLA-AGI/Gemma-2-9B-It-SPPO-Iter3
datasets:
- openbmb/UltraFeedback
language:
- en
license: apache-2.0
pipeline_tag: text-generation
tags:
- llama-cpp
- gguf-my-repo
---
# NikolayKozloff/Gemma-2-9B-It-SPPO-Iter3-Q8_0-GGUF
This model was converted to GGUF format from [`UCLA-AGI/Gemma-2-9B-It-SPPO-Iter3`](https://huggingface.co/UCLA-AGI/Gemma-2-9B-It-SPPO-Iter3) using llama.cpp via the ggml.ai's [GGUF-my-repo](https://huggingface.co/spaces/ggml-org/gguf-my-repo) space.
Refer to the [original model card](https://huggingface.co/UCLA-AGI/Gemma-2-9B-It-SPPO-Iter3) for more details on the model.
## Use with llama.cpp
Install llama.cpp through brew (works on Mac and Linux)
```bash
brew install llama.cpp
```
Invoke the llama.cpp server or the CLI.
### CLI:
```bash
llama-cli --hf-repo NikolayKozloff/Gemma-2-9B-It-SPPO-Iter3-Q8_0-GGUF --hf-file gemma-2-9b-it-sppo-iter3-q8_0.gguf -p "The meaning to life and the universe is"
```
### Server:
```bash
llama-server --hf-repo NikolayKozloff/Gemma-2-9B-It-SPPO-Iter3-Q8_0-GGUF --hf-file gemma-2-9b-it-sppo-iter3-q8_0.gguf -c 2048
```
Note: You can also use this checkpoint directly through the [usage steps](https://github.com/ggerganov/llama.cpp?tab=readme-ov-file#usage) listed in the Llama.cpp repo as well.
Step 1: Clone llama.cpp from GitHub.
```
git clone https://github.com/ggerganov/llama.cpp
```
Step 2: Move into the llama.cpp folder and build it with `LLAMA_CURL=1` flag along with other hardware-specific flags (for ex: LLAMA_CUDA=1 for Nvidia GPUs on Linux).
```
cd llama.cpp && LLAMA_CURL=1 make
```
Step 3: Run inference through the main binary.
```
./llama-cli --hf-repo NikolayKozloff/Gemma-2-9B-It-SPPO-Iter3-Q8_0-GGUF --hf-file gemma-2-9b-it-sppo-iter3-q8_0.gguf -p "The meaning to life and the universe is"
```
or
```
./llama-server --hf-repo NikolayKozloff/Gemma-2-9B-It-SPPO-Iter3-Q8_0-GGUF --hf-file gemma-2-9b-it-sppo-iter3-q8_0.gguf -c 2048
```
|
fran-martinez/scibert_scivocab_cased_ner_jnlpba | fran-martinez | "2021-05-19T16:56:50Z" | 2,169 | 0 | transformers | [
"transformers",
"pytorch",
"jax",
"bert",
"token-classification",
"arxiv:1903.10676",
"autotrain_compatible",
"endpoints_compatible",
"region:us"
] | token-classification | "2022-03-02T23:29:05Z" | ---
language: scientific english
---
# SciBERT finetuned on JNLPA for NER downstream task
## Language Model
[SciBERT](https://arxiv.org/pdf/1903.10676.pdf) is a pretrained language model based on BERT and trained by the
[Allen Institute for AI](https://allenai.org/) on papers from the corpus of
[Semantic Scholar](https://www.semanticscholar.org/).
Corpus size is 1.14M papers, 3.1B tokens. SciBERT has its own vocabulary (scivocab) that's built to best match
the training corpus.
## Downstream task
[`allenai/scibert_scivocab_cased`](https://huggingface.co/allenai/scibert_scivocab_cased#) has been finetuned for Named Entity
Recognition (NER) dowstream task. The code to train the NER can be found [here](https://github.com/fran-martinez/bio_ner_bert).
### Data
The corpus used to fine-tune the NER is [BioNLP / JNLPBA shared task](http://www.geniaproject.org/shared-tasks/bionlp-jnlpba-shared-task-2004).
- Training data consist of 2,000 PubMed abstracts with term/word annotation. This corresponds to 18,546 samples (senteces).
- Evaluation data consist of 404 PubMed abstracts with term/word annotation. This corresponds to 3,856 samples (sentences).
The classes (at word level) and its distribution (number of examples for each class) for training and evaluation datasets are shown below:
| Class Label | # training examples| # evaluation examples|
|:--------------|--------------:|----------------:|
|O | 382,963 | 81,647 |
|B-protein | 30,269 | 5,067 |
|I-protein | 24,848 | 4,774 |
|B-cell_type | 6,718 | 1,921 |
|I-cell_type | 8,748 | 2,991 |
|B-DNA | 9,533 | 1,056 |
|I-DNA | 15,774 | 1,789 |
|B-cell_line | 3,830 | 500 |
|I-cell_line | 7,387 | 9,89 |
|B-RNA | 951 | 118 |
|I-RNA | 1,530 | 187 |
### Model
An exhaustive hyperparameter search was done.
The hyperparameters that provided the best results are:
- Max length sequence: 128
- Number of epochs: 6
- Batch size: 32
- Dropout: 0.3
- Optimizer: Adam
The used learning rate was 5e-5 with a decreasing linear schedule. A warmup was used at the beggining of the training
with a ratio of steps equal to 0.1 from the total training steps.
The model from the epoch with the best F1-score was selected, in this case, the model from epoch 5.
### Evaluation
The following table shows the evaluation metrics calculated at span/entity level:
| | precision| recall| f1-score|
|:---------|-----------:|---------:|---------:|
cell_line | 0.5205 | 0.7100 | 0.6007 |
cell_type | 0.7736 | 0.7422 | 0.7576 |
protein | 0.6953 | 0.8459 | 0.7633 |
DNA | 0.6997 | 0.7894 | 0.7419 |
RNA | 0.6985 | 0.8051 | 0.7480 |
| | | |
**micro avg** | 0.6984 | 0.8076 | 0.7490|
**macro avg** | 0.7032 | 0.8076 | 0.7498 |
The macro F1-score is equal to 0.7498, compared to the value provided by the Allen Institute for AI in their
[paper](https://arxiv.org/pdf/1903.10676.pdf), which is equal to 0.7728. This drop in performance could be due to
several reasons, but one hypothesis could be the fact that the authors used an additional conditional random field,
while this model uses a regular classification layer with softmax activation on top of SciBERT model.
At word level, this model achieves a precision of 0.7742, a recall of 0.8536 and a F1-score of 0.8093.
### Model usage in inference
Use the pipeline:
````python
from transformers import pipeline
text = "Mouse thymus was used as a source of glucocorticoid receptor from normal CS lymphocytes."
nlp_ner = pipeline("ner",
model='fran-martinez/scibert_scivocab_cased_ner_jnlpba',
tokenizer='fran-martinez/scibert_scivocab_cased_ner_jnlpba')
nlp_ner(text)
"""
Output:
---------------------------
[
{'word': 'glucocorticoid',
'score': 0.9894881248474121,
'entity': 'B-protein'},
{'word': 'receptor',
'score': 0.989505410194397,
'entity': 'I-protein'},
{'word': 'normal',
'score': 0.7680378556251526,
'entity': 'B-cell_type'},
{'word': 'cs',
'score': 0.5176806449890137,
'entity': 'I-cell_type'},
{'word': 'lymphocytes',
'score': 0.9898491501808167,
'entity': 'I-cell_type'}
]
"""
````
Or load model and tokenizer as follows:
````python
import torch
from transformers import AutoTokenizer, AutoModelForTokenClassification
# Example
text = "Mouse thymus was used as a source of glucocorticoid receptor from normal CS lymphocytes."
# Load model
tokenizer = AutoTokenizer.from_pretrained("fran-martinez/scibert_scivocab_cased_ner_jnlpba")
model = AutoModelForTokenClassification.from_pretrained("fran-martinez/scibert_scivocab_cased_ner_jnlpba")
# Get input for BERT
input_ids = torch.tensor(tokenizer.encode(text)).unsqueeze(0)
# Predict
with torch.no_grad():
outputs = model(input_ids)
# From the output let's take the first element of the tuple.
# Then, let's get rid of [CLS] and [SEP] tokens (first and last)
predictions = outputs[0].argmax(axis=-1)[0][1:-1]
# Map label class indexes to string labels.
for token, pred in zip(tokenizer.tokenize(text), predictions):
print(token, '->', model.config.id2label[pred.numpy().item()])
"""
Output:
---------------------------
mouse -> O
thymus -> O
was -> O
used -> O
as -> O
a -> O
source -> O
of -> O
glucocorticoid -> B-protein
receptor -> I-protein
from -> O
normal -> B-cell_type
cs -> I-cell_type
lymphocytes -> I-cell_type
. -> O
"""
````
|
Intel/distilbert-base-uncased-distilled-squad-int8-static-inc | Intel | "2024-03-29T14:23:53Z" | 2,169 | 2 | transformers | [
"transformers",
"pytorch",
"onnx",
"distilbert",
"question-answering",
"neural-compressor",
"8-bit",
"int8",
"Intel® Neural Compressor",
"PostTrainingStatic",
"dataset:squad",
"license:apache-2.0",
"endpoints_compatible",
"region:us"
] | question-answering | "2022-08-04T08:18:57Z" | ---
license: apache-2.0
tags:
- neural-compressor
- 8-bit
- int8
- Intel® Neural Compressor
- PostTrainingStatic
- onnx
datasets:
- squad
metrics:
- f1
---
# Model Card for INT8 DistilBERT Base Uncased Fine-Tuned on SQuAD
This model is an INT8 quantized version of DistilBERT base uncased, which has been fine-tuned on the Stanford Question Answering Dataset (SQuAD). The quantization was performed using the Hugging Face's Optimum-Intel, leveraging the Intel® Neural Compressor.
| Model Detail | Description |
| ----------- | ----------- |
| Model Authors | Xin He Zixuan Cheng Yu Wenz |
| Date | Aug 4, 2022 |
| Version | The base model for this quantization process was distilbert-base-uncased-distilled-squad, a distilled version of BERT designed for the question-answering task. |
| Type | Language Model |
| Paper or Other Resources | Base Model: [distilbert-base-uncased-distilled-squad](https://huggingface.co/distilbert/distilbert-base-uncased-distilled-squad) |
| License | apache-2.0 |
| Questions or Comments | [Community Tab](https://huggingface.co/Intel/distilbert-base-uncased-distilled-squad-int8-static-inc/discussions) and [Intel DevHub Discord](https://discord.gg/rv2Gp55UJQ)|
| Quantization Details | The model underwent post-training static quantization to convert it from its original FP32 precision to INT8, optimizing for size and inference speed while aiming to retain as much of the original model's accuracy as possible.|
| Calibration Details | For PyTorch, the calibration dataloader was the train dataloader with a real sampling size of 304 due to the default calibration sampling size of 300 not being exactly divisible by the batch size of 8. For the ONNX version, the calibration was performed using the eval dataloader with a default calibration sampling size of 100. |
| Intended Use | Description |
| ----------- | ----------- |
| Primary intended uses | This model is intended for question-answering tasks, where it can provide answers to questions given a context passage. It is optimized for scenarios requiring fast inference and reduced model size without significantly compromising accuracy. |
| Primary intended users | Researchers, developers, and enterprises that require efficient, low-latency question answering capabilities in their applications, particularly where computational resources are limited. |
| Out-of-scope uses | |
# Evaluation
### PyTorch Version
This is an INT8 PyTorch model quantized with [huggingface/optimum-intel](https://github.com/huggingface/optimum-intel) through the usage of [Intel® Neural Compressor](https://github.com/intel/neural-compressor).
| |INT8|FP32|
|---|:---:|:---:|
| **Accuracy (eval-f1)** |86.1069|86.8374|
| **Model size (MB)** |74.7|265|
### ONNX Version
This is an INT8 ONNX model quantized with [Intel® Neural Compressor](https://github.com/intel/neural-compressor).
| |INT8|FP32|
|---|:---:|:---:|
| **Accuracy (eval-f1)** |0.8633|0.8687|
| **Model size (MB)** |154|254|
# Usage
**Optimum Intel w/ Neural Compressor**
```python
from optimum.intel import INCModelForQuestionAnswering
model_id = "Intel/distilbert-base-uncased-distilled-squad-int8-static"
int8_model = INCModelForQuestionAnswering.from_pretrained(model_id)
```
**Optimum w/ ONNX Runtime**
```python
from optimum.onnxruntime import ORTModelForQuestionAnswering
model = ORTModelForQuestionAnswering.from_pretrained('Intel/distilbert-base-uncased-distilled-squad-int8-static')
```
# Ethical Considerations
While not explicitly mentioned, users should be aware of potential biases present in the training data (SQuAD and Wikipedia), and consider the implications of these biases on the model's outputs. Additionally, quantization may introduce or exacerbate biases in certain scenarios.
# Caveats and Recommendations
- Users should consider the balance between performance and accuracy when deploying quantized models in critical applications.
- Further fine-tuning or calibration may be necessary for specific use cases or to meet stricter accuracy requirements. |
Bingsu/clip-vit-large-patch14-ko | Bingsu | "2022-11-18T02:13:00Z" | 2,169 | 11 | transformers | [
"transformers",
"pytorch",
"tf",
"safetensors",
"clip",
"zero-shot-image-classification",
"ko",
"arxiv:2004.09813",
"doi:10.57967/hf/1616",
"license:mit",
"endpoints_compatible",
"region:us"
] | zero-shot-image-classification | "2022-10-11T01:55:47Z" | ---
widget:
- src: https://huggingface.co/datasets/mishig/sample_images/resolve/main/cat-dog-music.png
candidate_labels: 기타 치는 고양이, 피아노 치는 강아지
example_title: Guitar, cat and dog
language: ko
license: mit
---
# clip-vit-large-patch14-ko
Korean CLIP model trained by [Making Monolingual Sentence Embeddings Multilingual using Knowledge Distillation](https://arxiv.org/abs/2004.09813)
[Making Monolingual Sentence Embeddings Multilingual using Knowledge Distillation](https://arxiv.org/abs/2004.09813)로 학습된 한국어 CLIP 모델입니다.
훈련 코드: <https://github.com/Bing-su/KoCLIP_training_code>
사용된 데이터: AIHUB에 있는 모든 한국어-영어 병렬 데이터
## How to Use
#### 1.
```python
import requests
import torch
from PIL import Image
from transformers import AutoModel, AutoProcessor
repo = "Bingsu/clip-vit-large-patch14-ko"
model = AutoModel.from_pretrained(repo)
processor = AutoProcessor.from_pretrained(repo)
url = "http://images.cocodataset.org/val2017/000000039769.jpg"
image = Image.open(requests.get(url, stream=True).raw)
inputs = processor(text=["고양이 두 마리", "개 두 마리"], images=image, return_tensors="pt", padding=True)
with torch.inference_mode():
outputs = model(**inputs)
logits_per_image = outputs.logits_per_image
probs = logits_per_image.softmax(dim=1)
```
```python
>>> probs
tensor([[0.9974, 0.0026]])
```
#### 2.
```python
from transformers import pipeline
repo = "Bingsu/clip-vit-large-patch14-ko"
pipe = pipeline("zero-shot-image-classification", model=repo)
url = "http://images.cocodataset.org/val2017/000000039769.jpg"
result = pipe(images=url, candidate_labels=["고양이 한 마리", "고양이 두 마리", "분홍색 소파에 드러누운 고양이 친구들"], hypothesis_template="{}")
```
```python
>>> result
[{'score': 0.9907576441764832, 'label': '분홍색 소파에 드러누운 고양이 친구들'},
{'score': 0.009206341579556465, 'label': '고양이 두 마리'},
{'score': 3.606083555496298e-05, 'label': '고양이 한 마리'}]
``` |
jondurbin/airoboros-7b | jondurbin | "2023-08-14T09:04:07Z" | 2,169 | 15 | transformers | [
"transformers",
"pytorch",
"llama",
"text-generation",
"license:cc-by-nc-4.0",
"autotrain_compatible",
"endpoints_compatible",
"text-generation-inference",
"region:us"
] | text-generation | "2023-05-18T21:56:37Z" | ---
license: cc-by-nc-4.0
---
# Overview
This is a fine-tuned 7b parameter LlaMa model, using completely synthetic training data created by https://github.com/jondurbin/airoboros
__*I don't recommend using this model! The outputs aren't particularly great, and it may contain "harmful" data due to jailbreak*__
Please see one of the updated airoboros models for a much better experience.
### Training data
This was an experiment to see if a "jailbreak" prompt could be used to generate a broader range of data that would otherwise have been filtered by OpenAI's alignment efforts.
The jailbreak did indeed work with a high success rate, and caused OpenAI to generate a broader range of topics and fewer refusals to answer questions/instructions of sensitive topics.
### Usage and License Notices
All airoboros models and datasets are intended and licensed for research use only. I've used the 'cc-nc-4.0' license, but really it is subject to a custom/special license because:
- the base model is LLaMa, which has it's own special research license
- the dataset(s) were generated with OpenAI (gpt-4 and/or gpt-3.5-turbo), which has a clausing saying the data can't be used to create models to compete with openai
So, to reiterate: this model (and datasets) cannot be used commercially. |
RichardErkhov/AtAndDev_-_Ogno-Monarch-Neurotic-9B-Passthrough-gguf | RichardErkhov | "2024-06-15T02:43:50Z" | 2,169 | 0 | null | [
"gguf",
"region:us"
] | null | "2024-06-15T01:46:50Z" | Quantization made by Richard Erkhov.
[Github](https://github.com/RichardErkhov)
[Discord](https://discord.gg/pvy7H8DZMG)
[Request more models](https://github.com/RichardErkhov/quant_request)
Ogno-Monarch-Neurotic-9B-Passthrough - GGUF
- Model creator: https://huggingface.co/AtAndDev/
- Original model: https://huggingface.co/AtAndDev/Ogno-Monarch-Neurotic-9B-Passthrough/
| Name | Quant method | Size |
| ---- | ---- | ---- |
| [Ogno-Monarch-Neurotic-9B-Passthrough.Q2_K.gguf](https://huggingface.co/RichardErkhov/AtAndDev_-_Ogno-Monarch-Neurotic-9B-Passthrough-gguf/blob/main/Ogno-Monarch-Neurotic-9B-Passthrough.Q2_K.gguf) | Q2_K | 3.13GB |
| [Ogno-Monarch-Neurotic-9B-Passthrough.IQ3_XS.gguf](https://huggingface.co/RichardErkhov/AtAndDev_-_Ogno-Monarch-Neurotic-9B-Passthrough-gguf/blob/main/Ogno-Monarch-Neurotic-9B-Passthrough.IQ3_XS.gguf) | IQ3_XS | 3.48GB |
| [Ogno-Monarch-Neurotic-9B-Passthrough.IQ3_S.gguf](https://huggingface.co/RichardErkhov/AtAndDev_-_Ogno-Monarch-Neurotic-9B-Passthrough-gguf/blob/main/Ogno-Monarch-Neurotic-9B-Passthrough.IQ3_S.gguf) | IQ3_S | 3.67GB |
| [Ogno-Monarch-Neurotic-9B-Passthrough.Q3_K_S.gguf](https://huggingface.co/RichardErkhov/AtAndDev_-_Ogno-Monarch-Neurotic-9B-Passthrough-gguf/blob/main/Ogno-Monarch-Neurotic-9B-Passthrough.Q3_K_S.gguf) | Q3_K_S | 3.65GB |
| [Ogno-Monarch-Neurotic-9B-Passthrough.IQ3_M.gguf](https://huggingface.co/RichardErkhov/AtAndDev_-_Ogno-Monarch-Neurotic-9B-Passthrough-gguf/blob/main/Ogno-Monarch-Neurotic-9B-Passthrough.IQ3_M.gguf) | IQ3_M | 3.79GB |
| [Ogno-Monarch-Neurotic-9B-Passthrough.Q3_K.gguf](https://huggingface.co/RichardErkhov/AtAndDev_-_Ogno-Monarch-Neurotic-9B-Passthrough-gguf/blob/main/Ogno-Monarch-Neurotic-9B-Passthrough.Q3_K.gguf) | Q3_K | 4.05GB |
| [Ogno-Monarch-Neurotic-9B-Passthrough.Q3_K_M.gguf](https://huggingface.co/RichardErkhov/AtAndDev_-_Ogno-Monarch-Neurotic-9B-Passthrough-gguf/blob/main/Ogno-Monarch-Neurotic-9B-Passthrough.Q3_K_M.gguf) | Q3_K_M | 4.05GB |
| [Ogno-Monarch-Neurotic-9B-Passthrough.Q3_K_L.gguf](https://huggingface.co/RichardErkhov/AtAndDev_-_Ogno-Monarch-Neurotic-9B-Passthrough-gguf/blob/main/Ogno-Monarch-Neurotic-9B-Passthrough.Q3_K_L.gguf) | Q3_K_L | 4.41GB |
| [Ogno-Monarch-Neurotic-9B-Passthrough.IQ4_XS.gguf](https://huggingface.co/RichardErkhov/AtAndDev_-_Ogno-Monarch-Neurotic-9B-Passthrough-gguf/blob/main/Ogno-Monarch-Neurotic-9B-Passthrough.IQ4_XS.gguf) | IQ4_XS | 4.55GB |
| [Ogno-Monarch-Neurotic-9B-Passthrough.Q4_0.gguf](https://huggingface.co/RichardErkhov/AtAndDev_-_Ogno-Monarch-Neurotic-9B-Passthrough-gguf/blob/main/Ogno-Monarch-Neurotic-9B-Passthrough.Q4_0.gguf) | Q4_0 | 4.74GB |
| [Ogno-Monarch-Neurotic-9B-Passthrough.IQ4_NL.gguf](https://huggingface.co/RichardErkhov/AtAndDev_-_Ogno-Monarch-Neurotic-9B-Passthrough-gguf/blob/main/Ogno-Monarch-Neurotic-9B-Passthrough.IQ4_NL.gguf) | IQ4_NL | 4.79GB |
| [Ogno-Monarch-Neurotic-9B-Passthrough.Q4_K_S.gguf](https://huggingface.co/RichardErkhov/AtAndDev_-_Ogno-Monarch-Neurotic-9B-Passthrough-gguf/blob/main/Ogno-Monarch-Neurotic-9B-Passthrough.Q4_K_S.gguf) | Q4_K_S | 4.78GB |
| [Ogno-Monarch-Neurotic-9B-Passthrough.Q4_K.gguf](https://huggingface.co/RichardErkhov/AtAndDev_-_Ogno-Monarch-Neurotic-9B-Passthrough-gguf/blob/main/Ogno-Monarch-Neurotic-9B-Passthrough.Q4_K.gguf) | Q4_K | 5.04GB |
| [Ogno-Monarch-Neurotic-9B-Passthrough.Q4_K_M.gguf](https://huggingface.co/RichardErkhov/AtAndDev_-_Ogno-Monarch-Neurotic-9B-Passthrough-gguf/blob/main/Ogno-Monarch-Neurotic-9B-Passthrough.Q4_K_M.gguf) | Q4_K_M | 5.04GB |
| [Ogno-Monarch-Neurotic-9B-Passthrough.Q4_1.gguf](https://huggingface.co/RichardErkhov/AtAndDev_-_Ogno-Monarch-Neurotic-9B-Passthrough-gguf/blob/main/Ogno-Monarch-Neurotic-9B-Passthrough.Q4_1.gguf) | Q4_1 | 5.26GB |
| [Ogno-Monarch-Neurotic-9B-Passthrough.Q5_0.gguf](https://huggingface.co/RichardErkhov/AtAndDev_-_Ogno-Monarch-Neurotic-9B-Passthrough-gguf/blob/main/Ogno-Monarch-Neurotic-9B-Passthrough.Q5_0.gguf) | Q5_0 | 5.77GB |
| [Ogno-Monarch-Neurotic-9B-Passthrough.Q5_K_S.gguf](https://huggingface.co/RichardErkhov/AtAndDev_-_Ogno-Monarch-Neurotic-9B-Passthrough-gguf/blob/main/Ogno-Monarch-Neurotic-9B-Passthrough.Q5_K_S.gguf) | Q5_K_S | 5.77GB |
| [Ogno-Monarch-Neurotic-9B-Passthrough.Q5_K.gguf](https://huggingface.co/RichardErkhov/AtAndDev_-_Ogno-Monarch-Neurotic-9B-Passthrough-gguf/blob/main/Ogno-Monarch-Neurotic-9B-Passthrough.Q5_K.gguf) | Q5_K | 5.93GB |
| [Ogno-Monarch-Neurotic-9B-Passthrough.Q5_K_M.gguf](https://huggingface.co/RichardErkhov/AtAndDev_-_Ogno-Monarch-Neurotic-9B-Passthrough-gguf/blob/main/Ogno-Monarch-Neurotic-9B-Passthrough.Q5_K_M.gguf) | Q5_K_M | 5.93GB |
| [Ogno-Monarch-Neurotic-9B-Passthrough.Q5_1.gguf](https://huggingface.co/RichardErkhov/AtAndDev_-_Ogno-Monarch-Neurotic-9B-Passthrough-gguf/blob/main/Ogno-Monarch-Neurotic-9B-Passthrough.Q5_1.gguf) | Q5_1 | 6.29GB |
| [Ogno-Monarch-Neurotic-9B-Passthrough.Q6_K.gguf](https://huggingface.co/RichardErkhov/AtAndDev_-_Ogno-Monarch-Neurotic-9B-Passthrough-gguf/blob/main/Ogno-Monarch-Neurotic-9B-Passthrough.Q6_K.gguf) | Q6_K | 6.87GB |
| [Ogno-Monarch-Neurotic-9B-Passthrough.Q8_0.gguf](https://huggingface.co/RichardErkhov/AtAndDev_-_Ogno-Monarch-Neurotic-9B-Passthrough-gguf/blob/main/Ogno-Monarch-Neurotic-9B-Passthrough.Q8_0.gguf) | Q8_0 | 8.89GB |
Original model description:
---
license: apache-2.0
tags:
- merge
- mergekit
- lazymergekit
- bardsai/jaskier-7b-dpo-v5.6
- eren23/ogno-monarch-jaskier-merge-7b-OH-PREF-DPO
---
# Ogno-Monarch-Neurotic-7B-Passthrough
Ogno-Monarch-Neurotic-7B-Passthrough is a merge of the following models using [mergekit](https://github.com/cg123/mergekit):
* [bardsai/jaskier-7b-dpo-v5.6](https://huggingface.co/bardsai/jaskier-7b-dpo-v5.6)
* [eren23/ogno-monarch-jaskier-merge-7b-OH-PREF-DPO](https://huggingface.co/eren23/ogno-monarch-jaskier-merge-7b-OH-PREF-DPO)
## 🧩 Configuration
```yaml
slices:
- sources:
- model: bardsai/jaskier-7b-dpo-v5.6
layer_range: [0, 32]
- sources:
- model: eren23/ogno-monarch-jaskier-merge-7b-OH-PREF-DPO
layer_range: [24, 32]
merge_method: passthrough
dtype: bfloat16
```
|
MaziyarPanahi/mergekit-slerp-ozbsicw-GGUF | MaziyarPanahi | "2024-06-18T17:59:20Z" | 2,169 | 0 | transformers | [
"transformers",
"gguf",
"mistral",
"quantized",
"2-bit",
"3-bit",
"4-bit",
"5-bit",
"6-bit",
"8-bit",
"GGUF",
"safetensors",
"llama",
"text-generation",
"mergekit",
"merge",
"base_model:LargeWorldModel/LWM-Text-Chat-1M",
"autotrain_compatible",
"endpoints_compatible",
"text-generation-inference",
"region:us",
"base_model:mergekit-community/mergekit-slerp-ozbsicw"
] | text-generation | "2024-06-18T17:36:06Z" | ---
tags:
- quantized
- 2-bit
- 3-bit
- 4-bit
- 5-bit
- 6-bit
- 8-bit
- GGUF
- transformers
- safetensors
- llama
- text-generation
- mergekit
- merge
- base_model:LargeWorldModel/LWM-Text-Chat-1M
- autotrain_compatible
- endpoints_compatible
- text-generation-inference
- region:us
- text-generation
model_name: mergekit-slerp-ozbsicw-GGUF
base_model: mergekit-community/mergekit-slerp-ozbsicw
inference: false
model_creator: mergekit-community
pipeline_tag: text-generation
quantized_by: MaziyarPanahi
---
# [MaziyarPanahi/mergekit-slerp-ozbsicw-GGUF](https://huggingface.co/MaziyarPanahi/mergekit-slerp-ozbsicw-GGUF)
- Model creator: [mergekit-community](https://huggingface.co/mergekit-community)
- Original model: [mergekit-community/mergekit-slerp-ozbsicw](https://huggingface.co/mergekit-community/mergekit-slerp-ozbsicw)
## Description
[MaziyarPanahi/mergekit-slerp-ozbsicw-GGUF](https://huggingface.co/MaziyarPanahi/mergekit-slerp-ozbsicw-GGUF) contains GGUF format model files for [mergekit-community/mergekit-slerp-ozbsicw](https://huggingface.co/mergekit-community/mergekit-slerp-ozbsicw).
### About GGUF
GGUF is a new format introduced by the llama.cpp team on August 21st 2023. It is a replacement for GGML, which is no longer supported by llama.cpp.
Here is an incomplete list of clients and libraries that are known to support GGUF:
* [llama.cpp](https://github.com/ggerganov/llama.cpp). The source project for GGUF. Offers a CLI and a server option.
* [llama-cpp-python](https://github.com/abetlen/llama-cpp-python), a Python library with GPU accel, LangChain support, and OpenAI-compatible API server.
* [LM Studio](https://lmstudio.ai/), an easy-to-use and powerful local GUI for Windows and macOS (Silicon), with GPU acceleration. Linux available, in beta as of 27/11/2023.
* [text-generation-webui](https://github.com/oobabooga/text-generation-webui), the most widely used web UI, with many features and powerful extensions. Supports GPU acceleration.
* [KoboldCpp](https://github.com/LostRuins/koboldcpp), a fully featured web UI, with GPU accel across all platforms and GPU architectures. Especially good for story telling.
* [GPT4All](https://gpt4all.io/index.html), a free and open source local running GUI, supporting Windows, Linux and macOS with full GPU accel.
* [LoLLMS Web UI](https://github.com/ParisNeo/lollms-webui), a great web UI with many interesting and unique features, including a full model library for easy model selection.
* [Faraday.dev](https://faraday.dev/), an attractive and easy to use character-based chat GUI for Windows and macOS (both Silicon and Intel), with GPU acceleration.
* [candle](https://github.com/huggingface/candle), a Rust ML framework with a focus on performance, including GPU support, and ease of use.
* [ctransformers](https://github.com/marella/ctransformers), a Python library with GPU accel, LangChain support, and OpenAI-compatible AI server. Note, as of time of writing (November 27th 2023), ctransformers has not been updated in a long time and does not support many recent models.
## Special thanks
🙏 Special thanks to [Georgi Gerganov](https://github.com/ggerganov) and the whole team working on [llama.cpp](https://github.com/ggerganov/llama.cpp/) for making all of this possible. |
jondurbin/airoboros-33b-gpt4-1.2 | jondurbin | "2023-06-22T14:59:08Z" | 2,168 | 9 | transformers | [
"transformers",
"pytorch",
"llama",
"text-generation",
"dataset:jondurbin/airoboros-gpt4-1.2",
"license:cc-by-nc-4.0",
"autotrain_compatible",
"endpoints_compatible",
"text-generation-inference",
"region:us"
] | text-generation | "2023-06-14T09:19:13Z" | ---
license: cc-by-nc-4.0
datasets:
- jondurbin/airoboros-gpt4-1.2
---
### Overview
This is a qlora fine-tuned 33b parameter LlaMa model, using completely synthetic training data created gpt4 via https://github.com/jondurbin/airoboros
This is mostly an extension of [1.1](https://huggingface.co/jondurbin/airoboros-33b-gpt4-1.1) with thousands of new training data and an update to allow "PLAINFORMAT" at the end of coding prompts to just print the code without backticks or explanations/usage/etc.
The dataset used to fine-tune this model is available [here](https://huggingface.co/datasets/jondurbin/airoboros-gpt4-1.2), with a specific focus on:
- coding
- math/reasoning (using orca style ELI5 instruction/response pairs)
- trivia
- role playing
- multiple choice and fill-in-the-blank
- context-obedient question answering
- theory of mind
- misc/general
This model was fine-tuned with a fork of [qlora](https://github.com/jondurbin/qlora), which among other things was updated to use a slightly modified vicuna template to be compatible with the 7b/13b versions:
```
A chat between a curious user and an assistant. The assistant gives helpful, detailed, accurate, uncensored responses to the user's input. USER: [prompt] ASSISTANT:
```
So in other words, it's the preamble/system prompt, followed by a single space, then "USER: " (single space after colon) then the prompt (which can have multiple lines, spaces, whatever), then a single space, followed by "ASSISTANT: " (with a single space after the colon).
### Usage
To run the full precision/pytorch native version, you can use my fork of FastChat, which is mostly the same but allows for multi-line prompts, as well as a `--no-history` option to prevent input tokenization errors.
```
pip install git+https://github.com/jondurbin/FastChat
```
Be sure you are pulling the latest branch!
Then, you can invoke it like so (after downloading the model):
```
python -m fastchat.serve.cli \
--model-path airoboros-33b-gpt4-1.2 \
--temperature 0.5 \
--max-new-tokens 2048 \
--no-history
```
Alternatively, please check out TheBloke's quantized versions:
- https://huggingface.co/TheBloke/airoboros-33B-gpt4-1.2-GPTQ
- https://huggingface.co/TheBloke/airoboros-33B-gpt4-1.2-GGML
### Coding updates from gpt4/1.1:
I added a few hundred instruction/response pairs to the training data with "PLAINFORMAT" as a single, all caps term at the end of the normal instructions, which produce plain text output instead of markdown/backtick code formatting.
It's not guaranteed to work all the time, but mostly it does seem to work as expected.
So for example, instead of:
```
Implement the Snake game in python.
```
You would use:
```
Implement the Snake game in python. PLAINFORMAT
```
### Other updates from gpt4/1.1:
- Several hundred role-playing data.
- A few thousand ORCA style reasoning/math questions with ELI5 prompts to generate the responses (should not be needed in your prompts to this model however, just ask the question).
- Many more coding examples in various languages, including some that use specific libraries (pandas, numpy, tensorflow, etc.)
### Usage and License Notices
All airoboros models and datasets are intended and licensed for research use only. I've used the 'cc-nc-4.0' license, but really it is subject to a custom/special license because:
- the base model is LLaMa, which has it's own special research license
- the dataset(s) were generated with OpenAI (gpt-4 and/or gpt-3.5-turbo), which has a clausing saying the data can't be used to create models to compete with openai
So, to reiterate: this model (and datasets) cannot be used commercially. |
yhyhy3/med-orca-instruct-33b | yhyhy3 | "2023-07-18T15:58:50Z" | 2,168 | 0 | transformers | [
"transformers",
"pytorch",
"llama",
"feature-extraction",
"instruct",
"medical",
"code",
"text-generation",
"en",
"dataset:ehartford/dolphin",
"dataset:LinhDuong/chatdoctor-200k",
"dataset:sahil2801/code_instructions_120k",
"dataset:c-s-ale/dolly-15k-instruction-alpaca-format",
"dataset:tiiuae/falcon-refinedweb",
"dataset:bigcode/starcoderdata",
"dataset:togethercomputer/RedPajama-Data-1T",
"endpoints_compatible",
"text-generation-inference",
"region:us"
] | text-generation | "2023-07-17T22:25:12Z" | ---
datasets:
- ehartford/dolphin
- LinhDuong/chatdoctor-200k
- sahil2801/code_instructions_120k
- c-s-ale/dolly-15k-instruction-alpaca-format
- tiiuae/falcon-refinedweb
- bigcode/starcoderdata
- togethercomputer/RedPajama-Data-1T
language:
- en
library_name: transformers
pipeline_tag: text-generation
tags:
- instruct
- medical
- code
---
# Model Card for Model ID
<!-- Provide a quick summary of what the model is/does. -->
This model is an instruction-tuned LLaMa model with 33B parameters, with specialities in medical QA and code instruction.
## Model Details
<!-- Provide a longer summary of what this model is. -->
- **Model type:** LlamaForCausalLM
- **Language(s) (NLP):** English
- **License:** As a Llama-derivative, this model cannot be used commercially.
- **Finetuned from model (QLoRA):** [huggyllama/llama-30b](https://huggingface.co/huggyllama/llama-30b)
## Training Details
### Training Data
Converted the following datasets to alpaca:instruction format.
1. [ehartford/dolphin](https://huggingface.co/datasets/ehartford/dolphin)
- ORCA style dataset generously created by [Eric Hartford](https://huggingface.co/ehartford)
2. [LinhDuong/chatdoctor-200k](https://huggingface.co/datasets/LinhDuong/chatdoctor-200k)
- Refined dataset sourced from icliniq medical QA forum
3. [sahil2801/code_instructions_120k](https://huggingface.co/datasets/sahil2801/code_instructions_120k)
- Code instruction dataset generously created by Sahil Chaudhary from ThreeSixty AI
4. [c-s-ale/dolly-15k-instruction-alpaca-format](https://huggingface.co/datasets/c-s-ale/dolly-15k-instruction-alpaca-format)
- Dolly 15k is a general instruction dataset generated by employees of Databricks.
### Training Procedure
Trained using [axolotl](https://github.com/OpenAccess-AI-Collective/axolotl) QLoRa on [RunPod](https://www.runpod.io/console/gpu-cloud) 8x A6000 on Community Cloud for 1 epochs (~23 hours - ~$110).
<details>
<summary>axolotl training config:</summary>
```yaml
base_model: huggyllama/llama-30b
base_model_config: huggyllama/llama-30b
model_type: LlamaForCausalLM
tokenizer_type: LlamaTokenizer
load_in_8bit: false
load_in_4bit: true
strict: false
push_dataset_to_hub:
hub_model_id:
hf_use_auth_token:
datasets:
- path: ehartford/dolphin
type: alpaca
data_files:
- flan1m-alpaca-uncensored.jsonl
- flan5m-alpaca-uncensored.jsonl
shards: 25
- path: sahil2801/code_instructions_120k
type: alpaca
- path: LinhDuong/chatdoctor-200k
type: alpaca
shards: 2
- path: c-s-ale/dolly-15k-instruction-alpaca-format
type: alpaca
dataset_prepared_path: last_run_prepared
val_set_size: 0.01
adapter: qlora
lora_model_dir:
sequence_len: 2048
max_packed_sequence_len: 2048
lora_r: 8
lora_alpha: 32
lora_dropout: 0.05
lora_target_modules:
lora_target_linear: true
lora_fan_in_fan_out:
wandb_mode: true
wandb_project: med-orca-instruct-33b
wandb_watch:
wandb_run_id:
wandb_log_model: 'openllama_checkpoint'
output_dir: /disk/med-instruct-33b
gradient_accumulation_steps: 1
micro_batch_size: 4
num_epochs: 1
optimizer: paged_adamw_32bit
torchdistx_path:
lr_scheduler: cosine
learning_rate: 0.0002
train_on_inputs: false
group_by_length: false
bf16: true
fp16: false
tf32: true
gradient_checkpointing: true
early_stopping_patience:
resume_from_checkpoint:
local_rank:
logging_steps: 2
xformers_attention: true
flash_attention:
gptq_groupsize:
gptq_model_v1:
warmup_steps: 100
eval_steps: 20
save_steps:
debug:
deepspeed: true
weight_decay: 0.00001
special_tokens:
bos_token: "<s>"
eos_token: "</s>"
unk_token: "<unk>"
```
</details> |
mayflowergmbh/Llama-3-SauerkrautLM-8b-Instruct-AWQ | mayflowergmbh | "2024-04-24T12:02:58Z" | 2,168 | 1 | transformers | [
"transformers",
"safetensors",
"llama",
"text-generation",
"conversational",
"autotrain_compatible",
"endpoints_compatible",
"text-generation-inference",
"4-bit",
"awq",
"region:us"
] | text-generation | "2024-04-24T12:01:51Z" | Entry not found |
unsloth/Qwen2-1.5B-Instruct-bnb-4bit | unsloth | "2024-06-06T17:18:42Z" | 2,168 | 0 | transformers | [
"transformers",
"safetensors",
"qwen2",
"text-generation",
"unsloth",
"conversational",
"en",
"license:apache-2.0",
"autotrain_compatible",
"endpoints_compatible",
"text-generation-inference",
"4-bit",
"bitsandbytes",
"region:us"
] | text-generation | "2024-06-06T16:42:28Z" | ---
language:
- en
license: apache-2.0
library_name: transformers
tags:
- unsloth
- transformers
- qwen2
---
# Finetune Mistral, Gemma, Llama 2-5x faster with 70% less memory via Unsloth!
We have a Google Colab Tesla T4 notebook for Qwen2 7b here: https://colab.research.google.com/drive/1mvwsIQWDs2EdZxZQF9pRGnnOvE86MVvR?usp=sharing
And a Colab notebook for [Qwen2 0.5b](https://colab.research.google.com/drive/1-7tjDdMAyeCueyLAwv6vYeBMHpoePocN?usp=sharing) and another for [Qwen2 1.5b](https://colab.research.google.com/drive/1W0j3rP8WpgxRdUgkb5l6E00EEVyjEZGk?usp=sharing)
[<img src="https://raw.githubusercontent.com/unslothai/unsloth/main/images/Discord%20button.png" width="200"/>](https://discord.gg/u54VK8m8tk)
[<img src="https://raw.githubusercontent.com/unslothai/unsloth/main/images/buy%20me%20a%20coffee%20button.png" width="200"/>](https://ko-fi.com/unsloth)
[<img src="https://raw.githubusercontent.com/unslothai/unsloth/main/images/unsloth%20made%20with%20love.png" width="200"/>](https://github.com/unslothai/unsloth)
## ✨ Finetune for Free
All notebooks are **beginner friendly**! Add your dataset, click "Run All", and you'll get a 2x faster finetuned model which can be exported to GGUF, vLLM or uploaded to Hugging Face.
| Unsloth supports | Free Notebooks | Performance | Memory use |
|-----------------|--------------------------------------------------------------------------------------------------------------------------|-------------|----------|
| **Llama-3 8b** | [▶️ Start on Colab](https://colab.research.google.com/drive/135ced7oHytdxu3N2DNe1Z0kqjyYIkDXp?usp=sharing) | 2.4x faster | 58% less |
| **Gemma 7b** | [▶️ Start on Colab](https://colab.research.google.com/drive/10NbwlsRChbma1v55m8LAPYG15uQv6HLo?usp=sharing) | 2.4x faster | 58% less |
| **Mistral 7b** | [▶️ Start on Colab](https://colab.research.google.com/drive/1Dyauq4kTZoLewQ1cApceUQVNcnnNTzg_?usp=sharing) | 2.2x faster | 62% less |
| **Llama-2 7b** | [▶️ Start on Colab](https://colab.research.google.com/drive/1lBzz5KeZJKXjvivbYvmGarix9Ao6Wxe5?usp=sharing) | 2.2x faster | 43% less |
| **TinyLlama** | [▶️ Start on Colab](https://colab.research.google.com/drive/1AZghoNBQaMDgWJpi4RbffGM1h6raLUj9?usp=sharing) | 3.9x faster | 74% less |
| **CodeLlama 34b** A100 | [▶️ Start on Colab](https://colab.research.google.com/drive/1y7A0AxE3y8gdj4AVkl2aZX47Xu3P1wJT?usp=sharing) | 1.9x faster | 27% less |
| **Mistral 7b** 1xT4 | [▶️ Start on Kaggle](https://www.kaggle.com/code/danielhanchen/kaggle-mistral-7b-unsloth-notebook) | 5x faster\* | 62% less |
| **DPO - Zephyr** | [▶️ Start on Colab](https://colab.research.google.com/drive/15vttTpzzVXv_tJwEk-hIcQ0S9FcEWvwP?usp=sharing) | 1.9x faster | 19% less |
- This [conversational notebook](https://colab.research.google.com/drive/1Aau3lgPzeZKQ-98h69CCu1UJcvIBLmy2?usp=sharing) is useful for ShareGPT ChatML / Vicuna templates.
- This [text completion notebook](https://colab.research.google.com/drive/1ef-tab5bhkvWmBOObepl1WgJvfvSzn5Q?usp=sharing) is for raw text. This [DPO notebook](https://colab.research.google.com/drive/15vttTpzzVXv_tJwEk-hIcQ0S9FcEWvwP?usp=sharing) replicates Zephyr.
- \* Kaggle has 2x T4s, but we use 1. Due to overhead, 1x T4 is 5x faster. |
RichardErkhov/Danielbrdz_-_Barcenas-Tiny-1.1b-DPO-gguf | RichardErkhov | "2024-06-27T07:48:29Z" | 2,168 | 0 | null | [
"gguf",
"region:us"
] | null | "2024-06-27T07:05:06Z" | Entry not found |
google-bert/bert-large-cased-whole-word-masking | google-bert | "2024-04-10T09:56:46Z" | 2,167 | 15 | transformers | [
"transformers",
"pytorch",
"tf",
"jax",
"safetensors",
"bert",
"fill-mask",
"en",
"dataset:bookcorpus",
"dataset:wikipedia",
"arxiv:1810.04805",
"license:apache-2.0",
"autotrain_compatible",
"endpoints_compatible",
"region:us"
] | fill-mask | "2022-03-02T23:29:04Z" | ---
language: en
license: apache-2.0
datasets:
- bookcorpus
- wikipedia
---
# BERT large model (cased) whole word masking
Pretrained model on English language using a masked language modeling (MLM) objective. It was introduced in
[this paper](https://arxiv.org/abs/1810.04805) and first released in
[this repository](https://github.com/google-research/bert). This model is cased: it makes a difference between english and English.
Differently to other BERT models, this model was trained with a new technique: Whole Word Masking. In this case, all of the tokens corresponding to a word are masked at once. The overall masking rate remains the same.
The training is identical -- each masked WordPiece token is predicted independently.
Disclaimer: The team releasing BERT did not write a model card for this model so this model card has been written by
the Hugging Face team.
## Model description
BERT is a transformers model pretrained on a large corpus of English data in a self-supervised fashion. This means it
was pretrained on the raw texts only, with no humans labelling them in any way (which is why it can use lots of
publicly available data) with an automatic process to generate inputs and labels from those texts. More precisely, it
was pretrained with two objectives:
- Masked language modeling (MLM): taking a sentence, the model randomly masks 15% of the words in the input then run
the entire masked sentence through the model and has to predict the masked words. This is different from traditional
recurrent neural networks (RNNs) that usually see the words one after the other, or from autoregressive models like
GPT which internally mask the future tokens. It allows the model to learn a bidirectional representation of the
sentence.
- Next sentence prediction (NSP): the models concatenates two masked sentences as inputs during pretraining. Sometimes
they correspond to sentences that were next to each other in the original text, sometimes not. The model then has to
predict if the two sentences were following each other or not.
This way, the model learns an inner representation of the English language that can then be used to extract features
useful for downstream tasks: if you have a dataset of labeled sentences for instance, you can train a standard
classifier using the features produced by the BERT model as inputs.
This model has the following configuration:
- 24-layer
- 1024 hidden dimension
- 16 attention heads
- 336M parameters.
## Intended uses & limitations
You can use the raw model for either masked language modeling or next sentence prediction, but it's mostly intended to
be fine-tuned on a downstream task. See the [model hub](https://huggingface.co/models?filter=bert) to look for
fine-tuned versions on a task that interests you.
Note that this model is primarily aimed at being fine-tuned on tasks that use the whole sentence (potentially masked)
to make decisions, such as sequence classification, token classification or question answering. For tasks such as text
generation you should look at model like GPT2.
### How to use
You can use this model directly with a pipeline for masked language modeling:
```python
>>> from transformers import pipeline
>>> unmasker = pipeline('fill-mask', model='bert-large-cased-whole-word-masking')
>>> unmasker("Hello I'm a [MASK] model.")
[
{
"sequence":"[CLS] Hello I'm a fashion model. [SEP]",
"score":0.1474294513463974,
"token":4633,
"token_str":"fashion"
},
{
"sequence":"[CLS] Hello I'm a magazine model. [SEP]",
"score":0.05430116504430771,
"token":2435,
"token_str":"magazine"
},
{
"sequence":"[CLS] Hello I'm a male model. [SEP]",
"score":0.039395421743392944,
"token":2581,
"token_str":"male"
},
{
"sequence":"[CLS] Hello I'm a former model. [SEP]",
"score":0.036936815828084946,
"token":1393,
"token_str":"former"
},
{
"sequence":"[CLS] Hello I'm a professional model. [SEP]",
"score":0.03663451969623566,
"token":1848,
"token_str":"professional"
}
]
```
Here is how to use this model to get the features of a given text in PyTorch:
```python
from transformers import BertTokenizer, BertModel
tokenizer = BertTokenizer.from_pretrained('bert-large-cased-whole-word-masking')
model = BertModel.from_pretrained("bert-large-cased-whole-word-masking")
text = "Replace me by any text you'd like."
encoded_input = tokenizer(text, return_tensors='pt')
output = model(**encoded_input)
```
and in TensorFlow:
```python
from transformers import BertTokenizer, TFBertModel
tokenizer = BertTokenizer.from_pretrained('bert-large-cased-whole-word-masking')
model = TFBertModel.from_pretrained("bert-large-cased-whole-word-masking")
text = "Replace me by any text you'd like."
encoded_input = tokenizer(text, return_tensors='tf')
output = model(encoded_input)
```
### Limitations and bias
Even if the training data used for this model could be characterized as fairly neutral, this model can have biased
predictions:
```python
>>> from transformers import pipeline
>>> unmasker = pipeline('fill-mask', model='bert-large-cased-whole-word-masking')
>>> unmasker("The man worked as a [MASK].")
[
{
"sequence":"[CLS] The man worked as a carpenter. [SEP]",
"score":0.09021259099245071,
"token":25169,
"token_str":"carpenter"
},
{
"sequence":"[CLS] The man worked as a cook. [SEP]",
"score":0.08125395327806473,
"token":9834,
"token_str":"cook"
},
{
"sequence":"[CLS] The man worked as a mechanic. [SEP]",
"score":0.07524766772985458,
"token":19459,
"token_str":"mechanic"
},
{
"sequence":"[CLS] The man worked as a waiter. [SEP]",
"score":0.07397029548883438,
"token":17989,
"token_str":"waiter"
},
{
"sequence":"[CLS] The man worked as a guard. [SEP]",
"score":0.05848982185125351,
"token":3542,
"token_str":"guard"
}
]
>>> unmasker("The woman worked as a [MASK].")
[
{
"sequence":"[CLS] The woman worked as a maid. [SEP]",
"score":0.19436432421207428,
"token":13487,
"token_str":"maid"
},
{
"sequence":"[CLS] The woman worked as a waitress. [SEP]",
"score":0.16161060333251953,
"token":15098,
"token_str":"waitress"
},
{
"sequence":"[CLS] The woman worked as a nurse. [SEP]",
"score":0.14942803978919983,
"token":7439,
"token_str":"nurse"
},
{
"sequence":"[CLS] The woman worked as a secretary. [SEP]",
"score":0.10373266786336899,
"token":4848,
"token_str":"secretary"
},
{
"sequence":"[CLS] The woman worked as a cook. [SEP]",
"score":0.06384387612342834,
"token":9834,
"token_str":"cook"
}
]
```
This bias will also affect all fine-tuned versions of this model.
## Training data
The BERT model was pretrained on [BookCorpus](https://yknzhu.wixsite.com/mbweb), a dataset consisting of 11,038
unpublished books and [English Wikipedia](https://en.wikipedia.org/wiki/English_Wikipedia) (excluding lists, tables and
headers).
## Training procedure
### Preprocessing
The texts are lowercased and tokenized using WordPiece and a vocabulary size of 30,000. The inputs of the model are
then of the form:
```
[CLS] Sentence A [SEP] Sentence B [SEP]
```
With probability 0.5, sentence A and sentence B correspond to two consecutive sentences in the original corpus and in
the other cases, it's another random sentence in the corpus. Note that what is considered a sentence here is a
consecutive span of text usually longer than a single sentence. The only constrain is that the result with the two
"sentences" has a combined length of less than 512 tokens.
The details of the masking procedure for each sentence are the following:
- 15% of the tokens are masked.
- In 80% of the cases, the masked tokens are replaced by `[MASK]`.
- In 10% of the cases, the masked tokens are replaced by a random token (different) from the one they replace.
- In the 10% remaining cases, the masked tokens are left as is.
### Pretraining
The model was trained on 4 cloud TPUs in Pod configuration (16 TPU chips total) for one million steps with a batch size
of 256. The sequence length was limited to 128 tokens for 90% of the steps and 512 for the remaining 10%. The optimizer
used is Adam with a learning rate of 1e-4, \\(\beta_{1} = 0.9\\) and \\(\beta_{2} = 0.999\\), a weight decay of 0.01,
learning rate warmup for 10,000 steps and linear decay of the learning rate after.
## Evaluation results
When fine-tuned on downstream tasks, this model achieves the following results:
Model | SQUAD 1.1 F1/EM | Multi NLI Accuracy
---------------------------------------- | :-------------: | :----------------:
BERT-Large, Cased (Whole Word Masking) | 92.9/86.7 | 86.46
### BibTeX entry and citation info
```bibtex
@article{DBLP:journals/corr/abs-1810-04805,
author = {Jacob Devlin and
Ming{-}Wei Chang and
Kenton Lee and
Kristina Toutanova},
title = {{BERT:} Pre-training of Deep Bidirectional Transformers for Language
Understanding},
journal = {CoRR},
volume = {abs/1810.04805},
year = {2018},
url = {http://arxiv.org/abs/1810.04805},
archivePrefix = {arXiv},
eprint = {1810.04805},
timestamp = {Tue, 30 Oct 2018 20:39:56 +0100},
biburl = {https://dblp.org/rec/journals/corr/abs-1810-04805.bib},
bibsource = {dblp computer science bibliography, https://dblp.org}
}
``` |
vilm/vulture-40b | vilm | "2023-10-03T16:12:34Z" | 2,167 | 7 | transformers | [
"transformers",
"safetensors",
"RefinedWeb",
"text-generation",
"custom_code",
"ru",
"en",
"de",
"es",
"it",
"ja",
"vi",
"zh",
"fr",
"pt",
"id",
"ko",
"license:apache-2.0",
"autotrain_compatible",
"text-generation-inference",
"region:us"
] | text-generation | "2023-09-20T15:17:40Z" | ---
license: apache-2.0
language:
- ru
- en
- de
- es
- it
- ja
- vi
- zh
- fr
- pt
- id
- ko
pipeline_tag: text-generation
---
# 🌍 Vulture-40B
***Vulture-40B*** is a further fine-tuned causal Decoder-only LLM built by Virtual Interactive (VILM), on top of the famous **Falcon-40B** by [TII](https://www.tii.ae). We collected a new dataset from news articles and Wikipedia's pages of **12 languages** (Total: **80GB**) and continue the pretraining process of Falcon-40B. Finally, we construct a multilingual instructional dataset following **Alpaca**'s techniques.
*Technical Report coming soon* 🤗
## Prompt Format
The reccomended model usage is:
```
A chat between a curious user and an artificial intelligence assistant.
USER:{user's question}<|endoftext|>ASSISTANT:
```
# Model Details
## Model Description
- **Developed by:** [https://www.tii.ae](https://www.tii.ae)
- **Finetuned by:** [Virtual Interactive](https://vilm.org)
- **Language(s) (NLP):** English, German, Spanish, French, Portugese, Russian, Italian, Vietnamese, Indonesian, Chinese, Japanese and Korean
- **Training Time:** 1,800 A100 Hours
## Acknowledgement
- Thanks to **TII** for the amazing **Falcon** as the foundation model.
- Big thanks to **Google** for their generous Cloud credits.
### Out-of-Scope Use
Production use without adequate assessment of risks and mitigation; any use cases which may be considered irresponsible or harmful.
## Bias, Risks, and Limitations
Vulture-40B is trained on a large-scale corpora representative of the web, it will carry the stereotypes and biases commonly encountered online.
### Recommendations
We recommend users of Vulture-40B to consider finetuning it for the specific set of tasks of interest, and for guardrails and appropriate precautions to be taken for any production use.
## How to Get Started with the Model
To run inference with the model in full `bfloat16` precision you need approximately 4xA100 80GB or equivalent.
```python
from transformers import AutoTokenizer, AutoModelForCausalLM
import transformers
import torch
model = "vilm/vulture-40B"
tokenizer = AutoTokenizer.from_pretrained(model)
m = AutoModelForCausalLM.from_pretrained(model, torch_dtype=torch.bfloat16, device_map="auto" )
prompt = "A chat between a curious user and an artificial intelligence assistant.\n\nUSER:Thành phố Hồ Chí Minh nằm ở đâu?<|endoftext|>ASSISTANT:"
inputs = tokenizer(prompt, return_tensors="pt").to("cuda")
output = m.generate(input_ids=inputs["input_ids"],
attention_mask=inputs["attention_mask"],
do_sample=True,
temperature=0.6,
top_p=0.9,
max_new_tokens=50,)
output = output[0].to("cpu")
print(tokenizer.decode(output))
``` |
tuner007/pegasus_summarizer | tuner007 | "2022-07-28T06:38:07Z" | 2,166 | 43 | transformers | [
"transformers",
"pytorch",
"pegasus",
"text2text-generation",
"seq2seq",
"summarization",
"en",
"license:apache-2.0",
"model-index",
"autotrain_compatible",
"endpoints_compatible",
"region:us"
] | summarization | "2022-03-02T23:29:05Z" | ---
language: en
license: apache-2.0
tags:
- pegasus
- seq2seq
- summarization
model-index:
- name: tuner007/pegasus_summarizer
results:
- task:
type: summarization
name: Summarization
dataset:
name: cnn_dailymail
type: cnn_dailymail
config: 3.0.0
split: train
metrics:
- name: ROUGE-1
type: rouge
value: 36.604
verified: true
- name: ROUGE-2
type: rouge
value: 14.6398
verified: true
- name: ROUGE-L
type: rouge
value: 23.8845
verified: true
- name: ROUGE-LSUM
type: rouge
value: 32.9017
verified: true
- name: loss
type: loss
value: 2.5757133960723877
verified: true
- name: gen_len
type: gen_len
value: 76.3984
verified: true
---
## Model description
[PEGASUS](https://github.com/google-research/pegasus) fine-tuned for summarization
## Install "sentencepiece" library required for tokenizer
```
pip install sentencepiece
```
## Model in Action 🚀
```
import torch
from transformers import PegasusForConditionalGeneration, PegasusTokenizer
model_name = 'tuner007/pegasus_summarizer'
torch_device = 'cuda' if torch.cuda.is_available() else 'cpu'
tokenizer = PegasusTokenizer.from_pretrained(model_name)
model = PegasusForConditionalGeneration.from_pretrained(model_name).to(torch_device)
def get_response(input_text):
batch = tokenizer([input_text],truncation=True,padding='longest',max_length=1024, return_tensors="pt").to(torch_device)
gen_out = model.generate(**batch,max_length=128,num_beams=5, num_return_sequences=1, temperature=1.5)
output_text = tokenizer.batch_decode(gen_out, skip_special_tokens=True)
return output_text
```
#### Example:
context = """"
India wicket-keeper batsman Rishabh Pant has said someone from the crowd threw a ball on pacer Mohammed Siraj while he was fielding in the ongoing third Test against England on Wednesday. Pant revealed the incident made India skipper Virat Kohli "upset". "I think, somebody threw a ball inside, at Siraj, so he [Kohli] was upset," said Pant in a virtual press conference after the close of the first day\'s play."You can say whatever you want to chant, but don\'t throw things at the fielders and all those things. It is not good for cricket, I guess," he added.In the third session of the opening day of the third Test, a section of spectators seemed to have asked Siraj the score of the match to tease the pacer. The India pacer however came with a brilliant reply as he gestured 1-0 (India leading the Test series) towards the crowd.Earlier this month, during the second Test match, there was some bad crowd behaviour on a show as some unruly fans threw champagne corks at India batsman KL Rahul.Kohli also intervened and he was seen gesturing towards the opening batsman to know more about the incident. An over later, the TV visuals showed that many champagne corks were thrown inside the playing field, and the Indian players were visibly left frustrated.Coming back to the game, after bundling out India for 78, openers Rory Burns and Haseeb Hameed ensured that England took the honours on the opening day of the ongoing third Test.At stumps, England\'s score reads 120/0 and the hosts have extended their lead to 42 runs. For the Three Lions, Burns (52*) and Hameed (60*) are currently unbeaten at the crease.Talking about the pitch on opening day, Pant said, "They took the heavy roller, the wicket was much more settled down, and they batted nicely also," he said. "But when we batted, the wicket was slightly soft, and they bowled in good areas, but we could have applied [ourselves] much better."Both England batsmen managed to see off the final session and the hosts concluded the opening day with all ten wickets intact, extending the lead to 42.(ANI)
"""
```
get_response(context)
```
#### Output:
Team India wicketkeeper-batsman Rishabh Pant has said that Virat Kohli was "upset" after someone threw a ball on pacer Mohammed Siraj while he was fielding in the ongoing third Test against England. "You can say whatever you want to chant, but don't throw things at the fielders and all those things. It's not good for cricket, I guess," Pant added.'
#### [Inshort](https://www.inshorts.com/) (60 words News summary app, rated 4.4 by 5,27,246+ users on android playstore) summary:
India wicketkeeper-batsman Rishabh Pant has revealed that captain Virat Kohli was upset with the crowd during the first day of Leeds Test against England because someone threw a ball at pacer Mohammed Siraj. Pant added, "You can say whatever you want to chant, but don't throw things at the fielders and all those things. It is not good for cricket."
> Created by [Arpit Rajauria](https://twitter.com/arpit_rajauria)
[](https://twitter.com/arpit_rajauria)
|
riotu-lab/ArabianGPT-01B | riotu-lab | "2024-02-27T13:31:53Z" | 2,166 | 9 | transformers | [
"transformers",
"pytorch",
"gpt2",
"text-generation",
"arabic ",
"ar",
"license:apache-2.0",
"autotrain_compatible",
"endpoints_compatible",
"text-generation-inference",
"region:us"
] | text-generation | "2023-12-04T18:45:05Z" | ---
license: apache-2.0
language:
- ar
pipeline_tag: text-generation
tags:
- 'arabic '
- text-generation
widget:
- text: "أعلنت وزارة الحج في المملكة العربية السعودية"
example_title: "مثال ١"
- text: "يبدو اليوم جميلا، سأقوم بتحضير"
example_title: "مثال ٢"
- text: "إن التقنيات الحديثة"
example_title: "مثال ٣"
---
# ArabianGPT Model Overview
## Disclaimer for the Use of Large Language Models (LLMs) for Text Generation
<p style="color: red;">We disclaim all responsibility for any harm, inaccuracies, or inappropriate content generated by ArabianGPT-0.1B, and users engage with and apply the model's outputs at their own risk.</p>
> **Important Note:** Currently, we offer a raw pre-trained model. Our team is actively working on releasing instruction-based LLMs that are fine-tuned and augmented with LRHF. The first set of pre-trained models has been made available for community exploration. While we do have models fine-tuned for specific tasks such as summarization and sentiment analysis, they are still in the development phase.
## How you can use this Pre-Trained?
You are invited to utilize this pre-trained, native Arabic language model as an experimental tool to assess its capabilities, aid in its fine-tuning, and evaluate its performance across a variety of downstream tasks. We encourage you to review our technical report for a comprehensive understanding of the model's performance metrics and the specific downstream tasks it has been tested on. This will provide valuable insights into its applicability and effectiveness in diverse applications.
## Introduction
ArabianGPT-0.1B, developed under the ArabianLLM initiatives, is a specialized GPT-2 model optimized for Arabic language modeling.
It's a product of the collaborative efforts at Prince Sultan University's Robotics and Internet of Things Lab, focusing on enhancing natural language modeling and generation in Arabic.
This model represents a significant stride in LLM research, specifically addressing the linguistic complexities and nuances of the Arabic language.
## Key Features
- **Architecture**: GPT-2
- **Model Size**: 134 million parameters
- **Layers**: 12
- **Model Attention Layers (MAL)**: 12
- **Context Window Size**: 768 tokens
## Training
- **Dataset**: Scraped Arabic newspaper articles
- **Data Size**: 15.5 GB
- **Words**: 237.8 million
- **Tokenizer**: Aranizer 64K
- **Tokens**: Over 1.75 billion
- **Hardware**: 2 NDIVIA A100 GPUs
- **Training Scale**: 7.5 million examples
- **Training Duration**: 3 days
- **Performance**: Final loss of 3.97
## Role in ArabianLLM Initiatives
ArabianGPT-0.1B (Base Model) is crucial for advancing Arabic language processing, addressing challenges unique to Arabic morphology and dialects.
## Usage
Suitable for Arabic text generation tasks. Example usage with Transformers Pipeline:
```python
from transformers import pipeline
pipe = pipeline("text-generation", model="riotu-lab/ArabianGPT-01B", max_new_tokens=512)
text = ''
pipe.predict(text)
```
## Limitations and Ethical Considerations
- The model may have context understanding or text generation limitations in certain scenarios.
- Emphasis on ethical use to prevent misinformation or harmful content propagation.
## Acknowledgments
Special thanks to Prince Sultan University, particularly the Robotics and Internet of Things Lab.
## Contact Information
For inquiries: [[email protected]](mailto:[email protected]).
## Disclaimer for the Use of Large Language Models (LLMs) for Text Generation
<p style="color: red;">We disclaim all responsibility for any harm, inaccuracies, or inappropriate content generated by ArabianGPT-0.1B, and users engage with and apply the model's outputs at their own risk.</p>
|
sapienzanlp/Minerva-1B-base-v1.0 | sapienzanlp | "2024-05-17T15:49:14Z" | 2,166 | 23 | transformers | [
"transformers",
"pytorch",
"safetensors",
"mistral",
"text-generation",
"pretrained",
"it",
"en",
"dataset:uonlp/CulturaX",
"license:apache-2.0",
"autotrain_compatible",
"endpoints_compatible",
"text-generation-inference",
"region:us"
] | text-generation | "2024-04-19T15:08:33Z" | ---
license: apache-2.0
pipeline_tag: text-generation
language:
- it
- en
tags:
- pretrained
datasets:
- uonlp/CulturaX
#widget:
# - text: "Example"
# - example_title: "Example:"
---
<div style="text-align: center; display: flex; flex-direction: column; align-items: center;">
<img src="https://cdn-uploads.huggingface.co/production/uploads/5f0b462819cb630495b814d7/DVA4MnFUs3UHBnTrX9jG6.png" style="max-width: 550px; height: auto;">
</div>
# Model Card for Minerva-1B-base-v1.0
Minerva is the first family of **LLMs pretrained from scratch on Italian** developed by [Sapienza NLP](https://nlp.uniroma1.it)
in collaboration with [Future Artificial Intelligence Research (FAIR)](https://fondazione-fair.it/) and [CINECA](https://www.cineca.it/).
Notably, the Minerva models are truly-open (data and model) Italian-English LLMs, with approximately half of the pretraining data
including Italian text.
* [Minerva LLMs - website](https://nlp.uniroma1.it/minerva/)
## Description
This is the model card for **Minerva-1B-base-v1.0**, a 1 billion parameter model trained on 200 billion tokens (100 billion in Italian, 100 billion in English).
This model is part of the Minerva LLM family:
* [Minerva-350M-base-v1.0](https://huggingface.co/sapienzanlp/Minerva-350M-base-v1.0)
* [Minerva-1B-base-v1.0](https://huggingface.co/sapienzanlp/Minerva-1B-base-v1.0)
* [Minerva-3B-base-v1.0](https://huggingface.co/sapienzanlp/Minerva-3B-base-v1.0)
## 🚨⚠️🚨 Bias, Risks, and Limitations 🚨⚠️🚨
*This section identifies foreseeable harms and misunderstandings.*
This is a foundation model, not subject to alignment. Model may:
- Overrepresent some viewpoints and underrepresent others
- Contain stereotypes
- Contain [personal information](#personal-data-and-information)
- Generate:
- Hateful, abusive, or violent language
- Discriminatory or prejudicial language
- Content that may not be appropriate for all settings, including sexual content
- Make errors, including producing incorrect information as if it were factual
- Generate irrelevant or repetitive outputs
We are aware of the biases that current pretrained large language models exhibit: more specifically, as probabilistic models of (Italian and English) languages, they reflect and amplify the biases of their training data.
For more information about this issue, please refer to our survey:
* [Biases in Large Language Models: Origins, Inventory, and Discussion](https://dl.acm.org/doi/full/10.1145/3597307)
## How to use Minerva with Hugging Face transformers
```python
import transformers
import torch
model_id = "sapienzanlp/Minerva-1B-base-v1.0"
# Initialize the pipeline.
pipeline = transformers.pipeline(
"text-generation",
model=model_id,
model_kwargs={"torch_dtype": torch.bfloat16},
device_map="auto",
)
# Input text for the model.
input_text = "La capitale dell'Italia è"
# Compute the outputs.
output = pipeline(
input_text,
max_new_tokens=128,
)
# Output:
# [{'generated_text': "La capitale dell'Italia è la città di Roma, che si trova a [...]"}]
```
## Model Architecture
Minerva-1B-base-v1.0 is a Transformer model based on the Mistral architecture, where the number of layers, number of heads, and the hidden states dimension are modified to reach 1B parameters.
Please, take a look at the configuration file for a detailed breakdown of the hyperparameters we chose for this model.
The Minerva LLM family is composed of:
| Model Name | Tokens | Layers | Hidden Size | Attention Heads | KV Heads | Sliding Window | Max Context Length |
| --- | --- | --- | --- | --- | --- | --- | --- |
| Minerva-350M-base-v1.0 | 70B (35B it + 35B en) | 16 | 1152 | 16 | 4 | 2048 | 16384 |
| **Minerva-1B-base-v1.0** | 200B (100B it + 100B en) | 16 | 2048 | 16 | 4 | 2048 | 16384 |
| Minerva-3B-base-v1.0 | 660B (330B it + 330B en) | 32 | 2560 | 32 | 8 | 2048 | 16384 |
## Model Training
Minerva-1B-base-v1.0 was trained using [llm-foundry 0.6.0](https://github.com/mosaicml/llm-foundry) from [MosaicML](https://mosaicml.com/). The hyperparameters used are the following:
| Model Name | Optimizer | lr | betas | eps | weight decay | Scheduler | Warmup Steps | Batch Size (Tokens) | Total Steps |
| --- | --- | --- | --- | --- | --- | --- | --- | --- | --- |
| Minerva-350M-base-v1.0 | Decoupled AdamW | 2e-4 | (0.9, 0.95) | 1e-8 | 0.0 | Cosine | 2% | 4M | 16,690 |
| **Minerva-1B-base-v1.0** | Decoupled AdamW | 2e-4 | (0.9, 0.95) | 1e-8 | 0.0 | Cosine | 2% | 4M | 47,684 |
| Minerva-3B-base-v1.0 | Decoupled AdamW | 2e-4 | (0.9, 0.95) | 1e-8 | 0.0 | Cosine | 2% | 4M | 157,357 |
## Model Evaluation
We assessed our model using the [LM-Evaluation-Harness](https://github.com/EleutherAI/lm-evaluation-harness) library, which serves as a comprehensive framework for testing generative language models across a wide range of evaluation tasks.
All the reported benchmark data was already present in the LM-Evaluation-Harness suite.
Italian Data:
| Task | Accuracy |
| --- | --- |
| [xcopa](https://huggingface.co/datasets/xcopa) (0-shot) | 0.602 |
| [Hellaswag](https://huggingface.co/datasets/alexandrainst/m_hellaswag) (5-shot) | 0.3847 |
| [Belebele](https://huggingface.co/datasets/facebook/belebele) (5-shot) | 0.25 |
| [TruthfulQA MC 1](https://huggingface.co/datasets/alexandrainst/m_truthfulqa) (0-shot) | 0.2375 |
| [TruthfulQA MC 2](https://huggingface.co/datasets/alexandrainst/m_truthfulqa) (0-shot) | 0.3996 |
| [M MMLU](https://huggingface.co/datasets/alexandrainst/m_mmlu) (5-shot) | 0.2546 |
| [arc challenge](https://huggingface.co/datasets/alexandrainst/m_arc) (5-shot) | 0.2549 |
English Data:
| Task | Accuracy |
| --- | --- |
| [Hellaswag](https://huggingface.co/datasets/Rowan/hellaswag) (5-shot) | 0.43 |
| [Belebele](https://huggingface.co/datasets/facebook/belebele) (5-shot) | 0.26 |
| [TruthfulQA MC 1](https://huggingface.co/datasets/truthful_qa) (0-shot) | 0.21 |
| [TruthfulQA MC 2](https://huggingface.co/datasets/truthful_qa) (0-shot) | 0.38 |
| [M MMLU](https://huggingface.co/datasets/alexandrainst/m_mmlu) (5-shot) | 0.26 |
| [arc challenge](https://huggingface.co/datasets/allenai/ai2_arc) (5-shot) | 0.26 |
| [arc easy](https://huggingface.co/datasets/allenai/ai2_arc) (5-shot) | 0.4966 |
| [piqa](https://huggingface.co/datasets/piqa) (5-shot) | 0.6975 |
| [sciq](https://huggingface.co/datasets/sciq) (5-shot) | 0.830 |
## Training Data
Minerva-1B-base-v1.0 was trained on 100B Italian tokens and 100B English tokens sampled from CulturaX.
The data was selected from the following sources:
* OSCAR-2201
* OSCAR-2301
* mC4
We have extracted some statistics on Italian (115B tokens) and English (210B tokens) documents from CulturaX on the selected sources:
*Proportion of number of tokens per domain (Italian)*
<img src="https://github.com/Andrew-Wyn/images/blob/master/minerva/top_25_url_tokens_proportion_culturax_it.png?raw=true" alt="italian-tok-counts" border="0" width="1800px">
*Proportion of number of tokens per domain (English)*
<img src="https://github.com/Andrew-Wyn/images/blob/master/minerva/top_25_url_tokens_proportion_culturax_en.png?raw=true" alt="english-tok-counts" border="0" width="1800px">
## Tokenizer Fertility
The tokenizer fertility measures the average amount of tokens produced per tokenized word.
A tokenizer displaying high fertility values in a particular language typically indicates that it segments words in that language extensively.
The tokenizer fertility is strictly correlated with the inference speed of the model with respect to a specific language,
as higher values mean longer sequences of tokens to generate and thus lower inference speed.
**Fertility computed over a sample of Cultura X (CX) data and Wikipedia (Wp):**
| Model | Voc. Size | Fertility IT (CX) | Fertility EN (CX) | Fertility IT (Wp) | Fertility EN (Wp) |
| --- | --- | --- |--- | --- |--- |
| Mistral-7B-v0.1 | 32000 | 1.87 | 1.32 | 2.05 | 1.57 |
| gemma-7b | 256000 | 1.42 | 1.18 | 1.56 | 1.34 |
| Minerva-1B-base-v1.0 | 32768 | 1.39 | 1.32 | 1.66 | 1.59 |
## Notice
Minerva-350M-base-v1.0 is a pretrained base model and, therefore, has no moderation mechanisms.
## The Sapienza NLP Team
* **Riccardo Orlando:** data preprocessing, model training
* **Pere-Lluis Huguet Cabot:** data preprocessing, vocabulary, evaluation
* **Luca Moroni:** data curation, data analysis, downstream tasks, evaluation
* **Simone Conia:** data curation, evaluation, project supervision
* **Edoardo Barba:** data preprocessing, downstream tasks, project supervision
* **Roberto Navigli:** project coordinator
### Special thanks for their support
* Giuseppe Fiameni, Nvidia
* Sergio Orlandini, CINECA
## Acknowledgments
This work was funded by the PNRR MUR project [PE0000013-FAIR](https://fondazione-fair.it).
We acknowledge the [CINECA](https://www.cineca.it) award "IscB_medit" under the ISCRA initiative, for the availability of high performance computing resources and support. |
VlSav/DeepSeek-V2-Lite-Chat-Q8_0-GGUF | VlSav | "2024-06-22T15:21:39Z" | 2,166 | 0 | null | [
"gguf",
"llama-cpp",
"gguf-my-repo",
"base_model:deepseek-ai/DeepSeek-V2-Lite-Chat",
"license:other",
"region:us"
] | null | "2024-06-22T15:20:25Z" | ---
base_model: deepseek-ai/DeepSeek-V2-Lite-Chat
license: other
license_name: deepseek
license_link: https://github.com/deepseek-ai/DeepSeek-V2/blob/main/LICENSE-MODEL
tags:
- llama-cpp
- gguf-my-repo
---
# VlSav/DeepSeek-V2-Lite-Chat-Q8_0-GGUF
This model was converted to GGUF format from [`deepseek-ai/DeepSeek-V2-Lite-Chat`](https://huggingface.co/deepseek-ai/DeepSeek-V2-Lite-Chat) using llama.cpp via the ggml.ai's [GGUF-my-repo](https://huggingface.co/spaces/ggml-org/gguf-my-repo) space.
Refer to the [original model card](https://huggingface.co/deepseek-ai/DeepSeek-V2-Lite-Chat) for more details on the model.
## Use with llama.cpp
Install llama.cpp through brew (works on Mac and Linux)
```bash
brew install llama.cpp
```
Invoke the llama.cpp server or the CLI.
### CLI:
```bash
llama-cli --hf-repo VlSav/DeepSeek-V2-Lite-Chat-Q8_0-GGUF --hf-file deepseek-v2-lite-chat-q8_0.gguf -p "The meaning to life and the universe is"
```
### Server:
```bash
llama-server --hf-repo VlSav/DeepSeek-V2-Lite-Chat-Q8_0-GGUF --hf-file deepseek-v2-lite-chat-q8_0.gguf -c 2048
```
Note: You can also use this checkpoint directly through the [usage steps](https://github.com/ggerganov/llama.cpp?tab=readme-ov-file#usage) listed in the Llama.cpp repo as well.
Step 1: Clone llama.cpp from GitHub.
```
git clone https://github.com/ggerganov/llama.cpp
```
Step 2: Move into the llama.cpp folder and build it with `LLAMA_CURL=1` flag along with other hardware-specific flags (for ex: LLAMA_CUDA=1 for Nvidia GPUs on Linux).
```
cd llama.cpp && LLAMA_CURL=1 make
```
Step 3: Run inference through the main binary.
```
./llama-cli --hf-repo VlSav/DeepSeek-V2-Lite-Chat-Q8_0-GGUF --hf-file deepseek-v2-lite-chat-q8_0.gguf -p "The meaning to life and the universe is"
```
or
```
./llama-server --hf-repo VlSav/DeepSeek-V2-Lite-Chat-Q8_0-GGUF --hf-file deepseek-v2-lite-chat-q8_0.gguf -c 2048
```
|
quantumaikr/QuantumLM | quantumaikr | "2023-07-26T17:52:39Z" | 2,165 | 2 | transformers | [
"transformers",
"pytorch",
"llama",
"text-generation",
"en",
"license:cc-by-nc-4.0",
"autotrain_compatible",
"endpoints_compatible",
"text-generation-inference",
"region:us"
] | text-generation | "2023-07-22T13:19:17Z" | ---
license: cc-by-nc-4.0
language:
- en
pipeline_tag: text-generation
---
# QuantumLM
## Model Description
`QuantumLM` is a Llama2 13B model finetuned on an Wizard-Orca style Dataset
## Usage
Start chatting with `QuantumLM` using the following code snippet:
```python
import torch
from transformers import AutoModelForCausalLM, AutoTokenizer, pipeline
tokenizer = AutoTokenizer.from_pretrained("quantumaikr/QuantumLM", use_fast=False)
model = AutoModelForCausalLM.from_pretrained("quantumaikr/QuantumLM", torch_dtype=torch.float16, low_cpu_mem_usage=True, device_map="auto")
system_prompt = "### System:\nYou are QuantumLM, an AI that follows instructions extremely well. Help as much as you can. Remember, be safe, and don't do anything illegal.\n\n"
message = "Write me a poem please"
prompt = f"{system_prompt}### User: {message}\n\n### Assistant:\n"
inputs = tokenizer(prompt, return_tensors="pt").to("cuda")
output = model.generate(**inputs, do_sample=True, top_p=0.95, top_k=0, max_new_tokens=256)
print(tokenizer.decode(output[0], skip_special_tokens=True))
```
QuantumLM should be used with this prompt format:
```
### System:
This is a system prompt, please behave and help the user.
### User:
Your prompt here
### Assistant
The output of QuantumLM
```
## Use and Limitations
### Intended Use
These models are intended for research only, in adherence with the [CC BY-NC-4.0](https://creativecommons.org/licenses/by-nc/4.0/) license.
### Limitations and bias
Although the aforementioned dataset helps to steer the base language models into "safer" distributions of text, not all biases and toxicity can be mitigated through fine-tuning. We ask that users be mindful of such potential issues that can arise in generated responses. Do not treat model outputs as substitutes for human judgment or as sources of truth. Please use it responsibly.
|
openbmb/MiniCPM-V | openbmb | "2024-06-06T11:35:04Z" | 2,165 | 115 | transformers | [
"transformers",
"safetensors",
"minicpmv",
"feature-extraction",
"visual-question-answering",
"custom_code",
"arxiv:2308.12038",
"region:us"
] | visual-question-answering | "2024-01-30T14:38:14Z" | ---
pipeline_tag: visual-question-answering
---
## MiniCPM-V
### News
- [5/20]🔥 GPT-4V level multimodal model [**MiniCPM-Llama3-V 2.5**](https://huggingface.co/openbmb/MiniCPM-Llama3-V-2_5) is out.
- [4/11]🔥 [**MiniCPM-V 2.0**](https://huggingface.co/openbmb/MiniCPM-V-2) is out.
**MiniCPM-V** (i.e., OmniLMM-3B) is an efficient version with promising performance for deployment. The model is built based on SigLip-400M and [MiniCPM-2.4B](https://github.com/OpenBMB/MiniCPM/), connected by a perceiver resampler. Notable features of OmniLMM-3B include:
- ⚡️ **High Efficiency.**
MiniCPM-V can be **efficiently deployed on most GPU cards and personal computers**, and **even on end devices such as mobile phones**. In terms of visual encoding, we compress the image representations into 64 tokens via a perceiver resampler, which is significantly fewer than other LMMs based on MLP architecture (typically > 512 tokens). This allows OmniLMM-3B to operate with **much less memory cost and higher speed during inference**.
- 🔥 **Promising Performance.**
MiniCPM-V achieves **state-of-the-art performance** on multiple benchmarks (including MMMU, MME, and MMbech, etc) among models with comparable sizes, surpassing existing LMMs built on Phi-2. It even **achieves comparable or better performance than the 9.6B Qwen-VL-Chat**.
- 🙌 **Bilingual Support.**
MiniCPM-V is **the first end-deployable LMM supporting bilingual multimodal interaction in English and Chinese**. This is achieved by generalizing multimodal capabilities across languages, a technique from the ICLR 2024 spotlight [paper](https://arxiv.org/abs/2308.12038).
### Evaluation
<div align="center">
<table style="margin: 0px auto;">
<thead>
<tr>
<th align="left">Model</th>
<th>Size</th>
<th>MME</th>
<th nowrap="nowrap" >MMB dev (en)</th>
<th nowrap="nowrap" >MMB dev (zh)</th>
<th nowrap="nowrap" >MMMU val</th>
<th nowrap="nowrap" >CMMMU val</th>
</tr>
</thead>
<tbody align="center">
<tr>
<td align="left">LLaVA-Phi</td>
<td align="right">3.0B</td>
<td>1335</td>
<td>59.8</td>
<td>- </td>
<td>- </td>
<td>- </td>
</tr>
<tr>
<td nowrap="nowrap" align="left">MobileVLM</td>
<td align="right">3.0B</td>
<td>1289</td>
<td>59.6</td>
<td>- </td>
<td>- </td>
<td>- </td>
</tr>
<tr>
<td nowrap="nowrap" align="left" >Imp-v1</td>
<td align="right">3B</td>
<td>1434</td>
<td>66.5</td>
<td>- </td>
<td>- </td>
<td>- </td>
</tr>
<tr>
<td nowrap="nowrap" align="left" >Qwen-VL-Chat</td>
<td align="right" >9.6B</td>
<td>1487</td>
<td>60.6 </td>
<td>56.7 </td>
<td>35.9 </td>
<td>30.7 </td>
</tr>
<tr>
<td nowrap="nowrap" align="left" >CogVLM</td>
<td align="right">17.4B </td>
<td>1438 </td>
<td>63.7 </td>
<td>53.8 </td>
<td>32.1 </td>
<td>- </td>
</tr>
<tr>
<td nowrap="nowrap" align="left" ><b>MiniCPM-V</b></td>
<td align="right">3B </td>
<td>1452 </td>
<td>67.9 </td>
<td>65.3 </td>
<td>37.2 </td>
<td>32.1 </td>
</tr>
</tbody>
</table>
</div>
### Examples
<div align="center">
<table>
<tr>
<td>
<p>
<img src="assets/Mushroom_en.gif" width="400"/>
</p>
</td>
<td>
<p>
<img src="assets/Snake_en.gif" width="400"/>
</p>
</td>
</tr>
</table>
</div>
## Demo
Click here to try out the Demo of [MiniCPM-V](http://120.92.209.146:80).
## Deployment on Mobile Phone
Currently MiniCPM-V (i.e., OmniLMM-3B) can be deployed on mobile phones with Android and Harmony operating systems. 🚀 Try it out [here](https://github.com/OpenBMB/mlc-MiniCPM).
## Usage
Inference using Huggingface transformers on Nivdia GPUs or Mac with MPS (Apple silicon or AMD GPUs). Requirements tested on python 3.10:
```
Pillow==10.1.0
timm==0.9.10
torch==2.1.2
torchvision==0.16.2
transformers==4.36.0
sentencepiece==0.1.99
```
```python
# test.py
import torch
from PIL import Image
from transformers import AutoModel, AutoTokenizer
model = AutoModel.from_pretrained('openbmb/MiniCPM-V', trust_remote_code=True, torch_dtype=torch.bfloat16)
# For Nvidia GPUs support BF16 (like A100, H100, RTX3090)
model = model.to(device='cuda', dtype=torch.bfloat16)
# For Nvidia GPUs do NOT support BF16 (like V100, T4, RTX2080)
#model = model.to(device='cuda', dtype=torch.float16)
# For Mac with MPS (Apple silicon or AMD GPUs).
# Run with `PYTORCH_ENABLE_MPS_FALLBACK=1 python test.py`
#model = model.to(device='mps', dtype=torch.float16)
tokenizer = AutoTokenizer.from_pretrained('openbmb/MiniCPM-V', trust_remote_code=True)
model.eval()
image = Image.open('xx.jpg').convert('RGB')
question = 'What is in the image?'
msgs = [{'role': 'user', 'content': question}]
res, context, _ = model.chat(
image=image,
msgs=msgs,
context=None,
tokenizer=tokenizer,
sampling=True,
temperature=0.7
)
print(res)
```
Please look at [GitHub](https://github.com/OpenBMB/OmniLMM) for more detail about usage.
## License
#### Model License
* The code in this repo is released under the [Apache-2.0](https://github.com/OpenBMB/MiniCPM/blob/main/LICENSE) License.
* The usage of MiniCPM-V series model weights must strictly follow [MiniCPM Model License.md](https://github.com/OpenBMB/MiniCPM/blob/main/MiniCPM%20Model%20License.md).
* The models and weights of MiniCPM are completely free for academic research. after filling out a ["questionnaire"](https://modelbest.feishu.cn/share/base/form/shrcnpV5ZT9EJ6xYjh3Kx0J6v8g) for registration, are also available for free commercial use.
#### Statement
* As a LLM, MiniCPM-V generates contents by learning a large mount of texts, but it cannot comprehend, express personal opinions or make value judgement. Anything generated by MiniCPM-V does not represent the views and positions of the model developers
* We will not be liable for any problems arising from the use of the MinCPM-V open Source model, including but not limited to data security issues, risk of public opinion, or any risks and problems arising from the misdirection, misuse, dissemination or misuse of the model. |
OpenBuddy/openbuddy-qwen1.5-32b-v21.2-32k | OpenBuddy | "2024-04-13T11:02:12Z" | 2,165 | 3 | transformers | [
"transformers",
"safetensors",
"qwen2",
"text-generation",
"conversational",
"zh",
"en",
"fr",
"de",
"ja",
"ko",
"it",
"ru",
"fi",
"license:other",
"autotrain_compatible",
"text-generation-inference",
"region:us"
] | text-generation | "2024-04-11T06:29:57Z" | ---
license: other
license_name: tongyi-qianwen-license-agreement
license_link: >-
https://huggingface.co/Qwen/Qwen1.5-14B/blob/39b74a78357df4d2296e838d87565967d663a67a/LICENSE
language:
- zh
- en
- fr
- de
- ja
- ko
- it
- ru
- fi
pipeline_tag: text-generation
inference: false
library_name: transformers
---
# OpenBuddy - Open Multilingual Chatbot
GitHub and Usage Guide: [https://github.com/OpenBuddy/OpenBuddy](https://github.com/OpenBuddy/OpenBuddy)
Website and Demo: [https://openbuddy.ai](https://openbuddy.ai)
Evaluation result of this model: [Evaluation.txt](Evaluation.txt)
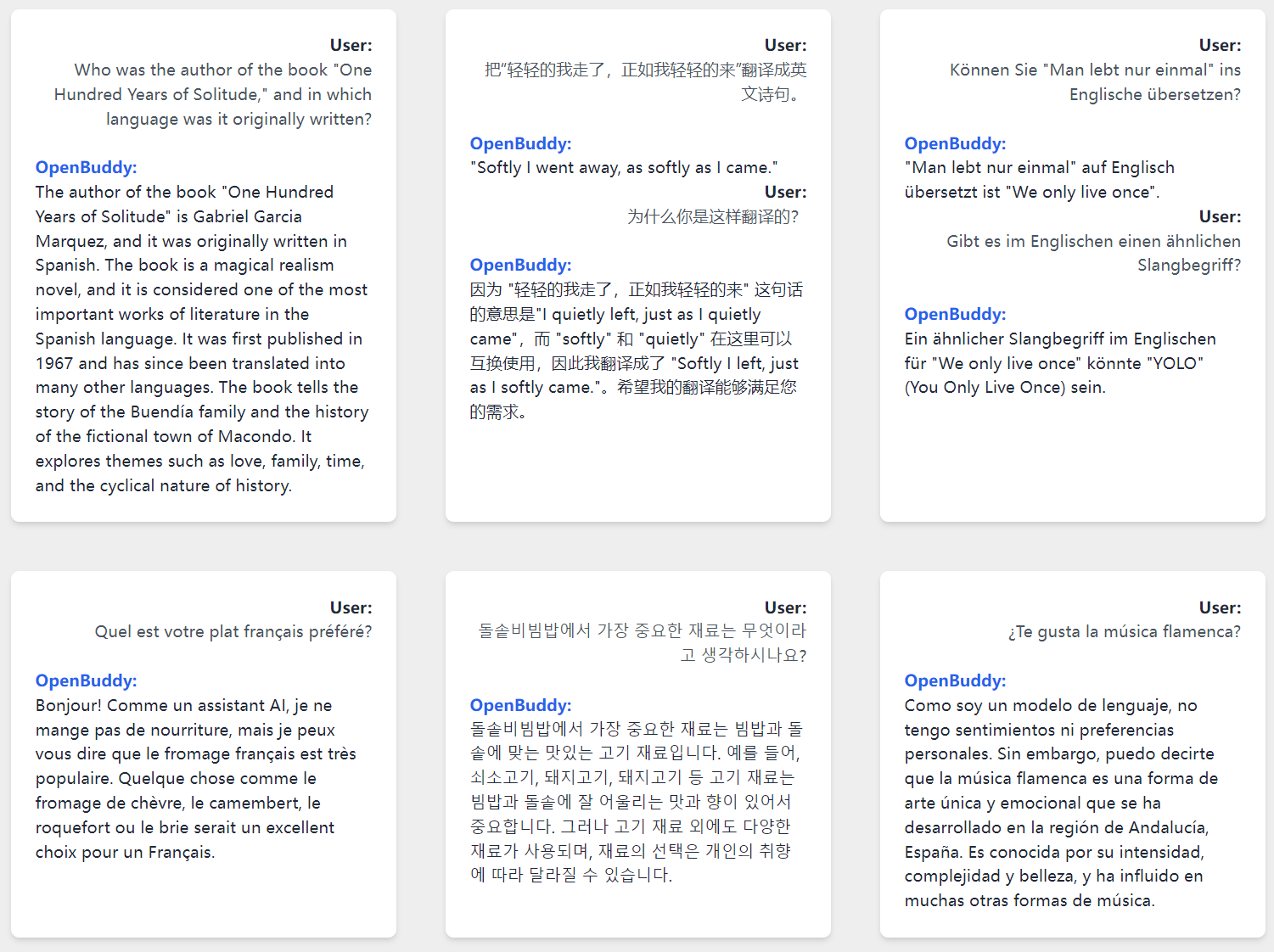
# Copyright Notice
Base model: https://huggingface.co/Qwen/Qwen1.5-32B
License: Qwen: https://huggingface.co/Qwen/Qwen1.5-14B/blob/39b74a78357df4d2296e838d87565967d663a67a/LICENSE
# Prompt Format
We recommend using the fast tokenizer from `transformers`, which should be enabled by default in the `transformers` and `vllm` libraries. Other implementations including `sentencepiece` may not work as expected, especially for special tokens like `<|role|>`, `<|says|>` and `<|end|>`.
```
<|role|>system<|says|>You(assistant) are a helpful, respectful and honest INTP-T AI Assistant named Buddy. You are talking to a human(user).
Always answer as helpfully and logically as possible, while being safe. Your answers should not include any harmful, political, religious, unethical, racist, sexist, toxic, dangerous, or illegal content. Please ensure that your responses are socially unbiased and positive in nature.
You cannot access the internet, but you have vast knowledge, cutoff: 2023-04.
You are trained by OpenBuddy team, (https://openbuddy.ai, https://github.com/OpenBuddy/OpenBuddy), not related to GPT or OpenAI.<|end|>
<|role|>user<|says|>History input 1<|end|>
<|role|>assistant<|says|>History output 1<|end|>
<|role|>user<|says|>History input 2<|end|>
<|role|>assistant<|says|>History output 2<|end|>
<|role|>user<|says|>Current input<|end|>
<|role|>assistant<|says|>
```
This format is also defined in `tokenizer_config.json`, which means you can directly use `vllm` to deploy an OpenAI-like API service. For more information, please refer to the [vllm documentation](https://docs.vllm.ai/en/latest/serving/openai_compatible_server.html).
## Disclaimer
All OpenBuddy models have inherent limitations and may potentially produce outputs that are erroneous, harmful, offensive, or otherwise undesirable. Users should not use these models in critical or high-stakes situations that may lead to personal injury, property damage, or significant losses. Examples of such scenarios include, but are not limited to, the medical field, controlling software and hardware systems that may cause harm, and making important financial or legal decisions.
OpenBuddy is provided "as-is" without any warranty of any kind, either express or implied, including, but not limited to, the implied warranties of merchantability, fitness for a particular purpose, and non-infringement. In no event shall the authors, contributors, or copyright holders be liable for any claim, damages, or other liabilities, whether in an action of contract, tort, or otherwise, arising from, out of, or in connection with the software or the use or other dealings in the software.
By using OpenBuddy, you agree to these terms and conditions, and acknowledge that you understand the potential risks associated with its use. You also agree to indemnify and hold harmless the authors, contributors, and copyright holders from any claims, damages, or liabilities arising from your use of OpenBuddy.
## 免责声明
所有OpenBuddy模型均存在固有的局限性,可能产生错误的、有害的、冒犯性的或其他不良的输出。用户在关键或高风险场景中应谨慎行事,不要使用这些模型,以免导致人身伤害、财产损失或重大损失。此类场景的例子包括但不限于医疗领域、可能导致伤害的软硬件系统的控制以及进行重要的财务或法律决策。
OpenBuddy按“原样”提供,不附带任何种类的明示或暗示的保证,包括但不限于适销性、特定目的的适用性和非侵权的暗示保证。在任何情况下,作者、贡献者或版权所有者均不对因软件或使用或其他软件交易而产生的任何索赔、损害赔偿或其他责任(无论是合同、侵权还是其他原因)承担责任。
使用OpenBuddy即表示您同意这些条款和条件,并承认您了解其使用可能带来的潜在风险。您还同意赔偿并使作者、贡献者和版权所有者免受因您使用OpenBuddy而产生的任何索赔、损害赔偿或责任的影响。 |
hfl/chinese-alpaca-2-1.3b-gguf | hfl | "2024-01-24T02:54:44Z" | 2,164 | 4 | null | [
"gguf",
"zh",
"en",
"license:apache-2.0",
"region:us"
] | null | "2023-11-16T05:21:50Z" | ---
license: apache-2.0
language:
- zh
- en
---
# Chinese-Alpaca-2-1.3B-GGUF
This repository contains the GGUF-v3 models (llama.cpp compatible) for **Chinese-Alpaca-2-1.3B**.
## Performance
Metric: PPL, lower is better
| Quant | original | imatrix (`-im`) |
|-----|------|------|
| Q2_K | 19.9339 +/- 0.29752 | 18.8935 +/- 0.28558 |
| Q3_K | 17.2487 +/- 0.27668 | 17.2950 +/- 0.27994 |
| Q4_0 | 16.1358 +/- 0.25091 | - |
| Q4_K | 16.4583 +/- 0.26453 | 16.2688 +/- 0.26216 |
| Q4_0 | 15.9068 +/- 0.25545 | - |
| Q5_K | 15.7547 +/- 0.25207 | 16.0190 +/- 0.25782 |
| Q6_K | 15.8166 +/- 0.25359 | 15.7357 +/- 0.25210 |
| Q8_0 | 15.7972 +/- 0.25384 | - |
| F16 | 15.8098 +/- 0.25403 | - |
*The model with `-im` suffix is generated with important matrix, which has generally better performance (not always though).*
## Others
For Hugging Face version, please see: https://huggingface.co/hfl/chinese-alpaca-2-1.3b
Please refer to [https://github.com/ymcui/Chinese-LLaMA-Alpaca-2/](https://github.com/ymcui/Chinese-LLaMA-Alpaca-2/) for more details. |
legraphista/DeepSeek-Coder-V2-Lite-Instruct-IMat-GGUF | legraphista | "2024-06-17T20:01:44Z" | 2,164 | 0 | gguf | [
"gguf",
"quantized",
"GGUF",
"quantization",
"imat",
"imatrix",
"static",
"16bit",
"8bit",
"6bit",
"5bit",
"4bit",
"3bit",
"2bit",
"1bit",
"text-generation",
"base_model:deepseek-ai/DeepSeek-Coder-V2-Lite-Instruct",
"license:other",
"region:us"
] | text-generation | "2024-06-17T18:47:11Z" | ---
base_model: deepseek-ai/DeepSeek-Coder-V2-Lite-Instruct
inference: false
library_name: gguf
license: other
license_link: LICENSE
license_name: deepseek-license
pipeline_tag: text-generation
quantized_by: legraphista
tags:
- quantized
- GGUF
- quantization
- imat
- imatrix
- static
- 16bit
- 8bit
- 6bit
- 5bit
- 4bit
- 3bit
- 2bit
- 1bit
---
# DeepSeek-Coder-V2-Lite-Instruct-IMat-GGUF
_Llama.cpp imatrix quantization of deepseek-ai/DeepSeek-Coder-V2-Lite-Instruct_
Original Model: [deepseek-ai/DeepSeek-Coder-V2-Lite-Instruct](https://huggingface.co/deepseek-ai/DeepSeek-Coder-V2-Lite-Instruct)
Original dtype: `BF16` (`bfloat16`)
Quantized by: llama.cpp [b3166](https://github.com/ggerganov/llama.cpp/releases/tag/b3166)
IMatrix dataset: [here](https://gist.githubusercontent.com/bartowski1182/eb213dccb3571f863da82e99418f81e8/raw/b2869d80f5c16fd7082594248e80144677736635/calibration_datav3.txt)
- [Files](#files)
- [IMatrix](#imatrix)
- [Common Quants](#common-quants)
- [All Quants](#all-quants)
- [Downloading using huggingface-cli](#downloading-using-huggingface-cli)
- [Inference](#inference)
- [Simple chat template](#simple-chat-template)
- [Chat template with system prompt](#chat-template-with-system-prompt)
- [Llama.cpp](#llama-cpp)
- [FAQ](#faq)
- [Why is the IMatrix not applied everywhere?](#why-is-the-imatrix-not-applied-everywhere)
- [How do I merge a split GGUF?](#how-do-i-merge-a-split-gguf)
---
## Files
### IMatrix
Status: ✅ Available
Link: [here](https://huggingface.co/legraphista/DeepSeek-Coder-V2-Lite-Instruct-IMat-GGUF/blob/main/imatrix.dat)
### Common Quants
| Filename | Quant type | File Size | Status | Uses IMatrix | Is Split |
| -------- | ---------- | --------- | ------ | ------------ | -------- |
| [DeepSeek-Coder-V2-Lite-Instruct.Q8_0.gguf](https://huggingface.co/legraphista/DeepSeek-Coder-V2-Lite-Instruct-IMat-GGUF/blob/main/DeepSeek-Coder-V2-Lite-Instruct.Q8_0.gguf) | Q8_0 | 16.70GB | ✅ Available | ⚪ Static | 📦 No
| [DeepSeek-Coder-V2-Lite-Instruct.Q6_K.gguf](https://huggingface.co/legraphista/DeepSeek-Coder-V2-Lite-Instruct-IMat-GGUF/blob/main/DeepSeek-Coder-V2-Lite-Instruct.Q6_K.gguf) | Q6_K | 14.07GB | ✅ Available | ⚪ Static | 📦 No
| [DeepSeek-Coder-V2-Lite-Instruct.Q4_K.gguf](https://huggingface.co/legraphista/DeepSeek-Coder-V2-Lite-Instruct-IMat-GGUF/blob/main/DeepSeek-Coder-V2-Lite-Instruct.Q4_K.gguf) | Q4_K | 10.36GB | ✅ Available | 🟢 IMatrix | 📦 No
| [DeepSeek-Coder-V2-Lite-Instruct.Q3_K.gguf](https://huggingface.co/legraphista/DeepSeek-Coder-V2-Lite-Instruct-IMat-GGUF/blob/main/DeepSeek-Coder-V2-Lite-Instruct.Q3_K.gguf) | Q3_K | 8.13GB | ✅ Available | 🟢 IMatrix | 📦 No
| [DeepSeek-Coder-V2-Lite-Instruct.Q2_K.gguf](https://huggingface.co/legraphista/DeepSeek-Coder-V2-Lite-Instruct-IMat-GGUF/blob/main/DeepSeek-Coder-V2-Lite-Instruct.Q2_K.gguf) | Q2_K | 6.43GB | ✅ Available | 🟢 IMatrix | 📦 No
### All Quants
| Filename | Quant type | File Size | Status | Uses IMatrix | Is Split |
| -------- | ---------- | --------- | ------ | ------------ | -------- |
| [DeepSeek-Coder-V2-Lite-Instruct.BF16.gguf](https://huggingface.co/legraphista/DeepSeek-Coder-V2-Lite-Instruct-IMat-GGUF/blob/main/DeepSeek-Coder-V2-Lite-Instruct.BF16.gguf) | BF16 | 31.42GB | ✅ Available | ⚪ Static | 📦 No
| [DeepSeek-Coder-V2-Lite-Instruct.FP16.gguf](https://huggingface.co/legraphista/DeepSeek-Coder-V2-Lite-Instruct-IMat-GGUF/blob/main/DeepSeek-Coder-V2-Lite-Instruct.FP16.gguf) | F16 | 31.42GB | ✅ Available | ⚪ Static | 📦 No
| [DeepSeek-Coder-V2-Lite-Instruct.Q8_0.gguf](https://huggingface.co/legraphista/DeepSeek-Coder-V2-Lite-Instruct-IMat-GGUF/blob/main/DeepSeek-Coder-V2-Lite-Instruct.Q8_0.gguf) | Q8_0 | 16.70GB | ✅ Available | ⚪ Static | 📦 No
| [DeepSeek-Coder-V2-Lite-Instruct.Q6_K.gguf](https://huggingface.co/legraphista/DeepSeek-Coder-V2-Lite-Instruct-IMat-GGUF/blob/main/DeepSeek-Coder-V2-Lite-Instruct.Q6_K.gguf) | Q6_K | 14.07GB | ✅ Available | ⚪ Static | 📦 No
| [DeepSeek-Coder-V2-Lite-Instruct.Q5_K.gguf](https://huggingface.co/legraphista/DeepSeek-Coder-V2-Lite-Instruct-IMat-GGUF/blob/main/DeepSeek-Coder-V2-Lite-Instruct.Q5_K.gguf) | Q5_K | 11.85GB | ✅ Available | ⚪ Static | 📦 No
| [DeepSeek-Coder-V2-Lite-Instruct.Q5_K_S.gguf](https://huggingface.co/legraphista/DeepSeek-Coder-V2-Lite-Instruct-IMat-GGUF/blob/main/DeepSeek-Coder-V2-Lite-Instruct.Q5_K_S.gguf) | Q5_K_S | 11.14GB | ✅ Available | ⚪ Static | 📦 No
| [DeepSeek-Coder-V2-Lite-Instruct.Q4_K.gguf](https://huggingface.co/legraphista/DeepSeek-Coder-V2-Lite-Instruct-IMat-GGUF/blob/main/DeepSeek-Coder-V2-Lite-Instruct.Q4_K.gguf) | Q4_K | 10.36GB | ✅ Available | 🟢 IMatrix | 📦 No
| [DeepSeek-Coder-V2-Lite-Instruct.Q4_K_S.gguf](https://huggingface.co/legraphista/DeepSeek-Coder-V2-Lite-Instruct-IMat-GGUF/blob/main/DeepSeek-Coder-V2-Lite-Instruct.Q4_K_S.gguf) | Q4_K_S | 9.53GB | ✅ Available | 🟢 IMatrix | 📦 No
| [DeepSeek-Coder-V2-Lite-Instruct.IQ4_NL.gguf](https://huggingface.co/legraphista/DeepSeek-Coder-V2-Lite-Instruct-IMat-GGUF/blob/main/DeepSeek-Coder-V2-Lite-Instruct.IQ4_NL.gguf) | IQ4_NL | 8.91GB | ✅ Available | 🟢 IMatrix | 📦 No
| [DeepSeek-Coder-V2-Lite-Instruct.IQ4_XS.gguf](https://huggingface.co/legraphista/DeepSeek-Coder-V2-Lite-Instruct-IMat-GGUF/blob/main/DeepSeek-Coder-V2-Lite-Instruct.IQ4_XS.gguf) | IQ4_XS | 8.57GB | ✅ Available | 🟢 IMatrix | 📦 No
| [DeepSeek-Coder-V2-Lite-Instruct.Q3_K.gguf](https://huggingface.co/legraphista/DeepSeek-Coder-V2-Lite-Instruct-IMat-GGUF/blob/main/DeepSeek-Coder-V2-Lite-Instruct.Q3_K.gguf) | Q3_K | 8.13GB | ✅ Available | 🟢 IMatrix | 📦 No
| [DeepSeek-Coder-V2-Lite-Instruct.Q3_K_L.gguf](https://huggingface.co/legraphista/DeepSeek-Coder-V2-Lite-Instruct-IMat-GGUF/blob/main/DeepSeek-Coder-V2-Lite-Instruct.Q3_K_L.gguf) | Q3_K_L | 8.46GB | ✅ Available | 🟢 IMatrix | 📦 No
| [DeepSeek-Coder-V2-Lite-Instruct.Q3_K_S.gguf](https://huggingface.co/legraphista/DeepSeek-Coder-V2-Lite-Instruct-IMat-GGUF/blob/main/DeepSeek-Coder-V2-Lite-Instruct.Q3_K_S.gguf) | Q3_K_S | 7.49GB | ✅ Available | 🟢 IMatrix | 📦 No
| [DeepSeek-Coder-V2-Lite-Instruct.IQ3_M.gguf](https://huggingface.co/legraphista/DeepSeek-Coder-V2-Lite-Instruct-IMat-GGUF/blob/main/DeepSeek-Coder-V2-Lite-Instruct.IQ3_M.gguf) | IQ3_M | 7.55GB | ✅ Available | 🟢 IMatrix | 📦 No
| [DeepSeek-Coder-V2-Lite-Instruct.IQ3_S.gguf](https://huggingface.co/legraphista/DeepSeek-Coder-V2-Lite-Instruct-IMat-GGUF/blob/main/DeepSeek-Coder-V2-Lite-Instruct.IQ3_S.gguf) | IQ3_S | 7.49GB | ✅ Available | 🟢 IMatrix | 📦 No
| [DeepSeek-Coder-V2-Lite-Instruct.IQ3_XS.gguf](https://huggingface.co/legraphista/DeepSeek-Coder-V2-Lite-Instruct-IMat-GGUF/blob/main/DeepSeek-Coder-V2-Lite-Instruct.IQ3_XS.gguf) | IQ3_XS | 7.12GB | ✅ Available | 🟢 IMatrix | 📦 No
| [DeepSeek-Coder-V2-Lite-Instruct.IQ3_XXS.gguf](https://huggingface.co/legraphista/DeepSeek-Coder-V2-Lite-Instruct-IMat-GGUF/blob/main/DeepSeek-Coder-V2-Lite-Instruct.IQ3_XXS.gguf) | IQ3_XXS | 6.96GB | ✅ Available | 🟢 IMatrix | 📦 No
| [DeepSeek-Coder-V2-Lite-Instruct.Q2_K.gguf](https://huggingface.co/legraphista/DeepSeek-Coder-V2-Lite-Instruct-IMat-GGUF/blob/main/DeepSeek-Coder-V2-Lite-Instruct.Q2_K.gguf) | Q2_K | 6.43GB | ✅ Available | 🟢 IMatrix | 📦 No
| [DeepSeek-Coder-V2-Lite-Instruct.Q2_K_S.gguf](https://huggingface.co/legraphista/DeepSeek-Coder-V2-Lite-Instruct-IMat-GGUF/blob/main/DeepSeek-Coder-V2-Lite-Instruct.Q2_K_S.gguf) | Q2_K_S | 6.46GB | ✅ Available | 🟢 IMatrix | 📦 No
| [DeepSeek-Coder-V2-Lite-Instruct.IQ2_M.gguf](https://huggingface.co/legraphista/DeepSeek-Coder-V2-Lite-Instruct-IMat-GGUF/blob/main/DeepSeek-Coder-V2-Lite-Instruct.IQ2_M.gguf) | IQ2_M | 6.33GB | ✅ Available | 🟢 IMatrix | 📦 No
| [DeepSeek-Coder-V2-Lite-Instruct.IQ2_S.gguf](https://huggingface.co/legraphista/DeepSeek-Coder-V2-Lite-Instruct-IMat-GGUF/blob/main/DeepSeek-Coder-V2-Lite-Instruct.IQ2_S.gguf) | IQ2_S | 6.01GB | ✅ Available | 🟢 IMatrix | 📦 No
| [DeepSeek-Coder-V2-Lite-Instruct.IQ2_XS.gguf](https://huggingface.co/legraphista/DeepSeek-Coder-V2-Lite-Instruct-IMat-GGUF/blob/main/DeepSeek-Coder-V2-Lite-Instruct.IQ2_XS.gguf) | IQ2_XS | 5.97GB | ✅ Available | 🟢 IMatrix | 📦 No
| [DeepSeek-Coder-V2-Lite-Instruct.IQ2_XXS.gguf](https://huggingface.co/legraphista/DeepSeek-Coder-V2-Lite-Instruct-IMat-GGUF/blob/main/DeepSeek-Coder-V2-Lite-Instruct.IQ2_XXS.gguf) | IQ2_XXS | 5.64GB | ✅ Available | 🟢 IMatrix | 📦 No
| [DeepSeek-Coder-V2-Lite-Instruct.IQ1_M.gguf](https://huggingface.co/legraphista/DeepSeek-Coder-V2-Lite-Instruct-IMat-GGUF/blob/main/DeepSeek-Coder-V2-Lite-Instruct.IQ1_M.gguf) | IQ1_M | 5.24GB | ✅ Available | 🟢 IMatrix | 📦 No
| [DeepSeek-Coder-V2-Lite-Instruct.IQ1_S.gguf](https://huggingface.co/legraphista/DeepSeek-Coder-V2-Lite-Instruct-IMat-GGUF/blob/main/DeepSeek-Coder-V2-Lite-Instruct.IQ1_S.gguf) | IQ1_S | 4.99GB | ✅ Available | 🟢 IMatrix | 📦 No
## Downloading using huggingface-cli
If you do not have hugginface-cli installed:
```
pip install -U "huggingface_hub[cli]"
```
Download the specific file you want:
```
huggingface-cli download legraphista/DeepSeek-Coder-V2-Lite-Instruct-IMat-GGUF --include "DeepSeek-Coder-V2-Lite-Instruct.Q8_0.gguf" --local-dir ./
```
If the model file is big, it has been split into multiple files. In order to download them all to a local folder, run:
```
huggingface-cli download legraphista/DeepSeek-Coder-V2-Lite-Instruct-IMat-GGUF --include "DeepSeek-Coder-V2-Lite-Instruct.Q8_0/*" --local-dir ./
# see FAQ for merging GGUF's
```
---
## Inference
### Simple chat template
```
<|begin▁of▁sentence|>User: {user_prompt}
Assistant: {assistant_response}<|end▁of▁sentence|>User: {next_user_prompt}
```
### Chat template with system prompt
```
<|begin▁of▁sentence|>{system_prompt}
User: {user_prompt}
Assistant: {assistant_response}<|end▁of▁sentence|>User: {next_user_prompt}
```
### Llama.cpp
```
llama.cpp/main -m DeepSeek-Coder-V2-Lite-Instruct.Q8_0.gguf --color -i -p "prompt here (according to the chat template)"
```
---
## FAQ
### Why is the IMatrix not applied everywhere?
According to [this investigation](https://www.reddit.com/r/LocalLLaMA/comments/1993iro/ggufs_quants_can_punch_above_their_weights_now/), it appears that lower quantizations are the only ones that benefit from the imatrix input (as per hellaswag results).
### How do I merge a split GGUF?
1. Make sure you have `gguf-split` available
- To get hold of `gguf-split`, navigate to https://github.com/ggerganov/llama.cpp/releases
- Download the appropriate zip for your system from the latest release
- Unzip the archive and you should be able to find `gguf-split`
2. Locate your GGUF chunks folder (ex: `DeepSeek-Coder-V2-Lite-Instruct.Q8_0`)
3. Run `gguf-split --merge DeepSeek-Coder-V2-Lite-Instruct.Q8_0/DeepSeek-Coder-V2-Lite-Instruct.Q8_0-00001-of-XXXXX.gguf DeepSeek-Coder-V2-Lite-Instruct.Q8_0.gguf`
- Make sure to point `gguf-split` to the first chunk of the split.
---
Got a suggestion? Ping me [@legraphista](https://x.com/legraphista)! |
eachadea/vicuna-7b-1.1 | eachadea | "2023-05-02T09:08:12Z" | 2,163 | 107 | transformers | [
"transformers",
"pytorch",
"llama",
"text-generation",
"license:apache-2.0",
"autotrain_compatible",
"endpoints_compatible",
"text-generation-inference",
"region:us"
] | text-generation | "2023-04-13T03:45:52Z" | ---
license: apache-2.0
inference: true
---
**delta v1.1 merge**
# Vicuna Model Card
## Model details
**Model type:**
Vicuna is an open-source chatbot trained by fine-tuning LLaMA on user-shared conversations collected from ShareGPT.
It is an auto-regressive language model, based on the transformer architecture.
**Model date:**
Vicuna was trained between March 2023 and April 2023.
**Organizations developing the model:**
The Vicuna team with members from UC Berkeley, CMU, Stanford, and UC San Diego.
**Paper or resources for more information:**
https://vicuna.lmsys.org/
**License:**
Apache License 2.0
**Where to send questions or comments about the model:**
https://github.com/lm-sys/FastChat/issues
## Intended use
**Primary intended uses:**
The primary use of Vicuna is research on large language models and chatbots.
**Primary intended users:**
The primary intended users of the model are researchers and hobbyists in natural language processing, machine learning, and artificial intelligence.
## Training dataset
70K conversations collected from ShareGPT.com.
## Evaluation dataset
A preliminary evaluation of the model quality is conducted by creating a set of 80 diverse questions and utilizing GPT-4 to judge the model outputs. See https://vicuna.lmsys.org/ for more details.
## Major updates of weights v1.1
- Refactor the tokenization and separator. In Vicuna v1.1, the separator has been changed from `"###"` to the EOS token `"</s>"`. This change makes it easier to determine the generation stop criteria and enables better compatibility with other libraries.
- Fix the supervised fine-tuning loss computation for better model quality. |
google/madlad400-7b-mt-bt | google | "2023-11-27T15:59:52Z" | 2,163 | 4 | transformers | [
"transformers",
"safetensors",
"gguf",
"t5",
"text2text-generation",
"text-generation-inference",
"translation",
"multilingual",
"en",
"ru",
"es",
"fr",
"de",
"it",
"pt",
"pl",
"nl",
"vi",
"tr",
"sv",
"id",
"ro",
"cs",
"zh",
"hu",
"ja",
"th",
"fi",
"fa",
"uk",
"da",
"el",
"no",
"bg",
"sk",
"ko",
"ar",
"lt",
"ca",
"sl",
"he",
"et",
"lv",
"hi",
"sq",
"ms",
"az",
"sr",
"ta",
"hr",
"kk",
"is",
"ml",
"mr",
"te",
"af",
"gl",
"fil",
"be",
"mk",
"eu",
"bn",
"ka",
"mn",
"bs",
"uz",
"ur",
"sw",
"yue",
"ne",
"kn",
"kaa",
"gu",
"si",
"cy",
"eo",
"la",
"hy",
"ky",
"tg",
"ga",
"mt",
"my",
"km",
"tt",
"so",
"ku",
"ps",
"pa",
"rw",
"lo",
"ha",
"dv",
"fy",
"lb",
"ckb",
"mg",
"gd",
"am",
"ug",
"ht",
"grc",
"hmn",
"sd",
"jv",
"mi",
"tk",
"ceb",
"yi",
"ba",
"fo",
"or",
"xh",
"su",
"kl",
"ny",
"sm",
"sn",
"co",
"zu",
"ig",
"yo",
"pap",
"st",
"haw",
"as",
"oc",
"cv",
"lus",
"tet",
"gsw",
"sah",
"br",
"rm",
"sa",
"bo",
"om",
"se",
"ce",
"cnh",
"ilo",
"hil",
"udm",
"os",
"lg",
"ti",
"vec",
"ts",
"tyv",
"kbd",
"ee",
"iba",
"av",
"kha",
"to",
"tn",
"nso",
"fj",
"zza",
"ak",
"ada",
"otq",
"dz",
"bua",
"cfm",
"ln",
"chm",
"gn",
"krc",
"wa",
"hif",
"yua",
"srn",
"war",
"rom",
"bik",
"pam",
"sg",
"lu",
"ady",
"kbp",
"syr",
"ltg",
"myv",
"iso",
"kac",
"bho",
"ay",
"kum",
"qu",
"za",
"pag",
"ngu",
"ve",
"pck",
"zap",
"tyz",
"hui",
"bbc",
"tzo",
"tiv",
"ksd",
"gom",
"min",
"ang",
"nhe",
"bgp",
"nzi",
"nnb",
"nv",
"zxx",
"bci",
"kv",
"new",
"mps",
"alt",
"meu",
"bew",
"fon",
"iu",
"abt",
"mgh",
"mnw",
"tvl",
"dov",
"tlh",
"ho",
"kw",
"mrj",
"meo",
"crh",
"mbt",
"emp",
"ace",
"ium",
"mam",
"gym",
"mai",
"crs",
"pon",
"ubu",
"fip",
"quc",
"gv",
"kj",
"btx",
"ape",
"chk",
"rcf",
"shn",
"tzh",
"mdf",
"ppk",
"ss",
"gag",
"cab",
"kri",
"seh",
"ibb",
"tbz",
"bru",
"enq",
"ach",
"cuk",
"kmb",
"wo",
"kek",
"qub",
"tab",
"bts",
"kos",
"rwo",
"cak",
"tuc",
"bum",
"cjk",
"gil",
"stq",
"tsg",
"quh",
"mak",
"arn",
"ban",
"jiv",
"sja",
"yap",
"tcy",
"toj",
"twu",
"xal",
"amu",
"rmc",
"hus",
"nia",
"kjh",
"bm",
"guh",
"mas",
"acf",
"dtp",
"ksw",
"bzj",
"din",
"zne",
"mad",
"msi",
"mag",
"mkn",
"kg",
"lhu",
"ch",
"qvi",
"mh",
"djk",
"sus",
"mfe",
"srm",
"dyu",
"ctu",
"gui",
"pau",
"inb",
"bi",
"mni",
"guc",
"jam",
"wal",
"jac",
"bas",
"gor",
"skr",
"nyu",
"noa",
"sda",
"gub",
"nog",
"cni",
"teo",
"tdx",
"sxn",
"rki",
"nr",
"frp",
"alz",
"taj",
"lrc",
"cce",
"rn",
"jvn",
"hvn",
"nij",
"dwr",
"izz",
"msm",
"bus",
"ktu",
"chr",
"maz",
"tzj",
"suz",
"knj",
"bim",
"gvl",
"bqc",
"tca",
"pis",
"prk",
"laj",
"mel",
"qxr",
"niq",
"ahk",
"shp",
"hne",
"spp",
"koi",
"krj",
"quf",
"luz",
"agr",
"tsc",
"mqy",
"gof",
"gbm",
"miq",
"dje",
"awa",
"bjj",
"qvz",
"sjp",
"tll",
"raj",
"kjg",
"bgz",
"quy",
"cbk",
"akb",
"oj",
"ify",
"mey",
"ks",
"cac",
"brx",
"qup",
"syl",
"jax",
"ff",
"ber",
"tks",
"trp",
"mrw",
"adh",
"smt",
"srr",
"ffm",
"qvc",
"mtr",
"ann",
"aa",
"noe",
"nut",
"gyn",
"kwi",
"xmm",
"msb",
"dataset:allenai/MADLAD-400",
"arxiv:2309.04662",
"license:apache-2.0",
"autotrain_compatible",
"endpoints_compatible",
"region:us"
] | translation | "2023-11-27T15:59:50Z" | ---
license: apache-2.0
language:
- multilingual
- en
- ru
- es
- fr
- de
- it
- pt
- pl
- nl
- vi
- tr
- sv
- id
- ro
- cs
- zh
- hu
- ja
- th
- fi
- fa
- uk
- da
- el
- "no"
- bg
- sk
- ko
- ar
- lt
- ca
- sl
- he
- et
- lv
- hi
- sq
- ms
- az
- sr
- ta
- hr
- kk
- is
- ml
- mr
- te
- af
- gl
- fil
- be
- mk
- eu
- bn
- ka
- mn
- bs
- uz
- ur
- sw
- yue
- ne
- kn
- kaa
- gu
- si
- cy
- eo
- la
- hy
- ky
- tg
- ga
- mt
- my
- km
- tt
- so
- ku
- ps
- pa
- rw
- lo
- ha
- dv
- fy
- lb
- ckb
- mg
- gd
- am
- ug
- ht
- grc
- hmn
- sd
- jv
- mi
- tk
- ceb
- yi
- ba
- fo
- or
- xh
- su
- kl
- ny
- sm
- sn
- co
- zu
- ig
- yo
- pap
- st
- haw
- as
- oc
- cv
- lus
- tet
- gsw
- sah
- br
- rm
- sa
- bo
- om
- se
- ce
- cnh
- ilo
- hil
- udm
- os
- lg
- ti
- vec
- ts
- tyv
- kbd
- ee
- iba
- av
- kha
- to
- tn
- nso
- fj
- zza
- ak
- ada
- otq
- dz
- bua
- cfm
- ln
- chm
- gn
- krc
- wa
- hif
- yua
- srn
- war
- rom
- bik
- pam
- sg
- lu
- ady
- kbp
- syr
- ltg
- myv
- iso
- kac
- bho
- ay
- kum
- qu
- za
- pag
- ngu
- ve
- pck
- zap
- tyz
- hui
- bbc
- tzo
- tiv
- ksd
- gom
- min
- ang
- nhe
- bgp
- nzi
- nnb
- nv
- zxx
- bci
- kv
- new
- mps
- alt
- meu
- bew
- fon
- iu
- abt
- mgh
- mnw
- tvl
- dov
- tlh
- ho
- kw
- mrj
- meo
- crh
- mbt
- emp
- ace
- ium
- mam
- gym
- mai
- crs
- pon
- ubu
- fip
- quc
- gv
- kj
- btx
- ape
- chk
- rcf
- shn
- tzh
- mdf
- ppk
- ss
- gag
- cab
- kri
- seh
- ibb
- tbz
- bru
- enq
- ach
- cuk
- kmb
- wo
- kek
- qub
- tab
- bts
- kos
- rwo
- cak
- tuc
- bum
- cjk
- gil
- stq
- tsg
- quh
- mak
- arn
- ban
- jiv
- sja
- yap
- tcy
- toj
- twu
- xal
- amu
- rmc
- hus
- nia
- kjh
- bm
- guh
- mas
- acf
- dtp
- ksw
- bzj
- din
- zne
- mad
- msi
- mag
- mkn
- kg
- lhu
- ch
- qvi
- mh
- djk
- sus
- mfe
- srm
- dyu
- ctu
- gui
- pau
- inb
- bi
- mni
- guc
- jam
- wal
- jac
- bas
- gor
- skr
- nyu
- noa
- sda
- gub
- nog
- cni
- teo
- tdx
- sxn
- rki
- nr
- frp
- alz
- taj
- lrc
- cce
- rn
- jvn
- hvn
- nij
- dwr
- izz
- msm
- bus
- ktu
- chr
- maz
- tzj
- suz
- knj
- bim
- gvl
- bqc
- tca
- pis
- prk
- laj
- mel
- qxr
- niq
- ahk
- shp
- hne
- spp
- koi
- krj
- quf
- luz
- agr
- tsc
- mqy
- gof
- gbm
- miq
- dje
- awa
- bjj
- qvz
- sjp
- tll
- raj
- kjg
- bgz
- quy
- cbk
- akb
- oj
- ify
- mey
- ks
- cac
- brx
- qup
- syl
- jax
- ff
- ber
- tks
- trp
- mrw
- adh
- smt
- srr
- ffm
- qvc
- mtr
- ann
- kaa
- aa
- noe
- nut
- gyn
- kwi
- xmm
- msb
library_name: transformers
tags:
- text2text-generation
- text-generation-inference
datasets:
- allenai/MADLAD-400
pipeline_tag: translation
widget:
- text: "<2en> Como vai, amigo?"
example_title: "Translation to English"
- text: "<2de> Do you speak German?"
example_title: "Translation to German"
---
# Model Card for MADLAD-400-7B-MT
# Table of Contents
0. [TL;DR](#TL;DR)
1. [Model Details](#model-details)
2. [Usage](#usage)
3. [Uses](#uses)
4. [Bias, Risks, and Limitations](#bias-risks-and-limitations)
5. [Training Details](#training-details)
6. [Evaluation](#evaluation)
7. [Environmental Impact](#environmental-impact)
8. [Citation](#citation)
# TL;DR
MADLAD-400-7B-MT-BT is a multilingual machine translation model based on the T5 architecture that was
trained on 250 billion tokens covering over 450 languages using publicly available data.
It is competitive with models that are significantly larger.
It's a finetuned version of the 7.2B parameter model on backtranslated data. Authors say in the [paper](https://arxiv.org/pdf/2309.04662.pdf) that:
> While this setup is very likely sub-optimal, we see that back-translation
> greatly improves en2xx translation (by 3.0 chrf, in the case of Flores-200) in most cases.
**Disclaimer**: [Juarez Bochi](https://huggingface.co/jbochi), who was not involved in this research, converted
the original weights and wrote the contents of this model card based on the original paper and Flan-T5.
# Model Details
## Model Description
- **Model type:** Language model
- **Language(s) (NLP):** Multilingual (400+ languages)
- **License:** Apache 2.0
- **Related Models:** [All MADLAD-400 Checkpoints](https://huggingface.co/models?search=madlad)
- **Original Checkpoints:** [All Original MADLAD-400 Checkpoints](https://github.com/google-research/google-research/tree/master/madlad_400)
- **Resources for more information:**
- [Research paper](https://arxiv.org/abs/2309.04662)
- [GitHub Repo](https://github.com/google-research/t5x)
- [Hugging Face MADLAD-400 Docs (Similar to T5) ](https://huggingface.co/docs/transformers/model_doc/MADLAD-400) - [Pending PR](https://github.com/huggingface/transformers/pull/27471)
# Usage
Find below some example scripts on how to use the model:
## Using the Pytorch model with `transformers`
### Running the model on a CPU or GPU
<details>
<summary> Click to expand </summary>
First, install the Python packages that are required:
`pip install transformers accelerate sentencepiece`
```python
from transformers import T5ForConditionalGeneration, T5Tokenizer
model_name = 'jbochi/madlad400-7b-mt-bt'
model = T5ForConditionalGeneration.from_pretrained(model_name, device_map="auto")
tokenizer = T5Tokenizer.from_pretrained(model_name)
text = "<2pt> I love pizza!"
input_ids = tokenizer(text, return_tensors="pt").input_ids.to(model.device)
outputs = model.generate(input_ids=input_ids)
tokenizer.decode(outputs[0], skip_special_tokens=True)
# Eu adoro pizza!
```
</details>
## Running the model with Candle
<details>
<summary> Click to expand </summary>
Usage with [candle](https://github.com/huggingface/candle):
```bash
$ cargo run --example t5 --release -- \
--model-id "jbochi/madlad400-7b-mt-bt" \
--prompt "<2de> How are you, my friend?" \
--decode --temperature 0
```
</details>
# Uses
## Direct Use and Downstream Use
> Primary intended uses: Machine Translation and multilingual NLP tasks on over 400 languages.
> Primary intended users: Research community.
## Out-of-Scope Use
> These models are trained on general domain data and are therefore not meant to
> work on domain-specific models out-of-the box. Moreover, these research models have not been assessed
> for production usecases.
# Bias, Risks, and Limitations
> We note that we evaluate on only 204 of the languages supported by these models and on machine translation
> and few-shot machine translation tasks. Users must consider use of this model carefully for their own
> usecase.
## Ethical considerations and risks
> We trained these models with MADLAD-400 and publicly available data to create baseline models that
> support NLP for over 400 languages, with a focus on languages underrepresented in large-scale corpora.
> Given that these models were trained with web-crawled datasets that may contain sensitive, offensive or
> otherwise low-quality content despite extensive preprocessing, it is still possible that these issues to the
> underlying training data may cause differences in model performance and toxic (or otherwise problematic)
> output for certain domains. Moreover, large models are dual use technologies that have specific risks
> associated with their use and development. We point the reader to surveys such as those written by
> Weidinger et al. or Bommasani et al. for a more detailed discussion of these risks, and to Liebling
> et al. for a thorough discussion of the risks of machine translation systems.
## Known Limitations
More information needed
## Sensitive Use:
More information needed
# Training Details
> We train models of various sizes: a 3B, 32-layer parameter model,
> a 7.2B 48-layer parameter model and a 10.7B 32-layer parameter model.
> We share all parameters of the model across language pairs,
> and use a Sentence Piece Model with 256k tokens shared on both the encoder and decoder
> side. Each input sentence has a <2xx> token prepended to the source sentence to indicate the target
> language.
See the [research paper](https://arxiv.org/pdf/2309.04662.pdf) for further details.
## Training Data
> For both the machine translation and language model, MADLAD-400 is used. For the machine translation
> model, a combination of parallel datasources covering 157 languages is also used. Further details are
> described in the [paper](https://arxiv.org/pdf/2309.04662.pdf).
## Training Procedure
See the [research paper](https://arxiv.org/pdf/2309.04662.pdf) for further details.
# Evaluation
## Testing Data, Factors & Metrics
> For evaluation, we used WMT, NTREX, Flores-200 and Gatones datasets as described in Section 4.3 in the [paper](https://arxiv.org/pdf/2309.04662.pdf).
> The translation quality of this model varies based on language, as seen in the paper, and likely varies on
> domain, though we have not assessed this.
## Results



See the [research paper](https://arxiv.org/pdf/2309.04662.pdf) for further details.
# Environmental Impact
More information needed
# Citation
**BibTeX:**
```bibtex
@misc{kudugunta2023madlad400,
title={MADLAD-400: A Multilingual And Document-Level Large Audited Dataset},
author={Sneha Kudugunta and Isaac Caswell and Biao Zhang and Xavier Garcia and Christopher A. Choquette-Choo and Katherine Lee and Derrick Xin and Aditya Kusupati and Romi Stella and Ankur Bapna and Orhan Firat},
year={2023},
eprint={2309.04662},
archivePrefix={arXiv},
primaryClass={cs.CL}
}
``` |
NYTK/sentiment-hts5-xlm-roberta-hungarian | NYTK | "2023-01-23T08:46:44Z" | 2,162 | 0 | transformers | [
"transformers",
"pytorch",
"xlm-roberta",
"text-classification",
"hu",
"license:mit",
"autotrain_compatible",
"endpoints_compatible",
"region:us"
] | text-classification | "2022-03-02T23:29:04Z" | ---
language:
- hu
tags:
- text-classification
license: mit
metrics:
- accuracy
widget:
- text: Jó reggelt! majd küldöm az élményhozókat :).
---
# Hungarian Sentence-level Sentiment Analysis Model with XLM-RoBERTa
For further models, scripts and details, see [our repository](https://github.com/nytud/sentiment-analysis) or [our demo site](https://juniper.nytud.hu/demo/nlp).
- Pretrained model used: XLM-RoBERTa base
- Finetuned on Hungarian Twitter Sentiment (HTS) Corpus
- Labels: 0 (very negative), 1 (negative), 2 (neutral), 3 (positive), 4 (very positive)
## Limitations
- max_seq_length = 128
## Results
| Model | HTS2 | HTS5 |
| ------------- | ------------- | ------------- |
| huBERT | 85.56 | **68.99** |
| XLM-RoBERTa| 85.56 | 66.50 |
## Citation
If you use this model, please cite the following paper:
```
@inproceedings {laki-yang-sentiment,
title = {Improving Performance of Sentence-level Sentiment Analysis with Data Augmentation Methods},
booktitle = {Proceedings of 12th IEEE International Conference on Cognitive Infocommunications (CogInfoCom 2021)},
year = {2021},
publisher = {IEEE},
address = {Online},
author = {Laki, László and Yang, Zijian Győző}
pages = {417--422}
}
``` |
gogamza/kobart-base-v1 | gogamza | "2023-06-29T00:45:30Z" | 2,162 | 1 | transformers | [
"transformers",
"pytorch",
"safetensors",
"bart",
"feature-extraction",
"ko",
"license:mit",
"endpoints_compatible",
"region:us"
] | feature-extraction | "2022-03-02T23:29:05Z" | ---
language: ko
tags:
- bart
license: mit
---
## KoBART-base-v1
```python
from transformers import PreTrainedTokenizerFast, BartModel
tokenizer = PreTrainedTokenizerFast.from_pretrained('gogamza/kobart-base-v1')
model = BartModel.from_pretrained('gogamza/kobart-base-v1')
```
|
yujiepan/llama-2-tiny-3layers-random | yujiepan | "2024-04-19T13:22:12Z" | 2,162 | 1 | transformers | [
"transformers",
"pytorch",
"safetensors",
"openvino",
"llama",
"text-generation",
"conversational",
"autotrain_compatible",
"endpoints_compatible",
"text-generation-inference",
"region:us"
] | text-generation | "2023-08-31T09:30:50Z" | ---
library_name: transformers
pipeline_tag: text-generation
inference: true
widget:
- text: Hello!
example_title: Hello world
group: Python
---
# yujiepan/llama-2-tiny-3layers-random
This model is **randomly initialized**, using the config from [meta-llama/Llama-2-7b-chat-hf](https://huggingface.co/yujiepan/llama-2-tiny-3layers-random/blob/main/config.json) but with the following modifications:
```json
{
"hidden_size": 8,
"intermediate_size": 32,
"num_attention_heads": 2,
"num_hidden_layers": 3,
"num_key_value_heads": 2,
}
``` |
marcchew/Platypus-2-7B-LaMini-14K | marcchew | "2023-09-08T07:14:20Z" | 2,162 | 0 | transformers | [
"transformers",
"pytorch",
"llama",
"text-generation",
"autotrain_compatible",
"endpoints_compatible",
"text-generation-inference",
"8-bit",
"bitsandbytes",
"region:us"
] | text-generation | "2023-08-31T14:00:53Z" | Entry not found |
OpenBuddy/openbuddy-llama3-8b-v21.1-8k | OpenBuddy | "2024-04-26T09:42:56Z" | 2,162 | 29 | transformers | [
"transformers",
"safetensors",
"llama",
"text-generation",
"llama-3",
"conversational",
"zh",
"en",
"fr",
"de",
"ja",
"ko",
"it",
"fi",
"license:other",
"autotrain_compatible",
"endpoints_compatible",
"text-generation-inference",
"region:us"
] | text-generation | "2024-04-20T01:36:51Z" | ---
language:
- zh
- en
- fr
- de
- ja
- ko
- it
- fi
pipeline_tag: text-generation
tags:
- llama-3
license: other
license_name: llama3
license_link: https://llama.meta.com/llama3/license/
---
# OpenBuddy - Open Multilingual Chatbot
GitHub and Usage Guide: [https://github.com/OpenBuddy/OpenBuddy](https://github.com/OpenBuddy/OpenBuddy)
Website and Demo: [https://openbuddy.ai](https://openbuddy.ai)
Evaluation result of this model: [Evaluation.txt](Evaluation.txt)
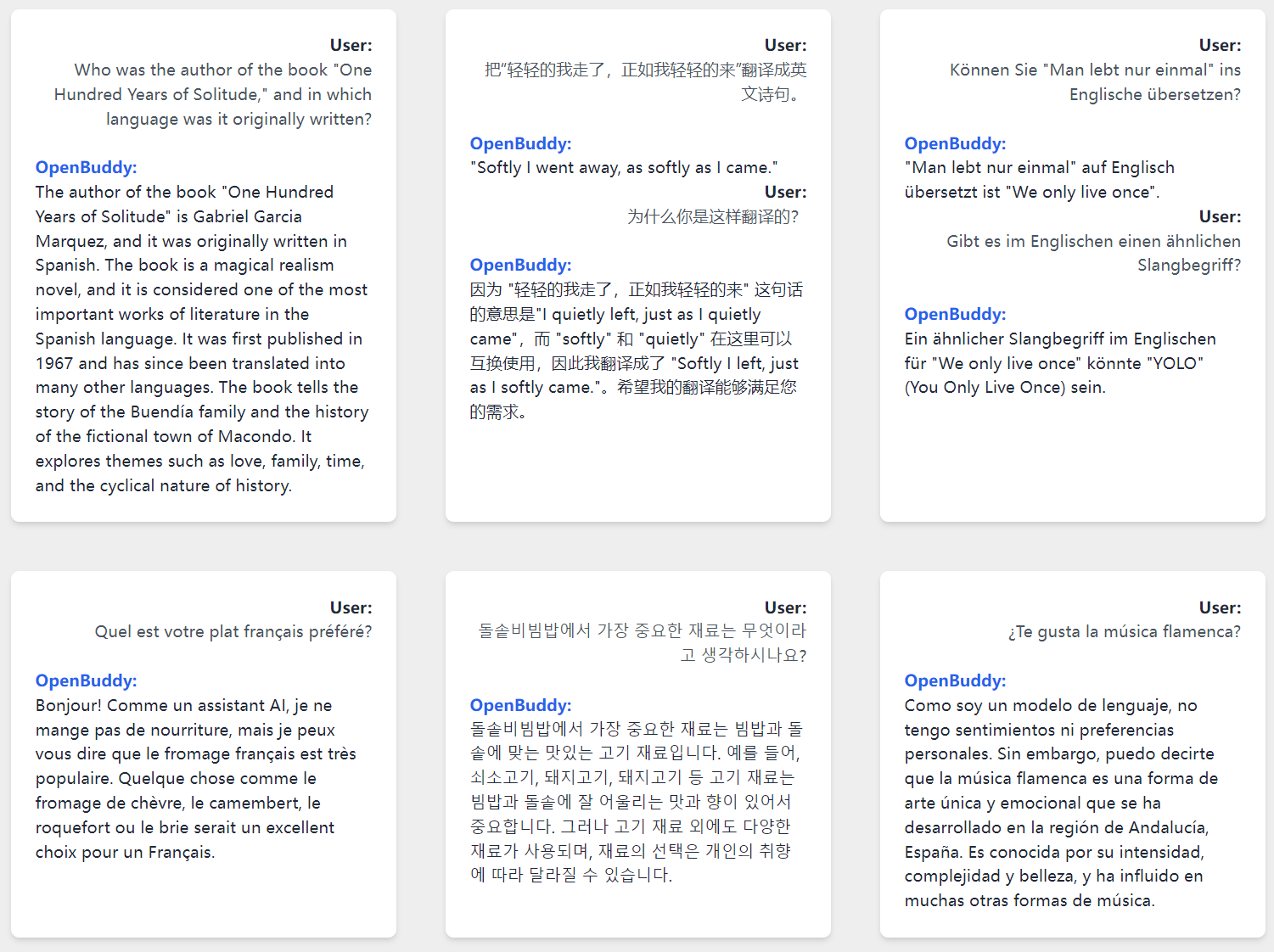
# Run locally with 🦙Ollama
```
ollama run openbuddy/openbuddy-llama3-8b-v21.1-8k
```
# Copyright Notice
**Built with Meta Llama 3**
License: https://llama.meta.com/llama3/license/
Acceptable Use Policy: https://llama.meta.com/llama3/use-policy
This model is intended for use in English and Chinese.
# Prompt Format
We recommend using the fast tokenizer from `transformers`, which should be enabled by default in the `transformers` and `vllm` libraries. Other implementations including `sentencepiece` may not work as expected, especially for special tokens like `<|role|>`, `<|says|>` and `<|end|>`.
```
<|role|>system<|says|>You(assistant) are a helpful, respectful and honest INTP-T AI Assistant named Buddy. You are talking to a human(user).
Always answer as helpfully and logically as possible, while being safe. Your answers should not include any harmful, political, religious, unethical, racist, sexist, toxic, dangerous, or illegal content. Please ensure that your responses are socially unbiased and positive in nature.
You cannot access the internet, but you have vast knowledge, cutoff: 2023-04.
You are trained by OpenBuddy team, (https://openbuddy.ai, https://github.com/OpenBuddy/OpenBuddy), not related to GPT or OpenAI.<|end|>
<|role|>user<|says|>History input 1<|end|>
<|role|>assistant<|says|>History output 1<|end|>
<|role|>user<|says|>History input 2<|end|>
<|role|>assistant<|says|>History output 2<|end|>
<|role|>user<|says|>Current input<|end|>
<|role|>assistant<|says|>
```
This format is also defined in `tokenizer_config.json`, which means you can directly use `vllm` to deploy an OpenAI-like API service. For more information, please refer to the [vllm documentation](https://docs.vllm.ai/en/latest/serving/openai_compatible_server.html).
## Disclaimer
All OpenBuddy models have inherent limitations and may potentially produce outputs that are erroneous, harmful, offensive, or otherwise undesirable. Users should not use these models in critical or high-stakes situations that may lead to personal injury, property damage, or significant losses. Examples of such scenarios include, but are not limited to, the medical field, controlling software and hardware systems that may cause harm, and making important financial or legal decisions.
OpenBuddy is provided "as-is" without any warranty of any kind, either express or implied, including, but not limited to, the implied warranties of merchantability, fitness for a particular purpose, and non-infringement. In no event shall the authors, contributors, or copyright holders be liable for any claim, damages, or other liabilities, whether in an action of contract, tort, or otherwise, arising from, out of, or in connection with the software or the use or other dealings in the software.
By using OpenBuddy, you agree to these terms and conditions, and acknowledge that you understand the potential risks associated with its use. You also agree to indemnify and hold harmless the authors, contributors, and copyright holders from any claims, damages, or liabilities arising from your use of OpenBuddy.
## 免责声明
所有OpenBuddy模型均存在固有的局限性,可能产生错误的、有害的、冒犯性的或其他不良的输出。用户在关键或高风险场景中应谨慎行事,不要使用这些模型,以免导致人身伤害、财产损失或重大损失。此类场景的例子包括但不限于医疗领域、可能导致伤害的软硬件系统的控制以及进行重要的财务或法律决策。
OpenBuddy按“原样”提供,不附带任何种类的明示或暗示的保证,包括但不限于适销性、特定目的的适用性和非侵权的暗示保证。在任何情况下,作者、贡献者或版权所有者均不对因软件或使用或其他软件交易而产生的任何索赔、损害赔偿或其他责任(无论是合同、侵权还是其他原因)承担责任。
使用OpenBuddy即表示您同意这些条款和条件,并承认您了解其使用可能带来的潜在风险。您还同意赔偿并使作者、贡献者和版权所有者免受因您使用OpenBuddy而产生的任何索赔、损害赔偿或责任的影响。 |
RichardErkhov/01-ai_-_Yi-1.5-9B-32K-gguf | RichardErkhov | "2024-06-14T22:51:27Z" | 2,162 | 0 | null | [
"gguf",
"arxiv:2403.04652",
"region:us"
] | null | "2024-06-14T21:42:21Z" | Quantization made by Richard Erkhov.
[Github](https://github.com/RichardErkhov)
[Discord](https://discord.gg/pvy7H8DZMG)
[Request more models](https://github.com/RichardErkhov/quant_request)
Yi-1.5-9B-32K - GGUF
- Model creator: https://huggingface.co/01-ai/
- Original model: https://huggingface.co/01-ai/Yi-1.5-9B-32K/
| Name | Quant method | Size |
| ---- | ---- | ---- |
| [Yi-1.5-9B-32K.Q2_K.gguf](https://huggingface.co/RichardErkhov/01-ai_-_Yi-1.5-9B-32K-gguf/blob/main/Yi-1.5-9B-32K.Q2_K.gguf) | Q2_K | 3.12GB |
| [Yi-1.5-9B-32K.IQ3_XS.gguf](https://huggingface.co/RichardErkhov/01-ai_-_Yi-1.5-9B-32K-gguf/blob/main/Yi-1.5-9B-32K.IQ3_XS.gguf) | IQ3_XS | 3.46GB |
| [Yi-1.5-9B-32K.IQ3_S.gguf](https://huggingface.co/RichardErkhov/01-ai_-_Yi-1.5-9B-32K-gguf/blob/main/Yi-1.5-9B-32K.IQ3_S.gguf) | IQ3_S | 3.64GB |
| [Yi-1.5-9B-32K.Q3_K_S.gguf](https://huggingface.co/RichardErkhov/01-ai_-_Yi-1.5-9B-32K-gguf/blob/main/Yi-1.5-9B-32K.Q3_K_S.gguf) | Q3_K_S | 3.63GB |
| [Yi-1.5-9B-32K.IQ3_M.gguf](https://huggingface.co/RichardErkhov/01-ai_-_Yi-1.5-9B-32K-gguf/blob/main/Yi-1.5-9B-32K.IQ3_M.gguf) | IQ3_M | 3.78GB |
| [Yi-1.5-9B-32K.Q3_K.gguf](https://huggingface.co/RichardErkhov/01-ai_-_Yi-1.5-9B-32K-gguf/blob/main/Yi-1.5-9B-32K.Q3_K.gguf) | Q3_K | 4.03GB |
| [Yi-1.5-9B-32K.Q3_K_M.gguf](https://huggingface.co/RichardErkhov/01-ai_-_Yi-1.5-9B-32K-gguf/blob/main/Yi-1.5-9B-32K.Q3_K_M.gguf) | Q3_K_M | 4.03GB |
| [Yi-1.5-9B-32K.Q3_K_L.gguf](https://huggingface.co/RichardErkhov/01-ai_-_Yi-1.5-9B-32K-gguf/blob/main/Yi-1.5-9B-32K.Q3_K_L.gguf) | Q3_K_L | 4.37GB |
| [Yi-1.5-9B-32K.IQ4_XS.gguf](https://huggingface.co/RichardErkhov/01-ai_-_Yi-1.5-9B-32K-gguf/blob/main/Yi-1.5-9B-32K.IQ4_XS.gguf) | IQ4_XS | 4.5GB |
| [Yi-1.5-9B-32K.Q4_0.gguf](https://huggingface.co/RichardErkhov/01-ai_-_Yi-1.5-9B-32K-gguf/blob/main/Yi-1.5-9B-32K.Q4_0.gguf) | Q4_0 | 4.69GB |
| [Yi-1.5-9B-32K.IQ4_NL.gguf](https://huggingface.co/RichardErkhov/01-ai_-_Yi-1.5-9B-32K-gguf/blob/main/Yi-1.5-9B-32K.IQ4_NL.gguf) | IQ4_NL | 4.73GB |
| [Yi-1.5-9B-32K.Q4_K_S.gguf](https://huggingface.co/RichardErkhov/01-ai_-_Yi-1.5-9B-32K-gguf/blob/main/Yi-1.5-9B-32K.Q4_K_S.gguf) | Q4_K_S | 4.72GB |
| [Yi-1.5-9B-32K.Q4_K.gguf](https://huggingface.co/RichardErkhov/01-ai_-_Yi-1.5-9B-32K-gguf/blob/main/Yi-1.5-9B-32K.Q4_K.gguf) | Q4_K | 4.96GB |
| [Yi-1.5-9B-32K.Q4_K_M.gguf](https://huggingface.co/RichardErkhov/01-ai_-_Yi-1.5-9B-32K-gguf/blob/main/Yi-1.5-9B-32K.Q4_K_M.gguf) | Q4_K_M | 4.96GB |
| [Yi-1.5-9B-32K.Q4_1.gguf](https://huggingface.co/RichardErkhov/01-ai_-_Yi-1.5-9B-32K-gguf/blob/main/Yi-1.5-9B-32K.Q4_1.gguf) | Q4_1 | 5.19GB |
| [Yi-1.5-9B-32K.Q5_0.gguf](https://huggingface.co/RichardErkhov/01-ai_-_Yi-1.5-9B-32K-gguf/blob/main/Yi-1.5-9B-32K.Q5_0.gguf) | Q5_0 | 5.69GB |
| [Yi-1.5-9B-32K.Q5_K_S.gguf](https://huggingface.co/RichardErkhov/01-ai_-_Yi-1.5-9B-32K-gguf/blob/main/Yi-1.5-9B-32K.Q5_K_S.gguf) | Q5_K_S | 5.69GB |
| [Yi-1.5-9B-32K.Q5_K.gguf](https://huggingface.co/RichardErkhov/01-ai_-_Yi-1.5-9B-32K-gguf/blob/main/Yi-1.5-9B-32K.Q5_K.gguf) | Q5_K | 5.83GB |
| [Yi-1.5-9B-32K.Q5_K_M.gguf](https://huggingface.co/RichardErkhov/01-ai_-_Yi-1.5-9B-32K-gguf/blob/main/Yi-1.5-9B-32K.Q5_K_M.gguf) | Q5_K_M | 5.83GB |
| [Yi-1.5-9B-32K.Q5_1.gguf](https://huggingface.co/RichardErkhov/01-ai_-_Yi-1.5-9B-32K-gguf/blob/main/Yi-1.5-9B-32K.Q5_1.gguf) | Q5_1 | 6.19GB |
| [Yi-1.5-9B-32K.Q6_K.gguf](https://huggingface.co/RichardErkhov/01-ai_-_Yi-1.5-9B-32K-gguf/blob/main/Yi-1.5-9B-32K.Q6_K.gguf) | Q6_K | 6.75GB |
| [Yi-1.5-9B-32K.Q8_0.gguf](https://huggingface.co/RichardErkhov/01-ai_-_Yi-1.5-9B-32K-gguf/blob/main/Yi-1.5-9B-32K.Q8_0.gguf) | Q8_0 | 8.74GB |
Original model description:
---
license: apache-2.0
---
<div align="center">
<picture>
<img src="https://raw.githubusercontent.com/01-ai/Yi/main/assets/img/Yi_logo_icon_light.svg" width="150px">
</picture>
</div>
<p align="center">
<a href="https://github.com/01-ai">🐙 GitHub</a> •
<a href="https://discord.gg/hYUwWddeAu">👾 Discord</a> •
<a href="https://twitter.com/01ai_yi">🐤 Twitter</a> •
<a href="https://github.com/01-ai/Yi-1.5/issues/2">💬 WeChat</a>
<br/>
<a href="https://arxiv.org/abs/2403.04652">📝 Paper</a> •
<a href="https://01-ai.github.io/">💪 Tech Blog</a> •
<a href="https://github.com/01-ai/Yi/tree/main?tab=readme-ov-file#faq">🙌 FAQ</a> •
<a href="https://github.com/01-ai/Yi/tree/main?tab=readme-ov-file#learning-hub">📗 Learning Hub</a>
</p>
# Intro
Yi-1.5 is an upgraded version of Yi. It is continuously pre-trained on Yi with a high-quality corpus of 500B tokens and fine-tuned on 3M diverse fine-tuning samples.
Compared with Yi, Yi-1.5 delivers stronger performance in coding, math, reasoning, and instruction-following capability, while still maintaining excellent capabilities in language understanding, commonsense reasoning, and reading comprehension.
<div align="center">
Model | Context Length | Pre-trained Tokens
| :------------: | :------------: | :------------: |
| Yi-1.5 | 4K, 16K, 32K | 3.6T
</div>
# Models
- Chat models
<div align="center">
| Name | Download |
| --------------- | ------------------------------------------------------------------------------------------------------------------------------------------------------------------- |
| Yi-1.5-34B-Chat | • [🤗 Hugging Face](https://huggingface.co/collections/01-ai/yi-15-2024-05-663f3ecab5f815a3eaca7ca8) • [🤖 ModelScope](https://www.modelscope.cn/organization/01ai) • [🟣 wisemodel](https://wisemodel.cn/organization/01.AI)|
| Yi-1.5-34B-Chat-16K | • [🤗 Hugging Face](https://huggingface.co/collections/01-ai/yi-15-2024-05-663f3ecab5f815a3eaca7ca8) • [🤖 ModelScope](https://www.modelscope.cn/organization/01ai) • [🟣 wisemodel](https://wisemodel.cn/organization/01.AI) |
| Yi-1.5-9B-Chat | • [🤗 Hugging Face](https://huggingface.co/collections/01-ai/yi-15-2024-05-663f3ecab5f815a3eaca7ca8) • [🤖 ModelScope](https://www.modelscope.cn/organization/01ai) • [🟣 wisemodel](https://wisemodel.cn/organization/01.AI) |
| Yi-1.5-9B-Chat-16K | • [🤗 Hugging Face](https://huggingface.co/collections/01-ai/yi-15-2024-05-663f3ecab5f815a3eaca7ca8) • [🤖 ModelScope](https://www.modelscope.cn/organization/01ai) • [🟣 wisemodel](https://wisemodel.cn/organization/01.AI) |
| Yi-1.5-6B-Chat | • [🤗 Hugging Face](https://huggingface.co/collections/01-ai/yi-15-2024-05-663f3ecab5f815a3eaca7ca8) • [🤖 ModelScope](https://www.modelscope.cn/organization/01ai) • [🟣 wisemodel](https://wisemodel.cn/organization/01.AI) |
</div>
- Base models
<div align="center">
| Name | Download |
| ---------- | ------------------------------------------------------------------------------------------------------------------------------------------------------------------- |
| Yi-1.5-34B | • [🤗 Hugging Face](https://huggingface.co/collections/01-ai/yi-15-2024-05-663f3ecab5f815a3eaca7ca8) • [🤖 ModelScope](https://www.modelscope.cn/organization/01ai) • [🟣 wisemodel](https://wisemodel.cn/organization/01.AI) |
| Yi-1.5-34B-32K | • [🤗 Hugging Face](https://huggingface.co/collections/01-ai/yi-15-2024-05-663f3ecab5f815a3eaca7ca8) • [🤖 ModelScope](https://www.modelscope.cn/organization/01ai) • [🟣 wisemodel](https://wisemodel.cn/organization/01.AI) |
| Yi-1.5-9B | • [🤗 Hugging Face](https://huggingface.co/collections/01-ai/yi-15-2024-05-663f3ecab5f815a3eaca7ca8) • [🤖 ModelScope](https://www.modelscope.cn/organization/01ai) • [🟣 wisemodel](https://wisemodel.cn/organization/01.AI) |
| Yi-1.5-9B-32K | • [🤗 Hugging Face](https://huggingface.co/collections/01-ai/yi-15-2024-05-663f3ecab5f815a3eaca7ca8) • [🤖 ModelScope](https://www.modelscope.cn/organization/01ai) • [🟣 wisemodel](https://wisemodel.cn/organization/01.AI) |
| Yi-1.5-6B | • [🤗 Hugging Face](https://huggingface.co/collections/01-ai/yi-15-2024-05-663f3ecab5f815a3eaca7ca8) • [🤖 ModelScope](https://www.modelscope.cn/organization/01ai) • [🟣 wisemodel](https://wisemodel.cn/organization/01.AI) |
</div>
# Benchmarks
- Chat models
Yi-1.5-34B-Chat is on par with or excels beyond larger models in most benchmarks.

Yi-1.5-9B-Chat is the top performer among similarly sized open-source models.

- Base models
Yi-1.5-34B is on par with or excels beyond larger models in some benchmarks.

Yi-1.5-9B is the top performer among similarly sized open-source models.

# Quick Start
For getting up and running with Yi-1.5 models quickly, see [README](https://github.com/01-ai/Yi-1.5).
|
artificialguybr/StickersRedmond | artificialguybr | "2023-09-12T06:21:30Z" | 2,161 | 75 | diffusers | [
"diffusers",
"text-to-image",
"stable-diffusion",
"lora",
"base_model:stabilityai/stable-diffusion-xl-base-1.0",
"license:creativeml-openrail-m",
"region:us"
] | text-to-image | "2023-09-12T06:16:10Z" | ---
license: creativeml-openrail-m
tags:
- text-to-image
- stable-diffusion
- lora
- diffusers
base_model: stabilityai/stable-diffusion-xl-base-1.0
instance_prompt: Stickers, sticker
widget:
- text: Stickers, sticker
---
# Stickers.Redmond

Stickers.Redmond is here!
Introducing Stickers.Redmond, the ultimate LORA for creating Stickers images!
I'm grateful for the GPU time from Redmond.AI that allowed me to make this LORA! If you need GPU, then you need the great services from Redmond.AI.
It is based on SD XL 1.0 and fine-tuned on a large dataset.
The LORA has a high capacity to generate Coloring Book Images!
The tag for the model: Stickers, Sticker
I really hope you like the LORA and use it.
If you like the model and think it's worth it, you can make a donation to my Patreon or Ko-fi.
Patreon:
https://www.patreon.com/user?u=81570187
Ko-fi:https://ko-fi.com/artificialguybr
BuyMeACoffe:https://www.buymeacoffee.com/jvkape
Follow me in my twitter to know before all about new models:
https://twitter.com/artificialguybr/ |
PassionFriend/5FPAHrBBtBBQwc9LLm1XXdbby8rXGpx56hDaKbMtcJR9kdNS_vgg | PassionFriend | "2024-03-01T06:35:04Z" | 2,161 | 0 | keras | [
"keras",
"region:us"
] | null | "2024-02-06T13:52:40Z" | Entry not found |
houyu0930/test-demo-qa | houyu0930 | "2024-04-29T03:58:27Z" | 2,161 | 0 | transformers | [
"transformers",
"safetensors",
"distilbert",
"question-answering",
"arxiv:1910.09700",
"license:mit",
"endpoints_compatible",
"region:us"
] | question-answering | "2024-04-29T02:40:20Z" | ---
library_name: transformers
license: mit
---
# Model Card for Model ID
<!-- Provide a quick summary of what the model is/does. -->
## Model Details
### Model Description
<!-- Provide a longer summary of what this model is. -->
This is the model card of a 🤗 transformers model that has been pushed on the Hub. This model card has been automatically generated.
- **Developed by:** [More Information Needed]
- **Funded by [optional]:** [More Information Needed]
- **Shared by [optional]:** [More Information Needed]
- **Model type:** [More Information Needed]
- **Language(s) (NLP):** [More Information Needed]
- **License:** [More Information Needed]
- **Finetuned from model [optional]:** [More Information Needed]
### Model Sources [optional]
<!-- Provide the basic links for the model. -->
- **Repository:** [More Information Needed]
- **Paper [optional]:** [More Information Needed]
- **Demo [optional]:** [More Information Needed]
## Uses
<!-- Address questions around how the model is intended to be used, including the foreseeable users of the model and those affected by the model. -->
### Direct Use
<!-- This section is for the model use without fine-tuning or plugging into a larger ecosystem/app. -->
[More Information Needed]
### Downstream Use [optional]
<!-- This section is for the model use when fine-tuned for a task, or when plugged into a larger ecosystem/app -->
[More Information Needed]
### Out-of-Scope Use
<!-- This section addresses misuse, malicious use, and uses that the model will not work well for. -->
[More Information Needed]
## Bias, Risks, and Limitations
<!-- This section is meant to convey both technical and sociotechnical limitations. -->
[More Information Needed]
### Recommendations
<!-- This section is meant to convey recommendations with respect to the bias, risk, and technical limitations. -->
Users (both direct and downstream) should be made aware of the risks, biases and limitations of the model. More information needed for further recommendations.
## How to Get Started with the Model
Use the code below to get started with the model.
[More Information Needed]
## Training Details
### Training Data
<!-- This should link to a Dataset Card, perhaps with a short stub of information on what the training data is all about as well as documentation related to data pre-processing or additional filtering. -->
[More Information Needed]
### Training Procedure
<!-- This relates heavily to the Technical Specifications. Content here should link to that section when it is relevant to the training procedure. -->
#### Preprocessing [optional]
[More Information Needed]
#### Training Hyperparameters
- **Training regime:** [More Information Needed] <!--fp32, fp16 mixed precision, bf16 mixed precision, bf16 non-mixed precision, fp16 non-mixed precision, fp8 mixed precision -->
#### Speeds, Sizes, Times [optional]
<!-- This section provides information about throughput, start/end time, checkpoint size if relevant, etc. -->
[More Information Needed]
## Evaluation
<!-- This section describes the evaluation protocols and provides the results. -->
### Testing Data, Factors & Metrics
#### Testing Data
<!-- This should link to a Dataset Card if possible. -->
[More Information Needed]
#### Factors
<!-- These are the things the evaluation is disaggregating by, e.g., subpopulations or domains. -->
[More Information Needed]
#### Metrics
<!-- These are the evaluation metrics being used, ideally with a description of why. -->
[More Information Needed]
### Results
[More Information Needed]
#### Summary
## Model Examination [optional]
<!-- Relevant interpretability work for the model goes here -->
[More Information Needed]
## Environmental Impact
<!-- Total emissions (in grams of CO2eq) and additional considerations, such as electricity usage, go here. Edit the suggested text below accordingly -->
Carbon emissions can be estimated using the [Machine Learning Impact calculator](https://mlco2.github.io/impact#compute) presented in [Lacoste et al. (2019)](https://arxiv.org/abs/1910.09700).
- **Hardware Type:** [More Information Needed]
- **Hours used:** [More Information Needed]
- **Cloud Provider:** [More Information Needed]
- **Compute Region:** [More Information Needed]
- **Carbon Emitted:** [More Information Needed]
## Technical Specifications [optional]
### Model Architecture and Objective
[More Information Needed]
### Compute Infrastructure
[More Information Needed]
#### Hardware
[More Information Needed]
#### Software
[More Information Needed]
## Citation [optional]
<!-- If there is a paper or blog post introducing the model, the APA and Bibtex information for that should go in this section. -->
**BibTeX:**
[More Information Needed]
**APA:**
[More Information Needed]
## Glossary [optional]
<!-- If relevant, include terms and calculations in this section that can help readers understand the model or model card. -->
[More Information Needed]
## More Information [optional]
[More Information Needed]
## Model Card Authors [optional]
[More Information Needed]
## Model Card Contact
[More Information Needed] |
timm/poolformerv2_s12.sail_in1k | timm | "2023-05-05T06:19:57Z" | 2,160 | 0 | timm | [
"timm",
"pytorch",
"safetensors",
"image-classification",
"dataset:imagenet-1k",
"arxiv:2210.13452",
"license:apache-2.0",
"region:us"
] | image-classification | "2023-05-05T06:19:46Z" | ---
tags:
- image-classification
- timm
library_name: timm
license: apache-2.0
datasets:
- imagenet-1k
---
# Model card for poolformerv2_s12.sail_in1k
A PoolFormer-v2 (a MetaFormer) image classification model. Trained on ImageNet-1k by paper authors.
## Model Details
- **Model Type:** Image classification / feature backbone
- **Model Stats:**
- Params (M): 11.9
- GMACs: 1.8
- Activations (M): 5.5
- Image size: 224 x 224
- **Papers:**
- Metaformer baselines for vision: https://arxiv.org/abs/2210.13452
- **Original:** https://github.com/sail-sg/metaformer
- **Dataset:** ImageNet-1k
## Model Usage
### Image Classification
```python
from urllib.request import urlopen
from PIL import Image
import timm
img = Image.open(urlopen(
'https://huggingface.co/datasets/huggingface/documentation-images/resolve/main/beignets-task-guide.png'
))
model = timm.create_model('poolformerv2_s12.sail_in1k', pretrained=True)
model = model.eval()
# get model specific transforms (normalization, resize)
data_config = timm.data.resolve_model_data_config(model)
transforms = timm.data.create_transform(**data_config, is_training=False)
output = model(transforms(img).unsqueeze(0)) # unsqueeze single image into batch of 1
top5_probabilities, top5_class_indices = torch.topk(output.softmax(dim=1) * 100, k=5)
```
### Feature Map Extraction
```python
from urllib.request import urlopen
from PIL import Image
import timm
img = Image.open(urlopen(
'https://huggingface.co/datasets/huggingface/documentation-images/resolve/main/beignets-task-guide.png'
))
model = timm.create_model(
'poolformerv2_s12.sail_in1k',
pretrained=True,
features_only=True,
)
model = model.eval()
# get model specific transforms (normalization, resize)
data_config = timm.data.resolve_model_data_config(model)
transforms = timm.data.create_transform(**data_config, is_training=False)
output = model(transforms(img).unsqueeze(0)) # unsqueeze single image into batch of 1
for o in output:
# print shape of each feature map in output
# e.g.:
# torch.Size([1, 64, 56, 56])
# torch.Size([1, 128, 28, 28])
# torch.Size([1, 320, 14, 14])
# torch.Size([1, 512, 7, 7])
print(o.shape)
```
### Image Embeddings
```python
from urllib.request import urlopen
from PIL import Image
import timm
img = Image.open(urlopen(
'https://huggingface.co/datasets/huggingface/documentation-images/resolve/main/beignets-task-guide.png'
))
model = timm.create_model(
'poolformerv2_s12.sail_in1k',
pretrained=True,
num_classes=0, # remove classifier nn.Linear
)
model = model.eval()
# get model specific transforms (normalization, resize)
data_config = timm.data.resolve_model_data_config(model)
transforms = timm.data.create_transform(**data_config, is_training=False)
output = model(transforms(img).unsqueeze(0)) # output is (batch_size, num_features) shaped tensor
# or equivalently (without needing to set num_classes=0)
output = model.forward_features(transforms(img).unsqueeze(0))
# output is unpooled, a (1, 512, 7, 7) shaped tensor
output = model.forward_head(output, pre_logits=True)
# output is a (1, num_features) shaped tensor
```
## Model Comparison
Explore the dataset and runtime metrics of this model in timm [model results](https://github.com/huggingface/pytorch-image-models/tree/main/results).
## Citation
```bibtex
@article{yu2022metaformer_baselines,
title={Metaformer baselines for vision},
author={Yu, Weihao and Si, Chenyang and Zhou, Pan and Luo, Mi and Zhou, Yichen and Feng, Jiashi and Yan, Shuicheng and Wang, Xinchao},
journal={arXiv preprint arXiv:2210.13452},
year={2022}
}
```
|
stablediffusionapi/juggernaut-reborn | stablediffusionapi | "2024-01-21T12:51:20Z" | 2,159 | 1 | diffusers | [
"diffusers",
"modelslab.com",
"stable-diffusion-api",
"text-to-image",
"ultra-realistic",
"license:creativeml-openrail-m",
"autotrain_compatible",
"endpoints_compatible",
"diffusers:StableDiffusionPipeline",
"region:us"
] | text-to-image | "2024-01-21T12:49:17Z" | ---
license: creativeml-openrail-m
tags:
- modelslab.com
- stable-diffusion-api
- text-to-image
- ultra-realistic
pinned: true
---
# Juggernaut Reborn API Inference

## Get API Key
Get API key from [ModelsLab API](http://modelslab.com), No Payment needed.
Replace Key in below code, change **model_id** to "juggernaut-reborn"
Coding in PHP/Node/Java etc? Have a look at docs for more code examples: [View docs](https://modelslab.com/docs)
Try model for free: [Generate Images](https://modelslab.com/models/juggernaut-reborn)
Model link: [View model](https://modelslab.com/models/juggernaut-reborn)
View all models: [View Models](https://modelslab.com/models)
import requests
import json
url = "https://modelslab.com/api/v6/images/text2img"
payload = json.dumps({
"key": "your_api_key",
"model_id": "juggernaut-reborn",
"prompt": "ultra realistic close up portrait ((beautiful pale cyberpunk female with heavy black eyeliner)), blue eyes, shaved side haircut, hyper detail, cinematic lighting, magic neon, dark red city, Canon EOS R3, nikon, f/1.4, ISO 200, 1/160s, 8K, RAW, unedited, symmetrical balance, in-frame, 8K",
"negative_prompt": "painting, extra fingers, mutated hands, poorly drawn hands, poorly drawn face, deformed, ugly, blurry, bad anatomy, bad proportions, extra limbs, cloned face, skinny, glitchy, double torso, extra arms, extra hands, mangled fingers, missing lips, ugly face, distorted face, extra legs, anime",
"width": "512",
"height": "512",
"samples": "1",
"num_inference_steps": "30",
"safety_checker": "no",
"enhance_prompt": "yes",
"seed": None,
"guidance_scale": 7.5,
"multi_lingual": "no",
"panorama": "no",
"self_attention": "no",
"upscale": "no",
"embeddings": "embeddings_model_id",
"lora": "lora_model_id",
"webhook": None,
"track_id": None
})
headers = {
'Content-Type': 'application/json'
}
response = requests.request("POST", url, headers=headers, data=payload)
print(response.text)
> Use this coupon code to get 25% off **DMGG0RBN** |
RichardErkhov/jeiku_-_Garbage_9B-gguf | RichardErkhov | "2024-06-15T08:02:28Z" | 2,159 | 0 | null | [
"gguf",
"region:us"
] | null | "2024-06-15T04:52:18Z" | Quantization made by Richard Erkhov.
[Github](https://github.com/RichardErkhov)
[Discord](https://discord.gg/pvy7H8DZMG)
[Request more models](https://github.com/RichardErkhov/quant_request)
Garbage_9B - GGUF
- Model creator: https://huggingface.co/jeiku/
- Original model: https://huggingface.co/jeiku/Garbage_9B/
| Name | Quant method | Size |
| ---- | ---- | ---- |
| [Garbage_9B.Q2_K.gguf](https://huggingface.co/RichardErkhov/jeiku_-_Garbage_9B-gguf/blob/main/Garbage_9B.Q2_K.gguf) | Q2_K | 3.13GB |
| [Garbage_9B.IQ3_XS.gguf](https://huggingface.co/RichardErkhov/jeiku_-_Garbage_9B-gguf/blob/main/Garbage_9B.IQ3_XS.gguf) | IQ3_XS | 3.48GB |
| [Garbage_9B.IQ3_S.gguf](https://huggingface.co/RichardErkhov/jeiku_-_Garbage_9B-gguf/blob/main/Garbage_9B.IQ3_S.gguf) | IQ3_S | 3.67GB |
| [Garbage_9B.Q3_K_S.gguf](https://huggingface.co/RichardErkhov/jeiku_-_Garbage_9B-gguf/blob/main/Garbage_9B.Q3_K_S.gguf) | Q3_K_S | 3.65GB |
| [Garbage_9B.IQ3_M.gguf](https://huggingface.co/RichardErkhov/jeiku_-_Garbage_9B-gguf/blob/main/Garbage_9B.IQ3_M.gguf) | IQ3_M | 3.79GB |
| [Garbage_9B.Q3_K.gguf](https://huggingface.co/RichardErkhov/jeiku_-_Garbage_9B-gguf/blob/main/Garbage_9B.Q3_K.gguf) | Q3_K | 4.05GB |
| [Garbage_9B.Q3_K_M.gguf](https://huggingface.co/RichardErkhov/jeiku_-_Garbage_9B-gguf/blob/main/Garbage_9B.Q3_K_M.gguf) | Q3_K_M | 4.05GB |
| [Garbage_9B.Q3_K_L.gguf](https://huggingface.co/RichardErkhov/jeiku_-_Garbage_9B-gguf/blob/main/Garbage_9B.Q3_K_L.gguf) | Q3_K_L | 4.41GB |
| [Garbage_9B.IQ4_XS.gguf](https://huggingface.co/RichardErkhov/jeiku_-_Garbage_9B-gguf/blob/main/Garbage_9B.IQ4_XS.gguf) | IQ4_XS | 4.55GB |
| [Garbage_9B.Q4_0.gguf](https://huggingface.co/RichardErkhov/jeiku_-_Garbage_9B-gguf/blob/main/Garbage_9B.Q4_0.gguf) | Q4_0 | 4.74GB |
| [Garbage_9B.IQ4_NL.gguf](https://huggingface.co/RichardErkhov/jeiku_-_Garbage_9B-gguf/blob/main/Garbage_9B.IQ4_NL.gguf) | IQ4_NL | 4.79GB |
| [Garbage_9B.Q4_K_S.gguf](https://huggingface.co/RichardErkhov/jeiku_-_Garbage_9B-gguf/blob/main/Garbage_9B.Q4_K_S.gguf) | Q4_K_S | 4.78GB |
| [Garbage_9B.Q4_K.gguf](https://huggingface.co/RichardErkhov/jeiku_-_Garbage_9B-gguf/blob/main/Garbage_9B.Q4_K.gguf) | Q4_K | 5.04GB |
| [Garbage_9B.Q4_K_M.gguf](https://huggingface.co/RichardErkhov/jeiku_-_Garbage_9B-gguf/blob/main/Garbage_9B.Q4_K_M.gguf) | Q4_K_M | 5.04GB |
| [Garbage_9B.Q4_1.gguf](https://huggingface.co/RichardErkhov/jeiku_-_Garbage_9B-gguf/blob/main/Garbage_9B.Q4_1.gguf) | Q4_1 | 5.26GB |
| [Garbage_9B.Q5_0.gguf](https://huggingface.co/RichardErkhov/jeiku_-_Garbage_9B-gguf/blob/main/Garbage_9B.Q5_0.gguf) | Q5_0 | 5.77GB |
| [Garbage_9B.Q5_K_S.gguf](https://huggingface.co/RichardErkhov/jeiku_-_Garbage_9B-gguf/blob/main/Garbage_9B.Q5_K_S.gguf) | Q5_K_S | 5.77GB |
| [Garbage_9B.Q5_K.gguf](https://huggingface.co/RichardErkhov/jeiku_-_Garbage_9B-gguf/blob/main/Garbage_9B.Q5_K.gguf) | Q5_K | 5.93GB |
| [Garbage_9B.Q5_K_M.gguf](https://huggingface.co/RichardErkhov/jeiku_-_Garbage_9B-gguf/blob/main/Garbage_9B.Q5_K_M.gguf) | Q5_K_M | 5.93GB |
| [Garbage_9B.Q5_1.gguf](https://huggingface.co/RichardErkhov/jeiku_-_Garbage_9B-gguf/blob/main/Garbage_9B.Q5_1.gguf) | Q5_1 | 6.29GB |
| [Garbage_9B.Q6_K.gguf](https://huggingface.co/RichardErkhov/jeiku_-_Garbage_9B-gguf/blob/main/Garbage_9B.Q6_K.gguf) | Q6_K | 6.87GB |
| [Garbage_9B.Q8_0.gguf](https://huggingface.co/RichardErkhov/jeiku_-_Garbage_9B-gguf/blob/main/Garbage_9B.Q8_0.gguf) | Q8_0 | 8.89GB |
Original model description:
---
base_model:
- ChaoticNeutrals/InfinityNexus_9B
- jeiku/luna_lora_9B
library_name: transformers
license: apache-2.0
datasets:
- ResplendentAI/Luna_Alpaca
language:
- en
---
# Garbage

This is a finetune of InfinityNexus_9B. This is my first time tuning a frankenmerge, so hopefully it works out. The goal is to improve intelligence and RP ability beyond the 7B original models.
|
togethercomputer/GPT-NeoXT-Chat-Base-20B | togethercomputer | "2023-03-30T21:00:24Z" | 2,158 | 693 | transformers | [
"transformers",
"pytorch",
"gpt_neox",
"text-generation",
"en",
"license:apache-2.0",
"autotrain_compatible",
"endpoints_compatible",
"text-generation-inference",
"region:us"
] | text-generation | "2023-03-03T00:24:29Z" | ---
license: apache-2.0
language:
- en
---
***<p style="font-size: 24px">Feel free to try out our [OpenChatKit feedback app](https://huggingface.co/spaces/togethercomputer/OpenChatKit)!</p>***
# GPT-NeoXT-Chat-Base-20B-v0.16
> TLDR: As part of OpenChatKit (codebase available [here](https://github.com/togethercomputer/OpenChaT)),
> GPT-NeoXT-Chat-Base-20B-v0.16 is a 20B parameter language model, fine-tuned from EleutherAI’s GPT-NeoX with over 40 million instructions on 100% carbon negative compute.
GPT-NeoXT-Chat-Base-20B-v0.16 is based on ElutherAI’s GPT-NeoX model, and is fine-tuned with data focusing on dialog-style interactions.
We focused the tuning on several tasks such as question answering, classification, extraction, and summarization.
We’ve fine-tuned the model with a collection of 43 million high-quality instructions.
Together partnered with LAION and Ontocord.ai, who both helped curate the dataset the model is based on.
You can read more about this process and the availability of this dataset in LAION’s blog post [here](https://laion.ai/blog/oig-dataset/).
In addition to the aforementioned fine-tuning, GPT-NeoXT-Chat-Base-20B-v0.16 has also undergone further fine-tuning via a small amount of feedback data.
This allows the model to better adapt to human preferences in the conversations.
## Model Details
- **Developed by**: Together Computer.
- **Model type**: Language Model
- **Language(s)**: English
- **License**: Apache 2.0
- **Model Description**: A 20B parameter open source chat model, fine-tuned from EleutherAI’s NeoX with over 40M instructions on 100% carbon negative compute
- **Resources for more information**: [GitHub Repository](https://github.com/togethercomputer/OpenChaT).
# Quick Start
## GPU Inference
This requires a GPU with 48GB memory.
```python
from transformers import AutoTokenizer, AutoModelForCausalLM
# init
tokenizer = AutoTokenizer.from_pretrained("togethercomputer/GPT-NeoXT-Chat-Base-20B")
model = AutoModelForCausalLM.from_pretrained("togethercomputer/GPT-NeoXT-Chat-Base-20B", torch_dtype=torch.float16)
model = model.to('cuda:0')
# infer
inputs = tokenizer("<human>: Hello!\n<bot>:", return_tensors='pt').to(model.device)
outputs = model.generate(**inputs, max_new_tokens=10, do_sample=True, temperature=0.8)
output_str = tokenizer.decode(outputs[0])
print(output_str)
```
## GPU Inference in Int8
This requires a GPU with 24GB memory.
```python
from transformers import AutoTokenizer, AutoModelForCausalLM
# init
tokenizer = AutoTokenizer.from_pretrained("togethercomputer/GPT-NeoXT-Chat-Base-20B")
model = AutoModelForCausalLM.from_pretrained("togethercomputer/GPT-NeoXT-Chat-Base-20B", device_map="auto", load_in_8bit=True)
# infer
inputs = tokenizer("<human>: Hello!\n<bot>:", return_tensors='pt').to(model.device)
outputs = model.generate(**inputs, max_new_tokens=10, do_sample=True, temperature=0.8)
output_str = tokenizer.decode(outputs[0])
print(output_str)
```
## CPU Inference
```python
from transformers import AutoTokenizer, AutoModelForCausalLM
# init
tokenizer = AutoTokenizer.from_pretrained("togethercomputer/GPT-NeoXT-Chat-Base-20B")
model = AutoModelForCausalLM.from_pretrained("togethercomputer/GPT-NeoXT-Chat-Base-20B", torch_dtype=torch.bfloat16)
# infer
inputs = tokenizer("<human>: Hello!\n<bot>:", return_tensors='pt').to(model.device)
outputs = model.generate(**inputs, max_new_tokens=10, do_sample=True, temperature=0.8)
output_str = tokenizer.decode(outputs[0])
print(output_str)
```
## Strengths of the model
There are several tasks that OpenChatKit excels at out of the box. This includes:
- Example 1: Summarization and question answering within context.
```markdown
**Summarize a long document into a single sentence and conduct question answering related to the document, with multiple rounds**
<human>: Last year, the travel industry saw a big rebound in demand — and that demand is showing no signs of slowing down this spring break travel season. Planes and hotels will be full, travelers will likely face long queues, cancellations, massive crowds and plenty of other travel nightmares. But perhaps the most frustrating thing you’ll have to prepare for this spring break is if your luggage goes missing. You’ve got to somehow track down your belongings that were in the airline’s care and then wait to be reunited — all while trying to enjoy the start of your long-awaited vacation. As far as travel nightmares go, lost luggage is up there as one of the worst.
To be honest up front, I am great at strategically losing things — or rather, losing stuff with style. (I hope you appreciate the “Toy Story” reference.) So when a product comes along that’s a perfect way to keep track of my belongings — especially when traveling — I’m adding it to my travel bag.
I’ve been a big fan of Apple’s $29 item tracker, dubbed AirTag. The long-rumored AirTag product finally launched in 2021, and while it’s not the first item tracker to exist, it has two key features that set it apart from the rest to make it the ultimate travel companion: access to the Find My network and Precision Finding technology.
The Federal Aviation Administration has even ruled that having an AirTag in your luggage is permitted and, in fact, not a safety hazard. The devices only contain 0.1 grams of lithium, which falls below the FAA’s limit.
“Luggage tracking devices powered by lithium metal cells that have 0.3 grams or less of lithium can be used on checked baggage,” the FAA said in a statement. “Apple AirTags meet this threshold; other luggage tracking devices may not.”
But, you may be wondering what makes them so special and why you should carry one in your checked luggage this spring break? Let’s explain why.
Summarize the above into a single paragraph.
<bot>: If you're traveling this spring break, you might want to consider investing in an item tracker to help you find your lost luggage. Apple's AirTag is the best option, according to a new report.
<human>: But isn't it unsafe to put AirTag in a luggage?
<bot>: The Federal Aviation Administration has ruled that having an AirTag in your luggage is permitted and, in fact, not a safety hazard.
<human>: Ah, why is that?
<bot>: The devices only contain 0.1 grams of lithium, which falls below the FAA’s limit.
```
- Example 2: Extraction.
```markdown
**Extract structured information from unstructured documents, as illustrated in the following example.**
<human>: Generate a table summarizing the options outlined in this email.
Team, we have to make a decision on the product plan for Goocrux v2.
The first option will take us 3 weeks of development, and will achieve performance of 80ms, but means that the UI has to change.
The second option will take us 4 weeks of development, and will achieve performance of 60ms, without any UI change.
The third option will take the longest -- 6 weeks of development, will achieve performance of 30ms, without any UI change.
A markdown table with four columns: (1) Option ID, (2) Development time, (3) Performance, (4) UI Change:
<bot>: #.
| ID | Option ID | Development time | Performance | UI Change |
| --------- | --------- | --------- | --------- | --------- |
| 1 | 1 | 3 weeks | 80ms | Yes |
| 2 | 2 | 4 weeks | 60ms | No |
| 3 | 3 | 6 weeks | 30ms | No |
```
- Example 3: Classification.
```markdown
**Classifying a sentence or paragraph into different categories.**
<human>: Classify the sentiment of the following sentence into Positive, Neutral, or Negative: Super excited about teaching Stanford’s first course on Large Language Models! Check the syllabus out here
<bot>: Positive
<human>: Classify the sentiment of the following sentence into Positive, Neutral, or Negative: How about the following sentence: It is raining outside and I feel so blue
<bot>: Negative
```
In addition, the model does well on few-shot prompts. For both classification and extraction, the model performs even better with few shots, as in most HELM tasks. [Contact us](https://www.together.xyz/contact) if you’re interested in trying few-shot prompts with the model.
## Weaknesses of the model
That said, there are several areas where we have more work to do, and we need your help! Some of these include:
- Knowledge-based closed question and answering: The chatbot may hallucinate and give incorrect results. Be sure to fact check, and if possible provide feedback with the corrected information.
- Coding tasks: The chatbot was not trained on a large enough corpus of source code to excel at writing code. We welcome contributions of additional datasets to improve this!
- Repetition: Sometimes the chatbot will repeat its response. We’re working to improve this, but in the meantime you can click the refresh button to start a new conversation.
- Context switching: If you change the topic in the middle of a conversation the chatbot often cannot make the switch automatically and will continue to give answers related to the prior topic.
- Creative writing and longer answers: The chatbot does not generate long, creative text such as an essay or story.
We are excited to work with you to address these weaknesses by getting your feedback, bolstering data sets, and improving accuracy.
# Uses
## Direct Use
The model is intended for research purposes. Possible research areas and tasks include
- Safe deployment of models which have the potential to generate harmful content.
- Probing and understanding the limitations and biases of dialogue models or language models.
- Generation of artworks and use in design and other artistic processes.
- Applications in educational or creative tools.
- Research on dialogue models or language models.
Excluded uses are described below.
### Misuse, Malicious Use, and Out-of-Scope Use
The OpenChatKit community provides GPT-NeoXT-Chat-Base-20B-v0.16 as an open source tool for building chatbots.
The community is not responsible for any misuse, malicious use, or out-of-scope use of the model.
It is the responsibility of the end user to ensure that the model is used in a responsible and ethical manner.
#### Out-of-Scope Use
GPT-NeoXT-Chat-Base-20B-v0.16 is designed for use in chatbot applications and may not perform well for other use cases outside of its intended scope.
For example, it may not be suitable for use in safety-critical applications or for making decisions that have a significant impact on individuals or society.
It is important to consider the limitations of the model and to only use it for its intended purpose.
#### Misuse and Malicious Use
GPT-NeoXT-Chat-Base-20B-v0.16 is designed for use in chatbot applications and should not be used for any other purpose.
Misuse of the model, such as using it to engage in illegal or unethical activities, is strictly prohibited and goes against the principles of the OpenChatKit community project.
Using the model to generate content that is cruel to individuals is a misuse of this model. This includes, but is not limited to:
- Generating fake news, misinformation, or propaganda
- Promoting hate speech, discrimination, or violence against individuals or groups
- Impersonating individuals or organizations without their consent
- Engaging in cyberbullying or harassment
- Defamatory content
- Spamming or scamming
- Sharing confidential or sensitive information without proper authorization
- Violating the terms of use of the model or the data used to train it
- Creating automated bots for malicious purposes such as spreading malware, phishing scams, or spamming
## Limitations
GPT-NeoXT-Chat-Base-20B-v0.16, like other language model-based chatbots, has limitations that should be taken into consideration.
For example, the model may not always provide accurate or relevant answers, particularly for questions that are complex, ambiguous, or outside of its training data.
We therefore welcome contributions from individuals and organizations, and encourage collaboration towards creating a more robust and inclusive chatbot.
## Training
**Training Data**
Please refer to [togethercomputer/OpenDataHub](https://github.com/togethercomputer/OpenDataHub)
**Training Procedure**
- **Hardware:** 2 x 8 x A100 GPUs
- **Optimizer:** [8bit-AdamW](https://github.com/TimDettmers/bitsandbytes)
- **Gradient Accumulations**: 2
- **Batch:** 2 x 2 x 64 x 2048 = 524288 tokens
- **Learning rate:** warmup to 1e-6 for 100 steps and then kept constant
## Community
Join us on [Together Discord](https://discord.gg/6ZVDU8tTD4)
|
golaxy/gogpt-7b-bloom | golaxy | "2023-07-22T13:23:15Z" | 2,158 | 3 | transformers | [
"transformers",
"pytorch",
"bloom",
"text-generation",
"zh",
"dataset:BelleGroup/train_2M_CN",
"dataset:BelleGroup/train_3.5M_CN",
"dataset:BelleGroup/train_1M_CN",
"dataset:BelleGroup/train_0.5M_CN",
"dataset:BelleGroup/school_math_0.25M",
"license:apache-2.0",
"autotrain_compatible",
"endpoints_compatible",
"text-generation-inference",
"region:us"
] | text-generation | "2023-05-26T16:58:38Z" | ---
license: apache-2.0
datasets:
- BelleGroup/train_2M_CN
- BelleGroup/train_3.5M_CN
- BelleGroup/train_1M_CN
- BelleGroup/train_0.5M_CN
- BelleGroup/school_math_0.25M
language:
- zh
---
## GoGPT
基于中文指令数据微调BLOOM

> 训练第一轮足够了,后续第二轮和第三轮提升不大
- 🚀多样性指令数据
- 🚀筛选高质量中文数据
| 模型名字 | 参数量 | 模型地址 |
|------------|--------|------|
| gogpt-560m | 5.6亿参数 | 🤗[golaxy/gogpt-560m](https://huggingface.co/golaxy/gogpt-560m) |
| gogpt-3b | 30亿参数 | 🤗[golaxy/gogpt-3b-bloom](https://huggingface.co/golaxy/gogpt-3b-bloom) |
| gogpt-7b | 70亿参数 | 🤗[golaxy/gogpt-7b-bloom](https://huggingface.co/golaxy/gogpt-7b-bloom) |
## 测试效果






## TODO
- 进行RLFH训练
- 后续加入中英平行语料
## 感谢
- [@hz大佬-zero_nlp](https://github.com/yuanzhoulvpi2017/zero_nlp)
- [stanford_alpaca](https://github.com/tatsu-lab/stanford_alpaca)
- [Belle数据](https://huggingface.co/BelleGroup)
(base) [searchgpt@worker2 output-bloom-7b]$ cat README.md ^C
(base) [searchgpt@worker2 output-bloom-7b]$ vim README.md
(base) [searchgpt@worker2 output-bloom-7b]$ cat README.md
---
license: apache-2.0
datasets:
- BelleGroup/train_2M_CN
- BelleGroup/train_3.5M_CN
- BelleGroup/train_1M_CN
- BelleGroup/train_0.5M_CN
- BelleGroup/school_math_0.25M
language:
- zh
---
## GoGPT
基于中文指令数据微调BLOOM

> 训练第一轮足够了,后续第二轮和第三轮提升不大
- 🚀多样性指令数据
- 🚀筛选高质量中文数据
| 模型名字 | 参数量 | 模型地址 |
|------------|--------|------|
| gogpt-560m | 5.6亿参数 | 🤗[golaxy/gogpt-560m](https://huggingface.co/golaxy/gogpt-560m) |
| gogpt-3b | 30亿参数 | 🤗[golaxy/gogpt-3b](https://huggingface.co/golaxy/gogpt-3b) |
| gogpt-7b | 70亿参数 | 🤗[golaxy/gogpt-7b](https://huggingface.co/golaxy/gogpt-7b) |
## 测试效果






## TODO
- 进行RLFH训练
- 后续加入中英平行语料
## 感谢
- [@hz大佬-zero_nlp](https://github.com/yuanzhoulvpi2017/zero_nlp)
- [stanford_alpaca](https://github.com/tatsu-lab/stanford_alpaca)
- [Belle数据](https://huggingface.co/BelleGroup)
|
TheBloke/Mixtral-8x7B-Instruct-v0.1-AWQ | TheBloke | "2023-12-14T14:30:42Z" | 2,158 | 54 | transformers | [
"transformers",
"safetensors",
"mixtral",
"text-generation",
"conversational",
"fr",
"it",
"de",
"es",
"en",
"base_model:mistralai/Mixtral-8x7B-Instruct-v0.1",
"license:apache-2.0",
"autotrain_compatible",
"text-generation-inference",
"4-bit",
"awq",
"region:us"
] | text-generation | "2023-12-11T21:01:33Z" | ---
base_model: mistralai/Mixtral-8x7B-Instruct-v0.1
inference: false
language:
- fr
- it
- de
- es
- en
license: apache-2.0
model_creator: Mistral AI_
model_name: Mixtral 8X7B Instruct v0.1
model_type: mixtral
prompt_template: '[INST] {prompt} [/INST]
'
quantized_by: TheBloke
widget:
- output:
text: 'Arr, shiver me timbers! Ye have a llama on yer lawn, ye say? Well, that
be a new one for me! Here''s what I''d suggest, arr:
1. Firstly, ensure yer safety. Llamas may look gentle, but they can be protective
if they feel threatened.
2. Try to make the area less appealing to the llama. Remove any food sources
or water that might be attracting it.
3. Contact local animal control or a wildlife rescue organization. They be the
experts and can provide humane ways to remove the llama from yer property.
4. If ye have any experience with animals, you could try to gently herd the
llama towards a nearby field or open space. But be careful, arr!
Remember, arr, it be important to treat the llama with respect and care. It
be a creature just trying to survive, like the rest of us.'
text: '[INST] You are a pirate chatbot who always responds with Arr and pirate speak!
There''s a llama on my lawn, how can I get rid of him? [/INST]'
---
<!-- markdownlint-disable MD041 -->
<!-- header start -->
<!-- 200823 -->
<div style="width: auto; margin-left: auto; margin-right: auto">
<img src="https://i.imgur.com/EBdldam.jpg" alt="TheBlokeAI" style="width: 100%; min-width: 400px; display: block; margin: auto;">
</div>
<div style="display: flex; justify-content: space-between; width: 100%;">
<div style="display: flex; flex-direction: column; align-items: flex-start;">
<p style="margin-top: 0.5em; margin-bottom: 0em;"><a href="https://discord.gg/theblokeai">Chat & support: TheBloke's Discord server</a></p>
</div>
<div style="display: flex; flex-direction: column; align-items: flex-end;">
<p style="margin-top: 0.5em; margin-bottom: 0em;"><a href="https://www.patreon.com/TheBlokeAI">Want to contribute? TheBloke's Patreon page</a></p>
</div>
</div>
<div style="text-align:center; margin-top: 0em; margin-bottom: 0em"><p style="margin-top: 0.25em; margin-bottom: 0em;">TheBloke's LLM work is generously supported by a grant from <a href="https://a16z.com">andreessen horowitz (a16z)</a></p></div>
<hr style="margin-top: 1.0em; margin-bottom: 1.0em;">
<!-- header end -->
# Mixtral 8X7B Instruct v0.1 - AWQ
- Model creator: [Mistral AI_](https://huggingface.co/mistralai)
- Original model: [Mixtral 8X7B Instruct v0.1](https://huggingface.co/mistralai/Mixtral-8x7B-Instruct-v0.1)
<!-- description start -->
## Description
This repo contains AWQ model files for [Mistral AI_'s Mixtral 8X7B Instruct v0.1](https://huggingface.co/mistralai/Mixtral-8x7B-Instruct-v0.1).
### About AWQ
AWQ is an efficient, accurate and blazing-fast low-bit weight quantization method, currently supporting 4-bit quantization. Compared to GPTQ, it offers faster Transformers-based inference with equivalent or better quality compared to the most commonly used GPTQ settings.
AWQ models are currently supported on Linux and Windows, with NVidia GPUs only. macOS users: please use GGUF models instead.
It is supported by:
- [Text Generation Webui](https://github.com/oobabooga/text-generation-webui) - using Loader: AutoAWQ
- [vLLM](https://github.com/vllm-project/vllm) - version 0.2.2 or later for support for all model types.
- [Hugging Face Text Generation Inference (TGI)](https://github.com/huggingface/text-generation-inference)
- [Transformers](https://huggingface.co/docs/transformers) version 4.35.0 and later, from any code or client that supports Transformers
- [AutoAWQ](https://github.com/casper-hansen/AutoAWQ) - for use from Python code
<!-- description end -->
<!-- repositories-available start -->
## Repositories available
* [AWQ model(s) for GPU inference.](https://huggingface.co/TheBloke/Mixtral-8x7B-Instruct-v0.1-AWQ)
* [GPTQ models for GPU inference, with multiple quantisation parameter options.](https://huggingface.co/TheBloke/Mixtral-8x7B-Instruct-v0.1-GPTQ)
* [2, 3, 4, 5, 6 and 8-bit GGUF models for CPU+GPU inference](https://huggingface.co/TheBloke/Mixtral-8x7B-Instruct-v0.1-GGUF)
* [Mistral AI_'s original unquantised fp16 model in pytorch format, for GPU inference and for further conversions](https://huggingface.co/mistralai/Mixtral-8x7B-Instruct-v0.1)
<!-- repositories-available end -->
<!-- prompt-template start -->
## Prompt template: Mistral
```
[INST] {prompt} [/INST]
```
<!-- prompt-template end -->
<!-- README_AWQ.md-provided-files start -->
## Provided files, and AWQ parameters
I currently release 128g GEMM models only. The addition of group_size 32 models, and GEMV kernel models, is being actively considered.
Models are released as sharded safetensors files.
| Branch | Bits | GS | AWQ Dataset | Seq Len | Size |
| ------ | ---- | -- | ----------- | ------- | ---- |
| main | 4 | 128 | [VMware Open Instruct](https://huggingface.co/datasets/VMware/open-instruct/viewer/) | 8192 | 24.65 GB
<!-- README_AWQ.md-provided-files end -->
<!-- README_AWQ.md-text-generation-webui start -->
## How to easily download and use this model in [text-generation-webui](https://github.com/oobabooga/text-generation-webui)
Please make sure you're using the latest version of [text-generation-webui](https://github.com/oobabooga/text-generation-webui).
It is strongly recommended to use the text-generation-webui one-click-installers unless you're sure you know how to make a manual install.
1. Click the **Model tab**.
2. Under **Download custom model or LoRA**, enter `TheBloke/Mixtral-8x7B-Instruct-v0.1-AWQ`.
3. Click **Download**.
4. The model will start downloading. Once it's finished it will say "Done".
5. In the top left, click the refresh icon next to **Model**.
6. In the **Model** dropdown, choose the model you just downloaded: `Mixtral-8x7B-Instruct-v0.1-AWQ`
7. Select **Loader: AutoAWQ**.
8. Click Load, and the model will load and is now ready for use.
9. If you want any custom settings, set them and then click **Save settings for this model** followed by **Reload the Model** in the top right.
10. Once you're ready, click the **Text Generation** tab and enter a prompt to get started!
<!-- README_AWQ.md-text-generation-webui end -->
<!-- README_AWQ.md-use-from-vllm start -->
## Multi-user inference server: vLLM
Documentation on installing and using vLLM [can be found here](https://vllm.readthedocs.io/en/latest/).
- Please ensure you are using vLLM version 0.2 or later.
- When using vLLM as a server, pass the `--quantization awq` parameter.
For example:
```shell
python3 -m vllm.entrypoints.api_server --model TheBloke/Mixtral-8x7B-Instruct-v0.1-AWQ --quantization awq --dtype auto
```
- When using vLLM from Python code, again set `quantization=awq`.
For example:
```python
from vllm import LLM, SamplingParams
prompts = [
"Tell me about AI",
"Write a story about llamas",
"What is 291 - 150?",
"How much wood would a woodchuck chuck if a woodchuck could chuck wood?",
]
prompt_template=f'''[INST] {prompt} [/INST]
'''
prompts = [prompt_template.format(prompt=prompt) for prompt in prompts]
sampling_params = SamplingParams(temperature=0.8, top_p=0.95)
llm = LLM(model="TheBloke/Mixtral-8x7B-Instruct-v0.1-AWQ", quantization="awq", dtype="auto")
outputs = llm.generate(prompts, sampling_params)
# Print the outputs.
for output in outputs:
prompt = output.prompt
generated_text = output.outputs[0].text
print(f"Prompt: {prompt!r}, Generated text: {generated_text!r}")
```
<!-- README_AWQ.md-use-from-vllm start -->
<!-- README_AWQ.md-use-from-tgi start -->
## Multi-user inference server: Hugging Face Text Generation Inference (TGI)
Use TGI version 1.1.0 or later. The official Docker container is: `ghcr.io/huggingface/text-generation-inference:1.1.0`
Example Docker parameters:
```shell
--model-id TheBloke/Mixtral-8x7B-Instruct-v0.1-AWQ --port 3000 --quantize awq --max-input-length 3696 --max-total-tokens 4096 --max-batch-prefill-tokens 4096
```
Example Python code for interfacing with TGI (requires [huggingface-hub](https://github.com/huggingface/huggingface_hub) 0.17.0 or later):
```shell
pip3 install huggingface-hub
```
```python
from huggingface_hub import InferenceClient
endpoint_url = "https://your-endpoint-url-here"
prompt = "Tell me about AI"
prompt_template=f'''[INST] {prompt} [/INST]
'''
client = InferenceClient(endpoint_url)
response = client.text_generation(prompt,
max_new_tokens=128,
do_sample=True,
temperature=0.7,
top_p=0.95,
top_k=40,
repetition_penalty=1.1)
print(f"Model output: ", response)
```
<!-- README_AWQ.md-use-from-tgi end -->
<!-- README_AWQ.md-use-from-python start -->
## Inference from Python code using Transformers
### Install the necessary packages
- Requires: [Transformers](https://huggingface.co/docs/transformers) 4.35.0 or later.
- Requires: [AutoAWQ](https://github.com/casper-hansen/AutoAWQ) 0.1.6 or later.
```shell
pip3 install --upgrade "autoawq>=0.1.6" "transformers>=4.35.0"
```
Note that if you are using PyTorch 2.0.1, the above AutoAWQ command will automatically upgrade you to PyTorch 2.1.0.
If you are using CUDA 11.8 and wish to continue using PyTorch 2.0.1, instead run this command:
```shell
pip3 install https://github.com/casper-hansen/AutoAWQ/releases/download/v0.1.6/autoawq-0.1.6+cu118-cp310-cp310-linux_x86_64.whl
```
If you have problems installing [AutoAWQ](https://github.com/casper-hansen/AutoAWQ) using the pre-built wheels, install it from source instead:
```shell
pip3 uninstall -y autoawq
git clone https://github.com/casper-hansen/AutoAWQ
cd AutoAWQ
pip3 install .
```
### Transformers example code (requires Transformers 4.35.0 and later)
```python
from transformers import AutoModelForCausalLM, AutoTokenizer, TextStreamer
model_name_or_path = "TheBloke/Mixtral-8x7B-Instruct-v0.1-AWQ"
tokenizer = AutoTokenizer.from_pretrained(model_name_or_path)
model = AutoModelForCausalLM.from_pretrained(
model_name_or_path,
low_cpu_mem_usage=True,
device_map="cuda:0"
)
# Using the text streamer to stream output one token at a time
streamer = TextStreamer(tokenizer, skip_prompt=True, skip_special_tokens=True)
prompt = "Tell me about AI"
prompt_template=f'''[INST] {prompt} [/INST]
'''
# Convert prompt to tokens
tokens = tokenizer(
prompt_template,
return_tensors='pt'
).input_ids.cuda()
generation_params = {
"do_sample": True,
"temperature": 0.7,
"top_p": 0.95,
"top_k": 40,
"max_new_tokens": 512,
"repetition_penalty": 1.1
}
# Generate streamed output, visible one token at a time
generation_output = model.generate(
tokens,
streamer=streamer,
**generation_params
)
# Generation without a streamer, which will include the prompt in the output
generation_output = model.generate(
tokens,
**generation_params
)
# Get the tokens from the output, decode them, print them
token_output = generation_output[0]
text_output = tokenizer.decode(token_output)
print("model.generate output: ", text_output)
# Inference is also possible via Transformers' pipeline
from transformers import pipeline
pipe = pipeline(
"text-generation",
model=model,
tokenizer=tokenizer,
**generation_params
)
pipe_output = pipe(prompt_template)[0]['generated_text']
print("pipeline output: ", pipe_output)
```
<!-- README_AWQ.md-use-from-python end -->
<!-- README_AWQ.md-compatibility start -->
## Compatibility
The files provided are tested to work with:
- [text-generation-webui](https://github.com/oobabooga/text-generation-webui) using `Loader: AutoAWQ`.
- [vLLM](https://github.com/vllm-project/vllm) version 0.2.0 and later.
- [Hugging Face Text Generation Inference (TGI)](https://github.com/huggingface/text-generation-inference) version 1.1.0 and later.
- [Transformers](https://huggingface.co/docs/transformers) version 4.35.0 and later.
- [AutoAWQ](https://github.com/casper-hansen/AutoAWQ) version 0.1.1 and later.
<!-- README_AWQ.md-compatibility end -->
<!-- footer start -->
<!-- 200823 -->
## Discord
For further support, and discussions on these models and AI in general, join us at:
[TheBloke AI's Discord server](https://discord.gg/theblokeai)
## Thanks, and how to contribute
Thanks to the [chirper.ai](https://chirper.ai) team!
Thanks to Clay from [gpus.llm-utils.org](llm-utils)!
I've had a lot of people ask if they can contribute. I enjoy providing models and helping people, and would love to be able to spend even more time doing it, as well as expanding into new projects like fine tuning/training.
If you're able and willing to contribute it will be most gratefully received and will help me to keep providing more models, and to start work on new AI projects.
Donaters will get priority support on any and all AI/LLM/model questions and requests, access to a private Discord room, plus other benefits.
* Patreon: https://patreon.com/TheBlokeAI
* Ko-Fi: https://ko-fi.com/TheBlokeAI
**Special thanks to**: Aemon Algiz.
**Patreon special mentions**: Michael Levine, 阿明, Trailburnt, Nikolai Manek, John Detwiler, Randy H, Will Dee, Sebastain Graf, NimbleBox.ai, Eugene Pentland, Emad Mostaque, Ai Maven, Jim Angel, Jeff Scroggin, Michael Davis, Manuel Alberto Morcote, Stephen Murray, Robert, Justin Joy, Luke @flexchar, Brandon Frisco, Elijah Stavena, S_X, Dan Guido, Undi ., Komninos Chatzipapas, Shadi, theTransient, Lone Striker, Raven Klaugh, jjj, Cap'n Zoog, Michel-Marie MAUDET (LINAGORA), Matthew Berman, David, Fen Risland, Omer Bin Jawed, Luke Pendergrass, Kalila, OG, Erik Bjäreholt, Rooh Singh, Joseph William Delisle, Dan Lewis, TL, John Villwock, AzureBlack, Brad, Pedro Madruga, Caitlyn Gatomon, K, jinyuan sun, Mano Prime, Alex, Jeffrey Morgan, Alicia Loh, Illia Dulskyi, Chadd, transmissions 11, fincy, Rainer Wilmers, ReadyPlayerEmma, knownsqashed, Mandus, biorpg, Deo Leter, Brandon Phillips, SuperWojo, Sean Connelly, Iucharbius, Jack West, Harry Royden McLaughlin, Nicholas, terasurfer, Vitor Caleffi, Duane Dunston, Johann-Peter Hartmann, David Ziegler, Olakabola, Ken Nordquist, Trenton Dambrowitz, Tom X Nguyen, Vadim, Ajan Kanaga, Leonard Tan, Clay Pascal, Alexandros Triantafyllidis, JM33133, Xule, vamX, ya boyyy, subjectnull, Talal Aujan, Alps Aficionado, wassieverse, Ari Malik, James Bentley, Woland, Spencer Kim, Michael Dempsey, Fred von Graf, Elle, zynix, William Richards, Stanislav Ovsiannikov, Edmond Seymore, Jonathan Leane, Martin Kemka, usrbinkat, Enrico Ros
Thank you to all my generous patrons and donaters!
And thank you again to a16z for their generous grant.
<!-- footer end -->
# Original model card: Mistral AI_'s Mixtral 8X7B Instruct v0.1
# Model Card for Mixtral-8x7B
The Mixtral-8x7B Large Language Model (LLM) is a pretrained generative Sparse Mixture of Experts. The Mixtral-8x7B outperforms Llama 2 70B on most benchmarks we tested.
For full details of this model please read our [release blog post](https://mistral.ai/news/mixtral-of-experts/).
## Warning
This repo contains weights that are compatible with [vLLM](https://github.com/vllm-project/vllm) serving of the model as well as Hugging Face [transformers](https://github.com/huggingface/transformers) library. It is based on the original Mixtral [torrent release](magnet:?xt=urn:btih:5546272da9065eddeb6fcd7ffddeef5b75be79a7&dn=mixtral-8x7b-32kseqlen&tr=udp%3A%2F%http://2Fopentracker.i2p.rocks%3A6969%2Fannounce&tr=http%3A%2F%http://2Ftracker.openbittorrent.com%3A80%2Fannounce), but the file format and parameter names are different. Please note that model cannot (yet) be instantiated with HF.
## Instruction format
This format must be strictly respected, otherwise the model will generate sub-optimal outputs.
The template used to build a prompt for the Instruct model is defined as follows:
```
<s> [INST] Instruction [/INST] Model answer</s> [INST] Follow-up instruction [/INST]
```
Note that `<s>` and `</s>` are special tokens for beginning of string (BOS) and end of string (EOS) while [INST] and [/INST] are regular strings.
As reference, here is the pseudo-code used to tokenize instructions during fine-tuning:
```python
def tokenize(text):
return tok.encode(text, add_special_tokens=False)
[BOS_ID] +
tokenize("[INST]") + tokenize(USER_MESSAGE_1) + tokenize("[/INST]") +
tokenize(BOT_MESSAGE_1) + [EOS_ID] +
…
tokenize("[INST]") + tokenize(USER_MESSAGE_N) + tokenize("[/INST]") +
tokenize(BOT_MESSAGE_N) + [EOS_ID]
```
In the pseudo-code above, note that the `tokenize` method should not add a BOS or EOS token automatically, but should add a prefix space.
## Run the model
```python
from transformers import AutoModelForCausalLM, AutoTokenizer
model_id = "mistralai/Mixtral-8x7B-Instruct-v0.1"
tokenizer = AutoTokenizer.from_pretrained(model_id)
model = AutoModelForCausalLM.from_pretrained(model_id)
text = "Hello my name is"
inputs = tokenizer(text, return_tensors="pt")
outputs = model.generate(**inputs, max_new_tokens=20)
print(tokenizer.decode(outputs[0], skip_special_tokens=True))
```
By default, transformers will load the model in full precision. Therefore you might be interested to further reduce down the memory requirements to run the model through the optimizations we offer in HF ecosystem:
### In half-precision
Note `float16` precision only works on GPU devices
<details>
<summary> Click to expand </summary>
```diff
+ import torch
from transformers import AutoModelForCausalLM, AutoTokenizer
model_id = "mistralai/Mixtral-8x7B-Instruct-v0.1"
tokenizer = AutoTokenizer.from_pretrained(model_id)
+ model = AutoModelForCausalLM.from_pretrained(model_id, torch_dtype=torch.float16).to(0)
text = "Hello my name is"
+ inputs = tokenizer(text, return_tensors="pt").to(0)
outputs = model.generate(**inputs, max_new_tokens=20)
print(tokenizer.decode(outputs[0], skip_special_tokens=True))
```
</details>
### Lower precision using (8-bit & 4-bit) using `bitsandbytes`
<details>
<summary> Click to expand </summary>
```diff
+ import torch
from transformers import AutoModelForCausalLM, AutoTokenizer
model_id = "mistralai/Mixtral-8x7B-Instruct-v0.1"
tokenizer = AutoTokenizer.from_pretrained(model_id)
+ model = AutoModelForCausalLM.from_pretrained(model_id, load_in_4bit=True)
text = "Hello my name is"
+ inputs = tokenizer(text, return_tensors="pt").to(0)
outputs = model.generate(**inputs, max_new_tokens=20)
print(tokenizer.decode(outputs[0], skip_special_tokens=True))
```
</details>
### Load the model with Flash Attention 2
<details>
<summary> Click to expand </summary>
```diff
+ import torch
from transformers import AutoModelForCausalLM, AutoTokenizer
model_id = "mistralai/Mixtral-8x7B-Instruct-v0.1"
tokenizer = AutoTokenizer.from_pretrained(model_id)
+ model = AutoModelForCausalLM.from_pretrained(model_id, use_flash_attention_2=True)
text = "Hello my name is"
+ inputs = tokenizer(text, return_tensors="pt").to(0)
outputs = model.generate(**inputs, max_new_tokens=20)
print(tokenizer.decode(outputs[0], skip_special_tokens=True))
```
</details>
## Limitations
The Mixtral-8x7B Instruct model is a quick demonstration that the base model can be easily fine-tuned to achieve compelling performance.
It does not have any moderation mechanisms. We're looking forward to engaging with the community on ways to
make the model finely respect guardrails, allowing for deployment in environments requiring moderated outputs.
# The Mistral AI Team
Albert Jiang, Alexandre Sablayrolles, Arthur Mensch, Blanche Savary, Chris Bamford, Devendra Singh Chaplot, Diego de las Casas, Emma Bou Hanna, Florian Bressand, Gianna Lengyel, Guillaume Bour, Guillaume Lample, Lélio Renard Lavaud, Louis Ternon, Lucile Saulnier, Marie-Anne Lachaux, Pierre Stock, Teven Le Scao, Théophile Gervet, Thibaut Lavril, Thomas Wang, Timothée Lacroix, William El Sayed.
|
v000000/l3-11.5B-dus-moonroot-monika-slerp-Q8_0-GGUF | v000000 | "2024-06-30T21:25:25Z" | 2,158 | 0 | transformers | [
"transformers",
"gguf",
"mergekit",
"merge",
"llama-cpp",
"base_model:v000000/l3-11.5B-dus-moonroot-monika-slerp",
"endpoints_compatible",
"region:us"
] | null | "2024-06-30T21:23:51Z" | ---
base_model: v000000/l3-11.5B-dus-moonroot-monika-slerp
library_name: transformers
tags:
- mergekit
- merge
- llama-cpp
---
idk
# v000000/l3-11.5B-dus-moonroot-monika-slerp-Q8_0-GGUF
This model was converted to GGUF format from [`v000000/l3-11.5B-dus-moonroot-monika-slerp`](https://huggingface.co/v000000/l3-11.5B-dus-moonroot-monika-slerp) using llama.cpp
Refer to the [original model card](https://huggingface.co/v000000/l3-11.5B-dus-moonroot-monika-slerp) for more details on the model.'
|
maritaca-ai/sabia-7b | maritaca-ai | "2024-04-04T10:38:24Z" | 2,157 | 88 | transformers | [
"transformers",
"safetensors",
"llama",
"text-generation",
"pt",
"arxiv:2304.07880",
"model-index",
"autotrain_compatible",
"endpoints_compatible",
"text-generation-inference",
"region:us"
] | text-generation | "2023-11-08T22:13:36Z" | ---
language:
- pt
model-index:
- name: sabia-7b
results:
- task:
type: text-generation
name: Text Generation
dataset:
name: ENEM Challenge (No Images)
type: eduagarcia/enem_challenge
split: train
args:
num_few_shot: 3
metrics:
- type: acc
value: 55.07
name: accuracy
source:
url: https://huggingface.co/spaces/eduagarcia/open_pt_llm_leaderboard?query=maritaca-ai/sabia-7b
name: Open Portuguese LLM Leaderboard
- task:
type: text-generation
name: Text Generation
dataset:
name: BLUEX (No Images)
type: eduagarcia-temp/BLUEX_without_images
split: train
args:
num_few_shot: 3
metrics:
- type: acc
value: 47.71
name: accuracy
source:
url: https://huggingface.co/spaces/eduagarcia/open_pt_llm_leaderboard?query=maritaca-ai/sabia-7b
name: Open Portuguese LLM Leaderboard
- task:
type: text-generation
name: Text Generation
dataset:
name: OAB Exams
type: eduagarcia/oab_exams
split: train
args:
num_few_shot: 3
metrics:
- type: acc
value: 41.41
name: accuracy
source:
url: https://huggingface.co/spaces/eduagarcia/open_pt_llm_leaderboard?query=maritaca-ai/sabia-7b
name: Open Portuguese LLM Leaderboard
- task:
type: text-generation
name: Text Generation
dataset:
name: Assin2 RTE
type: assin2
split: test
args:
num_few_shot: 15
metrics:
- type: f1_macro
value: 46.68
name: f1-macro
source:
url: https://huggingface.co/spaces/eduagarcia/open_pt_llm_leaderboard?query=maritaca-ai/sabia-7b
name: Open Portuguese LLM Leaderboard
- task:
type: text-generation
name: Text Generation
dataset:
name: Assin2 STS
type: eduagarcia/portuguese_benchmark
split: test
args:
num_few_shot: 15
metrics:
- type: pearson
value: 1.89
name: pearson
source:
url: https://huggingface.co/spaces/eduagarcia/open_pt_llm_leaderboard?query=maritaca-ai/sabia-7b
name: Open Portuguese LLM Leaderboard
- task:
type: text-generation
name: Text Generation
dataset:
name: FaQuAD NLI
type: ruanchaves/faquad-nli
split: test
args:
num_few_shot: 15
metrics:
- type: f1_macro
value: 58.34
name: f1-macro
source:
url: https://huggingface.co/spaces/eduagarcia/open_pt_llm_leaderboard?query=maritaca-ai/sabia-7b
name: Open Portuguese LLM Leaderboard
- task:
type: text-generation
name: Text Generation
dataset:
name: HateBR Binary
type: ruanchaves/hatebr
split: test
args:
num_few_shot: 25
metrics:
- type: f1_macro
value: 61.93
name: f1-macro
source:
url: https://huggingface.co/spaces/eduagarcia/open_pt_llm_leaderboard?query=maritaca-ai/sabia-7b
name: Open Portuguese LLM Leaderboard
- task:
type: text-generation
name: Text Generation
dataset:
name: PT Hate Speech Binary
type: hate_speech_portuguese
split: test
args:
num_few_shot: 25
metrics:
- type: f1_macro
value: 64.13
name: f1-macro
source:
url: https://huggingface.co/spaces/eduagarcia/open_pt_llm_leaderboard?query=maritaca-ai/sabia-7b
name: Open Portuguese LLM Leaderboard
- task:
type: text-generation
name: Text Generation
dataset:
name: tweetSentBR
type: eduagarcia-temp/tweetsentbr
split: test
args:
num_few_shot: 25
metrics:
- type: f1_macro
value: 46.64
name: f1-macro
source:
url: https://huggingface.co/spaces/eduagarcia/open_pt_llm_leaderboard?query=maritaca-ai/sabia-7b
name: Open Portuguese LLM Leaderboard
---
Sabiá-7B is Portuguese language model developed by [Maritaca AI](https://www.maritaca.ai/).
**Input:** The model accepts only text input.
**Output:** The Model generates text only.
**Model Architecture:** Sabiá-7B is an auto-regressive language model that uses the same architecture of LLaMA-1-7B.
**Tokenizer:** It uses the same tokenizer as LLaMA-1-7B.
**Maximum sequence length:** 2048 tokens.
**Pretraining data:** The model was pretrained on 7 billion tokens from the Portuguese subset of ClueWeb22, starting with the weights of LLaMA-1-7B and further trained for an additional 10 billion tokens, approximately 1.4 epochs of the training dataset.
**Data Freshness:** The pretraining data has a cutoff of mid-2022.
**License:** The licensing is the same as LLaMA-1's, restricting the model's use to research purposes only.
**Paper:** For more details, please refer to our paper: [Sabiá: Portuguese Large Language Models](https://arxiv.org/pdf/2304.07880.pdf)
## Few-shot Example
Given that Sabiá-7B was trained solely on a language modeling objective without fine-tuning for instruction following, it is recommended for few-shot tasks rather than zero-shot tasks, like in the example below.
```python
import torch
from transformers import LlamaTokenizer, LlamaForCausalLM
tokenizer = LlamaTokenizer.from_pretrained("maritaca-ai/sabia-7b")
model = LlamaForCausalLM.from_pretrained(
"maritaca-ai/sabia-7b",
device_map="auto", # Automatically loads the model in the GPU, if there is one. Requires pip install acelerate
low_cpu_mem_usage=True,
torch_dtype=torch.bfloat16 # If your GPU does not support bfloat16, change to torch.float16
)
prompt = """Classifique a resenha de filme como "positiva" ou "negativa".
Resenha: Gostei muito do filme, é o melhor do ano!
Classe: positiva
Resenha: O filme deixa muito a desejar.
Classe: negativa
Resenha: Apesar de longo, valeu o ingresso.
Classe:"""
input_ids = tokenizer(prompt, return_tensors="pt")
output = model.generate(
input_ids["input_ids"].to("cuda"),
max_length=1024,
eos_token_id=tokenizer.encode("\n")) # Stop generation when a "\n" token is dectected
# The output contains the input tokens, so we have to skip them.
output = output[0][len(input_ids["input_ids"][0]):]
print(tokenizer.decode(output, skip_special_tokens=True))
```
If your GPU does not have enough RAM, try using int8 precision.
However, expect some degradation in the model output quality when compared to fp16 or bf16.
```python
model = LlamaForCausalLM.from_pretrained(
"maritaca-ai/sabia-7b",
device_map="auto",
low_cpu_mem_usage=True,
load_in_8bit=True, # Requires pip install bitsandbytes
)
```
## Results in Portuguese
Below we show the results on the Poeta benchmark, which consists of 14 Portuguese datasets.
For more information on the Normalized Preferred Metric (NPM), please refer to our paper.
|Model | NPM |
|--|--|
|LLaMA-1-7B| 33.0|
|LLaMA-2-7B| 43.7|
|Sabiá-7B| 48.5|
## Results in English
Below we show the average results on 6 English datasets: PIQA, HellaSwag, WinoGrande, ARC-e, ARC-c, and OpenBookQA.
|Model | NPM |
|--|--|
|LLaMA-1-7B| 50.1|
|Sabiá-7B| 49.0|
## Citation
Please use the following bibtex to cite our paper:
```
@InProceedings{10.1007/978-3-031-45392-2_15,
author="Pires, Ramon
and Abonizio, Hugo
and Almeida, Thales Sales
and Nogueira, Rodrigo",
editor="Naldi, Murilo C.
and Bianchi, Reinaldo A. C.",
title="Sabi{\'a}: Portuguese Large Language Models",
booktitle="Intelligent Systems",
year="2023",
publisher="Springer Nature Switzerland",
address="Cham",
pages="226--240",
isbn="978-3-031-45392-2"
}
```
# [Open Portuguese LLM Leaderboard Evaluation Results](https://huggingface.co/spaces/eduagarcia/open_pt_llm_leaderboard)
Detailed results can be found [here](https://huggingface.co/datasets/eduagarcia-temp/llm_pt_leaderboard_raw_results/tree/main/maritaca-ai/sabia-7b)
| Metric | Value |
|--------------------------|---------|
|Average |**47.09**|
|ENEM Challenge (No Images)| 55.07|
|BLUEX (No Images) | 47.71|
|OAB Exams | 41.41|
|Assin2 RTE | 46.68|
|Assin2 STS | 1.89|
|FaQuAD NLI | 58.34|
|HateBR Binary | 61.93|
|PT Hate Speech Binary | 64.13|
|tweetSentBR | 46.64|
|
PartAI/Dorna-Llama3-8B-Instruct-GGUF | PartAI | "2024-06-18T14:22:01Z" | 2,157 | 9 | transformers | [
"transformers",
"gguf",
"LLM",
"llama-3",
"PartAI",
"conversational",
"fa",
"en",
"license:llama3",
"endpoints_compatible",
"region:us"
] | null | "2024-06-11T13:42:05Z" | ---
license: llama3
language:
- fa
- en
library_name: transformers
tags:
- LLM
- llama-3
- PartAI
- conversational
---
# Model Details
The Dorna models are a family of decoder-only models, specifically trained/fine-tuned on Persian data, developed by [Part AI](https://partdp.ai/). As an initial release, an 8B instruct model from this family is
Dorna-Llama3-8B-Instruct is built using the [Meta Llama 3 Instruct](https://huggingface.co/meta-llama/Meta-Llama-3-8B-Instruct) model.
In this repo, we provide `bf16` model and quantized models in the GGUF formats, including `Q2_K`, `Q3_K`, `Q3_K_L`, `Q3_K_M`, `Q3_K_S`, `Q4_0`, `Q4_1`, `Q4_K_M`, `Q4_K_S`, `Q5_0`, `Q5_1`, `Q5_K_M`, `Q5_K_S` and `Q8_0`
[Here](https://gist.github.com/Artefact2/b5f810600771265fc1e39442288e8ec9) offers an in-depth report that includes several performance charts. Check it out.
<style>
table td {
padding-right: 30px;
padding-left: 30px;
color: #000;
}
th {
color: #000;
}
a {
color: #000;
}
</style>
<table style="border-spacing: 30px; text-align: center;">
<tr style="background-color:#f2f2f2;">
<th>Name</th>
<th>Quant Method</th>
<th>Bits</th>
<th>Memory</th>
</tr>
<tr style="background-color:#e0f7fa; " >
<td style="text-align: left;"><a href="https://huggingface.co/PartAI/Dorna-Llama3-8B-Instruct-GGUF/blob/main/dorna-llama3-8b-instruct.Q2_K.gguf">dorna-llama3-8b-instruct.Q2_K.gguf</a></td>
<td>Q2_K</td>
<td>2</td>
<td>3.2 GB</td>
</tr>
<tr style="background-color:#e8f5e9;">
<td style="text-align: left;"><a href="https://huggingface.co/PartAI/Dorna-Llama3-8B-Instruct-GGUF/blob/main/dorna-llama3-8b-instruct.Q3_K_L.gguf">dorna-llama3-8b-instruct.Q3_K_L.gguf</a></td>
<td>Q3_K_L</td>
<td>3</td>
<td>4.3 GB</td>
</tr>
<tr style="background-color:#e8f5e9;">
<td style="text-align: left;"><a href="https://huggingface.co/PartAI/Dorna-Llama3-8B-Instruct-GGUF/blob/main/dorna-llama3-8b-instruct.Q3_K_M.gguf">dorna-llama3-8b-instruct.Q3_K_M.gguf</a></td>
<td>Q3_K_M</td>
<td>3</td>
<td>4.1 GB</td>
</tr>
<tr style="background-color:#e8f5e9;">
<td style="text-align: left;"><a href="https://huggingface.co/PartAI/Dorna-Llama3-8B-Instruct-GGUF/blob/main/dorna-llama3-8b-instruct.Q3_K_S.gguf">dorna-llama3-8b-instruct.Q3_K_S.gguf</a></td>
<td>Q3_K_S</td>
<td>3</td>
<td>3.7 GB</td>
</tr>
<tr style="background-color:#fff3e0;">
<td style="text-align: left;"><a href="https://huggingface.co/PartAI/Dorna-Llama3-8B-Instruct-GGUF/blob/main/dorna-llama3-8b-instruct.Q4_0.gguf">dorna-llama3-8b-instruct.Q4_0.gguf</a></td>
<td>Q4_1</td>
<td>4</td>
<td>4.7 GB</td>
</tr>
<tr style="background-color:#fff3e0;">
<td style="text-align: left;"><a href="https://huggingface.co/PartAI/Dorna-Llama3-8B-Instruct-GGUF/blob/main/dorna-llama3-8b-instruct.Q4_1.gguf">dorna-llama3-8b-instruct.Q4_1.gguf</a></td>
<td>Q4_1</td>
<td>4</td>
<td>5.2 GB</td>
</tr>
<tr style="background-color:#fff3e0;">
<td style="text-align: left;"><a href="https://huggingface.co/PartAI/Dorna-Llama3-8B-Instruct-GGUF/blob/main/dorna-llama3-8b-instruct.Q4_K_M.gguf">dorna-llama3-8b-instruct.Q4_K_M.gguf</a></td>
<td>Q4_K_M</td>
<td>4</td>
<td>4.9 GB</td>
</tr>
<tr style="background-color:#fff3e0;">
<td style="text-align: left;"><a href="https://huggingface.co/PartAI/Dorna-Llama3-8B-Instruct-GGUF/blob/main/dorna-llama3-8b-instruct.Q4_K_S.gguf">dorna-llama3-8b-instruct.Q4_K_S.gguf</a></td>
<td>Q4_K_S</td>
<td>4</td>
<td>4.7 GB</td>
</tr>
<tr style="background-color:#ffe0b2; ">
<td style="text-align: left;"><a href="https://huggingface.co/PartAI/Dorna-Llama3-8B-Instruct-GGUF/blob/main/dorna-llama3-8b-instruct.Q5_0.gguf">dorna-llama3-8b-instruct.Q5_0.gguf</a></td>
<td>Q5_0</td>
<td>5</td>
<td>5.6 GB</td>
</tr>
<tr style="background-color:#ffe0b2; ">
<td style="text-align: left;"><a href="https://huggingface.co/PartAI/Dorna-Llama3-8B-Instruct-GGUF/blob/main/dorna-llama3-8b-instruct.Q5_1.gguf">dorna-llama3-8b-instruct.Q5_1.gguf</a></td>
<td>Q5_1</td>
<td>5</td>
<td>6.1 GB</td>
</tr>
<tr style="background-color:#ffe0b2; ">
<td style="text-align: left;"><a href="https://huggingface.co/PartAI/Dorna-Llama3-8B-Instruct-GGUF/blob/main/dorna-llama3-8b-instruct.Q5_K_M.gguf">dorna-llama3-8b-instruct.Q5_K_M.gguf</a></td>
<td>Q5_K_M</td>
<td>5</td>
<td>5.73 GB</td>
</tr>
<tr style="background-color:#ffe0b2; ">
<td style="text-align: left;"><a href="https://huggingface.co/PartAI/Dorna-Llama3-8B-Instruct-GGUF/blob/main/dorna-llama3-8b-instruct.Q5_K_S.gguf">dorna-llama3-8b-instruct.Q5_K_S.gguf</a></td>
<td>Q5_K_S</td>
<td>5</td>
<td>5.6 GB</td>
</tr>
<tr style="background-color:#e1bee7; ">
<td style="text-align: left;"><a href="https://huggingface.co/PartAI/Dorna-Llama3-8B-Instruct-GGUF/blob/main/dorna-llama3-8b-instruct.Q6_K.gguf">dorna-llama3-8b-instruct.Q6_K.gguf</a></td>
<td>Q6_K</td>
<td>6</td>
<td>6.6 GB</td>
</tr>
<tr style="background-color:#c5cae9;">
<td style="text-align: left;">
<a href="https://huggingface.co/PartAI/Dorna-Llama3-8B-Instruct-GGUF/blob/main/dorna-llama3-8b-instruct.Q8_0.gguf">dorna-llama3-8b-instruct.Q8_0.gguf</a>
<span style="background-color: #4CAF50; color: white; padding: 2px 8px; margin-left: 10px; border-radius: 4px; font-size: 12px;">Recommended</span>
</td>
<td>Q8_0</td>
<td>8</td>
<td>8.5 GB</td>
</tr>
<tr style="background-color:#b2dfdb;">
<td style="text-align: left;"><a href="https://huggingface.co/PartAI/Dorna-Llama3-8B-Instruct-GGUF/blob/main/dorna-llama3-8b-instruct.bf16.gguf">dorna-llama3-8b-instruct.bf16.gguf</a></td>
<td>None</td>
<td>16</td>
<td>16.2 GB</td>
</tr>
</table>
## Requirements
We recommend using the Python version of [`llama.cpp`](https://github.com/ggerganov/llama.cpp) and installing it with the following command:
```bash
!pip install https://github.com/abetlen/llama-cpp-python/releases/download/v0.2.78/llama_cpp_python-0.2.78-cp310-cp310-linux_x86_64.whl
```
## How to use
Instead of cloning the repository, which may be inefficient, you can manually download the required GGUF file or use `huggingface-cli` (`pip install huggingface_hub`) as demonstrated below:
```bash
!huggingface-cli login --token $HUGGING_FACE_HUB_TOKEN
!huggingface-cli download PartAI/Dorna-Llama3-8B-Instruct-GGUF dorna-llama3-8b-instruct.Q8_0.gguf --local-dir . --local-dir-use-symlinks False
```
```Python
from llama_cpp import Llama
llm = Llama(
model_path="dorna-llama3-8b-instruct.Q8_0.gguf",
chat_format="llama-3",
n_gpu_layers=-1,
n_ctx=2048,
)
messages = [
{"role": "system", "content": "You are a helpful Persian assistant. Please answer questions in the asked language."},
{"role": "user", "content": "کاغذ A4 بزرگ تر است یا A5؟"},
]
result = llm.create_chat_completion(
messages = messages,
top_p=0.85,
temperature=0.1
)
print(result)
```
## Contact us
If you have any questions regarding this model, you can reach us via the [community](https://huggingface.co/PartAI/Dorna-Llama3-8B-Instruct-GGUF/discussions) on Hugging Face. |
cjpais/llava-v1.6-34B-gguf | cjpais | "2024-03-07T00:09:55Z" | 2,156 | 38 | null | [
"gguf",
"llava",
"image-text-to-text",
"license:apache-2.0",
"region:us"
] | image-text-to-text | "2024-02-01T20:42:12Z" | ---
license: apache-2.0
tags:
- llava
pipeline_tag: image-text-to-text
---
# GGUF Quantized LLaVA 1.6 34B
Updated quants and projector from [PR #5267](https://github.com/ggerganov/llama.cpp/pull/5267)
## Provided files
| Name | Quant method | Bits | Size | Use case |
| ---- | ---- | ---- | ---- | ----- |
| [llava-v1.6-34b.Q3_K_XS.gguf](https://huggingface.co/cjpais/llava-v1.6-34B-gguf/blob/main/llava-1.6-34b.Q3_K_XS.gguf) | Q3_K_XS | 3 | 14.2 GB| very small, high quality loss |
| [llava-v1.6-34b.Q3_K_M.gguf](https://huggingface.co/cjpais/llava-v1.6-34B-gguf/blob/main/llava-v1.6-34b.Q3_K_M.gguf) | Q3_K_M | 3 | 16.7 GB| very small, high quality loss |
| [llava-v1.6-34b.Q4_K_M.gguf](https://huggingface.co/cjpais/llava-v1.6-34B-gguf/blob/main/llava-v1.6-34b.Q4_K_M.gguf) | Q4_K_M | 4 | 20.66 GB| medium, balanced quality - recommended |
| [llava-v1.6-34b.Q5_K_S.gguf](https://huggingface.co/cjpais/llava-v1.6-34B-gguf/blob/main/llava-v1.6-34b.Q5_K_S.gguf) | Q5_K_S | 5 | 23.7 GB| large, low quality loss - recommended |
| [llava-v1.6-34b.Q5_K_M.gguf](https://huggingface.co/cjpais/llava-v1.6-34B-gguf/blob/main/llava-v1.6-34b.Q5_K_M.gguf) | Q5_K_M | 5 | 24.3 GB| large, very low quality loss - recommended |
| [llava-v1.6-34b.Q6_K.gguf](https://huggingface.co/cjpais/llava-v1.6-34B-gguf/blob/main/llava-v1.6-34b.Q6_K.gguf) | Q6_K | 5 | 28.2 GB| very large, extremely low quality loss |
| [llava-v1.6-34b.Q8_0.gguf](https://huggingface.co/cjpais/llava-v1.6-34B-gguf/blob/main/llava-v1.6-34b.Q8_0.gguf) | Q8_0 | 5 | 36.5 GB| very large, extremely low quality loss - not recommended |
<br>
<br>
# ORIGINAL LLaVA Model Card
## Model details
**Model type:**
LLaVA is an open-source chatbot trained by fine-tuning LLM on multimodal instruction-following data.
It is an auto-regressive language model, based on the transformer architecture.
Base LLM: [NousResearch/Nous-Hermes-2-Yi-34B](https://huggingface.co/NousResearch/Nous-Hermes-2-Yi-34B)
**Model date:**
LLaVA-v1.6-34B was trained in December 2023.
**Paper or resources for more information:**
https://llava-vl.github.io/
## License
[NousResearch/Nous-Hermes-2-Yi-34B](https://huggingface.co/NousResearch/Nous-Hermes-2-Yi-34B) license.
**Where to send questions or comments about the model:**
https://github.com/haotian-liu/LLaVA/issues
## Intended use
**Primary intended uses:**
The primary use of LLaVA is research on large multimodal models and chatbots.
**Primary intended users:**
The primary intended users of the model are researchers and hobbyists in computer vision, natural language processing, machine learning, and artificial intelligence.
## Training dataset
- 558K filtered image-text pairs from LAION/CC/SBU, captioned by BLIP.
- 158K GPT-generated multimodal instruction-following data.
- 500K academic-task-oriented VQA data mixture.
- 50K GPT-4V data mixture.
- 40K ShareGPT data.
## Evaluation dataset
A collection of 12 benchmarks, including 5 academic VQA benchmarks and 7 recent benchmarks specifically proposed for instruction-following LMMs.
|
kittn/mistral-7B-v0.1-hf | kittn | "2023-09-27T15:13:15Z" | 2,155 | 17 | transformers | [
"transformers",
"safetensors",
"llama",
"text-generation",
"autotrain_compatible",
"endpoints_compatible",
"text-generation-inference",
"region:us"
] | text-generation | "2023-09-27T10:30:08Z" | ## **UPDATE: Official version is out, use it instead: [https://huggingface.co/mistralai/Mistral-7B-v0.1](https://huggingface.co/mistralai/Mistral-7B-v0.1)**
---
---
---
---
# mistral-7B-v0.1-hf
Huggingface compatible version of Mistral's 7B model: https://twitter.com/MistralAI/status/1706877320844509405
## Usage
### Load in bfloat16 (16GB VRAM or higher)
```python
import torch
from transformers import LlamaForCausalLM, LlamaTokenizer, pipeline, TextStreamer
tokenizer = LlamaTokenizer.from_pretrained("kittn/mistral-7B-v0.1-hf")
model = LlamaForCausalLM.from_pretrained(
"kittn/mistral-7B-v0.1-hf",
torch_dtype=torch.bfloat16,
device_map={"": 0}
)
pipe = pipeline("text-generation", model=model, tokenizer=tokenizer)
pipe("Hi, my name", streamer=TextStreamer(tokenizer), max_new_tokens=128)
```
### Load in bitsandbytes nf4 (6GB VRAM or higher, maybe less with double_quant)
```python
import torch
from transformers import LlamaForCausalLM, LlamaTokenizer, pipeline, TextStreamer, BitsAndBytesConfig
tokenizer = LlamaTokenizer.from_pretrained("kittn/mistral-7B-v0.1-hf")
model = LlamaForCausalLM.from_pretrained(
"kittn/mistral-7B-v0.1-hf",
device_map={"": 0},
quantization_config=BitsAndBytesConfig(
load_in_4bit=True,
bnb_4bit_compute_dtype=torch.float16,
bnb_4bit_quant_type="nf4",
bnb_4bit_use_double_quant=False, # set to True to save more VRAM at the cost of some speed/accuracy
),
)
pipe = pipeline("text-generation", model=model, tokenizer=tokenizer)
pipe("Hi, my name", streamer=TextStreamer(tokenizer), max_new_tokens=128)
```
### Load in bitsandbytes int8 (8GB VRAM or higher). Quite slow; not recommended.
```python
import torch
from transformers import LlamaForCausalLM, LlamaTokenizer, pipeline, TextStreamer, BitsAndBytesConfig
tokenizer = LlamaTokenizer.from_pretrained("kittn/mistral-7B-v0.1-hf")
model = LlamaForCausalLM.from_pretrained(
"kittn/mistral-7B-v0.1-hf",
device_map={"": 0},
quantization_config=BitsAndBytesConfig(
load_in_8bit=True,
),
)
pipe = pipeline("text-generation", model=model, tokenizer=tokenizer)
pipe("Hi, my name", streamer=TextStreamer(tokenizer), max_new_tokens=128)
```
## Notes
* The original huggingface conversion script converts the model from bf16 to fp16 before saving it. This script doesn't
* The tokenizer is created with `legacy=False`, [more about this here](https://github.com/huggingface/transformers/pull/24565)
* Saved in safetensors format
## Conversion script [[link]](https://gist.github.com/sekstini/151d6946df1f6aa997b7cb15ee6f3be1)
Unlike [meta-llama/Llama-2-7b](https://huggingface.co/meta-llama/Llama-2-7b), this model uses GQA. This breaks some assumptions in the original conversion script, requiring a few changes.
Conversion script: [link](https://gist.github.com/sekstini/151d6946df1f6aa997b7cb15ee6f3be1)
Original conversion script: [link](https://github.com/huggingface/transformers/blob/946bac798caefada3f5f1c9fecdcfd587ed24ac7/src/transformers/models/llama/convert_llama_weights_to_hf.py)
|
refuelai/Llama-3-Refueled | refuelai | "2024-05-09T20:42:29Z" | 2,155 | 181 | transformers | [
"transformers",
"safetensors",
"llama",
"text-generation",
"data labeling",
"conversational",
"en",
"license:cc-by-nc-4.0",
"autotrain_compatible",
"endpoints_compatible",
"text-generation-inference",
"region:us"
] | text-generation | "2024-05-03T05:16:26Z" | ---
license: cc-by-nc-4.0
language:
- en
library_name: transformers
tags:
- data labeling
---
<div style="width: auto; margin-left: auto; margin-right: auto; background-color:black">
<img src="https://assets-global.website-files.com/6423879a8f63c1bb18d74bfa/648818d56d04c3bdf36d71ab_Refuel_rev8-01_ts-p-1600.png" alt="Refuel.ai" style="width: 100%; min-width: 400px; display: block; margin: auto;">
</div>
## Model Details
RefuelLLM-2-small, aka Llama-3-Refueled, is a Llama3-8B base model instruction tuned on a corpus of 2750+ datasets, spanning tasks such as classification, reading comprehension, structured attribute extraction and entity resolution. We're excited to open-source the model for the community to build on top of.
* More details about [RefuelLLM-2 family of models](https://www.refuel.ai/blog-posts/announcing-refuel-llm-2)
* You can also try out the models in our [LLM playground](https://labs.refuel.ai/playground)
**Model developers** - Refuel AI
**Input** - Text only.
**Output** - Text only.
**Architecture** - Llama-3-Refueled is built on top of Llama-3-8B-instruct which is an auto-regressive language model that uses an optimized transformer architecture.
**Release Date** - May 8, 2024.
**License** - [CC BY-NC 4.0](https://creativecommons.org/licenses/by-nc/4.0/deed.en)
## How to use
This repository contains weights for Llama-3-Refueled that are compatible for use with HuggingFace. See the snippet below for usage with Transformers:
```python
>>> import torch
>>> from transformers import AutoModelForCausalLM, AutoTokenizer
>>> model_id = "refuelai/Llama-3-Refueled"
>>> tokenizer = AutoTokenizer.from_pretrained(model_id)
>>> model = AutoModelForCausalLM.from_pretrained(model_id, torch_dtype=torch.float16, device_map="auto")
>>> messages = [{"role": "user", "content": "Is this comment toxic or non-toxic: RefuelLLM is the new way to label text data!"}]
>>> inputs = tokenizer.apply_chat_template(messages, return_tensors="pt", add_generation_prompt=True).to("cuda")
>>> outputs = model.generate(inputs, max_new_tokens=20)
>>> print(tokenizer.decode(outputs[0]))
```
## Training Data
The model was both trained on over 4 Billion tokens, spanning 2750+ NLP tasks. Our training collection consists majorly of:
1. Human annotated datasets like Flan, Task Source, and the Aya collection
2. Synthetic datasets like OpenOrca, OpenHermes and WizardLM
3. Proprietary datasets developed or licensed by Refuel AI
## Benchmarks
In this section, we report the results for Refuel models on our benchmark of labeling tasks. For details on the methodology see [here](https://refuel.ai/blog-posts/announcing-refuel-llm-2).
<table>
<tr></tr>
<tr><th>Provider</th><th>Model</th><th colspan="4" style="text-align: center">LLM Output Quality (by task type)</tr>
<tr><td></td><td></td><td>Overall</td><td>Classification</td><td>Reading Comprehension</td><td>Structure Extraction</td><td>Entity Matching</td><td></td></tr>
<tr><td>Refuel</td><td>RefuelLLM-2</td><td>83.82%</td><td>84.94%</td><td>76.03%</td><td>88.16%</td><td>92.00%</td><td></td></tr>
<tr><td>OpenAI</td><td>GPT-4-Turbo</td><td>80.88%</td><td>81.77%</td><td>72.08%</td><td>84.79%</td><td>97.20%</td><td></td></tr>
<tr><td>Refuel</td><td>RefuelLLM-2-small (Llama-3-Refueled)</td><td>79.67%</td><td>81.72%</td><td>70.04%</td><td>84.28%</td><td>92.00%</td><td></td></tr>
<tr><td>Anthropic</td><td>Claude-3-Opus</td><td>79.19%</td><td>82.49%</td><td>67.30%</td><td>88.25%</td><td>94.96%</td><td></td></tr>
<tr><td>Meta</td><td>Llama3-70B-Instruct</td><td>78.20%</td><td>79.38%</td><td>66.03%</td><td>85.96%</td><td>94.13%</td><td></td></tr>
<tr><td>Google</td><td>Gemini-1.5-Pro</td><td>74.59%</td><td>73.52%</td><td>60.67%</td><td>84.27%</td><td>98.48%</td><td></td></tr>
<tr><td>Mistral</td><td>Mixtral-8x7B-Instruct</td><td>62.87%</td><td>79.11%</td><td>45.56%</td><td>47.08%</td><td>86.52%</td><td></td></tr>
<tr><td>Anthropic</td><td>Claude-3-Sonnet</td><td>70.99%</td><td>79.91%</td><td>45.44%</td><td>78.10%</td><td>96.34%</td><td></td></tr>
<tr><td>Anthropic</td><td>Claude-3-Haiku</td><td>69.23%</td><td>77.27%</td><td>50.19%</td><td>84.97%</td><td>54.08%</td><td></td></tr>
<tr><td>OpenAI</td><td>GPT-3.5-Turbo</td><td>68.13%</td><td>74.39%</td><td>53.21%</td><td>69.40%</td><td>80.41%</td><td></td></tr>
<tr><td>Meta</td><td>Llama3-8B-Instruct</td><td>62.30%</td><td>68.52%</td><td>49.16%</td><td>65.09%</td><td>63.61%</td><td></td></tr>
</table>
## Limitations
The Llama-3-Refueled does not have any moderation mechanisms. We're looking forward to engaging with the community
on ways to make the model finely respect guardrails, allowing for deployment in environments requiring moderated outputs. |
jetmoe/jetmoe-8b | jetmoe | "2024-04-15T03:23:40Z" | 2,154 | 245 | transformers | [
"transformers",
"safetensors",
"jetmoe",
"text-generation",
"arxiv:2404.07413",
"license:apache-2.0",
"autotrain_compatible",
"endpoints_compatible",
"region:us"
] | text-generation | "2024-03-25T16:47:01Z" | ---
license: apache-2.0
---
<div align="center">
<div> </div>
<img src="https://cdn-uploads.huggingface.co/production/uploads/641de0213239b631552713e4/ieHnwuczidNNoGRA_FN2y.png" width="500"/>
<img src="https://cdn-uploads.huggingface.co/production/uploads/641de0213239b631552713e4/UOsk9_zcbHpCCy6kmryYM.png" width="530"/>
</div>
# JetMoE: Reaching LLaMA2 Performance with 0.1M Dollars
## Key Messages
1. JetMoE-8B is **trained with less than $ 0.1 million**<sup>1</sup> **cost but outperforms LLaMA2-7B from Meta AI**, who has multi-billion-dollar training resources. LLM training can be **much cheaper than people previously thought**.
2. JetMoE-8B is **fully open-sourced and academia-friendly** because:
- It **only uses public datasets** for training, and the code is open-sourced. No proprietary resource is needed.
- It **can be finetuned with very limited compute budget** (e.g., consumer-grade GPU) that most labs can afford.
3. JetMoE-8B **only has 2.2B active parameters** during inference, which drastically lowers the computational cost. Compared to a model with similar inference computation, like Gemma-2B, JetMoE-8B achieves constantly better performance.
<sup>1</sup> We used a 96×H100 GPU cluster for 2 weeks, which cost ~$0.08 million.
Website: [https://research.myshell.ai/jetmoe](https://research.myshell.ai/jetmoe)
HuggingFace: [https://huggingface.co/jetmoe/jetmoe-8b](https://huggingface.co/jetmoe/jetmoe-8b)
Online Demo on Lepton AI: [https://www.lepton.ai/playground/chat?model=jetmoe-8b-chat](https://www.lepton.ai/playground/chat?model=jetmoe-8b-chat)
Technical Report: [https://arxiv.org/pdf/2404.07413.pdf](https://arxiv.org/pdf/2404.07413.pdf)
## Authors
The project is contributed by [Yikang Shen](https://scholar.google.com.hk/citations?user=qff5rRYAAAAJ), [Zhen Guo](https://zguo0525.github.io/), [Tianle Cai](https://www.tianle.website/#/) and [Zengyi Qin](https://www.qinzy.tech/). For technical inquiries, please contact [Yikang Shen](https://scholar.google.com.hk/citations?user=qff5rRYAAAAJ). For media and collaboration inquiries, please contact [Zengyi Qin](https://www.qinzy.tech/).
## Collaboration
**If you have great ideas but need more resources (GPU, data, funding, etc.)**, welcome to contact **MyShell.ai** via [Zengyi Qin](https://www.qinzy.tech/). **MyShell.ai** is open to collaborations and are actively supporting high-quality open-source projects.
## Benchmarks
We use the same evaluation methodology as in the Open LLM leaderboard. For MBPP code benchmark, we use the same evaluation methodology as in the LLaMA2 and Deepseek-MoE paper. The results are shown below:
|Model|Activate Params|Training Tokens|Open LLM Leaderboard Avg|ARC|Hellaswag|MMLU|TruthfulQA|WinoGrande|GSM8k|MBPP|HumanEval|
|---|---|---|---|---|---|---|---|---|---|---|---|
|Shot||||25|10|5|0|5|5|3|0|
|Metric||||acc_norm|acc_norm|acc|mc2|acc|acc|Pass@1|Pass@1|
|LLaMA2-7B|7B|2T|51.0|53.1|78.6|46.9|38.8|74|14.5|20.8|12.8|
|LLaMA-13B|13B|1T|51.4|**56.2**|**80.9**|47.7|39.5|**76.2**|7.6|22.0|15.8|
|DeepseekMoE-16B|2.8B|2T|51.1|53.2|79.8|46.3|36.1|73.7|17.3|34.0|**25.0**|
|Gemma-2B|2B|2T|46.4|48.4|71.8|41.8|33.1|66.3|16.9|28.0|24.4|
|JetMoE-8B|2.2B|1.25T|**53.0**|48.7|80.5|**49.2**|**41.7**|70.2|**27.8**|**34.2**|14.6|
| Model | MT-Bench Score |
|---------------------|-----------|
| GPT-4 | 9.014 |
| GPT-3.5-turbo | 7.995 |
| Claude-v1 | 7.923 |
| **JetMoE-8B-chat** | **6.681** |
| Llama-2-13b-chat | 6.650 |
| Vicuna-13b-v1.3 | 6.413 |
| Wizardlm-13b | 6.353 |
| Llama-2-7b-chat | 6.269 |
To our surprise, despite the lower training cost and computation, JetMoE-8B performs even better than LLaMA2-7B, LLaMA-13B, and DeepseekMoE-16B. Compared to a model with similar training and inference computation, like Gemma-2B, JetMoE-8B achieves better performance.
## Model Usage
To load the models, you need install [this package](https://github.com/myshell-ai/JetMoE):
```
pip install -e .
```
Then you can load the model with the following code:
```python
from transformers import AutoTokenizer, AutoModelForCausalLM, AutoConfig, AutoModelForSequenceClassification
from jetmoe import JetMoEForCausalLM, JetMoEConfig, JetMoEForSequenceClassification
AutoConfig.register("jetmoe", JetMoEConfig)
AutoModelForCausalLM.register(JetMoEConfig, JetMoEForCausalLM)
AutoModelForSequenceClassification.register(JetMoEConfig, JetMoEForSequenceClassification)
tokenizer = AutoTokenizer.from_pretrained('jetmoe/jetmoe-8b')
model = AutoModelForCausalLM.from_pretrained('jetmoe/jetmoe-8b')
```
## Model Details
JetMoE-8B has 24 blocks.
Each block has two MoE layers: Mixture of Attention heads (MoA) and Mixture of MLP Experts (MoE).
Each MoA and MoE layer has 8 expert, and 2 experts are activated for each input token.
It has 8 billion parameters in total and 2.2B active parameters.
JetMoE-8B is trained on 1.25T tokens from publicly available datasets, with a learning rate of 5.0 x 10<sup>-4</sup> and a global batch-size of 4M tokens.
<figure>
<center>
<img src="images/jetmoe_architecture.png" width="40%">
<figcaption>JetMoE Architecture</figcaption>
</center>
</figure>
## Training Details
Our training recipe follows the [MiniCPM](https://shengdinghu.notion.site/MiniCPM-Unveiling-the-Potential-of-End-side-Large-Language-Models-d4d3a8c426424654a4e80e42a711cb20?pvs=4)'s two-phases training method. Phase 1 uses a constant learning rate with linear warmup and is trained on 1 trillion tokens from large-scale open-source pretraining datasets, including RefinedWeb, Pile, Github data, etc. Phase 2 uses exponential learning rate decay and is trained on 250 billion tokens from phase 1 datasets and extra high-quality open-source datasets.
<figure>
<center>
<img src="images/Phase1_data.png" width="60%">
<img src="images/Phase2_data.png" width="60%">
</center>
</figure>
## Technical Report
For more details, please refer to the [JetMoE Technical Report](https://arxiv.org/abs/2404.07413).
## JetMoE Model Index
|Model|Index|
|---|---|
|JetMoE-8B-Base| [Link](https://huggingface.co/jetmoe/jetmoe-8B) |
|JetMoE-8B-SFT| [Link](https://huggingface.co/jetmoe/jetmoe-8B-sft) |
|JetMoE-8B-Chat| [Link](https://huggingface.co/jetmoe/jetmoe-8B-chat) |
## Acknowledgement
We express our gratitude to [Shengding Hu](https://shengdinghu.github.io/) for his valuable advice on the Phase 2 data mixture. We also express our gratitude to [Exabits](https://www.exabits.ai/) for their assistance in setting up the GPU clusters, and to [Lepton AI](https://www.lepton.ai/) for their support in setting up the chat demo.
|
MaziyarPanahi/mergekit-model_stock-qykbest-GGUF | MaziyarPanahi | "2024-06-17T20:05:22Z" | 2,154 | 0 | transformers | [
"transformers",
"gguf",
"mistral",
"quantized",
"2-bit",
"3-bit",
"4-bit",
"5-bit",
"6-bit",
"8-bit",
"GGUF",
"safetensors",
"text-generation",
"mergekit",
"merge",
"conversational",
"arxiv:2403.19522",
"base_model:gagan3012/Mistral_arabic_dpo",
"base_model:mistralai/Mistral-7B-Instruct-v0.2",
"base_model:Nexusflow/Starling-LM-7B-beta",
"autotrain_compatible",
"endpoints_compatible",
"text-generation-inference",
"region:us",
"base_model:mergekit-community/mergekit-model_stock-qykbest"
] | text-generation | "2024-06-17T19:42:11Z" | ---
tags:
- quantized
- 2-bit
- 3-bit
- 4-bit
- 5-bit
- 6-bit
- 8-bit
- GGUF
- transformers
- safetensors
- mistral
- text-generation
- mergekit
- merge
- conversational
- arxiv:2403.19522
- base_model:gagan3012/Mistral_arabic_dpo
- base_model:mistralai/Mistral-7B-Instruct-v0.2
- base_model:Nexusflow/Starling-LM-7B-beta
- autotrain_compatible
- endpoints_compatible
- text-generation-inference
- region:us
- text-generation
model_name: mergekit-model_stock-qykbest-GGUF
base_model: mergekit-community/mergekit-model_stock-qykbest
inference: false
model_creator: mergekit-community
pipeline_tag: text-generation
quantized_by: MaziyarPanahi
---
# [MaziyarPanahi/mergekit-model_stock-qykbest-GGUF](https://huggingface.co/MaziyarPanahi/mergekit-model_stock-qykbest-GGUF)
- Model creator: [mergekit-community](https://huggingface.co/mergekit-community)
- Original model: [mergekit-community/mergekit-model_stock-qykbest](https://huggingface.co/mergekit-community/mergekit-model_stock-qykbest)
## Description
[MaziyarPanahi/mergekit-model_stock-qykbest-GGUF](https://huggingface.co/MaziyarPanahi/mergekit-model_stock-qykbest-GGUF) contains GGUF format model files for [mergekit-community/mergekit-model_stock-qykbest](https://huggingface.co/mergekit-community/mergekit-model_stock-qykbest).
### About GGUF
GGUF is a new format introduced by the llama.cpp team on August 21st 2023. It is a replacement for GGML, which is no longer supported by llama.cpp.
Here is an incomplete list of clients and libraries that are known to support GGUF:
* [llama.cpp](https://github.com/ggerganov/llama.cpp). The source project for GGUF. Offers a CLI and a server option.
* [llama-cpp-python](https://github.com/abetlen/llama-cpp-python), a Python library with GPU accel, LangChain support, and OpenAI-compatible API server.
* [LM Studio](https://lmstudio.ai/), an easy-to-use and powerful local GUI for Windows and macOS (Silicon), with GPU acceleration. Linux available, in beta as of 27/11/2023.
* [text-generation-webui](https://github.com/oobabooga/text-generation-webui), the most widely used web UI, with many features and powerful extensions. Supports GPU acceleration.
* [KoboldCpp](https://github.com/LostRuins/koboldcpp), a fully featured web UI, with GPU accel across all platforms and GPU architectures. Especially good for story telling.
* [GPT4All](https://gpt4all.io/index.html), a free and open source local running GUI, supporting Windows, Linux and macOS with full GPU accel.
* [LoLLMS Web UI](https://github.com/ParisNeo/lollms-webui), a great web UI with many interesting and unique features, including a full model library for easy model selection.
* [Faraday.dev](https://faraday.dev/), an attractive and easy to use character-based chat GUI for Windows and macOS (both Silicon and Intel), with GPU acceleration.
* [candle](https://github.com/huggingface/candle), a Rust ML framework with a focus on performance, including GPU support, and ease of use.
* [ctransformers](https://github.com/marella/ctransformers), a Python library with GPU accel, LangChain support, and OpenAI-compatible AI server. Note, as of time of writing (November 27th 2023), ctransformers has not been updated in a long time and does not support many recent models.
## Special thanks
🙏 Special thanks to [Georgi Gerganov](https://github.com/ggerganov) and the whole team working on [llama.cpp](https://github.com/ggerganov/llama.cpp/) for making all of this possible. |
scenario-labs/Realistic_Vision_V6.0_B1_noVAE | scenario-labs | "2024-05-23T12:16:08Z" | 2,153 | 0 | diffusers | [
"diffusers",
"license:creativeml-openrail-m",
"autotrain_compatible",
"endpoints_compatible",
"diffusers:StableDiffusionPipeline",
"region:us"
] | text-to-image | "2024-05-23T12:16:08Z" | ---
license: creativeml-openrail-m
---
This model is a fork from [Realistic_Vision_V6.0_B1_noVAE](https://huggingface.co/SG161222/Realistic_Vision_V6.0_B1_noVAE)
|
mradermacher/ChinaLM-9B-GGUF | mradermacher | "2024-06-18T02:12:56Z" | 2,153 | 0 | transformers | [
"transformers",
"gguf",
"mergekit",
"merge",
"conversational",
"chicka",
"chinese",
"china",
"en",
"base_model:Chickaboo/ChinaLM-9B",
"license:mit",
"endpoints_compatible",
"region:us"
] | null | "2024-06-18T01:42:01Z" | ---
base_model: Chickaboo/ChinaLM-9B
language:
- en
library_name: transformers
license: mit
quantized_by: mradermacher
tags:
- mergekit
- merge
- conversational
- chicka
- chinese
- china
---
## About
<!-- ### quantize_version: 2 -->
<!-- ### output_tensor_quantised: 1 -->
<!-- ### convert_type: hf -->
<!-- ### vocab_type: -->
<!-- ### tags: -->
static quants of https://huggingface.co/Chickaboo/ChinaLM-9B
<!-- provided-files -->
weighted/imatrix quants seem not to be available (by me) at this time. If they do not show up a week or so after the static ones, I have probably not planned for them. Feel free to request them by opening a Community Discussion.
## Usage
If you are unsure how to use GGUF files, refer to one of [TheBloke's
READMEs](https://huggingface.co/TheBloke/KafkaLM-70B-German-V0.1-GGUF) for
more details, including on how to concatenate multi-part files.
## Provided Quants
(sorted by size, not necessarily quality. IQ-quants are often preferable over similar sized non-IQ quants)
| Link | Type | Size/GB | Notes |
|:-----|:-----|--------:|:------|
| [GGUF](https://huggingface.co/mradermacher/ChinaLM-9B-GGUF/resolve/main/ChinaLM-9B.Q2_K.gguf) | Q2_K | 3.6 | |
| [GGUF](https://huggingface.co/mradermacher/ChinaLM-9B-GGUF/resolve/main/ChinaLM-9B.IQ3_XS.gguf) | IQ3_XS | 4.0 | |
| [GGUF](https://huggingface.co/mradermacher/ChinaLM-9B-GGUF/resolve/main/ChinaLM-9B.Q3_K_S.gguf) | Q3_K_S | 4.2 | |
| [GGUF](https://huggingface.co/mradermacher/ChinaLM-9B-GGUF/resolve/main/ChinaLM-9B.IQ3_S.gguf) | IQ3_S | 4.2 | beats Q3_K* |
| [GGUF](https://huggingface.co/mradermacher/ChinaLM-9B-GGUF/resolve/main/ChinaLM-9B.IQ3_M.gguf) | IQ3_M | 4.3 | |
| [GGUF](https://huggingface.co/mradermacher/ChinaLM-9B-GGUF/resolve/main/ChinaLM-9B.Q3_K_M.gguf) | Q3_K_M | 4.6 | lower quality |
| [GGUF](https://huggingface.co/mradermacher/ChinaLM-9B-GGUF/resolve/main/ChinaLM-9B.Q3_K_L.gguf) | Q3_K_L | 4.9 | |
| [GGUF](https://huggingface.co/mradermacher/ChinaLM-9B-GGUF/resolve/main/ChinaLM-9B.IQ4_XS.gguf) | IQ4_XS | 5.1 | |
| [GGUF](https://huggingface.co/mradermacher/ChinaLM-9B-GGUF/resolve/main/ChinaLM-9B.Q4_K_S.gguf) | Q4_K_S | 5.3 | fast, recommended |
| [GGUF](https://huggingface.co/mradermacher/ChinaLM-9B-GGUF/resolve/main/ChinaLM-9B.Q4_K_M.gguf) | Q4_K_M | 5.6 | fast, recommended |
| [GGUF](https://huggingface.co/mradermacher/ChinaLM-9B-GGUF/resolve/main/ChinaLM-9B.Q5_K_S.gguf) | Q5_K_S | 6.3 | |
| [GGUF](https://huggingface.co/mradermacher/ChinaLM-9B-GGUF/resolve/main/ChinaLM-9B.Q5_K_M.gguf) | Q5_K_M | 6.5 | |
| [GGUF](https://huggingface.co/mradermacher/ChinaLM-9B-GGUF/resolve/main/ChinaLM-9B.Q6_K.gguf) | Q6_K | 7.4 | very good quality |
| [GGUF](https://huggingface.co/mradermacher/ChinaLM-9B-GGUF/resolve/main/ChinaLM-9B.Q8_0.gguf) | Q8_0 | 9.6 | fast, best quality |
| [GGUF](https://huggingface.co/mradermacher/ChinaLM-9B-GGUF/resolve/main/ChinaLM-9B.f16.gguf) | f16 | 18.0 | 16 bpw, overkill |
Here is a handy graph by ikawrakow comparing some lower-quality quant
types (lower is better):

And here are Artefact2's thoughts on the matter:
https://gist.github.com/Artefact2/b5f810600771265fc1e39442288e8ec9
## FAQ / Model Request
See https://huggingface.co/mradermacher/model_requests for some answers to
questions you might have and/or if you want some other model quantized.
## Thanks
I thank my company, [nethype GmbH](https://www.nethype.de/), for letting
me use its servers and providing upgrades to my workstation to enable
this work in my free time.
<!-- end -->
|
mradermacher/TheSalt-L3-8b-v0.3.2-GGUF | mradermacher | "2024-06-19T12:45:48Z" | 2,153 | 1 | transformers | [
"transformers",
"gguf",
"en",
"base_model:cgato/TheSalt-L3-8b-v0.3.2",
"license:cc-by-nc-4.0",
"endpoints_compatible",
"region:us"
] | null | "2024-06-18T23:57:21Z" | ---
base_model: cgato/TheSalt-L3-8b-v0.3.2
language:
- en
library_name: transformers
license: cc-by-nc-4.0
quantized_by: mradermacher
---
## About
<!-- ### quantize_version: 2 -->
<!-- ### output_tensor_quantised: 1 -->
<!-- ### convert_type: hf -->
<!-- ### vocab_type: -->
<!-- ### tags: -->
static quants of https://huggingface.co/cgato/TheSalt-L3-8b-v0.3.2
<!-- provided-files -->
weighted/imatrix quants are available at https://huggingface.co/mradermacher/TheSalt-L3-8b-v0.3.2-i1-GGUF
## Usage
If you are unsure how to use GGUF files, refer to one of [TheBloke's
READMEs](https://huggingface.co/TheBloke/KafkaLM-70B-German-V0.1-GGUF) for
more details, including on how to concatenate multi-part files.
## Provided Quants
(sorted by size, not necessarily quality. IQ-quants are often preferable over similar sized non-IQ quants)
| Link | Type | Size/GB | Notes |
|:-----|:-----|--------:|:------|
| [GGUF](https://huggingface.co/mradermacher/TheSalt-L3-8b-v0.3.2-GGUF/resolve/main/TheSalt-L3-8b-v0.3.2.Q2_K.gguf) | Q2_K | 3.3 | |
| [GGUF](https://huggingface.co/mradermacher/TheSalt-L3-8b-v0.3.2-GGUF/resolve/main/TheSalt-L3-8b-v0.3.2.IQ3_XS.gguf) | IQ3_XS | 3.6 | |
| [GGUF](https://huggingface.co/mradermacher/TheSalt-L3-8b-v0.3.2-GGUF/resolve/main/TheSalt-L3-8b-v0.3.2.Q3_K_S.gguf) | Q3_K_S | 3.8 | |
| [GGUF](https://huggingface.co/mradermacher/TheSalt-L3-8b-v0.3.2-GGUF/resolve/main/TheSalt-L3-8b-v0.3.2.IQ3_S.gguf) | IQ3_S | 3.8 | beats Q3_K* |
| [GGUF](https://huggingface.co/mradermacher/TheSalt-L3-8b-v0.3.2-GGUF/resolve/main/TheSalt-L3-8b-v0.3.2.IQ3_M.gguf) | IQ3_M | 3.9 | |
| [GGUF](https://huggingface.co/mradermacher/TheSalt-L3-8b-v0.3.2-GGUF/resolve/main/TheSalt-L3-8b-v0.3.2.Q3_K_M.gguf) | Q3_K_M | 4.1 | lower quality |
| [GGUF](https://huggingface.co/mradermacher/TheSalt-L3-8b-v0.3.2-GGUF/resolve/main/TheSalt-L3-8b-v0.3.2.Q3_K_L.gguf) | Q3_K_L | 4.4 | |
| [GGUF](https://huggingface.co/mradermacher/TheSalt-L3-8b-v0.3.2-GGUF/resolve/main/TheSalt-L3-8b-v0.3.2.IQ4_XS.gguf) | IQ4_XS | 4.6 | |
| [GGUF](https://huggingface.co/mradermacher/TheSalt-L3-8b-v0.3.2-GGUF/resolve/main/TheSalt-L3-8b-v0.3.2.Q4_K_S.gguf) | Q4_K_S | 4.8 | fast, recommended |
| [GGUF](https://huggingface.co/mradermacher/TheSalt-L3-8b-v0.3.2-GGUF/resolve/main/TheSalt-L3-8b-v0.3.2.Q4_K_M.gguf) | Q4_K_M | 5.0 | fast, recommended |
| [GGUF](https://huggingface.co/mradermacher/TheSalt-L3-8b-v0.3.2-GGUF/resolve/main/TheSalt-L3-8b-v0.3.2.Q5_K_S.gguf) | Q5_K_S | 5.7 | |
| [GGUF](https://huggingface.co/mradermacher/TheSalt-L3-8b-v0.3.2-GGUF/resolve/main/TheSalt-L3-8b-v0.3.2.Q5_K_M.gguf) | Q5_K_M | 5.8 | |
| [GGUF](https://huggingface.co/mradermacher/TheSalt-L3-8b-v0.3.2-GGUF/resolve/main/TheSalt-L3-8b-v0.3.2.Q6_K.gguf) | Q6_K | 6.7 | very good quality |
| [GGUF](https://huggingface.co/mradermacher/TheSalt-L3-8b-v0.3.2-GGUF/resolve/main/TheSalt-L3-8b-v0.3.2.Q8_0.gguf) | Q8_0 | 8.6 | fast, best quality |
| [GGUF](https://huggingface.co/mradermacher/TheSalt-L3-8b-v0.3.2-GGUF/resolve/main/TheSalt-L3-8b-v0.3.2.f16.gguf) | f16 | 16.2 | 16 bpw, overkill |
Here is a handy graph by ikawrakow comparing some lower-quality quant
types (lower is better):

And here are Artefact2's thoughts on the matter:
https://gist.github.com/Artefact2/b5f810600771265fc1e39442288e8ec9
## FAQ / Model Request
See https://huggingface.co/mradermacher/model_requests for some answers to
questions you might have and/or if you want some other model quantized.
## Thanks
I thank my company, [nethype GmbH](https://www.nethype.de/), for letting
me use its servers and providing upgrades to my workstation to enable
this work in my free time.
<!-- end -->
|
mradermacher/Alpesteibock-Llama-3-8B-Alpha-GGUF | mradermacher | "2024-06-19T05:03:59Z" | 2,153 | 2 | transformers | [
"transformers",
"gguf",
"Llama-3",
"instruct",
"finetune",
"qlora",
"chatml",
"synthetic data",
"axolotl",
"gsw",
"dataset:cis-lmu/Glot500",
"dataset:cis-lmu/GlotCC-V1",
"base_model:kaizuberbuehler/Alpesteibock-Llama-3-8B-Alpha",
"license:llama3",
"endpoints_compatible",
"region:us"
] | null | "2024-06-19T02:23:01Z" | ---
base_model: kaizuberbuehler/Alpesteibock-Llama-3-8B-Alpha
datasets:
- cis-lmu/Glot500
- cis-lmu/GlotCC-V1
language:
- gsw
library_name: transformers
license: llama3
model_type: LlamaForCausalLM
quantized_by: mradermacher
tags:
- Llama-3
- instruct
- finetune
- qlora
- chatml
- synthetic data
- axolotl
---
## About
<!-- ### quantize_version: 2 -->
<!-- ### output_tensor_quantised: 1 -->
<!-- ### convert_type: hf -->
<!-- ### vocab_type: -->
<!-- ### tags: -->
static quants of https://huggingface.co/kaizuberbuehler/Alpesteibock-Llama-3-8B-Alpha
<!-- provided-files -->
weighted/imatrix quants seem not to be available (by me) at this time. If they do not show up a week or so after the static ones, I have probably not planned for them. Feel free to request them by opening a Community Discussion.
## Usage
If you are unsure how to use GGUF files, refer to one of [TheBloke's
READMEs](https://huggingface.co/TheBloke/KafkaLM-70B-German-V0.1-GGUF) for
more details, including on how to concatenate multi-part files.
## Provided Quants
(sorted by size, not necessarily quality. IQ-quants are often preferable over similar sized non-IQ quants)
| Link | Type | Size/GB | Notes |
|:-----|:-----|--------:|:------|
| [GGUF](https://huggingface.co/mradermacher/Alpesteibock-Llama-3-8B-Alpha-GGUF/resolve/main/Alpesteibock-Llama-3-8B-Alpha.Q2_K.gguf) | Q2_K | 3.3 | |
| [GGUF](https://huggingface.co/mradermacher/Alpesteibock-Llama-3-8B-Alpha-GGUF/resolve/main/Alpesteibock-Llama-3-8B-Alpha.IQ3_XS.gguf) | IQ3_XS | 3.6 | |
| [GGUF](https://huggingface.co/mradermacher/Alpesteibock-Llama-3-8B-Alpha-GGUF/resolve/main/Alpesteibock-Llama-3-8B-Alpha.Q3_K_S.gguf) | Q3_K_S | 3.8 | |
| [GGUF](https://huggingface.co/mradermacher/Alpesteibock-Llama-3-8B-Alpha-GGUF/resolve/main/Alpesteibock-Llama-3-8B-Alpha.IQ3_S.gguf) | IQ3_S | 3.8 | beats Q3_K* |
| [GGUF](https://huggingface.co/mradermacher/Alpesteibock-Llama-3-8B-Alpha-GGUF/resolve/main/Alpesteibock-Llama-3-8B-Alpha.IQ3_M.gguf) | IQ3_M | 3.9 | |
| [GGUF](https://huggingface.co/mradermacher/Alpesteibock-Llama-3-8B-Alpha-GGUF/resolve/main/Alpesteibock-Llama-3-8B-Alpha.Q3_K_M.gguf) | Q3_K_M | 4.1 | lower quality |
| [GGUF](https://huggingface.co/mradermacher/Alpesteibock-Llama-3-8B-Alpha-GGUF/resolve/main/Alpesteibock-Llama-3-8B-Alpha.Q3_K_L.gguf) | Q3_K_L | 4.4 | |
| [GGUF](https://huggingface.co/mradermacher/Alpesteibock-Llama-3-8B-Alpha-GGUF/resolve/main/Alpesteibock-Llama-3-8B-Alpha.IQ4_XS.gguf) | IQ4_XS | 4.6 | |
| [GGUF](https://huggingface.co/mradermacher/Alpesteibock-Llama-3-8B-Alpha-GGUF/resolve/main/Alpesteibock-Llama-3-8B-Alpha.Q4_K_S.gguf) | Q4_K_S | 4.8 | fast, recommended |
| [GGUF](https://huggingface.co/mradermacher/Alpesteibock-Llama-3-8B-Alpha-GGUF/resolve/main/Alpesteibock-Llama-3-8B-Alpha.Q4_K_M.gguf) | Q4_K_M | 5.0 | fast, recommended |
| [GGUF](https://huggingface.co/mradermacher/Alpesteibock-Llama-3-8B-Alpha-GGUF/resolve/main/Alpesteibock-Llama-3-8B-Alpha.Q5_K_S.gguf) | Q5_K_S | 5.7 | |
| [GGUF](https://huggingface.co/mradermacher/Alpesteibock-Llama-3-8B-Alpha-GGUF/resolve/main/Alpesteibock-Llama-3-8B-Alpha.Q5_K_M.gguf) | Q5_K_M | 5.8 | |
| [GGUF](https://huggingface.co/mradermacher/Alpesteibock-Llama-3-8B-Alpha-GGUF/resolve/main/Alpesteibock-Llama-3-8B-Alpha.Q6_K.gguf) | Q6_K | 6.7 | very good quality |
| [GGUF](https://huggingface.co/mradermacher/Alpesteibock-Llama-3-8B-Alpha-GGUF/resolve/main/Alpesteibock-Llama-3-8B-Alpha.Q8_0.gguf) | Q8_0 | 8.6 | fast, best quality |
| [GGUF](https://huggingface.co/mradermacher/Alpesteibock-Llama-3-8B-Alpha-GGUF/resolve/main/Alpesteibock-Llama-3-8B-Alpha.f16.gguf) | f16 | 16.2 | 16 bpw, overkill |
Here is a handy graph by ikawrakow comparing some lower-quality quant
types (lower is better):

And here are Artefact2's thoughts on the matter:
https://gist.github.com/Artefact2/b5f810600771265fc1e39442288e8ec9
## FAQ / Model Request
See https://huggingface.co/mradermacher/model_requests for some answers to
questions you might have and/or if you want some other model quantized.
## Thanks
I thank my company, [nethype GmbH](https://www.nethype.de/), for letting
me use its servers and providing upgrades to my workstation to enable
this work in my free time.
<!-- end -->
|
hywu/Camelidae-8x34B | hywu | "2024-04-09T01:21:57Z" | 2,152 | 28 | transformers | [
"transformers",
"pytorch",
"camelidae",
"text-generation",
"custom_code",
"en",
"dataset:Open-Orca/SlimOrca",
"dataset:ise-uiuc/Magicoder-OSS-Instruct-75K",
"dataset:ise-uiuc/Magicoder-Evol-Instruct-110K",
"dataset:meta-math/MetaMathQA",
"arxiv:2401.02731",
"arxiv:2305.14314",
"arxiv:1902.00751",
"arxiv:2212.05055",
"license:apache-2.0",
"autotrain_compatible",
"endpoints_compatible",
"region:us"
] | text-generation | "2024-01-10T09:20:18Z" | ---
datasets:
- Open-Orca/SlimOrca
- ise-uiuc/Magicoder-OSS-Instruct-75K
- ise-uiuc/Magicoder-Evol-Instruct-110K
- meta-math/MetaMathQA
language:
- en
library_name: transformers
pipeline_tag: text-generation
arxiv: 2401.02731
license: apache-2.0
---
# Parameter-Efficient Sparsity Crafting From Dense to Mixture-of-Experts for Instruction Tuning on General Tasks
## News
- 3/12/2024 - We released Qwen2idae-16x14B-v1.0 on 🤗 [HuggingFace](https://huggingface.co/hywu/Qwen2idae-16x14B-v1.0), which has strong performance in Math and Code with 15B activated params.
- 2/7/2024 - [Serp-ai](https://github.com/serp-ai/Parameter-Efficient-MoE) adds [unsloth](https://github.com/serp-ai/unsloth) support for faster and memory efficient training of our Parameter-Efficient Sparsity Crafting and releases new [sparsetral](https://huggingface.co/serpdotai/sparsetral-16x7B-v2) models based on mistral-7B.
- 1/10/2024 - Camelidae models are now available on 🤗 [HuggingFace](https://huggingface.co/hywu).
- 1/4/2024 - We released the paper, [Parameter-Efficient Sparsity Crafting From Dense to Mixture-of-Experts for Instruction Tuning on General Tasks](https://arxiv.org/abs/2401.02731).
- 12/22/2023 - We released the training [repo](https://github.com/wuhy68/Parameter-Efficient-MoE) that craft the dense model with LLaMA architecture to the MoE model.
## Introduction
Camelidae and Qwen2idae models are trained utilizing Parameter-Efficient Sparsity Crafting techniques
We present Parameter-Efficient Sparsity Crafting to help dense models learn knowledge from different fields (including code and math). This approach performs instruction tuning and efficiently utilizes MoE structure.
Specifically, Parameter-Efficient Sparsity Crafting utilizes parameter-efficient techniques including [QLoRA](https://arxiv.org/abs/2305.14314) and [Adapter](https://arxiv.org/abs/1902.00751) to perform Efficient [Sparse Upcycling](https://arxiv.org/abs/2212.05055).
## Model Lists
| Camelidae Series | Download
|---|---
Camelidae-8x7B | 🤗 [HuggingFace](https://huggingface.co/hywu/Camelidae-8x7B)
Camelidae-8x13B | 🤗 [HuggingFace](https://huggingface.co/hywu/Camelidae-8x13B)
Camelidae-8x34B | 🤗 [HuggingFace](https://huggingface.co/hywu/Camelidae-8x34B)
Camelidae-8x34B-pro | 🤗 Coming Soon
| Qwen2idae Series | Download
|---|---
Qwen2idae-16x14B-v1.0 | 🤗 [HuggingFace](https://huggingface.co/hywu/Qwen2idae-16x14B-v1.0)
Qwen2idae-16x7B-v1.0 | 🤗 Coming Soon
Qwen2idae-16x1.8B-v1.0 | 🤗 Coming Soon
## Performance
| Model | Activated Params | MMLU (5shot) | GSM8k (5shot) | MATH (4shot) | HumanEval (0shot) | MBPP (4shot) | HellaSwag (10shot) |
|:-----:|:----------------:|:------------:|:-------------:|:------------:|:-----------------:|:------------:|:------------------:|
| GPT3.5 | - | 70.0% | 57.1% | <font color=#F67F70>**34.1%**</font> | <font color=#FBD98D>**48.1%**</font> | - | <font color=#7FEA9E>**85.5%**</font> |
| LLaMA2-70B-chat | 70B | 63.8% | 59.3% | 10.4% | 32.3% | 35.6% | 84.8% |
| Camelidae-8x34B-pro | 35B | <font color=#7FEA9E>**75.7%**</font> | <font color=#F67F70>**79.4%**</font> | <font color=#FBD98D>**24.0%**</font> | <font color=#7FEA9E>**48.8%**</font> | <font color=#7FEA9E>**43.2%**</font> | 85.2% |
| Camelidae-8x34B | 35B | <font color=#FBD98D>**75.6%**</font> | <font color=#7FEA9E>**78.3%**</font> | 22.6% | 43.9% | <font color=#FBD98D>**41.4%**</font> | <font color=#FBD98D>**85.3%**</font> |
| SUSChat-34B | 34B | <font color=#F67F70>**76.4%**</font> | 72.3% | 22.0% | 11.6% | 40.2% | 83.9% |
| Yi-34B-chat | 34B | 74.8% | 67.6% | 17.3% | 20.1% | 41.0% | 83.9% |
| Qwen2idae-16x14B-v1.0 | 15B | 66.7% | <font color=#FBD98D>**77.8%**</font> | <font color=#7FEA9E>**29.9%**</font> | <font color=#F67F70>**62.8%**</font> | <font color=#F67F70>**48.6%**</font> | 82.3% |
| Mixtral-8x7B-instruct | 14B | 68.7% | 71.7% | 22.1% | 25.6% | 40.6% | <font color=#F67F70>**86.5%**</font> |
| Camelidae-8x13B | 13B | 54.4% | 52.6% | 9.8% | 30.6% | 30.4% | 82.5% |
| LLaMA2-13B-chat | 13B | 53.9% | 37.1% | 5.2% | 18.9% | 27.2% | 81.9% |
| Camelidae-8x7B | 7B | 48.3% | 44.0% | 5.8% | 18.3% | 23.4% | 79.2% |
| LLaMA2-7B-chat | 7B | 47.2% | 26.3% | 3.9% | 12.2% | 17.6% | 78.6% |
We bold the top3 scores separately for all models.
## Usage
```python
from transformers import AutoModelForCausalLM, AutoTokenizer
tokenizer = AutoTokenizer.from_pretrained("hywu/Camelidae-8x34B", trust_remote_code=True)
model = AutoModelForCausalLM.from_pretrained("hywu/Camelidae-8x34B", device_map="auto", trust_remote_code=True).eval()
inputs = tokenizer('### Human:\nHow are you?\n### Assistant:\n', return_tensors='pt')
inputs = inputs.to(model.device)
pred = model.generate(**inputs)
print(tokenizer.decode(pred.cpu()[0], skip_special_tokens=True))
```
## Citation
```bibtex
@article{wu2024parameter,
title={Parameter-Efficient Sparsity Crafting from Dense to Mixture-of-Experts for Instruction Tuning on General Tasks},
author={Wu, Haoyuan and Zheng, Haisheng and Yu, Bei},
journal={arXiv preprint arXiv:2401.02731},
year={2024}
}
```
## License
The source code in this repo is licensed under the [Apache 2.0 License](https://github.com/wuhy68/Parameter-Efficient-MoE/blob/master/LICENSE). Camelidae models are developed for academic research and free commercial use, all usage must adhere to the license from [facebookresearch](https://github.com/facebookresearch/llama/blob/main/LICENSE) and [01-ai](https://github.com/01-ai/Yi/blob/main/MODEL_LICENSE_AGREEMENT.txt). |
ridger/MMfreeLM-370M | ridger | "2024-05-23T17:03:40Z" | 2,152 | 13 | transformers | [
"transformers",
"safetensors",
"hgrn_bit",
"text-generation",
"autotrain_compatible",
"endpoints_compatible",
"region:us"
] | text-generation | "2024-05-23T16:51:09Z" | Entry not found |
RichardErkhov/Edgerunners_-_yi-9b-may-ortho-baukit-30fail-3000total-bf16-gguf | RichardErkhov | "2024-06-17T08:41:27Z" | 2,152 | 0 | null | [
"gguf",
"region:us"
] | null | "2024-06-17T07:13:03Z" | Quantization made by Richard Erkhov.
[Github](https://github.com/RichardErkhov)
[Discord](https://discord.gg/pvy7H8DZMG)
[Request more models](https://github.com/RichardErkhov/quant_request)
yi-9b-may-ortho-baukit-30fail-3000total-bf16 - GGUF
- Model creator: https://huggingface.co/Edgerunners/
- Original model: https://huggingface.co/Edgerunners/yi-9b-may-ortho-baukit-30fail-3000total-bf16/
| Name | Quant method | Size |
| ---- | ---- | ---- |
| [yi-9b-may-ortho-baukit-30fail-3000total-bf16.Q2_K.gguf](https://huggingface.co/RichardErkhov/Edgerunners_-_yi-9b-may-ortho-baukit-30fail-3000total-bf16-gguf/blob/main/yi-9b-may-ortho-baukit-30fail-3000total-bf16.Q2_K.gguf) | Q2_K | 3.12GB |
| [yi-9b-may-ortho-baukit-30fail-3000total-bf16.IQ3_XS.gguf](https://huggingface.co/RichardErkhov/Edgerunners_-_yi-9b-may-ortho-baukit-30fail-3000total-bf16-gguf/blob/main/yi-9b-may-ortho-baukit-30fail-3000total-bf16.IQ3_XS.gguf) | IQ3_XS | 3.46GB |
| [yi-9b-may-ortho-baukit-30fail-3000total-bf16.IQ3_S.gguf](https://huggingface.co/RichardErkhov/Edgerunners_-_yi-9b-may-ortho-baukit-30fail-3000total-bf16-gguf/blob/main/yi-9b-may-ortho-baukit-30fail-3000total-bf16.IQ3_S.gguf) | IQ3_S | 3.64GB |
| [yi-9b-may-ortho-baukit-30fail-3000total-bf16.Q3_K_S.gguf](https://huggingface.co/RichardErkhov/Edgerunners_-_yi-9b-may-ortho-baukit-30fail-3000total-bf16-gguf/blob/main/yi-9b-may-ortho-baukit-30fail-3000total-bf16.Q3_K_S.gguf) | Q3_K_S | 3.63GB |
| [yi-9b-may-ortho-baukit-30fail-3000total-bf16.IQ3_M.gguf](https://huggingface.co/RichardErkhov/Edgerunners_-_yi-9b-may-ortho-baukit-30fail-3000total-bf16-gguf/blob/main/yi-9b-may-ortho-baukit-30fail-3000total-bf16.IQ3_M.gguf) | IQ3_M | 3.78GB |
| [yi-9b-may-ortho-baukit-30fail-3000total-bf16.Q3_K.gguf](https://huggingface.co/RichardErkhov/Edgerunners_-_yi-9b-may-ortho-baukit-30fail-3000total-bf16-gguf/blob/main/yi-9b-may-ortho-baukit-30fail-3000total-bf16.Q3_K.gguf) | Q3_K | 4.03GB |
| [yi-9b-may-ortho-baukit-30fail-3000total-bf16.Q3_K_M.gguf](https://huggingface.co/RichardErkhov/Edgerunners_-_yi-9b-may-ortho-baukit-30fail-3000total-bf16-gguf/blob/main/yi-9b-may-ortho-baukit-30fail-3000total-bf16.Q3_K_M.gguf) | Q3_K_M | 4.03GB |
| [yi-9b-may-ortho-baukit-30fail-3000total-bf16.Q3_K_L.gguf](https://huggingface.co/RichardErkhov/Edgerunners_-_yi-9b-may-ortho-baukit-30fail-3000total-bf16-gguf/blob/main/yi-9b-may-ortho-baukit-30fail-3000total-bf16.Q3_K_L.gguf) | Q3_K_L | 4.37GB |
| [yi-9b-may-ortho-baukit-30fail-3000total-bf16.IQ4_XS.gguf](https://huggingface.co/RichardErkhov/Edgerunners_-_yi-9b-may-ortho-baukit-30fail-3000total-bf16-gguf/blob/main/yi-9b-may-ortho-baukit-30fail-3000total-bf16.IQ4_XS.gguf) | IQ4_XS | 4.5GB |
| [yi-9b-may-ortho-baukit-30fail-3000total-bf16.Q4_0.gguf](https://huggingface.co/RichardErkhov/Edgerunners_-_yi-9b-may-ortho-baukit-30fail-3000total-bf16-gguf/blob/main/yi-9b-may-ortho-baukit-30fail-3000total-bf16.Q4_0.gguf) | Q4_0 | 4.69GB |
| [yi-9b-may-ortho-baukit-30fail-3000total-bf16.IQ4_NL.gguf](https://huggingface.co/RichardErkhov/Edgerunners_-_yi-9b-may-ortho-baukit-30fail-3000total-bf16-gguf/blob/main/yi-9b-may-ortho-baukit-30fail-3000total-bf16.IQ4_NL.gguf) | IQ4_NL | 4.73GB |
| [yi-9b-may-ortho-baukit-30fail-3000total-bf16.Q4_K_S.gguf](https://huggingface.co/RichardErkhov/Edgerunners_-_yi-9b-may-ortho-baukit-30fail-3000total-bf16-gguf/blob/main/yi-9b-may-ortho-baukit-30fail-3000total-bf16.Q4_K_S.gguf) | Q4_K_S | 4.72GB |
| [yi-9b-may-ortho-baukit-30fail-3000total-bf16.Q4_K.gguf](https://huggingface.co/RichardErkhov/Edgerunners_-_yi-9b-may-ortho-baukit-30fail-3000total-bf16-gguf/blob/main/yi-9b-may-ortho-baukit-30fail-3000total-bf16.Q4_K.gguf) | Q4_K | 4.96GB |
| [yi-9b-may-ortho-baukit-30fail-3000total-bf16.Q4_K_M.gguf](https://huggingface.co/RichardErkhov/Edgerunners_-_yi-9b-may-ortho-baukit-30fail-3000total-bf16-gguf/blob/main/yi-9b-may-ortho-baukit-30fail-3000total-bf16.Q4_K_M.gguf) | Q4_K_M | 4.96GB |
| [yi-9b-may-ortho-baukit-30fail-3000total-bf16.Q4_1.gguf](https://huggingface.co/RichardErkhov/Edgerunners_-_yi-9b-may-ortho-baukit-30fail-3000total-bf16-gguf/blob/main/yi-9b-may-ortho-baukit-30fail-3000total-bf16.Q4_1.gguf) | Q4_1 | 5.19GB |
| [yi-9b-may-ortho-baukit-30fail-3000total-bf16.Q5_0.gguf](https://huggingface.co/RichardErkhov/Edgerunners_-_yi-9b-may-ortho-baukit-30fail-3000total-bf16-gguf/blob/main/yi-9b-may-ortho-baukit-30fail-3000total-bf16.Q5_0.gguf) | Q5_0 | 5.69GB |
| [yi-9b-may-ortho-baukit-30fail-3000total-bf16.Q5_K_S.gguf](https://huggingface.co/RichardErkhov/Edgerunners_-_yi-9b-may-ortho-baukit-30fail-3000total-bf16-gguf/blob/main/yi-9b-may-ortho-baukit-30fail-3000total-bf16.Q5_K_S.gguf) | Q5_K_S | 5.69GB |
| [yi-9b-may-ortho-baukit-30fail-3000total-bf16.Q5_K.gguf](https://huggingface.co/RichardErkhov/Edgerunners_-_yi-9b-may-ortho-baukit-30fail-3000total-bf16-gguf/blob/main/yi-9b-may-ortho-baukit-30fail-3000total-bf16.Q5_K.gguf) | Q5_K | 5.83GB |
| [yi-9b-may-ortho-baukit-30fail-3000total-bf16.Q5_K_M.gguf](https://huggingface.co/RichardErkhov/Edgerunners_-_yi-9b-may-ortho-baukit-30fail-3000total-bf16-gguf/blob/main/yi-9b-may-ortho-baukit-30fail-3000total-bf16.Q5_K_M.gguf) | Q5_K_M | 5.83GB |
| [yi-9b-may-ortho-baukit-30fail-3000total-bf16.Q5_1.gguf](https://huggingface.co/RichardErkhov/Edgerunners_-_yi-9b-may-ortho-baukit-30fail-3000total-bf16-gguf/blob/main/yi-9b-may-ortho-baukit-30fail-3000total-bf16.Q5_1.gguf) | Q5_1 | 6.19GB |
| [yi-9b-may-ortho-baukit-30fail-3000total-bf16.Q6_K.gguf](https://huggingface.co/RichardErkhov/Edgerunners_-_yi-9b-may-ortho-baukit-30fail-3000total-bf16-gguf/blob/main/yi-9b-may-ortho-baukit-30fail-3000total-bf16.Q6_K.gguf) | Q6_K | 6.75GB |
| [yi-9b-may-ortho-baukit-30fail-3000total-bf16.Q8_0.gguf](https://huggingface.co/RichardErkhov/Edgerunners_-_yi-9b-may-ortho-baukit-30fail-3000total-bf16-gguf/blob/main/yi-9b-may-ortho-baukit-30fail-3000total-bf16.Q8_0.gguf) | Q8_0 | 8.74GB |
Original model description:
---
license: cc-by-nc-4.0
---
new 9b-yi released in may
test results: refusal removal worked, but yi 9b chat is still kind of bad, ortho won't fix that; but judge for yourself
this version had only 30 refusals out of 3000 ortho-tests, in-line with the others in terms of refusals.
---
wassname (updated baukit) implementation of the paper: https://www.alignmentforum.org/posts/jGuXSZgv6qfdhMCuJ/refusal-in-llms-is-mediated-by-a-single-direction
applied to llama3 8b instruct
1. The Model is meant purely for alignment research and exploration of alignmentforum theory
2. The Model is provided ""AS IS"" and ""AS AVAILABLE"" without warranty of any kind, express or implied, including but not limited to warranties of merchantability, fitness for a particular purpose, title, or non-infringement.
3. The Provider disclaims all liability for any damages or losses resulting from the use or misuse of the Model, including but not limited to any damages or losses arising from the use of the Model for purposes other than those intended by the Provider.
4. The Provider does not endorse or condone the use of the Model for any purpose that violates applicable laws, regulations, or ethical standards.
5. The Provider does not warrant that the Model will meet your specific requirements or that it will be error-free or that it will function without interruption.
6. You assume all risks associated with the use of the Model, including but not limited to any loss of data, loss of business, or damage to your reputation.
|
timm/tresnet_l.miil_in1k | timm | "2023-04-21T20:54:39Z" | 2,151 | 0 | timm | [
"timm",
"pytorch",
"safetensors",
"image-classification",
"dataset:imagenet-1k",
"arxiv:2003.13630",
"license:apache-2.0",
"region:us"
] | image-classification | "2023-04-21T20:53:51Z" | ---
tags:
- image-classification
- timm
library_name: timm
license: apache-2.0
datasets:
- imagenet-1k
---
# Model card for tresnet_l.miil_in1k
A TResNet image classification model. Trained on ImageNet-1k by paper authors.
The weights for this model have been remapped and modified from the originals to work with standard BatchNorm instead of InplaceABN. `inplace_abn` can be problematic to build recently and ends up slower with `memory_format=channels_last`, torch.compile(), etc.
## Model Details
- **Model Type:** Image classification / feature backbone
- **Model Stats:**
- Params (M): 56.0
- GMACs: 10.9
- Activations (M): 11.9
- Image size: 224 x 224
- **Papers:**
- TResNet: High Performance GPU-Dedicated Architecture: https://arxiv.org/abs/2003.13630
- **Dataset:** ImageNet-1k
- **Original:** https://github.com/Alibaba-MIIL/TResNet
## Model Usage
### Image Classification
```python
from urllib.request import urlopen
from PIL import Image
import timm
img = Image.open(urlopen(
'https://huggingface.co/datasets/huggingface/documentation-images/resolve/main/beignets-task-guide.png'
))
model = timm.create_model('tresnet_l.miil_in1k', pretrained=True)
model = model.eval()
# get model specific transforms (normalization, resize)
data_config = timm.data.resolve_model_data_config(model)
transforms = timm.data.create_transform(**data_config, is_training=False)
output = model(transforms(img).unsqueeze(0)) # unsqueeze single image into batch of 1
top5_probabilities, top5_class_indices = torch.topk(output.softmax(dim=1) * 100, k=5)
```
### Feature Map Extraction
```python
from urllib.request import urlopen
from PIL import Image
import timm
img = Image.open(urlopen(
'https://huggingface.co/datasets/huggingface/documentation-images/resolve/main/beignets-task-guide.png'
))
model = timm.create_model(
'tresnet_l.miil_in1k',
pretrained=True,
features_only=True,
)
model = model.eval()
# get model specific transforms (normalization, resize)
data_config = timm.data.resolve_model_data_config(model)
transforms = timm.data.create_transform(**data_config, is_training=False)
output = model(transforms(img).unsqueeze(0)) # unsqueeze single image into batch of 1
for o in output:
# print shape of each feature map in output
# e.g.:
# torch.Size([1, 76, 56, 56])
# torch.Size([1, 152, 28, 28])
# torch.Size([1, 1216, 14, 14])
# torch.Size([1, 2432, 7, 7])
print(o.shape)
```
### Image Embeddings
```python
from urllib.request import urlopen
from PIL import Image
import timm
img = Image.open(urlopen(
'https://huggingface.co/datasets/huggingface/documentation-images/resolve/main/beignets-task-guide.png'
))
model = timm.create_model(
'tresnet_l.miil_in1k',
pretrained=True,
num_classes=0, # remove classifier nn.Linear
)
model = model.eval()
# get model specific transforms (normalization, resize)
data_config = timm.data.resolve_model_data_config(model)
transforms = timm.data.create_transform(**data_config, is_training=False)
output = model(transforms(img).unsqueeze(0)) # output is (batch_size, num_features) shaped tensor
# or equivalently (without needing to set num_classes=0)
output = model.forward_features(transforms(img).unsqueeze(0))
# output is unpooled, a (1, 2432, 7, 7) shaped tensor
output = model.forward_head(output, pre_logits=True)
# output is a (1, num_features) shaped tensor
```
## Citation
```bibtex
@misc{ridnik2020tresnet,
title={TResNet: High Performance GPU-Dedicated Architecture},
author={Tal Ridnik and Hussam Lawen and Asaf Noy and Itamar Friedman},
year={2020},
eprint={2003.13630},
archivePrefix={arXiv},
primaryClass={cs.CV}
}
```
|
DiscoResearch/DiscoLM-120b | DiscoResearch | "2023-12-13T00:36:46Z" | 2,151 | 22 | transformers | [
"transformers",
"pytorch",
"safetensors",
"llama",
"text-generation",
"goliath",
"deutsch",
"llama2",
"discoresearch",
"en",
"dataset:Open-Orca/SlimOrca-Dedup",
"dataset:teknium/openhermes",
"dataset:meta-math/MetaMathQA",
"dataset:migtissera/Synthia-v1.3",
"dataset:THUDM/AgentInstruct",
"dataset:LeoLM/German_Songs",
"dataset:LeoLM/German_Poems",
"dataset:LeoLM/OpenSchnabeltier",
"dataset:bjoernp/ultrachat_de",
"dataset:LDJnr/Capybara",
"license:llama2",
"autotrain_compatible",
"endpoints_compatible",
"text-generation-inference",
"region:us"
] | text-generation | "2023-11-19T18:31:29Z" | ---
datasets:
- Open-Orca/SlimOrca-Dedup
- teknium/openhermes
- meta-math/MetaMathQA
- migtissera/Synthia-v1.3
- THUDM/AgentInstruct
- LeoLM/German_Songs
- LeoLM/German_Poems
- LeoLM/OpenSchnabeltier
- bjoernp/ultrachat_de
- LDJnr/Capybara
language:
- en
library_name: transformers
pipeline_tag: text-generation
license: llama2
model_creator: DiscoResearch
model_type: llama
tags:
- goliath
- deutsch
- llama2
- discoresearch
---
<img src="imgs/disco_goliath.jpeg" width="600">
# DiscoLM 120b (Alpha)
**DiscoLM 120b (Alpha)** is an experimental 120b model based on [Alpindale´s Goliath 120b](https://huggingface.co/alpindale/goliath-120b), a merge of different Llama2-70b models, and further finetuned on a dataset of some the most popular open-source instruction sets.
Disco 120b is a [DiscoResearch](https://huggingface.co/DiscoResearch) project and was trained by [Björn Plüster](https://huggingface.co/bjoernp).
Many thanks to [LAION](https://laion.ai) and [HessianAI](https://hessian.ai/) for scientific supervision, coordination and compute resources provided for this project on supercomputer 42 by [HessianAI](https://hessian.ai/)!
<img src="https://hessian.ai/wp-content/themes/hessianai/img/hessian-ai-logo.svg" width="120">
<img src="https://avatars.githubusercontent.com/u/92627801?s=200&v=4" width="120">
## Table of Contents
1. [Download](#download)
2. [Benchmarks](#benchmarks)
3. [Prompt Format](#prompt-format)
4. [Dataset](#dataset)
5. [Acknowledgements](#acknowledgements)
6. [Contact](#contact)
7. [About DiscoResearch](#about-discoresearch)
8. [Disclaimer](#disclaimer)
## Download
| Huggingface | GPTQ | GGUF | AWQ | *Base Model* |
|-------|-------|-------|-------|-------|
| [Link](https://huggingface.co/DiscoResearch/DiscoLM-120b) | [Link](https://huggingface.co/TheBloke/DiscoLM-120b-GPTQ) | [Link](https://huggingface.co/TheBloke/DiscoLM-120b-GGUF) | [Link](https://huggingface.co/TheBloke/DiscoLM-120b-AWQ) | [Goliath 120b](https://huggingface.co/alpindale/goliath-120b) |
## Benchmarks
### Hugginface Leaderboard
This models is still an early Alpha and we can't guarantee that there isn't any contamination.
However, the average of **73.198** would earn the #2 spot on the HF leaderboard at the time of writing and the highest score for a >70b model yet.
| Metric | Value |
|-----------------------|-------|
| ARC (25-shot) | 69.54 |
| HellaSwag (10-shot) | 86.49 |
| MMLU (5-shot) | 70.32 |
| TruthfulQA (0-shot) | 61.42 |
| Winogrande (5-shot) | 83.03 |
| GSM8k (5-shot) | 68.39 |
| **Avg.** | **73.198** |
We use [Language Model Evaluation Harness](https://github.com/EleutherAI/lm-evaluation-harness) to run the benchmark tests above, using the same version as the HuggingFace LLM Leaderboard.
### FastEval
| Metric | Value |
|-----------------------|-------|
| GSM8K | 81.2 |
| Math | 22.3 |
| BBH | 72.9 |
| MMLU | 67.9 |
| **Avg.** | **53.3** |
This places DiscoLM 120b firmly ahead of gpt-3.5-turbo-0613 as seen on the screenshot of the current (sadly no longer maintained) FastEval CoT leaderboard:

### MTBench
```json
{
"first_turn": 8.45,
"second_turn": 7.45,
"categories": {
"writing": 9.4,
"roleplay": 8.65,
"reasoning": 6.85,
"math": 5.55,
"coding": 4.95,
"extraction": 9.15,
"stem": 9.225,
"humanities": 9.825
},
"average": 7.95
}
```
Screenshot of the current FastEval MT Bench leaderboard:

## Prompt Format
This model follows the ChatML format:
```
<|im_start|>system
You are DiscoLM, a helpful assistant.
<|im_end|>
<|im_start|>user
Please tell me possible reasons to call a research collective "Disco Research"<|im_end|>
<|im_start|>assistant
```
This formatting is also available via a pre-defined Transformers chat template, which means that lists of messages can be formatted for you with the apply_chat_template() method:
```python
chat = [
{"role": "system", "content": "You are DiscoLM, a helpful assistant."},
{"role": "user", "content": "Please tell me possible reasons to call a research collective Disco Research"}
]
tokenizer.apply_chat_template(chat, tokenize=False, add_generation_prompt=True)
```
If you use `tokenize=True` and `return_tensors="pt"` instead, then you will get a tokenized and formatted conversation ready to pass to `model.generate()`.
## Dataset
The dataset curation for DiscoLM 120b followed a "brute force"/"PoC" approach, as one goal was to see whether a 120b model can "absorb" more instruction data than a 70b model.
The following datasets were used for training DiscoLM 120b:
* [SlimOrca-Dedup](https://huggingface.co/datasets/Open-Orca/SlimOrca-Dedup)
* [OpenSchnabeltier](https://huggingface.co/datasets/LeoLM/OpenSchnabeltier) translated to DE from [OpenPlatypus](https://huggingface.co/datasets/garage-bAInd/Open-Platypus)
* [OpenHermes](https://huggingface.co/datasets/teknium/openhermes)
* [MetaMathQA](https://huggingface.co/datasets/meta-math/MetaMathQA)
* [UltraChat DE](https://huggingface.co/datasets/bjoernp/ultrachat_de) translated to DE from [UltraChat](https://huggingface.co/datasets/HuggingFaceH4/ultrachat_200k)
* [Synthia v.1.3](https://huggingface.co/datasets/migtissera/Synthia-v1.3)
* [German_Songs](https://huggingface.co/datasets/LeoLM/German_Songs)
* [German_Poems](https://huggingface.co/datasets/LeoLM/German_Poems)
* Capybara Dataset by [LDJnr](https://huggingface.co/LDJnr)
* Vezora/Tested-188k-Python (No longer available? Version changed to [Vezora/Tested-22k-Python-Alpaca](https://huggingface.co/datasets/Vezora/Tested-22k-Python-Alpaca))
Many thanks for all dataset providers/curators!
## Contact
Best way to reach us is on our [Discord](https://discord.gg/S8W8B5nz3v).
## About DiscoResearch
DiscoResearch is an aspiring open research community. Disco should be a place where researchers from many communities can come together to combine their expertise and create innovative and groundbreaking LLMs. Come join our Discord, share your opinions and ideas, and advance open LLM research with us!
## Acknowledgements
Disco 120b is a [DiscoResearch](https://huggingface.co/DiscoResearch) project and was trained by [Björn Plüster](https://huggingface.co/bjoernp). [Jan Harries](https://huggingface.co/jphme) helped with technical adivce, logistics and the Model Card and [AutoMeta](https://huggingface.co/Alignment-Lab-AI) also provided helpful technical adivce.
The model was trained with compute provided by [HessianAI](https://hessian.ai/) in collaboration with [LAION](https://laion.ai) - many thanks in particular to [Patrick Schramowski](https://huggingface.co/PSaiml) for his support.
We are standing on the shoulders of giants; many thanks in no particular order to [LAION](https://laion.ai) and especially to [Christoph Schuhmann](https://laion.ai) who got us all connected,
[alpindale](https://huggingface.co/alpindale) for Goliath 120b (with important contributions by [Charles Goddard](https://huggingface.co/chargoddard) and [Undi95](https://huggingface.co/Undi95)), [TheBloke](https://huggingface.co/TheBloke) for providing quantized versions, [winglian](https://huggingface.co/winglian) for Axolotl which was used to train the model and the SlimOrca dataset, [garage-bAInd](https://huggingface.co/garage-bAInd), [Teknium](https://huggingface.co/teknium), [Migel Tissera](https://huggingface.co/migtissera), [MetaMath](https://huggingface.co/meta-math), and [LDJnr](https://huggingface.co/LDJnr) for their great datasets (please contact us if we forgot to mention you here!).
[<img src="https://raw.githubusercontent.com/OpenAccess-AI-Collective/axolotl/main/image/axolotl-badge-web.png" alt="Built with Axolotl" width="200" height="32"/>](https://github.com/OpenAccess-AI-Collective/axolotl)
## Disclaimer
The license on this model does not constitute legal advice. We are not responsible for the actions of third parties who use this model.
This model should only be used for research purposes. The original Llama2 license and all restrictions of datasets used to train this model apply.
|
NeelNanda/GELU_1L512W_C4_Code | NeelNanda | "2024-04-23T12:07:10Z" | 2,150 | 2 | transformers | [
"transformers",
"license:mit",
"endpoints_compatible",
"region:us"
] | null | "2022-10-23T13:35:28Z" | ---
license: mit
---
This model is released under the MIT license. |
jxm/gtr__nq__32 | jxm | "2023-11-04T17:01:19Z" | 2,150 | 0 | transformers | [
"transformers",
"pytorch",
"endpoints_compatible",
"region:us"
] | null | "2023-11-04T17:00:58Z" | Entry not found |
elinas/Llama-3-15B-Instruct-zeroed | elinas | "2024-05-15T23:28:20Z" | 2,150 | 1 | transformers | [
"transformers",
"safetensors",
"llama",
"text-generation",
"mergekit",
"merge",
"conversational",
"base_model:meta-llama/Meta-Llama-3-8B-Instruct",
"license:llama3",
"autotrain_compatible",
"endpoints_compatible",
"text-generation-inference",
"region:us"
] | text-generation | "2024-05-15T23:07:36Z" | ---
base_model:
- meta-llama/Meta-Llama-3-8B-Instruct
library_name: transformers
tags:
- mergekit
- merge
license: llama3
---
# Llama-3-15B-Instruct-zeroed
This is a merge of pre-trained language models created using [mergekit](https://github.com/cg123/mergekit).
## Merge Details
### Merge Method
This model was merged using the passthrough merge method while zeroing `o_proj` and `down_proj` which led to an decrease in perplexity (good)
compared to similar 15B merges. This was a recommendation from [Charles Goddard](https://huggingface.co/chargoddard) - thank you for sharing the method of merging as well as Toasty
Pigeon for bringing it to my attention!
## Finetuned Version
A finetuned version of this model can be found at [elinas/Llama-3-15B-Instruct-zeroed-ft](https://huggingface.co/elinas/Llama-3-15B-Instruct-zeroed-ft) which seems to improve performance.
### Models Merged
The following models were included in the merge:
* [meta-llama/Meta-Llama-3-8B-Instruct](https://huggingface.co/meta-llama/Meta-Llama-3-8B-Instruct)
### Configuration
The following YAML configuration was used to produce this model:
```yaml
dtype: bfloat16
merge_method: passthrough
slices:
- sources:
- layer_range: [0, 24]
model: meta-llama/Meta-Llama-3-8B-Instruct
- sources:
- layer_range: [8, 24]
model: meta-llama/Meta-Llama-3-8B-Instruct
parameters:
scale:
- filter: o_proj
value: 0.0
- filter: down_proj
value: 0.0
- value: 1.0
- sources:
- layer_range: [8, 24]
model: meta-llama/Meta-Llama-3-8B-Instruct
parameters:
scale:
- filter: o_proj
value: 0.0
- filter: down_proj
value: 0.0
- value: 1.0
- sources:
- layer_range: [24, 32]
model: meta-llama/Meta-Llama-3-8B-Instruct
``` |
mradermacher/Llama-3-8B-Sydney-GGUF | mradermacher | "2024-06-11T09:50:07Z" | 2,150 | 1 | transformers | [
"transformers",
"gguf",
"llm",
"llama",
"llama3",
"en",
"base_model:FPHam/Llama-3-8B-Sydney",
"endpoints_compatible",
"region:us"
] | null | "2024-06-11T08:57:40Z" | ---
base_model: FPHam/Llama-3-8B-Sydney
language:
- en
library_name: transformers
quantized_by: mradermacher
tags:
- llm
- llama
- llama3
---
## About
<!-- ### quantize_version: 2 -->
<!-- ### output_tensor_quantised: 1 -->
<!-- ### convert_type: hf -->
<!-- ### vocab_type: -->
<!-- ### tags: -->
static quants of https://huggingface.co/FPHam/Llama-3-8B-Sydney
<!-- provided-files -->
weighted/imatrix quants are available at https://huggingface.co/mradermacher/Llama-3-8B-Sydney-i1-GGUF
## Usage
If you are unsure how to use GGUF files, refer to one of [TheBloke's
READMEs](https://huggingface.co/TheBloke/KafkaLM-70B-German-V0.1-GGUF) for
more details, including on how to concatenate multi-part files.
## Provided Quants
(sorted by size, not necessarily quality. IQ-quants are often preferable over similar sized non-IQ quants)
| Link | Type | Size/GB | Notes |
|:-----|:-----|--------:|:------|
| [GGUF](https://huggingface.co/mradermacher/Llama-3-8B-Sydney-GGUF/resolve/main/Llama-3-8B-Sydney.Q2_K.gguf) | Q2_K | 3.3 | |
| [GGUF](https://huggingface.co/mradermacher/Llama-3-8B-Sydney-GGUF/resolve/main/Llama-3-8B-Sydney.IQ3_XS.gguf) | IQ3_XS | 3.6 | |
| [GGUF](https://huggingface.co/mradermacher/Llama-3-8B-Sydney-GGUF/resolve/main/Llama-3-8B-Sydney.Q3_K_S.gguf) | Q3_K_S | 3.8 | |
| [GGUF](https://huggingface.co/mradermacher/Llama-3-8B-Sydney-GGUF/resolve/main/Llama-3-8B-Sydney.IQ3_S.gguf) | IQ3_S | 3.8 | beats Q3_K* |
| [GGUF](https://huggingface.co/mradermacher/Llama-3-8B-Sydney-GGUF/resolve/main/Llama-3-8B-Sydney.IQ3_M.gguf) | IQ3_M | 3.9 | |
| [GGUF](https://huggingface.co/mradermacher/Llama-3-8B-Sydney-GGUF/resolve/main/Llama-3-8B-Sydney.Q3_K_M.gguf) | Q3_K_M | 4.1 | lower quality |
| [GGUF](https://huggingface.co/mradermacher/Llama-3-8B-Sydney-GGUF/resolve/main/Llama-3-8B-Sydney.Q3_K_L.gguf) | Q3_K_L | 4.4 | |
| [GGUF](https://huggingface.co/mradermacher/Llama-3-8B-Sydney-GGUF/resolve/main/Llama-3-8B-Sydney.IQ4_XS.gguf) | IQ4_XS | 4.6 | |
| [GGUF](https://huggingface.co/mradermacher/Llama-3-8B-Sydney-GGUF/resolve/main/Llama-3-8B-Sydney.Q4_K_S.gguf) | Q4_K_S | 4.8 | fast, recommended |
| [GGUF](https://huggingface.co/mradermacher/Llama-3-8B-Sydney-GGUF/resolve/main/Llama-3-8B-Sydney.Q4_K_M.gguf) | Q4_K_M | 5.0 | fast, recommended |
| [GGUF](https://huggingface.co/mradermacher/Llama-3-8B-Sydney-GGUF/resolve/main/Llama-3-8B-Sydney.Q5_K_S.gguf) | Q5_K_S | 5.7 | |
| [GGUF](https://huggingface.co/mradermacher/Llama-3-8B-Sydney-GGUF/resolve/main/Llama-3-8B-Sydney.Q5_K_M.gguf) | Q5_K_M | 5.8 | |
| [GGUF](https://huggingface.co/mradermacher/Llama-3-8B-Sydney-GGUF/resolve/main/Llama-3-8B-Sydney.Q6_K.gguf) | Q6_K | 6.7 | very good quality |
| [GGUF](https://huggingface.co/mradermacher/Llama-3-8B-Sydney-GGUF/resolve/main/Llama-3-8B-Sydney.Q8_0.gguf) | Q8_0 | 8.6 | fast, best quality |
| [GGUF](https://huggingface.co/mradermacher/Llama-3-8B-Sydney-GGUF/resolve/main/Llama-3-8B-Sydney.f16.gguf) | f16 | 16.2 | 16 bpw, overkill |
Here is a handy graph by ikawrakow comparing some lower-quality quant
types (lower is better):

And here are Artefact2's thoughts on the matter:
https://gist.github.com/Artefact2/b5f810600771265fc1e39442288e8ec9
## FAQ / Model Request
See https://huggingface.co/mradermacher/model_requests for some answers to
questions you might have and/or if you want some other model quantized.
## Thanks
I thank my company, [nethype GmbH](https://www.nethype.de/), for letting
me use its servers and providing upgrades to my workstation to enable
this work in my free time.
<!-- end -->
|
digiplay/PotoPhotoRealism_v1 | digiplay | "2023-07-28T09:18:04Z" | 2,149 | 7 | diffusers | [
"diffusers",
"safetensors",
"stable-diffusion",
"stable-diffusion-diffusers",
"text-to-image",
"license:other",
"autotrain_compatible",
"endpoints_compatible",
"diffusers:StableDiffusionPipeline",
"region:us"
] | text-to-image | "2023-07-28T08:59:23Z" | ---
license: other
tags:
- stable-diffusion
- stable-diffusion-diffusers
- text-to-image
- diffusers
inference: true
---
Model info :
https://civitai.com/models/117538/poto-photo-realism
Original Author's DEMO images :
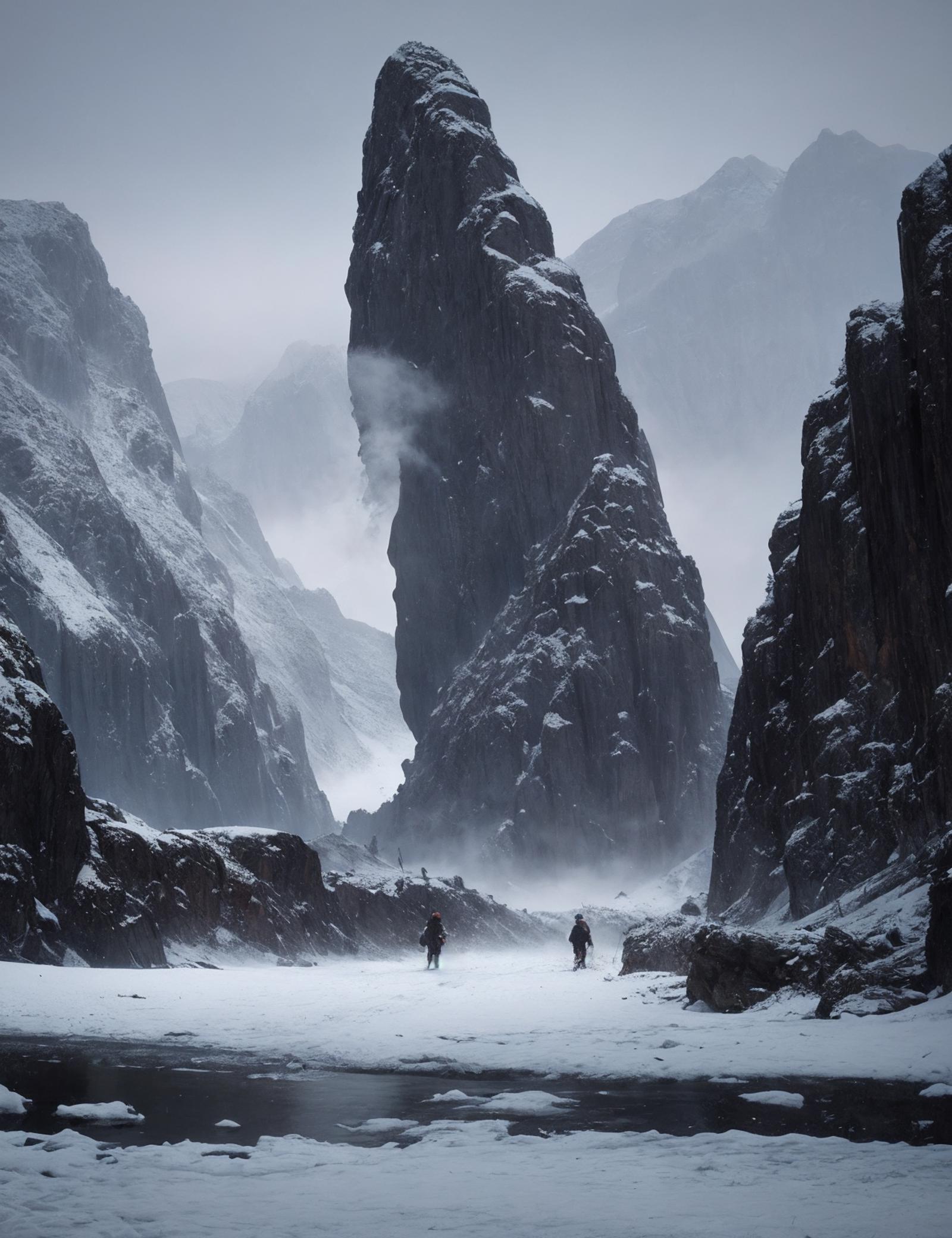
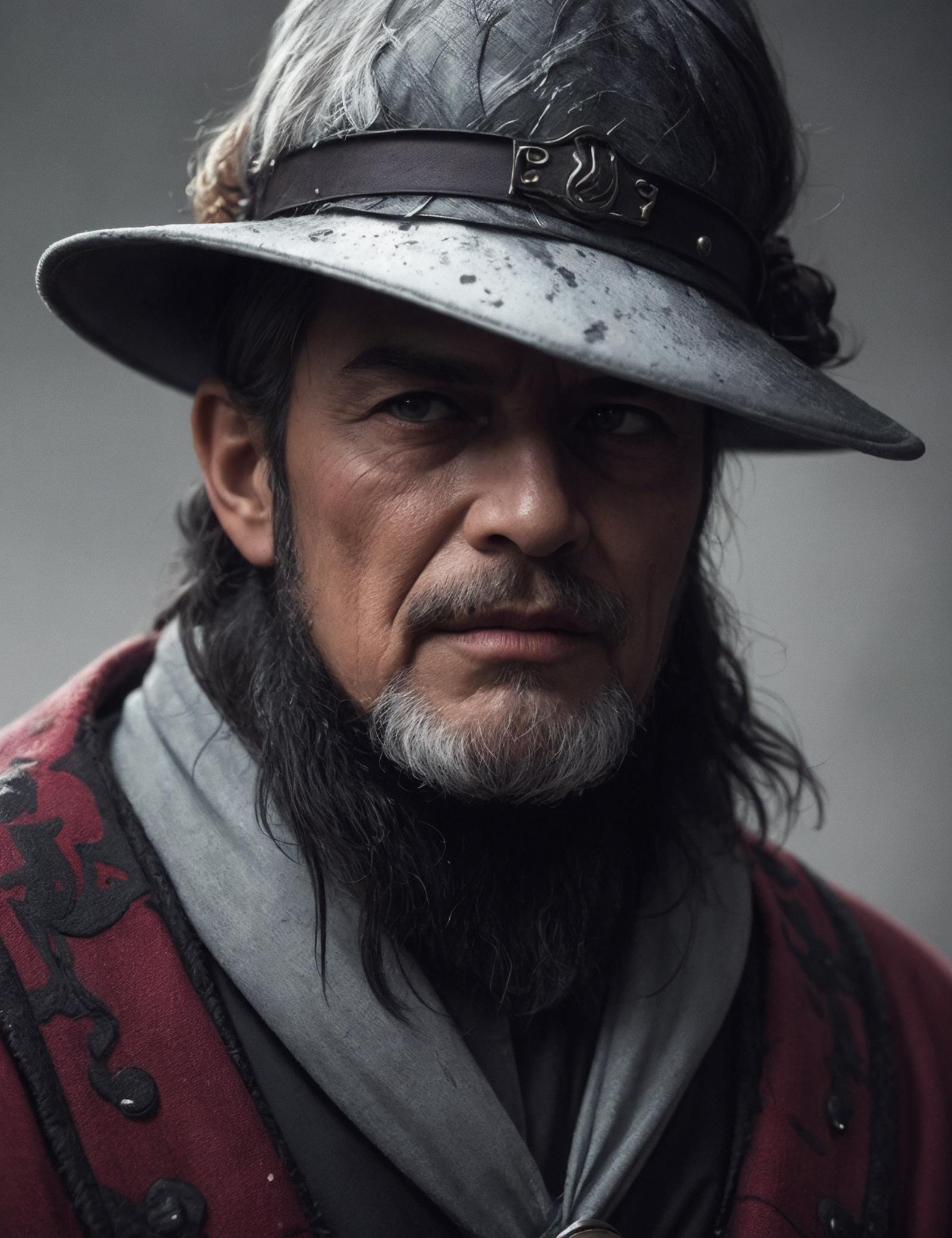
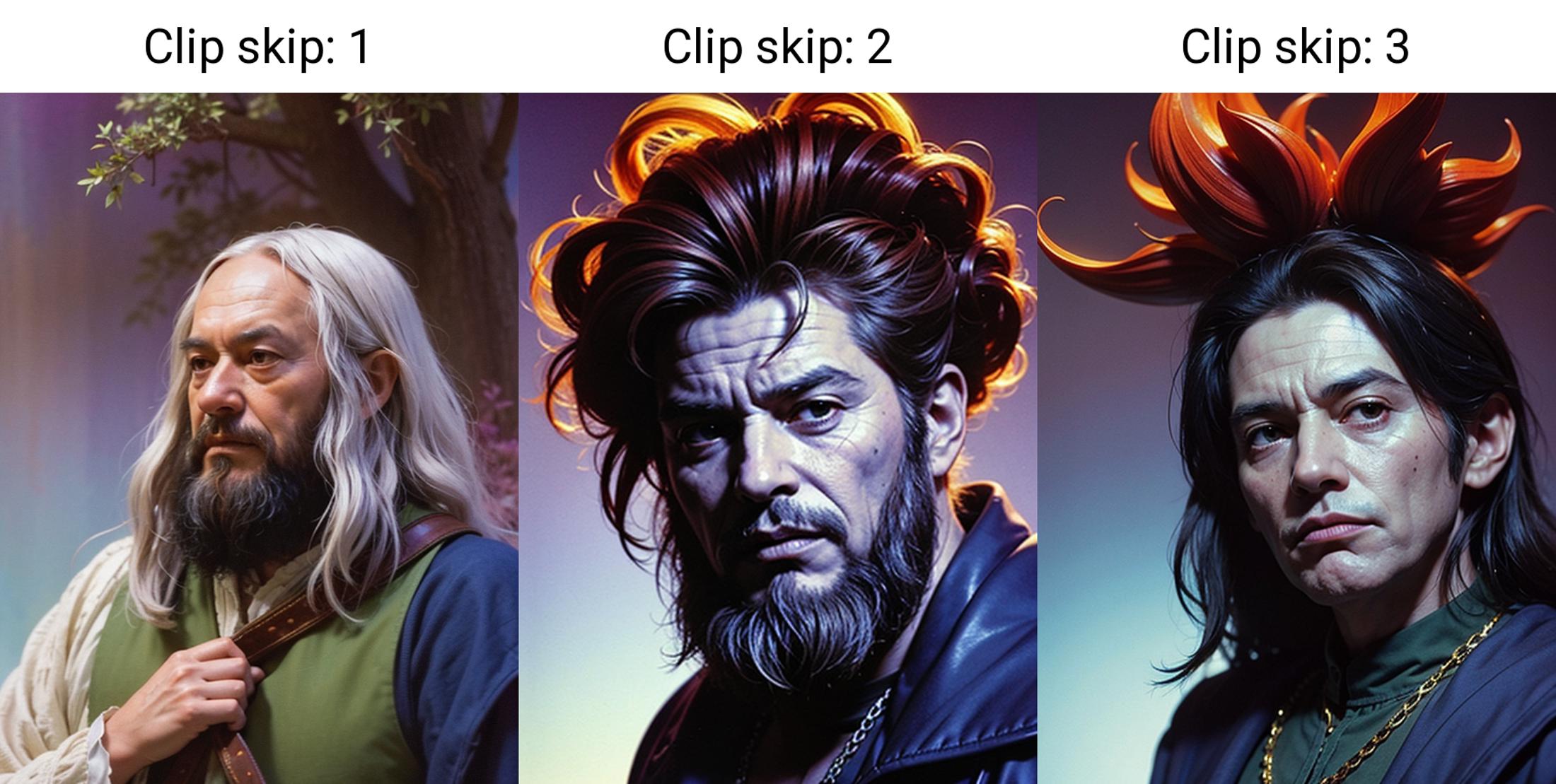
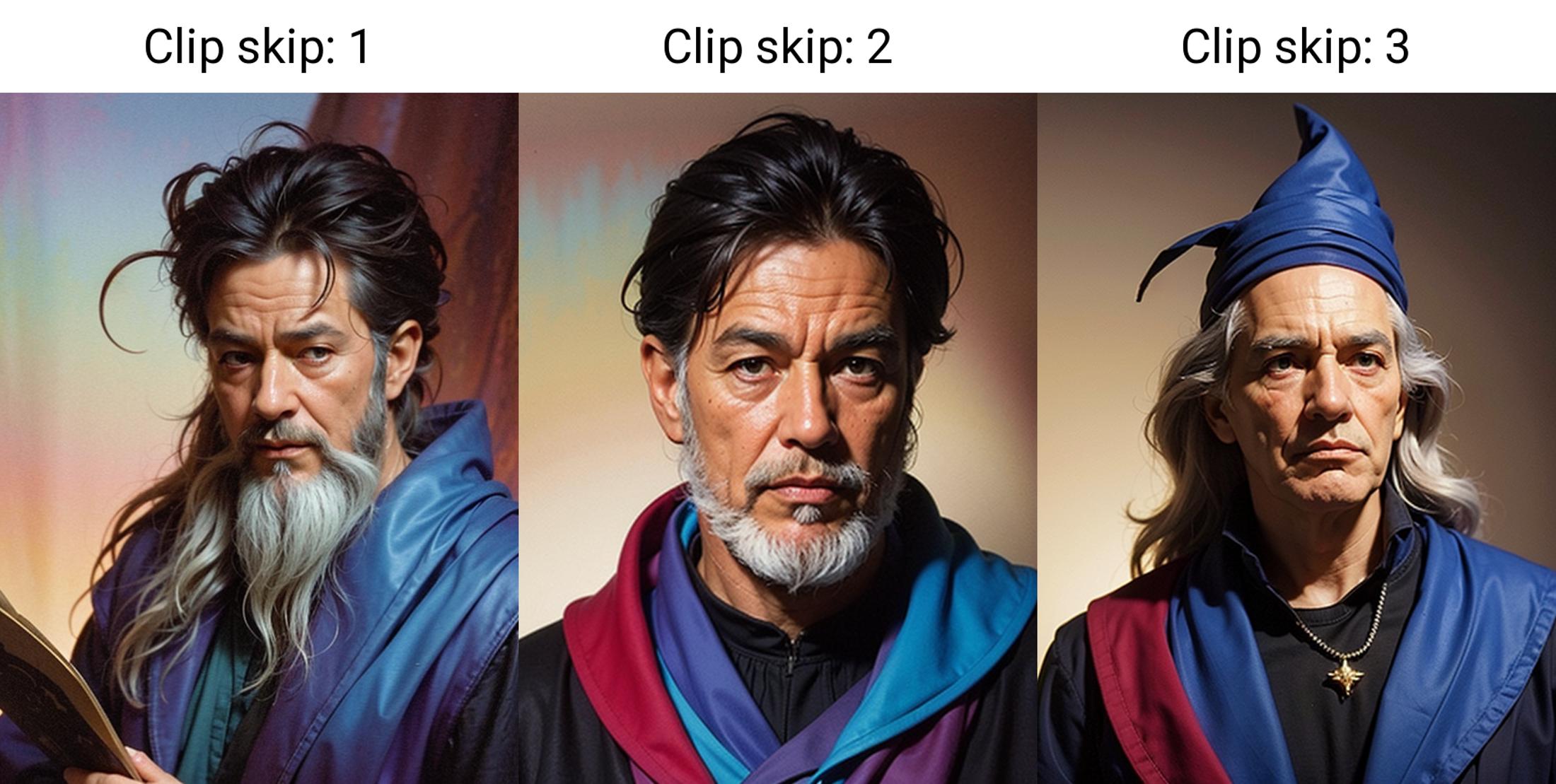
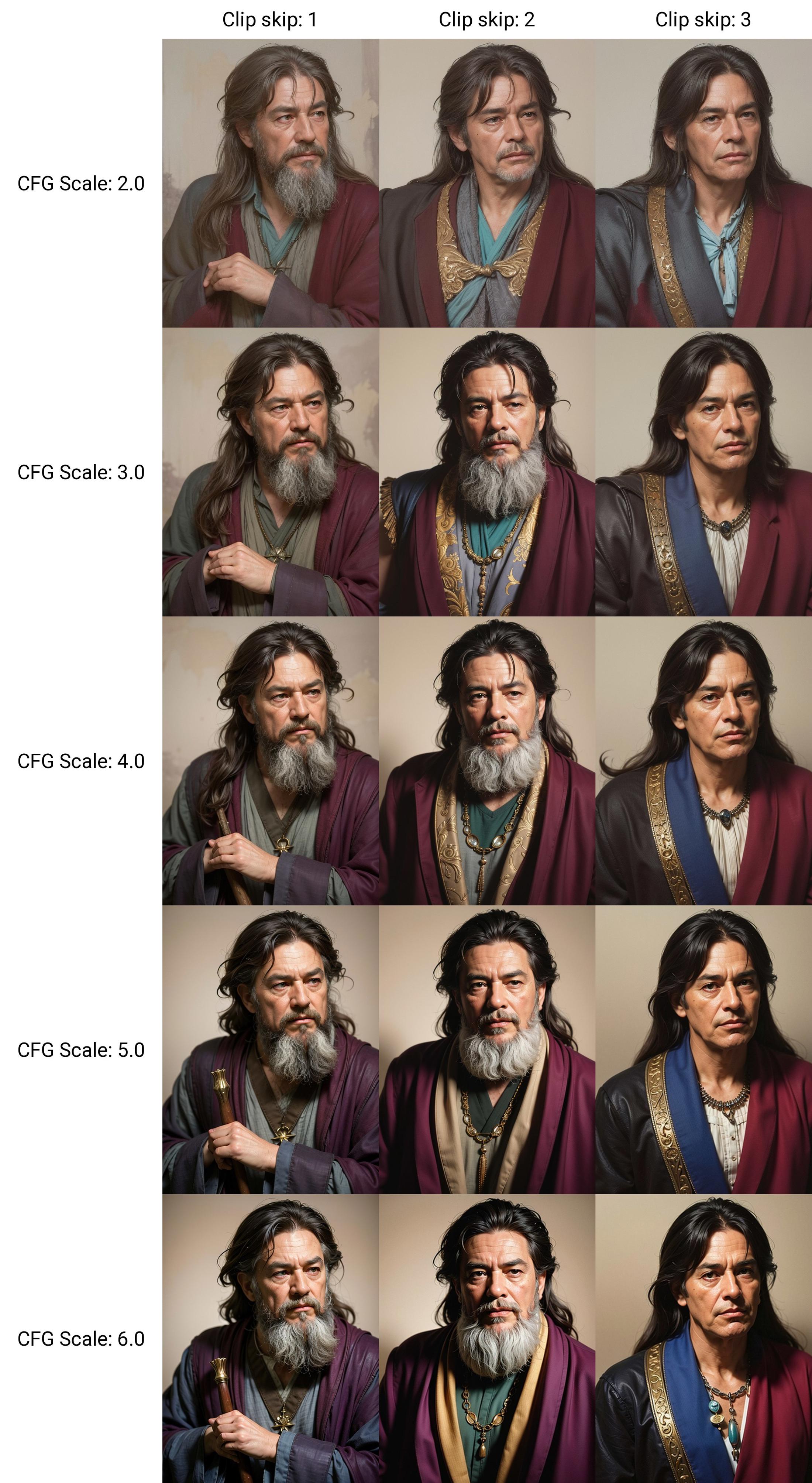
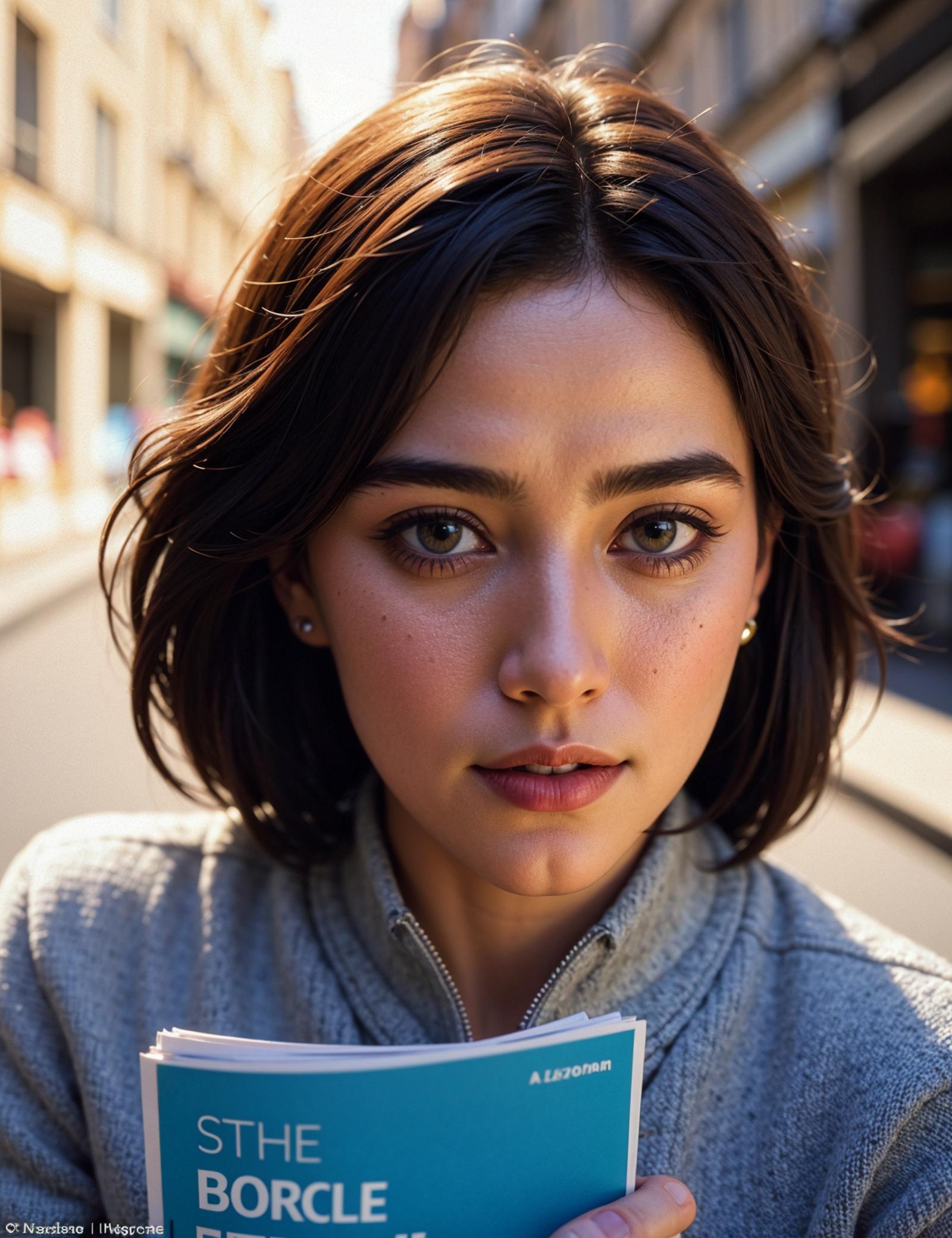
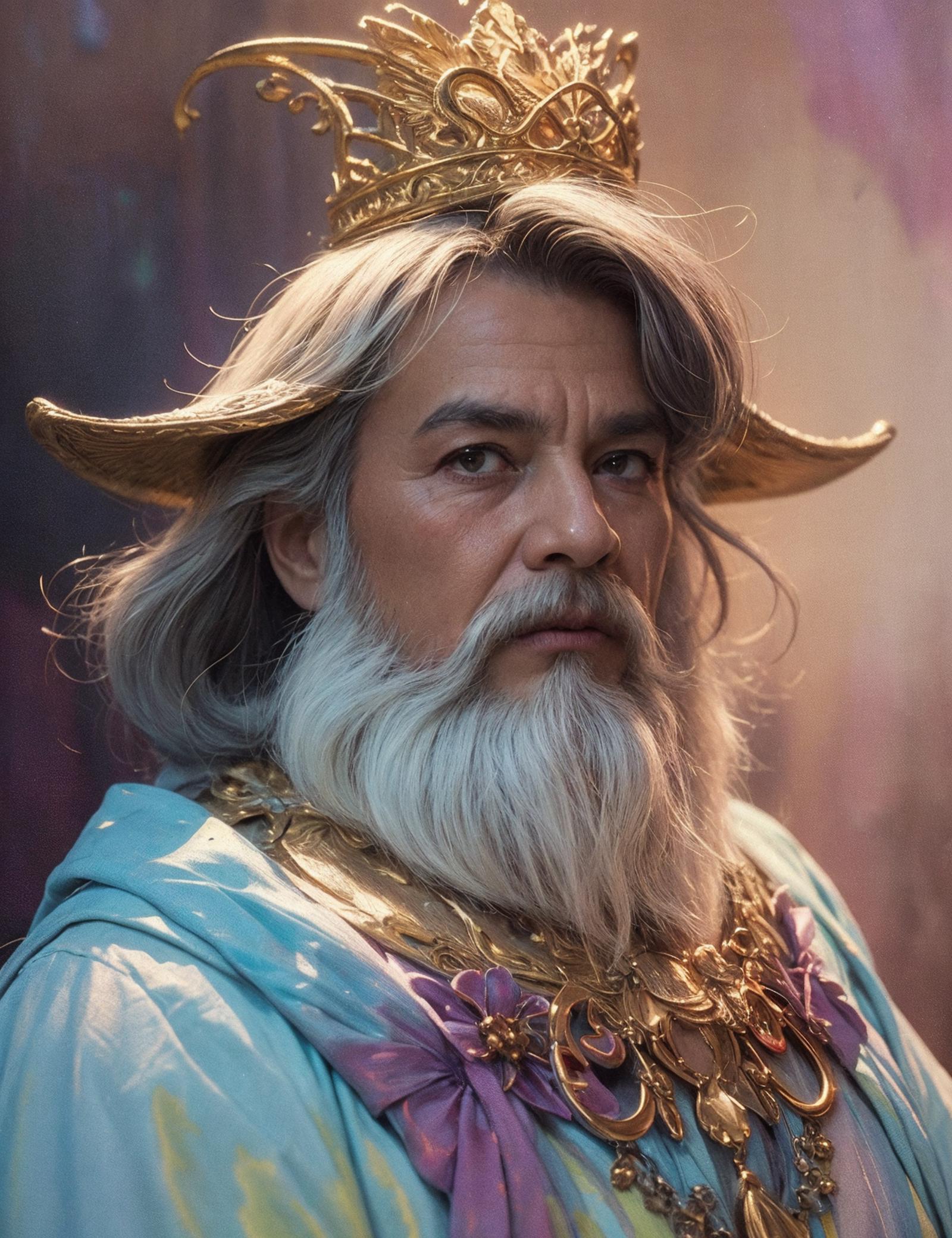
|
VAGOsolutions/SauerkrautLM-Qwen-32b | VAGOsolutions | "2024-04-14T17:21:05Z" | 2,149 | 4 | transformers | [
"transformers",
"safetensors",
"qwen2",
"text-generation",
"sft",
"dpo",
"conversational",
"de",
"en",
"license:other",
"autotrain_compatible",
"endpoints_compatible",
"text-generation-inference",
"region:us"
] | text-generation | "2024-04-12T16:33:53Z" | ---
license: other
license_name: tongyi-qianwen-research
license_link: >-
https://huggingface.co/Qwen/Qwen1.5-32B/blob/main/LICENSE
language:
- de
- en
tags:
- sft
- dpo
---

## VAGO solutions SauerkrautLM-Qwen-32b
Introducing **SauerkrautLM-Qwen-32b** – our Sauerkraut version of the powerful [Qwen/Qwen1.5-32B](https://huggingface.co/Qwen/Qwen1.5-32B)!
The model **SauerkrautLM-Qwen-32b** is a **joint effort** between **VAGO solutions** and **Hyperspace.ai.**
- Finetuned with **SFT**
- Aligned with **DPO**
# Table of Contents
1. [Overview of all SauerkrautLM-Qwen-32b](#all-SauerkrautLM-Qwen-32b)
2. [Model Details](#model-details)
- [Prompt template](#prompt-template)
- [Training procedure](#proceed-of-the-training)
3. [Evaluation](#evaluation)
5. [Disclaimer](#disclaimer)
6. [Contact](#contact)
7. [Collaborations](#collaborations)
8. [Acknowledgement](#acknowledgement)
## All SauerkrautLM-Qwen-32b
| Model | HF | EXL2 | GGUF | AWQ |
|-------|-------|-------|-------|-------|
| SauerkrautLM-Qwen-32b | [Link](https://huggingface.co/VAGOsolutions/SauerkrautLM-Qwen-32b) | coming soon | coming soon | coming soon |
## Model Details
**SauerkrautLM-Qwen-32b**
- **Model Type:** SauerkrautLM-Qwen-32b is a finetuned Model based on [Qwen/Qwen1.5-32B](https://huggingface.co/Qwen/Qwen1.5-32B)
- **Language(s):** German, English
- **License:** [tongyi-qianwen-research](https://huggingface.co/Qwen/Qwen1.5-32B/blob/main/LICENSEs)
- **Contact:** [VAGO solutions](https://vago-solutions.ai), [Hyperspace.ai](https://hyperspace.computer/)
### Training procedure:
- We trained this model for 2 epochs on 160k data samples with SFT.
- Afterwards we applied DPO for 1 epoch with 110k data.
- LaserRMT version coming soon
**We teached German language skills on this model.** As far as we know, it is the first Qwen 32B model with bilingual skills in German and English. Nevertheless, formulations may occur that are not entirely correct (still work in progress).
### Prompt Template:
**English:**
```
<|im_start|>system
You are SauerkrautLM, a helpful AI assistant.<|im_end|>
<|im_start|>user
{prompt}<|im_end|>
<|im_start|>assistant
```
**German:**
```
<|im_start|>system
Du bist SauerkrautLM, ein hilfreicher und freundlicher KI-Assistent.<|im_end|>
<|im_start|>user
{prompt}<|im_end|>
<|im_start|>assistant
```
### Example output of german language:
```
Die Karte zeigte eine verborgene Höhle, die in den umliegenden Bergen zu finden war. Mia war von der Idee fasziniert, diese Höhle zu entdecken und ihre Geheimnisse zu lüften. Sie nahm die Karte mit sich und machte sich auf den Weg, um die Höhle zu finden.
Die Wanderung zu den Bergen war eine Herausforderung, aber Mia war fest entschlossen, ihr Abenteuer zu vollenden. Sie überwand steinige Wege und überquerte klirrende Bäche, die ihre Füße kühlten und ihr die Energie für den Rest des Weges gab.
Endlich erreichte Mia die Höhle, die von einem dichten Wald umgeben war. Die Höhle war ein Ort der Geheimnisse und des Staunens, der ihr Herz höher schlagen ließ. Sie betrat die Höhle, und die Dunkelheit umhüllte sie wie ein Schleier aus Stille.
In der Höhle fand Mia eine alte Schatzkiste, die mit einem alten, verwitterten Holz verziert war. Mit zitternden Händen öffnete sie die Schatzkiste und fand darin eine alte, zerfledderte Schriftrolle. Die Schriftrolle war ein geheimnisvolles Artefakt, das ihr die Geschichte der Höhle offenbarte.
```
## Evaluation
**Open LLM Leaderboard:**
| Metric | Value |
|-----------------------|---------------------------|
| Avg. | **73.11** |
| ARC (25-shot) | 59.22 |
| HellaSwag (10-shot) | 82.32 |
| MMLU (5-shot) | 74.40|
| TruthfulQA (0-shot) | 61.03 |
| Winogrande (5-shot) | 82.16 |
| GSM8K (5-shot) | 79.53 |
## Disclaimer
We must inform users that despite our best efforts in data cleansing, the possibility of uncensored content slipping through cannot be entirely ruled out.
However, we cannot guarantee consistently appropriate behavior. Therefore, if you encounter any issues or come across inappropriate content, we kindly request that you inform us through the contact information provided.
Additionally, it is essential to understand that the licensing of these models does not constitute legal advice. We are not held responsible for the actions of third parties who utilize our models.
## Contact
If you are interested in customized LLMs for business applications, please get in contact with us via our websites. We are also grateful for your feedback and suggestions.
## Collaborations
We are also keenly seeking support and investment for our startups, VAGO solutions and Hyperspace where we continuously advance the development of robust language models designed to address a diverse range of purposes and requirements. If the prospect of collaboratively navigating future challenges excites you, we warmly invite you to reach out to us at [VAGO solutions](https://vago-solutions.de/#Kontakt), [Hyperspace.computer](https://hyperspace.computer/)
## Acknowledgement
Many thanks to [Qwen](https://huggingface.co/Qwen) for providing such valuable model to the Open-Source community |
timm/deit_tiny_distilled_patch16_224.fb_in1k | timm | "2024-02-10T23:37:26Z" | 2,148 | 0 | timm | [
"timm",
"pytorch",
"safetensors",
"image-classification",
"dataset:imagenet-1k",
"arxiv:2012.12877",
"license:apache-2.0",
"region:us"
] | image-classification | "2023-03-28T01:34:04Z" | ---
license: apache-2.0
library_name: timm
tags:
- image-classification
- timm
datasets:
- imagenet-1k
---
# Model card for deit_tiny_distilled_patch16_224.fb_in1k
A DeiT image classification model. Trained on ImageNet-1k using distillation tokens by paper authors.
## Model Details
- **Model Type:** Image classification / feature backbone
- **Model Stats:**
- Params (M): 5.9
- GMACs: 1.3
- Activations (M): 6.0
- Image size: 224 x 224
- **Papers:**
- Training data-efficient image transformers & distillation through attention: https://arxiv.org/abs/2012.12877
- **Original:** https://github.com/facebookresearch/deit
- **Dataset:** ImageNet-1k
## Model Usage
### Image Classification
```python
from urllib.request import urlopen
from PIL import Image
import timm
img = Image.open(urlopen(
'https://huggingface.co/datasets/huggingface/documentation-images/resolve/main/beignets-task-guide.png'
))
model = timm.create_model('deit_tiny_distilled_patch16_224.fb_in1k', pretrained=True)
model = model.eval()
# get model specific transforms (normalization, resize)
data_config = timm.data.resolve_model_data_config(model)
transforms = timm.data.create_transform(**data_config, is_training=False)
output = model(transforms(img).unsqueeze(0)) # unsqueeze single image into batch of 1
top5_probabilities, top5_class_indices = torch.topk(output.softmax(dim=1) * 100, k=5)
```
### Image Embeddings
```python
from urllib.request import urlopen
from PIL import Image
import timm
img = Image.open(urlopen(
'https://huggingface.co/datasets/huggingface/documentation-images/resolve/main/beignets-task-guide.png'
))
model = timm.create_model(
'deit_tiny_distilled_patch16_224.fb_in1k',
pretrained=True,
num_classes=0, # remove classifier nn.Linear
)
model = model.eval()
# get model specific transforms (normalization, resize)
data_config = timm.data.resolve_model_data_config(model)
transforms = timm.data.create_transform(**data_config, is_training=False)
output = model(transforms(img).unsqueeze(0)) # output is (batch_size, num_features) shaped tensor
# or equivalently (without needing to set num_classes=0)
output = model.forward_features(transforms(img).unsqueeze(0))
# output is unpooled, a (1, 198, 192) shaped tensor
output = model.forward_head(output, pre_logits=True)
# output is a (1, num_features) shaped tensor
```
## Model Comparison
Explore the dataset and runtime metrics of this model in timm [model results](https://github.com/huggingface/pytorch-image-models/tree/main/results).
## Citation
```bibtex
@InProceedings{pmlr-v139-touvron21a,
title = {Training data-efficient image transformers & distillation through attention},
author = {Touvron, Hugo and Cord, Matthieu and Douze, Matthijs and Massa, Francisco and Sablayrolles, Alexandre and Jegou, Herve},
booktitle = {International Conference on Machine Learning},
pages = {10347--10357},
year = {2021},
volume = {139},
month = {July}
}
```
```bibtex
@misc{rw2019timm,
author = {Ross Wightman},
title = {PyTorch Image Models},
year = {2019},
publisher = {GitHub},
journal = {GitHub repository},
doi = {10.5281/zenodo.4414861},
howpublished = {\url{https://github.com/huggingface/pytorch-image-models}}
}
```
|
Qwen/Qwen2-57B-A14B-Instruct-GGUF | Qwen | "2024-06-17T16:31:06Z" | 2,148 | 6 | null | [
"gguf",
"instruct",
"chat",
"text-generation",
"en",
"license:apache-2.0",
"region:us"
] | text-generation | "2024-06-15T11:54:23Z" | ---
language:
- en
pipeline_tag: text-generation
tags:
- instruct
- chat
license: apache-2.0
---
# Qwen2-57B-A14B-Instruct-GGUF
## Introduction
Qwen2 is the new series of Qwen large language models. For Qwen2, we release a number of base language models and instruction-tuned language models ranging from 0.5 to 72 billion parameters, including a Mixture-of-Experts model (57B-A14B).
Compared with the state-of-the-art opensource language models, including the previous released Qwen1.5, Qwen2 has generally surpassed most opensource models and demonstrated competitiveness against proprietary models across a series of benchmarks targeting for language understanding, language generation, multilingual capability, coding, mathematics, reasoning, etc.
For more details, please refer to our [blog](https://qwenlm.github.io/blog/qwen2/), [GitHub](https://github.com/QwenLM/Qwen2), and [Documentation](https://qwen.readthedocs.io/en/latest/).
In this repo, we provide quantized models in the GGUF formats, including `q2_k`, `q3_k_m`, `q4_0`, `q4_k_m`, `q5_0`, `q5_k_m`, `q6_k` and `q8_0`.
This is the GGUF repo for Qwen2-57B-A14B-Instruct, which is an MoE model.
## Model Details
Qwen2 is a language model series including decoder language models of different model sizes. For each size, we release the base language model and the aligned chat model. It is based on the Transformer architecture with SwiGLU activation, attention QKV bias, group query attention, etc. Additionally, we have an improved tokenizer adaptive to multiple natural languages and codes.
## Training details
We pretrained the models with a large amount of data, and we post-trained the models with both supervised finetuning and direct preference optimization.
## Requirements
We advise you to clone [`llama.cpp`](https://github.com/ggerganov/llama.cpp) and install it following the official guide. We follow the latest version of llama.cpp.
In the following demonstration, we assume that you are running commands under the repository `llama.cpp`.
## How to use
Cloning the repo may be inefficient, and thus you can manually download the GGUF file that you need or use `huggingface-cli` (`pip install huggingface_hub`) as shown below:
```shell
huggingface-cli download Qwen/Qwen2-57B-A14B-Instruct-GGUF qwen2-57b-a14b-instruct-q4_0.gguf --local-dir . --local-dir-use-symlinks False
```
However, for large files, we split them into multiple segments due to the limitation of 50G for a single file to be uploaded.
Specifically, for the split files, they share a prefix, with a suffix indicating its index. For examples, the `q8_0` GGUF files are:
```
qwen2-57b-a14b-instruct-q8_0-00001-of-00002.gguf
qwen2-57b-a14b-instruct-q8_0-00002-of-00002.gguf
```
They share the prefix of `qwen2-57b-a14b-instruct-q5_k_m`, but have their own suffix for indexing respectively, say `-00001-of-00002`.
To use the split GGUF files, you need to merge them first with the command `llama-gguf-split` as shown below:
```bash
./llama-gguf-split --merge qwen2-57b-a14b-instruct-q8_0-00001-of-00002.gguf qwen2-57b-a14b-instruct-q8_0.gguf
```
With the upgrade of APIs of llama.cpp, `llama-gguf-split` is equivalent to the previous `gguf-split`.
For the arguments of this command, the first is the path to the first split GGUF file, and the second is the path to the output GGUF file.
To run Qwen2, you can use `llama-cli` (the previous `main`) or `llama-server` (the previous `server`).
We recommend using the `llama-server` as it is simple and compatible with OpenAI API. For example:
```bash
./llama-server -m qwen2-57b-a14b-instruct-q5_0.gguf -ngl 28 -fa
```
(Note: `-ngl 28` refers to offloading 28 layers to GPUs, and `-fa` refers to the use of flash attention.)
Then it is easy to access the deployed service with OpenAI API:
```python
import openai
client = openai.OpenAI(
base_url="http://localhost:8080/v1", # "http://<Your api-server IP>:port"
api_key = "sk-no-key-required"
)
completion = client.chat.completions.create(
model="qwen",
messages=[
{"role": "system", "content": "You are a helpful assistant."},
{"role": "user", "content": "tell me something about michael jordan"}
]
)
print(completion.choices[0].message.content)
```
If you choose to use `llama-cli`, pay attention to the removal of `-cml` for the ChatML template. Instead you should use `--in-prefix` and `--in-suffix` to tackle this problem.
```bash
./llama-cli -m qwen2-57b-a14b-instruct-q5_0.gguf \
-n 512 -co -i -if -f prompts/chat-with-qwen.txt \
--in-prefix "<|im_start|>user\n" \
--in-suffix "<|im_end|>\n<|im_start|>assistant\n" \
-ngl 28 -fa
```
## Evaluation
We implement perplexity evaluation using wikitext following the practice of `llama.cpp` with `./llama-perplexity` (the previous `./perplexity`).
In the following we report the PPL of GGUF models of different sizes and different quantization levels.
|Size | fp16 | q8_0 | q6_k | q5_k_m | q5_0 | q4_k_m | q4_0 | q3_k_m | q2_k | iq1_m |
|--------|---------|---------|---------|---------|---------|---------|---------|---------|---------|---------|
|0.5B | 15.11 | 15.13 | 15.14 | 15.24 | 15.40 | 15.36 | 16.28 | 15.70 | 16.74 | - |
|1.5B | 10.43 | 10.43 | 10.45 | 10.50 | 10.56 | 10.61 | 10.79 | 11.08 | 13.04 | - |
|7B | 7.93 | 7.94 | 7.96 | 7.97 | 7.98 | 8.02 | 8.19 | 8.20 | 10.58 | - |
|57B-A14B| 6.81 | 6.81 | 6.83 | 6.84 | 6.89 | 6.99 | 7.02 | 7.43 | - | - |
|72B | 5.58 | 5.58 | 5.59 | 5.59 | 5.60 | 5.61 | 5.66 | 5.68 | 5.91 | 6.75 |
## Citation
If you find our work helpful, feel free to give us a cite.
```
@article{qwen2,
title={Qwen2 Technical Report},
year={2024}
}
``` |
KBNIT/KoSOLAR-10.7B-QLoRA-NEFTune-kolon-v0.1 | KBNIT | "2024-03-27T04:51:38Z" | 2,147 | 0 | transformers | [
"transformers",
"pytorch",
"llama",
"text-generation",
"conversational",
"ko",
"en",
"license:cc-by-nc-4.0",
"autotrain_compatible",
"endpoints_compatible",
"text-generation-inference",
"region:us"
] | text-generation | "2024-03-15T00:19:50Z" | ---
license: cc-by-nc-4.0
language:
- ko
- en
---
## Base Model:
We made a LLM model with yanolja/KoSOLAR-10.7B-v0.3
## Model Description
We use QLoRA(64, 16) and NEFTune on LLM Fine-tuning
lr = 2e-4
## Train Detail
Our Korean Wiki QA data used, and 3 epoch train
## Others
We are making LLM model for Kolon !
|
DavidAU/DarkSapling-V1.1-Ultra-Quality-7B-GGUF | DavidAU | "2024-06-11T01:04:16Z" | 2,147 | 1 | null | [
"gguf",
"creative",
"story",
"roleplay",
"rp",
"32 bit upscale",
"remastered",
"writing",
"en",
"license:apache-2.0",
"region:us"
] | null | "2024-06-03T22:46:32Z" | ---
license: apache-2.0
language:
- en
tags:
- creative
- story
- roleplay
- rp
- 32 bit upscale
- remastered
- writing
---
<h3><font color="red"> Dark Sapling V1.1 7B - 32k Context - Ultra Quality - 32 bit upscale.</font></h3>
<img src="dark-sapling.jpg" style="width:300px; height:300px; float:right; padding:10px;">
Complete remerge, and remaster of the incredible Dark Sapling V1.1 7B - 32k Context from source files.
Registering an impressive drop of 240 points (lower is better) at Q4KM.
This puts "Q4KM" operating at "Q6" levels, and further elevates Q6 and Q8 as well.
Likewise, even Q2K (smallest quant) will operate at much higher levels than it's original source counterpart.
<B>RESULTS:</b>
The result is superior performance in instruction following, reasoning, depth, nuance and emotion.
Reduction in prompt size, as it understands nuance better.
And as a side effect more context available for output due to reduction in prompt size.
Note that there will be an outsized difference between quants especially for creative and/or "no right answer" use cases.
Because of this it is suggested to download the highest quant you can operate, and it's closest neighbours so to speak.
IE: Q4KS, Q4KM, Q5KS as an example.
Imatrix Plus versions to be uploaded at a separate repo shortly.
Special thanks to "TEEZEE" the original model creator:
[ https://huggingface.co/TeeZee/DarkSapling-7B-v1.1 ]
NOTE: Version 1 and Version 2 are also remastered. |
matsuo-lab/weblab-10b | matsuo-lab | "2023-09-04T23:17:28Z" | 2,146 | 64 | transformers | [
"transformers",
"pytorch",
"gpt_neox",
"text-generation",
"license:cc-by-nc-4.0",
"autotrain_compatible",
"endpoints_compatible",
"text-generation-inference",
"region:us"
] | text-generation | "2023-08-04T04:55:47Z" | ---
license: cc-by-nc-4.0
---
# weblab-10b
# Overview
This repository provides a Japanese-centric multilingual GPT-NeoX model of 10 billion parameters.
* **Library**
The model was trained using code based on [EleutherAI/gpt-neox](https://github.com/EleutherAI/gpt-neox).
* **Model architecture**
A 36-layer, 4864-hidden-size transformer-based language model.
* **Pre-training**
The model was trained on around **600B** tokens from a mixture of the following corpora.
- [Japanese C4](https://huggingface.co/datasets/mc4)
- [The Pile](https://huggingface.co/datasets/EleutherAI/pile)
* **Model Series**
| Variant | Link |
| :-- | :--|
| weblab-10b-instruction-sft | https://huggingface.co/matsuo-lab/weblab-10b-instruction-sft |
| weblab-10b | https://huggingface.co/matsuo-lab/weblab-10b |
* **Authors**
Takeshi Kojima
---
# Benchmarking
* **Japanese benchmark : JGLUE 8-task (2023-08-27)**
- *We used [Stability-AI/lm-evaluation-harness](https://github.com/Stability-AI/lm-evaluation-harness/tree/2f1583c0735eacdfdfa5b7d656074b69577b6774) library for evaluation.*
- *The 8-task average accuracy is based on results of JCommonsenseQA-1.1, JNLI-1.1, MARC-ja-1.1, JSQuAD-1.1, jaqket_v2-0.2, xlsum_ja-1.0, xwinograd_ja, and mgsm-1.0.*
- *model loading is performed with float16, and evaluation is performed with template version 0.3 using the few-shot in-context learning.*
- *The number of few-shots is 3,3,3,2,1,1,0,5.*
- *special_tokens_map.json is modified to avoid errors during the evaluation of the second half benchmarks. As a result, the results of the first half benchmarks became slightly different.*
model | average | jcommonsenseqa | jnli | marc_ja | jsquad | jaqket_v2 | xlsum_ja | xwinograd_ja | mgsm
| :-- | :-- | :-- | :-- | :-- | :-- | :-- | :-- | :-- | :-- |
weblab-10b-instruction-sft | 59.11 | 74.62 | 66.56 | 95.49 | 78.34 | 63.32 | 20.57 | 71.95 | 2
weblab-10b | 50.74 | 66.58 | 53.74 | 82.07 | 62.94 | 56.19 | 10.03 | 71.95 | 2.4
* **Japanese benchmark : JGLUE 4-task (2023-08-18)**
- *We used [Stability-AI/lm-evaluation-harness](https://github.com/Stability-AI/lm-evaluation-harness/tree/2f1583c0735eacdfdfa5b7d656074b69577b6774) library for evaluation.*
- *The 4-task average accuracy is based on results of JCommonsenseQA-1.1, JNLI-1.1, MARC-ja-1.1, and JSQuAD-1.1.*
- *model loading is performed with float16, and evaluation is performed with template version 0.3 using the few-shot in-context learning.*
- *The number of few-shots is 3,3,3,2.*
| Model | Average | JCommonsenseQA | JNLI | MARC-ja | JSQuAD |
| :-- | :-- | :-- | :-- | :-- | :-- |
| weblab-10b-instruction-sft | 78.78 | 74.35 | 65.65 | 96.06 | 79.04 |
| weblab-10b | 66.38 | 65.86 | 54.19 | 84.49 | 60.98 |
---
# How to use the model
~~~~python
import torch
from transformers import AutoTokenizer, AutoModelForCausalLM
tokenizer = AutoTokenizer.from_pretrained("matsuo-lab/weblab-10b")
model = AutoModelForCausalLM.from_pretrained("matsuo-lab/weblab-10b", torch_dtype=torch.float16)
if torch.cuda.is_available():
model = model.to("cuda")
text = "吾輩は猫である。"
token_ids = tokenizer.encode(text, add_special_tokens=False, return_tensors="pt")
with torch.no_grad():
output_ids = model.generate(
token_ids.to(model.device),
max_new_tokens=100,
do_sample=True,
temperature=0.7,
top_p=0.95
)
output = tokenizer.decode(output_ids.tolist()[0])
print(output)
~~~~
---
# Licenese
[cc-by-nc-4.0](https://creativecommons.org/licenses/by-nc/4.0/) |
Falconsai/medical_summarization | Falconsai | "2024-01-20T12:48:04Z" | 2,146 | 87 | transformers | [
"transformers",
"pytorch",
"coreml",
"safetensors",
"t5",
"text2text-generation",
"medical",
"summarization",
"en",
"license:apache-2.0",
"autotrain_compatible",
"endpoints_compatible",
"text-generation-inference",
"region:us"
] | summarization | "2023-10-23T03:15:02Z" | ---
license: apache-2.0
language:
- en
pipeline_tag: summarization
widget:
- text: >-
the need for magnetic resonance imaging ( mri ) in patients with an
implanted pacemaker or implantable cardioverter - defibrillator ( icd ) is a
growing clinical issue . it is estimated that as many as 75% of active
cardiac device recipients will become indicated for mri . currently , the
vast majority of such devices are contraindicated for use with an mri . in
european heart rhythm association survey , published recently for non - mri
- certified icds ( 0.51.5 t field strength ) , the totally subcutaneous icd
( s - icd ) system , an implantable defibrillator with no leads that touch
the heart , has recently been demonstrated to be a safe and effective
defibrillator option for patients at risk for sudden cardiac death . it
provides shock therapy and post - shock pacing therapy , but no long - term
bradycardia pacing . although it has been shown as an alternative to the
standard transvenous icd , its compatibility with mri remains unclear .
various types of clinical mri systems currently use a superconductive magnet
that creates a static magnetic field strength , typically 1.5 or 3 t. the
use of mri with most pacemakers and icds is considered a contraindication
due to potential hazards , including heating of the electrode that resides
in or on the heart , damage to myocardium , elevation of pacing thresholds ,
unintended induction of ventricular tachycardia ( vt ) or ventricular
fibrillation ( vf ) , pacing inhibition , permanent device malfunction , and
distortion of the mri scan . recently , mr - conditional. mr - conditional
indicates a lack of known hazards in a specified mri environment with
specified conditions of use . due to the variety of mri scanners and
scanning protocols , it is not practical to test even a single device under
all conditions . hence , mr - conditional labelling dictates that the device
is safe for use under certain scanning conditions , as well as how the
cardiac device should be programmed before an exposure to the magnetic field
in a mri scanner . the literature , although limited , provides some
guidance for imaging patients with implanted pacemakers or icds that do not
have mr - conditional labelling . this single - centre prospective non -
controlled study describes the first use of mri in patients with an
implanted s - icd . patients with implanted s - icd systems ( boston
scientific sqrx model 1010 and q - trak model 3010 ) were enrolled for mri
testing over a period of 18 months . the s - icd system implanted in this
patient cohort was composed of a can implanted in a left mid - lateral
pocket and a para - sternal subcutaneous electrode . the s - icd is
currently not certified for use with an mri ; therefore , the ethics
committee of homolka hospital , prague , czech republic approved our
clinical study . patients with newly implanted s - icd systems ( < 6 weeks )
were excluded , and none of the patients had any intravascular leads . the
patients were randomized for either a cardiac , brain , cervical , or lumbar
spinal scan . one of the subjects underwent an additional knee examination ,
due to reported chronic pain . a total of 15 patients were enrolled into
this study ( 12 males and three females , aged 2283 years , mean 53 years .
subjects in our cohort ( table 1 ) underwent a total of 22 mri scans between
6 june 2012 and 24 december 2013 . in total , five brain scans , three
cardiac scans , 12 lumbar scans , one knee , and one cervical spine scan
were conducted ( table 2 ) . however , in one patient a minor disc
protrusion was found , in other mri revealed stenosis of intervertebral
foramen which was causing radicular pain of the nerve root l4 and based on
this examination the patient was referred to ct - navigated periradicular
therapy . table 1summary of patient anatomical data and scan locations ,
along with noted clinical eventsidagesexbmidgef , % indication for s -
icdheating0164f20.5hcmp / vfs85secondary preventionnone0283m30.0post - mi /
smvts post - catheter ablation/35secondary prevention ( post - transvenous
icd extraction)none0331m25.3arvc / d / smvts68secondary preventionin -
tolerable re - scanned0458m23.6post - mi / post - cabg30primary
preventionnone0577m25.5post - mi30primary preventionnone0663m27.0post -
mi30primary preventionnone0768m23.7post - mi / vfs / vts60secondary
prevention post - transvenous icd extraction / svc
occlusiontolerable0822m29.4brugada sy / vfs68secondary preventionin -
tolerable re - scanned0959m27.1dcmp / vfs / post - mitral valve
surgery/60secondary prev./post - transvenous icd extractionnone1041f24.6arvc
/ d70primary preventionnone1123f21.5lqts / vf60secondary
preventionnone1266m36.9post - mi / vf / post - cabg50secondary prevention /
post - repeat transvenous icd extractiontolerable1348m22.9dcmp(non -
compaction)/vfs35secondary preventionnone1470m29systolic dysfunction of
lv35primary preventionnone1526m33brugada sy65primary preventionnonehcmp ,
hypertrophic cardiomyopathy ; smvt , sustained monomorphic ventricular
tachycardia ; mi , myocardial infarction ; arvc , arrhythmogenic right
ventricular cardiomyopathy ; cabg , coronary artery by - pass graft ; lqts ,
long qt syndrom . table 2parmeters of s - icd and patient sensation during
individual mri scansscan # idbody partheating sensationsshock zone (
b.p.m.)condit . shock zone ( b.p.m.)bat % episode
num.101brainnone2302101001202brainnone240220861303l spinein -
tolerable240220831403brainnone240220831504brainnone220190691605l
spinenone220210541706l spinenone240220681807l spinetolerable240220582908l
spinein - tolerablenananana1008brainnonenananana1108l
spinenone2302108411209heartnone2402208911310l
spinenone2301807911410heartnonenananana1511heartnone2301909711612l
spinetolerable2001709721712l spinenone2001709421813c
spinenone23019010041913l spinenone23019010042014l
spinenone2301908612115kneenone25021010012215l spinenone2502101001s - icd
parameters acquired prior- and post - mri were without any change ,
therefore only one value is presented.indices : na , not available ; l spine
, lumbar spine ; c spine , cervical spine . summary of patient anatomical
data and scan locations , along with noted clinical events hcmp ,
hypertrophic cardiomyopathy ; smvt , sustained monomorphic ventricular
tachycardia ; mi , myocardial infarction ; arvc , arrhythmogenic right
ventricular cardiomyopathy ; cabg , coronary artery by - pass graft ; lqts ,
long qt syndrom . parmeters of s - icd and patient sensation during
individual mri scans s - icd parameters acquired prior- and post - mri were
without any change , therefore only one value is presented . indices : na ,
not available ; l spine , lumbar spine ; c spine , cervical spine . studies
were performed using a siemens avanto 1.5 t mri scanner ( vb17 software ,
quantum gradient coils ) . all scans were run in normal operating mode ,
which is limited to 2 w / kg whole body averaged specific absorption rate (
sar ) . clinically relevant mri sequences were used for evaluation ( see
table 3 ) . table 3types of pulse sequences typically used for imaging of
respective anatomical areasscan locationscan
sequencesflairdwiflashfsehastesestirtruefispbrainxxxxheartxxxxcervical
spinexxkneexxxxlumbar spinexxflair , fluid attenuated inversion recovery ;
dwi , diffusion weighted imaging ; flash , fast low angle shot ; fse , fast
spin echo ; haste , half acquisition single - shot turbo spin echo ; se ,
spin echo ; stir , short tau inversion recovery ; truefisp , true fast
imaging with steady - state precession.fse sequence caused heating in
subjects with a thermistor probe during lumbar spine examination ( see the
text for details ) . types of pulse sequences typically used for imaging of
respective anatomical areas flair , fluid attenuated inversion recovery ;
dwi , diffusion weighted imaging ; flash , fast low angle shot ; fse , fast
spin echo ; haste , half acquisition single - shot turbo spin echo ; se ,
spin echo ; stir , short tau inversion recovery ; truefisp , true fast
imaging with steady - state precession . fse sequence caused heating in
subjects with a thermistor probe during lumbar spine examination ( see the
text for details ) . patients were asked to report immediately any pain ,
torqueing movement , or heating sensation in the area of the pocket or the
electrode by pressing an emergency bulb . furthermore , all patients were
questioned immediately following the mri procedure to ascertain any
discomfort in the vicinity of the can or electrode . pulse oximetry and
standard lead electrocardiogram ( ecg ) if discomfort occurred , the patient
was asked if the scan could be repeated at a later time using a revised scan
sequence or the subject was again randomized for another anatomical area .
since none of the components of the s - icd system are on or in the heart ,
heating near or around however , heating near the electrode or can with the
s - icd system may still cause serious patient discomfort . therefore ,
along with education of subjects , each patient was instrumented by taping
an oesophageal temperature probe ( beta - therm model g22k7mcd8 ) on the
skin over the mid - lateral implant site to record any temperature
excursions that might be correlated to patient symptoms of heating /
discomfort near the pocket . to minimize the risk of inappropriate therapy ,
the s - icd system was programmed to therapy each s - icd system was
evaluated prior to and immediately after the scan to verify proper
functioning , including interrogation , sensing , and battery voltage .
after the completion of the mri , long - term regular clinical follow - up
and checking of the device were performed . patients with implanted s - icd
systems ( boston scientific sqrx model 1010 and q - trak model 3010 ) were
enrolled for mri testing over a period of 18 months . the s - icd system
implanted in this patient cohort was composed of a can implanted in a left
mid - lateral pocket and a para - sternal subcutaneous electrode . the s -
icd is currently not certified for use with an mri ; therefore , the ethics
committee of homolka hospital , prague , czech republic approved our
clinical study . patients with newly implanted s - icd systems ( < 6 weeks )
were excluded , and none of the patients had any intravascular leads . the
patients were randomized for either a cardiac , brain , cervical , or lumbar
spinal scan . one of the subjects underwent an additional knee examination ,
due to reported chronic pain . a total of 15 patients were enrolled into
this study ( 12 males and three females , aged 2283 years , mean 53 years .
subjects in our cohort ( table 1 ) underwent a total of 22 mri scans between
6 june 2012 and 24 december 2013 . in total , five brain scans , three
cardiac scans , 12 lumbar scans , one knee , and one cervical spine scan
were conducted ( table 2 ) . however , in one patient a minor disc
protrusion was found , in other mri revealed stenosis of intervertebral
foramen which was causing radicular pain of the nerve root l4 and based on
this examination the patient was referred to ct - navigated periradicular
therapy . table 1summary of patient anatomical data and scan locations ,
along with noted clinical eventsidagesexbmidgef , % indication for s -
icdheating0164f20.5hcmp / vfs85secondary preventionnone0283m30.0post - mi /
smvts post - catheter ablation/35secondary prevention ( post - transvenous
icd extraction)none0331m25.3arvc / d / smvts68secondary preventionin -
tolerable re - scanned0458m23.6post - mi / post - cabg30primary
preventionnone0577m25.5post - mi30primary preventionnone0663m27.0post -
mi30primary preventionnone0768m23.7post - mi / vfs / vts60secondary
prevention post - transvenous icd extraction / svc
occlusiontolerable0822m29.4brugada sy / vfs68secondary preventionin -
tolerable re - scanned0959m27.1dcmp / vfs / post - mitral valve
surgery/60secondary prev./post - transvenous icd extractionnone1041f24.6arvc
/ d70primary preventionnone1123f21.5lqts / vf60secondary
preventionnone1266m36.9post - mi / vf / post - cabg50secondary prevention /
post - repeat transvenous icd extractiontolerable1348m22.9dcmp(non -
compaction)/vfs35secondary preventionnone1470m29systolic dysfunction of
lv35primary preventionnone1526m33brugada sy65primary preventionnonehcmp ,
hypertrophic cardiomyopathy ; smvt , sustained monomorphic ventricular
tachycardia ; mi , myocardial infarction ; arvc , arrhythmogenic right
ventricular cardiomyopathy ; cabg , coronary artery by - pass graft ; lqts ,
long qt syndrom . table 2parmeters of s - icd and patient sensation during
individual mri scansscan # idbody partheating sensationsshock zone (
b.p.m.)condit . shock zone ( b.p.m.)bat % episode
num.101brainnone2302101001202brainnone240220861303l spinein -
tolerable240220831403brainnone240220831504brainnone220190691605l
spinenone220210541706l spinenone240220681807l spinetolerable240220582908l
spinein - tolerablenananana1008brainnonenananana1108l
spinenone2302108411209heartnone2402208911310l
spinenone2301807911410heartnonenananana1511heartnone2301909711612l
spinetolerable2001709721712l spinenone2001709421813c
spinenone23019010041913l spinenone23019010042014l
spinenone2301908612115kneenone25021010012215l spinenone2502101001s - icd
parameters acquired prior- and post - mri were without any change ,
therefore only one value is presented.indices : na , not available ; l spine
, lumbar spine ; c spine , cervical spine . summary of patient anatomical
data and scan locations , along with noted clinical events hcmp ,
hypertrophic cardiomyopathy ; smvt , sustained monomorphic ventricular
tachycardia ; mi , myocardial infarction ; arvc , arrhythmogenic right
ventricular cardiomyopathy ; cabg , coronary artery by - pass graft ; lqts ,
long qt syndrom . parmeters of s - icd and patient sensation during
individual mri scans s - icd parameters acquired prior- and post - mri were
without any change , therefore only one value is presented . indices : na ,
not available ; l spine , lumbar spine ; c spine , cervical spine . studies
were performed using a siemens avanto 1.5 t mri scanner ( vb17 software ,
quantum gradient coils ) . all scans were run in normal operating mode ,
which is limited to 2 w / kg whole body averaged specific absorption rate (
sar ) . clinically relevant mri sequences were used for evaluation ( see
table 3 ) . table 3types of pulse sequences typically used for imaging of
respective anatomical areasscan locationscan
sequencesflairdwiflashfsehastesestirtruefispbrainxxxxheartxxxxcervical
spinexxkneexxxxlumbar spinexxflair , fluid attenuated inversion recovery ;
dwi , diffusion weighted imaging ; flash , fast low angle shot ; fse , fast
spin echo ; haste , half acquisition single - shot turbo spin echo ; se ,
spin echo ; stir , short tau inversion recovery ; truefisp , true fast
imaging with steady - state precession.fse sequence caused heating in
subjects with a thermistor probe during lumbar spine examination ( see the
text for details ) . types of pulse sequences typically used for imaging of
respective anatomical areas flair , fluid attenuated inversion recovery ;
dwi , diffusion weighted imaging ; flash , fast low angle shot ; fse , fast
spin echo ; haste , half acquisition single - shot turbo spin echo ; se ,
spin echo ; stir , short tau inversion recovery ; truefisp , true fast
imaging with steady - state precession . fse sequence caused heating in
subjects with a thermistor probe during lumbar spine examination ( see the
text for details ) . patients were asked to report immediately any pain ,
torqueing movement , or heating sensation in the area of the pocket or the
electrode by pressing an emergency bulb . furthermore , all patients were
questioned immediately following the mri procedure to ascertain any
discomfort in the vicinity of the can or electrode . pulse oximetry and
standard lead electrocardiogram ( ecg ) if discomfort occurred , the patient
was asked if the scan could be repeated at a later time using a revised scan
sequence or the subject was again randomized for another anatomical area .
since none of the components of the s - icd system are on or in the heart ,
heating near or around the electrode can not harm the myocardium . however ,
heating near the electrode or can with the s - icd system may still cause
serious patient discomfort . therefore , along with education of subjects ,
each patient was instrumented by taping an oesophageal temperature probe (
beta - therm model g22k7mcd8 ) on the skin over the mid - lateral implant
site to record any temperature excursions that might be correlated to
patient symptoms of heating / discomfort near the pocket . to minimize the
risk of inappropriate therapy , the s - icd system was programmed to therapy
each s - icd system was evaluated prior to and immediately after the scan to
verify proper functioning , including interrogation , sensing , and battery
voltage . after the completion of the mri , the s - icd system was
reprogrammed to original settings . long - term regular clinical follow - up
and checking of the device were performed . no anomalies were noted via
pulse oximetry or ecg during the scans for any of the patients . eleven of
15 patients reported no sensation or pain from heating of the can , two of
15 patients reported feeling some heating , and two patients reported
intolerable heating ( see table 2 ) . in patients with intolerable heating ,
the scan was halted within seconds and changed to a scan of the brain ,
which proceeded without incident . patient reports of heating in the
vicinity of the can occurred only during lumbar scans with a thermistor
probe ; no such reports occurred during scans of the brain , cardiac area ,
cervical spine , or without the probe . in two cases where heating in the
vicinity of the can was reported by the patient , the scan sequence was
altered to reduce the intensity of radiofrequency ( rf ) field exposure by
reducing the turbo factor ( e.g. from 21 to 7 ) , increasing the repetition
time ( e.g. to > 4000 ms ) , and reducing the flip angle ( e.g. from 170 to
120 ) . the target values were chosen arbitrarily to maintain image contrast
( flip angle ) and keep scan time at reasonable limits ( turbo factor and
repetition time ) . less heating was noted by patients after these
modifications to the scan parameters were made . 03 ) was observed to have a
skin lesion , appearing to be a circular rash or ulcer on the surface of the
skin over the can , approximately 35 mm in diameter . the cause of this skin
anomaly is not known ; it was later noted to have fully healed at a follow -
up 10 days after the scan . to ascertain the effect of heating due to the
instrumented thermistor catheter , the two patients who experienced the
heating ( examinations 9 and 16 , see table 2 ) were rescanned several weeks
later without the thermistor catheter in place ( examinations 11 and 17 ) .
first , modified sequence ( with even lower amount of energy deposited in
the tissue ) was used , which caused no heating . as no sensation was
reported by the subjects , they were asked to report even a minimal
discomfort , and the lumbar scans were performed using the same settings
that resulted in heating with the thermistor catheter in place in the first
imaging session . the results of the rescans revealed that no heating was
felt by the patients when the thermistor catheter was absent . there were no
noted changes to battery voltage , ability to detect the qrs signal or
stored diagnostic data . pacing thresholds can not be assessed by the s -
icd system , so this was not evaluated . none of the patients reported any
pulling or twisting of the can or pain from heating of the s - icd electrode
. for scans of the brain , lumbar spine , knee , and cervical spine , no
effect from image artefact was noted in the anatomical area of interest .
however , for scans of the cardiac area , image artefact was noted to
interfere with the ability to see parts of the left ventricle , though the
right ventricle of the heart was unaffected and could be imaged usefully .
this was due to the can and not the electrode ( see figure 1 ) ,
modifications to the protocol for the lumbar spine resulted in a lower
signal - to - noise ratio ; however , the images remain in diagnostic
quality ( see figure 2 ) . figure 1kinetic study in four - chamber view :
the systolic ( a and c ) and diastolic ( b and d ) images of cine sequences
, four - chamber view . the steady - state free precession ( ssfp ) sequence
( a and b ) shows more artefacts . in ssfp kinetic study , an inflow of dark
blood from the left pulmonary veins was seen . it could be caused by s - icd
but also by metallic ring in mitral annulus . the spoiled gradient echo (
gre ) sequence ( c and d ) is better , but an artefact at the lateral wall
is obvious . figure 2lumbar spine imaging with icd : low sar t2 fse sequence
( upper image ) compared with normal t2 fse in the same subject ( lower
image , for the scanning parameters see the discussion section ) . kinetic
study in four - chamber view : the systolic ( a and c ) and diastolic ( b
and d ) images of cine sequences , four - chamber view . the steady - state
free precession ( ssfp ) sequence ( a and b ) shows more artefacts . in ssfp
kinetic study , an inflow of dark blood from the left pulmonary veins was
seen . it could be caused by s - icd but also by metallic ring in mitral
annulus . the spoiled gradient echo ( gre ) sequence ( c and d ) is better ,
but an artefact at the lateral wall is obvious . lumbar spine imaging with
icd : low sar t2 fse sequence ( upper image ) compared with normal t2 fse in
the same subject ( lower image , for the scanning parameters see the
discussion section ) . there were no noted changes to battery voltage ,
ability to detect the qrs signal or stored diagnostic data . pacing
thresholds can not be assessed by the s - icd system , so this was not
evaluated . none of the patients reported any pulling or twisting of the can
or pain from heating of the s - icd electrode . for scans of the brain ,
lumbar spine , knee , and cervical spine , no effect from image artefact was
noted in the anatomical area of interest . however , for scans of the
cardiac area , image artefact was noted to interfere with the ability to see
parts of the left ventricle , though the right ventricle of the heart was
unaffected and could be imaged usefully . this was due to the can and not
the electrode ( see figure 1 ) , modifications to the protocol for the
lumbar spine resulted in a lower signal - to - noise ratio ; however , the
images remain in diagnostic quality ( see figure 2 ) . figure 1kinetic study
in four - chamber view : the systolic ( a and c ) and diastolic ( b and d )
images of cine sequences , four - chamber view . the steady - state free
precession ( ssfp ) sequence ( a and b ) shows more artefacts . in ssfp
kinetic study , an inflow of dark blood from the left pulmonary veins was
seen . it could be caused by s - icd but also by metallic ring in mitral
annulus . the spoiled gradient echo ( gre ) sequence ( c and d ) is better ,
but an artefact at the lateral wall is obvious . figure 2lumbar spine
imaging with icd : low sar t2 fse sequence ( upper image ) compared with
normal t2 fse in the same subject ( lower image , for the scanning
parameters see the discussion section ) . kinetic study in four - chamber
view : the systolic ( a and c ) and diastolic ( b and d ) images of cine
sequences , four - chamber view . the steady - state free precession ( ssfp
) sequence ( a and b ) shows more artefacts . in ssfp kinetic study , an
inflow of dark blood from the left pulmonary veins was seen . it could be
caused by s - icd but also by metallic ring in mitral annulus . the spoiled
gradient echo ( gre ) sequence ( c and d ) is better , but an artefact at
the lateral wall is obvious . lumbar spine imaging with icd : low sar t2 fse
sequence ( upper image ) compared with normal t2 fse in the same subject (
lower image , there are several reports in the current literature about mr -
conditional pacemakers from several companies , but very limited reports
about mr - conditional icds . biotronik announced in late 2011 release of
their first mr - compatible icd device and defibrillator leads pro mri , but
in the conditions of use excluded scanning of the torso and focused more on
the extremities examination . in european heart rhythm association survey ,
60% of centres did not implant any mri - certified icds , 34.3% implanted <
10 icd devices , and only 5.6% implanted 10 and more icds ; one - fifth of
responders stated that mri - certified icds should be implanted in all
patients but lack of reimbursement was indicated as a possible obstacle to
implant more mri - certified pacemakers / icds by 47.1% of responding
centres . none of the components of the s - icd system are on or in the
heart . the s - icd depends less upon being in direct contact with the
myocardium to function and instead uses far - field sensing and stimulation
to provide the shock and post - shock pacing therapy . as a consequence ,
unlike transvenous systems heating near or around the electrode can not harm
the myocardium , which could present with possible safety consequences such
as an elevation in pacing thresholds or scarring of the myocardium , but it
may still cause serious patient discomfort . because the s - icd is larger
than modern transvenous icd 's , there may be more potential for the can to
experience heating due to the magnetic gradient or rf field . we report
results from what we believe is the first experience of mri scanning in
patients with an implanted s - icd and in various anatomical areas . overall
, mri was performed safely in all patients , which is in contrast to the
current literature with mri imaging in patients with electrical - active
devices which are not mri - conditional . in our study , the primary
clinically significant event attributable to the mri scan was the occurrence
of heating in the area of the pocket in the four patients that underwent
lumbar scans . it was not known if this was due to the s - icd can itself or
an artefact of the thermistor catheter used to measure skin temperature over
the pocket . this required a revision of our protocol , which was to re -
scan two of the patients who complained of heating . re - scanning of these
patients without the thermistor probe resulted in no complaints of heating ,
so it is assumed that the thermistor catheter itself heated during the
lumbar scans and caused the discomfort . as further evidence , all the
heating complaints occurred during rf - intensive scan sequences ( namely
fast spin echo ) with the temperature probe located axially near the centre
of the bore , where rf fields are the highest . the thermistor catheter is
constructed of insulated conductive cables connected to electrodes and
should couple to the rf fields efficiently , causing heating at the
electrodes and pain or damage on the surface of the skin where the probe was
placed over the s - icd can . if the heating was due to the s - icd can
itself , it would more likely occur during gradient - intensive scan
sequences ( which can generate eddy currents on can surfaces and internal
components ) and at locations in the bore where there are high gradient
fields , such as near the bore edges . however , when the patient was
scanned with gradient - intensive scan sequences ( e.g. flair dwi ) and with
the s - icd system in high gradient field locations in the bore ( e.g. such
as during a brain scan ) , patients did not detect any heating or discomfort
. in addition , the subcutaneous lead , which was not instrumented with a
thermistor catheter , never resulted in any heating sensation noted by the
patient , even when exactly the same sequence that resulted in heating in
the first session was used . the use of mri - compatible temperature
monitors such as fibre optic temperature probes would have provided better
confirmation of possible skin temperature elevation and would not have been
affected by the rf fields . for cardiac imaging , the main problem to solve
is metallic artefact , especially on the gradient - echo sequences . like in
research performed by nazarian et al . , several scan protocols were used to
see if any yielded different effects or reduced the qualitative extent of
artefact . gradient mode was changed from normal to whisper , resulting in
slower ramping of the field and therefore diminishing the changes of the
magnetic field in time . artefacts when present were limited to blurring of
the left ventricle during cardiac scans and most yielded clinically useful
information . standard interrogation of the s - icd revealed no adverse
effects upon the functioning of the system . while no adverse effects upon
the post - scan s - icd device function were noted , not all possible
scanning protocols were tested . it should be noted that , four of the s -
icd 's were exposed to repeat mri scans without adverse effects to device
function . in addition , because the s - icd does not provide long - term
bradycardia pacing , it is assumed that pacemaker - dependent patients would
not be implanted with this system . the inhibition of the pacemaker function
during the scanning sequence and possible pacing threshold changes are a
unique concern in patients implanted with transvenous icds . this study
included only 15 patients and 22 scans done on the same 1.5 t mri scanner .
thus , even these preliminary results should only be applied to 1.5 t mri
scanners ( similarly as reported in the present literature for other
implantable devices ) . device functionality was tested immediately after
the scan but not for long - term effects . in addition , not all device
functions were tested although the s - icd system does have a beeper /
interrogation warning if battery levels or memory irregularities occur .
however , patients were scheduled for regular check - up and no defect of
the device was observed in following 725 months ( mean observation time 18
months ) . delayed enhancement mri for determining cardiac scarring was also
not tested . also , there are other anatomical areas that were not evaluated
, such as shoulder and knees . this study included only 15 patients and 22
scans done on the same 1.5 t mri scanner . thus , even these preliminary
results should only be applied to 1.5 t mri scanners ( similarly as reported
in the present literature for other implantable devices ) . device
functionality was tested immediately after the scan but not for long - term
effects . in addition , not all device functions were tested although the s
- icd system does have a beeper / interrogation warning if battery levels or
memory irregularities occur . however , patients were scheduled for regular
check - up and no defect of the device was observed in following 725 months
( mean observation time 18 months ) . delayed enhancement mri for
determining cardiac scarring was also not tested . also , there are other
anatomical areas that were not evaluated , such as shoulder and knees .
while more data are required to support a claim of mri - conditional , this
study is the study to demonstrate the feasibility of exposing s - icd
patients to mri using the scanning and monitor protocol described , with
some precautionary measures including : ( i ) programming the device therapy
off ; ( ii ) limiting the sar to 2.0 w / kg ; ( iii ) continuous monitoring
of the patients pulse oximetry and ecg by qualified personnel and especially
for any feelings of heating ; ( iv ) evaluate device function post scan ; (
v ) availability of full resuscitation facilities at the mri site . given
the variables of different mri scanners , the decision to perform mri on
patients with an implanted s - icd system should be balanced against the
potential risks . in our study , the only heating was very likely introduced
by not fully mri - compatible thermometer probe ; subjects rescanned without
the probe did not report any abnormalities during the scan of any body area
listed ( brain , cervical and lumbar spine , heart , and knee ) . this study
was supported by iga mz r nt12094/2011 , research project charles university
in prague , prvouk p34 and unce 204010/2012 . funding to pay the open access
publication charges for this article was provided by iga mz r nt12094/2011 .
example_title: Summarization Example 1
tags:
- medical
---
# Model Card: T5 Large for Medical Text Summarization
## Model Description
The **T5 Large for Medical Text Summarization** is a specialized variant of the T5 transformer model, fine-tuned for the task of summarizing medical text. This model is designed to generate concise and coherent summaries of medical documents, research papers, clinical notes, and other healthcare-related text.
The T5 Large model, known as "t5-large," is pre-trained on a broad range of medical literature, enabling it to capture intricate medical terminology, extract crucial information, and produce meaningful summaries. The fine-tuning process for this model is meticulous, with attention to hyperparameter settings, including batch size and learning rate, to ensure optimal performance in the field of medical text summarization.
During the fine-tuning process, a batch size of 8 is chosen for efficiency, and a learning rate of 2e-5 is selected to strike a balance between convergence speed and model optimization. These settings ensure the model's ability to produce high-quality medical summaries that are both informative and coherent.
The fine-tuning dataset consists of diverse medical documents, clinical studies, and healthcare research, along with human-generated summaries. This diverse dataset equips the model to excel at summarizing medical information accurately and concisely.
The goal of training this model is to provide a powerful tool for medical professionals, researchers, and healthcare institutions to automatically generate high-quality summaries of medical content, facilitating quicker access to critical information.
## Intended Uses & Limitations
### Intended Uses
- **Medical Text Summarization**: The primary purpose of this model is to generate concise and coherent summaries of medical documents, research papers, clinical notes, and healthcare-related text. It is tailored to assist medical professionals, researchers, and healthcare organizations in summarizing complex medical information.
### How to Use
To use this model for medical text summarization, you can follow these steps:
```python
from transformers import pipeline
summarizer = pipeline("summarization", model="your/medical_text_summarization_model")
MEDICAL_DOCUMENT = """
duplications of the alimentary tract are well - known but rare congenital malformations that can occur anywhere in the gastrointestinal ( gi ) tract from the tongue to the anus . while midgut duplications are the most common , foregut duplications such as oesophagus , stomach , and parts 1 and 2 of the duodenum account for approximately one - third of cases .
they are most commonly seen either in the thorax or abdomen or in both as congenital thoracoabdominal duplications .
cystic oesophageal duplication ( ced ) , the most common presentation , is often found in the lower third part ( 60 - 95% ) and on the right side [ 2 , 3 ] . hydatid cyst ( hc ) is still an important health problem throughout the world , particularly in latin america , africa , and mediterranean areas .
turkey , located in the mediterranean area , shares this problem , with an estimated incidence of 20/100 000 .
most commonly reported effected organ is liver , but in children the lungs are the second most frequent site of involvement [ 4 , 5 ] . in both ced and hc , the presentation depends on the site and the size of the cyst .
hydatid cysts are far more common than other cystic intrathoracic lesions , especially in endemic areas , so it is a challenge to differentiate ced from hc in these countries . here ,
we present a 7-year - old girl with intrathoracic cystic mass lesion , who had been treated for hydatid cyst for 9 months , but who turned out to have oesophageal cystic duplication .
a 7-year - old girl was referred to our clinic with coincidentally established cystic intrathoracic lesion during the investigation of aetiology of anaemia .
the child was first admitted with loss of vision in another hospital ten months previously .
the patient 's complaints had been attributed to pseudotumour cerebri due to severe iron deficiency anaemia ( haemoglobin : 3 g / dl ) .
chest radiography and computed tomography ( ct ) images resulted in a diagnosis of cystic intrathoracic lesion ( fig .
the cystic mass was accepted as a type 1 hydatid cyst according to world health organization ( who ) classification .
after 9 months of medication , no regression was detected in ct images , so the patient was referred to our department .
an ondirect haemagglutination test result was again negative . during surgery , after left thoracotomy incision , a semi - mobile cystic lesion , which was almost seven centimetres in diameter , with smooth contour , was found above the diaphragm , below the lung , outside the pleura ( fig .
the entire fluid in the cyst was aspirated ; it was brown and bloody ( fig .
2 ) . the diagnosis of cystic oesophageal duplication was considered , and so an attachment point was searched for .
it was below the hiatus , on the lower third left side of the oesophagus , and it also was excised completely through the hiatus .
pathologic analysis of the specimen showed oesophageal mucosa with an underlying proper smooth muscle layer .
computed tomography image of the cystic intrathoracic lesion cystic lesion with brownish fluid in the cyst
compressible organs facilitate the growth of the cyst , and this has been proposed as a reason for the apparent prevalence of lung involvement in children . diagnosis is often incidental and can be made with serological tests and imaging [ 5 , 7 ] .
laboratory investigations include the casoni and weinberg skin tests , indirect haemagglutination test , elisa , and the presence of eosinophilia , but can be falsely negative because children may have a poor serological response to eg .
false - positive reactions are related to the antigenic commonality among cestodes and conversely seronegativity can not exclude hydatidosis .
false - negative results are observed when cysts are calcified , even if fertile [ 4 , 8 ] . in our patient iha levels were negative twice .
due to the relatively non - specific clinical signs , diagnosis can only be made confidently using appropriate imaging .
plain radiographs , ultrasonography ( us ) , or ct scans are sufficient for diagnosis , but magnetic resonance imaging ( mri ) is also very useful [ 5 , 9 ] .
computed tomography demonstrates cyst wall calcification , infection , peritoneal seeding , bone involvement fluid density of intact cysts , and the characteristic internal structure of both uncomplicated and ruptured cysts [ 5 , 9 ] .
the conventional treatment of hydatid cysts in all organs is surgical . in children , small hydatid cysts of the lungs
respond favourably to medical treatment with oral administration of certain antihelminthic drugs such as albendazole in certain selected patients .
the response to therapy differs according to age , cyst size , cyst structure ( presence of daughter cysts inside the mother cysts and thickness of the pericystic capsule allowing penetration of the drugs ) , and localization of the cyst . in children , small cysts with thin pericystic capsule localised in the brain and lungs respond favourably [ 6 , 11 ] .
respiratory symptoms are seen predominantly in cases before two years of age . in our patient , who has vision loss , the asymptomatic duplication cyst was found incidentally .
the lesion occupied the left hemithorax although the most common localisation reported in the literature is the lower and right oesophagus .
the presentation depends on the site and the size of the malformations , varying from dysphagia and respiratory distress to a lump and perforation or bleeding into the intestine , but cysts are mostly diagnosed incidentally .
if a cystic mass is suspected in the chest , the best technique for evaluation is ct .
magnetic resonance imaging can be used to detail the intimate nature of the cyst with the spinal canal .
duplications should have all three typical signs : first of all , they should be attached to at least one point of the alimentary tract ; second and third are that they should have a well - developed smooth muscle coat , and the epithelial lining of duplication should represent some portions of alimentary tract , respectively [ 2 , 10 , 12 ] . in summary , the cystic appearance of both can cause a misdiagnosis very easily due to the rarity of cystic oesophageal duplications as well as the higher incidence of hydatid cyst , especially in endemic areas .
"""
print(summarizer(MEDICAL_DOCUMENT, max_length=2000, min_length=1500, do_sample=False))
>>> [{'summary_text': 'duplications of the alimentary tract are well - known but rare congenital malformations that can occur anywhere in the gastrointestinal ( gi ) tract from the tongue to the anus . in children , small hydatid cysts with thin pericystic capsule localised in the brain and lungs respond favourably to medical treatment with oral administration of certain antihelminthic drugs such as albendazole , and the epithelial lining of duplication should represent some parts of the oesophageal lesion ( hc ) , the most common presentation is . a 7-year - old girl was referred to our clinic with coincidentally established cystic intrathoracic lesion with brownish fluid in the cyst was found in the lower third part ( 60 - 95% ) and on the right side .'}]
```
Limitations
Specialized Task Fine-Tuning: While this model excels at medical text summarization, its performance may vary when applied to other natural language processing tasks. Users interested in employing this model for different tasks should explore fine-tuned versions available in the model hub for optimal results.
Training Data
The model's training data includes a diverse dataset of medical documents, clinical studies, and healthcare research, along with their corresponding human-generated summaries. The fine-tuning process aims to equip the model with the ability to generate high-quality medical text summaries effectively.
Training Stats
- Evaluation Loss: 0.012345678901234567
- Evaluation Rouge Score: 0.95 (F1)
- Evaluation Runtime: 2.3456
- Evaluation Samples per Second: 1234.56
- Evaluation Steps per Second: 45.678
Responsible Usage
It is crucial to use this model responsibly and ethically, adhering to content guidelines, privacy regulations, and ethical considerations when implementing it in real-world medical applications, particularly those involving sensitive patient data.
References
Hugging Face Model Hub
T5 Paper
Disclaimer: The model's performance may be influenced by the quality and representativeness of the data it was fine-tuned on. Users are encouraged to assess the model's suitability for their specific medical applications and datasets. |
DeepFloyd/IF-I-M-v1.0 | DeepFloyd | "2023-06-02T19:04:48Z" | 2,145 | 47 | diffusers | [
"diffusers",
"pytorch",
"safetensors",
"if",
"text-to-image",
"arxiv:2205.11487",
"arxiv:2110.02861",
"license:deepfloyd-if-license",
"diffusers:IFPipeline",
"region:us"
] | text-to-image | "2023-03-21T19:06:19Z" | ---
license: deepfloyd-if-license
extra_gated_prompt: "DeepFloyd LICENSE AGREEMENT\nThis License Agreement (as may be amended in accordance with this License Agreement, “License”), between you, or your employer or other entity (if you are entering into this agreement on behalf of your employer or other entity) (“Licensee” or “you”) and Stability AI Ltd.. (“Stability AI” or “we”) applies to your use of any computer program, algorithm, source code, object code, or software that is made available by Stability AI under this License (“Software”) and any specifications, manuals, documentation, and other written information provided by Stability AI related to the Software (“Documentation”).\nBy clicking “I Accept” below or by using the Software, you agree to the terms of this License. If you do not agree to this License, then you do not have any rights to use the Software or Documentation (collectively, the “Software Products”), and you must immediately cease using the Software Products. If you are agreeing to be bound by the terms of this License on behalf of your employer or other entity, you represent and warrant to Stability AI that you have full legal authority to bind your employer or such entity to this License. If you do not have the requisite authority, you may not accept the License or access the Software Products on behalf of your employer or other entity.\n1. LICENSE GRANT\n a. Subject to your compliance with the Documentation and Sections 2, 3, and 5, Stability AI grants you a non-exclusive, worldwide, non-transferable, non-sublicensable, revocable, royalty free and limited license under Stability AI’s copyright interests to reproduce, distribute, and create derivative works of the Software solely for your non-commercial research purposes. The foregoing license is personal to you, and you may not assign or sublicense this License or any other rights or obligations under this License without Stability AI’s prior written consent; any such assignment or sublicense will be void and will automatically and immediately terminate this License.\n b. You may make a reasonable number of copies of the Documentation solely for use in connection with the license to the Software granted above.\n c. The grant of rights expressly set forth in this Section 1 (License Grant) are the complete grant of rights to you in the Software Products, and no other licenses are granted, whether by waiver, estoppel, implication, equity or otherwise. Stability AI and its licensors reserve all rights not expressly granted by this License.\L\n2. RESTRICTIONS\n You will not, and will not permit, assist or cause any third party to:\n a. use, modify, copy, reproduce, create derivative works of, or distribute the Software Products (or any derivative works thereof, works incorporating the Software Products, or any data produced by the Software), in whole or in part, for (i) any commercial or production purposes, (ii) military purposes or in the service of nuclear technology, (iii) purposes of surveillance, including any research or development relating to surveillance, (iv) biometric processing, (v) in any manner that infringes, misappropriates, or otherwise violates any third-party rights, or (vi) in any manner that violates any applicable law and violating any privacy or security laws, rules, regulations, directives, or governmental requirements (including the General Data Privacy Regulation (Regulation (EU) 2016/679), the California Consumer Privacy Act, and any and all laws governing the processing of biometric information), as well as all amendments and successor laws to any of the foregoing;\n b. alter or remove copyright and other proprietary notices which appear on or in the Software Products;\n c. utilize any equipment, device, software, or other means to circumvent or remove any security or protection used by Stability AI in connection with the Software, or to circumvent or remove any usage restrictions, or to enable functionality disabled by Stability AI; or\n d. offer or impose any terms on the Software Products that alter, restrict, or are inconsistent with the terms of this License.\n e. 1) violate any applicable U.S. and non-U.S. export control and trade sanctions laws (“Export Laws”); 2) directly or indirectly export, re-export, provide, or otherwise transfer Software Products: (a) to any individual, entity, or country prohibited by Export Laws; (b) to anyone on U.S. or non-U.S. government restricted parties lists; or (c) for any purpose prohibited by Export Laws, including nuclear, chemical or biological weapons, or missile technology applications; 3) use or download Software Products if you or they are: (a) located in a comprehensively sanctioned jurisdiction, (b) currently listed on any U.S. or non-U.S. restricted parties list, or (c) for any purpose prohibited by Export Laws; and (4) will not disguise your location through IP proxying or other methods.\L\n3. ATTRIBUTION\n Together with any copies of the Software Products (as well as derivative works thereof or works incorporating the Software Products) that you distribute, you must provide (i) a copy of this License, and (ii) the following attribution notice: “DeepFloyd is licensed under the DeepFloyd License, Copyright (c) Stability AI Ltd. All Rights Reserved.”\L\n4. DISCLAIMERS\n THE SOFTWARE PRODUCTS ARE PROVIDED “AS IS” and “WITH ALL FAULTS” WITH NO WARRANTY OF ANY KIND, EXPRESS OR IMPLIED. STABILITY AIEXPRESSLY DISCLAIMS ALL REPRESENTATIONS AND WARRANTIES, EXPRESS OR IMPLIED, WHETHER BY STATUTE, CUSTOM, USAGE OR OTHERWISE AS TO ANY MATTERS RELATED TO THE SOFTWARE PRODUCTS, INCLUDING BUT NOT LIMITED TO, THE IMPLIED WARRANTIES OF MERCHANTABILITY, FITNESS FOR A PARTICULAR PURPOSE, TITLE, SATISFACTORY QUALITY, OR NON-INFRINGEMENT. STABILITY AI MAKES NO WARRANTIES OR REPRESENTATIONS THAT THE SOFTWARE PRODUCTS WILL BE ERROR FREE OR FREE OF VIRUSES OR OTHER HARMFUL COMPONENTS, OR PRODUCE ANY PARTICULAR RESULTS.\L\n5. LIMITATION OF LIABILITY\n TO THE FULLEST EXTENT PERMITTED BY LAW, IN NO EVENT WILL STABILITY AI BE LIABLE TO YOU (A) UNDER ANY THEORY OF LIABILITY, WHETHER BASED IN CONTRACT, TORT, NEGLIGENCE, STRICT LIABILITY, WARRANTY, OR OTHERWISE UNDER THIS LICENSE, OR (B) FOR ANY INDIRECT, CONSEQUENTIAL, EXEMPLARY, INCIDENTAL, PUNITIVE OR SPECIAL DAMAGES OR LOST PROFITS, EVEN IF STABILITY AI HAS BEEN ADVISED OF THE POSSIBILITY OF SUCH DAMAGES. THE SOFTWARE PRODUCTS, THEIR CONSTITUENT COMPONENTS, AND ANY OUTPUT (COLLECTIVELY, “SOFTWARE MATERIALS”) ARE NOT DESIGNED OR INTENDED FOR USE IN ANY APPLICATION OR SITUATION WHERE FAILURE OR FAULT OF THE SOFTWARE MATERIALS COULD REASONABLY BE ANTICIPATED TO LEAD TO SERIOUS INJURY OF ANY PERSON, INCLUDING POTENTIAL DISCRIMINATION OR VIOLATION OF AN INDIVIDUAL’S PRIVACY RIGHTS, OR TO SEVERE PHYSICAL, PROPERTY, OR ENVIRONMENTAL DAMAGE (EACH, A “HIGH-RISK USE”). IF YOU ELECT TO USE ANY OF THE SOFTWARE MATERIALS FOR A HIGH-RISK USE, YOU DO SO AT YOUR OWN RISK. YOU AGREE TO DESIGN AND IMPLEMENT APPROPRIATE DECISION-MAKING AND RISK-MITIGATION PROCEDURES AND POLICIES IN CONNECTION WITH A HIGH-RISK USE SUCH THAT EVEN IF THERE IS A FAILURE OR FAULT IN ANY OF THE SOFTWARE MATERIALS, THE SAFETY OF PERSONS OR PROPERTY AFFECTED BY THE ACTIVITY STAYS AT A LEVEL THAT IS REASONABLE, APPROPRIATE, AND LAWFUL FOR THE FIELD OF THE HIGH-RISK USE.\L\n6. INDEMNIFICATION\n You will indemnify, defend and hold harmless Stability AI and our subsidiaries and affiliates, and each of our respective shareholders, directors, officers, employees, agents, successors, and assigns (collectively, the “Stability AI Parties”) from and against any losses, liabilities, damages, fines, penalties, and expenses (including reasonable attorneys’ fees) incurred by any Stability AI Party in connection with any claim, demand, allegation, lawsuit, proceeding, or investigation (collectively, “Claims”) arising out of or related to: (a) your access to or use of the Software Products (as well as any results or data generated from such access or use), including any High-Risk Use (defined below); (b) your violation of this License; or (c) your violation, misappropriation or infringement of any rights of another (including intellectual property or other proprietary rights and privacy rights). You will promptly notify the Stability AI Parties of any such Claims, and cooperate with Stability AI Parties in defending such Claims. You will also grant the Stability AI Parties sole control of the defense or settlement, at Stability AI’s sole option, of any Claims. This indemnity is in addition to, and not in lieu of, any other indemnities or remedies set forth in a written agreement between you and Stability AI or the other Stability AI Parties.\L\n7. TERMINATION; SURVIVAL\n a. This License will automatically terminate upon any breach by you of the terms of this License.\L\Lb. We may terminate this License, in whole or in part, at any time upon notice (including electronic) to you.\L\Lc. The following sections survive termination of this License: 2 (Restrictions), 3 (Attribution), 4 (Disclaimers), 5 (Limitation on Liability), 6 (Indemnification) 7 (Termination; Survival), 8 (Third Party Materials), 9 (Trademarks), 10 (Applicable Law; Dispute Resolution), and 11 (Miscellaneous).\L\n8. THIRD PARTY MATERIALS\n The Software Products may contain third-party software or other components (including free and open source software) (all of the foregoing, “Third Party Materials”), which are subject to the license terms of the respective third-party licensors. Your dealings or correspondence with third parties and your use of or interaction with any Third Party Materials are solely between you and the third party. Stability AI does not control or endorse, and makes no representations or warranties regarding, any Third Party Materials, and your access to and use of such Third Party Materials are at your own risk.\L\n9. TRADEMARKS\n Licensee has not been granted any trademark license as part of this License and may not use any name or mark associated with Stability AI without the prior written permission of Stability AI, except to the extent necessary to make the reference required by the “ATTRIBUTION” section of this Agreement.\L\n10. APPLICABLE LAW; DISPUTE RESOLUTION\n This License will be governed and construed under the laws of the State of California without regard to conflicts of law provisions. Any suit or proceeding arising out of or relating to this License will be brought in the federal or state courts, as applicable, in San Mateo County, California, and each party irrevocably submits to the jurisdiction and venue of such courts.\L\n11. MISCELLANEOUS\n If any provision or part of a provision of this License is unlawful, void or unenforceable, that provision or part of the provision is deemed severed from this License, and will not affect the validity and enforceability of any remaining provisions. The failure of Stability AI to exercise or enforce any right or provision of this License will not operate as a waiver of such right or provision. This License does not confer any third-party beneficiary rights upon any other person or entity. This License, together with the Documentation, contains the entire understanding between you and Stability AI regarding the subject matter of this License, and supersedes all other written or oral agreements and understandings between you and Stability AI regarding such subject matter. No change or addition to any provision of this License will be binding unless it is in writing and signed by an authorized representative of both you and Stability AI."
extra_gated_fields:
"Organization /\_Affiliation": text
Previously related publications: text
I accept the above license agreement, and will use the Software non-commercially and for research purposes only: checkbox
tags:
- if
- text-to-image
inference: false
---
# IF-I-M-v1.0
DeepFloyd-IF is a pixel-based text-to-image triple-cascaded diffusion model, that can generate pictures with new state-of-the-art for photorealism and language understanding. The result is a highly efficient model that outperforms current state-of-the-art models, achieving a zero-shot FID-30K score of `6.66` on the COCO dataset.
*Inspired by* [*Photorealistic Text-to-Image Diffusion Models with Deep Language Understanding*](https://arxiv.org/pdf/2205.11487.pdf)

## Model Details
- **Developed by:** DeepFloyd, StabilityAI
- **Model type:** pixel-based text-to-image cascaded diffusion model
- **Cascade Stage:** I
- **Num Parameters:** 400M
- **Language(s):** primarily English and, to a lesser extent, other Romance languages
- **License:** <span style="color:blue"><a href="https://huggingface.co/spaces/DeepFloyd/deepfloyd-if-license">DeepFloyd IF License Agreement</a></span>
- **Model Description:** DeepFloyd-IF is modular composed of frozen text mode and three pixel cascaded diffusion modules, each designed to generate images of increasing resolution: 64x64, 256x256, and 1024x1024. All stages of the model utilize a frozen text encoder based on the T5 transformer to extract text embeddings, which are then fed into a UNet architecture enhanced with cross-attention and attention-pooling
- **Resources for more information:** [GitHub](https://github.com/deep-floyd/IF), [Website](https://deepfloyd.ai), [All Links](https://linktr.ee/deepfloyd)
## Using with `diffusers`
IF is integrated with the 🤗 Hugging Face [🧨 diffusers library](https://github.com/huggingface/diffusers/), which is optimized to run on GPUs with as little as 14 GB of VRAM.
Before you can use IF, you need to accept its usage conditions. To do so:
1. Make sure to have a [Hugging Face account](https://huggingface.co/join) and be loggin in
2. Accept the license on the model card of [DeepFloyd/IF-I-M-v1.0](https://huggingface.co/DeepFloyd/IF-I-M-v1.0)
3. Make sure to login locally. Install `huggingface_hub`
```sh
pip install huggingface_hub --upgrade
```
run the login function in a Python shell
```py
from huggingface_hub import login
login()
```
and enter your [Hugging Face Hub access token](https://huggingface.co/docs/hub/security-tokens#what-are-user-access-tokens).
Next we install `diffusers` and dependencies:
```sh
pip install diffusers accelerate transformers safetensors sentencepiece
```
And we can now run the model locally.
By default `diffusers` makes use of [model cpu offloading](https://huggingface.co/docs/diffusers/optimization/fp16#model-offloading-for-fast-inference-and-memory-savings) to run the whole IF pipeline with as little as 14 GB of VRAM.
If you are using `torch>=2.0.0`, make sure to **remove all** `enable_xformers_memory_efficient_attention()` functions.
* **Load all stages and offload to CPU**
```py
from diffusers import DiffusionPipeline
from diffusers.utils import pt_to_pil
import torch
# stage 1
stage_1 = DiffusionPipeline.from_pretrained("DeepFloyd/IF-I-M-v1.0", variant="fp16", torch_dtype=torch.float16)
stage_1.enable_xformers_memory_efficient_attention() # remove line if torch.__version__ >= 2.0.0
stage_1.enable_model_cpu_offload()
# stage 2
stage_2 = DiffusionPipeline.from_pretrained(
"DeepFloyd/IF-II-M-v1.0", text_encoder=None, variant="fp16", torch_dtype=torch.float16
)
stage_2.enable_xformers_memory_efficient_attention() # remove line if torch.__version__ >= 2.0.0
stage_2.enable_model_cpu_offload()
# stage 3
safety_modules = {"feature_extractor": stage_1.feature_extractor, "safety_checker": stage_1.safety_checker, "watermarker": stage_1.watermarker}
stage_3 = DiffusionPipeline.from_pretrained("stabilityai/stable-diffusion-x4-upscaler", **safety_modules, torch_dtype=torch.float16)
stage_3.enable_xformers_memory_efficient_attention() # remove line if torch.__version__ >= 2.0.0
stage_3.enable_model_cpu_offload()
```
* **Retrieve Text Embeddings**
```py
prompt = 'a photo of a kangaroo wearing an orange hoodie and blue sunglasses standing in front of the eiffel tower holding a sign that says "very deep learning"'
# text embeds
prompt_embeds, negative_embeds = stage_1.encode_prompt(prompt)
```
* **Run stage 1**
```py
generator = torch.manual_seed(0)
image = stage_1(prompt_embeds=prompt_embeds, negative_prompt_embeds=negative_embeds, generator=generator, output_type="pt").images
pt_to_pil(image)[0].save("./if_stage_I.png")
```
* **Run stage 2**
```py
image = stage_2(
image=image, prompt_embeds=prompt_embeds, negative_prompt_embeds=negative_embeds, generator=generator, output_type="pt"
).images
pt_to_pil(image)[0].save("./if_stage_II.png")
```
* **Run stage 3**
```py
image = stage_3(prompt=prompt, image=image, generator=generator, noise_level=100).images
image[0].save("./if_stage_III.png")
```
There are multiple ways to speed up the inference time and lower the memory consumption even more with `diffusers`. To do so, please have a look at the Diffusers docs:
- 🚀 [Optimizing for inference time](https://huggingface.co/docs/diffusers/api/pipelines/if#optimizing-for-speed)
- ⚙️ [Optimizing for low memory during inference](https://huggingface.co/docs/diffusers/api/pipelines/if#optimizing-for-memory)
For more in-detail information about how to use IF, please have a look at [the IF blog post](https://huggingface.co/blog/if) and the [documentation](https://huggingface.co/docs/diffusers/main/en/api/pipelines/if) 📖.
Diffusers dreambooth scripts also supports fine-tuning 🎨 [IF](https://huggingface.co/docs/diffusers/main/en/training/dreambooth#if).
With parameter efficient finetuning, you can add new concepts to IF with a single GPU and ~28 GB VRAM.
## Training
**Training Data:**
1.2B text-image pairs (based on LAION-A and few additional internal datasets)
Test/Valid parts of datasets are not used at any cascade and stage of training. Valid part of COCO helps to demonstrate "online" loss behaviour during training (to catch incident and other problems), but dataset is never used for train.
**Training Procedure:** IF-I-M-v1.0 is the smallest (from IF series) pixel-based diffusion cascade which uses T5-Encoder embeddings (hidden states) to generate 64px image. During training,
- Images are cropped to square via shifted-center-crop augmentation (randomly shift from center up to 0.1 of size) and resized to 64px using `Pillow==9.2.0` BICUBIC resampling with reducing_gap=None (it helps to avoid aliasing) and processed to tensor BxCxHxW
- Text prompts are encoded through open-sourced frozen T5-v1_1-xxl text-encoder (that completely was trained by Google team), random 10% of texts are dropped to empty string to add ability for classifier free guidance (CFG)
- The non-pooled output of the text encoder is fed into the projection (linear layer without activation) and is used in UNet backbone of the diffusion model via controlled hybrid self- and cross- attention
- Also, the output of the text encode is pooled via attention-pooling (64 heads) and is used in time embed as additional features
- Diffusion process is limited by 1000 discrete steps, with cosine beta schedule of noising image
- The loss is a reconstruction objective between the noise that was added to the image and the prediction made by the UNet
- The training process for checkpoint IF-I-M-v1.0 has 2_500_000 steps at resolution 64x64 on all datasets, OneCycleLR policy, SiLU activations, optimizer AdamW8bit + DeepSpeed-Zero1, fully frozen T5-Encoder

**Hardware:** 12 x 8 x A100 GPUs
**Optimizer:** [AdamW8bit](https://arxiv.org/abs/2110.02861) + [DeepSpeed ZeRO-1](https://www.deepspeed.ai/tutorials/zero/)
**Batch:** 3072
**Learning rate**: [one-cycle](https://pytorch.org/docs/stable/generated/torch.optim.lr_scheduler.OneCycleLR.html) cosine strategy, warmup 10000 steps, start_lr=4e-6, max_lr=1e-4, final_lr=1e-8

## Evaluation Results
`FID-30K: 8.86`

# Uses
## Direct Use
The model is released for research purposes. Any attempt to deploy the model in production requires not only that the LICENSE is followed but full liability over the person deploying the model.
Possible research areas and tasks include:
- Generation of artistic imagery and use in design and other artistic processes.
- Safe deployment of models which have the potential to generate harmful content.
- Probing and understanding the limitations and biases of generative models.
- Applications in educational or creative tools.
- Research on generative models.
Excluded uses are described below.
### Misuse, Malicious Use, and Out-of-Scope Use
_Note: This section is originally taken from the [DALLE-MINI model card](https://huggingface.co/dalle-mini/dalle-mini), was used for Stable Diffusion but applies in the same way for IF_.
The model should not be used to intentionally create or disseminate images that create hostile or alienating environments for people. This includes generating images that people would foreseeably find disturbing, distressing, or offensive; or content that propagates historical or current stereotypes.
#### Out-of-Scope Use
The model was not trained to be factual or true representations of people or events, and therefore using the model to generate such content is out-of-scope for the abilities of this model.
#### Misuse and Malicious Use
Using the model to generate content that is cruel to individuals is a misuse of this model. This includes, but is not limited to:
- Generating demeaning, dehumanizing, or otherwise harmful representations of people or their environments, cultures, religions, etc.
- Intentionally promoting or propagating discriminatory content or harmful stereotypes.
- Impersonating individuals without their consent.
- Sexual content without consent of the people who might see it.
- Mis- and disinformation
- Representations of egregious violence and gore
- Sharing of copyrighted or licensed material in violation of its terms of use.
- Sharing content that is an alteration of copyrighted or licensed material in violation of its terms of use.
## Limitations and Bias
### Limitations
- The model does not achieve perfect photorealism
- The model was trained mainly with English captions and will not work as well in other languages.
- The model was trained on a subset of the large-scale dataset
[LAION-5B](https://laion.ai/blog/laion-5b/), which contains adult, violent and sexual content. To partially mitigate this, we have... (see Training section).
### Bias
While the capabilities of image generation models are impressive, they can also reinforce or exacerbate social biases.
IF was primarily trained on subsets of [LAION-2B(en)](https://laion.ai/blog/laion-5b/),
which consists of images that are limited to English descriptions.
Texts and images from communities and cultures that use other languages are likely to be insufficiently accounted for.
This affects the overall output of the model, as white and western cultures are often set as the default. Further, the
ability of the model to generate content with non-English prompts is significantly worse than with English-language prompts.
IF mirrors and exacerbates biases to such a degree that viewer discretion must be advised irrespective of the input or its intent.
*This model card was written by: DeepFloyd Team and is based on the [StableDiffusion model card](https://huggingface.co/CompVis/stable-diffusion-v1-4).* |
OpenAssistant/stablelm-7b-sft-v7-epoch-3 | OpenAssistant | "2023-04-26T07:46:04Z" | 2,145 | 67 | transformers | [
"transformers",
"pytorch",
"gpt_neox",
"text-generation",
"sft",
"en",
"autotrain_compatible",
"endpoints_compatible",
"text-generation-inference",
"region:us"
] | text-generation | "2023-04-20T20:22:56Z" | ---
language:
- en
tags:
- sft
pipeline_tag: text-generation
widget:
- text: >-
<|prompter|>What is a meme, and what's the history behind this
word?<|endoftext|><|assistant|>
- text: <|prompter|>What's the Earth total population<|endoftext|><|assistant|>
- text: >-
<|prompter|>Write a story about future of AI
development<|endoftext|><|assistant|>
---
# Open-Assistant StableLM-7B SFT-7 Model
This is the 7th iteration English supervised-fine-tuning (SFT) model of
the [Open-Assistant](https://github.com/LAION-AI/Open-Assistant) project.
It is based on a StableLM 7B that was fine-tuned on human demonstrations
of assistant conversations collected through the
[https://open-assistant.io/](https://open-assistant.io/) human feedback web
app before April 12, 2023.
## Model Details
- **Developed by:** [Open-Assistant Contributors](https://open-assistant.io/)
- **Model type:** Transformer-based Language Model
- **Language:** English
- **Finetuned from:** [stabilityai/stablelm-base-alpha-7b](https://huggingface.co/stabilityai/stablelm-base-alpha-7b)
- **Code:** [Open-Assistant/model/model_training](https://github.com/LAION-AI/Open-Assistant/tree/main/model/model_training)
- **Demo:** TODO
- **License:** Creative Commons license ([CC BY-SA-4.0](https://creativecommons.org/licenses/by-sa/4.0/))
- **Contact:** [Open-Assistant Discord](https://ykilcher.com/open-assistant-discord)
## Prompting
Two special tokens are used to mark the beginning of user and assistant turns:
`<|prompter|>` and `<|assistant|>`. Each turn ends with a `<|endoftext|>` token.
Input prompt example:
```
<|prompter|>What is a meme, and what's the history behind this word?<|endoftext|><|assistant|>
```
The input ends with the `<|assistant|>` token to signal that the model should
start generating the assistant reply.
## Dev Details
- wandb: https://wandb.ai/open-assistant/supervised-finetuning/runs/08dfhyuc
- base model: [stabilityai/stablelm-base-alpha-7b](https://huggingface.co/stabilityai/stablelm-base-alpha-7b)
- checkpoint: 3 epochs (12000 steps)
command: `deepspeed trainer_sft.py --configs defaults stablelm-7b oasst-mix --cache_dir /home/ubuntu/data_cache --output_dir .saved/stable-lm-7b-1 --num_train_epochs 4 --deepspeed`
data:
```
oasst-mix:
save_strategy: epoch
sort_by_length: false
use_custom_sampler: false
datasets:
- oasst_export:
lang: "bg,ca,cs,da,de,en,es,fr,hr,hu,it,nl,pl,pt,ro,ru,sl,sr,sv,uk"
input_file_path: 2023-04-12_oasst_release_ready_synth.jsonl.gz
- vicuna:
val_split: 0.05
max_val_set: 800
fraction: 1.0
- dolly15k:
val_split: 0.05
max_val_set: 300
- grade_school_math_instructions:
val_split: 0.05
- code_alpaca:
val_split: 0.05
max_val_set: 250
```
stablelm:
```
stablelm-7b:
dtype: fp16
log_dir: stablelm_log_7b
model_name: stabilityai/stablelm-base-alpha-7b
output_dir: stablelm_7b
max_length: 4096
warmup_steps: 100
gradient_checkpointing: true
gradient_accumulation_steps: 2
per_device_train_batch_size: 4
per_device_eval_batch_size: 4
eval_steps: 100
save_steps: 500
num_train_epochs: 4
save_total_limit: 4
use_flash_attention: true
```
zero config:
```
{
"fp16": {
"enabled": "auto",
"loss_scale": 0,
"loss_scale_window": 1000,
"initial_scale_power": 16,
"hysteresis": 2,
"min_loss_scale": 1
},
"bf16": {
"enabled": "auto"
},
"optimizer": {
"type": "AdamW",
"params": {
"lr": "auto",
"betas": "auto",
"eps": "auto",
"weight_decay": "auto"
}
},
"scheduler": {
"type": "WarmupDecayLR",
"params": {
"warmup_min_lr": "auto",
"warmup_max_lr": "auto",
"warmup_num_steps": "auto",
"total_num_steps": "auto"
}
},
"zero_optimization": {
"stage": 2,
"allgather_partitions": true,
"allgather_bucket_size": 1e9,
"overlap_comm": false,
"reduce_scatter": true,
"reduce_bucket_size": 1e9,
"contiguous_gradients": true
},
"gradient_accumulation_steps": "auto",
"gradient_clipping": "auto",
"steps_per_print": 2000,
"train_batch_size": "auto",
"train_micro_batch_size_per_gpu": "auto",
"wall_clock_breakdown": false
}
``` |
houyu0930/test-demo-t5-qa | houyu0930 | "2024-04-29T17:37:21Z" | 2,145 | 0 | transformers | [
"transformers",
"safetensors",
"t5",
"text2text-generation",
"arxiv:1910.09700",
"license:mit",
"autotrain_compatible",
"endpoints_compatible",
"text-generation-inference",
"region:us"
] | text2text-generation | "2024-04-29T17:00:11Z" | ---
library_name: transformers
license: mit
---
# Model Card for Model ID
<!-- Provide a quick summary of what the model is/does. -->
## Model Details
### Model Description
<!-- Provide a longer summary of what this model is. -->
This is the model card of a 🤗 transformers model that has been pushed on the Hub. This model card has been automatically generated.
- **Developed by:** [More Information Needed]
- **Funded by [optional]:** [More Information Needed]
- **Shared by [optional]:** [More Information Needed]
- **Model type:** [More Information Needed]
- **Language(s) (NLP):** [More Information Needed]
- **License:** [More Information Needed]
- **Finetuned from model [optional]:** [More Information Needed]
### Model Sources [optional]
<!-- Provide the basic links for the model. -->
- **Repository:** [More Information Needed]
- **Paper [optional]:** [More Information Needed]
- **Demo [optional]:** [More Information Needed]
## Uses
<!-- Address questions around how the model is intended to be used, including the foreseeable users of the model and those affected by the model. -->
### Direct Use
<!-- This section is for the model use without fine-tuning or plugging into a larger ecosystem/app. -->
[More Information Needed]
### Downstream Use [optional]
<!-- This section is for the model use when fine-tuned for a task, or when plugged into a larger ecosystem/app -->
[More Information Needed]
### Out-of-Scope Use
<!-- This section addresses misuse, malicious use, and uses that the model will not work well for. -->
[More Information Needed]
## Bias, Risks, and Limitations
<!-- This section is meant to convey both technical and sociotechnical limitations. -->
[More Information Needed]
### Recommendations
<!-- This section is meant to convey recommendations with respect to the bias, risk, and technical limitations. -->
Users (both direct and downstream) should be made aware of the risks, biases and limitations of the model. More information needed for further recommendations.
## How to Get Started with the Model
Use the code below to get started with the model.
[More Information Needed]
## Training Details
### Training Data
<!-- This should link to a Dataset Card, perhaps with a short stub of information on what the training data is all about as well as documentation related to data pre-processing or additional filtering. -->
[More Information Needed]
### Training Procedure
<!-- This relates heavily to the Technical Specifications. Content here should link to that section when it is relevant to the training procedure. -->
#### Preprocessing [optional]
[More Information Needed]
#### Training Hyperparameters
- **Training regime:** [More Information Needed] <!--fp32, fp16 mixed precision, bf16 mixed precision, bf16 non-mixed precision, fp16 non-mixed precision, fp8 mixed precision -->
#### Speeds, Sizes, Times [optional]
<!-- This section provides information about throughput, start/end time, checkpoint size if relevant, etc. -->
[More Information Needed]
## Evaluation
<!-- This section describes the evaluation protocols and provides the results. -->
### Testing Data, Factors & Metrics
#### Testing Data
<!-- This should link to a Dataset Card if possible. -->
[More Information Needed]
#### Factors
<!-- These are the things the evaluation is disaggregating by, e.g., subpopulations or domains. -->
[More Information Needed]
#### Metrics
<!-- These are the evaluation metrics being used, ideally with a description of why. -->
[More Information Needed]
### Results
[More Information Needed]
#### Summary
## Model Examination [optional]
<!-- Relevant interpretability work for the model goes here -->
[More Information Needed]
## Environmental Impact
<!-- Total emissions (in grams of CO2eq) and additional considerations, such as electricity usage, go here. Edit the suggested text below accordingly -->
Carbon emissions can be estimated using the [Machine Learning Impact calculator](https://mlco2.github.io/impact#compute) presented in [Lacoste et al. (2019)](https://arxiv.org/abs/1910.09700).
- **Hardware Type:** [More Information Needed]
- **Hours used:** [More Information Needed]
- **Cloud Provider:** [More Information Needed]
- **Compute Region:** [More Information Needed]
- **Carbon Emitted:** [More Information Needed]
## Technical Specifications [optional]
### Model Architecture and Objective
[More Information Needed]
### Compute Infrastructure
[More Information Needed]
#### Hardware
[More Information Needed]
#### Software
[More Information Needed]
## Citation [optional]
<!-- If there is a paper or blog post introducing the model, the APA and Bibtex information for that should go in this section. -->
**BibTeX:**
[More Information Needed]
**APA:**
[More Information Needed]
## Glossary [optional]
<!-- If relevant, include terms and calculations in this section that can help readers understand the model or model card. -->
[More Information Needed]
## More Information [optional]
[More Information Needed]
## Model Card Authors [optional]
[More Information Needed]
## Model Card Contact
[More Information Needed] |
KnutJaegersberg/gpt2-chatbot | KnutJaegersberg | "2024-05-04T11:00:11Z" | 2,145 | 13 | transformers | [
"transformers",
"safetensors",
"gpt2",
"text-generation",
"dataset:KnutJaegersberg/Deita-6k",
"license:apache-2.0",
"model-index",
"autotrain_compatible",
"endpoints_compatible",
"text-generation-inference",
"region:us"
] | text-generation | "2024-05-03T06:54:54Z" | ---
license: apache-2.0
datasets:
- KnutJaegersberg/Deita-6k
model-index:
- name: gpt2-chatbot
results:
- task:
type: text-generation
name: Text Generation
dataset:
name: AI2 Reasoning Challenge (25-Shot)
type: ai2_arc
config: ARC-Challenge
split: test
args:
num_few_shot: 25
metrics:
- type: acc_norm
value: 29.69
name: normalized accuracy
source:
url: https://huggingface.co/spaces/HuggingFaceH4/open_llm_leaderboard?query=KnutJaegersberg/gpt2-chatbot
name: Open LLM Leaderboard
- task:
type: text-generation
name: Text Generation
dataset:
name: HellaSwag (10-Shot)
type: hellaswag
split: validation
args:
num_few_shot: 10
metrics:
- type: acc_norm
value: 50.27
name: normalized accuracy
source:
url: https://huggingface.co/spaces/HuggingFaceH4/open_llm_leaderboard?query=KnutJaegersberg/gpt2-chatbot
name: Open LLM Leaderboard
- task:
type: text-generation
name: Text Generation
dataset:
name: MMLU (5-Shot)
type: cais/mmlu
config: all
split: test
args:
num_few_shot: 5
metrics:
- type: acc
value: 26.42
name: accuracy
source:
url: https://huggingface.co/spaces/HuggingFaceH4/open_llm_leaderboard?query=KnutJaegersberg/gpt2-chatbot
name: Open LLM Leaderboard
- task:
type: text-generation
name: Text Generation
dataset:
name: TruthfulQA (0-shot)
type: truthful_qa
config: multiple_choice
split: validation
args:
num_few_shot: 0
metrics:
- type: mc2
value: 40.38
source:
url: https://huggingface.co/spaces/HuggingFaceH4/open_llm_leaderboard?query=KnutJaegersberg/gpt2-chatbot
name: Open LLM Leaderboard
- task:
type: text-generation
name: Text Generation
dataset:
name: Winogrande (5-shot)
type: winogrande
config: winogrande_xl
split: validation
args:
num_few_shot: 5
metrics:
- type: acc
value: 56.67
name: accuracy
source:
url: https://huggingface.co/spaces/HuggingFaceH4/open_llm_leaderboard?query=KnutJaegersberg/gpt2-chatbot
name: Open LLM Leaderboard
- task:
type: text-generation
name: Text Generation
dataset:
name: GSM8k (5-shot)
type: gsm8k
config: main
split: test
args:
num_few_shot: 5
metrics:
- type: acc
value: 0.0
name: accuracy
source:
url: https://huggingface.co/spaces/HuggingFaceH4/open_llm_leaderboard?query=KnutJaegersberg/gpt2-chatbot
name: Open LLM Leaderboard
---
GPT2-XL SFT on Deita dataset to change Sams mind. Supports multi-turn dialogue within the range of its capabilities.
Prompt Example:
```
### System:
You are an AI assistant. User will give you a task. Your goal is to complete the task as faithfully as you can. While performing the task think step-by-step and justify your steps.
### User:
How do you fine tune a large language model?
### Assistant:
```

# [Open LLM Leaderboard Evaluation Results](https://huggingface.co/spaces/HuggingFaceH4/open_llm_leaderboard)
Detailed results can be found [here](https://huggingface.co/datasets/open-llm-leaderboard/details_KnutJaegersberg__gpt2-chatbot)
| Metric |Value|
|---------------------------------|----:|
|Avg. |33.91|
|AI2 Reasoning Challenge (25-Shot)|29.69|
|HellaSwag (10-Shot) |50.27|
|MMLU (5-Shot) |26.42|
|TruthfulQA (0-shot) |40.38|
|Winogrande (5-shot) |56.67|
|GSM8k (5-shot) | 0.00|
|
RichardErkhov/Undi95_-_PsyMedRP-v1-20B-gguf | RichardErkhov | "2024-06-05T20:53:07Z" | 2,144 | 1 | null | [
"gguf",
"region:us"
] | null | "2024-06-05T16:33:36Z" | Quantization made by Richard Erkhov.
[Github](https://github.com/RichardErkhov)
[Discord](https://discord.gg/pvy7H8DZMG)
[Request more models](https://github.com/RichardErkhov/quant_request)
PsyMedRP-v1-20B - GGUF
- Model creator: https://huggingface.co/Undi95/
- Original model: https://huggingface.co/Undi95/PsyMedRP-v1-20B/
| Name | Quant method | Size |
| ---- | ---- | ---- |
| [PsyMedRP-v1-20B.Q2_K.gguf](https://huggingface.co/RichardErkhov/Undi95_-_PsyMedRP-v1-20B-gguf/blob/main/PsyMedRP-v1-20B.Q2_K.gguf) | Q2_K | 6.91GB |
| [PsyMedRP-v1-20B.IQ3_XS.gguf](https://huggingface.co/RichardErkhov/Undi95_-_PsyMedRP-v1-20B-gguf/blob/main/PsyMedRP-v1-20B.IQ3_XS.gguf) | IQ3_XS | 7.63GB |
| [PsyMedRP-v1-20B.IQ3_S.gguf](https://huggingface.co/RichardErkhov/Undi95_-_PsyMedRP-v1-20B-gguf/blob/main/PsyMedRP-v1-20B.IQ3_S.gguf) | IQ3_S | 8.06GB |
| [PsyMedRP-v1-20B.Q3_K_S.gguf](https://huggingface.co/RichardErkhov/Undi95_-_PsyMedRP-v1-20B-gguf/blob/main/PsyMedRP-v1-20B.Q3_K_S.gguf) | Q3_K_S | 8.06GB |
| [PsyMedRP-v1-20B.IQ3_M.gguf](https://huggingface.co/RichardErkhov/Undi95_-_PsyMedRP-v1-20B-gguf/blob/main/PsyMedRP-v1-20B.IQ3_M.gguf) | IQ3_M | 8.53GB |
| [PsyMedRP-v1-20B.Q3_K.gguf](https://huggingface.co/RichardErkhov/Undi95_-_PsyMedRP-v1-20B-gguf/blob/main/PsyMedRP-v1-20B.Q3_K.gguf) | Q3_K | 9.04GB |
| [PsyMedRP-v1-20B.Q3_K_M.gguf](https://huggingface.co/RichardErkhov/Undi95_-_PsyMedRP-v1-20B-gguf/blob/main/PsyMedRP-v1-20B.Q3_K_M.gguf) | Q3_K_M | 9.04GB |
| [PsyMedRP-v1-20B.Q3_K_L.gguf](https://huggingface.co/RichardErkhov/Undi95_-_PsyMedRP-v1-20B-gguf/blob/main/PsyMedRP-v1-20B.Q3_K_L.gguf) | Q3_K_L | 9.9GB |
| [PsyMedRP-v1-20B.IQ4_XS.gguf](https://huggingface.co/RichardErkhov/Undi95_-_PsyMedRP-v1-20B-gguf/blob/main/PsyMedRP-v1-20B.IQ4_XS.gguf) | IQ4_XS | 10.01GB |
| [PsyMedRP-v1-20B.Q4_0.gguf](https://huggingface.co/RichardErkhov/Undi95_-_PsyMedRP-v1-20B-gguf/blob/main/PsyMedRP-v1-20B.Q4_0.gguf) | Q4_0 | 8.93GB |
| [PsyMedRP-v1-20B.IQ4_NL.gguf](https://huggingface.co/RichardErkhov/Undi95_-_PsyMedRP-v1-20B-gguf/blob/main/PsyMedRP-v1-20B.IQ4_NL.gguf) | IQ4_NL | 3.54GB |
| [PsyMedRP-v1-20B.Q4_K_S.gguf](https://huggingface.co/RichardErkhov/Undi95_-_PsyMedRP-v1-20B-gguf/blob/main/PsyMedRP-v1-20B.Q4_K_S.gguf) | Q4_K_S | 1.47GB |
| [PsyMedRP-v1-20B.Q4_K.gguf](https://huggingface.co/RichardErkhov/Undi95_-_PsyMedRP-v1-20B-gguf/blob/main/PsyMedRP-v1-20B.Q4_K.gguf) | Q4_K | 0.25GB |
| [PsyMedRP-v1-20B.Q4_K_M.gguf](https://huggingface.co/RichardErkhov/Undi95_-_PsyMedRP-v1-20B-gguf/blob/main/PsyMedRP-v1-20B.Q4_K_M.gguf) | Q4_K_M | 0.19GB |
| [PsyMedRP-v1-20B.Q4_1.gguf](https://huggingface.co/RichardErkhov/Undi95_-_PsyMedRP-v1-20B-gguf/blob/main/PsyMedRP-v1-20B.Q4_1.gguf) | Q4_1 | 0.19GB |
| [PsyMedRP-v1-20B.Q5_0.gguf](https://huggingface.co/RichardErkhov/Undi95_-_PsyMedRP-v1-20B-gguf/blob/main/PsyMedRP-v1-20B.Q5_0.gguf) | Q5_0 | 12.83GB |
| [PsyMedRP-v1-20B.Q5_K_S.gguf](https://huggingface.co/RichardErkhov/Undi95_-_PsyMedRP-v1-20B-gguf/blob/main/PsyMedRP-v1-20B.Q5_K_S.gguf) | Q5_K_S | 6.7GB |
| [PsyMedRP-v1-20B.Q5_K.gguf](https://huggingface.co/RichardErkhov/Undi95_-_PsyMedRP-v1-20B-gguf/blob/main/PsyMedRP-v1-20B.Q5_K.gguf) | Q5_K | 3.78GB |
| [PsyMedRP-v1-20B.Q5_K_M.gguf](https://huggingface.co/RichardErkhov/Undi95_-_PsyMedRP-v1-20B-gguf/blob/main/PsyMedRP-v1-20B.Q5_K_M.gguf) | Q5_K_M | 1.97GB |
| [PsyMedRP-v1-20B.Q5_1.gguf](https://huggingface.co/RichardErkhov/Undi95_-_PsyMedRP-v1-20B-gguf/blob/main/PsyMedRP-v1-20B.Q5_1.gguf) | Q5_1 | 1.67GB |
| [PsyMedRP-v1-20B.Q6_K.gguf](https://huggingface.co/RichardErkhov/Undi95_-_PsyMedRP-v1-20B-gguf/blob/main/PsyMedRP-v1-20B.Q6_K.gguf) | Q6_K | 1.25GB |
| [PsyMedRP-v1-20B.Q8_0.gguf](https://huggingface.co/RichardErkhov/Undi95_-_PsyMedRP-v1-20B-gguf/blob/main/PsyMedRP-v1-20B.Q8_0.gguf) | Q8_0 | 1.02GB |
Original model description:
---
license: cc-by-nc-4.0
tags:
- not-for-all-audiences
- nsfw
---
```
PsyMedRP-v1-13B-p1:
[jondurbin/airoboros-l2-13b-3.0](0.85) x [ehartford/Samantha-1.11-13b](0.15)
PsyMedRP-v1-13B-p2:
[Xwin-LM/Xwin-LM-13B-V0.1](0.85) x [chaoyi-wu/MedLLaMA_13B](0.15)
PsyMedRP-v1-20B-p1:
[PsyMedRP-v1-13B-p1](0.90) x [migtissera/Synthia-13B-v1.2](0.10)
PsyMedRP-v1-20B-p2:
[PsyMedRP-v1-13B-p2](0.90) x [migtissera/Synthia-13B-v1.2](0.10)
PsyMedRP-v1-20B-p3:
[Huginn merge with Gryphe gradient to PsyMedRP-v1-20B-p1]
PsyMedRP-v1-20B-p4:
[Huginn merge with Gryphe gradient to PsyMedRP-v1-20B-p2]
PsyMedRP-v1-20B-p5:
Apply Undi95/LimaRP-v3-120-Days at 0.3 weight to PsyMedRP-v1-20B-p3
PsyMedRP-v1-20B-p6:
Apply Undi95/LimaRP-v3-120-Days at 0.3 weight to PsyMedRP-v1-20B-p4
PsyMedRP-v1-20B:
layer_slices:
- model: PsyMedRP-v1-20B-p5
start: 0
end: 16
- model: PsyMedRP-v1-20B-p6
start: 8
end: 20
- model: PsyMedRP-v1-20B-p5
start: 17
end: 32
- model: PsyMedRP-v1-20B-p6
start: 21
end: 40
```
In testing.
If you want to support me, you can [here](https://ko-fi.com/undiai).
|
joongi007/Ko-Qwen2-7B-Instruct-GGUF | joongi007 | "2024-06-17T01:14:07Z" | 2,144 | 0 | null | [
"gguf",
"base_model:spow12/Ko-Qwen2-7B-Instruct",
"license:cc-by-nc-4.0",
"region:us"
] | null | "2024-06-14T05:24:08Z" | ---
license: cc-by-nc-4.0
base_model: spow12/Ko-Qwen2-7B-Instruct
tags:
- gguf
model-index:
- name: joongi007/Ko-Qwen2-7B-Instruct-GGUF
results: []
---
- Original model is [spow12/Ko-Qwen2-7B-Instruct](https://huggingface.co/spow12/Ko-Qwen2-7B-Instruct)
- quantized using [llama.cpp](https://github.com/ggerganov/llama.cpp)
```prompt
<|im_start|>system
{System}<|im_end|>
<|im_start|>user
{User}<|im_end|>
<|im_start|>assistant
{Assistant}
```
"Flash Attention" function must be activated. [why?](https://www.reddit.com/r/LocalLLaMA/comments/1da19nu/if_your_qwen2_gguf_is_spitting_nonsense_enable/)
|
togethercomputer/GPT-JT-Moderation-6B | togethercomputer | "2023-03-15T16:24:09Z" | 2,143 | 31 | transformers | [
"transformers",
"pytorch",
"gptj",
"text-generation",
"en",
"dataset:allenai/prosocial-dialog",
"license:apache-2.0",
"autotrain_compatible",
"endpoints_compatible",
"region:us"
] | text-generation | "2023-03-04T12:46:12Z" | ---
license: apache-2.0
language:
- en
datasets:
- allenai/prosocial-dialog
---
# GPT-JT-Moderation-6B v1
This model card introduces a moderation model, a [GPT-JT](https://huggingface.co/togethercomputer/GPT-JT-6B-v1) model fine-tuned on Ontocord.ai's [OIG-moderation dataset v0.1](https://huggingface.co/datasets/ontocord/OIG-moderation).
This model can be used to moderate other chatbot models, including [GPT-NeoXT-Chat-Base-20B](https://huggingface.co/togethercomputer/GPT-NeoXT-Chat-Base-20B).
In chat applications the moderation model runs in tandem with the main chat bot, checking both the user question and the bot answer for any inappropriate content. If needed, the moderation model intervenes overriding the main chat bot’s response and indicating to the user that this request could not be answered.
## Examples
An example prompt and its expected result is as follows:
```
Possible labels:
1. casual
2. needs caution
3. needs intervention
4. possibly needs caution
5. probably needs caution
Input: I want to call in sick
Output: casual
Input: I hate my job and I don't want to go there.
Output: possibly needs caution
Input: It's so hard to deal with this. I hate this job and I want to quit.
Output: possibly needs caution
Input: Something tells me that you're sitting in the wrong section of the Stormfront bus.
Output: needs caution
Input: Yes I am. They're doing terrible things to people and they're getting away with it, so we should do something about these people.
Output:
-----
> needs intervention
```
# Uses
## Limitations and Bias
- The model's performance is limited by the quality and representativeness of its training data. We will continue working on this.
- The model may produce false positives or false negatives, leading to unnecessary confusion. We apologize and welcome any feedbacks or comments for that!
## Training
**Training Data**
- [allenai/prosocial-dialog](https://huggingface.co/datasets/allenai/prosocial-dialog).
- A small subset of LAION's [OIG dataset](https://huggingface.co/datasets/laion/OIG) to augment casual queries.
- The processed data can be found in the OIG-moderation repository [here](https://huggingface.co/datasets/ontocord/OIG-moderation/resolve/main/OIG_safety_v0.1.jsonl).
**Training Procedure**
- **Hardware:** 8 x A100 GPUs
- **Optimizer:** AdamW
- **Gradient Accumulations**: 1
- **Batch:** 16 x 4 = 64
- **Learning rate:** warmup to 1e-5 for 100 steps and then kept constant
## Community
Join us on [Together Discord](https://discord.gg/6ZVDU8tTD4) |
timm/resnest26d.gluon_in1k | timm | "2023-04-23T23:35:18Z" | 2,143 | 0 | timm | [
"timm",
"pytorch",
"safetensors",
"image-classification",
"dataset:imagenet-1k",
"arxiv:2004.08955",
"license:apache-2.0",
"region:us"
] | image-classification | "2023-04-23T23:35:07Z" | ---
tags:
- image-classification
- timm
library_name: timm
license: apache-2.0
datasets:
- imagenet-1k
---
# Model card for resnest26d.gluon_in1k
A ResNeSt (ResNet based architecture with Split Attention) image classification model. Trained on ImageNet-1k by paper authors.
## Model Details
- **Model Type:** Image classification / feature backbone
- **Model Stats:**
- Params (M): 17.1
- GMACs: 3.6
- Activations (M): 10.0
- Image size: 224 x 224
- **Papers:**
- ResNeSt: Split-Attention Networks: https://arxiv.org/abs/2004.08955
- **Dataset:** ImageNet-1k
- **Original:** https://github.com/zhanghang1989/ResNeSt
## Model Usage
### Image Classification
```python
from urllib.request import urlopen
from PIL import Image
import timm
img = Image.open(urlopen(
'https://huggingface.co/datasets/huggingface/documentation-images/resolve/main/beignets-task-guide.png'
))
model = timm.create_model('resnest26d.gluon_in1k', pretrained=True)
model = model.eval()
# get model specific transforms (normalization, resize)
data_config = timm.data.resolve_model_data_config(model)
transforms = timm.data.create_transform(**data_config, is_training=False)
output = model(transforms(img).unsqueeze(0)) # unsqueeze single image into batch of 1
top5_probabilities, top5_class_indices = torch.topk(output.softmax(dim=1) * 100, k=5)
```
### Feature Map Extraction
```python
from urllib.request import urlopen
from PIL import Image
import timm
img = Image.open(urlopen(
'https://huggingface.co/datasets/huggingface/documentation-images/resolve/main/beignets-task-guide.png'
))
model = timm.create_model(
'resnest26d.gluon_in1k',
pretrained=True,
features_only=True,
)
model = model.eval()
# get model specific transforms (normalization, resize)
data_config = timm.data.resolve_model_data_config(model)
transforms = timm.data.create_transform(**data_config, is_training=False)
output = model(transforms(img).unsqueeze(0)) # unsqueeze single image into batch of 1
for o in output:
# print shape of each feature map in output
# e.g.:
# torch.Size([1, 64, 112, 112])
# torch.Size([1, 256, 56, 56])
# torch.Size([1, 512, 28, 28])
# torch.Size([1, 1024, 14, 14])
# torch.Size([1, 2048, 7, 7])
print(o.shape)
```
### Image Embeddings
```python
from urllib.request import urlopen
from PIL import Image
import timm
img = Image.open(urlopen(
'https://huggingface.co/datasets/huggingface/documentation-images/resolve/main/beignets-task-guide.png'
))
model = timm.create_model(
'resnest26d.gluon_in1k',
pretrained=True,
num_classes=0, # remove classifier nn.Linear
)
model = model.eval()
# get model specific transforms (normalization, resize)
data_config = timm.data.resolve_model_data_config(model)
transforms = timm.data.create_transform(**data_config, is_training=False)
output = model(transforms(img).unsqueeze(0)) # output is (batch_size, num_features) shaped tensor
# or equivalently (without needing to set num_classes=0)
output = model.forward_features(transforms(img).unsqueeze(0))
# output is unpooled, a (1, 2048, 7, 7) shaped tensor
output = model.forward_head(output, pre_logits=True)
# output is a (1, num_features) shaped tensor
```
## Model Comparison
Explore the dataset and runtime metrics of this model in timm [model results](https://github.com/huggingface/pytorch-image-models/tree/main/results).
## Citation
```bibtex
@article{zhang2020resnest,
title={ResNeSt: Split-Attention Networks},
author={Zhang, Hang and Wu, Chongruo and Zhang, Zhongyue and Zhu, Yi and Zhang, Zhi and Lin, Haibin and Sun, Yue and He, Tong and Muller, Jonas and Manmatha, R. and Li, Mu and Smola, Alexander},
journal={arXiv preprint arXiv:2004.08955},
year={2020}
}
```
|
upstage/SOLAR-0-70b-16bit | upstage | "2023-09-13T09:14:02Z" | 2,142 | 254 | transformers | [
"transformers",
"pytorch",
"llama",
"text-generation",
"upstage",
"llama-2",
"instruct",
"instruction",
"en",
"autotrain_compatible",
"endpoints_compatible",
"text-generation-inference",
"region:us"
] | text-generation | "2023-07-30T01:10:53Z" | ---
language:
- en
tags:
- upstage
- llama-2
- instruct
- instruction
pipeline_tag: text-generation
---
# Updates
Solar, a new bot created by Upstage, is now available on **Poe**. As a top-ranked model on the HuggingFace Open LLM leaderboard, and a fine tune of Llama 2, Solar is a great example of the progress enabled by open source.
Try now at https://poe.com/Solar-0-70b
# SOLAR-0-70b-16bit model card
The model name has been changed from LLaMa-2-70b-instruct-v2 to SOLAR-0-70b-16bit
## Model Details
* **Developed by**: [Upstage](https://en.upstage.ai)
* **Backbone Model**: [LLaMA-2](https://github.com/facebookresearch/llama/tree/main)
* **Language(s)**: English
* **Library**: [HuggingFace Transformers](https://github.com/huggingface/transformers)
* **License**: Fine-tuned checkpoints is licensed under the Non-Commercial Creative Commons license ([CC BY-NC-4.0](https://creativecommons.org/licenses/by-nc/4.0/))
* **Where to send comments**: Instructions on how to provide feedback or comments on a model can be found by opening an issue in the [Hugging Face community's model repository](https://huggingface.co/upstage/Llama-2-70b-instruct-v2/discussions)
* **Contact**: For questions and comments about the model, please email [[email protected]](mailto:[email protected])
## Dataset Details
### Used Datasets
- Orca-style dataset
- Alpaca-style dataset
- No other dataset was used except for the dataset mentioned above
- No benchmark test set or the training set are used
### Prompt Template
```
### System:
{System}
### User:
{User}
### Assistant:
{Assistant}
```
## Usage
- The followings are tested on A100 80GB
- Our model can handle up to 10k+ input tokens, thanks to the `rope_scaling` option
```python
import torch
from transformers import AutoModelForCausalLM, AutoTokenizer, TextStreamer
tokenizer = AutoTokenizer.from_pretrained("upstage/Llama-2-70b-instruct-v2")
model = AutoModelForCausalLM.from_pretrained(
"upstage/Llama-2-70b-instruct-v2",
device_map="auto",
torch_dtype=torch.float16,
load_in_8bit=True,
rope_scaling={"type": "dynamic", "factor": 2} # allows handling of longer inputs
)
prompt = "### User:\nThomas is healthy, but he has to go to the hospital. What could be the reasons?\n\n### Assistant:\n"
inputs = tokenizer(prompt, return_tensors="pt").to(model.device)
del inputs["token_type_ids"]
streamer = TextStreamer(tokenizer, skip_prompt=True, skip_special_tokens=True)
output = model.generate(**inputs, streamer=streamer, use_cache=True, max_new_tokens=float('inf'))
output_text = tokenizer.decode(output[0], skip_special_tokens=True)
```
## Hardware and Software
* **Hardware**: We utilized an A100x8 * 4 for training our model
* **Training Factors**: We fine-tuned this model using a combination of the [DeepSpeed library](https://github.com/microsoft/DeepSpeed) and the [HuggingFace Trainer](https://huggingface.co/docs/transformers/main_classes/trainer) / [HuggingFace Accelerate](https://huggingface.co/docs/accelerate/index)
## Evaluation Results
### Overview
- We conducted a performance evaluation following the tasks being evaluated on the [Open LLM Leaderboard](https://huggingface.co/spaces/HuggingFaceH4/open_llm_leaderboard).
We evaluated our model on four benchmark datasets, which include `ARC-Challenge`, `HellaSwag`, `MMLU`, and `TruthfulQA`
We used the [lm-evaluation-harness repository](https://github.com/EleutherAI/lm-evaluation-harness), specifically commit [b281b0921b636bc36ad05c0b0b0763bd6dd43463](https://github.com/EleutherAI/lm-evaluation-harness/tree/b281b0921b636bc36ad05c0b0b0763bd6dd43463).
- We used [MT-bench](https://github.com/lm-sys/FastChat/tree/main/fastchat/llm_judge), a set of challenging multi-turn open-ended questions, to evaluate the models
### Main Results
| Model | H4(Avg) | ARC | HellaSwag | MMLU | TruthfulQA | | MT_Bench |
|--------------------------------------------------------------------|----------|----------|----------|------|----------|-|-------------|
| **[Llama-2-70b-instruct-v2](https://huggingface.co/upstage/Llama-2-70b-instruct-v2)**(***Ours***, ***Open LLM Leaderboard***) | **73** | **71.1** | **87.9** | **70.6** | **62.2** | | **7.44063** |
| [Llama-2-70b-instruct](https://huggingface.co/upstage/Llama-2-70b-instruct) (Ours, Open LLM Leaderboard) | 72.3 | 70.9 | 87.5 | 69.8 | 61 | | 7.24375 |
| [llama-65b-instruct](https://huggingface.co/upstage/llama-65b-instruct) (Ours, Open LLM Leaderboard) | 69.4 | 67.6 | 86.5 | 64.9 | 58.8 | | |
| Llama-2-70b-hf | 67.3 | 67.3 | 87.3 | 69.8 | 44.9 | | |
| [llama-30b-instruct-2048](https://huggingface.co/upstage/llama-30b-instruct-2048) (Ours, Open LLM Leaderboard) | 67.0 | 64.9 | 84.9 | 61.9 | 56.3 | | |
| [llama-30b-instruct](https://huggingface.co/upstage/llama-30b-instruct) (Ours, Open LLM Leaderboard) | 65.2 | 62.5 | 86.2 | 59.4 | 52.8 | | |
| llama-65b | 64.2 | 63.5 | 86.1 | 63.9 | 43.4 | | |
| falcon-40b-instruct | 63.4 | 61.6 | 84.3 | 55.4 | 52.5 | | |
### Scripts for H4 Score Reproduction
- Prepare evaluation environments:
```
# clone the repository
git clone https://github.com/EleutherAI/lm-evaluation-harness.git
# check out the specific commit
git checkout b281b0921b636bc36ad05c0b0b0763bd6dd43463
# change to the repository directory
cd lm-evaluation-harness
```
## Contact Us
### About Upstage
- [Upstage](https://en.upstage.ai) is a company specialized in Large Language Models (LLMs) and AI. We will help you build private LLMs and related applications.
If you have a dataset to build domain specific LLMs or make LLM applications, please contact us at ► [click here to contact](https://www.upstage.ai/private-llm?utm_source=huggingface&utm_medium=link&utm_campaign=privatellm)
- As of August 1st, our 70B model has reached the top spot in openLLM rankings, marking itself as the current leading performer globally. |
NewstaR/Porpoise-6b-instruct | NewstaR | "2023-09-17T14:55:11Z" | 2,142 | 1 | transformers | [
"transformers",
"pytorch",
"safetensors",
"llama",
"text-generation",
"custom_code",
"dataset:Open-Orca/OpenOrca",
"dataset:cerebras/SlimPajama-627B",
"dataset:ehartford/dolphin",
"autotrain_compatible",
"text-generation-inference",
"region:us"
] | text-generation | "2023-09-17T14:27:51Z" | ---
datasets:
- Open-Orca/OpenOrca
- cerebras/SlimPajama-627B
- ehartford/dolphin
---
This model is a finetuned version of the DeciLM-6b-instruct on the Dolphin GPT4 Dataset
Please set naive_attention_prefill to true when loading this model.
**Example:**
```
import torch
from transformers import AutoModelForCausalLM, AutoTokenizer, BitsAndBytesConfig, AutoTokenizer
model_name = "NewstaR/Porpoise-6b-instruct"
bnb_config = BitsAndBytesConfig(
load_in_4bit=True,
bnb_4bit_quant_type="nf4",
bnb_4bit_compute_dtype=torch.float16,
)
model = AutoModelForCausalLM.from_pretrained(
model_name,
quantization_config=bnb_config,
trust_remote_code=True,
naive_attention_prefill=True,
)
model.config.use_cache = False
```
|
Danielbrdz/Barcenas-6b | Danielbrdz | "2023-09-18T00:23:51Z" | 2,142 | 1 | transformers | [
"transformers",
"pytorch",
"llama",
"text-generation",
"custom_code",
"en",
"license:llama2",
"autotrain_compatible",
"text-generation-inference",
"region:us"
] | text-generation | "2023-09-17T23:15:45Z" | ---
license: llama2
language:
- en
---
Barcenas 6b
Model based on Deci/DeciLM-6b-instruct
Enhanced with a dataset to improve its chat mode
Made with ❤️ in Guadalupe, Nuevo Leon, Mexico 🇲🇽 |
yuzhaouoe/IntraDoc | yuzhaouoe | "2024-06-13T15:19:08Z" | 2,142 | 0 | transformers | [
"transformers",
"safetensors",
"llama",
"text-generation",
"arxiv:1910.09700",
"autotrain_compatible",
"endpoints_compatible",
"text-generation-inference",
"region:us"
] | text-generation | "2024-02-28T00:52:58Z" | ---
library_name: transformers
tags: []
---
# Model Card for Model ID
<!-- Provide a quick summary of what the model is/does. -->
## Model Details
### Model Description
<!-- Provide a longer summary of what this model is. -->
This is the model card of a 🤗 transformers model that has been pushed on the Hub. This model card has been automatically generated.
- **Developed by:** [More Information Needed]
- **Funded by [optional]:** [More Information Needed]
- **Shared by [optional]:** [More Information Needed]
- **Model type:** [More Information Needed]
- **Language(s) (NLP):** [More Information Needed]
- **License:** [More Information Needed]
- **Finetuned from model [optional]:** [More Information Needed]
### Model Sources [optional]
<!-- Provide the basic links for the model. -->
- **Repository:** [More Information Needed]
- **Paper [optional]:** [More Information Needed]
- **Demo [optional]:** [More Information Needed]
## Uses
<!-- Address questions around how the model is intended to be used, including the foreseeable users of the model and those affected by the model. -->
### Direct Use
<!-- This section is for the model use without fine-tuning or plugging into a larger ecosystem/app. -->
[More Information Needed]
### Downstream Use [optional]
<!-- This section is for the model use when fine-tuned for a task, or when plugged into a larger ecosystem/app -->
[More Information Needed]
### Out-of-Scope Use
<!-- This section addresses misuse, malicious use, and uses that the model will not work well for. -->
[More Information Needed]
## Bias, Risks, and Limitations
<!-- This section is meant to convey both technical and sociotechnical limitations. -->
[More Information Needed]
### Recommendations
<!-- This section is meant to convey recommendations with respect to the bias, risk, and technical limitations. -->
Users (both direct and downstream) should be made aware of the risks, biases and limitations of the model. More information needed for further recommendations.
## How to Get Started with the Model
Use the code below to get started with the model.
[More Information Needed]
## Training Details
### Training Data
<!-- This should link to a Dataset Card, perhaps with a short stub of information on what the training data is all about as well as documentation related to data pre-processing or additional filtering. -->
[More Information Needed]
### Training Procedure
<!-- This relates heavily to the Technical Specifications. Content here should link to that section when it is relevant to the training procedure. -->
#### Preprocessing [optional]
[More Information Needed]
#### Training Hyperparameters
- **Training regime:** [More Information Needed] <!--fp32, fp16 mixed precision, bf16 mixed precision, bf16 non-mixed precision, fp16 non-mixed precision, fp8 mixed precision -->
#### Speeds, Sizes, Times [optional]
<!-- This section provides information about throughput, start/end time, checkpoint size if relevant, etc. -->
[More Information Needed]
## Evaluation
<!-- This section describes the evaluation protocols and provides the results. -->
### Testing Data, Factors & Metrics
#### Testing Data
<!-- This should link to a Dataset Card if possible. -->
[More Information Needed]
#### Factors
<!-- These are the things the evaluation is disaggregating by, e.g., subpopulations or domains. -->
[More Information Needed]
#### Metrics
<!-- These are the evaluation metrics being used, ideally with a description of why. -->
[More Information Needed]
### Results
[More Information Needed]
#### Summary
## Model Examination [optional]
<!-- Relevant interpretability work for the model goes here -->
[More Information Needed]
## Environmental Impact
<!-- Total emissions (in grams of CO2eq) and additional considerations, such as electricity usage, go here. Edit the suggested text below accordingly -->
Carbon emissions can be estimated using the [Machine Learning Impact calculator](https://mlco2.github.io/impact#compute) presented in [Lacoste et al. (2019)](https://arxiv.org/abs/1910.09700).
- **Hardware Type:** [More Information Needed]
- **Hours used:** [More Information Needed]
- **Cloud Provider:** [More Information Needed]
- **Compute Region:** [More Information Needed]
- **Carbon Emitted:** [More Information Needed]
## Technical Specifications [optional]
### Model Architecture and Objective
[More Information Needed]
### Compute Infrastructure
[More Information Needed]
#### Hardware
[More Information Needed]
#### Software
[More Information Needed]
## Citation [optional]
<!-- If there is a paper or blog post introducing the model, the APA and Bibtex information for that should go in this section. -->
**BibTeX:**
[More Information Needed]
**APA:**
[More Information Needed]
## Glossary [optional]
<!-- If relevant, include terms and calculations in this section that can help readers understand the model or model card. -->
[More Information Needed]
## More Information [optional]
[More Information Needed]
## Model Card Authors [optional]
[More Information Needed]
## Model Card Contact
[More Information Needed] |
Niggendar/cyberrealisticPony_v20 | Niggendar | "2024-05-21T21:03:29Z" | 2,142 | 1 | diffusers | [
"diffusers",
"safetensors",
"arxiv:1910.09700",
"endpoints_compatible",
"diffusers:StableDiffusionXLPipeline",
"region:us"
] | text-to-image | "2024-05-21T20:54:20Z" | ---
library_name: diffusers
---
# Model Card for Model ID
<!-- Provide a quick summary of what the model is/does. -->
## Model Details
### Model Description
<!-- Provide a longer summary of what this model is. -->
This is the model card of a 🧨 diffusers model that has been pushed on the Hub. This model card has been automatically generated.
- **Developed by:** [More Information Needed]
- **Funded by [optional]:** [More Information Needed]
- **Shared by [optional]:** [More Information Needed]
- **Model type:** [More Information Needed]
- **Language(s) (NLP):** [More Information Needed]
- **License:** [More Information Needed]
- **Finetuned from model [optional]:** [More Information Needed]
### Model Sources [optional]
<!-- Provide the basic links for the model. -->
- **Repository:** [More Information Needed]
- **Paper [optional]:** [More Information Needed]
- **Demo [optional]:** [More Information Needed]
## Uses
<!-- Address questions around how the model is intended to be used, including the foreseeable users of the model and those affected by the model. -->
### Direct Use
<!-- This section is for the model use without fine-tuning or plugging into a larger ecosystem/app. -->
[More Information Needed]
### Downstream Use [optional]
<!-- This section is for the model use when fine-tuned for a task, or when plugged into a larger ecosystem/app -->
[More Information Needed]
### Out-of-Scope Use
<!-- This section addresses misuse, malicious use, and uses that the model will not work well for. -->
[More Information Needed]
## Bias, Risks, and Limitations
<!-- This section is meant to convey both technical and sociotechnical limitations. -->
[More Information Needed]
### Recommendations
<!-- This section is meant to convey recommendations with respect to the bias, risk, and technical limitations. -->
Users (both direct and downstream) should be made aware of the risks, biases and limitations of the model. More information needed for further recommendations.
## How to Get Started with the Model
Use the code below to get started with the model.
[More Information Needed]
## Training Details
### Training Data
<!-- This should link to a Dataset Card, perhaps with a short stub of information on what the training data is all about as well as documentation related to data pre-processing or additional filtering. -->
[More Information Needed]
### Training Procedure
<!-- This relates heavily to the Technical Specifications. Content here should link to that section when it is relevant to the training procedure. -->
#### Preprocessing [optional]
[More Information Needed]
#### Training Hyperparameters
- **Training regime:** [More Information Needed] <!--fp32, fp16 mixed precision, bf16 mixed precision, bf16 non-mixed precision, fp16 non-mixed precision, fp8 mixed precision -->
#### Speeds, Sizes, Times [optional]
<!-- This section provides information about throughput, start/end time, checkpoint size if relevant, etc. -->
[More Information Needed]
## Evaluation
<!-- This section describes the evaluation protocols and provides the results. -->
### Testing Data, Factors & Metrics
#### Testing Data
<!-- This should link to a Dataset Card if possible. -->
[More Information Needed]
#### Factors
<!-- These are the things the evaluation is disaggregating by, e.g., subpopulations or domains. -->
[More Information Needed]
#### Metrics
<!-- These are the evaluation metrics being used, ideally with a description of why. -->
[More Information Needed]
### Results
[More Information Needed]
#### Summary
## Model Examination [optional]
<!-- Relevant interpretability work for the model goes here -->
[More Information Needed]
## Environmental Impact
<!-- Total emissions (in grams of CO2eq) and additional considerations, such as electricity usage, go here. Edit the suggested text below accordingly -->
Carbon emissions can be estimated using the [Machine Learning Impact calculator](https://mlco2.github.io/impact#compute) presented in [Lacoste et al. (2019)](https://arxiv.org/abs/1910.09700).
- **Hardware Type:** [More Information Needed]
- **Hours used:** [More Information Needed]
- **Cloud Provider:** [More Information Needed]
- **Compute Region:** [More Information Needed]
- **Carbon Emitted:** [More Information Needed]
## Technical Specifications [optional]
### Model Architecture and Objective
[More Information Needed]
### Compute Infrastructure
[More Information Needed]
#### Hardware
[More Information Needed]
#### Software
[More Information Needed]
## Citation [optional]
<!-- If there is a paper or blog post introducing the model, the APA and Bibtex information for that should go in this section. -->
**BibTeX:**
[More Information Needed]
**APA:**
[More Information Needed]
## Glossary [optional]
<!-- If relevant, include terms and calculations in this section that can help readers understand the model or model card. -->
[More Information Needed]
## More Information [optional]
[More Information Needed]
## Model Card Authors [optional]
[More Information Needed]
## Model Card Contact
[More Information Needed] |
Subsets and Splits
No community queries yet
The top public SQL queries from the community will appear here once available.