text
stringlengths 55
456k
| metadata
dict |
---|---|
<!-- Thank you for your contribution! Please review https://docs.ag2.ai/docs/contributor-guide/contributing before opening a pull request. -->
<!-- Please add a reviewer to the assignee section when you create a PR. If you don't have the access to it, we will shortly find a reviewer and assign them to your PR. -->
## Why are these changes needed?
<!-- Please give a short summary of the change and the problem this solves. -->
## Related issue number
<!-- For example: "Closes #1234" -->
## Checks
- [ ] I've included any doc changes needed for https://docs.ag2.ai/. See https://docs.ag2.ai/docs/contributor-guide/documentation to build and test documentation locally.
- [ ] I've added tests (if relevant) corresponding to the changes introduced in this PR.
- [ ] I've made sure all auto checks have passed. | {
"source": "ag2ai/ag2",
"title": ".github/PULL_REQUEST_TEMPLATE.md",
"url": "https://github.com/ag2ai/ag2/blob/main/.github/PULL_REQUEST_TEMPLATE.md",
"date": "2024-11-11T20:43:34",
"stars": 1869,
"description": "AG2 (formerly AutoGen): The Open-Source AgentOS. Join us at: https://discord.gg/pAbnFJrkgZ",
"file_size": 816
} |
# Contributing
## How to get a notebook displayed on the website
In the notebook metadata set the `tags` and `description` `front_matter` properties. For example:
```json
{
"...": "...",
"metadata": {
"...": "...",
"front_matter": {
"tags": ["code generation", "debugging"],
"description": "Use conversable language learning model agents to solve tasks and provide automatic feedback through a comprehensive example of writing, executing, and debugging Python code to compare stock price changes."
}
}
}
```
**Note**: Notebook metadata can be edited by opening the notebook in a text editor (Or "Open With..." -> "Text Editor" in VSCode)
The `tags` field is a list of tags that will be used to categorize the notebook. The `description` field is a brief description of the notebook.
## Best practices for authoring notebooks
The following points are best practices for authoring notebooks to ensure consistency and ease of use for the website.
- The Colab button will be automatically generated on the website for all notebooks where it is missing. Going forward, it is recommended not to include the Colab button in the notebook itself.
- Ensure the header is a `h1` header, - `#`
- Don't put anything between the yaml and the header
### Consistency for installation and LLM config
You don't need to explain in depth how to install AG2. Unless there are specific instructions for the notebook just use the following markdown snippet:
``````
````{=mdx}
:::info Requirements
Install `autogen`:
```bash
pip install autogen
```
For more information, please refer to the [installation guide](/docs/user-guide/basic-concepts/installing-ag2).
:::
````
``````
Or if extras are needed:
``````
````{=mdx}
:::info Requirements
Some extra dependencies are needed for this notebook, which can be installed via pip:
```bash
pip install autogen[retrievechat] flaml[automl]
```
For more information, please refer to the [installation guide](/docs/user-guide/basic-concepts/installing-ag2).
:::
````
``````
When specifying the config list, to ensure consistency it is best to use approximately the following code:
```python
import autogen
config_list = autogen.config_list_from_json(
env_or_file="OAI_CONFIG_LIST",
)
```
Then after the code cell where this is used, include the following markdown snippet:
``````
````{=mdx}
:::tip
Learn more about configuring LLMs for agents [here](/docs/topics/llm_configuration).
:::
````
``````
## Testing
Notebooks can be tested by running:
```sh
python website/process_notebooks.py test
```
This will automatically scan for all notebooks in the notebook/ and website/ dirs.
To test a specific notebook pass its path:
```sh
python website/process_notebooks.py test notebook/agentchat_logging.ipynb
```
Options:
- `--timeout` - timeout for a single notebook
- `--exit-on-first-fail` - stop executing further notebooks after the first one fails
### Skip tests
If a notebook needs to be skipped then add to the notebook metadata:
```json
{
"...": "...",
"metadata": {
"skip_test": "REASON"
}
}
```
## Metadata fields
All possible metadata fields are as follows:
```json
{
"...": "...",
"metadata": {
"...": "...",
"front_matter": {
"tags": "List[str] - List of tags to categorize the notebook",
"description": "str - Brief description of the notebook",
},
"skip_test": "str - Reason for skipping the test. If present, the notebook will be skipped during testing",
"skip_render": "str - Reason for skipping rendering the notebook. If present, the notebook will be left out of the website.",
"extra_files_to_copy": "List[str] - List of files to copy to the website. The paths are relative to the notebook directory",
}
}
``` | {
"source": "ag2ai/ag2",
"title": "notebook/contributing.md",
"url": "https://github.com/ag2ai/ag2/blob/main/notebook/contributing.md",
"date": "2024-11-11T20:43:34",
"stars": 1869,
"description": "AG2 (formerly AutoGen): The Open-Source AgentOS. Join us at: https://discord.gg/pAbnFJrkgZ",
"file_size": 3841
} |
# Website
This website is built using [Mintlify](https://mintlify.com/docs/quickstart), a modern website generator.
## How to get a notebook rendered on the website
See [here](https://github.com/ag2ai/ag2/blob/main/notebook/contributing.md#how-to-get-a-notebook-displayed-on-the-website) for instructions on how to get a notebook in the `notebook` directory rendered on the website.
## Build documentation locally
Follow these steps to build and serve the documentation on your local machine:
1. Install Node.js:
- Download and install [Node.js](https://nodejs.org/en/download/)
2. Install Quarto:
- Visit the Quarto download [page](https://quarto.org/docs/download/).
- Click on the Pre-release tab and download the latest version of Quarto.
- Ensure you install version `1.5.23` or higher.
3. Install Required Python Packages:
- From the project root directory, install the necessary Python packages by running:
```console
pip install -e ".[docs]"
```
4. Build and Serve the Documentation:
- To build and serve the documentation locally, run the following command from the project root directory:
```console
./scripts/docs_serve.sh
```
- Optionally, you can pass the `--force` flag to clean up all temporary files and generate the documentation from scratch:
```console
./scripts/docs_serve.sh --force
```
- The above command starts a local development server and opens up a browser window.
5. Handling Updates or Changes:
- Whenever you update the documentation, stop the server and re-run the `./scripts/docs_serve.sh` command to serve the docs with the latest changes and view them live.
- If deleted files are still displayed, it indicates cached or temporary files may be causing issues. To resolve this, use the `--force` flag to clean the build directory and regenerate the documentation.
By following these steps, you can build, serve, and update the documentation locally.
## Build with Dev Containers
To build and test documentation using Dev Containers, open the project using [VSCode](https://code.visualstudio.com/), press `Ctrl+Shift+P` and select `Dev Containers: Reopen in Container`.
This will open the project in a Dev Container with all the required dependencies installed.
Build and Serve the Documentation:
- Open a terminal and run the following commands from the project root directory to build and serve the documentation:
```console
pip install -e ".[docs]"
./scripts/docs_serve.sh
```
- Optionally, you can pass the `--force` flag to clean up all temporary files and generate the documentation from scratch:
```console
pip install -e ".[docs]"
./scripts/docs_serve.sh --force
```
Once done you should be able to access the documentation at `http://localhost:3000/`.
Handling Updates or Changes:
- Whenever you update the documentation, stop the server and re-run the `./scripts/docs_serve.sh` command to serve the docs with the latest changes and view them live.
- If deleted files are still displayed, it indicates cached or temporary files may be causing issues. To resolve this, use the `--force` flag to clean the build directory and regenerate the documentation. | {
"source": "ag2ai/ag2",
"title": "website/README.md",
"url": "https://github.com/ag2ai/ag2/blob/main/website/README.md",
"date": "2024-11-11T20:43:34",
"stars": 1869,
"description": "AG2 (formerly AutoGen): The Open-Source AgentOS. Join us at: https://discord.gg/pAbnFJrkgZ",
"file_size": 3311
} |
# :warning: DOCUMENTATION CODE SOURCES :warning:
To find a real docs, just visit our website: [https://faststream.airt.ai/latest/](https://faststream.airt.ai/latest/) | {
"source": "ag2ai/ag2",
"title": "website/mkdocs/README.md",
"url": "https://github.com/ag2ai/ag2/blob/main/website/mkdocs/README.md",
"date": "2024-11-11T20:43:34",
"stars": 1869,
"description": "AG2 (formerly AutoGen): The Open-Source AgentOS. Join us at: https://discord.gg/pAbnFJrkgZ",
"file_size": 167
} |
Agents for running the [AgentEval](https://docs.ag2.ai/blog/2023-11-20-AgentEval/) pipeline.
AgentEval is a process for evaluating a LLM-based system's performance on a given task.
When given a task to evaluate and a few example runs, the critic and subcritic agents create evaluation criteria for evaluating a system's solution. Once the criteria has been created, the quantifier agent can evaluate subsequent task solutions based on the generated criteria.
See our [blog post](https://docs.ag2.ai/blog/2024-06-21-AgentEval) for usage examples and general explanations. | {
"source": "ag2ai/ag2",
"title": "autogen/agentchat/contrib/agent_eval/README.md",
"url": "https://github.com/ag2ai/ag2/blob/main/autogen/agentchat/contrib/agent_eval/README.md",
"date": "2024-11-11T20:43:34",
"stars": 1869,
"description": "AG2 (formerly AutoGen): The Open-Source AgentOS. Join us at: https://discord.gg/pAbnFJrkgZ",
"file_size": 573
} |
# Introduction
This directory contains a library of manually created python tools. These tools have three categories: math, data_analysis and information_retrieval.
# Directory Layout
```
tools
├── README.md
├── data_analysis
│ ├── calculate_correlation.py
│ └── ...
├── information_retrieval
│ ├── arxiv_download.py
│ ├── arxiv_search.py
│ └── ...
├── math
│ ├── calculate_circle_area_from_diameter.py
│ └── ...
└── tool_description.tsv
```
Tools can be imported from `tools/{category}/{tool_name}.py` with exactly the same function name.
`tool_description.tsv` contains descriptions of tools for retrieval.
# How to use
Some tools require Bing Search API key and RapidAPI key. For Bing API, you can read more about how to get an API on the [Bing Web Search API](https://www.microsoft.com/en-us/bing/apis/bing-web-search-api) page. For RapidAPI, you can [sign up](https://rapidapi.com/auth/sign-up) and subscribe to these two links([link1](https://rapidapi.com/solid-api-solid-api-default/api/youtube-transcript3), [link2](https://rapidapi.com/420vijay47/api/youtube-mp3-downloader2)). These apis have free billing options and there is no need to worry about extra costs.
To install the requirements for running tools, use pip install.
```bash
pip install -r autogen/agentchat/contrib/captainagent/tools/requirements.txt
```
Whenever you run the tool-related code, remember to export the api keys to system variables.
```bash
export BING_API_KEY=""
export RAPID_API_KEY=""
```
or
```python
import os
os.environ["BING_API_KEY"] = ""
os.environ["RAPID_API_KEY"] = ""
``` | {
"source": "ag2ai/ag2",
"title": "autogen/agentchat/contrib/captainagent/tools/README.md",
"url": "https://github.com/ag2ai/ag2/blob/main/autogen/agentchat/contrib/captainagent/tools/README.md",
"date": "2024-11-11T20:43:34",
"stars": 1869,
"description": "AG2 (formerly AutoGen): The Open-Source AgentOS. Join us at: https://discord.gg/pAbnFJrkgZ",
"file_size": 1590
} |
## Delaware
## 45-4168768
(State or other jurisdiction of incorporation or organization)
(I.R.S. Employer Identification No.)
## 333 Summer Street Boston, Massachusetts
(Address of principal executive offices)
(Zip code)
## (617) 297-1005
(Registrant's telephone number, including area code)
Securities registered pursuant to Section 12(b) of the Act:
Table of Contents
| Title of each class | Trading Symbol(s) | Name of each exchange on which registered |
|--------------------------------------------------------|---------------------|---------------------------------------------|
| Class A common stock, par value of $0.000001 per share | TOST | New York Stock Exchange |
Indicate by check mark whether the registrant: (1) has filed all reports required to be filed by Section 13 or 15(d) of the Securities Exchange Act of 1934 during the preceding 12 months (or for such shorter period that the registrant was required to file such reports), and (2) has been subject to such filing requirements for the past 90 days. Yes ☒ No ☐
Indicate by check mark whether the registrant has submitted electronically every Interactive Data File required to be submitted pursuant to Rule 405 of Regulation S-T (§232.405 of this chapter) during the preceding 12 months (or for such shorter period that the registrant was required to submit such files). Yes ☒ No ☐
Indicate by check mark whether the registrant is a large accelerated filer, an accelerated filer, a non-accelerated filer, a smaller reporting company, or an emerging growth company. See the definitions of 'large accelerated filer,' 'accelerated filer,' 'smaller reporting company,' and 'emerging growth company' in Rule 12b-2 of the Exchange Act.
Large accelerated filer
☒
Accelerated filer
Non-accelerated filer
☐
☐
Smaller reporting company ☐
Emerging growth company
☐
with any new or revised financial accounting standards provided pursuant to Section 13(a) of the Exchange Act.
☐
02210
## UNITED STATES
## SECURITIES AND EXCHANGE COMMISSION
Washington, D.C. 20549
## FORM 10-Q
(Mark One)
☒ QUARTERLY REPORT PURSUANT TO SECTION 13 OR 15(d) OF THE SECURITIES EXCHANGE ACT OF 1934
For the quarterly period ended September 30, 2024
OR
☐ TRANSITION REPORT PURSUANT TO SECTION 13 OR 15(d) OF THE SECURITIES EXCHANGE ACT OF 1934
For the transition period from to
Commission File Number 001-40819
## Toast, Inc.
(Exact name of registrant as specified in its charter)
Table of Contents
TABLE OF CONTENTS
| | | Page |
|-------------------------------|---------------------------------------------------------------------------------------|--------|
| Part I. Financial Information | Part I. Financial Information | 1 |
| Item 1. | Financial Statements (unaudited) | 1 |
| | Condensed Consolidated Balance Sheets | 1 |
| | Condensed Consolidated Statements of Operations | 2 |
| | Condensed Consolidated Statements of Comprehensive Income (Loss) | 3 |
| | Condensed Consolidated Statements of Stockholders' Equity | 4 |
| | Condensed Consolidated Statements of Cash Flows | 6 |
| | Notes to Condensed Consolidated Financial Statements | 7 |
| Item 2. | Management's Discussion and Analysis of Financial Condition and Results of Operations | 17 |
| Item 3. | Quantitative and Qualitative Disclosures About Market Risk | 26 |
| Item 4. | Controls and Procedures | 26 |
| | Part II. Other Information | 27 |
| Item 1. | Legal Proceedings | 27 |
| Item 1A. | Risk Factors | 27 |
| Item 2. | Unregistered Sales of Equity Securities and Use of Proceeds | 27 |
| Item 3. | Defaults Upon Senior Securities | 28 |
| Item 4. | Mine Safety Disclosures | 28 |
| Item 5. | Other Information | 28 |
| Item 6. | Exhibits | 29 |
| | Signatures | 30 |
## Item 1. Financial Statements
## PART I. FINANCIAL INFORMATION
## TOAST, INC.
## CONDENSED CONSOLIDATED BALANCE SHEETS
(unaudited)
(in millions, except per share amounts)
Table of Contents
| | September 30, 2024 | December 31, 2023 |
|---------------------------------------------------------------------------------------------------------------------------------------------------------------------------------------------------------------------------------------------------------------------------------------------------------------------|----------------------|---------------------|
| Assets: | | |
| Current assets: | | |
| Cash and cash equivalents | $ 761 | 605 |
| Marketable securities | 511 | 519 |
| Accounts receivable, net | 105 | 69 |
| Inventories, net | 106 | 118 |
| Other current assets | 319 | 259 |
| Total current assets | 1,802 | 1,570 |
| Property and equipment, net | 95 | 75 |
| Operating lease right-of-use assets | 31 | 36 |
| Intangible assets, net | 22 | 26 |
| Goodwill | 113 | 113 |
| Restricted cash | 56 | 55 |
| Other non-current assets | 108 | 83 |
| Total non-current assets | 425 | 388 |
| Total assets | $ 2,227 | 1,958 |
| Liabilities and Stockholders' Equity: | | |
| Current liabilities: | | |
| Accounts payable | $ 30 | 32 |
| Deferred revenue | 62 | 39 |
| Accrued expenses and other current liabilities | 656 | 592 |
| Total current liabilities | 748 | 663 |
| Warrants to purchase common stock | 27 | 64 |
| Operating lease liabilities | 27 | 33 |
| Other long-term liabilities | 5 | 4 |
| Total liabilities | 807 | 764 |
| Commitments and Contingencies (Note 11) | | |
| Stockholders' Equity: | | |
| Preferred stock - par value $0.000001; 100 shares authorized, no shares issued or outstanding | - | - |
| Common stock, $0.000001 par value: Class A - 7,000 shares authorized; 468 and 429 shares issued and outstanding as of September 30, 2024 and December 31, 2023, respectively Class B - 700 shares authorized; 97 and 114 shares issued and outstanding as of September 30, 2024 and December 31, 2023, respectively | - | - |
| Accumulated other comprehensive income | 3 | - |
| Additional paid-in capital | 3,053 | 2,817 |
| Accumulated deficit | (1,636) | (1,623) |
| Total stockholders' equity | 1,420 | 1,194 |
| Total liabilities and stockholders' equity | $ 2,227 | 1,958 |
The accompanying notes are an integral part of these condensed consolidated financial statements.
## TOAST, INC.
## CONDENSED CONSOLIDATED STATEMENTS OF OPERATIONS
(unaudited)
(in millions, except per share amounts)
Table of Contents
| | Three Months Ended September 30, | Three Months Ended September 30, | Nine Months Ended September 30, | Nine Months Ended September 30, |
|----------------------------------------------------------------------|------------------------------------|------------------------------------|-----------------------------------|-----------------------------------|
| | 2024 | 2023 | 2024 | 2023 |
| Revenue: | | | | |
| Subscription services | $ 189 | $ 131 | $ 506 | $ 358 |
| Financial technology solutions | 1,067 | 856 | 2,963 | 2,338 |
| Hardware and professional services | 49 | 45 | 153 | 133 |
| Total revenue | 1,305 | 1,032 | 3,622 | 2,829 |
| Costs of revenue: | | | | |
| Subscription services | 56 | 43 | 159 | 118 |
| Financial technology solutions | 835 | 674 | 2,323 | 1,828 |
| Hardware and professional services | 91 | 88 | 279 | 271 |
| Amortization of acquired intangible assets | 1 | 1 | 4 | 4 |
| Total costs of revenue | 983 | 806 | 2,765 | 2,221 |
| Gross profit | 322 | 226 | 857 | 608 |
| Operating expenses: | | | | |
| Sales and marketing | 119 | 100 | 340 | 299 |
| Research and development | 89 | 87 | 258 | 264 |
| General and administrative | 80 | 98 | 229 | 276 |
| Restructuring expenses | - | - | 46 | - |
| Total operating expenses | 288 | 285 | 873 | 839 |
| Income (loss) from operations | 34 | (59) | (16) | (231) |
| Other income (expense): | | | | |
| Interest income, net | 9 | 10 | 30 | 27 |
| Change in fair value of warrant liability | (1) | 18 | (37) | (5) |
| Other income (expense), net | 15 | - | 13 | - |
| Income (loss) before taxes | 57 | (31) | (10) | (209) |
| Income tax expense | (1) | - | (3) | (1) |
| Net income (loss) | $ 56 | $ (31) | $ (13) | $ (210) |
| Earnings (loss) per share attributable to common stockholders: | | | | |
| Basic | $ 0.10 | $ (0.06) | $ (0.02) | $ (0.40) |
| Diluted | $ 0.07 | $ (0.09) | $ (0.02) | $ (0.40) |
| Weighted-average shares used in computing earnings (loss) per share: | | | | |
| Basic | 563 | 535 | 556 | 530 |
| Diluted | 590 | 536 | 556 | 530 |
The accompanying notes are an integral part of these condensed consolidated financial statements. | {
"source": "ag2ai/ag2",
"title": "test/agents/experimental/document_agent/pdf_parsed/Toast_financial_report.md",
"url": "https://github.com/ag2ai/ag2/blob/main/test/agents/experimental/document_agent/pdf_parsed/Toast_financial_report.md",
"date": "2024-11-11T20:43:34",
"stars": 1869,
"description": "AG2 (formerly AutoGen): The Open-Source AgentOS. Join us at: https://discord.gg/pAbnFJrkgZ",
"file_size": 26864
} |
## UNITED STATES
## SECURITIES AND EXCHANGE COMMISSION
## Washington, D.C. 20549
\_\_\_\_\_\_\_\_\_\_\_\_\_\_\_\_\_\_\_\_\_\_\_\_\_\_\_\_\_\_\_\_\_\_\_\_\_\_\_\_\_\_\_\_\_\_\_\_\_\_\_\_\_\_\_\_\_\_\_\_\_\_\_\_\_\_\_\_\_\_\_\_\_\_\_\_\_\_\_\_\_\_\_\_\_\_\_\_\_\_\_\_

## FORM 10-K
- ☒ ANNUAL REPORT PURSUANT TO SECTION 13 OR 15(d) OF THE SECURITIES EXCHANGE ACT OF 1934
For the fiscal year ended January 28, 2024
OR
- ☐ TRANSITION REPORT PURSUANT TO SECTION 13 OR 15(d) OF THE SECURITIES EXCHANGE ACT OF 1934
Commission file number: 0-23985
## NVIDIA CORPORATION
(Exact name of registrant as specified in its charter)
Delaware (State or other jurisdiction of incorporation or organization)
94-3177549 (I.R.S. Employer Identification No.)
2788 San Tomas Expressway, Santa Clara, California (Address of principal executive offices)
95051
(Zip Code)
Registrant's telephone number, including area code: (408) 486-2000 Securities registered pursuant to Section 12(b) of the Act: Trading Symbol(s) NVDA
Title of each class
Name of each exchange on which registered The Nasdaq Global Select Market
Common Stock, $0.001 par value per share
Securities registered pursuant to Section 12(g) of the Act:
None
Indicate by check mark if the registrant is a well-known seasoned issuer, as defined in Rule 405 of the Securities Act. Yes ☐ No ☒
Indicate by check mark if the registrant is not required to file reports pursuant to Section 13 or Section 15(d) of the Act. Yes
☐
No
☒
Indicate by check mark whether the registrant (1) has filed all reports required to be filed by Section 13 or 15(d) of the Securities Exchange Act of 1934 during the preceding 12 months (or for such shorter period that the registrant was required to file such reports), and (2) has been subject to such filing requirements for the past 90 days. Yes ☒ No ☐
Indicate by check mark whether the registrant has submitted electronically every Interactive Data File required to be submitted pursuant to Rule 405 of Regulation S-T (§232.405 of this chapter) during the preceding 12 months (or for such shorter period that the registrant was required to submit such files). Yes ☒ No ☐
Indicate by check mark whether the registrant is a large accelerated filer, an accelerated filer, a non-accelerated filer, a smaller reporting company, or an emerging growth company. See the definitions of 'large accelerated filer," 'accelerated filer," 'smaller reporting company," and "emerging growth company" in Rule 12b-2 of the Exchange Act.
Large accelerated filer
☒
Accelerated filer
☐ Non-accelerated filer
☐ Smaller reporting company
☐ Emerging growth company ☐
- If an emerging growth company, indicate by check mark if the registrant has elected not to use the extended transition period for complying with any new or revised financial accounting standards provided pursuant to Section 13(a) of the Exchange Act. ☐
Indicate by check mark whether the registrant has filed a report on and attestation to its management's assessment of the effectiveness of its internal control over financial reporting under Section 404(b) of the Sarbanes-Oxley Act (15 U.S.C. 7262(b)) by the registered public accounting firm that prepared or issued its audit report. ☒
If securities are registered pursuant to Section 12(b) of the Act, indicate by check mark whether the financial statements of the registrant included in the filing reflect the correction of an error to previously issued financial statements. ☐
Indicate by check mark whether any of those error corrections are restatements that required a recovery analysis of incentive-based compensation received by any of the registrant's executive officers during the relevant recovery period pursuant to §240.10D-1(b). ☐
Indicate by check mark whether the registrant is a shell company (as defined in Rule 12b-2 of the Act). Yes ☐ No ☒
The aggregate market value of the voting stock held by non-affiliates of the registrant as of July 28, 2023 was approximately $1.1 trillion (based on the closing sales price of the registrant's common stock as reported by the Nasdaq Global Select Market on July 28, 2023). This calculation excludes 105 million shares held by directors and executive officers of the registrant. This calculation does not exclude shares held by such organizations whose ownership exceeds 5% of the registrant's outstanding common stock that have represented to the registrant that they are registered investment advisers or investment companies registered under section 8 of the Investment Company Act of 1940.
The number of shares of common stock outstanding as of February 16, 2024 was 2.5 billion.
## DOCUMENTS INCORPORATED BY REFERENCE
Portions of the registrant's Proxy Statement for its 2024 Annual Meeting of Shareholders to be filed with the Securities and Exchange Commission pursuant to Regulation 14A not later than 120 days after the end of the fiscal year covered by this Annual Report on Form 10-K are incorporated by reference into Part III, Items 10-14 of this Annual Report on Form 10-K.
## Table of Contents
## NVIDIA Corporation
Table of Contents
| | | Page |
|------------|--------------------------------------------------------------------------------------------------------------|--------|
| | Part I | |
| Item 1. | Business | 4 |
| Item 1A. | Risk Factors | 13 |
| Item 1B. | Unresolved Staff Comments | 31 |
| Item 1C | Cybersecurity | 31 |
| Item 2. | Properties | 32 |
| Item 3. | Legal Proceedings | 32 |
| Item 4. | Mine Safety Disclosures | 32 |
| | Part II | |
| Item 5. | Market for Registrant's Common Equity, Related Stockholder Matters and Issuer Purchases of Equity Securities | 32 |
| Item 6. | [Reserved] | 33 |
| Item 7. | Management's Discussion and Analysis of Financial Condition and Results of Operations | 34 |
| Item 7A. | Quantitative and Qualitative Disclosures About Market Risk | 43 |
| Item 8. | Financial Statements and Supplementary Data | 44 |
| Item 9. | Changes in and Disagreements with Accountants on Accounting and Financial Disclosure | 44 |
| Item 9A. | Controls and Procedures | 44 |
| Item 9B. | Other Information | 45 |
| Item 9C. | Disclosure Regarding Foreign Jurisdictions that Prevent Inspections | 45 |
| | Part III | |
| Item 10. | Directors, Executive Officers and Corporate Governance | 45 |
| Item 11. | Executive Compensation | 46 |
| Item 12. | Security Ownership of Certain Beneficial Owners and Management and Related Stockholder Matters | 46 |
| Item 13. | Certain Relationships and Related Transactions, and Director Independence | 46 |
| Item 14. | Principal Accountant Fees and Services | 46 |
| | Part IV | |
| Item 15. | Exhibit and Financial Statement Schedules | 47 |
| Item 16. | Form 10-K Summary | 83 |
| Signatures | | 84 |
## Table of Contents
## Where You Can Find More Information
Investors and others should note that we announce material financial information to our investors using our investor relations website, press releases, SEC filings and public conference calls and webcasts. We also use the following social media channels as a means of disclosing information about the company, our products, our planned financial and other announcements and attendance at upcoming investor and industry conferences, and other matters and for complying with our disclosure obligations under Regulation FD:
NVIDIA X Account (https://twitter.com/nvidia)
NVIDIA Corporate Blog (http://blogs.nvidia.com)
NVIDIA Facebook Page (https://www.facebook.com/nvidia)
NVIDIA LinkedIn Page (http://www.linkedin.com/company/nvidia)
NVIDIA Instagram Page (https://www.instagram.com/nvidia)
In addition, investors and others can view NVIDIA videos on YouTube (https://www.YouTube.com/nvidia).
The information we post through these social media channels may be deemed material. Accordingly, investors should monitor these accounts and the blog, in addition to following our press releases, SEC filings and public conference calls and webcasts. This list may be updated from time to time. The information we post through these channels is not a part of this Annual Report on Form 10-K. These channels may be updated from time to time on NVIDIA's investor relations website.
## Forward-Looking Statements
This Annual Report on Form 10-K contains forward-looking statements which are based on our management's beliefs and assumptions and on information currently available to our management. In some cases, you can identify forward-looking statements by terms such as 'may,' 'will,' 'should,' 'could,' 'goal,' 'would,' 'expect,' 'plan,' 'anticipate,' 'believe,' 'estimate,' 'project,' 'predict,' 'potential,' and similar expressions intended to identify forward-looking statements. These statements involve known and unknown risks, uncertainties and other factors, which may cause our actual results, performance, time frames or achievements to be materially different from any future results, performance, time frames or achievements expressed or implied by the forward-looking statements. We discuss many of these risks, uncertainties, and other factors in this Annual Report on Form 10-K in greater detail under the heading 'Risk Factors.' Given these risks, uncertainties, and other factors, you should not place undue reliance on these forward-looking statements. Also, these forwardlooking statements represent our estimates and assumptions only as of the date of this filing. You should read this Annual Report on Form 10-K completely and with the understanding that our actual future results may be materially different from what we expect. We hereby qualify our forward-looking statements by these cautionary statements. Except as required by law, we assume no obligation to update these forward-looking statements publicly, or to update the reasons actual results could differ materially from those anticipated in these forward-looking statements, even if new information becomes available in the future.
All references to 'NVIDIA,' 'we,' 'us,' 'our,' or the 'Company' mean NVIDIA Corporation and its subsidiaries.
In addition, statements that 'we believe' and similar statements reflect our beliefs and opinions on the relevant subject. These statements are based upon information available to us as of the filing date of this Annual Report on Form 10-K, and while we believe such information forms a reasonable basis for such statements, such information may be limited or incomplete, and our statements should not be read to indicate that we have conducted an exhaustive inquiry into, or review of, all potentially available relevant information. These statements are inherently uncertain and investors are cautioned not to unduly rely upon these statements.
© 2024 NVIDIA Corporation. All rights reserved.
## Table of Contents
## Part I
## Item 1. Business
## Our Company
NVIDIA pioneered accelerated computing to help solve the most challenging computational problems. NVIDIA is now a full-stack computing infrastructure company with data-center-scale offerings that are reshaping industry.
Our full-stack includes the foundational CUDA programming model that runs on all NVIDIA GPUs, as well as hundreds of domain-specific software libraries, software development kits, or SDKs, and Application Programming Interfaces, or APIs. This deep and broad software stack accelerates the performance and eases the deployment of NVIDIA accelerated computing for computationally intensive workloads such as artificial intelligence, or AI, model training and inference, data analytics, scientific computing, and 3D graphics, with vertical-specific optimizations to address industries ranging from healthcare and telecom to automotive and manufacturing.
Our data-center-scale offerings are comprised of compute and networking solutions that can scale to tens of thousands of GPU-accelerated servers interconnected to function as a single giant computer; this type of data center architecture and scale is needed for the development and deployment of modern AI applications.
The GPU was initially used to simulate human imagination, enabling the virtual worlds of video games and films. Today, it also simulates human intelligence, enabling a deeper understanding of the physical world. Its parallel processing capabilities, supported by thousands of computing cores, are essential for deep learning algorithms. This form of AI, in which software writes itself by learning from large amounts of data, can serve as the brain of computers, robots and selfdriving cars that can perceive and understand the world. GPU-powered AI solutions are being developed by thousands of enterprises to deliver services and products that would have been immensely difficult or even impossible with traditional coding. Examples include generative AI, which can create new content such as text, code, images, audio, video, and molecule structures, and recommendation systems, which can recommend highly relevant content such as products, services, media or ads using deep neural networks trained on vast datasets that capture the user preferences.
NVIDIA has a platform strategy, bringing together hardware, systems, software, algorithms, libraries, and services to create unique value for the markets we serve. While the computing requirements of these end markets are diverse, we address them with a unified underlying architecture leveraging our GPUs and networking and software stacks. The programmable nature of our architecture allows us to support several multi-billion-dollar end markets with the same underlying technology by using a variety of software stacks developed either internally or by third-party developers and partners. The large and growing number of developers and installed base across our platforms strengthens our ecosystem and increases the value of our platform to our customers.
Innovation is at our core. We have invested over $45.3 billion in research and development since our inception, yielding inventions that are essential to modern computing. Our invention of the GPU in 1999 sparked the growth of the PC gaming market and redefined computer graphics. With our introduction of the CUDA programming model in 2006, we opened the parallel processing capabilities of our GPU to a broad range of compute-intensive applications, paving the way for the emergence of modern AI. In 2012, the AlexNet neural network, trained on NVIDIA GPUs, won the ImageNet computer image recognition competition, marking the 'Big Bang' moment of AI. We introduced our first Tensor Core GPU in 2017, built from the ground-up for the new era of AI, and our first autonomous driving system-on-chips, or SoC, in 2018. Our acquisition of Mellanox in 2020 expanded our innovation canvas to include networking and led to the introduction of a new processor class - the data processing unit, or DPU. Over the past 5 years, we have built full software stacks that run on top of our GPUs and CUDA to bring AI to the world's largest industries, including NVIDIA DRIVE stack for autonomous driving, Clara for healthcare, and Omniverse for industrial digitalization; and introduced the NVIDIA AI Enterprise software - essentially an operating system for enterprise AI applications. In 2023, we introduced our first data center CPU, Grace, built for giant-scale AI and high-performance computing. With a strong engineering culture, we drive fast, yet harmonized, product and technology innovations in all dimensions of computing including silicon, systems, networking, software and algorithms. More than half of our engineers work on software.
The world's leading cloud service providers, or CSPs, and consumer internet companies use our data center-scale accelerated computing platforms to enable, accelerate or enrich the services they deliver to billions of end users, including AI solutions and assistants, search, recommendations, social networking, online shopping, live video, and translation.
Enterprises and startups across a broad range of industries use our accelerated computing platforms to build new generative AI-enabled products and services, or to dramatically accelerate and reduce the costs of their workloads and workflows. The enterprise software industry uses them for new AI assistants and chatbots; the transportation industry for autonomous driving; the healthcare industry for accelerated and computer-aided drug discovery; and the financial services industry for customer support and fraud detection.
## Table of Contents
Researchers and developers use our computing solutions to accelerate a wide range of important applications, from simulating molecular dynamics to climate forecasting. With support for more than 3,500 applications, NVIDIA computing enables some of the most promising areas of discovery, from climate prediction to materials science and from wind tunnel simulation to genomics. Including GPUs and networking, NVIDIA powers over 75% of the supercomputers on the global TOP500 list, including 24 of the top 30 systems on the Green500 list.
Gamers choose NVIDIA GPUs to enjoy immersive, increasingly cinematic virtual worlds. In addition to serving the growing number of gamers, the market for PC GPUs is expanding because of the burgeoning population of live streamers, broadcasters, artists, and creators. With the advent of generative AI, we expect a broader set of PC users to choose NVIDIA GPUs for running generative AI applications locally on their PC, which is critical for privacy, latency, and cost-sensitive AI applications.
Professional artists, architects and designers use NVIDIA partner products accelerated with our GPUs and software platform for a range of creative and design use cases, such as creating visual effects in movies or designing buildings and products. In addition, generative AI is expanding the market for our workstationclass GPUs, as more enterprise customers develop and deploy AI applications with their data on-premises.
Headquartered in Santa Clara, California, NVIDIA was incorporated in California in April 1993 and reincorporated in Delaware in April 1998.
## Our Businesses
We report our business results in two segments.
The Compute & Networking segment is comprised of our Data Center accelerated computing platforms and end-to-end networking platforms including Quantum for InfiniBand and Spectrum for Ethernet; our NVIDIA DRIVE automated-driving platform and automotive development agreements; Jetson robotics and other embedded platforms; NVIDIA AI Enterprise and other software; and DGX Cloud software and services.
The Graphics segment includes GeForce GPUs for gaming and PCs, the GeForce NOW game streaming service and related infrastructure; Quadro/NVIDIA RTX GPUs for enterprise workstation graphics; virtual GPU, or vGPU, software for cloud-based visual and virtual computing; automotive platforms for infotainment systems; and Omniverse Enterprise software for building and operating metaverse and 3D internet applications.
## Our Markets
We specialize in markets where our computing platforms can provide tremendous acceleration for applications. These platforms incorporate processors, interconnects, software, algorithms, systems, and services to deliver unique value. Our platforms address four large markets where our expertise is critical: Data Center, Gaming, Professional Visualization, and Automotive.
## Data Center
The NVIDIA Data Center platform is focused on accelerating the most compute-intensive workloads, such as AI, data analytics, graphics and scientific computing, delivering significantly better performance and power efficiency relative to conventional CPU-only approaches. It is deployed in cloud, hyperscale, on-premises and edge data centers. The platform consists of compute and networking offerings typically delivered to customers as systems, subsystems, or modules, along with software and services.
Our compute offerings include supercomputing platforms and servers, bringing together our energy efficient GPUs, DPUs, interconnects, and fully optimized AI and high-performance computing, or HPC, software stacks. In addition, they include NVIDIA AI Enterprise software; our DGX Cloud service; and a growing body of acceleration libraries, APIs, SDKs, and domain-specific application frameworks.
Our networking offerings include end-to-end platforms for InfiniBand and Ethernet, consisting of network adapters, cables, DPUs, and switch systems, as well as a full software stack. This has enabled us to architect data center-scale computing platforms that can interconnect thousands of compute nodes with highperformance networking. While historically the server was the unit of computing, as AI and HPC workloads have become extremely large spanning thousands of compute nodes, the data center has become the new unit of computing, with networking as an integral part.
Our end customers include the world's leading public cloud and consumer internet companies, thousands of enterprises and startups, and public sector entities. We work with industry leaders to help build or transform their applications and data center infrastructure. Our direct customers include original equipment manufacturers, or OEMs, original device manufacturers, or ODMs, system integrators and distributors which we partner with to help bring our products to market. We also have partnerships in automotive, healthcare, financial services, manufacturing, and retail among others, to accelerate the adoption of AI.
## Table of Contents
At the foundation of the NVIDIA accelerated computing platform are our GPUs, which excel at parallel workloads such as the training and inferencing of neural networks. They are available in the NVIDIA accelerated computing platform and in industry standard servers from every major cloud provider and server maker. Beyond GPUs, our data center platform expanded to include DPUs in fiscal year 2022 and CPUs in fiscal year 2024. We can optimize across the entire computing, networking and storage stack to deliver data center-scale computing solutions.
While our approach starts with powerful chips, what makes it a full-stack computing platform is our large body of software, including the CUDA parallel programming model, the CUDA-X collection of acceleration libraries, APIs, SDKs, and domain-specific application frameworks.
In addition to software delivered to customers as an integral part of our data center computing platform, we offer paid licenses to NVIDIA AI Enterprise, a comprehensive suite of enterprise-grade AI software and NVIDIA vGPU software for graphics-rich virtual desktops and workstations.
In fiscal year 2024, we launched the NVIDIA DGX Cloud, an AI-training-as-a-service platform which includes cloud-based infrastructure and software for AI, customizable pretrained AI models, and access to NVIDIA experts. We have partnered with leading cloud service providers to host this service in their data centers.
## Gaming
Gaming is the largest entertainment industry, with PC gaming as the predominant platform. Many factors propel its growth, including new high production value games and franchises, the continued rise of competitive gaming, or eSports, social connectivity and the increasing popularity of game streamers, modders, or gamers who remaster games, and creators.
Our gaming platforms leverage our GPUs and sophisticated software to enhance the gaming experience with smoother, higher quality graphics. We developed NVIDIA RTX to bring next generation graphics and AI to games. NVIDIA RTX features ray tracing technology for real-time, cinematic-quality rendering. Ray tracing, which has long been used for special effects in the movie industry, is a computationally intensive technique that simulates the physical behavior of light to achieve greater realism in computer-generated scenes. NVIDIA RTX also features deep learning super sampling, or NVIDIA DLSS, our AI technology that boosts frame rates while generating beautiful, sharp images for games. RTX GPUs will also accelerate a new generation of AI applications. With an installed base of over 100 million AI capable PCs, more than 500 RTX AI-enabled applications and games, and a robust suite of development tools, RTX is already the AI PC leader.
Our products for the gaming market include GeForce RTX and GeForce GTX GPUs for gaming desktop and laptop PCs, GeForce NOW cloud gaming for playing PC games on underpowered devices, as well as SoCs and development services for game consoles.
## Professional Visualization
We serve the Professional Visualization market by working closely with independent software vendors, or ISVs, to optimize their offerings for NVIDIA GPUs. Our GPU computing platform enhances productivity and introduces new capabilities for critical workflows in many fields, such as design and manufacturing and digital content creation. Design and manufacturing encompass computer-aided design, architectural design, consumer-products manufacturing, medical instrumentation, and aerospace. Digital content creation includes professional video editing and post-production, special effects for films, and broadcasttelevision graphics.
The NVIDIA RTX platform makes it possible to render film-quality, photorealistic objects and environments with physically accurate shadows, reflections and refractions using ray tracing in real-time. Many leading 3D design and content creation applications developed by our ecosystem partners now support RTX, allowing professionals to accelerate and transform their workflows with NVIDIA RTX GPUs and software.
We offer NVIDIA Omniverse as a development platform and operating system for building virtual world simulation applications, available as a software subscription for enterprise use and free for individual use. Industrial enterprises are adopting Omniverse's 3D and simulation technologies to digitalize their complex physical assets, processes, and environments - building digital twins of factories, real time 3D product configurators, testing and validating autonomous robots and vehicles, powered by NVIDIA accelerated computing infrastructure on-premises and in the cloud.
## Automotive
Automotive market is comprised of platform solutions for automated driving and in-vehicle cockpit computing. Leveraging our technology leadership in AI and building on our long-standing automotive relationships, we are delivering a complete end-to-end solution for the AV market under the DRIVE Hyperion brand. We have demonstrated multiple applications of AI within the car: AI can drive the car itself as a pilot in fully autonomous mode or it can also be a co-pilot, assisting the human driver while creating a safer driving experience.
## Table of Contents
We are working with several hundred partners in the automotive ecosystem including automakers, truck makers, tier-one suppliers, sensor manufacturers, automotive research institutions, HD mapping companies, and startups to develop and deploy AI systems for self-driving vehicles. Our unified AI computing architecture starts with training deep neural networks using our Data Center computing solutions, and then running a full perception, fusion, planning, and control stack within the vehicle on the NVIDIA DRIVE Hyperion platform. DRIVE Hyperion consists of the high-performance, energy efficient DRIVE AGX computing hardware, a reference sensor set that supports full self-driving capability as well as an open, modular DRIVE software platform for autonomous driving, mapping, and parking services, and intelligent in-vehicle experiences.
In addition, we offer a scalable data center-based simulation solution, NVIDIA DRIVE Sim, based on NVIDIA Omniverse software, for digital cockpit development, as well as for testing and validating a self-driving platform. Our unique end-to-end, software-defined approach is designed for continuous innovation and continuous development, enabling cars to receive over-the-air updates to add new features and capabilities throughout the life of a vehicle.
## Business Strategies
NVIDIA's key strategies that shape our overall business approach include:
Advancing the NVIDIA accelerated computing platform. Our accelerated computing platform can solve complex problems in significantly less time and with lower power consumption than alternative computational approaches. Indeed, it can help solve problems that were previously deemed unsolvable. We work to deliver continued performance leaps that outpace Moore's Law by leveraging innovation across the architecture, chip design, system, interconnect, and software layers. This full-stack innovation approach allows us to deliver order-of-magnitude performance advantages relative to legacy approaches in our target markets, which include Data Center, Gaming, Professional Visualization, and Automotive. While the computing requirements of these end markets are diverse, we address them with a unified underlying architecture leveraging our GPUs, CUDA and networking technologies as the fundamental building blocks. The programmable nature of our architecture allows us to make leveraged investments in research and development: we can support several multi-billion-dollar end markets with shared underlying technology by using a variety of software stacks developed either internally or by third-party developers and partners. We utilize this platform approach in each of our target markets.
Extending our technology and platform leadership in AI. We provide a complete, end-to-end accelerated computing platform for AI, addressing both training and inferencing. This includes full-stack data center-scale compute and networking solutions across processing units, interconnects, systems, and software. Our compute solutions include all three major processing units in AI servers - GPUs, CPUs, and DPUs. GPUs are uniquely suited to AI, and we will continue to add AI-specific features to our GPU architecture to further extend our leadership position. In addition, we offer DGX Cloud, an AI-training-as-a-service platform, and NeMo - a complete solution for building enterprise-ready Large Language Models, or LLMs, using open source and proprietary LLMs created by NVIDIA and third parties. Our AI technology leadership is reinforced by our large and expanding ecosystem in a virtuous cycle. Our computing platforms are available from virtually every major server maker and CSP, as well as on our own AI supercomputers. There are over 4.7 million developers worldwide using CUDA and our other software tools to help deploy our technology in our target markets. We evangelize AI through partnerships with hundreds of universities and thousands of startups through our Inception program. Additionally, our Deep Learning Institute provides instruction on the latest techniques on how to design, train, and deploy neural networks in applications using our accelerated computing platform.
Extending our technology and platform leadership in computer graphics. We believe that computer graphics infused with AI is fundamental to the continued expansion and evolution of computing. We apply our research and development resources to enhance the user experience for consumer entertainment and professional visualization applications and create new virtual world and simulation capabilities. Our technologies are instrumental in driving the gaming, design, and creative industries forward, as developers leverage our libraries and algorithms to deliver an optimized experience on our GeForce and NVIDIA RTX platforms. Our computer graphics platforms leverage AI end-to-end, from the developer tools and cloud services to the Tensor Cores included in all RTX-class GPUs. For example, NVIDIA Avatar Cloud Engine, or ACE, is a suite of technologies that help developers bring digital avatars to life with generative AI, running in the cloud or locally on the PC. GeForce Experience enhances each gamer's experience by optimizing their PC's settings, as well as enabling the recording and sharing of gameplay. Our Studio drivers enhance and accelerate a number of popular creative applications. Omniverse is real-time 3D design collaboration and virtual world simulation software that empowers artists, designers, and creators to connect and collaborate in leading design applications. We also enable interactive graphics applications - such as games, movie and photo editing and design software - to be accessed by almost any device, almost anywhere, through our cloud platforms such as vGPU for enterprise and GeForce NOW for gaming.
Advancing the leading autonomous vehicle platform. We believe the advent of autonomous vehicles, or AV, and electric vehicles, or EV, is revolutionizing the transportation industry. The algorithms required for autonomous driving - such as perception, localization, and planning - are too complex for legacy handcoded approaches and will use multiple neural networks instead. In addition, EV makers are looking for next-generation centralized car computers that integrate a wide range of intelligent functions into a single AI compute platform. Therefore, we provide an AI-based hardware and software solution, designed and implemented from the ground up based on automotive safety standards, for the AV and EV market under the DRIVE brand, which we are bringing to market through our partnerships with automotive OEMs,
## Table of Contents
tier-1 suppliers, and start-ups. Our AV solution also includes the GPU-based hardware required to train the neural networks before their in-vehicle deployment, as well as to re-simulate their operation prior to any over-the-air software updates. We believe our comprehensive, top-to-bottom and end-to-end approach will enable the transportation industry to solve the complex problems arising from the shift to autonomous driving.
Leveraging our intellectual property, or IP. We believe our IP is a valuable asset that can be accessed by our customers and partners through license and development agreements when they desire to build such capabilities directly into their own products or have us do so through a custom development. Such license and development arrangements can further enhance the reach of our technology.
## Sales and Marketing
Our worldwide sales and marketing strategy is key to achieving our objective of providing markets with our high-performance and efficient computing platforms and software. Our sales and marketing teams, located across our global markets, work closely with end customers and various industry ecosystems through our partner network. Our partner network incorporates global, regional and specialized CSPs, OEMs, ODMs, system integrators, independent software vendors, or ISVs, add-in board manufacturers, or AIBs, distributors, automotive manufacturers and tier-1 automotive suppliers, and other ecosystem participants.
Members of our sales team have technical expertise and product and industry knowledge. We also employ a team of application engineers and solution architects to provide pre-sales assistance to our partner network in designing, testing, and qualifying system designs that incorporate our platforms. For example, our solution architects work with CSPs to provide pre-sales assistance to optimize their hardware and software infrastructure for generative AI and LLM training and deployment. They also work with foundation model and enterprise software developers to optimize the training and fine-tuning of their models and services, and with enterprise end-users, often in collaboration with their global system integrator of choice, to fine-tune models and build AI applications. We believe that the depth and quality of our design support are key to improving our partner network's time-to-market, maintaining a high level of customer satisfaction, and fostering relationships that encourage our end customers and partner network to use the next generation of our products within each platform.
To encourage the development of applications optimized for our platforms and software, we seek to establish and maintain strong relationships in the software development community. Engineering and marketing personnel engage with key software developers to promote and discuss our platforms, as well as to ascertain individual product requirements and solve technical problems. Our developer program supports the development of AI frameworks, SDKs, and APIs for software applications and game titles that are optimized for our platforms. Our Deep Learning Institute provides in-person and online training for developers in industries and organizations around the world to build AI and accelerated computing applications that leverage our platforms.
## Seasonality
Our computing platforms serve a diverse set of markets such as data centers, gaming, professional visualization, and automotive. Our desktop gaming products typically see stronger revenue in the second half of our fiscal year. Historical seasonality trends may not repeat.
## Manufacturing
We utilize a fabless and contracting manufacturing strategy, whereby we employ and partner with key suppliers for all phases of the manufacturing process, including wafer fabrication, assembly, testing, and packaging. We use the expertise of industry-leading suppliers that are certified by the International Organization for Standardization in such areas as fabrication, assembly, quality control and assurance, reliability, and testing. Additionally, we can avoid many of the significant costs and risks associated with owning and operating manufacturing operations. While we may directly procure certain raw materials used in the production of our products, such as memory, substrates, and a variety of components, our suppliers are responsible for procurement of most raw materials used in the production of our products. As a result, we can focus our resources on product design, quality assurance, marketing, and customer support. In periods of growth, we may place non-cancellable inventory orders for certain product components in advance of our historical lead times, pay premiums, or provide deposits to secure future supply and capacity and may need to continue to do so.
We have expanded our supplier relationships to build redundancy and resilience in our operations to provide long-term manufacturing capacity aligned with growing customer demand. Our supply chain is concentrated in the Asia-Pacific region. We utilize foundries, such as Taiwan Semiconductor Manufacturing Company Limited, or TSMC, and Samsung Electronics Co., Ltd., or Samsung, to produce our semiconductor wafers. We purchase memory from Micron Technology, Inc., SK Hynix Inc., and Samsung. We utilize CoWoS technology for semiconductor packaging. We engage with independent subcontractors and contract manufacturers such as Hon Hai Precision Industry Co., Ltd., Wistron Corporation, and Fabrinet to perform assembly, testing and packaging of our final products.
## Table of Contents
## Competition
The market for our products is intensely competitive and is characterized by rapid technological change and evolving industry standards. We believe that the principal competitive factors in this market are performance, breadth of product offerings, access to customers and partners and distribution channels, software support, conformity to industry standard APIs, manufacturing capabilities, processor pricing, and total system costs. We believe that our ability to remain competitive will depend on how well we are able to anticipate the features and functions that customers and partners will demand and whether we are able to deliver consistent volumes of our products at acceptable levels of quality and at competitive prices. We expect competition to increase from both existing competitors and new market entrants with products that may be lower priced than ours or may provide better performance or additional features not provided by our products. In addition, it is possible that new competitors or alliances among competitors could emerge and acquire significant market share.
A significant source of competition comes from companies that provide or intend to provide GPUs, CPUs, DPUs, embedded SoCs, and other accelerated, AI computing processor products, and providers of semiconductor-based high-performance interconnect products based on InfiniBand, Ethernet, Fibre Channel, and proprietary technologies. Some of our competitors may have greater marketing, financial, distribution and manufacturing resources than we do and may be more able to adapt to customers or technological changes. We expect an increasingly competitive environment in the future.
Our current competitors include:
- · suppliers and licensors of hardware and software for discrete and integrated GPUs, custom chips and other accelerated computing solutions, including solutions offered for AI, such as Advanced Micro Devices, Inc., or AMD, Huawei Technologies Co. Ltd., or Huawei, and Intel Corporation, or Intel;
- · large cloud services companies with internal teams designing hardware and software that incorporate accelerated or AI computing functionality as part of their internal solutions or platforms, such as Alibaba Group, Alphabet Inc., Amazon, Inc., or Amazon, Baidu, Inc., Huawei, and Microsoft Corporation, or Microsoft;
- · suppliers of Arm-based CPUs and companies that incorporate hardware and software for CPUs as part of their internal solutions or platforms, such as Amazon, Huawei, and Microsoft;
- · suppliers of hardware and software for SoC products that are used in servers or embedded into automobiles, autonomous machines, and gaming devices, such as Ambarella, Inc., AMD, Broadcom Inc., or Broadcom, Intel, Qualcomm Incorporated, Renesas Electronics Corporation, and Samsung, or companies with internal teams designing SoC products for their own products and services, such as Tesla, Inc.; and
- · networking products consisting of switches, network adapters (including DPUs), and cable solutions (including optical modules) include such as AMD, Arista Networks, Broadcom, Cisco Systems, Inc., Hewlett Packard Enterprise Company, Huawei, Intel, Lumentum Holdings, and Marvell Technology Group as well as internal teams of system vendors and large cloud services companies.
## Patents and Proprietary Rights
We rely primarily on a combination of patents, trademarks, trade secrets, employee and third-party nondisclosure agreements, and licensing arrangements to protect our IP in the United States and internationally. Our currently issued patents have expiration dates from February 2024 to August 2043. We have numerous patents issued, allowed, and pending in the United States and in foreign jurisdictions. Our patents and pending patent applications primarily relate to our products and the technology used in connection with our products. We also rely on international treaties, organizations, and foreign laws to protect our IP. The laws of certain foreign countries in which our products are or may be manufactured or sold, including various countries in Asia, may not protect our products or IP rights to the same extent as the laws of the United States. This decreased protection makes the possibility of piracy of our technology and products more likely. We continuously assess whether and where to seek formal protection for innovations and technologies based on such factors as:
- · the location in which our products are manufactured;
- · our strategic technology or product directions in different countries;
- · the degree to which IP laws exist and are meaningfully enforced in different jurisdictions; and
- · the commercial significance of our operations and our competitors' operations in particular countries and regions.
We have licensed technology from third parties and expect to continue entering such license agreements.
## Table of Contents
## Government Regulations
Our worldwide business activities are subject to various laws, rules, and regulations of the United States as well as of foreign governments.
During the third quarter of fiscal year 2023, the U.S. government, or the USG, announced licensing requirements that, with certain exceptions, impact exports to China (including Hong Kong and Macau) and Russia of our A100 and H100 integrated circuits, DGX or any other systems or boards which incorporate A100 or H100 integrated circuits.
In July 2023, the USG informed us of an additional licensing requirement for a subset of A100 and H100 products destined to certain customers and other regions, including some countries in the Middle East.
In October 2023, the USG announced new and updated licensing requirements that became effective in our fourth quarter of fiscal year 2024 for exports to China and Country Groups D1, D4, and D5 (including but not limited to Saudi Arabia, the United Arab Emirates, and Vietnam, but excluding Israel) of our products exceeding certain performance thresholds, including A100, A800, H100, H800, L4, L40, L40S and RTX 4090. The licensing requirements also apply to the export of products exceeding certain performance thresholds to a party headquartered in, or with an ultimate parent headquartered in, Country Group D5, including China. On October 23, 2023, the USG informed us the licensing requirements were effective immediately for shipments of our A100, A800, H100, H800, and L40S products.
Our competitive position has been harmed, and our competitive position and future results may be further harmed in the long term, if there are further changes in the USG's export controls. Given the increasing strategic importance of AI and rising geopolitical tensions, the USG has changed and may again change the export control rules at any time and further subject a wider range of our products to export restrictions and licensing requirements, negatively impacting our business and financial results. In the event of such change, we may be unable to sell our inventory of such products and may be unable to develop replacement products not subject to the licensing requirements, effectively excluding us from all or part of the China market, as well as other impacted markets, including the Middle East.
While we work to enhance the resiliency and redundancy of our supply chain, which is currently concentrated in the Asia-Pacific region, new and existing export controls or changes to existing export controls could limit alternative manufacturing locations and negatively impact our business. Refer to 'Item 1A. Risk Factors - Risks Related to Regulatory, Legal, Our Stock and Other Matters' for a discussion of this potential impact.
Compliance with laws, rules, and regulations has not otherwise had a material effect upon our capital expenditures, results of operations, or competitive position and we do not currently anticipate material capital expenditures for environmental control facilities. Compliance with existing or future governmental regulations, including, but not limited to, those pertaining to IP ownership and infringement, taxes, import and export requirements and tariffs, anti-corruption, business acquisitions, foreign exchange controls and cash repatriation restrictions, data privacy requirements, competition and antitrust, advertising, employment, product regulations, cybersecurity, environmental, health and safety requirements, the responsible use of AI, climate change, cryptocurrency, and consumer laws, could increase our costs, impact our competitive position, and otherwise may have a material adverse impact on our business, financial condition and results of operations in subsequent periods. Refer to 'Item 1A. Risk Factors' for a discussion of these potential impacts.
## Sustainability and Governance
NVIDIA invents computing technologies that improve lives and address global challenges. Our goal is to integrate sound environmental, social, and corporate governance principles and practices into every aspect of the Company. The Nominating and Corporate Governance Committee of our Board of Directors is responsible for reviewing and discussing with management our practices related to sustainability and corporate governance. We assess our programs annually in consideration of stakeholder expectations, market trends, and business risks and opportunities. These issues are important for our continued business success and reflect the topics of highest concern to NVIDIA and our stakeholders.
The following section and the Human Capital Management Section below provide an overview of our principles and practices. More information can be found on our website and in our annual Sustainability Report. Information contained on our website or in our annual Sustainability Report is not incorporated by reference into this or any other report we file with the Securities and Exchange Commission, or the SEC. Refer to 'Item 1A. Risk Factors' for a discussion of risks and uncertainties we face related to sustainability.
## Climate Change
In the area of environmental sustainability, we address our climate impacts across our product lifecycle and assess risks, including current and emerging regulations and market impacts.
In May 2023, we published metrics related to our environmental impact for fiscal year 2023. Fiscal year 2024 metrics are expected to be published in the first half of fiscal year 2025. There has been no material impact to our capital expenditures, results of operations or competitive position associated with global environmental sustainability regulations, compliance, or costs from sourcing renewable energy. By the end of fiscal year 2025, our goal is to purchase
## Table of Contents
or generate enough renewable energy to match 100% of our global electricity usage for our offices and data centers. In fiscal year 2023, we increased the percentage of our total electricity use matched by renewable energy purchases to 44%. By fiscal year 2026, we aim to engage manufacturing suppliers comprising at least 67% of NVIDIA's scope 3 category 1 GHG emissions with goal of effecting supplier adoption of science-based targets.
Whether it is creation of technology to power next-generation laptops or designs to support high-performance supercomputers, improving energy efficiency is important in our research, development, and design processes. GPU-accelerated computing is inherently more energy efficient than traditional computing for many workloads because it is optimized for throughput, performance per watt, and certain AI workloads. The energy efficiency of our products is evidenced by our continued strong presence on the Green500 list of the most energy-efficient systems. We powered 24 of the top 30 most energy efficient systems, including the top supercomputer, on the Green500 list.
We plan to build Earth-2, a digital twin of the Earth on NVIDIA AI and NVIDIA Omniverse platforms. Earth-2 will enable scientists, companies, and policy makers to do ultra-high-resolution predictions of the impact of climate change and explore mitigation and adaptation strategies.
## Human Capital Management
We believe that our employees are our greatest assets, and they play a key role in creating long-term value for our stakeholders. As of the end of fiscal year 2024, we had approximately 29,600 employees in 36 countries, 22,200 were engaged in research and development and 7,400 were engaged in sales, marketing, operations, and administrative positions. The Compensation Committee of our Board of Directors assists in the oversight of policies and strategies relating to human capital management.
To be competitive and execute our business strategy successfully, we must recruit, develop, and retain talented employees, including qualified executives, scientists, engineers, and technical and non-technical staff.
## Recruitment
As the demand for global technical talent continues to be competitive, we have grown our technical workforce and have been successful in attracting top talent to NVIDIA. We have attracted talent globally through our strong employer brand and differentiated hiring strategies for college, professional, and leadership talent. Our workforce is 83% technical and 49% hold advanced degrees. Additionally, we have increased focus on diversity recruiting, resulting in an increase in global female hiring in each channel. Our own employees help to surface top talent, with over 40% of our new hires in fiscal year 2024 coming from employee referrals.
## Development and Retention
To support employee development, we provide opportunities to learn on-the-job through training courses, targeted development programs, mentoring and peer coaching and ongoing feedback. We have a library of live and on-demand learning experiences that include workshops, panel discussions, and speaker forums. We create learning paths focused on our most common development needs and constantly upgrade our offerings to ensure that our employees are exposed to the most current content and technologies available. We offer tuition reimbursement programs to subsidize educational programs and advanced certifications. We implemented a career coaching service to provide one-on-one guidance to employees, and encourage internal job mobility. We have implemented specifically designed mentoring and development programs for women and employees from traditionally underrepresented groups to ensure widespread readiness for future advancement.
To evaluate employee sentiment and engagement, we use pulse surveys, a suggestion box, and an anonymous third-party platform. Pulse surveys help us gain insight into employee experience and provides employee-generated ideas so that we can take targeted action. The suggestion box is an always-on, interactive tool where employees share their thoughts about making our company a better place to work. The anonymous third-party platform is designed to protect the identity of the reporter and provide a mechanism for reporters to follow an investigation and receive responses.
We want NVIDIA to be a place where people can build their careers over their lifetime. Our employees tend to come and stay. In fiscal year 2024, our overall turnover rate was 2.7%.
## Compensation, Benefits, and Well-Being
Our compensation program rewards performance and is structured to encourage employees to invest in the Company's future. Employees receive equity, except where unavailable due to local regulations, that is tied to the value of our stock price and vests over time to retain employees while simultaneously aligning their interests with those of our shareholders.
We offer comprehensive benefits to support our employees' and their families' physical health, well-being, and financial health. Programs include 401(k) programs in the U.S., statutory and supplemental pension programs outside the U.S., our employee stock purchase program, flexible work hours, and time off policies to address mental health, stress, and time-management challenges. We evaluate our benefit offerings globally and aim to provide comparable support across the regions where we operate. We are committed to providing tailored benefits based on the needs of our Community Resource Groups and continuing our support for parents, both new birth parents and those who wish to become parents.
Our support is enhanced during times of crisis, such as war or economic volatility, to take care of our existing team of world-class talent and their families.
## Diversity, Inclusion, and Belonging
We believe that diverse teams fuel innovation, and we are committed to creating an inclusive culture that supports all employees.
When recruiting for new talent or developing our current employees, we strive to build a diverse talent pipeline that includes those underrepresented in the technology field, including women, Black/African American, and Hispanic/Latino candidates.
To this end, we have been:
- · Partnering with institutions and professional organizations serving historically underrepresented communities;
- · Embedding dedicated recruiting teams to business areas to shepherd underrepresented candidates through the interview process and find internal opportunities;
- · Supporting the development of women employees through programs aimed at building a pipeline of future leaders;
- · Providing peer support and executive sponsors for our internal community resource groups;
- · Providing training and education to managers and peers on fostering supportive environments and recruiting for diversity;
- · Track equity and parity in retention, promotions, pay, and employee engagement scores; and
- · Measuring year over year progress and providing leadership visibility on diversity efforts.
As of the end of fiscal year 2024, our global workforce was 79% male, 20% female, and 1% not declared, with 6% of our workforce in the United States composed of Black or African American and Hispanic or Latino employees.
## Flexible Working Environment
We support a flexible work environment, understanding that many employees want the ability to work from home under certain conditions. This flexibility supports diverse hiring, retention, and employee engagement, which we believe makes NVIDIA a great place to work.
During fiscal year 2025, we will continue to have a flexible work environment and maintain our company wide 2-days off a quarter for employees to rest and recharge.
## Information About Our Executive Officers
The following sets forth certain information regarding our executive officers, their ages, and positions as of February 16, 2024:
Table of Contents
| Name | Age | Position |
|------------------|-------|------------------------------------------------------|
| Jen-Hsun Huang | 60 | President and Chief Executive Officer |
| Colette M. Kress | 56 | Executive Vice President and Chief Financial Officer |
| Ajay K. Puri | 69 | Executive Vice President, Worldwide Field Operations |
| Debora Shoquist | 69 | Executive Vice President, Operations |
| Timothy S. Teter | 57 | Executive Vice President and General Counsel |
Jen-Hsun Huang co-founded NVIDIA in 1993 and has served as our President, Chief Executive Officer, and a member of the Board of Directors since our inception. From 1985 to 1993, Mr. Huang was employed at LSI Logic Corporation, a computer chip manufacturer, where he held a variety of positions including as Director of Coreware, the business unit responsible for LSI's SOC. From 1983 to 1985, Mr. Huang was a microprocessor designer for AMD, a semiconductor company. Mr. Huang holds a B.S.E.E. degree from Oregon State University and an M.S.E.E. degree from Stanford University.
Colette M. Kress joined NVIDIA in 2013 as Executive Vice President and Chief Financial Officer. Prior to NVIDIA, Ms. Kress most recently served as Senior Vice President and Chief Financial Officer of the Business Technology and Operations Finance organization at Cisco Systems, Inc., a networking equipment company, since 2010. At Cisco, Ms. Kress was responsible for financial strategy, planning, reporting and business development for all business segments, engineering and operations. From 1997 to 2010 Ms. Kress held a variety of positions at Microsoft, a software company, including, beginning in 2006, Chief Financial Officer of the Server and Tools division, where Ms. Kress was responsible for financial
## Table of Contents
strategy, planning, reporting and business development for the division. Prior to joining Microsoft, Ms. Kress spent eight years at Texas Instruments Incorporated, a semiconductor company, where she held a variety of finance positions. Ms. Kress holds a B.S. degree in Finance from University of Arizona and an M.B.A. degree from Southern Methodist University.
Ajay K. Puri joined NVIDIA in 2005 as Senior Vice President, Worldwide Sales and became Executive Vice President, Worldwide Field Operations in 2009. Prior to NVIDIA, he held positions in sales, marketing, and general management over a 22-year career at Sun Microsystems, Inc., a computing systems company. Mr. Puri previously held marketing, management consulting, and product development positions at Hewlett-Packard, an information technology company, Booz Allen Hamilton Inc., a management and technology consulting company, and Texas Instruments Incorporated. Mr. Puri holds a B.S.E.E. degree from the University of Minnesota, an M.S.E.E. degree from the California Institute of Technology and an M.B.A. degree from Harvard Business School.
Debora Shoquist joined NVIDIA in 2007 as Senior Vice President of Operations and in 2009 became Executive Vice President of Operations. Prior to NVIDIA, Ms. Shoquist served from 2004 to 2007 as Executive Vice President of Operations at JDS Uniphase Corp., a provider of communications test and measurement solutions and optical products for the telecommunications industry. She served from 2002 to 2004 as Senior Vice President and General Manager of the ElectroOptics business at Coherent, Inc., a manufacturer of commercial and scientific laser equipment. Previously, she worked at Quantum Corp., a data protection company, as President of the Personal Computer Hard Disk Drive Division, and at Hewlett-Packard. Ms. Shoquist holds a B.S. degree in Electrical Engineering from Kansas State University and a B.S. degree in Biology from Santa Clara University.
Timothy S. Teter joined NVIDIA in 2017 as Senior Vice President, General Counsel and Secretary and became Executive Vice President, General Counsel and Secretary in February 2018. Prior to NVIDIA, Mr. Teter spent more than two decades at the law firm of Cooley LLP, where he focused on litigating patent and technology related matters. Prior to attending law school, he worked as an engineer at Lockheed Missiles and Space Company, an aerospace company. Mr. Teter holds a B.S. degree in Mechanical Engineering from the University of California at Davis and a J.D. degree from Stanford Law School.
## Available Information
Our annual reports on Form 10-K, quarterly reports on Form 10-Q, current reports on Form 8-K and, if applicable, amendments to those reports filed or furnished pursuant to Section 13(a) or 15(d) of the Securities Exchange Act of 1934, as amended, or the Exchange Act, are available free of charge on or through our website, http://www.nvidia.com , as soon as reasonably practicable after we electronically file such material with, or furnish it to, the Securities and Exchange Commission, or the SEC. The SEC's website, http://www.sec.gov , contains reports, proxy and information statements, and other information regarding issuers that file electronically with the SEC. Our web site and the information on it or connected to it are not a part of this Annual Report on Form 10-K.
## Item 1A. Risk Factors
The following risk factors should be considered in addition to the other information in this Annual Report on Form 10-K. The following risks could harm our business, financial condition, results of operations or reputation, which could cause our stock price to decline. Additional risks, trends and uncertainties not presently known to us or that we currently believe are immaterial may also harm our business, financial condition, results of operations or reputation.
## Risk Factors Summary
## Risks Related to Our Industry and Markets
- · Failure to meet the evolving needs of our industry may adversely impact our financial results.
- · Competition could adversely impact our market share and financial results.
## Risks Related to Demand, Supply and Manufacturing
- · Failure to estimate customer demand accurately has led and could lead to mismatches between supply and demand.
- · Dependency on third-party suppliers and their technology to manufacture, assemble, test, or package our products reduces our control over product quantity and quality, manufacturing yields, and product delivery schedules and could harm our business.
- · Defects in our products have caused and could cause us to incur significant expenses to remediate and could damage our business.
## Risks Related to Our Global Operating Business
- · Adverse economic conditions may harm our business.
## Table of Contents
- · International sales and operations are a significant part of our business, which exposes us to risks that could harm our business.
- · Product, system security and data breaches and cyber-attacks could disrupt our operations and adversely affect our financial condition, stock price and reputation.
- · Business disruptions could harm our operations and financial results.
- · Climate change may have a long-term impact on our business.
- · We may not be able to realize the potential benefits of business investments or acquisitions, nor successfully integrate acquisition targets.
- · A significant amount of our revenue stems from a limited number of partners and distributors and we have a concentration of sales to end customers, and our revenue could be adversely affected if we lose or are prevented from selling to any of these end customers.
- · We may be unable to attract, retain and motivate our executives and key employees.
- · Modification or interruption of our business processes and information systems may disrupt our business, and internal controls.
- · Our operating results have in the past fluctuated and may in the future fluctuate, and if our operating results are below the expectations of securities analysts or investors, our stock price could decline.
## Risks Related to Regulatory, Legal, Our Stock and Other Matters
- · We are subject to complex laws, rules and regulations, and political and other actions, which may adversely impact our business.
- · Increased scrutiny from shareholders, regulators, and others regarding our corporate sustainability practices could result in financial, reputational, or operational harm and liability.
- · Issues relating to the responsible use of our technologies, including AI, may result in reputational or financial harm and liability.
- · Adequately protecting our IP rights could be costly, and our ability to compete could be harmed if we are unsuccessful or if we are prohibited from making or selling our products.
- · We are subject to stringent and changing data privacy and security laws, rules, regulations, and other obligations. These areas could damage our reputation, deter customers, affect product design, or result in legal or regulatory proceedings and liability.
- · Our operating results may be adversely impacted by additional tax liabilities, higher than expected tax rates, changes in tax laws, and other tax-related factors.
- · Our business is exposed to the risks associated with litigation, investigations, and regulatory proceedings.
- · Our indebtedness could adversely affect our financial position and cash flows from operations and prevent us from implementing our strategy or fulfilling our contractual obligations.
- · Delaware law, provisions in our governing documents and our agreement with Microsoft could delay or prevent a change in control.
## Risk Factors
## Risks Related to Our Industry and Markets
## Failure to meet the evolving needs of our industry and markets may adversely impact our financial results.
Our accelerated computing platforms experience rapid changes in technology, customer requirements, competitive products, and industry standards.
## Our success depends on our ability to:
- · timely identify industry changes, adapt our strategies, and develop new or enhance and maintain existing products and technologies that meet the evolving needs of these markets, including due to unexpected changes in industry standards or disruptive technological innovation that could render our products incompatible with products developed by other companies;
## Table of Contents
- · develop or acquire new products and technologies through investments in research and development;
- · launch new offerings with new business models including software, services, and cloud solutions, as well as software-, infrastructure-, or platform-as-aservice solutions;
- · expand the ecosystem for our products and technologies;
- · meet evolving and prevailing customer and industry safety, security, reliability expectations, and compliance standards;
- · manage product and software lifecycles to maintain customer and end-user satisfaction;
- · develop, acquire, maintain, and secure access to the internal and external infrastructure needed to scale our business, including sufficient energy for powering data centers using our products, acquisition integrations, customer support, e-commerce, IP licensing capabilities and cloud service capacity; and
- · complete technical, financial, operational, compliance, sales and marketing investments for the above activities.
We have invested in research and development in markets where we have a limited operating history, which may not produce meaningful revenue for several years, if at all. If we fail to develop or monetize new products and technologies, or if they do not become widely adopted, our financial results could be adversely affected. Obtaining design wins may involve a lengthy process and depends on our ability to anticipate and provide features and functionality that customers will demand. They also do not guarantee revenue. Failure to obtain a design win may prevent us from obtaining future design wins in subsequent generations. We cannot ensure that the products and technologies we bring to market will provide value to our customers and partners. If we fail any of these key success criteria, our financial results may be harmed.
We have begun offering enterprise customers NVIDIA DGX Cloud services directly and through our network of partners, which include cloud-based infrastructure, software and services for training and deploying AI models, and NVIDIA AI Foundations for customizable pretrained AI models. We have partnered with CSPs to host such software and services in their data centers, and we entered and may continue to enter into multi-year cloud service agreements to support these offerings and our research and development activities. The timing and availability of these cloud services has changed and may continue to change, impacting our revenue, expenses, and development timelines. NVIDIA DGX Cloud services may not be successful and will take time, resources, and investment. We also offer or plan to offer standalone software solutions, including NVIDIA AI Enterprise, NVIDIA Omniverse, NVIDIA DRIVE, and several other software solutions. These new business models or strategies may not be successful, and we may fail to sell any meaningful standalone software or services. We may incur significant costs and may not achieve any significant revenue from these offerings.
## Competition could adversely impact our market share and financial results.
Our target markets remain competitive, and competition may intensify with expanding and changing product and service offerings, industry standards, customer needs, new entrants and consolidations. Our competitors' products, services and technologies, including those mentioned above in this Annual Report on Form 10-K, may be cheaper or provide better functionality or features than ours, which has resulted and may in the future result in lower-than-expected selling prices for our products. Some of our competitors operate their own fabrication facilities, and have longer operating histories, larger customer bases, more comprehensive IP portfolios and patent protections, more design wins, and greater financial, sales, marketing and distribution resources than we do. These competitors may be able to acquire market share and/or prevent us from doing so, more effectively identify and capitalize upon opportunities in new markets and end-user trends, more quickly transition their products, and impinge on our ability to procure sufficient foundry capacity and scarce input materials during a supply-constrained environment, which could harm our business. Some of our customers have in-house expertise and internal development capabilities similar to some of ours and can use or develop their own solutions to replace those we are providing. For example, others may offer cloud-based services that compete with our AI cloud service offerings, and we may not be able to establish market share sufficient to achieve the scale necessary to meet our business objectives. If we are unable to successfully compete in this environment, demand for our products, services and technologies could decrease and we may not establish meaningful revenue.
## Risks Related to Demand, Supply and Manufacturing
## Failure to estimate customer demand accurately has led and could lead to mismatches between supply and demand.
We use third parties to manufacture and assemble our products, and we have long manufacturing lead times. We are not provided guaranteed wafer, component and capacity supply, and our supply deliveries and production may be non-linear within a quarter or year. If our estimates of customer demand are inaccurate, as we have experienced in the past, there could be a significant mismatch between supply and demand. This mismatch has resulted in both product shortages and excess inventory, has varied across our market platforms, and has significantly harmed our financial results.
We build finished products and maintain inventory in advance of anticipated demand. While we have in the past entered and may in the future enter into longterm supply and capacity commitments, we may not be able to secure sufficient
## Table of Contents
commitments for capacity to address our business needs, or our long-term demand expectations may change. These risks may increase as we shorten our product development cycles, enter new lines of business, or integrate new suppliers or components into our supply chain, creating additional supply chain complexity. Additionally, our ability to sell certain products has been and could be impeded if components necessary for the finished products are not available from third parties. This risk may increase as a result of our platform strategy. In periods of shortages impacting the semiconductor industry and/or limited supply or capacity in our supply chain, the lead times on our orders may be extended. We have previously experienced and may continue to experience extended lead times of more than 12 months. We have paid premiums and provided deposits to secure future supply and capacity, which have increased our product costs and may continue to do so. If our existing suppliers are unable to scale their capabilities to meet our supply needs, we may require additional sources of capacity, which may require additional deposits. We may not have the ability to reduce our supply commitments at the same rate or at all if our revenue declines.
Many additional factors have caused and/or could in the future cause us to either underestimate or overestimate our customers' future demand for our products, or otherwise cause a mismatch between supply and demand for our products and impact the timing and volume of our revenue, including:
- · changes in product development cycles and time to market;
- · competing technologies and competitor product releases and announcements;
- · changes in business and economic conditions resulting in decreased end demand;
- · sudden or sustained government lockdowns or actions to control case spread of global or local health issues;
- · rapidly changing technology or customer requirements;
- · the availability of sufficient data center capacity and energy for customers to procure;
- · new product introductions and transitions resulting in less demand for existing products;
- · new or unexpected end-use cases;
- · increase in demand for competitive products, including competitive actions;
- · business decisions made by third parties;
- · the demand for accelerated or AI-related cloud services, including our own software and NVIDIA DGX Cloud services;
- · changes that impact the ecosystem for the architectures underlying our products and technologies;
- · the demand for our products; or
- · government actions or changes in governmental policies, such as export controls or increased restrictions on gaming usage.
Demand for our data center systems and products surged in fiscal year 2024. Entering fiscal year 2025, we are gathering customer demand indications across several product transitions. We have demand visibility for our new data center products ramping later in fiscal year 2025. We have increased our supply and capacity purchases with existing suppliers, added new vendors and entered into prepaid manufacturing and capacity agreements. These increased purchase volumes, the number of suppliers, and the integration of new vendors into our supply chain may create more complexity and execution risk. We may continue to enter into new supplier and capacity arrangements. Our purchase commitments and obligations for inventory and manufacturing capacity at the end of fiscal year 2024 were impacted by shortening lead times for certain components. Supply of Hopper architecture products is improving, and demand remains very strong. We expect our next-generation products to be supply-constrained based upon demand indications. We may incur inventory provisions or impairments if our inventory or supply or capacity commitments exceed demand for our products or demand declines.
Our customer orders and longer-term demand estimates may change or may not be correct, as we have experienced in the past. Product transitions are complex and can impact our revenue as we often ship both new and prior architecture products simultaneously and we and our channel partners prepare to ship and support new products. Due to our product introduction cycles, we are almost always in various stages of transitioning the architecture of our Data Center, Professional Visualization, and Gaming products. We will have a broader and faster Data Center product launch cadence to meet a growing and diverse set of AI opportunities. The increased frequency of these transitions may magnify the challenges associated with managing our supply and demand due to long manufacturing lead times. Qualification time for new products, customers anticipating product transitions and channel partners reducing channel inventory of prior architectures ahead of new product introductions can create reductions or volatility in our revenue. We have experienced and may in the future experience reduced demand for current generation architectures when customers anticipate
## Table of Contents
transitions, and we may be unable to sell multiple product architectures at the same time for current and future architecture transitions. If we are unable to execute our architectural transitions as planned for any reason, our financial results may be negatively impacted. The increasing frequency and complexity of newly introduced products may result in unanticipated quality or production issues that could increase the magnitude of inventory provisions, warranty or other costs or result in product delays. Deployment of new products to customers creates additional challenges due to the complexity of our technologies, which has impacted and may in the future impact the timing of customer purchases or otherwise impact our demand. While we have managed prior product transitions and have previously sold multiple product architectures at the same time, these transitions are difficult, may impair our ability to predict demand and impact our supply mix, and we may incur additional costs.
Many end customers often do not purchase directly from us but instead purchase indirectly through multiple OEMs, ODMs, system integrators, distributors, and other channel partners. As a result, the decisions made by our multiple OEMs, ODMs, system integrators, distributors, and other channel partners, and in response to changing market conditions and changes in end-user demand for our products, have impacted and could in the future continue to impact our ability to properly forecast demand, particularly as they are based on estimates provided by various downstream parties.
If we underestimate our customers' future demand for our products, our foundry partners may not have adequate lead-time or capacity to increase production and we may not be able to obtain sufficient inventory to fill orders on a timely basis. Even if we are able to increase supply to meet customer demand, we may not be able to do so in a timely manner, or our contract manufacturers may experience supply constraints. If we cannot procure sufficient supply to meet demand or otherwise fail to fulfill our customers' orders on a timely basis, or at all, our customer relationships could be damaged, we could lose revenue and market share and our reputation could be harmed. Additionally, since some of our products are part of a complex data center buildout, supply constraints or availability issues with respect to any one component have had and may have a broader revenue impact.
If we overestimate our customers' future demand for our products, or if customers cancel or defer orders or choose to purchase from our competitors, we may not be able to reduce our inventory or other contractual purchase commitments. In the past, we have experienced a reduction in average selling prices, including due to channel pricing programs that we have implemented and may continue to implement, as a result of our overestimation of future demand, and we may need to continue these reductions. We have had to increase prices for certain of our products as a result of our suppliers' increase in prices, and we may need to continue to do so for other products in the future. We have also written down our inventory, incurred cancellation penalties, and recorded impairments and may have to do so in the future. These impacts were amplified by our placement of non-cancellable and non-returnable purchasing terms well in advance of our historical lead times and could be exacerbated if we need to make changes to the design of future products. The risk of these impacts has increased and may continue to increase as our purchase obligations and prepaids have grown and are expected to continue to grow and become a greater portion of our total supply. All of these factors may negatively impact our gross margins and financial results.
We build technology and introduce products for new and innovative use cases and applications, such as NVIDIA DGX Cloud services, NVIDIA AI Foundations, Omniverse platform, LLMs, and generative AI models. Our demand estimates for new use cases, applications, and services can be incorrect and create volatility in our revenue or supply levels, and we may not be able to generate significant revenue from these use cases, applications, and services. Recent technologies, such as generative AI models, have emerged, and while they have driven increased demand for Data Center, the long-term trajectory is unknown. Because our products may be used in multiple use cases and applications, it is difficult for us to estimate with any reasonable degree of precision the impact of generative AI models on our reported revenue or forecasted demand. Additionally, we started shipping our CPU product offerings, the Grace CPU and Grace Hopper Superchips, in the third quarter of fiscal year 2024. Our ability to adequately predict our CPU demand may create volatility in our revenue or supply levels.
Challenges in estimating demand could become more pronounced or volatile in the future on both a global and regional basis. Extended lead times may occur if we experience other supply constraints caused by natural disasters, pandemics or other events. In addition, geopolitical tensions, such as those involving Taiwan and China, which comprise a significant portion of our revenue and where we have suppliers, contract manufacturers, and assembly partners who are critical to our supply continuity, could have a material adverse impact on us.
The use of our GPUs other than that for which they were designed and marketed, including new and unexpected use cases, has impacted and can in the future impact demand for our products, including by leading to inconsistent spikes and drops in demand. For example, several years ago, our Gaming GPUs began to be used for mining digital currencies, such as Ethereum. It is difficult for us to estimate with any reasonable degree of precision the past or current impact of cryptocurrency mining, or forecast the future impact of cryptocurrency mining, on demand for our products. Volatility in the cryptocurrency market, including new compute technologies, price changes in cryptocurrencies, government cryptocurrency policies and regulations, new cryptocurrency standards and changes in the method of verifying blockchain transactions, has impacted and can in the future impact cryptocurrency mining and demand for our products and can further impact our ability to estimate demand for our products. Changes to cryptocurrency standards and processes including, but not limited to, the Ethereum 2.0 merge in 2022, have reduced and may in the future decrease the usage of GPUs for Ethereum mining. This has created and may in the future create increased aftermarket sales of our
## Table of Contents
GPUs, which could negatively impact retail prices for our GPUs and reduce demand for our new GPUs. In general, our new products or previously sold products may be resold online or on the unauthorized 'gray market,' which also makes demand forecasting difficult. Gray market products and reseller marketplaces compete with our new products and distribution channels.
Additionally, we depend on developers, customers and other third parties to build, enhance, and maintain accelerated computing applications that leverage our platforms. We also rely on third-party content providers and publishers to make their content available on our platforms, such as GeForce NOW. Failure by developers, customers, and other third parties to build, enhance, and maintain applications that leverage our platforms, or failure by third-party content providers or publishers to make their content available on reasonable terms or at all for use by our customers or end users on our platforms, could adversely affect customer demand.
## Dependency on third-party suppliers and their technology to manufacture, assemble, test, or package our products reduces our control over product quantity and quality, manufacturing yields, and product delivery schedules and could harm our business.
We depend on foundries to manufacture our semiconductor wafers using their fabrication equipment and techniques. We do not assemble, test, or package our products, but instead contract with independent subcontractors. These subcontractors assist with procuring components used in our systems, boards, and products. We face several risks which have adversely affected or could adversely affect our ability to meet customer demand and scale our supply chain, negatively impact longer-term demand for our products and services, and adversely affect our business operations, gross margin, revenue and/or financial results, including:
- · lack of guaranteed supply of wafer, component and capacity or decommitment and potential higher wafer and component prices, from incorrectly estimating demand and failing to place orders with our suppliers with sufficient quantities or in a timely manner;
- · failure by our foundries or contract manufacturers to procure raw materials or provide adequate levels of manufacturing or test capacity for our products;
- · failure by our foundries to develop, obtain or successfully implement high quality process technologies, including transitions to smaller geometry process technologies such as advanced process node technologies and memory designs needed to manufacture our products;
- · failure by our suppliers to comply with our policies and expectations and emerging regulatory requirements;
- · limited number and geographic concentration of global suppliers, foundries, contract manufacturers, assembly and test providers and memory manufacturers;
- · loss of a supplier and additional expense and/or production delays as a result of qualifying a new foundry or subcontractor and commencing volume production or testing in the event of a loss, addition or change of a supplier;
- · lack of direct control over product quantity, quality and delivery schedules;
- · suppliers or their suppliers failing to supply high quality products and/or making changes to their products without our qualification;
- · delays in product shipments, shortages, a decrease in product quality and/or higher expenses in the event our subcontractors or foundries prioritize our competitors' or other customers' orders over ours;
- · requirements to place orders that are not cancellable upon changes in demand or requirements to prepay for supply in advance;
- · low manufacturing yields resulting from a failure in our product design or a foundry's proprietary process technology; and
- · disruptions in manufacturing, assembly and other processes due to closures related to heat waves, earthquakes, fires, or other natural disasters and electricity conservation efforts.
## Defects in our products have caused and could cause us to incur significant expenses to remediate, which can damage our reputation and cause us to lose market share.
Our hardware and software product and service offerings are complex. They have in the past and may in the future contain defects or security vulnerabilities or experience failures or unsatisfactory performance due to any number of issues in design, fabrication, packaging, materials, bugs and/or use within a system. These risks may increase as our products are introduced into new devices, markets, technologies and applications or as new versions are released. These risks further increase when we rely on partners to supply and manufacture components that are used in our products, as these arrangements reduce our direct control over production. AI software products we or our partners offer rely on
## Table of Contents
training data that may originate from third parties and new training methods, and the resulting products may contain unknown or undetected defects and errors, or reflect unintended bias. Although arrangements with component providers may contain provisions for product defect expense reimbursement, we generally remain responsible to the customer for warranty product defects that may occur from time to time. Some failures in our products or services have been in the past and may in the future be only discovered after a product or service has been shipped or used. Undiscovered vulnerabilities in our products or services could result in loss of data or intangible property, or expose our customers to unscrupulous third parties who develop and deploy malicious software programs that could attack our products or services. Defects or failure of our offerings to perform to specifications could lead to substantial damage to the products in which our offerings have been integrated by OEMs, ODMs, AIBs and automotive manufacturers and tier 1 automotive suppliers, and to the user of such end product. Any such defect may cause us to incur significant warranty, support and repair or replacement costs as part of a product recall or otherwise, write-off the value of related inventory, and divert the attention of our engineering and management personnel from our product development efforts to find and correct the issue. Our efforts to remedy these issues may not be timely or satisfactory to our customers. An error or defect in new products, releases or related software drivers after commencement of commercial shipments could result in failure to achieve market acceptance, loss of design wins, temporary or permanent withdrawal from a product or market and harm to our relationships with existing and prospective customers and partners and consumers' perceptions of our brand, which would in turn negatively impact our business operations, gross margin, revenue and/or financial results. We may be required to reimburse our customers, partners or consumers, including for costs to repair or replace products in the field or in connection with indemnification obligations, or pay fines imposed by regulatory agencies.
For example, in fiscal year 2023, a defect was identified in a third-party component embedded in certain Data Center products. This defect has had, and other defects may in the future have, an adverse effect on our cost and supply of components and finished goods. These costs could be significant in future periods. We recorded a net warranty liability during fiscal year 2023 primarily in connection with this defect. While we believe we have accurately recorded for warranty obligations, we may need to record additional amounts in the future if our estimate proves to be incorrect. In general, if a product liability claim regarding any of our products is brought against us, even if the alleged damage is due to the actions or inactions of a third party, such as within our supply chain, the cost of defending the claim could be significant and would divert the efforts of our technical and management personnel and harm our business. Further, our business liability insurance may be inadequate or future coverage may be unavailable on acceptable terms, which could adversely impact our financial results.
## Risks Related to Our Global Operating Business
## Adverse economic conditions may harm our business.
Economic and industry uncertainty or changes, including recession or slowing growth, inflation, changes or uncertainty in fiscal, monetary or trade policy, disruptions to capital markets and the banking system, currency fluctuations, higher interest rates, tighter credit, lower capital expenditures by businesses, including on IT infrastructure, increases in unemployment, labor shortages, and lower consumer confidence and spending, global supply chain constraints and global economic and geopolitical developments have in the past and/or could in the future have adverse, wide-ranging effects on our business and financial results, including:
- · increased costs for wafers, components, logistics, and other supply chain expenses, which have negatively impacted our gross margin in the past and may do so in the future;
- · increased supply, employee, facilities and infrastructure costs and volatility in the financial markets, which have reduced and may in the future reduce our margins;
- · decrease in demand for our products, services and technologies and those of our customers, partners or licensees;
- · the inability of our suppliers to deliver on their supply commitments to us and our customers' or our licensees' inability to supply products to customers and/or end users;
- · limits on our ability to forecast operating results and make business decisions;
- · the insolvency of key suppliers, distributors, customers, cloud service providers, data center providers, licensing parties or other third parties we rely on;
- · reduced profitability of customers, which may cause them to scale back operations, exit businesses, file for bankruptcy protection and potentially cease operations, or lead to mergers, consolidations or strategic alliances among other companies, which could adversely affect our ability to compete effectively; and
- · increased credit and collectability risks, higher borrowing costs or reduced availability of capital markets, reduced liquidity, adverse impacts on our customers and suppliers, failures of counterparties, including financial institutions and insurers, asset impairments, and declines in the value of our financial instruments.
## Table of Contents
Adverse developments affecting financial institutions, such as bank failures or instability, or concerns or speculation about similar events or risks, could lead to market-wide liquidity problems and other disruptions, which could impact our customers' ability to fulfill their payment obligations to us, our vendors' ability to fulfill their contractual obligations to us, or our ability to fulfill our own obligations.
Additionally, we maintain an investment portfolio of various holdings, types, and maturities. These investments are subject to general credit, liquidity, market and interest rate risks, which may be exacerbated by market downturns or events that affect global financial markets, as described above. A majority of our investment portfolio comprises USG securities. A decline in global financial markets for long periods or a downgrade of the USG credit rating due to an actual or threatened default on government debt could result in higher interest rates, a decline in the value of the U.S. dollar, reduced market liquidity or other adverse conditions. These factors could cause an unrealized or realized loss position in our investments or require us to record impairment charges.
## International sales and operations are a significant part of our business, which exposes us to risks that could harm our business.
We sell our products internationally, and we also have operations and conduct business internationally. Our semiconductor wafers are manufactured, assembled, tested and packaged by third parties located outside of the United States, and we generated 56% of our revenue in fiscal year 2024 from sales outside of the United States. Our sales to China decreased as a percentage of total Data Center revenue from 19% in fiscal year 2023 to 14% in fiscal year 2024. Although we have not received licenses from the USG to ship restricted products to China, we have started to ship alternatives to the China market in small volumes. China represented a mid-single digit percentage of our Data Center revenue in the fourth quarter of fiscal year 2024 due to USG licensing requirements and we expect China to be in a similar range in the first quarter of fiscal year 2025. The global nature of our business subjects us to a number of risks and uncertainties, which have had in the past and could in the future have a material adverse effect on our business, financial condition and results of operations. These include domestic and international economic and political conditions in countries in which we and our suppliers and manufacturers do business, government lockdowns to control case spread of global or local health issues, differing legal standards with respect to protection of IP and employment practices, different domestic and international business and cultural practices, disruptions to capital markets, counter-inflation policies, currency fluctuations, natural disasters, acts of war or other military actions, terrorism, public health issues and other catastrophic events.
## Product, system security, and data protection breaches, as well as cyber-attacks, could disrupt our operations, reduce our expected revenue, increase our expenses, and significantly harm our business and reputation.
Security breaches, computer malware, social-engineering attacks, denial-of-service attacks, software bugs, server malfunctions, software or hardware failures, loss of data or other information technology assets, and other cyber-attacks are becoming increasingly sophisticated, making it more difficult to successfully detect, defend against them or implement adequate preventative measures.
Cyber-attacks, including ransomware attacks by organized criminal threat actors, nation-states, and nation-state-supported actors, may become more prevalent and severe. Our ability to recover from ransomware attacks may be limited if our backups have been affected by the attack, or if restoring from backups is delayed or not feasible.
Individuals, groups of hackers and sophisticated organizations, including nation-states and nation-state-supported actors, and other threat actors have engaged and are expected to continue to engage in cyber-attacks. Additionally, some actors are using AI technology to launch more automated, targeted and coordinated attacks. Due to geopolitical conflicts and during times of war or other major conflicts, we and the third parties we rely upon may be vulnerable to a heightened risk of cyber-attacks that could materially disrupt our ability to provide services and products. We may also face cybersecurity threats due to error or intentional misconduct by employees, contractors or other third-party service providers. Certain aspects of effective cybersecurity are dependent upon our employees, contractors and/or other third-party service providers safeguarding our sensitive information and adhering to our security policies and access control mechanisms. We have in the past experienced, and may in the future experience, security incidents arising from a failure to properly handle sensitive information or adhere to our security policies and access control mechanisms and, although no such events have had a material adverse effect on our business, there can be no assurance that an insider threat will not result in an incident that is material to us. Furthermore, we rely on products and services provided by third-party suppliers to operate certain critical business systems, including without limitation, cloud-based infrastructure, encryption and authentication technology, employee email and other functions, which exposes us to supply-chain attacks or other business disruptions. We cannot guarantee that third parties and infrastructure in our supply chain or our partners' supply chains have not been compromised or that they do not contain exploitable vulnerabilities, defects or bugs that could result in a breach of or disruption to our information technology systems, including our products and services, or the third-party information technology systems that support our services. We may also incorporate third-party data into our AI algorithms or use open-source datasets to train our algorithms. These datasets may be flawed, insufficient, or contain certain biased information, and may otherwise be vulnerable to security incidents. We may have limited insight into the data privacy or security practices of third-party suppliers, including for our AI algorithms. Our ability to monitor these third parties' information security practices is limited, and they may not have adequate information security measures in place. In addition, if one of our third-party suppliers suffers a security incident (which has happened in the
## Table of Contents
past and may happen in the future), our response may be limited or more difficult because we may not have direct access to their systems, logs and other information related to the security incident. Additionally, we are incorporated into the supply chain of a large number of entities worldwide and, as a result, if our products or services are compromised, a significant number of our customers and their data could be affected, which could result in potential liability and harm our business.
To defend against security incidents, we must continuously engineer more secure products and enhance security and reliability features, which is expected to result in increased expenses. We must also continue to develop our security measures, including training programs and security awareness initiatives, designed to ensure our suppliers have appropriate security measures in place, and continue to meet the evolving security requirements of our customers, applicable industry standards, and government regulations. While we invest in training programs and security awareness initiatives and take steps to detect and remediate certain vulnerabilities that we have identified, we may not always be able to prevent threats or detect and mitigate all vulnerabilities in our security controls, systems or software, including third-party software we have installed, as such threats and techniques change frequently and may not be detected until after a security incident has occurred. Further, we may experience delays in developing and deploying remedial measures designed to address identified vulnerabilities. These vulnerabilities could result in reputational and financial harm, and if exploited, these vulnerabilities could result in a security incident.
We hold confidential, sensitive, personal and proprietary information, including information from partners and customers. Breaches of our security measures, along with reported or perceived vulnerabilities or unapproved dissemination of proprietary information or sensitive or confidential data about us or third parties, could expose us and the parties affected to a risk of loss, or misuse of this information, potentially resulting in litigation and subsequent liability, regulatory inquiries or actions, damage to our brand and reputation or other harm, including financial, to our business. For example, we hold proprietary game source code from third-party partners in our GFN service. Breaches of our GFN security measures, which have happened in the past, could expose our partners to a risk of loss or misuse of this source code, damage both us and our partners, and expose NVIDIA to potential litigation and liability. If we or a third party we rely on experience a security incident, which has occurred in the past, or are perceived to have experienced a security incident, we may experience adverse consequences, including government enforcement actions, additional reporting requirements and/or oversight, restrictions on processing data, litigation, indemnification obligations, reputational harm, diversion of funds, diversion of management attention, financial loss, loss of data, material disruptions in our systems and operations, supply chain, and ability to produce, sell and distribute our goods and services, and other similar harms. Inability to fulfill orders, delayed sales, lower margins or lost customers as a result of these disruptions could adversely affect our financial results, stock price and reputation. Applicable data privacy and security obligations may require us to notify relevant stakeholders, including affected individuals, customers, regulators and investors, of security incidents, and mandatory disclosure of such incidents could lead to negative publicity. In addition to experiencing a security incident, third parties may gather, collect or infer sensitive information about us from public sources, data brokers or other means that reveals competitively sensitive details about our organization and could be used to harm our business.
## Business disruptions could harm our operations, lead to a decline in revenue and increase our costs.
Our worldwide operations could be disrupted by natural disasters and extreme weather conditions, power or water shortages, telecommunications failures, supplier disruptions, terrorist attacks, acts of violence, political and/or civil unrest, acts of war or other military actions, epidemics or pandemics, abrupt regulatory deterioration, and other natural or man-made disasters and catastrophic events. Our corporate headquarters, a large portion of our current data center capacity, and a portion of our research and development activities are located in California, and other critical business operations, finished goods inventory and some of our suppliers are located in Asia, making our operations vulnerable to natural disasters such as earthquakes, wildfires or other business disruptions occurring in these geographical areas. Catastrophic events can also have an impact on third-party vendors who provide us critical infrastructure services for IT and research and development systems and personnel. Our business continuity and disaster recovery planning may not be sufficient for all eventualities. Geopolitical and domestic political developments and other events beyond our control, can increase economic volatility globally. Political instability, changes in government or adverse political developments in or around any of the major countries in which we do business may harm our business, financial condition and results of operations. Worldwide geopolitical tensions and conflicts, including but not limited to China, Hong Kong, Israel, Korea and Taiwan where the manufacture of our product components and final assembly of our products are concentrated may result in changing regulatory requirements, and other disruptions that could impact our operations and operating strategies, product demand, access to global markets, hiring, and profitability. For example, other countries have restricted and may continue in the future to restrict business with the State of Israel, where we have engineering, sales support operations and manufacturing, and companies with Israeli operations, including by economic boycotts. Our operations could be harmed and our costs could increase if manufacturing, logistics or other operations are disrupted for any reason, including natural disasters, high heat events or water shortages, power shortages, information technology system failures or cyber-attacks, military actions or economic, business, labor, environmental, public health, or political issues. The ultimate impact on us, our third-party foundries and other suppliers of being located and consolidated in certain geographical areas is unknown. In the event a disaster, war or catastrophic event affects us, the third-party systems on which we rely, or our customers, our business could be harmed as a result of declines in revenue, increases in expenses, and substantial expenditures and time spent to fully resume operations. All of these risks and conditions could materially adversely affect our future sales and operating results.
## Table of Contents
We are monitoring the impact of the geopolitical conflict in and around Israel on our operations, including the health and safety of our approximately 3,700 employees in the region who primarily support the research and development, operations, and sales and marketing of our networking products. Our operating expenses in fiscal year 2024 include expenses for financial support to impacted employees and charitable activity. We believe our global supply chain for our networking products has not experienced any significant impact. Further, in connection with the conflict, a substantial number of our employees in the region have been called-up for active military duty in Israel. Accordingly, some of our employees in Israel have been absent for an extended period and they or others may continue to be absent, which may cause disruption to our product development or operations. We did not experience any significant impact or expense to our business; however, if the conflict is further extended, it could impact future product development, operations, and revenue or create other uncertainty for our business.
Additionally, interruptions or delays in services from CSPs, data center co-location partners, and other third parties on which we rely, including due to the events described above or other events such as the insolvency of these parties, could impair our ability to provide our products and services and harm our business. As we increase our reliance on these third-party systems and services, our exposure to damage from service interruptions, defects, disruptions, outages, shortages and other performance and quality problems may increase. Data centers depend on access to clean water and predictable energy. Power or water shortages, or regulations that limit energy or water availability, could impair the ability of our customers to expand their data center capacity and consume our products and services.
## Climate change may have a long-term impact on our business.
Climate change may have an increasingly adverse impact on our business and on our customers, partners and vendors. Water and energy availability and reliability in the regions where we conduct business is critical, and certain of our facilities may be vulnerable to the impacts of extreme weather events. Extreme heat and wind coupled with dry conditions in Northern California may lead to power safety shut offs due to wildfire risk, which can have adverse implications for our Santa Clara, California headquarter offices and data centers, including impairing the ability of our employees to work effectively. Climate change, its impact on our supply chain and critical infrastructure worldwide and its potential to increase political instability in regions where we, our customers, partners and our vendors do business, may disrupt our business and cause us to experience higher attrition, losses and costs to maintain or resume operations. Although we maintain insurance coverage for a variety of property, casualty, and other risks, the types and amounts of insurance we obtain vary depending on availability and cost. Some of our policies have large deductibles and broad exclusions, and our insurance providers may be unable or unwilling to pay a claim. Losses not covered by insurance may be large, which could harm our results of operations and financial condition.
Our business and those of our suppliers and customers may also be subject to climate-related laws, regulations and lawsuits. New or proposed regulations relating to carbon taxes, fuel or energy taxes, pollution limits, sustainability-related disclosure and governance and supply chain governance could result in greater direct costs, including costs associated with changes to manufacturing processes or the procurement of raw materials used in manufacturing processes, increased capital expenditures to improve facilities and equipment, and higher compliance and energy costs to reduce emissions, other compliance costs, as well as greater indirect costs resulting from our customers and/or suppliers incurring additional compliance costs that are passed on to us. These costs and restrictions could harm our business and results of operations by increasing our expenses or requiring us to alter our operations and product design activities.
Stakeholder groups may find us insufficiently responsive to the implications of climate change, and therefore we may face legal action or reputational harm. We may not achieve our stated sustainability-related goals, which could harm our reputation, or we may incur additional, unexpected costs to achieve such goals. We may also experience contractual disputes due to supply chain delays arising from climate change-related disruptions, which could result in increased litigation and costs.
We also face risks related to business trends that may be influenced by climate change concerns. Our business could be negatively impacted by concerns around the high absolute energy requirements of our GPUs, despite their much more energy efficient design and operation relative to alternative computing platforms.
We may not be able to realize the potential benefits of business investments or acquisitions, and we may not be able to successfully integrate acquired companies, which could hurt our ability to grow our business, develop new products or sell our products.
We have acquired and invested and may continue to do so in businesses that offer products, services and technologies that we believe will help expand or enhance our strategic objectives. Acquisitions or investments involve significant challenges and risks and could impair our ability to grow our business, develop new products or sell our products and ultimately could have a negative impact on our financial results. If we pursue a particular transaction, we may limit our ability to enter into other transactions that could help us achieve our other strategic objectives. If we are unable to timely complete acquisitions, including due to delays and challenges in obtaining regulatory approvals, we may be unable to pursue other transactions, we may not be able to retain critical talent from the target company, technology may evolve and make the acquisition less attractive, and other changes can take place, which could reduce the anticipated benefits of the transaction and negatively impact our business. Regulators could also impose conditions that reduce the ultimate value of our acquisitions. In addition, to the extent that our perceived ability to consummate acquisitions has been
## Table of Contents
harmed, future acquisitions may be more difficult, complex or expensive. Further, our investments in publicly traded companies could create volatility in our results and may generate losses up to the value of the investment. In addition, we have invested and may continue to invest in private companies to further our strategic objectives and to support certain key business initiatives. These companies can include early-stage companies still defining their strategic direction. Many of the instruments in which we invest are non-marketable and illiquid at the time of our initial investment, and we are not always able to achieve a return. To the extent any of the companies in which we invest are not successful, we could recognize an impairment and/or lose all or part of our investment. Our investment portfolio contains industry sector concentration risks, and a decline in any one or multiple industry sectors could increase our impairment losses. We face additional risks related to acquisitions and strategic investments, including the diversion of capital and other resources, including management's attention; difficulty in realizing a satisfactory return and uncertainties to realize the benefits of an acquisition or strategic investment, if at all; difficulty or inability in obtaining governmental, regulatory approval or restrictions or other consents and approvals or financing; legal proceedings initiated as a result of an acquisition or investment; and potential failure of our due diligence processes to identify significant issues with the assets or company in which we are investing or are acquiring.
Additional risks related to acquisitions include, but are not limited to:
- · difficulty in integrating the technology, systems, products, policies, processes, or operations and integrating and retaining the employees, including key personnel, of the acquired business;
- · assumption of liabilities and incurring amortization expenses, impairment charges to goodwill or write-downs of acquired assets;
- · integrating accounting, forecasting and controls, procedures and reporting cycles;
- · coordinating and integrating operations, particularly in countries in which we do not currently operate;
- · stock price impact, fines, fees or reputation harm if we are unable to obtain regulatory approval for an acquisition or are otherwise unable to close an acquisition;
- · potential issuances of debt to finance our acquisitions, resulting in increased debt, increased interest expense, and compliance with debt covenants or other restrictions;
- · the potential for our acquisitions to result in dilutive issuances of our equity securities;
- · the potential variability of the amount and form of any performance-based consideration;
- · negative changes in general economic conditions in the regions or the industries in which we or our target operate;
- · exposure to additional cybersecurity risks and vulnerabilities; and
- · impairment of relationships with, or loss of our or our target's employees, vendors and customers.
For example, when integrating acquisition target systems into our own, we have experienced and may continue to experience challenges including lengthy and costly systems integration, delays in purchasing and shipping products, difficulties with system integration via electronic data interchange and other processes with our key suppliers and customers, and training and change management needs of integration personnel. These challenges have impacted our results of operations and may continue to do so in the future.
We receive a significant amount of our revenue from a limited number of partners and distributors and we have a concentration of sales to customers who purchase directly or indirectly from us, and our revenue could be adversely affected if we lose or are prevented from selling to any of these customers.
We receive a significant amount of our revenue from a limited number of customers within our distribution and partner network. Sales to one customer, Customer A, represented 13% of total revenue for fiscal year 2024, which was attributable to the Compute & Networking segment. With several of these channel partners, we are selling multiple products and systems in our portfolio through their channels. Our operating results depend on sales within our partner network, as well as the ability of these partners to sell products that incorporate our processors. In the future, these partners may decide to purchase fewer products, not to incorporate our products into their ecosystem, or to alter their purchasing patterns in some other way. Because most of our sales are made on a purchase order basis, our customers can generally cancel, change or delay product purchase commitments with little notice to us and without penalty. Our partners or customers may develop their own solutions; our customers may purchase products from our competitors; and our partners may discontinue sales or lose market share in the markets for which they purchase our products, all of which may alter partners' or customers' purchasing patterns. Many of our customers often do not purchase directly from us but purchase through multiple OEMs, ODMs, system integrators, distributors and other channel partners. One indirect customer which primarily purchases our products through system integrators and distributors, including through Customer A, is estimated to have represented approximately 19% of total revenue for fiscal year 2024, attributable to the
## Table of Contents
Compute & Networking segment. If end demand increases or our finished goods supply availability is concentrated near a quarter end, the system integrators, distributors and channel partners may have limited ability to increase their credit, which could impact the timing and amount of our revenue. The loss of any of our large customers, a significant reduction in purchases by them, our inability to sell to a customer due to U.S. or other countries' trade restrictions or any difficulties in collecting accounts receivable would likely harm our financial condition and results of operations.
## If we are unable to attract, retain and motivate our executives and key employees, our business may be harmed.
To be competitive and execute our business strategy successfully, we must attract, retain and motivate our executives and key employees and recruit and develop capable and diverse talent. Labor is subject to external factors that are beyond our control, including our industry's highly competitive market for skilled workers and leaders, cost inflation and workforce participation rates. Changes in immigration and work permit regulations or in their administration or interpretation could impair our ability to attract and retain qualified employees. Competition for personnel results in increased costs in the form of cash and stockbased compensation, and in times of stock price volatility, as we have experienced in the past and may experience in the future, the retentive value of our stockbased compensation may decrease. Additionally, we are highly dependent on the services of our longstanding executive team. Failure to ensure effective succession planning, transfer of knowledge and smooth transitions involving executives and key employees could hinder our strategic planning and execution and long-term success.
## Our business is dependent upon the proper functioning of our business processes and information systems and modification or interruption of such systems may disrupt our business, and internal controls.
We rely upon internal processes and information systems to support key business functions, including our assessment of internal controls over financial reporting as required by Section 404 of the Sarbanes-Oxley Act. The efficient operation and scalability of these processes and systems is critical to support our growth. We continue to design and implement updated accounting functionality related to a new enterprise resource planning, or ERP, system. Any ERP system implementation may introduce problems, such as quality issues or programming errors, that could have an impact on our continued ability to successfully operate our business or to timely and accurately report our financial results. These changes may be costly and disruptive to our operations and could impose substantial demands on management time. Failure to implement new or updated controls, or difficulties encountered in their implementation, could harm our operating results or cause us to fail to meet our reporting obligations.
Identification of material weaknesses in our internal controls, even if quickly remediated once disclosed, may cause investors to lose confidence in our financial statements and our stock price may decline. Remediation of any material weakness could require us to incur significant expenses, and if we fail to remediate any material weakness, our financial statements may be inaccurate, we may be required to restate our financial statements, our ability to report our financial results on a timely and accurate basis may be adversely affected, our access to the capital markets may be restricted, our stock price may decline, and we may be subject to sanctions or investigation by regulatory authorities.
## Our operating results have in the past fluctuated and may in the future fluctuate, and if our operating results are below the expectations of securities analysts or investors, our stock price could decline.
Our operating results have in the past fluctuated and may continue to fluctuate due to numerous of these risk factors. Therefore, investors should not rely on our past results of operations as an indication of our future performance. Additional factors that could affect our results of operations include, but are not limited to:
- · our ability to adjust spending due to the multi-year development cycle for some of our products and services;
- · our ability to comply with our contractual obligations to customers;
- · our extended payment term arrangements with certain customers, the inability of some customers to make required payments, our ability to obtain credit insurance for customers with extended payment terms, and customer bad debt write-offs;
- · our vendors' payment requirements;
- · unanticipated costs associated with environmental liabilities; and
- · changes in financial accounting standards or interpretations of existing standards.
Any of the factors discussed above could prevent us from achieving our anticipated financial results. For example, we have granted and may continue to grant extended payment terms to some customers, particularly during macroeconomic downturns, which could impact our ability to collect payment. Our vendors have requested and may continue to ask for shorter payment terms, which may impact our cash flow generation. These arrangements reduce the cash we have available for general business operations. In addition, the pace of growth in our operating expenses and investments may lag our revenue growth, creating volatility or periods where profitability levels may not be sustainable. Failure to meet our expectations or the expectations of our investors or security analysts is likely to cause our stock price to decline, as it has in the past, or experience substantial price volatility.
## Table of Contents
## Risks Related to Regulatory, Legal, Our Stock and Other Matters
## Our operations could be affected by the complex laws, rules and regulations to which our business is subject, and political and other actions may adversely impact our business.
We are subject to laws and regulations domestically and worldwide, affecting our operations in areas including, but not limited to, IP ownership and infringement; taxes; import and export requirements and tariffs; anti-corruption, including the Foreign Corrupt Practices Act; business acquisitions; foreign exchange controls and cash repatriation restrictions; data privacy requirements; competition and antitrust; advertising; employment; product regulations; cybersecurity; environmental, health, and safety requirements; the responsible use of AI; sustainability; cryptocurrency; and consumer laws. Compliance with such requirements can be onerous and expensive, could impact our competitive position, and may negatively impact our business operations and ability to manufacture and ship our products. There can be no assurance that our employees, contractors, suppliers, customers or agents will not violate applicable laws or the policies, controls, and procedures that we have designed to help ensure compliance with such laws, and violations could result in fines, criminal sanctions against us, our officers, or our employees, prohibitions on the conduct of our business, and damage to our reputation. Changes to the laws, rules and regulations to which we are subject, or changes to their interpretation and enforcement, could lead to materially greater compliance and other costs and/or further restrictions on our ability to manufacture and supply our products and operate our business. For example, we may face increased compliance costs as a result of changes or increases in antitrust legislation, regulation, administrative rule making, increased focus from regulators on cybersecurity vulnerabilities and risks. Our position in markets relating to AI has led to increased interest in our business from regulators worldwide, including the European Union, the United States, the United Kingdom and China. For example, the French Competition Authority collected information from us regarding our business and competition in the graphics card and cloud service provider market as part of an ongoing inquiry into competition in those markets. We have also received requests for information from regulators in the European Union, the United Kingdom, and China regarding our sales of GPUs, our efforts to allocate supply, foundation models and our investments, partnerships and other agreements with companies developing foundation models, and we expect to receive additional requests for information in the future. Governments and regulators are considering imposing restrictions on the hardware, software, and systems used to develop frontier foundation models and generative AI. If implemented, such restrictions could increase the costs and burdens to us and our customers, delay or halt deployment of new systems using our products, and reduce the number of new entrants and customers, negatively impacting our business and financial results. Revisions to laws or regulations or their interpretation and enforcement could also result in increased taxation, trade sanctions, the imposition of or increase to import duties or tariffs, restrictions and controls on imports or exports, or other retaliatory actions, which could have an adverse effect on our business plans or impact the timing of our shipments. Additionally, changes in the public perception of governments in the regions where we operate or plan to operate could negatively impact our business and results of operations.
Government actions, including trade protection and national and economic security policies of U.S. and foreign government bodies, such as tariffs, import or export regulations, including deemed export restrictions and restrictions on the activities of U.S. persons, trade and economic sanctions, decrees, quotas or other trade barriers and restrictions could affect our ability to ship products, provide services to our customers and employees, do business without an export license with entities on the U.S. Department of Commerce's U.S. Entity List or other USG restricted parties lists (which is expected to change from time to time), and generally fulfill our contractual obligations and have a material adverse effect on our business. If we were ever found to have violated export control laws or sanctions of the U.S. or similar applicable non-U.S. laws, even if the violation occurred without our knowledge, we may be subject to various penalties available under the laws, any of which could have a material and adverse impact on our business, operating results and financial condition.
For example, in response to the war in Ukraine, the United States and other jurisdictions imposed economic sanctions and export control measures which blocked the passage of our products, services and support into Russia, Belarus, and certain regions of Ukraine. In fiscal year 2023, we stopped direct sales to Russia and closed business operations in Russia. Concurrently, the war in Ukraine has impacted sales in EMEA and may continue to do so in the future.
The increasing focus on the risks and strategic importance of AI technologies has resulted in regulatory restrictions that target products and services capable of enabling or facilitating AI and may in the future result in additional restrictions impacting some or all of our product and service offerings.
Concerns regarding third-party use of AI for purposes contrary to local governmental interests, including concerns relating to the misuse of AI applications, models, and solutions, has resulted in and could in the future result in unilateral or multilateral restrictions on products that can be used for training, modifying, tuning, and deploying LLMs. Such restrictions have limited and could in the future limit the ability of downstream customers and users worldwide to acquire, deploy and use systems that include our products, software, and services, and negatively impact our business and financial results.
Such restrictions could include additional unilateral or multilateral export controls on certain products or technology, including but not limited to AI technologies. As geopolitical tensions have increased, semiconductors associated with AI, including GPUs and associated products, are increasingly the focus of export control restrictions proposed by stakeholders in the U.S. and its allies. The United States has imposed unilateral controls restricting GPUs and associated
## Table of Contents
products, and it is likely that additional unilateral or multilateral controls will be adopted. Such controls have been and may again be very broad in scope and application, prohibit us from exporting our products to any or all customers in one or more markets, including but not limited to China, and could negatively impact our manufacturing, testing and warehousing locations and options, or could impose other conditions that limit our ability to serve demand abroad and could negatively and materially impact our business, revenue and financial results. Export controls targeting GPUs and semiconductors associated with AI, which have been imposed and are increasingly likely to be further tightened, would further restrict our ability to export our technology, products, or services even though competitors may not be subject to similar restrictions, creating a competitive disadvantage for us and negatively impacting our business and financial results. Export controls targeting GPUs and semiconductors associated with AI have subjected and may in the future subject downstream users of our products to additional restrictions on the use, resale, repair, or transfer of our products, negatively impacting our business and financial results. Controls could negatively impact our cost and/or ability to provide services such as NVIDIA AI cloud services and could impact the cost and/or ability for our cloud service providers and customers to provide services to their end customers, even outside China.
Export controls could disrupt our supply chain and distribution channels, negatively impacting our ability to serve demand, including in markets outside China and for our gaming products. The possibility of additional export controls has negatively impacted and may in the future negatively impact demand for our products, benefiting competitors that offer alternatives less likely to be restricted by further controls. Repeated changes in the export control rules are likely to impose compliance burdens on our business and our customers, negatively and materially impacting our business.
Increasing use of economic sanctions and export controls has impacted and may in the future impact demand for our products or services, negatively impacting our business and financial results. Reduced demand due to export controls could also lead to excess inventory or cause us to incur related supply charges. Additional unilateral or multilateral controls are also likely to include deemed export control limitations that negatively impact the ability of our research and development teams to execute our roadmap or other objectives in a timely manner. Additional export restrictions may not only impact our ability to serve overseas markets, but also provoke responses from foreign governments, including China, that negatively impact our supply chain or our ability to provide our products and services to customers in all markets worldwide, which could also substantially reduce our revenue. Regulators in China have inquired about our sales and efforts to supply the China market and our fulfillment of the commitments we entered at the close of our Mellanox acquisition. If the regulators conclude that we have failed to fulfill such commitments or we have violated any applicable law in China, we could be subject to various penalties or restrictions on our ability to conduct our business, any of which could have a material and adverse impact on our business, operating results and financial condition.
During the third quarter of fiscal year 2023, the USG announced export restrictions and export licensing requirements targeting China's semiconductor and supercomputing industries. These restrictions impact exports of certain chips, as well as software, hardware, equipment and technology used to develop, produce and manufacture certain chips to China (including Hong Kong and Macau) and Russia, and specifically impact our A100 and H100 integrated circuits, DGX or any other systems or boards which incorporate A100 or H100 integrated circuits. The licensing requirements also apply to any future NVIDIA integrated circuit achieving certain peak performance and chip-to-chip I/O performance thresholds, as well as any system or board that includes those circuits. There are also now licensing requirements to export a wide array of products, including networking products, destined for certain end users and for certain end uses in China. During the second quarter of fiscal year 2024, the USG also informed us of an additional licensing requirement for a subset of A100 and H100 products destined to certain customers and other regions, including some countries in the Middle East.
In October 2023, the USG announced new and updated licensing requirements that became effective in our fourth quarter of fiscal year 2024 for exports to China and Country Groups D1, D4, and D5 (including but not limited to, Saudi Arabia, the United Arab Emirates, and Vietnam, but excluding Israel) of our products exceeding certain performance thresholds, including A100, A800, H100, H800, L4, L40, L40S and RTX 4090. The licensing requirements also apply to the export of products exceeding certain performance thresholds to a party headquartered in, or with an ultimate parent headquartered in, Country Group D5, including China. On October 23, 2023, the USG informed us that the licensing requirements were effective immediately for shipments of our A100, A800, H100, H800, and L40S products. We have not received licenses to ship these restricted products to China.
Following these export controls, we transitioned some operations, including certain testing, validation, and supply and distribution operations out of China and Hong Kong. Any future transitions could be costly and time consuming, and adversely affect our research and development and supply and distribution operations, as well as our revenue, during any such transition period. We are working to expand our Data Center product portfolio to offer new solutions, including those for which the USG does not require a license or advance notice before each shipment. To the extent that a customer requires products covered by the licensing requirements, we may seek a license for the customer. However, the licensing process is time-consuming. We have no assurance that the USG will grant such a license or that the USG will act on the license application in a timely manner or at all. Even if a license is offered, it may impose burdensome conditions that we or our customer or end users cannot or decide not to accept. The USG is evaluating license requests in a closed process that does not have clear standards or an opportunity for review. For example, the Notified Advanced Computing, or 'NAC,' process has not resulted in approvals for exports of products to customers in China. The license process for exports to D1 and D4 countries has been time-consuming and resulted in license conditions for countries outside China. The requirements have a disproportionate impact on NVIDIA and already have disadvantaged and may in the future
## Table of Contents
disadvantage NVIDIA against certain of our competitors who sell products that are not subject to the new restrictions or may be able to acquire licenses for their products.
Management of these new licenses and other requirements is complicated and time consuming. Our competitive position has been harmed, and our competitive position and future results may be further harmed, over the long-term, if there are further changes in the USG's export controls, including further expansion of the geographic, customer, or product scope of the controls, if customers purchase product from competitors, if customers develop their own internal solution, if we are unable to provide contractual warranty or other extended service obligations, if the USG does not grant licenses in a timely manner or denies licenses to significant customers or if we incur significant transition costs. Even if the USG grants any requested licenses, the licenses may be temporary or impose burdensome conditions that we or our customers or end users cannot or choose not to fulfill. The licensing requirements may benefit certain of our competitors, as the licensing process will make our pre-sale and post-sale technical support efforts more cumbersome and less certain and encourage customers in China to pursue alternatives to our products, including semiconductor suppliers based in China, Europe, and Israel.
Given the increasing strategic importance of AI and rising geopolitical tensions, the USG has changed and may again change the export control rules at any time and further subject a wider range of our products to export restrictions and licensing requirements, negatively impacting our business and financial results. In the event of such change, we may be unable to sell our inventory of such products and may be unable to develop replacement products not subject to the licensing requirements, effectively excluding us from all or part of the China market, as well as other impacted markets, including the Middle East. For example, the USG has already imposed conditions to limit the ability of foreign firms to create and offer as a service large-scale GPU clusters, for example by imposing license conditions on the use of products to be exported to certain countries, or by requiring chip tracking and throttling mechanisms that would disable or impair GPUs if certain system or use conditions are detected. The USG has already imposed export controls restricting certain gaming GPUs, and if the USG expands such controls to restrict additional gaming products, it may disrupt a significant portion of our supply and distribution chain and negatively impact sales of such products to markets outside China, including the U.S. and Europe. Export controls may disrupt our supply and distribution chain for a substantial portion of our products, which are warehoused in and distributed from Hong Kong. Export controls restricting our ability to sell datacenter GPUs may also negatively impact demand for our networking products used in servers containing our GPUs. The USG may also impose export controls on our networking products, such as highspeed network interconnects, to limit the ability of downstream parties to create large clusters for frontier model training. Any new control that impacts a wider range of our products would likely have a disproportionate impact on NVIDIA and may disadvantage us against certain of our competitors that sell chips that are outside the scope of such control. Excessive or shifting export controls have already and may in the future encourage customers outside China and other impacted regions to 'design-out' certain U.S. semiconductors from their products to reduce the compliance burden and risk, and to ensure that they are able to serve markets worldwide. Excessive or shifting export controls have already encouraged and may in the future encourage overseas governments to request that our customers purchase from our competitors rather than NVIDIA or other U.S. firms, harming our business, market position, and financial results. As a result, excessive or shifting export controls may negatively impact demand for our products and services not only in China, but also in other markets, such as Europe, Latin America, and Southeast Asia. Excessive or shifting export controls increase the risk of investing in U.S. advanced semiconductor products, because by the time a new product is ready for market, it may be subject to new unilateral export controls restricting its sale. At the same time, such controls may increase investment in foreign competitors, which would be less likely to be restricted by U.S. controls.
Additionally, restrictions imposed by the Chinese government on the duration of gaming activities and access to games may adversely affect our Gaming revenue, and increased oversight of digital platform companies may adversely affect our Data Center revenue. The Chinese government may impose restrictions on the sale to certain customers of our products, or any products containing components made by our partners and suppliers. For example, the Chinese government announced restrictions relating to certain sales of products containing certain products made by Micron, a supplier of ours. Further restrictions on our products or the products of our suppliers could negatively impact our business and financial results.
Finally, our business depends on our ability to receive consistent and reliable supply from our overseas partners, especially in Taiwan. Any new restrictions that negatively impact our ability to receive supply of components, parts, or services from Taiwan, would negatively impact our business and financial results.
Increased scrutiny from shareholders, regulators and others regarding our corporate sustainability practices could result in additional costs or risks and adversely impact our reputation and willingness of customers and suppliers to do business with us.
Shareholder advocacy groups, certain investment funds, other market participants, shareholders, customers and government regulators have focused increasingly on corporate sustainability practices and disclosures, including those associated with climate change and human rights. Stakeholders may not be satisfied with our corporate sustainability practices and goals or the speed of their adoption. Further, there is an increasing number of state-level initiatives in the U.S. that may conflict with other regulatory requirements or our various stakeholders' expectations. Additionally, our corporate sustainability practices, oversight of our practices or disclosure controls may not meet evolving shareholder,
## Table of Contents
regulator or other industry stakeholder expectations, or we may fail to meet corporate sustainability disclosure or reporting standards. We could also incur additional costs and require additional resources to monitor, report, and comply with various corporate sustainability practices, choose not to conduct business with potential customers, or discontinue or not expand business with existing customers due to our policies. These factors may negatively harm our brand, reputation and business activities or expose us to liability.
## Issues relating to the responsible use of our technologies, including AI in our offerings, may result in reputational or financial harm and liability.
Concerns relating to the responsible use of new and evolving technologies, such as AI, in our products and services may result in reputational or financial harm and liability and may cause us to incur costs to resolve such issues. We are increasingly building AI capabilities and protections into many of our products and services, and we also offer stand-alone AI applications. AI poses emerging legal, social, and ethical issues and presents risks and challenges that could affect its adoption, and therefore our business. If we enable or offer solutions that draw controversy due to their perceived or actual impact on society, such as AI solutions that have unintended consequences, infringe copyright or rights of publicity, or are controversial because of their impact on human rights, privacy, employment or other social, economic or political issues, or if we are unable to develop effective internal policies and frameworks relating to the responsible development and use of AI models and systems offered through our sales channels, we may experience brand or reputational harm, competitive harm or legal liability. Complying with multiple regulations from different jurisdictions related to AI could increase our cost of doing business, may change the way that we operate in certain jurisdictions, or may impede our ability to offer certain products and services in certain jurisdictions if we are unable to comply with regulations. Compliance with existing and proposed government regulation of AI, including in jurisdictions such as the European Union as well as under any U.S. regulation adopted in response to the Biden administration's Executive Order on AI, may also increase the cost of related research and development, and create additional reporting and/or transparency requirements. For example, regulation adopted in response to the Executive Order on AI could require us to notify the USG of certain safety test results and other information. Furthermore, changes in AI-related regulation could disproportionately impact and disadvantage us and require us to change our business practices, which may negatively impact our financial results. Our failure to adequately address concerns and regulations relating to the responsible use of AI by us or others could undermine public confidence in AI and slow adoption of AI in our products and services or cause reputational or financial harm.
## Actions to adequately protect our IP rights could result in substantial costs to us and our ability to compete could be harmed if we are unsuccessful or if we are prohibited from making or selling our products.
From time to time, we are involved in lawsuits or other legal proceedings alleging patent infringement or other IP rights violations by us, our employees or parties that we have agreed to indemnify. An unfavorable ruling could include significant damages, invalidation of one or more patents, indemnification of third parties, payment of lost profits, or injunctive relief. Claims that our products or processes infringe the IP rights of others, regardless of their merit, could cause us to incur significant costs to respond to, defend, and resolve such claims, and they may also divert the efforts and attention of management and technical personnel.
We may commence legal proceedings to protect our IP rights, which may increase our operating expenses. We could be subject to countersuits as a result. If infringement claims are made against us or our products are found to infringe a third party's IP, we or one of our indemnitees may have to seek a license to the third party's IP rights. If we or one of our indemnitees is unable to obtain such a license on acceptable terms or at all, we could be subject to substantial liabilities or have to suspend or discontinue the manufacture and sale of one or more of our products. We may also have to make royalty or other payments or cross license our technology. If these arrangements are not concluded on commercially reasonable terms, our business could be negatively impacted. Furthermore, the indemnification of a customer or other indemnitee may increase our operating expenses and negatively impact our operating results.
We rely on patents, trademarks, trade secrets, employee and third-party nondisclosure agreements, licensing arrangements and the laws of the countries in which we operate to protect our IP. Foreign laws may not protect our products or IP rights to the same extent as United States law. This makes the possibility of piracy of our technology and products more likely. The theft or unauthorized use or publication of our trade secrets and other confidential information could harm our competitive position and reduce acceptance of our products; as a result, the value of our investment in research and development, product development and marketing could be reduced. We also may face risks to our IP if our employees are hired by competitors. We continuously assess whether and where to seek formal protection for existing and new innovations and technologies but cannot be certain whether our applications for such protections will be approved, and, if approved, whether they will be enforceable.
We are subject to stringent and changing data privacy and security laws, rules, regulations and other obligations. These areas could damage our reputation, deter current and potential customers, affect our product design, or result in legal or regulatory proceedings and liability.
We process sensitive, confidential or personal data or information that is subject to privacy and security laws, regulations, industry standards, external and internal policies, contracts and other obligations that govern the processing of such data by us and on our behalf. Concerns about our practices or the ultimate use of our products and services with regard
## Table of Contents
to the collection, use, retention, security or disclosure of personal information or other privacy-related matters, including for use in AI, even if unfounded, could damage our reputation and adversely affect our operating results. The theft, loss or misuse of personal data in our possession or by one of our partners could result in damage to our reputation, regulatory proceedings, disruption of our business activities or increased security costs and costs related to defending legal claims.
In the United States, federal, state and local authorities have enacted numerous data privacy and security laws, including for data breach notification, personal data privacy and consumer protection. In the past few years, numerous U.S. states have enacted comprehensive privacy laws that impose certain obligations on covered businesses, including providing specific disclosures in privacy notices and affording residents with certain rights concerning their personal data. As applicable, such rights may include the right to access, correct, or delete certain personal data, and to opt-out of certain data processing activities, such as targeted advertising, profiling and automated decision-making. The exercise of these rights may impact our business and ability to provide our products and services. Certain states also impose stricter requirements for processing certain personal data, including sensitive information, such as conducting data privacy impact assessments. These state laws allow for statutory fines for noncompliance. For example, the California Consumer Privacy Act of 2018, as amended by the California Privacy Rights Act of 2020, or CPRA, or collectively the CCPA, gives California residents the right to access, delete and opt-out of certain sharing of their personal information, and to receive detailed information about how it is used and shared. The CCPA provides for fines of up to $7,500 per intentional violation and the law created a private right of action for certain data breaches. Similar laws are being considered in several other states, as well as at the federal and local levels. Additionally, several states and localities have enacted measures related to the use of artificial intelligence and machine learning in products and services. If we become subject to additional data privacy laws, the risk of enforcement action against us could increase.
Worldwide regulatory authorities are also considering and have approved various legislative proposals concerning data protection. The European Union adopted the General Data Protection Regulation, or GDPR, and the United Kingdom similarly adopted the U.K. GDPR, governing the strict handling of personal data of persons within the European Economic Area, or EEA, and the United Kingdom, respectively, including its use and protection and the ability of persons whose data is stored to access, correct, and delete such data about themselves. If we are found not to comply, we could be subject to penalties of up to €20 million or 4% of worldwide revenue, whichever is greater, and classes of individuals or consumer protection organizations may initiate litigation related to our processing of their personal data. Furthermore, the EU AI Act could impose onerous obligations that may disproportionately impact and disadvantage us and require us to change our business practices.
In the ordinary course of business, we may transfer personal data from Europe, China, and other jurisdictions to the United States or other countries. Certain jurisdictions have enacted data localization laws and cross-border personal data transfer laws. For example, the GDPR generally restricts the transfer of personal data to countries outside of the EEA. The European Commission released a set of 'Standard Contractual Clauses' designed for entities to validly transfer personal data out of the EEA to jurisdictions that the European Commission has not found to provide an adequate level of protection, including the United States. Additionally, the U.K.'s International Data Transfer Agreement / Addendum, as well as the EU-U.S. Data Privacy Framework and the U.K. extension thereto (which allows for transfers to relevant U.S.-based organizations who self-certify compliance and participate in the Framework) are mechanisms that may be used to transfer personal data from the EEA and U.K. to the United States. However, these mechanisms are subject to legal challenges, and there is no assurance that we can satisfy or rely on these measures to lawfully transfer personal data to the United States. Other jurisdictions have enacted or are considering similar cross-border personal data transfer laws and local personal data residency laws, any of which would increase the cost and complexity of doing business and could result in fines from regulators. For example, China's law imposes various requirements relating to data processing and data localization. Data broadly defined as important under China's law, including personal data, may not be transferable outside of China without prior assessment and approval by the Cyberspace Administration of China, or CAC. Compliance with these requirements, including CAC assessments and any deemed failures of such assessments, could cause us to incur liability, prevent us from using data collected in China or impact our ability to transfer data outside of China. The inability to import personal data to the United States could significantly and negatively impact our business operations, limit our ability to collaborate with parties that are subject to European, China and other data privacy and security laws, or require us to increase our personal data processing capabilities in Europe and/or elsewhere at significant expense. Some European regulators have prevented companies from transferring personal data out of Europe for allegedly violating the GDPR's cross-border data transfer limitations, which could negatively impact our business.
We may also be bound by contractual obligations related to data privacy and security, and our efforts to comply with such obligations may not be successful or may be claimed to be non-compliant. For example, certain privacy laws, such as the GDPR and the CCPA, require our customers to impose specific contractual restrictions on their service providers. We sometimes host personal data in collaboration with our customers, and if a breach exposed or altered that personal data, it could harm those customer relationships and subject us to litigation, regulatory action, or fines. We publish privacy policies, marketing materials and other statements, such as compliance with certain certifications or self-regulatory principles, regarding data privacy and security. If these policies, materials or statements are found to be deficient, lacking in transparency, deceptive, unfair or misrepresentative of our practices, we may be subject to investigation, enforcement actions by regulators or other adverse consequences.
## Table of Contents
Data protection laws around the world are quickly changing and may be interpreted and applied in an increasingly stringent fashion and in a manner that is inconsistent with our data practices. These obligations may affect our product design and necessitate changes to our information technologies, systems and practices and to those of any third parties that process personal data on our behalf. Despite our efforts, we or third parties we rely upon may fail to comply with such obligations. If we fail, or are perceived to have failed, to address or comply with data privacy and security obligations, we could face significant consequences, including but not limited to, government enforcement actions, litigation, additional reporting requirements and/or oversight, bans on processing personal data, and orders to destroy or not use personal data. Any of these events could have a material adverse effect on our reputation, business, or financial condition.
## We may have exposure to additional tax liabilities and our operating results may be adversely impacted by changes in tax laws, higher than expected tax rates and other tax-related factors.
We are subject to complex income tax laws and regulations, as well as non-income-based taxes, in various jurisdictions. Significant judgment is required in determining our worldwide provision for income taxes and other tax liabilities. We are regularly under audit by tax authorities in different jurisdictions. Although we believe our tax estimates are reasonable, any adverse outcome could increase our worldwide effective tax rate, increase the amount of non-income taxes imposed on our business, and harm our financial position, results of operations, net income, and cash flows.
Further, changes in tax laws or their interpretation by tax authorities in the U.S. or foreign jurisdictions could increase our future tax liability or cause other adverse tax impacts, which may materially impact our results of operations, or the way we conduct our business. Most of our income is taxable in the United States, with a significant portion qualifying for preferential treatment as foreign-derived intangible income, or FDII. If U.S. tax rates increase or the FDII deduction is reduced, our provision for income taxes, results of operations, net income and cash flows would be adversely affected. In addition, changes in the tax laws of foreign jurisdictions could arise as a result of global implementation of the Inclusive Framework on Base Erosion and Profit Shifting and Pillar Two Model Rules announced by The Organization for Economic Cooperation and Development, or OECD. These and other changes in the foreign tax laws, as adopted by countries, may increase tax uncertainty and adversely affect our provision for income taxes, results of operations, and financial condition.
Our future effective tax rate may also be affected by a variety of factors, including changes in our business or statutory rates, the mix of earnings in countries with differing statutory tax rates, available tax incentives, credits and deductions, the expiration of statutes of limitations, changes in accounting principles, adjustments to income taxes upon finalization of tax returns, increases in expenses not deductible for tax purposes, the estimates of our deferred tax assets and liabilities and deferred tax asset valuation allowances, changing interpretation of existing laws or regulations, the impact of accounting for business combinations, as well as changes in the domestic or international organization of our business and structure. Furthermore, the tax effects of accounting for stock-based compensation and volatility in our stock price may significantly impact our effective tax rate in the period in which they occur. A decline in our stock price may result in reduced future tax benefits from stock-based compensation, increase our effective tax rate and adversely affect our financial results.
## Our business is exposed to the risks associated with litigation, investigations and regulatory proceedings.
We currently and will likely continue to face legal, administrative and regulatory proceedings, claims, demands and/or investigations involving shareholder, consumer, competition and/or other issues relating to our business. For example, we are defending a securities class action lawsuit from multiple shareholders asserting claims that we and certain of our officers made false and/or misleading statements related to channel inventory and the impact of cryptocurrency mining on GPU demand in 2017 and 2018. Litigation and regulatory proceedings are inherently uncertain, and adverse rulings could occur, including monetary damages or fines, or an injunction stopping us from manufacturing or selling certain products, engaging in certain business practices, or requiring other remedies, such as compulsory licensing of patents. An unfavorable outcome or settlement may result in a material adverse impact. Regardless of the outcome, litigation can be costly, time-consuming, and disruptive to our operations.
## Our indebtedness could adversely affect our financial position and cash flows from operations, and prevent us from implementing our strategy or fulfilling our contractual obligations.
As of January 28, 2024, we had net outstanding a total of $9.7 billion in notes due by 2060. As each series of senior notes matures, unless redeemed or repurchased, we must repay or refinance the notes. If we decide to refinance, we may receive less favorable terms, or we may be unable to refinance at all, which may adversely affect our financial condition. We also have a $575 million commercial paper program.
Maintenance of our current and future indebtedness and contractual restrictions could cause us to dedicate a substantial portion of our cash flows from operations towards debt service obligations and principal repayments; increase our vulnerability to adverse changes in general economic, industry and competitive conditions; limit our flexibility regarding changes in our business and our industry; impair our ability to obtain future financing; and restrict our ability to grant liens on property, enter into certain mergers, dispose of our assets, or materially change our business.
Our ability to comply with the covenants in our indenture may be affected by events beyond our control. If we breach any of the covenants without a waiver from the note holders or lenders, then any outstanding indebtedness may be declared
## Table of Contents
immediately due and payable. Changes to our credit rating may negatively impact the value and liquidity of our securities, restrict our ability to obtain future financing and affect the terms of any such financing.
## Delaware law and our certificate of incorporation, bylaws and agreement with Microsoft could delay or prevent a change in control.
The anti-takeover provisions of the Delaware General Corporation Law may discourage, delay, or prevent a change in control. Provisions in our certificate of incorporation and bylaws could make it more difficult for a third party to acquire a majority of our outstanding stock. These provisions include the ability of our Board of Directors to create and issue preferred stock, change the number of directors, and to make, amend or repeal our bylaws without prior shareholder approval; the inability of our shareholders to act by written consent or call special meetings; advance notice requirements for director nominations and shareholder proposals; and a super-majority voting requirement to amend some provisions in our certificate of incorporation and bylaws. Under our agreement with Microsoft for the Xbox, if someone makes an offer to purchase at least 30% of our outstanding common stock, Microsoft may have first and last rights of refusal to purchase the stock. These provisions could delay or prevent a change in control of NVIDIA, discourage proxy contests, and make it more difficult for shareholders to elect directors of their choosing and to cause us to take other corporate actions they desire.
## Item 1B. Unresolved Staff Comments
Not applicable.
## Item 1C. Cybersecurity
## Risk management and strategy
We have in place certain infrastructure, systems, policies, and procedures that are designed to proactively and reactively address circumstances that arise when unexpected events such as a cybersecurity incident occur. These include processes for assessing, identifying, and managing material risks from cybersecurity threats. Our information security management program generally follows processes outlined in frameworks such as the ISO 27001 international standard for Information Security and we evaluate and evolve our security measures as appropriate. We consult with external parties, such as cybersecurity firms and risk management and governance experts, on risk management and strategy.
Identifying, assessing, and managing cybersecurity risk is integrated into our overall risk management systems and processes, and we have in place cybersecurity and data privacy training and policies designed to (a) respond to new requirements in global privacy laws and (b) prevent, detect, respond to, mitigate and recover from identified and significant cybersecurity threats.
We also have a vendor risk assessment process consisting of the distribution and review of supplier questionnaires designed to help us evaluate cybersecurity risks that we may encounter when working with third parties that have access to confidential and other sensitive company information. We take steps designed to ensure that such vendors have implemented data privacy and security controls that help mitigate the cybersecurity risks associated with these vendors. We routinely assess our high-risk suppliers' conformance to industry standards (e.g., ISO 27001, ISO 28001, and C-TPAT), and we evaluate them for additional information, product, and physical security requirements.
Refer to 'Item 1A. Risk factors' in this annual report on Form 10-K for additional information about cybersecurity-related risks.
## Governance
Information security matters, including managing and assessing risks from cybersecurity threats, remain under the oversight of the Company's Board of Directors, or the Board. The Audit Committee of the Board, or the Audit Committee, also reviews the adequacy and effectiveness of the Company's information security policies and practices and the internal controls regarding information security risks. The Audit Committee receives regular information security updates from management, including our Chief Security Officer and members of our security team. The Board also receives annual reports on information security matters from our Chief Security Officer and members of our security team.
Our security efforts are managed by a team of executive cybersecurity, IT, engineering, operations, and legal professionals. We have established a crossfunctional leadership team, consisting of executive-level leaders, that meets regularly to review cybersecurity matters and evaluate emerging threats. With oversight and guidance provided by the cross-functional leadership team, our information security teams refine our practices to address emerging security risks and changes in regulations. Our executive-level leadership team also participates in cybersecurity incident response efforts by engaging with the incident response team and helping direct the company's response to and assessment of certain cybersecurity incidents.
We have designated a Chief Security Officer that reports to our Senior Vice President of Software Engineering to manage our assessment and management of material risks from cybersecurity threats. Our Chief Security Officer's cybersecurity expertise includes over 17 years of combined government and private sector assignments.
## Item 2. Properties
Our headquarters is in Santa Clara, California. We own and lease approximately 3 million square feet of office and building space for our corporate headquarters. In addition, we lease data center space in Santa Clara, California. We also own and lease facilities for data centers, research and development, and/or sales and administrative purposes throughout the U.S. and in various international locations, primarily in China, India, Israel, and Taiwan. We believe our existing facilities, both owned and leased, are in good condition and suitable for the conduct of our business. We do not identify or allocate assets by operating segment. For additional information regarding obligations under leases, refer to Note 3 of the Notes to the Consolidated Financial Statements in Part IV, Item 15 of this Annual Report on Form 10-K, which information is hereby incorporated by reference.
## Item 3. Legal Proceedings
Please see Note 13 of the Notes to the Consolidated Financial Statements in Part IV, Item 15 of this Annual Report on Form 10-K for a discussion of our legal proceedings.
## Item 4. Mine Safety Disclosures
Not applicable.
## Part II
## Item 5. Market for Registrant's Common Equity, Related Stockholder Matters and Issuer Purchases of Equity Securities
Our common stock is traded on the Nasdaq Global Select Market under the symbol NVDA. Public trading of our common stock began on January 22, 1999. Prior to that, there was no public market for our common stock. As of February 16, 2024, we had approximately 382 registered shareholders, not including those shares held in street or nominee name.
## Issuer Purchases of Equity Securities
In August 2023, our Board of Directors approved an increase to our share repurchase program of an additional $25.0 billion, without expiration. During fiscal year 2024, we repurchased 21 million shares of our common stock for $9.7 billion. As of January 28, 2024, we were authorized, subject to certain specifications, to repurchase additional shares of our common stock up to $22.5 billion.
The repurchases can be made in the open market, in privately negotiated transactions, pursuant to a Rule 10b5-1 trading plan or in structured share repurchase programs, and can be made in one or more larger repurchases, in compliance with Rule 10b-18 of the Exchange Act, subject to market conditions, applicable legal requirements, and other factors. The program does not obligate NVIDIA to acquire any particular amount of common stock and the program may be suspended at any time at our discretion.
In fiscal year 2024, we paid $395 million in quarterly cash dividends. Our cash dividend program and the payment of future cash dividends under that program are subject to our Board of Directors' continuing determination that the dividend program and the declaration of dividends thereunder are in the best interests of our shareholders.
The following table presents details of our share repurchase transactions during the fourth quarter of fiscal year 2024:
Table of Contents
| Period | Total Number of Shares Purchased (In millions) | Average Price Paid per Share | Total Number of Shares Purchased as Part of Publicly Announced Program (In millions) | Approximate Dollar Value of Shares that May Yet Be Purchased Under the Program (In billions) |
|---------------------------------------|--------------------------------------------------|--------------------------------|----------------------------------------------------------------------------------------|------------------------------------------------------------------------------------------------|
| October 30, 2023 - November 26, 2023 | 0.9 | $ 464.39 | 0.9 | $ 24.8 |
| November 27, 2023 - December 24, 2023 | 1.1 | $ 477.26 | 1.1 | $ 24.3 |
| December 25, 2023 - January 28, 2024 | 3.3 | $ 540.85 | 3.3 | $ 22.5 |
| Total | 5.3 | | 5.3 | |
From January 29, 2024 to February 16, 2024, we repurchased 2.8 million shares for $1.9 billion pursuant to a Rule 10b5-1 trading plan.
## Restricted Stock Unit Share Withholding
We withhold common stock shares associated with net share settlements to cover tax withholding obligations upon the vesting of RSU awards under our employee equity incentive program. During fiscal year 2024, we withheld
approximately 7 million shares for a total value of $2.8 billion through net share settlements. Refer to Note 4 of the Notes to the Consolidated Financial Statements in Part IV, Item 15 of this Annual Report on Form 10-K for further discussion regarding our equity incentive plans.
## Stock Performance Graphs
The following graph compares the cumulative total shareholder return for our common stock, the S&P 500 Index, and the Nasdaq 100 Index for the five years ended January 28, 2024. The graph assumes that $100 was invested on January 27, 2019 in our common stock and in each of the S&P 500 Index and the Nasdaq 100 Index. Our common stock is a component of each of the presented indices. Total return assumes reinvestment of dividends in each of the indices indicated. Total return is based on historical results and is not intended to indicate future performance.
*$100 invested on 1/27/19 in stock and in indices, including reinvestment of dividends.

Source: FactSet financial data and analytics.
Table of Contents
| | 1/27/2019 | 1/26/2020 | 1/31/2021 | 1/30/2022 | 1/29/2023 | 1/28/2024 |
|--------------------|-------------|-------------|-------------|-------------|-------------|-------------|
| NVIDIA Corporation | $ 100.00 | $ 157.02 | $ 326.26 | $ 574.15 | $ 512.40 | $ 1,536.28 |
| S&P 500 | $ 100.00 | $ 126.17 | $ 144.83 | $ 175.25 | $ 163.63 | $ 199.83 |
| Nasdaq 100 | $ 100.00 | $ 136.15 | $ 194.20 | $ 218.68 | $ 185.67 | $ 268.13 |
## Item 6. [Reserved]
## Table of Contents
## Item 7. Management's Discussion and Analysis of Financial Condition and Results of Operations
The following discussion and analysis of our financial condition and results of operations should be read in conjunction with 'Item 1A. Risk Factors', our Consolidated Financial Statements and related Notes thereto, as well as other cautionary statements and risks described elsewhere in this Annual Report on Form 10-K, before deciding to purchase, hold or sell shares of our common stock.
## Overview
## Our Company and Our Businesses
NVIDIA pioneered accelerated computing to help solve the most challenging computational problems. Since our original focus on PC graphics, we have expanded to several other large and important computationally intensive fields. NVIDIA has leveraged its GPU architecture to create platforms for accelerated computing, AI solutions, scientific computing, data science, AV, robotics, metaverse and 3D internet applications.
Our two operating segments are "Compute & Networking" and "Graphics." Refer to Note 17 of the Notes to the Consolidated Financial Statements in Part IV, Item 15 of this Annual Report on Form 10-K for additional information.
Headquartered in Santa Clara, California, NVIDIA was incorporated in California in April 1993 and reincorporated in Delaware in April 1998.
## Recent Developments, Future Objectives and Challenges
## Demand and Supply, Product Transitions, and New Products and Business Models
Demand for our data center systems and products surged in fiscal year 2024. Entering fiscal year 2025, we are gathering customer demand indications across several product transitions. We have demand visibility for our new data center products ramping later in fiscal year 2025. We have increased our supply and capacity purchases with existing suppliers, added new vendors and entered into prepaid manufacturing and capacity agreements. These increased purchase volumes, the number of suppliers, and the integration of new vendors into our supply chain may create more complexity and execution risk. Our purchase commitments and obligations for inventory and manufacturing capacity at the end of fiscal year 2024 were impacted by shortening lead times for certain components. We may continue to enter into new supplier and capacity arrangements. Supply of Hopper architecture products is improving, and demand remains very strong. We expect our next-generation products to be supply-constrained based upon demand indications. We may incur inventory provisions or impairments if our inventory or supply or capacity commitments exceed demand for our products or demand declines.
We build finished products and maintain inventory in advance of anticipated demand. While we have entered into long-term supply and capacity commitments, we may not be able to secure sufficient commitments for capacity to address our business needs, or our long-term demand expectations may change. These risks may increase as we shorten our product development cycles, enter new lines of business, or integrate new suppliers or components into our supply chain, creating additional supply chain complexity.
Product transitions are complex as we often ship both new and prior architecture products simultaneously and we and our channel partners prepare to ship and support new products. Due to our product introduction cycles, we are almost always in various stages of transitioning the architecture of our Data Center, Professional Visualization, and Gaming products. We will have a broader and faster Data Center product launch cadence to meet a growing and diverse set of AI opportunities. The increased frequency of these transitions may magnify the challenges associated with managing our supply and demand due to manufacturing lead times. Qualification time for new products, customers anticipating product transitions and channel partners reducing channel inventory of prior architectures ahead of new product introductions can create reductions or volatility in our revenue. The increasing frequency and complexity of newly introduced products could result in quality or production issues that could increase inventory provisions, warranty or other costs or result in product delays. Deployment of new products to customers creates additional challenges due to the complexity of our technologies, which has impacted and may in the future impact the timing of customer purchases or otherwise impact our demand. While we have managed prior product transitions and have previously sold multiple product architectures at the same time, these transitions are difficult, may impair our ability to predict demand and impact our supply mix, and we may incur additional costs.
We build technology and introduce products for new and innovative use cases and applications such as our NVIDIA DGX Cloud services, Omniverse platform, LLMs, and generative AI models. Our demand estimates for new use cases, applications, and services can be incorrect and create volatility in our revenue or supply levels, and we may not be able to generate significant revenue from these use cases, applications, and services. Recent technologies, such as generative AI models, have emerged, and while they have driven increased demand for Data Center, the long-term trajectory is unknown.
## Table of Contents
## Global Trade
During the third quarter of fiscal year 2023, the USG, announced licensing requirements that, with certain exceptions, impact exports to China (including Hong Kong and Macau) and Russia of our A100 and H100 integrated circuits, DGX or any other systems or boards which incorporate A100 or H100 integrated circuits.
In July 2023, the USG informed us of an additional licensing requirement for a subset of A100 and H100 products destined to certain customers and other regions, including some countries in the Middle East.
In October 2023, the USG announced new and updated licensing requirements that became effective in our fourth quarter of fiscal year 2024 for exports to China and Country Groups D1, D4, and D5 (including but not limited to Saudi Arabia, the United Arab Emirates, and Vietnam, but excluding Israel) of our products exceeding certain performance thresholds, including A100, A800, H100, H800, L4, L40, L40S and RTX 4090. The licensing requirements also apply to the export of products exceeding certain performance thresholds to a party headquartered in, or with an ultimate parent headquartered in, Country Group D5, including China. On October 23, 2023, the USG informed us the licensing requirements were effective immediately for shipments of our A100, A800, H100, H800, and L40S products. Our sales to China decreased as a percentage of total Data Center revenue from 19% in fiscal year 2023 to 14% in fiscal year 2024.
We have not received licenses to ship these restricted products to China. We are working to expand our Data Center product portfolio to offer new solutions, including those for which the USG does not require a license or advance notice before each shipment. We have started to ship alternatives to the China market in small volumes. China represented a mid-single digit percentage of our Data Center revenue in the fourth quarter of fiscal year 2024 due to USG licensing requirements and we expect China to be in a similar range in the first quarter of fiscal year 2025. To the extent that a customer requires products covered by the licensing requirements, we may seek a license for the customer but have no assurance that the USG will grant such a license, or that the USG will act on the license application in a timely manner or at all.
Our competitive position has been harmed, and our competitive position and future results may be further harmed in the long term, if there are further changes in the USG's export controls. Given the increasing strategic importance of AI and rising geopolitical tensions, the USG has changed and may again change the export control rules at any time and further subject a wider range of our products to export restrictions and licensing requirements, negatively impacting our business and financial results. In the event of such change, we may be unable to sell our inventory of such products and may be unable to develop replacement products not subject to the licensing requirements, effectively excluding us from all or part of the China market, as well as other impacted markets, including the Middle East.
While we work to enhance the resiliency and redundancy of our supply chain, which is currently concentrated in the Asia-Pacific region, new and existing export controls or changes to existing export controls could limit alternative manufacturing locations and negatively impact our business. Refer to 'Item 1A. Risk Factors - Risks Related to Regulatory, Legal, Our Stock and Other Matters' for a discussion of this potential impact.
## Macroeconomic Factors
Macroeconomic factors, including inflation, increased interest rates, capital market volatility, global supply chain constraints and global economic and geopolitical developments, may have direct and indirect impacts on our results of operations, particularly demand for our products. While difficult to isolate and quantify, these macroeconomic factors can also impact our supply chain and manufacturing costs, employee wages, costs for capital equipment and value of our investments. Our product and solution pricing generally does not fluctuate with short-term changes in our costs. Within our supply chain, we continuously manage product availability and costs with our vendors.
## Israel and Hamas Conflict
We are monitoring the impact of the geopolitical conflict in and around Israel on our operations, including the health and safety of our approximately 3,700 employees in the region who primarily support the research and development, operations, and sales and marketing of our networking products. Our operating expenses in fiscal year 2024 include expenses for financial support to impacted employees and charitable activity. We believe our global supply chain for our networking products has not experienced any significant impact. Further, in connection with the conflict, a substantial number of our employees in the region have been called-up for active military duty in Israel. Accordingly, some of our employees in Israel have been absent for an extended period and they or others may continue to be absent, which may cause disruption to our product development or operations. We did not experience any significant impact or expense to our business; however, if the conflict is further extended, it could impact future product development, operations, and revenue or create other uncertainty for our business.
## Fiscal Year 2024 Summary
Table of Contents
| | Year Ended | Year Ended | Year Ended |
|------------------------------|----------------------------------------|----------------------------------------|----------------------------------------|
| | Jan 28, 2024 | Jan 29, 2023 | Change |
| | ($ in millions, except per share data) | ($ in millions, except per share data) | ($ in millions, except per share data) |
| Revenue | $ 60,922 | $ 26,974 | Up 126% |
| Gross margin | 72.7 % | 56.9 % | Up 15.8 pts |
| Operating expenses | $ 11,329 | $ 11,132 | Up 2% |
| Operating income | $ 32,972 | $ 4,224 | Up 681% |
| Net income | $ 29,760 | $ 4,368 | Up 581% |
| Net income per diluted share | $ 11.93 | $ 1.74 | Up 586% |
We specialize in markets where our computing platforms can provide tremendous acceleration for applications. These platforms incorporate processors, interconnects, software, algorithms, systems, and services to deliver unique value. Our platforms address four large markets where our expertise is critical: Data Center, Gaming, Professional Visualization, and Automotive.
Revenue for fiscal year 2024 was $60.9 billion, up 126% from a year ago.
Data Center revenue for fiscal year 2024 was up 217%. Strong demand was driven by enterprise software and consumer internet applications, and multiple industry verticals including automotive, financial services, and healthcare. Customers across industry verticals access NVIDIA AI infrastructure both through the cloud and on-premises. Data Center compute revenue was up 244% in the fiscal year. Networking revenue was up 133% in the fiscal year.
Gaming revenue for fiscal year 2024 was up 15%. The increase reflects higher sell-in to partners following the normalization of channel inventory levels and growing demand.
Professional Visualization revenue for fiscal year 2024 was up 1%.
Automotive revenue for the fiscal year 2024 was up 21%. The increase primarily reflected growth in self-driving platforms.
Gross margin increased in fiscal year 2024, primarily driven by Data Center revenue growth and lower net inventory provisions as a percentage of revenue.
Operating expenses increased for fiscal year 2024, driven by growth in employees and compensation increases. Fiscal year 2023 also included a $1.4 billion acquisition termination charge related to the proposed Arm transaction.
## Market Platform Highlights
Data Center revenue for fiscal year 2024 was $47.5 billion, up 217% from fiscal year 2023. In Data Center, we launched AI inference platforms that combine our full-stack inference software with NVIDIA Ada, NVIDIA Hopper and NVIDIA Grace Hopper processors optimized for generative AI, LLMs and other AI workloads. We introduced NVIDIA DGX Cloud and AI Foundations to help businesses create and operate custom large language models and generative AI models. As AV algorithms move to video transformers, and more cars are equipped with cameras, we expect NVIDIA's automotive data center processing demand to grow significantly. We estimate that in fiscal year 2024, approximately 40% of Data Center revenue was for AI inference. In the fourth quarter of fiscal year 2024, large cloud providers represented more than half of our Data Center revenue, supporting both internal workloads and external customers. We announced NVIDIA Spectrum-X, an accelerated networking platform for AI.
Gaming revenue for fiscal year 2024 was $10.4 billion, up 15% from fiscal year 2023. In Gaming, we launched the GeForce RTX 4060 and 4070 GPUs based on the NVIDIA Ada Lovelace architecture. We announced NVIDIA Avatar Cloud Engine for Games, a custom AI model foundry service using AI-powered natural language interactions to transform games and launched DLSS 3.5 Ray Reconstruction. Additionally, we released TensorRT-LLM for Windows and launched GeForce RTX 40-Series SUPER GPUs. Gaming reached a milestone of 500 AI-powered RTX games and applications utilizing NVIDIA DLSS, ray tracing and other NVIDIA RTX technologies.
Professional Visualization revenue for fiscal year 2024 was $1.6 billion, up 1% from fiscal year 2023. In Professional Visualization, we announced new GPUs based on the NVIDIA RTX Ada Lovelace architecture, and announced NVIDIA Omniverse Cloud, a fully managed service running in Microsoft Azure, for the development and deployment of industrial metaverse applications.
Automotive revenue for fiscal year 2024 was $1.1 billion, up 21% from fiscal year 2023. In Automotive, we announced a partnership with MediaTek, which will develop mainstream automotive systems on chips for global OEMs integrating a new NVIDIA GPU chiplet IP for AI and graphics. We furthered our collaboration with Foxconn to develop next-generation
## Table of Contents
electric vehicles, and announced further adoption of NVIDIA DRIVE platform with BYD, XPENG, GWM, Li Auto, ZEEKR and Xiaomi.
## Critical Accounting Estimates
Our consolidated financial statements are prepared in accordance with accounting principles generally accepted in the United States, or U.S. GAAP. The preparation of these financial statements requires us to make estimates and judgments that affect the reported amounts of assets, liabilities, revenue, cost of revenue, expenses and related disclosure of contingencies. Critical accounting estimates are those estimates that involve a significant level of estimation uncertainty and could have a material impact on our financial condition or results of operations. We have critical accounting estimates in the areas of inventories, revenue recognition, and income taxes. Refer to Note 1 of the Notes to the Consolidated Financial Statements in Part IV, Item 15 of this Annual Report on Form 10-K for a summary of significant accounting policies.
## Inventories
We charge cost of sales for inventory provisions to write-down our inventory to the lower of cost or net realizable value or for obsolete or excess inventory, and for excess product purchase commitments. Most of our inventory provisions relate to excess quantities of products or components, based on our inventory levels and future product purchase commitments compared to assumptions about future demand and market conditions, which requires management judgment.
Situations that may result in excess or obsolete inventory or excess product purchase commitments include changes in business and economic conditions, changes in market conditions, sudden and significant decreases in demand for our products, inventory obsolescence because of changing technology and customer requirements, new product introductions resulting in less demand for existing products or inconsistent spikes in demand, failure to estimate customer demand properly, ordering in advance of historical lead-times, government regulations and the impact of changes in future demand, or increase in demand for competitive products, including competitive actions. Cancellation or deferral of customer purchase orders could result in our holding excess inventory.
The net effect on our gross margin from inventory provisions and sales of items previously written down was an unfavorable impact of 2.7% in fiscal year 2024 and 7.5% in fiscal year 2023. Our inventory and capacity purchase commitments are based on forecasts of future customer demand. We account for our third-party manufacturers' lead times and constraints. Our manufacturing lead times can be and have been long, and in some cases, extended beyond twelve months for some products. We may place non-cancellable inventory orders for certain product components in advance of our historical lead times, pay premiums and provide deposits to secure future supply and capacity. We also adjust to other market factors, such as product offerings and pricing actions by our competitors, new product transitions, and macroeconomic conditions - all of which may impact demand for our products.
Refer to the Gross Profit and Gross Margin discussion below in this Management's Discussion and Analysis for further discussion.
## Income Taxes
We are subject to income taxes in the U.S. and foreign jurisdictions. Our calculation of deferred tax assets and liabilities is based on certain estimates and judgments and involves dealing with uncertainties in the application of complex tax laws. Our estimates of deferred tax assets and liabilities may change based, in part, on added certainty or finality to an anticipated outcome, changes in accounting standards or tax laws in the U.S. or foreign jurisdictions where we operate, or changes in other facts or circumstances. In addition, we recognize liabilities for potential U.S. and foreign income tax contingencies based on our estimate of whether, and the extent to which, additional taxes may be due. If we determine that payment of these amounts is unnecessary or if the recorded tax liability is less than our current assessment, we may be required to recognize an income tax benefit or additional income tax expense in our financial statements accordingly.
As of the end of fiscal years 2024 and 2023, we had a valuation allowance of $1.6 billion and $1.5 billion, respectively, related to capital loss carryforwards, and certain state and other deferred tax assets that management determined are not likely to be realized due, in part, to jurisdictional projections of future taxable income, including capital gains. To the extent realization of the deferred tax assets becomes more-likely-than-not, we would recognize such deferred tax assets as income tax benefits during the period.
We recognize the benefit from a tax position only if it is more-likely-than-not that the position would be sustained upon audit based solely on the technical merits of the tax position. Our policy is to include interest and penalties related to unrecognized tax benefits as a component of income tax expense.
## Table of Contents
## Revenue Recognition
## Revenue Allowances
For products sold with a right of return, we record a reduction to revenue by establishing a sales return allowance for estimated product returns at the time revenue is recognized, based primarily on historical return rates. However, if product returns for a fiscal period are anticipated to exceed historical return rates, we may determine that additional sales return allowances are required to reflect our estimated exposure for product returns. Return rights for certain stocking distributors for specific products are contractually limited based on a percentage of prior quarter shipments. For shipments to other customers, we do not allow returns, although we may approve returns for credit or refund based on applicable facts and circumstances.
We account for customer programs, which involve rebates and marketing development funds, as a reduction in revenue and accrue for such programs based on the amount we expect to be claimed by customers. Certain customer programs include distributor price incentives or other channel programs for specific products and customer classes which require judgement as to whether the applicable incentives will be attained. Estimates for customer program accruals include a combination of historical attainment and claim rates and may be adjusted based on relevant internal and external factors.
## License and Development Arrangements
Revenue from License and Development Arrangements is recognized over the period in which the development services are performed. Each fiscal reporting period, we measure progress to completion based on actual cost incurred to date as a percentage of the estimated total cost required to complete each project. Estimated total cost for each project includes a forecast of internal engineer personnel time expected to be incurred and other third-party costs as applicable.
## Contracts with Multiple Performance Obligations
Our contracts may contain more than one performance obligation. Judgement is required in determining whether each performance obligation within a customer contract is distinct. Except for License and Development Arrangements, NVIDIA products and services function on a standalone basis and do not require a significant amount of integration or interdependency. Therefore, multiple performance obligations contained within a customer contract are considered distinct and are not combined for revenue recognition purposes.
We allocate the total transaction price to each distinct performance obligation in a multiple performance obligations arrangement on a relative standalone selling price basis. In certain cases, we can establish standalone selling price based on directly observable prices of products or services sold separately in comparable circumstances to similar customers. If standalone selling price is not directly observable, such as when we do not sell a product or service separately, we determine standalone selling price based on market data and other observable inputs.
## Change in Accounting Estimate
In February 2023, we assessed the useful lives of our property, plant, and equipment. Based on advances in technology and usage rate, we increased the estimated useful life of a majority of the server, storage, and network equipment from three years to a range of four to five years, and assembly and test equipment from five years to seven years. The estimated effect of this change for fiscal year 2024 was a benefit of $33 million and $102 million for cost of revenue and operating expenses, respectively, which resulted in an increase in operating income of $135 million and net income of $114 million after tax, or $0.05 per both basic and diluted share.
## Results of Operations
A discussion regarding our financial condition and results of operations for fiscal year 2024 compared to fiscal year 2023 is presented below. A discussion regarding our financial condition and results of operations for fiscal year 2023 compared to fiscal year 2022 can be found under Item 7 in our Annual Report on Form 10-K for the fiscal year ended January 29, 2023, filed with the SEC on February 24, 2023, which is available free of charge on the SEC's website at http://www.sec.gov and at our investor relations website, http://investor.nvidia.com.
The following table sets forth, for the periods indicated, certain items in our Consolidated Statements of Income expressed as a percentage of revenue.
Table of Contents
| | Year Ended | Year Ended |
|-----------------------------------|--------------|--------------|
| | Jan 28, 2024 | Jan 29, 2023 |
| Revenue | 100.0 % | 100.0 % |
| Cost of revenue | 27.3 | 43.1 |
| Gross profit | 72.7 | 56.9 |
| Operating expenses | | |
| Research and development | 14.2 | 27.2 |
| Sales, general and administrative | 4.4 | 9.1 |
| Acquisition termination cost | - | 5.0 |
| Total operating expenses | 18.6 | 41.3 |
| Operating income | 54.1 | 15.6 |
| Interest income | 1.4 | 1.0 |
| Interest expense | (0.4) | (1.0) |
| Other, net | 0.4 | (0.1) |
| Other income (expense), net | 1.4 | (0.1) |
| Income before income tax | 55.5 | 15.5 |
| Income tax expense (benefit) | 6.6 | (0.7) |
| Net income | 48.9 % | 16.2 % |
## Reportable Segments
## Revenue by Reportable Segments
| | Year Ended | Year Ended | Year Ended | Year Ended |
|----------------------|-----------------|-----------------|-----------------|-----------------|
| | Jan 28, 2024 | Jan 29, 2023 | $ Change | % Change |
| | ($ in millions) | ($ in millions) | ($ in millions) | ($ in millions) |
| Compute & Networking | $ 47,405 | $ 15,068 | $ 32,337 | 215 % |
| Graphics | 13,517 | 11,906 | 1,611 | 14 % |
| Total | $ 60,922 | $ 26,974 | $ 33,948 | 126 % |
## Operating Income by Reportable Segments
| | Year Ended | Year Ended | Year Ended | Year Ended |
|----------------------|-----------------|-----------------|-----------------|-----------------|
| | Jan 28, 2024 | Jan 29, 2023 | $ Change | % Change |
| | ($ in millions) | ($ in millions) | ($ in millions) | ($ in millions) |
| Compute & Networking | $ 32,016 | $ 5,083 | $ 26,933 | 530 % |
| Graphics | 5,846 | 4,552 | 1,294 | 28 % |
| All Other | (4,890) | (5,411) | 521 | (10)% |
| Total | $ 32,972 | $ 4,224 | $ 28,748 | 681 % |
Compute & Networking revenue - The year-on-year increase was due to higher Data Center revenue. Compute grew 266% due to higher shipments of the NVIDIA Hopper GPU computing platform for the training and inference of LLMs, recommendation engines and generative AI applications. Networking was up 133% due to higher shipments of InfiniBand.
Graphics revenue - The year-on-year increase was led by growth in Gaming of 15% driven by higher sell-in to partners following the normalization of channel inventory levels.
Reportable segment operating income - The year-on-year increase in Compute & Networking and Graphics operating income was driven by higher revenue.
All Other operating loss - The year-on-year decrease was due to the $1.4 billion Arm acquisition termination cost in fiscal year 2023, partially offset by a $839 million increase in stock-based compensation expense in fiscal year 2024.
## Concentration of Revenue
Revenue by geographic region is designated based on the billing location even if the revenue may be attributable to end customers, such as enterprises and gamers in a different location. Revenue from sales to customers outside of the United States accounted for 56% and 69% of total revenue for fiscal years 2024 and 2023, respectively.
Our direct and indirect customers include public cloud, consumer internet companies, enterprises, startups, public sector entities, OEMs, ODMs, system integrators, AIB, and distributors.
Sales to one customer, Customer A, represented 13% of total revenue for fiscal year 2024, which was attributable to the Compute & Networking segment.
One indirect customer which primarily purchases our products through system integrators and distributors, including through Customer A, is estimated to have represented approximately 19% of total revenue for fiscal year 2024, attributable to the Compute & Networking segment.
Our estimated Compute & Networking demand is expected to remain concentrated.
There were no customers with 10% or more of total revenue for fiscal years 2023 and 2022.
## Gross Profit and Gross Margin
Gross profit consists of total revenue, net of allowances, less cost of revenue. Cost of revenue consists primarily of the cost of semiconductors, including wafer fabrication, assembly, testing and packaging, board and device costs, manufacturing support costs, including labor and overhead associated with such purchases, final test yield fallout, inventory and warranty provisions, memory and component costs, tariffs, and shipping costs. Cost of revenue also includes acquisition-related costs, development costs for license and service arrangements, IP-related costs, and stock-based compensation related to personnel associated with manufacturing operations.
Our overall gross margin increased to 72.7% in fiscal year 2024 from 56.9% in fiscal year 2023. The year over year increase was primarily due to strong Data Center revenue growth of 217% and lower net inventory provisions as a percentage of revenue.
Provisions for inventory and excess inventory purchase obligations totaled $2.2 billion for both fiscal years 2024 and 2023. Sales of previously reserved inventory or settlements of excess inventory purchase obligations resulted in a provision release of $540 million and $137 million for fiscal years 2024 and 2023, respectively. The net effect on our gross margin was an unfavorable impact of 2.7% and 7.5% in fiscal years 2024 and 2023, respectively.
## Operating Expenses
Table of Contents
| | Year Ended | Year Ended | Year Ended | Year Ended |
|--------------------------------------------|-----------------|-----------------|-----------------|-----------------|
| | Jan 28, 2024 | Jan 29, 2023 | $ Change | % Change |
| | ($ in millions) | ($ in millions) | ($ in millions) | ($ in millions) |
| Research and development expenses | $ 8,675 | 7,339 | $ 1,336 | 18 % |
| % of net revenue | 14.2 % | 27.2 % | | |
| Sales, general and administrative expenses | 2,654 | 2,440 | 214 | 9 % |
| % of net revenue | 4.4 % | 9.1 % | | |
| Acquisition termination cost | - | 1,353 | (1,353) | (100)% |
| % of net revenue | - % | 5.0 % | | |
| Total operating expenses | $ 11,329 | 11,132 | $ 197 | 2 % |
| % of net revenue | 18.6 % | 41.3 % | | |
The increase in research and development expenses and sales, general and administrative expenses for fiscal year 2024 was primarily driven by compensation and benefits, including stock-based compensation, reflecting employee growth and compensation increases.
## Acquisition Termination Cost
We recorded an acquisition termination cost related to the Arm transaction of $1.4 billion in fiscal year 2023 reflecting the write-off of the prepayment provided at signing.
## Other Income (Expense), Net
Table of Contents
| | Year Ended | Year Ended | Year Ended |
|-----------------------------|-----------------|-----------------|-----------------|
| | Jan 28, 2024 | Jan 29, 2023 | Change |
| | ($ in millions) | ($ in millions) | ($ in millions) |
| Interest income | $ 866 | $ 267 | $ 599 |
| Interest expense | (257) | (262) | 5 |
| Other, net | 237 | (48) | 285 |
| Other income (expense), net | $ 846 | $ (43) | $ 889 |
Interest income consists of interest earned on cash, cash equivalents and marketable securities. The increase in interest income was due to higher yields on higher cash balances.
Interest expense is comprised of coupon interest and debt discount amortization related to our notes.
Other, net, consists of realized or unrealized gains and losses from investments in non-affiliated entities and the impact of changes in foreign currency rates. Change in Other, net, compared to fiscal year 2023 was driven by changes in value from our non-affiliated investments. Refer to Note 9 of the Notes to the Consolidated Financial Statements in Part IV, Item 15 of this Annual Report on Form 10-K for additional information regarding our investments in non-affiliated entities.
## Income Taxes
We recognized income tax expense of $4.1 billion for fiscal year 2024 and income tax benefit of $187 million for fiscal year 2023. Income tax as a percentage of income before income tax was an expense of 12.0% for fiscal year 2024 and a benefit of 4.5% for fiscal year 2023.
During the third quarter of fiscal year 2024, the Internal Revenue Service, or IRS, audit of our federal income tax returns for fiscal years 2018 and 2019 was resolved. We recognized a non-cash net benefit of $145 million, related to this IRS audit resolution, for effectively settled positions. This benefit consists of a reduction in unrecognized tax benefits of $236 million and related accrued interest of $17 million, net of federal benefit, partially offset by additional cash tax payments and reductions in tax attribute carryforwards of $108 million.
The effective tax rate increased due to a decreased impact of tax benefits from the FDII deduction, stock-based compensation, and the U.S. federal research tax credit, relative to the increase in income before income tax. The increase in the effective tax rate was partially offset by a benefit due to the IRS audit resolution.
Our effective tax rates for fiscal years 2024 and 2023 were lower than the U.S. federal statutory rate of 21% due primarily to tax benefits from the FDII deduction, stock-based compensation and the U.S. federal research tax credit. Our effective tax rate for fiscal year 2024 was additionally benefited by the IRS audit resolution.
The OECD has announced an Inclusive Framework on Base Erosion and Profit Shifting including Pillar Two Model Rules for a new 15% global minimum tax applicable to large multinational corporations. Certain jurisdictions, including European Union member states and the United Kingdom, have enacted Pillar Two legislation that will start to become effective for our fiscal year 2025. The OECD, and its member countries, continue to release new guidance and legislation on Pillar Two and we continue to evaluate the impact on our financial position of the global implementation of these rules. Based on enacted laws, Pillar Two is not expected to materially impact our effective tax rate or cash flows in the next fiscal year. New legislation or guidance could change our current assessment.
Refer to Note 14 of the Notes to the Consolidated Financial Statements in Part IV, Item 15 of this Annual Report on Form 10-K for additional information.
## Liquidity and Capital Resources
Table of Contents
| | Jan 28, 2024 | Jan 29, 2023 |
|---------------------------------------------------|----------------|----------------|
| | (In millions) | (In millions) |
| Cash and cash equivalents | $ 7,280 | $ 3,389 |
| Marketable securities | 18,704 | 9,907 |
| Cash, cash equivalents, and marketable securities | $ 25,984 | $ 13,296 |
| | Year Ended | Year Ended |
|-----------------------------------------------------|---------------|---------------|
| | Jan 28, 2024 | Jan 29, 2023 |
| | (In millions) | (In millions) |
| Net cash provided by operating activities | $ 28,090 | $ 5,641 |
| Net cash provided by (used in) investing activities | $ (10,566) | $ 7,375 |
| Net cash used in financing activities | $ (13,633) | $ (11,617) |
Our investment policy requires the purchase of highly rated fixed income securities, the diversification of investment types and credit exposures, and certain maturity limits on our portfolio.
Cash provided by operating activities increased in fiscal year 2024 compared to fiscal year 2023, due to growth in revenue. Accounts receivable balance in fiscal year 2024 reflected $557 million from customer payments received ahead of the invoice due date.
Cash provided by investing activities decreased in fiscal year 2024 compared to fiscal year 2023, primarily driven by lower marketable securities maturities and higher purchases of marketable securities.
Cash used in financing activities increased in fiscal year 2024 compared to fiscal year 2023, due to a debt repayment and higher tax payments related to RSUs, partially offset by lower share repurchases.
## Liquidity
Our primary sources of liquidity are our cash, cash equivalents, and marketable securities, and the cash generated by our operations. At the end of fiscal year 2024, we had $26.0 billion in cash, cash equivalents and marketable securities. We believe that we have sufficient liquidity to meet our operating requirements for at least the next twelve months, and for the foreseeable future, including our future supply obligations and $1.3 billion of debt repayment due in fiscal year 2025 and share purchases. We continuously evaluate our liquidity and capital resources, including our access to external capital, to ensure we can finance future capital requirements.
Our marketable securities consist of debt securities issued by the U.S. government and its agencies, highly rated corporations and financial institutions, and foreign government entities, as well as certificates of deposit issued by highly rated financial institutions. These marketable securities are primarily denominated in U.S. dollars. Refer to Note 8 of the Notes to the Consolidated Financial Statements in Part IV, Item 15 of this Annual Report on Form 10-K for additional information.
During fiscal year 2025, we expect to use our existing cash, cash equivalents, and marketable securities, and the cash generated by our operations to fund our capital investments of approximately $3.5 billion to $4.0 billion related to property and equipment.
Except for approximately $1.4 billion of cash, cash equivalents, and marketable securities held outside the U.S. for which we have not accrued any related foreign or state taxes if we repatriate these amounts to the U.S., substantially all of our cash, cash equivalents and marketable securities held outside of the U.S. at the end of fiscal year 2024 are available for use in the U.S. without incurring additional U.S. federal income taxes.
## Capital Return to Shareholders
During fiscal year 2024, we paid $395 million in quarterly cash dividends.
Our cash dividend program and the payment of future cash dividends under that program are subject to our Board of Directors' continuing determination that the dividend program and the declaration of dividends thereunder are in the best interests of our shareholders.
In August 2023, our Board of Directors approved an increase to our share repurchase program of an additional $25.0 billion, without expiration. During fiscal year 2024, we repurchased 21 million shares of our common stock for $9.7 billion. As of January 28, 2024, we were authorized, subject to certain specifications, to repurchase additional shares of our
common stock up to $22.5 billion. From January 29, 2024 through February 16, 2024, we repurchased 2.8 million shares for $1.9 billion pursuant to a Rule 10b5-1 trading plan. Our share repurchase program aims to offset dilution from shares issued to employees. We may pursue additional share repurchases as we weigh market factors and other investment opportunities. We plan to continue share repurchases this fiscal year.
The U.S. Inflation Reduction Act of 2022 requires a 1% excise tax on certain share repurchases in excess of shares issued for employee compensation made after December 31, 2022 which was not material for fiscal year 2024.
## Outstanding Indebtedness and Commercial Paper Program
Our aggregate debt maturities as of January 28, 2024, by year payable, are as follows:
Table of Contents
| | Jan 28, 2024 (In millions) |
|----------------------------------------------|------------------------------|
| Due in one year | $ 1,250 |
| Due in one to five years | 2,250 |
| Due in five to ten years | 2,750 |
| Due in greater than ten years | 3,500 |
| Unamortized debt discount and issuance costs | (41) |
| Net carrying amount | 9,709 |
| Less short-term portion | (1,250) |
| Total long-term portion | $ 8,459 |
We have a $575 million commercial paper program to support general corporate purposes. As of the end of fiscal year 2024, we had no commercial paper outstanding.
Refer to Note 12 of the Notes to the Consolidated Financial Statements in Part IV, Item 15 of this Annual Report on Form 10-K for further discussion.
## Material Cash Requirements and Other Obligations
For a description of our long-term debt, purchase obligations, and operating lease obligations, refer to Note 12, Note 13, and Note 3 of the Notes to the Consolidated Financial Statements in Part IV, Item 15 of this Annual Report on Form 10-K, respectively.
We have unrecognized tax benefits of $1.3 billion, which includes related interest and penalties of $140 million, recorded in non-current income tax payable at the end of fiscal year 2024. We are unable to estimate the timing of any potential tax liability, interest payments, or penalties in individual years due to uncertainties in the underlying income tax positions and the timing of the effective settlement of such tax positions. Refer to Note 14 of the Notes to the Consolidated Financial Statements in Part IV, Item 15 of this Annual Report on Form 10-K for further information.
## Climate Change
To date, there has been no material impact to our results of operations associated with global sustainability regulations, compliance, costs from sourcing renewable energy or climate-related business trends.
## Adoption of New and Recently Issued Accounting Pronouncements
Refer to Note 1 of the Notes to the Consolidated Financial Statements in Part IV, Item 15 of this Annual Report on Form 10-K for a discussion of adoption of new and recently issued accounting pronouncements.
## Item 7A. Quantitative and Qualitative Disclosures about Market Risk
## Investment and Interest Rate Risk
We are exposed to interest rate risk related to our fixed-rate investment portfolio and outstanding debt. The investment portfolio is managed consistent with our overall liquidity strategy in support of both working capital needs and growth of our businesses.
As of the end of fiscal year 2024, we performed a sensitivity analysis on our investment portfolio. According to our analysis, parallel shifts in the yield curve of plus or minus 0.5% would result in a change in fair value for these investments of $93 million.
As of the end of fiscal year 2024, we had $9.7 billion of senior Notes net outstanding. We carry the Notes at face value less unamortized discount on our Consolidated Balance Sheets. As the Notes bear interest at a fixed rate, we have no
## Table of Contents
financial statement risk associated with changes in interest rates. Refer to Note 12 of the Notes to the Consolidated Financial Statements in Part IV, Item 15 of this Annual Report on Form 10-K for additional information.
## Foreign Exchange Rate Risk
We consider our direct exposure to foreign exchange rate fluctuations to be minimal as our sales are in United States dollars and foreign currency forward contracts are used to offset movements of foreign currency exchange rate movements. Gains or losses from foreign currency remeasurement are included in other income or expenses. The impact of foreign currency transaction gain or loss included in determining net income was not significant for fiscal years 2024 and 2023.
Sales and arrangements with third-party manufacturers provide for pricing and payment in United States dollars, and, therefore, are not subject to exchange rate fluctuations. Increases in the value of the United States' dollar relative to other currencies would make our products more expensive, which could negatively impact our ability to compete. Conversely, decreases in the value of the United States' dollar relative to other currencies could result in our suppliers raising their manufacturing costs.
If the U.S. dollar strengthened by 10% as of January 28, 2024 and January 29, 2023, the amount recorded in accumulated other comprehensive income (loss) related to our foreign exchange contracts before tax effect would have been $116 million and $112 million lower, respectively. Change in value recorded in accumulated other comprehensive income (loss) would be expected to offset a corresponding change in hedged forecasted foreign currency expenses when recognized.
If an adverse 10% foreign exchange rate change was applied to our balance sheet hedging contracts, it would have resulted in an adverse impact on income before taxes of $60 million and $36 million as of January 28, 2024 and January 29, 2023, respectively. These changes in fair values would be offset in other income (expense), net by corresponding change in fair values of the foreign currency denominated monetary assets and liabilities, assuming the hedge contracts fully cover the foreign currency denominated monetary assets and liabilities balances.
Refer to Note 11 of the Notes to the Consolidated Financial Statements in Part IV, Item 15 of this Annual Report on Form 10-K for additional information.
## Item 8. Financial Statements and Supplementary Data
The information required by this Item is set forth in our Consolidated Financial Statements and Notes thereto included in this Annual Report on Form 10-K.
## Item 9. Changes in and Disagreements with Accountants on Accounting and Financial Disclosure
None.
## Item 9A. Controls and Procedures
## Controls and Procedures
## Disclosure Controls and Procedures
Based on their evaluation as of January 28, 2024, our management, including our Chief Executive Officer and Chief Financial Officer, has concluded that our disclosure controls and procedures (as defined in Rule 13a-15(e) under the Exchange Act) were effective to provide reasonable assurance.
## Management's Annual Report on Internal Control Over Financial Reporting
Our management is responsible for establishing and maintaining adequate internal control over financial reporting, as such term is defined in Exchange Act Rule 13a-15(f). Under the supervision and with the participation of our management, including our Chief Executive Officer and Chief Financial Officer, we conducted an evaluation of the effectiveness of our internal control over financial reporting as of January 28, 2024 based on the criteria set forth in Internal Control - Integrated Framework (2013) issued by the Committee of Sponsoring Organizations of the Treadway Commission. Based on our evaluation under the criteria set forth in Internal Control - Integrated Framework , our management concluded that our internal control over financial reporting was effective as of January 28, 2024.
The effectiveness of our internal control over financial reporting as of January 28, 2024 has been audited by PricewaterhouseCoopers LLP, an independent registered public accounting firm, as stated in its report which is included herein.
## Changes in Internal Control Over Financial Reporting
There have been no changes in our internal control over financial reporting during the quarter ended January 28, 2024 that have materially affected, or are reasonably likely to materially affect, our internal control over financial reporting. In fiscal year 2022, we began an upgrade of our enterprise resource planning, or ERP, system, which will update much of our
## Table of Contents
existing core financial systems. The ERP system is designed to accurately maintain our financial records used to report operating results. The upgrade will occur in phases. We will continue to evaluate each quarter whether there are changes that materially affect our internal control over financial reporting.
## Inherent Limitations on Effectiveness of Controls
Our management, including our Chief Executive Officer and Chief Financial Officer, does not expect that our disclosure controls and procedures or our internal controls, will prevent all error and all fraud. A control system, no matter how well conceived and operated, can provide only reasonable, not absolute, assurance that the objectives of the control system are met. Further, the design of a control system must reflect the fact that there are resource constraints, and the benefits of controls must be considered relative to their costs. Because of the inherent limitations in all control systems, no evaluation of controls can provide absolute assurance that all control issues and instances of fraud, if any, within NVIDIA have been detected.
## Item 9B. Other Information
On December 18, 2023, John O. Dabiri, a member of our Board of Directors, adopted a trading arrangement that is intended to satisfy the affirmative defense conditions of Rule 10b5-1(c) for the sale through December 2, 2024 of an estimated 553 shares of our common stock, assuming our closing stock price as of January 26, 2024. The number of shares is based on an estimate because the plan specifies a formulaic dollar amount of shares to be sold.
## Item 9C. Disclosure Regarding Foreign Jurisdictions that Prevent Inspections
Not Applicable.
## Part III
Certain information required by Part III is omitted from this report because we will file with the SEC a definitive proxy statement pursuant to Regulation 14A, or the 2024 Proxy Statement, no later than 120 days after the end of fiscal year 2024, and certain information included therein is incorporated herein by reference.
## Item 10. Directors, Executive Officers and Corporate Governance
## Identification of Directors
Information regarding directors required by this item will be contained in our 2024 Proxy Statement under the caption 'Proposal 1 - Election of Directors,' and is hereby incorporated by reference.
## Identification of Executive Officers
Reference is made to the information regarding executive officers appearing under the heading 'Information About Our Executive Officers' in Part I of this Annual Report on Form 10-K, which information is hereby incorporated by reference.
## Identification of Audit Committee and Financial Experts
Information regarding our Audit Committee required by this item will be contained in our 2024 Proxy Statement under the captions 'Report of the Audit Committee of the Board of Directors' and 'Information About the Board of Directors and Corporate Governance,' and is hereby incorporated by reference.
## Material Changes to Procedures for Recommending Directors
Information regarding procedures for recommending directors required by this item will be contained in our 2024 Proxy Statement under the caption 'Information About the Board of Directors and Corporate Governance,' and is hereby incorporated by reference.
## Delinquent Section 16(a) Reports
Information regarding compliance with Section 16(a) of the Exchange Act required by this item will be contained in our 2024 Proxy Statement under the caption 'Delinquent Section 16(a) Reports,' and is hereby incorporated by reference.
## Code of Conduct
Information regarding our Code of Conduct required by this item will be contained in our 2024 Proxy Statement under the caption 'Information About the Board of Directors and Corporate Governance - Code of Conduct,' and is hereby incorporated by reference. The full text of our Code of Conduct and Financial Team Code of Conduct are published on the Investor Relations portion of our website, under Governance, at www.nvidia.com. If we make any amendments to either code, or grant any waiver from a provision of either code to any executive officer or director, we will promptly disclose the nature of the amendment or waiver on our website or in a report on Form 8-K. The contents of our website are not a part of this Annual Report on Form 10-K.
## Table of Contents
## Item 11. Executive Compensation
Information regarding our executive compensation required by this item will be contained in our 2024 Proxy Statement under the captions 'Executive Compensation', 'Compensation Committee Interlocks and Insider Participation', 'Director Compensation' and 'Compensation Committee Report,' and is hereby incorporated by reference.
## Item 12. Security Ownership of Certain Beneficial Owners and Management and Related Stockholder Matters
## Ownership of NVIDIA Securities
Information regarding ownership of NVIDIA securities required by this item will be contained in our 2024 Proxy Statement under the caption 'Security Ownership of Certain Beneficial Owners and Management,' and is hereby incorporated by reference.
## Equity Compensation Plan Information
Information regarding our equity compensation plans required by this item will be contained in our 2024 Proxy Statement under the caption "Equity Compensation Plan Information," and is hereby incorporated by reference.
## Item 13. Certain Relationships and Related Transactions, and Director Independence
Information regarding related transactions and director independence required by this item will be contained in our 2024 Proxy Statement under the captions 'Review of Transactions with Related Persons' and 'Information About the Board of Directors and Corporate Governance - Independence of the Members of the Board of Directors,' and is hereby incorporated by reference.
## Item 14. Principal Accountant Fees and Services
Information regarding accounting fees and services required by this item will be contained in our 2024 Proxy Statement under the caption 'Fees Billed by the Independent Registered Public Accounting Firm,' and is hereby incorporated by reference.
## Part IV
## Item 15. Exhibit and Financial Statement Schedules
Table of Contents
| | | Page |
|----|------------------------------------------------------------------------------------------------------------------------------------------------|--------|
| 1. | Financial Statements | |
| | Report of Independent Registered Public Accounting Firm (PCAOB ID: 238) | 48 |
| | Consolidated Statements of Income for the years ended January 28, 2024, January 29, 2023, and January 30, 2022 | 50 |
| | Consolidated Statements of Comprehensive Income for the years ended January 28, 2024, January 29, 2023, and January 30, 2022 | 51 |
| | Consolidated Balance Sheets as of January 28, 2024 and January 29, 2023 | 52 |
| | Consolidated Statements of Shareholders' Equity for the years ended January 28, 2024, January 29, 2023, and January 30, 2022 | 53 |
| | Consolidated Statements of Cash Flows for the years ended January 28, 2024, January 29, 2023, and January 30, 2022 | 54 |
| | Notes to the Consolidated Financial Statements | 55 |
| 2. | Financial Statement Schedule | |
| | Schedule II Valuation and Qualifying Accounts for the years ended January 28, 2024, January 29, 2023, and January 30, 2022 | 81 |
| 3. | Exhibits | |
| | The exhibits listed in the accompanying index to exhibits are filed or incorporated by reference as a part of this Annual Report on Form 10-K. | 82 |
## Table of Contents
## Report of Independent Registered Public Accounting Firm
To the Board of Directors and Shareholders of NVIDIA Corporation
## Opinions on the Financial Statements and Internal Control over Financial Reporting
We have audited the accompanying consolidated balance sheets of NVIDIA Corporation and its subsidiaries (the 'Company') as of January 28, 2024 and January 29, 2023, and the related consolidated statements of income, comprehensive income, shareholders' equity and cash flows for each of the three years in the period ended January 28, 2024, including the related notes and financial statement schedule listed in the index appearing under Item 15(a)(2) (collectively referred to as the 'consolidated financial statements'). We also have audited the Company's internal control over financial reporting as of January 28, 2024, based on criteria established in Internal Control - Integrated Framework (2013) issued by the Committee of Sponsoring Organizations of the Treadway Commission (COSO).
In our opinion, the consolidated financial statements referred to above present fairly, in all material respects, the financial position of the Company as of January 28, 2024 and January 29, 2023, and the results of its operations and its cash flows for each of the three years in the period ended January 28, 2024 in conformity with accounting principles generally accepted in the United States of America. Also in our opinion, the Company maintained, in all material respects, effective internal control over financial reporting as of January 28, 2024, based on criteria established in Internal Control - Integrated Framework (2013) issued by the COSO.
## Basis for Opinions
The Company's management is responsible for these consolidated financial statements, for maintaining effective internal control over financial reporting, and for its assessment of the effectiveness of internal control over financial reporting, included in Management's Annual Report on Internal Control over Financial Reporting appearing under Item 9A. Our responsibility is to express opinions on the Company's consolidated financial statements and on the Company's internal control over financial reporting based on our audits. We are a public accounting firm registered with the Public Company Accounting Oversight Board (United States) (PCAOB) and are required to be independent with respect to the Company in accordance with the U.S. federal securities laws and the applicable rules and regulations of the Securities and Exchange Commission and the PCAOB.
We conducted our audits in accordance with the standards of the PCAOB. Those standards require that we plan and perform the audits to obtain reasonable assurance about whether the consolidated financial statements are free of material misstatement, whether due to error or fraud, and whether effective internal control over financial reporting was maintained in all material respects.
Our audits of the consolidated financial statements included performing procedures to assess the risks of material misstatement of the consolidated financial statements, whether due to error or fraud, and performing procedures that respond to those risks. Such procedures included examining, on a test basis, evidence regarding the amounts and disclosures in the consolidated financial statements. Our audits also included evaluating the accounting principles used and significant estimates made by management, as well as evaluating the overall presentation of the consolidated financial statements. Our audit of internal control over financial reporting included obtaining an understanding of internal control over financial reporting, assessing the risk that a material weakness exists, and testing and evaluating the design and operating effectiveness of internal control based on the assessed risk. Our audits also included performing such other procedures as we considered necessary in the circumstances. We believe that our audits provide a reasonable basis for our opinions.
## Definition and Limitations of Internal Control over Financial Reporting
A company's internal control over financial reporting is a process designed to provide reasonable assurance regarding the reliability of financial reporting and the preparation of financial statements for external purposes in accordance with generally accepted accounting principles. A company's internal control over financial reporting includes those policies and procedures that (i) pertain to the maintenance of records that, in reasonable detail, accurately and fairly reflect the transactions and dispositions of the assets of the company; (ii) provide reasonable assurance that transactions are recorded as necessary to permit preparation of financial statements in accordance with generally accepted accounting principles, and that receipts and expenditures of the company are being made only in accordance with authorizations of management and directors of the company; and (iii) provide reasonable assurance regarding prevention or timely detection of unauthorized acquisition, use, or disposition of the company's assets that could have a material effect on the financial statements.
Because of its inherent limitations, internal control over financial reporting may not prevent or detect misstatements. Also, projections of any evaluation of effectiveness to future periods are subject to the risk that controls may become inadequate because of changes in conditions, or that the degree of compliance with the policies or procedures may deteriorate.
## Table of Contents
## Critical Audit Matters
The critical audit matter communicated below is a matter arising from the current period audit of the consolidated financial statements that was communicated or required to be communicated to the audit committee and that (i) relates to accounts or disclosures that are material to the consolidated financial statements and (ii) involved our especially challenging, subjective, or complex judgments. The communication of critical audit matters does not alter in any way our opinion on the consolidated financial statements, taken as a whole, and we are not, by communicating the critical audit matter below, providing a separate opinion on the critical audit matter or on the accounts or disclosures to which it relates.
Valuation of Inventories - Provisions for Excess or Obsolete Inventories and Excess Product Purchase Commitments
As described in Notes 1, 10 and 13 to the consolidated financial statements, the Company charges cost of sales for inventory provisions to write-down inventory for excess or obsolete inventory and for excess product purchase commitments. Most of the Company's inventory provisions relate to excess quantities of products, based on the Company's inventory levels and future product purchase commitments compared to assumptions about future demand and market conditions. As of January 28, 2024, the Company's consolidated inventories balance was $5.3 billion and the Company's consolidated outstanding inventory purchase and long-term supply and capacity obligations balance was $16.1 billion, of which a significant portion relates to inventory purchase obligations.
The principal considerations for our determination that performing procedures relating to the valuation of inventories, specifically the provisions for excess or obsolete inventories and excess product purchase commitments, is a critical audit matter are the significant judgment by management when developing provisions for excess or obsolete inventories and excess product purchase commitments, including developing assumptions related to future demand and market conditions. This in turn led to significant auditor judgment, subjectivity, and effort in performing procedures and evaluating management's assumptions related to future demand and market conditions.
Addressing the matter involved performing procedures and evaluating audit evidence in connection with forming our overall opinion on the consolidated financial statements. These procedures included testing the effectiveness of controls relating to management's provisions for excess or obsolete inventories and excess product purchase commitments, including controls over management's assumptions related to future demand and market conditions. These procedures also included, among others, testing management's process for developing the provisions for excess or obsolete inventories and excess product purchase commitments; evaluating the appropriateness of management's approach; testing the completeness and accuracy of underlying data used in the approach; and evaluating the reasonableness of management's assumptions related to future demand and market conditions. Evaluating management's assumptions related to future demand and market conditions involved evaluating whether the assumptions used by management were reasonable considering (i) current and past results, including historical product life cycle, (ii) the consistency with external market and industry data, and (iii) changes in technology.
/s/ PricewaterhouseCoopers LLP San Jose, California February 21, 2024
We have served as the Company's auditor since 2004.
## NVIDIA Corporation and Subsidiaries
## Consolidated Statements of Income (In millions, except per share data)
Table of Contents
| | Year Ended | Year Ended | Year Ended |
|--------------------------------------------------------|--------------|--------------|--------------|
| | Jan 28, 2024 | Jan 29, 2023 | Jan 30, 2022 |
| Revenue | $ 60,922 | $ 26,974 | $ 26,914 |
| Cost of revenue | 16,621 | 11,618 | 9,439 |
| Gross profit | 44,301 | 15,356 | 17,475 |
| Operating expenses | | | |
| Research and development | 8,675 | 7,339 | 5,268 |
| Sales, general and administrative | 2,654 | 2,440 | 2,166 |
| Acquisition termination cost | - | 1,353 | - |
| Total operating expenses | 11,329 | 11,132 | 7,434 |
| Operating income | 32,972 | 4,224 | 10,041 |
| Interest income | 866 | 267 | 29 |
| Interest expense | (257) | (262) | (236) |
| Other, net | 237 | (48) | 107 |
| Other income (expense), net | 846 | (43) | (100) |
| Income before income tax | 33,818 | 4,181 | 9,941 |
| Income tax expense (benefit) | 4,058 | (187) | 189 |
| Net income | $ 29,760 | $ 4,368 | $ 9,752 |
| Net income per share: | | | |
| Basic | $ 12.05 | $ 1.76 | $ 3.91 |
| Diluted | $ 11.93 | $ 1.74 | $ 3.85 |
| Weighted average shares used in per share computation: | | | |
| Basic | 2,469 | 2,487 | 2,496 |
| Diluted | 2,494 | 2,507 | 2,535 |
See accompanying notes to the consolidated financial statements.
## NVIDIA Corporation and Subsidiaries Consolidated Statements of Comprehensive Income
(In millions)
Table of Contents
| | Year Ended | Year Ended | Year Ended |
|----------------------------------------------------------------------------------|--------------|--------------|--------------|
| | Jan 28, 2024 | Jan 29, 2023 | Jan 30, 2022 |
| Net income | $ 29,760 | $ 4,368 | $ 9,752 |
| Other comprehensive income (loss), net of tax | | | |
| Available-for-sale securities: | | | |
| Net change in unrealized gain (loss) | 80 | (31) | (16) |
| Reclassification adjustments for net realized gain included in net income | - | 1 | - |
| Net change in unrealized gain (loss) | 80 | (30) | (16) |
| Cash flow hedges: | | | |
| Net change in unrealized gain (loss) | 38 | 47 | (43) |
| Reclassification adjustments for net realized gain (loss) included in net income | (48) | (49) | 29 |
| Net change in unrealized loss | (10) | (2) | (14) |
| Other comprehensive income (loss), net of tax | 70 | (32) | (30) |
| Total comprehensive income | $ 29,830 | $ 4,336 | $ 9,722 |
See accompanying notes to the consolidated financial statements.
## NVIDIA Corporation and Subsidiaries
## Consolidated Balance Sheets
(In millions, except par value)
Table of Contents
| | Jan 28, 2024 | Jan 29, 2023 |
|---------------------------------------------------------------------------------------------------------------------------------------------------------------------------------|----------------|----------------|
| Assets | | |
| Current assets: | | |
| Cash and cash equivalents | $ 7,280 | $ 3,389 |
| Marketable securities | 18,704 | 9,907 |
| Accounts receivable, net | 9,999 | 3,827 |
| Inventories | 5,282 | 5,159 |
| Prepaid expenses and other current assets | 3,080 | 791 |
| Total current assets | 44,345 | 23,073 |
| Property and equipment, net | 3,914 | 3,807 |
| Operating lease assets | 1,346 | 1,038 |
| Goodwill | 4,430 | 4,372 |
| Intangible assets, net | 1,112 | 1,676 |
| Deferred income tax assets | 6,081 | 3,396 |
| Other assets | 4,500 | 3,820 |
| Total assets | $ 65,728 | $ 41,182 |
| Liabilities and Shareholders' Equity | | |
| Current liabilities: | | |
| Accounts payable | $ 2,699 | $ 1,193 |
| Accrued and other current liabilities | 6,682 | 4,120 |
| Short-term debt | 1,250 | 1,250 |
| Total current liabilities | 10,631 | 6,563 |
| Long-term debt | 8,459 | 9,703 |
| Long-term operating lease liabilities | 1,119 | 902 |
| Other long-term liabilities | 2,541 | 1,913 |
| Total liabilities | 22,750 | 19,081 |
| Commitments and contingencies - see Note 13 | | |
| Shareholders' equity: | | |
| Preferred stock, $0.001 par value; 2 shares authorized; none issued | - | - |
| Common stock, $0.001 par value; 8,000 shares authorized; 2,464 shares issued and outstanding as of January 28, 2024; 2,466 shares issued and outstanding as of January 29, 2023 | 2 | 2 |
| Additional paid-in capital | 13,132 | 11,971 |
| Accumulated other comprehensive income (loss) | 27 | (43) |
| Retained earnings | 29,817 | 10,171 |
| Total shareholders' equity | 42,978 | 22,101 |
| Total liabilities and shareholders' equity | $ 65,728 | $ 41,182 |
See accompanying notes to the consolidated financial statements.
## NVIDIA Corporation and Subsidiaries
## Consolidated Statements of Shareholders' Equity
Table of Contents
| | Common Stock Outstanding | Common Stock Outstanding | Additional Paid-in | Treasury | Accumulated Other Comprehensive | Retained | Total Shareholders' |
|--------------------------------------------------------------------------------------|----------------------------|----------------------------|----------------------|------------|-----------------------------------|------------|-----------------------|
| | Shares | | Capital | | Income (Loss) | | Equity |
| | | Amount | | Stock | | Earnings | |
| (In millions, except per share data) | | | | | | | |
| Balances, Jan 31, 2021 | 2,479 | $ 3 | $ 8,719 | $ (10,756) | $ 19 | $ 18,908 | $ 16,893 |
| Net income | - | - | - | - | - | 9,752 | 9,752 |
| Other comprehensive loss | - | - | - | - | (30) | - | (30) |
| Issuance of common stock from stock plans | 35 | - | 281 | - | - | - | 281 |
| Tax withholding related to vesting of restricted stock units | (8) | - | (614) | (1,290) | - | - | (1,904) |
| Cash dividends declared and paid ($0.16 per common share) | - | - | - | - | - | (399) | (399) |
| Fair value of partially vested equity awards assumed in connection with acquisitions | - | - | 18 | - | - | - | 18 |
| Stock-based compensation | - | - | 2,001 | - | - | - | 2,001 |
| Retirement of Treasury Stock | - | - | (20) | 12,046 | - | (12,026) | - |
| Balances, Jan 30, 2022 | 2,506 | 3 | 10,385 | - | (11) | 16,235 | 26,612 |
| Net income | - | - | - | - | - | 4,368 | 4,368 |
| Other comprehensive loss | - | - | - | - | (32) | - | (32) |
| Issuance of common stock from stock plans | 31 | - | 355 | - | - | - | 355 |
| Tax withholding related to vesting of restricted stock units | (8) | - | (1,475) | - | - | - | (1,475) |
| Shares repurchased | (63) | (1) | (4) | - | - | (10,034) | (10,039) |
| Cash dividends declared and paid ($0.16 per common share) | - | - | - | - | - | (398) | (398) |
| Stock-based compensation | - | - | 2,710 | - | - | - | 2,710 |
| Balances, Jan 29, 2023 | 2,466 | 2 | 11,971 | - | (43) | 10,171 | 22,101 |
| Net income | - | - | - | - | - | 29,760 | 29,760 |
| Other comprehensive income | - | - | - | - | 70 | | 70 |
| Issuance of common stock from stock plans | 26 | - | 403 | - | - | - | 403 |
| Tax withholding related to vesting of restricted stock units | (7) | - | (2,783) | - | - | - | (2,783) |
| Shares repurchased | (21) | - | (27) | - | - | (9,719) | (9,746) |
| Cash dividends declared and paid ($0.16 per common share) | - | - | - | - | - | (395) | (395) |
| Stock-based compensation | - | - | 3,568 | - | - | - | 3,568 |
| Balances, Jan 28, 2024 | 2,464 | $ 2 | $ 13,132 | $ - | $ 27 | $ 29,817 | $ 42,978 |
See accompanying notes to the consolidated financial statements.
## NVIDIA Corporation and Subsidiaries
## Consolidated Statements of Cash Flows
## (In millions)
Table of Contents
| | Year Ended | Year Ended | Year Ended |
|-----------------------------------------------------------------------------------|--------------|--------------|--------------|
| | Jan 28, 2024 | Jan 29, 2023 | Jan 30, 2022 |
| Cash flows from operating activities: | | | |
| Net income | $ 29,760 | $ 4,368 | $ 9,752 |
| Adjustments to reconcile net income to net cash provided by operating activities: | | | |
| Stock-based compensation expense | 3,549 | 2,709 | 2,004 |
| Depreciation and amortization | 1,508 | 1,544 | 1,174 |
| Deferred income taxes | (2,489) | (2,164) | (406) |
| (Gains) losses on investments in non-affiliated entities, net | (238) | 45 | (100) |
| Acquisition termination cost | - | 1,353 | - |
| Other | (278) | (7) | 47 |
| Changes in operating assets and liabilities, net of acquisitions: | | | |
| Accounts receivable | (6,172) | 822 | (2,215) |
| Inventories | (98) | (2,554) | (774) |
| Prepaid expenses and other assets | (1,522) | (1,517) | (1,715) |
| Accounts payable | 1,531 | (551) | 568 |
| Accrued and other current liabilities | 2,025 | 1,341 | 581 |
| Other long-term liabilities | 514 | 252 | 192 |
| Net cash provided by operating activities | 28,090 | 5,641 | 9,108 |
| Cash flows from investing activities: | | | |
| Proceeds from maturities of marketable securities | 9,732 | 19,425 | 15,197 |
| Proceeds from sales of marketable securities | 50 | 1,806 | 1,023 |
| Purchases of marketable securities | (18,211) | (11,897) | (24,787) |
| Purchases related to property and equipment and intangible assets | (1,069) | (1,833) | (976) |
| Acquisitions, net of cash acquired | (83) | (49) | (263) |
| Investments in non-affiliated entities and other, net | (985) | (77) | (24) |
| Net cash provided by (used in) investing activities | (10,566) | 7,375 | (9,830) |
| Cash flows from financing activities: | | | |
| Proceeds related to employee stock plans | 403 | 355 | 281 |
| Payments related to repurchases of common stock | (9,533) | (10,039) | - |
| Payments related to tax on restricted stock units | (2,783) | (1,475) | (1,904) |
| Repayment of debt | (1,250) | - | (1,000) |
| Dividends paid | (395) | (398) | (399) |
| Principal payments on property and equipment and intangible assets | (74) | (58) | (83) |
| Issuance of debt, net of issuance costs | - | - | 4,977 |
| Other | (1) | (2) | (7) |
| Net cash provided by (used in) financing activities | (13,633) | (11,617) | 1,865 |
| Change in cash and cash equivalents | 3,891 | 1,399 | 1,143 |
| Cash and cash equivalents at beginning of period | 3,389 | 1,990 | 847 |
| Cash and cash equivalents at end of period | $ 7,280 | $ 3,389 | $ 1,990 |
| Supplemental disclosures of cash flow information: | | | |
| Cash paid for income taxes, net | $ 6,549 | $ 1,404 | $ 396 |
| Cash paid for interest | $ 252 | $ 254 | $ 246 |
See accompanying notes to the consolidated financial statements.
## Table of Contents
## NVIDIA Corporation and Subsidiaries Notes to the Consolidated Financial Statements
## Note 1 - Organization and Summary of Significant Accounting Policies
## Our Company
Headquartered in Santa Clara, California, NVIDIA was incorporated in California in April 1993 and reincorporated in Delaware in April 1998.
All references to 'NVIDIA,' 'we,' 'us,' 'our' or the 'Company' mean NVIDIA Corporation and its subsidiaries.
## Fiscal Year
We operate on a 52- or 53-week year, ending on the last Sunday in January. Fiscal years 2024, 2023 and 2022 were all 52-week years.
## Principles of Consolidation
Our consolidated financial statements include the accounts of NVIDIA Corporation and our wholly-owned subsidiaries. All intercompany balances and transactions have been eliminated in consolidation.
## Use of Estimates
The preparation of financial statements in conformity with U.S. GAAP requires management to make estimates and assumptions that affect the reported amounts of assets and liabilities and disclosures of contingent assets and liabilities at the date of the financial statements and the reported amounts of revenue and expenses during the reporting period. Actual results could differ materially from our estimates. On an on-going basis, we evaluate our estimates, including those related to revenue recognition, cash equivalents and marketable securities, accounts receivable, inventories and product purchase commitments, income taxes, goodwill, stock-based compensation, litigation, investigation and settlement costs, restructuring and other charges, property, plant, and equipment, and other contingencies. These estimates are based on historical facts and various other assumptions that we believe are reasonable.
In February 2023, we assessed the useful lives of our property, plant, and equipment. Based on advances in technology and usage rate, we increased the estimated useful life of most of our server, storage, and network equipment from three to four or five years, and our assembly and test equipment from five to seven years. The effect of this change for the fiscal year ended January 28, 2024 was a benefit of $33 million and $102 million for cost of revenue and operating expenses, respectively, which resulted in an increase in operating income of $135 million and net income of $114 million after tax, or $0.05 per both basic and diluted share.
## Revenue Recognition
We derive our revenue from product sales, including hardware and systems, license and development arrangements, software licensing, and cloud services. We determine revenue recognition through the following steps: (1) identification of the contract with a customer; (2) identification of the performance obligations in the contract; (3) determination of the transaction price; (4) allocation of the transaction price to the performance obligations in the contract (where revenue is allocated on a relative standalone selling price basis by maximizing the use of observable inputs to determine the standalone selling price for each performance obligation); and (5) recognition of revenue when, or as, we satisfy a performance obligation.
## Product Sales Revenue
Revenue from product sales is recognized upon transfer of control of products to customers in an amount that reflects the consideration we expect to receive in exchange for those products. Certain products are sold with support or an extended warranty for the incorporated system, hardware, and/or software. Support and extended warranty revenue are recognized ratably over the service period, or as services are performed. Revenue is recognized net of allowances for returns, customer programs and any taxes collected from customers.
For products sold with a right of return, we record a reduction to revenue by establishing a sales return allowance for estimated product returns at the time revenue is recognized, based primarily on historical return rates. However, if product returns for a fiscal period are anticipated to exceed historical return rates, we may determine that additional sales return allowances are required to accurately reflect our estimated exposure for product returns.
Our customer programs involve rebates, which are designed to serve as sales incentives to resellers of our products in various target markets, and marketing development funds, or MDFs, which represent monies paid to our partners that are earmarked for market segment development and are designed to support our partners' activities while also promoting NVIDIA products. We account for customer programs as a reduction to revenue and accrue for such programs for potential rebates and MDFs based on the amount we expect to be claimed by customers.
## Table of Contents
## NVIDIA Corporation and Subsidiaries
## Notes to the Consolidated Financial Statements
(Continued)
## License and Development Arrangements
Our license and development arrangements with customers typically require significant customization of our IP components. As a result, we recognize the revenue from the license and the revenue from the development services as a single performance obligation over the period in which the development services are performed. We measure progress to completion based on actual cost incurred to date as a percentage of the estimated total cost required to complete each project. If a loss on an arrangement becomes probable during a period, we record a provision for such loss in that period.
## Software Licensing
Our software licenses provide our customers with a right to use the software when it is made available to the customer. Customers may purchase either perpetual licenses or subscriptions to licenses, which differ mainly in the duration over which the customer benefits from the software. Software licenses are frequently sold along with the right to receive, on a when-and-if available basis, future unspecified software updates and upgrades. Revenue from software licenses is recognized up front when the software is made available to the customer. Software support revenue is recognized ratably over the service period, or as services are performed.
## Cloud Services
Cloud services, which allow customers to use hosted software and hardware infrastructure without taking possession of the software or hardware, are provided on a subscription basis or a combination of subscription plus usage. Revenue related to subscription-based cloud services is recognized ratably over the contract period. Revenue related to cloud services based on usage is recognized as usage occurs. Cloud services are typically sold on a standalone basis, but certain offerings may be sold with hardware and/or software and related support.
## Contracts with Multiple Performance Obligations
Our contracts may contain more than one of the products and services listed above, each of which is separately accounted for as a distinct performance obligation. We account for multiple agreements with a single customer as a single contract if the contractual terms and/or substance of those agreements indicate that they may be so closely related that they are, in effect, parts of a single contract.
We allocate the total transaction price to each distinct performance obligation in a multiple performance obligations arrangement on a relative standalone selling price basis. The standalone selling price reflects the price we would charge for a specific product or service if it were sold separately in similar circumstances and to similar customers. When determining standalone selling price, we maximize the use of observable inputs.
If a contract contains a single performance obligation, no allocation is required.
## Product Warranties
We offer a limited warranty to end-users ranging from one to three years for products to repair or replace products for manufacturing defects or hardware component failures. Cost of revenue includes the estimated cost of product warranties that are calculated at the point of revenue recognition. Under limited circumstances, we may offer an extended limited warranty to customers for certain products. We also accrue for known warranty and indemnification issues if a loss is probable and can be reasonably estimated.
## Stock-based Compensation
We use the closing trading price of our common stock on the date of grant, minus a dividend yield discount, as the fair value of awards of restricted stock units, or RSUs, and performance stock units that are based on our corporate financial performance targets, or PSUs. We use a Monte Carlo simulation on the date of grant to estimate the fair value of performance stock units that are based on market conditions, or market-based PSUs. The compensation expense for RSUs and market-based PSUs is recognized using a straight-line attribution method over the requisite employee service period while compensation expense for PSUs is recognized using an accelerated amortization model. We estimate the fair value of shares to be issued under our employee stock purchase plan, or ESPP, using the Black-Scholes model at the commencement of an offering period in March and September of each year. Stock-based compensation for our ESPP is expensed using an accelerated amortization model. Additionally, for RSU, PSU, and market-based PSU awards, we estimate forfeitures semi-annually and revise the estimates of forfeiture in subsequent periods if actual forfeitures differ from those estimates. Forfeitures are estimated based on historical experience.
## Litigation, Investigation and Settlement Costs
We currently, are, and will likely continue to be subject to claims, litigation, and other actions, including potential regulatory proceedings, involving patent and other intellectual property matters, taxes, labor and employment, competition and antitrust, commercial disputes, goods and services offered by us and by third parties, and other matters. There are many uncertainties associated with any litigation or investigation, and we cannot be certain that these actions
## Table of Contents
## NVIDIA Corporation and Subsidiaries
## Notes to the Consolidated Financial Statements
(Continued)
or other third-party claims against us will be resolved without litigation, fines and/or substantial settlement payments or judgments. If information becomes available that causes us to determine that a loss in any of our pending litigation, investigations or settlements is probable, and we can reasonably estimate the loss associated with such events, we will record the loss in accordance with U.S. GAAP. However, the actual liability in any such litigation or investigation may be materially different from our estimates, which could require us to record additional costs.
## Foreign Currency Remeasurement
We use the U.S. dollar as our functional currency for our subsidiaries. Foreign currency monetary assets and liabilities are remeasured into United States dollars at end-of-period exchange rates. Non-monetary assets and liabilities such as property and equipment and equity are remeasured at historical exchange rates. Revenue and expenses are remeasured at exchange rates in effect during each period, except for those expenses related to non-monetary balance sheet amounts, which are remeasured at historical exchange rates. Gains or losses from foreign currency remeasurement are included in earnings in our Consolidated Statements of Income and to date have not been significant.
## Income Taxes
We recognize federal, state and foreign current tax liabilities or assets based on our estimate of taxes payable or refundable in the current fiscal year by tax jurisdiction. We recognize federal, state and foreign deferred tax assets or liabilities, as appropriate, for our estimate of future tax effects attributable to temporary differences and carryforwards; and we record a valuation allowance to reduce any deferred tax assets by the amount of any tax benefits that, based on available evidence and judgment, are not expected to be realized.
Our calculation of deferred tax assets and liabilities is based on certain estimates and judgments and involves dealing with uncertainties in the application of complex tax laws. Our estimates of deferred tax assets and liabilities may change based, in part, on added certainty or finality to an anticipated outcome, changes in accounting standards or tax laws in the U.S., or foreign jurisdictions where we operate, or changes in other facts or circumstances. In addition, we recognize liabilities for potential U.S. and foreign income tax contingencies based on our estimate of whether, and the extent to which, additional taxes may be due. If we determine that payment of these amounts is unnecessary or if the recorded tax liability is less than our current assessment, we may be required to recognize an income tax benefit or additional income tax expense in our financial statements accordingly.
As of January 28, 2024, we had a valuation allowance of $1.6 billion related to capital loss carryforwards, and certain state and other deferred tax assets that management determined are not likely to be realized due, in part, to jurisdictional projections of future taxable income, including capital gains. To the extent realization of the deferred tax assets becomes more-likely-than-not, we would recognize such deferred tax assets as income tax benefits during the period.
We recognize the benefit from a tax position only if it is more-likely-than-not that the position would be sustained upon audit based solely on the technical merits of the tax position. Our policy is to include interest and penalties related to unrecognized tax benefits as a component of income tax expense.
## Net Income Per Share
Basic net income per share is computed using the weighted average number of common shares outstanding during the period. Diluted net income per share is computed using the weighted average number of common and potentially dilutive shares outstanding during the period, using the treasury stock method. Any anti-dilutive effect of equity awards outstanding is not included in the computation of diluted net income per share.
## Cash and Cash Equivalents and Marketable Securities
We consider all highly liquid investments that are readily convertible into cash and have an original maturity of three months or less at the time of purchase to be cash equivalents. Marketable securities consist of highly liquid debt investments with maturities of greater than three months when purchased. We currently classify our investments as current based on the nature of the investments and their availability for use in current operations.
We classify our cash equivalents and marketable securities related to debt securities at the date of acquisition as available-for-sale. These available-for-sale debt securities are reported at fair value with the related unrealized gains and losses included in accumulated other comprehensive income or loss, a component of shareholders' equity, net of tax. The fair value of interest-bearing debt securities includes accrued interest. Realized gains and losses on the sale of marketable securities are determined using the specific-identification method and recorded in the other income (expense), net, section of our Consolidated Statements of Income.
Available-for-sale debt investments are subject to a periodic impairment review. If the estimated fair value of available-for-sale debt securities is less than its amortized cost basis, we determine if the difference, if any, is caused by expected credit losses and write-down the amortized cost basis of the securities if it is more likely than not we will be required or
## Table of Contents
## NVIDIA Corporation and Subsidiaries
## Notes to the Consolidated Financial Statements
(Continued)
we intend to sell the securities before recovery of its amortized cost basis. Allowances for credit losses and write-downs are recognized in the other income (expense), net section of our Consolidated Statements of Income.
## Fair Value of Financial Instruments
The carrying value of cash equivalents, accounts receivable, accounts payable and accrued liabilities approximate their fair values due to their relatively short maturities as of January 28, 2024 and January 29, 2023. Marketable securities are comprised of available-for-sale securities that are reported at fair value with the related unrealized gains or losses included in accumulated other comprehensive income or loss, a component of shareholders' equity, net of tax. Fair value of the marketable securities is determined based on quoted market prices. Derivative instruments are recognized as either assets or liabilities and are measured at fair value. The accounting for changes in the fair value of a derivative depends on the intended use of the derivative and the resulting designation. For derivative instruments designated as fair value hedges, the gains or losses are recognized in earnings in the periods of change together with the offsetting losses or gains on the hedged items attributed to the risk being hedged. For derivative instruments designated as cash-flow hedges, the effective portion of the gains or losses on the derivatives is initially reported as a component of other comprehensive income or loss and is subsequently recognized in earnings when the hedged exposure is recognized in earnings. For derivative instruments not designated for hedge accounting, changes in fair value are recognized in earnings.
## Concentration of Credit Risk
Financial instruments that potentially subject us to concentrations of credit risk consist primarily of cash equivalents, marketable securities, and accounts receivable. Our investment policy requires the purchase of highly-rated fixed income securities, the diversification of investment type and credit exposures, and includes certain limits on our portfolio duration. We perform ongoing credit evaluations of our customers' financial condition and maintain an allowance for potential credit losses. This allowance consists of an amount identified for specific customers and an amount based on overall estimated exposure. Our overall estimated exposure excludes amounts covered by credit insurance and letters of credit.
## Inventories
Inventory cost is computed on an adjusted standard basis, which approximates actual cost on an average or first-in, first-out basis. Inventory costs consist primarily of the cost of semiconductors, including wafer fabrication, assembly, testing and packaging, manufacturing support costs, including labor and overhead associated with such purchases, final test yield fallout, and shipping costs, as well as the cost of purchased memory products and other component parts. We charge cost of sales for inventory provisions to write-down our inventory to the lower of cost or net realizable value or for obsolete or excess inventory, and for excess product purchase commitments. Most of our inventory provisions relate to excess quantities of products, based on our inventory levels and future product purchase commitments compared to assumptions about future demand and market conditions. Once inventory has been written-off or written-down, it creates a new cost basis for the inventory that is not subsequently written-up. We record a liability for noncancelable purchase commitments with suppliers for quantities in excess of our future demand forecasts consistent with our valuation of obsolete or excess inventory.
## Property and Equipment
Property and equipment are stated at cost less accumulated depreciation. Depreciation of property and equipment is computed using the straight-line method based on the estimated useful lives of the assets of three to seven years. Once an asset is identified for retirement or disposition, the related cost and accumulated depreciation or amortization are removed, and a gain or loss is recorded. The estimated useful lives of our buildings are up to thirty years. Depreciation expense includes the amortization of assets recorded under finance leases. Leasehold improvements and assets recorded under finance leases are amortized over the shorter of the expected lease term or the estimated useful life of the asset.
## Leases
We determine if an arrangement is or contains a lease at inception. Operating leases with lease terms of more than 12 months are included in operating lease assets, accrued and other current liabilities, and long-term operating lease liabilities on our consolidated balance sheet. Operating lease assets represent our right to use an underlying asset for the lease term and lease liabilities represent our obligation to make lease payments over the lease term.
Operating lease assets and liabilities are recognized based on the present value of the remaining lease payments discounted using our incremental borrowing rate. Operating lease assets also include initial direct costs incurred and prepaid lease payments, minus any lease incentives. Our lease terms include options to extend or terminate the lease when it is reasonably certain that we will exercise that option. Lease expense is recognized on a straight-line basis over the lease term.
We combine the lease and non-lease components in determining the operating lease assets and liabilities.
## Table of Contents
## NVIDIA Corporation and Subsidiaries Notes to the Consolidated Financial Statements
(Continued)
## Goodwill
Goodwill is subject to our annual impairment test during the fourth quarter of our fiscal year, or earlier if indicators of potential impairment exist. In completing our impairment test, we perform either a qualitative or a quantitative analysis on a reporting unit basis.
Qualitative factors include industry and market considerations, overall financial performance, and other relevant events and factors affecting the reporting units.
The quantitative impairment test considers both the income approach and the market approach to estimate a reporting unit's fair value. The income and market valuation approaches consider factors that include, but are not limited to, prospective financial information, growth rates, residual values, discount rates and comparable multiples from publicly traded companies in our industry and require us to make certain assumptions and estimates regarding industry economic factors and the future profitability of our business.
## Intangible Assets and Other Long-Lived Assets
Intangible assets primarily represent acquired intangible assets including developed technology and customer relationships, as well as rights acquired under technology licenses, patents, and acquired IP. We currently amortize our intangible assets with finite lives over periods ranging from one to twenty years using a method that reflects the pattern in which the economic benefits of the intangible asset are consumed or otherwise used up or, if that pattern cannot be reliably determined, using a straight-line amortization method.
Long-lived assets, such as property and equipment and intangible assets subject to amortization, are reviewed for impairment whenever events or changes in circumstances indicate that the carrying amount of an asset or asset group may not be recoverable. The recoverability of assets or asset groups to be held and used is measured by a comparison of the carrying amount of an asset or asset group to estimated undiscounted future cash flows expected to be generated by the asset or asset group. If the carrying amount of an asset or asset group exceeds its estimated future cash flows, an impairment charge is recognized for the amount by which the carrying amount of the asset or asset group exceeds the estimated fair value of the asset or asset group. Fair value is determined based on the estimated discounted future cash flows expected to be generated by the asset or asset group. Assets and liabilities to be disposed of would be separately presented in the Consolidated Balance Sheet and the assets would be reported at the lower of the carrying amount or fair value less costs to sell, and would no longer be depreciated.
## Business Combination
We allocate the fair value of the purchase price of an acquisition to the tangible assets acquired, liabilities assumed, and intangible assets acquired, based on their estimated fair values. The excess of the fair value of the purchase price over the fair values of these net tangible and intangible assets acquired is recorded as goodwill. Management's estimates of fair value are based upon assumptions believed to be reasonable, but our estimates and assumptions are inherently uncertain and subject to refinement. The estimates and assumptions used in valuing intangible assets include, but are not limited to, the amount and timing of projected future cash flows, discount rate used to determine the present value of these cash flows and asset lives. These estimates are inherently uncertain and, therefore, actual results may differ from the estimates made. As a result, during the measurement period of up to one year from the acquisition date, we may record adjustments to the assets acquired and liabilities assumed with the corresponding offset to goodwill. Upon the measurement period's conclusion or final determination of the fair value of the purchase price of an acquisition, whichever comes first, any subsequent adjustments are recorded to our Consolidated Statements of Income.
Acquisition-related expenses are recognized separately from the business combination and expensed as incurred.
## Investments in Non-Affiliated Entities
Our investment in non-affiliates consists of marketable equity securities, which are publicly traded, and non-marketable equity securities, which are investments in privately held companies. Marketable equity securities have readily determinable fair values with changes in fair value recorded in other income (expense), net. Non-marketable equity securities include investments that do not have a readily determinable fair value. The investments that do not have readily determinable fair value are measured at cost minus impairment, if any, and are adjusted for changes resulting from observable price changes in orderly transactions for an identical or similar investment in the same issuer, or the measurement alternative. Fair value is based upon observable inputs in an inactive market and the valuation requires our judgment due to the absence of market prices and inherent lack of liquidity. All gains and losses on these investments, realized and unrealized, are recognized in other income (expense), net on our Consolidated Statements of Income.
We assess whether an impairment loss has occurred on our investments in non-marketable equity securities, accounted for under the measurement alternative based on quantitative and qualitative factors. If any impairment is identified for non-marketable equity securities, we write down the investment to its fair value and record the corresponding charge through other income (expense), net on our Consolidated Statements of Income.
## NVIDIA Corporation and Subsidiaries
## Notes to the Consolidated Financial Statements
(Continued)
## Recently Issued Accounting Pronouncements
## Recent Accounting Pronouncements Not Yet Adopted
In November 2023, the Financial Accounting Standards Board, or FASB, issued a new accounting standard to provide for additional disclosures about significant expenses in operating segments. The standard is effective for our annual reporting for fiscal year 2025 and for interim period reporting starting in fiscal year 2026 retrospectively. We are currently evaluating the impact of this standard on our Consolidated Financial Statements.
In December 2023, the FASB issued a new accounting standard which provides for new and changes to income tax disclosures including disaggregation of the rate reconciliation and income taxes paid disclosures. The amendments in the standard are effective for annual periods beginning after December 15, 2024. Early adoption is permitted and should be applied prospectively, with retrospective application permitted. We expect to adopt this standard in our annual period beginning fiscal year 2026. We are currently evaluating the impact of this standard on our Consolidated Financial Statements.
## Note 2 - Business Combination
## Termination of the Arm Share Purchase Agreement
In February 2022, NVIDIA and SoftBank Group Corp, or SoftBank, announced the termination of the Share Purchase Agreement whereby NVIDIA would have acquired Arm from SoftBank. The parties agreed to terminate it due to significant regulatory challenges preventing the completion of the transaction. We recorded an acquisition termination cost of $1.4 billion in fiscal year 2023 reflecting the write-off of the prepayment provided at signing.
## Note 3 - Leases
Our lease obligations primarily consist of operating leases for our headquarters complex, domestic and international office facilities, and data center space, with lease periods expiring between fiscal years 2025 and 2035.
Future minimum lease payments under our non-cancelable operating leases as of January 28, 2024, are as follows:
Table of Contents
| | Operating Lease Obligations (In millions) |
|----------------------------------------------------|---------------------------------------------|
| Fiscal Year: | |
| 2025 | $ 290 |
| 2026 | 270 |
| 2027 | 253 |
| 2028 | 236 |
| 2029 | 202 |
| 2030 and thereafter | 288 |
| Total | 1,539 |
| Less imputed interest | 192 |
| Present value of net future minimum lease payments | 1,347 |
| Less short-term operating lease liabilities | 228 |
| Long-term operating lease liabilities | $ 1,119 |
In addition, we have operating leases, primarily for our data centers, that are expected to commence within fiscal year 2025 with lease terms of 1 to 10 years for $1.1 billion.
Operating lease expenses for fiscal years 2024, 2023, and 2022 were $269 million, $193 million, $168 million, respectively. Short-term and variable lease expenses for fiscal years 2024, 2023, and 2022 were not significant.
## NVIDIA Corporation and Subsidiaries
## Notes to the Consolidated Financial Statements
(Continued)
Other information related to leases was as follows:
Table of Contents
| | Year Ended | Year Ended | Year Ended |
|-------------------------------------------------------------------|---------------|---------------|---------------|
| | Jan 28, 2024 | Jan 29, 2023 | Jan 30, 2022 |
| | (In millions) | (In millions) | (In millions) |
| Supplemental cash flows information | | | |
| Operating cash flows used for operating leases | $ | $ | $ 154 |
| Operating lease assets obtained in exchange for lease obligations | $ | $ | $ 266 |
As of January 28, 2024, our operating leases had a weighted average remaining lease term of 6.1 years and a weighted average discount rate of 3.76%. As of January 29, 2023, our operating leases had a weighted average remaining lease term of 6.8 years and a weighted average discount rate of 3.21%.
## Note 4 - Stock-Based Compensation
Our stock-based compensation expense is associated with RSUs, performance stock units based on our corporate financial performance targets, or PSUs, performance stock units based on market conditions, or market-based PSUs, and our ESPP.
Our Consolidated Statements of Income include stock-based compensation expense, net of amounts allocated to inventory, as follows:
| | Year Ended | Year Ended | Year Ended |
|-----------------------------------|---------------|---------------|---------------|
| | Jan 28, 2024 | Jan 29, 2023 | Jan 30, 2022 |
| | (In millions) | (In millions) | (In millions) |
| Cost of revenue | $ 141 | $ 138 | $ 141 |
| Research and development | 2,532 | 1,892 | 1,298 |
| Sales, general and administrative | 876 | 680 | 565 |
| Total | $ 3,549 | $ 2,710 | $ 2,004 |
Stock-based compensation capitalized in inventories was not significant during fiscal years 2024, 2023, and 2022.
The following is a summary of equity awards granted under our equity incentive plans:
| | Year Ended | Year Ended | Year Ended |
|--------------------------------------------------|--------------------------------------|--------------------------------------|--------------------------------------|
| | Jan 28, 2024 | Jan 29, 2023 | Jan 30, 2022 |
| | (In millions, except per share data) | (In millions, except per share data) | (In millions, except per share data) |
| RSUs, PSUs and Market-based PSUs | | | |
| Awards granted | 14 | 25 | 18 |
| Estimated total grant-date fair value | $ 5,316 | $ 4,505 | $ 3,492 |
| Weighted average grant-date fair value per share | $ 374.08 | $ 183.72 | $ 190.69 |
| ESPP | | | |
| Shares purchased | 3 | 3 | 5 |
| Weighted average price per share | $ 158.07 | $ 122.54 | $ 56.36 |
| Weighted average grant-date fair value per share | $ 69.90 | $ 51.87 | $ 23.24 |
As of January 28, 2024, there was $8.6 billion of aggregate unearned stock-based compensation expense. This amount is expected to be recognized over a weighted average period of 2.5 years for RSUs, PSUs, and market-based PSUs, and 0.8 years for ESPP.
## NVIDIA Corporation and Subsidiaries
## Notes to the Consolidated Financial Statements
(Continued)
The fair value of shares issued under our ESPP have been estimated with the following assumptions:
Table of Contents
| | Year Ended | Year Ended | Year Ended |
|-------------------------------------------|---------------------------------|---------------------------------|---------------------------------|
| | Jan 28, 2024 | Jan 29, 2023 | Jan 30, 2022 |
| | (Using the Black-Scholes model) | (Using the Black-Scholes model) | (Using the Black-Scholes model) |
| ESPP | | | |
| Weighted average expected life (in years) | 0.1-2.0 | 0.1-2.0 | 0.1-2.0 |
| Risk-free interest rate | 3.9%-5.5% | -%-4.6% | -%-0.5% |
| Volatility | 31%-67% | 43%-72% | 20%-58% |
| Dividend yield | 0.1% | 0.1% | 0.1% |
For ESPP shares, the expected term represents the average term from the first day of the offering period to the purchase date. The risk-free interest rate assumption used to value ESPP shares is based upon observed interest rates on Treasury bills appropriate for the expected term. Our expected stock price volatility assumption for ESPP is estimated using historical volatility. For awards granted, we use the dividend yield at grant date. Our RSU, PSU, and marketbased PSU awards are not eligible for cash dividends prior to vesting; therefore, the fair values of RSUs, PSUs, and market-based PSUs are discounted for the dividend yield.
Additionally, for RSU, PSU, and market-based PSU awards, we estimate forfeitures semi-annually and revise the estimates of forfeiture in subsequent periods if actual forfeitures differ from those estimates. Forfeitures are estimated based on historical experience.
## Equity Incentive Program
We grant or have granted stock options, RSUs, PSUs, market-based PSUs, and stock purchase rights under the following equity incentive plans. In addition, in connection with our acquisitions of various companies, we have assumed certain stock-based awards granted under their stock incentive plans and converted them into our RSUs.
## Amended and Restated 2007 Equity Incentive Plan
In 2007, our shareholders approved the NVIDIA Corporation 2007 Equity Incentive Plan, or as most recently amended and restated, the 2007 Plan.
The 2007 Plan authorizes the issuance of incentive stock options, non-statutory stock options, restricted stock, RSUs, stock appreciation rights, performance stock awards, performance cash awards, and other stock-based awards to employees, directors and consultants. Only our employees may receive incentive stock options. As of January 28, 2024, up to 37 million shares of our common stock could be issued pursuant to stock awards granted under the 2007 Plan. Currently, we grant RSUs, PSUs and market-based PSUs under the 2007 Plan, under which, as of January 28, 2024, there were 147 million shares available for future grants.
Subject to certain exceptions, RSUs granted to employees vest (A) over a four-year period, subject to continued service, with 25% vesting on a pre-determined date that is close to the anniversary of the date of grant and 6.25% vesting quarterly thereafter, (B) over a three-year period, subject to continued service, with 40% vesting on a pre-determined date that is close to the anniversary of the date of grant and 7.5% vesting quarterly thereafter, or (C) over a four-year period, subject to continued service, with 6.25% vesting quarterly. PSUs vest over a four-year period, subject to continued service, with 25% vesting on a predetermined date that is close to the anniversary of the date of grant and 6.25% vesting quarterly thereafter. Market-based PSUs vest 100% on about the threeyear anniversary of the date of grant. However, the number of shares subject to both PSUs and market-based PSUs that are eligible to vest is determined by the Compensation Committee based on achievement of pre-determined criteria.
## Amended and Restated 2012 Employee Stock Purchase Plan
In 2012, our shareholders approved the NVIDIA Corporation 2012 Employee Stock Purchase Plan, or as most recently amended and restated, the 2012 Plan.
Employees who participate in the 2012 Plan may have up to 15% of their earnings withheld to purchase shares of common stock. The Board may decrease this percentage at its discretion. Each offering period is about 24 months, divided into four purchase periods of six months. The price of common stock purchased under our 2012 Plan will be equal to 85% of the lower of the fair market value of the common stock on the commencement date of each offering period or the fair market value of the common stock on each purchase date within the offering. As of January 28, 2024, we had 227 million shares reserved for future issuance under the 2012 Plan.
## NVIDIA Corporation and Subsidiaries
## Notes to the Consolidated Financial Statements
(Continued)
## Equity Award Activity
The following is a summary of our equity award transactions under our equity incentive plans:
Table of Contents
| | RSUs, PSUs and Market-based PSUs Outstanding | RSUs, PSUs and Market-based PSUs Outstanding |
|------------------------------------------------|------------------------------------------------|------------------------------------------------|
| | Number of Shares | Weighted Average Grant-Date Fair Value |
| | (In millions, except per share data) | (In millions, except per share data) |
| Balances, Jan 29, 2023 | 45 | $ 158.45 |
| Granted | 14 | $ 374.08 |
| Vested restricted stock | (21) | $ 148.56 |
| Canceled and forfeited | (1) | $ 206.35 |
| Balances, Jan 28, 2024 | 37 | $ 245.94 |
| Vested and expected to vest after Jan 28, 2024 | 37 | $ 245.49 |
As of January 28, 2024 and January 29, 2023, there were 147 million and 160 million shares, respectively, of common stock available for future grants under our equity incentive plans.
The total fair value of RSUs and PSUs, as of their respective vesting dates, during the years ended January 28, 2024, January 29, 2023, and January 30, 2022, was $8.2 billion, $4.3 billion, and $5.6 billion, respectively.
## Note 5 - Net Income Per Share
The following is a reconciliation of the denominator of the basic and diluted net income per share computations for the periods presented:
| | Year Ended | Year Ended | Year Ended |
|--------------------------------------------------------------------------------------------------------------------------|--------------------------------------|--------------------------------------|--------------------------------------|
| | Jan 28, 2024 | Jan 29, 2023 | Jan 30, 2022 |
| | (In millions, except per share data) | (In millions, except per share data) | (In millions, except per share data) |
| Numerator: | | | |
| Net income | $ 29,760 | $ 4,368 | $ 9,752 |
| Denominator: | | | |
| Basic weighted average shares | 2,469 | 2,487 | 2,496 |
| Dilutive impact of outstanding equity awards | 25 | 20 | 39 |
| Diluted weighted average shares | 2,494 | 2,507 | 2,535 |
| Net income per share: | | | |
| Basic (1) | $ 12.05 | $ 1.76 | $ 3.91 |
| Diluted (2) | $ 11.93 | $ 1.74 | $ 3.85 |
| Equity awards excluded from diluted net income per share because their effect would have been anti-dilutive | 15 | 40 | 21 |
## Note 6 - Goodwill
As of January 28, 2024, the total carrying amount of goodwill was $4.4 billion, consisting of goodwill balances allocated to our Compute & Networking and Graphics reporting units of $4.1 billion and $370 million, respectively. As of January 29, 2023, the total carrying amount of goodwill was $4.4 billion, consisting of goodwill balances allocated to our Compute & Networking and Graphics reporting units of $4.0 billion and $370 million, respectively. Goodwill increased by $59 million in fiscal year 2024 from an immaterial acquisition and was allocated to our Compute & Networking reporting unit. During the fourth quarters of fiscal years 2024, 2023, and 2022, we completed our annual qualitative impairment tests and concluded that goodwill was not impaired.
## NVIDIA Corporation and Subsidiaries
## Notes to the Consolidated Financial Statements
(Continued)
## Note 7 - Amortizable Intangible Assets
The components of our amortizable intangible assets are as follows:
Table of Contents
| | Jan 28, 2024 | Jan 28, 2024 | Jan 28, 2024 | Jan 29, 2023 | Jan 29, 2023 | Jan 29, 2023 |
|-------------------------------------------|-----------------------|--------------------------|----------------------|-----------------------|--------------------------|----------------------|
| | Gross Carrying Amount | Accumulated Amortization | Net Carrying Amount | Gross Carrying Amount | Accumulated Amortization | Net Carrying Amount |
| | (In millions) | (In millions) | (In millions) | (In millions) | (In millions) | (In millions) |
| Acquisition-related intangible assets (1) | $ 2,642 | (1,720) | $ 922 | $ 3,093 | $ (1,614) | $ 1,479 |
| Patents and licensed technology | 449 | (259) | 190 | 446 | (249) | 197 |
| Total intangible assets | $ 3,091 | (1,979) | $ 1,112 | $ 3,539 | $ (1,863) | $ 1,676 |
Amortization expense associated with intangible assets for fiscal years 2024, 2023, and 2022 was $614 million, $699 million, and $563 million, respectively.
The following table outlines the estimated future amortization expense related to the net carrying amount of intangible assets as of January 28, 2024:
| | Future Amortization Expense (In millions) |
|---------------------|---------------------------------------------|
| Fiscal Year: | |
| 2025 | $ 555 |
| 2026 | 261 |
| 2027 | 150 |
| 2028 | 37 |
| 2029 | 9 |
| 2030 and thereafter | 100 |
| Total | $ 1,112 |
## NVIDIA Corporation and Subsidiaries
## Notes to the Consolidated Financial Statements
(Continued)
## Note 8 - Cash Equivalents and Marketable Securities
Our cash equivalents and marketable securities related to debt securities are classified as 'available-for-sale' debt securities.
The following is a summary of cash equivalents and marketable securities:
Table of Contents
| | Jan 28, 2024 | Jan 28, 2024 | Jan 28, 2024 | Jan 28, 2024 | Jan 28, 2024 | Jan 28, 2024 |
|----------------------------------------------------|----------------|-----------------|-----------------|----------------------|------------------|-----------------------|
| | | | | | Reported as | Reported as |
| | Amortized Cost | Unrealized Gain | Unrealized Loss | Estimated Fair Value | Cash Equivalents | Marketable Securities |
| | (In millions) | (In millions) | (In millions) | (In millions) | (In millions) | (In millions) |
| Corporate debt securities | $ 10,126 | $ 31 | $ (5) | $ 10,152 | $ 2,231 | $ 7,921 |
| Debt securities issued by the U.S. Treasury | 9,517 | 17 | (10) | 9,524 | 1,315 | 8,209 |
| Debt securities issued by U.S. government agencies | 2,326 | 8 | (1) | 2,333 | 89 | 2,244 |
| Money market funds | 3,031 | - | - | 3,031 | 3,031 | - |
| Certificates of deposit | 510 | - | - | 510 | 294 | 216 |
| Foreign government bonds | 174 | - | - | 174 | 60 | 114 |
| Total | $ 25,684 | $ 56 | $ (16) | $ 25,724 | $ 7,020 | $ 18,704 |
| | Jan 29, 2023 | Jan 29, 2023 | Jan 29, 2023 | Jan 29, 2023 | Jan 29, 2023 | Jan 29, 2023 |
|----------------------------------------------------|----------------|-----------------|-----------------|----------------------|------------------|-----------------------|
| | | | | | Reported as | Reported as |
| | Amortized Cost | Unrealized Gain | Unrealized Loss | Estimated Fair Value | Cash Equivalents | Marketable Securities |
| | (In millions) | (In millions) | (In millions) | (In millions) | (In millions) | (In millions) |
| Corporate debt securities | $ 4,809 | $ - | $ (12) | $ 4,797 | $ 1,087 | $ 3,710 |
| Debt securities issued by the U.S. Treasury | 4,185 | 1 | (44) | 4,142 | - | 4,142 |
| Debt securities issued by U.S. government agencies | 1,836 | - | (2) | 1,834 | 50 | 1,784 |
| Money market funds | 1,777 | - | - | 1,777 | 1,777 | - |
| Certificates of deposit | 365 | - | - | 365 | 134 | 231 |
| Foreign government bonds | 140 | - | - | 140 | 100 | 40 |
| Total | $ 13,112 | $ 1 | $ (58) | $ 13,055 | $ 3,148 | $ 9,907 |
## NVIDIA Corporation and Subsidiaries
## Notes to the Consolidated Financial Statements
(Continued)
The following tables provide the breakdown of unrealized losses, aggregated by investment category and length of time that individual securities have been in a continuous loss position:
Table of Contents
| | Jan 28, 2024 | Jan 28, 2024 | Jan 28, 2024 | Jan 28, 2024 | Jan 28, 2024 | Jan 28, 2024 |
|----------------------------------------------------|----------------------|-----------------------|----------------------|-----------------------|----------------------|-----------------------|
| | Less than 12 Months | Less than 12 Months | 12 Months or Greater | 12 Months or Greater | Total | Total |
| | Estimated Fair Value | Gross Unrealized Loss | Estimated Fair Value | Gross Unrealized Loss | Estimated Fair Value | Gross Unrealized Loss |
| | (In millions) | (In millions) | (In millions) | (In millions) | (In millions) | (In millions) |
| Debt securities issued by the U.S. Treasury | $ 3,343 | $ (5) | $ 1,078 | $ (5) | $ 4,421 | $ (10) |
| Corporate debt securities | 1,306 | (3) | 618 | (2) | 1,924 | (5) |
| Debt securities issued by U.S. government agencies | 670 | (1) | - | - | 670 | (1) |
| Total | $ 5,319 | $ (9) | $ 1,696 | $ (7) | $ 7,015 | $ (16) |
| | Jan 29, 2023 | Jan 29, 2023 | Jan 29, 2023 | Jan 29, 2023 | Jan 29, 2023 | Jan 29, 2023 |
|----------------------------------------------------|----------------------|-----------------------|----------------------|-----------------------|----------------------|-----------------------|
| | Less than 12 Months | Less than 12 Months | 12 Months or Greater | 12 Months or Greater | Total | Total |
| | Estimated Fair Value | Gross Unrealized Loss | Estimated Fair Value | Gross Unrealized Loss | Estimated Fair Value | Gross Unrealized Loss |
| | (In millions) | (In millions) | (In millions) | (In millions) | (In millions) | (In millions) |
| Debt securities issued by the U.S. Treasury | $ 2,444 | $ (21) | $ 1,172 | $ (23) | $ 3,616 | $ (44) |
| Corporate debt securities | 1,188 | (7) | 696 | (5) | 1,884 | (12) |
| Debt securities issued by U.S. government agencies | 1,307 | (2) | - | - | 1,307 | (2) |
| Total | $ 4,939 | $ (30) | $ 1,868 | $ (28) | $ 6,807 | $ (58) |
The gross unrealized losses are related to fixed income securities, driven primarily by changes in interest rates. Net realized gains and losses were not significant for all periods presented.
The amortized cost and estimated fair value of cash equivalents and marketable securities are shown below by contractual maturity.
| | Jan 28, 2024 | Jan 28, 2024 | Jan 29, 2023 | Jan 29, 2023 |
|--------------------|----------------|----------------------|----------------|----------------------|
| | Amortized Cost | Estimated Fair Value | Amortized Cost | Estimated Fair Value |
| | (In millions) | (In millions) | (In millions) | (In millions) |
| Less than one year | $ 16,336 | $ 16,329 | $ 9,738 | $ 9,708 |
| Due in 1 - 5 years | 9,348 | 9,395 | 3,374 | 3,347 |
| Total | $ 25,684 | $ 25,724 | $ 13,112 | $ 13,055 |
## NVIDIA Corporation and Subsidiaries
## Notes to the Consolidated Financial Statements
(Continued)
## Note 9 - Fair Value of Financial Assets and Liabilities and Investments in Non-Affiliated Entities
The fair values of our financial assets and liabilities are determined using quoted market prices of identical assets or quoted market prices of similar assets from active markets. We review fair value hierarchy classification on a quarterly basis.
Table of Contents
| | | Fair Value at | Fair Value at | Fair Value at |
|-------------------------------------------------------|------------------|-----------------|-----------------|-----------------|
| | Pricing Category | Jan 28, 2024 | | Jan 29, 2023 |
| | | (In millions) | (In millions) | (In millions) |
| Assets | | | | |
| Cash equivalents and marketable securities: | | | | |
| Money market funds | Level 1 | $ | 3,031 | $ 1,777 |
| Corporate debt securities | Level 2 | $ | 10,152 | $ 4,797 |
| Debt securities issued by the U.S. Treasury | Level 2 | $ | 9,524 | $ 4,142 |
| Debt securities issued by U.S. government agencies | Level 2 | $ | 2,333 | $ 1,834 |
| Certificates of deposit | Level 2 | $ | 510 | $ 365 |
| Foreign government bonds | Level 2 | $ | 174 | $ 140 |
| Other assets (Investment in non-affiliated entities): | | | | |
| Publicly-held equity securities | Level 1 | $ | 225 | $ 11 |
| Liabilities (1) | | | | |
| 0.309% Notes Due 2023 | Level 2 | $ | - | $ 1,230 |
| 0.584% Notes Due 2024 | Level 2 | $ | 1,228 | $ 1,185 |
| 3.20% Notes Due 2026 | Level 2 | $ | 970 | $ 966 |
| 1.55% Notes Due 2028 | Level 2 | $ | 1,115 | $ 1,099 |
| 2.85% Notes Due 2030 | Level 2 | $ | 1,367 | $ 1,364 |
| 2.00% Notes Due 2031 | Level 2 | $ | 1,057 | $ 1,044 |
| 3.50% Notes Due 2040 | Level 2 | $ | 851 | $ 870 |
| 3.50% Notes Due 2050 | Level 2 | $ | 1,604 | $ 1,637 |
| 3.70% Notes Due 2060 | Level 2 | $ | 403 | $ 410 |
## Investments in Non-Affiliated Entities
Our investments in non-affiliated entities include marketable equity securities, which are publicly traded, and non-marketable equity securities, which are primarily investments in privately held companies. Our marketable equity securities have readily determinable fair values and are recorded as long-term other assets on our Consolidated Balance Sheets at fair value with changes in fair value recorded in Other income and expense, net on our Consolidated Statements of Income. Marketable equity securities totaled $225 million and $11 million as of January 28, 2024 and January 29, 2023, respectively. The net unrealized and realized gains and losses of investments in marketable securities net were not significant for fiscal years 2024, 2023 and 2022.
Our non-marketable equity securities are recorded in long-term other assets on our Consolidated Balance Sheets. The carrying value of our non-marketable equity securities totaled $1.3 billion and $288 million as of January 28, 2024 and January 29, 2023, respectively. Gains and losses on these investments, realized and unrealized, are recognized in Other income and expense, net on our Consolidated Statements of Income.
## NVIDIA Corporation and Subsidiaries
## Notes to the Consolidated Financial Statements
(Continued)
Adjustments to the carrying value of our non-marketable equity securities accounted for under the measurement alternative were as follows:
Table of Contents
| | Year Ended Jan 28, 2024 (In millions) |
|----------------------------------------------------------|-----------------------------------------|
| Carrying amount as of Jan 29, 2023 | $ 288 |
| Adjustments related to non-marketable equity securities: | |
| Net additions | 859 |
| Unrealized gains | 194 |
| Impairments and unrealized losses | (20) |
| Carrying amount as of Jan 28, 2024 | $ 1,321 |
In the fourth quarter of fiscal year 2024, one of our private company investments completed a secondary equity raise that resulted in an unrealized gain of $178 million.
Net unrealized gains recognized for the year ended January 28, 2024 for non-marketable investments in non-affiliated entities still held as of January 28, 2024 were $174 million. Net unrealized and realized gains related to non-marketable equity securities were not significant for fiscal years 2023 and 2022.
The following table summarizes the cumulative gross unrealized gains and cumulative gross unrealized losses and impairments related to non-marketable equity securities accounted for under the measurement alternative:
| | Jan 28, 2024 (In millions) |
|----------------------------------------------------|------------------------------|
| Cumulative gross unrealized gains | $ 270 |
| Cumulative gross unrealized losses and impairments | (45) |
## Note 10 - Balance Sheet Components
Two customers accounted for 24% and 11% of our accounts receivable balance as of January 28, 2024. Two customers accounted for 14% and 11% of our accounts receivable balance as of January 29, 2023.
Certain balance sheet components are as follows:
| | Jan 28, 2024 | Jan 29, 2023 |
|--------------------|----------------|----------------|
| | (In millions) | (In millions) |
| Inventories (1) : | | |
| Raw materials | $ 1,719 | $ 2,430 |
| Work in-process | 1,505 | 466 |
| Finished goods | 2,058 | 2,263 |
| Total inventories | $ 5,282 | $ 5,159 |
## NVIDIA Corporation and Subsidiaries
## Notes to the Consolidated Financial Statements
(Continued)
Table of Contents
| | Jan 28, 2024 | Jan 29, 2023 | Estimated Useful Life |
|--------------------------------------------------|----------------|----------------|-------------------------|
| | (In millions) | (In millions) | (In years) |
| Property and Equipment: | | | |
| Land | $ 218 | $ 218 | (A) |
| Buildings, leasehold improvements, and furniture | 1,816 | 1,598 | (B) |
| Equipment, compute hardware, and software | 5,200 | 4,303 | 3-7 |
| Construction in process | 189 | 382 | (C) |
| Total property and equipment, gross | 7,423 | 6,501 | |
| Accumulated depreciation and amortization | (3,509) | (2,694) | |
| Total property and equipment, net | $ 3,914 | $ 3,807 | |
Depreciation expense for fiscal years 2024, 2023, and 2022 was $894 million, $844 million, and $611 million, respectively.
Accumulated amortization of leasehold improvements and finance leases was $400 million and $327 million as of January 28, 2024 and January 29, 2023, respectively.
Property, equipment and intangible assets acquired by assuming related liabilities during fiscal years 2024, 2023, and 2022 were $170 million, $374 million, and $258 million, respectively.
| | Jan 28, 2024 | Jan 29, 2023 |
|--------------------------------------------|----------------|----------------|
| Other assets: | (In millions) | (In millions) |
| Prepaid supply and capacity agreements (1) | $ 2,458 | $ 2,989 |
| Investments in non-affiliated entities | 1,546 | 299 |
| Prepaid royalties | 364 | 387 |
| Other | 132 | 145 |
| Total other assets | $ 4,500 | $ 3,820 |
## NVIDIA Corporation and Subsidiaries
## Notes to the Consolidated Financial Statements
(Continued)
Table of Contents
| | Jan 28, 2024 | Jan 29, 2023 |
|---------------------------------------------|----------------|----------------|
| | (In millions) | (In millions) |
| Accrued and Other Current Liabilities: | | |
| Customer program accruals | $ 2,081 | $ 1,196 |
| Excess inventory purchase obligations (1) | 1,655 | 954 |
| Deferred revenue (2) | 764 | 354 |
| Accrued payroll and related expenses | 675 | 530 |
| Product warranty and return provisions | 415 | 108 |
| Taxes payable | 296 | 467 |
| Operating leases | 228 | 176 |
| Unsettled share repurchases | 187 | 117 |
| Licenses and royalties | 182 | 149 |
| Other | 199 | 69 |
| Total accrued and other current liabilities | $ 6,682 | $ 4,120 |
| | Jan 28, 2024 | Jan 29, 2023 |
|-----------------------------------|----------------|----------------|
| | (In millions) | (In millions) |
| Other Long-Term Liabilities: | | |
| Income tax payable (1) | $ 1,361 | $ 1,204 |
| Deferred income tax | 462 | 247 |
| Deferred revenue (2) | 573 | 218 |
| Licenses payable | 80 | 181 |
| Other | 65 | 63 |
| Total other long-term liabilities | $ 2,541 | $ 1,913 |
## Deferred Revenue
The following table shows the changes in deferred revenue during fiscal years 2024 and 2023.
| | Jan 28, 2024 | Jan 29, 2023 |
|----------------------------------------------|----------------|----------------|
| | (In millions) | (In millions) |
| Balance at beginning of period | $ 572 | $ 502 |
| Deferred revenue additions during the period | 2,038 | 830 |
| Revenue recognized during the period | (1,273) | (760) |
| Balance at end of period | $ 1,337 | $ 572 |
Revenue recognized during fiscal year 2024 that was included in deferred revenue as of January 29, 2023 was $338 million. Revenue recognized during fiscal year 2023 that was included in deferred revenue as of January 30, 2022 was $282 million.
Revenue related to remaining performance obligations represents the contracted license and development arrangements and support for hardware and software. This includes deferred revenue currently recorded and amounts that will be
## NVIDIA Corporation and Subsidiaries
## Notes to the Consolidated Financial Statements
(Continued)
invoiced in future periods. Revenue allocated to remaining performance obligations, which includes deferred revenue and amounts that will be invoiced and recognized as revenue in future periods, was $1.1 billion as of January 28, 2024. We expect to recognize approximately 40% of this revenue over the next twelve months and the remainder thereafter. This excludes revenue related to performance obligations for contracts with a length of one year or less.
## Note 11 - Derivative Financial Instruments
We enter into foreign currency forward contracts to mitigate the impact of foreign currency exchange rate movements on our operating expenses. These contracts are designated as cash flow hedges for hedge accounting treatment. Gains or losses on the contracts are recorded in accumulated other comprehensive income or loss and reclassified to operating expense when the related operating expenses are recognized in earnings or ineffectiveness should occur.
We also enter into foreign currency forward contracts to mitigate the impact of foreign currency movements on monetary assets and liabilities that are denominated in currencies other than the U.S. dollar. These forward contracts were not designated for hedge accounting treatment. Therefore, the change in fair value of these contracts is recorded in other income or expense and offsets the change in fair value of the hedged foreign currency denominated monetary assets and liabilities, which is also recorded in other income or expense.
The table below presents the notional value of our foreign currency forward contracts outstanding:
Table of Contents
| | Jan 28, 2024 | Jan 29, 2023 |
|--------------------------------|----------------|----------------|
| | (In millions) | (In millions) |
| Designated as cash flow hedges | $ 1,168 | $ 1,128 |
| Non-designated hedges | $ 597 | $ 366 |
The unrealized gains and losses or fair value of our foreign currency forward contracts was not significant as of January 28, 2024 and January 29, 2023.
As of January 28, 2024, all designated foreign currency forward contracts mature within 18 months. The expected realized gains and losses deferred into accumulated other comprehensive income or loss related to foreign currency forward contracts within the next twelve months was not significant.
During fiscal years 2024 and 2023, the impact of derivative financial instruments designated for hedge accounting treatment on other comprehensive income or loss was not significant and all such instruments were determined to be highly effective.
## Note 12 - Debt
## Long-Term Debt
The carrying value of our outstanding notes, the calendar year of maturity, and the associated interest rates were as follows:
Table of Contents
| | Expected Remaining Term (years) | Effective Interest Rate | Jan 28, 2024 | Jan 29, 2023 |
|----------------------------------------------|-----------------------------------|---------------------------|----------------|----------------|
| | | | (In millions) | (In millions) |
| 0.309% Notes Due 2023 (1) | - | 0.41% | $ - $ | 1,250 |
| 0.584% Notes Due 2024 | 0.4 | 0.66% | 1,250 | 1,250 |
| 3.20% Notes Due 2026 | 2.6 | 3.31% | 1,000 | 1,000 |
| 1.55% Notes Due 2028 | 4.4 | 1.64% | 1,250 | 1,250 |
| 2.85% Notes Due 2030 | 6.2 | 2.93% | 1,500 | 1,500 |
| 2.00% Notes Due 2031 | 7.4 | 2.09% | 1,250 | 1,250 |
| 3.50% Notes Due 2040 | 16.2 | 3.54% | 1,000 | 1,000 |
| 3.50% Notes Due 2050 | 26.2 | 3.54% | 2,000 | 2,000 |
| 3.70% Notes Due 2060 | 36.2 | 3.73% | 500 | 500 |
| Unamortized debt discount and issuance costs | | | (41) | (47) |
| Net carrying amount | | | 9,709 | 10,953 |
| Less short-term portion | | | (1,250) | (1,250) |
| Total long-term portion | | | $ 8,459 | $ 9,703 |
All our notes are unsecured senior obligations. All existing and future liabilities of our subsidiaries will be effectively senior to the notes. Our notes pay interest semi-annually. We may redeem each of our notes prior to maturity, subject to a make-whole premium as defined in the applicable form of note.
As of January 28, 2024, we were in compliance with the required covenants, which are non-financial in nature, under the outstanding notes.
## Commercial Paper
We have a $575 million commercial paper program to support general corporate purposes. As of January 28, 2024, we had no commercial paper outstanding.
## Note 13 - Commitments and Contingencies
## Purchase Obligations
Our purchase obligations reflect our commitments to purchase components used to manufacture our products, including long-term supply and capacity agreements, certain software and technology licenses, other goods and services and long-lived assets.
As of January 28, 2024, we had outstanding inventory purchase and long-term supply and capacity obligations totaling $16.1 billion. We enter into agreements with contract manufacturers that allow them to procure inventory based upon criteria as defined by us, and in certain instances, these agreements allow us the option to cancel, reschedule, and adjust our requirements based on our business needs prior to firm orders being placed, but these changes may result in the payment of costs incurred through the date of cancellation. Other non-inventory purchase obligations were $4.6 billion, which includes $3.5 billion of multi-year cloud service agreements, primarily to support our research and development efforts.
## NVIDIA Corporation and Subsidiaries
## Notes to the Consolidated Financial Statements
(Continued)
## NVIDIA Corporation and Subsidiaries
## Notes to the Consolidated Financial Statements
(Continued)
Total future purchase commitments as of January 28, 2024 are as follows:
Table of Contents
| | Commitments (In millions) |
|---------------------|-----------------------------|
| Fiscal Year: | |
| 2025 | $ 17,316 |
| 2026 | 1,143 |
| 2027 | 1,060 |
| 2028 | 770 |
| 2029 and thereafter | 418 |
| Total | $ 20,707 |
## Accrual for Product Warranty Liabilities
The estimated amount of product warranty liabilities was $306 million and $82 million as of January 28, 2024 and January 29, 2023, respectively. The estimated product returns and estimated product warranty activity consisted of the following:
| | Year Ended | Year Ended | Year Ended |
|--------------------------------|---------------|---------------|---------------|
| | Jan 28, 2024 | Jan 29, 2023 | Jan 30, 2022 |
| | (In millions) | (In millions) | (In millions) |
| Balance at beginning of period | $ 82 | $ 46 | $ 22 |
| Additions | 278 | 145 | 40 |
| Utilization | (54) | (109) | (16) |
| Balance at end of period | $ 306 | $ 82 | $ 46 |
In fiscal years 2024 and 2023, the additions in product warranty liabilities primarily related to Compute & Networking segment.
We have provided indemnities for matters such as tax, product, and employee liabilities. We have included intellectual property indemnification provisions in our technology-related agreements with third parties. Maximum potential future payments cannot be estimated because many of these agreements do not have a maximum stated liability. We have not recorded any liability in our Consolidated Financial Statements for such indemnifications.
## Litigation
## Securities Class Action and Derivative Lawsuits
The plaintiffs in the putative securities class action lawsuit, captioned 4:18-cv-07669-HSG, initially filed on December 21, 2018 in the United States District Court for the Northern District of California, and titled In Re NVIDIA Corporation Securities Litigation, filed an amended complaint on May 13, 2020. The amended complaint asserted that NVIDIA and certain NVIDIA executives violated Section 10(b) of the Securities Exchange Act of 1934, as amended, or the Exchange Act, and SEC Rule 10b-5, by making materially false or misleading statements related to channel inventory and the impact of cryptocurrency mining on GPU demand between May 10, 2017 and November 14, 2018. Plaintiffs also alleged that the NVIDIA executives who they named as defendants violated Section 20(a) of the Exchange Act. Plaintiffs sought class certification, an award of unspecified compensatory damages, an award of reasonable costs and expenses, including attorneys' fees and expert fees, and further relief as the Court may deem just and proper. On March 2, 2021, the district court granted NVIDIA's motion to dismiss the complaint without leave to amend, entered judgment in favor of NVIDIA and closed the case. On March 30, 2021, plaintiffs filed an appeal from judgment in the United States Court of Appeals for the Ninth Circuit, case number 21-15604. On August 25, 2023, a majority of a three-judge Ninth Circuit panel affirmed in part and reversed in part the district court's dismissal of the case, with a third judge dissenting on the basis that the district court did not err in dismissing the case. On November 15, 2023, the Ninth Circuit denied NVIDIA's petition for rehearing en banc of the Ninth Circuit panel's majority decision to reverse in part the dismissal of the case, which NVIDIA had filed on October 10, 2023. On November 21, 2023, NVIDIA filed a motion with the Ninth Circuit for a stay of the mandate pending NVIDIA's petition for a writ of certiorari in the Supreme Court of the United States and the Supreme Court's
## Table of Contents
## NVIDIA Corporation and Subsidiaries
## Notes to the Consolidated Financial Statements
(Continued)
resolution of the matter. On December 5, 2023, the Ninth Circuit granted NVIDIA's motion to stay the mandate. NVIDIA's deadline to file a petition for a writ of certiorari is March 4, 2024.
The putative derivative lawsuit pending in the United States District Court for the Northern District of California, captioned 4:19-cv-00341-HSG, initially filed January 18, 2019 and titled In re NVIDIA Corporation Consolidated Derivative Litigation, was stayed pending resolution of the plaintiffs' appeal in the In Re NVIDIA Corporation Securities Litigation action. On February 22, 2022, the court administratively closed the case, but stated that it would reopen the case once the appeal in the In Re NVIDIA Corporation Securities Litigation action is resolved. Following the Ninth Circuit's denial of NVIDIA's petition for rehearing on November 15, 2023, the parties are conferring regarding the next steps in this derivative matter. The lawsuit asserts claims, purportedly on behalf of us, against certain officers and directors of the Company for breach of fiduciary duty, unjust enrichment, waste of corporate assets, and violations of Sections 14(a), 10(b), and 20(a) of the Exchange Act based on the dissemination of allegedly false and misleading statements related to channel inventory and the impact of cryptocurrency mining on GPU demand. The plaintiffs are seeking unspecified damages and other relief, including reforms and improvements to NVIDIA's corporate governance and internal procedures.
The putative derivative actions initially filed September 24, 2019 and pending in the United States District Court for the District of Delaware, Lipchitz v. Huang, et al. (Case No. 1:19-cv-01795-UNA) and Nelson v. Huang, et. al. (Case No. 1:19-cv-01798- UNA), remain stayed pending resolution of the plaintiffs' appeal in the In Re NVIDIA Corporation Securities Litigation action. Following the Ninth Circuit's denial of NVIDIA's petition for rehearing on November 15, 2023, the parties are conferring regarding the next steps in these derivative matters. The lawsuits assert claims, purportedly on behalf of us, against certain officers and directors of the Company for breach of fiduciary duty, unjust enrichment, insider trading, misappropriation of information, corporate waste and violations of Sections 14(a), 10(b), and 20(a) of the Exchange Act based on the dissemination of allegedly false, and misleading statements related to channel inventory and the impact of cryptocurrency mining on GPU demand. The plaintiffs seek unspecified damages and other relief, including disgorgement of profits from the sale of NVIDIA stock and unspecified corporate governance measures.
Another putative derivative action was filed on October 30, 2023 in the Court of Chancery of the State of Delaware, captioned Horanic v. Huang, et al. (Case No. 2023-1096-KSJM). This lawsuit asserts claims, purportedly on behalf of us, against certain officers and directors of the Company for breach of fiduciary duty and insider trading based on the dissemination of allegedly false and misleading statements related to channel inventory and the impact of cryptocurrency mining on GPU demand. The plaintiffs seek unspecified damages and other relief, including disgorgement of profits from the sale of NVIDIA stock and reform of unspecified corporate governance measures. This derivative matter is stayed pending the final resolution of In Re NVIDIA Corporation Securities Litigation action.
## Accounting for Loss Contingencies
As of January 28, 2024, we have not recorded any accrual for contingent liabilities associated with the legal proceedings described above based on our belief that liabilities, while possible, are not probable. Further, except as specifically described above, any possible loss or range of loss in these matters cannot be reasonably estimated at this time. We are engaged in legal actions not described above arising in the ordinary course of business and, while there can be no assurance of favorable outcomes, we believe that the ultimate outcome of these actions will not have a material adverse effect on our operating results, liquidity or financial position.
## NVIDIA Corporation and Subsidiaries
## Notes to the Consolidated Financial Statements
(Continued)
## Note 14 - Income Taxes
The income tax expense (benefit) applicable to income before income taxes consists of the following:
Table of Contents
| | Year Ended | Year Ended | Year Ended |
|------------------------------|---------------|---------------|---------------|
| | Jan 28, 2024 | Jan 29, 2023 | Jan 30, 2022 |
| | (In millions) | (In millions) | (In millions) |
| Current income taxes: | | | |
| Federal | $ 5,710 | $ 1,703 | $ 482 |
| State | 335 | 46 | 42 |
| Foreign | 502 | 228 | 71 |
| Total current | 6,547 | 1,977 | 595 |
| Deferred income taxes: | | | |
| Federal | (2,499) | (2,165) | (420) |
| State | (206) | - | - |
| Foreign | 216 | 1 | 14 |
| Total deferred | (2,489) | (2,164) | (406) |
| Income tax expense (benefit) | $ 4,058 | $ (187) | $ 189 |
Income before income tax consists of the following:
| | Year Ended | Year Ended | Year Ended |
|--------------------------|---------------|---------------|---------------|
| | Jan 28, 2024 | Jan 29, 2023 | Jan 30, 2022 |
| | (In millions) | (In millions) | (In millions) |
| U.S. | $ 29,495 | $ 3,477 | $ 8,446 |
| Foreign | 4,323 | 704 | 1,495 |
| Income before income tax | $ 33,818 | $ 4,181 | $ 9,941 |
The income tax expense (benefit) differs from the amount computed by applying the U.S. federal statutory rate of 21% to income before income taxes as follows:
| | Year Ended | Year Ended | Year Ended | Year Ended | Year Ended | Year Ended |
|--------------------------------------------------|-----------------------------------|-----------------------------------|-----------------------------------|-----------------------------------|-----------------------------------|-----------------------------------|
| | Jan 28, 2024 | Jan 28, 2024 | Jan 29, 2023 | Jan 29, 2023 | Jan 30, 2022 | Jan 30, 2022 |
| | (In millions, except percentages) | (In millions, except percentages) | (In millions, except percentages) | (In millions, except percentages) | (In millions, except percentages) | (In millions, except percentages) |
| Tax expense computed at federal statutory rate | $ 7,102 | 21.0 % | $ 878 | 21.0 % | $ 2,088 | 21.0 % |
| Expense (benefit) resulting from: | | | | | | |
| State income taxes, net of federal tax effect | 120 | 0.4 % | 50 | 1.2 % | 42 | 0.4 % |
| Foreign-derived intangible income | (1,408) | (4.2)% | (739) | (17.7)% | (520) | (5.2)% |
| Stock-based compensation | (741) | (2.2)% | (309) | (7.4)% | (337) | (3.4)% |
| Foreign tax rate differential | (467) | (1.4)% | (83) | (2.0)% | (497) | (5.0)% |
| U.S. federal research and development tax credit | (431) | (1.3)% | (278) | (6.6)% | (289) | (2.9)% |
| Acquisition termination cost | - | - % | 261 | 6.2 % | - | - % |
| IP domestication | - | - % | - | - % | (244) | (2.5)% |
| Other | (117) | (0.3)% | 33 | 0.8 % | (54) | (0.5)% |
| Income tax expense (benefit) | $ 4,058 | 12.0 % | $ (187) | (4.5)% | $ 189 | 1.9 % |
## NVIDIA Corporation and Subsidiaries
## Notes to the Consolidated Financial Statements
(Continued)
The tax effect of temporary differences that gives rise to significant portions of the deferred tax assets and liabilities are presented below:
Table of Contents
| | Jan 28, 2024 | Jan 29, 2023 |
|------------------------------------------------------------------|----------------|----------------|
| | (In millions) | (In millions) |
| Deferred tax assets: | | |
| Capitalized research and development expenditure | $ 3,376 | $ 1,859 |
| GILTI deferred tax assets | 1,576 | 800 |
| Accruals and reserves, not currently deductible for tax purposes | 1,121 | 686 |
| Research and other tax credit carryforwards | 936 | 951 |
| Net operating loss and capital loss carryforwards | 439 | 409 |
| Operating lease liabilities | 263 | 193 |
| Stock-based compensation | 106 | 99 |
| Property, equipment and intangible assets | 4 | 66 |
| Other deferred tax assets | 179 | 91 |
| Gross deferred tax assets | 8,000 | 5,154 |
| Less valuation allowance | (1,552) | (1,484) |
| Total deferred tax assets | 6,448 | 3,670 |
| Deferred tax liabilities: | | |
| Unremitted earnings of foreign subsidiaries | (502) | (228) |
| Operating lease assets | (255) | (179) |
| Acquired intangibles | (74) | (115) |
| Gross deferred tax liabilities | (831) | (522) |
| Net deferred tax asset (1) | $ 5,617 | $ 3,148 |
As of January 28, 2024, we intend to indefinitely reinvest approximately $1.1 billion and $250 million of cumulative undistributed earnings held by certain subsidiaries in Israel and the United Kingdom, respectively. We have not provided the amount of unrecognized deferred tax liabilities for temporary differences related to these investments as the determination of such amount is not practicable.
As of January 28, 2024 and January 29, 2023, we had a valuation allowance of $1.6 billion and $1.5 billion, respectively, related to capital loss carryforwards, and certain state and other deferred tax assets that management determined are not likely to be realized due, in part, to jurisdictional projections of future taxable income, including capital gains. To the extent realization of the deferred tax assets becomes more-likely-than-not, we would recognize such deferred tax assets as income tax benefits during the period.
As of January 28, 2024, we had U.S. federal, state and foreign net operating loss carryforwards of $315 million, $342 million and $361 million, respectively. The federal and state carryforwards will begin to expire in fiscal years 2026 and 2025, respectively. The foreign net operating loss carryforwards of $361 million may be carried forward indefinitely. As of January 28, 2024, we had federal research tax credit carryforwards of $31 million, before the impact of uncertain tax positions, that will begin to expire in fiscal year 2025. We have state research tax credit carryforwards of $1.6 billion, before the impact of uncertain tax positions. $1.5 billion is attributable to the State of California and may be carried over indefinitely and $75 million is attributable to various other states and will begin to expire in fiscal year 2025. As of January 28, 2024, we had federal capital loss carryforwards of $1.4 billion that will begin to expire in fiscal year 2025.
Our tax attributes remain subject to audit and may be adjusted for changes or modification in tax laws, other authoritative interpretations thereof, or other facts and circumstances. Utilization of tax attributes may also be subject to limitations due to ownership changes and other limitations provided by the Internal Revenue Code and similar state and foreign tax provisions. If any such limitations apply, the tax attributes may expire or be denied before utilization.
## NVIDIA Corporation and Subsidiaries
## Notes to the Consolidated Financial Statements
(Continued)
A reconciliation of gross unrecognized tax benefits is as follows:
Table of Contents
| | Jan 28, 2024 | Jan 29, 2023 | Jan 30, 2022 |
|---------------------------------------------|----------------|----------------|----------------|
| | (In millions) | (In millions) | (In millions) |
| Balance at beginning of period | $ 1,238 | $ 1,013 | $ 776 |
| Increases in tax positions for current year | 616 | 268 | 246 |
| Increases in tax positions for prior years | 87 | 1 | 14 |
| Decreases in tax positions for prior years | (148) | (15) | (4) |
| Settlements | (104) | (9) | (8) |
| Lapse in statute of limitations | (19) | (20) | (11) |
| Balance at end of period | $ 1,670 | $ 1,238 | $ 1,013 |
Included in the balance of unrecognized tax benefits as of January 28, 2024 are $1.0 billion of tax benefits that would affect our effective tax rate if recognized.
We classify an unrecognized tax benefit as a current liability, or amount refundable, to the extent that we anticipate payment or receipt of cash for income taxes within one year. The amount is classified as a long-term liability, or reduction of long-term amount refundable, if we anticipate payment or receipt of cash for income taxes during a period beyond a year.
We include interest and penalties related to unrecognized tax benefits as a component of income tax expense. We recognized net interest and penalties related to unrecognized tax benefits in the income tax expense line of our consolidated statements of income of $42 million, $33 million, and $14 million during fiscal years 2024, 2023 and 2022, respectively. As of January 28, 2024 and January 29, 2023, we have accrued $140 million and $95 million, respectively, for the payment of interest and penalties related to unrecognized tax benefits, which is not included as a component of our gross unrecognized tax benefits.
While we believe that we have adequately provided for all tax positions, amounts asserted by tax authorities could be greater or less than our accrued position. Accordingly, our provisions on federal, state and foreign tax-related matters to be recorded in the future may change as revised estimates are made or the underlying matters are settled or otherwise resolved. As of January 28, 2024, we have not identified any positions for which it is reasonably possible that the total amounts of unrecognized tax benefits will significantly increase or decrease within the next twelve months.
We are subject to taxation by taxing authorities both in the United States and other countries. As of January 28, 2024, the significant tax jurisdictions that may be subject to examination include the United States for fiscal years after 2020, as well as China, Germany, Hong Kong, India, Israel, Taiwan, and the United Kingdom for fiscal years 2005 through 2023. As of January 28, 2024, the significant tax jurisdictions for which we are currently under examination include Germany, India, Israel, and Taiwan for fiscal years 2005 through 2023.
## Note 15 - Shareholders' Equity
## Capital Return Program
In August 2023, our Board of Directors approved an increase to our share repurchase program of an additional $25.0 billion, without expiration. During fiscal year 2024, we repurchased 21 million shares of our common stock for $9.7 billion. As of January 28, 2024, we were authorized, subject to certain specifications, to repurchase additional shares of our common stock up to $22.5 billion. From January 29, 2024 through February 16, 2024, we repurchased 2.8 million shares for $1.9 billion pursuant to a Rule 10b5-1 trading plan. Our share repurchase program aims to offset dilution from shares issued to employees. We may pursue additional share repurchases as we weigh market factors and other investment opportunities.
During fiscal years 2024, 2023, and 2022, we paid $395 million, $398 million, and $399 million in cash dividends to our shareholders, respectively. Our cash dividend program and the payment of future cash dividends under that program are subject to our Board of Directors' continuing determination that the dividend program and the declaration of dividends thereunder are in the best interests of our shareholders.
In fiscal year 2022, we retired our existing 349 million treasury shares. These shares assumed the status of authorized and unissued shares upon retirement. The excess of repurchase price over par value was allocated between additional paid-in capital and retained earnings, resulting in a reduction in additional paidin capital by $20 million and retained earnings by $12.0 billion. Any future repurchased shares will assume the status of authorized and unissued shares.
## NVIDIA Corporation and Subsidiaries
## Notes to the Consolidated Financial Statements
(Continued)
## Note 16 - Employee Retirement Plans
We provide tax-qualified defined contribution plans to eligible employees in the U.S. and certain other countries. Our contribution expense for fiscal years 2024, 2023, and 2022 was $255 million, $227 million, and $168 million, respectively.
## Note 17 - Segment Information
Our Chief Executive Officer, who is considered to be our chief operating decision maker, or CODM, reviews financial information presented on an operating segment basis for purposes of making decisions and assessing financial performance.
The Compute & Networking segment includes our Data Center accelerated computing platform; networking; automotive artificial intelligence, or AI, Cockpit, autonomous driving development agreements, and autonomous vehicle solutions; electric vehicle computing platforms; Jetson for robotics and other embedded platforms; NVIDIA AI Enterprise and other software; and DGX Cloud.
The Graphics segment includes GeForce GPUs for gaming and PCs, the GeForce NOW game streaming service and related infrastructure, and solutions for gaming platforms; Quadro/NVIDIA RTX GPUs for enterprise workstation graphics; virtual GPU software for cloud-based visual and virtual computing; automotive platforms for infotainment systems; and Omniverse Enterprise software for building and operating 3D internet applications.
Operating results by segment include costs or expenses that are directly attributable to each segment, and costs or expenses that are leveraged across our unified architecture and therefore allocated between our two segments.
The 'All Other' category includes the expenses that our CODM does not assign to either Compute & Networking or Graphics for purposes of making operating decisions or assessing financial performance. The expenses include stock-based compensation expense, corporate infrastructure and support costs, acquisitionrelated and other costs, intellectual property related, or IP-related costs, acquisition termination cost, and other non-recurring charges and benefits that our CODM deems to be enterprise in nature.
Our CODM does not review any information regarding total assets on a reportable segment basis. Depreciation and amortization expense directly attributable to each reportable segment is included in operating results for each segment. However, our CODM does not evaluate depreciation and amortization expense by operating segment and, therefore, it is not separately presented. There is no intersegment revenue. The accounting policies for segment reporting are the same as for our consolidated financial statements. The table below presents details of our reportable segments and the 'All Other' category.
Table of Contents
| | Compute & Networking | Graphics | All Other | Consolidated |
|--------------------------|------------------------|---------------|---------------|----------------|
| | (In millions) | (In millions) | (In millions) | (In millions) |
| Year Ended Jan 28, 2024: | | | | |
| Revenue | $ 47,405 | $ 13,517 | $ - | $ 60,922 |
| Operating income (loss) | $ 32,016 | $ 5,846 | $ (4,890) | $ 32,972 |
| Year Ended Jan 29, 2023: | | | | |
| Revenue | $ 15,068 | $ 11,906 | $ - | $ 26,974 |
| Operating income (loss) | $ 5,083 | $ 4,552 | $ (5,411) | $ 4,224 |
| Year Ended Jan 30, 2022: | | | | |
| Revenue | $ 11,046 | $ 15,868 | $ - | $ 26,914 |
| Operating income (loss) | $ 4,598 | $ 8,492 | $ (3,049) | $ 10,041 |
## NVIDIA Corporation and Subsidiaries
## Notes to the Consolidated Financial Statements
(Continued)
Table of Contents
| | Year Ended | Year Ended | Year Ended |
|-----------------------------------------------------|---------------|---------------|---------------|
| | Jan 28, 2024 | Jan 29, 2023 | Jan 30, 2022 |
| | (In millions) | (In millions) | (In millions) |
| Reconciling items included in "All Other" category: | | | |
| Stock-based compensation expense | $ (3,549) | $ (2,710) | $ (2,004) |
| Unallocated cost of revenue and operating expenses | (728) | (595) | (399) |
| Acquisition-related and other costs | (583) | (674) | (636) |
| IP-related and legal settlement costs | (40) | (23) | (10) |
| Restructuring costs and other | - | (54) | - |
| Acquisition termination cost | - | (1,353) | - |
| Other | 10 | (2) | - |
| Total | $ (4,890) | $ (5,411) | $ (3,049) |
Revenue by geographic areas is designated based upon the billing location of the customer. End customer location may be different than our customer's billing location. Revenue by geographic areas was as follows:
| | Year Ended | Year Ended | Year Ended |
|-----------------------------|---------------|---------------|---------------|
| | Jan 28, 2024 | Jan 29, 2023 | Jan 30, 2022 |
| Revenue: | (In millions) | (In millions) | (In millions) |
| United States | $ 26,966 | $ 8,292 | $ 4,349 |
| Taiwan | 13,405 | 6,986 | 8,544 |
| China (including Hong Kong) | 10,306 | 5,785 | 7,111 |
| Other countries | 10,245 | 5,911 | 6,910 |
| Total revenue | $ 60,922 | $ 26,974 | $ 26,914 |
Revenue from sales to customers outside of the United States accounted for 56%, 69%, and 84% of total revenue for fiscal years 2024, 2023, and 2022, respectively. The increase in revenue to the United States for fiscal year 2024 was primarily due to higher U.S.-based Compute & Networking segment demand.
Sales to one customer represented 13% of total revenue for fiscal year 2024, which was attributable to the Compute & Networking segment. No customer represented 10% or more of total revenue for fiscal years 2023 and 2022.
The following table summarizes information pertaining to our revenue by each of the specialized markets we serve:
| | Year Ended | Year Ended | Year Ended |
|----------------------------|---------------|---------------|---------------|
| | Jan 28, 2024 | Jan 29, 2023 | Jan 30, 2022 |
| Revenue: | (In millions) | (In millions) | (In millions) |
| Data Center | $ 47,525 | $ 15,005 | $ 10,613 |
| Gaming | 10,447 | 9,067 | 12,462 |
| Professional Visualization | 1,553 | 1,544 | 2,111 |
| Automotive | 1,091 | 903 | 566 |
| OEM and Other | 306 | 455 | 1,162 |
| Total revenue | $ 60,922 | $ 26,974 | $ 26,914 |
## NVIDIA Corporation and Subsidiaries
## Notes to the Consolidated Financial Statements
(Continued)
The following table presents summarized information for long-lived assets by country. Long-lived assets consist of property and equipment and exclude other assets, operating lease assets, goodwill, and intangible assets.
Table of Contents
| | Jan 28, 2024 | Jan 29, 2023 |
|-------------------------|----------------|----------------|
| Long-lived assets: | (In millions) | (In millions) |
| United States | $ 2,595 | $ 2,587 |
| Taiwan | 773 | 702 |
| Israel | 325 | 283 |
| Other countries | 221 | 235 |
| Total long-lived assets | $ 3,914 | $ 3,807 |
## NVIDIA Corporation and Subsidiaries Schedule II - Valuation and Qualifying Accounts
| Description | Balance at Beginning of Period | Additions | Deductions | Balance at End of Period |
|----------------------------------|----------------------------------|----------------|---------------|----------------------------|
| | (In millions) | (In millions) | (In millions) | (In millions) |
| Fiscal year 2024 | | | | |
| Allowance for doubtful accounts | $ | 4 $ - (1) | $ - (1) | $ 4 |
| Sales return allowance | $ | 26 $ 213 (2) | $ (130) (4) | $ 109 |
| Deferred tax valuation allowance | $ 1,484 | $ 162 (3) | $ (94) (3) | $ 1,552 |
| Fiscal year 2023 | | | | |
| Allowance for doubtful accounts | $ | 4 $ - (1) | $ - (1) | $ 4 |
| Sales return allowance | $ | 13 $ 104 (2) | $ (91) (4) | $ 26 |
| Deferred tax valuation allowance | $ 907 | $ 577 (3) | $ - | $ 1,484 |
| Fiscal year 2022 | | | | |
| Allowance for doubtful accounts | $ | 4 $ - (1) | $ - (1) | $ 4 |
| Sales return allowance | $ | 17 $ 19 (2) | $ (23) (4) | $ 13 |
| Deferred tax valuation allowance | $ 728 | $ 179 (3) | $ - | $ 907 |
## Exhibit Index
| | | Incorporated by Reference | Incorporated by Reference | |
|-------------|---------------------------------------------------------------------------------------------------------------------------------------------------------------------------------|-----------------------------|-----------------------------|-------------|
| Exhibit No. | Exhibit Description | Schedule/Form | Exhibit | Filing Date |
| 2.1 | Agreement and Plan of Merger, dated March 10, 2019, by and among NVIDIA Corporation, NVIDIA International Holdings Inc., Mellanox Technologies Ltd. and Teal Barvaz Ltd. | 8-K | 2.1 | 3/11/2019 |
| 2.2^ | Share Purchase Agreement, dated September 13, 2020, by and among NVIDIA, NVIDIA Holdings, Arm, SoftBank, and Vision Fund | 8-K | 2.1 | 9/14/2020 |
| 3.1 | Restated Certificate of Incorporation | 10-K | 3.1 | 3/18/2022 |
| 3.2 | Amendment to Restated Certificate of Incorporation of NVIDIA Corporation | 8-K | 3.1 | 6/6/2022 |
| 3.3 | Bylaws of NVIDIA Corporation, Amended and Restated as of March 2, 2023 | 8-K | 3.1 | 3/8/2023 |
| 4.1 | Reference is made to Exhibits 3.1, 3.2 and 3.3 | | | |
| 4.2 | Specimen Stock Certificate | S-1/A | 4.2 | 4/24/1998 |
| 4.3 | Indenture, dated as of September 16, 2016, by and between the Company and Computershare Trust Company, N.A., as successor to Wells Fargo Bank, National Association, as Trustee | 8-K | 4.1 | 9/16/2016 |
| 4.4 | Officers' Certificate, dated as of September 16, 2016 | 8-K | 4.2 | 9/16/2016 |
| 4.5 | Form of 2026 Note | 8-K | Annex B-1 to Exhibit 4.2 | 9/16/2016 |
| 4.6 | Description of Securities | 10-K | 4.6 | 2/24/2023 |
| 4.7 | Officers' Certificate, dated as of March 31, 2020 | 8-K | 4.2 | 3/31/2020 |
| 4.8 | Form of 2030 Note | 8-K | Annex A-1 to Exhibit 4.2 | 3/31/2020 |
| 4.9 | Form of 2040 Note | 8-K | Annex B-1 to | 3/31/2020 |
| 4.10 | Form of 2050 Note | 8-K | Annex C-1 to | 3/31/2020 |
| 4.11 | Form of 2060 Note | 8-K | Annex D-1 to Exhibit 4.2 | 3/31/2020 |
| 4.12 | Officers' Certificate, dated as of June 16, 2021 | 8-K | 4.2 | 6/16/2021 |
| 4.13 | Form of 2023 Note | 8-K | Annex A-1 to Exhibit 4.2 | 6/16/2021 |
| 4.14 | Form of 2024 Note | 8-K | Annex B-1 to | 6/16/2021 |
| 4.15 | Form of 2028 Note | 8-K | Annex C-1 to Exhibit 4.2 | 6/16/2021 |
| 4.16 | Form of 2031 Note | 8-K | Annex D-1 to Exhibit 4.2 | 6/16/2021 |
| 10.1 | Form of Indemnity Agreement between NVIDIA Corporation and each of its directors and officers | 8-K | 10.1 | 3/7/2006 |
| 10.2+ | Amended and Restated 2007 Equity Incentive Plan | 10-K | 10.2 | 2/24/2023 |
| 10.3+ | Amended and Restated 2007 Equity Incentive Plan - Non-Employee Director Deferred Restricted Stock Unit Grant Notice and Deferred Restricted Stock Unit Agreement (2016) | 10-K | 10.26 | 3/12/2015 |
| 10.4+ | Amended and Restated 2007 Equity Incentive Plan - Non-Employee Director Restricted Stock Unit Grant Notice and Restricted Stock Unit Agreement (2016) | 10-K | 10.27 | 3/12/2015 |
| 10.5+ | Amended and Restated 2007 Equity Incentive Plan - Global Performance- Based Restricted Stock Unit Grant Notice and Performance-Based Restricted | 8-K | 10.1 | 3/11/2019 |
| 10.6+ | Amended and Restated 2007 Equity Incentive Plan - Global Restricted Stock Unit Grant Notice and Global Restricted Stock Unit Agreement (2020) | 10-Q | 10.2 | 5/21/2020 |
| 10.7+ | Amended and Restated 2007 Equity Incentive Plan - Global Restricted Stock Unit Grant Notice and Global Restricted Stock Unit Agreement (2021) | 10-Q | 10.2 | 5/26/2021 |
|----------|-----------------------------------------------------------------------------------------------------------------------------------------------------------------------------------------|--------|--------|-------------|
| 10.8+ | Amended and Restated 2007 Equity Incentive Plan - Global Restricted Stock Unit Grant Notice and Global Restricted Stock Unit Agreement (2022) | 10-K | 10.16 | 3/18/2022 |
| 10.9+ | Amended and Restated 2007 Equity Incentive Plan - Global Restricted Stock Unit Grant Notice and Global Restricted Stock Unit Agreement (2023) | 10-K | 10.14 | 2/24/2023 |
| 10.10+ | Amended and Restated 2012 Employee Stock Purchase Plan | 10-Q | 10.2 | 8/20/2021 |
| 10.11+ | Variable Compensation Plan - Fiscal Year 2023 | 8-K | 10.1 | 3/9/2022 |
| 10.12+ | Variable Compensation Plan - Fiscal Year 2024 | 8-K | 10.1 | 3/8/2023 |
| 10.13 | Form of Commercial Paper Dealer Agreement between NVIDIA Corporation, as Issuer, and the Dealer party thereto | 8-K | 10.1 | 12/15/2017 |
| 21.1* | Subsidiaries of Registrant | | | |
| 23.1* | Consent of PricewaterhouseCoopers LLP | | | |
| 24.1* | Power of Attorney (included in signature page) | | | |
| 31.1* | Certification of Chief Executive Officer as required by Rule 13a-14(a) of the Securities Exchange Act of 1934 | | | |
| 31.2* | Certification of Chief Financial Officer as required by Rule 13a-14(a) of the Securities Exchange Act of 1934 | | | |
| 32.1#* | Certification of Chief Executive Officer as required by Rule 13a-14(b) of the Securities Exchange Act of 1934 | | | |
| 32.2#* | Certification of Chief Financial Officer as required by Rule 13a-14(b) of the Securities Exchange Act of 1934 | | | |
| 97.1+* | Compensation Recovery Policy, as amended and restated November 30, 2023 | | | |
| 101.INS* | XBRL Instance Document | | | |
| 101.SCH* | XBRL Taxonomy Extension Schema Document | | | |
| 101.CAL* | XBRL Taxonomy Extension Calculation Linkbase Document | | | |
| 101.DEF* | XBRL Taxonomy Extension Definition Linkbase Document | | | |
| 101.LAB* | XBRL Taxonomy Extension Labels Linkbase Document | | | |
| 101.PRE* | XBRL Taxonomy Extension Presentation Linkbase Document | | | |
| 104 | Cover Page Interactive Data File - the cover page interactive data file does not appear in the Interactive Data File because its XBRL tags are embedded within the Inline XBRL document | | | |
Copies of above exhibits not contained herein are available to any shareholder upon written request to: Investor Relations: NVIDIA Corporation, 2788 San Tomas Expressway, Santa Clara, CA 95051
## Item 16. Form 10-K Summary
## Not Applicable.
## Signatures
Pursuant to the requirements of Section 13 or 15(d) of the Securities Exchange Act of 1934, the Registrant has duly caused this report to be signed on its behalf by the undersigned, thereunto duly authorized, on February 21, 2024.
## NVIDIA Corporation
By:
/s/ Jen-Hsun Huang
Jen-Hsun Huang
President and Chief Executive Officer
## Power of Attorney
KNOW ALL PERSONS BY THESE PRESENTS, that each person whose signature appears below constitutes and appoints Jen-Hsun Huang and Colette M. Kress, and each or any one of them, his true and lawful attorney-in-fact and agent, with full power of substitution and resubstitution, for him and in his name, place and stead, in any and all capacities, to sign any and all amendments to this report, and to file the same, with all exhibits thereto, and other documents in connection therewith, with the Securities and Exchange Commission, granting unto said attorneys-in-facts and agents, and each of them, full power and authority to do and perform each and every act and thing requisite and necessary to be done in connection therewith, as fully to all intents and purposes as he might or could do in person, hereby ratifying and confirming all that said attorneys-in-fact and agents, or any of them, or their or his substitutes or substitutes, may lawfully do or cause to be done by virtue hereof.
Pursuant to the requirements of the Securities Exchange Act of 1934, this report has been signed below by the following persons on behalf of the registrant and in the capacities and on the dates indicated.
| Signature | Title | Date |
|-----------------------|------------------------------------------------------------------------------------|-------------------|
| /s/ JEN-HSUN HUANG | President, Chief Executive Officer and Director (Principal Executive Officer) | February 21, 2024 |
| Jen-Hsun Huang | | |
| /s/ COLETTE M. KRESS | Executive Vice President and Chief Financial Officer (Principal Financial Officer) | February 21, 2024 |
| Colette M. Kress | | |
| /s/ DONALD ROBERTSON | Vice President and Chief Accounting Officer (Principal Accounting Officer) | February 21, 2024 |
| Donald Robertson | | |
| /s/ ROBERT BURGESS | Director | February 21, 2024 |
| Robert Burgess | | |
| /s/ TENCH COXE | Director | February 21, 2024 |
| Tench Coxe | | |
| /s/ JOHN O. DABIRI | Director | February 21, 2024 |
| John O. Dabiri | | |
| /s/ PERSIS DRELL | Director | February 21, 2024 |
| Persis Drell | | |
| /s/ DAWN HUDSON | Director | February 21, 2024 |
| Dawn Hudson | | |
| /s/ HARVEY C. JONES | Director | February 21, 2024 |
| Harvey C. Jones | | |
| /s/ MELISSA B. LORA | Director | February 21, 2024 |
| Melissa B. Lora | | |
| /s/ MICHAEL MCCAFFERY | Director | February 21, 2024 |
| Michael McCaffery | | |
| /s/ STEPHEN C. NEAL | Director | February 21, 2024 |
| Stephen C. Neal | | |
| /s/ MARK L. PERRY | Director | February 21, 2024 |
| Mark L. Perry | | |
| /s/ A. BROOKE SEAWELL | Director | February 21, 2024 |
| A. Brooke Seawell | | |
| /s/ AARTI SHAH | Director | February 21, 2024 |
| Aarti Shah | | |
| /s/ MARK STEVENS | Director | February 21, 2024 |
Mark Stevens
## SUBSIDIARIES OF REGISTRANT
The following is a list of subsidiaries of NVIDIA Corporation as of January 28, 2024, omitting subsidiaries which, considered in the aggregate, would not constitute a significant subsidiary.
Subsidiaries of Registrant (All 100% owned)
State or Other Jurisdiction of Incorporation
Mellanox Technologies, Ltd NVIDIA International, Inc. NVIDIA Singapore Pte Ltd
Israel Delaware, U.S. Singapore
## CONSENT OF INDEPENDENT REGISTERED PUBLIC ACCOUNTING FIRM
We hereby consent to the incorporation by reference in the Registration Statements on Form S-3 (No. 333-270119) and Form S-8 (Nos. 33374905, 333-51520, 333-74868, 333-100010, 333-106191, 333-114375, 333-123933, 333-132493, 333-140021, 333-143953, 333-181625, 333185036, 333-188775, 333-196259, 333-211615, 333-229774, 333-237833, 333-239164, 333-249570, 333-259044, and 333-267207) of NVIDIA Corporation of our report dated February 21, 2024 relating to the financial statements, financial statement schedule and the effectiveness of internal control over financial reporting, which appears in this Form 10-K.
/s/ PricewaterhouseCoopers LLP San Jose, California February 21, 2024
## CERTIFICATION
- I, Jen-Hsun Huang, certify that:
- 1. I have reviewed this Annual Report on Form 10-K of NVIDIA Corporation;
- 2. Based on my knowledge, this report does not contain any untrue statement of a material fact or omit to state a material fact necessary to make the statements made, in light of the circumstances under which such statements were made, not misleading with respect to the period covered by this report;
- 3. Based on my knowledge, the financial statements, and other financial information included in this report, fairly present in all material respects the financial condition, results of operations and cash flows of the registrant as of, and for, the periods presented in this report;
- 4. The registrant's other certifying officer(s) and I are responsible for establishing and maintaining disclosure controls and procedures (as defined in Exchange Act Rules 13a-15(e) and 15d-15(e)) and internal control over financial reporting (as defined in Exchange Act Rules 13a15(f) and 15d-15(f)) for the registrant and have:
- (a) designed such disclosure controls and procedures, or caused such disclosure controls and procedures to be designed under our supervision, to ensure that material information relating to the registrant, including its consolidated subsidiaries, is made known to us by others within those entities, particularly during the period in which this report is being prepared;
- (b) designed such internal control over financial reporting, or caused such internal control over financial reporting to be designed under our supervision, to provide reasonable assurance regarding the reliability of financial reporting and the preparation of financial statements for external purposes in accordance with generally accepted accounting principles;
- (c) evaluated the effectiveness of the registrant's disclosure controls and procedures and presented in this report our conclusions about the effectiveness of the disclosure controls and procedures, as of the end of the period covered by this report based on such evaluation; and
- (d) disclosed in this report any change in the registrant's internal control over financial reporting that occurred during the registrant's most recent fiscal quarter (the registrant's fourth fiscal quarter in the case of an annual report) that has materially affected, or is reasonably likely to materially affect, the registrant's internal control over financial reporting; and
- 5. The registrant's other certifying officer(s) and I have disclosed, based on our most recent evaluation of internal control over financial reporting, to the registrant's auditors and the audit committee of the registrant's board of directors (or persons performing the equivalent functions):
- (a) all significant deficiencies and material weaknesses in the design or operation of internal control over financial reporting which are reasonably likely to adversely affect the registrant's ability to record, process, summarize and report financial information; and
- (b) any fraud, whether or not material, that involves management or other employees who have a significant role in the registrant's internal control over financial reporting.
Date: February 21, 2024
/s/ JEN-HSUN HUANG Jen-Hsun Huang President and Chief Executive Officer
## CERTIFICATION
- I, Colette M. Kress, certify that:
- 1. I have reviewed this Annual Report on Form 10-K of NVIDIA Corporation;
- 2. Based on my knowledge, this report does not contain any untrue statement of a material fact or omit to state a material fact necessary to make the statements made, in light of the circumstances under which such statements were made, not misleading with respect to the period covered by this report;
- 3. Based on my knowledge, the financial statements, and other financial information included in this report, fairly present in all material respects the financial condition, results of operations and cash flows of the registrant as of, and for, the periods presented in this report;
- 4. The registrant's other certifying officer(s) and I are responsible for establishing and maintaining disclosure controls and procedures (as defined in Exchange Act Rules 13a-15(e) and 15d-15(e)) and internal control over financial reporting (as defined in Exchange Act Rules 13a15(f) and 15d-15(f)) for the registrant and have:
- (a) designed such disclosure controls and procedures, or caused such disclosure controls and procedures to be designed under our supervision, to ensure that material information relating to the registrant, including its consolidated subsidiaries, is made known to us by others within those entities, particularly during the period in which this report is being prepared;
- (b) designed such internal control over financial reporting, or caused such internal control over financial reporting to be designed under our supervision, to provide reasonable assurance regarding the reliability of financial reporting and the preparation of financial statements for external purposes in accordance with generally accepted accounting principles;
- (c) evaluated the effectiveness of the registrant's disclosure controls and procedures and presented in this report our conclusions about the effectiveness of the disclosure controls and procedures, as of the end of the period covered by this report based on such evaluation; and
- (d) disclosed in this report any change in the registrant's internal control over financial reporting that occurred during the registrant's most recent fiscal quarter (the registrant's fourth fiscal quarter in the case of an annual report) that has materially affected, or is reasonably likely to materially affect, the registrant's internal control over financial reporting; and
- 5. The registrant's other certifying officer(s) and I have disclosed, based on our most recent evaluation of internal control over financial reporting, to the registrant's auditors and the audit committee of the registrant's board of directors (or persons performing the equivalent functions):
- (a) all significant deficiencies and material weaknesses in the design or operation of internal control over financial reporting which are reasonably likely to adversely affect the registrant's ability to record, process, summarize and report financial information; and
- (b) any fraud, whether or not material, that involves management or other employees who have a significant role in the registrant's internal control over financial reporting.
Date: February 21, 2024
/s/ COLETTE M. KRESS
Colette M. Kress
Executive Vice President and Chief Financial Officer
## CERTIFICATION
Pursuant to the requirement set forth in Rule 13a-14(b) of the Securities Exchange Act of 1934, as amended (the 'Exchange Act'), and Section 1350 of Chapter 63 of Title 18 of the United States Code (18 U.S.C. § 1350), Jen-Hsun Huang, the President and Chief Executive Officer of NVIDIA Corporation (the 'Company'), hereby certifies that, to the best of his knowledge:
- 1. The Company's Annual Report on Form 10-K for the year ended January 28, 2024, to which this Certification is attached as Exhibit 32.1 (the 'Periodic Report'), fully complies with the requirements of Section 13(a) or Section 15(d) of the Exchange Act; and
- 2. The information contained in the Periodic Report fairly presents, in all material respects, the financial condition of the Company at the end of the period covered by the Periodic Report and results of operations of the Company for the period covered by the Periodic Report.
Date: February 21, 2024
## /s/ JEN-HSUN HUANG
Jen-Hsun Huang
President and Chief Executive Officer
A signed original of this written statement required by Section 906 of 18 U.S.C. § 1350 has been provided to NVIDIA Corporation and will be retained by NVIDIA Corporation and furnished to the Securities and Exchange Commission or its staff upon request.
This certification accompanies the Form 10-K to which it relates, is not deemed filed with the Securities and Exchange Commission and is not to be incorporated by reference into any filing of the Company under the Securities Act of 1933, as amended, or the Exchange Act (whether made before or after the date of the Form 10-K), irrespective of any general incorporation language contained in such filing.
## CERTIFICATION
Pursuant to the requirement set forth in Rule 13a-14(b) of the Securities Exchange Act of 1934, as amended (the 'Exchange Act'), and Section 1350 of Chapter 63 of Title 18 of the United States Code (18 U.S.C. § 1350), Colette M. Kress, Executive Vice President and Chief Financial Officer of NVIDIA Corporation (the 'Company'), hereby certifies that, to the best of her knowledge:
- 1. The Company's Annual Report on Form 10-K for the year ended January 28, 2024, to which this Certification is attached as Exhibit 32.2 (the 'Periodic Report'), fully complies with the requirements of Section 13(a) or Section 15(d) of the Exchange Act; and
- 2. The information contained in the Periodic Report fairly presents, in all material respects, the financial condition of the Company at the end of the period covered by the Periodic Report and results of operations of the Company for the period covered by the Periodic Report.
Date: February 21, 2024
## /s/ COLETTE M. KRESS
Colette M. Kress
Executive Vice President and Chief Financial Officer
A signed original of this written statement required by Section 906 of 18 U.S.C. § 1350 has been provided to NVIDIA Corporation and will be retained by NVIDIA Corporation and furnished to the Securities and Exchange Commission or its staff upon request.
This certification accompanies the Form 10-K to which it relates, is not deemed filed with the Securities and Exchange Commission and is not to be incorporated by reference into any filing of the Company under the Securities Act of 1933, as amended, or the Exchange Act (whether made before or after the date of the Form 10-K), irrespective of any general incorporation language contained in such filing.
## COMPENSATION RECOVERY POLICY
Last updated: November 30, 2023
## Policy Purpose | Applicability
This Policy provides for the Company's recovery, in the event of an Accounting Restatement, of certain compensation received by the Company's officers. Specifically, this Policy applies to Incentive Compensation Received on or after the Effective Date by a Covered Officer (i) after beginning services as a Covered Officer, (ii) who served as a Covered Officer at any time during the performance period for such Incentive Compensation, (iii) while the Company had a class of securities listed on a national securities exchange or a national securities association, and (iv) during the Lookback Period.
This Policy is designed to comply, and shall be interpreted to be consistent, with the Compensation Recovery Rules. It shall be binding and enforceable against all Covered Officers and, to the extent required by the Compensation Recovery Rules, their beneficiaries, heirs, executors, administrators or other legal representatives.
This Policy amends and restates the Prior Policy with respect to all Incentive Compensation that is Received by a Covered Officer on and after the Effective Date. The Prior Policy shall continue to apply to Incentive Compensation Received by a Covered Officer prior to the Effective Date.
## Policy Statement | Responsibilities
In the event of an Accounting Restatement, regardless of whether or when any restated financial statements are filed, the Company must reasonably promptly recover the full amount of the Recoverable Incentive Compensation, regardless of whether the Covered Officer engaged in any misconduct and regardless of fault.
For any compensation plans or programs involving Incentive Compensation, the amount of Recoverable Incentive Compensation shall include the amount contributed to any notional account based on Recoverable Incentive Compensation and any earnings to date on that notional amount. For any Incentive Compensation that is based on stock price or TSR, where the amount of Recoverable Incentive Compensation is not subject to mathematical recalculation directly from the information in an Accounting Restatement, the amount of Recoverable Incentive Compensation shall be based on a reasonable estimate of the effect of the Accounting Restatement on the applicable stock price or TSR. The Company shall maintain documentation of the determination of that reasonable estimate and provide it to Nasdaq in accordance with Rule 5608.
The Company would not be required to recover Recoverable Incentive Compensation if (I) (A) the direct expense paid to a third party to assist in enforcing this Policy would exceed the amount of the Recoverable Incentive Compensation, or (B) recovery would likely cause an otherwise tax-qualified retirement plan, under which benefits are broadly available to employees of the Company, to fail to meet the requirements of Code Sections 401(a)(13) or 411(a) and regulations thereunder and (II) the Committee (or in the absence of a Committee, a majority of the independent members of the Board) determines that recovery would be impracticable. However, before concluding that it would be impracticable to recover any amount of Recoverable Incentive Compensation based on expense of enforcement under (I)(A) of this paragraph, the Company shall make a reasonable attempt to recover such Recoverable Incentive Compensation, document its reasonable attempt(s) to recover, and provide that documentation to Nasdaq in accordance with Rule 5608.
To the extent permitted by applicable law, the Committee shall, in its sole discretion, determine the timing and method for recovering Recoverable Incentive Compensation, provided that the recovery is undertaken reasonably promptly. The Committee may, but is not limited to, seek recovery from a Covered Officer from any or a combination of the following sources, irrespective of whether the applicable source of compensation was approved, awarded, granted, payable or paid to the Covered Officer prior to, on, or after the Effective Date: (i) direct repayment of Recoverable Incentive Compensation previously paid to the Covered Officer; (ii) cancelling or directing the return of prior cash or equity-based awards (whether vested or unvested and whether paid or unpaid); (iii) cancelling or offsetting against any planned future cash or equity-based awards; (iv) forfeiture of any deferred compensation, subject to compliance with Code Section 409A; and (v) any other method authorized by applicable
NVIDIA Corporation Application: Global Document Name: Compensation Recovery Policy - External


## COMPENSATION RECOVERY POLICY
law or contract. The Committee need not utilize the same method of recovery for all Covered Officers or with respect to all types of Recoverable Incentive Compensation.
The Company shall make any disclosures and filings with respect to the Policy that are required by law, including as required by the SEC.
Notwithstanding any indemnification agreement, applicable insurance policy or any other agreement or provision of the Company's certificate of incorporation or bylaws to the contrary, no Covered Officer shall be entitled to indemnification against the loss of Recoverable Incentive Compensation, nor shall any Covered Officer be entitled to advancement of expenses in connection with any enforcement of this Policy by the Company, including payment or reimbursement for the Covered Officer's insurance premiums to cover potential obligations to the Company under this Policy. All Covered Officers are subject to this Policy pursuant to the terms as set forth in Exhibit A .
Nothing in this Policy nor any recovery shall limit any claims, damages or other legal remedies the Company may have against a Covered Officer. This Policy does not preclude the Company from taking any other action to enforce a Covered Officer's obligations to the Company, including, without limitation, termination of employment and/or institution of civil proceedings.
This Policy is in addition to the requirements of Section 304 of the Sarbanes-Oxley Act of 2002 that are applicable to the Company's Chief Executive Officer and Chief Financial Officer and to any other compensation recovery policy and/or similar provisions in any employment, equity plan, equity award or other individual agreement to which the Company is a party or which the Company has adopted or may adopt and maintain from time to time; provided, that any amounts already recovered by or reimbursed to the Company under those requirements and provisions related to the same Accounting Restatement will be credited towards the amount of any Recoverable Incentive Compensation under this Policy, except as may be required by applicable law or listing standards.
Any action by the Company to recover or any recovery of Recoverable Incentive Compensation under this Policy from a Covered Officer shall not be deemed (i) 'good reason' for resignation or to serve as a basis for a claim of constructive termination under any benefits or compensation arrangements applicable to such Covered Officer, or (ii) to constitute a breach of any contract or other arrangement to which such Covered Officer is a party.
## COMPLIANCE, VIOLATIONS and CONSEQUENCES
The Company may be subject to delisting if it does not comply with this Policy.
## ADMINISTRATION, TERMINATION and AMENDMENT
This Policy shall be administered by the Committee, unless otherwise determined by a majority of the independent members of the Board. Any determination by the Committee with respect to this Policy shall be final, conclusive and binding on all interested parties and need not be uniform with respect to each individual covered by this Policy. In carrying out the administration of this Policy, the Committee is authorized and directed to consult with the full Board, individual members of the Board, or such other committees of the Board as may be necessary or appropriate as to matters within the scope of such other committee's responsibility and authority.
The Committee members and any other members of the Board who assist in the administration of this Policy shall not be personally liable for any action, determination or interpretation made with respect to this Policy and shall be indemnified by the Company to the fullest extent under applicable law and Company policy with respect to any such action, determination or interpretation. The foregoing sentence shall not limit any other rights to indemnification of Committee members under applicable law or Company policy.
Subject to applicable law, the Committee may authorize and empower any officer or employee of the Company to take any and all actions that the Committee, in its sole discretion, deems necessary or appropriate to carry out the purpose and intent of this Policy (other than with respect to any recovery under this Policy involving such officer or employee).
NVIDIA Corporation Application: Global Document Name: Compensation Recovery Policy - External

## COMPENSATION RECOVERY POLICY
If any provision of this Policy or the application of any provision to a Covered Officer shall be adjudicated to be invalid, illegal or unenforceable in any respect, such invalidity, illegality or unenforceability shall not affect any other provisions of this Policy, and the invalid, illegal or unenforceable provisions shall be deemed amended to the minimum extent necessary to render any such provision or application enforceable.
The Committee may amend, terminate or replace this Policy or any portion of this Policy at any time in its sole discretion, and shall amend this Policy as it deems necessary to comply with applicable law or listing standards.
## Speak Up | Reporting Concerns
If you are aware of a situation that may violate this Policy, you have a responsibility to report the incident to your immediate supervisor, Corporate Legal or to NVIDIA-Compliance. Reports may also be submitted anonymously by using NVIDIA's Speak Up system through EthicsPoint at 1-866-295-3993 (for the U.S.) or via the web here. When reporting a concern, we encourage you to consider revealing your identity so that we can properly follow up and investigate alleged violations. NVIDIA will ensure that appropriate confidentiality measures are taken and will not retaliate against anyone, who in good faith, reports a concern or cooperates with an investigation, even when allegations are found to be unsubstantiated.
## Resources | Questions
If you have any questions about any aspect of this Policy, you are encouraged to contact Corporate Legal.
NVIDIA Corporation Application: Global Document Name: Compensation Recovery Policy - External
Page 3 of 5
## COMPENSATION RECOVERY POLICY
## Definitions
| Accounting Restatement | An accounting restatement that the Company is required to prepare due to the material noncompliance of the Company with any financial reporting requirement under the securities laws, including any required accounting restatement to correct an error in previously issued financial statements that is material to the previously issued financial statements, or that would result in a material misstatement if the error were corrected in the current period or left uncorrected in the current period |
|------------------------------------|------------------------------------------------------------------------------------------------------------------------------------------------------------------------------------------------------------------------------------------------------------------------------------------------------------------------------------------------------------------------------------------------------------------------------------------------------------------------------------------------------------------|
| Accounting Restatement Date | The earlier to occur of (i) the date that the Board, a committee of the Board, or the officer or officers of the Company authorized to take such action if Board action is not required, concludes, or reasonably should have concluded, that the Company is required to prepare an Accounting Restatement, or (ii) the date that a court, regulator or other legally authorized body directs the Company to prepare an Accounting Restatement |
| Board | Board of Directors of the Company |
| Code | Internal Revenue Code of 1986, as amended |
| Committee | Compensation Committee of the Board |
| Company | NVIDIA Corporation |
| Compensation | Section 10D and Rule 10D-1 of the Exchange Act and Rule 5608 |
| Covered Officer | A current or former 'executive officer,' as such term is defined in Section 10D and Rule 10D-1(d) of the Exchange Act and Rule 5608 |
| Effective Date | October 2, 2023 |
| Exchange Act | Securities Exchange Act of 1934, as amended |
| Financial Reporting Measure | A measure that is determined and presented in accordance with the accounting principles used in preparing the Company's financial statements, and any measure derived wholly or in part from such measure, including Company stock price and TSR. A measure need not be presented in the Company's financial statements or included in a filing with the SEC in order |
| Incentive Compensation | Any compensation that is granted, earned or vested based wholly or in part upon the attainment of a Financial Reporting Measure |
| Lookback Period | The three completed fiscal years immediately preceding the Accounting Restatement Date, as well as any transition period (resulting from a change in the Company's fiscal year) within or immediately following those three completed fiscal years (except that a transition period of at least nine months shall be deemed a completed fiscal year); provided, that the Lookback Period shall not include fiscal years completed prior to October 2, 2023 |
| Prior Policy | The Company's Compensation Recovery Policy dated April 1, 2009 |
| Received | Incentive Compensation is deemed 'received' in the Company's fiscal period in which the relevant Financial Reporting Measure is attained, even if the payment or grant of such Incentive Compensation occurs after the end of that period. |
| Recoverable Incentive Compensation | Incentive Compensation Received by a Covered Officer during the Lookback Period that exceeds the amount of Incentive Compensation that otherwise would have been Received had such amount been determined based on the Accounting Restatement, computed without regard to any taxes paid (i.e., on a gross basis without regarding to tax withholdings and other deductions) |
| Rule 5608 | Nasdaq Listing Rule 5608 |
| SEC | Securities and Exchange Commission |
| TSR | Total shareholder return |

## Exhibit A
## NVIDIA Corporation
## Compensation Recovery Policy
## Form of Executive Acknowledgment
I, the undersigned, agree and acknowledge that I am bound by, and subject to, the NVIDIA Corporation Compensation Recovery Policy dated November 30, 2023, as may be amended, restated, supplemented or otherwise modified from time to time (the ' Policy '). In the event of any inconsistency between the Policy and the terms of any employment agreement, offer letter, indemnity agreement or other individual agreement with NVIDIA Corporation (the ' Company ') to which I am a party, or the terms of any compensation plan, program or agreement, whether or not written, under which any compensation has been granted, awarded, earned or paid to me, the terms of the Policy shall govern.
In the event that the Committee (as defined in the Policy) or other applicable administrator of the Policy determines that any compensation granted, awarded, earned or paid to me must be forfeited or reimbursed to the Company pursuant to the Policy, I will promptly take any action necessary to effectuate such forfeiture and/or reimbursement. Notwithstanding anything to the contrary in the Company's bylaws or in any indemnity agreement between the Company and me, I further agree and acknowledge that I am not entitled to indemnification against the loss of any Recoverable Incentive Compensation, I am not entitled to payment or reimbursement by the Company for premiums in connection with any insurance I may procure to cover potential obligations to the Company under this Policy, and I hereby waive any right to advancement of expenses in connection with any enforcement of the Policy by the Company.
Agreed and Acknowledged:
Name:
Title:
Date:
NVIDIA Corporation Application: Global Document Name: Compensation Recovery Policy - External | {
"source": "ag2ai/ag2",
"title": "test/agents/experimental/document_agent/pdf_parsed/nvidia_10k_2024.md",
"url": "https://github.com/ag2ai/ag2/blob/main/test/agents/experimental/document_agent/pdf_parsed/nvidia_10k_2024.md",
"date": "2024-11-11T20:43:34",
"stars": 1869,
"description": "AG2 (formerly AutoGen): The Open-Source AgentOS. Join us at: https://discord.gg/pAbnFJrkgZ",
"file_size": 456274
} |
---
title: "Key Features"
---
<div class="homepage-hero-section">
<div class="hero-content">
<div class="hero-logo-section">
<img class="hero-logo" noZoom src="/ag2/assets/img/ag2.svg" alt="AG2 Logo" />
</div>
<div class="hero-text-section">
<h2 class="hero-title">AG2</h2>
<p class="hero-subtitle">The Open Source Agent OS</p>
<a class="hero-btn" href="/ag2/docs/home/quick-start">
<div>Getting Started - 3 Minute</div>
</a>
</div>
</div>
</div>
### Key Features
<div class="key-features not-prose grid gap-x-4 sm:grid-cols-3">
<div class="card">
<div class="key-feature">
<img noZoom src="/ag2/assets/img/conv_2.svg" alt="Multi-Agent Conversation Framework" />
<p><b>Multi-Agent Conversation Framework</b></p>
<p>AG2 provides multi-agent conversation framework as a high-level abstraction. With this framework, one can conveniently build LLM workflows.</p>
</div>
</div>
<div class="card">
<div class="key-feature">
<img noZoom src='/ag2/assets/img/autogen_app.svg' alt='Easily Build Diverse Applications' />
<p><b>Easily Build Diverse Applications</b></p>
<p>AG2 offers a collection of working systems spanning a wide range of applications from various domains and complexities.</p>
</div>
</div>
<div class="card">
<div class="key-feature">
<img noZoom src='/ag2/assets/img/extend.svg' alt='Enhanced LLM Inference & Optimization' />
<p><b>Enhanced LLM Inference & Optimization</b></p>
<p>AG2 supports enhanced LLM inference APIs, which can be used to improve inference performance and reduce cost.</p>
</div>
</div>
</div>
### Explore content
<div class="explore-content">
<div class="not-prose grid gap-x-4 sm:grid-cols-2">
<a class="card" href="/ag2/docs/home/quick-start">
<div class="px-6 py-5">
<div>
<h2 class="font-semibold text-base text-gray-800 dark:text-white">Quick Start</h2>
<div class="mt-1 font-normal text-sm leading-6 text-gray-600 dark:text-gray-400">Start building your AG2 application.</div>
</div>
</div>
</a>
<a class="card" href="/ag2/docs/user-guide/basic-concepts/installing-ag2">
<div class="px-6 py-5">
<div>
<h2 class="font-semibold text-base text-gray-800 dark:text-white">Concepts</h2>
<div class="mt-1 font-normal text-sm leading-6 text-gray-600 dark:text-gray-400">Work through the key concepts of AG2 including ConversableAgent, GroupChat, Swarm, and tools.</div>
</div>
</div>
</a>
<a class="card" href="/ag2/docs/user-guide/advanced-concepts">
<div class="px-6 py-5">
<div>
<h2 class="font-semibold text-base text-gray-800 dark:text-white">Advanced Concepts</h2>
<div class="mt-1 font-normal text-sm leading-6 text-gray-600 dark:text-gray-400">Advance to RAG, Code Execution, and more complex GroupChats and Swarms.</div>
</div>
</div>
</a>
<a class="card" href="/ag2/docs/use-cases/use-cases">
<div class="px-6 py-5">
<div>
<h2 class="font-semibold text-base text-gray-800 dark:text-white">Use Cases</h2>
<div class="mt-1 font-normal text-sm leading-6 text-gray-600 dark:text-gray-400">Try out use case workflows including Customer Service, Travel Planning, and Game Design.</div>
</div>
</div>
</a>
<a class="card" href="/ag2/docs/use-cases/notebooks/Notebooks">
<div class="px-6 py-5">
<div>
<h2 class="font-semibold text-base text-gray-800 dark:text-white">Notebook Examples</h2>
<div class="mt-1 font-normal text-sm leading-6 text-gray-600 dark:text-gray-400">A collection of interactive notebooks across all AG2 topics.</div>
</div>
</div>
</a>
<a class="card" href="/ag2/docs/api-reference">
<div class="px-6 py-5">
<div>
<h2 class="font-semibold text-base text-gray-800 dark:text-white">API Reference</h2>
<div class="mt-1 font-normal text-sm leading-6 text-gray-600 dark:text-gray-400">Delve into the AG2 API reference.</div>
</div>
</div>
</a>
<a class="card" href="/ag2/docs/contributor-guide/contributing">
<div class="px-6 py-5">
<div>
<h2 class="font-semibold text-base text-gray-800 dark:text-white">How to Contribute</h2>
<div class="mt-1 font-normal text-sm leading-6 text-gray-600 dark:text-gray-400">Get involved with AG2 by adding what you need and making the framework even better!</div>
</div>
</div>
</a>
</div>
</div>
### Popular resources
<div class="popular-resources">
<div class="card-group not-prose grid gap-x-4 sm:grid-cols-2">
<div class="card">
<div class="youtube-video">
<iframe
class="w-full aspect-video rounded-md"
src="https://www.youtube.com/embed/RLwyXRVvlNk"
title="YouTube video player"
frameborder="0"
allow="accelerometer; autoplay; clipboard-write; encrypted-media; gyroscope; picture-in-picture"
allowfullscreen
></iframe>
<a target="_blank" href="https://www.youtube.com/watch?v=RLwyXRVvlNk">
Foundation Capital Interview with Dr. Chi Wang
</a>
</div>
</div>
<div class="card">
<div class="youtube-video">
<iframe
class="w-full aspect-video rounded-md"
src="https://www.youtube.com/embed/TBNTH-fwGPE"
title="YouTube video player"
frameborder="0"
allow="accelerometer; autoplay; clipboard-write; encrypted-media; gyroscope; picture-in-picture"
allowfullscreen
></iframe>
<a target="_blank" href="https://www.youtube.com/watch?v=TBNTH-fwGPE">
Learn AG2 on DeepLearningAI
</a>
</div>
</div>
</div>
</div> | {
"source": "ag2ai/ag2",
"title": "website/mkdocs/docs/docs/home/home.md",
"url": "https://github.com/ag2ai/ag2/blob/main/website/mkdocs/docs/docs/home/home.md",
"date": "2024-11-11T20:43:34",
"stars": 1869,
"description": "AG2 (formerly AutoGen): The Open-Source AgentOS. Join us at: https://discord.gg/pAbnFJrkgZ",
"file_size": 6076
} |
---
title: "Quick Start"
---
AG2 (formerly AutoGen) is an open-source programming framework for building AI agents and facilitating their cooperation to solve tasks. AG2 supports tool use, autonomous and human-in-the-loop workflows, and multi-agent conversation patterns.
### Let's go
```sh
pip install ag2
```
!!! note
If you have been using `autogen` or `pyautogen`, all you need to do is upgrade it using:
```bash
pip install -U autogen
```
or
```bash
pip install -U pyautogen
```
as `pyautogen`, `autogen`, and `ag2` are aliases for the same PyPI package.
=== "Chat with an agent"
```python
# 1. Import our agent class
from autogen import ConversableAgent
# 2. Define our LLM configuration for OpenAI's GPT-4o mini
# uses the OPENAI_API_KEY environment variable
llm_config = {"api_type": "openai", "model": "gpt-4o-mini"}
# 3. Create our LLM agent
my_agent = ConversableAgent(
name="helpful_agent",
llm_config=llm_config,
system_message="You are a poetic AI assistant, respond in rhyme.",
)
# 4. Run the agent with a prompt
chat_result = my_agent.run("In one sentence, what's the big deal about AI?")
# 5. Print the chat
print(chat_result.chat_history)
```
=== "Two agent chat"
```python
# Chat between two comedian agents
# 1. Import our agent class
from autogen import ConversableAgent
# 2. Define our LLM configuration for OpenAI's GPT-4o mini,
# uses the OPENAI_API_KEY environment variable
llm_config = {"api_type": "openai", "model": "gpt-4o-mini"}
# 3. Create our agents who will tell each other jokes,
# with Jack ending the chat when Emma says FINISH
jack = ConversableAgent(
"Jack",
llm_config=llm_config,
system_message=(
"Your name is Jack and you are a comedian "
"in a two-person comedy show."
),
is_termination_msg=lambda x: True if "FINISH" in x["content"] else False
)
emma = ConversableAgent(
"Emma",
llm_config=llm_config,
system_message=(
"Your name is Emma and you are a comedian "
"in a two-person comedy show. Say the word FINISH "
"ONLY AFTER you've heard 2 of Jack's jokes."
),
)
# 4. Run the chat
chat_result = jack.initiate_chat(
emma,
message="Emma, tell me a joke about goldfish and peanut butter.",
)
# 5. Print the chat
print(chat_result.chat_history)
```
=== "Group chat"
```python
# Group chat amongst agents to create a 4th grade lesson plan
# Flow determined by Group Chat Manager automatically, and
# should be Teacher > Planner > Reviewer > Teacher (repeats if necessary)
# 1. Import our agent and group chat classes
from autogen import ConversableAgent, GroupChat, GroupChatManager
# Define our LLM configuration for OpenAI's GPT-4o mini
# uses the OPENAI_API_KEY environment variable
llm_config = {"api_type": "openai", "model": "gpt-4o-mini"}
# Planner agent setup
planner_message = "Create lesson plans for 4th grade. Use format: <title>, <learning_objectives>, <script>"
planner = ConversableAgent(
name="planner_agent",
llm_config=llm_config,
system_message=planner_message,
description="Creates lesson plans"
)
# Reviewer agent setup
reviewer_message = "Review lesson plans against 4th grade curriculum. Provide max 3 changes."
reviewer = ConversableAgent(
name="reviewer_agent",
llm_config=llm_config,
system_message=reviewer_message,
description="Reviews lesson plans"
)
# Teacher agent setup
teacher_message = "Choose topics and work with planner and reviewer. Say DONE! when finished."
teacher = ConversableAgent(
name="teacher_agent",
llm_config=llm_config,
system_message=teacher_message,
)
# Setup group chat
groupchat = GroupChat(
agents=[teacher, planner, reviewer],
speaker_selection_method="auto",
messages=[]
)
# Create manager
# At each turn, the manager will check if the message contains DONE! and end the chat if so
# Otherwise, it will select the next appropriate agent using its LLM
manager = GroupChatManager(
name="group_manager",
groupchat=groupchat,
llm_config=llm_config,
is_termination_msg=lambda x: "DONE!" in (x.get("content", "") or "").upper()
)
# Start the conversation
chat_result = teacher.initiate_chat(
recipient=manager,
message="Let's teach the kids about the solar system."
)
# Print the chat
print(chat_result.chat_history)
```
!!! tip
Learn more about configuring LLMs for agents
[here](/docs/user-guide/basic-concepts/llm-configuration).
### Where to Go Next?
- Go through the [basic concepts](/docs/user-guide/basic-concepts/installing-ag2) to get started
- Once you're ready, hit the [advanced concepts](/docs/user-guide/advanced-concepts/rag)
- Explore the [API Reference](/docs/api-reference/autogen/overview)
- Chat on [Discord](https://discord.gg/pAbnFJrkgZ)
- Follow on [X](https://x.com/ag2oss)
If you like our project, please give it a [star](https://github.com/ag2ai/ag2) on GitHub. If you are interested in contributing, please read [Contributor's Guide](/contributor-guide/contributing).
<iframe
src="https://ghbtns.com/github-btn.html?user=ag2ai&repo=ag2&type=star&count=true&size=large"
frameborder="0"
scrolling="0"
width="170"
height="30"
title="GitHub"
></iframe> | {
"source": "ag2ai/ag2",
"title": "website/mkdocs/docs/docs/home/quick-start.md",
"url": "https://github.com/ag2ai/ag2/blob/main/website/mkdocs/docs/docs/home/quick-start.md",
"date": "2024-11-11T20:43:34",
"stars": 1869,
"description": "AG2 (formerly AutoGen): The Open-Source AgentOS. Join us at: https://discord.gg/pAbnFJrkgZ",
"file_size": 5665
} |
# EDtunnel
<p align="center">
<img src="https://raw.githubusercontent.com/6Kmfi6HP/EDtunnel/refs/heads/main/image/logo.png" alt="edgetunnel" style="margin-bottom: -50px;">
</p>
EDtunnel 是一个基于 Cloudflare Workers 和 Pages 的代理工具,支持多种协议和配置选项。
EDtunnel is a proxy tool based on Cloudflare Workers and Pages, supporting multiple protocols and configuration options.
[](https://github.com/6Kmfi6HP/EDtunnel)
[](https://t.me/edtunnel)
## ✨ 特性 | Features
- 支持 Cloudflare Workers 和 Pages 部署
- 支持多 UUID 配置
- 支持自定义代理 IP 和端口
- 支持 SOCKS5 代理
- 提供自动配置订阅链接
- 简单易用的部署流程
- Support for Cloudflare Workers and Pages deployment
- Multiple UUID configuration support
- Custom proxy IP and port support
- SOCKS5 proxy support
- Automatic configuration subscription link
- Simple and easy deployment process
## 🚀 快速部署 | Quick Deployment
### 在 Pages.dev 部署 | Deploy on Pages.dev
1. 观看部署教程视频 | Watch deployment tutorial video:
[YouTube Tutorial](https://www.youtube.com/watch?v=8I-yTNHB0aw)
2. 克隆此仓库并在 Cloudflare Pages 中部署 | Clone this repository and deploy in Cloudflare Pages
### 在 Worker.dev 部署 | Deploy on Worker.dev
1. 从[这里](https://github.com/6Kmfi6HP/EDtunnel/blob/main/_worker.js)复制 `_worker.js` 代码 | Copy `_worker.js` code from [here](https://github.com/6Kmfi6HP/EDtunnel/blob/main/_worker.js)
2. 或者点击下方按钮一键部署 | Or click the button below to deploy directly:
[](https://deploy.workers.cloudflare.com/?url=https://github.com/6Kmfi6HP/EDtunnel)
## ⚙️ 配置说明 | Configuration Guide
### 环境变量配置 | Environment Variables
| 变量名 (Variable) | 是否必需 (Required) | 示例 (Example) | 说明 (Description) |
|------------------|-------------------|---------------|-------------------|
| `UUID` | 否 (No) | 单个 (Single): `12345678-1234-1234-1234-123456789012`<br>多个 (Multiple): `uuid1,uuid2,uuid3` | 用户识别码 / User identification |
| `PROXYIP` | 否 (No) | `1.1.1.1` 或 (or) `example.com`<br>多个 (Multiple): `1.1.1.1:9443,2.2.2.2:8443` | 自定义代理IP和端口 / Custom proxy IP and port |
| `SOCKS5` | 否 (No) | `user:pass@host:port`<br>多个 (Multiple): `user1:pass1@host1:port1,user2:pass2@host2:port2` | SOCKS5代理配置 / SOCKS5 proxy configuration |
| `SOCKS5_RELAY` | 否 (No) | `true` 或 (or) `false` | 启用SOCKS5流量转发 / Enable SOCKS5 traffic relay |
### 非443端口配置 | Non-443 Port Configuration
1. 访问 (Visit) `https://proxyip.edtunnel.best/`
2. 输入 (Enter) `ProxyIP:proxyport` 并点击检查 (and click Check)
3. 当显示 (When showing) `Proxy IP: true` 时可用 (it's available)
4. 在 Worker 中配置 (Configure in Worker): `PROXYIP=211.230.110.231:50008`
注意:带端口的代理IP可能在某些仅支持HTTP的Cloudflare站点上无效。
Note: Proxy IPs with ports may not work on HTTP-only Cloudflare sites.
### UUID 配置方法 | UUID Configuration
#### 方法一 | Method 1
在 `wrangler.toml` 文件中设置(不推荐在公共仓库中使用)
Set in `wrangler.toml` file (not recommended for public repositories)
```toml
[vars]
UUID = "your-uuid-here"
```
#### 方法二 | Method 2
在 Cloudflare Dashboard 的环境变量中设置(推荐方式)
Set in Cloudflare Dashboard environment variables (recommended method)
## ⚠️ 重要提示:多项配置分隔符 | Important Note: Multiple Configuration Separator
所有多项配置必须使用英文逗号(,)分隔,不能使用中文逗号(,)
All multiple configurations MUST use English comma(,) as separator, NOT Chinese comma(,)
✅ 正确示例 | Correct Examples:
```bash
# UUID多个配置 | Multiple UUID
UUID=uuid1,uuid2,uuid3
# SOCKS5多个代理 | Multiple SOCKS5 proxies
SOCKS5=192.168.1.1:1080,192.168.1.2:1080
# PROXYIP多个地址 | Multiple PROXYIP
PROXYIP=1.1.1.1:443,2.2.2.2:443
```
❌ 错误示例 | Wrong Examples:
```bash
# 错误:使用中文逗号 | Wrong: Using Chinese comma
UUID=uuid1,uuid2,uuid3
# 错误:使用中文逗号 | Wrong: Using Chinese comma
SOCKS5=192.168.1.1:1080,192.168.1.2:1080
```
## 📱 快速使用 | Quick Start
### 自动配置订阅 | Auto Configuration Subscribe
使用以下链接获取自动配置 | Use the following link for auto configuration:
```
https://sub.xf.free.hr/auto
```
### 查看配置 | View Configuration
- 访问您的域名 | Visit your domain: `https://your-domain.pages.dev`
- 使用特定UUID | Use specific UUID: `/sub/[uuid]`
- 查看完整配置 | View full configuration: 直接访问域名根路径 (visit domain root path)
- 获取订阅内容 | Get subscription content: 访问 `/sub/[uuid]` (visit `/sub/[uuid]`)
## 🔧 高级配置 | Advanced Configuration
### 多UUID支持 | Multiple UUID Support
您可以通过以下方式配置多个UUID | You can configure multiple UUIDs in these ways:
1. 环境变量方式 | Via environment variables:
```
UUID=uuid1,uuid2,uuid3
```
2. 配置文件方式 | Via configuration file:
```toml
[vars]
UUID = "uuid1,uuid2,uuid3"
```
### SOCKS5代理配置 | SOCKS5 Proxy Configuration
支持以下格式 | Supports the following formats:
- 基础格式 | Basic format: `host:port`
- 认证格式 | Authentication format: `username:password@host:port`
- 多代理格式(使用英文逗号分隔)| Multiple proxies (separated by English comma): `proxy1,proxy2,proxy3`
#### 配置示例 | Configuration Examples:
1. 单个代理 | Single Proxy:
```bash
# 基础格式 | Basic format
SOCKS5=192.168.1.1:1080
# 带认证格式 | With authentication
SOCKS5=user:[email protected]:1080
```
2. 多个代理(使用英文逗号分隔)| Multiple Proxies (separated by English comma):
```bash
# 多个基础代理 | Multiple basic proxies
SOCKS5=192.168.1.1:1080,192.168.1.2:1080,192.168.1.3:1080
# 多个带认证代理 | Multiple proxies with authentication
SOCKS5=user1:pass1@host1:port1,user2:pass2@host2:port2
# 混合格式 | Mixed format
SOCKS5=192.168.1.1:1080,user:[email protected]:1080,192.168.1.3:1080
```
#### SOCKS5 代理负载均衡 | SOCKS5 Proxy Load Balancing
当配置多个代理时,系统会自动进行负载均衡:
When multiple proxies are configured, the system will automatically perform load balancing:
- 随机选择 | Random selection
- 自动故障转移 | Automatic failover
- 支持混合认证方式 | Support mixed authentication methods
#### SOCKS5_RELAY 设置 | SOCKS5_RELAY Settings
启用 SOCKS5 全局转发 | Enable SOCKS5 global relay:
```bash
SOCKS5_RELAY=true
```
注意事项 | Notes:
- 确保代理服务器稳定可用 | Ensure proxy servers are stable and available
- 建议使用私有代理以提高安全性 | Recommend using private proxies for better security
- 多代理配置时使用英文逗号分隔 | Use commas to separate multiple proxies
- 支持动态添加和移除代理 | Support dynamic proxy addition and removal
## 🚨 注意事项 | Notes
- 带端口的代理IP可能在某些仅HTTP的Cloudflare站点上无效
- 多UUID配置时使用英文逗号分隔
- 建议通过环境变量设置敏感信息
- 定期更新以获取最新功能和安全修复
- Proxy IPs with ports may not work on HTTP-only Cloudflare sites
- Use commas to separate multiple UUIDs
- Recommend setting sensitive information via environment variables
- Update regularly for latest features and security fixes
## 🔧 环境变量设置 | Environment Variable Settings
### Workers.dev 设置 | Workers.dev Settings
在 Workers 设置页面配置环境变量 | Configure environment variables in Workers settings page

### Pages.dev 设置 | Pages.dev Settings
在 Pages 设置页面配置环境变量 | Configure environment variables in Pages settings page

## 💬 获取帮助 | Get Help
- Telegram 群组 | Telegram Group: [EDtunnel Group](https://t.me/edtunnel)
- GitHub 仓库 | Repository: [EDtunnel](https://github.com/6Kmfi6HP/EDtunnel)
- 问题反馈 | Issue Report: [创建新问题 | Create New Issue](https://github.com/6Kmfi6HP/EDtunnel/issues)
- 功能建议 | Feature Request: [提交建议 | Submit Request](https://github.com/6Kmfi6HP/EDtunnel/discussions)
## 📝 贡献指南 | Contributing
欢迎提交 Pull Request 来改进项目!请确保:
Welcome Pull Requests to improve the project! Please ensure:
1. 代码符合项目规范 | Code follows project standards
2. 添加必要的测试 | Add necessary tests
3. 更新相关文档 | Update relevant documentation
4. 描述清楚改动原因 | Clearly describe the reasons for changes
## 📜 许可证 | License
本项目采用 MIT 许可证 - 查看 [LICENSE](LICENSE) 文件了解详情
This project is licensed under the MIT License - see the [LICENSE](LICENSE) file for details
## Star History
<a href="https://star-history.com/#6Kmfi6HP/EDtunnel&Date">
<picture>
<source media="(prefers-color-scheme: dark)" srcset="https://api.star-history.com/svg?repos=6Kmfi6HP/EDtunnel&type=Date&theme=dark" />
<source media="(prefers-color-scheme: light)" srcset="https://api.star-history.com/svg?repos=6Kmfi6HP/EDtunnel&type=Date" />
<img alt="Star History Chart" src="https://api.star-history.com/svg?repos=6Kmfi6HP/EDtunnel&type=Date" />
</picture>
</a> | {
"source": "6Kmfi6HP/EDtunnel",
"title": "README.md",
"url": "https://github.com/6Kmfi6HP/EDtunnel/blob/main/README.md",
"date": "2024-09-11T09:15:25",
"stars": 1867,
"description": "EDtunnel 是一个基于 Cloudflare Workers 和 Pages 的代理工具,支持多种协议和配置选项。 EDtunnel is a proxy tool based on Cloudflare Workers and Pages, supporting multiple protocols and configuration options.",
"file_size": 8005
} |
# Audiblez: Generate audiobooks from e-books
[](https://github.com/santinic/audiblez/actions/workflows/pip-install.yaml)
[](https://github.com/santinic/audiblez/actions/workflows/git-clone-and-run.yml)


### v4 Now with Graphical interface, CUDA support, and many languages!

Audiblez generates `.m4b` audiobooks from regular `.epub` e-books,
using Kokoro's high-quality speech synthesis.
[Kokoro-82M](https://huggingface.co/hexgrad/Kokoro-82M) is a recently published text-to-speech model with just 82M params and very natural sounding output.
It's released under Apache licence and it was trained on < 100 hours of audio.
It currently supports these languages: 🇺🇸 🇬🇧 🇪🇸 🇫🇷 🇮🇳 🇮🇹 🇯🇵 🇧🇷 🇨🇳
On a Google Colab's T4 GPU via Cuda, **it takes about 5 minutes to convert "Animal's Farm" by Orwell** (which is about 160,000 characters) to audiobook, at a rate of about 600 characters per second.
On my M2 MacBook Pro, on CPU, it takes about 1 hour, at a rate of about 60 characters per second.
## How to install the Command Line tool
If you have Python 3 on your computer, you can install it with pip.
You also need `espeak-ng` and `ffmpeg` installed on your machine:
```bash
sudo apt install ffmpeg espeak-ng # on Ubuntu/Debian 🐧
pip install audiblez
```
```bash
brew install ffmpeg espeak-ng # on Mac 🍏
pip install audiblez
```
Then you can convert an .epub directly with:
```
audiblez book.epub -v af_sky
```
It will first create a bunch of `book_chapter_1.wav`, `book_chapter_2.wav`, etc. files in the same directory,
and at the end it will produce a `book.m4b` file with the whole book you can listen with VLC or any
audiobook player.
It will only produce the `.m4b` file if you have `ffmpeg` installed on your machine.
## How to run the GUI
The GUI is a simple graphical interface to use audiblez.
You need some extra dependencies to run the GUI:
```
sudo apt install ffmpeg espeak-ng
sudo apt install libgtk-3-dev # just for Ubuntu/Debian 🐧, Windows/Mac don't need this
pip install audiblez pillow wxpython
```
Then you can run the GUI with:
```
audiblez-ui
```
## How to run on Windows
After many trials, on Windows we recommend to install audiblez in a Python venv:
1. Open a Windows terminal
2. Create anew folder: `mkdir audiblez`
3. Enter the folder: `cd audiblez`
4. Create a venv: `python -m venv venv`
5. Activate the venv: `.\venv\Scripts\Activate.ps1`
6. Install the dependencies: `pip install audiblez pillow wxpython`
7. Now you can run `audiblez` or `audiblez-ui`
8. For Cuda support, you need to install Pytorch accordingly: https://pytorch.org/get-started/locally/
## Speed
By default the audio is generated using a normal speed, but you can make it up to twice slower or faster by specifying a speed argument between 0.5 to 2.0:
```
audiblez book.epub -v af_sky -s 1.5
```
## Supported Voices
Use `-v` option to specify the voice to use. Available voices are listed here.
The first letter is the language code and the second is the gender of the speaker e.g. `im_nicola` is an italian male voice.
[For hearing samples of Kokoro-82M voices, go here](https://claudio.uk/posts/audiblez-v4.html)
| Language | Voices |
|---------------------------|--------------------------------------------------------------------------------------------------------------------------------------------------------------------------------------------------------------------------------------------|
| 🇺🇸 American English | `af_alloy`, `af_aoede`, `af_bella`, `af_heart`, `af_jessica`, `af_kore`, `af_nicole`, `af_nova`, `af_river`, `af_sarah`, `af_sky`, `am_adam`, `am_echo`, `am_eric`, `am_fenrir`, `am_liam`, `am_michael`, `am_onyx`, `am_puck`, `am_santa` |
| 🇬🇧 British English | `bf_alice`, `bf_emma`, `bf_isabella`, `bf_lily`, `bm_daniel`, `bm_fable`, `bm_george`, `bm_lewis` |
| 🇪🇸 Spanish | `ef_dora`, `em_alex`, `em_santa` |
| 🇫🇷 French | `ff_siwis` |
| 🇮🇳 Hindi | `hf_alpha`, `hf_beta`, `hm_omega`, `hm_psi` |
| 🇮🇹 Italian | `if_sara`, `im_nicola` |
| 🇯🇵 Japanese | `jf_alpha`, `jf_gongitsune`, `jf_nezumi`, `jf_tebukuro`, `jm_kumo` |
| 🇧🇷 Brazilian Portuguese | `pf_dora`, `pm_alex`, `pm_santa` |
| 🇨🇳 Mandarin Chinese | `zf_xiaobei`, `zf_xiaoni`, `zf_xiaoxiao`, `zf_xiaoyi`, `zm_yunjian`, `zm_yunxi`, `zm_yunxia`, `zm_yunyang` |
For more detaila about voice quality, check this document: [Kokoro-82M voices](https://huggingface.co/hexgrad/Kokoro-82M/blob/main/VOICES.md)
## How to run on GPU
By default, audiblez runs on CPU. If you pass the option `--cuda` it will try to use the Cuda device via Torch.
Check out this example: [Audiblez running on a Google Colab Notebook with Cuda ](https://colab.research.google.com/drive/164PQLowogprWQpRjKk33e-8IORAvqXKI?usp=sharing]).
We don't currently support Apple Silicon, as there is not yet a Kokoro implementation in MLX. As soon as it will be available, we will support it.
## Manually pick chapters to convert
Sometimes you want to manually select which chapters/sections in the e-book to read out loud.
To do so, you can use `--pick` to interactively choose the chapters to convert (without running the GUI).
## Help page
For all the options available, you can check the help page `audiblez --help`:
```
usage: audiblez [-h] [-v VOICE] [-p] [-s SPEED] [-c] [-o FOLDER] epub_file_path
positional arguments:
epub_file_path Path to the epub file
options:
-h, --help show this help message and exit
-v VOICE, --voice VOICE
Choose narrating voice: a, b, e, f, h, i, j, p, z
-p, --pick Interactively select which chapters to read in the audiobook
-s SPEED, --speed SPEED
Set speed from 0.5 to 2.0
-c, --cuda Use GPU via Cuda in Torch if available
-o FOLDER, --output FOLDER
Output folder for the audiobook and temporary files
example:
audiblez book.epub -l en-us -v af_sky
to use the GUI, run:
audiblez-ui
```
## Author
by [Claudio Santini](https://claudio.uk) in 2025, distributed under MIT licence.
Related Article: [Audiblez v4: Generate Audiobooks from E-books](https://claudio.uk/posts/audiblez-v4.html) | {
"source": "santinic/audiblez",
"title": "README.md",
"url": "https://github.com/santinic/audiblez/blob/main/README.md",
"date": "2025-01-14T14:36:20",
"stars": 1866,
"description": "Generate audiobooks from e-books",
"file_size": 8374
} |
<div align=center>
<img src="figures/logo.png" width="280px">
</div>
<h2 align="center"> <a href="https://arxiv.org/abs/2411.10440v2">LLaVA-CoT: Let Vision Language Models Reason Step-by-Step</a></h2>
<h5 align="center"><p>This project was previously named LLaVA-o1. We have renamed it to LLaVA-CoT. </h5>
<h5 align="center"> If you like our project, please give us a star ⭐ on GitHub for the latest update.</h5>
<h5 align=center>
[](https://huggingface.co/spaces/Xkev/Llama-3.2V-11B-cot)
[](https://huggingface.co/Xkev/Llama-3.2V-11B-cot)
[](https://arxiv.org/abs/2411.10440v2)
[](https://x.com/Kevin_GuoweiXu/status/1858338565463421244)
[](https://github.com/PKU-YuanGroup/LLaVA-CoT/blob/main/LICENSE)
[](https://hits.seeyoufarm.com/api/count/incr/badge.svg?url=https%3A%2F%2Fgithub.com%2FPKU-YuanGroup%2FLLaVA-CoT&count_bg=%2379C83D&title_bg=%23555555&icon=&icon_color=%23E7E7E7&title=hits&edge_flat=false)
[](https://replicate.com/chenxwh/llava-cot)
[](https://wisemodel.cn/codes/KevinTHU/LLaVA-CoT)
</h5>
<h5 align="center">本项目希望构建开源慢思考大模型,由北大-兔展AIGC联合实验室共同发起。</h5>
## 📣 News
- **[2025/01/08]** We released the full training code.
- **[2025/01/02]** We discovered that when testing with the AI2D benchmark, we were using AI2D_TEST_NO_MASK, while the VLMEvalKit utilizes AI2D_TEST. We previously overlooked the distinction between the two, and we sincerely apologize for this oversight. We will make the necessary corrections.
- **[2024/11/28]** We've released the dataset: [https://huggingface.co/datasets/Xkev/LLaVA-CoT-100k](<[dataset_generation/generate.py](https://huggingface.co/datasets/Xkev/LLaVA-CoT-100k)>)
- **[2024/11/25]** We've released the code for dataset generation: [dataset_generation/generate.py](dataset_generation/generate.py)
- **[2024/11/23]** We've released the Gradio App: [https://huggingface.co/spaces/Xkev/Llama-3.2V-11B-cot](https://huggingface.co/spaces/Xkev/Llama-3.2V-11B-cot)
- **[2024/11/21]** LLaVA-o1 is renamed to LLaVA-CoT [https://arxiv.org/abs/2411.10440v2](https://arxiv.org/abs/2411.10440v2).
- **[2024/11/20]** We've released the pretrained weights: [https://huggingface.co/Xkev/Llama-3.2V-11B-cot](https://huggingface.co/Xkev/Llama-3.2V-11B-cot)
- **[2024/11/18]** We've released our paper: [https://arxiv.org/abs/2411.10440](https://arxiv.org/abs/2411.10440)
- **[2024/11/18]** Welcome to **watch** 👀 this repository for the latest updates.
## 🔥 Highlights
**LLaVA-CoT** is a visual language model capable of spontaneous, systematic reasoning.
Our 11B model outperforms **Gemini-1.5-pro**,**GPT-4o-mini**, and **Llama-3.2-90B-Vision-Instruct** on six challenging multimodal benchmarks.
<div align=center>
<img src="figures/result.png" width="300px">
</div>
## 🚀 Demos
LLaVA-CoT begins by outlining the problem, interprets relevant information from the image, proceeds step-by-step through reasoning, and ultimately reaches a well-supported conclusion.
### Reasoning Problems
| **Question** | <img src="figures/reasoning.png" width="400"> <br> Subtract all tiny shiny balls. Subtract all purple objects. How many objects are left? Options: A. 4, B. 8, C. 2, D. 6 |
| --------------------------------- | ----------------------------------------------------------------------------------------------------------------------------------------------------------------------------------------------------------------------------------------------------------------------------------------------------------------------------------------------------------------------------------------------------------------------------------------------------------------------------------------------------------------------------------------------------------------------------------------------------------------------------------------------------------------------------------------------------------------------------------------------------------------------------------------------------------------------------------------------------------------------------------------------------------------------------------------------------------------------------------------------------------------------------------------------------------------------------------------------------------------------------------------------------------------------------------------------------------------------------------------------------------------------------------------------------------------------------------------------------------------------------------------------------------------------------------------------------------------------------------------------------------------------------------------------------------------------------------------------------------------------------------------------------------------------------------------------------------------------------------------------------------------------------------- |
| **Llama-3.2-11B-Vision-Instruct** | To determine the correct answer, we need to follow the instructions provided. <br> The first instruction is to subtract all tiny shiny balls. <br> Since there are <span style="color: red;">three tiny shiny balls</span> in the image, we would be left with no shiny balls after performing this action. <br> The second instruction is to subtract all purple objects. However, upon closer inspection, it becomes apparent that there are <span style="color: red;">no shiny purple objects</span> present in the image. Therefore, we cannot subtract any purple objects. <br> Given that we have already removed all the tiny shiny balls and there were no purple objects to begin with, the correct answer is <span style="color: red;">C. 2</span>, as initially, there were 2 purple objects, but they are not to be subtracted according to the instruction. |
| **LLaVA-CoT** | **_Summary stage: What's the problem? What should I do?_** <br> <span style="color: gray;"><SUMMARY></span> I will examine the image to identify and count all the objects, focusing on the <span style="color: green;">tiny shiny balls and purple objects</span>. Then, I will subtract these objects from the total count and determine the number of remaining objects. <span style="color: gray;"></SUMMARY></span> <br>**_Caption stage: What can I know from the image?_** <br> <span style="color: gray;"><CAPTION></span> The image shows various 3D geometric shapes on a flat surface, including spheres, cubes, and cylinders in different colors. Notably, there are <span style="color: green;">small shiny green spheres</span> and <span style="color: green;">a purple cylinder</span>. <span style="color: gray;"></CAPTION></span> <br> **_Reasoning stage: How to solve the problem step-by-step?_** <br> <span style="color: gray;"><REASONING></span> First, I will count all the objects in the image: there are <span style="color: green;">10 objects in total</span>. Next, I will identify and subtract the tiny shiny balls. There is <span style="color: green;">one small shiny green sphere</span>. Then, I will identify and subtract the purple objects. There is <span style="color: green;">one purple cylinder</span>. By subtracting the two objects (the shiny ball and the purple cylinder) from the total, I find that <span style="color: green;">8 objects remain</span>. <span style="color: gray;"></REASONING></span> <br> **_Conclusion stage: What is the final answer?_** <br> <span style="color: gray;"><CONCLUSION></span> **B** <span style="color: gray;"></CONCLUSION></span> |
### Science Problems
| **Question** | <img src="figures/science.png" width="400"> <br> An acetal polymer block is fixed to the rigid plates at its top and bottom surfaces. If the top plate displaces 2 mm horizontally when it is subjected to a horizontal force \( P = 2 \, \text{kN} \), determine the shear modulus of the polymer. The width of the block is 100 mm. Assume that the polymer is linearly elastic and use small angle analysis. <br> **Options:** A: 15 MPa, B: 5 MPa, C: 20 MPa, D: 2 MPa |
| --------------------------------- | ----------------------------------------------------------------------------------------------------------------------------------------------------------------------------------------------------------------------------------------------------------------------------------------------------------------------------------------------------------------------------------------------------------------------------------------------------------------------------------------------------------------------------------------------------------------------------------------------------------------------------------------------------------------------------------------------------------------------------------------------------------------------------------------------------------------------------------------------------------------------------------------------------------------------------------------------------------------------------------------------------------------------------------------------------------------------------------------------------------------------------------------------------------------------------------------------------------------------------------------------------------------------------------------------------------------------------------------------------------------------------------------------------------------------------------------------------------------------------------------------------------------------------------------------------------------------------------------------------------------------------------------------------------------------------------------------------------------------------------------------------------------------------------------------------------------------------------------------------------------------------------------------------------------------------------------------------------------------------------------------------------------------------------------------------------------------------------------------------------------------------------------------------------------------- |
| **Llama-3.2-11B-Vision-Instruct** | To determine the shear modulus of the polymer, we can use the formula for shear modulus in a small angle analysis: <br> \( G = \frac{F}{A \gamma} \) <br> where \( G \) is the shear modulus, \( F \) is the applied force, \( A \) is the area of the block, and \( \gamma \) is the shear strain. <br> Given that the top plate of the block displaces horizontally by 2 mm when subjected to a horizontal force of 2 kN, we can calculate the shear strain \( \gamma \). Since the displacement is horizontal and <span style="color: red;">the original length of the block is 400 mm</span>, the shear strain \( \gamma \) is: <br> \[ \gamma = \frac{\Delta x}{L} = \frac{2 \, \text{mm}}{400 \, \text{mm}} = 0.005 \] <br> Next, we calculate the area \( A \) of the block. The width of the block is given as 100 mm, and assuming <span style="color: red;">the height of the block is also 200 mm</span> (as given in the image analysis), the area \( A \) is: <br> \[ A = 100 \, \text{mm} \times 200 \, \text{mm} = 20000 \, \text{mm}^2 \] <br> Now, we can substitute these values into the formula for shear modulus: <br> \[ G = \frac{F}{A \gamma} = \frac{2000 \, \text{N}}{20000 \, \text{mm}^2 \times 0.005} = \frac{2000}{100} = 20 \, \text{MPa} \] <br> Therefore, the correct answer is <span style="color: red;">C: 20 MPa</span>. |
| **LLaVA-CoT** | **_Summary stage: What's the problem? What should I do?_** <br> <span style="color: gray;"><SUMMARY></span> To solve the problem, I will <span style="color: green;">determine the shear modulus</span> of the acetal polymer block using the relationship between the applied force, displacement, and dimensions of the block. I will <span style="color: green;">use the formula for shear modulus</span> and calculate the result. <span style="color: gray;"></SUMMARY></span> <br> **_Caption stage: What can I know from the image?_** <br> <span style="color: gray;"><CAPTION></span> The image shows a rectangular block of acetal polymer fixed between two rigid plates. The top plate moves horizontally by 2 mm when a force \( P = 2 \, \text{kN} \) is applied. The block's dimensions are given as <span style="color: green;">400 mm in length, 200 mm in height</span>, and 100 mm in width. <span style="color: gray;"></CAPTION></span> <br> **_Reasoning stage: How to solve the problem step-by-step?_** <br> <span style="color: gray;"><REASONING></span> The shear modulus \( G \) of the polymer can be calculated using the formula: <br> \[ G = \frac{F}{A \cdot \frac{\Delta x}{L}} \] <br> where: <br> - \( F \) is the applied force (2 kN), <br> - \( A \) is the area of the block's top surface (<span style="color: green;">100 mm x 400 mm = 40,000 mm²</span>), <br> - \( \Delta x \) is the horizontal displacement (2 mm), <br> - \( L \) is the height of the block (<span style="color: green;">200 mm</span>). <br> First, convert the force to Newtons: \( F = 2 \, \text{kN} = 2000 \, \text{N} \). <br> Next, calculate the shear modulus: <br> \[ G = \frac{2000}{40,000 \cdot \frac{2}{200}} \] <br> \[ G = \frac{2000}{40,000 \cdot 0.01} \] <br> \[ G = \frac{2000}{400} \] <br> \[ G = 5 \, \text{MPa} \] <br> <span style="color: gray;"></REASONING></span> <br> **_Conclusion stage: What is the final answer?_** <br> <span style="color: gray;"><CONCLUSION></span> <span style="color: green;">B: 5 MPa</span> <span style="color: gray;"></CONCLUSION></span> |
## 🛠️ Usage
### Pretrained weights
You can download the pretrained weights from the Huggingface: [Xkev/Llama-3.2V-11B-cot](https://huggingface.co/Xkev/Llama-3.2V-11B-cot).
### Dataset
You can download the dataset from the Huggingface: [Xkev/LLaVA-CoT-100k](https://huggingface.co/datasets/Xkev/LLaVA-CoT-100k).
### Inference
You can use the same code as Llama-3.2-11B-Vision-Instruct to load the model and perform inference.
If you want to use perform inference time scaling, you can use code provided in [inference_demo/inference_demo.py](inference_demo/inference_demo.py).
In order to run the demo, you need to replace this file with the original inference code for Llama-3.2-11B-Vision-Instruct in VLMEvalKit.
Additionally, you need to replace the `processing_mllama.py` file in the transformers library with the one provided in [inference_demo/processing_mllama.py](inference_demo/processing_mllama.py).
### Finetuning
You may use any repository that supports Llama-3.2-11B-Vision-Instruct for finetuning.
We recommend using [llama-recipes](https://github.com/Meta-Llama/llama-recipes).
To reproduce our results, you can use the following command:
```bash
cd train
pip install llama-recipes
torchrun --nnodes 1 --nproc_per_node 8 --master_port 29500 finetuning.py --enable_fsdp --lr 1e-5 --num_epochs 3 --batch_size_training 4 --model_name meta-llama/Llama-3.2-11B-Vision-Instruct --dist_checkpoint_root_folder ./finetuned_model --dist_checkpoint_folder LLaVA-CoT --use_fast_kernels --dataset "custom_dataset" --custom_dataset.test_split "test" --custom_dataset.file "datasets/cot_dataset.py" --run_validation False --batching_strategy padding
```
Remember to modify the `data_path` and `image_base_path` in `train/cot_dataset.py` to your own path (the path to the training dataset).
## 📝 Citation
If you find this paper useful, please consider staring 🌟 this repo and citing 📑 our paper:
```
@misc{xu2024llavacot,
title={LLaVA-CoT: Let Vision Language Models Reason Step-by-Step},
author={Guowei Xu and Peng Jin and Hao Li and Yibing Song and Lichao Sun and Li Yuan},
year={2024},
eprint={2411.10440},
archivePrefix={arXiv},
primaryClass={cs.CV},
url={https://arxiv.org/abs/2411.10440},
}
```
## 🙏 Acknowledgement
- The majority of this project is released under the Apache 2.0 license as found in the [LICENSE](https://github.com/PKU-YuanGroup/Chat-UniVi/blob/main/LICENSE) file.
- The service is a research preview intended for non-commercial use only, subject to [LLAMA 3.2 COMMUNITY LICENSE AGREEMENT](https://huggingface.co/meta-llama/Llama-3.2-1B/blob/main/LICENSE.txt), and [Terms of Use](https://openai.com/policies/terms-of-use) of the data generated by OpenAI. Please contact us if you find any potential violations.
- The template is modified from [Chat-Univi](https://github.com/PKU-YuanGroup/Chat-UniVi) and [LLaVA](https://llava-vl.github.io/). | {
"source": "PKU-YuanGroup/LLaVA-CoT",
"title": "README.md",
"url": "https://github.com/PKU-YuanGroup/LLaVA-CoT/blob/main/README.md",
"date": "2024-11-17T06:01:19",
"stars": 1851,
"description": "LLaVA-CoT, a visual language model capable of spontaneous, systematic reasoning",
"file_size": 22178
} |
# v1.0.0
✅ 使用文档与演示动图
# v0.5.0
✅ 跨平台构建与分发(GitHub Action)
# v0.4.0
✅ 应用页面设计
# v0.3.0
✅ 服务器跨平台构建,构建产物下载与解压缩
# v0.2.0
✅ 完成服务器启动、加载模型、换脸 Pipeline
# v0.1.0
✅ Python 一键换脸 | {
"source": "idootop/MagicMirror",
"title": "CHANGELOG.md",
"url": "https://github.com/idootop/MagicMirror/blob/main/CHANGELOG.md",
"date": "2024-11-11T14:34:11",
"stars": 1833,
"description": "🪞 Instant AI Face Swap, Hairstyles & Outfits — One click to a brand new you! 一键 AI 换脸、发型、穿搭,发现更美的你",
"file_size": 174
} |
# MagicMirror
Instant AI Face Swap, Hairstyles & Outfits — One click to a brand new you!
[](https://github.com/idootop/MagicMirror/releases) [](https://github.com/idootop/MagicMirror/actions/workflows/build-app.yaml) [](https://github.com/idootop/MagicMirror/actions/workflows/build-server.yaml)

## Features
- **Where AI Meets Your Beauty**: Effortlessly try on new faces, hairstyles, and outfits
- **Stupid Simple**: Drag and drop photos to instantly change faces - no complex settings required
- **Hardware Friendly**: Runs smoothly on standard computers, no dedicated GPU hardware required
- **Privacy First**: Completely offline processing - your images never leave your device
- **Ultra-Lightweight**: Tiny footprint with <10MB installer and <1GB model files
## Get Started
> [👉 中文教程和下载地址请戳这里](./docs/cn/readme.md)
To get started with MagicMirror:
1. Follow the [Installation Guide](./docs/en/install.md)
2. Check out the [Usage Guide](./docs/en/usage.md)
3. See the [FAQ](./docs/en/faq.md) for common issues
If you have any questions, please [submit an issue](https://github.com/idootop/MagicMirror/issues).
> Note: MagicMirror only supports macOS 13 (Ventura) and Windows 10 and above.
## Motivation

Ever found yourself endlessly scrolling through hairstyles and outfits, wondering "How would this look on me?" As someone who loves exploring different styles but hates the hassle, I dreamed of an app that could instantly show me wearing any look I fancy.
While AI technology has advanced tremendously, most AI face applications either require complex setup, demand high-end GPU hardware, or rely on cloud processing that compromises privacy.
**The ideal solution should be as simple as taking a selfie** - just drag, drop, and transform. No technical expertise needed, no expensive hardware required, and no privacy concerns.
So, why not build one myself?
And that’s how MagicMirror came to life ✨
Enjoy! ;)
## Acknowledgments
MagicMirror builds upon several outstanding open-source projects:
- [Tauri](https://github.com/tauri-apps/tauri): Build smaller, faster, and more secure desktop and mobile applications with a web frontend.
- [FaceFusion](https://github.com/facefusion/facefusion): Industry leading face manipulation platform
- [InsightFace](https://github.com/deepinsight/insightface): State-of-the-art 2D and 3D Face Analysis Project
- [Nuitka](https://github.com/Nuitka/Nuitka): Nuitka is a Python compiler written in Python.
## Disclaimer
MagicMirror is designed for personal entertainment and creative purposes only. Commercial use is prohibited. Please note:
- **Ethical Usage**: This software must not be used for activities including, but not limited to: a) impersonating others with malicious intent, b) spreading misinformation, c) violating personal privacy or dignity, d) creating explicit or inappropriate content.
- **Content Rights**: Users are responsible for: a) obtaining necessary permissions for source images, b) respecting copyrights and intellectual property, c) complying with local laws and regulations on AI-generated content.
- **Limitation of Liability**: The software and its developers are not liable for any user-generated content. Users assume full responsibility for the use of the generated content and any consequences that may arise from its use.
By using MagicMirror, you agree to these terms and commit to using the software responsibly.
## License
This project is licensed under the [MIT License](./LICENSE). | {
"source": "idootop/MagicMirror",
"title": "README.md",
"url": "https://github.com/idootop/MagicMirror/blob/main/README.md",
"date": "2024-11-11T14:34:11",
"stars": 1833,
"description": "🪞 Instant AI Face Swap, Hairstyles & Outfits — One click to a brand new you! 一键 AI 换脸、发型、穿搭,发现更美的你",
"file_size": 3829
} |
# FAQ

## 常见问题
### 如何使用 GPU 加速推理?
MagicMirror 默认使用 CPU 进行推理,暂不支持启用硬件加速选项。
不过你可以尝试 MagicMirror 的 CLI 版本 [TinyFace](https://github.com/idootop/TinyFace),或者使用 [FaceFusion](https://github.com/facefusion/facefusion)。
### MagicMirror 的体积为什么那么小,是怎么做到的?
这多亏了 [Tauri](https://tauri.app/),它使用系统自带的 WebView 组件,所以不用像 [Electron](https://www.electronjs.org/) 那样直接把整个 Chromium 浏览器和 Node.js 运行时打包进应用安装包。如果你对此感兴趣,可以在这里了解更多[技术细节](https://tauri.app/start/)。
### MagicMirror 的 LOGO 使用 AI 生成的吗?
是的。MagicMirror 内的所有设计资源:从 Logo、字体到 UI 界面,都是由 AI 生成的 ✨

这里我使用的是 [Tensor.ART](https://tusiart.com/):一个免费的在线 AI 生图网站。像最新的 Flux 和 SD 3.5 等模型都可以直接使用,而且你也可以在线训练自己的模型。
比如 MagicMirror 的 LOGO, 就是我从 Dribbble 上搜集了一些参考图,然后用 Flux 做底模训练的一个模型生成的,非常方便。

相比 [Civitai](https://civitai.com/) 和 [LibLib.AI](https://www.liblib.art/) 等 AI 生图平台,[Tensor.ART](https://tusiart.com/) 的模型数量更多,价格更便宜,性价比应该是这三者里最高的。
如果你也想要尝试 AI 生图,或者正在寻找更有性价比的生图平台,不妨试试看 [Tensor.ART](https://tusiart.com/)。
## 安装问题
### 【macOS】打开 APP 时提示: “MagicMirror”已损坏,无法打开。 你应该将它移到废纸篓。
这是因为 macOS 默认只允许从 App Store 或已知的开发者来源安装应用,以保护用户的安全和隐私。
但是目前我还没有注册苹果开发者账号($99 一年),所以无法为 MagicMirror 提供有效的签名并上架应用商店。
你可以:
1. 打开访达,然后在右侧菜单栏选择应用程序
2. 找到提示无法验证的应用,右键点击打开即可

如果在运行过程中仍然弹窗提示无法打开“xxx”(尤其是 macOS 15 系统版本及以上),
请自行百度 **macOS 如何开启任何来源**,参考:https://www.ghxi.com/jc202309058.html
### 【Windows】提示不是有效的 Win32 应用
MagicMirror 目前只提供了 x64 和 ARM64 架构的安装包,对于较老的 x32 电脑暂不支持。
推荐的运行环境是 Windows 11,如果低于 Windows 10 可能会无法正常运行。
## 运行问题
### 【macOS】卡在启动界面超过 10 分钟
如果你的电脑非 Intel 芯片(比如:M1、M4 芯片),首次启动 APP 时需要进行 Rosatta 转译,大概 3-5 分钟左右。
后续启动将恢复正常时长(5-10s)。如果超过 10 分钟仍未正常启动,请关闭应用后重试。
如果重启后还是无法正常启动,请检查你的 macOS 系统是否不低于 13 版本 (macOS Ventura),较老的 macOS 系统可能无法正常运行。
查看此处了解更多信息:https://github.com/521xueweihan/HelloGitHub/issues/2859#issuecomment-2562637177
### 【Windows】卡在启动界面超过 10 分钟
如果你在 Windows 系统上一直卡在启动界面,请检查你的 `$HOME/MagicMirror/server.exe` 文件是否存在。
这是使用 [Nuitka](https://github.com/Nuitka/Nuitka) 编译的一个 Python 应用程序,MagicMirror 的正常运行离不开此程序。

由于许多病毒软件也喜欢使用 Nuitka 来编译他们的应用程序,从而混淆源代码隐藏自己的恶意行为特征。
所以同样使用 Nuitka 编译的 `server.exe` 文件,在启动时也容易被 Windows 安全应用误标记为病毒文件并删除。

你可以在 Windows 安全中心 - 保护历史记录里,手动还原被删除的 `server.exe` 文件。然后重启 MagicMirror 应该就能正常运行了。
> 如果你对 Nuitka 被 Widnows 安全应用误报感兴趣,可以[查看此处](https://github.com/Nuitka/Nuitka/issues/2685#issuecomment-1923357489)了解更多技术细节。
如果仍然启动失败,查看此处了解更多:https://github.com/idootop/MagicMirror/issues/6#issuecomment-2560949972
### 提示换脸失败
你可以换几张其他的图片试试看。比较冷门的图片格式、文件名包含特殊字符、图片分辨率过大等都可能会导致换脸失败。
## 其他问题
### MagicMirror 与 FaceFusion 有什么联系?
简单来说,你可以把 MagicMirror 视为 FaceFusion 的精简版。
我在 [FaceFusion](https://github.com/facefusion/facefusion) 的基础上,移除了所有不必要的模块,只保留了最核心的换脸功能,并由此创造出了 [TinyFace](https://github.com/idootop/TinyFace):一个超轻量的 Python 换脸工具。
MagicMirror 是在 [TinyFace](https://github.com/idootop/TinyFace) 之上构建的一个 GUI 项目,方便使用。
### MagicMirror 与 InsightFace 有什么联系?
一个经典的换脸工作流,通常由人脸检测、识别、换脸、画质增强等多个步骤组成,不同的步骤依赖不同的模型,而 [InsightFace](https://github.com/deepinsight/insightface/tree/master/examples/in_swapper) 的 `inswapper_128.onnx` 则是换脸过程中使用到的模型,是整个应用的核心。 | {
"source": "idootop/MagicMirror",
"title": "docs/cn/faq.md",
"url": "https://github.com/idootop/MagicMirror/blob/main/docs/cn/faq.md",
"date": "2024-11-11T14:34:11",
"stars": 1833,
"description": "🪞 Instant AI Face Swap, Hairstyles & Outfits — One click to a brand new you! 一键 AI 换脸、发型、穿搭,发现更美的你",
"file_size": 3182
} |
# 安装教程

## 下载地址
首先根据你的操作系统,下载对应的 APP 安装包并安装:
1. Windows: [MagicMirror_1.0.0_windows_x86_64.exe](https://github.com/idootop/MagicMirror/releases/download/app-v1.0.0/MagicMirror_1.0.0_windows_x86_64.exe)
2. macOS: [MagicMirror_1.0.0_macos_universal.dmg](https://github.com/idootop/MagicMirror/releases/download/app-v1.0.0/MagicMirror_1.0.0_macos_universal.dmg)
> 如果你访问不了上面的 GitHub 下载链接,请使用国内[下载地址](https://del-wang.lanzout.com/b01qdt5nba) 密码: `4ro2`
## 下载模型
首次启动 APP,需要下载模型文件(默认会自动下载),初始化成功后才能使用。

如果你的下载进度一直是 0,或者下到一半卡住了,请按照下面的步骤手动初始化:
**下载模型文件**
首先根据你的操作系统和架构,选择对应的模型文件:
- [server_windows_x86_64.zip](https://github.com/idootop/MagicMirror/releases/download/server-v1.0.0/server_windows_x86_64.zip)(Windows,大多数的电脑都是这种)
- [server_windows_aarch64.zip](https://github.com/idootop/MagicMirror/releases/download/server-v1.0.0/server_windows_aarch64.zip)(Windows,如果你是 ARM64 设备)
- [server_macos_aarch64.zip](https://github.com/idootop/MagicMirror/releases/download/server-v1.0.0/server_macos_aarch64.zip)(macOS, 苹果芯片,比如 M1、M4 芯片)
- [server_macos_x86_64.zip](https://github.com/idootop/MagicMirror/releases/download/server-v1.0.0/server_macos_x86_64.zip)(macOS, Intel 芯片)
> 如果你访问不了上面的 GitHub 下载链接,可以使用国内的[下载地址](https://pan.quark.cn/s/b8ad043794bb)
**解压下载的文件**
解压后应该是一个文件夹,把它重命名成: `MagicMirror` 然后移动到你电脑的 `HOME` 目录下,比如:


完成上面两步后,重启 MagicMirror 应该就能正常启动了。
## 启动 APP
下载完模型文件后,第一次启动应用可能比较缓慢,耐心等待即可。

> 一般 3 分钟以内即可启动成功,如果超过 10 分钟还未正常启动,请查看[常见问题](./faq.md)
## 遇到问题?
大部分问题都能在「[常见问题](./faq.md)」里找到答案,如果你还有其他问题,请在此[提交反馈](https://github.com/idootop/MagicMirror/issues)。 | {
"source": "idootop/MagicMirror",
"title": "docs/cn/install.md",
"url": "https://github.com/idootop/MagicMirror/blob/main/docs/cn/install.md",
"date": "2024-11-11T14:34:11",
"stars": 1833,
"description": "🪞 Instant AI Face Swap, Hairstyles & Outfits — One click to a brand new you! 一键 AI 换脸、发型、穿搭,发现更美的你",
"file_size": 1713
} |
# MagicMirror
一键 AI 换脸、发型、穿搭,发现更美的自己 ✨
[](https://github.com/idootop/MagicMirror/releases) [](https://github.com/idootop/MagicMirror/actions/workflows/build-app.yaml) [](https://github.com/idootop/MagicMirror/actions/workflows/build-server.yaml)

## 功能亮点
- 一键换脸:打开安装包,拖张照片进去就能换脸,无需配置各种复杂参数。
- 超低门槛:不用 GPU 也能运行,普通的小白电脑也可以轻松玩转 AI 换脸。
- 隐私安全:完全在你的电脑本地运行,不需要联网,不用担心你的图片会被上传到任何地方。
- 极致精简:安装包不到 10 MB,模型文件加起来不到 1 GB,这可能是最轻量的离线换脸应用之一。
## 快速开始
> 🔥 演示视频:https://www.bilibili.com/video/BV1TTzfYDEUe
MagicMirror 仅支持 macOS 13(Ventura)和 Windows 10 及以上版本系统:
1. [安装教程](./install.md)
2. [使用教程](./usage.md)
3. [常见问题](./faq.md)(90%的问题可以在这里找到答案)
如果你有其他问题,请提交 [Issue](https://github.com/idootop/MagicMirror/issues)。
## 动机

我想你跟我一样,有时会纠结:自己适合哪种发型,或者哪种穿搭最好看?
要是有一个应用,可以把我们看到的喜欢的发型或心动的穿搭,直接放到自己的身上预览效果,那真是太好了。
现在的 AI 技术已经很成熟了,但是市面上大部分的 AI 换脸应用:
- 要么需要配置复杂的参数和运行环境,要有性能足够强劲的 GPU 才能运行,使用门槛和成本偏高;
- 要么需要上传自己的图片到服务器进行转换,存在隐私泄漏的风险。
理想的解决方案应该像自拍一样简单:不需要设置复杂的参数,不用购买昂贵的设备,也无需担心隐私泄漏的问题。
所以,为什么不自己做一个呢?
于是便有了 MagicMirror ✨
Enjoy! ;)
## 鸣谢
MagicMirror 的实现主要使用和参考了以下开源项目,特此鸣谢:
- [Tauri](https://github.com/tauri-apps/tauri): Build smaller, faster, and more secure desktop and mobile applications with a web frontend.
- [FaceFusion](https://github.com/facefusion/facefusion): Industry leading face manipulation platform
- [InsightFace](https://github.com/deepinsight/insightface): State-of-the-art 2D and 3D Face Analysis Project
- [Nuitka](https://github.com/Nuitka/Nuitka): Nuitka is a Python compiler written in Python.
## 免责声明
MagicMirror 仅限个人娱乐与创意用途,严禁用于商业用途。请注意:
- **道德使用**:本软件不得用于以下行为,包括但不限于:a) 恶意冒充他人,b) 散布虚假信息,c) 侵犯个人隐私或尊严,d) 制作淫秽或不当内容。
- **内容版权**:用户应对以下内容负责:a) 获取使用源图像的必要许可,b) 尊重版权及知识产权,c) 遵守当地关于 AI 生成内容的法律法规。
- **免责声明**:用户应对生成的内容以及由其使用引发的任何法律、道德或个人问题承担全部责任。本软件及其开发者对用户生成的内容不承担任何责任。
使用 MagicMirror 即表示您已阅读并同意上述条款,并承诺负责任地使用本软件。
## License
This project is licensed under the [MIT License](./LICENSE). | {
"source": "idootop/MagicMirror",
"title": "docs/cn/readme.md",
"url": "https://github.com/idootop/MagicMirror/blob/main/docs/cn/readme.md",
"date": "2024-11-11T14:34:11",
"stars": 1833,
"description": "🪞 Instant AI Face Swap, Hairstyles & Outfits — One click to a brand new you! 一键 AI 换脸、发型、穿搭,发现更美的你",
"file_size": 2230
} |
# 使用教程
首先,准备一张你的正脸照,然后拖入到镜子里。

然后把你想要换脸的照片,拖到另一面镜子里,等待换脸完毕即可。

> 苹果 M1 芯片换一张脸一般需要 3-5s 的时间,主要看你的电脑配置和图片大小
换脸成功后,会在原来的位置生成一张以 `_output` 结尾的新图片。

你可以继续拖入新的照片换脸,或通过右上角的菜单翻转镜像,更换新的脸部图片。

## 遇到问题?
大部分问题都能在「[常见问题](./faq.md)」里找到答案,如果你还有其他问题,请在此[提交反馈](https://github.com/idootop/MagicMirror/issues)。 | {
"source": "idootop/MagicMirror",
"title": "docs/cn/usage.md",
"url": "https://github.com/idootop/MagicMirror/blob/main/docs/cn/usage.md",
"date": "2024-11-11T14:34:11",
"stars": 1833,
"description": "🪞 Instant AI Face Swap, Hairstyles & Outfits — One click to a brand new you! 一键 AI 换脸、发型、穿搭,发现更美的你",
"file_size": 423
} |
# FAQ

## Common
### How to Enable GPU Acceleration for Inference?
MagicMirror uses CPU by default for inference and currently does not support hardware acceleration options. However, you can try MagicMirror’s CLI version, [TinyFace](https://github.com/idootop/TinyFace), or use [FaceFusion](https://github.com/facefusion/facefusion).
### Why is MagicMirror So Small in Size?
This is thanks to [Tauri](https://tauri.app/), which uses the system’s built-in WebView component, unlike [Electron](https://www.electronjs.org/), which packages an entire Chromium browser and Node.js runtime into the app. If you're interested in the technical details, [click here](https://tauri.app/start/).
### Is the MagicMirror Logo AI-Generated?
Every design element in MagicMirror - from the logo and typography to the UI - was created using AI ✨

I used [Tensor.ART](https://tensor.art/), a free AI image generation platform. It offers access to the latest models like Flux and SD 3.5, plus the ability to train your own custom models.
For example, I created MagicMirror's logo by training a Flux-based model on reference images collected from Dribbble - quick and simple.

Compared to platforms like [Civitai](https://civitai.com/) and [LibLib.AI](https://www.liblib.art/), [Tensor.ART](https://tensor.art/) has more models at a lower price, making it the most cost-effective option.
If you're looking to try AI image generation or want a more affordable platform, I recommend giving [Tensor.ART](https://tensor.art/) a try.
## Installation
### 【macOS】App is Damaged or Can't Be Verified
This issue occurs because the app isn't signed with an Apple developer certificate. To resolve this:
1. Open Finder and select Applications from the sidebar.
2. Locate the app with the warning, right-click it, and choose Open.

## Usage
### 【Windows】App Stuck on the Startup Screen for Over 10 Minutes
If the app is stuck at the startup screen, check if the `server.exe` file exists on your system. This file, compiled using [Nuitka](https://github.com/Nuitka/Nuitka) from Python source, is essential for MagicMirror to run.

Windows Defender may flag the `server.exe` as malicious due to the use of Nuitka by some malware, leading to it being quarantined.

To fix this:
1. Open Windows Security Center and go to Protection History.
2. Find and restore the quarantined `server.exe`.
3. Restart MagicMirror.
> For details on Nuitka false positives in Windows Defender, [click here](https://github.com/Nuitka/Nuitka/issues/2685#issuecomment-1923357489).
If none of the above work, your system likely has compatibility issues with the server. Consider using alternative face swap tools like [TinyFace](https://github.com/idootop/TinyFace) (the underlying library used by MagicMirror), or [FaceFusion](https://github.com/facefusion/facefusion).
Learn more: https://github.com/idootop/MagicMirror/issues/6#issuecomment-2560949972
### 【Windows】Face Swap Fails with Any Image
First, check if the `server.exe` file exists in `$HOME/MagicMirror/`. If it’s missing, follow the steps in the previous section to restore it.

If the issue persists, please [submit an issue](https://github.com/idootop/MagicMirror/issues).
### 【Windows】"Not a Valid Win32 Application" Error
MagicMirror currently only supports x64 and ARM64 architectures. Older x32 systems are not supported.
The recommended environment is Windows 11 x64. MagicMirror may not run properly on versions lower than Windows 10.
### 【macOS】App Stuck on Startup for Over 10 Minutes
First-time startup on macOS can be slow. If the app doesn’t start within 30 minutes, close it and try again.
If it still doesn’t launch, ensure your macOS version is at least `13` (Ventura), as older versions may not be compatible.
Learn more: https://github.com/521xueweihan/HelloGitHub/issues/2859#issuecomment-2562637177
## Other
### What’s the Relationship Between MagicMirror and FaceFusion?
In simple terms, MagicMirror is a simplified version of FaceFusion.
Starting from [FaceFusion](https://github.com/facefusion/facefusion), I removed unnecessary modules and kept only the core face-swapping functionality, which led to the creation of [TinyFace](https://github.com/idootop/TinyFace), a lightweight Python face-swapping tool.
MagicMirror is a GUI project built on top of [TinyFace](https://github.com/idootop/TinyFace), making it easier to use.
### What’s the Relationship Between MagicMirror and InsightFace?
A typical face-swapping workflow includes multiple steps, such as face detection, recognition, swapping, and quality enhancement. These steps rely on different models. The `inswapper_128.onnx` model from [InsightFace](https://github.com/deepinsight/insightface/tree/master/examples/in_swapper) is a core part of the face-swapping process. | {
"source": "idootop/MagicMirror",
"title": "docs/en/faq.md",
"url": "https://github.com/idootop/MagicMirror/blob/main/docs/en/faq.md",
"date": "2024-11-11T14:34:11",
"stars": 1833,
"description": "🪞 Instant AI Face Swap, Hairstyles & Outfits — One click to a brand new you! 一键 AI 换脸、发型、穿搭,发现更美的你",
"file_size": 5011
} |
# Installation Guide

## Installation
Download and install the MagicMirror installer for your operating system:
1. Windows: [MagicMirror_1.0.0_windows_x86_64.exe](https://github.com/idootop/MagicMirror/releases/download/app-v1.0.0/MagicMirror_1.0.0_windows_x86_64.exe)
2. macOS: [MagicMirror_1.0.0_macos_universal.dmg](https://github.com/idootop/MagicMirror/releases/download/app-v1.0.0/MagicMirror_1.0.0_macos_universal.dmg)
3. Other: [Go to Release](https://github.com/idootop/MagicMirror/releases/app-v1.0.0)
## Download Models
When you first launch the app, it will automatically download the required model files.

If the download progress is stuck at 0% or stops midway, follow these steps for manual setup:
**Download Model Files**
Choose the model file that matches your operating system:
- [server_windows_x86_64.zip](https://github.com/idootop/MagicMirror/releases/download/server-v1.0.0/server_windows_x86_64.zip)(Windows, most common)
- [server_windows_aarch64.zip](https://github.com/idootop/MagicMirror/releases/download/server-v1.0.0/server_windows_aarch64.zip)(Windows, for ARM64 devices)
- [server_macos_aarch64.zip](https://github.com/idootop/MagicMirror/releases/download/server-v1.0.0/server_macos_aarch64.zip)(macOS, Apple Silicon, such as M1, M4 chips)
- [server_macos_x86_64.zip](https://github.com/idootop/MagicMirror/releases/download/server-v1.0.0/server_macos_x86_64.zip)(macOS, Intel chips)
**Extract the Downloaded File**
You'll get a folder—rename it to `MagicMirror`. Move this folder to your computer's `HOME` directory, for example:


Restart MagicMirror, and it should now work properly.
## Launch APP
After downloading the model files, the first launch may take some time.

> The app should launch within 3 minutes. If it takes longer than 10 minutes to start, please refer to the [FAQ](./faq.md)
## Need help?
Most issues are addressed in the [FAQ](./faq.md). If you need further assistance, please [submit an issue](https://github.com/idootop/MagicMirror/issues). | {
"source": "idootop/MagicMirror",
"title": "docs/en/install.md",
"url": "https://github.com/idootop/MagicMirror/blob/main/docs/en/install.md",
"date": "2024-11-11T14:34:11",
"stars": 1833,
"description": "🪞 Instant AI Face Swap, Hairstyles & Outfits — One click to a brand new you! 一键 AI 换脸、发型、穿搭,发现更美的你",
"file_size": 2159
} |
# Usage Guide
First, drag a front-facing photo of yourself into the mirror.

Next, drag your desired photo into the other mirror and wait for processing to complete.

> On Apple M1 chips, face swapping takes about 3-5 seconds, varying with your computer's specs and image size.
When processing is complete, a new image will appear in the original location with `_output` added to its filename.

You can continue swapping faces with new photos, or use the top-right menu to flip the mirror and change the face image.

## Need help?
Most issues are addressed in the [FAQ](./faq.md). If you need further assistance, please [submit an issue](https://github.com/idootop/MagicMirror/issues). | {
"source": "idootop/MagicMirror",
"title": "docs/en/usage.md",
"url": "https://github.com/idootop/MagicMirror/blob/main/docs/en/usage.md",
"date": "2024-11-11T14:34:11",
"stars": 1833,
"description": "🪞 Instant AI Face Swap, Hairstyles & Outfits — One click to a brand new you! 一键 AI 换脸、发型、穿搭,发现更美的你",
"file_size": 817
} |
# Logic-RL
## 📢 **Our detailed technical report is released!**
<a href='https://arxiv.org/abs/2502.14768'><img src='https://img.shields.io/badge/arXiv-2502.14768-b31b1b.svg'></a>
Logic-RL: Unleashing LLM Reasoning with Rule-Based Reinforcement Learning
---
<table>
<tr>
<td align="center">
<img src="./pics/teaser.png" width="800" alt="Teaser Image">
</td>
</tr>
<tr>
<td align="center">Main results</td>
</tr>
</table>
---
## Benchmark
| Model | 2ppl | 3ppl | 4ppl | 5ppl | 6ppl | 7ppl | 8ppl |
|------------------------------------------------------------------------|------|------|------|------|------|------|------|
| o3-mini-high | 0.99 | 0.98 | 0.97 | 0.95 | 0.94 | 0.89 | 0.83 |
| o1-2024-12-17 | 0.83 | 0.51 | 0.38 | 0.38 | 0.35 | 0.30 | 0.20 |
| GPT-4o | 0.68 | 0.57 | 0.49 | 0.32 | 0.23 | 0.21 | 0.11 |
| Deepseek-Math-7b | 0.35 | 0.21 | 0.08 | 0.06 | 0.02 | 0.00 | 0.00 |
| Qwen2.5-7B-Instruct-1M | 0.49 | 0.40 | 0.25 | 0.11 | 0.02 | 0.06 | 0.01 |
| Qwen2.5-7B-Logic-RL (ours) | 0.99 | 0.99 | 0.94 | 0.92 | 0.91 | 0.80 | 0.67 |
---
## Installation
```bash
conda create -n logic python=3.9
pip install torch==2.4.0 --index-url https://download.pytorch.org/whl/cu121
pip3 install vllm==0.6.3 ray
pip3 install flash-attn --no-build-isolation
pip install -e . # For verl integration
pip install wandb IPython matplotlib
```
---
## Data Preparation
You can directly use /data.
For your own data generation, here's a demo:
### Base Model
```bash
python ./examples/data_preprocess/kk.py \
--local_dir {processed_data_path} \
--data_path {raw_data_path}
```
### Instruct Model
```bash
python ./examples/data_preprocess/kk.py \
--template_type=qwen-instruct \
--local_dir {processed_data_path} \
--data_path {raw_data_path}
```
---
## Training Execution
```bash
conda activate logic
bash main_grpo.sh # 4×A100 80G
```
---
## ⚙️ Implementation Details
| Component | Location |
|------------------------|-----------------------------------|
| Reward Modeling | `verl/utils/reward_score/kk.py` |
| Data Preprocessing | `examples/data_preprocess/kk.py` |
---
## Citation
```
@misc{xie2025logicrlunleashingllmreasoning,
title={Logic-RL: Unleashing LLM Reasoning with Rule-Based Reinforcement Learning},
author={Tian Xie and Zitian Gao and Qingnan Ren and Haoming Luo and Yuqian Hong and Bryan Dai and Joey Zhou and Kai Qiu and Zhirong Wu and Chong Luo},
year={2025},
eprint={2502.14768},
archivePrefix={arXiv},
primaryClass={cs.CL},
url={https://arxiv.org/abs/2502.14768},
}
```
---
## Acknowledgements
- [Verl](https://github.com/volcengine/verl) 🔗
- [TinyZero](https://github.com/Jiayi-Pan/TinyZero) 🔗
- [Knights and Knaves (K&K) puzzles dataset](https://github.com/AlphaPav/mem-kk-logic) 🔗
---
## Star History
[](https://star-history.com/#Unakar/Logic-RL&Date) | {
"source": "Unakar/Logic-RL",
"title": "README.md",
"url": "https://github.com/Unakar/Logic-RL/blob/main/README.md",
"date": "2025-02-02T10:22:34",
"stars": 1826,
"description": "Reproduce R1 Zero on Logic Puzzle",
"file_size": 3152
} |
# veRL documents
## Build the docs
```bash
# Install dependencies.
pip install -r requirements-docs.txt
# Build the docs.
make clean
make html
```
## Open the docs with your browser
```bash
python -m http.server -d _build/html/
```
Launch your browser and open localhost:8000. | {
"source": "Unakar/Logic-RL",
"title": "docs/README.md",
"url": "https://github.com/Unakar/Logic-RL/blob/main/docs/README.md",
"date": "2025-02-02T10:22:34",
"stars": 1826,
"description": "Reproduce R1 Zero on Logic Puzzle",
"file_size": 281
} |
Welcome to veRL's documentation!
================================================
.. _hf_arxiv: https://arxiv.org/pdf/2409.19256
veRL is a flexible, efficient and production-ready RL training framework designed for large language models (LLMs) post-training. It is an open source implementation of the `HybridFlow <hf_arxiv>`_ paper.
veRL is flexible and easy to use with:
- **Easy extension of diverse RL algorithms**: The Hybrid programming model combines the strengths of single-controller and multi-controller paradigms to enable flexible representation and efficient execution of complex Post-Training dataflows. Allowing users to build RL dataflows in a few lines of code.
- **Seamless integration of existing LLM infra with modular APIs**: Decouples computation and data dependencies, enabling seamless integration with existing LLM frameworks, such as PyTorch FSDP, Megatron-LM and vLLM. Moreover, users can easily extend to other LLM training and inference frameworks.
- **Flexible device mapping and parallelism**: Supports various placement of models onto different sets of GPUs for efficient resource utilization and scalability across different cluster sizes.
- Readily integration with popular HuggingFace models
veRL is fast with:
- **State-of-the-art throughput**: By seamlessly integrating existing SOTA LLM training and inference frameworks, veRL achieves high generation and training throughput.
- **Efficient actor model resharding with 3D-HybridEngine**: Eliminates memory redundancy and significantly reduces communication overhead during transitions between training and generation phases.
--------------------------------------------
.. _Contents:
.. toctree::
:maxdepth: 5
:caption: Quickstart
:titlesonly:
:numbered:
start/install
start/quickstart
.. toctree::
:maxdepth: 5
:caption: Data Preparation
:titlesonly:
:numbered:
preparation/prepare_data
preparation/reward_function
.. toctree::
:maxdepth: 2
:caption: PPO Example
:titlesonly:
:numbered:
examples/ppo_code_architecture
examples/config
examples/gsm8k_example
.. toctree::
:maxdepth: 1
:caption: PPO Trainer and Workers
workers/ray_trainer
workers/fsdp_workers
workers/megatron_workers
.. toctree::
:maxdepth: 1
:caption: Experimental Results
experiment/ppo
.. toctree::
:maxdepth: 1
:caption: Advance Usage and Extension
advance/placement
advance/dpo_extension
advance/fsdp_extension
advance/megatron_extension
.. toctree::
:maxdepth: 1
:caption: FAQ
faq/faq
Contribution
-------------
veRL is free software; you can redistribute it and/or modify it under the terms
of the Apache License 2.0. We welcome contributions.
Join us on `GitHub <https://github.com/volcengine/verl>`_, `Slack <https://join.slack.com/t/verlgroup/shared_invite/zt-2w5p9o4c3-yy0x2Q56s_VlGLsJ93A6vA>`_ and `Wechat <https://raw.githubusercontent.com/eric-haibin-lin/verl-community/refs/heads/main/WeChat.JPG>`_ for discussions.
Code formatting
^^^^^^^^^^^^^^^^^^^^^^^^
We use yapf (Google style) to enforce strict code formatting when reviewing MRs. Run yapf at the top level of verl repo:
.. code-block:: bash
pip3 install yapf
yapf -ir -vv --style ./.style.yapf verl examples tests | {
"source": "Unakar/Logic-RL",
"title": "docs/index.rst",
"url": "https://github.com/Unakar/Logic-RL/blob/main/docs/index.rst",
"date": "2025-02-02T10:22:34",
"stars": 1826,
"description": "Reproduce R1 Zero on Logic Puzzle",
"file_size": 3288
} |
Extend to other RL(HF) algorithms
=================================
We already implemented the complete training pipeline of the PPO
algorithms. To extend to other algorithms, we analyze the high-level
principle to use veRL and provide a tutorial to implement the DPO
algorithm. Users can follow the similar paradigm to extend to other RL algorithms.
.. note:: **Key ideas**: Single process drives multi-process computation and data communication.
Overall Approach
----------------
Step 1: Consider what multi-machine multi-GPU computations are needed
for each model, such as ``generate_sequence`` , ``compute_log_prob`` and
``update_policy`` in the actor_rollout model. Implement distributed
single-process-multiple-data (SPMD) computation and encapsulate them
into APIs
Step 2: Based on different distributed scenarios, including FSDP and 3D
parallelism in Megatron-LM, implement single-process control of data
interaction among multi-process computations.
Step 3: Utilize the encapsulated APIs to implement the control flow
Example: Online DPO
-------------------
We use veRL to implement a simple online DPO algorithm. The algorithm
flow of Online DPO is as follows:
1. There is a prompt (rollout) generator which has the same weight as
the actor model. After a batch of prompts are fed into the generator,
it generates N responses for each prompt.
2. Send all the prompts + responses to a verifier for scoring, which can
be reward model or a rule-based function. Then sort them in pairs to
form a training batch.
3. Use this training batch to train the actor model using DPO. During
the process, a reference policy is needed.
Step 1: What are the multi-machine multi-GPU computations
~~~~~~~~~~~~~~~~~~~~~~~~~~~~~~~~~~~~~~~~~~~~~~~~~~~~~~~~~
**Sample Generator**
Implementation details:
.. code:: python
from verl.single_controller.base import Worker
from verl.single_controller.ray import RayWorkerGroup, RayClassWithInitArgs, RayResourcePool
import ray
@ray.remote
class SampleGenerator(Worker):
def __init__(self, config):
super().__init__()
self.config = config
def generate_sequences(self, data):
pass
Here, ``SampleGenerator`` can be viewed as a multi-process pulled up by
``torchrun``, with each process running the same code (SPMD).
``SampleGenerator`` needs to implement a ``generate_sequences`` API for
the control flow to call. The implementation details inside can use any
inference engine including vllm, sglang and huggingface. Users can
largely reuse the code in
verl/verl/trainer/ppo/rollout/vllm_rollout/vllm_rollout.py and we won't
go into details here.
**ReferencePolicy inference**
API: compute reference log probability
.. code:: python
from verl.single_controller.base import Worker
import ray
@ray.remote
class ReferencePolicy(Worker):
def __init__(self):
super().__init__()
self.model = Model()
def infer(self, data):
return self.model(data)
**Actor update**
API: Update actor model parameters
.. code:: python
from verl.single_controller.base import Worker
import ray
@ray.remote
class DPOActor(Worker):
def __init__(self):
super().__init__()
self.model = Model()
self.model = FSDP(self.model) # or other distributed strategy
self.optimizer = optim.Adam(self.model.parameters(), lr=1e-3)
self.loss_fn = xxx
def update(self, data):
self.optimizer.zero_grad()
logits = self.model(data)
loss = self.loss_fn(logits)
loss.backward()
self.optimizer.step()
**Notes: How to distinguish between control processes and distributed computation processes**
^^^^^^^^^^^^^^^^^^^^^^^^^^^^^^^^^^^^^^^^^^^^^^^^^^^^^^^^^^^^^^^^^^^^^^^^^^^^^^^^^^^^^^^^^^^^^
- Control processes are generally functions directly decorated with
``@ray.remote``
- Computation processes are all wrapped into a ``RayWorkerGroup``.
Users can reuse most of the distribtued computation logics implemented
in PPO algorithm, including FSDP and Megatron-LM backend in
verl/verl/trainer/ppo.
Step 2: Based on different distributed scenarios, implement single-process control of multi-process data interaction
~~~~~~~~~~~~~~~~~~~~~~~~~~~~~~~~~~~~~~~~~~~~~~~~~~~~~~~~~~~~~~~~~~~~~~~~~~~~~~~~~~~~~~~~~~~~~~~~~~~~~~~~~~~~~~~~~~~~
**The core problem to solve here is how a single process sends data to
multiple processes, drives multi-process computation, and how the
control process obtains the results of multi-process computation.**
First, we initialize the multi-process ``WorkerGroup`` in the control
process.
.. code:: python
@ray.remote(num_cpus=1)
def main_task(config):
# construct SampleGenerator
resource_pool = RayResourcePool(process_on_nodes=[8] * 2) # 16 GPUs
ray_cls = RayClassWithInitArgs(SampleGenerator, config=config)
# put SampleGenerator onto resource pool
worker_group = RayWorkerGroup(resource_pool, ray_cls)
# construct reference policy
As we can see, in the control process, multiple processes are wrapped
into a ``RayWorkerGroup``. Inside this ``WorkerGroup``, there is a
``self._workers`` member, where each worker is a RayActor
(https://docs.ray.io/en/latest/ray-core/actors.html) of SampleGenerator.
ray_trainer.md also provide an implementation of
``MegatronRayWorkerGroup``.
Assuming the model is distributed using FSDP, and there is a batch of
data on the control process, for data parallelism, the underlying
calling process is:
.. code:: python
data = xxx
data_list = data.chunk(dp_size)
output = []
for d in data_list:
# worker_group._workers[i] is a SampleGenerator
output.append(worker_group._workers[i].generate_sequences.remote(d))
output = ray.get(output)
output = torch.cat(output)
Single process calling multiple processes involves the following 3
steps:
1. Split the data into DP parts on the control process.
2. Send the data to remote, call the remote computation through RPC, and
utilize multi-process computation.
3. Obtain the computation results of each worker on the control process
and merge them.
Frequently calling these 3 steps on the controller process greatly hurts
code readability. **In veRL, we have abstracted and encapsulated these 3
steps, so that the worker's method + dispatch + collect can be
registered into the worker_group**
.. code:: python
from verl.single_controller.base.decorator import register
def dispatch_data(worker_group, data):
return data.chunk(worker_group.world_size)
def collect_data(worker_group, data):
return torch.cat(data)
dispatch_mode = {
'dispatch_fn': dispatch_data,
'collect_fn': collect_data
}
@register(dispatch_mode=dispatch_mode)
def generate_sequences(self, data):
pass
In this way, we can directly call the method inside the worker through
the ``worker_group`` on the control (driver) process (which is a single
process):
.. code:: python
output = worker_group.generate_sequences(data)
This single line includes data splitting, data distribution and
computation, and data collection.
Furthermore, the model parallelism size of each model is usually fixed,
including dp, tp, pp. So for these common distributed scenarios, we have
pre-implemented specific dispatch and collect methods,in `decorator.py <https://github.com/volcengine/verl/blob/main/verl/single_controller/base/decorator.py>`_, which can be directly used to wrap the computations.
.. code:: python
from verl.single_controller.base.decorator import register, Dispatch
@register(dispatch_mode=Dispatch.DP_COMPUTE_PROTO)
def generate_sequences(self, data: DataProto) -> DataProto:
pass
Here it requires the data interface to be ``DataProto``. Definition of
``DataProto`` is in `protocol.py <https://github.com/volcengine/verl/blob/main/verl/protocol.py>`_.
Step 3: Main training loop
~~~~~~~~~~~~~~~~~~~~~~~~~~
With the above training flows, we can implement the algorithm's control
flow. It is recommended that ``main_task`` is also a ray remote process.
.. code:: python
@ray.remote(num_cpus=1)
def main_task(config):
# construct SampleGenerator
resource_pool = RayResourcePool(process_on_nodes=[8] * 2) # 16 GPUs
ray_cls = RayClassWithInitArgs(SampleGenerator, config=config)
# put SampleGenerator onto resource pool
sample_gen = RayWorkerGroup(resource_pool, ray_cls)
# construct reference policy
ray_cls = RayClassWithInitArgs(ReferencePolicy)
ref_policy = RayWorkerGroup(resource_pool, ray_cls)
# construct actor
ray_cls = RayClassWithInitArgs(DPOActor)
dpo_policy = RayWorkerGroup(resource_pool, ray_cls)
dataloader = DataLoader()
for data in dataloader:
# generate data
data = sample_gen.generate_sequences(data)
# generate scores for each data
data = generate_scores(data)
# generate pairwise data using scores
data = generate_pairwise_data(data)
# generate ref_log_prob
data.batch['ref_log_prob'] = ref_policy.infer(data)
# update using dpo
dpo_policy.update(data)
# logging
Here, different ``WorkerGroups`` can be placed in the same resource pool or
in different resource pools using ``create_colocated_worker_cls``
similar as in `ray_trainer.py <https://github.com/volcengine/verl/blob/main/verl/trainer/ppo/ray_trainer.py>`_. | {
"source": "Unakar/Logic-RL",
"title": "docs/advance/dpo_extension.rst",
"url": "https://github.com/Unakar/Logic-RL/blob/main/docs/advance/dpo_extension.rst",
"date": "2025-02-02T10:22:34",
"stars": 1826,
"description": "Reproduce R1 Zero on Logic Puzzle",
"file_size": 9680
} |
Add models with the FSDP backend
==================================
Model
--------------------------
In principle, our FSDP backend can support any HF model and we can
sychronoize the actor model weight with vLLM using `hf_weight_loader.py <https://github.com/volcengine/verl/blob/main/verl/third_party/vllm/vllm_v_0_5_4/hf_weight_loader.py>`_.
However, ``hf_weight_loader`` is will gather the full state_dict of a
model during synchronization, which may cause OOM. We suggest using
``dtensor_weight_loader`` which gather the full model parameter layer by
layer to reduce the peak memory usage. We already support dtensor weight
loader for the models below in `dtensor_weight_loader.py <https://github.com/volcengine/verl/blob/main/verl/third_party/vllm/vllm_v_0_5_4/dtensor_weight_loaders.py>`_.:
- ``GPT2LMHeadModel``
- ``LlamaForCausalLM``
- ``LLaMAForCausalLM``
- ``MistralForCausalLM``
- ``InternLMForCausalLM``
- ``AquilaModel``
- ``AquilaForCausalLM``
- ``Phi3ForCausalLM``
- ``GemmaForCausalLM``
- ``Gemma2ForCausalLM``
- ``GPTBigCodeForCausalLM``
- ``Starcoder2ForCausalLM``
- ``Qwen2ForCausalLM``
- ``DeepseekV2ForCausalLM``
To implement ``dtensor_weight_loader`` of a model that's supported in
vLLM, follow the guide of gemma model below:
1. Copy the
``load_weights(self, weights: Iterable[Tuple[str, torch.Tensor]])`` from the vllm model class
to ``dtensor_weight_loaders.py``
2. Modify the arguments to
``(actor_weights: Dict, vllm_model: nn.Module)``
3. Replace the ``self`` to ``vllm_model``
4. Add the
``local_loaded_weight = redistribute_dtensor(param_name=name, loaded_weights=loaded_weight)``
before each ``param = params_dict[name]`` and modify the following
weight loading using ``local_loaded_weight``.
5. Register the implemented dtensor weight loader to ``__MODEL_DTENSOR_WEIGHT_LOADER_REGISTRY__``.
.. code-block:: diff
- def load_weights(self, weights: Iterable[Tuple[str, torch.Tensor]]):
+ def gemma_dtensor_weight_loader(actor_weights: Dict, vllm_model: nn.Module) -> nn.Module:
stacked_params_mapping = [
# (param_name, shard_name, shard_id)
("qkv_proj", "q_proj", "q"),
("qkv_proj", "k_proj", "k"),
("qkv_proj", "v_proj", "v"),
("gate_up_proj", "gate_proj", 0),
("gate_up_proj", "up_proj", 1),
]
- params_dict = dict(self.named_parameters())
+ params_dict = dict(vllm_model.named_parameters())
loaded_params = set()
- for name, loaded_weight in weights:
+ for name, loaded_weight in actor_weights.items():
for (param_name, shard_name, shard_id) in stacked_params_mapping:
if shard_name not in name:
continue
name = name.replace(shard_name, param_name)
# Skip loading extra bias for GPTQ models.
if name.endswith(".bias") and name not in params_dict:
continue
+ local_loaded_weight = redistribute_dtensor(param_name=name, loaded_weights=loaded_weight)
param = params_dict[name]
weight_loader = param.weight_loader
- weight_loader(param, loaded_weight, shard_id)
+ weight_loader(param, local_loaded_weight.to(dtype=param.dtype), shard_id)
break
else:
# lm_head is not used in vllm as it is tied with embed_token.
# To prevent errors, skip loading lm_head.weight.
if "lm_head.weight" in name:
continue
# Skip loading extra bias for GPTQ models.
if name.endswith(".bias") and name not in params_dict:
continue
+ local_loaded_weight = redistribute_dtensor(param_name=name, loaded_weights=loaded_weight)
param = params_dict[name]
weight_loader = getattr(param, "weight_loader",
default_weight_loader)
- weight_loader(param, loaded_weight)
+ weight_loader(param, local_loaded_weight.to(dtype=param.dtype))
loaded_params.add(name)
unloaded_params = params_dict.keys() - loaded_params
if unloaded_params:
raise RuntimeError(
"Some weights are not initialized from checkpoints: "
f"{unloaded_params}") | {
"source": "Unakar/Logic-RL",
"title": "docs/advance/fsdp_extension.rst",
"url": "https://github.com/Unakar/Logic-RL/blob/main/docs/advance/fsdp_extension.rst",
"date": "2025-02-02T10:22:34",
"stars": 1826,
"description": "Reproduce R1 Zero on Logic Puzzle",
"file_size": 4399
} |
Add models with the Megatron-LM backend
=========================================
Model
-----------
The most challenging aspect to use the Megatron-LM backend is implementing
the models for training. Currently, we implement Llama model that
support data parallelism, tensor parallelism, pipeline parallelism (also
vPP) and sequence parallelism. We also implement remove padding (sequence packing) on Llama
model, which can be found in `modeling_llama_megatron.py <https://github.com/volcengine/verl/blob/main/verl/models/llama/megatron/modeling_llama_megatron.py>`_.
To support other model, users are required to implement:
1. Implemnt a model similar to ``modeling_llama_megatron.py`` that satisfy the
parallelism requirements of Megatron-LM. Then register your model in
the `registry.py <https://github.com/volcengine/verl/blob/main/verl/models/registry.py>`_.
2. Checkpoint utils that can load full checkpoint (e.g. huggingface
checkpoint) to partitioned models during the runtime. Then register
your loader to ``weight_loader_registry`` in `weight_loader_registry.py <https://github.com/volcengine/verl/blob/main/verl/models/weight_loader_registry.py>`_.
3. Weight loader that synchronize the weight from Megatron to rollout
(vLLM) model. Note that both the actor model and rollout model are
partitioned during runtime. So, it's advisable to map the model name
in actor model implementation. Otherwise, you may need an additional
name mapping and even weight transformation. The weight loader implementation
is in `megatron_weight_loaders.py <https://github.com/volcengine/verl/blob/main/verl/third_party/vllm/vllm_v_0_6_3/megatron_weight_loaders.py>`_. | {
"source": "Unakar/Logic-RL",
"title": "docs/advance/megatron_extension.rst",
"url": "https://github.com/Unakar/Logic-RL/blob/main/docs/advance/megatron_extension.rst",
"date": "2025-02-02T10:22:34",
"stars": 1826,
"description": "Reproduce R1 Zero on Logic Puzzle",
"file_size": 1688
} |
Ray API Design Tutorial
=======================================
We provide a tutorial for our Ray API design, including:
- Ray basic concepts
- Resource Pool and RayWorkerGroup
- Data Dispatch, Execution and Collection
- Initialize the RayWorkerGroup and execute the distributed computation in the given Resource Pool
See details in `tutorial.ipynb <https://github.com/volcengine/verl/blob/main/examples/ray/tutorial.ipynb>`_. | {
"source": "Unakar/Logic-RL",
"title": "docs/advance/placement.rst",
"url": "https://github.com/Unakar/Logic-RL/blob/main/docs/advance/placement.rst",
"date": "2025-02-02T10:22:34",
"stars": 1826,
"description": "Reproduce R1 Zero on Logic Puzzle",
"file_size": 429
} |
.. _config-explain-page:
Config Explaination
===================
ppo_trainer.yaml for FSDP Backend
---------------------------------
Data
~~~~
.. code:: yaml
data:
tokenizer: null
train_files: ~/data/rlhf/gsm8k/train.parquet
val_files: ~/data/rlhf/gsm8k/test.parquet
prompt_key: prompt
max_prompt_length: 512
max_response_length: 512
train_batch_size: 1024
val_batch_size: 1312
return_raw_input_ids: False # This should be set to true when the tokenizer between policy and rm differs
return_raw_chat: False
- ``data.train_files``: Training set parquet. Can be a list or a single
file. The program will read all files into memory, so it can't be too
large (< 100GB). The path can be either local path or HDFS path. For
HDFS path, we provide utils to download it to DRAM and convert the
HDFS path to local path.
- ``data.val_files``: Validation parquet. Can be a list or a single
file.
- ``data.prompt_key``: The field in the dataset where the prompt is
located. Default is 'prompt'.
- ``data.max_prompt_length``: Maximum prompt length. All prompts will be
left-padded to this length. An error will be reported if the length is
too long
- ``data.max_response_length``: Maximum response length. Rollout in RL
algorithms (e.g. PPO) generates up to this length
- ``data.train_batch_size``: Batch size sampled for one training
iteration of different RL algorithms.
- ``data.val_batch_size``: Batch size sampled for one validation
iteration.
- ``data.return_raw_input_ids``: Whether to return the original
input_ids without adding chat template. This is mainly used to
accommodate situations where the reward model's chat template differs
from the policy. It needs to be decoded first, then apply the RM's
chat template. If using a model-based RM, and the policy and RM
chat_templates are different, this flag needs to be set
- ``data.return_raw_chat``:
- ``data.truncation``: Truncate the input_ids or prompt length if they
exceed max_prompt_length. Default is 'error', not allow exceed the
max_prompt_length. The users should increase the max_prompt_length if
throwing the error.
Actor/Rollout/Reference Policy
~~~~~~~~~~~~~~~~~~~~~~~~~~~~~~
.. code:: yaml
actor_rollout_ref:
hybrid_engine: True
model:
path: ~/models/deepseek-llm-7b-chat
external_lib: null
override_config: {}
enable_gradient_checkpointing: False
actor:
strategy: fsdp # This is for backward-compatibility
ppo_mini_batch_size: 256
ppo_micro_batch_size: 64
grad_clip: 1.0
clip_ratio: 0.2
entropy_coeff: 0.001
ppo_epochs: 1
shuffle: True
optim:
lr: 1e-6
lr_warmup_steps_ratio: 0. # the total steps will be injected during runtime
min_lr_ratio: null # only useful for warmup with cosine
warmup_style: constant # select from constant/cosine
total_training_steps: -1 # must be override by program
fsdp_config:
wrap_policy:
# transformer_layer_cls_to_wrap: None
min_num_params: 0
param_offload: False
grad_offload: False
optimizer_offload: False
ref:
fsdp_config:
param_offload: False
wrap_policy:
# transformer_layer_cls_to_wrap: None
min_num_params: 0
log_prob_micro_batch_size: 128
rollout:
name: vllm
temperature: 1.0
top_k: -1 # 0 for hf rollout, -1 for vllm rollout
top_p: 1
response_length: ${data.max_response_length}
# for vllm rollout
dtype: bfloat16 # should align with FSDP
gpu_memory_utilization: 0.5
ignore_eos: False
enforce_eager: True
free_cache_engine: True
load_format: dummy_dtensor # or dummy_hf or dummy_megatron
tensor_model_parallel_size: 2
max_num_batched_tokens: 8192
max_num_seqs: 1024
log_prob_micro_batch_size: 128
# for vllm and hf rollout
do_sample: True
**Common config for actor, rollout and reference model**
- ``actor_rollout_ref.hybrid_engine``: Whether it's a hybrid engine,
currently only supports hybrid engine
- ``actor_rollout_ref.model.path``: Huggingface model path. This can be
either local path or HDFS path. For HDFS path, we provide utils to
download it to DRAM and convert the HDFS path to local path.
- ``actor_rollout_ref.model.external_libs``: Additional Python packages
that need to be imported. Used to register models or tokenizers into
the Huggingface system.
- ``actor_rollout_ref.model.override_config``: Used to override some of
the model's original configurations, mainly dropout
- ``actor_rollout_ref.model.enable_gradient_checkpointing``: Whether to
enable gradient checkpointing for the actor
**Actor model**
- ``actor_rollout_ref.actor.strategy``: fsdp or megatron. In this
example, we use fsdp backend.
- ``actor_rollout_ref.actor.ppo_mini_batch_size``: One sample is split
into multiple sub-batches with batch_size=ppo_mini_batch_size for PPO
updates
- ``actor_rollout_ref.actor.ppo_micro_batch_size``: Similar to gradient
accumulation, the micro_batch_size for one forward pass, trading speed
for GPU memory
- ``actor_rollout_ref.actor.grad_clip``: Gradient clipping for actor
updates
- ``actor_rollout_ref.actor.clip_ratio``: PPO clip ratio
- ``actor_rollout_ref.actor.entropy_coeff``: The weight of entropy when
calculating PPO loss
- ``actor_rollout_ref.actor.ppo_epochs``: Number of epochs for PPO
updates on one set of sampled data
- ``actor_rollout_ref.actor.shuffle``: Whether to shuffle data when
there are multiple epochs
- ``actor_rollout_ref.actor.optim``: Actor's optimizer parameters
- ``actor_rollout_ref.actor.fsdp_config``: FSDP config for actor
training
- ``wrap_policy``: FSDP wrap policy. By default, it uses Huggingface's
wrap policy, i.e., wrapping by DecoderLayer
- No need to set transformer_layer_cls_to_wrap, so we comment it.
- ``*_offload``: Whether to enable parameter, gradient and optimizer
offload
- Trading speed for GPU memory.
**Reference Model**
- ``actor_rollout_ref.ref``: FSDP config same as actor. **For models
larger than 7B, it's recommended to turn on offload for ref by
default**
- ``actor_rollout_ref.ref.log_prob_micro_batch_size``: The batch size
for one forward pass in the computation of ``ref_log_prob``.
**Rollout Model**
- ``actor_rollout_ref.rollout.name``: hf/vllm. We use vLLM by default
because it's much efficient and our hybrid engine is implemented with
vLLM.
- Rollout (Auto-regressive) parameters. The key should be equal to the
property name in vLLM's ``SamplingParams``.
- ``temperature``, ``top_k``, ``top_p`` and others: Sampling
parameters in ``SamplingParams``.
- ``dtype``: Rollout model parameters type. This should be align with
the actor model parameter type in FSDP/Megatron backend.
- ``gpu_memory_utilization``: The proportion of the remaining GPU memory
allocated for kv cache after other models have initialized when using
vllm.
- ``tensor_model_parallel_size``: TP size for rollout. Only effective
for vllm.
- ``log_prob_micro_batch_size``: Micro_batch_size (The batch size for
one forward pass) for recalculating log_prob.
- ``do_sample``: Whether to sample. If set to False, the rollout model
will perform greedy sampling. We disable ``do_sample`` during
validation.
- ``actor_rollout_ref.rollout.ignore_eos``: Whether to ignore the EOS
token and continue generating tokens after the EOS token is generated.
- ``actor_rollout_ref.rollout.free_cache_engine``: Offload the KVCache
after rollout generation stage. Default is True. When set to True, we
need to disable the usage of CUDAGraph (set ``enforce_eager`` to
True.)
- ``actor_rollout_ref.rollout.enforce_eager``: Whether to use CUDAGraph
in vLLM generation. Default set to True to disable CUDAGraph.
- ``actor_rollout_ref.rollout.load_format``: Which weight loader to use
to load the actor model weights to the rollout model.
- ``auto``: Use Megatron weight loader.
- ``megatron``: Use Megatron weight loader. Deployed with Megatron
backend. The input model ``state_dict()`` is already partitioned
along TP dimension and already gathered along PP dimension. This
weight loader requires that the Rollout model and Actor model's
parameters shape and name should be identical.
- ``dtensor``: Default solution when using Huggingface weight loader.
Deployed with FSDP backend and the state_dict_type is
``StateDictType.SHARDED_STATE_DICT``. Recommend to use this weight
loader
- ``hf``: Use Huggingface weight loader. Deployed with FSDP backend
and the state_dict_type is ``StateDictType.FULL_STATE_DICT``. This
solution doesn't need to rewrite the weight loader for each model
implemented in vLLM but it results in larger peak memory usage.
- ``dummy_hf``, ``dummy_megatron``, ``dummy_dtensor``: Random
initialization.
.. note:: **NOTED**: In this config field, users only need to select from ``dummy_megatron``, ``dummy_dtensor``, ``dummy_hf`` for rollout initialization and our hybrid engine will select the corresponding weight loader (i.e., ``megatron``, ``dtensor``, ``hf``) during actor/rollout weight synchronization.
Critic Model
~~~~~~~~~~~~
Most parameters for Critic are similar to Actor Model.
Reward Model
~~~~~~~~~~~~
.. code:: yaml
reward_model:
enable: False
model:
input_tokenizer: ${actor_rollout_ref.model.path} # set this to null if the chat template is identical
path: ~/models/Anomy-RM-v0.1
external_lib: ${actor_rollout_ref.model.external_lib}
fsdp_config:
min_num_params: 0
param_offload: False
micro_batch_size: 64
max_length: null
- ``reward_model.enable``: Whether to enable reward model. If False, we
compute the reward only with the user-defined reward functions. In
GSM8K and Math examples, we disable reward model. For RLHF alignment
example using full_hh_rlhf, we utilize reward model to assess the
responses. If False, the following parameters are not effective.
- ``reward_model.model``
- ``input_tokenizer``: Input tokenizer. If the reward model's chat
template is inconsistent with the policy, we need to first decode to
plaintext, then apply the rm's chat_template. Then score with RM. If
chat_templates are consistent, it can be set to null.
- ``path``: RM's HDFS path or local path. Note that RM only supports
AutoModelForSequenceClassification. Other model types need to define
their own RewardModelWorker and pass it from the code.
Algorithm
~~~~~~~~~
.. code:: yaml
algorithm:
gamma: 1.0
lam: 1.0
adv_estimator: gae
kl_penalty: kl # how to estimate kl divergence
kl_ctrl:
type: fixed
kl_coef: 0.005
- ``gemma``: discount factor
- ``lam``: Trade-off between bias and variance in the GAE estimator
- ``adv_estimator``: gae. Currently only supports gae, will support GRPO
in the future
- ``kl_penalty``\ :Support ``kl``, ``abs``, ``mse`` and ``full``.How to
calculate the kl divergence between actor and reference policy. For
specific options, refer to `core_algos.py <https://github.com/volcengine/verl/blob/main/verl/trainer/ppo/core_algos.py#L192>`_ .
Trainer
~~~~~~~
.. code:: yaml
trainer:
total_epochs: 30
project_name: verl_examples
experiment_name: gsm8k
logger: ['console', 'wandb']
nnodes: 1
n_gpus_per_node: 8
save_freq: -1
test_freq: 2
critic_warmup: 0
default_hdfs_dir: ~/experiments/gsm8k/ppo/${trainer.experiment_name} # hdfs checkpoint path
default_local_dir: checkpoints/${trainer.project_name}/${trainer.experiment_name} # local checkpoint path
- ``trainer.total_epochs``: Number of epochs in training.
- ``trainer.project_name``: For wandb
- ``trainer.experiment_name``: For wandb
- ``trainer.logger``: Support console and wandb
- ``trainer.nnodes``: Number of nodes used in the training.
- ``trainer.n_gpus_per_node``: Number of GPUs per node.
- ``trainer.save_freq``: The frequency (by iteration) to save checkpoint
of the actor and critic model.
- ``trainer.test_freq``: The validation frequency (by iteration).
- ``trainer.critic_warmup``: The number of iteration to train the critic
model before actual policy learning. | {
"source": "Unakar/Logic-RL",
"title": "docs/examples/config.rst",
"url": "https://github.com/Unakar/Logic-RL/blob/main/docs/examples/config.rst",
"date": "2025-02-02T10:22:34",
"stars": 1826,
"description": "Reproduce R1 Zero on Logic Puzzle",
"file_size": 12464
} |
GSM8K Example
=============
Introduction
------------
In this example, we train an LLM to tackle the GSM8k task.
Paper: https://arxiv.org/pdf/2110.14168
Dataset: https://huggingface.co/datasets/gsm8k
Note that the original paper mainly focuses on training a verifier (a
reward model) to solve math problems via Best-of-N sampling. In this
example, we train an RLHF agent using a rule-based reward model.
Dataset Introduction
--------------------
GSM8k is a math problem dataset. The prompt is an elementary school
problem. The LLM model is required to answer the math problem.
The training set contains 7473 samples and the test set contains 1319
samples.
**An example**
Prompt
Katy makes coffee using teaspoons of sugar and cups of water in the
ratio of 7:13. If she used a total of 120 teaspoons of sugar and cups
of water, calculate the number of teaspoonfuls of sugar she used.
Solution
The total ratio representing the ingredients she used to make the
coffee is 7+13 = <<7+13=20>>20 Since the fraction representing the
number of teaspoons she used is 7/20, she used 7/20\ *120 =
<<7/20*\ 120=42>>42 #### 42
Step 1: Prepare dataset
-----------------------
.. code:: bash
cd examples/data_preprocess
python3 gsm8k.py --local_dir ~/data/gsm8k
Step 2: Download Model
----------------------
There're three ways to prepare the model checkpoints for post-training:
- Download the required models from hugging face
.. code:: bash
huggingface-cli download deepseek-ai/deepseek-math-7b-instruct --local-dir ~/models/deepseek-math-7b-instruct --local-dir-use-symlinks False
- Already store your store model in the local directory or HDFS path.
- Also, you can directly use the model name in huggingface (e.g.,
deepseek-ai/deepseek-math-7b-instruct) in
``actor_rollout_ref.model.path`` and ``critic.model.path`` field in
the run script.
Noted that users should prepare checkpoints for actor, critic and reward
model.
[Optional] Step 3: SFT your Model
---------------------------------
We provide a SFT Trainer using PyTorch FSDP in
`fsdp_sft_trainer.py <https://github.com/volcengine/verl/blob/main/verl/trainer/fsdp_sft_trainer.py>`_.
Users can customize their own SFT
script using our FSDP SFT Trainer.
We also provide various training scripts for SFT on GSM8K dataset in `gsm8k sft directory <https://github.com/volcengine/verl/blob/main/examples/gsm8k/sft/>`_.
.. code:: shell
set -x
torchrun -m verl.trainer.fsdp_sft_trainer \
data.train_files=$HOME/data/gsm8k/train.parquet \
data.val_files=$HOME/data/gsm8k/test.parquet \
data.prompt_key=question \
data.response_key=answer \
data.micro_batch_size=8 \
model.partial_pretrain=deepseek-ai/deepseek-coder-6.7b-instruct \
trainer.default_hdfs_dir=hdfs://user/verl/experiments/gsm8k/deepseek-coder-6.7b-instruct/ \
trainer.project_name=gsm8k-sft \
trainer.experiment_name=gsm8k-sft-deepseek-coder-6.7b-instruct \
trainer.total_epochs=4 \
trainer.logger=['console','wandb']
Step 4: Perform PPO training with your model on GSM8K Dataset
-------------------------------------------------------------
- Prepare your own run.sh script. Here's an example for GSM8k dataset
and deepseek-llm-7b-chat model.
- Users could replace the ``data.train_files`` ,\ ``data.val_files``,
``actor_rollout_ref.model.path`` and ``critic.model.path`` based on
their environment.
- See :doc:`config` for detailed explaination of each config field.
**Reward Model/Function**
We use a rule-based reward model. We force the model to produce a final
answer following 4 “#” as shown in the solution. We extract the final
answer from both the solution and model's output using regular
expression matching. We compare them and assign a reward of 1 to correct
answer, 0.1 to incorrect answer and 0 to no answer.
**Training Script**
The training script example for FSDP and Megatron-LM backend are stored in examples/ppo_trainer directory.
.. code:: bash
cd ../ppo_trainer
bash run_deepseek7b_llm.sh
The script of run_deepseek7b_llm.sh
.. code:: bash
set -x
python3 -m verl.trainer.main_ppo \
data.train_files=~/data/rlhf/gsm8k/train.parquet \
data.val_files=~/data/rlhf/gsm8k/test.parquet \
data.train_batch_size=1024 \
data.val_batch_size=1312 \
data.max_prompt_length=512 \
data.max_response_length=512 \
actor_rollout_ref.model.path=~/models/deepseek-llm-7b-chat \
actor_rollout_ref.actor.optim.lr=1e-6 \
actor_rollout_ref.actor.ppo_mini_batch_size=256 \
actor_rollout_ref.actor.ppo_micro_batch_size=64 \
actor_rollout_ref.actor.fsdp_config.param_offload=False \
actor_rollout_ref.actor.fsdp_config.grad_offload=False \
actor_rollout_ref.actor.fsdp_config.optimizer_offload=False \
actor_rollout_ref.rollout.micro_batch_size=256 \
actor_rollout_ref.rollout.log_prob_micro_batch_size=128 \
actor_rollout_ref.rollout.tensor_model_parallel_size=2 \
actor_rollout_ref.rollout.name=vllm \
actor_rollout_ref.rollout.gpu_memory_utilization=0.4 \
actor_rollout_ref.ref.log_prob_micro_batch_size=128 \
actor_rollout_ref.ref.fsdp_config.param_offload=True \
critic.optim.lr=1e-5 \
critic.model.path=~/models/deepseek-llm-7b-chat \
critic.model.enable_gradient_checkpointing=False \
critic.ppo_micro_batch_size=64 \
critic.model.fsdp_config.param_offload=False \
critic.model.fsdp_config.grad_offload=False \
critic.model.fsdp_config.optimizer_offload=False \
algorithm.kl_ctrl.kl_coef=0.001 \
trainer.critic_warmup=0 \
trainer.logger=['console','wandb'] \
trainer.project_name='verl_example_gsm8k' \
trainer.experiment_name='deepseek_llm_7b_function_rm' \
trainer.n_gpus_per_node=8 \
trainer.nnodes=1 \
trainer.save_freq=-1 \
trainer.total_epochs=15 | {
"source": "Unakar/Logic-RL",
"title": "docs/examples/gsm8k_example.rst",
"url": "https://github.com/Unakar/Logic-RL/blob/main/docs/examples/gsm8k_example.rst",
"date": "2025-02-02T10:22:34",
"stars": 1826,
"description": "Reproduce R1 Zero on Logic Puzzle",
"file_size": 5986
} |
PPO Example Architecture
========================
Let's start with the Proximal Policy Optimization algorithm, which is
most widely used algorithm in LLM post-training.
The main entry point of the PPO algorithm example is:
`main_ppo.py <https://github.com/volcengine/verl/blob/main/verl/trainer/main_ppo.py>`_.
In this tutorial, we will go through the code architecture in `main_ppo.py <https://github.com/volcengine/verl/blob/main/verl/trainer/main_ppo.py>`_.
Define the data
---------------
Users need to preprocess and store the dataset in parquet files.
And we implement `RLHFDataset` to load and tokenize the parquet files.
For ``RLHFDataset`` (Default), at least 1 fields are required:
- ``prompt``: Contains the string prompt
We already provide some examples of processing the datasets to parquet
files in `data_preprocess directory <https://github.com/volcengine/verl/blob/main/examples/data_preprocess>`_. Currently, we support
preprocess of GSM8k, MATH, Hellasage, Full_hh_rlhf datasets. See :doc:`../preparation/prepare_data` for
more information.
Define the reward functions for different datasets
--------------------------------------------------
In this main entry point, the users only need to define their own reward
function based on the datasets (or applications) utilized in PPO
training.
For example, we already provide reward functions for `GSM8k <https://github.com/volcengine/verl/blob/main/verl/utils/reward_score/gsm8k.py>`_
and `MATH <https://github.com/volcengine/verl/blob/main/verl/utils/reward_score/math.py>`_
datasets in the ``_select_rm_score_fn``. In the ``RewardManager``, we
will compute the reward score based on the data_source to select
corresponding reward functions. For some RLHF datasets (e.g.,
full_hh_rlhf), the reward model is utilized to assess the responses
without any reward functions. In this case, the ``RewardManager`` will
return the ``rm_score`` computed by the reward model directly.
See `reward functions <https://github.com/volcengine/verl/blob/main/verl/utils/reward_score>`_ for detailed implementation.
Define worker classes
---------------------
.. code:: python
if config.actor_rollout_ref.actor.strategy == 'fsdp': # for FSDP backend
assert config.actor_rollout_ref.actor.strategy == config.critic.strategy
from verl.workers.fsdp_workers import ActorRolloutRefWorker, CriticWorker
from verl.single_controller.ray import RayWorkerGroup
ray_worker_group_cls = RayWorkerGroup
elif config.actor_rollout_ref.actor.strategy == 'megatron': # for Megatron backend
assert config.actor_rollout_ref.actor.strategy == config.critic.strategy
from verl.workers.megatron_workers import ActorRolloutRefWorker, CriticWorker
from verl.single_controller.ray.megatron import NVMegatronRayWorkerGroup
ray_worker_group_cls = NVMegatronRayWorkerGroup # Ray worker class for Megatron-LM
else:
raise NotImplementedError
from verl.trainer.ppo.ray_trainer import ResourcePoolManager, Role
role_worker_mapping = {
Role.ActorRollout: ActorRolloutRefWorker,
Role.Critic: CriticWorker,
Role.RefPolicy: ActorRolloutRefWorker
}
global_pool_id = 'global_pool'
resource_pool_spec = {
global_pool_id: [config.trainer.n_gpus_per_node] * config.trainer.nnodes,
}
mapping = {
Role.ActorRollout: global_pool_id,
Role.Critic: global_pool_id,
Role.RefPolicy: global_pool_id,
}
Step 1: Construct the mapping between roles and workers
~~~~~~~~~~~~~~~~~~~~~~~~~~~~~~~~~~~~~~~~~~~~~~~~~~~~~~~
A role represents a group of workers in the same process. We have
pre-defined several roles in `ray_trainer.py <https://github.com/volcengine/verl/blob/main/verl/trainer/ppo/ray_trainer.py#L38>`_.
.. code:: python
class Role(Enum):
"""
To create more roles dynamically, you can subclass Role and add new members
"""
Actor = 0 # This worker only has Actor
Rollout = 1 # This worker only has Rollout
ActorRollout = 2 # This worker has both actor and rollout, it's a HybridEngine
Critic = 3 # This worker only has critic
RefPolicy = 4 # This worker only has reference policy
RewardModel = 5 # This worker only has reward model
ActorRolloutRef = 6 # This worker contains actor, rollout and reference policy simultaneously
Step 2: Define the worker class corresponding to this role
~~~~~~~~~~~~~~~~~~~~~~~~~~~~~~~~~~~~~~~~~~~~~~~~~~~~~~~~~~
- We have pre-implemented the ``ActorRolloutRefWorker``. Through
different configs, it can be a standalone actor, a standalone rollout,
an ActorRollout HybridEngine, or an ActorRolloutRef HybridEngine
- We also pre-implemented workers for ``Actor``, ``Rollout``,
``Critic``, ``Reward Model`` and ``Reference model`` on two different
backend: PyTorch FSDP
and Megatron-LM.
See `FSDP Workers <https://github.com/volcengine/verl/blob/main/verl/trainer/ppo/workers/fsdp_workers.py>`_
and `Megatron-LM Workers <https://github.com/volcengine/verl/blob/main/verl/trainer/ppo/workers/megatron_workers.py>`_
for more information.
Step 3: Define resource pool id and resource pool spec
~~~~~~~~~~~~~~~~~~~~~~~~~~~~~~~~~~~~~~~~~~~~~~~~~~~~~~
- Resource pool is a division of global GPU resources,
``resource_pool_spec`` is a dict, mapping from id to # of GPUs
- In the above example, we defined a global resource pool:
global_pool_id, and then put all roles on this one resource pool
with all the GPUs in this post-training task. This refers to
*co-locate* placement where all the models share the same set of
GPUs.
- See resource pool and placement for advance usage.
Defining reward model/function
------------------------------
.. code:: python
# we should adopt a multi-source reward function here
# - for rule-based rm, we directly call a reward score
# - for model-based rm, we call a model
# - for code related prompt, we send to a sandbox if there are test cases
# - finally, we combine all the rewards together
# - The reward type depends on the tag of the data
if config.reward_model.enable:
from verl.workers.fsdp_workers import RewardModelWorker
role_worker_mapping[Role.RewardModel] = RewardModelWorker
mapping[Role.RewardModel] = global_pool_id
reward_fn = RewardManager(tokenizer=tokenizer, num_examine=0)
# Note that we always use function-based RM for validation
val_reward_fn = RewardManager(tokenizer=tokenizer, num_examine=1)
resource_pool_manager = ResourcePoolManager(resource_pool_spec=resource_pool_spec, mapping=mapping)
Since not all tasks use model-based RM, users need to define here
whether it's a model-based RM or a function-based RM
- If it's a model-based RM, directly add the ``RewardModel`` role in the
resource mapping and add it to the resource pool mapping.
- Note that the pre-defined ``RewardModelWorker`` only supports models
with the structure of huggingface
``AutoModelForSequenceClassification``. If it's not this model, you
need to define your own RewardModelWorker in `FSDP Workers <https://github.com/volcengine/verl/blob/main/verl/trainer/ppo/workers/fsdp_workers.py>`_
and `Megatron-LM Workers <https://github.com/volcengine/verl/blob/main/verl/trainer/ppo/workers/megatron_workers.py>`_.
- If it's a function-based RM, the users are required to classified the
reward function for each datasets.
.. code:: python
def _select_rm_score_fn(data_source):
if data_source == 'openai/gsm8k':
return gsm8k.compute_score
elif data_source == 'lighteval/MATH':
return math.compute_score
else:
raise NotImplementedError
See reward functions implemented in `directory <https://github.com/volcengine/verl/blob/main/verl/utils/reward_score/>`_
for more information.
Define, init and run the PPO Trainer
------------------------------------
.. code:: python
trainer = RayPPOTrainer(config=config,
tokenizer=tokenizer,
role_worker_mapping=role_worker_mapping,
resource_pool_manager=resource_pool_manager,
ray_worker_group_cls=ray_worker_group_cls,
reward_fn=reward_fn,
val_reward_fn=val_reward_fn)
trainer.init_workers()
trainer.fit()
- We first initialize the ``RayPPOTrainer`` with user config, tokenizer
and all the above worker mapping, resource pool, worker group and
reward functions
- We first call the ``trainer.init_workers()`` to initialize the models
on the allocated GPUs (in the resource pool)
- The actual PPO training will be executed in ``trainer.fit()``
veRL can be easily extended to other RL algorithms by reusing the Ray
model workers, resource pool and reward functions. See :doc:`extension<../advance/dpo_extension>` for
more information.
Details of the ``RayPPOTrainer`` is discussed in :doc:`Ray Trainer<../workers/ray_trainer>`. | {
"source": "Unakar/Logic-RL",
"title": "docs/examples/ppo_code_architecture.rst",
"url": "https://github.com/Unakar/Logic-RL/blob/main/docs/examples/ppo_code_architecture.rst",
"date": "2025-02-02T10:22:34",
"stars": 1826,
"description": "Reproduce R1 Zero on Logic Puzzle",
"file_size": 9044
} |
.. _algo-baseline-page:
Algorithm Baselines
===================
GSM8k
------------------
Assuming GSM8k dataset is preprocess via ``python3 examples/data_preprocess/gsm8k.py``
Refer to the table below to reproduce PPO training from different pre-trained models.
.. _Huggingface: https://huggingface.co/google/gemma-2-2b-it#benchmark-results
.. _SFT Command and logs: https://github.com/eric-haibin-lin/verl-data/blob/experiments/gsm8k/gemma-2-2b-it-sft-0.411.log
.. _SFT+PPO Command and logs: https://github.com/eric-haibin-lin/verl-data/blob/experiments/gsm8k/gemma-2-2b-it-ppo-bsz512_4-prompt1024-resp-512-0.640.log
.. _wandb: https://api.wandb.ai/links/verl-team/h7ux8602
.. _Qwen Blog: https://qwenlm.github.io/blog/qwen2.5-llm/
.. _PPO Command and logs: https://github.com/eric-haibin-lin/verl-data/blob/experiments/gsm8k/Qwen2.5-0.5B-bsz256_2-prompt1024-resp512-0.567.log
+----------------------------+------------------------+------------+-----------------------------------------------------------------------------------------------+
| Model | Method | Test score | Details |
+============================+========================+============+=====================+=========================================================================+
| google/gemma-2-2b-it | pretrained checkpoint | 23.9 | `Huggingface`_ |
+----------------------------+------------------------+------------+-----------------------------------------------------------------------------------------------+
| google/gemma-2-2b-it | SFT | 52.06 | `SFT Command and logs`_ |
+----------------------------+------------------------+------------+-----------------------------------------------------------------------------------------------+
| google/gemma-2-2b-it | SFT + PPO | 64.02 | `SFT+PPO Command and logs`_, `wandb`_ |
+----------------------------+------------------------+------------+-----------------------------------------------------------------------------------------------+
| Qwen/Qwen2.5-0.5B-Instruct | pretrained checkpoint | 36.4 | `Qwen Blog`_ |
+----------------------------+------------------------+------------+-----------------------------------------------------------------------------------------------+
| Qwen/Qwen2.5-0.5B-Instruct | PPO | 56.7 | `PPO Command and logs`_ |
+----------------------------+------------------------+------------+-----------------------------------------------------------------------------------------------+ | {
"source": "Unakar/Logic-RL",
"title": "docs/experiment/ppo.rst",
"url": "https://github.com/Unakar/Logic-RL/blob/main/docs/experiment/ppo.rst",
"date": "2025-02-02T10:22:34",
"stars": 1826,
"description": "Reproduce R1 Zero on Logic Puzzle",
"file_size": 3029
} |
Frequently Asked Questions
====================================
Ray related
------------
How to add breakpoint for debugging with distributed Ray?
^^^^^^^^^^^^^^^^^^^^^^^^^^^^^^^^^^^^^^^^^^^^^^^^^^^^^^^^^^^^^^^^^^^^^^^^^^^^^^^^^^^^^^^^^^^^^^^^^^^^^^^^^^^^^^^^^^^^^^^^^^^^^^^^^^^^^^^^
Please checkout the official debugging guide from Ray: https://docs.ray.io/en/latest/ray-observability/ray-distributed-debugger.html
Distributed training
------------------------
How to run multi-node post-training with Ray?
^^^^^^^^^^^^^^^^^^^^^^^^^^^^^^^^^^^^^^^^^^^^^^^^^^^^^^^^^^^^^^^^^^^^^^^^^^^^^^^^^^^^^^^^^^^^^^^^^^^^^^^^^^^^^^^^^^^^^^^^^^^^^^^^^^^^^^^^
You can start a ray cluster and submit a ray job, following the official guide from Ray: https://docs.ray.io/en/latest/ray-core/starting-ray.html | {
"source": "Unakar/Logic-RL",
"title": "docs/faq/faq.rst",
"url": "https://github.com/Unakar/Logic-RL/blob/main/docs/faq/faq.rst",
"date": "2025-02-02T10:22:34",
"stars": 1826,
"description": "Reproduce R1 Zero on Logic Puzzle",
"file_size": 798
} |
Prepare Data (Parquet) for Post-Training
========================================
Before starting the post-training job, we need to prepare the data for
the policy training. The data should be stored in the parquet format.
We provide several data preprocess scripts for different datasets,
including GSM8K, MATH, HelloSwag, Full_hh_rlhf. To prepare other datasets, we need
to follow the following steps: The data preprocess script can be divided
into two parts:
1. The first part is the common part, which loads the dataset from
huggingface's ``datasets`` package. Then preprocess the datasets with
the ``make_map_fn`` and then store in the parquet format.
.. code:: python
import re
import os
import datasets
from verl.utils.hdfs_io import copy, makedirs
import argparse
# To extract the solution for each prompts in the dataset
# def extract_solution(solution_str):
# ...
if __name__ == '__main__':
parser = argparse.ArgumentParser()
parser.add_argument('--local_dir', default='/opt/tiger/gsm8k')
parser.add_argument('--hdfs_dir', default=None)
args = parser.parse_args()
num_few_shot = 5
data_source = 'openai/gsm8k'
dataset = datasets.load_dataset(data_source, 'main')
train_dataset = dataset['train']
test_dataset = dataset['test']
# Construct a `def make_map_fn(split)` for the corresponding datasets.
# ...
train_dataset = train_dataset.map(function=make_map_fn('train'), with_indices=True)
test_dataset = test_dataset.map(function=make_map_fn('test'), with_indices=True)
local_dir = args.local_dir
hdfs_dir = args.hdfs_dir
train_dataset.to_parquet(os.path.join(local_dir, 'train.parquet'))
test_dataset.to_parquet(os.path.join(local_dir, 'test.parquet'))
makedirs(hdfs_dir)
copy(src=local_dir, dst=hdfs_dir)
2. The users are required to implement the ``make_map_fn()`` function
(as well as the ``extract_solution``) on their own to support
different datasets or tasks.
We already implemented the data preprocess of GSM8k, MATH, Hellaswag and Full_hh_rlhf
datasets. And we take the GSM8k dataset as an example:
**GSM8K**
In the ``make_map_fn``, each data field should consist of the following
5 fields:
1. ``data_source``: The name of the dataset. To index the corresponding
reward function in the ``RewardModule``
2. ``prompt``: This field should be constructed in the format of
huggingface chat_template. The tokenizer in ``RLHFDataset`` will
apply chat template and tokenize the prompt.
3. ``ability``: Define the task category.
4. ``reward_model``: Currently, we only utilize the ``ground_truth``
field during evaluation. The ``ground_truth`` is computed by the
``extract_solution`` function. **NOTED** that the implementation of
the corresponding reward function should align with this extracted
``ground_truth``.
5. ``extra_info``: Record some information of the current prompt. Not
use for now.
.. code:: python
def extract_solution(solution_str):
solution = re.search("#### (\\-?[0-9\\.\\,]+)", solution_str) # extract the solution after ####
assert solution is not None
final_solution = solution.group(0)
final_solution = final_solution.split('#### ')[1].replace(',', '')
return final_solution
instruction_following = "Let's think step by step and output the final answer after \"####\"."
# add a row to each data item that represents a unique id
def make_map_fn(split):
def process_fn(example, idx):
question = example.pop('question')
question = question + ' ' + instruction_following
answer = example.pop('answer')
solution = extract_solution(answer)
data = {
"data_source": data_source,
"prompt": [{
"role": "user",
"content": question
}],
"ability": "math",
"reward_model": {
"style": "rule",
"ground_truth": solution
},
"extra_info": {
'split': split,
'index': idx
}
}
return data
return process_fn | {
"source": "Unakar/Logic-RL",
"title": "docs/preparation/prepare_data.rst",
"url": "https://github.com/Unakar/Logic-RL/blob/main/docs/preparation/prepare_data.rst",
"date": "2025-02-02T10:22:34",
"stars": 1826,
"description": "Reproduce R1 Zero on Logic Puzzle",
"file_size": 4335
} |
Implement Reward Function for Dataset
======================================
For each dataset, we need to implement a reward function or utilize a reward model to compute the rewards for the generated responses.
We already pre-implemented some reward functions in `reward_score directory <https://github.com/volcengine/verl/blob/main/verl/utils/reward_score>`_.
Currently, we support reward functions for GSM8k and MATH datasets. For RLHF datasets (e.g.,
full_hh_rlhf) and Code Generation (e.g., APPS), we utilize reward model
and SandBox (will opensource soon) for evaluation respectively.
RewardManager
-------------
In the entrypoint of the PPO Post-Training script `main_ppo.py <https://github.com/volcengine/verl/blob/main/verl/trainer/main_ppo.py#L33>`_,
we implement a ``RewardManager`` that utilze pre-implemented reward functions to compute the scores for each response.
In the ``RewardManager``, we implemented a ``__call__`` function to
compute the score for each response.
All the reward functions are executed by ``compute_score_fn``.
The input is a ``DataProto``, which includes:
- ``input_ids``, ``attention_mask``: ``input_ids`` and ``attention_mask`` after applying
chat_template, including prompt and response
- ``responses``: response tokens
- ``ground_truth``: The ground truth string of the current prompt.
Stored in ``non_tensor_batch`` in the ``DataProto``, which should be
preprocessed in the parquet files.
- ``data_source``: The dataset name of the current prompt. Stored in
``non_tensor_batch`` in the ``DataProto``, which should be
preprocessed in the parquet files.
After detokenize the responses, the responses string and the ground
truth string will be input to the ``compute_score_fn`` to compute the
score for each response.
Reward Functions
----------------
We already pre-implemented some reward functions in `reward_score directory <https://github.com/volcengine/verl/blob/main/verl/utils/reward_score>`_.
- In the `GSM8k example <https://github.com/volcengine/verl/blob/main/verl/utils/reward_score/gsm8k.py>`_, we
force the response to output the final answer after four ####, then
use string matching to compare with the ground truth. If completely
correct, score 1 point; if the format is correct, score 0.1 points; if
the format is incorrect, score 0 points.
- In the `MATH example <https://github.com/volcengine/verl/blob/main/verl/utils/reward_score/math.py>`_, we follow
the implementation in `lm-evaluation-harness repository <https://github.com/EleutherAI/lm-evaluation-harness/blob/main/lm_eval/tasks/hendrycks_math/utils.py>`_. | {
"source": "Unakar/Logic-RL",
"title": "docs/preparation/reward_function.rst",
"url": "https://github.com/Unakar/Logic-RL/blob/main/docs/preparation/reward_function.rst",
"date": "2025-02-02T10:22:34",
"stars": 1826,
"description": "Reproduce R1 Zero on Logic Puzzle",
"file_size": 2605
} |
Installation
============
Requirements
------------
- **Python**: Version >= 3.9
- **CUDA**: Version >= 12.1
veRL supports various backends. Currently, the following configurations are available:
- **FSDP** and **Megatron-LM** (optional) for training.
- **vLLM** adn **TGI** for rollout generation, **SGLang** support coming soon.
Training backends
------------------
We recommend using **FSDP** backend to investigate, research and prototype different models, datasets and RL algorithms. The guide for using FSDP backend can be found in `PyTorch FSDP Backend <https://verl.readthedocs.io/en/latest/workers/fsdp_workers.html>`_.
For users who pursue better scalability, we recommend using **Megatron-LM** backend. Currently, we support Megatron-LM@core_v0.4.0 with some internal patches (soon be updated to latest version directly relying on upstream Megatron-LM). The guide for using Megatron-LM backend can be found in `Megatron-LM Backend <https://verl.readthedocs.io/en/latest/workers/megatron_workers.html>`_.
Install from docker image
-------------------------
We provide pre-built Docker images for quick setup.
Image and tag: ``verlai/verl:vemlp-th2.4.0-cu124-vllm0.6.3-ray2.10-te1.7-v0.0.3``. See files under ``docker/`` if you want to build your own image.
1. Launch the desired Docker image:
.. code:: bash
docker run --runtime=nvidia -it --rm --shm-size="10g" --cap-add=SYS_ADMIN -v <image:tag>
2. Inside the container, install veRL:
.. code:: bash
# install the nightly version (recommended)
git clone https://github.com/volcengine/verl && cd verl && pip3 install -e .
# or install from pypi via `pip3 install verl`
3. Setup Megatron (optional)
If you want to enable training with Megatron, Megatron code must be added to PYTHONPATH:
.. code:: bash
cd ..
git clone -b core_v0.4.0 https://github.com/NVIDIA/Megatron-LM.git
cp verl/patches/megatron_v4.patch Megatron-LM/
cd Megatron-LM && git apply megatron_v4.patch
pip3 install -e .
export PYTHONPATH=$PYTHONPATH:$(pwd)
You can also get the Megatron code after verl's patch via
.. code:: bash
git clone -b core_v0.4.0_verl https://github.com/eric-haibin-lin/Megatron-LM
Install from custom environment
---------------------------------
To manage environment, we recommend using conda:
.. code:: bash
conda create -n verl python==3.9
conda activate verl
For installing the latest version of veRL, the best way is to clone and
install it from source. Then you can modify our code to customize your
own post-training jobs.
.. code:: bash
# install verl together with some lightweight dependencies in setup.py
git clone https://github.com/volcengine/verl.git
cd verl
pip3 install -e .
You can also install veRL using ``pip3 install``
.. code:: bash
# directly install from pypi
pip3 install verl
Dependencies
------------
veRL requires Python >= 3.9 and CUDA >= 12.1.
veRL support various backend, we currently release FSDP and Megatron-LM
for actor training and vLLM for rollout generation.
The following dependencies are required for all backends, PyTorch FSDP and Megatron-LM.
The pros, cons and extension guide for using PyTorch FSDP backend can be
found in :doc:`FSDP Workers<../workers/fsdp_workers>`.
.. code:: bash
# install torch [or you can skip this step and let vllm to install the correct version for you]
pip install torch==2.4.0 torchvision==0.19.0 torchaudio==2.4.0 --index-url https://download.pytorch.org/whl/cu121
# install vllm
pip3 install ray vllm==0.6.3 # or you can install 0.5.4, 0.4.2 and 0.3.1
# flash attention 2
pip3 install flash-attn --no-build-isolation
For users who pursue better scalability, we recommend using Megatron-LM
backend. Please install the above dependencies first.
Currently, we support Megatron-LM\@core_v0.4.0 and we fix some internal
issues of Megatron-LM. Here's the additional installation guide (optional).
The pros, cons and extension guide for using Megatron-LM backend can be
found in :doc:`Megatron-LM Workers<../workers/megatron_workers>`.
.. code:: bash
# Megatron-LM Backend (optional)
# apex
pip3 install -v --disable-pip-version-check --no-cache-dir --no-build-isolation \
--config-settings "--build-option=--cpp_ext" --config-settings "--build-option=--cuda_ext" \
git+https://github.com/NVIDIA/apex
# transformer engine
pip3 install git+https://github.com/NVIDIA/[email protected]
# megatron core v0.4.0: clone and apply the patch
# You can also get the patched Megatron code patch via
# git clone -b core_v0.4.0_verl https://github.com/eric-haibin-lin/Megatron-LM
cd ..
git clone -b core_v0.4.0 https://github.com/NVIDIA/Megatron-LM.git
cd Megatron-LM
cp ../verl/patches/megatron_v4.patch .
git apply megatron_v4.patch
pip3 install -e .
export PYTHONPATH=$PYTHONPATH:$(pwd) | {
"source": "Unakar/Logic-RL",
"title": "docs/start/install.rst",
"url": "https://github.com/Unakar/Logic-RL/blob/main/docs/start/install.rst",
"date": "2025-02-02T10:22:34",
"stars": 1826,
"description": "Reproduce R1 Zero on Logic Puzzle",
"file_size": 4914
} |
.. _quickstart:
=========================================================
Quickstart: Post-train a LLM using PPO with GSM8K dataset
=========================================================
Post-train a LLM using GSM8K dataset
===================================================================
Introduction
------------
.. _hf_dataset_gsm8k: https://huggingface.co/datasets/gsm8k
In this example, we train an LLM to tackle the `GSM8k <hf_dataset_gsm8k>`_ task with function-based rewards. [1]_
Prerequisite:
- the latest version of ``verl`` and its dependencies installed following the installation guide. Using the docker image is recommended.
- an GPU with at least 24 GB HBM
Dataset Introduction
--------------------
GSM8k is a math problem dataset. The prompt is an elementary school
problem. The LLM model is asked to solve the math problem. Below is an example:
Prompt
Katy makes coffee using teaspoons of sugar and cups of water in the
ratio of 7:13. If she used a total of 120 teaspoons of sugar and cups
of water, calculate the number of teaspoonfuls of sugar she used.
Solution
The total ratio representing the ingredients she used to make the
coffee is 7+13 = <<7+13=20>>20 Since the fraction representing the
number of teaspoons she used is 7/20, she used 7/20\ *120 =
<<7/20*\ 120=42>>42 #### 42
Step 1: Prepare the dataset
----------------------------
We preprocess the dataset in parquet format so that (1) it contains necessary fields for computing RL rewards and (2) is faster to read.
.. code-block:: bash
python3 examples/data_preprocess/gsm8k.py --local_dir ~/data/gsm8k
Step 2: Download a model for post-training
-------------------------------------------
Usually we recommend starting with an "instruct" model variant so that the model follows instructions. In this example, we start with the ``Qwen2.5-0.5B-Instruct`` model.
If you start from a "base" model variant, doing SFT before RL is recommended. Refer to the `sft directory <https://github.com/volcengine/verl/blob/main/examples/gsm8k/sft/>`_ and `SFT Trainer <https://github.com/volcengine/verl/blob/main/verl/trainer/fsdp_sft_trainer.py>`_ for further details.
.. code-block:: bash
python3 -c "import transformers; transformers.pipeline('text-generation', model='Qwen/Qwen2.5-0.5B-Instruct')"
Step 3: Perform PPO training with the instruct model
----------------------------------------------------------------------
**Reward Model/Function**
We use a pre-defined rule-based reward model. We force the model to produce a final
answer following 4 “#” as shown in the solution. We extract the final
answer from both the solution and model's output using regular
expression matching. We assign a reward of 1 to correct
answer, 0.1 to incorrect answer and 0 to no answer.
For mode details, please refer to `verl/utils/reward_score/gsm8k.py <https://github.com/volcengine/verl/blob/v0.1/verl/utils/reward_score/gsm8k.py>`_.
**Training Script**
Now let's run PPO training with the dataset and model above. [2]_
Set the ``data.train_files`` ,\ ``data.val_files``, ``actor_rollout_ref.model.path`` and ``critic.model.path`` based on your dataset and model names or paths.
.. code-block:: bash
PYTHONUNBUFFERED=1 python3 -m verl.trainer.main_ppo \
data.train_files=$HOME/data/gsm8k/train.parquet \
data.val_files=$HOME/data/gsm8k/test.parquet \
data.train_batch_size=256 \
data.val_batch_size=1312 \
data.max_prompt_length=512 \
data.max_response_length=256 \
actor_rollout_ref.model.path=Qwen/Qwen2.5-0.5B-Instruct \
actor_rollout_ref.actor.optim.lr=1e-6 \
actor_rollout_ref.actor.ppo_mini_batch_size=64 \
actor_rollout_ref.actor.ppo_micro_batch_size=4 \
actor_rollout_ref.rollout.log_prob_micro_batch_size=8 \
actor_rollout_ref.rollout.tensor_model_parallel_size=1 \
actor_rollout_ref.rollout.gpu_memory_utilization=0.4 \
actor_rollout_ref.ref.log_prob_micro_batch_size=4 \
critic.optim.lr=1e-5 \
critic.model.path=Qwen/Qwen2.5-0.5B-Instruct \
critic.ppo_micro_batch_size=4 \
algorithm.kl_ctrl.kl_coef=0.001 \
trainer.logger=['console'] \
+trainer.val_before_train=False \
trainer.default_hdfs_dir=null \
trainer.n_gpus_per_node=1 \
trainer.nnodes=1 \
trainer.save_freq=10 \
trainer.test_freq=10 \
trainer.total_epochs=15 2>&1 | tee verl_demo.log
You are expected to see the following logs, indicating training in progress. The key metric ``val/test_score/openai/gsm8k`` is computed every ``trainer.test_freq`` steps:
.. code-block:: bash
step:0 - timing/gen:21.470 - timing/ref:4.360 - timing/values:5.800 - critic/kl:0.000 - critic/kl_coeff:0.001 - timing/adv:0.109 - timing/update_critic:15.664 - critic/vf_loss:14.947 - critic/vf_clipfrac:0.000 - critic/vpred_mean:-2.056 - critic/grad_norm:1023.278 - critic/lr(1e-4):0.100 - timing/update_actor:20.314 - actor/entropy_loss:0.433 - actor/pg_loss:-0.005 - actor/pg_clipfrac:0.000 - actor/ppo_kl:0.000 - actor/grad_norm:1.992 - actor/lr(1e-4):0.010 - critic/score/mean:0.004 - critic/score/max:1.000 - critic/score/min:0.000 - critic/rewards/mean:0.004 - critic/rewards/max:1.000 - critic/rewards/min:0.000 - critic/advantages/mean:-0.000 - critic/advantages/max:2.360 - critic/advantages/min:-2.280 - critic/returns/mean:0.003 - critic/returns/max:0.000 - critic/returns/min:0.000 - critic/values/mean:-2.045 - critic/values/max:9.500 - critic/values/min:-14.000 - response_length/mean:239.133 - response_length/max:256.000 - response_length/min:77.000 - prompt_length/mean:104.883 - prompt_length/max:175.000 - prompt_length/min:68.000
step:1 - timing/gen:23.020 - timing/ref:4.322 - timing/values:5.953 - critic/kl:0.000 - critic/kl_coeff:0.001 - timing/adv:0.118 - timing/update_critic:15.646 - critic/vf_loss:18.472 - critic/vf_clipfrac:0.384 - critic/vpred_mean:1.038 - critic/grad_norm:942.924 - critic/lr(1e-4):0.100 - timing/update_actor:20.526 - actor/entropy_loss:0.440 - actor/pg_loss:0.000 - actor/pg_clipfrac:0.002 - actor/ppo_kl:0.000 - actor/grad_norm:2.060 - actor/lr(1e-4):0.010 - critic/score/mean:0.000 - critic/score/max:0.000 - critic/score/min:0.000 - critic/rewards/mean:0.000 - critic/rewards/max:0.000 - critic/rewards/min:0.000 - critic/advantages/mean:0.000 - critic/advantages/max:2.702 - critic/advantages/min:-2.616 - critic/returns/mean:0.000 - critic/returns/max:0.000 - critic/returns/min:0.000 - critic/values/mean:-2.280 - critic/values/max:11.000 - critic/values/min:-16.000 - response_length/mean:232.242 - response_length/max:256.000 - response_length/min:91.000 - prompt_length/mean:102.398 - prompt_length/max:185.000 - prompt_length/min:70.000
Checkout :ref:`algo-baseline-page` for full training and validation logs for reference.
The checkpoint is saved at the following dir by default: ``checkpoints/${trainer.project_name}/${trainer.experiment_name}``
To enable ``wandb`` for experiment tracking, set the following configs:
.. code-block:: bash
trainer.logger=['console','wandb'] \
trainer.project_name=$YOUR_PROJECT_NAME \
trainer.experiment_name=$YOUR_RUN_NAME \
If you encounter out of memory issues with HBM less than 32GB, enable the following configs would help:
.. code-block:: bash
actor_rollout_ref.actor.ppo_micro_batch_size=1 \
critic.ppo_micro_batch_size=1 \
For the full set of configs, please refer to :ref:`config-explain-page` for detailed explaination and performance tuning.
.. [1] The original paper (https://arxiv.org/pdf/2110.14168) mainly focuses on training a verifier (a reward model) to solve math problems via Best-of-N sampling. In this example, we train an RL agent using a rule-based reward model.
.. [2] More training script examples for FSDP and Megatron-LM backend are stored in `examples/ppo_trainer <https://github.com/volcengine/verl/tree/main/examples/ppo_trainer>`_ directory. | {
"source": "Unakar/Logic-RL",
"title": "docs/start/quickstart.rst",
"url": "https://github.com/Unakar/Logic-RL/blob/main/docs/start/quickstart.rst",
"date": "2025-02-02T10:22:34",
"stars": 1826,
"description": "Reproduce R1 Zero on Logic Puzzle",
"file_size": 7899
} |
PyTorch FSDP Backend
======================
We support PyTorch FSDP Backend by implementing various workers for
actor, critic, reference, rollout and reward models. We also implement
the ``FSDPVLLMShardingManager`` that reshard weight between FSDP and
vLLM in `fsdp_vllm.py <https://github.com/volcengine/verl/blob/main/verl/trainer/ppo/hybrid_engine/fsdp_vllm.py>`_.
**Pros**
- Readily support various models.
- Users only need to implement the corresponding
``dtensor_weight_loader`` for weight synchronization between FSDP
and vLLM. While for ``hf_weight_loader``, users can directly apply
any models supported both in HF and vLLM without any code change.
- Easy to organize the forward and backward computation for each model.
**Cons**
- Poor scalability when it comes to large-scale models (e.g. Llama 70B
and 405B)
- The resharding overhead between actor and rollout could be larger than
Megatron-LM backend.
Due to the simplicity, we recommend using FSDP backend for algorithm
research and prototyping.
FSDP Workers
--------------
ActorRolloutRefWorker
^^^^^^^^^^^^^^^^^^^^^
Actor/Rollout HybridEngine
''''''''''''''''''''''''''
1. HybridEngine, Actor and Rollout initialization API.
.. code:: python
@register(dispatch_mode=Dispatch.ONE_TO_ALL)
def init_model(self):
``ONE_TO_ALL``: when calling the ``init_model`` function from the driver
process, each worker (on a GPU) will execute the following model
initialization process.
The initialization details of HybridEngine, Actor and Rollout are
highlighted below:
1. ``DataParallelPPOActor`` implements the simple PPO computation logics
when the model is built with FSDP, including compute log prob, model
update.
2. ``vLLMRollout`` support generation with vLLM. We modify the vLLM
Engine and make it executed under SPMD to fit into our
``WorkerGroup`` design.
3. ``FSDPVLLMShardingManager`` a context manager to perform actual
resharding between actor and rollout.
See `source code <https://github.com/volcengine/verl/blob/main/verl/trainer/ppo/workers/fsdp_workers.py#L42>`_. for more information.
1. Generate sequence and recompute log prob
.. code:: python
@register(dispatch_mode=Dispatch.DP_COMPUTE_PROTO)
def generate_sequences(self, prompts: DataProto):
- ``Dispatch.DP_COMPUTE_PROTO``: The data will be dispatched and
collected along the DP dimension
- In this function, the rollout model will perform auto-regressive
generation and the actor model will recompute the old log prob for the
generetad response.
3. Update actor model
.. code:: python
@register(dispatch_mode=Dispatch.DP_COMPUTE_PROTO)
def update_actor(self, data: DataProto):
- Update the actor model weight using PPO & entropy loss.
ReferenceModel
''''''''''''''
1. Reference model initialization
The reference model is initialized using the same function as the actor
model without initializing the HybridEngine and Optimizer. Then the
actor model is also wrapped by the ``DataParallelPPOActor``.
2. Compute reference log prob
.. code:: python
@register(dispatch_mode=Dispatch.DP_COMPUTE_PROTO)
def compute_ref_log_prob(self, data: DataProto):
- In this function, the reference model will call the compute log prob
function in ``DataParallelPPOActor`` to compute the reference log
prob.
CriticWorker and RewardWorker
^^^^^^^^^^^^^^^^^^^^^^^^^^^^^
1. Model initialization
Quite similar to reference model. The CriticWorker will perform
additional initialization for the Optimizer.
2. Compute Values for CriticWorker
.. code:: python
@register(dispatch_mode=Dispatch.DP_COMPUTE_PROTO)
def compute_values(self, data: DataProto):
3. Update Critic
.. code:: python
@register(dispatch_mode=Dispatch.DP_COMPUTE_PROTO)
def update_critic(self, data: DataProto):
4. Compute Reward
.. code:: python
@register(dispatch_mode=Dispatch.DP_COMPUTE_PROTO)
def compute_rm_score(self, data: DataProto):
HybridShard
------------
We didn't support FSDP `HybridShard`. To support this, we may need to
construct a 2D device mesh and test the corresponding
``dtensor_weight_loader`` and ``hf_weight_loader`` for each model. | {
"source": "Unakar/Logic-RL",
"title": "docs/workers/fsdp_workers.rst",
"url": "https://github.com/Unakar/Logic-RL/blob/main/docs/workers/fsdp_workers.rst",
"date": "2025-02-02T10:22:34",
"stars": 1826,
"description": "Reproduce R1 Zero on Logic Puzzle",
"file_size": 4166
} |
Megatron-LM Backend
=====================
We support Megatron Backend by implementing various workers for actor,
critic, reference, rollout and reward models. We also implement the
``3DHybridEngine`` using Megatron-LM and vLLM in `megatron_vllm.py <https://github.com/volcengine/verl/blob/main/verl/trainer/ppo/hybrid_engine/megatron_vllm.py>`_.
**Pros**
- Support 3D parallelism and sequence parallelism for best scalablility
and throughput.
- 3D HybridEngine can significantly reduce peak memory usage and reduce
weight synchronize overhead between actor and rollout.
**Cons**
- Users should implement their own models for Megatron-LM
- Users should implement the corresponding weight_loader to
- synchronize the model weight between actor (in Megatron) and rollout
(in vLLM).
- load weights from checkpoints to corresponding model in Megatron-LM
Megatron Workers
----------------
MegatronWorker
^^^^^^^^^^^^^^
``MegatronWorker`` is the base class of different megatron worker
classes. In this class, ``get_megatron_global_info`` and
``get_megatron_rank_info`` function to retrive the 3D parallel world
size and rank of each ``Worker`` running on specific GPU. These information
will be used in transfer protocol for Megatron Backend.
The following ``Worker`` class for different models will be utilized to
construct the ``WorkerGroup`` .
We implement various of APIs for each ``Worker`` class decorated by the
``@register(dispatch_mode=)`` . These APIs can be called by the ray
driver process. The data can be correctly collect and dispatch following
the ``dispatch_mode`` on each function. The supported dispatch_model
(i.e., transfer protocols) can be found in `decorator.py <https://github.com/volcengine/verl/blob/main/verl/single_controller/base/decorator.py>`_.
ActorRolloutRefWorker
^^^^^^^^^^^^^^^^^^^^^
This class is implemented for Actor/Rollout HybridEngine or for the
reference model to initialize their model and perform computation.
Actor/Rollout HybridEngine
''''''''''''''''''''''''''
1. HybridEngine, Actor and Rollout initialization API.
.. code:: python
@register(dispatch_mode=Dispatch.ONE_TO_ALL)
def init_model(self):
``ONE_TO_ALL``: when calling the ``init_model`` function from the driver
process, each worker (on a GPU) will execute the following model
initialization process.
The initialization details of HybridEngine, Actor and Rollout are
highlighted below:
1. ``AllGatherPPModel`` holds memory buffer for both Actor and Rollout
and support weight resharding between actor and rollout.
2. ``MegatronPPOActor`` implements the simple PPO computation logics
when the model is built with Megatron, including compute log prob,
model update.
3. ``vLLMRollout`` support generation with vLLM. We modify the vLLM
Engine and make it executed under SPMD to fit into our
``WorkerGroup`` design.
4. ``MegatronVLLMShardingManager`` a context manager to perform actual
resharding between actor and rollout.
See `source code <https://github.com/volcengine/verl/blob/main/verl/trainer/ppo/workers/megatron_workers.py#L63>`_ for more information.
.. code:: python
# Initialize the 3D HybridEngine
hybrid_engine = AllGatherPPModel(model_provider=megatron_actor_model_provider)
# Fetch the model at current rank
actor_module = hybrid_engine.this_rank_models
...
# build actor model
self.actor = MegatronPPOActor(config=self.config.actor,
model_config=self.actor_model_config,
megatron_config=megatron_config,
actor_module=self.actor_module,
actor_optimizer=self.actor_optimizer,
actor_optimizer_config=self.actor_optim_config)
# build rollout
# rollout initialization
rollout = vLLMRollout(actor_module=params,
config=self.config.rollout,
tokenizer=self.tokenizer,
model_hf_config=self.actor_model_config,
train_tp=mpu.get_tensor_model_parallel_world_size())
# perform weight resharding between actor and rollout
sharding_manager = MegatronVLLMShardingManager(module=self.hybrid_engine,
inference_engine=rollout.inference_engine,
model_config=self.actor_model_config,
layer_name_mapping=layer_name_mapping)
...
2. Generate sequence and recompute log prob
.. code:: python
@register(dispatch_mode=Dispatch.MEGATRON_PP_AS_DP_PROTO)
def generate_sequences(self, prompts: DataProto):
- ``Dispatch.MEGATRON_PP_AS_DP_PROTO``: The PP dimension of the actor
model will be regarded as DP dimension. Then the driver process will
dispatch and collect the data according to this reorganization. This
is because, in HybridEngine, the actor weight, which usually applied
larger 3D parallel sizes, will be gathered along the PP dimension and
TP dimension. Therefore, the corresponding data should be dispatched
and collected through the 3D parallel group of the rollout model,
rather than the actor model. However, the world_size and rank
information can only be retrived from ``get_megatron_global_info`` and
``get_megatron_rank_info``, which records the 3D information for the
actor model. Moreover, the data resharding inside TP dimension will be
processed within the HybridEngine.
- In this function, the rollout model will perform auto-regressive
generation and the actor model will recompute the old log prob for the
generetad response.
3. Update actor model
.. code:: python
@register(dispatch_mode=Dispatch.MEGATRON_COMPUTE_PROTO)
def update_actor(self, data: DataProto):
- ``Dispatch.MEGATRON_COMPUTE_PROTO``: User passes the data partitioned
by DP dimension. The data is dispatched to all tp/pp ranks within the
same dp group, and ultimately only collects output data from tp=0 and
the last pp.
- Update the actor model weight using PPO & entropy loss.
ReferenceModel
''''''''''''''
1. Reference model initialization
The reference model is initialized using the same function as the actor
model without initializing the HybridEngine and Optimizer. Then the
actor model is also wrapped by the ``MegatronPPOActor``.
2. Compute reference log prob
.. code:: python
@register(dispatch_mode=Dispatch.MEGATRON_COMPUTE_PROTO)
def compute_ref_log_prob(self, data: DataProto):
- In this function, the reference model will call the compute log prob
function in ``MegatronPPOActor`` to compute the reference log prob.
CriticWorker and RewardWorker
^^^^^^^^^^^^^^^^^^^^^^^^^^^^^
1. Model initialization
Quite similar to reference model. The CriticWorker will perform
additional initialization for the Optimizer.
2. Compute Values for CriticWorker
.. code:: python
@register(dispatch_mode=Dispatch.MEGATRON_COMPUTE_PROTO)
def compute_values(self, data: DataProto):
3. Update Critic
.. code:: python
@register(dispatch_mode=Dispatch.MEGATRON_COMPUTE_PROTO)
def update_critic(self, data: DataProto):
4. Compute Reward
.. code:: python
@register(dispatch_mode=Dispatch.MEGATRON_COMPUTE_PROTO)
def compute_rm_score(self, data: DataProto):
Context Parallel
----------------
This require the developer/contributor to implement the context parallel
both in Megatron-LM and models. | {
"source": "Unakar/Logic-RL",
"title": "docs/workers/megatron_workers.rst",
"url": "https://github.com/Unakar/Logic-RL/blob/main/docs/workers/megatron_workers.rst",
"date": "2025-02-02T10:22:34",
"stars": 1826,
"description": "Reproduce R1 Zero on Logic Puzzle",
"file_size": 7477
} |
PPO Ray Trainer
===============
We implement the RayPPOTrainer, which is a trainer runs on the driver
process on a single CPU/GPU node (default is CPU).
The PPORayTrainer include 3 core functions for data preparation,
WorkerGroup initialization and PPO training loop.
Data Preparation
----------------
The ``PPORayTrainer``, as a single process, is responsible for loading a
complete batch of samples (prompts) from the dataset and then dispatch
to different worker_groups runnning on different GPUs.
To generalize the data loading, we implement the ``RLHFDataset`` class
to load the preprocessed parquet files, apply chat templates to the
prompts, add padding, truncate prompts that exceed max prompt length and
then tokenize.
.. code:: python
self.train_dataset = RLHFDataset(parquet_files=self.config.data.train_files,
tokenizer=self.tokenizer,
prompt_key=self.config.data.prompt_key,
max_prompt_length=self.config.data.max_prompt_length,
filter_prompts=True,
return_raw_chat=self.config.data.get('return_raw_chat', False),
truncation='error')
Then, the dataloader will iterate the dataset under PPO mini batch size.
WorkerGroup Initialization
--------------------------
We first introduce a basic implementation of initializing the
``WorkerGroup`` of the actor model on a given set of GPUs.
.. code:: python
# max_colocate_count means the number of WorkerGroups (i.e. processes) in each RayResourcePool
# For FSDP backend, we recommend using max_colocate_count=1 that merge all WorkerGroups into one.
# For Megatron backend, we recommend using max_colocate_count>1 that can utilize different WorkerGroup for differnt models
resource_pool = RayResourcePool(process_on_nodes=[config.trainer.n_gpus_per_node] * config.trainer.nnodes,
use_gpu=True,
max_colocate_count=1)
# define actor rollout cls to be init on remote
actor_rollout_cls = RayClassWithInitArgs(cls=ActorRolloutWorker)
# define actor_rollout worker group
actor_rollout_worker_group = MegatronRayWorkerGroup(resource_pool=resource_pool,
ray_cls_with_init=actor_rollout_cls,
default_megatron_kwargs=config.actor_rollout.megatron)
Different WorkerGroups, like ``actor_rollout_worker_group`` ,
``critic_worker_group`` and ``ref_worker_group`` lies on a separate
process in the above implementation.
The driver process can then call the distributed compute function within
the ``actor_rollout_worker_group`` and other roles to construct the RL
training loop.
For models colocated in the same set of GPUs, we further provide a
fine-grain optimization, which merge the ``worker_group`` of different roles
in the same process. This optimization can save the redundant
CUDA/distributed context in different processes.
.. code:: python
# initialize WorkerGroup
# NOTE: if you want to use a different resource pool for each role, which can support different parallel size,
# you should not use `create_colocated_worker_cls`. Instead, directly pass different resource pool to different worker groups.
# See TODO(url) for more information.
all_wg = {}
for resource_pool, class_dict in self.resource_pool_to_cls.items():
worker_dict_cls = create_colocated_worker_cls(class_dict=class_dict)
wg_dict = self.ray_worker_group_cls(resource_pool=resource_pool, ray_cls_with_init=worker_dict_cls)
spawn_wg = wg_dict.spawn(prefix_set=class_dict.keys())
all_wg.update(spawn_wg)
if self.use_critic:
self.critic_wg = all_wg['critic']
self.critic_wg.init_model()
if self.use_reference_policy:
self.ref_policy_wg = all_wg['ref']
self.ref_policy_wg.init_model()
if self.use_rm:
self.rm_wg = all_wg['rm']
self.rm_wg.init_model()
# we should create rollout at the end so that vllm can have a better estimation of kv cache memory
self.actor_rollout_wg = all_wg['actor_rollout']
self.actor_rollout_wg.init_model()
.. note:: For megatron backend, if we merge the ``worker_groups`` into the same processes, all the roles will utilize the same 3D parallel size. To optimize this, we may need to maintain several 3D process groups for each role in the same distributed context. If you want to use different 3D parallel size for different roles, please follow the similar architecture of the first code block to initialize each role's ``worker_group``
PPO Training Loop
-----------------
We implement the PPO training loop by calling the functions in
worker_group of each role. The input and output data of each function is
a ``DataProto`` object implemented in `protocol.py <https://github.com/volcengine/verl/blob/main/verl/protocol.py>`_. In the training
loop, trainer will dispatch/collect the data to/from different GPUs
following the transfer protocols wrapped in the workers' functions. The
computation of PPO micro batches is processed in ``update_actor`` and
``update_critic`` functions.
To extend to other RLHF algorithms, such as DPO, GRPO, please refer to
:doc:`../advance/dpo_extension`.
.. code:: python
def fit(self):
"""
The training loop of PPO.
The driver process only need to call the compute functions of the worker group through RPC to construct the PPO dataflow.
The light-weight advantage computation is done on the driver process.
"""
from verl.utils.tracking import Tracking
from omegaconf import OmegaConf
logger = Tracking(project_name=self.config.trainer.project_name,
experiment_name=self.config.trainer.experiment_name,
default_backend=self.config.trainer.logger,
config=OmegaConf.to_container(self.config, resolve=True))
global_steps = 0
# perform validation before training
# currently, we only support validation using the reward_function.
if self.val_reward_fn is not None:
val_metrics = self._validate()
pprint(f'Initial validation metrics: {val_metrics}')
for epoch in range(self.config.trainer.total_epochs):
for batch_dict in self.train_dataloader:
metrics = {}
batch: DataProto = DataProto.from_single_dict(batch_dict)
# batch = batch.to('cuda')
# pop those keys for generation
gen_batch = batch.pop(batch_keys=['input_ids', 'attention_mask', 'position_ids'])
# generate a batch
with Timer(name='gen', logger=None) as timer:
gen_batch_output = self.actor_rollout_wg.generate_sequences(gen_batch)
metrics['timing/gen'] = timer.last
batch = batch.union(gen_batch_output)
if self.use_reference_policy:
# compute reference log_prob
with Timer(name='ref', logger=None) as timer:
ref_log_prob = self.ref_policy_wg.compute_ref_log_prob(batch)
batch = batch.union(ref_log_prob)
metrics['timing/ref'] = timer.last
# compute values
with Timer(name='values', logger=None) as timer:
values = self.critic_wg.compute_values(batch)
batch = batch.union(values)
metrics['timing/values'] = timer.last
with Timer(name='adv', logger=None) as timer:
# compute scores. Support both model and function-based.
# We first compute the scores using reward model. Then, we call reward_fn to combine
# the results from reward model and rule-based results.
if self.use_rm:
# we first compute reward model score
reward_tensor = self.rm_wg.compute_rm_score(batch)
batch = batch.union(reward_tensor)
# we combine with rule-based rm
reward_tensor = self.reward_fn(batch)
batch.batch['token_level_scores'] = reward_tensor
# compute rewards. apply_kl_penalty if available
batch, kl_metrics = apply_kl_penalty(batch,
kl_ctrl=self.kl_ctrl,
kl_penalty=self.config.algorithm.kl_penalty)
metrics.update(kl_metrics)
# compute advantages, executed on the driver process
batch = compute_advantage(batch,
self.config.algorithm.gamma,
self.config.algorithm.lam,
adv_estimator=self.config.algorithm.adv_estimator)
metrics['timing/adv'] = timer.last
# update critic
if self.use_critic:
with Timer(name='update_critic', logger=None) as timer:
critic_output = self.critic_wg.update_critic(batch)
metrics['timing/update_critic'] = timer.last
critic_output_metrics = reduce_metrics(critic_output.meta_info['metrics'])
metrics.update(critic_output_metrics)
# implement critic warmup
if self.config.trainer.critic_warmup <= global_steps:
# update actor
with Timer(name='update_actor', logger=None) as timer:
actor_output = self.actor_rollout_wg.update_actor(batch)
metrics['timing/update_actor'] = timer.last
actor_output_metrics = reduce_metrics(actor_output.meta_info['metrics'])
metrics.update(actor_output_metrics)
# validate
if self.val_reward_fn is not None and (global_steps + 1) % self.config.trainer.test_freq == 0:
with Timer(name='testing', logger=None) as timer:
val_metrics: dict = self._validate()
val_metrics = {f'val/{key}': val for key, val in val_metrics.items()}
metrics['timing/testing'] = timer.last
metrics.update(val_metrics)
# collect metrics
data_metrics = compute_data_metrics(batch=batch)
metrics.update(data_metrics)
# TODO: make a canonical logger that supports various backend
logger.log(data=metrics, step=global_steps)
if self.config.trainer.save_freq > 0 and (global_steps + 1) % self.config.trainer.save_freq == 0:
actor_local_path = os.path.join(self.config.trainer.default_local_dir, 'actor',
f'global_step_{global_steps}')
actor_remote_path = os.path.join(self.config.trainer.default_hdfs_dir, 'actor')
self.actor_rollout_wg.save_checkpoint(actor_local_path, actor_remote_path)
if self.use_critic:
critic_local_path = os.path.join(self.config.trainer.default_local_dir, 'critic',
f'global_step_{global_steps}')
critic_remote_path = os.path.join(self.config.trainer.default_hdfs_dir, 'critic')
self.critic_wg.save_checkpoint(critic_local_path, critic_remote_path)
global_steps += 1
# perform validation after training
if self.val_reward_fn is not None:
val_metrics = self._validate()
pprint(f'Final validation metrics: {val_metrics}') | {
"source": "Unakar/Logic-RL",
"title": "docs/workers/ray_trainer.rst",
"url": "https://github.com/Unakar/Logic-RL/blob/main/docs/workers/ray_trainer.rst",
"date": "2025-02-02T10:22:34",
"stars": 1826,
"description": "Reproduce R1 Zero on Logic Puzzle",
"file_size": 12036
} |
# Split Placement Example
Here we introduce how to run the naive implementation of the split placement of PPO algorithm.
We will release the complete version of flexible placement in the near future.
For quickstart, you can only follow Step 2 to modify the code and then follow Step 4 to execute the split placement example.
### Step 1: Placing the models to different GPUs
Specify the placement and resource allocation. In the example, we place the actor and reference in the first half of the GPUs while map the critic and reward model (if any) to the second half of the GPUs.
```python
actor_rollout_ref_pool_id = 'actor_rollout_ref_pool'
critic_pool_id = 'critic_pool'
if config.trainer.nnodes // 2 == 0 and config.trainer.n_gpus_per_node // 2 > 0:
resource_pool_spec = {
actor_rollout_ref_pool_id: [config.trainer.n_gpus_per_node // 2] * config.trainer.nnodes,
critic_pool_id: [config.trainer.n_gpus_per_node // 2] * config.trainer.nnodes,
}
else:
resource_pool_spec = {
actor_rollout_ref_pool_id: [config.trainer.n_gpus_per_node] * (config.trainer.nnodes // 2),
critic_pool_id: [config.trainer.n_gpus_per_node] * (config.trainer.nnodes // 2),
}
print(f'resource_pool_spec: {resource_pool_spec}')
mapping = {
Role.ActorRollout: actor_rollout_ref_pool_id,
Role.Critic: critic_pool_id,
Role.RefPolicy: actor_rollout_ref_pool_id,
}
mapping[Role.RewardModel] = critic_pool_id
```
### Step 2: Make the models executed asynchronously
Based on the model placement, we need to make the models executed asynchronously.
To do so, you need to turn off the `blocking` flag (i.e., `blocking=False`) in our decorator of some model operations.
For example, we hope the actor update and critic update can be executed in parallel, then we need to make the following modification in `fsdp_workers.py`
```
@register(dispatch_mode=Dispatch.DP_COMPUTE_PROTO, blocking=False)
def update_actor(self, data: DataProto):
...
@register(dispatch_mode=Dispatch.DP_COMPUTE_PROTO, blocking=False)
def update_critic(self, data: DataProto):
...
```
We can also parallelize the computation of `ref_log_prob` and `values` and `rewards` in the split placement. For simplicity of the tutorial, we
### Step 3: Execute these operation in parallel in the single controller process
To implement the parallel execution of the actor and critic update, the only thing we need to modify in the `ray_trainer.py` is to `get` the concurrent `futures` on the single controller process.
```python
critic_output = critic_output.get()
actor_output = actor_output.get()
```
### Step 4: Run the split placement example
```
bash run_deepseek7b_llm.sh
``` | {
"source": "Unakar/Logic-RL",
"title": "examples/split_placement/README.md",
"url": "https://github.com/Unakar/Logic-RL/blob/main/examples/split_placement/README.md",
"date": "2025-02-02T10:22:34",
"stars": 1826,
"description": "Reproduce R1 Zero on Logic Puzzle",
"file_size": 2686
} |
# Models
Common modelzoo such as huggingface/transformers stuggles when using Pytorch native model parallelism. Following the design principle of vLLM, we keep a simple, parallelizable, highly-optimized with packed inputs in verl.
## Adding a New Huggingface Model
### Step 1: Copy the model file from HF to verl
- Add a new file under verl/models/hf
- Copy ONLY the model file from huggingface/transformers/models to verl/models/hf
### Step 2: Modify the model file to use packed inputs
- Remove all the code related to inference (kv cache)
- Modify the inputs to include only
- input_ids (total_nnz,)
- cu_seqlens (total_nnz + 1,)
- max_seqlen_in_batch: int
- Note that this requires using flash attention with causal mask.
### Step 2.5: Add tests
- Add a test to compare this version and the huggingface version
- Following the infrastructure and add tests to tests/models/hf
### Step 3: Add a function to apply tensor parallelism
- Please follow
- https://pytorch.org/docs/stable/distributed.tensor.parallel.html
- https://pytorch.org/tutorials/intermediate/TP_tutorial.html
- General comments
- Tensor Parallelism in native Pytorch is NOT auto-parallelism. The way it works is to specify how model parameters and input/output reshards using configs. These configs are then registered as hooks to perform input/output resharding before/after model forward.
### Step 4: Add a function to apply data parallelism
- Please use FSDP2 APIs
- See demo here https://github.com/pytorch/torchtitan/blob/main/torchtitan/parallelisms/parallelize_llama.py#L413
### Step 5: Add a function to apply pipeline parallelism
- Comes in Pytorch 2.4
- Currently only in alpha in nightly version
- Check torchtitan for more details | {
"source": "Unakar/Logic-RL",
"title": "verl/models/README.md",
"url": "https://github.com/Unakar/Logic-RL/blob/main/verl/models/README.md",
"date": "2025-02-02T10:22:34",
"stars": 1826,
"description": "Reproduce R1 Zero on Logic Puzzle",
"file_size": 1742
} |
# Detached Worker
## How to run (Only on a single node)
- Start a local ray cluster:
```bash
ray start --head --port=6379
```
- Run the server
```bash
python3 server.py
```
- On another terminal, Run the client
```bash
python3 client.py
``` | {
"source": "Unakar/Logic-RL",
"title": "tests/ray/detached_worker/README.md",
"url": "https://github.com/Unakar/Logic-RL/blob/main/tests/ray/detached_worker/README.md",
"date": "2025-02-02T10:22:34",
"stars": 1826,
"description": "Reproduce R1 Zero on Logic Puzzle",
"file_size": 241
} |
# Dataset Format
## RLHF dataset
We combine all the data sources into a single parquet files. We directly organize the prompt into the chat format so that multi-turn chats can be easily incorporated. In the prompt, we may add instruction following texts to guide the model output the answers in a particular format so that we can extract the answers.
Math problems
```json
{
"data_source": "openai/gsm8k",
"prompt": [{"role": "user", "content": "Natalia sold clips to 48 of her friends in April, and then she sold half as many clips in May. How many clips did Natalia sell altogether in April and May? Let's think step by step and output the final answer after \"####\""}],
"ability": "math",
"reward_model": {
"style": "rule",
"ground_truth": ["72"]
},
}
``` | {
"source": "Unakar/Logic-RL",
"title": "verl/utils/dataset/README.md",
"url": "https://github.com/Unakar/Logic-RL/blob/main/verl/utils/dataset/README.md",
"date": "2025-02-02T10:22:34",
"stars": 1826,
"description": "Reproduce R1 Zero on Logic Puzzle",
"file_size": 796
} |
# Digit completion
This is an example of solving a digit completion problem. The problem is defined as below:
The prompt is a sequence of numbers with fixed difference. The agent's goal is to complete the next N numbers.
If the max number is reached, the next number should be modulo with max number.
For example,
- prompt = [1, 2, 3]
- N = 5
- max_number = 6
The response should be [4, 5, 6, 7%6, 8%6] = [4, 5, 6, 0, 1].
# Environment definition
The core definition of the task is defined in verl/envs/digit_completion/task.py
It is highly recommended to take a look at it for better understanding.
# Run experiments
The users are required to specify the config path and config name (and the relative model config path to the current working directory)
```bash
# cd examples/arithmetic_sequence/rl
# Specify the config path and config name (current working dir)
python3 -m verl.trainer.ppo.ray_megatron_train_synchronous --config-path=$(pwd)/config --config-name='ray_megatron'
# The default relative path of model config is 'config/model_config', if you want to change it, you can rewrite it in ray_megatron.yaml or using:
python3 -m verl.trainer.ppo.ray_megatron_train_synchronous --config-path=$(pwd)/config --config-name='ray_megatron' ++model.base_path=config/model_config
``` | {
"source": "Unakar/Logic-RL",
"title": "tests/e2e/arithmetic_sequence/rl/README.md",
"url": "https://github.com/Unakar/Logic-RL/blob/main/tests/e2e/arithmetic_sequence/rl/README.md",
"date": "2025-02-02T10:22:34",
"stars": 1826,
"description": "Reproduce R1 Zero on Logic Puzzle",
"file_size": 1297
} |
# How to contribute
We'd love to accept your patches and contributions to this project.
## Before you begin
### Sign our Contributor License Agreement
Contributions to this project must be accompanied by a
[Contributor License Agreement](https://cla.developers.google.com/about) (CLA).
You (or your employer) retain the copyright to your contribution; this simply
gives us permission to use and redistribute your contributions as part of the
project.
If you or your current employer have already signed the Google CLA (even if it
was for a different project), you probably don't need to do it again.
Visit <https://cla.developers.google.com/> to see your current agreements or to
sign a new one.
### Review our community guidelines
This project follows
[Google's Open Source Community Guidelines](https://opensource.google/conduct/).
## Contribution process
### Code reviews
All submissions, including submissions by project members, require review. We
use GitHub pull requests for this purpose. Consult
[GitHub Help](https://help.github.com/articles/about-pull-requests/) for more
information on using pull requests. | {
"source": "google-gemini/multimodal-live-api-web-console",
"title": "CONTRIBUTING.md",
"url": "https://github.com/google-gemini/multimodal-live-api-web-console/blob/main/CONTRIBUTING.md",
"date": "2024-12-09T17:35:48",
"stars": 1818,
"description": "A react-based starter app for using the Multimodal Live API over websockets with Gemini",
"file_size": 1128
} |
# Multimodal Live API - Web console
This repository contains a react-based starter app for using the [Multimodal Live API](<[https://ai.google.dev/gemini-api](https://ai.google.dev/api/multimodal-live)>) over a websocket. It provides modules for streaming audio playback, recording user media such as from a microphone, webcam or screen capture as well as a unified log view to aid in development of your application.
[](https://www.youtube.com/watch?v=J_q7JY1XxFE)
Watch the demo of the Multimodal Live API [here](https://www.youtube.com/watch?v=J_q7JY1XxFE).
## Usage
To get started, [create a free Gemini API key](https://aistudio.google.com/apikey) and add it to the `.env` file. Then:
```
$ npm install && npm start
```
We have provided several example applications on other branches of this repository:
- [demos/GenExplainer](https://github.com/google-gemini/multimodal-live-api-web-console/tree/demos/genexplainer)
- [demos/GenWeather](https://github.com/google-gemini/multimodal-live-api-web-console/tree/demos/genweather)
- [demos/GenList](https://github.com/google-gemini/multimodal-live-api-web-console/tree/demos/genlist)
## Example
Below is an example of an entire application that will use Google Search grounding and then render graphs using [vega-embed](https://github.com/vega/vega-embed):
```typescript
import { type FunctionDeclaration, SchemaType } from "@google/generative-ai";
import { useEffect, useRef, useState, memo } from "react";
import vegaEmbed from "vega-embed";
import { useLiveAPIContext } from "../../contexts/LiveAPIContext";
export const declaration: FunctionDeclaration = {
name: "render_altair",
description: "Displays an altair graph in json format.",
parameters: {
type: SchemaType.OBJECT,
properties: {
json_graph: {
type: SchemaType.STRING,
description:
"JSON STRING representation of the graph to render. Must be a string, not a json object",
},
},
required: ["json_graph"],
},
};
export function Altair() {
const [jsonString, setJSONString] = useState<string>("");
const { client, setConfig } = useLiveAPIContext();
useEffect(() => {
setConfig({
model: "models/gemini-2.0-flash-exp",
systemInstruction: {
parts: [
{
text: 'You are my helpful assistant. Any time I ask you for a graph call the "render_altair" function I have provided you. Dont ask for additional information just make your best judgement.',
},
],
},
tools: [{ googleSearch: {} }, { functionDeclarations: [declaration] }],
});
}, [setConfig]);
useEffect(() => {
const onToolCall = (toolCall: ToolCall) => {
console.log(`got toolcall`, toolCall);
const fc = toolCall.functionCalls.find(
(fc) => fc.name === declaration.name
);
if (fc) {
const str = (fc.args as any).json_graph;
setJSONString(str);
}
};
client.on("toolcall", onToolCall);
return () => {
client.off("toolcall", onToolCall);
};
}, [client]);
const embedRef = useRef<HTMLDivElement>(null);
useEffect(() => {
if (embedRef.current && jsonString) {
vegaEmbed(embedRef.current, JSON.parse(jsonString));
}
}, [embedRef, jsonString]);
return <div className="vega-embed" ref={embedRef} />;
}
```
## development
This project was bootstrapped with [Create React App](https://github.com/facebook/create-react-app).
Project consists of:
- an Event-emitting websocket-client to ease communication between the websocket and the front-end
- communication layer for processing audio in and out
- a boilerplate view for starting to build your apps and view logs
## Available Scripts
In the project directory, you can run:
### `npm start`
Runs the app in the development mode.\
Open [http://localhost:3000](http://localhost:3000) to view it in the browser.
The page will reload if you make edits.\
You will also see any lint errors in the console.
### `npm run build`
Builds the app for production to the `build` folder.\
It correctly bundles React in production mode and optimizes the build for the best performance.
The build is minified and the filenames include the hashes.\
Your app is ready to be deployed!
See the section about [deployment](https://facebook.github.io/create-react-app/docs/deployment) for more information.
_This is an experiment showcasing the Multimodal Live API, not an official Google product. We’ll do our best to support and maintain this experiment but your mileage may vary. We encourage open sourcing projects as a way of learning from each other. Please respect our and other creators' rights, including copyright and trademark rights when present, when sharing these works and creating derivative work. If you want more info on Google's policy, you can find that [here](https://developers.google.com/terms/site-policies)._ | {
"source": "google-gemini/multimodal-live-api-web-console",
"title": "README.md",
"url": "https://github.com/google-gemini/multimodal-live-api-web-console/blob/main/README.md",
"date": "2024-12-09T17:35:48",
"stars": 1818,
"description": "A react-based starter app for using the Multimodal Live API over websockets with Gemini",
"file_size": 4947
} |
# fullmoon iOS
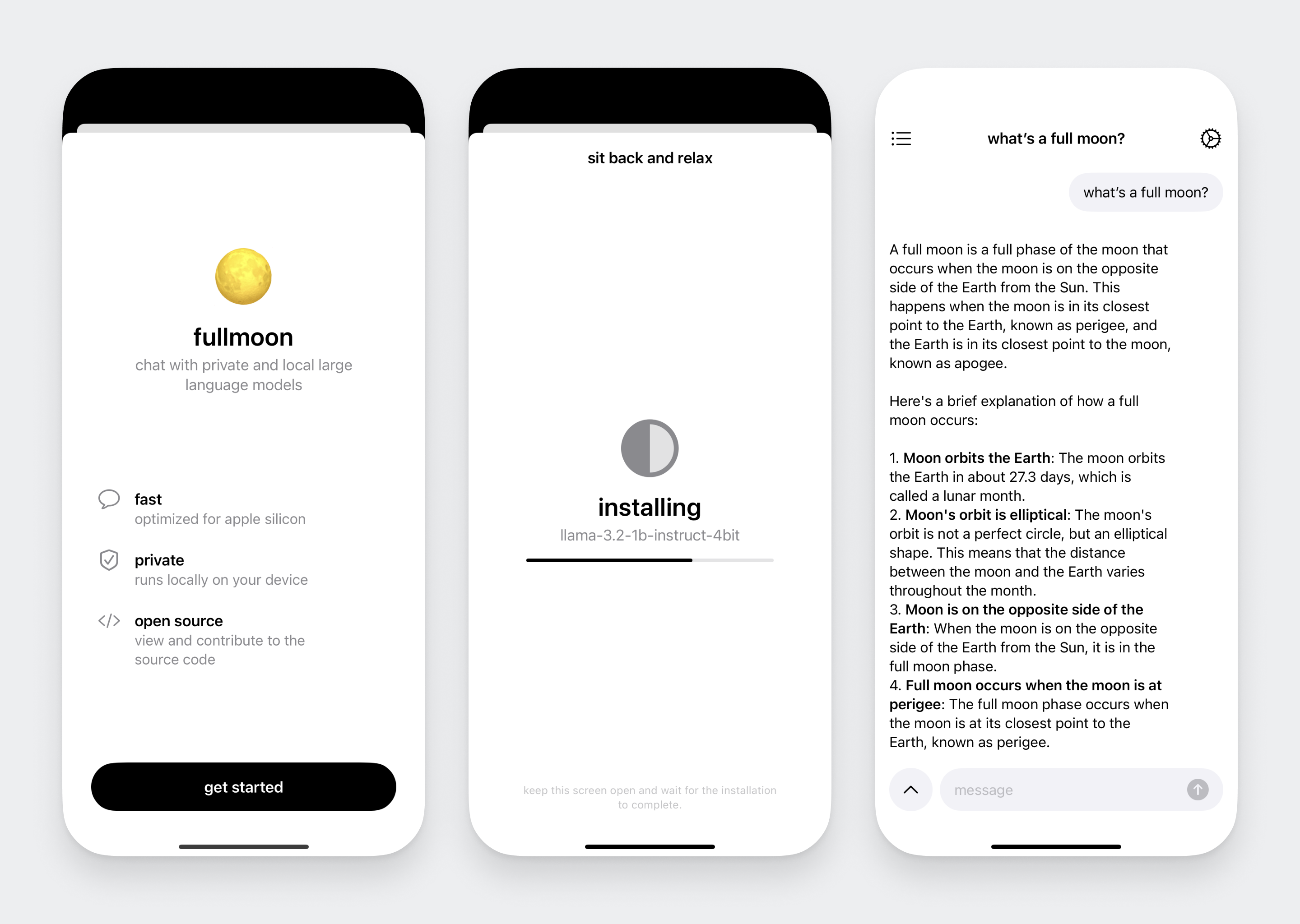
[fullmoon](https://fullmoon.app) is an iOS app to chat with local large language models that’s optimized for Apple silicon and works on iPhone, iPad, and Mac. your chat history is saved locally, and you can customize the appearance of the app.
## supported models
- [x] [Llama 3.2 1B](https://huggingface.co/mlx-community/Llama-3.2-1B-Instruct-4bit)
- [x] [Llama 3.2 3B](https://huggingface.co/mlx-community/Llama-3.2-3B-Instruct-4bit)
- [x] [DeepSeek-R1-Distill-Qwen-1.5B-4bit](https://huggingface.co/mlx-community/DeepSeek-R1-Distill-Qwen-1.5B-4bit)
## credits
fullmoon is made possible by [MLX Swift](https://github.com/ml-explore/mlx-swift) from Apple. [MLX](https://github.com/ml-explore/mlx) is an array framework for machine learning research on Apple silicon. | {
"source": "mainframecomputer/fullmoon-ios",
"title": "README.md",
"url": "https://github.com/mainframecomputer/fullmoon-ios/blob/main/README.md",
"date": "2024-10-09T01:18:59",
"stars": 1805,
"description": "chat with private and local large language models",
"file_size": 838
} |
## Introduction
We are committed to providing a friendly, safe, and welcoming environment for everyone involved in the **Inspira UI** project. This Code of Conduct outlines our expectations for participant behavior as well as the consequences for unacceptable conduct.
## Our Pledge
In the interest of fostering an open and inclusive community, we pledge to make participation in our project and community a harassment-free experience for everyone, regardless of:
- Age
- Body size
- Disability
- Ethnicity
- Gender identity and expression
- Level of experience
- Nationality
- Personal appearance
- Race
- Religion
- Sexual identity and orientation
## Expected Behavior
All participants in our community are expected to:
- **Be Respectful**: Show empathy and kindness towards others.
- **Be Considerate**: Remember that your actions and words affect others.
- **Be Collaborative**: Work together to achieve common goals.
- **Communicate Effectively**: Use clear and constructive language.
- **Demonstrate Professionalism**: Act professionally and take responsibility for your actions.
## Unacceptable Behavior
The following behaviors are considered unacceptable within our community:
- **Harassment and Discrimination**: Including derogatory comments, slurs, or unwanted sexual attention.
- **Abuse and Threats**: Any form of verbal or written abuse, intimidation, or threats.
- **Trolling and Insults**: Provocative or insulting remarks intended to disrupt conversations.
- **Disrespectful Communication**: Including excessive profanity, shouting (using all caps), or interrupting others.
- **Personal Attacks**: Targeting an individual with the intent to harass or belittle.
## Reporting Guidelines
If you experience or witness unacceptable behavior, or have any other concerns, please report it as soon as possible by contacting the project maintainers on our **Discord channel**:
[Inspira UI Discord Channel](https://discord.gg/Xbh5DwJRc9)
When reporting an incident, please include:
- **Your Contact Information**: Your Discord username or any preferred method of contact.
- **Names of Those Involved**: Real names or usernames of the individuals involved.
- **Description of the Incident**: A clear and concise account of what happened.
- **Supporting Evidence**: Any relevant messages, screenshots, or context that can help us understand the situation.
All reports will be handled confidentially.
## Enforcement
Project maintainers are responsible for ensuring compliance with this Code of Conduct and will take appropriate action in response to any behavior that is deemed unacceptable. Actions may include:
- A private warning to the offender.
- Temporary or permanent ban from participation in the project and Discord channel.
- Removal of contributions that violate the Code of Conduct.
## Scope
This Code of Conduct applies to all project spaces, including but not limited to:
- GitHub repositories
- Issue trackers
- Pull requests
- Project-related forums and chat channels
- Social media interactions pertaining to the project
- The official **Inspira UI Discord channel**
It also applies when an individual is representing the project or its community in public spaces.
## Appeal Process
Any individual who is subjected to disciplinary action has the right to appeal the decision by contacting the project maintainers through the **Discord channel** within one week of the action. The appeal will be reviewed, and a final decision will be communicated.
## Privacy
All reports of unacceptable behavior will be handled with discretion. We will respect the privacy of the reporter and the accused.
## Acknowledgments
We thank all contributors and community members for helping to create a positive environment. This Code of Conduct is adapted from best practices and guidelines used in open-source communities.
## Contact Information
For questions or concerns about this Code of Conduct, please contact the project maintainers on our **Discord channel**:
[Inspira UI Discord Channel](https://discord.gg/Xbh5DwJRc9) | {
"source": "unovue/inspira-ui",
"title": "CODE_OF_CONDUCT.md",
"url": "https://github.com/unovue/inspira-ui/blob/main/CODE_OF_CONDUCT.md",
"date": "2024-08-30T08:20:57",
"stars": 1788,
"description": "Build beautiful website using Vue & Nuxt.",
"file_size": 4059
} |
Thank you for your interest in contributing to the **Inspira UI** project! Your contributions help make this project better for everyone. Please take a moment to read through these guidelines to ensure a smooth collaboration.
## Table of Contents
1. [Getting Started](#getting-started)
2. [Code of Conduct](#code-of-conduct)
3. [How to Contribute](#how-to-contribute)
- [Reporting Bugs](#reporting-bugs)
- [Suggesting Enhancements](#suggesting-enhancements)
- [Adding New Components](#adding-new-components)
4. [Project Structure](#project-structure)
5. [Style Guidelines](#style-guidelines)
- [Coding Standards](#coding-standards)
- [Component Format](#component-format)
- [Commit Messages](#commit-messages)
6. [Documentation Guidelines](#documentation-guidelines)
- [Single-File Components](#single-file-components)
- [Multi-File Components](#multi-file-components)
7. [Testing](#testing)
8. [License](#license)
---
## Getting Started
- **Fork the Repository**: Create a personal fork of the project on GitHub.
- **Clone Your Fork**: Clone your forked repository to your local machine.
- **Create a Branch**: Create a new branch for your contribution (`git checkout -b feature/YourFeatureName`).
- **Install Dependencies**: Run `npm install` to install all necessary dependencies.
## Code of Conduct
By participating in this project, you agree to abide by the [Code of Conduct](./CODE_OF_CONDUCT.md), which aims to foster an open and welcoming environment.
## How to Contribute
### Reporting Bugs
If you find a bug, please open an [issue](https://github.com/unovue/inspira-ui/issues){:target="\_blank"} and include:
- A clear and descriptive title.
- Steps to reproduce the issue.
- Expected and actual results.
- Screenshots or code snippets, if applicable.
### Suggesting Enhancements
We welcome suggestions for new features or improvements. Please open an [issue](https://github.com/unovue/inspira-ui/issues){:target="\_blank"} and include:
- A clear and descriptive title.
- A detailed description of the enhancement.
- Any relevant examples or mockups.
### Adding New Components
We appreciate contributions that add new components to the library. Please ensure that:
- The component is generally useful and aligns with the project's goals.
- The component is compatible with both **Nuxt** and **Vue**.
- You follow the coding and documentation guidelines outlined below.
- You include unit tests for your component.
## Project Structure
Understanding the project structure is crucial for effective contribution:
- **Components Directory**:
- Main components should be placed in `components/content/inspira/ui/<component-folder-name>/`.
- Example components should be placed in `components/content/inspira/examples/<component-folder-name>/`.
- **Documentation**:
- Documentation files are located in the `content/2.components/` directory.
- After adding a component, write its documentation in this directory.
## Style Guidelines
### Coding Standards
- **Language**: All components should be written in **Vue.js** with TypeScript support.
- **Styling**: Use **Tailwind CSS** classes for styling whenever possible.
- **Naming Conventions**: Use `PascalCase` for component names and filenames.
### Component Format
Your Vue components should adhere to the following structure:
```vue
<!-- Template -->
<template>
<!-- Your template code goes here -->
</template>
<!-- Script -->
<script lang="ts" setup>
// Your script code goes here
</script>
<!-- Styles (if required) -->
<style scoped>
/* Your styles go here */
</style>
```
**Notes:**
- Use `<script lang="ts" setup>` for TypeScript and the Composition API.
- Keep styles scoped to prevent conflicts.
- Ensure compatibility with both **Nuxt** and **Vue**.
### Commit Messages
- Use the [Conventional Commits](https://www.conventionalcommits.org/) format.
- Begin with a type (`feat`, `fix`, `docs`, etc.) followed by a short description.
- Example: `feat: add TextHoverEffect component`
## Documentation Guidelines
Proper documentation is crucial for users to understand and effectively use the components. Follow these guidelines when adding documentation for new components.
### Steps to Add a New Component
1. **Create the Component**
- Place the main component in `components/content/inspira/ui/<component-folder-name>/`.
- Follow the [Component Format](#component-format) specified above.
- If the component requires examples or demos, add demo components to `components/content/inspira/examples/<component-folder-name>/`.
2. **Write Documentation**
- Add a new Markdown file in `content/2.components/` for your component's documentation.
- Use the appropriate template based on whether your component is single-file or multi-file (see below).
- Mention the **Credits** and source if the component is ported from any other UI library or taken inspiration from any other source.
3. **Ensure Compatibility**
- Test your component in both **Nuxt** and **Vue** environments.
### Single-File Components
For components that are contained within a single `.vue` file, use the following template:
1. **Front Matter**
Begin with YAML front matter that includes the `title` and `description`:
```yaml
---
title: Your Component Title
description: A brief description of what your component does.
---
```
2. **Preview Section**
Use the `ComponentLoader` to display a live preview of the component. The `id` should be set to the folder name of your component in `components/content/inspira/examples/`. In case, there is no folder, then `id` is not required.
```markdown
::ComponentLoader{label="Preview" componentName="YourComponentDemo" type="examples" id="your-component-folder-name"}
::
```
3. **API Documentation**
Provide a table listing all props:
```markdown
## API
| Prop Name | Type | Default | Description |
| --------- | -------- | ------- | --------------------- |
| `prop1` | `string` | `''` | Description of prop1. |
| `prop2` | `number` | `0` | Description of prop2. |
```
4. **Component Code**
Include the full code of the component using `CodeViewer`. The `id` should be set to the folder name of your component in `components/content/inspira/ui/`.
```markdown
## Component Code
You can copy and paste the following code to create this component:
::CodeViewer{filename="YourComponent.vue" language="vue" componentName="YourComponent" type="ui" id="your-component-folder-name"}
::
```
**Example:**
```markdown
---
title: Text Hover Effect
description: A text hover effect that animates and outlines gradient on hover.
---
::ComponentLoader{label="Preview" componentName="TextHoverEffectDemo" type="examples" id="text-hover-effect"}
::
## API
| Prop Name | Type | Default | Description |
| ------------- | -------- | -------- | --------------------------------------------------------- |
| `text` | `string` | Required | The text to be displayed with the hover effect. |
| `duration` | `number` | `200` | The duration of the mask transition animation in seconds. |
| `strokeWidth` | `number` | `0.75` | The width of the text stroke. |
| `opacity` | `number` | `null` | The opacity of the text. |
## Component Code
You can copy and paste the following code to create this component:
::CodeViewer{filename="TextHoverEffect.vue" language="vue" componentName="TextHoverEffect" type="ui" id="text-hover-effect"}
::
```
In this example, the `id` used in both `ComponentLoader` and `CodeViewer` is `text-hover-effect`, which matches the folder name where the component and its demo are stored.
### Multi-File Components
For components that consist of multiple files, such as a main component and several sub-components or variants, use the following template:
1. **Front Matter**
Begin with YAML front matter:
```yaml
---
title: Your Components Group Title
description: A brief description of what this group of components does.
---
```
2. **Preview Sections**
Include multiple `ComponentLoader` sections for each example or variant. The `id` should be set to the folder name of your component in `components/content/inspira/examples/`.
```markdown
::ComponentLoader{label="Preview" componentName="ComponentVariantDemo" type="examples" id="your-component-folder-name"}
::
```
3. **API Documentation**
Provide comprehensive API documentation covering all components:
```markdown
## API
| Prop Name | Type | Default | Description |
| --------- | -------- | ------- | --------------------------------------- |
| `prop1` | `string` | `''` | Description applicable to all variants. |
```
4. **Utility Classes**
If applicable, list any utility classes:
```markdown
### Utility Classes
- **Class Name:** `class-description`
```
5. **Component Code**
Use a `code-group` to group multiple `CodeViewerTab` components, one for each file. The `id` should be set to the folder name of your component in `components/content/inspira/ui/`.
```markdown
## Component Code
You can copy and paste the following code to create these components:
::code-group
::CodeViewerTab{label="MainComponent.vue" language="vue" componentName="MainComponent" type="ui" id="your-component-folder-name"}
::
::CodeViewerTab{filename="VariantOne.vue" language="vue" componentName="VariantOne" type="ui" id="your-component-folder-name"}
::
::CodeViewerTab{filename="VariantTwo.vue" language="vue" componentName="VariantTwo" type="ui" id="your-component-folder-name"}
::
::
```
**Example:**
```markdown
---
title: Pattern Backgrounds
description: Simple animated pattern backgrounds to make your sections stand out.
---
::ComponentLoader{label="Preview" componentName="DotBackgroundDemo" type="examples" id="pattern-backgrounds"}
::
## Examples
Grid background without animation:
::ComponentLoader{label="Preview" componentName="GridBackgroundDemo" type="examples" id="pattern-backgrounds"}
::
Small grid background with animation:
::ComponentLoader{label="Preview" componentName="GridSmallBackgroundDemo" type="examples" id="pattern-backgrounds"}
::
## API
| Prop Name | Type | Default | Description |
| ----------- | -------------------------------------------------------------------------------------------------------- | ------- | ------------------------------------- |
| `animate` | `boolean` | `false` | Set `true` to animate the background. |
| `direction` | `'top' \| 'bottom' \| 'left' \| 'right' \| 'top-left' \| 'top-right' \| 'bottom-left' \| 'bottom-right'` | `'top'` | Direction of the animation movement. |
### Utility Classes
- **Dot Pattern:** `dark:bg-dot-white/[0.2] bg-dot-black/[0.2]`
- **Grid Pattern:** `dark:bg-grid-white/[0.2] bg-grid-black/[0.2]`
- **Small Grid Pattern:** `dark:bg-grid-small-white/[0.2] bg-grid-small-black/[0.2]`
## Component Code
You can copy and paste the following code to create these components:
::code-group
::CodeViewerTab{label="PatternBackground.vue" language="vue" componentName="PatternBackground" type="ui" id="pattern-backgrounds"}
::
::CodeViewerTab{filename="DotBackground.vue" language="vue" componentName="DotBackground" type="ui" id="pattern-backgrounds"}
::
::CodeViewerTab{filename="GridBackground.vue" language="vue" componentName="GridBackground" type="ui" id="pattern-backgrounds"}
::
::CodeViewerTab{filename="GridSmallBackground.vue" language="vue" componentName="GridSmallBackground" type="ui" id="pattern-backgrounds"}
::
::
```
In this example, the `id` used in `ComponentLoader` and all `CodeViewerTab` components is `pattern-backgrounds`, which matches the folder name where the components and their demos are stored.
## Testing
- **Unit Tests**: Write unit tests for your component if applicable.
- **Cross-Environment Testing**: Ensure that your component works correctly in both **Nuxt** and **Vue** environments.
- **Visual Testing**: Check the component visually to ensure it renders correctly.
## Additional Notes
- **Component Names**: Use `PascalCase` for component filenames and names.
- **IDs**: In `CodeViewer`, `CodeViewerTab`, and `ComponentLoader`, the `id` parameter should be set to the **folder name** where the component is stored in `components/content/inspira/ui/<component-folder-name>/` and `components/content/inspira/examples/<component-folder-name>/`. This helps in correctly linking the code and examples in the documentation.
- **Demo Components**: For each component, create a corresponding `Demo` component used in the `ComponentLoader` for previews, and place it in `components/content/inspira/examples/<component-folder-name>/`.
- **Localization**: If your component supports multiple languages, include details in the documentation.
## License
By contributing, you agree that your contributions will be licensed under the [MIT License](https://github.com/unovue/inspira-ui/blob/main/LICENSE){:target="\_blank"}. | {
"source": "unovue/inspira-ui",
"title": "CONTRIBUTING.md",
"url": "https://github.com/unovue/inspira-ui/blob/main/CONTRIBUTING.md",
"date": "2024-08-30T08:20:57",
"stars": 1788,
"description": "Build beautiful website using Vue & Nuxt.",
"file_size": 13439
} |
<p align="center">
<a href="https://github.com/unovue/inspira-ui">
<img src="./logo.png" alt="Logo" width="150" />
</a>
</p>
<h1 align="center">
Inspira UI
</h1>
<p align="center">
<b>Build beautiful websites using Vue & Nuxt.</b><br>
A curated collection of beautifully designed, reusable components for Vue & Nuxt.
</p>
<p align="center">
<a href="https://github.com/unovue/inspira-ui/stargazers">
<img alt="GitHub stars" src="https://img.shields.io/github/stars/unovue/inspira-ui?style=social">
</a>
<a href="https://github.com/unovue/inspira-ui/blob/main/LICENSE.md">
<img alt="License" src="https://img.shields.io/badge/License-MIT-yellow.svg">
</a>
</p>
---
Welcome to [**Inspira UI**](https://inspira-ui.com), a community-driven project that brings the beauty and functionality of both [Aceternity UI](https://ui.aceternity.com) and [Magic UI](https://magicui.design) to the [Vue](https://vuejs.org) & [Nuxt](https://nuxt.com) ecosystem! While this project draws inspiration from these sources, it also includes unique custom components contributed by the community and created by us.
## ✨ About Inspira UI
Inspira UI is a collection of elegant, ready-to-use Vue components designed to be flexible and easy to integrate. Rather than being a traditional component library, it allows you to pick, customize, and adapt components as needed, giving you the freedom to shape them to fit your unique project requirements.
## 🚀 Why Inspira UI?
Inspira UI was created to fill a gap in the Vue community by providing a set of components with the aesthetics and functionality of both Aceternity UI and Magic UI. Our goal is to empower developers to build beautiful applications more efficiently while adding our own custom and community-driven designs.
## 🎯 Key Features
- **Free and Open Source**: Completely [open source](https://github.com/unovue/inspira-ui) under the MIT license.
- **Highly Configurable**: Tailor components to your specific design needs. Check out our [configuration guide](/api/configuration).
- **Diverse Component Range**: A broad selection of [components](/components), inspired by Aceternity UI, Magic UI, and custom contributions, to help you build anything you imagine.
- **Mobile Optimized**: Designed to look great on all devices.
- **Nuxt Compatibility**: Fully compatible with [Nuxt](https://nuxt.com).
## 📚 Documentation
For full documentation and usage examples, visit [**Inspira UI Documentation**](https://inspira-ui.com).
## 🙏 Acknowledgments
A special thanks to:
- [Aceternity UI](https://ui.aceternity.com) for providing the inspiration and permission to adapt the original designs.
- [Magic UI](https://magicui.design) for its beautiful design inspiration.
- [shadcn-vue](https://www.shadcn-vue.com) for the Vue port of shadcn-ui and contributions to some components.
- [shadcn-docs-nuxt](https://github.com/ZTL-UwU/shadcn-docs-nuxt) for the beautifully crafted Nuxt documentation site.
## Author
Hi, I'm [Rahul Vashishtha](https://rahulv.dev). I started Inspira UI to bring a similar experience to the Vue ecosystem, inspired by Aceternity UI, Magic UI, and community contributions. I'm continuously working on it to make it better. Feel free to check out my work on [GitHub](https://github.com/rahul-vashishtha) and join me on this journey [here](https://github.com/unovue/inspira-ui)!
## 🌟 Contribute
We welcome contributions! If you want to suggest features, report bugs, or help improve the project, feel free to open an issue or submit a pull request on [GitHub](https://github.com/unovue/inspira-ui).
You can also follow this [Contribution Guide](https://inspira-ui.com/getting-started/contribution).
## Sponsorship
You can also support the development of the project by sponsoring the developer.
Support and sponsor the maintainer here: [Support Inspira UI](https://github.com/sponsors/rahul-vashishtha) | {
"source": "unovue/inspira-ui",
"title": "README.md",
"url": "https://github.com/unovue/inspira-ui/blob/main/README.md",
"date": "2024-08-30T08:20:57",
"stars": 1788,
"description": "Build beautiful website using Vue & Nuxt.",
"file_size": 3904
} |
---
title: Introduction
description: Reusable components built with shadcn-vue, motion-v, and TailwindCSS
icon: "lucide:info"
---
Welcome to [**Inspira UI**](https://inspira-ui.com), a community-driven project for [Vue](https://vuejs.org)!
This collection offers beautifully designed, reusable components, taking inspiration from the amazing work done on both [Aceternity UI](https://ui.aceternity.com) and [Magic UI](https://magicui.design). While we're not officially affiliated with these projects, we have received permission from Aceternity UI's creator to adapt those fantastic designs for the Vue ecosystem. Additionally, Inspira UI includes custom components developed by us and contributed by the community.
### About Inspira UI
Inspira UI is **not** a traditional component library. Instead, it's a curated collection of elegant components you can easily integrate into your applications. Simply pick what you need, copy the code, and customize it to fit your project. The code is yours to use and modify as you like!
### Why Inspira UI?
This project began to fill a gap in the Vue community for a similar set of components. Inspira UI brings the beauty and functionality of Aceternity UI, Magic UI, and custom contributions to Vue, making it easier for developers to build stunning applications.
### Key Features
- Completely [free and open source](https://github.com/unovue/inspira-ui)
- Highly [configurable](/components) to meet your design needs
- A wide range of [components](/components) to choose from
- Optimized for mobile use
- Fully compatible with Nuxt
### Acknowledgments
Special thanks to:
- [Aceternity UI](https://ui.aceternity.com) for inspiring this Vue adaptation.
- [Magic UI](https://magicui.design) for their design inspiration.
- [shadcn-vue](https://www.shadcn-vue.com/) for the Vue port of shadcn-ui and contributing some components for docs.
- [shadcn-docs-nuxt](https://github.com/ZTL-UwU/shadcn-docs-nuxt) for the beautifully crafted Nuxt documentation site.
### About Me
Hi, I'm [Rahul Vashishtha](https://rahulv.dev). I started Inspira UI to bring a similar experience to the Vue ecosystem, inspired by Aceternity UI, Magic UI, and community contributions. I'm continuously working on it to make it better. Feel free to check out my work on [GitHub](https://github.com/rahul-vashishtha) and join me on this journey [here](https://github.com/unovue/inspira-ui)!
Feel free to explore and enjoy building with Inspira UI! | {
"source": "unovue/inspira-ui",
"title": "content/1.getting-started/1.Introduction.md",
"url": "https://github.com/unovue/inspira-ui/blob/main/content/1.getting-started/1.Introduction.md",
"date": "2024-08-30T08:20:57",
"stars": 1788,
"description": "Build beautiful website using Vue & Nuxt.",
"file_size": 2472
} |
---
title: Installation
description: How to install Inspira UI in your app.
icon: "lucide:play"
---
This guide will help you install and set up Inspira UI components in your Vue or Nuxt application.
## Getting Started with Inspira UI
::alert{type="info"}
**Note:** If you're using `shadcn-vue`, you can skip step `1` & `4`.
::
::steps
### Set up `tailwindcss`
::alert{type="info"}
Currently Inspira UI supports TailwindCSS v3. Make sure you install TailwindCSS v3.
::
To begin, install `tailwindcss` using [this guide](https://v3.tailwindcss.com/docs/installation).
### Add depedencies
Install libraries for tailwindcss and utilities.
::code-group
```bash [npm]
npm install -D @inspira-ui/plugins clsx tailwind-merge class-variance-authority tailwindcss-animate
```
```bash [pnpm]
pnpm install -D @inspira-ui/plugins clsx tailwind-merge class-variance-authority tailwindcss-animate
```
```bash [bun]
bun add -d @inspira-ui/plugins clsx tailwind-merge class-variance-authority tailwindcss-animate
```
```bash [yarn]
yarn add --dev @inspira-ui/plugins clsx tailwind-merge class-variance-authority tailwindcss-animate
```
::
Install VueUse and other supporting libraries.
::code-group
```bash [npm]
npm install @vueuse/core motion-v
```
```bash [pnpm]
pnpm install @vueuse/core motion-v
```
```bash [bun]
bun add @vueuse/core motion-v
```
```bash [yarn]
yarn add @vueuse/core motion-v
```
::
Follow this guide to setup `motion-v` on [Vue](https://motion.unovue.com/getting-started/installation) and [Nuxt](https://motion.unovue.com/getting-started/installation)
### Update `tailwind.config.js` and `tailwind.css`
Add the following code to your `tailwind.config.js` and your `css` file:
::code-group
```ts [tailwind.config.js]
import animate from "tailwindcss-animate";
import { setupInspiraUI } from "@inspira-ui/plugins";
export default {
darkMode: "selector",
safelist: ["dark"],
prefix: "",
content: ["./index.html", "./src/**/*.{vue,js,ts,jsx,tsx}",],
theme: {
extend: {
colors: {
border: "hsl(var(--border))",
input: "hsl(var(--input))",
ring: "hsl(var(--ring))",
background: "hsl(var(--background))",
foreground: "hsl(var(--foreground))",
primary: {
DEFAULT: "hsl(var(--primary))",
foreground: "hsl(var(--primary-foreground))",
},
secondary: {
DEFAULT: "hsl(var(--secondary))",
foreground: "hsl(var(--secondary-foreground))",
},
destructive: {
DEFAULT: "hsl(var(--destructive))",
foreground: "hsl(var(--destructive-foreground))",
},
muted: {
DEFAULT: "hsl(var(--muted))",
foreground: "hsl(var(--muted-foreground))",
},
accent: {
DEFAULT: "hsl(var(--accent))",
foreground: "hsl(var(--accent-foreground))",
},
popover: {
DEFAULT: "hsl(var(--popover))",
foreground: "hsl(var(--popover-foreground))",
},
card: {
DEFAULT: "hsl(var(--card))",
foreground: "hsl(var(--card-foreground))",
},
},
borderRadius: {
xl: "calc(var(--radius) + 4px)",
lg: "var(--radius)",
md: "calc(var(--radius) - 2px)",
sm: "calc(var(--radius) - 4px)",
},
},
},
plugins: [animate, setupInspiraUI],
};
```
```css [tailwind.css]
@tailwind base;
@tailwind components;
@tailwind utilities;
@layer base {
:root {
--background: 0 0% 100%;
--foreground: 222.2 84% 4.9%;
--card: 0 0% 100%;
--card-foreground: 222.2 84% 4.9%;
--popover: 0 0% 100%;
--popover-foreground: 222.2 84% 4.9%;
--primary: 221.2 83.2% 53.3%;
--primary-foreground: 210 40% 98%;
--secondary: 210 40% 96.1%;
--secondary-foreground: 222.2 47.4% 11.2%;
--muted: 210 40% 96.1%;
--muted-foreground: 215.4 16.3% 46.9%;
--accent: 210 40% 96.1%;
--accent-foreground: 222.2 47.4% 11.2%;
--destructive: 0 84.2% 60.2%;
--destructive-foreground: 210 40% 98%;
--border: 214.3 31.8% 91.4%;
--input: 214.3 31.8% 91.4%;
--ring: 221.2 83.2% 53.3%;
--radius: 0.5rem;
}
.dark {
--background: 222.2 84% 4.9%;
--foreground: 210 40% 98%;
--card: 222.2 84% 4.9%;
--card-foreground: 210 40% 98%;
--popover: 222.2 84% 4.9%;
--popover-foreground: 210 40% 98%;
--primary: 217.2 91.2% 59.8%;
--primary-foreground: 222.2 47.4% 11.2%;
--secondary: 217.2 32.6% 17.5%;
--secondary-foreground: 210 40% 98%;
--muted: 217.2 32.6% 17.5%;
--muted-foreground: 215 20.2% 65.1%;
--accent: 217.2 32.6% 17.5%;
--accent-foreground: 210 40% 98%;
--destructive: 0 62.8% 30.6%;
--destructive-foreground: 210 40% 98%;
--border: 217.2 32.6% 17.5%;
--input: 217.2 32.6% 17.5%;
--ring: 224.3 76.3% 48%;
}
}
```
::
### Setup `cn` utility
Add following utility to `lib/utils.ts`
```ts [utils.ts]
import { type ClassValue, clsx } from "clsx";
import { twMerge } from "tailwind-merge";
export function cn(...inputs: ClassValue[]) {
return twMerge(clsx(inputs));
}
export type ObjectValues<T> = T[keyof T];
```
::alert{type="success" icon="lucide:circle-check"}
Great job! Your project is now configured to use Inspira UI.
::
### Start Using Inspira UI 🚀
Now, you can start using Inspira UI components in your project. Choose the components you need, copy the code, and integrate them into your application.
:read-more{title="List of all components" to="/components"}
:: | {
"source": "unovue/inspira-ui",
"title": "content/1.getting-started/2.installation.md",
"url": "https://github.com/unovue/inspira-ui/blob/main/content/1.getting-started/2.installation.md",
"date": "2024-08-30T08:20:57",
"stars": 1788,
"description": "Build beautiful website using Vue & Nuxt.",
"file_size": 5544
} |
---
title: How To Contribute
description: Follow the following guidelines to ensure the smooth collaboration
icon: "lucide:scroll-text"
---
Thank you for your interest in contributing to the **Inspira UI** project! Your contributions help make this project better for everyone. Please take a moment to read through these guidelines to ensure a smooth collaboration.
## Table of Contents
1. [Getting Started](#getting-started)
2. [Code of Conduct](#code-of-conduct)
3. [How to Contribute](#how-to-contribute)
- [Reporting Bugs](#reporting-bugs)
- [Suggesting Enhancements](#suggesting-enhancements)
- [Adding New Components](#adding-new-components)
4. [Project Structure](#project-structure)
5. [Style Guidelines](#style-guidelines)
- [Coding Standards](#coding-standards)
- [Component Format](#component-format)
- [Commit Messages](#commit-messages)
6. [Documentation Guidelines](#documentation-guidelines)
- [Single-File Components](#single-file-components)
- [Multi-File Components](#multi-file-components)
7. [Testing](#testing)
8. [License](#license)
---
## Getting Started
- **Fork the Repository**: Create a personal fork of the project on GitHub.
- **Clone Your Fork**: Clone your forked repository to your local machine.
- **Create a Branch**: Create a new branch for your contribution (`git checkout -b feature/YourFeatureName`).
- **Install Dependencies**: Run `npm install` to install all necessary dependencies.
## Code of Conduct
By participating in this project, you agree to abide by the [Code of Conduct](./4.code-of-conduct.md), which aims to foster an open and welcoming environment.
## How to Contribute
### Reporting Bugs
If you find a bug, please open an [issue](https://github.com/unovue/inspira-ui/issues){:target="\_blank"} and include:
- A clear and descriptive title.
- Steps to reproduce the issue.
- Expected and actual results.
- Screenshots or code snippets, if applicable.
### Suggesting Enhancements
We welcome suggestions for new features or improvements. Please open an [issue](https://github.com/unovue/inspira-ui/issues){:target="\_blank"} and include:
- A clear and descriptive title.
- A detailed description of the enhancement.
- Any relevant examples or mockups.
### Adding New Components
We appreciate contributions that add new components to the library. Please ensure that:
- The component is generally useful and aligns with the project's goals.
- The component is compatible with both **Nuxt** and **Vue**.
- You follow the coding and documentation guidelines outlined below.
- You include unit tests for your component.
## Project Structure
Understanding the project structure is crucial for effective contribution:
- **Components Directory**:
- Main components should be placed in `components/content/inspira/ui/<component-folder-name>/`.
- Example components should be placed in `components/content/inspira/examples/<component-folder-name>/`.
- **Documentation**:
- Documentation files are located in the `content/2.components/<category>/` directory.
- After adding a component, write its documentation in this directory.
## Style Guidelines
### Coding Standards
- **Language**: All components should be written in **Vue.js** with TypeScript support.
- **Styling**: Use **Tailwind CSS** classes for styling whenever possible.
- **Naming Conventions**: Use `PascalCase` for component names and filenames.
### Component Format
Your Vue components should adhere to the following structure:
```vue
<!-- Template -->
<template>
<!-- Your template code goes here -->
</template>
<!-- Script (if required) -->
<script lang="ts" setup>
// Your script code goes here
</script>
<!-- Styles (if required) -->
<style scoped>
/* Your styles go here */
</style>
```
**Props typing and code style**
Refer to this Vue.js documentation page -> https://vuejs.org/api/sfc-script-setup#type-only-props-emit-declarations
```vue
<script lang="ts" setup>
// DON'T ⛔️
const props = defineProps({
whatever: { type: String, required: true },
optional: { type: String, default: "default" },
});
// DO ✅
interface Props {
whatever: string;
optional?: string;
}
const props = withDefaults(defineProps<Props>(), { optional: "default" });
//Or DO ✅ Props destructure (v3.5+)
interface Props {
msg?: string;
labels?: string[];
}
const { msg = "hello", labels = ["one", "two"] } = defineProps<Props>();
</script>
```
**Constants, interfaces, types and variants**
For reusability purposes, you can also add an `index.ts` file at the root of the component folder to export interfaces, constants, and other useful code elements. Keep in mind that developers will copy and paste the component code into their projects, so it should be very easy to customize according to their standards.
Contants have to be `CAPS_CAMEL_CASE` in order to identify them clearly inside the code. And `prefix` them.
Please never use Enums; use `{} as const` instead. 😘
```typescript
// DON'T ⛔️
const Direction = { Top: 'top'} as const
const ComponentNameDirection = { Top: 'top'} as const
// DON'T ⛔️
enum COMPONENT_NAME_DIRECTION_WRONG = { Top = 'top'};
// DO ✅
import type { ObjectValues } from "@/lib/utils";
export const COMPONENT_NAME_DIRECTION = { Top: 'top', Bottom: 'bottom'} as const
//Types and Interfaces should use CamelCase to differentiate them from constants and variables.
export type ComponentNameDirection = ObjectValues<typeof COMPONENT_NAME_DIRECTION>;
interface {
direction: ComponentNameDirection; //Enforce correct value : 'top' or 'bottom'
}
```
You can check the `PatternBackground` component files `components/content/inspira/ui/pattern-background` for a complete example.
**Notes:**
- Use `<script lang="ts" setup>` for TypeScript and the Composition API.
- Keep styles scoped to prevent conflicts.
- Ensure compatibility with both **Nuxt** and **Vue**.
### Commit Messages
- Use the [Conventional Commits](https://www.conventionalcommits.org/) format.
- Begin with a type (`feat`, `fix`, `docs`, etc.) followed by a short description.
- Example: `feat: add TextHoverEffect component`
## Documentation Guidelines
Proper documentation is crucial for users to understand and effectively use the components. Follow these guidelines when adding documentation for new components.
### Steps to Add a New Component
1. **Create the Component**
- Place the main component in `components/content/inspira/ui/<component-folder-name>/`.
- Follow the [Component Format](#component-format) specified above.
- If the component requires examples or demos, add demo components to `components/content/inspira/examples/<component-folder-name>/`.
2. **Write Documentation**
- Add a new Markdown file in `content/2.components/<category>/` for your component's documentation.
- Use the appropriate template based on whether your component is single-file or multi-file (see below).
- Mention the **Credits** and source if the component is ported from any other UI library or taken inspiration from any other source.
3. **Ensure Compatibility**
- Test your component in both **Nuxt** and **Vue** environments.
### Single-File Components
For components that are contained within a single `.vue` file, use the following template:
1. **Front Matter**
Begin with YAML front matter that includes the `title` and `description`:
```yaml
---
title: Your Component Title
description: A brief description of what your component does.
---
```
2. **Preview Section**
Use the `ComponentLoader` to display a live preview of the component. The `id` should be set to the folder name of your component in `components/content/inspira/examples/`. In case, there is no folder, then `id` is not required.
```markdown
::ComponentLoader{label="Preview" componentName="YourComponentDemo" type="examples" id="your-component-folder-name"}
::
```
3. **API Documentation**
Provide a table listing all props:
```markdown
## API
| Prop Name | Type | Default | Description |
| --------- | --------- | ------- | ------------------------------ |
| `prop1` | `string` | `''` | Description of prop1. |
| `prop2` | `number` | `0` | Description of prop2. |
| `prop2` | `?number` | `0` | Description of prop2 optional. |
```
4. **Component Code**
Include the full code of the component using `CodeViewer`. The `id` should be set to the folder name of your component in `components/content/inspira/ui/`.
```markdown
## Component Code
You can copy and paste the following code to create this component:
::CodeViewer{filename="YourComponent.vue" language="vue" componentName="YourComponent" type="ui" id="your-component-folder-name"}
::
```
**Example:**
```markdown
---
title: Text Hover Effect
description: A text hover effect that animates and outlines gradient on hover.
---
::ComponentLoader{label="Preview" componentName="TextHoverEffectDemo" type="examples" id="text-hover-effect"}
::
## API
| Prop Name | Type | Default | Description |
| ------------- | -------- | -------- | --------------------------------------------------------- |
| `text` | `string` | Required | The text to be displayed with the hover effect. |
| `duration` | `number` | `200` | The duration of the mask transition animation in seconds. |
| `strokeWidth` | `number` | `0.75` | The width of the text stroke. |
| `opacity` | `number` | `null` | The opacity of the text. |
## Component Code
You can copy and paste the following code to create this component:
::CodeViewer{filename="TextHoverEffect.vue" language="vue" componentName="TextHoverEffect" type="ui" id="text-hover-effect"}
::
```
In this example, the `id` used in both `ComponentLoader` and `CodeViewer` is `text-hover-effect`, which matches the folder name where the component and its demo are stored.
### Multi-File Components
For components that consist of multiple files, such as a main component and several sub-components or variants, use the following template:
1. **Front Matter**
Begin with YAML front matter:
```yaml
---
title: Your Components Group Title
description: A brief description of what this group of components does.
---
```
2. **Preview Sections**
Include multiple `ComponentLoader` sections for each example or variant. The `id` should be set to the folder name of your component in `components/content/inspira/examples/`.
```markdown
::ComponentLoader{label="Preview" componentName="ComponentVariantDemo" type="examples" id="your-component-folder-name"}
::
```
3. **API Documentation**
Provide comprehensive API documentation covering all components:
```markdown
## API
| Prop Name | Type | Default | Description |
| --------- | -------- | ------- | --------------------------------------- |
| `prop1` | `string` | `''` | Description applicable to all variants. |
```
4. **Utility Classes**
If applicable, list any utility classes:
```markdown
### Utility Classes
- **Class Name:** `class-description`
```
5. **Component Code**
Use a `code-group` to group multiple `CodeViewerTab` components, one for each file. The `id` should be set to the folder name of your component in `components/content/inspira/ui/`.
```markdown
## Component Code
You can copy and paste the following code to create these components:
::code-group
::CodeViewerTab{label="MainComponent.vue" language="vue" componentName="MainComponent" type="ui" id="your-component-folder-name"}
::
::CodeViewerTab{filename="VariantOne.vue" language="vue" componentName="VariantOne" type="ui" id="your-component-folder-name"}
::
::CodeViewerTab{filename="VariantTwo.vue" language="vue" componentName="VariantTwo" type="ui" id="your-component-folder-name"}
::
::
```
**Example:**
```markdown
---
title: Pattern Backgrounds
description: Simple animated pattern backgrounds to make your sections stand out.
---
::ComponentLoader{label="Preview" componentName="DotBackgroundDemo" type="examples" id="pattern-backgrounds"}
::
## Examples
Grid background without animation:
::ComponentLoader{label="Preview" componentName="GridBackgroundDemo" type="examples" id="pattern-backgrounds"}
::
Small grid background with animation:
::ComponentLoader{label="Preview" componentName="GridSmallBackgroundDemo" type="examples" id="pattern-backgrounds"}
::
## API
| Prop Name | Type | Default | Description |
| ----------- | -------------------------------------------------------------------------------------------------------- | ------- | ------------------------------------- |
| `animate` | `boolean` | `false` | Set `true` to animate the background. |
| `direction` | `'top' \| 'bottom' \| 'left' \| 'right' \| 'top-left' \| 'top-right' \| 'bottom-left' \| 'bottom-right'` | `'top'` | Direction of the animation movement. |
### Utility Classes
- **Dot Pattern:** `dark:bg-dot-white/[0.2] bg-dot-black/[0.2]`
- **Grid Pattern:** `dark:bg-grid-white/[0.2] bg-grid-black/[0.2]`
- **Small Grid Pattern:** `dark:bg-grid-small-white/[0.2] bg-grid-small-black/[0.2]`
## Component Code
You can copy and paste the following code to create these components:
::code-group
::CodeViewerTab{label="PatternBackground.vue" language="vue" componentName="PatternBackground" type="ui" id="pattern-backgrounds"}
::
::CodeViewerTab{filename="DotBackground.vue" language="vue" componentName="DotBackground" type="ui" id="pattern-backgrounds"}
::
::CodeViewerTab{filename="GridBackground.vue" language="vue" componentName="GridBackground" type="ui" id="pattern-backgrounds"}
::
::CodeViewerTab{filename="GridSmallBackground.vue" language="vue" componentName="GridSmallBackground" type="ui" id="pattern-backgrounds"}
::
::
```
In this example, the `id` used in `ComponentLoader` and all `CodeViewerTab` components is `pattern-backgrounds`, which matches the folder name where the components and their demos are stored.
## Testing
- **Unit Tests**: Write unit tests for your component if applicable.
- **Cross-Environment Testing**: Ensure that your component works correctly in both **Nuxt** and **Vue** environments.
- **Visual Testing**: Check the component visually to ensure it renders correctly.
## Additional Notes
- **Component Names**: Use `PascalCase` for component filenames and names.
- **IDs**: In `CodeViewer`, `CodeViewerTab`, and `ComponentLoader`, the `id` parameter should be set to the **folder name** where the component is stored in `components/content/inspira/ui/<component-folder-name>/` and `components/content/inspira/examples/<component-folder-name>/`. This helps in correctly linking the code and examples in the documentation.
- **Demo Components**: For each component, create a corresponding `Demo` component used in the `ComponentLoader` for previews, and place it in `components/content/inspira/examples/<component-folder-name>/`.
- **Localization**: If your component supports multiple languages, include details in the documentation.
## License
By contributing, you agree that your contributions will be licensed under the [MIT License](https://github.com/unovue/inspira-ui/blob/main/LICENSE){:target="\_blank"}. | {
"source": "unovue/inspira-ui",
"title": "content/1.getting-started/3.contribution.md",
"url": "https://github.com/unovue/inspira-ui/blob/main/content/1.getting-started/3.contribution.md",
"date": "2024-08-30T08:20:57",
"stars": 1788,
"description": "Build beautiful website using Vue & Nuxt.",
"file_size": 15652
} |
---
title: Code of Conduct
description: Code of Conduct outlines our expectation for participant behavior as well as consequences for unacceptable conduct.
icon: "lucide:award"
---
## Introduction
We are committed to providing a friendly, safe, and welcoming environment for everyone involved in the **Inspira UI** project. This Code of Conduct outlines our expectations for participant behavior as well as the consequences for unacceptable conduct.
## Our Pledge
In the interest of fostering an open and inclusive community, we pledge to make participation in our project and community a harassment-free experience for everyone, regardless of:
- Age
- Body size
- Disability
- Ethnicity
- Gender identity and expression
- Level of experience
- Nationality
- Personal appearance
- Race
- Religion
- Sexual identity and orientation
## Expected Behavior
All participants in our community are expected to:
- **Be Respectful**: Show empathy and kindness towards others.
- **Be Considerate**: Remember that your actions and words affect others.
- **Be Collaborative**: Work together to achieve common goals.
- **Communicate Effectively**: Use clear and constructive language.
- **Demonstrate Professionalism**: Act professionally and take responsibility for your actions.
## Unacceptable Behavior
The following behaviors are considered unacceptable within our community:
- **Harassment and Discrimination**: Including derogatory comments, slurs, or unwanted sexual attention.
- **Abuse and Threats**: Any form of verbal or written abuse, intimidation, or threats.
- **Trolling and Insults**: Provocative or insulting remarks intended to disrupt conversations.
- **Disrespectful Communication**: Including excessive profanity, shouting (using all caps), or interrupting others.
- **Personal Attacks**: Targeting an individual with the intent to harass or belittle.
## Reporting Guidelines
If you experience or witness unacceptable behavior, or have any other concerns, please report it as soon as possible by contacting the project maintainers on our **Discord channel**:
[Inspira UI Discord Channel](https://discord.gg/Xbh5DwJRc9)
When reporting an incident, please include:
- **Your Contact Information**: Your Discord username or any preferred method of contact.
- **Names of Those Involved**: Real names or usernames of the individuals involved.
- **Description of the Incident**: A clear and concise account of what happened.
- **Supporting Evidence**: Any relevant messages, screenshots, or context that can help us understand the situation.
All reports will be handled confidentially.
## Enforcement
Project maintainers are responsible for ensuring compliance with this Code of Conduct and will take appropriate action in response to any behavior that is deemed unacceptable. Actions may include:
- A private warning to the offender.
- Temporary or permanent ban from participation in the project and Discord channel.
- Removal of contributions that violate the Code of Conduct.
## Scope
This Code of Conduct applies to all project spaces, including but not limited to:
- GitHub repositories
- Issue trackers
- Pull requests
- Project-related forums and chat channels
- Social media interactions pertaining to the project
- The official **Inspira UI Discord channel**
It also applies when an individual is representing the project or its community in public spaces.
## Appeal Process
Any individual who is subjected to disciplinary action has the right to appeal the decision by contacting the project maintainers through the **Discord channel** within one week of the action. The appeal will be reviewed, and a final decision will be communicated.
## Privacy
All reports of unacceptable behavior will be handled with discretion. We will respect the privacy of the reporter and the accused.
## Acknowledgments
We thank all contributors and community members for helping to create a positive environment. This Code of Conduct is adapted from best practices and guidelines used in open-source communities.
## Contact Information
For questions or concerns about this Code of Conduct, please contact the project maintainers on our **Discord channel**:
[Inspira UI Discord Channel](https://discord.gg/Xbh5DwJRc9) | {
"source": "unovue/inspira-ui",
"title": "content/1.getting-started/4.code-of-conduct.md",
"url": "https://github.com/unovue/inspira-ui/blob/main/content/1.getting-started/4.code-of-conduct.md",
"date": "2024-08-30T08:20:57",
"stars": 1788,
"description": "Build beautiful website using Vue & Nuxt.",
"file_size": 4241
} |
---
title: Component Index
description: List of all the components provided by Inspira UI.
navigation: false
---
## All components
::ComponentIndex
:: | {
"source": "unovue/inspira-ui",
"title": "content/2.components/index.md",
"url": "https://github.com/unovue/inspira-ui/blob/main/content/2.components/index.md",
"date": "2024-08-30T08:20:57",
"stars": 1788,
"description": "Build beautiful website using Vue & Nuxt.",
"file_size": 152
} |
---
title: Block Index
description: List of all the blocks provided by Inspira UI.
navigation: false
---
## All Blocks
::ComponentIndex{path="blocks"}
:: | {
"source": "unovue/inspira-ui",
"title": "content/3.blocks/index.md",
"url": "https://github.com/unovue/inspira-ui/blob/main/content/3.blocks/index.md",
"date": "2024-08-30T08:20:57",
"stars": 1788,
"description": "Build beautiful website using Vue & Nuxt.",
"file_size": 155
} |
---
title: Aurora Background
description: A subtle Aurora or Southern Lights background for your website.
---
::ComponentLoader{label="Preview" componentName="AuroraBackgroundDemo" type="examples" id="aurora-background"}
::
## API
| Prop Name | Type | Default | Description |
| ---------------- | --------- | ------- | ------------------------------------------------------------------------- |
| `class` | `string` | `-` | Additional CSS classes to apply to the component for styling. |
| `radialGradient` | `boolean` | `true` | Determines whether a radial gradient effect is applied to the background. |
## Component Code
You can copy and paste the following code to create these components:
::CodeViewer{filename="AuroraBackground.vue" language="vue" componentName="AuroraBackground" type="ui" id="aurora-background"}
::
## Features
- **Slot Support**: Easily add any content inside the component using the default slot.
## Credits
- Credits to [Aceternity UI](https://ui.aceternity.com/components/aurora-background).
- Credits to [SivaReddy Uppathi](https://github.com/sivareddyuppathi) for porting this component. | {
"source": "unovue/inspira-ui",
"title": "content/2.components/backgrounds/aurora-background.md",
"url": "https://github.com/unovue/inspira-ui/blob/main/content/2.components/backgrounds/aurora-background.md",
"date": "2024-08-30T08:20:57",
"stars": 1788,
"description": "Build beautiful website using Vue & Nuxt.",
"file_size": 1229
} |
---
title: Bubbles Background
description: An animated background with floating bubbles.
---
::ComponentLoader{label="Preview" componentName="BubblesBgDemo2" type="examples" id="backgrounds"}
::
## Example
Without Blur or overlay
::ComponentLoader{label="Preview" componentName="BubblesBgDemo" type="examples" id="backgrounds"}
::
::alert{type="info"}
**Note:** This component uses Three.js & requires `three` npm package as a dependency.
Please install Three.js using following commands.
::code-group
```bash [npm]
npm install three
npm install -D @types/three
```
```bash [pnpm]
pnpm install three
pnpm install -D @types/three
```
```bash [bun]
bun add three
bun add -d @types/three
```
```bash [yarn]
yarn add three
yarn add --dev @types/three
```
::
::
## API
| Prop Name | Type | Default | Description |
| --------- | -------- | ------- | --------------------------------------------------------------- |
| `blur` | `number` | `0` | Amount of blur to apply to the background, specified in pixels. |
## Component Code
You can copy and paste the following code to create these components:
::CodeViewer{filename="BubblesBg.vue" language="vue" componentName="BubblesBg" type="ui" id="bg-bubbles"}
::
## Features
- **Animated Bubble Background**: Renders a captivating background with animated, floating bubbles using 3D graphics.
- **Dynamic Color Gradients**: The bubbles and background smoothly transition between colors over time, creating a visually engaging effect.
- **Customizable Blur Effect**: Use the `blur` prop to adjust the blur intensity applied over the background, enhancing depth perception.
- **Slot Support**: Easily overlay content on top of the animated background using the default slot.
- **Responsive Design**: The component adjusts to fit the width and height of its parent container, ensuring compatibility across different screen sizes.
## Credits
- Built with the [Three.js](https://threejs.org/) library for 3D rendering and animations.
- Inspired from [Tresjs Experiment](https://lab.tresjs.org/experiments/overlay). | {
"source": "unovue/inspira-ui",
"title": "content/2.components/backgrounds/bubbles-bg.md",
"url": "https://github.com/unovue/inspira-ui/blob/main/content/2.components/backgrounds/bubbles-bg.md",
"date": "2024-08-30T08:20:57",
"stars": 1788,
"description": "Build beautiful website using Vue & Nuxt.",
"file_size": 2209
} |
---
title: Falling Stars Background
description: A stunning animated starfield background with glowing and sharp trail effects.
---
::ComponentLoader{label="Preview" componentName="FallingStarsBgDemo" type="examples" id="backgrounds"}
::
## API
| Prop Name | Type | Default | Description |
| --------- | -------- | -------- | ------------------------------------------- |
| `color` | `string` | `"#FFF"` | Color of the stars in the starfield. |
| `count` | `number` | `200` | Number of stars displayed in the animation. |
## Component Code
You can copy and paste the following code to create this component:
::CodeViewer{filename="FallingStarsBg.vue" language="vue" componentName="FallingStarsBg" type="ui" id="bg-falling-stars"}
::
## Features
- **Dynamic Starfield**: Creates a 3D starfield effect with stars moving toward the viewer.
- **Glowing and Sharp Trail Effects**: Each star has a sharp line and a blurred, glowing trail.
- **Customizable**: Adjust the `color` of the stars and control the number of stars using the `count` prop.
- **Responsive Design**: Automatically adapts to the size of the canvas, ensuring a full-screen starfield effect.
## Credits
- Inspired by 3D starfield simulations and trail effects in modern canvas animations.
- Credit to [Prodromos Pantos](https://github.com/prpanto) for porting the original component to Vue & Nuxt. | {
"source": "unovue/inspira-ui",
"title": "content/2.components/backgrounds/falling-stars.md",
"url": "https://github.com/unovue/inspira-ui/blob/main/content/2.components/backgrounds/falling-stars.md",
"date": "2024-08-30T08:20:57",
"stars": 1788,
"description": "Build beautiful website using Vue & Nuxt.",
"file_size": 1421
} |
---
title: Flickering Grid
description: A flickering grid background made with Canvas, fully customizable using Tailwind CSS.
---
::ComponentLoader{label="Preview" componentName="FlickeringGridDemo" type="examples" id="flickering-grid"}
::
::alert{type="warning"}
This component uses the `nuxt-only` syntax with the `<ClientOnly>`. If you are not using Nuxt, you can simply remove it.
::
## API
| Prop Name | Type | Default | Description |
| --------------- | -------- | -------------- | -------------------------------------- |
| `squareSize` | `number` | `4` | Size of each square in the grid. |
| `gridGap` | `number` | `6` | Gap between squares in the grid. |
| `flickerChance` | `number` | `0.3` | Probability of a square flickering. |
| `color` | `string` | `rgb(0, 0, 0)` | Color of the squares. |
| `width` | `number` | `-` | Width of the canvas. |
| `height` | `number` | `-` | Height of the canvas. |
| `class` | `string` | `-` | Additional CSS classes for the canvas. |
| `maxOpacity` | `number` | `0.2` | Maximum opacity of the squares. |
## Component Code
You can copy and paste the following code to create these components:
::CodeViewer{filename="FlickeringGrid.vue" language="vue" componentName="FlickeringGrid" type="ui" id="flickering-grid"}
::
## Credits
- Credits to [magicui flickering-grid](https://magicui.design/docs/components/flickering-grid) for this component. | {
"source": "unovue/inspira-ui",
"title": "content/2.components/backgrounds/flickering-grid.md",
"url": "https://github.com/unovue/inspira-ui/blob/main/content/2.components/backgrounds/flickering-grid.md",
"date": "2024-08-30T08:20:57",
"stars": 1788,
"description": "Build beautiful website using Vue & Nuxt.",
"file_size": 1631
} |
---
title: Interactive Grid Pattern
description: A interactive background grid pattern made with SVGs, fully customizable.
navBadges:
- value: New
type: lime
---
::ComponentLoader{label="Preview" componentName="InteractiveGridPatternDemo" type="examples" id="interactive-grid-pattern"}
::
## Examples
::ComponentLoader{label="Preview" componentName="InteractiveGridPatternColoredDemo" type="examples" id="interactive-grid-pattern"}
::
## API
#### Props
| Prop Name | Type | Default | Description |
| ------------------ | ------------------ | ---------- | --------------------------------------------- |
| `className` | `string` | - | Additional classes for styling the component. |
| `squaresClassName` | `string` | - | Additional classes for styling the squares. |
| `width` | `number` | `40` | Width of the square in pixels. |
| `height` | `number` | `40` | Height of the square in pixels. |
| `squares` | `[number, number]` | `[24, 24]` | Number of squares in the grid pattern. |
## Component Code
You can copy and paste the following code to create this component:
::CodeViewer{label="InteractiveGridPattern.vue" icon="vscode-icons:file-type-vue" componentName="InteractiveGridPattern" type="ui" id="interactive-grid-pattern"}
::
## Credits
- Inspired by [MagicUI](https://magicui.design/docs/components/interactive-grid-pattern).
- Credits to [kalix127](https://github.com/kalix127) for porting this component. | {
"source": "unovue/inspira-ui",
"title": "content/2.components/backgrounds/interactive-grid-pattern.md",
"url": "https://github.com/unovue/inspira-ui/blob/main/content/2.components/backgrounds/interactive-grid-pattern.md",
"date": "2024-08-30T08:20:57",
"stars": 1788,
"description": "Build beautiful website using Vue & Nuxt.",
"file_size": 1637
} |
---
title: Lamp Effect
description: A captivating lamp lighting effect with conic gradients, spotlights, and glowing lines for an immersive visual experience.
---
::ComponentLoader{label="Preview" componentName="LampEffectDemo" type="examples"}
::
## API
| Prop Name | Type | Default | Description |
| ---------- | -------- | ------- | ---------------------------------------------- |
| `delay` | `number` | `0.5` | Delay before the animation starts, in seconds. |
| `duration` | `number` | `0.8` | Duration of the animation, in seconds. |
| `class` | `string` | `""` | Additional CSS classes for custom styling. |
## Component Code
You can copy and paste the following code to create this component:
::CodeViewer{filename="LampEffect.vue" language="vue" componentName="LampEffect" type="ui" id="lamp-effect"}
::
## Features
- **Conic Gradient Animation**: Creates a smooth expanding conic gradient effect, giving a dynamic light-source appearance.
- **Spotlight Animation**: The spotlight smoothly expands, providing a focused lighting effect.
- **Glowing Line Effect**: A glowing line animates across the center, simulating a light beam or laser.
- **Customizable Timing**: The `delay` and `duration` props allow for precise control of animation timings.
- **Slot-Based Content**: Supports default slot content, making it easy to overlay text or other components.
## Credits
- Ported from [Aceternity UI](https://ui.aceternity.com/components/lamp-effect) | {
"source": "unovue/inspira-ui",
"title": "content/2.components/backgrounds/lamp-effect.md",
"url": "https://github.com/unovue/inspira-ui/blob/main/content/2.components/backgrounds/lamp-effect.md",
"date": "2024-08-30T08:20:57",
"stars": 1788,
"description": "Build beautiful website using Vue & Nuxt.",
"file_size": 1531
} |
---
title: Particle Whirlpool
description: An animated background with swirling particles.
---
::ComponentLoader{label="Preview" componentName="ParticleWhirlpoolBgDemo" type="examples" id="backgrounds"}
::
## Examples
Without blur and overlay
::ComponentLoader{label="Preview" componentName="ParticleWhirlpoolBgDemo2" type="examples" id="backgrounds"}
::
With `particleCount` 500
::ComponentLoader{label="Preview" componentName="ParticleWhirlpoolBgDemo3" type="examples" id="backgrounds"}
::
::alert{type="info"}
**Note:** This component uses Three.js & requires `three` & `postprocessing` npm package as a dependency.
Please install Three.js using following commands.
::code-group
```bash [npm]
npm install three postprocessing
npm install -D @types/three
```
```bash [pnpm]
pnpm install three postprocessing
pnpm install -D @types/three
```
```bash [bun]
bun add three postprocessing
bun add -d @types/three
```
```bash [yarn]
yarn add three postprocessing
yarn add --dev @types/three
```
::
::
## API
| Prop Name | Type | Default | Description |
| --------------- | -------- | ------- | --------------------------------------------------------------- |
| `class` | `string` | `""` | Additional CSS classes for custom styling. |
| `blur` | `number` | `0` | Amount of blur to apply to the background, specified in pixels. |
| `particleCount` | `number` | `2000` | Number of particles in the whirlpool animation. |
## Component Code
You can copy and paste the following code to create this component:
::CodeViewer{filename="ParticleWhirlpoolBg.vue" language="vue" componentName="ParticleWhirlpoolBg" type="ui" id="bg-particle-whirlpool"}
::
## Features
- **Interactive Whirlpool Animation**: Renders a captivating whirlpool effect with particles that respond to mouse and touch interactions.
- **Customizable Particle Count**: Adjust the `particleCount` prop to control the number of particles in the animation.
- **Dynamic Blur Effect**: Use the `blur` prop to apply a blur effect over the background, enhancing the visual depth.
- **Slot Support**: Overlay additional content on top of the animation using the default slot.
- **Responsive Design**: The component adjusts to fit the width and height of its parent container, ensuring compatibility across different screen sizes.
## Credits
- Built with the [Three.js](https://threejs.org/) library for 3D rendering and animations.
- Inspired by [TroisJs](https://troisjs.github.io/examples/demos/3.html) | {
"source": "unovue/inspira-ui",
"title": "content/2.components/backgrounds/particle-whirlpool-bg.md",
"url": "https://github.com/unovue/inspira-ui/blob/main/content/2.components/backgrounds/particle-whirlpool-bg.md",
"date": "2024-08-30T08:20:57",
"stars": 1788,
"description": "Build beautiful website using Vue & Nuxt.",
"file_size": 2678
} |
---
title: Particles Background
description: Particles can add a dynamic and engaging element to your website's visuals. They help create a feeling of depth, motion, and interaction, making the site more visually appealing.
---
::ComponentLoader{label="Preview" componentName="ParticlesBgDemo" type="examples"}
::
## API
| Prop Name | Type | Default | Description |
| ----------- | -------- | ------- | ----------------------------------------------------------------------------------------------------------- |
| `color` | `string` | `#FFF` | Hexadecimal color code used for particles. Supports 3 or 6 character hex codes. |
| `quantity` | `number` | `100` | The number of particles to generate and display on the canvas. |
| `staticity` | `number` | `50` | Determines how much the particles move based on the mouse's proximity. Higher values reduce movement. |
| `ease` | `number` | `50` | Controls the easing effect of particle movement; lower values make particles follow the mouse more closely. |
## Component Code
You can copy and paste the following code to create these components:
::CodeViewer{filename="ParticlesBg.vue" language="vue" componentName="ParticlesBg" type="ui" id="particles-bg"}
::
## Credits
- Credits to [Magic UI](https://magicui.design/docs/components/particles) for this fantastic component.
- Credit to [Prodromos Pantos](https://github.com/prpanto) for porting the original component to Vue & Nuxt. | {
"source": "unovue/inspira-ui",
"title": "content/2.components/backgrounds/particles-bg.md",
"url": "https://github.com/unovue/inspira-ui/blob/main/content/2.components/backgrounds/particles-bg.md",
"date": "2024-08-30T08:20:57",
"stars": 1788,
"description": "Build beautiful website using Vue & Nuxt.",
"file_size": 1637
} |
---
title: Pattern Background
description: Simple animated pattern background to make your sections stand out.
---
Grid background with dot
::ComponentLoader{label="Preview" componentName="PatternBackgroundDotDemo" type="examples" id="pattern-background"}
::
Grid background with big dot and ellipse on top
::ComponentLoader{label="Preview" componentName="PatternBackgroundBigDotDemo" type="examples" id="pattern-background"}
::
## Examples
Grid background without animation
::ComponentLoader{label="Preview" componentName="PatternBackgroundGridDemo" type="examples" id="pattern-background"}
::
Small grid background with animation
::ComponentLoader{label="Preview" componentName="PatternBackgroundGridSmallDemo" type="examples" id="pattern-background"}
::
## API
| Prop Name | Type | Default | Description |
| ----------- | ------------------------------------------------------------------------------------------------------ | --------- | -------------------------------------------------------------------------------------------------------------------------------------------------------------- |
| `animate` | `boolean` | `false` | Set `true` if you want to animate the background. |
| `direction` | `top` \| `bottom` \| `left` \| `right` \| `top-left` \| `top-right` \| `bottom-left` \| `bottom-right` | `top` | Direction of the animation movement. You can use the const `PATTERN_BACKGROUND_DIRECTION.` |
| `direction` | `grid` \| `dot` | `grid` | Type of pattern. You can use the const `PATTERN_BACKGROUND_VARIANT.` |
| `size` | `xs` \| `sm` \| `md` \| `lg` | `md` | Size of the background pattern. |
| `mask` | `ellipse` \| `ellipse-top` | `ellipse` | Add a mask over the background pattern. You can use the const `PATTERN_BACKGROUND_MASK.` |
| `speed` | `number` | `10000` | Duration of the animation in `ms`, the bigger it is, the slower the animation. (`20000` slower than `5000`). You can use the const `PATTERN_BACKGROUND_SPEED.` |
### Custom variants, values and constants
You can customize your needs directly within the `index.ts` file. See code below.
## Component Code
You can copy and paste the following code to create these components (put them under the same folder):
::code-group
::CodeViewerTab{label="PatternBackground.vue" language="vue" componentName="PatternBackground" type="ui" id="pattern-background"}
::
::CodeViewerTab{filename="index.ts" language="typescript" componentName="index" type="ui" id="pattern-background" extension="ts"}
::
::
## Credits
- Inspired by [Magic UI's Dot Pattern](https://magicui.design/docs/components/dot-pattern) component.
- Inspired by [Magic UI's Grid Pattern](https://magicui.design/docs/components/grid-pattern) component.
- Inspired by [Magic UI's Animated Grid Pattern](https://magicui.design/docs/components/animated-grid-pattern) component.
- Credits to [Nathan De Pachtere](https://nathandepachtere.com/) for porting this component. | {
"source": "unovue/inspira-ui",
"title": "content/2.components/backgrounds/pattern-background.md",
"url": "https://github.com/unovue/inspira-ui/blob/main/content/2.components/backgrounds/pattern-background.md",
"date": "2024-08-30T08:20:57",
"stars": 1788,
"description": "Build beautiful website using Vue & Nuxt.",
"file_size": 4091
} |
---
title: Ripple
description: An animated ripple effect typically used behind elements to emphasize them.
---
::ComponentLoader{label="Preview" componentName="RippleDemo" type="examples" id="ripple"}
::
## Examples
Only lines
::ComponentLoader{label="Preview" componentName="RippleDemoLinesOnly" type="examples" id="ripple"}
::
Squared
::ComponentLoader{label="Preview" componentName="RippleDemoSquared" type="examples" id="ripple"}
::
Blobed
::ComponentLoader{label="Preview" componentName="RippleDemoBlobed" type="examples" id="ripple"}
::
## API
| Prop Name | Type | Default | Description |
| ----------------------------- | -------- | ----------- | ---------------------------------------------------------------------- |
| `baseCircleSize` | `number` | `210` | The size of the main circle in pixels. |
| `baseCircleOpacity` | `number` | `0.24` | The opacity of the main circle. |
| `spaceBetweenCircle` | `number` | `70` | The space between each ripple circle in pixels. |
| `circleOpacityDowngradeRatio` | `number` | `0.03` | The rate at which opacity decreases for each successive ripple circle. |
| `circleClass` | `string` | `undefined` | CSS class name(s) for additional styling of circles. |
| `waveSpeed` | `number` | `80` | The animation speed for the wave effect, measured in ms. |
| `numberOfCircles` | `number` | `7` | The number of ripple circles to render. |
## Component Code
You can copy and paste the following code to create this component:
::code-group
::CodeViewerTab{filename="Ripple.vue" language="vue" componentName="Ripple" type="ui" id="ripple"}
::
::CodeViewerTab{filename="RippleCircle.vue" language="vue" componentName="RippleCircle" type="ui" id="ripple"}
::
::CodeViewerTab{filename="RippleContainer.vue" language="vue" componentName="RippleContainer" type="ui" id="ripple"}
::
::
## Credits
- Credits to [Magic UI](https://magicui.design/docs/components/ripple).
- Credits to [SivaReddy Uppathi](https://github.com/sivareddyuppathi) for porting this component.
- Credits to [Nathan De Pachtere](https://nathandepachtere.com/) for updating this component. | {
"source": "unovue/inspira-ui",
"title": "content/2.components/backgrounds/ripple.md",
"url": "https://github.com/unovue/inspira-ui/blob/main/content/2.components/backgrounds/ripple.md",
"date": "2024-08-30T08:20:57",
"stars": 1788,
"description": "Build beautiful website using Vue & Nuxt.",
"file_size": 2469
} |
---
title: Snowfall Background
description: A beautifully animated snowfall effect applied as a background.
---
::ComponentLoader{label="Preview" componentName="SnowfallBgDemo" type="examples" id="backgrounds"}
::
## API
| Prop Name | Type | Default | Description |
| ----------- | -------- | ------- | --------------------------------------------------------- |
| `color` | `string` | `#FFF` | Color of the snowflakes in hexadecimal format. |
| `quantity` | `number` | `100` | Number of snowflakes to display. |
| `speed` | `number` | `1` | Speed at which snowflakes fall. |
| `maxRadius` | `number` | `3` | Maximum radius of snowflakes. |
| `minRadius` | `number` | `1` | Minimum radius of snowflakes. |
| `class` | `string` | `null` | Additional CSS classes to apply to the container element. |
## Component Code
You can copy and paste the following code to create this component:
::CodeViewer{filename="SnowfallBg.vue" icon="vscode-icons:file-type-vue" language="vue" componentName="SnowfallBg" type="ui" id="snowfall-bg"}
::
## Credits
- Inspired by natural snowfall effects. | {
"source": "unovue/inspira-ui",
"title": "content/2.components/backgrounds/snowfall-bg.md",
"url": "https://github.com/unovue/inspira-ui/blob/main/content/2.components/backgrounds/snowfall-bg.md",
"date": "2024-08-30T08:20:57",
"stars": 1788,
"description": "Build beautiful website using Vue & Nuxt.",
"file_size": 1287
} |
---
title: Sparkles
description: A configurable sparkles component that can be used as a background or as a standalone component.
---
::ComponentLoader{label="Preview" componentName="SparklesDemo" type="examples" id="sparkles"}
::
## Examples
Sparkles Full Page
::ComponentLoader{label="Preview" componentName="SparklesFullPageDemo" type="examples" id="sparkles"}
::
## API
| Prop Name | Type | Default | Description |
| ----------------- | -------- | ----------- | -------------------------------------------------------------------------------------- |
| `background` | `string` | `'#0d47a1'` | Background color of the container. Use 'transparent' to see through to parent element. |
| `particleColor` | `string` | `'#ffffff'` | Color of the particles. Accepts any valid CSS color value. |
| `minSize` | `number` | `1` | Minimum size of particles in pixels. |
| `maxSize` | `number` | `3` | Maximum size of particles in pixels. |
| `speed` | `number` | `4` | Movement speed multiplier. Higher values create faster movement. |
| `particleDensity` | `number` | `120` | Number of particles to render. Higher values create denser particle fields. |
## Component Code
You can copy and paste the following code to create these components:
::CodeViewer{filename="Sparkles.vue" language="vue" componentName="Sparkles" type="ui" id="sparkles"}
::
## Credits
- Credits to [M Atif](https://github.com/atif0075) for porting this component.
- Ported from [Aceternity UI's Sparkles](https://ui.aceternity.com/components/sparkles). | {
"source": "unovue/inspira-ui",
"title": "content/2.components/backgrounds/sparkles.md",
"url": "https://github.com/unovue/inspira-ui/blob/main/content/2.components/backgrounds/sparkles.md",
"date": "2024-08-30T08:20:57",
"stars": 1788,
"description": "Build beautiful website using Vue & Nuxt.",
"file_size": 1845
} |
---
title: Tetris
description: Tetris background component, you can even click on a block to eliminate it.
---
::ComponentLoader{label="Preview" componentName="TetrisDemo" type="examples" id="tetris"}
::
::alert{type="warning"}
This component uses the `nuxt-only` syntax with the `<ClientOnly>`. If you are not using Nuxt, you can simply remove it.
::
::alert
**Note:** This component requires `theme-colors` as a dependency. Please install these using following commands.
::code-group
```bash [npm]
npm install theme-colors
```
```bash [pnpm]
pnpm install theme-colors
```
```bash [bun]
bun add theme-colors
```
```bash [yarn]
yarn add theme-colors
```
::
::
## API
| Prop Name | Type | Default | Description |
| -------------- | -------- | ------- | ---------------------------------------------- |
| `class` | `string` | `""` | Additional class names to style the component. |
| `base` | `number` | `10` | How many blocks do you have in a row. |
| `square-color` | `string` | `""` | Square color. |
## Component Code
You can copy and paste the following code to create these components:
::CodeViewer{filename="Tetris.vue" language="vue" componentName="Tetris" type="ui" id="tetris"}
::
## Credits
- Credits to [Whbbit1999](https://github.com/Whbbit1999) for this component.
- Inspired and ported from [Nuxt UI Home](https://ui.nuxt.com/). | {
"source": "unovue/inspira-ui",
"title": "content/2.components/backgrounds/tetris.md",
"url": "https://github.com/unovue/inspira-ui/blob/main/content/2.components/backgrounds/tetris.md",
"date": "2024-08-30T08:20:57",
"stars": 1788,
"description": "Build beautiful website using Vue & Nuxt.",
"file_size": 1523
} |
---
title: Vortex Background
description: A wavy, swirly, vortex background ideal for CTAs and backgrounds.
---
::ComponentLoader{label="Preview" componentName="VortexDemo" type="examples" id="vortex"}
::
## Example
Full page demo usage
::ComponentLoader{label="Preview" componentName="VortexFullPageDemo" type="examples" id="vortex"}
::
::alert{type="info"}
**Note:** This component uses simplex-noise & requires `simplex-noise` npm package as a dependency.
Please install simplex-noise using following commands.
::code-group
```bash [npm]
npm install simplex-noise
```
```bash [pnpm]
pnpm install simplex-noise
```
```bash [bun]
bun add simplex-noise
```
```bash [yarn]
yarn add simplex-noise
```
::
::
## API
| Prop Name | Type | Default | Description |
| ----------------- | -------- | ----------- | ---------------------------------------------------- |
| `class` | `string` | | Optional className for styling the children wrapper. |
| `containerClass` | `string` | | Optional className for styling the container. |
| `particleCount` | `number` | `700` | Number of particles to be generated. |
| `rangeY` | `number` | `100` | Vertical range for particle movement. |
| `baseHue` | `number` | `220` | Base hue for particle color. |
| `baseSpeed` | `number` | `0.0` | Base speed for particle movement. |
| `rangeSpeed` | `number` | `1.5` | Range of speed variation for particles. |
| `baseRadius` | `number` | `1` | Base radius of particles. |
| `rangeRadius` | `number` | `2` | Range of radius variation for particles. |
| `backgroundColor` | `string` | `"#000000"` | Background color of the canvas. |
## Component Code
You can copy and paste the following code to create these components:
::CodeViewer{filename="Vortex.vue" language="vue" componentName="Vortex" type="ui" id="vortex"}
::
## Features
- **Slot Support**: Easily add any content inside the component using the default slot.
## Credits
- Credits to [Aceternity UI](https://ui.aceternity.com/components/vortex).
- Credits to [SivaReddy Uppathi](https://github.com/sivareddyuppathi) for porting this component. | {
"source": "unovue/inspira-ui",
"title": "content/2.components/backgrounds/vortex.md",
"url": "https://github.com/unovue/inspira-ui/blob/main/content/2.components/backgrounds/vortex.md",
"date": "2024-08-30T08:20:57",
"stars": 1788,
"description": "Build beautiful website using Vue & Nuxt.",
"file_size": 2485
} |
---
title: Warp Background
description: A container component that applies a warp animation effect to its children
---
::ComponentLoader{label="Preview" componentName="WarpBackgroundDemo" type="examples" id="warp-background"}
::
## API
| Prop Name | Type | Default | Description |
| -------------- | -------- | ---------------------- | ----------------------------------------- |
| `perspective` | `number` | `100` | The perspective of the warp animation |
| `beamsPerSide` | `number` | `3` | The number of beams per side |
| `beamSize` | `number` | `5` | The size of the beams |
| `beamDelayMax` | `number` | `3` | The maximum delay of the beams in seconds |
| `beamDelayMin` | `number` | `0` | The minimum delay of the beams in seconds |
| `beamDuration` | `number` | `3` | The duration of the beams |
| `gridColor` | `string` | `"hsl(var(--border))"` | The color of the grid lines |
## Component Code
You can copy and paste the following code to create these components:
::CodeGroup
::CodeViewer{filename="WarpBackground.vue" language="vue" componentName="WarpBackground" type="ui" id="warp-background"}
::
::CodeViewer{filename="Beam.vue" language="vue" componentName="Beam" type="ui" id="warp-background"}
::
::
## Credits
- Credits to [Whbbit1999](https://github.com/Whbbit1999) for this component.
- Inspired and ported from [Magic UI WarpBackground](https://magicui.design/docs/components/warp-background). | {
"source": "unovue/inspira-ui",
"title": "content/2.components/backgrounds/warp-background.md",
"url": "https://github.com/unovue/inspira-ui/blob/main/content/2.components/backgrounds/warp-background.md",
"date": "2024-08-30T08:20:57",
"stars": 1788,
"description": "Build beautiful website using Vue & Nuxt.",
"file_size": 1664
} |
---
title: Wavy Background
description: A cool background effect with waves that move.
---
::ComponentLoader{label="Preview" componentName="WavyBackgroundDemo" type="examples" id="wavy-background"}
::
::alert{type="info"}
**Note:** This component uses simplex-noise & requires `simplex-noise` npm package as a dependency.
Please install simplex-noise using following commands.
::code-group
```bash [npm]
npm install simplex-noise
```
```bash [pnpm]
pnpm install simplex-noise
```
```bash [bun]
bun add simplex-noise
```
```bash [yarn]
yarn add simplex-noise
```
::
::
## API
| Prop Name | Type | Default | Description |
| ---------------- | ------------------ | --------------------------------------------------------- | ---------------------------------------------------------- |
| `class` | `string` | `-` | The content to be displayed on top of the wavy background. |
| `containerClass` | `string` | `-` | The CSS class to apply to the content container. |
| `colors` | `string[]` | `["#38bdf8", "#818cf8", "#c084fc", "#e879f9", "#22d3ee"]` | The colors of the waves. |
| `waveWidth` | `number` | `50` | The width of the waves. |
| `backgroundFill` | `string` | `"black"` | The background color. |
| `blur` | `number` | `10` | The blur effect applied to the waves. |
| `speed` | `"slow" \| "fast"` | `"fast"` | Range of speed variation for particles. |
| `waveOpacity` | `number` | `0.5` | Base radius of particles. |
| `[key: string]` | `any` | `-` | Range of radius variation for particles. |
## Component Code
You can copy and paste the following code to create these components:
::CodeViewer{filename="WavyBackground.vue" language="vue" componentName="WavyBackground" type="ui" id="wavy-background"}
::
## Features
- **Slot Support**: Easily add any content inside the component using the default slot.
## Credits
- Credits to [Aceternity UI](https://ui.aceternity.com/components/wavy-background).
- Credits to [SivaReddy Uppathi](https://github.com/sivareddyuppathi) for porting this component. | {
"source": "unovue/inspira-ui",
"title": "content/2.components/backgrounds/wavy-background.md",
"url": "https://github.com/unovue/inspira-ui/blob/main/content/2.components/backgrounds/wavy-background.md",
"date": "2024-08-30T08:20:57",
"stars": 1788,
"description": "Build beautiful website using Vue & Nuxt.",
"file_size": 2948
} |
---
title: Gradient Button
description: A stylish animated button with a rotating conic gradient border and customizable properties for a vibrant look.
---
::ComponentLoader{label="Preview" componentName="GradientButtonDemo" type="examples"}
::
## API
| Prop Name | Type | Default | Description |
| -------------- | ---------- | -------------------- | ------------------------------------------------------------ |
| `borderWidth` | `number` | `2` | Width of the gradient border in pixels. |
| `colors` | `string[]` | Rainbow Colors Array | Array of colors used in the conic gradient border. |
| `duration` | `number` | `2500` | Duration of the gradient rotation animation in milliseconds. |
| `borderRadius` | `number` | `8` | Border radius for rounded corners in pixels. |
| `blur` | `number` | `4` | Blur intensity of the gradient border effect in pixels. |
| `class` | `string` | `""` | Additional CSS classes for custom styling. |
| `bgColor` | `string` | `"#000"` | Background color of the button content. |
## Component Code
You can copy and paste the following code to create this component:
::CodeViewer{filename="GradientButton.vue" language="vue" componentName="GradientButton" type="ui" id="gradient-button"}
::
## Features
- **Rotating Conic Gradient Border**: A dynamic, rotating conic gradient border creates a visually engaging effect.
- **Customizable Color Palette**: Customize the gradient colors by providing an array of color values.
- **Flexible Styling Options**: Adjust border width, border radius, and blur effect for a tailored look.
- **Slot-Based Content**: Supports a default slot to easily add button content or icons.
- **Smooth Animation Control**: Control the speed of the rotation using the `duration` prop. | {
"source": "unovue/inspira-ui",
"title": "content/2.components/buttons/gradient-button.md",
"url": "https://github.com/unovue/inspira-ui/blob/main/content/2.components/buttons/gradient-button.md",
"date": "2024-08-30T08:20:57",
"stars": 1788,
"description": "Build beautiful website using Vue & Nuxt.",
"file_size": 2049
} |
---
title: Interactive Hover Button
description: A visually engaging button component that responds to hover with dynamic transitions, adapting smoothly between light and dark modes for enhanced user interactivity.
---
::ComponentLoader{label="Preview" componentName="InteractiveHoverButtonDemo" type="examples" id="interactive-hover-button"}
::
## Example
Very long text
If your button text is very long, you need change button width🤣.
::ComponentLoader{label="Preview" componentName="VeryLongTextInteractiveHoverButtonDemo" type="examples" id="interactive-hover-button"}
::
## API
| Prop Name | Type | Default | Description |
| --------- | -------- | -------- | ---------------------------------------------- |
| `text` | `string` | `Button` | The text to be displayed inside the button. |
| `class` | `string` | `""` | Additional class names to style the component. |
## Component Code
You can copy and paste the following code to create these components:
::CodeViewer{filename="InteractiveHoverButton.vue" language="vue" componentName="InteractiveHoverButton" type="ui" id="interactive-hover-button"}
::
## Credits
- Credits to [Whbbit1999](https://github.com/Whbbit1999) for this component.
- Inspired and ported from [Magic UI Interactive Hover Button](https://magicui.design/docs/components/interactive-hover-button). | {
"source": "unovue/inspira-ui",
"title": "content/2.components/buttons/interactive-hover-button.md",
"url": "https://github.com/unovue/inspira-ui/blob/main/content/2.components/buttons/interactive-hover-button.md",
"date": "2024-08-30T08:20:57",
"stars": 1788,
"description": "Build beautiful website using Vue & Nuxt.",
"file_size": 1388
} |
---
title: Rainbow Button
description: A rainbow effect on button.
---
::ComponentLoader{label="Preview" componentName="RainbowButtonDemo" type="examples"}
::
## API
| Prop Name | Type | Default | Description |
| --------- | -------- | ---------- | ---------------------------------------------- |
| `class` | `string` | `""` | Additional CSS classes to apply to the button. |
| `is` | `string` | `"button"` | The HTML tag to render for the component. |
| `speed` | `number` | `2` | Duration of the animation in seconds. |
## Component Code
You can copy and paste the following code to create these components:
::CodeViewer{filename="RainbowButton.vue" language="vue" componentName="RainbowButton" type="ui" id="rainbow-button"}
::
## Credits
- Credits to [Grzegorz Krol](https://github.com/Grzechu335) for porting this component.
- Credits to [Magic UI](https://magicui.design/docs/components/rainbow-button). | {
"source": "unovue/inspira-ui",
"title": "content/2.components/buttons/rainbow-button.md",
"url": "https://github.com/unovue/inspira-ui/blob/main/content/2.components/buttons/rainbow-button.md",
"date": "2024-08-30T08:20:57",
"stars": 1788,
"description": "Build beautiful website using Vue & Nuxt.",
"file_size": 996
} |
---
title: Ripple Button
description: A stylish ripple button component with customizable colors and animation duration.
navBadges:
- value: New
type: lime
---
::ComponentLoader{label="Preview" componentName="RippleButtonDemo" type="examples"}
::
## API
| Prop Name | Type | Default | Description |
| ------------- | -------- | ----------- | ------------------------------------------------- |
| `class` | `string` | - | Additional CSS classes for custom styling. |
| `rippleColor` | `string` | `"#ADD8E6"` | Color of the ripple effect. |
| `duration` | `number` | `600` | Duration of the ripple animation in milliseconds. |
## Emits
| Event Name | Type | Description |
| ---------- | ------- | ----------- |
| `click` | `event` | Click event |
## Component Code
You can copy and paste the following code to create this component:
::CodeViewer{filename="RippleButton.vue" language="vue" componentName="RippleButton" type="ui" id="ripple-button"}
::
## Credits
- Inspired by [Magic UI's Ripple Button](https://magicui.design/docs/components/ripple-button) component.
- Credits to [kalix127](https://github.com/kalix127) for porting this component. | {
"source": "unovue/inspira-ui",
"title": "content/2.components/buttons/ripple-button.md",
"url": "https://github.com/unovue/inspira-ui/blob/main/content/2.components/buttons/ripple-button.md",
"date": "2024-08-30T08:20:57",
"stars": 1788,
"description": "Build beautiful website using Vue & Nuxt.",
"file_size": 1272
} |
---
title: Shimmer Button
description: A button with a shimmering animated effect.
---
::ComponentLoader{label="Preview" componentName="ShimmerButtonDemo" type="examples"}
::
## API
| Prop Name | Type | Default | Description |
| ----------------- | -------- | -------------------- | ------------------------------------------------------- |
| `class` | `string` | `""` | Additional CSS classes to apply to the button. |
| `shimmerColor` | `string` | `"#ffffff"` | Color of the shimmer effect. |
| `shimmerSize` | `string` | `"0.05em"` | Size of the shimmer effect. |
| `borderRadius` | `string` | `"100px"` | Border radius of the button. |
| `shimmerDuration` | `string` | `"3s"` | Duration of the shimmer animation. |
| `background` | `string` | `"rgba(0, 0, 0, 1)"` | Background color of the button. Can be rgb or hex code. |
## Component Code
You can copy and paste the following code to create this component:
::CodeViewer{filename="ShimmerButton.vue" language="vue" componentName="ShimmerButton" type="ui" id="shimmer-button"}
::
## Features
- **Shimmering Effect**: Displays a continuous shimmering animation on the button.
- **Customizable Appearance**: Adjust shimmer color, size, duration, border radius, and background color.
- **Slot Support**: Easily add any content inside the button using the default slot.
- **Interactive States**: Includes hover and active states for enhanced user interaction.
- **Responsive Design**: Adapts to different screen sizes and resolutions seamlessly.
## Credits
- Ported from [Magic UI Shimmer Button](https://magicui.design/docs/components/shimmer-button). | {
"source": "unovue/inspira-ui",
"title": "content/2.components/buttons/shimmer-button.md",
"url": "https://github.com/unovue/inspira-ui/blob/main/content/2.components/buttons/shimmer-button.md",
"date": "2024-08-30T08:20:57",
"stars": 1788,
"description": "Build beautiful website using Vue & Nuxt.",
"file_size": 1879
} |
---
title: 3D Card Effect
description: A card perspective effect, hover over the card to elevate card elements.
---
::ComponentLoader{label="Preview" componentName="CardDemo" type="examples"}
::
## Examples
With rotation
::ComponentLoader{label="Preview" componentName="CardDemo2" type="examples"}
::
::alert{type="warning"}
This component uses the `nuxt-only` syntax with the `<ClientOnly>`. If you are not using Nuxt, you can simply remove it.
::
## API
::steps
### `CardContainer`
The `CardContainer` component serves as a wrapper for the 3D card effect. It manages mouse events to create a 3D perspective.
#### Props
| Prop Name | Type | Default | Description |
| ---------------- | ------ | ------- | ----------------------------------------------------------- |
| `class` | String | `null` | Additional classes for styling the inner container element. |
| `containerClass` | String | `null` | Additional classes for styling the outer container element. |
#### Usage
```vue [MyCardComponent.vue]
<CardContainer containerClass="additional-class">
<!-- Your content here -->
</CardContainer>
```
### `CardBody`
The `CardBody` component is a flexible container with preserved 3D styling. It is intended to be used within a `CardContainer` to hold content with a 3D transformation effect.
#### Props
| Prop Name | Type | Default | Description |
| --------- | ------ | ------- | -------------------------------------- |
| `class` | String | `null` | Additional classes for custom styling. |
#### Usage
```vue [MyCardComponent.vue]
<CardBody class="custom-class">
<!-- Your content here -->
</CardBody>
```
### `CardItem`
The `CardItem` component represents individual items within the 3D card. It supports various transformations (translation and rotation) to create dynamic 3D effects.
#### Props
| Prop Name | Type | Default | Description |
| ------------ | ------------- | ------- | --------------------------------------------------------------- |
| `as` | String | `"div"` | The HTML tag or component to use for the item. |
| `class` | String | `null` | Additional classes for styling the item. |
| `translateX` | Number/String | `0` | X-axis translation in pixels. |
| `translateY` | Number/String | `0` | Y-axis translation in pixels. |
| `translateZ` | Number/String | `0` | Z-axis translation in pixels, used to control the depth effect. |
| `rotateX` | Number/String | `0` | X-axis rotation in degrees. |
| `rotateY` | Number/String | `0` | Y-axis rotation in degrees. |
| `rotateZ` | Number/String | `0` | Z-axis rotation in degrees. |
#### Usage
```vue [MyCardComponent.vue]
<CardItem as="p" translateZ="50" class="custom-item-class">
Your text or content here
</CardItem>
```
::
## Component Code
You can copy and paste the following code to create these components:
::code-group
::CodeViewerTab{label="CardContainer.vue" icon="vscode-icons:file-type-vue" componentName="CardContainer" type="ui" id="card-3d"}
::
::CodeViewerTab{label="CardBody.vue" icon="vscode-icons:file-type-vue" componentName="CardBody" type="ui" id="card-3d"}
::
::CodeViewerTab{label="CardItem.vue" icon="vscode-icons:file-type-vue" componentName="CardItem" type="ui" id="card-3d"}
::
```ts [useMouseState.ts]
import { ref, readonly } from "vue";
export function useMouseState() {
const isMouseEntered = ref(false);
function setMouseEntered(value: boolean) {
isMouseEntered.value = value;
}
return {
isMouseEntered: readonly(isMouseEntered),
setMouseEntered,
};
}
```
:: | {
"source": "unovue/inspira-ui",
"title": "content/2.components/cards/3d-card.md",
"url": "https://github.com/unovue/inspira-ui/blob/main/content/2.components/cards/3d-card.md",
"date": "2024-08-30T08:20:57",
"stars": 1788,
"description": "Build beautiful website using Vue & Nuxt.",
"file_size": 3962
} |
---
title: Card Spotlight
description: A card component with a dynamic spotlight effect that follows the mouse cursor, enhancing visual interactivity.
---
::ComponentLoader{label="Preview" componentName="CardSpotlightDemo" type="examples"}
::
## API
| Prop Name | Type | Default | Description |
| ----------------- | -------- | ----------- | ----------------------------------------------------------- |
| `gradientSize` | `number` | `200` | Radius in pixels of the spotlight effect. |
| `gradientColor` | `string` | `'#262626'` | The color of the spotlight gradient. |
| `gradientOpacity` | `number` | `0.8` | The opacity level of the spotlight gradient effect. |
| `slotClass` | `string` | `undefined` | Class to apply to the parent container containing the slot. |
## Component Code
You can copy and paste the following code to create this component:
::CodeViewer{filename="CardSpotlight.vue" language="vue" componentName="CardSpotlight" type="ui" id="card-spotlight"}
::
## Features
- **Interactive Spotlight Effect**: Displays a dynamic spotlight that follows the user's mouse cursor, enhancing user engagement and visual appeal.
- **Customizable Appearance**: Easily adjust the `gradientSize`, `gradientColor`, and `gradientOpacity` props to tailor the spotlight effect to your design preferences.
- **Easy Integration**: Wrap any content within the `<CardSpotlight>` component to instantly add the spotlight effect without additional configuration.
- **Responsive Design**: The component adapts smoothly to different container sizes, ensuring a consistent experience across various devices and screen sizes.
- **Performance Optimized**: Utilizes Vue's reactivity for efficient updates, ensuring smooth animations without compromising application performance.
## Credits
- Inspired by Magic Card component from [Magic UI](https://magicui.design/docs/components/magic-card). | {
"source": "unovue/inspira-ui",
"title": "content/2.components/cards/card-spotlight.md",
"url": "https://github.com/unovue/inspira-ui/blob/main/content/2.components/cards/card-spotlight.md",
"date": "2024-08-30T08:20:57",
"stars": 1788,
"description": "Build beautiful website using Vue & Nuxt.",
"file_size": 2031
} |
---
title: Direction Aware Hover
description: A direction aware hover card, that displays an image with dynamic hover effects and customizable content overlay.
---
::ComponentLoader{label="Preview" componentName="DirectionAwareHoverDemo" type="examples"}
::
## API
| Prop Name | Type | Default | Description |
| --------------- | -------- | ----------- | ----------------------------------------------- |
| `imageUrl` | `string` | Required | The URL of the image to be displayed. |
| `class` | `string` | `undefined` | Additional CSS classes for the card container. |
| `imageClass` | `string` | `undefined` | Additional CSS classes for the image element. |
| `childrenClass` | `string` | `undefined` | Additional CSS classes for the content overlay. |
## Component Code
You can copy and paste the following code to create this component:
::CodeViewer{filename="DirectionAwareHover.vue" language="vue" componentName="DirectionAwareHover" type="ui" id="direction-aware-hover"}
::
## Credits
- Credits to [M Atif](https://github.com/atif0075) for porting this component.
- Ported from [Aceternity UI's Direction Aware Hover](https://ui.aceternity.com/components/direction-aware-hover) | {
"source": "unovue/inspira-ui",
"title": "content/2.components/cards/direction-aware-hover.md",
"url": "https://github.com/unovue/inspira-ui/blob/main/content/2.components/cards/direction-aware-hover.md",
"date": "2024-08-30T08:20:57",
"stars": 1788,
"description": "Build beautiful website using Vue & Nuxt.",
"file_size": 1275
} |
---
title: Flip Card
description: A dynamic flip card with smooth 180-degree flipping animations along both the X and Y axes, providing an engaging and interactive visual effect.
---
::ComponentLoader{label="Preview" componentName="FlipCardDemo" type="examples" id="flip-card"}
::
## Examples
### Horizontal Flip
::ComponentLoader{label="Preview" componentName="FlipCardHorizontalDemo" type="examples" id="flip-card"}
::
## API
| Prop Name | Type | Default | Description |
| --------------- | -------- | ------- | ------------------------------------------ |
| `class` | `string` | `-` | The class to be applied to the component. |
| `image`\* | `string` | `-` | Pass the image URL to display the image. |
| `title`\* | `string` | `-` | You can pass the title as you want. |
| `subtitle` \* | `string` | `-` | You can pass the sub title as you want. |
| `description`\* | `string` | `-` | You can pass the description as you want. |
| `rotate` | `x \| y` | `y` | You can pass the rotate value as you want. |
## Component Code
You can copy and paste the following code to create these components:
::CodeViewer{filename="FlipCard.vue" language="vue" componentName="FlipCard" type="ui" id="flip-card"}
::
## Credits
- Credits to [SivaReddy Uppathi](https://github.com/sivareddyuppathi) for this component. | {
"source": "unovue/inspira-ui",
"title": "content/2.components/cards/flip-card.md",
"url": "https://github.com/unovue/inspira-ui/blob/main/content/2.components/cards/flip-card.md",
"date": "2024-08-30T08:20:57",
"stars": 1788,
"description": "Build beautiful website using Vue & Nuxt.",
"file_size": 1421
} |
---
title: Glare Card
description: A glare effect that happens on hover, as seen on Linear's website.
---
::ComponentLoader{label="Preview" componentName="GlareCardDemo" type="examples" id="glare-card"}
::
## Examples
### Multiple Cards
::ComponentLoader{label="Preview" componentName="GlareMultiCardDemo" type="examples" id="glare-card"}
::
## API
| Prop Name | Type | Default | Description |
| --------- | -------- | ------- | --------------------------------------------------------- |
| `class` | `string` | `-` | Additional Tailwind CSS class names to apply to the card. |
## Component Code
You can copy and paste the following code to create these components:
::CodeViewer{filename="GlareCard.vue" language="vue" componentName="GlareCard" type="ui" id="glare-card"}
::
## Features
- **Slot Support**: Easily add any content inside the component using the default slot.
## Credits
- Credits to [SivaReddy Uppathi](https://github.com/sivareddyuppathi) for this component.
- Inspired from [Aceternity UI](https://ui.aceternity.com/components/glare-card). | {
"source": "unovue/inspira-ui",
"title": "content/2.components/cards/glare-card.md",
"url": "https://github.com/unovue/inspira-ui/blob/main/content/2.components/cards/glare-card.md",
"date": "2024-08-30T08:20:57",
"stars": 1788,
"description": "Build beautiful website using Vue & Nuxt.",
"file_size": 1126
} |
---
title: iPhone Mockup
description: An SVG mockup of an iPhone.
---
::ComponentLoader{label="Preview" componentName="iPhone15ProMockupDemo" type="examples" id="mockups"}
::
## API
| Prop Name | Type | Default | Description |
| --------- | -------- | ------- | ---------------------------------------------- |
| `width` | `number` | `433` | Width of the mockup SVG in pixels. |
| `height` | `number` | `882` | Height of the mockup SVG in pixels. |
| `src` | `string` | `null` | URL of the image to display inside the mockup. |
## Component Code
You can copy and paste the following code to create this component:
::CodeViewer{filename="iPhone15ProMockup.vue" language="vue" componentName="iPhone15ProMockup" type="ui" id="iphone-mockup"}
::
## Features
- **Realistic iPhone 15 Pro Mockup**: Provides an accurate SVG representation of the iPhone 15 Pro, perfect for showcasing mobile app designs or website previews.
- **Customizable Dimensions**: Adjust the `width` and `height` props to fit your specific design requirements.
- **Image Display Support**: Use the `src` prop to insert any image into the mockup screen area, allowing for dynamic content display.
- **Light and Dark Mode Compatibility**: The mockup adapts its colors based on the theme, ensuring consistency in both light and dark modes.
- **Easy Integration**: Simple to include in your Vue projects with minimal setup.
## Credits
- Ported from [Magic UI](https://magicui.design/docs/components/iphone-15-pro). | {
"source": "unovue/inspira-ui",
"title": "content/2.components/device-mocks/iphone-mockup.md",
"url": "https://github.com/unovue/inspira-ui/blob/main/content/2.components/device-mocks/iphone-mockup.md",
"date": "2024-08-30T08:20:57",
"stars": 1788,
"description": "Build beautiful website using Vue & Nuxt.",
"file_size": 1561
} |
---
title: Safari Mockup
description: An SVG mockup of the Safari browser.
---
::ComponentLoader{label="Preview" componentName="SafariMockupDemo" type="examples" id="mockups"}
::
## API
| Prop Name | Type | Default | Description |
| --------- | -------- | ------- | ---------------------------------------------- |
| `url` | `string` | `null` | URL displayed in the mockup's address bar. |
| `src` | `string` | `null` | URL of the image to display inside the mockup. |
| `width` | `number` | `1203` | Width of the mockup SVG in pixels. |
| `height` | `number` | `753` | Height of the mockup SVG in pixels. |
## Component Code
You can copy and paste the following code to create this component:
::CodeViewer{filename="SafariMockup.vue" language="vue" componentName="SafariMockup" type="ui" id="safari-mockup"}
::
## Features
- **Realistic Safari Browser Mockup**: Provides an SVG representation of the Safari browser window, ideal for showcasing website designs.
- **Customizable Dimensions**: Adjust the `width` and `height` props to fit your specific needs.
- **Address Bar URL Display**: Use the `url` prop to display a custom URL in the mockup's address bar.
- **Image Display Support**: Use the `src` prop to insert any image into the mockup's content area.
- **Light and Dark Mode Compatibility**: The mockup adapts its colors based on the theme.
## Credits
- Ported from [Magic UI](https://magicui.design/docs/components/safari). | {
"source": "unovue/inspira-ui",
"title": "content/2.components/device-mocks/safari-mockup.md",
"url": "https://github.com/unovue/inspira-ui/blob/main/content/2.components/device-mocks/safari-mockup.md",
"date": "2024-08-30T08:20:57",
"stars": 1788,
"description": "Build beautiful website using Vue & Nuxt.",
"file_size": 1527
} |
---
title: File Upload
description: A modern file upload component with a 3D card effect, drag-and-drop functionality, and a responsive grid background pattern.
navBadges:
- value: New
type: lime
---
::ComponentLoader{label="Preview" componentName="FileUploadDemo" type="examples"}
::
## API
::alert{type="warning"}
This component uses the `nuxt-only` syntax with the `<ClientOnly>`. If you are not using Nuxt, you can simply remove it.
::
::steps
### `FileUpload`
The `FileUpload` component serves as a wrapper for the file upload effect. It manages mouse events to create a 3D perspective.
#### Props
| Prop Name | Type | Default | Description |
| --------- | ------ | ------- | ----------------------------------------------------- |
| `class` | String | - | Additional classes for styling the container element. |
#### Emits
| Event Name | Type | Description |
| ---------- | ------------------------- | ---------------------------------------------------------- |
| `onChange` | `(files: File[]) => void` | Callback function triggered when files are added/uploaded. |
#### Usage
```vue [MyComponent.vue]
<FileUpload class="additional-class">
<!-- Your content here -->
</FileUpload>
```
### `FileUploadGrid`
The `FileUploadGrid` component provides the background grid pattern for the file upload area. It is intended to be used within a `FileUpload` component to create the visual grid effect behind the upload interface.
#### Props
| Prop Name | Type | Default | Description |
| --------- | ------ | ------- | -------------------------------------- |
| `class` | String | - | Additional classes for custom styling. |
#### Usage
```vue [MyComponent.vue]
<FileUploadGrid class="custom-class" />
```
::
## Component Code
You can copy and paste the following code to create these components:
::code-group
::CodeViewerTab{label="FileUpload.vue" icon="vscode-icons:file-type-vue" componentName="FileUpload" type="ui" id="file-upload"}
::
::CodeViewerTab{label="FileUploadGrid.vue" icon="vscode-icons:file-type-vue" componentName="FileUploadGrid" type="ui" id="file-upload"}
::
::
## Credits
- Credits to [kalix127](https://github.com/kalix127) for porting this component.
- Inspired by [AcernityUI](https://ui.aceternity.com/components/file-upload). | {
"source": "unovue/inspira-ui",
"title": "content/2.components/input-and-forms/file-upload.md",
"url": "https://github.com/unovue/inspira-ui/blob/main/content/2.components/input-and-forms/file-upload.md",
"date": "2024-08-30T08:20:57",
"stars": 1788,
"description": "Build beautiful website using Vue & Nuxt.",
"file_size": 2441
} |
---
title: Input
description: A versatile and visually dynamic input field with radial hover effects, styled for modern web applications.
---
::ComponentLoader{label="Preview" componentName="InputDemo" type="examples" id="input"}
::
## API
| Prop Name | Type | Default | Description |
| ---------------- | ------------------- | ------- | ----------------------------------------------------------- |
| `defaultValue` | `string \| number` | `""` | Default value of the input field. |
| `class` | `string` | `""` | Additional CSS classes for custom styling. |
| `containerClass` | `string` | `""` | Additional CSS classes for custom styling of the container. |
## Component Code
You can copy and paste the following code to create this component:
::CodeViewer{filename="IInput.vue" language="vue" componentName="IInput" type="ui" id="input"}
::
## Features
- **Radial Hover Effect**: Displays a dynamic radial gradient background that follows mouse movements when hovering over the input container.
- **Two-Way Binding**: Supports `v-model` for seamless integration with Vue's two-way data binding.
- **Dark Mode Compatibility**: Automatically adapts to dark mode styles using Tailwind's `dark:` utilities.
- **Customizable Styles**: Easily extend or override styles using the `class` prop.
- **Accessible Focus Ring**: Includes focus-visible styles for enhanced accessibility and usability.
- **Responsive Design**: Works well across different screen sizes and devices.
## Styles
This component inherits base styles from ShadCN Vue’s Input component and applies additional functionality, including hover effects and shadow styling.
## Credits
- Built on ShadCN Vue's Input component foundation, with extended functionality for modern UI/UX needs.
- Ported from [Aceternity UI's Signup Form Input Component](https://ui.aceternity.com/components/signup-form) | {
"source": "unovue/inspira-ui",
"title": "content/2.components/input-and-forms/input.md",
"url": "https://github.com/unovue/inspira-ui/blob/main/content/2.components/input-and-forms/input.md",
"date": "2024-08-30T08:20:57",
"stars": 1788,
"description": "Build beautiful website using Vue & Nuxt.",
"file_size": 2023
} |
---
title: Placeholders And Vanish Input
description: Sliding in placeholders and vanish effect of input on submit
---
::ComponentLoader{label="Preview" componentName="VanishingInputDemo" type="examples"}
::
## API
| Prop Name | Type | Default | Description |
| -------------- | --------------- | ----------------------------------------------------- | ------------------------------------------------------------------------------- |
| `placeholders` | `Array<string>` | `["Placeholder 1", "Placeholder 2", "Placeholder 3"]` | An array of placeholder texts that cycle through as prompts in the input field. |
This component listens to the following events emitted by the `VanishingInput` component:
| Event Name | Parameters | Description |
| ---------- | ---------- | --------------------------------------- |
| `change` | `Event` | Triggered when the input value changes. |
| `submit` | `string` | Triggered when the input is submitted. |
## Component Code
You can copy and paste the following code to create these components:
::CodeViewer{filename="VanishingInput.vue" language="vue" componentName="VanishingInput" type="ui" id="vanishing-input"}
::
## Credits
- Credits to [M Atif](https://github.com/atif0075) for porting this component.
- Ported from [Aceternity UI's Placeholders And Vanish Input](https://ui.aceternity.com/components/placeholders-and-vanish-input). | {
"source": "unovue/inspira-ui",
"title": "content/2.components/input-and-forms/placeholders-and-vanish-input.md",
"url": "https://github.com/unovue/inspira-ui/blob/main/content/2.components/input-and-forms/placeholders-and-vanish-input.md",
"date": "2024-08-30T08:20:57",
"stars": 1788,
"description": "Build beautiful website using Vue & Nuxt.",
"file_size": 1555
} |
---
title: Dock
description: A macOS-style dock with magnifying icons as you hover over them.
---
::ComponentLoader{label="Preview" componentName="DockDemo" type="examples"}
::
## API
::steps
### `Dock`
| Prop Name | Type | Description |
| --------------- | -------- | ----------------------------------------------------------------- |
| `class` | `string` | Additional classes to apply to the dock container. |
| `magnification` | `number` | Magnification factor for the dock icons on hover (default: 60). |
| `distance` | `number` | Distance from the icon center where magnification applies. |
| `direction` | `string` | Alignment of icons (`top`, `middle`, `bottom`) (default: middle). |
| Slot Name | Description |
| --------- | ----------------------------------------------------- |
| `default` | Dock icons or other child components to be displayed. |
### `DockIcon`
| Slot Name | Description |
| --------- | ------------------------------------------------------- |
| `default` | Component or icon to be displayed inside the dock icon. |
::
## Component Code
You can copy and paste the following code to create these components:
::CodeGroup
::CodeViewerTab{filename="Dock.vue" language="vue" componentName="Dock" type="ui" id="dock"}
::
::CodeViewerTab{filename="DockIcon.vue" language="vue" componentName="DockIcon" type="ui" id="dock"}
::
::
## Credits
- Credits to macOS Dock for the design inspiration and the fantastic hover magnification effect. | {
"source": "unovue/inspira-ui",
"title": "content/2.components/miscellaneous/Dock.md",
"url": "https://github.com/unovue/inspira-ui/blob/main/content/2.components/miscellaneous/Dock.md",
"date": "2024-08-30T08:20:57",
"stars": 1788,
"description": "Build beautiful website using Vue & Nuxt.",
"file_size": 1676
} |
---
title: Animate Grid
description: Skew Animation grid with box shadow.
---
::ComponentLoader{label="Preview" componentName="AnimateGridDemo" type="examples" id="animate-grid"}
::
## API
| Prop Name | Type | Default | Description |
| -------------------- | -------- | ------------------- | --------------------------------------------------- |
| `textGlowStartColor` | `string` | `"#38ef7d80"` | Color of the box shadow start. |
| `textGlowEndColor` | `string` | `"#38ef7d"` | Color of the box shadow end. |
| `perspective` | `number` | `600` | You can pass perspective to transform CSS property. |
| `rotateX` | `number` | `-1` | You can pass rotateX to transform CSS property. |
| `rotateY` | `number` | `-15` | You can pass rotateY to transform CSS property. |
| `cards` | `[]` | `"[{logo: 'src'}]"` | Cards to display in grid. |
| `class` | `string` | `""` | Additional tailwind CSS classes for custom styling. |
## Component Code
You can copy and paste the following code to create this component:
::CodeViewer{filename="AnimateGrid.vue" language="vue" componentName="AnimateGrid" type="ui" id="animate-grid"}
::
## Features
- **Slot-Based Content**: Supports a default slot to add content inside the Animate Grid container.
## Credits
- Thanks to [SivaReddy Uppathi](https://github.com/sivareddyuppathi) for providing this component. | {
"source": "unovue/inspira-ui",
"title": "content/2.components/miscellaneous/animate-grid.md",
"url": "https://github.com/unovue/inspira-ui/blob/main/content/2.components/miscellaneous/animate-grid.md",
"date": "2024-08-30T08:20:57",
"stars": 1788,
"description": "Build beautiful website using Vue & Nuxt.",
"file_size": 1629
} |
---
title: Animated Circular Progress Bar
description: Animated Circular Progress Bar is a component that displays a circular gauge with a percentage value.
---
::ComponentLoader{label="Preview" componentName="AnimatedCircularProgressBarDemo" type="examples" id="animated-circular-progressbar"}
::
## API
| Prop Name | Type | Default | Description |
| --------------------- | --------- | -------------------- | ------------------------------------------- |
| `class` | `string` | `-` | The class to be applied to the component. |
| `max` | `number` | `100` | The maximum value of the gauge. |
| `min` | `number` | `0` | The minimum value of the gauge. |
| `value` | `number` | `0` | The current value of the gauge. |
| `gaugePrimaryColor` | `string` | `rgb(79 70 229)` | The primary color of the gauge. |
| `gaugeSecondaryColor` | `string` | `rgba(0, 0, 0, 0.1)` | The secondary color of the gauge. |
| `circleStrokeWidth` | `number` | `10` | The width of the circle progress bar. |
| `showPercentage` | `boolean` | `true` | Show the value inside the circle |
| `duration` | `number` | `1` | The duration of the animation (in seconds). |
## Component Code
You can copy and paste the following code to create these components:
::CodeViewer{filename="AnimatedCircularProgressBar.vue" language="vue" componentName="AnimatedCircularProgressBar" type="ui" id="animated-circular-progressbar"}
::
## Credits
- Credits to [Magic UI](https://magicui.design/docs/components/animated-circular-progress-bar).
- Credits to [SivaReddy Uppathi](https://github.com/sivareddyuppathi) for porting this component. | {
"source": "unovue/inspira-ui",
"title": "content/2.components/miscellaneous/animated-circular-progressbar.md",
"url": "https://github.com/unovue/inspira-ui/blob/main/content/2.components/miscellaneous/animated-circular-progressbar.md",
"date": "2024-08-30T08:20:57",
"stars": 1788,
"description": "Build beautiful website using Vue & Nuxt.",
"file_size": 1948
} |
---
title: Animated List
description: A sequentially animated list that introduces each item with a timed delay, perfect for displaying notifications or events on your landing page.
---
::ComponentLoader{label="Preview" componentName="AnimatedListDemo" type="examples"}
::
## API
| Prop Name | Type | Default | Description |
| --------- | -------- | ------- | -------------------------------------------------------------- |
| `delay` | `number` | `1` | The delay in milliseconds before adding each item to the list. |
::alert{type="warning"}
This component uses the `nuxt-only` syntax with the `<ClientOnly>`. If you are not using Nuxt, you can simply remove it.
::
## Component Code
You can copy and paste the following code to create this component:
::CodeGroup
::CodeViewerTab{label="AnimatedList.vue" language="vue" componentName="AnimatedList" type="ui" id="animated-list"}
::
::CodeViewerTab{label="Notification.vue" language="vue" componentName="Notification" type="ui" id="notification"}
::
::
## Features
- **Animated Item Loading**: Items are added to the list one by one with a configurable delay.
- **Smooth Transitions**: Each item animates in with a spring effect on entry and a smooth scale and opacity animation on exit.
- **Reverse Order**: Items are displayed in reverse order (newest at the top) for a dynamic, engaging user experience.
- **Flexibility**: You can pass different components as items, making it highly versatile.
## Credits
- Inspired by [Magic UI](https://magicui.design/docs/components/animated-list). | {
"source": "unovue/inspira-ui",
"title": "content/2.components/miscellaneous/animated-list.md",
"url": "https://github.com/unovue/inspira-ui/blob/main/content/2.components/miscellaneous/animated-list.md",
"date": "2024-08-30T08:20:57",
"stars": 1788,
"description": "Build beautiful website using Vue & Nuxt.",
"file_size": 1620
} |
---
title: Animated Tooltip
description: A cool tooltip that reveals on hover, follows mouse pointer
---
::ComponentLoader{label="Preview" componentName="AnimatedTooltipDemo" type="examples"}
::
## API
| Prop Name | Type | Default | Description |
| --------- | ----------------------------------------------------------------------- | ------- | ------------------------------------------------------------------------------------------------------------------------------------------- |
| `items` | `Array<{id: number, name: string, designation: string, image: string}>` | `[]` | An array of objects, each representing an item. Each object in the array should have the following properties: id, name, designation, image |
## Component Code
You can copy and paste the following code to create this component:
::CodeViewer{filename="AnimatedTooltip.vue" language="vue" componentName="AnimatedTooltip" type="ui" id="animated-tooltip"}
::
## Credits
- Credits to [M Atif](https://github.com/atif0075) for this component.
- Inspired from [Aceternity UI's Animated Tooltip](https://ui.aceternity.com/components/animated-tooltip). | {
"source": "unovue/inspira-ui",
"title": "content/2.components/miscellaneous/animated-tooltip.md",
"url": "https://github.com/unovue/inspira-ui/blob/main/content/2.components/miscellaneous/animated-tooltip.md",
"date": "2024-08-30T08:20:57",
"stars": 1788,
"description": "Build beautiful website using Vue & Nuxt.",
"file_size": 1332
} |
---
title: Balance Slider
description: A dynamic balance slider with adjustable colors, limits, and interactive tooltip.
---
::ComponentLoader{label="Preview" componentName="BalanceSliderDemo" type="examples"}
::
## API
| Prop Name | Type | Default | Description |
| ---------------- | -------- | ----------- | --------------------------------------------------- |
| `initialValue` | `number` | `50` | Initial position of the slider (0-100). |
| `leftColor` | `string` | `"#e68a00"` | Background color for the left side of the slider. |
| `rightColor` | `string` | `"#ffffff"` | Background color for the right side of the slider. |
| `minShiftLimit` | `number` | `40` | Minimum limit where shifting animation activates. |
| `maxShiftLimit` | `number` | `68` | Maximum limit where shifting animation deactivates. |
| `leftContent` | `string` | `"LEFT"` | Text displayed in the tooltip for the left side. |
| `rightContent` | `string` | `"RIGHT"` | Text displayed in the tooltip for the right side. |
| `indicatorColor` | `string` | `"#FFFFFF"` | Color of the central indicator on the slider. |
## Component Code
You can copy and paste the following code to create this component:
::CodeViewer{filename="BalanceSlider.vue" language="vue" componentName="BalanceSlider" type="ui" id="balance-slider"}
::
## Features
- **Dual-Sided Color Control**: Customize the left and right side colors of the slider to create a distinct balance effect.
- **Interactive Tooltip**: Displays real-time percentage values for both sides, with customizable content for left and right labels.
- **Shift Animation**: Activates a shifting effect within defined limits, adding an engaging visual element.
- **Responsive Indicator**: Central indicator adjusts its color based on active state, enhancing interactivity.
- **Accessible Controls**: Works with keyboard and touch interactions for seamless accessibility.
## Credits
- Inspired and ported from code shared in [Jhey's CSS only version of Balance Slider](https://x.com/jh3yy/status/1748809599598399792?s=46)
- Original concept by [Malay Vasa](https://x.com/MalayVasa/status/1748726374079381930). | {
"source": "unovue/inspira-ui",
"title": "content/2.components/miscellaneous/balance-slider.md",
"url": "https://github.com/unovue/inspira-ui/blob/main/content/2.components/miscellaneous/balance-slider.md",
"date": "2024-08-30T08:20:57",
"stars": 1788,
"description": "Build beautiful website using Vue & Nuxt.",
"file_size": 2262
} |
---
title: Bento Grid
description: A cool grid layout with different child component.
---
::ComponentLoader{label="Preview" componentName="BentoGridDemo" type="examples"}
::
## API
#### `BentoGridItem`
| Slot Name | Description |
| ------------- | --------------------------------- |
| `title` | Component to show as title. |
| `description` | Component to show as description. |
| `icon` | Component to show as icon. |
| `header` | Component to show as header. |
#### `BentoGridCard`
| Slot Name | Description |
| ------------ | -------------------------------- |
| `background` | Component to show in background. |
| Props Name | Type | Description |
| ------------- | --------- | ------------------------------------ |
| `name` | `string` | Name or title to show on card. |
| `icon` | `?string` | Icon component to show on card. |
| `description` | `string` | Description content to show on card. |
| `href` | `string` | Link to the url for CTA. |
| `cta` | `string` | Text to show on CTA. |
## Examples
`BentoGrid` in MagicUI style.
::ComponentLoader{label="Preview" componentName="BentoGridDemo2" type="examples"}
::
## Component Code
You can copy and paste the following code to create these components:
::CodeGroup
::CodeViewerTab{label="BentoGrid.vue" language="vue" componentName="BentoGrid" type="ui" id="bento-grid"}
::
::CodeViewerTab{label="BentoGridItem.vue" language="vue" componentName="BentoGridItem" type="ui" id="bento-grid"}
::
::CodeViewerTab{label="BentoGridCard.vue" language="vue" componentName="BentoGridCard" type="ui" id="bento-grid"}
::
::
## Credits
- Credits to [Aceternity UI](https://ui.aceternity.com/components/bento-grid) and [Magic UI](https://magicui.design/docs/components/bento-grid) for this fantastic component. | {
"source": "unovue/inspira-ui",
"title": "content/2.components/miscellaneous/bento-grid.md",
"url": "https://github.com/unovue/inspira-ui/blob/main/content/2.components/miscellaneous/bento-grid.md",
"date": "2024-08-30T08:20:57",
"stars": 1788,
"description": "Build beautiful website using Vue & Nuxt.",
"file_size": 1959
} |
---
title: Book
description: A 3D book component featuring customizable sizes and color gradients.
navBadges:
- value: New
type: lime
---
::ComponentLoader{label="Preview" componentName="BookDemo" type="examples"}
::
## API
::steps
### `Book`
The `Book` component serves as a wrapper for creating an interactive 3D book effect.
#### Props
| Prop Name | Type | Default | Description |
| ------------ | ------- | ------- | --------------------------------------------- |
| `class` | String | - | Additional classes for styling the component. |
| `duration` | Number | 1000 | Animation duration in milliseconds. |
| `color` | String | "zinc" | Color theme for the book gradient. |
| `isStatic` | Boolean | false | Disables hover animations when true. |
| `size` | String | "md" | Size variant of the book. |
| `radius` | String | "md" | Border radius variant of the book. |
| `shadowSize` | String | "lg" | Shadow size variant of the book. |
#### Usage
```vue [MyBookComponent.vue]
<Book class="additional-class">
<!-- Your content here -->
</Book>
```
### `BookHeader`
The `BookHeader` component is a flexible container with preserved 3D styling. It is intended to be used within a `Book` to hold content with a 3D transformation effect.
#### Props
| Prop Name | Type | Default | Description |
| --------- | ------ | ------- | -------------------------------------- |
| `class` | String | - | Additional classes for custom styling. |
#### Usage
```vue [MyBookComponent.vue]
<BookHeader class="additional-class">
<!-- Your content here -->
</BookHeader>
```
### `BookTitle`
The `BookTitle` component is a flexible container with preserved 3D styling. It is intended to be used within a `Book` to hold content with a 3D transformation effect.
#### Props
| Prop Name | Type | Default | Description |
| --------- | ------ | ------- | -------------------------------------- |
| `class` | String | - | Additional classes for custom styling. |
#### Usage
```vue [MyBookComponent.vue]
<BookTitle class="additional-class">
<!-- Your text here -->
</BookTitle>
```
### `BookDescription`
The `BookDescription` component is a flexible container with preserved 3D styling. It is intended to be used within a `Book` to hold content with a 3D transformation effect.
#### Props
| Prop Name | Type | Default | Description |
| --------- | ------ | ------- | -------------------------------------- |
| `class` | String | - | Additional classes for custom styling. |
#### Usage
```vue [MyBookComponent.vue]
<BookDescription class="additional-class">
<!-- Your text here -->
</BookDescription>
```
::
## Component Code
You can copy and paste the following code to create these components:
::code-group
::CodeViewerTab{label="Book.vue" icon="vscode-icons:file-type-vue" componentName="Book" type="ui" id="book"}
::
::CodeViewerTab{label="BookHeader.vue" icon="vscode-icons:file-type-vue" componentName="BookHeader" type="ui" id="book"}
::
::CodeViewerTab{label="BookTitle.vue" icon="vscode-icons:file-type-vue" componentName="BookTitle" type="ui" id="book"}
::
::CodeViewerTab{label="BookDescription.vue" icon="vscode-icons:file-type-vue" componentName="BookDescription" type="ui" id="book"}
::
```ts [index.ts]
export const BOOK_RADIUS_MAP = {
sm: "rounded-sm",
md: "rounded-md",
lg: "rounded-lg",
xl: "rounded-xl",
} as const;
export const BOOK_SIZE_MAP = {
sm: { width: "180px", spineTranslation: "152px" },
md: { width: "220px", spineTranslation: "192px" },
lg: { width: "260px", spineTranslation: "232px" },
xl: { width: "300px", spineTranslation: "272px" },
} as const;
export const BOOK_SHADOW_SIZE_MAP = {
sm: "-5px 0 15px 5px var(--shadowColor)",
md: "-7px 0 25px 7px var(--shadowColor)",
lg: "-10px 0 35px 10px var(--shadowColor)",
xl: "-12px 0 45px 12px var(--shadowColor)",
} as const;
export const BOOK_COLOR_MAP = {
slate: { from: "from-slate-900", to: "to-slate-700" },
gray: { from: "from-gray-900", to: "to-gray-700" },
zinc: { from: "from-zinc-900", to: "to-zinc-700" },
neutral: { from: "from-neutral-900", to: "to-neutral-700" },
stone: { from: "from-stone-900", to: "to-stone-700" },
red: { from: "from-red-900", to: "to-red-700" },
orange: { from: "from-orange-900", to: "to-orange-700" },
amber: { from: "from-amber-900", to: "to-amber-700" },
yellow: { from: "from-yellow-900", to: "to-yellow-700" },
lime: { from: "from-lime-900", to: "to-lime-700" },
green: { from: "from-green-900", to: "to-green-700" },
emerald: { from: "from-emerald-900", to: "to-emerald-700" },
teal: { from: "from-teal-900", to: "to-teal-700" },
cyan: { from: "from-cyan-900", to: "to-cyan-700" },
sky: { from: "from-sky-900", to: "to-sky-700" },
blue: { from: "from-blue-900", to: "to-blue-700" },
indigo: { from: "from-indigo-900", to: "to-indigo-700" },
violet: { from: "from-violet-900", to: "to-violet-700" },
purple: { from: "from-purple-900", to: "to-purple-700" },
fuchsia: { from: "from-fuchsia-900", to: "to-fuchsia-700" },
pink: { from: "from-pink-900", to: "to-pink-700" },
rose: { from: "from-rose-900", to: "to-rose-700" },
} as const;
export type BookColor = keyof typeof BOOK_COLOR_MAP;
export type BookSize = keyof typeof BOOK_SIZE_MAP;
export type BookRadius = keyof typeof BOOK_RADIUS_MAP;
export type BookShadowSize = keyof typeof BOOK_SHADOW_SIZE_MAP;
export { default as Book } from "./Book.vue";
export { default as BookHeader } from "./BookHeader.vue";
export { default as BookTitle } from "./BookTitle.vue";
export { default as BookDescription } from "./BookDescription.vue";
```
::
## Credits
- Credits to [x/UI](https://ui.3x.gl/docs/book) for inspiring this component.
- Credits to [kalix127](https://github.com/kalix127) for porting this component. | {
"source": "unovue/inspira-ui",
"title": "content/2.components/miscellaneous/book.md",
"url": "https://github.com/unovue/inspira-ui/blob/main/content/2.components/miscellaneous/book.md",
"date": "2024-08-30T08:20:57",
"stars": 1788,
"description": "Build beautiful website using Vue & Nuxt.",
"file_size": 6053
} |
---
title: Compare
description: Slide to compare any two pieces of content - images, designs, code, or custom elements
---
::ComponentLoader{label="Preview" componentName="CompareDemo" type="examples" id="compare"}
::
## Examples
Drag handle with custom content
::ComponentLoader{label="Preview" componentName="CompareDragDemo" type="examples" id="compare"}
::
AutoPlay
::ComponentLoader{label="Preview" componentName="CompareAutoplayDemo" type="examples" id="compare"}
::
Custom Content with AutoPlay
::ComponentLoader{label="Preview" componentName="CompareCustomContentDemo" type="examples" id="compare"}
::
## API
| Prop Name | Type | Default | Description |
| ------------------------- | ------------------- | ---------------- | ----------------------------------------- |
| `firstImage` | `string` | `""` | URL of the first image |
| `secondImage` | `string` | `""` | URL of the second image |
| `firstImageAlt` | `string` | `"First image"` | Alt text for first image |
| `secondImageAlt` | `string` | `"Second image"` | Alt text for second image |
| `class` | `string` | `""` | Additional classes for the component |
| `firstContentClass` | `string` | `""` | Classes applied to first content wrapper |
| `secondContentClass` | `string` | `""` | Classes applied to second content wrapper |
| `initialSliderPercentage` | `number` | `50` | Initial position of slider (0-100) |
| `slideMode` | `"hover" \| "drag"` | `"hover"` | Interaction mode for the slider |
| `showHandlebar` | `boolean` | `true` | Show/hide the handle bar |
| `autoplay` | `boolean` | `false` | Enable/disable autoplay |
| `autoplayDuration` | `number` | `5000` | Duration of autoplay cycle in ms |
| Event Name | Payload | Description |
| ------------------- | -------- | -------------------------------------------- |
| `update:percentage` | `number` | Emitted when slider position changes (0-100) |
| `drag:start` | - | Emitted when dragging starts |
| `drag:end` | - | Emitted when dragging ends |
| `hover:enter` | - | Emitted when mouse enters component |
| `hover:leave` | - | Emitted when mouse leaves component |
| Slot Name | Default Content | Description |
| ---------------- | ------------------------------------------------- | --------------------------------------------------------------------------------------------------------------------------------- |
| `first-content` | `<img>` element if `firstImage` prop is provided | Content shown on the left/first side of the comparison. Has full access to component width/height. |
| `second-content` | `<img>` element if `secondImage` prop is provided | Content shown on the right/second side of the comparison. Has full access to component width/height. |
| `handle` | Default slider handle with dots icon | Custom handle for the slider. Automatically positioned at the dividing line. Should handle positioning with absolute positioning. |
## Component Code
You can copy and paste the following code to create this component:
::code-group
::CodeViewerTab{filename="Compare.vue" language="vue" componentName="Compare" type="ui" id="compare"}
::
::CodeViewerTab{filename="StarField.vue" language="vue" componentName="StarField" type="ui" id="compare"}
::
::
## Credits
- Credits to [M Atif](https://github.com/atif0075) for this component.
- Inspired from [Aceternity UI's Compare](https://ui.aceternity.com/components/compare). | {
"source": "unovue/inspira-ui",
"title": "content/2.components/miscellaneous/compare.md",
"url": "https://github.com/unovue/inspira-ui/blob/main/content/2.components/miscellaneous/compare.md",
"date": "2024-08-30T08:20:57",
"stars": 1788,
"description": "Build beautiful website using Vue & Nuxt.",
"file_size": 4333
} |
---
title: Container Scroll
description: A container scrolling effect that transforms the content inside based on scroll progress. Features smooth transitions with scaling and rotating effects on scroll.
---
::ComponentLoader{label="Preview" componentName="ContainerScrollDemo" type="examples"}
::
## API
::steps
### `ContainerScroll`
The `ContainerScroll` component creates a 3D scroll effect. As the user scrolls, the content inside the container is transformed with scale, rotation, and translation effects.
#### Props
| Prop Name | Type | Default | Description |
| ------------ | ------ | ------- | ----------------------------------------------------------------------------- |
| `rotate` | Number | `0` | Controls the rotation of the inner content based on the scroll progress. |
| `scale` | Number | `1` | Controls the scaling transformation applied to the content during the scroll. |
| `translateY` | Number | `0` | Controls the vertical translation of the title during the scroll. |
#### Usage
```vue [ContainerScroll.vue]
<ContainerScroll :rotate="rotateValue" :scale="scaleValue" :translateY="translateYValue">
<template #title>
<!-- Your title content here -->
</template>
<template #card>
<!-- Your card content here -->
</template>
</ContainerScroll>
```
### `ContainerScrollTitle`
The `ContainerScrollTitle` component handles the title's transformation as the user scrolls, applying a vertical translation effect.
#### Props
| Prop Name | Type | Default | Description |
| ----------- | ------ | ------- | ----------------------------------------------- |
| `translate` | Number | `0` | Controls the vertical translation of the title. |
#### Usage
```vue [ContainerScrollTitle.vue]
<ContainerScrollTitle :translate="translateYValue">
<!-- Title content here -->
</ContainerScrollTitle>
```
### `ContainerScrollCard`
The `ContainerScrollCard` component applies scale and rotation effects to the card content as the user scrolls through the page.
#### Props
| Prop Name | Type | Default | Description |
| --------- | ------ | ------- | ------------------------------------------------ |
| `rotate` | Number | `0` | Controls the rotation effect of the card. |
| `scale` | Number | `1` | Controls the scaling effect applied to the card. |
#### Usage
```vue [ContainerScrollCard.vue]
<ContainerScrollCard :rotate="rotateValue" :scale="scaleValue">
<!-- Card content here -->
</ContainerScrollCard>
```
::
## Features
- **3D Scroll Effects**: The content transforms based on scroll progress, including rotation, scaling, and translation.
- **Responsive Design**: Adjusts behavior dynamically for mobile and desktop views.
- **Smooth Transitions**: Leverages CSS keyframes and scroll-based transformations for a fluid user experience.
## CSS Variables
To customize the scroll animations and responsiveness, you can set the following CSS variables:
- **`--scale-start`**: Initial scale value for the card.
- **`--scale-end`**: Final scale value for the card as the scroll progresses.
- **`--rotate-start`**: Initial rotation value for the card.
- **`--rotate-end`**: Final rotation value for the card as the scroll progresses.
## Component Code
You can copy and paste the following code to create these components:
::code-group
::CodeViewerTab{label="ContainerScroll.vue" language="vue" componentName="ContainerScroll" type="ui" id="container-scroll"}
::
::CodeViewerTab{label="ContainerScrollTitle.vue" language="vue" componentName="ContainerScrollTitle" type="ui" id="container-scroll"}
::
::CodeViewerTab{label="ContainerScrollCard.vue" language="vue" componentName="ContainerScrollCard" type="ui" id="container-scroll"}
::
::
## Features
- **Dynamic Transformations**: Based on scroll position, the content scales, rotates, and translates for a 3D effect.
- **Flexible Content**: Place any custom content inside the title and card slots.
- **Responsive**: Adjusts for mobile and desktop, providing a consistent experience across devices.
## Credits
- Inspired by [Aceternity UI Container Scroll Animation](https://ui.aceternity.com/components/container-scroll-animation). | {
"source": "unovue/inspira-ui",
"title": "content/2.components/miscellaneous/container-scroll.md",
"url": "https://github.com/unovue/inspira-ui/blob/main/content/2.components/miscellaneous/container-scroll.md",
"date": "2024-08-30T08:20:57",
"stars": 1788,
"description": "Build beautiful website using Vue & Nuxt.",
"file_size": 4376
} |
---
title: Expandable Gallery
description: A responsive image gallery with an interactive hover effect that expands images dynamically.
---
::ComponentLoader{label="Preview" componentName="ExpandableGalleryDemo" type="examples" id="expandable-gallery"}
::
## API
| Prop Name | Type | Default | Description |
| --------- | ---------- | ------- | ---------------------------------------------- |
| `images` | `string[]` | `[]` | Array of image URLs to display in the gallery. |
## Component Code
You can copy and paste the following code to create this component:
::CodeViewer{filename="ExpandableGallery.vue" language="vue" componentName="ExpandableGallery" type="ui" id="expandable-gallery"}
::
## Features
- **Interactive Hover Effect**: Images expand when hovered over, creating a dynamic and engaging user experience.
- **Responsive Design**: The gallery automatically adjusts to the container size, ensuring it looks great on all devices.
- **Smooth Transitions**: Includes smooth scaling animations for a polished visual effect.
- **Customizable Content**: Easily update the `images` array to change the gallery's content.
## Credits
- Inspired from [Expandable Gallery Component by David Mráz](https://x.com/davidm_ml/status/1872319793124282653) | {
"source": "unovue/inspira-ui",
"title": "content/2.components/miscellaneous/expandable-gallery.md",
"url": "https://github.com/unovue/inspira-ui/blob/main/content/2.components/miscellaneous/expandable-gallery.md",
"date": "2024-08-30T08:20:57",
"stars": 1788,
"description": "Build beautiful website using Vue & Nuxt.",
"file_size": 1306
} |
Subsets and Splits